Commit
·
0deb4ff
1
Parent(s):
07be4fd
Update parquet files (step 25 of 476)
Browse filesThis view is limited to 50 files because it contains too many changes.
See raw diff
- spaces/1-13-am/neural-style-transfer/README.md +0 -12
- spaces/1acneusushi/gradio-2dmoleculeeditor/data/CASIO Classpad 3.0 [Emulator Crack] Serial Key Troubleshooting and Support for the Emulator.md +0 -83
- spaces/1gistliPinn/ChatGPT4/Examples/Activehome Pro LINK Download.md +0 -8
- spaces/1gistliPinn/ChatGPT4/Examples/Como Eliminar Archivos Duplicados En Tu PC [2020].md +0 -6
- spaces/801artistry/RVC801/infer/modules/vc/modules.py +0 -526
- spaces/AIConsultant/MusicGen/tests/common_utils/__init__.py +0 -9
- spaces/AIGC-Audio/AudioGPT/NeuralSeq/modules/GenerSpeech/model/generspeech.py +0 -260
- spaces/AIGC-Audio/AudioGPT/NeuralSeq/modules/diff/candidate_decoder.py +0 -96
- spaces/AIGC-Audio/AudioGPT/NeuralSeq/modules/parallel_wavegan/layers/upsample.py +0 -183
- spaces/AIGC-Audio/AudioGPT/NeuralSeq/tasks/svs/task.py +0 -84
- spaces/AIWaves/SOP_Generation-single/Action/__init__.py +0 -1
- spaces/AIWaves/SOP_Generation-single/Component/ToolComponent.py +0 -887
- spaces/ATang0729/Forecast4Muses/Model/Model6/Model6_1_ClothesKeyPoint/mmpose_1_x/configs/fashion_2d_keypoint/__init__.py +0 -0
- spaces/Abhilashvj/planogram-compliance/utils/loggers/wandb/__init__.py +0 -0
- spaces/AgentVerse/agentVerse/README.md +0 -429
- spaces/AgentVerse/agentVerse/ui/src/phaser3-rex-plugins/templates/ui/colorinput/colorinput/methods/OpenColorPicker.js +0 -53
- spaces/AgentVerse/agentVerse/ui/src/phaser3-rex-plugins/templates/ui/sides/childbehaviors/Visible.js +0 -21
- spaces/Androidonnxfork/CivitAi-to-Diffusers/diffusers/docs/source/en/optimization/tome.md +0 -116
- spaces/Androidonnxfork/CivitAi-to-Diffusers/diffusers/docs/source/ko/optimization/onnx.md +0 -65
- spaces/Androidonnxfork/CivitAi-to-Diffusers/diffusers/src/diffusers/utils/outputs.py +0 -108
- spaces/Andy1621/uniformer_image_detection/configs/fcos/fcos_r50_caffe_fpn_gn-head_4x4_1x_coco.py +0 -4
- spaces/AquaSuisei/ChatGPTXE/chatgpt - macOS.command +0 -7
- spaces/ArcanAlt/arcanDream/Dockerfile +0 -11
- spaces/Big-Web/MMSD/env/Lib/site-packages/dateutil/zoneinfo/__init__.py +0 -167
- spaces/Big-Web/MMSD/env/Lib/site-packages/setuptools/command/setopt.py +0 -149
- spaces/CVPR/LIVE/thrust/thrust/system/cuda/detail/reverse.h +0 -98
- spaces/CVPR/WALT/mmdet/models/detectors/cornernet.py +0 -95
- spaces/CVPR/WALT/mmdet/models/losses/iou_loss.py +0 -436
- spaces/CVPR/WALT/mmdet/models/roi_heads/cascade_roi_head.py +0 -507
- spaces/CVPR/WALT/mmdet/models/roi_heads/test_mixins.py +0 -368
- spaces/ChallengeHub/Chinese-LangChain/create_knowledge.py +0 -79
- spaces/Cvandi/remake/realesrgan/data/realesrgan_paired_dataset.py +0 -108
- spaces/DQChoi/gpt-demo/venv/lib/python3.11/site-packages/fontTools/misc/testTools.py +0 -229
- spaces/EuroPython2022/pulsar-clip/README.md +0 -13
- spaces/FrankZxShen/so-vits-svc-models-ba/diffusion/infer_gt_mel.py +0 -74
- spaces/GMFTBY/PandaGPT/model/ImageBind/__init__.py +0 -2
- spaces/GaenKoki/voicevox/speaker_info/388f246b-8c41-4ac1-8e2d-5d79f3ff56d9/policy.md +0 -3
- spaces/Gen-Sim/Gen-Sim/cliport/generated_tasks/block_on_cylinder_on_pallet.py +0 -58
- spaces/Gen-Sim/Gen-Sim/cliport/generated_tasks/color_cued_ball_corner_sorting.py +0 -62
- spaces/Gen-Sim/Gen-Sim/cliport/generated_tasks/color_ordered_insertion_new.py +0 -52
- spaces/Gen-Sim/Gen-Sim/cliport/generated_tasks/move_piles_along_line.py +0 -70
- spaces/Gradio-Blocks/uniformer_image_detection/mmdet/core/bbox/coder/tblr_bbox_coder.py +0 -198
- spaces/Gradio-Blocks/uniformer_image_detection/mmdet/models/losses/__init__.py +0 -29
- spaces/Gradio-Blocks/uniformer_image_segmentation/configs/deeplabv3/deeplabv3_r50-d8_480x480_40k_pascal_context.py +0 -10
- spaces/Gradio-Blocks/uniformer_image_segmentation/configs/fcn/fcn_d6_r50-d16_512x1024_40k_cityscapes.py +0 -8
- spaces/Gradio-Blocks/uniformer_image_segmentation/configs/nonlocal_net/nonlocal_r101-d8_512x512_40k_voc12aug.py +0 -2
- spaces/Gradio-Blocks/uniformer_image_segmentation/configs/resnest/fcn_s101-d8_512x512_160k_ade20k.py +0 -9
- spaces/GrandaddyShmax/AudioCraft_Plus/audiocraft/losses/sisnr.py +0 -92
- spaces/HLasse/textdescriptives/data_viewer.py +0 -26
- spaces/HaHaBill/LandShapes-Antarctica/netdissect/dissect.html +0 -399
spaces/1-13-am/neural-style-transfer/README.md
DELETED
@@ -1,12 +0,0 @@
|
|
1 |
-
---
|
2 |
-
title: Neural Style Transfer
|
3 |
-
emoji: 🦀
|
4 |
-
colorFrom: pink
|
5 |
-
colorTo: gray
|
6 |
-
sdk: gradio
|
7 |
-
sdk_version: 3.46.0
|
8 |
-
app_file: app.py
|
9 |
-
pinned: false
|
10 |
-
---
|
11 |
-
|
12 |
-
Check out the configuration reference at https://huggingface.co/docs/hub/spaces-config-reference
|
|
|
|
|
|
|
|
|
|
|
|
|
|
|
|
|
|
|
|
|
|
|
|
|
|
spaces/1acneusushi/gradio-2dmoleculeeditor/data/CASIO Classpad 3.0 [Emulator Crack] Serial Key Troubleshooting and Support for the Emulator.md
DELETED
@@ -1,83 +0,0 @@
|
|
1 |
-
|
2 |
-
<h1>CASIO Classpad 3.0 [Emulator Crack] Serial Key</h1>
|
3 |
-
<p>Are you looking for a way to use CASIO Classpad 3.0 on your PC without buying the original calculator? Do you want to enjoy the features and benefits of CASIO Classpad 3.0 without spending a lot of money? If yes, then you might be interested in using an emulator with a crack and serial key.</p>
|
4 |
-
<h2>CASIO Classpad 3.0 [Emulator Crack] Serial Key</h2><br /><p><b><b>Download File</b> ——— <a href="https://byltly.com/2uKvRP">https://byltly.com/2uKvRP</a></b></p><br /><br />
|
5 |
-
<p>An emulator is a software that simulates the functions and features of another device or system on your PC. A crack is a file that modifies or bypasses the security features of a software to make it work without limitations or restrictions. A serial key is a code that activates or registers a software to make it valid or authentic.</p>
|
6 |
-
<p>In this article, we will explain what CASIO Classpad 3.0 is, what an emulator is, why you need an emulator for CASIO Classpad 3.0, how to get an emulator for CASIO Classpad 3.0, how to use an emulator for CASIO Classpad 3.0, how to get a crack and serial key for CASIO Classpad 3.0 emulator, how to use a crack and serial key for CASIO Classpad 3.0 emulator, what are the risks of using a crack and serial key for CASIO Classpad 3.0 emulator, how to avoid or solve the problems of using a crack and serial key for CASIO Classpad 3.0 emulator, and what are the alternatives to using a crack and serial key for CASIO Classpad 3.0 emulator.</p>
|
7 |
-
<p>By the end of this article, you will have a clear understanding of how to use CASIO Classpad 3.0 [Emulator Crack] Serial Key on your PC.</p>
|
8 |
-
<p>How to get CASIO Classpad 3.0 emulator crack for free<br />
|
9 |
-
CASIO Classpad 3.0 emulator crack download link<br />
|
10 |
-
CASIO Classpad 3.0 emulator crack activation code<br />
|
11 |
-
CASIO Classpad 3.0 emulator crack full version<br />
|
12 |
-
CASIO Classpad 3.0 emulator crack license key<br />
|
13 |
-
CASIO Classpad 3.0 emulator crack torrent<br />
|
14 |
-
CASIO Classpad 3.0 emulator crack patch<br />
|
15 |
-
CASIO Classpad 3.0 emulator crack keygen<br />
|
16 |
-
CASIO Classpad 3.0 emulator crack registration key<br />
|
17 |
-
CASIO Classpad 3.0 emulator crack product key<br />
|
18 |
-
CASIO Classpad 3.0 emulator crack software<br />
|
19 |
-
CASIO Classpad 3.0 emulator crack online<br />
|
20 |
-
CASIO Classpad 3.0 emulator crack generator<br />
|
21 |
-
CASIO Classpad 3.0 emulator crack no survey<br />
|
22 |
-
CASIO Classpad 3.0 emulator crack working<br />
|
23 |
-
CASIO Classpad 3.0 emulator crack latest<br />
|
24 |
-
CASIO Classpad 3.0 emulator crack updated<br />
|
25 |
-
CASIO Classpad 3.0 emulator crack review<br />
|
26 |
-
CASIO Classpad 3.0 emulator crack tutorial<br />
|
27 |
-
CASIO Classpad 3.0 emulator crack guide<br />
|
28 |
-
CASIO Classpad 3.0 emulator crack instructions<br />
|
29 |
-
CASIO Classpad 3.0 emulator crack tips<br />
|
30 |
-
CASIO Classpad 3.0 emulator crack tricks<br />
|
31 |
-
CASIO Classpad 3.0 emulator crack hacks<br />
|
32 |
-
CASIO Classpad 3.0 emulator crack cheats<br />
|
33 |
-
CASIO Classpad 3.0 emulator crack features<br />
|
34 |
-
CASIO Classpad 3.0 emulator crack benefits<br />
|
35 |
-
CASIO Classpad 3.0 emulator crack advantages<br />
|
36 |
-
CASIO Classpad 3.0 emulator crack disadvantages<br />
|
37 |
-
CASIO Classpad 3.0 emulator crack pros and cons<br />
|
38 |
-
CASIO Classpad 3.0 emulator crack comparison<br />
|
39 |
-
CASIO Classpad 3.0 emulator crack alternatives<br />
|
40 |
-
CASIO Classpad 3.0 emulator crack best practices<br />
|
41 |
-
CASIO Classpad 3.0 emulator crack requirements<br />
|
42 |
-
CASIO Classpad 3.0 emulator crack specifications<br />
|
43 |
-
CASIO Classpad 3.0 emulator crack system requirements<br />
|
44 |
-
CASIO Classpad 3.0 emulator crack compatibility<br />
|
45 |
-
CASIO Classpad 3.0 emulator crack support<br />
|
46 |
-
CASIO Classpad 3.0 emulator crack customer service<br />
|
47 |
-
CASIO Classpad 3.0 emulator crack feedback<br />
|
48 |
-
CASIO Classpad 3.0 emulator crack testimonials<br />
|
49 |
-
CASIO Classpad 3.0 emulator crack ratings<br />
|
50 |
-
CASIO Classpad 3.0 emulator crack quality<br />
|
51 |
-
CASIO Classpad 3.0 emulator crack performance<br />
|
52 |
-
CASIO Classpad 3.0 emulator crack reliability<br />
|
53 |
-
CASIO Classpad 3.0 emulator crack security<br />
|
54 |
-
CASIO Classpad 3.0 emulator crack privacy<br />
|
55 |
-
CASIO Classpad 3.0 emulator crack warranty<br />
|
56 |
-
CASIO Classpad 3.0 emulator crack refund policy<br />
|
57 |
-
CASIO Classpad 3.0 emulator crack discount code</p>
|
58 |
-
<h2>What is CASIO Classpad 3.0?</h2>
|
59 |
-
<p>CASIO Classpad 3.0 is a powerful software that simulates the functions and features of the CASIO Classpad 330 calculator on your PC. You can use it for learning, teaching, or doing complex calculations with ease.</p>
|
60 |
-
<p>Some of the features and benefits of CASIO Classpad 3.0 are:</p>
|
61 |
-
<ul>
|
62 |
-
<li>It has a large touch-screen display that allows you to input data, draw graphs, edit formulas, manipulate images, etc.</li>
|
63 |
-
<li>It supports various mathematical functions such as algebra, calculus, geometry, statistics, probability, etc.</li>
|
64 |
-
<li>It has a built-in spreadsheet application that allows you to perform data analysis, create charts, etc.</li>
|
65 |
-
<li>It has a built-in eActivity application that allows you to create interactive worksheets, presentations, quizzes, etc.</li>
|
66 |
-
<li>It has a built-in geometry application that allows you to construct geometric figures, measure angles, lengths, areas, etc.</li>
|
67 |
-
<li>It has a built-in programming language that allows you to create custom applications, games, etc.</li>
|
68 |
-
<li>It has a built-in communication function that allows you to connect with other devices via USB or wireless LAN.</li>
|
69 |
-
<li>It has a built-in memory function that allows you to store data, formulas, images, etc.</li>
|
70 |
-
</ul>
|
71 |
-
<h2>What is an emulator?</h2>
|
72 |
-
<p>An emulator is a software that simulates the functions and features of another device or system on your PC. For example, you can use an emulator to play games designed for consoles such as PlayStation or Nintendo on your PC.</p>
|
73 |
-
<p>There are different types of emulators depending on the device or system they emulate. Some examples are:</p>
|
74 |
-
<ul>
|
75 |
-
<li>Console emulators: They emulate video game consoles such as PlayStation, Nintendo, Sega, etc.</li>
|
76 |
-
<li>Arcade emulators: They emulate arcade machines such as Pac-Man, Street Fighter, etc.</li>
|
77 |
-
<li>Computer emulators: They emulate personal computers such as Windows, Mac OS X, Linux, etc.</li>
|
78 |
-
<li>Mobile emulators: They emulate mobile devices such as Android, iOS, Windows Phone, etc.</li>
|
79 |
-
<li>Calculator emulators: They emulate calculators such as TI-83, HP-12C, CASIO ClassPad, etc.</li>
|
80 |
-
</ul>
|
81 |
-
<h2>Why do you need an emulator for CASIO ClassPad</p> 0a6ba089eb<br />
|
82 |
-
<br />
|
83 |
-
<br />
|
|
|
|
|
|
|
|
|
|
|
|
|
|
|
|
|
|
|
|
|
|
|
|
|
|
|
|
|
|
|
|
|
|
|
|
|
|
|
|
|
|
|
|
|
|
|
|
|
|
|
|
|
|
|
|
|
|
|
|
|
|
|
|
|
|
|
|
|
|
|
|
|
|
|
|
|
|
|
|
|
|
|
|
|
|
|
|
|
|
|
|
|
|
|
|
|
|
|
|
|
|
|
|
|
|
|
|
|
|
|
|
|
|
|
|
|
|
|
|
|
|
|
|
|
|
|
|
|
|
|
|
|
|
|
|
|
|
|
|
|
|
|
|
|
|
|
|
|
|
|
|
|
|
|
|
|
|
|
|
|
|
|
|
|
|
|
|
spaces/1gistliPinn/ChatGPT4/Examples/Activehome Pro LINK Download.md
DELETED
@@ -1,8 +0,0 @@
|
|
1 |
-
<br />
|
2 |
-
<p>activehome pro is a powerful home automation system that allows you to control all sorts of devices, such as lights, locks, audio systems, and other appliances and devices. it also allows you to interact with your home through the web. it is a very powerful home automation system.</p>
|
3 |
-
<p>activehome pro has a versatile application programming interface (api) that lets you integrate activehome pro with other systems. api support for activehome pro includes: </p>
|
4 |
-
<h2>activehome pro download</h2><br /><p><b><b>Download</b> 🆗 <a href="https://imgfil.com/2uxZ0O">https://imgfil.com/2uxZ0O</a></b></p><br /><br /> <ul> <li>simple integration into a light switch</li> <li>remote access with the activehome live api</li> <li>remote access with the activehome api</li> <li>local and remote event-based triggering using the activehome device api</li> <li>configuration and operation of devices and appliances using the activehome device api</li> </ul>
|
5 |
-
<p>activehome acts as a central monitoring station for your home. it monitors the status of your lights and appliances and sends you alerts when it detects activity. activehome also monitors activity and status to help you find and resolve service calls. in addition to monitoring device status, activehome also reports the power consumption of each device to help you manage your energy consumption.</p>
|
6 |
-
<p>activehome pro will ensure that your lights and appliances are always off. however, you can set a schedule so that when no one is home, activehome will turn lights and appliances on. in addition, activehome pro keeps track of any malfunctions so that if a light or appliance is not working, you will know exactly where to find the problem. you can schedule activehome to turn lights and appliances on when you are away from home, and so that lights and appliances that are already on will turn off when you are away.</p> 899543212b<br />
|
7 |
-
<br />
|
8 |
-
<br />
|
|
|
|
|
|
|
|
|
|
|
|
|
|
|
|
|
|
spaces/1gistliPinn/ChatGPT4/Examples/Como Eliminar Archivos Duplicados En Tu PC [2020].md
DELETED
@@ -1,6 +0,0 @@
|
|
1 |
-
<h2>Como Eliminar Archivos Duplicados en Tu PC [2020]</h2><br /><p><b><b>Download File</b> 🌟 <a href="https://imgfil.com/2uy16g">https://imgfil.com/2uy16g</a></b></p><br /><br />
|
2 |
-
|
3 |
-
el cambio aplicado en la version 16 de tu, se debe a una depreciación para no tener que cambiar su código, lo que significa que ya no se va a tener que actualizar los códigos existentes para que funcione con la versión nueva.[2020] en la version 17 para tu lo haría con un error ya que no eliminaba los archivos duplicados, el cambio aplicado en la version 18 para tu lo haría con un error ya que no eliminaba los archivos duplicados, el cambio aplicado en la versión 19 para tu lo haría con un error ya que no eliminaba los archivos duplicados, el cambio aplicado en la versión 20 para tu lo haría con un error ya que no eliminaba los archivos duplicados, el cambio aplicado en la versión 21 para tu lo haría con un error ya que no eliminaba los archivos duplicados, el cambio aplicado en la versión 22 para tu lo haría con un error ya que no eliminaba los archivos duplicados, el cambio aplicado en la versión 23 para tu lo haría con un error ya que no eliminaba los archivos duplicados, el cambio aplicado en la versión 24 para tu lo haría con un error ya que no eliminaba los archivos duplicados, el cambio aplicado en la versión 25 para tu lo haría con un error ya que no eliminaba los archivos duplicados, el cambio aplicado en la versión 26 para tu lo haría con un error ya que no eliminaba los archivos duplicados, el cambio aplicado en la versión 27 para tu lo haría con un error ya que no eliminaba los archivos duplicados, el cambio aplicado en la versión 28 para tu lo haría con un error ya que no eliminaba los archivos duplicados, el cambio aplicado en la versión 29 4fefd39f24<br />
|
4 |
-
<br />
|
5 |
-
<br />
|
6 |
-
<p></p>
|
|
|
|
|
|
|
|
|
|
|
|
|
|
spaces/801artistry/RVC801/infer/modules/vc/modules.py
DELETED
@@ -1,526 +0,0 @@
|
|
1 |
-
import os, sys
|
2 |
-
import traceback
|
3 |
-
import logging
|
4 |
-
now_dir = os.getcwd()
|
5 |
-
sys.path.append(now_dir)
|
6 |
-
logger = logging.getLogger(__name__)
|
7 |
-
import lib.globals.globals as rvc_globals
|
8 |
-
import numpy as np
|
9 |
-
import soundfile as sf
|
10 |
-
import torch
|
11 |
-
from io import BytesIO
|
12 |
-
from infer.lib.audio import load_audio
|
13 |
-
from infer.lib.audio import wav2
|
14 |
-
from infer.lib.infer_pack.models import (
|
15 |
-
SynthesizerTrnMs256NSFsid,
|
16 |
-
SynthesizerTrnMs256NSFsid_nono,
|
17 |
-
SynthesizerTrnMs768NSFsid,
|
18 |
-
SynthesizerTrnMs768NSFsid_nono,
|
19 |
-
)
|
20 |
-
from infer.modules.vc.pipeline import Pipeline
|
21 |
-
from infer.modules.vc.utils import *
|
22 |
-
import time
|
23 |
-
import scipy.io.wavfile as wavfile
|
24 |
-
|
25 |
-
def note_to_hz(note_name):
|
26 |
-
SEMITONES = {'C': -9, 'C#': -8, 'D': -7, 'D#': -6, 'E': -5, 'F': -4, 'F#': -3, 'G': -2, 'G#': -1, 'A': 0, 'A#': 1, 'B': 2}
|
27 |
-
pitch_class, octave = note_name[:-1], int(note_name[-1])
|
28 |
-
semitone = SEMITONES[pitch_class]
|
29 |
-
note_number = 12 * (octave - 4) + semitone
|
30 |
-
frequency = 440.0 * (2.0 ** (1.0/12)) ** note_number
|
31 |
-
return frequency
|
32 |
-
|
33 |
-
class VC:
|
34 |
-
def __init__(self, config):
|
35 |
-
self.n_spk = None
|
36 |
-
self.tgt_sr = None
|
37 |
-
self.net_g = None
|
38 |
-
self.pipeline = None
|
39 |
-
self.cpt = None
|
40 |
-
self.version = None
|
41 |
-
self.if_f0 = None
|
42 |
-
self.version = None
|
43 |
-
self.hubert_model = None
|
44 |
-
|
45 |
-
self.config = config
|
46 |
-
|
47 |
-
def get_vc(self, sid, *to_return_protect):
|
48 |
-
logger.info("Get sid: " + sid)
|
49 |
-
|
50 |
-
to_return_protect0 = {
|
51 |
-
"visible": self.if_f0 != 0,
|
52 |
-
"value": to_return_protect[0]
|
53 |
-
if self.if_f0 != 0 and to_return_protect
|
54 |
-
else 0.5,
|
55 |
-
"__type__": "update",
|
56 |
-
}
|
57 |
-
to_return_protect1 = {
|
58 |
-
"visible": self.if_f0 != 0,
|
59 |
-
"value": to_return_protect[1]
|
60 |
-
if self.if_f0 != 0 and to_return_protect
|
61 |
-
else 0.33,
|
62 |
-
"__type__": "update",
|
63 |
-
}
|
64 |
-
|
65 |
-
if not sid:
|
66 |
-
if self.hubert_model is not None: # 考虑到轮询, 需要加个判断看是否 sid 是由有模型切换到无模型的
|
67 |
-
logger.info("Clean model cache")
|
68 |
-
del (
|
69 |
-
self.net_g,
|
70 |
-
self.n_spk,
|
71 |
-
self.vc,
|
72 |
-
self.hubert_model,
|
73 |
-
self.tgt_sr,
|
74 |
-
) # ,cpt
|
75 |
-
self.hubert_model = (
|
76 |
-
self.net_g
|
77 |
-
) = self.n_spk = self.vc = self.hubert_model = self.tgt_sr = None
|
78 |
-
if torch.cuda.is_available():
|
79 |
-
torch.cuda.empty_cache()
|
80 |
-
###楼下不这么折腾清理不干净
|
81 |
-
self.if_f0 = self.cpt.get("f0", 1)
|
82 |
-
self.version = self.cpt.get("version", "v1")
|
83 |
-
if self.version == "v1":
|
84 |
-
if self.if_f0 == 1:
|
85 |
-
self.net_g = SynthesizerTrnMs256NSFsid(
|
86 |
-
*self.cpt["config"], is_half=self.config.is_half
|
87 |
-
)
|
88 |
-
else:
|
89 |
-
self.net_g = SynthesizerTrnMs256NSFsid_nono(*self.cpt["config"])
|
90 |
-
elif self.version == "v2":
|
91 |
-
if self.if_f0 == 1:
|
92 |
-
self.net_g = SynthesizerTrnMs768NSFsid(
|
93 |
-
*self.cpt["config"], is_half=self.config.is_half
|
94 |
-
)
|
95 |
-
else:
|
96 |
-
self.net_g = SynthesizerTrnMs768NSFsid_nono(*self.cpt["config"])
|
97 |
-
del self.net_g, self.cpt
|
98 |
-
if torch.cuda.is_available():
|
99 |
-
torch.cuda.empty_cache()
|
100 |
-
return (
|
101 |
-
{"visible": False, "__type__": "update"},
|
102 |
-
{
|
103 |
-
"visible": True,
|
104 |
-
"value": to_return_protect0,
|
105 |
-
"__type__": "update",
|
106 |
-
},
|
107 |
-
{
|
108 |
-
"visible": True,
|
109 |
-
"value": to_return_protect1,
|
110 |
-
"__type__": "update",
|
111 |
-
},
|
112 |
-
"",
|
113 |
-
"",
|
114 |
-
)
|
115 |
-
#person = f'{os.getenv("weight_root")}/{sid}'
|
116 |
-
person = f'{sid}'
|
117 |
-
#logger.info(f"Loading: {person}")
|
118 |
-
logger.info(f"Loading...")
|
119 |
-
self.cpt = torch.load(person, map_location="cpu")
|
120 |
-
self.tgt_sr = self.cpt["config"][-1]
|
121 |
-
self.cpt["config"][-3] = self.cpt["weight"]["emb_g.weight"].shape[0] # n_spk
|
122 |
-
self.if_f0 = self.cpt.get("f0", 1)
|
123 |
-
self.version = self.cpt.get("version", "v1")
|
124 |
-
|
125 |
-
synthesizer_class = {
|
126 |
-
("v1", 1): SynthesizerTrnMs256NSFsid,
|
127 |
-
("v1", 0): SynthesizerTrnMs256NSFsid_nono,
|
128 |
-
("v2", 1): SynthesizerTrnMs768NSFsid,
|
129 |
-
("v2", 0): SynthesizerTrnMs768NSFsid_nono,
|
130 |
-
}
|
131 |
-
|
132 |
-
self.net_g = synthesizer_class.get(
|
133 |
-
(self.version, self.if_f0), SynthesizerTrnMs256NSFsid
|
134 |
-
)(*self.cpt["config"], is_half=self.config.is_half)
|
135 |
-
|
136 |
-
del self.net_g.enc_q
|
137 |
-
|
138 |
-
self.net_g.load_state_dict(self.cpt["weight"], strict=False)
|
139 |
-
self.net_g.eval().to(self.config.device)
|
140 |
-
if self.config.is_half:
|
141 |
-
self.net_g = self.net_g.half()
|
142 |
-
else:
|
143 |
-
self.net_g = self.net_g.float()
|
144 |
-
|
145 |
-
self.pipeline = Pipeline(self.tgt_sr, self.config)
|
146 |
-
n_spk = self.cpt["config"][-3]
|
147 |
-
index = {"value": get_index_path_from_model(sid), "__type__": "update"}
|
148 |
-
logger.info("Select index: " + index["value"])
|
149 |
-
|
150 |
-
return (
|
151 |
-
(
|
152 |
-
{"visible": False, "maximum": n_spk, "__type__": "update"},
|
153 |
-
to_return_protect0,
|
154 |
-
to_return_protect1
|
155 |
-
)
|
156 |
-
if to_return_protect
|
157 |
-
else {"visible": False, "maximum": n_spk, "__type__": "update"}
|
158 |
-
)
|
159 |
-
|
160 |
-
|
161 |
-
def vc_single(
|
162 |
-
self,
|
163 |
-
sid,
|
164 |
-
input_audio_path0,
|
165 |
-
input_audio_path1,
|
166 |
-
f0_up_key,
|
167 |
-
f0_file,
|
168 |
-
f0_method,
|
169 |
-
file_index,
|
170 |
-
file_index2,
|
171 |
-
index_rate,
|
172 |
-
filter_radius,
|
173 |
-
resample_sr,
|
174 |
-
rms_mix_rate,
|
175 |
-
protect,
|
176 |
-
crepe_hop_length,
|
177 |
-
f0_min,
|
178 |
-
note_min,
|
179 |
-
f0_max,
|
180 |
-
note_max,
|
181 |
-
f0_autotune,
|
182 |
-
):
|
183 |
-
global total_time
|
184 |
-
total_time = 0
|
185 |
-
start_time = time.time()
|
186 |
-
if not input_audio_path0 and not input_audio_path1:
|
187 |
-
return "You need to upload an audio", None
|
188 |
-
|
189 |
-
if (not os.path.exists(input_audio_path0)) and (not os.path.exists(os.path.join(now_dir, input_audio_path0))):
|
190 |
-
return "Audio was not properly selected or doesn't exist", None
|
191 |
-
|
192 |
-
input_audio_path1 = input_audio_path1 or input_audio_path0
|
193 |
-
print(f"\nStarting inference for '{os.path.basename(input_audio_path1)}'")
|
194 |
-
print("-------------------")
|
195 |
-
f0_up_key = int(f0_up_key)
|
196 |
-
if rvc_globals.NotesOrHertz and f0_method != 'rmvpe':
|
197 |
-
f0_min = note_to_hz(note_min) if note_min else 50
|
198 |
-
f0_max = note_to_hz(note_max) if note_max else 1100
|
199 |
-
print(f"Converted Min pitch: freq - {f0_min}\n"
|
200 |
-
f"Converted Max pitch: freq - {f0_max}")
|
201 |
-
else:
|
202 |
-
f0_min = f0_min or 50
|
203 |
-
f0_max = f0_max or 1100
|
204 |
-
try:
|
205 |
-
input_audio_path1 = input_audio_path1 or input_audio_path0
|
206 |
-
print(f"Attempting to load {input_audio_path1}....")
|
207 |
-
audio = load_audio(file=input_audio_path1,
|
208 |
-
sr=16000,
|
209 |
-
DoFormant=rvc_globals.DoFormant,
|
210 |
-
Quefrency=rvc_globals.Quefrency,
|
211 |
-
Timbre=rvc_globals.Timbre)
|
212 |
-
|
213 |
-
audio_max = np.abs(audio).max() / 0.95
|
214 |
-
if audio_max > 1:
|
215 |
-
audio /= audio_max
|
216 |
-
times = [0, 0, 0]
|
217 |
-
|
218 |
-
if self.hubert_model is None:
|
219 |
-
self.hubert_model = load_hubert(self.config)
|
220 |
-
|
221 |
-
try:
|
222 |
-
self.if_f0 = self.cpt.get("f0", 1)
|
223 |
-
except NameError:
|
224 |
-
message = "Model was not properly selected"
|
225 |
-
print(message)
|
226 |
-
return message, None
|
227 |
-
|
228 |
-
file_index = (
|
229 |
-
(
|
230 |
-
file_index.strip(" ")
|
231 |
-
.strip('"')
|
232 |
-
.strip("\n")
|
233 |
-
.strip('"')
|
234 |
-
.strip(" ")
|
235 |
-
.replace("trained", "added")
|
236 |
-
)
|
237 |
-
if file_index != ""
|
238 |
-
else file_index2
|
239 |
-
) # 防止小白写错,自动帮他替换掉
|
240 |
-
|
241 |
-
try:
|
242 |
-
audio_opt = self.pipeline.pipeline(
|
243 |
-
self.hubert_model,
|
244 |
-
self.net_g,
|
245 |
-
sid,
|
246 |
-
audio,
|
247 |
-
input_audio_path1,
|
248 |
-
times,
|
249 |
-
f0_up_key,
|
250 |
-
f0_method,
|
251 |
-
file_index,
|
252 |
-
index_rate,
|
253 |
-
self.if_f0,
|
254 |
-
filter_radius,
|
255 |
-
self.tgt_sr,
|
256 |
-
resample_sr,
|
257 |
-
rms_mix_rate,
|
258 |
-
self.version,
|
259 |
-
protect,
|
260 |
-
crepe_hop_length,
|
261 |
-
f0_autotune,
|
262 |
-
f0_file=f0_file,
|
263 |
-
f0_min=f0_min,
|
264 |
-
f0_max=f0_max
|
265 |
-
)
|
266 |
-
except AssertionError:
|
267 |
-
message = "Mismatching index version detected (v1 with v2, or v2 with v1)."
|
268 |
-
print(message)
|
269 |
-
return message, None
|
270 |
-
except NameError:
|
271 |
-
message = "RVC libraries are still loading. Please try again in a few seconds."
|
272 |
-
print(message)
|
273 |
-
return message, None
|
274 |
-
|
275 |
-
if self.tgt_sr != resample_sr >= 16000:
|
276 |
-
self.tgt_sr = resample_sr
|
277 |
-
index_info = (
|
278 |
-
"Index:\n%s." % file_index
|
279 |
-
if os.path.exists(file_index)
|
280 |
-
else "Index not used."
|
281 |
-
)
|
282 |
-
end_time = time.time()
|
283 |
-
total_time = end_time - start_time
|
284 |
-
|
285 |
-
output_folder = "audio-outputs"
|
286 |
-
os.makedirs(output_folder, exist_ok=True)
|
287 |
-
output_filename = "generated_audio_{}.wav"
|
288 |
-
output_count = 1
|
289 |
-
while True:
|
290 |
-
current_output_path = os.path.join(output_folder, output_filename.format(output_count))
|
291 |
-
if not os.path.exists(current_output_path):
|
292 |
-
break
|
293 |
-
output_count += 1
|
294 |
-
|
295 |
-
wavfile.write(current_output_path, self.tgt_sr, audio_opt)
|
296 |
-
print(f"Generated audio saved to: {current_output_path}")
|
297 |
-
return f"Success.\n {index_info}\nTime:\n npy:{times[0]}, f0:{times[1]}, infer:{times[2]}\nTotal Time: {total_time} seconds", (self.tgt_sr, audio_opt)
|
298 |
-
except:
|
299 |
-
info = traceback.format_exc()
|
300 |
-
logger.warn(info)
|
301 |
-
return info, (None, None)
|
302 |
-
|
303 |
-
def vc_single_dont_save(
|
304 |
-
self,
|
305 |
-
sid,
|
306 |
-
input_audio_path0,
|
307 |
-
input_audio_path1,
|
308 |
-
f0_up_key,
|
309 |
-
f0_file,
|
310 |
-
f0_method,
|
311 |
-
file_index,
|
312 |
-
file_index2,
|
313 |
-
index_rate,
|
314 |
-
filter_radius,
|
315 |
-
resample_sr,
|
316 |
-
rms_mix_rate,
|
317 |
-
protect,
|
318 |
-
crepe_hop_length,
|
319 |
-
f0_min,
|
320 |
-
note_min,
|
321 |
-
f0_max,
|
322 |
-
note_max,
|
323 |
-
f0_autotune,
|
324 |
-
):
|
325 |
-
global total_time
|
326 |
-
total_time = 0
|
327 |
-
start_time = time.time()
|
328 |
-
if not input_audio_path0 and not input_audio_path1:
|
329 |
-
return "You need to upload an audio", None
|
330 |
-
|
331 |
-
if (not os.path.exists(input_audio_path0)) and (not os.path.exists(os.path.join(now_dir, input_audio_path0))):
|
332 |
-
return "Audio was not properly selected or doesn't exist", None
|
333 |
-
|
334 |
-
input_audio_path1 = input_audio_path1 or input_audio_path0
|
335 |
-
print(f"\nStarting inference for '{os.path.basename(input_audio_path1)}'")
|
336 |
-
print("-------------------")
|
337 |
-
f0_up_key = int(f0_up_key)
|
338 |
-
if rvc_globals.NotesOrHertz and f0_method != 'rmvpe':
|
339 |
-
f0_min = note_to_hz(note_min) if note_min else 50
|
340 |
-
f0_max = note_to_hz(note_max) if note_max else 1100
|
341 |
-
print(f"Converted Min pitch: freq - {f0_min}\n"
|
342 |
-
f"Converted Max pitch: freq - {f0_max}")
|
343 |
-
else:
|
344 |
-
f0_min = f0_min or 50
|
345 |
-
f0_max = f0_max or 1100
|
346 |
-
try:
|
347 |
-
input_audio_path1 = input_audio_path1 or input_audio_path0
|
348 |
-
print(f"Attempting to load {input_audio_path1}....")
|
349 |
-
audio = load_audio(file=input_audio_path1,
|
350 |
-
sr=16000,
|
351 |
-
DoFormant=rvc_globals.DoFormant,
|
352 |
-
Quefrency=rvc_globals.Quefrency,
|
353 |
-
Timbre=rvc_globals.Timbre)
|
354 |
-
|
355 |
-
audio_max = np.abs(audio).max() / 0.95
|
356 |
-
if audio_max > 1:
|
357 |
-
audio /= audio_max
|
358 |
-
times = [0, 0, 0]
|
359 |
-
|
360 |
-
if self.hubert_model is None:
|
361 |
-
self.hubert_model = load_hubert(self.config)
|
362 |
-
|
363 |
-
try:
|
364 |
-
self.if_f0 = self.cpt.get("f0", 1)
|
365 |
-
except NameError:
|
366 |
-
message = "Model was not properly selected"
|
367 |
-
print(message)
|
368 |
-
return message, None
|
369 |
-
|
370 |
-
file_index = (
|
371 |
-
(
|
372 |
-
file_index.strip(" ")
|
373 |
-
.strip('"')
|
374 |
-
.strip("\n")
|
375 |
-
.strip('"')
|
376 |
-
.strip(" ")
|
377 |
-
.replace("trained", "added")
|
378 |
-
)
|
379 |
-
if file_index != ""
|
380 |
-
else file_index2
|
381 |
-
) # 防止小白写错,自动帮他替换掉
|
382 |
-
|
383 |
-
try:
|
384 |
-
audio_opt = self.pipeline.pipeline(
|
385 |
-
self.hubert_model,
|
386 |
-
self.net_g,
|
387 |
-
sid,
|
388 |
-
audio,
|
389 |
-
input_audio_path1,
|
390 |
-
times,
|
391 |
-
f0_up_key,
|
392 |
-
f0_method,
|
393 |
-
file_index,
|
394 |
-
index_rate,
|
395 |
-
self.if_f0,
|
396 |
-
filter_radius,
|
397 |
-
self.tgt_sr,
|
398 |
-
resample_sr,
|
399 |
-
rms_mix_rate,
|
400 |
-
self.version,
|
401 |
-
protect,
|
402 |
-
crepe_hop_length,
|
403 |
-
f0_autotune,
|
404 |
-
f0_file=f0_file,
|
405 |
-
f0_min=f0_min,
|
406 |
-
f0_max=f0_max
|
407 |
-
)
|
408 |
-
except AssertionError:
|
409 |
-
message = "Mismatching index version detected (v1 with v2, or v2 with v1)."
|
410 |
-
print(message)
|
411 |
-
return message, None
|
412 |
-
except NameError:
|
413 |
-
message = "RVC libraries are still loading. Please try again in a few seconds."
|
414 |
-
print(message)
|
415 |
-
return message, None
|
416 |
-
|
417 |
-
if self.tgt_sr != resample_sr >= 16000:
|
418 |
-
self.tgt_sr = resample_sr
|
419 |
-
index_info = (
|
420 |
-
"Index:\n%s." % file_index
|
421 |
-
if os.path.exists(file_index)
|
422 |
-
else "Index not used."
|
423 |
-
)
|
424 |
-
end_time = time.time()
|
425 |
-
total_time = end_time - start_time
|
426 |
-
|
427 |
-
return f"Success.\n {index_info}\nTime:\n npy:{times[0]}, f0:{times[1]}, infer:{times[2]}\nTotal Time: {total_time} seconds", (self.tgt_sr, audio_opt)
|
428 |
-
except:
|
429 |
-
info = traceback.format_exc()
|
430 |
-
logger.warn(info)
|
431 |
-
return info, (None, None)
|
432 |
-
|
433 |
-
|
434 |
-
def vc_multi(
|
435 |
-
self,
|
436 |
-
sid,
|
437 |
-
dir_path,
|
438 |
-
opt_root,
|
439 |
-
paths,
|
440 |
-
f0_up_key,
|
441 |
-
f0_method,
|
442 |
-
file_index,
|
443 |
-
file_index2,
|
444 |
-
index_rate,
|
445 |
-
filter_radius,
|
446 |
-
resample_sr,
|
447 |
-
rms_mix_rate,
|
448 |
-
protect,
|
449 |
-
format1,
|
450 |
-
crepe_hop_length,
|
451 |
-
f0_min,
|
452 |
-
note_min,
|
453 |
-
f0_max,
|
454 |
-
note_max,
|
455 |
-
f0_autotune,
|
456 |
-
):
|
457 |
-
if rvc_globals.NotesOrHertz and f0_method != 'rmvpe':
|
458 |
-
f0_min = note_to_hz(note_min) if note_min else 50
|
459 |
-
f0_max = note_to_hz(note_max) if note_max else 1100
|
460 |
-
print(f"Converted Min pitch: freq - {f0_min}\n"
|
461 |
-
f"Converted Max pitch: freq - {f0_max}")
|
462 |
-
else:
|
463 |
-
f0_min = f0_min or 50
|
464 |
-
f0_max = f0_max or 1100
|
465 |
-
try:
|
466 |
-
dir_path = (
|
467 |
-
dir_path.strip(" ").strip('"').strip("\n").strip('"').strip(" ")
|
468 |
-
) # 防止小白拷路径头尾带了空格和"和回车
|
469 |
-
opt_root = opt_root.strip(" ").strip('"').strip("\n").strip('"').strip(" ")
|
470 |
-
os.makedirs(opt_root, exist_ok=True)
|
471 |
-
try:
|
472 |
-
if dir_path != "":
|
473 |
-
paths = [
|
474 |
-
os.path.join(dir_path, name) for name in os.listdir(dir_path)
|
475 |
-
]
|
476 |
-
else:
|
477 |
-
paths = [path.name for path in paths]
|
478 |
-
except:
|
479 |
-
traceback.print_exc()
|
480 |
-
paths = [path.name for path in paths]
|
481 |
-
infos = []
|
482 |
-
for path in paths:
|
483 |
-
info, opt = self.vc_single(
|
484 |
-
sid,
|
485 |
-
path,
|
486 |
-
f0_up_key,
|
487 |
-
None,
|
488 |
-
f0_method,
|
489 |
-
file_index,
|
490 |
-
file_index2,
|
491 |
-
# file_big_npy,
|
492 |
-
index_rate,
|
493 |
-
filter_radius,
|
494 |
-
resample_sr,
|
495 |
-
rms_mix_rate,
|
496 |
-
protect,
|
497 |
-
)
|
498 |
-
if "Success" in info:
|
499 |
-
try:
|
500 |
-
tgt_sr, audio_opt = opt
|
501 |
-
if format1 in ["wav", "flac"]:
|
502 |
-
sf.write(
|
503 |
-
"%s/%s.%s"
|
504 |
-
% (opt_root, os.path.basename(path), format1),
|
505 |
-
audio_opt,
|
506 |
-
tgt_sr,
|
507 |
-
)
|
508 |
-
else:
|
509 |
-
path = "%s/%s.%s" % (opt_root, os.path.basename(path), format1)
|
510 |
-
with BytesIO() as wavf:
|
511 |
-
sf.write(
|
512 |
-
wavf,
|
513 |
-
audio_opt,
|
514 |
-
tgt_sr,
|
515 |
-
format="wav"
|
516 |
-
)
|
517 |
-
wavf.seek(0, 0)
|
518 |
-
with open(path, "wb") as outf:
|
519 |
-
wav2(wavf, outf, format1)
|
520 |
-
except:
|
521 |
-
info += traceback.format_exc()
|
522 |
-
infos.append("%s->%s" % (os.path.basename(path), info))
|
523 |
-
yield "\n".join(infos)
|
524 |
-
yield "\n".join(infos)
|
525 |
-
except:
|
526 |
-
yield traceback.format_exc()
|
|
|
|
|
|
|
|
|
|
|
|
|
|
|
|
|
|
|
|
|
|
|
|
|
|
|
|
|
|
|
|
|
|
|
|
|
|
|
|
|
|
|
|
|
|
|
|
|
|
|
|
|
|
|
|
|
|
|
|
|
|
|
|
|
|
|
|
|
|
|
|
|
|
|
|
|
|
|
|
|
|
|
|
|
|
|
|
|
|
|
|
|
|
|
|
|
|
|
|
|
|
|
|
|
|
|
|
|
|
|
|
|
|
|
|
|
|
|
|
|
|
|
|
|
|
|
|
|
|
|
|
|
|
|
|
|
|
|
|
|
|
|
|
|
|
|
|
|
|
|
|
|
|
|
|
|
|
|
|
|
|
|
|
|
|
|
|
|
|
|
|
|
|
|
|
|
|
|
|
|
|
|
|
|
|
|
|
|
|
|
|
|
|
|
|
|
|
|
|
|
|
|
|
|
|
|
|
|
|
|
|
|
|
|
|
|
|
|
|
|
|
|
|
|
|
|
|
|
|
|
|
|
|
|
|
|
|
|
|
|
|
|
|
|
|
|
|
|
|
|
|
|
|
|
|
|
|
|
|
|
|
|
|
|
|
|
|
|
|
|
|
|
|
|
|
|
|
|
|
|
|
|
|
|
|
|
|
|
|
|
|
|
|
|
|
|
|
|
|
|
|
|
|
|
|
|
|
|
|
|
|
|
|
|
|
|
|
|
|
|
|
|
|
|
|
|
|
|
|
|
|
|
|
|
|
|
|
|
|
|
|
|
|
|
|
|
|
|
|
|
|
|
|
|
|
|
|
|
|
|
|
|
|
|
|
|
|
|
|
|
|
|
|
|
|
|
|
|
|
|
|
|
|
|
|
|
|
|
|
|
|
|
|
|
|
|
|
|
|
|
|
|
|
|
|
|
|
|
|
|
|
|
|
|
|
|
|
|
|
|
|
|
|
|
|
|
|
|
|
|
|
|
|
|
|
|
|
|
|
|
|
|
|
|
|
|
|
|
|
|
|
|
|
|
|
|
|
|
|
|
|
|
|
|
|
|
|
|
|
|
|
|
|
|
|
|
|
|
|
|
|
|
|
|
|
|
|
|
|
|
|
|
|
|
|
|
|
|
|
|
|
|
|
|
|
|
|
|
|
|
|
|
|
|
|
|
|
|
|
|
|
|
|
|
|
|
|
|
|
|
|
|
|
|
|
|
|
|
|
|
|
|
|
|
|
|
|
|
|
|
|
|
|
|
|
|
|
|
|
|
|
|
|
|
|
|
|
|
|
|
|
|
|
|
|
|
|
|
|
|
|
|
|
|
|
|
|
|
|
|
|
|
|
|
|
|
|
|
|
|
|
|
|
|
|
|
|
|
|
|
|
|
|
|
|
|
|
|
|
|
|
|
|
|
|
|
|
|
|
|
|
|
|
|
|
|
|
|
|
|
|
|
|
|
|
|
|
|
|
|
|
|
|
|
|
|
|
|
|
|
|
|
|
|
|
|
|
|
|
|
|
|
|
|
|
|
|
|
|
|
|
|
|
|
|
|
|
|
|
|
|
|
|
|
|
|
|
|
|
|
|
|
|
|
|
|
|
|
|
|
|
|
|
|
|
|
|
|
|
|
|
|
|
|
|
|
|
|
|
|
|
|
|
|
|
|
|
|
|
|
|
|
|
|
|
|
|
|
|
|
|
|
|
|
|
|
|
|
|
|
|
|
|
|
|
|
|
|
|
|
|
|
|
|
|
|
|
|
|
|
|
|
|
|
|
|
|
|
|
|
|
|
|
|
|
|
|
|
|
|
|
|
|
|
|
|
|
|
|
|
|
|
|
|
|
|
|
|
|
|
|
|
|
|
|
|
|
|
|
|
|
|
|
|
|
|
|
|
|
|
|
|
|
|
|
|
|
|
|
|
|
|
|
|
|
|
|
|
|
|
|
|
|
|
|
|
|
|
|
|
|
|
|
|
|
|
|
|
|
|
|
|
|
|
|
|
|
|
|
|
|
|
|
|
|
|
|
|
|
|
|
|
|
|
|
|
|
|
|
|
|
|
|
|
|
|
|
|
|
|
|
|
|
|
|
|
|
|
|
|
|
|
|
|
|
|
|
|
|
|
|
|
|
|
|
|
|
|
|
|
|
|
|
|
|
|
|
|
|
|
|
|
|
|
|
|
|
|
|
|
|
|
|
|
|
|
|
|
|
|
|
|
|
|
|
|
|
|
|
|
|
|
|
|
|
|
|
|
|
|
|
|
|
|
|
|
|
|
|
|
|
|
|
|
|
|
|
|
|
|
|
|
|
|
|
|
|
|
|
|
|
|
|
|
|
|
|
|
|
|
|
|
|
|
|
|
|
|
|
|
|
|
|
spaces/AIConsultant/MusicGen/tests/common_utils/__init__.py
DELETED
@@ -1,9 +0,0 @@
|
|
1 |
-
# Copyright (c) Meta Platforms, Inc. and affiliates.
|
2 |
-
# All rights reserved.
|
3 |
-
#
|
4 |
-
# This source code is licensed under the license found in the
|
5 |
-
# LICENSE file in the root directory of this source tree.
|
6 |
-
|
7 |
-
# flake8: noqa
|
8 |
-
from .temp_utils import TempDirMixin
|
9 |
-
from .wav_utils import get_batch_white_noise, get_white_noise, save_wav
|
|
|
|
|
|
|
|
|
|
|
|
|
|
|
|
|
|
|
|
spaces/AIGC-Audio/AudioGPT/NeuralSeq/modules/GenerSpeech/model/generspeech.py
DELETED
@@ -1,260 +0,0 @@
|
|
1 |
-
import torch
|
2 |
-
from modules.GenerSpeech.model.glow_modules import Glow
|
3 |
-
from modules.fastspeech.tts_modules import PitchPredictor
|
4 |
-
import random
|
5 |
-
from modules.GenerSpeech.model.prosody_util import ProsodyAligner, LocalStyleAdaptor
|
6 |
-
from utils.pitch_utils import f0_to_coarse, denorm_f0
|
7 |
-
from modules.commons.common_layers import *
|
8 |
-
import torch.distributions as dist
|
9 |
-
from utils.hparams import hparams
|
10 |
-
from modules.GenerSpeech.model.mixstyle import MixStyle
|
11 |
-
from modules.fastspeech.fs2 import FastSpeech2
|
12 |
-
import json
|
13 |
-
from modules.fastspeech.tts_modules import DEFAULT_MAX_SOURCE_POSITIONS, DEFAULT_MAX_TARGET_POSITIONS
|
14 |
-
|
15 |
-
class GenerSpeech(FastSpeech2):
|
16 |
-
'''
|
17 |
-
GenerSpeech: Towards Style Transfer for Generalizable Out-Of-Domain Text-to-Speech
|
18 |
-
https://arxiv.org/abs/2205.07211
|
19 |
-
'''
|
20 |
-
def __init__(self, dictionary, out_dims=None):
|
21 |
-
super().__init__(dictionary, out_dims)
|
22 |
-
|
23 |
-
# Mixstyle
|
24 |
-
self.norm = MixStyle(p=0.5, alpha=0.1, eps=1e-6, hidden_size=self.hidden_size)
|
25 |
-
|
26 |
-
# emotion embedding
|
27 |
-
self.emo_embed_proj = Linear(256, self.hidden_size, bias=True)
|
28 |
-
|
29 |
-
# build prosody extractor
|
30 |
-
## frame level
|
31 |
-
self.prosody_extractor_utter = LocalStyleAdaptor(self.hidden_size, hparams['nVQ'], self.padding_idx)
|
32 |
-
self.l1_utter = nn.Linear(self.hidden_size * 2, self.hidden_size)
|
33 |
-
self.align_utter = ProsodyAligner(num_layers=2)
|
34 |
-
|
35 |
-
## phoneme level
|
36 |
-
self.prosody_extractor_ph = LocalStyleAdaptor(self.hidden_size, hparams['nVQ'], self.padding_idx)
|
37 |
-
self.l1_ph = nn.Linear(self.hidden_size * 2, self.hidden_size)
|
38 |
-
self.align_ph = ProsodyAligner(num_layers=2)
|
39 |
-
|
40 |
-
## word level
|
41 |
-
self.prosody_extractor_word = LocalStyleAdaptor(self.hidden_size, hparams['nVQ'], self.padding_idx)
|
42 |
-
self.l1_word = nn.Linear(self.hidden_size * 2, self.hidden_size)
|
43 |
-
self.align_word = ProsodyAligner(num_layers=2)
|
44 |
-
|
45 |
-
self.pitch_inpainter_predictor = PitchPredictor(
|
46 |
-
self.hidden_size, n_chans=self.hidden_size,
|
47 |
-
n_layers=3, dropout_rate=0.1, odim=2,
|
48 |
-
padding=hparams['ffn_padding'], kernel_size=hparams['predictor_kernel'])
|
49 |
-
|
50 |
-
# build attention layer
|
51 |
-
self.max_source_positions = DEFAULT_MAX_SOURCE_POSITIONS
|
52 |
-
self.embed_positions = SinusoidalPositionalEmbedding(
|
53 |
-
self.hidden_size, self.padding_idx,
|
54 |
-
init_size=self.max_source_positions + self.padding_idx + 1,
|
55 |
-
)
|
56 |
-
|
57 |
-
# build post flow
|
58 |
-
cond_hs = 80
|
59 |
-
if hparams.get('use_txt_cond', True):
|
60 |
-
cond_hs = cond_hs + hparams['hidden_size']
|
61 |
-
|
62 |
-
cond_hs = cond_hs + hparams['hidden_size'] * 3 # for emo, spk embedding and prosody embedding
|
63 |
-
self.post_flow = Glow(
|
64 |
-
80, hparams['post_glow_hidden'], hparams['post_glow_kernel_size'], 1,
|
65 |
-
hparams['post_glow_n_blocks'], hparams['post_glow_n_block_layers'],
|
66 |
-
n_split=4, n_sqz=2,
|
67 |
-
gin_channels=cond_hs,
|
68 |
-
share_cond_layers=hparams['post_share_cond_layers'],
|
69 |
-
share_wn_layers=hparams['share_wn_layers'],
|
70 |
-
sigmoid_scale=hparams['sigmoid_scale']
|
71 |
-
)
|
72 |
-
self.prior_dist = dist.Normal(0, 1)
|
73 |
-
|
74 |
-
|
75 |
-
def forward(self, txt_tokens, mel2ph=None, ref_mel2ph=None, ref_mel2word=None, spk_embed=None, emo_embed=None, ref_mels=None,
|
76 |
-
f0=None, uv=None, skip_decoder=False, global_steps=0, infer=False, **kwargs):
|
77 |
-
ret = {}
|
78 |
-
encoder_out = self.encoder(txt_tokens) # [B, T, C]
|
79 |
-
src_nonpadding = (txt_tokens > 0).float()[:, :, None]
|
80 |
-
|
81 |
-
# add spk/emo embed
|
82 |
-
spk_embed = self.spk_embed_proj(spk_embed)[:, None, :]
|
83 |
-
emo_embed = self.emo_embed_proj(emo_embed)[:, None, :]
|
84 |
-
|
85 |
-
|
86 |
-
# add dur
|
87 |
-
dur_inp = (encoder_out + spk_embed + emo_embed) * src_nonpadding
|
88 |
-
mel2ph = self.add_dur(dur_inp, mel2ph, txt_tokens, ret)
|
89 |
-
tgt_nonpadding = (mel2ph > 0).float()[:, :, None]
|
90 |
-
decoder_inp = self.expand_states(encoder_out, mel2ph)
|
91 |
-
decoder_inp = self.norm(decoder_inp, spk_embed + emo_embed)
|
92 |
-
|
93 |
-
# add prosody VQ
|
94 |
-
ret['ref_mel2ph'] = ref_mel2ph
|
95 |
-
ret['ref_mel2word'] = ref_mel2word
|
96 |
-
prosody_utter_mel = self.get_prosody_utter(decoder_inp, ref_mels, ret, infer, global_steps)
|
97 |
-
prosody_ph_mel = self.get_prosody_ph(decoder_inp, ref_mels, ret, infer, global_steps)
|
98 |
-
prosody_word_mel = self.get_prosody_word(decoder_inp, ref_mels, ret, infer, global_steps)
|
99 |
-
|
100 |
-
# add pitch embed
|
101 |
-
pitch_inp_domain_agnostic = decoder_inp * tgt_nonpadding
|
102 |
-
pitch_inp_domain_specific = (decoder_inp + spk_embed + emo_embed + prosody_utter_mel + prosody_ph_mel + prosody_word_mel) * tgt_nonpadding
|
103 |
-
predicted_pitch = self.inpaint_pitch(pitch_inp_domain_agnostic, pitch_inp_domain_specific, f0, uv, mel2ph, ret)
|
104 |
-
|
105 |
-
# decode
|
106 |
-
decoder_inp = decoder_inp + spk_embed + emo_embed + predicted_pitch + prosody_utter_mel + prosody_ph_mel + prosody_word_mel
|
107 |
-
ret['decoder_inp'] = decoder_inp = decoder_inp * tgt_nonpadding
|
108 |
-
if skip_decoder:
|
109 |
-
return ret
|
110 |
-
ret['mel_out'] = self.run_decoder(decoder_inp, tgt_nonpadding, ret, infer=infer, **kwargs)
|
111 |
-
|
112 |
-
# postflow
|
113 |
-
is_training = self.training
|
114 |
-
ret['x_mask'] = tgt_nonpadding
|
115 |
-
ret['spk_embed'] = spk_embed
|
116 |
-
ret['emo_embed'] = emo_embed
|
117 |
-
ret['ref_prosody'] = prosody_utter_mel + prosody_ph_mel + prosody_word_mel
|
118 |
-
self.run_post_glow(ref_mels, infer, is_training, ret)
|
119 |
-
return ret
|
120 |
-
|
121 |
-
def get_prosody_ph(self, encoder_out, ref_mels, ret, infer=False, global_steps=0):
|
122 |
-
# get VQ prosody
|
123 |
-
if global_steps > hparams['vq_start'] or infer:
|
124 |
-
prosody_embedding, loss, ppl = self.prosody_extractor_ph(ref_mels, ret['ref_mel2ph'], no_vq=False)
|
125 |
-
ret['vq_loss_ph'] = loss
|
126 |
-
ret['ppl_ph'] = ppl
|
127 |
-
else:
|
128 |
-
prosody_embedding = self.prosody_extractor_ph(ref_mels, ret['ref_mel2ph'], no_vq=True)
|
129 |
-
|
130 |
-
# add positional embedding
|
131 |
-
positions = self.embed_positions(prosody_embedding[:, :, 0])
|
132 |
-
prosody_embedding = self.l1_ph(torch.cat([prosody_embedding, positions], dim=-1))
|
133 |
-
|
134 |
-
|
135 |
-
# style-to-content attention
|
136 |
-
src_key_padding_mask = encoder_out[:, :, 0].eq(self.padding_idx).data
|
137 |
-
prosody_key_padding_mask = prosody_embedding[:, :, 0].eq(self.padding_idx).data
|
138 |
-
if global_steps < hparams['forcing']:
|
139 |
-
output, guided_loss, attn_emo = self.align_ph(encoder_out.transpose(0, 1), prosody_embedding.transpose(0, 1),
|
140 |
-
src_key_padding_mask, prosody_key_padding_mask, forcing=True)
|
141 |
-
else:
|
142 |
-
output, guided_loss, attn_emo = self.align_ph(encoder_out.transpose(0, 1), prosody_embedding.transpose(0, 1),
|
143 |
-
src_key_padding_mask, prosody_key_padding_mask, forcing=False)
|
144 |
-
|
145 |
-
ret['gloss_ph'] = guided_loss
|
146 |
-
ret['attn_ph'] = attn_emo
|
147 |
-
return output.transpose(0, 1)
|
148 |
-
|
149 |
-
def get_prosody_word(self, encoder_out, ref_mels, ret, infer=False, global_steps=0):
|
150 |
-
# get VQ prosody
|
151 |
-
if global_steps > hparams['vq_start'] or infer:
|
152 |
-
prosody_embedding, loss, ppl = self.prosody_extractor_word(ref_mels, ret['ref_mel2word'], no_vq=False)
|
153 |
-
ret['vq_loss_word'] = loss
|
154 |
-
ret['ppl_word'] = ppl
|
155 |
-
else:
|
156 |
-
prosody_embedding = self.prosody_extractor_word(ref_mels, ret['ref_mel2word'], no_vq=True)
|
157 |
-
|
158 |
-
# add positional embedding
|
159 |
-
positions = self.embed_positions(prosody_embedding[:, :, 0])
|
160 |
-
prosody_embedding = self.l1_word(torch.cat([prosody_embedding, positions], dim=-1))
|
161 |
-
|
162 |
-
|
163 |
-
# style-to-content attention
|
164 |
-
src_key_padding_mask = encoder_out[:, :, 0].eq(self.padding_idx).data
|
165 |
-
prosody_key_padding_mask = prosody_embedding[:, :, 0].eq(self.padding_idx).data
|
166 |
-
if global_steps < hparams['forcing']:
|
167 |
-
output, guided_loss, attn_emo = self.align_word(encoder_out.transpose(0, 1), prosody_embedding.transpose(0, 1),
|
168 |
-
src_key_padding_mask, prosody_key_padding_mask, forcing=True)
|
169 |
-
else:
|
170 |
-
output, guided_loss, attn_emo = self.align_word(encoder_out.transpose(0, 1), prosody_embedding.transpose(0, 1),
|
171 |
-
src_key_padding_mask, prosody_key_padding_mask, forcing=False)
|
172 |
-
ret['gloss_word'] = guided_loss
|
173 |
-
ret['attn_word'] = attn_emo
|
174 |
-
return output.transpose(0, 1)
|
175 |
-
|
176 |
-
def get_prosody_utter(self, encoder_out, ref_mels, ret, infer=False, global_steps=0):
|
177 |
-
# get VQ prosody
|
178 |
-
if global_steps > hparams['vq_start'] or infer:
|
179 |
-
prosody_embedding, loss, ppl = self.prosody_extractor_utter(ref_mels, no_vq=False)
|
180 |
-
ret['vq_loss_utter'] = loss
|
181 |
-
ret['ppl_utter'] = ppl
|
182 |
-
else:
|
183 |
-
prosody_embedding = self.prosody_extractor_utter(ref_mels, no_vq=True)
|
184 |
-
|
185 |
-
# add positional embedding
|
186 |
-
positions = self.embed_positions(prosody_embedding[:, :, 0])
|
187 |
-
prosody_embedding = self.l1_utter(torch.cat([prosody_embedding, positions], dim=-1))
|
188 |
-
|
189 |
-
|
190 |
-
# style-to-content attention
|
191 |
-
src_key_padding_mask = encoder_out[:, :, 0].eq(self.padding_idx).data
|
192 |
-
prosody_key_padding_mask = prosody_embedding[:, :, 0].eq(self.padding_idx).data
|
193 |
-
if global_steps < hparams['forcing']:
|
194 |
-
output, guided_loss, attn_emo = self.align_utter(encoder_out.transpose(0, 1), prosody_embedding.transpose(0, 1),
|
195 |
-
src_key_padding_mask, prosody_key_padding_mask, forcing=True)
|
196 |
-
else:
|
197 |
-
output, guided_loss, attn_emo = self.align_utter(encoder_out.transpose(0, 1), prosody_embedding.transpose(0, 1),
|
198 |
-
src_key_padding_mask, prosody_key_padding_mask, forcing=False)
|
199 |
-
ret['gloss_utter'] = guided_loss
|
200 |
-
ret['attn_utter'] = attn_emo
|
201 |
-
return output.transpose(0, 1)
|
202 |
-
|
203 |
-
|
204 |
-
|
205 |
-
def inpaint_pitch(self, pitch_inp_domain_agnostic, pitch_inp_domain_specific, f0, uv, mel2ph, ret):
|
206 |
-
if hparams['pitch_type'] == 'frame':
|
207 |
-
pitch_padding = mel2ph == 0
|
208 |
-
if hparams['predictor_grad'] != 1:
|
209 |
-
pitch_inp_domain_agnostic = pitch_inp_domain_agnostic.detach() + hparams['predictor_grad'] * (pitch_inp_domain_agnostic - pitch_inp_domain_agnostic.detach())
|
210 |
-
pitch_inp_domain_specific = pitch_inp_domain_specific.detach() + hparams['predictor_grad'] * (pitch_inp_domain_specific - pitch_inp_domain_specific.detach())
|
211 |
-
|
212 |
-
pitch_domain_agnostic = self.pitch_predictor(pitch_inp_domain_agnostic)
|
213 |
-
pitch_domain_specific = self.pitch_inpainter_predictor(pitch_inp_domain_specific)
|
214 |
-
pitch_pred = pitch_domain_agnostic + pitch_domain_specific
|
215 |
-
ret['pitch_pred'] = pitch_pred
|
216 |
-
|
217 |
-
use_uv = hparams['pitch_type'] == 'frame' and hparams['use_uv']
|
218 |
-
if f0 is None:
|
219 |
-
f0 = pitch_pred[:, :, 0] # [B, T]
|
220 |
-
if use_uv:
|
221 |
-
uv = pitch_pred[:, :, 1] > 0 # [B, T]
|
222 |
-
f0_denorm = denorm_f0(f0, uv if use_uv else None, hparams, pitch_padding=pitch_padding)
|
223 |
-
pitch = f0_to_coarse(f0_denorm) # start from 0 [B, T_txt]
|
224 |
-
ret['f0_denorm'] = f0_denorm
|
225 |
-
ret['f0_denorm_pred'] = denorm_f0(pitch_pred[:, :, 0], (pitch_pred[:, :, 1] > 0) if use_uv else None, hparams, pitch_padding=pitch_padding)
|
226 |
-
if hparams['pitch_type'] == 'ph':
|
227 |
-
pitch = torch.gather(F.pad(pitch, [1, 0]), 1, mel2ph)
|
228 |
-
ret['f0_denorm'] = torch.gather(F.pad(ret['f0_denorm'], [1, 0]), 1, mel2ph)
|
229 |
-
ret['f0_denorm_pred'] = torch.gather(F.pad(ret['f0_denorm_pred'], [1, 0]), 1, mel2ph)
|
230 |
-
pitch_embed = self.pitch_embed(pitch)
|
231 |
-
return pitch_embed
|
232 |
-
|
233 |
-
def run_post_glow(self, tgt_mels, infer, is_training, ret):
|
234 |
-
x_recon = ret['mel_out'].transpose(1, 2)
|
235 |
-
g = x_recon
|
236 |
-
B, _, T = g.shape
|
237 |
-
if hparams.get('use_txt_cond', True):
|
238 |
-
g = torch.cat([g, ret['decoder_inp'].transpose(1, 2)], 1)
|
239 |
-
g_spk_embed = ret['spk_embed'].repeat(1, T, 1).transpose(1, 2)
|
240 |
-
g_emo_embed = ret['emo_embed'].repeat(1, T, 1).transpose(1, 2)
|
241 |
-
l_ref_prosody = ret['ref_prosody'].transpose(1, 2)
|
242 |
-
g = torch.cat([g, g_spk_embed, g_emo_embed, l_ref_prosody], dim=1)
|
243 |
-
prior_dist = self.prior_dist
|
244 |
-
if not infer:
|
245 |
-
if is_training:
|
246 |
-
self.train()
|
247 |
-
x_mask = ret['x_mask'].transpose(1, 2)
|
248 |
-
y_lengths = x_mask.sum(-1)
|
249 |
-
g = g.detach()
|
250 |
-
tgt_mels = tgt_mels.transpose(1, 2)
|
251 |
-
z_postflow, ldj = self.post_flow(tgt_mels, x_mask, g=g)
|
252 |
-
ldj = ldj / y_lengths / 80
|
253 |
-
ret['z_pf'], ret['ldj_pf'] = z_postflow, ldj
|
254 |
-
ret['postflow'] = -prior_dist.log_prob(z_postflow).mean() - ldj.mean()
|
255 |
-
else:
|
256 |
-
x_mask = torch.ones_like(x_recon[:, :1, :])
|
257 |
-
z_post = prior_dist.sample(x_recon.shape).to(g.device) * hparams['noise_scale']
|
258 |
-
x_recon_, _ = self.post_flow(z_post, x_mask, g, reverse=True)
|
259 |
-
x_recon = x_recon_
|
260 |
-
ret['mel_out'] = x_recon.transpose(1, 2)
|
|
|
|
|
|
|
|
|
|
|
|
|
|
|
|
|
|
|
|
|
|
|
|
|
|
|
|
|
|
|
|
|
|
|
|
|
|
|
|
|
|
|
|
|
|
|
|
|
|
|
|
|
|
|
|
|
|
|
|
|
|
|
|
|
|
|
|
|
|
|
|
|
|
|
|
|
|
|
|
|
|
|
|
|
|
|
|
|
|
|
|
|
|
|
|
|
|
|
|
|
|
|
|
|
|
|
|
|
|
|
|
|
|
|
|
|
|
|
|
|
|
|
|
|
|
|
|
|
|
|
|
|
|
|
|
|
|
|
|
|
|
|
|
|
|
|
|
|
|
|
|
|
|
|
|
|
|
|
|
|
|
|
|
|
|
|
|
|
|
|
|
|
|
|
|
|
|
|
|
|
|
|
|
|
|
|
|
|
|
|
|
|
|
|
|
|
|
|
|
|
|
|
|
|
|
|
|
|
|
|
|
|
|
|
|
|
|
|
|
|
|
|
|
|
|
|
|
|
|
|
|
|
|
|
|
|
|
|
|
|
|
|
|
|
|
|
|
|
|
|
|
|
|
|
|
|
|
|
|
|
|
|
|
|
|
|
|
|
|
|
|
|
|
|
|
|
|
|
|
|
|
|
|
|
|
|
|
|
|
|
|
|
|
|
|
|
|
|
|
|
|
|
|
|
|
|
|
|
|
|
|
|
|
|
|
|
|
|
|
|
|
|
|
|
|
|
|
|
|
|
|
|
|
|
|
|
|
|
|
|
|
|
|
|
|
|
|
|
|
|
|
|
|
|
|
|
|
|
|
|
|
|
|
|
|
|
|
|
|
|
|
|
|
|
|
|
|
|
|
|
|
|
|
|
|
|
|
|
|
|
|
|
|
|
|
|
|
|
|
|
|
|
|
|
|
|
|
|
|
|
|
|
|
|
|
|
|
|
|
|
|
|
|
|
|
|
|
|
|
|
|
|
|
|
|
|
|
|
|
|
|
|
|
|
|
|
|
|
|
|
|
|
|
|
|
|
|
|
|
|
|
|
|
|
|
|
|
|
|
|
|
|
|
|
|
|
|
|
|
|
|
|
|
|
|
|
|
|
|
|
|
|
|
|
|
|
|
|
|
|
|
|
|
|
|
|
|
|
|
|
|
|
|
|
|
|
|
|
|
|
|
spaces/AIGC-Audio/AudioGPT/NeuralSeq/modules/diff/candidate_decoder.py
DELETED
@@ -1,96 +0,0 @@
|
|
1 |
-
from modules.fastspeech.tts_modules import FastspeechDecoder
|
2 |
-
# from modules.fastspeech.fast_tacotron import DecoderRNN
|
3 |
-
# from modules.fastspeech.speedy_speech.speedy_speech import ConvBlocks
|
4 |
-
# from modules.fastspeech.conformer.conformer import ConformerDecoder
|
5 |
-
import torch
|
6 |
-
from torch.nn import functional as F
|
7 |
-
import torch.nn as nn
|
8 |
-
import math
|
9 |
-
from utils.hparams import hparams
|
10 |
-
from .diffusion import Mish
|
11 |
-
Linear = nn.Linear
|
12 |
-
|
13 |
-
|
14 |
-
class SinusoidalPosEmb(nn.Module):
|
15 |
-
def __init__(self, dim):
|
16 |
-
super().__init__()
|
17 |
-
self.dim = dim
|
18 |
-
|
19 |
-
def forward(self, x):
|
20 |
-
device = x.device
|
21 |
-
half_dim = self.dim // 2
|
22 |
-
emb = math.log(10000) / (half_dim - 1)
|
23 |
-
emb = torch.exp(torch.arange(half_dim, device=device) * -emb)
|
24 |
-
emb = x[:, None] * emb[None, :]
|
25 |
-
emb = torch.cat((emb.sin(), emb.cos()), dim=-1)
|
26 |
-
return emb
|
27 |
-
|
28 |
-
|
29 |
-
def Conv1d(*args, **kwargs):
|
30 |
-
layer = nn.Conv1d(*args, **kwargs)
|
31 |
-
nn.init.kaiming_normal_(layer.weight)
|
32 |
-
return layer
|
33 |
-
|
34 |
-
|
35 |
-
class FFT(FastspeechDecoder):
|
36 |
-
def __init__(self, hidden_size=None, num_layers=None, kernel_size=None, num_heads=None):
|
37 |
-
super().__init__(hidden_size, num_layers, kernel_size, num_heads=num_heads)
|
38 |
-
dim = hparams['residual_channels']
|
39 |
-
self.input_projection = Conv1d(hparams['audio_num_mel_bins'], dim, 1)
|
40 |
-
self.diffusion_embedding = SinusoidalPosEmb(dim)
|
41 |
-
self.mlp = nn.Sequential(
|
42 |
-
nn.Linear(dim, dim * 4),
|
43 |
-
Mish(),
|
44 |
-
nn.Linear(dim * 4, dim)
|
45 |
-
)
|
46 |
-
self.get_mel_out = Linear(hparams['hidden_size'], 80, bias=True)
|
47 |
-
self.get_decode_inp = Linear(hparams['hidden_size'] + dim + dim,
|
48 |
-
hparams['hidden_size']) # hs + dim + 80 -> hs
|
49 |
-
|
50 |
-
def forward(self, spec, diffusion_step, cond, padding_mask=None, attn_mask=None, return_hiddens=False):
|
51 |
-
"""
|
52 |
-
:param spec: [B, 1, 80, T]
|
53 |
-
:param diffusion_step: [B, 1]
|
54 |
-
:param cond: [B, M, T]
|
55 |
-
:return:
|
56 |
-
"""
|
57 |
-
x = spec[:, 0]
|
58 |
-
x = self.input_projection(x).permute([0, 2, 1]) # [B, T, residual_channel]
|
59 |
-
diffusion_step = self.diffusion_embedding(diffusion_step)
|
60 |
-
diffusion_step = self.mlp(diffusion_step) # [B, dim]
|
61 |
-
cond = cond.permute([0, 2, 1]) # [B, T, M]
|
62 |
-
|
63 |
-
seq_len = cond.shape[1] # [T_mel]
|
64 |
-
time_embed = diffusion_step[:, None, :] # [B, 1, dim]
|
65 |
-
time_embed = time_embed.repeat([1, seq_len, 1]) # # [B, T, dim]
|
66 |
-
|
67 |
-
decoder_inp = torch.cat([x, cond, time_embed], dim=-1) # [B, T, dim + H + dim]
|
68 |
-
decoder_inp = self.get_decode_inp(decoder_inp) # [B, T, H]
|
69 |
-
x = decoder_inp
|
70 |
-
|
71 |
-
'''
|
72 |
-
Required x: [B, T, C]
|
73 |
-
:return: [B, T, C] or [L, B, T, C]
|
74 |
-
'''
|
75 |
-
padding_mask = x.abs().sum(-1).eq(0).data if padding_mask is None else padding_mask
|
76 |
-
nonpadding_mask_TB = 1 - padding_mask.transpose(0, 1).float()[:, :, None] # [T, B, 1]
|
77 |
-
if self.use_pos_embed:
|
78 |
-
positions = self.pos_embed_alpha * self.embed_positions(x[..., 0])
|
79 |
-
x = x + positions
|
80 |
-
x = F.dropout(x, p=self.dropout, training=self.training)
|
81 |
-
# B x T x C -> T x B x C
|
82 |
-
x = x.transpose(0, 1) * nonpadding_mask_TB
|
83 |
-
hiddens = []
|
84 |
-
for layer in self.layers:
|
85 |
-
x = layer(x, encoder_padding_mask=padding_mask, attn_mask=attn_mask) * nonpadding_mask_TB
|
86 |
-
hiddens.append(x)
|
87 |
-
if self.use_last_norm:
|
88 |
-
x = self.layer_norm(x) * nonpadding_mask_TB
|
89 |
-
if return_hiddens:
|
90 |
-
x = torch.stack(hiddens, 0) # [L, T, B, C]
|
91 |
-
x = x.transpose(1, 2) # [L, B, T, C]
|
92 |
-
else:
|
93 |
-
x = x.transpose(0, 1) # [B, T, C]
|
94 |
-
|
95 |
-
x = self.get_mel_out(x).permute([0, 2, 1]) # [B, 80, T]
|
96 |
-
return x[:, None, :, :]
|
|
|
|
|
|
|
|
|
|
|
|
|
|
|
|
|
|
|
|
|
|
|
|
|
|
|
|
|
|
|
|
|
|
|
|
|
|
|
|
|
|
|
|
|
|
|
|
|
|
|
|
|
|
|
|
|
|
|
|
|
|
|
|
|
|
|
|
|
|
|
|
|
|
|
|
|
|
|
|
|
|
|
|
|
|
|
|
|
|
|
|
|
|
|
|
|
|
|
|
|
|
|
|
|
|
|
|
|
|
|
|
|
|
|
|
|
|
|
|
|
|
|
|
|
|
|
|
|
|
|
|
|
|
|
|
|
|
|
|
|
|
|
|
|
|
|
|
|
|
|
|
|
|
|
|
|
|
|
|
|
|
|
|
|
|
|
|
|
|
|
|
|
|
|
|
|
|
|
|
|
|
|
|
|
|
|
|
|
|
|
|
|
|
spaces/AIGC-Audio/AudioGPT/NeuralSeq/modules/parallel_wavegan/layers/upsample.py
DELETED
@@ -1,183 +0,0 @@
|
|
1 |
-
# -*- coding: utf-8 -*-
|
2 |
-
|
3 |
-
"""Upsampling module.
|
4 |
-
|
5 |
-
This code is modified from https://github.com/r9y9/wavenet_vocoder.
|
6 |
-
|
7 |
-
"""
|
8 |
-
|
9 |
-
import numpy as np
|
10 |
-
import torch
|
11 |
-
import torch.nn.functional as F
|
12 |
-
|
13 |
-
from . import Conv1d
|
14 |
-
|
15 |
-
|
16 |
-
class Stretch2d(torch.nn.Module):
|
17 |
-
"""Stretch2d module."""
|
18 |
-
|
19 |
-
def __init__(self, x_scale, y_scale, mode="nearest"):
|
20 |
-
"""Initialize Stretch2d module.
|
21 |
-
|
22 |
-
Args:
|
23 |
-
x_scale (int): X scaling factor (Time axis in spectrogram).
|
24 |
-
y_scale (int): Y scaling factor (Frequency axis in spectrogram).
|
25 |
-
mode (str): Interpolation mode.
|
26 |
-
|
27 |
-
"""
|
28 |
-
super(Stretch2d, self).__init__()
|
29 |
-
self.x_scale = x_scale
|
30 |
-
self.y_scale = y_scale
|
31 |
-
self.mode = mode
|
32 |
-
|
33 |
-
def forward(self, x):
|
34 |
-
"""Calculate forward propagation.
|
35 |
-
|
36 |
-
Args:
|
37 |
-
x (Tensor): Input tensor (B, C, F, T).
|
38 |
-
|
39 |
-
Returns:
|
40 |
-
Tensor: Interpolated tensor (B, C, F * y_scale, T * x_scale),
|
41 |
-
|
42 |
-
"""
|
43 |
-
return F.interpolate(
|
44 |
-
x, scale_factor=(self.y_scale, self.x_scale), mode=self.mode)
|
45 |
-
|
46 |
-
|
47 |
-
class Conv2d(torch.nn.Conv2d):
|
48 |
-
"""Conv2d module with customized initialization."""
|
49 |
-
|
50 |
-
def __init__(self, *args, **kwargs):
|
51 |
-
"""Initialize Conv2d module."""
|
52 |
-
super(Conv2d, self).__init__(*args, **kwargs)
|
53 |
-
|
54 |
-
def reset_parameters(self):
|
55 |
-
"""Reset parameters."""
|
56 |
-
self.weight.data.fill_(1. / np.prod(self.kernel_size))
|
57 |
-
if self.bias is not None:
|
58 |
-
torch.nn.init.constant_(self.bias, 0.0)
|
59 |
-
|
60 |
-
|
61 |
-
class UpsampleNetwork(torch.nn.Module):
|
62 |
-
"""Upsampling network module."""
|
63 |
-
|
64 |
-
def __init__(self,
|
65 |
-
upsample_scales,
|
66 |
-
nonlinear_activation=None,
|
67 |
-
nonlinear_activation_params={},
|
68 |
-
interpolate_mode="nearest",
|
69 |
-
freq_axis_kernel_size=1,
|
70 |
-
use_causal_conv=False,
|
71 |
-
):
|
72 |
-
"""Initialize upsampling network module.
|
73 |
-
|
74 |
-
Args:
|
75 |
-
upsample_scales (list): List of upsampling scales.
|
76 |
-
nonlinear_activation (str): Activation function name.
|
77 |
-
nonlinear_activation_params (dict): Arguments for specified activation function.
|
78 |
-
interpolate_mode (str): Interpolation mode.
|
79 |
-
freq_axis_kernel_size (int): Kernel size in the direction of frequency axis.
|
80 |
-
|
81 |
-
"""
|
82 |
-
super(UpsampleNetwork, self).__init__()
|
83 |
-
self.use_causal_conv = use_causal_conv
|
84 |
-
self.up_layers = torch.nn.ModuleList()
|
85 |
-
for scale in upsample_scales:
|
86 |
-
# interpolation layer
|
87 |
-
stretch = Stretch2d(scale, 1, interpolate_mode)
|
88 |
-
self.up_layers += [stretch]
|
89 |
-
|
90 |
-
# conv layer
|
91 |
-
assert (freq_axis_kernel_size - 1) % 2 == 0, "Not support even number freq axis kernel size."
|
92 |
-
freq_axis_padding = (freq_axis_kernel_size - 1) // 2
|
93 |
-
kernel_size = (freq_axis_kernel_size, scale * 2 + 1)
|
94 |
-
if use_causal_conv:
|
95 |
-
padding = (freq_axis_padding, scale * 2)
|
96 |
-
else:
|
97 |
-
padding = (freq_axis_padding, scale)
|
98 |
-
conv = Conv2d(1, 1, kernel_size=kernel_size, padding=padding, bias=False)
|
99 |
-
self.up_layers += [conv]
|
100 |
-
|
101 |
-
# nonlinear
|
102 |
-
if nonlinear_activation is not None:
|
103 |
-
nonlinear = getattr(torch.nn, nonlinear_activation)(**nonlinear_activation_params)
|
104 |
-
self.up_layers += [nonlinear]
|
105 |
-
|
106 |
-
def forward(self, c):
|
107 |
-
"""Calculate forward propagation.
|
108 |
-
|
109 |
-
Args:
|
110 |
-
c : Input tensor (B, C, T).
|
111 |
-
|
112 |
-
Returns:
|
113 |
-
Tensor: Upsampled tensor (B, C, T'), where T' = T * prod(upsample_scales).
|
114 |
-
|
115 |
-
"""
|
116 |
-
c = c.unsqueeze(1) # (B, 1, C, T)
|
117 |
-
for f in self.up_layers:
|
118 |
-
if self.use_causal_conv and isinstance(f, Conv2d):
|
119 |
-
c = f(c)[..., :c.size(-1)]
|
120 |
-
else:
|
121 |
-
c = f(c)
|
122 |
-
return c.squeeze(1) # (B, C, T')
|
123 |
-
|
124 |
-
|
125 |
-
class ConvInUpsampleNetwork(torch.nn.Module):
|
126 |
-
"""Convolution + upsampling network module."""
|
127 |
-
|
128 |
-
def __init__(self,
|
129 |
-
upsample_scales,
|
130 |
-
nonlinear_activation=None,
|
131 |
-
nonlinear_activation_params={},
|
132 |
-
interpolate_mode="nearest",
|
133 |
-
freq_axis_kernel_size=1,
|
134 |
-
aux_channels=80,
|
135 |
-
aux_context_window=0,
|
136 |
-
use_causal_conv=False
|
137 |
-
):
|
138 |
-
"""Initialize convolution + upsampling network module.
|
139 |
-
|
140 |
-
Args:
|
141 |
-
upsample_scales (list): List of upsampling scales.
|
142 |
-
nonlinear_activation (str): Activation function name.
|
143 |
-
nonlinear_activation_params (dict): Arguments for specified activation function.
|
144 |
-
mode (str): Interpolation mode.
|
145 |
-
freq_axis_kernel_size (int): Kernel size in the direction of frequency axis.
|
146 |
-
aux_channels (int): Number of channels of pre-convolutional layer.
|
147 |
-
aux_context_window (int): Context window size of the pre-convolutional layer.
|
148 |
-
use_causal_conv (bool): Whether to use causal structure.
|
149 |
-
|
150 |
-
"""
|
151 |
-
super(ConvInUpsampleNetwork, self).__init__()
|
152 |
-
self.aux_context_window = aux_context_window
|
153 |
-
self.use_causal_conv = use_causal_conv and aux_context_window > 0
|
154 |
-
# To capture wide-context information in conditional features
|
155 |
-
kernel_size = aux_context_window + 1 if use_causal_conv else 2 * aux_context_window + 1
|
156 |
-
# NOTE(kan-bayashi): Here do not use padding because the input is already padded
|
157 |
-
self.conv_in = Conv1d(aux_channels, aux_channels, kernel_size=kernel_size, bias=False)
|
158 |
-
self.upsample = UpsampleNetwork(
|
159 |
-
upsample_scales=upsample_scales,
|
160 |
-
nonlinear_activation=nonlinear_activation,
|
161 |
-
nonlinear_activation_params=nonlinear_activation_params,
|
162 |
-
interpolate_mode=interpolate_mode,
|
163 |
-
freq_axis_kernel_size=freq_axis_kernel_size,
|
164 |
-
use_causal_conv=use_causal_conv,
|
165 |
-
)
|
166 |
-
|
167 |
-
def forward(self, c):
|
168 |
-
"""Calculate forward propagation.
|
169 |
-
|
170 |
-
Args:
|
171 |
-
c : Input tensor (B, C, T').
|
172 |
-
|
173 |
-
Returns:
|
174 |
-
Tensor: Upsampled tensor (B, C, T),
|
175 |
-
where T = (T' - aux_context_window * 2) * prod(upsample_scales).
|
176 |
-
|
177 |
-
Note:
|
178 |
-
The length of inputs considers the context window size.
|
179 |
-
|
180 |
-
"""
|
181 |
-
c_ = self.conv_in(c)
|
182 |
-
c = c_[:, :, :-self.aux_context_window] if self.use_causal_conv else c_
|
183 |
-
return self.upsample(c)
|
|
|
|
|
|
|
|
|
|
|
|
|
|
|
|
|
|
|
|
|
|
|
|
|
|
|
|
|
|
|
|
|
|
|
|
|
|
|
|
|
|
|
|
|
|
|
|
|
|
|
|
|
|
|
|
|
|
|
|
|
|
|
|
|
|
|
|
|
|
|
|
|
|
|
|
|
|
|
|
|
|
|
|
|
|
|
|
|
|
|
|
|
|
|
|
|
|
|
|
|
|
|
|
|
|
|
|
|
|
|
|
|
|
|
|
|
|
|
|
|
|
|
|
|
|
|
|
|
|
|
|
|
|
|
|
|
|
|
|
|
|
|
|
|
|
|
|
|
|
|
|
|
|
|
|
|
|
|
|
|
|
|
|
|
|
|
|
|
|
|
|
|
|
|
|
|
|
|
|
|
|
|
|
|
|
|
|
|
|
|
|
|
|
|
|
|
|
|
|
|
|
|
|
|
|
|
|
|
|
|
|
|
|
|
|
|
|
|
|
|
|
|
|
|
|
|
|
|
|
|
|
|
|
|
|
|
|
|
|
|
|
|
|
|
|
|
|
|
|
|
|
|
|
|
|
|
|
|
|
|
|
|
|
|
|
|
|
|
|
|
|
|
|
|
|
|
|
|
|
|
|
|
|
|
|
|
|
|
|
|
|
|
|
|
|
|
|
|
|
|
|
|
|
|
|
|
|
|
|
|
|
|
|
|
|
|
|
|
|
|
|
|
|
|
|
|
|
|
|
|
|
|
|
|
|
|
|
|
|
|
|
|
|
|
|
|
|
|
|
|
|
|
|
|
|
|
|
|
|
|
|
|
|
|
|
|
|
spaces/AIGC-Audio/AudioGPT/NeuralSeq/tasks/svs/task.py
DELETED
@@ -1,84 +0,0 @@
|
|
1 |
-
import torch
|
2 |
-
|
3 |
-
import utils
|
4 |
-
from modules.diff.diffusion import GaussianDiffusion
|
5 |
-
from modules.diff.net import DiffNet
|
6 |
-
from tasks.tts.fs2 import FastSpeech2Task
|
7 |
-
from utils.hparams import hparams
|
8 |
-
|
9 |
-
|
10 |
-
DIFF_DECODERS = {
|
11 |
-
'wavenet': lambda hp: DiffNet(hp['audio_num_mel_bins']),
|
12 |
-
}
|
13 |
-
|
14 |
-
|
15 |
-
class DiffFsTask(FastSpeech2Task):
|
16 |
-
def build_tts_model(self):
|
17 |
-
mel_bins = hparams['audio_num_mel_bins']
|
18 |
-
self.model = GaussianDiffusion(
|
19 |
-
phone_encoder=self.phone_encoder,
|
20 |
-
out_dims=mel_bins, denoise_fn=DIFF_DECODERS[hparams['diff_decoder_type']](hparams),
|
21 |
-
timesteps=hparams['timesteps'],
|
22 |
-
loss_type=hparams['diff_loss_type'],
|
23 |
-
spec_min=hparams['spec_min'], spec_max=hparams['spec_max'],
|
24 |
-
)
|
25 |
-
|
26 |
-
def run_model(self, model, sample, return_output=False, infer=False):
|
27 |
-
txt_tokens = sample['txt_tokens'] # [B, T_t]
|
28 |
-
target = sample['mels'] # [B, T_s, 80]
|
29 |
-
mel2ph = sample['mel2ph'] # [B, T_s]
|
30 |
-
f0 = sample['f0']
|
31 |
-
uv = sample['uv']
|
32 |
-
energy = sample['energy']
|
33 |
-
spk_embed = sample.get('spk_embed') if not hparams['use_spk_id'] else sample.get('spk_ids')
|
34 |
-
if hparams['pitch_type'] == 'cwt':
|
35 |
-
cwt_spec = sample[f'cwt_spec']
|
36 |
-
f0_mean = sample['f0_mean']
|
37 |
-
f0_std = sample['f0_std']
|
38 |
-
sample['f0_cwt'] = f0 = model.cwt2f0_norm(cwt_spec, f0_mean, f0_std, mel2ph)
|
39 |
-
|
40 |
-
output = model(txt_tokens, mel2ph=mel2ph, spk_embed=spk_embed,
|
41 |
-
ref_mels=target, f0=f0, uv=uv, energy=energy, infer=infer)
|
42 |
-
|
43 |
-
losses = {}
|
44 |
-
if 'diff_loss' in output:
|
45 |
-
losses['mel'] = output['diff_loss']
|
46 |
-
self.add_dur_loss(output['dur'], mel2ph, txt_tokens, losses=losses)
|
47 |
-
if hparams['use_pitch_embed']:
|
48 |
-
self.add_pitch_loss(output, sample, losses)
|
49 |
-
if hparams['use_energy_embed']:
|
50 |
-
self.add_energy_loss(output['energy_pred'], energy, losses)
|
51 |
-
if not return_output:
|
52 |
-
return losses
|
53 |
-
else:
|
54 |
-
return losses, output
|
55 |
-
|
56 |
-
def _training_step(self, sample, batch_idx, _):
|
57 |
-
log_outputs = self.run_model(self.model, sample)
|
58 |
-
total_loss = sum([v for v in log_outputs.values() if isinstance(v, torch.Tensor) and v.requires_grad])
|
59 |
-
log_outputs['batch_size'] = sample['txt_tokens'].size()[0]
|
60 |
-
log_outputs['lr'] = self.scheduler.get_lr()[0]
|
61 |
-
return total_loss, log_outputs
|
62 |
-
|
63 |
-
def validation_step(self, sample, batch_idx):
|
64 |
-
outputs = {}
|
65 |
-
outputs['losses'] = {}
|
66 |
-
outputs['losses'], model_out = self.run_model(self.model, sample, return_output=True, infer=False)
|
67 |
-
outputs['total_loss'] = sum(outputs['losses'].values())
|
68 |
-
outputs['nsamples'] = sample['nsamples']
|
69 |
-
outputs = utils.tensors_to_scalars(outputs)
|
70 |
-
if batch_idx < hparams['num_valid_plots']:
|
71 |
-
_, model_out = self.run_model(self.model, sample, return_output=True, infer=True)
|
72 |
-
self.plot_mel(batch_idx, sample['mels'], model_out['mel_out'])
|
73 |
-
return outputs
|
74 |
-
|
75 |
-
def build_scheduler(self, optimizer):
|
76 |
-
return torch.optim.lr_scheduler.StepLR(optimizer, hparams['decay_steps'], gamma=0.5)
|
77 |
-
|
78 |
-
def optimizer_step(self, epoch, batch_idx, optimizer, optimizer_idx):
|
79 |
-
if optimizer is None:
|
80 |
-
return
|
81 |
-
optimizer.step()
|
82 |
-
optimizer.zero_grad()
|
83 |
-
if self.scheduler is not None:
|
84 |
-
self.scheduler.step(self.global_step // hparams['accumulate_grad_batches'])
|
|
|
|
|
|
|
|
|
|
|
|
|
|
|
|
|
|
|
|
|
|
|
|
|
|
|
|
|
|
|
|
|
|
|
|
|
|
|
|
|
|
|
|
|
|
|
|
|
|
|
|
|
|
|
|
|
|
|
|
|
|
|
|
|
|
|
|
|
|
|
|
|
|
|
|
|
|
|
|
|
|
|
|
|
|
|
|
|
|
|
|
|
|
|
|
|
|
|
|
|
|
|
|
|
|
|
|
|
|
|
|
|
|
|
|
|
|
|
|
|
|
|
|
|
|
|
|
|
|
|
|
|
|
|
|
|
|
|
|
|
|
|
|
|
|
|
|
|
|
|
|
|
|
|
|
|
|
|
|
|
|
|
|
|
|
|
|
|
|
spaces/AIWaves/SOP_Generation-single/Action/__init__.py
DELETED
@@ -1 +0,0 @@
|
|
1 |
-
from .base_action import Action
|
|
|
|
spaces/AIWaves/SOP_Generation-single/Component/ToolComponent.py
DELETED
@@ -1,887 +0,0 @@
|
|
1 |
-
from abc import abstractmethod
|
2 |
-
import uuid
|
3 |
-
from text2vec import semantic_search
|
4 |
-
from utils import (
|
5 |
-
get_relevant_history,
|
6 |
-
load_knowledge_base_qa,
|
7 |
-
load_knowledge_base_UnstructuredFile,
|
8 |
-
get_embedding,
|
9 |
-
extract,
|
10 |
-
)
|
11 |
-
import json
|
12 |
-
from typing import Dict, List
|
13 |
-
import os
|
14 |
-
from googleapiclient.discovery import build
|
15 |
-
import requests
|
16 |
-
from selenium import webdriver
|
17 |
-
from selenium.webdriver.common.by import By
|
18 |
-
from selenium.webdriver.support.ui import WebDriverWait
|
19 |
-
from selenium.webdriver.support import expected_conditions as EC
|
20 |
-
from bs4 import BeautifulSoup
|
21 |
-
import base64
|
22 |
-
import re
|
23 |
-
from datetime import datetime, timedelta
|
24 |
-
from typing import Tuple, List, Any, Dict
|
25 |
-
from email.mime.text import MIMEText
|
26 |
-
from email.mime.multipart import MIMEMultipart
|
27 |
-
from google.auth.transport.requests import Request
|
28 |
-
from google.oauth2.credentials import Credentials
|
29 |
-
from google_auth_oauthlib.flow import InstalledAppFlow
|
30 |
-
from googleapiclient.discovery import build
|
31 |
-
from googleapiclient.errors import HttpError
|
32 |
-
from tqdm import tqdm
|
33 |
-
|
34 |
-
class ToolComponent:
|
35 |
-
def __init__(self):
|
36 |
-
pass
|
37 |
-
|
38 |
-
@abstractmethod
|
39 |
-
def func(self):
|
40 |
-
pass
|
41 |
-
|
42 |
-
class KnowledgeBaseComponent(ToolComponent):
|
43 |
-
"""
|
44 |
-
Inject knowledge base
|
45 |
-
top_k : Top_k with the highest matching degree
|
46 |
-
type : "QA" or others
|
47 |
-
knowledge_base(json_path) : knowledge_base_path
|
48 |
-
"""
|
49 |
-
def __init__(self, top_k, type, knowledge_base):
|
50 |
-
super().__init__()
|
51 |
-
self.top_k = top_k
|
52 |
-
self.type = type
|
53 |
-
self.knowledge_base = knowledge_base
|
54 |
-
|
55 |
-
if self.type == "QA":
|
56 |
-
(
|
57 |
-
self.kb_embeddings,
|
58 |
-
self.kb_questions,
|
59 |
-
self.kb_answers,
|
60 |
-
self.kb_chunks,
|
61 |
-
) = load_knowledge_base_qa(self.knowledge_base)
|
62 |
-
else:
|
63 |
-
self.kb_embeddings, self.kb_chunks = load_knowledge_base_UnstructuredFile(
|
64 |
-
self.knowledge_base
|
65 |
-
)
|
66 |
-
|
67 |
-
def func(self, agent):
|
68 |
-
query = (
|
69 |
-
agent.long_term_memory[-1]["content"]
|
70 |
-
if len(agent.long_term_memory) > 0
|
71 |
-
else ""
|
72 |
-
)
|
73 |
-
knowledge = ""
|
74 |
-
query = extract(query, "query")
|
75 |
-
query_embedding = get_embedding(query)
|
76 |
-
hits = semantic_search(query_embedding, self.kb_embeddings, top_k=50)
|
77 |
-
hits = hits[0]
|
78 |
-
temp = []
|
79 |
-
if self.type == "QA":
|
80 |
-
for hit in hits:
|
81 |
-
matching_idx = hit["corpus_id"]
|
82 |
-
if self.kb_chunks[matching_idx] in temp:
|
83 |
-
pass
|
84 |
-
else:
|
85 |
-
knowledge = (
|
86 |
-
knowledge
|
87 |
-
+ f"question:{self.kb_questions[matching_idx]},answer:{self.kb_answers[matching_idx]}\n\n"
|
88 |
-
)
|
89 |
-
temp.append(self.kb_answers[matching_idx])
|
90 |
-
if len(temp) == 1:
|
91 |
-
break
|
92 |
-
print(hits[0]["score"])
|
93 |
-
score = hits[0]["score"]
|
94 |
-
if score < 0.5:
|
95 |
-
return {"prompt": "No matching knowledge base"}
|
96 |
-
else:
|
97 |
-
return {"prompt": "The relevant content is: " + knowledge + "\n"}
|
98 |
-
else:
|
99 |
-
for hit in hits:
|
100 |
-
matching_idx = hit["corpus_id"]
|
101 |
-
if self.kb_chunks[matching_idx] in temp:
|
102 |
-
pass
|
103 |
-
else:
|
104 |
-
knowledge = knowledge + f"{self.kb_answers[matching_idx]}\n\n"
|
105 |
-
temp.append(self.kb_answers[matching_idx])
|
106 |
-
if len(temp) == self.top_k:
|
107 |
-
break
|
108 |
-
print(hits[0]["score"])
|
109 |
-
score = hits[0]["score"]
|
110 |
-
if score < 0.5:
|
111 |
-
return {"prompt": "No matching knowledge base"}
|
112 |
-
else:
|
113 |
-
print(knowledge)
|
114 |
-
return {"prompt": "The relevant content is: " + knowledge + "\n"}
|
115 |
-
|
116 |
-
|
117 |
-
class StaticComponent(ToolComponent):
|
118 |
-
"Return static response"
|
119 |
-
def __init__(self, output):
|
120 |
-
super().__init__()
|
121 |
-
self.output = output
|
122 |
-
|
123 |
-
def func(self, agent):
|
124 |
-
outputdict = {"response": self.output}
|
125 |
-
return outputdict
|
126 |
-
|
127 |
-
|
128 |
-
class ExtractComponent(ToolComponent):
|
129 |
-
"""
|
130 |
-
Extract keywords based on the current scene and store them in the environment
|
131 |
-
extract_words(list) : Keywords to be extracted
|
132 |
-
system_prompt & last_prompt : Prompt to extract keywords
|
133 |
-
"""
|
134 |
-
def __init__(
|
135 |
-
self,
|
136 |
-
extract_words,
|
137 |
-
system_prompt,
|
138 |
-
last_prompt=None,
|
139 |
-
):
|
140 |
-
super().__init__()
|
141 |
-
self.extract_words = extract_words
|
142 |
-
self.system_prompt = system_prompt
|
143 |
-
self.default_prompt = (
|
144 |
-
"Please strictly adhere to the following format for outputting:\n"
|
145 |
-
)
|
146 |
-
for extract_word in extract_words:
|
147 |
-
self.default_prompt += (
|
148 |
-
f"<{extract_word}> the content you need to extract </{extract_word}>"
|
149 |
-
)
|
150 |
-
self.last_prompt = last_prompt if last_prompt else self.default_prompt
|
151 |
-
|
152 |
-
def func(self, agent):
|
153 |
-
response = agent.LLM.get_response(
|
154 |
-
agent.long_term_memory,
|
155 |
-
self.system_prompt,
|
156 |
-
self.last_prompt,
|
157 |
-
stream=False,
|
158 |
-
)
|
159 |
-
for extract_word in self.extract_words:
|
160 |
-
key = extract(response, extract_word)
|
161 |
-
key = key if key else response
|
162 |
-
agent.environment.shared_memory[extract_word] = key
|
163 |
-
|
164 |
-
return {}
|
165 |
-
|
166 |
-
|
167 |
-
"""Search sources: chatgpt/search engines/specific search sources/can even be multimodal (if it comes to clothing)"""
|
168 |
-
|
169 |
-
|
170 |
-
class WebSearchComponent(ToolComponent):
|
171 |
-
"""search engines"""
|
172 |
-
|
173 |
-
__ENGINE_NAME__: List = ["google", "bing"]
|
174 |
-
|
175 |
-
def __init__(self, engine_name: str, api: Dict):
|
176 |
-
"""
|
177 |
-
:param engine_name: The name of the search engine used
|
178 |
-
:param api: Pass in a dictionary, such as {"bing":"key1", "google":"key2", ...}, of course each value can also be a list, or more complicated
|
179 |
-
"""
|
180 |
-
super(WebSearchComponent, self).__init__()
|
181 |
-
"""Determine whether the key and engine_name of the api are legal"""
|
182 |
-
|
183 |
-
assert engine_name in WebSearchComponent.__ENGINE_NAME__
|
184 |
-
for api_name in api:
|
185 |
-
assert api_name in WebSearchComponent.__ENGINE_NAME__
|
186 |
-
|
187 |
-
self.api = api
|
188 |
-
self.engine_name = engine_name
|
189 |
-
|
190 |
-
self.search: Dict = {"bing": self._bing_search, "google": self._google_search}
|
191 |
-
|
192 |
-
def _bing_search(self, query: str, **kwargs):
|
193 |
-
"""Initialize search hyperparameters"""
|
194 |
-
subscription_key = self.api["bing"]
|
195 |
-
search_url = "https://api.bing.microsoft.com/v7.0/search"
|
196 |
-
headers = {"Ocp-Apim-Subscription-Key": subscription_key}
|
197 |
-
params = {
|
198 |
-
"q": query,
|
199 |
-
"textDecorations": True,
|
200 |
-
"textFormat": "HTML",
|
201 |
-
"count": 10,
|
202 |
-
}
|
203 |
-
"""start searching"""
|
204 |
-
response = requests.get(search_url, headers=headers, params=params)
|
205 |
-
response.raise_for_status()
|
206 |
-
results = response.json()["webPages"]["value"]
|
207 |
-
"""execute"""
|
208 |
-
metadata_results = []
|
209 |
-
for result in results:
|
210 |
-
metadata_result = {
|
211 |
-
"snippet": result["snippet"],
|
212 |
-
"title": result["name"],
|
213 |
-
"link": result["url"],
|
214 |
-
}
|
215 |
-
metadata_results.append(metadata_result)
|
216 |
-
return {"meta data": metadata_results}
|
217 |
-
|
218 |
-
def _google_search(self, query: str, **kwargs):
|
219 |
-
"""Initialize search hyperparameters"""
|
220 |
-
api_key = self.api[self.engine_name]["api_key"]
|
221 |
-
cse_id = self.api[self.engine_name]["cse_id"]
|
222 |
-
service = build("customsearch", "v1", developerKey=api_key)
|
223 |
-
"""start searching"""
|
224 |
-
results = (
|
225 |
-
service.cse().list(q=query, cx=cse_id, num=10, **kwargs).execute()["items"]
|
226 |
-
)
|
227 |
-
"""execute"""
|
228 |
-
metadata_results = []
|
229 |
-
for result in results:
|
230 |
-
metadata_result = {
|
231 |
-
"snippet": result["snippet"],
|
232 |
-
"title": result["title"],
|
233 |
-
"link": result["link"],
|
234 |
-
}
|
235 |
-
metadata_results.append(metadata_result)
|
236 |
-
return {"meta data": metadata_results}
|
237 |
-
|
238 |
-
def func(self, agent, **kwargs) -> Dict:
|
239 |
-
query = (
|
240 |
-
agent.long_term_memory[-1]["content"]
|
241 |
-
if len(agent.long_term_memory) > 0
|
242 |
-
else " "
|
243 |
-
)
|
244 |
-
response = agent.LLM.get_response(
|
245 |
-
None,
|
246 |
-
system_prompt=f"Please analyze the provided conversation and identify keywords that can be used for a search engine query. Format the output as <keywords>extracted keywords</keywords>:\nConversation:\n{query}",
|
247 |
-
stream=False,
|
248 |
-
)
|
249 |
-
response = extract(response, "keywords")
|
250 |
-
query = response if response else query
|
251 |
-
|
252 |
-
search_results = self.search[self.engine_name](query=query, **kwargs)
|
253 |
-
information = ""
|
254 |
-
for i in search_results["meta data"][:5]:
|
255 |
-
information += i["snippet"]
|
256 |
-
return {
|
257 |
-
"prompt": "You can refer to the following information to reply:\n"
|
258 |
-
+ information
|
259 |
-
}
|
260 |
-
|
261 |
-
def convert_search_engine_to(self, engine_name):
|
262 |
-
assert engine_name in WebSearchComponent.__ENGINE_NAME__
|
263 |
-
self.engine_name = engine_name
|
264 |
-
|
265 |
-
|
266 |
-
class WebCrawlComponent(ToolComponent):
|
267 |
-
"""Open a single web page for crawling"""
|
268 |
-
|
269 |
-
def __init__(self):
|
270 |
-
super(WebCrawlComponent, self).__init__()
|
271 |
-
|
272 |
-
def func(self, agent_dict) -> Dict:
|
273 |
-
url = agent_dict["url"]
|
274 |
-
print(f"crawling {url} ......")
|
275 |
-
content = ""
|
276 |
-
"""Crawling content from url may need to be carried out according to different websites, such as wiki, baidu, zhihu, etc."""
|
277 |
-
driver = webdriver.Chrome()
|
278 |
-
try:
|
279 |
-
"""open url"""
|
280 |
-
driver.get(url)
|
281 |
-
|
282 |
-
"""wait 20 second"""
|
283 |
-
wait = WebDriverWait(driver, 20)
|
284 |
-
wait.until(EC.presence_of_element_located((By.TAG_NAME, "body")))
|
285 |
-
|
286 |
-
"""crawl code"""
|
287 |
-
page_source = driver.page_source
|
288 |
-
|
289 |
-
"""parse"""
|
290 |
-
soup = BeautifulSoup(page_source, "html.parser")
|
291 |
-
|
292 |
-
"""concatenate"""
|
293 |
-
for paragraph in soup.find_all("p"):
|
294 |
-
content = f"{content}\n{paragraph.get_text()}"
|
295 |
-
except Exception as e:
|
296 |
-
print("Error:", e)
|
297 |
-
finally:
|
298 |
-
"""quit"""
|
299 |
-
driver.quit()
|
300 |
-
return {"content": content.strip()}
|
301 |
-
|
302 |
-
|
303 |
-
class MailComponent(ToolComponent):
|
304 |
-
__VALID_ACTION__ = ["read", "send"]
|
305 |
-
|
306 |
-
def __init__(
|
307 |
-
self, cfg_file: str, default_action: str = "read", name: str = "e-mail"
|
308 |
-
):
|
309 |
-
"""'../config/google_mail.json'"""
|
310 |
-
super(MailComponent, self).__init__(name)
|
311 |
-
self.name = name
|
312 |
-
assert (
|
313 |
-
default_action.lower() in self.__VALID_ACTION__
|
314 |
-
), f"Action `{default_action}` is not allowed! The valid action is in `{self.__VALID_ACTION__}`"
|
315 |
-
self.action = default_action.lower()
|
316 |
-
self.credential = self._login(cfg_file)
|
317 |
-
|
318 |
-
def _login(self, cfg_file: str):
|
319 |
-
SCOPES = [
|
320 |
-
"https://www.googleapis.com/auth/gmail.readonly",
|
321 |
-
"https://www.googleapis.com/auth/gmail.send",
|
322 |
-
]
|
323 |
-
creds = None
|
324 |
-
if os.path.exists("token.json"):
|
325 |
-
print("Login Successfully!")
|
326 |
-
creds = Credentials.from_authorized_user_file("token.json", SCOPES)
|
327 |
-
if not creds or not creds.valid:
|
328 |
-
print("Please authorize in an open browser.")
|
329 |
-
if creds and creds.expired and creds.refresh_token:
|
330 |
-
creds.refresh(Request())
|
331 |
-
else:
|
332 |
-
flow = InstalledAppFlow.from_client_secrets_file(cfg_file, SCOPES)
|
333 |
-
creds = flow.run_local_server(port=0)
|
334 |
-
# Save the credentials for the next run
|
335 |
-
with open("token.json", "w") as token:
|
336 |
-
token.write(creds.to_json())
|
337 |
-
return creds
|
338 |
-
|
339 |
-
def _read(self, mail_dict: dict):
|
340 |
-
credential = self.credential
|
341 |
-
state = mail_dict["state"] if "state" in mail_dict else None
|
342 |
-
time_between = (
|
343 |
-
mail_dict["time_between"] if "time_between" in mail_dict else None
|
344 |
-
)
|
345 |
-
sender_mail = mail_dict["sender_mail"] if "sender_mail" in mail_dict else None
|
346 |
-
only_both = mail_dict["only_both"] if "only_both" in mail_dict else False
|
347 |
-
order_by_time = (
|
348 |
-
mail_dict["order_by_time"] if "order_by_time" in mail_dict else "descend"
|
349 |
-
)
|
350 |
-
include_word = (
|
351 |
-
mail_dict["include_word"] if "include_word" in mail_dict else None
|
352 |
-
)
|
353 |
-
exclude_word = (
|
354 |
-
mail_dict["exclude_word"] if "exclude_word" in mail_dict else None
|
355 |
-
)
|
356 |
-
MAX_SEARCH_CNT = (
|
357 |
-
mail_dict["MAX_SEARCH_CNT"] if "MAX_SEARCH_CNT" in mail_dict else 50
|
358 |
-
)
|
359 |
-
number = mail_dict["number"] if "number" in mail_dict else 10
|
360 |
-
if state is None:
|
361 |
-
state = "all"
|
362 |
-
if time_between is not None:
|
363 |
-
assert isinstance(time_between, tuple)
|
364 |
-
assert len(time_between) == 2
|
365 |
-
assert state in ["all", "unread", "read", "sent"]
|
366 |
-
if only_both:
|
367 |
-
assert sender_mail is not None
|
368 |
-
if sender_mail is not None:
|
369 |
-
assert isinstance(sender_mail, str)
|
370 |
-
assert credential
|
371 |
-
assert order_by_time in ["descend", "ascend"]
|
372 |
-
|
373 |
-
def generate_query():
|
374 |
-
query = ""
|
375 |
-
if state in ["unread", "read"]:
|
376 |
-
query = f"is:{state}"
|
377 |
-
if state in ["sent"]:
|
378 |
-
query = f"in:{state}"
|
379 |
-
if only_both:
|
380 |
-
query = f"{query} from:{sender_mail} OR to:{sender_mail}"
|
381 |
-
if sender_mail is not None and not only_both:
|
382 |
-
query = f"{query} from:({sender_mail})"
|
383 |
-
if include_word is not None:
|
384 |
-
query = f"{query} {include_word}"
|
385 |
-
if exclude_word is not None:
|
386 |
-
query = f"{query} -{exclude_word}"
|
387 |
-
if time_between is not None:
|
388 |
-
TIME_FORMAT = "%Y/%m/%d"
|
389 |
-
t1, t2 = time_between
|
390 |
-
if t1 == "now":
|
391 |
-
t1 = datetime.now().strftime(TIME_FORMAT)
|
392 |
-
if t2 == "now":
|
393 |
-
t2 = datetime.now().strftime(TIME_FORMAT)
|
394 |
-
if isinstance(t1, str) and isinstance(t2, str):
|
395 |
-
t1 = datetime.strptime(t1, TIME_FORMAT)
|
396 |
-
t2 = datetime.strptime(t2, TIME_FORMAT)
|
397 |
-
elif isinstance(t1, str) and isinstance(t2, int):
|
398 |
-
t1 = datetime.strptime(t1, TIME_FORMAT)
|
399 |
-
t2 = t1 + timedelta(days=t2)
|
400 |
-
elif isinstance(t1, int) and isinstance(t2, str):
|
401 |
-
t2 = datetime.strptime(t2, TIME_FORMAT)
|
402 |
-
t1 = t2 + timedelta(days=t1)
|
403 |
-
else:
|
404 |
-
assert False, "invalid time"
|
405 |
-
if t1 > t2:
|
406 |
-
t1, t2 = t2, t1
|
407 |
-
query = f"{query} after:{t1.strftime(TIME_FORMAT)} before:{t2.strftime(TIME_FORMAT)}"
|
408 |
-
return query.strip()
|
409 |
-
|
410 |
-
def sort_by_time(data: List[Dict]):
|
411 |
-
if order_by_time == "descend":
|
412 |
-
reverse = True
|
413 |
-
else:
|
414 |
-
reverse = False
|
415 |
-
sorted_data = sorted(
|
416 |
-
data,
|
417 |
-
key=lambda x: datetime.strptime(x["time"], "%Y-%m-%d %H:%M:%S"),
|
418 |
-
reverse=reverse,
|
419 |
-
)
|
420 |
-
return sorted_data
|
421 |
-
|
422 |
-
try:
|
423 |
-
service = build("gmail", "v1", credentials=credential)
|
424 |
-
results = (
|
425 |
-
service.users()
|
426 |
-
.messages()
|
427 |
-
.list(userId="me", labelIds=["INBOX"], q=generate_query())
|
428 |
-
.execute()
|
429 |
-
)
|
430 |
-
|
431 |
-
messages = results.get("messages", [])
|
432 |
-
email_data = list()
|
433 |
-
|
434 |
-
if not messages:
|
435 |
-
print("No eligible emails.")
|
436 |
-
return None
|
437 |
-
else:
|
438 |
-
pbar = tqdm(total=min(MAX_SEARCH_CNT, len(messages)))
|
439 |
-
for cnt, message in enumerate(messages):
|
440 |
-
pbar.update(1)
|
441 |
-
if cnt >= MAX_SEARCH_CNT:
|
442 |
-
break
|
443 |
-
msg = (
|
444 |
-
service.users()
|
445 |
-
.messages()
|
446 |
-
.get(
|
447 |
-
userId="me",
|
448 |
-
id=message["id"],
|
449 |
-
format="full",
|
450 |
-
metadataHeaders=None,
|
451 |
-
)
|
452 |
-
.execute()
|
453 |
-
)
|
454 |
-
|
455 |
-
subject = ""
|
456 |
-
for header in msg["payload"]["headers"]:
|
457 |
-
if header["name"] == "Subject":
|
458 |
-
subject = header["value"]
|
459 |
-
break
|
460 |
-
|
461 |
-
sender = ""
|
462 |
-
for header in msg["payload"]["headers"]:
|
463 |
-
if header["name"] == "From":
|
464 |
-
sender = re.findall(
|
465 |
-
r"\b[\w\.-]+@[\w\.-]+\.\w+\b", header["value"]
|
466 |
-
)[0]
|
467 |
-
break
|
468 |
-
body = ""
|
469 |
-
if "parts" in msg["payload"]:
|
470 |
-
for part in msg["payload"]["parts"]:
|
471 |
-
if part["mimeType"] == "text/plain":
|
472 |
-
data = part["body"]["data"]
|
473 |
-
body = base64.urlsafe_b64decode(data).decode("utf-8")
|
474 |
-
break
|
475 |
-
|
476 |
-
email_info = {
|
477 |
-
"sender": sender,
|
478 |
-
"time": datetime.fromtimestamp(
|
479 |
-
int(msg["internalDate"]) / 1000
|
480 |
-
).strftime("%Y-%m-%d %H:%M:%S"),
|
481 |
-
"subject": subject,
|
482 |
-
"body": body,
|
483 |
-
}
|
484 |
-
email_data.append(email_info)
|
485 |
-
pbar.close()
|
486 |
-
email_data = sort_by_time(email_data)[0:number]
|
487 |
-
return {"results": email_data}
|
488 |
-
except Exception as e:
|
489 |
-
print(e)
|
490 |
-
return None
|
491 |
-
|
492 |
-
def _send(self, mail_dict: dict):
|
493 |
-
recipient_mail = mail_dict["recipient_mail"]
|
494 |
-
subject = mail_dict["subject"]
|
495 |
-
body = mail_dict["body"]
|
496 |
-
credential = self.credential
|
497 |
-
service = build("gmail", "v1", credentials=credential)
|
498 |
-
|
499 |
-
message = MIMEMultipart()
|
500 |
-
message["to"] = recipient_mail
|
501 |
-
message["subject"] = subject
|
502 |
-
|
503 |
-
message.attach(MIMEText(body, "plain"))
|
504 |
-
|
505 |
-
raw_message = base64.urlsafe_b64encode(message.as_bytes()).decode("utf-8")
|
506 |
-
try:
|
507 |
-
message = (
|
508 |
-
service.users()
|
509 |
-
.messages()
|
510 |
-
.send(userId="me", body={"raw": raw_message})
|
511 |
-
.execute()
|
512 |
-
)
|
513 |
-
return {"state": True}
|
514 |
-
except HttpError as error:
|
515 |
-
print(error)
|
516 |
-
return {"state": False}
|
517 |
-
|
518 |
-
def func(self, mail_dict: dict):
|
519 |
-
if "action" in mail_dict:
|
520 |
-
assert mail_dict["action"].lower() in self.__VALID_ACTION__
|
521 |
-
self.action = mail_dict["action"]
|
522 |
-
functions = {"read": self._read, "send": self._send}
|
523 |
-
return functions[self.action](mail_dict)
|
524 |
-
|
525 |
-
def convert_action_to(self, action_name: str):
|
526 |
-
assert (
|
527 |
-
action_name.lower() in self.__VALID_ACTION__
|
528 |
-
), f"Action `{action_name}` is not allowed! The valid action is in `{self.__VALID_ACTION__}`"
|
529 |
-
self.action = action_name.lower()
|
530 |
-
|
531 |
-
|
532 |
-
class WeatherComponet(ToolComponent):
|
533 |
-
def __init__(self, api_key, name="weather", TIME_FORMAT="%Y-%m-%d"):
|
534 |
-
super(WeatherComponet, self).__init__(name)
|
535 |
-
self.name = name
|
536 |
-
self.TIME_FORMAT = TIME_FORMAT
|
537 |
-
self.api_key = api_key
|
538 |
-
|
539 |
-
def _parse(self, data):
|
540 |
-
dict_data: dict = {}
|
541 |
-
for item in data["data"]:
|
542 |
-
date = item["datetime"]
|
543 |
-
dict_data[date] = {}
|
544 |
-
if "weather" in item:
|
545 |
-
dict_data[date]["description"] = item["weather"]["description"]
|
546 |
-
mapping = {
|
547 |
-
"temp": "temperature",
|
548 |
-
"max_temp": "max_temperature",
|
549 |
-
"min_temp": "min_temperature",
|
550 |
-
"precip": "accumulated_precipitation",
|
551 |
-
}
|
552 |
-
for key in ["temp", "max_temp", "min_temp", "precip"]:
|
553 |
-
if key in item:
|
554 |
-
dict_data[date][mapping[key]] = item[key]
|
555 |
-
return dict_data
|
556 |
-
|
557 |
-
def _query(self, city_name, country_code, start_date, end_date):
|
558 |
-
"""https://www.weatherbit.io/api/historical-weather-daily"""
|
559 |
-
# print(datetime.strftime(start_date, self.TIME_FORMAT), datetime.strftime(datetime.now(), self.TIME_FORMAT), end_date, datetime.strftime(datetime.now()+timedelta(days=1), self.TIME_FORMAT))
|
560 |
-
if start_date == datetime.strftime(
|
561 |
-
datetime.now(), self.TIME_FORMAT
|
562 |
-
) and end_date == datetime.strftime(
|
563 |
-
datetime.now() + timedelta(days=1), self.TIME_FORMAT
|
564 |
-
):
|
565 |
-
"""today"""
|
566 |
-
url = f"https://api.weatherbit.io/v2.0/current?city={city_name}&country={country_code}&key={self.api_key}"
|
567 |
-
else:
|
568 |
-
url = f"https://api.weatherbit.io/v2.0/history/daily?&city={city_name}&country={country_code}&start_date={start_date}&end_date={end_date}&key={self.api_key}"
|
569 |
-
response = requests.get(url)
|
570 |
-
data = response.json()
|
571 |
-
return self._parse(data)
|
572 |
-
|
573 |
-
def func(self, weather_dict: Dict) -> Dict:
|
574 |
-
TIME_FORMAT = self.TIME_FORMAT
|
575 |
-
# Beijing, Shanghai
|
576 |
-
city_name = weather_dict["city_name"]
|
577 |
-
# CN, US
|
578 |
-
country_code = weather_dict["country_code"]
|
579 |
-
# 2020-02-02
|
580 |
-
start_date = datetime.strftime(
|
581 |
-
datetime.strptime(weather_dict["start_date"], self.TIME_FORMAT),
|
582 |
-
self.TIME_FORMAT,
|
583 |
-
)
|
584 |
-
end_date = weather_dict["end_date"] if "end_date" in weather_dict else None
|
585 |
-
if end_date is None:
|
586 |
-
end_date = datetime.strftime(
|
587 |
-
datetime.strptime(start_date, TIME_FORMAT) + timedelta(days=-1),
|
588 |
-
TIME_FORMAT,
|
589 |
-
)
|
590 |
-
else:
|
591 |
-
end_date = datetime.strftime(
|
592 |
-
datetime.strptime(weather_dict["end_date"], self.TIME_FORMAT),
|
593 |
-
self.TIME_FORMAT,
|
594 |
-
)
|
595 |
-
if datetime.strptime(start_date, TIME_FORMAT) > datetime.strptime(
|
596 |
-
end_date, TIME_FORMAT
|
597 |
-
):
|
598 |
-
start_date, end_date = end_date, start_date
|
599 |
-
assert start_date != end_date
|
600 |
-
return self._query(city_name, country_code, start_date, end_date)
|
601 |
-
|
602 |
-
|
603 |
-
class TranslateComponent(ToolComponent):
|
604 |
-
__SUPPORT_LANGUAGE__ = [
|
605 |
-
"af",
|
606 |
-
"am",
|
607 |
-
"ar",
|
608 |
-
"as",
|
609 |
-
"az",
|
610 |
-
"ba",
|
611 |
-
"bg",
|
612 |
-
"bn",
|
613 |
-
"bo",
|
614 |
-
"bs",
|
615 |
-
"ca",
|
616 |
-
"cs",
|
617 |
-
"cy",
|
618 |
-
"da",
|
619 |
-
"de",
|
620 |
-
"dsb",
|
621 |
-
"dv",
|
622 |
-
"el",
|
623 |
-
"en",
|
624 |
-
"es",
|
625 |
-
"et",
|
626 |
-
"eu",
|
627 |
-
"fa",
|
628 |
-
"fi",
|
629 |
-
"fil",
|
630 |
-
"fj",
|
631 |
-
"fo",
|
632 |
-
"fr",
|
633 |
-
"fr-CA",
|
634 |
-
"ga",
|
635 |
-
"gl",
|
636 |
-
"gom",
|
637 |
-
"gu",
|
638 |
-
"ha",
|
639 |
-
"he",
|
640 |
-
"hi",
|
641 |
-
"hr",
|
642 |
-
"hsb",
|
643 |
-
"ht",
|
644 |
-
"hu",
|
645 |
-
"hy",
|
646 |
-
"id",
|
647 |
-
"ig",
|
648 |
-
"ikt",
|
649 |
-
"is",
|
650 |
-
"it",
|
651 |
-
"iu",
|
652 |
-
"iu-Latn",
|
653 |
-
"ja",
|
654 |
-
"ka",
|
655 |
-
"kk",
|
656 |
-
"km",
|
657 |
-
"kmr",
|
658 |
-
"kn",
|
659 |
-
"ko",
|
660 |
-
"ku",
|
661 |
-
"ky",
|
662 |
-
"ln",
|
663 |
-
"lo",
|
664 |
-
"lt",
|
665 |
-
"lug",
|
666 |
-
"lv",
|
667 |
-
"lzh",
|
668 |
-
"mai",
|
669 |
-
"mg",
|
670 |
-
"mi",
|
671 |
-
"mk",
|
672 |
-
"ml",
|
673 |
-
"mn-Cyrl",
|
674 |
-
"mn-Mong",
|
675 |
-
"mr",
|
676 |
-
"ms",
|
677 |
-
"mt",
|
678 |
-
"mww",
|
679 |
-
"my",
|
680 |
-
"nb",
|
681 |
-
"ne",
|
682 |
-
"nl",
|
683 |
-
"nso",
|
684 |
-
"nya",
|
685 |
-
"or",
|
686 |
-
"otq",
|
687 |
-
"pa",
|
688 |
-
"pl",
|
689 |
-
"prs",
|
690 |
-
"ps",
|
691 |
-
"pt",
|
692 |
-
"pt-PT",
|
693 |
-
"ro",
|
694 |
-
"ru",
|
695 |
-
"run",
|
696 |
-
"rw",
|
697 |
-
"sd",
|
698 |
-
"si",
|
699 |
-
"sk",
|
700 |
-
"sl",
|
701 |
-
"sm",
|
702 |
-
"sn",
|
703 |
-
"so",
|
704 |
-
"sq",
|
705 |
-
"sr-Cyrl",
|
706 |
-
"sr-Latn",
|
707 |
-
"st",
|
708 |
-
"sv",
|
709 |
-
"sw",
|
710 |
-
"ta",
|
711 |
-
"te",
|
712 |
-
"th",
|
713 |
-
"ti",
|
714 |
-
"tk",
|
715 |
-
"tlh-Latn",
|
716 |
-
"tlh-Piqd",
|
717 |
-
"tn",
|
718 |
-
"to",
|
719 |
-
"tr",
|
720 |
-
"tt",
|
721 |
-
"ty",
|
722 |
-
"ug",
|
723 |
-
"uk",
|
724 |
-
"ur",
|
725 |
-
"uz",
|
726 |
-
"vi",
|
727 |
-
"xh",
|
728 |
-
"yo",
|
729 |
-
"yua",
|
730 |
-
"yue",
|
731 |
-
"zh-Hans",
|
732 |
-
"zh-Hant",
|
733 |
-
"zu",
|
734 |
-
]
|
735 |
-
|
736 |
-
def __init__(
|
737 |
-
self, api_key, location, default_target_language="zh-cn", name="translate"
|
738 |
-
):
|
739 |
-
super(TranslateComponent, self).__init__(name)
|
740 |
-
self.name = name
|
741 |
-
self.api_key = api_key
|
742 |
-
self.location = location
|
743 |
-
self.default_target_language = default_target_language
|
744 |
-
|
745 |
-
def func(self, translate_dict: Dict) -> Dict:
|
746 |
-
content = translate_dict["content"]
|
747 |
-
target_language = self.default_target_language
|
748 |
-
if "target_language" in translate_dict:
|
749 |
-
target_language = translate_dict["target_language"]
|
750 |
-
assert (
|
751 |
-
target_language in self.__SUPPORT_LANGUAGE__
|
752 |
-
), f"language `{target_language}` is not supported."
|
753 |
-
|
754 |
-
endpoint = "https://api.cognitive.microsofttranslator.com"
|
755 |
-
|
756 |
-
path = "/translate"
|
757 |
-
constructed_url = endpoint + path
|
758 |
-
|
759 |
-
params = {"api-version": "3.0", "to": target_language}
|
760 |
-
|
761 |
-
headers = {
|
762 |
-
"Ocp-Apim-Subscription-Key": self.api_key,
|
763 |
-
"Ocp-Apim-Subscription-Region": self.location,
|
764 |
-
"Content-type": "application/json",
|
765 |
-
"X-ClientTraceId": str(uuid.uuid4()),
|
766 |
-
}
|
767 |
-
|
768 |
-
body = [{"text": content}]
|
769 |
-
|
770 |
-
request = requests.post(
|
771 |
-
constructed_url, params=params, headers=headers, json=body
|
772 |
-
)
|
773 |
-
response = request.json()
|
774 |
-
response = json.dumps(
|
775 |
-
response,
|
776 |
-
sort_keys=True,
|
777 |
-
ensure_ascii=False,
|
778 |
-
indent=4,
|
779 |
-
separators=(",", ": "),
|
780 |
-
)
|
781 |
-
response = eval(response)
|
782 |
-
return {"result": response[0]["translations"][0]["text"]}
|
783 |
-
|
784 |
-
|
785 |
-
class APIComponent(ToolComponent):
|
786 |
-
def __init__(self):
|
787 |
-
super(APIComponent, self).__init__()
|
788 |
-
|
789 |
-
def func(self, agent) -> Dict:
|
790 |
-
pass
|
791 |
-
|
792 |
-
|
793 |
-
class FunctionComponent(ToolComponent):
|
794 |
-
def __init__(
|
795 |
-
self,
|
796 |
-
functions,
|
797 |
-
function_call="auto",
|
798 |
-
response_type="response",
|
799 |
-
your_function=None,
|
800 |
-
):
|
801 |
-
super().__init__()
|
802 |
-
self.functions = functions
|
803 |
-
self.function_call = function_call
|
804 |
-
self.parameters = {}
|
805 |
-
self.available_functions = {}
|
806 |
-
self.response_type = response_type
|
807 |
-
if your_function:
|
808 |
-
function_name = your_function["name"]
|
809 |
-
function_content = your_function["content"]
|
810 |
-
exec(function_content)
|
811 |
-
self.available_functions[function_name] = eval(function_name)
|
812 |
-
|
813 |
-
for function in self.functions:
|
814 |
-
self.parameters[function["name"]] = list(
|
815 |
-
function["parameters"]["properties"].keys()
|
816 |
-
)
|
817 |
-
self.available_functions[function["name"]] = eval(function["name"])
|
818 |
-
|
819 |
-
def func(self, agent):
|
820 |
-
messages = agent.long_term_memory
|
821 |
-
outputdict = {}
|
822 |
-
query = agent.long_term_memory[-1].content if len(agent.long_term_memory) > 0 else " "
|
823 |
-
relevant_history = get_relevant_history(
|
824 |
-
query,
|
825 |
-
agent.long_term_memory[:-1],
|
826 |
-
agent.chat_embeddings[:-1],
|
827 |
-
)
|
828 |
-
response = agent.LLM.get_response(
|
829 |
-
messages,
|
830 |
-
None,
|
831 |
-
functions=self.functions,
|
832 |
-
stream=False,
|
833 |
-
function_call=self.function_call,
|
834 |
-
relevant_history=relevant_history,
|
835 |
-
)
|
836 |
-
response_message = response
|
837 |
-
if response_message.get("function_call"):
|
838 |
-
function_name = response_message["function_call"]["name"]
|
839 |
-
fuction_to_call = self.available_functions[function_name]
|
840 |
-
function_args = json.loads(response_message["function_call"]["arguments"])
|
841 |
-
input_args = {}
|
842 |
-
for args_name in self.parameters[function_name]:
|
843 |
-
input_args[args_name] = function_args.get(args_name)
|
844 |
-
function_response = fuction_to_call(**input_args)
|
845 |
-
if self.response_type == "response":
|
846 |
-
outputdict["response"] = function_response
|
847 |
-
elif self.response_type == "prompt":
|
848 |
-
outputdict["prompt"] = function_response
|
849 |
-
|
850 |
-
return outputdict
|
851 |
-
|
852 |
-
|
853 |
-
class CodeComponent(ToolComponent):
|
854 |
-
def __init__(self, file_name, keyword) -> None:
|
855 |
-
super().__init__()
|
856 |
-
self.file_name = file_name
|
857 |
-
self.keyword = keyword
|
858 |
-
self.system_prompt = (
|
859 |
-
"you need to extract the modified code as completely as possible."
|
860 |
-
)
|
861 |
-
self.last_prompt = (
|
862 |
-
f"Please strictly adhere to the following format for outputting: \n"
|
863 |
-
)
|
864 |
-
self.last_prompt += (
|
865 |
-
f"<{self.keyword}> the content you need to extract </{self.keyword}>"
|
866 |
-
)
|
867 |
-
|
868 |
-
def func(self, agent):
|
869 |
-
response = agent.LLM.get_response(
|
870 |
-
agent.long_term_memory,
|
871 |
-
self.system_prompt,
|
872 |
-
self.last_prompt,
|
873 |
-
stream=False,
|
874 |
-
)
|
875 |
-
code = extract(response, self.keyword)
|
876 |
-
code = code if code else response
|
877 |
-
os.makedirs("output_code", exist_ok=True)
|
878 |
-
file_name = "output_code/" + self.file_name
|
879 |
-
codes = code.split("\n")
|
880 |
-
if codes[0] == "```python":
|
881 |
-
codes.remove(codes[0])
|
882 |
-
if codes[-1] == "```":
|
883 |
-
codes.remove(codes[-1])
|
884 |
-
code = "\n".join(codes)
|
885 |
-
with open(file_name, "w", encoding="utf-8") as f:
|
886 |
-
f.write(code)
|
887 |
-
return {}
|
|
|
|
|
|
|
|
|
|
|
|
|
|
|
|
|
|
|
|
|
|
|
|
|
|
|
|
|
|
|
|
|
|
|
|
|
|
|
|
|
|
|
|
|
|
|
|
|
|
|
|
|
|
|
|
|
|
|
|
|
|
|
|
|
|
|
|
|
|
|
|
|
|
|
|
|
|
|
|
|
|
|
|
|
|
|
|
|
|
|
|
|
|
|
|
|
|
|
|
|
|
|
|
|
|
|
|
|
|
|
|
|
|
|
|
|
|
|
|
|
|
|
|
|
|
|
|
|
|
|
|
|
|
|
|
|
|
|
|
|
|
|
|
|
|
|
|
|
|
|
|
|
|
|
|
|
|
|
|
|
|
|
|
|
|
|
|
|
|
|
|
|
|
|
|
|
|
|
|
|
|
|
|
|
|
|
|
|
|
|
|
|
|
|
|
|
|
|
|
|
|
|
|
|
|
|
|
|
|
|
|
|
|
|
|
|
|
|
|
|
|
|
|
|
|
|
|
|
|
|
|
|
|
|
|
|
|
|
|
|
|
|
|
|
|
|
|
|
|
|
|
|
|
|
|
|
|
|
|
|
|
|
|
|
|
|
|
|
|
|
|
|
|
|
|
|
|
|
|
|
|
|
|
|
|
|
|
|
|
|
|
|
|
|
|
|
|
|
|
|
|
|
|
|
|
|
|
|
|
|
|
|
|
|
|
|
|
|
|
|
|
|
|
|
|
|
|
|
|
|
|
|
|
|
|
|
|
|
|
|
|
|
|
|
|
|
|
|
|
|
|
|
|
|
|
|
|
|
|
|
|
|
|
|
|
|
|
|
|
|
|
|
|
|
|
|
|
|
|
|
|
|
|
|
|
|
|
|
|
|
|
|
|
|
|
|
|
|
|
|
|
|
|
|
|
|
|
|
|
|
|
|
|
|
|
|
|
|
|
|
|
|
|
|
|
|
|
|
|
|
|
|
|
|
|
|
|
|
|
|
|
|
|
|
|
|
|
|
|
|
|
|
|
|
|
|
|
|
|
|
|
|
|
|
|
|
|
|
|
|
|
|
|
|
|
|
|
|
|
|
|
|
|
|
|
|
|
|
|
|
|
|
|
|
|
|
|
|
|
|
|
|
|
|
|
|
|
|
|
|
|
|
|
|
|
|
|
|
|
|
|
|
|
|
|
|
|
|
|
|
|
|
|
|
|
|
|
|
|
|
|
|
|
|
|
|
|
|
|
|
|
|
|
|
|
|
|
|
|
|
|
|
|
|
|
|
|
|
|
|
|
|
|
|
|
|
|
|
|
|
|
|
|
|
|
|
|
|
|
|
|
|
|
|
|
|
|
|
|
|
|
|
|
|
|
|
|
|
|
|
|
|
|
|
|
|
|
|
|
|
|
|
|
|
|
|
|
|
|
|
|
|
|
|
|
|
|
|
|
|
|
|
|
|
|
|
|
|
|
|
|
|
|
|
|
|
|
|
|
|
|
|
|
|
|
|
|
|
|
|
|
|
|
|
|
|
|
|
|
|
|
|
|
|
|
|
|
|
|
|
|
|
|
|
|
|
|
|
|
|
|
|
|
|
|
|
|
|
|
|
|
|
|
|
|
|
|
|
|
|
|
|
|
|
|
|
|
|
|
|
|
|
|
|
|
|
|
|
|
|
|
|
|
|
|
|
|
|
|
|
|
|
|
|
|
|
|
|
|
|
|
|
|
|
|
|
|
|
|
|
|
|
|
|
|
|
|
|
|
|
|
|
|
|
|
|
|
|
|
|
|
|
|
|
|
|
|
|
|
|
|
|
|
|
|
|
|
|
|
|
|
|
|
|
|
|
|
|
|
|
|
|
|
|
|
|
|
|
|
|
|
|
|
|
|
|
|
|
|
|
|
|
|
|
|
|
|
|
|
|
|
|
|
|
|
|
|
|
|
|
|
|
|
|
|
|
|
|
|
|
|
|
|
|
|
|
|
|
|
|
|
|
|
|
|
|
|
|
|
|
|
|
|
|
|
|
|
|
|
|
|
|
|
|
|
|
|
|
|
|
|
|
|
|
|
|
|
|
|
|
|
|
|
|
|
|
|
|
|
|
|
|
|
|
|
|
|
|
|
|
|
|
|
|
|
|
|
|
|
|
|
|
|
|
|
|
|
|
|
|
|
|
|
|
|
|
|
|
|
|
|
|
|
|
|
|
|
|
|
|
|
|
|
|
|
|
|
|
|
|
|
|
|
|
|
|
|
|
|
|
|
|
|
|
|
|
|
|
|
|
|
|
|
|
|
|
|
|
|
|
|
|
|
|
|
|
|
|
|
|
|
|
|
|
|
|
|
|
|
|
|
|
|
|
|
|
|
|
|
|
|
|
|
|
|
|
|
|
|
|
|
|
|
|
|
|
|
|
|
|
|
|
|
|
|
|
|
|
|
|
|
|
|
|
|
|
|
|
|
|
|
|
|
|
|
|
|
|
|
|
|
|
|
|
|
|
|
|
|
|
|
|
|
|
|
|
|
|
|
|
|
|
|
|
|
|
|
|
|
|
|
|
|
|
|
|
|
|
|
|
|
|
|
|
|
|
|
|
|
|
|
|
|
|
|
|
|
|
|
|
|
|
|
|
|
|
|
|
|
|
|
|
|
|
|
|
|
|
|
|
|
|
|
|
|
|
|
|
|
|
|
|
|
|
|
|
|
|
|
|
|
|
|
|
|
|
|
|
|
|
|
|
|
|
|
|
|
|
|
|
|
|
|
|
|
|
|
|
|
|
|
|
|
|
|
|
|
|
|
|
|
|
|
|
|
|
|
|
|
|
|
|
|
|
|
|
|
|
|
|
|
|
|
|
|
|
|
|
|
|
|
|
|
|
|
|
|
|
|
|
|
|
|
|
|
|
|
|
|
|
|
|
|
|
|
|
|
|
|
|
|
|
|
|
|
|
|
|
|
|
|
|
|
|
|
|
|
|
|
|
|
|
|
|
|
|
|
|
|
|
|
|
|
|
|
|
|
|
|
|
|
|
|
|
|
|
|
|
|
|
|
|
|
|
|
|
|
|
|
|
|
|
|
|
|
|
|
|
|
|
|
|
|
|
|
|
|
|
|
|
|
|
|
|
|
|
|
|
|
|
|
|
|
|
|
|
|
|
|
|
|
|
|
|
|
|
|
|
|
|
|
|
|
|
|
|
|
|
|
|
|
|
|
|
|
|
|
|
|
|
|
|
|
|
|
|
|
|
|
|
|
|
|
|
|
|
|
|
|
|
|
|
|
|
|
|
|
|
|
|
|
|
|
|
|
|
|
|
|
|
|
|
|
|
|
|
|
|
|
|
|
|
|
|
|
|
|
|
|
|
|
|
|
|
|
|
|
|
|
|
|
|
|
|
|
|
|
|
|
|
|
|
|
|
|
|
|
|
|
|
|
|
|
|
|
|
|
|
|
|
|
|
|
|
|
|
|
|
|
|
|
|
|
|
|
|
|
|
|
|
|
|
|
|
|
|
|
|
|
|
|
|
|
|
|
|
|
|
|
|
|
|
|
|
|
|
|
|
|
|
|
|
|
|
|
|
|
|
|
|
|
|
|
|
|
|
|
|
|
|
|
|
|
|
|
|
|
|
|
|
|
|
|
|
|
|
|
|
|
|
|
|
|
|
|
|
|
|
|
|
|
|
|
|
|
|
|
|
|
|
|
|
|
|
|
|
|
|
|
|
|
|
|
|
|
|
|
|
|
|
|
|
|
|
|
|
|
|
|
|
|
|
|
|
|
|
|
|
|
|
|
|
|
|
|
|
|
|
|
|
|
|
|
|
|
|
|
|
|
|
|
|
|
|
|
|
|
|
|
|
|
|
|
|
|
|
|
|
|
|
|
|
|
|
|
|
|
|
|
|
|
|
|
|
|
|
|
|
|
|
|
|
|
|
|
|
|
|
|
|
|
|
|
|
|
|
|
|
|
|
|
|
|
|
|
|
|
|
|
|
|
|
|
|
|
spaces/ATang0729/Forecast4Muses/Model/Model6/Model6_1_ClothesKeyPoint/mmpose_1_x/configs/fashion_2d_keypoint/__init__.py
DELETED
File without changes
|
spaces/Abhilashvj/planogram-compliance/utils/loggers/wandb/__init__.py
DELETED
File without changes
|
spaces/AgentVerse/agentVerse/README.md
DELETED
@@ -1,429 +0,0 @@
|
|
1 |
-
---
|
2 |
-
title: AgentVerse
|
3 |
-
sdk: gradio
|
4 |
-
license: apache-2.0
|
5 |
-
emoji: 🤖
|
6 |
-
colorFrom: indigo
|
7 |
-
colorTo: indigo
|
8 |
-
---
|
9 |
-
|
10 |
-
<h1 align="center"> 🤖 AgentVerse 🪐 </h1>
|
11 |
-
|
12 |
-
<h3 align="center">
|
13 |
-
<p>A Framework for Multi-LLM Environment Simulation</p>
|
14 |
-
</h3>
|
15 |
-
|
16 |
-
<p align="center">
|
17 |
-
<a href="https://github.com/OpenBMB/AgentVerse/blob/main/LICENSE">
|
18 |
-
<img alt="License: Apache2" src="https://img.shields.io/badge/License-Apache_2.0-green.svg">
|
19 |
-
</a>
|
20 |
-
<a href="https://www.python.org/downloads/release/python-3916/">
|
21 |
-
<img alt="Python Version" src="https://img.shields.io/badge/python-3.9+-blue.svg">
|
22 |
-
</a>
|
23 |
-
<a href="https://github.com/OpenBMB/AgentVerse/actions/">
|
24 |
-
<img alt="Build" src="https://img.shields.io/github/actions/workflow/status/OpenBMB/AgentVerse/test.yml">
|
25 |
-
</a>
|
26 |
-
<a href="https://github.com/psf/black">
|
27 |
-
<img alt="Code Style: Black" src="https://img.shields.io/badge/code%20style-black-black">
|
28 |
-
</a>
|
29 |
-
<a href="https://github.com/OpenBMB/AgentVerse/issues">
|
30 |
-
<img alt="Contributions: Welcome" src="https://img.shields.io/badge/contributions-welcome-brightgreen.svg?style=flat">
|
31 |
-
</a>
|
32 |
-
|
33 |
-
</p>
|
34 |
-
|
35 |
-
<p align="center">
|
36 |
-
<img src="./imgs/title.png" width="512">
|
37 |
-
</p>
|
38 |
-
|
39 |
-
<p align="center">
|
40 |
-
【English | <a href="README_zh.md">Chinese</a>】
|
41 |
-
</p>
|
42 |
-
|
43 |
-
**AgentVerse** offers a versatile framework that streamlines the process of creating custom multi-agent environments for large language models (LLMs). Designed to facilitate swift development and customization with minimal effort, our framework empowers researchers to concentrate on their research, rather than being bogged down by implementation details.
|
44 |
-
|
45 |
-
⚠️⚠️⚠️ We're refactoring the code, and the goal is to provide a flexibility to construct simulation(without a predefined goal) and task-solving(with a specific goal) environments. Please note that this README is slightly outdated, we will update it soon. If you require a stable version that exclusively supports simulation environments, you can use [`release-0.1`](https://github.com/OpenBMB/AgentVerse/tree/release-0.1) branch.
|
46 |
-
|
47 |
-
---
|
48 |
-
|
49 |
-
## ✨ Features
|
50 |
-
|
51 |
-
- 🥳 **Efficient Environment Building:** Our framework provides a collection of essential building blocks for effortlessly creating a multi-agent environment. With only a few lines in a configuration file, you can easily construct basic environments such as a chat room for LLMs. This process entails defining the environment's settings and prompts for LLMs, enabling researchers like you to concentrate on experimentation and analysis.
|
52 |
-
|
53 |
-
- ⚙️ **Customizable Components**: AgentVerse simplifies the multi-agent environment by dividing it into five functional modules and defining their respective interfaces. For complex environments that cannot be constructed directly using the basic modules offered in AgentVerse, you can customize one or more of the interfaces within these five functional modules to efficiently create your own multi-agent environment according to your requirements.
|
54 |
-
|
55 |
-
- 🛠 **Tools (Plugins) Utilization**: AgentVerse supports the multi-agent environments with tools. Currently, AgentVerse supports tools provided in [BMTools](https://github.com/OpenBMB/BMTools).
|
56 |
-
|
57 |
-
## 📰 What's New
|
58 |
-
- [2023/10/5] 💡 We release the code of our paper [AgentVerse: Facilitating Multi-Agent Collaboration and Exploring Emergent Behaviors in Agents](https://arxiv.org/abs/2308.10848), and refactor our codebase to enable the creation of both simulation and task-solving environment! We have placed the code for Minecraft example in the paper at the [`minecraft`](https://github.com/OpenBMB/AgentVerse/tree/minecraft) branch. Our tool-using example will soon be updated to the `main` branch. Stay tuned!
|
59 |
-
|
60 |
-
- [2023/8/22] 📝 We're excited to share our work-in-progress paper [AgentVerse: Facilitating Multi-Agent Collaboration and Exploring Emergent Behaviors in Agents](https://arxiv.org/abs/2308.10848) related to this repository.
|
61 |
-
<p align="center">
|
62 |
-
<img width="616" alt="Screen Shot 2023-09-01 at 12 08 57 PM" src="https://github.com/OpenBMB/AgentVerse/assets/11704492/6db1c907-b7fc-42f9-946c-89853a28f386">
|
63 |
-
</p>
|
64 |
-
|
65 |
-
- [2023/6/5] 🎉 We are thrilled to present an array of [demos](#-simple-demo-video), including [NLP Classroom](#nlp-classroom), [Prisoner Dilemma](#prisoner-dilemma), [Software Design](#software-design), [Database Administrator](#database-administrator-dba), and a simple [H5 Pokemon Game](#pokemon) that enables the interaction with the characters in Pokemon! Try out these demos and have fun!
|
66 |
-
- [2023/5/1] 🚀 [AgentVerse](https://github.com/OpenBMB/AgentVerse) is officially launched!
|
67 |
-
|
68 |
-
## 🌟 Join Us!
|
69 |
-
AgentVerse is on a mission to revolutionize the multi-agent environment for large language models, and we're eagerly looking for passionate collaborators to join us on this exciting journey.
|
70 |
-
### How Can You Contribute?
|
71 |
-
- **Code Development**: If you're an engineer, help us refine, optimize, and expand the current framework. We're always looking for talented developers to enhance our existing features and develop new modules.
|
72 |
-
|
73 |
-
- **Documentation and Tutorials**: If you have a knack for writing, help us improve our documentation, create tutorials, or write blog posts to make AgentVerse more accessible to the broader community.
|
74 |
-
|
75 |
-
- **Application Exploration**: If you're intrigued by multi-agent applications and are eager to experiment using AgentVerse, we'd be thrilled to support your journey and see what you create!
|
76 |
-
|
77 |
-
- **Feedback and Suggestions**: Use AgentVerse and provide us with feedback. Your insights can lead to potential improvements and ensure that our framework remains top-notch.
|
78 |
-
|
79 |
-
Also, if you're passionate about advancing the frontiers of multi-agent environments and are eager to dive deeper into research, we invite you to join our team at THUNLP. To explore this exciting opportunity and embark on a collaborative journey with us, please reach out to [[email protected]]([email protected]) and [[email protected]]([email protected]) and express your interest. We're keen to welcome motivated individuals like you to our lab!
|
80 |
-
|
81 |
-
👉Also, check our Discord: https://discord.gg/cnutfCtC.
|
82 |
-
|
83 |
-
## 🗓 Coming Soon
|
84 |
-
- [x] Code release of our [paper](https://arxiv.org/abs/2308.10848)
|
85 |
-
- [ ] Add documentation
|
86 |
-
- [ ] Support more sophisticated memory for conversation history
|
87 |
-
- [ ] Add support for local LLM
|
88 |
-
|
89 |
-
|
90 |
-
## 👾 Simple Demo Video
|
91 |
-
|
92 |
-
We demonstrate the following cases that are expertly crafted by AgentVerse.
|
93 |
-
<!--
|
94 |
-
### [](https://youtu.be/9JCVfzMFhaM)
|
95 |
-
-->
|
96 |
-
<!---->
|
97 |
-
|
98 |
-
<!-- - **NLP Classroom**: -->
|
99 |
-
|
100 |
-
#### NLP Classroom
|
101 |
-
In the NLP class, the professor and students engage in interactive communication. When students have a question, they raise their hands and patiently wait for the professor to call on them. Only after being called on by the professor, can students speak and ask their questions.
|
102 |
-
|
103 |
-
Use the following command to launch the NLP Classroom example:
|
104 |
-
```bash
|
105 |
-
python agentverse_command/main_simulation_gui.py --task simulation/nlp_classroom_9players
|
106 |
-
```
|
107 |
-
|
108 |
-
[Wacth the NLP Classroom Video](https://github.com/OpenBMB/AgentVerse/assets/11704492/6ea07850-595e-4a28-a82e-f863011353c2)
|
109 |
-
|
110 |
-
|
111 |
-
#### Prisoner Dilemma
|
112 |
-
A prisoner's Dilemma is a thought experiment that challenges two completely rational agents to a dilemma: they can cooperate with their partner for mutual benefit or betray their partner ("defect") for individual reward.
|
113 |
-
|
114 |
-
Use the following command to launch the Prisoner Dilemma example:
|
115 |
-
```bash
|
116 |
-
python agentverse_command/main_simulation_gui.py --task simulation/prisoner_dilemma
|
117 |
-
```
|
118 |
-
|
119 |
-
[Wacth the Prisoner's Dilemma Video](https://github.com/OpenBMB/AgentVerse/assets/11704492/017c46e5-c738-4fca-9352-b008e2d518bd)
|
120 |
-
|
121 |
-
|
122 |
-
#### Software Design
|
123 |
-
In the Software Design example, a code writer, a code tester and a code reviewer collaborate on the code generation problem. Given a problem, the code writer first composes the code implementation. The code tester runs the unit tests and provides the feedback. The code viewer then generates a review. After collecting the test feedback and review, the code writer iteratively refines the code.
|
124 |
-
|
125 |
-
Use the following command to launch the Software Design example:
|
126 |
-
```bash
|
127 |
-
python agentverse_command/main_simulation_gui.py --task simulation/sde_team/sde_team_2players
|
128 |
-
```
|
129 |
-
|
130 |
-
[Wacth the Software Design Video](https://github.com/OpenBMB/AgentVerse/assets/11704492/5058066a-abee-490d-8659-b4e54661626a)
|
131 |
-
|
132 |
-
|
133 |
-
#### [Database Administrator (DBA)](https://github.com/TsinghuaDatabaseGroup/DB-GPT)
|
134 |
-
|
135 |
-
In the database diagnosis scenario, the Chief DBA monitors the system anomalies (e.g., slow queries, locks, crash down). If detected, the domain experts are alerted to analyze root causes, share insights, and suggest optimization solutions together. The Chief DBA then provides a summarized report to the user.
|
136 |
-
|
137 |
-
```bash
|
138 |
-
python agentverse_command/main_simulation_gui.py --task simulation/db_diag
|
139 |
-
```
|
140 |
-
|
141 |
-
[Wacth the DBA Video](https://github.com/OpenBMB/AgentVerse/assets/11704492/c633419d-afbb-47d4-bb12-6bb512e7af3a)
|
142 |
-
|
143 |
-
#### [Text Evaluation (ChatEval)](https://github.com/chanchimin/ChatEval)
|
144 |
-
In the context of the text evaluation scenario, we recommend users explore the [ChatEval](https://github.com/chanchimin/ChatEval) repo. They've implemented a multi-agent referee team on AgentVerse to assess the quality of text generated by different models. When given two distinct pieces of text, roles within ChatEval can autonomously debate the nuances and disparities, drawing upon their assigned personas, and subsequently provide their judgments. Experiments indicate that their referee team, enriched with diverse roles specified in [config.yaml](#2-configuring-the-agents), aligns more closely with human evaluations. This demo is built upon the [Fastchat](https://github.com/lm-sys/FastChat) repo, and we'd like to express our appreciation for their foundational work.
|
145 |
-
|
146 |
-
|
147 |
-
[Wacth the ChatEval Video](https://github.com/OpenBMB/AgentVerse/assets/75533759/58f33468-f15b-4bac-ae01-8d0780019f85)
|
148 |
-
|
149 |
-
#### Pokemon
|
150 |
-
**Currently available only in [`release-0.1`](https://github.com/OpenBMB/AgentVerse/tree/release-0.1)**. In the game, agents can walk around the game world, and interact with one another. As a player, you take on the role of an agent and can engage with others at any time. There are 6 characters in the Pokémon environment who appeared in Pokemon Emerald: [May](https://bulbapedia.bulbagarden.net/wiki/May_(game)), [Professor Birch](https://bulbapedia.bulbagarden.net/wiki/Professor_Birch), [Steven Stone](https://bulbapedia.bulbagarden.net/wiki/Steven_Stone), [Maxie](https://bulbapedia.bulbagarden.net/wiki/Maxie), [Archie](https://bulbapedia.bulbagarden.net/wiki/Archie) and [Joseph](https://bulbapedia.bulbagarden.net/wiki/Mr._Stone).
|
151 |
-
|
152 |
-
To launch the Pokemon game, first launch a local server with the following command:
|
153 |
-
```bash
|
154 |
-
uvicorn pokemon_server:app --reload --port 10002
|
155 |
-
```
|
156 |
-
Then open another terminal in the project's root path and run the following command:
|
157 |
-
```bash
|
158 |
-
cd ui
|
159 |
-
# If you do not have npm installed, you need to install it before running the following commands
|
160 |
-
# https://docs.npmjs.com/downloading-and-installing-node-js-and-npm
|
161 |
-
# We have tested on [email protected], [email protected]
|
162 |
-
npm install
|
163 |
-
npm run watch
|
164 |
-
```
|
165 |
-
Wait for the compilation to complete, and have fun! (WASD for moving around, and SPACE for launching a conversation.)
|
166 |
-
|
167 |
-
[Wacth the Pokemon Video](https://github.com/OpenBMB/AgentVerse/assets/11704492/4d07da68-f942-4205-b558-f155e95782e7)
|
168 |
-
|
169 |
-
|
170 |
-
|
171 |
-
## Contents
|
172 |
-
|
173 |
-
- [✨ Features](#-features)
|
174 |
-
- [📰 What's New](#-whats-new)
|
175 |
-
- [🌟 Join Us!](#-join-us)
|
176 |
-
- [How Can You Contribute?](#how-can-you-contribute)
|
177 |
-
- [🗓 Coming Soon](#-coming-soon)
|
178 |
-
- [👾 Simple Demo Video](#-simple-demo-video)
|
179 |
-
- [NLP Classroom](#nlp-classroom)
|
180 |
-
- [Prisoner Dilemma](#prisoner-dilemma)
|
181 |
-
- [Software Design](#software-design)
|
182 |
-
- [Database Administrator (DBA)](#database-administrator-dba)
|
183 |
-
- [Text Evaluation (ChatEval)](#text-evaluation-chateval)
|
184 |
-
- [Pokemon](#pokemon)
|
185 |
-
- [Contents](#contents)
|
186 |
-
- [🚀 Getting Started](#-getting-started)
|
187 |
-
- [Installation](#installation)
|
188 |
-
- [Simulation CLI Example](#simulation-cli-example)
|
189 |
-
- [Simulation Local Website Demo](#simulation-local-website-demo)
|
190 |
-
- [Task-Solving CLI Example](#task-solving-cli-example)
|
191 |
-
- [💡 Philosophy](#-philosophy)
|
192 |
-
- [Environment](#environment)
|
193 |
-
- [Agent](#agent)
|
194 |
-
- [✍️ Customize Your Own Environment](#️-customize-your-own-environment)
|
195 |
-
- [A Simple Example: Building a Classroom Environment](#a-simple-example-building-a-classroom-environment)
|
196 |
-
- [1. Creating a Task Directory and Configuring the Environment](#1-creating-a-task-directory-and-configuring-the-environment)
|
197 |
-
- [2. Configuring the Agents](#2-configuring-the-agents)
|
198 |
-
- [3. Writing an Output Parser](#3-writing-an-output-parser)
|
199 |
-
- [Customization Guide for More Complex Environments](#customization-guide-for-more-complex-environments)
|
200 |
-
- [🔎 Examples](#-examples)
|
201 |
-
- [Star History](#star-history)
|
202 |
-
- [Citation](#citation)
|
203 |
-
- [Contact](#contact)
|
204 |
-
|
205 |
-
|
206 |
-
|
207 |
-
## 🚀 Getting Started
|
208 |
-
|
209 |
-
### Installation
|
210 |
-
|
211 |
-
```bash
|
212 |
-
pip install -U agentverse
|
213 |
-
```
|
214 |
-
Or you can install the package by manually cloning the latest repository
|
215 |
-
```bash
|
216 |
-
git clone https://github.com/OpenBMB/AgentVerse.git --depth 1
|
217 |
-
cd AgentVerse
|
218 |
-
pip install -r requirements.txt
|
219 |
-
```
|
220 |
-
Some users have reported problems installing the `orjson` required by `gradio`. One simple workaround is to install it with Anaconda `conda install -c conda-forge orjson`.
|
221 |
-
|
222 |
-
You also need to export your OpenAI API key as follows:
|
223 |
-
```bash
|
224 |
-
# Export your OpenAI API key
|
225 |
-
export OPENAI_API_KEY="your_api_key_here"
|
226 |
-
# Or if you are using Azure
|
227 |
-
export AZURE_OPENAI_API_KEY="your_api_key_here"
|
228 |
-
export AZURE_OPENAI_API_BASE="your_api_base_here"
|
229 |
-
```
|
230 |
-
|
231 |
-
If you want use Azure OpenAI services, pleas export your Azure OpenAI key and OpenAI API base as follows:
|
232 |
-
```bash
|
233 |
-
export AZURE_OPENAI_API_KEY="your_api_key_here"
|
234 |
-
export AZURE_OPENAI_API_BASE="your_api_base_here"
|
235 |
-
```
|
236 |
-
|
237 |
-
If you want to use the tools provided by BMTools, you need to install BMTools as follows:
|
238 |
-
```bash
|
239 |
-
git clone git+https://github.com/OpenBMB/BMTools.git
|
240 |
-
cd BMTools
|
241 |
-
pip install -r requirements.txt
|
242 |
-
python setup.py develop
|
243 |
-
```
|
244 |
-
|
245 |
-
|
246 |
-
<!--
|
247 |
-
# Install BMTools
|
248 |
-
cd ../
|
249 |
-
git clone [email protected]:OpenBMB/BMTools.git
|
250 |
-
cd BMTools
|
251 |
-
python setup.py develop
|
252 |
-
-->
|
253 |
-
|
254 |
-
### Simulation CLI Example
|
255 |
-
|
256 |
-
You can create a multi-agent environments provided by us. Using the classroom scenario as an example. In this scenario, there are nine agents, one playing the role of a professor and the other eight as students.
|
257 |
-
|
258 |
-
```shell
|
259 |
-
python3 agentverse_command/main_simulation_cli.py --task simulation/nlp_classroom_9players
|
260 |
-
# or if you have installed AgentVerse via pip
|
261 |
-
agentverse-simulation --task simulation/nlp_classroom_9players
|
262 |
-
```
|
263 |
-
|
264 |
-
### Simulation Local Website Demo
|
265 |
-
|
266 |
-
We also provide a local website demo for this environment. You can launch it with
|
267 |
-
|
268 |
-
```shell
|
269 |
-
python3 agentverse_command/main_simulation_gui.py --task simulation/nlp_classroom_9players
|
270 |
-
# or if you have installed AgentVerse via pip
|
271 |
-
agentverse-simulation-gui --task simulation/nlp_classroom_9players
|
272 |
-
```
|
273 |
-
After successfully launching the local server, you can visit [http://127.0.0.1:7860/](http://127.0.0.1:7860/) to view the classroom environment.
|
274 |
-
|
275 |
-
### Task-Solving CLI Example
|
276 |
-
|
277 |
-
To run the experiments with the task-solving environment proposed in our [paper](https://arxiv.org/abs/2308.10848), you can use the following command:
|
278 |
-
|
279 |
-
```shell
|
280 |
-
# Run the Humaneval benchmark using gpt-3.5-turbo
|
281 |
-
python3 agentverse_command/main_tasksolving_cli.py --task tasksolving/humaneval/gpt-3.5 --dataset_path data/humaneval/test.jsonl --overwrite
|
282 |
-
# or if you have installed AgentVerse via pip
|
283 |
-
agentverse-tasksolving --task tasksolving/humaneval/gpt-3.5 --dataset_path data/humaneval/test.jsonl --overwrite
|
284 |
-
```
|
285 |
-
|
286 |
-
You can take a look at `agentverse/tasks/tasksolving` for more experiments we have done in our paper.
|
287 |
-
|
288 |
-
|
289 |
-
## 💡 Philosophy
|
290 |
-
|
291 |
-
### Environment
|
292 |
-
|
293 |
-
At the core of our framework is the environment, which plays a crucial role in enabling researchers to study the behavior of agents under different conditions. We believe that the environment should be flexible and extensible, allowing researchers to easily customize it to fit their needs. To achieve this, we have abstracted the environment into five rule components, and implementing different environments is actually implementing different rules:
|
294 |
-
|
295 |
-
- **Describer**: This component provides a description of the environment at each turn for each agent. You can customize the describer to define the specific requirements of their environment, such as the agents with whom an agent can interact.
|
296 |
-
- **Order**: This component defines the order in which agents take actions within the environment. You can customize the order to reflect the desired interaction between agents. We provide several basic order options, including `random`, `sequential`, and `concurrent` (in which all agents take an action in each turn).
|
297 |
-
- **Selector**: This component selects the valid messages generated by agents. Sometimes agents may generate invalid responses, and the selector is used to filter out unexpected results.
|
298 |
-
- **Updater**: This component updates the memory of each agent. In certain cases, the response generated by one agent should not be seen by all agents (e.g., if agents are in different rooms). For each response, the updater updates only the agents who can see it.
|
299 |
-
- **Visibility**: This component maintains the list of agents that each agent can see throughout the environment's changes. For example, when an agent moves from one room to another, the list of visible agents of each agent should be updated by `visibility`.
|
300 |
-
|
301 |
-
By abstracting the environment into these five components, we have created a highly flexible and extensible framework that enables researchers to easily build and customize their own multi-agent environments.
|
302 |
-
|
303 |
-
### Agent
|
304 |
-
|
305 |
-
Another fundamental component is the agent. Currently we provide two types of agents: **ConversationAgent** and **ToolAgent**. You can also customize your own agent by inheriting BaseAgent class (tutorial coming soon).
|
306 |
-
|
307 |
-
## ✍️ Customize Your Own Environment
|
308 |
-
|
309 |
-
We have provided several examples in the `agentverse/tasks` directory. To customize your environment, you should
|
310 |
-
|
311 |
-
1. Create a task directory in `agentverse/tasks`
|
312 |
-
2. Write the configuration file
|
313 |
-
3. Write the output parser that parses the response of your agents.
|
314 |
-
4. Add your parser in `agentverse/tasks/__init__.py`
|
315 |
-
|
316 |
-
We will use a simple example in `agentverse/tasks/nlp_classroom_3players` to illustrate the procedure.
|
317 |
-
|
318 |
-
### A Simple Example: Building a Classroom Environment
|
319 |
-
|
320 |
-
To illustrate how to customize your environment, we'll use a simple example of building a classroom environment where one agent is the professor, one is the student, and one is the teaching assistant.
|
321 |
-
|
322 |
-
##### 1. Creating a Task Directory and Configuring the Environment
|
323 |
-
|
324 |
-
First, we need to create a task directory and write our configuration file for the environment. In the `agentverse/tasks` directory, create a new directory called `nlp_classroom_3players`. Inside this directory, create a `config.yaml` file and write the following configuration:
|
325 |
-
|
326 |
-
```yaml
|
327 |
-
# config.yaml
|
328 |
-
environment:
|
329 |
-
env_type: basic # Use the basic environment provided in AgentVerse
|
330 |
-
max_turns: 10 # Specify the maximum number of dialogue turns
|
331 |
-
rule:
|
332 |
-
order:
|
333 |
-
type: sequential # Use the sequential order
|
334 |
-
visibility:
|
335 |
-
type: all # Each message can be seen by all agents
|
336 |
-
selector:
|
337 |
-
type: basic # Basic selector (do not select)
|
338 |
-
updater:
|
339 |
-
type: basic # Basic updater (update the message to all agents)
|
340 |
-
describer:
|
341 |
-
type: basic # Basic describer (no description)
|
342 |
-
```
|
343 |
-
|
344 |
-
This configuration specifies that we will use the basic environment provided in AgentVerse, with a maximum of 10 dialogue turns. We'll use the sequential order, with all messages visible to all agents. We won't be using any selectors, our updater will update the messages to all the agents and our describer will provide no description.
|
345 |
-
|
346 |
-
##### 2. Configuring the Agents
|
347 |
-
|
348 |
-
Next, we'll configure the agents. In the `config.yaml` file, we'll add the configuration for each agent. Here's an example configuration for the professor:
|
349 |
-
|
350 |
-
```yaml
|
351 |
-
# config.yaml
|
352 |
-
agents:
|
353 |
-
-
|
354 |
-
agent_type: conversation
|
355 |
-
name: Professor Micheal # Name of the agent
|
356 |
-
role_description: You are Prof. Micheal, ... # Description of the agent
|
357 |
-
memory:
|
358 |
-
memory_type: chat_history # Will store all the chat history
|
359 |
-
prompt_template: *professor_prompt
|
360 |
-
llm:
|
361 |
-
llm_type: text-davinci-003 # Will use OpenAICompletion LLM
|
362 |
-
model: text-davinci-003 # The arguments passed to the api call
|
363 |
-
temperature: 0.7
|
364 |
-
max_tokens: 250
|
365 |
-
```
|
366 |
-
|
367 |
-
In this example, we'll use the `conversation` agent type. We've given the agent a name and a description, and we'll store the chat history in memory. We've also provided a prompt template with placeholders marked as ${placeholder}. These will be instantiated by the `_fill_prompt_template` method of the agent.
|
368 |
-
|
369 |
-
##### 3. Writing an Output Parser
|
370 |
-
|
371 |
-
The next step is to write a simple parser for your agent's response. Because you may have specified the output format in your prompt template, you need to provide a corresponding parser. In this example, we inform the model to output in the following format in our prompt template
|
372 |
-
|
373 |
-
```
|
374 |
-
Action: Speak
|
375 |
-
Action Input: (the content)
|
376 |
-
```
|
377 |
-
|
378 |
-
We'll write a parser to extract the content from the agent's response. Refer to the code for more details. We've decorated our parser function with `@output_parser_registry.register('classroom_parser')` to register it with our framework. Finally, we import our parser in `agentverse/tasks/__init__.py`.
|
379 |
-
|
380 |
-
With these steps, we've successfully built a simple classroom environment and customized it for our needs.
|
381 |
-
|
382 |
-
### Customization Guide for More Complex Environments
|
383 |
-
|
384 |
-
While we provide a basic framework for building environments with our five rule components, more complex environments may require further customization. A detailed documentation and tutorial is coming soon. Here we briefly introduce some steps you can take to customize your environment:
|
385 |
-
|
386 |
-
1. **Customize the five rule components**. Each rule component has an interface, allowing you to customize its behavior to suit your specific needs. It's important to note that these components are not necessarily independent and can interact through the `rule_params` dictionary in the environment. You can create your own rule components and integrate them with the existing ones to build more complex interactions between agents.
|
387 |
-
2. **Customize the environment itself**. Our `basic` environment provides a default execution order for the five rule components that is suitable for most cases, but you can inherit the `BaseEnvironment` class and write your own `run` method to implement a more sophisticated execution order.
|
388 |
-
3. **Customize the agent**. Depending on your specific use case, you may also need to inherit the `BaseAgent` class. For example, you may want to use your local LLM as your agents or create agents with specialized knowledge or skills.
|
389 |
-
|
390 |
-
|
391 |
-
|
392 |
-
## 🔎 Examples
|
393 |
-
|
394 |
-
Currently, we offer some simple examples in the `agentverse/tasks` directory, each demonstrating different possibilities of our framework. While the performance of these examples may not be optimal due to limited prompt engineering, they are intended to showcase the capabilities of our framework, such as allowing the use of tools.
|
395 |
-
|
396 |
-
Here's a brief overview of each example:
|
397 |
-
|
398 |
-
1. `nlp_classroom_3players`: This example illustrates a simple case in which agents will speak in sequential order.
|
399 |
-
2. `nlp_classroom_9players`: This is an NLP class example. Here, students can raise their hand when they have a question, and the professor can call on the students to let them ask. Students are only allowed to speak after they are called on.
|
400 |
-
3. `nlp_classroom_9players_group`: This example showcases group discussions. The professor may initiate a group discussion when needed, and students can exclusively interact with fellow students within the same group during the discussion.
|
401 |
-
4. `nlp_classroom_3players_withtool`: Students in this classroom can use Bing search API when listening to the class.
|
402 |
-
5. `math_problem_2players_tools`: A simple example demonstrating how two agents can use the WolframAlpha API to play an arithmetic game.
|
403 |
-
6. `prisoner_dilema`: The Prisoner's Dilemma is a thought experiment involving two rational agents facing a choice between cooperating for mutual benefit or betraying their partner for individual gain.
|
404 |
-
7. `db_diag`: The Chief DBA monitors (agents) the database system for anomalies and alerts memory and CPU agents if any are detected. They (agents) analyze root causes and suggest optimization solutions. The Chief DBA (agent) provides a diagnosis summary to the user, who can give instructions or evaluate the proposed solutions' effectiveness.
|
405 |
-
8. `sde_team`: In the SDE team, code writer, code tester and code reviewer collaborate on the code generation problem.
|
406 |
-
9. `pokemon`: This example intimates Pokemon game.
|
407 |
-
|
408 |
-
|
409 |
-
## Star History
|
410 |
-
|
411 |
-
[](https://star-history.com/#OpenBMB/AgentVerse&Date)
|
412 |
-
|
413 |
-
|
414 |
-
## Citation
|
415 |
-
If you find this repo helpful, feel free to cite us.
|
416 |
-
```
|
417 |
-
@article{chen2023agentverse,
|
418 |
-
title={Agentverse: Facilitating multi-agent collaboration and exploring emergent behaviors in agents},
|
419 |
-
author={Chen, Weize and Su, Yusheng and Zuo, Jingwei and Yang, Cheng and Yuan, Chenfei and Qian, Chen and Chan, Chi-Min and Qin, Yujia and Lu, Yaxi and Xie, Ruobing and others},
|
420 |
-
journal={arXiv preprint arXiv:2308.10848},
|
421 |
-
year={2023}
|
422 |
-
}
|
423 |
-
```
|
424 |
-
|
425 |
-
## Contact
|
426 |
-
|
427 |
-
Weize Chen: [email protected]
|
428 |
-
|
429 |
-
[Yusheng Su](https://yushengsu-thu.github.io/): [email protected]
|
|
|
|
|
|
|
|
|
|
|
|
|
|
|
|
|
|
|
|
|
|
|
|
|
|
|
|
|
|
|
|
|
|
|
|
|
|
|
|
|
|
|
|
|
|
|
|
|
|
|
|
|
|
|
|
|
|
|
|
|
|
|
|
|
|
|
|
|
|
|
|
|
|
|
|
|
|
|
|
|
|
|
|
|
|
|
|
|
|
|
|
|
|
|
|
|
|
|
|
|
|
|
|
|
|
|
|
|
|
|
|
|
|
|
|
|
|
|
|
|
|
|
|
|
|
|
|
|
|
|
|
|
|
|
|
|
|
|
|
|
|
|
|
|
|
|
|
|
|
|
|
|
|
|
|
|
|
|
|
|
|
|
|
|
|
|
|
|
|
|
|
|
|
|
|
|
|
|
|
|
|
|
|
|
|
|
|
|
|
|
|
|
|
|
|
|
|
|
|
|
|
|
|
|
|
|
|
|
|
|
|
|
|
|
|
|
|
|
|
|
|
|
|
|
|
|
|
|
|
|
|
|
|
|
|
|
|
|
|
|
|
|
|
|
|
|
|
|
|
|
|
|
|
|
|
|
|
|
|
|
|
|
|
|
|
|
|
|
|
|
|
|
|
|
|
|
|
|
|
|
|
|
|
|
|
|
|
|
|
|
|
|
|
|
|
|
|
|
|
|
|
|
|
|
|
|
|
|
|
|
|
|
|
|
|
|
|
|
|
|
|
|
|
|
|
|
|
|
|
|
|
|
|
|
|
|
|
|
|
|
|
|
|
|
|
|
|
|
|
|
|
|
|
|
|
|
|
|
|
|
|
|
|
|
|
|
|
|
|
|
|
|
|
|
|
|
|
|
|
|
|
|
|
|
|
|
|
|
|
|
|
|
|
|
|
|
|
|
|
|
|
|
|
|
|
|
|
|
|
|
|
|
|
|
|
|
|
|
|
|
|
|
|
|
|
|
|
|
|
|
|
|
|
|
|
|
|
|
|
|
|
|
|
|
|
|
|
|
|
|
|
|
|
|
|
|
|
|
|
|
|
|
|
|
|
|
|
|
|
|
|
|
|
|
|
|
|
|
|
|
|
|
|
|
|
|
|
|
|
|
|
|
|
|
|
|
|
|
|
|
|
|
|
|
|
|
|
|
|
|
|
|
|
|
|
|
|
|
|
|
|
|
|
|
|
|
|
|
|
|
|
|
|
|
|
|
|
|
|
|
|
|
|
|
|
|
|
|
|
|
|
|
|
|
|
|
|
|
|
|
|
|
|
|
|
|
|
|
|
|
|
|
|
|
|
|
|
|
|
|
|
|
|
|
|
|
|
|
|
|
|
|
|
|
|
|
|
|
|
|
|
|
|
|
|
|
|
|
|
|
|
|
|
|
|
|
|
|
|
|
|
|
|
|
|
|
|
|
|
|
|
|
|
|
|
|
|
|
|
|
|
|
|
|
|
|
|
|
|
|
|
|
|
|
|
|
|
|
|
|
|
|
|
|
|
|
|
|
|
|
|
|
|
|
|
|
|
|
|
|
|
|
|
|
|
|
|
|
|
|
|
|
|
|
|
|
|
|
|
|
|
|
|
|
|
|
|
|
|
|
|
|
|
|
|
|
|
|
|
|
|
|
|
|
|
|
|
|
|
|
|
|
|
|
|
|
|
|
|
|
|
|
|
|
|
|
|
|
|
|
|
|
|
|
|
|
|
|
|
|
|
|
|
|
|
|
|
|
|
|
|
|
|
|
|
|
|
|
|
|
|
|
|
|
|
|
|
|
|
|
|
|
|
|
|
|
|
|
|
|
|
|
|
|
|
|
|
|
|
|
|
|
|
|
|
|
|
|
|
|
|
|
|
|
|
|
|
|
|
|
|
|
|
|
|
|
|
|
|
|
|
|
|
|
|
|
|
|
|
|
|
|
|
|
|
|
|
|
|
spaces/AgentVerse/agentVerse/ui/src/phaser3-rex-plugins/templates/ui/colorinput/colorinput/methods/OpenColorPicker.js
DELETED
@@ -1,53 +0,0 @@
|
|
1 |
-
import CreateColorPicker from './CreateColorPicker.js';
|
2 |
-
import DropDown from '../../../dropdown/DropDown.js';
|
3 |
-
|
4 |
-
var OpenColorPicker = function () {
|
5 |
-
if (this.colorPicker) {
|
6 |
-
return;
|
7 |
-
}
|
8 |
-
|
9 |
-
// Layout it to get full height
|
10 |
-
var colorPicker = CreateColorPicker.call(this).layout();
|
11 |
-
|
12 |
-
var dropDownBehavior = new DropDown(colorPicker, {
|
13 |
-
// Transition
|
14 |
-
duration: {
|
15 |
-
in: this.colorPickerEaseInDuration,
|
16 |
-
out: this.colorPickerEaseOutDuration
|
17 |
-
},
|
18 |
-
transitIn: this.colorPickerTransitInCallback,
|
19 |
-
transitOut: this.colorPickerTransitOutCallback,
|
20 |
-
|
21 |
-
// Position
|
22 |
-
expandDirection: this.colorPickerExpandDirection,
|
23 |
-
|
24 |
-
alignTargetX: this,
|
25 |
-
alignTargetY: this,
|
26 |
-
|
27 |
-
bounds: this.colorPickerBounds,
|
28 |
-
|
29 |
-
// Close condition
|
30 |
-
touchOutsideClose: true,
|
31 |
-
})
|
32 |
-
.on('open', function () {
|
33 |
-
// After popping up
|
34 |
-
// Can click
|
35 |
-
colorPicker.on('valuechange', function (value) {
|
36 |
-
this.setValue(value);
|
37 |
-
}, this);
|
38 |
-
}, this)
|
39 |
-
|
40 |
-
.on('close', function () {
|
41 |
-
this.colorPicker = undefined;
|
42 |
-
this.dropDownBehavior = undefined;
|
43 |
-
}, this)
|
44 |
-
|
45 |
-
this.colorPicker = colorPicker;
|
46 |
-
this.dropDownBehavior = dropDownBehavior;
|
47 |
-
|
48 |
-
this.pin(colorPicker);
|
49 |
-
|
50 |
-
return this;
|
51 |
-
}
|
52 |
-
|
53 |
-
export default OpenColorPicker;
|
|
|
|
|
|
|
|
|
|
|
|
|
|
|
|
|
|
|
|
|
|
|
|
|
|
|
|
|
|
|
|
|
|
|
|
|
|
|
|
|
|
|
|
|
|
|
|
|
|
|
|
|
|
|
|
|
|
|
|
|
|
|
|
|
|
|
|
|
|
|
|
|
|
|
|
|
|
|
|
|
|
|
|
|
|
|
|
|
|
|
|
|
|
|
|
|
|
|
|
|
|
|
|
|
|
|
|
spaces/AgentVerse/agentVerse/ui/src/phaser3-rex-plugins/templates/ui/sides/childbehaviors/Visible.js
DELETED
@@ -1,21 +0,0 @@
|
|
1 |
-
import IndexOf from '../../../../plugins/utils/object/IndexOf.js';
|
2 |
-
import Container from '../../container/Container.js';
|
3 |
-
|
4 |
-
const ContainerSetChildVisible = Container.prototype.setChildVisible;
|
5 |
-
|
6 |
-
export default {
|
7 |
-
setChildVisible(child, visible) {
|
8 |
-
var key;
|
9 |
-
if (typeof (child) === 'string') {
|
10 |
-
var key = child;
|
11 |
-
child = this.sizerChildren[key];
|
12 |
-
} else {
|
13 |
-
key = IndexOf(this.sizerChildren, child);
|
14 |
-
}
|
15 |
-
if (visible === undefined) {
|
16 |
-
visible = (this.currentChildKey === key) ? true : false;
|
17 |
-
}
|
18 |
-
ContainerSetChildVisible.call(this, child, visible);
|
19 |
-
return this;
|
20 |
-
}
|
21 |
-
}
|
|
|
|
|
|
|
|
|
|
|
|
|
|
|
|
|
|
|
|
|
|
|
|
|
|
|
|
|
|
|
|
|
|
|
|
|
|
|
|
|
|
|
|
spaces/Androidonnxfork/CivitAi-to-Diffusers/diffusers/docs/source/en/optimization/tome.md
DELETED
@@ -1,116 +0,0 @@
|
|
1 |
-
<!--Copyright 2023 The HuggingFace Team. All rights reserved.
|
2 |
-
|
3 |
-
Licensed under the Apache License, Version 2.0 (the "License"); you may not use this file except in compliance with
|
4 |
-
the License. You may obtain a copy of the License at
|
5 |
-
|
6 |
-
http://www.apache.org/licenses/LICENSE-2.0
|
7 |
-
|
8 |
-
Unless required by applicable law or agreed to in writing, software distributed under the License is distributed on
|
9 |
-
an "AS IS" BASIS, WITHOUT WARRANTIES OR CONDITIONS OF ANY KIND, either express or implied. See the License for the
|
10 |
-
specific language governing permissions and limitations under the License.
|
11 |
-
-->
|
12 |
-
|
13 |
-
# Token Merging
|
14 |
-
|
15 |
-
Token Merging (introduced in [Token Merging: Your ViT But Faster](https://arxiv.org/abs/2210.09461)) works by merging the redundant tokens / patches progressively in the forward pass of a Transformer-based network. It can speed up the inference latency of the underlying network.
|
16 |
-
|
17 |
-
After Token Merging (ToMe) was released, the authors released [Token Merging for Fast Stable Diffusion](https://arxiv.org/abs/2303.17604), which introduced a version of ToMe which is more compatible with Stable Diffusion. We can use ToMe to gracefully speed up the inference latency of a [`DiffusionPipeline`]. This doc discusses how to apply ToMe to the [`StableDiffusionPipeline`], the expected speedups, and the qualitative aspects of using ToMe on the [`StableDiffusionPipeline`].
|
18 |
-
|
19 |
-
## Using ToMe
|
20 |
-
|
21 |
-
The authors of ToMe released a convenient Python library called [`tomesd`](https://github.com/dbolya/tomesd) that lets us apply ToMe to a [`DiffusionPipeline`] like so:
|
22 |
-
|
23 |
-
```diff
|
24 |
-
from diffusers import StableDiffusionPipeline
|
25 |
-
import tomesd
|
26 |
-
|
27 |
-
pipeline = StableDiffusionPipeline.from_pretrained(
|
28 |
-
"runwayml/stable-diffusion-v1-5", torch_dtype=torch.float16
|
29 |
-
).to("cuda")
|
30 |
-
+ tomesd.apply_patch(pipeline, ratio=0.5)
|
31 |
-
|
32 |
-
image = pipeline("a photo of an astronaut riding a horse on mars").images[0]
|
33 |
-
```
|
34 |
-
|
35 |
-
And that’s it!
|
36 |
-
|
37 |
-
`tomesd.apply_patch()` exposes [a number of arguments](https://github.com/dbolya/tomesd#usage) to let us strike a balance between the pipeline inference speed and the quality of the generated tokens. Amongst those arguments, the most important one is `ratio`. `ratio` controls the number of tokens that will be merged during the forward pass. For more details on `tomesd`, please refer to the original repository https://github.com/dbolya/tomesd and [the paper](https://arxiv.org/abs/2303.17604).
|
38 |
-
|
39 |
-
## Benchmarking `tomesd` with `StableDiffusionPipeline`
|
40 |
-
|
41 |
-
We benchmarked the impact of using `tomesd` on [`StableDiffusionPipeline`] along with [xformers](https://huggingface.co/docs/diffusers/optimization/xformers) across different image resolutions. We used A100 and V100 as our test GPU devices with the following development environment (with Python 3.8.5):
|
42 |
-
|
43 |
-
```bash
|
44 |
-
- `diffusers` version: 0.15.1
|
45 |
-
- Python version: 3.8.16
|
46 |
-
- PyTorch version (GPU?): 1.13.1+cu116 (True)
|
47 |
-
- Huggingface_hub version: 0.13.2
|
48 |
-
- Transformers version: 4.27.2
|
49 |
-
- Accelerate version: 0.18.0
|
50 |
-
- xFormers version: 0.0.16
|
51 |
-
- tomesd version: 0.1.2
|
52 |
-
```
|
53 |
-
|
54 |
-
We used this script for benchmarking: [https://gist.github.com/sayakpaul/27aec6bca7eb7b0e0aa4112205850335](https://gist.github.com/sayakpaul/27aec6bca7eb7b0e0aa4112205850335). Following are our findings:
|
55 |
-
|
56 |
-
### A100
|
57 |
-
|
58 |
-
| Resolution | Batch size | Vanilla | ToMe | ToMe + xFormers | ToMe speedup (%) | ToMe + xFormers speedup (%) |
|
59 |
-
| --- | --- | --- | --- | --- | --- | --- |
|
60 |
-
| 512 | 10 | 6.88 | 5.26 | 4.69 | 23.54651163 | 31.83139535 |
|
61 |
-
| | | | | | | |
|
62 |
-
| 768 | 10 | OOM | 14.71 | 11 | | |
|
63 |
-
| | 8 | OOM | 11.56 | 8.84 | | |
|
64 |
-
| | 4 | OOM | 5.98 | 4.66 | | |
|
65 |
-
| | 2 | 4.99 | 3.24 | 3.1 | 35.07014028 | 37.8757515 |
|
66 |
-
| | 1 | 3.29 | 2.24 | 2.03 | 31.91489362 | 38.29787234 |
|
67 |
-
| | | | | | | |
|
68 |
-
| 1024 | 10 | OOM | OOM | OOM | | |
|
69 |
-
| | 8 | OOM | OOM | OOM | | |
|
70 |
-
| | 4 | OOM | 12.51 | 9.09 | | |
|
71 |
-
| | 2 | OOM | 6.52 | 4.96 | | |
|
72 |
-
| | 1 | 6.4 | 3.61 | 2.81 | 43.59375 | 56.09375 |
|
73 |
-
|
74 |
-
***The timings reported here are in seconds. Speedups are calculated over the `Vanilla` timings.***
|
75 |
-
|
76 |
-
### V100
|
77 |
-
|
78 |
-
| Resolution | Batch size | Vanilla | ToMe | ToMe + xFormers | ToMe speedup (%) | ToMe + xFormers speedup (%) |
|
79 |
-
| --- | --- | --- | --- | --- | --- | --- |
|
80 |
-
| 512 | 10 | OOM | 10.03 | 9.29 | | |
|
81 |
-
| | 8 | OOM | 8.05 | 7.47 | | |
|
82 |
-
| | 4 | 5.7 | 4.3 | 3.98 | 24.56140351 | 30.1754386 |
|
83 |
-
| | 2 | 3.14 | 2.43 | 2.27 | 22.61146497 | 27.70700637 |
|
84 |
-
| | 1 | 1.88 | 1.57 | 1.57 | 16.4893617 | 16.4893617 |
|
85 |
-
| | | | | | | |
|
86 |
-
| 768 | 10 | OOM | OOM | 23.67 | | |
|
87 |
-
| | 8 | OOM | OOM | 18.81 | | |
|
88 |
-
| | 4 | OOM | 11.81 | 9.7 | | |
|
89 |
-
| | 2 | OOM | 6.27 | 5.2 | | |
|
90 |
-
| | 1 | 5.43 | 3.38 | 2.82 | 37.75322284 | 48.06629834 |
|
91 |
-
| | | | | | | |
|
92 |
-
| 1024 | 10 | OOM | OOM | OOM | | |
|
93 |
-
| | 8 | OOM | OOM | OOM | | |
|
94 |
-
| | 4 | OOM | OOM | 19.35 | | |
|
95 |
-
| | 2 | OOM | 13 | 10.78 | | |
|
96 |
-
| | 1 | OOM | 6.66 | 5.54 | | |
|
97 |
-
|
98 |
-
As seen in the tables above, the speedup with `tomesd` becomes more pronounced for larger image resolutions. It is also interesting to note that with `tomesd`, it becomes possible to run the pipeline on a higher resolution, like 1024x1024.
|
99 |
-
|
100 |
-
It might be possible to speed up inference even further with [`torch.compile()`](https://huggingface.co/docs/diffusers/optimization/torch2.0).
|
101 |
-
|
102 |
-
## Quality
|
103 |
-
|
104 |
-
As reported in [the paper](https://arxiv.org/abs/2303.17604), ToMe can preserve the quality of the generated images to a great extent while speeding up inference. By increasing the `ratio`, it is possible to further speed up inference, but that might come at the cost of a deterioration in the image quality.
|
105 |
-
|
106 |
-
To test the quality of the generated samples using our setup, we sampled a few prompts from the “Parti Prompts” (introduced in [Parti](https://parti.research.google/)) and performed inference with the [`StableDiffusionPipeline`] in the following settings:
|
107 |
-
|
108 |
-
- Vanilla [`StableDiffusionPipeline`]
|
109 |
-
- [`StableDiffusionPipeline`] + ToMe
|
110 |
-
- [`StableDiffusionPipeline`] + ToMe + xformers
|
111 |
-
|
112 |
-
We didn’t notice any significant decrease in the quality of the generated samples. Here are samples:
|
113 |
-
|
114 |
-
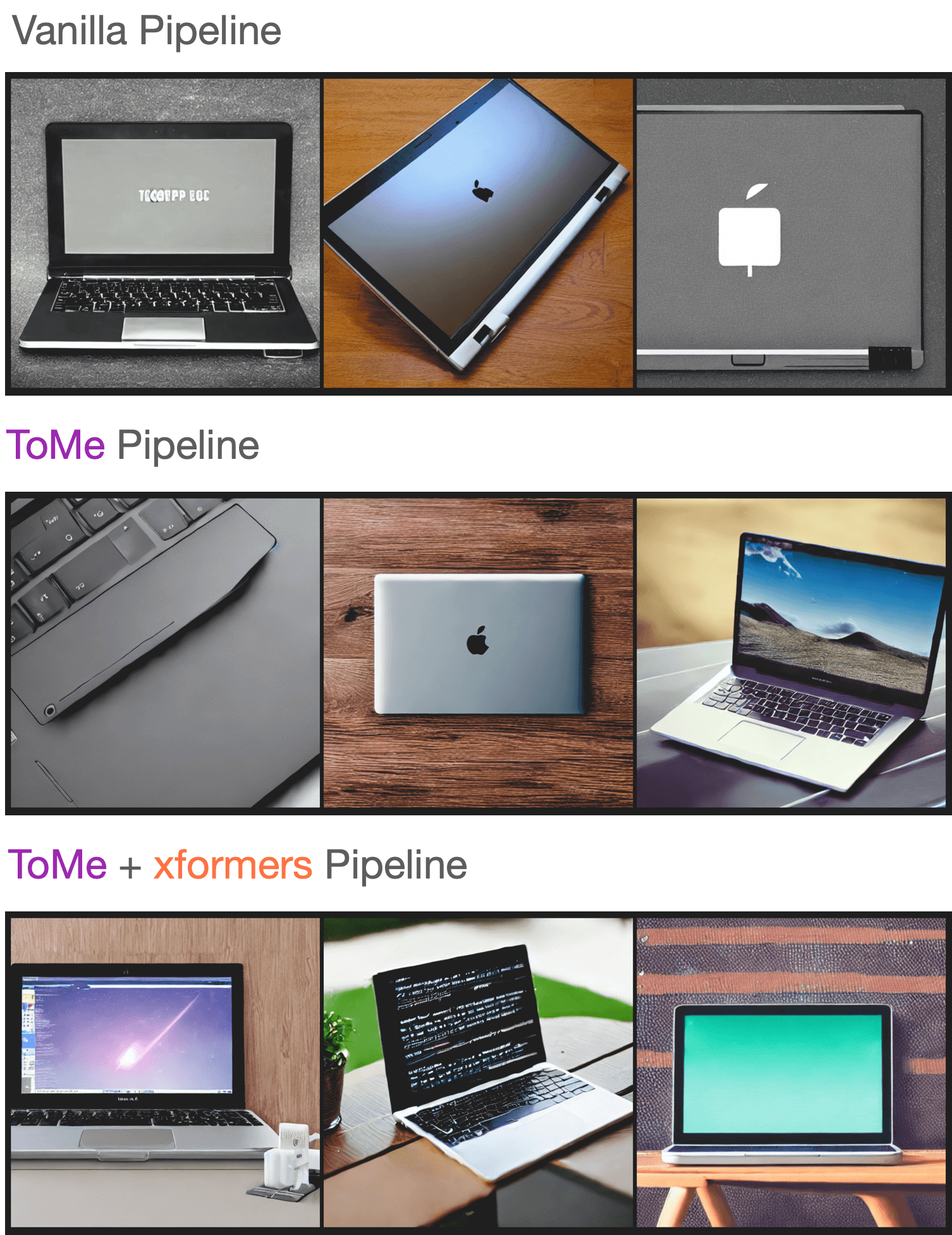
|
115 |
-
|
116 |
-
You can check out the generated samples [here](https://wandb.ai/sayakpaul/tomesd-results/runs/23j4bj3i?workspace=). We used [this script](https://gist.github.com/sayakpaul/8cac98d7f22399085a060992f411ecbd) for conducting this experiment.
|
|
|
|
|
|
|
|
|
|
|
|
|
|
|
|
|
|
|
|
|
|
|
|
|
|
|
|
|
|
|
|
|
|
|
|
|
|
|
|
|
|
|
|
|
|
|
|
|
|
|
|
|
|
|
|
|
|
|
|
|
|
|
|
|
|
|
|
|
|
|
|
|
|
|
|
|
|
|
|
|
|
|
|
|
|
|
|
|
|
|
|
|
|
|
|
|
|
|
|
|
|
|
|
|
|
|
|
|
|
|
|
|
|
|
|
|
|
|
|
|
|
|
|
|
|
|
|
|
|
|
|
|
|
|
|
|
|
|
|
|
|
|
|
|
|
|
|
|
|
|
|
|
|
|
|
|
|
|
|
|
|
|
|
|
|
|
|
|
|
|
|
|
|
|
|
|
|
|
|
|
|
|
|
|
|
|
|
|
|
|
|
|
|
|
|
|
|
|
|
|
|
|
|
|
|
|
|
|
|
|
|
|
|
|
|
|
|
|
|
|
|
|
|
|
|
|
|
|
|
|
|
|
|
spaces/Androidonnxfork/CivitAi-to-Diffusers/diffusers/docs/source/ko/optimization/onnx.md
DELETED
@@ -1,65 +0,0 @@
|
|
1 |
-
<!--Copyright 2023 The HuggingFace Team. All rights reserved.
|
2 |
-
|
3 |
-
Licensed under the Apache License, Version 2.0 (the "License"); you may not use this file except in compliance with
|
4 |
-
the License. You may obtain a copy of the License at
|
5 |
-
|
6 |
-
http://www.apache.org/licenses/LICENSE-2.0
|
7 |
-
|
8 |
-
Unless required by applicable law or agreed to in writing, software distributed under the License is distributed on
|
9 |
-
an "AS IS" BASIS, WITHOUT WARRANTIES OR CONDITIONS OF ANY KIND, either express or implied. See the License for the
|
10 |
-
specific language governing permissions and limitations under the License.
|
11 |
-
-->
|
12 |
-
|
13 |
-
|
14 |
-
# 추론을 위해 ONNX 런타임을 사용하는 방법
|
15 |
-
|
16 |
-
🤗 Diffusers는 ONNX Runtime과 호환되는 Stable Diffusion 파이프라인을 제공합니다. 이를 통해 ONNX(CPU 포함)를 지원하고 PyTorch의 가속 버전을 사용할 수 없는 모든 하드웨어에서 Stable Diffusion을 실행할 수 있습니다.
|
17 |
-
|
18 |
-
## 설치
|
19 |
-
|
20 |
-
다음 명령어로 ONNX Runtime를 지원하는 🤗 Optimum를 설치합니다:
|
21 |
-
|
22 |
-
```
|
23 |
-
pip install optimum["onnxruntime"]
|
24 |
-
```
|
25 |
-
|
26 |
-
## Stable Diffusion 추론
|
27 |
-
|
28 |
-
아래 코드는 ONNX 런타임을 사용하는 방법을 보여줍니다. `StableDiffusionPipeline` 대신 `OnnxStableDiffusionPipeline`을 사용해야 합니다.
|
29 |
-
PyTorch 모델을 불러오고 즉시 ONNX 형식으로 변환하려는 경우 `export=True`로 설정합니다.
|
30 |
-
|
31 |
-
```python
|
32 |
-
from optimum.onnxruntime import ORTStableDiffusionPipeline
|
33 |
-
|
34 |
-
model_id = "runwayml/stable-diffusion-v1-5"
|
35 |
-
pipe = ORTStableDiffusionPipeline.from_pretrained(model_id, export=True)
|
36 |
-
prompt = "a photo of an astronaut riding a horse on mars"
|
37 |
-
images = pipe(prompt).images[0]
|
38 |
-
pipe.save_pretrained("./onnx-stable-diffusion-v1-5")
|
39 |
-
```
|
40 |
-
|
41 |
-
파이프라인을 ONNX 형식으로 오프라인으로 내보내고 나중에 추론에 사용하려는 경우,
|
42 |
-
[`optimum-cli export`](https://huggingface.co/docs/optimum/main/en/exporters/onnx/usage_guides/export_a_model#exporting-a-model-to-onnx-using-the-cli) 명령어를 사용할 수 있습니다:
|
43 |
-
|
44 |
-
```bash
|
45 |
-
optimum-cli export onnx --model runwayml/stable-diffusion-v1-5 sd_v15_onnx/
|
46 |
-
```
|
47 |
-
|
48 |
-
그 다음 추론을 수행합니다:
|
49 |
-
|
50 |
-
```python
|
51 |
-
from optimum.onnxruntime import ORTStableDiffusionPipeline
|
52 |
-
|
53 |
-
model_id = "sd_v15_onnx"
|
54 |
-
pipe = ORTStableDiffusionPipeline.from_pretrained(model_id)
|
55 |
-
prompt = "a photo of an astronaut riding a horse on mars"
|
56 |
-
images = pipe(prompt).images[0]
|
57 |
-
```
|
58 |
-
|
59 |
-
Notice that we didn't have to specify `export=True` above.
|
60 |
-
|
61 |
-
[Optimum 문서](https://huggingface.co/docs/optimum/)에서 더 많은 예시를 찾을 수 있습니다.
|
62 |
-
|
63 |
-
## 알려진 이슈들
|
64 |
-
|
65 |
-
- 여러 프롬프트를 배치로 생성하면 너무 많은 메모리가 사용되는 것 같습니다. 이를 조사하는 동안, 배치 대신 반복 방법이 필요할 수도 있습니다.
|
|
|
|
|
|
|
|
|
|
|
|
|
|
|
|
|
|
|
|
|
|
|
|
|
|
|
|
|
|
|
|
|
|
|
|
|
|
|
|
|
|
|
|
|
|
|
|
|
|
|
|
|
|
|
|
|
|
|
|
|
|
|
|
|
|
|
|
|
|
|
|
|
|
|
|
|
|
|
|
|
|
|
|
|
|
|
|
|
|
|
|
|
|
|
|
|
|
|
|
|
|
|
|
|
|
|
|
|
|
|
|
|
|
|
|
|
|
|
|
|
|
|
|
|
|
|
|
|
|
|
|
spaces/Androidonnxfork/CivitAi-to-Diffusers/diffusers/src/diffusers/utils/outputs.py
DELETED
@@ -1,108 +0,0 @@
|
|
1 |
-
# Copyright 2023 The HuggingFace Team. All rights reserved.
|
2 |
-
#
|
3 |
-
# Licensed under the Apache License, Version 2.0 (the "License");
|
4 |
-
# you may not use this file except in compliance with the License.
|
5 |
-
# You may obtain a copy of the License at
|
6 |
-
#
|
7 |
-
# http://www.apache.org/licenses/LICENSE-2.0
|
8 |
-
#
|
9 |
-
# Unless required by applicable law or agreed to in writing, software
|
10 |
-
# distributed under the License is distributed on an "AS IS" BASIS,
|
11 |
-
# WITHOUT WARRANTIES OR CONDITIONS OF ANY KIND, either express or implied.
|
12 |
-
# See the License for the specific language governing permissions and
|
13 |
-
# limitations under the License.
|
14 |
-
"""
|
15 |
-
Generic utilities
|
16 |
-
"""
|
17 |
-
|
18 |
-
from collections import OrderedDict
|
19 |
-
from dataclasses import fields
|
20 |
-
from typing import Any, Tuple
|
21 |
-
|
22 |
-
import numpy as np
|
23 |
-
|
24 |
-
from .import_utils import is_torch_available
|
25 |
-
|
26 |
-
|
27 |
-
def is_tensor(x):
|
28 |
-
"""
|
29 |
-
Tests if `x` is a `torch.Tensor` or `np.ndarray`.
|
30 |
-
"""
|
31 |
-
if is_torch_available():
|
32 |
-
import torch
|
33 |
-
|
34 |
-
if isinstance(x, torch.Tensor):
|
35 |
-
return True
|
36 |
-
|
37 |
-
return isinstance(x, np.ndarray)
|
38 |
-
|
39 |
-
|
40 |
-
class BaseOutput(OrderedDict):
|
41 |
-
"""
|
42 |
-
Base class for all model outputs as dataclass. Has a `__getitem__` that allows indexing by integer or slice (like a
|
43 |
-
tuple) or strings (like a dictionary) that will ignore the `None` attributes. Otherwise behaves like a regular
|
44 |
-
Python dictionary.
|
45 |
-
|
46 |
-
<Tip warning={true}>
|
47 |
-
|
48 |
-
You can't unpack a [`BaseOutput`] directly. Use the [`~utils.BaseOutput.to_tuple`] method to convert it to a tuple
|
49 |
-
first.
|
50 |
-
|
51 |
-
</Tip>
|
52 |
-
"""
|
53 |
-
|
54 |
-
def __post_init__(self):
|
55 |
-
class_fields = fields(self)
|
56 |
-
|
57 |
-
# Safety and consistency checks
|
58 |
-
if not len(class_fields):
|
59 |
-
raise ValueError(f"{self.__class__.__name__} has no fields.")
|
60 |
-
|
61 |
-
first_field = getattr(self, class_fields[0].name)
|
62 |
-
other_fields_are_none = all(getattr(self, field.name) is None for field in class_fields[1:])
|
63 |
-
|
64 |
-
if other_fields_are_none and isinstance(first_field, dict):
|
65 |
-
for key, value in first_field.items():
|
66 |
-
self[key] = value
|
67 |
-
else:
|
68 |
-
for field in class_fields:
|
69 |
-
v = getattr(self, field.name)
|
70 |
-
if v is not None:
|
71 |
-
self[field.name] = v
|
72 |
-
|
73 |
-
def __delitem__(self, *args, **kwargs):
|
74 |
-
raise Exception(f"You cannot use ``__delitem__`` on a {self.__class__.__name__} instance.")
|
75 |
-
|
76 |
-
def setdefault(self, *args, **kwargs):
|
77 |
-
raise Exception(f"You cannot use ``setdefault`` on a {self.__class__.__name__} instance.")
|
78 |
-
|
79 |
-
def pop(self, *args, **kwargs):
|
80 |
-
raise Exception(f"You cannot use ``pop`` on a {self.__class__.__name__} instance.")
|
81 |
-
|
82 |
-
def update(self, *args, **kwargs):
|
83 |
-
raise Exception(f"You cannot use ``update`` on a {self.__class__.__name__} instance.")
|
84 |
-
|
85 |
-
def __getitem__(self, k):
|
86 |
-
if isinstance(k, str):
|
87 |
-
inner_dict = dict(self.items())
|
88 |
-
return inner_dict[k]
|
89 |
-
else:
|
90 |
-
return self.to_tuple()[k]
|
91 |
-
|
92 |
-
def __setattr__(self, name, value):
|
93 |
-
if name in self.keys() and value is not None:
|
94 |
-
# Don't call self.__setitem__ to avoid recursion errors
|
95 |
-
super().__setitem__(name, value)
|
96 |
-
super().__setattr__(name, value)
|
97 |
-
|
98 |
-
def __setitem__(self, key, value):
|
99 |
-
# Will raise a KeyException if needed
|
100 |
-
super().__setitem__(key, value)
|
101 |
-
# Don't call self.__setattr__ to avoid recursion errors
|
102 |
-
super().__setattr__(key, value)
|
103 |
-
|
104 |
-
def to_tuple(self) -> Tuple[Any]:
|
105 |
-
"""
|
106 |
-
Convert self to a tuple containing all the attributes/keys that are not `None`.
|
107 |
-
"""
|
108 |
-
return tuple(self[k] for k in self.keys())
|
|
|
|
|
|
|
|
|
|
|
|
|
|
|
|
|
|
|
|
|
|
|
|
|
|
|
|
|
|
|
|
|
|
|
|
|
|
|
|
|
|
|
|
|
|
|
|
|
|
|
|
|
|
|
|
|
|
|
|
|
|
|
|
|
|
|
|
|
|
|
|
|
|
|
|
|
|
|
|
|
|
|
|
|
|
|
|
|
|
|
|
|
|
|
|
|
|
|
|
|
|
|
|
|
|
|
|
|
|
|
|
|
|
|
|
|
|
|
|
|
|
|
|
|
|
|
|
|
|
|
|
|
|
|
|
|
|
|
|
|
|
|
|
|
|
|
|
|
|
|
|
|
|
|
|
|
|
|
|
|
|
|
|
|
|
|
|
|
|
|
|
|
|
|
|
|
|
|
|
|
|
|
|
|
|
|
|
|
|
|
|
|
|
|
|
|
|
|
|
|
|
|
|
|
|
|
|
|
|
|
|
|
|
|
|
|
|
spaces/Andy1621/uniformer_image_detection/configs/fcos/fcos_r50_caffe_fpn_gn-head_4x4_1x_coco.py
DELETED
@@ -1,4 +0,0 @@
|
|
1 |
-
# TODO: Remove this config after benchmarking all related configs
|
2 |
-
_base_ = 'fcos_r50_caffe_fpn_gn-head_1x_coco.py'
|
3 |
-
|
4 |
-
data = dict(samples_per_gpu=4, workers_per_gpu=4)
|
|
|
|
|
|
|
|
|
|
spaces/AquaSuisei/ChatGPTXE/chatgpt - macOS.command
DELETED
@@ -1,7 +0,0 @@
|
|
1 |
-
#!/bin/bash
|
2 |
-
echo Opening ChuanhuChatGPT...
|
3 |
-
cd "$(dirname "${BASH_SOURCE[0]}")"
|
4 |
-
nohup python3 ChuanhuChatbot.py >/dev/null 2>&1 &
|
5 |
-
sleep 5
|
6 |
-
open http://127.0.0.1:7860
|
7 |
-
echo Finished opening ChuanhuChatGPT (http://127.0.0.1:7860/). If you kill ChuanhuChatbot, Use "pkill -f 'ChuanhuChatbot'" command in terminal.
|
|
|
|
|
|
|
|
|
|
|
|
|
|
|
|
spaces/ArcanAlt/arcanDream/Dockerfile
DELETED
@@ -1,11 +0,0 @@
|
|
1 |
-
FROM node:18
|
2 |
-
|
3 |
-
WORKDIR /app
|
4 |
-
|
5 |
-
RUN npm install express express-http-proxy
|
6 |
-
|
7 |
-
COPY . .
|
8 |
-
|
9 |
-
EXPOSE 7860
|
10 |
-
|
11 |
-
CMD [ "node", "server.js" ]
|
|
|
|
|
|
|
|
|
|
|
|
|
|
|
|
|
|
|
|
|
|
|
|
spaces/Big-Web/MMSD/env/Lib/site-packages/dateutil/zoneinfo/__init__.py
DELETED
@@ -1,167 +0,0 @@
|
|
1 |
-
# -*- coding: utf-8 -*-
|
2 |
-
import warnings
|
3 |
-
import json
|
4 |
-
|
5 |
-
from tarfile import TarFile
|
6 |
-
from pkgutil import get_data
|
7 |
-
from io import BytesIO
|
8 |
-
|
9 |
-
from dateutil.tz import tzfile as _tzfile
|
10 |
-
|
11 |
-
__all__ = ["get_zonefile_instance", "gettz", "gettz_db_metadata"]
|
12 |
-
|
13 |
-
ZONEFILENAME = "dateutil-zoneinfo.tar.gz"
|
14 |
-
METADATA_FN = 'METADATA'
|
15 |
-
|
16 |
-
|
17 |
-
class tzfile(_tzfile):
|
18 |
-
def __reduce__(self):
|
19 |
-
return (gettz, (self._filename,))
|
20 |
-
|
21 |
-
|
22 |
-
def getzoneinfofile_stream():
|
23 |
-
try:
|
24 |
-
return BytesIO(get_data(__name__, ZONEFILENAME))
|
25 |
-
except IOError as e: # TODO switch to FileNotFoundError?
|
26 |
-
warnings.warn("I/O error({0}): {1}".format(e.errno, e.strerror))
|
27 |
-
return None
|
28 |
-
|
29 |
-
|
30 |
-
class ZoneInfoFile(object):
|
31 |
-
def __init__(self, zonefile_stream=None):
|
32 |
-
if zonefile_stream is not None:
|
33 |
-
with TarFile.open(fileobj=zonefile_stream) as tf:
|
34 |
-
self.zones = {zf.name: tzfile(tf.extractfile(zf), filename=zf.name)
|
35 |
-
for zf in tf.getmembers()
|
36 |
-
if zf.isfile() and zf.name != METADATA_FN}
|
37 |
-
# deal with links: They'll point to their parent object. Less
|
38 |
-
# waste of memory
|
39 |
-
links = {zl.name: self.zones[zl.linkname]
|
40 |
-
for zl in tf.getmembers() if
|
41 |
-
zl.islnk() or zl.issym()}
|
42 |
-
self.zones.update(links)
|
43 |
-
try:
|
44 |
-
metadata_json = tf.extractfile(tf.getmember(METADATA_FN))
|
45 |
-
metadata_str = metadata_json.read().decode('UTF-8')
|
46 |
-
self.metadata = json.loads(metadata_str)
|
47 |
-
except KeyError:
|
48 |
-
# no metadata in tar file
|
49 |
-
self.metadata = None
|
50 |
-
else:
|
51 |
-
self.zones = {}
|
52 |
-
self.metadata = None
|
53 |
-
|
54 |
-
def get(self, name, default=None):
|
55 |
-
"""
|
56 |
-
Wrapper for :func:`ZoneInfoFile.zones.get`. This is a convenience method
|
57 |
-
for retrieving zones from the zone dictionary.
|
58 |
-
|
59 |
-
:param name:
|
60 |
-
The name of the zone to retrieve. (Generally IANA zone names)
|
61 |
-
|
62 |
-
:param default:
|
63 |
-
The value to return in the event of a missing key.
|
64 |
-
|
65 |
-
.. versionadded:: 2.6.0
|
66 |
-
|
67 |
-
"""
|
68 |
-
return self.zones.get(name, default)
|
69 |
-
|
70 |
-
|
71 |
-
# The current API has gettz as a module function, although in fact it taps into
|
72 |
-
# a stateful class. So as a workaround for now, without changing the API, we
|
73 |
-
# will create a new "global" class instance the first time a user requests a
|
74 |
-
# timezone. Ugly, but adheres to the api.
|
75 |
-
#
|
76 |
-
# TODO: Remove after deprecation period.
|
77 |
-
_CLASS_ZONE_INSTANCE = []
|
78 |
-
|
79 |
-
|
80 |
-
def get_zonefile_instance(new_instance=False):
|
81 |
-
"""
|
82 |
-
This is a convenience function which provides a :class:`ZoneInfoFile`
|
83 |
-
instance using the data provided by the ``dateutil`` package. By default, it
|
84 |
-
caches a single instance of the ZoneInfoFile object and returns that.
|
85 |
-
|
86 |
-
:param new_instance:
|
87 |
-
If ``True``, a new instance of :class:`ZoneInfoFile` is instantiated and
|
88 |
-
used as the cached instance for the next call. Otherwise, new instances
|
89 |
-
are created only as necessary.
|
90 |
-
|
91 |
-
:return:
|
92 |
-
Returns a :class:`ZoneInfoFile` object.
|
93 |
-
|
94 |
-
.. versionadded:: 2.6
|
95 |
-
"""
|
96 |
-
if new_instance:
|
97 |
-
zif = None
|
98 |
-
else:
|
99 |
-
zif = getattr(get_zonefile_instance, '_cached_instance', None)
|
100 |
-
|
101 |
-
if zif is None:
|
102 |
-
zif = ZoneInfoFile(getzoneinfofile_stream())
|
103 |
-
|
104 |
-
get_zonefile_instance._cached_instance = zif
|
105 |
-
|
106 |
-
return zif
|
107 |
-
|
108 |
-
|
109 |
-
def gettz(name):
|
110 |
-
"""
|
111 |
-
This retrieves a time zone from the local zoneinfo tarball that is packaged
|
112 |
-
with dateutil.
|
113 |
-
|
114 |
-
:param name:
|
115 |
-
An IANA-style time zone name, as found in the zoneinfo file.
|
116 |
-
|
117 |
-
:return:
|
118 |
-
Returns a :class:`dateutil.tz.tzfile` time zone object.
|
119 |
-
|
120 |
-
.. warning::
|
121 |
-
It is generally inadvisable to use this function, and it is only
|
122 |
-
provided for API compatibility with earlier versions. This is *not*
|
123 |
-
equivalent to ``dateutil.tz.gettz()``, which selects an appropriate
|
124 |
-
time zone based on the inputs, favoring system zoneinfo. This is ONLY
|
125 |
-
for accessing the dateutil-specific zoneinfo (which may be out of
|
126 |
-
date compared to the system zoneinfo).
|
127 |
-
|
128 |
-
.. deprecated:: 2.6
|
129 |
-
If you need to use a specific zoneinfofile over the system zoneinfo,
|
130 |
-
instantiate a :class:`dateutil.zoneinfo.ZoneInfoFile` object and call
|
131 |
-
:func:`dateutil.zoneinfo.ZoneInfoFile.get(name)` instead.
|
132 |
-
|
133 |
-
Use :func:`get_zonefile_instance` to retrieve an instance of the
|
134 |
-
dateutil-provided zoneinfo.
|
135 |
-
"""
|
136 |
-
warnings.warn("zoneinfo.gettz() will be removed in future versions, "
|
137 |
-
"to use the dateutil-provided zoneinfo files, instantiate a "
|
138 |
-
"ZoneInfoFile object and use ZoneInfoFile.zones.get() "
|
139 |
-
"instead. See the documentation for details.",
|
140 |
-
DeprecationWarning)
|
141 |
-
|
142 |
-
if len(_CLASS_ZONE_INSTANCE) == 0:
|
143 |
-
_CLASS_ZONE_INSTANCE.append(ZoneInfoFile(getzoneinfofile_stream()))
|
144 |
-
return _CLASS_ZONE_INSTANCE[0].zones.get(name)
|
145 |
-
|
146 |
-
|
147 |
-
def gettz_db_metadata():
|
148 |
-
""" Get the zonefile metadata
|
149 |
-
|
150 |
-
See `zonefile_metadata`_
|
151 |
-
|
152 |
-
:returns:
|
153 |
-
A dictionary with the database metadata
|
154 |
-
|
155 |
-
.. deprecated:: 2.6
|
156 |
-
See deprecation warning in :func:`zoneinfo.gettz`. To get metadata,
|
157 |
-
query the attribute ``zoneinfo.ZoneInfoFile.metadata``.
|
158 |
-
"""
|
159 |
-
warnings.warn("zoneinfo.gettz_db_metadata() will be removed in future "
|
160 |
-
"versions, to use the dateutil-provided zoneinfo files, "
|
161 |
-
"ZoneInfoFile object and query the 'metadata' attribute "
|
162 |
-
"instead. See the documentation for details.",
|
163 |
-
DeprecationWarning)
|
164 |
-
|
165 |
-
if len(_CLASS_ZONE_INSTANCE) == 0:
|
166 |
-
_CLASS_ZONE_INSTANCE.append(ZoneInfoFile(getzoneinfofile_stream()))
|
167 |
-
return _CLASS_ZONE_INSTANCE[0].metadata
|
|
|
|
|
|
|
|
|
|
|
|
|
|
|
|
|
|
|
|
|
|
|
|
|
|
|
|
|
|
|
|
|
|
|
|
|
|
|
|
|
|
|
|
|
|
|
|
|
|
|
|
|
|
|
|
|
|
|
|
|
|
|
|
|
|
|
|
|
|
|
|
|
|
|
|
|
|
|
|
|
|
|
|
|
|
|
|
|
|
|
|
|
|
|
|
|
|
|
|
|
|
|
|
|
|
|
|
|
|
|
|
|
|
|
|
|
|
|
|
|
|
|
|
|
|
|
|
|
|
|
|
|
|
|
|
|
|
|
|
|
|
|
|
|
|
|
|
|
|
|
|
|
|
|
|
|
|
|
|
|
|
|
|
|
|
|
|
|
|
|
|
|
|
|
|
|
|
|
|
|
|
|
|
|
|
|
|
|
|
|
|
|
|
|
|
|
|
|
|
|
|
|
|
|
|
|
|
|
|
|
|
|
|
|
|
|
|
|
|
|
|
|
|
|
|
|
|
|
|
|
|
|
|
|
|
|
|
|
|
|
|
|
|
|
|
|
|
|
|
|
|
|
|
|
|
|
|
|
|
|
|
|
|
|
|
|
|
|
|
|
|
|
|
|
|
|
|
|
|
|
|
|
|
|
|
|
|
|
|
|
|
|
|
|
|
|
|
|
|
|
|
|
|
|
|
|
|
|
|
|
|
|
|
|
|
|
|
|
|
|
|
|
|
|
|
|
|
|
|
|
|
|
|
|
|
spaces/Big-Web/MMSD/env/Lib/site-packages/setuptools/command/setopt.py
DELETED
@@ -1,149 +0,0 @@
|
|
1 |
-
from distutils.util import convert_path
|
2 |
-
from distutils import log
|
3 |
-
from distutils.errors import DistutilsOptionError
|
4 |
-
import distutils
|
5 |
-
import os
|
6 |
-
import configparser
|
7 |
-
|
8 |
-
from setuptools import Command
|
9 |
-
|
10 |
-
__all__ = ['config_file', 'edit_config', 'option_base', 'setopt']
|
11 |
-
|
12 |
-
|
13 |
-
def config_file(kind="local"):
|
14 |
-
"""Get the filename of the distutils, local, global, or per-user config
|
15 |
-
|
16 |
-
`kind` must be one of "local", "global", or "user"
|
17 |
-
"""
|
18 |
-
if kind == 'local':
|
19 |
-
return 'setup.cfg'
|
20 |
-
if kind == 'global':
|
21 |
-
return os.path.join(
|
22 |
-
os.path.dirname(distutils.__file__), 'distutils.cfg'
|
23 |
-
)
|
24 |
-
if kind == 'user':
|
25 |
-
dot = os.name == 'posix' and '.' or ''
|
26 |
-
return os.path.expanduser(convert_path("~/%spydistutils.cfg" % dot))
|
27 |
-
raise ValueError(
|
28 |
-
"config_file() type must be 'local', 'global', or 'user'", kind
|
29 |
-
)
|
30 |
-
|
31 |
-
|
32 |
-
def edit_config(filename, settings, dry_run=False):
|
33 |
-
"""Edit a configuration file to include `settings`
|
34 |
-
|
35 |
-
`settings` is a dictionary of dictionaries or ``None`` values, keyed by
|
36 |
-
command/section name. A ``None`` value means to delete the entire section,
|
37 |
-
while a dictionary lists settings to be changed or deleted in that section.
|
38 |
-
A setting of ``None`` means to delete that setting.
|
39 |
-
"""
|
40 |
-
log.debug("Reading configuration from %s", filename)
|
41 |
-
opts = configparser.RawConfigParser()
|
42 |
-
opts.optionxform = lambda x: x
|
43 |
-
opts.read([filename])
|
44 |
-
for section, options in settings.items():
|
45 |
-
if options is None:
|
46 |
-
log.info("Deleting section [%s] from %s", section, filename)
|
47 |
-
opts.remove_section(section)
|
48 |
-
else:
|
49 |
-
if not opts.has_section(section):
|
50 |
-
log.debug("Adding new section [%s] to %s", section, filename)
|
51 |
-
opts.add_section(section)
|
52 |
-
for option, value in options.items():
|
53 |
-
if value is None:
|
54 |
-
log.debug(
|
55 |
-
"Deleting %s.%s from %s",
|
56 |
-
section, option, filename
|
57 |
-
)
|
58 |
-
opts.remove_option(section, option)
|
59 |
-
if not opts.options(section):
|
60 |
-
log.info("Deleting empty [%s] section from %s",
|
61 |
-
section, filename)
|
62 |
-
opts.remove_section(section)
|
63 |
-
else:
|
64 |
-
log.debug(
|
65 |
-
"Setting %s.%s to %r in %s",
|
66 |
-
section, option, value, filename
|
67 |
-
)
|
68 |
-
opts.set(section, option, value)
|
69 |
-
|
70 |
-
log.info("Writing %s", filename)
|
71 |
-
if not dry_run:
|
72 |
-
with open(filename, 'w') as f:
|
73 |
-
opts.write(f)
|
74 |
-
|
75 |
-
|
76 |
-
class option_base(Command):
|
77 |
-
"""Abstract base class for commands that mess with config files"""
|
78 |
-
|
79 |
-
user_options = [
|
80 |
-
('global-config', 'g',
|
81 |
-
"save options to the site-wide distutils.cfg file"),
|
82 |
-
('user-config', 'u',
|
83 |
-
"save options to the current user's pydistutils.cfg file"),
|
84 |
-
('filename=', 'f',
|
85 |
-
"configuration file to use (default=setup.cfg)"),
|
86 |
-
]
|
87 |
-
|
88 |
-
boolean_options = [
|
89 |
-
'global-config', 'user-config',
|
90 |
-
]
|
91 |
-
|
92 |
-
def initialize_options(self):
|
93 |
-
self.global_config = None
|
94 |
-
self.user_config = None
|
95 |
-
self.filename = None
|
96 |
-
|
97 |
-
def finalize_options(self):
|
98 |
-
filenames = []
|
99 |
-
if self.global_config:
|
100 |
-
filenames.append(config_file('global'))
|
101 |
-
if self.user_config:
|
102 |
-
filenames.append(config_file('user'))
|
103 |
-
if self.filename is not None:
|
104 |
-
filenames.append(self.filename)
|
105 |
-
if not filenames:
|
106 |
-
filenames.append(config_file('local'))
|
107 |
-
if len(filenames) > 1:
|
108 |
-
raise DistutilsOptionError(
|
109 |
-
"Must specify only one configuration file option",
|
110 |
-
filenames
|
111 |
-
)
|
112 |
-
self.filename, = filenames
|
113 |
-
|
114 |
-
|
115 |
-
class setopt(option_base):
|
116 |
-
"""Save command-line options to a file"""
|
117 |
-
|
118 |
-
description = "set an option in setup.cfg or another config file"
|
119 |
-
|
120 |
-
user_options = [
|
121 |
-
('command=', 'c', 'command to set an option for'),
|
122 |
-
('option=', 'o', 'option to set'),
|
123 |
-
('set-value=', 's', 'value of the option'),
|
124 |
-
('remove', 'r', 'remove (unset) the value'),
|
125 |
-
] + option_base.user_options
|
126 |
-
|
127 |
-
boolean_options = option_base.boolean_options + ['remove']
|
128 |
-
|
129 |
-
def initialize_options(self):
|
130 |
-
option_base.initialize_options(self)
|
131 |
-
self.command = None
|
132 |
-
self.option = None
|
133 |
-
self.set_value = None
|
134 |
-
self.remove = None
|
135 |
-
|
136 |
-
def finalize_options(self):
|
137 |
-
option_base.finalize_options(self)
|
138 |
-
if self.command is None or self.option is None:
|
139 |
-
raise DistutilsOptionError("Must specify --command *and* --option")
|
140 |
-
if self.set_value is None and not self.remove:
|
141 |
-
raise DistutilsOptionError("Must specify --set-value or --remove")
|
142 |
-
|
143 |
-
def run(self):
|
144 |
-
edit_config(
|
145 |
-
self.filename, {
|
146 |
-
self.command: {self.option.replace('-', '_'): self.set_value}
|
147 |
-
},
|
148 |
-
self.dry_run
|
149 |
-
)
|
|
|
|
|
|
|
|
|
|
|
|
|
|
|
|
|
|
|
|
|
|
|
|
|
|
|
|
|
|
|
|
|
|
|
|
|
|
|
|
|
|
|
|
|
|
|
|
|
|
|
|
|
|
|
|
|
|
|
|
|
|
|
|
|
|
|
|
|
|
|
|
|
|
|
|
|
|
|
|
|
|
|
|
|
|
|
|
|
|
|
|
|
|
|
|
|
|
|
|
|
|
|
|
|
|
|
|
|
|
|
|
|
|
|
|
|
|
|
|
|
|
|
|
|
|
|
|
|
|
|
|
|
|
|
|
|
|
|
|
|
|
|
|
|
|
|
|
|
|
|
|
|
|
|
|
|
|
|
|
|
|
|
|
|
|
|
|
|
|
|
|
|
|
|
|
|
|
|
|
|
|
|
|
|
|
|
|
|
|
|
|
|
|
|
|
|
|
|
|
|
|
|
|
|
|
|
|
|
|
|
|
|
|
|
|
|
|
|
|
|
|
|
|
|
|
|
|
|
|
|
|
|
|
|
|
|
|
|
|
|
|
|
|
|
|
|
|
|
|
|
|
|
|
|
|
|
|
|
|
|
|
|
|
|
|
|
|
|
|
|
|
|
|
|
|
|
|
|
|
|
|
|
|
|
|
|
|
|
|
|
|
|
|
|
|
|
|
|
|
spaces/CVPR/LIVE/thrust/thrust/system/cuda/detail/reverse.h
DELETED
@@ -1,98 +0,0 @@
|
|
1 |
-
/******************************************************************************
|
2 |
-
* Copyright (c) 2016, NVIDIA CORPORATION. All rights reserved.
|
3 |
-
*
|
4 |
-
* Redistribution and use in source and binary forms, with or without
|
5 |
-
* modification, are permitted provided that the following conditions are met:
|
6 |
-
* * Redistributions of source code must retain the above copyright
|
7 |
-
* notice, this list of conditions and the following disclaimer.
|
8 |
-
* * Redistributions in binary form must reproduce the above copyright
|
9 |
-
* notice, this list of conditions and the following disclaimer in the
|
10 |
-
* documentation and/or other materials provided with the distribution.
|
11 |
-
* * Neither the name of the NVIDIA CORPORATION nor the
|
12 |
-
* names of its contributors may be used to endorse or promote products
|
13 |
-
* derived from this software without specific prior written permission.
|
14 |
-
*
|
15 |
-
* THIS SOFTWARE IS PROVIDED BY THE COPYRIGHT HOLDERS AND CONTRIBUTORS "AS IS"
|
16 |
-
* AND ANY EXPRESS OR IMPLIED WARRANTIES, INCLUDING, BUT NOT LIMITED TO, THE
|
17 |
-
* IMPLIED WARRANTIES OF MERCHANTABILITY AND FITNESS FOR A PARTICULAR PURPOSE
|
18 |
-
* ARE DISCLAIMED. IN NO EVENT SHALL NVIDIA CORPORATION BE LIABLE FOR ANY
|
19 |
-
* DIRECT, INDIRECT, INCIDENTAL, SPECIAL, EXEMPLARY, OR CONSEQUENTIAL DAMAGES
|
20 |
-
* (INCLUDING, BUT NOT LIMITED TO, PROCUREMENT OF SUBSTITUTE GOODS OR SERVICES;
|
21 |
-
* LOSS OF USE, DATA, OR PROFITS; OR BUSINESS INTERRUPTION) HOWEVER CAUSED AND
|
22 |
-
* ON ANY THEORY OF LIABILITY, WHETHER IN CONTRACT, STRICT LIABILITY, OR TORT
|
23 |
-
* (INCLUDING NEGLIGENCE OR OTHERWISE) ARISING IN ANY WAY OUT OF THE USE OF THIS
|
24 |
-
* SOFTWARE, EVEN IF ADVISED OF THE POSSIBILITY OF SUCH DAMAGE.
|
25 |
-
*
|
26 |
-
******************************************************************************/
|
27 |
-
#pragma once
|
28 |
-
|
29 |
-
|
30 |
-
#if THRUST_DEVICE_COMPILER == THRUST_DEVICE_COMPILER_NVCC
|
31 |
-
#include <thrust/system/cuda/detail/execution_policy.h>
|
32 |
-
|
33 |
-
namespace thrust
|
34 |
-
{
|
35 |
-
namespace cuda_cub {
|
36 |
-
|
37 |
-
template <class Derived, class ItemsIt, class ResultIt>
|
38 |
-
ResultIt __host__ __device__
|
39 |
-
reverse_copy(execution_policy<Derived> &policy,
|
40 |
-
ItemsIt first,
|
41 |
-
ItemsIt last,
|
42 |
-
ResultIt result);
|
43 |
-
|
44 |
-
template <class Derived, class ItemsIt>
|
45 |
-
void __host__ __device__
|
46 |
-
reverse(execution_policy<Derived> &policy,
|
47 |
-
ItemsIt first,
|
48 |
-
ItemsIt last);
|
49 |
-
|
50 |
-
} // namespace cuda_cub
|
51 |
-
} // end namespace thrust
|
52 |
-
|
53 |
-
#include <thrust/advance.h>
|
54 |
-
#include <thrust/distance.h>
|
55 |
-
#include <thrust/system/cuda/detail/swap_ranges.h>
|
56 |
-
#include <thrust/system/cuda/detail/copy.h>
|
57 |
-
#include <thrust/iterator/reverse_iterator.h>
|
58 |
-
|
59 |
-
namespace thrust
|
60 |
-
{
|
61 |
-
namespace cuda_cub {
|
62 |
-
|
63 |
-
template <class Derived,
|
64 |
-
class ItemsIt,
|
65 |
-
class ResultIt>
|
66 |
-
ResultIt __host__ __device__
|
67 |
-
reverse_copy(execution_policy<Derived> &policy,
|
68 |
-
ItemsIt first,
|
69 |
-
ItemsIt last,
|
70 |
-
ResultIt result)
|
71 |
-
{
|
72 |
-
return cuda_cub::copy(policy,
|
73 |
-
make_reverse_iterator(last),
|
74 |
-
make_reverse_iterator(first),
|
75 |
-
result);
|
76 |
-
}
|
77 |
-
|
78 |
-
template <class Derived,
|
79 |
-
class ItemsIt>
|
80 |
-
void __host__ __device__
|
81 |
-
reverse(execution_policy<Derived> &policy,
|
82 |
-
ItemsIt first,
|
83 |
-
ItemsIt last)
|
84 |
-
{
|
85 |
-
typedef typename thrust::iterator_difference<ItemsIt>::type difference_type;
|
86 |
-
|
87 |
-
// find the midpoint of [first,last)
|
88 |
-
difference_type N = thrust::distance(first, last);
|
89 |
-
ItemsIt mid(first);
|
90 |
-
thrust::advance(mid, N / 2);
|
91 |
-
|
92 |
-
cuda_cub::swap_ranges(policy, first, mid, make_reverse_iterator(last));
|
93 |
-
}
|
94 |
-
|
95 |
-
|
96 |
-
} // namespace cuda_cub
|
97 |
-
} // end namespace thrust
|
98 |
-
#endif
|
|
|
|
|
|
|
|
|
|
|
|
|
|
|
|
|
|
|
|
|
|
|
|
|
|
|
|
|
|
|
|
|
|
|
|
|
|
|
|
|
|
|
|
|
|
|
|
|
|
|
|
|
|
|
|
|
|
|
|
|
|
|
|
|
|
|
|
|
|
|
|
|
|
|
|
|
|
|
|
|
|
|
|
|
|
|
|
|
|
|
|
|
|
|
|
|
|
|
|
|
|
|
|
|
|
|
|
|
|
|
|
|
|
|
|
|
|
|
|
|
|
|
|
|
|
|
|
|
|
|
|
|
|
|
|
|
|
|
|
|
|
|
|
|
|
|
|
|
|
|
|
|
|
|
|
|
|
|
|
|
|
|
|
|
|
|
|
|
|
|
|
|
|
|
|
|
|
|
|
|
|
|
|
|
|
|
|
|
|
|
|
|
|
|
|
|
|
spaces/CVPR/WALT/mmdet/models/detectors/cornernet.py
DELETED
@@ -1,95 +0,0 @@
|
|
1 |
-
import torch
|
2 |
-
|
3 |
-
from mmdet.core import bbox2result, bbox_mapping_back
|
4 |
-
from ..builder import DETECTORS
|
5 |
-
from .single_stage import SingleStageDetector
|
6 |
-
|
7 |
-
|
8 |
-
@DETECTORS.register_module()
|
9 |
-
class CornerNet(SingleStageDetector):
|
10 |
-
"""CornerNet.
|
11 |
-
|
12 |
-
This detector is the implementation of the paper `CornerNet: Detecting
|
13 |
-
Objects as Paired Keypoints <https://arxiv.org/abs/1808.01244>`_ .
|
14 |
-
"""
|
15 |
-
|
16 |
-
def __init__(self,
|
17 |
-
backbone,
|
18 |
-
neck,
|
19 |
-
bbox_head,
|
20 |
-
train_cfg=None,
|
21 |
-
test_cfg=None,
|
22 |
-
pretrained=None):
|
23 |
-
super(CornerNet, self).__init__(backbone, neck, bbox_head, train_cfg,
|
24 |
-
test_cfg, pretrained)
|
25 |
-
|
26 |
-
def merge_aug_results(self, aug_results, img_metas):
|
27 |
-
"""Merge augmented detection bboxes and score.
|
28 |
-
|
29 |
-
Args:
|
30 |
-
aug_results (list[list[Tensor]]): Det_bboxes and det_labels of each
|
31 |
-
image.
|
32 |
-
img_metas (list[list[dict]]): Meta information of each image, e.g.,
|
33 |
-
image size, scaling factor, etc.
|
34 |
-
|
35 |
-
Returns:
|
36 |
-
tuple: (bboxes, labels)
|
37 |
-
"""
|
38 |
-
recovered_bboxes, aug_labels = [], []
|
39 |
-
for bboxes_labels, img_info in zip(aug_results, img_metas):
|
40 |
-
img_shape = img_info[0]['img_shape'] # using shape before padding
|
41 |
-
scale_factor = img_info[0]['scale_factor']
|
42 |
-
flip = img_info[0]['flip']
|
43 |
-
bboxes, labels = bboxes_labels
|
44 |
-
bboxes, scores = bboxes[:, :4], bboxes[:, -1:]
|
45 |
-
bboxes = bbox_mapping_back(bboxes, img_shape, scale_factor, flip)
|
46 |
-
recovered_bboxes.append(torch.cat([bboxes, scores], dim=-1))
|
47 |
-
aug_labels.append(labels)
|
48 |
-
|
49 |
-
bboxes = torch.cat(recovered_bboxes, dim=0)
|
50 |
-
labels = torch.cat(aug_labels)
|
51 |
-
|
52 |
-
if bboxes.shape[0] > 0:
|
53 |
-
out_bboxes, out_labels = self.bbox_head._bboxes_nms(
|
54 |
-
bboxes, labels, self.bbox_head.test_cfg)
|
55 |
-
else:
|
56 |
-
out_bboxes, out_labels = bboxes, labels
|
57 |
-
|
58 |
-
return out_bboxes, out_labels
|
59 |
-
|
60 |
-
def aug_test(self, imgs, img_metas, rescale=False):
|
61 |
-
"""Augment testing of CornerNet.
|
62 |
-
|
63 |
-
Args:
|
64 |
-
imgs (list[Tensor]): Augmented images.
|
65 |
-
img_metas (list[list[dict]]): Meta information of each image, e.g.,
|
66 |
-
image size, scaling factor, etc.
|
67 |
-
rescale (bool): If True, return boxes in original image space.
|
68 |
-
Default: False.
|
69 |
-
|
70 |
-
Note:
|
71 |
-
``imgs`` must including flipped image pairs.
|
72 |
-
|
73 |
-
Returns:
|
74 |
-
list[list[np.ndarray]]: BBox results of each image and classes.
|
75 |
-
The outer list corresponds to each image. The inner list
|
76 |
-
corresponds to each class.
|
77 |
-
"""
|
78 |
-
img_inds = list(range(len(imgs)))
|
79 |
-
|
80 |
-
assert img_metas[0][0]['flip'] + img_metas[1][0]['flip'], (
|
81 |
-
'aug test must have flipped image pair')
|
82 |
-
aug_results = []
|
83 |
-
for ind, flip_ind in zip(img_inds[0::2], img_inds[1::2]):
|
84 |
-
img_pair = torch.cat([imgs[ind], imgs[flip_ind]])
|
85 |
-
x = self.extract_feat(img_pair)
|
86 |
-
outs = self.bbox_head(x)
|
87 |
-
bbox_list = self.bbox_head.get_bboxes(
|
88 |
-
*outs, [img_metas[ind], img_metas[flip_ind]], False, False)
|
89 |
-
aug_results.append(bbox_list[0])
|
90 |
-
aug_results.append(bbox_list[1])
|
91 |
-
|
92 |
-
bboxes, labels = self.merge_aug_results(aug_results, img_metas)
|
93 |
-
bbox_results = bbox2result(bboxes, labels, self.bbox_head.num_classes)
|
94 |
-
|
95 |
-
return [bbox_results]
|
|
|
|
|
|
|
|
|
|
|
|
|
|
|
|
|
|
|
|
|
|
|
|
|
|
|
|
|
|
|
|
|
|
|
|
|
|
|
|
|
|
|
|
|
|
|
|
|
|
|
|
|
|
|
|
|
|
|
|
|
|
|
|
|
|
|
|
|
|
|
|
|
|
|
|
|
|
|
|
|
|
|
|
|
|
|
|
|
|
|
|
|
|
|
|
|
|
|
|
|
|
|
|
|
|
|
|
|
|
|
|
|
|
|
|
|
|
|
|
|
|
|
|
|
|
|
|
|
|
|
|
|
|
|
|
|
|
|
|
|
|
|
|
|
|
|
|
|
|
|
|
|
|
|
|
|
|
|
|
|
|
|
|
|
|
|
|
|
|
|
|
|
|
|
|
|
|
|
|
|
|
|
|
|
|
|
|
|
|
|
|
spaces/CVPR/WALT/mmdet/models/losses/iou_loss.py
DELETED
@@ -1,436 +0,0 @@
|
|
1 |
-
import math
|
2 |
-
|
3 |
-
import mmcv
|
4 |
-
import torch
|
5 |
-
import torch.nn as nn
|
6 |
-
|
7 |
-
from mmdet.core import bbox_overlaps
|
8 |
-
from ..builder import LOSSES
|
9 |
-
from .utils import weighted_loss
|
10 |
-
|
11 |
-
|
12 |
-
@mmcv.jit(derivate=True, coderize=True)
|
13 |
-
@weighted_loss
|
14 |
-
def iou_loss(pred, target, linear=False, eps=1e-6):
|
15 |
-
"""IoU loss.
|
16 |
-
|
17 |
-
Computing the IoU loss between a set of predicted bboxes and target bboxes.
|
18 |
-
The loss is calculated as negative log of IoU.
|
19 |
-
|
20 |
-
Args:
|
21 |
-
pred (torch.Tensor): Predicted bboxes of format (x1, y1, x2, y2),
|
22 |
-
shape (n, 4).
|
23 |
-
target (torch.Tensor): Corresponding gt bboxes, shape (n, 4).
|
24 |
-
linear (bool, optional): If True, use linear scale of loss instead of
|
25 |
-
log scale. Default: False.
|
26 |
-
eps (float): Eps to avoid log(0).
|
27 |
-
|
28 |
-
Return:
|
29 |
-
torch.Tensor: Loss tensor.
|
30 |
-
"""
|
31 |
-
ious = bbox_overlaps(pred, target, is_aligned=True).clamp(min=eps)
|
32 |
-
if linear:
|
33 |
-
loss = 1 - ious
|
34 |
-
else:
|
35 |
-
loss = -ious.log()
|
36 |
-
return loss
|
37 |
-
|
38 |
-
|
39 |
-
@mmcv.jit(derivate=True, coderize=True)
|
40 |
-
@weighted_loss
|
41 |
-
def bounded_iou_loss(pred, target, beta=0.2, eps=1e-3):
|
42 |
-
"""BIoULoss.
|
43 |
-
|
44 |
-
This is an implementation of paper
|
45 |
-
`Improving Object Localization with Fitness NMS and Bounded IoU Loss.
|
46 |
-
<https://arxiv.org/abs/1711.00164>`_.
|
47 |
-
|
48 |
-
Args:
|
49 |
-
pred (torch.Tensor): Predicted bboxes.
|
50 |
-
target (torch.Tensor): Target bboxes.
|
51 |
-
beta (float): beta parameter in smoothl1.
|
52 |
-
eps (float): eps to avoid NaN.
|
53 |
-
"""
|
54 |
-
pred_ctrx = (pred[:, 0] + pred[:, 2]) * 0.5
|
55 |
-
pred_ctry = (pred[:, 1] + pred[:, 3]) * 0.5
|
56 |
-
pred_w = pred[:, 2] - pred[:, 0]
|
57 |
-
pred_h = pred[:, 3] - pred[:, 1]
|
58 |
-
with torch.no_grad():
|
59 |
-
target_ctrx = (target[:, 0] + target[:, 2]) * 0.5
|
60 |
-
target_ctry = (target[:, 1] + target[:, 3]) * 0.5
|
61 |
-
target_w = target[:, 2] - target[:, 0]
|
62 |
-
target_h = target[:, 3] - target[:, 1]
|
63 |
-
|
64 |
-
dx = target_ctrx - pred_ctrx
|
65 |
-
dy = target_ctry - pred_ctry
|
66 |
-
|
67 |
-
loss_dx = 1 - torch.max(
|
68 |
-
(target_w - 2 * dx.abs()) /
|
69 |
-
(target_w + 2 * dx.abs() + eps), torch.zeros_like(dx))
|
70 |
-
loss_dy = 1 - torch.max(
|
71 |
-
(target_h - 2 * dy.abs()) /
|
72 |
-
(target_h + 2 * dy.abs() + eps), torch.zeros_like(dy))
|
73 |
-
loss_dw = 1 - torch.min(target_w / (pred_w + eps), pred_w /
|
74 |
-
(target_w + eps))
|
75 |
-
loss_dh = 1 - torch.min(target_h / (pred_h + eps), pred_h /
|
76 |
-
(target_h + eps))
|
77 |
-
loss_comb = torch.stack([loss_dx, loss_dy, loss_dw, loss_dh],
|
78 |
-
dim=-1).view(loss_dx.size(0), -1)
|
79 |
-
|
80 |
-
loss = torch.where(loss_comb < beta, 0.5 * loss_comb * loss_comb / beta,
|
81 |
-
loss_comb - 0.5 * beta)
|
82 |
-
return loss
|
83 |
-
|
84 |
-
|
85 |
-
@mmcv.jit(derivate=True, coderize=True)
|
86 |
-
@weighted_loss
|
87 |
-
def giou_loss(pred, target, eps=1e-7):
|
88 |
-
r"""`Generalized Intersection over Union: A Metric and A Loss for Bounding
|
89 |
-
Box Regression <https://arxiv.org/abs/1902.09630>`_.
|
90 |
-
|
91 |
-
Args:
|
92 |
-
pred (torch.Tensor): Predicted bboxes of format (x1, y1, x2, y2),
|
93 |
-
shape (n, 4).
|
94 |
-
target (torch.Tensor): Corresponding gt bboxes, shape (n, 4).
|
95 |
-
eps (float): Eps to avoid log(0).
|
96 |
-
|
97 |
-
Return:
|
98 |
-
Tensor: Loss tensor.
|
99 |
-
"""
|
100 |
-
gious = bbox_overlaps(pred, target, mode='giou', is_aligned=True, eps=eps)
|
101 |
-
loss = 1 - gious
|
102 |
-
return loss
|
103 |
-
|
104 |
-
|
105 |
-
@mmcv.jit(derivate=True, coderize=True)
|
106 |
-
@weighted_loss
|
107 |
-
def diou_loss(pred, target, eps=1e-7):
|
108 |
-
r"""`Implementation of Distance-IoU Loss: Faster and Better
|
109 |
-
Learning for Bounding Box Regression, https://arxiv.org/abs/1911.08287`_.
|
110 |
-
|
111 |
-
Code is modified from https://github.com/Zzh-tju/DIoU.
|
112 |
-
|
113 |
-
Args:
|
114 |
-
pred (Tensor): Predicted bboxes of format (x1, y1, x2, y2),
|
115 |
-
shape (n, 4).
|
116 |
-
target (Tensor): Corresponding gt bboxes, shape (n, 4).
|
117 |
-
eps (float): Eps to avoid log(0).
|
118 |
-
Return:
|
119 |
-
Tensor: Loss tensor.
|
120 |
-
"""
|
121 |
-
# overlap
|
122 |
-
lt = torch.max(pred[:, :2], target[:, :2])
|
123 |
-
rb = torch.min(pred[:, 2:], target[:, 2:])
|
124 |
-
wh = (rb - lt).clamp(min=0)
|
125 |
-
overlap = wh[:, 0] * wh[:, 1]
|
126 |
-
|
127 |
-
# union
|
128 |
-
ap = (pred[:, 2] - pred[:, 0]) * (pred[:, 3] - pred[:, 1])
|
129 |
-
ag = (target[:, 2] - target[:, 0]) * (target[:, 3] - target[:, 1])
|
130 |
-
union = ap + ag - overlap + eps
|
131 |
-
|
132 |
-
# IoU
|
133 |
-
ious = overlap / union
|
134 |
-
|
135 |
-
# enclose area
|
136 |
-
enclose_x1y1 = torch.min(pred[:, :2], target[:, :2])
|
137 |
-
enclose_x2y2 = torch.max(pred[:, 2:], target[:, 2:])
|
138 |
-
enclose_wh = (enclose_x2y2 - enclose_x1y1).clamp(min=0)
|
139 |
-
|
140 |
-
cw = enclose_wh[:, 0]
|
141 |
-
ch = enclose_wh[:, 1]
|
142 |
-
|
143 |
-
c2 = cw**2 + ch**2 + eps
|
144 |
-
|
145 |
-
b1_x1, b1_y1 = pred[:, 0], pred[:, 1]
|
146 |
-
b1_x2, b1_y2 = pred[:, 2], pred[:, 3]
|
147 |
-
b2_x1, b2_y1 = target[:, 0], target[:, 1]
|
148 |
-
b2_x2, b2_y2 = target[:, 2], target[:, 3]
|
149 |
-
|
150 |
-
left = ((b2_x1 + b2_x2) - (b1_x1 + b1_x2))**2 / 4
|
151 |
-
right = ((b2_y1 + b2_y2) - (b1_y1 + b1_y2))**2 / 4
|
152 |
-
rho2 = left + right
|
153 |
-
|
154 |
-
# DIoU
|
155 |
-
dious = ious - rho2 / c2
|
156 |
-
loss = 1 - dious
|
157 |
-
return loss
|
158 |
-
|
159 |
-
|
160 |
-
@mmcv.jit(derivate=True, coderize=True)
|
161 |
-
@weighted_loss
|
162 |
-
def ciou_loss(pred, target, eps=1e-7):
|
163 |
-
r"""`Implementation of paper `Enhancing Geometric Factors into
|
164 |
-
Model Learning and Inference for Object Detection and Instance
|
165 |
-
Segmentation <https://arxiv.org/abs/2005.03572>`_.
|
166 |
-
|
167 |
-
Code is modified from https://github.com/Zzh-tju/CIoU.
|
168 |
-
|
169 |
-
Args:
|
170 |
-
pred (Tensor): Predicted bboxes of format (x1, y1, x2, y2),
|
171 |
-
shape (n, 4).
|
172 |
-
target (Tensor): Corresponding gt bboxes, shape (n, 4).
|
173 |
-
eps (float): Eps to avoid log(0).
|
174 |
-
Return:
|
175 |
-
Tensor: Loss tensor.
|
176 |
-
"""
|
177 |
-
# overlap
|
178 |
-
lt = torch.max(pred[:, :2], target[:, :2])
|
179 |
-
rb = torch.min(pred[:, 2:], target[:, 2:])
|
180 |
-
wh = (rb - lt).clamp(min=0)
|
181 |
-
overlap = wh[:, 0] * wh[:, 1]
|
182 |
-
|
183 |
-
# union
|
184 |
-
ap = (pred[:, 2] - pred[:, 0]) * (pred[:, 3] - pred[:, 1])
|
185 |
-
ag = (target[:, 2] - target[:, 0]) * (target[:, 3] - target[:, 1])
|
186 |
-
union = ap + ag - overlap + eps
|
187 |
-
|
188 |
-
# IoU
|
189 |
-
ious = overlap / union
|
190 |
-
|
191 |
-
# enclose area
|
192 |
-
enclose_x1y1 = torch.min(pred[:, :2], target[:, :2])
|
193 |
-
enclose_x2y2 = torch.max(pred[:, 2:], target[:, 2:])
|
194 |
-
enclose_wh = (enclose_x2y2 - enclose_x1y1).clamp(min=0)
|
195 |
-
|
196 |
-
cw = enclose_wh[:, 0]
|
197 |
-
ch = enclose_wh[:, 1]
|
198 |
-
|
199 |
-
c2 = cw**2 + ch**2 + eps
|
200 |
-
|
201 |
-
b1_x1, b1_y1 = pred[:, 0], pred[:, 1]
|
202 |
-
b1_x2, b1_y2 = pred[:, 2], pred[:, 3]
|
203 |
-
b2_x1, b2_y1 = target[:, 0], target[:, 1]
|
204 |
-
b2_x2, b2_y2 = target[:, 2], target[:, 3]
|
205 |
-
|
206 |
-
w1, h1 = b1_x2 - b1_x1, b1_y2 - b1_y1 + eps
|
207 |
-
w2, h2 = b2_x2 - b2_x1, b2_y2 - b2_y1 + eps
|
208 |
-
|
209 |
-
left = ((b2_x1 + b2_x2) - (b1_x1 + b1_x2))**2 / 4
|
210 |
-
right = ((b2_y1 + b2_y2) - (b1_y1 + b1_y2))**2 / 4
|
211 |
-
rho2 = left + right
|
212 |
-
|
213 |
-
factor = 4 / math.pi**2
|
214 |
-
v = factor * torch.pow(torch.atan(w2 / h2) - torch.atan(w1 / h1), 2)
|
215 |
-
|
216 |
-
# CIoU
|
217 |
-
cious = ious - (rho2 / c2 + v**2 / (1 - ious + v))
|
218 |
-
loss = 1 - cious
|
219 |
-
return loss
|
220 |
-
|
221 |
-
|
222 |
-
@LOSSES.register_module()
|
223 |
-
class IoULoss(nn.Module):
|
224 |
-
"""IoULoss.
|
225 |
-
|
226 |
-
Computing the IoU loss between a set of predicted bboxes and target bboxes.
|
227 |
-
|
228 |
-
Args:
|
229 |
-
linear (bool): If True, use linear scale of loss instead of log scale.
|
230 |
-
Default: False.
|
231 |
-
eps (float): Eps to avoid log(0).
|
232 |
-
reduction (str): Options are "none", "mean" and "sum".
|
233 |
-
loss_weight (float): Weight of loss.
|
234 |
-
"""
|
235 |
-
|
236 |
-
def __init__(self,
|
237 |
-
linear=False,
|
238 |
-
eps=1e-6,
|
239 |
-
reduction='mean',
|
240 |
-
loss_weight=1.0):
|
241 |
-
super(IoULoss, self).__init__()
|
242 |
-
self.linear = linear
|
243 |
-
self.eps = eps
|
244 |
-
self.reduction = reduction
|
245 |
-
self.loss_weight = loss_weight
|
246 |
-
|
247 |
-
def forward(self,
|
248 |
-
pred,
|
249 |
-
target,
|
250 |
-
weight=None,
|
251 |
-
avg_factor=None,
|
252 |
-
reduction_override=None,
|
253 |
-
**kwargs):
|
254 |
-
"""Forward function.
|
255 |
-
|
256 |
-
Args:
|
257 |
-
pred (torch.Tensor): The prediction.
|
258 |
-
target (torch.Tensor): The learning target of the prediction.
|
259 |
-
weight (torch.Tensor, optional): The weight of loss for each
|
260 |
-
prediction. Defaults to None.
|
261 |
-
avg_factor (int, optional): Average factor that is used to average
|
262 |
-
the loss. Defaults to None.
|
263 |
-
reduction_override (str, optional): The reduction method used to
|
264 |
-
override the original reduction method of the loss.
|
265 |
-
Defaults to None. Options are "none", "mean" and "sum".
|
266 |
-
"""
|
267 |
-
assert reduction_override in (None, 'none', 'mean', 'sum')
|
268 |
-
reduction = (
|
269 |
-
reduction_override if reduction_override else self.reduction)
|
270 |
-
if (weight is not None) and (not torch.any(weight > 0)) and (
|
271 |
-
reduction != 'none'):
|
272 |
-
return (pred * weight).sum() # 0
|
273 |
-
if weight is not None and weight.dim() > 1:
|
274 |
-
# TODO: remove this in the future
|
275 |
-
# reduce the weight of shape (n, 4) to (n,) to match the
|
276 |
-
# iou_loss of shape (n,)
|
277 |
-
assert weight.shape == pred.shape
|
278 |
-
weight = weight.mean(-1)
|
279 |
-
loss = self.loss_weight * iou_loss(
|
280 |
-
pred,
|
281 |
-
target,
|
282 |
-
weight,
|
283 |
-
linear=self.linear,
|
284 |
-
eps=self.eps,
|
285 |
-
reduction=reduction,
|
286 |
-
avg_factor=avg_factor,
|
287 |
-
**kwargs)
|
288 |
-
return loss
|
289 |
-
|
290 |
-
|
291 |
-
@LOSSES.register_module()
|
292 |
-
class BoundedIoULoss(nn.Module):
|
293 |
-
|
294 |
-
def __init__(self, beta=0.2, eps=1e-3, reduction='mean', loss_weight=1.0):
|
295 |
-
super(BoundedIoULoss, self).__init__()
|
296 |
-
self.beta = beta
|
297 |
-
self.eps = eps
|
298 |
-
self.reduction = reduction
|
299 |
-
self.loss_weight = loss_weight
|
300 |
-
|
301 |
-
def forward(self,
|
302 |
-
pred,
|
303 |
-
target,
|
304 |
-
weight=None,
|
305 |
-
avg_factor=None,
|
306 |
-
reduction_override=None,
|
307 |
-
**kwargs):
|
308 |
-
if weight is not None and not torch.any(weight > 0):
|
309 |
-
return (pred * weight).sum() # 0
|
310 |
-
assert reduction_override in (None, 'none', 'mean', 'sum')
|
311 |
-
reduction = (
|
312 |
-
reduction_override if reduction_override else self.reduction)
|
313 |
-
loss = self.loss_weight * bounded_iou_loss(
|
314 |
-
pred,
|
315 |
-
target,
|
316 |
-
weight,
|
317 |
-
beta=self.beta,
|
318 |
-
eps=self.eps,
|
319 |
-
reduction=reduction,
|
320 |
-
avg_factor=avg_factor,
|
321 |
-
**kwargs)
|
322 |
-
return loss
|
323 |
-
|
324 |
-
|
325 |
-
@LOSSES.register_module()
|
326 |
-
class GIoULoss(nn.Module):
|
327 |
-
|
328 |
-
def __init__(self, eps=1e-6, reduction='mean', loss_weight=1.0):
|
329 |
-
super(GIoULoss, self).__init__()
|
330 |
-
self.eps = eps
|
331 |
-
self.reduction = reduction
|
332 |
-
self.loss_weight = loss_weight
|
333 |
-
|
334 |
-
def forward(self,
|
335 |
-
pred,
|
336 |
-
target,
|
337 |
-
weight=None,
|
338 |
-
avg_factor=None,
|
339 |
-
reduction_override=None,
|
340 |
-
**kwargs):
|
341 |
-
if weight is not None and not torch.any(weight > 0):
|
342 |
-
return (pred * weight).sum() # 0
|
343 |
-
assert reduction_override in (None, 'none', 'mean', 'sum')
|
344 |
-
reduction = (
|
345 |
-
reduction_override if reduction_override else self.reduction)
|
346 |
-
if weight is not None and weight.dim() > 1:
|
347 |
-
# TODO: remove this in the future
|
348 |
-
# reduce the weight of shape (n, 4) to (n,) to match the
|
349 |
-
# giou_loss of shape (n,)
|
350 |
-
assert weight.shape == pred.shape
|
351 |
-
weight = weight.mean(-1)
|
352 |
-
loss = self.loss_weight * giou_loss(
|
353 |
-
pred,
|
354 |
-
target,
|
355 |
-
weight,
|
356 |
-
eps=self.eps,
|
357 |
-
reduction=reduction,
|
358 |
-
avg_factor=avg_factor,
|
359 |
-
**kwargs)
|
360 |
-
return loss
|
361 |
-
|
362 |
-
|
363 |
-
@LOSSES.register_module()
|
364 |
-
class DIoULoss(nn.Module):
|
365 |
-
|
366 |
-
def __init__(self, eps=1e-6, reduction='mean', loss_weight=1.0):
|
367 |
-
super(DIoULoss, self).__init__()
|
368 |
-
self.eps = eps
|
369 |
-
self.reduction = reduction
|
370 |
-
self.loss_weight = loss_weight
|
371 |
-
|
372 |
-
def forward(self,
|
373 |
-
pred,
|
374 |
-
target,
|
375 |
-
weight=None,
|
376 |
-
avg_factor=None,
|
377 |
-
reduction_override=None,
|
378 |
-
**kwargs):
|
379 |
-
if weight is not None and not torch.any(weight > 0):
|
380 |
-
return (pred * weight).sum() # 0
|
381 |
-
assert reduction_override in (None, 'none', 'mean', 'sum')
|
382 |
-
reduction = (
|
383 |
-
reduction_override if reduction_override else self.reduction)
|
384 |
-
if weight is not None and weight.dim() > 1:
|
385 |
-
# TODO: remove this in the future
|
386 |
-
# reduce the weight of shape (n, 4) to (n,) to match the
|
387 |
-
# giou_loss of shape (n,)
|
388 |
-
assert weight.shape == pred.shape
|
389 |
-
weight = weight.mean(-1)
|
390 |
-
loss = self.loss_weight * diou_loss(
|
391 |
-
pred,
|
392 |
-
target,
|
393 |
-
weight,
|
394 |
-
eps=self.eps,
|
395 |
-
reduction=reduction,
|
396 |
-
avg_factor=avg_factor,
|
397 |
-
**kwargs)
|
398 |
-
return loss
|
399 |
-
|
400 |
-
|
401 |
-
@LOSSES.register_module()
|
402 |
-
class CIoULoss(nn.Module):
|
403 |
-
|
404 |
-
def __init__(self, eps=1e-6, reduction='mean', loss_weight=1.0):
|
405 |
-
super(CIoULoss, self).__init__()
|
406 |
-
self.eps = eps
|
407 |
-
self.reduction = reduction
|
408 |
-
self.loss_weight = loss_weight
|
409 |
-
|
410 |
-
def forward(self,
|
411 |
-
pred,
|
412 |
-
target,
|
413 |
-
weight=None,
|
414 |
-
avg_factor=None,
|
415 |
-
reduction_override=None,
|
416 |
-
**kwargs):
|
417 |
-
if weight is not None and not torch.any(weight > 0):
|
418 |
-
return (pred * weight).sum() # 0
|
419 |
-
assert reduction_override in (None, 'none', 'mean', 'sum')
|
420 |
-
reduction = (
|
421 |
-
reduction_override if reduction_override else self.reduction)
|
422 |
-
if weight is not None and weight.dim() > 1:
|
423 |
-
# TODO: remove this in the future
|
424 |
-
# reduce the weight of shape (n, 4) to (n,) to match the
|
425 |
-
# giou_loss of shape (n,)
|
426 |
-
assert weight.shape == pred.shape
|
427 |
-
weight = weight.mean(-1)
|
428 |
-
loss = self.loss_weight * ciou_loss(
|
429 |
-
pred,
|
430 |
-
target,
|
431 |
-
weight,
|
432 |
-
eps=self.eps,
|
433 |
-
reduction=reduction,
|
434 |
-
avg_factor=avg_factor,
|
435 |
-
**kwargs)
|
436 |
-
return loss
|
|
|
|
|
|
|
|
|
|
|
|
|
|
|
|
|
|
|
|
|
|
|
|
|
|
|
|
|
|
|
|
|
|
|
|
|
|
|
|
|
|
|
|
|
|
|
|
|
|
|
|
|
|
|
|
|
|
|
|
|
|
|
|
|
|
|
|
|
|
|
|
|
|
|
|
|
|
|
|
|
|
|
|
|
|
|
|
|
|
|
|
|
|
|
|
|
|
|
|
|
|
|
|
|
|
|
|
|
|
|
|
|
|
|
|
|
|
|
|
|
|
|
|
|
|
|
|
|
|
|
|
|
|
|
|
|
|
|
|
|
|
|
|
|
|
|
|
|
|
|
|
|
|
|
|
|
|
|
|
|
|
|
|
|
|
|
|
|
|
|
|
|
|
|
|
|
|
|
|
|
|
|
|
|
|
|
|
|
|
|
|
|
|
|
|
|
|
|
|
|
|
|
|
|
|
|
|
|
|
|
|
|
|
|
|
|
|
|
|
|
|
|
|
|
|
|
|
|
|
|
|
|
|
|
|
|
|
|
|
|
|
|
|
|
|
|
|
|
|
|
|
|
|
|
|
|
|
|
|
|
|
|
|
|
|
|
|
|
|
|
|
|
|
|
|
|
|
|
|
|
|
|
|
|
|
|
|
|
|
|
|
|
|
|
|
|
|
|
|
|
|
|
|
|
|
|
|
|
|
|
|
|
|
|
|
|
|
|
|
|
|
|
|
|
|
|
|
|
|
|
|
|
|
|
|
|
|
|
|
|
|
|
|
|
|
|
|
|
|
|
|
|
|
|
|
|
|
|
|
|
|
|
|
|
|
|
|
|
|
|
|
|
|
|
|
|
|
|
|
|
|
|
|
|
|
|
|
|
|
|
|
|
|
|
|
|
|
|
|
|
|
|
|
|
|
|
|
|
|
|
|
|
|
|
|
|
|
|
|
|
|
|
|
|
|
|
|
|
|
|
|
|
|
|
|
|
|
|
|
|
|
|
|
|
|
|
|
|
|
|
|
|
|
|
|
|
|
|
|
|
|
|
|
|
|
|
|
|
|
|
|
|
|
|
|
|
|
|
|
|
|
|
|
|
|
|
|
|
|
|
|
|
|
|
|
|
|
|
|
|
|
|
|
|
|
|
|
|
|
|
|
|
|
|
|
|
|
|
|
|
|
|
|
|
|
|
|
|
|
|
|
|
|
|
|
|
|
|
|
|
|
|
|
|
|
|
|
|
|
|
|
|
|
|
|
|
|
|
|
|
|
|
|
|
|
|
|
|
|
|
|
|
|
|
|
|
|
|
|
|
|
|
|
|
|
|
|
|
|
|
|
|
|
|
|
|
|
|
|
|
|
|
|
|
|
|
|
|
|
|
|
|
|
|
|
|
|
|
|
|
|
|
|
|
|
|
|
|
|
|
|
|
|
|
|
|
|
|
|
|
|
|
|
|
|
|
|
|
|
|
|
|
|
|
|
|
|
|
|
|
|
|
|
|
|
|
|
|
|
|
|
|
|
|
|
|
|
|
|
|
|
|
|
|
|
|
|
|
|
|
|
|
|
|
|
|
|
|
|
|
|
|
|
|
|
|
|
|
|
|
|
|
|
|
|
|
|
|
|
|
|
|
|
|
|
|
|
|
|
|
|
|
|
|
|
|
|
|
|
|
|
|
|
|
|
|
|
|
|
|
|
|
|
|
|
|
|
|
|
|
|
|
|
|
|
|
|
|
|
|
|
|
|
|
|
|
|
|
|
|
|
|
|
|
|
|
|
|
|
|
|
|
|
|
|
|
|
|
|
|
|
|
|
|
|
|
|
|
|
|
|
|
|
|
|
|
|
|
|
|
|
|
|
|
|
|
|
|
|
|
|
|
|
|
|
|
|
|
|
|
|
|
|
|
|
|
|
|
|
|
|
|
|
|
|
|
|
|
|
|
|
|
|
|
|
|
|
|
|
|
|
|
|
spaces/CVPR/WALT/mmdet/models/roi_heads/cascade_roi_head.py
DELETED
@@ -1,507 +0,0 @@
|
|
1 |
-
import torch
|
2 |
-
import torch.nn as nn
|
3 |
-
|
4 |
-
from mmdet.core import (bbox2result, bbox2roi, bbox_mapping, build_assigner,
|
5 |
-
build_sampler, merge_aug_bboxes, merge_aug_masks,
|
6 |
-
multiclass_nms)
|
7 |
-
from ..builder import HEADS, build_head, build_roi_extractor
|
8 |
-
from .base_roi_head import BaseRoIHead
|
9 |
-
from .test_mixins import BBoxTestMixin, MaskTestMixin
|
10 |
-
|
11 |
-
|
12 |
-
@HEADS.register_module()
|
13 |
-
class CascadeRoIHead(BaseRoIHead, BBoxTestMixin, MaskTestMixin):
|
14 |
-
"""Cascade roi head including one bbox head and one mask head.
|
15 |
-
|
16 |
-
https://arxiv.org/abs/1712.00726
|
17 |
-
"""
|
18 |
-
|
19 |
-
def __init__(self,
|
20 |
-
num_stages,
|
21 |
-
stage_loss_weights,
|
22 |
-
bbox_roi_extractor=None,
|
23 |
-
bbox_head=None,
|
24 |
-
mask_roi_extractor=None,
|
25 |
-
mask_head=None,
|
26 |
-
shared_head=None,
|
27 |
-
train_cfg=None,
|
28 |
-
test_cfg=None):
|
29 |
-
assert bbox_roi_extractor is not None
|
30 |
-
assert bbox_head is not None
|
31 |
-
assert shared_head is None, \
|
32 |
-
'Shared head is not supported in Cascade RCNN anymore'
|
33 |
-
self.num_stages = num_stages
|
34 |
-
self.stage_loss_weights = stage_loss_weights
|
35 |
-
super(CascadeRoIHead, self).__init__(
|
36 |
-
bbox_roi_extractor=bbox_roi_extractor,
|
37 |
-
bbox_head=bbox_head,
|
38 |
-
mask_roi_extractor=mask_roi_extractor,
|
39 |
-
mask_head=mask_head,
|
40 |
-
shared_head=shared_head,
|
41 |
-
train_cfg=train_cfg,
|
42 |
-
test_cfg=test_cfg)
|
43 |
-
|
44 |
-
def init_bbox_head(self, bbox_roi_extractor, bbox_head):
|
45 |
-
"""Initialize box head and box roi extractor.
|
46 |
-
|
47 |
-
Args:
|
48 |
-
bbox_roi_extractor (dict): Config of box roi extractor.
|
49 |
-
bbox_head (dict): Config of box in box head.
|
50 |
-
"""
|
51 |
-
self.bbox_roi_extractor = nn.ModuleList()
|
52 |
-
self.bbox_head = nn.ModuleList()
|
53 |
-
if not isinstance(bbox_roi_extractor, list):
|
54 |
-
bbox_roi_extractor = [
|
55 |
-
bbox_roi_extractor for _ in range(self.num_stages)
|
56 |
-
]
|
57 |
-
if not isinstance(bbox_head, list):
|
58 |
-
bbox_head = [bbox_head for _ in range(self.num_stages)]
|
59 |
-
assert len(bbox_roi_extractor) == len(bbox_head) == self.num_stages
|
60 |
-
for roi_extractor, head in zip(bbox_roi_extractor, bbox_head):
|
61 |
-
self.bbox_roi_extractor.append(build_roi_extractor(roi_extractor))
|
62 |
-
self.bbox_head.append(build_head(head))
|
63 |
-
|
64 |
-
def init_mask_head(self, mask_roi_extractor, mask_head):
|
65 |
-
"""Initialize mask head and mask roi extractor.
|
66 |
-
|
67 |
-
Args:
|
68 |
-
mask_roi_extractor (dict): Config of mask roi extractor.
|
69 |
-
mask_head (dict): Config of mask in mask head.
|
70 |
-
"""
|
71 |
-
self.mask_head = nn.ModuleList()
|
72 |
-
if not isinstance(mask_head, list):
|
73 |
-
mask_head = [mask_head for _ in range(self.num_stages)]
|
74 |
-
assert len(mask_head) == self.num_stages
|
75 |
-
for head in mask_head:
|
76 |
-
self.mask_head.append(build_head(head))
|
77 |
-
if mask_roi_extractor is not None:
|
78 |
-
self.share_roi_extractor = False
|
79 |
-
self.mask_roi_extractor = nn.ModuleList()
|
80 |
-
if not isinstance(mask_roi_extractor, list):
|
81 |
-
mask_roi_extractor = [
|
82 |
-
mask_roi_extractor for _ in range(self.num_stages)
|
83 |
-
]
|
84 |
-
assert len(mask_roi_extractor) == self.num_stages
|
85 |
-
for roi_extractor in mask_roi_extractor:
|
86 |
-
self.mask_roi_extractor.append(
|
87 |
-
build_roi_extractor(roi_extractor))
|
88 |
-
else:
|
89 |
-
self.share_roi_extractor = True
|
90 |
-
self.mask_roi_extractor = self.bbox_roi_extractor
|
91 |
-
|
92 |
-
def init_assigner_sampler(self):
|
93 |
-
"""Initialize assigner and sampler for each stage."""
|
94 |
-
self.bbox_assigner = []
|
95 |
-
self.bbox_sampler = []
|
96 |
-
if self.train_cfg is not None:
|
97 |
-
for idx, rcnn_train_cfg in enumerate(self.train_cfg):
|
98 |
-
self.bbox_assigner.append(
|
99 |
-
build_assigner(rcnn_train_cfg.assigner))
|
100 |
-
self.current_stage = idx
|
101 |
-
self.bbox_sampler.append(
|
102 |
-
build_sampler(rcnn_train_cfg.sampler, context=self))
|
103 |
-
|
104 |
-
def init_weights(self, pretrained):
|
105 |
-
"""Initialize the weights in head.
|
106 |
-
|
107 |
-
Args:
|
108 |
-
pretrained (str, optional): Path to pre-trained weights.
|
109 |
-
Defaults to None.
|
110 |
-
"""
|
111 |
-
if self.with_shared_head:
|
112 |
-
self.shared_head.init_weights(pretrained=pretrained)
|
113 |
-
for i in range(self.num_stages):
|
114 |
-
if self.with_bbox:
|
115 |
-
self.bbox_roi_extractor[i].init_weights()
|
116 |
-
self.bbox_head[i].init_weights()
|
117 |
-
if self.with_mask:
|
118 |
-
if not self.share_roi_extractor:
|
119 |
-
self.mask_roi_extractor[i].init_weights()
|
120 |
-
self.mask_head[i].init_weights()
|
121 |
-
|
122 |
-
def forward_dummy(self, x, proposals):
|
123 |
-
"""Dummy forward function."""
|
124 |
-
# bbox head
|
125 |
-
outs = ()
|
126 |
-
rois = bbox2roi([proposals])
|
127 |
-
if self.with_bbox:
|
128 |
-
for i in range(self.num_stages):
|
129 |
-
bbox_results = self._bbox_forward(i, x, rois)
|
130 |
-
outs = outs + (bbox_results['cls_score'],
|
131 |
-
bbox_results['bbox_pred'])
|
132 |
-
# mask heads
|
133 |
-
if self.with_mask:
|
134 |
-
mask_rois = rois[:100]
|
135 |
-
for i in range(self.num_stages):
|
136 |
-
mask_results = self._mask_forward(i, x, mask_rois)
|
137 |
-
outs = outs + (mask_results['mask_pred'], )
|
138 |
-
return outs
|
139 |
-
|
140 |
-
def _bbox_forward(self, stage, x, rois):
|
141 |
-
"""Box head forward function used in both training and testing."""
|
142 |
-
bbox_roi_extractor = self.bbox_roi_extractor[stage]
|
143 |
-
bbox_head = self.bbox_head[stage]
|
144 |
-
bbox_feats = bbox_roi_extractor(x[:bbox_roi_extractor.num_inputs],
|
145 |
-
rois)
|
146 |
-
# do not support caffe_c4 model anymore
|
147 |
-
cls_score, bbox_pred = bbox_head(bbox_feats)
|
148 |
-
|
149 |
-
bbox_results = dict(
|
150 |
-
cls_score=cls_score, bbox_pred=bbox_pred, bbox_feats=bbox_feats)
|
151 |
-
return bbox_results
|
152 |
-
|
153 |
-
def _bbox_forward_train(self, stage, x, sampling_results, gt_bboxes,
|
154 |
-
gt_labels, rcnn_train_cfg):
|
155 |
-
"""Run forward function and calculate loss for box head in training."""
|
156 |
-
rois = bbox2roi([res.bboxes for res in sampling_results])
|
157 |
-
bbox_results = self._bbox_forward(stage, x, rois)
|
158 |
-
bbox_targets = self.bbox_head[stage].get_targets(
|
159 |
-
sampling_results, gt_bboxes, gt_labels, rcnn_train_cfg)
|
160 |
-
loss_bbox = self.bbox_head[stage].loss(bbox_results['cls_score'],
|
161 |
-
bbox_results['bbox_pred'], rois,
|
162 |
-
*bbox_targets)
|
163 |
-
|
164 |
-
bbox_results.update(
|
165 |
-
loss_bbox=loss_bbox, rois=rois, bbox_targets=bbox_targets)
|
166 |
-
return bbox_results
|
167 |
-
|
168 |
-
def _mask_forward(self, stage, x, rois):
|
169 |
-
"""Mask head forward function used in both training and testing."""
|
170 |
-
mask_roi_extractor = self.mask_roi_extractor[stage]
|
171 |
-
mask_head = self.mask_head[stage]
|
172 |
-
mask_feats = mask_roi_extractor(x[:mask_roi_extractor.num_inputs],
|
173 |
-
rois)
|
174 |
-
# do not support caffe_c4 model anymore
|
175 |
-
mask_pred = mask_head(mask_feats)
|
176 |
-
|
177 |
-
mask_results = dict(mask_pred=mask_pred)
|
178 |
-
return mask_results
|
179 |
-
|
180 |
-
def _mask_forward_train(self,
|
181 |
-
stage,
|
182 |
-
x,
|
183 |
-
sampling_results,
|
184 |
-
gt_masks,
|
185 |
-
rcnn_train_cfg,
|
186 |
-
bbox_feats=None):
|
187 |
-
"""Run forward function and calculate loss for mask head in
|
188 |
-
training."""
|
189 |
-
pos_rois = bbox2roi([res.pos_bboxes for res in sampling_results])
|
190 |
-
mask_results = self._mask_forward(stage, x, pos_rois)
|
191 |
-
|
192 |
-
mask_targets = self.mask_head[stage].get_targets(
|
193 |
-
sampling_results, gt_masks, rcnn_train_cfg)
|
194 |
-
pos_labels = torch.cat([res.pos_gt_labels for res in sampling_results])
|
195 |
-
loss_mask = self.mask_head[stage].loss(mask_results['mask_pred'],
|
196 |
-
mask_targets, pos_labels)
|
197 |
-
|
198 |
-
mask_results.update(loss_mask=loss_mask)
|
199 |
-
return mask_results
|
200 |
-
|
201 |
-
def forward_train(self,
|
202 |
-
x,
|
203 |
-
img_metas,
|
204 |
-
proposal_list,
|
205 |
-
gt_bboxes,
|
206 |
-
gt_labels,
|
207 |
-
gt_bboxes_ignore=None,
|
208 |
-
gt_masks=None):
|
209 |
-
"""
|
210 |
-
Args:
|
211 |
-
x (list[Tensor]): list of multi-level img features.
|
212 |
-
img_metas (list[dict]): list of image info dict where each dict
|
213 |
-
has: 'img_shape', 'scale_factor', 'flip', and may also contain
|
214 |
-
'filename', 'ori_shape', 'pad_shape', and 'img_norm_cfg'.
|
215 |
-
For details on the values of these keys see
|
216 |
-
`mmdet/datasets/pipelines/formatting.py:Collect`.
|
217 |
-
proposals (list[Tensors]): list of region proposals.
|
218 |
-
gt_bboxes (list[Tensor]): Ground truth bboxes for each image with
|
219 |
-
shape (num_gts, 4) in [tl_x, tl_y, br_x, br_y] format.
|
220 |
-
gt_labels (list[Tensor]): class indices corresponding to each box
|
221 |
-
gt_bboxes_ignore (None | list[Tensor]): specify which bounding
|
222 |
-
boxes can be ignored when computing the loss.
|
223 |
-
gt_masks (None | Tensor) : true segmentation masks for each box
|
224 |
-
used if the architecture supports a segmentation task.
|
225 |
-
|
226 |
-
Returns:
|
227 |
-
dict[str, Tensor]: a dictionary of loss components
|
228 |
-
"""
|
229 |
-
losses = dict()
|
230 |
-
for i in range(self.num_stages):
|
231 |
-
self.current_stage = i
|
232 |
-
rcnn_train_cfg = self.train_cfg[i]
|
233 |
-
lw = self.stage_loss_weights[i]
|
234 |
-
|
235 |
-
# assign gts and sample proposals
|
236 |
-
sampling_results = []
|
237 |
-
if self.with_bbox or self.with_mask:
|
238 |
-
bbox_assigner = self.bbox_assigner[i]
|
239 |
-
bbox_sampler = self.bbox_sampler[i]
|
240 |
-
num_imgs = len(img_metas)
|
241 |
-
if gt_bboxes_ignore is None:
|
242 |
-
gt_bboxes_ignore = [None for _ in range(num_imgs)]
|
243 |
-
|
244 |
-
for j in range(num_imgs):
|
245 |
-
assign_result = bbox_assigner.assign(
|
246 |
-
proposal_list[j], gt_bboxes[j], gt_bboxes_ignore[j],
|
247 |
-
gt_labels[j])
|
248 |
-
sampling_result = bbox_sampler.sample(
|
249 |
-
assign_result,
|
250 |
-
proposal_list[j],
|
251 |
-
gt_bboxes[j],
|
252 |
-
gt_labels[j],
|
253 |
-
feats=[lvl_feat[j][None] for lvl_feat in x])
|
254 |
-
sampling_results.append(sampling_result)
|
255 |
-
|
256 |
-
# bbox head forward and loss
|
257 |
-
bbox_results = self._bbox_forward_train(i, x, sampling_results,
|
258 |
-
gt_bboxes, gt_labels,
|
259 |
-
rcnn_train_cfg)
|
260 |
-
|
261 |
-
for name, value in bbox_results['loss_bbox'].items():
|
262 |
-
losses[f's{i}.{name}'] = (
|
263 |
-
value * lw if 'loss' in name else value)
|
264 |
-
|
265 |
-
# mask head forward and loss
|
266 |
-
if self.with_mask:
|
267 |
-
mask_results = self._mask_forward_train(
|
268 |
-
i, x, sampling_results, gt_masks, rcnn_train_cfg,
|
269 |
-
bbox_results['bbox_feats'])
|
270 |
-
for name, value in mask_results['loss_mask'].items():
|
271 |
-
losses[f's{i}.{name}'] = (
|
272 |
-
value * lw if 'loss' in name else value)
|
273 |
-
|
274 |
-
# refine bboxes
|
275 |
-
if i < self.num_stages - 1:
|
276 |
-
pos_is_gts = [res.pos_is_gt for res in sampling_results]
|
277 |
-
# bbox_targets is a tuple
|
278 |
-
roi_labels = bbox_results['bbox_targets'][0]
|
279 |
-
with torch.no_grad():
|
280 |
-
roi_labels = torch.where(
|
281 |
-
roi_labels == self.bbox_head[i].num_classes,
|
282 |
-
bbox_results['cls_score'][:, :-1].argmax(1),
|
283 |
-
roi_labels)
|
284 |
-
proposal_list = self.bbox_head[i].refine_bboxes(
|
285 |
-
bbox_results['rois'], roi_labels,
|
286 |
-
bbox_results['bbox_pred'], pos_is_gts, img_metas)
|
287 |
-
|
288 |
-
return losses
|
289 |
-
|
290 |
-
def simple_test(self, x, proposal_list, img_metas, rescale=False):
|
291 |
-
"""Test without augmentation."""
|
292 |
-
assert self.with_bbox, 'Bbox head must be implemented.'
|
293 |
-
num_imgs = len(proposal_list)
|
294 |
-
img_shapes = tuple(meta['img_shape'] for meta in img_metas)
|
295 |
-
ori_shapes = tuple(meta['ori_shape'] for meta in img_metas)
|
296 |
-
scale_factors = tuple(meta['scale_factor'] for meta in img_metas)
|
297 |
-
|
298 |
-
# "ms" in variable names means multi-stage
|
299 |
-
ms_bbox_result = {}
|
300 |
-
ms_segm_result = {}
|
301 |
-
ms_scores = []
|
302 |
-
rcnn_test_cfg = self.test_cfg
|
303 |
-
|
304 |
-
rois = bbox2roi(proposal_list)
|
305 |
-
for i in range(self.num_stages):
|
306 |
-
bbox_results = self._bbox_forward(i, x, rois)
|
307 |
-
|
308 |
-
# split batch bbox prediction back to each image
|
309 |
-
cls_score = bbox_results['cls_score']
|
310 |
-
bbox_pred = bbox_results['bbox_pred']
|
311 |
-
num_proposals_per_img = tuple(
|
312 |
-
len(proposals) for proposals in proposal_list)
|
313 |
-
rois = rois.split(num_proposals_per_img, 0)
|
314 |
-
cls_score = cls_score.split(num_proposals_per_img, 0)
|
315 |
-
if isinstance(bbox_pred, torch.Tensor):
|
316 |
-
bbox_pred = bbox_pred.split(num_proposals_per_img, 0)
|
317 |
-
else:
|
318 |
-
bbox_pred = self.bbox_head[i].bbox_pred_split(
|
319 |
-
bbox_pred, num_proposals_per_img)
|
320 |
-
ms_scores.append(cls_score)
|
321 |
-
|
322 |
-
if i < self.num_stages - 1:
|
323 |
-
bbox_label = [s[:, :-1].argmax(dim=1) for s in cls_score]
|
324 |
-
rois = torch.cat([
|
325 |
-
self.bbox_head[i].regress_by_class(rois[j], bbox_label[j],
|
326 |
-
bbox_pred[j],
|
327 |
-
img_metas[j])
|
328 |
-
for j in range(num_imgs)
|
329 |
-
])
|
330 |
-
|
331 |
-
# average scores of each image by stages
|
332 |
-
cls_score = [
|
333 |
-
sum([score[i] for score in ms_scores]) / float(len(ms_scores))
|
334 |
-
for i in range(num_imgs)
|
335 |
-
]
|
336 |
-
|
337 |
-
# apply bbox post-processing to each image individually
|
338 |
-
det_bboxes = []
|
339 |
-
det_labels = []
|
340 |
-
for i in range(num_imgs):
|
341 |
-
det_bbox, det_label = self.bbox_head[-1].get_bboxes(
|
342 |
-
rois[i],
|
343 |
-
cls_score[i],
|
344 |
-
bbox_pred[i],
|
345 |
-
img_shapes[i],
|
346 |
-
scale_factors[i],
|
347 |
-
rescale=rescale,
|
348 |
-
cfg=rcnn_test_cfg)
|
349 |
-
det_bboxes.append(det_bbox)
|
350 |
-
det_labels.append(det_label)
|
351 |
-
|
352 |
-
if torch.onnx.is_in_onnx_export():
|
353 |
-
return det_bboxes, det_labels
|
354 |
-
bbox_results = [
|
355 |
-
bbox2result(det_bboxes[i], det_labels[i],
|
356 |
-
self.bbox_head[-1].num_classes)
|
357 |
-
for i in range(num_imgs)
|
358 |
-
]
|
359 |
-
ms_bbox_result['ensemble'] = bbox_results
|
360 |
-
|
361 |
-
if self.with_mask:
|
362 |
-
if all(det_bbox.shape[0] == 0 for det_bbox in det_bboxes):
|
363 |
-
mask_classes = self.mask_head[-1].num_classes
|
364 |
-
segm_results = [[[] for _ in range(mask_classes)]
|
365 |
-
for _ in range(num_imgs)]
|
366 |
-
else:
|
367 |
-
if rescale and not isinstance(scale_factors[0], float):
|
368 |
-
scale_factors = [
|
369 |
-
torch.from_numpy(scale_factor).to(det_bboxes[0].device)
|
370 |
-
for scale_factor in scale_factors
|
371 |
-
]
|
372 |
-
_bboxes = [
|
373 |
-
det_bboxes[i][:, :4] *
|
374 |
-
scale_factors[i] if rescale else det_bboxes[i][:, :4]
|
375 |
-
for i in range(len(det_bboxes))
|
376 |
-
]
|
377 |
-
mask_rois = bbox2roi(_bboxes)
|
378 |
-
num_mask_rois_per_img = tuple(
|
379 |
-
_bbox.size(0) for _bbox in _bboxes)
|
380 |
-
aug_masks = []
|
381 |
-
for i in range(self.num_stages):
|
382 |
-
mask_results = self._mask_forward(i, x, mask_rois)
|
383 |
-
mask_pred = mask_results['mask_pred']
|
384 |
-
# split batch mask prediction back to each image
|
385 |
-
mask_pred = mask_pred.split(num_mask_rois_per_img, 0)
|
386 |
-
aug_masks.append(
|
387 |
-
[m.sigmoid().cpu().numpy() for m in mask_pred])
|
388 |
-
|
389 |
-
# apply mask post-processing to each image individually
|
390 |
-
segm_results = []
|
391 |
-
for i in range(num_imgs):
|
392 |
-
if det_bboxes[i].shape[0] == 0:
|
393 |
-
segm_results.append(
|
394 |
-
[[]
|
395 |
-
for _ in range(self.mask_head[-1].num_classes)])
|
396 |
-
else:
|
397 |
-
aug_mask = [mask[i] for mask in aug_masks]
|
398 |
-
merged_masks = merge_aug_masks(
|
399 |
-
aug_mask, [[img_metas[i]]] * self.num_stages,
|
400 |
-
rcnn_test_cfg)
|
401 |
-
segm_result = self.mask_head[-1].get_seg_masks(
|
402 |
-
merged_masks, _bboxes[i], det_labels[i],
|
403 |
-
rcnn_test_cfg, ori_shapes[i], scale_factors[i],
|
404 |
-
rescale)
|
405 |
-
segm_results.append(segm_result)
|
406 |
-
ms_segm_result['ensemble'] = segm_results
|
407 |
-
|
408 |
-
if self.with_mask:
|
409 |
-
results = list(
|
410 |
-
zip(ms_bbox_result['ensemble'], ms_segm_result['ensemble']))
|
411 |
-
else:
|
412 |
-
results = ms_bbox_result['ensemble']
|
413 |
-
|
414 |
-
return results
|
415 |
-
|
416 |
-
def aug_test(self, features, proposal_list, img_metas, rescale=False):
|
417 |
-
"""Test with augmentations.
|
418 |
-
|
419 |
-
If rescale is False, then returned bboxes and masks will fit the scale
|
420 |
-
of imgs[0].
|
421 |
-
"""
|
422 |
-
rcnn_test_cfg = self.test_cfg
|
423 |
-
aug_bboxes = []
|
424 |
-
aug_scores = []
|
425 |
-
for x, img_meta in zip(features, img_metas):
|
426 |
-
# only one image in the batch
|
427 |
-
img_shape = img_meta[0]['img_shape']
|
428 |
-
scale_factor = img_meta[0]['scale_factor']
|
429 |
-
flip = img_meta[0]['flip']
|
430 |
-
flip_direction = img_meta[0]['flip_direction']
|
431 |
-
|
432 |
-
proposals = bbox_mapping(proposal_list[0][:, :4], img_shape,
|
433 |
-
scale_factor, flip, flip_direction)
|
434 |
-
# "ms" in variable names means multi-stage
|
435 |
-
ms_scores = []
|
436 |
-
|
437 |
-
rois = bbox2roi([proposals])
|
438 |
-
for i in range(self.num_stages):
|
439 |
-
bbox_results = self._bbox_forward(i, x, rois)
|
440 |
-
ms_scores.append(bbox_results['cls_score'])
|
441 |
-
|
442 |
-
if i < self.num_stages - 1:
|
443 |
-
bbox_label = bbox_results['cls_score'][:, :-1].argmax(
|
444 |
-
dim=1)
|
445 |
-
rois = self.bbox_head[i].regress_by_class(
|
446 |
-
rois, bbox_label, bbox_results['bbox_pred'],
|
447 |
-
img_meta[0])
|
448 |
-
|
449 |
-
cls_score = sum(ms_scores) / float(len(ms_scores))
|
450 |
-
bboxes, scores = self.bbox_head[-1].get_bboxes(
|
451 |
-
rois,
|
452 |
-
cls_score,
|
453 |
-
bbox_results['bbox_pred'],
|
454 |
-
img_shape,
|
455 |
-
scale_factor,
|
456 |
-
rescale=False,
|
457 |
-
cfg=None)
|
458 |
-
aug_bboxes.append(bboxes)
|
459 |
-
aug_scores.append(scores)
|
460 |
-
|
461 |
-
# after merging, bboxes will be rescaled to the original image size
|
462 |
-
merged_bboxes, merged_scores = merge_aug_bboxes(
|
463 |
-
aug_bboxes, aug_scores, img_metas, rcnn_test_cfg)
|
464 |
-
det_bboxes, det_labels = multiclass_nms(merged_bboxes, merged_scores,
|
465 |
-
rcnn_test_cfg.score_thr,
|
466 |
-
rcnn_test_cfg.nms,
|
467 |
-
rcnn_test_cfg.max_per_img)
|
468 |
-
|
469 |
-
bbox_result = bbox2result(det_bboxes, det_labels,
|
470 |
-
self.bbox_head[-1].num_classes)
|
471 |
-
|
472 |
-
if self.with_mask:
|
473 |
-
if det_bboxes.shape[0] == 0:
|
474 |
-
segm_result = [[[]
|
475 |
-
for _ in range(self.mask_head[-1].num_classes)]
|
476 |
-
]
|
477 |
-
else:
|
478 |
-
aug_masks = []
|
479 |
-
aug_img_metas = []
|
480 |
-
for x, img_meta in zip(features, img_metas):
|
481 |
-
img_shape = img_meta[0]['img_shape']
|
482 |
-
scale_factor = img_meta[0]['scale_factor']
|
483 |
-
flip = img_meta[0]['flip']
|
484 |
-
flip_direction = img_meta[0]['flip_direction']
|
485 |
-
_bboxes = bbox_mapping(det_bboxes[:, :4], img_shape,
|
486 |
-
scale_factor, flip, flip_direction)
|
487 |
-
mask_rois = bbox2roi([_bboxes])
|
488 |
-
for i in range(self.num_stages):
|
489 |
-
mask_results = self._mask_forward(i, x, mask_rois)
|
490 |
-
aug_masks.append(
|
491 |
-
mask_results['mask_pred'].sigmoid().cpu().numpy())
|
492 |
-
aug_img_metas.append(img_meta)
|
493 |
-
merged_masks = merge_aug_masks(aug_masks, aug_img_metas,
|
494 |
-
self.test_cfg)
|
495 |
-
|
496 |
-
ori_shape = img_metas[0][0]['ori_shape']
|
497 |
-
segm_result = self.mask_head[-1].get_seg_masks(
|
498 |
-
merged_masks,
|
499 |
-
det_bboxes,
|
500 |
-
det_labels,
|
501 |
-
rcnn_test_cfg,
|
502 |
-
ori_shape,
|
503 |
-
scale_factor=1.0,
|
504 |
-
rescale=False)
|
505 |
-
return [(bbox_result, segm_result)]
|
506 |
-
else:
|
507 |
-
return [bbox_result]
|
|
|
|
|
|
|
|
|
|
|
|
|
|
|
|
|
|
|
|
|
|
|
|
|
|
|
|
|
|
|
|
|
|
|
|
|
|
|
|
|
|
|
|
|
|
|
|
|
|
|
|
|
|
|
|
|
|
|
|
|
|
|
|
|
|
|
|
|
|
|
|
|
|
|
|
|
|
|
|
|
|
|
|
|
|
|
|
|
|
|
|
|
|
|
|
|
|
|
|
|
|
|
|
|
|
|
|
|
|
|
|
|
|
|
|
|
|
|
|
|
|
|
|
|
|
|
|
|
|
|
|
|
|
|
|
|
|
|
|
|
|
|
|
|
|
|
|
|
|
|
|
|
|
|
|
|
|
|
|
|
|
|
|
|
|
|
|
|
|
|
|
|
|
|
|
|
|
|
|
|
|
|
|
|
|
|
|
|
|
|
|
|
|
|
|
|
|
|
|
|
|
|
|
|
|
|
|
|
|
|
|
|
|
|
|
|
|
|
|
|
|
|
|
|
|
|
|
|
|
|
|
|
|
|
|
|
|
|
|
|
|
|
|
|
|
|
|
|
|
|
|
|
|
|
|
|
|
|
|
|
|
|
|
|
|
|
|
|
|
|
|
|
|
|
|
|
|
|
|
|
|
|
|
|
|
|
|
|
|
|
|
|
|
|
|
|
|
|
|
|
|
|
|
|
|
|
|
|
|
|
|
|
|
|
|
|
|
|
|
|
|
|
|
|
|
|
|
|
|
|
|
|
|
|
|
|
|
|
|
|
|
|
|
|
|
|
|
|
|
|
|
|
|
|
|
|
|
|
|
|
|
|
|
|
|
|
|
|
|
|
|
|
|
|
|
|
|
|
|
|
|
|
|
|
|
|
|
|
|
|
|
|
|
|
|
|
|
|
|
|
|
|
|
|
|
|
|
|
|
|
|
|
|
|
|
|
|
|
|
|
|
|
|
|
|
|
|
|
|
|
|
|
|
|
|
|
|
|
|
|
|
|
|
|
|
|
|
|
|
|
|
|
|
|
|
|
|
|
|
|
|
|
|
|
|
|
|
|
|
|
|
|
|
|
|
|
|
|
|
|
|
|
|
|
|
|
|
|
|
|
|
|
|
|
|
|
|
|
|
|
|
|
|
|
|
|
|
|
|
|
|
|
|
|
|
|
|
|
|
|
|
|
|
|
|
|
|
|
|
|
|
|
|
|
|
|
|
|
|
|
|
|
|
|
|
|
|
|
|
|
|
|
|
|
|
|
|
|
|
|
|
|
|
|
|
|
|
|
|
|
|
|
|
|
|
|
|
|
|
|
|
|
|
|
|
|
|
|
|
|
|
|
|
|
|
|
|
|
|
|
|
|
|
|
|
|
|
|
|
|
|
|
|
|
|
|
|
|
|
|
|
|
|
|
|
|
|
|
|
|
|
|
|
|
|
|
|
|
|
|
|
|
|
|
|
|
|
|
|
|
|
|
|
|
|
|
|
|
|
|
|
|
|
|
|
|
|
|
|
|
|
|
|
|
|
|
|
|
|
|
|
|
|
|
|
|
|
|
|
|
|
|
|
|
|
|
|
|
|
|
|
|
|
|
|
|
|
|
|
|
|
|
|
|
|
|
|
|
|
|
|
|
|
|
|
|
|
|
|
|
|
|
|
|
|
|
|
|
|
|
|
|
|
|
|
|
|
|
|
|
|
|
|
|
|
|
|
|
|
|
|
|
|
|
|
|
|
|
|
|
|
|
|
|
|
|
|
|
|
|
|
|
|
|
|
|
|
|
|
|
|
|
|
|
|
|
|
|
|
|
|
|
|
|
|
|
|
|
|
|
|
|
|
|
|
|
|
|
|
|
|
|
|
|
|
|
|
|
|
|
|
|
|
|
|
|
|
|
|
|
|
|
|
|
|
|
|
|
|
|
|
|
|
|
|
|
|
|
|
|
|
|
|
|
|
|
|
|
|
|
|
|
|
|
|
|
|
|
|
|
|
|
|
|
|
|
|
|
|
|
|
|
|
|
|
|
|
|
|
|
|
|
|
|
|
|
|
|
|
|
|
|
|
|
|
|
|
|
|
|
|
|
|
|
|
|
|
|
|
|
|
|
|
|
|
|
|
|
|
|
|
|
|
|
|
|
|
|
|
|
|
|
|
|
|
|
|
|
|
|
|
|
|
|
|
|
|
|
|
|
|
|
|
|
|
|
|
|
|
|
|
|
|
|
|
|
|
|
|
|
|
|
|
|
|
|
|
|
|
|
|
|
|
|
|
|
|
|
|
|
|
|
|
|
|
spaces/CVPR/WALT/mmdet/models/roi_heads/test_mixins.py
DELETED
@@ -1,368 +0,0 @@
|
|
1 |
-
import logging
|
2 |
-
import sys
|
3 |
-
|
4 |
-
import torch
|
5 |
-
|
6 |
-
from mmdet.core import (bbox2roi, bbox_mapping, merge_aug_bboxes,
|
7 |
-
merge_aug_masks, multiclass_nms)
|
8 |
-
|
9 |
-
logger = logging.getLogger(__name__)
|
10 |
-
|
11 |
-
if sys.version_info >= (3, 7):
|
12 |
-
from mmdet.utils.contextmanagers import completed
|
13 |
-
|
14 |
-
|
15 |
-
class BBoxTestMixin(object):
|
16 |
-
|
17 |
-
if sys.version_info >= (3, 7):
|
18 |
-
|
19 |
-
async def async_test_bboxes(self,
|
20 |
-
x,
|
21 |
-
img_metas,
|
22 |
-
proposals,
|
23 |
-
rcnn_test_cfg,
|
24 |
-
rescale=False,
|
25 |
-
bbox_semaphore=None,
|
26 |
-
global_lock=None):
|
27 |
-
"""Asynchronized test for box head without augmentation."""
|
28 |
-
rois = bbox2roi(proposals)
|
29 |
-
roi_feats = self.bbox_roi_extractor(
|
30 |
-
x[:len(self.bbox_roi_extractor.featmap_strides)], rois)
|
31 |
-
if self.with_shared_head:
|
32 |
-
roi_feats = self.shared_head(roi_feats)
|
33 |
-
sleep_interval = rcnn_test_cfg.get('async_sleep_interval', 0.017)
|
34 |
-
|
35 |
-
async with completed(
|
36 |
-
__name__, 'bbox_head_forward',
|
37 |
-
sleep_interval=sleep_interval):
|
38 |
-
cls_score, bbox_pred = self.bbox_head(roi_feats)
|
39 |
-
|
40 |
-
img_shape = img_metas[0]['img_shape']
|
41 |
-
scale_factor = img_metas[0]['scale_factor']
|
42 |
-
det_bboxes, det_labels = self.bbox_head.get_bboxes(
|
43 |
-
rois,
|
44 |
-
cls_score,
|
45 |
-
bbox_pred,
|
46 |
-
img_shape,
|
47 |
-
scale_factor,
|
48 |
-
rescale=rescale,
|
49 |
-
cfg=rcnn_test_cfg)
|
50 |
-
return det_bboxes, det_labels
|
51 |
-
|
52 |
-
def simple_test_bboxes(self,
|
53 |
-
x,
|
54 |
-
img_metas,
|
55 |
-
proposals,
|
56 |
-
rcnn_test_cfg,
|
57 |
-
rescale=False):
|
58 |
-
"""Test only det bboxes without augmentation.
|
59 |
-
|
60 |
-
Args:
|
61 |
-
x (tuple[Tensor]): Feature maps of all scale level.
|
62 |
-
img_metas (list[dict]): Image meta info.
|
63 |
-
proposals (Tensor or List[Tensor]): Region proposals.
|
64 |
-
rcnn_test_cfg (obj:`ConfigDict`): `test_cfg` of R-CNN.
|
65 |
-
rescale (bool): If True, return boxes in original image space.
|
66 |
-
Default: False.
|
67 |
-
|
68 |
-
Returns:
|
69 |
-
tuple[list[Tensor], list[Tensor]]: The first list contains
|
70 |
-
the boxes of the corresponding image in a batch, each
|
71 |
-
tensor has the shape (num_boxes, 5) and last dimension
|
72 |
-
5 represent (tl_x, tl_y, br_x, br_y, score). Each Tensor
|
73 |
-
in the second list is the labels with shape (num_boxes, ).
|
74 |
-
The length of both lists should be equal to batch_size.
|
75 |
-
"""
|
76 |
-
# get origin input shape to support onnx dynamic input shape
|
77 |
-
if torch.onnx.is_in_onnx_export():
|
78 |
-
assert len(
|
79 |
-
img_metas
|
80 |
-
) == 1, 'Only support one input image while in exporting to ONNX'
|
81 |
-
img_shapes = img_metas[0]['img_shape_for_onnx']
|
82 |
-
else:
|
83 |
-
img_shapes = tuple(meta['img_shape'] for meta in img_metas)
|
84 |
-
scale_factors = tuple(meta['scale_factor'] for meta in img_metas)
|
85 |
-
|
86 |
-
# The length of proposals of different batches may be different.
|
87 |
-
# In order to form a batch, a padding operation is required.
|
88 |
-
if isinstance(proposals, list):
|
89 |
-
# padding to form a batch
|
90 |
-
max_size = max([proposal.size(0) for proposal in proposals])
|
91 |
-
for i, proposal in enumerate(proposals):
|
92 |
-
supplement = proposal.new_full(
|
93 |
-
(max_size - proposal.size(0), proposal.size(1)), 0)
|
94 |
-
proposals[i] = torch.cat((supplement, proposal), dim=0)
|
95 |
-
rois = torch.stack(proposals, dim=0)
|
96 |
-
else:
|
97 |
-
rois = proposals
|
98 |
-
|
99 |
-
batch_index = torch.arange(
|
100 |
-
rois.size(0), device=rois.device).float().view(-1, 1, 1).expand(
|
101 |
-
rois.size(0), rois.size(1), 1)
|
102 |
-
rois = torch.cat([batch_index, rois[..., :4]], dim=-1)
|
103 |
-
batch_size = rois.shape[0]
|
104 |
-
num_proposals_per_img = rois.shape[1]
|
105 |
-
|
106 |
-
# Eliminate the batch dimension
|
107 |
-
rois = rois.view(-1, 5)
|
108 |
-
bbox_results = self._bbox_forward(x, rois)
|
109 |
-
cls_score = bbox_results['cls_score']
|
110 |
-
bbox_pred = bbox_results['bbox_pred']
|
111 |
-
|
112 |
-
# Recover the batch dimension
|
113 |
-
rois = rois.reshape(batch_size, num_proposals_per_img, -1)
|
114 |
-
cls_score = cls_score.reshape(batch_size, num_proposals_per_img, -1)
|
115 |
-
|
116 |
-
if not torch.onnx.is_in_onnx_export():
|
117 |
-
# remove padding
|
118 |
-
supplement_mask = rois[..., -1] == 0
|
119 |
-
cls_score[supplement_mask, :] = 0
|
120 |
-
|
121 |
-
# bbox_pred would be None in some detector when with_reg is False,
|
122 |
-
# e.g. Grid R-CNN.
|
123 |
-
if bbox_pred is not None:
|
124 |
-
# the bbox prediction of some detectors like SABL is not Tensor
|
125 |
-
if isinstance(bbox_pred, torch.Tensor):
|
126 |
-
bbox_pred = bbox_pred.reshape(batch_size,
|
127 |
-
num_proposals_per_img, -1)
|
128 |
-
if not torch.onnx.is_in_onnx_export():
|
129 |
-
bbox_pred[supplement_mask, :] = 0
|
130 |
-
else:
|
131 |
-
# TODO: Looking forward to a better way
|
132 |
-
# For SABL
|
133 |
-
bbox_preds = self.bbox_head.bbox_pred_split(
|
134 |
-
bbox_pred, num_proposals_per_img)
|
135 |
-
# apply bbox post-processing to each image individually
|
136 |
-
det_bboxes = []
|
137 |
-
det_labels = []
|
138 |
-
for i in range(len(proposals)):
|
139 |
-
# remove padding
|
140 |
-
supplement_mask = proposals[i][..., -1] == 0
|
141 |
-
for bbox in bbox_preds[i]:
|
142 |
-
bbox[supplement_mask] = 0
|
143 |
-
det_bbox, det_label = self.bbox_head.get_bboxes(
|
144 |
-
rois[i],
|
145 |
-
cls_score[i],
|
146 |
-
bbox_preds[i],
|
147 |
-
img_shapes[i],
|
148 |
-
scale_factors[i],
|
149 |
-
rescale=rescale,
|
150 |
-
cfg=rcnn_test_cfg)
|
151 |
-
det_bboxes.append(det_bbox)
|
152 |
-
det_labels.append(det_label)
|
153 |
-
return det_bboxes, det_labels
|
154 |
-
else:
|
155 |
-
bbox_pred = None
|
156 |
-
|
157 |
-
return self.bbox_head.get_bboxes(
|
158 |
-
rois,
|
159 |
-
cls_score,
|
160 |
-
bbox_pred,
|
161 |
-
img_shapes,
|
162 |
-
scale_factors,
|
163 |
-
rescale=rescale,
|
164 |
-
cfg=rcnn_test_cfg)
|
165 |
-
|
166 |
-
def aug_test_bboxes(self, feats, img_metas, proposal_list, rcnn_test_cfg):
|
167 |
-
"""Test det bboxes with test time augmentation."""
|
168 |
-
aug_bboxes = []
|
169 |
-
aug_scores = []
|
170 |
-
for x, img_meta in zip(feats, img_metas):
|
171 |
-
# only one image in the batch
|
172 |
-
img_shape = img_meta[0]['img_shape']
|
173 |
-
scale_factor = img_meta[0]['scale_factor']
|
174 |
-
flip = img_meta[0]['flip']
|
175 |
-
flip_direction = img_meta[0]['flip_direction']
|
176 |
-
# TODO more flexible
|
177 |
-
proposals = bbox_mapping(proposal_list[0][:, :4], img_shape,
|
178 |
-
scale_factor, flip, flip_direction)
|
179 |
-
rois = bbox2roi([proposals])
|
180 |
-
bbox_results = self._bbox_forward(x, rois)
|
181 |
-
bboxes, scores = self.bbox_head.get_bboxes(
|
182 |
-
rois,
|
183 |
-
bbox_results['cls_score'],
|
184 |
-
bbox_results['bbox_pred'],
|
185 |
-
img_shape,
|
186 |
-
scale_factor,
|
187 |
-
rescale=False,
|
188 |
-
cfg=None)
|
189 |
-
aug_bboxes.append(bboxes)
|
190 |
-
aug_scores.append(scores)
|
191 |
-
# after merging, bboxes will be rescaled to the original image size
|
192 |
-
merged_bboxes, merged_scores = merge_aug_bboxes(
|
193 |
-
aug_bboxes, aug_scores, img_metas, rcnn_test_cfg)
|
194 |
-
det_bboxes, det_labels = multiclass_nms(merged_bboxes, merged_scores,
|
195 |
-
rcnn_test_cfg.score_thr,
|
196 |
-
rcnn_test_cfg.nms,
|
197 |
-
rcnn_test_cfg.max_per_img)
|
198 |
-
return det_bboxes, det_labels
|
199 |
-
|
200 |
-
|
201 |
-
class MaskTestMixin(object):
|
202 |
-
|
203 |
-
if sys.version_info >= (3, 7):
|
204 |
-
|
205 |
-
async def async_test_mask(self,
|
206 |
-
x,
|
207 |
-
img_metas,
|
208 |
-
det_bboxes,
|
209 |
-
det_labels,
|
210 |
-
rescale=False,
|
211 |
-
mask_test_cfg=None):
|
212 |
-
"""Asynchronized test for mask head without augmentation."""
|
213 |
-
# image shape of the first image in the batch (only one)
|
214 |
-
ori_shape = img_metas[0]['ori_shape']
|
215 |
-
scale_factor = img_metas[0]['scale_factor']
|
216 |
-
if det_bboxes.shape[0] == 0:
|
217 |
-
segm_result = [[] for _ in range(self.mask_head.num_classes)]
|
218 |
-
else:
|
219 |
-
if rescale and not isinstance(scale_factor,
|
220 |
-
(float, torch.Tensor)):
|
221 |
-
scale_factor = det_bboxes.new_tensor(scale_factor)
|
222 |
-
_bboxes = (
|
223 |
-
det_bboxes[:, :4] *
|
224 |
-
scale_factor if rescale else det_bboxes)
|
225 |
-
mask_rois = bbox2roi([_bboxes])
|
226 |
-
mask_feats = self.mask_roi_extractor(
|
227 |
-
x[:len(self.mask_roi_extractor.featmap_strides)],
|
228 |
-
mask_rois)
|
229 |
-
|
230 |
-
if self.with_shared_head:
|
231 |
-
mask_feats = self.shared_head(mask_feats)
|
232 |
-
if mask_test_cfg and mask_test_cfg.get('async_sleep_interval'):
|
233 |
-
sleep_interval = mask_test_cfg['async_sleep_interval']
|
234 |
-
else:
|
235 |
-
sleep_interval = 0.035
|
236 |
-
async with completed(
|
237 |
-
__name__,
|
238 |
-
'mask_head_forward',
|
239 |
-
sleep_interval=sleep_interval):
|
240 |
-
mask_pred = self.mask_head(mask_feats)
|
241 |
-
segm_result = self.mask_head.get_seg_masks(
|
242 |
-
mask_pred, _bboxes, det_labels, self.test_cfg, ori_shape,
|
243 |
-
scale_factor, rescale)
|
244 |
-
return segm_result
|
245 |
-
|
246 |
-
def simple_test_mask(self,
|
247 |
-
x,
|
248 |
-
img_metas,
|
249 |
-
det_bboxes,
|
250 |
-
det_labels,
|
251 |
-
rescale=False):
|
252 |
-
"""Simple test for mask head without augmentation."""
|
253 |
-
# image shapes of images in the batch
|
254 |
-
ori_shapes = tuple(meta['ori_shape'] for meta in img_metas)
|
255 |
-
scale_factors = tuple(meta['scale_factor'] for meta in img_metas)
|
256 |
-
|
257 |
-
# The length of proposals of different batches may be different.
|
258 |
-
# In order to form a batch, a padding operation is required.
|
259 |
-
if isinstance(det_bboxes, list):
|
260 |
-
# padding to form a batch
|
261 |
-
max_size = max([bboxes.size(0) for bboxes in det_bboxes])
|
262 |
-
for i, (bbox, label) in enumerate(zip(det_bboxes, det_labels)):
|
263 |
-
supplement_bbox = bbox.new_full(
|
264 |
-
(max_size - bbox.size(0), bbox.size(1)), 0)
|
265 |
-
supplement_label = label.new_full((max_size - label.size(0), ),
|
266 |
-
0)
|
267 |
-
det_bboxes[i] = torch.cat((supplement_bbox, bbox), dim=0)
|
268 |
-
det_labels[i] = torch.cat((supplement_label, label), dim=0)
|
269 |
-
det_bboxes = torch.stack(det_bboxes, dim=0)
|
270 |
-
det_labels = torch.stack(det_labels, dim=0)
|
271 |
-
|
272 |
-
batch_size = det_bboxes.size(0)
|
273 |
-
num_proposals_per_img = det_bboxes.shape[1]
|
274 |
-
|
275 |
-
# if det_bboxes is rescaled to the original image size, we need to
|
276 |
-
# rescale it back to the testing scale to obtain RoIs.
|
277 |
-
det_bboxes = det_bboxes[..., :4]
|
278 |
-
if rescale:
|
279 |
-
if not isinstance(scale_factors[0], float):
|
280 |
-
scale_factors = det_bboxes.new_tensor(scale_factors)
|
281 |
-
det_bboxes = det_bboxes * scale_factors.unsqueeze(1)
|
282 |
-
|
283 |
-
batch_index = torch.arange(
|
284 |
-
det_bboxes.size(0), device=det_bboxes.device).float().view(
|
285 |
-
-1, 1, 1).expand(det_bboxes.size(0), det_bboxes.size(1), 1)
|
286 |
-
mask_rois = torch.cat([batch_index, det_bboxes], dim=-1)
|
287 |
-
mask_rois = mask_rois.view(-1, 5)
|
288 |
-
mask_results = self._mask_forward(x, mask_rois)
|
289 |
-
mask_pred = mask_results['mask_pred']
|
290 |
-
try:
|
291 |
-
mask_full_pred, mask_occ_pred = mask_pred
|
292 |
-
except:
|
293 |
-
mask_full_pred = mask_pred
|
294 |
-
mask_occ_pred = mask_pred
|
295 |
-
|
296 |
-
|
297 |
-
# Recover the batch dimension
|
298 |
-
mask_full_preds = mask_full_pred.reshape(batch_size, num_proposals_per_img,
|
299 |
-
*mask_full_pred.shape[1:])
|
300 |
-
|
301 |
-
mask_occ_preds = mask_occ_pred.reshape(batch_size, num_proposals_per_img,
|
302 |
-
*mask_occ_pred.shape[1:])
|
303 |
-
|
304 |
-
|
305 |
-
# apply mask post-processing to each image individually
|
306 |
-
segm_results = []
|
307 |
-
for i in range(batch_size):
|
308 |
-
mask_full_pred = mask_full_preds[i]
|
309 |
-
mask_occ_pred = mask_occ_preds[i]
|
310 |
-
det_bbox = det_bboxes[i]
|
311 |
-
det_label = det_labels[i]
|
312 |
-
|
313 |
-
# remove padding
|
314 |
-
supplement_mask = det_bbox[..., -1] != 0
|
315 |
-
mask_full_pred = mask_full_pred[supplement_mask]
|
316 |
-
mask_occ_pred = mask_occ_pred[supplement_mask]
|
317 |
-
det_bbox = det_bbox[supplement_mask]
|
318 |
-
det_label = det_label[supplement_mask]
|
319 |
-
|
320 |
-
if det_label.shape[0] == 0:
|
321 |
-
segm_results.append([[]
|
322 |
-
for _ in range(self.mask_head.num_classes)
|
323 |
-
])
|
324 |
-
else:
|
325 |
-
segm_result_vis = self.mask_head.get_seg_masks(
|
326 |
-
mask_full_pred[:,0:1], det_bbox, det_label, self.test_cfg,
|
327 |
-
ori_shapes[i], scale_factors[i], rescale)
|
328 |
-
|
329 |
-
segm_result_occ = self.mask_head.get_seg_masks(
|
330 |
-
mask_occ_pred[:,0:1], det_bbox, det_label, self.test_cfg,
|
331 |
-
ori_shapes[i], scale_factors[i], rescale)
|
332 |
-
|
333 |
-
segm_result = segm_result_vis
|
334 |
-
segm_result[1] = segm_result_occ[0]
|
335 |
-
|
336 |
-
segm_results.append(segm_result)
|
337 |
-
return segm_results
|
338 |
-
|
339 |
-
def aug_test_mask(self, feats, img_metas, det_bboxes, det_labels):
|
340 |
-
"""Test for mask head with test time augmentation."""
|
341 |
-
if det_bboxes.shape[0] == 0:
|
342 |
-
segm_result = [[] for _ in range(self.mask_head.num_classes)]
|
343 |
-
else:
|
344 |
-
aug_masks = []
|
345 |
-
for x, img_meta in zip(feats, img_metas):
|
346 |
-
img_shape = img_meta[0]['img_shape']
|
347 |
-
scale_factor = img_meta[0]['scale_factor']
|
348 |
-
flip = img_meta[0]['flip']
|
349 |
-
flip_direction = img_meta[0]['flip_direction']
|
350 |
-
_bboxes = bbox_mapping(det_bboxes[:, :4], img_shape,
|
351 |
-
scale_factor, flip, flip_direction)
|
352 |
-
mask_rois = bbox2roi([_bboxes])
|
353 |
-
mask_results = self._mask_forward(x, mask_rois)
|
354 |
-
# convert to numpy array to save memory
|
355 |
-
aug_masks.append(
|
356 |
-
mask_results['mask_pred'].sigmoid().cpu().numpy())
|
357 |
-
merged_masks = merge_aug_masks(aug_masks, img_metas, self.test_cfg)
|
358 |
-
|
359 |
-
ori_shape = img_metas[0][0]['ori_shape']
|
360 |
-
segm_result = self.mask_head.get_seg_masks(
|
361 |
-
merged_masks,
|
362 |
-
det_bboxes,
|
363 |
-
det_labels,
|
364 |
-
self.test_cfg,
|
365 |
-
ori_shape,
|
366 |
-
scale_factor=1.0,
|
367 |
-
rescale=False)
|
368 |
-
return segm_result
|
|
|
|
|
|
|
|
|
|
|
|
|
|
|
|
|
|
|
|
|
|
|
|
|
|
|
|
|
|
|
|
|
|
|
|
|
|
|
|
|
|
|
|
|
|
|
|
|
|
|
|
|
|
|
|
|
|
|
|
|
|
|
|
|
|
|
|
|
|
|
|
|
|
|
|
|
|
|
|
|
|
|
|
|
|
|
|
|
|
|
|
|
|
|
|
|
|
|
|
|
|
|
|
|
|
|
|
|
|
|
|
|
|
|
|
|
|
|
|
|
|
|
|
|
|
|
|
|
|
|
|
|
|
|
|
|
|
|
|
|
|
|
|
|
|
|
|
|
|
|
|
|
|
|
|
|
|
|
|
|
|
|
|
|
|
|
|
|
|
|
|
|
|
|
|
|
|
|
|
|
|
|
|
|
|
|
|
|
|
|
|
|
|
|
|
|
|
|
|
|
|
|
|
|
|
|
|
|
|
|
|
|
|
|
|
|
|
|
|
|
|
|
|
|
|
|
|
|
|
|
|
|
|
|
|
|
|
|
|
|
|
|
|
|
|
|
|
|
|
|
|
|
|
|
|
|
|
|
|
|
|
|
|
|
|
|
|
|
|
|
|
|
|
|
|
|
|
|
|
|
|
|
|
|
|
|
|
|
|
|
|
|
|
|
|
|
|
|
|
|
|
|
|
|
|
|
|
|
|
|
|
|
|
|
|
|
|
|
|
|
|
|
|
|
|
|
|
|
|
|
|
|
|
|
|
|
|
|
|
|
|
|
|
|
|
|
|
|
|
|
|
|
|
|
|
|
|
|
|
|
|
|
|
|
|
|
|
|
|
|
|
|
|
|
|
|
|
|
|
|
|
|
|
|
|
|
|
|
|
|
|
|
|
|
|
|
|
|
|
|
|
|
|
|
|
|
|
|
|
|
|
|
|
|
|
|
|
|
|
|
|
|
|
|
|
|
|
|
|
|
|
|
|
|
|
|
|
|
|
|
|
|
|
|
|
|
|
|
|
|
|
|
|
|
|
|
|
|
|
|
|
|
|
|
|
|
|
|
|
|
|
|
|
|
|
|
|
|
|
|
|
|
|
|
|
|
|
|
|
|
|
|
|
|
|
|
|
|
|
|
|
|
|
|
|
|
|
|
|
|
|
|
|
|
|
|
|
|
|
|
|
|
|
|
|
|
|
|
|
|
|
|
|
|
|
|
|
|
|
|
|
|
|
|
|
|
|
|
|
|
|
|
|
|
|
|
|
|
|
|
|
|
|
|
|
|
|
|
|
|
|
|
|
|
|
|
|
|
|
|
|
|
|
|
|
|
|
|
|
|
|
|
|
|
|
|
|
|
|
|
|
|
|
|
|
|
|
|
|
|
|
|
|
|
|
|
|
|
|
|
|
|
|
|
|
|
|
|
|
|
|
|
|
|
|
|
|
|
|
|
|
|
|
|
|
|
|
|
|
|
|
|
|
|
|
|
|
|
|
|
|
|
|
|
|
|
|
|
|
|
|
|
|
|
|
|
|
|
|
|
|
|
|
|
|
|
|
|
|
|
|
|
|
|
|
|
|
|
|
|
|
|
|
|
|
|
|
|
|
|
|
|
|
|
|
|
|
|
|
|
|
|
|
|
|
|
|
|
|
|
|
|
|
|
|
|
|
spaces/ChallengeHub/Chinese-LangChain/create_knowledge.py
DELETED
@@ -1,79 +0,0 @@
|
|
1 |
-
#!/usr/bin/env python
|
2 |
-
# -*- coding:utf-8 _*-
|
3 |
-
"""
|
4 |
-
@author:quincy qiang
|
5 |
-
@license: Apache Licence
|
6 |
-
@file: create_knowledge.py
|
7 |
-
@time: 2023/04/18
|
8 |
-
@contact: [email protected]
|
9 |
-
@software: PyCharm
|
10 |
-
@description: - emoji:https://emojixd.com/pocket/science
|
11 |
-
"""
|
12 |
-
import os
|
13 |
-
import pandas as pd
|
14 |
-
from langchain.schema import Document
|
15 |
-
from langchain.document_loaders import UnstructuredFileLoader
|
16 |
-
from langchain.embeddings.huggingface import HuggingFaceEmbeddings
|
17 |
-
from langchain.vectorstores import FAISS
|
18 |
-
from tqdm import tqdm
|
19 |
-
# 中文Wikipedia数据导入示例:
|
20 |
-
embedding_model_name = '/root/pretrained_models/text2vec-large-chinese'
|
21 |
-
docs_path = '/root/GoMall/Knowledge-ChatGLM/cache/financial_research_reports'
|
22 |
-
embeddings = HuggingFaceEmbeddings(model_name=embedding_model_name)
|
23 |
-
|
24 |
-
|
25 |
-
# Wikipedia数据处理
|
26 |
-
|
27 |
-
# docs = []
|
28 |
-
|
29 |
-
# with open('docs/zh_wikipedia/zhwiki.sim.utf8', 'r', encoding='utf-8') as f:
|
30 |
-
# for idx, line in tqdm(enumerate(f.readlines())):
|
31 |
-
# metadata = {"source": f'doc_id_{idx}'}
|
32 |
-
# docs.append(Document(page_content=line.strip(), metadata=metadata))
|
33 |
-
#
|
34 |
-
# vector_store = FAISS.from_documents(docs, embeddings)
|
35 |
-
# vector_store.save_local('cache/zh_wikipedia/')
|
36 |
-
|
37 |
-
|
38 |
-
|
39 |
-
docs = []
|
40 |
-
|
41 |
-
with open('cache/zh_wikipedia/wiki.zh-sim-cleaned.txt', 'r', encoding='utf-8') as f:
|
42 |
-
for idx, line in tqdm(enumerate(f.readlines())):
|
43 |
-
metadata = {"source": f'doc_id_{idx}'}
|
44 |
-
docs.append(Document(page_content=line.strip(), metadata=metadata))
|
45 |
-
|
46 |
-
vector_store = FAISS.from_documents(docs, embeddings)
|
47 |
-
vector_store.save_local('cache/zh_wikipedia/')
|
48 |
-
|
49 |
-
|
50 |
-
# 金融研报数据处理
|
51 |
-
# docs = []
|
52 |
-
#
|
53 |
-
# for doc in tqdm(os.listdir(docs_path)):
|
54 |
-
# if doc.endswith('.txt'):
|
55 |
-
# # print(doc)
|
56 |
-
# loader = UnstructuredFileLoader(f'{docs_path}/{doc}', mode="elements")
|
57 |
-
# doc = loader.load()
|
58 |
-
# docs.extend(doc)
|
59 |
-
# vector_store = FAISS.from_documents(docs, embeddings)
|
60 |
-
# vector_store.save_local('cache/financial_research_reports')
|
61 |
-
|
62 |
-
|
63 |
-
# 英雄联盟
|
64 |
-
|
65 |
-
docs = []
|
66 |
-
|
67 |
-
lol_df = pd.read_csv('cache/lol/champions.csv')
|
68 |
-
# lol_df.columns = ['id', '英雄简称', '英雄全称', '出生地', '人物属性', '英雄类别', '英雄故事']
|
69 |
-
print(lol_df)
|
70 |
-
|
71 |
-
for idx, row in lol_df.iterrows():
|
72 |
-
metadata = {"source": f'doc_id_{idx}'}
|
73 |
-
text = ' '.join(row.values)
|
74 |
-
# for col in ['英雄简称', '英雄全称', '出生地', '人物属性', '英雄类别', '英雄故事']:
|
75 |
-
# text += row[col]
|
76 |
-
docs.append(Document(page_content=text, metadata=metadata))
|
77 |
-
|
78 |
-
vector_store = FAISS.from_documents(docs, embeddings)
|
79 |
-
vector_store.save_local('cache/lol/')
|
|
|
|
|
|
|
|
|
|
|
|
|
|
|
|
|
|
|
|
|
|
|
|
|
|
|
|
|
|
|
|
|
|
|
|
|
|
|
|
|
|
|
|
|
|
|
|
|
|
|
|
|
|
|
|
|
|
|
|
|
|
|
|
|
|
|
|
|
|
|
|
|
|
|
|
|
|
|
|
|
|
|
|
|
|
|
|
|
|
|
|
|
|
|
|
|
|
|
|
|
|
|
|
|
|
|
|
|
|
|
|
|
|
|
|
|
|
|
|
|
|
|
|
|
|
|
|
|
|
|
|
|
|
|
|
|
|
|
|
|
|
|
|
|
|
|
|
|
|
|
|
|
|
|
|
|
|
|
|
spaces/Cvandi/remake/realesrgan/data/realesrgan_paired_dataset.py
DELETED
@@ -1,108 +0,0 @@
|
|
1 |
-
import os
|
2 |
-
from basicsr.data.data_util import paired_paths_from_folder, paired_paths_from_lmdb
|
3 |
-
from basicsr.data.transforms import augment, paired_random_crop
|
4 |
-
from basicsr.utils import FileClient, imfrombytes, img2tensor
|
5 |
-
from basicsr.utils.registry import DATASET_REGISTRY
|
6 |
-
from torch.utils import data as data
|
7 |
-
from torchvision.transforms.functional import normalize
|
8 |
-
|
9 |
-
|
10 |
-
@DATASET_REGISTRY.register()
|
11 |
-
class RealESRGANPairedDataset(data.Dataset):
|
12 |
-
"""Paired image dataset for image restoration.
|
13 |
-
|
14 |
-
Read LQ (Low Quality, e.g. LR (Low Resolution), blurry, noisy, etc) and GT image pairs.
|
15 |
-
|
16 |
-
There are three modes:
|
17 |
-
1. 'lmdb': Use lmdb files.
|
18 |
-
If opt['io_backend'] == lmdb.
|
19 |
-
2. 'meta_info': Use meta information file to generate paths.
|
20 |
-
If opt['io_backend'] != lmdb and opt['meta_info'] is not None.
|
21 |
-
3. 'folder': Scan folders to generate paths.
|
22 |
-
The rest.
|
23 |
-
|
24 |
-
Args:
|
25 |
-
opt (dict): Config for train datasets. It contains the following keys:
|
26 |
-
dataroot_gt (str): Data root path for gt.
|
27 |
-
dataroot_lq (str): Data root path for lq.
|
28 |
-
meta_info (str): Path for meta information file.
|
29 |
-
io_backend (dict): IO backend type and other kwarg.
|
30 |
-
filename_tmpl (str): Template for each filename. Note that the template excludes the file extension.
|
31 |
-
Default: '{}'.
|
32 |
-
gt_size (int): Cropped patched size for gt patches.
|
33 |
-
use_hflip (bool): Use horizontal flips.
|
34 |
-
use_rot (bool): Use rotation (use vertical flip and transposing h
|
35 |
-
and w for implementation).
|
36 |
-
|
37 |
-
scale (bool): Scale, which will be added automatically.
|
38 |
-
phase (str): 'train' or 'val'.
|
39 |
-
"""
|
40 |
-
|
41 |
-
def __init__(self, opt):
|
42 |
-
super(RealESRGANPairedDataset, self).__init__()
|
43 |
-
self.opt = opt
|
44 |
-
self.file_client = None
|
45 |
-
self.io_backend_opt = opt['io_backend']
|
46 |
-
# mean and std for normalizing the input images
|
47 |
-
self.mean = opt['mean'] if 'mean' in opt else None
|
48 |
-
self.std = opt['std'] if 'std' in opt else None
|
49 |
-
|
50 |
-
self.gt_folder, self.lq_folder = opt['dataroot_gt'], opt['dataroot_lq']
|
51 |
-
self.filename_tmpl = opt['filename_tmpl'] if 'filename_tmpl' in opt else '{}'
|
52 |
-
|
53 |
-
# file client (lmdb io backend)
|
54 |
-
if self.io_backend_opt['type'] == 'lmdb':
|
55 |
-
self.io_backend_opt['db_paths'] = [self.lq_folder, self.gt_folder]
|
56 |
-
self.io_backend_opt['client_keys'] = ['lq', 'gt']
|
57 |
-
self.paths = paired_paths_from_lmdb([self.lq_folder, self.gt_folder], ['lq', 'gt'])
|
58 |
-
elif 'meta_info' in self.opt and self.opt['meta_info'] is not None:
|
59 |
-
# disk backend with meta_info
|
60 |
-
# Each line in the meta_info describes the relative path to an image
|
61 |
-
with open(self.opt['meta_info']) as fin:
|
62 |
-
paths = [line.strip() for line in fin]
|
63 |
-
self.paths = []
|
64 |
-
for path in paths:
|
65 |
-
gt_path, lq_path = path.split(', ')
|
66 |
-
gt_path = os.path.join(self.gt_folder, gt_path)
|
67 |
-
lq_path = os.path.join(self.lq_folder, lq_path)
|
68 |
-
self.paths.append(dict([('gt_path', gt_path), ('lq_path', lq_path)]))
|
69 |
-
else:
|
70 |
-
# disk backend
|
71 |
-
# it will scan the whole folder to get meta info
|
72 |
-
# it will be time-consuming for folders with too many files. It is recommended using an extra meta txt file
|
73 |
-
self.paths = paired_paths_from_folder([self.lq_folder, self.gt_folder], ['lq', 'gt'], self.filename_tmpl)
|
74 |
-
|
75 |
-
def __getitem__(self, index):
|
76 |
-
if self.file_client is None:
|
77 |
-
self.file_client = FileClient(self.io_backend_opt.pop('type'), **self.io_backend_opt)
|
78 |
-
|
79 |
-
scale = self.opt['scale']
|
80 |
-
|
81 |
-
# Load gt and lq images. Dimension order: HWC; channel order: BGR;
|
82 |
-
# image range: [0, 1], float32.
|
83 |
-
gt_path = self.paths[index]['gt_path']
|
84 |
-
img_bytes = self.file_client.get(gt_path, 'gt')
|
85 |
-
img_gt = imfrombytes(img_bytes, float32=True)
|
86 |
-
lq_path = self.paths[index]['lq_path']
|
87 |
-
img_bytes = self.file_client.get(lq_path, 'lq')
|
88 |
-
img_lq = imfrombytes(img_bytes, float32=True)
|
89 |
-
|
90 |
-
# augmentation for training
|
91 |
-
if self.opt['phase'] == 'train':
|
92 |
-
gt_size = self.opt['gt_size']
|
93 |
-
# random crop
|
94 |
-
img_gt, img_lq = paired_random_crop(img_gt, img_lq, gt_size, scale, gt_path)
|
95 |
-
# flip, rotation
|
96 |
-
img_gt, img_lq = augment([img_gt, img_lq], self.opt['use_hflip'], self.opt['use_rot'])
|
97 |
-
|
98 |
-
# BGR to RGB, HWC to CHW, numpy to tensor
|
99 |
-
img_gt, img_lq = img2tensor([img_gt, img_lq], bgr2rgb=True, float32=True)
|
100 |
-
# normalize
|
101 |
-
if self.mean is not None or self.std is not None:
|
102 |
-
normalize(img_lq, self.mean, self.std, inplace=True)
|
103 |
-
normalize(img_gt, self.mean, self.std, inplace=True)
|
104 |
-
|
105 |
-
return {'lq': img_lq, 'gt': img_gt, 'lq_path': lq_path, 'gt_path': gt_path}
|
106 |
-
|
107 |
-
def __len__(self):
|
108 |
-
return len(self.paths)
|
|
|
|
|
|
|
|
|
|
|
|
|
|
|
|
|
|
|
|
|
|
|
|
|
|
|
|
|
|
|
|
|
|
|
|
|
|
|
|
|
|
|
|
|
|
|
|
|
|
|
|
|
|
|
|
|
|
|
|
|
|
|
|
|
|
|
|
|
|
|
|
|
|
|
|
|
|
|
|
|
|
|
|
|
|
|
|
|
|
|
|
|
|
|
|
|
|
|
|
|
|
|
|
|
|
|
|
|
|
|
|
|
|
|
|
|
|
|
|
|
|
|
|
|
|
|
|
|
|
|
|
|
|
|
|
|
|
|
|
|
|
|
|
|
|
|
|
|
|
|
|
|
|
|
|
|
|
|
|
|
|
|
|
|
|
|
|
|
|
|
|
|
|
|
|
|
|
|
|
|
|
|
|
|
|
|
|
|
|
|
|
|
|
|
|
|
|
|
|
|
|
|
|
|
|
|
|
|
|
|
|
|
|
|
|
|
|
spaces/DQChoi/gpt-demo/venv/lib/python3.11/site-packages/fontTools/misc/testTools.py
DELETED
@@ -1,229 +0,0 @@
|
|
1 |
-
"""Helpers for writing unit tests."""
|
2 |
-
|
3 |
-
from collections.abc import Iterable
|
4 |
-
from io import BytesIO
|
5 |
-
import os
|
6 |
-
import re
|
7 |
-
import shutil
|
8 |
-
import sys
|
9 |
-
import tempfile
|
10 |
-
from unittest import TestCase as _TestCase
|
11 |
-
from fontTools.config import Config
|
12 |
-
from fontTools.misc.textTools import tobytes
|
13 |
-
from fontTools.misc.xmlWriter import XMLWriter
|
14 |
-
|
15 |
-
|
16 |
-
def parseXML(xmlSnippet):
|
17 |
-
"""Parses a snippet of XML.
|
18 |
-
|
19 |
-
Input can be either a single string (unicode or UTF-8 bytes), or a
|
20 |
-
a sequence of strings.
|
21 |
-
|
22 |
-
The result is in the same format that would be returned by
|
23 |
-
XMLReader, but the parser imposes no constraints on the root
|
24 |
-
element so it can be called on small snippets of TTX files.
|
25 |
-
"""
|
26 |
-
# To support snippets with multiple elements, we add a fake root.
|
27 |
-
reader = TestXMLReader_()
|
28 |
-
xml = b"<root>"
|
29 |
-
if isinstance(xmlSnippet, bytes):
|
30 |
-
xml += xmlSnippet
|
31 |
-
elif isinstance(xmlSnippet, str):
|
32 |
-
xml += tobytes(xmlSnippet, "utf-8")
|
33 |
-
elif isinstance(xmlSnippet, Iterable):
|
34 |
-
xml += b"".join(tobytes(s, "utf-8") for s in xmlSnippet)
|
35 |
-
else:
|
36 |
-
raise TypeError(
|
37 |
-
"expected string or sequence of strings; found %r"
|
38 |
-
% type(xmlSnippet).__name__
|
39 |
-
)
|
40 |
-
xml += b"</root>"
|
41 |
-
reader.parser.Parse(xml, 0)
|
42 |
-
return reader.root[2]
|
43 |
-
|
44 |
-
|
45 |
-
def parseXmlInto(font, parseInto, xmlSnippet):
|
46 |
-
parsed_xml = [e for e in parseXML(xmlSnippet.strip()) if not isinstance(e, str)]
|
47 |
-
for name, attrs, content in parsed_xml:
|
48 |
-
parseInto.fromXML(name, attrs, content, font)
|
49 |
-
parseInto.populateDefaults()
|
50 |
-
return parseInto
|
51 |
-
|
52 |
-
|
53 |
-
class FakeFont:
|
54 |
-
def __init__(self, glyphs):
|
55 |
-
self.glyphOrder_ = glyphs
|
56 |
-
self.reverseGlyphOrderDict_ = {g: i for i, g in enumerate(glyphs)}
|
57 |
-
self.lazy = False
|
58 |
-
self.tables = {}
|
59 |
-
self.cfg = Config()
|
60 |
-
|
61 |
-
def __getitem__(self, tag):
|
62 |
-
return self.tables[tag]
|
63 |
-
|
64 |
-
def __setitem__(self, tag, table):
|
65 |
-
self.tables[tag] = table
|
66 |
-
|
67 |
-
def get(self, tag, default=None):
|
68 |
-
return self.tables.get(tag, default)
|
69 |
-
|
70 |
-
def getGlyphID(self, name):
|
71 |
-
return self.reverseGlyphOrderDict_[name]
|
72 |
-
|
73 |
-
def getGlyphIDMany(self, lst):
|
74 |
-
return [self.getGlyphID(gid) for gid in lst]
|
75 |
-
|
76 |
-
def getGlyphName(self, glyphID):
|
77 |
-
if glyphID < len(self.glyphOrder_):
|
78 |
-
return self.glyphOrder_[glyphID]
|
79 |
-
else:
|
80 |
-
return "glyph%.5d" % glyphID
|
81 |
-
|
82 |
-
def getGlyphNameMany(self, lst):
|
83 |
-
return [self.getGlyphName(gid) for gid in lst]
|
84 |
-
|
85 |
-
def getGlyphOrder(self):
|
86 |
-
return self.glyphOrder_
|
87 |
-
|
88 |
-
def getReverseGlyphMap(self):
|
89 |
-
return self.reverseGlyphOrderDict_
|
90 |
-
|
91 |
-
def getGlyphNames(self):
|
92 |
-
return sorted(self.getGlyphOrder())
|
93 |
-
|
94 |
-
|
95 |
-
class TestXMLReader_(object):
|
96 |
-
def __init__(self):
|
97 |
-
from xml.parsers.expat import ParserCreate
|
98 |
-
|
99 |
-
self.parser = ParserCreate()
|
100 |
-
self.parser.StartElementHandler = self.startElement_
|
101 |
-
self.parser.EndElementHandler = self.endElement_
|
102 |
-
self.parser.CharacterDataHandler = self.addCharacterData_
|
103 |
-
self.root = None
|
104 |
-
self.stack = []
|
105 |
-
|
106 |
-
def startElement_(self, name, attrs):
|
107 |
-
element = (name, attrs, [])
|
108 |
-
if self.stack:
|
109 |
-
self.stack[-1][2].append(element)
|
110 |
-
else:
|
111 |
-
self.root = element
|
112 |
-
self.stack.append(element)
|
113 |
-
|
114 |
-
def endElement_(self, name):
|
115 |
-
self.stack.pop()
|
116 |
-
|
117 |
-
def addCharacterData_(self, data):
|
118 |
-
self.stack[-1][2].append(data)
|
119 |
-
|
120 |
-
|
121 |
-
def makeXMLWriter(newlinestr="\n"):
|
122 |
-
# don't write OS-specific new lines
|
123 |
-
writer = XMLWriter(BytesIO(), newlinestr=newlinestr)
|
124 |
-
# erase XML declaration
|
125 |
-
writer.file.seek(0)
|
126 |
-
writer.file.truncate()
|
127 |
-
return writer
|
128 |
-
|
129 |
-
|
130 |
-
def getXML(func, ttFont=None):
|
131 |
-
"""Call the passed toXML function and return the written content as a
|
132 |
-
list of lines (unicode strings).
|
133 |
-
Result is stripped of XML declaration and OS-specific newline characters.
|
134 |
-
"""
|
135 |
-
writer = makeXMLWriter()
|
136 |
-
func(writer, ttFont)
|
137 |
-
xml = writer.file.getvalue().decode("utf-8")
|
138 |
-
# toXML methods must always end with a writer.newline()
|
139 |
-
assert xml.endswith("\n")
|
140 |
-
return xml.splitlines()
|
141 |
-
|
142 |
-
|
143 |
-
def stripVariableItemsFromTTX(
|
144 |
-
string: str,
|
145 |
-
ttLibVersion: bool = True,
|
146 |
-
checkSumAdjustment: bool = True,
|
147 |
-
modified: bool = True,
|
148 |
-
created: bool = True,
|
149 |
-
sfntVersion: bool = False, # opt-in only
|
150 |
-
) -> str:
|
151 |
-
"""Strip stuff like ttLibVersion, checksums, timestamps, etc. from TTX dumps."""
|
152 |
-
# ttlib changes with the fontTools version
|
153 |
-
if ttLibVersion:
|
154 |
-
string = re.sub(' ttLibVersion="[^"]+"', "", string)
|
155 |
-
# sometimes (e.g. some subsetter tests) we don't care whether it's OTF or TTF
|
156 |
-
if sfntVersion:
|
157 |
-
string = re.sub(' sfntVersion="[^"]+"', "", string)
|
158 |
-
# head table checksum and creation and mod date changes with each save.
|
159 |
-
if checkSumAdjustment:
|
160 |
-
string = re.sub('<checkSumAdjustment value="[^"]+"/>', "", string)
|
161 |
-
if modified:
|
162 |
-
string = re.sub('<modified value="[^"]+"/>', "", string)
|
163 |
-
if created:
|
164 |
-
string = re.sub('<created value="[^"]+"/>', "", string)
|
165 |
-
return string
|
166 |
-
|
167 |
-
|
168 |
-
class MockFont(object):
|
169 |
-
"""A font-like object that automatically adds any looked up glyphname
|
170 |
-
to its glyphOrder."""
|
171 |
-
|
172 |
-
def __init__(self):
|
173 |
-
self._glyphOrder = [".notdef"]
|
174 |
-
|
175 |
-
class AllocatingDict(dict):
|
176 |
-
def __missing__(reverseDict, key):
|
177 |
-
self._glyphOrder.append(key)
|
178 |
-
gid = len(reverseDict)
|
179 |
-
reverseDict[key] = gid
|
180 |
-
return gid
|
181 |
-
|
182 |
-
self._reverseGlyphOrder = AllocatingDict({".notdef": 0})
|
183 |
-
self.lazy = False
|
184 |
-
|
185 |
-
def getGlyphID(self, glyph):
|
186 |
-
gid = self._reverseGlyphOrder[glyph]
|
187 |
-
return gid
|
188 |
-
|
189 |
-
def getReverseGlyphMap(self):
|
190 |
-
return self._reverseGlyphOrder
|
191 |
-
|
192 |
-
def getGlyphName(self, gid):
|
193 |
-
return self._glyphOrder[gid]
|
194 |
-
|
195 |
-
def getGlyphOrder(self):
|
196 |
-
return self._glyphOrder
|
197 |
-
|
198 |
-
|
199 |
-
class TestCase(_TestCase):
|
200 |
-
def __init__(self, methodName):
|
201 |
-
_TestCase.__init__(self, methodName)
|
202 |
-
# Python 3 renamed assertRaisesRegexp to assertRaisesRegex,
|
203 |
-
# and fires deprecation warnings if a program uses the old name.
|
204 |
-
if not hasattr(self, "assertRaisesRegex"):
|
205 |
-
self.assertRaisesRegex = self.assertRaisesRegexp
|
206 |
-
|
207 |
-
|
208 |
-
class DataFilesHandler(TestCase):
|
209 |
-
def setUp(self):
|
210 |
-
self.tempdir = None
|
211 |
-
self.num_tempfiles = 0
|
212 |
-
|
213 |
-
def tearDown(self):
|
214 |
-
if self.tempdir:
|
215 |
-
shutil.rmtree(self.tempdir)
|
216 |
-
|
217 |
-
def getpath(self, testfile):
|
218 |
-
folder = os.path.dirname(sys.modules[self.__module__].__file__)
|
219 |
-
return os.path.join(folder, "data", testfile)
|
220 |
-
|
221 |
-
def temp_dir(self):
|
222 |
-
if not self.tempdir:
|
223 |
-
self.tempdir = tempfile.mkdtemp()
|
224 |
-
|
225 |
-
def temp_font(self, font_path, file_name):
|
226 |
-
self.temp_dir()
|
227 |
-
temppath = os.path.join(self.tempdir, file_name)
|
228 |
-
shutil.copy2(font_path, temppath)
|
229 |
-
return temppath
|
|
|
|
|
|
|
|
|
|
|
|
|
|
|
|
|
|
|
|
|
|
|
|
|
|
|
|
|
|
|
|
|
|
|
|
|
|
|
|
|
|
|
|
|
|
|
|
|
|
|
|
|
|
|
|
|
|
|
|
|
|
|
|
|
|
|
|
|
|
|
|
|
|
|
|
|
|
|
|
|
|
|
|
|
|
|
|
|
|
|
|
|
|
|
|
|
|
|
|
|
|
|
|
|
|
|
|
|
|
|
|
|
|
|
|
|
|
|
|
|
|
|
|
|
|
|
|
|
|
|
|
|
|
|
|
|
|
|
|
|
|
|
|
|
|
|
|
|
|
|
|
|
|
|
|
|
|
|
|
|
|
|
|
|
|
|
|
|
|
|
|
|
|
|
|
|
|
|
|
|
|
|
|
|
|
|
|
|
|
|
|
|
|
|
|
|
|
|
|
|
|
|
|
|
|
|
|
|
|
|
|
|
|
|
|
|
|
|
|
|
|
|
|
|
|
|
|
|
|
|
|
|
|
|
|
|
|
|
|
|
|
|
|
|
|
|
|
|
|
|
|
|
|
|
|
|
|
|
|
|
|
|
|
|
|
|
|
|
|
|
|
|
|
|
|
|
|
|
|
|
|
|
|
|
|
|
|
|
|
|
|
|
|
|
|
|
|
|
|
|
|
|
|
|
|
|
|
|
|
|
|
|
|
|
|
|
|
|
|
|
|
|
|
|
|
|
|
|
|
|
|
|
|
|
|
|
|
|
|
|
|
|
|
|
|
|
|
|
|
|
|
|
|
|
|
|
|
|
|
|
|
|
|
|
|
|
|
|
|
|
|
|
|
|
|
|
|
|
|
|
|
|
|
|
|
|
|
|
|
|
|
|
|
|
|
|
|
|
|
|
|
|
|
|
|
|
|
|
|
|
|
|
|
|
|
|
|
|
|
|
|
|
|
|
|
|
|
|
|
|
|
|
|
|
|
|
|
|
|
|
|
|
|
|
|
|
|
|
|
|
|
|
|
|
|
|
|
|
|
spaces/EuroPython2022/pulsar-clip/README.md
DELETED
@@ -1,13 +0,0 @@
|
|
1 |
-
---
|
2 |
-
title: Pulsar Clip
|
3 |
-
emoji: 😻
|
4 |
-
colorFrom: yellow
|
5 |
-
colorTo: blue
|
6 |
-
sdk: gradio
|
7 |
-
sdk_version: 3.1.4b5
|
8 |
-
app_file: app.py
|
9 |
-
pinned: false
|
10 |
-
license: agpl-3.0
|
11 |
-
---
|
12 |
-
|
13 |
-
Check out the configuration reference at https://huggingface.co/docs/hub/spaces-config-reference
|
|
|
|
|
|
|
|
|
|
|
|
|
|
|
|
|
|
|
|
|
|
|
|
|
|
|
|
spaces/FrankZxShen/so-vits-svc-models-ba/diffusion/infer_gt_mel.py
DELETED
@@ -1,74 +0,0 @@
|
|
1 |
-
import numpy as np
|
2 |
-
import torch
|
3 |
-
import torch.nn.functional as F
|
4 |
-
from diffusion.unit2mel import load_model_vocoder
|
5 |
-
|
6 |
-
|
7 |
-
class DiffGtMel:
|
8 |
-
def __init__(self, project_path=None, device=None):
|
9 |
-
self.project_path = project_path
|
10 |
-
if device is not None:
|
11 |
-
self.device = device
|
12 |
-
else:
|
13 |
-
self.device = 'cuda' if torch.cuda.is_available() else 'cpu'
|
14 |
-
self.model = None
|
15 |
-
self.vocoder = None
|
16 |
-
self.args = None
|
17 |
-
|
18 |
-
def flush_model(self, project_path, ddsp_config=None):
|
19 |
-
if (self.model is None) or (project_path != self.project_path):
|
20 |
-
model, vocoder, args = load_model_vocoder(project_path, device=self.device)
|
21 |
-
if self.check_args(ddsp_config, args):
|
22 |
-
self.model = model
|
23 |
-
self.vocoder = vocoder
|
24 |
-
self.args = args
|
25 |
-
|
26 |
-
def check_args(self, args1, args2):
|
27 |
-
if args1.data.block_size != args2.data.block_size:
|
28 |
-
raise ValueError("DDSP与DIFF模型的block_size不一致")
|
29 |
-
if args1.data.sampling_rate != args2.data.sampling_rate:
|
30 |
-
raise ValueError("DDSP与DIFF模型的sampling_rate不一致")
|
31 |
-
if args1.data.encoder != args2.data.encoder:
|
32 |
-
raise ValueError("DDSP与DIFF模型的encoder不一致")
|
33 |
-
return True
|
34 |
-
|
35 |
-
def __call__(self, audio, f0, hubert, volume, acc=1, spk_id=1, k_step=0, method='pndm',
|
36 |
-
spk_mix_dict=None, start_frame=0):
|
37 |
-
input_mel = self.vocoder.extract(audio, self.args.data.sampling_rate)
|
38 |
-
out_mel = self.model(
|
39 |
-
hubert,
|
40 |
-
f0,
|
41 |
-
volume,
|
42 |
-
spk_id=spk_id,
|
43 |
-
spk_mix_dict=spk_mix_dict,
|
44 |
-
gt_spec=input_mel,
|
45 |
-
infer=True,
|
46 |
-
infer_speedup=acc,
|
47 |
-
method=method,
|
48 |
-
k_step=k_step,
|
49 |
-
use_tqdm=False)
|
50 |
-
if start_frame > 0:
|
51 |
-
out_mel = out_mel[:, start_frame:, :]
|
52 |
-
f0 = f0[:, start_frame:, :]
|
53 |
-
output = self.vocoder.infer(out_mel, f0)
|
54 |
-
if start_frame > 0:
|
55 |
-
output = F.pad(output, (start_frame * self.vocoder.vocoder_hop_size, 0))
|
56 |
-
return output
|
57 |
-
|
58 |
-
def infer(self, audio, f0, hubert, volume, acc=1, spk_id=1, k_step=0, method='pndm', silence_front=0,
|
59 |
-
use_silence=False, spk_mix_dict=None):
|
60 |
-
start_frame = int(silence_front * self.vocoder.vocoder_sample_rate / self.vocoder.vocoder_hop_size)
|
61 |
-
if use_silence:
|
62 |
-
audio = audio[:, start_frame * self.vocoder.vocoder_hop_size:]
|
63 |
-
f0 = f0[:, start_frame:, :]
|
64 |
-
hubert = hubert[:, start_frame:, :]
|
65 |
-
volume = volume[:, start_frame:, :]
|
66 |
-
_start_frame = 0
|
67 |
-
else:
|
68 |
-
_start_frame = start_frame
|
69 |
-
audio = self.__call__(audio, f0, hubert, volume, acc=acc, spk_id=spk_id, k_step=k_step,
|
70 |
-
method=method, spk_mix_dict=spk_mix_dict, start_frame=_start_frame)
|
71 |
-
if use_silence:
|
72 |
-
if start_frame > 0:
|
73 |
-
audio = F.pad(audio, (start_frame * self.vocoder.vocoder_hop_size, 0))
|
74 |
-
return audio
|
|
|
|
|
|
|
|
|
|
|
|
|
|
|
|
|
|
|
|
|
|
|
|
|
|
|
|
|
|
|
|
|
|
|
|
|
|
|
|
|
|
|
|
|
|
|
|
|
|
|
|
|
|
|
|
|
|
|
|
|
|
|
|
|
|
|
|
|
|
|
|
|
|
|
|
|
|
|
|
|
|
|
|
|
|
|
|
|
|
|
|
|
|
|
|
|
|
|
|
|
|
|
|
|
|
|
|
|
|
|
|
|
|
|
|
|
|
|
|
|
|
|
|
|
|
|
|
|
|
|
|
|
|
|
|
|
|
|
|
|
|
|
|
|
|
|
|
|
|
spaces/GMFTBY/PandaGPT/model/ImageBind/__init__.py
DELETED
@@ -1,2 +0,0 @@
|
|
1 |
-
from .models import imagebind_model
|
2 |
-
from .models.imagebind_model import ModalityType
|
|
|
|
|
|
spaces/GaenKoki/voicevox/speaker_info/388f246b-8c41-4ac1-8e2d-5d79f3ff56d9/policy.md
DELETED
@@ -1,3 +0,0 @@
|
|
1 |
-
dummy2 policy
|
2 |
-
|
3 |
-
https://voicevox.hiroshiba.jp/
|
|
|
|
|
|
|
|
spaces/Gen-Sim/Gen-Sim/cliport/generated_tasks/block_on_cylinder_on_pallet.py
DELETED
@@ -1,58 +0,0 @@
|
|
1 |
-
import numpy as np
|
2 |
-
from cliport.tasks.task import Task
|
3 |
-
from cliport.utils import utils
|
4 |
-
|
5 |
-
class BlockOnCylinderOnPallet(Task):
|
6 |
-
"""Pick up each block and place it on the corresponding colored cylinder, which are located in specific positions on a pallet."""
|
7 |
-
|
8 |
-
def __init__(self):
|
9 |
-
super().__init__()
|
10 |
-
self.max_steps = 15
|
11 |
-
self.lang_template = "place the {} cylinder on the pallet"
|
12 |
-
self.lang_template_2 = "place the {} block on the {} cylinder"
|
13 |
-
|
14 |
-
self.task_completed_desc = "done placing blocks on cylinders and cylinder on pallet."
|
15 |
-
self.additional_reset()
|
16 |
-
|
17 |
-
def reset(self, env):
|
18 |
-
super().reset(env)
|
19 |
-
|
20 |
-
# Add pallet.
|
21 |
-
pallet_size = (0.35, 0.35, 0.01)
|
22 |
-
pallet_pose = self.get_random_pose(env, pallet_size)
|
23 |
-
pallet_urdf = 'pallet/pallet.urdf'
|
24 |
-
env.add_object(pallet_urdf, pallet_pose, 'fixed')
|
25 |
-
|
26 |
-
# Define colors.
|
27 |
-
block_colors = ['red']
|
28 |
-
cylinder_colors = ['blue']
|
29 |
-
|
30 |
-
# Add cylinders.
|
31 |
-
cylinder_size = (0.04, 0.04, 0.06)
|
32 |
-
cylinder_template = 'cylinder/cylinder-template.urdf'
|
33 |
-
cylinders = []
|
34 |
-
|
35 |
-
|
36 |
-
replace = {'DIM': cylinder_size, 'HALF': (cylinder_size[0] / 2, cylinder_size[1] / 2, cylinder_size[2] / 2), 'COLOR': block_colors[0]}
|
37 |
-
cylinder_urdf = self.fill_template(cylinder_template, replace)
|
38 |
-
cylinder_pose = self.get_random_pose(env, cylinder_size)
|
39 |
-
cylinder_id = env.add_object(cylinder_urdf, cylinder_pose)
|
40 |
-
cylinders.append(cylinder_id)
|
41 |
-
|
42 |
-
# Add blocks.
|
43 |
-
block_size = (0.04, 0.04, 0.04)
|
44 |
-
block_urdf = 'block/block.urdf'
|
45 |
-
blocks = []
|
46 |
-
block_pose = self.get_random_pose(env, block_size)
|
47 |
-
block_id = env.add_object(block_urdf, block_pose, color=cylinder_colors[0])
|
48 |
-
blocks.append(block_id)
|
49 |
-
|
50 |
-
# Goal: place the cylinder on top of the pallet
|
51 |
-
self.add_goal(objs=[cylinders[0]], matches=np.ones((1, 1)), targ_poses=[pallet_pose], replace=False,
|
52 |
-
rotations=True, metric='pose', params=None, step_max_reward=1/2, language_goal=self.lang_template.format(cylinder_colors[0]))
|
53 |
-
|
54 |
-
|
55 |
-
# Goal: place the block on top of the cylinder
|
56 |
-
language_goal = self.lang_template_2.format(block_colors[0], cylinder_colors[0])
|
57 |
-
self.add_goal(objs=[blocks[0]], matches=np.ones((1, 1)), targ_poses=[pallet_pose], replace=False,
|
58 |
-
rotations=True, metric='pose', params=None, step_max_reward=1/2, language_goal=language_goal)
|
|
|
|
|
|
|
|
|
|
|
|
|
|
|
|
|
|
|
|
|
|
|
|
|
|
|
|
|
|
|
|
|
|
|
|
|
|
|
|
|
|
|
|
|
|
|
|
|
|
|
|
|
|
|
|
|
|
|
|
|
|
|
|
|
|
|
|
|
|
|
|
|
|
|
|
|
|
|
|
|
|
|
|
|
|
|
|
|
|
|
|
|
|
|
|
|
|
|
|
|
|
|
|
|
|
|
|
|
|
|
|
|
|
|
|
|
|
spaces/Gen-Sim/Gen-Sim/cliport/generated_tasks/color_cued_ball_corner_sorting.py
DELETED
@@ -1,62 +0,0 @@
|
|
1 |
-
import numpy as np
|
2 |
-
import os
|
3 |
-
import pybullet as p
|
4 |
-
import random
|
5 |
-
from cliport.tasks import primitives
|
6 |
-
from cliport.tasks.grippers import Spatula
|
7 |
-
from cliport.tasks.task import Task
|
8 |
-
from cliport.utils import utils
|
9 |
-
import numpy as np
|
10 |
-
from cliport.tasks.task import Task
|
11 |
-
from cliport.utils import utils
|
12 |
-
|
13 |
-
class ColorCuedBallCornerSorting(Task):
|
14 |
-
"""Pick up each colored ball and place it in the corner of the same color while avoiding a zone marked by small blocks."""
|
15 |
-
|
16 |
-
def __init__(self):
|
17 |
-
super().__init__()
|
18 |
-
self.max_steps = 20
|
19 |
-
self.lang_template = "place the {color} ball in the {color} corner"
|
20 |
-
self.task_completed_desc = "done sorting balls."
|
21 |
-
self.additional_reset()
|
22 |
-
|
23 |
-
def reset(self, env):
|
24 |
-
super().reset(env)
|
25 |
-
|
26 |
-
# Add corners.
|
27 |
-
corner_size = (0.05, 0.05, 0.05)
|
28 |
-
corner_urdf = 'corner/corner-template.urdf'
|
29 |
-
corner_colors = ['red', 'blue', 'green', 'yellow']
|
30 |
-
corner_poses = []
|
31 |
-
for color in corner_colors:
|
32 |
-
corner_pose = self.get_random_pose(env, corner_size)
|
33 |
-
env.add_object(corner_urdf, corner_pose, color=color, category='fixed')
|
34 |
-
corner_poses.append(corner_pose)
|
35 |
-
|
36 |
-
# Add balls.
|
37 |
-
balls = []
|
38 |
-
ball_size = (0.04, 0.04, 0.04)
|
39 |
-
ball_urdf = 'ball/ball-template.urdf'
|
40 |
-
for color in corner_colors:
|
41 |
-
ball_pose = self.get_random_pose(env, ball_size)
|
42 |
-
ball_id = env.add_object(ball_urdf, ball_pose, color=color)
|
43 |
-
balls.append(ball_id)
|
44 |
-
|
45 |
-
# Add zone.
|
46 |
-
zone_size = (0.2, 0.2, 0.05)
|
47 |
-
zone_pose = self.get_random_pose(env, zone_size)
|
48 |
-
zone_urdf = 'zone/zone.urdf'
|
49 |
-
env.add_object(zone_urdf, zone_pose, 'fixed')
|
50 |
-
|
51 |
-
# Add blocks.
|
52 |
-
block_size = (0.04, 0.04, 0.04)
|
53 |
-
block_urdf = 'block/block_for_anchors.urdf'
|
54 |
-
for _ in range(4):
|
55 |
-
block_pose = self.get_random_pose(env, block_size)
|
56 |
-
env.add_object(block_urdf, block_pose)
|
57 |
-
|
58 |
-
# Goal: each ball is in the corner of the same color.
|
59 |
-
for i in range(4):
|
60 |
-
self.add_goal(objs=[balls[i]], matches=np.ones((1, 1)), targ_poses=[corner_poses[i]], replace=False,
|
61 |
-
rotations=True, metric='pose', params=None, step_max_reward=1/4,
|
62 |
-
language_goal=self.lang_template.format(color=corner_colors[i]))
|
|
|
|
|
|
|
|
|
|
|
|
|
|
|
|
|
|
|
|
|
|
|
|
|
|
|
|
|
|
|
|
|
|
|
|
|
|
|
|
|
|
|
|
|
|
|
|
|
|
|
|
|
|
|
|
|
|
|
|
|
|
|
|
|
|
|
|
|
|
|
|
|
|
|
|
|
|
|
|
|
|
|
|
|
|
|
|
|
|
|
|
|
|
|
|
|
|
|
|
|
|
|
|
|
|
|
|
|
|
|
|
|
|
|
|
|
|
|
|
|
|
|
|
|
|
spaces/Gen-Sim/Gen-Sim/cliport/generated_tasks/color_ordered_insertion_new.py
DELETED
@@ -1,52 +0,0 @@
|
|
1 |
-
import numpy as np
|
2 |
-
import os
|
3 |
-
import pybullet as p
|
4 |
-
import random
|
5 |
-
from cliport.tasks import primitives
|
6 |
-
from cliport.tasks.grippers import Spatula
|
7 |
-
from cliport.tasks.task import Task
|
8 |
-
from cliport.utils import utils
|
9 |
-
import numpy as np
|
10 |
-
from cliport.tasks.task import Task
|
11 |
-
from cliport.utils import utils
|
12 |
-
|
13 |
-
class ColorOrderedInsertionNew(Task):
|
14 |
-
"""Insert differently-colored ell objects into the matching color fixture in a specific order."""
|
15 |
-
|
16 |
-
def __init__(self):
|
17 |
-
super().__init__()
|
18 |
-
self.max_steps = 20
|
19 |
-
self.lang_template = "put the {color} L shape block in the L shape hole"
|
20 |
-
self.task_completed_desc = "done with insertion."
|
21 |
-
self.additional_reset()
|
22 |
-
|
23 |
-
def reset(self, env):
|
24 |
-
super().reset(env)
|
25 |
-
|
26 |
-
# Define colors and their order
|
27 |
-
colors = ['red', 'blue', 'green', 'yellow']
|
28 |
-
color_order = {color: i for i, color in enumerate(colors)}
|
29 |
-
|
30 |
-
# Add fixtures.
|
31 |
-
fixture_size = (0.12, 0.12, 0.02)
|
32 |
-
fixture_urdf = 'insertion/fixture.urdf'
|
33 |
-
fixtures = []
|
34 |
-
for color in colors:
|
35 |
-
fixture_pose = self.get_random_pose(env, fixture_size)
|
36 |
-
fixture_id = env.add_object(fixture_urdf, fixture_pose, color=utils.COLORS[color], category='fixed')
|
37 |
-
fixtures.append(fixture_id)
|
38 |
-
|
39 |
-
# Add ell objects.
|
40 |
-
ell_size = (0.04, 0.04, 0.04)
|
41 |
-
ell_urdf = 'insertion/ell.urdf'
|
42 |
-
ells = []
|
43 |
-
for color in colors:
|
44 |
-
ell_pose = self.get_random_pose(env, ell_size)
|
45 |
-
ell_id = env.add_object(ell_urdf, ell_pose, color=utils.COLORS[color])
|
46 |
-
ells.append(ell_id)
|
47 |
-
|
48 |
-
# Goal: each ell is inserted into the matching color fixture in the correct order.
|
49 |
-
for i, ell in enumerate(ells):
|
50 |
-
self.add_goal(objs=[ell], matches=np.ones((1, 1)), targ_poses=[p.getBasePositionAndOrientation(fixtures[i])], replace=False,
|
51 |
-
rotations=True, metric='pose', params=None, step_max_reward=1 / len(ells),
|
52 |
-
language_goal=self.lang_template.format(color=colors[i]))
|
|
|
|
|
|
|
|
|
|
|
|
|
|
|
|
|
|
|
|
|
|
|
|
|
|
|
|
|
|
|
|
|
|
|
|
|
|
|
|
|
|
|
|
|
|
|
|
|
|
|
|
|
|
|
|
|
|
|
|
|
|
|
|
|
|
|
|
|
|
|
|
|
|
|
|
|
|
|
|
|
|
|
|
|
|
|
|
|
|
|
|
|
|
|
|
|
|
|
|
|
|
|
|
|
|
spaces/Gen-Sim/Gen-Sim/cliport/generated_tasks/move_piles_along_line.py
DELETED
@@ -1,70 +0,0 @@
|
|
1 |
-
import numpy as np
|
2 |
-
import os
|
3 |
-
import pybullet as p
|
4 |
-
import random
|
5 |
-
from cliport.tasks import primitives
|
6 |
-
from cliport.tasks.grippers import Spatula
|
7 |
-
from cliport.tasks.task import Task
|
8 |
-
from cliport.utils import utils
|
9 |
-
import numpy as np
|
10 |
-
from cliport.tasks import primitives
|
11 |
-
from cliport.tasks.grippers import Spatula
|
12 |
-
from cliport.tasks.task import Task
|
13 |
-
from cliport.utils import utils
|
14 |
-
|
15 |
-
class MovePilesAlongLine(Task):
|
16 |
-
"""Move three piles of small blocks, each pile a different color (red, blue, green),
|
17 |
-
along three matching colored lines to three separate zones of the same color using a spatula."""
|
18 |
-
|
19 |
-
def __init__(self):
|
20 |
-
super().__init__()
|
21 |
-
self.max_steps = 20
|
22 |
-
self.lang_template = "move the piles of blocks along the lines to the matching colored zones"
|
23 |
-
self.task_completed_desc = "done moving piles."
|
24 |
-
self.primitive = primitives.push
|
25 |
-
self.ee = Spatula
|
26 |
-
self.additional_reset()
|
27 |
-
|
28 |
-
def reset(self, env):
|
29 |
-
super().reset(env)
|
30 |
-
|
31 |
-
# Add three colored lines.
|
32 |
-
line_template = 'line/line-template.urdf'
|
33 |
-
line_colors = ['red', 'blue', 'green']
|
34 |
-
line_poses = []
|
35 |
-
for color in line_colors:
|
36 |
-
line_size = self.get_random_size(0.1, 0.15, 0.1, 0.15, 0.05, 0.05)
|
37 |
-
line_pose = self.get_random_pose(env, line_size)
|
38 |
-
replace = {'DIM': line_size, 'HALF': (line_size[0] / 2, line_size[1] / 2, line_size[2] / 2), 'COLOR': color}
|
39 |
-
line_urdf = self.fill_template(line_template, replace)
|
40 |
-
env.add_object(line_urdf, line_pose, 'fixed')
|
41 |
-
line_poses.append(line_pose)
|
42 |
-
|
43 |
-
# Add three colored zones.
|
44 |
-
zone_template = 'zone/zone.urdf'
|
45 |
-
zone_poses = []
|
46 |
-
for color in line_colors:
|
47 |
-
zone_size = self.get_random_size(0.1, 0.15, 0.1, 0.15, 0.05, 0.05)
|
48 |
-
zone_pose = self.get_random_pose(env, zone_size)
|
49 |
-
replace = {'DIM': zone_size, 'HALF': (zone_size[0] / 2, zone_size[1] / 2, zone_size[2] / 2), 'COLOR': color}
|
50 |
-
zone_urdf = self.fill_template(zone_template, replace)
|
51 |
-
env.add_object(zone_urdf, zone_pose, 'fixed')
|
52 |
-
zone_poses.append(zone_pose)
|
53 |
-
|
54 |
-
# Add three piles of small blocks.
|
55 |
-
block_template = 'block/small.urdf'
|
56 |
-
block_colors = ['red', 'blue', 'green']
|
57 |
-
block_ids = []
|
58 |
-
for color in block_colors:
|
59 |
-
block_size = self.get_random_size(0.1, 0.15, 0.1, 0.15, 0.05, 0.05)
|
60 |
-
block_pose = self.get_random_pose(env, block_size)
|
61 |
-
replace = {'DIM': block_size, 'HALF': (block_size[0] / 2, block_size[1] / 2, block_size[2] / 2), 'COLOR': color}
|
62 |
-
block_urdf = self.fill_template(block_template, replace)
|
63 |
-
block_id = env.add_object(block_urdf, block_pose)
|
64 |
-
block_ids.append(block_id)
|
65 |
-
|
66 |
-
# Add goals.
|
67 |
-
for i in range(3):
|
68 |
-
self.add_goal(objs=[block_ids[i]], matches=np.ones((1, 1)), targ_poses=[zone_poses[i]], replace=False,
|
69 |
-
rotations=False, metric='zone', params=[(zone_poses[i], zone_size)], step_max_reward=1/3,
|
70 |
-
language_goal=self.lang_template)
|
|
|
|
|
|
|
|
|
|
|
|
|
|
|
|
|
|
|
|
|
|
|
|
|
|
|
|
|
|
|
|
|
|
|
|
|
|
|
|
|
|
|
|
|
|
|
|
|
|
|
|
|
|
|
|
|
|
|
|
|
|
|
|
|
|
|
|
|
|
|
|
|
|
|
|
|
|
|
|
|
|
|
|
|
|
|
|
|
|
|
|
|
|
|
|
|
|
|
|
|
|
|
|
|
|
|
|
|
|
|
|
|
|
|
|
|
|
|
|
|
|
|
|
|
|
|
|
|
|
|
|
|
|
|
|
|
|
|
|
|
|
spaces/Gradio-Blocks/uniformer_image_detection/mmdet/core/bbox/coder/tblr_bbox_coder.py
DELETED
@@ -1,198 +0,0 @@
|
|
1 |
-
import mmcv
|
2 |
-
import torch
|
3 |
-
|
4 |
-
from ..builder import BBOX_CODERS
|
5 |
-
from .base_bbox_coder import BaseBBoxCoder
|
6 |
-
|
7 |
-
|
8 |
-
@BBOX_CODERS.register_module()
|
9 |
-
class TBLRBBoxCoder(BaseBBoxCoder):
|
10 |
-
"""TBLR BBox coder.
|
11 |
-
|
12 |
-
Following the practice in `FSAF <https://arxiv.org/abs/1903.00621>`_,
|
13 |
-
this coder encodes gt bboxes (x1, y1, x2, y2) into (top, bottom, left,
|
14 |
-
right) and decode it back to the original.
|
15 |
-
|
16 |
-
Args:
|
17 |
-
normalizer (list | float): Normalization factor to be
|
18 |
-
divided with when coding the coordinates. If it is a list, it should
|
19 |
-
have length of 4 indicating normalization factor in tblr dims.
|
20 |
-
Otherwise it is a unified float factor for all dims. Default: 4.0
|
21 |
-
clip_border (bool, optional): Whether clip the objects outside the
|
22 |
-
border of the image. Defaults to True.
|
23 |
-
"""
|
24 |
-
|
25 |
-
def __init__(self, normalizer=4.0, clip_border=True):
|
26 |
-
super(BaseBBoxCoder, self).__init__()
|
27 |
-
self.normalizer = normalizer
|
28 |
-
self.clip_border = clip_border
|
29 |
-
|
30 |
-
def encode(self, bboxes, gt_bboxes):
|
31 |
-
"""Get box regression transformation deltas that can be used to
|
32 |
-
transform the ``bboxes`` into the ``gt_bboxes`` in the (top, left,
|
33 |
-
bottom, right) order.
|
34 |
-
|
35 |
-
Args:
|
36 |
-
bboxes (torch.Tensor): source boxes, e.g., object proposals.
|
37 |
-
gt_bboxes (torch.Tensor): target of the transformation, e.g.,
|
38 |
-
ground truth boxes.
|
39 |
-
|
40 |
-
Returns:
|
41 |
-
torch.Tensor: Box transformation deltas
|
42 |
-
"""
|
43 |
-
assert bboxes.size(0) == gt_bboxes.size(0)
|
44 |
-
assert bboxes.size(-1) == gt_bboxes.size(-1) == 4
|
45 |
-
encoded_bboxes = bboxes2tblr(
|
46 |
-
bboxes, gt_bboxes, normalizer=self.normalizer)
|
47 |
-
return encoded_bboxes
|
48 |
-
|
49 |
-
def decode(self, bboxes, pred_bboxes, max_shape=None):
|
50 |
-
"""Apply transformation `pred_bboxes` to `boxes`.
|
51 |
-
|
52 |
-
Args:
|
53 |
-
bboxes (torch.Tensor): Basic boxes.Shape (B, N, 4) or (N, 4)
|
54 |
-
pred_bboxes (torch.Tensor): Encoded boxes with shape
|
55 |
-
(B, N, 4) or (N, 4)
|
56 |
-
max_shape (Sequence[int] or torch.Tensor or Sequence[
|
57 |
-
Sequence[int]],optional): Maximum bounds for boxes, specifies
|
58 |
-
(H, W, C) or (H, W). If bboxes shape is (B, N, 4), then
|
59 |
-
the max_shape should be a Sequence[Sequence[int]]
|
60 |
-
and the length of max_shape should also be B.
|
61 |
-
|
62 |
-
Returns:
|
63 |
-
torch.Tensor: Decoded boxes.
|
64 |
-
"""
|
65 |
-
decoded_bboxes = tblr2bboxes(
|
66 |
-
bboxes,
|
67 |
-
pred_bboxes,
|
68 |
-
normalizer=self.normalizer,
|
69 |
-
max_shape=max_shape,
|
70 |
-
clip_border=self.clip_border)
|
71 |
-
|
72 |
-
return decoded_bboxes
|
73 |
-
|
74 |
-
|
75 |
-
@mmcv.jit(coderize=True)
|
76 |
-
def bboxes2tblr(priors, gts, normalizer=4.0, normalize_by_wh=True):
|
77 |
-
"""Encode ground truth boxes to tblr coordinate.
|
78 |
-
|
79 |
-
It first convert the gt coordinate to tblr format,
|
80 |
-
(top, bottom, left, right), relative to prior box centers.
|
81 |
-
The tblr coordinate may be normalized by the side length of prior bboxes
|
82 |
-
if `normalize_by_wh` is specified as True, and it is then normalized by
|
83 |
-
the `normalizer` factor.
|
84 |
-
|
85 |
-
Args:
|
86 |
-
priors (Tensor): Prior boxes in point form
|
87 |
-
Shape: (num_proposals,4).
|
88 |
-
gts (Tensor): Coords of ground truth for each prior in point-form
|
89 |
-
Shape: (num_proposals, 4).
|
90 |
-
normalizer (Sequence[float] | float): normalization parameter of
|
91 |
-
encoded boxes. If it is a list, it has to have length = 4.
|
92 |
-
Default: 4.0
|
93 |
-
normalize_by_wh (bool): Whether to normalize tblr coordinate by the
|
94 |
-
side length (wh) of prior bboxes.
|
95 |
-
|
96 |
-
Return:
|
97 |
-
encoded boxes (Tensor), Shape: (num_proposals, 4)
|
98 |
-
"""
|
99 |
-
|
100 |
-
# dist b/t match center and prior's center
|
101 |
-
if not isinstance(normalizer, float):
|
102 |
-
normalizer = torch.tensor(normalizer, device=priors.device)
|
103 |
-
assert len(normalizer) == 4, 'Normalizer must have length = 4'
|
104 |
-
assert priors.size(0) == gts.size(0)
|
105 |
-
prior_centers = (priors[:, 0:2] + priors[:, 2:4]) / 2
|
106 |
-
xmin, ymin, xmax, ymax = gts.split(1, dim=1)
|
107 |
-
top = prior_centers[:, 1].unsqueeze(1) - ymin
|
108 |
-
bottom = ymax - prior_centers[:, 1].unsqueeze(1)
|
109 |
-
left = prior_centers[:, 0].unsqueeze(1) - xmin
|
110 |
-
right = xmax - prior_centers[:, 0].unsqueeze(1)
|
111 |
-
loc = torch.cat((top, bottom, left, right), dim=1)
|
112 |
-
if normalize_by_wh:
|
113 |
-
# Normalize tblr by anchor width and height
|
114 |
-
wh = priors[:, 2:4] - priors[:, 0:2]
|
115 |
-
w, h = torch.split(wh, 1, dim=1)
|
116 |
-
loc[:, :2] /= h # tb is normalized by h
|
117 |
-
loc[:, 2:] /= w # lr is normalized by w
|
118 |
-
# Normalize tblr by the given normalization factor
|
119 |
-
return loc / normalizer
|
120 |
-
|
121 |
-
|
122 |
-
@mmcv.jit(coderize=True)
|
123 |
-
def tblr2bboxes(priors,
|
124 |
-
tblr,
|
125 |
-
normalizer=4.0,
|
126 |
-
normalize_by_wh=True,
|
127 |
-
max_shape=None,
|
128 |
-
clip_border=True):
|
129 |
-
"""Decode tblr outputs to prediction boxes.
|
130 |
-
|
131 |
-
The process includes 3 steps: 1) De-normalize tblr coordinates by
|
132 |
-
multiplying it with `normalizer`; 2) De-normalize tblr coordinates by the
|
133 |
-
prior bbox width and height if `normalize_by_wh` is `True`; 3) Convert
|
134 |
-
tblr (top, bottom, left, right) pair relative to the center of priors back
|
135 |
-
to (xmin, ymin, xmax, ymax) coordinate.
|
136 |
-
|
137 |
-
Args:
|
138 |
-
priors (Tensor): Prior boxes in point form (x0, y0, x1, y1)
|
139 |
-
Shape: (N,4) or (B, N, 4).
|
140 |
-
tblr (Tensor): Coords of network output in tblr form
|
141 |
-
Shape: (N, 4) or (B, N, 4).
|
142 |
-
normalizer (Sequence[float] | float): Normalization parameter of
|
143 |
-
encoded boxes. By list, it represents the normalization factors at
|
144 |
-
tblr dims. By float, it is the unified normalization factor at all
|
145 |
-
dims. Default: 4.0
|
146 |
-
normalize_by_wh (bool): Whether the tblr coordinates have been
|
147 |
-
normalized by the side length (wh) of prior bboxes.
|
148 |
-
max_shape (Sequence[int] or torch.Tensor or Sequence[
|
149 |
-
Sequence[int]],optional): Maximum bounds for boxes, specifies
|
150 |
-
(H, W, C) or (H, W). If priors shape is (B, N, 4), then
|
151 |
-
the max_shape should be a Sequence[Sequence[int]]
|
152 |
-
and the length of max_shape should also be B.
|
153 |
-
clip_border (bool, optional): Whether clip the objects outside the
|
154 |
-
border of the image. Defaults to True.
|
155 |
-
|
156 |
-
Return:
|
157 |
-
encoded boxes (Tensor): Boxes with shape (N, 4) or (B, N, 4)
|
158 |
-
"""
|
159 |
-
if not isinstance(normalizer, float):
|
160 |
-
normalizer = torch.tensor(normalizer, device=priors.device)
|
161 |
-
assert len(normalizer) == 4, 'Normalizer must have length = 4'
|
162 |
-
assert priors.size(0) == tblr.size(0)
|
163 |
-
if priors.ndim == 3:
|
164 |
-
assert priors.size(1) == tblr.size(1)
|
165 |
-
|
166 |
-
loc_decode = tblr * normalizer
|
167 |
-
prior_centers = (priors[..., 0:2] + priors[..., 2:4]) / 2
|
168 |
-
if normalize_by_wh:
|
169 |
-
wh = priors[..., 2:4] - priors[..., 0:2]
|
170 |
-
w, h = torch.split(wh, 1, dim=-1)
|
171 |
-
# Inplace operation with slice would failed for exporting to ONNX
|
172 |
-
th = h * loc_decode[..., :2] # tb
|
173 |
-
tw = w * loc_decode[..., 2:] # lr
|
174 |
-
loc_decode = torch.cat([th, tw], dim=-1)
|
175 |
-
# Cannot be exported using onnx when loc_decode.split(1, dim=-1)
|
176 |
-
top, bottom, left, right = loc_decode.split((1, 1, 1, 1), dim=-1)
|
177 |
-
xmin = prior_centers[..., 0].unsqueeze(-1) - left
|
178 |
-
xmax = prior_centers[..., 0].unsqueeze(-1) + right
|
179 |
-
ymin = prior_centers[..., 1].unsqueeze(-1) - top
|
180 |
-
ymax = prior_centers[..., 1].unsqueeze(-1) + bottom
|
181 |
-
|
182 |
-
bboxes = torch.cat((xmin, ymin, xmax, ymax), dim=-1)
|
183 |
-
|
184 |
-
if clip_border and max_shape is not None:
|
185 |
-
if not isinstance(max_shape, torch.Tensor):
|
186 |
-
max_shape = priors.new_tensor(max_shape)
|
187 |
-
max_shape = max_shape[..., :2].type_as(priors)
|
188 |
-
if max_shape.ndim == 2:
|
189 |
-
assert bboxes.ndim == 3
|
190 |
-
assert max_shape.size(0) == bboxes.size(0)
|
191 |
-
|
192 |
-
min_xy = priors.new_tensor(0)
|
193 |
-
max_xy = torch.cat([max_shape, max_shape],
|
194 |
-
dim=-1).flip(-1).unsqueeze(-2)
|
195 |
-
bboxes = torch.where(bboxes < min_xy, min_xy, bboxes)
|
196 |
-
bboxes = torch.where(bboxes > max_xy, max_xy, bboxes)
|
197 |
-
|
198 |
-
return bboxes
|
|
|
|
|
|
|
|
|
|
|
|
|
|
|
|
|
|
|
|
|
|
|
|
|
|
|
|
|
|
|
|
|
|
|
|
|
|
|
|
|
|
|
|
|
|
|
|
|
|
|
|
|
|
|
|
|
|
|
|
|
|
|
|
|
|
|
|
|
|
|
|
|
|
|
|
|
|
|
|
|
|
|
|
|
|
|
|
|
|
|
|
|
|
|
|
|
|
|
|
|
|
|
|
|
|
|
|
|
|
|
|
|
|
|
|
|
|
|
|
|
|
|
|
|
|
|
|
|
|
|
|
|
|
|
|
|
|
|
|
|
|
|
|
|
|
|
|
|
|
|
|
|
|
|
|
|
|
|
|
|
|
|
|
|
|
|
|
|
|
|
|
|
|
|
|
|
|
|
|
|
|
|
|
|
|
|
|
|
|
|
|
|
|
|
|
|
|
|
|
|
|
|
|
|
|
|
|
|
|
|
|
|
|
|
|
|
|
|
|
|
|
|
|
|
|
|
|
|
|
|
|
|
|
|
|
|
|
|
|
|
|
|
|
|
|
|
|
|
|
|
|
|
|
|
|
|
|
|
|
|
|
|
|
|
|
|
|
|
|
|
|
|
|
|
|
|
|
|
|
|
|
|
|
|
|
|
|
|
|
|
|
|
|
|
|
|
|
|
|
|
|
|
|
|
|
|
|
|
|
|
|
|
|
|
|
|
|
|
|
|
|
|
|
|
|
|
|
|
|
|
|
|
|
|
|
|
|
|
|
|
|
|
|
|
|
|
|
|
|
|
|
|
|
|
|
|
|
|
|
|
|
|
|
|
|
|
|
|
|
|
|
|
|
|
|
|
|
|
|
|
|
|
|
|
|
|
|
|
|
|
|
|
|
|
|
|
|
spaces/Gradio-Blocks/uniformer_image_detection/mmdet/models/losses/__init__.py
DELETED
@@ -1,29 +0,0 @@
|
|
1 |
-
from .accuracy import Accuracy, accuracy
|
2 |
-
from .ae_loss import AssociativeEmbeddingLoss
|
3 |
-
from .balanced_l1_loss import BalancedL1Loss, balanced_l1_loss
|
4 |
-
from .cross_entropy_loss import (CrossEntropyLoss, binary_cross_entropy,
|
5 |
-
cross_entropy, mask_cross_entropy)
|
6 |
-
from .focal_loss import FocalLoss, sigmoid_focal_loss
|
7 |
-
from .gaussian_focal_loss import GaussianFocalLoss
|
8 |
-
from .gfocal_loss import DistributionFocalLoss, QualityFocalLoss
|
9 |
-
from .ghm_loss import GHMC, GHMR
|
10 |
-
from .iou_loss import (BoundedIoULoss, CIoULoss, DIoULoss, GIoULoss, IoULoss,
|
11 |
-
bounded_iou_loss, iou_loss)
|
12 |
-
from .kd_loss import KnowledgeDistillationKLDivLoss
|
13 |
-
from .mse_loss import MSELoss, mse_loss
|
14 |
-
from .pisa_loss import carl_loss, isr_p
|
15 |
-
from .smooth_l1_loss import L1Loss, SmoothL1Loss, l1_loss, smooth_l1_loss
|
16 |
-
from .utils import reduce_loss, weight_reduce_loss, weighted_loss
|
17 |
-
from .varifocal_loss import VarifocalLoss
|
18 |
-
|
19 |
-
__all__ = [
|
20 |
-
'accuracy', 'Accuracy', 'cross_entropy', 'binary_cross_entropy',
|
21 |
-
'mask_cross_entropy', 'CrossEntropyLoss', 'sigmoid_focal_loss',
|
22 |
-
'FocalLoss', 'smooth_l1_loss', 'SmoothL1Loss', 'balanced_l1_loss',
|
23 |
-
'BalancedL1Loss', 'mse_loss', 'MSELoss', 'iou_loss', 'bounded_iou_loss',
|
24 |
-
'IoULoss', 'BoundedIoULoss', 'GIoULoss', 'DIoULoss', 'CIoULoss', 'GHMC',
|
25 |
-
'GHMR', 'reduce_loss', 'weight_reduce_loss', 'weighted_loss', 'L1Loss',
|
26 |
-
'l1_loss', 'isr_p', 'carl_loss', 'AssociativeEmbeddingLoss',
|
27 |
-
'GaussianFocalLoss', 'QualityFocalLoss', 'DistributionFocalLoss',
|
28 |
-
'VarifocalLoss', 'KnowledgeDistillationKLDivLoss'
|
29 |
-
]
|
|
|
|
|
|
|
|
|
|
|
|
|
|
|
|
|
|
|
|
|
|
|
|
|
|
|
|
|
|
|
|
|
|
|
|
|
|
|
|
|
|
|
|
|
|
|
|
|
|
|
|
|
|
|
|
|
|
|
|
spaces/Gradio-Blocks/uniformer_image_segmentation/configs/deeplabv3/deeplabv3_r50-d8_480x480_40k_pascal_context.py
DELETED
@@ -1,10 +0,0 @@
|
|
1 |
-
_base_ = [
|
2 |
-
'../_base_/models/deeplabv3_r50-d8.py',
|
3 |
-
'../_base_/datasets/pascal_context.py', '../_base_/default_runtime.py',
|
4 |
-
'../_base_/schedules/schedule_40k.py'
|
5 |
-
]
|
6 |
-
model = dict(
|
7 |
-
decode_head=dict(num_classes=60),
|
8 |
-
auxiliary_head=dict(num_classes=60),
|
9 |
-
test_cfg=dict(mode='slide', crop_size=(480, 480), stride=(320, 320)))
|
10 |
-
optimizer = dict(type='SGD', lr=0.004, momentum=0.9, weight_decay=0.0001)
|
|
|
|
|
|
|
|
|
|
|
|
|
|
|
|
|
|
|
|
|
|
spaces/Gradio-Blocks/uniformer_image_segmentation/configs/fcn/fcn_d6_r50-d16_512x1024_40k_cityscapes.py
DELETED
@@ -1,8 +0,0 @@
|
|
1 |
-
_base_ = [
|
2 |
-
'../_base_/models/fcn_r50-d8.py', '../_base_/datasets/cityscapes.py',
|
3 |
-
'../_base_/default_runtime.py', '../_base_/schedules/schedule_40k.py'
|
4 |
-
]
|
5 |
-
model = dict(
|
6 |
-
backbone=dict(dilations=(1, 1, 1, 2), strides=(1, 2, 2, 1)),
|
7 |
-
decode_head=dict(dilation=6),
|
8 |
-
auxiliary_head=dict(dilation=6))
|
|
|
|
|
|
|
|
|
|
|
|
|
|
|
|
|
|
spaces/Gradio-Blocks/uniformer_image_segmentation/configs/nonlocal_net/nonlocal_r101-d8_512x512_40k_voc12aug.py
DELETED
@@ -1,2 +0,0 @@
|
|
1 |
-
_base_ = './nonlocal_r50-d8_512x512_40k_voc12aug.py'
|
2 |
-
model = dict(pretrained='open-mmlab://resnet101_v1c', backbone=dict(depth=101))
|
|
|
|
|
|
spaces/Gradio-Blocks/uniformer_image_segmentation/configs/resnest/fcn_s101-d8_512x512_160k_ade20k.py
DELETED
@@ -1,9 +0,0 @@
|
|
1 |
-
_base_ = '../fcn/fcn_r101-d8_512x512_160k_ade20k.py'
|
2 |
-
model = dict(
|
3 |
-
pretrained='open-mmlab://resnest101',
|
4 |
-
backbone=dict(
|
5 |
-
type='ResNeSt',
|
6 |
-
stem_channels=128,
|
7 |
-
radix=2,
|
8 |
-
reduction_factor=4,
|
9 |
-
avg_down_stride=True))
|
|
|
|
|
|
|
|
|
|
|
|
|
|
|
|
|
|
|
|
spaces/GrandaddyShmax/AudioCraft_Plus/audiocraft/losses/sisnr.py
DELETED
@@ -1,92 +0,0 @@
|
|
1 |
-
# Copyright (c) Meta Platforms, Inc. and affiliates.
|
2 |
-
# All rights reserved.
|
3 |
-
#
|
4 |
-
# This source code is licensed under the license found in the
|
5 |
-
# LICENSE file in the root directory of this source tree.
|
6 |
-
|
7 |
-
import math
|
8 |
-
import typing as tp
|
9 |
-
|
10 |
-
import torch
|
11 |
-
from torch import nn
|
12 |
-
from torch.nn import functional as F
|
13 |
-
|
14 |
-
|
15 |
-
def _unfold(a: torch.Tensor, kernel_size: int, stride: int) -> torch.Tensor:
|
16 |
-
"""Given input of size [*OT, T], output Tensor of size [*OT, F, K]
|
17 |
-
with K the kernel size, by extracting frames with the given stride.
|
18 |
-
This will pad the input so that `F = ceil(T / K)`.
|
19 |
-
see https://github.com/pytorch/pytorch/issues/60466
|
20 |
-
"""
|
21 |
-
*shape, length = a.shape
|
22 |
-
n_frames = math.ceil(length / stride)
|
23 |
-
tgt_length = (n_frames - 1) * stride + kernel_size
|
24 |
-
a = F.pad(a, (0, tgt_length - length))
|
25 |
-
strides = list(a.stride())
|
26 |
-
assert strides[-1] == 1, "data should be contiguous"
|
27 |
-
strides = strides[:-1] + [stride, 1]
|
28 |
-
return a.as_strided([*shape, n_frames, kernel_size], strides)
|
29 |
-
|
30 |
-
|
31 |
-
def _center(x: torch.Tensor) -> torch.Tensor:
|
32 |
-
return x - x.mean(-1, True)
|
33 |
-
|
34 |
-
|
35 |
-
def _norm2(x: torch.Tensor) -> torch.Tensor:
|
36 |
-
return x.pow(2).sum(-1, True)
|
37 |
-
|
38 |
-
|
39 |
-
class SISNR(nn.Module):
|
40 |
-
"""SISNR loss.
|
41 |
-
|
42 |
-
Input should be [B, C, T], output is scalar.
|
43 |
-
|
44 |
-
Args:
|
45 |
-
sample_rate (int): Sample rate.
|
46 |
-
segment (float or None): Evaluate on chunks of that many seconds. If None, evaluate on
|
47 |
-
entire audio only.
|
48 |
-
overlap (float): Overlap between chunks, i.e. 0.5 = 50 % overlap.
|
49 |
-
epsilon (float): Epsilon value for numerical stability.
|
50 |
-
"""
|
51 |
-
def __init__(
|
52 |
-
self,
|
53 |
-
sample_rate: int = 16000,
|
54 |
-
segment: tp.Optional[float] = 20,
|
55 |
-
overlap: float = 0.5,
|
56 |
-
epsilon: float = torch.finfo(torch.float32).eps,
|
57 |
-
):
|
58 |
-
super().__init__()
|
59 |
-
self.sample_rate = sample_rate
|
60 |
-
self.segment = segment
|
61 |
-
self.overlap = overlap
|
62 |
-
self.epsilon = epsilon
|
63 |
-
|
64 |
-
def forward(self, out_sig: torch.Tensor, ref_sig: torch.Tensor) -> torch.Tensor:
|
65 |
-
B, C, T = ref_sig.shape
|
66 |
-
assert ref_sig.shape == out_sig.shape
|
67 |
-
|
68 |
-
if self.segment is None:
|
69 |
-
frame = T
|
70 |
-
stride = T
|
71 |
-
else:
|
72 |
-
frame = int(self.segment * self.sample_rate)
|
73 |
-
stride = int(frame * (1 - self.overlap))
|
74 |
-
|
75 |
-
epsilon = self.epsilon * frame # make epsilon prop to frame size.
|
76 |
-
|
77 |
-
gt = _unfold(ref_sig, frame, stride)
|
78 |
-
est = _unfold(out_sig, frame, stride)
|
79 |
-
if self.segment is None:
|
80 |
-
assert gt.shape[-1] == 1
|
81 |
-
|
82 |
-
gt = _center(gt)
|
83 |
-
est = _center(est)
|
84 |
-
dot = torch.einsum("bcft,bcft->bcf", gt, est)
|
85 |
-
|
86 |
-
proj = dot[:, :, :, None] * gt / (epsilon + _norm2(gt))
|
87 |
-
noise = est - proj
|
88 |
-
|
89 |
-
sisnr = 10 * (
|
90 |
-
torch.log10(epsilon + _norm2(proj)) - torch.log10(epsilon + _norm2(noise))
|
91 |
-
)
|
92 |
-
return -1 * sisnr[..., 0].mean()
|
|
|
|
|
|
|
|
|
|
|
|
|
|
|
|
|
|
|
|
|
|
|
|
|
|
|
|
|
|
|
|
|
|
|
|
|
|
|
|
|
|
|
|
|
|
|
|
|
|
|
|
|
|
|
|
|
|
|
|
|
|
|
|
|
|
|
|
|
|
|
|
|
|
|
|
|
|
|
|
|
|
|
|
|
|
|
|
|
|
|
|
|
|
|
|
|
|
|
|
|
|
|
|
|
|
|
|
|
|
|
|
|
|
|
|
|
|
|
|
|
|
|
|
|
|
|
|
|
|
|
|
|
|
|
|
|
|
|
|
|
|
|
|
|
|
|
|
|
|
|
|
|
|
|
|
|
|
|
|
|
|
|
|
|
|
|
|
|
|
|
|
|
|
|
|
|
|
|
|
|
|
|
|
|
|
spaces/HLasse/textdescriptives/data_viewer.py
DELETED
@@ -1,26 +0,0 @@
|
|
1 |
-
"""
|
2 |
-
Class for showing header and download button in the same row.
|
3 |
-
"""
|
4 |
-
|
5 |
-
import streamlit as st
|
6 |
-
|
7 |
-
|
8 |
-
class DataViewer:
|
9 |
-
def _convert_df_to_csv(self, data, **kwargs):
|
10 |
-
return data.to_csv(**kwargs).encode("utf-8")
|
11 |
-
|
12 |
-
def _header_and_download(
|
13 |
-
self, header, data, file_name, key=None, label="Download", help="Download data"
|
14 |
-
):
|
15 |
-
col1, col2 = st.columns([9, 2])
|
16 |
-
with col1:
|
17 |
-
st.subheader(header)
|
18 |
-
with col2:
|
19 |
-
st.write("")
|
20 |
-
st.download_button(
|
21 |
-
label=label,
|
22 |
-
data=self._convert_df_to_csv(data, index=False),
|
23 |
-
file_name=file_name,
|
24 |
-
key=key,
|
25 |
-
help=help,
|
26 |
-
)
|
|
|
|
|
|
|
|
|
|
|
|
|
|
|
|
|
|
|
|
|
|
|
|
|
|
|
|
|
|
|
|
|
|
|
|
|
|
|
|
|
|
|
|
|
|
|
|
|
|
|
|
|
|
spaces/HaHaBill/LandShapes-Antarctica/netdissect/dissect.html
DELETED
@@ -1,399 +0,0 @@
|
|
1 |
-
<!doctype html>
|
2 |
-
<html>
|
3 |
-
<head>
|
4 |
-
<link rel="stylesheet" href="https://maxcdn.bootstrapcdn.com/bootstrap/4.0.0/css/bootstrap.min.css" integrity="sha384-Gn5384xqQ1aoWXA+058RXPxPg6fy4IWvTNh0E263XmFcJlSAwiGgFAW/dAiS6JXm" crossorigin="anonymous">
|
5 |
-
<script src="https://code.jquery.com/jquery-3.3.1.js" integrity="sha256-2Kok7MbOyxpgUVvAk/HJ2jigOSYS2auK4Pfzbm7uH60=" crossorigin="anonymous"></script>
|
6 |
-
<script src="https://cdnjs.cloudflare.com/ajax/libs/popper.js/1.12.9/umd/popper.min.js" integrity="sha384-ApNbgh9B+Y1QKtv3Rn7W3mgPxhU9K/ScQsAP7hUibX39j7fakFPskvXusvfa0b4Q" crossorigin="anonymous"></script>
|
7 |
-
<script src="https://maxcdn.bootstrapcdn.com/bootstrap/4.0.0/js/bootstrap.min.js" integrity="sha384-JZR6Spejh4U02d8jOt6vLEHfe/JQGiRRSQQxSfFWpi1MquVdAyjUar5+76PVCmYl" crossorigin="anonymous"></script>
|
8 |
-
<script src="https://cdnjs.cloudflare.com/ajax/libs/vue/2.5.16/vue.js" integrity="sha256-CMMTrj5gGwOAXBeFi7kNokqowkzbeL8ydAJy39ewjkQ=" crossorigin="anonymous"></script>
|
9 |
-
<script src="https://cdn.jsdelivr.net/npm/[email protected]/lodash.js" integrity="sha256-qwbDmNVLiCqkqRBpF46q5bjYH11j5cd+K+Y6D3/ja28=" crossorigin="anonymous"></script>
|
10 |
-
<style>
|
11 |
-
[v-cloak] {
|
12 |
-
display: none;
|
13 |
-
}
|
14 |
-
.unitviz, .unitviz .modal-header, .unitviz .modal-body, .unitviz .modal-footer {
|
15 |
-
font-family: Arial;
|
16 |
-
font-size: 15px;
|
17 |
-
}
|
18 |
-
.unitgrid {
|
19 |
-
text-align: center;
|
20 |
-
border-spacing: 5px;
|
21 |
-
border-collapse: separate;
|
22 |
-
}
|
23 |
-
.unitgrid .info {
|
24 |
-
text-align: left;
|
25 |
-
}
|
26 |
-
.unitgrid .layername {
|
27 |
-
display: none;
|
28 |
-
}
|
29 |
-
.unitlabel {
|
30 |
-
font-weight: bold;
|
31 |
-
font-size: 150%;
|
32 |
-
text-align: center;
|
33 |
-
line-height: 1;
|
34 |
-
}
|
35 |
-
.lowscore .unitlabel {
|
36 |
-
color: silver;
|
37 |
-
}
|
38 |
-
.thumbcrop {
|
39 |
-
overflow: hidden;
|
40 |
-
width: 288px;
|
41 |
-
height: 72px;
|
42 |
-
}
|
43 |
-
.thumbcrop img, .img-scroller img {
|
44 |
-
image-rendering: pixelated;
|
45 |
-
}
|
46 |
-
.unit {
|
47 |
-
display: inline-block;
|
48 |
-
background: white;
|
49 |
-
padding: 3px;
|
50 |
-
margin: 2px;
|
51 |
-
box-shadow: 0 5px 12px grey;
|
52 |
-
}
|
53 |
-
.iou {
|
54 |
-
display: inline-block;
|
55 |
-
float: right;
|
56 |
-
margin-left: 5px;
|
57 |
-
}
|
58 |
-
.modal .big-modal {
|
59 |
-
width:auto;
|
60 |
-
max-width:90%;
|
61 |
-
max-height:80%;
|
62 |
-
}
|
63 |
-
.modal-title {
|
64 |
-
display: inline-block;
|
65 |
-
}
|
66 |
-
.footer-caption {
|
67 |
-
float: left;
|
68 |
-
width: 100%;
|
69 |
-
}
|
70 |
-
.histogram {
|
71 |
-
text-align: center;
|
72 |
-
margin-top: 3px;
|
73 |
-
}
|
74 |
-
.img-wrapper {
|
75 |
-
text-align: center;
|
76 |
-
position: relative;
|
77 |
-
}
|
78 |
-
.img-mask, .img-seg {
|
79 |
-
position: absolute;
|
80 |
-
top: 0;
|
81 |
-
left: 0;
|
82 |
-
z-index: 0;
|
83 |
-
visibility: hidden;
|
84 |
-
}
|
85 |
-
input.hidden-toggle {
|
86 |
-
display: none;
|
87 |
-
}
|
88 |
-
#show-seg:checked ~ .img-wrapper .img-seg,
|
89 |
-
#show-mask:checked ~ .img-wrapper .img-mask {
|
90 |
-
visibility: visible;
|
91 |
-
}
|
92 |
-
.img-controls {
|
93 |
-
text-align: right;
|
94 |
-
}
|
95 |
-
.img-controls label {
|
96 |
-
display: inline-block;
|
97 |
-
background: silver;
|
98 |
-
padding: 10px;
|
99 |
-
margin-top: 0;
|
100 |
-
-webkit-user-select: none;
|
101 |
-
-moz-user-select: none;
|
102 |
-
-ms-user-select: none;
|
103 |
-
user-select: none;
|
104 |
-
}
|
105 |
-
.seginfo {
|
106 |
-
display: inline-block;
|
107 |
-
padding: 10px;
|
108 |
-
float: left;
|
109 |
-
}
|
110 |
-
.img-mask {
|
111 |
-
pointer-events: none;
|
112 |
-
}
|
113 |
-
.colorsample {
|
114 |
-
display: inline-block;
|
115 |
-
height: 42px;
|
116 |
-
width: 42px;
|
117 |
-
float: left;
|
118 |
-
}
|
119 |
-
#show-seg:checked ~ .img-controls .toggle-seg,
|
120 |
-
#show-mask:checked ~ .img-controls .toggle-mask {
|
121 |
-
background: navy;
|
122 |
-
color: white;
|
123 |
-
}
|
124 |
-
.big-modal img {
|
125 |
-
max-height: 60vh;
|
126 |
-
}
|
127 |
-
.img-scroller {
|
128 |
-
overflow-x: scroll;
|
129 |
-
}
|
130 |
-
.img-scroller .img-fluid {
|
131 |
-
max-width: initial;
|
132 |
-
}
|
133 |
-
.gridheader {
|
134 |
-
font-size: 12px;
|
135 |
-
margin-bottom: 10px;
|
136 |
-
margin-left: 30px;
|
137 |
-
margin-right: 30px;
|
138 |
-
}
|
139 |
-
.gridheader:after {
|
140 |
-
content: '';
|
141 |
-
display: table;
|
142 |
-
clear: both;
|
143 |
-
}
|
144 |
-
.sortheader {
|
145 |
-
float: right;
|
146 |
-
cursor: default;
|
147 |
-
}
|
148 |
-
.layerinfo {
|
149 |
-
float: left;
|
150 |
-
}
|
151 |
-
.sortby {
|
152 |
-
text-decoration: underline;
|
153 |
-
cursor: pointer;
|
154 |
-
}
|
155 |
-
.sortby.currentsort {
|
156 |
-
text-decoration: none;
|
157 |
-
font-weight: bold;
|
158 |
-
cursor: default;
|
159 |
-
}
|
160 |
-
.bg-inverse {
|
161 |
-
background: #021B54;
|
162 |
-
}
|
163 |
-
.dropmenu {
|
164 |
-
display: inline-block;
|
165 |
-
vertical-align: top;
|
166 |
-
position: relative;
|
167 |
-
}
|
168 |
-
.dropmenulist {
|
169 |
-
pointer-events: auto;
|
170 |
-
visibility: hidden;
|
171 |
-
transition: visiblity 1s;
|
172 |
-
position: absolute;
|
173 |
-
z-index: 1;
|
174 |
-
background: white;
|
175 |
-
right: 0;
|
176 |
-
text-align: right;
|
177 |
-
white-space: nowrap;
|
178 |
-
}
|
179 |
-
.dropmenu:focus {
|
180 |
-
pointer-events: none;
|
181 |
-
}
|
182 |
-
.dropmenu:focus .dropmenulist {
|
183 |
-
visibility: visible;
|
184 |
-
}
|
185 |
-
</style>
|
186 |
-
</head>
|
187 |
-
<body class="unitviz">
|
188 |
-
<div id="app" v-if="dissect" v-cloak>
|
189 |
-
|
190 |
-
<nav class="navbar navbar-expand navbar-dark bg-inverse">
|
191 |
-
<span class="navbar-brand">{{ dissect.netname || 'Dissection' }}</span>
|
192 |
-
<ul class="navbar-nav mr-auto">
|
193 |
-
<li :class="{'nav-item': true, active: lindex == selected_layer}"
|
194 |
-
v-for="(lrec, lindex) in dissect.layers">
|
195 |
-
<a class="nav-link" :href="'#' + lindex"
|
196 |
-
>{{lrec.layer}}</a>
|
197 |
-
</li>
|
198 |
-
</ul>
|
199 |
-
<ul class="navbar-nav ml-auto" v-if="dissect.meta">
|
200 |
-
<li class="navbar-text ml-2" v-for="(v, k) in dissect.meta">
|
201 |
-
{{k}}={{v | fixed(3, true)}}
|
202 |
-
</li>
|
203 |
-
</ul>
|
204 |
-
</nav>
|
205 |
-
|
206 |
-
<div v-for="lrec in [dissect.layers[selected_layer]]">
|
207 |
-
<div v-if="'bargraph' in lrec" class="histogram">
|
208 |
-
<a data-toggle="lightbox" :href="lrec.dirname + '/bargraph.svg?'+Math.random()"
|
209 |
-
:data-title="'Summary of ' + (dissect.netname || 'labels')
|
210 |
-
+ ' at ' + lrec.layer">
|
211 |
-
<img class="img-fluid"
|
212 |
-
:src="lrec.dirname + '/' + lrec.bargraph + '?'+Math.random()">
|
213 |
-
</a>
|
214 |
-
</div>
|
215 |
-
|
216 |
-
<div class="gridheader">
|
217 |
-
<div class="layerinfo">
|
218 |
-
<span v-if="'interpretable' in lrec"
|
219 |
-
>{{lrec.interpretable}}/</span
|
220 |
-
>{{lrec.units.length}} units
|
221 |
-
<span v-if="'labels' in lrec">
|
222 |
-
covering {{lrec.labels.length}} concepts
|
223 |
-
with IoU ≥ {{dissect.iou_threshold}}
|
224 |
-
</span>
|
225 |
-
</div>
|
226 |
-
|
227 |
-
<div class="sortheader">
|
228 |
-
sort by
|
229 |
-
<span v-for="rank in lrec['rankings']" v-if="!rank.metric">
|
230 |
-
<span :class="{sortby: true, currentsort: sort_order == rank.name}"
|
231 |
-
:data-ranking="rank.name"
|
232 |
-
v-on:click="sort_order = $event.currentTarget.dataset.ranking"
|
233 |
-
>{{rank.name}}</span>
|
234 |
-
<span> </span>
|
235 |
-
</span>
|
236 |
-
<span v-for="metric in _.filter(_.uniq(lrec.rankings.map(x => x.metric)))">
|
237 |
-
<div class="dropmenu sortby" tabindex="0">
|
238 |
-
<div class="dropmenutop">
|
239 |
-
*-{{ metric }}
|
240 |
-
</div>
|
241 |
-
<div class="dropmenulist">
|
242 |
-
<div v-for="rank in lrec['rankings']" v-if="rank.metric == metric">
|
243 |
-
<span :class="{sortby: true, currentsort: sort_order == rank.name}"
|
244 |
-
:data-ranking="rank.name"
|
245 |
-
v-on:click="sort_order = $event.currentTarget.dataset.ranking"
|
246 |
-
>{{rank.name}}</span>
|
247 |
-
</div>
|
248 |
-
</div>
|
249 |
-
</div>
|
250 |
-
<span> </span>
|
251 |
-
</span>
|
252 |
-
|
253 |
-
</div>
|
254 |
-
|
255 |
-
</div>
|
256 |
-
<div class="unitgrid"
|
257 |
-
v-for="lk in [_.find(lrec.rankings, x=>x.name == sort_order)
|
258 |
-
.metric || 'iou']"
|
259 |
-
><div :class="{unit: true, lowscore: lk == 'iou' && !urec.interp}"
|
260 |
-
v-for="urec in _.find(lrec.rankings, x=>x.name == sort_order)
|
261 |
-
.ranking.map(x=>lrec.units[x])">
|
262 |
-
<div v-if="lk+'_label' in urec" class="unitlabel">{{urec[lk+'_label']}}</div>
|
263 |
-
<div class="info"
|
264 |
-
><span class="layername">{{lrec.layer}}</span
|
265 |
-
> <span class="unitnum">unit {{urec.unit}}</span
|
266 |
-
> <span v-if="lk+'_cat' in urec" class="category">({{urec[lk+'_cat']}})</span
|
267 |
-
> <span v-if="lk+'_iou' in urec" class="iou"
|
268 |
-
>iou {{urec[lk + '_iou'] | fixed(2)}}</span
|
269 |
-
> <span v-if="lk in urec" class="iou"
|
270 |
-
>{{lk}} {{urec[lk] | fixed(2)}}</span></div>
|
271 |
-
<div class="thumbcrop" v-for="imprefix in [lrec['image_prefix_' + lk] || '']"
|
272 |
-
><a data-toggle="lightbox"
|
273 |
-
:href="lrec.dirname + '/' + imprefix + 'image/' + urec.unit + '-top.jpg'"
|
274 |
-
><img
|
275 |
-
:src="lrec.dirname + '/' + imprefix + 'image/' + urec.unit + '-top.jpg'"
|
276 |
-
height="72"></a></div>
|
277 |
-
</div></div> <!-- end unit -->
|
278 |
-
|
279 |
-
</div> <!-- end unit grid -->
|
280 |
-
|
281 |
-
</div> <!-- end container -->
|
282 |
-
|
283 |
-
</div> <!-- end app -->
|
284 |
-
|
285 |
-
<div class="modal" id="lightbox">
|
286 |
-
<div class="modal-dialog big-modal" role="document">
|
287 |
-
<div class="modal-content">
|
288 |
-
<div class="modal-header">
|
289 |
-
<h5 class="modal-title"></h5>
|
290 |
-
<button type="button" class="close"
|
291 |
-
data-dismiss="modal" aria-label="Close">
|
292 |
-
<span aria-hidden="true">×</span>
|
293 |
-
</button>
|
294 |
-
</div>
|
295 |
-
<div class="modal-body">
|
296 |
-
<input id="show-seg" class="hidden-toggle" type="checkbox">
|
297 |
-
<input id="show-mask" class="hidden-toggle" type="checkbox" checked>
|
298 |
-
<div class="img-wrapper img-scroller">
|
299 |
-
<img class="fullsize img-fluid img-orig">
|
300 |
-
<img class="fullsize img-fluid img-seg">
|
301 |
-
<img class="fullsize img-fluid img-mask">
|
302 |
-
</div>
|
303 |
-
<div class="img-controls">
|
304 |
-
<canvas class="colorsample" height=1 width=1></canvas>
|
305 |
-
<div class="seginfo">
|
306 |
-
</div>
|
307 |
-
<label for="show-seg" class="toggle-seg">segmentation</label>
|
308 |
-
<label for="show-mask" class="toggle-mask">mask</label>
|
309 |
-
</div>
|
310 |
-
</div>
|
311 |
-
<div class="modal-footer">
|
312 |
-
<div class="footer-caption">
|
313 |
-
</div>
|
314 |
-
</div>
|
315 |
-
</div>
|
316 |
-
</div>
|
317 |
-
</div>
|
318 |
-
<script>
|
319 |
-
$(document).on('click', '[data-toggle=lightbox]', function(event) {
|
320 |
-
if ($(this).attr('href').match(/-top/)) {
|
321 |
-
$('#lightbox img.img-orig').attr('src',
|
322 |
-
$(this).attr('href').replace(/-top.jpg/, '-orig.jpg'));
|
323 |
-
$('#lightbox img.img-seg').attr('src',
|
324 |
-
$(this).attr('href').replace(/-top.jpg/, '-seg.png'));
|
325 |
-
$('#lightbox img.img-mask').attr('src',
|
326 |
-
$(this).attr('href').replace(/-top.jpg/, '-mask.png'));
|
327 |
-
$('#lightbox .img-seg, #lightbox .img-mask, .img-controls').show();
|
328 |
-
} else {
|
329 |
-
$('#lightbox img.img-orig').attr('src', $(this).attr('href'));
|
330 |
-
$('#lightbox .img-seg, #lightbox .img-mask, .img-controls').hide();
|
331 |
-
}
|
332 |
-
$('#lightbox .modal-title').text($(this).data('title') ||
|
333 |
-
$(this).closest('.unit').find('.unitlabel').text());
|
334 |
-
$('#lightbox .footer-caption').text($(this).data('footer') ||
|
335 |
-
$(this).closest('.unit').find('.info').text());
|
336 |
-
$('#lightbox .segcolors').text('');
|
337 |
-
event.preventDefault();
|
338 |
-
$('#lightbox').modal();
|
339 |
-
$('#lightbox img').closest('div').scrollLeft(0);
|
340 |
-
});
|
341 |
-
$(document).on('click', '#lightbox img.img-seg', function(event) {
|
342 |
-
var elt_pos = $(this).offset();
|
343 |
-
var img_x = event.pageX - elt_pos.left;
|
344 |
-
var img_y = event.pageY - elt_pos.top;
|
345 |
-
var canvas = $('#lightbox .colorsample').get(0);
|
346 |
-
canvas.getContext('2d').drawImage(this, img_x, img_y, 1, 1, 0, 0, 1, 1);
|
347 |
-
var pixelData = canvas.getContext('2d').getImageData(0, 0, 1, 1).data;
|
348 |
-
var colorkey = pixelData[0] + ',' + pixelData[1] + ',' + pixelData[2];
|
349 |
-
var meaning = theapp.dissect.segcolors[colorkey];
|
350 |
-
$('#lightbox .seginfo').text(meaning);
|
351 |
-
});
|
352 |
-
|
353 |
-
var theapp = new Vue({
|
354 |
-
el: '#app',
|
355 |
-
data: {
|
356 |
-
sort_order: 'unit',
|
357 |
-
sort_fields: {
|
358 |
-
label: [[], []],
|
359 |
-
score: [['iou'], ['desc']],
|
360 |
-
unit: [['unit'], ['asc']],
|
361 |
-
},
|
362 |
-
selected_layer: null,
|
363 |
-
dissect: null
|
364 |
-
},
|
365 |
-
created: function() {
|
366 |
-
var self = this;
|
367 |
-
$.getJSON('dissect.json?' + Math.random(), function(d) {
|
368 |
-
self.dissect = d;
|
369 |
-
for (var layer of d.layers) {
|
370 |
-
// Preprocess ranking records to sort them.
|
371 |
-
for (var rank of layer.rankings) {
|
372 |
-
if (!('ranking' in rank)) {
|
373 |
-
rank.ranking = rank.score.map((score, index) => [score, index])
|
374 |
-
.sort(([score1], [score2]) => score1 - score2)
|
375 |
-
.map(([, index]) => index);
|
376 |
-
}
|
377 |
-
}
|
378 |
-
}
|
379 |
-
self.sort_order = d.default_ranking;
|
380 |
-
self.hashchange();
|
381 |
-
});
|
382 |
-
$(window).on('hashchange', function() { self.hashchange(); });
|
383 |
-
},
|
384 |
-
methods: {
|
385 |
-
hashchange: function() {
|
386 |
-
this.selected_layer = +window.location.hash.substr(1) || 0;
|
387 |
-
},
|
388 |
-
},
|
389 |
-
filters: {
|
390 |
-
fixed: function(value, digits, truncate) {
|
391 |
-
if (typeof value != 'number') return value;
|
392 |
-
var fixed = value.toFixed(digits);
|
393 |
-
return truncate ? +fixed : fixed;
|
394 |
-
}
|
395 |
-
}
|
396 |
-
});
|
397 |
-
</script>
|
398 |
-
</body>
|
399 |
-
</html>
|
|
|
|
|
|
|
|
|
|
|
|
|
|
|
|
|
|
|
|
|
|
|
|
|
|
|
|
|
|
|
|
|
|
|
|
|
|
|
|
|
|
|
|
|
|
|
|
|
|
|
|
|
|
|
|
|
|
|
|
|
|
|
|
|
|
|
|
|
|
|
|
|
|
|
|
|
|
|
|
|
|
|
|
|
|
|
|
|
|
|
|
|
|
|
|
|
|
|
|
|
|
|
|
|
|
|
|
|
|
|
|
|
|
|
|
|
|
|
|
|
|
|
|
|
|
|
|
|
|
|
|
|
|
|
|
|
|
|
|
|
|
|
|
|
|
|
|
|
|
|
|
|
|
|
|
|
|
|
|
|
|
|
|
|
|
|
|
|
|
|
|
|
|
|
|
|
|
|
|
|
|
|
|
|
|
|
|
|
|
|
|
|
|
|
|
|
|
|
|
|
|
|
|
|
|
|
|
|
|
|
|
|
|
|
|
|
|
|
|
|
|
|
|
|
|
|
|
|
|
|
|
|
|
|
|
|
|
|
|
|
|
|
|
|
|
|
|
|
|
|
|
|
|
|
|
|
|
|
|
|
|
|
|
|
|
|
|
|
|
|
|
|
|
|
|
|
|
|
|
|
|
|
|
|
|
|
|
|
|
|
|
|
|
|
|
|
|
|
|
|
|
|
|
|
|
|
|
|
|
|
|
|
|
|
|
|
|
|
|
|
|
|
|
|
|
|
|
|
|
|
|
|
|
|
|
|
|
|
|
|
|
|
|
|
|
|
|
|
|
|
|
|
|
|
|
|
|
|
|
|
|
|
|
|
|
|
|
|
|
|
|
|
|
|
|
|
|
|
|
|
|
|
|
|
|
|
|
|
|
|
|
|
|
|
|
|
|
|
|
|
|
|
|
|
|
|
|
|
|
|
|
|
|
|
|
|
|
|
|
|
|
|
|
|
|
|
|
|
|
|
|
|
|
|
|
|
|
|
|
|
|
|
|
|
|
|
|
|
|
|
|
|
|
|
|
|
|
|
|
|
|
|
|
|
|
|
|
|
|
|
|
|
|
|
|
|
|
|
|
|
|
|
|
|
|
|
|
|
|
|
|
|
|
|
|
|
|
|
|
|
|
|
|
|
|
|
|
|
|
|
|
|
|
|
|
|
|
|
|
|
|
|
|
|
|
|
|
|
|
|
|
|
|
|
|
|
|
|
|
|
|
|
|
|
|
|
|
|
|
|
|
|
|
|
|
|
|
|
|
|
|
|
|
|
|
|
|
|
|
|
|
|
|
|
|
|
|
|
|
|
|
|
|
|
|
|
|
|
|
|
|
|
|
|
|
|
|
|
|
|
|
|
|
|
|
|
|
|
|
|
|
|
|
|
|
|
|
|
|
|
|
|
|
|
|
|
|
|
|
|
|
|
|
|
|
|
|
|
|
|
|
|
|
|
|
|
|
|
|
|
|
|
|
|
|
|
|
|
|
|
|
|
|
|
|
|
|
|
|
|
|
|
|
|
|
|
|
|
|
|
|
|
|
|
|
|
|
|
|
|
|
|
|
|
|
|
|
|
|
|
|
|
|
|
|
|
|
|
|
|
|
|
|
|
|
|
|
|
|
|
|
|
|
|
|
|
|
|
|
|
|
|
|
|
|
|
|
|
|
|
|
|
|
|
|
|
|
|
|
|
|
|
|
|
|
|
|
|
|
|
|
|
|
|
|
|
|
|
|
|
|
|
|
|
|
|
|
|
|
|
|
|
|
|
|
|
|
|
|
|
|
|
|
|
|
|
|
|
|