-
-Select a license to activate the product
-The Microsoft Software License Agreement provides for a choice of one of several available versions:
-â–º Product Key License - to install the program on one computer or on one computer and on several computers if it is connected to the Internet.
-â–º Product License on Demand - a license to install the product on multiple computers if it is connected to the Internet.
-â–º Product Code License - For installing the program on a single computer. 8a78ff9644
-
-
-
diff --git a/spaces/1gistliPinn/ChatGPT4/Examples/Download Cs 1.6 Hack Aimbot.md b/spaces/1gistliPinn/ChatGPT4/Examples/Download Cs 1.6 Hack Aimbot.md
deleted file mode 100644
index d2d4272589e55719853e3dc90a2193e3da7b540f..0000000000000000000000000000000000000000
--- a/spaces/1gistliPinn/ChatGPT4/Examples/Download Cs 1.6 Hack Aimbot.md
+++ /dev/null
@@ -1,15 +0,0 @@
-
-
-Features: Server hack that works under linux and windows servers. Damage/health, aimbot, teleport, etc. skins player, cs 1.6 ... Type: Client / Online-mode
-Genre: First person shooter
-Publication type: License
-Platform: PC
-Developer: Jedidos
-Year of release: 2012
-Interface language: Russian
-Tablet: Sewn
-System requirements: Operating system: Windows XP, Windows Vista, Windows 7 Processor: Pentium 4 2 GHz RAM: 512 MB Video card: 128 MB VRAM Sound card: DirectX 9.0c compatible
-Description: Counter-Strike 1.6 by Jedidos is the most popular among cyber teams. 8a78ff9644
-
-
-
diff --git a/spaces/1pelhydcardo/ChatGPT-prompt-generator/assets/Download 8 Ball Pool Mod APK 2022 and Play with Legends on Android.md b/spaces/1pelhydcardo/ChatGPT-prompt-generator/assets/Download 8 Ball Pool Mod APK 2022 and Play with Legends on Android.md
deleted file mode 100644
index e7cb2e8e5d7eb98b16f7c434f702d2edbd145b1e..0000000000000000000000000000000000000000
--- a/spaces/1pelhydcardo/ChatGPT-prompt-generator/assets/Download 8 Ball Pool Mod APK 2022 and Play with Legends on Android.md
+++ /dev/null
@@ -1,115 +0,0 @@
-
-
8 Ball Pool Mod APK 2022 _Apkpure: Everything You Need to Know
-
If you are a fan of pool games, you might have heard of 8 Ball Pool, one of the most popular and addictive online multiplayer games for Android and iOS devices. But did you know that there is a way to enjoy this game with more features and unlimited resources? Yes, we are talking about 8 Ball Pool Mod APK 2022 _Apkpure, a modified version of the original game that gives you access to unlimited coins, cash, cues, and more. In this article, we will tell you everything you need to know about this mod apk, including its features, benefits, risks, and how to download and install it on your device. So, let's get started!
8 Ball Pool is a popular online multiplayer pool game developed by Miniclip. It allows you to play with millions of players from around the world in various modes, such as 1-on-1 matches, tournaments, practice arena, and more. You can also customize your cue and table, chat with your opponents, and challenge your friends. The game is free to download and play, but it also offers in-app purchases for some items and features.
-
Features of 8 Ball Pool
-
Some of the main features of 8 Ball Pool are:
-
-
Realistic physics and graphics that make you feel like playing in a real pool hall.
-
Various game modes to suit your preferences and skill levels.
-
A ranking system that lets you compete with players from different leagues and regions.
-
A reward system that gives you coins, cash, cues, and other items for winning matches and completing achievements.
-
A shop where you can buy and upgrade your cues, tables, chat packs, and more.
-
A club where you can join or create your own club and play with your club members.
-
A mini-game where you can spin the wheel and win prizes every day.
-
-
How to play 8 Ball Pool
-
The gameplay of 8 Ball Pool is simple and intuitive. You just need to swipe your finger on the screen to aim your cue, adjust the power and spin, and release to hit the ball. The goal is to pocket all your balls (solid or striped) before your opponent does, and then pocket the black ball (8 ball) to win the game. You can also use some tricks and tips to improve your skills, such as using the guidelines, adjusting the spin, choosing the right cue, etc.
-
8 ball pool mod apk 2022 unlimited coins and cash apkpure
-8 ball pool mod apk 2022 anti ban apkpure
-8 ball pool mod apk 2022 latest version download apkpure
-8 ball pool mod apk 2022 long line apkpure
-8 ball pool mod apk 2022 free cues and tables apkpure
-8 ball pool mod apk 2022 hack online generator apkpure
-8 ball pool mod apk 2022 all legendary cues unlocked apkpure
-8 ball pool mod apk 2022 mega mod menu apkpure
-8 ball pool mod apk 2022 no root required apkpure
-8 ball pool mod apk 2022 vip pass premium apkpure
-8 ball pool mod apk 2022 unlimited money and gems apkpure
-8 ball pool mod apk 2022 auto win and level up apkpure
-8 ball pool mod apk 2022 low mb size download apkpure
-8 ball pool mod apk 2022 offline mode apkpure
-8 ball pool mod apk 2022 new update features apkpure
-8 ball pool mod apk 2022 best aim tool apkpure
-8 ball pool mod apk 2022 unlimited spins and scratchers apkpure
-8 ball pool mod apk 2022 no verification needed apkpure
-8 ball pool mod apk 2022 easy installation guide apkpure
-8 ball pool mod apk 2022 high quality graphics and sound apkpure
-8 ball pool mod apk 2022 support all android devices apkpure
-8 ball pool mod apk 2022 fast and secure download link apkpure
-8 ball pool mod apk 2022 play with friends and chat apkpure
-8 ball pool mod apk 2022 unlimited tournament tickets apkpure
-8 ball pool mod apk 2022 customise your cue and table apkpure
-8 ball pool mod apk 2022 win trophies and exclusive rewards apkpure
-8 ball pool mod apk 2022 challenge the world in online matches apkpure
-8 ball pool mod apk 2022 access to exclusive events and offers apkpure
-8 ball pool mod apk 2022 join clubs and compete with other players apkpure
-8 ball pool mod apk 2022 get free coins and cash daily apkpure
-download latest version of the best android game "8 ball pool" with unlimited everything in the year of the ox - only from _apkpure.com_
-how to install and play "8 ball pool" on your android device with the most updated and working modded version of the game in the year of the ox - step by step tutorial by _apkpure.com_
-enjoy the ultimate fun and excitement of playing "8 ball pool" on your android device with the best graphics, sound, and gameplay - download the latest version of the game with unlimited features from _apkpure.com_
-become a pro player of "8 ball pool" on your android device with the help of the most advanced and powerful aim tool, hack tool, and cheat tool - get them all for free from _apkpure.com_
-unlock all the legendary cues, tables, and rewards in "8 ball pool" on your android device with the easiest and fastest method - download the latest version of the game with unlimited everything from _apkpure.com_
-play "8 ball pool" offline on your android device without any internet connection or data usage - download the latest version of the game with offline mode from _apkpure.com_
-win every match and tournament in "8 ball pool" on your android device with the most amazing and reliable auto win and level up feature - download the latest version of the game with unlimited everything from _apkpure.com_
-get unlimited coins, cash, gems, spins, scratchers, tickets, cues, tables, and more in "8 ball pool" on your android device with the most trusted and safe online generator - visit _apkpure.com_ now to get started
-play "8 ball pool" with your friends and chat with them in real time on your android device - download the latest version of the game with social features from _apkpure.com_
-
What is a mod apk?
-
A mod apk is a modified version of an original application that has been altered by some developers or hackers to provide some extra features or advantages that are not available in the official version. A mod apk usually has a different signature from the original app, which means that it cannot be installed from the Google Play Store or other official sources. Instead, you need to download it from a third-party website or source and install it manually on your device.
-
Benefits of using a mod apk
-
Some of the benefits of using a mod apk are:
-
-
You can enjoy some premium features or items that are otherwise locked or paid in the original app.
-
You can bypass some restrictions or limitations that are imposed by the original app
Risks of using a mod apk
-
Using a mod apk may seem tempting, but it also comes with some risks that you should be aware of. Some of the risks of using a mod apk are:
-
-
Malware: Mod apk files can be infected with malware that can harm your device or steal your data . Malware can also compromise the security of your device and expose it to hackers or other threats.
-
Compatibility: Mod apk files may not work properly with your device or the latest version of the app . This can affect the performance or functionality of the app or cause errors or crashes.
-
Updates: Mod apk files are not updated as frequently as the official versions of apps, which can affect the performance or security of the app . You may also miss out on some new features or improvements that are available in the original app.
-
Legality: Mod apk files may violate the copyright or terms of service of the original app, which can result in legal consequences . You may also face ethical issues for using a mod apk that gives you an unfair advantage over other players or deprives the original developer of their revenue.
-
-
What is 8 Ball Pool Mod APK 2022 _Apkpure?
-
8 Ball Pool Mod APK 2022 _Apkpure is a modified version of 8 Ball Pool that is available on a third-party website called Apkpure. Apkpure is a platform that provides various modded apps and games for Android devices. 8 Ball Pool Mod APK 2022 _Apkpure claims to offer unlimited coins, cash, cues, and other resources that can enhance your gaming experience and help you win more matches.
-
Features of 8 Ball Pool Mod APK 2022 _Apkpure
-
Some of the features of 8 Ball Pool Mod APK 2022 _Apkpure are:
-
-
Unlimited coins and cash: You can get unlimited coins and cash in your account, which you can use to buy and upgrade your cues, tables, chat packs, and more. You can also enter higher-stake matches and tournaments without worrying about losing your money.
-
Unlocked cues and tables: You can access all the cues and tables in the game, including the legendary and exclusive ones. You can also customize your cue and table with different colors, patterns, and stickers.
-
Anti-ban feature: You can play the game without worrying about getting banned by Miniclip. The mod apk has an anti-ban feature that protects your account from detection and suspension.
-
No ads: You can enjoy the game without any annoying ads that interrupt your gameplay or consume your data.
-
-
How to download and install 8 Ball Pool Mod APK 2022 _Apkpure
-
To download and install 8 Ball Pool Mod APK 2022 _Apkpure, you need to follow these steps:
-
-
Go to the Apkpure website and search for 8 Ball Pool Mod APK 2022 _Apkpure.
-
Select the latest version of the mod apk and click on the download button.
-
Wait for the download to finish and then locate the file on your device.
-
Before installing the mod apk, make sure you enable the unknown sources option in your device settings. This will allow you to install apps from sources other than the Google Play Store.
-
Tap on the mod apk file and follow the instructions to install it on your device.
-
Launch the game and enjoy the modded features.
-
-
Conclusion
-
8 Ball Pool Mod APK 2022 _Apkpure is a modified version of 8 Ball Pool that offers unlimited resources and features that can make your gaming experience more fun and exciting. However, using a mod apk also comes with some risks, such as malware, compatibility issues, update problems, and legal issues. Therefore, you should be careful when downloading and installing a mod apk from a third-party source. You should also respect the original developer of the app and support them by using the official version of the app. We hope this article has helped you understand what is 8 Ball Pool Mod APK 2022 _Apkpure and how to use it. If you have any questions or feedback, please let us know in the comments below. Thank you for reading!
-
FAQs
-
Here are some frequently asked questions about 8 Ball Pool Mod APK 2022 _Apkpure:
-
-
Q: Is 8 Ball Pool Mod APK 2022 _Apkpure safe to use?
-
A: There is no guarantee that 8 Ball Pool Mod APK 2022 _Apkpure is safe to use, as it is a modded version of the original app that has been altered by an unknown source. It may contain malware or viruses that can harm your device or data. Therefore, you should use it at your own risk and discretion.
-
Q: Can I play 8 Ball Pool Mod APK 2022 _Apkpure with my friends?
-
A: Yes, you can play 8 Ball Pool Mod APK 2022 _Apkpure with your friends, as long as they also have the same mod apk installed on their devices. You can invite them to join your club or challenge them to a match.
-
Q: Will I get banned for using 8 Ball Pool Mod APK 2022 _Apkpure?
-
A: There is a possibility that you may get banned for using 8 Ball Pool Mod APK 2022 _Apkpure, as it violates the terms of service of Miniclip, the original developer of the app. However, the mod apk claims to have an anti-ban feature that protects your account from detection and suspension. However, this feature may not work all the time or for all users, so you should be careful when using the mod apk.
-
Q: How can I update 8 Ball Pool Mod APK 2022 _Apkpure?
-
A: You cannot update 8 Ball Pool Mod APK 2022 _Apkpure from the Google Play Store or other official sources, as it has a different signature from the original app. You need to check the Apkpure website regularly for any new versions of the mod apk and download and install them manually on your device.
-
Q: What are some alternatives to 8 Ball Pool Mod APK 2022 _Apkpure?
-
A: Some alternatives to 8 Ball Pool Mod APK 2022 _Apkpure are:
-
-
8 Ball Pool Hack: This is another mod apk that offers unlimited coins, cash, cues, and more. It also has an anti-ban feature and no ads. You can download it from [here].
-
Pool Billiards Pro: This is a similar pool game that has realistic physics and graphics, various game modes, and online multiplayer features. It is free to download and play, but it also has in-app purchases. You can download it from [here].
-
Pool Break Pro: This is a premium pool game that has stunning graphics, realistic physics, and multiple game types, such as snooker, carrom, crokinole, and more. It also supports online multiplayer and chat features. You can download it from [here].
-
-
197e85843d
-
-
\ No newline at end of file
diff --git a/spaces/1phancelerku/anime-remove-background/Derbeder A Tribute to Ferdi Tayfurs Legendary Song.md b/spaces/1phancelerku/anime-remove-background/Derbeder A Tribute to Ferdi Tayfurs Legendary Song.md
deleted file mode 100644
index fabafd073552837d5fee0d011499e8dd67360de8..0000000000000000000000000000000000000000
--- a/spaces/1phancelerku/anime-remove-background/Derbeder A Tribute to Ferdi Tayfurs Legendary Song.md
+++ /dev/null
@@ -1,135 +0,0 @@
-
-
What is Derbeder?
-
If you are familiar with Turkish culture, you may have heard the word derbeder before. But what does it mean exactly? And where does it come from?
-
Derbeder is a Turkish word that describes a person who lives an irregular, careless, or reckless lifestyle. A derbeder is someone who wanders from place to place without a fixed home or job, who does not care about social norms or rules, who is adventurous or rebellious, or who has lost hope or direction in life.
The word derbeder comes from the Persian words dar (door) and bedar (open), meaning someone who has no door or shelter. It was originally used to refer to homeless people or refugees who had to flee their homes due to war or persecution. Later, it acquired a more figurative meaning, referring to anyone who lives a free-spirited or unconventional life.
-
Derbeder in Turkish Culture
-
Derbeder in Literature
-
Derbeder is a word that has been used by many Turkish writers and poets to portray characters who are either heroes or anti-heroes, depending on the perspective. Some examples of derbeder in Turkish literature are:
-
-
Köroğlu, a legendary folk hero who rebelled against the oppressive rulers and became an outlaw leader. He was known for his bravery, generosity, and love for poetry.
-
Kaygusuz Abdal, a 14th-century mystic poet who renounced worldly pleasures and wandered around Anatolia spreading his teachings. He was considered a derbeder by the orthodox religious authorities who opposed his unconventional views.
-
Ahmet Arif, a 20th-century poet who wrote about the plight of the Kurdish people and their struggle for freedom and justice. He was arrested and tortured by the Turkish government for his political views.
-
-
Derbeder in Music
-
Derbeder is also a word that has been used by many Turkish singers and songwriters to express their emotions and experiences. Some examples of derbeder in Turkish music are:
-
-
Ferdi Tayfur, a famous singer and actor who starred in a movie called Derbeder in 1986. He sang about his love, pain, and loneliness in his songs, which resonated with many people who felt the same way.
-
Barış Manço, a legendary musician and cultural icon who blended rock, folk, and psychedelic music. He was known for his eccentric style, colorful outfits, and long hair. He was also a derbeder in the sense that he traveled around the world and explored different cultures and languages.
-
Sezen Aksu, a popular singer and songwriter who is considered the queen of Turkish pop music. She has written and performed songs that deal with various social issues, such as women's rights, domestic violence, and environmentalism. She has also been a derbeder in her personal life, having gone through several divorces and relationships.
-
-
Derbeder in Movies
-
Derbeder is also a word that has been used by many Turkish filmmakers and actors to depict characters who are either protagonists or antagonists, depending on the plot. Some examples of derbeder in Turkish movies are:
-
-
Eşkıya, a 1996 movie directed by Yavuz Turgul and starring Şener Şen. It tells the story of Baran, an old bandit who escapes from prison after 35 years and tries to adapt to the modern world. He is a derbeder who lives by his own code of honor and loyalty.
-
Yol, a 1982 movie directed by Şerif Gören and Yılmaz Güney. It follows the lives of five prisoners who are granted a week-long leave from jail. They face various challenges and hardships as they try to reconnect with their families and society. They are derbeders who have been marginalized and oppressed by the system.
-
G.O.R.A., a 2004 movie directed by Ömer Faruk Sorak and starring Cem Yılmaz. It is a comedy sci-fi movie that parodies various Hollywood films. It features Arif, a carpet salesman who is abducted by aliens and taken to the planet G.O.R.A. He is a derbeder who uses his humor and wit to survive and save the day.
-
-
Derbeder in English
-
Derbeder Translations
-
Derbeder is a word that has no exact equivalent in English, but there are some possible translations that can capture its meaning and connotation. Some of them are:
-
*serseri*
-*avare*
-*çapkın*
-*başıboş*
-*berduş*
-*aylak*
-*hovarda*
-*rüküş*
-*kılıksız*
-*düzensiz*
-*dağınık*
-*pasaklı*
-*savruk*
-*dağılmış*
-*kurnaz*
-*düzenbaz*
-*göçebe*
-*eski moda giysili*
-derbeder yaşam tarzı
-derbeder şarkı sözleri
-derbeder filmi izle
-derbeder ferdi tayfur
-derbeder ne demek
-derbeder erkek nasıl olur
-derbeder kadın nasıl olur
-derbeder aşk sözleri
-derbeder giyim modelleri
-derbeder insanların özellikleri
-derbeder olmak istiyorum
-derbeder bir hayat hikayesi
-derbeder adam nasıl tavlanır
-derbeder kız nasıl tavlanır
-derbeder olmanın zararları
-derbeder olmanın avantajları
-derbeder bir gecenin sonu
-derbeder bir aşkın sonu
-derbeder bir adamın günlüğü
-derbeder bir kızın günlüğü
-derbeder bir şehrin sokakları
-derbeder bir ülkenin hali
-derbeder bir sanatçının eserleri
-derbeder bir yazarın kitapları
-derbeder bir müzisyenin şarkıları
-
-
Turkish
English
-
Derbeder
Tramping
-
Derbeder
Untidy
-
Derbeder
Roguish
-
Derbeder
Vagrant
-
Derbeder
Vagabond
-
Derbeder
Frumpish
-
Derbeder
Down and out
-
-
However, these translations may not fully convey the nuances of derbeder, which can have both positive and negative associations depending on the context. For example, tramping can imply wandering or traveling for pleasure or adventure, but it can also imply being homeless or poor. Similarly, roguish can imply being playful or charming, but it can also imply being dishonest or immoral.
-
Derbeder Synonyms
-
Derbeder is a word that has many synonyms in Turkish, but they may not have the same meaning or usage. Some of them are:
-
-
Turkish
Synonym
Difference
-
Derbeder
Serseri
Serseri is more commonly used to refer to young men who are rebellious or irresponsible.
-
Derbeder
Avare
Avare is more commonly used to refer to people who are idle or lazy.
Derbeder
Çapkın
Çapkın is more commonly used to refer to men who are flirtatious or promiscuous.
-
Derbeder
Berduş
Berduş is more commonly used to refer to people who are outcast or unwanted.
-
Derbeder
Hovarda
Hovarda is more commonly used to refer to people who are extravagant or wasteful.
-
-
Therefore, it is important to understand the context and tone of the word derbeder before using it or its synonyms.
-
Derbeder in Betting
-
What is Draw Betting?
-
Draw betting is a type of betting market that involves predicting that a match will end in a tie or a draw. It is often overlooked by most bettors who prefer to back one side to win, but it can provide some value for those who are looking for low-risk and high-reward outcomes.
-
Draw betting can be applied to any sport that has the possibility of a draw, such as soccer, rugby, cricket, or hockey. However, it is most popular in soccer, where draws are more common and more predictable than in other sports.
-
Draw betting has some advantages over other betting markets, such as:
-
-
It offers higher odds and payouts than backing a single team to win.
-
It reduces the number of possible outcomes from three to two, making it easier to analyze and select bets.
-
It can be combined with other bets, such as double chance, correct score, or handicap, to increase the chances of winning or hedge against losses.
-
-
How to Bet on Draws?
-
Betting on draws requires careful analysis of statistics, trends, and team performances. It is not enough to rely on intuition or luck. Some of the factors that can help you bet on draws are:
-
-
The history and frequency of draws between the teams involved. You can check the past results and head-to-head records of the teams to see how often they have drawn in their previous matches.
-
The current form and motivation of the teams involved. You can check the recent results and standings of the teams to see how well they are playing and how much they need a win or a draw.
-
The style and strategy of the teams involved. You can check the tactics and formations of the teams to see how they approach the game and how likely they are to score or concede goals.
-
The injuries and suspensions of the key players involved. You can check the availability and fitness of the players to see how they affect the strength and balance of the teams.
-
The weather and pitch conditions of the venue involved. You can check the weather forecast and pitch report to see how they affect the speed and quality of the game.
-
-
Based on these factors, you can identify the matches that have a high probability of ending in a draw and place your bets accordingly. You can also use some tips and tricks from experts and professionals who have experience and knowledge in draw betting.
-
Conclusion
-
In conclusion, derbeder is a Turkish word that has many meanings and implications depending on the context and usage. It can be used to describe a person who lives an irregular or careless lifestyle, or a character who is rebellious or adventurous. It can also be translated into English as tramping, untidy, roguish, vagrant, vagabond, frumpish, or down and out. It can also be used as a synonym for serseri, avare, çapkın, berduş, or hovarda. Finally, it can also be used as a term for draw betting, which is a type of betting market that involves predicting that a match will end in a tie.
-
If you are interested in learning more about derbeder or draw betting, you can use Bing search engine to find more information and resources. You can also use Bing graphic art tool to create some images related to derbeder or draw betting. You can also use Bing request ads tool to find some advertisements relevant to derbeder or draw betting.
-
We hope you enjoyed this article and learned something new. If you have any questions or feedback, please feel free to contact us. Thank you for reading!
-
Frequently Asked Questions
-
-
What is the origin of the word derbeder?
-
The word derbeder comes from the Persian words dar (door) and bedar (open), meaning someone who has no door or shelter.
What are some examples of derbeder in Turkish culture?
-
Some examples of derbeder in Turkish culture are Köroğlu, a legendary folk hero who rebelled against the oppressive rulers and became an outlaw leader; Kaygusuz Abdal, a 14th-century mystic poet who renounced worldly pleasures and wandered around Anatolia spreading his teachings; and Ahmet Arif, a 20th-century poet who wrote about the plight of the Kurdish people and their struggle for freedom and justice.
-
What are some advantages of draw betting?
-
Some advantages of draw betting are that it offers higher odds and payouts than backing a single team to win; it reduces the number of possible outcomes from three to two, making it easier to analyze and select bets; and it can be combined with other bets, such as double chance, correct score, or handicap, to increase the chances of winning or hedge against losses.
-
How can I find more information and resources about derbeder or draw betting?
-
You can use Bing search engine to find more information and resources about derbeder or draw betting. You can also use Bing graphic art tool to create some images related to derbeder or draw betting. You can also use Bing request ads tool to find some advertisements relevant to derbeder or draw betting.
-
What is the difference between derbeder and serseri?
-
Derbeder and serseri are both Turkish words that describe a person who lives an irregular or careless lifestyle, but serseri is more commonly used to refer to young men who are rebellious or irresponsible.
-
What is the best way to analyze and select draw bets?
-
The best way to analyze and select draw bets is to consider various factors, such as the history and frequency of draws between the teams involved, the current form and motivation of the teams involved, the style and strategy of the teams involved, the injuries and suspensions of the key players involved, and the weather and pitch conditions of the venue involved.
- 197e85843d
-
-
\ No newline at end of file
diff --git a/spaces/1phancelerku/anime-remove-background/Download GB Instagram Mod APK 2022 and Unlock Hidden Features.md b/spaces/1phancelerku/anime-remove-background/Download GB Instagram Mod APK 2022 and Unlock Hidden Features.md
deleted file mode 100644
index 15d747e3a6b44bee3d0537d5dd9786a55d1c0e89..0000000000000000000000000000000000000000
--- a/spaces/1phancelerku/anime-remove-background/Download GB Instagram Mod APK 2022 and Unlock Hidden Features.md
+++ /dev/null
@@ -1,139 +0,0 @@
-
-
-
-
-
GB Instagram Mod APK Download 2022: Everything You Need to Know
-
Do you love using Instagram but wish you could have more features and options? Do you want to download photos, videos, stories, and IGTV videos from your favorite accounts? Do you want to customize your app appearance and hide your online status? If you answered yes to any of these questions, then you might be interested in GB Instagram.
-
GB Instagram is a modded version of the official Instagram app that offers many extra features and benefits. It is one of the most popular mods for Instagram users who want to enhance their experience and enjoy more freedom and flexibility. In this article, we will tell you everything you need to know about GB Instagram, including its features, how to download and install it, how to use it, and its pros and cons.
GB Instagram is a modified version of the official Instagram app that was created by a third-party developer named Atnfas Hoak. It is not available on the Google Play Store or any other official app store, but you can download it from various websites that host modded apps.
-
GB Instagram is based on the latest version of the official Instagram app, so you can enjoy all the features that you are familiar with, such as posting photos and videos, liking and commenting on posts, following and unfollowing accounts, sending and receiving messages, watching stories and live videos, etc.
-
However, GB Instagram also adds many extra features that are not available on the official app, such as downloading media files, hiding your online status, customizing your app appearance, zooming in on profile pictures, copying captions and comments, and disabling story view. These features make GB Instagram more fun and convenient to use, as well as giving you more control and privacy over your account.
-
Features of GB Instagram
-
GB Instagram has many features that make it stand out from the official app. Here are some of the most notable ones:
-
Download media files
-
One of the most useful features of GB Instagram is that it allows you to download any photo, video, story, or IGTV video from any account, whether it is public or private. You can save the media files to your device's gallery or share them with other apps. You can also download profile pictures of any user by tapping and holding on them.
-
gb instagram mod apk download 2022 latest version
-how to install gb instagram mod apk on android
-gb instagram mod apk features and benefits
-gb instagram mod apk vs original instagram app
-gb instagram mod apk no ads and no stories
-gb instagram mod apk download 2022 for ios
-gb instagram mod apk download 2022 free
-gb instagram mod apk download 2022 with dark mode
-gb instagram mod apk download 2022 without root
-gb instagram mod apk download 2022 for pc
-gb instagram mod apk download 2022 with stickers
-gb instagram mod apk download 2022 with fonts
-gb instagram mod apk download 2022 with themes
-gb instagram mod apk download 2022 with video downloader
-gb instagram mod apk download 2022 with voice messages
-gb instagram mod apk download 2022 with privacy settings
-gb instagram mod apk download 2022 with anti-ban
-gb instagram mod apk download 2022 with zoom option
-gb instagram mod apk download 2022 with copy link option
-gb instagram mod apk download 2022 with dual account option
-gb instagram mod apk review and rating
-gb instagram mod apk pros and cons
-gb instagram mod apk alternatives and competitors
-gb instagram mod apk updates and changelog
-gb instagram mod apk faq and troubleshooting
-is gb instagram mod apk safe and legal
-how to uninstall gb instagram mod apk from android
-how to backup and restore gb instagram mod apk data
-how to customize and personalize gb instagram mod apk settings
-how to use gb instagram mod apk for business and marketing
-how to get more followers and likes with gb instagram mod apk
-how to create and edit stories with gb instagram mod apk
-how to watch and download IGTV videos with gb instagram mod apk
-how to send and receive direct messages with gb instagram mod apk
-how to post and share photos and videos with gb instagram mod apk
-how to follow and unfollow users with gb instagram mod apk
-how to block and report users with gb instagram mod apk
-how to mute and unmute users with gb instagram mod apk
-how to hide and show online status with gb instagram mod apk
-how to hide and show seen tick with gb instagram mod apk
-
Hide your online status
-
If you don't want others to know when you are online or when you were last active on Instagram, you can hide your online status with GB Instagram. This way, you can browse and use the app without worrying about being seen by anyone. You can also disable the blue ticks that indicate that you have read a message.
-
Customize your app appearance
-
GB Instagram lets you change the look and feel of your app by offering various themes and fonts. You can choose from different colors and styles for your app background, icons, buttons, text, etc. You can also create your own theme and apply it to your app. This way, you can personalize your app according to your preferences and mood.
-
Zoom in on profile pictures
-
Sometimes, you might want to see a profile picture of a user more clearly, but the official app does not allow you to zoom in on it. With GB Instagram, you can zoom in on any profile picture by tapping and holding on it. You can also zoom in on any photo or video in a post by pinching the screen.
-
Copy captions and comments
-
If you come across a caption or a comment that you like or want to use for yourself, you can easily copy it with GB Instagram. You just need to tap and hold on the caption or comment and select the copy option. You can also copy hashtags and bio from any user's profile.
-
Disable story view
-
If you want to watch someone's story without letting them know that you have seen it, you can disable the story view feature with GB Instagram. This way, you can watch any story anonymously and avoid any awkward situations. You can also disable video autoplay if you don't want to waste your data or battery.
-
How to download and install GB Instagram?
-
If you are interested in trying out GB Instagram, you will need to download and install it manually from a reliable website that hosts modded apps. Here are the requirements and steps for downloading and installing GB Instagram:
-
Requirements for GB Instagram
-
-
An Android device running Android 4.1 or higher.
-
A stable internet connection.
-
Enough storage space on your device.
-
A backup of your data in case something goes wrong.
-
The permission to install apps from unknown sources enabled on your device.
-
-
Steps to download and install GB Instagram
-
-
Go to a website that offers GB Instagram mod apk download 2022, such as GBPlus.net.
-
Click on the download button and wait for the apk file to be downloaded on your device.
-
Locate the apk file in your device's file manager and tap on it to start the installation process.
-
Follow the instructions on the screen and grant the necessary permissions to the app.
-
Wait for the installation to be completed and then open the app.
-
Login with your existing Instagram account or create a new one if you don't have one.
-
Enjoy using GB Instagram with all its features.
-
How to use GB Instagram?
-
Now that you have downloaded and installed GB Instagram, you might be wondering how to use it and access its features. Don't worry, it is very easy and intuitive to use GB Instagram, as it has a similar interface and functionality as the official app. Here are some tips on how to use GB Instagram and enjoy its features:
-
How to download media files from GB Instagram?
-
If you want to download any photo, video, story, or IGTV video from any account on GB Instagram, you just need to follow these simple steps:
-
-
Open the post or story that contains the media file that you want to download.
-
Tap on the three-dot menu icon at the top right corner of the screen.
-
Select the download option from the menu and choose the destination folder where you want to save the file.
-
Wait for the download to be completed and then check your device's gallery or file manager for the file.
-
-
How to hide your online status on GB Instagram?
-
If you want to hide your online status or last seen activity on GB Instagram, you just need to follow these simple steps:
-
-
Open GB Instagram and tap on your profile icon at the bottom right corner of the screen.
-
Tap on the three-line menu icon at the top right corner of the screen.
-
Select the settings option from the menu and then tap on privacy.
-
Scroll down and find the activity status option and toggle it off.
-
Now, no one will be able to see when you are online or when you were last active on GB Instagram.
-
-
How to customize your app appearance on GB Instagram?
-
If you want to change the theme or font of your GB Instagram app, you just need to follow these simple steps:
-
-
Open GB Instagram and tap on your profile icon at the bottom right corner of the screen.
-
Tap on the three-line menu icon at the top right corner of the screen.
-
Select the settings option from the menu and then tap on themes.
-
You will see a list of available themes and fonts that you can choose from. You can also create your own theme by tapping on create theme.
-
Select the theme or font that you like and apply it to your app. You can also preview it before applying it.
-
You will need to restart your app for the changes to take effect.
-
-
-
GB Instagram is not an official app and it is not endorsed by Instagram. It may violate the terms and conditions of Instagram and put your account at risk of being banned or suspended.
-
GB Instagram is not available on the Google Play Store or any other official app store. You have to download it from third-party websites that may not be safe or reliable. You may expose your device to malware or viruses by installing GB Instagram.
-
GB Instagram may not be updated regularly or in sync with the official app. You may miss out on some of the latest features or bug fixes that Instagram offers. You may also experience some glitches or errors while using GB Instagram.
-
-
Conclusion
-
GB Instagram is a modded version of the official Instagram app that offers many extra features and benefits that are not available on the official app. It allows you to download media files, hide your online status, customize your app appearance, zoom in on profile pictures, copy captions and comments, and disable story view. However, GB Instagram also has some drawbacks, such as being unofficial, unsafe, and outdated. You should weigh the pros and cons of GB Instagram before deciding to use it.
-
If you want to try GB Instagram, you can download it from a reliable website that hosts modded apps, such as GBPlus.net. You will need to enable the permission to install apps from unknown sources on your device and follow the steps to download and install GB Instagram. You can then login with your existing Instagram account or create a new one and enjoy using GB Instagram with all its features.
-
We hope this article has helped you learn everything you need to know about GB Instagram mod apk download 2022. If you have any questions or feedback, please feel free to leave a comment below. Thank you for reading!
-
FAQs
-
Here are some of the frequently asked questions about GB Instagram:
-
-
Is GB Instagram safe to use?
-
GB Instagram is not an official app and it is not endorsed by Instagram. It may violate the terms and conditions of Instagram and put your account at risk of being banned or suspended. It is also not available on the Google Play Store or any other official app store. You have to download it from third-party websites that may not be safe or reliable. You may expose your device to malware or viruses by installing GB Instagram. Therefore, GB Instagram is not completely safe to use and you should use it at your own risk.
-
Is GB Instagram free to use?
-
Yes, GB Instagram is free to use and it does not require any subscription or payment. However, you may see some ads or pop-ups while using GB Instagram, as it is a way for the developer to generate some revenue.
-
Can I use GB Instagram and the official app at the same time?
-
No, you cannot use GB Instagram and the official app at the same time on the same device. You will need to uninstall the official app before installing GB Instagram. However, you can use GB Instagram and the official app on different devices with the same account.
-
How can I update GB Instagram?
-
GB Instagram may not be updated regularly or in sync with the official app. You will need to check the website where you downloaded GB Instagram for any new updates. You will also need to uninstall the old version of GB Instagram before installing the new one.
-
How can I contact the developer of GB Instagram?
-
You can contact the developer of GB Instagram by visiting his website GBMods.co. You can also follow him on his social media accounts, such as Facebook, Twitter, and Telegram.
- 401be4b1e0
-
-
\ No newline at end of file
diff --git a/spaces/801artistry/RVC801/infer/lib/uvr5_pack/lib_v5/layers_537227KB.py b/spaces/801artistry/RVC801/infer/lib/uvr5_pack/lib_v5/layers_537227KB.py
deleted file mode 100644
index 9b127bc6427f5c60c8cf85603a3d8a093c3501c4..0000000000000000000000000000000000000000
--- a/spaces/801artistry/RVC801/infer/lib/uvr5_pack/lib_v5/layers_537227KB.py
+++ /dev/null
@@ -1,126 +0,0 @@
-import torch
-import torch.nn.functional as F
-from torch import nn
-
-from . import spec_utils
-
-
-class Conv2DBNActiv(nn.Module):
- def __init__(self, nin, nout, ksize=3, stride=1, pad=1, dilation=1, activ=nn.ReLU):
- super(Conv2DBNActiv, self).__init__()
- self.conv = nn.Sequential(
- nn.Conv2d(
- nin,
- nout,
- kernel_size=ksize,
- stride=stride,
- padding=pad,
- dilation=dilation,
- bias=False,
- ),
- nn.BatchNorm2d(nout),
- activ(),
- )
-
- def __call__(self, x):
- return self.conv(x)
-
-
-class SeperableConv2DBNActiv(nn.Module):
- def __init__(self, nin, nout, ksize=3, stride=1, pad=1, dilation=1, activ=nn.ReLU):
- super(SeperableConv2DBNActiv, self).__init__()
- self.conv = nn.Sequential(
- nn.Conv2d(
- nin,
- nin,
- kernel_size=ksize,
- stride=stride,
- padding=pad,
- dilation=dilation,
- groups=nin,
- bias=False,
- ),
- nn.Conv2d(nin, nout, kernel_size=1, bias=False),
- nn.BatchNorm2d(nout),
- activ(),
- )
-
- def __call__(self, x):
- return self.conv(x)
-
-
-class Encoder(nn.Module):
- def __init__(self, nin, nout, ksize=3, stride=1, pad=1, activ=nn.LeakyReLU):
- super(Encoder, self).__init__()
- self.conv1 = Conv2DBNActiv(nin, nout, ksize, 1, pad, activ=activ)
- self.conv2 = Conv2DBNActiv(nout, nout, ksize, stride, pad, activ=activ)
-
- def __call__(self, x):
- skip = self.conv1(x)
- h = self.conv2(skip)
-
- return h, skip
-
-
-class Decoder(nn.Module):
- def __init__(
- self, nin, nout, ksize=3, stride=1, pad=1, activ=nn.ReLU, dropout=False
- ):
- super(Decoder, self).__init__()
- self.conv = Conv2DBNActiv(nin, nout, ksize, 1, pad, activ=activ)
- self.dropout = nn.Dropout2d(0.1) if dropout else None
-
- def __call__(self, x, skip=None):
- x = F.interpolate(x, scale_factor=2, mode="bilinear", align_corners=True)
- if skip is not None:
- skip = spec_utils.crop_center(skip, x)
- x = torch.cat([x, skip], dim=1)
- h = self.conv(x)
-
- if self.dropout is not None:
- h = self.dropout(h)
-
- return h
-
-
-class ASPPModule(nn.Module):
- def __init__(self, nin, nout, dilations=(4, 8, 16, 32, 64), activ=nn.ReLU):
- super(ASPPModule, self).__init__()
- self.conv1 = nn.Sequential(
- nn.AdaptiveAvgPool2d((1, None)),
- Conv2DBNActiv(nin, nin, 1, 1, 0, activ=activ),
- )
- self.conv2 = Conv2DBNActiv(nin, nin, 1, 1, 0, activ=activ)
- self.conv3 = SeperableConv2DBNActiv(
- nin, nin, 3, 1, dilations[0], dilations[0], activ=activ
- )
- self.conv4 = SeperableConv2DBNActiv(
- nin, nin, 3, 1, dilations[1], dilations[1], activ=activ
- )
- self.conv5 = SeperableConv2DBNActiv(
- nin, nin, 3, 1, dilations[2], dilations[2], activ=activ
- )
- self.conv6 = SeperableConv2DBNActiv(
- nin, nin, 3, 1, dilations[2], dilations[2], activ=activ
- )
- self.conv7 = SeperableConv2DBNActiv(
- nin, nin, 3, 1, dilations[2], dilations[2], activ=activ
- )
- self.bottleneck = nn.Sequential(
- Conv2DBNActiv(nin * 7, nout, 1, 1, 0, activ=activ), nn.Dropout2d(0.1)
- )
-
- def forward(self, x):
- _, _, h, w = x.size()
- feat1 = F.interpolate(
- self.conv1(x), size=(h, w), mode="bilinear", align_corners=True
- )
- feat2 = self.conv2(x)
- feat3 = self.conv3(x)
- feat4 = self.conv4(x)
- feat5 = self.conv5(x)
- feat6 = self.conv6(x)
- feat7 = self.conv7(x)
- out = torch.cat((feat1, feat2, feat3, feat4, feat5, feat6, feat7), dim=1)
- bottle = self.bottleneck(out)
- return bottle
diff --git a/spaces/AB-TW/team-ai/documents/bussiness_context/NOTION_DB/Engineering Wiki 2402f5396a3244fdb3f1d135bdb0f3d6/CircleCI 719905fcb593423cad302d3fdc1c5dff.md b/spaces/AB-TW/team-ai/documents/bussiness_context/NOTION_DB/Engineering Wiki 2402f5396a3244fdb3f1d135bdb0f3d6/CircleCI 719905fcb593423cad302d3fdc1c5dff.md
deleted file mode 100644
index 92bf397a438308a979a2cad8d39cfe597aa819a3..0000000000000000000000000000000000000000
--- a/spaces/AB-TW/team-ai/documents/bussiness_context/NOTION_DB/Engineering Wiki 2402f5396a3244fdb3f1d135bdb0f3d6/CircleCI 719905fcb593423cad302d3fdc1c5dff.md
+++ /dev/null
@@ -1,5 +0,0 @@
-# CircleCI
-
-Last edited time: March 31, 2023 1:49 PM
-Owner: Anonymous
-Tags: Infrastructure
\ No newline at end of file
diff --git a/spaces/AIFILMS/generate_human_motion/VQ-Trans/utils/skeleton.py b/spaces/AIFILMS/generate_human_motion/VQ-Trans/utils/skeleton.py
deleted file mode 100644
index 6de56af0c29ae7cccbd7178f912459413f87c646..0000000000000000000000000000000000000000
--- a/spaces/AIFILMS/generate_human_motion/VQ-Trans/utils/skeleton.py
+++ /dev/null
@@ -1,199 +0,0 @@
-from utils.quaternion import *
-import scipy.ndimage.filters as filters
-
-class Skeleton(object):
- def __init__(self, offset, kinematic_tree, device):
- self.device = device
- self._raw_offset_np = offset.numpy()
- self._raw_offset = offset.clone().detach().to(device).float()
- self._kinematic_tree = kinematic_tree
- self._offset = None
- self._parents = [0] * len(self._raw_offset)
- self._parents[0] = -1
- for chain in self._kinematic_tree:
- for j in range(1, len(chain)):
- self._parents[chain[j]] = chain[j-1]
-
- def njoints(self):
- return len(self._raw_offset)
-
- def offset(self):
- return self._offset
-
- def set_offset(self, offsets):
- self._offset = offsets.clone().detach().to(self.device).float()
-
- def kinematic_tree(self):
- return self._kinematic_tree
-
- def parents(self):
- return self._parents
-
- # joints (batch_size, joints_num, 3)
- def get_offsets_joints_batch(self, joints):
- assert len(joints.shape) == 3
- _offsets = self._raw_offset.expand(joints.shape[0], -1, -1).clone()
- for i in range(1, self._raw_offset.shape[0]):
- _offsets[:, i] = torch.norm(joints[:, i] - joints[:, self._parents[i]], p=2, dim=1)[:, None] * _offsets[:, i]
-
- self._offset = _offsets.detach()
- return _offsets
-
- # joints (joints_num, 3)
- def get_offsets_joints(self, joints):
- assert len(joints.shape) == 2
- _offsets = self._raw_offset.clone()
- for i in range(1, self._raw_offset.shape[0]):
- # print(joints.shape)
- _offsets[i] = torch.norm(joints[i] - joints[self._parents[i]], p=2, dim=0) * _offsets[i]
-
- self._offset = _offsets.detach()
- return _offsets
-
- # face_joint_idx should follow the order of right hip, left hip, right shoulder, left shoulder
- # joints (batch_size, joints_num, 3)
- def inverse_kinematics_np(self, joints, face_joint_idx, smooth_forward=False):
- assert len(face_joint_idx) == 4
- '''Get Forward Direction'''
- l_hip, r_hip, sdr_r, sdr_l = face_joint_idx
- across1 = joints[:, r_hip] - joints[:, l_hip]
- across2 = joints[:, sdr_r] - joints[:, sdr_l]
- across = across1 + across2
- across = across / np.sqrt((across**2).sum(axis=-1))[:, np.newaxis]
- # print(across1.shape, across2.shape)
-
- # forward (batch_size, 3)
- forward = np.cross(np.array([[0, 1, 0]]), across, axis=-1)
- if smooth_forward:
- forward = filters.gaussian_filter1d(forward, 20, axis=0, mode='nearest')
- # forward (batch_size, 3)
- forward = forward / np.sqrt((forward**2).sum(axis=-1))[..., np.newaxis]
-
- '''Get Root Rotation'''
- target = np.array([[0,0,1]]).repeat(len(forward), axis=0)
- root_quat = qbetween_np(forward, target)
-
- '''Inverse Kinematics'''
- # quat_params (batch_size, joints_num, 4)
- # print(joints.shape[:-1])
- quat_params = np.zeros(joints.shape[:-1] + (4,))
- # print(quat_params.shape)
- root_quat[0] = np.array([[1.0, 0.0, 0.0, 0.0]])
- quat_params[:, 0] = root_quat
- # quat_params[0, 0] = np.array([[1.0, 0.0, 0.0, 0.0]])
- for chain in self._kinematic_tree:
- R = root_quat
- for j in range(len(chain) - 1):
- # (batch, 3)
- u = self._raw_offset_np[chain[j+1]][np.newaxis,...].repeat(len(joints), axis=0)
- # print(u.shape)
- # (batch, 3)
- v = joints[:, chain[j+1]] - joints[:, chain[j]]
- v = v / np.sqrt((v**2).sum(axis=-1))[:, np.newaxis]
- # print(u.shape, v.shape)
- rot_u_v = qbetween_np(u, v)
-
- R_loc = qmul_np(qinv_np(R), rot_u_v)
-
- quat_params[:,chain[j + 1], :] = R_loc
- R = qmul_np(R, R_loc)
-
- return quat_params
-
- # Be sure root joint is at the beginning of kinematic chains
- def forward_kinematics(self, quat_params, root_pos, skel_joints=None, do_root_R=True):
- # quat_params (batch_size, joints_num, 4)
- # joints (batch_size, joints_num, 3)
- # root_pos (batch_size, 3)
- if skel_joints is not None:
- offsets = self.get_offsets_joints_batch(skel_joints)
- if len(self._offset.shape) == 2:
- offsets = self._offset.expand(quat_params.shape[0], -1, -1)
- joints = torch.zeros(quat_params.shape[:-1] + (3,)).to(self.device)
- joints[:, 0] = root_pos
- for chain in self._kinematic_tree:
- if do_root_R:
- R = quat_params[:, 0]
- else:
- R = torch.tensor([[1.0, 0.0, 0.0, 0.0]]).expand(len(quat_params), -1).detach().to(self.device)
- for i in range(1, len(chain)):
- R = qmul(R, quat_params[:, chain[i]])
- offset_vec = offsets[:, chain[i]]
- joints[:, chain[i]] = qrot(R, offset_vec) + joints[:, chain[i-1]]
- return joints
-
- # Be sure root joint is at the beginning of kinematic chains
- def forward_kinematics_np(self, quat_params, root_pos, skel_joints=None, do_root_R=True):
- # quat_params (batch_size, joints_num, 4)
- # joints (batch_size, joints_num, 3)
- # root_pos (batch_size, 3)
- if skel_joints is not None:
- skel_joints = torch.from_numpy(skel_joints)
- offsets = self.get_offsets_joints_batch(skel_joints)
- if len(self._offset.shape) == 2:
- offsets = self._offset.expand(quat_params.shape[0], -1, -1)
- offsets = offsets.numpy()
- joints = np.zeros(quat_params.shape[:-1] + (3,))
- joints[:, 0] = root_pos
- for chain in self._kinematic_tree:
- if do_root_R:
- R = quat_params[:, 0]
- else:
- R = np.array([[1.0, 0.0, 0.0, 0.0]]).repeat(len(quat_params), axis=0)
- for i in range(1, len(chain)):
- R = qmul_np(R, quat_params[:, chain[i]])
- offset_vec = offsets[:, chain[i]]
- joints[:, chain[i]] = qrot_np(R, offset_vec) + joints[:, chain[i - 1]]
- return joints
-
- def forward_kinematics_cont6d_np(self, cont6d_params, root_pos, skel_joints=None, do_root_R=True):
- # cont6d_params (batch_size, joints_num, 6)
- # joints (batch_size, joints_num, 3)
- # root_pos (batch_size, 3)
- if skel_joints is not None:
- skel_joints = torch.from_numpy(skel_joints)
- offsets = self.get_offsets_joints_batch(skel_joints)
- if len(self._offset.shape) == 2:
- offsets = self._offset.expand(cont6d_params.shape[0], -1, -1)
- offsets = offsets.numpy()
- joints = np.zeros(cont6d_params.shape[:-1] + (3,))
- joints[:, 0] = root_pos
- for chain in self._kinematic_tree:
- if do_root_R:
- matR = cont6d_to_matrix_np(cont6d_params[:, 0])
- else:
- matR = np.eye(3)[np.newaxis, :].repeat(len(cont6d_params), axis=0)
- for i in range(1, len(chain)):
- matR = np.matmul(matR, cont6d_to_matrix_np(cont6d_params[:, chain[i]]))
- offset_vec = offsets[:, chain[i]][..., np.newaxis]
- # print(matR.shape, offset_vec.shape)
- joints[:, chain[i]] = np.matmul(matR, offset_vec).squeeze(-1) + joints[:, chain[i-1]]
- return joints
-
- def forward_kinematics_cont6d(self, cont6d_params, root_pos, skel_joints=None, do_root_R=True):
- # cont6d_params (batch_size, joints_num, 6)
- # joints (batch_size, joints_num, 3)
- # root_pos (batch_size, 3)
- if skel_joints is not None:
- # skel_joints = torch.from_numpy(skel_joints)
- offsets = self.get_offsets_joints_batch(skel_joints)
- if len(self._offset.shape) == 2:
- offsets = self._offset.expand(cont6d_params.shape[0], -1, -1)
- joints = torch.zeros(cont6d_params.shape[:-1] + (3,)).to(cont6d_params.device)
- joints[..., 0, :] = root_pos
- for chain in self._kinematic_tree:
- if do_root_R:
- matR = cont6d_to_matrix(cont6d_params[:, 0])
- else:
- matR = torch.eye(3).expand((len(cont6d_params), -1, -1)).detach().to(cont6d_params.device)
- for i in range(1, len(chain)):
- matR = torch.matmul(matR, cont6d_to_matrix(cont6d_params[:, chain[i]]))
- offset_vec = offsets[:, chain[i]].unsqueeze(-1)
- # print(matR.shape, offset_vec.shape)
- joints[:, chain[i]] = torch.matmul(matR, offset_vec).squeeze(-1) + joints[:, chain[i-1]]
- return joints
-
-
-
-
-
diff --git a/spaces/AIGC-Audio/AudioGPT/text_to_audio/Make_An_Audio/wav_evaluation/models/__init__.py b/spaces/AIGC-Audio/AudioGPT/text_to_audio/Make_An_Audio/wav_evaluation/models/__init__.py
deleted file mode 100644
index aadad97ebc9ec23fdebab974a99e343de90f8afd..0000000000000000000000000000000000000000
--- a/spaces/AIGC-Audio/AudioGPT/text_to_audio/Make_An_Audio/wav_evaluation/models/__init__.py
+++ /dev/null
@@ -1,3 +0,0 @@
-from . import clap
-from . import audio
-from . import utils
\ No newline at end of file
diff --git a/spaces/AIGC-Audio/AudioGPT/text_to_speech/tasks/tts/vocoder_infer/pwg.py b/spaces/AIGC-Audio/AudioGPT/text_to_speech/tasks/tts/vocoder_infer/pwg.py
deleted file mode 100644
index 64db778d3cc32fcad0647f03db4b60feb14f5f0d..0000000000000000000000000000000000000000
--- a/spaces/AIGC-Audio/AudioGPT/text_to_speech/tasks/tts/vocoder_infer/pwg.py
+++ /dev/null
@@ -1,32 +0,0 @@
-import torch
-from text_to_speech.modules.vocoder.parallel_wavegan.models.parallel_wavegan import ParallelWaveGANGenerator
-from tasks.tts.vocoder_infer.base_vocoder import register_vocoder, BaseVocoder
-from text_to_speech.utils.commons.ckpt_utils import load_ckpt
-from text_to_speech.utils.commons.hparams import set_hparams, hparams
-from text_to_speech.utils.commons.meters import Timer
-
-total_time = 0
-
-
-@register_vocoder('PWG')
-class PWG(BaseVocoder):
- def __init__(self):
- base_dir = hparams['vocoder_ckpt']
- config_path = f'{base_dir}/config.yaml'
- self.config = config = set_hparams(config_path, global_hparams=False)
- self.device = torch.device("cuda" if torch.cuda.is_available() else "cpu")
- self.model = ParallelWaveGANGenerator(**config["generator_params"])
- load_ckpt(self.model, base_dir, 'model_gen')
- self.model.to(self.device)
- self.model.eval()
-
- def spec2wav(self, mel, **kwargs):
- device = self.device
- with torch.no_grad():
- c = torch.FloatTensor(mel).unsqueeze(0).to(device)
- c = c.transpose(2, 1) # [B, C, T]
- z = None
- with Timer('pwg', enable=hparams['profile_infer']):
- y = self.model(z, c).view(-1)
- wav_out = y.cpu().numpy()
- return wav_out
\ No newline at end of file
diff --git a/spaces/AIGC-Audio/Make_An_Audio/ldm/modules/encoders/open_clap/utils.py b/spaces/AIGC-Audio/Make_An_Audio/ldm/modules/encoders/open_clap/utils.py
deleted file mode 100644
index ad9801c0ac819473f738e2c1fbbdf711006ea440..0000000000000000000000000000000000000000
--- a/spaces/AIGC-Audio/Make_An_Audio/ldm/modules/encoders/open_clap/utils.py
+++ /dev/null
@@ -1,369 +0,0 @@
-import numpy as np
-import torch
-from torch import nn as nn
-from torchvision.ops.misc import FrozenBatchNorm2d
-import logging
-import h5py
-from tqdm import tqdm
-import random
-import json
-import os
-import pathlib
-
-# TODO: (yusong) this not a good place to store those information and does not scale. Need to be fixed later.
-dataset_split = {
- "audiocaps": ["train", "valid", "test"],
- "audioset": ["balanced_train", "unbalanced_train", "eval"],
- "BBCSoundEffects": ["train", "test"],
- "Clotho": ["train", "test", "valid"],
- "free_to_use_sounds": ["train", "test"],
- "paramount_motion": ["train", "test"],
- "sonniss_game_effects": ["train", "test"],
- "wesoundeffects": ["train", "test"],
- "MACS": ["train", "test"],
- "freesound": ["train", "test"],
- "FSD50K": ["train", "test", "valid"],
- "fsd50k_class_label": ["train", "test", "valid"],
- "esc50": ["train", "test"],
- "audiostock": ["train", "test"],
- "freesound_no_overlap_noesc50": ["train", "test"],
- "epidemic_sound_effects": ["train", "test"],
- "VGGSound": ["train", "test"],
- "urbansound8k_class_label": ["train", "test"],
- "audioset_t5": ["balanced_train", "unbalanced_train", "eval"],
- "epidemic_sound_effects_t5": ["train", "test"],
- "WavText5K": ["train", "test"],
- "esc50_no_overlap": ["train", "test"],
- "usd8k_no_overlap": ["train", "test"],
- "fsd50k_200_class_label": ["train", "test", "valid"]
-}
-
-
-def freeze_batch_norm_2d(module, module_match={}, name=""):
- """
- Converts all `BatchNorm2d` and `SyncBatchNorm` layers of provided module into `FrozenBatchNorm2d`. If `module` is
- itself an instance of either `BatchNorm2d` or `SyncBatchNorm`, it is converted into `FrozenBatchNorm2d` and
- returned. Otherwise, the module is walked recursively and submodules are converted in place.
-
- Args:
- module (torch.nn.Module): Any PyTorch module.
- module_match (dict): Dictionary of full module names to freeze (all if empty)
- name (str): Full module name (prefix)
-
- Returns:
- torch.nn.Module: Resulting module
-
- Inspired by https://github.com/pytorch/pytorch/blob/a5895f85be0f10212791145bfedc0261d364f103/torch/nn/modules/batchnorm.py#L762
- """
- res = module
- is_match = True
- if module_match:
- is_match = name in module_match
- if is_match and isinstance(
- module, (nn.modules.batchnorm.BatchNorm2d, nn.modules.batchnorm.SyncBatchNorm)
- ):
- res = FrozenBatchNorm2d(module.num_features)
- res.num_features = module.num_features
- res.affine = module.affine
- if module.affine:
- res.weight.data = module.weight.data.clone().detach()
- res.bias.data = module.bias.data.clone().detach()
- res.running_mean.data = module.running_mean.data
- res.running_var.data = module.running_var.data
- res.eps = module.eps
- else:
- for child_name, child in module.named_children():
- full_child_name = ".".join([name, child_name]) if name else child_name
- new_child = freeze_batch_norm_2d(child, module_match, full_child_name)
- if new_child is not child:
- res.add_module(child_name, new_child)
- return res
-
-
-def exist(dataset_name, dataset_type):
- """
- Check if dataset exists
- """
- if dataset_type in dataset_split[dataset_name]:
- return True
- else:
- return False
-
-
-def get_tar_path_from_dataset_name(
- dataset_names,
- dataset_types,
- islocal,
- dataset_path,
- proportion=1,
- full_dataset=None
-):
- """
- Get tar path from dataset name and type
- """
- output = []
- for n in dataset_names:
- if full_dataset is not None and n in full_dataset:
- current_dataset_types = dataset_split[n]
- else:
- current_dataset_types = dataset_types
- for s in current_dataset_types:
- tmp = []
- if islocal:
- sizefilepath_ = f"{dataset_path}/{n}/{s}/sizes.json"
- if not os.path.exists(sizefilepath_):
- sizefilepath_ = f"./json_files/{n}/{s}/sizes.json"
- else:
- sizefilepath_ = f"./json_files/{n}/{s}/sizes.json"
- if not os.path.exists(sizefilepath_):
- continue
- sizes = json.load(open(sizefilepath_, "r"))
- for k in sizes.keys():
- if islocal:
- tmp.append(f"{dataset_path}/{n}/{s}/{k}")
- else:
- tmp.append(
- f"pipe:aws s3 --cli-connect-timeout 0 cp s3://s-laion-audio/webdataset_tar/{n}/{s}/{k} -"
- )
- if proportion != 1:
- tmp = random.sample(tmp, int(proportion * len(tmp)))
- output.append(tmp)
- return sum(output, [])
-
-
-def get_tar_path_from_txts(txt_path, islocal, proportion=1):
- """
- Get tar path from txt path
- """
- if isinstance(txt_path, (list, tuple)):
- return sum(
- [
- get_tar_path_from_txts(
- txt_path[i], islocal=islocal, proportion=proportion
- )
- for i in range(len(txt_path))
- ],
- [],
- )
- if isinstance(txt_path, str):
- with open(txt_path) as f:
- lines = f.readlines()
- if islocal:
- lines = [
- lines[i]
- .split("\n")[0]
- .replace("pipe:aws s3 cp s3://s-laion-audio/", "/mnt/audio_clip/")
- for i in range(len(lines))
- ]
- else:
- lines = [
- lines[i].split("\n")[0].replace(".tar", ".tar -")
- for i in range(len(lines))
- ]
- if proportion != 1:
- print("Sampling tars with proportion of {}".format(proportion))
- lines = random.sample(lines, int(proportion * len(lines)))
- return lines
-
-
-def get_mix_lambda(mixup_alpha, batch_size):
- mixup_lambdas = [
- np.random.beta(mixup_alpha, mixup_alpha, 1)[0] for _ in range(batch_size)
- ]
- return np.array(mixup_lambdas).astype(np.float32)
-
-
-def do_mixup(x, mixup_lambda):
- """
- Args:
- x: (batch_size , ...)
- mixup_lambda: (batch_size,)
- Returns:
- out: (batch_size, ...)
- """
- out = (
- x.transpose(0, -1) * mixup_lambda
- + torch.flip(x, dims=[0]).transpose(0, -1) * (1 - mixup_lambda)
- ).transpose(0, -1)
- return out
-
-
-def interpolate(x, ratio):
- """Interpolate data in time domain. This is used to compensate the
- resolution reduction in downsampling of a CNN.
-
- Args:
- x: (batch_size, time_steps, classes_num)
- ratio: int, ratio to interpolate
- Returns:
- upsampled: (batch_size, time_steps * ratio, classes_num)
- """
- (batch_size, time_steps, classes_num) = x.shape
- upsampled = x[:, :, None, :].repeat(1, 1, ratio, 1)
- upsampled = upsampled.reshape(batch_size, time_steps * ratio, classes_num)
- return upsampled
-
-
-def pad_framewise_output(framewise_output, frames_num):
- """Pad framewise_output to the same length as input frames. The pad value
- is the same as the value of the last frame.
- Args:
- framewise_output: (batch_size, frames_num, classes_num)
- frames_num: int, number of frames to pad
- Outputs:
- output: (batch_size, frames_num, classes_num)
- """
- pad = framewise_output[:, -1:, :].repeat(
- 1, frames_num - framewise_output.shape[1], 1
- )
- """tensor for padding"""
-
- output = torch.cat((framewise_output, pad), dim=1)
- """(batch_size, frames_num, classes_num)"""
-
-
-def process_ipc(index_path, classes_num, filename):
- # load data
- logging.info("Load Data...............")
- ipc = [[] for _ in range(classes_num)]
- with h5py.File(index_path, "r") as f:
- for i in tqdm(range(len(f["target"]))):
- t_class = np.where(f["target"][i])[0]
- for t in t_class:
- ipc[t].append(i)
- print(ipc)
- np.save(filename, ipc)
- logging.info("Load Data Succeed...............")
-
-
-def save_to_dict(s, o_={}):
- sp = s.split(": ")
- o_.update({sp[0]: float(sp[1])})
- return o_
-
-
-def get_data_from_log(txt_path):
- """
- Output dictionary from out.txt log file
- """
- with open(txt_path) as f:
- lines = f.readlines()
- val_data = {}
- train_data = {}
- train_losses = []
- train_losses_epoch = []
- for i in range(len(lines)):
- if "| INFO |" in lines[i]:
- if "Eval Epoch" in lines[i]:
- if "val_loss" in lines[i]:
- # float(regex.sub("", lines[310].split(" ")[-1]).replace(" ", ""))
- line = lines[i].split("Eval Epoch: ")[-1]
- num_epoch = int(line.split(" ")[0].split(" ")[0])
- d = {
- line.split(" ")[0]
- .split(" ")[1]
- .replace(":", ""): float(line.split(" ")[0].split(" ")[-1])
- }
- for i in range(1, len(line.split(" "))):
- d = save_to_dict(line.split(" ")[i], d)
- val_data[num_epoch] = d
- elif "Train Epoch" in lines[i]:
- num_epoch = int(lines[i].split("Train Epoch: ")[1][0])
- loss = float(lines[i].split("Loss: ")[-1].split(" (")[0])
- train_losses.append(loss)
- train_losses_epoch.append(num_epoch)
- for i in range(len(train_losses)):
- train_data[i] = {
- "num_epoch": train_losses_epoch[i],
- "train_loss": train_losses[i],
- }
- return train_data, val_data
-
-
-def save_p(obj, filename):
- import pickle
-
- try:
- from deepdiff import DeepDiff
- except:
- os.system("pip install deepdiff")
- from deepdiff import DeepDiff
- with open(filename, "wb") as file:
- pickle.dump(obj, file, protocol=pickle.HIGHEST_PROTOCOL) # highest protocol
- with open(filename, "rb") as file:
- z = pickle.load(file)
- assert (
- DeepDiff(obj, z, ignore_string_case=True) == {}
- ), "there is something wrong with the saving process"
- return
-
-
-def load_p(filename):
- import pickle
-
- with open(filename, "rb") as file:
- z = pickle.load(file)
- return z
-
-
-def save_json(data, name="data.json"):
- import json
- with open(name, 'w') as fp:
- json.dump(data, fp)
- return
-
-
-def load_json(name):
- import json
- with open(name, 'r') as fp:
- data = json.load(fp)
- return data
-
-
-from multiprocessing import Process, Manager
-from multiprocessing import Process, Value, Array
-from ctypes import c_wchar
-
-
-def load_class_label(path):
- # https://stackoverflow.com/questions/48004243/how-to-share-large-read-only-dictionary-list-across-processes-in-multiprocessing
- # https://stackoverflow.com/questions/45693949/storing-strings-in-a-multiprocessing-sharedctypes-array
- out = None
- if path is not None:
- if pathlib.Path(path).suffix in [".pkl", ".pickle"]:
- out = load_p(path)
- elif pathlib.Path(path).suffix in [".json", ".txt"]:
- out = load_json(path)
- elif pathlib.Path(path).suffix in [".npy", ".npz"]:
- out = np.load(path)
- elif pathlib.Path(path).suffix in [".csv"]:
- import pandas as pd
- out = pd.read_csv(path)
- return out
- # if out is None:
- # return None
- # else:
- # key = Array(c_wchar, '\n'.join(list(out.keys())), lock=False)
- # val = Array('i', out.values(), lock=False)
- # return (key, val)
-
-
-from torch import optim
-
-
-def get_optimizer(params, lr, betas, eps, momentum, optimizer_name):
- if optimizer_name.lower() == "adamw":
- optimizer = optim.AdamW(
- params, lr=lr, betas=betas, eps=eps
- )
- elif optimizer_name.lower() == "sgd":
- optimizer = optim.SGD(
- params, lr=lr, momentum=momentum
- )
- elif optimizer_name.lower() == "adam":
- optimizer = optim.Adam(
- params, lr=lr, betas=betas, eps=eps
- )
- else:
- raise ValueError("optimizer name is not correct")
- return optimizer
diff --git a/spaces/ANLPRL/NER_On_Oral_Medicine/README.md b/spaces/ANLPRL/NER_On_Oral_Medicine/README.md
deleted file mode 100644
index e06585d9007ac0813d34d763bd694d11b1efa049..0000000000000000000000000000000000000000
--- a/spaces/ANLPRL/NER_On_Oral_Medicine/README.md
+++ /dev/null
@@ -1,12 +0,0 @@
----
-title: NER On Oral Medicine
-emoji: 😻
-colorFrom: green
-colorTo: gray
-sdk: streamlit
-sdk_version: 1.19.0
-app_file: app.py
-pinned: false
----
-
-Check out the configuration reference at https://huggingface.co/docs/hub/spaces-config-reference
diff --git a/spaces/ATang0729/Forecast4Muses/Model/Model6/Model6_1_ClothesKeyPoint/work_dirs_1-x/td_hm_res50_4xb64-60e_deepfashion2_trousers_256x192/__init__.py b/spaces/ATang0729/Forecast4Muses/Model/Model6/Model6_1_ClothesKeyPoint/work_dirs_1-x/td_hm_res50_4xb64-60e_deepfashion2_trousers_256x192/__init__.py
deleted file mode 100644
index e69de29bb2d1d6434b8b29ae775ad8c2e48c5391..0000000000000000000000000000000000000000
diff --git a/spaces/AgentVerse/agentVerse/ui/src/phaser3-rex-plugins/templates/ui/easemove/EaseMove.js b/spaces/AgentVerse/agentVerse/ui/src/phaser3-rex-plugins/templates/ui/easemove/EaseMove.js
deleted file mode 100644
index 8782c417d6922bc2efe8f486c42572908f4fdaf0..0000000000000000000000000000000000000000
--- a/spaces/AgentVerse/agentVerse/ui/src/phaser3-rex-plugins/templates/ui/easemove/EaseMove.js
+++ /dev/null
@@ -1,2 +0,0 @@
-import { EaseMove, EaseMoveTo, EaseMoveFrom } from '../../../plugins/easemove.js';
-export { EaseMove, EaseMoveTo, EaseMoveFrom };
\ No newline at end of file
diff --git a/spaces/AgentVerse/agentVerse/ui/src/phaser3-rex-plugins/templates/ui/gridtable/GridTable.d.ts b/spaces/AgentVerse/agentVerse/ui/src/phaser3-rex-plugins/templates/ui/gridtable/GridTable.d.ts
deleted file mode 100644
index 671601c0ca68e57b7cde20775ed6fef346f25209..0000000000000000000000000000000000000000
--- a/spaces/AgentVerse/agentVerse/ui/src/phaser3-rex-plugins/templates/ui/gridtable/GridTable.d.ts
+++ /dev/null
@@ -1,63 +0,0 @@
-// import * as Phaser from 'phaser';
-import Scrollable from '../utils/scrollable/Scrollable';
-import GridTableCore from '../../../plugins/gridtable'
-
-export default GridTable;
-
-declare namespace GridTable {
-
- type CreateCellContainerCallbackType = (
- cell: GridTableCore.CellData,
- cellContainer: Phaser.GameObjects.GameObject | null
- ) => Phaser.GameObjects.GameObject | null;
-
- interface IConfig extends Scrollable.IConfig {
- space?: {
- left?: number, right?: number, top?: number, bottom?: number,
-
- table?: number | {
- left?: number, right?: number, top?: number, bottom?: number,
- },
-
- header?: number,
- footer?: number,
- },
-
- scrollMode?: GridTableCore.ScrollModeType,
-
- table: {
- width?: number | undefined,
- height?: number | undefined,
-
- cellWidth?: number | undefined,
- cellHeight?: number | undefined,
- columns?: number,
- mask?: GridTableCore.MaskConfig,
- interactive?: boolean,
- reuseCellContainer?: boolean,
- },
-
- createCellContainerCallback: CreateCellContainerCallbackType,
-
- items: unknown[]
- }
-
-}
-
-declare class GridTable extends Scrollable {
- constructor(
- scene: Phaser.Scene,
- config?: GridTable.IConfig
- );
-
- setItems(items?: unknown[]): this;
- refresh(): this;
- updateVisibleCell(cellIndex: number): this;
-
- getCell(cellIndex: number): GridTableCore.CellData;
- getCellContainer(cellIndex: number): Phaser.GameObjects.GameObject | null;
- startRowIndex: number;
-
- scrollToRow(rowIndex: number): this;
- scrollToNextRow(rowCount?: number): this;
-}
\ No newline at end of file
diff --git a/spaces/Aloento/9Nine-PITS/text/frontend/zh_normalization/__init__.py b/spaces/Aloento/9Nine-PITS/text/frontend/zh_normalization/__init__.py
deleted file mode 100644
index 6e9d9f7d05913afabadc3d3730f6e51e9e09502c..0000000000000000000000000000000000000000
--- a/spaces/Aloento/9Nine-PITS/text/frontend/zh_normalization/__init__.py
+++ /dev/null
@@ -1,14 +0,0 @@
-# Copyright (c) 2020 PaddlePaddle Authors. All Rights Reserved.
-#
-# Licensed under the Apache License, Version 2.0 (the "License");
-# you may not use this file except in compliance with the License.
-# You may obtain a copy of the License at
-#
-# http://www.apache.org/licenses/LICENSE-2.0
-#
-# Unless required by applicable law or agreed to in writing, software
-# distributed under the License is distributed on an "AS IS" BASIS,
-# WITHOUT WARRANTIES OR CONDITIONS OF ANY KIND, either express or implied.
-# See the License for the specific language governing permissions and
-# limitations under the License.
-from text.frontend.zh_normalization.text_normlization import *
diff --git a/spaces/Alpaca233/SadTalker/scripts/extension.py b/spaces/Alpaca233/SadTalker/scripts/extension.py
deleted file mode 100644
index c90ec25c2811d87a00a2e2a14e270c75d07d713d..0000000000000000000000000000000000000000
--- a/spaces/Alpaca233/SadTalker/scripts/extension.py
+++ /dev/null
@@ -1,189 +0,0 @@
-import os, sys
-from pathlib import Path
-import tempfile
-import gradio as gr
-from modules.call_queue import wrap_gradio_gpu_call, wrap_queued_call
-from modules.shared import opts, OptionInfo
-from modules import shared, paths, script_callbacks
-import launch
-import glob
-from huggingface_hub import snapshot_download
-
-
-
-def check_all_files_safetensor(current_dir):
- kv = {
- "SadTalker_V0.0.2_256.safetensors": "sadtalker-256",
- "SadTalker_V0.0.2_512.safetensors": "sadtalker-512",
- "mapping_00109-model.pth.tar" : "mapping-109" ,
- "mapping_00229-model.pth.tar" : "mapping-229" ,
- }
-
- if not os.path.isdir(current_dir):
- return False
-
- dirs = os.listdir(current_dir)
-
- for f in dirs:
- if f in kv.keys():
- del kv[f]
-
- return len(kv.keys()) == 0
-
-def check_all_files(current_dir):
- kv = {
- "auido2exp_00300-model.pth": "audio2exp",
- "auido2pose_00140-model.pth": "audio2pose",
- "epoch_20.pth": "face_recon",
- "facevid2vid_00189-model.pth.tar": "face-render",
- "mapping_00109-model.pth.tar" : "mapping-109" ,
- "mapping_00229-model.pth.tar" : "mapping-229" ,
- "wav2lip.pth": "wav2lip",
- "shape_predictor_68_face_landmarks.dat": "dlib",
- }
-
- if not os.path.isdir(current_dir):
- return False
-
- dirs = os.listdir(current_dir)
-
- for f in dirs:
- if f in kv.keys():
- del kv[f]
-
- return len(kv.keys()) == 0
-
-
-
-def download_model(local_dir='./checkpoints'):
- REPO_ID = 'vinthony/SadTalker'
- snapshot_download(repo_id=REPO_ID, local_dir=local_dir, local_dir_use_symlinks=False)
-
-def get_source_image(image):
- return image
-
-def get_img_from_txt2img(x):
- talker_path = Path(paths.script_path) / "outputs"
- imgs_from_txt_dir = str(talker_path / "txt2img-images/")
- imgs = glob.glob(imgs_from_txt_dir+'/*/*.png')
- imgs.sort(key=lambda x:os.path.getmtime(os.path.join(imgs_from_txt_dir, x)))
- img_from_txt_path = os.path.join(imgs_from_txt_dir, imgs[-1])
- return img_from_txt_path, img_from_txt_path
-
-def get_img_from_img2img(x):
- talker_path = Path(paths.script_path) / "outputs"
- imgs_from_img_dir = str(talker_path / "img2img-images/")
- imgs = glob.glob(imgs_from_img_dir+'/*/*.png')
- imgs.sort(key=lambda x:os.path.getmtime(os.path.join(imgs_from_img_dir, x)))
- img_from_img_path = os.path.join(imgs_from_img_dir, imgs[-1])
- return img_from_img_path, img_from_img_path
-
-def get_default_checkpoint_path():
- # check the path of models/checkpoints and extensions/
- checkpoint_path = Path(paths.script_path) / "models"/ "SadTalker"
- extension_checkpoint_path = Path(paths.script_path) / "extensions"/ "SadTalker" / "checkpoints"
-
- if check_all_files_safetensor(checkpoint_path):
- # print('founding sadtalker checkpoint in ' + str(checkpoint_path))
- return checkpoint_path
-
- if check_all_files_safetensor(extension_checkpoint_path):
- # print('founding sadtalker checkpoint in ' + str(extension_checkpoint_path))
- return extension_checkpoint_path
-
- if check_all_files(checkpoint_path):
- # print('founding sadtalker checkpoint in ' + str(checkpoint_path))
- return checkpoint_path
-
- if check_all_files(extension_checkpoint_path):
- # print('founding sadtalker checkpoint in ' + str(extension_checkpoint_path))
- return extension_checkpoint_path
-
- return None
-
-
-
-def install():
-
- kv = {
- "face_alignment": "face-alignment==1.3.5",
- "imageio": "imageio==2.19.3",
- "imageio_ffmpeg": "imageio-ffmpeg==0.4.7",
- "librosa":"librosa==0.8.0",
- "pydub":"pydub==0.25.1",
- "scipy":"scipy==1.8.1",
- "tqdm": "tqdm",
- "yacs":"yacs==0.1.8",
- "yaml": "pyyaml",
- "av":"av",
- "gfpgan": "gfpgan",
- }
-
- # # dlib is not necessary currently
- # if 'darwin' in sys.platform:
- # kv['dlib'] = "dlib"
- # else:
- # kv['dlib'] = 'dlib-bin'
-
- # #### we need to have a newer version of imageio for our method.
- # launch.run_pip("install imageio==2.19.3", "requirements for SadTalker")
-
- for k,v in kv.items():
- if not launch.is_installed(k):
- print(k, launch.is_installed(k))
- launch.run_pip("install "+ v, "requirements for SadTalker")
-
- if os.getenv('SADTALKER_CHECKPOINTS'):
- print('load Sadtalker Checkpoints from '+ os.getenv('SADTALKER_CHECKPOINTS'))
-
- elif get_default_checkpoint_path() is not None:
- os.environ['SADTALKER_CHECKPOINTS'] = str(get_default_checkpoint_path())
- else:
-
- print(
- """"
- SadTalker will not support download all the files from hugging face, which will take a long time.
-
- please manually set the SADTALKER_CHECKPOINTS in `webui_user.bat`(windows) or `webui_user.sh`(linux)
- """
- )
-
- # python = sys.executable
-
- # launch.run(f'"{python}" -m pip uninstall -y huggingface_hub', live=True)
- # launch.run(f'"{python}" -m pip install --upgrade git+https://github.com/huggingface/huggingface_hub@main', live=True)
- # ### run the scripts to downlod models to correct localtion.
- # # print('download models for SadTalker')
- # # launch.run("cd " + paths.script_path+"/extensions/SadTalker && bash ./scripts/download_models.sh", live=True)
- # # print('SadTalker is successfully installed!')
- # download_model(paths.script_path+'/extensions/SadTalker/checkpoints')
-
-
-def on_ui_tabs():
- install()
-
- sys.path.extend([paths.script_path+'/extensions/SadTalker'])
-
- repo_dir = paths.script_path+'/extensions/SadTalker/'
-
- result_dir = opts.sadtalker_result_dir
- os.makedirs(result_dir, exist_ok=True)
-
- from app_sadtalker import sadtalker_demo
-
- if os.getenv('SADTALKER_CHECKPOINTS'):
- checkpoint_path = os.getenv('SADTALKER_CHECKPOINTS')
- else:
- checkpoint_path = repo_dir+'checkpoints/'
-
- audio_to_video = sadtalker_demo(checkpoint_path=checkpoint_path, config_path=repo_dir+'src/config', warpfn = wrap_queued_call)
-
- return [(audio_to_video, "SadTalker", "extension")]
-
-def on_ui_settings():
- talker_path = Path(paths.script_path) / "outputs"
- section = ('extension', "SadTalker")
- opts.add_option("sadtalker_result_dir", OptionInfo(str(talker_path / "SadTalker/"), "Path to save results of sadtalker", section=section))
-
-script_callbacks.on_ui_settings(on_ui_settings)
-script_callbacks.on_ui_tabs(on_ui_tabs)
diff --git a/spaces/Amrrs/DragGan-Inversion/PTI/torch_utils/ops/fma.py b/spaces/Amrrs/DragGan-Inversion/PTI/torch_utils/ops/fma.py
deleted file mode 100644
index 2eeac58a626c49231e04122b93e321ada954c5d3..0000000000000000000000000000000000000000
--- a/spaces/Amrrs/DragGan-Inversion/PTI/torch_utils/ops/fma.py
+++ /dev/null
@@ -1,60 +0,0 @@
-# Copyright (c) 2021, NVIDIA CORPORATION. All rights reserved.
-#
-# NVIDIA CORPORATION and its licensors retain all intellectual property
-# and proprietary rights in and to this software, related documentation
-# and any modifications thereto. Any use, reproduction, disclosure or
-# distribution of this software and related documentation without an express
-# license agreement from NVIDIA CORPORATION is strictly prohibited.
-
-"""Fused multiply-add, with slightly faster gradients than `torch.addcmul()`."""
-
-import torch
-
-#----------------------------------------------------------------------------
-
-def fma(a, b, c): # => a * b + c
- return _FusedMultiplyAdd.apply(a, b, c)
-
-#----------------------------------------------------------------------------
-
-class _FusedMultiplyAdd(torch.autograd.Function): # a * b + c
- @staticmethod
- def forward(ctx, a, b, c): # pylint: disable=arguments-differ
- out = torch.addcmul(c, a, b)
- ctx.save_for_backward(a, b)
- ctx.c_shape = c.shape
- return out
-
- @staticmethod
- def backward(ctx, dout): # pylint: disable=arguments-differ
- a, b = ctx.saved_tensors
- c_shape = ctx.c_shape
- da = None
- db = None
- dc = None
-
- if ctx.needs_input_grad[0]:
- da = _unbroadcast(dout * b, a.shape)
-
- if ctx.needs_input_grad[1]:
- db = _unbroadcast(dout * a, b.shape)
-
- if ctx.needs_input_grad[2]:
- dc = _unbroadcast(dout, c_shape)
-
- return da, db, dc
-
-#----------------------------------------------------------------------------
-
-def _unbroadcast(x, shape):
- extra_dims = x.ndim - len(shape)
- assert extra_dims >= 0
- dim = [i for i in range(x.ndim) if x.shape[i] > 1 and (i < extra_dims or shape[i - extra_dims] == 1)]
- if len(dim):
- x = x.sum(dim=dim, keepdim=True)
- if extra_dims:
- x = x.reshape(-1, *x.shape[extra_dims+1:])
- assert x.shape == shape
- return x
-
-#----------------------------------------------------------------------------
diff --git a/spaces/Androidonnxfork/CivitAi-to-Diffusers/diffusers/docs/source/en/api/pipelines/stable_diffusion/adapter.md b/spaces/Androidonnxfork/CivitAi-to-Diffusers/diffusers/docs/source/en/api/pipelines/stable_diffusion/adapter.md
deleted file mode 100644
index 19351e1713b65f7c6e31cc8bb985c5d5abfcd096..0000000000000000000000000000000000000000
--- a/spaces/Androidonnxfork/CivitAi-to-Diffusers/diffusers/docs/source/en/api/pipelines/stable_diffusion/adapter.md
+++ /dev/null
@@ -1,187 +0,0 @@
-
-
-# Text-to-Image Generation with Adapter Conditioning
-
-## Overview
-
-[T2I-Adapter: Learning Adapters to Dig out More Controllable Ability for Text-to-Image Diffusion Models](https://arxiv.org/abs/2302.08453) by Chong Mou, Xintao Wang, Liangbin Xie, Jian Zhang, Zhongang Qi, Ying Shan, Xiaohu Qie.
-
-Using the pretrained models we can provide control images (for example, a depth map) to control Stable Diffusion text-to-image generation so that it follows the structure of the depth image and fills in the details.
-
-The abstract of the paper is the following:
-
-*The incredible generative ability of large-scale text-to-image (T2I) models has demonstrated strong power of learning complex structures and meaningful semantics. However, relying solely on text prompts cannot fully take advantage of the knowledge learned by the model, especially when flexible and accurate structure control is needed. In this paper, we aim to ``dig out" the capabilities that T2I models have implicitly learned, and then explicitly use them to control the generation more granularly. Specifically, we propose to learn simple and small T2I-Adapters to align internal knowledge in T2I models with external control signals, while freezing the original large T2I models. In this way, we can train various adapters according to different conditions, and achieve rich control and editing effects. Further, the proposed T2I-Adapters have attractive properties of practical value, such as composability and generalization ability. Extensive experiments demonstrate that our T2I-Adapter has promising generation quality and a wide range of applications.*
-
-This model was contributed by the community contributor [HimariO](https://github.com/HimariO) ❤️ .
-
-## Available Pipelines:
-
-| Pipeline | Tasks | Demo
-|---|---|:---:|
-| [StableDiffusionAdapterPipeline](https://github.com/huggingface/diffusers/blob/main/src/diffusers/pipelines/stable_diffusion/pipeline_stable_diffusion_adapter.py) | *Text-to-Image Generation with T2I-Adapter Conditioning* | -
-
-## Usage example
-
-In the following we give a simple example of how to use a *T2IAdapter* checkpoint with Diffusers for inference.
-All adapters use the same pipeline.
-
- 1. Images are first converted into the appropriate *control image* format.
- 2. The *control image* and *prompt* are passed to the [`StableDiffusionAdapterPipeline`].
-
-Let's have a look at a simple example using the [Color Adapter](https://huggingface.co/TencentARC/t2iadapter_color_sd14v1).
-
-```python
-from diffusers.utils import load_image
-
-image = load_image("https://huggingface.co/datasets/diffusers/docs-images/resolve/main/t2i-adapter/color_ref.png")
-```
-
-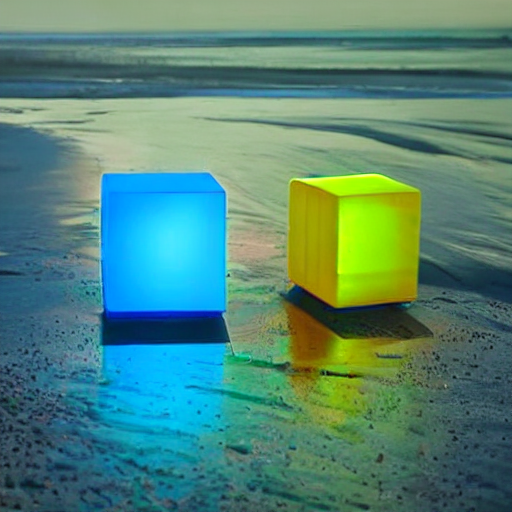
-
-
-Then we can create our color palette by simply resizing it to 8 by 8 pixels and then scaling it back to original size.
-
-```python
-from PIL import Image
-
-color_palette = image.resize((8, 8))
-color_palette = color_palette.resize((512, 512), resample=Image.Resampling.NEAREST)
-```
-
-Let's take a look at the processed image.
-
-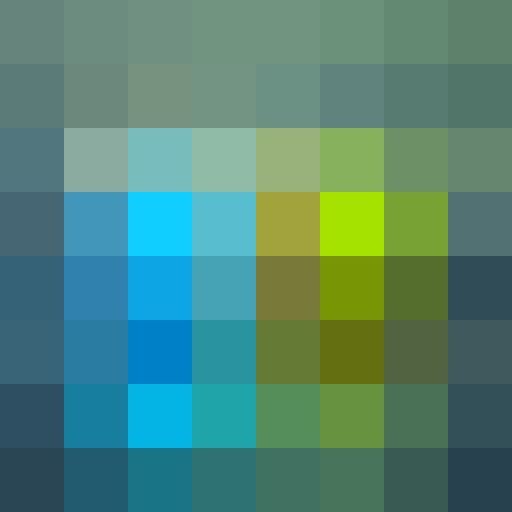
-
-
-Next, create the adapter pipeline
-
-```py
-import torch
-from diffusers import StableDiffusionAdapterPipeline, T2IAdapter
-
-adapter = T2IAdapter.from_pretrained("TencentARC/t2iadapter_color_sd14v1")
-pipe = StableDiffusionAdapterPipeline.from_pretrained(
- "CompVis/stable-diffusion-v1-4",
- adapter=adapter,
- torch_dtype=torch.float16,
-)
-pipe.to("cuda")
-```
-
-Finally, pass the prompt and control image to the pipeline
-
-```py
-# fix the random seed, so you will get the same result as the example
-generator = torch.manual_seed(7)
-
-out_image = pipe(
- "At night, glowing cubes in front of the beach",
- image=color_palette,
- generator=generator,
-).images[0]
-```
-
-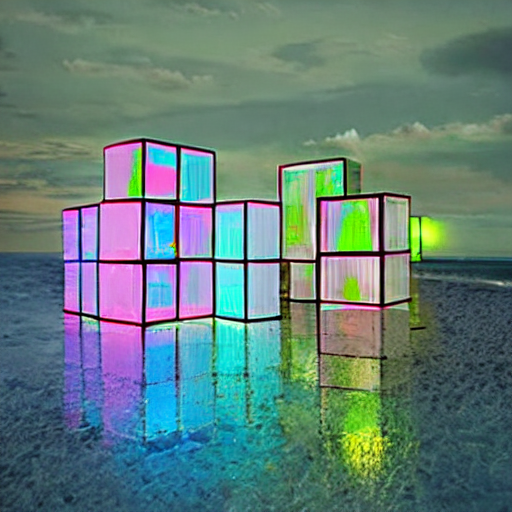
-
-
-## Available checkpoints
-
-Non-diffusers checkpoints can be found under [TencentARC/T2I-Adapter](https://huggingface.co/TencentARC/T2I-Adapter/tree/main/models).
-
-### T2I-Adapter with Stable Diffusion 1.4
-
-| Model Name | Control Image Overview| Control Image Example | Generated Image Example |
-|---|---|---|---|
-|[TencentARC/t2iadapter_color_sd14v1](https://huggingface.co/TencentARC/t2iadapter_color_sd14v1) *Trained with spatial color palette* | A image with 8x8 color palette.|||
-|[TencentARC/t2iadapter_canny_sd14v1](https://huggingface.co/TencentARC/t2iadapter_canny_sd14v1) *Trained with canny edge detection* | A monochrome image with white edges on a black background.|||
-|[TencentARC/t2iadapter_sketch_sd14v1](https://huggingface.co/TencentARC/t2iadapter_sketch_sd14v1) *Trained with [PidiNet](https://github.com/zhuoinoulu/pidinet) edge detection* | A hand-drawn monochrome image with white outlines on a black background.|||
-|[TencentARC/t2iadapter_depth_sd14v1](https://huggingface.co/TencentARC/t2iadapter_depth_sd14v1) *Trained with Midas depth estimation* | A grayscale image with black representing deep areas and white representing shallow areas.|||
-|[TencentARC/t2iadapter_openpose_sd14v1](https://huggingface.co/TencentARC/t2iadapter_openpose_sd14v1) *Trained with OpenPose bone image* | A [OpenPose bone](https://github.com/CMU-Perceptual-Computing-Lab/openpose) image.|||
-|[TencentARC/t2iadapter_keypose_sd14v1](https://huggingface.co/TencentARC/t2iadapter_keypose_sd14v1) *Trained with mmpose skeleton image* | A [mmpose skeleton](https://github.com/open-mmlab/mmpose) image.|||
-|[TencentARC/t2iadapter_seg_sd14v1](https://huggingface.co/TencentARC/t2iadapter_seg_sd14v1) *Trained with semantic segmentation* | An [custom](https://github.com/TencentARC/T2I-Adapter/discussions/25) segmentation protocol image.|| |
-|[TencentARC/t2iadapter_canny_sd15v2](https://huggingface.co/TencentARC/t2iadapter_canny_sd15v2)||
-|[TencentARC/t2iadapter_depth_sd15v2](https://huggingface.co/TencentARC/t2iadapter_depth_sd15v2)||
-|[TencentARC/t2iadapter_sketch_sd15v2](https://huggingface.co/TencentARC/t2iadapter_sketch_sd15v2)||
-|[TencentARC/t2iadapter_zoedepth_sd15v1](https://huggingface.co/TencentARC/t2iadapter_zoedepth_sd15v1)||
-
-## Combining multiple adapters
-
-[`MultiAdapter`] can be used for applying multiple conditionings at once.
-
-Here we use the keypose adapter for the character posture and the depth adapter for creating the scene.
-
-```py
-import torch
-from PIL import Image
-from diffusers.utils import load_image
-
-cond_keypose = load_image(
- "https://huggingface.co/datasets/diffusers/docs-images/resolve/main/t2i-adapter/keypose_sample_input.png"
-)
-cond_depth = load_image(
- "https://huggingface.co/datasets/diffusers/docs-images/resolve/main/t2i-adapter/depth_sample_input.png"
-)
-cond = [[cond_keypose, cond_depth]]
-
-prompt = ["A man walking in an office room with a nice view"]
-```
-
-The two control images look as such:
-
-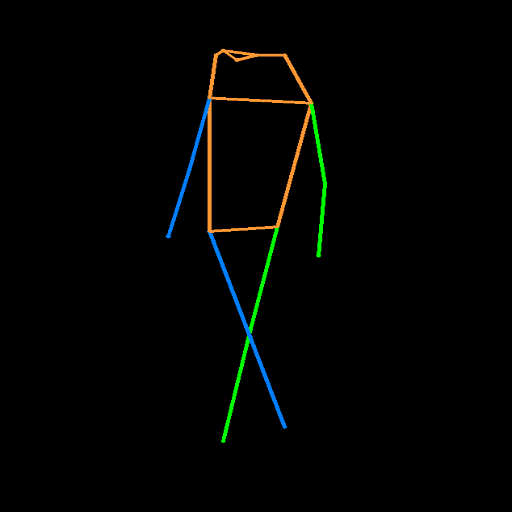
-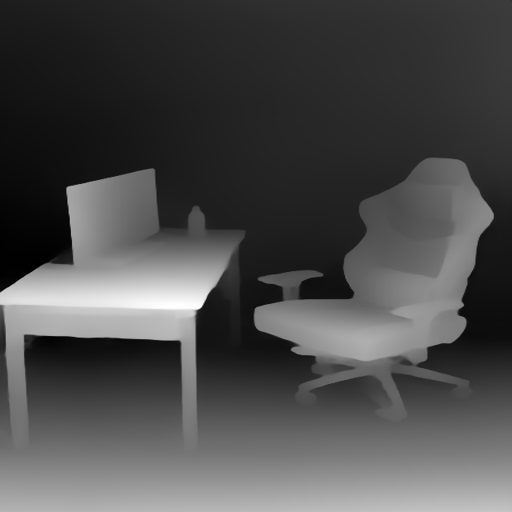
-
-
-`MultiAdapter` combines keypose and depth adapters.
-
-`adapter_conditioning_scale` balances the relative influence of the different adapters.
-
-```py
-from diffusers import StableDiffusionAdapterPipeline, MultiAdapter
-
-adapters = MultiAdapter(
- [
- T2IAdapter.from_pretrained("TencentARC/t2iadapter_keypose_sd14v1"),
- T2IAdapter.from_pretrained("TencentARC/t2iadapter_depth_sd14v1"),
- ]
-)
-adapters = adapters.to(torch.float16)
-
-pipe = StableDiffusionAdapterPipeline.from_pretrained(
- "CompVis/stable-diffusion-v1-4",
- torch_dtype=torch.float16,
- adapter=adapters,
-)
-
-images = pipe(prompt, cond, adapter_conditioning_scale=[0.8, 0.8])
-```
-
-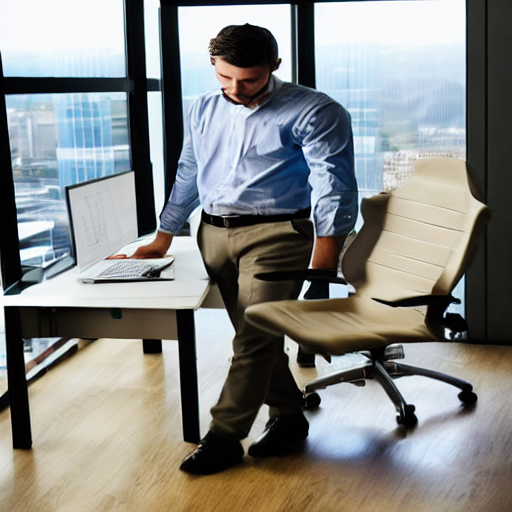
-
-
-## T2I Adapter vs ControlNet
-
-T2I-Adapter is similar to [ControlNet](https://huggingface.co/docs/diffusers/main/en/api/pipelines/controlnet).
-T2i-Adapter uses a smaller auxiliary network which is only run once for the entire diffusion process.
-However, T2I-Adapter performs slightly worse than ControlNet.
-
-## StableDiffusionAdapterPipeline
-[[autodoc]] StableDiffusionAdapterPipeline
- - all
- - __call__
- - enable_attention_slicing
- - disable_attention_slicing
- - enable_vae_slicing
- - disable_vae_slicing
- - enable_xformers_memory_efficient_attention
- - disable_xformers_memory_efficient_attention
diff --git a/spaces/Androidonnxfork/CivitAi-to-Diffusers/diffusers/tests/pipelines/dance_diffusion/test_dance_diffusion.py b/spaces/Androidonnxfork/CivitAi-to-Diffusers/diffusers/tests/pipelines/dance_diffusion/test_dance_diffusion.py
deleted file mode 100644
index fadb3ead7a78ffdebb514be461953a90a7a74bd0..0000000000000000000000000000000000000000
--- a/spaces/Androidonnxfork/CivitAi-to-Diffusers/diffusers/tests/pipelines/dance_diffusion/test_dance_diffusion.py
+++ /dev/null
@@ -1,162 +0,0 @@
-# coding=utf-8
-# Copyright 2023 HuggingFace Inc.
-#
-# Licensed under the Apache License, Version 2.0 (the "License");
-# you may not use this file except in compliance with the License.
-# You may obtain a copy of the License at
-#
-# http://www.apache.org/licenses/LICENSE-2.0
-#
-# Unless required by applicable law or agreed to in writing, software
-# distributed under the License is distributed on an "AS IS" BASIS,
-# WITHOUT WARRANTIES OR CONDITIONS OF ANY KIND, either express or implied.
-# See the License for the specific language governing permissions and
-# limitations under the License.
-
-import gc
-import unittest
-
-import numpy as np
-import torch
-
-from diffusers import DanceDiffusionPipeline, IPNDMScheduler, UNet1DModel
-from diffusers.utils import slow, torch_device
-from diffusers.utils.testing_utils import enable_full_determinism, require_torch_gpu, skip_mps
-
-from ..pipeline_params import UNCONDITIONAL_AUDIO_GENERATION_BATCH_PARAMS, UNCONDITIONAL_AUDIO_GENERATION_PARAMS
-from ..test_pipelines_common import PipelineTesterMixin
-
-
-enable_full_determinism()
-
-
-class DanceDiffusionPipelineFastTests(PipelineTesterMixin, unittest.TestCase):
- pipeline_class = DanceDiffusionPipeline
- params = UNCONDITIONAL_AUDIO_GENERATION_PARAMS
- required_optional_params = PipelineTesterMixin.required_optional_params - {
- "callback",
- "latents",
- "callback_steps",
- "output_type",
- "num_images_per_prompt",
- }
- batch_params = UNCONDITIONAL_AUDIO_GENERATION_BATCH_PARAMS
- test_attention_slicing = False
-
- def get_dummy_components(self):
- torch.manual_seed(0)
- unet = UNet1DModel(
- block_out_channels=(32, 32, 64),
- extra_in_channels=16,
- sample_size=512,
- sample_rate=16_000,
- in_channels=2,
- out_channels=2,
- flip_sin_to_cos=True,
- use_timestep_embedding=False,
- time_embedding_type="fourier",
- mid_block_type="UNetMidBlock1D",
- down_block_types=("DownBlock1DNoSkip", "DownBlock1D", "AttnDownBlock1D"),
- up_block_types=("AttnUpBlock1D", "UpBlock1D", "UpBlock1DNoSkip"),
- )
- scheduler = IPNDMScheduler()
-
- components = {
- "unet": unet,
- "scheduler": scheduler,
- }
- return components
-
- def get_dummy_inputs(self, device, seed=0):
- if str(device).startswith("mps"):
- generator = torch.manual_seed(seed)
- else:
- generator = torch.Generator(device=device).manual_seed(seed)
- inputs = {
- "batch_size": 1,
- "generator": generator,
- "num_inference_steps": 4,
- }
- return inputs
-
- def test_dance_diffusion(self):
- device = "cpu" # ensure determinism for the device-dependent torch.Generator
- components = self.get_dummy_components()
- pipe = DanceDiffusionPipeline(**components)
- pipe = pipe.to(device)
- pipe.set_progress_bar_config(disable=None)
-
- inputs = self.get_dummy_inputs(device)
- output = pipe(**inputs)
- audio = output.audios
-
- audio_slice = audio[0, -3:, -3:]
-
- assert audio.shape == (1, 2, components["unet"].sample_size)
- expected_slice = np.array([-0.7265, 1.0000, -0.8388, 0.1175, 0.9498, -1.0000])
- assert np.abs(audio_slice.flatten() - expected_slice).max() < 1e-2
-
- @skip_mps
- def test_save_load_local(self):
- return super().test_save_load_local()
-
- @skip_mps
- def test_dict_tuple_outputs_equivalent(self):
- return super().test_dict_tuple_outputs_equivalent(expected_max_difference=3e-3)
-
- @skip_mps
- def test_save_load_optional_components(self):
- return super().test_save_load_optional_components()
-
- @skip_mps
- def test_attention_slicing_forward_pass(self):
- return super().test_attention_slicing_forward_pass()
-
- def test_inference_batch_single_identical(self):
- super().test_inference_batch_single_identical(expected_max_diff=3e-3)
-
-
-@slow
-@require_torch_gpu
-class PipelineIntegrationTests(unittest.TestCase):
- def tearDown(self):
- # clean up the VRAM after each test
- super().tearDown()
- gc.collect()
- torch.cuda.empty_cache()
-
- def test_dance_diffusion(self):
- device = torch_device
-
- pipe = DanceDiffusionPipeline.from_pretrained("harmonai/maestro-150k")
- pipe = pipe.to(device)
- pipe.set_progress_bar_config(disable=None)
-
- generator = torch.manual_seed(0)
- output = pipe(generator=generator, num_inference_steps=100, audio_length_in_s=4.096)
- audio = output.audios
-
- audio_slice = audio[0, -3:, -3:]
-
- assert audio.shape == (1, 2, pipe.unet.sample_size)
- expected_slice = np.array([-0.0192, -0.0231, -0.0318, -0.0059, 0.0002, -0.0020])
-
- assert np.abs(audio_slice.flatten() - expected_slice).max() < 1e-2
-
- def test_dance_diffusion_fp16(self):
- device = torch_device
-
- pipe = DanceDiffusionPipeline.from_pretrained("harmonai/maestro-150k", torch_dtype=torch.float16)
- pipe = pipe.to(device)
- pipe.set_progress_bar_config(disable=None)
-
- generator = torch.manual_seed(0)
- output = pipe(generator=generator, num_inference_steps=100, audio_length_in_s=4.096)
- audio = output.audios
-
- audio_slice = audio[0, -3:, -3:]
-
- assert audio.shape == (1, 2, pipe.unet.sample_size)
- expected_slice = np.array([-0.0367, -0.0488, -0.0771, -0.0525, -0.0444, -0.0341])
-
- assert np.abs(audio_slice.flatten() - expected_slice).max() < 1e-2
diff --git a/spaces/Anish13/characterGPT/app.py b/spaces/Anish13/characterGPT/app.py
deleted file mode 100644
index 1890bc910cc8e6224078b86f530d8cf157c378dc..0000000000000000000000000000000000000000
--- a/spaces/Anish13/characterGPT/app.py
+++ /dev/null
@@ -1,187 +0,0 @@
-import gradio as gr
-
-import torch
-import torch.nn as nn
-import torch.nn.functional as F
-
-batch_size = 64 # how many independent sequences will we process in parallel?
-block_size = 256 # what is the maximum context length for predictions?
-max_iters = 5000
-eval_interval = 500
-learning_rate = 3e-4
-device = 'cuda' if torch.cuda.is_available() else 'cpu'
-print(f"The code is running on {device}")
-eval_iters = 200
-n_embd = 384
-n_head = 6
-n_layer = 6
-dropout = 0.2
-
-
-torch.manual_seed(1337)
-
-# wget https://raw.githubusercontent.com/karpathy/char-rnn/master/data/tinyshakespeare/input.txt
-with open('input.txt', 'r', encoding='utf-8') as f:
- text = f.read()
-
-# here are all the unique characters that occur in this text
-chars = sorted(list(set(text)))
-vocab_size = len(chars)
-# create a mapping from characters to integers
-stoi = { ch:i for i,ch in enumerate(chars) }
-itos = { i:ch for i,ch in enumerate(chars) }
-encode = lambda s: [stoi[c] for c in s] # encoder: take a string, output a list of integers
-decode = lambda l: ''.join([itos[i] for i in l]) # decoder: take a list of integers, output a string
-
-
-class Head(nn.Module):
- """ one head of self-attention """
-
- def __init__(self, head_size):
- super().__init__()
- self.key = nn.Linear(n_embd, head_size, bias=False)
- self.query = nn.Linear(n_embd, head_size, bias=False)
- self.value = nn.Linear(n_embd, head_size, bias=False)
- self.register_buffer('tril', torch.tril(torch.ones(block_size, block_size))) # create lower triangular matrix
-
- self.dropout = nn.Dropout(dropout)
-
- def forward(self, x):
- B,T,C = x.shape
- k = self.key(x) # B, T, C
- q = self.query(x) # B, T, C
- # compute attention scores = ("affinities")
- wei = q @ k.transpose(-2, -1) * C**-0.5 # (B, T, C) @ (B, C, T) -> (B, T, T)
- #wei = wei.masked_fill(self.tril[:T, :T]==0, float('-inf')) # (B, T, T)
- tril = torch.tril(torch.ones(T, T)).to(device)
- wei = wei.masked_fill(tril == 0, float('-inf'))
- wei = F.softmax(wei, dim=-1) # (B, T, T)
- wei = self.dropout(wei)
- # perform the weighted aggregation of the values
- v = self.value(x) # (B, T, C)
- out = wei @ v
- return out
-
-
-class MultiHeadAttention(nn.Module):
- """ multiple heads of self-attention in parallel """
-
- def __init__(self, num_heads, head_size):
- super().__init__()
- self.heads = nn.ModuleList([Head(head_size) for _ in range(num_heads)])
- self.proj = nn.Linear(n_embd, n_embd)
- self.dropout = nn.Dropout(dropout)
-
- def forward(self, x):
- out = torch.cat([h(x) for h in self.heads], dim=-1) # h(x) call forward function is Head class
- out = self.dropout(self.proj(out))
- return out
-
-class FeedForward(nn.Module): # per token level, every token does this independently, its allowing tokens to think on data provided by self attention
- """ a simple linear layer followed by a non-linearity"""
-
- def __init__(self, n_embd):
- super().__init__()
- self.net = nn.Sequential(
- nn.Linear(n_embd, 4 * n_embd), # we multiply by 4 cause the paper says so
- nn.ReLU(),
- nn.Linear(4 * n_embd, n_embd),
- nn.Dropout(dropout)
- )
-
- def forward(self, x):
- return self.net(x)
-
-class Block(nn.Module):
- """Transformer block: communication followed by computation """
-
- def __init__(self, n_embed, n_head):
- # n_embd: embedding dimension, n_head: the number of heads we'd like
- super().__init__()
- head_size = n_embd // n_head
- self.sa = MultiHeadAttention(n_head, head_size)
- self.ffwd = FeedForward(n_embd)
- self.ln1 = nn.LayerNorm(n_embd)
- self.ln2 = nn.LayerNorm(n_embd)
-
- def forward(self, x):
- x = x + self.sa(self.ln1(x)) # x = x + self .. is residual connection
- x = x + self.ffwd(self.ln2(x))
- return x
-
-
-class BigramLanguageModel(nn.Module):
-
- def __init__(self):
- super().__init__()
- # each token directly reads off the logits for the next token from a lookup table
- self.token_embedding_table = nn.Embedding(vocab_size, n_embd)
- self.position_embedding_table = nn.Embedding(block_size, n_embd) # so each position from 0 to block_size - 1 will also get its own embedding vector
- self.blocks = nn.Sequential(*[Block(n_embd, n_head=n_head) for _ in range(n_layer)])
- self.ln_f = nn.LayerNorm(n_embd) # final layer Norm
- self.lm_head = nn.Linear(n_embd, vocab_size)
-
- def forward(self, idx, targets=None):
- B, T = idx.shape
-
- # idx and targets are both (B,T) tensor of integers
- tok_emb = self.token_embedding_table(idx) # (B,T,C=n_embed)
- pos_emb = self.position_embedding_table(torch.arange(T, device=device)) # (T, C)
- # pos_emb tensor will be a (block_size, n_emb) tensor # block_size is max context length for predictions
- # each row represents the embedding vector for the corresponding position
- # so 0th row will represent the vector for 0th position
- x = tok_emb + pos_emb # (B, T, C)
- x = self.blocks(x) # (B, T, C)
- logits = self.lm_head(x) # (B, T, C=vocab_size)
-
- if targets is None:
- loss = None
- else:
- B, T, C = logits.shape
- logits = logits.view(B*T, C)
- targets = targets.view(B*T)
- loss = F.cross_entropy(logits, targets)
-
- return logits, loss
-
- def generate(self, idx, max_new_tokens):
- # idx is (B, T) array of indices in the current context
- for _ in range(max_new_tokens):
- # crop idx to the last block_size tokens
- idx_cond = idx[:, -block_size:]
- # get the predictions
- logits, loss = self.forward(idx_cond)
- # focus only on the last time step
- logits = logits[:, -1, :] # becomes (B, C)
- # apply softmax to get probabilities
- probs = F.softmax(logits, dim=-1) # (B, C)
- # sample from the distribution
- idx_next = torch.multinomial(probs, num_samples=1) # (B, 1)
- # append sampled index to the running sequence
- idx = torch.cat((idx, idx_next), dim=1) # (B, T+1)
- return idx
-
-
-# Instantiate the model
-model = BigramLanguageModel()
-
-# Specify the path to the pre-trained model checkpoint
-checkpoint_path = 'checkpoint.pth'
-
-# Load the model checkpoint
-checkpoint = torch.load(checkpoint_path, map_location=torch.device(device))
-model.load_state_dict(checkpoint['model_state_dict'])
-model.eval()
-model.to(device)
-
-
-# generate from the model
-context = torch.zeros((1, 1), dtype=torch.long, device=device)
-
-def greet(number_of_tokens, start_character):
- context[0][0] = encode(start_character)[0]
- max_new_tokens = number_of_tokens
- return decode(model.generate(context, max_new_tokens=int(max_new_tokens))[0].tolist())
-
-iface = gr.Interface(fn=greet, inputs=["number", "text"], outputs="text")
-iface.launch()
\ No newline at end of file
diff --git a/spaces/AnishKumbhar/ChatBot/text-generation-webui-main/wsl.sh b/spaces/AnishKumbhar/ChatBot/text-generation-webui-main/wsl.sh
deleted file mode 100644
index 32ee585dfe74d801e56e60b983e06126fc3f1550..0000000000000000000000000000000000000000
--- a/spaces/AnishKumbhar/ChatBot/text-generation-webui-main/wsl.sh
+++ /dev/null
@@ -1,112 +0,0 @@
-#!/bin/bash
-
-# detect if build-essential is missing or broken
-if ! dpkg-query -W -f'${Status}' "build-essential" 2>/dev/null | grep -q "ok installed"; then
-echo "build-essential not found or broken!
-
-A C++ compiler is required to build needed Python packages!
-To install one, run cmd_wsl.bat and enter these commands:
-
-sudo apt-get update
-sudo apt-get install build-essential
-"
-read -n1 -p "Continue the installer anyway? [y,n]" EXIT_PROMPT
-# only continue if user inputs 'y' else exit
-if ! [[ $EXIT_PROMPT == "Y" || $EXIT_PROMPT == "y" ]]; then exit; fi
-fi
-
-# deactivate existing conda envs as needed to avoid conflicts
-{ conda deactivate && conda deactivate && conda deactivate; } 2> /dev/null
-
-# config unlike other scripts, can't use current directory due to file IO bug in WSL, needs to be in virtual drive
-INSTALL_DIR_PREFIX="$HOME/text-gen-install"
-if [[ ! $(realpath "$(pwd)/..") = /mnt/* ]]; then
- INSTALL_DIR_PREFIX="$(realpath "$(pwd)/..")" && INSTALL_INPLACE=1
-fi
-INSTALL_DIR="$INSTALL_DIR_PREFIX/text-generation-webui"
-CONDA_ROOT_PREFIX="$INSTALL_DIR/installer_files/conda"
-INSTALL_ENV_DIR="$INSTALL_DIR/installer_files/env"
-MINICONDA_DOWNLOAD_URL="https://repo.anaconda.com/miniconda/Miniconda3-py310_23.3.1-0-Linux-x86_64.sh"
-conda_exists="F"
-
-# environment isolation
-export PYTHONNOUSERSITE=1
-unset PYTHONPATH
-unset PYTHONHOME
-export CUDA_PATH="$INSTALL_ENV_DIR"
-export CUDA_HOME="$CUDA_PATH"
-
-# /usr/lib/wsl/lib needs to be added to LD_LIBRARY_PATH to fix years-old bug in WSL where GPU drivers aren't linked properly
-export LD_LIBRARY_PATH="$CUDA_HOME/lib:/usr/lib/wsl/lib:$LD_LIBRARY_PATH"
-
-# open bash cli if called with 'wsl.sh cmd' with workarounds for existing conda
-if [ "$1" == "cmd" ]; then
- exec bash --init-file <(echo ". ~/.bashrc; conda deactivate 2> /dev/null; cd $INSTALL_DIR || cd $HOME; source $CONDA_ROOT_PREFIX/etc/profile.d/conda.sh; conda activate $INSTALL_ENV_DIR")
- exit
-fi
-
-if [[ "$INSTALL_DIR" =~ " " ]]; then echo This script relies on Miniconda which can not be silently installed under a path with spaces. && exit; fi
-
-# create install dir if missing
-if [ ! -d "$INSTALL_DIR" ]; then mkdir -p "$INSTALL_DIR" || exit; fi
-
-# figure out whether git and conda needs to be installed
-if "$CONDA_ROOT_PREFIX/bin/conda" --version &>/dev/null; then conda_exists="T"; fi
-
-# (if necessary) install git and conda into a contained environment
-# download miniconda
-if [ "$conda_exists" == "F" ]; then
- echo "Downloading Miniconda from $MINICONDA_DOWNLOAD_URL to $INSTALL_DIR/miniconda_installer.sh"
-
- curl -Lk "$MINICONDA_DOWNLOAD_URL" > "$INSTALL_DIR/miniconda_installer.sh"
-
- chmod u+x "$INSTALL_DIR/miniconda_installer.sh"
- bash "$INSTALL_DIR/miniconda_installer.sh" -b -p $CONDA_ROOT_PREFIX
-
- # test the conda binary
- echo "Miniconda version:"
- "$CONDA_ROOT_PREFIX/bin/conda" --version
-fi
-
-# create the installer env
-if [ ! -e "$INSTALL_ENV_DIR" ]; then
- "$CONDA_ROOT_PREFIX/bin/conda" create -y -k --prefix "$INSTALL_ENV_DIR" python=3.10 git
-fi
-
-# check if conda environment was actually created
-if [ ! -e "$INSTALL_ENV_DIR/bin/python" ]; then
- echo "Conda environment is empty."
- exit
-fi
-
-# activate installer env
-source "$CONDA_ROOT_PREFIX/etc/profile.d/conda.sh" # otherwise conda complains about 'shell not initialized' (needed when running in a script)
-conda activate "$INSTALL_ENV_DIR"
-
-pushd $INSTALL_DIR 1> /dev/null || exit
-
-if [ ! -f "./server.py" ]; then
- git init -b main
- git remote add origin https://github.com/oobabooga/text-generation-webui
- git fetch
- git remote set-head origin -a
- git reset origin/HEAD --hard
- git branch --set-upstream-to=origin/HEAD
- git restore -- . :!./CMD_FLAGS.txt
-fi
-
-# copy CMD_FLAGS.txt to install dir to allow edits within Windows
-if [[ $INSTALL_INPLACE != 1 ]]; then
- # workaround for old install migration
- if [ ! -f "./wsl.sh" ]; then
- git pull || exit
- [ -f "../webui.py" ] && mv "../webui.py" "../webui-old.py"
- fi
- if [ -f "$(dirs +1)/CMD_FLAGS.txt" ] && [ -f "./CMD_FLAGS.txt" ]; then cp -u "$(dirs +1)/CMD_FLAGS.txt" "$INSTALL_DIR"; fi
-fi
-
-# setup installer env update env if called with 'wsl.sh update'
-case "$1" in
-("update") python one_click.py --update;;
-(*) python one_click.py $@;;
-esac
diff --git a/spaces/Anonymous-sub/Rerender/ControlNet/annotator/uniformer/mmcv/image/misc.py b/spaces/Anonymous-sub/Rerender/ControlNet/annotator/uniformer/mmcv/image/misc.py
deleted file mode 100644
index 3e61f05e3b05e4c7b40de4eb6c8eb100e6da41d0..0000000000000000000000000000000000000000
--- a/spaces/Anonymous-sub/Rerender/ControlNet/annotator/uniformer/mmcv/image/misc.py
+++ /dev/null
@@ -1,44 +0,0 @@
-# Copyright (c) OpenMMLab. All rights reserved.
-import numpy as np
-
-import annotator.uniformer.mmcv as mmcv
-
-try:
- import torch
-except ImportError:
- torch = None
-
-
-def tensor2imgs(tensor, mean=(0, 0, 0), std=(1, 1, 1), to_rgb=True):
- """Convert tensor to 3-channel images.
-
- Args:
- tensor (torch.Tensor): Tensor that contains multiple images, shape (
- N, C, H, W).
- mean (tuple[float], optional): Mean of images. Defaults to (0, 0, 0).
- std (tuple[float], optional): Standard deviation of images.
- Defaults to (1, 1, 1).
- to_rgb (bool, optional): Whether the tensor was converted to RGB
- format in the first place. If so, convert it back to BGR.
- Defaults to True.
-
- Returns:
- list[np.ndarray]: A list that contains multiple images.
- """
-
- if torch is None:
- raise RuntimeError('pytorch is not installed')
- assert torch.is_tensor(tensor) and tensor.ndim == 4
- assert len(mean) == 3
- assert len(std) == 3
-
- num_imgs = tensor.size(0)
- mean = np.array(mean, dtype=np.float32)
- std = np.array(std, dtype=np.float32)
- imgs = []
- for img_id in range(num_imgs):
- img = tensor[img_id, ...].cpu().numpy().transpose(1, 2, 0)
- img = mmcv.imdenormalize(
- img, mean, std, to_bgr=to_rgb).astype(np.uint8)
- imgs.append(np.ascontiguousarray(img))
- return imgs
diff --git a/spaces/ArtificialArtist007/Rate-my-Aiart/app.py b/spaces/ArtificialArtist007/Rate-my-Aiart/app.py
deleted file mode 100644
index 5d1568109f87a00104797cdbc96b5f7ab95a6f07..0000000000000000000000000000000000000000
--- a/spaces/ArtificialArtist007/Rate-my-Aiart/app.py
+++ /dev/null
@@ -1,37 +0,0 @@
-import gradio as gr
-import tensorflow as tf
-from tensorflow.compat.v2.experimental import dtensor
-
-import numpy as np
-from PIL import Image
-
-# Load pre-trained MobileNetV2 model
-model = tf.keras.applications.MobileNetV2(weights='imagenet')
-
-def predict_difficulty_score(image):
- # Load image and preprocess it for the model
- img = Image.fromarray(image.astype('uint8'), 'RGB')
- img = img.resize((224, 224))
- img_array = tf.keras.preprocessing.image.img_to_array(img)
- img_array = tf.keras.applications.mobilenet_v2.preprocess_input(img_array[np.newaxis,...])
-
- # Use the model to predict the image class probabilities
- preds = model.predict(img_array)
-
- # Get the index of the top predicted class
- class_idx = np.argmax(preds[0])
-
- # Get the difficulty score based on the class index
- difficulty_score = round((class_idx / 999) * 99000) + 1000
-
- # Return the difficulty score
- return difficulty_score
-
-# Create a Gradio interface
-inputs = gr.inputs.Image(shape=(224, 224))
-outputs = gr.outputs.Textbox(label="Difficulty Score")
-interface = gr.Interface(fn=predict_difficulty_score, inputs=inputs, outputs=outputs,
- title="AI Art Difficulty Score", description="Upload an AI art image and get its difficulty score.")
-
-# Launch the interface
-interface.launch()
diff --git a/spaces/BAAI/vid2vid-zero/vid2vid_zero/util.py b/spaces/BAAI/vid2vid-zero/vid2vid_zero/util.py
deleted file mode 100644
index 33d7eab97f28a2ea165a5ac08bc9e0a62cd804c3..0000000000000000000000000000000000000000
--- a/spaces/BAAI/vid2vid-zero/vid2vid_zero/util.py
+++ /dev/null
@@ -1,114 +0,0 @@
-import os
-import imageio
-import tempfile
-import numpy as np
-from PIL import Image
-from typing import Union
-
-import torch
-import torchvision
-
-from tqdm import tqdm
-from einops import rearrange
-
-
-def save_videos_as_images(videos: torch.Tensor, path: str, rescale=False, n_rows=4, fps=2):
- dir_name = os.path.dirname(path)
- videos = rearrange(videos, "b c t h w -> t b h w c")
-
- os.makedirs(os.path.join(dir_name, "vis_images"), exist_ok=True)
- for frame_idx, x in enumerate(videos):
- if rescale:
- x = (x + 1.0) / 2.0
- x = (x * 255).numpy().astype(np.uint8)
-
- for batch_idx, image in enumerate(x):
- save_dir = os.path.join(dir_name, "vis_images", f"batch_{batch_idx}")
- os.makedirs(save_dir, exist_ok=True)
- save_path = os.path.join(save_dir, f"frame_{frame_idx}.png")
- image = Image.fromarray(image)
- image.save(save_path)
-
-
-def save_videos_grid(videos: torch.Tensor, path: str, rescale=False, n_rows=4, fps=2):
- videos = rearrange(videos, "b c t h w -> t b c h w")
- outputs = []
- for x in videos:
- x = torchvision.utils.make_grid(x, nrow=n_rows)
- x = x.transpose(0, 1).transpose(1, 2).squeeze(-1)
- if rescale:
- x = (x + 1.0) / 2.0 # -1,1 -> 0,1
- x = (x * 255).numpy().astype(np.uint8)
- outputs.append(x)
-
- os.makedirs(os.path.dirname(path), exist_ok=True)
- imageio.mimsave(path, outputs, fps=8)
-
- # save for gradio demo
- out_file = tempfile.NamedTemporaryFile(suffix='.mp4', delete=False)
- out_file.name = path.replace('.gif', '.mp4')
- writer = imageio.get_writer(out_file.name, fps=fps)
- for frame in outputs:
- writer.append_data(frame)
- writer.close()
-
-
-@torch.no_grad()
-def init_prompt(prompt, pipeline):
- uncond_input = pipeline.tokenizer(
- [""], padding="max_length", max_length=pipeline.tokenizer.model_max_length,
- return_tensors="pt"
- )
- uncond_embeddings = pipeline.text_encoder(uncond_input.input_ids.to(pipeline.device))[0]
- text_input = pipeline.tokenizer(
- [prompt],
- padding="max_length",
- max_length=pipeline.tokenizer.model_max_length,
- truncation=True,
- return_tensors="pt",
- )
- text_embeddings = pipeline.text_encoder(text_input.input_ids.to(pipeline.device))[0]
- context = torch.cat([uncond_embeddings, text_embeddings])
-
- return context
-
-
-def next_step(model_output: Union[torch.FloatTensor, np.ndarray], timestep: int,
- sample: Union[torch.FloatTensor, np.ndarray], ddim_scheduler):
- timestep, next_timestep = min(
- timestep - ddim_scheduler.config.num_train_timesteps // ddim_scheduler.num_inference_steps, 999), timestep
- alpha_prod_t = ddim_scheduler.alphas_cumprod[timestep] if timestep >= 0 else ddim_scheduler.final_alpha_cumprod
- alpha_prod_t_next = ddim_scheduler.alphas_cumprod[next_timestep]
- beta_prod_t = 1 - alpha_prod_t
- next_original_sample = (sample - beta_prod_t ** 0.5 * model_output) / alpha_prod_t ** 0.5
- next_sample_direction = (1 - alpha_prod_t_next) ** 0.5 * model_output
- next_sample = alpha_prod_t_next ** 0.5 * next_original_sample + next_sample_direction
- return next_sample
-
-
-def get_noise_pred_single(latents, t, context, unet, normal_infer=False):
- bs = latents.shape[0] # (b*f, c, h, w) or (b, c, f, h, w)
- if bs != context.shape[0]:
- context = context.repeat(bs, 1, 1) # (b*f, len, dim)
- noise_pred = unet(latents, t, encoder_hidden_states=context, normal_infer=normal_infer)["sample"]
- return noise_pred
-
-
-@torch.no_grad()
-def ddim_loop(pipeline, ddim_scheduler, latent, num_inv_steps, prompt, normal_infer=False):
- context = init_prompt(prompt, pipeline)
- uncond_embeddings, cond_embeddings = context.chunk(2)
- all_latent = [latent]
- latent = latent.clone().detach()
- for i in tqdm(range(num_inv_steps)):
- t = ddim_scheduler.timesteps[len(ddim_scheduler.timesteps) - i - 1]
- noise_pred = get_noise_pred_single(latent, t, cond_embeddings, pipeline.unet, normal_infer=normal_infer)
- latent = next_step(noise_pred, t, latent, ddim_scheduler)
- all_latent.append(latent)
- return all_latent
-
-
-@torch.no_grad()
-def ddim_inversion(pipeline, ddim_scheduler, video_latent, num_inv_steps, prompt="", normal_infer=False):
- ddim_latents = ddim_loop(pipeline, ddim_scheduler, video_latent, num_inv_steps, prompt, normal_infer=normal_infer)
- return ddim_latents
diff --git a/spaces/Babelscape/rebel-demo/README.md b/spaces/Babelscape/rebel-demo/README.md
deleted file mode 100644
index 41894a2bcd412dcf1fe45b7038d11df50576c8a7..0000000000000000000000000000000000000000
--- a/spaces/Babelscape/rebel-demo/README.md
+++ /dev/null
@@ -1,37 +0,0 @@
----
-title: Rebel Demo
-emoji: 🌍
-colorFrom: purple
-colorTo: pink
-sdk: streamlit
-app_file: app.py
-pinned: false
----
-
-# Configuration
-
-`title`: _string_
-Display title for the Space
-
-`emoji`: _string_
-Space emoji (emoji-only character allowed)
-
-`colorFrom`: _string_
-Color for Thumbnail gradient (red, yellow, green, blue, indigo, purple, pink, gray)
-
-`colorTo`: _string_
-Color for Thumbnail gradient (red, yellow, green, blue, indigo, purple, pink, gray)
-
-`sdk`: _string_
-Can be either `gradio` or `streamlit`
-
-`sdk_version` : _string_
-Only applicable for `streamlit` SDK.
-See [doc](https://hf.co/docs/hub/spaces) for more info on supported versions.
-
-`app_file`: _string_
-Path to your main application file (which contains either `gradio` or `streamlit` Python code).
-Path is relative to the root of the repository.
-
-`pinned`: _boolean_
-Whether the Space stays on top of your list.
diff --git a/spaces/Banbri/zcvzcv/src/lib/cleanJson.ts b/spaces/Banbri/zcvzcv/src/lib/cleanJson.ts
deleted file mode 100644
index 8e914d329008deae4e14679597a76ca352b64925..0000000000000000000000000000000000000000
--- a/spaces/Banbri/zcvzcv/src/lib/cleanJson.ts
+++ /dev/null
@@ -1,19 +0,0 @@
-import { dirtyLLMResponseCleaner } from "./dirtyLLMResponseCleaner"
-
-export function cleanJson(input: string) {
-
- if (input.includes('```')) {
- input = input.split('```')[0]
- }
- let tmp = dirtyLLMResponseCleaner(input)
-
- // we only keep what's after the first [
- tmp = `[${tmp.split("[").pop() || ""}`
-
- // and before the first ]
- tmp = `${tmp.split("]").shift() || ""}]`
-
- tmp = dirtyLLMResponseCleaner(tmp)
-
- return tmp
-}
\ No newline at end of file
diff --git a/spaces/Bart92/RVC_HF/audioEffects.py b/spaces/Bart92/RVC_HF/audioEffects.py
deleted file mode 100644
index 1830b19e1a5e3ec1f431388d8444ef3a2c9ed91f..0000000000000000000000000000000000000000
--- a/spaces/Bart92/RVC_HF/audioEffects.py
+++ /dev/null
@@ -1,37 +0,0 @@
-from pedalboard import Pedalboard, Compressor, Reverb, NoiseGate
-from pedalboard.io import AudioFile
-import sys
-import os
-now_dir = os.getcwd()
-sys.path.append(now_dir)
-from i18n import I18nAuto
-i18n = I18nAuto()
-from pydub import AudioSegment
-import numpy as np
-import soundfile as sf
-from pydub.playback import play
-
-def process_audio(input_path, output_path, reverb_enabled, compressor_enabled, noise_gate_enabled, ):
- print(reverb_enabled)
- print(compressor_enabled)
- print(noise_gate_enabled)
- effects = []
- if reverb_enabled:
- effects.append(Reverb(room_size=0.01))
- if compressor_enabled:
- effects.append(Compressor(threshold_db=-10, ratio=25))
- if noise_gate_enabled:
- effects.append(NoiseGate(threshold_db=-16, ratio=1.5, release_ms=250))
-
- board = Pedalboard(effects)
-
- with AudioFile(input_path) as f:
- with AudioFile(output_path, 'w', f.samplerate, f.num_channels) as o:
- while f.tell() < f.frames:
- chunk = f.read(f.samplerate)
- effected = board(chunk, f.samplerate, reset=False)
- o.write(effected)
-
- result = i18n("Processed audio saved at: ") + output_path
- print(result)
- return output_path
\ No newline at end of file
diff --git a/spaces/Bart92/RVC_HF/infer/lib/infer_pack/modules/F0Predictor/HarvestF0Predictor.py b/spaces/Bart92/RVC_HF/infer/lib/infer_pack/modules/F0Predictor/HarvestF0Predictor.py
deleted file mode 100644
index f9664fb1f89ef068e923211179e1c7e1ce7fdbd2..0000000000000000000000000000000000000000
--- a/spaces/Bart92/RVC_HF/infer/lib/infer_pack/modules/F0Predictor/HarvestF0Predictor.py
+++ /dev/null
@@ -1,87 +0,0 @@
-import numpy as np
-import pyworld
-
-from infer.lib.infer_pack.modules.F0Predictor.F0Predictor import F0Predictor
-
-
-class HarvestF0Predictor(F0Predictor):
- def __init__(self, hop_length=512, f0_min=50, f0_max=1100, sampling_rate=44100):
- self.hop_length = hop_length
- self.f0_min = f0_min
- self.f0_max = f0_max
- self.sampling_rate = sampling_rate
-
- def interpolate_f0(self, f0):
- """
- 对F0进行插值处理
- """
-
- data = np.reshape(f0, (f0.size, 1))
-
- vuv_vector = np.zeros((data.size, 1), dtype=np.float32)
- vuv_vector[data > 0.0] = 1.0
- vuv_vector[data <= 0.0] = 0.0
-
- ip_data = data
-
- frame_number = data.size
- last_value = 0.0
- for i in range(frame_number):
- if data[i] <= 0.0:
- j = i + 1
- for j in range(i + 1, frame_number):
- if data[j] > 0.0:
- break
- if j < frame_number - 1:
- if last_value > 0.0:
- step = (data[j] - data[i - 1]) / float(j - i)
- for k in range(i, j):
- ip_data[k] = data[i - 1] + step * (k - i + 1)
- else:
- for k in range(i, j):
- ip_data[k] = data[j]
- else:
- for k in range(i, frame_number):
- ip_data[k] = last_value
- else:
- ip_data[i] = data[i] # 这里可能存在一个没有必要的拷贝
- last_value = data[i]
-
- return ip_data[:, 0], vuv_vector[:, 0]
-
- def resize_f0(self, x, target_len):
- source = np.array(x)
- source[source < 0.001] = np.nan
- target = np.interp(
- np.arange(0, len(source) * target_len, len(source)) / target_len,
- np.arange(0, len(source)),
- source,
- )
- res = np.nan_to_num(target)
- return res
-
- def compute_f0(self, wav, p_len=None):
- if p_len is None:
- p_len = wav.shape[0] // self.hop_length
- f0, t = pyworld.harvest(
- wav.astype(np.double),
- fs=self.hop_length,
- f0_ceil=self.f0_max,
- f0_floor=self.f0_min,
- frame_period=1000 * self.hop_length / self.sampling_rate,
- )
- f0 = pyworld.stonemask(wav.astype(np.double), f0, t, self.fs)
- return self.interpolate_f0(self.resize_f0(f0, p_len))[0]
-
- def compute_f0_uv(self, wav, p_len=None):
- if p_len is None:
- p_len = wav.shape[0] // self.hop_length
- f0, t = pyworld.harvest(
- wav.astype(np.double),
- fs=self.sampling_rate,
- f0_floor=self.f0_min,
- f0_ceil=self.f0_max,
- frame_period=1000 * self.hop_length / self.sampling_rate,
- )
- f0 = pyworld.stonemask(wav.astype(np.double), f0, t, self.sampling_rate)
- return self.interpolate_f0(self.resize_f0(f0, p_len))
diff --git a/spaces/Benson/text-generation/Examples/Cmo Descargar Videos De Google Drive.md b/spaces/Benson/text-generation/Examples/Cmo Descargar Videos De Google Drive.md
deleted file mode 100644
index 0baee9e6ac6a8e4f96f6b2c103753157c84db51a..0000000000000000000000000000000000000000
--- a/spaces/Benson/text-generation/Examples/Cmo Descargar Videos De Google Drive.md
+++ /dev/null
@@ -1,157 +0,0 @@
-
-
Cómo descargar videos de Google Drive
-
Google Drive es un servicio de almacenamiento en la nube popular que le permite almacenar y acceder a sus archivos en línea. Puede usarlo para almacenar sus videos y verlos en cualquier momento, en cualquier lugar y en cualquier dispositivo. Pero lo que si desea descargar sus vídeos de Google Drive a su ordenador o teléfono? Tal vez quieras liberar algo de espacio en tu Drive, o crear una copia de seguridad de tus videos, o verlos sin conexión. Cualquiera que sea la razón, la descarga de vídeo desde Google Drive es fácil y rápido. En este artículo, le mostraremos cómo descargar videos de Google Drive a diferentes dispositivos, incluyendo PC con Windows, Mac, Android y iPhone.
¿Qué es Google Drive y por qué usarlo para vídeos?
-
Google Drive es un servicio de almacenamiento en la nube que te permite almacenar hasta 15 GB de archivos de forma gratuita. Puede cargar cualquier tipo de archivo, incluidos documentos, fotos, música y videos. También puede crear y editar archivos con Google Docs, Hojas y Diapositivas. Puede acceder a sus archivos desde cualquier dispositivo que tenga una conexión a Internet y un navegador web. También puede usar la aplicación Google Drive en su computadora o teléfono para sincronizar sus archivos y acceder a ellos sin conexión.
-
Uno de los beneficios de usar Google Drive para videos es que puedes verlos en línea sin descargarlos. También puede compartirlos con otros enviando un enlace o invitándolos a ver o editar sus archivos. También puede colaborar en videos con otros mediante comentarios y sugerencias. También puede organizar sus vídeos en carpetas y subcarpetas, y buscarlos usando palabras clave o filtros.
-
Cómo descargar video de Google Drive a diferentes dispositivos
-
Descargar video de Google Drive es simple y sencillo. Solo tiene que seguir estos pasos:
-
-
Vaya a drive.google.com e inicie sesión con su cuenta de Google.
-
Seleccione el vídeo o vídeos que desea descargar.
-
-
Elija una ubicación en su dispositivo donde desea guardar el vídeo o vídeos descargados.
-
-
Los pasos exactos pueden variar ligeramente dependiendo del dispositivo que esté utilizando. Explicaremos las diferencias en las siguientes secciones.
-
Descargar vídeo de Google Drive a Windows PC
-
Descargar vídeos individuales
-
Si desea descargar un solo video de Google Drive a su PC con Windows, puede seguir estos pasos:
-
-
-
Vaya a drive.google.com e inicie sesión con su cuenta de Google.
-
Haga clic en el vídeo que desea descargar.
-
Haga clic en el icono de menú de tres puntos en la esquina superior derecha y elija "Descargar".
-
El vídeo se descargará como un archivo MP4 a su carpeta de descarga predeterminada. Puede cambiar esta carpeta en la configuración de su navegador.
-
-
Descargar múltiples vídeos
-
Si desea descargar más de un video de Google Drive a su PC con Windows, puede seguir estos pasos:
-
-
Vaya a drive.google.com e inicie sesión con su cuenta de Google.
-
Mantenga presionada la tecla Ctrl y haga clic en cada video que desea descargar.
-
Haga clic en el icono de menú de tres puntos en la esquina superior derecha y elija "Descargar".
-
Los vídeos se descargarán como un archivo ZIP en la carpeta de descarga predeterminada. Puede cambiar esta carpeta en la configuración de su navegador.
-
Extraer el archivo ZIP para acceder a los archivos MP4 individuales.
-
-
Sincronizar Google Drive a PC
-
Si desea sincronizar sus videos de Google Drive a su PC con Windows, puede seguir estos pasos:
Inicie sesión con su cuenta de Google y elija una carpeta en su PC donde desea sincronizar sus archivos de Google Drive.
-
Haga clic en el icono de Google Drive en la bandeja del sistema y seleccione "Preferencias".
-
-
Haga clic en "OK" y espere a que se complete la sincronización.
-
-
Una vez que se realiza la sincronización, puede acceder a sus videos de Google Drive desde la carpeta que eligió en su PC. También puede verlos sin conexión, editarlos o eliminarlos. Cualquier cambio que realice se reflejará en su Google Drive en línea.
-
Descargar vídeo de Google Drive a Mac
-
Descargar vídeos individuales
-
Si desea descargar un solo video de Google Drive a su Mac, puede seguir estos pasos:
Inicie sesión con su cuenta de Google y elija una carpeta en su Mac donde desea sincronizar sus archivos de Google Drive.
-
Haga clic en el icono de Google Drive en la barra de menú y seleccione "Preferencias".
-
-
Haga clic en "OK" y espere a que se complete la sincronización.
-
-
Una vez que se realiza la sincronización, puede acceder a sus videos de Google Drive desde la carpeta que eligió en su Mac. También puede verlos sin conexión, editarlos o eliminarlos. Cualquier cambio que realice se reflejará en su Google Drive en línea.
-
Descargar vídeo de Google Drive para Android o iPhone
-
Descargar vídeos individuales
-
Si desea descargar un solo video de Google Drive a su Android o iPhone, puede seguir estos pasos:
Abra la aplicación e inicie sesión con su cuenta de Google.
-
Toque en el vídeo que desea descargar.
-
Toque en el icono de menú de tres puntos en la esquina inferior derecha y elija "Descargar".
-
El video se descargará como un archivo MP4 en el almacenamiento de su dispositivo. Puede encontrarlo en la carpeta "Descargas" o en la aplicación "Fotos".
-
-
Descargar múltiples vídeos
-
Si quieres descargar más de un video de Google Drive a tu Android o iPhone, puedes seguir estos pasos:
Abra la aplicación e inicie sesión con su cuenta de Google.
-
Mantenga pulsado el primer vídeo que desea descargar, luego pulse sobre los otros vídeos que desea descargar.
-
Toque en el icono de menú de tres puntos en la esquina superior derecha y elija "Descargar".
-
Los vídeos se descargarán como archivos MP4 en el almacenamiento de su dispositivo. Puede encontrarlos en la carpeta "Descargas" o en la aplicación "Fotos".
-
-
Sincronizar Google Drive al teléfono
-
Si quieres sincronizar tus vídeos de Google Drive con tu Android o iPhone, puedes seguir estos pasos:
Abra la aplicación e inicie sesión con su cuenta de Google.
-
-
Toque en el icono de menú de tres líneas en la esquina superior izquierda y elija "Configuración".
-
Toque en "Copia de seguridad y sincronización".
-
Activar la palanca para "Copia de seguridad y sincronización".
-
Seleccione las carpetas que desea sincronizar. También puede optar por sincronizar todo en su Google Drive o solo archivos específicos.
-
Toque en "Hecho" y espere a que la sincronización se complete.
-
-
Una vez que se realiza la sincronización, puede acceder a sus videos de Google Drive desde la pestaña "Archivos" en la aplicación. También puede verlos sin conexión, editarlos o eliminarlos. Cualquier cambio que realice se reflejará en su Google Drive en línea.
-
Conclusión
-
Resumen de los puntos principales
-
En este artículo, le hemos mostrado cómo descargar video de Google Drive a diferentes dispositivos, incluyendo PC con Windows, Mac, Android y iPhone. También hemos explicado cómo sincronizar tus vídeos de Google Drive con tus dispositivos, para que puedas acceder a ellos sin conexión y mantenerlos actualizados. Descargar video desde Google Drive es fácil y rápido, y puede ayudarlo a ahorrar espacio en su unidad, crear copias de seguridad de sus videos o verlos sin una conexión a Internet.
-
Llamada a la acción
-
Esperamos que haya encontrado este artículo útil e informativo. Si tiene alguna pregunta o comentario, deje un comentario a continuación. Si te gustó este artículo, por favor compártelo con tus amigos y familiares. Y si desea obtener más información sobre Google Drive y otros servicios de almacenamiento en la nube, suscríbase a nuestro boletín y síganos en las redes sociales. ¡Gracias por leer!
-
Preguntas frecuentes
-
¿Cómo puedo descargar un video de Google Drive que es demasiado grande?
-
Si intenta descargar un video de Google Drive que es mayor que 2 GB, puede encontrar un mensaje de error que dice "Este archivo es demasiado grande para que Google lo escanee en busca de virus". Esto no significa que el archivo esté infectado, sino que Google no puede verificar su seguridad. Para descargar este archivo, debe hacer lo siguiente:
-
-
-
Haga clic en el icono de menú de tres puntos en la esquina superior derecha y elija "Descargar de todos modos".
-
El vídeo se descargará como un archivo MP4 a su carpeta de descarga predeterminada. Puede cambiar esta carpeta en la configuración de su navegador.
-
-
¿Cómo puedo descargar un video de Google Drive que se comparte conmigo?
-
Si alguien ha compartido un video contigo en Google Drive, puedes descargarlo siguiendo estos pasos:
¿Cómo puedo descargar un video de Google Drive que está en un formato diferente?
-
-
-
Descargar el video de Google Drive como un archivo MP4 utilizando los pasos anteriores.
-
Ir a la página web de la herramienta en línea que desea utilizar y cargar el archivo MP4.
-
Seleccione el formato de salida al que desea convertir el vídeo, como AVI, MOV, WMV, etc.
-
Haga clic en "Convertir" o "Iniciar" y espere a que la conversión termine.
-
Descargar el archivo de vídeo convertido a su dispositivo.
-
-
¿Cómo puedo descargar un video de Google Drive que está incrustado en un sitio web?
-
Si desea descargar un video de Google Drive que está incrustado en un sitio web, puede usar una extensión del navegador o una herramienta de raspado web para extraer la URL del video. Algunos ejemplos de estas herramientas son Video DownloadHelper, Flash Video Downloader, o Video Downloader Professional. Puedes usar estas herramientas siguiendo estos pasos:
-
-
Descargue e instale la extensión del navegador o la herramienta de raspador web que desea usar.
-
Ir al sitio web que tiene el video incrustado de Google Drive.
-
Haga clic en el icono de la herramienta que instaló en la barra de herramientas del navegador y elija "Descargar" o "Extraer".
-
La herramienta le mostrará la URL del video y le permitirá descargarlo como un archivo MP4.
-
-
-
Este es el final del artículo. Espero que hayas disfrutado leyéndolo y hayas aprendido algo nuevo. Si tiene alguna pregunta o comentario, por favor deje un comentario abajo. ¡Gracias por su atención!
64aa2da5cf
-
-
\ No newline at end of file
diff --git a/spaces/Big-Web/MMSD/env/Lib/site-packages/pip/_vendor/urllib3/util/timeout.py b/spaces/Big-Web/MMSD/env/Lib/site-packages/pip/_vendor/urllib3/util/timeout.py
deleted file mode 100644
index 78e18a6272482e3946de83c0274badc4a5cfcdfa..0000000000000000000000000000000000000000
--- a/spaces/Big-Web/MMSD/env/Lib/site-packages/pip/_vendor/urllib3/util/timeout.py
+++ /dev/null
@@ -1,271 +0,0 @@
-from __future__ import absolute_import
-
-import time
-
-# The default socket timeout, used by httplib to indicate that no timeout was; specified by the user
-from socket import _GLOBAL_DEFAULT_TIMEOUT, getdefaulttimeout
-
-from ..exceptions import TimeoutStateError
-
-# A sentinel value to indicate that no timeout was specified by the user in
-# urllib3
-_Default = object()
-
-
-# Use time.monotonic if available.
-current_time = getattr(time, "monotonic", time.time)
-
-
-class Timeout(object):
- """Timeout configuration.
-
- Timeouts can be defined as a default for a pool:
-
- .. code-block:: python
-
- timeout = Timeout(connect=2.0, read=7.0)
- http = PoolManager(timeout=timeout)
- response = http.request('GET', 'http://example.com/')
-
- Or per-request (which overrides the default for the pool):
-
- .. code-block:: python
-
- response = http.request('GET', 'http://example.com/', timeout=Timeout(10))
-
- Timeouts can be disabled by setting all the parameters to ``None``:
-
- .. code-block:: python
-
- no_timeout = Timeout(connect=None, read=None)
- response = http.request('GET', 'http://example.com/, timeout=no_timeout)
-
-
- :param total:
- This combines the connect and read timeouts into one; the read timeout
- will be set to the time leftover from the connect attempt. In the
- event that both a connect timeout and a total are specified, or a read
- timeout and a total are specified, the shorter timeout will be applied.
-
- Defaults to None.
-
- :type total: int, float, or None
-
- :param connect:
- The maximum amount of time (in seconds) to wait for a connection
- attempt to a server to succeed. Omitting the parameter will default the
- connect timeout to the system default, probably `the global default
- timeout in socket.py
- `_.
- None will set an infinite timeout for connection attempts.
-
- :type connect: int, float, or None
-
- :param read:
- The maximum amount of time (in seconds) to wait between consecutive
- read operations for a response from the server. Omitting the parameter
- will default the read timeout to the system default, probably `the
- global default timeout in socket.py
- `_.
- None will set an infinite timeout.
-
- :type read: int, float, or None
-
- .. note::
-
- Many factors can affect the total amount of time for urllib3 to return
- an HTTP response.
-
- For example, Python's DNS resolver does not obey the timeout specified
- on the socket. Other factors that can affect total request time include
- high CPU load, high swap, the program running at a low priority level,
- or other behaviors.
-
- In addition, the read and total timeouts only measure the time between
- read operations on the socket connecting the client and the server,
- not the total amount of time for the request to return a complete
- response. For most requests, the timeout is raised because the server
- has not sent the first byte in the specified time. This is not always
- the case; if a server streams one byte every fifteen seconds, a timeout
- of 20 seconds will not trigger, even though the request will take
- several minutes to complete.
-
- If your goal is to cut off any request after a set amount of wall clock
- time, consider having a second "watcher" thread to cut off a slow
- request.
- """
-
- #: A sentinel object representing the default timeout value
- DEFAULT_TIMEOUT = _GLOBAL_DEFAULT_TIMEOUT
-
- def __init__(self, total=None, connect=_Default, read=_Default):
- self._connect = self._validate_timeout(connect, "connect")
- self._read = self._validate_timeout(read, "read")
- self.total = self._validate_timeout(total, "total")
- self._start_connect = None
-
- def __repr__(self):
- return "%s(connect=%r, read=%r, total=%r)" % (
- type(self).__name__,
- self._connect,
- self._read,
- self.total,
- )
-
- # __str__ provided for backwards compatibility
- __str__ = __repr__
-
- @classmethod
- def resolve_default_timeout(cls, timeout):
- return getdefaulttimeout() if timeout is cls.DEFAULT_TIMEOUT else timeout
-
- @classmethod
- def _validate_timeout(cls, value, name):
- """Check that a timeout attribute is valid.
-
- :param value: The timeout value to validate
- :param name: The name of the timeout attribute to validate. This is
- used to specify in error messages.
- :return: The validated and casted version of the given value.
- :raises ValueError: If it is a numeric value less than or equal to
- zero, or the type is not an integer, float, or None.
- """
- if value is _Default:
- return cls.DEFAULT_TIMEOUT
-
- if value is None or value is cls.DEFAULT_TIMEOUT:
- return value
-
- if isinstance(value, bool):
- raise ValueError(
- "Timeout cannot be a boolean value. It must "
- "be an int, float or None."
- )
- try:
- float(value)
- except (TypeError, ValueError):
- raise ValueError(
- "Timeout value %s was %s, but it must be an "
- "int, float or None." % (name, value)
- )
-
- try:
- if value <= 0:
- raise ValueError(
- "Attempted to set %s timeout to %s, but the "
- "timeout cannot be set to a value less "
- "than or equal to 0." % (name, value)
- )
- except TypeError:
- # Python 3
- raise ValueError(
- "Timeout value %s was %s, but it must be an "
- "int, float or None." % (name, value)
- )
-
- return value
-
- @classmethod
- def from_float(cls, timeout):
- """Create a new Timeout from a legacy timeout value.
-
- The timeout value used by httplib.py sets the same timeout on the
- connect(), and recv() socket requests. This creates a :class:`Timeout`
- object that sets the individual timeouts to the ``timeout`` value
- passed to this function.
-
- :param timeout: The legacy timeout value.
- :type timeout: integer, float, sentinel default object, or None
- :return: Timeout object
- :rtype: :class:`Timeout`
- """
- return Timeout(read=timeout, connect=timeout)
-
- def clone(self):
- """Create a copy of the timeout object
-
- Timeout properties are stored per-pool but each request needs a fresh
- Timeout object to ensure each one has its own start/stop configured.
-
- :return: a copy of the timeout object
- :rtype: :class:`Timeout`
- """
- # We can't use copy.deepcopy because that will also create a new object
- # for _GLOBAL_DEFAULT_TIMEOUT, which socket.py uses as a sentinel to
- # detect the user default.
- return Timeout(connect=self._connect, read=self._read, total=self.total)
-
- def start_connect(self):
- """Start the timeout clock, used during a connect() attempt
-
- :raises urllib3.exceptions.TimeoutStateError: if you attempt
- to start a timer that has been started already.
- """
- if self._start_connect is not None:
- raise TimeoutStateError("Timeout timer has already been started.")
- self._start_connect = current_time()
- return self._start_connect
-
- def get_connect_duration(self):
- """Gets the time elapsed since the call to :meth:`start_connect`.
-
- :return: Elapsed time in seconds.
- :rtype: float
- :raises urllib3.exceptions.TimeoutStateError: if you attempt
- to get duration for a timer that hasn't been started.
- """
- if self._start_connect is None:
- raise TimeoutStateError(
- "Can't get connect duration for timer that has not started."
- )
- return current_time() - self._start_connect
-
- @property
- def connect_timeout(self):
- """Get the value to use when setting a connection timeout.
-
- This will be a positive float or integer, the value None
- (never timeout), or the default system timeout.
-
- :return: Connect timeout.
- :rtype: int, float, :attr:`Timeout.DEFAULT_TIMEOUT` or None
- """
- if self.total is None:
- return self._connect
-
- if self._connect is None or self._connect is self.DEFAULT_TIMEOUT:
- return self.total
-
- return min(self._connect, self.total)
-
- @property
- def read_timeout(self):
- """Get the value for the read timeout.
-
- This assumes some time has elapsed in the connection timeout and
- computes the read timeout appropriately.
-
- If self.total is set, the read timeout is dependent on the amount of
- time taken by the connect timeout. If the connection time has not been
- established, a :exc:`~urllib3.exceptions.TimeoutStateError` will be
- raised.
-
- :return: Value to use for the read timeout.
- :rtype: int, float, :attr:`Timeout.DEFAULT_TIMEOUT` or None
- :raises urllib3.exceptions.TimeoutStateError: If :meth:`start_connect`
- has not yet been called on this object.
- """
- if (
- self.total is not None
- and self.total is not self.DEFAULT_TIMEOUT
- and self._read is not None
- and self._read is not self.DEFAULT_TIMEOUT
- ):
- # In case the connect timeout has not yet been established.
- if self._start_connect is None:
- return self._read
- return max(0, min(self.total - self.get_connect_duration(), self._read))
- elif self.total is not None and self.total is not self.DEFAULT_TIMEOUT:
- return max(0, self.total - self.get_connect_duration())
- else:
- return self._read
diff --git a/spaces/CC123123/blip2_t/index.html b/spaces/CC123123/blip2_t/index.html
deleted file mode 100644
index 58275de3b1c343a98420342baa076b9baaafa157..0000000000000000000000000000000000000000
--- a/spaces/CC123123/blip2_t/index.html
+++ /dev/null
@@ -1,19 +0,0 @@
-
-
-
-
-
- My static Space
-
-
-
-
-
Welcome to your static Space!
-
You can modify this app directly by editing index.html in the Files and versions tab.
[Recommended to use google colab for more features](https://colab.research.google.com/drive/1hx6kKvIuv5XNY1Gai2PEuZhpO5z6xpVh?usp=sharing)\n"
- "[](https://colab.research.google.com/drive/1hx6kKvIuv5XNY1Gai2PEuZhpO5z6xpVh?usp=sharing)\n\n"
- "[](https://github.com/RVC-Project/Retrieval-based-Voice-Conversion-WebUI)"
- )
- with gr.Tabs():
- for (name, title, author, cover, vc_fn) in models:
- with gr.TabItem(name):
- with gr.Row():
- gr.Markdown(
- '
'
- f'
{title}
\n'+
- (f'
Model author: {author}
' if author else "")+
- (f'' if cover else "")+
- '
'
- )
- with gr.Row():
- with gr.Column():
- vc_input = gr.Audio(label="Input audio"+' (less than 20 seconds)' if limitation else '')
- vc_transpose = gr.Number(label="Transpose", value=0)
- vc_f0method = gr.Radio(
- label="Pitch extraction algorithm, PM is fast but Harvest is better for low frequencies",
- choices=["pm", "harvest"],
- value="pm",
- interactive=True,
- )
- vc_index_ratio = gr.Slider(
- minimum=0,
- maximum=1,
- label="Retrieval feature ratio",
- value=0.6,
- interactive=True,
- )
- tts_mode = gr.Checkbox(label="tts (use edge-tts as input)", value=False)
- tts_text = gr.Textbox(visible=False,label="TTS text (100 words limitation)" if limitation else "TTS text")
- tts_voice = gr.Dropdown(label="Edge-tts speaker", choices=voices, visible=False, allow_custom_value=False, value="en-US-AnaNeural-Female")
- vc_submit = gr.Button("Generate", variant="primary")
- with gr.Column():
- vc_output1 = gr.Textbox(label="Output Message")
- vc_output2 = gr.Audio(label="Output Audio")
- vc_submit.click(vc_fn, [vc_input, vc_transpose, vc_f0method, vc_index_ratio, tts_mode, tts_text, tts_voice], [vc_output1, vc_output2])
- tts_mode.change(change_to_tts_mode, [tts_mode], [vc_input, tts_text, tts_voice])
- app.queue(concurrency_count=1, max_size=20, api_open=args.api).launch(share=args.colab)
\ No newline at end of file
diff --git a/spaces/DemoLou/moe-tts/README.md b/spaces/DemoLou/moe-tts/README.md
deleted file mode 100644
index 6f67c1368b0673f2d0d88e1ded980b6637e0fa4e..0000000000000000000000000000000000000000
--- a/spaces/DemoLou/moe-tts/README.md
+++ /dev/null
@@ -1,14 +0,0 @@
----
-title: Moe TTS
-emoji: 😊🎙️
-colorFrom: red
-colorTo: pink
-sdk: gradio
-sdk_version: 3.22.1
-app_file: test.py
-pinned: false
-license: mit
-duplicated_from: skytnt/moe-tts
----
-
-Check out the configuration reference at https://huggingface.co/docs/hub/spaces-config-reference
diff --git a/spaces/Dinoking/Guccio-AI-Designer/netdissect/tool/allunitsample.py b/spaces/Dinoking/Guccio-AI-Designer/netdissect/tool/allunitsample.py
deleted file mode 100644
index 9f86e196ce63ebfcad1fcee8bd2b7358463ff3d1..0000000000000000000000000000000000000000
--- a/spaces/Dinoking/Guccio-AI-Designer/netdissect/tool/allunitsample.py
+++ /dev/null
@@ -1,199 +0,0 @@
-'''
-A simple tool to generate sample of output of a GAN,
-subject to filtering, sorting, or intervention.
-'''
-
-import torch, numpy, os, argparse, sys, shutil, errno, numbers
-from PIL import Image
-from torch.utils.data import TensorDataset
-from netdissect.zdataset import standard_z_sample
-from netdissect.progress import default_progress, verbose_progress
-from netdissect.autoeval import autoimport_eval
-from netdissect.workerpool import WorkerBase, WorkerPool
-from netdissect.nethook import retain_layers
-from netdissect.runningstats import RunningTopK
-
-def main():
- parser = argparse.ArgumentParser(description='GAN sample making utility')
- parser.add_argument('--model', type=str, default=None,
- help='constructor for the model to test')
- parser.add_argument('--pthfile', type=str, default=None,
- help='filename of .pth file for the model')
- parser.add_argument('--outdir', type=str, default='images',
- help='directory for image output')
- parser.add_argument('--size', type=int, default=100,
- help='number of images to output')
- parser.add_argument('--test_size', type=int, default=None,
- help='number of images to test')
- parser.add_argument('--layer', type=str, default=None,
- help='layer to inspect')
- parser.add_argument('--seed', type=int, default=1,
- help='seed')
- parser.add_argument('--quiet', action='store_true', default=False,
- help='silences console output')
- if len(sys.argv) == 1:
- parser.print_usage(sys.stderr)
- sys.exit(1)
- args = parser.parse_args()
- verbose_progress(not args.quiet)
-
- # Instantiate the model
- model = autoimport_eval(args.model)
- if args.pthfile is not None:
- data = torch.load(args.pthfile)
- if 'state_dict' in data:
- meta = {}
- for key in data:
- if isinstance(data[key], numbers.Number):
- meta[key] = data[key]
- data = data['state_dict']
- model.load_state_dict(data)
- # Unwrap any DataParallel-wrapped model
- if isinstance(model, torch.nn.DataParallel):
- model = next(model.children())
- # Examine first conv in model to determine input feature size.
- first_layer = [c for c in model.modules()
- if isinstance(c, (torch.nn.Conv2d, torch.nn.ConvTranspose2d,
- torch.nn.Linear))][0]
- # 4d input if convolutional, 2d input if first layer is linear.
- if isinstance(first_layer, (torch.nn.Conv2d, torch.nn.ConvTranspose2d)):
- z_channels = first_layer.in_channels
- spatialdims = (1, 1)
- else:
- z_channels = first_layer.in_features
- spatialdims = ()
- # Instrument the model
- retain_layers(model, [args.layer])
- model.cuda()
-
- if args.test_size is None:
- args.test_size = args.size * 20
- z_universe = standard_z_sample(args.test_size, z_channels,
- seed=args.seed)
- z_universe = z_universe.view(tuple(z_universe.shape) + spatialdims)
- indexes = get_all_highest_znums(
- model, z_universe, args.size, seed=args.seed)
- save_chosen_unit_images(args.outdir, model, z_universe, indexes,
- lightbox=True)
-
-
-def get_all_highest_znums(model, z_universe, size,
- batch_size=10, seed=1):
- # The model should have been instrumented already
- retained_items = list(model.retained.items())
- assert len(retained_items) == 1
- layer = retained_items[0][0]
- # By default, a 10% sample
- progress = default_progress()
- num_units = None
- with torch.no_grad():
- # Pass 1: collect max activation stats
- z_loader = torch.utils.data.DataLoader(TensorDataset(z_universe),
- batch_size=batch_size, num_workers=2,
- pin_memory=True)
- rtk = RunningTopK(k=size)
- for [z] in progress(z_loader, desc='Finding max activations'):
- z = z.cuda()
- model(z)
- feature = model.retained[layer]
- num_units = feature.shape[1]
- max_feature = feature.view(
- feature.shape[0], num_units, -1).max(2)[0]
- rtk.add(max_feature)
- td, ti = rtk.result()
- highest = ti.sort(1)[0]
- return highest
-
-def save_chosen_unit_images(dirname, model, z_universe, indices,
- shared_dir="shared_images",
- unitdir_template="unit_{}",
- name_template="image_{}.jpg",
- lightbox=False, batch_size=50, seed=1):
- all_indices = torch.unique(indices.view(-1), sorted=True)
- z_sample = z_universe[all_indices]
- progress = default_progress()
- sdir = os.path.join(dirname, shared_dir)
- created_hashdirs = set()
- for index in range(len(z_universe)):
- hd = hashdir(index)
- if hd not in created_hashdirs:
- created_hashdirs.add(hd)
- os.makedirs(os.path.join(sdir, hd), exist_ok=True)
- with torch.no_grad():
- # Pass 2: now generate images
- z_loader = torch.utils.data.DataLoader(TensorDataset(z_sample),
- batch_size=batch_size, num_workers=2,
- pin_memory=True)
- saver = WorkerPool(SaveImageWorker)
- for batch_num, [z] in enumerate(progress(z_loader,
- desc='Saving images')):
- z = z.cuda()
- start_index = batch_num * batch_size
- im = ((model(z) + 1) / 2 * 255).clamp(0, 255).byte().permute(
- 0, 2, 3, 1).cpu()
- for i in range(len(im)):
- index = all_indices[i + start_index].item()
- filename = os.path.join(sdir, hashdir(index),
- name_template.format(index))
- saver.add(im[i].numpy(), filename)
- saver.join()
- linker = WorkerPool(MakeLinkWorker)
- for u in progress(range(len(indices)), desc='Making links'):
- udir = os.path.join(dirname, unitdir_template.format(u))
- os.makedirs(udir, exist_ok=True)
- for r in range(indices.shape[1]):
- index = indices[u,r].item()
- fn = name_template.format(index)
- # sourcename = os.path.join('..', shared_dir, fn)
- sourcename = os.path.join(sdir, hashdir(index), fn)
- targname = os.path.join(udir, fn)
- linker.add(sourcename, targname)
- if lightbox:
- copy_lightbox_to(udir)
- linker.join()
-
-def copy_lightbox_to(dirname):
- srcdir = os.path.realpath(
- os.path.join(os.getcwd(), os.path.dirname(__file__)))
- shutil.copy(os.path.join(srcdir, 'lightbox.html'),
- os.path.join(dirname, '+lightbox.html'))
-
-def hashdir(index):
- # To keep the number of files the shared directory lower, split it
- # into 100 subdirectories named as follows.
- return '%02d' % (index % 100)
-
-class SaveImageWorker(WorkerBase):
- # Saving images can be sped up by sending jpeg encoding and
- # file-writing work to a pool.
- def work(self, data, filename):
- Image.fromarray(data).save(filename, optimize=True, quality=100)
-
-class MakeLinkWorker(WorkerBase):
- # Creating symbolic links is a bit slow and can be done faster
- # in parallel rather than waiting for each to be created.
- def work(self, sourcename, targname):
- try:
- os.link(sourcename, targname)
- except OSError as e:
- if e.errno == errno.EEXIST:
- os.remove(targname)
- os.link(sourcename, targname)
- else:
- raise
-
-class MakeSyminkWorker(WorkerBase):
- # Creating symbolic links is a bit slow and can be done faster
- # in parallel rather than waiting for each to be created.
- def work(self, sourcename, targname):
- try:
- os.symlink(sourcename, targname)
- except OSError as e:
- if e.errno == errno.EEXIST:
- os.remove(targname)
- os.symlink(sourcename, targname)
- else:
- raise
-
-if __name__ == '__main__':
- main()
diff --git a/spaces/Dralkkin/Lorule-Proxy/Dockerfile b/spaces/Dralkkin/Lorule-Proxy/Dockerfile
deleted file mode 100644
index eef259fa372a804549fb0af0913718a13344da34..0000000000000000000000000000000000000000
--- a/spaces/Dralkkin/Lorule-Proxy/Dockerfile
+++ /dev/null
@@ -1,11 +0,0 @@
-FROM node:18-bullseye-slim
-RUN apt-get update && \
- apt-get install -y git
-RUN git clone https://gitgud.io/khanon/oai-reverse-proxy.git /app
-WORKDIR /app
-RUN npm install
-COPY Dockerfile greeting.md* .env* ./
-RUN npm run build
-EXPOSE 7860
-ENV NODE_ENV=production
-CMD [ "npm", "start" ]
diff --git a/spaces/Dusan/clickbaitonator/fudge/predict_clickbait.py b/spaces/Dusan/clickbaitonator/fudge/predict_clickbait.py
deleted file mode 100644
index 38f9fc0d6121db14c1083335dab6ba9ac8e5290d..0000000000000000000000000000000000000000
--- a/spaces/Dusan/clickbaitonator/fudge/predict_clickbait.py
+++ /dev/null
@@ -1,199 +0,0 @@
-import os
-import random
-import time
-import pickle
-import math
-from argparse import ArgumentParser
-
-from typing import Iterable, List, Optional, Tuple
-
-from tqdm import tqdm
-import numpy as np
-import torch
-import torch.nn as nn
-import torch.nn.functional as F
-from transformers import AutoTokenizer, AutoModelWithLMHead
-from torch import Tensor
-
-from fudge.data import Dataset
-from fudge.model import Model
-from fudge.util import num_params
-from fudge.constants import *
-
-
-
-tokenizer = AutoTokenizer.from_pretrained('google/pegasus-xsum')
-classifier_tokenizer = AutoTokenizer.from_pretrained('sentence-transformers/all-mpnet-base-v2')
-
-
-def main(args):
- with open(args.dataset_info, 'rb') as rf:
- dataset_info = pickle.load(rf)
-
- article_content = """Australian actor Guy Pearce will return for the iconic soap Neighbours finale on August 1 to reprise his role as Mike Young.
- Guy, 54, played the troubled Mike from 1986 to 1989, and is now set to make a comeback on the show after 33 years, Metro.co.uk reports.
- The star's character arcs explored the implications of domestic abuse, student-teacher relationships and dealing with loss of loved ones.
- Speaking to Metro.co.uk, Guy said: 'It is very exciting and surreal at the same time being back on set again, however it feels like coming home.
- 'It's where it all started for me professionally. I've been asked to come back on occasions over the years and wondered if it was the right thing
- to do, but once I knew the show was finishing, I knew I had to do it.'He added that there is 'nothing like being here all together again'
- , even though he's had a chance to catch-up with other cast members."""
-
- tokenizer.add_special_tokens({'pad_token': PAD_TOKEN})
- pad_id = tokenizer.encode(PAD_TOKEN)[0]
-
- #For loading Clickbait summarizer
- model = AutoModelWithLMHead.from_pretrained(args.model_string, return_dict=True).to(args.device)
-
- model.eval()
-
- checkpoint = torch.load(args.ckpt, map_location=args.device)
- model_args = checkpoint['args']
- conditioning_model = Model(model_args, pad_id, len(dataset_info.index2word)) # no need to get the glove embeddings when reloading since they're saved in model ckpt anyway
- conditioning_model.load_state_dict(checkpoint['state_dict'])
- conditioning_model = conditioning_model.to(args.device)
- conditioning_model.eval()
- print("=> loaded checkpoint '{}' (epoch {})"
- .format(args.ckpt, checkpoint['epoch']))
- print('num params', num_params(conditioning_model))
-
- while True:
- results = generate_clickbait(model,
- tokenizer,
- conditioning_model,
- [args.input_text],
- dataset_info,
- precondition_topk=args.precondition_topk,
- do_sample=args.do_sample,
- length_cutoff=args.length_cutoff,
- condition_lambda=args.condition_lambda,
- article_content=article_content,
- device=args.device)
- # print(results)
- import pdb; pdb.set_trace()
-
-
-def generate_clickbait(model,
- tokenizer,
- conditioning_model,
- input_text,
- dataset_info,
- precondition_topk,
- length_cutoff,
- condition_lambda=1.0,
- article_content=None,
- device='cuda'):
- with torch.no_grad():
- batch_size = len(input_text)
- # encoded_input_article = [tokenizer.encode(article_content, return_tensors='pt',add_special_tokens=False).to(device)] # batch x seq
- max_input_length = 512
- encoded_input_article = tokenizer(article_content, return_tensors='pt',add_special_tokens=False, max_length = max_input_length).to(device) # batch x seq
- # encoded_input_article = torch.cat(encoded_input_article, dim=0)
- # attention_mask = encoded_input_article.new_ones(encoded_input_article.shape).to(device)
-
- # CHANGE=ko
- encoded_input = tokenizer('', return_tensors='pt',add_special_tokens=False).to(device) # batch x seq
- # encoded_input = tokenizer(''+ input_text[0], return_tensors='pt',add_special_tokens=False).to(device) # batch x seq
- # encoded_input = torch.cat(encoded_input, dim=0)
- encoded_input = encoded_input['input_ids']
-
-
- lengths = torch.LongTensor([encoded_input.shape[1]]).to(device)
- # lengths = 1
-
- past = None
- use_cache = True
-
- # CHANGE
- # model_kwargs = {'encoder_outputs': model.get_encoder()(encoded_input_article, attention_mask=attention_mask)}
- model_kwargs = {'encoder_outputs': model.get_encoder()(input_ids=encoded_input_article['input_ids'],
- attention_mask=encoded_input_article['attention_mask'],
- return_dict=True,
- output_attentions=False,
- output_hidden_states=False),
- }
-
- while lengths.max() < length_cutoff:
- model_inputs = model.prepare_inputs_for_generation(
- input_ids = encoded_input_article['input_ids'],
- decoder_input_ids=encoded_input,
- # past=past,
- attention_mask=encoded_input_article['attention_mask'],
- use_cache=use_cache,
- **model_kwargs
- )
-
- outputs = model(**model_inputs, return_dict=True)
- logits = outputs.logits[:, -1, :]
-
- if "past_key_values" in outputs:
- model_kwargs["past"] = outputs.past_key_values
-
- # logits = model(encoded_input)[0][:, -1, :] # batch x vocab
- top_logits, top_indices = logits.topk(precondition_topk, dim=1) # batch x topk
- new_input_candidates = torch.cat([encoded_input.unsqueeze(1).expand(-1, precondition_topk, -1), top_indices.unsqueeze(2)], dim=2) # batch x topk x seq+1
- expanded_lengths = (lengths + 1).unsqueeze(1).expand(batch_size, precondition_topk) # batch x topk
-
- if condition_lambda == 0:
- condition_logits = torch.zeros_like(top_logits).float()
- condition_logits = condition_logits.view(batch_size, precondition_topk, -1) # batch x topk x N
- else:
- decoded_outputs = tokenizer.batch_decode(new_input_candidates.view(-1, new_input_candidates.size(-1)), clean_up_tokenization_spaces=False)
- resulting_tokenization = classifier_tokenizer(decoded_outputs, add_special_tokens=False, padding='longest')
- encoded_with_classifier = resulting_tokenization['input_ids']
- attention_mask = torch.tensor(resulting_tokenization['attention_mask']).to(model.device)
- tplus1_candidates_classifier = torch.tensor(encoded_with_classifier).view(batch_size, precondition_topk, -1).to(model.device)
-
- condition_logits = conditioning_model(tplus1_candidates_classifier.flatten(0, 1), # batch*topk x seq+1
- expanded_lengths.flatten(0, 1), # batch*topk
- None,
- None,
- None,
- attention_mask=attention_mask
- )
- condition_logits = condition_logits.view(batch_size, precondition_topk, -1) # batch x topk x N
- condition_logits = condition_logits - torch.log(1 + torch.exp(condition_logits)) # get correct log probs
-
- condition_logits = torch.mean(condition_logits, dim=2)
- full_logits = top_logits + condition_logits * condition_lambda # batch x topk
- post_logits, post_indices = full_logits.topk(precondition_topk, dim=1)
- post_probs = F.softmax(post_logits, dim=1)
- # index_into_top_indices = post_indices[torch.arange(batch_size).to(post_indices.device), torch.multinomial(post_probs, 1).flatten()] # batch
- index_into_top_indices = post_indices[:, torch.multinomial(post_probs, 1).flatten()] # batch
-
- # next_indices = top_indices[torch.arange(batch_size).to(top_indices.device), index_into_top_indices] # batch
- next_indices = top_indices[:, index_into_top_indices] # batch
-
- # encoded_input = torch.cat([encoded_input, next_indices.unsqueeze(1)], dim=1) # batch x seq+1
- encoded_input = torch.cat([encoded_input, next_indices.squeeze(1)], dim=1)
- lengths = lengths + 1 # batch
-
-# print(tokenizer.decode(encoded_input[0], add_special_tokens=False))
- return [tokenizer.decode(s) for s in encoded_input]
-
-
-if __name__=='__main__':
- parser = ArgumentParser()
-
- # DATA
- parser.add_argument('--ckpt', type=str, required=True)
- parser.add_argument('--dataset_info', type=str, required=True, help='saved dataset info')
- parser.add_argument('--model_string', type=str, default='Helsinki-NLP/opus-mt-es-en')
-
- parser.add_argument('--in_file', type=str, default=None, required=True, help='text to run pred on')
-
- parser.add_argument('--precondition_topk', type=int, default=200, help='consider top k outputs from text generation at each step before conditioning and re-pruning')
- parser.add_argument('--do_sample', action='store_true', default=False, help='sample instead of greedy')
- parser.add_argument('--condition_lambda', type=float, default=1.0, help='lambda weight on conditioning model')
- parser.add_argument('--length_cutoff', type=int, default=512, help='max length')
-
- parser.add_argument('--seed', type=int, default=1, help='random seed')
- parser.add_argument('--device', type=str, default='cuda', choices=['cpu', 'cuda'])
- parser.add_argument('--debug', action='store_true', default=False)
-
- args = parser.parse_args()
-
- random.seed(args.seed)
- np.random.seed(args.seed)
- torch.manual_seed(args.seed)
-
- main(args)
diff --git a/spaces/Duskfallcrew/Duskfallcrew-duskfallai/README.md b/spaces/Duskfallcrew/Duskfallcrew-duskfallai/README.md
deleted file mode 100644
index 3b620928a938e9baaa99406717764daac5795ae8..0000000000000000000000000000000000000000
--- a/spaces/Duskfallcrew/Duskfallcrew-duskfallai/README.md
+++ /dev/null
@@ -1,13 +0,0 @@
----
-title: Duskfallcrew Duskfallai
-emoji: 🏢
-colorFrom: blue
-colorTo: green
-sdk: gradio
-sdk_version: 3.16.2
-app_file: app.py
-pinned: false
-license: creativeml-openrail-m
----
-
-Check out the configuration reference at https://huggingface.co/docs/hub/spaces-config-reference
diff --git a/spaces/EPFL-VILAB/MultiMAE/dpt/base_model.py b/spaces/EPFL-VILAB/MultiMAE/dpt/base_model.py
deleted file mode 100644
index 5c2e0e93b0495f48a3405546b6fe1969be3480a2..0000000000000000000000000000000000000000
--- a/spaces/EPFL-VILAB/MultiMAE/dpt/base_model.py
+++ /dev/null
@@ -1,16 +0,0 @@
-import torch
-
-
-class BaseModel(torch.nn.Module):
- def load(self, path):
- """Load model from file.
-
- Args:
- path (str): file path
- """
- parameters = torch.load(path, map_location=torch.device("cpu"))
-
- if "optimizer" in parameters:
- parameters = parameters["model"]
-
- self.load_state_dict(parameters)
diff --git a/spaces/EXPOSUREEE/Ai-Image-Enhancer/realesrgan/models/realesrnet_model.py b/spaces/EXPOSUREEE/Ai-Image-Enhancer/realesrgan/models/realesrnet_model.py
deleted file mode 100644
index d11668f3712bffcd062c57db14d22ca3a0e1e59d..0000000000000000000000000000000000000000
--- a/spaces/EXPOSUREEE/Ai-Image-Enhancer/realesrgan/models/realesrnet_model.py
+++ /dev/null
@@ -1,188 +0,0 @@
-import numpy as np
-import random
-import torch
-from basicsr.data.degradations import random_add_gaussian_noise_pt, random_add_poisson_noise_pt
-from basicsr.data.transforms import paired_random_crop
-from basicsr.models.sr_model import SRModel
-from basicsr.utils import DiffJPEG, USMSharp
-from basicsr.utils.img_process_util import filter2D
-from basicsr.utils.registry import MODEL_REGISTRY
-from torch.nn import functional as F
-
-
-@MODEL_REGISTRY.register()
-class RealESRNetModel(SRModel):
- """RealESRNet Model for Real-ESRGAN: Training Real-World Blind Super-Resolution with Pure Synthetic Data.
-
- It is trained without GAN losses.
- It mainly performs:
- 1. randomly synthesize LQ images in GPU tensors
- 2. optimize the networks with GAN training.
- """
-
- def __init__(self, opt):
- super(RealESRNetModel, self).__init__(opt)
- self.jpeger = DiffJPEG(differentiable=False).cuda() # simulate JPEG compression artifacts
- self.usm_sharpener = USMSharp().cuda() # do usm sharpening
- self.queue_size = opt.get('queue_size', 180)
-
- @torch.no_grad()
- def _dequeue_and_enqueue(self):
- """It is the training pair pool for increasing the diversity in a batch.
-
- Batch processing limits the diversity of synthetic degradations in a batch. For example, samples in a
- batch could not have different resize scaling factors. Therefore, we employ this training pair pool
- to increase the degradation diversity in a batch.
- """
- # initialize
- b, c, h, w = self.lq.size()
- if not hasattr(self, 'queue_lr'):
- assert self.queue_size % b == 0, f'queue size {self.queue_size} should be divisible by batch size {b}'
- self.queue_lr = torch.zeros(self.queue_size, c, h, w).cuda()
- _, c, h, w = self.gt.size()
- self.queue_gt = torch.zeros(self.queue_size, c, h, w).cuda()
- self.queue_ptr = 0
- if self.queue_ptr == self.queue_size: # the pool is full
- # do dequeue and enqueue
- # shuffle
- idx = torch.randperm(self.queue_size)
- self.queue_lr = self.queue_lr[idx]
- self.queue_gt = self.queue_gt[idx]
- # get first b samples
- lq_dequeue = self.queue_lr[0:b, :, :, :].clone()
- gt_dequeue = self.queue_gt[0:b, :, :, :].clone()
- # update the queue
- self.queue_lr[0:b, :, :, :] = self.lq.clone()
- self.queue_gt[0:b, :, :, :] = self.gt.clone()
-
- self.lq = lq_dequeue
- self.gt = gt_dequeue
- else:
- # only do enqueue
- self.queue_lr[self.queue_ptr:self.queue_ptr + b, :, :, :] = self.lq.clone()
- self.queue_gt[self.queue_ptr:self.queue_ptr + b, :, :, :] = self.gt.clone()
- self.queue_ptr = self.queue_ptr + b
-
- @torch.no_grad()
- def feed_data(self, data):
- """Accept data from dataloader, and then add two-order degradations to obtain LQ images.
- """
- if self.is_train and self.opt.get('high_order_degradation', True):
- # training data synthesis
- self.gt = data['gt'].to(self.device)
- # USM sharpen the GT images
- if self.opt['gt_usm'] is True:
- self.gt = self.usm_sharpener(self.gt)
-
- self.kernel1 = data['kernel1'].to(self.device)
- self.kernel2 = data['kernel2'].to(self.device)
- self.sinc_kernel = data['sinc_kernel'].to(self.device)
-
- ori_h, ori_w = self.gt.size()[2:4]
-
- # ----------------------- The first degradation process ----------------------- #
- # blur
- out = filter2D(self.gt, self.kernel1)
- # random resize
- updown_type = random.choices(['up', 'down', 'keep'], self.opt['resize_prob'])[0]
- if updown_type == 'up':
- scale = np.random.uniform(1, self.opt['resize_range'][1])
- elif updown_type == 'down':
- scale = np.random.uniform(self.opt['resize_range'][0], 1)
- else:
- scale = 1
- mode = random.choice(['area', 'bilinear', 'bicubic'])
- out = F.interpolate(out, scale_factor=scale, mode=mode)
- # add noise
- gray_noise_prob = self.opt['gray_noise_prob']
- if np.random.uniform() < self.opt['gaussian_noise_prob']:
- out = random_add_gaussian_noise_pt(
- out, sigma_range=self.opt['noise_range'], clip=True, rounds=False, gray_prob=gray_noise_prob)
- else:
- out = random_add_poisson_noise_pt(
- out,
- scale_range=self.opt['poisson_scale_range'],
- gray_prob=gray_noise_prob,
- clip=True,
- rounds=False)
- # JPEG compression
- jpeg_p = out.new_zeros(out.size(0)).uniform_(*self.opt['jpeg_range'])
- out = torch.clamp(out, 0, 1) # clamp to [0, 1], otherwise JPEGer will result in unpleasant artifacts
- out = self.jpeger(out, quality=jpeg_p)
-
- # ----------------------- The second degradation process ----------------------- #
- # blur
- if np.random.uniform() < self.opt['second_blur_prob']:
- out = filter2D(out, self.kernel2)
- # random resize
- updown_type = random.choices(['up', 'down', 'keep'], self.opt['resize_prob2'])[0]
- if updown_type == 'up':
- scale = np.random.uniform(1, self.opt['resize_range2'][1])
- elif updown_type == 'down':
- scale = np.random.uniform(self.opt['resize_range2'][0], 1)
- else:
- scale = 1
- mode = random.choice(['area', 'bilinear', 'bicubic'])
- out = F.interpolate(
- out, size=(int(ori_h / self.opt['scale'] * scale), int(ori_w / self.opt['scale'] * scale)), mode=mode)
- # add noise
- gray_noise_prob = self.opt['gray_noise_prob2']
- if np.random.uniform() < self.opt['gaussian_noise_prob2']:
- out = random_add_gaussian_noise_pt(
- out, sigma_range=self.opt['noise_range2'], clip=True, rounds=False, gray_prob=gray_noise_prob)
- else:
- out = random_add_poisson_noise_pt(
- out,
- scale_range=self.opt['poisson_scale_range2'],
- gray_prob=gray_noise_prob,
- clip=True,
- rounds=False)
-
- # JPEG compression + the final sinc filter
- # We also need to resize images to desired sizes. We group [resize back + sinc filter] together
- # as one operation.
- # We consider two orders:
- # 1. [resize back + sinc filter] + JPEG compression
- # 2. JPEG compression + [resize back + sinc filter]
- # Empirically, we find other combinations (sinc + JPEG + Resize) will introduce twisted lines.
- if np.random.uniform() < 0.5:
- # resize back + the final sinc filter
- mode = random.choice(['area', 'bilinear', 'bicubic'])
- out = F.interpolate(out, size=(ori_h // self.opt['scale'], ori_w // self.opt['scale']), mode=mode)
- out = filter2D(out, self.sinc_kernel)
- # JPEG compression
- jpeg_p = out.new_zeros(out.size(0)).uniform_(*self.opt['jpeg_range2'])
- out = torch.clamp(out, 0, 1)
- out = self.jpeger(out, quality=jpeg_p)
- else:
- # JPEG compression
- jpeg_p = out.new_zeros(out.size(0)).uniform_(*self.opt['jpeg_range2'])
- out = torch.clamp(out, 0, 1)
- out = self.jpeger(out, quality=jpeg_p)
- # resize back + the final sinc filter
- mode = random.choice(['area', 'bilinear', 'bicubic'])
- out = F.interpolate(out, size=(ori_h // self.opt['scale'], ori_w // self.opt['scale']), mode=mode)
- out = filter2D(out, self.sinc_kernel)
-
- # clamp and round
- self.lq = torch.clamp((out * 255.0).round(), 0, 255) / 255.
-
- # random crop
- gt_size = self.opt['gt_size']
- self.gt, self.lq = paired_random_crop(self.gt, self.lq, gt_size, self.opt['scale'])
-
- # training pair pool
- self._dequeue_and_enqueue()
- self.lq = self.lq.contiguous() # for the warning: grad and param do not obey the gradient layout contract
- else:
- # for paired training or validation
- self.lq = data['lq'].to(self.device)
- if 'gt' in data:
- self.gt = data['gt'].to(self.device)
- self.gt_usm = self.usm_sharpener(self.gt)
-
- def nondist_validation(self, dataloader, current_iter, tb_logger, save_img):
- # do not use the synthetic process during validation
- self.is_train = False
- super(RealESRNetModel, self).nondist_validation(dataloader, current_iter, tb_logger, save_img)
- self.is_train = True
diff --git a/spaces/EagleLoveAI/ChatGPT_Application_Robot/README.md b/spaces/EagleLoveAI/ChatGPT_Application_Robot/README.md
deleted file mode 100644
index 68dc36be652165af711087d14ed83fe8fea4664d..0000000000000000000000000000000000000000
--- a/spaces/EagleLoveAI/ChatGPT_Application_Robot/README.md
+++ /dev/null
@@ -1,13 +0,0 @@
----
-title: ChatGPT Application Robot
-emoji: 💩
-colorFrom: indigo
-colorTo: purple
-sdk: gradio
-sdk_version: 3.27.0
-app_file: app.py
-pinned: false
-license: mit
----
-
-Check out the configuration reference at https://huggingface.co/docs/hub/spaces-config-reference
diff --git a/spaces/Eddycrack864/Applio-Inference/lib/infer_pack/modules/F0Predictor/F0Predictor.py b/spaces/Eddycrack864/Applio-Inference/lib/infer_pack/modules/F0Predictor/F0Predictor.py
deleted file mode 100644
index f56e49e7f0e6eab3babf0711cae2933371b9f9cc..0000000000000000000000000000000000000000
--- a/spaces/Eddycrack864/Applio-Inference/lib/infer_pack/modules/F0Predictor/F0Predictor.py
+++ /dev/null
@@ -1,16 +0,0 @@
-class F0Predictor(object):
- def compute_f0(self, wav, p_len):
- """
- input: wav:[signal_length]
- p_len:int
- output: f0:[signal_length//hop_length]
- """
- pass
-
- def compute_f0_uv(self, wav, p_len):
- """
- input: wav:[signal_length]
- p_len:int
- output: f0:[signal_length//hop_length],uv:[signal_length//hop_length]
- """
- pass
diff --git a/spaces/ElainaFanBoy/MusicGen/audiocraft/quantization/vq.py b/spaces/ElainaFanBoy/MusicGen/audiocraft/quantization/vq.py
deleted file mode 100644
index f67c3a0cd30d4b8993a36c587f00dc8a451d926f..0000000000000000000000000000000000000000
--- a/spaces/ElainaFanBoy/MusicGen/audiocraft/quantization/vq.py
+++ /dev/null
@@ -1,116 +0,0 @@
-# Copyright (c) Meta Platforms, Inc. and affiliates.
-# All rights reserved.
-#
-# This source code is licensed under the license found in the
-# LICENSE file in the root directory of this source tree.
-
-import math
-import typing as tp
-
-import torch
-
-from .base import BaseQuantizer, QuantizedResult
-from .core_vq import ResidualVectorQuantization
-
-
-class ResidualVectorQuantizer(BaseQuantizer):
- """Residual Vector Quantizer.
-
- Args:
- dimension (int): Dimension of the codebooks.
- n_q (int): Number of residual vector quantizers used.
- q_dropout (bool): Random quantizer drop out at train time.
- bins (int): Codebook size.
- decay (float): Decay for exponential moving average over the codebooks.
- kmeans_init (bool): Whether to use kmeans to initialize the codebooks.
- kmeans_iters (int): Number of iterations used for kmeans initialization.
- threshold_ema_dead_code (int): Threshold for dead code expiration. Replace any codes
- that have an exponential moving average cluster size less than the specified threshold with
- randomly selected vector from the current batch.
- orthogonal_reg_weight (float): Orthogonal regularization weights.
- orthogonal_reg_active_codes_only (bool): Apply orthogonal regularization only on active codes.
- orthogonal_reg_max_codes (optional int): Maximum number of codes to consider.
- for orthogonal regulariation.
- """
- def __init__(
- self,
- dimension: int = 256,
- n_q: int = 8,
- q_dropout: bool = False,
- bins: int = 1024,
- decay: float = 0.99,
- kmeans_init: bool = True,
- kmeans_iters: int = 10,
- threshold_ema_dead_code: int = 2,
- orthogonal_reg_weight: float = 0.0,
- orthogonal_reg_active_codes_only: bool = False,
- orthogonal_reg_max_codes: tp.Optional[int] = None,
- ):
- super().__init__()
- self.max_n_q = n_q
- self.n_q = n_q
- self.q_dropout = q_dropout
- self.dimension = dimension
- self.bins = bins
- self.decay = decay
- self.kmeans_init = kmeans_init
- self.kmeans_iters = kmeans_iters
- self.threshold_ema_dead_code = threshold_ema_dead_code
- self.orthogonal_reg_weight = orthogonal_reg_weight
- self.orthogonal_reg_active_codes_only = orthogonal_reg_active_codes_only
- self.orthogonal_reg_max_codes = orthogonal_reg_max_codes
- self.vq = ResidualVectorQuantization(
- dim=self.dimension,
- codebook_size=self.bins,
- num_quantizers=self.n_q,
- decay=self.decay,
- kmeans_init=self.kmeans_init,
- kmeans_iters=self.kmeans_iters,
- threshold_ema_dead_code=self.threshold_ema_dead_code,
- orthogonal_reg_weight=self.orthogonal_reg_weight,
- orthogonal_reg_active_codes_only=self.orthogonal_reg_active_codes_only,
- orthogonal_reg_max_codes=self.orthogonal_reg_max_codes,
- channels_last=False
- )
-
- def forward(self, x: torch.Tensor, frame_rate: int):
- n_q = self.n_q
- if self.training and self.q_dropout:
- n_q = int(torch.randint(1, self.n_q + 1, (1,)).item())
- bw_per_q = math.log2(self.bins) * frame_rate / 1000
- quantized, codes, commit_loss = self.vq(x, n_q=n_q)
- codes = codes.transpose(0, 1)
- # codes is [B, K, T], with T frames, K nb of codebooks.
- bw = torch.tensor(n_q * bw_per_q).to(x)
- return QuantizedResult(quantized, codes, bw, penalty=torch.mean(commit_loss))
-
- def encode(self, x: torch.Tensor) -> torch.Tensor:
- """Encode a given input tensor with the specified frame rate at the given bandwidth.
- The RVQ encode method sets the appropriate number of quantizer to use
- and returns indices for each quantizer.
- """
- n_q = self.n_q
- codes = self.vq.encode(x, n_q=n_q)
- codes = codes.transpose(0, 1)
- # codes is [B, K, T], with T frames, K nb of codebooks.
- return codes
-
- def decode(self, codes: torch.Tensor) -> torch.Tensor:
- """Decode the given codes to the quantized representation.
- """
- # codes is [B, K, T], with T frames, K nb of codebooks, vq.decode expects [K, B, T].
- codes = codes.transpose(0, 1)
- quantized = self.vq.decode(codes)
- return quantized
-
- @property
- def total_codebooks(self):
- return self.max_n_q
-
- @property
- def num_codebooks(self):
- return self.n_q
-
- def set_num_codebooks(self, n: int):
- assert n > 0 and n <= self.max_n_q
- self.n_q = n
diff --git a/spaces/Endercat126/anything-v5-testing/app.py b/spaces/Endercat126/anything-v5-testing/app.py
deleted file mode 100644
index 6db423fde2b7e32c68e8be737dfc7c6175cd67a4..0000000000000000000000000000000000000000
--- a/spaces/Endercat126/anything-v5-testing/app.py
+++ /dev/null
@@ -1,3 +0,0 @@
-import gradio as gr
-
-gr.Interface.load("models/stablediffusionapi/anything-v5").launch()
\ No newline at end of file
diff --git a/spaces/Faridmaruf/RVCV2MODEL/lib/infer_pack/modules/F0Predictor/DioF0Predictor.py b/spaces/Faridmaruf/RVCV2MODEL/lib/infer_pack/modules/F0Predictor/DioF0Predictor.py
deleted file mode 100644
index ee3171bcb7c4a5066560723108b56e055f18be45..0000000000000000000000000000000000000000
--- a/spaces/Faridmaruf/RVCV2MODEL/lib/infer_pack/modules/F0Predictor/DioF0Predictor.py
+++ /dev/null
@@ -1,90 +0,0 @@
-from lib.infer_pack.modules.F0Predictor.F0Predictor import F0Predictor
-import pyworld
-import numpy as np
-
-
-class DioF0Predictor(F0Predictor):
- def __init__(self, hop_length=512, f0_min=50, f0_max=1100, sampling_rate=44100):
- self.hop_length = hop_length
- self.f0_min = f0_min
- self.f0_max = f0_max
- self.sampling_rate = sampling_rate
-
- def interpolate_f0(self, f0):
- """
- 对F0进行插值处理
- """
-
- data = np.reshape(f0, (f0.size, 1))
-
- vuv_vector = np.zeros((data.size, 1), dtype=np.float32)
- vuv_vector[data > 0.0] = 1.0
- vuv_vector[data <= 0.0] = 0.0
-
- ip_data = data
-
- frame_number = data.size
- last_value = 0.0
- for i in range(frame_number):
- if data[i] <= 0.0:
- j = i + 1
- for j in range(i + 1, frame_number):
- if data[j] > 0.0:
- break
- if j < frame_number - 1:
- if last_value > 0.0:
- step = (data[j] - data[i - 1]) / float(j - i)
- for k in range(i, j):
- ip_data[k] = data[i - 1] + step * (k - i + 1)
- else:
- for k in range(i, j):
- ip_data[k] = data[j]
- else:
- for k in range(i, frame_number):
- ip_data[k] = last_value
- else:
- ip_data[i] = data[i] # 这里可能存在一个没有必要的拷贝
- last_value = data[i]
-
- return ip_data[:, 0], vuv_vector[:, 0]
-
- def resize_f0(self, x, target_len):
- source = np.array(x)
- source[source < 0.001] = np.nan
- target = np.interp(
- np.arange(0, len(source) * target_len, len(source)) / target_len,
- np.arange(0, len(source)),
- source,
- )
- res = np.nan_to_num(target)
- return res
-
- def compute_f0(self, wav, p_len=None):
- if p_len is None:
- p_len = wav.shape[0] // self.hop_length
- f0, t = pyworld.dio(
- wav.astype(np.double),
- fs=self.sampling_rate,
- f0_floor=self.f0_min,
- f0_ceil=self.f0_max,
- frame_period=1000 * self.hop_length / self.sampling_rate,
- )
- f0 = pyworld.stonemask(wav.astype(np.double), f0, t, self.sampling_rate)
- for index, pitch in enumerate(f0):
- f0[index] = round(pitch, 1)
- return self.interpolate_f0(self.resize_f0(f0, p_len))[0]
-
- def compute_f0_uv(self, wav, p_len=None):
- if p_len is None:
- p_len = wav.shape[0] // self.hop_length
- f0, t = pyworld.dio(
- wav.astype(np.double),
- fs=self.sampling_rate,
- f0_floor=self.f0_min,
- f0_ceil=self.f0_max,
- frame_period=1000 * self.hop_length / self.sampling_rate,
- )
- f0 = pyworld.stonemask(wav.astype(np.double), f0, t, self.sampling_rate)
- for index, pitch in enumerate(f0):
- f0[index] = round(pitch, 1)
- return self.interpolate_f0(self.resize_f0(f0, p_len))
diff --git a/spaces/FauziNL/Voice_anime2/infer_pack/models.py b/spaces/FauziNL/Voice_anime2/infer_pack/models.py
deleted file mode 100644
index 96165f73644e6fb92d0ffedb4a3c9e1a457cb989..0000000000000000000000000000000000000000
--- a/spaces/FauziNL/Voice_anime2/infer_pack/models.py
+++ /dev/null
@@ -1,982 +0,0 @@
-import math, pdb, os
-from time import time as ttime
-import torch
-from torch import nn
-from torch.nn import functional as F
-from infer_pack import modules
-from infer_pack import attentions
-from infer_pack import commons
-from infer_pack.commons import init_weights, get_padding
-from torch.nn import Conv1d, ConvTranspose1d, AvgPool1d, Conv2d
-from torch.nn.utils import weight_norm, remove_weight_norm, spectral_norm
-from infer_pack.commons import init_weights
-import numpy as np
-from infer_pack import commons
-
-
-class TextEncoder256(nn.Module):
- def __init__(
- self,
- out_channels,
- hidden_channels,
- filter_channels,
- n_heads,
- n_layers,
- kernel_size,
- p_dropout,
- f0=True,
- ):
- super().__init__()
- self.out_channels = out_channels
- self.hidden_channels = hidden_channels
- self.filter_channels = filter_channels
- self.n_heads = n_heads
- self.n_layers = n_layers
- self.kernel_size = kernel_size
- self.p_dropout = p_dropout
- self.emb_phone = nn.Linear(256, hidden_channels)
- self.lrelu = nn.LeakyReLU(0.1, inplace=True)
- if f0 == True:
- self.emb_pitch = nn.Embedding(256, hidden_channels) # pitch 256
- self.encoder = attentions.Encoder(
- hidden_channels, filter_channels, n_heads, n_layers, kernel_size, p_dropout
- )
- self.proj = nn.Conv1d(hidden_channels, out_channels * 2, 1)
-
- def forward(self, phone, pitch, lengths):
- if pitch == None:
- x = self.emb_phone(phone)
- else:
- x = self.emb_phone(phone) + self.emb_pitch(pitch)
- x = x * math.sqrt(self.hidden_channels) # [b, t, h]
- x = self.lrelu(x)
- x = torch.transpose(x, 1, -1) # [b, h, t]
- x_mask = torch.unsqueeze(commons.sequence_mask(lengths, x.size(2)), 1).to(
- x.dtype
- )
- x = self.encoder(x * x_mask, x_mask)
- stats = self.proj(x) * x_mask
-
- m, logs = torch.split(stats, self.out_channels, dim=1)
- return m, logs, x_mask
-
-
-class TextEncoder256Sim(nn.Module):
- def __init__(
- self,
- out_channels,
- hidden_channels,
- filter_channels,
- n_heads,
- n_layers,
- kernel_size,
- p_dropout,
- f0=True,
- ):
- super().__init__()
- self.out_channels = out_channels
- self.hidden_channels = hidden_channels
- self.filter_channels = filter_channels
- self.n_heads = n_heads
- self.n_layers = n_layers
- self.kernel_size = kernel_size
- self.p_dropout = p_dropout
- self.emb_phone = nn.Linear(256, hidden_channels)
- self.lrelu = nn.LeakyReLU(0.1, inplace=True)
- if f0 == True:
- self.emb_pitch = nn.Embedding(256, hidden_channels) # pitch 256
- self.encoder = attentions.Encoder(
- hidden_channels, filter_channels, n_heads, n_layers, kernel_size, p_dropout
- )
- self.proj = nn.Conv1d(hidden_channels, out_channels, 1)
-
- def forward(self, phone, pitch, lengths):
- if pitch == None:
- x = self.emb_phone(phone)
- else:
- x = self.emb_phone(phone) + self.emb_pitch(pitch)
- x = x * math.sqrt(self.hidden_channels) # [b, t, h]
- x = self.lrelu(x)
- x = torch.transpose(x, 1, -1) # [b, h, t]
- x_mask = torch.unsqueeze(commons.sequence_mask(lengths, x.size(2)), 1).to(
- x.dtype
- )
- x = self.encoder(x * x_mask, x_mask)
- x = self.proj(x) * x_mask
- return x, x_mask
-
-
-class ResidualCouplingBlock(nn.Module):
- def __init__(
- self,
- channels,
- hidden_channels,
- kernel_size,
- dilation_rate,
- n_layers,
- n_flows=4,
- gin_channels=0,
- ):
- super().__init__()
- self.channels = channels
- self.hidden_channels = hidden_channels
- self.kernel_size = kernel_size
- self.dilation_rate = dilation_rate
- self.n_layers = n_layers
- self.n_flows = n_flows
- self.gin_channels = gin_channels
-
- self.flows = nn.ModuleList()
- for i in range(n_flows):
- self.flows.append(
- modules.ResidualCouplingLayer(
- channels,
- hidden_channels,
- kernel_size,
- dilation_rate,
- n_layers,
- gin_channels=gin_channels,
- mean_only=True,
- )
- )
- self.flows.append(modules.Flip())
-
- def forward(self, x, x_mask, g=None, reverse=False):
- if not reverse:
- for flow in self.flows:
- x, _ = flow(x, x_mask, g=g, reverse=reverse)
- else:
- for flow in reversed(self.flows):
- x = flow(x, x_mask, g=g, reverse=reverse)
- return x
-
- def remove_weight_norm(self):
- for i in range(self.n_flows):
- self.flows[i * 2].remove_weight_norm()
-
-
-class PosteriorEncoder(nn.Module):
- def __init__(
- self,
- in_channels,
- out_channels,
- hidden_channels,
- kernel_size,
- dilation_rate,
- n_layers,
- gin_channels=0,
- ):
- super().__init__()
- self.in_channels = in_channels
- self.out_channels = out_channels
- self.hidden_channels = hidden_channels
- self.kernel_size = kernel_size
- self.dilation_rate = dilation_rate
- self.n_layers = n_layers
- self.gin_channels = gin_channels
-
- self.pre = nn.Conv1d(in_channels, hidden_channels, 1)
- self.enc = modules.WN(
- hidden_channels,
- kernel_size,
- dilation_rate,
- n_layers,
- gin_channels=gin_channels,
- )
- self.proj = nn.Conv1d(hidden_channels, out_channels * 2, 1)
-
- def forward(self, x, x_lengths, g=None):
- x_mask = torch.unsqueeze(commons.sequence_mask(x_lengths, x.size(2)), 1).to(
- x.dtype
- )
- x = self.pre(x) * x_mask
- x = self.enc(x, x_mask, g=g)
- stats = self.proj(x) * x_mask
- m, logs = torch.split(stats, self.out_channels, dim=1)
- z = (m + torch.randn_like(m) * torch.exp(logs)) * x_mask
- return z, m, logs, x_mask
-
- def remove_weight_norm(self):
- self.enc.remove_weight_norm()
-
-
-class Generator(torch.nn.Module):
- def __init__(
- self,
- initial_channel,
- resblock,
- resblock_kernel_sizes,
- resblock_dilation_sizes,
- upsample_rates,
- upsample_initial_channel,
- upsample_kernel_sizes,
- gin_channels=0,
- ):
- super(Generator, self).__init__()
- self.num_kernels = len(resblock_kernel_sizes)
- self.num_upsamples = len(upsample_rates)
- self.conv_pre = Conv1d(
- initial_channel, upsample_initial_channel, 7, 1, padding=3
- )
- resblock = modules.ResBlock1 if resblock == "1" else modules.ResBlock2
-
- self.ups = nn.ModuleList()
- for i, (u, k) in enumerate(zip(upsample_rates, upsample_kernel_sizes)):
- self.ups.append(
- weight_norm(
- ConvTranspose1d(
- upsample_initial_channel // (2**i),
- upsample_initial_channel // (2 ** (i + 1)),
- k,
- u,
- padding=(k - u) // 2,
- )
- )
- )
-
- self.resblocks = nn.ModuleList()
- for i in range(len(self.ups)):
- ch = upsample_initial_channel // (2 ** (i + 1))
- for j, (k, d) in enumerate(
- zip(resblock_kernel_sizes, resblock_dilation_sizes)
- ):
- self.resblocks.append(resblock(ch, k, d))
-
- self.conv_post = Conv1d(ch, 1, 7, 1, padding=3, bias=False)
- self.ups.apply(init_weights)
-
- if gin_channels != 0:
- self.cond = nn.Conv1d(gin_channels, upsample_initial_channel, 1)
-
- def forward(self, x, g=None):
- x = self.conv_pre(x)
- if g is not None:
- x = x + self.cond(g)
-
- for i in range(self.num_upsamples):
- x = F.leaky_relu(x, modules.LRELU_SLOPE)
- x = self.ups[i](x)
- xs = None
- for j in range(self.num_kernels):
- if xs is None:
- xs = self.resblocks[i * self.num_kernels + j](x)
- else:
- xs += self.resblocks[i * self.num_kernels + j](x)
- x = xs / self.num_kernels
- x = F.leaky_relu(x)
- x = self.conv_post(x)
- x = torch.tanh(x)
-
- return x
-
- def remove_weight_norm(self):
- for l in self.ups:
- remove_weight_norm(l)
- for l in self.resblocks:
- l.remove_weight_norm()
-
-
-class SineGen(torch.nn.Module):
- """Definition of sine generator
- SineGen(samp_rate, harmonic_num = 0,
- sine_amp = 0.1, noise_std = 0.003,
- voiced_threshold = 0,
- flag_for_pulse=False)
- samp_rate: sampling rate in Hz
- harmonic_num: number of harmonic overtones (default 0)
- sine_amp: amplitude of sine-wavefrom (default 0.1)
- noise_std: std of Gaussian noise (default 0.003)
- voiced_thoreshold: F0 threshold for U/V classification (default 0)
- flag_for_pulse: this SinGen is used inside PulseGen (default False)
- Note: when flag_for_pulse is True, the first time step of a voiced
- segment is always sin(np.pi) or cos(0)
- """
-
- def __init__(
- self,
- samp_rate,
- harmonic_num=0,
- sine_amp=0.1,
- noise_std=0.003,
- voiced_threshold=0,
- flag_for_pulse=False,
- ):
- super(SineGen, self).__init__()
- self.sine_amp = sine_amp
- self.noise_std = noise_std
- self.harmonic_num = harmonic_num
- self.dim = self.harmonic_num + 1
- self.sampling_rate = samp_rate
- self.voiced_threshold = voiced_threshold
-
- def _f02uv(self, f0):
- # generate uv signal
- uv = torch.ones_like(f0)
- uv = uv * (f0 > self.voiced_threshold)
- return uv
-
- def forward(self, f0, upp):
- """sine_tensor, uv = forward(f0)
- input F0: tensor(batchsize=1, length, dim=1)
- f0 for unvoiced steps should be 0
- output sine_tensor: tensor(batchsize=1, length, dim)
- output uv: tensor(batchsize=1, length, 1)
- """
- with torch.no_grad():
- f0 = f0[:, None].transpose(1, 2)
- f0_buf = torch.zeros(f0.shape[0], f0.shape[1], self.dim, device=f0.device)
- # fundamental component
- f0_buf[:, :, 0] = f0[:, :, 0]
- for idx in np.arange(self.harmonic_num):
- f0_buf[:, :, idx + 1] = f0_buf[:, :, 0] * (
- idx + 2
- ) # idx + 2: the (idx+1)-th overtone, (idx+2)-th harmonic
- rad_values = (f0_buf / self.sampling_rate) % 1 ###%1意味着n_har的乘积无法后处理优化
- rand_ini = torch.rand(
- f0_buf.shape[0], f0_buf.shape[2], device=f0_buf.device
- )
- rand_ini[:, 0] = 0
- rad_values[:, 0, :] = rad_values[:, 0, :] + rand_ini
- tmp_over_one = torch.cumsum(rad_values, 1) # % 1 #####%1意味着后面的cumsum无法再优化
- tmp_over_one *= upp
- tmp_over_one = F.interpolate(
- tmp_over_one.transpose(2, 1),
- scale_factor=upp,
- mode="linear",
- align_corners=True,
- ).transpose(2, 1)
- rad_values = F.interpolate(
- rad_values.transpose(2, 1), scale_factor=upp, mode="nearest"
- ).transpose(
- 2, 1
- ) #######
- tmp_over_one %= 1
- tmp_over_one_idx = (tmp_over_one[:, 1:, :] - tmp_over_one[:, :-1, :]) < 0
- cumsum_shift = torch.zeros_like(rad_values)
- cumsum_shift[:, 1:, :] = tmp_over_one_idx * -1.0
- sine_waves = torch.sin(
- torch.cumsum(rad_values + cumsum_shift, dim=1) * 2 * np.pi
- )
- sine_waves = sine_waves * self.sine_amp
- uv = self._f02uv(f0)
- uv = F.interpolate(
- uv.transpose(2, 1), scale_factor=upp, mode="nearest"
- ).transpose(2, 1)
- noise_amp = uv * self.noise_std + (1 - uv) * self.sine_amp / 3
- noise = noise_amp * torch.randn_like(sine_waves)
- sine_waves = sine_waves * uv + noise
- return sine_waves, uv, noise
-
-
-class SourceModuleHnNSF(torch.nn.Module):
- """SourceModule for hn-nsf
- SourceModule(sampling_rate, harmonic_num=0, sine_amp=0.1,
- add_noise_std=0.003, voiced_threshod=0)
- sampling_rate: sampling_rate in Hz
- harmonic_num: number of harmonic above F0 (default: 0)
- sine_amp: amplitude of sine source signal (default: 0.1)
- add_noise_std: std of additive Gaussian noise (default: 0.003)
- note that amplitude of noise in unvoiced is decided
- by sine_amp
- voiced_threshold: threhold to set U/V given F0 (default: 0)
- Sine_source, noise_source = SourceModuleHnNSF(F0_sampled)
- F0_sampled (batchsize, length, 1)
- Sine_source (batchsize, length, 1)
- noise_source (batchsize, length 1)
- uv (batchsize, length, 1)
- """
-
- def __init__(
- self,
- sampling_rate,
- harmonic_num=0,
- sine_amp=0.1,
- add_noise_std=0.003,
- voiced_threshod=0,
- is_half=True,
- ):
- super(SourceModuleHnNSF, self).__init__()
-
- self.sine_amp = sine_amp
- self.noise_std = add_noise_std
- self.is_half = is_half
- # to produce sine waveforms
- self.l_sin_gen = SineGen(
- sampling_rate, harmonic_num, sine_amp, add_noise_std, voiced_threshod
- )
-
- # to merge source harmonics into a single excitation
- self.l_linear = torch.nn.Linear(harmonic_num + 1, 1)
- self.l_tanh = torch.nn.Tanh()
-
- def forward(self, x, upp=None):
- sine_wavs, uv, _ = self.l_sin_gen(x, upp)
- if self.is_half:
- sine_wavs = sine_wavs.half()
- sine_merge = self.l_tanh(self.l_linear(sine_wavs))
- return sine_merge, None, None # noise, uv
-
-
-class GeneratorNSF(torch.nn.Module):
- def __init__(
- self,
- initial_channel,
- resblock,
- resblock_kernel_sizes,
- resblock_dilation_sizes,
- upsample_rates,
- upsample_initial_channel,
- upsample_kernel_sizes,
- gin_channels,
- sr,
- is_half=False,
- ):
- super(GeneratorNSF, self).__init__()
- self.num_kernels = len(resblock_kernel_sizes)
- self.num_upsamples = len(upsample_rates)
-
- self.f0_upsamp = torch.nn.Upsample(scale_factor=np.prod(upsample_rates))
- self.m_source = SourceModuleHnNSF(
- sampling_rate=sr, harmonic_num=0, is_half=is_half
- )
- self.noise_convs = nn.ModuleList()
- self.conv_pre = Conv1d(
- initial_channel, upsample_initial_channel, 7, 1, padding=3
- )
- resblock = modules.ResBlock1 if resblock == "1" else modules.ResBlock2
-
- self.ups = nn.ModuleList()
- for i, (u, k) in enumerate(zip(upsample_rates, upsample_kernel_sizes)):
- c_cur = upsample_initial_channel // (2 ** (i + 1))
- self.ups.append(
- weight_norm(
- ConvTranspose1d(
- upsample_initial_channel // (2**i),
- upsample_initial_channel // (2 ** (i + 1)),
- k,
- u,
- padding=(k - u) // 2,
- )
- )
- )
- if i + 1 < len(upsample_rates):
- stride_f0 = np.prod(upsample_rates[i + 1 :])
- self.noise_convs.append(
- Conv1d(
- 1,
- c_cur,
- kernel_size=stride_f0 * 2,
- stride=stride_f0,
- padding=stride_f0 // 2,
- )
- )
- else:
- self.noise_convs.append(Conv1d(1, c_cur, kernel_size=1))
-
- self.resblocks = nn.ModuleList()
- for i in range(len(self.ups)):
- ch = upsample_initial_channel // (2 ** (i + 1))
- for j, (k, d) in enumerate(
- zip(resblock_kernel_sizes, resblock_dilation_sizes)
- ):
- self.resblocks.append(resblock(ch, k, d))
-
- self.conv_post = Conv1d(ch, 1, 7, 1, padding=3, bias=False)
- self.ups.apply(init_weights)
-
- if gin_channels != 0:
- self.cond = nn.Conv1d(gin_channels, upsample_initial_channel, 1)
-
- self.upp = np.prod(upsample_rates)
-
- def forward(self, x, f0, g=None):
- har_source, noi_source, uv = self.m_source(f0, self.upp)
- har_source = har_source.transpose(1, 2)
- x = self.conv_pre(x)
- if g is not None:
- x = x + self.cond(g)
-
- for i in range(self.num_upsamples):
- x = F.leaky_relu(x, modules.LRELU_SLOPE)
- x = self.ups[i](x)
- x_source = self.noise_convs[i](har_source)
- x = x + x_source
- xs = None
- for j in range(self.num_kernels):
- if xs is None:
- xs = self.resblocks[i * self.num_kernels + j](x)
- else:
- xs += self.resblocks[i * self.num_kernels + j](x)
- x = xs / self.num_kernels
- x = F.leaky_relu(x)
- x = self.conv_post(x)
- x = torch.tanh(x)
- return x
-
- def remove_weight_norm(self):
- for l in self.ups:
- remove_weight_norm(l)
- for l in self.resblocks:
- l.remove_weight_norm()
-
-
-sr2sr = {
- "32k": 32000,
- "40k": 40000,
- "48k": 48000,
-}
-
-
-class SynthesizerTrnMs256NSFsid(nn.Module):
- def __init__(
- self,
- spec_channels,
- segment_size,
- inter_channels,
- hidden_channels,
- filter_channels,
- n_heads,
- n_layers,
- kernel_size,
- p_dropout,
- resblock,
- resblock_kernel_sizes,
- resblock_dilation_sizes,
- upsample_rates,
- upsample_initial_channel,
- upsample_kernel_sizes,
- spk_embed_dim,
- gin_channels,
- sr,
- **kwargs
- ):
- super().__init__()
- if type(sr) == type("strr"):
- sr = sr2sr[sr]
- self.spec_channels = spec_channels
- self.inter_channels = inter_channels
- self.hidden_channels = hidden_channels
- self.filter_channels = filter_channels
- self.n_heads = n_heads
- self.n_layers = n_layers
- self.kernel_size = kernel_size
- self.p_dropout = p_dropout
- self.resblock = resblock
- self.resblock_kernel_sizes = resblock_kernel_sizes
- self.resblock_dilation_sizes = resblock_dilation_sizes
- self.upsample_rates = upsample_rates
- self.upsample_initial_channel = upsample_initial_channel
- self.upsample_kernel_sizes = upsample_kernel_sizes
- self.segment_size = segment_size
- self.gin_channels = gin_channels
- # self.hop_length = hop_length#
- self.spk_embed_dim = spk_embed_dim
- self.enc_p = TextEncoder256(
- inter_channels,
- hidden_channels,
- filter_channels,
- n_heads,
- n_layers,
- kernel_size,
- p_dropout,
- )
- self.dec = GeneratorNSF(
- inter_channels,
- resblock,
- resblock_kernel_sizes,
- resblock_dilation_sizes,
- upsample_rates,
- upsample_initial_channel,
- upsample_kernel_sizes,
- gin_channels=gin_channels,
- sr=sr,
- is_half=kwargs["is_half"],
- )
- self.enc_q = PosteriorEncoder(
- spec_channels,
- inter_channels,
- hidden_channels,
- 5,
- 1,
- 16,
- gin_channels=gin_channels,
- )
- self.flow = ResidualCouplingBlock(
- inter_channels, hidden_channels, 5, 1, 3, gin_channels=gin_channels
- )
- self.emb_g = nn.Embedding(self.spk_embed_dim, gin_channels)
- print("gin_channels:", gin_channels, "self.spk_embed_dim:", self.spk_embed_dim)
-
- def remove_weight_norm(self):
- self.dec.remove_weight_norm()
- self.flow.remove_weight_norm()
- self.enc_q.remove_weight_norm()
-
- def forward(
- self, phone, phone_lengths, pitch, pitchf, y, y_lengths, ds
- ): # 这里ds是id,[bs,1]
- # print(1,pitch.shape)#[bs,t]
- g = self.emb_g(ds).unsqueeze(-1) # [b, 256, 1]##1是t,广播的
- m_p, logs_p, x_mask = self.enc_p(phone, pitch, phone_lengths)
- z, m_q, logs_q, y_mask = self.enc_q(y, y_lengths, g=g)
- z_p = self.flow(z, y_mask, g=g)
- z_slice, ids_slice = commons.rand_slice_segments(
- z, y_lengths, self.segment_size
- )
- # print(-1,pitchf.shape,ids_slice,self.segment_size,self.hop_length,self.segment_size//self.hop_length)
- pitchf = commons.slice_segments2(pitchf, ids_slice, self.segment_size)
- # print(-2,pitchf.shape,z_slice.shape)
- o = self.dec(z_slice, pitchf, g=g)
- return o, ids_slice, x_mask, y_mask, (z, z_p, m_p, logs_p, m_q, logs_q)
-
- def infer(self, phone, phone_lengths, pitch, nsff0, sid, max_len=None):
- g = self.emb_g(sid).unsqueeze(-1)
- m_p, logs_p, x_mask = self.enc_p(phone, pitch, phone_lengths)
- z_p = (m_p + torch.exp(logs_p) * torch.randn_like(m_p) * 0.66666) * x_mask
- z = self.flow(z_p, x_mask, g=g, reverse=True)
- o = self.dec((z * x_mask)[:, :, :max_len], nsff0, g=g)
- return o, x_mask, (z, z_p, m_p, logs_p)
-
-
-class SynthesizerTrnMs256NSFsid_nono(nn.Module):
- def __init__(
- self,
- spec_channels,
- segment_size,
- inter_channels,
- hidden_channels,
- filter_channels,
- n_heads,
- n_layers,
- kernel_size,
- p_dropout,
- resblock,
- resblock_kernel_sizes,
- resblock_dilation_sizes,
- upsample_rates,
- upsample_initial_channel,
- upsample_kernel_sizes,
- spk_embed_dim,
- gin_channels,
- sr=None,
- **kwargs
- ):
- super().__init__()
- self.spec_channels = spec_channels
- self.inter_channels = inter_channels
- self.hidden_channels = hidden_channels
- self.filter_channels = filter_channels
- self.n_heads = n_heads
- self.n_layers = n_layers
- self.kernel_size = kernel_size
- self.p_dropout = p_dropout
- self.resblock = resblock
- self.resblock_kernel_sizes = resblock_kernel_sizes
- self.resblock_dilation_sizes = resblock_dilation_sizes
- self.upsample_rates = upsample_rates
- self.upsample_initial_channel = upsample_initial_channel
- self.upsample_kernel_sizes = upsample_kernel_sizes
- self.segment_size = segment_size
- self.gin_channels = gin_channels
- # self.hop_length = hop_length#
- self.spk_embed_dim = spk_embed_dim
- self.enc_p = TextEncoder256(
- inter_channels,
- hidden_channels,
- filter_channels,
- n_heads,
- n_layers,
- kernel_size,
- p_dropout,
- f0=False,
- )
- self.dec = Generator(
- inter_channels,
- resblock,
- resblock_kernel_sizes,
- resblock_dilation_sizes,
- upsample_rates,
- upsample_initial_channel,
- upsample_kernel_sizes,
- gin_channels=gin_channels,
- )
- self.enc_q = PosteriorEncoder(
- spec_channels,
- inter_channels,
- hidden_channels,
- 5,
- 1,
- 16,
- gin_channels=gin_channels,
- )
- self.flow = ResidualCouplingBlock(
- inter_channels, hidden_channels, 5, 1, 3, gin_channels=gin_channels
- )
- self.emb_g = nn.Embedding(self.spk_embed_dim, gin_channels)
- print("gin_channels:", gin_channels, "self.spk_embed_dim:", self.spk_embed_dim)
-
- def remove_weight_norm(self):
- self.dec.remove_weight_norm()
- self.flow.remove_weight_norm()
- self.enc_q.remove_weight_norm()
-
- def forward(self, phone, phone_lengths, y, y_lengths, ds): # 这里ds是id,[bs,1]
- g = self.emb_g(ds).unsqueeze(-1) # [b, 256, 1]##1是t,广播的
- m_p, logs_p, x_mask = self.enc_p(phone, None, phone_lengths)
- z, m_q, logs_q, y_mask = self.enc_q(y, y_lengths, g=g)
- z_p = self.flow(z, y_mask, g=g)
- z_slice, ids_slice = commons.rand_slice_segments(
- z, y_lengths, self.segment_size
- )
- o = self.dec(z_slice, g=g)
- return o, ids_slice, x_mask, y_mask, (z, z_p, m_p, logs_p, m_q, logs_q)
-
- def infer(self, phone, phone_lengths, sid, max_len=None):
- g = self.emb_g(sid).unsqueeze(-1)
- m_p, logs_p, x_mask = self.enc_p(phone, None, phone_lengths)
- z_p = (m_p + torch.exp(logs_p) * torch.randn_like(m_p) * 0.66666) * x_mask
- z = self.flow(z_p, x_mask, g=g, reverse=True)
- o = self.dec((z * x_mask)[:, :, :max_len], g=g)
- return o, x_mask, (z, z_p, m_p, logs_p)
-
-
-class SynthesizerTrnMs256NSFsid_sim(nn.Module):
- """
- Synthesizer for Training
- """
-
- def __init__(
- self,
- spec_channels,
- segment_size,
- inter_channels,
- hidden_channels,
- filter_channels,
- n_heads,
- n_layers,
- kernel_size,
- p_dropout,
- resblock,
- resblock_kernel_sizes,
- resblock_dilation_sizes,
- upsample_rates,
- upsample_initial_channel,
- upsample_kernel_sizes,
- spk_embed_dim,
- # hop_length,
- gin_channels=0,
- use_sdp=True,
- **kwargs
- ):
- super().__init__()
- self.spec_channels = spec_channels
- self.inter_channels = inter_channels
- self.hidden_channels = hidden_channels
- self.filter_channels = filter_channels
- self.n_heads = n_heads
- self.n_layers = n_layers
- self.kernel_size = kernel_size
- self.p_dropout = p_dropout
- self.resblock = resblock
- self.resblock_kernel_sizes = resblock_kernel_sizes
- self.resblock_dilation_sizes = resblock_dilation_sizes
- self.upsample_rates = upsample_rates
- self.upsample_initial_channel = upsample_initial_channel
- self.upsample_kernel_sizes = upsample_kernel_sizes
- self.segment_size = segment_size
- self.gin_channels = gin_channels
- # self.hop_length = hop_length#
- self.spk_embed_dim = spk_embed_dim
- self.enc_p = TextEncoder256Sim(
- inter_channels,
- hidden_channels,
- filter_channels,
- n_heads,
- n_layers,
- kernel_size,
- p_dropout,
- )
- self.dec = GeneratorNSF(
- inter_channels,
- resblock,
- resblock_kernel_sizes,
- resblock_dilation_sizes,
- upsample_rates,
- upsample_initial_channel,
- upsample_kernel_sizes,
- gin_channels=gin_channels,
- is_half=kwargs["is_half"],
- )
-
- self.flow = ResidualCouplingBlock(
- inter_channels, hidden_channels, 5, 1, 3, gin_channels=gin_channels
- )
- self.emb_g = nn.Embedding(self.spk_embed_dim, gin_channels)
- print("gin_channels:", gin_channels, "self.spk_embed_dim:", self.spk_embed_dim)
-
- def remove_weight_norm(self):
- self.dec.remove_weight_norm()
- self.flow.remove_weight_norm()
- self.enc_q.remove_weight_norm()
-
- def forward(
- self, phone, phone_lengths, pitch, pitchf, y_lengths, ds
- ): # y是spec不需要了现在
- g = self.emb_g(ds).unsqueeze(-1) # [b, 256, 1]##1是t,广播的
- x, x_mask = self.enc_p(phone, pitch, phone_lengths)
- x = self.flow(x, x_mask, g=g, reverse=True)
- z_slice, ids_slice = commons.rand_slice_segments(
- x, y_lengths, self.segment_size
- )
-
- pitchf = commons.slice_segments2(pitchf, ids_slice, self.segment_size)
- o = self.dec(z_slice, pitchf, g=g)
- return o, ids_slice
-
- def infer(
- self, phone, phone_lengths, pitch, pitchf, ds, max_len=None
- ): # y是spec不需要了现在
- g = self.emb_g(ds).unsqueeze(-1) # [b, 256, 1]##1是t,广播的
- x, x_mask = self.enc_p(phone, pitch, phone_lengths)
- x = self.flow(x, x_mask, g=g, reverse=True)
- o = self.dec((x * x_mask)[:, :, :max_len], pitchf, g=g)
- return o, o
-
-
-class MultiPeriodDiscriminator(torch.nn.Module):
- def __init__(self, use_spectral_norm=False):
- super(MultiPeriodDiscriminator, self).__init__()
- periods = [2, 3, 5, 7, 11, 17]
- # periods = [3, 5, 7, 11, 17, 23, 37]
-
- discs = [DiscriminatorS(use_spectral_norm=use_spectral_norm)]
- discs = discs + [
- DiscriminatorP(i, use_spectral_norm=use_spectral_norm) for i in periods
- ]
- self.discriminators = nn.ModuleList(discs)
-
- def forward(self, y, y_hat):
- y_d_rs = [] #
- y_d_gs = []
- fmap_rs = []
- fmap_gs = []
- for i, d in enumerate(self.discriminators):
- y_d_r, fmap_r = d(y)
- y_d_g, fmap_g = d(y_hat)
- # for j in range(len(fmap_r)):
- # print(i,j,y.shape,y_hat.shape,fmap_r[j].shape,fmap_g[j].shape)
- y_d_rs.append(y_d_r)
- y_d_gs.append(y_d_g)
- fmap_rs.append(fmap_r)
- fmap_gs.append(fmap_g)
-
- return y_d_rs, y_d_gs, fmap_rs, fmap_gs
-
-
-class DiscriminatorS(torch.nn.Module):
- def __init__(self, use_spectral_norm=False):
- super(DiscriminatorS, self).__init__()
- norm_f = weight_norm if use_spectral_norm == False else spectral_norm
- self.convs = nn.ModuleList(
- [
- norm_f(Conv1d(1, 16, 15, 1, padding=7)),
- norm_f(Conv1d(16, 64, 41, 4, groups=4, padding=20)),
- norm_f(Conv1d(64, 256, 41, 4, groups=16, padding=20)),
- norm_f(Conv1d(256, 1024, 41, 4, groups=64, padding=20)),
- norm_f(Conv1d(1024, 1024, 41, 4, groups=256, padding=20)),
- norm_f(Conv1d(1024, 1024, 5, 1, padding=2)),
- ]
- )
- self.conv_post = norm_f(Conv1d(1024, 1, 3, 1, padding=1))
-
- def forward(self, x):
- fmap = []
-
- for l in self.convs:
- x = l(x)
- x = F.leaky_relu(x, modules.LRELU_SLOPE)
- fmap.append(x)
- x = self.conv_post(x)
- fmap.append(x)
- x = torch.flatten(x, 1, -1)
-
- return x, fmap
-
-
-class DiscriminatorP(torch.nn.Module):
- def __init__(self, period, kernel_size=5, stride=3, use_spectral_norm=False):
- super(DiscriminatorP, self).__init__()
- self.period = period
- self.use_spectral_norm = use_spectral_norm
- norm_f = weight_norm if use_spectral_norm == False else spectral_norm
- self.convs = nn.ModuleList(
- [
- norm_f(
- Conv2d(
- 1,
- 32,
- (kernel_size, 1),
- (stride, 1),
- padding=(get_padding(kernel_size, 1), 0),
- )
- ),
- norm_f(
- Conv2d(
- 32,
- 128,
- (kernel_size, 1),
- (stride, 1),
- padding=(get_padding(kernel_size, 1), 0),
- )
- ),
- norm_f(
- Conv2d(
- 128,
- 512,
- (kernel_size, 1),
- (stride, 1),
- padding=(get_padding(kernel_size, 1), 0),
- )
- ),
- norm_f(
- Conv2d(
- 512,
- 1024,
- (kernel_size, 1),
- (stride, 1),
- padding=(get_padding(kernel_size, 1), 0),
- )
- ),
- norm_f(
- Conv2d(
- 1024,
- 1024,
- (kernel_size, 1),
- 1,
- padding=(get_padding(kernel_size, 1), 0),
- )
- ),
- ]
- )
- self.conv_post = norm_f(Conv2d(1024, 1, (3, 1), 1, padding=(1, 0)))
-
- def forward(self, x):
- fmap = []
-
- # 1d to 2d
- b, c, t = x.shape
- if t % self.period != 0: # pad first
- n_pad = self.period - (t % self.period)
- x = F.pad(x, (0, n_pad), "reflect")
- t = t + n_pad
- x = x.view(b, c, t // self.period, self.period)
-
- for l in self.convs:
- x = l(x)
- x = F.leaky_relu(x, modules.LRELU_SLOPE)
- fmap.append(x)
- x = self.conv_post(x)
- fmap.append(x)
- x = torch.flatten(x, 1, -1)
-
- return x, fmap
diff --git a/spaces/FrankZxShen/so-vits-svc-models-pcr/vencoder/WhisperPPG.py b/spaces/FrankZxShen/so-vits-svc-models-pcr/vencoder/WhisperPPG.py
deleted file mode 100644
index aa988b0a6d05696ea519d1652e5801302ba8a6c6..0000000000000000000000000000000000000000
--- a/spaces/FrankZxShen/so-vits-svc-models-pcr/vencoder/WhisperPPG.py
+++ /dev/null
@@ -1,30 +0,0 @@
-from vencoder.encoder import SpeechEncoder
-import torch
-
-from vencoder.whisper.model import Whisper, ModelDimensions
-from vencoder.whisper.audio import pad_or_trim, log_mel_spectrogram
-
-
-class WhisperPPG(SpeechEncoder):
- def __init__(self,vec_path = "pretrain/medium.pt",device=None):
- if device is None:
- self.dev = torch.device("cuda" if torch.cuda.is_available() else "cpu")
- else:
- self.dev = torch.device(device)
- checkpoint = torch.load(vec_path, map_location=device)
- dims = ModelDimensions(**checkpoint["dims"])
- model = Whisper(dims)
- model.load_state_dict(checkpoint["model_state_dict"])
- self.hidden_dim = dims
- self.model = model.to(self.dev)
-
- def encoder(self, wav):
- audio = wav
- audln = audio.shape[0]
- ppgln = audln // 320
- audio = pad_or_trim(audio)
- mel = log_mel_spectrogram(audio).to(self.dev)
- with torch.no_grad():
- ppg = self.model.encoder(mel.unsqueeze(0)).squeeze().data.cpu().float().numpy()
- ppg = torch.FloatTensor(ppg[:ppgln,]).to(self.dev)
- return ppg[None,:,:].transpose(1, 2)
diff --git a/spaces/Gabriel/Swe_summarizer/app.py b/spaces/Gabriel/Swe_summarizer/app.py
deleted file mode 100644
index 0bcd356af899921a5e73c17db7e0c5c88a0a216c..0000000000000000000000000000000000000000
--- a/spaces/Gabriel/Swe_summarizer/app.py
+++ /dev/null
@@ -1,138 +0,0 @@
-import gradio as gr
-from transformers import pipeline
-import pandas as pd
-import json
-import nltk
-from sentence_transformers import SentenceTransformer, util
-import numpy as np
-from LexRank import *
-from text import *
-
-nltk.download('punkt')
-
-
-def lex_rank(in_text, threshold=None , ex_sent=4 ,model_in = 'KBLab/sentence-bert-swedish-cased', language='swedish' ):
- if threshold == 'None':
- threshold=None
-
- model = SentenceTransformer(model_in)
- #Split the document into sentences
- sentences = nltk.sent_tokenize(in_text, language=language)
-
- #Compute the sentence embeddings
- embeddings = model.encode(sentences, convert_to_tensor=True)
- cos_scores = util.cos_sim(embeddings, embeddings).cpu().numpy()
-
- #Compute the centrality for each sentence
- centrality_scores = degree_centrality_scores(cos_scores, threshold=threshold)
-
- most_central_sentence_indices = np.argsort(-centrality_scores)
- sent_list= []
- for idx in most_central_sentence_indices[0:ex_sent]:
- sent_list.append(sentences[idx])
- return ' '.join(sent_list)
-
-
-def generate(in_text, num_beams, min_len, max_len, model_in):
- print(in_text)
- pipe = pipeline("summarization", model=model_in)
- answer = pipe(in_text, num_beams=num_beams ,min_length=min_len, max_length=max_len)
- print(answer)
- return answer[0]["summary_text"]
-
-
-def update_history(df, in_text, gen_text ,model_in, sum_typ, parameters):
- # get rid of first seed phrase
- new_row = [{"In_text": in_text,
- "Gen_text": gen_text,
- "Sum_type": sum_typ ,
- "Gen_model": model_in,
- "Parameters": json.dumps(parameters)}]
- return pd.concat([df, pd.DataFrame(new_row)])
-
-def generate_transformer(in_text, num_beams, min_len, max_len, model_in, history):
- gen_text= generate(in_text,num_beams, min_len, max_len, model_in)
- return gen_text, update_history(history, in_text, gen_text, "Abstractive" ,model_in, {"num_beams": num_beams,
- "min_len": min_len,
- "max_len": max_len})
-
-def generate_lexrank(in_text, threshold, model_in, ex_sent ,language, history):
- gen_text= lex_rank(in_text, threshold, ex_sent ,model_in, language)
- return gen_text, update_history(history, in_text, gen_text, "Extractive" ,model_in, {"threshold": threshold,
- "Nr_sent": ex_sent,
- "language": language})
-
-with gr.Blocks() as demo:
- gr.Markdown("
Swedish Summarization Engine!
")
- with gr.Accordion("Read here for details about the app", open=False):
- with gr.Row():
- with gr.Column(css=".gr-prose img {margin-bottom: 0em !important;}"):
- gr.Markdown(sum_app_text_tab_1)
- with gr.Column(css=".gr-prose img {margin-bottom: 0em !important;}"):
- gr.Markdown(sum_app_text_tab_2)
-
- with gr.Tabs():
- with gr.TabItem("Abstractive Generation for Summarization"):
- gr.Markdown(
- """The default parameters for this transformer based model work well to generate summarization.
- Use this tab to experiment summarization task of text for different types Abstractive models.""")
- with gr.Row():
- with gr.Column(scale=4):
- text_baseline_transformer= gr.TextArea(label="Input text to summarize", placeholder="Input summarization")
-
- with gr.Row():
- transformer_button_clear = gr.Button("Clear", variant='secondary')
- transformer_button = gr.Button("Summarize!", variant='primary')
-
- with gr.Column(scale=3):
- with gr.Row():
- num_beams = gr.Slider(minimum=2, maximum=10, value=2, step=1, label="Number of Beams")
- min_len = gr.Slider(minimum=10, maximum=50, value=25, step=5, label="Min length")
- max_len = gr.Slider(minimum=50, maximum=130, value=120, step=10, label="Max length")
- model_in = gr.Dropdown(["Gabriel/bart-base-cnn-swe", "Gabriel/bart-base-cnn-xsum-swe", "Gabriel/bart-base-cnn-xsum-wiki-swe"], value="Gabriel/bart-base-cnn-xsum-swe", label="Model")
- output_basline_transformer = gr.Textbox(label="Output Text")
-
- with gr.Row():
- with gr.Accordion("Here are some examples you can use:", open=False):
- gr.Markdown("
Press one of the test examples below.
")
- gr.Markdown("NOTE: First time inference for a new model will take time, since a new model has to downloaded before inference.")
- gr.Examples([[abstractive_example_text_1
- , 5,25,120, "Gabriel/bart-base-cnn-swe"],
- [abstractive_example_text_2
- , 5,25,120, "Gabriel/bart-base-cnn-xsum-swe"]
- ], [text_baseline_transformer, num_beams, min_len, max_len, model_in])
-
- with gr.TabItem("Extractive Ranking Graph for Summarization"):
- gr.Markdown(
- """Use this tab to experiment summarization task of text with a graph based method (LexRank).""")
- with gr.Row():
- with gr.Column(scale=4):
- text_extract= gr.TextArea(label="Input text to summarize", placeholder="Input text")
- with gr.Row():
- extract_button_clear = gr.Button("Clear", variant='secondary')
- extract_button = gr.Button("Summarize!", variant='primary')
- with gr.Column(scale=3):
- with gr.Row():
- ex_sent =gr.Slider(minimum=1, maximum=7, value=4, step=1, label="Sentences to return")
- ex_threshold = gr.Dropdown(['None',0.1,0.2,0.3,0.4,0.5], value='None', label="Similar Threshold")
- ex_language = gr.Dropdown(["swedish","english"], value="swedish", label="Language")
- model_in_ex = gr.Dropdown(["KBLab/sentence-bert-swedish-cased","sentence-transformers/all-MiniLM-L6-v2"], value="KBLab/sentence-bert-swedish-cased", label="Model")
- output_extract = gr.Textbox(label="Output Text")
-
- with gr.Row():
- with gr.Accordion("Here are some examples you can use:", open=False):
- gr.Markdown("
Press one of the test examples below.
")
- gr.Markdown("NOTE: First time inference for a new model will take time, since a new model has to downloaded before inference.")
- gr.Examples([[extractive_example_text_1
- , 'None', 4,'swedish', "KBLab/sentence-bert-swedish-cased"]], [text_extract, ex_threshold, ex_sent ,ex_language, model_in_ex])
-
- with gr.Box():
- gr.Markdown("
- )
-}
diff --git a/spaces/NeuroSenko/tts-silero/README.md b/spaces/NeuroSenko/tts-silero/README.md
deleted file mode 100644
index 8679d5639cb146d77bda35834f2218ae3779ac9e..0000000000000000000000000000000000000000
--- a/spaces/NeuroSenko/tts-silero/README.md
+++ /dev/null
@@ -1,15 +0,0 @@
----
-title: Tts Silero
-emoji: 📊
-colorFrom: gray
-colorTo: indigo
-sdk: gradio
-sdk_version: 3.39.0
-app_file: app.py
-pinned: false
----
-
-How to run locally using Windows:
-1. Clone the repo: `git clone https://huggingface.co/spaces/NeuroSenko/tts-silero`
-2. Run `install.bat`
-3. Run `start.bat`
diff --git a/spaces/Nixtla/chatgpt-forecast/README.md b/spaces/Nixtla/chatgpt-forecast/README.md
deleted file mode 100644
index f236e4bd350cfb5740026ceaab3d3cc968cbf24f..0000000000000000000000000000000000000000
--- a/spaces/Nixtla/chatgpt-forecast/README.md
+++ /dev/null
@@ -1,13 +0,0 @@
----
-title: Chatgpt Forecast
-emoji: 🌖
-colorFrom: green
-colorTo: purple
-sdk: streamlit
-sdk_version: 1.21.0
-app_file: app.py
-pinned: false
-license: apache-2.0
----
-
-Check out the configuration reference at https://huggingface.co/docs/hub/spaces-config-reference
diff --git a/spaces/OFA-Sys/OFA-Image_Caption/fairseq/examples/speech_to_text/docs/mustc_example.md b/spaces/OFA-Sys/OFA-Image_Caption/fairseq/examples/speech_to_text/docs/mustc_example.md
deleted file mode 100644
index c95ef3e15660107c3384f87c1680f005044e7f3b..0000000000000000000000000000000000000000
--- a/spaces/OFA-Sys/OFA-Image_Caption/fairseq/examples/speech_to_text/docs/mustc_example.md
+++ /dev/null
@@ -1,155 +0,0 @@
-[[Back]](..)
-
-# S2T Example: Speech Translation (ST) on MuST-C
-
-[MuST-C](https://www.aclweb.org/anthology/N19-1202) is multilingual speech-to-text translation corpus with
-8-language translations on English TED talks. We match the state-of-the-art performance in
-[ESPNet-ST](https://arxiv.org/pdf/2004.10234.pdf) with a simpler model training pipeline.
-
-## Data Preparation
-[Download](https://ict.fbk.eu/must-c) and unpack MuST-C data to a path
-`${MUSTC_ROOT}/en-${TARGET_LANG_ID}`, then preprocess it with
-```bash
-# additional Python packages for S2T data processing/model training
-pip install pandas torchaudio soundfile sentencepiece
-
-# Generate TSV manifests, features, vocabulary
-# and configuration for each language
-python examples/speech_to_text/prep_mustc_data.py \
- --data-root ${MUSTC_ROOT} --task asr \
- --vocab-type unigram --vocab-size 5000
-python examples/speech_to_text/prep_mustc_data.py \
- --data-root ${MUSTC_ROOT} --task st \
- --vocab-type unigram --vocab-size 8000
-
-# Add vocabulary and configuration for joint data
-# (based on the manifests and features generated above)
-python examples/speech_to_text/prep_mustc_data.py \
- --data-root ${MUSTC_ROOT} --task asr --joint \
- --vocab-type unigram --vocab-size 10000
-python examples/speech_to_text/prep_mustc_data.py \
- --data-root ${MUSTC_ROOT} --task st --joint \
- --vocab-type unigram --vocab-size 10000
-```
-The generated files (manifest, features, vocabulary and data configuration) will be added to
-`${MUSTC_ROOT}/en-${TARGET_LANG_ID}` (per-language data) and `MUSTC_ROOT` (joint data).
-
-Download our vocabulary files if you want to use our pre-trained models:
-- ASR: [En-De](https://dl.fbaipublicfiles.com/fairseq/s2t/mustc_de_asr_vocab_unigram5000.zip), [En-Nl](https://dl.fbaipublicfiles.com/fairseq/s2t/mustc_nl_asr_vocab_unigram5000.zip), [En-Es](https://dl.fbaipublicfiles.com/fairseq/s2t/mustc_es_asr_vocab_unigram5000.zip), [En-Fr](https://dl.fbaipublicfiles.com/fairseq/s2t/mustc_fr_asr_vocab_unigram5000.zip), [En-It](https://dl.fbaipublicfiles.com/fairseq/s2t/mustc_it_asr_vocab_unigram5000.zip), [En-Pt](https://dl.fbaipublicfiles.com/fairseq/s2t/mustc_pt_asr_vocab_unigram5000.zip), [En-Ro](https://dl.fbaipublicfiles.com/fairseq/s2t/mustc_ro_asr_vocab_unigram5000.zip), [En-Ru](https://dl.fbaipublicfiles.com/fairseq/s2t/mustc_ru_asr_vocab_unigram5000.zip), [Joint](https://dl.fbaipublicfiles.com/fairseq/s2t/mustc_joint_asr_vocab_unigram10000.zip)
-- ST: [En-De](https://dl.fbaipublicfiles.com/fairseq/s2t/mustc_de_st_vocab_unigram8000.zip), [En-Nl](https://dl.fbaipublicfiles.com/fairseq/s2t/mustc_nl_st_vocab_unigram8000.zip), [En-Es](https://dl.fbaipublicfiles.com/fairseq/s2t/mustc_es_st_vocab_unigram8000.zip), [En-Fr](https://dl.fbaipublicfiles.com/fairseq/s2t/mustc_fr_st_vocab_unigram8000.zip), [En-It](https://dl.fbaipublicfiles.com/fairseq/s2t/mustc_it_st_vocab_unigram8000.zip), [En-Pt](https://dl.fbaipublicfiles.com/fairseq/s2t/mustc_pt_st_vocab_unigram8000.zip), [En-Ro](https://dl.fbaipublicfiles.com/fairseq/s2t/mustc_ro_st_vocab_unigram8000.zip), [En-Ru](https://dl.fbaipublicfiles.com/fairseq/s2t/mustc_ru_st_vocab_unigram8000.zip), [Multilingual](https://dl.fbaipublicfiles.com/fairseq/s2t/mustc_multilingual_st_vocab_unigram10000.zip)
-
-## ASR
-#### Training
-En-De as example:
-```bash
-fairseq-train ${MUSTC_ROOT}/en-de \
- --config-yaml config_asr.yaml --train-subset train_asr --valid-subset dev_asr \
- --save-dir ${ASR_SAVE_DIR} --num-workers 4 --max-tokens 40000 --max-update 100000 \
- --task speech_to_text --criterion label_smoothed_cross_entropy --label-smoothing 0.1 --report-accuracy \
- --arch s2t_transformer_s --optimizer adam --lr 1e-3 --lr-scheduler inverse_sqrt \
- --warmup-updates 10000 --clip-norm 10.0 --seed 1 --update-freq 8
-```
-For joint model (using ASR data from all 8 directions):
-```bash
-fairseq-train ${MUSTC_ROOT} \
- --config-yaml config_asr.yaml \
- --train-subset train_de_asr,train_nl_asr,train_es_asr,train_fr_asr,train_it_asr,train_pt_asr,train_ro_asr,train_ru_asr \
- --valid-subset dev_de_asr,dev_nl_asr,dev_es_asr,dev_fr_asr,dev_it_asr,dev_pt_asr,dev_ro_asr,dev_ru_asr \
- --save-dir ${JOINT_ASR_SAVE_DIR} --num-workers 4 --max-tokens 40000 --max-update 100000 \
- --task speech_to_text --criterion label_smoothed_cross_entropy --label-smoothing 0.1 --report-accuracy \
- --arch s2t_transformer_s --optimizer adam --lr 1e-3 --lr-scheduler inverse_sqrt \
- --warmup-updates 10000 --clip-norm 10.0 --seed 1 --update-freq 8
-```
-where `ASR_SAVE_DIR` (`JOINT_ASR_SAVE_DIR`) is the checkpoint root path. We set `--update-freq 8` to simulate 8 GPUs
-with 1 GPU. You may want to update it accordingly when using more than 1 GPU.
-
-#### Inference & Evaluation
-```bash
-CHECKPOINT_FILENAME=avg_last_10_checkpoint.pt
-python scripts/average_checkpoints.py \
- --inputs ${ASR_SAVE_DIR} --num-epoch-checkpoints 10 \
- --output "${ASR_SAVE_DIR}/${CHECKPOINT_FILENAME}"
-fairseq-generate ${MUSTC_ROOT}/en-de \
- --config-yaml config_asr.yaml --gen-subset tst-COMMON_asr --task speech_to_text \
- --path ${ASR_SAVE_DIR}/${CHECKPOINT_FILENAME} --max-tokens 50000 --beam 5 \
- --scoring wer --wer-tokenizer 13a --wer-lowercase --wer-remove-punct
-
-# For models trained on joint data
-python scripts/average_checkpoints.py \
- --inputs ${JOINT_ASR_SAVE_DIR} --num-epoch-checkpoints 10 \
- --output "${JOINT_ASR_SAVE_DIR}/${CHECKPOINT_FILENAME}"
-for LANG in de nl es fr it pt ro ru; do
- fairseq-generate ${MUSTC_ROOT} \
- --config-yaml config_asr.yaml --gen-subset tst-COMMON_${LANG}_asr --task speech_to_text \
- --path ${JOINT_ASR_SAVE_DIR}/${CHECKPOINT_FILENAME} --max-tokens 50000 --beam 5 \
- --scoring wer --wer-tokenizer 13a --wer-lowercase --wer-remove-punct
-done
-```
-#### Results
-| Data | --arch | Params | En-De | En-Nl | En-Es | En-Fr | En-It | En-Pt | En-Ro | En-Ru | Model |
-|---|---|---|---|---|---|---|---|---|---|---|---|
-| Single | s2t_transformer_s | 31M | [18.2](https://dl.fbaipublicfiles.com/fairseq/s2t/mustc_de_asr_transformer_s.pt) | [17.6](https://dl.fbaipublicfiles.com/fairseq/s2t/mustc_nl_asr_transformer_s.pt) | [17.7](https://dl.fbaipublicfiles.com/fairseq/s2t/mustc_es_asr_transformer_s.pt) | [17.2](https://dl.fbaipublicfiles.com/fairseq/s2t/mustc_fr_asr_transformer_s.pt) | [17.9](https://dl.fbaipublicfiles.com/fairseq/s2t/mustc_it_asr_transformer_s.pt) | [19.1](https://dl.fbaipublicfiles.com/fairseq/s2t/mustc_pt_asr_transformer_s.pt) | [18.1](https://dl.fbaipublicfiles.com/fairseq/s2t/mustc_ro_asr_transformer_s.pt) | [17.7](https://dl.fbaipublicfiles.com/fairseq/s2t/mustc_ru_asr_transformer_s.pt) | (<-Download) |
-| Joint | s2t_transformer_m | 76M | 16.8 | 16.7 | 16.9 | 16.9 | 17.0 | 17.4 | 17.0 | 16.9 | [Download](https://dl.fbaipublicfiles.com/fairseq/s2t/mustc_joint_asr_transformer_m.pt) |
-
-## ST
-#### Training
-En-De as example:
-```bash
-fairseq-train ${MUSTC_ROOT}/en-de \
- --config-yaml config_st.yaml --train-subset train_st --valid-subset dev_st \
- --save-dir ${ST_SAVE_DIR} --num-workers 4 --max-tokens 40000 --max-update 100000 \
- --task speech_to_text --criterion label_smoothed_cross_entropy --label-smoothing 0.1 --report-accuracy \
- --arch s2t_transformer_s --optimizer adam --lr 2e-3 --lr-scheduler inverse_sqrt \
- --warmup-updates 10000 --clip-norm 10.0 --seed 1 --update-freq 8 \
- --load-pretrained-encoder-from ${ASR_SAVE_DIR}/${CHECKPOINT_FILENAME}
-```
-For multilingual model (all 8 directions):
-```bash
-fairseq-train ${MUSTC_ROOT} \
- --config-yaml config_st.yaml \
- --train-subset train_de_st,train_nl_st,train_es_st,train_fr_st,train_it_st,train_pt_st,train_ro_st,train_ru_st \
- --valid-subset dev_de_st,dev_nl_st,dev_es_st,dev_fr_st,dev_it_st,dev_pt_st,dev_ro_st,dev_ru_st \
- --save-dir ${MULTILINGUAL_ST_SAVE_DIR} --num-workers 4 --max-tokens 40000 --max-update 100000 \
- --task speech_to_text --criterion label_smoothed_cross_entropy --label-smoothing 0.1 --report-accuracy \
- --arch s2t_transformer_s --ignore-prefix-size 1 --optimizer adam --lr 2e-3 --lr-scheduler inverse_sqrt \
- --warmup-updates 10000 --clip-norm 10.0 --seed 1 --update-freq 8 \
- --load-pretrained-encoder-from ${JOINT_ASR_SAVE_DIR}/${CHECKPOINT_FILENAME}
-```
-where `ST_SAVE_DIR` (`MULTILINGUAL_ST_SAVE_DIR`) is the checkpoint root path. The ST encoder is pre-trained by ASR
-for faster training and better performance: `--load-pretrained-encoder-from <(JOINT_)ASR checkpoint path>`. We set
-`--update-freq 8` to simulate 8 GPUs with 1 GPU. You may want to update it accordingly when using more than 1 GPU.
-For multilingual models, we prepend target language ID token as target BOS, which should be excluded from
-the training loss via `--ignore-prefix-size 1`.
-
-#### Inference & Evaluation
-Average the last 10 checkpoints and evaluate on the `tst-COMMON` split:
-```bash
-CHECKPOINT_FILENAME=avg_last_10_checkpoint.pt
-python scripts/average_checkpoints.py \
- --inputs ${ST_SAVE_DIR} --num-epoch-checkpoints 10 \
- --output "${ST_SAVE_DIR}/${CHECKPOINT_FILENAME}"
-fairseq-generate ${MUSTC_ROOT}/en-de \
- --config-yaml config_st.yaml --gen-subset tst-COMMON_st --task speech_to_text \
- --path ${ST_SAVE_DIR}/${CHECKPOINT_FILENAME} \
- --max-tokens 50000 --beam 5 --scoring sacrebleu
-
-# For multilingual models
-python scripts/average_checkpoints.py \
- --inputs ${MULTILINGUAL_ST_SAVE_DIR} --num-epoch-checkpoints 10 \
- --output "${MULTILINGUAL_ST_SAVE_DIR}/${CHECKPOINT_FILENAME}"
-for LANG in de nl es fr it pt ro ru; do
- fairseq-generate ${MUSTC_ROOT} \
- --config-yaml config_st.yaml --gen-subset tst-COMMON_${LANG}_st --task speech_to_text \
- --prefix-size 1 --path ${MULTILINGUAL_ST_SAVE_DIR}/${CHECKPOINT_FILENAME} \
- --max-tokens 50000 --beam 5 --scoring sacrebleu
-done
-```
-For multilingual models, we force decoding from the target language ID token (as BOS) via `--prefix-size 1`.
-
-#### Results
-| Data | --arch | Params | En-De | En-Nl | En-Es | En-Fr | En-It | En-Pt | En-Ro | En-Ru | Model |
-|---|---|---|---|---|---|---|---|---|---|---|---|
-| Bilingual | s2t_transformer_s | 31M | [22.7](https://dl.fbaipublicfiles.com/fairseq/s2t/mustc_de_st_transformer_s.pt) | [27.3](https://dl.fbaipublicfiles.com/fairseq/s2t/mustc_nl_st_transformer_s.pt) | [27.2](https://dl.fbaipublicfiles.com/fairseq/s2t/mustc_es_st_transformer_s.pt) | [32.9](https://dl.fbaipublicfiles.com/fairseq/s2t/mustc_fr_st_transformer_s.pt) | [22.7](https://dl.fbaipublicfiles.com/fairseq/s2t/mustc_it_st_transformer_s.pt) | [28.1](https://dl.fbaipublicfiles.com/fairseq/s2t/mustc_pt_st_transformer_s.pt) | [21.9](https://dl.fbaipublicfiles.com/fairseq/s2t/mustc_ro_st_transformer_s.pt) | [15.3](https://dl.fbaipublicfiles.com/fairseq/s2t/mustc_ru_st_transformer_s.pt) | (<-Download) |
-| Multilingual | s2t_transformer_m | 76M | 24.5 | 28.6 | 28.2 | 34.9 | 24.6 | 31.1 | 23.8 | 16.0 | [Download](https://dl.fbaipublicfiles.com/fairseq/s2t/mustc_multilingual_st_transformer_m.pt) |
-
-[[Back]](..)
diff --git a/spaces/OFA-Sys/OFA-Image_Caption/fairseq/tests/test_valid_subset_checks.py b/spaces/OFA-Sys/OFA-Image_Caption/fairseq/tests/test_valid_subset_checks.py
deleted file mode 100644
index 3e9191bda66fccfebba34920f88bf7b1efea5f7e..0000000000000000000000000000000000000000
--- a/spaces/OFA-Sys/OFA-Image_Caption/fairseq/tests/test_valid_subset_checks.py
+++ /dev/null
@@ -1,138 +0,0 @@
-import os
-import shutil
-import tempfile
-import unittest
-
-from fairseq import options
-from fairseq.dataclass.utils import convert_namespace_to_omegaconf
-from fairseq.data.data_utils import raise_if_valid_subsets_unintentionally_ignored
-from .utils import create_dummy_data, preprocess_lm_data, train_language_model
-
-
-def make_lm_config(
- data_dir=None,
- extra_flags=None,
- task="language_modeling",
- arch="transformer_lm_gpt2_tiny",
-):
- task_args = [task]
- if data_dir is not None:
- task_args += [data_dir]
- train_parser = options.get_training_parser()
- train_args = options.parse_args_and_arch(
- train_parser,
- [
- "--task",
- *task_args,
- "--arch",
- arch,
- "--optimizer",
- "adam",
- "--lr",
- "0.0001",
- "--max-tokens",
- "500",
- "--tokens-per-sample",
- "500",
- "--save-dir",
- data_dir,
- "--max-epoch",
- "1",
- ]
- + (extra_flags or []),
- )
- cfg = convert_namespace_to_omegaconf(train_args)
- return cfg
-
-
-def write_empty_file(path):
- with open(path, "w"):
- pass
- assert os.path.exists(path)
-
-
-class TestValidSubsetsErrors(unittest.TestCase):
- """Test various filesystem, clarg combinations and ensure that error raising happens as expected"""
-
- def _test_case(self, paths, extra_flags):
- with tempfile.TemporaryDirectory() as data_dir:
- [
- write_empty_file(os.path.join(data_dir, f"{p}.bin"))
- for p in paths + ["train"]
- ]
- cfg = make_lm_config(data_dir, extra_flags=extra_flags)
- raise_if_valid_subsets_unintentionally_ignored(cfg)
-
- def test_default_raises(self):
- with self.assertRaises(ValueError):
- self._test_case(["valid", "valid1"], [])
- with self.assertRaises(ValueError):
- self._test_case(
- ["valid", "valid1", "valid2"], ["--valid-subset", "valid,valid1"]
- )
-
- def partially_specified_valid_subsets(self):
- with self.assertRaises(ValueError):
- self._test_case(
- ["valid", "valid1", "valid2"], ["--valid-subset", "valid,valid1"]
- )
- # Fix with ignore unused
- self._test_case(
- ["valid", "valid1", "valid2"],
- ["--valid-subset", "valid,valid1", "--ignore-unused-valid-subsets"],
- )
-
- def test_legal_configs(self):
- self._test_case(["valid"], [])
- self._test_case(["valid", "valid1"], ["--ignore-unused-valid-subsets"])
- self._test_case(["valid", "valid1"], ["--combine-val"])
- self._test_case(["valid", "valid1"], ["--valid-subset", "valid,valid1"])
- self._test_case(["valid", "valid1"], ["--valid-subset", "valid1"])
- self._test_case(
- ["valid", "valid1"], ["--combine-val", "--ignore-unused-valid-subsets"]
- )
- self._test_case(
- ["valid1"], ["--valid-subset", "valid1"]
- ) # valid.bin doesn't need to be ignored.
-
- def test_disable_validation(self):
- self._test_case([], ["--disable-validation"])
- self._test_case(["valid", "valid1"], ["--disable-validation"])
-
- def test_dummy_task(self):
- cfg = make_lm_config(task="dummy_lm")
- raise_if_valid_subsets_unintentionally_ignored(cfg)
-
- def test_masked_dummy_task(self):
- cfg = make_lm_config(task="dummy_masked_lm")
- raise_if_valid_subsets_unintentionally_ignored(cfg)
-
-
-class TestCombineValidSubsets(unittest.TestCase):
- def _train(self, extra_flags):
- with self.assertLogs() as logs:
- with tempfile.TemporaryDirectory("test_transformer_lm") as data_dir:
- create_dummy_data(data_dir, num_examples=20)
- preprocess_lm_data(data_dir)
-
- shutil.copyfile(f"{data_dir}/valid.bin", f"{data_dir}/valid1.bin")
- shutil.copyfile(f"{data_dir}/valid.idx", f"{data_dir}/valid1.idx")
- train_language_model(
- data_dir,
- "transformer_lm",
- ["--max-update", "0", "--log-format", "json"] + extra_flags,
- run_validation=False,
- )
- return [x.message for x in logs.records]
-
- def test_combined(self):
- flags = ["--combine-valid-subsets"]
- logs = self._train(flags)
- assert any(["valid1" in x for x in logs]) # loaded 100 examples from valid1
- assert not any(["valid1_ppl" in x for x in logs]) # metrics are combined
-
- def test_subsets(self):
- flags = ["--valid-subset", "valid,valid1"]
- logs = self._train(flags)
- assert any(["valid_ppl" in x for x in logs]) # loaded 100 examples from valid1
- assert any(["valid1_ppl" in x for x in logs]) # metrics are combined
diff --git a/spaces/OFA-Sys/OFA-Visual_Grounding/fairseq/.github/ISSUE_TEMPLATE/feature_request.md b/spaces/OFA-Sys/OFA-Visual_Grounding/fairseq/.github/ISSUE_TEMPLATE/feature_request.md
deleted file mode 100644
index 93c8668041f8a7af29e4c11e905d8b56b946dd51..0000000000000000000000000000000000000000
--- a/spaces/OFA-Sys/OFA-Visual_Grounding/fairseq/.github/ISSUE_TEMPLATE/feature_request.md
+++ /dev/null
@@ -1,24 +0,0 @@
----
-name: 🚀 Feature Request
-about: Submit a proposal/request for a new feature
-labels: 'enhancement, help wanted, needs triage'
----
-
-## 🚀 Feature Request
-
-
-### Motivation
-
-
-
-### Pitch
-
-
-
-### Alternatives
-
-
-
-### Additional context
-
-
diff --git a/spaces/OFA-Sys/OFA-vqa/fairseq/examples/multilingual/data_scripts/utils/strip_sgm.sh b/spaces/OFA-Sys/OFA-vqa/fairseq/examples/multilingual/data_scripts/utils/strip_sgm.sh
deleted file mode 100644
index 7f4f61d7b1a46f51a1221de6b336cb70b5a0b8b3..0000000000000000000000000000000000000000
--- a/spaces/OFA-Sys/OFA-vqa/fairseq/examples/multilingual/data_scripts/utils/strip_sgm.sh
+++ /dev/null
@@ -1 +0,0 @@
-grep "seg id" | sed 's///g' | sed 's/<\/seg>//g'
diff --git a/spaces/OFA-Sys/OFA-vqa/fairseq/fairseq/models/fairseq_model.py b/spaces/OFA-Sys/OFA-vqa/fairseq/fairseq/models/fairseq_model.py
deleted file mode 100644
index e55c7ba1ad90f4e2f12db6c814d04a90c4e3b77c..0000000000000000000000000000000000000000
--- a/spaces/OFA-Sys/OFA-vqa/fairseq/fairseq/models/fairseq_model.py
+++ /dev/null
@@ -1,569 +0,0 @@
-# Copyright (c) Facebook, Inc. and its affiliates.
-#
-# This source code is licensed under the MIT license found in the
-# LICENSE file in the root directory of this source tree.
-"""
-Base classes for various fairseq models.
-"""
-
-import logging
-from argparse import Namespace
-from typing import Dict, List, Optional, Tuple
-
-import torch
-import torch.nn as nn
-import torch.nn.functional as F
-from fairseq import utils
-from fairseq.data import Dictionary
-from fairseq.dataclass.utils import (
- convert_namespace_to_omegaconf,
- gen_parser_from_dataclass,
-)
-from fairseq.models import FairseqDecoder, FairseqEncoder
-from omegaconf import DictConfig
-from torch import Tensor
-
-
-logger = logging.getLogger(__name__)
-
-
-def check_type(module, expected_type):
- if hasattr(module, "unwrapped_module"):
- assert isinstance(module.unwrapped_module, expected_type), \
- f"{type(module.unwrapped_module)} != {expected_type}"
- else:
- assert isinstance(module, expected_type), f"{type(module)} != {expected_type}"
-
-
-class BaseFairseqModel(nn.Module):
- """Base class for fairseq models."""
-
- def __init__(self):
- super().__init__()
- self._is_generation_fast = False
-
- @classmethod
- def add_args(cls, parser):
- """Add model-specific arguments to the parser."""
- dc = getattr(cls, "__dataclass", None)
- if dc is not None:
- # do not set defaults so that settings defaults from various architectures still works
- gen_parser_from_dataclass(parser, dc(), delete_default=True)
-
- @classmethod
- def build_model(cls, args, task):
- """Build a new model instance."""
- raise NotImplementedError("Model must implement the build_model method")
-
- def get_targets(self, sample, net_output):
- """Get targets from either the sample or the net's output."""
- return sample["target"]
-
- def get_normalized_probs(
- self,
- net_output: Tuple[Tensor, Optional[Dict[str, List[Optional[Tensor]]]]],
- log_probs: bool,
- sample: Optional[Dict[str, Tensor]] = None,
- ):
- """Get normalized probabilities (or log probs) from a net's output."""
- return self.get_normalized_probs_scriptable(net_output, log_probs, sample)
-
- # TorchScript doesn't support super() method so that the scriptable Subclass
- # can't access the base class model in Torchscript.
- # Current workaround is to add a helper function with different name and
- # call the helper function from scriptable Subclass.
- def get_normalized_probs_scriptable(
- self,
- net_output: Tuple[Tensor, Optional[Dict[str, List[Optional[Tensor]]]]],
- log_probs: bool,
- sample: Optional[Dict[str, Tensor]] = None,
- ):
- """Scriptable helper function for get_normalized_probs in ~BaseFairseqModel"""
- if hasattr(self, "decoder"):
- return self.decoder.get_normalized_probs(net_output, log_probs, sample)
- elif torch.is_tensor(net_output):
- # syntactic sugar for simple models which don't have a decoder
- # (e.g., the classification tutorial)
- logits = net_output.float()
- if log_probs:
- return F.log_softmax(logits, dim=-1)
- else:
- return F.softmax(logits, dim=-1)
- raise NotImplementedError
-
- def extract_features(self, *args, **kwargs):
- """Similar to *forward* but only return features."""
- return self(*args, **kwargs)
-
- def max_positions(self):
- """Maximum length supported by the model."""
- return None
-
- def load_state_dict(
- self,
- state_dict,
- strict=True,
- model_cfg: Optional[DictConfig] = None,
- args: Optional[Namespace] = None,
- ):
- """Copies parameters and buffers from *state_dict* into this module and
- its descendants.
-
- Overrides the method in :class:`nn.Module`. Compared with that method
- this additionally "upgrades" *state_dicts* from old checkpoints.
- """
-
- if model_cfg is None and args is not None:
- logger.warn("using 'args' is deprecated, please update your code to use dataclass config")
- model_cfg = convert_namespace_to_omegaconf(args).model
-
- self.upgrade_state_dict(state_dict)
-
- from fairseq.checkpoint_utils import prune_state_dict
-
- new_state_dict = prune_state_dict(state_dict, model_cfg)
- return super().load_state_dict(new_state_dict, strict)
-
- def upgrade_state_dict(self, state_dict):
- """Upgrade old state dicts to work with newer code."""
- self.upgrade_state_dict_named(state_dict, "")
-
- def upgrade_state_dict_named(self, state_dict, name):
- """Upgrade old state dicts to work with newer code.
-
- Args:
- state_dict (dict): state dictionary to upgrade, in place
- name (str): the state dict key corresponding to the current module
- """
- assert state_dict is not None
-
- def do_upgrade(m, prefix):
- if len(prefix) > 0:
- prefix += "."
-
- for n, c in m.named_children():
- name = prefix + n
- if hasattr(c, "upgrade_state_dict_named"):
- c.upgrade_state_dict_named(state_dict, name)
- elif hasattr(c, "upgrade_state_dict"):
- c.upgrade_state_dict(state_dict)
- do_upgrade(c, name)
-
- do_upgrade(self, name)
-
- def set_num_updates(self, num_updates):
- """State from trainer to pass along to model at every update."""
- for m in self.modules():
- if hasattr(m, "set_num_updates") and m != self:
- m.set_num_updates(num_updates)
-
- def prepare_for_inference_(self, cfg: DictConfig):
- """Prepare model for inference."""
- kwargs = {}
- kwargs["beamable_mm_beam_size"] = (
- None
- if getattr(cfg.generation, "no_beamable_mm", False)
- else getattr(cfg.generation, "beam", 5)
- )
- kwargs["need_attn"] = getattr(cfg.generation, "print_alignment", False)
- if getattr(cfg.generation, "retain_dropout", False):
- kwargs["retain_dropout"] = cfg.generation.retain_dropout
- kwargs["retain_dropout_modules"] = cfg.generation.retain_dropout_modules
- self.make_generation_fast_(**kwargs)
-
- def make_generation_fast_(self, **kwargs):
- """
- Legacy entry point to optimize model for faster generation.
- Prefer prepare_for_inference_.
- """
- if self._is_generation_fast:
- return # only apply once
- self._is_generation_fast = True
-
- # remove weight norm from all modules in the network
- def apply_remove_weight_norm(module):
- try:
- nn.utils.remove_weight_norm(module)
- except (AttributeError, ValueError): # this module didn't have weight norm
- return
-
- self.apply(apply_remove_weight_norm)
-
- def apply_make_generation_fast_(module, prefix):
- if len(prefix) > 0:
- prefix += "."
-
- base_func = BaseFairseqModel.make_generation_fast_
- for n, m in module.named_modules():
- if (
- m != self
- and hasattr(m, "make_generation_fast_")
- # don't call this implementation again, e.g., if
- # children modules also inherit from BaseFairseqModel
- and m.make_generation_fast_.__func__ is not base_func
- ):
- name = prefix + n
- m.make_generation_fast_(name=name, **kwargs)
-
- apply_make_generation_fast_(self, "")
-
- def train(mode=True):
- if mode:
- raise RuntimeError("cannot train after make_generation_fast")
-
- # this model should no longer be used for training
- self.eval()
- self.train = train
-
- def prepare_for_onnx_export_(self, **kwargs):
- """Make model exportable via ONNX trace."""
- seen = set()
-
- def apply_prepare_for_onnx_export_(module):
- if (
- module != self
- and hasattr(module, "prepare_for_onnx_export_")
- and module not in seen
- ):
- seen.add(module)
- module.prepare_for_onnx_export_(**kwargs)
-
- self.apply(apply_prepare_for_onnx_export_)
-
- @classmethod
- def from_pretrained(
- cls,
- model_name_or_path,
- checkpoint_file="model.pt",
- data_name_or_path=".",
- **kwargs,
- ):
- """
- Load a :class:`~fairseq.models.FairseqModel` from a pre-trained model
- file. Downloads and caches the pre-trained model file if needed.
-
- The base implementation returns a
- :class:`~fairseq.hub_utils.GeneratorHubInterface`, which can be used to
- generate translations or sample from language models. The underlying
- :class:`~fairseq.models.FairseqModel` can be accessed via the
- *generator.models* attribute.
-
- Other models may override this to implement custom hub interfaces.
-
- Args:
- model_name_or_path (str): either the name of a pre-trained model to
- load or a path/URL to a pre-trained model state dict
- checkpoint_file (str, optional): colon-separated list of checkpoint
- files in the model archive to ensemble (default: 'model.pt')
- data_name_or_path (str, optional): point args.data to the archive
- at the given path/URL. Can start with '.' or './' to reuse the
- model archive path.
- """
- from fairseq import hub_utils
-
- x = hub_utils.from_pretrained(
- model_name_or_path,
- checkpoint_file,
- data_name_or_path,
- archive_map=cls.hub_models(),
- **kwargs,
- )
- logger.info(x["args"])
- return hub_utils.GeneratorHubInterface(x["args"], x["task"], x["models"])
-
- @classmethod
- def hub_models(cls):
- return {}
-
-
-class FairseqEncoderDecoderModel(BaseFairseqModel):
- """Base class for encoder-decoder models.
-
- Args:
- encoder (FairseqEncoder): the encoder
- decoder (FairseqDecoder): the decoder
- """
-
- def __init__(self, encoder, decoder):
- super().__init__()
-
- self.encoder = encoder
- self.decoder = decoder
-
- check_type(self.encoder, FairseqEncoder)
- check_type(self.decoder, FairseqDecoder)
-
- def forward(self, src_tokens, src_lengths, prev_output_tokens, **kwargs):
- """
- Run the forward pass for an encoder-decoder model.
-
- First feed a batch of source tokens through the encoder. Then, feed the
- encoder output and previous decoder outputs (i.e., teacher forcing) to
- the decoder to produce the next outputs::
-
- encoder_out = self.encoder(src_tokens, src_lengths)
- return self.decoder(prev_output_tokens, encoder_out)
-
- Args:
- src_tokens (LongTensor): tokens in the source language of shape
- `(batch, src_len)`
- src_lengths (LongTensor): source sentence lengths of shape `(batch)`
- prev_output_tokens (LongTensor): previous decoder outputs of shape
- `(batch, tgt_len)`, for teacher forcing
-
- Returns:
- tuple:
- - the decoder's output of shape `(batch, tgt_len, vocab)`
- - a dictionary with any model-specific outputs
- """
- encoder_out = self.encoder(src_tokens, src_lengths=src_lengths, **kwargs)
- decoder_out = self.decoder(
- prev_output_tokens, encoder_out=encoder_out, **kwargs
- )
- return decoder_out
-
- def forward_decoder(self, prev_output_tokens, **kwargs):
- return self.decoder(prev_output_tokens, **kwargs)
-
- def extract_features(self, src_tokens, src_lengths, prev_output_tokens, **kwargs):
- """
- Similar to *forward* but only return features.
-
- Returns:
- tuple:
- - the decoder's features of shape `(batch, tgt_len, embed_dim)`
- - a dictionary with any model-specific outputs
- """
- encoder_out = self.encoder(src_tokens, src_lengths=src_lengths, **kwargs)
- features = self.decoder.extract_features(
- prev_output_tokens, encoder_out=encoder_out, **kwargs
- )
- return features
-
- def output_layer(self, features, **kwargs):
- """Project features to the default output size (typically vocabulary size)."""
- return self.decoder.output_layer(features, **kwargs)
-
- def max_positions(self):
- """Maximum length supported by the model."""
- return (self.encoder.max_positions(), self.decoder.max_positions())
-
- def max_decoder_positions(self):
- """Maximum length supported by the decoder."""
- return self.decoder.max_positions()
-
-
-class FairseqModel(FairseqEncoderDecoderModel):
- def __init__(self, *args, **kwargs):
- super().__init__(*args, **kwargs)
- utils.deprecation_warning(
- "FairseqModel is deprecated, please use FairseqEncoderDecoderModel "
- "or BaseFairseqModel instead",
- stacklevel=4,
- )
-
-
-class FairseqMultiModel(BaseFairseqModel):
- """Base class for combining multiple encoder-decoder models."""
-
- def __init__(self, encoders, decoders):
- super().__init__()
- assert encoders.keys() == decoders.keys()
- self.keys = list(encoders.keys())
- for key in self.keys:
- check_type(encoders[key], FairseqEncoder)
- check_type(decoders[key], FairseqDecoder)
-
- self.models = nn.ModuleDict(
- {
- key: FairseqEncoderDecoderModel(encoders[key], decoders[key])
- for key in self.keys
- }
- )
-
- @staticmethod
- def build_shared_embeddings(
- dicts: Dict[str, Dictionary],
- langs: List[str],
- embed_dim: int,
- build_embedding: callable,
- pretrained_embed_path: Optional[str] = None,
- ):
- """
- Helper function to build shared embeddings for a set of languages after
- checking that all dicts corresponding to those languages are equivalent.
-
- Args:
- dicts: Dict of lang_id to its corresponding Dictionary
- langs: languages that we want to share embeddings for
- embed_dim: embedding dimension
- build_embedding: callable function to actually build the embedding
- pretrained_embed_path: Optional path to load pretrained embeddings
- """
- shared_dict = dicts[langs[0]]
- if any(dicts[lang] != shared_dict for lang in langs):
- raise ValueError(
- "--share-*-embeddings requires a joined dictionary: "
- "--share-encoder-embeddings requires a joined source "
- "dictionary, --share-decoder-embeddings requires a joined "
- "target dictionary, and --share-all-embeddings requires a "
- "joint source + target dictionary."
- )
- return build_embedding(shared_dict, embed_dim, pretrained_embed_path)
-
- def forward(self, src_tokens, src_lengths, prev_output_tokens, **kwargs):
- raise NotImplementedError
-
- def max_positions(self):
- """Maximum length supported by the model."""
- return {
- key: (
- self.models[key].encoder.max_positions(),
- self.models[key].decoder.max_positions(),
- )
- for key in self.keys
- }
-
- def max_decoder_positions(self):
- """Maximum length supported by the decoder."""
- return min(model.decoder.max_positions() for model in self.models.values())
-
- @property
- def encoder(self):
- return self.models[self.keys[0]].encoder
-
- @property
- def decoder(self):
- return self.models[self.keys[0]].decoder
-
- def forward_decoder(self, prev_output_tokens, **kwargs):
- return self.decoder(prev_output_tokens, **kwargs)
-
- def load_state_dict(
- self,
- state_dict,
- strict=True,
- model_cfg=None,
- args: Optional[Namespace] = None,
- ):
- """Copies parameters and buffers from *state_dict* into this module and
- its descendants.
-
- Overrides the method in :class:`nn.Module`. Compared with that method
- this additionally "upgrades" *state_dicts* from old checkpoints.
- """
-
- if model_cfg is None and args is not None:
- logger.warn("using 'args' is deprecated, please update your code to use dataclass config")
- model_cfg = convert_namespace_to_omegaconf(args).model
-
- self.upgrade_state_dict(state_dict)
-
- from fairseq.checkpoint_utils import prune_state_dict
-
- new_state_dict = prune_state_dict(state_dict, model_cfg)
- return super().load_state_dict(new_state_dict, strict)
-
-
-class FairseqLanguageModel(BaseFairseqModel):
- """Base class for decoder-only models.
-
- Args:
- decoder (FairseqDecoder): the decoder
- """
-
- def __init__(self, decoder):
- super().__init__()
- self.decoder = decoder
- check_type(self.decoder, FairseqDecoder)
-
- def forward(self, src_tokens, **kwargs):
- """
- Run the forward pass for a decoder-only model.
-
- Feeds a batch of tokens through the decoder to predict the next tokens.
-
- Args:
- src_tokens (LongTensor): tokens on which to condition the decoder,
- of shape `(batch, tgt_len)`
- src_lengths (LongTensor): source sentence lengths of shape `(batch)`
-
- Returns:
- tuple:
- - the decoder's output of shape `(batch, seq_len, vocab)`
- - a dictionary with any model-specific outputs
- """
- return self.decoder(src_tokens, **kwargs)
-
- def forward_decoder(self, prev_output_tokens, **kwargs):
- return self.decoder(prev_output_tokens, **kwargs)
-
- def extract_features(self, src_tokens, **kwargs):
- """
- Similar to *forward* but only return features.
-
- Returns:
- tuple:
- - the decoder's features of shape `(batch, seq_len, embed_dim)`
- - a dictionary with any model-specific outputs
- """
- return self.decoder.extract_features(src_tokens, **kwargs)
-
- def output_layer(self, features, **kwargs):
- """Project features to the default output size (typically vocabulary size)."""
- return self.decoder.output_layer(features, **kwargs)
-
- def max_positions(self):
- """Maximum length supported by the model."""
- return self.decoder.max_positions()
-
- def max_decoder_positions(self):
- """Maximum length supported by the decoder."""
- return self.decoder.max_positions()
-
- @property
- def supported_targets(self):
- return {"future"}
-
-
-class FairseqEncoderModel(BaseFairseqModel):
- """Base class for encoder-only models.
-
- Args:
- encoder (FairseqEncoder): the encoder
- """
-
- def __init__(self, encoder):
- super().__init__()
- self.encoder = encoder
- check_type(self.encoder, FairseqEncoder)
-
- def forward(self, src_tokens, src_lengths, **kwargs):
- """
- Run the forward pass for a encoder-only model.
-
- Feeds a batch of tokens through the encoder to generate features.
-
- Args:
- src_tokens (LongTensor): input tokens of shape `(batch, src_len)`
- src_lengths (LongTensor): source sentence lengths of shape `(batch)`
-
- Returns:
- the encoder's output, typically of shape `(batch, src_len, features)`
- """
- return self.encoder(src_tokens, src_lengths, **kwargs)
-
- def get_normalized_probs(self, net_output, log_probs, sample=None):
- """Get normalized probabilities (or log probs) from a net's output."""
- encoder_out = net_output["encoder_out"]
- if torch.is_tensor(encoder_out):
- logits = encoder_out.float()
- if log_probs:
- return F.log_softmax(logits, dim=-1)
- else:
- return F.softmax(logits, dim=-1)
- raise NotImplementedError
-
- def max_positions(self):
- """Maximum length supported by the model."""
- return self.encoder.max_positions()
diff --git a/spaces/OIUGLK/bingo/src/components/ui/badge.tsx b/spaces/OIUGLK/bingo/src/components/ui/badge.tsx
deleted file mode 100644
index d9a84b394090e5b4b3bd34f6135b9a2f2ead0aa2..0000000000000000000000000000000000000000
--- a/spaces/OIUGLK/bingo/src/components/ui/badge.tsx
+++ /dev/null
@@ -1,36 +0,0 @@
-import * as React from 'react'
-import { cva, type VariantProps } from 'class-variance-authority'
-
-import { cn } from '@/lib/utils'
-
-const badgeVariants = cva(
- 'inline-flex items-center rounded-full border px-2.5 py-0.5 text-xs font-semibold transition-colors focus:outline-none focus:ring-2 focus:ring-ring focus:ring-offset-2',
- {
- variants: {
- variant: {
- default:
- 'border-transparent bg-primary text-primary-foreground hover:bg-primary/80',
- secondary:
- 'border-transparent bg-secondary text-secondary-foreground hover:bg-secondary/80',
- destructive:
- 'border-transparent bg-destructive text-destructive-foreground hover:bg-destructive/80',
- outline: 'text-foreground'
- }
- },
- defaultVariants: {
- variant: 'default'
- }
- }
-)
-
-export interface BadgeProps
- extends React.HTMLAttributes,
- VariantProps {}
-
-function Badge({ className, variant, ...props }: BadgeProps) {
- return (
-
- )
-}
-
-export { Badge, badgeVariants }
diff --git a/spaces/OpenDILabCommunity/LLMRiddlesChatGPTCN/llmriddles/llms/base.py b/spaces/OpenDILabCommunity/LLMRiddlesChatGPTCN/llmriddles/llms/base.py
deleted file mode 100644
index 358bb552a0fd8d511ee76f59d6712162832b6bc1..0000000000000000000000000000000000000000
--- a/spaces/OpenDILabCommunity/LLMRiddlesChatGPTCN/llmriddles/llms/base.py
+++ /dev/null
@@ -1,11 +0,0 @@
-from typing import Callable, Dict
-
-_LLMS: Dict[str, Callable] = {}
-
-
-def register_llm(name: str, llm_ask_fn: Callable):
- _LLMS[name] = llm_ask_fn
-
-
-def get_llm_fn(name: str) -> Callable:
- return _LLMS[name]
diff --git a/spaces/OpenGVLab/InternGPT/iGPT/models/grit_src/third_party/CenterNet2/detectron2/checkpoint/detection_checkpoint.py b/spaces/OpenGVLab/InternGPT/iGPT/models/grit_src/third_party/CenterNet2/detectron2/checkpoint/detection_checkpoint.py
deleted file mode 100644
index 82fd3b2d40054573917a445b138d29a6dabfb907..0000000000000000000000000000000000000000
--- a/spaces/OpenGVLab/InternGPT/iGPT/models/grit_src/third_party/CenterNet2/detectron2/checkpoint/detection_checkpoint.py
+++ /dev/null
@@ -1,120 +0,0 @@
-# Copyright (c) Facebook, Inc. and its affiliates.
-import logging
-import os
-import pickle
-import torch
-from fvcore.common.checkpoint import Checkpointer
-from torch.nn.parallel import DistributedDataParallel
-
-import detectron2.utils.comm as comm
-from detectron2.utils.file_io import PathManager
-
-from .c2_model_loading import align_and_update_state_dicts
-
-
-class DetectionCheckpointer(Checkpointer):
- """
- Same as :class:`Checkpointer`, but is able to:
- 1. handle models in detectron & detectron2 model zoo, and apply conversions for legacy models.
- 2. correctly load checkpoints that are only available on the master worker
- """
-
- def __init__(self, model, save_dir="", *, save_to_disk=None, **checkpointables):
- is_main_process = comm.is_main_process()
- super().__init__(
- model,
- save_dir,
- save_to_disk=is_main_process if save_to_disk is None else save_to_disk,
- **checkpointables,
- )
- self.path_manager = PathManager
-
- def load(self, path, *args, **kwargs):
- need_sync = False
-
- if path and isinstance(self.model, DistributedDataParallel):
- logger = logging.getLogger(__name__)
- path = self.path_manager.get_local_path(path)
- has_file = os.path.isfile(path)
- all_has_file = comm.all_gather(has_file)
- if not all_has_file[0]:
- raise OSError(f"File {path} not found on main worker.")
- if not all(all_has_file):
- logger.warning(
- f"Not all workers can read checkpoint {path}. "
- "Training may fail to fully resume."
- )
- # TODO: broadcast the checkpoint file contents from main
- # worker, and load from it instead.
- need_sync = True
- if not has_file:
- path = None # don't load if not readable
- ret = super().load(path, *args, **kwargs)
-
- if need_sync:
- logger.info("Broadcasting model states from main worker ...")
- self.model._sync_params_and_buffers()
- return ret
-
- def _load_file(self, filename):
- if filename.endswith(".pkl"):
- with PathManager.open(filename, "rb") as f:
- data = pickle.load(f, encoding="latin1")
- if "model" in data and "__author__" in data:
- # file is in Detectron2 model zoo format
- self.logger.info("Reading a file from '{}'".format(data["__author__"]))
- return data
- else:
- # assume file is from Caffe2 / Detectron1 model zoo
- if "blobs" in data:
- # Detection models have "blobs", but ImageNet models don't
- data = data["blobs"]
- data = {k: v for k, v in data.items() if not k.endswith("_momentum")}
- return {"model": data, "__author__": "Caffe2", "matching_heuristics": True}
- elif filename.endswith(".pyth"):
- # assume file is from pycls; no one else seems to use the ".pyth" extension
- with PathManager.open(filename, "rb") as f:
- data = torch.load(f)
- assert (
- "model_state" in data
- ), f"Cannot load .pyth file {filename}; pycls checkpoints must contain 'model_state'."
- model_state = {
- k: v
- for k, v in data["model_state"].items()
- if not k.endswith("num_batches_tracked")
- }
- return {"model": model_state, "__author__": "pycls", "matching_heuristics": True}
-
- loaded = super()._load_file(filename) # load native pth checkpoint
- if "model" not in loaded:
- loaded = {"model": loaded}
- return loaded
-
- def _load_model(self, checkpoint):
- if checkpoint.get("matching_heuristics", False):
- self._convert_ndarray_to_tensor(checkpoint["model"])
- # convert weights by name-matching heuristics
- checkpoint["model"] = align_and_update_state_dicts(
- self.model.state_dict(),
- checkpoint["model"],
- c2_conversion=checkpoint.get("__author__", None) == "Caffe2",
- )
- # for non-caffe2 models, use standard ways to load it
- incompatible = super()._load_model(checkpoint)
-
- model_buffers = dict(self.model.named_buffers(recurse=False))
- for k in ["pixel_mean", "pixel_std"]:
- # Ignore missing key message about pixel_mean/std.
- # Though they may be missing in old checkpoints, they will be correctly
- # initialized from config anyway.
- if k in model_buffers:
- try:
- incompatible.missing_keys.remove(k)
- except ValueError:
- pass
- for k in incompatible.unexpected_keys[:]:
- # Ignore unexpected keys about cell anchors. They exist in old checkpoints
- # but now they are non-persistent buffers and will not be in new checkpoints.
- if "anchor_generator.cell_anchors" in k:
- incompatible.unexpected_keys.remove(k)
- return incompatible
diff --git a/spaces/OpenGVLab/InternGPT/iGPT/models/grit_src/third_party/CenterNet2/detectron2/data/datasets/lvis_v1_categories.py b/spaces/OpenGVLab/InternGPT/iGPT/models/grit_src/third_party/CenterNet2/detectron2/data/datasets/lvis_v1_categories.py
deleted file mode 100644
index 7374e6968bb006f5d8c49e75d9d3b31ea3d77d05..0000000000000000000000000000000000000000
--- a/spaces/OpenGVLab/InternGPT/iGPT/models/grit_src/third_party/CenterNet2/detectron2/data/datasets/lvis_v1_categories.py
+++ /dev/null
@@ -1,16 +0,0 @@
-# Copyright (c) Facebook, Inc. and its affiliates.
-# Autogen with
-# with open("lvis_v1_val.json", "r") as f:
-# a = json.load(f)
-# c = a["categories"]
-# for x in c:
-# del x["image_count"]
-# del x["instance_count"]
-# LVIS_CATEGORIES = repr(c) + " # noqa"
-# with open("/tmp/lvis_categories.py", "wt") as f:
-# f.write(f"LVIS_CATEGORIES = {LVIS_CATEGORIES}")
-# Then paste the contents of that file below
-
-# fmt: off
-LVIS_CATEGORIES = [{'frequency': 'c', 'synset': 'aerosol.n.02', 'synonyms': ['aerosol_can', 'spray_can'], 'id': 1, 'def': 'a dispenser that holds a substance under pressure', 'name': 'aerosol_can'}, {'frequency': 'f', 'synset': 'air_conditioner.n.01', 'synonyms': ['air_conditioner'], 'id': 2, 'def': 'a machine that keeps air cool and dry', 'name': 'air_conditioner'}, {'frequency': 'f', 'synset': 'airplane.n.01', 'synonyms': ['airplane', 'aeroplane'], 'id': 3, 'def': 'an aircraft that has a fixed wing and is powered by propellers or jets', 'name': 'airplane'}, {'frequency': 'f', 'synset': 'alarm_clock.n.01', 'synonyms': ['alarm_clock'], 'id': 4, 'def': 'a clock that wakes a sleeper at some preset time', 'name': 'alarm_clock'}, {'frequency': 'c', 'synset': 'alcohol.n.01', 'synonyms': ['alcohol', 'alcoholic_beverage'], 'id': 5, 'def': 'a liquor or brew containing alcohol as the active agent', 'name': 'alcohol'}, {'frequency': 'c', 'synset': 'alligator.n.02', 'synonyms': ['alligator', 'gator'], 'id': 6, 'def': 'amphibious reptiles related to crocodiles but with shorter broader snouts', 'name': 'alligator'}, {'frequency': 'c', 'synset': 'almond.n.02', 'synonyms': ['almond'], 'id': 7, 'def': 'oval-shaped edible seed of the almond tree', 'name': 'almond'}, {'frequency': 'c', 'synset': 'ambulance.n.01', 'synonyms': ['ambulance'], 'id': 8, 'def': 'a vehicle that takes people to and from hospitals', 'name': 'ambulance'}, {'frequency': 'c', 'synset': 'amplifier.n.01', 'synonyms': ['amplifier'], 'id': 9, 'def': 'electronic equipment that increases strength of signals', 'name': 'amplifier'}, {'frequency': 'c', 'synset': 'anklet.n.03', 'synonyms': ['anklet', 'ankle_bracelet'], 'id': 10, 'def': 'an ornament worn around the ankle', 'name': 'anklet'}, {'frequency': 'f', 'synset': 'antenna.n.01', 'synonyms': ['antenna', 'aerial', 'transmitting_aerial'], 'id': 11, 'def': 'an electrical device that sends or receives radio or television signals', 'name': 'antenna'}, {'frequency': 'f', 'synset': 'apple.n.01', 'synonyms': ['apple'], 'id': 12, 'def': 'fruit with red or yellow or green skin and sweet to tart crisp whitish flesh', 'name': 'apple'}, {'frequency': 'r', 'synset': 'applesauce.n.01', 'synonyms': ['applesauce'], 'id': 13, 'def': 'puree of stewed apples usually sweetened and spiced', 'name': 'applesauce'}, {'frequency': 'r', 'synset': 'apricot.n.02', 'synonyms': ['apricot'], 'id': 14, 'def': 'downy yellow to rosy-colored fruit resembling a small peach', 'name': 'apricot'}, {'frequency': 'f', 'synset': 'apron.n.01', 'synonyms': ['apron'], 'id': 15, 'def': 'a garment of cloth that is tied about the waist and worn to protect clothing', 'name': 'apron'}, {'frequency': 'c', 'synset': 'aquarium.n.01', 'synonyms': ['aquarium', 'fish_tank'], 'id': 16, 'def': 'a tank/pool/bowl filled with water for keeping live fish and underwater animals', 'name': 'aquarium'}, {'frequency': 'r', 'synset': 'arctic.n.02', 'synonyms': ['arctic_(type_of_shoe)', 'galosh', 'golosh', 'rubber_(type_of_shoe)', 'gumshoe'], 'id': 17, 'def': 'a waterproof overshoe that protects shoes from water or snow', 'name': 'arctic_(type_of_shoe)'}, {'frequency': 'c', 'synset': 'armband.n.02', 'synonyms': ['armband'], 'id': 18, 'def': 'a band worn around the upper arm', 'name': 'armband'}, {'frequency': 'f', 'synset': 'armchair.n.01', 'synonyms': ['armchair'], 'id': 19, 'def': 'chair with a support on each side for arms', 'name': 'armchair'}, {'frequency': 'r', 'synset': 'armoire.n.01', 'synonyms': ['armoire'], 'id': 20, 'def': 'a large wardrobe or cabinet', 'name': 'armoire'}, {'frequency': 'r', 'synset': 'armor.n.01', 'synonyms': ['armor', 'armour'], 'id': 21, 'def': 'protective covering made of metal and used in combat', 'name': 'armor'}, {'frequency': 'c', 'synset': 'artichoke.n.02', 'synonyms': ['artichoke'], 'id': 22, 'def': 'a thistlelike flower head with edible fleshy leaves and heart', 'name': 'artichoke'}, {'frequency': 'f', 'synset': 'ashcan.n.01', 'synonyms': ['trash_can', 'garbage_can', 'wastebin', 'dustbin', 'trash_barrel', 'trash_bin'], 'id': 23, 'def': 'a bin that holds rubbish until it is collected', 'name': 'trash_can'}, {'frequency': 'c', 'synset': 'ashtray.n.01', 'synonyms': ['ashtray'], 'id': 24, 'def': "a receptacle for the ash from smokers' cigars or cigarettes", 'name': 'ashtray'}, {'frequency': 'c', 'synset': 'asparagus.n.02', 'synonyms': ['asparagus'], 'id': 25, 'def': 'edible young shoots of the asparagus plant', 'name': 'asparagus'}, {'frequency': 'c', 'synset': 'atomizer.n.01', 'synonyms': ['atomizer', 'atomiser', 'spray', 'sprayer', 'nebulizer', 'nebuliser'], 'id': 26, 'def': 'a dispenser that turns a liquid (such as perfume) into a fine mist', 'name': 'atomizer'}, {'frequency': 'f', 'synset': 'avocado.n.01', 'synonyms': ['avocado'], 'id': 27, 'def': 'a pear-shaped fruit with green or blackish skin and rich yellowish pulp enclosing a single large seed', 'name': 'avocado'}, {'frequency': 'c', 'synset': 'award.n.02', 'synonyms': ['award', 'accolade'], 'id': 28, 'def': 'a tangible symbol signifying approval or distinction', 'name': 'award'}, {'frequency': 'f', 'synset': 'awning.n.01', 'synonyms': ['awning'], 'id': 29, 'def': 'a canopy made of canvas to shelter people or things from rain or sun', 'name': 'awning'}, {'frequency': 'r', 'synset': 'ax.n.01', 'synonyms': ['ax', 'axe'], 'id': 30, 'def': 'an edge tool with a heavy bladed head mounted across a handle', 'name': 'ax'}, {'frequency': 'r', 'synset': 'baboon.n.01', 'synonyms': ['baboon'], 'id': 31, 'def': 'large terrestrial monkeys having doglike muzzles', 'name': 'baboon'}, {'frequency': 'f', 'synset': 'baby_buggy.n.01', 'synonyms': ['baby_buggy', 'baby_carriage', 'perambulator', 'pram', 'stroller'], 'id': 32, 'def': 'a small vehicle with four wheels in which a baby or child is pushed around', 'name': 'baby_buggy'}, {'frequency': 'c', 'synset': 'backboard.n.01', 'synonyms': ['basketball_backboard'], 'id': 33, 'def': 'a raised vertical board with basket attached; used to play basketball', 'name': 'basketball_backboard'}, {'frequency': 'f', 'synset': 'backpack.n.01', 'synonyms': ['backpack', 'knapsack', 'packsack', 'rucksack', 'haversack'], 'id': 34, 'def': 'a bag carried by a strap on your back or shoulder', 'name': 'backpack'}, {'frequency': 'f', 'synset': 'bag.n.04', 'synonyms': ['handbag', 'purse', 'pocketbook'], 'id': 35, 'def': 'a container used for carrying money and small personal items or accessories', 'name': 'handbag'}, {'frequency': 'f', 'synset': 'bag.n.06', 'synonyms': ['suitcase', 'baggage', 'luggage'], 'id': 36, 'def': 'cases used to carry belongings when traveling', 'name': 'suitcase'}, {'frequency': 'c', 'synset': 'bagel.n.01', 'synonyms': ['bagel', 'beigel'], 'id': 37, 'def': 'glazed yeast-raised doughnut-shaped roll with hard crust', 'name': 'bagel'}, {'frequency': 'r', 'synset': 'bagpipe.n.01', 'synonyms': ['bagpipe'], 'id': 38, 'def': 'a tubular wind instrument; the player blows air into a bag and squeezes it out', 'name': 'bagpipe'}, {'frequency': 'r', 'synset': 'baguet.n.01', 'synonyms': ['baguet', 'baguette'], 'id': 39, 'def': 'narrow French stick loaf', 'name': 'baguet'}, {'frequency': 'r', 'synset': 'bait.n.02', 'synonyms': ['bait', 'lure'], 'id': 40, 'def': 'something used to lure fish or other animals into danger so they can be trapped or killed', 'name': 'bait'}, {'frequency': 'f', 'synset': 'ball.n.06', 'synonyms': ['ball'], 'id': 41, 'def': 'a spherical object used as a plaything', 'name': 'ball'}, {'frequency': 'r', 'synset': 'ballet_skirt.n.01', 'synonyms': ['ballet_skirt', 'tutu'], 'id': 42, 'def': 'very short skirt worn by ballerinas', 'name': 'ballet_skirt'}, {'frequency': 'f', 'synset': 'balloon.n.01', 'synonyms': ['balloon'], 'id': 43, 'def': 'large tough nonrigid bag filled with gas or heated air', 'name': 'balloon'}, {'frequency': 'c', 'synset': 'bamboo.n.02', 'synonyms': ['bamboo'], 'id': 44, 'def': 'woody tropical grass having hollow woody stems', 'name': 'bamboo'}, {'frequency': 'f', 'synset': 'banana.n.02', 'synonyms': ['banana'], 'id': 45, 'def': 'elongated crescent-shaped yellow fruit with soft sweet flesh', 'name': 'banana'}, {'frequency': 'c', 'synset': 'band_aid.n.01', 'synonyms': ['Band_Aid'], 'id': 46, 'def': 'trade name for an adhesive bandage to cover small cuts or blisters', 'name': 'Band_Aid'}, {'frequency': 'c', 'synset': 'bandage.n.01', 'synonyms': ['bandage'], 'id': 47, 'def': 'a piece of soft material that covers and protects an injured part of the body', 'name': 'bandage'}, {'frequency': 'f', 'synset': 'bandanna.n.01', 'synonyms': ['bandanna', 'bandana'], 'id': 48, 'def': 'large and brightly colored handkerchief; often used as a neckerchief', 'name': 'bandanna'}, {'frequency': 'r', 'synset': 'banjo.n.01', 'synonyms': ['banjo'], 'id': 49, 'def': 'a stringed instrument of the guitar family with a long neck and circular body', 'name': 'banjo'}, {'frequency': 'f', 'synset': 'banner.n.01', 'synonyms': ['banner', 'streamer'], 'id': 50, 'def': 'long strip of cloth or paper used for decoration or advertising', 'name': 'banner'}, {'frequency': 'r', 'synset': 'barbell.n.01', 'synonyms': ['barbell'], 'id': 51, 'def': 'a bar to which heavy discs are attached at each end; used in weightlifting', 'name': 'barbell'}, {'frequency': 'r', 'synset': 'barge.n.01', 'synonyms': ['barge'], 'id': 52, 'def': 'a flatbottom boat for carrying heavy loads (especially on canals)', 'name': 'barge'}, {'frequency': 'f', 'synset': 'barrel.n.02', 'synonyms': ['barrel', 'cask'], 'id': 53, 'def': 'a cylindrical container that holds liquids', 'name': 'barrel'}, {'frequency': 'c', 'synset': 'barrette.n.01', 'synonyms': ['barrette'], 'id': 54, 'def': "a pin for holding women's hair in place", 'name': 'barrette'}, {'frequency': 'c', 'synset': 'barrow.n.03', 'synonyms': ['barrow', 'garden_cart', 'lawn_cart', 'wheelbarrow'], 'id': 55, 'def': 'a cart for carrying small loads; has handles and one or more wheels', 'name': 'barrow'}, {'frequency': 'f', 'synset': 'base.n.03', 'synonyms': ['baseball_base'], 'id': 56, 'def': 'a place that the runner must touch before scoring', 'name': 'baseball_base'}, {'frequency': 'f', 'synset': 'baseball.n.02', 'synonyms': ['baseball'], 'id': 57, 'def': 'a ball used in playing baseball', 'name': 'baseball'}, {'frequency': 'f', 'synset': 'baseball_bat.n.01', 'synonyms': ['baseball_bat'], 'id': 58, 'def': 'an implement used in baseball by the batter', 'name': 'baseball_bat'}, {'frequency': 'f', 'synset': 'baseball_cap.n.01', 'synonyms': ['baseball_cap', 'jockey_cap', 'golf_cap'], 'id': 59, 'def': 'a cap with a bill', 'name': 'baseball_cap'}, {'frequency': 'f', 'synset': 'baseball_glove.n.01', 'synonyms': ['baseball_glove', 'baseball_mitt'], 'id': 60, 'def': 'the handwear used by fielders in playing baseball', 'name': 'baseball_glove'}, {'frequency': 'f', 'synset': 'basket.n.01', 'synonyms': ['basket', 'handbasket'], 'id': 61, 'def': 'a container that is usually woven and has handles', 'name': 'basket'}, {'frequency': 'c', 'synset': 'basketball.n.02', 'synonyms': ['basketball'], 'id': 62, 'def': 'an inflated ball used in playing basketball', 'name': 'basketball'}, {'frequency': 'r', 'synset': 'bass_horn.n.01', 'synonyms': ['bass_horn', 'sousaphone', 'tuba'], 'id': 63, 'def': 'the lowest brass wind instrument', 'name': 'bass_horn'}, {'frequency': 'c', 'synset': 'bat.n.01', 'synonyms': ['bat_(animal)'], 'id': 64, 'def': 'nocturnal mouselike mammal with forelimbs modified to form membranous wings', 'name': 'bat_(animal)'}, {'frequency': 'f', 'synset': 'bath_mat.n.01', 'synonyms': ['bath_mat'], 'id': 65, 'def': 'a heavy towel or mat to stand on while drying yourself after a bath', 'name': 'bath_mat'}, {'frequency': 'f', 'synset': 'bath_towel.n.01', 'synonyms': ['bath_towel'], 'id': 66, 'def': 'a large towel; to dry yourself after a bath', 'name': 'bath_towel'}, {'frequency': 'c', 'synset': 'bathrobe.n.01', 'synonyms': ['bathrobe'], 'id': 67, 'def': 'a loose-fitting robe of towelling; worn after a bath or swim', 'name': 'bathrobe'}, {'frequency': 'f', 'synset': 'bathtub.n.01', 'synonyms': ['bathtub', 'bathing_tub'], 'id': 68, 'def': 'a large open container that you fill with water and use to wash the body', 'name': 'bathtub'}, {'frequency': 'r', 'synset': 'batter.n.02', 'synonyms': ['batter_(food)'], 'id': 69, 'def': 'a liquid or semiliquid mixture, as of flour, eggs, and milk, used in cooking', 'name': 'batter_(food)'}, {'frequency': 'c', 'synset': 'battery.n.02', 'synonyms': ['battery'], 'id': 70, 'def': 'a portable device that produces electricity', 'name': 'battery'}, {'frequency': 'r', 'synset': 'beach_ball.n.01', 'synonyms': ['beachball'], 'id': 71, 'def': 'large and light ball; for play at the seaside', 'name': 'beachball'}, {'frequency': 'c', 'synset': 'bead.n.01', 'synonyms': ['bead'], 'id': 72, 'def': 'a small ball with a hole through the middle used for ornamentation, jewellery, etc.', 'name': 'bead'}, {'frequency': 'c', 'synset': 'bean_curd.n.01', 'synonyms': ['bean_curd', 'tofu'], 'id': 73, 'def': 'cheeselike food made of curdled soybean milk', 'name': 'bean_curd'}, {'frequency': 'c', 'synset': 'beanbag.n.01', 'synonyms': ['beanbag'], 'id': 74, 'def': 'a bag filled with dried beans or similar items; used in games or to sit on', 'name': 'beanbag'}, {'frequency': 'f', 'synset': 'beanie.n.01', 'synonyms': ['beanie', 'beany'], 'id': 75, 'def': 'a small skullcap; formerly worn by schoolboys and college freshmen', 'name': 'beanie'}, {'frequency': 'f', 'synset': 'bear.n.01', 'synonyms': ['bear'], 'id': 76, 'def': 'large carnivorous or omnivorous mammals with shaggy coats and claws', 'name': 'bear'}, {'frequency': 'f', 'synset': 'bed.n.01', 'synonyms': ['bed'], 'id': 77, 'def': 'a piece of furniture that provides a place to sleep', 'name': 'bed'}, {'frequency': 'r', 'synset': 'bedpan.n.01', 'synonyms': ['bedpan'], 'id': 78, 'def': 'a shallow vessel used by a bedridden patient for defecation and urination', 'name': 'bedpan'}, {'frequency': 'f', 'synset': 'bedspread.n.01', 'synonyms': ['bedspread', 'bedcover', 'bed_covering', 'counterpane', 'spread'], 'id': 79, 'def': 'decorative cover for a bed', 'name': 'bedspread'}, {'frequency': 'f', 'synset': 'beef.n.01', 'synonyms': ['cow'], 'id': 80, 'def': 'cattle/cow', 'name': 'cow'}, {'frequency': 'f', 'synset': 'beef.n.02', 'synonyms': ['beef_(food)', 'boeuf_(food)'], 'id': 81, 'def': 'meat from an adult domestic bovine', 'name': 'beef_(food)'}, {'frequency': 'r', 'synset': 'beeper.n.01', 'synonyms': ['beeper', 'pager'], 'id': 82, 'def': 'an device that beeps when the person carrying it is being paged', 'name': 'beeper'}, {'frequency': 'f', 'synset': 'beer_bottle.n.01', 'synonyms': ['beer_bottle'], 'id': 83, 'def': 'a bottle that holds beer', 'name': 'beer_bottle'}, {'frequency': 'c', 'synset': 'beer_can.n.01', 'synonyms': ['beer_can'], 'id': 84, 'def': 'a can that holds beer', 'name': 'beer_can'}, {'frequency': 'r', 'synset': 'beetle.n.01', 'synonyms': ['beetle'], 'id': 85, 'def': 'insect with hard wing covers', 'name': 'beetle'}, {'frequency': 'f', 'synset': 'bell.n.01', 'synonyms': ['bell'], 'id': 86, 'def': 'a hollow device made of metal that makes a ringing sound when struck', 'name': 'bell'}, {'frequency': 'f', 'synset': 'bell_pepper.n.02', 'synonyms': ['bell_pepper', 'capsicum'], 'id': 87, 'def': 'large bell-shaped sweet pepper in green or red or yellow or orange or black varieties', 'name': 'bell_pepper'}, {'frequency': 'f', 'synset': 'belt.n.02', 'synonyms': ['belt'], 'id': 88, 'def': 'a band to tie or buckle around the body (usually at the waist)', 'name': 'belt'}, {'frequency': 'f', 'synset': 'belt_buckle.n.01', 'synonyms': ['belt_buckle'], 'id': 89, 'def': 'the buckle used to fasten a belt', 'name': 'belt_buckle'}, {'frequency': 'f', 'synset': 'bench.n.01', 'synonyms': ['bench'], 'id': 90, 'def': 'a long seat for more than one person', 'name': 'bench'}, {'frequency': 'c', 'synset': 'beret.n.01', 'synonyms': ['beret'], 'id': 91, 'def': 'a cap with no brim or bill; made of soft cloth', 'name': 'beret'}, {'frequency': 'c', 'synset': 'bib.n.02', 'synonyms': ['bib'], 'id': 92, 'def': 'a napkin tied under the chin of a child while eating', 'name': 'bib'}, {'frequency': 'r', 'synset': 'bible.n.01', 'synonyms': ['Bible'], 'id': 93, 'def': 'the sacred writings of the Christian religions', 'name': 'Bible'}, {'frequency': 'f', 'synset': 'bicycle.n.01', 'synonyms': ['bicycle', 'bike_(bicycle)'], 'id': 94, 'def': 'a wheeled vehicle that has two wheels and is moved by foot pedals', 'name': 'bicycle'}, {'frequency': 'f', 'synset': 'bill.n.09', 'synonyms': ['visor', 'vizor'], 'id': 95, 'def': 'a brim that projects to the front to shade the eyes', 'name': 'visor'}, {'frequency': 'f', 'synset': 'billboard.n.01', 'synonyms': ['billboard'], 'id': 96, 'def': 'large outdoor signboard', 'name': 'billboard'}, {'frequency': 'c', 'synset': 'binder.n.03', 'synonyms': ['binder', 'ring-binder'], 'id': 97, 'def': 'holds loose papers or magazines', 'name': 'binder'}, {'frequency': 'c', 'synset': 'binoculars.n.01', 'synonyms': ['binoculars', 'field_glasses', 'opera_glasses'], 'id': 98, 'def': 'an optical instrument designed for simultaneous use by both eyes', 'name': 'binoculars'}, {'frequency': 'f', 'synset': 'bird.n.01', 'synonyms': ['bird'], 'id': 99, 'def': 'animal characterized by feathers and wings', 'name': 'bird'}, {'frequency': 'c', 'synset': 'bird_feeder.n.01', 'synonyms': ['birdfeeder'], 'id': 100, 'def': 'an outdoor device that supplies food for wild birds', 'name': 'birdfeeder'}, {'frequency': 'c', 'synset': 'birdbath.n.01', 'synonyms': ['birdbath'], 'id': 101, 'def': 'an ornamental basin (usually in a garden) for birds to bathe in', 'name': 'birdbath'}, {'frequency': 'c', 'synset': 'birdcage.n.01', 'synonyms': ['birdcage'], 'id': 102, 'def': 'a cage in which a bird can be kept', 'name': 'birdcage'}, {'frequency': 'c', 'synset': 'birdhouse.n.01', 'synonyms': ['birdhouse'], 'id': 103, 'def': 'a shelter for birds', 'name': 'birdhouse'}, {'frequency': 'f', 'synset': 'birthday_cake.n.01', 'synonyms': ['birthday_cake'], 'id': 104, 'def': 'decorated cake served at a birthday party', 'name': 'birthday_cake'}, {'frequency': 'r', 'synset': 'birthday_card.n.01', 'synonyms': ['birthday_card'], 'id': 105, 'def': 'a card expressing a birthday greeting', 'name': 'birthday_card'}, {'frequency': 'r', 'synset': 'black_flag.n.01', 'synonyms': ['pirate_flag'], 'id': 106, 'def': 'a flag usually bearing a white skull and crossbones on a black background', 'name': 'pirate_flag'}, {'frequency': 'c', 'synset': 'black_sheep.n.02', 'synonyms': ['black_sheep'], 'id': 107, 'def': 'sheep with a black coat', 'name': 'black_sheep'}, {'frequency': 'c', 'synset': 'blackberry.n.01', 'synonyms': ['blackberry'], 'id': 108, 'def': 'large sweet black or very dark purple edible aggregate fruit', 'name': 'blackberry'}, {'frequency': 'f', 'synset': 'blackboard.n.01', 'synonyms': ['blackboard', 'chalkboard'], 'id': 109, 'def': 'sheet of slate; for writing with chalk', 'name': 'blackboard'}, {'frequency': 'f', 'synset': 'blanket.n.01', 'synonyms': ['blanket'], 'id': 110, 'def': 'bedding that keeps a person warm in bed', 'name': 'blanket'}, {'frequency': 'c', 'synset': 'blazer.n.01', 'synonyms': ['blazer', 'sport_jacket', 'sport_coat', 'sports_jacket', 'sports_coat'], 'id': 111, 'def': 'lightweight jacket; often striped in the colors of a club or school', 'name': 'blazer'}, {'frequency': 'f', 'synset': 'blender.n.01', 'synonyms': ['blender', 'liquidizer', 'liquidiser'], 'id': 112, 'def': 'an electrically powered mixer that mix or chop or liquefy foods', 'name': 'blender'}, {'frequency': 'r', 'synset': 'blimp.n.02', 'synonyms': ['blimp'], 'id': 113, 'def': 'a small nonrigid airship used for observation or as a barrage balloon', 'name': 'blimp'}, {'frequency': 'f', 'synset': 'blinker.n.01', 'synonyms': ['blinker', 'flasher'], 'id': 114, 'def': 'a light that flashes on and off; used as a signal or to send messages', 'name': 'blinker'}, {'frequency': 'f', 'synset': 'blouse.n.01', 'synonyms': ['blouse'], 'id': 115, 'def': 'a top worn by women', 'name': 'blouse'}, {'frequency': 'f', 'synset': 'blueberry.n.02', 'synonyms': ['blueberry'], 'id': 116, 'def': 'sweet edible dark-blue berries of blueberry plants', 'name': 'blueberry'}, {'frequency': 'r', 'synset': 'board.n.09', 'synonyms': ['gameboard'], 'id': 117, 'def': 'a flat portable surface (usually rectangular) designed for board games', 'name': 'gameboard'}, {'frequency': 'f', 'synset': 'boat.n.01', 'synonyms': ['boat', 'ship_(boat)'], 'id': 118, 'def': 'a vessel for travel on water', 'name': 'boat'}, {'frequency': 'r', 'synset': 'bob.n.05', 'synonyms': ['bob', 'bobber', 'bobfloat'], 'id': 119, 'def': 'a small float usually made of cork; attached to a fishing line', 'name': 'bob'}, {'frequency': 'c', 'synset': 'bobbin.n.01', 'synonyms': ['bobbin', 'spool', 'reel'], 'id': 120, 'def': 'a thing around which thread/tape/film or other flexible materials can be wound', 'name': 'bobbin'}, {'frequency': 'c', 'synset': 'bobby_pin.n.01', 'synonyms': ['bobby_pin', 'hairgrip'], 'id': 121, 'def': 'a flat wire hairpin used to hold bobbed hair in place', 'name': 'bobby_pin'}, {'frequency': 'c', 'synset': 'boiled_egg.n.01', 'synonyms': ['boiled_egg', 'coddled_egg'], 'id': 122, 'def': 'egg cooked briefly in the shell in gently boiling water', 'name': 'boiled_egg'}, {'frequency': 'r', 'synset': 'bolo_tie.n.01', 'synonyms': ['bolo_tie', 'bolo', 'bola_tie', 'bola'], 'id': 123, 'def': 'a cord fastened around the neck with an ornamental clasp and worn as a necktie', 'name': 'bolo_tie'}, {'frequency': 'c', 'synset': 'bolt.n.03', 'synonyms': ['deadbolt'], 'id': 124, 'def': 'the part of a lock that is engaged or withdrawn with a key', 'name': 'deadbolt'}, {'frequency': 'f', 'synset': 'bolt.n.06', 'synonyms': ['bolt'], 'id': 125, 'def': 'a screw that screws into a nut to form a fastener', 'name': 'bolt'}, {'frequency': 'r', 'synset': 'bonnet.n.01', 'synonyms': ['bonnet'], 'id': 126, 'def': 'a hat tied under the chin', 'name': 'bonnet'}, {'frequency': 'f', 'synset': 'book.n.01', 'synonyms': ['book'], 'id': 127, 'def': 'a written work or composition that has been published', 'name': 'book'}, {'frequency': 'c', 'synset': 'bookcase.n.01', 'synonyms': ['bookcase'], 'id': 128, 'def': 'a piece of furniture with shelves for storing books', 'name': 'bookcase'}, {'frequency': 'c', 'synset': 'booklet.n.01', 'synonyms': ['booklet', 'brochure', 'leaflet', 'pamphlet'], 'id': 129, 'def': 'a small book usually having a paper cover', 'name': 'booklet'}, {'frequency': 'r', 'synset': 'bookmark.n.01', 'synonyms': ['bookmark', 'bookmarker'], 'id': 130, 'def': 'a marker (a piece of paper or ribbon) placed between the pages of a book', 'name': 'bookmark'}, {'frequency': 'r', 'synset': 'boom.n.04', 'synonyms': ['boom_microphone', 'microphone_boom'], 'id': 131, 'def': 'a pole carrying an overhead microphone projected over a film or tv set', 'name': 'boom_microphone'}, {'frequency': 'f', 'synset': 'boot.n.01', 'synonyms': ['boot'], 'id': 132, 'def': 'footwear that covers the whole foot and lower leg', 'name': 'boot'}, {'frequency': 'f', 'synset': 'bottle.n.01', 'synonyms': ['bottle'], 'id': 133, 'def': 'a glass or plastic vessel used for storing drinks or other liquids', 'name': 'bottle'}, {'frequency': 'c', 'synset': 'bottle_opener.n.01', 'synonyms': ['bottle_opener'], 'id': 134, 'def': 'an opener for removing caps or corks from bottles', 'name': 'bottle_opener'}, {'frequency': 'c', 'synset': 'bouquet.n.01', 'synonyms': ['bouquet'], 'id': 135, 'def': 'an arrangement of flowers that is usually given as a present', 'name': 'bouquet'}, {'frequency': 'r', 'synset': 'bow.n.04', 'synonyms': ['bow_(weapon)'], 'id': 136, 'def': 'a weapon for shooting arrows', 'name': 'bow_(weapon)'}, {'frequency': 'f', 'synset': 'bow.n.08', 'synonyms': ['bow_(decorative_ribbons)'], 'id': 137, 'def': 'a decorative interlacing of ribbons', 'name': 'bow_(decorative_ribbons)'}, {'frequency': 'f', 'synset': 'bow_tie.n.01', 'synonyms': ['bow-tie', 'bowtie'], 'id': 138, 'def': "a man's tie that ties in a bow", 'name': 'bow-tie'}, {'frequency': 'f', 'synset': 'bowl.n.03', 'synonyms': ['bowl'], 'id': 139, 'def': 'a dish that is round and open at the top for serving foods', 'name': 'bowl'}, {'frequency': 'r', 'synset': 'bowl.n.08', 'synonyms': ['pipe_bowl'], 'id': 140, 'def': 'a small round container that is open at the top for holding tobacco', 'name': 'pipe_bowl'}, {'frequency': 'c', 'synset': 'bowler_hat.n.01', 'synonyms': ['bowler_hat', 'bowler', 'derby_hat', 'derby', 'plug_hat'], 'id': 141, 'def': 'a felt hat that is round and hard with a narrow brim', 'name': 'bowler_hat'}, {'frequency': 'r', 'synset': 'bowling_ball.n.01', 'synonyms': ['bowling_ball'], 'id': 142, 'def': 'a large ball with finger holes used in the sport of bowling', 'name': 'bowling_ball'}, {'frequency': 'f', 'synset': 'box.n.01', 'synonyms': ['box'], 'id': 143, 'def': 'a (usually rectangular) container; may have a lid', 'name': 'box'}, {'frequency': 'r', 'synset': 'boxing_glove.n.01', 'synonyms': ['boxing_glove'], 'id': 144, 'def': 'large glove coverings the fists of a fighter worn for the sport of boxing', 'name': 'boxing_glove'}, {'frequency': 'c', 'synset': 'brace.n.06', 'synonyms': ['suspenders'], 'id': 145, 'def': 'elastic straps that hold trousers up (usually used in the plural)', 'name': 'suspenders'}, {'frequency': 'f', 'synset': 'bracelet.n.02', 'synonyms': ['bracelet', 'bangle'], 'id': 146, 'def': 'jewelry worn around the wrist for decoration', 'name': 'bracelet'}, {'frequency': 'r', 'synset': 'brass.n.07', 'synonyms': ['brass_plaque'], 'id': 147, 'def': 'a memorial made of brass', 'name': 'brass_plaque'}, {'frequency': 'c', 'synset': 'brassiere.n.01', 'synonyms': ['brassiere', 'bra', 'bandeau'], 'id': 148, 'def': 'an undergarment worn by women to support their breasts', 'name': 'brassiere'}, {'frequency': 'c', 'synset': 'bread-bin.n.01', 'synonyms': ['bread-bin', 'breadbox'], 'id': 149, 'def': 'a container used to keep bread or cake in', 'name': 'bread-bin'}, {'frequency': 'f', 'synset': 'bread.n.01', 'synonyms': ['bread'], 'id': 150, 'def': 'food made from dough of flour or meal and usually raised with yeast or baking powder and then baked', 'name': 'bread'}, {'frequency': 'r', 'synset': 'breechcloth.n.01', 'synonyms': ['breechcloth', 'breechclout', 'loincloth'], 'id': 151, 'def': 'a garment that provides covering for the loins', 'name': 'breechcloth'}, {'frequency': 'f', 'synset': 'bridal_gown.n.01', 'synonyms': ['bridal_gown', 'wedding_gown', 'wedding_dress'], 'id': 152, 'def': 'a gown worn by the bride at a wedding', 'name': 'bridal_gown'}, {'frequency': 'c', 'synset': 'briefcase.n.01', 'synonyms': ['briefcase'], 'id': 153, 'def': 'a case with a handle; for carrying papers or files or books', 'name': 'briefcase'}, {'frequency': 'f', 'synset': 'broccoli.n.01', 'synonyms': ['broccoli'], 'id': 154, 'def': 'plant with dense clusters of tight green flower buds', 'name': 'broccoli'}, {'frequency': 'r', 'synset': 'brooch.n.01', 'synonyms': ['broach'], 'id': 155, 'def': 'a decorative pin worn by women', 'name': 'broach'}, {'frequency': 'c', 'synset': 'broom.n.01', 'synonyms': ['broom'], 'id': 156, 'def': 'bundle of straws or twigs attached to a long handle; used for cleaning', 'name': 'broom'}, {'frequency': 'c', 'synset': 'brownie.n.03', 'synonyms': ['brownie'], 'id': 157, 'def': 'square or bar of very rich chocolate cake usually with nuts', 'name': 'brownie'}, {'frequency': 'c', 'synset': 'brussels_sprouts.n.01', 'synonyms': ['brussels_sprouts'], 'id': 158, 'def': 'the small edible cabbage-like buds growing along a stalk', 'name': 'brussels_sprouts'}, {'frequency': 'r', 'synset': 'bubble_gum.n.01', 'synonyms': ['bubble_gum'], 'id': 159, 'def': 'a kind of chewing gum that can be blown into bubbles', 'name': 'bubble_gum'}, {'frequency': 'f', 'synset': 'bucket.n.01', 'synonyms': ['bucket', 'pail'], 'id': 160, 'def': 'a roughly cylindrical vessel that is open at the top', 'name': 'bucket'}, {'frequency': 'r', 'synset': 'buggy.n.01', 'synonyms': ['horse_buggy'], 'id': 161, 'def': 'a small lightweight carriage; drawn by a single horse', 'name': 'horse_buggy'}, {'frequency': 'c', 'synset': 'bull.n.11', 'synonyms': ['horned_cow'], 'id': 162, 'def': 'a cow with horns', 'name': 'bull'}, {'frequency': 'c', 'synset': 'bulldog.n.01', 'synonyms': ['bulldog'], 'id': 163, 'def': 'a thickset short-haired dog with a large head and strong undershot lower jaw', 'name': 'bulldog'}, {'frequency': 'r', 'synset': 'bulldozer.n.01', 'synonyms': ['bulldozer', 'dozer'], 'id': 164, 'def': 'large powerful tractor; a large blade in front flattens areas of ground', 'name': 'bulldozer'}, {'frequency': 'c', 'synset': 'bullet_train.n.01', 'synonyms': ['bullet_train'], 'id': 165, 'def': 'a high-speed passenger train', 'name': 'bullet_train'}, {'frequency': 'c', 'synset': 'bulletin_board.n.02', 'synonyms': ['bulletin_board', 'notice_board'], 'id': 166, 'def': 'a board that hangs on a wall; displays announcements', 'name': 'bulletin_board'}, {'frequency': 'r', 'synset': 'bulletproof_vest.n.01', 'synonyms': ['bulletproof_vest'], 'id': 167, 'def': 'a vest capable of resisting the impact of a bullet', 'name': 'bulletproof_vest'}, {'frequency': 'c', 'synset': 'bullhorn.n.01', 'synonyms': ['bullhorn', 'megaphone'], 'id': 168, 'def': 'a portable loudspeaker with built-in microphone and amplifier', 'name': 'bullhorn'}, {'frequency': 'f', 'synset': 'bun.n.01', 'synonyms': ['bun', 'roll'], 'id': 169, 'def': 'small rounded bread either plain or sweet', 'name': 'bun'}, {'frequency': 'c', 'synset': 'bunk_bed.n.01', 'synonyms': ['bunk_bed'], 'id': 170, 'def': 'beds built one above the other', 'name': 'bunk_bed'}, {'frequency': 'f', 'synset': 'buoy.n.01', 'synonyms': ['buoy'], 'id': 171, 'def': 'a float attached by rope to the seabed to mark channels in a harbor or underwater hazards', 'name': 'buoy'}, {'frequency': 'r', 'synset': 'burrito.n.01', 'synonyms': ['burrito'], 'id': 172, 'def': 'a flour tortilla folded around a filling', 'name': 'burrito'}, {'frequency': 'f', 'synset': 'bus.n.01', 'synonyms': ['bus_(vehicle)', 'autobus', 'charabanc', 'double-decker', 'motorbus', 'motorcoach'], 'id': 173, 'def': 'a vehicle carrying many passengers; used for public transport', 'name': 'bus_(vehicle)'}, {'frequency': 'c', 'synset': 'business_card.n.01', 'synonyms': ['business_card'], 'id': 174, 'def': "a card on which are printed the person's name and business affiliation", 'name': 'business_card'}, {'frequency': 'f', 'synset': 'butter.n.01', 'synonyms': ['butter'], 'id': 175, 'def': 'an edible emulsion of fat globules made by churning milk or cream; for cooking and table use', 'name': 'butter'}, {'frequency': 'c', 'synset': 'butterfly.n.01', 'synonyms': ['butterfly'], 'id': 176, 'def': 'insect typically having a slender body with knobbed antennae and broad colorful wings', 'name': 'butterfly'}, {'frequency': 'f', 'synset': 'button.n.01', 'synonyms': ['button'], 'id': 177, 'def': 'a round fastener sewn to shirts and coats etc to fit through buttonholes', 'name': 'button'}, {'frequency': 'f', 'synset': 'cab.n.03', 'synonyms': ['cab_(taxi)', 'taxi', 'taxicab'], 'id': 178, 'def': 'a car that takes passengers where they want to go in exchange for money', 'name': 'cab_(taxi)'}, {'frequency': 'r', 'synset': 'cabana.n.01', 'synonyms': ['cabana'], 'id': 179, 'def': 'a small tent used as a dressing room beside the sea or a swimming pool', 'name': 'cabana'}, {'frequency': 'c', 'synset': 'cabin_car.n.01', 'synonyms': ['cabin_car', 'caboose'], 'id': 180, 'def': 'a car on a freight train for use of the train crew; usually the last car on the train', 'name': 'cabin_car'}, {'frequency': 'f', 'synset': 'cabinet.n.01', 'synonyms': ['cabinet'], 'id': 181, 'def': 'a piece of furniture resembling a cupboard with doors and shelves and drawers', 'name': 'cabinet'}, {'frequency': 'r', 'synset': 'cabinet.n.03', 'synonyms': ['locker', 'storage_locker'], 'id': 182, 'def': 'a storage compartment for clothes and valuables; usually it has a lock', 'name': 'locker'}, {'frequency': 'f', 'synset': 'cake.n.03', 'synonyms': ['cake'], 'id': 183, 'def': 'baked goods made from or based on a mixture of flour, sugar, eggs, and fat', 'name': 'cake'}, {'frequency': 'c', 'synset': 'calculator.n.02', 'synonyms': ['calculator'], 'id': 184, 'def': 'a small machine that is used for mathematical calculations', 'name': 'calculator'}, {'frequency': 'f', 'synset': 'calendar.n.02', 'synonyms': ['calendar'], 'id': 185, 'def': 'a list or register of events (appointments/social events/court cases, etc)', 'name': 'calendar'}, {'frequency': 'c', 'synset': 'calf.n.01', 'synonyms': ['calf'], 'id': 186, 'def': 'young of domestic cattle', 'name': 'calf'}, {'frequency': 'c', 'synset': 'camcorder.n.01', 'synonyms': ['camcorder'], 'id': 187, 'def': 'a portable television camera and videocassette recorder', 'name': 'camcorder'}, {'frequency': 'c', 'synset': 'camel.n.01', 'synonyms': ['camel'], 'id': 188, 'def': 'cud-chewing mammal used as a draft or saddle animal in desert regions', 'name': 'camel'}, {'frequency': 'f', 'synset': 'camera.n.01', 'synonyms': ['camera'], 'id': 189, 'def': 'equipment for taking photographs', 'name': 'camera'}, {'frequency': 'c', 'synset': 'camera_lens.n.01', 'synonyms': ['camera_lens'], 'id': 190, 'def': 'a lens that focuses the image in a camera', 'name': 'camera_lens'}, {'frequency': 'c', 'synset': 'camper.n.02', 'synonyms': ['camper_(vehicle)', 'camping_bus', 'motor_home'], 'id': 191, 'def': 'a recreational vehicle equipped for camping out while traveling', 'name': 'camper_(vehicle)'}, {'frequency': 'f', 'synset': 'can.n.01', 'synonyms': ['can', 'tin_can'], 'id': 192, 'def': 'airtight sealed metal container for food or drink or paint etc.', 'name': 'can'}, {'frequency': 'c', 'synset': 'can_opener.n.01', 'synonyms': ['can_opener', 'tin_opener'], 'id': 193, 'def': 'a device for cutting cans open', 'name': 'can_opener'}, {'frequency': 'f', 'synset': 'candle.n.01', 'synonyms': ['candle', 'candlestick'], 'id': 194, 'def': 'stick of wax with a wick in the middle', 'name': 'candle'}, {'frequency': 'f', 'synset': 'candlestick.n.01', 'synonyms': ['candle_holder'], 'id': 195, 'def': 'a holder with sockets for candles', 'name': 'candle_holder'}, {'frequency': 'r', 'synset': 'candy_bar.n.01', 'synonyms': ['candy_bar'], 'id': 196, 'def': 'a candy shaped as a bar', 'name': 'candy_bar'}, {'frequency': 'c', 'synset': 'candy_cane.n.01', 'synonyms': ['candy_cane'], 'id': 197, 'def': 'a hard candy in the shape of a rod (usually with stripes)', 'name': 'candy_cane'}, {'frequency': 'c', 'synset': 'cane.n.01', 'synonyms': ['walking_cane'], 'id': 198, 'def': 'a stick that people can lean on to help them walk', 'name': 'walking_cane'}, {'frequency': 'c', 'synset': 'canister.n.02', 'synonyms': ['canister', 'cannister'], 'id': 199, 'def': 'metal container for storing dry foods such as tea or flour', 'name': 'canister'}, {'frequency': 'c', 'synset': 'canoe.n.01', 'synonyms': ['canoe'], 'id': 200, 'def': 'small and light boat; pointed at both ends; propelled with a paddle', 'name': 'canoe'}, {'frequency': 'c', 'synset': 'cantaloup.n.02', 'synonyms': ['cantaloup', 'cantaloupe'], 'id': 201, 'def': 'the fruit of a cantaloup vine; small to medium-sized melon with yellowish flesh', 'name': 'cantaloup'}, {'frequency': 'r', 'synset': 'canteen.n.01', 'synonyms': ['canteen'], 'id': 202, 'def': 'a flask for carrying water; used by soldiers or travelers', 'name': 'canteen'}, {'frequency': 'f', 'synset': 'cap.n.01', 'synonyms': ['cap_(headwear)'], 'id': 203, 'def': 'a tight-fitting headwear', 'name': 'cap_(headwear)'}, {'frequency': 'f', 'synset': 'cap.n.02', 'synonyms': ['bottle_cap', 'cap_(container_lid)'], 'id': 204, 'def': 'a top (as for a bottle)', 'name': 'bottle_cap'}, {'frequency': 'c', 'synset': 'cape.n.02', 'synonyms': ['cape'], 'id': 205, 'def': 'a sleeveless garment like a cloak but shorter', 'name': 'cape'}, {'frequency': 'c', 'synset': 'cappuccino.n.01', 'synonyms': ['cappuccino', 'coffee_cappuccino'], 'id': 206, 'def': 'equal parts of espresso and steamed milk', 'name': 'cappuccino'}, {'frequency': 'f', 'synset': 'car.n.01', 'synonyms': ['car_(automobile)', 'auto_(automobile)', 'automobile'], 'id': 207, 'def': 'a motor vehicle with four wheels', 'name': 'car_(automobile)'}, {'frequency': 'f', 'synset': 'car.n.02', 'synonyms': ['railcar_(part_of_a_train)', 'railway_car_(part_of_a_train)', 'railroad_car_(part_of_a_train)'], 'id': 208, 'def': 'a wheeled vehicle adapted to the rails of railroad (mark each individual railcar separately)', 'name': 'railcar_(part_of_a_train)'}, {'frequency': 'r', 'synset': 'car.n.04', 'synonyms': ['elevator_car'], 'id': 209, 'def': 'where passengers ride up and down', 'name': 'elevator_car'}, {'frequency': 'r', 'synset': 'car_battery.n.01', 'synonyms': ['car_battery', 'automobile_battery'], 'id': 210, 'def': 'a battery in a motor vehicle', 'name': 'car_battery'}, {'frequency': 'c', 'synset': 'card.n.02', 'synonyms': ['identity_card'], 'id': 211, 'def': 'a card certifying the identity of the bearer', 'name': 'identity_card'}, {'frequency': 'c', 'synset': 'card.n.03', 'synonyms': ['card'], 'id': 212, 'def': 'a rectangular piece of paper used to send messages (e.g. greetings or pictures)', 'name': 'card'}, {'frequency': 'c', 'synset': 'cardigan.n.01', 'synonyms': ['cardigan'], 'id': 213, 'def': 'knitted jacket that is fastened up the front with buttons or a zipper', 'name': 'cardigan'}, {'frequency': 'r', 'synset': 'cargo_ship.n.01', 'synonyms': ['cargo_ship', 'cargo_vessel'], 'id': 214, 'def': 'a ship designed to carry cargo', 'name': 'cargo_ship'}, {'frequency': 'r', 'synset': 'carnation.n.01', 'synonyms': ['carnation'], 'id': 215, 'def': 'plant with pink to purple-red spice-scented usually double flowers', 'name': 'carnation'}, {'frequency': 'c', 'synset': 'carriage.n.02', 'synonyms': ['horse_carriage'], 'id': 216, 'def': 'a vehicle with wheels drawn by one or more horses', 'name': 'horse_carriage'}, {'frequency': 'f', 'synset': 'carrot.n.01', 'synonyms': ['carrot'], 'id': 217, 'def': 'deep orange edible root of the cultivated carrot plant', 'name': 'carrot'}, {'frequency': 'f', 'synset': 'carryall.n.01', 'synonyms': ['tote_bag'], 'id': 218, 'def': 'a capacious bag or basket', 'name': 'tote_bag'}, {'frequency': 'c', 'synset': 'cart.n.01', 'synonyms': ['cart'], 'id': 219, 'def': 'a heavy open wagon usually having two wheels and drawn by an animal', 'name': 'cart'}, {'frequency': 'c', 'synset': 'carton.n.02', 'synonyms': ['carton'], 'id': 220, 'def': 'a container made of cardboard for holding food or drink', 'name': 'carton'}, {'frequency': 'c', 'synset': 'cash_register.n.01', 'synonyms': ['cash_register', 'register_(for_cash_transactions)'], 'id': 221, 'def': 'a cashbox with an adding machine to register transactions', 'name': 'cash_register'}, {'frequency': 'r', 'synset': 'casserole.n.01', 'synonyms': ['casserole'], 'id': 222, 'def': 'food cooked and served in a casserole', 'name': 'casserole'}, {'frequency': 'r', 'synset': 'cassette.n.01', 'synonyms': ['cassette'], 'id': 223, 'def': 'a container that holds a magnetic tape used for recording or playing sound or video', 'name': 'cassette'}, {'frequency': 'c', 'synset': 'cast.n.05', 'synonyms': ['cast', 'plaster_cast', 'plaster_bandage'], 'id': 224, 'def': 'bandage consisting of a firm covering that immobilizes broken bones while they heal', 'name': 'cast'}, {'frequency': 'f', 'synset': 'cat.n.01', 'synonyms': ['cat'], 'id': 225, 'def': 'a domestic house cat', 'name': 'cat'}, {'frequency': 'f', 'synset': 'cauliflower.n.02', 'synonyms': ['cauliflower'], 'id': 226, 'def': 'edible compact head of white undeveloped flowers', 'name': 'cauliflower'}, {'frequency': 'c', 'synset': 'cayenne.n.02', 'synonyms': ['cayenne_(spice)', 'cayenne_pepper_(spice)', 'red_pepper_(spice)'], 'id': 227, 'def': 'ground pods and seeds of pungent red peppers of the genus Capsicum', 'name': 'cayenne_(spice)'}, {'frequency': 'c', 'synset': 'cd_player.n.01', 'synonyms': ['CD_player'], 'id': 228, 'def': 'electronic equipment for playing compact discs (CDs)', 'name': 'CD_player'}, {'frequency': 'f', 'synset': 'celery.n.01', 'synonyms': ['celery'], 'id': 229, 'def': 'widely cultivated herb with aromatic leaf stalks that are eaten raw or cooked', 'name': 'celery'}, {'frequency': 'f', 'synset': 'cellular_telephone.n.01', 'synonyms': ['cellular_telephone', 'cellular_phone', 'cellphone', 'mobile_phone', 'smart_phone'], 'id': 230, 'def': 'a hand-held mobile telephone', 'name': 'cellular_telephone'}, {'frequency': 'r', 'synset': 'chain_mail.n.01', 'synonyms': ['chain_mail', 'ring_mail', 'chain_armor', 'chain_armour', 'ring_armor', 'ring_armour'], 'id': 231, 'def': '(Middle Ages) flexible armor made of interlinked metal rings', 'name': 'chain_mail'}, {'frequency': 'f', 'synset': 'chair.n.01', 'synonyms': ['chair'], 'id': 232, 'def': 'a seat for one person, with a support for the back', 'name': 'chair'}, {'frequency': 'r', 'synset': 'chaise_longue.n.01', 'synonyms': ['chaise_longue', 'chaise', 'daybed'], 'id': 233, 'def': 'a long chair; for reclining', 'name': 'chaise_longue'}, {'frequency': 'r', 'synset': 'chalice.n.01', 'synonyms': ['chalice'], 'id': 234, 'def': 'a bowl-shaped drinking vessel; especially the Eucharistic cup', 'name': 'chalice'}, {'frequency': 'f', 'synset': 'chandelier.n.01', 'synonyms': ['chandelier'], 'id': 235, 'def': 'branched lighting fixture; often ornate; hangs from the ceiling', 'name': 'chandelier'}, {'frequency': 'r', 'synset': 'chap.n.04', 'synonyms': ['chap'], 'id': 236, 'def': 'leather leggings without a seat; worn over trousers by cowboys to protect their legs', 'name': 'chap'}, {'frequency': 'r', 'synset': 'checkbook.n.01', 'synonyms': ['checkbook', 'chequebook'], 'id': 237, 'def': 'a book issued to holders of checking accounts', 'name': 'checkbook'}, {'frequency': 'r', 'synset': 'checkerboard.n.01', 'synonyms': ['checkerboard'], 'id': 238, 'def': 'a board having 64 squares of two alternating colors', 'name': 'checkerboard'}, {'frequency': 'c', 'synset': 'cherry.n.03', 'synonyms': ['cherry'], 'id': 239, 'def': 'a red fruit with a single hard stone', 'name': 'cherry'}, {'frequency': 'r', 'synset': 'chessboard.n.01', 'synonyms': ['chessboard'], 'id': 240, 'def': 'a checkerboard used to play chess', 'name': 'chessboard'}, {'frequency': 'c', 'synset': 'chicken.n.02', 'synonyms': ['chicken_(animal)'], 'id': 241, 'def': 'a domestic fowl bred for flesh or eggs', 'name': 'chicken_(animal)'}, {'frequency': 'c', 'synset': 'chickpea.n.01', 'synonyms': ['chickpea', 'garbanzo'], 'id': 242, 'def': 'the seed of the chickpea plant; usually dried', 'name': 'chickpea'}, {'frequency': 'c', 'synset': 'chili.n.02', 'synonyms': ['chili_(vegetable)', 'chili_pepper_(vegetable)', 'chilli_(vegetable)', 'chilly_(vegetable)', 'chile_(vegetable)'], 'id': 243, 'def': 'very hot and finely tapering pepper of special pungency', 'name': 'chili_(vegetable)'}, {'frequency': 'r', 'synset': 'chime.n.01', 'synonyms': ['chime', 'gong'], 'id': 244, 'def': 'an instrument consisting of a set of bells that are struck with a hammer', 'name': 'chime'}, {'frequency': 'r', 'synset': 'chinaware.n.01', 'synonyms': ['chinaware'], 'id': 245, 'def': 'dishware made of high quality porcelain', 'name': 'chinaware'}, {'frequency': 'c', 'synset': 'chip.n.04', 'synonyms': ['crisp_(potato_chip)', 'potato_chip'], 'id': 246, 'def': 'a thin crisp slice of potato fried in deep fat', 'name': 'crisp_(potato_chip)'}, {'frequency': 'r', 'synset': 'chip.n.06', 'synonyms': ['poker_chip'], 'id': 247, 'def': 'a small disk-shaped counter used to represent money when gambling', 'name': 'poker_chip'}, {'frequency': 'c', 'synset': 'chocolate_bar.n.01', 'synonyms': ['chocolate_bar'], 'id': 248, 'def': 'a bar of chocolate candy', 'name': 'chocolate_bar'}, {'frequency': 'c', 'synset': 'chocolate_cake.n.01', 'synonyms': ['chocolate_cake'], 'id': 249, 'def': 'cake containing chocolate', 'name': 'chocolate_cake'}, {'frequency': 'r', 'synset': 'chocolate_milk.n.01', 'synonyms': ['chocolate_milk'], 'id': 250, 'def': 'milk flavored with chocolate syrup', 'name': 'chocolate_milk'}, {'frequency': 'r', 'synset': 'chocolate_mousse.n.01', 'synonyms': ['chocolate_mousse'], 'id': 251, 'def': 'dessert mousse made with chocolate', 'name': 'chocolate_mousse'}, {'frequency': 'f', 'synset': 'choker.n.03', 'synonyms': ['choker', 'collar', 'neckband'], 'id': 252, 'def': 'shirt collar, animal collar, or tight-fitting necklace', 'name': 'choker'}, {'frequency': 'f', 'synset': 'chopping_board.n.01', 'synonyms': ['chopping_board', 'cutting_board', 'chopping_block'], 'id': 253, 'def': 'a wooden board where meats or vegetables can be cut', 'name': 'chopping_board'}, {'frequency': 'f', 'synset': 'chopstick.n.01', 'synonyms': ['chopstick'], 'id': 254, 'def': 'one of a pair of slender sticks used as oriental tableware to eat food with', 'name': 'chopstick'}, {'frequency': 'f', 'synset': 'christmas_tree.n.05', 'synonyms': ['Christmas_tree'], 'id': 255, 'def': 'an ornamented evergreen used as a Christmas decoration', 'name': 'Christmas_tree'}, {'frequency': 'c', 'synset': 'chute.n.02', 'synonyms': ['slide'], 'id': 256, 'def': 'sloping channel through which things can descend', 'name': 'slide'}, {'frequency': 'r', 'synset': 'cider.n.01', 'synonyms': ['cider', 'cyder'], 'id': 257, 'def': 'a beverage made from juice pressed from apples', 'name': 'cider'}, {'frequency': 'r', 'synset': 'cigar_box.n.01', 'synonyms': ['cigar_box'], 'id': 258, 'def': 'a box for holding cigars', 'name': 'cigar_box'}, {'frequency': 'f', 'synset': 'cigarette.n.01', 'synonyms': ['cigarette'], 'id': 259, 'def': 'finely ground tobacco wrapped in paper; for smoking', 'name': 'cigarette'}, {'frequency': 'c', 'synset': 'cigarette_case.n.01', 'synonyms': ['cigarette_case', 'cigarette_pack'], 'id': 260, 'def': 'a small flat case for holding cigarettes', 'name': 'cigarette_case'}, {'frequency': 'f', 'synset': 'cistern.n.02', 'synonyms': ['cistern', 'water_tank'], 'id': 261, 'def': 'a tank that holds the water used to flush a toilet', 'name': 'cistern'}, {'frequency': 'r', 'synset': 'clarinet.n.01', 'synonyms': ['clarinet'], 'id': 262, 'def': 'a single-reed instrument with a straight tube', 'name': 'clarinet'}, {'frequency': 'c', 'synset': 'clasp.n.01', 'synonyms': ['clasp'], 'id': 263, 'def': 'a fastener (as a buckle or hook) that is used to hold two things together', 'name': 'clasp'}, {'frequency': 'c', 'synset': 'cleansing_agent.n.01', 'synonyms': ['cleansing_agent', 'cleanser', 'cleaner'], 'id': 264, 'def': 'a preparation used in cleaning something', 'name': 'cleansing_agent'}, {'frequency': 'r', 'synset': 'cleat.n.02', 'synonyms': ['cleat_(for_securing_rope)'], 'id': 265, 'def': 'a fastener (usually with two projecting horns) around which a rope can be secured', 'name': 'cleat_(for_securing_rope)'}, {'frequency': 'r', 'synset': 'clementine.n.01', 'synonyms': ['clementine'], 'id': 266, 'def': 'a variety of mandarin orange', 'name': 'clementine'}, {'frequency': 'c', 'synset': 'clip.n.03', 'synonyms': ['clip'], 'id': 267, 'def': 'any of various small fasteners used to hold loose articles together', 'name': 'clip'}, {'frequency': 'c', 'synset': 'clipboard.n.01', 'synonyms': ['clipboard'], 'id': 268, 'def': 'a small writing board with a clip at the top for holding papers', 'name': 'clipboard'}, {'frequency': 'r', 'synset': 'clipper.n.03', 'synonyms': ['clippers_(for_plants)'], 'id': 269, 'def': 'shears for cutting grass or shrubbery (often used in the plural)', 'name': 'clippers_(for_plants)'}, {'frequency': 'r', 'synset': 'cloak.n.02', 'synonyms': ['cloak'], 'id': 270, 'def': 'a loose outer garment', 'name': 'cloak'}, {'frequency': 'f', 'synset': 'clock.n.01', 'synonyms': ['clock', 'timepiece', 'timekeeper'], 'id': 271, 'def': 'a timepiece that shows the time of day', 'name': 'clock'}, {'frequency': 'f', 'synset': 'clock_tower.n.01', 'synonyms': ['clock_tower'], 'id': 272, 'def': 'a tower with a large clock visible high up on an outside face', 'name': 'clock_tower'}, {'frequency': 'c', 'synset': 'clothes_hamper.n.01', 'synonyms': ['clothes_hamper', 'laundry_basket', 'clothes_basket'], 'id': 273, 'def': 'a hamper that holds dirty clothes to be washed or wet clothes to be dried', 'name': 'clothes_hamper'}, {'frequency': 'c', 'synset': 'clothespin.n.01', 'synonyms': ['clothespin', 'clothes_peg'], 'id': 274, 'def': 'wood or plastic fastener; for holding clothes on a clothesline', 'name': 'clothespin'}, {'frequency': 'r', 'synset': 'clutch_bag.n.01', 'synonyms': ['clutch_bag'], 'id': 275, 'def': "a woman's strapless purse that is carried in the hand", 'name': 'clutch_bag'}, {'frequency': 'f', 'synset': 'coaster.n.03', 'synonyms': ['coaster'], 'id': 276, 'def': 'a covering (plate or mat) that protects the surface of a table', 'name': 'coaster'}, {'frequency': 'f', 'synset': 'coat.n.01', 'synonyms': ['coat'], 'id': 277, 'def': 'an outer garment that has sleeves and covers the body from shoulder down', 'name': 'coat'}, {'frequency': 'c', 'synset': 'coat_hanger.n.01', 'synonyms': ['coat_hanger', 'clothes_hanger', 'dress_hanger'], 'id': 278, 'def': "a hanger that is shaped like a person's shoulders", 'name': 'coat_hanger'}, {'frequency': 'c', 'synset': 'coatrack.n.01', 'synonyms': ['coatrack', 'hatrack'], 'id': 279, 'def': 'a rack with hooks for temporarily holding coats and hats', 'name': 'coatrack'}, {'frequency': 'c', 'synset': 'cock.n.04', 'synonyms': ['cock', 'rooster'], 'id': 280, 'def': 'adult male chicken', 'name': 'cock'}, {'frequency': 'r', 'synset': 'cockroach.n.01', 'synonyms': ['cockroach'], 'id': 281, 'def': 'any of numerous chiefly nocturnal insects; some are domestic pests', 'name': 'cockroach'}, {'frequency': 'r', 'synset': 'cocoa.n.01', 'synonyms': ['cocoa_(beverage)', 'hot_chocolate_(beverage)', 'drinking_chocolate'], 'id': 282, 'def': 'a beverage made from cocoa powder and milk and sugar; usually drunk hot', 'name': 'cocoa_(beverage)'}, {'frequency': 'c', 'synset': 'coconut.n.02', 'synonyms': ['coconut', 'cocoanut'], 'id': 283, 'def': 'large hard-shelled brown oval nut with a fibrous husk', 'name': 'coconut'}, {'frequency': 'f', 'synset': 'coffee_maker.n.01', 'synonyms': ['coffee_maker', 'coffee_machine'], 'id': 284, 'def': 'a kitchen appliance for brewing coffee automatically', 'name': 'coffee_maker'}, {'frequency': 'f', 'synset': 'coffee_table.n.01', 'synonyms': ['coffee_table', 'cocktail_table'], 'id': 285, 'def': 'low table where magazines can be placed and coffee or cocktails are served', 'name': 'coffee_table'}, {'frequency': 'c', 'synset': 'coffeepot.n.01', 'synonyms': ['coffeepot'], 'id': 286, 'def': 'tall pot in which coffee is brewed', 'name': 'coffeepot'}, {'frequency': 'r', 'synset': 'coil.n.05', 'synonyms': ['coil'], 'id': 287, 'def': 'tubing that is wound in a spiral', 'name': 'coil'}, {'frequency': 'c', 'synset': 'coin.n.01', 'synonyms': ['coin'], 'id': 288, 'def': 'a flat metal piece (usually a disc) used as money', 'name': 'coin'}, {'frequency': 'c', 'synset': 'colander.n.01', 'synonyms': ['colander', 'cullender'], 'id': 289, 'def': 'bowl-shaped strainer; used to wash or drain foods', 'name': 'colander'}, {'frequency': 'c', 'synset': 'coleslaw.n.01', 'synonyms': ['coleslaw', 'slaw'], 'id': 290, 'def': 'basically shredded cabbage', 'name': 'coleslaw'}, {'frequency': 'r', 'synset': 'coloring_material.n.01', 'synonyms': ['coloring_material', 'colouring_material'], 'id': 291, 'def': 'any material used for its color', 'name': 'coloring_material'}, {'frequency': 'r', 'synset': 'combination_lock.n.01', 'synonyms': ['combination_lock'], 'id': 292, 'def': 'lock that can be opened only by turning dials in a special sequence', 'name': 'combination_lock'}, {'frequency': 'c', 'synset': 'comforter.n.04', 'synonyms': ['pacifier', 'teething_ring'], 'id': 293, 'def': 'device used for an infant to suck or bite on', 'name': 'pacifier'}, {'frequency': 'r', 'synset': 'comic_book.n.01', 'synonyms': ['comic_book'], 'id': 294, 'def': 'a magazine devoted to comic strips', 'name': 'comic_book'}, {'frequency': 'r', 'synset': 'compass.n.01', 'synonyms': ['compass'], 'id': 295, 'def': 'navigational instrument for finding directions', 'name': 'compass'}, {'frequency': 'f', 'synset': 'computer_keyboard.n.01', 'synonyms': ['computer_keyboard', 'keyboard_(computer)'], 'id': 296, 'def': 'a keyboard that is a data input device for computers', 'name': 'computer_keyboard'}, {'frequency': 'f', 'synset': 'condiment.n.01', 'synonyms': ['condiment'], 'id': 297, 'def': 'a preparation (a sauce or relish or spice) to enhance flavor or enjoyment', 'name': 'condiment'}, {'frequency': 'f', 'synset': 'cone.n.01', 'synonyms': ['cone', 'traffic_cone'], 'id': 298, 'def': 'a cone-shaped object used to direct traffic', 'name': 'cone'}, {'frequency': 'f', 'synset': 'control.n.09', 'synonyms': ['control', 'controller'], 'id': 299, 'def': 'a mechanism that controls the operation of a machine', 'name': 'control'}, {'frequency': 'r', 'synset': 'convertible.n.01', 'synonyms': ['convertible_(automobile)'], 'id': 300, 'def': 'a car that has top that can be folded or removed', 'name': 'convertible_(automobile)'}, {'frequency': 'r', 'synset': 'convertible.n.03', 'synonyms': ['sofa_bed'], 'id': 301, 'def': 'a sofa that can be converted into a bed', 'name': 'sofa_bed'}, {'frequency': 'r', 'synset': 'cooker.n.01', 'synonyms': ['cooker'], 'id': 302, 'def': 'a utensil for cooking', 'name': 'cooker'}, {'frequency': 'f', 'synset': 'cookie.n.01', 'synonyms': ['cookie', 'cooky', 'biscuit_(cookie)'], 'id': 303, 'def': "any of various small flat sweet cakes (`biscuit' is the British term)", 'name': 'cookie'}, {'frequency': 'r', 'synset': 'cooking_utensil.n.01', 'synonyms': ['cooking_utensil'], 'id': 304, 'def': 'a kitchen utensil made of material that does not melt easily; used for cooking', 'name': 'cooking_utensil'}, {'frequency': 'f', 'synset': 'cooler.n.01', 'synonyms': ['cooler_(for_food)', 'ice_chest'], 'id': 305, 'def': 'an insulated box for storing food often with ice', 'name': 'cooler_(for_food)'}, {'frequency': 'f', 'synset': 'cork.n.04', 'synonyms': ['cork_(bottle_plug)', 'bottle_cork'], 'id': 306, 'def': 'the plug in the mouth of a bottle (especially a wine bottle)', 'name': 'cork_(bottle_plug)'}, {'frequency': 'r', 'synset': 'corkboard.n.01', 'synonyms': ['corkboard'], 'id': 307, 'def': 'a sheet consisting of cork granules', 'name': 'corkboard'}, {'frequency': 'c', 'synset': 'corkscrew.n.01', 'synonyms': ['corkscrew', 'bottle_screw'], 'id': 308, 'def': 'a bottle opener that pulls corks', 'name': 'corkscrew'}, {'frequency': 'f', 'synset': 'corn.n.03', 'synonyms': ['edible_corn', 'corn', 'maize'], 'id': 309, 'def': 'ears or kernels of corn that can be prepared and served for human food (only mark individual ears or kernels)', 'name': 'edible_corn'}, {'frequency': 'r', 'synset': 'cornbread.n.01', 'synonyms': ['cornbread'], 'id': 310, 'def': 'bread made primarily of cornmeal', 'name': 'cornbread'}, {'frequency': 'c', 'synset': 'cornet.n.01', 'synonyms': ['cornet', 'horn', 'trumpet'], 'id': 311, 'def': 'a brass musical instrument with a narrow tube and a flared bell and many valves', 'name': 'cornet'}, {'frequency': 'c', 'synset': 'cornice.n.01', 'synonyms': ['cornice', 'valance', 'valance_board', 'pelmet'], 'id': 312, 'def': 'a decorative framework to conceal curtain fixtures at the top of a window casing', 'name': 'cornice'}, {'frequency': 'r', 'synset': 'cornmeal.n.01', 'synonyms': ['cornmeal'], 'id': 313, 'def': 'coarsely ground corn', 'name': 'cornmeal'}, {'frequency': 'c', 'synset': 'corset.n.01', 'synonyms': ['corset', 'girdle'], 'id': 314, 'def': "a woman's close-fitting foundation garment", 'name': 'corset'}, {'frequency': 'c', 'synset': 'costume.n.04', 'synonyms': ['costume'], 'id': 315, 'def': 'the attire characteristic of a country or a time or a social class', 'name': 'costume'}, {'frequency': 'r', 'synset': 'cougar.n.01', 'synonyms': ['cougar', 'puma', 'catamount', 'mountain_lion', 'panther'], 'id': 316, 'def': 'large American feline resembling a lion', 'name': 'cougar'}, {'frequency': 'r', 'synset': 'coverall.n.01', 'synonyms': ['coverall'], 'id': 317, 'def': 'a loose-fitting protective garment that is worn over other clothing', 'name': 'coverall'}, {'frequency': 'c', 'synset': 'cowbell.n.01', 'synonyms': ['cowbell'], 'id': 318, 'def': 'a bell hung around the neck of cow so that the cow can be easily located', 'name': 'cowbell'}, {'frequency': 'f', 'synset': 'cowboy_hat.n.01', 'synonyms': ['cowboy_hat', 'ten-gallon_hat'], 'id': 319, 'def': 'a hat with a wide brim and a soft crown; worn by American ranch hands', 'name': 'cowboy_hat'}, {'frequency': 'c', 'synset': 'crab.n.01', 'synonyms': ['crab_(animal)'], 'id': 320, 'def': 'decapod having eyes on short stalks and a broad flattened shell and pincers', 'name': 'crab_(animal)'}, {'frequency': 'r', 'synset': 'crab.n.05', 'synonyms': ['crabmeat'], 'id': 321, 'def': 'the edible flesh of any of various crabs', 'name': 'crabmeat'}, {'frequency': 'c', 'synset': 'cracker.n.01', 'synonyms': ['cracker'], 'id': 322, 'def': 'a thin crisp wafer', 'name': 'cracker'}, {'frequency': 'r', 'synset': 'crape.n.01', 'synonyms': ['crape', 'crepe', 'French_pancake'], 'id': 323, 'def': 'small very thin pancake', 'name': 'crape'}, {'frequency': 'f', 'synset': 'crate.n.01', 'synonyms': ['crate'], 'id': 324, 'def': 'a rugged box (usually made of wood); used for shipping', 'name': 'crate'}, {'frequency': 'c', 'synset': 'crayon.n.01', 'synonyms': ['crayon', 'wax_crayon'], 'id': 325, 'def': 'writing or drawing implement made of a colored stick of composition wax', 'name': 'crayon'}, {'frequency': 'r', 'synset': 'cream_pitcher.n.01', 'synonyms': ['cream_pitcher'], 'id': 326, 'def': 'a small pitcher for serving cream', 'name': 'cream_pitcher'}, {'frequency': 'c', 'synset': 'crescent_roll.n.01', 'synonyms': ['crescent_roll', 'croissant'], 'id': 327, 'def': 'very rich flaky crescent-shaped roll', 'name': 'crescent_roll'}, {'frequency': 'c', 'synset': 'crib.n.01', 'synonyms': ['crib', 'cot'], 'id': 328, 'def': 'baby bed with high sides made of slats', 'name': 'crib'}, {'frequency': 'c', 'synset': 'crock.n.03', 'synonyms': ['crock_pot', 'earthenware_jar'], 'id': 329, 'def': 'an earthen jar (made of baked clay) or a modern electric crockpot', 'name': 'crock_pot'}, {'frequency': 'f', 'synset': 'crossbar.n.01', 'synonyms': ['crossbar'], 'id': 330, 'def': 'a horizontal bar that goes across something', 'name': 'crossbar'}, {'frequency': 'r', 'synset': 'crouton.n.01', 'synonyms': ['crouton'], 'id': 331, 'def': 'a small piece of toasted or fried bread; served in soup or salads', 'name': 'crouton'}, {'frequency': 'c', 'synset': 'crow.n.01', 'synonyms': ['crow'], 'id': 332, 'def': 'black birds having a raucous call', 'name': 'crow'}, {'frequency': 'r', 'synset': 'crowbar.n.01', 'synonyms': ['crowbar', 'wrecking_bar', 'pry_bar'], 'id': 333, 'def': 'a heavy iron lever with one end forged into a wedge', 'name': 'crowbar'}, {'frequency': 'c', 'synset': 'crown.n.04', 'synonyms': ['crown'], 'id': 334, 'def': 'an ornamental jeweled headdress signifying sovereignty', 'name': 'crown'}, {'frequency': 'c', 'synset': 'crucifix.n.01', 'synonyms': ['crucifix'], 'id': 335, 'def': 'representation of the cross on which Jesus died', 'name': 'crucifix'}, {'frequency': 'c', 'synset': 'cruise_ship.n.01', 'synonyms': ['cruise_ship', 'cruise_liner'], 'id': 336, 'def': 'a passenger ship used commercially for pleasure cruises', 'name': 'cruise_ship'}, {'frequency': 'c', 'synset': 'cruiser.n.01', 'synonyms': ['police_cruiser', 'patrol_car', 'police_car', 'squad_car'], 'id': 337, 'def': 'a car in which policemen cruise the streets', 'name': 'police_cruiser'}, {'frequency': 'f', 'synset': 'crumb.n.03', 'synonyms': ['crumb'], 'id': 338, 'def': 'small piece of e.g. bread or cake', 'name': 'crumb'}, {'frequency': 'c', 'synset': 'crutch.n.01', 'synonyms': ['crutch'], 'id': 339, 'def': 'a wooden or metal staff that fits under the armpit and reaches to the ground', 'name': 'crutch'}, {'frequency': 'c', 'synset': 'cub.n.03', 'synonyms': ['cub_(animal)'], 'id': 340, 'def': 'the young of certain carnivorous mammals such as the bear or wolf or lion', 'name': 'cub_(animal)'}, {'frequency': 'c', 'synset': 'cube.n.05', 'synonyms': ['cube', 'square_block'], 'id': 341, 'def': 'a block in the (approximate) shape of a cube', 'name': 'cube'}, {'frequency': 'f', 'synset': 'cucumber.n.02', 'synonyms': ['cucumber', 'cuke'], 'id': 342, 'def': 'cylindrical green fruit with thin green rind and white flesh eaten as a vegetable', 'name': 'cucumber'}, {'frequency': 'c', 'synset': 'cufflink.n.01', 'synonyms': ['cufflink'], 'id': 343, 'def': 'jewelry consisting of linked buttons used to fasten the cuffs of a shirt', 'name': 'cufflink'}, {'frequency': 'f', 'synset': 'cup.n.01', 'synonyms': ['cup'], 'id': 344, 'def': 'a small open container usually used for drinking; usually has a handle', 'name': 'cup'}, {'frequency': 'c', 'synset': 'cup.n.08', 'synonyms': ['trophy_cup'], 'id': 345, 'def': 'a metal award or cup-shaped vessel with handles that is awarded as a trophy to a competition winner', 'name': 'trophy_cup'}, {'frequency': 'f', 'synset': 'cupboard.n.01', 'synonyms': ['cupboard', 'closet'], 'id': 346, 'def': 'a small room (or recess) or cabinet used for storage space', 'name': 'cupboard'}, {'frequency': 'f', 'synset': 'cupcake.n.01', 'synonyms': ['cupcake'], 'id': 347, 'def': 'small cake baked in a muffin tin', 'name': 'cupcake'}, {'frequency': 'r', 'synset': 'curler.n.01', 'synonyms': ['hair_curler', 'hair_roller', 'hair_crimper'], 'id': 348, 'def': 'a cylindrical tube around which the hair is wound to curl it', 'name': 'hair_curler'}, {'frequency': 'r', 'synset': 'curling_iron.n.01', 'synonyms': ['curling_iron'], 'id': 349, 'def': 'a cylindrical home appliance that heats hair that has been curled around it', 'name': 'curling_iron'}, {'frequency': 'f', 'synset': 'curtain.n.01', 'synonyms': ['curtain', 'drapery'], 'id': 350, 'def': 'hanging cloth used as a blind (especially for a window)', 'name': 'curtain'}, {'frequency': 'f', 'synset': 'cushion.n.03', 'synonyms': ['cushion'], 'id': 351, 'def': 'a soft bag filled with air or padding such as feathers or foam rubber', 'name': 'cushion'}, {'frequency': 'r', 'synset': 'cylinder.n.04', 'synonyms': ['cylinder'], 'id': 352, 'def': 'a cylindrical container', 'name': 'cylinder'}, {'frequency': 'r', 'synset': 'cymbal.n.01', 'synonyms': ['cymbal'], 'id': 353, 'def': 'a percussion instrument consisting of a concave brass disk', 'name': 'cymbal'}, {'frequency': 'r', 'synset': 'dagger.n.01', 'synonyms': ['dagger'], 'id': 354, 'def': 'a short knife with a pointed blade used for piercing or stabbing', 'name': 'dagger'}, {'frequency': 'r', 'synset': 'dalmatian.n.02', 'synonyms': ['dalmatian'], 'id': 355, 'def': 'a large breed having a smooth white coat with black or brown spots', 'name': 'dalmatian'}, {'frequency': 'c', 'synset': 'dartboard.n.01', 'synonyms': ['dartboard'], 'id': 356, 'def': 'a circular board of wood or cork used as the target in the game of darts', 'name': 'dartboard'}, {'frequency': 'r', 'synset': 'date.n.08', 'synonyms': ['date_(fruit)'], 'id': 357, 'def': 'sweet edible fruit of the date palm with a single long woody seed', 'name': 'date_(fruit)'}, {'frequency': 'f', 'synset': 'deck_chair.n.01', 'synonyms': ['deck_chair', 'beach_chair'], 'id': 358, 'def': 'a folding chair for use outdoors; a wooden frame supports a length of canvas', 'name': 'deck_chair'}, {'frequency': 'c', 'synset': 'deer.n.01', 'synonyms': ['deer', 'cervid'], 'id': 359, 'def': "distinguished from Bovidae by the male's having solid deciduous antlers", 'name': 'deer'}, {'frequency': 'c', 'synset': 'dental_floss.n.01', 'synonyms': ['dental_floss', 'floss'], 'id': 360, 'def': 'a soft thread for cleaning the spaces between the teeth', 'name': 'dental_floss'}, {'frequency': 'f', 'synset': 'desk.n.01', 'synonyms': ['desk'], 'id': 361, 'def': 'a piece of furniture with a writing surface and usually drawers or other compartments', 'name': 'desk'}, {'frequency': 'r', 'synset': 'detergent.n.01', 'synonyms': ['detergent'], 'id': 362, 'def': 'a surface-active chemical widely used in industry and laundering', 'name': 'detergent'}, {'frequency': 'c', 'synset': 'diaper.n.01', 'synonyms': ['diaper'], 'id': 363, 'def': 'garment consisting of a folded cloth drawn up between the legs and fastened at the waist', 'name': 'diaper'}, {'frequency': 'r', 'synset': 'diary.n.01', 'synonyms': ['diary', 'journal'], 'id': 364, 'def': 'yearly planner book', 'name': 'diary'}, {'frequency': 'r', 'synset': 'die.n.01', 'synonyms': ['die', 'dice'], 'id': 365, 'def': 'a small cube with 1 to 6 spots on the six faces; used in gambling', 'name': 'die'}, {'frequency': 'r', 'synset': 'dinghy.n.01', 'synonyms': ['dinghy', 'dory', 'rowboat'], 'id': 366, 'def': 'a small boat of shallow draft with seats and oars with which it is propelled', 'name': 'dinghy'}, {'frequency': 'f', 'synset': 'dining_table.n.01', 'synonyms': ['dining_table'], 'id': 367, 'def': 'a table at which meals are served', 'name': 'dining_table'}, {'frequency': 'r', 'synset': 'dinner_jacket.n.01', 'synonyms': ['tux', 'tuxedo'], 'id': 368, 'def': 'semiformal evening dress for men', 'name': 'tux'}, {'frequency': 'f', 'synset': 'dish.n.01', 'synonyms': ['dish'], 'id': 369, 'def': 'a piece of dishware normally used as a container for holding or serving food', 'name': 'dish'}, {'frequency': 'c', 'synset': 'dish.n.05', 'synonyms': ['dish_antenna'], 'id': 370, 'def': 'directional antenna consisting of a parabolic reflector', 'name': 'dish_antenna'}, {'frequency': 'c', 'synset': 'dishrag.n.01', 'synonyms': ['dishrag', 'dishcloth'], 'id': 371, 'def': 'a cloth for washing dishes or cleaning in general', 'name': 'dishrag'}, {'frequency': 'f', 'synset': 'dishtowel.n.01', 'synonyms': ['dishtowel', 'tea_towel'], 'id': 372, 'def': 'a towel for drying dishes', 'name': 'dishtowel'}, {'frequency': 'f', 'synset': 'dishwasher.n.01', 'synonyms': ['dishwasher', 'dishwashing_machine'], 'id': 373, 'def': 'a machine for washing dishes', 'name': 'dishwasher'}, {'frequency': 'r', 'synset': 'dishwasher_detergent.n.01', 'synonyms': ['dishwasher_detergent', 'dishwashing_detergent', 'dishwashing_liquid', 'dishsoap'], 'id': 374, 'def': 'dishsoap or dish detergent designed for use in dishwashers', 'name': 'dishwasher_detergent'}, {'frequency': 'f', 'synset': 'dispenser.n.01', 'synonyms': ['dispenser'], 'id': 375, 'def': 'a container so designed that the contents can be used in prescribed amounts', 'name': 'dispenser'}, {'frequency': 'r', 'synset': 'diving_board.n.01', 'synonyms': ['diving_board'], 'id': 376, 'def': 'a springboard from which swimmers can dive', 'name': 'diving_board'}, {'frequency': 'f', 'synset': 'dixie_cup.n.01', 'synonyms': ['Dixie_cup', 'paper_cup'], 'id': 377, 'def': 'a disposable cup made of paper; for holding drinks', 'name': 'Dixie_cup'}, {'frequency': 'f', 'synset': 'dog.n.01', 'synonyms': ['dog'], 'id': 378, 'def': 'a common domesticated dog', 'name': 'dog'}, {'frequency': 'f', 'synset': 'dog_collar.n.01', 'synonyms': ['dog_collar'], 'id': 379, 'def': 'a collar for a dog', 'name': 'dog_collar'}, {'frequency': 'f', 'synset': 'doll.n.01', 'synonyms': ['doll'], 'id': 380, 'def': 'a toy replica of a HUMAN (NOT AN ANIMAL)', 'name': 'doll'}, {'frequency': 'r', 'synset': 'dollar.n.02', 'synonyms': ['dollar', 'dollar_bill', 'one_dollar_bill'], 'id': 381, 'def': 'a piece of paper money worth one dollar', 'name': 'dollar'}, {'frequency': 'r', 'synset': 'dollhouse.n.01', 'synonyms': ['dollhouse', "doll's_house"], 'id': 382, 'def': "a house so small that it is likened to a child's plaything", 'name': 'dollhouse'}, {'frequency': 'c', 'synset': 'dolphin.n.02', 'synonyms': ['dolphin'], 'id': 383, 'def': 'any of various small toothed whales with a beaklike snout; larger than porpoises', 'name': 'dolphin'}, {'frequency': 'c', 'synset': 'domestic_ass.n.01', 'synonyms': ['domestic_ass', 'donkey'], 'id': 384, 'def': 'domestic beast of burden descended from the African wild ass; patient but stubborn', 'name': 'domestic_ass'}, {'frequency': 'f', 'synset': 'doorknob.n.01', 'synonyms': ['doorknob', 'doorhandle'], 'id': 385, 'def': "a knob used to open a door (often called `doorhandle' in Great Britain)", 'name': 'doorknob'}, {'frequency': 'c', 'synset': 'doormat.n.02', 'synonyms': ['doormat', 'welcome_mat'], 'id': 386, 'def': 'a mat placed outside an exterior door for wiping the shoes before entering', 'name': 'doormat'}, {'frequency': 'f', 'synset': 'doughnut.n.02', 'synonyms': ['doughnut', 'donut'], 'id': 387, 'def': 'a small ring-shaped friedcake', 'name': 'doughnut'}, {'frequency': 'r', 'synset': 'dove.n.01', 'synonyms': ['dove'], 'id': 388, 'def': 'any of numerous small pigeons', 'name': 'dove'}, {'frequency': 'r', 'synset': 'dragonfly.n.01', 'synonyms': ['dragonfly'], 'id': 389, 'def': 'slender-bodied non-stinging insect having iridescent wings that are outspread at rest', 'name': 'dragonfly'}, {'frequency': 'f', 'synset': 'drawer.n.01', 'synonyms': ['drawer'], 'id': 390, 'def': 'a boxlike container in a piece of furniture; made so as to slide in and out', 'name': 'drawer'}, {'frequency': 'c', 'synset': 'drawers.n.01', 'synonyms': ['underdrawers', 'boxers', 'boxershorts'], 'id': 391, 'def': 'underpants worn by men', 'name': 'underdrawers'}, {'frequency': 'f', 'synset': 'dress.n.01', 'synonyms': ['dress', 'frock'], 'id': 392, 'def': 'a one-piece garment for a woman; has skirt and bodice', 'name': 'dress'}, {'frequency': 'c', 'synset': 'dress_hat.n.01', 'synonyms': ['dress_hat', 'high_hat', 'opera_hat', 'silk_hat', 'top_hat'], 'id': 393, 'def': "a man's hat with a tall crown; usually covered with silk or with beaver fur", 'name': 'dress_hat'}, {'frequency': 'f', 'synset': 'dress_suit.n.01', 'synonyms': ['dress_suit'], 'id': 394, 'def': 'formalwear consisting of full evening dress for men', 'name': 'dress_suit'}, {'frequency': 'f', 'synset': 'dresser.n.05', 'synonyms': ['dresser'], 'id': 395, 'def': 'a cabinet with shelves', 'name': 'dresser'}, {'frequency': 'c', 'synset': 'drill.n.01', 'synonyms': ['drill'], 'id': 396, 'def': 'a tool with a sharp rotating point for making holes in hard materials', 'name': 'drill'}, {'frequency': 'r', 'synset': 'drone.n.04', 'synonyms': ['drone'], 'id': 397, 'def': 'an aircraft without a pilot that is operated by remote control', 'name': 'drone'}, {'frequency': 'r', 'synset': 'dropper.n.01', 'synonyms': ['dropper', 'eye_dropper'], 'id': 398, 'def': 'pipet consisting of a small tube with a vacuum bulb at one end for drawing liquid in and releasing it a drop at a time', 'name': 'dropper'}, {'frequency': 'c', 'synset': 'drum.n.01', 'synonyms': ['drum_(musical_instrument)'], 'id': 399, 'def': 'a musical percussion instrument; usually consists of a hollow cylinder with a membrane stretched across each end', 'name': 'drum_(musical_instrument)'}, {'frequency': 'r', 'synset': 'drumstick.n.02', 'synonyms': ['drumstick'], 'id': 400, 'def': 'a stick used for playing a drum', 'name': 'drumstick'}, {'frequency': 'f', 'synset': 'duck.n.01', 'synonyms': ['duck'], 'id': 401, 'def': 'small web-footed broad-billed swimming bird', 'name': 'duck'}, {'frequency': 'c', 'synset': 'duckling.n.02', 'synonyms': ['duckling'], 'id': 402, 'def': 'young duck', 'name': 'duckling'}, {'frequency': 'c', 'synset': 'duct_tape.n.01', 'synonyms': ['duct_tape'], 'id': 403, 'def': 'a wide silvery adhesive tape', 'name': 'duct_tape'}, {'frequency': 'f', 'synset': 'duffel_bag.n.01', 'synonyms': ['duffel_bag', 'duffle_bag', 'duffel', 'duffle'], 'id': 404, 'def': 'a large cylindrical bag of heavy cloth (does not include suitcases)', 'name': 'duffel_bag'}, {'frequency': 'r', 'synset': 'dumbbell.n.01', 'synonyms': ['dumbbell'], 'id': 405, 'def': 'an exercising weight with two ball-like ends connected by a short handle', 'name': 'dumbbell'}, {'frequency': 'c', 'synset': 'dumpster.n.01', 'synonyms': ['dumpster'], 'id': 406, 'def': 'a container designed to receive and transport and dump waste', 'name': 'dumpster'}, {'frequency': 'r', 'synset': 'dustpan.n.02', 'synonyms': ['dustpan'], 'id': 407, 'def': 'a short-handled receptacle into which dust can be swept', 'name': 'dustpan'}, {'frequency': 'c', 'synset': 'eagle.n.01', 'synonyms': ['eagle'], 'id': 408, 'def': 'large birds of prey noted for their broad wings and strong soaring flight', 'name': 'eagle'}, {'frequency': 'f', 'synset': 'earphone.n.01', 'synonyms': ['earphone', 'earpiece', 'headphone'], 'id': 409, 'def': 'device for listening to audio that is held over or inserted into the ear', 'name': 'earphone'}, {'frequency': 'r', 'synset': 'earplug.n.01', 'synonyms': ['earplug'], 'id': 410, 'def': 'a soft plug that is inserted into the ear canal to block sound', 'name': 'earplug'}, {'frequency': 'f', 'synset': 'earring.n.01', 'synonyms': ['earring'], 'id': 411, 'def': 'jewelry to ornament the ear', 'name': 'earring'}, {'frequency': 'c', 'synset': 'easel.n.01', 'synonyms': ['easel'], 'id': 412, 'def': "an upright tripod for displaying something (usually an artist's canvas)", 'name': 'easel'}, {'frequency': 'r', 'synset': 'eclair.n.01', 'synonyms': ['eclair'], 'id': 413, 'def': 'oblong cream puff', 'name': 'eclair'}, {'frequency': 'r', 'synset': 'eel.n.01', 'synonyms': ['eel'], 'id': 414, 'def': 'an elongate fish with fatty flesh', 'name': 'eel'}, {'frequency': 'f', 'synset': 'egg.n.02', 'synonyms': ['egg', 'eggs'], 'id': 415, 'def': 'oval reproductive body of a fowl (especially a hen) used as food', 'name': 'egg'}, {'frequency': 'r', 'synset': 'egg_roll.n.01', 'synonyms': ['egg_roll', 'spring_roll'], 'id': 416, 'def': 'minced vegetables and meat wrapped in a pancake and fried', 'name': 'egg_roll'}, {'frequency': 'c', 'synset': 'egg_yolk.n.01', 'synonyms': ['egg_yolk', 'yolk_(egg)'], 'id': 417, 'def': 'the yellow spherical part of an egg', 'name': 'egg_yolk'}, {'frequency': 'c', 'synset': 'eggbeater.n.02', 'synonyms': ['eggbeater', 'eggwhisk'], 'id': 418, 'def': 'a mixer for beating eggs or whipping cream', 'name': 'eggbeater'}, {'frequency': 'c', 'synset': 'eggplant.n.01', 'synonyms': ['eggplant', 'aubergine'], 'id': 419, 'def': 'egg-shaped vegetable having a shiny skin typically dark purple', 'name': 'eggplant'}, {'frequency': 'r', 'synset': 'electric_chair.n.01', 'synonyms': ['electric_chair'], 'id': 420, 'def': 'a chair-shaped instrument of execution by electrocution', 'name': 'electric_chair'}, {'frequency': 'f', 'synset': 'electric_refrigerator.n.01', 'synonyms': ['refrigerator'], 'id': 421, 'def': 'a refrigerator in which the coolant is pumped around by an electric motor', 'name': 'refrigerator'}, {'frequency': 'f', 'synset': 'elephant.n.01', 'synonyms': ['elephant'], 'id': 422, 'def': 'a common elephant', 'name': 'elephant'}, {'frequency': 'c', 'synset': 'elk.n.01', 'synonyms': ['elk', 'moose'], 'id': 423, 'def': 'large northern deer with enormous flattened antlers in the male', 'name': 'elk'}, {'frequency': 'c', 'synset': 'envelope.n.01', 'synonyms': ['envelope'], 'id': 424, 'def': 'a flat (usually rectangular) container for a letter, thin package, etc.', 'name': 'envelope'}, {'frequency': 'c', 'synset': 'eraser.n.01', 'synonyms': ['eraser'], 'id': 425, 'def': 'an implement used to erase something', 'name': 'eraser'}, {'frequency': 'r', 'synset': 'escargot.n.01', 'synonyms': ['escargot'], 'id': 426, 'def': 'edible snail usually served in the shell with a sauce of melted butter and garlic', 'name': 'escargot'}, {'frequency': 'r', 'synset': 'eyepatch.n.01', 'synonyms': ['eyepatch'], 'id': 427, 'def': 'a protective cloth covering for an injured eye', 'name': 'eyepatch'}, {'frequency': 'r', 'synset': 'falcon.n.01', 'synonyms': ['falcon'], 'id': 428, 'def': 'birds of prey having long pointed powerful wings adapted for swift flight', 'name': 'falcon'}, {'frequency': 'f', 'synset': 'fan.n.01', 'synonyms': ['fan'], 'id': 429, 'def': 'a device for creating a current of air by movement of a surface or surfaces', 'name': 'fan'}, {'frequency': 'f', 'synset': 'faucet.n.01', 'synonyms': ['faucet', 'spigot', 'tap'], 'id': 430, 'def': 'a regulator for controlling the flow of a liquid from a reservoir', 'name': 'faucet'}, {'frequency': 'r', 'synset': 'fedora.n.01', 'synonyms': ['fedora'], 'id': 431, 'def': 'a hat made of felt with a creased crown', 'name': 'fedora'}, {'frequency': 'r', 'synset': 'ferret.n.02', 'synonyms': ['ferret'], 'id': 432, 'def': 'domesticated albino variety of the European polecat bred for hunting rats and rabbits', 'name': 'ferret'}, {'frequency': 'c', 'synset': 'ferris_wheel.n.01', 'synonyms': ['Ferris_wheel'], 'id': 433, 'def': 'a large wheel with suspended seats that remain upright as the wheel rotates', 'name': 'Ferris_wheel'}, {'frequency': 'c', 'synset': 'ferry.n.01', 'synonyms': ['ferry', 'ferryboat'], 'id': 434, 'def': 'a boat that transports people or vehicles across a body of water and operates on a regular schedule', 'name': 'ferry'}, {'frequency': 'r', 'synset': 'fig.n.04', 'synonyms': ['fig_(fruit)'], 'id': 435, 'def': 'fleshy sweet pear-shaped yellowish or purple fruit eaten fresh or preserved or dried', 'name': 'fig_(fruit)'}, {'frequency': 'c', 'synset': 'fighter.n.02', 'synonyms': ['fighter_jet', 'fighter_aircraft', 'attack_aircraft'], 'id': 436, 'def': 'a high-speed military or naval airplane designed to destroy enemy targets', 'name': 'fighter_jet'}, {'frequency': 'f', 'synset': 'figurine.n.01', 'synonyms': ['figurine'], 'id': 437, 'def': 'a small carved or molded figure', 'name': 'figurine'}, {'frequency': 'c', 'synset': 'file.n.03', 'synonyms': ['file_cabinet', 'filing_cabinet'], 'id': 438, 'def': 'office furniture consisting of a container for keeping papers in order', 'name': 'file_cabinet'}, {'frequency': 'r', 'synset': 'file.n.04', 'synonyms': ['file_(tool)'], 'id': 439, 'def': 'a steel hand tool with small sharp teeth on some or all of its surfaces; used for smoothing wood or metal', 'name': 'file_(tool)'}, {'frequency': 'f', 'synset': 'fire_alarm.n.02', 'synonyms': ['fire_alarm', 'smoke_alarm'], 'id': 440, 'def': 'an alarm that is tripped off by fire or smoke', 'name': 'fire_alarm'}, {'frequency': 'f', 'synset': 'fire_engine.n.01', 'synonyms': ['fire_engine', 'fire_truck'], 'id': 441, 'def': 'large trucks that carry firefighters and equipment to the site of a fire', 'name': 'fire_engine'}, {'frequency': 'f', 'synset': 'fire_extinguisher.n.01', 'synonyms': ['fire_extinguisher', 'extinguisher'], 'id': 442, 'def': 'a manually operated device for extinguishing small fires', 'name': 'fire_extinguisher'}, {'frequency': 'c', 'synset': 'fire_hose.n.01', 'synonyms': ['fire_hose'], 'id': 443, 'def': 'a large hose that carries water from a fire hydrant to the site of the fire', 'name': 'fire_hose'}, {'frequency': 'f', 'synset': 'fireplace.n.01', 'synonyms': ['fireplace'], 'id': 444, 'def': 'an open recess in a wall at the base of a chimney where a fire can be built', 'name': 'fireplace'}, {'frequency': 'f', 'synset': 'fireplug.n.01', 'synonyms': ['fireplug', 'fire_hydrant', 'hydrant'], 'id': 445, 'def': 'an upright hydrant for drawing water to use in fighting a fire', 'name': 'fireplug'}, {'frequency': 'r', 'synset': 'first-aid_kit.n.01', 'synonyms': ['first-aid_kit'], 'id': 446, 'def': 'kit consisting of a set of bandages and medicines for giving first aid', 'name': 'first-aid_kit'}, {'frequency': 'f', 'synset': 'fish.n.01', 'synonyms': ['fish'], 'id': 447, 'def': 'any of various mostly cold-blooded aquatic vertebrates usually having scales and breathing through gills', 'name': 'fish'}, {'frequency': 'c', 'synset': 'fish.n.02', 'synonyms': ['fish_(food)'], 'id': 448, 'def': 'the flesh of fish used as food', 'name': 'fish_(food)'}, {'frequency': 'r', 'synset': 'fishbowl.n.02', 'synonyms': ['fishbowl', 'goldfish_bowl'], 'id': 449, 'def': 'a transparent bowl in which small fish are kept', 'name': 'fishbowl'}, {'frequency': 'c', 'synset': 'fishing_rod.n.01', 'synonyms': ['fishing_rod', 'fishing_pole'], 'id': 450, 'def': 'a rod that is used in fishing to extend the fishing line', 'name': 'fishing_rod'}, {'frequency': 'f', 'synset': 'flag.n.01', 'synonyms': ['flag'], 'id': 451, 'def': 'emblem usually consisting of a rectangular piece of cloth of distinctive design (do not include pole)', 'name': 'flag'}, {'frequency': 'f', 'synset': 'flagpole.n.02', 'synonyms': ['flagpole', 'flagstaff'], 'id': 452, 'def': 'a tall staff or pole on which a flag is raised', 'name': 'flagpole'}, {'frequency': 'c', 'synset': 'flamingo.n.01', 'synonyms': ['flamingo'], 'id': 453, 'def': 'large pink web-footed bird with down-bent bill', 'name': 'flamingo'}, {'frequency': 'c', 'synset': 'flannel.n.01', 'synonyms': ['flannel'], 'id': 454, 'def': 'a soft light woolen fabric; used for clothing', 'name': 'flannel'}, {'frequency': 'c', 'synset': 'flap.n.01', 'synonyms': ['flap'], 'id': 455, 'def': 'any broad thin covering attached at one edge, such as a mud flap next to a wheel or a flap on an airplane wing', 'name': 'flap'}, {'frequency': 'r', 'synset': 'flash.n.10', 'synonyms': ['flash', 'flashbulb'], 'id': 456, 'def': 'a lamp for providing momentary light to take a photograph', 'name': 'flash'}, {'frequency': 'c', 'synset': 'flashlight.n.01', 'synonyms': ['flashlight', 'torch'], 'id': 457, 'def': 'a small portable battery-powered electric lamp', 'name': 'flashlight'}, {'frequency': 'r', 'synset': 'fleece.n.03', 'synonyms': ['fleece'], 'id': 458, 'def': 'a soft bulky fabric with deep pile; used chiefly for clothing', 'name': 'fleece'}, {'frequency': 'f', 'synset': 'flip-flop.n.02', 'synonyms': ['flip-flop_(sandal)'], 'id': 459, 'def': 'a backless sandal held to the foot by a thong between two toes', 'name': 'flip-flop_(sandal)'}, {'frequency': 'c', 'synset': 'flipper.n.01', 'synonyms': ['flipper_(footwear)', 'fin_(footwear)'], 'id': 460, 'def': 'a shoe to aid a person in swimming', 'name': 'flipper_(footwear)'}, {'frequency': 'f', 'synset': 'flower_arrangement.n.01', 'synonyms': ['flower_arrangement', 'floral_arrangement'], 'id': 461, 'def': 'a decorative arrangement of flowers', 'name': 'flower_arrangement'}, {'frequency': 'c', 'synset': 'flute.n.02', 'synonyms': ['flute_glass', 'champagne_flute'], 'id': 462, 'def': 'a tall narrow wineglass', 'name': 'flute_glass'}, {'frequency': 'c', 'synset': 'foal.n.01', 'synonyms': ['foal'], 'id': 463, 'def': 'a young horse', 'name': 'foal'}, {'frequency': 'c', 'synset': 'folding_chair.n.01', 'synonyms': ['folding_chair'], 'id': 464, 'def': 'a chair that can be folded flat for storage', 'name': 'folding_chair'}, {'frequency': 'c', 'synset': 'food_processor.n.01', 'synonyms': ['food_processor'], 'id': 465, 'def': 'a kitchen appliance for shredding, blending, chopping, or slicing food', 'name': 'food_processor'}, {'frequency': 'c', 'synset': 'football.n.02', 'synonyms': ['football_(American)'], 'id': 466, 'def': 'the inflated oblong ball used in playing American football', 'name': 'football_(American)'}, {'frequency': 'r', 'synset': 'football_helmet.n.01', 'synonyms': ['football_helmet'], 'id': 467, 'def': 'a padded helmet with a face mask to protect the head of football players', 'name': 'football_helmet'}, {'frequency': 'c', 'synset': 'footstool.n.01', 'synonyms': ['footstool', 'footrest'], 'id': 468, 'def': 'a low seat or a stool to rest the feet of a seated person', 'name': 'footstool'}, {'frequency': 'f', 'synset': 'fork.n.01', 'synonyms': ['fork'], 'id': 469, 'def': 'cutlery used for serving and eating food', 'name': 'fork'}, {'frequency': 'c', 'synset': 'forklift.n.01', 'synonyms': ['forklift'], 'id': 470, 'def': 'an industrial vehicle with a power operated fork in front that can be inserted under loads to lift and move them', 'name': 'forklift'}, {'frequency': 'c', 'synset': 'freight_car.n.01', 'synonyms': ['freight_car'], 'id': 471, 'def': 'a railway car that carries freight', 'name': 'freight_car'}, {'frequency': 'c', 'synset': 'french_toast.n.01', 'synonyms': ['French_toast'], 'id': 472, 'def': 'bread slice dipped in egg and milk and fried', 'name': 'French_toast'}, {'frequency': 'c', 'synset': 'freshener.n.01', 'synonyms': ['freshener', 'air_freshener'], 'id': 473, 'def': 'anything that freshens air by removing or covering odor', 'name': 'freshener'}, {'frequency': 'f', 'synset': 'frisbee.n.01', 'synonyms': ['frisbee'], 'id': 474, 'def': 'a light, plastic disk propelled with a flip of the wrist for recreation or competition', 'name': 'frisbee'}, {'frequency': 'c', 'synset': 'frog.n.01', 'synonyms': ['frog', 'toad', 'toad_frog'], 'id': 475, 'def': 'a tailless stout-bodied amphibians with long hind limbs for leaping', 'name': 'frog'}, {'frequency': 'c', 'synset': 'fruit_juice.n.01', 'synonyms': ['fruit_juice'], 'id': 476, 'def': 'drink produced by squeezing or crushing fruit', 'name': 'fruit_juice'}, {'frequency': 'f', 'synset': 'frying_pan.n.01', 'synonyms': ['frying_pan', 'frypan', 'skillet'], 'id': 477, 'def': 'a pan used for frying foods', 'name': 'frying_pan'}, {'frequency': 'r', 'synset': 'fudge.n.01', 'synonyms': ['fudge'], 'id': 478, 'def': 'soft creamy candy', 'name': 'fudge'}, {'frequency': 'r', 'synset': 'funnel.n.02', 'synonyms': ['funnel'], 'id': 479, 'def': 'a cone-shaped utensil used to channel a substance into a container with a small mouth', 'name': 'funnel'}, {'frequency': 'r', 'synset': 'futon.n.01', 'synonyms': ['futon'], 'id': 480, 'def': 'a pad that is used for sleeping on the floor or on a raised frame', 'name': 'futon'}, {'frequency': 'r', 'synset': 'gag.n.02', 'synonyms': ['gag', 'muzzle'], 'id': 481, 'def': "restraint put into a person's mouth to prevent speaking or shouting", 'name': 'gag'}, {'frequency': 'r', 'synset': 'garbage.n.03', 'synonyms': ['garbage'], 'id': 482, 'def': 'a receptacle where waste can be discarded', 'name': 'garbage'}, {'frequency': 'c', 'synset': 'garbage_truck.n.01', 'synonyms': ['garbage_truck'], 'id': 483, 'def': 'a truck for collecting domestic refuse', 'name': 'garbage_truck'}, {'frequency': 'c', 'synset': 'garden_hose.n.01', 'synonyms': ['garden_hose'], 'id': 484, 'def': 'a hose used for watering a lawn or garden', 'name': 'garden_hose'}, {'frequency': 'c', 'synset': 'gargle.n.01', 'synonyms': ['gargle', 'mouthwash'], 'id': 485, 'def': 'a medicated solution used for gargling and rinsing the mouth', 'name': 'gargle'}, {'frequency': 'r', 'synset': 'gargoyle.n.02', 'synonyms': ['gargoyle'], 'id': 486, 'def': 'an ornament consisting of a grotesquely carved figure of a person or animal', 'name': 'gargoyle'}, {'frequency': 'c', 'synset': 'garlic.n.02', 'synonyms': ['garlic', 'ail'], 'id': 487, 'def': 'aromatic bulb used as seasoning', 'name': 'garlic'}, {'frequency': 'r', 'synset': 'gasmask.n.01', 'synonyms': ['gasmask', 'respirator', 'gas_helmet'], 'id': 488, 'def': 'a protective face mask with a filter', 'name': 'gasmask'}, {'frequency': 'c', 'synset': 'gazelle.n.01', 'synonyms': ['gazelle'], 'id': 489, 'def': 'small swift graceful antelope of Africa and Asia having lustrous eyes', 'name': 'gazelle'}, {'frequency': 'c', 'synset': 'gelatin.n.02', 'synonyms': ['gelatin', 'jelly'], 'id': 490, 'def': 'an edible jelly made with gelatin and used as a dessert or salad base or a coating for foods', 'name': 'gelatin'}, {'frequency': 'r', 'synset': 'gem.n.02', 'synonyms': ['gemstone'], 'id': 491, 'def': 'a crystalline rock that can be cut and polished for jewelry', 'name': 'gemstone'}, {'frequency': 'r', 'synset': 'generator.n.02', 'synonyms': ['generator'], 'id': 492, 'def': 'engine that converts mechanical energy into electrical energy by electromagnetic induction', 'name': 'generator'}, {'frequency': 'c', 'synset': 'giant_panda.n.01', 'synonyms': ['giant_panda', 'panda', 'panda_bear'], 'id': 493, 'def': 'large black-and-white herbivorous mammal of bamboo forests of China and Tibet', 'name': 'giant_panda'}, {'frequency': 'c', 'synset': 'gift_wrap.n.01', 'synonyms': ['gift_wrap'], 'id': 494, 'def': 'attractive wrapping paper suitable for wrapping gifts', 'name': 'gift_wrap'}, {'frequency': 'c', 'synset': 'ginger.n.03', 'synonyms': ['ginger', 'gingerroot'], 'id': 495, 'def': 'the root of the common ginger plant; used fresh as a seasoning', 'name': 'ginger'}, {'frequency': 'f', 'synset': 'giraffe.n.01', 'synonyms': ['giraffe'], 'id': 496, 'def': 'tall animal having a spotted coat and small horns and very long neck and legs', 'name': 'giraffe'}, {'frequency': 'c', 'synset': 'girdle.n.02', 'synonyms': ['cincture', 'sash', 'waistband', 'waistcloth'], 'id': 497, 'def': 'a band of material around the waist that strengthens a skirt or trousers', 'name': 'cincture'}, {'frequency': 'f', 'synset': 'glass.n.02', 'synonyms': ['glass_(drink_container)', 'drinking_glass'], 'id': 498, 'def': 'a container for holding liquids while drinking', 'name': 'glass_(drink_container)'}, {'frequency': 'c', 'synset': 'globe.n.03', 'synonyms': ['globe'], 'id': 499, 'def': 'a sphere on which a map (especially of the earth) is represented', 'name': 'globe'}, {'frequency': 'f', 'synset': 'glove.n.02', 'synonyms': ['glove'], 'id': 500, 'def': 'handwear covering the hand', 'name': 'glove'}, {'frequency': 'c', 'synset': 'goat.n.01', 'synonyms': ['goat'], 'id': 501, 'def': 'a common goat', 'name': 'goat'}, {'frequency': 'f', 'synset': 'goggles.n.01', 'synonyms': ['goggles'], 'id': 502, 'def': 'tight-fitting spectacles worn to protect the eyes', 'name': 'goggles'}, {'frequency': 'r', 'synset': 'goldfish.n.01', 'synonyms': ['goldfish'], 'id': 503, 'def': 'small golden or orange-red freshwater fishes used as pond or aquarium pets', 'name': 'goldfish'}, {'frequency': 'c', 'synset': 'golf_club.n.02', 'synonyms': ['golf_club', 'golf-club'], 'id': 504, 'def': 'golf equipment used by a golfer to hit a golf ball', 'name': 'golf_club'}, {'frequency': 'c', 'synset': 'golfcart.n.01', 'synonyms': ['golfcart'], 'id': 505, 'def': 'a small motor vehicle in which golfers can ride between shots', 'name': 'golfcart'}, {'frequency': 'r', 'synset': 'gondola.n.02', 'synonyms': ['gondola_(boat)'], 'id': 506, 'def': 'long narrow flat-bottomed boat propelled by sculling; traditionally used on canals of Venice', 'name': 'gondola_(boat)'}, {'frequency': 'c', 'synset': 'goose.n.01', 'synonyms': ['goose'], 'id': 507, 'def': 'loud, web-footed long-necked aquatic birds usually larger than ducks', 'name': 'goose'}, {'frequency': 'r', 'synset': 'gorilla.n.01', 'synonyms': ['gorilla'], 'id': 508, 'def': 'largest ape', 'name': 'gorilla'}, {'frequency': 'r', 'synset': 'gourd.n.02', 'synonyms': ['gourd'], 'id': 509, 'def': 'any of numerous inedible fruits with hard rinds', 'name': 'gourd'}, {'frequency': 'f', 'synset': 'grape.n.01', 'synonyms': ['grape'], 'id': 510, 'def': 'any of various juicy fruit with green or purple skins; grow in clusters', 'name': 'grape'}, {'frequency': 'c', 'synset': 'grater.n.01', 'synonyms': ['grater'], 'id': 511, 'def': 'utensil with sharp perforations for shredding foods (as vegetables or cheese)', 'name': 'grater'}, {'frequency': 'c', 'synset': 'gravestone.n.01', 'synonyms': ['gravestone', 'headstone', 'tombstone'], 'id': 512, 'def': 'a stone that is used to mark a grave', 'name': 'gravestone'}, {'frequency': 'r', 'synset': 'gravy_boat.n.01', 'synonyms': ['gravy_boat', 'gravy_holder'], 'id': 513, 'def': 'a dish (often boat-shaped) for serving gravy or sauce', 'name': 'gravy_boat'}, {'frequency': 'f', 'synset': 'green_bean.n.02', 'synonyms': ['green_bean'], 'id': 514, 'def': 'a common bean plant cultivated for its slender green edible pods', 'name': 'green_bean'}, {'frequency': 'f', 'synset': 'green_onion.n.01', 'synonyms': ['green_onion', 'spring_onion', 'scallion'], 'id': 515, 'def': 'a young onion before the bulb has enlarged', 'name': 'green_onion'}, {'frequency': 'r', 'synset': 'griddle.n.01', 'synonyms': ['griddle'], 'id': 516, 'def': 'cooking utensil consisting of a flat heated surface on which food is cooked', 'name': 'griddle'}, {'frequency': 'f', 'synset': 'grill.n.02', 'synonyms': ['grill', 'grille', 'grillwork', 'radiator_grille'], 'id': 517, 'def': 'a framework of metal bars used as a partition or a grate', 'name': 'grill'}, {'frequency': 'r', 'synset': 'grits.n.01', 'synonyms': ['grits', 'hominy_grits'], 'id': 518, 'def': 'coarsely ground corn boiled as a breakfast dish', 'name': 'grits'}, {'frequency': 'c', 'synset': 'grizzly.n.01', 'synonyms': ['grizzly', 'grizzly_bear'], 'id': 519, 'def': 'powerful brownish-yellow bear of the uplands of western North America', 'name': 'grizzly'}, {'frequency': 'c', 'synset': 'grocery_bag.n.01', 'synonyms': ['grocery_bag'], 'id': 520, 'def': "a sack for holding customer's groceries", 'name': 'grocery_bag'}, {'frequency': 'f', 'synset': 'guitar.n.01', 'synonyms': ['guitar'], 'id': 521, 'def': 'a stringed instrument usually having six strings; played by strumming or plucking', 'name': 'guitar'}, {'frequency': 'c', 'synset': 'gull.n.02', 'synonyms': ['gull', 'seagull'], 'id': 522, 'def': 'mostly white aquatic bird having long pointed wings and short legs', 'name': 'gull'}, {'frequency': 'c', 'synset': 'gun.n.01', 'synonyms': ['gun'], 'id': 523, 'def': 'a weapon that discharges a bullet at high velocity from a metal tube', 'name': 'gun'}, {'frequency': 'f', 'synset': 'hairbrush.n.01', 'synonyms': ['hairbrush'], 'id': 524, 'def': "a brush used to groom a person's hair", 'name': 'hairbrush'}, {'frequency': 'c', 'synset': 'hairnet.n.01', 'synonyms': ['hairnet'], 'id': 525, 'def': 'a small net that someone wears over their hair to keep it in place', 'name': 'hairnet'}, {'frequency': 'c', 'synset': 'hairpin.n.01', 'synonyms': ['hairpin'], 'id': 526, 'def': "a double pronged pin used to hold women's hair in place", 'name': 'hairpin'}, {'frequency': 'r', 'synset': 'halter.n.03', 'synonyms': ['halter_top'], 'id': 527, 'def': "a woman's top that fastens behind the back and neck leaving the back and arms uncovered", 'name': 'halter_top'}, {'frequency': 'f', 'synset': 'ham.n.01', 'synonyms': ['ham', 'jambon', 'gammon'], 'id': 528, 'def': 'meat cut from the thigh of a hog (usually smoked)', 'name': 'ham'}, {'frequency': 'c', 'synset': 'hamburger.n.01', 'synonyms': ['hamburger', 'beefburger', 'burger'], 'id': 529, 'def': 'a sandwich consisting of a patty of minced beef served on a bun', 'name': 'hamburger'}, {'frequency': 'c', 'synset': 'hammer.n.02', 'synonyms': ['hammer'], 'id': 530, 'def': 'a hand tool with a heavy head and a handle; used to deliver an impulsive force by striking', 'name': 'hammer'}, {'frequency': 'c', 'synset': 'hammock.n.02', 'synonyms': ['hammock'], 'id': 531, 'def': 'a hanging bed of canvas or rope netting (usually suspended between two trees)', 'name': 'hammock'}, {'frequency': 'r', 'synset': 'hamper.n.02', 'synonyms': ['hamper'], 'id': 532, 'def': 'a basket usually with a cover', 'name': 'hamper'}, {'frequency': 'c', 'synset': 'hamster.n.01', 'synonyms': ['hamster'], 'id': 533, 'def': 'short-tailed burrowing rodent with large cheek pouches', 'name': 'hamster'}, {'frequency': 'f', 'synset': 'hand_blower.n.01', 'synonyms': ['hair_dryer'], 'id': 534, 'def': 'a hand-held electric blower that can blow warm air onto the hair', 'name': 'hair_dryer'}, {'frequency': 'r', 'synset': 'hand_glass.n.01', 'synonyms': ['hand_glass', 'hand_mirror'], 'id': 535, 'def': 'a mirror intended to be held in the hand', 'name': 'hand_glass'}, {'frequency': 'f', 'synset': 'hand_towel.n.01', 'synonyms': ['hand_towel', 'face_towel'], 'id': 536, 'def': 'a small towel used to dry the hands or face', 'name': 'hand_towel'}, {'frequency': 'c', 'synset': 'handcart.n.01', 'synonyms': ['handcart', 'pushcart', 'hand_truck'], 'id': 537, 'def': 'wheeled vehicle that can be pushed by a person', 'name': 'handcart'}, {'frequency': 'r', 'synset': 'handcuff.n.01', 'synonyms': ['handcuff'], 'id': 538, 'def': 'shackle that consists of a metal loop that can be locked around the wrist', 'name': 'handcuff'}, {'frequency': 'c', 'synset': 'handkerchief.n.01', 'synonyms': ['handkerchief'], 'id': 539, 'def': 'a square piece of cloth used for wiping the eyes or nose or as a costume accessory', 'name': 'handkerchief'}, {'frequency': 'f', 'synset': 'handle.n.01', 'synonyms': ['handle', 'grip', 'handgrip'], 'id': 540, 'def': 'the appendage to an object that is designed to be held in order to use or move it', 'name': 'handle'}, {'frequency': 'r', 'synset': 'handsaw.n.01', 'synonyms': ['handsaw', "carpenter's_saw"], 'id': 541, 'def': 'a saw used with one hand for cutting wood', 'name': 'handsaw'}, {'frequency': 'r', 'synset': 'hardback.n.01', 'synonyms': ['hardback_book', 'hardcover_book'], 'id': 542, 'def': 'a book with cardboard or cloth or leather covers', 'name': 'hardback_book'}, {'frequency': 'r', 'synset': 'harmonium.n.01', 'synonyms': ['harmonium', 'organ_(musical_instrument)', 'reed_organ_(musical_instrument)'], 'id': 543, 'def': 'a free-reed instrument in which air is forced through the reeds by bellows', 'name': 'harmonium'}, {'frequency': 'f', 'synset': 'hat.n.01', 'synonyms': ['hat'], 'id': 544, 'def': 'headwear that protects the head from bad weather, sun, or worn for fashion', 'name': 'hat'}, {'frequency': 'r', 'synset': 'hatbox.n.01', 'synonyms': ['hatbox'], 'id': 545, 'def': 'a round piece of luggage for carrying hats', 'name': 'hatbox'}, {'frequency': 'c', 'synset': 'head_covering.n.01', 'synonyms': ['veil'], 'id': 546, 'def': 'a garment that covers the head OR face', 'name': 'veil'}, {'frequency': 'f', 'synset': 'headband.n.01', 'synonyms': ['headband'], 'id': 547, 'def': 'a band worn around or over the head', 'name': 'headband'}, {'frequency': 'f', 'synset': 'headboard.n.01', 'synonyms': ['headboard'], 'id': 548, 'def': 'a vertical board or panel forming the head of a bedstead', 'name': 'headboard'}, {'frequency': 'f', 'synset': 'headlight.n.01', 'synonyms': ['headlight', 'headlamp'], 'id': 549, 'def': 'a powerful light with reflector; attached to the front of an automobile or locomotive', 'name': 'headlight'}, {'frequency': 'c', 'synset': 'headscarf.n.01', 'synonyms': ['headscarf'], 'id': 550, 'def': 'a kerchief worn over the head and tied under the chin', 'name': 'headscarf'}, {'frequency': 'r', 'synset': 'headset.n.01', 'synonyms': ['headset'], 'id': 551, 'def': 'receiver consisting of a pair of headphones', 'name': 'headset'}, {'frequency': 'c', 'synset': 'headstall.n.01', 'synonyms': ['headstall_(for_horses)', 'headpiece_(for_horses)'], 'id': 552, 'def': "the band that is the part of a bridle that fits around a horse's head", 'name': 'headstall_(for_horses)'}, {'frequency': 'c', 'synset': 'heart.n.02', 'synonyms': ['heart'], 'id': 553, 'def': 'a muscular organ; its contractions move the blood through the body', 'name': 'heart'}, {'frequency': 'c', 'synset': 'heater.n.01', 'synonyms': ['heater', 'warmer'], 'id': 554, 'def': 'device that heats water or supplies warmth to a room', 'name': 'heater'}, {'frequency': 'c', 'synset': 'helicopter.n.01', 'synonyms': ['helicopter'], 'id': 555, 'def': 'an aircraft without wings that obtains its lift from the rotation of overhead blades', 'name': 'helicopter'}, {'frequency': 'f', 'synset': 'helmet.n.02', 'synonyms': ['helmet'], 'id': 556, 'def': 'a protective headgear made of hard material to resist blows', 'name': 'helmet'}, {'frequency': 'r', 'synset': 'heron.n.02', 'synonyms': ['heron'], 'id': 557, 'def': 'grey or white wading bird with long neck and long legs and (usually) long bill', 'name': 'heron'}, {'frequency': 'c', 'synset': 'highchair.n.01', 'synonyms': ['highchair', 'feeding_chair'], 'id': 558, 'def': 'a chair for feeding a very young child', 'name': 'highchair'}, {'frequency': 'f', 'synset': 'hinge.n.01', 'synonyms': ['hinge'], 'id': 559, 'def': 'a joint that holds two parts together so that one can swing relative to the other', 'name': 'hinge'}, {'frequency': 'r', 'synset': 'hippopotamus.n.01', 'synonyms': ['hippopotamus'], 'id': 560, 'def': 'massive thick-skinned animal living in or around rivers of tropical Africa', 'name': 'hippopotamus'}, {'frequency': 'r', 'synset': 'hockey_stick.n.01', 'synonyms': ['hockey_stick'], 'id': 561, 'def': 'sports implement consisting of a stick used by hockey players to move the puck', 'name': 'hockey_stick'}, {'frequency': 'c', 'synset': 'hog.n.03', 'synonyms': ['hog', 'pig'], 'id': 562, 'def': 'domestic swine', 'name': 'hog'}, {'frequency': 'f', 'synset': 'home_plate.n.01', 'synonyms': ['home_plate_(baseball)', 'home_base_(baseball)'], 'id': 563, 'def': '(baseball) a rubber slab where the batter stands; it must be touched by a base runner in order to score', 'name': 'home_plate_(baseball)'}, {'frequency': 'c', 'synset': 'honey.n.01', 'synonyms': ['honey'], 'id': 564, 'def': 'a sweet yellow liquid produced by bees', 'name': 'honey'}, {'frequency': 'f', 'synset': 'hood.n.06', 'synonyms': ['fume_hood', 'exhaust_hood'], 'id': 565, 'def': 'metal covering leading to a vent that exhausts smoke or fumes', 'name': 'fume_hood'}, {'frequency': 'f', 'synset': 'hook.n.05', 'synonyms': ['hook'], 'id': 566, 'def': 'a curved or bent implement for suspending or pulling something', 'name': 'hook'}, {'frequency': 'r', 'synset': 'hookah.n.01', 'synonyms': ['hookah', 'narghile', 'nargileh', 'sheesha', 'shisha', 'water_pipe'], 'id': 567, 'def': 'a tobacco pipe with a long flexible tube connected to a container where the smoke is cooled by passing through water', 'name': 'hookah'}, {'frequency': 'r', 'synset': 'hornet.n.01', 'synonyms': ['hornet'], 'id': 568, 'def': 'large stinging wasp', 'name': 'hornet'}, {'frequency': 'f', 'synset': 'horse.n.01', 'synonyms': ['horse'], 'id': 569, 'def': 'a common horse', 'name': 'horse'}, {'frequency': 'f', 'synset': 'hose.n.03', 'synonyms': ['hose', 'hosepipe'], 'id': 570, 'def': 'a flexible pipe for conveying a liquid or gas', 'name': 'hose'}, {'frequency': 'r', 'synset': 'hot-air_balloon.n.01', 'synonyms': ['hot-air_balloon'], 'id': 571, 'def': 'balloon for travel through the air in a basket suspended below a large bag of heated air', 'name': 'hot-air_balloon'}, {'frequency': 'r', 'synset': 'hot_plate.n.01', 'synonyms': ['hotplate'], 'id': 572, 'def': 'a portable electric appliance for heating or cooking or keeping food warm', 'name': 'hotplate'}, {'frequency': 'c', 'synset': 'hot_sauce.n.01', 'synonyms': ['hot_sauce'], 'id': 573, 'def': 'a pungent peppery sauce', 'name': 'hot_sauce'}, {'frequency': 'r', 'synset': 'hourglass.n.01', 'synonyms': ['hourglass'], 'id': 574, 'def': 'a sandglass timer that runs for sixty minutes', 'name': 'hourglass'}, {'frequency': 'r', 'synset': 'houseboat.n.01', 'synonyms': ['houseboat'], 'id': 575, 'def': 'a barge that is designed and equipped for use as a dwelling', 'name': 'houseboat'}, {'frequency': 'c', 'synset': 'hummingbird.n.01', 'synonyms': ['hummingbird'], 'id': 576, 'def': 'tiny American bird having brilliant iridescent plumage and long slender bills', 'name': 'hummingbird'}, {'frequency': 'r', 'synset': 'hummus.n.01', 'synonyms': ['hummus', 'humus', 'hommos', 'hoummos', 'humous'], 'id': 577, 'def': 'a thick spread made from mashed chickpeas', 'name': 'hummus'}, {'frequency': 'f', 'synset': 'ice_bear.n.01', 'synonyms': ['polar_bear'], 'id': 578, 'def': 'white bear of Arctic regions', 'name': 'polar_bear'}, {'frequency': 'c', 'synset': 'ice_cream.n.01', 'synonyms': ['icecream'], 'id': 579, 'def': 'frozen dessert containing cream and sugar and flavoring', 'name': 'icecream'}, {'frequency': 'r', 'synset': 'ice_lolly.n.01', 'synonyms': ['popsicle'], 'id': 580, 'def': 'ice cream or water ice on a small wooden stick', 'name': 'popsicle'}, {'frequency': 'c', 'synset': 'ice_maker.n.01', 'synonyms': ['ice_maker'], 'id': 581, 'def': 'an appliance included in some electric refrigerators for making ice cubes', 'name': 'ice_maker'}, {'frequency': 'r', 'synset': 'ice_pack.n.01', 'synonyms': ['ice_pack', 'ice_bag'], 'id': 582, 'def': 'a waterproof bag filled with ice: applied to the body (especially the head) to cool or reduce swelling', 'name': 'ice_pack'}, {'frequency': 'r', 'synset': 'ice_skate.n.01', 'synonyms': ['ice_skate'], 'id': 583, 'def': 'skate consisting of a boot with a steel blade fitted to the sole', 'name': 'ice_skate'}, {'frequency': 'c', 'synset': 'igniter.n.01', 'synonyms': ['igniter', 'ignitor', 'lighter'], 'id': 584, 'def': 'a substance or device used to start a fire', 'name': 'igniter'}, {'frequency': 'r', 'synset': 'inhaler.n.01', 'synonyms': ['inhaler', 'inhalator'], 'id': 585, 'def': 'a dispenser that produces a chemical vapor to be inhaled through mouth or nose', 'name': 'inhaler'}, {'frequency': 'f', 'synset': 'ipod.n.01', 'synonyms': ['iPod'], 'id': 586, 'def': 'a pocket-sized device used to play music files', 'name': 'iPod'}, {'frequency': 'c', 'synset': 'iron.n.04', 'synonyms': ['iron_(for_clothing)', 'smoothing_iron_(for_clothing)'], 'id': 587, 'def': 'home appliance consisting of a flat metal base that is heated and used to smooth cloth', 'name': 'iron_(for_clothing)'}, {'frequency': 'c', 'synset': 'ironing_board.n.01', 'synonyms': ['ironing_board'], 'id': 588, 'def': 'narrow padded board on collapsible supports; used for ironing clothes', 'name': 'ironing_board'}, {'frequency': 'f', 'synset': 'jacket.n.01', 'synonyms': ['jacket'], 'id': 589, 'def': 'a waist-length coat', 'name': 'jacket'}, {'frequency': 'c', 'synset': 'jam.n.01', 'synonyms': ['jam'], 'id': 590, 'def': 'preserve of crushed fruit', 'name': 'jam'}, {'frequency': 'f', 'synset': 'jar.n.01', 'synonyms': ['jar'], 'id': 591, 'def': 'a vessel (usually cylindrical) with a wide mouth and without handles', 'name': 'jar'}, {'frequency': 'f', 'synset': 'jean.n.01', 'synonyms': ['jean', 'blue_jean', 'denim'], 'id': 592, 'def': '(usually plural) close-fitting trousers of heavy denim for manual work or casual wear', 'name': 'jean'}, {'frequency': 'c', 'synset': 'jeep.n.01', 'synonyms': ['jeep', 'landrover'], 'id': 593, 'def': 'a car suitable for traveling over rough terrain', 'name': 'jeep'}, {'frequency': 'r', 'synset': 'jelly_bean.n.01', 'synonyms': ['jelly_bean', 'jelly_egg'], 'id': 594, 'def': 'sugar-glazed jellied candy', 'name': 'jelly_bean'}, {'frequency': 'f', 'synset': 'jersey.n.03', 'synonyms': ['jersey', 'T-shirt', 'tee_shirt'], 'id': 595, 'def': 'a close-fitting pullover shirt', 'name': 'jersey'}, {'frequency': 'c', 'synset': 'jet.n.01', 'synonyms': ['jet_plane', 'jet-propelled_plane'], 'id': 596, 'def': 'an airplane powered by one or more jet engines', 'name': 'jet_plane'}, {'frequency': 'r', 'synset': 'jewel.n.01', 'synonyms': ['jewel', 'gem', 'precious_stone'], 'id': 597, 'def': 'a precious or semiprecious stone incorporated into a piece of jewelry', 'name': 'jewel'}, {'frequency': 'c', 'synset': 'jewelry.n.01', 'synonyms': ['jewelry', 'jewellery'], 'id': 598, 'def': 'an adornment (as a bracelet or ring or necklace) made of precious metals and set with gems (or imitation gems)', 'name': 'jewelry'}, {'frequency': 'r', 'synset': 'joystick.n.02', 'synonyms': ['joystick'], 'id': 599, 'def': 'a control device for computers consisting of a vertical handle that can move freely in two directions', 'name': 'joystick'}, {'frequency': 'c', 'synset': 'jump_suit.n.01', 'synonyms': ['jumpsuit'], 'id': 600, 'def': "one-piece garment fashioned after a parachutist's uniform", 'name': 'jumpsuit'}, {'frequency': 'c', 'synset': 'kayak.n.01', 'synonyms': ['kayak'], 'id': 601, 'def': 'a small canoe consisting of a light frame made watertight with animal skins', 'name': 'kayak'}, {'frequency': 'r', 'synset': 'keg.n.02', 'synonyms': ['keg'], 'id': 602, 'def': 'small cask or barrel', 'name': 'keg'}, {'frequency': 'r', 'synset': 'kennel.n.01', 'synonyms': ['kennel', 'doghouse'], 'id': 603, 'def': 'outbuilding that serves as a shelter for a dog', 'name': 'kennel'}, {'frequency': 'c', 'synset': 'kettle.n.01', 'synonyms': ['kettle', 'boiler'], 'id': 604, 'def': 'a metal pot for stewing or boiling; usually has a lid', 'name': 'kettle'}, {'frequency': 'f', 'synset': 'key.n.01', 'synonyms': ['key'], 'id': 605, 'def': 'metal instrument used to unlock a lock', 'name': 'key'}, {'frequency': 'r', 'synset': 'keycard.n.01', 'synonyms': ['keycard'], 'id': 606, 'def': 'a plastic card used to gain access typically to a door', 'name': 'keycard'}, {'frequency': 'c', 'synset': 'kilt.n.01', 'synonyms': ['kilt'], 'id': 607, 'def': 'a knee-length pleated tartan skirt worn by men as part of the traditional dress in the Highlands of northern Scotland', 'name': 'kilt'}, {'frequency': 'c', 'synset': 'kimono.n.01', 'synonyms': ['kimono'], 'id': 608, 'def': 'a loose robe; imitated from robes originally worn by Japanese', 'name': 'kimono'}, {'frequency': 'f', 'synset': 'kitchen_sink.n.01', 'synonyms': ['kitchen_sink'], 'id': 609, 'def': 'a sink in a kitchen', 'name': 'kitchen_sink'}, {'frequency': 'r', 'synset': 'kitchen_table.n.01', 'synonyms': ['kitchen_table'], 'id': 610, 'def': 'a table in the kitchen', 'name': 'kitchen_table'}, {'frequency': 'f', 'synset': 'kite.n.03', 'synonyms': ['kite'], 'id': 611, 'def': 'plaything consisting of a light frame covered with tissue paper; flown in wind at end of a string', 'name': 'kite'}, {'frequency': 'c', 'synset': 'kitten.n.01', 'synonyms': ['kitten', 'kitty'], 'id': 612, 'def': 'young domestic cat', 'name': 'kitten'}, {'frequency': 'c', 'synset': 'kiwi.n.03', 'synonyms': ['kiwi_fruit'], 'id': 613, 'def': 'fuzzy brown egg-shaped fruit with slightly tart green flesh', 'name': 'kiwi_fruit'}, {'frequency': 'f', 'synset': 'knee_pad.n.01', 'synonyms': ['knee_pad'], 'id': 614, 'def': 'protective garment consisting of a pad worn by football or baseball or hockey players', 'name': 'knee_pad'}, {'frequency': 'f', 'synset': 'knife.n.01', 'synonyms': ['knife'], 'id': 615, 'def': 'tool with a blade and point used as a cutting instrument', 'name': 'knife'}, {'frequency': 'r', 'synset': 'knitting_needle.n.01', 'synonyms': ['knitting_needle'], 'id': 616, 'def': 'needle consisting of a slender rod with pointed ends; usually used in pairs', 'name': 'knitting_needle'}, {'frequency': 'f', 'synset': 'knob.n.02', 'synonyms': ['knob'], 'id': 617, 'def': 'a round handle often found on a door', 'name': 'knob'}, {'frequency': 'r', 'synset': 'knocker.n.05', 'synonyms': ['knocker_(on_a_door)', 'doorknocker'], 'id': 618, 'def': 'a device (usually metal and ornamental) attached by a hinge to a door', 'name': 'knocker_(on_a_door)'}, {'frequency': 'r', 'synset': 'koala.n.01', 'synonyms': ['koala', 'koala_bear'], 'id': 619, 'def': 'sluggish tailless Australian marsupial with grey furry ears and coat', 'name': 'koala'}, {'frequency': 'r', 'synset': 'lab_coat.n.01', 'synonyms': ['lab_coat', 'laboratory_coat'], 'id': 620, 'def': 'a light coat worn to protect clothing from substances used while working in a laboratory', 'name': 'lab_coat'}, {'frequency': 'f', 'synset': 'ladder.n.01', 'synonyms': ['ladder'], 'id': 621, 'def': 'steps consisting of two parallel members connected by rungs', 'name': 'ladder'}, {'frequency': 'c', 'synset': 'ladle.n.01', 'synonyms': ['ladle'], 'id': 622, 'def': 'a spoon-shaped vessel with a long handle frequently used to transfer liquids', 'name': 'ladle'}, {'frequency': 'c', 'synset': 'ladybug.n.01', 'synonyms': ['ladybug', 'ladybeetle', 'ladybird_beetle'], 'id': 623, 'def': 'small round bright-colored and spotted beetle, typically red and black', 'name': 'ladybug'}, {'frequency': 'f', 'synset': 'lamb.n.01', 'synonyms': ['lamb_(animal)'], 'id': 624, 'def': 'young sheep', 'name': 'lamb_(animal)'}, {'frequency': 'r', 'synset': 'lamb_chop.n.01', 'synonyms': ['lamb-chop', 'lambchop'], 'id': 625, 'def': 'chop cut from a lamb', 'name': 'lamb-chop'}, {'frequency': 'f', 'synset': 'lamp.n.02', 'synonyms': ['lamp'], 'id': 626, 'def': 'a piece of furniture holding one or more electric light bulbs', 'name': 'lamp'}, {'frequency': 'f', 'synset': 'lamppost.n.01', 'synonyms': ['lamppost'], 'id': 627, 'def': 'a metal post supporting an outdoor lamp (such as a streetlight)', 'name': 'lamppost'}, {'frequency': 'f', 'synset': 'lampshade.n.01', 'synonyms': ['lampshade'], 'id': 628, 'def': 'a protective ornamental shade used to screen a light bulb from direct view', 'name': 'lampshade'}, {'frequency': 'c', 'synset': 'lantern.n.01', 'synonyms': ['lantern'], 'id': 629, 'def': 'light in a transparent protective case', 'name': 'lantern'}, {'frequency': 'f', 'synset': 'lanyard.n.02', 'synonyms': ['lanyard', 'laniard'], 'id': 630, 'def': 'a cord worn around the neck to hold a knife or whistle, etc.', 'name': 'lanyard'}, {'frequency': 'f', 'synset': 'laptop.n.01', 'synonyms': ['laptop_computer', 'notebook_computer'], 'id': 631, 'def': 'a portable computer small enough to use in your lap', 'name': 'laptop_computer'}, {'frequency': 'r', 'synset': 'lasagna.n.01', 'synonyms': ['lasagna', 'lasagne'], 'id': 632, 'def': 'baked dish of layers of lasagna pasta with sauce and cheese and meat or vegetables', 'name': 'lasagna'}, {'frequency': 'f', 'synset': 'latch.n.02', 'synonyms': ['latch'], 'id': 633, 'def': 'a bar that can be lowered or slid into a groove to fasten a door or gate', 'name': 'latch'}, {'frequency': 'r', 'synset': 'lawn_mower.n.01', 'synonyms': ['lawn_mower'], 'id': 634, 'def': 'garden tool for mowing grass on lawns', 'name': 'lawn_mower'}, {'frequency': 'r', 'synset': 'leather.n.01', 'synonyms': ['leather'], 'id': 635, 'def': 'an animal skin made smooth and flexible by removing the hair and then tanning', 'name': 'leather'}, {'frequency': 'c', 'synset': 'legging.n.01', 'synonyms': ['legging_(clothing)', 'leging_(clothing)', 'leg_covering'], 'id': 636, 'def': 'a garment covering the leg (usually extending from the knee to the ankle)', 'name': 'legging_(clothing)'}, {'frequency': 'c', 'synset': 'lego.n.01', 'synonyms': ['Lego', 'Lego_set'], 'id': 637, 'def': "a child's plastic construction set for making models from blocks", 'name': 'Lego'}, {'frequency': 'r', 'synset': 'legume.n.02', 'synonyms': ['legume'], 'id': 638, 'def': 'the fruit or seed of bean or pea plants', 'name': 'legume'}, {'frequency': 'f', 'synset': 'lemon.n.01', 'synonyms': ['lemon'], 'id': 639, 'def': 'yellow oval fruit with juicy acidic flesh', 'name': 'lemon'}, {'frequency': 'r', 'synset': 'lemonade.n.01', 'synonyms': ['lemonade'], 'id': 640, 'def': 'sweetened beverage of diluted lemon juice', 'name': 'lemonade'}, {'frequency': 'f', 'synset': 'lettuce.n.02', 'synonyms': ['lettuce'], 'id': 641, 'def': 'leafy plant commonly eaten in salad or on sandwiches', 'name': 'lettuce'}, {'frequency': 'f', 'synset': 'license_plate.n.01', 'synonyms': ['license_plate', 'numberplate'], 'id': 642, 'def': "a plate mounted on the front and back of car and bearing the car's registration number", 'name': 'license_plate'}, {'frequency': 'f', 'synset': 'life_buoy.n.01', 'synonyms': ['life_buoy', 'lifesaver', 'life_belt', 'life_ring'], 'id': 643, 'def': 'a ring-shaped life preserver used to prevent drowning (NOT a life-jacket or vest)', 'name': 'life_buoy'}, {'frequency': 'f', 'synset': 'life_jacket.n.01', 'synonyms': ['life_jacket', 'life_vest'], 'id': 644, 'def': 'life preserver consisting of a sleeveless jacket of buoyant or inflatable design', 'name': 'life_jacket'}, {'frequency': 'f', 'synset': 'light_bulb.n.01', 'synonyms': ['lightbulb'], 'id': 645, 'def': 'lightblub/source of light', 'name': 'lightbulb'}, {'frequency': 'r', 'synset': 'lightning_rod.n.02', 'synonyms': ['lightning_rod', 'lightning_conductor'], 'id': 646, 'def': 'a metallic conductor that is attached to a high point and leads to the ground', 'name': 'lightning_rod'}, {'frequency': 'f', 'synset': 'lime.n.06', 'synonyms': ['lime'], 'id': 647, 'def': 'the green acidic fruit of any of various lime trees', 'name': 'lime'}, {'frequency': 'r', 'synset': 'limousine.n.01', 'synonyms': ['limousine'], 'id': 648, 'def': 'long luxurious car; usually driven by a chauffeur', 'name': 'limousine'}, {'frequency': 'c', 'synset': 'lion.n.01', 'synonyms': ['lion'], 'id': 649, 'def': 'large gregarious predatory cat of Africa and India', 'name': 'lion'}, {'frequency': 'c', 'synset': 'lip_balm.n.01', 'synonyms': ['lip_balm'], 'id': 650, 'def': 'a balm applied to the lips', 'name': 'lip_balm'}, {'frequency': 'r', 'synset': 'liquor.n.01', 'synonyms': ['liquor', 'spirits', 'hard_liquor', 'liqueur', 'cordial'], 'id': 651, 'def': 'liquor or beer', 'name': 'liquor'}, {'frequency': 'c', 'synset': 'lizard.n.01', 'synonyms': ['lizard'], 'id': 652, 'def': 'a reptile with usually two pairs of legs and a tapering tail', 'name': 'lizard'}, {'frequency': 'f', 'synset': 'log.n.01', 'synonyms': ['log'], 'id': 653, 'def': 'a segment of the trunk of a tree when stripped of branches', 'name': 'log'}, {'frequency': 'c', 'synset': 'lollipop.n.02', 'synonyms': ['lollipop'], 'id': 654, 'def': 'hard candy on a stick', 'name': 'lollipop'}, {'frequency': 'f', 'synset': 'loudspeaker.n.01', 'synonyms': ['speaker_(stero_equipment)'], 'id': 655, 'def': 'electronic device that produces sound often as part of a stereo system', 'name': 'speaker_(stero_equipment)'}, {'frequency': 'c', 'synset': 'love_seat.n.01', 'synonyms': ['loveseat'], 'id': 656, 'def': 'small sofa that seats two people', 'name': 'loveseat'}, {'frequency': 'r', 'synset': 'machine_gun.n.01', 'synonyms': ['machine_gun'], 'id': 657, 'def': 'a rapidly firing automatic gun', 'name': 'machine_gun'}, {'frequency': 'f', 'synset': 'magazine.n.02', 'synonyms': ['magazine'], 'id': 658, 'def': 'a paperback periodic publication', 'name': 'magazine'}, {'frequency': 'f', 'synset': 'magnet.n.01', 'synonyms': ['magnet'], 'id': 659, 'def': 'a device that attracts iron and produces a magnetic field', 'name': 'magnet'}, {'frequency': 'c', 'synset': 'mail_slot.n.01', 'synonyms': ['mail_slot'], 'id': 660, 'def': 'a slot (usually in a door) through which mail can be delivered', 'name': 'mail_slot'}, {'frequency': 'f', 'synset': 'mailbox.n.01', 'synonyms': ['mailbox_(at_home)', 'letter_box_(at_home)'], 'id': 661, 'def': 'a private box for delivery of mail', 'name': 'mailbox_(at_home)'}, {'frequency': 'r', 'synset': 'mallard.n.01', 'synonyms': ['mallard'], 'id': 662, 'def': 'wild dabbling duck from which domestic ducks are descended', 'name': 'mallard'}, {'frequency': 'r', 'synset': 'mallet.n.01', 'synonyms': ['mallet'], 'id': 663, 'def': 'a sports implement with a long handle and a hammer-like head used to hit a ball', 'name': 'mallet'}, {'frequency': 'r', 'synset': 'mammoth.n.01', 'synonyms': ['mammoth'], 'id': 664, 'def': 'any of numerous extinct elephants widely distributed in the Pleistocene', 'name': 'mammoth'}, {'frequency': 'r', 'synset': 'manatee.n.01', 'synonyms': ['manatee'], 'id': 665, 'def': 'sirenian mammal of tropical coastal waters of America', 'name': 'manatee'}, {'frequency': 'c', 'synset': 'mandarin.n.05', 'synonyms': ['mandarin_orange'], 'id': 666, 'def': 'a somewhat flat reddish-orange loose skinned citrus of China', 'name': 'mandarin_orange'}, {'frequency': 'c', 'synset': 'manger.n.01', 'synonyms': ['manger', 'trough'], 'id': 667, 'def': 'a container (usually in a barn or stable) from which cattle or horses feed', 'name': 'manger'}, {'frequency': 'f', 'synset': 'manhole.n.01', 'synonyms': ['manhole'], 'id': 668, 'def': 'a hole (usually with a flush cover) through which a person can gain access to an underground structure', 'name': 'manhole'}, {'frequency': 'f', 'synset': 'map.n.01', 'synonyms': ['map'], 'id': 669, 'def': "a diagrammatic representation of the earth's surface (or part of it)", 'name': 'map'}, {'frequency': 'f', 'synset': 'marker.n.03', 'synonyms': ['marker'], 'id': 670, 'def': 'a writing implement for making a mark', 'name': 'marker'}, {'frequency': 'r', 'synset': 'martini.n.01', 'synonyms': ['martini'], 'id': 671, 'def': 'a cocktail made of gin (or vodka) with dry vermouth', 'name': 'martini'}, {'frequency': 'r', 'synset': 'mascot.n.01', 'synonyms': ['mascot'], 'id': 672, 'def': 'a person or animal that is adopted by a team or other group as a symbolic figure', 'name': 'mascot'}, {'frequency': 'c', 'synset': 'mashed_potato.n.01', 'synonyms': ['mashed_potato'], 'id': 673, 'def': 'potato that has been peeled and boiled and then mashed', 'name': 'mashed_potato'}, {'frequency': 'r', 'synset': 'masher.n.02', 'synonyms': ['masher'], 'id': 674, 'def': 'a kitchen utensil used for mashing (e.g. potatoes)', 'name': 'masher'}, {'frequency': 'f', 'synset': 'mask.n.04', 'synonyms': ['mask', 'facemask'], 'id': 675, 'def': 'a protective covering worn over the face', 'name': 'mask'}, {'frequency': 'f', 'synset': 'mast.n.01', 'synonyms': ['mast'], 'id': 676, 'def': 'a vertical spar for supporting sails', 'name': 'mast'}, {'frequency': 'c', 'synset': 'mat.n.03', 'synonyms': ['mat_(gym_equipment)', 'gym_mat'], 'id': 677, 'def': 'sports equipment consisting of a piece of thick padding on the floor for gymnastics', 'name': 'mat_(gym_equipment)'}, {'frequency': 'r', 'synset': 'matchbox.n.01', 'synonyms': ['matchbox'], 'id': 678, 'def': 'a box for holding matches', 'name': 'matchbox'}, {'frequency': 'f', 'synset': 'mattress.n.01', 'synonyms': ['mattress'], 'id': 679, 'def': 'a thick pad filled with resilient material used as a bed or part of a bed', 'name': 'mattress'}, {'frequency': 'c', 'synset': 'measuring_cup.n.01', 'synonyms': ['measuring_cup'], 'id': 680, 'def': 'graduated cup used to measure liquid or granular ingredients', 'name': 'measuring_cup'}, {'frequency': 'c', 'synset': 'measuring_stick.n.01', 'synonyms': ['measuring_stick', 'ruler_(measuring_stick)', 'measuring_rod'], 'id': 681, 'def': 'measuring instrument having a sequence of marks at regular intervals', 'name': 'measuring_stick'}, {'frequency': 'c', 'synset': 'meatball.n.01', 'synonyms': ['meatball'], 'id': 682, 'def': 'ground meat formed into a ball and fried or simmered in broth', 'name': 'meatball'}, {'frequency': 'c', 'synset': 'medicine.n.02', 'synonyms': ['medicine'], 'id': 683, 'def': 'something that treats or prevents or alleviates the symptoms of disease', 'name': 'medicine'}, {'frequency': 'c', 'synset': 'melon.n.01', 'synonyms': ['melon'], 'id': 684, 'def': 'fruit of the gourd family having a hard rind and sweet juicy flesh', 'name': 'melon'}, {'frequency': 'f', 'synset': 'microphone.n.01', 'synonyms': ['microphone'], 'id': 685, 'def': 'device for converting sound waves into electrical energy', 'name': 'microphone'}, {'frequency': 'r', 'synset': 'microscope.n.01', 'synonyms': ['microscope'], 'id': 686, 'def': 'magnifier of the image of small objects', 'name': 'microscope'}, {'frequency': 'f', 'synset': 'microwave.n.02', 'synonyms': ['microwave_oven'], 'id': 687, 'def': 'kitchen appliance that cooks food by passing an electromagnetic wave through it', 'name': 'microwave_oven'}, {'frequency': 'r', 'synset': 'milestone.n.01', 'synonyms': ['milestone', 'milepost'], 'id': 688, 'def': 'stone post at side of a road to show distances', 'name': 'milestone'}, {'frequency': 'f', 'synset': 'milk.n.01', 'synonyms': ['milk'], 'id': 689, 'def': 'a white nutritious liquid secreted by mammals and used as food by human beings', 'name': 'milk'}, {'frequency': 'r', 'synset': 'milk_can.n.01', 'synonyms': ['milk_can'], 'id': 690, 'def': 'can for transporting milk', 'name': 'milk_can'}, {'frequency': 'r', 'synset': 'milkshake.n.01', 'synonyms': ['milkshake'], 'id': 691, 'def': 'frothy drink of milk and flavoring and sometimes fruit or ice cream', 'name': 'milkshake'}, {'frequency': 'f', 'synset': 'minivan.n.01', 'synonyms': ['minivan'], 'id': 692, 'def': 'a small box-shaped passenger van', 'name': 'minivan'}, {'frequency': 'r', 'synset': 'mint.n.05', 'synonyms': ['mint_candy'], 'id': 693, 'def': 'a candy that is flavored with a mint oil', 'name': 'mint_candy'}, {'frequency': 'f', 'synset': 'mirror.n.01', 'synonyms': ['mirror'], 'id': 694, 'def': 'polished surface that forms images by reflecting light', 'name': 'mirror'}, {'frequency': 'c', 'synset': 'mitten.n.01', 'synonyms': ['mitten'], 'id': 695, 'def': 'glove that encases the thumb separately and the other four fingers together', 'name': 'mitten'}, {'frequency': 'c', 'synset': 'mixer.n.04', 'synonyms': ['mixer_(kitchen_tool)', 'stand_mixer'], 'id': 696, 'def': 'a kitchen utensil that is used for mixing foods', 'name': 'mixer_(kitchen_tool)'}, {'frequency': 'c', 'synset': 'money.n.03', 'synonyms': ['money'], 'id': 697, 'def': 'the official currency issued by a government or national bank', 'name': 'money'}, {'frequency': 'f', 'synset': 'monitor.n.04', 'synonyms': ['monitor_(computer_equipment) computer_monitor'], 'id': 698, 'def': 'a computer monitor', 'name': 'monitor_(computer_equipment) computer_monitor'}, {'frequency': 'c', 'synset': 'monkey.n.01', 'synonyms': ['monkey'], 'id': 699, 'def': 'any of various long-tailed primates', 'name': 'monkey'}, {'frequency': 'f', 'synset': 'motor.n.01', 'synonyms': ['motor'], 'id': 700, 'def': 'machine that converts other forms of energy into mechanical energy and so imparts motion', 'name': 'motor'}, {'frequency': 'f', 'synset': 'motor_scooter.n.01', 'synonyms': ['motor_scooter', 'scooter'], 'id': 701, 'def': 'a wheeled vehicle with small wheels and a low-powered engine', 'name': 'motor_scooter'}, {'frequency': 'r', 'synset': 'motor_vehicle.n.01', 'synonyms': ['motor_vehicle', 'automotive_vehicle'], 'id': 702, 'def': 'a self-propelled wheeled vehicle that does not run on rails', 'name': 'motor_vehicle'}, {'frequency': 'f', 'synset': 'motorcycle.n.01', 'synonyms': ['motorcycle'], 'id': 703, 'def': 'a motor vehicle with two wheels and a strong frame', 'name': 'motorcycle'}, {'frequency': 'f', 'synset': 'mound.n.01', 'synonyms': ['mound_(baseball)', "pitcher's_mound"], 'id': 704, 'def': '(baseball) the slight elevation on which the pitcher stands', 'name': 'mound_(baseball)'}, {'frequency': 'f', 'synset': 'mouse.n.04', 'synonyms': ['mouse_(computer_equipment)', 'computer_mouse'], 'id': 705, 'def': 'a computer input device that controls an on-screen pointer (does not include trackpads / touchpads)', 'name': 'mouse_(computer_equipment)'}, {'frequency': 'f', 'synset': 'mousepad.n.01', 'synonyms': ['mousepad'], 'id': 706, 'def': 'a small portable pad that provides an operating surface for a computer mouse', 'name': 'mousepad'}, {'frequency': 'c', 'synset': 'muffin.n.01', 'synonyms': ['muffin'], 'id': 707, 'def': 'a sweet quick bread baked in a cup-shaped pan', 'name': 'muffin'}, {'frequency': 'f', 'synset': 'mug.n.04', 'synonyms': ['mug'], 'id': 708, 'def': 'with handle and usually cylindrical', 'name': 'mug'}, {'frequency': 'f', 'synset': 'mushroom.n.02', 'synonyms': ['mushroom'], 'id': 709, 'def': 'a common mushroom', 'name': 'mushroom'}, {'frequency': 'r', 'synset': 'music_stool.n.01', 'synonyms': ['music_stool', 'piano_stool'], 'id': 710, 'def': 'a stool for piano players; usually adjustable in height', 'name': 'music_stool'}, {'frequency': 'c', 'synset': 'musical_instrument.n.01', 'synonyms': ['musical_instrument', 'instrument_(musical)'], 'id': 711, 'def': 'any of various devices or contrivances that can be used to produce musical tones or sounds', 'name': 'musical_instrument'}, {'frequency': 'r', 'synset': 'nailfile.n.01', 'synonyms': ['nailfile'], 'id': 712, 'def': 'a small flat file for shaping the nails', 'name': 'nailfile'}, {'frequency': 'f', 'synset': 'napkin.n.01', 'synonyms': ['napkin', 'table_napkin', 'serviette'], 'id': 713, 'def': 'a small piece of table linen or paper that is used to wipe the mouth and to cover the lap in order to protect clothing', 'name': 'napkin'}, {'frequency': 'r', 'synset': 'neckerchief.n.01', 'synonyms': ['neckerchief'], 'id': 714, 'def': 'a kerchief worn around the neck', 'name': 'neckerchief'}, {'frequency': 'f', 'synset': 'necklace.n.01', 'synonyms': ['necklace'], 'id': 715, 'def': 'jewelry consisting of a cord or chain (often bearing gems) worn about the neck as an ornament', 'name': 'necklace'}, {'frequency': 'f', 'synset': 'necktie.n.01', 'synonyms': ['necktie', 'tie_(necktie)'], 'id': 716, 'def': 'neckwear consisting of a long narrow piece of material worn under a collar and tied in knot at the front', 'name': 'necktie'}, {'frequency': 'c', 'synset': 'needle.n.03', 'synonyms': ['needle'], 'id': 717, 'def': 'a sharp pointed implement (usually metal)', 'name': 'needle'}, {'frequency': 'c', 'synset': 'nest.n.01', 'synonyms': ['nest'], 'id': 718, 'def': 'a structure in which animals lay eggs or give birth to their young', 'name': 'nest'}, {'frequency': 'f', 'synset': 'newspaper.n.01', 'synonyms': ['newspaper', 'paper_(newspaper)'], 'id': 719, 'def': 'a daily or weekly publication on folded sheets containing news, articles, and advertisements', 'name': 'newspaper'}, {'frequency': 'c', 'synset': 'newsstand.n.01', 'synonyms': ['newsstand'], 'id': 720, 'def': 'a stall where newspapers and other periodicals are sold', 'name': 'newsstand'}, {'frequency': 'c', 'synset': 'nightwear.n.01', 'synonyms': ['nightshirt', 'nightwear', 'sleepwear', 'nightclothes'], 'id': 721, 'def': 'garments designed to be worn in bed', 'name': 'nightshirt'}, {'frequency': 'r', 'synset': 'nosebag.n.01', 'synonyms': ['nosebag_(for_animals)', 'feedbag'], 'id': 722, 'def': 'a canvas bag that is used to feed an animal (such as a horse); covers the muzzle and fastens at the top of the head', 'name': 'nosebag_(for_animals)'}, {'frequency': 'c', 'synset': 'noseband.n.01', 'synonyms': ['noseband_(for_animals)', 'nosepiece_(for_animals)'], 'id': 723, 'def': "a strap that is the part of a bridle that goes over the animal's nose", 'name': 'noseband_(for_animals)'}, {'frequency': 'f', 'synset': 'notebook.n.01', 'synonyms': ['notebook'], 'id': 724, 'def': 'a book with blank pages for recording notes or memoranda', 'name': 'notebook'}, {'frequency': 'c', 'synset': 'notepad.n.01', 'synonyms': ['notepad'], 'id': 725, 'def': 'a pad of paper for keeping notes', 'name': 'notepad'}, {'frequency': 'f', 'synset': 'nut.n.03', 'synonyms': ['nut'], 'id': 726, 'def': 'a small metal block (usually square or hexagonal) with internal screw thread to be fitted onto a bolt', 'name': 'nut'}, {'frequency': 'r', 'synset': 'nutcracker.n.01', 'synonyms': ['nutcracker'], 'id': 727, 'def': 'a hand tool used to crack nuts open', 'name': 'nutcracker'}, {'frequency': 'f', 'synset': 'oar.n.01', 'synonyms': ['oar'], 'id': 728, 'def': 'an implement used to propel or steer a boat', 'name': 'oar'}, {'frequency': 'r', 'synset': 'octopus.n.01', 'synonyms': ['octopus_(food)'], 'id': 729, 'def': 'tentacles of octopus prepared as food', 'name': 'octopus_(food)'}, {'frequency': 'r', 'synset': 'octopus.n.02', 'synonyms': ['octopus_(animal)'], 'id': 730, 'def': 'bottom-living cephalopod having a soft oval body with eight long tentacles', 'name': 'octopus_(animal)'}, {'frequency': 'c', 'synset': 'oil_lamp.n.01', 'synonyms': ['oil_lamp', 'kerosene_lamp', 'kerosine_lamp'], 'id': 731, 'def': 'a lamp that burns oil (as kerosine) for light', 'name': 'oil_lamp'}, {'frequency': 'c', 'synset': 'olive_oil.n.01', 'synonyms': ['olive_oil'], 'id': 732, 'def': 'oil from olives', 'name': 'olive_oil'}, {'frequency': 'r', 'synset': 'omelet.n.01', 'synonyms': ['omelet', 'omelette'], 'id': 733, 'def': 'beaten eggs cooked until just set; may be folded around e.g. ham or cheese or jelly', 'name': 'omelet'}, {'frequency': 'f', 'synset': 'onion.n.01', 'synonyms': ['onion'], 'id': 734, 'def': 'the bulb of an onion plant', 'name': 'onion'}, {'frequency': 'f', 'synset': 'orange.n.01', 'synonyms': ['orange_(fruit)'], 'id': 735, 'def': 'orange (FRUIT of an orange tree)', 'name': 'orange_(fruit)'}, {'frequency': 'c', 'synset': 'orange_juice.n.01', 'synonyms': ['orange_juice'], 'id': 736, 'def': 'bottled or freshly squeezed juice of oranges', 'name': 'orange_juice'}, {'frequency': 'c', 'synset': 'ostrich.n.02', 'synonyms': ['ostrich'], 'id': 737, 'def': 'fast-running African flightless bird with two-toed feet; largest living bird', 'name': 'ostrich'}, {'frequency': 'f', 'synset': 'ottoman.n.03', 'synonyms': ['ottoman', 'pouf', 'pouffe', 'hassock'], 'id': 738, 'def': 'a thick standalone cushion used as a seat or footrest, often next to a chair', 'name': 'ottoman'}, {'frequency': 'f', 'synset': 'oven.n.01', 'synonyms': ['oven'], 'id': 739, 'def': 'kitchen appliance used for baking or roasting', 'name': 'oven'}, {'frequency': 'c', 'synset': 'overall.n.01', 'synonyms': ['overalls_(clothing)'], 'id': 740, 'def': 'work clothing consisting of denim trousers usually with a bib and shoulder straps', 'name': 'overalls_(clothing)'}, {'frequency': 'c', 'synset': 'owl.n.01', 'synonyms': ['owl'], 'id': 741, 'def': 'nocturnal bird of prey with hawk-like beak and claws and large head with front-facing eyes', 'name': 'owl'}, {'frequency': 'c', 'synset': 'packet.n.03', 'synonyms': ['packet'], 'id': 742, 'def': 'a small package or bundle', 'name': 'packet'}, {'frequency': 'r', 'synset': 'pad.n.03', 'synonyms': ['inkpad', 'inking_pad', 'stamp_pad'], 'id': 743, 'def': 'absorbent material saturated with ink used to transfer ink evenly to a rubber stamp', 'name': 'inkpad'}, {'frequency': 'c', 'synset': 'pad.n.04', 'synonyms': ['pad'], 'id': 744, 'def': 'mostly arm/knee pads labeled', 'name': 'pad'}, {'frequency': 'f', 'synset': 'paddle.n.04', 'synonyms': ['paddle', 'boat_paddle'], 'id': 745, 'def': 'a short light oar used without an oarlock to propel a canoe or small boat', 'name': 'paddle'}, {'frequency': 'c', 'synset': 'padlock.n.01', 'synonyms': ['padlock'], 'id': 746, 'def': 'a detachable, portable lock', 'name': 'padlock'}, {'frequency': 'c', 'synset': 'paintbrush.n.01', 'synonyms': ['paintbrush'], 'id': 747, 'def': 'a brush used as an applicator to apply paint', 'name': 'paintbrush'}, {'frequency': 'f', 'synset': 'painting.n.01', 'synonyms': ['painting'], 'id': 748, 'def': 'graphic art consisting of an artistic composition made by applying paints to a surface', 'name': 'painting'}, {'frequency': 'f', 'synset': 'pajama.n.02', 'synonyms': ['pajamas', 'pyjamas'], 'id': 749, 'def': 'loose-fitting nightclothes worn for sleeping or lounging', 'name': 'pajamas'}, {'frequency': 'c', 'synset': 'palette.n.02', 'synonyms': ['palette', 'pallet'], 'id': 750, 'def': 'board that provides a flat surface on which artists mix paints and the range of colors used', 'name': 'palette'}, {'frequency': 'f', 'synset': 'pan.n.01', 'synonyms': ['pan_(for_cooking)', 'cooking_pan'], 'id': 751, 'def': 'cooking utensil consisting of a wide metal vessel', 'name': 'pan_(for_cooking)'}, {'frequency': 'r', 'synset': 'pan.n.03', 'synonyms': ['pan_(metal_container)'], 'id': 752, 'def': 'shallow container made of metal', 'name': 'pan_(metal_container)'}, {'frequency': 'c', 'synset': 'pancake.n.01', 'synonyms': ['pancake'], 'id': 753, 'def': 'a flat cake of thin batter fried on both sides on a griddle', 'name': 'pancake'}, {'frequency': 'r', 'synset': 'pantyhose.n.01', 'synonyms': ['pantyhose'], 'id': 754, 'def': "a woman's tights consisting of underpants and stockings", 'name': 'pantyhose'}, {'frequency': 'r', 'synset': 'papaya.n.02', 'synonyms': ['papaya'], 'id': 755, 'def': 'large oval melon-like tropical fruit with yellowish flesh', 'name': 'papaya'}, {'frequency': 'f', 'synset': 'paper_plate.n.01', 'synonyms': ['paper_plate'], 'id': 756, 'def': 'a disposable plate made of cardboard', 'name': 'paper_plate'}, {'frequency': 'f', 'synset': 'paper_towel.n.01', 'synonyms': ['paper_towel'], 'id': 757, 'def': 'a disposable towel made of absorbent paper', 'name': 'paper_towel'}, {'frequency': 'r', 'synset': 'paperback_book.n.01', 'synonyms': ['paperback_book', 'paper-back_book', 'softback_book', 'soft-cover_book'], 'id': 758, 'def': 'a book with paper covers', 'name': 'paperback_book'}, {'frequency': 'r', 'synset': 'paperweight.n.01', 'synonyms': ['paperweight'], 'id': 759, 'def': 'a weight used to hold down a stack of papers', 'name': 'paperweight'}, {'frequency': 'c', 'synset': 'parachute.n.01', 'synonyms': ['parachute'], 'id': 760, 'def': 'rescue equipment consisting of a device that fills with air and retards your fall', 'name': 'parachute'}, {'frequency': 'c', 'synset': 'parakeet.n.01', 'synonyms': ['parakeet', 'parrakeet', 'parroket', 'paraquet', 'paroquet', 'parroquet'], 'id': 761, 'def': 'any of numerous small slender long-tailed parrots', 'name': 'parakeet'}, {'frequency': 'c', 'synset': 'parasail.n.01', 'synonyms': ['parasail_(sports)'], 'id': 762, 'def': 'parachute that will lift a person up into the air when it is towed by a motorboat or a car', 'name': 'parasail_(sports)'}, {'frequency': 'c', 'synset': 'parasol.n.01', 'synonyms': ['parasol', 'sunshade'], 'id': 763, 'def': 'a handheld collapsible source of shade', 'name': 'parasol'}, {'frequency': 'r', 'synset': 'parchment.n.01', 'synonyms': ['parchment'], 'id': 764, 'def': 'a superior paper resembling sheepskin', 'name': 'parchment'}, {'frequency': 'c', 'synset': 'parka.n.01', 'synonyms': ['parka', 'anorak'], 'id': 765, 'def': "a kind of heavy jacket (`windcheater' is a British term)", 'name': 'parka'}, {'frequency': 'f', 'synset': 'parking_meter.n.01', 'synonyms': ['parking_meter'], 'id': 766, 'def': 'a coin-operated timer located next to a parking space', 'name': 'parking_meter'}, {'frequency': 'c', 'synset': 'parrot.n.01', 'synonyms': ['parrot'], 'id': 767, 'def': 'usually brightly colored tropical birds with short hooked beaks and the ability to mimic sounds', 'name': 'parrot'}, {'frequency': 'c', 'synset': 'passenger_car.n.01', 'synonyms': ['passenger_car_(part_of_a_train)', 'coach_(part_of_a_train)'], 'id': 768, 'def': 'a railcar where passengers ride', 'name': 'passenger_car_(part_of_a_train)'}, {'frequency': 'r', 'synset': 'passenger_ship.n.01', 'synonyms': ['passenger_ship'], 'id': 769, 'def': 'a ship built to carry passengers', 'name': 'passenger_ship'}, {'frequency': 'c', 'synset': 'passport.n.02', 'synonyms': ['passport'], 'id': 770, 'def': 'a document issued by a country to a citizen allowing that person to travel abroad and re-enter the home country', 'name': 'passport'}, {'frequency': 'f', 'synset': 'pastry.n.02', 'synonyms': ['pastry'], 'id': 771, 'def': 'any of various baked foods made of dough or batter', 'name': 'pastry'}, {'frequency': 'r', 'synset': 'patty.n.01', 'synonyms': ['patty_(food)'], 'id': 772, 'def': 'small flat mass of chopped food', 'name': 'patty_(food)'}, {'frequency': 'c', 'synset': 'pea.n.01', 'synonyms': ['pea_(food)'], 'id': 773, 'def': 'seed of a pea plant used for food', 'name': 'pea_(food)'}, {'frequency': 'c', 'synset': 'peach.n.03', 'synonyms': ['peach'], 'id': 774, 'def': 'downy juicy fruit with sweet yellowish or whitish flesh', 'name': 'peach'}, {'frequency': 'c', 'synset': 'peanut_butter.n.01', 'synonyms': ['peanut_butter'], 'id': 775, 'def': 'a spread made from ground peanuts', 'name': 'peanut_butter'}, {'frequency': 'f', 'synset': 'pear.n.01', 'synonyms': ['pear'], 'id': 776, 'def': 'sweet juicy gritty-textured fruit available in many varieties', 'name': 'pear'}, {'frequency': 'c', 'synset': 'peeler.n.03', 'synonyms': ['peeler_(tool_for_fruit_and_vegetables)'], 'id': 777, 'def': 'a device for peeling vegetables or fruits', 'name': 'peeler_(tool_for_fruit_and_vegetables)'}, {'frequency': 'r', 'synset': 'peg.n.04', 'synonyms': ['wooden_leg', 'pegleg'], 'id': 778, 'def': 'a prosthesis that replaces a missing leg', 'name': 'wooden_leg'}, {'frequency': 'r', 'synset': 'pegboard.n.01', 'synonyms': ['pegboard'], 'id': 779, 'def': 'a board perforated with regularly spaced holes into which pegs can be fitted', 'name': 'pegboard'}, {'frequency': 'c', 'synset': 'pelican.n.01', 'synonyms': ['pelican'], 'id': 780, 'def': 'large long-winged warm-water seabird having a large bill with a distensible pouch for fish', 'name': 'pelican'}, {'frequency': 'f', 'synset': 'pen.n.01', 'synonyms': ['pen'], 'id': 781, 'def': 'a writing implement with a point from which ink flows', 'name': 'pen'}, {'frequency': 'f', 'synset': 'pencil.n.01', 'synonyms': ['pencil'], 'id': 782, 'def': 'a thin cylindrical pointed writing implement made of wood and graphite', 'name': 'pencil'}, {'frequency': 'r', 'synset': 'pencil_box.n.01', 'synonyms': ['pencil_box', 'pencil_case'], 'id': 783, 'def': 'a box for holding pencils', 'name': 'pencil_box'}, {'frequency': 'r', 'synset': 'pencil_sharpener.n.01', 'synonyms': ['pencil_sharpener'], 'id': 784, 'def': 'a rotary implement for sharpening the point on pencils', 'name': 'pencil_sharpener'}, {'frequency': 'r', 'synset': 'pendulum.n.01', 'synonyms': ['pendulum'], 'id': 785, 'def': 'an apparatus consisting of an object mounted so that it swings freely under the influence of gravity', 'name': 'pendulum'}, {'frequency': 'c', 'synset': 'penguin.n.01', 'synonyms': ['penguin'], 'id': 786, 'def': 'short-legged flightless birds of cold southern regions having webbed feet and wings modified as flippers', 'name': 'penguin'}, {'frequency': 'r', 'synset': 'pennant.n.02', 'synonyms': ['pennant'], 'id': 787, 'def': 'a flag longer than it is wide (and often tapering)', 'name': 'pennant'}, {'frequency': 'r', 'synset': 'penny.n.02', 'synonyms': ['penny_(coin)'], 'id': 788, 'def': 'a coin worth one-hundredth of the value of the basic unit', 'name': 'penny_(coin)'}, {'frequency': 'f', 'synset': 'pepper.n.03', 'synonyms': ['pepper', 'peppercorn'], 'id': 789, 'def': 'pungent seasoning from the berry of the common pepper plant; whole or ground', 'name': 'pepper'}, {'frequency': 'c', 'synset': 'pepper_mill.n.01', 'synonyms': ['pepper_mill', 'pepper_grinder'], 'id': 790, 'def': 'a mill for grinding pepper', 'name': 'pepper_mill'}, {'frequency': 'c', 'synset': 'perfume.n.02', 'synonyms': ['perfume'], 'id': 791, 'def': 'a toiletry that emits and diffuses a fragrant odor', 'name': 'perfume'}, {'frequency': 'r', 'synset': 'persimmon.n.02', 'synonyms': ['persimmon'], 'id': 792, 'def': 'orange fruit resembling a plum; edible when fully ripe', 'name': 'persimmon'}, {'frequency': 'f', 'synset': 'person.n.01', 'synonyms': ['person', 'baby', 'child', 'boy', 'girl', 'man', 'woman', 'human'], 'id': 793, 'def': 'a human being', 'name': 'person'}, {'frequency': 'c', 'synset': 'pet.n.01', 'synonyms': ['pet'], 'id': 794, 'def': 'a domesticated animal kept for companionship or amusement', 'name': 'pet'}, {'frequency': 'c', 'synset': 'pew.n.01', 'synonyms': ['pew_(church_bench)', 'church_bench'], 'id': 795, 'def': 'long bench with backs; used in church by the congregation', 'name': 'pew_(church_bench)'}, {'frequency': 'r', 'synset': 'phonebook.n.01', 'synonyms': ['phonebook', 'telephone_book', 'telephone_directory'], 'id': 796, 'def': 'a directory containing an alphabetical list of telephone subscribers and their telephone numbers', 'name': 'phonebook'}, {'frequency': 'c', 'synset': 'phonograph_record.n.01', 'synonyms': ['phonograph_record', 'phonograph_recording', 'record_(phonograph_recording)'], 'id': 797, 'def': 'sound recording consisting of a typically black disk with a continuous groove', 'name': 'phonograph_record'}, {'frequency': 'f', 'synset': 'piano.n.01', 'synonyms': ['piano'], 'id': 798, 'def': 'a keyboard instrument that is played by depressing keys that cause hammers to strike tuned strings and produce sounds', 'name': 'piano'}, {'frequency': 'f', 'synset': 'pickle.n.01', 'synonyms': ['pickle'], 'id': 799, 'def': 'vegetables (especially cucumbers) preserved in brine or vinegar', 'name': 'pickle'}, {'frequency': 'f', 'synset': 'pickup.n.01', 'synonyms': ['pickup_truck'], 'id': 800, 'def': 'a light truck with an open body and low sides and a tailboard', 'name': 'pickup_truck'}, {'frequency': 'c', 'synset': 'pie.n.01', 'synonyms': ['pie'], 'id': 801, 'def': 'dish baked in pastry-lined pan often with a pastry top', 'name': 'pie'}, {'frequency': 'c', 'synset': 'pigeon.n.01', 'synonyms': ['pigeon'], 'id': 802, 'def': 'wild and domesticated birds having a heavy body and short legs', 'name': 'pigeon'}, {'frequency': 'r', 'synset': 'piggy_bank.n.01', 'synonyms': ['piggy_bank', 'penny_bank'], 'id': 803, 'def': "a child's coin bank (often shaped like a pig)", 'name': 'piggy_bank'}, {'frequency': 'f', 'synset': 'pillow.n.01', 'synonyms': ['pillow'], 'id': 804, 'def': 'a cushion to support the head of a sleeping person', 'name': 'pillow'}, {'frequency': 'r', 'synset': 'pin.n.09', 'synonyms': ['pin_(non_jewelry)'], 'id': 805, 'def': 'a small slender (often pointed) piece of wood or metal used to support or fasten or attach things', 'name': 'pin_(non_jewelry)'}, {'frequency': 'f', 'synset': 'pineapple.n.02', 'synonyms': ['pineapple'], 'id': 806, 'def': 'large sweet fleshy tropical fruit with a tuft of stiff leaves', 'name': 'pineapple'}, {'frequency': 'c', 'synset': 'pinecone.n.01', 'synonyms': ['pinecone'], 'id': 807, 'def': 'the seed-producing cone of a pine tree', 'name': 'pinecone'}, {'frequency': 'r', 'synset': 'ping-pong_ball.n.01', 'synonyms': ['ping-pong_ball'], 'id': 808, 'def': 'light hollow ball used in playing table tennis', 'name': 'ping-pong_ball'}, {'frequency': 'r', 'synset': 'pinwheel.n.03', 'synonyms': ['pinwheel'], 'id': 809, 'def': 'a toy consisting of vanes of colored paper or plastic that is pinned to a stick and spins when it is pointed into the wind', 'name': 'pinwheel'}, {'frequency': 'r', 'synset': 'pipe.n.01', 'synonyms': ['tobacco_pipe'], 'id': 810, 'def': 'a tube with a small bowl at one end; used for smoking tobacco', 'name': 'tobacco_pipe'}, {'frequency': 'f', 'synset': 'pipe.n.02', 'synonyms': ['pipe', 'piping'], 'id': 811, 'def': 'a long tube made of metal or plastic that is used to carry water or oil or gas etc.', 'name': 'pipe'}, {'frequency': 'r', 'synset': 'pistol.n.01', 'synonyms': ['pistol', 'handgun'], 'id': 812, 'def': 'a firearm that is held and fired with one hand', 'name': 'pistol'}, {'frequency': 'c', 'synset': 'pita.n.01', 'synonyms': ['pita_(bread)', 'pocket_bread'], 'id': 813, 'def': 'usually small round bread that can open into a pocket for filling', 'name': 'pita_(bread)'}, {'frequency': 'f', 'synset': 'pitcher.n.02', 'synonyms': ['pitcher_(vessel_for_liquid)', 'ewer'], 'id': 814, 'def': 'an open vessel with a handle and a spout for pouring', 'name': 'pitcher_(vessel_for_liquid)'}, {'frequency': 'r', 'synset': 'pitchfork.n.01', 'synonyms': ['pitchfork'], 'id': 815, 'def': 'a long-handled hand tool with sharp widely spaced prongs for lifting and pitching hay', 'name': 'pitchfork'}, {'frequency': 'f', 'synset': 'pizza.n.01', 'synonyms': ['pizza'], 'id': 816, 'def': 'Italian open pie made of thin bread dough spread with a spiced mixture of e.g. tomato sauce and cheese', 'name': 'pizza'}, {'frequency': 'f', 'synset': 'place_mat.n.01', 'synonyms': ['place_mat'], 'id': 817, 'def': 'a mat placed on a table for an individual place setting', 'name': 'place_mat'}, {'frequency': 'f', 'synset': 'plate.n.04', 'synonyms': ['plate'], 'id': 818, 'def': 'dish on which food is served or from which food is eaten', 'name': 'plate'}, {'frequency': 'c', 'synset': 'platter.n.01', 'synonyms': ['platter'], 'id': 819, 'def': 'a large shallow dish used for serving food', 'name': 'platter'}, {'frequency': 'r', 'synset': 'playpen.n.01', 'synonyms': ['playpen'], 'id': 820, 'def': 'a portable enclosure in which babies may be left to play', 'name': 'playpen'}, {'frequency': 'c', 'synset': 'pliers.n.01', 'synonyms': ['pliers', 'plyers'], 'id': 821, 'def': 'a gripping hand tool with two hinged arms and (usually) serrated jaws', 'name': 'pliers'}, {'frequency': 'r', 'synset': 'plow.n.01', 'synonyms': ['plow_(farm_equipment)', 'plough_(farm_equipment)'], 'id': 822, 'def': 'a farm tool having one or more heavy blades to break the soil and cut a furrow prior to sowing', 'name': 'plow_(farm_equipment)'}, {'frequency': 'r', 'synset': 'plume.n.02', 'synonyms': ['plume'], 'id': 823, 'def': 'a feather or cluster of feathers worn as an ornament', 'name': 'plume'}, {'frequency': 'r', 'synset': 'pocket_watch.n.01', 'synonyms': ['pocket_watch'], 'id': 824, 'def': 'a watch that is carried in a small watch pocket', 'name': 'pocket_watch'}, {'frequency': 'c', 'synset': 'pocketknife.n.01', 'synonyms': ['pocketknife'], 'id': 825, 'def': 'a knife with a blade that folds into the handle; suitable for carrying in the pocket', 'name': 'pocketknife'}, {'frequency': 'c', 'synset': 'poker.n.01', 'synonyms': ['poker_(fire_stirring_tool)', 'stove_poker', 'fire_hook'], 'id': 826, 'def': 'fire iron consisting of a metal rod with a handle; used to stir a fire', 'name': 'poker_(fire_stirring_tool)'}, {'frequency': 'f', 'synset': 'pole.n.01', 'synonyms': ['pole', 'post'], 'id': 827, 'def': 'a long (usually round) rod of wood or metal or plastic', 'name': 'pole'}, {'frequency': 'f', 'synset': 'polo_shirt.n.01', 'synonyms': ['polo_shirt', 'sport_shirt'], 'id': 828, 'def': 'a shirt with short sleeves designed for comfort and casual wear', 'name': 'polo_shirt'}, {'frequency': 'r', 'synset': 'poncho.n.01', 'synonyms': ['poncho'], 'id': 829, 'def': 'a blanket-like cloak with a hole in the center for the head', 'name': 'poncho'}, {'frequency': 'c', 'synset': 'pony.n.05', 'synonyms': ['pony'], 'id': 830, 'def': 'any of various breeds of small gentle horses usually less than five feet high at the shoulder', 'name': 'pony'}, {'frequency': 'r', 'synset': 'pool_table.n.01', 'synonyms': ['pool_table', 'billiard_table', 'snooker_table'], 'id': 831, 'def': 'game equipment consisting of a heavy table on which pool is played', 'name': 'pool_table'}, {'frequency': 'f', 'synset': 'pop.n.02', 'synonyms': ['pop_(soda)', 'soda_(pop)', 'tonic', 'soft_drink'], 'id': 832, 'def': 'a sweet drink containing carbonated water and flavoring', 'name': 'pop_(soda)'}, {'frequency': 'c', 'synset': 'postbox.n.01', 'synonyms': ['postbox_(public)', 'mailbox_(public)'], 'id': 833, 'def': 'public box for deposit of mail', 'name': 'postbox_(public)'}, {'frequency': 'c', 'synset': 'postcard.n.01', 'synonyms': ['postcard', 'postal_card', 'mailing-card'], 'id': 834, 'def': 'a card for sending messages by post without an envelope', 'name': 'postcard'}, {'frequency': 'f', 'synset': 'poster.n.01', 'synonyms': ['poster', 'placard'], 'id': 835, 'def': 'a sign posted in a public place as an advertisement', 'name': 'poster'}, {'frequency': 'f', 'synset': 'pot.n.01', 'synonyms': ['pot'], 'id': 836, 'def': 'metal or earthenware cooking vessel that is usually round and deep; often has a handle and lid', 'name': 'pot'}, {'frequency': 'f', 'synset': 'pot.n.04', 'synonyms': ['flowerpot'], 'id': 837, 'def': 'a container in which plants are cultivated', 'name': 'flowerpot'}, {'frequency': 'f', 'synset': 'potato.n.01', 'synonyms': ['potato'], 'id': 838, 'def': 'an edible tuber native to South America', 'name': 'potato'}, {'frequency': 'c', 'synset': 'potholder.n.01', 'synonyms': ['potholder'], 'id': 839, 'def': 'an insulated pad for holding hot pots', 'name': 'potholder'}, {'frequency': 'c', 'synset': 'pottery.n.01', 'synonyms': ['pottery', 'clayware'], 'id': 840, 'def': 'ceramic ware made from clay and baked in a kiln', 'name': 'pottery'}, {'frequency': 'c', 'synset': 'pouch.n.01', 'synonyms': ['pouch'], 'id': 841, 'def': 'a small or medium size container for holding or carrying things', 'name': 'pouch'}, {'frequency': 'c', 'synset': 'power_shovel.n.01', 'synonyms': ['power_shovel', 'excavator', 'digger'], 'id': 842, 'def': 'a machine for excavating', 'name': 'power_shovel'}, {'frequency': 'c', 'synset': 'prawn.n.01', 'synonyms': ['prawn', 'shrimp'], 'id': 843, 'def': 'any of various edible decapod crustaceans', 'name': 'prawn'}, {'frequency': 'c', 'synset': 'pretzel.n.01', 'synonyms': ['pretzel'], 'id': 844, 'def': 'glazed and salted cracker typically in the shape of a loose knot', 'name': 'pretzel'}, {'frequency': 'f', 'synset': 'printer.n.03', 'synonyms': ['printer', 'printing_machine'], 'id': 845, 'def': 'a machine that prints', 'name': 'printer'}, {'frequency': 'c', 'synset': 'projectile.n.01', 'synonyms': ['projectile_(weapon)', 'missile'], 'id': 846, 'def': 'a weapon that is forcibly thrown or projected at a targets', 'name': 'projectile_(weapon)'}, {'frequency': 'c', 'synset': 'projector.n.02', 'synonyms': ['projector'], 'id': 847, 'def': 'an optical instrument that projects an enlarged image onto a screen', 'name': 'projector'}, {'frequency': 'f', 'synset': 'propeller.n.01', 'synonyms': ['propeller', 'propellor'], 'id': 848, 'def': 'a mechanical device that rotates to push against air or water', 'name': 'propeller'}, {'frequency': 'r', 'synset': 'prune.n.01', 'synonyms': ['prune'], 'id': 849, 'def': 'dried plum', 'name': 'prune'}, {'frequency': 'r', 'synset': 'pudding.n.01', 'synonyms': ['pudding'], 'id': 850, 'def': 'any of various soft thick unsweetened baked dishes', 'name': 'pudding'}, {'frequency': 'r', 'synset': 'puffer.n.02', 'synonyms': ['puffer_(fish)', 'pufferfish', 'blowfish', 'globefish'], 'id': 851, 'def': 'fishes whose elongated spiny body can inflate itself with water or air to form a globe', 'name': 'puffer_(fish)'}, {'frequency': 'r', 'synset': 'puffin.n.01', 'synonyms': ['puffin'], 'id': 852, 'def': 'seabirds having short necks and brightly colored compressed bills', 'name': 'puffin'}, {'frequency': 'r', 'synset': 'pug.n.01', 'synonyms': ['pug-dog'], 'id': 853, 'def': 'small compact smooth-coated breed of Asiatic origin having a tightly curled tail and broad flat wrinkled muzzle', 'name': 'pug-dog'}, {'frequency': 'c', 'synset': 'pumpkin.n.02', 'synonyms': ['pumpkin'], 'id': 854, 'def': 'usually large pulpy deep-yellow round fruit of the squash family maturing in late summer or early autumn', 'name': 'pumpkin'}, {'frequency': 'r', 'synset': 'punch.n.03', 'synonyms': ['puncher'], 'id': 855, 'def': 'a tool for making holes or indentations', 'name': 'puncher'}, {'frequency': 'r', 'synset': 'puppet.n.01', 'synonyms': ['puppet', 'marionette'], 'id': 856, 'def': 'a small figure of a person operated from above with strings by a puppeteer', 'name': 'puppet'}, {'frequency': 'c', 'synset': 'puppy.n.01', 'synonyms': ['puppy'], 'id': 857, 'def': 'a young dog', 'name': 'puppy'}, {'frequency': 'r', 'synset': 'quesadilla.n.01', 'synonyms': ['quesadilla'], 'id': 858, 'def': 'a tortilla that is filled with cheese and heated', 'name': 'quesadilla'}, {'frequency': 'r', 'synset': 'quiche.n.02', 'synonyms': ['quiche'], 'id': 859, 'def': 'a tart filled with rich unsweetened custard; often contains other ingredients (as cheese or ham or seafood or vegetables)', 'name': 'quiche'}, {'frequency': 'f', 'synset': 'quilt.n.01', 'synonyms': ['quilt', 'comforter'], 'id': 860, 'def': 'bedding made of two layers of cloth filled with stuffing and stitched together', 'name': 'quilt'}, {'frequency': 'c', 'synset': 'rabbit.n.01', 'synonyms': ['rabbit'], 'id': 861, 'def': 'any of various burrowing animals of the family Leporidae having long ears and short tails', 'name': 'rabbit'}, {'frequency': 'r', 'synset': 'racer.n.02', 'synonyms': ['race_car', 'racing_car'], 'id': 862, 'def': 'a fast car that competes in races', 'name': 'race_car'}, {'frequency': 'c', 'synset': 'racket.n.04', 'synonyms': ['racket', 'racquet'], 'id': 863, 'def': 'a sports implement used to strike a ball in various games', 'name': 'racket'}, {'frequency': 'r', 'synset': 'radar.n.01', 'synonyms': ['radar'], 'id': 864, 'def': 'measuring instrument in which the echo of a pulse of microwave radiation is used to detect and locate distant objects', 'name': 'radar'}, {'frequency': 'f', 'synset': 'radiator.n.03', 'synonyms': ['radiator'], 'id': 865, 'def': 'a mechanism consisting of a metal honeycomb through which hot fluids circulate', 'name': 'radiator'}, {'frequency': 'c', 'synset': 'radio_receiver.n.01', 'synonyms': ['radio_receiver', 'radio_set', 'radio', 'tuner_(radio)'], 'id': 866, 'def': 'an electronic receiver that detects and demodulates and amplifies transmitted radio signals', 'name': 'radio_receiver'}, {'frequency': 'c', 'synset': 'radish.n.03', 'synonyms': ['radish', 'daikon'], 'id': 867, 'def': 'pungent edible root of any of various cultivated radish plants', 'name': 'radish'}, {'frequency': 'c', 'synset': 'raft.n.01', 'synonyms': ['raft'], 'id': 868, 'def': 'a flat float (usually made of logs or planks) that can be used for transport or as a platform for swimmers', 'name': 'raft'}, {'frequency': 'r', 'synset': 'rag_doll.n.01', 'synonyms': ['rag_doll'], 'id': 869, 'def': 'a cloth doll that is stuffed and (usually) painted', 'name': 'rag_doll'}, {'frequency': 'c', 'synset': 'raincoat.n.01', 'synonyms': ['raincoat', 'waterproof_jacket'], 'id': 870, 'def': 'a water-resistant coat', 'name': 'raincoat'}, {'frequency': 'c', 'synset': 'ram.n.05', 'synonyms': ['ram_(animal)'], 'id': 871, 'def': 'uncastrated adult male sheep', 'name': 'ram_(animal)'}, {'frequency': 'c', 'synset': 'raspberry.n.02', 'synonyms': ['raspberry'], 'id': 872, 'def': 'red or black edible aggregate berries usually smaller than the related blackberries', 'name': 'raspberry'}, {'frequency': 'r', 'synset': 'rat.n.01', 'synonyms': ['rat'], 'id': 873, 'def': 'any of various long-tailed rodents similar to but larger than a mouse', 'name': 'rat'}, {'frequency': 'c', 'synset': 'razorblade.n.01', 'synonyms': ['razorblade'], 'id': 874, 'def': 'a blade that has very sharp edge', 'name': 'razorblade'}, {'frequency': 'c', 'synset': 'reamer.n.01', 'synonyms': ['reamer_(juicer)', 'juicer', 'juice_reamer'], 'id': 875, 'def': 'a squeezer with a conical ridged center that is used for squeezing juice from citrus fruit', 'name': 'reamer_(juicer)'}, {'frequency': 'f', 'synset': 'rearview_mirror.n.01', 'synonyms': ['rearview_mirror'], 'id': 876, 'def': 'vehicle mirror (side or rearview)', 'name': 'rearview_mirror'}, {'frequency': 'c', 'synset': 'receipt.n.02', 'synonyms': ['receipt'], 'id': 877, 'def': 'an acknowledgment (usually tangible) that payment has been made', 'name': 'receipt'}, {'frequency': 'c', 'synset': 'recliner.n.01', 'synonyms': ['recliner', 'reclining_chair', 'lounger_(chair)'], 'id': 878, 'def': 'an armchair whose back can be lowered and foot can be raised to allow the sitter to recline in it', 'name': 'recliner'}, {'frequency': 'c', 'synset': 'record_player.n.01', 'synonyms': ['record_player', 'phonograph_(record_player)', 'turntable'], 'id': 879, 'def': 'machine in which rotating records cause a stylus to vibrate and the vibrations are amplified acoustically or electronically', 'name': 'record_player'}, {'frequency': 'f', 'synset': 'reflector.n.01', 'synonyms': ['reflector'], 'id': 880, 'def': 'device that reflects light, radiation, etc.', 'name': 'reflector'}, {'frequency': 'f', 'synset': 'remote_control.n.01', 'synonyms': ['remote_control'], 'id': 881, 'def': 'a device that can be used to control a machine or apparatus from a distance', 'name': 'remote_control'}, {'frequency': 'c', 'synset': 'rhinoceros.n.01', 'synonyms': ['rhinoceros'], 'id': 882, 'def': 'massive powerful herbivorous odd-toed ungulate of southeast Asia and Africa having very thick skin and one or two horns on the snout', 'name': 'rhinoceros'}, {'frequency': 'r', 'synset': 'rib.n.03', 'synonyms': ['rib_(food)'], 'id': 883, 'def': 'cut of meat including one or more ribs', 'name': 'rib_(food)'}, {'frequency': 'c', 'synset': 'rifle.n.01', 'synonyms': ['rifle'], 'id': 884, 'def': 'a shoulder firearm with a long barrel', 'name': 'rifle'}, {'frequency': 'f', 'synset': 'ring.n.08', 'synonyms': ['ring'], 'id': 885, 'def': 'jewelry consisting of a circlet of precious metal (often set with jewels) worn on the finger', 'name': 'ring'}, {'frequency': 'r', 'synset': 'river_boat.n.01', 'synonyms': ['river_boat'], 'id': 886, 'def': 'a boat used on rivers or to ply a river', 'name': 'river_boat'}, {'frequency': 'r', 'synset': 'road_map.n.02', 'synonyms': ['road_map'], 'id': 887, 'def': '(NOT A ROAD) a MAP showing roads (for automobile travel)', 'name': 'road_map'}, {'frequency': 'c', 'synset': 'robe.n.01', 'synonyms': ['robe'], 'id': 888, 'def': 'any loose flowing garment', 'name': 'robe'}, {'frequency': 'c', 'synset': 'rocking_chair.n.01', 'synonyms': ['rocking_chair'], 'id': 889, 'def': 'a chair mounted on rockers', 'name': 'rocking_chair'}, {'frequency': 'r', 'synset': 'rodent.n.01', 'synonyms': ['rodent'], 'id': 890, 'def': 'relatively small placental mammals having a single pair of constantly growing incisor teeth specialized for gnawing', 'name': 'rodent'}, {'frequency': 'r', 'synset': 'roller_skate.n.01', 'synonyms': ['roller_skate'], 'id': 891, 'def': 'a shoe with pairs of rollers (small hard wheels) fixed to the sole', 'name': 'roller_skate'}, {'frequency': 'r', 'synset': 'rollerblade.n.01', 'synonyms': ['Rollerblade'], 'id': 892, 'def': 'an in-line variant of a roller skate', 'name': 'Rollerblade'}, {'frequency': 'c', 'synset': 'rolling_pin.n.01', 'synonyms': ['rolling_pin'], 'id': 893, 'def': 'utensil consisting of a cylinder (usually of wood) with a handle at each end; used to roll out dough', 'name': 'rolling_pin'}, {'frequency': 'r', 'synset': 'root_beer.n.01', 'synonyms': ['root_beer'], 'id': 894, 'def': 'carbonated drink containing extracts of roots and herbs', 'name': 'root_beer'}, {'frequency': 'c', 'synset': 'router.n.02', 'synonyms': ['router_(computer_equipment)'], 'id': 895, 'def': 'a device that forwards data packets between computer networks', 'name': 'router_(computer_equipment)'}, {'frequency': 'f', 'synset': 'rubber_band.n.01', 'synonyms': ['rubber_band', 'elastic_band'], 'id': 896, 'def': 'a narrow band of elastic rubber used to hold things (such as papers) together', 'name': 'rubber_band'}, {'frequency': 'c', 'synset': 'runner.n.08', 'synonyms': ['runner_(carpet)'], 'id': 897, 'def': 'a long narrow carpet', 'name': 'runner_(carpet)'}, {'frequency': 'f', 'synset': 'sack.n.01', 'synonyms': ['plastic_bag', 'paper_bag'], 'id': 898, 'def': "a bag made of paper or plastic for holding customer's purchases", 'name': 'plastic_bag'}, {'frequency': 'f', 'synset': 'saddle.n.01', 'synonyms': ['saddle_(on_an_animal)'], 'id': 899, 'def': 'a seat for the rider of a horse or camel', 'name': 'saddle_(on_an_animal)'}, {'frequency': 'f', 'synset': 'saddle_blanket.n.01', 'synonyms': ['saddle_blanket', 'saddlecloth', 'horse_blanket'], 'id': 900, 'def': 'stable gear consisting of a blanket placed under the saddle', 'name': 'saddle_blanket'}, {'frequency': 'c', 'synset': 'saddlebag.n.01', 'synonyms': ['saddlebag'], 'id': 901, 'def': 'a large bag (or pair of bags) hung over a saddle', 'name': 'saddlebag'}, {'frequency': 'r', 'synset': 'safety_pin.n.01', 'synonyms': ['safety_pin'], 'id': 902, 'def': 'a pin in the form of a clasp; has a guard so the point of the pin will not stick the user', 'name': 'safety_pin'}, {'frequency': 'f', 'synset': 'sail.n.01', 'synonyms': ['sail'], 'id': 903, 'def': 'a large piece of fabric by means of which wind is used to propel a sailing vessel', 'name': 'sail'}, {'frequency': 'f', 'synset': 'salad.n.01', 'synonyms': ['salad'], 'id': 904, 'def': 'food mixtures either arranged on a plate or tossed and served with a moist dressing; usually consisting of or including greens', 'name': 'salad'}, {'frequency': 'r', 'synset': 'salad_plate.n.01', 'synonyms': ['salad_plate', 'salad_bowl'], 'id': 905, 'def': 'a plate or bowl for individual servings of salad', 'name': 'salad_plate'}, {'frequency': 'c', 'synset': 'salami.n.01', 'synonyms': ['salami'], 'id': 906, 'def': 'highly seasoned fatty sausage of pork and beef usually dried', 'name': 'salami'}, {'frequency': 'c', 'synset': 'salmon.n.01', 'synonyms': ['salmon_(fish)'], 'id': 907, 'def': 'any of various large food and game fishes of northern waters', 'name': 'salmon_(fish)'}, {'frequency': 'r', 'synset': 'salmon.n.03', 'synonyms': ['salmon_(food)'], 'id': 908, 'def': 'flesh of any of various marine or freshwater fish of the family Salmonidae', 'name': 'salmon_(food)'}, {'frequency': 'c', 'synset': 'salsa.n.01', 'synonyms': ['salsa'], 'id': 909, 'def': 'spicy sauce of tomatoes and onions and chili peppers to accompany Mexican foods', 'name': 'salsa'}, {'frequency': 'f', 'synset': 'saltshaker.n.01', 'synonyms': ['saltshaker'], 'id': 910, 'def': 'a shaker with a perforated top for sprinkling salt', 'name': 'saltshaker'}, {'frequency': 'f', 'synset': 'sandal.n.01', 'synonyms': ['sandal_(type_of_shoe)'], 'id': 911, 'def': 'a shoe consisting of a sole fastened by straps to the foot', 'name': 'sandal_(type_of_shoe)'}, {'frequency': 'f', 'synset': 'sandwich.n.01', 'synonyms': ['sandwich'], 'id': 912, 'def': 'two (or more) slices of bread with a filling between them', 'name': 'sandwich'}, {'frequency': 'r', 'synset': 'satchel.n.01', 'synonyms': ['satchel'], 'id': 913, 'def': 'luggage consisting of a small case with a flat bottom and (usually) a shoulder strap', 'name': 'satchel'}, {'frequency': 'r', 'synset': 'saucepan.n.01', 'synonyms': ['saucepan'], 'id': 914, 'def': 'a deep pan with a handle; used for stewing or boiling', 'name': 'saucepan'}, {'frequency': 'f', 'synset': 'saucer.n.02', 'synonyms': ['saucer'], 'id': 915, 'def': 'a small shallow dish for holding a cup at the table', 'name': 'saucer'}, {'frequency': 'f', 'synset': 'sausage.n.01', 'synonyms': ['sausage'], 'id': 916, 'def': 'highly seasoned minced meat stuffed in casings', 'name': 'sausage'}, {'frequency': 'r', 'synset': 'sawhorse.n.01', 'synonyms': ['sawhorse', 'sawbuck'], 'id': 917, 'def': 'a framework for holding wood that is being sawed', 'name': 'sawhorse'}, {'frequency': 'r', 'synset': 'sax.n.02', 'synonyms': ['saxophone'], 'id': 918, 'def': "a wind instrument with a `J'-shaped form typically made of brass", 'name': 'saxophone'}, {'frequency': 'f', 'synset': 'scale.n.07', 'synonyms': ['scale_(measuring_instrument)'], 'id': 919, 'def': 'a measuring instrument for weighing; shows amount of mass', 'name': 'scale_(measuring_instrument)'}, {'frequency': 'r', 'synset': 'scarecrow.n.01', 'synonyms': ['scarecrow', 'strawman'], 'id': 920, 'def': 'an effigy in the shape of a man to frighten birds away from seeds', 'name': 'scarecrow'}, {'frequency': 'f', 'synset': 'scarf.n.01', 'synonyms': ['scarf'], 'id': 921, 'def': 'a garment worn around the head or neck or shoulders for warmth or decoration', 'name': 'scarf'}, {'frequency': 'c', 'synset': 'school_bus.n.01', 'synonyms': ['school_bus'], 'id': 922, 'def': 'a bus used to transport children to or from school', 'name': 'school_bus'}, {'frequency': 'f', 'synset': 'scissors.n.01', 'synonyms': ['scissors'], 'id': 923, 'def': 'a tool having two crossed pivoting blades with looped handles', 'name': 'scissors'}, {'frequency': 'f', 'synset': 'scoreboard.n.01', 'synonyms': ['scoreboard'], 'id': 924, 'def': 'a large board for displaying the score of a contest (and some other information)', 'name': 'scoreboard'}, {'frequency': 'r', 'synset': 'scraper.n.01', 'synonyms': ['scraper'], 'id': 925, 'def': 'any of various hand tools for scraping', 'name': 'scraper'}, {'frequency': 'c', 'synset': 'screwdriver.n.01', 'synonyms': ['screwdriver'], 'id': 926, 'def': 'a hand tool for driving screws; has a tip that fits into the head of a screw', 'name': 'screwdriver'}, {'frequency': 'f', 'synset': 'scrub_brush.n.01', 'synonyms': ['scrubbing_brush'], 'id': 927, 'def': 'a brush with short stiff bristles for heavy cleaning', 'name': 'scrubbing_brush'}, {'frequency': 'c', 'synset': 'sculpture.n.01', 'synonyms': ['sculpture'], 'id': 928, 'def': 'a three-dimensional work of art', 'name': 'sculpture'}, {'frequency': 'c', 'synset': 'seabird.n.01', 'synonyms': ['seabird', 'seafowl'], 'id': 929, 'def': 'a bird that frequents coastal waters and the open ocean: gulls; pelicans; gannets; cormorants; albatrosses; petrels; etc.', 'name': 'seabird'}, {'frequency': 'c', 'synset': 'seahorse.n.02', 'synonyms': ['seahorse'], 'id': 930, 'def': 'small fish with horse-like heads bent sharply downward and curled tails', 'name': 'seahorse'}, {'frequency': 'r', 'synset': 'seaplane.n.01', 'synonyms': ['seaplane', 'hydroplane'], 'id': 931, 'def': 'an airplane that can land on or take off from water', 'name': 'seaplane'}, {'frequency': 'c', 'synset': 'seashell.n.01', 'synonyms': ['seashell'], 'id': 932, 'def': 'the shell of a marine organism', 'name': 'seashell'}, {'frequency': 'c', 'synset': 'sewing_machine.n.01', 'synonyms': ['sewing_machine'], 'id': 933, 'def': 'a textile machine used as a home appliance for sewing', 'name': 'sewing_machine'}, {'frequency': 'c', 'synset': 'shaker.n.03', 'synonyms': ['shaker'], 'id': 934, 'def': 'a container in which something can be shaken', 'name': 'shaker'}, {'frequency': 'c', 'synset': 'shampoo.n.01', 'synonyms': ['shampoo'], 'id': 935, 'def': 'cleansing agent consisting of soaps or detergents used for washing the hair', 'name': 'shampoo'}, {'frequency': 'c', 'synset': 'shark.n.01', 'synonyms': ['shark'], 'id': 936, 'def': 'typically large carnivorous fishes with sharpe teeth', 'name': 'shark'}, {'frequency': 'r', 'synset': 'sharpener.n.01', 'synonyms': ['sharpener'], 'id': 937, 'def': 'any implement that is used to make something (an edge or a point) sharper', 'name': 'sharpener'}, {'frequency': 'r', 'synset': 'sharpie.n.03', 'synonyms': ['Sharpie'], 'id': 938, 'def': 'a pen with indelible ink that will write on any surface', 'name': 'Sharpie'}, {'frequency': 'r', 'synset': 'shaver.n.03', 'synonyms': ['shaver_(electric)', 'electric_shaver', 'electric_razor'], 'id': 939, 'def': 'a razor powered by an electric motor', 'name': 'shaver_(electric)'}, {'frequency': 'c', 'synset': 'shaving_cream.n.01', 'synonyms': ['shaving_cream', 'shaving_soap'], 'id': 940, 'def': 'toiletry consisting that forms a rich lather for softening the beard before shaving', 'name': 'shaving_cream'}, {'frequency': 'r', 'synset': 'shawl.n.01', 'synonyms': ['shawl'], 'id': 941, 'def': 'cloak consisting of an oblong piece of cloth used to cover the head and shoulders', 'name': 'shawl'}, {'frequency': 'r', 'synset': 'shears.n.01', 'synonyms': ['shears'], 'id': 942, 'def': 'large scissors with strong blades', 'name': 'shears'}, {'frequency': 'f', 'synset': 'sheep.n.01', 'synonyms': ['sheep'], 'id': 943, 'def': 'woolly usually horned ruminant mammal related to the goat', 'name': 'sheep'}, {'frequency': 'r', 'synset': 'shepherd_dog.n.01', 'synonyms': ['shepherd_dog', 'sheepdog'], 'id': 944, 'def': 'any of various usually long-haired breeds of dog reared to herd and guard sheep', 'name': 'shepherd_dog'}, {'frequency': 'r', 'synset': 'sherbert.n.01', 'synonyms': ['sherbert', 'sherbet'], 'id': 945, 'def': 'a frozen dessert made primarily of fruit juice and sugar', 'name': 'sherbert'}, {'frequency': 'c', 'synset': 'shield.n.02', 'synonyms': ['shield'], 'id': 946, 'def': 'armor carried on the arm to intercept blows', 'name': 'shield'}, {'frequency': 'f', 'synset': 'shirt.n.01', 'synonyms': ['shirt'], 'id': 947, 'def': 'a garment worn on the upper half of the body', 'name': 'shirt'}, {'frequency': 'f', 'synset': 'shoe.n.01', 'synonyms': ['shoe', 'sneaker_(type_of_shoe)', 'tennis_shoe'], 'id': 948, 'def': 'common footwear covering the foot', 'name': 'shoe'}, {'frequency': 'f', 'synset': 'shopping_bag.n.01', 'synonyms': ['shopping_bag'], 'id': 949, 'def': 'a bag made of plastic or strong paper (often with handles); used to transport goods after shopping', 'name': 'shopping_bag'}, {'frequency': 'c', 'synset': 'shopping_cart.n.01', 'synonyms': ['shopping_cart'], 'id': 950, 'def': 'a handcart that holds groceries or other goods while shopping', 'name': 'shopping_cart'}, {'frequency': 'f', 'synset': 'short_pants.n.01', 'synonyms': ['short_pants', 'shorts_(clothing)', 'trunks_(clothing)'], 'id': 951, 'def': 'trousers that end at or above the knee', 'name': 'short_pants'}, {'frequency': 'r', 'synset': 'shot_glass.n.01', 'synonyms': ['shot_glass'], 'id': 952, 'def': 'a small glass adequate to hold a single swallow of whiskey', 'name': 'shot_glass'}, {'frequency': 'f', 'synset': 'shoulder_bag.n.01', 'synonyms': ['shoulder_bag'], 'id': 953, 'def': 'a large handbag that can be carried by a strap looped over the shoulder', 'name': 'shoulder_bag'}, {'frequency': 'c', 'synset': 'shovel.n.01', 'synonyms': ['shovel'], 'id': 954, 'def': 'a hand tool for lifting loose material such as snow, dirt, etc.', 'name': 'shovel'}, {'frequency': 'f', 'synset': 'shower.n.01', 'synonyms': ['shower_head'], 'id': 955, 'def': 'a plumbing fixture that sprays water over you', 'name': 'shower_head'}, {'frequency': 'r', 'synset': 'shower_cap.n.01', 'synonyms': ['shower_cap'], 'id': 956, 'def': 'a tight cap worn to keep hair dry while showering', 'name': 'shower_cap'}, {'frequency': 'f', 'synset': 'shower_curtain.n.01', 'synonyms': ['shower_curtain'], 'id': 957, 'def': 'a curtain that keeps water from splashing out of the shower area', 'name': 'shower_curtain'}, {'frequency': 'r', 'synset': 'shredder.n.01', 'synonyms': ['shredder_(for_paper)'], 'id': 958, 'def': 'a device that shreds documents', 'name': 'shredder_(for_paper)'}, {'frequency': 'f', 'synset': 'signboard.n.01', 'synonyms': ['signboard'], 'id': 959, 'def': 'structure displaying a board on which advertisements can be posted', 'name': 'signboard'}, {'frequency': 'c', 'synset': 'silo.n.01', 'synonyms': ['silo'], 'id': 960, 'def': 'a cylindrical tower used for storing goods', 'name': 'silo'}, {'frequency': 'f', 'synset': 'sink.n.01', 'synonyms': ['sink'], 'id': 961, 'def': 'plumbing fixture consisting of a water basin fixed to a wall or floor and having a drainpipe', 'name': 'sink'}, {'frequency': 'f', 'synset': 'skateboard.n.01', 'synonyms': ['skateboard'], 'id': 962, 'def': 'a board with wheels that is ridden in a standing or crouching position and propelled by foot', 'name': 'skateboard'}, {'frequency': 'c', 'synset': 'skewer.n.01', 'synonyms': ['skewer'], 'id': 963, 'def': 'a long pin for holding meat in position while it is being roasted', 'name': 'skewer'}, {'frequency': 'f', 'synset': 'ski.n.01', 'synonyms': ['ski'], 'id': 964, 'def': 'sports equipment for skiing on snow', 'name': 'ski'}, {'frequency': 'f', 'synset': 'ski_boot.n.01', 'synonyms': ['ski_boot'], 'id': 965, 'def': 'a stiff boot that is fastened to a ski with a ski binding', 'name': 'ski_boot'}, {'frequency': 'f', 'synset': 'ski_parka.n.01', 'synonyms': ['ski_parka', 'ski_jacket'], 'id': 966, 'def': 'a parka to be worn while skiing', 'name': 'ski_parka'}, {'frequency': 'f', 'synset': 'ski_pole.n.01', 'synonyms': ['ski_pole'], 'id': 967, 'def': 'a pole with metal points used as an aid in skiing', 'name': 'ski_pole'}, {'frequency': 'f', 'synset': 'skirt.n.02', 'synonyms': ['skirt'], 'id': 968, 'def': 'a garment hanging from the waist; worn mainly by girls and women', 'name': 'skirt'}, {'frequency': 'r', 'synset': 'skullcap.n.01', 'synonyms': ['skullcap'], 'id': 969, 'def': 'rounded brimless cap fitting the crown of the head', 'name': 'skullcap'}, {'frequency': 'c', 'synset': 'sled.n.01', 'synonyms': ['sled', 'sledge', 'sleigh'], 'id': 970, 'def': 'a vehicle or flat object for transportation over snow by sliding or pulled by dogs, etc.', 'name': 'sled'}, {'frequency': 'c', 'synset': 'sleeping_bag.n.01', 'synonyms': ['sleeping_bag'], 'id': 971, 'def': 'large padded bag designed to be slept in outdoors', 'name': 'sleeping_bag'}, {'frequency': 'r', 'synset': 'sling.n.05', 'synonyms': ['sling_(bandage)', 'triangular_bandage'], 'id': 972, 'def': 'bandage to support an injured forearm; slung over the shoulder or neck', 'name': 'sling_(bandage)'}, {'frequency': 'c', 'synset': 'slipper.n.01', 'synonyms': ['slipper_(footwear)', 'carpet_slipper_(footwear)'], 'id': 973, 'def': 'low footwear that can be slipped on and off easily; usually worn indoors', 'name': 'slipper_(footwear)'}, {'frequency': 'r', 'synset': 'smoothie.n.02', 'synonyms': ['smoothie'], 'id': 974, 'def': 'a thick smooth drink consisting of fresh fruit pureed with ice cream or yoghurt or milk', 'name': 'smoothie'}, {'frequency': 'r', 'synset': 'snake.n.01', 'synonyms': ['snake', 'serpent'], 'id': 975, 'def': 'limbless scaly elongate reptile; some are venomous', 'name': 'snake'}, {'frequency': 'f', 'synset': 'snowboard.n.01', 'synonyms': ['snowboard'], 'id': 976, 'def': 'a board that resembles a broad ski or a small surfboard; used in a standing position to slide down snow-covered slopes', 'name': 'snowboard'}, {'frequency': 'c', 'synset': 'snowman.n.01', 'synonyms': ['snowman'], 'id': 977, 'def': 'a figure of a person made of packed snow', 'name': 'snowman'}, {'frequency': 'c', 'synset': 'snowmobile.n.01', 'synonyms': ['snowmobile'], 'id': 978, 'def': 'tracked vehicle for travel on snow having skis in front', 'name': 'snowmobile'}, {'frequency': 'f', 'synset': 'soap.n.01', 'synonyms': ['soap'], 'id': 979, 'def': 'a cleansing agent made from the salts of vegetable or animal fats', 'name': 'soap'}, {'frequency': 'f', 'synset': 'soccer_ball.n.01', 'synonyms': ['soccer_ball'], 'id': 980, 'def': "an inflated ball used in playing soccer (called `football' outside of the United States)", 'name': 'soccer_ball'}, {'frequency': 'f', 'synset': 'sock.n.01', 'synonyms': ['sock'], 'id': 981, 'def': 'cloth covering for the foot; worn inside the shoe; reaches to between the ankle and the knee', 'name': 'sock'}, {'frequency': 'f', 'synset': 'sofa.n.01', 'synonyms': ['sofa', 'couch', 'lounge'], 'id': 982, 'def': 'an upholstered seat for more than one person', 'name': 'sofa'}, {'frequency': 'r', 'synset': 'softball.n.01', 'synonyms': ['softball'], 'id': 983, 'def': 'ball used in playing softball', 'name': 'softball'}, {'frequency': 'c', 'synset': 'solar_array.n.01', 'synonyms': ['solar_array', 'solar_battery', 'solar_panel'], 'id': 984, 'def': 'electrical device consisting of a large array of connected solar cells', 'name': 'solar_array'}, {'frequency': 'r', 'synset': 'sombrero.n.02', 'synonyms': ['sombrero'], 'id': 985, 'def': 'a straw hat with a tall crown and broad brim; worn in American southwest and in Mexico', 'name': 'sombrero'}, {'frequency': 'f', 'synset': 'soup.n.01', 'synonyms': ['soup'], 'id': 986, 'def': 'liquid food especially of meat or fish or vegetable stock often containing pieces of solid food', 'name': 'soup'}, {'frequency': 'r', 'synset': 'soup_bowl.n.01', 'synonyms': ['soup_bowl'], 'id': 987, 'def': 'a bowl for serving soup', 'name': 'soup_bowl'}, {'frequency': 'c', 'synset': 'soupspoon.n.01', 'synonyms': ['soupspoon'], 'id': 988, 'def': 'a spoon with a rounded bowl for eating soup', 'name': 'soupspoon'}, {'frequency': 'c', 'synset': 'sour_cream.n.01', 'synonyms': ['sour_cream', 'soured_cream'], 'id': 989, 'def': 'soured light cream', 'name': 'sour_cream'}, {'frequency': 'r', 'synset': 'soya_milk.n.01', 'synonyms': ['soya_milk', 'soybean_milk', 'soymilk'], 'id': 990, 'def': 'a milk substitute containing soybean flour and water; used in some infant formulas and in making tofu', 'name': 'soya_milk'}, {'frequency': 'r', 'synset': 'space_shuttle.n.01', 'synonyms': ['space_shuttle'], 'id': 991, 'def': "a reusable spacecraft with wings for a controlled descent through the Earth's atmosphere", 'name': 'space_shuttle'}, {'frequency': 'r', 'synset': 'sparkler.n.02', 'synonyms': ['sparkler_(fireworks)'], 'id': 992, 'def': 'a firework that burns slowly and throws out a shower of sparks', 'name': 'sparkler_(fireworks)'}, {'frequency': 'f', 'synset': 'spatula.n.02', 'synonyms': ['spatula'], 'id': 993, 'def': 'a hand tool with a thin flexible blade used to mix or spread soft substances', 'name': 'spatula'}, {'frequency': 'r', 'synset': 'spear.n.01', 'synonyms': ['spear', 'lance'], 'id': 994, 'def': 'a long pointed rod used as a tool or weapon', 'name': 'spear'}, {'frequency': 'f', 'synset': 'spectacles.n.01', 'synonyms': ['spectacles', 'specs', 'eyeglasses', 'glasses'], 'id': 995, 'def': 'optical instrument consisting of a frame that holds a pair of lenses for correcting defective vision', 'name': 'spectacles'}, {'frequency': 'c', 'synset': 'spice_rack.n.01', 'synonyms': ['spice_rack'], 'id': 996, 'def': 'a rack for displaying containers filled with spices', 'name': 'spice_rack'}, {'frequency': 'c', 'synset': 'spider.n.01', 'synonyms': ['spider'], 'id': 997, 'def': 'predatory arachnid with eight legs, two poison fangs, two feelers, and usually two silk-spinning organs at the back end of the body', 'name': 'spider'}, {'frequency': 'r', 'synset': 'spiny_lobster.n.02', 'synonyms': ['crawfish', 'crayfish'], 'id': 998, 'def': 'large edible marine crustacean having a spiny carapace but lacking the large pincers of true lobsters', 'name': 'crawfish'}, {'frequency': 'c', 'synset': 'sponge.n.01', 'synonyms': ['sponge'], 'id': 999, 'def': 'a porous mass usable to absorb water typically used for cleaning', 'name': 'sponge'}, {'frequency': 'f', 'synset': 'spoon.n.01', 'synonyms': ['spoon'], 'id': 1000, 'def': 'a piece of cutlery with a shallow bowl-shaped container and a handle', 'name': 'spoon'}, {'frequency': 'c', 'synset': 'sportswear.n.01', 'synonyms': ['sportswear', 'athletic_wear', 'activewear'], 'id': 1001, 'def': 'attire worn for sport or for casual wear', 'name': 'sportswear'}, {'frequency': 'c', 'synset': 'spotlight.n.02', 'synonyms': ['spotlight'], 'id': 1002, 'def': 'a lamp that produces a strong beam of light to illuminate a restricted area; used to focus attention of a stage performer', 'name': 'spotlight'}, {'frequency': 'r', 'synset': 'squid.n.01', 'synonyms': ['squid_(food)', 'calamari', 'calamary'], 'id': 1003, 'def': '(Italian cuisine) squid prepared as food', 'name': 'squid_(food)'}, {'frequency': 'c', 'synset': 'squirrel.n.01', 'synonyms': ['squirrel'], 'id': 1004, 'def': 'a kind of arboreal rodent having a long bushy tail', 'name': 'squirrel'}, {'frequency': 'r', 'synset': 'stagecoach.n.01', 'synonyms': ['stagecoach'], 'id': 1005, 'def': 'a large coach-and-four formerly used to carry passengers and mail on regular routes between towns', 'name': 'stagecoach'}, {'frequency': 'c', 'synset': 'stapler.n.01', 'synonyms': ['stapler_(stapling_machine)'], 'id': 1006, 'def': 'a machine that inserts staples into sheets of paper in order to fasten them together', 'name': 'stapler_(stapling_machine)'}, {'frequency': 'c', 'synset': 'starfish.n.01', 'synonyms': ['starfish', 'sea_star'], 'id': 1007, 'def': 'echinoderms characterized by five arms extending from a central disk', 'name': 'starfish'}, {'frequency': 'f', 'synset': 'statue.n.01', 'synonyms': ['statue_(sculpture)'], 'id': 1008, 'def': 'a sculpture representing a human or animal', 'name': 'statue_(sculpture)'}, {'frequency': 'c', 'synset': 'steak.n.01', 'synonyms': ['steak_(food)'], 'id': 1009, 'def': 'a slice of meat cut from the fleshy part of an animal or large fish', 'name': 'steak_(food)'}, {'frequency': 'r', 'synset': 'steak_knife.n.01', 'synonyms': ['steak_knife'], 'id': 1010, 'def': 'a sharp table knife used in eating steak', 'name': 'steak_knife'}, {'frequency': 'f', 'synset': 'steering_wheel.n.01', 'synonyms': ['steering_wheel'], 'id': 1011, 'def': 'a handwheel that is used for steering', 'name': 'steering_wheel'}, {'frequency': 'r', 'synset': 'step_ladder.n.01', 'synonyms': ['stepladder'], 'id': 1012, 'def': 'a folding portable ladder hinged at the top', 'name': 'stepladder'}, {'frequency': 'c', 'synset': 'step_stool.n.01', 'synonyms': ['step_stool'], 'id': 1013, 'def': 'a stool that has one or two steps that fold under the seat', 'name': 'step_stool'}, {'frequency': 'c', 'synset': 'stereo.n.01', 'synonyms': ['stereo_(sound_system)'], 'id': 1014, 'def': 'electronic device for playing audio', 'name': 'stereo_(sound_system)'}, {'frequency': 'r', 'synset': 'stew.n.02', 'synonyms': ['stew'], 'id': 1015, 'def': 'food prepared by stewing especially meat or fish with vegetables', 'name': 'stew'}, {'frequency': 'r', 'synset': 'stirrer.n.02', 'synonyms': ['stirrer'], 'id': 1016, 'def': 'an implement used for stirring', 'name': 'stirrer'}, {'frequency': 'f', 'synset': 'stirrup.n.01', 'synonyms': ['stirrup'], 'id': 1017, 'def': "support consisting of metal loops into which rider's feet go", 'name': 'stirrup'}, {'frequency': 'f', 'synset': 'stool.n.01', 'synonyms': ['stool'], 'id': 1018, 'def': 'a simple seat without a back or arms', 'name': 'stool'}, {'frequency': 'f', 'synset': 'stop_sign.n.01', 'synonyms': ['stop_sign'], 'id': 1019, 'def': 'a traffic sign to notify drivers that they must come to a complete stop', 'name': 'stop_sign'}, {'frequency': 'f', 'synset': 'stoplight.n.01', 'synonyms': ['brake_light'], 'id': 1020, 'def': 'a red light on the rear of a motor vehicle that signals when the brakes are applied', 'name': 'brake_light'}, {'frequency': 'f', 'synset': 'stove.n.01', 'synonyms': ['stove', 'kitchen_stove', 'range_(kitchen_appliance)', 'kitchen_range', 'cooking_stove'], 'id': 1021, 'def': 'a kitchen appliance used for cooking food', 'name': 'stove'}, {'frequency': 'c', 'synset': 'strainer.n.01', 'synonyms': ['strainer'], 'id': 1022, 'def': 'a filter to retain larger pieces while smaller pieces and liquids pass through', 'name': 'strainer'}, {'frequency': 'f', 'synset': 'strap.n.01', 'synonyms': ['strap'], 'id': 1023, 'def': 'an elongated strip of material for binding things together or holding', 'name': 'strap'}, {'frequency': 'f', 'synset': 'straw.n.04', 'synonyms': ['straw_(for_drinking)', 'drinking_straw'], 'id': 1024, 'def': 'a thin paper or plastic tube used to suck liquids into the mouth', 'name': 'straw_(for_drinking)'}, {'frequency': 'f', 'synset': 'strawberry.n.01', 'synonyms': ['strawberry'], 'id': 1025, 'def': 'sweet fleshy red fruit', 'name': 'strawberry'}, {'frequency': 'f', 'synset': 'street_sign.n.01', 'synonyms': ['street_sign'], 'id': 1026, 'def': 'a sign visible from the street', 'name': 'street_sign'}, {'frequency': 'f', 'synset': 'streetlight.n.01', 'synonyms': ['streetlight', 'street_lamp'], 'id': 1027, 'def': 'a lamp supported on a lamppost; for illuminating a street', 'name': 'streetlight'}, {'frequency': 'r', 'synset': 'string_cheese.n.01', 'synonyms': ['string_cheese'], 'id': 1028, 'def': 'cheese formed in long strings twisted together', 'name': 'string_cheese'}, {'frequency': 'r', 'synset': 'stylus.n.02', 'synonyms': ['stylus'], 'id': 1029, 'def': 'a pointed tool for writing or drawing or engraving, including pens', 'name': 'stylus'}, {'frequency': 'r', 'synset': 'subwoofer.n.01', 'synonyms': ['subwoofer'], 'id': 1030, 'def': 'a loudspeaker that is designed to reproduce very low bass frequencies', 'name': 'subwoofer'}, {'frequency': 'r', 'synset': 'sugar_bowl.n.01', 'synonyms': ['sugar_bowl'], 'id': 1031, 'def': 'a dish in which sugar is served', 'name': 'sugar_bowl'}, {'frequency': 'r', 'synset': 'sugarcane.n.01', 'synonyms': ['sugarcane_(plant)'], 'id': 1032, 'def': 'juicy canes whose sap is a source of molasses and commercial sugar; fresh canes are sometimes chewed for the juice', 'name': 'sugarcane_(plant)'}, {'frequency': 'f', 'synset': 'suit.n.01', 'synonyms': ['suit_(clothing)'], 'id': 1033, 'def': 'a set of garments (usually including a jacket and trousers or skirt) for outerwear all of the same fabric and color', 'name': 'suit_(clothing)'}, {'frequency': 'c', 'synset': 'sunflower.n.01', 'synonyms': ['sunflower'], 'id': 1034, 'def': 'any plant of the genus Helianthus having large flower heads with dark disk florets and showy yellow rays', 'name': 'sunflower'}, {'frequency': 'f', 'synset': 'sunglasses.n.01', 'synonyms': ['sunglasses'], 'id': 1035, 'def': 'spectacles that are darkened or polarized to protect the eyes from the glare of the sun', 'name': 'sunglasses'}, {'frequency': 'c', 'synset': 'sunhat.n.01', 'synonyms': ['sunhat'], 'id': 1036, 'def': 'a hat with a broad brim that protects the face from direct exposure to the sun', 'name': 'sunhat'}, {'frequency': 'f', 'synset': 'surfboard.n.01', 'synonyms': ['surfboard'], 'id': 1037, 'def': 'a narrow buoyant board for riding surf', 'name': 'surfboard'}, {'frequency': 'c', 'synset': 'sushi.n.01', 'synonyms': ['sushi'], 'id': 1038, 'def': 'rice (with raw fish) wrapped in seaweed', 'name': 'sushi'}, {'frequency': 'c', 'synset': 'swab.n.02', 'synonyms': ['mop'], 'id': 1039, 'def': 'cleaning implement consisting of absorbent material fastened to a handle; for cleaning floors', 'name': 'mop'}, {'frequency': 'c', 'synset': 'sweat_pants.n.01', 'synonyms': ['sweat_pants'], 'id': 1040, 'def': 'loose-fitting trousers with elastic cuffs; worn by athletes', 'name': 'sweat_pants'}, {'frequency': 'c', 'synset': 'sweatband.n.02', 'synonyms': ['sweatband'], 'id': 1041, 'def': 'a band of material tied around the forehead or wrist to absorb sweat', 'name': 'sweatband'}, {'frequency': 'f', 'synset': 'sweater.n.01', 'synonyms': ['sweater'], 'id': 1042, 'def': 'a crocheted or knitted garment covering the upper part of the body', 'name': 'sweater'}, {'frequency': 'f', 'synset': 'sweatshirt.n.01', 'synonyms': ['sweatshirt'], 'id': 1043, 'def': 'cotton knit pullover with long sleeves worn during athletic activity', 'name': 'sweatshirt'}, {'frequency': 'c', 'synset': 'sweet_potato.n.02', 'synonyms': ['sweet_potato'], 'id': 1044, 'def': 'the edible tuberous root of the sweet potato vine', 'name': 'sweet_potato'}, {'frequency': 'f', 'synset': 'swimsuit.n.01', 'synonyms': ['swimsuit', 'swimwear', 'bathing_suit', 'swimming_costume', 'bathing_costume', 'swimming_trunks', 'bathing_trunks'], 'id': 1045, 'def': 'garment worn for swimming', 'name': 'swimsuit'}, {'frequency': 'c', 'synset': 'sword.n.01', 'synonyms': ['sword'], 'id': 1046, 'def': 'a cutting or thrusting weapon that has a long metal blade', 'name': 'sword'}, {'frequency': 'r', 'synset': 'syringe.n.01', 'synonyms': ['syringe'], 'id': 1047, 'def': 'a medical instrument used to inject or withdraw fluids', 'name': 'syringe'}, {'frequency': 'r', 'synset': 'tabasco.n.02', 'synonyms': ['Tabasco_sauce'], 'id': 1048, 'def': 'very spicy sauce (trade name Tabasco) made from fully-aged red peppers', 'name': 'Tabasco_sauce'}, {'frequency': 'r', 'synset': 'table-tennis_table.n.01', 'synonyms': ['table-tennis_table', 'ping-pong_table'], 'id': 1049, 'def': 'a table used for playing table tennis', 'name': 'table-tennis_table'}, {'frequency': 'f', 'synset': 'table.n.02', 'synonyms': ['table'], 'id': 1050, 'def': 'a piece of furniture having a smooth flat top that is usually supported by one or more vertical legs', 'name': 'table'}, {'frequency': 'c', 'synset': 'table_lamp.n.01', 'synonyms': ['table_lamp'], 'id': 1051, 'def': 'a lamp that sits on a table', 'name': 'table_lamp'}, {'frequency': 'f', 'synset': 'tablecloth.n.01', 'synonyms': ['tablecloth'], 'id': 1052, 'def': 'a covering spread over a dining table', 'name': 'tablecloth'}, {'frequency': 'r', 'synset': 'tachometer.n.01', 'synonyms': ['tachometer'], 'id': 1053, 'def': 'measuring instrument for indicating speed of rotation', 'name': 'tachometer'}, {'frequency': 'r', 'synset': 'taco.n.02', 'synonyms': ['taco'], 'id': 1054, 'def': 'a small tortilla cupped around a filling', 'name': 'taco'}, {'frequency': 'f', 'synset': 'tag.n.02', 'synonyms': ['tag'], 'id': 1055, 'def': 'a label associated with something for the purpose of identification or information', 'name': 'tag'}, {'frequency': 'f', 'synset': 'taillight.n.01', 'synonyms': ['taillight', 'rear_light'], 'id': 1056, 'def': 'lamp (usually red) mounted at the rear of a motor vehicle', 'name': 'taillight'}, {'frequency': 'r', 'synset': 'tambourine.n.01', 'synonyms': ['tambourine'], 'id': 1057, 'def': 'a shallow drum with a single drumhead and with metallic disks in the sides', 'name': 'tambourine'}, {'frequency': 'r', 'synset': 'tank.n.01', 'synonyms': ['army_tank', 'armored_combat_vehicle', 'armoured_combat_vehicle'], 'id': 1058, 'def': 'an enclosed armored military vehicle; has a cannon and moves on caterpillar treads', 'name': 'army_tank'}, {'frequency': 'f', 'synset': 'tank.n.02', 'synonyms': ['tank_(storage_vessel)', 'storage_tank'], 'id': 1059, 'def': 'a large (usually metallic) vessel for holding gases or liquids', 'name': 'tank_(storage_vessel)'}, {'frequency': 'f', 'synset': 'tank_top.n.01', 'synonyms': ['tank_top_(clothing)'], 'id': 1060, 'def': 'a tight-fitting sleeveless shirt with wide shoulder straps and low neck and no front opening', 'name': 'tank_top_(clothing)'}, {'frequency': 'f', 'synset': 'tape.n.01', 'synonyms': ['tape_(sticky_cloth_or_paper)'], 'id': 1061, 'def': 'a long thin piece of cloth or paper as used for binding or fastening', 'name': 'tape_(sticky_cloth_or_paper)'}, {'frequency': 'c', 'synset': 'tape.n.04', 'synonyms': ['tape_measure', 'measuring_tape'], 'id': 1062, 'def': 'measuring instrument consisting of a narrow strip (cloth or metal) marked in inches or centimeters and used for measuring lengths', 'name': 'tape_measure'}, {'frequency': 'c', 'synset': 'tapestry.n.02', 'synonyms': ['tapestry'], 'id': 1063, 'def': 'a heavy textile with a woven design; used for curtains and upholstery', 'name': 'tapestry'}, {'frequency': 'f', 'synset': 'tarpaulin.n.01', 'synonyms': ['tarp'], 'id': 1064, 'def': 'waterproofed canvas', 'name': 'tarp'}, {'frequency': 'c', 'synset': 'tartan.n.01', 'synonyms': ['tartan', 'plaid'], 'id': 1065, 'def': 'a cloth having a crisscross design', 'name': 'tartan'}, {'frequency': 'c', 'synset': 'tassel.n.01', 'synonyms': ['tassel'], 'id': 1066, 'def': 'adornment consisting of a bunch of cords fastened at one end', 'name': 'tassel'}, {'frequency': 'c', 'synset': 'tea_bag.n.01', 'synonyms': ['tea_bag'], 'id': 1067, 'def': 'a measured amount of tea in a bag for an individual serving of tea', 'name': 'tea_bag'}, {'frequency': 'c', 'synset': 'teacup.n.02', 'synonyms': ['teacup'], 'id': 1068, 'def': 'a cup from which tea is drunk', 'name': 'teacup'}, {'frequency': 'c', 'synset': 'teakettle.n.01', 'synonyms': ['teakettle'], 'id': 1069, 'def': 'kettle for boiling water to make tea', 'name': 'teakettle'}, {'frequency': 'f', 'synset': 'teapot.n.01', 'synonyms': ['teapot'], 'id': 1070, 'def': 'pot for brewing tea; usually has a spout and handle', 'name': 'teapot'}, {'frequency': 'f', 'synset': 'teddy.n.01', 'synonyms': ['teddy_bear'], 'id': 1071, 'def': "plaything consisting of a child's toy bear (usually plush and stuffed with soft materials)", 'name': 'teddy_bear'}, {'frequency': 'f', 'synset': 'telephone.n.01', 'synonyms': ['telephone', 'phone', 'telephone_set'], 'id': 1072, 'def': 'electronic device for communicating by voice over long distances (includes wired and wireless/cell phones)', 'name': 'telephone'}, {'frequency': 'c', 'synset': 'telephone_booth.n.01', 'synonyms': ['telephone_booth', 'phone_booth', 'call_box', 'telephone_box', 'telephone_kiosk'], 'id': 1073, 'def': 'booth for using a telephone', 'name': 'telephone_booth'}, {'frequency': 'f', 'synset': 'telephone_pole.n.01', 'synonyms': ['telephone_pole', 'telegraph_pole', 'telegraph_post'], 'id': 1074, 'def': 'tall pole supporting telephone wires', 'name': 'telephone_pole'}, {'frequency': 'r', 'synset': 'telephoto_lens.n.01', 'synonyms': ['telephoto_lens', 'zoom_lens'], 'id': 1075, 'def': 'a camera lens that magnifies the image', 'name': 'telephoto_lens'}, {'frequency': 'c', 'synset': 'television_camera.n.01', 'synonyms': ['television_camera', 'tv_camera'], 'id': 1076, 'def': 'television equipment for capturing and recording video', 'name': 'television_camera'}, {'frequency': 'f', 'synset': 'television_receiver.n.01', 'synonyms': ['television_set', 'tv', 'tv_set'], 'id': 1077, 'def': 'an electronic device that receives television signals and displays them on a screen', 'name': 'television_set'}, {'frequency': 'f', 'synset': 'tennis_ball.n.01', 'synonyms': ['tennis_ball'], 'id': 1078, 'def': 'ball about the size of a fist used in playing tennis', 'name': 'tennis_ball'}, {'frequency': 'f', 'synset': 'tennis_racket.n.01', 'synonyms': ['tennis_racket'], 'id': 1079, 'def': 'a racket used to play tennis', 'name': 'tennis_racket'}, {'frequency': 'r', 'synset': 'tequila.n.01', 'synonyms': ['tequila'], 'id': 1080, 'def': 'Mexican liquor made from fermented juices of an agave plant', 'name': 'tequila'}, {'frequency': 'c', 'synset': 'thermometer.n.01', 'synonyms': ['thermometer'], 'id': 1081, 'def': 'measuring instrument for measuring temperature', 'name': 'thermometer'}, {'frequency': 'c', 'synset': 'thermos.n.01', 'synonyms': ['thermos_bottle'], 'id': 1082, 'def': 'vacuum flask that preserves temperature of hot or cold drinks', 'name': 'thermos_bottle'}, {'frequency': 'f', 'synset': 'thermostat.n.01', 'synonyms': ['thermostat'], 'id': 1083, 'def': 'a regulator for automatically regulating temperature by starting or stopping the supply of heat', 'name': 'thermostat'}, {'frequency': 'r', 'synset': 'thimble.n.02', 'synonyms': ['thimble'], 'id': 1084, 'def': 'a small metal cap to protect the finger while sewing; can be used as a small container', 'name': 'thimble'}, {'frequency': 'c', 'synset': 'thread.n.01', 'synonyms': ['thread', 'yarn'], 'id': 1085, 'def': 'a fine cord of twisted fibers (of cotton or silk or wool or nylon etc.) used in sewing and weaving', 'name': 'thread'}, {'frequency': 'c', 'synset': 'thumbtack.n.01', 'synonyms': ['thumbtack', 'drawing_pin', 'pushpin'], 'id': 1086, 'def': 'a tack for attaching papers to a bulletin board or drawing board', 'name': 'thumbtack'}, {'frequency': 'c', 'synset': 'tiara.n.01', 'synonyms': ['tiara'], 'id': 1087, 'def': 'a jeweled headdress worn by women on formal occasions', 'name': 'tiara'}, {'frequency': 'c', 'synset': 'tiger.n.02', 'synonyms': ['tiger'], 'id': 1088, 'def': 'large feline of forests in most of Asia having a tawny coat with black stripes', 'name': 'tiger'}, {'frequency': 'c', 'synset': 'tights.n.01', 'synonyms': ['tights_(clothing)', 'leotards'], 'id': 1089, 'def': 'skintight knit hose covering the body from the waist to the feet worn by acrobats and dancers and as stockings by women and girls', 'name': 'tights_(clothing)'}, {'frequency': 'c', 'synset': 'timer.n.01', 'synonyms': ['timer', 'stopwatch'], 'id': 1090, 'def': 'a timepiece that measures a time interval and signals its end', 'name': 'timer'}, {'frequency': 'f', 'synset': 'tinfoil.n.01', 'synonyms': ['tinfoil'], 'id': 1091, 'def': 'foil made of tin or an alloy of tin and lead', 'name': 'tinfoil'}, {'frequency': 'c', 'synset': 'tinsel.n.01', 'synonyms': ['tinsel'], 'id': 1092, 'def': 'a showy decoration that is basically valueless', 'name': 'tinsel'}, {'frequency': 'f', 'synset': 'tissue.n.02', 'synonyms': ['tissue_paper'], 'id': 1093, 'def': 'a soft thin (usually translucent) paper', 'name': 'tissue_paper'}, {'frequency': 'c', 'synset': 'toast.n.01', 'synonyms': ['toast_(food)'], 'id': 1094, 'def': 'slice of bread that has been toasted', 'name': 'toast_(food)'}, {'frequency': 'f', 'synset': 'toaster.n.02', 'synonyms': ['toaster'], 'id': 1095, 'def': 'a kitchen appliance (usually electric) for toasting bread', 'name': 'toaster'}, {'frequency': 'f', 'synset': 'toaster_oven.n.01', 'synonyms': ['toaster_oven'], 'id': 1096, 'def': 'kitchen appliance consisting of a small electric oven for toasting or warming food', 'name': 'toaster_oven'}, {'frequency': 'f', 'synset': 'toilet.n.02', 'synonyms': ['toilet'], 'id': 1097, 'def': 'a plumbing fixture for defecation and urination', 'name': 'toilet'}, {'frequency': 'f', 'synset': 'toilet_tissue.n.01', 'synonyms': ['toilet_tissue', 'toilet_paper', 'bathroom_tissue'], 'id': 1098, 'def': 'a soft thin absorbent paper for use in toilets', 'name': 'toilet_tissue'}, {'frequency': 'f', 'synset': 'tomato.n.01', 'synonyms': ['tomato'], 'id': 1099, 'def': 'mildly acid red or yellow pulpy fruit eaten as a vegetable', 'name': 'tomato'}, {'frequency': 'f', 'synset': 'tongs.n.01', 'synonyms': ['tongs'], 'id': 1100, 'def': 'any of various devices for taking hold of objects; usually have two hinged legs with handles above and pointed hooks below', 'name': 'tongs'}, {'frequency': 'c', 'synset': 'toolbox.n.01', 'synonyms': ['toolbox'], 'id': 1101, 'def': 'a box or chest or cabinet for holding hand tools', 'name': 'toolbox'}, {'frequency': 'f', 'synset': 'toothbrush.n.01', 'synonyms': ['toothbrush'], 'id': 1102, 'def': 'small brush; has long handle; used to clean teeth', 'name': 'toothbrush'}, {'frequency': 'f', 'synset': 'toothpaste.n.01', 'synonyms': ['toothpaste'], 'id': 1103, 'def': 'a dentifrice in the form of a paste', 'name': 'toothpaste'}, {'frequency': 'f', 'synset': 'toothpick.n.01', 'synonyms': ['toothpick'], 'id': 1104, 'def': 'pick consisting of a small strip of wood or plastic; used to pick food from between the teeth', 'name': 'toothpick'}, {'frequency': 'f', 'synset': 'top.n.09', 'synonyms': ['cover'], 'id': 1105, 'def': 'covering for a hole (especially a hole in the top of a container)', 'name': 'cover'}, {'frequency': 'c', 'synset': 'tortilla.n.01', 'synonyms': ['tortilla'], 'id': 1106, 'def': 'thin unleavened pancake made from cornmeal or wheat flour', 'name': 'tortilla'}, {'frequency': 'c', 'synset': 'tow_truck.n.01', 'synonyms': ['tow_truck'], 'id': 1107, 'def': 'a truck equipped to hoist and pull wrecked cars (or to remove cars from no-parking zones)', 'name': 'tow_truck'}, {'frequency': 'f', 'synset': 'towel.n.01', 'synonyms': ['towel'], 'id': 1108, 'def': 'a rectangular piece of absorbent cloth (or paper) for drying or wiping', 'name': 'towel'}, {'frequency': 'f', 'synset': 'towel_rack.n.01', 'synonyms': ['towel_rack', 'towel_rail', 'towel_bar'], 'id': 1109, 'def': 'a rack consisting of one or more bars on which towels can be hung', 'name': 'towel_rack'}, {'frequency': 'f', 'synset': 'toy.n.03', 'synonyms': ['toy'], 'id': 1110, 'def': 'a device regarded as providing amusement', 'name': 'toy'}, {'frequency': 'c', 'synset': 'tractor.n.01', 'synonyms': ['tractor_(farm_equipment)'], 'id': 1111, 'def': 'a wheeled vehicle with large wheels; used in farming and other applications', 'name': 'tractor_(farm_equipment)'}, {'frequency': 'f', 'synset': 'traffic_light.n.01', 'synonyms': ['traffic_light'], 'id': 1112, 'def': 'a device to control vehicle traffic often consisting of three or more lights', 'name': 'traffic_light'}, {'frequency': 'c', 'synset': 'trail_bike.n.01', 'synonyms': ['dirt_bike'], 'id': 1113, 'def': 'a lightweight motorcycle equipped with rugged tires and suspension for off-road use', 'name': 'dirt_bike'}, {'frequency': 'f', 'synset': 'trailer_truck.n.01', 'synonyms': ['trailer_truck', 'tractor_trailer', 'trucking_rig', 'articulated_lorry', 'semi_truck'], 'id': 1114, 'def': 'a truck consisting of a tractor and trailer together', 'name': 'trailer_truck'}, {'frequency': 'f', 'synset': 'train.n.01', 'synonyms': ['train_(railroad_vehicle)', 'railroad_train'], 'id': 1115, 'def': 'public or private transport provided by a line of railway cars coupled together and drawn by a locomotive', 'name': 'train_(railroad_vehicle)'}, {'frequency': 'r', 'synset': 'trampoline.n.01', 'synonyms': ['trampoline'], 'id': 1116, 'def': 'gymnastic apparatus consisting of a strong canvas sheet attached with springs to a metal frame', 'name': 'trampoline'}, {'frequency': 'f', 'synset': 'tray.n.01', 'synonyms': ['tray'], 'id': 1117, 'def': 'an open receptacle for holding or displaying or serving articles or food', 'name': 'tray'}, {'frequency': 'r', 'synset': 'trench_coat.n.01', 'synonyms': ['trench_coat'], 'id': 1118, 'def': 'a military style raincoat; belted with deep pockets', 'name': 'trench_coat'}, {'frequency': 'r', 'synset': 'triangle.n.05', 'synonyms': ['triangle_(musical_instrument)'], 'id': 1119, 'def': 'a percussion instrument consisting of a metal bar bent in the shape of an open triangle', 'name': 'triangle_(musical_instrument)'}, {'frequency': 'c', 'synset': 'tricycle.n.01', 'synonyms': ['tricycle'], 'id': 1120, 'def': 'a vehicle with three wheels that is moved by foot pedals', 'name': 'tricycle'}, {'frequency': 'f', 'synset': 'tripod.n.01', 'synonyms': ['tripod'], 'id': 1121, 'def': 'a three-legged rack used for support', 'name': 'tripod'}, {'frequency': 'f', 'synset': 'trouser.n.01', 'synonyms': ['trousers', 'pants_(clothing)'], 'id': 1122, 'def': 'a garment extending from the waist to the knee or ankle, covering each leg separately', 'name': 'trousers'}, {'frequency': 'f', 'synset': 'truck.n.01', 'synonyms': ['truck'], 'id': 1123, 'def': 'an automotive vehicle suitable for hauling', 'name': 'truck'}, {'frequency': 'r', 'synset': 'truffle.n.03', 'synonyms': ['truffle_(chocolate)', 'chocolate_truffle'], 'id': 1124, 'def': 'creamy chocolate candy', 'name': 'truffle_(chocolate)'}, {'frequency': 'c', 'synset': 'trunk.n.02', 'synonyms': ['trunk'], 'id': 1125, 'def': 'luggage consisting of a large strong case used when traveling or for storage', 'name': 'trunk'}, {'frequency': 'r', 'synset': 'tub.n.02', 'synonyms': ['vat'], 'id': 1126, 'def': 'a large vessel for holding or storing liquids', 'name': 'vat'}, {'frequency': 'c', 'synset': 'turban.n.01', 'synonyms': ['turban'], 'id': 1127, 'def': 'a traditional headdress consisting of a long scarf wrapped around the head', 'name': 'turban'}, {'frequency': 'c', 'synset': 'turkey.n.04', 'synonyms': ['turkey_(food)'], 'id': 1128, 'def': 'flesh of large domesticated fowl usually roasted', 'name': 'turkey_(food)'}, {'frequency': 'r', 'synset': 'turnip.n.01', 'synonyms': ['turnip'], 'id': 1129, 'def': 'widely cultivated plant having a large fleshy edible white or yellow root', 'name': 'turnip'}, {'frequency': 'c', 'synset': 'turtle.n.02', 'synonyms': ['turtle'], 'id': 1130, 'def': 'any of various aquatic and land reptiles having a bony shell and flipper-like limbs for swimming', 'name': 'turtle'}, {'frequency': 'c', 'synset': 'turtleneck.n.01', 'synonyms': ['turtleneck_(clothing)', 'polo-neck'], 'id': 1131, 'def': 'a sweater or jersey with a high close-fitting collar', 'name': 'turtleneck_(clothing)'}, {'frequency': 'c', 'synset': 'typewriter.n.01', 'synonyms': ['typewriter'], 'id': 1132, 'def': 'hand-operated character printer for printing written messages one character at a time', 'name': 'typewriter'}, {'frequency': 'f', 'synset': 'umbrella.n.01', 'synonyms': ['umbrella'], 'id': 1133, 'def': 'a lightweight handheld collapsible canopy', 'name': 'umbrella'}, {'frequency': 'f', 'synset': 'underwear.n.01', 'synonyms': ['underwear', 'underclothes', 'underclothing', 'underpants'], 'id': 1134, 'def': 'undergarment worn next to the skin and under the outer garments', 'name': 'underwear'}, {'frequency': 'r', 'synset': 'unicycle.n.01', 'synonyms': ['unicycle'], 'id': 1135, 'def': 'a vehicle with a single wheel that is driven by pedals', 'name': 'unicycle'}, {'frequency': 'f', 'synset': 'urinal.n.01', 'synonyms': ['urinal'], 'id': 1136, 'def': 'a plumbing fixture (usually attached to the wall) used by men to urinate', 'name': 'urinal'}, {'frequency': 'c', 'synset': 'urn.n.01', 'synonyms': ['urn'], 'id': 1137, 'def': 'a large vase that usually has a pedestal or feet', 'name': 'urn'}, {'frequency': 'c', 'synset': 'vacuum.n.04', 'synonyms': ['vacuum_cleaner'], 'id': 1138, 'def': 'an electrical home appliance that cleans by suction', 'name': 'vacuum_cleaner'}, {'frequency': 'f', 'synset': 'vase.n.01', 'synonyms': ['vase'], 'id': 1139, 'def': 'an open jar of glass or porcelain used as an ornament or to hold flowers', 'name': 'vase'}, {'frequency': 'c', 'synset': 'vending_machine.n.01', 'synonyms': ['vending_machine'], 'id': 1140, 'def': 'a slot machine for selling goods', 'name': 'vending_machine'}, {'frequency': 'f', 'synset': 'vent.n.01', 'synonyms': ['vent', 'blowhole', 'air_vent'], 'id': 1141, 'def': 'a hole for the escape of gas or air', 'name': 'vent'}, {'frequency': 'f', 'synset': 'vest.n.01', 'synonyms': ['vest', 'waistcoat'], 'id': 1142, 'def': "a man's sleeveless garment worn underneath a coat", 'name': 'vest'}, {'frequency': 'c', 'synset': 'videotape.n.01', 'synonyms': ['videotape'], 'id': 1143, 'def': 'a video recording made on magnetic tape', 'name': 'videotape'}, {'frequency': 'r', 'synset': 'vinegar.n.01', 'synonyms': ['vinegar'], 'id': 1144, 'def': 'sour-tasting liquid produced usually by oxidation of the alcohol in wine or cider and used as a condiment or food preservative', 'name': 'vinegar'}, {'frequency': 'r', 'synset': 'violin.n.01', 'synonyms': ['violin', 'fiddle'], 'id': 1145, 'def': 'bowed stringed instrument that is the highest member of the violin family', 'name': 'violin'}, {'frequency': 'r', 'synset': 'vodka.n.01', 'synonyms': ['vodka'], 'id': 1146, 'def': 'unaged colorless liquor originating in Russia', 'name': 'vodka'}, {'frequency': 'c', 'synset': 'volleyball.n.02', 'synonyms': ['volleyball'], 'id': 1147, 'def': 'an inflated ball used in playing volleyball', 'name': 'volleyball'}, {'frequency': 'r', 'synset': 'vulture.n.01', 'synonyms': ['vulture'], 'id': 1148, 'def': 'any of various large birds of prey having naked heads and weak claws and feeding chiefly on carrion', 'name': 'vulture'}, {'frequency': 'c', 'synset': 'waffle.n.01', 'synonyms': ['waffle'], 'id': 1149, 'def': 'pancake batter baked in a waffle iron', 'name': 'waffle'}, {'frequency': 'r', 'synset': 'waffle_iron.n.01', 'synonyms': ['waffle_iron'], 'id': 1150, 'def': 'a kitchen appliance for baking waffles', 'name': 'waffle_iron'}, {'frequency': 'c', 'synset': 'wagon.n.01', 'synonyms': ['wagon'], 'id': 1151, 'def': 'any of various kinds of wheeled vehicles drawn by an animal or a tractor', 'name': 'wagon'}, {'frequency': 'c', 'synset': 'wagon_wheel.n.01', 'synonyms': ['wagon_wheel'], 'id': 1152, 'def': 'a wheel of a wagon', 'name': 'wagon_wheel'}, {'frequency': 'c', 'synset': 'walking_stick.n.01', 'synonyms': ['walking_stick'], 'id': 1153, 'def': 'a stick carried in the hand for support in walking', 'name': 'walking_stick'}, {'frequency': 'c', 'synset': 'wall_clock.n.01', 'synonyms': ['wall_clock'], 'id': 1154, 'def': 'a clock mounted on a wall', 'name': 'wall_clock'}, {'frequency': 'f', 'synset': 'wall_socket.n.01', 'synonyms': ['wall_socket', 'wall_plug', 'electric_outlet', 'electrical_outlet', 'outlet', 'electric_receptacle'], 'id': 1155, 'def': 'receptacle providing a place in a wiring system where current can be taken to run electrical devices', 'name': 'wall_socket'}, {'frequency': 'f', 'synset': 'wallet.n.01', 'synonyms': ['wallet', 'billfold'], 'id': 1156, 'def': 'a pocket-size case for holding papers and paper money', 'name': 'wallet'}, {'frequency': 'r', 'synset': 'walrus.n.01', 'synonyms': ['walrus'], 'id': 1157, 'def': 'either of two large northern marine mammals having ivory tusks and tough hide over thick blubber', 'name': 'walrus'}, {'frequency': 'r', 'synset': 'wardrobe.n.01', 'synonyms': ['wardrobe'], 'id': 1158, 'def': 'a tall piece of furniture that provides storage space for clothes; has a door and rails or hooks for hanging clothes', 'name': 'wardrobe'}, {'frequency': 'r', 'synset': 'washbasin.n.01', 'synonyms': ['washbasin', 'basin_(for_washing)', 'washbowl', 'washstand', 'handbasin'], 'id': 1159, 'def': 'a bathroom sink that is permanently installed and connected to a water supply and drainpipe; where you can wash your hands and face', 'name': 'washbasin'}, {'frequency': 'c', 'synset': 'washer.n.03', 'synonyms': ['automatic_washer', 'washing_machine'], 'id': 1160, 'def': 'a home appliance for washing clothes and linens automatically', 'name': 'automatic_washer'}, {'frequency': 'f', 'synset': 'watch.n.01', 'synonyms': ['watch', 'wristwatch'], 'id': 1161, 'def': 'a small, portable timepiece', 'name': 'watch'}, {'frequency': 'f', 'synset': 'water_bottle.n.01', 'synonyms': ['water_bottle'], 'id': 1162, 'def': 'a bottle for holding water', 'name': 'water_bottle'}, {'frequency': 'c', 'synset': 'water_cooler.n.01', 'synonyms': ['water_cooler'], 'id': 1163, 'def': 'a device for cooling and dispensing drinking water', 'name': 'water_cooler'}, {'frequency': 'c', 'synset': 'water_faucet.n.01', 'synonyms': ['water_faucet', 'water_tap', 'tap_(water_faucet)'], 'id': 1164, 'def': 'a faucet for drawing water from a pipe or cask', 'name': 'water_faucet'}, {'frequency': 'r', 'synset': 'water_heater.n.01', 'synonyms': ['water_heater', 'hot-water_heater'], 'id': 1165, 'def': 'a heater and storage tank to supply heated water', 'name': 'water_heater'}, {'frequency': 'c', 'synset': 'water_jug.n.01', 'synonyms': ['water_jug'], 'id': 1166, 'def': 'a jug that holds water', 'name': 'water_jug'}, {'frequency': 'r', 'synset': 'water_pistol.n.01', 'synonyms': ['water_gun', 'squirt_gun'], 'id': 1167, 'def': 'plaything consisting of a toy pistol that squirts water', 'name': 'water_gun'}, {'frequency': 'c', 'synset': 'water_scooter.n.01', 'synonyms': ['water_scooter', 'sea_scooter', 'jet_ski'], 'id': 1168, 'def': 'a motorboat resembling a motor scooter (NOT A SURFBOARD OR WATER SKI)', 'name': 'water_scooter'}, {'frequency': 'c', 'synset': 'water_ski.n.01', 'synonyms': ['water_ski'], 'id': 1169, 'def': 'broad ski for skimming over water towed by a speedboat (DO NOT MARK WATER)', 'name': 'water_ski'}, {'frequency': 'c', 'synset': 'water_tower.n.01', 'synonyms': ['water_tower'], 'id': 1170, 'def': 'a large reservoir for water', 'name': 'water_tower'}, {'frequency': 'c', 'synset': 'watering_can.n.01', 'synonyms': ['watering_can'], 'id': 1171, 'def': 'a container with a handle and a spout with a perforated nozzle; used to sprinkle water over plants', 'name': 'watering_can'}, {'frequency': 'f', 'synset': 'watermelon.n.02', 'synonyms': ['watermelon'], 'id': 1172, 'def': 'large oblong or roundish melon with a hard green rind and sweet watery red or occasionally yellowish pulp', 'name': 'watermelon'}, {'frequency': 'f', 'synset': 'weathervane.n.01', 'synonyms': ['weathervane', 'vane_(weathervane)', 'wind_vane'], 'id': 1173, 'def': 'mechanical device attached to an elevated structure; rotates freely to show the direction of the wind', 'name': 'weathervane'}, {'frequency': 'c', 'synset': 'webcam.n.01', 'synonyms': ['webcam'], 'id': 1174, 'def': 'a digital camera designed to take digital photographs and transmit them over the internet', 'name': 'webcam'}, {'frequency': 'c', 'synset': 'wedding_cake.n.01', 'synonyms': ['wedding_cake', 'bridecake'], 'id': 1175, 'def': 'a rich cake with two or more tiers and covered with frosting and decorations; served at a wedding reception', 'name': 'wedding_cake'}, {'frequency': 'c', 'synset': 'wedding_ring.n.01', 'synonyms': ['wedding_ring', 'wedding_band'], 'id': 1176, 'def': 'a ring given to the bride and/or groom at the wedding', 'name': 'wedding_ring'}, {'frequency': 'f', 'synset': 'wet_suit.n.01', 'synonyms': ['wet_suit'], 'id': 1177, 'def': 'a close-fitting garment made of a permeable material; worn in cold water to retain body heat', 'name': 'wet_suit'}, {'frequency': 'f', 'synset': 'wheel.n.01', 'synonyms': ['wheel'], 'id': 1178, 'def': 'a circular frame with spokes (or a solid disc) that can rotate on a shaft or axle', 'name': 'wheel'}, {'frequency': 'c', 'synset': 'wheelchair.n.01', 'synonyms': ['wheelchair'], 'id': 1179, 'def': 'a movable chair mounted on large wheels', 'name': 'wheelchair'}, {'frequency': 'c', 'synset': 'whipped_cream.n.01', 'synonyms': ['whipped_cream'], 'id': 1180, 'def': 'cream that has been beaten until light and fluffy', 'name': 'whipped_cream'}, {'frequency': 'c', 'synset': 'whistle.n.03', 'synonyms': ['whistle'], 'id': 1181, 'def': 'a small wind instrument that produces a whistling sound by blowing into it', 'name': 'whistle'}, {'frequency': 'c', 'synset': 'wig.n.01', 'synonyms': ['wig'], 'id': 1182, 'def': 'hairpiece covering the head and made of real or synthetic hair', 'name': 'wig'}, {'frequency': 'c', 'synset': 'wind_chime.n.01', 'synonyms': ['wind_chime'], 'id': 1183, 'def': 'a decorative arrangement of pieces of metal or glass or pottery that hang together loosely so the wind can cause them to tinkle', 'name': 'wind_chime'}, {'frequency': 'c', 'synset': 'windmill.n.01', 'synonyms': ['windmill'], 'id': 1184, 'def': 'A mill or turbine that is powered by wind', 'name': 'windmill'}, {'frequency': 'c', 'synset': 'window_box.n.01', 'synonyms': ['window_box_(for_plants)'], 'id': 1185, 'def': 'a container for growing plants on a windowsill', 'name': 'window_box_(for_plants)'}, {'frequency': 'f', 'synset': 'windshield_wiper.n.01', 'synonyms': ['windshield_wiper', 'windscreen_wiper', 'wiper_(for_windshield/screen)'], 'id': 1186, 'def': 'a mechanical device that cleans the windshield', 'name': 'windshield_wiper'}, {'frequency': 'c', 'synset': 'windsock.n.01', 'synonyms': ['windsock', 'air_sock', 'air-sleeve', 'wind_sleeve', 'wind_cone'], 'id': 1187, 'def': 'a truncated cloth cone mounted on a mast/pole; shows wind direction', 'name': 'windsock'}, {'frequency': 'f', 'synset': 'wine_bottle.n.01', 'synonyms': ['wine_bottle'], 'id': 1188, 'def': 'a bottle for holding wine', 'name': 'wine_bottle'}, {'frequency': 'c', 'synset': 'wine_bucket.n.01', 'synonyms': ['wine_bucket', 'wine_cooler'], 'id': 1189, 'def': 'a bucket of ice used to chill a bottle of wine', 'name': 'wine_bucket'}, {'frequency': 'f', 'synset': 'wineglass.n.01', 'synonyms': ['wineglass'], 'id': 1190, 'def': 'a glass that has a stem and in which wine is served', 'name': 'wineglass'}, {'frequency': 'f', 'synset': 'winker.n.02', 'synonyms': ['blinder_(for_horses)'], 'id': 1191, 'def': 'blinds that prevent a horse from seeing something on either side', 'name': 'blinder_(for_horses)'}, {'frequency': 'c', 'synset': 'wok.n.01', 'synonyms': ['wok'], 'id': 1192, 'def': 'pan with a convex bottom; used for frying in Chinese cooking', 'name': 'wok'}, {'frequency': 'r', 'synset': 'wolf.n.01', 'synonyms': ['wolf'], 'id': 1193, 'def': 'a wild carnivorous mammal of the dog family, living and hunting in packs', 'name': 'wolf'}, {'frequency': 'c', 'synset': 'wooden_spoon.n.02', 'synonyms': ['wooden_spoon'], 'id': 1194, 'def': 'a spoon made of wood', 'name': 'wooden_spoon'}, {'frequency': 'c', 'synset': 'wreath.n.01', 'synonyms': ['wreath'], 'id': 1195, 'def': 'an arrangement of flowers, leaves, or stems fastened in a ring', 'name': 'wreath'}, {'frequency': 'c', 'synset': 'wrench.n.03', 'synonyms': ['wrench', 'spanner'], 'id': 1196, 'def': 'a hand tool that is used to hold or twist a nut or bolt', 'name': 'wrench'}, {'frequency': 'f', 'synset': 'wristband.n.01', 'synonyms': ['wristband'], 'id': 1197, 'def': 'band consisting of a part of a sleeve that covers the wrist', 'name': 'wristband'}, {'frequency': 'f', 'synset': 'wristlet.n.01', 'synonyms': ['wristlet', 'wrist_band'], 'id': 1198, 'def': 'a band or bracelet worn around the wrist', 'name': 'wristlet'}, {'frequency': 'c', 'synset': 'yacht.n.01', 'synonyms': ['yacht'], 'id': 1199, 'def': 'an expensive vessel propelled by sail or power and used for cruising or racing', 'name': 'yacht'}, {'frequency': 'c', 'synset': 'yogurt.n.01', 'synonyms': ['yogurt', 'yoghurt', 'yoghourt'], 'id': 1200, 'def': 'a custard-like food made from curdled milk', 'name': 'yogurt'}, {'frequency': 'c', 'synset': 'yoke.n.07', 'synonyms': ['yoke_(animal_equipment)'], 'id': 1201, 'def': 'gear joining two animals at the neck; NOT egg yolk', 'name': 'yoke_(animal_equipment)'}, {'frequency': 'f', 'synset': 'zebra.n.01', 'synonyms': ['zebra'], 'id': 1202, 'def': 'any of several fleet black-and-white striped African equines', 'name': 'zebra'}, {'frequency': 'c', 'synset': 'zucchini.n.02', 'synonyms': ['zucchini', 'courgette'], 'id': 1203, 'def': 'small cucumber-shaped vegetable marrow; typically dark green', 'name': 'zucchini'}] # noqa
-# fmt: on
diff --git a/spaces/OpenGVLab/InternGPT/iGPT/models/grit_src/third_party/CenterNet2/detectron2/layers/roi_align.py b/spaces/OpenGVLab/InternGPT/iGPT/models/grit_src/third_party/CenterNet2/detectron2/layers/roi_align.py
deleted file mode 100644
index 163462e1f194e1e4100da92d76d9516f7cc22e35..0000000000000000000000000000000000000000
--- a/spaces/OpenGVLab/InternGPT/iGPT/models/grit_src/third_party/CenterNet2/detectron2/layers/roi_align.py
+++ /dev/null
@@ -1,74 +0,0 @@
-# Copyright (c) Facebook, Inc. and its affiliates.
-from torch import nn
-from torchvision.ops import roi_align
-
-
-# NOTE: torchvision's RoIAlign has a different default aligned=False
-class ROIAlign(nn.Module):
- def __init__(self, output_size, spatial_scale, sampling_ratio, aligned=True):
- """
- Args:
- output_size (tuple): h, w
- spatial_scale (float): scale the input boxes by this number
- sampling_ratio (int): number of inputs samples to take for each output
- sample. 0 to take samples densely.
- aligned (bool): if False, use the legacy implementation in
- Detectron. If True, align the results more perfectly.
-
- Note:
- The meaning of aligned=True:
-
- Given a continuous coordinate c, its two neighboring pixel indices (in our
- pixel model) are computed by floor(c - 0.5) and ceil(c - 0.5). For example,
- c=1.3 has pixel neighbors with discrete indices [0] and [1] (which are sampled
- from the underlying signal at continuous coordinates 0.5 and 1.5). But the original
- roi_align (aligned=False) does not subtract the 0.5 when computing neighboring
- pixel indices and therefore it uses pixels with a slightly incorrect alignment
- (relative to our pixel model) when performing bilinear interpolation.
-
- With `aligned=True`,
- we first appropriately scale the ROI and then shift it by -0.5
- prior to calling roi_align. This produces the correct neighbors; see
- detectron2/tests/test_roi_align.py for verification.
-
- The difference does not make a difference to the model's performance if
- ROIAlign is used together with conv layers.
- """
- super().__init__()
- self.output_size = output_size
- self.spatial_scale = spatial_scale
- self.sampling_ratio = sampling_ratio
- self.aligned = aligned
-
- from torchvision import __version__
-
- version = tuple(int(x) for x in __version__.split(".")[:2])
- # https://github.com/pytorch/vision/pull/2438
- assert version >= (0, 7), "Require torchvision >= 0.7"
-
- def forward(self, input, rois):
- """
- Args:
- input: NCHW images
- rois: Bx5 boxes. First column is the index into N. The other 4 columns are xyxy.
- """
- assert rois.dim() == 2 and rois.size(1) == 5
- if input.is_quantized:
- input = input.dequantize()
- return roi_align(
- input,
- rois.to(dtype=input.dtype),
- self.output_size,
- self.spatial_scale,
- self.sampling_ratio,
- self.aligned,
- )
-
- def __repr__(self):
- tmpstr = self.__class__.__name__ + "("
- tmpstr += "output_size=" + str(self.output_size)
- tmpstr += ", spatial_scale=" + str(self.spatial_scale)
- tmpstr += ", sampling_ratio=" + str(self.sampling_ratio)
- tmpstr += ", aligned=" + str(self.aligned)
- tmpstr += ")"
- return tmpstr
diff --git a/spaces/PAIR/Text2Video-Zero/annotator/uniformer/mmcv/ops/ball_query.py b/spaces/PAIR/Text2Video-Zero/annotator/uniformer/mmcv/ops/ball_query.py
deleted file mode 100644
index d0466847c6e5c1239e359a0397568413ebc1504a..0000000000000000000000000000000000000000
--- a/spaces/PAIR/Text2Video-Zero/annotator/uniformer/mmcv/ops/ball_query.py
+++ /dev/null
@@ -1,55 +0,0 @@
-# Copyright (c) OpenMMLab. All rights reserved.
-import torch
-from torch.autograd import Function
-
-from ..utils import ext_loader
-
-ext_module = ext_loader.load_ext('_ext', ['ball_query_forward'])
-
-
-class BallQuery(Function):
- """Find nearby points in spherical space."""
-
- @staticmethod
- def forward(ctx, min_radius: float, max_radius: float, sample_num: int,
- xyz: torch.Tensor, center_xyz: torch.Tensor) -> torch.Tensor:
- """
- Args:
- min_radius (float): minimum radius of the balls.
- max_radius (float): maximum radius of the balls.
- sample_num (int): maximum number of features in the balls.
- xyz (Tensor): (B, N, 3) xyz coordinates of the features.
- center_xyz (Tensor): (B, npoint, 3) centers of the ball query.
-
- Returns:
- Tensor: (B, npoint, nsample) tensor with the indices of
- the features that form the query balls.
- """
- assert center_xyz.is_contiguous()
- assert xyz.is_contiguous()
- assert min_radius < max_radius
-
- B, N, _ = xyz.size()
- npoint = center_xyz.size(1)
- idx = xyz.new_zeros(B, npoint, sample_num, dtype=torch.int)
-
- ext_module.ball_query_forward(
- center_xyz,
- xyz,
- idx,
- b=B,
- n=N,
- m=npoint,
- min_radius=min_radius,
- max_radius=max_radius,
- nsample=sample_num)
- if torch.__version__ != 'parrots':
- ctx.mark_non_differentiable(idx)
- return idx
-
- @staticmethod
- def backward(ctx, a=None):
- return None, None, None, None
-
-
-ball_query = BallQuery.apply
diff --git a/spaces/PSLD/PSLD/stable-diffusion/run/inverse_mb_ldm.sh b/spaces/PSLD/PSLD/stable-diffusion/run/inverse_mb_ldm.sh
deleted file mode 100644
index 33080325d6ef2a4b1701fceb3bf97e9366a0056b..0000000000000000000000000000000000000000
--- a/spaces/PSLD/PSLD/stable-diffusion/run/inverse_mb_ldm.sh
+++ /dev/null
@@ -1,14 +0,0 @@
-export CUDA_VISIBLE_DEVICES='1'
-python scripts/inverse.py \
- --file_id='00015.png' \
- --task_config='configs/motion_deblur_config.yaml' \
- --inpainting=0 \
- --general_inverse=1 \
- --gamma=1e-1 \
- --omega=1e-1 \
- --ffhq256 \
- --W=256 \
- --H=256 \
- --C=3 \
- --f=4 \
- --outdir='outputs/psld-ldm-samples-mb'
\ No newline at end of file
diff --git a/spaces/PaSathees/Vehicle_Tyre_Quality_Checker/model.py b/spaces/PaSathees/Vehicle_Tyre_Quality_Checker/model.py
deleted file mode 100644
index 485ef57d230c16a3f4cb856436fb26e79e26a672..0000000000000000000000000000000000000000
--- a/spaces/PaSathees/Vehicle_Tyre_Quality_Checker/model.py
+++ /dev/null
@@ -1,41 +0,0 @@
-import torch
-import torchvision
-
-from torch import nn
-
-def create_effnet_v2_l_model(num_classes:int=2, seed:int=42):
- """Creates an EfficientNet_V2_L feature extractor model and transforms.
-
- Args:
- num_classes (int, optional): number of classes in the classifier head.
- Defaults to 2.
- seed (int, optional): random seed value. Defaults to 42.
-
- Returns:
- model (torch.nn.Module): EfficientNet_V2_L feature extractor model.
- transforms (torchvision.transforms): EfficientNet_V2_L image transforms.
- """
- # Getting pre-trained model
- weights = torchvision.models.EfficientNet_V2_L_Weights.DEFAULT
- model = torchvision.models.efficientnet_v2_l(weights=weights)
-
- # Get transforms
- transforms = weights.transforms()
-
- # Freeze features
- for param in model.features.parameters():
- param.requires_grad = False
-
- # Changing head
- torch.manual_seed(seed)
- torch.cuda.manual_seed(seed)
-
- model.classifier = torch.nn.Sequential(
- torch.nn.Dropout(p=0.2, inplace=True),
- torch.nn.Linear(in_features=1280,
- out_features=1,
- bias=True),
- torch.nn.Sigmoid()
- )
-
- return model, transforms
diff --git a/spaces/Pattr/DrumClassification/lilypond-2.24.2/lib/guile/2.2/ccache/language/cps/renumber.go b/spaces/Pattr/DrumClassification/lilypond-2.24.2/lib/guile/2.2/ccache/language/cps/renumber.go
deleted file mode 100644
index 07b44f86333637550029a43fb8abe774fdb55e22..0000000000000000000000000000000000000000
Binary files a/spaces/Pattr/DrumClassification/lilypond-2.24.2/lib/guile/2.2/ccache/language/cps/renumber.go and /dev/null differ
diff --git a/spaces/Pie31415/control-animation/annotator/uniformer/mmcv/cnn/bricks/scale.py b/spaces/Pie31415/control-animation/annotator/uniformer/mmcv/cnn/bricks/scale.py
deleted file mode 100644
index c905fffcc8bf998d18d94f927591963c428025e2..0000000000000000000000000000000000000000
--- a/spaces/Pie31415/control-animation/annotator/uniformer/mmcv/cnn/bricks/scale.py
+++ /dev/null
@@ -1,21 +0,0 @@
-# Copyright (c) OpenMMLab. All rights reserved.
-import torch
-import torch.nn as nn
-
-
-class Scale(nn.Module):
- """A learnable scale parameter.
-
- This layer scales the input by a learnable factor. It multiplies a
- learnable scale parameter of shape (1,) with input of any shape.
-
- Args:
- scale (float): Initial value of scale factor. Default: 1.0
- """
-
- def __init__(self, scale=1.0):
- super(Scale, self).__init__()
- self.scale = nn.Parameter(torch.tensor(scale, dtype=torch.float))
-
- def forward(self, x):
- return x * self.scale
diff --git a/spaces/Plachta/VALL-E-X/examples.py b/spaces/Plachta/VALL-E-X/examples.py
deleted file mode 100644
index 205210e0d03f1203648c8fc327da713f9db5eb4e..0000000000000000000000000000000000000000
--- a/spaces/Plachta/VALL-E-X/examples.py
+++ /dev/null
@@ -1,24 +0,0 @@
-infer_from_audio_examples = [
- ["This is how this machine has taken my voice.", 'English', 'no-accent', "prompts/en-2.wav", None, "Wow, look at that! That's no ordinary Teddy bear!"],
- ["我喜欢抽电子烟,尤其是锐刻五代。", '中文', 'no-accent', "prompts/zh-1.wav", None, "今天我很荣幸,"],
- ["私の声を真似するのはそんなに面白いですか?", '日本語', 'no-accent', "prompts/ja-2.ogg", None, "初めまして、朝武よしのです。"],
- ["你可以听得出来我有多困。", '中文', 'no-accent', "prompts/en-1.wav", None, ""],
- ["この文は、クロスリンガル合成の例です。", '日本語', 'no-accent', "prompts/zh-2.wav", None, ""],
- ["Actually, I can't speak English, but this machine helped me do it.", 'English', 'no-accent', "prompts/ja-1.wav", None, ""],
-]
-
-make_npz_prompt_examples = [
- ["Gem-trader", "prompts/en-2.wav", None, "Wow, look at that! That's no ordinary Teddy bear!"],
- ["Ding Zhen", "prompts/zh-1.wav", None, "今天我很荣幸,"],
- ["Yoshino", "prompts/ja-2.ogg", None, "初めまして、朝武よしのです。"],
- ["Sleepy-woman", "prompts/en-1.wav", None, ""],
- ["Yae", "prompts/zh-2.wav", None, ""],
- ["Cafe", "prompts/ja-1.wav", None, ""],
-]
-
-infer_from_prompt_examples = [
- ["A prompt contains voice, prosody and emotion information of a certain speaker.", "English", "no-accent", "vctk_1", None],
- ["This prompt is made with an audio of three seconds.", "English", "no-accent", "librispeech_1", None],
- ["This prompt is made with Chinese speech", "English", "no-accent", "seel", None],
-]
-
diff --git a/spaces/Plachta/VALL-E-X/utils/g2p/symbols.py b/spaces/Plachta/VALL-E-X/utils/g2p/symbols.py
deleted file mode 100644
index 789e9df25d3d93d1976ef22d15d77f51d170ed00..0000000000000000000000000000000000000000
--- a/spaces/Plachta/VALL-E-X/utils/g2p/symbols.py
+++ /dev/null
@@ -1,76 +0,0 @@
-'''
-Defines the set of symbols used in text input to the model.
-'''
-
-# japanese_cleaners
-# _pad = '_'
-# _punctuation = ',.!?-'
-# _letters = 'AEINOQUabdefghijkmnoprstuvwyzʃʧ↓↑ '
-
-
-'''# japanese_cleaners2
-_pad = '_'
-_punctuation = ',.!?-~…'
-_letters = 'AEINOQUabdefghijkmnoprstuvwyzʃʧʦ↓↑ '
-'''
-
-
-'''# korean_cleaners
-_pad = '_'
-_punctuation = ',.!?…~'
-_letters = 'ㄱㄴㄷㄹㅁㅂㅅㅇㅈㅊㅋㅌㅍㅎㄲㄸㅃㅆㅉㅏㅓㅗㅜㅡㅣㅐㅔ '
-'''
-
-'''# chinese_cleaners
-_pad = '_'
-_punctuation = ',。!?—…'
-_letters = 'ㄅㄆㄇㄈㄉㄊㄋㄌㄍㄎㄏㄐㄑㄒㄓㄔㄕㄖㄗㄘㄙㄚㄛㄜㄝㄞㄟㄠㄡㄢㄣㄤㄥㄦㄧㄨㄩˉˊˇˋ˙ '
-'''
-
-# # zh_ja_mixture_cleaners
-# _pad = '_'
-# _punctuation = ',.!?-~…'
-# _letters = 'AEINOQUabdefghijklmnoprstuvwyzʃʧʦɯɹəɥ⁼ʰ`→↓↑ '
-
-
-'''# sanskrit_cleaners
-_pad = '_'
-_punctuation = '।'
-_letters = 'ँंःअआइईउऊऋएऐओऔकखगघङचछजझञटठडढणतथदधनपफबभमयरलळवशषसहऽािीुूृॄेैोौ्ॠॢ '
-'''
-
-'''# cjks_cleaners
-_pad = '_'
-_punctuation = ',.!?-~…'
-_letters = 'NQabdefghijklmnopstuvwxyzʃʧʥʦɯɹəɥçɸɾβŋɦː⁼ʰ`^#*=→↓↑ '
-'''
-
-'''# thai_cleaners
-_pad = '_'
-_punctuation = '.!? '
-_letters = 'กขฃคฆงจฉชซฌญฎฏฐฑฒณดตถทธนบปผฝพฟภมยรฤลวศษสหฬอฮฯะัาำิีึืุูเแโใไๅๆ็่้๊๋์'
-'''
-
-# # cjke_cleaners2
-_pad = '_'
-_punctuation = ',.!?-~…'
-_letters = 'NQabdefghijklmnopstuvwxyzɑæʃʑçɯɪɔɛɹðəɫɥɸʊɾʒθβŋɦ⁼ʰ`^#*=ˈˌ→↓↑ '
-
-
-'''# shanghainese_cleaners
-_pad = '_'
-_punctuation = ',.!?…'
-_letters = 'abdfghiklmnopstuvyzøŋȵɑɔɕəɤɦɪɿʑʔʰ̩̃ᴀᴇ15678 '
-'''
-
-'''# chinese_dialect_cleaners
-_pad = '_'
-_punctuation = ',.!?~…─'
-_letters = '#Nabdefghijklmnoprstuvwxyzæçøŋœȵɐɑɒɓɔɕɗɘəɚɛɜɣɤɦɪɭɯɵɷɸɻɾɿʂʅʊʋʌʏʑʔʦʮʰʷˀː˥˦˧˨˩̥̩̃̚ᴀᴇ↑↓∅ⱼ '
-'''
-
-# Export all symbols:
-symbols = [_pad] + list(_punctuation) + list(_letters)
-
-# Special symbol ids
-SPACE_ID = symbols.index(" ")
diff --git a/spaces/Pranay009/FACE2COMIC/app.py b/spaces/Pranay009/FACE2COMIC/app.py
deleted file mode 100644
index ed8af8bdb00379385d22f274f84495394b5b121c..0000000000000000000000000000000000000000
--- a/spaces/Pranay009/FACE2COMIC/app.py
+++ /dev/null
@@ -1,48 +0,0 @@
-import gradio as gr
-from src.Generator import Generator
-import cv2
-import numpy as np
-from src.Generator import Generator
-import tensorflow as tf
-def image_preprocessing(img):
- img=cv2.resize(img,(256,256))#resize image to 128*128
- img=cv2.cvtColor(img,cv2.COLOR_BGR2RGB)#BGR to RGB
- return img/255.0
-
-def output_processing(img):
- kernel = np.array([[-1,-1,-1], [-1,9,-1], [-1,-1,-1]])
- im = cv2.filter2D(img, -1, kernel)
- im = cv2.blur(im,(3,3))
- return im
-def generated_image(gen,data):
- if len(data.shape)==3:
- data=np.expand_dims(data,axis=0)
- predict=gen.predict(data)
- return predict
-
-
-def comic_generator(image_path):
- image=cv2.imread(image_path)
- image=image_preprocessing(image)
- model=Generator(256,256,3)
- model.load_weights(r"./src/saved_model_v2/model.ckpt")
-
- prediction=generated_image(model,image)
- pred=output_processing(prediction[0])
- #ret_img=cv2.cvtColor(prediction[0],cv2.COLOR_BGR2RGB)
- return prediction[0]
-
-
-#inputs = gr.inputs.Image(label="Input Image")
-#outputs = gr.outputs.Image(label="Output Image")
-title = "FACE2COMIC"
-description = "Face to Comic Avatar Translation"
-examples = [[r"./sampe_images/0.jpg"], [r"./sampe_images/1.jpg"], [r"./sampe_images/1001.jpg"],[r"./sampe_images/1009.jpg"],[r"./sampe_images/1037.jpg"],[r"./sampe_images/man_052.jpeg"],[r"./sampe_images/woman_0.58.jpeg"]]
-gr.Interface(fn=comic_generator,
- inputs=gr.Image(type="filepath",label="Input"),
- outputs=gr.Image(type="numpy",label="Output").style(height=256),
- title=title,
- description=description,
- examples=examples,
- max_output_size=(256,256),
- layout="horizontal").launch(share=False)
\ No newline at end of file
diff --git a/spaces/RamAnanth1/T2I-Adapter/ldm/models/diffusion/__init__.py b/spaces/RamAnanth1/T2I-Adapter/ldm/models/diffusion/__init__.py
deleted file mode 100644
index e69de29bb2d1d6434b8b29ae775ad8c2e48c5391..0000000000000000000000000000000000000000
diff --git a/spaces/RamAnanth1/videocrafter/extralibs/midas/midas/midas_net_custom.py b/spaces/RamAnanth1/videocrafter/extralibs/midas/midas/midas_net_custom.py
deleted file mode 100644
index 50e4acb5e53d5fabefe3dde16ab49c33c2b7797c..0000000000000000000000000000000000000000
--- a/spaces/RamAnanth1/videocrafter/extralibs/midas/midas/midas_net_custom.py
+++ /dev/null
@@ -1,128 +0,0 @@
-"""MidashNet: Network for monocular depth estimation trained by mixing several datasets.
-This file contains code that is adapted from
-https://github.com/thomasjpfan/pytorch_refinenet/blob/master/pytorch_refinenet/refinenet/refinenet_4cascade.py
-"""
-import torch
-import torch.nn as nn
-
-from .base_model import BaseModel
-from .blocks import FeatureFusionBlock, FeatureFusionBlock_custom, Interpolate, _make_encoder
-
-
-class MidasNet_small(BaseModel):
- """Network for monocular depth estimation.
- """
-
- def __init__(self, path=None, features=64, backbone="efficientnet_lite3", non_negative=True, exportable=True, channels_last=False, align_corners=True,
- blocks={'expand': True}):
- """Init.
-
- Args:
- path (str, optional): Path to saved model. Defaults to None.
- features (int, optional): Number of features. Defaults to 256.
- backbone (str, optional): Backbone network for encoder. Defaults to resnet50
- """
- print("Loading weights: ", path)
-
- super(MidasNet_small, self).__init__()
-
- use_pretrained = False if path else True
-
- self.channels_last = channels_last
- self.blocks = blocks
- self.backbone = backbone
-
- self.groups = 1
-
- features1=features
- features2=features
- features3=features
- features4=features
- self.expand = False
- if "expand" in self.blocks and self.blocks['expand'] == True:
- self.expand = True
- features1=features
- features2=features*2
- features3=features*4
- features4=features*8
-
- self.pretrained, self.scratch = _make_encoder(self.backbone, features, use_pretrained, groups=self.groups, expand=self.expand, exportable=exportable)
-
- self.scratch.activation = nn.ReLU(False)
-
- self.scratch.refinenet4 = FeatureFusionBlock_custom(features4, self.scratch.activation, deconv=False, bn=False, expand=self.expand, align_corners=align_corners)
- self.scratch.refinenet3 = FeatureFusionBlock_custom(features3, self.scratch.activation, deconv=False, bn=False, expand=self.expand, align_corners=align_corners)
- self.scratch.refinenet2 = FeatureFusionBlock_custom(features2, self.scratch.activation, deconv=False, bn=False, expand=self.expand, align_corners=align_corners)
- self.scratch.refinenet1 = FeatureFusionBlock_custom(features1, self.scratch.activation, deconv=False, bn=False, align_corners=align_corners)
-
-
- self.scratch.output_conv = nn.Sequential(
- nn.Conv2d(features, features//2, kernel_size=3, stride=1, padding=1, groups=self.groups),
- Interpolate(scale_factor=2, mode="bilinear"),
- nn.Conv2d(features//2, 32, kernel_size=3, stride=1, padding=1),
- self.scratch.activation,
- nn.Conv2d(32, 1, kernel_size=1, stride=1, padding=0),
- nn.ReLU(True) if non_negative else nn.Identity(),
- nn.Identity(),
- )
-
- if path:
- self.load(path)
-
-
- def forward(self, x):
- """Forward pass.
-
- Args:
- x (tensor): input data (image)
-
- Returns:
- tensor: depth
- """
- if self.channels_last==True:
- print("self.channels_last = ", self.channels_last)
- x.contiguous(memory_format=torch.channels_last)
-
-
- layer_1 = self.pretrained.layer1(x)
- layer_2 = self.pretrained.layer2(layer_1)
- layer_3 = self.pretrained.layer3(layer_2)
- layer_4 = self.pretrained.layer4(layer_3)
-
- layer_1_rn = self.scratch.layer1_rn(layer_1)
- layer_2_rn = self.scratch.layer2_rn(layer_2)
- layer_3_rn = self.scratch.layer3_rn(layer_3)
- layer_4_rn = self.scratch.layer4_rn(layer_4)
-
-
- path_4 = self.scratch.refinenet4(layer_4_rn)
- path_3 = self.scratch.refinenet3(path_4, layer_3_rn)
- path_2 = self.scratch.refinenet2(path_3, layer_2_rn)
- path_1 = self.scratch.refinenet1(path_2, layer_1_rn)
-
- out = self.scratch.output_conv(path_1)
-
- return torch.squeeze(out, dim=1)
-
-
-
-def fuse_model(m):
- prev_previous_type = nn.Identity()
- prev_previous_name = ''
- previous_type = nn.Identity()
- previous_name = ''
- for name, module in m.named_modules():
- if prev_previous_type == nn.Conv2d and previous_type == nn.BatchNorm2d and type(module) == nn.ReLU:
- # print("FUSED ", prev_previous_name, previous_name, name)
- torch.quantization.fuse_modules(m, [prev_previous_name, previous_name, name], inplace=True)
- elif prev_previous_type == nn.Conv2d and previous_type == nn.BatchNorm2d:
- # print("FUSED ", prev_previous_name, previous_name)
- torch.quantization.fuse_modules(m, [prev_previous_name, previous_name], inplace=True)
- # elif previous_type == nn.Conv2d and type(module) == nn.ReLU:
- # print("FUSED ", previous_name, name)
- # torch.quantization.fuse_modules(m, [previous_name, name], inplace=True)
-
- prev_previous_type = previous_type
- prev_previous_name = previous_name
- previous_type = type(module)
- previous_name = name
\ No newline at end of file
diff --git a/spaces/Raspberry-ai/main/.env/lib/python3.11/site-packages/pip/_vendor/requests/models.py b/spaces/Raspberry-ai/main/.env/lib/python3.11/site-packages/pip/_vendor/requests/models.py
deleted file mode 100644
index b45e8103258df99bc56826f2b9741a811cf4c030..0000000000000000000000000000000000000000
--- a/spaces/Raspberry-ai/main/.env/lib/python3.11/site-packages/pip/_vendor/requests/models.py
+++ /dev/null
@@ -1,1034 +0,0 @@
-"""
-requests.models
-~~~~~~~~~~~~~~~
-
-This module contains the primary objects that power Requests.
-"""
-
-import datetime
-
-# Import encoding now, to avoid implicit import later.
-# Implicit import within threads may cause LookupError when standard library is in a ZIP,
-# such as in Embedded Python. See https://github.com/psf/requests/issues/3578.
-import encodings.idna # noqa: F401
-from io import UnsupportedOperation
-
-from pip._vendor.urllib3.exceptions import (
- DecodeError,
- LocationParseError,
- ProtocolError,
- ReadTimeoutError,
- SSLError,
-)
-from pip._vendor.urllib3.fields import RequestField
-from pip._vendor.urllib3.filepost import encode_multipart_formdata
-from pip._vendor.urllib3.util import parse_url
-
-from ._internal_utils import to_native_string, unicode_is_ascii
-from .auth import HTTPBasicAuth
-from .compat import (
- Callable,
- JSONDecodeError,
- Mapping,
- basestring,
- builtin_str,
- chardet,
- cookielib,
-)
-from .compat import json as complexjson
-from .compat import urlencode, urlsplit, urlunparse
-from .cookies import _copy_cookie_jar, cookiejar_from_dict, get_cookie_header
-from .exceptions import (
- ChunkedEncodingError,
- ConnectionError,
- ContentDecodingError,
- HTTPError,
- InvalidJSONError,
- InvalidURL,
-)
-from .exceptions import JSONDecodeError as RequestsJSONDecodeError
-from .exceptions import MissingSchema
-from .exceptions import SSLError as RequestsSSLError
-from .exceptions import StreamConsumedError
-from .hooks import default_hooks
-from .status_codes import codes
-from .structures import CaseInsensitiveDict
-from .utils import (
- check_header_validity,
- get_auth_from_url,
- guess_filename,
- guess_json_utf,
- iter_slices,
- parse_header_links,
- requote_uri,
- stream_decode_response_unicode,
- super_len,
- to_key_val_list,
-)
-
-#: The set of HTTP status codes that indicate an automatically
-#: processable redirect.
-REDIRECT_STATI = (
- codes.moved, # 301
- codes.found, # 302
- codes.other, # 303
- codes.temporary_redirect, # 307
- codes.permanent_redirect, # 308
-)
-
-DEFAULT_REDIRECT_LIMIT = 30
-CONTENT_CHUNK_SIZE = 10 * 1024
-ITER_CHUNK_SIZE = 512
-
-
-class RequestEncodingMixin:
- @property
- def path_url(self):
- """Build the path URL to use."""
-
- url = []
-
- p = urlsplit(self.url)
-
- path = p.path
- if not path:
- path = "/"
-
- url.append(path)
-
- query = p.query
- if query:
- url.append("?")
- url.append(query)
-
- return "".join(url)
-
- @staticmethod
- def _encode_params(data):
- """Encode parameters in a piece of data.
-
- Will successfully encode parameters when passed as a dict or a list of
- 2-tuples. Order is retained if data is a list of 2-tuples but arbitrary
- if parameters are supplied as a dict.
- """
-
- if isinstance(data, (str, bytes)):
- return data
- elif hasattr(data, "read"):
- return data
- elif hasattr(data, "__iter__"):
- result = []
- for k, vs in to_key_val_list(data):
- if isinstance(vs, basestring) or not hasattr(vs, "__iter__"):
- vs = [vs]
- for v in vs:
- if v is not None:
- result.append(
- (
- k.encode("utf-8") if isinstance(k, str) else k,
- v.encode("utf-8") if isinstance(v, str) else v,
- )
- )
- return urlencode(result, doseq=True)
- else:
- return data
-
- @staticmethod
- def _encode_files(files, data):
- """Build the body for a multipart/form-data request.
-
- Will successfully encode files when passed as a dict or a list of
- tuples. Order is retained if data is a list of tuples but arbitrary
- if parameters are supplied as a dict.
- The tuples may be 2-tuples (filename, fileobj), 3-tuples (filename, fileobj, contentype)
- or 4-tuples (filename, fileobj, contentype, custom_headers).
- """
- if not files:
- raise ValueError("Files must be provided.")
- elif isinstance(data, basestring):
- raise ValueError("Data must not be a string.")
-
- new_fields = []
- fields = to_key_val_list(data or {})
- files = to_key_val_list(files or {})
-
- for field, val in fields:
- if isinstance(val, basestring) or not hasattr(val, "__iter__"):
- val = [val]
- for v in val:
- if v is not None:
- # Don't call str() on bytestrings: in Py3 it all goes wrong.
- if not isinstance(v, bytes):
- v = str(v)
-
- new_fields.append(
- (
- field.decode("utf-8")
- if isinstance(field, bytes)
- else field,
- v.encode("utf-8") if isinstance(v, str) else v,
- )
- )
-
- for (k, v) in files:
- # support for explicit filename
- ft = None
- fh = None
- if isinstance(v, (tuple, list)):
- if len(v) == 2:
- fn, fp = v
- elif len(v) == 3:
- fn, fp, ft = v
- else:
- fn, fp, ft, fh = v
- else:
- fn = guess_filename(v) or k
- fp = v
-
- if isinstance(fp, (str, bytes, bytearray)):
- fdata = fp
- elif hasattr(fp, "read"):
- fdata = fp.read()
- elif fp is None:
- continue
- else:
- fdata = fp
-
- rf = RequestField(name=k, data=fdata, filename=fn, headers=fh)
- rf.make_multipart(content_type=ft)
- new_fields.append(rf)
-
- body, content_type = encode_multipart_formdata(new_fields)
-
- return body, content_type
-
-
-class RequestHooksMixin:
- def register_hook(self, event, hook):
- """Properly register a hook."""
-
- if event not in self.hooks:
- raise ValueError(f'Unsupported event specified, with event name "{event}"')
-
- if isinstance(hook, Callable):
- self.hooks[event].append(hook)
- elif hasattr(hook, "__iter__"):
- self.hooks[event].extend(h for h in hook if isinstance(h, Callable))
-
- def deregister_hook(self, event, hook):
- """Deregister a previously registered hook.
- Returns True if the hook existed, False if not.
- """
-
- try:
- self.hooks[event].remove(hook)
- return True
- except ValueError:
- return False
-
-
-class Request(RequestHooksMixin):
- """A user-created :class:`Request ` object.
-
- Used to prepare a :class:`PreparedRequest `, which is sent to the server.
-
- :param method: HTTP method to use.
- :param url: URL to send.
- :param headers: dictionary of headers to send.
- :param files: dictionary of {filename: fileobject} files to multipart upload.
- :param data: the body to attach to the request. If a dictionary or
- list of tuples ``[(key, value)]`` is provided, form-encoding will
- take place.
- :param json: json for the body to attach to the request (if files or data is not specified).
- :param params: URL parameters to append to the URL. If a dictionary or
- list of tuples ``[(key, value)]`` is provided, form-encoding will
- take place.
- :param auth: Auth handler or (user, pass) tuple.
- :param cookies: dictionary or CookieJar of cookies to attach to this request.
- :param hooks: dictionary of callback hooks, for internal usage.
-
- Usage::
-
- >>> import requests
- >>> req = requests.Request('GET', 'https://httpbin.org/get')
- >>> req.prepare()
-
- """
-
- def __init__(
- self,
- method=None,
- url=None,
- headers=None,
- files=None,
- data=None,
- params=None,
- auth=None,
- cookies=None,
- hooks=None,
- json=None,
- ):
-
- # Default empty dicts for dict params.
- data = [] if data is None else data
- files = [] if files is None else files
- headers = {} if headers is None else headers
- params = {} if params is None else params
- hooks = {} if hooks is None else hooks
-
- self.hooks = default_hooks()
- for (k, v) in list(hooks.items()):
- self.register_hook(event=k, hook=v)
-
- self.method = method
- self.url = url
- self.headers = headers
- self.files = files
- self.data = data
- self.json = json
- self.params = params
- self.auth = auth
- self.cookies = cookies
-
- def __repr__(self):
- return f""
-
- def prepare(self):
- """Constructs a :class:`PreparedRequest ` for transmission and returns it."""
- p = PreparedRequest()
- p.prepare(
- method=self.method,
- url=self.url,
- headers=self.headers,
- files=self.files,
- data=self.data,
- json=self.json,
- params=self.params,
- auth=self.auth,
- cookies=self.cookies,
- hooks=self.hooks,
- )
- return p
-
-
-class PreparedRequest(RequestEncodingMixin, RequestHooksMixin):
- """The fully mutable :class:`PreparedRequest ` object,
- containing the exact bytes that will be sent to the server.
-
- Instances are generated from a :class:`Request ` object, and
- should not be instantiated manually; doing so may produce undesirable
- effects.
-
- Usage::
-
- >>> import requests
- >>> req = requests.Request('GET', 'https://httpbin.org/get')
- >>> r = req.prepare()
- >>> r
-
-
- >>> s = requests.Session()
- >>> s.send(r)
-
- """
-
- def __init__(self):
- #: HTTP verb to send to the server.
- self.method = None
- #: HTTP URL to send the request to.
- self.url = None
- #: dictionary of HTTP headers.
- self.headers = None
- # The `CookieJar` used to create the Cookie header will be stored here
- # after prepare_cookies is called
- self._cookies = None
- #: request body to send to the server.
- self.body = None
- #: dictionary of callback hooks, for internal usage.
- self.hooks = default_hooks()
- #: integer denoting starting position of a readable file-like body.
- self._body_position = None
-
- def prepare(
- self,
- method=None,
- url=None,
- headers=None,
- files=None,
- data=None,
- params=None,
- auth=None,
- cookies=None,
- hooks=None,
- json=None,
- ):
- """Prepares the entire request with the given parameters."""
-
- self.prepare_method(method)
- self.prepare_url(url, params)
- self.prepare_headers(headers)
- self.prepare_cookies(cookies)
- self.prepare_body(data, files, json)
- self.prepare_auth(auth, url)
-
- # Note that prepare_auth must be last to enable authentication schemes
- # such as OAuth to work on a fully prepared request.
-
- # This MUST go after prepare_auth. Authenticators could add a hook
- self.prepare_hooks(hooks)
-
- def __repr__(self):
- return f""
-
- def copy(self):
- p = PreparedRequest()
- p.method = self.method
- p.url = self.url
- p.headers = self.headers.copy() if self.headers is not None else None
- p._cookies = _copy_cookie_jar(self._cookies)
- p.body = self.body
- p.hooks = self.hooks
- p._body_position = self._body_position
- return p
-
- def prepare_method(self, method):
- """Prepares the given HTTP method."""
- self.method = method
- if self.method is not None:
- self.method = to_native_string(self.method.upper())
-
- @staticmethod
- def _get_idna_encoded_host(host):
- from pip._vendor import idna
-
- try:
- host = idna.encode(host, uts46=True).decode("utf-8")
- except idna.IDNAError:
- raise UnicodeError
- return host
-
- def prepare_url(self, url, params):
- """Prepares the given HTTP URL."""
- #: Accept objects that have string representations.
- #: We're unable to blindly call unicode/str functions
- #: as this will include the bytestring indicator (b'')
- #: on python 3.x.
- #: https://github.com/psf/requests/pull/2238
- if isinstance(url, bytes):
- url = url.decode("utf8")
- else:
- url = str(url)
-
- # Remove leading whitespaces from url
- url = url.lstrip()
-
- # Don't do any URL preparation for non-HTTP schemes like `mailto`,
- # `data` etc to work around exceptions from `url_parse`, which
- # handles RFC 3986 only.
- if ":" in url and not url.lower().startswith("http"):
- self.url = url
- return
-
- # Support for unicode domain names and paths.
- try:
- scheme, auth, host, port, path, query, fragment = parse_url(url)
- except LocationParseError as e:
- raise InvalidURL(*e.args)
-
- if not scheme:
- raise MissingSchema(
- f"Invalid URL {url!r}: No scheme supplied. "
- f"Perhaps you meant http://{url}?"
- )
-
- if not host:
- raise InvalidURL(f"Invalid URL {url!r}: No host supplied")
-
- # In general, we want to try IDNA encoding the hostname if the string contains
- # non-ASCII characters. This allows users to automatically get the correct IDNA
- # behaviour. For strings containing only ASCII characters, we need to also verify
- # it doesn't start with a wildcard (*), before allowing the unencoded hostname.
- if not unicode_is_ascii(host):
- try:
- host = self._get_idna_encoded_host(host)
- except UnicodeError:
- raise InvalidURL("URL has an invalid label.")
- elif host.startswith(("*", ".")):
- raise InvalidURL("URL has an invalid label.")
-
- # Carefully reconstruct the network location
- netloc = auth or ""
- if netloc:
- netloc += "@"
- netloc += host
- if port:
- netloc += f":{port}"
-
- # Bare domains aren't valid URLs.
- if not path:
- path = "/"
-
- if isinstance(params, (str, bytes)):
- params = to_native_string(params)
-
- enc_params = self._encode_params(params)
- if enc_params:
- if query:
- query = f"{query}&{enc_params}"
- else:
- query = enc_params
-
- url = requote_uri(urlunparse([scheme, netloc, path, None, query, fragment]))
- self.url = url
-
- def prepare_headers(self, headers):
- """Prepares the given HTTP headers."""
-
- self.headers = CaseInsensitiveDict()
- if headers:
- for header in headers.items():
- # Raise exception on invalid header value.
- check_header_validity(header)
- name, value = header
- self.headers[to_native_string(name)] = value
-
- def prepare_body(self, data, files, json=None):
- """Prepares the given HTTP body data."""
-
- # Check if file, fo, generator, iterator.
- # If not, run through normal process.
-
- # Nottin' on you.
- body = None
- content_type = None
-
- if not data and json is not None:
- # urllib3 requires a bytes-like body. Python 2's json.dumps
- # provides this natively, but Python 3 gives a Unicode string.
- content_type = "application/json"
-
- try:
- body = complexjson.dumps(json, allow_nan=False)
- except ValueError as ve:
- raise InvalidJSONError(ve, request=self)
-
- if not isinstance(body, bytes):
- body = body.encode("utf-8")
-
- is_stream = all(
- [
- hasattr(data, "__iter__"),
- not isinstance(data, (basestring, list, tuple, Mapping)),
- ]
- )
-
- if is_stream:
- try:
- length = super_len(data)
- except (TypeError, AttributeError, UnsupportedOperation):
- length = None
-
- body = data
-
- if getattr(body, "tell", None) is not None:
- # Record the current file position before reading.
- # This will allow us to rewind a file in the event
- # of a redirect.
- try:
- self._body_position = body.tell()
- except OSError:
- # This differentiates from None, allowing us to catch
- # a failed `tell()` later when trying to rewind the body
- self._body_position = object()
-
- if files:
- raise NotImplementedError(
- "Streamed bodies and files are mutually exclusive."
- )
-
- if length:
- self.headers["Content-Length"] = builtin_str(length)
- else:
- self.headers["Transfer-Encoding"] = "chunked"
- else:
- # Multi-part file uploads.
- if files:
- (body, content_type) = self._encode_files(files, data)
- else:
- if data:
- body = self._encode_params(data)
- if isinstance(data, basestring) or hasattr(data, "read"):
- content_type = None
- else:
- content_type = "application/x-www-form-urlencoded"
-
- self.prepare_content_length(body)
-
- # Add content-type if it wasn't explicitly provided.
- if content_type and ("content-type" not in self.headers):
- self.headers["Content-Type"] = content_type
-
- self.body = body
-
- def prepare_content_length(self, body):
- """Prepare Content-Length header based on request method and body"""
- if body is not None:
- length = super_len(body)
- if length:
- # If length exists, set it. Otherwise, we fallback
- # to Transfer-Encoding: chunked.
- self.headers["Content-Length"] = builtin_str(length)
- elif (
- self.method not in ("GET", "HEAD")
- and self.headers.get("Content-Length") is None
- ):
- # Set Content-Length to 0 for methods that can have a body
- # but don't provide one. (i.e. not GET or HEAD)
- self.headers["Content-Length"] = "0"
-
- def prepare_auth(self, auth, url=""):
- """Prepares the given HTTP auth data."""
-
- # If no Auth is explicitly provided, extract it from the URL first.
- if auth is None:
- url_auth = get_auth_from_url(self.url)
- auth = url_auth if any(url_auth) else None
-
- if auth:
- if isinstance(auth, tuple) and len(auth) == 2:
- # special-case basic HTTP auth
- auth = HTTPBasicAuth(*auth)
-
- # Allow auth to make its changes.
- r = auth(self)
-
- # Update self to reflect the auth changes.
- self.__dict__.update(r.__dict__)
-
- # Recompute Content-Length
- self.prepare_content_length(self.body)
-
- def prepare_cookies(self, cookies):
- """Prepares the given HTTP cookie data.
-
- This function eventually generates a ``Cookie`` header from the
- given cookies using cookielib. Due to cookielib's design, the header
- will not be regenerated if it already exists, meaning this function
- can only be called once for the life of the
- :class:`PreparedRequest ` object. Any subsequent calls
- to ``prepare_cookies`` will have no actual effect, unless the "Cookie"
- header is removed beforehand.
- """
- if isinstance(cookies, cookielib.CookieJar):
- self._cookies = cookies
- else:
- self._cookies = cookiejar_from_dict(cookies)
-
- cookie_header = get_cookie_header(self._cookies, self)
- if cookie_header is not None:
- self.headers["Cookie"] = cookie_header
-
- def prepare_hooks(self, hooks):
- """Prepares the given hooks."""
- # hooks can be passed as None to the prepare method and to this
- # method. To prevent iterating over None, simply use an empty list
- # if hooks is False-y
- hooks = hooks or []
- for event in hooks:
- self.register_hook(event, hooks[event])
-
-
-class Response:
- """The :class:`Response ` object, which contains a
- server's response to an HTTP request.
- """
-
- __attrs__ = [
- "_content",
- "status_code",
- "headers",
- "url",
- "history",
- "encoding",
- "reason",
- "cookies",
- "elapsed",
- "request",
- ]
-
- def __init__(self):
- self._content = False
- self._content_consumed = False
- self._next = None
-
- #: Integer Code of responded HTTP Status, e.g. 404 or 200.
- self.status_code = None
-
- #: Case-insensitive Dictionary of Response Headers.
- #: For example, ``headers['content-encoding']`` will return the
- #: value of a ``'Content-Encoding'`` response header.
- self.headers = CaseInsensitiveDict()
-
- #: File-like object representation of response (for advanced usage).
- #: Use of ``raw`` requires that ``stream=True`` be set on the request.
- #: This requirement does not apply for use internally to Requests.
- self.raw = None
-
- #: Final URL location of Response.
- self.url = None
-
- #: Encoding to decode with when accessing r.text.
- self.encoding = None
-
- #: A list of :class:`Response ` objects from
- #: the history of the Request. Any redirect responses will end
- #: up here. The list is sorted from the oldest to the most recent request.
- self.history = []
-
- #: Textual reason of responded HTTP Status, e.g. "Not Found" or "OK".
- self.reason = None
-
- #: A CookieJar of Cookies the server sent back.
- self.cookies = cookiejar_from_dict({})
-
- #: The amount of time elapsed between sending the request
- #: and the arrival of the response (as a timedelta).
- #: This property specifically measures the time taken between sending
- #: the first byte of the request and finishing parsing the headers. It
- #: is therefore unaffected by consuming the response content or the
- #: value of the ``stream`` keyword argument.
- self.elapsed = datetime.timedelta(0)
-
- #: The :class:`PreparedRequest ` object to which this
- #: is a response.
- self.request = None
-
- def __enter__(self):
- return self
-
- def __exit__(self, *args):
- self.close()
-
- def __getstate__(self):
- # Consume everything; accessing the content attribute makes
- # sure the content has been fully read.
- if not self._content_consumed:
- self.content
-
- return {attr: getattr(self, attr, None) for attr in self.__attrs__}
-
- def __setstate__(self, state):
- for name, value in state.items():
- setattr(self, name, value)
-
- # pickled objects do not have .raw
- setattr(self, "_content_consumed", True)
- setattr(self, "raw", None)
-
- def __repr__(self):
- return f""
-
- def __bool__(self):
- """Returns True if :attr:`status_code` is less than 400.
-
- This attribute checks if the status code of the response is between
- 400 and 600 to see if there was a client error or a server error. If
- the status code, is between 200 and 400, this will return True. This
- is **not** a check to see if the response code is ``200 OK``.
- """
- return self.ok
-
- def __nonzero__(self):
- """Returns True if :attr:`status_code` is less than 400.
-
- This attribute checks if the status code of the response is between
- 400 and 600 to see if there was a client error or a server error. If
- the status code, is between 200 and 400, this will return True. This
- is **not** a check to see if the response code is ``200 OK``.
- """
- return self.ok
-
- def __iter__(self):
- """Allows you to use a response as an iterator."""
- return self.iter_content(128)
-
- @property
- def ok(self):
- """Returns True if :attr:`status_code` is less than 400, False if not.
-
- This attribute checks if the status code of the response is between
- 400 and 600 to see if there was a client error or a server error. If
- the status code is between 200 and 400, this will return True. This
- is **not** a check to see if the response code is ``200 OK``.
- """
- try:
- self.raise_for_status()
- except HTTPError:
- return False
- return True
-
- @property
- def is_redirect(self):
- """True if this Response is a well-formed HTTP redirect that could have
- been processed automatically (by :meth:`Session.resolve_redirects`).
- """
- return "location" in self.headers and self.status_code in REDIRECT_STATI
-
- @property
- def is_permanent_redirect(self):
- """True if this Response one of the permanent versions of redirect."""
- return "location" in self.headers and self.status_code in (
- codes.moved_permanently,
- codes.permanent_redirect,
- )
-
- @property
- def next(self):
- """Returns a PreparedRequest for the next request in a redirect chain, if there is one."""
- return self._next
-
- @property
- def apparent_encoding(self):
- """The apparent encoding, provided by the charset_normalizer or chardet libraries."""
- return chardet.detect(self.content)["encoding"]
-
- def iter_content(self, chunk_size=1, decode_unicode=False):
- """Iterates over the response data. When stream=True is set on the
- request, this avoids reading the content at once into memory for
- large responses. The chunk size is the number of bytes it should
- read into memory. This is not necessarily the length of each item
- returned as decoding can take place.
-
- chunk_size must be of type int or None. A value of None will
- function differently depending on the value of `stream`.
- stream=True will read data as it arrives in whatever size the
- chunks are received. If stream=False, data is returned as
- a single chunk.
-
- If decode_unicode is True, content will be decoded using the best
- available encoding based on the response.
- """
-
- def generate():
- # Special case for urllib3.
- if hasattr(self.raw, "stream"):
- try:
- yield from self.raw.stream(chunk_size, decode_content=True)
- except ProtocolError as e:
- raise ChunkedEncodingError(e)
- except DecodeError as e:
- raise ContentDecodingError(e)
- except ReadTimeoutError as e:
- raise ConnectionError(e)
- except SSLError as e:
- raise RequestsSSLError(e)
- else:
- # Standard file-like object.
- while True:
- chunk = self.raw.read(chunk_size)
- if not chunk:
- break
- yield chunk
-
- self._content_consumed = True
-
- if self._content_consumed and isinstance(self._content, bool):
- raise StreamConsumedError()
- elif chunk_size is not None and not isinstance(chunk_size, int):
- raise TypeError(
- f"chunk_size must be an int, it is instead a {type(chunk_size)}."
- )
- # simulate reading small chunks of the content
- reused_chunks = iter_slices(self._content, chunk_size)
-
- stream_chunks = generate()
-
- chunks = reused_chunks if self._content_consumed else stream_chunks
-
- if decode_unicode:
- chunks = stream_decode_response_unicode(chunks, self)
-
- return chunks
-
- def iter_lines(
- self, chunk_size=ITER_CHUNK_SIZE, decode_unicode=False, delimiter=None
- ):
- """Iterates over the response data, one line at a time. When
- stream=True is set on the request, this avoids reading the
- content at once into memory for large responses.
-
- .. note:: This method is not reentrant safe.
- """
-
- pending = None
-
- for chunk in self.iter_content(
- chunk_size=chunk_size, decode_unicode=decode_unicode
- ):
-
- if pending is not None:
- chunk = pending + chunk
-
- if delimiter:
- lines = chunk.split(delimiter)
- else:
- lines = chunk.splitlines()
-
- if lines and lines[-1] and chunk and lines[-1][-1] == chunk[-1]:
- pending = lines.pop()
- else:
- pending = None
-
- yield from lines
-
- if pending is not None:
- yield pending
-
- @property
- def content(self):
- """Content of the response, in bytes."""
-
- if self._content is False:
- # Read the contents.
- if self._content_consumed:
- raise RuntimeError("The content for this response was already consumed")
-
- if self.status_code == 0 or self.raw is None:
- self._content = None
- else:
- self._content = b"".join(self.iter_content(CONTENT_CHUNK_SIZE)) or b""
-
- self._content_consumed = True
- # don't need to release the connection; that's been handled by urllib3
- # since we exhausted the data.
- return self._content
-
- @property
- def text(self):
- """Content of the response, in unicode.
-
- If Response.encoding is None, encoding will be guessed using
- ``charset_normalizer`` or ``chardet``.
-
- The encoding of the response content is determined based solely on HTTP
- headers, following RFC 2616 to the letter. If you can take advantage of
- non-HTTP knowledge to make a better guess at the encoding, you should
- set ``r.encoding`` appropriately before accessing this property.
- """
-
- # Try charset from content-type
- content = None
- encoding = self.encoding
-
- if not self.content:
- return ""
-
- # Fallback to auto-detected encoding.
- if self.encoding is None:
- encoding = self.apparent_encoding
-
- # Decode unicode from given encoding.
- try:
- content = str(self.content, encoding, errors="replace")
- except (LookupError, TypeError):
- # A LookupError is raised if the encoding was not found which could
- # indicate a misspelling or similar mistake.
- #
- # A TypeError can be raised if encoding is None
- #
- # So we try blindly encoding.
- content = str(self.content, errors="replace")
-
- return content
-
- def json(self, **kwargs):
- r"""Returns the json-encoded content of a response, if any.
-
- :param \*\*kwargs: Optional arguments that ``json.loads`` takes.
- :raises requests.exceptions.JSONDecodeError: If the response body does not
- contain valid json.
- """
-
- if not self.encoding and self.content and len(self.content) > 3:
- # No encoding set. JSON RFC 4627 section 3 states we should expect
- # UTF-8, -16 or -32. Detect which one to use; If the detection or
- # decoding fails, fall back to `self.text` (using charset_normalizer to make
- # a best guess).
- encoding = guess_json_utf(self.content)
- if encoding is not None:
- try:
- return complexjson.loads(self.content.decode(encoding), **kwargs)
- except UnicodeDecodeError:
- # Wrong UTF codec detected; usually because it's not UTF-8
- # but some other 8-bit codec. This is an RFC violation,
- # and the server didn't bother to tell us what codec *was*
- # used.
- pass
- except JSONDecodeError as e:
- raise RequestsJSONDecodeError(e.msg, e.doc, e.pos)
-
- try:
- return complexjson.loads(self.text, **kwargs)
- except JSONDecodeError as e:
- # Catch JSON-related errors and raise as requests.JSONDecodeError
- # This aliases json.JSONDecodeError and simplejson.JSONDecodeError
- raise RequestsJSONDecodeError(e.msg, e.doc, e.pos)
-
- @property
- def links(self):
- """Returns the parsed header links of the response, if any."""
-
- header = self.headers.get("link")
-
- resolved_links = {}
-
- if header:
- links = parse_header_links(header)
-
- for link in links:
- key = link.get("rel") or link.get("url")
- resolved_links[key] = link
-
- return resolved_links
-
- def raise_for_status(self):
- """Raises :class:`HTTPError`, if one occurred."""
-
- http_error_msg = ""
- if isinstance(self.reason, bytes):
- # We attempt to decode utf-8 first because some servers
- # choose to localize their reason strings. If the string
- # isn't utf-8, we fall back to iso-8859-1 for all other
- # encodings. (See PR #3538)
- try:
- reason = self.reason.decode("utf-8")
- except UnicodeDecodeError:
- reason = self.reason.decode("iso-8859-1")
- else:
- reason = self.reason
-
- if 400 <= self.status_code < 500:
- http_error_msg = (
- f"{self.status_code} Client Error: {reason} for url: {self.url}"
- )
-
- elif 500 <= self.status_code < 600:
- http_error_msg = (
- f"{self.status_code} Server Error: {reason} for url: {self.url}"
- )
-
- if http_error_msg:
- raise HTTPError(http_error_msg, response=self)
-
- def close(self):
- """Releases the connection back to the pool. Once this method has been
- called the underlying ``raw`` object must not be accessed again.
-
- *Note: Should not normally need to be called explicitly.*
- """
- if not self._content_consumed:
- self.raw.close()
-
- release_conn = getattr(self.raw, "release_conn", None)
- if release_conn is not None:
- release_conn()
diff --git a/spaces/RealKintaro/Offensive-Speech-Detection-From-Arabic-Dialects/README.md b/spaces/RealKintaro/Offensive-Speech-Detection-From-Arabic-Dialects/README.md
deleted file mode 100644
index 9bf613e579298ec43186c28247dbb649283d6acb..0000000000000000000000000000000000000000
--- a/spaces/RealKintaro/Offensive-Speech-Detection-From-Arabic-Dialects/README.md
+++ /dev/null
@@ -1,12 +0,0 @@
----
-title: NLP Project
-emoji: 🚀
-colorFrom: red
-colorTo: purple
-sdk: streamlit
-sdk_version: 1.15.2
-app_file: Deployment/app.py
-pinned: false
----
-
-Check out the configuration reference at https://huggingface.co/docs/hub/spaces-config-reference
\ No newline at end of file
diff --git a/spaces/Realcat/image-matching-webui/third_party/TopicFM/src/models/modules/__init__.py b/spaces/Realcat/image-matching-webui/third_party/TopicFM/src/models/modules/__init__.py
deleted file mode 100644
index 59cf36da37104dcf080e1b2c119c8123fa8d147f..0000000000000000000000000000000000000000
--- a/spaces/Realcat/image-matching-webui/third_party/TopicFM/src/models/modules/__init__.py
+++ /dev/null
@@ -1,2 +0,0 @@
-from .transformer import LocalFeatureTransformer, TopicFormer
-from .fine_preprocess import FinePreprocess
diff --git a/spaces/Ritori/Ritori-Yura_GPT2/README.md b/spaces/Ritori/Ritori-Yura_GPT2/README.md
deleted file mode 100644
index b60bcf20fd354ecc47095d2a0a27fa0f6c6f0603..0000000000000000000000000000000000000000
--- a/spaces/Ritori/Ritori-Yura_GPT2/README.md
+++ /dev/null
@@ -1,12 +0,0 @@
----
-title: Ritori-Yura GPT2
-emoji: 📚
-colorFrom: green
-colorTo: blue
-sdk: gradio
-sdk_version: 3.39.0
-app_file: app.py
-pinned: false
----
-
-Check out the configuration reference at https://huggingface.co/docs/hub/spaces-config-reference
diff --git a/spaces/Robert001/UniControl-Demo/annotator/uniformer/mmdet/core/bbox/coder/delta_xywh_bbox_coder.py b/spaces/Robert001/UniControl-Demo/annotator/uniformer/mmdet/core/bbox/coder/delta_xywh_bbox_coder.py
deleted file mode 100644
index da317184a6eb6f87b0b658e9ff8be289794a0cb2..0000000000000000000000000000000000000000
--- a/spaces/Robert001/UniControl-Demo/annotator/uniformer/mmdet/core/bbox/coder/delta_xywh_bbox_coder.py
+++ /dev/null
@@ -1,237 +0,0 @@
-import mmcv
-import numpy as np
-import torch
-
-from ..builder import BBOX_CODERS
-from .base_bbox_coder import BaseBBoxCoder
-
-
-@BBOX_CODERS.register_module()
-class DeltaXYWHBBoxCoder(BaseBBoxCoder):
- """Delta XYWH BBox coder.
-
- Following the practice in `R-CNN `_,
- this coder encodes bbox (x1, y1, x2, y2) into delta (dx, dy, dw, dh) and
- decodes delta (dx, dy, dw, dh) back to original bbox (x1, y1, x2, y2).
-
- Args:
- target_means (Sequence[float]): Denormalizing means of target for
- delta coordinates
- target_stds (Sequence[float]): Denormalizing standard deviation of
- target for delta coordinates
- clip_border (bool, optional): Whether clip the objects outside the
- border of the image. Defaults to True.
- """
-
- def __init__(self,
- target_means=(0., 0., 0., 0.),
- target_stds=(1., 1., 1., 1.),
- clip_border=True):
- super(BaseBBoxCoder, self).__init__()
- self.means = target_means
- self.stds = target_stds
- self.clip_border = clip_border
-
- def encode(self, bboxes, gt_bboxes):
- """Get box regression transformation deltas that can be used to
- transform the ``bboxes`` into the ``gt_bboxes``.
-
- Args:
- bboxes (torch.Tensor): Source boxes, e.g., object proposals.
- gt_bboxes (torch.Tensor): Target of the transformation, e.g.,
- ground-truth boxes.
-
- Returns:
- torch.Tensor: Box transformation deltas
- """
-
- assert bboxes.size(0) == gt_bboxes.size(0)
- assert bboxes.size(-1) == gt_bboxes.size(-1) == 4
- encoded_bboxes = bbox2delta(bboxes, gt_bboxes, self.means, self.stds)
- return encoded_bboxes
-
- def decode(self,
- bboxes,
- pred_bboxes,
- max_shape=None,
- wh_ratio_clip=16 / 1000):
- """Apply transformation `pred_bboxes` to `boxes`.
-
- Args:
- bboxes (torch.Tensor): Basic boxes. Shape (B, N, 4) or (N, 4)
- pred_bboxes (Tensor): Encoded offsets with respect to each roi.
- Has shape (B, N, num_classes * 4) or (B, N, 4) or
- (N, num_classes * 4) or (N, 4). Note N = num_anchors * W * H
- when rois is a grid of anchors.Offset encoding follows [1]_.
- max_shape (Sequence[int] or torch.Tensor or Sequence[
- Sequence[int]],optional): Maximum bounds for boxes, specifies
- (H, W, C) or (H, W). If bboxes shape is (B, N, 4), then
- the max_shape should be a Sequence[Sequence[int]]
- and the length of max_shape should also be B.
- wh_ratio_clip (float, optional): The allowed ratio between
- width and height.
-
- Returns:
- torch.Tensor: Decoded boxes.
- """
-
- assert pred_bboxes.size(0) == bboxes.size(0)
- if pred_bboxes.ndim == 3:
- assert pred_bboxes.size(1) == bboxes.size(1)
- decoded_bboxes = delta2bbox(bboxes, pred_bboxes, self.means, self.stds,
- max_shape, wh_ratio_clip, self.clip_border)
-
- return decoded_bboxes
-
-
-@mmcv.jit(coderize=True)
-def bbox2delta(proposals, gt, means=(0., 0., 0., 0.), stds=(1., 1., 1., 1.)):
- """Compute deltas of proposals w.r.t. gt.
-
- We usually compute the deltas of x, y, w, h of proposals w.r.t ground
- truth bboxes to get regression target.
- This is the inverse function of :func:`delta2bbox`.
-
- Args:
- proposals (Tensor): Boxes to be transformed, shape (N, ..., 4)
- gt (Tensor): Gt bboxes to be used as base, shape (N, ..., 4)
- means (Sequence[float]): Denormalizing means for delta coordinates
- stds (Sequence[float]): Denormalizing standard deviation for delta
- coordinates
-
- Returns:
- Tensor: deltas with shape (N, 4), where columns represent dx, dy,
- dw, dh.
- """
- assert proposals.size() == gt.size()
-
- proposals = proposals.float()
- gt = gt.float()
- px = (proposals[..., 0] + proposals[..., 2]) * 0.5
- py = (proposals[..., 1] + proposals[..., 3]) * 0.5
- pw = proposals[..., 2] - proposals[..., 0]
- ph = proposals[..., 3] - proposals[..., 1]
-
- gx = (gt[..., 0] + gt[..., 2]) * 0.5
- gy = (gt[..., 1] + gt[..., 3]) * 0.5
- gw = gt[..., 2] - gt[..., 0]
- gh = gt[..., 3] - gt[..., 1]
-
- dx = (gx - px) / pw
- dy = (gy - py) / ph
- dw = torch.log(gw / pw)
- dh = torch.log(gh / ph)
- deltas = torch.stack([dx, dy, dw, dh], dim=-1)
-
- means = deltas.new_tensor(means).unsqueeze(0)
- stds = deltas.new_tensor(stds).unsqueeze(0)
- deltas = deltas.sub_(means).div_(stds)
-
- return deltas
-
-
-@mmcv.jit(coderize=True)
-def delta2bbox(rois,
- deltas,
- means=(0., 0., 0., 0.),
- stds=(1., 1., 1., 1.),
- max_shape=None,
- wh_ratio_clip=16 / 1000,
- clip_border=True):
- """Apply deltas to shift/scale base boxes.
-
- Typically the rois are anchor or proposed bounding boxes and the deltas are
- network outputs used to shift/scale those boxes.
- This is the inverse function of :func:`bbox2delta`.
-
- Args:
- rois (Tensor): Boxes to be transformed. Has shape (N, 4) or (B, N, 4)
- deltas (Tensor): Encoded offsets with respect to each roi.
- Has shape (B, N, num_classes * 4) or (B, N, 4) or
- (N, num_classes * 4) or (N, 4). Note N = num_anchors * W * H
- when rois is a grid of anchors.Offset encoding follows [1]_.
- means (Sequence[float]): Denormalizing means for delta coordinates
- stds (Sequence[float]): Denormalizing standard deviation for delta
- coordinates
- max_shape (Sequence[int] or torch.Tensor or Sequence[
- Sequence[int]],optional): Maximum bounds for boxes, specifies
- (H, W, C) or (H, W). If rois shape is (B, N, 4), then
- the max_shape should be a Sequence[Sequence[int]]
- and the length of max_shape should also be B.
- wh_ratio_clip (float): Maximum aspect ratio for boxes.
- clip_border (bool, optional): Whether clip the objects outside the
- border of the image. Defaults to True.
-
- Returns:
- Tensor: Boxes with shape (B, N, num_classes * 4) or (B, N, 4) or
- (N, num_classes * 4) or (N, 4), where 4 represent
- tl_x, tl_y, br_x, br_y.
-
- References:
- .. [1] https://arxiv.org/abs/1311.2524
-
- Example:
- >>> rois = torch.Tensor([[ 0., 0., 1., 1.],
- >>> [ 0., 0., 1., 1.],
- >>> [ 0., 0., 1., 1.],
- >>> [ 5., 5., 5., 5.]])
- >>> deltas = torch.Tensor([[ 0., 0., 0., 0.],
- >>> [ 1., 1., 1., 1.],
- >>> [ 0., 0., 2., -1.],
- >>> [ 0.7, -1.9, -0.5, 0.3]])
- >>> delta2bbox(rois, deltas, max_shape=(32, 32, 3))
- tensor([[0.0000, 0.0000, 1.0000, 1.0000],
- [0.1409, 0.1409, 2.8591, 2.8591],
- [0.0000, 0.3161, 4.1945, 0.6839],
- [5.0000, 5.0000, 5.0000, 5.0000]])
- """
- means = deltas.new_tensor(means).view(1,
- -1).repeat(1,
- deltas.size(-1) // 4)
- stds = deltas.new_tensor(stds).view(1, -1).repeat(1, deltas.size(-1) // 4)
- denorm_deltas = deltas * stds + means
- dx = denorm_deltas[..., 0::4]
- dy = denorm_deltas[..., 1::4]
- dw = denorm_deltas[..., 2::4]
- dh = denorm_deltas[..., 3::4]
- max_ratio = np.abs(np.log(wh_ratio_clip))
- dw = dw.clamp(min=-max_ratio, max=max_ratio)
- dh = dh.clamp(min=-max_ratio, max=max_ratio)
- x1, y1 = rois[..., 0], rois[..., 1]
- x2, y2 = rois[..., 2], rois[..., 3]
- # Compute center of each roi
- px = ((x1 + x2) * 0.5).unsqueeze(-1).expand_as(dx)
- py = ((y1 + y2) * 0.5).unsqueeze(-1).expand_as(dy)
- # Compute width/height of each roi
- pw = (x2 - x1).unsqueeze(-1).expand_as(dw)
- ph = (y2 - y1).unsqueeze(-1).expand_as(dh)
- # Use exp(network energy) to enlarge/shrink each roi
- gw = pw * dw.exp()
- gh = ph * dh.exp()
- # Use network energy to shift the center of each roi
- gx = px + pw * dx
- gy = py + ph * dy
- # Convert center-xy/width/height to top-left, bottom-right
- x1 = gx - gw * 0.5
- y1 = gy - gh * 0.5
- x2 = gx + gw * 0.5
- y2 = gy + gh * 0.5
-
- bboxes = torch.stack([x1, y1, x2, y2], dim=-1).view(deltas.size())
-
- if clip_border and max_shape is not None:
- if not isinstance(max_shape, torch.Tensor):
- max_shape = x1.new_tensor(max_shape)
- max_shape = max_shape[..., :2].type_as(x1)
- if max_shape.ndim == 2:
- assert bboxes.ndim == 3
- assert max_shape.size(0) == bboxes.size(0)
-
- min_xy = x1.new_tensor(0)
- max_xy = torch.cat(
- [max_shape] * (deltas.size(-1) // 2),
- dim=-1).flip(-1).unsqueeze(-2)
- bboxes = torch.where(bboxes < min_xy, min_xy, bboxes)
- bboxes = torch.where(bboxes > max_xy, max_xy, bboxes)
-
- return bboxes
diff --git a/spaces/Robert001/UniControl-Demo/annotator/uniformer/mmdet_null/models/roi_heads/mask_heads/htc_mask_head.py b/spaces/Robert001/UniControl-Demo/annotator/uniformer/mmdet_null/models/roi_heads/mask_heads/htc_mask_head.py
deleted file mode 100644
index 330b778ebad8d48d55d09ddd42baa70ec10ae463..0000000000000000000000000000000000000000
--- a/spaces/Robert001/UniControl-Demo/annotator/uniformer/mmdet_null/models/roi_heads/mask_heads/htc_mask_head.py
+++ /dev/null
@@ -1,43 +0,0 @@
-from mmcv.cnn import ConvModule
-
-from mmdet.models.builder import HEADS
-from .fcn_mask_head import FCNMaskHead
-
-
-@HEADS.register_module()
-class HTCMaskHead(FCNMaskHead):
-
- def __init__(self, with_conv_res=True, *args, **kwargs):
- super(HTCMaskHead, self).__init__(*args, **kwargs)
- self.with_conv_res = with_conv_res
- if self.with_conv_res:
- self.conv_res = ConvModule(
- self.conv_out_channels,
- self.conv_out_channels,
- 1,
- conv_cfg=self.conv_cfg,
- norm_cfg=self.norm_cfg)
-
- def init_weights(self):
- super(HTCMaskHead, self).init_weights()
- if self.with_conv_res:
- self.conv_res.init_weights()
-
- def forward(self, x, res_feat=None, return_logits=True, return_feat=True):
- if res_feat is not None:
- assert self.with_conv_res
- res_feat = self.conv_res(res_feat)
- x = x + res_feat
- for conv in self.convs:
- x = conv(x)
- res_feat = x
- outs = []
- if return_logits:
- x = self.upsample(x)
- if self.upsample_method == 'deconv':
- x = self.relu(x)
- mask_pred = self.conv_logits(x)
- outs.append(mask_pred)
- if return_feat:
- outs.append(res_feat)
- return outs if len(outs) > 1 else outs[0]
diff --git a/spaces/Robert001/UniControl-Demo/annotator/uniformer_base/mmseg/core/__init__.py b/spaces/Robert001/UniControl-Demo/annotator/uniformer_base/mmseg/core/__init__.py
deleted file mode 100644
index 965605587211b7bf0bd6bc3acdbb33dd49cab023..0000000000000000000000000000000000000000
--- a/spaces/Robert001/UniControl-Demo/annotator/uniformer_base/mmseg/core/__init__.py
+++ /dev/null
@@ -1,3 +0,0 @@
-from .evaluation import * # noqa: F401, F403
-from .seg import * # noqa: F401, F403
-from .utils import * # noqa: F401, F403
diff --git a/spaces/Rongjiehuang/GenerSpeech/utils/trainer.py b/spaces/Rongjiehuang/GenerSpeech/utils/trainer.py
deleted file mode 100644
index 7df4cbc119b39aca580e61e3e9069fedd7e0d142..0000000000000000000000000000000000000000
--- a/spaces/Rongjiehuang/GenerSpeech/utils/trainer.py
+++ /dev/null
@@ -1,518 +0,0 @@
-import random
-from torch.cuda.amp import GradScaler, autocast
-from utils import move_to_cuda
-import subprocess
-import numpy as np
-import torch.optim
-import torch.utils.data
-import copy
-import logging
-import os
-import re
-import sys
-import torch
-import torch.distributed as dist
-import torch.multiprocessing as mp
-import tqdm
-
-from utils.ckpt_utils import get_last_checkpoint, get_all_ckpts
-from utils.ddp_utils import DDP
-from utils.hparams import hparams
-
-
-class Trainer:
- def __init__(
- self,
- work_dir,
- default_save_path=None,
- accumulate_grad_batches=1,
- max_updates=160000,
- print_nan_grads=False,
- val_check_interval=2000,
- num_sanity_val_steps=5,
- amp=False,
- # tb logger
- log_save_interval=100,
- tb_log_interval=10,
- # checkpoint
- monitor_key='val_loss',
- monitor_mode='min',
- num_ckpt_keep=5,
- save_best=True,
- resume_from_checkpoint=0,
- seed=1234,
- debug=False,
- ):
- os.makedirs(work_dir, exist_ok=True)
- self.work_dir = work_dir
- self.accumulate_grad_batches = accumulate_grad_batches
- self.max_updates = max_updates
- self.num_sanity_val_steps = num_sanity_val_steps
- self.print_nan_grads = print_nan_grads
- self.default_save_path = default_save_path
- self.resume_from_checkpoint = resume_from_checkpoint if resume_from_checkpoint > 0 else None
- self.seed = seed
- self.debug = debug
- # model and optm
- self.task = None
- self.optimizers = []
-
- # trainer state
- self.testing = False
- self.global_step = 0
- self.current_epoch = 0
- self.total_batches = 0
-
- # configure checkpoint
- self.monitor_key = monitor_key
- self.num_ckpt_keep = num_ckpt_keep
- self.save_best = save_best
- self.monitor_op = np.less if monitor_mode == 'min' else np.greater
- self.best_val_results = np.Inf if monitor_mode == 'min' else -np.Inf
- self.mode = 'min'
-
- # allow int, string and gpu list
- self.all_gpu_ids = [
- int(x) for x in os.environ.get("CUDA_VISIBLE_DEVICES", "").split(",") if x != '']
- self.num_gpus = len(self.all_gpu_ids)
- self.on_gpu = self.num_gpus > 0
- self.root_gpu = 0
- logging.info(f'GPU available: {torch.cuda.is_available()}, GPU used: {self.all_gpu_ids}')
- self.use_ddp = self.num_gpus > 1
- self.proc_rank = 0
- # Tensorboard logging
- self.log_save_interval = log_save_interval
- self.val_check_interval = val_check_interval
- self.tb_log_interval = tb_log_interval
- self.amp = amp
- self.amp_scalar = GradScaler()
-
- def test(self, task_cls):
- self.testing = True
- self.fit(task_cls)
-
- def fit(self, task_cls):
- if len(self.all_gpu_ids) > 1:
- mp.spawn(self.ddp_run, nprocs=self.num_gpus, args=(task_cls, copy.deepcopy(hparams)))
- else:
- self.task = task_cls()
- self.task.trainer = self
- self.run_single_process(self.task)
- return 1
-
- def ddp_run(self, gpu_idx, task_cls, hparams_):
- hparams.update(hparams_)
- task = task_cls()
- self.ddp_init(gpu_idx, task)
- self.run_single_process(task)
-
- def run_single_process(self, task):
- """Sanity check a few things before starting actual training.
-
- :param task:
- """
- # build model, optm and load checkpoint
- model = task.build_model()
- if model is not None:
- task.model = model
- checkpoint, _ = get_last_checkpoint(self.work_dir, self.resume_from_checkpoint)
- if checkpoint is not None:
- self.restore_weights(checkpoint)
- elif self.on_gpu:
- task.cuda(self.root_gpu)
- if not self.testing:
- self.optimizers = task.configure_optimizers()
- self.fisrt_epoch = True
- if checkpoint is not None:
- self.restore_opt_state(checkpoint)
- del checkpoint
- # clear cache after restore
- if self.on_gpu:
- torch.cuda.empty_cache()
-
- if self.use_ddp:
- self.task = self.configure_ddp(self.task)
- dist.barrier()
-
- task_ref = self.get_task_ref()
- task_ref.trainer = self
- task_ref.testing = self.testing
- # link up experiment object
- if self.proc_rank == 0:
- task_ref.build_tensorboard(save_dir=self.work_dir, name='lightning_logs', version='lastest')
- else:
- os.makedirs('tmp', exist_ok=True)
- task_ref.build_tensorboard(save_dir='tmp', name='tb_tmp', version='lastest')
- self.logger = task_ref.logger
- try:
- if self.testing:
- self.run_evaluation(test=True)
- else:
- self.train()
- except KeyboardInterrupt as e:
- task_ref.on_keyboard_interrupt()
-
- ####################
- # valid and test
- ####################
- def run_evaluation(self, test=False):
- eval_results = self.evaluate(self.task, test, tqdm_desc='Valid' if not test else 'test')
- if eval_results is not None and 'tb_log' in eval_results:
- tb_log_output = eval_results['tb_log']
- self.log_metrics_to_tb(tb_log_output)
- if self.proc_rank == 0 and not test:
- self.save_checkpoint(epoch=self.current_epoch, logs=eval_results)
-
- def evaluate(self, task, test=False, tqdm_desc='Valid', max_batches=None):
- # enable eval mode
- task.zero_grad()
- task.eval()
- torch.set_grad_enabled(False)
-
- task_ref = self.get_task_ref()
- if test:
- ret = task_ref.test_start()
- if ret == 'EXIT':
- return
-
- outputs = []
- dataloader = task_ref.test_dataloader() if test else task_ref.val_dataloader()
- pbar = tqdm.tqdm(dataloader, desc=tqdm_desc, total=max_batches, dynamic_ncols=True, unit='step',
- disable=self.root_gpu > 0)
- for batch_idx, batch in enumerate(pbar):
- if batch is None: # pragma: no cover
- continue
- # stop short when on fast_dev_run (sets max_batch=1)
- if max_batches is not None and batch_idx >= max_batches:
- break
-
- # make dataloader_idx arg in validation_step optional
- if self.on_gpu:
- batch = move_to_cuda(batch, self.root_gpu)
- args = [batch, batch_idx]
- if self.use_ddp:
- output = task(*args)
- else:
- if test:
- output = task_ref.test_step(*args)
- else:
- output = task_ref.validation_step(*args)
- # track outputs for collation
- outputs.append(output)
- # give model a chance to do something with the outputs (and method defined)
- if test:
- eval_results = task_ref.test_end(outputs)
- else:
- eval_results = task_ref.validation_end(outputs)
- # enable train mode again
- task.train()
- torch.set_grad_enabled(True)
- return eval_results
-
- ####################
- # train
- ####################
- def train(self):
- task_ref = self.get_task_ref()
- task_ref.on_train_start()
- if self.num_sanity_val_steps > 0:
- # run tiny validation (if validation defined) to make sure program won't crash during val
- self.evaluate(self.task, False, 'Sanity Val', max_batches=self.num_sanity_val_steps)
- # clear cache before training
- if self.on_gpu:
- torch.cuda.empty_cache()
- dataloader = task_ref.train_dataloader()
- epoch = self.current_epoch
- # run all epochs
- while True:
- # set seed for distributed sampler (enables shuffling for each epoch)
- if self.use_ddp and hasattr(dataloader.sampler, 'set_epoch'):
- dataloader.sampler.set_epoch(epoch)
- # update training progress in trainer and model
- task_ref.current_epoch = epoch
- self.current_epoch = epoch
- # total batches includes multiple val checks
- self.batch_loss_value = 0 # accumulated grads
- # before epoch hook
- task_ref.on_epoch_start()
-
- # run epoch
- train_pbar = tqdm.tqdm(dataloader, initial=self.global_step, total=float('inf'),
- dynamic_ncols=True, unit='step', disable=self.root_gpu > 0)
- for batch_idx, batch in enumerate(train_pbar):
- pbar_metrics, tb_metrics = self.run_training_batch(batch_idx, batch)
- train_pbar.set_postfix(**pbar_metrics)
- should_check_val = (self.global_step % self.val_check_interval == 0
- and not self.fisrt_epoch)
- if should_check_val:
- self.run_evaluation()
- self.fisrt_epoch = False
- # when metrics should be logged
- if (self.global_step + 1) % self.tb_log_interval == 0:
- # logs user requested information to logger
- self.log_metrics_to_tb(tb_metrics)
-
- self.global_step += 1
- task_ref.global_step = self.global_step
- if self.global_step > self.max_updates:
- print("| Training end..")
- break
- # epoch end hook
- task_ref.on_epoch_end()
- epoch += 1
- if self.global_step > self.max_updates:
- break
- task_ref.on_train_end()
-
- def run_training_batch(self, batch_idx, batch):
- if batch is None:
- return {}
- all_progress_bar_metrics = []
- all_log_metrics = []
- task_ref = self.get_task_ref()
- for opt_idx, optimizer in enumerate(self.optimizers):
- if optimizer is None:
- continue
- # make sure only the gradients of the current optimizer's paramaters are calculated
- # in the training step to prevent dangling gradients in multiple-optimizer setup.
- if len(self.optimizers) > 1:
- for param in task_ref.parameters():
- param.requires_grad = False
- for group in optimizer.param_groups:
- for param in group['params']:
- param.requires_grad = True
-
- # forward pass
- with autocast(enabled=self.amp):
- if self.on_gpu:
- batch = move_to_cuda(copy.copy(batch), self.root_gpu)
- args = [batch, batch_idx, opt_idx]
- if self.use_ddp:
- output = self.task(*args)
- else:
- output = task_ref.training_step(*args)
- loss = output['loss']
- if loss is None:
- continue
- progress_bar_metrics = output['progress_bar']
- log_metrics = output['tb_log']
- # accumulate loss
- loss = loss / self.accumulate_grad_batches
-
- # backward pass
- if loss.requires_grad:
- if self.amp:
- self.amp_scalar.scale(loss).backward()
- else:
- loss.backward()
-
- # track progress bar metrics
- all_log_metrics.append(log_metrics)
- all_progress_bar_metrics.append(progress_bar_metrics)
-
- if loss is None:
- continue
-
- # nan grads
- if self.print_nan_grads:
- has_nan_grad = False
- for name, param in task_ref.named_parameters():
- if (param.grad is not None) and torch.isnan(param.grad.float()).any():
- print("| NaN params: ", name, param, param.grad)
- has_nan_grad = True
- if has_nan_grad:
- exit(0)
-
- # gradient update with accumulated gradients
- if (self.global_step + 1) % self.accumulate_grad_batches == 0:
- task_ref.on_before_optimization(opt_idx)
- if self.amp:
- self.amp_scalar.step(optimizer)
- self.amp_scalar.update()
- else:
- optimizer.step()
- optimizer.zero_grad()
- task_ref.on_after_optimization(self.current_epoch, batch_idx, optimizer, opt_idx)
-
- # collapse all metrics into one dict
- all_progress_bar_metrics = {k: v for d in all_progress_bar_metrics for k, v in d.items()}
- all_log_metrics = {k: v for d in all_log_metrics for k, v in d.items()}
- return all_progress_bar_metrics, all_log_metrics
-
- ####################
- # load and save checkpoint
- ####################
- def restore_weights(self, checkpoint):
- # load model state
- task_ref = self.get_task_ref()
-
- if len([k for k in checkpoint['state_dict'].keys() if '.' in k]) > 0:
- task_ref.load_state_dict(checkpoint['state_dict'])
- else:
- for k, v in checkpoint['state_dict'].items():
- getattr(task_ref, k).load_state_dict(v)
-
- if self.on_gpu:
- task_ref.cuda(self.root_gpu)
- # load training state (affects trainer only)
- self.best_val_results = checkpoint['checkpoint_callback_best']
- self.global_step = checkpoint['global_step']
- self.current_epoch = checkpoint['epoch']
- task_ref.global_step = self.global_step
-
- # wait for all model to restore weights
- if self.use_ddp:
- # wait for all processes to catch up
- dist.barrier()
-
- def restore_opt_state(self, checkpoint):
- if self.testing:
- return
- # restore the optimizers
- optimizer_states = checkpoint['optimizer_states']
- for optimizer, opt_state in zip(self.optimizers, optimizer_states):
- if optimizer is None:
- return
- try:
- optimizer.load_state_dict(opt_state)
- # move optimizer to GPU 1 weight at a time
- if self.on_gpu:
- for state in optimizer.state.values():
- for k, v in state.items():
- if isinstance(v, torch.Tensor):
- state[k] = v.cuda(self.root_gpu)
- except ValueError:
- print("| WARMING: optimizer parameters not match !!!")
- try:
- if dist.is_initialized() and dist.get_rank() > 0:
- return
- except Exception as e:
- print(e)
- return
- did_restore = True
- return did_restore
-
- def save_checkpoint(self, epoch, logs=None):
- monitor_op = np.less
- ckpt_path = f'{self.work_dir}/model_ckpt_steps_{self.global_step}.ckpt'
- logging.info(f'Epoch {epoch:05d}@{self.global_step}: saving model to {ckpt_path}')
- self._atomic_save(ckpt_path)
- for old_ckpt in get_all_ckpts(self.work_dir)[self.num_ckpt_keep:]:
- subprocess.check_call(f'rm -rf "{old_ckpt}"', shell=True)
- logging.info(f'Delete ckpt: {os.path.basename(old_ckpt)}')
- current = None
- if logs is not None and self.monitor_key in logs:
- current = logs[self.monitor_key]
- if current is not None and self.save_best:
- if monitor_op(current, self.best_val_results):
- best_filepath = f'{self.work_dir}/model_ckpt_best.pt'
- self.best_val_results = current
- logging.info(
- f'Epoch {epoch:05d}@{self.global_step}: {self.monitor_key} reached {current:0.5f}. '
- f'Saving model to {best_filepath}')
- self._atomic_save(best_filepath)
-
- def _atomic_save(self, filepath):
- checkpoint = self.dump_checkpoint()
- tmp_path = str(filepath) + ".part"
- torch.save(checkpoint, tmp_path, _use_new_zipfile_serialization=False)
- os.replace(tmp_path, filepath)
-
- def dump_checkpoint(self):
- checkpoint = {'epoch': self.current_epoch, 'global_step': self.global_step,
- 'checkpoint_callback_best': self.best_val_results}
- # save optimizers
- optimizer_states = []
- for i, optimizer in enumerate(self.optimizers):
- if optimizer is not None:
- optimizer_states.append(optimizer.state_dict())
-
- checkpoint['optimizer_states'] = optimizer_states
- task_ref = self.get_task_ref()
- checkpoint['state_dict'] = {
- k: v.state_dict() for k, v in task_ref.named_children() if len(list(v.parameters())) > 0}
- return checkpoint
-
- ####################
- # DDP
- ####################
- def ddp_init(self, gpu_idx, task):
- # determine which process we are and world size
- self.proc_rank = gpu_idx
- task.trainer = self
- self.init_ddp_connection(self.proc_rank, self.num_gpus)
-
- # copy model to each gpu
- torch.cuda.set_device(gpu_idx)
- # override root GPU
- self.root_gpu = gpu_idx
- self.task = task
-
- def configure_ddp(self, task):
- task = DDP(task, device_ids=[self.root_gpu], find_unused_parameters=True)
- if dist.get_rank() != 0 and not self.debug:
- sys.stdout = open(os.devnull, "w")
- sys.stderr = open(os.devnull, "w")
- random.seed(self.seed)
- np.random.seed(self.seed)
- return task
-
- def init_ddp_connection(self, proc_rank, world_size):
- root_node = '127.0.0.1'
- root_node = self.resolve_root_node_address(root_node)
- os.environ['MASTER_ADDR'] = root_node
- dist.init_process_group('nccl', rank=proc_rank, world_size=world_size)
-
- def resolve_root_node_address(self, root_node):
- if '[' in root_node:
- name = root_node.split('[')[0]
- number = root_node.split(',')[0]
- if '-' in number:
- number = number.split('-')[0]
- number = re.sub('[^0-9]', '', number)
- root_node = name + number
- return root_node
-
- ####################
- # utils
- ####################
- def get_task_ref(self):
- from tasks.base_task import BaseTask
- task: BaseTask = self.task.module if isinstance(self.task, DDP) else self.task
- return task
-
- def log_metrics_to_tb(self, metrics, step=None):
- """Logs the metric dict passed in.
-
- :param metrics:
- """
- # added metrics by Lightning for convenience
- metrics['epoch'] = self.current_epoch
-
- # turn all tensors to scalars
- scalar_metrics = self.metrics_to_scalars(metrics)
-
- step = step if step is not None else self.global_step
- # log actual metrics
- if self.proc_rank == 0:
- self.log_metrics(self.logger, scalar_metrics, step=step)
-
- @staticmethod
- def log_metrics(logger, metrics, step=None):
- for k, v in metrics.items():
- if isinstance(v, torch.Tensor):
- v = v.item()
- logger.add_scalar(k, v, step)
-
- def metrics_to_scalars(self, metrics):
- new_metrics = {}
- for k, v in metrics.items():
- if isinstance(v, torch.Tensor):
- v = v.item()
-
- if type(v) is dict:
- v = self.metrics_to_scalars(v)
-
- new_metrics[k] = v
-
- return new_metrics
diff --git a/spaces/Rongjiehuang/ProDiff/modules/ProDiff/task/ProDiff_teacher_task.py b/spaces/Rongjiehuang/ProDiff/modules/ProDiff/task/ProDiff_teacher_task.py
deleted file mode 100644
index 8e4f89645ce09c0486c18429b0571b5bf6605b79..0000000000000000000000000000000000000000
--- a/spaces/Rongjiehuang/ProDiff/modules/ProDiff/task/ProDiff_teacher_task.py
+++ /dev/null
@@ -1,101 +0,0 @@
-import torch
-
-import utils
-from utils.hparams import hparams
-from modules.ProDiff.model.ProDiff_teacher import GaussianDiffusion
-from usr.diff.net import DiffNet
-from tasks.tts.fs2 import FastSpeech2Task
-from vocoders.base_vocoder import get_vocoder_cls, BaseVocoder
-from utils.pitch_utils import denorm_f0
-from tasks.tts.fs2_utils import FastSpeechDataset
-
-DIFF_DECODERS = {
- 'wavenet': lambda hp: DiffNet(hp['audio_num_mel_bins']),
-}
-
-
-class ProDiff_teacher_Task(FastSpeech2Task):
- def __init__(self):
- super(ProDiff_teacher_Task, self).__init__()
- self.dataset_cls = FastSpeechDataset
- self.vocoder: BaseVocoder = get_vocoder_cls(hparams)()
-
- def build_model(self):
- self.build_tts_model()
- utils.num_params(self.model)
- return self.model
-
- def build_tts_model(self):
- self.model = GaussianDiffusion(
- phone_encoder=self.phone_encoder,
- out_dims=hparams['audio_num_mel_bins'], denoise_fn=DIFF_DECODERS[hparams['diff_decoder_type']](hparams),
- timesteps=hparams['timesteps'], time_scale=hparams['timescale'],
- loss_type=hparams['diff_loss_type'],
- spec_min=hparams['spec_min'], spec_max=hparams['spec_max'],
- )
-
-
- def run_model(self, model, sample, return_output=False, infer=False):
- txt_tokens = sample['txt_tokens'] # [B, T_t]
- target = sample['mels'] # [B, T_s, 80]
- mel2ph = sample['mel2ph']
- f0 = sample['f0']
- uv = sample['uv']
- energy = sample['energy']
- spk_embed = sample.get('spk_embed') if not hparams['use_spk_id'] else sample.get('spk_ids')
- output = model(txt_tokens, mel2ph=mel2ph, spk_embed=spk_embed,
- ref_mels=target, f0=f0, uv=uv, energy=energy, infer=infer)
-
- losses = {}
- self.add_mel_loss(output['mel_out'], target, losses)
- self.add_dur_loss(output['dur'], mel2ph, txt_tokens, losses=losses)
- if hparams['use_pitch_embed']:
- self.add_pitch_loss(output, sample, losses)
- if hparams['use_energy_embed']:
- self.add_energy_loss(output['energy_pred'], energy, losses)
- if not return_output:
- return losses
- else:
- return losses, output
-
- def validation_step(self, sample, batch_idx):
- outputs = {}
- txt_tokens = sample['txt_tokens'] # [B, T_t]
-
- energy = sample['energy']
- spk_embed = sample.get('spk_embed') if not hparams['use_spk_id'] else sample.get('spk_ids')
- mel2ph = sample['mel2ph']
- f0 = sample['f0']
- uv = sample['uv']
-
- outputs['losses'] = {}
- outputs['losses'], model_out = self.run_model(self.model, sample, return_output=True, infer=False)
-
- outputs['total_loss'] = sum(outputs['losses'].values())
- outputs['nsamples'] = sample['nsamples']
- outputs = utils.tensors_to_scalars(outputs)
- if batch_idx < hparams['num_valid_plots']:
- # model_out = self.model(
- # txt_tokens, spk_embed=spk_embed, mel2ph=None, f0=None, uv=None, energy=None, ref_mels=None, inference=True)
- # self.plot_mel(batch_idx, model_out['mel_out'], model_out['fs2_mel'], name=f'diffspeech_vs_fs2_{batch_idx}')
- model_out = self.model(
- txt_tokens, spk_embed=spk_embed, mel2ph=mel2ph, f0=f0, uv=uv, energy=energy, ref_mels=None, infer=True)
- gt_f0 = denorm_f0(sample['f0'], sample['uv'], hparams)
- self.plot_wav(batch_idx, sample['mels'], model_out['mel_out'], is_mel=True, gt_f0=gt_f0, f0=model_out.get('f0_denorm'))
- self.plot_mel(batch_idx, sample['mels'], model_out['mel_out'])
- return outputs
-
- ############
- # validation plots
- ############
- def plot_wav(self, batch_idx, gt_wav, wav_out, is_mel=False, gt_f0=None, f0=None, name=None):
- gt_wav = gt_wav[0].cpu().numpy()
- wav_out = wav_out[0].cpu().numpy()
- gt_f0 = gt_f0[0].cpu().numpy()
- f0 = f0[0].cpu().numpy()
- if is_mel:
- gt_wav = self.vocoder.spec2wav(gt_wav, f0=gt_f0)
- wav_out = self.vocoder.spec2wav(wav_out, f0=f0)
- self.logger.add_audio(f'gt_{batch_idx}', gt_wav, sample_rate=hparams['audio_sample_rate'], global_step=self.global_step)
- self.logger.add_audio(f'wav_{batch_idx}', wav_out, sample_rate=hparams['audio_sample_rate'], global_step=self.global_step)
-
diff --git a/spaces/SERER/VITS-Umamusume-voice-synthesizer/transforms.py b/spaces/SERER/VITS-Umamusume-voice-synthesizer/transforms.py
deleted file mode 100644
index 4793d67ca5a5630e0ffe0f9fb29445c949e64dae..0000000000000000000000000000000000000000
--- a/spaces/SERER/VITS-Umamusume-voice-synthesizer/transforms.py
+++ /dev/null
@@ -1,193 +0,0 @@
-import torch
-from torch.nn import functional as F
-
-import numpy as np
-
-
-DEFAULT_MIN_BIN_WIDTH = 1e-3
-DEFAULT_MIN_BIN_HEIGHT = 1e-3
-DEFAULT_MIN_DERIVATIVE = 1e-3
-
-
-def piecewise_rational_quadratic_transform(inputs,
- unnormalized_widths,
- unnormalized_heights,
- unnormalized_derivatives,
- inverse=False,
- tails=None,
- tail_bound=1.,
- min_bin_width=DEFAULT_MIN_BIN_WIDTH,
- min_bin_height=DEFAULT_MIN_BIN_HEIGHT,
- min_derivative=DEFAULT_MIN_DERIVATIVE):
-
- if tails is None:
- spline_fn = rational_quadratic_spline
- spline_kwargs = {}
- else:
- spline_fn = unconstrained_rational_quadratic_spline
- spline_kwargs = {
- 'tails': tails,
- 'tail_bound': tail_bound
- }
-
- outputs, logabsdet = spline_fn(
- inputs=inputs,
- unnormalized_widths=unnormalized_widths,
- unnormalized_heights=unnormalized_heights,
- unnormalized_derivatives=unnormalized_derivatives,
- inverse=inverse,
- min_bin_width=min_bin_width,
- min_bin_height=min_bin_height,
- min_derivative=min_derivative,
- **spline_kwargs
- )
- return outputs, logabsdet
-
-
-def searchsorted(bin_locations, inputs, eps=1e-6):
- bin_locations[..., -1] += eps
- return torch.sum(
- inputs[..., None] >= bin_locations,
- dim=-1
- ) - 1
-
-
-def unconstrained_rational_quadratic_spline(inputs,
- unnormalized_widths,
- unnormalized_heights,
- unnormalized_derivatives,
- inverse=False,
- tails='linear',
- tail_bound=1.,
- min_bin_width=DEFAULT_MIN_BIN_WIDTH,
- min_bin_height=DEFAULT_MIN_BIN_HEIGHT,
- min_derivative=DEFAULT_MIN_DERIVATIVE):
- inside_interval_mask = (inputs >= -tail_bound) & (inputs <= tail_bound)
- outside_interval_mask = ~inside_interval_mask
-
- outputs = torch.zeros_like(inputs)
- logabsdet = torch.zeros_like(inputs)
-
- if tails == 'linear':
- unnormalized_derivatives = F.pad(unnormalized_derivatives, pad=(1, 1))
- constant = np.log(np.exp(1 - min_derivative) - 1)
- unnormalized_derivatives[..., 0] = constant
- unnormalized_derivatives[..., -1] = constant
-
- outputs[outside_interval_mask] = inputs[outside_interval_mask]
- logabsdet[outside_interval_mask] = 0
- else:
- raise RuntimeError('{} tails are not implemented.'.format(tails))
-
- outputs[inside_interval_mask], logabsdet[inside_interval_mask] = rational_quadratic_spline(
- inputs=inputs[inside_interval_mask],
- unnormalized_widths=unnormalized_widths[inside_interval_mask, :],
- unnormalized_heights=unnormalized_heights[inside_interval_mask, :],
- unnormalized_derivatives=unnormalized_derivatives[inside_interval_mask, :],
- inverse=inverse,
- left=-tail_bound, right=tail_bound, bottom=-tail_bound, top=tail_bound,
- min_bin_width=min_bin_width,
- min_bin_height=min_bin_height,
- min_derivative=min_derivative
- )
-
- return outputs, logabsdet
-
-def rational_quadratic_spline(inputs,
- unnormalized_widths,
- unnormalized_heights,
- unnormalized_derivatives,
- inverse=False,
- left=0., right=1., bottom=0., top=1.,
- min_bin_width=DEFAULT_MIN_BIN_WIDTH,
- min_bin_height=DEFAULT_MIN_BIN_HEIGHT,
- min_derivative=DEFAULT_MIN_DERIVATIVE):
- if torch.min(inputs) < left or torch.max(inputs) > right:
- raise ValueError('Input to a transform is not within its domain')
-
- num_bins = unnormalized_widths.shape[-1]
-
- if min_bin_width * num_bins > 1.0:
- raise ValueError('Minimal bin width too large for the number of bins')
- if min_bin_height * num_bins > 1.0:
- raise ValueError('Minimal bin height too large for the number of bins')
-
- widths = F.softmax(unnormalized_widths, dim=-1)
- widths = min_bin_width + (1 - min_bin_width * num_bins) * widths
- cumwidths = torch.cumsum(widths, dim=-1)
- cumwidths = F.pad(cumwidths, pad=(1, 0), mode='constant', value=0.0)
- cumwidths = (right - left) * cumwidths + left
- cumwidths[..., 0] = left
- cumwidths[..., -1] = right
- widths = cumwidths[..., 1:] - cumwidths[..., :-1]
-
- derivatives = min_derivative + F.softplus(unnormalized_derivatives)
-
- heights = F.softmax(unnormalized_heights, dim=-1)
- heights = min_bin_height + (1 - min_bin_height * num_bins) * heights
- cumheights = torch.cumsum(heights, dim=-1)
- cumheights = F.pad(cumheights, pad=(1, 0), mode='constant', value=0.0)
- cumheights = (top - bottom) * cumheights + bottom
- cumheights[..., 0] = bottom
- cumheights[..., -1] = top
- heights = cumheights[..., 1:] - cumheights[..., :-1]
-
- if inverse:
- bin_idx = searchsorted(cumheights, inputs)[..., None]
- else:
- bin_idx = searchsorted(cumwidths, inputs)[..., None]
-
- input_cumwidths = cumwidths.gather(-1, bin_idx)[..., 0]
- input_bin_widths = widths.gather(-1, bin_idx)[..., 0]
-
- input_cumheights = cumheights.gather(-1, bin_idx)[..., 0]
- delta = heights / widths
- input_delta = delta.gather(-1, bin_idx)[..., 0]
-
- input_derivatives = derivatives.gather(-1, bin_idx)[..., 0]
- input_derivatives_plus_one = derivatives[..., 1:].gather(-1, bin_idx)[..., 0]
-
- input_heights = heights.gather(-1, bin_idx)[..., 0]
-
- if inverse:
- a = (((inputs - input_cumheights) * (input_derivatives
- + input_derivatives_plus_one
- - 2 * input_delta)
- + input_heights * (input_delta - input_derivatives)))
- b = (input_heights * input_derivatives
- - (inputs - input_cumheights) * (input_derivatives
- + input_derivatives_plus_one
- - 2 * input_delta))
- c = - input_delta * (inputs - input_cumheights)
-
- discriminant = b.pow(2) - 4 * a * c
- assert (discriminant >= 0).all()
-
- root = (2 * c) / (-b - torch.sqrt(discriminant))
- outputs = root * input_bin_widths + input_cumwidths
-
- theta_one_minus_theta = root * (1 - root)
- denominator = input_delta + ((input_derivatives + input_derivatives_plus_one - 2 * input_delta)
- * theta_one_minus_theta)
- derivative_numerator = input_delta.pow(2) * (input_derivatives_plus_one * root.pow(2)
- + 2 * input_delta * theta_one_minus_theta
- + input_derivatives * (1 - root).pow(2))
- logabsdet = torch.log(derivative_numerator) - 2 * torch.log(denominator)
-
- return outputs, -logabsdet
- else:
- theta = (inputs - input_cumwidths) / input_bin_widths
- theta_one_minus_theta = theta * (1 - theta)
-
- numerator = input_heights * (input_delta * theta.pow(2)
- + input_derivatives * theta_one_minus_theta)
- denominator = input_delta + ((input_derivatives + input_derivatives_plus_one - 2 * input_delta)
- * theta_one_minus_theta)
- outputs = input_cumheights + numerator / denominator
-
- derivative_numerator = input_delta.pow(2) * (input_derivatives_plus_one * theta.pow(2)
- + 2 * input_delta * theta_one_minus_theta
- + input_derivatives * (1 - theta).pow(2))
- logabsdet = torch.log(derivative_numerator) - 2 * torch.log(denominator)
-
- return outputs, logabsdet
diff --git a/spaces/SeViLA/SeViLA/lavis/datasets/datasets/gqa_datasets.py b/spaces/SeViLA/SeViLA/lavis/datasets/datasets/gqa_datasets.py
deleted file mode 100644
index 073c57040d7852bffc273ce6177c246a4fce1ab8..0000000000000000000000000000000000000000
--- a/spaces/SeViLA/SeViLA/lavis/datasets/datasets/gqa_datasets.py
+++ /dev/null
@@ -1,101 +0,0 @@
-"""
- Copyright (c) 2022, salesforce.com, inc.
- All rights reserved.
- SPDX-License-Identifier: BSD-3-Clause
- For full license text, see the LICENSE file in the repo root or https://opensource.org/licenses/BSD-3-Clause
-"""
-
-import os
-import json
-
-from PIL import Image
-
-from lavis.datasets.datasets.vqa_datasets import VQADataset, VQAEvalDataset
-
-from collections import OrderedDict
-
-
-class __DisplMixin:
- def displ_item(self, index):
- sample, ann = self.__getitem__(index), self.annotation[index]
-
- return OrderedDict(
- {
- "file": ann["image"],
- "question": ann["question"],
- "question_id": ann["question_id"],
- "answers": "; ".join(ann["answer"]),
- "image": sample["image"],
- }
- )
-
-
-class GQADataset(VQADataset, __DisplMixin):
- def __init__(self, vis_processor, text_processor, vis_root, ann_paths):
- super().__init__(vis_processor, text_processor, vis_root, ann_paths)
-
- def __getitem__(self, index):
- ann = self.annotation[index]
-
- image_path = os.path.join(self.vis_root, ann["image"])
- image = Image.open(image_path).convert("RGB")
-
- image = self.vis_processor(image)
- question = self.text_processor(ann["question"])
-
- answers = [ann["answer"]]
- weights = [1]
-
- return {
- "image": image,
- "text_input": question,
- "answers": answers,
- "weights": weights,
- }
-
-
-class GQAEvalDataset(VQAEvalDataset, __DisplMixin):
- def __init__(self, vis_processor, text_processor, vis_root, ann_paths):
- """
- vis_root (string): Root directory of images (e.g. gqa/images/)
- ann_root (string): directory to store the annotation file
- """
-
- self.vis_root = vis_root
-
- self.annotation = json.load(open(ann_paths[0]))
-
- ## TODO: support inference method == 'ranking'
- answer_list_path = ann_paths[1] if len(ann_paths) > 1 else ''
- if os.path.exists(answer_list_path):
- self.answer_list = json.load(open(answer_list_path))
- else:
- self.answer_list = None
-
- self.vis_processor = vis_processor
- self.text_processor = text_processor
-
- self._add_instance_ids()
-
- def __getitem__(self, index):
- ann = self.annotation[index]
-
- image_path = os.path.join(self.vis_root, ann["image"])
- image = Image.open(image_path).convert("RGB")
-
- image = self.vis_processor(image)
- question = self.text_processor(ann["question"])
-
- if "answer" in ann:
- # answer is a string
- answer = ann["answer"]
- else:
- answer = None
-
- return {
- "image": image,
- "text_input": question,
- "answer": answer,
- "question_id": ann["question_id"],
- "instance_id": ann["instance_id"],
- }
diff --git a/spaces/SeyedAli/Image-Similarity/src/similarity/similarity.py b/spaces/SeyedAli/Image-Similarity/src/similarity/similarity.py
deleted file mode 100644
index 14786ca8ab8289c5b556d3f9391a3ff5e78cf953..0000000000000000000000000000000000000000
--- a/spaces/SeyedAli/Image-Similarity/src/similarity/similarity.py
+++ /dev/null
@@ -1,37 +0,0 @@
-from src.model import simlarity_model as model
-from src.util import image as image_util
-from src.util import matrix
-from .model_implements.mobilenet_v3 import ModelnetV3
-from .model_implements.vit_base import VitBase
-from .model_implements.ViTMSN import ViTMS
-from .model_implements.bit import BigTransfer
-
-
-class Similarity:
- def get_models(self):
- return [
- model.SimilarityModel(name= 'Mobilenet V3', image_size= 224, model_cls = ModelnetV3()),
- model.SimilarityModel(name= 'Big Transfer (BiT)', image_size= 224, model_cls = BigTransfer()),
- model.SimilarityModel(name= 'Vision Transformer', image_size= 224, model_cls = VitBase(), image_input_type='pil'),
- model.SimilarityModel(name= 'Masked Siamese Networks for Label-Efficient Learning', image_size= 224, model_cls = ViTMS(), image_input_type='pil')
- ]
-
- def check_similarity(self, img_urls, model):
- imgs = []
- for url in img_urls:
- if url == "": continue
- imgs.append(image_util.load_image_url(url, required_size=(model.image_size, model.image_size), image_type=model.image_input_type))
-
- features = model.model_cls.extract_feature(imgs)
- results = []
- for i, v in enumerate(features):
- if i == 0: continue
- dist = matrix.cosine(features[0], v)
- print(f'{i} -- distance: {dist}')
- # results.append((imgs[i], f'similarity: {int(dist*100)}%'))
- original_img = image_util.load_image_url(img_urls[i], required_size=None, image_type='pil')
- results.append((original_img, f'شباهت: {int(dist*100)}%'))
-
- return results
-
-
\ No newline at end of file
diff --git a/spaces/StarFox7/Llama-2-ko-7B-chat-ggml/app.py b/spaces/StarFox7/Llama-2-ko-7B-chat-ggml/app.py
deleted file mode 100644
index 361beaab96171a42b9321fbbf2ee7d07d1566554..0000000000000000000000000000000000000000
--- a/spaces/StarFox7/Llama-2-ko-7B-chat-ggml/app.py
+++ /dev/null
@@ -1,332 +0,0 @@
-"""Run codes."""
-# pylint: disable=line-too-long, broad-exception-caught, invalid-name, missing-function-docstring, too-many-instance-attributes, missing-class-docstring
-# ruff: noqa: E501
-import os
-import platform
-import random
-import time
-from dataclasses import asdict, dataclass
-from pathlib import Path
-
-# from types import SimpleNamespace
-import gradio as gr
-import psutil
-from about_time import about_time
-from ctransformers import AutoModelForCausalLM
-from dl_hf_model import dl_hf_model
-from loguru import logger
-
-filename_list = [
- "Llama-2-ko-7B-chat-ggml-q4_0.bin"
-]
-
-url = "https://huggingface.co/StarFox7/Llama-2-ko-7B-chat-ggml/blob/main/Llama-2-ko-7B-chat-ggml-q4_0.bin"
-
-prompt_template = "Q: {question}. A: "
-
-stop_string = ["Q:", "\n"]
-
-logger.debug(f"{stop_string=} not used")
-
-_ = psutil.cpu_count(logical=False) - 1
-cpu_count: int = int(_) if _ else 1
-logger.debug(f"{cpu_count=}")
-
-LLM = None
-
-try:
- model_loc, file_size = dl_hf_model(url)
-except Exception as exc_:
- logger.error(exc_)
- raise SystemExit(1) from exc_
-
-LLM = AutoModelForCausalLM.from_pretrained(
- model_loc,
- model_type="llama",
- # threads=cpu_count,
-)
-
-logger.info(f"done load llm {model_loc=} {file_size=}G")
-
-os.environ["TZ"] = "Asia/Seoul"
-try:
- time.tzset() # type: ignore # pylint: disable=no-member
-except Exception:
- # Windows
- logger.warning("Windows, cant run time.tzset()")
-
-_ = """
-ns = SimpleNamespace(
- response="",
- generator=(_ for _ in []),
-)
-# """
-
-@dataclass
-class GenerationConfig:
- temperature: float = 0.7
- top_k: int = 50
- top_p: float = 0.9
- repetition_penalty: float = 1.0
- max_new_tokens: int = 1024
- seed: int = 42
- reset: bool = False
- stream: bool = True
- # threads: int = cpu_count
- # stop: list[str] = field(default_factory=lambda: [stop_string])
-
-
-def generate(
- question: str,
- llm=LLM,
- config: GenerationConfig = GenerationConfig(),
-):
- """Run model inference, will return a Generator if streaming is true."""
- # _ = prompt_template.format(question=question)
- # print(_)
-
- prompt = prompt_template.format(question=question)
-
- return llm(
- prompt,
- **asdict(config),
- )
-
-
-logger.debug(f"{asdict(GenerationConfig())=}")
-
-
-def user(user_message, history):
- # return user_message, history + [[user_message, None]]
- history.append([user_message, None])
- return user_message, history # keep user_message
-
-
-def user1(user_message, history):
- # return user_message, history + [[user_message, None]]
- history.append([user_message, None])
- return "", history # clear user_message
-
-
-def bot_(history):
- user_message = history[-1][0]
- resp = random.choice(["How are you?", "I love you", "I'm very hungry"])
- bot_message = user_message + ": " + resp
- history[-1][1] = ""
- for character in bot_message:
- history[-1][1] += character
- time.sleep(0.02)
- yield history
-
- history[-1][1] = resp
- yield history
-
-
-def bot(history):
- user_message = history[-1][0]
- response = []
-
- logger.debug(f"{user_message=}")
-
- with about_time() as atime: # type: ignore
- flag = 1
- prefix = ""
- then = time.time()
-
- logger.debug("about to generate")
-
- config = GenerationConfig(reset=True)
- for elm in generate(user_message, config=config):
- if flag == 1:
- logger.debug("in the loop")
- prefix = f"({time.time() - then:.2f}s) "
- flag = 0
- print(prefix, end="", flush=True)
- logger.debug(f"{prefix=}")
- print(elm, end="", flush=True)
- # logger.debug(f"{elm}")
-
- temp_str = "".join(response).replace("▁"," ")
- if len(temp_str) > 2:
- if temp_str[-2:] in stop_string:
- response = response[:-2]
- break
- response.append(elm)
- history[-1][1] = prefix + "".join(response).replace("▁"," ")
- yield history
-
- _ = (
- f"(time elapsed: {atime.duration_human}, " # type: ignore
- f"{atime.duration/len(''.join(response)):.2f}s/char)" # type: ignore
- )
-
- history[-1][1] = "".join(response).replace("▁"," ") + f"\n{_}"
- yield history
-
-
-def predict_api(prompt):
- logger.debug(f"{prompt=}")
- try:
- # user_prompt = prompt
- config = GenerationConfig(
- temperature=0.2,
- top_k=10,
- top_p=0.9,
- repetition_penalty=1.0,
- max_new_tokens=512, # adjust as needed
- seed=42,
- reset=True, # reset history (cache)
- stream=False,
- # threads=cpu_count,
- # stop=prompt_prefix[1:2],
- )
-
- response = generate(
- prompt,
- config=config,
- )
-
- logger.debug(f"api: {response=}")
- except Exception as exc:
- logger.error(exc)
- response = f"{exc=}"
- # bot = {"inputs": [response]}
- # bot = [(prompt, response)]
-
- return response
-
-
-css = """
- .importantButton {
- background: linear-gradient(45deg, #7e0570,#5d1c99, #6e00ff) !important;
- border: none !important;
- }
- .importantButton:hover {
- background: linear-gradient(45deg, #ff00e0,#8500ff, #6e00ff) !important;
- border: none !important;
- }
- .disclaimer {font-variant-caps: all-small-caps; font-size: xx-small;}
- .xsmall {font-size: x-small;}
-"""
-
-examples_list = [
- ["인생이란 뭘까요?"],
-]
-
-logger.info("start block")
-
-with gr.Blocks(
- title=f"{Path(model_loc).name}",
- theme=gr.themes.Soft(text_size="sm", spacing_size="sm"),
- css=css,
-) as block:
- # buff_var = gr.State("")
- with gr.Accordion("🎈 Info", open=False):
- # gr.HTML(
- # """
- )
-}
diff --git a/spaces/artificialguybr/video-dubbing/TTS/setup.py b/spaces/artificialguybr/video-dubbing/TTS/setup.py
deleted file mode 100644
index df14b41adcdda02932894e0bdad8acad9bc05c27..0000000000000000000000000000000000000000
--- a/spaces/artificialguybr/video-dubbing/TTS/setup.py
+++ /dev/null
@@ -1,141 +0,0 @@
-#!/usr/bin/env python
-# ,*++++++*, ,*++++++*,
-# *++. .+++ *++. .++*
-# *+* ,++++* *+* *+* ,++++, *+*
-# ,+, .++++++++++* ,++,,,,*+, ,++++++++++. *+,
-# *+. .++++++++++++..++ *+.,++++++++++++. .+*
-# .+* ++++++++++++.*+, .+*.++++++++++++ *+,
-# .++ *++++++++* ++, .++.*++++++++* ++,
-# ,+++*. . .*++, ,++*. .*+++*
-# *+, .,*++**. .**++**. ,+*
-# .+* *+,
-# *+. Coqui .+*
-# *+* +++ TTS +++ *+*
-# .+++*. . . *+++.
-# ,+* *+++*... ...*+++* *+,
-# .++. .""""+++++++****+++++++"""". ++.
-# ,++. .++,
-# .++* *++.
-# *+++, ,+++*
-# .,*++++::::::++++*,.
-# ``````
-
-import os
-import subprocess
-import sys
-from packaging.version import Version
-
-import numpy
-import setuptools.command.build_py
-import setuptools.command.develop
-from Cython.Build import cythonize
-from setuptools import Extension, find_packages, setup
-
-python_version = sys.version.split()[0]
-if Version(python_version) < Version("3.9") or Version(python_version) >= Version("3.12"):
- raise RuntimeError("TTS requires python >= 3.9 and < 3.12 " "but your Python version is {}".format(sys.version))
-
-
-cwd = os.path.dirname(os.path.abspath(__file__))
-with open(os.path.join(cwd, "TTS", "VERSION")) as fin:
- version = fin.read().strip()
-
-
-class build_py(setuptools.command.build_py.build_py): # pylint: disable=too-many-ancestors
- def run(self):
- setuptools.command.build_py.build_py.run(self)
-
-
-class develop(setuptools.command.develop.develop):
- def run(self):
- setuptools.command.develop.develop.run(self)
-
-
-# The documentation for this feature is in server/README.md
-package_data = ["TTS/server/templates/*"]
-
-
-def pip_install(package_name):
- subprocess.call([sys.executable, "-m", "pip", "install", package_name])
-
-
-requirements = open(os.path.join(cwd, "requirements.txt"), "r").readlines()
-with open(os.path.join(cwd, "requirements.notebooks.txt"), "r") as f:
- requirements_notebooks = f.readlines()
-with open(os.path.join(cwd, "requirements.dev.txt"), "r") as f:
- requirements_dev = f.readlines()
-with open(os.path.join(cwd, "requirements.ja.txt"), "r") as f:
- requirements_ja = f.readlines()
-requirements_all = requirements_dev + requirements_notebooks + requirements_ja
-
-with open("README.md", "r", encoding="utf-8") as readme_file:
- README = readme_file.read()
-
-exts = [
- Extension(
- name="TTS.tts.utils.monotonic_align.core",
- sources=["TTS/tts/utils/monotonic_align/core.pyx"],
- )
-]
-setup(
- name="TTS",
- version=version,
- url="https://github.com/coqui-ai/TTS",
- author="Eren Gölge",
- author_email="egolge@coqui.ai",
- description="Deep learning for Text to Speech by Coqui.",
- long_description=README,
- long_description_content_type="text/markdown",
- license="MPL-2.0",
- # cython
- include_dirs=numpy.get_include(),
- ext_modules=cythonize(exts, language_level=3),
- # ext_modules=find_cython_extensions(),
- # package
- include_package_data=True,
- packages=find_packages(include=["TTS"], exclude=["*.tests", "*tests.*", "tests.*", "*tests", "tests"]),
- package_data={
- "TTS": [
- "VERSION",
- ]
- },
- project_urls={
- "Documentation": "https://github.com/coqui-ai/TTS/wiki",
- "Tracker": "https://github.com/coqui-ai/TTS/issues",
- "Repository": "https://github.com/coqui-ai/TTS",
- "Discussions": "https://github.com/coqui-ai/TTS/discussions",
- },
- cmdclass={
- "build_py": build_py,
- "develop": develop,
- # 'build_ext': build_ext
- },
- install_requires=requirements,
- extras_require={
- "all": requirements_all,
- "dev": requirements_dev,
- "notebooks": requirements_notebooks,
- "ja": requirements_ja,
- },
- python_requires=">=3.9.0, <3.12",
- entry_points={"console_scripts": ["tts=TTS.bin.synthesize:main", "tts-server = TTS.server.server:main"]},
- classifiers=[
- "Programming Language :: Python",
- "Programming Language :: Python :: 3",
- "Programming Language :: Python :: 3.9",
- "Programming Language :: Python :: 3.10",
- "Programming Language :: Python :: 3.11",
- "Development Status :: 3 - Alpha",
- "Intended Audience :: Science/Research",
- "Intended Audience :: Developers",
- "Operating System :: POSIX :: Linux",
- "License :: OSI Approved :: Mozilla Public License 2.0 (MPL 2.0)",
- "Topic :: Software Development",
- "Topic :: Software Development :: Libraries :: Python Modules",
- "Topic :: Multimedia :: Sound/Audio :: Speech",
- "Topic :: Multimedia :: Sound/Audio",
- "Topic :: Multimedia",
- "Topic :: Scientific/Engineering :: Artificial Intelligence",
- ],
- zip_safe=False,
-)
diff --git a/spaces/artificialguybr/video-dubbing/TTS/tests/zoo_tests/test_models.py b/spaces/artificialguybr/video-dubbing/TTS/tests/zoo_tests/test_models.py
deleted file mode 100644
index d1c6b67c3924cbb7a937b77ceb3430875f60311a..0000000000000000000000000000000000000000
--- a/spaces/artificialguybr/video-dubbing/TTS/tests/zoo_tests/test_models.py
+++ /dev/null
@@ -1,242 +0,0 @@
-#!/usr/bin/env python3`
-import glob
-import os
-import shutil
-
-import torch
-
-from tests import get_tests_data_path, get_tests_output_path, run_cli
-from TTS.tts.utils.languages import LanguageManager
-from TTS.tts.utils.speakers import SpeakerManager
-from TTS.utils.generic_utils import get_user_data_dir
-from TTS.utils.manage import ModelManager
-
-MODELS_WITH_SEP_TESTS = [
- "tts_models/multilingual/multi-dataset/bark",
- "tts_models/en/multi-dataset/tortoise-v2",
- "tts_models/multilingual/multi-dataset/xtts_v1.1",
- "tts_models/multilingual/multi-dataset/xtts_v2",
-]
-
-
-def run_models(offset=0, step=1):
- """Check if all the models are downloadable and tts models run correctly."""
- print(" > Run synthesizer with all the models.")
- output_path = os.path.join(get_tests_output_path(), "output.wav")
- manager = ModelManager(output_prefix=get_tests_output_path(), progress_bar=False)
- model_names = [name for name in manager.list_models() if name not in MODELS_WITH_SEP_TESTS]
- print("Model names:", model_names)
- for model_name in model_names[offset::step]:
- print(f"\n > Run - {model_name}")
- model_path, _, _ = manager.download_model(model_name)
- if "tts_models" in model_name:
- local_download_dir = os.path.dirname(model_path)
- # download and run the model
- speaker_files = glob.glob(local_download_dir + "/speaker*")
- language_files = glob.glob(local_download_dir + "/language*")
- language_id = ""
- if len(speaker_files) > 0:
- # multi-speaker model
- if "speaker_ids" in speaker_files[0]:
- speaker_manager = SpeakerManager(speaker_id_file_path=speaker_files[0])
- elif "speakers" in speaker_files[0]:
- speaker_manager = SpeakerManager(d_vectors_file_path=speaker_files[0])
-
- # multi-lingual model - Assuming multi-lingual models are also multi-speaker
- if len(language_files) > 0 and "language_ids" in language_files[0]:
- language_manager = LanguageManager(language_ids_file_path=language_files[0])
- language_id = language_manager.language_names[0]
-
- speaker_id = list(speaker_manager.name_to_id.keys())[0]
- run_cli(
- f"tts --model_name {model_name} "
- f'--text "This is an example." --out_path "{output_path}" --speaker_idx "{speaker_id}" --language_idx "{language_id}" --progress_bar False'
- )
- else:
- # single-speaker model
- run_cli(
- f"tts --model_name {model_name} "
- f'--text "This is an example." --out_path "{output_path}" --progress_bar False'
- )
- # remove downloaded models
- shutil.rmtree(local_download_dir)
- shutil.rmtree(get_user_data_dir("tts"))
- elif "voice_conversion_models" in model_name:
- speaker_wav = os.path.join(get_tests_data_path(), "ljspeech", "wavs", "LJ001-0001.wav")
- reference_wav = os.path.join(get_tests_data_path(), "ljspeech", "wavs", "LJ001-0032.wav")
- run_cli(
- f"tts --model_name {model_name} "
- f'--out_path "{output_path}" --source_wav "{speaker_wav}" --target_wav "{reference_wav}" --progress_bar False'
- )
- else:
- # only download the model
- manager.download_model(model_name)
- print(f" | > OK: {model_name}")
-
-
-def test_xtts():
- """XTTS is too big to run on github actions. We need to test it locally"""
- output_path = os.path.join(get_tests_output_path(), "output.wav")
- speaker_wav = os.path.join(get_tests_data_path(), "ljspeech", "wavs", "LJ001-0001.wav")
- use_gpu = torch.cuda.is_available()
- if use_gpu:
- run_cli(
- "yes | "
- f"tts --model_name tts_models/multilingual/multi-dataset/xtts_v1.1 "
- f'--text "This is an example." --out_path "{output_path}" --progress_bar False --use_cuda True '
- f'--speaker_wav "{speaker_wav}" --language_idx "en"'
- )
- else:
- run_cli(
- "yes | "
- f"tts --model_name tts_models/multilingual/multi-dataset/xtts_v1.1 "
- f'--text "This is an example." --out_path "{output_path}" --progress_bar False '
- f'--speaker_wav "{speaker_wav}" --language_idx "en"'
- )
-
-
-def test_xtts_streaming():
- """Testing the new inference_stream method"""
- from TTS.tts.configs.xtts_config import XttsConfig
- from TTS.tts.models.xtts import Xtts
-
- speaker_wav = [os.path.join(get_tests_data_path(), "ljspeech", "wavs", "LJ001-0001.wav")]
- speaker_wav_2 = os.path.join(get_tests_data_path(), "ljspeech", "wavs", "LJ001-0002.wav")
- speaker_wav.append(speaker_wav_2)
- model_path = os.path.join(get_user_data_dir("tts"), "tts_models--multilingual--multi-dataset--xtts_v1.1")
- config = XttsConfig()
- config.load_json(os.path.join(model_path, "config.json"))
- model = Xtts.init_from_config(config)
- model.load_checkpoint(config, checkpoint_dir=model_path)
- model.to(torch.device("cuda" if torch.cuda.is_available() else "cpu"))
-
- print("Computing speaker latents...")
- gpt_cond_latent, _, speaker_embedding = model.get_conditioning_latents(audio_path=speaker_wav)
-
- print("Inference...")
- chunks = model.inference_stream(
- "It took me quite a long time to develop a voice and now that I have it I am not going to be silent.",
- "en",
- gpt_cond_latent,
- speaker_embedding,
- )
- wav_chuncks = []
- for i, chunk in enumerate(chunks):
- if i == 0:
- assert chunk.shape[-1] > 5000
- wav_chuncks.append(chunk)
- assert len(wav_chuncks) > 1
-
-
-def test_xtts_v2():
- """XTTS is too big to run on github actions. We need to test it locally"""
- output_path = os.path.join(get_tests_output_path(), "output.wav")
- speaker_wav = os.path.join(get_tests_data_path(), "ljspeech", "wavs", "LJ001-0001.wav")
- speaker_wav_2 = os.path.join(get_tests_data_path(), "ljspeech", "wavs", "LJ001-0002.wav")
- use_gpu = torch.cuda.is_available()
- if use_gpu:
- run_cli(
- "yes | "
- f"tts --model_name tts_models/multilingual/multi-dataset/xtts_v2 "
- f'--text "This is an example." --out_path "{output_path}" --progress_bar False --use_cuda True '
- f'--speaker_wav "{speaker_wav}" "{speaker_wav_2}" "--language_idx "en"'
- )
- else:
- run_cli(
- "yes | "
- f"tts --model_name tts_models/multilingual/multi-dataset/xtts_v2 "
- f'--text "This is an example." --out_path "{output_path}" --progress_bar False '
- f'--speaker_wav "{speaker_wav}" "{speaker_wav_2}" --language_idx "en"'
- )
-
-
-def test_xtts_v2_streaming():
- """Testing the new inference_stream method"""
- from TTS.tts.configs.xtts_config import XttsConfig
- from TTS.tts.models.xtts import Xtts
-
- speaker_wav = [os.path.join(get_tests_data_path(), "ljspeech", "wavs", "LJ001-0001.wav")]
- model_path = os.path.join(get_user_data_dir("tts"), "tts_models--multilingual--multi-dataset--xtts_v2")
- config = XttsConfig()
- config.load_json(os.path.join(model_path, "config.json"))
- model = Xtts.init_from_config(config)
- model.load_checkpoint(config, checkpoint_dir=model_path)
- model.to(torch.device("cuda" if torch.cuda.is_available() else "cpu"))
-
- print("Computing speaker latents...")
- gpt_cond_latent, _, speaker_embedding = model.get_conditioning_latents(audio_path=speaker_wav)
-
- print("Inference...")
- chunks = model.inference_stream(
- "It took me quite a long time to develop a voice and now that I have it I am not going to be silent.",
- "en",
- gpt_cond_latent,
- speaker_embedding,
- )
- wav_chuncks = []
- for i, chunk in enumerate(chunks):
- if i == 0:
- assert chunk.shape[-1] > 5000
- wav_chuncks.append(chunk)
- assert len(wav_chuncks) > 1
-
-
-def test_tortoise():
- output_path = os.path.join(get_tests_output_path(), "output.wav")
- use_gpu = torch.cuda.is_available()
- if use_gpu:
- run_cli(
- f" tts --model_name tts_models/en/multi-dataset/tortoise-v2 "
- f'--text "This is an example." --out_path "{output_path}" --progress_bar False --use_cuda True'
- )
- else:
- run_cli(
- f" tts --model_name tts_models/en/multi-dataset/tortoise-v2 "
- f'--text "This is an example." --out_path "{output_path}" --progress_bar False'
- )
-
-
-def test_bark():
- """Bark is too big to run on github actions. We need to test it locally"""
- output_path = os.path.join(get_tests_output_path(), "output.wav")
- use_gpu = torch.cuda.is_available()
- if use_gpu:
- run_cli(
- f" tts --model_name tts_models/multilingual/multi-dataset/bark "
- f'--text "This is an example." --out_path "{output_path}" --progress_bar False --use_cuda True'
- )
- else:
- run_cli(
- f" tts --model_name tts_models/multilingual/multi-dataset/bark "
- f'--text "This is an example." --out_path "{output_path}" --progress_bar False'
- )
-
-
-def test_voice_conversion():
- print(" > Run voice conversion inference using YourTTS model.")
- model_name = "tts_models/multilingual/multi-dataset/your_tts"
- language_id = "en"
- speaker_wav = os.path.join(get_tests_data_path(), "ljspeech", "wavs", "LJ001-0001.wav")
- reference_wav = os.path.join(get_tests_data_path(), "ljspeech", "wavs", "LJ001-0032.wav")
- output_path = os.path.join(get_tests_output_path(), "output.wav")
- run_cli(
- f"tts --model_name {model_name}"
- f" --out_path {output_path} --speaker_wav {speaker_wav} --reference_wav {reference_wav} --language_idx {language_id} --progress_bar False"
- )
-
-
-"""
-These are used to split tests into different actions on Github.
-"""
-
-
-def test_models_offset_0_step_3():
- run_models(offset=0, step=3)
-
-
-def test_models_offset_1_step_3():
- run_models(offset=1, step=3)
-
-
-def test_models_offset_2_step_3():
- run_models(offset=2, step=3)
diff --git a/spaces/arxify/RVC-beta-v2-0618/runtime/Lib/site-packages/Crypto/Hash/MD4.py b/spaces/arxify/RVC-beta-v2-0618/runtime/Lib/site-packages/Crypto/Hash/MD4.py
deleted file mode 100644
index be12b192a155b65455f1be27c0669e2fcc70b9c4..0000000000000000000000000000000000000000
--- a/spaces/arxify/RVC-beta-v2-0618/runtime/Lib/site-packages/Crypto/Hash/MD4.py
+++ /dev/null
@@ -1,185 +0,0 @@
-# ===================================================================
-#
-# Copyright (c) 2014, Legrandin
-# All rights reserved.
-#
-# Redistribution and use in source and binary forms, with or without
-# modification, are permitted provided that the following conditions
-# are met:
-#
-# 1. Redistributions of source code must retain the above copyright
-# notice, this list of conditions and the following disclaimer.
-# 2. Redistributions in binary form must reproduce the above copyright
-# notice, this list of conditions and the following disclaimer in
-# the documentation and/or other materials provided with the
-# distribution.
-#
-# THIS SOFTWARE IS PROVIDED BY THE COPYRIGHT HOLDERS AND CONTRIBUTORS
-# "AS IS" AND ANY EXPRESS OR IMPLIED WARRANTIES, INCLUDING, BUT NOT
-# LIMITED TO, THE IMPLIED WARRANTIES OF MERCHANTABILITY AND FITNESS
-# FOR A PARTICULAR PURPOSE ARE DISCLAIMED. IN NO EVENT SHALL THE
-# COPYRIGHT HOLDER OR CONTRIBUTORS BE LIABLE FOR ANY DIRECT, INDIRECT,
-# INCIDENTAL, SPECIAL, EXEMPLARY, OR CONSEQUENTIAL DAMAGES (INCLUDING,
-# BUT NOT LIMITED TO, PROCUREMENT OF SUBSTITUTE GOODS OR SERVICES;
-# LOSS OF USE, DATA, OR PROFITS; OR BUSINESS INTERRUPTION) HOWEVER
-# CAUSED AND ON ANY THEORY OF LIABILITY, WHETHER IN CONTRACT, STRICT
-# LIABILITY, OR TORT (INCLUDING NEGLIGENCE OR OTHERWISE) ARISING IN
-# ANY WAY OUT OF THE USE OF THIS SOFTWARE, EVEN IF ADVISED OF THE
-# POSSIBILITY OF SUCH DAMAGE.
-# ===================================================================
-
-"""
-MD4 is specified in RFC1320_ and produces the 128 bit digest of a message.
-
- >>> from Crypto.Hash import MD4
- >>>
- >>> h = MD4.new()
- >>> h.update(b'Hello')
- >>> print h.hexdigest()
-
-MD4 stand for Message Digest version 4, and it was invented by Rivest in 1990.
-This algorithm is insecure. Do not use it for new designs.
-
-.. _RFC1320: http://tools.ietf.org/html/rfc1320
-"""
-
-from Crypto.Util.py3compat import bord
-
-from Crypto.Util._raw_api import (load_pycryptodome_raw_lib,
- VoidPointer, SmartPointer,
- create_string_buffer,
- get_raw_buffer, c_size_t,
- c_uint8_ptr)
-
-_raw_md4_lib = load_pycryptodome_raw_lib(
- "Crypto.Hash._MD4",
- """
- int md4_init(void **shaState);
- int md4_destroy(void *shaState);
- int md4_update(void *hs,
- const uint8_t *buf,
- size_t len);
- int md4_digest(const void *shaState,
- uint8_t digest[20]);
- int md4_copy(const void *src, void *dst);
- """)
-
-
-class MD4Hash(object):
- """Class that implements an MD4 hash
- """
-
- #: The size of the resulting hash in bytes.
- digest_size = 16
- #: The internal block size of the hash algorithm in bytes.
- block_size = 64
- #: ASN.1 Object ID
- oid = "1.2.840.113549.2.4"
-
- def __init__(self, data=None):
- state = VoidPointer()
- result = _raw_md4_lib.md4_init(state.address_of())
- if result:
- raise ValueError("Error %d while instantiating MD4"
- % result)
- self._state = SmartPointer(state.get(),
- _raw_md4_lib.md4_destroy)
- if data:
- self.update(data)
-
- def update(self, data):
- """Continue hashing of a message by consuming the next chunk of data.
-
- Repeated calls are equivalent to a single call with the concatenation
- of all the arguments. In other words:
-
- >>> m.update(a); m.update(b)
-
- is equivalent to:
-
- >>> m.update(a+b)
-
- :Parameters:
- data : byte string/byte array/memoryview
- The next chunk of the message being hashed.
- """
-
- result = _raw_md4_lib.md4_update(self._state.get(),
- c_uint8_ptr(data),
- c_size_t(len(data)))
- if result:
- raise ValueError("Error %d while instantiating MD4"
- % result)
-
- def digest(self):
- """Return the **binary** (non-printable) digest of the message that
- has been hashed so far.
-
- This method does not change the state of the hash object.
- You can continue updating the object after calling this function.
-
- :Return: A byte string of `digest_size` bytes. It may contain non-ASCII
- characters, including null bytes.
- """
-
- bfr = create_string_buffer(self.digest_size)
- result = _raw_md4_lib.md4_digest(self._state.get(),
- bfr)
- if result:
- raise ValueError("Error %d while instantiating MD4"
- % result)
-
- return get_raw_buffer(bfr)
-
- def hexdigest(self):
- """Return the **printable** digest of the message that has been
- hashed so far.
-
- This method does not change the state of the hash object.
-
- :Return: A string of 2* `digest_size` characters. It contains only
- hexadecimal ASCII digits.
- """
-
- return "".join(["%02x" % bord(x) for x in self.digest()])
-
- def copy(self):
- """Return a copy ("clone") of the hash object.
-
- The copy will have the same internal state as the original hash
- object.
- This can be used to efficiently compute the digests of strings that
- share a common initial substring.
-
- :Return: A hash object of the same type
- """
-
- clone = MD4Hash()
- result = _raw_md4_lib.md4_copy(self._state.get(),
- clone._state.get())
- if result:
- raise ValueError("Error %d while copying MD4" % result)
- return clone
-
- def new(self, data=None):
- return MD4Hash(data)
-
-
-def new(data=None):
- """Return a fresh instance of the hash object.
-
- :Parameters:
- data : byte string/byte array/memoryview
- The very first chunk of the message to hash.
- It is equivalent to an early call to `MD4Hash.update()`.
- Optional.
-
- :Return: A `MD4Hash` object
- """
- return MD4Hash().new(data)
-
-#: The size of the resulting hash in bytes.
-digest_size = MD4Hash.digest_size
-
-#: The internal block size of the hash algorithm in bytes.
-block_size = MD4Hash.block_size
diff --git a/spaces/arxify/RVC-beta-v2-0618/runtime/Lib/site-packages/PIL/ImageOps.py b/spaces/arxify/RVC-beta-v2-0618/runtime/Lib/site-packages/PIL/ImageOps.py
deleted file mode 100644
index 443c540b61a1676e43b42706b7a360949e3ec44e..0000000000000000000000000000000000000000
--- a/spaces/arxify/RVC-beta-v2-0618/runtime/Lib/site-packages/PIL/ImageOps.py
+++ /dev/null
@@ -1,616 +0,0 @@
-#
-# The Python Imaging Library.
-# $Id$
-#
-# standard image operations
-#
-# History:
-# 2001-10-20 fl Created
-# 2001-10-23 fl Added autocontrast operator
-# 2001-12-18 fl Added Kevin's fit operator
-# 2004-03-14 fl Fixed potential division by zero in equalize
-# 2005-05-05 fl Fixed equalize for low number of values
-#
-# Copyright (c) 2001-2004 by Secret Labs AB
-# Copyright (c) 2001-2004 by Fredrik Lundh
-#
-# See the README file for information on usage and redistribution.
-#
-
-import functools
-import operator
-import re
-
-from . import Image, ImagePalette
-
-#
-# helpers
-
-
-def _border(border):
- if isinstance(border, tuple):
- if len(border) == 2:
- left, top = right, bottom = border
- elif len(border) == 4:
- left, top, right, bottom = border
- else:
- left = top = right = bottom = border
- return left, top, right, bottom
-
-
-def _color(color, mode):
- if isinstance(color, str):
- from . import ImageColor
-
- color = ImageColor.getcolor(color, mode)
- return color
-
-
-def _lut(image, lut):
- if image.mode == "P":
- # FIXME: apply to lookup table, not image data
- raise NotImplementedError("mode P support coming soon")
- elif image.mode in ("L", "RGB"):
- if image.mode == "RGB" and len(lut) == 256:
- lut = lut + lut + lut
- return image.point(lut)
- else:
- raise OSError("not supported for this image mode")
-
-
-#
-# actions
-
-
-def autocontrast(image, cutoff=0, ignore=None, mask=None, preserve_tone=False):
- """
- Maximize (normalize) image contrast. This function calculates a
- histogram of the input image (or mask region), removes ``cutoff`` percent of the
- lightest and darkest pixels from the histogram, and remaps the image
- so that the darkest pixel becomes black (0), and the lightest
- becomes white (255).
-
- :param image: The image to process.
- :param cutoff: The percent to cut off from the histogram on the low and
- high ends. Either a tuple of (low, high), or a single
- number for both.
- :param ignore: The background pixel value (use None for no background).
- :param mask: Histogram used in contrast operation is computed using pixels
- within the mask. If no mask is given the entire image is used
- for histogram computation.
- :param preserve_tone: Preserve image tone in Photoshop-like style autocontrast.
-
- .. versionadded:: 8.2.0
-
- :return: An image.
- """
- if preserve_tone:
- histogram = image.convert("L").histogram(mask)
- else:
- histogram = image.histogram(mask)
-
- lut = []
- for layer in range(0, len(histogram), 256):
- h = histogram[layer : layer + 256]
- if ignore is not None:
- # get rid of outliers
- try:
- h[ignore] = 0
- except TypeError:
- # assume sequence
- for ix in ignore:
- h[ix] = 0
- if cutoff:
- # cut off pixels from both ends of the histogram
- if not isinstance(cutoff, tuple):
- cutoff = (cutoff, cutoff)
- # get number of pixels
- n = 0
- for ix in range(256):
- n = n + h[ix]
- # remove cutoff% pixels from the low end
- cut = n * cutoff[0] // 100
- for lo in range(256):
- if cut > h[lo]:
- cut = cut - h[lo]
- h[lo] = 0
- else:
- h[lo] -= cut
- cut = 0
- if cut <= 0:
- break
- # remove cutoff% samples from the high end
- cut = n * cutoff[1] // 100
- for hi in range(255, -1, -1):
- if cut > h[hi]:
- cut = cut - h[hi]
- h[hi] = 0
- else:
- h[hi] -= cut
- cut = 0
- if cut <= 0:
- break
- # find lowest/highest samples after preprocessing
- for lo in range(256):
- if h[lo]:
- break
- for hi in range(255, -1, -1):
- if h[hi]:
- break
- if hi <= lo:
- # don't bother
- lut.extend(list(range(256)))
- else:
- scale = 255.0 / (hi - lo)
- offset = -lo * scale
- for ix in range(256):
- ix = int(ix * scale + offset)
- if ix < 0:
- ix = 0
- elif ix > 255:
- ix = 255
- lut.append(ix)
- return _lut(image, lut)
-
-
-def colorize(image, black, white, mid=None, blackpoint=0, whitepoint=255, midpoint=127):
- """
- Colorize grayscale image.
- This function calculates a color wedge which maps all black pixels in
- the source image to the first color and all white pixels to the
- second color. If ``mid`` is specified, it uses three-color mapping.
- The ``black`` and ``white`` arguments should be RGB tuples or color names;
- optionally you can use three-color mapping by also specifying ``mid``.
- Mapping positions for any of the colors can be specified
- (e.g. ``blackpoint``), where these parameters are the integer
- value corresponding to where the corresponding color should be mapped.
- These parameters must have logical order, such that
- ``blackpoint <= midpoint <= whitepoint`` (if ``mid`` is specified).
-
- :param image: The image to colorize.
- :param black: The color to use for black input pixels.
- :param white: The color to use for white input pixels.
- :param mid: The color to use for midtone input pixels.
- :param blackpoint: an int value [0, 255] for the black mapping.
- :param whitepoint: an int value [0, 255] for the white mapping.
- :param midpoint: an int value [0, 255] for the midtone mapping.
- :return: An image.
- """
-
- # Initial asserts
- assert image.mode == "L"
- if mid is None:
- assert 0 <= blackpoint <= whitepoint <= 255
- else:
- assert 0 <= blackpoint <= midpoint <= whitepoint <= 255
-
- # Define colors from arguments
- black = _color(black, "RGB")
- white = _color(white, "RGB")
- if mid is not None:
- mid = _color(mid, "RGB")
-
- # Empty lists for the mapping
- red = []
- green = []
- blue = []
-
- # Create the low-end values
- for i in range(0, blackpoint):
- red.append(black[0])
- green.append(black[1])
- blue.append(black[2])
-
- # Create the mapping (2-color)
- if mid is None:
-
- range_map = range(0, whitepoint - blackpoint)
-
- for i in range_map:
- red.append(black[0] + i * (white[0] - black[0]) // len(range_map))
- green.append(black[1] + i * (white[1] - black[1]) // len(range_map))
- blue.append(black[2] + i * (white[2] - black[2]) // len(range_map))
-
- # Create the mapping (3-color)
- else:
-
- range_map1 = range(0, midpoint - blackpoint)
- range_map2 = range(0, whitepoint - midpoint)
-
- for i in range_map1:
- red.append(black[0] + i * (mid[0] - black[0]) // len(range_map1))
- green.append(black[1] + i * (mid[1] - black[1]) // len(range_map1))
- blue.append(black[2] + i * (mid[2] - black[2]) // len(range_map1))
- for i in range_map2:
- red.append(mid[0] + i * (white[0] - mid[0]) // len(range_map2))
- green.append(mid[1] + i * (white[1] - mid[1]) // len(range_map2))
- blue.append(mid[2] + i * (white[2] - mid[2]) // len(range_map2))
-
- # Create the high-end values
- for i in range(0, 256 - whitepoint):
- red.append(white[0])
- green.append(white[1])
- blue.append(white[2])
-
- # Return converted image
- image = image.convert("RGB")
- return _lut(image, red + green + blue)
-
-
-def contain(image, size, method=Image.Resampling.BICUBIC):
- """
- Returns a resized version of the image, set to the maximum width and height
- within the requested size, while maintaining the original aspect ratio.
-
- :param image: The image to resize and crop.
- :param size: The requested output size in pixels, given as a
- (width, height) tuple.
- :param method: Resampling method to use. Default is
- :py:attr:`PIL.Image.BICUBIC`. See :ref:`concept-filters`.
- :return: An image.
- """
-
- im_ratio = image.width / image.height
- dest_ratio = size[0] / size[1]
-
- if im_ratio != dest_ratio:
- if im_ratio > dest_ratio:
- new_height = round(image.height / image.width * size[0])
- if new_height != size[1]:
- size = (size[0], new_height)
- else:
- new_width = round(image.width / image.height * size[1])
- if new_width != size[0]:
- size = (new_width, size[1])
- return image.resize(size, resample=method)
-
-
-def pad(image, size, method=Image.Resampling.BICUBIC, color=None, centering=(0.5, 0.5)):
- """
- Returns a resized and padded version of the image, expanded to fill the
- requested aspect ratio and size.
-
- :param image: The image to resize and crop.
- :param size: The requested output size in pixels, given as a
- (width, height) tuple.
- :param method: Resampling method to use. Default is
- :py:attr:`PIL.Image.BICUBIC`. See :ref:`concept-filters`.
- :param color: The background color of the padded image.
- :param centering: Control the position of the original image within the
- padded version.
-
- (0.5, 0.5) will keep the image centered
- (0, 0) will keep the image aligned to the top left
- (1, 1) will keep the image aligned to the bottom
- right
- :return: An image.
- """
-
- resized = contain(image, size, method)
- if resized.size == size:
- out = resized
- else:
- out = Image.new(image.mode, size, color)
- if resized.palette:
- out.putpalette(resized.getpalette())
- if resized.width != size[0]:
- x = round((size[0] - resized.width) * max(0, min(centering[0], 1)))
- out.paste(resized, (x, 0))
- else:
- y = round((size[1] - resized.height) * max(0, min(centering[1], 1)))
- out.paste(resized, (0, y))
- return out
-
-
-def crop(image, border=0):
- """
- Remove border from image. The same amount of pixels are removed
- from all four sides. This function works on all image modes.
-
- .. seealso:: :py:meth:`~PIL.Image.Image.crop`
-
- :param image: The image to crop.
- :param border: The number of pixels to remove.
- :return: An image.
- """
- left, top, right, bottom = _border(border)
- return image.crop((left, top, image.size[0] - right, image.size[1] - bottom))
-
-
-def scale(image, factor, resample=Image.Resampling.BICUBIC):
- """
- Returns a rescaled image by a specific factor given in parameter.
- A factor greater than 1 expands the image, between 0 and 1 contracts the
- image.
-
- :param image: The image to rescale.
- :param factor: The expansion factor, as a float.
- :param resample: Resampling method to use. Default is
- :py:attr:`PIL.Image.BICUBIC`. See :ref:`concept-filters`.
- :returns: An :py:class:`~PIL.Image.Image` object.
- """
- if factor == 1:
- return image.copy()
- elif factor <= 0:
- raise ValueError("the factor must be greater than 0")
- else:
- size = (round(factor * image.width), round(factor * image.height))
- return image.resize(size, resample)
-
-
-def deform(image, deformer, resample=Image.Resampling.BILINEAR):
- """
- Deform the image.
-
- :param image: The image to deform.
- :param deformer: A deformer object. Any object that implements a
- ``getmesh`` method can be used.
- :param resample: An optional resampling filter. Same values possible as
- in the PIL.Image.transform function.
- :return: An image.
- """
- return image.transform(
- image.size, Image.Transform.MESH, deformer.getmesh(image), resample
- )
-
-
-def equalize(image, mask=None):
- """
- Equalize the image histogram. This function applies a non-linear
- mapping to the input image, in order to create a uniform
- distribution of grayscale values in the output image.
-
- :param image: The image to equalize.
- :param mask: An optional mask. If given, only the pixels selected by
- the mask are included in the analysis.
- :return: An image.
- """
- if image.mode == "P":
- image = image.convert("RGB")
- h = image.histogram(mask)
- lut = []
- for b in range(0, len(h), 256):
- histo = [_f for _f in h[b : b + 256] if _f]
- if len(histo) <= 1:
- lut.extend(list(range(256)))
- else:
- step = (functools.reduce(operator.add, histo) - histo[-1]) // 255
- if not step:
- lut.extend(list(range(256)))
- else:
- n = step // 2
- for i in range(256):
- lut.append(n // step)
- n = n + h[i + b]
- return _lut(image, lut)
-
-
-def expand(image, border=0, fill=0):
- """
- Add border to the image
-
- :param image: The image to expand.
- :param border: Border width, in pixels.
- :param fill: Pixel fill value (a color value). Default is 0 (black).
- :return: An image.
- """
- left, top, right, bottom = _border(border)
- width = left + image.size[0] + right
- height = top + image.size[1] + bottom
- color = _color(fill, image.mode)
- if image.palette:
- palette = ImagePalette.ImagePalette(palette=image.getpalette())
- if isinstance(color, tuple):
- color = palette.getcolor(color)
- else:
- palette = None
- out = Image.new(image.mode, (width, height), color)
- if palette:
- out.putpalette(palette.palette)
- out.paste(image, (left, top))
- return out
-
-
-def fit(image, size, method=Image.Resampling.BICUBIC, bleed=0.0, centering=(0.5, 0.5)):
- """
- Returns a resized and cropped version of the image, cropped to the
- requested aspect ratio and size.
-
- This function was contributed by Kevin Cazabon.
-
- :param image: The image to resize and crop.
- :param size: The requested output size in pixels, given as a
- (width, height) tuple.
- :param method: Resampling method to use. Default is
- :py:attr:`PIL.Image.BICUBIC`. See :ref:`concept-filters`.
- :param bleed: Remove a border around the outside of the image from all
- four edges. The value is a decimal percentage (use 0.01 for
- one percent). The default value is 0 (no border).
- Cannot be greater than or equal to 0.5.
- :param centering: Control the cropping position. Use (0.5, 0.5) for
- center cropping (e.g. if cropping the width, take 50% off
- of the left side, and therefore 50% off the right side).
- (0.0, 0.0) will crop from the top left corner (i.e. if
- cropping the width, take all of the crop off of the right
- side, and if cropping the height, take all of it off the
- bottom). (1.0, 0.0) will crop from the bottom left
- corner, etc. (i.e. if cropping the width, take all of the
- crop off the left side, and if cropping the height take
- none from the top, and therefore all off the bottom).
- :return: An image.
- """
-
- # by Kevin Cazabon, Feb 17/2000
- # kevin@cazabon.com
- # https://www.cazabon.com
-
- # ensure centering is mutable
- centering = list(centering)
-
- if not 0.0 <= centering[0] <= 1.0:
- centering[0] = 0.5
- if not 0.0 <= centering[1] <= 1.0:
- centering[1] = 0.5
-
- if not 0.0 <= bleed < 0.5:
- bleed = 0.0
-
- # calculate the area to use for resizing and cropping, subtracting
- # the 'bleed' around the edges
-
- # number of pixels to trim off on Top and Bottom, Left and Right
- bleed_pixels = (bleed * image.size[0], bleed * image.size[1])
-
- live_size = (
- image.size[0] - bleed_pixels[0] * 2,
- image.size[1] - bleed_pixels[1] * 2,
- )
-
- # calculate the aspect ratio of the live_size
- live_size_ratio = live_size[0] / live_size[1]
-
- # calculate the aspect ratio of the output image
- output_ratio = size[0] / size[1]
-
- # figure out if the sides or top/bottom will be cropped off
- if live_size_ratio == output_ratio:
- # live_size is already the needed ratio
- crop_width = live_size[0]
- crop_height = live_size[1]
- elif live_size_ratio >= output_ratio:
- # live_size is wider than what's needed, crop the sides
- crop_width = output_ratio * live_size[1]
- crop_height = live_size[1]
- else:
- # live_size is taller than what's needed, crop the top and bottom
- crop_width = live_size[0]
- crop_height = live_size[0] / output_ratio
-
- # make the crop
- crop_left = bleed_pixels[0] + (live_size[0] - crop_width) * centering[0]
- crop_top = bleed_pixels[1] + (live_size[1] - crop_height) * centering[1]
-
- crop = (crop_left, crop_top, crop_left + crop_width, crop_top + crop_height)
-
- # resize the image and return it
- return image.resize(size, method, box=crop)
-
-
-def flip(image):
- """
- Flip the image vertically (top to bottom).
-
- :param image: The image to flip.
- :return: An image.
- """
- return image.transpose(Image.Transpose.FLIP_TOP_BOTTOM)
-
-
-def grayscale(image):
- """
- Convert the image to grayscale.
-
- :param image: The image to convert.
- :return: An image.
- """
- return image.convert("L")
-
-
-def invert(image):
- """
- Invert (negate) the image.
-
- :param image: The image to invert.
- :return: An image.
- """
- lut = []
- for i in range(256):
- lut.append(255 - i)
- return image.point(lut) if image.mode == "1" else _lut(image, lut)
-
-
-def mirror(image):
- """
- Flip image horizontally (left to right).
-
- :param image: The image to mirror.
- :return: An image.
- """
- return image.transpose(Image.Transpose.FLIP_LEFT_RIGHT)
-
-
-def posterize(image, bits):
- """
- Reduce the number of bits for each color channel.
-
- :param image: The image to posterize.
- :param bits: The number of bits to keep for each channel (1-8).
- :return: An image.
- """
- lut = []
- mask = ~(2 ** (8 - bits) - 1)
- for i in range(256):
- lut.append(i & mask)
- return _lut(image, lut)
-
-
-def solarize(image, threshold=128):
- """
- Invert all pixel values above a threshold.
-
- :param image: The image to solarize.
- :param threshold: All pixels above this greyscale level are inverted.
- :return: An image.
- """
- lut = []
- for i in range(256):
- if i < threshold:
- lut.append(i)
- else:
- lut.append(255 - i)
- return _lut(image, lut)
-
-
-def exif_transpose(image):
- """
- If an image has an EXIF Orientation tag, other than 1, return a new image
- that is transposed accordingly. The new image will have the orientation
- data removed.
-
- Otherwise, return a copy of the image.
-
- :param image: The image to transpose.
- :return: An image.
- """
- exif = image.getexif()
- orientation = exif.get(0x0112)
- method = {
- 2: Image.Transpose.FLIP_LEFT_RIGHT,
- 3: Image.Transpose.ROTATE_180,
- 4: Image.Transpose.FLIP_TOP_BOTTOM,
- 5: Image.Transpose.TRANSPOSE,
- 6: Image.Transpose.ROTATE_270,
- 7: Image.Transpose.TRANSVERSE,
- 8: Image.Transpose.ROTATE_90,
- }.get(orientation)
- if method is not None:
- transposed_image = image.transpose(method)
- transposed_exif = transposed_image.getexif()
- if 0x0112 in transposed_exif:
- del transposed_exif[0x0112]
- if "exif" in transposed_image.info:
- transposed_image.info["exif"] = transposed_exif.tobytes()
- elif "Raw profile type exif" in transposed_image.info:
- transposed_image.info[
- "Raw profile type exif"
- ] = transposed_exif.tobytes().hex()
- elif "XML:com.adobe.xmp" in transposed_image.info:
- for pattern in (
- r'tiff:Orientation="([0-9])"',
- r"([0-9])",
- ):
- transposed_image.info["XML:com.adobe.xmp"] = re.sub(
- pattern, "", transposed_image.info["XML:com.adobe.xmp"]
- )
- return transposed_image
- return image.copy()
diff --git a/spaces/arxify/RVC-beta-v2-0618/runtime/Lib/site-packages/_soundfile.py b/spaces/arxify/RVC-beta-v2-0618/runtime/Lib/site-packages/_soundfile.py
deleted file mode 100644
index 336659651c31eaf4d3d4b709a6a8de2113a798ca..0000000000000000000000000000000000000000
--- a/spaces/arxify/RVC-beta-v2-0618/runtime/Lib/site-packages/_soundfile.py
+++ /dev/null
@@ -1,11 +0,0 @@
-# auto-generated file
-import _cffi_backend
-
-ffi = _cffi_backend.FFI('_soundfile',
- _version = 0x2601,
- _types = b'\x00\x00\x17\x0D\x00\x00\x6D\x03\x00\x00\x07\x01\x00\x00\x6C\x03\x00\x00\x7A\x03\x00\x00\x00\x0F\x00\x00\x17\x0D\x00\x00\x6F\x03\x00\x00\x07\x01\x00\x00\x03\x11\x00\x00\x00\x0F\x00\x00\x17\x0D\x00\x00\x07\x01\x00\x00\x07\x01\x00\x00\x03\x11\x00\x00\x07\x01\x00\x00\x00\x0F\x00\x00\x17\x0D\x00\x00\x7B\x03\x00\x00\x07\x01\x00\x00\x03\x11\x00\x00\x00\x0F\x00\x00\x07\x0D\x00\x00\x6E\x03\x00\x00\x00\x0F\x00\x00\x07\x0D\x00\x00\x17\x11\x00\x00\x07\x01\x00\x00\x00\x0F\x00\x00\x07\x0D\x00\x00\x07\x01\x00\x00\x00\x0F\x00\x00\x07\x0D\x00\x00\x00\x0F\x00\x00\x02\x0D\x00\x00\x6C\x03\x00\x00\x00\x0F\x00\x00\x02\x0D\x00\x00\x17\x11\x00\x00\x00\x0F\x00\x00\x02\x0D\x00\x00\x17\x11\x00\x00\x6F\x03\x00\x00\x1C\x01\x00\x00\x00\x0F\x00\x00\x02\x0D\x00\x00\x17\x11\x00\x00\x07\x01\x00\x00\x07\x11\x00\x00\x00\x0F\x00\x00\x02\x0D\x00\x00\x17\x11\x00\x00\x07\x01\x00\x00\x04\x11\x00\x00\x07\x01\x00\x00\x00\x0F\x00\x00\x3B\x0D\x00\x00\x17\x11\x00\x00\x70\x03\x00\x00\x17\x01\x00\x00\x00\x0F\x00\x00\x3B\x0D\x00\x00\x17\x11\x00\x00\x74\x03\x00\x00\x17\x01\x00\x00\x00\x0F\x00\x00\x3B\x0D\x00\x00\x17\x11\x00\x00\x02\x03\x00\x00\x17\x01\x00\x00\x00\x0F\x00\x00\x3B\x0D\x00\x00\x17\x11\x00\x00\x17\x01\x00\x00\x07\x01\x00\x00\x00\x0F\x00\x00\x3B\x0D\x00\x00\x17\x11\x00\x00\x79\x03\x00\x00\x17\x01\x00\x00\x00\x0F\x00\x00\x3B\x0D\x00\x00\x17\x11\x00\x00\x04\x11\x00\x00\x17\x01\x00\x00\x00\x0F\x00\x00\x3B\x0D\x00\x00\x17\x01\x00\x00\x07\x01\x00\x00\x04\x11\x00\x00\x00\x0F\x00\x00\x3B\x0D\x00\x00\x04\x11\x00\x00\x00\x0F\x00\x00\x3B\x0D\x00\x00\x04\x11\x00\x00\x17\x01\x00\x00\x04\x11\x00\x00\x00\x0F\x00\x00\x3B\x0D\x00\x00\x7A\x03\x00\x00\x17\x01\x00\x00\x04\x11\x00\x00\x00\x0F\x00\x00\x7A\x0D\x00\x00\x17\x11\x00\x00\x00\x0F\x00\x00\x00\x09\x00\x00\x01\x09\x00\x00\x02\x09\x00\x00\x03\x09\x00\x00\x02\x01\x00\x00\x0E\x01\x00\x00\x00\x0B\x00\x00\x01\x0B\x00\x00\x02\x0B\x00\x00\x0D\x01\x00\x00\x56\x03\x00\x00\x5B\x03\x00\x00\x5E\x03\x00\x00\x63\x03\x00\x00\x05\x01\x00\x00\x00\x01\x00\x00\x10\x01',
- _globals = (b'\xFF\xFF\xFF\x0BSFC_FILE_TRUNCATE',4224,b'\xFF\xFF\xFF\x0BSFC_GET_FORMAT_INFO',4136,b'\xFF\xFF\xFF\x0BSFC_GET_FORMAT_MAJOR',4145,b'\xFF\xFF\xFF\x0BSFC_GET_FORMAT_MAJOR_COUNT',4144,b'\xFF\xFF\xFF\x0BSFC_GET_FORMAT_SUBTYPE',4147,b'\xFF\xFF\xFF\x0BSFC_GET_FORMAT_SUBTYPE_COUNT',4146,b'\xFF\xFF\xFF\x0BSFC_GET_LIB_VERSION',4096,b'\xFF\xFF\xFF\x0BSFC_GET_LOG_INFO',4097,b'\xFF\xFF\xFF\x0BSFC_SET_CLIPPING',4288,b'\xFF\xFF\xFF\x0BSFC_SET_SCALE_FLOAT_INT_READ',4116,b'\xFF\xFF\xFF\x0BSFC_SET_SCALE_INT_FLOAT_WRITE',4117,b'\xFF\xFF\xFF\x0BSFM_RDWR',48,b'\xFF\xFF\xFF\x0BSFM_READ',16,b'\xFF\xFF\xFF\x0BSFM_WRITE',32,b'\xFF\xFF\xFF\x0BSF_FALSE',0,b'\xFF\xFF\xFF\x0BSF_FORMAT_ENDMASK',805306368,b'\xFF\xFF\xFF\x0BSF_FORMAT_SUBMASK',65535,b'\xFF\xFF\xFF\x0BSF_FORMAT_TYPEMASK',268369920,b'\xFF\xFF\xFF\x0BSF_TRUE',1,b'\x00\x00\x25\x23sf_close',0,b'\x00\x00\x32\x23sf_command',0,b'\x00\x00\x25\x23sf_error',0,b'\x00\x00\x1D\x23sf_error_number',0,b'\x00\x00\x28\x23sf_error_str',0,b'\x00\x00\x22\x23sf_format_check',0,b'\x00\x00\x19\x23sf_get_string',0,b'\x00\x00\x06\x23sf_open',0,b'\x00\x00\x0B\x23sf_open_fd',0,b'\x00\x00\x00\x23sf_open_virtual',0,b'\x00\x00\x25\x23sf_perror',0,b'\x00\x00\x38\x23sf_read_double',0,b'\x00\x00\x3D\x23sf_read_float',0,b'\x00\x00\x42\x23sf_read_int',0,b'\x00\x00\x51\x23sf_read_raw',0,b'\x00\x00\x4C\x23sf_read_short',0,b'\x00\x00\x51\x23sf_readf_double',0,b'\x00\x00\x51\x23sf_readf_float',0,b'\x00\x00\x51\x23sf_readf_int',0,b'\x00\x00\x51\x23sf_readf_short',0,b'\x00\x00\x47\x23sf_seek',0,b'\x00\x00\x2D\x23sf_set_string',0,b'\x00\x00\x16\x23sf_strerror',0,b'\x00\x00\x20\x23sf_version_string',0,b'\x00\x00\x11\x23sf_wchar_open',0,b'\x00\x00\x38\x23sf_write_double',0,b'\x00\x00\x3D\x23sf_write_float',0,b'\x00\x00\x42\x23sf_write_int',0,b'\x00\x00\x51\x23sf_write_raw',0,b'\x00\x00\x4C\x23sf_write_short',0,b'\x00\x00\x68\x23sf_write_sync',0,b'\x00\x00\x51\x23sf_writef_double',0,b'\x00\x00\x51\x23sf_writef_float',0,b'\x00\x00\x51\x23sf_writef_int',0,b'\x00\x00\x51\x23sf_writef_short',0),
- _struct_unions = ((b'\x00\x00\x00\x6B\x00\x00\x00\x02SF_FORMAT_INFO',b'\x00\x00\x02\x11format',b'\x00\x00\x07\x11name',b'\x00\x00\x07\x11extension'),(b'\x00\x00\x00\x6C\x00\x00\x00\x02SF_INFO',b'\x00\x00\x3B\x11frames',b'\x00\x00\x02\x11samplerate',b'\x00\x00\x02\x11channels',b'\x00\x00\x02\x11format',b'\x00\x00\x02\x11sections',b'\x00\x00\x02\x11seekable'),(b'\x00\x00\x00\x6D\x00\x00\x00\x02SF_VIRTUAL_IO',b'\x00\x00\x76\x11get_filelen',b'\x00\x00\x75\x11seek',b'\x00\x00\x77\x11read',b'\x00\x00\x78\x11write',b'\x00\x00\x76\x11tell'),(b'\x00\x00\x00\x6E\x00\x00\x00\x10SNDFILE_tag',)),
- _enums = (b'\x00\x00\x00\x71\x00\x00\x00\x16$1\x00SF_FORMAT_SUBMASK,SF_FORMAT_TYPEMASK,SF_FORMAT_ENDMASK',b'\x00\x00\x00\x72\x00\x00\x00\x16$2\x00SFC_GET_LIB_VERSION,SFC_GET_LOG_INFO,SFC_GET_FORMAT_INFO,SFC_GET_FORMAT_MAJOR_COUNT,SFC_GET_FORMAT_MAJOR,SFC_GET_FORMAT_SUBTYPE_COUNT,SFC_GET_FORMAT_SUBTYPE,SFC_FILE_TRUNCATE,SFC_SET_CLIPPING,SFC_SET_SCALE_FLOAT_INT_READ,SFC_SET_SCALE_INT_FLOAT_WRITE',b'\x00\x00\x00\x73\x00\x00\x00\x16$3\x00SF_FALSE,SF_TRUE,SFM_READ,SFM_WRITE,SFM_RDWR'),
- _typenames = (b'\x00\x00\x00\x6BSF_FORMAT_INFO',b'\x00\x00\x00\x6CSF_INFO',b'\x00\x00\x00\x6DSF_VIRTUAL_IO',b'\x00\x00\x00\x6ESNDFILE',b'\x00\x00\x00\x3Bsf_count_t',b'\x00\x00\x00\x76sf_vio_get_filelen',b'\x00\x00\x00\x77sf_vio_read',b'\x00\x00\x00\x75sf_vio_seek',b'\x00\x00\x00\x76sf_vio_tell',b'\x00\x00\x00\x78sf_vio_write'),
-)
diff --git a/spaces/auto-academic/auto-draft/app.py b/spaces/auto-academic/auto-draft/app.py
deleted file mode 100644
index b8857a6d549f3ef62a4bd288f99446249dc7b3db..0000000000000000000000000000000000000000
--- a/spaces/auto-academic/auto-draft/app.py
+++ /dev/null
@@ -1,323 +0,0 @@
-import uuid
-import gradio as gr
-import os
-import openai
-import yaml
-from utils.file_operations import list_folders, urlify
-from huggingface_hub import snapshot_download
-from wrapper import generator_wrapper
-
-# todo:
-# 6. get logs when the procedure is not completed. *
-# 7. 自己的文件库; 更多的prompts
-# 2. 实现别的功能
-# future:
-# generation.log sometimes disappears (ignore this)
-# 1. Check if there are any duplicated citations
-# 2. Remove potential thebibliography and bibitem in .tex file
-
-#######################################################################################################################
-# Environment Variables
-#######################################################################################################################
-# OPENAI_API_KEY: OpenAI API key for GPT models
-# OPENAI_API_BASE: (Optional) Support alternative OpenAI minors
-# GPT4_ENABLE: (Optional) Set it to 1 to enable GPT-4 model.
-
-# AWS_ACCESS_KEY_ID: (Optional)
-# Access AWS cloud storage (you need to edit `BUCKET_NAME` in `utils/storage.py` if you need to use this function)
-# AWS_SECRET_ACCESS_KEY: (Optional)
-# Access AWS cloud storage (you need to edit `BUCKET_NAME` in `utils/storage.py` if you need to use this function)
-# KDB_REPO: (Optional) A Huggingface dataset hosting Knowledge Databases
-# HF_TOKEN: (Optional) Access to KDB_REPO
-
-#######################################################################################################################
-# Check if openai and cloud storage available
-#######################################################################################################################
-openai_key = os.getenv("OPENAI_API_KEY")
-openai_api_base = os.getenv("OPENAI_API_BASE")
-if openai_api_base is not None:
- openai.api_base = openai_api_base
-GPT4_ENABLE = os.getenv("GPT4_ENABLE") # disable GPT-4 for public repo
-
-access_key_id = os.getenv('AWS_ACCESS_KEY_ID')
-secret_access_key = os.getenv('AWS_SECRET_ACCESS_KEY')
-
-if access_key_id is None or secret_access_key is None:
- print("Access keys are not provided. Outputs cannot be saved to AWS Cloud Storage.\n")
- IS_CACHE_AVAILABLE = False
-else:
- IS_CACHE_AVAILABLE = True
-
-if openai_key is None:
- print("OPENAI_API_KEY is not found in environment variables. The output may not be generated.\n")
- IS_OPENAI_API_KEY_AVAILABLE = False
-else:
- openai.api_key = openai_key
- try:
- openai.Model.list()
- IS_OPENAI_API_KEY_AVAILABLE = True
- # except Exception as e:
- except openai.error.AuthenticationError:
- IS_OPENAI_API_KEY_AVAILABLE = False
-
-DEFAULT_MODEL = "gpt-4" if GPT4_ENABLE else 'gpt-3.5-turbo-16k'
-GPT4_INTERACTIVE = True if GPT4_ENABLE else False
-DEFAULT_SECTIONS = ["introduction", "related works", "backgrounds", "methodology", "experiments",
- "conclusion", "abstract"] if GPT4_ENABLE \
- else ["introduction", "related works"]
-
-MODEL_LIST = ['gpt-4', 'gpt-3.5-turbo', 'gpt-3.5-turbo-16k']
-
-HF_TOKEN = os.getenv("HF_TOKEN")
-REPO_ID = os.getenv("KDB_REPO")
-if HF_TOKEN is not None and REPO_ID is not None:
- snapshot_download(REPO_ID, repo_type="dataset", local_dir="knowledge_databases/",
- local_dir_use_symlinks=False, token=HF_TOKEN)
- KDB_LIST = ["(None)"] + list_folders("knowledge_databases")
-
-#######################################################################################################################
-# Load the list of templates & knowledge databases
-#######################################################################################################################
-ALL_TEMPLATES = list_folders("latex_templates")
-ALL_DATABASES = ["(None)"] + list_folders("knowledge_databases")
-
-#######################################################################################################################
-# Gradio UI
-#######################################################################################################################
-theme = gr.themes.Default(font=gr.themes.GoogleFont("Questrial"))
-# .set(
-# background_fill_primary='#E5E4E2',
-# background_fill_secondary = '#F6F6F6',
-# button_primary_background_fill="#281A39"
-# )
-ANNOUNCEMENT = """
-# Auto-Draft: 学术写作辅助工具
-
-本Demo提供对[Auto-Draft](https://github.com/CCCBora/auto-draft)的学术论文模板生成功能的测试. 学术综述和Github文档功能正在开发中.
-
-## 主要功能
-通过输入想要生成的论文名称(比如Playing atari with deep reinforcement learning),即可由AI辅助生成论文模板.
-
-***2023-06-13 Update***:
-- 增加了最新的gpt-3.5-turbo-16k模型的支持.
-
-***2023-06-13 Update***:
-1. 新增‘高级选项-Prompts模式’. 这个模式仅会输出用于生成论文的Prompts而不会生成论文本身. 可以根据自己的需求修改Prompts, 也可以把Prompts复制给其他语言模型.
-2. 把默认的ICLR 2022模板改成了Default模板. 不再显示ICLR的页眉页尾.
-3. 中文支持: 暂不支持. 建议使用英文生成论文, 然后把输出结果送入[GPT 学术优化](https://github.com/binary-husky/gpt_academic)中的Latex全文翻译、润色功能即可.
-4. 使用GPT-4模型:
- - 点击Duplicate this Space, 进入Settings-> Repository secrets, 点击New Secret添加OPENAI_API_KEY为自己的OpenAI API Key. 添加GPT4_ENBALE为1.
- - 或者可以访问[Auto-Draft-Private](https://huggingface.co/spaces/auto-academic/auto-draft-private).
-
-如果有更多想法和建议欢迎加入QQ群里交流, 如果我在Space里更新了Key我会第一时间通知大家. 群号: ***249738228***."""
-
-ACADEMIC_PAPER = """## 一键生成论文初稿
-1. 在Title文本框中输入想要生成的论文名称(比如Playing Atari with Deep Reinforcement Learning).
-2. 点击Submit. 等待大概十五分钟(全文).
-3. 在右侧下载.zip格式的输出,在Overleaf上编译浏览.
-"""
-
-REFERENCES = """## 一键搜索相关论文
-(此功能已经被整合进一键生成论文初稿)
-1. 在Title文本框中输入想要搜索文献的论文(比如Playing Atari with Deep Reinforcement Learning).
-2. 点击Submit. 等待大概十分钟.
-3. 在右侧JSON处会显示相关文献.
-"""
-
-REFERENCES_INSTRUCTION = """### References
-这一栏用于定义AI如何选取参考文献. 目前是两种方式混合:
-1. GPT自动根据标题生成关键字,使用Semantic Scholar搜索引擎搜索文献,利用Specter获取Paper Embedding来自动选取最相关的文献作为GPT的参考资料.
-2. 用户通过输入文章标题(用英文逗号隔开), AI会自动搜索文献作为参考资料.
-关于有希望利用本地文件来供GPT参考的功能将在未来实装.
-"""
-
-DOMAIN_KNOWLEDGE_INSTRUCTION = """### Domain Knowledge
-这一栏用于定义AI的知识库. 将提供两种选择:
-1. 各个领域内由专家预先收集资料并构建的的FAISS向量数据库. 目前实装的数据库
-* (None): 不使用任何知识库
-* ml_textbook_test: 包含两本机器学习教材The Elements of Statistical Learning和Reinforcement Learning Theory and Algorithms. 仅用于测试知识库Pipeline.
-2. 自行构建的使用OpenAI text-embedding-ada-002模型创建的FAISS向量数据库. (暂未实装)
-"""
-
-OUTPUTS_INSTRUCTION = """### Outputs
-这一栏用于定义输出的内容:
-* Template: 用于填装内容的LaTeX模板.
-* Models: 使用GPT-4或者GPT-3.5-Turbo生成内容.
-* Prompts模式: 不生成内容, 而是生成用于生成内容的Prompts. 可以手动复制到网页版或者其他语言模型中进行使用. (放在输出的ZIP文件的prompts.json文件中)
-"""
-
-OTHERS_INSTRUCTION = """### Others
-
-"""
-
-style_mapping = {True: "color:white;background-color:green",
- False: "color:white;background-color:red"} # todo: to match website's style
-availability_mapping = {True: "AVAILABLE", False: "NOT AVAILABLE"}
-STATUS = f'''## Huggingface Space Status
- 当`OpenAI API`显示AVAILABLE的时候这个Space可以直接使用.
- 当`OpenAI API`显示NOT AVAILABLE的时候这个Space可以通过在左侧输入OPENAI KEY来使用. 需要有GPT-4的API权限.
- 当`Cache`显示AVAILABLE的时候, 所有的输入和输出会被备份到我的云储存中. 显示NOT AVAILABLE的时候不影响实际使用.
-`OpenAI API`: {availability_mapping[IS_OPENAI_API_KEY_AVAILABLE]}. `Cache`: {availability_mapping[IS_CACHE_AVAILABLE]}.'''
-
-
-def clear_inputs(*args):
- return "", ""
-
-
-def clear_inputs_refs(*args):
- return "", 5
-
-
-def wrapped_generator(
- paper_title, paper_description, # main input
- openai_api_key=None, # key
- tldr=True, max_kw_refs=10, refs=None, max_tokens_ref=2048, # references
- knowledge_database=None, max_tokens_kd=2048, query_counts=10, # domain knowledge
- paper_template="ICLR2022", selected_sections=None, model="gpt-4", prompts_mode=False, # outputs parameters
- cache_mode=IS_CACHE_AVAILABLE # handle cache mode
-):
- file_name_upload = urlify(paper_title) + "_" + uuid.uuid1().hex + ".zip"
-
- # load the default configuration file
- with open("configurations/default.yaml", 'r') as file:
- config = yaml.safe_load(file)
- config["paper"]["title"] = paper_title
- config["paper"]["description"] = paper_description
- config["references"]["tldr"] = tldr
- config["references"]["max_kw_refs"] = max_kw_refs
- config["references"]["refs"] = refs
- config["references"]["max_tokens_ref"] = max_tokens_ref
- config["domain_knowledge"]["knowledge_database"] = knowledge_database
- config["domain_knowledge"]["max_tokens_kd"] = max_tokens_kd
- config["domain_knowledge"]["query_counts"] = query_counts
- config["output"]["selected_sections"] = selected_sections
- config["output"]["model"] = model
- config["output"]["template"] = paper_template
- config["output"]["prompts_mode"] = prompts_mode
-
- if openai_api_key is not None:
- openai.api_key = openai_api_key
- try:
- openai.Model.list()
- except Exception as e:
- raise gr.Error(f"Key错误. Error: {e}")
- try:
- output = generator_wrapper(config)
- if cache_mode:
- from utils.storage import upload_file
- upload_file(output, target_name=file_name_upload)
- except Exception as e:
- raise gr.Error(f"生成失败. Error: {e}")
- return output
-
-
-with gr.Blocks(theme=theme) as demo:
- gr.Markdown(ANNOUNCEMENT)
-
- with gr.Row():
- with gr.Column(scale=2):
- key = gr.Textbox(value=openai_key, lines=1, max_lines=1, label="OpenAI Key",
- visible=not IS_OPENAI_API_KEY_AVAILABLE)
- # 每个功能做一个tab
- with gr.Tab("学术论文"):
- gr.Markdown(ACADEMIC_PAPER)
-
- title = gr.Textbox(value="Playing Atari with Deep Reinforcement Learning", lines=1, max_lines=1,
- label="Title", info="论文标题")
-
- description_pp = gr.Textbox(lines=5, label="Description (Optional)", visible=True,
- info="这篇论文的主要贡献和创新点. (生成所有章节时共享这个信息, 保持生成的一致性.)")
-
- with gr.Accordion("高级设置", open=False):
- with gr.Row():
- with gr.Column(scale=1):
- gr.Markdown(OUTPUTS_INSTRUCTION)
- with gr.Column(scale=2):
- with gr.Row():
- template = gr.Dropdown(label="Template", choices=ALL_TEMPLATES, value="Default",
- interactive=True,
- info="生成论文的模板.")
- model_selection = gr.Dropdown(label="Model", choices=MODEL_LIST,
- value=DEFAULT_MODEL,
- interactive=GPT4_INTERACTIVE,
- info="生成论文用到的语言模型.")
- prompts_mode = gr.Checkbox(value=False, visible=True, interactive=True,
- label="Prompts模式",
- info="只输出用于生成论文的Prompts, 可以复制到别的地方生成论文.")
-
- sections = gr.CheckboxGroup(
- choices=["introduction", "related works", "backgrounds", "methodology", "experiments",
- "conclusion", "abstract"],
- type="value", label="生成章节", interactive=True, info="选择生成论文的哪些章节.",
- value=DEFAULT_SECTIONS)
-
- with gr.Row():
- with gr.Column(scale=1):
- gr.Markdown(REFERENCES_INSTRUCTION)
-
- with gr.Column(scale=2):
- max_kw_ref_slider = gr.Slider(minimum=1, maximum=20, value=10, step=1,
- interactive=True, label="MAX_KW_REFS",
- info="每个Keyword搜索几篇参考文献", visible=False)
-
- max_tokens_ref_slider = gr.Slider(minimum=256, maximum=8192, value=2048, step=2,
- interactive=True, label="MAX_TOKENS",
- info="参考文献内容占用Prompts中的Token数")
-
- tldr_checkbox = gr.Checkbox(value=True, label="TLDR;",
- info="选择此筐表示将使用Semantic Scholar的TLDR作为文献的总结.",
- interactive=True)
-
- text_ref = gr.Textbox(lines=5, label="References (Optional)", visible=True,
- info="交给AI参考的文献的标题, 用英文逗号`,`隔开.")
-
- gr.Examples(
- examples = ["Understanding the Impact of Model Incoherence on Convergence of Incremental SGD with Random Reshuffle,"
- "Variance-Reduced Off-Policy TDC Learning: Non-Asymptotic Convergence Analysis,"
- "Greedy-GQ with Variance Reduction: Finite-time Analysis and Improved Complexity"],
- inputs=text_ref,
- cache_examples=False
- )
-
- with gr.Row():
- with gr.Column(scale=1):
- gr.Markdown(DOMAIN_KNOWLEDGE_INSTRUCTION)
-
- with gr.Column(scale=2):
- query_counts_slider = gr.Slider(minimum=1, maximum=20, value=10, step=1,
- interactive=True, label="QUERY_COUNTS",
- info="从知识库内检索多少条内容", visible=False)
- max_tokens_kd_slider = gr.Slider(minimum=256, maximum=8192, value=2048, step=2,
- interactive=True, label="MAX_TOKENS",
- info="知识库内容占用Prompts中的Token数")
- domain_knowledge = gr.Dropdown(label="预载知识库",
- choices=ALL_DATABASES,
- value="(None)",
- interactive=True,
- info="使用预先构建的知识库.")
- local_domain_knowledge = gr.File(label="本地知识库 (暂未实装)", interactive=False)
- with gr.Row():
- clear_button_pp = gr.Button("Clear")
- submit_button_pp = gr.Button("Submit", variant="primary")
- with gr.Tab("文献综述 (Coming soon!)"):
- gr.Markdown('''
-
Coming soon!
- ''')
- with gr.Tab("Github文档 (Coming soon!)"):
- gr.Markdown('''
-
Coming soon!
- ''')
-
- with gr.Column(scale=1):
- gr.Markdown(STATUS)
- file_output = gr.File(label="Output")
- json_output = gr.JSON(label="References")
- clear_button_pp.click(fn=clear_inputs, inputs=[title, description_pp], outputs=[title, description_pp])
- submit_button_pp.click(fn=wrapped_generator,
- inputs=[title, description_pp, key,
- tldr_checkbox, max_kw_ref_slider, text_ref, max_tokens_ref_slider,
- domain_knowledge, max_tokens_kd_slider, query_counts_slider,
- template, sections, model_selection, prompts_mode], outputs=file_output)
-
-demo.queue(concurrency_count=1, max_size=5, api_open=False)
-demo.launch(show_error=True)
diff --git a/spaces/autoevaluate/error-analysis/app.py b/spaces/autoevaluate/error-analysis/app.py
deleted file mode 100644
index dfe69779e3f48d4b8408e03da1151ed358eba390..0000000000000000000000000000000000000000
--- a/spaces/autoevaluate/error-analysis/app.py
+++ /dev/null
@@ -1,283 +0,0 @@
-## LIBRARIES ###
-## Data
-import numpy as np
-import pandas as pd
-import torch
-import json
-from tqdm import tqdm
-from math import floor
-from datasets import load_dataset
-from collections import defaultdict
-from transformers import AutoTokenizer
-pd.options.display.float_format = '${:,.2f}'.format
-
-# Analysis
-# from gensim.models.doc2vec import Doc2Vec
-# from sklearn.metrics import accuracy_score, f1_score, precision_score, recall_score
-import nltk
-from nltk.cluster import KMeansClusterer
-import scipy.spatial.distance as sdist
-from scipy.spatial import distance_matrix
-# nltk.download('punkt') #make sure that punkt is downloaded
-
-# App & Visualization
-import streamlit as st
-import altair as alt
-import plotly.graph_objects as go
-from streamlit_vega_lite import altair_component
-
-
-
-# utils
-from random import sample
-from error_analysis import utils as ut
-
-
-def down_samp(embedding):
- """Down sample a data frame for altiar visualization """
- # total number of positive and negative sentiments in the class
- #embedding = embedding.groupby('slice').apply(lambda x: x.sample(frac=0.3))
- total_size = embedding.groupby(['slice','label'], as_index=False).count()
-
- user_data = 0
- # if 'Your Sentences' in str(total_size['slice']):
- # tmp = embedding.groupby(['slice'], as_index=False).count()
- # val = int(tmp[tmp['slice'] == "Your Sentences"]['source'])
- # user_data = val
-
- max_sample = total_size.groupby('slice').max()['content']
-
- # # down sample to meeting altair's max values
- # # but keep the proportional representation of groups
- down_samp = 1/(sum(max_sample.astype(float))/(1000-user_data))
-
- max_samp = max_sample.apply(lambda x: floor(x*down_samp)).astype(int).to_dict()
- max_samp['Your Sentences'] = user_data
-
- # # sample down for each group in the data frame
- embedding = embedding.groupby('slice').apply(lambda x: x.sample(n=max_samp.get(x.name))).reset_index(drop=True)
-
- # # order the embedding
- return(embedding)
-
-
-def data_comparison(df):
- selection = alt.selection_multi(fields=['cluster:N','label:O'])
- color = alt.condition(alt.datum.slice == 'high-loss', alt.Color('cluster:N', scale = alt.Scale(domain=df.cluster.unique().tolist())), alt.value("lightgray"))
- opacity = alt.condition(selection, alt.value(0.7), alt.value(0.25))
-
- # basic chart
- scatter = alt.Chart(df).mark_point(size=100, filled=True).encode(
- x=alt.X('x:Q', axis=None),
- y=alt.Y('y:Q', axis=None),
- color=color,
- shape=alt.Shape('label:O', scale=alt.Scale(range=['circle', 'diamond'])),
- tooltip=['cluster:N','slice:N','content:N','label:O','pred:O'],
- opacity=opacity
- ).properties(
- width=1000,
- height=800
- ).interactive()
-
- legend = alt.Chart(df).mark_point(size=100, filled=True).encode(
- x=alt.X("label:O"),
- y=alt.Y('cluster:N', axis=alt.Axis(orient='right'), title=""),
- shape=alt.Shape('label:O', scale=alt.Scale(
- range=['circle', 'diamond']), legend=None),
- color=color,
- ).add_selection(
- selection
- )
- layered = scatter | legend
- layered = layered.configure_axis(
- grid=False
- ).configure_view(
- strokeOpacity=0
- )
- return layered
-
-def quant_panel(embedding_df):
- """ Quantitative Panel Layout"""
- all_metrics = {}
- st.warning("**Error slice visualization**")
- with st.expander("How to read this chart:"):
- st.markdown("* Each **point** is an input example.")
- st.markdown("* Gray points have low-loss and the colored have high-loss. High-loss instances are clustered using **kmeans** and each color represents a cluster.")
- st.markdown("* The **shape** of each point reflects the label category -- positive (diamond) or negative sentiment (circle).")
- st.altair_chart(data_comparison(down_samp(embedding_df)), use_container_width=True)
-
-
-def frequent_tokens(data, tokenizer, loss_quantile=0.95, top_k=200, smoothing=0.005):
- unique_tokens = []
- tokens = []
- for row in tqdm(data['content']):
- tokenized = tokenizer(row,padding=True, return_tensors='pt')
- tokens.append(tokenized['input_ids'].flatten())
- unique_tokens.append(torch.unique(tokenized['input_ids']))
- losses = data['loss'].astype(float)
- high_loss = losses.quantile(loss_quantile)
- loss_weights = (losses > high_loss)
- loss_weights = loss_weights / loss_weights.sum()
- token_frequencies = defaultdict(float)
- token_frequencies_error = defaultdict(float)
-
- weights_uniform = np.full_like(loss_weights, 1 / len(loss_weights))
-
- num_examples = len(data)
- for i in tqdm(range(num_examples)):
- for token in unique_tokens[i]:
- token_frequencies[token.item()] += weights_uniform[i]
- token_frequencies_error[token.item()] += loss_weights[i]
-
- token_lrs = {k: (smoothing+token_frequencies_error[k]) / (smoothing+token_frequencies[k]) for k in token_frequencies}
- tokens_sorted = list(map(lambda x: x[0], sorted(token_lrs.items(), key=lambda x: x[1])[::-1]))
-
- top_tokens = []
- for i, (token) in enumerate(tokens_sorted[:top_k]):
- top_tokens.append(['%10s' % (tokenizer.decode(token)), '%.4f' % (token_frequencies[token]), '%.4f' % (
- token_frequencies_error[token]), '%4.2f' % (token_lrs[token])])
- return pd.DataFrame(top_tokens, columns=['Token', 'Freq', 'Freq error slice', 'lrs'])
-
-
-@st.cache(ttl=600)
-def get_data(inference, emb):
- preds = inference.outputs.numpy()
- losses = inference.losses.numpy()
- embeddings = pd.DataFrame(emb, columns=['x', 'y'])
- num_examples = len(losses)
- # dataset_labels = [dataset[i]['label'] for i in range(num_examples)]
- return pd.concat([pd.DataFrame(np.transpose(np.vstack([dataset[:num_examples]['content'],
- dataset[:num_examples]['label'], preds, losses])), columns=['content', 'label', 'pred', 'loss']), embeddings], axis=1)
-
-def clustering(data,num_clusters):
- X = np.array(data['embedding'].tolist())
- kclusterer = KMeansClusterer(
- num_clusters, distance=nltk.cluster.util.cosine_distance,
- repeats=25,avoid_empty_clusters=True)
- assigned_clusters = kclusterer.cluster(X, assign_clusters=True)
- data['cluster'] = pd.Series(assigned_clusters, index=data.index).astype('int')
- data['centroid'] = data['cluster'].apply(lambda x: kclusterer.means()[x])
- return data, assigned_clusters
-
-def kmeans(df, num_clusters=3):
- data_hl = df.loc[df['slice'] == 'high-loss']
- data_kmeans,clusters = clustering(data_hl,num_clusters)
- merged = pd.merge(df, data_kmeans, left_index=True, right_index=True, how='outer', suffixes=('', '_y'))
- merged.drop(merged.filter(regex='_y$').columns.tolist(),axis=1,inplace=True)
- merged['cluster'] = merged['cluster'].fillna(num_clusters).astype('int')
- return merged
-
-def distance_from_centroid(row):
- return sdist.norm(row['embedding'] - row['centroid'].tolist())
-
-@st.cache(ttl=600)
-def topic_distribution(weights, smoothing=0.01):
- topic_frequencies = defaultdict(float)
- topic_frequencies_spotlight = defaultdict(float)
- weights_uniform = np.full_like(weights, 1 / len(weights))
- num_examples = len(weights)
- for i in range(num_examples):
- example = dataset[i]
- category = example['title']
- topic_frequencies[category] += weights_uniform[i]
- topic_frequencies_spotlight[category] += weights[i]
-
- topic_ratios = {c: (smoothing + topic_frequencies_spotlight[c]) / (
- smoothing + topic_frequencies[c]) for c in topic_frequencies}
-
- categories_sorted = map(lambda x: x[0], sorted(
- topic_ratios.items(), key=lambda x: x[1], reverse=True))
-
- topic_distr = []
- for category in categories_sorted:
- topic_distr.append(['%.3f' % topic_frequencies[category], '%.3f' %
- topic_frequencies_spotlight[category], '%.2f' % topic_ratios[category], '%s' % category])
-
- return pd.DataFrame(topic_distr, columns=['Overall frequency', 'Error frequency', 'Ratio', 'Category'])
- # for category in categories_sorted:
- # return(topic_frequencies[category], topic_frequencies_spotlight[category], topic_ratios[category], category)
-
-def populate_session(dataset,model):
- data_df = read_file_to_df('./assets/data/'+dataset+ '_'+ model+'.parquet')
- if model == 'albert-base-v2-yelp-polarity':
- tokenizer = AutoTokenizer.from_pretrained('textattack/'+model)
- else:
- tokenizer = AutoTokenizer.from_pretrained(model)
- if "user_data" not in st.session_state:
- st.session_state["user_data"] = data_df
- if "selected_slice" not in st.session_state:
- st.session_state["selected_slice"] = None
-
-@st.cache(allow_output_mutation=True)
-def read_file_to_df(file):
- return pd.read_parquet(file)
-
-if __name__ == "__main__":
- ### STREAMLIT APP CONGFIG ###
- st.set_page_config(layout="wide", page_title="Interactive Error Analysis")
-
- ut.init_style()
-
- lcol, rcol = st.columns([2, 2])
- # ******* loading the mode and the data
- #st.sidebar.mardown("
Interactive Error Analysis
", unsafe_allow_html=True)
-
- dataset = st.sidebar.selectbox(
- "Dataset",
- ["amazon_polarity", "yelp_polarity"],
- index = 1
- )
-
- model = st.sidebar.selectbox(
- "Model",
- ["distilbert-base-uncased-finetuned-sst-2-english",
- "albert-base-v2-yelp-polarity"],
- )
-
- ### LOAD DATA AND SESSION VARIABLES ###
- ##uncomment the next next line to run dynamically and not from file
- #populate_session(dataset, model)
- data_df = read_file_to_df('./assets/data/'+dataset+ '_'+ model+'.parquet')
- loss_quantile = st.sidebar.slider(
- "Loss Quantile", min_value=0.5, max_value=1.0,step=0.01,value=0.95
- )
- data_df['loss'] = data_df['loss'].astype(float)
- losses = data_df['loss']
- high_loss = losses.quantile(loss_quantile)
- data_df['slice'] = 'high-loss'
- data_df['slice'] = data_df['slice'].where(data_df['loss'] > high_loss, 'low-loss')
-
- with rcol:
- with st.spinner(text='loading...'):
- st.markdown('
Word Distribution in Error Slice
', unsafe_allow_html=True)
- #uncomment the next two lines to run dynamically and not from file
- #commontokens = frequent_tokens(data_df, tokenizer, loss_quantile=loss_quantile)
- commontokens = read_file_to_df('./assets/data/'+dataset+ '_'+ model+'_commontokens.parquet')
- with st.expander("How to read the table:"):
- st.markdown("* The table displays the most frequent tokens in error slices, relative to their frequencies in the val set.")
- st.write(commontokens)
-
- run_kmeans = st.sidebar.radio("Cluster error slice?", ('True', 'False'), index=0)
-
- num_clusters = st.sidebar.slider("# clusters", min_value=1, max_value=20, step=1, value=3)
-
- if run_kmeans == 'True':
- with st.spinner(text='running kmeans...'):
- merged = kmeans(data_df,num_clusters=num_clusters)
- with lcol:
- st.markdown('
Error Slices
',unsafe_allow_html=True)
- with st.expander("How to read the table:"):
- st.markdown("* *Error slice* refers to the subset of evaluation dataset the model performs poorly on.")
- st.markdown("* The table displays model error slices on the evaluation dataset, sorted by loss.")
- st.markdown("* Each row is an input example that includes the label, model pred, loss, and error cluster.")
- with st.spinner(text='loading error slice...'):
- dataframe=read_file_to_df('./assets/data/'+dataset+ '_'+ model+'_error-slices.parquet')
- #uncomment the next next line to run dynamically and not from file
- # dataframe = merged[['content', 'label', 'pred', 'loss', 'cluster']].sort_values(
- # by=['loss'], ascending=False)
- # table_html = dataframe.to_html(
- # columns=['content', 'label', 'pred', 'loss', 'cluster'], max_rows=50)
- # table_html = table_html.replace("
-
- aaccfb2cb3
-
-
-
diff --git a/spaces/bioriAsaeru/text-to-voice/Beyond the Stars 1989 How a Teenage Boy Befriended a Retired Astronaut.md b/spaces/bioriAsaeru/text-to-voice/Beyond the Stars 1989 How a Teenage Boy Befriended a Retired Astronaut.md
deleted file mode 100644
index c7326dbc336039d61c300c33ab2b1ea39f52a9e7..0000000000000000000000000000000000000000
--- a/spaces/bioriAsaeru/text-to-voice/Beyond the Stars 1989 How a Teenage Boy Befriended a Retired Astronaut.md
+++ /dev/null
@@ -1,23 +0,0 @@
-
-
The Quarterly Census of Employment and Wages (QCEW) program provides several different types of data files. These files are available for download. Data classified using the North American Industry Classification System (NAICS) are available from 1990 forward, and on a more limited basis from 1975 to 1989. NAICS-based data files from 1990 to 2000 were re-constructed from data classified under the Standard Industrial Classification (SIC) system. NAICS-based data files from 1975 to 1989 contain only totals by-ownership. NAICS data can be downloaded from the NAICS-Based Data Files table below.
This table contains links to data classified using the North American Industry Classification System (NAICS). The data are stored in several different formats. Each format is listed at the top of the table. By Industry files from 1975 to 1989 contain only ownership totals. If data are available, then the year will be visible as a link. Annual averages are provided only when an entire year's data are available. An "N/A" is present when an entire year is not available.
-
"Out of the Woods" is a song by American singer-songwriter Taylor Swift, taken from her fifth studio album, 1989 (2014). Swift wrote and produced the song with Jack Antonoff. With lyrics inspired by a failed relationship and the ensuing anxieties that Swift experienced, "Out of the Woods" is a synth-pop song with elements of indietronica and features heavy synthesizers, looping drums, and layered background vocals.
-
Big Machine Records made the song available for download on October 14, 2014, as a promotional single for 1989. Swift premiered the music video for "Out of the Woods" on ABC's Dick Clark's New Year's Rockin' Eve on December 31, 2015; the video depicts Swift struggling to escape from a magical forest. The song was released to US pop and hot adult contemporary radio as the album's sixth single on January 18, 2016, by Big Machine in partnership with Republic Records.
-
Music critics praised "Out of the Woods" for its 1980s-influenced production and narrative lyrics offering emotional engagement. The song peaked at number 18 on the US Billboard Hot 100 and was certified platinum by the Recording Industry Association of America (RIAA). It also reached the top 20 of charts in Australia, Canada, and New Zealand. Swift performed the song on television shows such as Good Morning America, and included it in the set list of the 1989 World Tour (2015).
-
For "Out of the Woods", Antonoff envisioned the song to feature a 1980s sound with a modern twist. He used a Yamaha DX7 synthesizer to create most parts of the song, and a Minimoog Voyager for the refrain, which brought forth an "extremely modern" sound that he desired.[11] He edited his background vocals and layered them over looping drums.[11] After completing the instrumental, Antonoff sent it to Swift when she was on a plane.[12] Swift sent him a voice memo containing the lyrics roughly 30 minutes later; it was the first time Swift wrote the lyrics to an existing track.[5][11] According to the liner notes of 1989, "Out of the Woods" was recorded by Laura Sisk, assisted by Brendan Morawski, at Jungle City Studios in New York City; and Sam Holland, assisted by Cory Bice, at Conway Recording Studios in Los Angeles. Swift's vocals were produced by Max Martin.[8]
-
-
Music critics described "Out of the Woods" as a 1980s-influenced synth-pop song.[9][13][14] Hannah Mylrea from NME noted influences of indietronica.[15] The song features pulsing synthesizers, loud drums, and echoing background vocals that gradually build up towards the end.[16][17][18] Compared to other tracks of 1989, "Out of the Woods" features a denser production.[19][20] Antonoff took inspiration from the music of rock band My Morning Jacket: "every sound is louder than the last ... It started out big, and then I think the obvious move would have been to do a down chorus, but the idea was to keep pushing."[9]
-
The lyrics are about a fragile romance, inspired by the anxieties Swift experienced from a tumultuous relationship.[21][22] In the refrain, Swift repeats the line, "Are we out of the woods yet?" over and over, indicating her desire to stabilize the relationship.[23] Swift ponders over its inevitable end: "Your necklace hanging from my neck the night we couldn't quite forget / When we decided to move the furniture so we could dance / Baby, like we stood a chance."[24] The bridge narrates an accident that requires one of the couple to undergo a surgery: "Remember when you hit the brakes too soon / Twenty stitches in a hospital room."[25][26] The accident in the bridge was inspired by a snowmobile accident that she and an ex-lover had suffered when they were on a ski trip; she had persuaded the tabloid media to not publicize it.[22] Besides its literal sense, the accident is a metaphor for the relationship's fragility and how the two have to deal with its aftermath.[27][28] When promoting 1989 in October 2014, Swift remarked that "Out of the Woods" was the song that "best represents [the album]".[29]
-
On October 13, 2014, Swift premiered 15 seconds of "Out of the Woods" on Good Morning America.[30] Big Machine Records made the song available for download on October 14, 2014, as a promotional single for 1989.[31] It is track number four on 1989, which was released on October 27, 2014, by Big Machine.[32]
-
Swift premiered the music video for "Out of the Woods" on Dick Clark's New Year's Rockin' Eve, broadcast on December 31, 2015.[33] Big Machine and Republic Records released the song to US pop and hot adult contemporary radio stations on January 19, 2016;[34][35] it was the sixth single from 1989.[36][37] In Italy, "Out of the Woods" was released to radio on February 5, 2016, by Universal Music Group.[38]
-
Upon the release of 1989, music critics compared the 1980s-influenced production of "Out of the Woods" to the music of 1980s musicians including Phil Collins and Madonna.[49] Sam Lansky from Time,[50] Jason Lipshutz from Billboard,[51] Brian Mansfield from USA Today,[13] and Lindsay Zoladz from Vulture praised the production for showcasing Swift's expanding artistry beyond her previous country styles.[52] In a review of 1989 for the Los Angeles Times, Mikael Wood deemed "Out of the Woods" one of the album's highlights, describing it as the most authentic tribute to the 1980s synth-pop sound that Swift tried to recreate on the album.[23]
-
Other reviews complimented Swift's lyrical craftsmanship and storytelling, which she had honed on her previous country songs.[24][53] Lipshutz remarked that although the song was a musical departure for Swift, it was a reminder of her abilities to present "striking, instantly unforgettable images".[51] Writing for The Independent, Andy Gill argued that the intricate lyrics capturing "dramatic emotional change in a few striking lines" of "Out of the Woods" were rare for a pop song.[26] Carl Wilson, in a 1989 review for Slate, picked it as his favorite off the album, highlighting both the detailed lyrics and the production.[20] Esther Zuckerman of Entertainment Weekly deemed the production generic, but highlighted the lyrics as a testament to Swift's ability to offer emotional engagement in her songs.[54]
-
The video shows Swift battling to get out of a forest, interpreting the title literally.[37] Swift is seen struggling to escape a magical forest while being chased by a pack of wolves as animate roots constantly follow her. She then finds herself in different natural settings like snowy mountains, an ocean, a barren landscape, a muddy location, and a burning forest. At the end of the video, the woods disappear as she finds a beach, where another version of her is standing by the shore as she reaches for her.[62] The video ends with the caption "She lost him, but she found herself, and somehow that was everything," which is a hidden message written in the booklet of 1989.[37]
-
During promotion of 1989, Swift performed "Out of the Woods" on televised shows including Jimmy Kimmel Live!,[67] The Ellen DeGeneres Show,[68] and Good Morning America.[69] She performed the song as part of the "1989 Secret Sessions", live streamed by iHeartRadio and Yahoo! on October 27, 2014, the same day the album was released.[70] On the 1989 World Tour in 2015, Swift included the song as the penultimate number on the regular set list.[71] Swift played a stripped-down rendition of "Out of the Woods" on piano at the Grammy Museum in Los Angeles on September 30, 2015; John Blistein from Rolling Stone praised this version over the synth-pop production for better conveying the emotional sentiments of the lyrics.[72]
-
Rock singer Ryan Adams recorded a country folk-oriented cover of "Out of the Woods" for his track-by-track cover of Swift's 1989.[78] Yahoo! writer Oscar Gracey said that the cover "makes us want to hike through a forest, find a clearing, and mourn the relationships that didn't quite work out",[79] and The A.V. Club's Annie Zaleski viewed that Adams's acoustic production "exacerbates the song's uncertainty about a relationship's status".[80]
-
There are currently 68 Dividend Aristocrats. You can download an Excel spreadsheet of all 68 (with metrics that matter such as dividend yields and price-to-earnings ratios) by clicking the link below:
-
SDI has been used in broadcast productions since 1989 and is supported on most legacy studio hardware devices. Hardware devices from AJA Video Systems and Blackmagic Design provide connectivity to legacy broadcast devices that use SDI.
-
Justice William J. Brennan Jr. revealed a streak of romantic individualism, as well as a belief in personal liberty, in his defense of boycotts, demonstrations, and other forms of civil disobedience as valid means of expression. Examples are his opinions in NAACP v. Button (1963), Edwards v. Aguillard (1987), and Texas v. Johnson (1989).
aaccfb2cb3
-
-
\ No newline at end of file
diff --git a/spaces/bioriAsaeru/text-to-voice/Django Unchained In Dual Audio Enghindi 720p Learn More About the Cast and Crew of the Film.md b/spaces/bioriAsaeru/text-to-voice/Django Unchained In Dual Audio Enghindi 720p Learn More About the Cast and Crew of the Film.md
deleted file mode 100644
index cebafa5f24b2a9e8ae9977c7107c3776cd96355b..0000000000000000000000000000000000000000
--- a/spaces/bioriAsaeru/text-to-voice/Django Unchained In Dual Audio Enghindi 720p Learn More About the Cast and Crew of the Film.md
+++ /dev/null
@@ -1,6 +0,0 @@
-
-
- aaccfb2cb3
-
-
-
diff --git a/spaces/bioriAsaeru/text-to-voice/File Scavenger 3.2.22.20100719 Incl Keygen [vokeon] Tips and Tricks for Using This Software Effectively.md b/spaces/bioriAsaeru/text-to-voice/File Scavenger 3.2.22.20100719 Incl Keygen [vokeon] Tips and Tricks for Using This Software Effectively.md
deleted file mode 100644
index 6aa3751858cb36a93207d570b9a6be61e9751603..0000000000000000000000000000000000000000
--- a/spaces/bioriAsaeru/text-to-voice/File Scavenger 3.2.22.20100719 Incl Keygen [vokeon] Tips and Tricks for Using This Software Effectively.md
+++ /dev/null
@@ -1,6 +0,0 @@
-
-
- aaccfb2cb3
-
-
-
diff --git a/spaces/bioriAsaeru/text-to-voice/Free Download Blue Line Backgrounds and Wallpapers in High Quality.md b/spaces/bioriAsaeru/text-to-voice/Free Download Blue Line Backgrounds and Wallpapers in High Quality.md
deleted file mode 100644
index 5fd3037f5861c062d031f9ba2d8af606d1dfd712..0000000000000000000000000000000000000000
--- a/spaces/bioriAsaeru/text-to-voice/Free Download Blue Line Backgrounds and Wallpapers in High Quality.md
+++ /dev/null
@@ -1,13 +0,0 @@
-
-
Blue Letter Bible is a free, searchable online Bible program providing access to many different Bible translations including:KJV, NKJV, NLT, ESV, NASB20, NASB95 and many others. In addition, in-depth study tools are provided on the site with access tocommentaries, encyclopedias, dictionaries, and other theological resources. Browse the site to see all of the Biblestudy tools available.
-
Get free Blue line icons in iOS, Material, Windows and other design styles for web, mobile, and graphic design projects. These free images are pixel perfect to fit your design and available in both PNG and vector. Download icons in all formats or edit them for your designs.
Complete the form below to receive a free download of Building The Business Case for Immersive Learning. As soon as you click Submit, the download will be available in your email inbox. Be sure to add [email protected] to your whitelist to avoid any deliverability issues.
-
This design consists of nine layers. For the project pictured above, I used foam tabs between all layers except the second and third layer. I glued those two layers together as it allowed the blue line to show. This design will download in SVG, DXF, EPS, and PNG formats.
-
University shuttles are outfitted with real-time tracking equipment. Simply go to the TransLoc site on your computer or smartphone, or download the free smartphone app to see the buses on a map. Live tracking, arrival predictions, real-time capacity, and proximity alerts are also available for all University bus lines.
-
Blue Lines Professional PowerPoint Template is a free abstract PowerPoint slide design that you can use to customize your presentations in Microsoft PowerPoint 2010 and 2007. You can also download this free lines PPT template for PowerPoint 2013 presentations and other widescreen PowerPoint templates for the new version of MS Office and MS PowerPoint.
-
Free templates for PowerPoint like this free blue lines PowerPoint background can be used to make serious and formal business presentations and impress your audience with a quite simple but powerful and original template for presentations. You can download this free business PowerPoint template for presentations on start-ups, Keynotes as well as other presentations for example to prepare a new business unit or developing a new product for Microsoft PowerPoint 2010 and 2013. Alternatively, you may combine the blue lines background design with other presentation designs or elements to make presentations on topics such as Starbucks or Pepsi.
-
-
There are numerous transportation options available at LAX, including: airport buses, door-to-door shuttle vans, local buses, light rail, taxicabs and rental cars. A free shuttle bus connects LAX with the Metro Rail Green Line light rail, and free shuttle buses transport passengers between airline terminals. The LAX FlyAway® bus service provides frequent non-stop transportation between LAX and several locations throughout the city. For detailed information on ground transportation to and from the airport, visit the LAX Ground Transportation page.
aaccfb2cb3
-
-
\ No newline at end of file
diff --git a/spaces/birsardar/stable-diffusion-mat-outpainting-primer/training/__init__.py b/spaces/birsardar/stable-diffusion-mat-outpainting-primer/training/__init__.py
deleted file mode 100644
index e1e1a5ba99e56a56ecaa14f7d4fa41777789c0cf..0000000000000000000000000000000000000000
--- a/spaces/birsardar/stable-diffusion-mat-outpainting-primer/training/__init__.py
+++ /dev/null
@@ -1,9 +0,0 @@
-# Copyright (c) 2021, NVIDIA CORPORATION. All rights reserved.
-#
-# NVIDIA CORPORATION and its licensors retain all intellectual property
-# and proprietary rights in and to this software, related documentation
-# and any modifications thereto. Any use, reproduction, disclosure or
-# distribution of this software and related documentation without an express
-# license agreement from NVIDIA CORPORATION is strictly prohibited.
-
-# empty
diff --git a/spaces/brogelio/air_draw/app.py b/spaces/brogelio/air_draw/app.py
deleted file mode 100644
index d626d6fe97d6165261cffe6ad03d64324d85a988..0000000000000000000000000000000000000000
--- a/spaces/brogelio/air_draw/app.py
+++ /dev/null
@@ -1,232 +0,0 @@
-import cv2
-import numpy as np
-from PIL import Image
-from PIL import ImageColor
-import mediapipe as mp
-import time
-import gradio as gr
-import glob
-import os
-
-width_, height_ = 144, 96
-
-drawing_flag = False
-sleepy_time = time.time()
-
-output_frames = []
-
-
-def is_hex(hexq):
- valid = ['0','1','2','3','4','5','6',
- '7','8','9','A','a','B','b',
- 'C','c','D','d','E','e','F',
- 'f']
- hexq = str(hexq)
- if len(hexq) == 7:
- if hexq[0] == '#':
- for h in hexq[1:]:
- if h in valid:
- return True
- else:
- return False
- else:
- return False
- else:
- return False
-
-
-def hex2rgb(hex):
- if is_hex(hex):
- return ImageColor.getcolor(hex, "RGB")
- else:
- return (0, 0, 0)
-
-
-def find_hands(brain, img):
- if img is not None:
- img_rgb = cv2.cvtColor(img, cv2.COLOR_BGR2RGB) # opencv image is in BGR form but mp is trained with RGB
- results = brain.process(img_rgb) # process finds the hands and outputs classification and 21 landmarks for each hand
- all_hands = [] # initializing array to hold the dictionary for the hands
- h, w, _ = img.shape # get height and width of image for scaling
- if results.multi_hand_landmarks:
- for hand_type, hand_lms in zip(results.multi_handedness,
- results.multi_hand_landmarks): # elegant solution for mp list object traversal
- hand = {} # initializing dict for each hand
- lm_list = [] # landmarks array for all 21 point of the hand
- for lm in hand_lms.landmark:
- px, py, pz = int(lm.x * w), int(lm.y * h), int(
- lm.z * w) # scaling landmark points to image size for frame coordinates
- lm_list.append([px, py, pz])
-
- hand["lm_list"] = lm_list # add "lm_list" key for all landmark points of the hand
- hand["type"] = hand_type.classification[0].label # adds the label (left/right) for the hand
- all_hands.append(hand) # appends the dict
- return all_hands
-
- else:
- return 0
-
-
-def is_drawing(index, thumb): # proximity function with arbitrary threshold
- npindex = np.array((index[0], index[1]))
- npthumb = np.array((thumb[0], thumb[1]))
- if np.linalg.norm(npindex - npthumb) < 20:
- return True
- else:
- return False
-
-
-def save(landmarks): # brute force finger orientation checking
- if landmarks[8][1] < landmarks[6][1]:
- if landmarks[12][1] < landmarks[10][1]:
- if landmarks[16][1] < landmarks[14][1]:
- if landmarks[20][1] < landmarks[18][1]:
- return True
- else:
- return False
-
-
-def clear(landmarks): # brute force finger orientation checking
- if landmarks[4][1] < landmarks[3][1] < landmarks[2][1] < landmarks[8][1]:
- return True
- else:
- return False
-
-
-def show(video, dominant_hand, hex_color='#FFFFFF'): # main
- cam = cv2.VideoCapture(video) # get the video file from path
- width = cam.get(cv2.CAP_PROP_FRAME_WIDTH)
- height = cam.get(cv2.CAP_PROP_FRAME_HEIGHT)
-
- detector = mp.solutions.hands.Hands(min_detection_confidence=0.8) # initialize detector
- # paper = np.zeros((width, height, 4), np.uint8)
- paper = np.zeros((int(height), int(width), 3), dtype=np.uint8) # create blank page
- paper.fill(255)
-
- color = hex2rgb(hex_color)
- past_holder = () # hold previous index coordinates
- palette = cv2.imread('palette_small.jpg')
-
- page_num = 0 # iterating for saving (not a viable function for gradio)
-
- global sleepy_time # get sleep time for multiple gestures
-
- while cam.isOpened():
- x, rgb_image = cam.read()
- rgb_image_f = cv2.flip(rgb_image, 1) # mirrored video
-
- hands = find_hands(detector, rgb_image_f)
-
- if x: # return flag for cv2
- try: # for error handling
- if hands:
- hand1 = hands[0] if hands[0]["type"] == dominant_hand else hands[1]
- lm_list1 = hand1["lm_list"] # List of 21 Landmarks
- handedness = hand1["type"]
-
- if handedness == dominant_hand:
- idx_coords = lm_list1[8][0], lm_list1[8][1] # 0 is width (bigger)
- # print(idx_coords)
- cv2.circle(rgb_image_f, idx_coords, 5, color, cv2.FILLED)
-
- ### Discontinued function due to gradio limitations ###
- # if idx_coords[1] < 72: # brute force but should be extremely marginally faster lol
- # if idx_coords[0] < 71: # red
- # color = (0, 0, 255)
- # if 71 < idx_coords[0] < 142: # orange
- # color = (0, 115, 255)
- # if 142 < idx_coords[0] < 213: # yellow
- # color = (0, 229, 255)
- # if 213 < idx_coords[0] < 284: # green
- # color = (0, 195, 88)
- # if 284 < idx_coords[0] < 356: # blue
- # color = (195, 85, 0)
- # if 356 < idx_coords[0] < 427: # indigo
- # color = (195, 0, 68)
- # if 427 < idx_coords[0] < 498: # violet
- # color = (195, 0, 143)
- # if 498 < idx_coords[0] < 569: # black
- # color = (0, 0, 0)
- # if 569 < idx_coords[0]: # white / eraser
- # color = (255, 255, 255)
-
- if len(past_holder) and drawing_flag: # start drawing
- cv2.line(paper, past_holder, idx_coords, color, 5)
- cv2.line(rgb_image_f, past_holder, idx_coords, color, 5)
- # paper[idx_coords[0]][idx_coords[1]][0] = 255
- # paper[idx_coords[0]][idx_coords[1]][3] = 255
- cv2.circle(rgb_image_f, idx_coords, 5, color, cv2.FILLED)
-
- ### Discontinued function due to gradio limitations ###
- # if save(lm_list1) and time.time() - sleepy_time > 3: # save / output
- # paper[0:height_, w - width_: w] = 255 # presenter eraser
- # paper = cv2.cvtColor(paper, cv2.COLOR_BGR2RGB)
- # im = Image.fromarray(paper)
- # im.save("paper%s.png" % page_num)
- # print("saved")
- # sleepy_time = time.time()
- # paper = cv2.cvtColor(paper, cv2.COLOR_RGB2BGR)
- # page_num += 1
-
- if clear(lm_list1) and time.time() - sleepy_time > 3: # reset paper
- paper = np.zeros((height, width, 3), dtype=np.uint8)
- paper.fill(255)
- print("page cleared")
- sleepy_time = time.time()
-
- past_holder = idx_coords
-
- if is_drawing(idx_coords, lm_list1[4]): # 4 is thumb
- drawing_flag = True
- else:
- drawing_flag = False
-
- except:
- pass
-
- finally:
- if True:
- presenter = cv2.resize(rgb_image_f, (width_, height_))
- h, w, _ = rgb_image_f.shape
- paper[0:height_, w - width_: w] = presenter
-
- else:
- break
-
- paper = cv2.cvtColor(paper, cv2.COLOR_RGB2BGR)
- im = Image.fromarray(paper)
- output_frames.append(paper)
- im.save("paper%s.png" % page_num)
- page_num += 1
-
- img_array = []
- for filename in glob.glob('*.png'):
- imggg = cv2.imread(filename)
- img_array.append(imggg)
- os.remove(filename)
-
- video_output = cv2.VideoWriter('any.webm', cv2.VideoWriter_fourcc(*'VP80'), 30, (640, 480))
-
- for i in range(len(img_array)):
- video_output.write(img_array[i])
- video_output.release()
-
- return 'any.webm'
-
-
-title = 'Air Draw'
-desc = 'A mediapipe hands wrapper for drawing in the air. Draw holding an invisible pen, ' \
- 'and open up your hand to lift the "pen" from the "paper". Use a "thumbs up" gesture to clear the drawing ' \
- 'paper. '
-iface = gr.Interface(
- fn=show,
- inputs=[
- gr.inputs.Video(source="webcam", label="Record yourself drawing in the air!"),
- gr.inputs.Radio(['Right', 'Left'], label="Dominant Hand"),
- gr.inputs.Textbox(placeholder="#355C7D", label="Hex Color")
- ],
- outputs='video',
- title=title,
- description=desc)
-
-iface.launch(share=True, enable_queue=True)
diff --git a/spaces/charles0519/ChuanhuChatGPT/run_Windows.bat b/spaces/charles0519/ChuanhuChatGPT/run_Windows.bat
deleted file mode 100644
index 4c18f9ccaeea0af972301ffdf48778641221f76d..0000000000000000000000000000000000000000
--- a/spaces/charles0519/ChuanhuChatGPT/run_Windows.bat
+++ /dev/null
@@ -1,5 +0,0 @@
-@echo off
-echo Opening ChuanhuChatGPT...
-
-REM Open powershell via bat
-start powershell.exe -NoExit -Command "python ./ChuanhuChatbot.py"
diff --git a/spaces/chendl/compositional_test/multimodal/YOLOX/yolox/models/darknet.py b/spaces/chendl/compositional_test/multimodal/YOLOX/yolox/models/darknet.py
deleted file mode 100644
index b3e053f163ade7b69979bcec86532466ab67eedf..0000000000000000000000000000000000000000
--- a/spaces/chendl/compositional_test/multimodal/YOLOX/yolox/models/darknet.py
+++ /dev/null
@@ -1,179 +0,0 @@
-#!/usr/bin/env python
-# -*- encoding: utf-8 -*-
-# Copyright (c) Megvii Inc. All rights reserved.
-
-from torch import nn
-
-from .network_blocks import BaseConv, CSPLayer, DWConv, Focus, ResLayer, SPPBottleneck
-
-
-class Darknet(nn.Module):
- # number of blocks from dark2 to dark5.
- depth2blocks = {21: [1, 2, 2, 1], 53: [2, 8, 8, 4]}
-
- def __init__(
- self,
- depth,
- in_channels=3,
- stem_out_channels=32,
- out_features=("dark3", "dark4", "dark5"),
- ):
- """
- Args:
- depth (int): depth of darknet used in model, usually use [21, 53] for this param.
- in_channels (int): number of input channels, for example, use 3 for RGB image.
- stem_out_channels (int): number of output channels of darknet stem.
- It decides channels of darknet layer2 to layer5.
- out_features (Tuple[str]): desired output layer name.
- """
- super().__init__()
- assert out_features, "please provide output features of Darknet"
- self.out_features = out_features
- self.stem = nn.Sequential(
- BaseConv(in_channels, stem_out_channels, ksize=3, stride=1, act="lrelu"),
- *self.make_group_layer(stem_out_channels, num_blocks=1, stride=2),
- )
- in_channels = stem_out_channels * 2 # 64
-
- num_blocks = Darknet.depth2blocks[depth]
- # create darknet with `stem_out_channels` and `num_blocks` layers.
- # to make model structure more clear, we don't use `for` statement in python.
- self.dark2 = nn.Sequential(
- *self.make_group_layer(in_channels, num_blocks[0], stride=2)
- )
- in_channels *= 2 # 128
- self.dark3 = nn.Sequential(
- *self.make_group_layer(in_channels, num_blocks[1], stride=2)
- )
- in_channels *= 2 # 256
- self.dark4 = nn.Sequential(
- *self.make_group_layer(in_channels, num_blocks[2], stride=2)
- )
- in_channels *= 2 # 512
-
- self.dark5 = nn.Sequential(
- *self.make_group_layer(in_channels, num_blocks[3], stride=2),
- *self.make_spp_block([in_channels, in_channels * 2], in_channels * 2),
- )
-
- def make_group_layer(self, in_channels: int, num_blocks: int, stride: int = 1):
- "starts with conv layer then has `num_blocks` `ResLayer`"
- return [
- BaseConv(in_channels, in_channels * 2, ksize=3, stride=stride, act="lrelu"),
- *[(ResLayer(in_channels * 2)) for _ in range(num_blocks)],
- ]
-
- def make_spp_block(self, filters_list, in_filters):
- m = nn.Sequential(
- *[
- BaseConv(in_filters, filters_list[0], 1, stride=1, act="lrelu"),
- BaseConv(filters_list[0], filters_list[1], 3, stride=1, act="lrelu"),
- SPPBottleneck(
- in_channels=filters_list[1],
- out_channels=filters_list[0],
- activation="lrelu",
- ),
- BaseConv(filters_list[0], filters_list[1], 3, stride=1, act="lrelu"),
- BaseConv(filters_list[1], filters_list[0], 1, stride=1, act="lrelu"),
- ]
- )
- return m
-
- def forward(self, x):
- outputs = {}
- x = self.stem(x)
- outputs["stem"] = x
- x = self.dark2(x)
- outputs["dark2"] = x
- x = self.dark3(x)
- outputs["dark3"] = x
- x = self.dark4(x)
- outputs["dark4"] = x
- x = self.dark5(x)
- outputs["dark5"] = x
- return {k: v for k, v in outputs.items() if k in self.out_features}
-
-
-class CSPDarknet(nn.Module):
- def __init__(
- self,
- dep_mul,
- wid_mul,
- out_features=("dark3", "dark4", "dark5"),
- depthwise=False,
- act="silu",
- ):
- super().__init__()
- assert out_features, "please provide output features of Darknet"
- self.out_features = out_features
- Conv = DWConv if depthwise else BaseConv
-
- base_channels = int(wid_mul * 64) # 64
- base_depth = max(round(dep_mul * 3), 1) # 3
-
- # stem
- self.stem = Focus(3, base_channels, ksize=3, act=act)
-
- # dark2
- self.dark2 = nn.Sequential(
- Conv(base_channels, base_channels * 2, 3, 2, act=act),
- CSPLayer(
- base_channels * 2,
- base_channels * 2,
- n=base_depth,
- depthwise=depthwise,
- act=act,
- ),
- )
-
- # dark3
- self.dark3 = nn.Sequential(
- Conv(base_channels * 2, base_channels * 4, 3, 2, act=act),
- CSPLayer(
- base_channels * 4,
- base_channels * 4,
- n=base_depth * 3,
- depthwise=depthwise,
- act=act,
- ),
- )
-
- # dark4
- self.dark4 = nn.Sequential(
- Conv(base_channels * 4, base_channels * 8, 3, 2, act=act),
- CSPLayer(
- base_channels * 8,
- base_channels * 8,
- n=base_depth * 3,
- depthwise=depthwise,
- act=act,
- ),
- )
-
- # dark5
- self.dark5 = nn.Sequential(
- Conv(base_channels * 8, base_channels * 16, 3, 2, act=act),
- SPPBottleneck(base_channels * 16, base_channels * 16, activation=act),
- CSPLayer(
- base_channels * 16,
- base_channels * 16,
- n=base_depth,
- shortcut=False,
- depthwise=depthwise,
- act=act,
- ),
- )
-
- def forward(self, x):
- outputs = {}
- x = self.stem(x)
- outputs["stem"] = x
- x = self.dark2(x)
- outputs["dark2"] = x
- x = self.dark3(x)
- outputs["dark3"] = x
- x = self.dark4(x)
- outputs["dark4"] = x
- x = self.dark5(x)
- outputs["dark5"] = x
- return {k: v for k, v in outputs.items() if k in self.out_features}
diff --git a/spaces/chendl/compositional_test/transformers/src/transformers/data/processors/__init__.py b/spaces/chendl/compositional_test/transformers/src/transformers/data/processors/__init__.py
deleted file mode 100644
index a26ab5776d74715428b10c4d9cd943e53b253785..0000000000000000000000000000000000000000
--- a/spaces/chendl/compositional_test/transformers/src/transformers/data/processors/__init__.py
+++ /dev/null
@@ -1,18 +0,0 @@
-# Copyright 2020 The HuggingFace Team. All rights reserved.
-#
-# Licensed under the Apache License, Version 2.0 (the "License");
-# you may not use this file except in compliance with the License.
-# You may obtain a copy of the License at
-#
-# http://www.apache.org/licenses/LICENSE-2.0
-#
-# Unless required by applicable law or agreed to in writing, software
-# distributed under the License is distributed on an "AS IS" BASIS,
-# WITHOUT WARRANTIES OR CONDITIONS OF ANY KIND, either express or implied.
-# See the License for the specific language governing permissions and
-# limitations under the License.
-
-from .glue import glue_convert_examples_to_features, glue_output_modes, glue_processors, glue_tasks_num_labels
-from .squad import SquadExample, SquadFeatures, SquadV1Processor, SquadV2Processor, squad_convert_examples_to_features
-from .utils import DataProcessor, InputExample, InputFeatures, SingleSentenceClassificationProcessor
-from .xnli import xnli_output_modes, xnli_processors, xnli_tasks_num_labels
diff --git a/spaces/chuan-hd/law-assistant-chatbot/.venv/lib/python3.11/site-packages/clickhouse_connect/driver/exceptions.py b/spaces/chuan-hd/law-assistant-chatbot/.venv/lib/python3.11/site-packages/clickhouse_connect/driver/exceptions.py
deleted file mode 100644
index 1cd41f993337aee8ba9a636f20f3e7f673f7637f..0000000000000000000000000000000000000000
--- a/spaces/chuan-hd/law-assistant-chatbot/.venv/lib/python3.11/site-packages/clickhouse_connect/driver/exceptions.py
+++ /dev/null
@@ -1,84 +0,0 @@
-"""
-The driver exception classes here include all named exceptions required by th DB API 2.0 specification. It's not clear
-how useful that naming convention is, but the convention is used for potential improved compatibility with other
-libraries. In most cases docstring are taken from the DBIApi 2.0 documentation
-"""
-
-
-class ClickHouseError(Exception):
- """Exception related to operation with ClickHouse."""
-
-
-# pylint: disable=redefined-builtin
-class Warning(Warning, ClickHouseError):
- """Exception raised for important warnings like data truncations
- while inserting, etc."""
-
-
-class Error(ClickHouseError):
- """Exception that is the base class of all other error exceptions
- (not Warning)."""
-
-
-class InterfaceError(Error):
- """Exception raised for errors that are related to the database
- interface rather than the database itself."""
-
-
-class DatabaseError(Error):
- """Exception raised for errors that are related to the
- database."""
-
-
-class DataError(DatabaseError):
- """Exception raised for errors that are due to problems with the
- processed data like division by zero, numeric value out of range,
- etc."""
-
-
-class OperationalError(DatabaseError):
- """Exception raised for errors that are related to the database's
- operation and not necessarily under the control of the programmer,
- e.g. an unexpected disconnect occurs, the data source name is not
- found, a transaction could not be processed, a memory allocation
- error occurred during processing, etc."""
-
-
-class IntegrityError(DatabaseError):
- """Exception raised when the relational integrity of the database
- is affected, e.g. a foreign key check fails, duplicate key,
- etc."""
-
-
-class InternalError(DatabaseError):
- """Exception raised when the database encounters an internal
- error, e.g. the cursor is not valid anymore, the transaction is
- out of sync, etc."""
-
-
-class ProgrammingError(DatabaseError):
- """Exception raised for programming errors, e.g. table not found
- or already exists, syntax error in the SQL statement, wrong number
- of parameters specified, etc."""
-
-
-class NotSupportedError(DatabaseError):
- """Exception raised in case a method or database API was used
- which is not supported by the database, e.g. requesting a
- .rollback() on a connection that does not support transaction or
- has transactions turned off."""
-
-
-class StreamClosedError(ProgrammingError):
- """Exception raised when a stream operation is executed on a closed stream."""
-
- def __init__(self):
- super().__init__('Executing a streaming operation on a closed stream')
-
-
-class StreamCompleteException(Exception):
- """ Internal exception used to indicate the end of a ClickHouse query result stream."""
-
-
-class StreamFailureError(Exception):
- """ Stream failed unexpectedly """
diff --git a/spaces/chuan-hd/law-assistant-chatbot/.venv/lib/python3.11/site-packages/docx/opc/packuri.py b/spaces/chuan-hd/law-assistant-chatbot/.venv/lib/python3.11/site-packages/docx/opc/packuri.py
deleted file mode 100644
index 621ed92e5eeca147eb96c8de689914f54f1cbfd5..0000000000000000000000000000000000000000
--- a/spaces/chuan-hd/law-assistant-chatbot/.venv/lib/python3.11/site-packages/docx/opc/packuri.py
+++ /dev/null
@@ -1,117 +0,0 @@
-# encoding: utf-8
-
-"""
-Provides the PackURI value type along with some useful known pack URI strings
-such as PACKAGE_URI.
-"""
-
-import posixpath
-import re
-
-
-class PackURI(str):
- """
- Provides access to pack URI components such as the baseURI and the
- filename slice. Behaves as |str| otherwise.
- """
- _filename_re = re.compile('([a-zA-Z]+)([1-9][0-9]*)?')
-
- def __new__(cls, pack_uri_str):
- if not pack_uri_str[0] == '/':
- tmpl = "PackURI must begin with slash, got '%s'"
- raise ValueError(tmpl % pack_uri_str)
- return str.__new__(cls, pack_uri_str)
-
- @staticmethod
- def from_rel_ref(baseURI, relative_ref):
- """
- Return a |PackURI| instance containing the absolute pack URI formed by
- translating *relative_ref* onto *baseURI*.
- """
- joined_uri = posixpath.join(baseURI, relative_ref)
- abs_uri = posixpath.abspath(joined_uri)
- return PackURI(abs_uri)
-
- @property
- def baseURI(self):
- """
- The base URI of this pack URI, the directory portion, roughly
- speaking. E.g. ``'/ppt/slides'`` for ``'/ppt/slides/slide1.xml'``.
- For the package pseudo-partname '/', baseURI is '/'.
- """
- return posixpath.split(self)[0]
-
- @property
- def ext(self):
- """
- The extension portion of this pack URI, e.g. ``'xml'`` for
- ``'/word/document.xml'``. Note the period is not included.
- """
- # raw_ext is either empty string or starts with period, e.g. '.xml'
- raw_ext = posixpath.splitext(self)[1]
- return raw_ext[1:] if raw_ext.startswith('.') else raw_ext
-
- @property
- def filename(self):
- """
- The "filename" portion of this pack URI, e.g. ``'slide1.xml'`` for
- ``'/ppt/slides/slide1.xml'``. For the package pseudo-partname '/',
- filename is ''.
- """
- return posixpath.split(self)[1]
-
- @property
- def idx(self):
- """
- Return partname index as integer for tuple partname or None for
- singleton partname, e.g. ``21`` for ``'/ppt/slides/slide21.xml'`` and
- |None| for ``'/ppt/presentation.xml'``.
- """
- filename = self.filename
- if not filename:
- return None
- name_part = posixpath.splitext(filename)[0] # filename w/ext removed
- match = self._filename_re.match(name_part)
- if match is None:
- return None
- if match.group(2):
- return int(match.group(2))
- return None
-
- @property
- def membername(self):
- """
- The pack URI with the leading slash stripped off, the form used as
- the Zip file membername for the package item. Returns '' for the
- package pseudo-partname '/'.
- """
- return self[1:]
-
- def relative_ref(self, baseURI):
- """
- Return string containing relative reference to package item from
- *baseURI*. E.g. PackURI('/ppt/slideLayouts/slideLayout1.xml') would
- return '../slideLayouts/slideLayout1.xml' for baseURI '/ppt/slides'.
- """
- # workaround for posixpath bug in 2.6, doesn't generate correct
- # relative path when *start* (second) parameter is root ('/')
- if baseURI == '/':
- relpath = self[1:]
- else:
- relpath = posixpath.relpath(self, baseURI)
- return relpath
-
- @property
- def rels_uri(self):
- """
- The pack URI of the .rels part corresponding to the current pack URI.
- Only produces sensible output if the pack URI is a partname or the
- package pseudo-partname '/'.
- """
- rels_filename = '%s.rels' % self.filename
- rels_uri_str = posixpath.join(self.baseURI, '_rels', rels_filename)
- return PackURI(rels_uri_str)
-
-
-PACKAGE_URI = PackURI('/')
-CONTENT_TYPES_URI = PackURI('/[Content_Types].xml')
diff --git a/spaces/chuan-hd/law-assistant-chatbot/.venv/lib/python3.11/site-packages/fontTools/ttLib/tables/_p_r_o_p.py b/spaces/chuan-hd/law-assistant-chatbot/.venv/lib/python3.11/site-packages/fontTools/ttLib/tables/_p_r_o_p.py
deleted file mode 100644
index aead9d72062e878d5e497f263a4f08eddbb048f6..0000000000000000000000000000000000000000
--- a/spaces/chuan-hd/law-assistant-chatbot/.venv/lib/python3.11/site-packages/fontTools/ttLib/tables/_p_r_o_p.py
+++ /dev/null
@@ -1,6 +0,0 @@
-from .otBase import BaseTTXConverter
-
-
-# https://developer.apple.com/fonts/TrueType-Reference-Manual/RM06/Chap6prop.html
-class table__p_r_o_p(BaseTTXConverter):
- pass
diff --git a/spaces/cihyFjudo/fairness-paper-search/Bias Fx Torrent The Ultimate Review of BIAS FX 2 Elite - Features Pros and Cons.md b/spaces/cihyFjudo/fairness-paper-search/Bias Fx Torrent The Ultimate Review of BIAS FX 2 Elite - Features Pros and Cons.md
deleted file mode 100644
index d61994dc57901f5f7ffca1a8353a46d4bdaed50e..0000000000000000000000000000000000000000
--- a/spaces/cihyFjudo/fairness-paper-search/Bias Fx Torrent The Ultimate Review of BIAS FX 2 Elite - Features Pros and Cons.md
+++ /dev/null
@@ -1,5 +0,0 @@
-
-
BIAS FX, BIAS Amp & BIAS Pedal\n","icon":"hidden","bgColor":"","color":"#ffffff","slug":"4/desktop-guitar-software","parentCid":0,"topic_count":1862,"post_count":7480,"disabled":0,"order":5,"link":"","numRecentReplies":1,"class":"col-md-3 col-xs-6","imageClass":"cover","undefined":0,"backgroundImage":"/assets/uploads/category/category-4.png","minTags":0,"maxTags":0,"postQueue":0,"isSection":0,"totalPostCount":7480,"totalTopicCount":1862},"tagWhitelist":[],"minTags":0,"maxTags":0,"thread_tools":[],"isFollowing":false,"isNotFollowing":true,"isIgnoring":false,"bookmark":null,"postSharing":["id":"facebook","name":"Facebook","class":"fa-facebook","activated":true,"id":"twitter","name":"Twitter","class":"fa-twitter","activated":true],"deleter":null,"merger":null,"related":[],"unreplied":false,"icons":[],"privileges":"topics:reply":false,"topics:read":true,"topics:tag":false,"topics:delete":false,"posts:edit":false,"posts:history":false,"posts:delete":false,"posts:view_deleted":false,"read":true,"purge":false,"view_thread_tools":false,"editable":false,"deletable":false,"view_deleted":false,"isAdminOrMod":false,"disabled":0,"tid":"1871","uid":0,"topicStaleDays":60,"reputation:disabled":0,"downvote:disabled":1,"feeds:disableRSS":0,"bookmarkThreshold":5,"necroThreshold":7,"postEditDuration":0,"postDeleteDuration":0,"scrollToMyPost":true,"updateUrlWithPostIndex":true,"allowMultipleBadges":false,"privateUploads":false,"rssFeedUrl":"/topic/1871.rss","postIndex":1,"breadcrumbs":["text":"[[global:home]]","url":"/","text":"Desktop guitar software","url":"/category/4/desktop-guitar-software","cid":4,"text":"Popping and crackling in Bias FX"],"pagination":"prev":"page":1,"active":false,"next":"page":1,"active":false,"first":"page":1,"active":true,"last":"page":1,"active":true,"rel":[],"pages":[],"currentPage":1,"pageCount":1,"loggedIn":false,"relative_path":"","template":"name":"topic","topic":true,"url":"/topic/1871/popping-and-crackling-in-bias-fx","bodyClass":"page-topic page-topic-1871 page-topic-popping-and-crackling-in-bias-fx page-topic-category-4 page-topic-category-desktop-guitar-software parent-category-4 page-status-200","loggedInUser":"uid":0,"username":"[[global:guest]]","picture":"/assets/uploads/system/avatar-default.png","icon:text":"?","icon:bgColor":"#aaa","_header":"tags":"meta":["name":"viewport","content":"width=device-width, initial-scale=1.0","name":"content-type","content":"text/html; charset=UTF-8","noEscape":true,"name":"apple-mobile-web-app-capable","content":"yes","name":"mobile-web-app-capable","content":"yes","property":"og:site_name","content":"NodeBB","name":"msapplication-badge","content":"frequency=30; polling-uri= ","noEscape":true,"name":"theme-color","content":"#ffffff","name":"msapplication-square150x150logo","content":"/assets/uploads/system/site-logo.png","noEscape":true,"name":"title","content":"Popping and crackling in Bias FX","name":"description","content":"Hello, I have just bought Bias FX, and there is loads of popping and cracking through my headphones. It does not happen in Bias Amp, just the FX, and it does also happen through the speakers, so its not the headphones. I wonder if anyone has any idea what...","property":"og:title","content":"Popping and crackling in Bias FX","property":"og:description","content":"Hello, I have just bought Bias FX, and there is loads of popping and cracking through my headphones. It does not happen in Bias Amp, just the FX, and it does also happen through the speakers, so its not the headphones. I wonder if anyone has any idea what...","property":"og:type","content":"article","property":"article:published_time","content":"2018-08-09T16:03:59.594Z","property":"article:modified_time","content":"2018-08-19T00:36:16.700Z","property":"article:section","content":"Desktop guitar software","property":"og:image","content":" -4.png","noEscape":true,"property":"og:image:url","content":" -4.png","noEscape":true,"property":"og:image","content":" =large","noEscape":true,"property":"og:image:url","content":" =large","noEscape":true,"property":"og:image","content":" -logo.png","noEscape":true,"property":"og:image:url","content":" -logo.png","noEscape":true,"content":" -and-crackling-in-bias-fx","property":"og:url"],"link":["rel":"icon","type":"image/x-icon","href":"/favicon.ico?v=no4c6ta2ksc","rel":"manifest","href":"/manifest.webmanifest","rel":"search","type":"application/opensearchdescription+xml","title":"Positive Grid Community Forum","href":"/osd.xml","rel":"apple-touch-icon","href":"/assets/images/touch/512.png","rel":"icon","sizes":"36x36","href":"/assets/images/touch/192.png","rel":"icon","sizes":"48x48","href":"/assets/images/touch/144.png","rel":"icon","sizes":"72x72","href":"/assets/images/touch/96.png","rel":"icon","sizes":"96x96","href":"/assets/images/touch/72.png","rel":"icon","sizes":"144x144","href":"/assets/images/touch/48.png","rel":"icon","sizes":"192x192","href":"/assets/images/touch/36.png","rel":"icon","sizes":"512x512","href":"/assets/images/touch/512.png","rel":"prefetch","href":"/assets/src/modules/composer.js?v=no4c6ta2ksc","rel":"prefetch","href":"/assets/src/modules/composer/uploads.js?v=no4c6ta2ksc","rel":"prefetch","href":"/assets/src/modules/composer/drafts.js?v=no4c6ta2ksc","rel":"prefetch","href":"/assets/src/modules/composer/tags.js?v=no4c6ta2ksc","rel":"prefetch","href":"/assets/src/modules/composer/categoryList.js?v=no4c6ta2ksc","rel":"prefetch","href":"/assets/src/modules/composer/resize.js?v=no4c6ta2ksc","rel":"prefetch","href":"/assets/src/modules/composer/autocomplete.js?v=no4c6ta2ksc","rel":"prefetch","href":"/assets/templates/composer.tpl?v=no4c6ta2ksc","rel":"prefetch","href":"/assets/language/en-US/topic.json?v=no4c6ta2ksc","rel":"prefetch","href":"/assets/language/en-US/modules.json?v=no4c6ta2ksc","rel":"prefetch","href":"/assets/language/en-US/tags.json?v=no4c6ta2ksc","rel":"prefetch stylesheet","type":"","href":"/plugins/nodebb-plugin-markdown/styles/railscasts.css","rel":"prefetch","href":"/assets/src/modules/highlight.js?v=no4c6ta2ksc","rel":"prefetch","href":"/assets/language/en-US/markdown.json?v=no4c6ta2ksc","rel":"canonical","href":" -and-crackling-in-bias-fx","rel":"alternate","type":"application/rss+xml","href":"/topic/1871.rss","rel":"up","href":" -guitar-software"],"widgets":,"_locals":"useragent":"isYaBrowser":false,"isAuthoritative":true,"isMobile":false,"isMobileNative":false,"isTablet":false,"isiPad":false,"isiPod":false,"isiPhone":false,"isiPhoneNative":false,"isAndroid":false,"isAndroidNative":false,"isBlackberry":false,"isOpera":false,"isIE":false,"isEdge":false,"isIECompatibilityMode":false,"isSafari":false,"isFirefox":false,"isWebkit":false,"isChrome":true,"isKonqueror":false,"isOmniWeb":false,"isSeaMonkey":false,"isFlock":false,"isAmaya":false,"isPhantomJS":false,"isEpiphany":false,"isDesktop":true,"isWindows":true,"isLinux":false,"isLinux64":false,"isMac":false,"isChromeOS":false,"isBada":false,"isSamsung":false,"isRaspberry":false,"isBot":false,"isCurl":false,"isAndroidTablet":false,"isWinJs":false,"isKindleFire":false,"isSilk":false,"isCaptive":false,"isSmartTV":false,"isUC":false,"isFacebook":false,"isAlamoFire":false,"isElectron":false,"silkAccelerated":false,"browser":"Chrome","version":"101.0.0.0","os":"Windows 10.0","platform":"Microsoft Windows","geoIp":,"source":"Mozilla/5.0 (Windows NT 10.0; Win64; x64) AppleWebKit/537.36 (KHTML, like Gecko) Chrome/101.0.0.0 Safari/537.36","isWechat":false,"renderHeader":true,"isAPI":false,"config": browserTitle","showSiteTitle":false,"minimumTitleLength":3,"maximumTitleLength":255,"minimumPostLength":8,"maximumPostLength":32767,"minimumTagsPerTopic":0,"maximumTagsPerTopic":5,"minimumTagLength":3,"maximumTagLength":15,"useOutgoingLinksPage":false,"allowGuestHandles":false,"allowTopicsThumbnail":false,"usePagination":true,"disableChat":false,"disableChatMessageEditing":false,"maximumChatMessageLength":1000,"socketioTransports":["polling","websocket"],"socketioOrigins":"*:*","websocketAddress":"","maxReconnectionAttempts":5,"reconnectionDelay":1500,"topicsPerPage":20,"postsPerPage":20,"maximumFileSize":2048,"theme:id":"nodebb-theme-persona","theme:src":"","defaultLang":"en-US","userLang":"en-US","loggedIn":false,"uid":0,"cache-buster":"v=no4c6ta2ksc","requireEmailConfirmation":false,"topicPostSort":"oldest_to_newest","categoryTopicSort":"newest_to_oldest","csrf_token":"lokgUgXR-2GAs7fGWDqqEsKY5mhiCm-jR-KQ","searchEnabled":true,"bootswatchSkin":"noskin","enablePostHistory":true,"timeagoCutoff":30,"timeagoCodes":["af","am","ar","az-short","az","be","bg","bs","ca","cs","cy","da","de-short","de","dv","el","en-short","en","es-short","es","et","eu","fa-short","fa","fi","fr-short","fr","gl","he","hr","hu","hy","id","is","it-short","it","ja","jv","ko","ky","lt","lv","mk","nl","no","pl","pt-br-short","pt-br","pt-short","pt","ro","rs","ru","rw","si","sk","sl","sq","sr","sv","th","tr-short","tr","uk","ur","uz","vi","zh-CN","zh-TW"],"cookies":"enabled":false,"message":"[[global:cookies.message]]","dismiss":"[[global:cookies.accept]]","link":"[[global:cookies.learn_more]]","link_url":" ","thumbs":"size":512,"acpLang":"en-US","topicSearchEnabled":false,"hideSubCategories":false,"hideCategoryLastPost":false,"enableQuickReply":false,"composer-default":,"markdown":"highlight":1,"highlightLinesLanguageList":[],"theme":"railscasts.css","spam-be-gone":,"metaTags":["name":"title","content":"Popping and crackling in Bias FX","name":"description","content":"Hello, I have just bought Bias FX, and there is loads of popping and cracking through my headphones. It does not happen in Bias Amp, just the FX, and it does also happen through the speakers, so its not the headphones. I wonder if anyone has any idea what...","property":"og:title","content":"Popping and crackling in Bias FX","property":"og:description","content":"Hello, I have just bought Bias FX, and there is loads of popping and cracking through my headphones. It does not happen in Bias Amp, just the FX, and it does also happen through the speakers, so its not the headphones. I wonder if anyone has any idea what...","property":"og:type","content":"article","property":"article:published_time","content":"2018-08-09T16:03:59.594Z","property":"article:modified_time","content":"2018-08-19T00:36:16.700Z","property":"article:section","content":"Desktop guitar software","property":"og:image","content":" -4.png","noEscape":true,"property":"og:image:url","content":" -4.png","noEscape":true,"property":"og:image","content":" =large","noEscape":true,"property":"og:image:url","content":" =large","noEscape":true],"linkTags":["rel":"canonical","href":" -and-crackling-in-bias-fx","rel":"alternate","type":"application/rss+xml","href":"/topic/1871.rss","rel":"up","href":" -guitar-software"],"template":"topic","scripts":["src":" "],"useCustomJS":1,"customJS":"+function replaceCategoriesParagraphToH1() \n var container = document.getElementById('content').querySelector('.category');\n var p = container.querySelector(':scope > p');\n var h1 = document.createElement(\"h1\"); \n h1.appendChild(document.createTextNode(p.innerText)); \n container.insertBefore(h1, p);\n container.removeChild(p);\n();","isSpider":false}×Looks like your connection to Positive Grid Community Forum was lost, please wait while we try to reconnect.
aaccfb2cb3
-
-
\ No newline at end of file
diff --git a/spaces/cihyFjudo/fairness-paper-search/Proteus 8 Portable The Best Solution for Circuit Design and Simulation.md b/spaces/cihyFjudo/fairness-paper-search/Proteus 8 Portable The Best Solution for Circuit Design and Simulation.md
deleted file mode 100644
index 28d65fc0fb575df24de8ecd4a5587cc90fa1eb0c..0000000000000000000000000000000000000000
--- a/spaces/cihyFjudo/fairness-paper-search/Proteus 8 Portable The Best Solution for Circuit Design and Simulation.md
+++ /dev/null
@@ -1,10 +0,0 @@
-
-
Blood flow measurement using Doppler ultrasound has become a useful tool for diagnosing cardiovascular diseases and as a physiological monitor. Recently, pocket-sized ultrasound scanners have been introduced for portable diagnosis. The present paper reports the implementation of a portable ultrasound pulsed-wave (PW) Doppler flowmeter using a smartphone. A 10-MHz ultrasonic surface transducer was designed for the dynamic monitoring of blood flow velocity. The directional baseband Doppler shift signals were obtained using a portable analog circuit system. After hardware processing, the Doppler signals were fed directly to a smartphone for Doppler spectrogram analysis and display in real time. To the best of our knowledge, this is the first report of the use of this system for medical ultrasound Doppler signal processing. A Couette flow phantom, consisting of two parallel disks with a 2-mm gap, was used to evaluate and calibrate the device. Doppler spectrograms of porcine blood flow were measured using this stand-alone portable device under the pulsatile condition. Subsequently, in vivo portable system verification was performed by measuring the arterial blood flow of a rat and comparing the results with the measurement from a commercial ultrasound duplex scanner. All of the results demonstrated the potential for using a smartphone as a novel embedded system for portable medical ultrasound applications.
I always love to drink chilled Coke. But when I go for a outing, no more any chance to get the chilled Coke.So I seriously wanted to have a portable mini refrigerator, so that I can carry, wherever I go.
-
Health insurance coverage for migrant workers who are U.S. citizens with Medicare transfers no matter the state in which a patient resides. But U.S. citizen workers with low incomes, and with Medicaid coverage, have limited access to health insurance while moving from state to state because Medicaid is not portable. Rather, the federal government partners with individual states, each of which has its own Medicaid rules for enrollment and portability.
-
AppNee provides the Proteus Professional Edition full installers, database installers, license key files and unlock patches, pre-activated setups, as well as portable full registered versions for Windows 32-bit & 64-bit.
-
-
Perhaps nowhere is the music blasting more quietly than in Silicon Valley, where tech workers with ears plugged into iPods or other portable music players are a common sight. Here in the valley that gave birth to the technology fueling the trend, music is endemic.
aaccfb2cb3
-
-
\ No newline at end of file
diff --git a/spaces/cihyFjudo/fairness-paper-search/Rappelz 138 Quest Line.md b/spaces/cihyFjudo/fairness-paper-search/Rappelz 138 Quest Line.md
deleted file mode 100644
index 6965e582ca1a7acf1911b327f468657f9e9320db..0000000000000000000000000000000000000000
--- a/spaces/cihyFjudo/fairness-paper-search/Rappelz 138 Quest Line.md
+++ /dev/null
@@ -1,18 +0,0 @@
-
-
Note: Reinke quest will not be cleared no matter how many times you complete it. This quest is bugged, after getting the next quest from Luci you will have nothing else to do with this dog ever again. Simply ignore the shining yellow pointer on his head. **Thanks to Seethe/thelunarsorcerer for reminding me of this**
4. Quest: History of the Land: Prelude of Blood Once activated, it will automatically port you to Charmer Jeina in Pyre site. Just talk to her to complete the quest and be teleported back to Luci. Talk to Luci again for next quest.
-
6. Quest: History of the Land: Witch Hunt Once activated, it will automatically port you to Desert Keeper Eleno in Ceriu Desert. Just talk to him to complete the quest and be teleported back to Luci. Talk to Luci again for next quest.
-
8. Quest: History of the Land: Fanatics Once activated, it will automatically port you to Elohim Siahpe in the Coast. Just talk to him to complete the quest and be teleported back to Luci. Talk to Luci again for next quest.
-
14. Quest: Fanatic Assassin: Rondo When you are done with the three cities. You will need to head to Rondo and speak to Guild Official Resha to get next quest. Again killing some more ambushers found north, south and east of rondo gates. Screenshot below only shows where I found the ambushers South of Rondo. Once completed go back to Resha and you will be then ask to proceed to Templar Headquarters.
-
-
17. Quest: Traces of the Witch: Sirag Ruins Instructor Bansky will now give you the next quest. You will be asked to to kill some mobs, I forgot the name. But they spawn in the middle of the ruins where there is a cross. Once completed, talk to Bansky again for next quest.
-
19. Quest: Traces of the Witch: Magic Test Ground Speak to Heingel to activate quest. You will be asked to kill some more fanatics. See screenshot below. Complete then speak to Heingel for next quest.
-
As soon you accept this quest you will be teleported to the Experimental Magic Field in Laksy. You gotta kill some Fanatics for Hector. The fanatics will spawn where-ever you are - every minute or so.
-
When you accept this quest you will be teleported to Sirag Ruins, and mobs will spawn just like at the Expirmental Field, ya know the drill, kill em then hit a return scroll and report back to Hector.
-
From now on AND UNTIL FURTHER NOTICE, you wont have to return to deliver the quests anywhere, just open the Quest window (press Q) and hit the "Complete" button, you will automatically get the next quest.
-
The Witch Quest Part 2 continues Beneath Templar Headquarters, this whole place is also called The Vault of Lies. Just complete the quest, and you should be lv 140 now! GZ!! You will (as used) get automatically the next quest.
-
Kill mobs there in the Mental Probing Chamber until one drops a Needle Fragment. If you fail to find the Needle Fragment within the allotted 10 minutes, merely give up the (failed) quest, find the crystal/feather item in you inventory, and double click it to regain the 2nd quest. If this doesn't work, try running around in the Vault of lies (nearby).
-
If you fail to kill Brighton's Dark Prophet (2nd boss in this room) within 14 minutes, merely give up the (failed) quest, find the crystal/feather item in you inventory, and double click it to regain the 2nd quest.
aaccfb2cb3
-
-
\ No newline at end of file
diff --git a/spaces/cihyFjudo/fairness-paper-search/The Expendables 4 Full Movie in Hindi Free Download 1035 Tips and Tricks for a Smooth Streaming Experience.md b/spaces/cihyFjudo/fairness-paper-search/The Expendables 4 Full Movie in Hindi Free Download 1035 Tips and Tricks for a Smooth Streaming Experience.md
deleted file mode 100644
index 21f9426ff9a7e4e2470bd09ca7654e01a009e616..0000000000000000000000000000000000000000
--- a/spaces/cihyFjudo/fairness-paper-search/The Expendables 4 Full Movie in Hindi Free Download 1035 Tips and Tricks for a Smooth Streaming Experience.md
+++ /dev/null
@@ -1,6 +0,0 @@
-
the expendables 4 full movie in hindi free download 1035
-
- aaccfb2cb3
-
-
-
diff --git a/spaces/cjayic/soft-vc-widowmaker/hifigan/__init__.py b/spaces/cjayic/soft-vc-widowmaker/hifigan/__init__.py
deleted file mode 100644
index e594d6628e9cb232b2a807234f8351339a3fe086..0000000000000000000000000000000000000000
--- a/spaces/cjayic/soft-vc-widowmaker/hifigan/__init__.py
+++ /dev/null
@@ -1 +0,0 @@
-from .generator import hifigan, hifigan_hubert_discrete, hifigan_hubert_soft
\ No newline at end of file
diff --git a/spaces/cloudtheboi/Lofi4All/.pythonlibs/lib/python3.10/site-packages/altair/utils/html.py b/spaces/cloudtheboi/Lofi4All/.pythonlibs/lib/python3.10/site-packages/altair/utils/html.py
deleted file mode 100644
index a7a29fc748cf0a72e9ccdcb66c38b79aa5d1ebba..0000000000000000000000000000000000000000
--- a/spaces/cloudtheboi/Lofi4All/.pythonlibs/lib/python3.10/site-packages/altair/utils/html.py
+++ /dev/null
@@ -1,314 +0,0 @@
-import json
-import jinja2
-
-
-HTML_TEMPLATE = jinja2.Template(
- """
-{%- if fullhtml -%}
-
-
-
-{%- endif %}
-
-{%- if not requirejs %}
-
- {%- if mode == 'vega-lite' %}
-
- {%- endif %}
-
-{%- endif %}
-{%- if fullhtml %}
-{%- if requirejs %}
-
-
-{%- endif %}
-
-
-{%- endif %}
-
-
-{%- if fullhtml %}
-
-
-{%- endif %}
-"""
-)
-
-
-HTML_TEMPLATE_UNIVERSAL = jinja2.Template(
- """
-
-
-
-"""
-)
-
-
-# This is like the HTML_TEMPLATE template, but includes vega javascript inline
-# so that the resulting file is not dependent on external resources. This was
-# ported over from altair_saver.
-#
-# implies requirejs=False and full_html=True
-INLINE_HTML_TEMPLATE = jinja2.Template(
- """\
-
-
-
-
-
-
-
-
-
-
-
-"""
-)
-
-
-TEMPLATES = {
- "standard": HTML_TEMPLATE,
- "universal": HTML_TEMPLATE_UNIVERSAL,
- "inline": INLINE_HTML_TEMPLATE,
-}
-
-
-def spec_to_html(
- spec,
- mode,
- vega_version,
- vegaembed_version,
- vegalite_version=None,
- base_url="https://cdn.jsdelivr.net/npm",
- output_div="vis",
- embed_options=None,
- json_kwds=None,
- fullhtml=True,
- requirejs=False,
- template="standard",
-):
- """Embed a Vega/Vega-Lite spec into an HTML page
-
- Parameters
- ----------
- spec : dict
- a dictionary representing a vega-lite plot spec.
- mode : string {'vega' | 'vega-lite'}
- The rendering mode. This value is overridden by embed_options['mode'],
- if it is present.
- vega_version : string
- For html output, the version of vega.js to use.
- vegalite_version : string
- For html output, the version of vegalite.js to use.
- vegaembed_version : string
- For html output, the version of vegaembed.js to use.
- base_url : string (optional)
- The base url from which to load the javascript libraries.
- output_div : string (optional)
- The id of the div element where the plot will be shown.
- embed_options : dict (optional)
- Dictionary of options to pass to the vega-embed script. Default
- entry is {'mode': mode}.
- json_kwds : dict (optional)
- Dictionary of keywords to pass to json.dumps().
- fullhtml : boolean (optional)
- If True (default) then return a full html page. If False, then return
- an HTML snippet that can be embedded into an HTML page.
- requirejs : boolean (optional)
- If False (default) then load libraries from base_url using
-
-
-
-
-
-
-
-
-
-
-
-
-
-
-
Loading…
-
█▒▒▒▒▒▒▒▒▒
-
-
-
This is a fork of Huggingface’s diffuse-the-rest, with the additional ability to change the strength, and other just miscellaneous tweaks.
The model is licensed with a CreativeML Open RAIL-M license. The authors claim no rights on the outputs you generate, you are free to use them and are accountable for their use which must not go against the provisions set in this license. The license forbids you from sharing any content that violates any laws, produce any harm to a person, disseminate any personal information that would be meant for harm, spread misinformation and target vulnerable groups. For the full list of restrictions please read the license
-
Biases and content acknowledgment
-
Despite how impressive being able to turn text into image is, beware to the fact that this model may output content that reinforces or exacerbates societal biases, as well as realistic faces, pornography and violence. The model was trained on the LAION-5B dataset, which scraped non-curated image-text-pairs from the internet (the exception being the removal of illegal content) and is meant for research purposes. You can read more in the model card