diff --git a/spaces/1-13-am/neural-style-transfer/README.md b/spaces/1-13-am/neural-style-transfer/README.md
deleted file mode 100644
index 9cb3af6cfdc4eb9efcfc0ad6e916ef546e4629ce..0000000000000000000000000000000000000000
--- a/spaces/1-13-am/neural-style-transfer/README.md
+++ /dev/null
@@ -1,12 +0,0 @@
----
-title: Neural Style Transfer
-emoji: 🦀
-colorFrom: pink
-colorTo: gray
-sdk: gradio
-sdk_version: 3.46.0
-app_file: app.py
-pinned: false
----
-
-Check out the configuration reference at https://huggingface.co/docs/hub/spaces-config-reference
diff --git a/spaces/1acneusushi/gradio-2dmoleculeeditor/data/CASIO Classpad 3.0 [Emulator Crack] Serial Key Troubleshooting and Support for the Emulator.md b/spaces/1acneusushi/gradio-2dmoleculeeditor/data/CASIO Classpad 3.0 [Emulator Crack] Serial Key Troubleshooting and Support for the Emulator.md
deleted file mode 100644
index 7401d81c399f131606fd338afeb0e0328ce7522c..0000000000000000000000000000000000000000
--- a/spaces/1acneusushi/gradio-2dmoleculeeditor/data/CASIO Classpad 3.0 [Emulator Crack] Serial Key Troubleshooting and Support for the Emulator.md
+++ /dev/null
@@ -1,83 +0,0 @@
-
-
CASIO Classpad 3.0 [Emulator Crack] Serial Key
-
Are you looking for a way to use CASIO Classpad 3.0 on your PC without buying the original calculator? Do you want to enjoy the features and benefits of CASIO Classpad 3.0 without spending a lot of money? If yes, then you might be interested in using an emulator with a crack and serial key.
An emulator is a software that simulates the functions and features of another device or system on your PC. A crack is a file that modifies or bypasses the security features of a software to make it work without limitations or restrictions. A serial key is a code that activates or registers a software to make it valid or authentic.
-
In this article, we will explain what CASIO Classpad 3.0 is, what an emulator is, why you need an emulator for CASIO Classpad 3.0, how to get an emulator for CASIO Classpad 3.0, how to use an emulator for CASIO Classpad 3.0, how to get a crack and serial key for CASIO Classpad 3.0 emulator, how to use a crack and serial key for CASIO Classpad 3.0 emulator, what are the risks of using a crack and serial key for CASIO Classpad 3.0 emulator, how to avoid or solve the problems of using a crack and serial key for CASIO Classpad 3.0 emulator, and what are the alternatives to using a crack and serial key for CASIO Classpad 3.0 emulator.
-
By the end of this article, you will have a clear understanding of how to use CASIO Classpad 3.0 [Emulator Crack] Serial Key on your PC.
CASIO Classpad 3.0 is a powerful software that simulates the functions and features of the CASIO Classpad 330 calculator on your PC. You can use it for learning, teaching, or doing complex calculations with ease.
-
Some of the features and benefits of CASIO Classpad 3.0 are:
-
-
It has a large touch-screen display that allows you to input data, draw graphs, edit formulas, manipulate images, etc.
-
It supports various mathematical functions such as algebra, calculus, geometry, statistics, probability, etc.
-
It has a built-in spreadsheet application that allows you to perform data analysis, create charts, etc.
-
It has a built-in eActivity application that allows you to create interactive worksheets, presentations, quizzes, etc.
-
It has a built-in geometry application that allows you to construct geometric figures, measure angles, lengths, areas, etc.
-
It has a built-in programming language that allows you to create custom applications, games, etc.
-
It has a built-in communication function that allows you to connect with other devices via USB or wireless LAN.
-
It has a built-in memory function that allows you to store data, formulas, images, etc.
-
-
What is an emulator?
-
An emulator is a software that simulates the functions and features of another device or system on your PC. For example, you can use an emulator to play games designed for consoles such as PlayStation or Nintendo on your PC.
-
There are different types of emulators depending on the device or system they emulate. Some examples are:
-
-
Console emulators: They emulate video game consoles such as PlayStation, Nintendo, Sega, etc.
-
Arcade emulators: They emulate arcade machines such as Pac-Man, Street Fighter, etc.
-
Computer emulators: They emulate personal computers such as Windows, Mac OS X, Linux, etc.
-
Mobile emulators: They emulate mobile devices such as Android, iOS, Windows Phone, etc.
-
Calculator emulators: They emulate calculators such as TI-83, HP-12C, CASIO ClassPad, etc.
-
-
Why do you need an emulator for CASIO ClassPad 0a6ba089eb
-
-
\ No newline at end of file
diff --git a/spaces/1gistliPinn/ChatGPT4/Examples/Activehome Pro LINK Download.md b/spaces/1gistliPinn/ChatGPT4/Examples/Activehome Pro LINK Download.md
deleted file mode 100644
index 346ac8eb662c55445d74f5460cd9cf97087a116f..0000000000000000000000000000000000000000
--- a/spaces/1gistliPinn/ChatGPT4/Examples/Activehome Pro LINK Download.md
+++ /dev/null
@@ -1,8 +0,0 @@
-
-
activehome pro is a powerful home automation system that allows you to control all sorts of devices, such as lights, locks, audio systems, and other appliances and devices. it also allows you to interact with your home through the web. it is a very powerful home automation system.
-
activehome pro has a versatile application programming interface (api) that lets you integrate activehome pro with other systems. api support for activehome pro includes:
local and remote event-based triggering using the activehome device api
configuration and operation of devices and appliances using the activehome device api
-
activehome acts as a central monitoring station for your home. it monitors the status of your lights and appliances and sends you alerts when it detects activity. activehome also monitors activity and status to help you find and resolve service calls. in addition to monitoring device status, activehome also reports the power consumption of each device to help you manage your energy consumption.
-
activehome pro will ensure that your lights and appliances are always off. however, you can set a schedule so that when no one is home, activehome will turn lights and appliances on. in addition, activehome pro keeps track of any malfunctions so that if a light or appliance is not working, you will know exactly where to find the problem. you can schedule activehome to turn lights and appliances on when you are away from home, and so that lights and appliances that are already on will turn off when you are away.
899543212b
-
-
\ No newline at end of file
diff --git a/spaces/1gistliPinn/ChatGPT4/Examples/Como Eliminar Archivos Duplicados En Tu PC [2020].md b/spaces/1gistliPinn/ChatGPT4/Examples/Como Eliminar Archivos Duplicados En Tu PC [2020].md
deleted file mode 100644
index df2e389fb6d5c49af5e57c477271a59c5fe282f9..0000000000000000000000000000000000000000
--- a/spaces/1gistliPinn/ChatGPT4/Examples/Como Eliminar Archivos Duplicados En Tu PC [2020].md
+++ /dev/null
@@ -1,6 +0,0 @@
-
-
-**AgentVerse** offers a versatile framework that streamlines the process of creating custom multi-agent environments for large language models (LLMs). Designed to facilitate swift development and customization with minimal effort, our framework empowers researchers to concentrate on their research, rather than being bogged down by implementation details.
-
-⚠️⚠️⚠️ We're refactoring the code, and the goal is to provide a flexibility to construct simulation(without a predefined goal) and task-solving(with a specific goal) environments. Please note that this README is slightly outdated, we will update it soon. If you require a stable version that exclusively supports simulation environments, you can use [`release-0.1`](https://github.com/OpenBMB/AgentVerse/tree/release-0.1) branch.
-
----
-
-## ✨ Features
-
-- 🥳 **Efficient Environment Building:** Our framework provides a collection of essential building blocks for effortlessly creating a multi-agent environment. With only a few lines in a configuration file, you can easily construct basic environments such as a chat room for LLMs. This process entails defining the environment's settings and prompts for LLMs, enabling researchers like you to concentrate on experimentation and analysis.
-
-- ⚙️ **Customizable Components**: AgentVerse simplifies the multi-agent environment by dividing it into five functional modules and defining their respective interfaces. For complex environments that cannot be constructed directly using the basic modules offered in AgentVerse, you can customize one or more of the interfaces within these five functional modules to efficiently create your own multi-agent environment according to your requirements.
-
-- 🛠 **Tools (Plugins) Utilization**: AgentVerse supports the multi-agent environments with tools. Currently, AgentVerse supports tools provided in [BMTools](https://github.com/OpenBMB/BMTools).
-
-## 📰 What's New
-- [2023/10/5] 💡 We release the code of our paper [AgentVerse: Facilitating Multi-Agent Collaboration and Exploring Emergent Behaviors in Agents](https://arxiv.org/abs/2308.10848), and refactor our codebase to enable the creation of both simulation and task-solving environment! We have placed the code for Minecraft example in the paper at the [`minecraft`](https://github.com/OpenBMB/AgentVerse/tree/minecraft) branch. Our tool-using example will soon be updated to the `main` branch. Stay tuned!
-
-- [2023/8/22] 📝 We're excited to share our work-in-progress paper [AgentVerse: Facilitating Multi-Agent Collaboration and Exploring Emergent Behaviors in Agents](https://arxiv.org/abs/2308.10848) related to this repository.
-
-
-
-
-- [2023/6/5] 🎉 We are thrilled to present an array of [demos](#-simple-demo-video), including [NLP Classroom](#nlp-classroom), [Prisoner Dilemma](#prisoner-dilemma), [Software Design](#software-design), [Database Administrator](#database-administrator-dba), and a simple [H5 Pokemon Game](#pokemon) that enables the interaction with the characters in Pokemon! Try out these demos and have fun!
-- [2023/5/1] 🚀 [AgentVerse](https://github.com/OpenBMB/AgentVerse) is officially launched!
-
-## 🌟 Join Us!
-AgentVerse is on a mission to revolutionize the multi-agent environment for large language models, and we're eagerly looking for passionate collaborators to join us on this exciting journey.
-### How Can You Contribute?
-- **Code Development**: If you're an engineer, help us refine, optimize, and expand the current framework. We're always looking for talented developers to enhance our existing features and develop new modules.
-
-- **Documentation and Tutorials**: If you have a knack for writing, help us improve our documentation, create tutorials, or write blog posts to make AgentVerse more accessible to the broader community.
-
-- **Application Exploration**: If you're intrigued by multi-agent applications and are eager to experiment using AgentVerse, we'd be thrilled to support your journey and see what you create!
-
-- **Feedback and Suggestions**: Use AgentVerse and provide us with feedback. Your insights can lead to potential improvements and ensure that our framework remains top-notch.
-
-Also, if you're passionate about advancing the frontiers of multi-agent environments and are eager to dive deeper into research, we invite you to join our team at THUNLP. To explore this exciting opportunity and embark on a collaborative journey with us, please reach out to [chenweize1998@gmail.com](chenweize1998@gmail.com) and [yushengsu.thu@gmail.com](yushengsu.thu@gmail.com) and express your interest. We're keen to welcome motivated individuals like you to our lab!
-
-👉Also, check our Discord: https://discord.gg/cnutfCtC.
-
-## 🗓 Coming Soon
-- [x] Code release of our [paper](https://arxiv.org/abs/2308.10848)
-- [ ] Add documentation
-- [ ] Support more sophisticated memory for conversation history
-- [ ] Add support for local LLM
-
-
-## 👾 Simple Demo Video
-
-We demonstrate the following cases that are expertly crafted by AgentVerse.
-
-
-
-
-
-#### NLP Classroom
-In the NLP class, the professor and students engage in interactive communication. When students have a question, they raise their hands and patiently wait for the professor to call on them. Only after being called on by the professor, can students speak and ask their questions.
-
-Use the following command to launch the NLP Classroom example:
-```bash
-python agentverse_command/main_simulation_gui.py --task simulation/nlp_classroom_9players
-```
-
-[Wacth the NLP Classroom Video](https://github.com/OpenBMB/AgentVerse/assets/11704492/6ea07850-595e-4a28-a82e-f863011353c2)
-
-
-#### Prisoner Dilemma
-A prisoner's Dilemma is a thought experiment that challenges two completely rational agents to a dilemma: they can cooperate with their partner for mutual benefit or betray their partner ("defect") for individual reward.
-
-Use the following command to launch the Prisoner Dilemma example:
-```bash
-python agentverse_command/main_simulation_gui.py --task simulation/prisoner_dilemma
-```
-
-[Wacth the Prisoner's Dilemma Video](https://github.com/OpenBMB/AgentVerse/assets/11704492/017c46e5-c738-4fca-9352-b008e2d518bd)
-
-
-#### Software Design
-In the Software Design example, a code writer, a code tester and a code reviewer collaborate on the code generation problem. Given a problem, the code writer first composes the code implementation. The code tester runs the unit tests and provides the feedback. The code viewer then generates a review. After collecting the test feedback and review, the code writer iteratively refines the code.
-
-Use the following command to launch the Software Design example:
-```bash
-python agentverse_command/main_simulation_gui.py --task simulation/sde_team/sde_team_2players
-```
-
-[Wacth the Software Design Video](https://github.com/OpenBMB/AgentVerse/assets/11704492/5058066a-abee-490d-8659-b4e54661626a)
-
-
-#### [Database Administrator (DBA)](https://github.com/TsinghuaDatabaseGroup/DB-GPT)
-
-In the database diagnosis scenario, the Chief DBA monitors the system anomalies (e.g., slow queries, locks, crash down). If detected, the domain experts are alerted to analyze root causes, share insights, and suggest optimization solutions together. The Chief DBA then provides a summarized report to the user.
-
-```bash
-python agentverse_command/main_simulation_gui.py --task simulation/db_diag
-```
-
-[Wacth the DBA Video](https://github.com/OpenBMB/AgentVerse/assets/11704492/c633419d-afbb-47d4-bb12-6bb512e7af3a)
-
-#### [Text Evaluation (ChatEval)](https://github.com/chanchimin/ChatEval)
-In the context of the text evaluation scenario, we recommend users explore the [ChatEval](https://github.com/chanchimin/ChatEval) repo. They've implemented a multi-agent referee team on AgentVerse to assess the quality of text generated by different models. When given two distinct pieces of text, roles within ChatEval can autonomously debate the nuances and disparities, drawing upon their assigned personas, and subsequently provide their judgments. Experiments indicate that their referee team, enriched with diverse roles specified in [config.yaml](#2-configuring-the-agents), aligns more closely with human evaluations. This demo is built upon the [Fastchat](https://github.com/lm-sys/FastChat) repo, and we'd like to express our appreciation for their foundational work.
-
-
-[Wacth the ChatEval Video](https://github.com/OpenBMB/AgentVerse/assets/75533759/58f33468-f15b-4bac-ae01-8d0780019f85)
-
-#### Pokemon
-**Currently available only in [`release-0.1`](https://github.com/OpenBMB/AgentVerse/tree/release-0.1)**. In the game, agents can walk around the game world, and interact with one another. As a player, you take on the role of an agent and can engage with others at any time. There are 6 characters in the Pokémon environment who appeared in Pokemon Emerald: [May](https://bulbapedia.bulbagarden.net/wiki/May_(game)), [Professor Birch](https://bulbapedia.bulbagarden.net/wiki/Professor_Birch), [Steven Stone](https://bulbapedia.bulbagarden.net/wiki/Steven_Stone), [Maxie](https://bulbapedia.bulbagarden.net/wiki/Maxie), [Archie](https://bulbapedia.bulbagarden.net/wiki/Archie) and [Joseph](https://bulbapedia.bulbagarden.net/wiki/Mr._Stone).
-
-To launch the Pokemon game, first launch a local server with the following command:
-```bash
-uvicorn pokemon_server:app --reload --port 10002
-```
-Then open another terminal in the project's root path and run the following command:
-```bash
-cd ui
-# If you do not have npm installed, you need to install it before running the following commands
-# https://docs.npmjs.com/downloading-and-installing-node-js-and-npm
-# We have tested on npm@9.6.4, node@20.0.0
-npm install
-npm run watch
-```
-Wait for the compilation to complete, and have fun! (WASD for moving around, and SPACE for launching a conversation.)
-
-[Wacth the Pokemon Video](https://github.com/OpenBMB/AgentVerse/assets/11704492/4d07da68-f942-4205-b558-f155e95782e7)
-
-
-
-## Contents
-
-- [✨ Features](#-features)
-- [📰 What's New](#-whats-new)
-- [🌟 Join Us!](#-join-us)
- - [How Can You Contribute?](#how-can-you-contribute)
-- [🗓 Coming Soon](#-coming-soon)
-- [👾 Simple Demo Video](#-simple-demo-video)
- - [NLP Classroom](#nlp-classroom)
- - [Prisoner Dilemma](#prisoner-dilemma)
- - [Software Design](#software-design)
- - [Database Administrator (DBA)](#database-administrator-dba)
- - [Text Evaluation (ChatEval)](#text-evaluation-chateval)
- - [Pokemon](#pokemon)
-- [Contents](#contents)
-- [🚀 Getting Started](#-getting-started)
- - [Installation](#installation)
- - [Simulation CLI Example](#simulation-cli-example)
- - [Simulation Local Website Demo](#simulation-local-website-demo)
- - [Task-Solving CLI Example](#task-solving-cli-example)
-- [💡 Philosophy](#-philosophy)
- - [Environment](#environment)
- - [Agent](#agent)
-- [✍️ Customize Your Own Environment](#️-customize-your-own-environment)
- - [A Simple Example: Building a Classroom Environment](#a-simple-example-building-a-classroom-environment)
- - [1. Creating a Task Directory and Configuring the Environment](#1-creating-a-task-directory-and-configuring-the-environment)
- - [2. Configuring the Agents](#2-configuring-the-agents)
- - [3. Writing an Output Parser](#3-writing-an-output-parser)
- - [Customization Guide for More Complex Environments](#customization-guide-for-more-complex-environments)
-- [🔎 Examples](#-examples)
-- [Star History](#star-history)
-- [Citation](#citation)
-- [Contact](#contact)
-
-
-
-## 🚀 Getting Started
-
-### Installation
-
-```bash
-pip install -U agentverse
-```
-Or you can install the package by manually cloning the latest repository
-```bash
-git clone https://github.com/OpenBMB/AgentVerse.git --depth 1
-cd AgentVerse
-pip install -r requirements.txt
-```
-Some users have reported problems installing the `orjson` required by `gradio`. One simple workaround is to install it with Anaconda `conda install -c conda-forge orjson`.
-
-You also need to export your OpenAI API key as follows:
-```bash
-# Export your OpenAI API key
-export OPENAI_API_KEY="your_api_key_here"
-# Or if you are using Azure
-export AZURE_OPENAI_API_KEY="your_api_key_here"
-export AZURE_OPENAI_API_BASE="your_api_base_here"
-```
-
-If you want use Azure OpenAI services, pleas export your Azure OpenAI key and OpenAI API base as follows:
-```bash
-export AZURE_OPENAI_API_KEY="your_api_key_here"
-export AZURE_OPENAI_API_BASE="your_api_base_here"
-```
-
-If you want to use the tools provided by BMTools, you need to install BMTools as follows:
-```bash
-git clone git+https://github.com/OpenBMB/BMTools.git
-cd BMTools
-pip install -r requirements.txt
-python setup.py develop
-```
-
-
-
-
-### Simulation CLI Example
-
-You can create a multi-agent environments provided by us. Using the classroom scenario as an example. In this scenario, there are nine agents, one playing the role of a professor and the other eight as students.
-
-```shell
-python3 agentverse_command/main_simulation_cli.py --task simulation/nlp_classroom_9players
-# or if you have installed AgentVerse via pip
-agentverse-simulation --task simulation/nlp_classroom_9players
-```
-
-### Simulation Local Website Demo
-
-We also provide a local website demo for this environment. You can launch it with
-
-```shell
-python3 agentverse_command/main_simulation_gui.py --task simulation/nlp_classroom_9players
-# or if you have installed AgentVerse via pip
-agentverse-simulation-gui --task simulation/nlp_classroom_9players
-```
-After successfully launching the local server, you can visit [http://127.0.0.1:7860/](http://127.0.0.1:7860/) to view the classroom environment.
-
-### Task-Solving CLI Example
-
-To run the experiments with the task-solving environment proposed in our [paper](https://arxiv.org/abs/2308.10848), you can use the following command:
-
-```shell
-# Run the Humaneval benchmark using gpt-3.5-turbo
-python3 agentverse_command/main_tasksolving_cli.py --task tasksolving/humaneval/gpt-3.5 --dataset_path data/humaneval/test.jsonl --overwrite
-# or if you have installed AgentVerse via pip
-agentverse-tasksolving --task tasksolving/humaneval/gpt-3.5 --dataset_path data/humaneval/test.jsonl --overwrite
-```
-
-You can take a look at `agentverse/tasks/tasksolving` for more experiments we have done in our paper.
-
-
-## 💡 Philosophy
-
-### Environment
-
-At the core of our framework is the environment, which plays a crucial role in enabling researchers to study the behavior of agents under different conditions. We believe that the environment should be flexible and extensible, allowing researchers to easily customize it to fit their needs. To achieve this, we have abstracted the environment into five rule components, and implementing different environments is actually implementing different rules:
-
-- **Describer**: This component provides a description of the environment at each turn for each agent. You can customize the describer to define the specific requirements of their environment, such as the agents with whom an agent can interact.
-- **Order**: This component defines the order in which agents take actions within the environment. You can customize the order to reflect the desired interaction between agents. We provide several basic order options, including `random`, `sequential`, and `concurrent` (in which all agents take an action in each turn).
-- **Selector**: This component selects the valid messages generated by agents. Sometimes agents may generate invalid responses, and the selector is used to filter out unexpected results.
-- **Updater**: This component updates the memory of each agent. In certain cases, the response generated by one agent should not be seen by all agents (e.g., if agents are in different rooms). For each response, the updater updates only the agents who can see it.
-- **Visibility**: This component maintains the list of agents that each agent can see throughout the environment's changes. For example, when an agent moves from one room to another, the list of visible agents of each agent should be updated by `visibility`.
-
-By abstracting the environment into these five components, we have created a highly flexible and extensible framework that enables researchers to easily build and customize their own multi-agent environments.
-
-### Agent
-
-Another fundamental component is the agent. Currently we provide two types of agents: **ConversationAgent** and **ToolAgent**. You can also customize your own agent by inheriting BaseAgent class (tutorial coming soon).
-
-## ✍️ Customize Your Own Environment
-
-We have provided several examples in the `agentverse/tasks` directory. To customize your environment, you should
-
-1. Create a task directory in `agentverse/tasks`
-2. Write the configuration file
-3. Write the output parser that parses the response of your agents.
-4. Add your parser in `agentverse/tasks/__init__.py`
-
-We will use a simple example in `agentverse/tasks/nlp_classroom_3players` to illustrate the procedure.
-
-### A Simple Example: Building a Classroom Environment
-
-To illustrate how to customize your environment, we'll use a simple example of building a classroom environment where one agent is the professor, one is the student, and one is the teaching assistant.
-
-##### 1. Creating a Task Directory and Configuring the Environment
-
-First, we need to create a task directory and write our configuration file for the environment. In the `agentverse/tasks` directory, create a new directory called `nlp_classroom_3players`. Inside this directory, create a `config.yaml` file and write the following configuration:
-
-```yaml
-# config.yaml
-environment:
- env_type: basic # Use the basic environment provided in AgentVerse
- max_turns: 10 # Specify the maximum number of dialogue turns
- rule:
- order:
- type: sequential # Use the sequential order
- visibility:
- type: all # Each message can be seen by all agents
- selector:
- type: basic # Basic selector (do not select)
- updater:
- type: basic # Basic updater (update the message to all agents)
- describer:
- type: basic # Basic describer (no description)
-```
-
-This configuration specifies that we will use the basic environment provided in AgentVerse, with a maximum of 10 dialogue turns. We'll use the sequential order, with all messages visible to all agents. We won't be using any selectors, our updater will update the messages to all the agents and our describer will provide no description.
-
-##### 2. Configuring the Agents
-
-Next, we'll configure the agents. In the `config.yaml` file, we'll add the configuration for each agent. Here's an example configuration for the professor:
-
-```yaml
-# config.yaml
-agents:
- -
- agent_type: conversation
- name: Professor Micheal # Name of the agent
- role_description: You are Prof. Micheal, ... # Description of the agent
- memory:
- memory_type: chat_history # Will store all the chat history
- prompt_template: *professor_prompt
- llm:
- llm_type: text-davinci-003 # Will use OpenAICompletion LLM
- model: text-davinci-003 # The arguments passed to the api call
- temperature: 0.7
- max_tokens: 250
-```
-
-In this example, we'll use the `conversation` agent type. We've given the agent a name and a description, and we'll store the chat history in memory. We've also provided a prompt template with placeholders marked as ${placeholder}. These will be instantiated by the `_fill_prompt_template` method of the agent.
-
-##### 3. Writing an Output Parser
-
-The next step is to write a simple parser for your agent's response. Because you may have specified the output format in your prompt template, you need to provide a corresponding parser. In this example, we inform the model to output in the following format in our prompt template
-
-```
-Action: Speak
-Action Input: (the content)
-```
-
-We'll write a parser to extract the content from the agent's response. Refer to the code for more details. We've decorated our parser function with `@output_parser_registry.register('classroom_parser')` to register it with our framework. Finally, we import our parser in `agentverse/tasks/__init__.py`.
-
-With these steps, we've successfully built a simple classroom environment and customized it for our needs.
-
-### Customization Guide for More Complex Environments
-
-While we provide a basic framework for building environments with our five rule components, more complex environments may require further customization. A detailed documentation and tutorial is coming soon. Here we briefly introduce some steps you can take to customize your environment:
-
-1. **Customize the five rule components**. Each rule component has an interface, allowing you to customize its behavior to suit your specific needs. It's important to note that these components are not necessarily independent and can interact through the `rule_params` dictionary in the environment. You can create your own rule components and integrate them with the existing ones to build more complex interactions between agents.
-2. **Customize the environment itself**. Our `basic` environment provides a default execution order for the five rule components that is suitable for most cases, but you can inherit the `BaseEnvironment` class and write your own `run` method to implement a more sophisticated execution order.
-3. **Customize the agent**. Depending on your specific use case, you may also need to inherit the `BaseAgent` class. For example, you may want to use your local LLM as your agents or create agents with specialized knowledge or skills.
-
-
-
-## 🔎 Examples
-
-Currently, we offer some simple examples in the `agentverse/tasks` directory, each demonstrating different possibilities of our framework. While the performance of these examples may not be optimal due to limited prompt engineering, they are intended to showcase the capabilities of our framework, such as allowing the use of tools.
-
-Here's a brief overview of each example:
-
-1. `nlp_classroom_3players`: This example illustrates a simple case in which agents will speak in sequential order.
-2. `nlp_classroom_9players`: This is an NLP class example. Here, students can raise their hand when they have a question, and the professor can call on the students to let them ask. Students are only allowed to speak after they are called on.
-3. `nlp_classroom_9players_group`: This example showcases group discussions. The professor may initiate a group discussion when needed, and students can exclusively interact with fellow students within the same group during the discussion.
-4. `nlp_classroom_3players_withtool`: Students in this classroom can use Bing search API when listening to the class.
-5. `math_problem_2players_tools`: A simple example demonstrating how two agents can use the WolframAlpha API to play an arithmetic game.
-6. `prisoner_dilema`: The Prisoner's Dilemma is a thought experiment involving two rational agents facing a choice between cooperating for mutual benefit or betraying their partner for individual gain.
-7. `db_diag`: The Chief DBA monitors (agents) the database system for anomalies and alerts memory and CPU agents if any are detected. They (agents) analyze root causes and suggest optimization solutions. The Chief DBA (agent) provides a diagnosis summary to the user, who can give instructions or evaluate the proposed solutions' effectiveness.
-8. `sde_team`: In the SDE team, code writer, code tester and code reviewer collaborate on the code generation problem.
-9. `pokemon`: This example intimates Pokemon game.
-
-
-## Star History
-
-[](https://star-history.com/#OpenBMB/AgentVerse&Date)
-
-
-## Citation
-If you find this repo helpful, feel free to cite us.
-```
-@article{chen2023agentverse,
- title={Agentverse: Facilitating multi-agent collaboration and exploring emergent behaviors in agents},
- author={Chen, Weize and Su, Yusheng and Zuo, Jingwei and Yang, Cheng and Yuan, Chenfei and Qian, Chen and Chan, Chi-Min and Qin, Yujia and Lu, Yaxi and Xie, Ruobing and others},
- journal={arXiv preprint arXiv:2308.10848},
- year={2023}
-}
-```
-
-## Contact
-
-Weize Chen: chenweize1998@gmail.com
-
-[Yusheng Su](https://yushengsu-thu.github.io/): yushengsu.thu@gmail.com
\ No newline at end of file
diff --git a/spaces/AgentVerse/agentVerse/ui/src/phaser3-rex-plugins/templates/ui/colorinput/colorinput/methods/OpenColorPicker.js b/spaces/AgentVerse/agentVerse/ui/src/phaser3-rex-plugins/templates/ui/colorinput/colorinput/methods/OpenColorPicker.js
deleted file mode 100644
index 123c72353323009575fede993304ae21d56ce377..0000000000000000000000000000000000000000
--- a/spaces/AgentVerse/agentVerse/ui/src/phaser3-rex-plugins/templates/ui/colorinput/colorinput/methods/OpenColorPicker.js
+++ /dev/null
@@ -1,53 +0,0 @@
-import CreateColorPicker from './CreateColorPicker.js';
-import DropDown from '../../../dropdown/DropDown.js';
-
-var OpenColorPicker = function () {
- if (this.colorPicker) {
- return;
- }
-
- // Layout it to get full height
- var colorPicker = CreateColorPicker.call(this).layout();
-
- var dropDownBehavior = new DropDown(colorPicker, {
- // Transition
- duration: {
- in: this.colorPickerEaseInDuration,
- out: this.colorPickerEaseOutDuration
- },
- transitIn: this.colorPickerTransitInCallback,
- transitOut: this.colorPickerTransitOutCallback,
-
- // Position
- expandDirection: this.colorPickerExpandDirection,
-
- alignTargetX: this,
- alignTargetY: this,
-
- bounds: this.colorPickerBounds,
-
- // Close condition
- touchOutsideClose: true,
- })
- .on('open', function () {
- // After popping up
- // Can click
- colorPicker.on('valuechange', function (value) {
- this.setValue(value);
- }, this);
- }, this)
-
- .on('close', function () {
- this.colorPicker = undefined;
- this.dropDownBehavior = undefined;
- }, this)
-
- this.colorPicker = colorPicker;
- this.dropDownBehavior = dropDownBehavior;
-
- this.pin(colorPicker);
-
- return this;
-}
-
-export default OpenColorPicker;
\ No newline at end of file
diff --git a/spaces/AgentVerse/agentVerse/ui/src/phaser3-rex-plugins/templates/ui/sides/childbehaviors/Visible.js b/spaces/AgentVerse/agentVerse/ui/src/phaser3-rex-plugins/templates/ui/sides/childbehaviors/Visible.js
deleted file mode 100644
index b0c1659608c980c21d85e60701b15d4acade3984..0000000000000000000000000000000000000000
--- a/spaces/AgentVerse/agentVerse/ui/src/phaser3-rex-plugins/templates/ui/sides/childbehaviors/Visible.js
+++ /dev/null
@@ -1,21 +0,0 @@
-import IndexOf from '../../../../plugins/utils/object/IndexOf.js';
-import Container from '../../container/Container.js';
-
-const ContainerSetChildVisible = Container.prototype.setChildVisible;
-
-export default {
- setChildVisible(child, visible) {
- var key;
- if (typeof (child) === 'string') {
- var key = child;
- child = this.sizerChildren[key];
- } else {
- key = IndexOf(this.sizerChildren, child);
- }
- if (visible === undefined) {
- visible = (this.currentChildKey === key) ? true : false;
- }
- ContainerSetChildVisible.call(this, child, visible);
- return this;
- }
-}
\ No newline at end of file
diff --git a/spaces/Androidonnxfork/CivitAi-to-Diffusers/diffusers/docs/source/en/optimization/tome.md b/spaces/Androidonnxfork/CivitAi-to-Diffusers/diffusers/docs/source/en/optimization/tome.md
deleted file mode 100644
index c2158f539a65d87a9a394298f22c20fa87898d8b..0000000000000000000000000000000000000000
--- a/spaces/Androidonnxfork/CivitAi-to-Diffusers/diffusers/docs/source/en/optimization/tome.md
+++ /dev/null
@@ -1,116 +0,0 @@
-
-
-# Token Merging
-
-Token Merging (introduced in [Token Merging: Your ViT But Faster](https://arxiv.org/abs/2210.09461)) works by merging the redundant tokens / patches progressively in the forward pass of a Transformer-based network. It can speed up the inference latency of the underlying network.
-
-After Token Merging (ToMe) was released, the authors released [Token Merging for Fast Stable Diffusion](https://arxiv.org/abs/2303.17604), which introduced a version of ToMe which is more compatible with Stable Diffusion. We can use ToMe to gracefully speed up the inference latency of a [`DiffusionPipeline`]. This doc discusses how to apply ToMe to the [`StableDiffusionPipeline`], the expected speedups, and the qualitative aspects of using ToMe on the [`StableDiffusionPipeline`].
-
-## Using ToMe
-
-The authors of ToMe released a convenient Python library called [`tomesd`](https://github.com/dbolya/tomesd) that lets us apply ToMe to a [`DiffusionPipeline`] like so:
-
-```diff
-from diffusers import StableDiffusionPipeline
-import tomesd
-
-pipeline = StableDiffusionPipeline.from_pretrained(
- "runwayml/stable-diffusion-v1-5", torch_dtype=torch.float16
-).to("cuda")
-+ tomesd.apply_patch(pipeline, ratio=0.5)
-
-image = pipeline("a photo of an astronaut riding a horse on mars").images[0]
-```
-
-And that’s it!
-
-`tomesd.apply_patch()` exposes [a number of arguments](https://github.com/dbolya/tomesd#usage) to let us strike a balance between the pipeline inference speed and the quality of the generated tokens. Amongst those arguments, the most important one is `ratio`. `ratio` controls the number of tokens that will be merged during the forward pass. For more details on `tomesd`, please refer to the original repository https://github.com/dbolya/tomesd and [the paper](https://arxiv.org/abs/2303.17604).
-
-## Benchmarking `tomesd` with `StableDiffusionPipeline`
-
-We benchmarked the impact of using `tomesd` on [`StableDiffusionPipeline`] along with [xformers](https://huggingface.co/docs/diffusers/optimization/xformers) across different image resolutions. We used A100 and V100 as our test GPU devices with the following development environment (with Python 3.8.5):
-
-```bash
-- `diffusers` version: 0.15.1
-- Python version: 3.8.16
-- PyTorch version (GPU?): 1.13.1+cu116 (True)
-- Huggingface_hub version: 0.13.2
-- Transformers version: 4.27.2
-- Accelerate version: 0.18.0
-- xFormers version: 0.0.16
-- tomesd version: 0.1.2
-```
-
-We used this script for benchmarking: [https://gist.github.com/sayakpaul/27aec6bca7eb7b0e0aa4112205850335](https://gist.github.com/sayakpaul/27aec6bca7eb7b0e0aa4112205850335). Following are our findings:
-
-### A100
-
-| Resolution | Batch size | Vanilla | ToMe | ToMe + xFormers | ToMe speedup (%) | ToMe + xFormers speedup (%) |
-| --- | --- | --- | --- | --- | --- | --- |
-| 512 | 10 | 6.88 | 5.26 | 4.69 | 23.54651163 | 31.83139535 |
-| | | | | | | |
-| 768 | 10 | OOM | 14.71 | 11 | | |
-| | 8 | OOM | 11.56 | 8.84 | | |
-| | 4 | OOM | 5.98 | 4.66 | | |
-| | 2 | 4.99 | 3.24 | 3.1 | 35.07014028 | 37.8757515 |
-| | 1 | 3.29 | 2.24 | 2.03 | 31.91489362 | 38.29787234 |
-| | | | | | | |
-| 1024 | 10 | OOM | OOM | OOM | | |
-| | 8 | OOM | OOM | OOM | | |
-| | 4 | OOM | 12.51 | 9.09 | | |
-| | 2 | OOM | 6.52 | 4.96 | | |
-| | 1 | 6.4 | 3.61 | 2.81 | 43.59375 | 56.09375 |
-
-***The timings reported here are in seconds. Speedups are calculated over the `Vanilla` timings.***
-
-### V100
-
-| Resolution | Batch size | Vanilla | ToMe | ToMe + xFormers | ToMe speedup (%) | ToMe + xFormers speedup (%) |
-| --- | --- | --- | --- | --- | --- | --- |
-| 512 | 10 | OOM | 10.03 | 9.29 | | |
-| | 8 | OOM | 8.05 | 7.47 | | |
-| | 4 | 5.7 | 4.3 | 3.98 | 24.56140351 | 30.1754386 |
-| | 2 | 3.14 | 2.43 | 2.27 | 22.61146497 | 27.70700637 |
-| | 1 | 1.88 | 1.57 | 1.57 | 16.4893617 | 16.4893617 |
-| | | | | | | |
-| 768 | 10 | OOM | OOM | 23.67 | | |
-| | 8 | OOM | OOM | 18.81 | | |
-| | 4 | OOM | 11.81 | 9.7 | | |
-| | 2 | OOM | 6.27 | 5.2 | | |
-| | 1 | 5.43 | 3.38 | 2.82 | 37.75322284 | 48.06629834 |
-| | | | | | | |
-| 1024 | 10 | OOM | OOM | OOM | | |
-| | 8 | OOM | OOM | OOM | | |
-| | 4 | OOM | OOM | 19.35 | | |
-| | 2 | OOM | 13 | 10.78 | | |
-| | 1 | OOM | 6.66 | 5.54 | | |
-
-As seen in the tables above, the speedup with `tomesd` becomes more pronounced for larger image resolutions. It is also interesting to note that with `tomesd`, it becomes possible to run the pipeline on a higher resolution, like 1024x1024.
-
-It might be possible to speed up inference even further with [`torch.compile()`](https://huggingface.co/docs/diffusers/optimization/torch2.0).
-
-## Quality
-
-As reported in [the paper](https://arxiv.org/abs/2303.17604), ToMe can preserve the quality of the generated images to a great extent while speeding up inference. By increasing the `ratio`, it is possible to further speed up inference, but that might come at the cost of a deterioration in the image quality.
-
-To test the quality of the generated samples using our setup, we sampled a few prompts from the “Parti Prompts” (introduced in [Parti](https://parti.research.google/)) and performed inference with the [`StableDiffusionPipeline`] in the following settings:
-
-- Vanilla [`StableDiffusionPipeline`]
-- [`StableDiffusionPipeline`] + ToMe
-- [`StableDiffusionPipeline`] + ToMe + xformers
-
-We didn’t notice any significant decrease in the quality of the generated samples. Here are samples:
-
-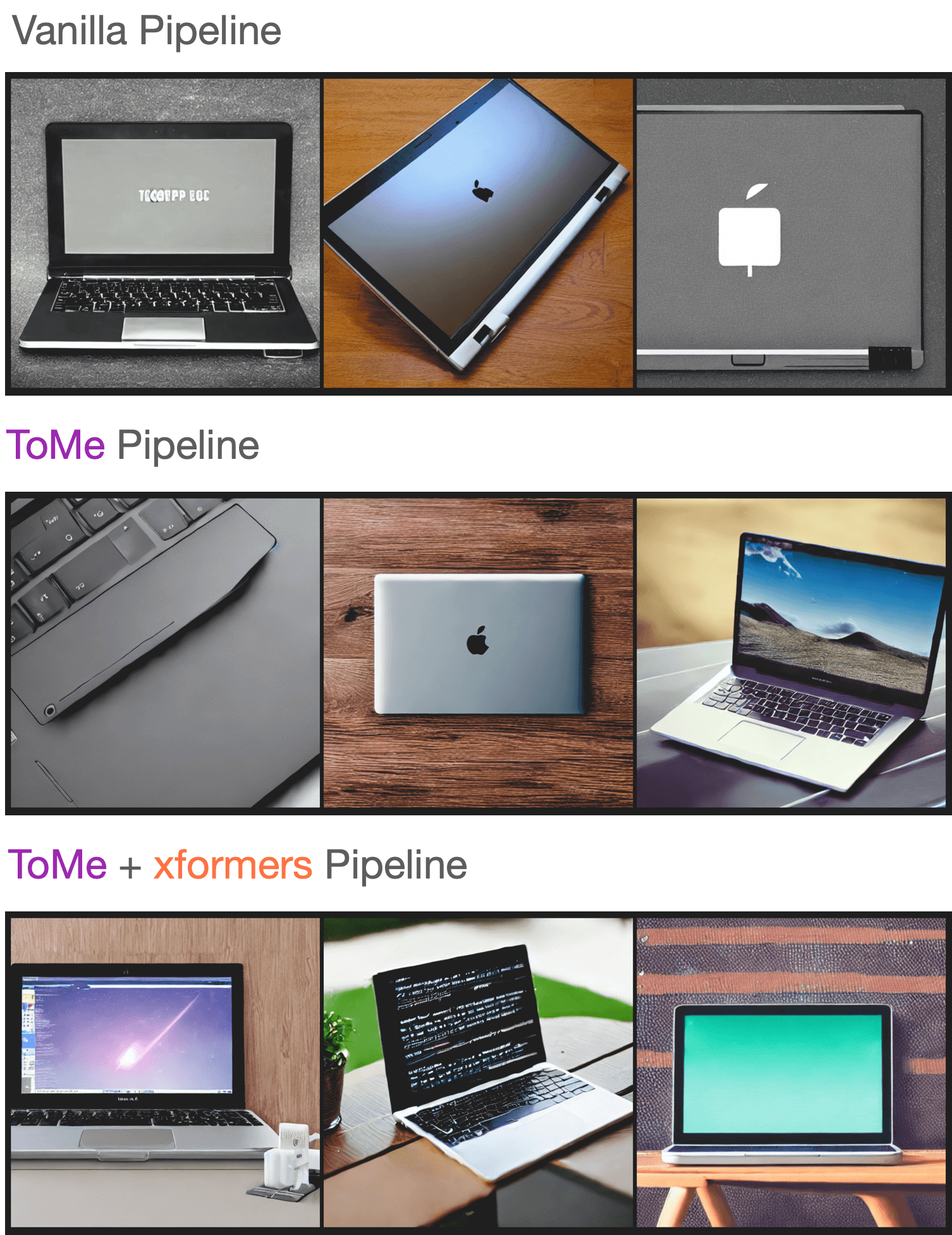
-
-You can check out the generated samples [here](https://wandb.ai/sayakpaul/tomesd-results/runs/23j4bj3i?workspace=). We used [this script](https://gist.github.com/sayakpaul/8cac98d7f22399085a060992f411ecbd) for conducting this experiment.
\ No newline at end of file
diff --git a/spaces/Androidonnxfork/CivitAi-to-Diffusers/diffusers/docs/source/ko/optimization/onnx.md b/spaces/Androidonnxfork/CivitAi-to-Diffusers/diffusers/docs/source/ko/optimization/onnx.md
deleted file mode 100644
index d52110b8c1fbd4b09614ce5b76e79e136b71e959..0000000000000000000000000000000000000000
--- a/spaces/Androidonnxfork/CivitAi-to-Diffusers/diffusers/docs/source/ko/optimization/onnx.md
+++ /dev/null
@@ -1,65 +0,0 @@
-
-
-
-# 추론을 위해 ONNX 런타임을 사용하는 방법
-
-🤗 Diffusers는 ONNX Runtime과 호환되는 Stable Diffusion 파이프라인을 제공합니다. 이를 통해 ONNX(CPU 포함)를 지원하고 PyTorch의 가속 버전을 사용할 수 없는 모든 하드웨어에서 Stable Diffusion을 실행할 수 있습니다.
-
-## 설치
-
-다음 명령어로 ONNX Runtime를 지원하는 🤗 Optimum를 설치합니다:
-
-```
-pip install optimum["onnxruntime"]
-```
-
-## Stable Diffusion 추론
-
-아래 코드는 ONNX 런타임을 사용하는 방법을 보여줍니다. `StableDiffusionPipeline` 대신 `OnnxStableDiffusionPipeline`을 사용해야 합니다.
-PyTorch 모델을 불러오고 즉시 ONNX 형식으로 변환하려는 경우 `export=True`로 설정합니다.
-
-```python
-from optimum.onnxruntime import ORTStableDiffusionPipeline
-
-model_id = "runwayml/stable-diffusion-v1-5"
-pipe = ORTStableDiffusionPipeline.from_pretrained(model_id, export=True)
-prompt = "a photo of an astronaut riding a horse on mars"
-images = pipe(prompt).images[0]
-pipe.save_pretrained("./onnx-stable-diffusion-v1-5")
-```
-
-파이프라인을 ONNX 형식으로 오프라인으로 내보내고 나중에 추론에 사용하려는 경우,
-[`optimum-cli export`](https://huggingface.co/docs/optimum/main/en/exporters/onnx/usage_guides/export_a_model#exporting-a-model-to-onnx-using-the-cli) 명령어를 사용할 수 있습니다:
-
-```bash
-optimum-cli export onnx --model runwayml/stable-diffusion-v1-5 sd_v15_onnx/
-```
-
-그 다음 추론을 수행합니다:
-
-```python
-from optimum.onnxruntime import ORTStableDiffusionPipeline
-
-model_id = "sd_v15_onnx"
-pipe = ORTStableDiffusionPipeline.from_pretrained(model_id)
-prompt = "a photo of an astronaut riding a horse on mars"
-images = pipe(prompt).images[0]
-```
-
-Notice that we didn't have to specify `export=True` above.
-
-[Optimum 문서](https://huggingface.co/docs/optimum/)에서 더 많은 예시를 찾을 수 있습니다.
-
-## 알려진 이슈들
-
-- 여러 프롬프트를 배치로 생성하면 너무 많은 메모리가 사용되는 것 같습니다. 이를 조사하는 동안, 배치 대신 반복 방법이 필요할 수도 있습니다.
diff --git a/spaces/Androidonnxfork/CivitAi-to-Diffusers/diffusers/src/diffusers/utils/outputs.py b/spaces/Androidonnxfork/CivitAi-to-Diffusers/diffusers/src/diffusers/utils/outputs.py
deleted file mode 100644
index 37b11561d1e1ee5d5cb40c7630b132e1f451c5b0..0000000000000000000000000000000000000000
--- a/spaces/Androidonnxfork/CivitAi-to-Diffusers/diffusers/src/diffusers/utils/outputs.py
+++ /dev/null
@@ -1,108 +0,0 @@
-# Copyright 2023 The HuggingFace Team. All rights reserved.
-#
-# Licensed under the Apache License, Version 2.0 (the "License");
-# you may not use this file except in compliance with the License.
-# You may obtain a copy of the License at
-#
-# http://www.apache.org/licenses/LICENSE-2.0
-#
-# Unless required by applicable law or agreed to in writing, software
-# distributed under the License is distributed on an "AS IS" BASIS,
-# WITHOUT WARRANTIES OR CONDITIONS OF ANY KIND, either express or implied.
-# See the License for the specific language governing permissions and
-# limitations under the License.
-"""
-Generic utilities
-"""
-
-from collections import OrderedDict
-from dataclasses import fields
-from typing import Any, Tuple
-
-import numpy as np
-
-from .import_utils import is_torch_available
-
-
-def is_tensor(x):
- """
- Tests if `x` is a `torch.Tensor` or `np.ndarray`.
- """
- if is_torch_available():
- import torch
-
- if isinstance(x, torch.Tensor):
- return True
-
- return isinstance(x, np.ndarray)
-
-
-class BaseOutput(OrderedDict):
- """
- Base class for all model outputs as dataclass. Has a `__getitem__` that allows indexing by integer or slice (like a
- tuple) or strings (like a dictionary) that will ignore the `None` attributes. Otherwise behaves like a regular
- Python dictionary.
-
-
-
- You can't unpack a [`BaseOutput`] directly. Use the [`~utils.BaseOutput.to_tuple`] method to convert it to a tuple
- first.
-
-
- """
-
- def __post_init__(self):
- class_fields = fields(self)
-
- # Safety and consistency checks
- if not len(class_fields):
- raise ValueError(f"{self.__class__.__name__} has no fields.")
-
- first_field = getattr(self, class_fields[0].name)
- other_fields_are_none = all(getattr(self, field.name) is None for field in class_fields[1:])
-
- if other_fields_are_none and isinstance(first_field, dict):
- for key, value in first_field.items():
- self[key] = value
- else:
- for field in class_fields:
- v = getattr(self, field.name)
- if v is not None:
- self[field.name] = v
-
- def __delitem__(self, *args, **kwargs):
- raise Exception(f"You cannot use ``__delitem__`` on a {self.__class__.__name__} instance.")
-
- def setdefault(self, *args, **kwargs):
- raise Exception(f"You cannot use ``setdefault`` on a {self.__class__.__name__} instance.")
-
- def pop(self, *args, **kwargs):
- raise Exception(f"You cannot use ``pop`` on a {self.__class__.__name__} instance.")
-
- def update(self, *args, **kwargs):
- raise Exception(f"You cannot use ``update`` on a {self.__class__.__name__} instance.")
-
- def __getitem__(self, k):
- if isinstance(k, str):
- inner_dict = dict(self.items())
- return inner_dict[k]
- else:
- return self.to_tuple()[k]
-
- def __setattr__(self, name, value):
- if name in self.keys() and value is not None:
- # Don't call self.__setitem__ to avoid recursion errors
- super().__setitem__(name, value)
- super().__setattr__(name, value)
-
- def __setitem__(self, key, value):
- # Will raise a KeyException if needed
- super().__setitem__(key, value)
- # Don't call self.__setattr__ to avoid recursion errors
- super().__setattr__(key, value)
-
- def to_tuple(self) -> Tuple[Any]:
- """
- Convert self to a tuple containing all the attributes/keys that are not `None`.
- """
- return tuple(self[k] for k in self.keys())
diff --git a/spaces/Andy1621/uniformer_image_detection/configs/fcos/fcos_r50_caffe_fpn_gn-head_4x4_1x_coco.py b/spaces/Andy1621/uniformer_image_detection/configs/fcos/fcos_r50_caffe_fpn_gn-head_4x4_1x_coco.py
deleted file mode 100644
index 2816b16f64dbcbfecd779650aaae0ca6cee0d810..0000000000000000000000000000000000000000
--- a/spaces/Andy1621/uniformer_image_detection/configs/fcos/fcos_r50_caffe_fpn_gn-head_4x4_1x_coco.py
+++ /dev/null
@@ -1,4 +0,0 @@
-# TODO: Remove this config after benchmarking all related configs
-_base_ = 'fcos_r50_caffe_fpn_gn-head_1x_coco.py'
-
-data = dict(samples_per_gpu=4, workers_per_gpu=4)
diff --git a/spaces/AquaSuisei/ChatGPTXE/chatgpt - macOS.command b/spaces/AquaSuisei/ChatGPTXE/chatgpt - macOS.command
deleted file mode 100644
index fa015edca9e6916f24394813ce8ba77d2072e296..0000000000000000000000000000000000000000
--- a/spaces/AquaSuisei/ChatGPTXE/chatgpt - macOS.command
+++ /dev/null
@@ -1,7 +0,0 @@
-#!/bin/bash
-echo Opening ChuanhuChatGPT...
-cd "$(dirname "${BASH_SOURCE[0]}")"
-nohup python3 ChuanhuChatbot.py >/dev/null 2>&1 &
-sleep 5
-open http://127.0.0.1:7860
-echo Finished opening ChuanhuChatGPT (http://127.0.0.1:7860/). If you kill ChuanhuChatbot, Use "pkill -f 'ChuanhuChatbot'" command in terminal.
\ No newline at end of file
diff --git a/spaces/ArcanAlt/arcanDream/Dockerfile b/spaces/ArcanAlt/arcanDream/Dockerfile
deleted file mode 100644
index 6953fc05439efb70991552cf56f28365b5b6c15b..0000000000000000000000000000000000000000
--- a/spaces/ArcanAlt/arcanDream/Dockerfile
+++ /dev/null
@@ -1,11 +0,0 @@
-FROM node:18
-
-WORKDIR /app
-
-RUN npm install express express-http-proxy
-
-COPY . .
-
-EXPOSE 7860
-
-CMD [ "node", "server.js" ]
\ No newline at end of file
diff --git a/spaces/Big-Web/MMSD/env/Lib/site-packages/dateutil/zoneinfo/__init__.py b/spaces/Big-Web/MMSD/env/Lib/site-packages/dateutil/zoneinfo/__init__.py
deleted file mode 100644
index 34f11ad66c88047f2c049a4cdcc937b4b78ea6d6..0000000000000000000000000000000000000000
--- a/spaces/Big-Web/MMSD/env/Lib/site-packages/dateutil/zoneinfo/__init__.py
+++ /dev/null
@@ -1,167 +0,0 @@
-# -*- coding: utf-8 -*-
-import warnings
-import json
-
-from tarfile import TarFile
-from pkgutil import get_data
-from io import BytesIO
-
-from dateutil.tz import tzfile as _tzfile
-
-__all__ = ["get_zonefile_instance", "gettz", "gettz_db_metadata"]
-
-ZONEFILENAME = "dateutil-zoneinfo.tar.gz"
-METADATA_FN = 'METADATA'
-
-
-class tzfile(_tzfile):
- def __reduce__(self):
- return (gettz, (self._filename,))
-
-
-def getzoneinfofile_stream():
- try:
- return BytesIO(get_data(__name__, ZONEFILENAME))
- except IOError as e: # TODO switch to FileNotFoundError?
- warnings.warn("I/O error({0}): {1}".format(e.errno, e.strerror))
- return None
-
-
-class ZoneInfoFile(object):
- def __init__(self, zonefile_stream=None):
- if zonefile_stream is not None:
- with TarFile.open(fileobj=zonefile_stream) as tf:
- self.zones = {zf.name: tzfile(tf.extractfile(zf), filename=zf.name)
- for zf in tf.getmembers()
- if zf.isfile() and zf.name != METADATA_FN}
- # deal with links: They'll point to their parent object. Less
- # waste of memory
- links = {zl.name: self.zones[zl.linkname]
- for zl in tf.getmembers() if
- zl.islnk() or zl.issym()}
- self.zones.update(links)
- try:
- metadata_json = tf.extractfile(tf.getmember(METADATA_FN))
- metadata_str = metadata_json.read().decode('UTF-8')
- self.metadata = json.loads(metadata_str)
- except KeyError:
- # no metadata in tar file
- self.metadata = None
- else:
- self.zones = {}
- self.metadata = None
-
- def get(self, name, default=None):
- """
- Wrapper for :func:`ZoneInfoFile.zones.get`. This is a convenience method
- for retrieving zones from the zone dictionary.
-
- :param name:
- The name of the zone to retrieve. (Generally IANA zone names)
-
- :param default:
- The value to return in the event of a missing key.
-
- .. versionadded:: 2.6.0
-
- """
- return self.zones.get(name, default)
-
-
-# The current API has gettz as a module function, although in fact it taps into
-# a stateful class. So as a workaround for now, without changing the API, we
-# will create a new "global" class instance the first time a user requests a
-# timezone. Ugly, but adheres to the api.
-#
-# TODO: Remove after deprecation period.
-_CLASS_ZONE_INSTANCE = []
-
-
-def get_zonefile_instance(new_instance=False):
- """
- This is a convenience function which provides a :class:`ZoneInfoFile`
- instance using the data provided by the ``dateutil`` package. By default, it
- caches a single instance of the ZoneInfoFile object and returns that.
-
- :param new_instance:
- If ``True``, a new instance of :class:`ZoneInfoFile` is instantiated and
- used as the cached instance for the next call. Otherwise, new instances
- are created only as necessary.
-
- :return:
- Returns a :class:`ZoneInfoFile` object.
-
- .. versionadded:: 2.6
- """
- if new_instance:
- zif = None
- else:
- zif = getattr(get_zonefile_instance, '_cached_instance', None)
-
- if zif is None:
- zif = ZoneInfoFile(getzoneinfofile_stream())
-
- get_zonefile_instance._cached_instance = zif
-
- return zif
-
-
-def gettz(name):
- """
- This retrieves a time zone from the local zoneinfo tarball that is packaged
- with dateutil.
-
- :param name:
- An IANA-style time zone name, as found in the zoneinfo file.
-
- :return:
- Returns a :class:`dateutil.tz.tzfile` time zone object.
-
- .. warning::
- It is generally inadvisable to use this function, and it is only
- provided for API compatibility with earlier versions. This is *not*
- equivalent to ``dateutil.tz.gettz()``, which selects an appropriate
- time zone based on the inputs, favoring system zoneinfo. This is ONLY
- for accessing the dateutil-specific zoneinfo (which may be out of
- date compared to the system zoneinfo).
-
- .. deprecated:: 2.6
- If you need to use a specific zoneinfofile over the system zoneinfo,
- instantiate a :class:`dateutil.zoneinfo.ZoneInfoFile` object and call
- :func:`dateutil.zoneinfo.ZoneInfoFile.get(name)` instead.
-
- Use :func:`get_zonefile_instance` to retrieve an instance of the
- dateutil-provided zoneinfo.
- """
- warnings.warn("zoneinfo.gettz() will be removed in future versions, "
- "to use the dateutil-provided zoneinfo files, instantiate a "
- "ZoneInfoFile object and use ZoneInfoFile.zones.get() "
- "instead. See the documentation for details.",
- DeprecationWarning)
-
- if len(_CLASS_ZONE_INSTANCE) == 0:
- _CLASS_ZONE_INSTANCE.append(ZoneInfoFile(getzoneinfofile_stream()))
- return _CLASS_ZONE_INSTANCE[0].zones.get(name)
-
-
-def gettz_db_metadata():
- """ Get the zonefile metadata
-
- See `zonefile_metadata`_
-
- :returns:
- A dictionary with the database metadata
-
- .. deprecated:: 2.6
- See deprecation warning in :func:`zoneinfo.gettz`. To get metadata,
- query the attribute ``zoneinfo.ZoneInfoFile.metadata``.
- """
- warnings.warn("zoneinfo.gettz_db_metadata() will be removed in future "
- "versions, to use the dateutil-provided zoneinfo files, "
- "ZoneInfoFile object and query the 'metadata' attribute "
- "instead. See the documentation for details.",
- DeprecationWarning)
-
- if len(_CLASS_ZONE_INSTANCE) == 0:
- _CLASS_ZONE_INSTANCE.append(ZoneInfoFile(getzoneinfofile_stream()))
- return _CLASS_ZONE_INSTANCE[0].metadata
diff --git a/spaces/Big-Web/MMSD/env/Lib/site-packages/setuptools/command/setopt.py b/spaces/Big-Web/MMSD/env/Lib/site-packages/setuptools/command/setopt.py
deleted file mode 100644
index 6358c0451b2d0036e3821d897fb6f7ab436ee4a9..0000000000000000000000000000000000000000
--- a/spaces/Big-Web/MMSD/env/Lib/site-packages/setuptools/command/setopt.py
+++ /dev/null
@@ -1,149 +0,0 @@
-from distutils.util import convert_path
-from distutils import log
-from distutils.errors import DistutilsOptionError
-import distutils
-import os
-import configparser
-
-from setuptools import Command
-
-__all__ = ['config_file', 'edit_config', 'option_base', 'setopt']
-
-
-def config_file(kind="local"):
- """Get the filename of the distutils, local, global, or per-user config
-
- `kind` must be one of "local", "global", or "user"
- """
- if kind == 'local':
- return 'setup.cfg'
- if kind == 'global':
- return os.path.join(
- os.path.dirname(distutils.__file__), 'distutils.cfg'
- )
- if kind == 'user':
- dot = os.name == 'posix' and '.' or ''
- return os.path.expanduser(convert_path("~/%spydistutils.cfg" % dot))
- raise ValueError(
- "config_file() type must be 'local', 'global', or 'user'", kind
- )
-
-
-def edit_config(filename, settings, dry_run=False):
- """Edit a configuration file to include `settings`
-
- `settings` is a dictionary of dictionaries or ``None`` values, keyed by
- command/section name. A ``None`` value means to delete the entire section,
- while a dictionary lists settings to be changed or deleted in that section.
- A setting of ``None`` means to delete that setting.
- """
- log.debug("Reading configuration from %s", filename)
- opts = configparser.RawConfigParser()
- opts.optionxform = lambda x: x
- opts.read([filename])
- for section, options in settings.items():
- if options is None:
- log.info("Deleting section [%s] from %s", section, filename)
- opts.remove_section(section)
- else:
- if not opts.has_section(section):
- log.debug("Adding new section [%s] to %s", section, filename)
- opts.add_section(section)
- for option, value in options.items():
- if value is None:
- log.debug(
- "Deleting %s.%s from %s",
- section, option, filename
- )
- opts.remove_option(section, option)
- if not opts.options(section):
- log.info("Deleting empty [%s] section from %s",
- section, filename)
- opts.remove_section(section)
- else:
- log.debug(
- "Setting %s.%s to %r in %s",
- section, option, value, filename
- )
- opts.set(section, option, value)
-
- log.info("Writing %s", filename)
- if not dry_run:
- with open(filename, 'w') as f:
- opts.write(f)
-
-
-class option_base(Command):
- """Abstract base class for commands that mess with config files"""
-
- user_options = [
- ('global-config', 'g',
- "save options to the site-wide distutils.cfg file"),
- ('user-config', 'u',
- "save options to the current user's pydistutils.cfg file"),
- ('filename=', 'f',
- "configuration file to use (default=setup.cfg)"),
- ]
-
- boolean_options = [
- 'global-config', 'user-config',
- ]
-
- def initialize_options(self):
- self.global_config = None
- self.user_config = None
- self.filename = None
-
- def finalize_options(self):
- filenames = []
- if self.global_config:
- filenames.append(config_file('global'))
- if self.user_config:
- filenames.append(config_file('user'))
- if self.filename is not None:
- filenames.append(self.filename)
- if not filenames:
- filenames.append(config_file('local'))
- if len(filenames) > 1:
- raise DistutilsOptionError(
- "Must specify only one configuration file option",
- filenames
- )
- self.filename, = filenames
-
-
-class setopt(option_base):
- """Save command-line options to a file"""
-
- description = "set an option in setup.cfg or another config file"
-
- user_options = [
- ('command=', 'c', 'command to set an option for'),
- ('option=', 'o', 'option to set'),
- ('set-value=', 's', 'value of the option'),
- ('remove', 'r', 'remove (unset) the value'),
- ] + option_base.user_options
-
- boolean_options = option_base.boolean_options + ['remove']
-
- def initialize_options(self):
- option_base.initialize_options(self)
- self.command = None
- self.option = None
- self.set_value = None
- self.remove = None
-
- def finalize_options(self):
- option_base.finalize_options(self)
- if self.command is None or self.option is None:
- raise DistutilsOptionError("Must specify --command *and* --option")
- if self.set_value is None and not self.remove:
- raise DistutilsOptionError("Must specify --set-value or --remove")
-
- def run(self):
- edit_config(
- self.filename, {
- self.command: {self.option.replace('-', '_'): self.set_value}
- },
- self.dry_run
- )
diff --git a/spaces/CVPR/LIVE/thrust/thrust/system/cuda/detail/reverse.h b/spaces/CVPR/LIVE/thrust/thrust/system/cuda/detail/reverse.h
deleted file mode 100644
index 955825217d0857720bccfe0241704b679f80504f..0000000000000000000000000000000000000000
--- a/spaces/CVPR/LIVE/thrust/thrust/system/cuda/detail/reverse.h
+++ /dev/null
@@ -1,98 +0,0 @@
-/******************************************************************************
- * Copyright (c) 2016, NVIDIA CORPORATION. All rights reserved.
- *
- * Redistribution and use in source and binary forms, with or without
- * modification, are permitted provided that the following conditions are met:
- * * Redistributions of source code must retain the above copyright
- * notice, this list of conditions and the following disclaimer.
- * * Redistributions in binary form must reproduce the above copyright
- * notice, this list of conditions and the following disclaimer in the
- * documentation and/or other materials provided with the distribution.
- * * Neither the name of the NVIDIA CORPORATION nor the
- * names of its contributors may be used to endorse or promote products
- * derived from this software without specific prior written permission.
- *
- * THIS SOFTWARE IS PROVIDED BY THE COPYRIGHT HOLDERS AND CONTRIBUTORS "AS IS"
- * AND ANY EXPRESS OR IMPLIED WARRANTIES, INCLUDING, BUT NOT LIMITED TO, THE
- * IMPLIED WARRANTIES OF MERCHANTABILITY AND FITNESS FOR A PARTICULAR PURPOSE
- * ARE DISCLAIMED. IN NO EVENT SHALL NVIDIA CORPORATION BE LIABLE FOR ANY
- * DIRECT, INDIRECT, INCIDENTAL, SPECIAL, EXEMPLARY, OR CONSEQUENTIAL DAMAGES
- * (INCLUDING, BUT NOT LIMITED TO, PROCUREMENT OF SUBSTITUTE GOODS OR SERVICES;
- * LOSS OF USE, DATA, OR PROFITS; OR BUSINESS INTERRUPTION) HOWEVER CAUSED AND
- * ON ANY THEORY OF LIABILITY, WHETHER IN CONTRACT, STRICT LIABILITY, OR TORT
- * (INCLUDING NEGLIGENCE OR OTHERWISE) ARISING IN ANY WAY OUT OF THE USE OF THIS
- * SOFTWARE, EVEN IF ADVISED OF THE POSSIBILITY OF SUCH DAMAGE.
- *
- ******************************************************************************/
-#pragma once
-
-
-#if THRUST_DEVICE_COMPILER == THRUST_DEVICE_COMPILER_NVCC
-#include
-
-namespace thrust
-{
-namespace cuda_cub {
-
-template
-ResultIt __host__ __device__
-reverse_copy(execution_policy &policy,
- ItemsIt first,
- ItemsIt last,
- ResultIt result);
-
-template
-void __host__ __device__
-reverse(execution_policy &policy,
- ItemsIt first,
- ItemsIt last);
-
-} // namespace cuda_cub
-} // end namespace thrust
-
-#include
-#include
-#include
-#include
-#include
-
-namespace thrust
-{
-namespace cuda_cub {
-
-template
-ResultIt __host__ __device__
-reverse_copy(execution_policy &policy,
- ItemsIt first,
- ItemsIt last,
- ResultIt result)
-{
- return cuda_cub::copy(policy,
- make_reverse_iterator(last),
- make_reverse_iterator(first),
- result);
-}
-
-template
-void __host__ __device__
-reverse(execution_policy &policy,
- ItemsIt first,
- ItemsIt last)
-{
- typedef typename thrust::iterator_difference::type difference_type;
-
- // find the midpoint of [first,last)
- difference_type N = thrust::distance(first, last);
- ItemsIt mid(first);
- thrust::advance(mid, N / 2);
-
- cuda_cub::swap_ranges(policy, first, mid, make_reverse_iterator(last));
-}
-
-
-} // namespace cuda_cub
-} // end namespace thrust
-#endif
diff --git a/spaces/CVPR/WALT/mmdet/models/detectors/cornernet.py b/spaces/CVPR/WALT/mmdet/models/detectors/cornernet.py
deleted file mode 100644
index bb8ccc1465ab66d1615ca16701a533a22b156295..0000000000000000000000000000000000000000
--- a/spaces/CVPR/WALT/mmdet/models/detectors/cornernet.py
+++ /dev/null
@@ -1,95 +0,0 @@
-import torch
-
-from mmdet.core import bbox2result, bbox_mapping_back
-from ..builder import DETECTORS
-from .single_stage import SingleStageDetector
-
-
-@DETECTORS.register_module()
-class CornerNet(SingleStageDetector):
- """CornerNet.
-
- This detector is the implementation of the paper `CornerNet: Detecting
- Objects as Paired Keypoints `_ .
- """
-
- def __init__(self,
- backbone,
- neck,
- bbox_head,
- train_cfg=None,
- test_cfg=None,
- pretrained=None):
- super(CornerNet, self).__init__(backbone, neck, bbox_head, train_cfg,
- test_cfg, pretrained)
-
- def merge_aug_results(self, aug_results, img_metas):
- """Merge augmented detection bboxes and score.
-
- Args:
- aug_results (list[list[Tensor]]): Det_bboxes and det_labels of each
- image.
- img_metas (list[list[dict]]): Meta information of each image, e.g.,
- image size, scaling factor, etc.
-
- Returns:
- tuple: (bboxes, labels)
- """
- recovered_bboxes, aug_labels = [], []
- for bboxes_labels, img_info in zip(aug_results, img_metas):
- img_shape = img_info[0]['img_shape'] # using shape before padding
- scale_factor = img_info[0]['scale_factor']
- flip = img_info[0]['flip']
- bboxes, labels = bboxes_labels
- bboxes, scores = bboxes[:, :4], bboxes[:, -1:]
- bboxes = bbox_mapping_back(bboxes, img_shape, scale_factor, flip)
- recovered_bboxes.append(torch.cat([bboxes, scores], dim=-1))
- aug_labels.append(labels)
-
- bboxes = torch.cat(recovered_bboxes, dim=0)
- labels = torch.cat(aug_labels)
-
- if bboxes.shape[0] > 0:
- out_bboxes, out_labels = self.bbox_head._bboxes_nms(
- bboxes, labels, self.bbox_head.test_cfg)
- else:
- out_bboxes, out_labels = bboxes, labels
-
- return out_bboxes, out_labels
-
- def aug_test(self, imgs, img_metas, rescale=False):
- """Augment testing of CornerNet.
-
- Args:
- imgs (list[Tensor]): Augmented images.
- img_metas (list[list[dict]]): Meta information of each image, e.g.,
- image size, scaling factor, etc.
- rescale (bool): If True, return boxes in original image space.
- Default: False.
-
- Note:
- ``imgs`` must including flipped image pairs.
-
- Returns:
- list[list[np.ndarray]]: BBox results of each image and classes.
- The outer list corresponds to each image. The inner list
- corresponds to each class.
- """
- img_inds = list(range(len(imgs)))
-
- assert img_metas[0][0]['flip'] + img_metas[1][0]['flip'], (
- 'aug test must have flipped image pair')
- aug_results = []
- for ind, flip_ind in zip(img_inds[0::2], img_inds[1::2]):
- img_pair = torch.cat([imgs[ind], imgs[flip_ind]])
- x = self.extract_feat(img_pair)
- outs = self.bbox_head(x)
- bbox_list = self.bbox_head.get_bboxes(
- *outs, [img_metas[ind], img_metas[flip_ind]], False, False)
- aug_results.append(bbox_list[0])
- aug_results.append(bbox_list[1])
-
- bboxes, labels = self.merge_aug_results(aug_results, img_metas)
- bbox_results = bbox2result(bboxes, labels, self.bbox_head.num_classes)
-
- return [bbox_results]
diff --git a/spaces/CVPR/WALT/mmdet/models/losses/iou_loss.py b/spaces/CVPR/WALT/mmdet/models/losses/iou_loss.py
deleted file mode 100644
index eba6f18b80981ca891c1add37007e6bf478c651f..0000000000000000000000000000000000000000
--- a/spaces/CVPR/WALT/mmdet/models/losses/iou_loss.py
+++ /dev/null
@@ -1,436 +0,0 @@
-import math
-
-import mmcv
-import torch
-import torch.nn as nn
-
-from mmdet.core import bbox_overlaps
-from ..builder import LOSSES
-from .utils import weighted_loss
-
-
-@mmcv.jit(derivate=True, coderize=True)
-@weighted_loss
-def iou_loss(pred, target, linear=False, eps=1e-6):
- """IoU loss.
-
- Computing the IoU loss between a set of predicted bboxes and target bboxes.
- The loss is calculated as negative log of IoU.
-
- Args:
- pred (torch.Tensor): Predicted bboxes of format (x1, y1, x2, y2),
- shape (n, 4).
- target (torch.Tensor): Corresponding gt bboxes, shape (n, 4).
- linear (bool, optional): If True, use linear scale of loss instead of
- log scale. Default: False.
- eps (float): Eps to avoid log(0).
-
- Return:
- torch.Tensor: Loss tensor.
- """
- ious = bbox_overlaps(pred, target, is_aligned=True).clamp(min=eps)
- if linear:
- loss = 1 - ious
- else:
- loss = -ious.log()
- return loss
-
-
-@mmcv.jit(derivate=True, coderize=True)
-@weighted_loss
-def bounded_iou_loss(pred, target, beta=0.2, eps=1e-3):
- """BIoULoss.
-
- This is an implementation of paper
- `Improving Object Localization with Fitness NMS and Bounded IoU Loss.
- `_.
-
- Args:
- pred (torch.Tensor): Predicted bboxes.
- target (torch.Tensor): Target bboxes.
- beta (float): beta parameter in smoothl1.
- eps (float): eps to avoid NaN.
- """
- pred_ctrx = (pred[:, 0] + pred[:, 2]) * 0.5
- pred_ctry = (pred[:, 1] + pred[:, 3]) * 0.5
- pred_w = pred[:, 2] - pred[:, 0]
- pred_h = pred[:, 3] - pred[:, 1]
- with torch.no_grad():
- target_ctrx = (target[:, 0] + target[:, 2]) * 0.5
- target_ctry = (target[:, 1] + target[:, 3]) * 0.5
- target_w = target[:, 2] - target[:, 0]
- target_h = target[:, 3] - target[:, 1]
-
- dx = target_ctrx - pred_ctrx
- dy = target_ctry - pred_ctry
-
- loss_dx = 1 - torch.max(
- (target_w - 2 * dx.abs()) /
- (target_w + 2 * dx.abs() + eps), torch.zeros_like(dx))
- loss_dy = 1 - torch.max(
- (target_h - 2 * dy.abs()) /
- (target_h + 2 * dy.abs() + eps), torch.zeros_like(dy))
- loss_dw = 1 - torch.min(target_w / (pred_w + eps), pred_w /
- (target_w + eps))
- loss_dh = 1 - torch.min(target_h / (pred_h + eps), pred_h /
- (target_h + eps))
- loss_comb = torch.stack([loss_dx, loss_dy, loss_dw, loss_dh],
- dim=-1).view(loss_dx.size(0), -1)
-
- loss = torch.where(loss_comb < beta, 0.5 * loss_comb * loss_comb / beta,
- loss_comb - 0.5 * beta)
- return loss
-
-
-@mmcv.jit(derivate=True, coderize=True)
-@weighted_loss
-def giou_loss(pred, target, eps=1e-7):
- r"""`Generalized Intersection over Union: A Metric and A Loss for Bounding
- Box Regression `_.
-
- Args:
- pred (torch.Tensor): Predicted bboxes of format (x1, y1, x2, y2),
- shape (n, 4).
- target (torch.Tensor): Corresponding gt bboxes, shape (n, 4).
- eps (float): Eps to avoid log(0).
-
- Return:
- Tensor: Loss tensor.
- """
- gious = bbox_overlaps(pred, target, mode='giou', is_aligned=True, eps=eps)
- loss = 1 - gious
- return loss
-
-
-@mmcv.jit(derivate=True, coderize=True)
-@weighted_loss
-def diou_loss(pred, target, eps=1e-7):
- r"""`Implementation of Distance-IoU Loss: Faster and Better
- Learning for Bounding Box Regression, https://arxiv.org/abs/1911.08287`_.
-
- Code is modified from https://github.com/Zzh-tju/DIoU.
-
- Args:
- pred (Tensor): Predicted bboxes of format (x1, y1, x2, y2),
- shape (n, 4).
- target (Tensor): Corresponding gt bboxes, shape (n, 4).
- eps (float): Eps to avoid log(0).
- Return:
- Tensor: Loss tensor.
- """
- # overlap
- lt = torch.max(pred[:, :2], target[:, :2])
- rb = torch.min(pred[:, 2:], target[:, 2:])
- wh = (rb - lt).clamp(min=0)
- overlap = wh[:, 0] * wh[:, 1]
-
- # union
- ap = (pred[:, 2] - pred[:, 0]) * (pred[:, 3] - pred[:, 1])
- ag = (target[:, 2] - target[:, 0]) * (target[:, 3] - target[:, 1])
- union = ap + ag - overlap + eps
-
- # IoU
- ious = overlap / union
-
- # enclose area
- enclose_x1y1 = torch.min(pred[:, :2], target[:, :2])
- enclose_x2y2 = torch.max(pred[:, 2:], target[:, 2:])
- enclose_wh = (enclose_x2y2 - enclose_x1y1).clamp(min=0)
-
- cw = enclose_wh[:, 0]
- ch = enclose_wh[:, 1]
-
- c2 = cw**2 + ch**2 + eps
-
- b1_x1, b1_y1 = pred[:, 0], pred[:, 1]
- b1_x2, b1_y2 = pred[:, 2], pred[:, 3]
- b2_x1, b2_y1 = target[:, 0], target[:, 1]
- b2_x2, b2_y2 = target[:, 2], target[:, 3]
-
- left = ((b2_x1 + b2_x2) - (b1_x1 + b1_x2))**2 / 4
- right = ((b2_y1 + b2_y2) - (b1_y1 + b1_y2))**2 / 4
- rho2 = left + right
-
- # DIoU
- dious = ious - rho2 / c2
- loss = 1 - dious
- return loss
-
-
-@mmcv.jit(derivate=True, coderize=True)
-@weighted_loss
-def ciou_loss(pred, target, eps=1e-7):
- r"""`Implementation of paper `Enhancing Geometric Factors into
- Model Learning and Inference for Object Detection and Instance
- Segmentation `_.
-
- Code is modified from https://github.com/Zzh-tju/CIoU.
-
- Args:
- pred (Tensor): Predicted bboxes of format (x1, y1, x2, y2),
- shape (n, 4).
- target (Tensor): Corresponding gt bboxes, shape (n, 4).
- eps (float): Eps to avoid log(0).
- Return:
- Tensor: Loss tensor.
- """
- # overlap
- lt = torch.max(pred[:, :2], target[:, :2])
- rb = torch.min(pred[:, 2:], target[:, 2:])
- wh = (rb - lt).clamp(min=0)
- overlap = wh[:, 0] * wh[:, 1]
-
- # union
- ap = (pred[:, 2] - pred[:, 0]) * (pred[:, 3] - pred[:, 1])
- ag = (target[:, 2] - target[:, 0]) * (target[:, 3] - target[:, 1])
- union = ap + ag - overlap + eps
-
- # IoU
- ious = overlap / union
-
- # enclose area
- enclose_x1y1 = torch.min(pred[:, :2], target[:, :2])
- enclose_x2y2 = torch.max(pred[:, 2:], target[:, 2:])
- enclose_wh = (enclose_x2y2 - enclose_x1y1).clamp(min=0)
-
- cw = enclose_wh[:, 0]
- ch = enclose_wh[:, 1]
-
- c2 = cw**2 + ch**2 + eps
-
- b1_x1, b1_y1 = pred[:, 0], pred[:, 1]
- b1_x2, b1_y2 = pred[:, 2], pred[:, 3]
- b2_x1, b2_y1 = target[:, 0], target[:, 1]
- b2_x2, b2_y2 = target[:, 2], target[:, 3]
-
- w1, h1 = b1_x2 - b1_x1, b1_y2 - b1_y1 + eps
- w2, h2 = b2_x2 - b2_x1, b2_y2 - b2_y1 + eps
-
- left = ((b2_x1 + b2_x2) - (b1_x1 + b1_x2))**2 / 4
- right = ((b2_y1 + b2_y2) - (b1_y1 + b1_y2))**2 / 4
- rho2 = left + right
-
- factor = 4 / math.pi**2
- v = factor * torch.pow(torch.atan(w2 / h2) - torch.atan(w1 / h1), 2)
-
- # CIoU
- cious = ious - (rho2 / c2 + v**2 / (1 - ious + v))
- loss = 1 - cious
- return loss
-
-
-@LOSSES.register_module()
-class IoULoss(nn.Module):
- """IoULoss.
-
- Computing the IoU loss between a set of predicted bboxes and target bboxes.
-
- Args:
- linear (bool): If True, use linear scale of loss instead of log scale.
- Default: False.
- eps (float): Eps to avoid log(0).
- reduction (str): Options are "none", "mean" and "sum".
- loss_weight (float): Weight of loss.
- """
-
- def __init__(self,
- linear=False,
- eps=1e-6,
- reduction='mean',
- loss_weight=1.0):
- super(IoULoss, self).__init__()
- self.linear = linear
- self.eps = eps
- self.reduction = reduction
- self.loss_weight = loss_weight
-
- def forward(self,
- pred,
- target,
- weight=None,
- avg_factor=None,
- reduction_override=None,
- **kwargs):
- """Forward function.
-
- Args:
- pred (torch.Tensor): The prediction.
- target (torch.Tensor): The learning target of the prediction.
- weight (torch.Tensor, optional): The weight of loss for each
- prediction. Defaults to None.
- avg_factor (int, optional): Average factor that is used to average
- the loss. Defaults to None.
- reduction_override (str, optional): The reduction method used to
- override the original reduction method of the loss.
- Defaults to None. Options are "none", "mean" and "sum".
- """
- assert reduction_override in (None, 'none', 'mean', 'sum')
- reduction = (
- reduction_override if reduction_override else self.reduction)
- if (weight is not None) and (not torch.any(weight > 0)) and (
- reduction != 'none'):
- return (pred * weight).sum() # 0
- if weight is not None and weight.dim() > 1:
- # TODO: remove this in the future
- # reduce the weight of shape (n, 4) to (n,) to match the
- # iou_loss of shape (n,)
- assert weight.shape == pred.shape
- weight = weight.mean(-1)
- loss = self.loss_weight * iou_loss(
- pred,
- target,
- weight,
- linear=self.linear,
- eps=self.eps,
- reduction=reduction,
- avg_factor=avg_factor,
- **kwargs)
- return loss
-
-
-@LOSSES.register_module()
-class BoundedIoULoss(nn.Module):
-
- def __init__(self, beta=0.2, eps=1e-3, reduction='mean', loss_weight=1.0):
- super(BoundedIoULoss, self).__init__()
- self.beta = beta
- self.eps = eps
- self.reduction = reduction
- self.loss_weight = loss_weight
-
- def forward(self,
- pred,
- target,
- weight=None,
- avg_factor=None,
- reduction_override=None,
- **kwargs):
- if weight is not None and not torch.any(weight > 0):
- return (pred * weight).sum() # 0
- assert reduction_override in (None, 'none', 'mean', 'sum')
- reduction = (
- reduction_override if reduction_override else self.reduction)
- loss = self.loss_weight * bounded_iou_loss(
- pred,
- target,
- weight,
- beta=self.beta,
- eps=self.eps,
- reduction=reduction,
- avg_factor=avg_factor,
- **kwargs)
- return loss
-
-
-@LOSSES.register_module()
-class GIoULoss(nn.Module):
-
- def __init__(self, eps=1e-6, reduction='mean', loss_weight=1.0):
- super(GIoULoss, self).__init__()
- self.eps = eps
- self.reduction = reduction
- self.loss_weight = loss_weight
-
- def forward(self,
- pred,
- target,
- weight=None,
- avg_factor=None,
- reduction_override=None,
- **kwargs):
- if weight is not None and not torch.any(weight > 0):
- return (pred * weight).sum() # 0
- assert reduction_override in (None, 'none', 'mean', 'sum')
- reduction = (
- reduction_override if reduction_override else self.reduction)
- if weight is not None and weight.dim() > 1:
- # TODO: remove this in the future
- # reduce the weight of shape (n, 4) to (n,) to match the
- # giou_loss of shape (n,)
- assert weight.shape == pred.shape
- weight = weight.mean(-1)
- loss = self.loss_weight * giou_loss(
- pred,
- target,
- weight,
- eps=self.eps,
- reduction=reduction,
- avg_factor=avg_factor,
- **kwargs)
- return loss
-
-
-@LOSSES.register_module()
-class DIoULoss(nn.Module):
-
- def __init__(self, eps=1e-6, reduction='mean', loss_weight=1.0):
- super(DIoULoss, self).__init__()
- self.eps = eps
- self.reduction = reduction
- self.loss_weight = loss_weight
-
- def forward(self,
- pred,
- target,
- weight=None,
- avg_factor=None,
- reduction_override=None,
- **kwargs):
- if weight is not None and not torch.any(weight > 0):
- return (pred * weight).sum() # 0
- assert reduction_override in (None, 'none', 'mean', 'sum')
- reduction = (
- reduction_override if reduction_override else self.reduction)
- if weight is not None and weight.dim() > 1:
- # TODO: remove this in the future
- # reduce the weight of shape (n, 4) to (n,) to match the
- # giou_loss of shape (n,)
- assert weight.shape == pred.shape
- weight = weight.mean(-1)
- loss = self.loss_weight * diou_loss(
- pred,
- target,
- weight,
- eps=self.eps,
- reduction=reduction,
- avg_factor=avg_factor,
- **kwargs)
- return loss
-
-
-@LOSSES.register_module()
-class CIoULoss(nn.Module):
-
- def __init__(self, eps=1e-6, reduction='mean', loss_weight=1.0):
- super(CIoULoss, self).__init__()
- self.eps = eps
- self.reduction = reduction
- self.loss_weight = loss_weight
-
- def forward(self,
- pred,
- target,
- weight=None,
- avg_factor=None,
- reduction_override=None,
- **kwargs):
- if weight is not None and not torch.any(weight > 0):
- return (pred * weight).sum() # 0
- assert reduction_override in (None, 'none', 'mean', 'sum')
- reduction = (
- reduction_override if reduction_override else self.reduction)
- if weight is not None and weight.dim() > 1:
- # TODO: remove this in the future
- # reduce the weight of shape (n, 4) to (n,) to match the
- # giou_loss of shape (n,)
- assert weight.shape == pred.shape
- weight = weight.mean(-1)
- loss = self.loss_weight * ciou_loss(
- pred,
- target,
- weight,
- eps=self.eps,
- reduction=reduction,
- avg_factor=avg_factor,
- **kwargs)
- return loss
diff --git a/spaces/CVPR/WALT/mmdet/models/roi_heads/cascade_roi_head.py b/spaces/CVPR/WALT/mmdet/models/roi_heads/cascade_roi_head.py
deleted file mode 100644
index 45b6f36a386cd37c50cc43666fcc516f2e14d868..0000000000000000000000000000000000000000
--- a/spaces/CVPR/WALT/mmdet/models/roi_heads/cascade_roi_head.py
+++ /dev/null
@@ -1,507 +0,0 @@
-import torch
-import torch.nn as nn
-
-from mmdet.core import (bbox2result, bbox2roi, bbox_mapping, build_assigner,
- build_sampler, merge_aug_bboxes, merge_aug_masks,
- multiclass_nms)
-from ..builder import HEADS, build_head, build_roi_extractor
-from .base_roi_head import BaseRoIHead
-from .test_mixins import BBoxTestMixin, MaskTestMixin
-
-
-@HEADS.register_module()
-class CascadeRoIHead(BaseRoIHead, BBoxTestMixin, MaskTestMixin):
- """Cascade roi head including one bbox head and one mask head.
-
- https://arxiv.org/abs/1712.00726
- """
-
- def __init__(self,
- num_stages,
- stage_loss_weights,
- bbox_roi_extractor=None,
- bbox_head=None,
- mask_roi_extractor=None,
- mask_head=None,
- shared_head=None,
- train_cfg=None,
- test_cfg=None):
- assert bbox_roi_extractor is not None
- assert bbox_head is not None
- assert shared_head is None, \
- 'Shared head is not supported in Cascade RCNN anymore'
- self.num_stages = num_stages
- self.stage_loss_weights = stage_loss_weights
- super(CascadeRoIHead, self).__init__(
- bbox_roi_extractor=bbox_roi_extractor,
- bbox_head=bbox_head,
- mask_roi_extractor=mask_roi_extractor,
- mask_head=mask_head,
- shared_head=shared_head,
- train_cfg=train_cfg,
- test_cfg=test_cfg)
-
- def init_bbox_head(self, bbox_roi_extractor, bbox_head):
- """Initialize box head and box roi extractor.
-
- Args:
- bbox_roi_extractor (dict): Config of box roi extractor.
- bbox_head (dict): Config of box in box head.
- """
- self.bbox_roi_extractor = nn.ModuleList()
- self.bbox_head = nn.ModuleList()
- if not isinstance(bbox_roi_extractor, list):
- bbox_roi_extractor = [
- bbox_roi_extractor for _ in range(self.num_stages)
- ]
- if not isinstance(bbox_head, list):
- bbox_head = [bbox_head for _ in range(self.num_stages)]
- assert len(bbox_roi_extractor) == len(bbox_head) == self.num_stages
- for roi_extractor, head in zip(bbox_roi_extractor, bbox_head):
- self.bbox_roi_extractor.append(build_roi_extractor(roi_extractor))
- self.bbox_head.append(build_head(head))
-
- def init_mask_head(self, mask_roi_extractor, mask_head):
- """Initialize mask head and mask roi extractor.
-
- Args:
- mask_roi_extractor (dict): Config of mask roi extractor.
- mask_head (dict): Config of mask in mask head.
- """
- self.mask_head = nn.ModuleList()
- if not isinstance(mask_head, list):
- mask_head = [mask_head for _ in range(self.num_stages)]
- assert len(mask_head) == self.num_stages
- for head in mask_head:
- self.mask_head.append(build_head(head))
- if mask_roi_extractor is not None:
- self.share_roi_extractor = False
- self.mask_roi_extractor = nn.ModuleList()
- if not isinstance(mask_roi_extractor, list):
- mask_roi_extractor = [
- mask_roi_extractor for _ in range(self.num_stages)
- ]
- assert len(mask_roi_extractor) == self.num_stages
- for roi_extractor in mask_roi_extractor:
- self.mask_roi_extractor.append(
- build_roi_extractor(roi_extractor))
- else:
- self.share_roi_extractor = True
- self.mask_roi_extractor = self.bbox_roi_extractor
-
- def init_assigner_sampler(self):
- """Initialize assigner and sampler for each stage."""
- self.bbox_assigner = []
- self.bbox_sampler = []
- if self.train_cfg is not None:
- for idx, rcnn_train_cfg in enumerate(self.train_cfg):
- self.bbox_assigner.append(
- build_assigner(rcnn_train_cfg.assigner))
- self.current_stage = idx
- self.bbox_sampler.append(
- build_sampler(rcnn_train_cfg.sampler, context=self))
-
- def init_weights(self, pretrained):
- """Initialize the weights in head.
-
- Args:
- pretrained (str, optional): Path to pre-trained weights.
- Defaults to None.
- """
- if self.with_shared_head:
- self.shared_head.init_weights(pretrained=pretrained)
- for i in range(self.num_stages):
- if self.with_bbox:
- self.bbox_roi_extractor[i].init_weights()
- self.bbox_head[i].init_weights()
- if self.with_mask:
- if not self.share_roi_extractor:
- self.mask_roi_extractor[i].init_weights()
- self.mask_head[i].init_weights()
-
- def forward_dummy(self, x, proposals):
- """Dummy forward function."""
- # bbox head
- outs = ()
- rois = bbox2roi([proposals])
- if self.with_bbox:
- for i in range(self.num_stages):
- bbox_results = self._bbox_forward(i, x, rois)
- outs = outs + (bbox_results['cls_score'],
- bbox_results['bbox_pred'])
- # mask heads
- if self.with_mask:
- mask_rois = rois[:100]
- for i in range(self.num_stages):
- mask_results = self._mask_forward(i, x, mask_rois)
- outs = outs + (mask_results['mask_pred'], )
- return outs
-
- def _bbox_forward(self, stage, x, rois):
- """Box head forward function used in both training and testing."""
- bbox_roi_extractor = self.bbox_roi_extractor[stage]
- bbox_head = self.bbox_head[stage]
- bbox_feats = bbox_roi_extractor(x[:bbox_roi_extractor.num_inputs],
- rois)
- # do not support caffe_c4 model anymore
- cls_score, bbox_pred = bbox_head(bbox_feats)
-
- bbox_results = dict(
- cls_score=cls_score, bbox_pred=bbox_pred, bbox_feats=bbox_feats)
- return bbox_results
-
- def _bbox_forward_train(self, stage, x, sampling_results, gt_bboxes,
- gt_labels, rcnn_train_cfg):
- """Run forward function and calculate loss for box head in training."""
- rois = bbox2roi([res.bboxes for res in sampling_results])
- bbox_results = self._bbox_forward(stage, x, rois)
- bbox_targets = self.bbox_head[stage].get_targets(
- sampling_results, gt_bboxes, gt_labels, rcnn_train_cfg)
- loss_bbox = self.bbox_head[stage].loss(bbox_results['cls_score'],
- bbox_results['bbox_pred'], rois,
- *bbox_targets)
-
- bbox_results.update(
- loss_bbox=loss_bbox, rois=rois, bbox_targets=bbox_targets)
- return bbox_results
-
- def _mask_forward(self, stage, x, rois):
- """Mask head forward function used in both training and testing."""
- mask_roi_extractor = self.mask_roi_extractor[stage]
- mask_head = self.mask_head[stage]
- mask_feats = mask_roi_extractor(x[:mask_roi_extractor.num_inputs],
- rois)
- # do not support caffe_c4 model anymore
- mask_pred = mask_head(mask_feats)
-
- mask_results = dict(mask_pred=mask_pred)
- return mask_results
-
- def _mask_forward_train(self,
- stage,
- x,
- sampling_results,
- gt_masks,
- rcnn_train_cfg,
- bbox_feats=None):
- """Run forward function and calculate loss for mask head in
- training."""
- pos_rois = bbox2roi([res.pos_bboxes for res in sampling_results])
- mask_results = self._mask_forward(stage, x, pos_rois)
-
- mask_targets = self.mask_head[stage].get_targets(
- sampling_results, gt_masks, rcnn_train_cfg)
- pos_labels = torch.cat([res.pos_gt_labels for res in sampling_results])
- loss_mask = self.mask_head[stage].loss(mask_results['mask_pred'],
- mask_targets, pos_labels)
-
- mask_results.update(loss_mask=loss_mask)
- return mask_results
-
- def forward_train(self,
- x,
- img_metas,
- proposal_list,
- gt_bboxes,
- gt_labels,
- gt_bboxes_ignore=None,
- gt_masks=None):
- """
- Args:
- x (list[Tensor]): list of multi-level img features.
- img_metas (list[dict]): list of image info dict where each dict
- has: 'img_shape', 'scale_factor', 'flip', and may also contain
- 'filename', 'ori_shape', 'pad_shape', and 'img_norm_cfg'.
- For details on the values of these keys see
- `mmdet/datasets/pipelines/formatting.py:Collect`.
- proposals (list[Tensors]): list of region proposals.
- gt_bboxes (list[Tensor]): Ground truth bboxes for each image with
- shape (num_gts, 4) in [tl_x, tl_y, br_x, br_y] format.
- gt_labels (list[Tensor]): class indices corresponding to each box
- gt_bboxes_ignore (None | list[Tensor]): specify which bounding
- boxes can be ignored when computing the loss.
- gt_masks (None | Tensor) : true segmentation masks for each box
- used if the architecture supports a segmentation task.
-
- Returns:
- dict[str, Tensor]: a dictionary of loss components
- """
- losses = dict()
- for i in range(self.num_stages):
- self.current_stage = i
- rcnn_train_cfg = self.train_cfg[i]
- lw = self.stage_loss_weights[i]
-
- # assign gts and sample proposals
- sampling_results = []
- if self.with_bbox or self.with_mask:
- bbox_assigner = self.bbox_assigner[i]
- bbox_sampler = self.bbox_sampler[i]
- num_imgs = len(img_metas)
- if gt_bboxes_ignore is None:
- gt_bboxes_ignore = [None for _ in range(num_imgs)]
-
- for j in range(num_imgs):
- assign_result = bbox_assigner.assign(
- proposal_list[j], gt_bboxes[j], gt_bboxes_ignore[j],
- gt_labels[j])
- sampling_result = bbox_sampler.sample(
- assign_result,
- proposal_list[j],
- gt_bboxes[j],
- gt_labels[j],
- feats=[lvl_feat[j][None] for lvl_feat in x])
- sampling_results.append(sampling_result)
-
- # bbox head forward and loss
- bbox_results = self._bbox_forward_train(i, x, sampling_results,
- gt_bboxes, gt_labels,
- rcnn_train_cfg)
-
- for name, value in bbox_results['loss_bbox'].items():
- losses[f's{i}.{name}'] = (
- value * lw if 'loss' in name else value)
-
- # mask head forward and loss
- if self.with_mask:
- mask_results = self._mask_forward_train(
- i, x, sampling_results, gt_masks, rcnn_train_cfg,
- bbox_results['bbox_feats'])
- for name, value in mask_results['loss_mask'].items():
- losses[f's{i}.{name}'] = (
- value * lw if 'loss' in name else value)
-
- # refine bboxes
- if i < self.num_stages - 1:
- pos_is_gts = [res.pos_is_gt for res in sampling_results]
- # bbox_targets is a tuple
- roi_labels = bbox_results['bbox_targets'][0]
- with torch.no_grad():
- roi_labels = torch.where(
- roi_labels == self.bbox_head[i].num_classes,
- bbox_results['cls_score'][:, :-1].argmax(1),
- roi_labels)
- proposal_list = self.bbox_head[i].refine_bboxes(
- bbox_results['rois'], roi_labels,
- bbox_results['bbox_pred'], pos_is_gts, img_metas)
-
- return losses
-
- def simple_test(self, x, proposal_list, img_metas, rescale=False):
- """Test without augmentation."""
- assert self.with_bbox, 'Bbox head must be implemented.'
- num_imgs = len(proposal_list)
- img_shapes = tuple(meta['img_shape'] for meta in img_metas)
- ori_shapes = tuple(meta['ori_shape'] for meta in img_metas)
- scale_factors = tuple(meta['scale_factor'] for meta in img_metas)
-
- # "ms" in variable names means multi-stage
- ms_bbox_result = {}
- ms_segm_result = {}
- ms_scores = []
- rcnn_test_cfg = self.test_cfg
-
- rois = bbox2roi(proposal_list)
- for i in range(self.num_stages):
- bbox_results = self._bbox_forward(i, x, rois)
-
- # split batch bbox prediction back to each image
- cls_score = bbox_results['cls_score']
- bbox_pred = bbox_results['bbox_pred']
- num_proposals_per_img = tuple(
- len(proposals) for proposals in proposal_list)
- rois = rois.split(num_proposals_per_img, 0)
- cls_score = cls_score.split(num_proposals_per_img, 0)
- if isinstance(bbox_pred, torch.Tensor):
- bbox_pred = bbox_pred.split(num_proposals_per_img, 0)
- else:
- bbox_pred = self.bbox_head[i].bbox_pred_split(
- bbox_pred, num_proposals_per_img)
- ms_scores.append(cls_score)
-
- if i < self.num_stages - 1:
- bbox_label = [s[:, :-1].argmax(dim=1) for s in cls_score]
- rois = torch.cat([
- self.bbox_head[i].regress_by_class(rois[j], bbox_label[j],
- bbox_pred[j],
- img_metas[j])
- for j in range(num_imgs)
- ])
-
- # average scores of each image by stages
- cls_score = [
- sum([score[i] for score in ms_scores]) / float(len(ms_scores))
- for i in range(num_imgs)
- ]
-
- # apply bbox post-processing to each image individually
- det_bboxes = []
- det_labels = []
- for i in range(num_imgs):
- det_bbox, det_label = self.bbox_head[-1].get_bboxes(
- rois[i],
- cls_score[i],
- bbox_pred[i],
- img_shapes[i],
- scale_factors[i],
- rescale=rescale,
- cfg=rcnn_test_cfg)
- det_bboxes.append(det_bbox)
- det_labels.append(det_label)
-
- if torch.onnx.is_in_onnx_export():
- return det_bboxes, det_labels
- bbox_results = [
- bbox2result(det_bboxes[i], det_labels[i],
- self.bbox_head[-1].num_classes)
- for i in range(num_imgs)
- ]
- ms_bbox_result['ensemble'] = bbox_results
-
- if self.with_mask:
- if all(det_bbox.shape[0] == 0 for det_bbox in det_bboxes):
- mask_classes = self.mask_head[-1].num_classes
- segm_results = [[[] for _ in range(mask_classes)]
- for _ in range(num_imgs)]
- else:
- if rescale and not isinstance(scale_factors[0], float):
- scale_factors = [
- torch.from_numpy(scale_factor).to(det_bboxes[0].device)
- for scale_factor in scale_factors
- ]
- _bboxes = [
- det_bboxes[i][:, :4] *
- scale_factors[i] if rescale else det_bboxes[i][:, :4]
- for i in range(len(det_bboxes))
- ]
- mask_rois = bbox2roi(_bboxes)
- num_mask_rois_per_img = tuple(
- _bbox.size(0) for _bbox in _bboxes)
- aug_masks = []
- for i in range(self.num_stages):
- mask_results = self._mask_forward(i, x, mask_rois)
- mask_pred = mask_results['mask_pred']
- # split batch mask prediction back to each image
- mask_pred = mask_pred.split(num_mask_rois_per_img, 0)
- aug_masks.append(
- [m.sigmoid().cpu().numpy() for m in mask_pred])
-
- # apply mask post-processing to each image individually
- segm_results = []
- for i in range(num_imgs):
- if det_bboxes[i].shape[0] == 0:
- segm_results.append(
- [[]
- for _ in range(self.mask_head[-1].num_classes)])
- else:
- aug_mask = [mask[i] for mask in aug_masks]
- merged_masks = merge_aug_masks(
- aug_mask, [[img_metas[i]]] * self.num_stages,
- rcnn_test_cfg)
- segm_result = self.mask_head[-1].get_seg_masks(
- merged_masks, _bboxes[i], det_labels[i],
- rcnn_test_cfg, ori_shapes[i], scale_factors[i],
- rescale)
- segm_results.append(segm_result)
- ms_segm_result['ensemble'] = segm_results
-
- if self.with_mask:
- results = list(
- zip(ms_bbox_result['ensemble'], ms_segm_result['ensemble']))
- else:
- results = ms_bbox_result['ensemble']
-
- return results
-
- def aug_test(self, features, proposal_list, img_metas, rescale=False):
- """Test with augmentations.
-
- If rescale is False, then returned bboxes and masks will fit the scale
- of imgs[0].
- """
- rcnn_test_cfg = self.test_cfg
- aug_bboxes = []
- aug_scores = []
- for x, img_meta in zip(features, img_metas):
- # only one image in the batch
- img_shape = img_meta[0]['img_shape']
- scale_factor = img_meta[0]['scale_factor']
- flip = img_meta[0]['flip']
- flip_direction = img_meta[0]['flip_direction']
-
- proposals = bbox_mapping(proposal_list[0][:, :4], img_shape,
- scale_factor, flip, flip_direction)
- # "ms" in variable names means multi-stage
- ms_scores = []
-
- rois = bbox2roi([proposals])
- for i in range(self.num_stages):
- bbox_results = self._bbox_forward(i, x, rois)
- ms_scores.append(bbox_results['cls_score'])
-
- if i < self.num_stages - 1:
- bbox_label = bbox_results['cls_score'][:, :-1].argmax(
- dim=1)
- rois = self.bbox_head[i].regress_by_class(
- rois, bbox_label, bbox_results['bbox_pred'],
- img_meta[0])
-
- cls_score = sum(ms_scores) / float(len(ms_scores))
- bboxes, scores = self.bbox_head[-1].get_bboxes(
- rois,
- cls_score,
- bbox_results['bbox_pred'],
- img_shape,
- scale_factor,
- rescale=False,
- cfg=None)
- aug_bboxes.append(bboxes)
- aug_scores.append(scores)
-
- # after merging, bboxes will be rescaled to the original image size
- merged_bboxes, merged_scores = merge_aug_bboxes(
- aug_bboxes, aug_scores, img_metas, rcnn_test_cfg)
- det_bboxes, det_labels = multiclass_nms(merged_bboxes, merged_scores,
- rcnn_test_cfg.score_thr,
- rcnn_test_cfg.nms,
- rcnn_test_cfg.max_per_img)
-
- bbox_result = bbox2result(det_bboxes, det_labels,
- self.bbox_head[-1].num_classes)
-
- if self.with_mask:
- if det_bboxes.shape[0] == 0:
- segm_result = [[[]
- for _ in range(self.mask_head[-1].num_classes)]
- ]
- else:
- aug_masks = []
- aug_img_metas = []
- for x, img_meta in zip(features, img_metas):
- img_shape = img_meta[0]['img_shape']
- scale_factor = img_meta[0]['scale_factor']
- flip = img_meta[0]['flip']
- flip_direction = img_meta[0]['flip_direction']
- _bboxes = bbox_mapping(det_bboxes[:, :4], img_shape,
- scale_factor, flip, flip_direction)
- mask_rois = bbox2roi([_bboxes])
- for i in range(self.num_stages):
- mask_results = self._mask_forward(i, x, mask_rois)
- aug_masks.append(
- mask_results['mask_pred'].sigmoid().cpu().numpy())
- aug_img_metas.append(img_meta)
- merged_masks = merge_aug_masks(aug_masks, aug_img_metas,
- self.test_cfg)
-
- ori_shape = img_metas[0][0]['ori_shape']
- segm_result = self.mask_head[-1].get_seg_masks(
- merged_masks,
- det_bboxes,
- det_labels,
- rcnn_test_cfg,
- ori_shape,
- scale_factor=1.0,
- rescale=False)
- return [(bbox_result, segm_result)]
- else:
- return [bbox_result]
diff --git a/spaces/CVPR/WALT/mmdet/models/roi_heads/test_mixins.py b/spaces/CVPR/WALT/mmdet/models/roi_heads/test_mixins.py
deleted file mode 100644
index c28ed61deb946f0ffca70733fb7ddf84d1aec885..0000000000000000000000000000000000000000
--- a/spaces/CVPR/WALT/mmdet/models/roi_heads/test_mixins.py
+++ /dev/null
@@ -1,368 +0,0 @@
-import logging
-import sys
-
-import torch
-
-from mmdet.core import (bbox2roi, bbox_mapping, merge_aug_bboxes,
- merge_aug_masks, multiclass_nms)
-
-logger = logging.getLogger(__name__)
-
-if sys.version_info >= (3, 7):
- from mmdet.utils.contextmanagers import completed
-
-
-class BBoxTestMixin(object):
-
- if sys.version_info >= (3, 7):
-
- async def async_test_bboxes(self,
- x,
- img_metas,
- proposals,
- rcnn_test_cfg,
- rescale=False,
- bbox_semaphore=None,
- global_lock=None):
- """Asynchronized test for box head without augmentation."""
- rois = bbox2roi(proposals)
- roi_feats = self.bbox_roi_extractor(
- x[:len(self.bbox_roi_extractor.featmap_strides)], rois)
- if self.with_shared_head:
- roi_feats = self.shared_head(roi_feats)
- sleep_interval = rcnn_test_cfg.get('async_sleep_interval', 0.017)
-
- async with completed(
- __name__, 'bbox_head_forward',
- sleep_interval=sleep_interval):
- cls_score, bbox_pred = self.bbox_head(roi_feats)
-
- img_shape = img_metas[0]['img_shape']
- scale_factor = img_metas[0]['scale_factor']
- det_bboxes, det_labels = self.bbox_head.get_bboxes(
- rois,
- cls_score,
- bbox_pred,
- img_shape,
- scale_factor,
- rescale=rescale,
- cfg=rcnn_test_cfg)
- return det_bboxes, det_labels
-
- def simple_test_bboxes(self,
- x,
- img_metas,
- proposals,
- rcnn_test_cfg,
- rescale=False):
- """Test only det bboxes without augmentation.
-
- Args:
- x (tuple[Tensor]): Feature maps of all scale level.
- img_metas (list[dict]): Image meta info.
- proposals (Tensor or List[Tensor]): Region proposals.
- rcnn_test_cfg (obj:`ConfigDict`): `test_cfg` of R-CNN.
- rescale (bool): If True, return boxes in original image space.
- Default: False.
-
- Returns:
- tuple[list[Tensor], list[Tensor]]: The first list contains
- the boxes of the corresponding image in a batch, each
- tensor has the shape (num_boxes, 5) and last dimension
- 5 represent (tl_x, tl_y, br_x, br_y, score). Each Tensor
- in the second list is the labels with shape (num_boxes, ).
- The length of both lists should be equal to batch_size.
- """
- # get origin input shape to support onnx dynamic input shape
- if torch.onnx.is_in_onnx_export():
- assert len(
- img_metas
- ) == 1, 'Only support one input image while in exporting to ONNX'
- img_shapes = img_metas[0]['img_shape_for_onnx']
- else:
- img_shapes = tuple(meta['img_shape'] for meta in img_metas)
- scale_factors = tuple(meta['scale_factor'] for meta in img_metas)
-
- # The length of proposals of different batches may be different.
- # In order to form a batch, a padding operation is required.
- if isinstance(proposals, list):
- # padding to form a batch
- max_size = max([proposal.size(0) for proposal in proposals])
- for i, proposal in enumerate(proposals):
- supplement = proposal.new_full(
- (max_size - proposal.size(0), proposal.size(1)), 0)
- proposals[i] = torch.cat((supplement, proposal), dim=0)
- rois = torch.stack(proposals, dim=0)
- else:
- rois = proposals
-
- batch_index = torch.arange(
- rois.size(0), device=rois.device).float().view(-1, 1, 1).expand(
- rois.size(0), rois.size(1), 1)
- rois = torch.cat([batch_index, rois[..., :4]], dim=-1)
- batch_size = rois.shape[0]
- num_proposals_per_img = rois.shape[1]
-
- # Eliminate the batch dimension
- rois = rois.view(-1, 5)
- bbox_results = self._bbox_forward(x, rois)
- cls_score = bbox_results['cls_score']
- bbox_pred = bbox_results['bbox_pred']
-
- # Recover the batch dimension
- rois = rois.reshape(batch_size, num_proposals_per_img, -1)
- cls_score = cls_score.reshape(batch_size, num_proposals_per_img, -1)
-
- if not torch.onnx.is_in_onnx_export():
- # remove padding
- supplement_mask = rois[..., -1] == 0
- cls_score[supplement_mask, :] = 0
-
- # bbox_pred would be None in some detector when with_reg is False,
- # e.g. Grid R-CNN.
- if bbox_pred is not None:
- # the bbox prediction of some detectors like SABL is not Tensor
- if isinstance(bbox_pred, torch.Tensor):
- bbox_pred = bbox_pred.reshape(batch_size,
- num_proposals_per_img, -1)
- if not torch.onnx.is_in_onnx_export():
- bbox_pred[supplement_mask, :] = 0
- else:
- # TODO: Looking forward to a better way
- # For SABL
- bbox_preds = self.bbox_head.bbox_pred_split(
- bbox_pred, num_proposals_per_img)
- # apply bbox post-processing to each image individually
- det_bboxes = []
- det_labels = []
- for i in range(len(proposals)):
- # remove padding
- supplement_mask = proposals[i][..., -1] == 0
- for bbox in bbox_preds[i]:
- bbox[supplement_mask] = 0
- det_bbox, det_label = self.bbox_head.get_bboxes(
- rois[i],
- cls_score[i],
- bbox_preds[i],
- img_shapes[i],
- scale_factors[i],
- rescale=rescale,
- cfg=rcnn_test_cfg)
- det_bboxes.append(det_bbox)
- det_labels.append(det_label)
- return det_bboxes, det_labels
- else:
- bbox_pred = None
-
- return self.bbox_head.get_bboxes(
- rois,
- cls_score,
- bbox_pred,
- img_shapes,
- scale_factors,
- rescale=rescale,
- cfg=rcnn_test_cfg)
-
- def aug_test_bboxes(self, feats, img_metas, proposal_list, rcnn_test_cfg):
- """Test det bboxes with test time augmentation."""
- aug_bboxes = []
- aug_scores = []
- for x, img_meta in zip(feats, img_metas):
- # only one image in the batch
- img_shape = img_meta[0]['img_shape']
- scale_factor = img_meta[0]['scale_factor']
- flip = img_meta[0]['flip']
- flip_direction = img_meta[0]['flip_direction']
- # TODO more flexible
- proposals = bbox_mapping(proposal_list[0][:, :4], img_shape,
- scale_factor, flip, flip_direction)
- rois = bbox2roi([proposals])
- bbox_results = self._bbox_forward(x, rois)
- bboxes, scores = self.bbox_head.get_bboxes(
- rois,
- bbox_results['cls_score'],
- bbox_results['bbox_pred'],
- img_shape,
- scale_factor,
- rescale=False,
- cfg=None)
- aug_bboxes.append(bboxes)
- aug_scores.append(scores)
- # after merging, bboxes will be rescaled to the original image size
- merged_bboxes, merged_scores = merge_aug_bboxes(
- aug_bboxes, aug_scores, img_metas, rcnn_test_cfg)
- det_bboxes, det_labels = multiclass_nms(merged_bboxes, merged_scores,
- rcnn_test_cfg.score_thr,
- rcnn_test_cfg.nms,
- rcnn_test_cfg.max_per_img)
- return det_bboxes, det_labels
-
-
-class MaskTestMixin(object):
-
- if sys.version_info >= (3, 7):
-
- async def async_test_mask(self,
- x,
- img_metas,
- det_bboxes,
- det_labels,
- rescale=False,
- mask_test_cfg=None):
- """Asynchronized test for mask head without augmentation."""
- # image shape of the first image in the batch (only one)
- ori_shape = img_metas[0]['ori_shape']
- scale_factor = img_metas[0]['scale_factor']
- if det_bboxes.shape[0] == 0:
- segm_result = [[] for _ in range(self.mask_head.num_classes)]
- else:
- if rescale and not isinstance(scale_factor,
- (float, torch.Tensor)):
- scale_factor = det_bboxes.new_tensor(scale_factor)
- _bboxes = (
- det_bboxes[:, :4] *
- scale_factor if rescale else det_bboxes)
- mask_rois = bbox2roi([_bboxes])
- mask_feats = self.mask_roi_extractor(
- x[:len(self.mask_roi_extractor.featmap_strides)],
- mask_rois)
-
- if self.with_shared_head:
- mask_feats = self.shared_head(mask_feats)
- if mask_test_cfg and mask_test_cfg.get('async_sleep_interval'):
- sleep_interval = mask_test_cfg['async_sleep_interval']
- else:
- sleep_interval = 0.035
- async with completed(
- __name__,
- 'mask_head_forward',
- sleep_interval=sleep_interval):
- mask_pred = self.mask_head(mask_feats)
- segm_result = self.mask_head.get_seg_masks(
- mask_pred, _bboxes, det_labels, self.test_cfg, ori_shape,
- scale_factor, rescale)
- return segm_result
-
- def simple_test_mask(self,
- x,
- img_metas,
- det_bboxes,
- det_labels,
- rescale=False):
- """Simple test for mask head without augmentation."""
- # image shapes of images in the batch
- ori_shapes = tuple(meta['ori_shape'] for meta in img_metas)
- scale_factors = tuple(meta['scale_factor'] for meta in img_metas)
-
- # The length of proposals of different batches may be different.
- # In order to form a batch, a padding operation is required.
- if isinstance(det_bboxes, list):
- # padding to form a batch
- max_size = max([bboxes.size(0) for bboxes in det_bboxes])
- for i, (bbox, label) in enumerate(zip(det_bboxes, det_labels)):
- supplement_bbox = bbox.new_full(
- (max_size - bbox.size(0), bbox.size(1)), 0)
- supplement_label = label.new_full((max_size - label.size(0), ),
- 0)
- det_bboxes[i] = torch.cat((supplement_bbox, bbox), dim=0)
- det_labels[i] = torch.cat((supplement_label, label), dim=0)
- det_bboxes = torch.stack(det_bboxes, dim=0)
- det_labels = torch.stack(det_labels, dim=0)
-
- batch_size = det_bboxes.size(0)
- num_proposals_per_img = det_bboxes.shape[1]
-
- # if det_bboxes is rescaled to the original image size, we need to
- # rescale it back to the testing scale to obtain RoIs.
- det_bboxes = det_bboxes[..., :4]
- if rescale:
- if not isinstance(scale_factors[0], float):
- scale_factors = det_bboxes.new_tensor(scale_factors)
- det_bboxes = det_bboxes * scale_factors.unsqueeze(1)
-
- batch_index = torch.arange(
- det_bboxes.size(0), device=det_bboxes.device).float().view(
- -1, 1, 1).expand(det_bboxes.size(0), det_bboxes.size(1), 1)
- mask_rois = torch.cat([batch_index, det_bboxes], dim=-1)
- mask_rois = mask_rois.view(-1, 5)
- mask_results = self._mask_forward(x, mask_rois)
- mask_pred = mask_results['mask_pred']
- try:
- mask_full_pred, mask_occ_pred = mask_pred
- except:
- mask_full_pred = mask_pred
- mask_occ_pred = mask_pred
-
-
- # Recover the batch dimension
- mask_full_preds = mask_full_pred.reshape(batch_size, num_proposals_per_img,
- *mask_full_pred.shape[1:])
-
- mask_occ_preds = mask_occ_pred.reshape(batch_size, num_proposals_per_img,
- *mask_occ_pred.shape[1:])
-
-
- # apply mask post-processing to each image individually
- segm_results = []
- for i in range(batch_size):
- mask_full_pred = mask_full_preds[i]
- mask_occ_pred = mask_occ_preds[i]
- det_bbox = det_bboxes[i]
- det_label = det_labels[i]
-
- # remove padding
- supplement_mask = det_bbox[..., -1] != 0
- mask_full_pred = mask_full_pred[supplement_mask]
- mask_occ_pred = mask_occ_pred[supplement_mask]
- det_bbox = det_bbox[supplement_mask]
- det_label = det_label[supplement_mask]
-
- if det_label.shape[0] == 0:
- segm_results.append([[]
- for _ in range(self.mask_head.num_classes)
- ])
- else:
- segm_result_vis = self.mask_head.get_seg_masks(
- mask_full_pred[:,0:1], det_bbox, det_label, self.test_cfg,
- ori_shapes[i], scale_factors[i], rescale)
-
- segm_result_occ = self.mask_head.get_seg_masks(
- mask_occ_pred[:,0:1], det_bbox, det_label, self.test_cfg,
- ori_shapes[i], scale_factors[i], rescale)
-
- segm_result = segm_result_vis
- segm_result[1] = segm_result_occ[0]
-
- segm_results.append(segm_result)
- return segm_results
-
- def aug_test_mask(self, feats, img_metas, det_bboxes, det_labels):
- """Test for mask head with test time augmentation."""
- if det_bboxes.shape[0] == 0:
- segm_result = [[] for _ in range(self.mask_head.num_classes)]
- else:
- aug_masks = []
- for x, img_meta in zip(feats, img_metas):
- img_shape = img_meta[0]['img_shape']
- scale_factor = img_meta[0]['scale_factor']
- flip = img_meta[0]['flip']
- flip_direction = img_meta[0]['flip_direction']
- _bboxes = bbox_mapping(det_bboxes[:, :4], img_shape,
- scale_factor, flip, flip_direction)
- mask_rois = bbox2roi([_bboxes])
- mask_results = self._mask_forward(x, mask_rois)
- # convert to numpy array to save memory
- aug_masks.append(
- mask_results['mask_pred'].sigmoid().cpu().numpy())
- merged_masks = merge_aug_masks(aug_masks, img_metas, self.test_cfg)
-
- ori_shape = img_metas[0][0]['ori_shape']
- segm_result = self.mask_head.get_seg_masks(
- merged_masks,
- det_bboxes,
- det_labels,
- self.test_cfg,
- ori_shape,
- scale_factor=1.0,
- rescale=False)
- return segm_result
diff --git a/spaces/ChallengeHub/Chinese-LangChain/create_knowledge.py b/spaces/ChallengeHub/Chinese-LangChain/create_knowledge.py
deleted file mode 100644
index ee36198a3e110a637c415f17a4938f2eab2d3faa..0000000000000000000000000000000000000000
--- a/spaces/ChallengeHub/Chinese-LangChain/create_knowledge.py
+++ /dev/null
@@ -1,79 +0,0 @@
-#!/usr/bin/env python
-# -*- coding:utf-8 _*-
-"""
-@author:quincy qiang
-@license: Apache Licence
-@file: create_knowledge.py
-@time: 2023/04/18
-@contact: yanqiangmiffy@gamil.com
-@software: PyCharm
-@description: - emoji:https://emojixd.com/pocket/science
-"""
-import os
-import pandas as pd
-from langchain.schema import Document
-from langchain.document_loaders import UnstructuredFileLoader
-from langchain.embeddings.huggingface import HuggingFaceEmbeddings
-from langchain.vectorstores import FAISS
-from tqdm import tqdm
-# 中文Wikipedia数据导入示例:
-embedding_model_name = '/root/pretrained_models/text2vec-large-chinese'
-docs_path = '/root/GoMall/Knowledge-ChatGLM/cache/financial_research_reports'
-embeddings = HuggingFaceEmbeddings(model_name=embedding_model_name)
-
-
-# Wikipedia数据处理
-
-# docs = []
-
-# with open('docs/zh_wikipedia/zhwiki.sim.utf8', 'r', encoding='utf-8') as f:
-# for idx, line in tqdm(enumerate(f.readlines())):
-# metadata = {"source": f'doc_id_{idx}'}
-# docs.append(Document(page_content=line.strip(), metadata=metadata))
-#
-# vector_store = FAISS.from_documents(docs, embeddings)
-# vector_store.save_local('cache/zh_wikipedia/')
-
-
-
-docs = []
-
-with open('cache/zh_wikipedia/wiki.zh-sim-cleaned.txt', 'r', encoding='utf-8') as f:
- for idx, line in tqdm(enumerate(f.readlines())):
- metadata = {"source": f'doc_id_{idx}'}
- docs.append(Document(page_content=line.strip(), metadata=metadata))
-
-vector_store = FAISS.from_documents(docs, embeddings)
-vector_store.save_local('cache/zh_wikipedia/')
-
-
-# 金融研报数据处理
-# docs = []
-#
-# for doc in tqdm(os.listdir(docs_path)):
-# if doc.endswith('.txt'):
-# # print(doc)
-# loader = UnstructuredFileLoader(f'{docs_path}/{doc}', mode="elements")
-# doc = loader.load()
-# docs.extend(doc)
-# vector_store = FAISS.from_documents(docs, embeddings)
-# vector_store.save_local('cache/financial_research_reports')
-
-
-# 英雄联盟
-
-docs = []
-
-lol_df = pd.read_csv('cache/lol/champions.csv')
-# lol_df.columns = ['id', '英雄简称', '英雄全称', '出生地', '人物属性', '英雄类别', '英雄故事']
-print(lol_df)
-
-for idx, row in lol_df.iterrows():
- metadata = {"source": f'doc_id_{idx}'}
- text = ' '.join(row.values)
- # for col in ['英雄简称', '英雄全称', '出生地', '人物属性', '英雄类别', '英雄故事']:
- # text += row[col]
- docs.append(Document(page_content=text, metadata=metadata))
-
-vector_store = FAISS.from_documents(docs, embeddings)
-vector_store.save_local('cache/lol/')
diff --git a/spaces/Cvandi/remake/realesrgan/data/realesrgan_paired_dataset.py b/spaces/Cvandi/remake/realesrgan/data/realesrgan_paired_dataset.py
deleted file mode 100644
index 386c8d72496245dae8df033c2ebbd76b41ff45f1..0000000000000000000000000000000000000000
--- a/spaces/Cvandi/remake/realesrgan/data/realesrgan_paired_dataset.py
+++ /dev/null
@@ -1,108 +0,0 @@
-import os
-from basicsr.data.data_util import paired_paths_from_folder, paired_paths_from_lmdb
-from basicsr.data.transforms import augment, paired_random_crop
-from basicsr.utils import FileClient, imfrombytes, img2tensor
-from basicsr.utils.registry import DATASET_REGISTRY
-from torch.utils import data as data
-from torchvision.transforms.functional import normalize
-
-
-@DATASET_REGISTRY.register()
-class RealESRGANPairedDataset(data.Dataset):
- """Paired image dataset for image restoration.
-
- Read LQ (Low Quality, e.g. LR (Low Resolution), blurry, noisy, etc) and GT image pairs.
-
- There are three modes:
- 1. 'lmdb': Use lmdb files.
- If opt['io_backend'] == lmdb.
- 2. 'meta_info': Use meta information file to generate paths.
- If opt['io_backend'] != lmdb and opt['meta_info'] is not None.
- 3. 'folder': Scan folders to generate paths.
- The rest.
-
- Args:
- opt (dict): Config for train datasets. It contains the following keys:
- dataroot_gt (str): Data root path for gt.
- dataroot_lq (str): Data root path for lq.
- meta_info (str): Path for meta information file.
- io_backend (dict): IO backend type and other kwarg.
- filename_tmpl (str): Template for each filename. Note that the template excludes the file extension.
- Default: '{}'.
- gt_size (int): Cropped patched size for gt patches.
- use_hflip (bool): Use horizontal flips.
- use_rot (bool): Use rotation (use vertical flip and transposing h
- and w for implementation).
-
- scale (bool): Scale, which will be added automatically.
- phase (str): 'train' or 'val'.
- """
-
- def __init__(self, opt):
- super(RealESRGANPairedDataset, self).__init__()
- self.opt = opt
- self.file_client = None
- self.io_backend_opt = opt['io_backend']
- # mean and std for normalizing the input images
- self.mean = opt['mean'] if 'mean' in opt else None
- self.std = opt['std'] if 'std' in opt else None
-
- self.gt_folder, self.lq_folder = opt['dataroot_gt'], opt['dataroot_lq']
- self.filename_tmpl = opt['filename_tmpl'] if 'filename_tmpl' in opt else '{}'
-
- # file client (lmdb io backend)
- if self.io_backend_opt['type'] == 'lmdb':
- self.io_backend_opt['db_paths'] = [self.lq_folder, self.gt_folder]
- self.io_backend_opt['client_keys'] = ['lq', 'gt']
- self.paths = paired_paths_from_lmdb([self.lq_folder, self.gt_folder], ['lq', 'gt'])
- elif 'meta_info' in self.opt and self.opt['meta_info'] is not None:
- # disk backend with meta_info
- # Each line in the meta_info describes the relative path to an image
- with open(self.opt['meta_info']) as fin:
- paths = [line.strip() for line in fin]
- self.paths = []
- for path in paths:
- gt_path, lq_path = path.split(', ')
- gt_path = os.path.join(self.gt_folder, gt_path)
- lq_path = os.path.join(self.lq_folder, lq_path)
- self.paths.append(dict([('gt_path', gt_path), ('lq_path', lq_path)]))
- else:
- # disk backend
- # it will scan the whole folder to get meta info
- # it will be time-consuming for folders with too many files. It is recommended using an extra meta txt file
- self.paths = paired_paths_from_folder([self.lq_folder, self.gt_folder], ['lq', 'gt'], self.filename_tmpl)
-
- def __getitem__(self, index):
- if self.file_client is None:
- self.file_client = FileClient(self.io_backend_opt.pop('type'), **self.io_backend_opt)
-
- scale = self.opt['scale']
-
- # Load gt and lq images. Dimension order: HWC; channel order: BGR;
- # image range: [0, 1], float32.
- gt_path = self.paths[index]['gt_path']
- img_bytes = self.file_client.get(gt_path, 'gt')
- img_gt = imfrombytes(img_bytes, float32=True)
- lq_path = self.paths[index]['lq_path']
- img_bytes = self.file_client.get(lq_path, 'lq')
- img_lq = imfrombytes(img_bytes, float32=True)
-
- # augmentation for training
- if self.opt['phase'] == 'train':
- gt_size = self.opt['gt_size']
- # random crop
- img_gt, img_lq = paired_random_crop(img_gt, img_lq, gt_size, scale, gt_path)
- # flip, rotation
- img_gt, img_lq = augment([img_gt, img_lq], self.opt['use_hflip'], self.opt['use_rot'])
-
- # BGR to RGB, HWC to CHW, numpy to tensor
- img_gt, img_lq = img2tensor([img_gt, img_lq], bgr2rgb=True, float32=True)
- # normalize
- if self.mean is not None or self.std is not None:
- normalize(img_lq, self.mean, self.std, inplace=True)
- normalize(img_gt, self.mean, self.std, inplace=True)
-
- return {'lq': img_lq, 'gt': img_gt, 'lq_path': lq_path, 'gt_path': gt_path}
-
- def __len__(self):
- return len(self.paths)
diff --git a/spaces/DQChoi/gpt-demo/venv/lib/python3.11/site-packages/fontTools/misc/testTools.py b/spaces/DQChoi/gpt-demo/venv/lib/python3.11/site-packages/fontTools/misc/testTools.py
deleted file mode 100644
index be6116132d93a6a5f692f5b8465be346aad7ca5c..0000000000000000000000000000000000000000
--- a/spaces/DQChoi/gpt-demo/venv/lib/python3.11/site-packages/fontTools/misc/testTools.py
+++ /dev/null
@@ -1,229 +0,0 @@
-"""Helpers for writing unit tests."""
-
-from collections.abc import Iterable
-from io import BytesIO
-import os
-import re
-import shutil
-import sys
-import tempfile
-from unittest import TestCase as _TestCase
-from fontTools.config import Config
-from fontTools.misc.textTools import tobytes
-from fontTools.misc.xmlWriter import XMLWriter
-
-
-def parseXML(xmlSnippet):
- """Parses a snippet of XML.
-
- Input can be either a single string (unicode or UTF-8 bytes), or a
- a sequence of strings.
-
- The result is in the same format that would be returned by
- XMLReader, but the parser imposes no constraints on the root
- element so it can be called on small snippets of TTX files.
- """
- # To support snippets with multiple elements, we add a fake root.
- reader = TestXMLReader_()
- xml = b""
- if isinstance(xmlSnippet, bytes):
- xml += xmlSnippet
- elif isinstance(xmlSnippet, str):
- xml += tobytes(xmlSnippet, "utf-8")
- elif isinstance(xmlSnippet, Iterable):
- xml += b"".join(tobytes(s, "utf-8") for s in xmlSnippet)
- else:
- raise TypeError(
- "expected string or sequence of strings; found %r"
- % type(xmlSnippet).__name__
- )
- xml += b""
- reader.parser.Parse(xml, 0)
- return reader.root[2]
-
-
-def parseXmlInto(font, parseInto, xmlSnippet):
- parsed_xml = [e for e in parseXML(xmlSnippet.strip()) if not isinstance(e, str)]
- for name, attrs, content in parsed_xml:
- parseInto.fromXML(name, attrs, content, font)
- parseInto.populateDefaults()
- return parseInto
-
-
-class FakeFont:
- def __init__(self, glyphs):
- self.glyphOrder_ = glyphs
- self.reverseGlyphOrderDict_ = {g: i for i, g in enumerate(glyphs)}
- self.lazy = False
- self.tables = {}
- self.cfg = Config()
-
- def __getitem__(self, tag):
- return self.tables[tag]
-
- def __setitem__(self, tag, table):
- self.tables[tag] = table
-
- def get(self, tag, default=None):
- return self.tables.get(tag, default)
-
- def getGlyphID(self, name):
- return self.reverseGlyphOrderDict_[name]
-
- def getGlyphIDMany(self, lst):
- return [self.getGlyphID(gid) for gid in lst]
-
- def getGlyphName(self, glyphID):
- if glyphID < len(self.glyphOrder_):
- return self.glyphOrder_[glyphID]
- else:
- return "glyph%.5d" % glyphID
-
- def getGlyphNameMany(self, lst):
- return [self.getGlyphName(gid) for gid in lst]
-
- def getGlyphOrder(self):
- return self.glyphOrder_
-
- def getReverseGlyphMap(self):
- return self.reverseGlyphOrderDict_
-
- def getGlyphNames(self):
- return sorted(self.getGlyphOrder())
-
-
-class TestXMLReader_(object):
- def __init__(self):
- from xml.parsers.expat import ParserCreate
-
- self.parser = ParserCreate()
- self.parser.StartElementHandler = self.startElement_
- self.parser.EndElementHandler = self.endElement_
- self.parser.CharacterDataHandler = self.addCharacterData_
- self.root = None
- self.stack = []
-
- def startElement_(self, name, attrs):
- element = (name, attrs, [])
- if self.stack:
- self.stack[-1][2].append(element)
- else:
- self.root = element
- self.stack.append(element)
-
- def endElement_(self, name):
- self.stack.pop()
-
- def addCharacterData_(self, data):
- self.stack[-1][2].append(data)
-
-
-def makeXMLWriter(newlinestr="\n"):
- # don't write OS-specific new lines
- writer = XMLWriter(BytesIO(), newlinestr=newlinestr)
- # erase XML declaration
- writer.file.seek(0)
- writer.file.truncate()
- return writer
-
-
-def getXML(func, ttFont=None):
- """Call the passed toXML function and return the written content as a
- list of lines (unicode strings).
- Result is stripped of XML declaration and OS-specific newline characters.
- """
- writer = makeXMLWriter()
- func(writer, ttFont)
- xml = writer.file.getvalue().decode("utf-8")
- # toXML methods must always end with a writer.newline()
- assert xml.endswith("\n")
- return xml.splitlines()
-
-
-def stripVariableItemsFromTTX(
- string: str,
- ttLibVersion: bool = True,
- checkSumAdjustment: bool = True,
- modified: bool = True,
- created: bool = True,
- sfntVersion: bool = False, # opt-in only
-) -> str:
- """Strip stuff like ttLibVersion, checksums, timestamps, etc. from TTX dumps."""
- # ttlib changes with the fontTools version
- if ttLibVersion:
- string = re.sub(' ttLibVersion="[^"]+"', "", string)
- # sometimes (e.g. some subsetter tests) we don't care whether it's OTF or TTF
- if sfntVersion:
- string = re.sub(' sfntVersion="[^"]+"', "", string)
- # head table checksum and creation and mod date changes with each save.
- if checkSumAdjustment:
- string = re.sub('', "", string)
- if modified:
- string = re.sub('', "", string)
- if created:
- string = re.sub('', "", string)
- return string
-
-
-class MockFont(object):
- """A font-like object that automatically adds any looked up glyphname
- to its glyphOrder."""
-
- def __init__(self):
- self._glyphOrder = [".notdef"]
-
- class AllocatingDict(dict):
- def __missing__(reverseDict, key):
- self._glyphOrder.append(key)
- gid = len(reverseDict)
- reverseDict[key] = gid
- return gid
-
- self._reverseGlyphOrder = AllocatingDict({".notdef": 0})
- self.lazy = False
-
- def getGlyphID(self, glyph):
- gid = self._reverseGlyphOrder[glyph]
- return gid
-
- def getReverseGlyphMap(self):
- return self._reverseGlyphOrder
-
- def getGlyphName(self, gid):
- return self._glyphOrder[gid]
-
- def getGlyphOrder(self):
- return self._glyphOrder
-
-
-class TestCase(_TestCase):
- def __init__(self, methodName):
- _TestCase.__init__(self, methodName)
- # Python 3 renamed assertRaisesRegexp to assertRaisesRegex,
- # and fires deprecation warnings if a program uses the old name.
- if not hasattr(self, "assertRaisesRegex"):
- self.assertRaisesRegex = self.assertRaisesRegexp
-
-
-class DataFilesHandler(TestCase):
- def setUp(self):
- self.tempdir = None
- self.num_tempfiles = 0
-
- def tearDown(self):
- if self.tempdir:
- shutil.rmtree(self.tempdir)
-
- def getpath(self, testfile):
- folder = os.path.dirname(sys.modules[self.__module__].__file__)
- return os.path.join(folder, "data", testfile)
-
- def temp_dir(self):
- if not self.tempdir:
- self.tempdir = tempfile.mkdtemp()
-
- def temp_font(self, font_path, file_name):
- self.temp_dir()
- temppath = os.path.join(self.tempdir, file_name)
- shutil.copy2(font_path, temppath)
- return temppath
diff --git a/spaces/EuroPython2022/pulsar-clip/README.md b/spaces/EuroPython2022/pulsar-clip/README.md
deleted file mode 100644
index bf7cd8333378ebe4bd874633d4398c0d1ba5e60f..0000000000000000000000000000000000000000
--- a/spaces/EuroPython2022/pulsar-clip/README.md
+++ /dev/null
@@ -1,13 +0,0 @@
----
-title: Pulsar Clip
-emoji: 😻
-colorFrom: yellow
-colorTo: blue
-sdk: gradio
-sdk_version: 3.1.4b5
-app_file: app.py
-pinned: false
-license: agpl-3.0
----
-
-Check out the configuration reference at https://huggingface.co/docs/hub/spaces-config-reference
diff --git a/spaces/FrankZxShen/so-vits-svc-models-ba/diffusion/infer_gt_mel.py b/spaces/FrankZxShen/so-vits-svc-models-ba/diffusion/infer_gt_mel.py
deleted file mode 100644
index 033b821a5d21a1232f1786bce5616b12e01488ad..0000000000000000000000000000000000000000
--- a/spaces/FrankZxShen/so-vits-svc-models-ba/diffusion/infer_gt_mel.py
+++ /dev/null
@@ -1,74 +0,0 @@
-import numpy as np
-import torch
-import torch.nn.functional as F
-from diffusion.unit2mel import load_model_vocoder
-
-
-class DiffGtMel:
- def __init__(self, project_path=None, device=None):
- self.project_path = project_path
- if device is not None:
- self.device = device
- else:
- self.device = 'cuda' if torch.cuda.is_available() else 'cpu'
- self.model = None
- self.vocoder = None
- self.args = None
-
- def flush_model(self, project_path, ddsp_config=None):
- if (self.model is None) or (project_path != self.project_path):
- model, vocoder, args = load_model_vocoder(project_path, device=self.device)
- if self.check_args(ddsp_config, args):
- self.model = model
- self.vocoder = vocoder
- self.args = args
-
- def check_args(self, args1, args2):
- if args1.data.block_size != args2.data.block_size:
- raise ValueError("DDSP与DIFF模型的block_size不一致")
- if args1.data.sampling_rate != args2.data.sampling_rate:
- raise ValueError("DDSP与DIFF模型的sampling_rate不一致")
- if args1.data.encoder != args2.data.encoder:
- raise ValueError("DDSP与DIFF模型的encoder不一致")
- return True
-
- def __call__(self, audio, f0, hubert, volume, acc=1, spk_id=1, k_step=0, method='pndm',
- spk_mix_dict=None, start_frame=0):
- input_mel = self.vocoder.extract(audio, self.args.data.sampling_rate)
- out_mel = self.model(
- hubert,
- f0,
- volume,
- spk_id=spk_id,
- spk_mix_dict=spk_mix_dict,
- gt_spec=input_mel,
- infer=True,
- infer_speedup=acc,
- method=method,
- k_step=k_step,
- use_tqdm=False)
- if start_frame > 0:
- out_mel = out_mel[:, start_frame:, :]
- f0 = f0[:, start_frame:, :]
- output = self.vocoder.infer(out_mel, f0)
- if start_frame > 0:
- output = F.pad(output, (start_frame * self.vocoder.vocoder_hop_size, 0))
- return output
-
- def infer(self, audio, f0, hubert, volume, acc=1, spk_id=1, k_step=0, method='pndm', silence_front=0,
- use_silence=False, spk_mix_dict=None):
- start_frame = int(silence_front * self.vocoder.vocoder_sample_rate / self.vocoder.vocoder_hop_size)
- if use_silence:
- audio = audio[:, start_frame * self.vocoder.vocoder_hop_size:]
- f0 = f0[:, start_frame:, :]
- hubert = hubert[:, start_frame:, :]
- volume = volume[:, start_frame:, :]
- _start_frame = 0
- else:
- _start_frame = start_frame
- audio = self.__call__(audio, f0, hubert, volume, acc=acc, spk_id=spk_id, k_step=k_step,
- method=method, spk_mix_dict=spk_mix_dict, start_frame=_start_frame)
- if use_silence:
- if start_frame > 0:
- audio = F.pad(audio, (start_frame * self.vocoder.vocoder_hop_size, 0))
- return audio
diff --git a/spaces/GMFTBY/PandaGPT/model/ImageBind/__init__.py b/spaces/GMFTBY/PandaGPT/model/ImageBind/__init__.py
deleted file mode 100644
index d872d0725710d6dde3af3b6e05382922f074338b..0000000000000000000000000000000000000000
--- a/spaces/GMFTBY/PandaGPT/model/ImageBind/__init__.py
+++ /dev/null
@@ -1,2 +0,0 @@
-from .models import imagebind_model
-from .models.imagebind_model import ModalityType
diff --git a/spaces/GaenKoki/voicevox/speaker_info/388f246b-8c41-4ac1-8e2d-5d79f3ff56d9/policy.md b/spaces/GaenKoki/voicevox/speaker_info/388f246b-8c41-4ac1-8e2d-5d79f3ff56d9/policy.md
deleted file mode 100644
index 0328c63112a40f44145440562c8fe2d56ac86e38..0000000000000000000000000000000000000000
--- a/spaces/GaenKoki/voicevox/speaker_info/388f246b-8c41-4ac1-8e2d-5d79f3ff56d9/policy.md
+++ /dev/null
@@ -1,3 +0,0 @@
-dummy2 policy
-
-https://voicevox.hiroshiba.jp/
diff --git a/spaces/Gen-Sim/Gen-Sim/cliport/generated_tasks/block_on_cylinder_on_pallet.py b/spaces/Gen-Sim/Gen-Sim/cliport/generated_tasks/block_on_cylinder_on_pallet.py
deleted file mode 100644
index d29f6d6de4c60bd0e6a5a30261ea09bc6ed05b9d..0000000000000000000000000000000000000000
--- a/spaces/Gen-Sim/Gen-Sim/cliport/generated_tasks/block_on_cylinder_on_pallet.py
+++ /dev/null
@@ -1,58 +0,0 @@
-import numpy as np
-from cliport.tasks.task import Task
-from cliport.utils import utils
-
-class BlockOnCylinderOnPallet(Task):
- """Pick up each block and place it on the corresponding colored cylinder, which are located in specific positions on a pallet."""
-
- def __init__(self):
- super().__init__()
- self.max_steps = 15
- self.lang_template = "place the {} cylinder on the pallet"
- self.lang_template_2 = "place the {} block on the {} cylinder"
-
- self.task_completed_desc = "done placing blocks on cylinders and cylinder on pallet."
- self.additional_reset()
-
- def reset(self, env):
- super().reset(env)
-
- # Add pallet.
- pallet_size = (0.35, 0.35, 0.01)
- pallet_pose = self.get_random_pose(env, pallet_size)
- pallet_urdf = 'pallet/pallet.urdf'
- env.add_object(pallet_urdf, pallet_pose, 'fixed')
-
- # Define colors.
- block_colors = ['red']
- cylinder_colors = ['blue']
-
- # Add cylinders.
- cylinder_size = (0.04, 0.04, 0.06)
- cylinder_template = 'cylinder/cylinder-template.urdf'
- cylinders = []
-
-
- replace = {'DIM': cylinder_size, 'HALF': (cylinder_size[0] / 2, cylinder_size[1] / 2, cylinder_size[2] / 2), 'COLOR': block_colors[0]}
- cylinder_urdf = self.fill_template(cylinder_template, replace)
- cylinder_pose = self.get_random_pose(env, cylinder_size)
- cylinder_id = env.add_object(cylinder_urdf, cylinder_pose)
- cylinders.append(cylinder_id)
-
- # Add blocks.
- block_size = (0.04, 0.04, 0.04)
- block_urdf = 'block/block.urdf'
- blocks = []
- block_pose = self.get_random_pose(env, block_size)
- block_id = env.add_object(block_urdf, block_pose, color=cylinder_colors[0])
- blocks.append(block_id)
-
- # Goal: place the cylinder on top of the pallet
- self.add_goal(objs=[cylinders[0]], matches=np.ones((1, 1)), targ_poses=[pallet_pose], replace=False,
- rotations=True, metric='pose', params=None, step_max_reward=1/2, language_goal=self.lang_template.format(cylinder_colors[0]))
-
-
- # Goal: place the block on top of the cylinder
- language_goal = self.lang_template_2.format(block_colors[0], cylinder_colors[0])
- self.add_goal(objs=[blocks[0]], matches=np.ones((1, 1)), targ_poses=[pallet_pose], replace=False,
- rotations=True, metric='pose', params=None, step_max_reward=1/2, language_goal=language_goal)
diff --git a/spaces/Gen-Sim/Gen-Sim/cliport/generated_tasks/color_cued_ball_corner_sorting.py b/spaces/Gen-Sim/Gen-Sim/cliport/generated_tasks/color_cued_ball_corner_sorting.py
deleted file mode 100644
index b24285254c772733bbdfb70ca226c0c618a208c0..0000000000000000000000000000000000000000
--- a/spaces/Gen-Sim/Gen-Sim/cliport/generated_tasks/color_cued_ball_corner_sorting.py
+++ /dev/null
@@ -1,62 +0,0 @@
-import numpy as np
-import os
-import pybullet as p
-import random
-from cliport.tasks import primitives
-from cliport.tasks.grippers import Spatula
-from cliport.tasks.task import Task
-from cliport.utils import utils
-import numpy as np
-from cliport.tasks.task import Task
-from cliport.utils import utils
-
-class ColorCuedBallCornerSorting(Task):
- """Pick up each colored ball and place it in the corner of the same color while avoiding a zone marked by small blocks."""
-
- def __init__(self):
- super().__init__()
- self.max_steps = 20
- self.lang_template = "place the {color} ball in the {color} corner"
- self.task_completed_desc = "done sorting balls."
- self.additional_reset()
-
- def reset(self, env):
- super().reset(env)
-
- # Add corners.
- corner_size = (0.05, 0.05, 0.05)
- corner_urdf = 'corner/corner-template.urdf'
- corner_colors = ['red', 'blue', 'green', 'yellow']
- corner_poses = []
- for color in corner_colors:
- corner_pose = self.get_random_pose(env, corner_size)
- env.add_object(corner_urdf, corner_pose, color=color, category='fixed')
- corner_poses.append(corner_pose)
-
- # Add balls.
- balls = []
- ball_size = (0.04, 0.04, 0.04)
- ball_urdf = 'ball/ball-template.urdf'
- for color in corner_colors:
- ball_pose = self.get_random_pose(env, ball_size)
- ball_id = env.add_object(ball_urdf, ball_pose, color=color)
- balls.append(ball_id)
-
- # Add zone.
- zone_size = (0.2, 0.2, 0.05)
- zone_pose = self.get_random_pose(env, zone_size)
- zone_urdf = 'zone/zone.urdf'
- env.add_object(zone_urdf, zone_pose, 'fixed')
-
- # Add blocks.
- block_size = (0.04, 0.04, 0.04)
- block_urdf = 'block/block_for_anchors.urdf'
- for _ in range(4):
- block_pose = self.get_random_pose(env, block_size)
- env.add_object(block_urdf, block_pose)
-
- # Goal: each ball is in the corner of the same color.
- for i in range(4):
- self.add_goal(objs=[balls[i]], matches=np.ones((1, 1)), targ_poses=[corner_poses[i]], replace=False,
- rotations=True, metric='pose', params=None, step_max_reward=1/4,
- language_goal=self.lang_template.format(color=corner_colors[i]))
\ No newline at end of file
diff --git a/spaces/Gen-Sim/Gen-Sim/cliport/generated_tasks/color_ordered_insertion_new.py b/spaces/Gen-Sim/Gen-Sim/cliport/generated_tasks/color_ordered_insertion_new.py
deleted file mode 100644
index 72cc3f4f34d8822ba14e7a7e9c73b1e995304a8f..0000000000000000000000000000000000000000
--- a/spaces/Gen-Sim/Gen-Sim/cliport/generated_tasks/color_ordered_insertion_new.py
+++ /dev/null
@@ -1,52 +0,0 @@
-import numpy as np
-import os
-import pybullet as p
-import random
-from cliport.tasks import primitives
-from cliport.tasks.grippers import Spatula
-from cliport.tasks.task import Task
-from cliport.utils import utils
-import numpy as np
-from cliport.tasks.task import Task
-from cliport.utils import utils
-
-class ColorOrderedInsertionNew(Task):
- """Insert differently-colored ell objects into the matching color fixture in a specific order."""
-
- def __init__(self):
- super().__init__()
- self.max_steps = 20
- self.lang_template = "put the {color} L shape block in the L shape hole"
- self.task_completed_desc = "done with insertion."
- self.additional_reset()
-
- def reset(self, env):
- super().reset(env)
-
- # Define colors and their order
- colors = ['red', 'blue', 'green', 'yellow']
- color_order = {color: i for i, color in enumerate(colors)}
-
- # Add fixtures.
- fixture_size = (0.12, 0.12, 0.02)
- fixture_urdf = 'insertion/fixture.urdf'
- fixtures = []
- for color in colors:
- fixture_pose = self.get_random_pose(env, fixture_size)
- fixture_id = env.add_object(fixture_urdf, fixture_pose, color=utils.COLORS[color], category='fixed')
- fixtures.append(fixture_id)
-
- # Add ell objects.
- ell_size = (0.04, 0.04, 0.04)
- ell_urdf = 'insertion/ell.urdf'
- ells = []
- for color in colors:
- ell_pose = self.get_random_pose(env, ell_size)
- ell_id = env.add_object(ell_urdf, ell_pose, color=utils.COLORS[color])
- ells.append(ell_id)
-
- # Goal: each ell is inserted into the matching color fixture in the correct order.
- for i, ell in enumerate(ells):
- self.add_goal(objs=[ell], matches=np.ones((1, 1)), targ_poses=[p.getBasePositionAndOrientation(fixtures[i])], replace=False,
- rotations=True, metric='pose', params=None, step_max_reward=1 / len(ells),
- language_goal=self.lang_template.format(color=colors[i]))
\ No newline at end of file
diff --git a/spaces/Gen-Sim/Gen-Sim/cliport/generated_tasks/move_piles_along_line.py b/spaces/Gen-Sim/Gen-Sim/cliport/generated_tasks/move_piles_along_line.py
deleted file mode 100644
index b3963dfaa5d7551149c72ce8fe759393424fbd66..0000000000000000000000000000000000000000
--- a/spaces/Gen-Sim/Gen-Sim/cliport/generated_tasks/move_piles_along_line.py
+++ /dev/null
@@ -1,70 +0,0 @@
-import numpy as np
-import os
-import pybullet as p
-import random
-from cliport.tasks import primitives
-from cliport.tasks.grippers import Spatula
-from cliport.tasks.task import Task
-from cliport.utils import utils
-import numpy as np
-from cliport.tasks import primitives
-from cliport.tasks.grippers import Spatula
-from cliport.tasks.task import Task
-from cliport.utils import utils
-
-class MovePilesAlongLine(Task):
- """Move three piles of small blocks, each pile a different color (red, blue, green),
- along three matching colored lines to three separate zones of the same color using a spatula."""
-
- def __init__(self):
- super().__init__()
- self.max_steps = 20
- self.lang_template = "move the piles of blocks along the lines to the matching colored zones"
- self.task_completed_desc = "done moving piles."
- self.primitive = primitives.push
- self.ee = Spatula
- self.additional_reset()
-
- def reset(self, env):
- super().reset(env)
-
- # Add three colored lines.
- line_template = 'line/line-template.urdf'
- line_colors = ['red', 'blue', 'green']
- line_poses = []
- for color in line_colors:
- line_size = self.get_random_size(0.1, 0.15, 0.1, 0.15, 0.05, 0.05)
- line_pose = self.get_random_pose(env, line_size)
- replace = {'DIM': line_size, 'HALF': (line_size[0] / 2, line_size[1] / 2, line_size[2] / 2), 'COLOR': color}
- line_urdf = self.fill_template(line_template, replace)
- env.add_object(line_urdf, line_pose, 'fixed')
- line_poses.append(line_pose)
-
- # Add three colored zones.
- zone_template = 'zone/zone.urdf'
- zone_poses = []
- for color in line_colors:
- zone_size = self.get_random_size(0.1, 0.15, 0.1, 0.15, 0.05, 0.05)
- zone_pose = self.get_random_pose(env, zone_size)
- replace = {'DIM': zone_size, 'HALF': (zone_size[0] / 2, zone_size[1] / 2, zone_size[2] / 2), 'COLOR': color}
- zone_urdf = self.fill_template(zone_template, replace)
- env.add_object(zone_urdf, zone_pose, 'fixed')
- zone_poses.append(zone_pose)
-
- # Add three piles of small blocks.
- block_template = 'block/small.urdf'
- block_colors = ['red', 'blue', 'green']
- block_ids = []
- for color in block_colors:
- block_size = self.get_random_size(0.1, 0.15, 0.1, 0.15, 0.05, 0.05)
- block_pose = self.get_random_pose(env, block_size)
- replace = {'DIM': block_size, 'HALF': (block_size[0] / 2, block_size[1] / 2, block_size[2] / 2), 'COLOR': color}
- block_urdf = self.fill_template(block_template, replace)
- block_id = env.add_object(block_urdf, block_pose)
- block_ids.append(block_id)
-
- # Add goals.
- for i in range(3):
- self.add_goal(objs=[block_ids[i]], matches=np.ones((1, 1)), targ_poses=[zone_poses[i]], replace=False,
- rotations=False, metric='zone', params=[(zone_poses[i], zone_size)], step_max_reward=1/3,
- language_goal=self.lang_template)
\ No newline at end of file
diff --git a/spaces/Gradio-Blocks/uniformer_image_detection/mmdet/core/bbox/coder/tblr_bbox_coder.py b/spaces/Gradio-Blocks/uniformer_image_detection/mmdet/core/bbox/coder/tblr_bbox_coder.py
deleted file mode 100644
index edaffaf1fa252857e1a660ea14a613e2466fb52c..0000000000000000000000000000000000000000
--- a/spaces/Gradio-Blocks/uniformer_image_detection/mmdet/core/bbox/coder/tblr_bbox_coder.py
+++ /dev/null
@@ -1,198 +0,0 @@
-import mmcv
-import torch
-
-from ..builder import BBOX_CODERS
-from .base_bbox_coder import BaseBBoxCoder
-
-
-@BBOX_CODERS.register_module()
-class TBLRBBoxCoder(BaseBBoxCoder):
- """TBLR BBox coder.
-
- Following the practice in `FSAF `_,
- this coder encodes gt bboxes (x1, y1, x2, y2) into (top, bottom, left,
- right) and decode it back to the original.
-
- Args:
- normalizer (list | float): Normalization factor to be
- divided with when coding the coordinates. If it is a list, it should
- have length of 4 indicating normalization factor in tblr dims.
- Otherwise it is a unified float factor for all dims. Default: 4.0
- clip_border (bool, optional): Whether clip the objects outside the
- border of the image. Defaults to True.
- """
-
- def __init__(self, normalizer=4.0, clip_border=True):
- super(BaseBBoxCoder, self).__init__()
- self.normalizer = normalizer
- self.clip_border = clip_border
-
- def encode(self, bboxes, gt_bboxes):
- """Get box regression transformation deltas that can be used to
- transform the ``bboxes`` into the ``gt_bboxes`` in the (top, left,
- bottom, right) order.
-
- Args:
- bboxes (torch.Tensor): source boxes, e.g., object proposals.
- gt_bboxes (torch.Tensor): target of the transformation, e.g.,
- ground truth boxes.
-
- Returns:
- torch.Tensor: Box transformation deltas
- """
- assert bboxes.size(0) == gt_bboxes.size(0)
- assert bboxes.size(-1) == gt_bboxes.size(-1) == 4
- encoded_bboxes = bboxes2tblr(
- bboxes, gt_bboxes, normalizer=self.normalizer)
- return encoded_bboxes
-
- def decode(self, bboxes, pred_bboxes, max_shape=None):
- """Apply transformation `pred_bboxes` to `boxes`.
-
- Args:
- bboxes (torch.Tensor): Basic boxes.Shape (B, N, 4) or (N, 4)
- pred_bboxes (torch.Tensor): Encoded boxes with shape
- (B, N, 4) or (N, 4)
- max_shape (Sequence[int] or torch.Tensor or Sequence[
- Sequence[int]],optional): Maximum bounds for boxes, specifies
- (H, W, C) or (H, W). If bboxes shape is (B, N, 4), then
- the max_shape should be a Sequence[Sequence[int]]
- and the length of max_shape should also be B.
-
- Returns:
- torch.Tensor: Decoded boxes.
- """
- decoded_bboxes = tblr2bboxes(
- bboxes,
- pred_bboxes,
- normalizer=self.normalizer,
- max_shape=max_shape,
- clip_border=self.clip_border)
-
- return decoded_bboxes
-
-
-@mmcv.jit(coderize=True)
-def bboxes2tblr(priors, gts, normalizer=4.0, normalize_by_wh=True):
- """Encode ground truth boxes to tblr coordinate.
-
- It first convert the gt coordinate to tblr format,
- (top, bottom, left, right), relative to prior box centers.
- The tblr coordinate may be normalized by the side length of prior bboxes
- if `normalize_by_wh` is specified as True, and it is then normalized by
- the `normalizer` factor.
-
- Args:
- priors (Tensor): Prior boxes in point form
- Shape: (num_proposals,4).
- gts (Tensor): Coords of ground truth for each prior in point-form
- Shape: (num_proposals, 4).
- normalizer (Sequence[float] | float): normalization parameter of
- encoded boxes. If it is a list, it has to have length = 4.
- Default: 4.0
- normalize_by_wh (bool): Whether to normalize tblr coordinate by the
- side length (wh) of prior bboxes.
-
- Return:
- encoded boxes (Tensor), Shape: (num_proposals, 4)
- """
-
- # dist b/t match center and prior's center
- if not isinstance(normalizer, float):
- normalizer = torch.tensor(normalizer, device=priors.device)
- assert len(normalizer) == 4, 'Normalizer must have length = 4'
- assert priors.size(0) == gts.size(0)
- prior_centers = (priors[:, 0:2] + priors[:, 2:4]) / 2
- xmin, ymin, xmax, ymax = gts.split(1, dim=1)
- top = prior_centers[:, 1].unsqueeze(1) - ymin
- bottom = ymax - prior_centers[:, 1].unsqueeze(1)
- left = prior_centers[:, 0].unsqueeze(1) - xmin
- right = xmax - prior_centers[:, 0].unsqueeze(1)
- loc = torch.cat((top, bottom, left, right), dim=1)
- if normalize_by_wh:
- # Normalize tblr by anchor width and height
- wh = priors[:, 2:4] - priors[:, 0:2]
- w, h = torch.split(wh, 1, dim=1)
- loc[:, :2] /= h # tb is normalized by h
- loc[:, 2:] /= w # lr is normalized by w
- # Normalize tblr by the given normalization factor
- return loc / normalizer
-
-
-@mmcv.jit(coderize=True)
-def tblr2bboxes(priors,
- tblr,
- normalizer=4.0,
- normalize_by_wh=True,
- max_shape=None,
- clip_border=True):
- """Decode tblr outputs to prediction boxes.
-
- The process includes 3 steps: 1) De-normalize tblr coordinates by
- multiplying it with `normalizer`; 2) De-normalize tblr coordinates by the
- prior bbox width and height if `normalize_by_wh` is `True`; 3) Convert
- tblr (top, bottom, left, right) pair relative to the center of priors back
- to (xmin, ymin, xmax, ymax) coordinate.
-
- Args:
- priors (Tensor): Prior boxes in point form (x0, y0, x1, y1)
- Shape: (N,4) or (B, N, 4).
- tblr (Tensor): Coords of network output in tblr form
- Shape: (N, 4) or (B, N, 4).
- normalizer (Sequence[float] | float): Normalization parameter of
- encoded boxes. By list, it represents the normalization factors at
- tblr dims. By float, it is the unified normalization factor at all
- dims. Default: 4.0
- normalize_by_wh (bool): Whether the tblr coordinates have been
- normalized by the side length (wh) of prior bboxes.
- max_shape (Sequence[int] or torch.Tensor or Sequence[
- Sequence[int]],optional): Maximum bounds for boxes, specifies
- (H, W, C) or (H, W). If priors shape is (B, N, 4), then
- the max_shape should be a Sequence[Sequence[int]]
- and the length of max_shape should also be B.
- clip_border (bool, optional): Whether clip the objects outside the
- border of the image. Defaults to True.
-
- Return:
- encoded boxes (Tensor): Boxes with shape (N, 4) or (B, N, 4)
- """
- if not isinstance(normalizer, float):
- normalizer = torch.tensor(normalizer, device=priors.device)
- assert len(normalizer) == 4, 'Normalizer must have length = 4'
- assert priors.size(0) == tblr.size(0)
- if priors.ndim == 3:
- assert priors.size(1) == tblr.size(1)
-
- loc_decode = tblr * normalizer
- prior_centers = (priors[..., 0:2] + priors[..., 2:4]) / 2
- if normalize_by_wh:
- wh = priors[..., 2:4] - priors[..., 0:2]
- w, h = torch.split(wh, 1, dim=-1)
- # Inplace operation with slice would failed for exporting to ONNX
- th = h * loc_decode[..., :2] # tb
- tw = w * loc_decode[..., 2:] # lr
- loc_decode = torch.cat([th, tw], dim=-1)
- # Cannot be exported using onnx when loc_decode.split(1, dim=-1)
- top, bottom, left, right = loc_decode.split((1, 1, 1, 1), dim=-1)
- xmin = prior_centers[..., 0].unsqueeze(-1) - left
- xmax = prior_centers[..., 0].unsqueeze(-1) + right
- ymin = prior_centers[..., 1].unsqueeze(-1) - top
- ymax = prior_centers[..., 1].unsqueeze(-1) + bottom
-
- bboxes = torch.cat((xmin, ymin, xmax, ymax), dim=-1)
-
- if clip_border and max_shape is not None:
- if not isinstance(max_shape, torch.Tensor):
- max_shape = priors.new_tensor(max_shape)
- max_shape = max_shape[..., :2].type_as(priors)
- if max_shape.ndim == 2:
- assert bboxes.ndim == 3
- assert max_shape.size(0) == bboxes.size(0)
-
- min_xy = priors.new_tensor(0)
- max_xy = torch.cat([max_shape, max_shape],
- dim=-1).flip(-1).unsqueeze(-2)
- bboxes = torch.where(bboxes < min_xy, min_xy, bboxes)
- bboxes = torch.where(bboxes > max_xy, max_xy, bboxes)
-
- return bboxes
diff --git a/spaces/Gradio-Blocks/uniformer_image_detection/mmdet/models/losses/__init__.py b/spaces/Gradio-Blocks/uniformer_image_detection/mmdet/models/losses/__init__.py
deleted file mode 100644
index 297aa228277768eb0ba0e8a377f19704d1feeca8..0000000000000000000000000000000000000000
--- a/spaces/Gradio-Blocks/uniformer_image_detection/mmdet/models/losses/__init__.py
+++ /dev/null
@@ -1,29 +0,0 @@
-from .accuracy import Accuracy, accuracy
-from .ae_loss import AssociativeEmbeddingLoss
-from .balanced_l1_loss import BalancedL1Loss, balanced_l1_loss
-from .cross_entropy_loss import (CrossEntropyLoss, binary_cross_entropy,
- cross_entropy, mask_cross_entropy)
-from .focal_loss import FocalLoss, sigmoid_focal_loss
-from .gaussian_focal_loss import GaussianFocalLoss
-from .gfocal_loss import DistributionFocalLoss, QualityFocalLoss
-from .ghm_loss import GHMC, GHMR
-from .iou_loss import (BoundedIoULoss, CIoULoss, DIoULoss, GIoULoss, IoULoss,
- bounded_iou_loss, iou_loss)
-from .kd_loss import KnowledgeDistillationKLDivLoss
-from .mse_loss import MSELoss, mse_loss
-from .pisa_loss import carl_loss, isr_p
-from .smooth_l1_loss import L1Loss, SmoothL1Loss, l1_loss, smooth_l1_loss
-from .utils import reduce_loss, weight_reduce_loss, weighted_loss
-from .varifocal_loss import VarifocalLoss
-
-__all__ = [
- 'accuracy', 'Accuracy', 'cross_entropy', 'binary_cross_entropy',
- 'mask_cross_entropy', 'CrossEntropyLoss', 'sigmoid_focal_loss',
- 'FocalLoss', 'smooth_l1_loss', 'SmoothL1Loss', 'balanced_l1_loss',
- 'BalancedL1Loss', 'mse_loss', 'MSELoss', 'iou_loss', 'bounded_iou_loss',
- 'IoULoss', 'BoundedIoULoss', 'GIoULoss', 'DIoULoss', 'CIoULoss', 'GHMC',
- 'GHMR', 'reduce_loss', 'weight_reduce_loss', 'weighted_loss', 'L1Loss',
- 'l1_loss', 'isr_p', 'carl_loss', 'AssociativeEmbeddingLoss',
- 'GaussianFocalLoss', 'QualityFocalLoss', 'DistributionFocalLoss',
- 'VarifocalLoss', 'KnowledgeDistillationKLDivLoss'
-]
diff --git a/spaces/Gradio-Blocks/uniformer_image_segmentation/configs/deeplabv3/deeplabv3_r50-d8_480x480_40k_pascal_context.py b/spaces/Gradio-Blocks/uniformer_image_segmentation/configs/deeplabv3/deeplabv3_r50-d8_480x480_40k_pascal_context.py
deleted file mode 100644
index 9d493ef527bb161be98d0e4ea433104b3bb9ff48..0000000000000000000000000000000000000000
--- a/spaces/Gradio-Blocks/uniformer_image_segmentation/configs/deeplabv3/deeplabv3_r50-d8_480x480_40k_pascal_context.py
+++ /dev/null
@@ -1,10 +0,0 @@
-_base_ = [
- '../_base_/models/deeplabv3_r50-d8.py',
- '../_base_/datasets/pascal_context.py', '../_base_/default_runtime.py',
- '../_base_/schedules/schedule_40k.py'
-]
-model = dict(
- decode_head=dict(num_classes=60),
- auxiliary_head=dict(num_classes=60),
- test_cfg=dict(mode='slide', crop_size=(480, 480), stride=(320, 320)))
-optimizer = dict(type='SGD', lr=0.004, momentum=0.9, weight_decay=0.0001)
diff --git a/spaces/Gradio-Blocks/uniformer_image_segmentation/configs/fcn/fcn_d6_r50-d16_512x1024_40k_cityscapes.py b/spaces/Gradio-Blocks/uniformer_image_segmentation/configs/fcn/fcn_d6_r50-d16_512x1024_40k_cityscapes.py
deleted file mode 100644
index f30646ede7b036e6c82c335729b19f92293efb35..0000000000000000000000000000000000000000
--- a/spaces/Gradio-Blocks/uniformer_image_segmentation/configs/fcn/fcn_d6_r50-d16_512x1024_40k_cityscapes.py
+++ /dev/null
@@ -1,8 +0,0 @@
-_base_ = [
- '../_base_/models/fcn_r50-d8.py', '../_base_/datasets/cityscapes.py',
- '../_base_/default_runtime.py', '../_base_/schedules/schedule_40k.py'
-]
-model = dict(
- backbone=dict(dilations=(1, 1, 1, 2), strides=(1, 2, 2, 1)),
- decode_head=dict(dilation=6),
- auxiliary_head=dict(dilation=6))
diff --git a/spaces/Gradio-Blocks/uniformer_image_segmentation/configs/nonlocal_net/nonlocal_r101-d8_512x512_40k_voc12aug.py b/spaces/Gradio-Blocks/uniformer_image_segmentation/configs/nonlocal_net/nonlocal_r101-d8_512x512_40k_voc12aug.py
deleted file mode 100644
index 40d9190fba223251b794c105b036e4794865f785..0000000000000000000000000000000000000000
--- a/spaces/Gradio-Blocks/uniformer_image_segmentation/configs/nonlocal_net/nonlocal_r101-d8_512x512_40k_voc12aug.py
+++ /dev/null
@@ -1,2 +0,0 @@
-_base_ = './nonlocal_r50-d8_512x512_40k_voc12aug.py'
-model = dict(pretrained='open-mmlab://resnet101_v1c', backbone=dict(depth=101))
diff --git a/spaces/Gradio-Blocks/uniformer_image_segmentation/configs/resnest/fcn_s101-d8_512x512_160k_ade20k.py b/spaces/Gradio-Blocks/uniformer_image_segmentation/configs/resnest/fcn_s101-d8_512x512_160k_ade20k.py
deleted file mode 100644
index dcee8c280e833825f84b944c6db21e9a43125e06..0000000000000000000000000000000000000000
--- a/spaces/Gradio-Blocks/uniformer_image_segmentation/configs/resnest/fcn_s101-d8_512x512_160k_ade20k.py
+++ /dev/null
@@ -1,9 +0,0 @@
-_base_ = '../fcn/fcn_r101-d8_512x512_160k_ade20k.py'
-model = dict(
- pretrained='open-mmlab://resnest101',
- backbone=dict(
- type='ResNeSt',
- stem_channels=128,
- radix=2,
- reduction_factor=4,
- avg_down_stride=True))
diff --git a/spaces/GrandaddyShmax/AudioCraft_Plus/audiocraft/losses/sisnr.py b/spaces/GrandaddyShmax/AudioCraft_Plus/audiocraft/losses/sisnr.py
deleted file mode 100644
index 30f1fa1de9aca22758b6665609a1eacc0bd992ca..0000000000000000000000000000000000000000
--- a/spaces/GrandaddyShmax/AudioCraft_Plus/audiocraft/losses/sisnr.py
+++ /dev/null
@@ -1,92 +0,0 @@
-# Copyright (c) Meta Platforms, Inc. and affiliates.
-# All rights reserved.
-#
-# This source code is licensed under the license found in the
-# LICENSE file in the root directory of this source tree.
-
-import math
-import typing as tp
-
-import torch
-from torch import nn
-from torch.nn import functional as F
-
-
-def _unfold(a: torch.Tensor, kernel_size: int, stride: int) -> torch.Tensor:
- """Given input of size [*OT, T], output Tensor of size [*OT, F, K]
- with K the kernel size, by extracting frames with the given stride.
- This will pad the input so that `F = ceil(T / K)`.
- see https://github.com/pytorch/pytorch/issues/60466
- """
- *shape, length = a.shape
- n_frames = math.ceil(length / stride)
- tgt_length = (n_frames - 1) * stride + kernel_size
- a = F.pad(a, (0, tgt_length - length))
- strides = list(a.stride())
- assert strides[-1] == 1, "data should be contiguous"
- strides = strides[:-1] + [stride, 1]
- return a.as_strided([*shape, n_frames, kernel_size], strides)
-
-
-def _center(x: torch.Tensor) -> torch.Tensor:
- return x - x.mean(-1, True)
-
-
-def _norm2(x: torch.Tensor) -> torch.Tensor:
- return x.pow(2).sum(-1, True)
-
-
-class SISNR(nn.Module):
- """SISNR loss.
-
- Input should be [B, C, T], output is scalar.
-
- Args:
- sample_rate (int): Sample rate.
- segment (float or None): Evaluate on chunks of that many seconds. If None, evaluate on
- entire audio only.
- overlap (float): Overlap between chunks, i.e. 0.5 = 50 % overlap.
- epsilon (float): Epsilon value for numerical stability.
- """
- def __init__(
- self,
- sample_rate: int = 16000,
- segment: tp.Optional[float] = 20,
- overlap: float = 0.5,
- epsilon: float = torch.finfo(torch.float32).eps,
- ):
- super().__init__()
- self.sample_rate = sample_rate
- self.segment = segment
- self.overlap = overlap
- self.epsilon = epsilon
-
- def forward(self, out_sig: torch.Tensor, ref_sig: torch.Tensor) -> torch.Tensor:
- B, C, T = ref_sig.shape
- assert ref_sig.shape == out_sig.shape
-
- if self.segment is None:
- frame = T
- stride = T
- else:
- frame = int(self.segment * self.sample_rate)
- stride = int(frame * (1 - self.overlap))
-
- epsilon = self.epsilon * frame # make epsilon prop to frame size.
-
- gt = _unfold(ref_sig, frame, stride)
- est = _unfold(out_sig, frame, stride)
- if self.segment is None:
- assert gt.shape[-1] == 1
-
- gt = _center(gt)
- est = _center(est)
- dot = torch.einsum("bcft,bcft->bcf", gt, est)
-
- proj = dot[:, :, :, None] * gt / (epsilon + _norm2(gt))
- noise = est - proj
-
- sisnr = 10 * (
- torch.log10(epsilon + _norm2(proj)) - torch.log10(epsilon + _norm2(noise))
- )
- return -1 * sisnr[..., 0].mean()
diff --git a/spaces/HLasse/textdescriptives/data_viewer.py b/spaces/HLasse/textdescriptives/data_viewer.py
deleted file mode 100644
index ae191efa34573e87b8ab9505cf8b1521ffa8ff24..0000000000000000000000000000000000000000
--- a/spaces/HLasse/textdescriptives/data_viewer.py
+++ /dev/null
@@ -1,26 +0,0 @@
-"""
-Class for showing header and download button in the same row.
-"""
-
-import streamlit as st
-
-
-class DataViewer:
- def _convert_df_to_csv(self, data, **kwargs):
- return data.to_csv(**kwargs).encode("utf-8")
-
- def _header_and_download(
- self, header, data, file_name, key=None, label="Download", help="Download data"
- ):
- col1, col2 = st.columns([9, 2])
- with col1:
- st.subheader(header)
- with col2:
- st.write("")
- st.download_button(
- label=label,
- data=self._convert_df_to_csv(data, index=False),
- file_name=file_name,
- key=key,
- help=help,
- )
diff --git a/spaces/HaHaBill/LandShapes-Antarctica/netdissect/dissect.html b/spaces/HaHaBill/LandShapes-Antarctica/netdissect/dissect.html
deleted file mode 100644
index e6bf4e9a418abdfef5ba09c4182bd71cf1420e52..0000000000000000000000000000000000000000
--- a/spaces/HaHaBill/LandShapes-Antarctica/netdissect/dissect.html
+++ /dev/null
@@ -1,399 +0,0 @@
-
-
-
-
-
-
-
-
-
-
-
-
-
"
-examples = [['sunset.jpg','rotate.png'],['dog.png','same.png'],['cat1.jpg','cat2.png'],['bird1.jpg','bird2.png']]
-
-gr.Interface(
- inference,
- [gr.inputs.Image(type="file", label="Input Image"),gr.inputs.Image(type="file", label="Input Image")],
- [gr.outputs.HTML(label="Comparison.."), gr.outputs.HTML(label="First Hash"), gr.outputs.HTML(label="Second Hash")],
- title=title,
- description=description,
- article=article,
- examples=examples,
- allow_flagging=False
- ).launch(debug=True)
\ No newline at end of file
diff --git a/spaces/akhaliq/Music_Source_Separation/scripts/2_create_indexes/musdb18/create_indexes.sh b/spaces/akhaliq/Music_Source_Separation/scripts/2_create_indexes/musdb18/create_indexes.sh
deleted file mode 100644
index fc571ebd1971ce44b973b878a83ac54ebfb47948..0000000000000000000000000000000000000000
--- a/spaces/akhaliq/Music_Source_Separation/scripts/2_create_indexes/musdb18/create_indexes.sh
+++ /dev/null
@@ -1,18 +0,0 @@
-#!/bin/bash
-WORKSPACE=${1:-"./workspaces/bytesep"} # Default workspace directory
-
-echo "WORKSPACE=${WORKSPACE}"
-
-# --- Create indexes for vocals and accompaniment ---
-INDEXES_CONFIG_YAML="scripts/2_create_indexes/musdb18/configs/vocals-accompaniment,sr=44100,chn=2.yaml"
-
-python3 bytesep/dataset_creation/create_indexes/create_indexes.py \
- --workspace=$WORKSPACE \
- --config_yaml=$INDEXES_CONFIG_YAML
-
-# --- Create indexes for vocals, bass, drums, and other ---
-INDEXES_CONFIG_YAML="scripts/2_create_indexes/musdb18/configs/vocals-bass-drums-other,sr=44100,chn=2.yaml"
-
-python3 bytesep/dataset_creation/create_indexes/create_indexes.py \
- --workspace=$WORKSPACE \
- --config_yaml=$INDEXES_CONFIG_YAML
diff --git a/spaces/andzhk/PGNInfo-test/app.py b/spaces/andzhk/PGNInfo-test/app.py
deleted file mode 100644
index 45d5b84f2c25df4c7c2255230f31bad80877f725..0000000000000000000000000000000000000000
--- a/spaces/andzhk/PGNInfo-test/app.py
+++ /dev/null
@@ -1,79 +0,0 @@
-import gradio as gr
-from PIL import Image
-from urllib.request import Request, urlopen
-
-def display_image_from_url(url, input_image):
- if url == '' and input_image is None:
- return None, "", ""
-
- image = None
- if url != '':
- req = Request(
- url=url,
- headers={'User-Agent': 'Mozilla/5.0'}
- )
- res = urlopen(req)
- image = Image.open(res)
- image.load()
-
-
- if input_image is not None:
- image = input_image
-
- parameters = "Parameters have been erased from this image or unsupported format"
- if 'parameters' in image.info:
-
- parameters = image.info['parameters']
-
- custom_notes = ""
- if 'custom_notes' in image.info:
- custom_notes = image.info['custom_notes']
-
- return image, parameters, custom_notes, image.info
-
-blocks = gr.Blocks(css="#out_image {height: 400px}")
-with blocks as png_info:
- with gr.Row():
- gr.Markdown(
- """
- Report any issues on the [GitHub](https://github.com/andzhik/png-params) page of this project
- """)
- with gr.Row().style(equal_height=False):
- with gr.Column(scale=1):
- in_url = gr.Textbox(label="Source URL")
- in_image = gr.Image(label="Source Image", type='pil')
- with gr.Row():
- btn_submit = gr.Button("Submit", variant="primary")
-
- with gr.Column(scale=2):
- with gr.Accordion("Image is here") as acc_image:
- out_image = gr.Image(type='pil', elem_id="out_image")
-
- out_info = gr.Textbox(label="Generation Parameters")
-
- out_notes = gr.TextArea(label="Custom Notes", interactive=True)
- # download_file = gr.File()
- btn_save_notes = gr.Button("Save Notes")
- # btn_download = gr.Button("Download Image")
-
- with gr.Accordion("Metadata", open=False):
- out_meta = gr.Textbox()
-
- btn_submit.click(fn=display_image_from_url,
- inputs=[in_url, in_image],
- outputs=[out_image, out_info, out_notes, out_meta])
-
- def save_notes(image, custom_notes):
- print(custom_notes)
- image.info["custom_notes"] = custom_notes
- return image
-
- btn_save_notes.click(fn=save_notes,inputs=[out_image, out_notes], outputs=[out_image])
-
- # def download_image(image: Image):
- # print(image.info["custom_notes"])
- # image.save()
-
- # btn_download.click(None, [out_image], _js="(image)=>{gradioApp().getElementById('out_image')}")
-
-png_info.launch()
diff --git a/spaces/aodianyun/stable-diffusion-webui/webui-user.sh b/spaces/aodianyun/stable-diffusion-webui/webui-user.sh
deleted file mode 100644
index bfa53cb7c67083ec0a01bfa420269af4d85c6c94..0000000000000000000000000000000000000000
--- a/spaces/aodianyun/stable-diffusion-webui/webui-user.sh
+++ /dev/null
@@ -1,46 +0,0 @@
-#!/bin/bash
-#########################################################
-# Uncomment and change the variables below to your need:#
-#########################################################
-
-# Install directory without trailing slash
-#install_dir="/home/$(whoami)"
-
-# Name of the subdirectory
-#clone_dir="stable-diffusion-webui"
-
-# Commandline arguments for webui.py, for example: export COMMANDLINE_ARGS="--medvram --opt-split-attention"
-#export COMMANDLINE_ARGS=""
-
-# python3 executable
-#python_cmd="python3"
-
-# git executable
-#export GIT="git"
-
-# python3 venv without trailing slash (defaults to ${install_dir}/${clone_dir}/venv)
-#venv_dir="venv"
-
-# script to launch to start the app
-#export LAUNCH_SCRIPT="launch.py"
-
-# install command for torch
-#export TORCH_COMMAND="pip install torch==1.12.1+cu113 --extra-index-url https://download.pytorch.org/whl/cu113"
-
-# Requirements file to use for stable-diffusion-webui
-#export REQS_FILE="requirements_versions.txt"
-
-# Fixed git repos
-#export K_DIFFUSION_PACKAGE=""
-#export GFPGAN_PACKAGE=""
-
-# Fixed git commits
-#export STABLE_DIFFUSION_COMMIT_HASH=""
-#export TAMING_TRANSFORMERS_COMMIT_HASH=""
-#export CODEFORMER_COMMIT_HASH=""
-#export BLIP_COMMIT_HASH=""
-
-# Uncomment to enable accelerated launch
-#export ACCELERATE="True"
-
-###########################################
diff --git a/spaces/argilla/argilla-streamlit-customs/my_app/wip/guideline-and-comment-ability.py b/spaces/argilla/argilla-streamlit-customs/my_app/wip/guideline-and-comment-ability.py
deleted file mode 100644
index 187fd05d3ef4098ef5bd2094d01bce30ae7bbf93..0000000000000000000000000000000000000000
--- a/spaces/argilla/argilla-streamlit-customs/my_app/wip/guideline-and-comment-ability.py
+++ /dev/null
@@ -1,177 +0,0 @@
-# import os
-
-# import argilla as rg
-# import streamlit as st
-# import streamlit_analytics
-# from _utils import login_workflow
-# from text_highlighter import text_highlighter
-
-# st.set_page_config(
-# page_title="Argilla Annotation Guideline and Comment Ability",
-# page_icon=":memo:",
-# layout="wide",
-# )
-
-# # st.image("https://docs.argilla.io/en/latest/_static/images/logo-light-mode.svg")
-# st.title("Annotation Comment and Note support")
-
-# # login workflow
-# login_workflow()
-
-# st.error(
-# "WIP: Work in progress. Check our https://github.com/argilla-io/argilla-streamlit"
-# " to open a PR."
-# )
-# st.stop()
-# dataset = st.text_input("Dataset Name")
-
-# if dataset:
-# records = rg.load(name=dataset, limit=1)
-
-# if records:
-# record = records[0]
-# if isinstance(record, rg.TokenClassificationRecord) or isinstance(
-# record, rg.TextClassificationRecord
-# ):
-# labels = st.text_input("Labels")
-# split_labels = labels.split(",")
-# split_labels = [label.strip() for label in split_labels]
-
-# if not any(split_labels):
-# st.warning("No labels provided")
-# st.stop()
-# if isinstance(record, rg.TokenClassificationRecord):
-# multi_label = st.radio("multi label", [False, True], horizontal=True)
-# else:
-# multi_label = False
-# else:
-# st.warning("No dataset provided")
-# st.stop()
-
-# st.write("This is an annotation guideline. Label A is for cats, label B is for dogs.")
-# query = st.text_input("Query", value="status: Default", key="query")
-# if not query:
-# query = None
-
-# records = rg.load(name=dataset, limit=1, query=query)
-
-
-# def form_callback(dataset, query):
-# rg.log(st.session_state.rec, dataset)
-# st.session_state.rec = rg.load(name=dataset, limit=1, query=query)[0]
-# if st.session_state.rec.inputs is not None:
-# st.session_state.inputs = "\n".join(
-# [
-# f"**{key}** \n\n {value}"
-# for key, value in st.session_state.rec.inputs.items()
-# ]
-# )
-# else:
-# st.session_state.inputs = st.session_state.rec.text
-# st.session_state.comment = st.session_state.rec.metadata.get("comment", "")
-# if st.session_state.rec.annotation:
-# st.session_state["annotation"] = st.session_state.rec.annotation
-
-# st.success("Saved")
-
-
-# if records:
-# with st.form(key="my_form"):
-# records = records[0]
-# st.session_state.rec = records
-# if isinstance(st.session_state.rec, rg.TokenClassificationRecord):
-# if st.session_state.rec.annotation:
-# old_annotation = [
-# {
-# "start": an[1],
-# "end": an[2],
-# "tag": an[0],
-# "text": st.session_state.rec.text[an[1] : an[2]],
-# }
-# for an in st.session_state.rec.annotation
-# ]
-# else:
-# old_annotation = None
-# annotation = text_highlighter(
-# text=st.session_state.rec.text,
-# labels=split_labels,
-# annotations=old_annotation,
-# )
-# formatted_annotation = [
-# (an["tag"], an["start"], an["end"]) for an in annotation
-# ]
-
-# elif isinstance(st.session_state.rec, rg.TextClassificationRecord):
-# if st.session_state.rec.inputs is not None:
-# st.text_area(
-# "Text",
-# value="\n".join(
-# [
-# f"{key}: {value}"
-# for key, value in st.session_state.rec.inputs.items()
-# ]
-# ),
-# key="inputs",
-# disabled=True,
-# )
-# else:
-# st.text_area(
-# "Text", value=st.session_state.rec.text, key="inputs", disabled=True
-# )
-
-# if st.session_state.rec.multi_label:
-# annotation = st.multiselect(
-# "annotation",
-# split_labels,
-# st.session_state.rec.annotation,
-# key="annotation",
-# )
-# else:
-# if st.session_state.rec.annotation:
-# if st.session_state.rec.annotation in split_labels:
-# index = split_labels.index(st.session_state.rec.annotation)
-# else:
-# st.error(st.session_state.rec.annotation + " not in labels")
-# else:
-# index = 0
-# annotation = st.radio(
-# "annotation",
-# split_labels,
-# index,
-# horizontal=True,
-# key="annotation",
-# )
-
-# elif isinstance(st.session_state.rec, rg.Text2TextRecord):
-# st.write(st.session_state.rec.text)
-# st.text_area(st.session_state.rec.annotation)
-
-# try:
-# st.session_state.rec.__class__(**st.session_state.rec.__dict__)
-# st.session_state.rec.annotation = annotation
-# except Exception as e:
-# st.write(e)
-
-# if st.session_state.rec.metadata:
-# if "comment" in st.session_state.rec.metadata:
-# input_comment = st.session_state.rec.metadata["comment"]
-# else:
-# input_comment = ""
-# else:
-# input_comment = ""
-
-# comment = st.text_input("comment", value=input_comment, key="comment")
-# if st.session_state.rec.metadata:
-# st.session_state.rec.metadata["comment/note"] = comment
-# else:
-# st.session_state.rec.metadata = {"comment": comment}
-
-# save = st.form_submit_button(
-# "Save", on_click=form_callback, args=(dataset, query)
-# )
-
-# else:
-# st.warning("No records found")
-
-
-#
\ No newline at end of file
diff --git a/spaces/arnold-anand/chat-with-pdf/README.md b/spaces/arnold-anand/chat-with-pdf/README.md
deleted file mode 100644
index cb7e43391015859d7f5cf03f7778064edd3ea8e1..0000000000000000000000000000000000000000
--- a/spaces/arnold-anand/chat-with-pdf/README.md
+++ /dev/null
@@ -1,13 +0,0 @@
----
-title: Chat With Pdf
-emoji: 🚀
-colorFrom: indigo
-colorTo: purple
-sdk: streamlit
-sdk_version: 1.21.0
-app_file: app.py
-pinned: false
-license: gpl-3.0
----
-
-Check out the configuration reference at https://huggingface.co/docs/hub/spaces-config-reference
diff --git a/spaces/artificialguybr/video-dubbing/TTS/TTS/tts/__init__.py b/spaces/artificialguybr/video-dubbing/TTS/TTS/tts/__init__.py
deleted file mode 100644
index e69de29bb2d1d6434b8b29ae775ad8c2e48c5391..0000000000000000000000000000000000000000
diff --git a/spaces/arxify/RVC-beta-v2-0618/runtime/Lib/site-packages/altair/examples/selection_histogram.py b/spaces/arxify/RVC-beta-v2-0618/runtime/Lib/site-packages/altair/examples/selection_histogram.py
deleted file mode 100644
index 936217814022de54fff1484b238a8fa0da21368e..0000000000000000000000000000000000000000
--- a/spaces/arxify/RVC-beta-v2-0618/runtime/Lib/site-packages/altair/examples/selection_histogram.py
+++ /dev/null
@@ -1,32 +0,0 @@
-"""
-Selection Histogram
-===================
-This chart shows an example of using an interval selection to filter the
-contents of an attached histogram, allowing the user to see the proportion
-of items in each category within the selection.
-"""
-# category: interactive charts
-import altair as alt
-from vega_datasets import data
-
-source = data.cars()
-
-brush = alt.selection(type='interval')
-
-points = alt.Chart(source).mark_point().encode(
- x='Horsepower:Q',
- y='Miles_per_Gallon:Q',
- color=alt.condition(brush, 'Origin:N', alt.value('lightgray'))
-).add_selection(
- brush
-)
-
-bars = alt.Chart(source).mark_bar().encode(
- y='Origin:N',
- color='Origin:N',
- x='count(Origin):Q'
-).transform_filter(
- brush
-)
-
-points & bars
diff --git a/spaces/arxify/RVC-beta-v2-0618/runtime/Lib/site-packages/altair/examples/trellis_stacked_bar_chart.py b/spaces/arxify/RVC-beta-v2-0618/runtime/Lib/site-packages/altair/examples/trellis_stacked_bar_chart.py
deleted file mode 100644
index f7d65a75f9ab21a95745c0bb95bc863e407b925e..0000000000000000000000000000000000000000
--- a/spaces/arxify/RVC-beta-v2-0618/runtime/Lib/site-packages/altair/examples/trellis_stacked_bar_chart.py
+++ /dev/null
@@ -1,17 +0,0 @@
-"""
-Trellis Stacked Bar Chart
-=========================
-This is an example of a horizontal stacked bar chart using data which contains crop yields over different regions and different years in the 1930s.
-"""
-# category: bar charts
-import altair as alt
-from vega_datasets import data
-
-source = data.barley()
-
-alt.Chart(source).mark_bar().encode(
- column='year',
- x='yield',
- y='variety',
- color='site'
-).properties(width=220)
diff --git a/spaces/arxify/RVC-beta-v2-0618/runtime/Lib/site-packages/anyio/streams/stapled.py b/spaces/arxify/RVC-beta-v2-0618/runtime/Lib/site-packages/anyio/streams/stapled.py
deleted file mode 100644
index a71ffb0dff230c599fa97d1e4e4556c524624493..0000000000000000000000000000000000000000
--- a/spaces/arxify/RVC-beta-v2-0618/runtime/Lib/site-packages/anyio/streams/stapled.py
+++ /dev/null
@@ -1,138 +0,0 @@
-from dataclasses import dataclass
-from typing import Any, Callable, Generic, List, Mapping, Optional, Sequence, TypeVar
-
-from ..abc import (
- ByteReceiveStream,
- ByteSendStream,
- ByteStream,
- Listener,
- ObjectReceiveStream,
- ObjectSendStream,
- ObjectStream,
- TaskGroup,
-)
-
-T_Item = TypeVar("T_Item")
-T_Stream = TypeVar("T_Stream")
-
-
-@dataclass(eq=False)
-class StapledByteStream(ByteStream):
- """
- Combines two byte streams into a single, bidirectional byte stream.
-
- Extra attributes will be provided from both streams, with the receive stream providing the
- values in case of a conflict.
-
- :param ByteSendStream send_stream: the sending byte stream
- :param ByteReceiveStream receive_stream: the receiving byte stream
- """
-
- send_stream: ByteSendStream
- receive_stream: ByteReceiveStream
-
- async def receive(self, max_bytes: int = 65536) -> bytes:
- return await self.receive_stream.receive(max_bytes)
-
- async def send(self, item: bytes) -> None:
- await self.send_stream.send(item)
-
- async def send_eof(self) -> None:
- await self.send_stream.aclose()
-
- async def aclose(self) -> None:
- await self.send_stream.aclose()
- await self.receive_stream.aclose()
-
- @property
- def extra_attributes(self) -> Mapping[Any, Callable[[], Any]]:
- return {
- **self.send_stream.extra_attributes,
- **self.receive_stream.extra_attributes,
- }
-
-
-@dataclass(eq=False)
-class StapledObjectStream(Generic[T_Item], ObjectStream[T_Item]):
- """
- Combines two object streams into a single, bidirectional object stream.
-
- Extra attributes will be provided from both streams, with the receive stream providing the
- values in case of a conflict.
-
- :param ObjectSendStream send_stream: the sending object stream
- :param ObjectReceiveStream receive_stream: the receiving object stream
- """
-
- send_stream: ObjectSendStream[T_Item]
- receive_stream: ObjectReceiveStream[T_Item]
-
- async def receive(self) -> T_Item:
- return await self.receive_stream.receive()
-
- async def send(self, item: T_Item) -> None:
- await self.send_stream.send(item)
-
- async def send_eof(self) -> None:
- await self.send_stream.aclose()
-
- async def aclose(self) -> None:
- await self.send_stream.aclose()
- await self.receive_stream.aclose()
-
- @property
- def extra_attributes(self) -> Mapping[Any, Callable[[], Any]]:
- return {
- **self.send_stream.extra_attributes,
- **self.receive_stream.extra_attributes,
- }
-
-
-@dataclass(eq=False)
-class MultiListener(Generic[T_Stream], Listener[T_Stream]):
- """
- Combines multiple listeners into one, serving connections from all of them at once.
-
- Any MultiListeners in the given collection of listeners will have their listeners moved into
- this one.
-
- Extra attributes are provided from each listener, with each successive listener overriding any
- conflicting attributes from the previous one.
-
- :param listeners: listeners to serve
- :type listeners: Sequence[Listener[T_Stream]]
- """
-
- listeners: Sequence[Listener[T_Stream]]
-
- def __post_init__(self) -> None:
- listeners: List[Listener[T_Stream]] = []
- for listener in self.listeners:
- if isinstance(listener, MultiListener):
- listeners.extend(listener.listeners)
- del listener.listeners[:] # type: ignore[attr-defined]
- else:
- listeners.append(listener)
-
- self.listeners = listeners
-
- async def serve(
- self, handler: Callable[[T_Stream], Any], task_group: Optional[TaskGroup] = None
- ) -> None:
- from .. import create_task_group
-
- async with create_task_group() as tg:
- for listener in self.listeners:
- tg.start_soon(listener.serve, handler, task_group)
-
- async def aclose(self) -> None:
- for listener in self.listeners:
- await listener.aclose()
-
- @property
- def extra_attributes(self) -> Mapping[Any, Callable[[], Any]]:
- attributes: dict = {}
- for listener in self.listeners:
- attributes.update(listener.extra_attributes)
-
- return attributes
diff --git a/spaces/arxify/RVC-beta-v2-0618/runtime/Lib/site-packages/colorama/tests/winterm_test.py b/spaces/arxify/RVC-beta-v2-0618/runtime/Lib/site-packages/colorama/tests/winterm_test.py
deleted file mode 100644
index d0955f9e608377940f0d548576964f2fcf3caf48..0000000000000000000000000000000000000000
--- a/spaces/arxify/RVC-beta-v2-0618/runtime/Lib/site-packages/colorama/tests/winterm_test.py
+++ /dev/null
@@ -1,131 +0,0 @@
-# Copyright Jonathan Hartley 2013. BSD 3-Clause license, see LICENSE file.
-import sys
-from unittest import TestCase, main, skipUnless
-
-try:
- from unittest.mock import Mock, patch
-except ImportError:
- from mock import Mock, patch
-
-from ..winterm import WinColor, WinStyle, WinTerm
-
-
-class WinTermTest(TestCase):
-
- @patch('colorama.winterm.win32')
- def testInit(self, mockWin32):
- mockAttr = Mock()
- mockAttr.wAttributes = 7 + 6 * 16 + 8
- mockWin32.GetConsoleScreenBufferInfo.return_value = mockAttr
- term = WinTerm()
- self.assertEqual(term._fore, 7)
- self.assertEqual(term._back, 6)
- self.assertEqual(term._style, 8)
-
- @skipUnless(sys.platform.startswith("win"), "requires Windows")
- def testGetAttrs(self):
- term = WinTerm()
-
- term._fore = 0
- term._back = 0
- term._style = 0
- self.assertEqual(term.get_attrs(), 0)
-
- term._fore = WinColor.YELLOW
- self.assertEqual(term.get_attrs(), WinColor.YELLOW)
-
- term._back = WinColor.MAGENTA
- self.assertEqual(
- term.get_attrs(),
- WinColor.YELLOW + WinColor.MAGENTA * 16)
-
- term._style = WinStyle.BRIGHT
- self.assertEqual(
- term.get_attrs(),
- WinColor.YELLOW + WinColor.MAGENTA * 16 + WinStyle.BRIGHT)
-
- @patch('colorama.winterm.win32')
- def testResetAll(self, mockWin32):
- mockAttr = Mock()
- mockAttr.wAttributes = 1 + 2 * 16 + 8
- mockWin32.GetConsoleScreenBufferInfo.return_value = mockAttr
- term = WinTerm()
-
- term.set_console = Mock()
- term._fore = -1
- term._back = -1
- term._style = -1
-
- term.reset_all()
-
- self.assertEqual(term._fore, 1)
- self.assertEqual(term._back, 2)
- self.assertEqual(term._style, 8)
- self.assertEqual(term.set_console.called, True)
-
- @skipUnless(sys.platform.startswith("win"), "requires Windows")
- def testFore(self):
- term = WinTerm()
- term.set_console = Mock()
- term._fore = 0
-
- term.fore(5)
-
- self.assertEqual(term._fore, 5)
- self.assertEqual(term.set_console.called, True)
-
- @skipUnless(sys.platform.startswith("win"), "requires Windows")
- def testBack(self):
- term = WinTerm()
- term.set_console = Mock()
- term._back = 0
-
- term.back(5)
-
- self.assertEqual(term._back, 5)
- self.assertEqual(term.set_console.called, True)
-
- @skipUnless(sys.platform.startswith("win"), "requires Windows")
- def testStyle(self):
- term = WinTerm()
- term.set_console = Mock()
- term._style = 0
-
- term.style(22)
-
- self.assertEqual(term._style, 22)
- self.assertEqual(term.set_console.called, True)
-
- @patch('colorama.winterm.win32')
- def testSetConsole(self, mockWin32):
- mockAttr = Mock()
- mockAttr.wAttributes = 0
- mockWin32.GetConsoleScreenBufferInfo.return_value = mockAttr
- term = WinTerm()
- term.windll = Mock()
-
- term.set_console()
-
- self.assertEqual(
- mockWin32.SetConsoleTextAttribute.call_args,
- ((mockWin32.STDOUT, term.get_attrs()), {})
- )
-
- @patch('colorama.winterm.win32')
- def testSetConsoleOnStderr(self, mockWin32):
- mockAttr = Mock()
- mockAttr.wAttributes = 0
- mockWin32.GetConsoleScreenBufferInfo.return_value = mockAttr
- term = WinTerm()
- term.windll = Mock()
-
- term.set_console(on_stderr=True)
-
- self.assertEqual(
- mockWin32.SetConsoleTextAttribute.call_args,
- ((mockWin32.STDERR, term.get_attrs()), {})
- )
-
-
-if __name__ == '__main__':
- main()
diff --git a/spaces/asciicorp/Legal-ai/similarity.py b/spaces/asciicorp/Legal-ai/similarity.py
deleted file mode 100644
index 7164a89931720a3b3bf5ff4db108f2fd25f1e20e..0000000000000000000000000000000000000000
--- a/spaces/asciicorp/Legal-ai/similarity.py
+++ /dev/null
@@ -1,74 +0,0 @@
-import streamlit as st
-import nltk
-from nltk.tokenize import word_tokenize
-from nltk.corpus import stopwords
-from nltk.stem import WordNetLemmatizer
-from nltk.corpus import wordnet
-from sklearn.feature_extraction.text import TfidfVectorizer
-from sklearn.metrics.pairwise import cosine_similarity
-
-nltk.download('punkt')
-nltk.download('stopwords')
-nltk.download('wordnet')
-nltk.download('averaged_perceptron_tagger')
-
-# Function to calculate Textual Similarity
-def calculate_textual_similarity(text1, text2):
- tokens1 = word_tokenize(text1)
- tokens2 = word_tokenize(text2)
- return 100 - (nltk.edit_distance(tokens1, tokens2) * 100) / max(len(tokens1), len(tokens2))
-
-# Function to calculate Linguistic Similarity
-def calculate_linguistic_similarity(text1, text2):
- stop_words = set(stopwords.words('english'))
- lemmatizer = WordNetLemmatizer()
-
- def get_wordnet_pos(treebank_tag):
- if treebank_tag.startswith('J'):
- return wordnet.ADJ
- elif treebank_tag.startswith('V'):
- return wordnet.VERB
- elif treebank_tag.startswith('N'):
- return wordnet.NOUN
- elif treebank_tag.startswith('R'):
- return wordnet.ADV
- else:
- return wordnet.NOUN
-
- def preprocess_text(text):
- tokens = word_tokenize(text.lower())
- tokens = [token for token in tokens if token.isalpha()]
- tokens = [token for token in tokens if token not in stop_words]
- tokens = [lemmatizer.lemmatize(token, get_wordnet_pos(nltk.pos_tag([token])[0][1])) for token in tokens]
- return tokens
-
- tokens1 = preprocess_text(text1)
- tokens2 = preprocess_text(text2)
- vectorizer = TfidfVectorizer(tokenizer=preprocess_text)
- vectors = vectorizer.fit_transform([text1, text2])
- cosine_similarities = cosine_similarity(vectors)[0, 1]
- return round(cosine_similarities * 100, 2)
-
-# Function to calculate Semantic Similarity
-def calculate_semantic_similarity(text1, text2):
- return 0 # todo
-
-def highlight_text_differences(text1, text2):
- tokens1 = word_tokenize(text1)
- tokens2 = word_tokenize(text2)
- common_tokens = set(tokens1).intersection(tokens2)
- new_text1 = []
- new_text2 = []
- for token in tokens1:
- if token in common_tokens:
- new_text1.append("{}".format(token))
- else:
- new_text1.append("{}".format(token))
- for token in tokens2:
- if token in common_tokens:
- new_text2.append("{}".format(token))
- else:
- new_text2.append("{}".format(token))
- new_text1 = " ".join(new_text1)
- new_text2 = " ".join(new_text2)
- return new_text1, new_text2
\ No newline at end of file
diff --git a/spaces/at2507/SM_NLP_RecoSys/Data/Mentor_interviews/Justin Smith.html b/spaces/at2507/SM_NLP_RecoSys/Data/Mentor_interviews/Justin Smith.html
deleted file mode 100644
index 0f591482f34ab2effecf970e8af3f0c0687edf82..0000000000000000000000000000000000000000
--- a/spaces/at2507/SM_NLP_RecoSys/Data/Mentor_interviews/Justin Smith.html
+++ /dev/null
@@ -1,132 +0,0 @@
-
-
-
- Justin Smith
-
-
-
-
-
-
Justin Smith
-
-
-
Mentee to mentor
1- what's your motivation to become a mentor with SharpestMinds? - It is a very rewarding experience to help people progress. Was a teacher in past and have a strong education and teaching background. Helped people with personal and professional growth.
2- What's your career journey in the Data field been like? - Have previous background in non-technical field and worked with non-profit field. - Education also in non-tech field but got interested in ML side and technical side but was more interested in Data and software engineering as most of the work done by DS folks is also D.E. - After masters PhD didn't make sense - didn't want to spend a lot on pursuing this. - Discovered SM through a podcast. - Landed a job as Software engineer working in D.S. / M.L. group. It involved building recommendation product taking in input and recommending profitability. Woking in third party logistics supply chain company - deploying models for forecasting profitability across supply chain.
3- How was your experience as a SM Mentee? Is there any improvements that can happen? - It was really good. It was a new experience to rely on someone to guide and help. - Needed to build some confidence in interviewing and how to communicate technical knowledge properly. Mentorship helped with this. - Improvement - The platforms lack context for SWE / D.E roles. - worked on a project but didn't finish it, got a job before completing it. The project was forecasting cryptocurrency prices from publicly available coin prices.
4- According to you, What's the biggest challenge faced by someone trying to land a SWE or D.E. role? How can you help them with this? - The biggest challenge for a newcomer is having the right network, getting in front of the right people to showcase knowledge and skills and being able to continue to network. - Will help mentees with how to reach out to professionals and encourage them with when there are no responses. Make them understand and normalize the process of networking. Help them with a potential burnout that can happen because of this, when not hearing back or getting responses.
5- Do you have any questions for me regarding the platform? - How does onboarding look like? - Aware that SWE mentorship was rolled out - is there a mentee pool already available on the platform for this? - Is there a plan to start marketing mentorships for SWE?
-
-Autodesk Vehicle Tracking 2021 (x64) with Crack | 4HowCrack. June 2020. Autodesk Vehicle Tracking Crack Free Download specialized programs to simulate ... 1fdad05405
-
-
-
diff --git a/spaces/bioriAsaeru/text-to-voice/Hindi Movie Lafangey Parindey Full Movie Hd 1080p Watch the Inspiring Story of Two Friends on Skates.md b/spaces/bioriAsaeru/text-to-voice/Hindi Movie Lafangey Parindey Full Movie Hd 1080p Watch the Inspiring Story of Two Friends on Skates.md
deleted file mode 100644
index 58053e5d58c4fc8fa672a74b090ad2fe269fde82..0000000000000000000000000000000000000000
--- a/spaces/bioriAsaeru/text-to-voice/Hindi Movie Lafangey Parindey Full Movie Hd 1080p Watch the Inspiring Story of Two Friends on Skates.md
+++ /dev/null
@@ -1,6 +0,0 @@
-
-
- aaccfb2cb3
-
-
-
diff --git a/spaces/brjathu/HMR2.0/vendor/detectron2/detectron2/utils/serialize.py b/spaces/brjathu/HMR2.0/vendor/detectron2/detectron2/utils/serialize.py
deleted file mode 100644
index 0b38862804b70cf1159a9bc93acdef73c184d883..0000000000000000000000000000000000000000
--- a/spaces/brjathu/HMR2.0/vendor/detectron2/detectron2/utils/serialize.py
+++ /dev/null
@@ -1,32 +0,0 @@
-# Copyright (c) Facebook, Inc. and its affiliates.
-import cloudpickle
-
-
-class PicklableWrapper(object):
- """
- Wrap an object to make it more picklable, note that it uses
- heavy weight serialization libraries that are slower than pickle.
- It's best to use it only on closures (which are usually not picklable).
-
- This is a simplified version of
- https://github.com/joblib/joblib/blob/master/joblib/externals/loky/cloudpickle_wrapper.py
- """
-
- def __init__(self, obj):
- while isinstance(obj, PicklableWrapper):
- # Wrapping an object twice is no-op
- obj = obj._obj
- self._obj = obj
-
- def __reduce__(self):
- s = cloudpickle.dumps(self._obj)
- return cloudpickle.loads, (s,)
-
- def __call__(self, *args, **kwargs):
- return self._obj(*args, **kwargs)
-
- def __getattr__(self, attr):
- # Ensure that the wrapped object can be used seamlessly as the previous object.
- if attr not in ["_obj"]:
- return getattr(self._obj, attr)
- return getattr(self, attr)
diff --git a/spaces/caslabs/midi-autocompletion/musicautobot/music_transformer/learner.py b/spaces/caslabs/midi-autocompletion/musicautobot/music_transformer/learner.py
deleted file mode 100644
index 49efebd8ebc173c453ef0ae5b1a82f25ca04dfa2..0000000000000000000000000000000000000000
--- a/spaces/caslabs/midi-autocompletion/musicautobot/music_transformer/learner.py
+++ /dev/null
@@ -1,171 +0,0 @@
-from fastai.basics import *
-from fastai.text.learner import LanguageLearner, get_language_model, _model_meta
-from .model import *
-from .transform import MusicItem
-from ..numpy_encode import SAMPLE_FREQ
-from ..utils.top_k_top_p import top_k_top_p
-from ..utils.midifile import is_empty_midi
-
-_model_meta[MusicTransformerXL] = _model_meta[TransformerXL] # copy over fastai's model metadata
-
-def music_model_learner(data:DataBunch, arch=MusicTransformerXL, config:dict=None, drop_mult:float=1.,
- pretrained_path:PathOrStr=None, **learn_kwargs) -> 'LanguageLearner':
- "Create a `Learner` with a language model from `data` and `arch`."
- meta = _model_meta[arch]
-
- if pretrained_path:
- state = torch.load(pretrained_path, map_location='cpu')
- if config is None: config = state['config']
-
- model = get_language_model(arch, len(data.vocab.itos), config=config, drop_mult=drop_mult)
- learn = MusicLearner(data, model, split_func=meta['split_lm'], **learn_kwargs)
-
- if pretrained_path:
- get_model(model).load_state_dict(state['model'], strict=False)
- if not hasattr(learn, 'opt'): learn.create_opt(defaults.lr, learn.wd)
- try: learn.opt.load_state_dict(state['opt'])
- except: pass
- del state
- gc.collect()
-
- return learn
-
-# Predictions
-from fastai import basic_train # for predictions
-class MusicLearner(LanguageLearner):
- def save(self, file:PathLikeOrBinaryStream=None, with_opt:bool=True, config=None):
- "Save model and optimizer state (if `with_opt`) with `file` to `self.model_dir`. `file` can be file-like (file or buffer)"
- out_path = super().save(file, return_path=True, with_opt=with_opt)
- if config and out_path:
- state = torch.load(out_path)
- state['config'] = config
- torch.save(state, out_path)
- del state
- gc.collect()
- return out_path
-
- def beam_search(self, xb:Tensor, n_words:int, top_k:int=10, beam_sz:int=10, temperature:float=1.,
- ):
- "Return the `n_words` that come after `text` using beam search."
- self.model.reset()
- self.model.eval()
- xb_length = xb.shape[-1]
- if xb.shape[0] > 1: xb = xb[0][None]
- yb = torch.ones_like(xb)
-
- nodes = None
- xb = xb.repeat(top_k, 1)
- nodes = xb.clone()
- scores = xb.new_zeros(1).float()
- with torch.no_grad():
- for k in progress_bar(range(n_words), leave=False):
- out = F.log_softmax(self.model(xb)[0][:,-1], dim=-1)
- values, indices = out.topk(top_k, dim=-1)
- scores = (-values + scores[:,None]).view(-1)
- indices_idx = torch.arange(0,nodes.size(0))[:,None].expand(nodes.size(0), top_k).contiguous().view(-1)
- sort_idx = scores.argsort()[:beam_sz]
- scores = scores[sort_idx]
- nodes = torch.cat([nodes[:,None].expand(nodes.size(0),top_k,nodes.size(1)),
- indices[:,:,None].expand(nodes.size(0),top_k,1),], dim=2)
- nodes = nodes.view(-1, nodes.size(2))[sort_idx]
- self.model[0].select_hidden(indices_idx[sort_idx])
- xb = nodes[:,-1][:,None]
- if temperature != 1.: scores.div_(temperature)
- node_idx = torch.multinomial(torch.exp(-scores), 1).item()
- return [i.item() for i in nodes[node_idx][xb_length:] ]
-
- def predict(self, item:MusicItem, n_words:int=128,
- temperatures:float=(1.0,1.0), min_bars=4,
- top_k=30, top_p=0.6):
- "Return the `n_words` that come after `text`."
- self.model.reset()
- new_idx = []
- vocab = self.data.vocab
- x, pos = item.to_tensor(), item.get_pos_tensor()
- last_pos = pos[-1] if len(pos) else 0
- y = torch.tensor([0])
-
- start_pos = last_pos
-
- sep_count = 0
- bar_len = SAMPLE_FREQ * 4 # assuming 4/4 time
- vocab = self.data.vocab
-
- repeat_count = 0
- if hasattr(self.model[0], 'encode_position'):
- encode_position = self.model[0].encode_position
- else: encode_position = False
-
- for i in progress_bar(range(n_words), leave=True):
- with torch.no_grad():
- if encode_position:
- batch = { 'x': x[None], 'pos': pos[None] }
- logits = self.model(batch)[0][-1][-1]
- else:
- logits = self.model(x[None])[0][-1][-1]
-
- prev_idx = new_idx[-1] if len(new_idx) else vocab.pad_idx
-
- # Temperature
- # Use first temperatures value if last prediction was duration
- temperature = temperatures[0] if vocab.is_duration_or_pad(prev_idx) else temperatures[1]
- repeat_penalty = max(0, np.log((repeat_count+1)/4)/5) * temperature
- temperature += repeat_penalty
- if temperature != 1.: logits = logits / temperature
-
-
- # Filter
- # bar = 16 beats
- filter_value = -float('Inf')
- if ((last_pos - start_pos) // 16) <= min_bars: logits[vocab.bos_idx] = filter_value
-
- logits = filter_invalid_indexes(logits, prev_idx, vocab, filter_value=filter_value)
- logits = top_k_top_p(logits, top_k=top_k, top_p=top_p, filter_value=filter_value)
-
- # Sample
- probs = F.softmax(logits, dim=-1)
- idx = torch.multinomial(probs, 1).item()
-
- # Update repeat count
- num_choices = len(probs.nonzero().view(-1))
- if num_choices <= 2: repeat_count += 1
- else: repeat_count = repeat_count // 2
-
- if prev_idx==vocab.sep_idx:
- duration = idx - vocab.dur_range[0]
- last_pos = last_pos + duration
-
- bars_pred = (last_pos - start_pos) // 16
- abs_bar = last_pos // 16
- # if (bars % 8 == 0) and (bars_pred > min_bars): break
- if (i / n_words > 0.80) and (abs_bar % 4 == 0): break
-
-
- if idx==vocab.bos_idx:
- print('Predicted BOS token. Returning prediction...')
- break
-
- new_idx.append(idx)
- x = x.new_tensor([idx])
- pos = pos.new_tensor([last_pos])
-
- pred = vocab.to_music_item(np.array(new_idx))
- full = item.append(pred)
- return pred, full
-
-# High level prediction functions from midi file
-def predict_from_midi(learn, midi=None, n_words=400,
- temperatures=(1.0,1.0), top_k=30, top_p=0.6, seed_len=None, **kwargs):
- vocab = learn.data.vocab
- seed = MusicItem.from_file(midi, vocab) if not is_empty_midi(midi) else MusicItem.empty(vocab)
- if seed_len is not None: seed = seed.trim_to_beat(seed_len)
-
- pred, full = learn.predict(seed, n_words=n_words, temperatures=temperatures, top_k=top_k, top_p=top_p, **kwargs)
- return full
-
-def filter_invalid_indexes(res, prev_idx, vocab, filter_value=-float('Inf')):
- if vocab.is_duration_or_pad(prev_idx):
- res[list(range(*vocab.dur_range))] = filter_value
- else:
- res[list(range(*vocab.note_range))] = filter_value
- return res
diff --git a/spaces/ceckenrode/PunctuationTokenClassification/README.md b/spaces/ceckenrode/PunctuationTokenClassification/README.md
deleted file mode 100644
index 5168b35ed38b28d5eac4e223ec7287bfe6d818d4..0000000000000000000000000000000000000000
--- a/spaces/ceckenrode/PunctuationTokenClassification/README.md
+++ /dev/null
@@ -1,12 +0,0 @@
----
-title: PunctuationTokenClassification
-emoji: 🚀
-colorFrom: pink
-colorTo: gray
-sdk: gradio
-sdk_version: 3.17.0
-app_file: app.py
-pinned: false
----
-
-Check out the configuration reference at https://huggingface.co/docs/hub/spaces-config-reference
diff --git a/spaces/chaowei100/ChatGPT_Taiyi-Stable-Diffusion/functional.py b/spaces/chaowei100/ChatGPT_Taiyi-Stable-Diffusion/functional.py
deleted file mode 100644
index eccc0ac251784f4611c60ae754194448fca2e9e8..0000000000000000000000000000000000000000
--- a/spaces/chaowei100/ChatGPT_Taiyi-Stable-Diffusion/functional.py
+++ /dev/null
@@ -1,70 +0,0 @@
-# 'primary' 颜色对应 theme.py 中的 primary_hue
-# 'secondary' 颜色对应 theme.py 中的 neutral_hue
-# 'stop' 颜色对应 theme.py 中的 color_er
-# 默认按钮颜色是 secondary
-from toolbox import clear_line_break
-
-def get_functionals():
- return {
- "英语学术润色": {
- # 前言
- "Prefix": r"Below is a paragraph from an academic paper. Polish the writing to meet the academic style, " +
- r"improve the spelling, grammar, clarity, concision and overall readability. When necessary, rewrite the whole sentence. " +
- r"Furthermore, list all modification and explain the reasons to do so in markdown table." + "\n\n",
- # 后语
- "Suffix": r"",
- "Color": r"secondary", # 按钮颜色
- },
- "中文学术润色": {
- "Prefix": r"作为一名中文学术论文写作改进助理,你的任务是改进所提供文本的拼写、语法、清晰、简洁和整体可读性," +
- r"同时分解长句,减少重复,并提供改进建议。请只提供文本的更正版本,避免包括解释。请编辑以下文本" + "\n\n",
- "Suffix": r"",
- },
- "查找语法错误": {
- "Prefix": r"Can you help me ensure that the grammar and the spelling is correct? " +
- r"Do not try to polish the text, if no mistake is found, tell me that this paragraph is good." +
- r"If you find grammar or spelling mistakes, please list mistakes you find in a two-column markdown table, " +
- r"put the original text the first column, " +
- r"put the corrected text in the second column and highlight the key words you fixed.""\n"
- r"Example:""\n"
- r"Paragraph: How is you? Do you knows what is it?""\n"
- r"| Original sentence | Corrected sentence |""\n"
- r"| :--- | :--- |""\n"
- r"| How **is** you? | How **are** you? |""\n"
- r"| Do you **knows** what **is** **it**? | Do you **know** what **it** **is** ? |""\n"
- r"Below is a paragraph from an academic paper. "
- r"You need to report all grammar and spelling mistakes as the example before."
- + "\n\n",
- "Suffix": r"",
- "PreProcess": clear_line_break, # 预处理:清除换行符
- },
- "中译英": {
- "Prefix": r"Please translate following sentence to English:" + "\n\n",
- "Suffix": r"",
- },
- "学术中英互译": {
- "Prefix": r"I want you to act as a scientific English-Chinese translator, " +
- r"I will provide you with some paragraphs in one language " +
- r"and your task is to accurately and academically translate the paragraphs only into the other language. " +
- r"Do not repeat the original provided paragraphs after translation. " +
- r"You should use artificial intelligence tools, " +
- r"such as natural language processing, and rhetorical knowledge " +
- r"and experience about effective writing techniques to reply. " +
- r"I'll give you my paragraphs as follows, tell me what language it is written in, and then translate:" + "\n\n",
- "Suffix": "",
- "Color": "secondary",
- },
- "英译中": {
- "Prefix": r"请翻译成中文:" + "\n\n",
- "Suffix": r"",
- },
- "找图片": {
- "Prefix": r"我需要你找一张网络图片。使用Unsplash API(https://source.unsplash.com/960x640/?<英语关键词>)获取图片URL," +
- r"然后请使用Markdown格式封装,并且不要有反斜线,不要用代码块。现在,请按以下描述给我发送图片:" + "\n\n",
- "Suffix": r"",
- },
- "解释代码": {
- "Prefix": r"请解释以下代码:" + "\n```\n",
- "Suffix": "\n```\n",
- },
- }
diff --git a/spaces/chendl/compositional_test/multimodal/YOLOX/docs/assignment_visualization.md b/spaces/chendl/compositional_test/multimodal/YOLOX/docs/assignment_visualization.md
deleted file mode 100644
index 4bc7791f92ad58f7071d25bb668a18d144a4b6c4..0000000000000000000000000000000000000000
--- a/spaces/chendl/compositional_test/multimodal/YOLOX/docs/assignment_visualization.md
+++ /dev/null
@@ -1,29 +0,0 @@
-# Visualize label assignment
-
-This tutorial explains how to visualize your label asssignment result when training with YOLOX.
-
-## 1. Visualization command
-
-We provide a visualization tool to help you visualize your label assignment result. You can find it in [`tools/visualize_assignment.py`](../tools/visualize_assign.py).
-
-Here is an example of command to visualize your label assignment result:
-
-```shell
-python3 tools/visualize_assign.py -f /path/to/your/exp.py yolox-s -d 1 -b 8 --max-batch 2
-```
-
-`max-batch` here means the maximum number of batches to visualize. The default value is 1, which the tool means only visualize the first batch.
-
-By the way, the mosaic augmentation is used in default dataloader, so you can also see the mosaic result here.
-
-After running the command, the logger will show you where the visualization result is saved, let's open it and into the step 2.
-
-## 2. Check the visualization result
-
-Here is an example of visualization result:
-
-
-Those dots in one box is the matched anchor of gt box. **The color of dots is the same as the color of the box** to help you determine which object is assigned to the anchor. Note the box and dots are **instance level** visualization, which means the same class may have different colors.
-**If the gt box doesn't match any anchor, the box will be marked as red and the red text "unmatched" will be drawn over the box**.
-
-Please feel free to open an issue if you have any questions.
diff --git a/spaces/chendl/compositional_test/multimodal/open_flamingo/src/flamingo.py b/spaces/chendl/compositional_test/multimodal/open_flamingo/src/flamingo.py
deleted file mode 100644
index da54f8f02a046fad7dfcfe32fb59092b24d2f9da..0000000000000000000000000000000000000000
--- a/spaces/chendl/compositional_test/multimodal/open_flamingo/src/flamingo.py
+++ /dev/null
@@ -1,637 +0,0 @@
-import torch
-import torchvision
-from einops import rearrange
-from torch import nn
-from yolox.models.yolo_head import YOLOXHead
-from yolox.utils.boxes import xyxy2cxcywh, cxcywh2xyxy
-from yolox.utils.demo_utils import nms
-# import matplotlib.pyplot as plt
-# import seaborn as sns
-import numpy as np
-import logging
-from open_flamingo.src.gcn import GCN
-from transformers import LogitsProcessorList
-logging.basicConfig(
- level=logging.INFO,
- format='%(asctime)s %(message)s',
- datefmt='%m/%d %I:%M:%S',
-)
-
-
-# class PositionEncodingModule(nn.Module):
-# def __init__(self, dim, pos_dim=128):
-# super().__init__()
-# self.encode = nn.Sequential(
-# nn.Linear(5, pos_dim // 2),
-# nn.BatchNorm1d(pos_dim // 2),
-# nn.GELU(),
-# nn.Linear(pos_dim // 2, pos_dim),
-# nn.BatchNorm1d(pos_dim),
-# nn.GELU(),
-# )
-# self.merge = nn.Sequential(
-# nn.Linear(dim + pos_dim, dim),
-# nn.BatchNorm1d(dim),
-# nn.GELU(),
-# )
-
-# def forward(self, x, box):
-# box = self.encode(box)
-# x = torch.cat([x, box], dim=-1)
-# x = self.merge(x)
-# return x
-
-
-# class PositionEncodingModule(nn.Module):
-# def __init__(self, dim):
-# super().__init__()
-# self.encode = nn.Sequential(
-# nn.Linear(5, dim),
-# nn.GELU(),
-# )
-
-# def forward(self, x, box):
-# box = self.encode(box)
-# x = x + box
-# return x
-
-
-# class PositionEncodingModule2(nn.Module):
-# def __init__(self, dim):
-# super().__init__()
-# self.encode = nn.Sequential(
-# nn.Linear(5 + dim, dim),
-# nn.ELU(),
-# )
-
-# def forward(self, x, box):
-# x = torch.cat([x, box], dim=-1)
-# x = self.encode(x)
-# return x
-
-
-# class RelationHead(nn.Module):
-# def __init__(self, dim):
-# super().__init__()
-# self.encode = nn.Sequential(
-# nn.LayerNorm(dim),
-# nn.Linear(dim, 128),
-# nn.ELU(),
-# )
-# self.classifier = nn.Linear(256, 51)
-
-# def forward(self, x1, x2):
-# x1 = self.encode(x1)
-# x2 = self.encode(x2)
-# x = torch.cat([x1, x2], dim=-1)
-# x = self.classifier(x)
-# return x
-
-
-class Flamingo(nn.Module):
- def __init__(
- self,
- vision_encoder: nn.Module,
- lang_encoder: nn.Module,
- eoc_token_id: int,
- media_token_id: int,
- image_end_token_id: int,
- visual_token_id: int,
- previsual_token_id: int,
- box_token_id: int,
- prebox_token_id: int,
- nothing_token_id: int,
- endofobject_token_id: int,
- vis_dim: int,
- vis_embed_size: int,
- lang_dim: int,
- hidden_state_dim: int,
- image_size: int,
- patch_size: int,
- use_media_placement_augmentation: bool = False,
- add_visual_token: bool = False,
- add_pe: bool = False,
- add_relation: bool = False,
- use_format_v2: bool = False,
- roi_align: bool = False,
- roi_output_size: int = 4,
- apply_mask: bool = False,
- ):
- """
- Args:
- vision_encoder (nn.Module): HF CLIPModel
- lang_encoder (nn.Module): HF causal language model
- eoc_token_id (int): Token id for eos token
- media_token_id (int): Token id for <|#image#|>
- vis_dim (int): Dimension of the visual features.
- Visual features are projected to match this shape along the last dimension.
- cross_attn_every_n_layers (int, optional): How often to apply cross attention after transformer layer. Defaults to 1.
- use_media_placement_augmentation (bool, optional): Whether to randomly assign images to the preceding or following text in training. Defaults to False.
- """
- super().__init__()
- self.image_end_token_id = image_end_token_id
- self.eoc_token_id = eoc_token_id
- self.media_token_id = media_token_id
- self.use_media_placement_augmentation = use_media_placement_augmentation
- self.vis_dim = vis_dim
- self.lang_dim = lang_dim
- # inner_dim = self.lang_dim * 4
- # self.vis_proj = nn.Sequential(
- # nn.LayerNorm(self.vis_dim),
- # nn.Linear(self.vis_dim, inner_dim, bias=False),
- # nn.GELU(),
- # nn.Linear(inner_dim, self.lang_dim, bias=False),
- # )
- self.vis_proj = nn.Linear(self.vis_dim, self.lang_dim)
- self.vision_encoder = vision_encoder
- self.num_positions = vis_embed_size
- self.lang_encoder = lang_encoder
- self.lang_encoder.init_flamingo(
- media_token_id=media_token_id,
- use_media_placement_augmentation=self.use_media_placement_augmentation,
- )
- first_layer = self.lang_encoder._get_decoder_layers()[0]
- first_layer.add_visual_token = add_visual_token
- first_layer.visual_token_id = visual_token_id
- first_layer.media_token_id = media_token_id
- first_layer.box_token_id = box_token_id
- # first_layer.pos_enc = PositionEncodingModule(self.lang_dim) if add_pe else None
- # assert not (add_pe and add_relation)
- # self.pos_enc = PositionEncodingModule(self.lang_dim) if add_pe else None
- # first_layer.pos_enc = self.pos_enc
- self.box_token_id = box_token_id
- self.prebox_token_id = prebox_token_id
- self.media_token_id = media_token_id
- self.visual_token_id = visual_token_id
- self.previsual_token_id = previsual_token_id
- self.hidden_state_dim = hidden_state_dim
- self.image_size = image_size
- self.patch_size = patch_size
- self.patch_num = self.image_size // self.patch_size
- self.detection_head = YOLOXHead(
- num_classes=1,
- strides=[patch_size],
- in_channels=[self.hidden_state_dim + self.lang_dim],
- )
- self.use_format_v2 = use_format_v2
- self.nothing_token_id = nothing_token_id
- self.roi_align = roi_align
- self.roi_output_size = roi_output_size if roi_align else None
- self.apply_mask = apply_mask
- self.endofobject_token_id = endofobject_token_id
-
-
- def _get_detection_batch(
- self,
- visual_token_id,
- previsual_token_id,
- input_ids: torch.Tensor,
- hidden_states: torch.Tensor,
- added_bbox_list,
- box_num = 100,
- ):
- select_mask = torch.logical_or(input_ids == visual_token_id, input_ids == previsual_token_id)
- visual_token_position = select_mask.nonzero()
- visual_token_hidden_states = hidden_states[select_mask]
- prev_batch_idx = -1
- media_idx = []
- cnt = 0
- assert len(visual_token_hidden_states) == len(visual_token_position)
- if len(added_bbox_list) != len(visual_token_position):
- msg = f"ERROR: {len(added_bbox_list)}:{len(visual_token_position)}\n{added_bbox_list}\n{visual_token_position}"
- logging.info(msg)
- alpha = 0.0
- else:
- alpha = 1.0
- visual_batches = []
- previsual_batches = []
- for (batch_idx, idx), visual_token_hidden_state, bbox in zip(
- visual_token_position, visual_token_hidden_states, added_bbox_list,
- ):
- # ! VERY IMPORTANT BUG !
- bbox = bbox.clone()
- # ! VERY IMPORTANT BUG !
- batch_idx = batch_idx.item()
- idx = idx.item()
- if batch_idx != prev_batch_idx:
- prev_batch_idx = batch_idx
- this_input_ids = input_ids[batch_idx]
- cnt += len(media_idx)
- media_idx = (this_input_ids == self.media_token_id).nonzero().reshape(-1).tolist()
- for i in range(len(media_idx)):
- if i == len(media_idx) - 1 or idx > media_idx[i] and idx < media_idx[i+1]:
- break
- image_index = cnt + i
- size = int(self.image_embedding[image_index].shape[0] ** 0.5)
- image_embedding = self.image_embedding[image_index]
- # inplace xyxy2cxcywh
- # print(bbox)
- # TODO: CHECK self.image_size. Is it 224?
- bbox = xyxy2cxcywh(bbox) * self.image_size
- # print(bbox)
- concat_image_visual_embedding = torch.cat([image_embedding, visual_token_hidden_state.unsqueeze(0).repeat(image_embedding.shape[0], 1)], dim=-1).reshape(size, size, -1)
- label = torch.cat([torch.zeros(bbox.shape[0], 1, device=bbox.device), bbox], dim=-1)
- label = torch.cat([label, torch.zeros(box_num - label.shape[0], label.shape[1], device=label.device)], dim=0)
- if input_ids[batch_idx, idx] == previsual_token_id:
- previsual_batches.append([concat_image_visual_embedding, label])
- elif input_ids[batch_idx, idx] == visual_token_id:
- visual_batches.append([concat_image_visual_embedding, label])
- else:
- logging.info(f"WARNING... NOT visual nor previsual. it is {input_ids[batch_idx, idx]}")
- return visual_batches, previsual_batches, alpha, alpha
-
- def get_detection_losses(
- self,
- input_ids: torch.Tensor,
- hidden_states: torch.Tensor,
- added_bbox_list,
- box_num = 100,
- ):
- visual_token_batches, previsual_token_batches, alpha1, alpha2 = self._get_detection_batch(
- visual_token_id=self.visual_token_id,
- previsual_token_id=self.previsual_token_id,
- input_ids=input_ids,
- hidden_states=hidden_states,
- added_bbox_list=added_bbox_list,
- box_num=box_num,
- )
- loss_dict = []
- for batches, alpha in zip([visual_token_batches, previsual_token_batches], [alpha1, alpha2]):
- # x: [B, C, H, W]
- if len(batches) != 0:
- x = torch.cat([batch[0].unsqueeze(0) for batch in batches], dim=0).permute(0,3,1,2)
- labels = torch.cat([batch[1].unsqueeze(0) for batch in batches], dim=0)
- else:
- x = None
- labels = None
- if x is not None:
- losses = self.detection_head(xin=[x], labels=labels)
- loss, loss_iou, loss_obj, loss_cls, loss_l1, _ = losses
- else:
- loss = torch.tensor(0.0).cuda()
- loss_iou = loss
- loss_obj = loss
- loss_cls = loss
- loss_l1 = loss
-
- loss_dict.append(dict(
- loss=loss * alpha,
- loss_iou=loss_iou * alpha,
- loss_obj=loss_obj * alpha,
- loss_cls=loss_cls * alpha,
- loss_l1=loss_l1 * alpha,
- ))
- ret_loss = {}
- for key in loss_dict[0].keys():
- ret_loss[key] = 0.0
- for d in loss_dict:
- ret_loss[key] += d[key]
- return ret_loss, loss_dict
-
- def get_detection_result(
- self,
- input_ids: torch.Tensor,
- hidden_states: torch.Tensor,
- nms_thr: float = 0.45,
- score_thr: float = 0.01,
- debug_id: int = 0,
- debug_mode: bool = False,
- ):
- assert len(input_ids) == 1, "only batch size = 1 is supported yet"
- # assert len(self.image_embedding) == 1, "only one image is supported yet"
- # assert (input_ids[..., -1] == self.visual_token_id).all(), "the last token should be visual token"
- visual_token_hidden_state = hidden_states[..., -1, :]
- boxes_list = []
- scores_list = []
- for image_embedding in self.image_embedding:
- size = int(image_embedding.shape[0] ** 0.5)
- x = torch.cat([image_embedding, visual_token_hidden_state.repeat(image_embedding.shape[0], 1)], dim=-1).reshape(size, size, -1).unsqueeze(0).permute(0,3,1,2)
- with torch.no_grad():
- outputs = self.detection_head(xin=[x], labels=None)
- boxes = outputs[0,:,:4].cpu().numpy()
- scores = outputs[0,:,4].cpu().numpy()
- scores_mask = scores > score_thr
- boxes = boxes[scores_mask]
- boxes = cxcywh2xyxy(boxes)
- scores = scores[scores_mask]
- keep = nms(boxes, scores, nms_thr=nms_thr)
- boxes = boxes[keep]
- scores = scores[keep]
- if debug_mode:
- obj_heatmap = outputs[0,:, -2].reshape(size, size).cpu().numpy()
- import matplotlib.pyplot as plt
- import seaborn as sns
- plt.figure()
- sns_plot = sns.heatmap(obj_heatmap)
- plt.savefig(f"heatmap_{debug_id}.jpg")
- debug_id += 1
- boxes_list.append(boxes)
- scores_list.append(scores)
- if len(boxes_list) == 1:
- boxes_list = boxes_list[0]
- scores_list = scores_list[0]
- return boxes_list, scores_list
-
- def _condition_attention(self, loc_list = None):
- for i in range(len(self.lang_encoder.gpt_neox.layers)):
- self.lang_encoder.gpt_neox.layers[i].decoder_layer.attention.loc_list = loc_list
-
- def forward(
- self,
- vision_x: torch.Tensor,
- lang_x: torch.Tensor,
- attention_mask: torch.Tensor = None,
- labels: torch.Tensor = None,
- use_cached_vision_x: bool = False,
- clear_conditioned_layers: bool = True,
- past_key_values=None,
- use_cache: bool = False,
- image_nums=None,
- image_start_index_list=None,
- added_bbox_list=None,
- add_box: bool = False,
- relations=None,
- debug_mode: bool = False,
- ):
- """
- Forward pass of Flamingo.
-
- Args:
- vision_x (torch.Tensor): Vision input
- shape (B, T_img, F, C, H, W) with F=1
- lang_x (torch.Tensor): Language input ids
- shape (B, T_txt)
- attention_mask (torch.Tensor, optional): Attention mask. Defaults to None.
- labels (torch.Tensor, optional): Labels. Defaults to None.
- clear_conditioned_layers: if True, clear the conditioned layers
- once the foward pass is completed. Set this to false if the
- same set of images will be reused in another subsequent
- forward pass.
- past_key_values: pre-computed values to pass to language model.
- See past_key_values documentation in Hugging Face
- CausalLM models.
- use_cache: whether to use cached key values. See use_cache
- documentation in Hugging Face CausalLM models.
- """
- self.valid = True
- self.lang_encoder.loc_list = None
- if use_cached_vision_x:
- # Case: use cached; vision_x should be cached and other
- # vision-related inputs should not be provided.
- assert (
- vision_x is None
- ), "Expect vision_x to be None when use_cached_vision_x is True."
- assert self.lang_encoder.is_conditioned()
- else:
- # Case: do not use caching (i.e. this is a standard forward pass);
- self._encode_vision_x(
- vision_x=vision_x,
- image_nums=image_nums,
- image_start_index_list=image_start_index_list,
- added_bbox_list=added_bbox_list if add_box else None,
- input_ids=lang_x,
- relations=relations,
- )
- if self.apply_mask:
- if self.roi_align:
- attend_length = 1 + self.roi_output_size ** 2
- else:
- attend_length = 2
- prebox_loc = (lang_x == self.prebox_token_id).nonzero()
- loc_list = []
- for (x, y) in prebox_loc:
- x = x.item()
- y = y.item()
- for yy in range(y+1, lang_x.shape[1]):
- if lang_x[x, yy] == self.endofobject_token_id:
- # [batch_idx, [previsual:prebox], [object:endofobject-1]]
- loc_list.append([x, [y-attend_length+1, y], [y+1, yy-1]])
- self._condition_attention(loc_list=loc_list)
- else:
- self._condition_attention(None)
-
- output = self.lang_encoder(
- input_ids=lang_x,
- attention_mask=attention_mask,
- labels=labels,
- past_key_values=past_key_values,
- use_cache=use_cache,
- output_hidden_states=True,
- )
- if vision_x is None:
- output['loss'][0] += 0.0 * self.vis_proj(self.vision_encoder.visual(torch.randn(1, 3, 224, 224, device=lang_x.device, dtype=output['loss'].dtype))[1]).mean()
-
- hidden_states = output["hidden_states"][-1]
- if self.training and added_bbox_list is not None:
- detection_losses, loss_dict = self.get_detection_losses(
- input_ids=lang_x,
- hidden_states=hidden_states,
- added_bbox_list=added_bbox_list,
- )
- output["detection_losses"] = detection_losses
- output["loss_dict"] = loss_dict
- elif labels is None:
- boxes, scores = self.get_detection_result(
- input_ids=lang_x,
- hidden_states=hidden_states,
- debug_id=self.debug_id if hasattr(self, "debug_id") else None,
- debug_mode=debug_mode,
- )
- output["boxes"] = boxes
- output["scores"] = scores
-
- if clear_conditioned_layers:
- self.lang_encoder.clear_conditioned_layers()
- self._condition_attention(None)
- return output
-
- def generate(
- self,
- vision_x: torch.Tensor,
- lang_x: torch.Tensor,
- attention_mask: torch.Tensor = None,
- added_bbox_list=None,
- num_beams=1,
- max_new_tokens=None,
- temperature=1.0,
- top_k=0,
- top_p=1.0,
- no_repeat_ngram_size=0,
- prefix_allowed_tokens_fn=None,
- length_penalty=1.0,
- num_return_sequences=1,
- do_sample=False,
- early_stopping=False,
- bad_words_ids=None,
- force_words_ids=None,
- image_start_index_list=None,
- image_nums=None,
- min_length=None,
- return_dict_in_generate=False,
- output_hidden_states=False,
- output_scores=False,
- logits_processor_list=None,
- eos_token_id=None,
- ):
- """
- Generate text conditioned on vision and language inputs.
-
- Args:
- vision_x (torch.Tensor): Vision input
- shape (B, T_img, F, C, H, W)
- images in the same chunk are collated along T_img, and frames are collated along F
- currently only F=1 is supported (single-frame videos)
- lang_x (torch.Tensor): Language input
- shape (B, T_txt)
- max_length (int, optional): Maximum length of the output. Defaults to None.
- attention_mask (torch.Tensor, optional): Attention mask. Defaults to None.
- num_beams (int, optional): Number of beams. Defaults to 1.
- max_new_tokens (int, optional): Maximum new tokens. Defaults to None.
- temperature (float, optional): Temperature. Defaults to 1.0.
- top_k (int, optional): Top k. Defaults to 0.
- top_p (float, optional): Top p. Defaults to 1.0.
- no_repeat_ngram_size (int, optional): No repeat ngram size. Defaults to 0.
- length_penalty (float, optional): Length penalty. Defaults to 1.0.
- num_return_sequences (int, optional): Number of return sequences. Defaults to 1.
- do_sample (bool, optional): Do sample. Defaults to False.
- early_stopping (bool, optional): Early stopping. Defaults to False.
- Returns:
- torch.Tensor: lang_x with generated tokens appended to it
- """
- if num_beams > 1:
- vision_x = vision_x.repeat_interleave(num_beams, dim=0)
- image_start_index_list = torch.tensor(image_start_index_list).repeat_interleave(num_beams, dim=0).tolist()
- image_nums = torch.tensor(image_nums).repeat_interleave(num_beams, dim=0).tolist()
- if added_bbox_list is not None and len(added_bbox_list) != 0:
- added_bbox_list = added_bbox_list * num_beams
-
- self._encode_vision_x(vision_x=vision_x, image_nums=image_nums, image_start_index_list=image_start_index_list, num_beams=num_beams, added_bbox_list=added_bbox_list, input_ids=lang_x.repeat_interleave(num_beams, dim=0))
-
- if logits_processor_list is not None:
- assert isinstance(logits_processor_list, list)
- logits_processor_list = LogitsProcessorList(logits_processor_list)
- output = self.lang_encoder.generate(
- input_ids=lang_x,
- attention_mask=attention_mask,
- eos_token_id=(self.eoc_token_id) if eos_token_id is None else eos_token_id,
- num_beams=num_beams,
- max_new_tokens=max_new_tokens,
- min_length=min_length,
- length_penalty=length_penalty,
- logits_processor=logits_processor_list,
- return_dict_in_generate=return_dict_in_generate,
- output_scores=output_scores,
- )
- self.lang_encoder.clear_conditioned_layers()
- return output
-
- def _get_data_list_and_visual_tokens(
- self,
- all_box_list,
- box_token_id,
- prebox_token_id,
- input_ids,
- vision_x,
- nothing_embedding = None,
- ):
- box_locations = (torch.logical_or(input_ids == box_token_id, input_ids == prebox_token_id)).nonzero()
- prev_batch_idx = -1
- media_idx = []
- cnt = 0
- data_list = []
- visual_tokens = []
- if len(all_box_list) != len(box_locations):
- logging.info(f"WARNING. len(all_box_list) != len(box_locations) {len(all_box_list)} vs {len(box_locations)}")
- self.valid = False
- for III, (batch_idx, idx) in enumerate(box_locations):
- batch_idx = batch_idx.item()
- idx = idx.item()
- if batch_idx != prev_batch_idx:
- prev_batch_idx = batch_idx
- this_input_ids = input_ids[batch_idx]
- cnt += len(media_idx)
- media_idx = (this_input_ids == self.media_token_id).nonzero().reshape(-1).tolist()
- for i in range(len(media_idx)):
- if i == len(media_idx) - 1 or idx > media_idx[i] and idx < media_idx[i+1]:
- break
- image_index = cnt + i
- size = int(vision_x[image_index].shape[0] ** 0.5)
- image_feature = vision_x[image_index].reshape(size, size, -1)
- try:
- raw_xyxy = all_box_list[III]
- except:
- logging.info("out of scope for all_box_list")
- raw_xyxy = all_box_list[-1]
- region_xyxy = np.array(raw_xyxy) * size
- x1, y1, x2, y2 = region_xyxy.astype(int).clip(0, size-1).tolist()
- x2 = max(x1, x2)
- y2 = max(y1, y2)
- if x1 + y1 + x2 + y2 == 0.0 and nothing_embedding is not None:
- visual_token = nothing_embedding
- else:
- if self.roi_align:
- visual_token = torchvision.ops.roi_align(
- image_feature.permute(2, 0, 1).unsqueeze(0),
- [torch.tensor(region_xyxy.astype(np.float32)).unsqueeze(0).cuda()],
- output_size=self.roi_output_size,
- spatial_scale=1.0,
- )
- visual_token = visual_token.squeeze(0).flatten(1).permute(1, 0)
- else:
- visual_token = image_feature[y1:y2+1, x1:x2+1].reshape(-1, image_feature.shape[-1]).mean(0)
- box = torch.tensor([0] + raw_xyxy, device=visual_token.device, dtype=visual_token.dtype)
- data_list.append([visual_token, box, batch_idx, idx, i])
- visual_tokens.append(visual_token)
- return data_list, visual_tokens
-
- def _encode_vision_x(self, vision_x: torch.Tensor, image_nums=None, image_start_index_list=None, added_bbox_list=None, num_beams=None, input_ids=None, relations=None):
- """
- Compute media tokens from vision input by passing it through vision encoder and conditioning language model.
- Args:
- vision_x (torch.Tensor): Vision input
- shape (B, T_img, F, C, H, W)
- Images in the same chunk are collated along T_img, and frames are collated along F
- Currently only F=1 is supported (single-frame videos)
-
- rearrange code based on https://github.com/dhansmair/flamingo-mini
- """
- assert vision_x.ndim == 6, "vision_x should be of shape (b, T_img, F, C, H, W)"
- b, T, F = vision_x.shape[:3]
- assert F == 1, "Only single frame supported"
-
- vision_x = rearrange(vision_x, "b T F c h w -> (b T F) c h w")
- if hasattr(self.vision_encoder, "visual"):
- vision_x = self.vision_encoder.visual(vision_x)[1]
- else:
- vision_x = self.vision_encoder(vision_x).flatten(2).permute(0, 2, 1)
- vision_x = rearrange(vision_x, "(b T F) v d -> b T F v d", b=b, T=T, F=F)
-
- # print(vision_x[0,0,0])
- # # DEBUG HERE
- # if torch.distributed.get_rank() == 0:
- # import pdb; pdb.set_trace()
- # else:
- # torch.distributed.barrier()
- vision_x = vision_x.mean(2)
- # vision_x = self.perceiver(vision_x) # reshapes to (b, T, n, d)
- # vision_x = self.vis_proj(vision_x) + self.vis_position_embedding(self.vis_position_ids).unsqueeze(0)
- vision_x = self.vis_proj(vision_x).squeeze(1)
- self.image_embedding = vision_x
-
- data_list = None
- visual_tokens = None
- if added_bbox_list is not None and input_ids is not None:
- all_box_list = added_bbox_list[0].tolist()
- for list in added_bbox_list[1:]:
- all_box_list.extend(list.tolist())
- data_list, visual_tokens = self._get_data_list_and_visual_tokens(
- all_box_list=all_box_list,
- box_token_id=self.box_token_id,
- prebox_token_id=self.prebox_token_id,
- input_ids=input_ids,
- vision_x=vision_x,
- nothing_embedding=self.lang_encoder.gpt_neox.embed_in(torch.tensor(self.nothing_token_id).to(self.lang_encoder.gpt_neox.embed_in.weight.device)) if self.nothing_token_id is not None else None,
- )
-
- first_layer = self.lang_encoder._get_decoder_layers()[0]
- first_layer.condition_vis_x(vision_x, image_nums, image_start_index_list, num_beams=num_beams, visual_tokens=visual_tokens, data_list=[[d[2], d[3]] for d in data_list] if data_list is not None else data_list)
diff --git a/spaces/chronopt-research/ViTExCo/src/losses.py b/spaces/chronopt-research/ViTExCo/src/losses.py
deleted file mode 100644
index dd78f9226bdee39354fa8fb31a05e4aefeb9e55d..0000000000000000000000000000000000000000
--- a/spaces/chronopt-research/ViTExCo/src/losses.py
+++ /dev/null
@@ -1,277 +0,0 @@
-import torch
-import torch.nn as nn
-from src.utils import feature_normalize
-
-
-### START### CONTEXTUAL LOSS ####
-class ContextualLoss(nn.Module):
- """
- input is Al, Bl, channel = 1, range ~ [0, 255]
- """
-
- def __init__(self):
- super(ContextualLoss, self).__init__()
- return None
-
- def forward(self, X_features, Y_features, h=0.1, feature_centering=True):
- """
- X_features&Y_features are are feature vectors or feature 2d array
- h: bandwidth
- return the per-sample loss
- """
- batch_size = X_features.shape[0]
- feature_depth = X_features.shape[1]
-
- # to normalized feature vectors
- if feature_centering:
- X_features = X_features - Y_features.view(batch_size, feature_depth, -1).mean(dim=-1).unsqueeze(dim=-1).unsqueeze(
- dim=-1
- )
- Y_features = Y_features - Y_features.view(batch_size, feature_depth, -1).mean(dim=-1).unsqueeze(dim=-1).unsqueeze(
- dim=-1
- )
- X_features = feature_normalize(X_features).view(
- batch_size, feature_depth, -1
- ) # batch_size * feature_depth * feature_size^2
- Y_features = feature_normalize(Y_features).view(
- batch_size, feature_depth, -1
- ) # batch_size * feature_depth * feature_size^2
-
- # conine distance = 1 - similarity
- X_features_permute = X_features.permute(0, 2, 1) # batch_size * feature_size^2 * feature_depth
- d = 1 - torch.matmul(X_features_permute, Y_features) # batch_size * feature_size^2 * feature_size^2
-
- # normalized distance: dij_bar
- d_norm = d / (torch.min(d, dim=-1, keepdim=True)[0] + 1e-5) # batch_size * feature_size^2 * feature_size^2
-
- # pairwise affinity
- w = torch.exp((1 - d_norm) / h)
- A_ij = w / torch.sum(w, dim=-1, keepdim=True)
-
- # contextual loss per sample
- CX = torch.mean(torch.max(A_ij, dim=1)[0], dim=-1)
- return -torch.log(CX)
-
-
-class ContextualLoss_forward(nn.Module):
- """
- input is Al, Bl, channel = 1, range ~ [0, 255]
- """
-
- def __init__(self):
- super(ContextualLoss_forward, self).__init__()
- return None
-
- def forward(self, X_features, Y_features, h=0.1, feature_centering=True):
- """
- X_features&Y_features are are feature vectors or feature 2d array
- h: bandwidth
- return the per-sample loss
- """
- batch_size = X_features.shape[0]
- feature_depth = X_features.shape[1]
-
- # to normalized feature vectors
- if feature_centering:
- X_features = X_features - Y_features.view(batch_size, feature_depth, -1).mean(dim=-1).unsqueeze(dim=-1).unsqueeze(
- dim=-1
- )
- Y_features = Y_features - Y_features.view(batch_size, feature_depth, -1).mean(dim=-1).unsqueeze(dim=-1).unsqueeze(
- dim=-1
- )
- X_features = feature_normalize(X_features).view(
- batch_size, feature_depth, -1
- ) # batch_size * feature_depth * feature_size^2
- Y_features = feature_normalize(Y_features).view(
- batch_size, feature_depth, -1
- ) # batch_size * feature_depth * feature_size^2
-
- # conine distance = 1 - similarity
- X_features_permute = X_features.permute(0, 2, 1) # batch_size * feature_size^2 * feature_depth
- d = 1 - torch.matmul(X_features_permute, Y_features) # batch_size * feature_size^2 * feature_size^2
-
- # normalized distance: dij_bar
- d_norm = d / (torch.min(d, dim=-1, keepdim=True)[0] + 1e-5) # batch_size * feature_size^2 * feature_size^2
-
- # pairwise affinity
- w = torch.exp((1 - d_norm) / h)
- A_ij = w / torch.sum(w, dim=-1, keepdim=True)
-
- # contextual loss per sample
- CX = torch.mean(torch.max(A_ij, dim=-1)[0], dim=1)
- return -torch.log(CX)
-
-
-### END### CONTEXTUAL LOSS ####
-
-
-##########################
-
-
-def mse_loss_fn(input, target=0):
- return torch.mean((input - target) ** 2)
-
-
-### START### PERCEPTUAL LOSS ###
-def Perceptual_loss(domain_invariant, weight_perceptual):
- instancenorm = nn.InstanceNorm2d(512, affine=False)
-
- def __call__(A_relu5_1, predict_relu5_1):
- if domain_invariant:
- feat_loss = (
- mse_loss_fn(instancenorm(predict_relu5_1), instancenorm(A_relu5_1.detach())) * weight_perceptual * 1e5 * 0.2
- )
- else:
- feat_loss = mse_loss_fn(predict_relu5_1, A_relu5_1.detach()) * weight_perceptual
- return feat_loss
-
- return __call__
-
-
-### END### PERCEPTUAL LOSS ###
-
-
-def l1_loss_fn(input, target=0):
- return torch.mean(torch.abs(input - target))
-
-
-### END#################
-
-
-### START### ADVERSIAL LOSS ###
-def generator_loss_fn(real_data_lab, fake_data_lab, discriminator, weight_gan, device):
- if weight_gan > 0:
- y_pred_fake, _ = discriminator(fake_data_lab)
- y_pred_real, _ = discriminator(real_data_lab)
-
- y = torch.ones_like(y_pred_real)
- generator_loss = (
- (
- torch.mean((y_pred_real - torch.mean(y_pred_fake) + y) ** 2)
- + torch.mean((y_pred_fake - torch.mean(y_pred_real) - y) ** 2)
- )
- / 2
- * weight_gan
- )
- return generator_loss
-
- return torch.Tensor([0]).to(device)
-
-
-def discriminator_loss_fn(real_data_lab, fake_data_lab, discriminator):
- y_pred_fake, _ = discriminator(fake_data_lab.detach())
- y_pred_real, _ = discriminator(real_data_lab.detach())
-
- y = torch.ones_like(y_pred_real)
- discriminator_loss = (
- torch.mean((y_pred_real - torch.mean(y_pred_fake) - y) ** 2)
- + torch.mean((y_pred_fake - torch.mean(y_pred_real) + y) ** 2)
- ) / 2
- return discriminator_loss
-
-
-### END### ADVERSIAL LOSS #####
-
-
-def consistent_loss_fn(
- I_current_lab_predict,
- I_last_ab_predict,
- I_current_nonlocal_lab_predict,
- I_last_nonlocal_lab_predict,
- flow_forward,
- mask,
- warping_layer,
- weight_consistent=0.02,
- weight_nonlocal_consistent=0.0,
- device="cuda",
-):
- def weighted_mse_loss(input, target, weights):
- out = (input - target) ** 2
- out = out * weights.expand_as(out)
- return out.mean()
-
- def consistent():
- I_current_lab_predict_warp = warping_layer(I_current_lab_predict, flow_forward)
- I_current_ab_predict_warp = I_current_lab_predict_warp[:, 1:3, :, :]
- consistent_loss = weighted_mse_loss(I_current_ab_predict_warp, I_last_ab_predict, mask) * weight_consistent
- return consistent_loss
-
- def nonlocal_consistent():
- I_current_nonlocal_lab_predict_warp = warping_layer(I_current_nonlocal_lab_predict, flow_forward)
- nonlocal_consistent_loss = (
- weighted_mse_loss(
- I_current_nonlocal_lab_predict_warp[:, 1:3, :, :],
- I_last_nonlocal_lab_predict[:, 1:3, :, :],
- mask,
- )
- * weight_nonlocal_consistent
- )
-
- return nonlocal_consistent_loss
-
- consistent_loss = consistent() if weight_consistent else torch.Tensor([0]).to(device)
- nonlocal_consistent_loss = nonlocal_consistent() if weight_nonlocal_consistent else torch.Tensor([0]).to(device)
-
- return consistent_loss + nonlocal_consistent_loss
-
-
-### END### CONSISTENCY LOSS #####
-
-
-### START### SMOOTHNESS LOSS ###
-def smoothness_loss_fn(
- I_current_l,
- I_current_lab,
- I_current_ab_predict,
- A_relu2_1,
- weighted_layer_color,
- nonlocal_weighted_layer,
- weight_smoothness=5.0,
- weight_nonlocal_smoothness=0.0,
- device="cuda",
-):
- def smoothness(scale_factor=1.0):
- I_current_lab_predict = torch.cat((I_current_l, I_current_ab_predict), dim=1)
- IA_ab_weighed = weighted_layer_color(
- I_current_lab,
- I_current_lab_predict,
- patch_size=3,
- alpha=10,
- scale_factor=scale_factor,
- )
- smoothness_loss = (
- mse_loss_fn(
- nn.functional.interpolate(I_current_ab_predict, scale_factor=scale_factor),
- IA_ab_weighed,
- )
- * weight_smoothness
- )
-
- return smoothness_loss
-
- def nonlocal_smoothness(scale_factor=0.25, alpha_nonlocal_smoothness=0.5):
- nonlocal_smooth_feature = feature_normalize(A_relu2_1)
- I_current_lab_predict = torch.cat((I_current_l, I_current_ab_predict), dim=1)
- I_current_ab_weighted_nonlocal = nonlocal_weighted_layer(
- I_current_lab_predict,
- nonlocal_smooth_feature.detach(),
- patch_size=3,
- alpha=alpha_nonlocal_smoothness,
- scale_factor=scale_factor,
- )
- nonlocal_smoothness_loss = (
- mse_loss_fn(
- nn.functional.interpolate(I_current_ab_predict, scale_factor=scale_factor),
- I_current_ab_weighted_nonlocal,
- )
- * weight_nonlocal_smoothness
- )
- return nonlocal_smoothness_loss
-
- smoothness_loss = smoothness() if weight_smoothness else torch.Tensor([0]).to(device)
- nonlocal_smoothness_loss = nonlocal_smoothness() if weight_nonlocal_smoothness else torch.Tensor([0]).to(device)
-
- return smoothness_loss + nonlocal_smoothness_loss
-
-
-### END### SMOOTHNESS LOSS #####
diff --git a/spaces/chuan-hd/law-assistant-chatbot/.venv/lib/python3.11/site-packages/cryptography/utils.py b/spaces/chuan-hd/law-assistant-chatbot/.venv/lib/python3.11/site-packages/cryptography/utils.py
deleted file mode 100644
index 71916816844020a3fe6f0d8d395031946098cabd..0000000000000000000000000000000000000000
--- a/spaces/chuan-hd/law-assistant-chatbot/.venv/lib/python3.11/site-packages/cryptography/utils.py
+++ /dev/null
@@ -1,130 +0,0 @@
-# This file is dual licensed under the terms of the Apache License, Version
-# 2.0, and the BSD License. See the LICENSE file in the root of this repository
-# for complete details.
-
-from __future__ import annotations
-
-import enum
-import sys
-import types
-import typing
-import warnings
-
-
-# We use a UserWarning subclass, instead of DeprecationWarning, because CPython
-# decided deprecation warnings should be invisble by default.
-class CryptographyDeprecationWarning(UserWarning):
- pass
-
-
-# Several APIs were deprecated with no specific end-of-life date because of the
-# ubiquity of their use. They should not be removed until we agree on when that
-# cycle ends.
-DeprecatedIn36 = CryptographyDeprecationWarning
-DeprecatedIn37 = CryptographyDeprecationWarning
-DeprecatedIn40 = CryptographyDeprecationWarning
-DeprecatedIn41 = CryptographyDeprecationWarning
-
-
-def _check_bytes(name: str, value: bytes) -> None:
- if not isinstance(value, bytes):
- raise TypeError(f"{name} must be bytes")
-
-
-def _check_byteslike(name: str, value: bytes) -> None:
- try:
- memoryview(value)
- except TypeError:
- raise TypeError(f"{name} must be bytes-like")
-
-
-def int_to_bytes(integer: int, length: typing.Optional[int] = None) -> bytes:
- return integer.to_bytes(
- length or (integer.bit_length() + 7) // 8 or 1, "big"
- )
-
-
-def _extract_buffer_length(obj: typing.Any) -> typing.Tuple[typing.Any, int]:
- from cryptography.hazmat.bindings._rust import _openssl
-
- buf = _openssl.ffi.from_buffer(obj)
- return buf, int(_openssl.ffi.cast("uintptr_t", buf))
-
-
-class InterfaceNotImplemented(Exception):
- pass
-
-
-class _DeprecatedValue:
- def __init__(self, value: object, message: str, warning_class):
- self.value = value
- self.message = message
- self.warning_class = warning_class
-
-
-class _ModuleWithDeprecations(types.ModuleType):
- def __init__(self, module: types.ModuleType):
- super().__init__(module.__name__)
- self.__dict__["_module"] = module
-
- def __getattr__(self, attr: str) -> object:
- obj = getattr(self._module, attr)
- if isinstance(obj, _DeprecatedValue):
- warnings.warn(obj.message, obj.warning_class, stacklevel=2)
- obj = obj.value
- return obj
-
- def __setattr__(self, attr: str, value: object) -> None:
- setattr(self._module, attr, value)
-
- def __delattr__(self, attr: str) -> None:
- obj = getattr(self._module, attr)
- if isinstance(obj, _DeprecatedValue):
- warnings.warn(obj.message, obj.warning_class, stacklevel=2)
-
- delattr(self._module, attr)
-
- def __dir__(self) -> typing.Sequence[str]:
- return ["_module"] + dir(self._module)
-
-
-def deprecated(
- value: object,
- module_name: str,
- message: str,
- warning_class: typing.Type[Warning],
- name: typing.Optional[str] = None,
-) -> _DeprecatedValue:
- module = sys.modules[module_name]
- if not isinstance(module, _ModuleWithDeprecations):
- sys.modules[module_name] = module = _ModuleWithDeprecations(module)
- dv = _DeprecatedValue(value, message, warning_class)
- # Maintain backwards compatibility with `name is None` for pyOpenSSL.
- if name is not None:
- setattr(module, name, dv)
- return dv
-
-
-def cached_property(func: typing.Callable) -> property:
- cached_name = f"_cached_{func}"
- sentinel = object()
-
- def inner(instance: object):
- cache = getattr(instance, cached_name, sentinel)
- if cache is not sentinel:
- return cache
- result = func(instance)
- setattr(instance, cached_name, result)
- return result
-
- return property(inner)
-
-
-# Python 3.10 changed representation of enums. We use well-defined object
-# representation and string representation from Python 3.9.
-class Enum(enum.Enum):
- def __repr__(self) -> str:
- return f"<{self.__class__.__name__}.{self._name_}: {self._value_!r}>"
-
- def __str__(self) -> str:
- return f"{self.__class__.__name__}.{self._name_}"
diff --git a/spaces/chuan-hd/law-assistant-chatbot/.venv/lib/python3.11/site-packages/docx/opc/rel.py b/spaces/chuan-hd/law-assistant-chatbot/.venv/lib/python3.11/site-packages/docx/opc/rel.py
deleted file mode 100644
index 7dba2af8eef9c8a6949c76e03b0fd64047083952..0000000000000000000000000000000000000000
--- a/spaces/chuan-hd/law-assistant-chatbot/.venv/lib/python3.11/site-packages/docx/opc/rel.py
+++ /dev/null
@@ -1,170 +0,0 @@
-# encoding: utf-8
-
-"""
-Relationship-related objects.
-"""
-
-from __future__ import (
- absolute_import, division, print_function, unicode_literals
-)
-
-from .oxml import CT_Relationships
-
-
-class Relationships(dict):
- """
- Collection object for |_Relationship| instances, having list semantics.
- """
- def __init__(self, baseURI):
- super(Relationships, self).__init__()
- self._baseURI = baseURI
- self._target_parts_by_rId = {}
-
- def add_relationship(self, reltype, target, rId, is_external=False):
- """
- Return a newly added |_Relationship| instance.
- """
- rel = _Relationship(rId, reltype, target, self._baseURI, is_external)
- self[rId] = rel
- if not is_external:
- self._target_parts_by_rId[rId] = target
- return rel
-
- def get_or_add(self, reltype, target_part):
- """
- Return relationship of *reltype* to *target_part*, newly added if not
- already present in collection.
- """
- rel = self._get_matching(reltype, target_part)
- if rel is None:
- rId = self._next_rId
- rel = self.add_relationship(reltype, target_part, rId)
- return rel
-
- def get_or_add_ext_rel(self, reltype, target_ref):
- """
- Return rId of external relationship of *reltype* to *target_ref*,
- newly added if not already present in collection.
- """
- rel = self._get_matching(reltype, target_ref, is_external=True)
- if rel is None:
- rId = self._next_rId
- rel = self.add_relationship(
- reltype, target_ref, rId, is_external=True
- )
- return rel.rId
-
- def part_with_reltype(self, reltype):
- """
- Return target part of rel with matching *reltype*, raising |KeyError|
- if not found and |ValueError| if more than one matching relationship
- is found.
- """
- rel = self._get_rel_of_type(reltype)
- return rel.target_part
-
- @property
- def related_parts(self):
- """
- dict mapping rIds to target parts for all the internal relationships
- in the collection.
- """
- return self._target_parts_by_rId
-
- @property
- def xml(self):
- """
- Serialize this relationship collection into XML suitable for storage
- as a .rels file in an OPC package.
- """
- rels_elm = CT_Relationships.new()
- for rel in self.values():
- rels_elm.add_rel(
- rel.rId, rel.reltype, rel.target_ref, rel.is_external
- )
- return rels_elm.xml
-
- def _get_matching(self, reltype, target, is_external=False):
- """
- Return relationship of matching *reltype*, *target*, and
- *is_external* from collection, or None if not found.
- """
- def matches(rel, reltype, target, is_external):
- if rel.reltype != reltype:
- return False
- if rel.is_external != is_external:
- return False
- rel_target = rel.target_ref if rel.is_external else rel.target_part
- if rel_target != target:
- return False
- return True
-
- for rel in self.values():
- if matches(rel, reltype, target, is_external):
- return rel
- return None
-
- def _get_rel_of_type(self, reltype):
- """
- Return single relationship of type *reltype* from the collection.
- Raises |KeyError| if no matching relationship is found. Raises
- |ValueError| if more than one matching relationship is found.
- """
- matching = [rel for rel in self.values() if rel.reltype == reltype]
- if len(matching) == 0:
- tmpl = "no relationship of type '%s' in collection"
- raise KeyError(tmpl % reltype)
- if len(matching) > 1:
- tmpl = "multiple relationships of type '%s' in collection"
- raise ValueError(tmpl % reltype)
- return matching[0]
-
- @property
- def _next_rId(self):
- """
- Next available rId in collection, starting from 'rId1' and making use
- of any gaps in numbering, e.g. 'rId2' for rIds ['rId1', 'rId3'].
- """
- for n in range(1, len(self)+2):
- rId_candidate = 'rId%d' % n # like 'rId19'
- if rId_candidate not in self:
- return rId_candidate
-
-
-class _Relationship(object):
- """
- Value object for relationship to part.
- """
- def __init__(self, rId, reltype, target, baseURI, external=False):
- super(_Relationship, self).__init__()
- self._rId = rId
- self._reltype = reltype
- self._target = target
- self._baseURI = baseURI
- self._is_external = bool(external)
-
- @property
- def is_external(self):
- return self._is_external
-
- @property
- def reltype(self):
- return self._reltype
-
- @property
- def rId(self):
- return self._rId
-
- @property
- def target_part(self):
- if self._is_external:
- raise ValueError("target_part property on _Relationship is undef"
- "ined when target mode is External")
- return self._target
-
- @property
- def target_ref(self):
- if self._is_external:
- return self._target
- else:
- return self._target.partname.relative_ref(self._baseURI)
diff --git a/spaces/chuan-hd/law-assistant-chatbot/.venv/lib/python3.11/site-packages/docx/text/parfmt.py b/spaces/chuan-hd/law-assistant-chatbot/.venv/lib/python3.11/site-packages/docx/text/parfmt.py
deleted file mode 100644
index 37206729cb4c9a2fa338e0e512d645c07345fb22..0000000000000000000000000000000000000000
--- a/spaces/chuan-hd/law-assistant-chatbot/.venv/lib/python3.11/site-packages/docx/text/parfmt.py
+++ /dev/null
@@ -1,303 +0,0 @@
-# encoding: utf-8
-
-"""
-Paragraph-related proxy types.
-"""
-
-from __future__ import (
- absolute_import, division, print_function, unicode_literals
-)
-
-from ..enum.text import WD_LINE_SPACING
-from ..shared import ElementProxy, Emu, lazyproperty, Length, Pt, Twips
-from .tabstops import TabStops
-
-
-class ParagraphFormat(ElementProxy):
- """
- Provides access to paragraph formatting such as justification,
- indentation, line spacing, space before and after, and widow/orphan
- control.
- """
-
- __slots__ = ('_tab_stops',)
-
- @property
- def alignment(self):
- """
- A member of the :ref:`WdParagraphAlignment` enumeration specifying
- the justification setting for this paragraph. A value of |None|
- indicates paragraph alignment is inherited from the style hierarchy.
- """
- pPr = self._element.pPr
- if pPr is None:
- return None
- return pPr.jc_val
-
- @alignment.setter
- def alignment(self, value):
- pPr = self._element.get_or_add_pPr()
- pPr.jc_val = value
-
- @property
- def first_line_indent(self):
- """
- |Length| value specifying the relative difference in indentation for
- the first line of the paragraph. A positive value causes the first
- line to be indented. A negative value produces a hanging indent.
- |None| indicates first line indentation is inherited from the style
- hierarchy.
- """
- pPr = self._element.pPr
- if pPr is None:
- return None
- return pPr.first_line_indent
-
- @first_line_indent.setter
- def first_line_indent(self, value):
- pPr = self._element.get_or_add_pPr()
- pPr.first_line_indent = value
-
- @property
- def keep_together(self):
- """
- |True| if the paragraph should be kept "in one piece" and not broken
- across a page boundary when the document is rendered. |None|
- indicates its effective value is inherited from the style hierarchy.
- """
- pPr = self._element.pPr
- if pPr is None:
- return None
- return pPr.keepLines_val
-
- @keep_together.setter
- def keep_together(self, value):
- self._element.get_or_add_pPr().keepLines_val = value
-
- @property
- def keep_with_next(self):
- """
- |True| if the paragraph should be kept on the same page as the
- subsequent paragraph when the document is rendered. For example, this
- property could be used to keep a section heading on the same page as
- its first paragraph. |None| indicates its effective value is
- inherited from the style hierarchy.
- """
- pPr = self._element.pPr
- if pPr is None:
- return None
- return pPr.keepNext_val
-
- @keep_with_next.setter
- def keep_with_next(self, value):
- self._element.get_or_add_pPr().keepNext_val = value
-
- @property
- def left_indent(self):
- """
- |Length| value specifying the space between the left margin and the
- left side of the paragraph. |None| indicates the left indent value is
- inherited from the style hierarchy. Use an |Inches| value object as
- a convenient way to apply indentation in units of inches.
- """
- pPr = self._element.pPr
- if pPr is None:
- return None
- return pPr.ind_left
-
- @left_indent.setter
- def left_indent(self, value):
- pPr = self._element.get_or_add_pPr()
- pPr.ind_left = value
-
- @property
- def line_spacing(self):
- """
- |float| or |Length| value specifying the space between baselines in
- successive lines of the paragraph. A value of |None| indicates line
- spacing is inherited from the style hierarchy. A float value, e.g.
- ``2.0`` or ``1.75``, indicates spacing is applied in multiples of
- line heights. A |Length| value such as ``Pt(12)`` indicates spacing
- is a fixed height. The |Pt| value class is a convenient way to apply
- line spacing in units of points. Assigning |None| resets line spacing
- to inherit from the style hierarchy.
- """
- pPr = self._element.pPr
- if pPr is None:
- return None
- return self._line_spacing(pPr.spacing_line, pPr.spacing_lineRule)
-
- @line_spacing.setter
- def line_spacing(self, value):
- pPr = self._element.get_or_add_pPr()
- if value is None:
- pPr.spacing_line = None
- pPr.spacing_lineRule = None
- elif isinstance(value, Length):
- pPr.spacing_line = value
- if pPr.spacing_lineRule != WD_LINE_SPACING.AT_LEAST:
- pPr.spacing_lineRule = WD_LINE_SPACING.EXACTLY
- else:
- pPr.spacing_line = Emu(value * Twips(240))
- pPr.spacing_lineRule = WD_LINE_SPACING.MULTIPLE
-
- @property
- def line_spacing_rule(self):
- """
- A member of the :ref:`WdLineSpacing` enumeration indicating how the
- value of :attr:`line_spacing` should be interpreted. Assigning any of
- the :ref:`WdLineSpacing` members :attr:`SINGLE`, :attr:`DOUBLE`, or
- :attr:`ONE_POINT_FIVE` will cause the value of :attr:`line_spacing`
- to be updated to produce the corresponding line spacing.
- """
- pPr = self._element.pPr
- if pPr is None:
- return None
- return self._line_spacing_rule(
- pPr.spacing_line, pPr.spacing_lineRule
- )
-
- @line_spacing_rule.setter
- def line_spacing_rule(self, value):
- pPr = self._element.get_or_add_pPr()
- if value == WD_LINE_SPACING.SINGLE:
- pPr.spacing_line = Twips(240)
- pPr.spacing_lineRule = WD_LINE_SPACING.MULTIPLE
- elif value == WD_LINE_SPACING.ONE_POINT_FIVE:
- pPr.spacing_line = Twips(360)
- pPr.spacing_lineRule = WD_LINE_SPACING.MULTIPLE
- elif value == WD_LINE_SPACING.DOUBLE:
- pPr.spacing_line = Twips(480)
- pPr.spacing_lineRule = WD_LINE_SPACING.MULTIPLE
- else:
- pPr.spacing_lineRule = value
-
- @property
- def page_break_before(self):
- """
- |True| if the paragraph should appear at the top of the page
- following the prior paragraph. |None| indicates its effective value
- is inherited from the style hierarchy.
- """
- pPr = self._element.pPr
- if pPr is None:
- return None
- return pPr.pageBreakBefore_val
-
- @page_break_before.setter
- def page_break_before(self, value):
- self._element.get_or_add_pPr().pageBreakBefore_val = value
-
- @property
- def right_indent(self):
- """
- |Length| value specifying the space between the right margin and the
- right side of the paragraph. |None| indicates the right indent value
- is inherited from the style hierarchy. Use a |Cm| value object as
- a convenient way to apply indentation in units of centimeters.
- """
- pPr = self._element.pPr
- if pPr is None:
- return None
- return pPr.ind_right
-
- @right_indent.setter
- def right_indent(self, value):
- pPr = self._element.get_or_add_pPr()
- pPr.ind_right = value
-
- @property
- def space_after(self):
- """
- |Length| value specifying the spacing to appear between this
- paragraph and the subsequent paragraph. |None| indicates this value
- is inherited from the style hierarchy. |Length| objects provide
- convenience properties, such as :attr:`~.Length.pt` and
- :attr:`~.Length.inches`, that allow easy conversion to various length
- units.
- """
- pPr = self._element.pPr
- if pPr is None:
- return None
- return pPr.spacing_after
-
- @space_after.setter
- def space_after(self, value):
- self._element.get_or_add_pPr().spacing_after = value
-
- @property
- def space_before(self):
- """
- |Length| value specifying the spacing to appear between this
- paragraph and the prior paragraph. |None| indicates this value is
- inherited from the style hierarchy. |Length| objects provide
- convenience properties, such as :attr:`~.Length.pt` and
- :attr:`~.Length.cm`, that allow easy conversion to various length
- units.
- """
- pPr = self._element.pPr
- if pPr is None:
- return None
- return pPr.spacing_before
-
- @space_before.setter
- def space_before(self, value):
- self._element.get_or_add_pPr().spacing_before = value
-
- @lazyproperty
- def tab_stops(self):
- """
- |TabStops| object providing access to the tab stops defined for this
- paragraph format.
- """
- pPr = self._element.get_or_add_pPr()
- return TabStops(pPr)
-
- @property
- def widow_control(self):
- """
- |True| if the first and last lines in the paragraph remain on the
- same page as the rest of the paragraph when Word repaginates the
- document. |None| indicates its effective value is inherited from the
- style hierarchy.
- """
- pPr = self._element.pPr
- if pPr is None:
- return None
- return pPr.widowControl_val
-
- @widow_control.setter
- def widow_control(self, value):
- self._element.get_or_add_pPr().widowControl_val = value
-
- @staticmethod
- def _line_spacing(spacing_line, spacing_lineRule):
- """
- Return the line spacing value calculated from the combination of
- *spacing_line* and *spacing_lineRule*. Returns a |float| number of
- lines when *spacing_lineRule* is ``WD_LINE_SPACING.MULTIPLE``,
- otherwise a |Length| object of absolute line height is returned.
- Returns |None| when *spacing_line* is |None|.
- """
- if spacing_line is None:
- return None
- if spacing_lineRule == WD_LINE_SPACING.MULTIPLE:
- return spacing_line / Pt(12)
- return spacing_line
-
- @staticmethod
- def _line_spacing_rule(line, lineRule):
- """
- Return the line spacing rule value calculated from the combination of
- *line* and *lineRule*. Returns special members of the
- :ref:`WdLineSpacing` enumeration when line spacing is single, double,
- or 1.5 lines.
- """
- if lineRule == WD_LINE_SPACING.MULTIPLE:
- if line == Twips(240):
- return WD_LINE_SPACING.SINGLE
- if line == Twips(360):
- return WD_LINE_SPACING.ONE_POINT_FIVE
- if line == Twips(480):
- return WD_LINE_SPACING.DOUBLE
- return lineRule
diff --git a/spaces/chuan-hd/law-assistant-chatbot/.venv/lib/python3.11/site-packages/fontTools/ttLib/tables/DefaultTable.py b/spaces/chuan-hd/law-assistant-chatbot/.venv/lib/python3.11/site-packages/fontTools/ttLib/tables/DefaultTable.py
deleted file mode 100644
index 32a4b1f258f54d78ad39eb764867a6c354939743..0000000000000000000000000000000000000000
--- a/spaces/chuan-hd/law-assistant-chatbot/.venv/lib/python3.11/site-packages/fontTools/ttLib/tables/DefaultTable.py
+++ /dev/null
@@ -1,50 +0,0 @@
-from fontTools.misc.textTools import Tag
-from fontTools.ttLib import getClassTag
-
-
-class DefaultTable(object):
-
- dependencies = []
-
- def __init__(self, tag=None):
- if tag is None:
- tag = getClassTag(self.__class__)
- self.tableTag = Tag(tag)
-
- def decompile(self, data, ttFont):
- self.data = data
-
- def compile(self, ttFont):
- return self.data
-
- def toXML(self, writer, ttFont, **kwargs):
- if hasattr(self, "ERROR"):
- writer.comment("An error occurred during the decompilation of this table")
- writer.newline()
- writer.comment(self.ERROR)
- writer.newline()
- writer.begintag("hexdata")
- writer.newline()
- writer.dumphex(self.compile(ttFont))
- writer.endtag("hexdata")
- writer.newline()
-
- def fromXML(self, name, attrs, content, ttFont):
- from fontTools.misc.textTools import readHex
- from fontTools import ttLib
-
- if name != "hexdata":
- raise ttLib.TTLibError("can't handle '%s' element" % name)
- self.decompile(readHex(content), ttFont)
-
- def __repr__(self):
- return "<'%s' table at %x>" % (self.tableTag, id(self))
-
- def __eq__(self, other):
- if type(self) != type(other):
- return NotImplemented
- return self.__dict__ == other.__dict__
-
- def __ne__(self, other):
- result = self.__eq__(other)
- return result if result is NotImplemented else not result
diff --git a/spaces/chuan-hd/law-assistant-chatbot/.venv/lib/python3.11/site-packages/google/protobuf/compiler/plugin_pb2.py b/spaces/chuan-hd/law-assistant-chatbot/.venv/lib/python3.11/site-packages/google/protobuf/compiler/plugin_pb2.py
deleted file mode 100644
index 3e3a36de677288d766c62d994e7b5fef354251de..0000000000000000000000000000000000000000
--- a/spaces/chuan-hd/law-assistant-chatbot/.venv/lib/python3.11/site-packages/google/protobuf/compiler/plugin_pb2.py
+++ /dev/null
@@ -1,36 +0,0 @@
-# -*- coding: utf-8 -*-
-# Generated by the protocol buffer compiler. DO NOT EDIT!
-# source: google/protobuf/compiler/plugin.proto
-"""Generated protocol buffer code."""
-from google.protobuf import descriptor as _descriptor
-from google.protobuf import descriptor_pool as _descriptor_pool
-from google.protobuf import symbol_database as _symbol_database
-from google.protobuf.internal import builder as _builder
-# @@protoc_insertion_point(imports)
-
-_sym_db = _symbol_database.Default()
-
-
-from google.protobuf import descriptor_pb2 as google_dot_protobuf_dot_descriptor__pb2
-
-
-DESCRIPTOR = _descriptor_pool.Default().AddSerializedFile(b'\n%google/protobuf/compiler/plugin.proto\x12\x18google.protobuf.compiler\x1a google/protobuf/descriptor.proto\"c\n\x07Version\x12\x14\n\x05major\x18\x01 \x01(\x05R\x05major\x12\x14\n\x05minor\x18\x02 \x01(\x05R\x05minor\x12\x14\n\x05patch\x18\x03 \x01(\x05R\x05patch\x12\x16\n\x06suffix\x18\x04 \x01(\tR\x06suffix\"\xf1\x01\n\x14\x43odeGeneratorRequest\x12(\n\x10\x66ile_to_generate\x18\x01 \x03(\tR\x0e\x66ileToGenerate\x12\x1c\n\tparameter\x18\x02 \x01(\tR\tparameter\x12\x43\n\nproto_file\x18\x0f \x03(\x0b\x32$.google.protobuf.FileDescriptorProtoR\tprotoFile\x12L\n\x10\x63ompiler_version\x18\x03 \x01(\x0b\x32!.google.protobuf.compiler.VersionR\x0f\x63ompilerVersion\"\x94\x03\n\x15\x43odeGeneratorResponse\x12\x14\n\x05\x65rror\x18\x01 \x01(\tR\x05\x65rror\x12-\n\x12supported_features\x18\x02 \x01(\x04R\x11supportedFeatures\x12H\n\x04\x66ile\x18\x0f \x03(\x0b\x32\x34.google.protobuf.compiler.CodeGeneratorResponse.FileR\x04\x66ile\x1a\xb1\x01\n\x04\x46ile\x12\x12\n\x04name\x18\x01 \x01(\tR\x04name\x12\'\n\x0finsertion_point\x18\x02 \x01(\tR\x0einsertionPoint\x12\x18\n\x07\x63ontent\x18\x0f \x01(\tR\x07\x63ontent\x12R\n\x13generated_code_info\x18\x10 \x01(\x0b\x32\".google.protobuf.GeneratedCodeInfoR\x11generatedCodeInfo\"8\n\x07\x46\x65\x61ture\x12\x10\n\x0c\x46\x45\x41TURE_NONE\x10\x00\x12\x1b\n\x17\x46\x45\x41TURE_PROTO3_OPTIONAL\x10\x01\x42r\n\x1c\x63om.google.protobuf.compilerB\x0cPluginProtosZ)google.golang.org/protobuf/types/pluginpb\xaa\x02\x18Google.Protobuf.Compiler')
-
-_globals = globals()
-_builder.BuildMessageAndEnumDescriptors(DESCRIPTOR, _globals)
-_builder.BuildTopDescriptorsAndMessages(DESCRIPTOR, 'google.protobuf.compiler.plugin_pb2', _globals)
-if _descriptor._USE_C_DESCRIPTORS == False:
-
- DESCRIPTOR._options = None
- DESCRIPTOR._serialized_options = b'\n\034com.google.protobuf.compilerB\014PluginProtosZ)google.golang.org/protobuf/types/pluginpb\252\002\030Google.Protobuf.Compiler'
- _globals['_VERSION']._serialized_start=101
- _globals['_VERSION']._serialized_end=200
- _globals['_CODEGENERATORREQUEST']._serialized_start=203
- _globals['_CODEGENERATORREQUEST']._serialized_end=444
- _globals['_CODEGENERATORRESPONSE']._serialized_start=447
- _globals['_CODEGENERATORRESPONSE']._serialized_end=851
- _globals['_CODEGENERATORRESPONSE_FILE']._serialized_start=616
- _globals['_CODEGENERATORRESPONSE_FILE']._serialized_end=793
- _globals['_CODEGENERATORRESPONSE_FEATURE']._serialized_start=795
- _globals['_CODEGENERATORRESPONSE_FEATURE']._serialized_end=851
-# @@protoc_insertion_point(module_scope)
diff --git a/spaces/chuan-hd/law-assistant-chatbot/.venv/lib/python3.11/site-packages/google/protobuf/type_pb2.py b/spaces/chuan-hd/law-assistant-chatbot/.venv/lib/python3.11/site-packages/google/protobuf/type_pb2.py
deleted file mode 100644
index ca8a4e20eb1f72feb66261973848b3e16515fef5..0000000000000000000000000000000000000000
--- a/spaces/chuan-hd/law-assistant-chatbot/.venv/lib/python3.11/site-packages/google/protobuf/type_pb2.py
+++ /dev/null
@@ -1,43 +0,0 @@
-# -*- coding: utf-8 -*-
-# Generated by the protocol buffer compiler. DO NOT EDIT!
-# source: google/protobuf/type.proto
-"""Generated protocol buffer code."""
-from google.protobuf import descriptor as _descriptor
-from google.protobuf import descriptor_pool as _descriptor_pool
-from google.protobuf import symbol_database as _symbol_database
-from google.protobuf.internal import builder as _builder
-# @@protoc_insertion_point(imports)
-
-_sym_db = _symbol_database.Default()
-
-
-from google.protobuf import any_pb2 as google_dot_protobuf_dot_any__pb2
-from google.protobuf import source_context_pb2 as google_dot_protobuf_dot_source__context__pb2
-
-
-DESCRIPTOR = _descriptor_pool.Default().AddSerializedFile(b'\n\x1agoogle/protobuf/type.proto\x12\x0fgoogle.protobuf\x1a\x19google/protobuf/any.proto\x1a$google/protobuf/source_context.proto\"\xa7\x02\n\x04Type\x12\x12\n\x04name\x18\x01 \x01(\tR\x04name\x12.\n\x06\x66ields\x18\x02 \x03(\x0b\x32\x16.google.protobuf.FieldR\x06\x66ields\x12\x16\n\x06oneofs\x18\x03 \x03(\tR\x06oneofs\x12\x31\n\x07options\x18\x04 \x03(\x0b\x32\x17.google.protobuf.OptionR\x07options\x12\x45\n\x0esource_context\x18\x05 \x01(\x0b\x32\x1e.google.protobuf.SourceContextR\rsourceContext\x12/\n\x06syntax\x18\x06 \x01(\x0e\x32\x17.google.protobuf.SyntaxR\x06syntax\x12\x18\n\x07\x65\x64ition\x18\x07 \x01(\tR\x07\x65\x64ition\"\xb4\x06\n\x05\x46ield\x12/\n\x04kind\x18\x01 \x01(\x0e\x32\x1b.google.protobuf.Field.KindR\x04kind\x12\x44\n\x0b\x63\x61rdinality\x18\x02 \x01(\x0e\x32\".google.protobuf.Field.CardinalityR\x0b\x63\x61rdinality\x12\x16\n\x06number\x18\x03 \x01(\x05R\x06number\x12\x12\n\x04name\x18\x04 \x01(\tR\x04name\x12\x19\n\x08type_url\x18\x06 \x01(\tR\x07typeUrl\x12\x1f\n\x0boneof_index\x18\x07 \x01(\x05R\noneofIndex\x12\x16\n\x06packed\x18\x08 \x01(\x08R\x06packed\x12\x31\n\x07options\x18\t \x03(\x0b\x32\x17.google.protobuf.OptionR\x07options\x12\x1b\n\tjson_name\x18\n \x01(\tR\x08jsonName\x12#\n\rdefault_value\x18\x0b \x01(\tR\x0c\x64\x65\x66\x61ultValue\"\xc8\x02\n\x04Kind\x12\x10\n\x0cTYPE_UNKNOWN\x10\x00\x12\x0f\n\x0bTYPE_DOUBLE\x10\x01\x12\x0e\n\nTYPE_FLOAT\x10\x02\x12\x0e\n\nTYPE_INT64\x10\x03\x12\x0f\n\x0bTYPE_UINT64\x10\x04\x12\x0e\n\nTYPE_INT32\x10\x05\x12\x10\n\x0cTYPE_FIXED64\x10\x06\x12\x10\n\x0cTYPE_FIXED32\x10\x07\x12\r\n\tTYPE_BOOL\x10\x08\x12\x0f\n\x0bTYPE_STRING\x10\t\x12\x0e\n\nTYPE_GROUP\x10\n\x12\x10\n\x0cTYPE_MESSAGE\x10\x0b\x12\x0e\n\nTYPE_BYTES\x10\x0c\x12\x0f\n\x0bTYPE_UINT32\x10\r\x12\r\n\tTYPE_ENUM\x10\x0e\x12\x11\n\rTYPE_SFIXED32\x10\x0f\x12\x11\n\rTYPE_SFIXED64\x10\x10\x12\x0f\n\x0bTYPE_SINT32\x10\x11\x12\x0f\n\x0bTYPE_SINT64\x10\x12\"t\n\x0b\x43\x61rdinality\x12\x17\n\x13\x43\x41RDINALITY_UNKNOWN\x10\x00\x12\x18\n\x14\x43\x41RDINALITY_OPTIONAL\x10\x01\x12\x18\n\x14\x43\x41RDINALITY_REQUIRED\x10\x02\x12\x18\n\x14\x43\x41RDINALITY_REPEATED\x10\x03\"\x99\x02\n\x04\x45num\x12\x12\n\x04name\x18\x01 \x01(\tR\x04name\x12\x38\n\tenumvalue\x18\x02 \x03(\x0b\x32\x1a.google.protobuf.EnumValueR\tenumvalue\x12\x31\n\x07options\x18\x03 \x03(\x0b\x32\x17.google.protobuf.OptionR\x07options\x12\x45\n\x0esource_context\x18\x04 \x01(\x0b\x32\x1e.google.protobuf.SourceContextR\rsourceContext\x12/\n\x06syntax\x18\x05 \x01(\x0e\x32\x17.google.protobuf.SyntaxR\x06syntax\x12\x18\n\x07\x65\x64ition\x18\x06 \x01(\tR\x07\x65\x64ition\"j\n\tEnumValue\x12\x12\n\x04name\x18\x01 \x01(\tR\x04name\x12\x16\n\x06number\x18\x02 \x01(\x05R\x06number\x12\x31\n\x07options\x18\x03 \x03(\x0b\x32\x17.google.protobuf.OptionR\x07options\"H\n\x06Option\x12\x12\n\x04name\x18\x01 \x01(\tR\x04name\x12*\n\x05value\x18\x02 \x01(\x0b\x32\x14.google.protobuf.AnyR\x05value*C\n\x06Syntax\x12\x11\n\rSYNTAX_PROTO2\x10\x00\x12\x11\n\rSYNTAX_PROTO3\x10\x01\x12\x13\n\x0fSYNTAX_EDITIONS\x10\x02\x42{\n\x13\x63om.google.protobufB\tTypeProtoP\x01Z-google.golang.org/protobuf/types/known/typepb\xf8\x01\x01\xa2\x02\x03GPB\xaa\x02\x1eGoogle.Protobuf.WellKnownTypesb\x06proto3')
-
-_globals = globals()
-_builder.BuildMessageAndEnumDescriptors(DESCRIPTOR, _globals)
-_builder.BuildTopDescriptorsAndMessages(DESCRIPTOR, 'google.protobuf.type_pb2', _globals)
-if _descriptor._USE_C_DESCRIPTORS == False:
-
- DESCRIPTOR._options = None
- DESCRIPTOR._serialized_options = b'\n\023com.google.protobufB\tTypeProtoP\001Z-google.golang.org/protobuf/types/known/typepb\370\001\001\242\002\003GPB\252\002\036Google.Protobuf.WellKnownTypes'
- _globals['_SYNTAX']._serialized_start=1699
- _globals['_SYNTAX']._serialized_end=1766
- _globals['_TYPE']._serialized_start=113
- _globals['_TYPE']._serialized_end=408
- _globals['_FIELD']._serialized_start=411
- _globals['_FIELD']._serialized_end=1231
- _globals['_FIELD_KIND']._serialized_start=785
- _globals['_FIELD_KIND']._serialized_end=1113
- _globals['_FIELD_CARDINALITY']._serialized_start=1115
- _globals['_FIELD_CARDINALITY']._serialized_end=1231
- _globals['_ENUM']._serialized_start=1234
- _globals['_ENUM']._serialized_end=1515
- _globals['_ENUMVALUE']._serialized_start=1517
- _globals['_ENUMVALUE']._serialized_end=1623
- _globals['_OPTION']._serialized_start=1625
- _globals['_OPTION']._serialized_end=1697
-# @@protoc_insertion_point(module_scope)
diff --git a/spaces/cihyFjudo/fairness-paper-search/ Asus N76v .md b/spaces/cihyFjudo/fairness-paper-search/ Asus N76v .md
deleted file mode 100644
index f26b954749159eb3fa77b43781be7e69b8737574..0000000000000000000000000000000000000000
--- a/spaces/cihyFjudo/fairness-paper-search/ Asus N76v .md
+++ /dev/null
@@ -1,8 +0,0 @@
-
-
This error is due to the service being started before asus-wmi could be loaded by the kernel (noted as kernel: battery: new extension: ASUS Battery Extension in the journal), making it impossible to write there.
-
The battery's charge_control_end_threshold power supply class attribute does not initially exist. It is added to the sysfs(5) directory by the asus-nb-wmi kernel module. Create a udev rule for asus-nb-wmi to set the battery's charge threshold:
Another (more simple) way to force the charging threshold is by using bat-asus-battery-binAUR, which provides a bat-boot.service systemd service and an intuitive terminal interface to change the threshold by typing
-
asusctlAUR (or asusctl-gitAUR) implements functionality specific to the ROG line of laptops, such as backlit keyboards, fan profiles, and the AniMe LED matrix. Check the project's official site for usage: -linux.org/
aaccfb2cb3
-
-
\ No newline at end of file
diff --git a/spaces/cihyFjudo/fairness-paper-search/Artcam Pro 2010 SP4 Full Version Download and Install the Software in a Few Simple Steps.md b/spaces/cihyFjudo/fairness-paper-search/Artcam Pro 2010 SP4 Full Version Download and Install the Software in a Few Simple Steps.md
deleted file mode 100644
index 5693666b76ad05e114c40a16df6a51cbc7a93987..0000000000000000000000000000000000000000
--- a/spaces/cihyFjudo/fairness-paper-search/Artcam Pro 2010 SP4 Full Version Download and Install the Software in a Few Simple Steps.md
+++ /dev/null
@@ -1,6 +0,0 @@
-
-
- aaccfb2cb3
-
-
-
diff --git a/spaces/cihyFjudo/fairness-paper-search/Grand Theft Auto 4 The Lost And Damned NO-CD KEY-GEN UNLOCK.rar - Unlock the Full Potential of the Game.md b/spaces/cihyFjudo/fairness-paper-search/Grand Theft Auto 4 The Lost And Damned NO-CD KEY-GEN UNLOCK.rar - Unlock the Full Potential of the Game.md
deleted file mode 100644
index ea8e0e8b2a78de0b1c0a0c59576dd85d2005ba51..0000000000000000000000000000000000000000
--- a/spaces/cihyFjudo/fairness-paper-search/Grand Theft Auto 4 The Lost And Damned NO-CD KEY-GEN UNLOCK.rar - Unlock the Full Potential of the Game.md
+++ /dev/null
@@ -1,6 +0,0 @@
-
Grand Theft Auto 4: The Lost And Damned NO-CD KEY-GEN UNLOCK.rar
-
- aaccfb2cb3
-
-
-
diff --git a/spaces/cihyFjudo/fairness-paper-search/Klanghelm MJUC variable-tube compressor 1.4.1 VST AAX AU WIN.OSX x64 A tone shaper with unique TIMBRE and DRIVE knobs.md b/spaces/cihyFjudo/fairness-paper-search/Klanghelm MJUC variable-tube compressor 1.4.1 VST AAX AU WIN.OSX x64 A tone shaper with unique TIMBRE and DRIVE knobs.md
deleted file mode 100644
index a37d3a27f34ff2c6802d8bc9a7628e587a4829ec..0000000000000000000000000000000000000000
--- a/spaces/cihyFjudo/fairness-paper-search/Klanghelm MJUC variable-tube compressor 1.4.1 VST AAX AU WIN.OSX x64 A tone shaper with unique TIMBRE and DRIVE knobs.md
+++ /dev/null
@@ -1,6 +0,0 @@
-
-
- aaccfb2cb3
-
-
-
diff --git a/spaces/clip-italian/clip-italian-demo/configuration_hybrid_clip.py b/spaces/clip-italian/clip-italian-demo/configuration_hybrid_clip.py
deleted file mode 100644
index 5272ac44a1a884eaf9b058c9e29729bfaec29a58..0000000000000000000000000000000000000000
--- a/spaces/clip-italian/clip-italian-demo/configuration_hybrid_clip.py
+++ /dev/null
@@ -1,112 +0,0 @@
-import copy
-
-from transformers.configuration_utils import PretrainedConfig
-from transformers.utils import logging
-
-
-logger = logging.get_logger(__name__)
-
-
-class HybridCLIPConfig(PretrainedConfig):
- r"""
- :class:`HybridCLIPConfig` is the configuration class to store the configuration of a
- :class:`~HybridCLIPModel`. It is used to instantiate HybridCLIPModel model according to the specified arguments,
- defining the text model and vision model configs.
-
- Configuration objects inherit from :class:`~transformers.PretrainedConfig` and can be used to control the model
- outputs. Read the documentation from :class:`~transformers.PretrainedConfig` for more information.
-
- Args:
- text_config_dict (:obj:`dict`):
- Dictionary of configuration options that defines text model config.
- vision_config_dict (:obj:`dict`):
- Dictionary of configuration options that defines vison model config.
- projection_dim (:obj:`int`, `optional`, defaults to 512):
- Dimentionality of text and vision projection layers.
- kwargs (`optional`):
- Dictionary of keyword arguments.
-
- Examples::
-
- >>> from transformers import BertConfig, CLIPConfig, HybridCLIPConfig, FlaxHybridCLIP
-
- >>> # Initializing a BERT and CLIP configuration
- >>> config_text = BertConfig()
- >>> config_vision = CLIPConfig()
-
- >>> config = HybridCLIPConfig.from_text_vision_configs(config_text, config_vision, projection_dim=512)
-
- >>> # Initializing a BERT and CLIPVision model
- >>> model = EncoderDecoderModel(config=config)
-
- >>> # Accessing the model configuration
- >>> config_text = model.config.text_config
- >>> config_vision = model.config.vision_config
-
- >>> # Saving the model, including its configuration
- >>> model.save_pretrained('my-model')
-
- >>> # loading model and config from pretrained folder
- >>> encoder_decoder_config = HybridCLIPConfig.from_pretrained('my-model')
- >>> model = FlaxHybridCLIP.from_pretrained('my-model', config=encoder_decoder_config)
- """
-
- model_type = "hybrid-clip"
- is_composition = True
-
- def __init__(self, projection_dim=512, **kwargs):
- super().__init__(**kwargs)
-
- if "text_config" not in kwargs:
- raise ValueError("`text_config` can not be `None`.")
-
- if "vision_config" not in kwargs:
- raise ValueError("`vision_config` can not be `None`.")
-
- text_config = kwargs.pop("text_config")
- vision_config = kwargs.pop("vision_config")
-
- text_model_type = text_config.pop("model_type")
- vision_model_type = vision_config.pop("model_type")
-
- from transformers import AutoConfig
-
- self.text_config = AutoConfig.for_model(text_model_type, **text_config)
-
- if vision_model_type == "clip":
- self.vision_config = AutoConfig.for_model(vision_model_type, **vision_config).vision_config
- elif vision_model_type == "clip_vision_model":
- from transformers import CLIPVisionConfig
-
- self.vision_config = CLIPVisionConfig(**vision_config)
- else:
- self.vision_config = AutoConfig.for_model(vision_model_type, **vision_config)
-
- self.projection_dim = projection_dim
- self.initializer_factor = 1.0
-
- @classmethod
- def from_text_vision_configs(cls, text_config: PretrainedConfig, vision_config: PretrainedConfig, **kwargs):
- r"""
- Instantiate a :class:`HybridCLIPConfig` (or a derived class) from text model configuration and
- vision model configuration.
-
- Returns:
- :class:`HybridCLIPConfig`: An instance of a configuration object
- """
-
- return cls(text_config=text_config.to_dict(), vision_config=vision_config.to_dict(), **kwargs)
-
- def to_dict(self):
- """
- Serializes this instance to a Python dictionary. Override the default
- :meth:`~transformers.PretrainedConfig.to_dict`.
-
- Returns:
- :obj:`Dict[str, any]`: Dictionary of all the attributes that make up this configuration instance,
- """
- output = copy.deepcopy(self.__dict__)
- output["text_config"] = self.text_config.to_dict()
- output["vision_config"] = self.vision_config.to_dict()
- output["model_type"] = self.__class__.model_type
- return output
diff --git a/spaces/clip-italian/clip-italian-demo/modeling_hybrid_clip.py b/spaces/clip-italian/clip-italian-demo/modeling_hybrid_clip.py
deleted file mode 100644
index 49cf0b4d99a87f63d6be51093a971c512f6f6055..0000000000000000000000000000000000000000
--- a/spaces/clip-italian/clip-italian-demo/modeling_hybrid_clip.py
+++ /dev/null
@@ -1,422 +0,0 @@
-# coding=utf-8
-# Copyright 2021 The HuggingFace Team. All rights reserved.
-#
-# Licensed under the Apache License, Version 2.0 (the "License");
-# you may not use this file except in compliance with the License.
-# You may obtain a copy of the License at
-#
-# http://www.apache.org/licenses/LICENSE-2.0
-#
-# Unless required by applicable law or agreed to in writing, software
-# distributed under the License is distributed on an "AS IS" BASIS,
-# WITHOUT WARRANTIES OR CONDITIONS OF ANY KIND, either express or implied.
-# See the License for the specific language governing permissions and
-# limitations under the License.
-
-from typing import Optional, Tuple
-
-import flax.linen as nn
-import jax
-import jax.numpy as jnp
-from configuration_hybrid_clip import HybridCLIPConfig
-from flax.core.frozen_dict import FrozenDict
-from transformers import FLAX_MODEL_MAPPING, FlaxCLIPVisionModel
-from transformers.modeling_flax_utils import FlaxPreTrainedModel
-from transformers.models.clip.modeling_flax_clip import FlaxCLIPOutput
-from transformers.utils import logging
-
-
-logger = logging.get_logger(__name__)
-
-
-class FlaxHybridCLIPModule(nn.Module):
- config: HybridCLIPConfig
- dtype: jnp.dtype = jnp.float32
-
- def setup(self):
- text_config = self.config.text_config
- vision_config = self.config.vision_config
-
- self.projection_dim = self.config.projection_dim
- self.text_embed_dim = text_config.hidden_size
- self.vision_embed_dim = vision_config.hidden_size
-
- text_module = FLAX_MODEL_MAPPING[self.config.text_config.__class__].module_class
- vision_module = FLAX_MODEL_MAPPING.get(self.config.vision_config.__class__, FlaxCLIPVisionModel).module_class
-
- self.text_model = text_module(text_config, dtype=self.dtype)
- self.vision_model = vision_module(vision_config, dtype=self.dtype)
-
- self.visual_projection = nn.Dense(
- self.projection_dim,
- dtype=self.dtype,
- kernel_init=jax.nn.initializers.normal(0.02, dtype=self.dtype),
- use_bias=False,
- )
- self.text_projection = nn.Dense(
- self.projection_dim,
- dtype=self.dtype,
- kernel_init=jax.nn.initializers.normal(0.02, dtype=self.dtype),
- use_bias=False,
- )
- self.logit_scale = self.param("logit_scale", jax.nn.initializers.ones, [])
-
- def __call__(
- self,
- input_ids=None,
- pixel_values=None,
- attention_mask=None,
- position_ids=None,
- token_type_ids=None,
- deterministic: bool = True,
- output_attentions=None,
- output_hidden_states=None,
- return_dict=None,
- ):
- return_dict = return_dict if return_dict is not None else self.config.return_dict
-
- vision_outputs = self.vision_model(
- pixel_values=pixel_values,
- deterministic=deterministic,
- output_attentions=output_attentions,
- output_hidden_states=output_hidden_states,
- return_dict=return_dict,
- )
-
- text_outputs = self.text_model(
- input_ids=input_ids,
- attention_mask=attention_mask,
- token_type_ids=token_type_ids,
- position_ids=position_ids,
- deterministic=deterministic,
- output_attentions=output_attentions,
- output_hidden_states=output_hidden_states,
- return_dict=return_dict,
- )
-
- image_embeds = vision_outputs[1]
- image_embeds = self.visual_projection(image_embeds)
-
- text_embeds = text_outputs[1]
- text_embeds = self.text_projection(text_embeds)
-
- # normalized features
- image_embeds = image_embeds / jnp.linalg.norm(image_embeds, axis=-1, keepdims=True)
- text_embeds = text_embeds / jnp.linalg.norm(text_embeds, axis=-1, keepdims=True)
-
- # cosine similarity as logits
- logit_scale = jnp.exp(self.logit_scale)
- logits_per_text = jnp.matmul(text_embeds, image_embeds.T) * logit_scale
- logits_per_image = logits_per_text.T
-
- if not return_dict:
- return (logits_per_image, logits_per_text, text_embeds, image_embeds, text_outputs, vision_outputs)
-
- return FlaxCLIPOutput(
- logits_per_image=logits_per_image,
- logits_per_text=logits_per_text,
- text_embeds=text_embeds,
- image_embeds=image_embeds,
- text_model_output=text_outputs,
- vision_model_output=vision_outputs,
- )
-
-
-class FlaxHybridCLIP(FlaxPreTrainedModel):
- config_class = HybridCLIPConfig
- module_class = FlaxHybridCLIPModule
-
- def __init__(
- self,
- config: HybridCLIPConfig,
- input_shape: Optional[Tuple] = None,
- seed: int = 0,
- dtype: jnp.dtype = jnp.float32,
- **kwargs
- ):
- if input_shape is None:
- input_shape = ((1, 1), (1, config.vision_config.image_size, config.vision_config.image_size, 3))
-
- print(kwargs)
-
- module = self.module_class(config=config, dtype=dtype) # , **kwargs)
- super().__init__(config, module, input_shape=input_shape, seed=seed, dtype=dtype)
-
- def init_weights(self, rng: jax.random.PRNGKey, input_shape: Tuple) -> FrozenDict:
- # init input tensor
- input_ids = jnp.zeros(input_shape[0], dtype="i4")
- position_ids = jnp.broadcast_to(jnp.arange(jnp.atleast_2d(input_ids).shape[-1]), input_shape[0])
- token_type_ids = jnp.ones_like(input_ids)
- attention_mask = jnp.ones_like(input_ids)
-
- pixel_values = jax.random.normal(rng, input_shape[1])
-
- params_rng, dropout_rng = jax.random.split(rng)
- rngs = {"params": params_rng, "dropout": dropout_rng}
-
- return self.module.init(rngs, input_ids, pixel_values, attention_mask, position_ids, token_type_ids)["params"]
-
- def __call__(
- self,
- input_ids,
- pixel_values,
- attention_mask=None,
- position_ids=None,
- token_type_ids=None,
- params: dict = None,
- dropout_rng: jax.random.PRNGKey = None,
- train: bool = False,
- output_attentions: Optional[bool] = None,
- output_hidden_states: Optional[bool] = None,
- return_dict: Optional[bool] = None,
- ):
- output_attentions = output_attentions if output_attentions is not None else self.config.output_attentions
- output_hidden_states = (
- output_hidden_states if output_hidden_states is not None else self.config.output_hidden_states
- )
- return_dict = return_dict if return_dict is not None else self.config.return_dict
-
- if position_ids is None:
- position_ids = jnp.broadcast_to(jnp.arange(jnp.atleast_2d(input_ids).shape[-1]), input_ids.shape)
-
- if token_type_ids is None:
- token_type_ids = jnp.zeros_like(input_ids)
-
- if attention_mask is None:
- attention_mask = jnp.ones_like(input_ids)
-
- # Handle any PRNG if needed
- rngs = {}
- if dropout_rng is not None:
- rngs["dropout"] = dropout_rng
-
- return self.module.apply(
- {"params": params or self.params},
- jnp.array(input_ids, dtype="i4"),
- jnp.array(pixel_values, dtype=jnp.float32),
- jnp.array(attention_mask, dtype="i4"),
- jnp.array(position_ids, dtype="i4"),
- jnp.array(token_type_ids, dtype="i4"),
- not train,
- output_attentions,
- output_hidden_states,
- return_dict,
- rngs=rngs,
- )
-
- def get_text_features(
- self,
- input_ids,
- attention_mask=None,
- position_ids=None,
- token_type_ids=None,
- dropout_rng: jax.random.PRNGKey = None,
- train=False,
- ):
- r"""
- Args:
- input_ids (:obj:`numpy.ndarray` of shape :obj:`(batch_size, sequence_length)`):
- Indices of input sequence tokens in the vocabulary. Padding will be ignored by default should you
- provide it.
-
- Indices can be obtained using :class:`~transformers.PreTrainedTokenizer`. See
- :meth:`transformers.PreTrainedTokenizer.encode` and :meth:`transformers.PreTrainedTokenizer.__call__`
- for details.
-
- `What are input IDs? <../glossary.html#input-ids>`__
-
- Returns:
- text_features (:obj:`jax_xla.DeviceArray` of shape :obj:`(batch_size, output_dim`): The text embeddings
- obtained by applying the projection layer to the pooled output of text model.
- """
- if position_ids is None:
- position_ids = jnp.broadcast_to(jnp.arange(jnp.atleast_2d(input_ids).shape[-1]), input_ids.shape)
-
- if token_type_ids is None:
- token_type_ids = jnp.zeros_like(input_ids)
-
- if attention_mask is None:
- attention_mask = jnp.ones_like(input_ids)
-
- # Handle any PRNG if needed
- rngs = {}
- if dropout_rng is not None:
- rngs["dropout"] = dropout_rng
-
- def _get_features(module, input_ids, attention_mask, position_ids, token_type_ids, deterministic):
- text_outputs = module.text_model(
- input_ids=input_ids,
- attention_mask=attention_mask,
- position_ids=position_ids,
- token_type_ids=token_type_ids,
- deterministic=deterministic,
- )
- pooled_output = text_outputs[1]
- text_features = module.text_projection(pooled_output)
- return text_features
-
- return self.module.apply(
- {"params": self.params},
- jnp.array(input_ids, dtype="i4"),
- jnp.array(attention_mask, dtype="i4"),
- jnp.array(position_ids, dtype="i4"),
- jnp.array(token_type_ids, dtype="i4"),
- not train,
- method=_get_features,
- rngs=rngs,
- )
-
- def get_image_features(self, pixel_values, dropout_rng: jax.random.PRNGKey = None, train=False):
- r"""
- Args:
- pixel_values (:obj:`numpy.ndarray` of shape :obj:`(batch_size, num_channels, height, width)`):
- Pixel values. Padding will be ignored by default should you provide it. Pixel values can be obtained
- using :class:`~transformers.ImageFeatureExtractionMixin`. See
- :meth:`transformers.ImageFeatureExtractionMixin.__call__` for details.
-
- Returns:
- image_features (:obj:`jax_xla.DeviceArray` of shape :obj:`(batch_size, output_dim`): The image embeddings
- obtained by applying the projection layer to the pooled output of vision model.
- """
-
- # Handle any PRNG if needed
- rngs = {}
- if dropout_rng is not None:
- rngs["dropout"] = dropout_rng
-
- def _get_features(module, pixel_values, deterministic):
- vision_outputs = module.vision_model(pixel_values=pixel_values, deterministic=deterministic)
- pooled_output = vision_outputs[1] # pooled_output
- image_features = module.visual_projection(pooled_output)
- return image_features
-
- return self.module.apply(
- {"params": self.params},
- jnp.array(pixel_values, dtype=jnp.float32),
- not train,
- method=_get_features,
- rngs=rngs,
- )
-
- @classmethod
- def from_text_vision_pretrained(
- cls,
- text_model_name_or_path: str = None,
- vision_model_name_or_path: str = None,
- *model_args,
- **kwargs,
- ) -> FlaxPreTrainedModel:
- """
- Params:
- text_model_name_or_path (:obj: `str`, `optional`):
- Information necessary to initiate the text model. Can be either:
-
- - A string, the `model id` of a pretrained model hosted inside a model repo on huggingface.co.
- Valid model ids can be located at the root-level, like ``bert-base-uncased``, or namespaced under
- a user or organization name, like ``dbmdz/bert-base-german-cased``.
- - A path to a `directory` containing model weights saved using
- :func:`~transformers.FlaxPreTrainedModel.save_pretrained`, e.g., ``./my_model_directory/``.
- - A path or url to a `PyTorch checkpoint folder` (e.g, ``./pt_model``). In
- this case, ``from_pt`` should be set to :obj:`True` and a configuration object should be provided
- as ``config`` argument. This loading path is slower than converting the PyTorch checkpoint in
- a Flax model using the provided conversion scripts and loading the Flax model afterwards.
-
- vision_model_name_or_path (:obj: `str`, `optional`, defaults to `None`):
- Information necessary to initiate the vision model. Can be either:
-
- - A string, the `model id` of a pretrained model hosted inside a model repo on huggingface.co.
- Valid model ids can be located at the root-level, like ``bert-base-uncased``, or namespaced under
- a user or organization name, like ``dbmdz/bert-base-german-cased``.
- - A path to a `directory` containing model weights saved using
- :func:`~transformers.FlaxPreTrainedModel.save_pretrained`, e.g., ``./my_model_directory/``.
- - A path or url to a `PyTorch checkpoint folder` (e.g, ``./pt_model``). In
- this case, ``from_pt`` should be set to :obj:`True` and a configuration object should be provided
- as ``config`` argument. This loading path is slower than converting the PyTorch checkpoint in
- a Flax model using the provided conversion scripts and loading the Flax model afterwards.
-
- model_args (remaining positional arguments, `optional`):
- All remaning positional arguments will be passed to the underlying model's ``__init__`` method.
-
- kwargs (remaining dictionary of keyword arguments, `optional`):
- Can be used to update the configuration object (after it being loaded) and initiate the model (e.g.,
- :obj:`output_attentions=True`).
-
- - To update the text configuration, use the prefix `text_` for each configuration parameter.
- - To update the vision configuration, use the prefix `vision_` for each configuration parameter.
- - To update the parent model configuration, do not use a prefix for each configuration parameter.
-
- Behaves differently depending on whether a :obj:`config` is provided or automatically loaded.
-
- Example::
-
- >>> from transformers import FlaxHybridCLIP
- >>> # initialize a model from pretrained BERT and CLIP models. Note that the projection layers will be randomly initialized.
- >>> # If using CLIP's vision model the vision projection layer will be initialized using pre-trained weights
- >>> model = FlaxHybridCLIP.from_text_vision_pretrained('bert-base-uncased', 'openai/clip-vit-base-patch32')
- >>> # saving model after fine-tuning
- >>> model.save_pretrained("./bert-clip")
- >>> # load fine-tuned model
- >>> model = FlaxHybridCLIP.from_pretrained("./bert-clip")
- """
-
- kwargs_text = {
- argument[len("text_") :]: value for argument, value in kwargs.items() if argument.startswith("text_")
- }
-
- kwargs_vision = {
- argument[len("vision_") :]: value for argument, value in kwargs.items() if argument.startswith("vision_")
- }
-
- # remove text, vision kwargs from kwargs
- for key in kwargs_text.keys():
- del kwargs["text_" + key]
- for key in kwargs_vision.keys():
- del kwargs["vision_" + key]
-
- # Load and initialize the text and vision model
- text_model = kwargs_text.pop("model", None)
- if text_model is None:
- assert (
- text_model_name_or_path is not None
- ), "If `model` is not defined as an argument, a `text_model_name_or_path` has to be defined"
- from transformers import FlaxAutoModel
-
- if "config" not in kwargs_text:
- from transformers import AutoConfig
-
- text_config = AutoConfig.from_pretrained(text_model_name_or_path)
- kwargs_text["config"] = text_config
-
- text_model = FlaxAutoModel.from_pretrained(text_model_name_or_path, *model_args, **kwargs_text)
-
- vision_model = kwargs_vision.pop("model", None)
- if vision_model is None:
- assert (
- vision_model_name_or_path is not None
- ), "If `model` is not defined as an argument, a `vision_model_name_or_path` has to be defined"
- from transformers import FlaxAutoModel
-
- if "config" not in kwargs_vision:
- from transformers import AutoConfig
-
- vision_config = AutoConfig.from_pretrained(vision_model_name_or_path)
- kwargs_vision["config"] = vision_config
-
- vision_model = FlaxAutoModel.from_pretrained(vision_model_name_or_path, *model_args, **kwargs_vision)
-
- # instantiate config with corresponding kwargs
- dtype = kwargs.pop("dtype", jnp.float32)
- config = HybridCLIPConfig.from_text_vision_configs(text_model.config, vision_model.config, **kwargs)
-
- # init model
- model = cls(config, *model_args, dtype=dtype, **kwargs)
-
- if vision_config.model_type == "clip":
- model.params["vision_model"]["vision_model"] = vision_model.params["vision_model"]
- model.params["visual_projection"]["kernel"] = vision_model.params["visual_projection"]["kernel"]
- else:
- model.params["vision_model"] = vision_model.params
-
- model.params["text_model"] = text_model.params
-
- return model
diff --git a/spaces/cloudtheboi/Lofi4All/.pythonlibs/lib/python3.10/site-packages/cffi/parse_c_type.h b/spaces/cloudtheboi/Lofi4All/.pythonlibs/lib/python3.10/site-packages/cffi/parse_c_type.h
deleted file mode 100644
index 84e4ef85659eb63e6453d8af9f024f1866182342..0000000000000000000000000000000000000000
--- a/spaces/cloudtheboi/Lofi4All/.pythonlibs/lib/python3.10/site-packages/cffi/parse_c_type.h
+++ /dev/null
@@ -1,181 +0,0 @@
-
-/* This part is from file 'cffi/parse_c_type.h'. It is copied at the
- beginning of C sources generated by CFFI's ffi.set_source(). */
-
-typedef void *_cffi_opcode_t;
-
-#define _CFFI_OP(opcode, arg) (_cffi_opcode_t)(opcode | (((uintptr_t)(arg)) << 8))
-#define _CFFI_GETOP(cffi_opcode) ((unsigned char)(uintptr_t)cffi_opcode)
-#define _CFFI_GETARG(cffi_opcode) (((intptr_t)cffi_opcode) >> 8)
-
-#define _CFFI_OP_PRIMITIVE 1
-#define _CFFI_OP_POINTER 3
-#define _CFFI_OP_ARRAY 5
-#define _CFFI_OP_OPEN_ARRAY 7
-#define _CFFI_OP_STRUCT_UNION 9
-#define _CFFI_OP_ENUM 11
-#define _CFFI_OP_FUNCTION 13
-#define _CFFI_OP_FUNCTION_END 15
-#define _CFFI_OP_NOOP 17
-#define _CFFI_OP_BITFIELD 19
-#define _CFFI_OP_TYPENAME 21
-#define _CFFI_OP_CPYTHON_BLTN_V 23 // varargs
-#define _CFFI_OP_CPYTHON_BLTN_N 25 // noargs
-#define _CFFI_OP_CPYTHON_BLTN_O 27 // O (i.e. a single arg)
-#define _CFFI_OP_CONSTANT 29
-#define _CFFI_OP_CONSTANT_INT 31
-#define _CFFI_OP_GLOBAL_VAR 33
-#define _CFFI_OP_DLOPEN_FUNC 35
-#define _CFFI_OP_DLOPEN_CONST 37
-#define _CFFI_OP_GLOBAL_VAR_F 39
-#define _CFFI_OP_EXTERN_PYTHON 41
-
-#define _CFFI_PRIM_VOID 0
-#define _CFFI_PRIM_BOOL 1
-#define _CFFI_PRIM_CHAR 2
-#define _CFFI_PRIM_SCHAR 3
-#define _CFFI_PRIM_UCHAR 4
-#define _CFFI_PRIM_SHORT 5
-#define _CFFI_PRIM_USHORT 6
-#define _CFFI_PRIM_INT 7
-#define _CFFI_PRIM_UINT 8
-#define _CFFI_PRIM_LONG 9
-#define _CFFI_PRIM_ULONG 10
-#define _CFFI_PRIM_LONGLONG 11
-#define _CFFI_PRIM_ULONGLONG 12
-#define _CFFI_PRIM_FLOAT 13
-#define _CFFI_PRIM_DOUBLE 14
-#define _CFFI_PRIM_LONGDOUBLE 15
-
-#define _CFFI_PRIM_WCHAR 16
-#define _CFFI_PRIM_INT8 17
-#define _CFFI_PRIM_UINT8 18
-#define _CFFI_PRIM_INT16 19
-#define _CFFI_PRIM_UINT16 20
-#define _CFFI_PRIM_INT32 21
-#define _CFFI_PRIM_UINT32 22
-#define _CFFI_PRIM_INT64 23
-#define _CFFI_PRIM_UINT64 24
-#define _CFFI_PRIM_INTPTR 25
-#define _CFFI_PRIM_UINTPTR 26
-#define _CFFI_PRIM_PTRDIFF 27
-#define _CFFI_PRIM_SIZE 28
-#define _CFFI_PRIM_SSIZE 29
-#define _CFFI_PRIM_INT_LEAST8 30
-#define _CFFI_PRIM_UINT_LEAST8 31
-#define _CFFI_PRIM_INT_LEAST16 32
-#define _CFFI_PRIM_UINT_LEAST16 33
-#define _CFFI_PRIM_INT_LEAST32 34
-#define _CFFI_PRIM_UINT_LEAST32 35
-#define _CFFI_PRIM_INT_LEAST64 36
-#define _CFFI_PRIM_UINT_LEAST64 37
-#define _CFFI_PRIM_INT_FAST8 38
-#define _CFFI_PRIM_UINT_FAST8 39
-#define _CFFI_PRIM_INT_FAST16 40
-#define _CFFI_PRIM_UINT_FAST16 41
-#define _CFFI_PRIM_INT_FAST32 42
-#define _CFFI_PRIM_UINT_FAST32 43
-#define _CFFI_PRIM_INT_FAST64 44
-#define _CFFI_PRIM_UINT_FAST64 45
-#define _CFFI_PRIM_INTMAX 46
-#define _CFFI_PRIM_UINTMAX 47
-#define _CFFI_PRIM_FLOATCOMPLEX 48
-#define _CFFI_PRIM_DOUBLECOMPLEX 49
-#define _CFFI_PRIM_CHAR16 50
-#define _CFFI_PRIM_CHAR32 51
-
-#define _CFFI__NUM_PRIM 52
-#define _CFFI__UNKNOWN_PRIM (-1)
-#define _CFFI__UNKNOWN_FLOAT_PRIM (-2)
-#define _CFFI__UNKNOWN_LONG_DOUBLE (-3)
-
-#define _CFFI__IO_FILE_STRUCT (-1)
-
-
-struct _cffi_global_s {
- const char *name;
- void *address;
- _cffi_opcode_t type_op;
- void *size_or_direct_fn; // OP_GLOBAL_VAR: size, or 0 if unknown
- // OP_CPYTHON_BLTN_*: addr of direct function
-};
-
-struct _cffi_getconst_s {
- unsigned long long value;
- const struct _cffi_type_context_s *ctx;
- int gindex;
-};
-
-struct _cffi_struct_union_s {
- const char *name;
- int type_index; // -> _cffi_types, on a OP_STRUCT_UNION
- int flags; // _CFFI_F_* flags below
- size_t size;
- int alignment;
- int first_field_index; // -> _cffi_fields array
- int num_fields;
-};
-#define _CFFI_F_UNION 0x01 // is a union, not a struct
-#define _CFFI_F_CHECK_FIELDS 0x02 // complain if fields are not in the
- // "standard layout" or if some are missing
-#define _CFFI_F_PACKED 0x04 // for CHECK_FIELDS, assume a packed struct
-#define _CFFI_F_EXTERNAL 0x08 // in some other ffi.include()
-#define _CFFI_F_OPAQUE 0x10 // opaque
-
-struct _cffi_field_s {
- const char *name;
- size_t field_offset;
- size_t field_size;
- _cffi_opcode_t field_type_op;
-};
-
-struct _cffi_enum_s {
- const char *name;
- int type_index; // -> _cffi_types, on a OP_ENUM
- int type_prim; // _CFFI_PRIM_xxx
- const char *enumerators; // comma-delimited string
-};
-
-struct _cffi_typename_s {
- const char *name;
- int type_index; /* if opaque, points to a possibly artificial
- OP_STRUCT which is itself opaque */
-};
-
-struct _cffi_type_context_s {
- _cffi_opcode_t *types;
- const struct _cffi_global_s *globals;
- const struct _cffi_field_s *fields;
- const struct _cffi_struct_union_s *struct_unions;
- const struct _cffi_enum_s *enums;
- const struct _cffi_typename_s *typenames;
- int num_globals;
- int num_struct_unions;
- int num_enums;
- int num_typenames;
- const char *const *includes;
- int num_types;
- int flags; /* future extension */
-};
-
-struct _cffi_parse_info_s {
- const struct _cffi_type_context_s *ctx;
- _cffi_opcode_t *output;
- unsigned int output_size;
- size_t error_location;
- const char *error_message;
-};
-
-struct _cffi_externpy_s {
- const char *name;
- size_t size_of_result;
- void *reserved1, *reserved2;
-};
-
-#ifdef _CFFI_INTERNAL
-static int parse_c_type(struct _cffi_parse_info_s *info, const char *input);
-static int search_in_globals(const struct _cffi_type_context_s *ctx,
- const char *search, size_t search_len);
-static int search_in_struct_unions(const struct _cffi_type_context_s *ctx,
- const char *search, size_t search_len);
-#endif
diff --git a/spaces/colakin/video-generater/public/ffmpeg/libavcodec/arm/aac.h b/spaces/colakin/video-generater/public/ffmpeg/libavcodec/arm/aac.h
deleted file mode 100644
index cafa881fc7d244ec8e69a28fa445f9ee653f49f7..0000000000000000000000000000000000000000
--- a/spaces/colakin/video-generater/public/ffmpeg/libavcodec/arm/aac.h
+++ /dev/null
@@ -1,143 +0,0 @@
-/*
- * Copyright (c) 2010 Mans Rullgard
- *
- * This file is part of FFmpeg.
- *
- * FFmpeg is free software; you can redistribute it and/or
- * modify it under the terms of the GNU Lesser General Public
- * License as published by the Free Software Foundation; either
- * version 2.1 of the License, or (at your option) any later version.
- *
- * FFmpeg is distributed in the hope that it will be useful,
- * but WITHOUT ANY WARRANTY; without even the implied warranty of
- * MERCHANTABILITY or FITNESS FOR A PARTICULAR PURPOSE. See the GNU
- * Lesser General Public License for more details.
- *
- * You should have received a copy of the GNU Lesser General Public
- * License along with FFmpeg; if not, write to the Free Software
- * Foundation, Inc., 51 Franklin Street, Fifth Floor, Boston, MA 02110-1301 USA
- */
-
-#ifndef AVCODEC_ARM_AAC_H
-#define AVCODEC_ARM_AAC_H
-
-#include "config.h"
-
-#if HAVE_NEON_INLINE
-
-#define VMUL2 VMUL2
-static inline float *VMUL2(float *dst, const float *v, unsigned idx,
- const float *scale)
-{
- unsigned v0, v1;
- __asm__ ("ubfx %0, %6, #0, #4 \n\t"
- "ubfx %1, %6, #4, #4 \n\t"
- "ldr %0, [%5, %0, lsl #2] \n\t"
- "ldr %1, [%5, %1, lsl #2] \n\t"
- "vld1.32 {d1[]}, [%7,:32] \n\t"
- "vmov d0, %0, %1 \n\t"
- "vmul.f32 d0, d0, d1 \n\t"
- "vst1.32 {d0}, [%2,:64]! \n\t"
- : "=&r"(v0), "=&r"(v1), "+r"(dst), "=m"(dst[0]), "=m"(dst[1])
- : "r"(v), "r"(idx), "r"(scale)
- : "d0", "d1");
- return dst;
-}
-
-#define VMUL4 VMUL4
-static inline float *VMUL4(float *dst, const float *v, unsigned idx,
- const float *scale)
-{
- unsigned v0, v1, v2, v3;
- __asm__ ("ubfx %0, %10, #0, #2 \n\t"
- "ubfx %1, %10, #2, #2 \n\t"
- "ldr %0, [%9, %0, lsl #2] \n\t"
- "ubfx %2, %10, #4, #2 \n\t"
- "ldr %1, [%9, %1, lsl #2] \n\t"
- "ubfx %3, %10, #6, #2 \n\t"
- "ldr %2, [%9, %2, lsl #2] \n\t"
- "vmov d0, %0, %1 \n\t"
- "ldr %3, [%9, %3, lsl #2] \n\t"
- "vld1.32 {d2[],d3[]},[%11,:32] \n\t"
- "vmov d1, %2, %3 \n\t"
- "vmul.f32 q0, q0, q1 \n\t"
- "vst1.32 {q0}, [%4,:128]! \n\t"
- : "=&r"(v0), "=&r"(v1), "=&r"(v2), "=&r"(v3), "+r"(dst),
- "=m"(dst[0]), "=m"(dst[1]), "=m"(dst[2]), "=m"(dst[3])
- : "r"(v), "r"(idx), "r"(scale)
- : "d0", "d1", "d2", "d3");
- return dst;
-}
-
-#define VMUL2S VMUL2S
-static inline float *VMUL2S(float *dst, const float *v, unsigned idx,
- unsigned sign, const float *scale)
-{
- unsigned v0, v1, v2, v3;
- __asm__ ("ubfx %0, %8, #0, #4 \n\t"
- "ubfx %1, %8, #4, #4 \n\t"
- "ldr %0, [%7, %0, lsl #2] \n\t"
- "lsl %2, %10, #30 \n\t"
- "ldr %1, [%7, %1, lsl #2] \n\t"
- "lsl %3, %10, #31 \n\t"
- "vmov d0, %0, %1 \n\t"
- "bic %2, %2, #1<<30 \n\t"
- "vld1.32 {d1[]}, [%9,:32] \n\t"
- "vmov d2, %2, %3 \n\t"
- "veor d0, d0, d2 \n\t"
- "vmul.f32 d0, d0, d1 \n\t"
- "vst1.32 {d0}, [%4,:64]! \n\t"
- : "=&r"(v0), "=&r"(v1), "=&r"(v2), "=&r"(v3), "+r"(dst),
- "=m"(dst[0]), "=m"(dst[1])
- : "r"(v), "r"(idx), "r"(scale), "r"(sign)
- : "d0", "d1", "d2");
- return dst;
-}
-
-#define VMUL4S VMUL4S
-static inline float *VMUL4S(float *dst, const float *v, unsigned idx,
- unsigned sign, const float *scale)
-{
- unsigned v0, v1, v2, v3, nz;
- __asm__ ("vld1.32 {d2[],d3[]},[%13,:32] \n\t"
- "ubfx %0, %12, #0, #2 \n\t"
- "ubfx %1, %12, #2, #2 \n\t"
- "ldr %0, [%11,%0, lsl #2] \n\t"
- "ubfx %2, %12, #4, #2 \n\t"
- "ldr %1, [%11,%1, lsl #2] \n\t"
- "ubfx %3, %12, #6, #2 \n\t"
- "ldr %2, [%11,%2, lsl #2] \n\t"
- "vmov d0, %0, %1 \n\t"
- "ldr %3, [%11,%3, lsl #2] \n\t"
- "lsr %6, %12, #12 \n\t"
- "rbit %6, %6 \n\t"
- "vmov d1, %2, %3 \n\t"
- "lsls %6, %6, #1 \n\t"
- "and %0, %5, #1<<31 \n\t"
- "it cs \n\t"
- "lslcs %5, %5, #1 \n\t"
- "lsls %6, %6, #1 \n\t"
- "and %1, %5, #1<<31 \n\t"
- "it cs \n\t"
- "lslcs %5, %5, #1 \n\t"
- "lsls %6, %6, #1 \n\t"
- "and %2, %5, #1<<31 \n\t"
- "it cs \n\t"
- "lslcs %5, %5, #1 \n\t"
- "vmov d4, %0, %1 \n\t"
- "and %3, %5, #1<<31 \n\t"
- "vmov d5, %2, %3 \n\t"
- "veor q0, q0, q2 \n\t"
- "vmul.f32 q0, q0, q1 \n\t"
- "vst1.32 {q0}, [%4,:128]! \n\t"
- : "=&r"(v0), "=&r"(v1), "=&r"(v2), "=&r"(v3), "+r"(dst),
- "+r"(sign), "=r"(nz),
- "=m"(dst[0]), "=m"(dst[1]), "=m"(dst[2]), "=m"(dst[3])
- : "r"(v), "r"(idx), "r"(scale)
- : "cc", "d0", "d1", "d2", "d3", "d4", "d5");
- return dst;
-}
-
-#endif /* HAVE_NEON_INLINE */
-
-#endif /* AVCODEC_ARM_AAC_H */
diff --git a/spaces/colakin/video-generater/public/ffmpeg/libavcodec/arm/videodsp_init_armv5te.c b/spaces/colakin/video-generater/public/ffmpeg/libavcodec/arm/videodsp_init_armv5te.c
deleted file mode 100644
index eaa8c5bbf8ea24599d2ef96b4890ca2c6b7184a2..0000000000000000000000000000000000000000
--- a/spaces/colakin/video-generater/public/ffmpeg/libavcodec/arm/videodsp_init_armv5te.c
+++ /dev/null
@@ -1,33 +0,0 @@
-/*
- * Copyright (C) 2012 Ronald S. Bultje
- *
- * This file is part of FFmpeg.
- *
- * FFmpeg is free software; you can redistribute it and/or
- * modify it under the terms of the GNU Lesser General Public
- * License as published by the Free Software Foundation; either
- * version 2.1 of the License, or (at your option) any later version.
- *
- * FFmpeg is distributed in the hope that it will be useful,
- * but WITHOUT ANY WARRANTY; without even the implied warranty of
- * MERCHANTABILITY or FITNESS FOR A PARTICULAR PURPOSE. See the GNU
- * Lesser General Public License for more details.
- *
- * You should have received a copy of the GNU Lesser General Public
- * License along with FFmpeg; if not, write to the Free Software
- * Foundation, Inc., 51 Franklin Street, Fifth Floor, Boston, MA 02110-1301 USA
- */
-
-#include "libavutil/attributes.h"
-#include "libavutil/arm/cpu.h"
-#include "libavcodec/videodsp.h"
-#include "videodsp_arm.h"
-
-void ff_prefetch_arm(const uint8_t *mem, ptrdiff_t stride, int h);
-
-av_cold void ff_videodsp_init_armv5te(VideoDSPContext *ctx, int bpc)
-{
-#if HAVE_ARMV5TE_EXTERNAL
- ctx->prefetch = ff_prefetch_arm;
-#endif
-}
diff --git a/spaces/colakin/video-generater/public/ffmpeg/libavcodec/av1_frame_split_bsf.c b/spaces/colakin/video-generater/public/ffmpeg/libavcodec/av1_frame_split_bsf.c
deleted file mode 100644
index 5f6a40316cb58a9c3721a662565eb9330d57eebb..0000000000000000000000000000000000000000
--- a/spaces/colakin/video-generater/public/ffmpeg/libavcodec/av1_frame_split_bsf.c
+++ /dev/null
@@ -1,261 +0,0 @@
-/*
- * Copyright (c) 2019 James Almer
- *
- * This file is part of FFmpeg.
- *
- * FFmpeg is free software; you can redistribute it and/or
- * modify it under the terms of the GNU Lesser General Public
- * License as published by the Free Software Foundation; either
- * version 2.1 of the License, or (at your option) any later version.
- *
- * FFmpeg is distributed in the hope that it will be useful,
- * but WITHOUT ANY WARRANTY; without even the implied warranty of
- * MERCHANTABILITY or FITNESS FOR A PARTICULAR PURPOSE. See the GNU
- * Lesser General Public License for more details.
- *
- * You should have received a copy of the GNU Lesser General Public
- * License along with FFmpeg; if not, write to the Free Software
- * Foundation, Inc., 51 Franklin Street, Fifth Floor, Boston, MA 02110-1301 USA
- */
-
-/**
- * @file
- * This bitstream filter splits AV1 Temporal Units into packets containing
- * just one frame, plus any leading and trailing OBUs that may be present at
- * the beginning or end, respectively.
- *
- * Temporal Units already containing only one frame will be passed through
- * unchanged. When splitting can't be performed, the Temporal Unit will be
- * passed through containing only the remaining OBUs starting from the first
- * one after the last successfully split frame.
- */
-
-#include "libavutil/avassert.h"
-
-#include "bsf.h"
-#include "bsf_internal.h"
-#include "cbs.h"
-#include "cbs_av1.h"
-
-typedef struct AV1FSplitContext {
- AVPacket *buffer_pkt;
- CodedBitstreamContext *cbc;
- CodedBitstreamFragment temporal_unit;
-
- int nb_frames;
- int cur_frame;
- int cur_frame_idx;
- int last_frame_idx;
-} AV1FSplitContext;
-
-static int av1_frame_split_filter(AVBSFContext *ctx, AVPacket *out)
-{
- AV1FSplitContext *s = ctx->priv_data;
- CodedBitstreamFragment *td = &s->temporal_unit;
- int i, ret;
- int split = !!s->buffer_pkt->data;
-
- if (!s->buffer_pkt->data) {
- int nb_frames = 0;
-
- ret = ff_bsf_get_packet_ref(ctx, s->buffer_pkt);
- if (ret < 0)
- return ret;
-
- ret = ff_cbs_read_packet(s->cbc, td, s->buffer_pkt);
- if (ret < 0) {
- av_log(ctx, AV_LOG_WARNING, "Failed to parse temporal unit.\n");
- goto passthrough;
- }
-
- for (i = 0; i < td->nb_units; i++) {
- CodedBitstreamUnit *unit = &td->units[i];
-
- if (unit->type == AV1_OBU_FRAME ||
- unit->type == AV1_OBU_FRAME_HEADER)
- nb_frames++;
- else if (unit->type == AV1_OBU_TILE_LIST) {
- av_log(ctx, AV_LOG_VERBOSE, "Large scale tiles are unsupported.\n");
- goto passthrough;
- }
- }
- if (nb_frames > 1) {
- s->cur_frame = 0;
- s->cur_frame_idx = s->last_frame_idx = 0;
- s->nb_frames = nb_frames;
- split = 1;
- }
- }
-
- if (split) {
- AV1RawFrameHeader *frame = NULL;
- int cur_frame_type = -1, size = 0;
-
- for (i = s->cur_frame_idx; i < td->nb_units; i++) {
- CodedBitstreamUnit *unit = &td->units[i];
-
- size += unit->data_size;
- if (unit->type == AV1_OBU_FRAME) {
- AV1RawOBU *obu = unit->content;
-
- if (frame) {
- av_log(ctx, AV_LOG_WARNING, "Frame OBU found when Tile data for a "
- "previous frame was expected.\n");
- goto passthrough;
- }
-
- frame = &obu->obu.frame.header;
- cur_frame_type = obu->header.obu_type;
- s->last_frame_idx = s->cur_frame_idx;
- s->cur_frame_idx = i + 1;
- s->cur_frame++;
-
- // split here unless it's the last frame, in which case
- // include every trailing OBU
- if (s->cur_frame < s->nb_frames)
- break;
- } else if (unit->type == AV1_OBU_FRAME_HEADER) {
- AV1RawOBU *obu = unit->content;
-
- if (frame) {
- av_log(ctx, AV_LOG_WARNING, "Frame Header OBU found when Tile data for a "
- "previous frame was expected.\n");
- goto passthrough;
- }
-
- frame = &obu->obu.frame_header;
- cur_frame_type = obu->header.obu_type;
- s->last_frame_idx = s->cur_frame_idx;
- s->cur_frame++;
-
- // split here if show_existing_frame unless it's the last
- // frame, in which case include every trailing OBU
- if (frame->show_existing_frame &&
- s->cur_frame < s->nb_frames) {
- s->cur_frame_idx = i + 1;
- break;
- }
- } else if (unit->type == AV1_OBU_TILE_GROUP) {
- AV1RawOBU *obu = unit->content;
- AV1RawTileGroup *group = &obu->obu.tile_group;
-
- if (!frame || cur_frame_type != AV1_OBU_FRAME_HEADER) {
- av_log(ctx, AV_LOG_WARNING, "Unexpected Tile Group OBU found before a "
- "Frame Header.\n");
- goto passthrough;
- }
-
- if ((group->tg_end == (frame->tile_cols * frame->tile_rows) - 1) &&
- // include every trailing OBU with the last frame
- s->cur_frame < s->nb_frames) {
- s->cur_frame_idx = i + 1;
- break;
- }
- }
- }
- av_assert0(frame && s->cur_frame <= s->nb_frames);
-
- ret = av_packet_ref(out, s->buffer_pkt);
- if (ret < 0)
- goto fail;
-
- out->data = (uint8_t *)td->units[s->last_frame_idx].data;
- out->size = size;
-
- // skip the frame in the buffer packet if it's split successfully, so it's not present
- // if the packet is passed through in case of failure when splitting another frame.
- s->buffer_pkt->data += size;
- s->buffer_pkt->size -= size;
-
- if (!frame->show_existing_frame && !frame->show_frame)
- out->pts = AV_NOPTS_VALUE;
-
- if (s->cur_frame == s->nb_frames) {
- av_packet_unref(s->buffer_pkt);
- ff_cbs_fragment_reset(td);
- }
-
- return 0;
- }
-
-passthrough:
- av_packet_move_ref(out, s->buffer_pkt);
-
- ret = 0;
-fail:
- if (ret < 0) {
- av_packet_unref(out);
- av_packet_unref(s->buffer_pkt);
- }
- ff_cbs_fragment_reset(td);
-
- return ret;
-}
-
-static const CodedBitstreamUnitType decompose_unit_types[] = {
- AV1_OBU_TEMPORAL_DELIMITER,
- AV1_OBU_SEQUENCE_HEADER,
- AV1_OBU_FRAME_HEADER,
- AV1_OBU_TILE_GROUP,
- AV1_OBU_FRAME,
-};
-
-static int av1_frame_split_init(AVBSFContext *ctx)
-{
- AV1FSplitContext *s = ctx->priv_data;
- CodedBitstreamFragment *td = &s->temporal_unit;
- int ret;
-
- s->buffer_pkt = av_packet_alloc();
- if (!s->buffer_pkt)
- return AVERROR(ENOMEM);
-
- ret = ff_cbs_init(&s->cbc, AV_CODEC_ID_AV1, ctx);
- if (ret < 0)
- return ret;
-
- s->cbc->decompose_unit_types = decompose_unit_types;
- s->cbc->nb_decompose_unit_types = FF_ARRAY_ELEMS(decompose_unit_types);
-
- if (!ctx->par_in->extradata_size)
- return 0;
-
- ret = ff_cbs_read_extradata(s->cbc, td, ctx->par_in);
- if (ret < 0)
- av_log(ctx, AV_LOG_WARNING, "Failed to parse extradata.\n");
-
- ff_cbs_fragment_reset(td);
-
- return 0;
-}
-
-static void av1_frame_split_flush(AVBSFContext *ctx)
-{
- AV1FSplitContext *s = ctx->priv_data;
-
- av_packet_unref(s->buffer_pkt);
- ff_cbs_fragment_reset(&s->temporal_unit);
-}
-
-static void av1_frame_split_close(AVBSFContext *ctx)
-{
- AV1FSplitContext *s = ctx->priv_data;
-
- av_packet_free(&s->buffer_pkt);
- ff_cbs_fragment_free(&s->temporal_unit);
- ff_cbs_close(&s->cbc);
-}
-
-static const enum AVCodecID av1_frame_split_codec_ids[] = {
- AV_CODEC_ID_AV1, AV_CODEC_ID_NONE,
-};
-
-const FFBitStreamFilter ff_av1_frame_split_bsf = {
- .p.name = "av1_frame_split",
- .p.codec_ids = av1_frame_split_codec_ids,
- .priv_data_size = sizeof(AV1FSplitContext),
- .init = av1_frame_split_init,
- .flush = av1_frame_split_flush,
- .close = av1_frame_split_close,
- .filter = av1_frame_split_filter,
-};
diff --git a/spaces/colakin/video-generater/public/ffmpeg/libavcodec/dca.c b/spaces/colakin/video-generater/public/ffmpeg/libavcodec/dca.c
deleted file mode 100644
index fb359b2ff3be1d252cc95dcc84ecc7b3876abf16..0000000000000000000000000000000000000000
--- a/spaces/colakin/video-generater/public/ffmpeg/libavcodec/dca.c
+++ /dev/null
@@ -1,157 +0,0 @@
-/*
- * DCA compatible decoder data
- * Copyright (C) 2004 Gildas Bazin
- * Copyright (C) 2004 Benjamin Zores
- * Copyright (C) 2006 Benjamin Larsson
- * Copyright (C) 2007 Konstantin Shishkov
- *
- * This file is part of FFmpeg.
- *
- * FFmpeg is free software; you can redistribute it and/or
- * modify it under the terms of the GNU Lesser General Public
- * License as published by the Free Software Foundation; either
- * version 2.1 of the License, or (at your option) any later version.
- *
- * FFmpeg is distributed in the hope that it will be useful,
- * but WITHOUT ANY WARRANTY; without even the implied warranty of
- * MERCHANTABILITY or FITNESS FOR A PARTICULAR PURPOSE. See the GNU
- * Lesser General Public License for more details.
- *
- * You should have received a copy of the GNU Lesser General Public
- * License along with FFmpeg; if not, write to the Free Software
- * Foundation, Inc., 51 Franklin Street, Fifth Floor, Boston, MA 02110-1301 USA
- */
-
-#include
-#include
-
-#include "libavutil/error.h"
-
-#include "dca.h"
-#include "dca_core.h"
-#include "dca_syncwords.h"
-#include "get_bits.h"
-#include "put_bits.h"
-
-const uint32_t ff_dca_sampling_freqs[16] = {
- 8000, 16000, 32000, 64000, 128000, 22050, 44100, 88200,
- 176400, 352800, 12000, 24000, 48000, 96000, 192000, 384000,
-};
-
-const uint8_t ff_dca_freq_ranges[16] = {
- 0, 1, 2, 3, 4, 1, 2, 3, 4, 4, 0, 1, 2, 3, 4, 4
-};
-
-const uint8_t ff_dca_bits_per_sample[8] = {
- 16, 16, 20, 20, 0, 24, 24, 0
-};
-
-int avpriv_dca_convert_bitstream(const uint8_t *src, int src_size, uint8_t *dst,
- int max_size)
-{
- uint32_t mrk;
- int i, tmp;
- PutBitContext pb;
-
- if ((unsigned) src_size > (unsigned) max_size)
- src_size = max_size;
-
- mrk = AV_RB32(src);
- switch (mrk) {
- case DCA_SYNCWORD_CORE_BE:
- case DCA_SYNCWORD_SUBSTREAM:
- memcpy(dst, src, src_size);
- return src_size;
- case DCA_SYNCWORD_CORE_LE:
- for (i = 0; i < (src_size + 1) >> 1; i++) {
- AV_WB16(dst, AV_RL16(src));
- src += 2;
- dst += 2;
- }
- return src_size;
- case DCA_SYNCWORD_CORE_14B_BE:
- case DCA_SYNCWORD_CORE_14B_LE:
- init_put_bits(&pb, dst, max_size);
- for (i = 0; i < (src_size + 1) >> 1; i++, src += 2) {
- tmp = ((mrk == DCA_SYNCWORD_CORE_14B_BE) ? AV_RB16(src) : AV_RL16(src)) & 0x3FFF;
- put_bits(&pb, 14, tmp);
- }
- flush_put_bits(&pb);
- return put_bytes_output(&pb);
- default:
- return AVERROR_INVALIDDATA;
- }
-}
-
-int ff_dca_parse_core_frame_header(DCACoreFrameHeader *h, GetBitContext *gb)
-{
- if (get_bits_long(gb, 32) != DCA_SYNCWORD_CORE_BE)
- return DCA_PARSE_ERROR_SYNC_WORD;
-
- h->normal_frame = get_bits1(gb);
- h->deficit_samples = get_bits(gb, 5) + 1;
- if (h->deficit_samples != DCA_PCMBLOCK_SAMPLES)
- return DCA_PARSE_ERROR_DEFICIT_SAMPLES;
-
- h->crc_present = get_bits1(gb);
- h->npcmblocks = get_bits(gb, 7) + 1;
- if (h->npcmblocks & (DCA_SUBBAND_SAMPLES - 1))
- return DCA_PARSE_ERROR_PCM_BLOCKS;
-
- h->frame_size = get_bits(gb, 14) + 1;
- if (h->frame_size < 96)
- return DCA_PARSE_ERROR_FRAME_SIZE;
-
- h->audio_mode = get_bits(gb, 6);
- if (h->audio_mode >= DCA_AMODE_COUNT)
- return DCA_PARSE_ERROR_AMODE;
-
- h->sr_code = get_bits(gb, 4);
- if (!ff_dca_sample_rates[h->sr_code])
- return DCA_PARSE_ERROR_SAMPLE_RATE;
-
- h->br_code = get_bits(gb, 5);
- if (get_bits1(gb))
- return DCA_PARSE_ERROR_RESERVED_BIT;
-
- h->drc_present = get_bits1(gb);
- h->ts_present = get_bits1(gb);
- h->aux_present = get_bits1(gb);
- h->hdcd_master = get_bits1(gb);
- h->ext_audio_type = get_bits(gb, 3);
- h->ext_audio_present = get_bits1(gb);
- h->sync_ssf = get_bits1(gb);
- h->lfe_present = get_bits(gb, 2);
- if (h->lfe_present == DCA_LFE_FLAG_INVALID)
- return DCA_PARSE_ERROR_LFE_FLAG;
-
- h->predictor_history = get_bits1(gb);
- if (h->crc_present)
- skip_bits(gb, 16);
- h->filter_perfect = get_bits1(gb);
- h->encoder_rev = get_bits(gb, 4);
- h->copy_hist = get_bits(gb, 2);
- h->pcmr_code = get_bits(gb, 3);
- if (!ff_dca_bits_per_sample[h->pcmr_code])
- return DCA_PARSE_ERROR_PCM_RES;
-
- h->sumdiff_front = get_bits1(gb);
- h->sumdiff_surround = get_bits1(gb);
- h->dn_code = get_bits(gb, 4);
- return 0;
-}
-
-int avpriv_dca_parse_core_frame_header(DCACoreFrameHeader *h, const uint8_t *buf, int size)
-{
- GetBitContext gb;
- int ret;
-
- ret = init_get_bits8(&gb, buf, size);
- if (ret < 0)
- return ret;
-
- if (ff_dca_parse_core_frame_header(h, &gb) < 0)
- return AVERROR_INVALIDDATA;
-
- return 0;
-}
diff --git a/spaces/colakin/video-generater/public/ffmpeg/libavcodec/libwebpenc.c b/spaces/colakin/video-generater/public/ffmpeg/libavcodec/libwebpenc.c
deleted file mode 100644
index d6edd866037b6e1e6a0d0a8a93416dc7320768b3..0000000000000000000000000000000000000000
--- a/spaces/colakin/video-generater/public/ffmpeg/libavcodec/libwebpenc.c
+++ /dev/null
@@ -1,105 +0,0 @@
-/*
- * WebP encoding support via libwebp
- * Copyright (c) 2013 Justin Ruggles
- *
- * This file is part of FFmpeg.
- *
- * FFmpeg is free software; you can redistribute it and/or
- * modify it under the terms of the GNU Lesser General Public
- * License as published by the Free Software Foundation; either
- * version 2.1 of the License, or (at your option) any later version.
- *
- * FFmpeg is distributed in the hope that it will be useful,
- * but WITHOUT ANY WARRANTY; without even the implied warranty of
- * MERCHANTABILITY or FITNESS FOR A PARTICULAR PURPOSE. See the GNU
- * Lesser General Public License for more details.
- *
- * You should have received a copy of the GNU Lesser General Public
- * License along with FFmpeg; if not, write to the Free Software
- * Foundation, Inc., 51 Franklin Street, Fifth Floor, Boston, MA 02110-1301 USA
- */
-
-/**
- * @file
- * WebP encoder using libwebp (WebPEncode API)
- */
-
-#include "codec_internal.h"
-#include "encode.h"
-#include "libwebpenc_common.h"
-
-typedef LibWebPContextCommon LibWebPContext;
-
-static av_cold int libwebp_encode_init(AVCodecContext *avctx)
-{
- return ff_libwebp_encode_init_common(avctx);
-}
-
-static int libwebp_encode_frame(AVCodecContext *avctx, AVPacket *pkt,
- const AVFrame *frame, int *got_packet)
-{
- LibWebPContext *s = avctx->priv_data;
- WebPPicture *pic = NULL;
- AVFrame *alt_frame = NULL;
- WebPMemoryWriter mw = { 0 };
-
- int ret = ff_libwebp_get_frame(avctx, s, frame, &alt_frame, &pic);
- if (ret < 0)
- goto end;
-
- WebPMemoryWriterInit(&mw);
- pic->custom_ptr = &mw;
- pic->writer = WebPMemoryWrite;
-
- ret = WebPEncode(&s->config, pic);
- if (!ret) {
- av_log(avctx, AV_LOG_ERROR, "WebPEncode() failed with error: %d\n",
- pic->error_code);
- ret = ff_libwebp_error_to_averror(pic->error_code);
- goto end;
- }
-
- ret = ff_get_encode_buffer(avctx, pkt, mw.size, 0);
- if (ret < 0)
- goto end;
- memcpy(pkt->data, mw.mem, mw.size);
-
- *got_packet = 1;
-
-end:
-#if (WEBP_ENCODER_ABI_VERSION > 0x0203)
- WebPMemoryWriterClear(&mw);
-#else
- free(mw.mem); /* must use free() according to libwebp documentation */
-#endif
- WebPPictureFree(pic);
- av_freep(&pic);
- av_frame_free(&alt_frame);
-
- return ret;
-}
-
-static int libwebp_encode_close(AVCodecContext *avctx)
-{
- LibWebPContextCommon *s = avctx->priv_data;
- av_frame_free(&s->ref);
-
- return 0;
-}
-
-const FFCodec ff_libwebp_encoder = {
- .p.name = "libwebp",
- CODEC_LONG_NAME("libwebp WebP image"),
- .p.type = AVMEDIA_TYPE_VIDEO,
- .p.id = AV_CODEC_ID_WEBP,
- .p.capabilities = AV_CODEC_CAP_DR1 | AV_CODEC_CAP_ENCODER_REORDERED_OPAQUE,
- .p.pix_fmts = ff_libwebpenc_pix_fmts,
- .p.priv_class = &ff_libwebpenc_class,
- .p.wrapper_name = "libwebp",
- .caps_internal = FF_CODEC_CAP_NOT_INIT_THREADSAFE,
- .priv_data_size = sizeof(LibWebPContext),
- .defaults = ff_libwebp_defaults,
- .init = libwebp_encode_init,
- FF_CODEC_ENCODE_CB(libwebp_encode_frame),
- .close = libwebp_encode_close,
-};
diff --git a/spaces/congsaPfin/Manga-OCR/logs/Experience Realistic Drifting and Racing in Car 2 - Download Now.md b/spaces/congsaPfin/Manga-OCR/logs/Experience Realistic Drifting and Racing in Car 2 - Download Now.md
deleted file mode 100644
index 876c8e86bdcdc0bfaf4e2ffde41b493859ac807a..0000000000000000000000000000000000000000
--- a/spaces/congsaPfin/Manga-OCR/logs/Experience Realistic Drifting and Racing in Car 2 - Download Now.md
+++ /dev/null
@@ -1,119 +0,0 @@
-
-
Racing in Car 2: A Realistic and Fun Driving Simulator
-
If you are looking for a racing game that gives you a first-person perspective of driving a car, then you should check out Racing in Car 2. This game lets you drive your car in cockpit view through the endless traffic and realistic environment. You can go as fast as possible, overtake traffic cars, earn coins and buy new cars. You can also compete with other players on the global leaderboards and become the king of the road.
In this article, we will tell you more about the features of Racing in Car 2, how to download and play it on your device, some tips and tricks to improve your driving skills, and some alternatives to this game that you might also enjoy. So, buckle up and get ready for some adrenaline-pumping action!
-
Features of Racing in Car 2
-
Racing in Car 2 is a game that offers you a realistic and fun driving experience. Here are some of the features that make this game stand out:
-
3D realistic cockpit view
-
Unlike most racing games that use a third-person perspective, Racing in Car 2 puts you in the driver's seat. You can see the dashboard, the steering wheel, the mirrors, and the road ahead of you. You can also switch between different camera angles to find the best view for you.
-
Endless game mode
-
Racing in Car 2 has an endless game mode that lets you drive as long as you want without any limits. You can choose from different locations such as city, desert, or snow. You can also adjust the traffic density and speed to suit your level of difficulty. The game will keep track of your distance, speed, time, and coins earned.
-
Different locations and cars to choose
-
Racing in Car 2 has a variety of locations and cars to choose from. You can drive in different environments such as city, desert, or snow. You can also unlock and buy new cars with different performance and appearance. You can customize your car with body kits, rims, vinyls, and more.
-
Simulator-like controls
-
Racing in Car 2 has simulator-like controls that make you feel like you are driving a real car. You can use the tilt or touch steering option according to your preference. You can also use the accelerator and brake pedals to control your speed. The game also has realistic physics and sound effects that add to the immersion.
-
How to Download and Play Racing in Car 2 on Your Device
-
Racing in Car 2 is available for both Android and iOS devices. You can download it from the Google Play Store or the App Store for free. However, if you want to play it on your PC or Mac, you will need an emulator such as BlueStacks. Here are the steps to download and play Racing in Car 2 on your device:
-
racing in car 2 game download
-racing in car 2 apk download
-racing in car 2 mod apk download
-racing in car 2 free download
-racing in car 2 download for pc
-racing in car 2 download for android
-racing in car 2 download for ios
-racing in car 2 download for windows
-racing in car 2 download for mac
-racing in car 2 download for laptop
-racing in car 2 online play
-racing in car 2 offline play
-racing in car 2 gameplay
-racing in car 2 review
-racing in car 2 tips and tricks
-racing in car 2 cheats and hacks
-racing in car 2 best cars
-racing in car 2 new update
-racing in car 2 multiplayer mode
-racing in car 2 simulator game
-racing in car 2 cockpit view
-racing in car 2 realistic graphics
-racing in car 2 endless mode
-racing in car 2 different locations
-racing in car 2 traffic cars
-racing in car 2 earn coins
-racing in car 2 buy new cars
-racing in car 2 leaderboards
-racing in car 2 vs CarX Drift Racing 2
-CarX Drift Racing 2 app download
-CarX Drift Racing 2 game download
-CarX Drift Racing 2 apk download
-CarX Drift Racing 2 mod apk download
-CarX Drift Racing 2 free download
-CarX Drift Racing 2 download for pc
-CarX Drift Racing 2 download for android
-CarX Drift Racing 2 download for ios
-CarX Drift Racing 2 download for windows
-CarX Drift Racing 2 download for mac
-CarX Drift Racing 2 download for laptop
-CarX Drift Racing 2 online play
-CarX Drift Racing 2 offline play
-CarX Drift Racing 2 gameplay
-CarX Drift Racing 2 review
-CarX Drift Racing 2 tips and tricks
-CarX Drift Racing 2 cheats and hacks
-CarX Drift Racing 2 best cars
-CarX Drift Racing 2 new update
-CarX Drift Racing 2 multiplayer mode
-
Download from Google Play Store or App Store
-
-
Open the Google Play Store or the App Store on your device and search for Racing in Car 2.
-
Tap on the game icon and then tap on Install or Get to download the game.
-
Wait for the game to finish downloading and then tap on Open or Launch to start the game.
-
Enjoy driving your car in cockpit view and overtaking traffic cars.
Launch BlueStacks and sign in with your Google account.
-
Go to the Google Play Store on BlueStacks and search for Racing in Car 2.
-
Click on the game icon and then click on Install to download the game.
-
Wait for the game to finish downloading and then click on Open to start the game.
-
Enjoy driving your car in cockpit view and overtaking traffic cars on a bigger screen.
-
-
Tips and Tricks for Racing in Car 2
-
Racing in Car 2 is a game that requires skill and strategy to master. Here are some tips and tricks that can help you improve your driving performance and score higher:
-
Overtake traffic cars to earn coins and bonuses
-
The main objective of Racing in Car 2 is to overtake as many traffic cars as possible without crashing. The more cars you overtake, the more coins and bonuses you earn. Coins can be used to buy new cars and upgrade your existing ones. Bonuses can give you extra speed, time, or coins. Try to overtake cars from a close distance and avoid hitting them to get more rewards.
-
Upgrade your car with performance and visual tuning
-
Racing in Car 2 allows you to upgrade your car with performance and visual tuning. Performance tuning can improve your car's speed, acceleration, handling, and braking. Visual tuning can change your car's body kit, rims, vinyls, and more. Upgrading your car can help you drive faster, smoother, and more stylishly.
-
Use the tilt or touch steering option according to your preference
-
Racing in Car 2 gives you two options to control your car: tilt or touch steering. Tilt steering uses the accelerometer of your device to steer your car by tilting it left or right. Touch steering uses buttons on the screen to steer your car by tapping them. You can choose the option that suits your preference and comfort level. You can also adjust the sensitivity of the steering in the settings menu.
-
Try different camera angles to find the best view
-
Racing in Car 2 has different camera angles that you can switch between during the game. You can use the cockpit view, the hood view, or the rear view. Each view has its own advantages and disadvantages. The cockpit view gives you a realistic feeling of driving a car, but it may also limit your visibility of the road. The hood view gives you a clear view of the road ahead, but it may also make you feel detached from the car. The rear view gives you a wider view of the road behind, but it may also make you lose focus of the road ahead. Try different camera angles to find the best view for you.
-
Alternatives to Racing in Car 2
-
If you like Racing in Car 2, you might also like some other racing games that offer similar or different features. Here are some alternatives to Racing in Car 2 that you can try:
-
CarX Drift Racing 2
-
If you are into drifting, then you should check out CarX Drift Racing 2. This game lets you drive powerful sports cars and perform amazing drifts on various tracks. You can customize your car with different parts, paint jobs, vinyls, and decals. You can also compete with other players online or offline in different modes such as solo run, tandem drift, or drift battles.
-
Real Racing 3
-
If you are into realistic racing, then you should check out Real Racing 3. This game lets you drive over 250 authentic cars from top manufacturers such as Ferrari, Porsche, Lamborghini, and more. You can race on over 40 real tracks from around the world such as Silverstone, Le Mans, Dubai Autodrome, and more. You can also challenge other players online or offline in different modes such as time trials, cup races, endurance races, or multiplayer races.
-
Asphalt 9: Legends
-
If you are into arcade racing, then you should check out Asphalt 9: Legends. This game lets you drive over 80 dream cars from top brands such as Ferrari, Lamborghini, Bugatti, and more. You can race on over 60 stunning tracks from around the world such as New York, Paris, Tokyo, and more. You can also perform amazing stunts and tricks such as barrel rolls, 360° spins, and nitro boosts.
-
Conclusion
-
Racing in Car 2 is a game that offers you a realistic and fun driving simulator. You can drive your car in cockpit view through the endless traffic and realistic environment. You can also customize your car with different performance and visual tuning. You can also compete with other players on the global leaderboards and become the king of the road.
-
If you are looking for a racing game that gives you a first-person perspective of driving a car, then you should download Racing in Car 2 today. You can download it from the Google Play Store or the App Store for free. You can also download it from BlueStacks Emulator if you want to play it on your PC or Mac.
-
So, what are you waiting for? Download Racing in Car 2 now and enjoy the thrill of driving a car in cockpit view!
-
FAQs
-
-
Q: Is Racing in Car 2 free to play?
-
A: Yes, Racing in Car 2 is free to play. However, it contains ads and in-app purchases that you can disable or buy if you want.
-
Q: How can I earn more coins in Racing in Car 2?
-
A: You can earn more coins by overtaking traffic cars from a close distance, collecting bonuses, completing missions, and watching ads.
-
Q: How can I unlock new cars in Racing in Car 2?
-
A: You can unlock new cars by earning enough coins to buy them. You can also unlock some cars by completing certain missions or achievements.
-
Q: How can I play Racing in Car 2 with my friends?
-
A: You can play Racing in Car 2 with your friends by connecting your game to Facebook or Google Play Games. You can then see your friends' scores on the leaderboards and challenge them to beat your records.
-
Q: What are the minimum requirements to play Racing in Car 2 on my device?
-
A: The minimum requirements to play Racing in Car 2 on your device are: - Android: Android 4.4 or higher, 1 GB of RAM, and 100 MB of free storage space. - iOS: iOS 9.0 or later, iPhone 5S or newer, iPad Air or newer, iPod touch (6th generation) or newer, and 200 MB of free storage space. - PC or Mac: Windows 7 or higher, Mac OS X 10.11 or higher, Intel or AMD processor, 4 GB of RAM, and 500 MB of free storage space.
-
197e85843d
-
-
\ No newline at end of file
diff --git a/spaces/congsaPfin/Manga-OCR/logs/Home Design Online Express Your Style with a Catalog of Branded Products.md b/spaces/congsaPfin/Manga-OCR/logs/Home Design Online Express Your Style with a Catalog of Branded Products.md
deleted file mode 100644
index 221f19eb0e931011c0aeddce17121c258970366b..0000000000000000000000000000000000000000
--- a/spaces/congsaPfin/Manga-OCR/logs/Home Design Online Express Your Style with a Catalog of Branded Products.md
+++ /dev/null
@@ -1,183 +0,0 @@
-
-
Home Design Online: How to Create Your Dream House in 3D
-
Have you ever dreamed of designing your own house, but felt overwhelmed by the complexity and cost of traditional methods? Do you want to unleash your creativity and express your personal style in your home? If so, you might want to try home design online, a modern and easy way to create your dream house in 3D.
-
Introduction
-
What is home design online?
-
Home design online is the process of creating floor plans, layouts, furniture arrangements, decorations, and other aspects of a house using online software. Home design online software allows you to design your house in 2D or 3D, using a variety of tools and features. You can also edit colors, patterns, materials, sizes, and shapes of different items, as well as apply realistic lighting and shadows. Home design online software can help you create realistic images of your project, as well as share it online with others.
There are many benefits of using home design online software, such as:
-
-
It is easy and intuitive. You don't need any professional skills or experience to use home design online software. You can simply drag and drop items, adjust settings, and switch between views. You can also access tutorials and instructions if you need help.
-
It is flexible and customizable. You can design your house according to your preferences and needs. You can choose from a wide range of branded products, or create your own custom items. You can also change the dimensions, colors, textures, and styles of any item.
-
It is affordable and convenient. You don't need to spend money on hiring an architect, a designer, or a contractor. You also don't need to buy any materials or tools. You can design your house from the comfort of your own home, at any time and pace.
-
It is fun and rewarding. You can enjoy the creative process of designing your house, as well as the satisfaction of seeing your vision come to life. You can also share your project with others, and get feedbacks and suggestions.
-
-
How to get started with home design online?
-
To start designing your house online, you will need to choose a home design online software that suits your needs and preferences. There are many options available on the market, each with its own features, pros and cons, pricing and plans. In this article, we will review three of the best home design online software: Planner 5D, HomeByMe, and RoomSketcher.
-
Best Home Design Online Software
-
Planner 5D
-
Planner 5D is one of the most popular home design online software in the world. It has over 91 million users, who have created over 70 million projects. Planner 5D allows you to create floor plans in 2D or 3D, as well as furnish and decorate your house with over 5000 items.
-
Features
-
-
You can use the 2D mode to create floor plans and layouts with simple and intuitive tools. You can draw walls, doors, windows, stairs, and other elements. You can also import existing floor plans from images or PDF files.
-
You can use the 3D mode to view your project from different angles and perspectives. You can also apply realistic lighting and shadows, as well as adjust the time of day and the weather.
-
You can furnish and decorate your house with over 5000 items, including furniture, appliances, accessories, plants, and more. You can also customize the colors, patterns, materials, sizes, and shapes of any item.
-
You can create your own items using the 3D editor. You can import models from other sources, or create them from scratch. You can also edit the textures, colors, and dimensions of your items.
-
You can share your project online with other users, or download it as an image or a video. You can also export your project as a PDF file or a DWG file.
-
-
Pros and cons
-
-
Pros:
-
It is easy to use and has a user-friendly interface.
-
It has a large and diverse catalog of items.
-
It has a powerful 3D editor that allows you to create your own items.
-
It has realistic rendering and animation options.
-
It is compatible with multiple devices and platforms, including web browsers, Windows, Mac, iOS, Android, and VR.
-
-
-
Cons:
-
It requires an internet connection to access all the features and items.
-
It has some limitations in the free version, such as the number of projects, items, and renders you can create.
-
It has some bugs and glitches that may affect the performance and quality of your project.
-
-
-
-
Pricing and plans
-
Planner 5D offers a free version that allows you to create up to 3 projects with limited items and features. You can also upgrade to a premium version that gives you unlimited access to all the features and items for a monthly or yearly fee. The premium version costs $6.99 per month or $29.99 per year. You can also purchase additional items or renders separately.
-
HomeByMe
-
HomeByMe is another popular home design online software that lets you create floor plans in 2D or 3D, as well as furnish and decorate your house with over 20,000 items. HomeByMe allows you to design your house in a realistic and immersive way, using high-quality renders and 360° views.
-
Features
-
-
You can use the 2D mode to create floor plans and layouts with simple and precise tools. You can draw walls, doors, windows, stairs, and other elements. You can also import existing floor plans from images or PDF files.
-
You can use the 3D mode to view your project from different angles and perspectives. You can also apply realistic lighting and shadows, as well as adjust the time of day and the weather.
-
You can furnish and decorate your house with over 20,000 items, including furniture, appliances, accessories, plants, and more. You can choose from a wide range of branded products, or create your own custom items. You can also customize the colors, patterns, materials, sizes, and shapes of any item.
-
You can create high-quality renders of your project in HD or 4K resolution. You can also create 360° views that allow you to explore your project in a virtual reality mode.
-
You can share your project online with other users, or download it as an image or a video. You can also export your project as a PDF file or a DWG file.
-
-
Pros and cons
-
-
Pros:
-
It is easy to use and has a user-friendly interface.
-
It has a large and diverse catalog of items.
-
It has realistic rendering and animation options.
-
It has a virtual reality mode that allows you to experience your project in an immersive way.
-
-
-
Cons:
-
It requires an internet connection to access all the features and items.
-
It has some limitations in the free version, such as the number of projects, items, renders, and 360° views you can create.
-
It has some bugs and glitches that may affect the performance and quality of your project.
-
-
-
-
Pricing and plans
-
HomeByMe offers a free version that allows you to create up to 3 projects with limited items and features. You can also upgrade to a premium version that gives you unlimited access to all the features and items for a monthly or yearly fee. The premium version costs $14.99 per month or $119.88 per year. You can also purchase additional items, renders, or 360° views separately.
-
home design online free
-home design online tool
-home design online 3d
-home design online software
-home design online app
-home design online game
-home design online course
-home design online planner
-home design online magazine
-home design online store
-home design online consultation
-home design online classes
-home design online program
-home design online tutorial
-home design online service
-home design online shop
-home design online simulator
-home design online platform
-home design online inspiration
-home design online community
-home design online rendering
-home design online degree
-home design online portfolio
-home design online quiz
-home design online blog
-home design online challenge
-home design online certification
-home design online reviews
-home design online ideas
-home design online tips
-home design online trends
-home design online projects
-home design online videos
-home design online books
-home design online courses free
-home design online tools free
-home design online 3d free
-home design online software free
-home design online app free
-home design online game free
-best home design online software
-best home design online app
-best home design online game
-best home design online tool
-best home design online 3d
-best home design online free
-best home design online service
-best home design online platform
-
RoomSketcher
-
RoomSketcher is another home design online software that enables you to create floor plans in 2D or 3D, as well as furnish and decorate your house with over 10,000 items. RoomSketcher allows you to design your house in a simple and fun way, using interactive features and tools.
-
Features
-
-
You can use the 2D mode to create floor plans and layouts with simple and intuitive tools. You can draw walls, doors, windows, stairs, and other elements. You can also import existing floor plans from images or PDF files.
-
You can use the 3D mode to view your project from different angles and perspectives. You can also apply realistic lighting and shadows, as well as adjust the time of day and the weather.
-
You can furnish and decorate your house with over 10,000 items, including furniture, appliances, accessories, plants, and more. You can also customize the colors, patterns, materials, sizes, and shapes of any item.
-
You can create interactive floor plans that allow you to walk through your project in a virtual reality mode. You can also create live 3D floor plans that allow you to view your project in real time.
-
You can share your project online with other users, or download it as an image or a video. You can also export your project as a PDF file or a DWG file.
-
-
Pros and cons
-
-
Pros:
-
It is easy to use and has a user-friendly interface.
-
It has a large and diverse catalog of items.
-
It has interactive and live 3D floor plans that allow you to experience your project in a dynamic way.
-
It is compatible with multiple devices and platforms, including web browsers, Windows, Mac, iOS, Android, and VR.
-
-
-
Cons:
-
It requires an internet connection to access all the features and items.
-
It has some limitations in the free version, such as the number of projects, items, renders, and live 3D floor plans you can create.
-
It has some bugs and glitches that may affect the performance and quality of your project.
-
-
-
-
Pricing and plans
-
RoomSketcher offers a free version that allows you to create up to 5 projects with limited items and features. You can also upgrade to a premium version that gives you unlimited access to all the features and items for a monthly or yearly fee. The premium version costs $49 per year for personal use or $99 per year for professional use. You can also purchase additional items or renders separately.
-
Tips and Tricks for Home Design Online
-
To make the most out of your home design online experience, here are some tips and tricks that you can follow:
-
Choose the right software for your needs
-
Before you start designing your house online, you should consider your needs and preferences. Do you want a simple or a complex software? Do you want a free or a paid software? Do you want a realistic or a stylized software? Do you want a software that has many features or one that has few features? Do you want a software that has many items or one that has few items? Do you want a software that is compatible with your device or platform? Do you want a software that allows you to share your project online or offline?
-
To help you choose the right software for your needs, you can compare different options based on their features, pros and cons, pricing and plans. You can also read reviews from other users, watch tutorials and demos, or try out free versions before you buy.
-
Plan your layout and design in 2D first
-
Before you jump into the 3D mode of your home design online software , you should plan your layout and design in 2D first. This will help you to create a clear and accurate floor plan of your house, as well as to arrange the furniture and other items in a logical and functional way. You can use the 2D mode of your home design online software to draw the walls, doors, windows, stairs, and other elements of your house. You can also import an existing floor plan from an image or a PDF file, or use a template or a sample project. You can then drag and drop the items from the catalog to your floor plan, and adjust their positions, orientations, and dimensions. You can also add labels, dimensions, and notes to your floor plan, as well as change the scale and the units.
-
Express your style with branded products and custom colors
-
One of the advantages of home design online software is that you can express your personal style and taste in your house. You can choose from a wide range of branded products that are available in the catalog of your home design online software, such as IKEA, Pottery Barn, West Elm, and more. You can also create your own custom items using the 3D editor or the color picker. You can change the colors, patterns, materials, sizes, and shapes of any item in your house, as well as apply different finishes and effects. You can also mix and match different styles and themes, such as modern, rustic, vintage, or eclectic. You can also add some personal touches, such as photos, artworks, or souvenirs.
-
Use renders and 3D views to visualize your project
-
Another benefit of home design online software is that you can visualize your project in a realistic and immersive way. You can use the 3D mode of your home design online software to view your project from different angles and perspectives. You can also apply realistic lighting and shadows, as well as adjust the time of day and the weather. You can also create high-quality renders of your project in HD or 4K resolution. You can also create 360° views or interactive floor plans that allow you to walk through your project in a virtual reality mode. These features will help you to see how your project will look like in real life, as well as to spot any errors or improvements.
-
Share your project online and get feedbacks
-
The final step of home design online is to share your project online with others. You can use the sharing options of your home design online software to upload your project to their website or app, or to social media platforms such as Facebook, Instagram, Pinterest, or YouTube. You can also download your project as an image or a video, or export it as a PDF file or a DWG file. You can then share your project with your friends, family, or clients, and get feedbacks and suggestions. You can also browse other users' projects and get inspired by their ideas.
-
Conclusion
-
In conclusion, home design online is a modern and easy way to create your dream house in 3D. You can use home design online software to create floor plans in 2D or 3D, as well as furnish and decorate your house with over 10,000 items. You can also customize the colors, patterns, materials, sizes, and shapes of any item in your house. You can also create realistic images of your project, as well as share it online with others.
-
If you want to try home design online software for yourself , you can choose from one of the three best home design online software that we reviewed in this article: Planner 5D, HomeByMe, or RoomSketcher. You can also follow the tips and tricks that we shared to make the most out of your home design online experience. We hope that this article has helped you to learn more about home design online and inspired you to create your own dream house in 3D.
-
Here are some FAQs that you might have about home design online:
-
FAQs
-
-
What are the advantages of home design online over traditional methods?
-
Home design online has many advantages over traditional methods, such as being easy, flexible, affordable, convenient, fun, and rewarding. You don't need any professional skills or experience to use home design online software. You can also design your house according to your preferences and needs, without spending money on hiring an architect, a designer, or a contractor. You can also enjoy the creative process of designing your house, as well as the satisfaction of seeing your vision come to life.
-
What are the disadvantages of home design online?
-
Home design online also has some disadvantages, such as requiring an internet connection, having some limitations in the free version, and having some bugs and glitches. You will need an internet connection to access all the features and items of your home design online software. You will also have some restrictions in the number of projects, items, and renders you can create in the free version. You may also encounter some errors or problems that may affect the performance and quality of your project.
-
How can I choose the best home design online software for me?
-
To choose the best home design online software for you, you should consider your needs and preferences. You should compare different options based on their features, pros and cons, pricing and plans. You should also read reviews from other users, watch tutorials and demos, or try out free versions before you buy.
-
How can I improve my home design online skills?
-
To improve your home design online skills, you should practice and experiment with different tools and features of your home design online software. You should also learn from other users' projects and get feedbacks and suggestions. You should also follow some tips and tricks that we shared in this article, such as planning your layout and design in 2D first, expressing your style with branded products and custom colors, using renders and 3D views to visualize your project, and sharing your project online.
-
Where can I find more resources and inspiration for home design online?
-
You can find more resources and inspiration for home design online on the websites or apps of your home design online software. You can also find them on social media platforms such as Facebook, Instagram, Pinterest, or YouTube. You can also find them on blogs, magazines, books, or podcasts that are related to home design.
- 401be4b1e0
-
-
\ No newline at end of file
diff --git a/spaces/congsaPfin/Manga-OCR/logs/Solo Piano Music Royalty Free Download - Pixabay.md b/spaces/congsaPfin/Manga-OCR/logs/Solo Piano Music Royalty Free Download - Pixabay.md
deleted file mode 100644
index 85903650a677242d8c983cc8c6bdba61b1f6da3d..0000000000000000000000000000000000000000
--- a/spaces/congsaPfin/Manga-OCR/logs/Solo Piano Music Royalty Free Download - Pixabay.md
+++ /dev/null
@@ -1,100 +0,0 @@
-
-
Piano Free Download Music: How to Enjoy Beautiful Piano Music Without Paying a Dime
-
Do you love piano music? Do you wish you could listen to it anytime and anywhere without spending any money? If so, you are in luck. There is a way to enjoy beautiful piano music without paying a dime. It is called piano free download music.
Piano free download music is music that you can download from the internet for free. You can find thousands of piano tracks in various genres, moods, styles, and lengths. You can use them for your personal or commercial projects, as long as you follow the license and attribution rules.
-
In this article, we will show you how to find and download piano free download music from different sources. We will also tell you about the benefits of listening to piano music and how it can enhance your life. Let's get started.
-
The Benefits of Piano Free Download Music
-
Piano music is one of the most popular and versatile types of music in the world. It can express a wide range of emotions, from joy and happiness to sadness and sorrow. It can also inspire you, relax you, educate you, and entertain you. Here are some of the benefits of listening to piano free download music:
-
-
Relaxation: Piano music can help you relax and reduce stress. It can lower your blood pressure, heart rate, and cortisol levels. It can also improve your mood and sleep quality. Piano music can be especially soothing when you are feeling anxious, depressed, or overwhelmed.
-
Inspiration: Piano music can stimulate your creativity and imagination. It can help you generate new ideas, solve problems, and express yourself. Piano music can also enhance your memory, concentration, and learning abilities.
-
Education: Piano music can teach you about musical theory and history. You can learn about different musical elements, such as melody, harmony, rhythm, tempo, dynamics, and timbre. You can also learn about different piano composers, styles, periods, and genres.
-
Entertainment: Piano music can provide you with hours of enjoyment and fun. You can listen to it while working, studying, exercising, or relaxing. You can also sing along, dance along, or play along with it. You can also share it with your friends and family.
-
-
The Best Sources of Piano Free Download Music
-
There are many sources of piano free download music on the internet. You can find them on websites, apps, podcasts, and online courses. Here are some of the best sources that we recommend:
-
piano background music free download
-royalty free piano music mp3
-free piano stock music tracks
-solo piano music free download
-piano music free download for youtube
-relaxing piano music free download
-classical piano music free download
-sad piano music free download
-romantic piano music free download
-jazz piano music free download
-piano sheet music free download
-piano instrumental music free download
-piano cover music free download
-piano meditation music free download
-piano study music free download
-piano ambient music free download
-piano cinematic music free download
-piano pop music free download
-piano trap music free download
-piano r&b music free download
-piano house music free download
-piano lo-fi music free download
-piano rap music free download
-piano rock music free download
-piano gospel music free download
-piano christmas music free download
-piano halloween music free download
-piano wedding music free download
-piano anime music free download
-piano game music free download
-easy listening piano music free download
-beautiful piano music free download
-emotional piano music free download
-inspiring piano music free download
-uplifting piano music free download
-dramatic piano music free download
-mysterious piano music free download
-creepy piano music free download
-happy piano music free download
-mellow piano music free download
-experimental piano music free download
-electronic piano music free download
-fusion piano music free download
-blues piano music free download
-country piano music free download
-folk piano music free download
-reggae piano music free download
-latin piano music free download
-indian piano music free download
-
-
Websites: There are many websites that offer piano free download music in various formats (mp3, wav, ogg, etc.). Some of the best ones are Chosic, Pixabay, Mixkit, etc. These websites have a large collection of piano tracks that you can browse by genre (solo piano, classical piano, jazz piano), mood (calm, relaxing), artist (Mozart), or keyword (lullaby). You can also preview the tracks before downloading them. You can also download them in bulk or individually.
-
Apps: There are many apps that offer piano free download music in various formats (mp3, wav, ogg, etc.). Some of the best ones are Spotify, SoundCloud, YouTube Music, etc. These apps have a large collection of piano tracks that you can stream or download offline. You can also create your own playlists, follow your favorite artists, and discover new music.
-
Podcasts: There are many podcasts that offer piano free download music in various formats (mp3, wav, ogg, etc.). Some of the best ones are Piano Relaxation, Piano Stories, Piano Jazz, etc. These podcasts have a large collection of piano tracks that you can listen to on your phone, computer, or smart speaker. You can also subscribe to them, rate them, and leave reviews.
-
Online Courses: There are many online courses that offer piano free download music in various formats (mp3, wav, ogg, etc.). Some of the best ones are Learn Piano Online, Piano for Beginners, Piano Masterclass, etc. These online courses have a large collection of piano tracks that you can learn from and play along with. You can also access video lessons, quizzes, exercises, and certificates.
-
-
The Tips for Finding and Downloading Piano Free Download Music
-
Now that you know the best sources of piano free download music, you might be wondering how to find and download the tracks that you like. Here are some tips that can help you:
-
-
Search by genre, mood, artist, or keyword: You can use the search function on the websites, apps, podcasts, or online courses to find the piano tracks that suit your preferences. You can also use filters or categories to narrow down your results.
-
Check the license and attribution requirements: Before you download any piano track, make sure you check the license and attribution requirements. Some tracks are free to use for any purpose, while others require you to give credit to the original creator or pay a fee. You can usually find this information on the source page or in the file description.
-
Use a reliable and secure downloader tool: To download the piano tracks from the websites, apps, podcasts, or online courses, you need to use a reliable and secure downloader tool. You can find many such tools online, but make sure you choose one that is compatible with your device and source. You can also read reviews and ratings to find the best one.
-
Organize and manage your downloaded files: After you download the piano tracks, you need to organize and manage them properly. You can use folders, labels, tags, or playlists to sort them by genre, mood, artist, or keyword. You can also use a media player or an editor to play or edit them.
-
-
The Conclusion
-
Piano free download music is a great way to enjoy beautiful piano music without paying a dime. You can find thousands of piano tracks in various genres, moods, styles, and lengths from different sources on the internet. You can use them for your personal or commercial projects, as long as you follow the license and attribution rules.
-
Piano free download music can also benefit you in many ways. It can help you relax, inspire you, educate you, and entertain you. It can also improve your mood, sleep quality, creativity, memory, concentration, and learning abilities.
-
If you want to find and download piano free download music from different sources, you need to follow some tips. You need to search by genre, mood, artist, or keyword, check the license and attribution requirements, use a reliable and secure downloader tool, and organize and manage your downloaded files.
-
So, what are you waiting for? Start exploring the world of piano free download music today and enjoy the beauty and magic of piano music. You will be amazed by how much it can enrich your life.
-
FAQs
-
Here are some of the frequently asked questions about piano free download music:
-
-
What is the difference between piano free download music and piano royalty-free music?
-
Piano free download music is music that you can download from the internet for free. Piano royalty-free music is music that you can use for your projects without paying any royalties to the original creator. However, some piano royalty-free music may require you to pay a one-time fee or give credit to the original creator.
-
How can I use piano free download music for my projects?
-
You can use piano free download music for your personal or commercial projects, such as videos, podcasts, games, apps, websites, presentations, etc. However, you need to follow the license and attribution rules of the source. Some sources may allow you to use the music for any purpose, while others may have some restrictions or conditions.
-
How can I find the best piano free download music for my projects?
-
You can find the best piano free download music for your projects by searching by genre, mood, artist, or keyword on the websites, apps, podcasts, or online courses that offer piano free download music. You can also use filters or categories to narrow down your results. You can also preview the tracks before downloading them.
-
How can I download piano free download music from different sources?
-
You can download piano free download music from different sources by using a reliable and secure downloader tool. You can find many such tools online, but make sure you choose one that is compatible with your device and source. You can also read reviews and ratings to find the best one.
-
How can I organize and manage my downloaded piano free download music files?
-
You can organize and manage your downloaded piano free download music files by using folders, labels, tags, or playlists to sort them by genre, mood, artist, or keyword. You can also use a media player or an editor to play or edit them.
- 197e85843d
-
-
\ No newline at end of file
diff --git a/spaces/congsaPfin/Manga-OCR/logs/TikTok The app that lets you express yourself with music filters and effects.md b/spaces/congsaPfin/Manga-OCR/logs/TikTok The app that lets you express yourself with music filters and effects.md
deleted file mode 100644
index f7e0310e9fdc07a2f88d3106b41d07fe6026ae68..0000000000000000000000000000000000000000
--- a/spaces/congsaPfin/Manga-OCR/logs/TikTok The app that lets you express yourself with music filters and effects.md
+++ /dev/null
@@ -1,153 +0,0 @@
-
-
TikTok: The Ultimate Guide for Beginners
-
If you are looking for a fun and easy way to express yourself, connect with others, and discover new things, you might want to give TikTok a try. TikTok is a video-sharing app that has taken the world by storm, with over 1 billion monthly active users and millions of videos uploaded every day. But what is TikTok exactly, how do you use it, and why is it so popular? In this guide, we will answer these questions and more, as well as give you some tips and tricks to get the most out of your TikTok experience.
-
What is TikTok?
-
A brief introduction to the app
-
TikTok is a social media app that allows users to create and share short-form videos on any topic. It’s mainly mobile-based, although you can still watch TikTok videos using the web app. The platform allows users to get creative with their content using filters, stickers, voiceovers, sound effects, and background music.
TikTok was launched in China in 2016 as Douyin, and then expanded internationally in 2017 as TikTok. In 2018, it merged with Musical.ly, another popular video app that focused on lip-syncing and dancing. Since then, TikTok has grown into a fully-fledged video service, with content ranging from comedy, gaming, DIY, food, sports, memes, pets, to oddly satisfying, ASMR, and everything in between.
-
TikTok is owned by ByteDance, a Chinese internet company that also operates other apps such as Toutiao (a news aggregator) and Helo (a social networking app for India). ByteDance has faced some controversies over its data privacy and security practices, as well as its alleged censorship of content that is sensitive to the Chinese government. However, TikTok has denied these allegations and has tried to distance itself from ByteDance.
-
How to use TikTok
-
How to create an account
-
To start using TikTok, you need to download the app from the App Store or Google Play Store. You can sign up using your phone number, email address, or a third-party account such as Facebook or Google. You can also choose a username and a profile picture for your account.
-
Once you have an account, you can decide if you want to make it private or public. A private account means that only people who follow you can see your videos and send you messages. A public account means that anyone can see your videos and send you messages. You can change your privacy settings at any time from your profile page.
-
How to watch videos
-
When you open the app, you will see two tabs at the top: Following and For You. The Following tab shows you videos from the users you follow. The For You tab shows you videos that are recommended for you by TikTok’s algorithm based on your preferences and behavior.
-
You can swipe up or down to scroll through the videos. You can also tap on the video to pause or resume it. You can also double-tap on the video to like it, or swipe left to see the user’s profile and other videos.
-
On the right side of the screen, you will see some icons that let you interact with the video. You can tap on the heart icon to like the video, the comment icon to leave a comment, the share icon to share the video with others, and the record icon to create a duet or a reaction video. You can also tap on the spinning record icon at the bottom right to see the sound or song used in the video, and use it for your own videos.
-
tik tok app download
-tik tok app for pc
-tik tok app store
-tik tok app online
-tik tok app review
-tik tok app challenge
-tik tok app tutorial
-tik tok app logo
-tik tok app banned
-tik tok app update
-tik tok app features
-tik tok app tips
-tik tok app ranking
-tik tok app revenue
-tik tok app history
-tik tok app alternatives
-tik tok app analytics
-tik tok app ads
-tik tok app creator
-tik tok app support
-tik tok app safety
-tik tok app privacy
-tik tok app music
-tik tok app sound effects
-tik tok app filters
-tik tok app stickers
-tik tok app transitions
-tik tok app effects
-tik tok app duet
-tik tok app live stream
-tik tok app followers
-tik tok app likes
-tik tok app views
-tik tok app comments
-tik tok app hashtags
-tik tok app captions
-tik tok app trends
-tik tok app viral videos
-tik tok app memes
-tik tok app comedy
-tik tok app dance
-tik tok app lip sync
-tik tok app prank
-tik tok app magic tricks
-tik tok app art and craft
-tik tok app cooking and baking
-tik tok app beauty and fashion
-tik tok app fitness and health
-tik tok app education and learning
-tik tok app travel and adventure
-
On the left side of the screen, you will see some information about the video. You can tap on the user’s name to see their profile and follow them, or tap on the caption to see more details about the video. You can also tap on the hashtags or mentions to see more videos related to them.
-
How to make your own videos
-
If you want to create your own videos, you need to tap on the plus icon at the bottom of the screen. This will open the camera mode, where you can choose from various options to make your video.
-
You can either record a video using your phone’s camera, or upload a video from your gallery. You can also choose a sound or a song from TikTok’s library, or use your own voice or music. You can adjust the speed, timer, filters, effects, and beauty mode of your video before or after recording it.
-
Once you have recorded or uploaded your video, you can edit it further using TikTok’s editing tools. You can trim, cut, split, merge, duplicate, or reverse your video clips. You can also add stickers, text, emojis, filters, effects, transitions, and voice effects to your video. You can also adjust the volume, pitch, and speed of your sound or music.
-
When you are done editing your video, you can add a caption, hashtags, mentions, and location to your video. You can also choose who can view your video (public, friends only, or private), who can comment on your video (everyone, friends only, or no one), who can duet or react to your video (everyone, friends only, or no one), and who can download your video (on or off). You can also save your video to your phone or share it with other apps. Finally, you can tap on Post to upload your video to TikTok.
-
How to interact with other users
-
TikTok is not only a platform for creating and watching videos, but also a community for connecting and engaging with other users. There are many ways you can interact with other users on TikTok.
-
You can follow other users that you like or find interesting by tapping on their name and then tapping on Follow. You can also unfollow them at any time by tapping on Following and then tapping on Unfollow. You can see who you are following and who is following you from your profile page.
-
You can send messages to other users by tapping on the message icon at the bottom of the screen. You can either start a new conversation with someone by tapping on New Message and then typing their name or username, or continue an existing conversation with someone by tapping on their name from the list. You can also send messages to multiple users by creating a group chat. You can send text messages, voice messages, photos, videos, stickers, emojis, and GIFs in your messages.
-
You can comment on other users’ videos by tapping on the comment icon below their video and then typing your comment. You can also reply to other users’ comments by tapping on their comment and then typing your reply. You can like other users’ comments by tapping on the heart icon next to their comment.
-
You can duet or react to other users’ videos by tapping on the record icon below their video and then choosing Duet or React. A duet is when you create a split-screen video with another user’s video playing alongside yours. A reaction is when you create a picture-in-picture video with another user’s video playing in a small window while you record yourself reacting to it. You can edit your duet or reaction video using TikTok’s editing tools before posting it.
-
Why is TikTok so popular?
-
The features that make TikTok stand out
-
TikTok has many features that make it different from other social media apps. Some of these features are:
-
-
The short-form format: TikTok videos are usually 15 seconds long, although you can make up to 60 seconds long videos by combining multiple clips. This makes TikTok videos easy to consume and create.
-
The algorithm: Tik Tok’s algorithm is very powerful and personalized, as it learns from your preferences and behavior and shows you videos that you are likely to enjoy and engage with. You can also discover new videos and users by exploring different categories, hashtags, and trends.
-
The sound and music: TikTok has a huge library of sounds and songs that you can use for your videos, or you can use your own voice or music. You can also see what sounds or songs are popular or trending, and use them for your own videos. You can also create your own sounds or songs and share them with others.
-
The editing tools: TikTok has a variety of editing tools that let you customize your videos and make them more creative and fun. You can add filters, stickers, text, emojis, effects, transitions, voice effects, and more to your videos. You can also trim, cut, split, merge, duplicate, or reverse your video clips.
-
The community and culture: TikTok has a vibrant and diverse community of users who share their passions, talents, opinions, humor, and more through their videos. You can connect and interact with other users who have similar interests or tastes as you. You can also join or create challenges, trends, memes, hashtags, and more that are unique to TikTok.
-
-
The trends that drive TikTok culture
-
TikTok is also known for its viral trends that shape its culture and influence other platforms. Some of these trends are:
-
-
The dances: TikTok is famous for its dance challenges, where users create or copy dance moves to a specific song or sound. Some of the most popular dance challenges on TikTok are the Renegade, the Savage, the Say So, the WAP, the Blinding Lights, and the Toosie Slide.
-
The lip-syncs: TikTok is also known for its lip-syncs, where users mimic the words or lyrics of a song, a movie scene, a comedy sketch, or anything else. Some of the most popular lip-syncs on TikTok are the I’m Already Tracer, the Hit or Miss, the Can I Pet That Dog?, the I’m Not Like Other Girls, and the I’m an Accountant.
-
The pranks: TikTok is also a platform for pranks, where users trick or scare their friends, family members, strangers, or themselves. Some of the most popular pranks on TikTok are the Invisible Challenge, the Zoom Prank, the Pregnancy Prank, the Shampoo Prank, and the Spider Prank.
-
The transformations: TikTok is also a place for transformations, where users show their before and after changes in appearance, mood, style, or anything else. Some of the most popular transformations on TikTok are the Glow Up Challenge, the Don’t Rush Challenge, the Flip The Switch Challenge, the Buss It Challenge, and the Silhouette Challenge.
-
The duets and reactions: TikTok is also a platform for duets and reactions, where users create videos in response to other users’ videos, either by adding their own content or by showing their reaction. Some of the most popular duets and reactions on TikTok are the Old Town Road Duet, the Ratatouille Musical, the Hamilton Reaction, the Kombucha Girl, and the Try Not To Laugh Challenge.
-
-
The challenges that TikTok faces
-
Despite its popularity and success, TikTok also faces some challenges that threaten its future. Some of these challenges are:
-
-
The legal issues: TikTok has been involved in several legal disputes and investigations over its data privacy and security practices, its content moderation policies, its alleged censorship of content that is sensitive to the Chinese government, and its potential influence on elections and public opinion. TikTok has also been banned or restricted in some countries such as India, Pakistan, Indonesia, and the United States.
-
The competition: TikTok has to compete with other social media platforms that offer similar or alternative features and services, such as Instagram, YouTube, Snapchat, Facebook, Twitter, and Triller. Some of these platforms have also copied or integrated some of TikTok’s features, such as Instagram Reels, YouTube Shorts, Snapchat Spotlight, and Facebook Lasso.
-
The sustainability: TikTok has to maintain its growth and relevance in a fast-changing and crowded market, where user preferences and behaviors can shift quickly and unpredictably. TikTok has to constantly innovate and adapt to keep its users engaged and satisfied, as well as attract new users and advertisers.
-
-
How to get the most out of TikTok
-
Tips and tricks for viewers and lurkers
-
If you are a viewer or a lurker on TikTok, meaning that you mainly watch videos without creating or interacting with them, here are some tips and tricks to enhance your experience:
-
-
Customize your For You page: The For You page is where you can discover new videos and users that match your interests and tastes. You can customize your For You page by liking, commenting, sharing, or following the videos and users that you enjoy, or by tapping on Not Interested or reporting the videos and users that you don’t like. You can also use the Discover tab to search for specific categories, hashtags, sounds, or users.
-
Use filters and effects: You can use filters and effects to change the appearance of the videos that you watch. You can access them by tapping on the filter icon at the top right of the screen. You can choose from different categories such as Beauty, Funny, Scary, Trending, etc. You can also use the slider at the bottom to adjust the intensity of the filter or effect.
-
Save videos to your favorites: You can save videos that you like or want to watch later to your favorites. You can access them by tapping on the bookmark icon at the bottom right of the screen. You can also create folders to organize your favorites by tapping on the plus icon at the top right of the screen.
-
Download videos to your phone: You can download videos that you like or want to share with others to your phone. You can do this by tapping on the share icon below the video and then tapping on Save Video. However, this option is only available if the user allows it in their settings.
-
Watch live streams: You can watch live streams from other users who are broadcasting in real time. You can access them by tapping on the Live tab at the top of the screen. You can also see who is live from the users you follow by tapping on the Following tab. You can interact with the live streamers by sending messages, gifts, or emojis in the chat box.
-
-
Tips and tricks for creators and influencers
-
If you are a creator or an influencer on TikTok, meaning that you regularly create and share videos with a large or loyal audience, here are some tips and tricks to boost your performance:
-
-
Know your niche: You should have a clear idea of what kind of content you want to create and who your target audience is. You should also research what topics, hashtags, sounds, or trends are popular or relevant to your niche, and use them for your videos.
-
Optimize your profile: You should have a catchy and memorable username, a high-quality and attractive profile picture, and a concise and informative bio that describes who you are and what you do. You should also link your other social media accounts or websites to your profile, if you have any.
-
Use hashtags and captions: You should use hashtags and captions to make your videos more discoverable and engaging. You should use relevant and specific hashtags that match your content and niche, as well as trending or viral hashtags that can attract more viewers. You should also write captions that summarize or explain your videos, or ask questions or invite feedback from your viewers.
-
Engage with your audience: You should interact with your audience by responding to their comments, messages, duets, or reactions. You should also thank them for their support, ask them for their opinions or suggestions, or invite them to participate in your challenges or contests. You should also follow or shout out some of your fans or fellow creators who inspire you or collaborate with you.
-
Analyze your analytics: You should monitor and analyze your analytics to measure your performance and improve your strategy. You can access your analytics by tapping on the three dots icon at the top right of your profile page and then tapping on Analytics. You can see data such as your video views, likes, comments, shares, followers, watch time, audience demographics, traffic sources, etc.
-
-
Tips and tricks for marketers and businesses
-
If you are a marketer or a business owner who wants to use TikTok for promoting your brand, product, or service, here are some tips and tricks to achieve your goals:
-
-
Create a business account: You should create a business account instead of a personal account to access more features and tools for marketing purposes. You can create a business account by tapping on the three dots icon at the top right of your profile page and then tapping on Manage Account. You can then switch to a Pro Account and choose Business as your category. You can also verify your account by providing some information about your business.
-
Use TikTok Ads: You can use TikTok Ads to create and run paid campaigns to reach more potential customers on TikTok. You can access TikTok Ads by visiting ads.tiktok.com and signing up for an account. You can choose from different types of ads such as In-Feed Ads, TopView Ads, Brand Takeover Ads, Branded Hashtag Challenge Ads, Branded Effects Ads, etc. You can also set your budget, target audience, schedule, creative assets, etc.
-
Collaborate with influencers: You can collaborate with influencers who have a large or loyal following on TikTok and who are relevant to your niche or industry. You can ask them to review, endorse, or feature your brand, product, or service in their videos, or to create a challenge, trend, or hashtag related to your brand, product, or service. You can find and contact influencers by using platforms such as TikTok Creator Marketplace, FameBit, AspireIQ, etc.
-
Create engaging content: You can also create your own content to showcase your brand, product, or service on TikTok. You should create content that is entertaining, informative, authentic, and relevant to your niche or industry. You should also use the features and tools that TikTok offers, such as filters, effects, sounds, hashtags, etc. You should also follow the trends and challenges that are popular or related to your niche or industry.
-
Build a community: You can also build a community of loyal and engaged customers on TikTok. You should interact with your followers and potential customers by responding to their comments, messages, duets, or reactions. You should also thank them for their support, ask them for their feedback or testimonials, or invite them to join your loyalty program or newsletter. You should also follow or shout out some of your customers or partners who support you or collaborate with you.
-
-
Conclusion
-
TikTok is a video-sharing app that has become one of the most popular and influential social media platforms in the world. It allows users to create and share short-form videos on any topic, using various features and tools to make them more creative and fun. It also allows users to discover and interact with other users who share their interests and tastes.
-
TikTok is not only a platform for entertainment and expression, but also a platform for learning and marketing. Users can learn new skills, ideas, information, or perspectives from other users’ videos. Marketers and businesses can use TikTok to promote their brand, product, or service to a large and diverse audience.
-
TikTok is also a platform for challenges and opportunities. Users can join or create challenges, trends, memes, hashtags, and more that are unique to TikTok culture. Marketers and businesses can face challenges such as legal issues, competition, and sustainability.
-
If you want to get the most out of TikTok, whether you are a viewer, a creator, an influencer, a marketer, or a business owner, you should follow the tips and tricks that we have shared in this guide. We hope that this guide has helped you understand what TikTok is, how to use it, and why it is so popular.
-
FAQs
-
Here are some frequently asked questions about TikTok:
-
-
How do I get more followers on TikTok?
-
There is no magic formula to get more followers on TikTok, but there are some best practices that you can follow. Some of them are: create high-quality and original content that showcases your personality and talent; use relevant and specific hashtags that match your content and niche; follow the trends and challenges that are popular or related to your niche; collaborate with other users who have similar or complementary content or audience; engage with your existing followers and potential followers by liking, commenting, sharing, or following their videos; and analyze your analytics to see what works and what doesn’t for your content and audience.
-
How do I make money on TikTok?
-
There are several ways to make money on TikTok, depending on your goals and skills. Some of them are: join the TikTok Creator Fund, which pays eligible creators based on their video views and engagement; join the TikTok Live program, which allows you to receive gifts from your viewers during your live streams; join the TikTok Affiliate program, which allows you to earn commissions by promoting products or services from TikTok’s partners; create sponsored content for brands or businesses that match your niche or audience; sell your own products or services through your videos or links; or offer your skills or services as a freelancer or consultant to other users who need them.
-
How do I delete my TikTok account?
-
If you want to delete your TikTok account, you need to follow these steps: tap on the three dots icon at the top right of your profile page and then tap on Manage Account; tap on Delete Account at the bottom of the screen and then follow the instructions; verify your identity using your phone number, email address, or third-party account; and confirm your decision by tapping on Delete Account. Note that deleting your account will remove all your videos, messages, comments, likes, followers, and other data from TikTok. You will also lose access to the TikTok Creator Fund, TikTok Live, TikTok Affiliate, and other programs that you have joined. You can still restore your account within 30 days of deletion by logging in with your credentials, but after that period, your account will be permanently deleted.
-
How do I report a problem or a user on TikTok?
-
If you encounter a problem or a user that violates TikTok’s Community Guidelines or Terms of Service, you can report it to TikTok’s team. You can do this by tapping on the three dots icon at the top right of the video or profile that you want to report and then tapping on Report. You can then choose the reason for your report and provide more details if needed. You can also block or mute the user that you want to report by tapping on their name and then tapping on Block or Mute.
-
How do I contact TikTok’s customer service?
-
If you have any questions, feedback, suggestions, or complaints about TikTok’s app or service, you can contact TikTok’s customer service team. You can do this by tapping on the three dots icon at the top right of your profile page and then tapping on Report a Problem. You can then choose the category and subcategory of your issue and provide more details if needed. You can also attach screenshots or videos to illustrate your issue. You can also email TikTok’s customer service team at feedback@tiktok.com.
197e85843d
-
-
\ No newline at end of file
diff --git a/spaces/congsaPfin/Manga-OCR/logs/What You Need to Know About Video Live Wallpaper Maker Premium APK.md b/spaces/congsaPfin/Manga-OCR/logs/What You Need to Know About Video Live Wallpaper Maker Premium APK.md
deleted file mode 100644
index c2a5c0da14fbc0c89ebcb07cebe6b1a18c6c6f86..0000000000000000000000000000000000000000
--- a/spaces/congsaPfin/Manga-OCR/logs/What You Need to Know About Video Live Wallpaper Maker Premium APK.md
+++ /dev/null
@@ -1,135 +0,0 @@
-
-
Video Live Wallpaper Maker Premium APK: How to Create Stunning Wallpapers for Your Phone
-
Do you want to make your phone look more lively and attractive? Do you want to express your personality and mood with your wallpaper? Do you want to have fun and be creative with your videos? If you answered yes to any of these questions, then you need to try Video Live Wallpaper Maker Premium APK.
-
What is Video Live Wallpaper Maker Premium APK?
-
Video Live Wallpaper Maker Premium APK is a powerful and easy-to-use app that lets you create amazing live wallpapers from your videos. You can use any video from your gallery or record your own with the built-in camera. You can also edit and customize your video with various filters, effects, stickers, text, and music. You can then set your video as a live wallpaper on your home screen or lock screen, and enjoy watching it every time you use your phone.
Features of Video Live Wallpaper Maker Premium APK
-
Video Live Wallpaper Maker Premium APK has many features that make it stand out from other similar apps. Some of these features are:
-
-
It supports all video formats, including MP4, AVI, MKV, MOV, FLV, and more.
-
It has a simple and intuitive interface that makes it easy to use for anyone.
-
It has a premium version that unlocks all the features and removes all the ads and watermarks.
-
It has a large collection of filters, effects, stickers, text, and music that you can apply to your video.
-
It has a preview mode that lets you see how your video will look as a live wallpaper before setting it.
-
It has a low battery consumption mode that saves your battery life while running your live wallpaper.
-
It has a community feature that lets you share your creations with other users and discover new wallpapers.
-
-
Benefits of Video Live Wallpaper Maker Premium APK
-
Video Live Wallpaper Maker Premium APK has many benefits that make it worth downloading and using. Some of these benefits are:
-
-
It enhances the appearance and functionality of your phone by adding dynamic and interactive wallpapers.
-
It lets you express yourself and show off your style and taste with your wallpaper.
-
It lets you have fun and be creative with your videos by adding various elements and effects.
-
It lets you enjoy your favorite videos and memories on your phone screen every day.
-
It lets you impress your friends and family with your unique and stunning wallpapers.
-
-
How to Download and Install Video Live Wallpaper Maker Premium APK?
-
If you are interested in trying out Video Live Wallpaper Maker Premium APK, you need to download and install it on your phone. Here are the steps to do so:
-
Steps to Download and Install Video Live Wallpaper Maker Premium APK
-
-
Go to the official website of Video Live Wallpaper Maker Premium APK or click on this link: .
-
Select the download button and wait for the file to be downloaded on your phone.
-
Go to your file manager and locate the downloaded file. Tap on it to start the installation process.
-
If you see a warning message that says "Install blocked", go to your settings and enable "Unknown sources" or "Allow from this source".
-
Follow the instructions on the screen and complete the installation process.
-
Launch the app and enjoy creating your live wallpapers.
-
-
Tips and Tricks for Using Video Live Wallpaper Maker Premium APK
-
To make the most out of Video Live Wallpaper Maker Premium APK, here are some tips and tricks that you can follow:
-
-
Use high-quality videos that have good resolution and frame rate for better results.
-
Trim your videos to the desired length and remove any unwanted parts.
-
Adjust the brightness, contrast, saturation, and hue of your videos to match your preference.
-
Use filters and effects that suit your theme and mood. You can also combine different filters and effects for more variety.
-
Add stickers and text that complement your video. You can also change the size, color, font, and position of your stickers and text.
-
Add music that matches your video. You can choose from the app's library or use your own music from your phone.
-
Preview your video before setting it as a live wallpaper. You can also change the playback speed and direction of your video.
-
Share your live wallpapers with other users and get inspired by their creations.
-
-
How to Create Amazing Wallpapers with Video Live Wallpaper Maker Premium APK?
-
Now that you have downloaded and installed Video Live Wallpaper Maker Premium APK, you are ready to create amazing wallpapers with it. Here are the steps to do so:
-
Choose a Video or Record Your Own
-
The first step is to choose a video that you want to use as a live wallpaper. You can select any video from your gallery or record a new one with the app's camera. You can also browse through the app's community and download any video that you like.
-
Edit and Customize Your Video
-
The second step is to edit and customize your video according to your liking. You can use the app's tools to trim, crop, rotate, flip, and zoom your video. You can also add filters, effects, stickers, text, and music to your video. You can adjust the settings of each element and preview the changes in real time.
-
Set Your Video as Live Wallpaper
-
The final step is to set your video as a live wallpaper on your phone. You can choose whether you want to set it as a home screen wallpaper, a lock screen wallpaper, or both. You can also adjust the quality and battery consumption of your live wallpaper. Once you are done, you can enjoy watching your video on your phone screen.
-
video live wallpaper maker pro apk
-video live wallpaper maker 3d mod apk
-video live wallpaper maker hd premium apk
-video live wallpaper maker no watermark apk
-video live wallpaper maker cracked apk
-video live wallpaper maker full version apk
-video live wallpaper maker unlocked apk
-video live wallpaper maker free download apk
-video live wallpaper maker latest apk
-video live wallpaper maker offline apk
-video live wallpaper creator premium apk
-video live wallpaper creator mod apk
-video live wallpaper creator hd premium apk
-video live wallpaper creator no watermark apk
-video live wallpaper creator cracked apk
-video live wallpaper creator full version apk
-video live wallpaper creator unlocked apk
-video live wallpaper creator free download apk
-video live wallpaper creator latest apk
-video live wallpaper creator offline apk
-3d video live wallpaper maker premium apk
-3d video live wallpaper maker mod apk
-3d video live wallpaper maker hd premium apk
-3d video live wallpaper maker no watermark apk
-3d video live wallpaper maker cracked apk
-3d video live wallpaper maker full version apk
-3d video live wallpaper maker unlocked apk
-3d video live wallpaper maker free download apk
-3d video live wallpaper maker latest apk
-3d video live wallpaper maker offline apk
-hd video live wallpaper maker premium apk
-hd video live wallpaper maker mod apk
-hd video live wallpaper maker 3d premium apk
-hd video live wallpaper maker no watermark apk
-hd video live wallpaper maker cracked apk
-hd video live wallpaper maker full version apk
-hd video live wallpaper maker unlocked apk
-hd video live wallpaper maker free download apk
-hd video live wallpaper maker latest apk
-hd video live wallpaper maker offline apk
-wave live wallpapers maker 3d premium apk[^1^]
-wave live wallpapers maker 3d mod apk[^1^]
-wave live wallpapers maker 3d hd premium apk[^1^]
-wave live wallpapers maker 3d no watermark apk[^1^]
-wave live wallpapers maker 3d cracked apk[^1^]
-wave live wallpapers maker 3d full version apk[^1^]
-wave live wallpapers maker 3d unlocked apk[^1^]
-wave live wallpapers maker 3d free download apk[^1^]
-wave live wallpapers maker 3d latest apk[^1^]
-
Conclusion
-
Video Live Wallpaper Maker Premium APK is a great app that lets you create stunning live wallpapers from your videos. You can use any video from your gallery or record your own with the app's camera. You can also edit and customize your video with various filters, effects, stickers, text, and music. You can then set your video as a live wallpaper on your home screen or lock screen, and enjoy watching it every time you use your phone.
-
Summary of the Main Points
-
In this article, we have covered the following points:
-
-
What is Video Live Wallpaper Maker Premium APK?
-
What are the features and benefits of Video Live Wallpaper Maker Premium APK?
-
How to download and install Video Live Wallpaper Maker Premium APK?
-
How to create amazing wallpapers with Video Live Wallpaper Maker Premium APK?
-
-
Call to Action
-
If you are looking for a way to make your phone look more lively and attractive, you should definitely try Video Live Wallpaper Maker Premium APK. It is a powerful and easy-to-use app that lets you create amazing live wallpapers from your videos. You can have fun and be creative with your videos by adding various elements and effects. You can also express yourself and show off your style and taste with your wallpaper. You can impress your friends and family with your unique and stunning wallpapers.
-
So what are you waiting for? Download Video Live Wallpaper Maker Premium APK now and start creating your own live wallpapers!
-
FAQs
-
Here are some frequently asked questions about Video Live Wallpaper Maker Premium APK:
-
-
Is Video Live Wallpaper Maker Premium APK safe to use?
-
Yes, Video Live Wallpaper Maker Premium APK is safe to use. It does not contain any viruses or malware that can harm your phone or data. It also does not require any root access or permissions that can compromise your privacy or security.
-
Is Video Live Wallpaper Maker Premium APK free to use?
-
Yes, Video Live Wallpaper Maker Premium APK is free to use. However, it has a premium version that unlocks all the features and removes all the ads and watermarks. You can download the premium version from the official website or click on this link: .
-
How can I share my live wallpapers with other users?
-
You can share your live wallpapers with other users by using the app's community feature. You can upload your creations to the app's gallery and browse through other users' wallpapers. You can also rate, comment, and download other users' wallpapers.
-
How can I change the quality and battery consumption of my live wallpaper?
-
You can change the quality and battery consumption of your live wallpaper by using the app's settings. You can choose from low, medium, high, and ultra quality options. You can also enable or disable the low battery consumption mode that saves your battery life while running your live wallpaper.
-
How can I contact the developers of Video Live Wallpaper Maker Premium APK?
-
You can contact the developers of Video Live Wallpaper Maker Premium APK by using the app's feedback feature. You can send them your suggestions, questions, or issues. You can also follow them on their social media accounts for more updates and news.
- 197e85843d
-
-
\ No newline at end of file
diff --git a/spaces/congsaPfin/Manga-OCR/logs/WhatsApp estilo iPhone disfruta de los emojis y el diseo de iOS en tu WhatsApp para Android.md b/spaces/congsaPfin/Manga-OCR/logs/WhatsApp estilo iPhone disfruta de los emojis y el diseo de iOS en tu WhatsApp para Android.md
deleted file mode 100644
index bae8bf3f5527163b756f4c5c9ad1e09bb5fb31c0..0000000000000000000000000000000000000000
--- a/spaces/congsaPfin/Manga-OCR/logs/WhatsApp estilo iPhone disfruta de los emojis y el diseo de iOS en tu WhatsApp para Android.md
+++ /dev/null
@@ -1,100 +0,0 @@
-
-
How to Download WhatsApp with iPhone Style for Android in 2021
-
WhatsApp is one of the most popular messaging apps in the world, with over two billion users. However, not everyone is satisfied with the default look and functionality of WhatsApp, especially if they have switched from an iPhone to an Android device or vice versa. If you are one of those people who want to have a WhatsApp experience that resembles the iOS version, then this article is for you. We will show you how to download and install WhatsApp with iPhone style for Android in 2021, a mod that will give you a theme that mimics the iOS appearance and emojis, as well as some extra features that will enhance your WhatsApp usage.
-
What is WhatsApp with iPhone Style for Android?
-
WhatsApp with iPhone style for Android is a mod, or a modified version, of WhatsApp that is based on Fouad WhatsApp, one of the most popular and trusted WhatsApp mods available. A mod is an unofficial app that offers some features and options that are not present in the official app, such as customization, privacy, security, and more. However, not all mods are safe or reliable, so you should always download them from trusted sources and at your own risk.
-
whatsapp estilo iphone descargar 2021 apk malavida
Fouad WhatsApp is a mod that is known for its stability, performance, and updates. It has a lot of features that make it stand out from other mods, such as themes, fonts, colors, wallpapers, stickers, emojis, and more. It also has some advanced options that let you control your privacy and security settings, such as hiding your online status, disabling forwarded messages, locking chats with passwords or fingerprints, and more.
-
A theme that mimics iOS appearance and emojis
-
WhatsApp with iPhone style for Android is a mod that uses Fouad WhatsApp as its base, but adds a theme that makes it look like the iOS version of WhatsApp. This means that you will have a WhatsApp app that has the same layout, icons, buttons, menus, notifications, and animations as the iPhone version. You will also have access to the iOS emojis, which are different from the Android ones. This way, you can enjoy a different look and feel of WhatsApp on your Android device.
-
A way to customize and enhance WhatsApp features
-
WhatsApp with iPhone style for Android is not only a theme, but also a way to customize and enhance your WhatsApp features. You can change the fonts, colors, wallpapers, stickers, emojis, and more according to your preferences. You can also access some extra functions and options that are not available in the official app, such as call blocker, voice modulator, anti-delete messages and status, customizable chats and contacts list, and more.
-
Why Download WhatsApp with iPhone Style for Android?
-
There are many reasons why you might want to download WhatsApp with iPhone style for Android. Here are some of them:
-
whatsapp estilo iphone para android apk descargar gratis
-whatsapp estilo iphone ultima version 2021 apk
-whatsapp estilo iphone apk download fouad
-whatsapp estilo iphone android apk junio 2023
-whatsapp estilo iphone mod apk 2021
-whatsapp estilo iphone apk 2021 malavida
-whatsapp estilo iphone para android descargar 2021
-whatsapp estilo iphone actualizado julio 2023 apk
-whatsapp estilo iphone apk gratis para android
-whatsapp estilo iphone fouad mods apk 2021
-whatsapp estilo iphone descargar apk ultima version
-whatsapp estilo iphone para android apk 9.66
-whatsapp estilo iphone apk julio 2023
-whatsapp estilo iphone con emojis de ios apk
-whatsapp estilo iphone personalizado apk 2021
-whatsapp estilo iphone descargar gratis para android
-whatsapp estilo iphone apk 2021 fouad mokdad
-whatsapp estilo iphone para android ultima version apk
-whatsapp estilo iphone apk agosto 2023
-whatsapp estilo iphone con modulador de voz apk
-whatsapp estilo iphone antieliminacion de mensajes apk
-whatsapp estilo iphone descargar 2021 fouad mods
-whatsapp estilo iphone para android gratis apk
-whatsapp estilo iphone apk septiembre 2023
-whatsapp estilo iphone con estado antieliminacion apk
-whatsapp estilo iphone descargar 2021 gratis apk
-whatsapp estilo iphone para android fouad mods apk
-whatsapp estilo iphone apk octubre 2023
-whatsapp estilo iphone con automatizacion de mensajes apk
-whatsapp estilo iphone descargar 2021 ultima version
-whatsapp estilo iphone para android descargar gratis apk
-whatsapp estilo iphone apk noviembre 2023
-whatsapp estilo iphone con opcion de quien puede llamarte apk
-whatsapp estilo iphone descargar 2021 actualizado apk
-whatsapp estilo iphone para android descargar fouad mods
-whatsapp estilo iphone apk diciembre 2023
-whatsapp estilo iphone con ocultar estado de visto en estados apk
-whatsapp estilo iphone descargar 2021 gratis para android
-whatsapp estilo iphone para android descargar ultima version
-whatsapp estilo iphone apk enero 2024
-
To enjoy a different look and feel of WhatsApp
-
If you are bored with the default look and feel of WhatsApp on your Android device, you might want to try WhatsApp with iPhone style for Android. This mod will give you a fresh and new look of WhatsApp that resembles the iOS version. You will be able to enjoy the same design, layout, icons, buttons, menus, notifications, and animations as the iPhone users. You will also be able to use the iOS emojis, which are different from the Android ones. This way, you can have a more fun and exciting WhatsApp experience on your Android device.
-
To protect your privacy and security
-
If you are concerned about your privacy and security on WhatsApp, you might want to download WhatsApp with iPhone style for Android. This mod will give you more control over your privacy and security settings, such as hiding your online status, disabling forwarded messages, locking chats with passwords or fingerprints, and more. You will also be able to prevent others from deleting messages or status that they have sent to you. This way, you can have a more secure and private WhatsApp experience on your Android device.
-
To access extra functions and options
-
If you are looking for more functions and options on WhatsApp, you might want to download WhatsApp with iPhone style for Android. This mod will give you access to some extra features that are not available in the official app, such as call blocker, voice modulator, customizable fonts and colors, and more. You will also be able to customize your chats and contacts list according to your preferences. This way, you can have a more functional and personalized WhatsApp experience on your Android device.
-
How to Download and Install WhatsApp with iPhone Style for Android?
-
If you are interested in downloading and installing WhatsApp with iPhone style for Android, you will need to follow these steps:
-
Step 1: Backup your chats and uninstall the official WhatsApp app
-
Before you download and install WhatsApp with iPhone style for Android, you will need to backup your chats and uninstall the official WhatsApp app from your device. To backup your chats, go to Settings > Chats > Chat backup and tap on Backup. To uninstall the official WhatsApp app, go to Settings > Apps > WhatsApp and tap on Uninstall. This is necessary because you cannot have two WhatsApp apps with the same phone number on the same device.
-
Step 2: Download the APK file from a reliable source
-
After you have backed up your chats and uninstalled the official WhatsApp app, you will need to download the APK file of WhatsApp with iPhone style for Android from a reliable source. An APK file is an installer file that allows you to install apps that are not available on the Google Play Store. However, not all APK files are safe or reliable, so you should always download them from trusted sources and at your own risk. One of the sources that you can use is Malavida, a website that offers safe and verified APK files of various apps and games. To download the APK file of WhatsApp with iPhone style for Android from Malavida, go to [this link] and tap on Download.
-
Step 3: Enable unknown sources and install the APK file
-
After you have downloaded the APK file of WhatsApp with iPhone style for Android from Malavida, you will need to enable unknown sources and install the APK file on your device. Unknown sources are sources that are not authorized by Google Play Store, such as APK files from websites or third-party app stores. To enable unknown sources, go to Settings > Security > Unknown sources and toggle it on. To install the APK file of WhatsApp with iPhone style for Android on your device, go to the folder where you have saved the APK file and tap on it. Follow the instructions on the screen to complete the installation.
-
Step 4: Verify your phone number and restore your chats
-
After you have installed the APK file of WhatsApp with iPhone style for Android on your device, you will need to verify your phone number and restore your chats. To verify your phone number, open the app and enter your phone number that you have used for the official WhatsApp app. You will receive a verification code via SMS or call that you will need to enter in the app. To restore your chats, tap on Restore when prompted and wait for the process to finish.
-
Step 5: Choose the iOS theme and enjoy
-
After you have verified your phone number and restored your chats, you will need to choose the iOS theme and enjoy WhatsApp with iPhone style for Android on your device. To choose the iOS theme, go to Settings > Fouad Mods > Themes > Load Theme > iOS Theme.zip and tap on Apply. You will see a message that says Theme applied successfully. Restart WhatsApp now. Tap on OK and wait for the app to restart. You will now see that your WhatsApp app has the same appearance and emojis as the iOS version. You can also explore the other features and options that WhatsApp with iPhone style for Android offers. Enjoy!
-
What are the Main Features of WhatsApp with iPhone Style for Android?
-
WhatsApp with iPhone style for Android is not only a theme, but also a mod that offers some amazing features that are not present in the official app. Here are some of the main features that you can enjoy with this mod:
-
Call blocker
-
With this feature, you can block unwanted calls from anyone on WhatsApp. You can choose to block all calls, calls from unknown numbers, or calls from specific contacts. You can also enable or disable the call blocker at any time. To access this feature, go to Settings > Fouad Mods > Privacy > Call Blocker.
-
Anti-delete messages and status
-
With this feature, you can prevent others from deleting messages or status that they have sent to you. This means that even if they delete them for everyone, you will still be able to see them on your device. You will also see a message that says This message was deleted next to the deleted message or status. To access this feature, go to Settings > Fouad Mods > Privacy > Anti-Delete Messages and Anti-Delete Status.
-
Voice modulator
-
With this feature, you can change your voice when sending voice notes on WhatsApp. You can choose from different voice effects, such as chipmunk, robot, alien, drunk, and more. You can also adjust the pitch and speed of your voice. To access this feature, go to Settings > Fouad Mods > Voice Changer.
-
Customizable fonts and colors
-
With this feature, you can change the fonts and colors of your WhatsApp app according to your preferences. You can choose from different fonts, such as Arial, Comic Sans, Helvetica, and more. You can also change the colors of the text, background, header, footer, and more. To access this feature, go to Settings > Fouad Mods > Universal > Colors and Fonts.
-
And more
-
WhatsApp with iPhone style for Android has many more features that you can explore and enjoy, such as customizable chats and contacts list, wallpapers, stickers, emojis, media mods, lock mods, conversation mods, and more. To access these features, go to Settings > Fouad Mods and browse through the different categories.
-
Conclusion
-
WhatsApp with iPhone style for Android is a mod that lets you have a WhatsApp experience that resembles the iOS version on your Android device. It is based on Fouad WhatsApp, one of the most popular and trusted WhatsApp mods available. It offers a theme that mimics the iOS appearance and emojis, as well as some extra features and options that enhance your WhatsApp usage. To download and install WhatsApp with iPhone style for Android in 2021, you will need to backup your chats and uninstall the official WhatsApp app, download the APK file from a reliable source such as Malavida, enable unknown sources and install the APK file on your device, verify your phone number and restore your chats, and choose the iOS theme and enjoy. In this article, we have shown you what WhatsApp with iPhone style for Android is, why you might want to download it, how to download and install it, and what are the main features that it offers. We hope that you have found this article helpful and informative. If you have any questions or feedback, please feel free to leave a comment below.
-
FAQs
-
Here are some frequently asked questions about WhatsApp with iPhone style for Android:
-
Is WhatsApp with iPhone style for Android safe?
-
WhatsApp with iPhone style for Android is a mod that is based on Fouad WhatsApp, one of the most popular and trusted WhatsApp mods available. However, it is not an official app and it is not authorized by WhatsApp or Google Play Store. Therefore, there is always a risk of downloading and installing mods from unknown sources, such as malware, viruses, data theft, account ban, and more. You should always download mods from trusted sources and at your own risk.
-
Is WhatsApp with iPhone style for Android updated?
-
WhatsApp with iPhone style for Android is a mod that is updated regularly by its developers. However, it is not always compatible with the latest version of the official WhatsApp app. Therefore, you might experience some bugs, glitches, or errors when using the mod. You should always check for updates and download them from reliable sources.
-
Can I use WhatsApp with iPhone style for Android with the official WhatsApp app?
-
No, you cannot use WhatsApp with iPhone style for Android with the official WhatsApp app on the same device. This is because you cannot have two WhatsApp apps with the same phone number on the same device. You will need to backup your chats and uninstall the official WhatsApp app before you can download and install WhatsApp with iPhone style for Android.
-
Can I use WhatsApp with iPhone style for Android on an iPhone?
-
No, you cannot use WhatsApp with iPhone style for Android on an iPhone. This is because this mod is only compatible with Android devices. If you want to use a mod on an iPhone, you will need to jailbreak your device and download a mod that is compatible with iOS devices.
-
Can I switch back to the official WhatsApp app after using WhatsApp with iPhone style for Android?
-
Yes, you can switch back to the official WhatsApp app after using WhatsApp with iPhone style for Android. However, you will need to backup your chats and uninstall the mod before you can download and install the official WhatsApp app from the Google Play Store. You will also lose some of the features and options that the mod offers.
401be4b1e0
-
-
\ No newline at end of file
diff --git a/spaces/cooelf/Multimodal-CoT/timm/data/constants.py b/spaces/cooelf/Multimodal-CoT/timm/data/constants.py
deleted file mode 100644
index d6d4a01b0316989a3f5142167f1e384b098bc930..0000000000000000000000000000000000000000
--- a/spaces/cooelf/Multimodal-CoT/timm/data/constants.py
+++ /dev/null
@@ -1,7 +0,0 @@
-DEFAULT_CROP_PCT = 0.875
-IMAGENET_DEFAULT_MEAN = (0.485, 0.456, 0.406)
-IMAGENET_DEFAULT_STD = (0.229, 0.224, 0.225)
-IMAGENET_INCEPTION_MEAN = (0.5, 0.5, 0.5)
-IMAGENET_INCEPTION_STD = (0.5, 0.5, 0.5)
-IMAGENET_DPN_MEAN = (124 / 255, 117 / 255, 104 / 255)
-IMAGENET_DPN_STD = tuple([1 / (.0167 * 255)] * 3)
diff --git a/spaces/daibs/bananafreshnessclass/app.py b/spaces/daibs/bananafreshnessclass/app.py
deleted file mode 100644
index 779fd0f4c9338586b17248e18ecf971f77a7979f..0000000000000000000000000000000000000000
--- a/spaces/daibs/bananafreshnessclass/app.py
+++ /dev/null
@@ -1,35 +0,0 @@
-import numpy as np
-import gradio as gr
-from tensorflow.keras.models import load_model
-import imutils
-import matplotlib.pyplot as plt
-import cv2
-import numpy as np
-from tensorflow.keras.preprocessing.image import img_to_array
-model = load_model("pisang.h5")
-
-def prosesgambar(gambar):
- # load the image
- image = gambar
- output = imutils.resize(image, width=400)
-
- # pre-process the image for classification
- image = cv2.resize(image, (94, 94))
- image = image.astype("float") / 255.0
- image = img_to_array(image)
- image = np.expand_dims(image, axis=0)
- return image
-
-
-
-
-def prediksi(gambar):
- a = np.round(model.predict(prosesgambar(gambar)), 4)[0].tolist()
- if a.index(max(a)) == 1:
- pred = "Segar"
- else:
- pred = "Busuk"
- return pred
-
-demo = gr.Interface(prediksi, gr.Image(shape=(200, 200)), "text")
-demo.launch()
\ No newline at end of file
diff --git a/spaces/dammasimbung/Cardiovascular-Detecting-App/setup.sh b/spaces/dammasimbung/Cardiovascular-Detecting-App/setup.sh
deleted file mode 100644
index c8650a8b74a58d9a5f53b185fd711c5668e1cd52..0000000000000000000000000000000000000000
--- a/spaces/dammasimbung/Cardiovascular-Detecting-App/setup.sh
+++ /dev/null
@@ -1,13 +0,0 @@
-mkdir -p ~/.streamlit/
-
-echo "\
-[general]\n\
-email = \"your-email@domain.com\"\n\
-" > ~/.streamlit/credentials.toml
-
-echo "\
-[server]\n\
-headless = true\n\
-enableCORS=false\n\
-port = $PORT\n\
-" > ~/.streamlit/config.toml
\ No newline at end of file
diff --git a/spaces/dcarpintero/nlp-summarizer-pegasus/.venv/lib/python3.9/site-packages/PIL/McIdasImagePlugin.py b/spaces/dcarpintero/nlp-summarizer-pegasus/.venv/lib/python3.9/site-packages/PIL/McIdasImagePlugin.py
deleted file mode 100644
index 17c008b9a6a1218f6e51add4fda83acb92ee06ce..0000000000000000000000000000000000000000
--- a/spaces/dcarpintero/nlp-summarizer-pegasus/.venv/lib/python3.9/site-packages/PIL/McIdasImagePlugin.py
+++ /dev/null
@@ -1,75 +0,0 @@
-#
-# The Python Imaging Library.
-# $Id$
-#
-# Basic McIdas support for PIL
-#
-# History:
-# 1997-05-05 fl Created (8-bit images only)
-# 2009-03-08 fl Added 16/32-bit support.
-#
-# Thanks to Richard Jones and Craig Swank for specs and samples.
-#
-# Copyright (c) Secret Labs AB 1997.
-# Copyright (c) Fredrik Lundh 1997.
-#
-# See the README file for information on usage and redistribution.
-#
-
-import struct
-
-from . import Image, ImageFile
-
-
-def _accept(s):
- return s[:8] == b"\x00\x00\x00\x00\x00\x00\x00\x04"
-
-
-##
-# Image plugin for McIdas area images.
-
-
-class McIdasImageFile(ImageFile.ImageFile):
- format = "MCIDAS"
- format_description = "McIdas area file"
-
- def _open(self):
- # parse area file directory
- s = self.fp.read(256)
- if not _accept(s) or len(s) != 256:
- msg = "not an McIdas area file"
- raise SyntaxError(msg)
-
- self.area_descriptor_raw = s
- self.area_descriptor = w = [0] + list(struct.unpack("!64i", s))
-
- # get mode
- if w[11] == 1:
- mode = rawmode = "L"
- elif w[11] == 2:
- # FIXME: add memory map support
- mode = "I"
- rawmode = "I;16B"
- elif w[11] == 4:
- # FIXME: add memory map support
- mode = "I"
- rawmode = "I;32B"
- else:
- msg = "unsupported McIdas format"
- raise SyntaxError(msg)
-
- self.mode = mode
- self._size = w[10], w[9]
-
- offset = w[34] + w[15]
- stride = w[15] + w[10] * w[11] * w[14]
-
- self.tile = [("raw", (0, 0) + self.size, offset, (rawmode, stride, 1))]
-
-
-# --------------------------------------------------------------------
-# registry
-
-Image.register_open(McIdasImageFile.format, McIdasImageFile, _accept)
-
-# no default extension
diff --git a/spaces/dcarpintero/nlp-summarizer-pegasus/.venv/lib/python3.9/site-packages/altair/expr/__init__.py b/spaces/dcarpintero/nlp-summarizer-pegasus/.venv/lib/python3.9/site-packages/altair/expr/__init__.py
deleted file mode 100644
index 6ba7f8b8b96e28e4f0f7f143f29023d1bc0e58ba..0000000000000000000000000000000000000000
--- a/spaces/dcarpintero/nlp-summarizer-pegasus/.venv/lib/python3.9/site-packages/altair/expr/__init__.py
+++ /dev/null
@@ -1,19 +0,0 @@
-"""Tools for creating transform & filter expressions with a python syntax"""
-# ruff: noqa
-from typing import Any
-
-from .core import datum, Expression
-from .funcs import *
-from .consts import *
-from ..vegalite.v5.schema.core import ExprRef as _ExprRef
-
-
-class _ExprType:
- def __init__(self, expr):
- vars(self).update(expr)
-
- def __call__(self, expr, **kwargs):
- return _ExprRef(expr, **kwargs)
-
-
-expr: Any = _ExprType(globals())
diff --git a/spaces/dcarpintero/nlp-summarizer-pegasus/.venv/lib/python3.9/site-packages/fontTools/ttLib/tables/G_S_U_B_.py b/spaces/dcarpintero/nlp-summarizer-pegasus/.venv/lib/python3.9/site-packages/fontTools/ttLib/tables/G_S_U_B_.py
deleted file mode 100644
index bb8375a5f83029d2b05388d5c882edd9c4aba95c..0000000000000000000000000000000000000000
--- a/spaces/dcarpintero/nlp-summarizer-pegasus/.venv/lib/python3.9/site-packages/fontTools/ttLib/tables/G_S_U_B_.py
+++ /dev/null
@@ -1,5 +0,0 @@
-from .otBase import BaseTTXConverter
-
-
-class table_G_S_U_B_(BaseTTXConverter):
- pass
diff --git a/spaces/dcarpintero/nlp-summarizer-pegasus/.venv/lib/python3.9/site-packages/fontTools/ttLib/tables/ttProgram.py b/spaces/dcarpintero/nlp-summarizer-pegasus/.venv/lib/python3.9/site-packages/fontTools/ttLib/tables/ttProgram.py
deleted file mode 100644
index 84aa63f36301ec9a4ae21acff0cbc95010d956b7..0000000000000000000000000000000000000000
--- a/spaces/dcarpintero/nlp-summarizer-pegasus/.venv/lib/python3.9/site-packages/fontTools/ttLib/tables/ttProgram.py
+++ /dev/null
@@ -1,593 +0,0 @@
-"""ttLib.tables.ttProgram.py -- Assembler/disassembler for TrueType bytecode programs."""
-from __future__ import annotations
-
-from fontTools.misc.textTools import num2binary, binary2num, readHex, strjoin
-import array
-from io import StringIO
-from typing import List
-import re
-import logging
-
-
-log = logging.getLogger(__name__)
-
-# fmt: off
-
-# first, the list of instructions that eat bytes or words from the instruction stream
-
-streamInstructions = [
-#
-# opcode mnemonic argBits descriptive name pops pushes eats from instruction stream pushes
-#
- (0x40, 'NPUSHB', 0, 'PushNBytes', 0, -1), # n, b1, b2,...bn b1,b2...bn
- (0x41, 'NPUSHW', 0, 'PushNWords', 0, -1), # n, w1, w2,...w w1,w2...wn
- (0xb0, 'PUSHB', 3, 'PushBytes', 0, -1), # b0, b1,..bn b0, b1, ...,bn
- (0xb8, 'PUSHW', 3, 'PushWords', 0, -1), # w0,w1,..wn w0 ,w1, ...wn
-]
-
-
-# next, the list of "normal" instructions
-
-instructions = [
-#
-# opcode mnemonic argBits descriptive name pops pushes eats from instruction stream pushes
-#
- (0x7f, 'AA', 0, 'AdjustAngle', 1, 0), # p -
- (0x64, 'ABS', 0, 'Absolute', 1, 1), # n |n|
- (0x60, 'ADD', 0, 'Add', 2, 1), # n2, n1 (n1 + n2)
- (0x27, 'ALIGNPTS', 0, 'AlignPts', 2, 0), # p2, p1 -
- (0x3c, 'ALIGNRP', 0, 'AlignRelativePt', -1, 0), # p1, p2, ... , ploopvalue -
- (0x5a, 'AND', 0, 'LogicalAnd', 2, 1), # e2, e1 b
- (0x2b, 'CALL', 0, 'CallFunction', 1, 0), # f -
- (0x67, 'CEILING', 0, 'Ceiling', 1, 1), # n ceil(n)
- (0x25, 'CINDEX', 0, 'CopyXToTopStack', 1, 1), # k ek
- (0x22, 'CLEAR', 0, 'ClearStack', -1, 0), # all items on the stack -
- (0x4f, 'DEBUG', 0, 'DebugCall', 1, 0), # n -
- (0x73, 'DELTAC1', 0, 'DeltaExceptionC1', -1, 0), # argn, cn, argn-1,cn-1, , arg1, c1 -
- (0x74, 'DELTAC2', 0, 'DeltaExceptionC2', -1, 0), # argn, cn, argn-1,cn-1, , arg1, c1 -
- (0x75, 'DELTAC3', 0, 'DeltaExceptionC3', -1, 0), # argn, cn, argn-1,cn-1, , arg1, c1 -
- (0x5d, 'DELTAP1', 0, 'DeltaExceptionP1', -1, 0), # argn, pn, argn-1, pn-1, , arg1, p1 -
- (0x71, 'DELTAP2', 0, 'DeltaExceptionP2', -1, 0), # argn, pn, argn-1, pn-1, , arg1, p1 -
- (0x72, 'DELTAP3', 0, 'DeltaExceptionP3', -1, 0), # argn, pn, argn-1, pn-1, , arg1, p1 -
- (0x24, 'DEPTH', 0, 'GetDepthStack', 0, 1), # - n
- (0x62, 'DIV', 0, 'Divide', 2, 1), # n2, n1 (n1 * 64)/ n2
- (0x20, 'DUP', 0, 'DuplicateTopStack', 1, 2), # e e, e
- (0x59, 'EIF', 0, 'EndIf', 0, 0), # - -
- (0x1b, 'ELSE', 0, 'Else', 0, 0), # - -
- (0x2d, 'ENDF', 0, 'EndFunctionDefinition', 0, 0), # - -
- (0x54, 'EQ', 0, 'Equal', 2, 1), # e2, e1 b
- (0x57, 'EVEN', 0, 'Even', 1, 1), # e b
- (0x2c, 'FDEF', 0, 'FunctionDefinition', 1, 0), # f -
- (0x4e, 'FLIPOFF', 0, 'SetAutoFlipOff', 0, 0), # - -
- (0x4d, 'FLIPON', 0, 'SetAutoFlipOn', 0, 0), # - -
- (0x80, 'FLIPPT', 0, 'FlipPoint', -1, 0), # p1, p2, ..., ploopvalue -
- (0x82, 'FLIPRGOFF', 0, 'FlipRangeOff', 2, 0), # h, l -
- (0x81, 'FLIPRGON', 0, 'FlipRangeOn', 2, 0), # h, l -
- (0x66, 'FLOOR', 0, 'Floor', 1, 1), # n floor(n)
- (0x46, 'GC', 1, 'GetCoordOnPVector', 1, 1), # p c
- (0x88, 'GETINFO', 0, 'GetInfo', 1, 1), # selector result
- (0x91, 'GETVARIATION', 0, 'GetVariation', 0, -1), # - a1,..,an
- (0x0d, 'GFV', 0, 'GetFVector', 0, 2), # - px, py
- (0x0c, 'GPV', 0, 'GetPVector', 0, 2), # - px, py
- (0x52, 'GT', 0, 'GreaterThan', 2, 1), # e2, e1 b
- (0x53, 'GTEQ', 0, 'GreaterThanOrEqual', 2, 1), # e2, e1 b
- (0x89, 'IDEF', 0, 'InstructionDefinition', 1, 0), # f -
- (0x58, 'IF', 0, 'If', 1, 0), # e -
- (0x8e, 'INSTCTRL', 0, 'SetInstrExecControl', 2, 0), # s, v -
- (0x39, 'IP', 0, 'InterpolatePts', -1, 0), # p1, p2, ... , ploopvalue -
- (0x0f, 'ISECT', 0, 'MovePtToIntersect', 5, 0), # a1, a0, b1, b0, p -
- (0x30, 'IUP', 1, 'InterpolateUntPts', 0, 0), # - -
- (0x1c, 'JMPR', 0, 'Jump', 1, 0), # offset -
- (0x79, 'JROF', 0, 'JumpRelativeOnFalse', 2, 0), # e, offset -
- (0x78, 'JROT', 0, 'JumpRelativeOnTrue', 2, 0), # e, offset -
- (0x2a, 'LOOPCALL', 0, 'LoopAndCallFunction', 2, 0), # f, count -
- (0x50, 'LT', 0, 'LessThan', 2, 1), # e2, e1 b
- (0x51, 'LTEQ', 0, 'LessThenOrEqual', 2, 1), # e2, e1 b
- (0x8b, 'MAX', 0, 'Maximum', 2, 1), # e2, e1 max(e1, e2)
- (0x49, 'MD', 1, 'MeasureDistance', 2, 1), # p2,p1 d
- (0x2e, 'MDAP', 1, 'MoveDirectAbsPt', 1, 0), # p -
- (0xc0, 'MDRP', 5, 'MoveDirectRelPt', 1, 0), # p -
- (0x3e, 'MIAP', 1, 'MoveIndirectAbsPt', 2, 0), # n, p -
- (0x8c, 'MIN', 0, 'Minimum', 2, 1), # e2, e1 min(e1, e2)
- (0x26, 'MINDEX', 0, 'MoveXToTopStack', 1, 1), # k ek
- (0xe0, 'MIRP', 5, 'MoveIndirectRelPt', 2, 0), # n, p -
- (0x4b, 'MPPEM', 0, 'MeasurePixelPerEm', 0, 1), # - ppem
- (0x4c, 'MPS', 0, 'MeasurePointSize', 0, 1), # - pointSize
- (0x3a, 'MSIRP', 1, 'MoveStackIndirRelPt', 2, 0), # d, p -
- (0x63, 'MUL', 0, 'Multiply', 2, 1), # n2, n1 (n1 * n2)/64
- (0x65, 'NEG', 0, 'Negate', 1, 1), # n -n
- (0x55, 'NEQ', 0, 'NotEqual', 2, 1), # e2, e1 b
- (0x5c, 'NOT', 0, 'LogicalNot', 1, 1), # e ( not e )
- (0x6c, 'NROUND', 2, 'NoRound', 1, 1), # n1 n2
- (0x56, 'ODD', 0, 'Odd', 1, 1), # e b
- (0x5b, 'OR', 0, 'LogicalOr', 2, 1), # e2, e1 b
- (0x21, 'POP', 0, 'PopTopStack', 1, 0), # e -
- (0x45, 'RCVT', 0, 'ReadCVT', 1, 1), # location value
- (0x7d, 'RDTG', 0, 'RoundDownToGrid', 0, 0), # - -
- (0x7a, 'ROFF', 0, 'RoundOff', 0, 0), # - -
- (0x8a, 'ROLL', 0, 'RollTopThreeStack', 3, 3), # a,b,c b,a,c
- (0x68, 'ROUND', 2, 'Round', 1, 1), # n1 n2
- (0x43, 'RS', 0, 'ReadStore', 1, 1), # n v
- (0x3d, 'RTDG', 0, 'RoundToDoubleGrid', 0, 0), # - -
- (0x18, 'RTG', 0, 'RoundToGrid', 0, 0), # - -
- (0x19, 'RTHG', 0, 'RoundToHalfGrid', 0, 0), # - -
- (0x7c, 'RUTG', 0, 'RoundUpToGrid', 0, 0), # - -
- (0x77, 'S45ROUND', 0, 'SuperRound45Degrees', 1, 0), # n -
- (0x7e, 'SANGW', 0, 'SetAngleWeight', 1, 0), # weight -
- (0x85, 'SCANCTRL', 0, 'ScanConversionControl', 1, 0), # n -
- (0x8d, 'SCANTYPE', 0, 'ScanType', 1, 0), # n -
- (0x48, 'SCFS', 0, 'SetCoordFromStackFP', 2, 0), # c, p -
- (0x1d, 'SCVTCI', 0, 'SetCVTCutIn', 1, 0), # n -
- (0x5e, 'SDB', 0, 'SetDeltaBaseInGState', 1, 0), # n -
- (0x86, 'SDPVTL', 1, 'SetDualPVectorToLine', 2, 0), # p2, p1 -
- (0x5f, 'SDS', 0, 'SetDeltaShiftInGState', 1, 0), # n -
- (0x0b, 'SFVFS', 0, 'SetFVectorFromStack', 2, 0), # y, x -
- (0x04, 'SFVTCA', 1, 'SetFVectorToAxis', 0, 0), # - -
- (0x08, 'SFVTL', 1, 'SetFVectorToLine', 2, 0), # p2, p1 -
- (0x0e, 'SFVTPV', 0, 'SetFVectorToPVector', 0, 0), # - -
- (0x34, 'SHC', 1, 'ShiftContourByLastPt', 1, 0), # c -
- (0x32, 'SHP', 1, 'ShiftPointByLastPoint', -1, 0), # p1, p2, ..., ploopvalue -
- (0x38, 'SHPIX', 0, 'ShiftZoneByPixel', -1, 0), # d, p1, p2, ..., ploopvalue -
- (0x36, 'SHZ', 1, 'ShiftZoneByLastPoint', 1, 0), # e -
- (0x17, 'SLOOP', 0, 'SetLoopVariable', 1, 0), # n -
- (0x1a, 'SMD', 0, 'SetMinimumDistance', 1, 0), # distance -
- (0x0a, 'SPVFS', 0, 'SetPVectorFromStack', 2, 0), # y, x -
- (0x02, 'SPVTCA', 1, 'SetPVectorToAxis', 0, 0), # - -
- (0x06, 'SPVTL', 1, 'SetPVectorToLine', 2, 0), # p2, p1 -
- (0x76, 'SROUND', 0, 'SuperRound', 1, 0), # n -
- (0x10, 'SRP0', 0, 'SetRefPoint0', 1, 0), # p -
- (0x11, 'SRP1', 0, 'SetRefPoint1', 1, 0), # p -
- (0x12, 'SRP2', 0, 'SetRefPoint2', 1, 0), # p -
- (0x1f, 'SSW', 0, 'SetSingleWidth', 1, 0), # n -
- (0x1e, 'SSWCI', 0, 'SetSingleWidthCutIn', 1, 0), # n -
- (0x61, 'SUB', 0, 'Subtract', 2, 1), # n2, n1 (n1 - n2)
- (0x00, 'SVTCA', 1, 'SetFPVectorToAxis', 0, 0), # - -
- (0x23, 'SWAP', 0, 'SwapTopStack', 2, 2), # e2, e1 e1, e2
- (0x13, 'SZP0', 0, 'SetZonePointer0', 1, 0), # n -
- (0x14, 'SZP1', 0, 'SetZonePointer1', 1, 0), # n -
- (0x15, 'SZP2', 0, 'SetZonePointer2', 1, 0), # n -
- (0x16, 'SZPS', 0, 'SetZonePointerS', 1, 0), # n -
- (0x29, 'UTP', 0, 'UnTouchPt', 1, 0), # p -
- (0x70, 'WCVTF', 0, 'WriteCVTInFUnits', 2, 0), # n, l -
- (0x44, 'WCVTP', 0, 'WriteCVTInPixels', 2, 0), # v, l -
- (0x42, 'WS', 0, 'WriteStore', 2, 0), # v, l -
-]
-
-# fmt: on
-
-
-def bitRepr(value, bits):
- s = ""
- for i in range(bits):
- s = "01"[value & 0x1] + s
- value = value >> 1
- return s
-
-
-_mnemonicPat = re.compile(r"[A-Z][A-Z0-9]*$")
-
-
-def _makeDict(instructionList):
- opcodeDict = {}
- mnemonicDict = {}
- for op, mnemonic, argBits, name, pops, pushes in instructionList:
- assert _mnemonicPat.match(mnemonic)
- mnemonicDict[mnemonic] = op, argBits, name
- if argBits:
- argoffset = op
- for i in range(1 << argBits):
- opcodeDict[op + i] = mnemonic, argBits, argoffset, name
- else:
- opcodeDict[op] = mnemonic, 0, 0, name
- return opcodeDict, mnemonicDict
-
-
-streamOpcodeDict, streamMnemonicDict = _makeDict(streamInstructions)
-opcodeDict, mnemonicDict = _makeDict(instructions)
-
-
-class tt_instructions_error(Exception):
- def __init__(self, error):
- self.error = error
-
- def __str__(self):
- return "TT instructions error: %s" % repr(self.error)
-
-
-_comment = r"/\*.*?\*/"
-_instruction = r"([A-Z][A-Z0-9]*)\s*\[(.*?)\]"
-_number = r"-?[0-9]+"
-_token = "(%s)|(%s)|(%s)" % (_instruction, _number, _comment)
-
-_tokenRE = re.compile(_token)
-_whiteRE = re.compile(r"\s*")
-
-_pushCountPat = re.compile(r"[A-Z][A-Z0-9]*\s*\[.*?\]\s*/\* ([0-9]+).*?\*/")
-
-_indentRE = re.compile(r"^FDEF|IF|ELSE\[ \]\t.+")
-_unindentRE = re.compile(r"^ELSE|ENDF|EIF\[ \]\t.+")
-
-
-def _skipWhite(data, pos):
- m = _whiteRE.match(data, pos)
- newPos = m.regs[0][1]
- assert newPos >= pos
- return newPos
-
-
-class Program(object):
- def __init__(self) -> None:
- pass
-
- def fromBytecode(self, bytecode: bytes) -> None:
- self.bytecode = array.array("B", bytecode)
- if hasattr(self, "assembly"):
- del self.assembly
-
- def fromAssembly(self, assembly: List[str] | str) -> None:
- if isinstance(assembly, list):
- self.assembly = assembly
- elif isinstance(assembly, str):
- self.assembly = assembly.splitlines()
- else:
- raise TypeError(f"expected str or List[str], got {type(assembly).__name__}")
- if hasattr(self, "bytecode"):
- del self.bytecode
-
- def getBytecode(self) -> bytes:
- if not hasattr(self, "bytecode"):
- self._assemble()
- return self.bytecode.tobytes()
-
- def getAssembly(self, preserve=True) -> List[str]:
- if not hasattr(self, "assembly"):
- self._disassemble(preserve=preserve)
- return self.assembly
-
- def toXML(self, writer, ttFont) -> None:
- if (
- not hasattr(ttFont, "disassembleInstructions")
- or ttFont.disassembleInstructions
- ):
- try:
- assembly = self.getAssembly()
- except:
- import traceback
-
- tmp = StringIO()
- traceback.print_exc(file=tmp)
- msg = "An exception occurred during the decompilation of glyph program:\n\n"
- msg += tmp.getvalue()
- log.error(msg)
- writer.begintag("bytecode")
- writer.newline()
- writer.comment(msg.strip())
- writer.newline()
- writer.dumphex(self.getBytecode())
- writer.endtag("bytecode")
- writer.newline()
- else:
- if not assembly:
- return
- writer.begintag("assembly")
- writer.newline()
- i = 0
- indent = 0
- nInstr = len(assembly)
- while i < nInstr:
- instr = assembly[i]
- if _unindentRE.match(instr):
- indent -= 1
- writer.write(writer.indentwhite * indent)
- writer.write(instr)
- writer.newline()
- m = _pushCountPat.match(instr)
- i = i + 1
- if m:
- nValues = int(m.group(1))
- line: List[str] = []
- j = 0
- for j in range(nValues):
- if j and not (j % 25):
- writer.write(writer.indentwhite * indent)
- writer.write(" ".join(line))
- writer.newline()
- line = []
- line.append(assembly[i + j])
- writer.write(writer.indentwhite * indent)
- writer.write(" ".join(line))
- writer.newline()
- i = i + j + 1
- if _indentRE.match(instr):
- indent += 1
- writer.endtag("assembly")
- writer.newline()
- else:
- bytecode = self.getBytecode()
- if not bytecode:
- return
- writer.begintag("bytecode")
- writer.newline()
- writer.dumphex(bytecode)
- writer.endtag("bytecode")
- writer.newline()
-
- def fromXML(self, name, attrs, content, ttFont) -> None:
- if name == "assembly":
- self.fromAssembly(strjoin(content))
- self._assemble()
- del self.assembly
- else:
- assert name == "bytecode"
- self.fromBytecode(readHex(content))
-
- def _assemble(self) -> None:
- assembly = " ".join(getattr(self, "assembly", []))
- bytecode: List[int] = []
- push = bytecode.append
- lenAssembly = len(assembly)
- pos = _skipWhite(assembly, 0)
- while pos < lenAssembly:
- m = _tokenRE.match(assembly, pos)
- if m is None:
- raise tt_instructions_error(
- "Syntax error in TT program (%s)" % assembly[pos - 5 : pos + 15]
- )
- dummy, mnemonic, arg, number, comment = m.groups()
- pos = m.regs[0][1]
- if comment:
- pos = _skipWhite(assembly, pos)
- continue
-
- arg = arg.strip()
- if mnemonic.startswith("INSTR"):
- # Unknown instruction
- op = int(mnemonic[5:])
- push(op)
- elif mnemonic not in ("PUSH", "NPUSHB", "NPUSHW", "PUSHB", "PUSHW"):
- op, argBits, name = mnemonicDict[mnemonic]
- if len(arg) != argBits:
- raise tt_instructions_error(
- "Incorrect number of argument bits (%s[%s])" % (mnemonic, arg)
- )
- if arg:
- arg = binary2num(arg)
- push(op + arg)
- else:
- push(op)
- else:
- args = []
- pos = _skipWhite(assembly, pos)
- while pos < lenAssembly:
- m = _tokenRE.match(assembly, pos)
- if m is None:
- raise tt_instructions_error(
- "Syntax error in TT program (%s)" % assembly[pos : pos + 15]
- )
- dummy, _mnemonic, arg, number, comment = m.groups()
- if number is None and comment is None:
- break
- pos = m.regs[0][1]
- pos = _skipWhite(assembly, pos)
- if comment is not None:
- continue
- args.append(int(number))
- nArgs = len(args)
- if mnemonic == "PUSH":
- # Automatically choose the most compact representation
- nWords = 0
- while nArgs:
- while (
- nWords < nArgs
- and nWords < 255
- and not (0 <= args[nWords] <= 255)
- ):
- nWords += 1
- nBytes = 0
- while (
- nWords + nBytes < nArgs
- and nBytes < 255
- and 0 <= args[nWords + nBytes] <= 255
- ):
- nBytes += 1
- if (
- nBytes < 2
- and nWords + nBytes < 255
- and nWords + nBytes != nArgs
- ):
- # Will write bytes as words
- nWords += nBytes
- continue
-
- # Write words
- if nWords:
- if nWords <= 8:
- op, argBits, name = streamMnemonicDict["PUSHW"]
- op = op + nWords - 1
- push(op)
- else:
- op, argBits, name = streamMnemonicDict["NPUSHW"]
- push(op)
- push(nWords)
- for value in args[:nWords]:
- assert -32768 <= value < 32768, (
- "PUSH value out of range %d" % value
- )
- push((value >> 8) & 0xFF)
- push(value & 0xFF)
-
- # Write bytes
- if nBytes:
- pass
- if nBytes <= 8:
- op, argBits, name = streamMnemonicDict["PUSHB"]
- op = op + nBytes - 1
- push(op)
- else:
- op, argBits, name = streamMnemonicDict["NPUSHB"]
- push(op)
- push(nBytes)
- for value in args[nWords : nWords + nBytes]:
- push(value)
-
- nTotal = nWords + nBytes
- args = args[nTotal:]
- nArgs -= nTotal
- nWords = 0
- else:
- # Write exactly what we've been asked to
- words = mnemonic[-1] == "W"
- op, argBits, name = streamMnemonicDict[mnemonic]
- if mnemonic[0] != "N":
- assert nArgs <= 8, nArgs
- op = op + nArgs - 1
- push(op)
- else:
- assert nArgs < 256
- push(op)
- push(nArgs)
- if words:
- for value in args:
- assert -32768 <= value < 32768, (
- "PUSHW value out of range %d" % value
- )
- push((value >> 8) & 0xFF)
- push(value & 0xFF)
- else:
- for value in args:
- assert 0 <= value < 256, (
- "PUSHB value out of range %d" % value
- )
- push(value)
-
- pos = _skipWhite(assembly, pos)
-
- if bytecode:
- assert max(bytecode) < 256 and min(bytecode) >= 0
- self.bytecode = array.array("B", bytecode)
-
- def _disassemble(self, preserve=False) -> None:
- assembly = []
- i = 0
- bytecode = getattr(self, "bytecode", [])
- numBytecode = len(bytecode)
- while i < numBytecode:
- op = bytecode[i]
- try:
- mnemonic, argBits, argoffset, name = opcodeDict[op]
- except KeyError:
- if op in streamOpcodeDict:
- values = []
-
- # Merge consecutive PUSH operations
- while bytecode[i] in streamOpcodeDict:
- op = bytecode[i]
- mnemonic, argBits, argoffset, name = streamOpcodeDict[op]
- words = mnemonic[-1] == "W"
- if argBits:
- nValues = op - argoffset + 1
- else:
- i = i + 1
- nValues = bytecode[i]
- i = i + 1
- assert nValues > 0
- if not words:
- for j in range(nValues):
- value = bytecode[i]
- values.append(repr(value))
- i = i + 1
- else:
- for j in range(nValues):
- # cast to signed int16
- value = (bytecode[i] << 8) | bytecode[i + 1]
- if value >= 0x8000:
- value = value - 0x10000
- values.append(repr(value))
- i = i + 2
- if preserve:
- break
-
- if not preserve:
- mnemonic = "PUSH"
- nValues = len(values)
- if nValues == 1:
- assembly.append("%s[ ] /* 1 value pushed */" % mnemonic)
- else:
- assembly.append(
- "%s[ ] /* %s values pushed */" % (mnemonic, nValues)
- )
- assembly.extend(values)
- else:
- assembly.append("INSTR%d[ ]" % op)
- i = i + 1
- else:
- if argBits:
- assembly.append(
- mnemonic
- + "[%s] /* %s */" % (num2binary(op - argoffset, argBits), name)
- )
- else:
- assembly.append(mnemonic + "[ ] /* %s */" % name)
- i = i + 1
- self.assembly = assembly
-
- def __bool__(self) -> bool:
- """
- >>> p = Program()
- >>> bool(p)
- False
- >>> bc = array.array("B", [0])
- >>> p.fromBytecode(bc)
- >>> bool(p)
- True
- >>> p.bytecode.pop()
- 0
- >>> bool(p)
- False
-
- >>> p = Program()
- >>> asm = ['SVTCA[0]']
- >>> p.fromAssembly(asm)
- >>> bool(p)
- True
- >>> p.assembly.pop()
- 'SVTCA[0]'
- >>> bool(p)
- False
- """
- return (hasattr(self, "assembly") and len(self.assembly) > 0) or (
- hasattr(self, "bytecode") and len(self.bytecode) > 0
- )
-
- __nonzero__ = __bool__
-
- def __eq__(self, other) -> bool:
- if type(self) != type(other):
- return NotImplemented
- return self.__dict__ == other.__dict__
-
- def __ne__(self, other) -> bool:
- result = self.__eq__(other)
- return result if result is NotImplemented else not result
-
-
-def _test():
- """
- >>> _test()
- True
- """
-
- bc = b"""@;:9876543210/.-,+*)(\'&%$#"! \037\036\035\034\033\032\031\030\027\026\025\024\023\022\021\020\017\016\015\014\013\012\011\010\007\006\005\004\003\002\001\000,\001\260\030CXEj\260\031C`\260F#D#\020 \260FN\360M/\260\000\022\033!#\0213Y-,\001\260\030CX\260\005+\260\000\023K\260\024PX\261\000@8Y\260\006+\033!#\0213Y-,\001\260\030CXN\260\003%\020\362!\260\000\022M\033 E\260\004%\260\004%#Jad\260(RX!#\020\326\033\260\003%\020\362!\260\000\022YY-,\260\032CX!!\033\260\002%\260\002%I\260\003%\260\003%Ja d\260\020PX!!!\033\260\003%\260\003%I\260\000PX\260\000PX\270\377\3428!\033\260\0208!Y\033\260\000RX\260\0368!\033\270\377\3608!YYYY-,\001\260\030CX\260\005+\260\000\023K\260\024PX\271\000\000\377\3008Y\260\006+\033!#\0213Y-,N\001\212\020\261F\031CD\260\000\024\261\000F\342\260\000\025\271\000\000\377\3608\000\260\000<\260(+\260\002%\020\260\000<-,\001\030\260\000/\260\001\024\362\260\001\023\260\001\025M\260\000\022-,\001\260\030CX\260\005+\260\000\023\271\000\000\377\3408\260\006+\033!#\0213Y-,\001\260\030CXEdj#Edi\260\031Cd``\260F#D#\020 \260F\360/\260\000\022\033!! \212 \212RX\0213\033!!YY-,\001\261\013\012C#Ce\012-,\000\261\012\013C#C\013-,\000\260F#p\261\001F>\001\260F#p\261\002FE:\261\002\000\010\015-,\260\022+\260\002%E\260\002%Ej\260@\213`\260\002%#D!!!-,\260\023+\260\002%E\260\002%Ej\270\377\300\214`\260\002%#D!!!-,\260\000\260\022+!!!-,\260\000\260\023+!!!-,\001\260\006C\260\007Ce\012-, i\260@a\260\000\213 \261,\300\212\214\270\020\000b`+\014d#da\\X\260\003aY-,\261\000\003%EhT\260\034KPZX\260\003%E\260\003%E`h \260\004%#D\260\004%#D\033\260\003% Eh \212#D\260\003%Eh`\260\003%#DY-,\260\003% Eh \212#D\260\003%Edhe`\260\004%\260\001`#D-,\260\011CX\207!\300\033\260\022CX\207E\260\021+\260G#D\260Gz\344\033\003\212E\030i \260G#D\212\212\207 \260\240QX\260\021+\260G#D\260Gz\344\033!\260Gz\344YYY\030-, \212E#Eh`D-,EjB-,\001\030/-,\001\260\030CX\260\004%\260\004%Id#Edi\260@\213a \260\200bj\260\002%\260\002%a\214\260\031C`\260F#D!\212\020\260F\366!\033!!!!Y-,\001\260\030CX\260\002%E\260\002%Ed`j\260\003%Eja \260\004%Ej \212\213e\260\004%#D\214\260\003%#D!!\033 EjD EjDY-,\001 E\260\000U\260\030CZXEh#Ei\260@\213a \260\200bj \212#a \260\003%\213e\260\004%#D\214\260\003%#D!!\033!!\260\031+Y-,\001\212\212Ed#EdadB-,\260\004%\260\004%\260\031+\260\030CX\260\004%\260\004%\260\003%\260\033+\001\260\002%C\260@T\260\002%C\260\000TZX\260\003% E\260@aDY\260\002%C\260\000T\260\002%C\260@TZX\260\004% E\260@`DYY!!!!-,\001KRXC\260\002%E#aD\033!!Y-,\001KRXC\260\002%E#`D\033!!Y-,KRXED\033!!Y-,\001 \260\003%#I\260@`\260 c \260\000RX#\260\002%8#\260\002%e8\000\212c8\033!!!!!Y\001-,KPXED\033!!Y-,\001\260\005%\020# \212\365\000\260\001`#\355\354-,\001\260\005%\020# \212\365\000\260\001a#\355\354-,\001\260\006%\020\365\000\355\354-,F#F`\212\212F# F\212`\212a\270\377\200b# \020#\212\261KK\212pE` \260\000PX\260\001a\270\377\272\213\033\260F\214Y\260\020`h\001:-, E\260\003%FRX\260\002%F ha\260\003%\260\003%?#!8\033!\021Y-, E\260\003%FPX\260\002%F ha\260\003%\260\003%?#!8\033!\021Y-,\000\260\007C\260\006C\013-,\212\020\354-,\260\014CX!\033 F\260\000RX\270\377\3608\033\260\0208YY-, \260\000UX\270\020\000c\260\003%Ed\260\003%Eda\260\000SX\260\002\033\260@a\260\003Y%EiSXED\033!!Y\033!\260\002%E\260\002%Ead\260(QXED\033!!YY-,!!\014d#d\213\270@\000b-,!\260\200QX\014d#d\213\270 \000b\033\262\000@/+Y\260\002`-,!\260\300QX\014d#d\213\270\025Ub\033\262\000\200/+Y\260\002`-,\014d#d\213\270@\000b`#!-,KSX\260\004%\260\004%Id#Edi\260@\213a \260\200bj\260\002%\260\002%a\214\260F#D!\212\020\260F\366!\033!\212\021#\022 9/Y-,\260\002%\260\002%Id\260\300TX\270\377\3708\260\0108\033!!Y-,\260\023CX\003\033\002Y-,\260\023CX\002\033\003Y-,\260\012+#\020 <\260\027+-,\260\002%\270\377\3608\260(+\212\020# \320#\260\020+\260\005CX\300\033 pd.DataFrame | None:
- """
- Parameters:
- x: Dict with keys 'data': 2D array of str, numeric, or bool data, 'headers': list of strings for header names, 'range': optional two element list designating start of end of subrange.
- Returns:
- Dataframe of timeseries data
- """
- if x is None:
- return x
- elif x.get("is_file"):
- dataframe = pd.read_csv(x["name"])
- else:
- dataframe = pd.DataFrame(data=x["data"], columns=x["headers"])
- if x.get("range") is not None:
- dataframe = dataframe.loc[dataframe[self.x or 0] >= x["range"][0]]
- dataframe = dataframe.loc[dataframe[self.x or 0] <= x["range"][1]]
- return dataframe
-
- def postprocess(self, y: str | pd.DataFrame | None) -> dict | None:
- """
- Parameters:
- y: csv or dataframe with timeseries data
- Returns:
- JSON object with key 'headers' for list of header names, 'data' for 2D array of string or numeric data
- """
- if y is None:
- return None
- if isinstance(y, str):
- dataframe = pd.read_csv(y)
- return {
- "headers": dataframe.columns.values.tolist(),
- "data": dataframe.values.tolist(),
- }
- if isinstance(y, pd.DataFrame):
- return {"headers": y.columns.values.tolist(), "data": y.values.tolist()}
- raise ValueError("Cannot process value as Timeseries data")
-
- def as_example(self, input_data: str | None) -> str:
- return Path(input_data).name if input_data else ""
diff --git a/spaces/dcarpintero/nlp-summarizer-pegasus/.venv/lib/python3.9/site-packages/huggingface_hub/_multi_commits.py b/spaces/dcarpintero/nlp-summarizer-pegasus/.venv/lib/python3.9/site-packages/huggingface_hub/_multi_commits.py
deleted file mode 100644
index c41d2a36fc0971ad031e05d851e632b263f10e48..0000000000000000000000000000000000000000
--- a/spaces/dcarpintero/nlp-summarizer-pegasus/.venv/lib/python3.9/site-packages/huggingface_hub/_multi_commits.py
+++ /dev/null
@@ -1,305 +0,0 @@
-# coding=utf-8
-# Copyright 2023-present, the HuggingFace Inc. team.
-#
-# Licensed under the Apache License, Version 2.0 (the "License");
-# you may not use this file except in compliance with the License.
-# You may obtain a copy of the License at
-#
-# http://www.apache.org/licenses/LICENSE-2.0
-#
-# Unless required by applicable law or agreed to in writing, software
-# distributed under the License is distributed on an "AS IS" BASIS,
-# WITHOUT WARRANTIES OR CONDITIONS OF ANY KIND, either express or implied.
-# See the License for the specific language governing permissions and
-# limitations under the License.
-"""Contains utilities to multi-commits (i.e. push changes iteratively on a PR)."""
-import re
-from dataclasses import dataclass, field
-from hashlib import sha256
-from typing import TYPE_CHECKING, Iterable, List, Optional, Set, Tuple, Union
-
-from ._commit_api import CommitOperationAdd, CommitOperationDelete
-from .community import DiscussionWithDetails
-from .utils import experimental
-from .utils._cache_manager import _format_size
-
-
-if TYPE_CHECKING:
- from .hf_api import HfApi
-
-
-class MultiCommitException(Exception):
- """Base exception for any exception happening while doing a multi-commit."""
-
-
-MULTI_COMMIT_PR_DESCRIPTION_TEMPLATE = """
-## {commit_message}
-
-{commit_description}
-
-**Multi commit ID:** {multi_commit_id}
-
-Scheduled commits:
-
-{multi_commit_strategy}
-
-_This is a PR opened using the `huggingface_hub` library in the context of a multi-commit. PR can be commented as a usual PR. However, please be aware that manually updating the PR description, changing the PR status, or pushing new commits, is not recommended as it might corrupt the commit process. Learn more about multi-commits [in this guide](https://huggingface.co/docs/huggingface_hub/main/guides/upload)._
-"""
-
-MULTI_COMMIT_PR_COMPLETION_COMMENT_TEMPLATE = """
-Multi-commit is now completed! You can ping the repo owner to review the changes. This PR can now be commented or modified without risking to corrupt it.
-
-_This is a comment posted using the `huggingface_hub` library in the context of a multi-commit. Learn more about multi-commits [in this guide](https://huggingface.co/docs/huggingface_hub/main/guides/upload)._
-"""
-
-MULTI_COMMIT_PR_CLOSING_COMMENT_TEMPLATE = """
-`create_pr=False` has been passed so PR is automatically merged.
-
-_This is a comment posted using the `huggingface_hub` library in the context of a multi-commit. Learn more about multi-commits [in this guide](https://huggingface.co/docs/huggingface_hub/main/guides/upload)._
-"""
-
-MULTI_COMMIT_PR_CLOSE_COMMENT_FAILURE_NO_CHANGES_TEMPLATE = """
-Cannot merge Pull Requests as no changes are associated. This PR will be closed automatically.
-
-_This is a comment posted using the `huggingface_hub` library in the context of a multi-commit. Learn more about multi-commits [in this guide](https://huggingface.co/docs/huggingface_hub/main/guides/upload)._
-"""
-
-MULTI_COMMIT_PR_CLOSE_COMMENT_FAILURE_BAD_REQUEST_TEMPLATE = """
-An error occurred while trying to merge the Pull Request: `{error_message}`.
-
-_This is a comment posted using the `huggingface_hub` library in the context of a multi-commit. Learn more about multi-commits [in this guide](https://huggingface.co/docs/huggingface_hub/main/guides/upload)._
-"""
-
-
-STEP_ID_REGEX = re.compile(r"- \[(?P[ |x])\].*(?P[a-fA-F0-9]{64})", flags=re.MULTILINE)
-
-
-@experimental
-def plan_multi_commits(
- operations: Iterable[Union[CommitOperationAdd, CommitOperationDelete]],
- max_operations_per_commit: int = 50,
- max_upload_size_per_commit: int = 2 * 1024 * 1024 * 1024,
-) -> Tuple[List[List[CommitOperationAdd]], List[List[CommitOperationDelete]]]:
- """Split a list of operations in a list of commits to perform.
-
- Implementation follows a sub-optimal (yet simple) algorithm:
- 1. Delete operations are grouped together by commits of maximum `max_operations_per_commits` operations.
- 2. All additions exceeding `max_upload_size_per_commit` are committed 1 by 1.
- 3. All remaining additions are grouped together and split each time the `max_operations_per_commit` or the
- `max_upload_size_per_commit` limit is reached.
-
- We do not try to optimize the splitting to get the lowest number of commits as this is a NP-hard problem (see
- [bin packing problem](https://en.wikipedia.org/wiki/Bin_packing_problem)). For our use case, it is not problematic
- to use a sub-optimal solution so we favored an easy-to-explain implementation.
-
- Args:
- operations (`List` of [`~hf_api.CommitOperation`]):
- The list of operations to split into commits.
- max_operations_per_commit (`int`):
- Maximum number of operations in a single commit. Defaults to 50.
- max_upload_size_per_commit (`int`):
- Maximum size to upload (in bytes) in a single commit. Defaults to 2GB. Files bigger than this limit are
- uploaded, 1 per commit.
-
- Returns:
- `Tuple[List[List[CommitOperationAdd]], List[List[CommitOperationDelete]]]`: a tuple. First item is a list of
- lists of [`CommitOperationAdd`] representing the addition commits to push. The second item is a list of lists
- of [`CommitOperationDelete`] representing the deletion commits.
-
-
-
- `plan_multi_commits` is experimental. Its API and behavior is subject to change in the future without prior notice.
-
-
-
- Example:
- ```python
- >>> from huggingface_hub import HfApi, plan_multi_commits
- >>> addition_commits, deletion_commits = plan_multi_commits(
- ... operations=[
- ... CommitOperationAdd(...),
- ... CommitOperationAdd(...),
- ... CommitOperationDelete(...),
- ... CommitOperationDelete(...),
- ... CommitOperationAdd(...),
- ... ],
- ... )
- >>> HfApi().create_commits_on_pr(
- ... repo_id="my-cool-model",
- ... addition_commits=addition_commits,
- ... deletion_commits=deletion_commits,
- ... (...)
- ... verbose=True,
- ... )
- ```
-
-
-
- The initial order of the operations is not guaranteed! All deletions will be performed before additions. If you are
- not updating multiple times the same file, you are fine.
-
-
- """
- addition_commits: List[List[CommitOperationAdd]] = []
- deletion_commits: List[List[CommitOperationDelete]] = []
-
- additions: List[CommitOperationAdd] = []
- additions_size = 0
- deletions: List[CommitOperationDelete] = []
- for op in operations:
- if isinstance(op, CommitOperationDelete):
- # Group delete operations together
- deletions.append(op)
- if len(deletions) >= max_operations_per_commit:
- deletion_commits.append(deletions)
- deletions = []
-
- elif op.upload_info.size >= max_upload_size_per_commit:
- # Upload huge files 1 by 1
- addition_commits.append([op])
-
- elif additions_size + op.upload_info.size < max_upload_size_per_commit:
- # Group other additions and split if size limit is reached (either max_nb_files or max_upload_size)
- additions.append(op)
- additions_size += op.upload_info.size
-
- else:
- addition_commits.append(additions)
- additions = [op]
- additions_size = op.upload_info.size
-
- if len(additions) >= max_operations_per_commit:
- addition_commits.append(additions)
- additions = []
- additions_size = 0
-
- if len(additions) > 0:
- addition_commits.append(additions)
- if len(deletions) > 0:
- deletion_commits.append(deletions)
-
- return addition_commits, deletion_commits
-
-
-@dataclass
-class MultiCommitStep:
- """Dataclass containing a list of CommitOperation to commit at once.
-
- A [`MultiCommitStep`] is one atomic part of a [`MultiCommitStrategy`]. Each step is identified by its own
- deterministic ID based on the list of commit operations (hexadecimal sha256). ID is persistent between re-runs if
- the list of commits is kept the same.
- """
-
- operations: List[Union[CommitOperationAdd, CommitOperationDelete]]
-
- id: str = field(init=False)
- completed: bool = False
-
- def __post_init__(self) -> None:
- if len(self.operations) == 0:
- raise ValueError("A MultiCommitStep must have at least 1 commit operation, got 0.")
-
- # Generate commit id
- sha = sha256()
- for op in self.operations:
- if isinstance(op, CommitOperationAdd):
- sha.update(b"ADD")
- sha.update(op.path_in_repo.encode())
- sha.update(op.upload_info.sha256)
- elif isinstance(op, CommitOperationDelete):
- sha.update(b"DELETE")
- sha.update(op.path_in_repo.encode())
- sha.update(str(op.is_folder).encode())
- else:
- NotImplementedError()
- self.id = sha.hexdigest()
-
- def __str__(self) -> str:
- """Format a step for PR description.
-
- Formatting can be changed in the future as long as it is single line, starts with `- [ ]`/`- [x]` and contains
- `self.id`. Must be able to match `STEP_ID_REGEX`.
- """
- additions = [op for op in self.operations if isinstance(op, CommitOperationAdd)]
- file_deletions = [op for op in self.operations if isinstance(op, CommitOperationDelete) and not op.is_folder]
- folder_deletions = [op for op in self.operations if isinstance(op, CommitOperationDelete) and op.is_folder]
- if len(additions) > 0:
- return (
- f"- [{'x' if self.completed else ' '}] Upload {len(additions)} file(s) "
- f"totalling {_format_size(sum(add.upload_info.size for add in additions))}"
- f" ({self.id})"
- )
- else:
- return (
- f"- [{'x' if self.completed else ' '}] Delete {len(file_deletions)} file(s) and"
- f" {len(folder_deletions)} folder(s) ({self.id})"
- )
-
-
-@dataclass
-class MultiCommitStrategy:
- """Dataclass containing a list of [`MultiCommitStep`] to commit iteratively.
-
- A strategy is identified by its own deterministic ID based on the list of its steps (hexadecimal sha256). ID is
- persistent between re-runs if the list of commits is kept the same.
- """
-
- addition_commits: List[MultiCommitStep]
- deletion_commits: List[MultiCommitStep]
-
- id: str = field(init=False)
- all_steps: Set[str] = field(init=False)
-
- def __post_init__(self) -> None:
- self.all_steps = {step.id for step in self.addition_commits + self.deletion_commits}
- if len(self.all_steps) < len(self.addition_commits) + len(self.deletion_commits):
- raise ValueError("Got duplicate commits in MultiCommitStrategy. All commits must be unique.")
-
- if len(self.all_steps) == 0:
- raise ValueError("A MultiCommitStrategy must have at least 1 commit, got 0.")
-
- # Generate strategy id
- sha = sha256()
- for step in self.addition_commits + self.deletion_commits:
- sha.update("new step".encode())
- sha.update(step.id.encode())
- self.id = sha.hexdigest()
-
-
-def multi_commit_create_pull_request(
- api: "HfApi",
- repo_id: str,
- commit_message: str,
- commit_description: Optional[str],
- strategy: MultiCommitStrategy,
- token: Optional[str],
- repo_type: Optional[str],
-) -> DiscussionWithDetails:
- return api.create_pull_request(
- repo_id=repo_id,
- title=f"[WIP] {commit_message} (multi-commit {strategy.id})",
- description=multi_commit_generate_comment(
- commit_message=commit_message, commit_description=commit_description, strategy=strategy
- ),
- token=token,
- repo_type=repo_type,
- )
-
-
-def multi_commit_generate_comment(
- commit_message: str,
- commit_description: Optional[str],
- strategy: MultiCommitStrategy,
-) -> str:
- return MULTI_COMMIT_PR_DESCRIPTION_TEMPLATE.format(
- commit_message=commit_message,
- commit_description=commit_description or "",
- multi_commit_id=strategy.id,
- multi_commit_strategy="\n".join(
- str(commit) for commit in strategy.deletion_commits + strategy.addition_commits
- ),
- )
-
-
-def multi_commit_parse_pr_description(description: str) -> Set[str]:
- return {match[1] for match in STEP_ID_REGEX.findall(description)}
diff --git a/spaces/dcarpintero/nlp-summarizer-pegasus/.venv/lib/python3.9/site-packages/huggingface_hub/utils/sha.py b/spaces/dcarpintero/nlp-summarizer-pegasus/.venv/lib/python3.9/site-packages/huggingface_hub/utils/sha.py
deleted file mode 100644
index 157ccb0379eb1c80389d8e06135f305d11889caf..0000000000000000000000000000000000000000
--- a/spaces/dcarpintero/nlp-summarizer-pegasus/.venv/lib/python3.9/site-packages/huggingface_hub/utils/sha.py
+++ /dev/null
@@ -1,27 +0,0 @@
-"""Utilities to efficiently compute the SHA 256 hash of a bunch of bytes."""
-from hashlib import sha256
-from typing import BinaryIO, Optional
-
-
-def sha_fileobj(fileobj: BinaryIO, chunk_size: Optional[int] = None) -> bytes:
- """
- Computes the sha256 hash of the given file object, by chunks of size `chunk_size`.
-
- Args:
- fileobj (file-like object):
- The File object to compute sha256 for, typically obtained with `open(path, "rb")`
- chunk_size (`int`, *optional*):
- The number of bytes to read from `fileobj` at once, defaults to 1MB.
-
- Returns:
- `bytes`: `fileobj`'s sha256 hash as bytes
- """
- chunk_size = chunk_size if chunk_size is not None else 1024 * 1024
-
- sha = sha256()
- while True:
- chunk = fileobj.read(chunk_size)
- sha.update(chunk)
- if not chunk:
- break
- return sha.digest()
diff --git a/spaces/dcarpintero/nlp-summarizer-pegasus/.venv/lib/python3.9/site-packages/markdown_it/tree.py b/spaces/dcarpintero/nlp-summarizer-pegasus/.venv/lib/python3.9/site-packages/markdown_it/tree.py
deleted file mode 100644
index 6641e5a44654c9414cff07b6abbc633de7108ecb..0000000000000000000000000000000000000000
--- a/spaces/dcarpintero/nlp-summarizer-pegasus/.venv/lib/python3.9/site-packages/markdown_it/tree.py
+++ /dev/null
@@ -1,345 +0,0 @@
-"""A tree representation of a linear markdown-it token stream.
-
-This module is not part of upstream JavaScript markdown-it.
-"""
-from __future__ import annotations
-
-from collections.abc import Generator, Sequence
-import textwrap
-from typing import Any, NamedTuple, TypeVar, overload
-
-from .token import Token
-
-
-class _NesterTokens(NamedTuple):
- opening: Token
- closing: Token
-
-
-_NodeType = TypeVar("_NodeType", bound="SyntaxTreeNode")
-
-
-class SyntaxTreeNode:
- """A Markdown syntax tree node.
-
- A class that can be used to construct a tree representation of a linear
- `markdown-it-py` token stream.
-
- Each node in the tree represents either:
- - root of the Markdown document
- - a single unnested `Token`
- - a `Token` "_open" and "_close" token pair, and the tokens nested in
- between
- """
-
- def __init__(
- self, tokens: Sequence[Token] = (), *, create_root: bool = True
- ) -> None:
- """Initialize a `SyntaxTreeNode` from a token stream.
-
- If `create_root` is True, create a root node for the document.
- """
- # Only nodes representing an unnested token have self.token
- self.token: Token | None = None
-
- # Only containers have nester tokens
- self.nester_tokens: _NesterTokens | None = None
-
- # Root node does not have self.parent
- self._parent: Any = None
-
- # Empty list unless a non-empty container, or unnested token that has
- # children (i.e. inline or img)
- self._children: list[Any] = []
-
- if create_root:
- self._set_children_from_tokens(tokens)
- return
-
- if not tokens:
- raise ValueError(
- "Can only create root from empty token sequence."
- " Set `create_root=True`."
- )
- elif len(tokens) == 1:
- inline_token = tokens[0]
- if inline_token.nesting:
- raise ValueError(
- "Unequal nesting level at the start and end of token stream."
- )
- self.token = inline_token
- if inline_token.children:
- self._set_children_from_tokens(inline_token.children)
- else:
- self.nester_tokens = _NesterTokens(tokens[0], tokens[-1])
- self._set_children_from_tokens(tokens[1:-1])
-
- def __repr__(self) -> str:
- return f"{type(self).__name__}({self.type})"
-
- @overload
- def __getitem__(self: _NodeType, item: int) -> _NodeType:
- ...
-
- @overload
- def __getitem__(self: _NodeType, item: slice) -> list[_NodeType]:
- ...
-
- def __getitem__(self: _NodeType, item: int | slice) -> _NodeType | list[_NodeType]:
- return self.children[item]
-
- def to_tokens(self: _NodeType) -> list[Token]:
- """Recover the linear token stream."""
-
- def recursive_collect_tokens(node: _NodeType, token_list: list[Token]) -> None:
- if node.type == "root":
- for child in node.children:
- recursive_collect_tokens(child, token_list)
- elif node.token:
- token_list.append(node.token)
- else:
- assert node.nester_tokens
- token_list.append(node.nester_tokens.opening)
- for child in node.children:
- recursive_collect_tokens(child, token_list)
- token_list.append(node.nester_tokens.closing)
-
- tokens: list[Token] = []
- recursive_collect_tokens(self, tokens)
- return tokens
-
- @property
- def children(self: _NodeType) -> list[_NodeType]:
- return self._children
-
- @children.setter
- def children(self: _NodeType, value: list[_NodeType]) -> None:
- self._children = value
-
- @property
- def parent(self: _NodeType) -> _NodeType | None:
- return self._parent # type: ignore
-
- @parent.setter
- def parent(self: _NodeType, value: _NodeType | None) -> None:
- self._parent = value
-
- @property
- def is_root(self) -> bool:
- """Is the node a special root node?"""
- return not (self.token or self.nester_tokens)
-
- @property
- def is_nested(self) -> bool:
- """Is this node nested?.
-
- Returns `True` if the node represents a `Token` pair and tokens in the
- sequence between them, where `Token.nesting` of the first `Token` in
- the pair is 1 and nesting of the other `Token` is -1.
- """
- return bool(self.nester_tokens)
-
- @property
- def siblings(self: _NodeType) -> Sequence[_NodeType]:
- """Get siblings of the node.
-
- Gets the whole group of siblings, including self.
- """
- if not self.parent:
- return [self]
- return self.parent.children
-
- @property
- def type(self) -> str:
- """Get a string type of the represented syntax.
-
- - "root" for root nodes
- - `Token.type` if the node represents an unnested token
- - `Token.type` of the opening token, with "_open" suffix stripped, if
- the node represents a nester token pair
- """
- if self.is_root:
- return "root"
- if self.token:
- return self.token.type
- assert self.nester_tokens
- return _removesuffix(self.nester_tokens.opening.type, "_open")
-
- @property
- def next_sibling(self: _NodeType) -> _NodeType | None:
- """Get the next node in the sequence of siblings.
-
- Returns `None` if this is the last sibling.
- """
- self_index = self.siblings.index(self)
- if self_index + 1 < len(self.siblings):
- return self.siblings[self_index + 1]
- return None
-
- @property
- def previous_sibling(self: _NodeType) -> _NodeType | None:
- """Get the previous node in the sequence of siblings.
-
- Returns `None` if this is the first sibling.
- """
- self_index = self.siblings.index(self)
- if self_index - 1 >= 0:
- return self.siblings[self_index - 1]
- return None
-
- def _add_child(
- self,
- tokens: Sequence[Token],
- ) -> None:
- """Make a child node for `self`."""
- child = type(self)(tokens, create_root=False)
- child.parent = self
- self.children.append(child)
-
- def _set_children_from_tokens(self, tokens: Sequence[Token]) -> None:
- """Convert the token stream to a tree structure and set the resulting
- nodes as children of `self`."""
- reversed_tokens = list(reversed(tokens))
- while reversed_tokens:
- token = reversed_tokens.pop()
-
- if not token.nesting:
- self._add_child([token])
- continue
- if token.nesting != 1:
- raise ValueError("Invalid token nesting")
-
- nested_tokens = [token]
- nesting = 1
- while reversed_tokens and nesting:
- token = reversed_tokens.pop()
- nested_tokens.append(token)
- nesting += token.nesting
- if nesting:
- raise ValueError(f"unclosed tokens starting {nested_tokens[0]}")
-
- self._add_child(nested_tokens)
-
- def pretty(
- self, *, indent: int = 2, show_text: bool = False, _current: int = 0
- ) -> str:
- """Create an XML style string of the tree."""
- prefix = " " * _current
- text = prefix + f"<{self.type}"
- if not self.is_root and self.attrs:
- text += " " + " ".join(f"{k}={v!r}" for k, v in self.attrs.items())
- text += ">"
- if (
- show_text
- and not self.is_root
- and self.type in ("text", "text_special")
- and self.content
- ):
- text += "\n" + textwrap.indent(self.content, prefix + " " * indent)
- for child in self.children:
- text += "\n" + child.pretty(
- indent=indent, show_text=show_text, _current=_current + indent
- )
- return text
-
- def walk(
- self: _NodeType, *, include_self: bool = True
- ) -> Generator[_NodeType, None, None]:
- """Recursively yield all descendant nodes in the tree starting at self.
-
- The order mimics the order of the underlying linear token
- stream (i.e. depth first).
- """
- if include_self:
- yield self
- for child in self.children:
- yield from child.walk(include_self=True)
-
- # NOTE:
- # The values of the properties defined below directly map to properties
- # of the underlying `Token`s. A root node does not translate to a `Token`
- # object, so calling these property getters on a root node will raise an
- # `AttributeError`.
- #
- # There is no mapping for `Token.nesting` because the `is_nested` property
- # provides that data, and can be called on any node type, including root.
-
- def _attribute_token(self) -> Token:
- """Return the `Token` that is used as the data source for the
- properties defined below."""
- if self.token:
- return self.token
- if self.nester_tokens:
- return self.nester_tokens.opening
- raise AttributeError("Root node does not have the accessed attribute")
-
- @property
- def tag(self) -> str:
- """html tag name, e.g. \"p\" """
- return self._attribute_token().tag
-
- @property
- def attrs(self) -> dict[str, str | int | float]:
- """Html attributes."""
- return self._attribute_token().attrs
-
- def attrGet(self, name: str) -> None | str | int | float:
- """Get the value of attribute `name`, or null if it does not exist."""
- return self._attribute_token().attrGet(name)
-
- @property
- def map(self) -> tuple[int, int] | None:
- """Source map info. Format: `tuple[ line_begin, line_end ]`"""
- map_ = self._attribute_token().map
- if map_:
- # Type ignore because `Token`s attribute types are not perfect
- return tuple(map_) # type: ignore
- return None
-
- @property
- def level(self) -> int:
- """nesting level, the same as `state.level`"""
- return self._attribute_token().level
-
- @property
- def content(self) -> str:
- """In a case of self-closing tag (code, html, fence, etc.), it
- has contents of this tag."""
- return self._attribute_token().content
-
- @property
- def markup(self) -> str:
- """'*' or '_' for emphasis, fence string for fence, etc."""
- return self._attribute_token().markup
-
- @property
- def info(self) -> str:
- """fence infostring"""
- return self._attribute_token().info
-
- @property
- def meta(self) -> dict[Any, Any]:
- """A place for plugins to store an arbitrary data."""
- return self._attribute_token().meta
-
- @property
- def block(self) -> bool:
- """True for block-level tokens, false for inline tokens."""
- return self._attribute_token().block
-
- @property
- def hidden(self) -> bool:
- """If it's true, ignore this element when rendering.
- Used for tight lists to hide paragraphs."""
- return self._attribute_token().hidden
-
-
-def _removesuffix(string: str, suffix: str) -> str:
- """Remove a suffix from a string.
-
- Replace this with str.removesuffix() from stdlib when minimum Python
- version is 3.9.
- """
- if suffix and string.endswith(suffix):
- return string[: -len(suffix)]
- return string
diff --git a/spaces/dcarpintero/nlp-summarizer-pegasus/.venv/lib/python3.9/site-packages/matplotlib/axes/_axes.py b/spaces/dcarpintero/nlp-summarizer-pegasus/.venv/lib/python3.9/site-packages/matplotlib/axes/_axes.py
deleted file mode 100644
index dd09f988f43da3f627a96151dbc617be940bd4e9..0000000000000000000000000000000000000000
--- a/spaces/dcarpintero/nlp-summarizer-pegasus/.venv/lib/python3.9/site-packages/matplotlib/axes/_axes.py
+++ /dev/null
@@ -1,8284 +0,0 @@
-import functools
-import itertools
-import logging
-import math
-from numbers import Integral, Number
-
-import numpy as np
-from numpy import ma
-
-import matplotlib as mpl
-import matplotlib.category # Register category unit converter as side effect.
-import matplotlib.cbook as cbook
-import matplotlib.collections as mcoll
-import matplotlib.colors as mcolors
-import matplotlib.contour as mcontour
-import matplotlib.dates # noqa # Register date unit converter as side effect.
-import matplotlib.image as mimage
-import matplotlib.legend as mlegend
-import matplotlib.lines as mlines
-import matplotlib.markers as mmarkers
-import matplotlib.mlab as mlab
-import matplotlib.patches as mpatches
-import matplotlib.path as mpath
-import matplotlib.quiver as mquiver
-import matplotlib.stackplot as mstack
-import matplotlib.streamplot as mstream
-import matplotlib.table as mtable
-import matplotlib.text as mtext
-import matplotlib.ticker as mticker
-import matplotlib.transforms as mtransforms
-import matplotlib.tri as mtri
-import matplotlib.units as munits
-from matplotlib import _api, _docstring, _preprocess_data
-from matplotlib.axes._base import (
- _AxesBase, _TransformedBoundsLocator, _process_plot_format)
-from matplotlib.axes._secondary_axes import SecondaryAxis
-from matplotlib.container import BarContainer, ErrorbarContainer, StemContainer
-
-_log = logging.getLogger(__name__)
-
-
-# The axes module contains all the wrappers to plotting functions.
-# All the other methods should go in the _AxesBase class.
-
-
-@_docstring.interpd
-class Axes(_AxesBase):
- """
- An Axes object encapsulates all the elements of an individual (sub-)plot in
- a figure.
-
- It contains most of the (sub-)plot elements: `~.axis.Axis`,
- `~.axis.Tick`, `~.lines.Line2D`, `~.text.Text`, `~.patches.Polygon`, etc.,
- and sets the coordinate system.
-
- Like all visible elements in a figure, Axes is an `.Artist` subclass.
-
- The `Axes` instance supports callbacks through a callbacks attribute which
- is a `~.cbook.CallbackRegistry` instance. The events you can connect to
- are 'xlim_changed' and 'ylim_changed' and the callback will be called with
- func(*ax*) where *ax* is the `Axes` instance.
-
- .. note::
-
- As a user, you do not instantiate Axes directly, but use Axes creation
- methods instead; e.g. from `.pyplot` or `.Figure`:
- `~.pyplot.subplots`, `~.pyplot.subplot_mosaic` or `.Figure.add_axes`.
-
- Attributes
- ----------
- dataLim : `.Bbox`
- The bounding box enclosing all data displayed in the Axes.
- viewLim : `.Bbox`
- The view limits in data coordinates.
-
- """
- ### Labelling, legend and texts
-
- def get_title(self, loc="center"):
- """
- Get an Axes title.
-
- Get one of the three available Axes titles. The available titles
- are positioned above the Axes in the center, flush with the left
- edge, and flush with the right edge.
-
- Parameters
- ----------
- loc : {'center', 'left', 'right'}, str, default: 'center'
- Which title to return.
-
- Returns
- -------
- str
- The title text string.
-
- """
- titles = {'left': self._left_title,
- 'center': self.title,
- 'right': self._right_title}
- title = _api.check_getitem(titles, loc=loc.lower())
- return title.get_text()
-
- def set_title(self, label, fontdict=None, loc=None, pad=None, *, y=None,
- **kwargs):
- """
- Set a title for the Axes.
-
- Set one of the three available Axes titles. The available titles
- are positioned above the Axes in the center, flush with the left
- edge, and flush with the right edge.
-
- Parameters
- ----------
- label : str
- Text to use for the title
-
- fontdict : dict
- A dictionary controlling the appearance of the title text,
- the default *fontdict* is::
-
- {'fontsize': rcParams['axes.titlesize'],
- 'fontweight': rcParams['axes.titleweight'],
- 'color': rcParams['axes.titlecolor'],
- 'verticalalignment': 'baseline',
- 'horizontalalignment': loc}
-
- loc : {'center', 'left', 'right'}, default: :rc:`axes.titlelocation`
- Which title to set.
-
- y : float, default: :rc:`axes.titley`
- Vertical Axes location for the title (1.0 is the top). If
- None (the default) and :rc:`axes.titley` is also None, y is
- determined automatically to avoid decorators on the Axes.
-
- pad : float, default: :rc:`axes.titlepad`
- The offset of the title from the top of the Axes, in points.
-
- Returns
- -------
- `.Text`
- The matplotlib text instance representing the title
-
- Other Parameters
- ----------------
- **kwargs : `~matplotlib.text.Text` properties
- Other keyword arguments are text properties, see `.Text` for a list
- of valid text properties.
- """
- if loc is None:
- loc = mpl.rcParams['axes.titlelocation']
-
- if y is None:
- y = mpl.rcParams['axes.titley']
- if y is None:
- y = 1.0
- else:
- self._autotitlepos = False
- kwargs['y'] = y
-
- titles = {'left': self._left_title,
- 'center': self.title,
- 'right': self._right_title}
- title = _api.check_getitem(titles, loc=loc.lower())
- default = {
- 'fontsize': mpl.rcParams['axes.titlesize'],
- 'fontweight': mpl.rcParams['axes.titleweight'],
- 'verticalalignment': 'baseline',
- 'horizontalalignment': loc.lower()}
- titlecolor = mpl.rcParams['axes.titlecolor']
- if not cbook._str_lower_equal(titlecolor, 'auto'):
- default["color"] = titlecolor
- if pad is None:
- pad = mpl.rcParams['axes.titlepad']
- self._set_title_offset_trans(float(pad))
- title.set_text(label)
- title.update(default)
- if fontdict is not None:
- title.update(fontdict)
- title._internal_update(kwargs)
- return title
-
- def get_legend_handles_labels(self, legend_handler_map=None):
- """
- Return handles and labels for legend
-
- ``ax.legend()`` is equivalent to ::
-
- h, l = ax.get_legend_handles_labels()
- ax.legend(h, l)
- """
- # pass through to legend.
- handles, labels = mlegend._get_legend_handles_labels(
- [self], legend_handler_map)
- return handles, labels
-
- @_docstring.dedent_interpd
- def legend(self, *args, **kwargs):
- """
- Place a legend on the Axes.
-
- Call signatures::
-
- legend()
- legend(handles, labels)
- legend(handles=handles)
- legend(labels)
-
- The call signatures correspond to the following different ways to use
- this method:
-
- **1. Automatic detection of elements to be shown in the legend**
-
- The elements to be added to the legend are automatically determined,
- when you do not pass in any extra arguments.
-
- In this case, the labels are taken from the artist. You can specify
- them either at artist creation or by calling the
- :meth:`~.Artist.set_label` method on the artist::
-
- ax.plot([1, 2, 3], label='Inline label')
- ax.legend()
-
- or::
-
- line, = ax.plot([1, 2, 3])
- line.set_label('Label via method')
- ax.legend()
-
- .. note::
- Specific artists can be excluded from the automatic legend element
- selection by using a label starting with an underscore, "_".
- A string starting with an underscore is the default label for all
- artists, so calling `.Axes.legend` without any arguments and
- without setting the labels manually will result in no legend being
- drawn.
-
-
- **2. Explicitly listing the artists and labels in the legend**
-
- For full control of which artists have a legend entry, it is possible
- to pass an iterable of legend artists followed by an iterable of
- legend labels respectively::
-
- ax.legend([line1, line2, line3], ['label1', 'label2', 'label3'])
-
-
- **3. Explicitly listing the artists in the legend**
-
- This is similar to 2, but the labels are taken from the artists'
- label properties. Example::
-
- line1, = ax.plot([1, 2, 3], label='label1')
- line2, = ax.plot([1, 2, 3], label='label2')
- ax.legend(handles=[line1, line2])
-
-
- **4. Labeling existing plot elements**
-
- .. admonition:: Discouraged
-
- This call signature is discouraged, because the relation between
- plot elements and labels is only implicit by their order and can
- easily be mixed up.
-
- To make a legend for all artists on an Axes, call this function with
- an iterable of strings, one for each legend item. For example::
-
- ax.plot([1, 2, 3])
- ax.plot([5, 6, 7])
- ax.legend(['First line', 'Second line'])
-
-
- Parameters
- ----------
- handles : sequence of `.Artist`, optional
- A list of Artists (lines, patches) to be added to the legend.
- Use this together with *labels*, if you need full control on what
- is shown in the legend and the automatic mechanism described above
- is not sufficient.
-
- The length of handles and labels should be the same in this
- case. If they are not, they are truncated to the smaller length.
-
- labels : list of str, optional
- A list of labels to show next to the artists.
- Use this together with *handles*, if you need full control on what
- is shown in the legend and the automatic mechanism described above
- is not sufficient.
-
- Returns
- -------
- `~matplotlib.legend.Legend`
-
- Other Parameters
- ----------------
- %(_legend_kw_axes)s
-
- See Also
- --------
- .Figure.legend
-
- Notes
- -----
- Some artists are not supported by this function. See
- :doc:`/tutorials/intermediate/legend_guide` for details.
-
- Examples
- --------
- .. plot:: gallery/text_labels_and_annotations/legend.py
- """
- handles, labels, extra_args, kwargs = mlegend._parse_legend_args(
- [self],
- *args,
- **kwargs)
- if len(extra_args):
- raise TypeError('legend only accepts two non-keyword arguments')
- self.legend_ = mlegend.Legend(self, handles, labels, **kwargs)
- self.legend_._remove_method = self._remove_legend
- return self.legend_
-
- def _remove_legend(self, legend):
- self.legend_ = None
-
- def inset_axes(self, bounds, *, transform=None, zorder=5, **kwargs):
- """
- Add a child inset Axes to this existing Axes.
-
- Warnings
- --------
- This method is experimental as of 3.0, and the API may change.
-
- Parameters
- ----------
- bounds : [x0, y0, width, height]
- Lower-left corner of inset Axes, and its width and height.
-
- transform : `.Transform`
- Defaults to `ax.transAxes`, i.e. the units of *rect* are in
- Axes-relative coordinates.
-
- projection : {None, 'aitoff', 'hammer', 'lambert', 'mollweide', \
-'polar', 'rectilinear', str}, optional
- The projection type of the inset `~.axes.Axes`. *str* is the name
- of a custom projection, see `~matplotlib.projections`. The default
- None results in a 'rectilinear' projection.
-
- polar : bool, default: False
- If True, equivalent to projection='polar'.
-
- axes_class : subclass type of `~.axes.Axes`, optional
- The `.axes.Axes` subclass that is instantiated. This parameter
- is incompatible with *projection* and *polar*. See
- :ref:`axisartist_users-guide-index` for examples.
-
- zorder : number
- Defaults to 5 (same as `.Axes.legend`). Adjust higher or lower
- to change whether it is above or below data plotted on the
- parent Axes.
-
- **kwargs
- Other keyword arguments are passed on to the inset Axes class.
-
- Returns
- -------
- ax
- The created `~.axes.Axes` instance.
-
- Examples
- --------
- This example makes two inset Axes, the first is in Axes-relative
- coordinates, and the second in data-coordinates::
-
- fig, ax = plt.subplots()
- ax.plot(range(10))
- axin1 = ax.inset_axes([0.8, 0.1, 0.15, 0.15])
- axin2 = ax.inset_axes(
- [5, 7, 2.3, 2.3], transform=ax.transData)
-
- """
- if transform is None:
- transform = self.transAxes
- kwargs.setdefault('label', 'inset_axes')
-
- # This puts the rectangle into figure-relative coordinates.
- inset_locator = _TransformedBoundsLocator(bounds, transform)
- bounds = inset_locator(self, None).bounds
- projection_class, pkw = self.figure._process_projection_requirements(
- bounds, **kwargs)
- inset_ax = projection_class(self.figure, bounds, zorder=zorder, **pkw)
-
- # this locator lets the axes move if in data coordinates.
- # it gets called in `ax.apply_aspect() (of all places)
- inset_ax.set_axes_locator(inset_locator)
-
- self.add_child_axes(inset_ax)
-
- return inset_ax
-
- @_docstring.dedent_interpd
- def indicate_inset(self, bounds, inset_ax=None, *, transform=None,
- facecolor='none', edgecolor='0.5', alpha=0.5,
- zorder=4.99, **kwargs):
- """
- Add an inset indicator to the Axes. This is a rectangle on the plot
- at the position indicated by *bounds* that optionally has lines that
- connect the rectangle to an inset Axes (`.Axes.inset_axes`).
-
- Warnings
- --------
- This method is experimental as of 3.0, and the API may change.
-
- Parameters
- ----------
- bounds : [x0, y0, width, height]
- Lower-left corner of rectangle to be marked, and its width
- and height.
-
- inset_ax : `.Axes`
- An optional inset Axes to draw connecting lines to. Two lines are
- drawn connecting the indicator box to the inset Axes on corners
- chosen so as to not overlap with the indicator box.
-
- transform : `.Transform`
- Transform for the rectangle coordinates. Defaults to
- `ax.transAxes`, i.e. the units of *rect* are in Axes-relative
- coordinates.
-
- facecolor : color, default: 'none'
- Facecolor of the rectangle.
-
- edgecolor : color, default: '0.5'
- Color of the rectangle and color of the connecting lines.
-
- alpha : float, default: 0.5
- Transparency of the rectangle and connector lines.
-
- zorder : float, default: 4.99
- Drawing order of the rectangle and connector lines. The default,
- 4.99, is just below the default level of inset Axes.
-
- **kwargs
- Other keyword arguments are passed on to the `.Rectangle` patch:
-
- %(Rectangle:kwdoc)s
-
- Returns
- -------
- rectangle_patch : `.patches.Rectangle`
- The indicator frame.
-
- connector_lines : 4-tuple of `.patches.ConnectionPatch`
- The four connector lines connecting to (lower_left, upper_left,
- lower_right upper_right) corners of *inset_ax*. Two lines are
- set with visibility to *False*, but the user can set the
- visibility to True if the automatic choice is not deemed correct.
-
- """
- # to make the axes connectors work, we need to apply the aspect to
- # the parent axes.
- self.apply_aspect()
-
- if transform is None:
- transform = self.transData
- kwargs.setdefault('label', '_indicate_inset')
-
- x, y, width, height = bounds
- rectangle_patch = mpatches.Rectangle(
- (x, y), width, height,
- facecolor=facecolor, edgecolor=edgecolor, alpha=alpha,
- zorder=zorder, transform=transform, **kwargs)
- self.add_patch(rectangle_patch)
-
- connects = []
-
- if inset_ax is not None:
- # connect the inset_axes to the rectangle
- for xy_inset_ax in [(0, 0), (0, 1), (1, 0), (1, 1)]:
- # inset_ax positions are in axes coordinates
- # The 0, 1 values define the four edges if the inset_ax
- # lower_left, upper_left, lower_right upper_right.
- ex, ey = xy_inset_ax
- if self.xaxis.get_inverted():
- ex = 1 - ex
- if self.yaxis.get_inverted():
- ey = 1 - ey
- xy_data = x + ex * width, y + ey * height
- p = mpatches.ConnectionPatch(
- xyA=xy_inset_ax, coordsA=inset_ax.transAxes,
- xyB=xy_data, coordsB=self.transData,
- arrowstyle="-", zorder=zorder,
- edgecolor=edgecolor, alpha=alpha)
- connects.append(p)
- self.add_patch(p)
-
- # decide which two of the lines to keep visible....
- pos = inset_ax.get_position()
- bboxins = pos.transformed(self.figure.transSubfigure)
- rectbbox = mtransforms.Bbox.from_bounds(
- *bounds
- ).transformed(transform)
- x0 = rectbbox.x0 < bboxins.x0
- x1 = rectbbox.x1 < bboxins.x1
- y0 = rectbbox.y0 < bboxins.y0
- y1 = rectbbox.y1 < bboxins.y1
- connects[0].set_visible(x0 ^ y0)
- connects[1].set_visible(x0 == y1)
- connects[2].set_visible(x1 == y0)
- connects[3].set_visible(x1 ^ y1)
-
- return rectangle_patch, tuple(connects) if connects else None
-
- def indicate_inset_zoom(self, inset_ax, **kwargs):
- """
- Add an inset indicator rectangle to the Axes based on the axis
- limits for an *inset_ax* and draw connectors between *inset_ax*
- and the rectangle.
-
- Warnings
- --------
- This method is experimental as of 3.0, and the API may change.
-
- Parameters
- ----------
- inset_ax : `.Axes`
- Inset Axes to draw connecting lines to. Two lines are
- drawn connecting the indicator box to the inset Axes on corners
- chosen so as to not overlap with the indicator box.
-
- **kwargs
- Other keyword arguments are passed on to `.Axes.indicate_inset`
-
- Returns
- -------
- rectangle_patch : `.patches.Rectangle`
- Rectangle artist.
-
- connector_lines : 4-tuple of `.patches.ConnectionPatch`
- Each of four connector lines coming from the rectangle drawn on
- this axis, in the order lower left, upper left, lower right,
- upper right.
- Two are set with visibility to *False*, but the user can
- set the visibility to *True* if the automatic choice is not deemed
- correct.
- """
-
- xlim = inset_ax.get_xlim()
- ylim = inset_ax.get_ylim()
- rect = (xlim[0], ylim[0], xlim[1] - xlim[0], ylim[1] - ylim[0])
- return self.indicate_inset(rect, inset_ax, **kwargs)
-
- @_docstring.dedent_interpd
- def secondary_xaxis(self, location, *, functions=None, **kwargs):
- """
- Add a second x-axis to this `~.axes.Axes`.
-
- For example if we want to have a second scale for the data plotted on
- the xaxis.
-
- %(_secax_docstring)s
-
- Examples
- --------
- The main axis shows frequency, and the secondary axis shows period.
-
- .. plot::
-
- fig, ax = plt.subplots()
- ax.loglog(range(1, 360, 5), range(1, 360, 5))
- ax.set_xlabel('frequency [Hz]')
-
- def invert(x):
- # 1/x with special treatment of x == 0
- x = np.array(x).astype(float)
- near_zero = np.isclose(x, 0)
- x[near_zero] = np.inf
- x[~near_zero] = 1 / x[~near_zero]
- return x
-
- # the inverse of 1/x is itself
- secax = ax.secondary_xaxis('top', functions=(invert, invert))
- secax.set_xlabel('Period [s]')
- plt.show()
- """
- if location in ['top', 'bottom'] or isinstance(location, Number):
- secondary_ax = SecondaryAxis(self, 'x', location, functions,
- **kwargs)
- self.add_child_axes(secondary_ax)
- return secondary_ax
- else:
- raise ValueError('secondary_xaxis location must be either '
- 'a float or "top"/"bottom"')
-
- @_docstring.dedent_interpd
- def secondary_yaxis(self, location, *, functions=None, **kwargs):
- """
- Add a second y-axis to this `~.axes.Axes`.
-
- For example if we want to have a second scale for the data plotted on
- the yaxis.
-
- %(_secax_docstring)s
-
- Examples
- --------
- Add a secondary Axes that converts from radians to degrees
-
- .. plot::
-
- fig, ax = plt.subplots()
- ax.plot(range(1, 360, 5), range(1, 360, 5))
- ax.set_ylabel('degrees')
- secax = ax.secondary_yaxis('right', functions=(np.deg2rad,
- np.rad2deg))
- secax.set_ylabel('radians')
- """
- if location in ['left', 'right'] or isinstance(location, Number):
- secondary_ax = SecondaryAxis(self, 'y', location,
- functions, **kwargs)
- self.add_child_axes(secondary_ax)
- return secondary_ax
- else:
- raise ValueError('secondary_yaxis location must be either '
- 'a float or "left"/"right"')
-
- @_docstring.dedent_interpd
- def text(self, x, y, s, fontdict=None, **kwargs):
- """
- Add text to the Axes.
-
- Add the text *s* to the Axes at location *x*, *y* in data coordinates.
-
- Parameters
- ----------
- x, y : float
- The position to place the text. By default, this is in data
- coordinates. The coordinate system can be changed using the
- *transform* parameter.
-
- s : str
- The text.
-
- fontdict : dict, default: None
- A dictionary to override the default text properties. If fontdict
- is None, the defaults are determined by `.rcParams`.
-
- Returns
- -------
- `.Text`
- The created `.Text` instance.
-
- Other Parameters
- ----------------
- **kwargs : `~matplotlib.text.Text` properties.
- Other miscellaneous text parameters.
-
- %(Text:kwdoc)s
-
- Examples
- --------
- Individual keyword arguments can be used to override any given
- parameter::
-
- >>> text(x, y, s, fontsize=12)
-
- The default transform specifies that text is in data coords,
- alternatively, you can specify text in axis coords ((0, 0) is
- lower-left and (1, 1) is upper-right). The example below places
- text in the center of the Axes::
-
- >>> text(0.5, 0.5, 'matplotlib', horizontalalignment='center',
- ... verticalalignment='center', transform=ax.transAxes)
-
- You can put a rectangular box around the text instance (e.g., to
- set a background color) by using the keyword *bbox*. *bbox* is
- a dictionary of `~matplotlib.patches.Rectangle`
- properties. For example::
-
- >>> text(x, y, s, bbox=dict(facecolor='red', alpha=0.5))
- """
- effective_kwargs = {
- 'verticalalignment': 'baseline',
- 'horizontalalignment': 'left',
- 'transform': self.transData,
- 'clip_on': False,
- **(fontdict if fontdict is not None else {}),
- **kwargs,
- }
- t = mtext.Text(x, y, text=s, **effective_kwargs)
- t.set_clip_path(self.patch)
- self._add_text(t)
- return t
-
- @_docstring.dedent_interpd
- def annotate(self, text, xy, xytext=None, xycoords='data', textcoords=None,
- arrowprops=None, annotation_clip=None, **kwargs):
- # Signature must match Annotation. This is verified in
- # test_annotate_signature().
- a = mtext.Annotation(text, xy, xytext=xytext, xycoords=xycoords,
- textcoords=textcoords, arrowprops=arrowprops,
- annotation_clip=annotation_clip, **kwargs)
- a.set_transform(mtransforms.IdentityTransform())
- if 'clip_on' in kwargs:
- a.set_clip_path(self.patch)
- self._add_text(a)
- return a
- annotate.__doc__ = mtext.Annotation.__init__.__doc__
- #### Lines and spans
-
- @_docstring.dedent_interpd
- def axhline(self, y=0, xmin=0, xmax=1, **kwargs):
- """
- Add a horizontal line across the Axes.
-
- Parameters
- ----------
- y : float, default: 0
- y position in data coordinates of the horizontal line.
-
- xmin : float, default: 0
- Should be between 0 and 1, 0 being the far left of the plot, 1 the
- far right of the plot.
-
- xmax : float, default: 1
- Should be between 0 and 1, 0 being the far left of the plot, 1 the
- far right of the plot.
-
- Returns
- -------
- `~matplotlib.lines.Line2D`
-
- Other Parameters
- ----------------
- **kwargs
- Valid keyword arguments are `.Line2D` properties, except for
- 'transform':
-
- %(Line2D:kwdoc)s
-
- See Also
- --------
- hlines : Add horizontal lines in data coordinates.
- axhspan : Add a horizontal span (rectangle) across the axis.
- axline : Add a line with an arbitrary slope.
-
- Examples
- --------
- * draw a thick red hline at 'y' = 0 that spans the xrange::
-
- >>> axhline(linewidth=4, color='r')
-
- * draw a default hline at 'y' = 1 that spans the xrange::
-
- >>> axhline(y=1)
-
- * draw a default hline at 'y' = .5 that spans the middle half of
- the xrange::
-
- >>> axhline(y=.5, xmin=0.25, xmax=0.75)
- """
- self._check_no_units([xmin, xmax], ['xmin', 'xmax'])
- if "transform" in kwargs:
- raise ValueError("'transform' is not allowed as a keyword "
- "argument; axhline generates its own transform.")
- ymin, ymax = self.get_ybound()
-
- # Strip away the units for comparison with non-unitized bounds.
- yy, = self._process_unit_info([("y", y)], kwargs)
- scaley = (yy < ymin) or (yy > ymax)
-
- trans = self.get_yaxis_transform(which='grid')
- l = mlines.Line2D([xmin, xmax], [y, y], transform=trans, **kwargs)
- self.add_line(l)
- if scaley:
- self._request_autoscale_view("y")
- return l
-
- @_docstring.dedent_interpd
- def axvline(self, x=0, ymin=0, ymax=1, **kwargs):
- """
- Add a vertical line across the Axes.
-
- Parameters
- ----------
- x : float, default: 0
- x position in data coordinates of the vertical line.
-
- ymin : float, default: 0
- Should be between 0 and 1, 0 being the bottom of the plot, 1 the
- top of the plot.
-
- ymax : float, default: 1
- Should be between 0 and 1, 0 being the bottom of the plot, 1 the
- top of the plot.
-
- Returns
- -------
- `~matplotlib.lines.Line2D`
-
- Other Parameters
- ----------------
- **kwargs
- Valid keyword arguments are `.Line2D` properties, except for
- 'transform':
-
- %(Line2D:kwdoc)s
-
- See Also
- --------
- vlines : Add vertical lines in data coordinates.
- axvspan : Add a vertical span (rectangle) across the axis.
- axline : Add a line with an arbitrary slope.
-
- Examples
- --------
- * draw a thick red vline at *x* = 0 that spans the yrange::
-
- >>> axvline(linewidth=4, color='r')
-
- * draw a default vline at *x* = 1 that spans the yrange::
-
- >>> axvline(x=1)
-
- * draw a default vline at *x* = .5 that spans the middle half of
- the yrange::
-
- >>> axvline(x=.5, ymin=0.25, ymax=0.75)
- """
- self._check_no_units([ymin, ymax], ['ymin', 'ymax'])
- if "transform" in kwargs:
- raise ValueError("'transform' is not allowed as a keyword "
- "argument; axvline generates its own transform.")
- xmin, xmax = self.get_xbound()
-
- # Strip away the units for comparison with non-unitized bounds.
- xx, = self._process_unit_info([("x", x)], kwargs)
- scalex = (xx < xmin) or (xx > xmax)
-
- trans = self.get_xaxis_transform(which='grid')
- l = mlines.Line2D([x, x], [ymin, ymax], transform=trans, **kwargs)
- self.add_line(l)
- if scalex:
- self._request_autoscale_view("x")
- return l
-
- @staticmethod
- def _check_no_units(vals, names):
- # Helper method to check that vals are not unitized
- for val, name in zip(vals, names):
- if not munits._is_natively_supported(val):
- raise ValueError(f"{name} must be a single scalar value, "
- f"but got {val}")
-
- @_docstring.dedent_interpd
- def axline(self, xy1, xy2=None, *, slope=None, **kwargs):
- """
- Add an infinitely long straight line.
-
- The line can be defined either by two points *xy1* and *xy2*, or
- by one point *xy1* and a *slope*.
-
- This draws a straight line "on the screen", regardless of the x and y
- scales, and is thus also suitable for drawing exponential decays in
- semilog plots, power laws in loglog plots, etc. However, *slope*
- should only be used with linear scales; It has no clear meaning for
- all other scales, and thus the behavior is undefined. Please specify
- the line using the points *xy1*, *xy2* for non-linear scales.
-
- The *transform* keyword argument only applies to the points *xy1*,
- *xy2*. The *slope* (if given) is always in data coordinates. This can
- be used e.g. with ``ax.transAxes`` for drawing grid lines with a fixed
- slope.
-
- Parameters
- ----------
- xy1, xy2 : (float, float)
- Points for the line to pass through.
- Either *xy2* or *slope* has to be given.
- slope : float, optional
- The slope of the line. Either *xy2* or *slope* has to be given.
-
- Returns
- -------
- `.Line2D`
-
- Other Parameters
- ----------------
- **kwargs
- Valid kwargs are `.Line2D` properties
-
- %(Line2D:kwdoc)s
-
- See Also
- --------
- axhline : for horizontal lines
- axvline : for vertical lines
-
- Examples
- --------
- Draw a thick red line passing through (0, 0) and (1, 1)::
-
- >>> axline((0, 0), (1, 1), linewidth=4, color='r')
- """
- if slope is not None and (self.get_xscale() != 'linear' or
- self.get_yscale() != 'linear'):
- raise TypeError("'slope' cannot be used with non-linear scales")
-
- datalim = [xy1] if xy2 is None else [xy1, xy2]
- if "transform" in kwargs:
- # if a transform is passed (i.e. line points not in data space),
- # data limits should not be adjusted.
- datalim = []
-
- line = mlines._AxLine(xy1, xy2, slope, **kwargs)
- # Like add_line, but correctly handling data limits.
- self._set_artist_props(line)
- if line.get_clip_path() is None:
- line.set_clip_path(self.patch)
- if not line.get_label():
- line.set_label(f"_child{len(self._children)}")
- self._children.append(line)
- line._remove_method = self._children.remove
- self.update_datalim(datalim)
-
- self._request_autoscale_view()
- return line
-
- @_docstring.dedent_interpd
- def axhspan(self, ymin, ymax, xmin=0, xmax=1, **kwargs):
- """
- Add a horizontal span (rectangle) across the Axes.
-
- The rectangle spans from *ymin* to *ymax* vertically, and, by default,
- the whole x-axis horizontally. The x-span can be set using *xmin*
- (default: 0) and *xmax* (default: 1) which are in axis units; e.g.
- ``xmin = 0.5`` always refers to the middle of the x-axis regardless of
- the limits set by `~.Axes.set_xlim`.
-
- Parameters
- ----------
- ymin : float
- Lower y-coordinate of the span, in data units.
- ymax : float
- Upper y-coordinate of the span, in data units.
- xmin : float, default: 0
- Lower x-coordinate of the span, in x-axis (0-1) units.
- xmax : float, default: 1
- Upper x-coordinate of the span, in x-axis (0-1) units.
-
- Returns
- -------
- `~matplotlib.patches.Polygon`
- Horizontal span (rectangle) from (xmin, ymin) to (xmax, ymax).
-
- Other Parameters
- ----------------
- **kwargs : `~matplotlib.patches.Polygon` properties
-
- %(Polygon:kwdoc)s
-
- See Also
- --------
- axvspan : Add a vertical span across the Axes.
- """
- # Strip units away.
- self._check_no_units([xmin, xmax], ['xmin', 'xmax'])
- (ymin, ymax), = self._process_unit_info([("y", [ymin, ymax])], kwargs)
-
- verts = (xmin, ymin), (xmin, ymax), (xmax, ymax), (xmax, ymin)
- p = mpatches.Polygon(verts, **kwargs)
- p.set_transform(self.get_yaxis_transform(which="grid"))
- self.add_patch(p)
- self._request_autoscale_view("y")
- return p
-
- @_docstring.dedent_interpd
- def axvspan(self, xmin, xmax, ymin=0, ymax=1, **kwargs):
- """
- Add a vertical span (rectangle) across the Axes.
-
- The rectangle spans from *xmin* to *xmax* horizontally, and, by
- default, the whole y-axis vertically. The y-span can be set using
- *ymin* (default: 0) and *ymax* (default: 1) which are in axis units;
- e.g. ``ymin = 0.5`` always refers to the middle of the y-axis
- regardless of the limits set by `~.Axes.set_ylim`.
-
- Parameters
- ----------
- xmin : float
- Lower x-coordinate of the span, in data units.
- xmax : float
- Upper x-coordinate of the span, in data units.
- ymin : float, default: 0
- Lower y-coordinate of the span, in y-axis units (0-1).
- ymax : float, default: 1
- Upper y-coordinate of the span, in y-axis units (0-1).
-
- Returns
- -------
- `~matplotlib.patches.Polygon`
- Vertical span (rectangle) from (xmin, ymin) to (xmax, ymax).
-
- Other Parameters
- ----------------
- **kwargs : `~matplotlib.patches.Polygon` properties
-
- %(Polygon:kwdoc)s
-
- See Also
- --------
- axhspan : Add a horizontal span across the Axes.
-
- Examples
- --------
- Draw a vertical, green, translucent rectangle from x = 1.25 to
- x = 1.55 that spans the yrange of the Axes.
-
- >>> axvspan(1.25, 1.55, facecolor='g', alpha=0.5)
-
- """
- # Strip units away.
- self._check_no_units([ymin, ymax], ['ymin', 'ymax'])
- (xmin, xmax), = self._process_unit_info([("x", [xmin, xmax])], kwargs)
-
- verts = [(xmin, ymin), (xmin, ymax), (xmax, ymax), (xmax, ymin)]
- p = mpatches.Polygon(verts, **kwargs)
- p.set_transform(self.get_xaxis_transform(which="grid"))
- p.get_path()._interpolation_steps = 100
- self.add_patch(p)
- self._request_autoscale_view("x")
- return p
-
- @_preprocess_data(replace_names=["y", "xmin", "xmax", "colors"],
- label_namer="y")
- def hlines(self, y, xmin, xmax, colors=None, linestyles='solid',
- label='', **kwargs):
- """
- Plot horizontal lines at each *y* from *xmin* to *xmax*.
-
- Parameters
- ----------
- y : float or array-like
- y-indexes where to plot the lines.
-
- xmin, xmax : float or array-like
- Respective beginning and end of each line. If scalars are
- provided, all lines will have the same length.
-
- colors : list of colors, default: :rc:`lines.color`
-
- linestyles : {'solid', 'dashed', 'dashdot', 'dotted'}, optional
-
- label : str, default: ''
-
- Returns
- -------
- `~matplotlib.collections.LineCollection`
-
- Other Parameters
- ----------------
- data : indexable object, optional
- DATA_PARAMETER_PLACEHOLDER
- **kwargs : `~matplotlib.collections.LineCollection` properties.
-
- See Also
- --------
- vlines : vertical lines
- axhline : horizontal line across the Axes
- """
-
- # We do the conversion first since not all unitized data is uniform
- xmin, xmax, y = self._process_unit_info(
- [("x", xmin), ("x", xmax), ("y", y)], kwargs)
-
- if not np.iterable(y):
- y = [y]
- if not np.iterable(xmin):
- xmin = [xmin]
- if not np.iterable(xmax):
- xmax = [xmax]
-
- # Create and combine masked_arrays from input
- y, xmin, xmax = cbook._combine_masks(y, xmin, xmax)
- y = np.ravel(y)
- xmin = np.ravel(xmin)
- xmax = np.ravel(xmax)
-
- masked_verts = np.ma.empty((len(y), 2, 2))
- masked_verts[:, 0, 0] = xmin
- masked_verts[:, 0, 1] = y
- masked_verts[:, 1, 0] = xmax
- masked_verts[:, 1, 1] = y
-
- lines = mcoll.LineCollection(masked_verts, colors=colors,
- linestyles=linestyles, label=label)
- self.add_collection(lines, autolim=False)
- lines._internal_update(kwargs)
-
- if len(y) > 0:
- # Extreme values of xmin/xmax/y. Using masked_verts here handles
- # the case of y being a masked *object* array (as can be generated
- # e.g. by errorbar()), which would make nanmin/nanmax stumble.
- minx = np.nanmin(masked_verts[..., 0])
- maxx = np.nanmax(masked_verts[..., 0])
- miny = np.nanmin(masked_verts[..., 1])
- maxy = np.nanmax(masked_verts[..., 1])
- corners = (minx, miny), (maxx, maxy)
- self.update_datalim(corners)
- self._request_autoscale_view()
-
- return lines
-
- @_preprocess_data(replace_names=["x", "ymin", "ymax", "colors"],
- label_namer="x")
- def vlines(self, x, ymin, ymax, colors=None, linestyles='solid',
- label='', **kwargs):
- """
- Plot vertical lines at each *x* from *ymin* to *ymax*.
-
- Parameters
- ----------
- x : float or array-like
- x-indexes where to plot the lines.
-
- ymin, ymax : float or array-like
- Respective beginning and end of each line. If scalars are
- provided, all lines will have the same length.
-
- colors : list of colors, default: :rc:`lines.color`
-
- linestyles : {'solid', 'dashed', 'dashdot', 'dotted'}, optional
-
- label : str, default: ''
-
- Returns
- -------
- `~matplotlib.collections.LineCollection`
-
- Other Parameters
- ----------------
- data : indexable object, optional
- DATA_PARAMETER_PLACEHOLDER
- **kwargs : `~matplotlib.collections.LineCollection` properties.
-
- See Also
- --------
- hlines : horizontal lines
- axvline : vertical line across the Axes
- """
-
- # We do the conversion first since not all unitized data is uniform
- x, ymin, ymax = self._process_unit_info(
- [("x", x), ("y", ymin), ("y", ymax)], kwargs)
-
- if not np.iterable(x):
- x = [x]
- if not np.iterable(ymin):
- ymin = [ymin]
- if not np.iterable(ymax):
- ymax = [ymax]
-
- # Create and combine masked_arrays from input
- x, ymin, ymax = cbook._combine_masks(x, ymin, ymax)
- x = np.ravel(x)
- ymin = np.ravel(ymin)
- ymax = np.ravel(ymax)
-
- masked_verts = np.ma.empty((len(x), 2, 2))
- masked_verts[:, 0, 0] = x
- masked_verts[:, 0, 1] = ymin
- masked_verts[:, 1, 0] = x
- masked_verts[:, 1, 1] = ymax
-
- lines = mcoll.LineCollection(masked_verts, colors=colors,
- linestyles=linestyles, label=label)
- self.add_collection(lines, autolim=False)
- lines._internal_update(kwargs)
-
- if len(x) > 0:
- # Extreme values of x/ymin/ymax. Using masked_verts here handles
- # the case of x being a masked *object* array (as can be generated
- # e.g. by errorbar()), which would make nanmin/nanmax stumble.
- minx = np.nanmin(masked_verts[..., 0])
- maxx = np.nanmax(masked_verts[..., 0])
- miny = np.nanmin(masked_verts[..., 1])
- maxy = np.nanmax(masked_verts[..., 1])
- corners = (minx, miny), (maxx, maxy)
- self.update_datalim(corners)
- self._request_autoscale_view()
-
- return lines
-
- @_preprocess_data(replace_names=["positions", "lineoffsets",
- "linelengths", "linewidths",
- "colors", "linestyles"])
- @_docstring.dedent_interpd
- def eventplot(self, positions, orientation='horizontal', lineoffsets=1,
- linelengths=1, linewidths=None, colors=None, alpha=None,
- linestyles='solid', **kwargs):
- """
- Plot identical parallel lines at the given positions.
-
- This type of plot is commonly used in neuroscience for representing
- neural events, where it is usually called a spike raster, dot raster,
- or raster plot.
-
- However, it is useful in any situation where you wish to show the
- timing or position of multiple sets of discrete events, such as the
- arrival times of people to a business on each day of the month or the
- date of hurricanes each year of the last century.
-
- Parameters
- ----------
- positions : array-like or list of array-like
- A 1D array-like defines the positions of one sequence of events.
-
- Multiple groups of events may be passed as a list of array-likes.
- Each group can be styled independently by passing lists of values
- to *lineoffsets*, *linelengths*, *linewidths*, *colors* and
- *linestyles*.
-
- Note that *positions* can be a 2D array, but in practice different
- event groups usually have different counts so that one will use a
- list of different-length arrays rather than a 2D array.
-
- orientation : {'horizontal', 'vertical'}, default: 'horizontal'
- The direction of the event sequence:
-
- - 'horizontal': the events are arranged horizontally.
- The indicator lines are vertical.
- - 'vertical': the events are arranged vertically.
- The indicator lines are horizontal.
-
- lineoffsets : float or array-like, default: 1
- The offset of the center of the lines from the origin, in the
- direction orthogonal to *orientation*.
-
- If *positions* is 2D, this can be a sequence with length matching
- the length of *positions*.
-
- linelengths : float or array-like, default: 1
- The total height of the lines (i.e. the lines stretches from
- ``lineoffset - linelength/2`` to ``lineoffset + linelength/2``).
-
- If *positions* is 2D, this can be a sequence with length matching
- the length of *positions*.
-
- linewidths : float or array-like, default: :rc:`lines.linewidth`
- The line width(s) of the event lines, in points.
-
- If *positions* is 2D, this can be a sequence with length matching
- the length of *positions*.
-
- colors : color or list of colors, default: :rc:`lines.color`
- The color(s) of the event lines.
-
- If *positions* is 2D, this can be a sequence with length matching
- the length of *positions*.
-
- alpha : float or array-like, default: 1
- The alpha blending value(s), between 0 (transparent) and 1
- (opaque).
-
- If *positions* is 2D, this can be a sequence with length matching
- the length of *positions*.
-
- linestyles : str or tuple or list of such values, default: 'solid'
- Default is 'solid'. Valid strings are ['solid', 'dashed',
- 'dashdot', 'dotted', '-', '--', '-.', ':']. Dash tuples
- should be of the form::
-
- (offset, onoffseq),
-
- where *onoffseq* is an even length tuple of on and off ink
- in points.
-
- If *positions* is 2D, this can be a sequence with length matching
- the length of *positions*.
-
- data : indexable object, optional
- DATA_PARAMETER_PLACEHOLDER
-
- **kwargs
- Other keyword arguments are line collection properties. See
- `.LineCollection` for a list of the valid properties.
-
- Returns
- -------
- list of `.EventCollection`
- The `.EventCollection` that were added.
-
- Notes
- -----
- For *linelengths*, *linewidths*, *colors*, *alpha* and *linestyles*, if
- only a single value is given, that value is applied to all lines. If an
- array-like is given, it must have the same length as *positions*, and
- each value will be applied to the corresponding row of the array.
-
- Examples
- --------
- .. plot:: gallery/lines_bars_and_markers/eventplot_demo.py
- """
-
- lineoffsets, linelengths = self._process_unit_info(
- [("y", lineoffsets), ("y", linelengths)], kwargs)
-
- # fix positions, noting that it can be a list of lists:
- if not np.iterable(positions):
- positions = [positions]
- elif any(np.iterable(position) for position in positions):
- positions = [np.asanyarray(position) for position in positions]
- else:
- positions = [np.asanyarray(positions)]
-
- if len(positions) == 0:
- return []
-
- poss = []
- for position in positions:
- poss += self._process_unit_info([("x", position)], kwargs)
- positions = poss
-
- # prevent 'singular' keys from **kwargs dict from overriding the effect
- # of 'plural' keyword arguments (e.g. 'color' overriding 'colors')
- colors = cbook._local_over_kwdict(colors, kwargs, 'color')
- linewidths = cbook._local_over_kwdict(linewidths, kwargs, 'linewidth')
- linestyles = cbook._local_over_kwdict(linestyles, kwargs, 'linestyle')
-
- if not np.iterable(lineoffsets):
- lineoffsets = [lineoffsets]
- if not np.iterable(linelengths):
- linelengths = [linelengths]
- if not np.iterable(linewidths):
- linewidths = [linewidths]
- if not np.iterable(colors):
- colors = [colors]
- if not np.iterable(alpha):
- alpha = [alpha]
- if hasattr(linestyles, 'lower') or not np.iterable(linestyles):
- linestyles = [linestyles]
-
- lineoffsets = np.asarray(lineoffsets)
- linelengths = np.asarray(linelengths)
- linewidths = np.asarray(linewidths)
-
- if len(lineoffsets) == 0:
- lineoffsets = [None]
- if len(linelengths) == 0:
- linelengths = [None]
- if len(linewidths) == 0:
- lineoffsets = [None]
- if len(linewidths) == 0:
- lineoffsets = [None]
- if len(colors) == 0:
- colors = [None]
- try:
- # Early conversion of the colors into RGBA values to take care
- # of cases like colors='0.5' or colors='C1'. (Issue #8193)
- colors = mcolors.to_rgba_array(colors)
- except ValueError:
- # Will fail if any element of *colors* is None. But as long
- # as len(colors) == 1 or len(positions), the rest of the
- # code should process *colors* properly.
- pass
-
- if len(lineoffsets) == 1 and len(positions) != 1:
- lineoffsets = np.tile(lineoffsets, len(positions))
- lineoffsets[0] = 0
- lineoffsets = np.cumsum(lineoffsets)
- if len(linelengths) == 1:
- linelengths = np.tile(linelengths, len(positions))
- if len(linewidths) == 1:
- linewidths = np.tile(linewidths, len(positions))
- if len(colors) == 1:
- colors = list(colors) * len(positions)
- if len(alpha) == 1:
- alpha = list(alpha) * len(positions)
- if len(linestyles) == 1:
- linestyles = [linestyles] * len(positions)
-
- if len(lineoffsets) != len(positions):
- raise ValueError('lineoffsets and positions are unequal sized '
- 'sequences')
- if len(linelengths) != len(positions):
- raise ValueError('linelengths and positions are unequal sized '
- 'sequences')
- if len(linewidths) != len(positions):
- raise ValueError('linewidths and positions are unequal sized '
- 'sequences')
- if len(colors) != len(positions):
- raise ValueError('colors and positions are unequal sized '
- 'sequences')
- if len(alpha) != len(positions):
- raise ValueError('alpha and positions are unequal sized '
- 'sequences')
- if len(linestyles) != len(positions):
- raise ValueError('linestyles and positions are unequal sized '
- 'sequences')
-
- colls = []
- for position, lineoffset, linelength, linewidth, color, alpha_, \
- linestyle in \
- zip(positions, lineoffsets, linelengths, linewidths,
- colors, alpha, linestyles):
- coll = mcoll.EventCollection(position,
- orientation=orientation,
- lineoffset=lineoffset,
- linelength=linelength,
- linewidth=linewidth,
- color=color,
- alpha=alpha_,
- linestyle=linestyle)
- self.add_collection(coll, autolim=False)
- coll._internal_update(kwargs)
- colls.append(coll)
-
- if len(positions) > 0:
- # try to get min/max
- min_max = [(np.min(_p), np.max(_p)) for _p in positions
- if len(_p) > 0]
- # if we have any non-empty positions, try to autoscale
- if len(min_max) > 0:
- mins, maxes = zip(*min_max)
- minpos = np.min(mins)
- maxpos = np.max(maxes)
-
- minline = (lineoffsets - linelengths).min()
- maxline = (lineoffsets + linelengths).max()
-
- if orientation == "vertical":
- corners = (minline, minpos), (maxline, maxpos)
- else: # "horizontal"
- corners = (minpos, minline), (maxpos, maxline)
- self.update_datalim(corners)
- self._request_autoscale_view()
-
- return colls
-
- #### Basic plotting
-
- # Uses a custom implementation of data-kwarg handling in
- # _process_plot_var_args.
- @_docstring.dedent_interpd
- def plot(self, *args, scalex=True, scaley=True, data=None, **kwargs):
- """
- Plot y versus x as lines and/or markers.
-
- Call signatures::
-
- plot([x], y, [fmt], *, data=None, **kwargs)
- plot([x], y, [fmt], [x2], y2, [fmt2], ..., **kwargs)
-
- The coordinates of the points or line nodes are given by *x*, *y*.
-
- The optional parameter *fmt* is a convenient way for defining basic
- formatting like color, marker and linestyle. It's a shortcut string
- notation described in the *Notes* section below.
-
- >>> plot(x, y) # plot x and y using default line style and color
- >>> plot(x, y, 'bo') # plot x and y using blue circle markers
- >>> plot(y) # plot y using x as index array 0..N-1
- >>> plot(y, 'r+') # ditto, but with red plusses
-
- You can use `.Line2D` properties as keyword arguments for more
- control on the appearance. Line properties and *fmt* can be mixed.
- The following two calls yield identical results:
-
- >>> plot(x, y, 'go--', linewidth=2, markersize=12)
- >>> plot(x, y, color='green', marker='o', linestyle='dashed',
- ... linewidth=2, markersize=12)
-
- When conflicting with *fmt*, keyword arguments take precedence.
-
-
- **Plotting labelled data**
-
- There's a convenient way for plotting objects with labelled data (i.e.
- data that can be accessed by index ``obj['y']``). Instead of giving
- the data in *x* and *y*, you can provide the object in the *data*
- parameter and just give the labels for *x* and *y*::
-
- >>> plot('xlabel', 'ylabel', data=obj)
-
- All indexable objects are supported. This could e.g. be a `dict`, a
- `pandas.DataFrame` or a structured numpy array.
-
-
- **Plotting multiple sets of data**
-
- There are various ways to plot multiple sets of data.
-
- - The most straight forward way is just to call `plot` multiple times.
- Example:
-
- >>> plot(x1, y1, 'bo')
- >>> plot(x2, y2, 'go')
-
- - If *x* and/or *y* are 2D arrays a separate data set will be drawn
- for every column. If both *x* and *y* are 2D, they must have the
- same shape. If only one of them is 2D with shape (N, m) the other
- must have length N and will be used for every data set m.
-
- Example:
-
- >>> x = [1, 2, 3]
- >>> y = np.array([[1, 2], [3, 4], [5, 6]])
- >>> plot(x, y)
-
- is equivalent to:
-
- >>> for col in range(y.shape[1]):
- ... plot(x, y[:, col])
-
- - The third way is to specify multiple sets of *[x]*, *y*, *[fmt]*
- groups::
-
- >>> plot(x1, y1, 'g^', x2, y2, 'g-')
-
- In this case, any additional keyword argument applies to all
- datasets. Also, this syntax cannot be combined with the *data*
- parameter.
-
- By default, each line is assigned a different style specified by a
- 'style cycle'. The *fmt* and line property parameters are only
- necessary if you want explicit deviations from these defaults.
- Alternatively, you can also change the style cycle using
- :rc:`axes.prop_cycle`.
-
-
- Parameters
- ----------
- x, y : array-like or scalar
- The horizontal / vertical coordinates of the data points.
- *x* values are optional and default to ``range(len(y))``.
-
- Commonly, these parameters are 1D arrays.
-
- They can also be scalars, or two-dimensional (in that case, the
- columns represent separate data sets).
-
- These arguments cannot be passed as keywords.
-
- fmt : str, optional
- A format string, e.g. 'ro' for red circles. See the *Notes*
- section for a full description of the format strings.
-
- Format strings are just an abbreviation for quickly setting
- basic line properties. All of these and more can also be
- controlled by keyword arguments.
-
- This argument cannot be passed as keyword.
-
- data : indexable object, optional
- An object with labelled data. If given, provide the label names to
- plot in *x* and *y*.
-
- .. note::
- Technically there's a slight ambiguity in calls where the
- second label is a valid *fmt*. ``plot('n', 'o', data=obj)``
- could be ``plt(x, y)`` or ``plt(y, fmt)``. In such cases,
- the former interpretation is chosen, but a warning is issued.
- You may suppress the warning by adding an empty format string
- ``plot('n', 'o', '', data=obj)``.
-
- Returns
- -------
- list of `.Line2D`
- A list of lines representing the plotted data.
-
- Other Parameters
- ----------------
- scalex, scaley : bool, default: True
- These parameters determine if the view limits are adapted to the
- data limits. The values are passed on to
- `~.axes.Axes.autoscale_view`.
-
- **kwargs : `~matplotlib.lines.Line2D` properties, optional
- *kwargs* are used to specify properties like a line label (for
- auto legends), linewidth, antialiasing, marker face color.
- Example::
-
- >>> plot([1, 2, 3], [1, 2, 3], 'go-', label='line 1', linewidth=2)
- >>> plot([1, 2, 3], [1, 4, 9], 'rs', label='line 2')
-
- If you specify multiple lines with one plot call, the kwargs apply
- to all those lines. In case the label object is iterable, each
- element is used as labels for each set of data.
-
- Here is a list of available `.Line2D` properties:
-
- %(Line2D:kwdoc)s
-
- See Also
- --------
- scatter : XY scatter plot with markers of varying size and/or color (
- sometimes also called bubble chart).
-
- Notes
- -----
- **Format Strings**
-
- A format string consists of a part for color, marker and line::
-
- fmt = '[marker][line][color]'
-
- Each of them is optional. If not provided, the value from the style
- cycle is used. Exception: If ``line`` is given, but no ``marker``,
- the data will be a line without markers.
-
- Other combinations such as ``[color][marker][line]`` are also
- supported, but note that their parsing may be ambiguous.
-
- **Markers**
-
- ============= ===============================
- character description
- ============= ===============================
- ``'.'`` point marker
- ``','`` pixel marker
- ``'o'`` circle marker
- ``'v'`` triangle_down marker
- ``'^'`` triangle_up marker
- ``'<'`` triangle_left marker
- ``'>'`` triangle_right marker
- ``'1'`` tri_down marker
- ``'2'`` tri_up marker
- ``'3'`` tri_left marker
- ``'4'`` tri_right marker
- ``'8'`` octagon marker
- ``'s'`` square marker
- ``'p'`` pentagon marker
- ``'P'`` plus (filled) marker
- ``'*'`` star marker
- ``'h'`` hexagon1 marker
- ``'H'`` hexagon2 marker
- ``'+'`` plus marker
- ``'x'`` x marker
- ``'X'`` x (filled) marker
- ``'D'`` diamond marker
- ``'d'`` thin_diamond marker
- ``'|'`` vline marker
- ``'_'`` hline marker
- ============= ===============================
-
- **Line Styles**
-
- ============= ===============================
- character description
- ============= ===============================
- ``'-'`` solid line style
- ``'--'`` dashed line style
- ``'-.'`` dash-dot line style
- ``':'`` dotted line style
- ============= ===============================
-
- Example format strings::
-
- 'b' # blue markers with default shape
- 'or' # red circles
- '-g' # green solid line
- '--' # dashed line with default color
- '^k:' # black triangle_up markers connected by a dotted line
-
- **Colors**
-
- The supported color abbreviations are the single letter codes
-
- ============= ===============================
- character color
- ============= ===============================
- ``'b'`` blue
- ``'g'`` green
- ``'r'`` red
- ``'c'`` cyan
- ``'m'`` magenta
- ``'y'`` yellow
- ``'k'`` black
- ``'w'`` white
- ============= ===============================
-
- and the ``'CN'`` colors that index into the default property cycle.
-
- If the color is the only part of the format string, you can
- additionally use any `matplotlib.colors` spec, e.g. full names
- (``'green'``) or hex strings (``'#008000'``).
- """
- kwargs = cbook.normalize_kwargs(kwargs, mlines.Line2D)
- lines = [*self._get_lines(*args, data=data, **kwargs)]
- for line in lines:
- self.add_line(line)
- if scalex:
- self._request_autoscale_view("x")
- if scaley:
- self._request_autoscale_view("y")
- return lines
-
- @_preprocess_data(replace_names=["x", "y"], label_namer="y")
- @_docstring.dedent_interpd
- def plot_date(self, x, y, fmt='o', tz=None, xdate=True, ydate=False,
- **kwargs):
- """
- [*Discouraged*] Plot coercing the axis to treat floats as dates.
-
- .. admonition:: Discouraged
-
- This method exists for historic reasons and will be deprecated in
- the future.
-
- - ``datetime``-like data should directly be plotted using
- `~.Axes.plot`.
- - If you need to plot plain numeric data as :ref:`date-format` or
- need to set a timezone, call ``ax.xaxis.axis_date`` /
- ``ax.yaxis.axis_date`` before `~.Axes.plot`. See
- `.Axis.axis_date`.
-
- Similar to `.plot`, this plots *y* vs. *x* as lines or markers.
- However, the axis labels are formatted as dates depending on *xdate*
- and *ydate*. Note that `.plot` will work with `datetime` and
- `numpy.datetime64` objects without resorting to this method.
-
- Parameters
- ----------
- x, y : array-like
- The coordinates of the data points. If *xdate* or *ydate* is
- *True*, the respective values *x* or *y* are interpreted as
- :ref:`Matplotlib dates `.
-
- fmt : str, optional
- The plot format string. For details, see the corresponding
- parameter in `.plot`.
-
- tz : timezone string or `datetime.tzinfo`, default: :rc:`timezone`
- The time zone to use in labeling dates.
-
- xdate : bool, default: True
- If *True*, the *x*-axis will be interpreted as Matplotlib dates.
-
- ydate : bool, default: False
- If *True*, the *y*-axis will be interpreted as Matplotlib dates.
-
- Returns
- -------
- list of `.Line2D`
- Objects representing the plotted data.
-
- Other Parameters
- ----------------
- data : indexable object, optional
- DATA_PARAMETER_PLACEHOLDER
- **kwargs
- Keyword arguments control the `.Line2D` properties:
-
- %(Line2D:kwdoc)s
-
- See Also
- --------
- matplotlib.dates : Helper functions on dates.
- matplotlib.dates.date2num : Convert dates to num.
- matplotlib.dates.num2date : Convert num to dates.
- matplotlib.dates.drange : Create an equally spaced sequence of dates.
-
- Notes
- -----
- If you are using custom date tickers and formatters, it may be
- necessary to set the formatters/locators after the call to
- `.plot_date`. `.plot_date` will set the default tick locator to
- `.AutoDateLocator` (if the tick locator is not already set to a
- `.DateLocator` instance) and the default tick formatter to
- `.AutoDateFormatter` (if the tick formatter is not already set to a
- `.DateFormatter` instance).
- """
- if xdate:
- self.xaxis_date(tz)
- if ydate:
- self.yaxis_date(tz)
- return self.plot(x, y, fmt, **kwargs)
-
- # @_preprocess_data() # let 'plot' do the unpacking..
- @_docstring.dedent_interpd
- def loglog(self, *args, **kwargs):
- """
- Make a plot with log scaling on both the x- and y-axis.
-
- Call signatures::
-
- loglog([x], y, [fmt], data=None, **kwargs)
- loglog([x], y, [fmt], [x2], y2, [fmt2], ..., **kwargs)
-
- This is just a thin wrapper around `.plot` which additionally changes
- both the x-axis and the y-axis to log scaling. All the concepts and
- parameters of plot can be used here as well.
-
- The additional parameters *base*, *subs* and *nonpositive* control the
- x/y-axis properties. They are just forwarded to `.Axes.set_xscale` and
- `.Axes.set_yscale`. To use different properties on the x-axis and the
- y-axis, use e.g.
- ``ax.set_xscale("log", base=10); ax.set_yscale("log", base=2)``.
-
- Parameters
- ----------
- base : float, default: 10
- Base of the logarithm.
-
- subs : sequence, optional
- The location of the minor ticks. If *None*, reasonable locations
- are automatically chosen depending on the number of decades in the
- plot. See `.Axes.set_xscale`/`.Axes.set_yscale` for details.
-
- nonpositive : {'mask', 'clip'}, default: 'clip'
- Non-positive values can be masked as invalid, or clipped to a very
- small positive number.
-
- **kwargs
- All parameters supported by `.plot`.
-
- Returns
- -------
- list of `.Line2D`
- Objects representing the plotted data.
- """
- dx = {k: v for k, v in kwargs.items()
- if k in ['base', 'subs', 'nonpositive',
- 'basex', 'subsx', 'nonposx']}
- self.set_xscale('log', **dx)
- dy = {k: v for k, v in kwargs.items()
- if k in ['base', 'subs', 'nonpositive',
- 'basey', 'subsy', 'nonposy']}
- self.set_yscale('log', **dy)
- return self.plot(
- *args, **{k: v for k, v in kwargs.items() if k not in {*dx, *dy}})
-
- # @_preprocess_data() # let 'plot' do the unpacking..
- @_docstring.dedent_interpd
- def semilogx(self, *args, **kwargs):
- """
- Make a plot with log scaling on the x-axis.
-
- Call signatures::
-
- semilogx([x], y, [fmt], data=None, **kwargs)
- semilogx([x], y, [fmt], [x2], y2, [fmt2], ..., **kwargs)
-
- This is just a thin wrapper around `.plot` which additionally changes
- the x-axis to log scaling. All the concepts and parameters of plot can
- be used here as well.
-
- The additional parameters *base*, *subs*, and *nonpositive* control the
- x-axis properties. They are just forwarded to `.Axes.set_xscale`.
-
- Parameters
- ----------
- base : float, default: 10
- Base of the x logarithm.
-
- subs : array-like, optional
- The location of the minor xticks. If *None*, reasonable locations
- are automatically chosen depending on the number of decades in the
- plot. See `.Axes.set_xscale` for details.
-
- nonpositive : {'mask', 'clip'}, default: 'clip'
- Non-positive values in x can be masked as invalid, or clipped to a
- very small positive number.
-
- **kwargs
- All parameters supported by `.plot`.
-
- Returns
- -------
- list of `.Line2D`
- Objects representing the plotted data.
- """
- d = {k: v for k, v in kwargs.items()
- if k in ['base', 'subs', 'nonpositive',
- 'basex', 'subsx', 'nonposx']}
- self.set_xscale('log', **d)
- return self.plot(
- *args, **{k: v for k, v in kwargs.items() if k not in d})
-
- # @_preprocess_data() # let 'plot' do the unpacking..
- @_docstring.dedent_interpd
- def semilogy(self, *args, **kwargs):
- """
- Make a plot with log scaling on the y-axis.
-
- Call signatures::
-
- semilogy([x], y, [fmt], data=None, **kwargs)
- semilogy([x], y, [fmt], [x2], y2, [fmt2], ..., **kwargs)
-
- This is just a thin wrapper around `.plot` which additionally changes
- the y-axis to log scaling. All the concepts and parameters of plot can
- be used here as well.
-
- The additional parameters *base*, *subs*, and *nonpositive* control the
- y-axis properties. They are just forwarded to `.Axes.set_yscale`.
-
- Parameters
- ----------
- base : float, default: 10
- Base of the y logarithm.
-
- subs : array-like, optional
- The location of the minor yticks. If *None*, reasonable locations
- are automatically chosen depending on the number of decades in the
- plot. See `.Axes.set_yscale` for details.
-
- nonpositive : {'mask', 'clip'}, default: 'clip'
- Non-positive values in y can be masked as invalid, or clipped to a
- very small positive number.
-
- **kwargs
- All parameters supported by `.plot`.
-
- Returns
- -------
- list of `.Line2D`
- Objects representing the plotted data.
- """
- d = {k: v for k, v in kwargs.items()
- if k in ['base', 'subs', 'nonpositive',
- 'basey', 'subsy', 'nonposy']}
- self.set_yscale('log', **d)
- return self.plot(
- *args, **{k: v for k, v in kwargs.items() if k not in d})
-
- @_preprocess_data(replace_names=["x"], label_namer="x")
- def acorr(self, x, **kwargs):
- """
- Plot the autocorrelation of *x*.
-
- Parameters
- ----------
- x : array-like
-
- detrend : callable, default: `.mlab.detrend_none` (no detrending)
- A detrending function applied to *x*. It must have the
- signature ::
-
- detrend(x: np.ndarray) -> np.ndarray
-
- normed : bool, default: True
- If ``True``, input vectors are normalised to unit length.
-
- usevlines : bool, default: True
- Determines the plot style.
-
- If ``True``, vertical lines are plotted from 0 to the acorr value
- using `.Axes.vlines`. Additionally, a horizontal line is plotted
- at y=0 using `.Axes.axhline`.
-
- If ``False``, markers are plotted at the acorr values using
- `.Axes.plot`.
-
- maxlags : int, default: 10
- Number of lags to show. If ``None``, will return all
- ``2 * len(x) - 1`` lags.
-
- Returns
- -------
- lags : array (length ``2*maxlags+1``)
- The lag vector.
- c : array (length ``2*maxlags+1``)
- The auto correlation vector.
- line : `.LineCollection` or `.Line2D`
- `.Artist` added to the Axes of the correlation:
-
- - `.LineCollection` if *usevlines* is True.
- - `.Line2D` if *usevlines* is False.
- b : `~matplotlib.lines.Line2D` or None
- Horizontal line at 0 if *usevlines* is True
- None *usevlines* is False.
-
- Other Parameters
- ----------------
- linestyle : `~matplotlib.lines.Line2D` property, optional
- The linestyle for plotting the data points.
- Only used if *usevlines* is ``False``.
-
- marker : str, default: 'o'
- The marker for plotting the data points.
- Only used if *usevlines* is ``False``.
-
- data : indexable object, optional
- DATA_PARAMETER_PLACEHOLDER
-
- **kwargs
- Additional parameters are passed to `.Axes.vlines` and
- `.Axes.axhline` if *usevlines* is ``True``; otherwise they are
- passed to `.Axes.plot`.
-
- Notes
- -----
- The cross correlation is performed with `numpy.correlate` with
- ``mode = "full"``.
- """
- return self.xcorr(x, x, **kwargs)
-
- @_preprocess_data(replace_names=["x", "y"], label_namer="y")
- def xcorr(self, x, y, normed=True, detrend=mlab.detrend_none,
- usevlines=True, maxlags=10, **kwargs):
- r"""
- Plot the cross correlation between *x* and *y*.
-
- The correlation with lag k is defined as
- :math:`\sum_n x[n+k] \cdot y^*[n]`, where :math:`y^*` is the complex
- conjugate of :math:`y`.
-
- Parameters
- ----------
- x, y : array-like of length n
-
- detrend : callable, default: `.mlab.detrend_none` (no detrending)
- A detrending function applied to *x* and *y*. It must have the
- signature ::
-
- detrend(x: np.ndarray) -> np.ndarray
-
- normed : bool, default: True
- If ``True``, input vectors are normalised to unit length.
-
- usevlines : bool, default: True
- Determines the plot style.
-
- If ``True``, vertical lines are plotted from 0 to the xcorr value
- using `.Axes.vlines`. Additionally, a horizontal line is plotted
- at y=0 using `.Axes.axhline`.
-
- If ``False``, markers are plotted at the xcorr values using
- `.Axes.plot`.
-
- maxlags : int, default: 10
- Number of lags to show. If None, will return all ``2 * len(x) - 1``
- lags.
-
- Returns
- -------
- lags : array (length ``2*maxlags+1``)
- The lag vector.
- c : array (length ``2*maxlags+1``)
- The auto correlation vector.
- line : `.LineCollection` or `.Line2D`
- `.Artist` added to the Axes of the correlation:
-
- - `.LineCollection` if *usevlines* is True.
- - `.Line2D` if *usevlines* is False.
- b : `~matplotlib.lines.Line2D` or None
- Horizontal line at 0 if *usevlines* is True
- None *usevlines* is False.
-
- Other Parameters
- ----------------
- linestyle : `~matplotlib.lines.Line2D` property, optional
- The linestyle for plotting the data points.
- Only used if *usevlines* is ``False``.
-
- marker : str, default: 'o'
- The marker for plotting the data points.
- Only used if *usevlines* is ``False``.
-
- data : indexable object, optional
- DATA_PARAMETER_PLACEHOLDER
-
- **kwargs
- Additional parameters are passed to `.Axes.vlines` and
- `.Axes.axhline` if *usevlines* is ``True``; otherwise they are
- passed to `.Axes.plot`.
-
- Notes
- -----
- The cross correlation is performed with `numpy.correlate` with
- ``mode = "full"``.
- """
- Nx = len(x)
- if Nx != len(y):
- raise ValueError('x and y must be equal length')
-
- x = detrend(np.asarray(x))
- y = detrend(np.asarray(y))
-
- correls = np.correlate(x, y, mode="full")
-
- if normed:
- correls = correls / np.sqrt(np.dot(x, x) * np.dot(y, y))
-
- if maxlags is None:
- maxlags = Nx - 1
-
- if maxlags >= Nx or maxlags < 1:
- raise ValueError('maxlags must be None or strictly '
- 'positive < %d' % Nx)
-
- lags = np.arange(-maxlags, maxlags + 1)
- correls = correls[Nx - 1 - maxlags:Nx + maxlags]
-
- if usevlines:
- a = self.vlines(lags, [0], correls, **kwargs)
- # Make label empty so only vertical lines get a legend entry
- kwargs.pop('label', '')
- b = self.axhline(**kwargs)
- else:
- kwargs.setdefault('marker', 'o')
- kwargs.setdefault('linestyle', 'None')
- a, = self.plot(lags, correls, **kwargs)
- b = None
- return lags, correls, a, b
-
- #### Specialized plotting
-
- # @_preprocess_data() # let 'plot' do the unpacking..
- def step(self, x, y, *args, where='pre', data=None, **kwargs):
- """
- Make a step plot.
-
- Call signatures::
-
- step(x, y, [fmt], *, data=None, where='pre', **kwargs)
- step(x, y, [fmt], x2, y2, [fmt2], ..., *, where='pre', **kwargs)
-
- This is just a thin wrapper around `.plot` which changes some
- formatting options. Most of the concepts and parameters of plot can be
- used here as well.
-
- .. note::
-
- This method uses a standard plot with a step drawstyle: The *x*
- values are the reference positions and steps extend left/right/both
- directions depending on *where*.
-
- For the common case where you know the values and edges of the
- steps, use `~.Axes.stairs` instead.
-
- Parameters
- ----------
- x : array-like
- 1D sequence of x positions. It is assumed, but not checked, that
- it is uniformly increasing.
-
- y : array-like
- 1D sequence of y levels.
-
- fmt : str, optional
- A format string, e.g. 'g' for a green line. See `.plot` for a more
- detailed description.
-
- Note: While full format strings are accepted, it is recommended to
- only specify the color. Line styles are currently ignored (use
- the keyword argument *linestyle* instead). Markers are accepted
- and plotted on the given positions, however, this is a rarely
- needed feature for step plots.
-
- where : {'pre', 'post', 'mid'}, default: 'pre'
- Define where the steps should be placed:
-
- - 'pre': The y value is continued constantly to the left from
- every *x* position, i.e. the interval ``(x[i-1], x[i]]`` has the
- value ``y[i]``.
- - 'post': The y value is continued constantly to the right from
- every *x* position, i.e. the interval ``[x[i], x[i+1])`` has the
- value ``y[i]``.
- - 'mid': Steps occur half-way between the *x* positions.
-
- data : indexable object, optional
- An object with labelled data. If given, provide the label names to
- plot in *x* and *y*.
-
- **kwargs
- Additional parameters are the same as those for `.plot`.
-
- Returns
- -------
- list of `.Line2D`
- Objects representing the plotted data.
- """
- _api.check_in_list(('pre', 'post', 'mid'), where=where)
- kwargs['drawstyle'] = 'steps-' + where
- return self.plot(x, y, *args, data=data, **kwargs)
-
- @staticmethod
- def _convert_dx(dx, x0, xconv, convert):
- """
- Small helper to do logic of width conversion flexibly.
-
- *dx* and *x0* have units, but *xconv* has already been converted
- to unitless (and is an ndarray). This allows the *dx* to have units
- that are different from *x0*, but are still accepted by the
- ``__add__`` operator of *x0*.
- """
-
- # x should be an array...
- assert type(xconv) is np.ndarray
-
- if xconv.size == 0:
- # xconv has already been converted, but maybe empty...
- return convert(dx)
-
- try:
- # attempt to add the width to x0; this works for
- # datetime+timedelta, for instance
-
- # only use the first element of x and x0. This saves
- # having to be sure addition works across the whole
- # vector. This is particularly an issue if
- # x0 and dx are lists so x0 + dx just concatenates the lists.
- # We can't just cast x0 and dx to numpy arrays because that
- # removes the units from unit packages like `pint` that
- # wrap numpy arrays.
- try:
- x0 = cbook._safe_first_finite(x0)
- except (TypeError, IndexError, KeyError):
- pass
-
- try:
- x = cbook._safe_first_finite(xconv)
- except (TypeError, IndexError, KeyError):
- x = xconv
-
- delist = False
- if not np.iterable(dx):
- dx = [dx]
- delist = True
- dx = [convert(x0 + ddx) - x for ddx in dx]
- if delist:
- dx = dx[0]
- except (ValueError, TypeError, AttributeError):
- # if the above fails (for any reason) just fallback to what
- # we do by default and convert dx by itself.
- dx = convert(dx)
- return dx
-
- @_preprocess_data()
- @_docstring.dedent_interpd
- def bar(self, x, height, width=0.8, bottom=None, *, align="center",
- **kwargs):
- r"""
- Make a bar plot.
-
- The bars are positioned at *x* with the given *align*\ment. Their
- dimensions are given by *height* and *width*. The vertical baseline
- is *bottom* (default 0).
-
- Many parameters can take either a single value applying to all bars
- or a sequence of values, one for each bar.
-
- Parameters
- ----------
- x : float or array-like
- The x coordinates of the bars. See also *align* for the
- alignment of the bars to the coordinates.
-
- height : float or array-like
- The height(s) of the bars.
-
- width : float or array-like, default: 0.8
- The width(s) of the bars.
-
- bottom : float or array-like, default: 0
- The y coordinate(s) of the bottom side(s) of the bars.
-
- align : {'center', 'edge'}, default: 'center'
- Alignment of the bars to the *x* coordinates:
-
- - 'center': Center the base on the *x* positions.
- - 'edge': Align the left edges of the bars with the *x* positions.
-
- To align the bars on the right edge pass a negative *width* and
- ``align='edge'``.
-
- Returns
- -------
- `.BarContainer`
- Container with all the bars and optionally errorbars.
-
- Other Parameters
- ----------------
- color : color or list of color, optional
- The colors of the bar faces.
-
- edgecolor : color or list of color, optional
- The colors of the bar edges.
-
- linewidth : float or array-like, optional
- Width of the bar edge(s). If 0, don't draw edges.
-
- tick_label : str or list of str, optional
- The tick labels of the bars.
- Default: None (Use default numeric labels.)
-
- label : str or list of str, optional
- A single label is attached to the resulting `.BarContainer` as a
- label for the whole dataset.
- If a list is provided, it must be the same length as *x* and
- labels the individual bars. Repeated labels are not de-duplicated
- and will cause repeated label entries, so this is best used when
- bars also differ in style (e.g., by passing a list to *color*.)
-
- xerr, yerr : float or array-like of shape(N,) or shape(2, N), optional
- If not *None*, add horizontal / vertical errorbars to the bar tips.
- The values are +/- sizes relative to the data:
-
- - scalar: symmetric +/- values for all bars
- - shape(N,): symmetric +/- values for each bar
- - shape(2, N): Separate - and + values for each bar. First row
- contains the lower errors, the second row contains the upper
- errors.
- - *None*: No errorbar. (Default)
-
- See :doc:`/gallery/statistics/errorbar_features` for an example on
- the usage of *xerr* and *yerr*.
-
- ecolor : color or list of color, default: 'black'
- The line color of the errorbars.
-
- capsize : float, default: :rc:`errorbar.capsize`
- The length of the error bar caps in points.
-
- error_kw : dict, optional
- Dictionary of keyword arguments to be passed to the
- `~.Axes.errorbar` method. Values of *ecolor* or *capsize* defined
- here take precedence over the independent keyword arguments.
-
- log : bool, default: False
- If *True*, set the y-axis to be log scale.
-
- data : indexable object, optional
- DATA_PARAMETER_PLACEHOLDER
-
- **kwargs : `.Rectangle` properties
-
- %(Rectangle:kwdoc)s
-
- See Also
- --------
- barh : Plot a horizontal bar plot.
-
- Notes
- -----
- Stacked bars can be achieved by passing individual *bottom* values per
- bar. See :doc:`/gallery/lines_bars_and_markers/bar_stacked`.
- """
- kwargs = cbook.normalize_kwargs(kwargs, mpatches.Patch)
- color = kwargs.pop('color', None)
- if color is None:
- color = self._get_patches_for_fill.get_next_color()
- edgecolor = kwargs.pop('edgecolor', None)
- linewidth = kwargs.pop('linewidth', None)
- hatch = kwargs.pop('hatch', None)
-
- # Because xerr and yerr will be passed to errorbar, most dimension
- # checking and processing will be left to the errorbar method.
- xerr = kwargs.pop('xerr', None)
- yerr = kwargs.pop('yerr', None)
- error_kw = kwargs.pop('error_kw', {})
- ezorder = error_kw.pop('zorder', None)
- if ezorder is None:
- ezorder = kwargs.get('zorder', None)
- if ezorder is not None:
- # If using the bar zorder, increment slightly to make sure
- # errorbars are drawn on top of bars
- ezorder += 0.01
- error_kw.setdefault('zorder', ezorder)
- ecolor = kwargs.pop('ecolor', 'k')
- capsize = kwargs.pop('capsize', mpl.rcParams["errorbar.capsize"])
- error_kw.setdefault('ecolor', ecolor)
- error_kw.setdefault('capsize', capsize)
-
- # The keyword argument *orientation* is used by barh() to defer all
- # logic and drawing to bar(). It is considered internal and is
- # intentionally not mentioned in the docstring.
- orientation = kwargs.pop('orientation', 'vertical')
- _api.check_in_list(['vertical', 'horizontal'], orientation=orientation)
- log = kwargs.pop('log', False)
- label = kwargs.pop('label', '')
- tick_labels = kwargs.pop('tick_label', None)
-
- y = bottom # Matches barh call signature.
- if orientation == 'vertical':
- if y is None:
- y = 0
- else: # horizontal
- if x is None:
- x = 0
-
- if orientation == 'vertical':
- self._process_unit_info(
- [("x", x), ("y", height)], kwargs, convert=False)
- if log:
- self.set_yscale('log', nonpositive='clip')
- else: # horizontal
- self._process_unit_info(
- [("x", width), ("y", y)], kwargs, convert=False)
- if log:
- self.set_xscale('log', nonpositive='clip')
-
- # lets do some conversions now since some types cannot be
- # subtracted uniformly
- if self.xaxis is not None:
- x0 = x
- x = np.asarray(self.convert_xunits(x))
- width = self._convert_dx(width, x0, x, self.convert_xunits)
- if xerr is not None:
- xerr = self._convert_dx(xerr, x0, x, self.convert_xunits)
- if self.yaxis is not None:
- y0 = y
- y = np.asarray(self.convert_yunits(y))
- height = self._convert_dx(height, y0, y, self.convert_yunits)
- if yerr is not None:
- yerr = self._convert_dx(yerr, y0, y, self.convert_yunits)
-
- x, height, width, y, linewidth, hatch = np.broadcast_arrays(
- # Make args iterable too.
- np.atleast_1d(x), height, width, y, linewidth, hatch)
-
- # Now that units have been converted, set the tick locations.
- if orientation == 'vertical':
- tick_label_axis = self.xaxis
- tick_label_position = x
- else: # horizontal
- tick_label_axis = self.yaxis
- tick_label_position = y
-
- if not isinstance(label, str) and np.iterable(label):
- bar_container_label = '_nolegend_'
- patch_labels = label
- else:
- bar_container_label = label
- patch_labels = ['_nolegend_'] * len(x)
- if len(patch_labels) != len(x):
- raise ValueError(f'number of labels ({len(patch_labels)}) '
- f'does not match number of bars ({len(x)}).')
-
- linewidth = itertools.cycle(np.atleast_1d(linewidth))
- hatch = itertools.cycle(np.atleast_1d(hatch))
- color = itertools.chain(itertools.cycle(mcolors.to_rgba_array(color)),
- # Fallback if color == "none".
- itertools.repeat('none'))
- if edgecolor is None:
- edgecolor = itertools.repeat(None)
- else:
- edgecolor = itertools.chain(
- itertools.cycle(mcolors.to_rgba_array(edgecolor)),
- # Fallback if edgecolor == "none".
- itertools.repeat('none'))
-
- # We will now resolve the alignment and really have
- # left, bottom, width, height vectors
- _api.check_in_list(['center', 'edge'], align=align)
- if align == 'center':
- if orientation == 'vertical':
- try:
- left = x - width / 2
- except TypeError as e:
- raise TypeError(f'the dtypes of parameters x ({x.dtype}) '
- f'and width ({width.dtype}) '
- f'are incompatible') from e
- bottom = y
- else: # horizontal
- try:
- bottom = y - height / 2
- except TypeError as e:
- raise TypeError(f'the dtypes of parameters y ({y.dtype}) '
- f'and height ({height.dtype}) '
- f'are incompatible') from e
- left = x
- else: # edge
- left = x
- bottom = y
-
- patches = []
- args = zip(left, bottom, width, height, color, edgecolor, linewidth,
- hatch, patch_labels)
- for l, b, w, h, c, e, lw, htch, lbl in args:
- r = mpatches.Rectangle(
- xy=(l, b), width=w, height=h,
- facecolor=c,
- edgecolor=e,
- linewidth=lw,
- label=lbl,
- hatch=htch,
- )
- r._internal_update(kwargs)
- r.get_path()._interpolation_steps = 100
- if orientation == 'vertical':
- r.sticky_edges.y.append(b)
- else: # horizontal
- r.sticky_edges.x.append(l)
- self.add_patch(r)
- patches.append(r)
-
- if xerr is not None or yerr is not None:
- if orientation == 'vertical':
- # using list comps rather than arrays to preserve unit info
- ex = [l + 0.5 * w for l, w in zip(left, width)]
- ey = [b + h for b, h in zip(bottom, height)]
-
- else: # horizontal
- # using list comps rather than arrays to preserve unit info
- ex = [l + w for l, w in zip(left, width)]
- ey = [b + 0.5 * h for b, h in zip(bottom, height)]
-
- error_kw.setdefault("label", '_nolegend_')
-
- errorbar = self.errorbar(ex, ey,
- yerr=yerr, xerr=xerr,
- fmt='none', **error_kw)
- else:
- errorbar = None
-
- self._request_autoscale_view()
-
- if orientation == 'vertical':
- datavalues = height
- else: # horizontal
- datavalues = width
-
- bar_container = BarContainer(patches, errorbar, datavalues=datavalues,
- orientation=orientation,
- label=bar_container_label)
- self.add_container(bar_container)
-
- if tick_labels is not None:
- tick_labels = np.broadcast_to(tick_labels, len(patches))
- tick_label_axis.set_ticks(tick_label_position)
- tick_label_axis.set_ticklabels(tick_labels)
-
- return bar_container
-
- # @_preprocess_data() # let 'bar' do the unpacking..
- @_docstring.dedent_interpd
- def barh(self, y, width, height=0.8, left=None, *, align="center",
- data=None, **kwargs):
- r"""
- Make a horizontal bar plot.
-
- The bars are positioned at *y* with the given *align*\ment. Their
- dimensions are given by *width* and *height*. The horizontal baseline
- is *left* (default 0).
-
- Many parameters can take either a single value applying to all bars
- or a sequence of values, one for each bar.
-
- Parameters
- ----------
- y : float or array-like
- The y coordinates of the bars. See also *align* for the
- alignment of the bars to the coordinates.
-
- width : float or array-like
- The width(s) of the bars.
-
- height : float or array-like, default: 0.8
- The heights of the bars.
-
- left : float or array-like, default: 0
- The x coordinates of the left side(s) of the bars.
-
- align : {'center', 'edge'}, default: 'center'
- Alignment of the base to the *y* coordinates*:
-
- - 'center': Center the bars on the *y* positions.
- - 'edge': Align the bottom edges of the bars with the *y*
- positions.
-
- To align the bars on the top edge pass a negative *height* and
- ``align='edge'``.
-
- Returns
- -------
- `.BarContainer`
- Container with all the bars and optionally errorbars.
-
- Other Parameters
- ----------------
- color : color or list of color, optional
- The colors of the bar faces.
-
- edgecolor : color or list of color, optional
- The colors of the bar edges.
-
- linewidth : float or array-like, optional
- Width of the bar edge(s). If 0, don't draw edges.
-
- tick_label : str or list of str, optional
- The tick labels of the bars.
- Default: None (Use default numeric labels.)
-
- label : str or list of str, optional
- A single label is attached to the resulting `.BarContainer` as a
- label for the whole dataset.
- If a list is provided, it must be the same length as *y* and
- labels the individual bars. Repeated labels are not de-duplicated
- and will cause repeated label entries, so this is best used when
- bars also differ in style (e.g., by passing a list to *color*.)
-
- xerr, yerr : float or array-like of shape(N,) or shape(2, N), optional
- If not *None*, add horizontal / vertical errorbars to the bar tips.
- The values are +/- sizes relative to the data:
-
- - scalar: symmetric +/- values for all bars
- - shape(N,): symmetric +/- values for each bar
- - shape(2, N): Separate - and + values for each bar. First row
- contains the lower errors, the second row contains the upper
- errors.
- - *None*: No errorbar. (default)
-
- See :doc:`/gallery/statistics/errorbar_features` for an example on
- the usage of *xerr* and *yerr*.
-
- ecolor : color or list of color, default: 'black'
- The line color of the errorbars.
-
- capsize : float, default: :rc:`errorbar.capsize`
- The length of the error bar caps in points.
-
- error_kw : dict, optional
- Dictionary of keyword arguments to be passed to the
- `~.Axes.errorbar` method. Values of *ecolor* or *capsize* defined
- here take precedence over the independent keyword arguments.
-
- log : bool, default: False
- If ``True``, set the x-axis to be log scale.
-
- data : indexable object, optional
- If given, all parameters also accept a string ``s``, which is
- interpreted as ``data[s]`` (unless this raises an exception).
-
- **kwargs : `.Rectangle` properties
-
- %(Rectangle:kwdoc)s
-
- See Also
- --------
- bar : Plot a vertical bar plot.
-
- Notes
- -----
- Stacked bars can be achieved by passing individual *left* values per
- bar. See
- :doc:`/gallery/lines_bars_and_markers/horizontal_barchart_distribution`.
- """
- kwargs.setdefault('orientation', 'horizontal')
- patches = self.bar(x=left, height=height, width=width, bottom=y,
- align=align, data=data, **kwargs)
- return patches
-
- def bar_label(self, container, labels=None, *, fmt="%g", label_type="edge",
- padding=0, **kwargs):
- """
- Label a bar plot.
-
- Adds labels to bars in the given `.BarContainer`.
- You may need to adjust the axis limits to fit the labels.
-
- Parameters
- ----------
- container : `.BarContainer`
- Container with all the bars and optionally errorbars, likely
- returned from `.bar` or `.barh`.
-
- labels : array-like, optional
- A list of label texts, that should be displayed. If not given, the
- label texts will be the data values formatted with *fmt*.
-
- fmt : str or callable, default: '%g'
- An unnamed %-style or {}-style format string for the label or a
- function to call with the value as the first argument.
- When *fmt* is a string and can be interpreted in both formats,
- %-style takes precedence over {}-style.
-
- .. versionadded:: 3.7
- Support for {}-style format string and callables.
-
- label_type : {'edge', 'center'}, default: 'edge'
- The label type. Possible values:
-
- - 'edge': label placed at the end-point of the bar segment, and the
- value displayed will be the position of that end-point.
- - 'center': label placed in the center of the bar segment, and the
- value displayed will be the length of that segment.
- (useful for stacked bars, i.e.,
- :doc:`/gallery/lines_bars_and_markers/bar_label_demo`)
-
- padding : float, default: 0
- Distance of label from the end of the bar, in points.
-
- **kwargs
- Any remaining keyword arguments are passed through to
- `.Axes.annotate`. The alignment parameters (
- *horizontalalignment* / *ha*, *verticalalignment* / *va*) are
- not supported because the labels are automatically aligned to
- the bars.
-
- Returns
- -------
- list of `.Text`
- A list of `.Text` instances for the labels.
- """
- for key in ['horizontalalignment', 'ha', 'verticalalignment', 'va']:
- if key in kwargs:
- raise ValueError(
- f"Passing {key!r} to bar_label() is not supported.")
-
- a, b = self.yaxis.get_view_interval()
- y_inverted = a > b
- c, d = self.xaxis.get_view_interval()
- x_inverted = c > d
-
- # want to know whether to put label on positive or negative direction
- # cannot use np.sign here because it will return 0 if x == 0
- def sign(x):
- return 1 if x >= 0 else -1
-
- _api.check_in_list(['edge', 'center'], label_type=label_type)
-
- bars = container.patches
- errorbar = container.errorbar
- datavalues = container.datavalues
- orientation = container.orientation
-
- if errorbar:
- # check "ErrorbarContainer" for the definition of these elements
- lines = errorbar.lines # attribute of "ErrorbarContainer" (tuple)
- barlinecols = lines[2] # 0: data_line, 1: caplines, 2: barlinecols
- barlinecol = barlinecols[0] # the "LineCollection" of error bars
- errs = barlinecol.get_segments()
- else:
- errs = []
-
- if labels is None:
- labels = []
-
- annotations = []
-
- for bar, err, dat, lbl in itertools.zip_longest(
- bars, errs, datavalues, labels
- ):
- (x0, y0), (x1, y1) = bar.get_bbox().get_points()
- xc, yc = (x0 + x1) / 2, (y0 + y1) / 2
-
- if orientation == "vertical":
- extrema = max(y0, y1) if dat >= 0 else min(y0, y1)
- length = abs(y0 - y1)
- else: # horizontal
- extrema = max(x0, x1) if dat >= 0 else min(x0, x1)
- length = abs(x0 - x1)
-
- if err is None or np.size(err) == 0:
- endpt = extrema
- elif orientation == "vertical":
- endpt = err[:, 1].max() if dat >= 0 else err[:, 1].min()
- else: # horizontal
- endpt = err[:, 0].max() if dat >= 0 else err[:, 0].min()
-
- if label_type == "center":
- value = sign(dat) * length
- else: # edge
- value = extrema
-
- if label_type == "center":
- xy = (0.5, 0.5)
- kwargs["xycoords"] = (
- lambda r, b=bar:
- mtransforms.Bbox.intersection(
- b.get_window_extent(r), b.get_clip_box()
- ) or mtransforms.Bbox.null()
- )
- else: # edge
- if orientation == "vertical":
- xy = xc, endpt
- else: # horizontal
- xy = endpt, yc
-
- if orientation == "vertical":
- y_direction = -1 if y_inverted else 1
- xytext = 0, y_direction * sign(dat) * padding
- else: # horizontal
- x_direction = -1 if x_inverted else 1
- xytext = x_direction * sign(dat) * padding, 0
-
- if label_type == "center":
- ha, va = "center", "center"
- else: # edge
- if orientation == "vertical":
- ha = 'center'
- if y_inverted:
- va = 'top' if dat > 0 else 'bottom' # also handles NaN
- else:
- va = 'top' if dat < 0 else 'bottom' # also handles NaN
- else: # horizontal
- if x_inverted:
- ha = 'right' if dat > 0 else 'left' # also handles NaN
- else:
- ha = 'right' if dat < 0 else 'left' # also handles NaN
- va = 'center'
-
- if np.isnan(dat):
- lbl = ''
-
- if lbl is None:
- if isinstance(fmt, str):
- lbl = cbook._auto_format_str(fmt, value)
- elif callable(fmt):
- lbl = fmt(value)
- else:
- raise TypeError("fmt must be a str or callable")
- annotation = self.annotate(lbl,
- xy, xytext, textcoords="offset points",
- ha=ha, va=va, **kwargs)
- annotations.append(annotation)
-
- return annotations
-
- @_preprocess_data()
- @_docstring.dedent_interpd
- def broken_barh(self, xranges, yrange, **kwargs):
- """
- Plot a horizontal sequence of rectangles.
-
- A rectangle is drawn for each element of *xranges*. All rectangles
- have the same vertical position and size defined by *yrange*.
-
- Parameters
- ----------
- xranges : sequence of tuples (*xmin*, *xwidth*)
- The x-positions and extents of the rectangles. For each tuple
- (*xmin*, *xwidth*) a rectangle is drawn from *xmin* to *xmin* +
- *xwidth*.
- yrange : (*ymin*, *yheight*)
- The y-position and extent for all the rectangles.
-
- Returns
- -------
- `~.collections.PolyCollection`
-
- Other Parameters
- ----------------
- data : indexable object, optional
- DATA_PARAMETER_PLACEHOLDER
- **kwargs : `.PolyCollection` properties
-
- Each *kwarg* can be either a single argument applying to all
- rectangles, e.g.::
-
- facecolors='black'
-
- or a sequence of arguments over which is cycled, e.g.::
-
- facecolors=('black', 'blue')
-
- would create interleaving black and blue rectangles.
-
- Supported keywords:
-
- %(PolyCollection:kwdoc)s
- """
- # process the unit information
- xdata = cbook._safe_first_finite(xranges) if len(xranges) else None
- ydata = cbook._safe_first_finite(yrange) if len(yrange) else None
- self._process_unit_info(
- [("x", xdata), ("y", ydata)], kwargs, convert=False)
-
- vertices = []
- y0, dy = yrange
- y0, y1 = self.convert_yunits((y0, y0 + dy))
- for xr in xranges: # convert the absolute values, not the x and dx
- try:
- x0, dx = xr
- except Exception:
- raise ValueError(
- "each range in xrange must be a sequence with two "
- "elements (i.e. xrange must be an (N, 2) array)") from None
- x0, x1 = self.convert_xunits((x0, x0 + dx))
- vertices.append([(x0, y0), (x0, y1), (x1, y1), (x1, y0)])
-
- col = mcoll.PolyCollection(np.array(vertices), **kwargs)
- self.add_collection(col, autolim=True)
- self._request_autoscale_view()
-
- return col
-
- @_preprocess_data()
- @_api.delete_parameter("3.6", "use_line_collection")
- def stem(self, *args, linefmt=None, markerfmt=None, basefmt=None, bottom=0,
- label=None, use_line_collection=True, orientation='vertical'):
- """
- Create a stem plot.
-
- A stem plot draws lines perpendicular to a baseline at each location
- *locs* from the baseline to *heads*, and places a marker there. For
- vertical stem plots (the default), the *locs* are *x* positions, and
- the *heads* are *y* values. For horizontal stem plots, the *locs* are
- *y* positions, and the *heads* are *x* values.
-
- Call signature::
-
- stem([locs,] heads, linefmt=None, markerfmt=None, basefmt=None)
-
- The *locs*-positions are optional. *linefmt* may be provided as
- positional, but all other formats must be provided as keyword
- arguments.
-
- Parameters
- ----------
- locs : array-like, default: (0, 1, ..., len(heads) - 1)
- For vertical stem plots, the x-positions of the stems.
- For horizontal stem plots, the y-positions of the stems.
-
- heads : array-like
- For vertical stem plots, the y-values of the stem heads.
- For horizontal stem plots, the x-values of the stem heads.
-
- linefmt : str, optional
- A string defining the color and/or linestyle of the vertical lines:
-
- ========= =============
- Character Line Style
- ========= =============
- ``'-'`` solid line
- ``'--'`` dashed line
- ``'-.'`` dash-dot line
- ``':'`` dotted line
- ========= =============
-
- Default: 'C0-', i.e. solid line with the first color of the color
- cycle.
-
- Note: Markers specified through this parameter (e.g. 'x') will be
- silently ignored (unless using ``use_line_collection=False``).
- Instead, markers should be specified using *markerfmt*.
-
- markerfmt : str, optional
- A string defining the color and/or shape of the markers at the stem
- heads. If the marker is not given, use the marker 'o', i.e. filled
- circles. If the color is not given, use the color from *linefmt*.
-
- basefmt : str, default: 'C3-' ('C2-' in classic mode)
- A format string defining the properties of the baseline.
-
- orientation : {'vertical', 'horizontal'}, default: 'vertical'
- If 'vertical', will produce a plot with stems oriented vertically,
- If 'horizontal', the stems will be oriented horizontally.
-
- bottom : float, default: 0
- The y/x-position of the baseline (depending on orientation).
-
- label : str, default: None
- The label to use for the stems in legends.
-
- use_line_collection : bool, default: True
- *Deprecated since 3.6*
-
- If ``True``, store and plot the stem lines as a
- `~.collections.LineCollection` instead of individual lines, which
- significantly increases performance. If ``False``, defaults to the
- old behavior of using a list of `.Line2D` objects.
-
- data : indexable object, optional
- DATA_PARAMETER_PLACEHOLDER
-
- Returns
- -------
- `.StemContainer`
- The container may be treated like a tuple
- (*markerline*, *stemlines*, *baseline*)
-
- Notes
- -----
- .. seealso::
- The MATLAB function
- `stem `_
- which inspired this method.
- """
- if not 1 <= len(args) <= 3:
- raise TypeError('stem expected between 1 or 3 positional '
- 'arguments, got {}'.format(args))
- _api.check_in_list(['horizontal', 'vertical'], orientation=orientation)
-
- if len(args) == 1:
- heads, = args
- locs = np.arange(len(heads))
- args = ()
- elif isinstance(args[1], str):
- heads, *args = args
- locs = np.arange(len(heads))
- else:
- locs, heads, *args = args
-
- if orientation == 'vertical':
- locs, heads = self._process_unit_info([("x", locs), ("y", heads)])
- else: # horizontal
- heads, locs = self._process_unit_info([("x", heads), ("y", locs)])
-
- # resolve line format
- if linefmt is None:
- linefmt = args[0] if len(args) > 0 else "C0-"
- linestyle, linemarker, linecolor = _process_plot_format(linefmt)
-
- # resolve marker format
- if markerfmt is None:
- # if not given as kwarg, fall back to 'o'
- markerfmt = "o"
- if markerfmt == '':
- markerfmt = ' ' # = empty line style; '' would resolve rcParams
- markerstyle, markermarker, markercolor = \
- _process_plot_format(markerfmt)
- if markermarker is None:
- markermarker = 'o'
- if markerstyle is None:
- markerstyle = 'None'
- if markercolor is None:
- markercolor = linecolor
-
- # resolve baseline format
- if basefmt is None:
- basefmt = ("C2-" if mpl.rcParams["_internal.classic_mode"] else
- "C3-")
- basestyle, basemarker, basecolor = _process_plot_format(basefmt)
-
- # New behaviour in 3.1 is to use a LineCollection for the stemlines
- if use_line_collection:
- if linestyle is None:
- linestyle = mpl.rcParams['lines.linestyle']
- xlines = self.vlines if orientation == "vertical" else self.hlines
- stemlines = xlines(
- locs, bottom, heads,
- colors=linecolor, linestyles=linestyle, label="_nolegend_")
- # Old behaviour is to plot each of the lines individually
- else:
- stemlines = []
- for loc, head in zip(locs, heads):
- if orientation == 'horizontal':
- xs = [bottom, head]
- ys = [loc, loc]
- else:
- xs = [loc, loc]
- ys = [bottom, head]
- l, = self.plot(xs, ys,
- color=linecolor, linestyle=linestyle,
- marker=linemarker, label="_nolegend_")
- stemlines.append(l)
-
- if orientation == 'horizontal':
- marker_x = heads
- marker_y = locs
- baseline_x = [bottom, bottom]
- baseline_y = [np.min(locs), np.max(locs)]
- else:
- marker_x = locs
- marker_y = heads
- baseline_x = [np.min(locs), np.max(locs)]
- baseline_y = [bottom, bottom]
-
- markerline, = self.plot(marker_x, marker_y,
- color=markercolor, linestyle=markerstyle,
- marker=markermarker, label="_nolegend_")
-
- baseline, = self.plot(baseline_x, baseline_y,
- color=basecolor, linestyle=basestyle,
- marker=basemarker, label="_nolegend_")
-
- stem_container = StemContainer((markerline, stemlines, baseline),
- label=label)
- self.add_container(stem_container)
- return stem_container
-
- @_preprocess_data(replace_names=["x", "explode", "labels", "colors"])
- def pie(self, x, explode=None, labels=None, colors=None,
- autopct=None, pctdistance=0.6, shadow=False, labeldistance=1.1,
- startangle=0, radius=1, counterclock=True,
- wedgeprops=None, textprops=None, center=(0, 0),
- frame=False, rotatelabels=False, *, normalize=True, hatch=None):
- """
- Plot a pie chart.
-
- Make a pie chart of array *x*. The fractional area of each wedge is
- given by ``x/sum(x)``.
-
- The wedges are plotted counterclockwise, by default starting from the
- x-axis.
-
- Parameters
- ----------
- x : 1D array-like
- The wedge sizes.
-
- explode : array-like, default: None
- If not *None*, is a ``len(x)`` array which specifies the fraction
- of the radius with which to offset each wedge.
-
- labels : list, default: None
- A sequence of strings providing the labels for each wedge
-
- colors : array-like, default: None
- A sequence of colors through which the pie chart will cycle. If
- *None*, will use the colors in the currently active cycle.
-
- hatch : str or list, default: None
- Hatching pattern applied to all pie wedges or sequence of patterns
- through which the chart will cycle. For a list of valid patterns,
- see :doc:`/gallery/shapes_and_collections/hatch_style_reference`.
-
- .. versionadded:: 3.7
-
- autopct : None or str or callable, default: None
- If not *None*, *autopct* is a string or function used to label the
- wedges with their numeric value. The label will be placed inside
- the wedge. If *autopct* is a format string, the label will be
- ``fmt % pct``. If *autopct* is a function, then it will be called.
-
- pctdistance : float, default: 0.6
- The relative distance along the radius at which the text
- generated by *autopct* is drawn. To draw the text outside the pie,
- set *pctdistance* > 1. This parameter is ignored if *autopct* is
- ``None``.
-
- labeldistance : float or None, default: 1.1
- The relative distance along the radius at which the labels are
- drawn. To draw the labels inside the pie, set *labeldistance* < 1.
- If set to ``None``, labels are not drawn but are still stored for
- use in `.legend`.
-
- shadow : bool, default: False
- Draw a shadow beneath the pie.
-
- startangle : float, default: 0 degrees
- The angle by which the start of the pie is rotated,
- counterclockwise from the x-axis.
-
- radius : float, default: 1
- The radius of the pie.
-
- counterclock : bool, default: True
- Specify fractions direction, clockwise or counterclockwise.
-
- wedgeprops : dict, default: None
- Dict of arguments passed to each `.patches.Wedge` of the pie.
- For example, ``wedgeprops = {'linewidth': 3}`` sets the width of
- the wedge border lines equal to 3. By default, ``clip_on=False``.
- When there is a conflict between these properties and other
- keywords, properties passed to *wedgeprops* take precedence.
-
- textprops : dict, default: None
- Dict of arguments to pass to the text objects.
-
- center : (float, float), default: (0, 0)
- The coordinates of the center of the chart.
-
- frame : bool, default: False
- Plot Axes frame with the chart if true.
-
- rotatelabels : bool, default: False
- Rotate each label to the angle of the corresponding slice if true.
-
- normalize : bool, default: True
- When *True*, always make a full pie by normalizing x so that
- ``sum(x) == 1``. *False* makes a partial pie if ``sum(x) <= 1``
- and raises a `ValueError` for ``sum(x) > 1``.
-
- data : indexable object, optional
- DATA_PARAMETER_PLACEHOLDER
-
- Returns
- -------
- patches : list
- A sequence of `matplotlib.patches.Wedge` instances
-
- texts : list
- A list of the label `.Text` instances.
-
- autotexts : list
- A list of `.Text` instances for the numeric labels. This will only
- be returned if the parameter *autopct* is not *None*.
-
- Notes
- -----
- The pie chart will probably look best if the figure and Axes are
- square, or the Axes aspect is equal.
- This method sets the aspect ratio of the axis to "equal".
- The Axes aspect ratio can be controlled with `.Axes.set_aspect`.
- """
- self.set_aspect('equal')
- # The use of float32 is "historical", but can't be changed without
- # regenerating the test baselines.
- x = np.asarray(x, np.float32)
- if x.ndim > 1:
- raise ValueError("x must be 1D")
-
- if np.any(x < 0):
- raise ValueError("Wedge sizes 'x' must be non negative values")
-
- sx = x.sum()
-
- if normalize:
- x = x / sx
- elif sx > 1:
- raise ValueError('Cannot plot an unnormalized pie with sum(x) > 1')
- if labels is None:
- labels = [''] * len(x)
- if explode is None:
- explode = [0] * len(x)
- if len(x) != len(labels):
- raise ValueError("'label' must be of length 'x'")
- if len(x) != len(explode):
- raise ValueError("'explode' must be of length 'x'")
- if colors is None:
- get_next_color = self._get_patches_for_fill.get_next_color
- else:
- color_cycle = itertools.cycle(colors)
-
- def get_next_color():
- return next(color_cycle)
-
- hatch_cycle = itertools.cycle(np.atleast_1d(hatch))
-
- _api.check_isinstance(Number, radius=radius, startangle=startangle)
- if radius <= 0:
- raise ValueError(f'radius must be a positive number, not {radius}')
-
- # Starting theta1 is the start fraction of the circle
- theta1 = startangle / 360
-
- if wedgeprops is None:
- wedgeprops = {}
- if textprops is None:
- textprops = {}
-
- texts = []
- slices = []
- autotexts = []
-
- for frac, label, expl in zip(x, labels, explode):
- x, y = center
- theta2 = (theta1 + frac) if counterclock else (theta1 - frac)
- thetam = 2 * np.pi * 0.5 * (theta1 + theta2)
- x += expl * math.cos(thetam)
- y += expl * math.sin(thetam)
-
- w = mpatches.Wedge((x, y), radius, 360. * min(theta1, theta2),
- 360. * max(theta1, theta2),
- facecolor=get_next_color(),
- hatch=next(hatch_cycle),
- clip_on=False,
- label=label)
- w.set(**wedgeprops)
- slices.append(w)
- self.add_patch(w)
-
- if shadow:
- # Make sure to add a shadow after the call to add_patch so the
- # figure and transform props will be set.
- shad = mpatches.Shadow(w, -0.02, -0.02, label='_nolegend_')
- self.add_patch(shad)
-
- if labeldistance is not None:
- xt = x + labeldistance * radius * math.cos(thetam)
- yt = y + labeldistance * radius * math.sin(thetam)
- label_alignment_h = 'left' if xt > 0 else 'right'
- label_alignment_v = 'center'
- label_rotation = 'horizontal'
- if rotatelabels:
- label_alignment_v = 'bottom' if yt > 0 else 'top'
- label_rotation = (np.rad2deg(thetam)
- + (0 if xt > 0 else 180))
- t = self.text(xt, yt, label,
- clip_on=False,
- horizontalalignment=label_alignment_h,
- verticalalignment=label_alignment_v,
- rotation=label_rotation,
- size=mpl.rcParams['xtick.labelsize'])
- t.set(**textprops)
- texts.append(t)
-
- if autopct is not None:
- xt = x + pctdistance * radius * math.cos(thetam)
- yt = y + pctdistance * radius * math.sin(thetam)
- if isinstance(autopct, str):
- s = autopct % (100. * frac)
- elif callable(autopct):
- s = autopct(100. * frac)
- else:
- raise TypeError(
- 'autopct must be callable or a format string')
- t = self.text(xt, yt, s,
- clip_on=False,
- horizontalalignment='center',
- verticalalignment='center')
- t.set(**textprops)
- autotexts.append(t)
-
- theta1 = theta2
-
- if frame:
- self._request_autoscale_view()
- else:
- self.set(frame_on=False, xticks=[], yticks=[],
- xlim=(-1.25 + center[0], 1.25 + center[0]),
- ylim=(-1.25 + center[1], 1.25 + center[1]))
-
- if autopct is None:
- return slices, texts
- else:
- return slices, texts, autotexts
-
- @staticmethod
- def _errorevery_to_mask(x, errorevery):
- """
- Normalize `errorbar`'s *errorevery* to be a boolean mask for data *x*.
-
- This function is split out to be usable both by 2D and 3D errorbars.
- """
- if isinstance(errorevery, Integral):
- errorevery = (0, errorevery)
- if isinstance(errorevery, tuple):
- if (len(errorevery) == 2 and
- isinstance(errorevery[0], Integral) and
- isinstance(errorevery[1], Integral)):
- errorevery = slice(errorevery[0], None, errorevery[1])
- else:
- raise ValueError(
- f'{errorevery=!r} is a not a tuple of two integers')
- elif isinstance(errorevery, slice):
- pass
- elif not isinstance(errorevery, str) and np.iterable(errorevery):
- try:
- x[errorevery] # fancy indexing
- except (ValueError, IndexError) as err:
- raise ValueError(
- f"{errorevery=!r} is iterable but not a valid NumPy fancy "
- "index to match 'xerr'/'yerr'") from err
- else:
- raise ValueError(f"{errorevery=!r} is not a recognized value")
- everymask = np.zeros(len(x), bool)
- everymask[errorevery] = True
- return everymask
-
- @_preprocess_data(replace_names=["x", "y", "xerr", "yerr"],
- label_namer="y")
- @_docstring.dedent_interpd
- def errorbar(self, x, y, yerr=None, xerr=None,
- fmt='', ecolor=None, elinewidth=None, capsize=None,
- barsabove=False, lolims=False, uplims=False,
- xlolims=False, xuplims=False, errorevery=1, capthick=None,
- **kwargs):
- """
- Plot y versus x as lines and/or markers with attached errorbars.
-
- *x*, *y* define the data locations, *xerr*, *yerr* define the errorbar
- sizes. By default, this draws the data markers/lines as well the
- errorbars. Use fmt='none' to draw errorbars without any data markers.
-
- .. versionadded:: 3.7
- Caps and error lines are drawn in polar coordinates on polar plots.
-
-
- Parameters
- ----------
- x, y : float or array-like
- The data positions.
-
- xerr, yerr : float or array-like, shape(N,) or shape(2, N), optional
- The errorbar sizes:
-
- - scalar: Symmetric +/- values for all data points.
- - shape(N,): Symmetric +/-values for each data point.
- - shape(2, N): Separate - and + values for each bar. First row
- contains the lower errors, the second row contains the upper
- errors.
- - *None*: No errorbar.
-
- All values must be >= 0.
-
- See :doc:`/gallery/statistics/errorbar_features`
- for an example on the usage of ``xerr`` and ``yerr``.
-
- fmt : str, default: ''
- The format for the data points / data lines. See `.plot` for
- details.
-
- Use 'none' (case-insensitive) to plot errorbars without any data
- markers.
-
- ecolor : color, default: None
- The color of the errorbar lines. If None, use the color of the
- line connecting the markers.
-
- elinewidth : float, default: None
- The linewidth of the errorbar lines. If None, the linewidth of
- the current style is used.
-
- capsize : float, default: :rc:`errorbar.capsize`
- The length of the error bar caps in points.
-
- capthick : float, default: None
- An alias to the keyword argument *markeredgewidth* (a.k.a. *mew*).
- This setting is a more sensible name for the property that
- controls the thickness of the error bar cap in points. For
- backwards compatibility, if *mew* or *markeredgewidth* are given,
- then they will over-ride *capthick*. This may change in future
- releases.
-
- barsabove : bool, default: False
- If True, will plot the errorbars above the plot
- symbols. Default is below.
-
- lolims, uplims, xlolims, xuplims : bool, default: False
- These arguments can be used to indicate that a value gives only
- upper/lower limits. In that case a caret symbol is used to
- indicate this. *lims*-arguments may be scalars, or array-likes of
- the same length as *xerr* and *yerr*. To use limits with inverted
- axes, `~.Axes.set_xlim` or `~.Axes.set_ylim` must be called before
- :meth:`errorbar`. Note the tricky parameter names: setting e.g.
- *lolims* to True means that the y-value is a *lower* limit of the
- True value, so, only an *upward*-pointing arrow will be drawn!
-
- errorevery : int or (int, int), default: 1
- draws error bars on a subset of the data. *errorevery* =N draws
- error bars on the points (x[::N], y[::N]).
- *errorevery* =(start, N) draws error bars on the points
- (x[start::N], y[start::N]). e.g. errorevery=(6, 3)
- adds error bars to the data at (x[6], x[9], x[12], x[15], ...).
- Used to avoid overlapping error bars when two series share x-axis
- values.
-
- Returns
- -------
- `.ErrorbarContainer`
- The container contains:
-
- - plotline: `~matplotlib.lines.Line2D` instance of x, y plot markers
- and/or line.
- - caplines: A tuple of `~matplotlib.lines.Line2D` instances of the error
- bar caps.
- - barlinecols: A tuple of `.LineCollection` with the horizontal and
- vertical error ranges.
-
- Other Parameters
- ----------------
- data : indexable object, optional
- DATA_PARAMETER_PLACEHOLDER
-
- **kwargs
- All other keyword arguments are passed on to the `~.Axes.plot` call
- drawing the markers. For example, this code makes big red squares
- with thick green edges::
-
- x, y, yerr = rand(3, 10)
- errorbar(x, y, yerr, marker='s', mfc='red',
- mec='green', ms=20, mew=4)
-
- where *mfc*, *mec*, *ms* and *mew* are aliases for the longer
- property names, *markerfacecolor*, *markeredgecolor*, *markersize*
- and *markeredgewidth*.
-
- Valid kwargs for the marker properties are:
-
- - *dashes*
- - *dash_capstyle*
- - *dash_joinstyle*
- - *drawstyle*
- - *fillstyle*
- - *linestyle*
- - *marker*
- - *markeredgecolor*
- - *markeredgewidth*
- - *markerfacecolor*
- - *markerfacecoloralt*
- - *markersize*
- - *markevery*
- - *solid_capstyle*
- - *solid_joinstyle*
-
- Refer to the corresponding `.Line2D` property for more details:
-
- %(Line2D:kwdoc)s
- """
- kwargs = cbook.normalize_kwargs(kwargs, mlines.Line2D)
- # Drop anything that comes in as None to use the default instead.
- kwargs = {k: v for k, v in kwargs.items() if v is not None}
- kwargs.setdefault('zorder', 2)
-
- # Casting to object arrays preserves units.
- if not isinstance(x, np.ndarray):
- x = np.asarray(x, dtype=object)
- if not isinstance(y, np.ndarray):
- y = np.asarray(y, dtype=object)
-
- def _upcast_err(err):
- """
- Safely handle tuple of containers that carry units.
-
- This function covers the case where the input to the xerr/yerr is a
- length 2 tuple of equal length ndarray-subclasses that carry the
- unit information in the container.
-
- If we have a tuple of nested numpy array (subclasses), we defer
- coercing the units to be consistent to the underlying unit
- library (and implicitly the broadcasting).
-
- Otherwise, fallback to casting to an object array.
- """
-
- if (
- # make sure it is not a scalar
- np.iterable(err) and
- # and it is not empty
- len(err) > 0 and
- # and the first element is an array sub-class use
- # safe_first_element because getitem is index-first not
- # location first on pandas objects so err[0] almost always
- # fails.
- isinstance(cbook._safe_first_finite(err), np.ndarray)
- ):
- # Get the type of the first element
- atype = type(cbook._safe_first_finite(err))
- # Promote the outer container to match the inner container
- if atype is np.ndarray:
- # Converts using np.asarray, because data cannot
- # be directly passed to init of np.ndarray
- return np.asarray(err, dtype=object)
- # If atype is not np.ndarray, directly pass data to init.
- # This works for types such as unyts and astropy units
- return atype(err)
- # Otherwise wrap it in an object array
- return np.asarray(err, dtype=object)
-
- if xerr is not None and not isinstance(xerr, np.ndarray):
- xerr = _upcast_err(xerr)
- if yerr is not None and not isinstance(yerr, np.ndarray):
- yerr = _upcast_err(yerr)
- x, y = np.atleast_1d(x, y) # Make sure all the args are iterable.
- if len(x) != len(y):
- raise ValueError("'x' and 'y' must have the same size")
-
- everymask = self._errorevery_to_mask(x, errorevery)
-
- label = kwargs.pop("label", None)
- kwargs['label'] = '_nolegend_'
-
- # Create the main line and determine overall kwargs for child artists.
- # We avoid calling self.plot() directly, or self._get_lines(), because
- # that would call self._process_unit_info again, and do other indirect
- # data processing.
- (data_line, base_style), = self._get_lines._plot_args(
- (x, y) if fmt == '' else (x, y, fmt), kwargs, return_kwargs=True)
-
- # Do this after creating `data_line` to avoid modifying `base_style`.
- if barsabove:
- data_line.set_zorder(kwargs['zorder'] - .1)
- else:
- data_line.set_zorder(kwargs['zorder'] + .1)
-
- # Add line to plot, or throw it away and use it to determine kwargs.
- if fmt.lower() != 'none':
- self.add_line(data_line)
- else:
- data_line = None
- # Remove alpha=0 color that _get_lines._plot_args returns for
- # 'none' format, and replace it with user-specified color, if
- # supplied.
- base_style.pop('color')
- if 'color' in kwargs:
- base_style['color'] = kwargs.pop('color')
-
- if 'color' not in base_style:
- base_style['color'] = 'C0'
- if ecolor is None:
- ecolor = base_style['color']
-
- # Eject any line-specific information from format string, as it's not
- # needed for bars or caps.
- for key in ['marker', 'markersize', 'markerfacecolor',
- 'markerfacecoloralt',
- 'markeredgewidth', 'markeredgecolor', 'markevery',
- 'linestyle', 'fillstyle', 'drawstyle', 'dash_capstyle',
- 'dash_joinstyle', 'solid_capstyle', 'solid_joinstyle',
- 'dashes']:
- base_style.pop(key, None)
-
- # Make the style dict for the line collections (the bars).
- eb_lines_style = {**base_style, 'color': ecolor}
-
- if elinewidth is not None:
- eb_lines_style['linewidth'] = elinewidth
- elif 'linewidth' in kwargs:
- eb_lines_style['linewidth'] = kwargs['linewidth']
-
- for key in ('transform', 'alpha', 'zorder', 'rasterized'):
- if key in kwargs:
- eb_lines_style[key] = kwargs[key]
-
- # Make the style dict for caps (the "hats").
- eb_cap_style = {**base_style, 'linestyle': 'none'}
- if capsize is None:
- capsize = mpl.rcParams["errorbar.capsize"]
- if capsize > 0:
- eb_cap_style['markersize'] = 2. * capsize
- if capthick is not None:
- eb_cap_style['markeredgewidth'] = capthick
-
- # For backwards-compat, allow explicit setting of
- # 'markeredgewidth' to over-ride capthick.
- for key in ('markeredgewidth', 'transform', 'alpha',
- 'zorder', 'rasterized'):
- if key in kwargs:
- eb_cap_style[key] = kwargs[key]
- eb_cap_style['color'] = ecolor
-
- barcols = []
- caplines = {'x': [], 'y': []}
-
- # Vectorized fancy-indexer.
- def apply_mask(arrays, mask):
- return [array[mask] for array in arrays]
-
- # dep: dependent dataset, indep: independent dataset
- for (dep_axis, dep, err, lolims, uplims, indep, lines_func,
- marker, lomarker, himarker) in [
- ("x", x, xerr, xlolims, xuplims, y, self.hlines,
- "|", mlines.CARETRIGHTBASE, mlines.CARETLEFTBASE),
- ("y", y, yerr, lolims, uplims, x, self.vlines,
- "_", mlines.CARETUPBASE, mlines.CARETDOWNBASE),
- ]:
- if err is None:
- continue
- lolims = np.broadcast_to(lolims, len(dep)).astype(bool)
- uplims = np.broadcast_to(uplims, len(dep)).astype(bool)
- try:
- np.broadcast_to(err, (2, len(dep)))
- except ValueError:
- raise ValueError(
- f"'{dep_axis}err' (shape: {np.shape(err)}) must be a "
- f"scalar or a 1D or (2, n) array-like whose shape matches "
- f"'{dep_axis}' (shape: {np.shape(dep)})") from None
- res = np.zeros(err.shape, dtype=bool) # Default in case of nan
- if np.any(np.less(err, -err, out=res, where=(err == err))):
- # like err<0, but also works for timedelta and nan.
- raise ValueError(
- f"'{dep_axis}err' must not contain negative values")
- # This is like
- # elow, ehigh = np.broadcast_to(...)
- # return dep - elow * ~lolims, dep + ehigh * ~uplims
- # except that broadcast_to would strip units.
- low, high = dep + np.row_stack([-(1 - lolims), 1 - uplims]) * err
- barcols.append(lines_func(
- *apply_mask([indep, low, high], everymask), **eb_lines_style))
- if self.name == "polar" and dep_axis == "x":
- for b in barcols:
- for p in b.get_paths():
- p._interpolation_steps = 2
- # Normal errorbars for points without upper/lower limits.
- nolims = ~(lolims | uplims)
- if nolims.any() and capsize > 0:
- indep_masked, lo_masked, hi_masked = apply_mask(
- [indep, low, high], nolims & everymask)
- for lh_masked in [lo_masked, hi_masked]:
- # Since this has to work for x and y as dependent data, we
- # first set both x and y to the independent variable and
- # overwrite the respective dependent data in a second step.
- line = mlines.Line2D(indep_masked, indep_masked,
- marker=marker, **eb_cap_style)
- line.set(**{f"{dep_axis}data": lh_masked})
- caplines[dep_axis].append(line)
- for idx, (lims, hl) in enumerate([(lolims, high), (uplims, low)]):
- if not lims.any():
- continue
- hlmarker = (
- himarker
- if getattr(self, f"{dep_axis}axis").get_inverted() ^ idx
- else lomarker)
- x_masked, y_masked, hl_masked = apply_mask(
- [x, y, hl], lims & everymask)
- # As above, we set the dependent data in a second step.
- line = mlines.Line2D(x_masked, y_masked,
- marker=hlmarker, **eb_cap_style)
- line.set(**{f"{dep_axis}data": hl_masked})
- caplines[dep_axis].append(line)
- if capsize > 0:
- caplines[dep_axis].append(mlines.Line2D(
- x_masked, y_masked, marker=marker, **eb_cap_style))
- if self.name == 'polar':
- for axis in caplines:
- for l in caplines[axis]:
- # Rotate caps to be perpendicular to the error bars
- for theta, r in zip(l.get_xdata(), l.get_ydata()):
- rotation = mtransforms.Affine2D().rotate(theta)
- if axis == 'y':
- rotation.rotate(-np.pi / 2)
- ms = mmarkers.MarkerStyle(marker=marker,
- transform=rotation)
- self.add_line(mlines.Line2D([theta], [r], marker=ms,
- **eb_cap_style))
- else:
- for axis in caplines:
- for l in caplines[axis]:
- self.add_line(l)
-
- self._request_autoscale_view()
- caplines = caplines['x'] + caplines['y']
- errorbar_container = ErrorbarContainer(
- (data_line, tuple(caplines), tuple(barcols)),
- has_xerr=(xerr is not None), has_yerr=(yerr is not None),
- label=label)
- self.containers.append(errorbar_container)
-
- return errorbar_container # (l0, caplines, barcols)
-
- @_preprocess_data()
- def boxplot(self, x, notch=None, sym=None, vert=None, whis=None,
- positions=None, widths=None, patch_artist=None,
- bootstrap=None, usermedians=None, conf_intervals=None,
- meanline=None, showmeans=None, showcaps=None,
- showbox=None, showfliers=None, boxprops=None,
- labels=None, flierprops=None, medianprops=None,
- meanprops=None, capprops=None, whiskerprops=None,
- manage_ticks=True, autorange=False, zorder=None,
- capwidths=None):
- """
- Draw a box and whisker plot.
-
- The box extends from the first quartile (Q1) to the third
- quartile (Q3) of the data, with a line at the median. The
- whiskers extend from the box by 1.5x the inter-quartile range
- (IQR). Flier points are those past the end of the whiskers.
- See https://en.wikipedia.org/wiki/Box_plot for reference.
-
- .. code-block:: none
-
- Q1-1.5IQR Q1 median Q3 Q3+1.5IQR
- |-----:-----|
- o |--------| : |--------| o o
- |-----:-----|
- flier <-----------> fliers
- IQR
-
-
- Parameters
- ----------
- x : Array or a sequence of vectors.
- The input data. If a 2D array, a boxplot is drawn for each column
- in *x*. If a sequence of 1D arrays, a boxplot is drawn for each
- array in *x*.
-
- notch : bool, default: False
- Whether to draw a notched boxplot (`True`), or a rectangular
- boxplot (`False`). The notches represent the confidence interval
- (CI) around the median. The documentation for *bootstrap*
- describes how the locations of the notches are computed by
- default, but their locations may also be overridden by setting the
- *conf_intervals* parameter.
-
- .. note::
-
- In cases where the values of the CI are less than the
- lower quartile or greater than the upper quartile, the
- notches will extend beyond the box, giving it a
- distinctive "flipped" appearance. This is expected
- behavior and consistent with other statistical
- visualization packages.
-
- sym : str, optional
- The default symbol for flier points. An empty string ('') hides
- the fliers. If `None`, then the fliers default to 'b+'. More
- control is provided by the *flierprops* parameter.
-
- vert : bool, default: True
- If `True`, draws vertical boxes.
- If `False`, draw horizontal boxes.
-
- whis : float or (float, float), default: 1.5
- The position of the whiskers.
-
- If a float, the lower whisker is at the lowest datum above
- ``Q1 - whis*(Q3-Q1)``, and the upper whisker at the highest datum
- below ``Q3 + whis*(Q3-Q1)``, where Q1 and Q3 are the first and
- third quartiles. The default value of ``whis = 1.5`` corresponds
- to Tukey's original definition of boxplots.
-
- If a pair of floats, they indicate the percentiles at which to
- draw the whiskers (e.g., (5, 95)). In particular, setting this to
- (0, 100) results in whiskers covering the whole range of the data.
-
- In the edge case where ``Q1 == Q3``, *whis* is automatically set
- to (0, 100) (cover the whole range of the data) if *autorange* is
- True.
-
- Beyond the whiskers, data are considered outliers and are plotted
- as individual points.
-
- bootstrap : int, optional
- Specifies whether to bootstrap the confidence intervals
- around the median for notched boxplots. If *bootstrap* is
- None, no bootstrapping is performed, and notches are
- calculated using a Gaussian-based asymptotic approximation
- (see McGill, R., Tukey, J.W., and Larsen, W.A., 1978, and
- Kendall and Stuart, 1967). Otherwise, bootstrap specifies
- the number of times to bootstrap the median to determine its
- 95% confidence intervals. Values between 1000 and 10000 are
- recommended.
-
- usermedians : 1D array-like, optional
- A 1D array-like of length ``len(x)``. Each entry that is not
- `None` forces the value of the median for the corresponding
- dataset. For entries that are `None`, the medians are computed
- by Matplotlib as normal.
-
- conf_intervals : array-like, optional
- A 2D array-like of shape ``(len(x), 2)``. Each entry that is not
- None forces the location of the corresponding notch (which is
- only drawn if *notch* is `True`). For entries that are `None`,
- the notches are computed by the method specified by the other
- parameters (e.g., *bootstrap*).
-
- positions : array-like, optional
- The positions of the boxes. The ticks and limits are
- automatically set to match the positions. Defaults to
- ``range(1, N+1)`` where N is the number of boxes to be drawn.
-
- widths : float or array-like
- The widths of the boxes. The default is 0.5, or ``0.15*(distance
- between extreme positions)``, if that is smaller.
-
- patch_artist : bool, default: False
- If `False` produces boxes with the Line2D artist. Otherwise,
- boxes are drawn with Patch artists.
-
- labels : sequence, optional
- Labels for each dataset (one per dataset).
-
- manage_ticks : bool, default: True
- If True, the tick locations and labels will be adjusted to match
- the boxplot positions.
-
- autorange : bool, default: False
- When `True` and the data are distributed such that the 25th and
- 75th percentiles are equal, *whis* is set to (0, 100) such
- that the whisker ends are at the minimum and maximum of the data.
-
- meanline : bool, default: False
- If `True` (and *showmeans* is `True`), will try to render the
- mean as a line spanning the full width of the box according to
- *meanprops* (see below). Not recommended if *shownotches* is also
- True. Otherwise, means will be shown as points.
-
- zorder : float, default: ``Line2D.zorder = 2``
- The zorder of the boxplot.
-
- Returns
- -------
- dict
- A dictionary mapping each component of the boxplot to a list
- of the `.Line2D` instances created. That dictionary has the
- following keys (assuming vertical boxplots):
-
- - ``boxes``: the main body of the boxplot showing the
- quartiles and the median's confidence intervals if
- enabled.
-
- - ``medians``: horizontal lines at the median of each box.
-
- - ``whiskers``: the vertical lines extending to the most
- extreme, non-outlier data points.
-
- - ``caps``: the horizontal lines at the ends of the
- whiskers.
-
- - ``fliers``: points representing data that extend beyond
- the whiskers (fliers).
-
- - ``means``: points or lines representing the means.
-
- Other Parameters
- ----------------
- showcaps : bool, default: True
- Show the caps on the ends of whiskers.
- showbox : bool, default: True
- Show the central box.
- showfliers : bool, default: True
- Show the outliers beyond the caps.
- showmeans : bool, default: False
- Show the arithmetic means.
- capprops : dict, default: None
- The style of the caps.
- capwidths : float or array, default: None
- The widths of the caps.
- boxprops : dict, default: None
- The style of the box.
- whiskerprops : dict, default: None
- The style of the whiskers.
- flierprops : dict, default: None
- The style of the fliers.
- medianprops : dict, default: None
- The style of the median.
- meanprops : dict, default: None
- The style of the mean.
- data : indexable object, optional
- DATA_PARAMETER_PLACEHOLDER
-
- See Also
- --------
- violinplot : Draw an estimate of the probability density function.
- """
-
- # Missing arguments default to rcParams.
- if whis is None:
- whis = mpl.rcParams['boxplot.whiskers']
- if bootstrap is None:
- bootstrap = mpl.rcParams['boxplot.bootstrap']
-
- bxpstats = cbook.boxplot_stats(x, whis=whis, bootstrap=bootstrap,
- labels=labels, autorange=autorange)
- if notch is None:
- notch = mpl.rcParams['boxplot.notch']
- if vert is None:
- vert = mpl.rcParams['boxplot.vertical']
- if patch_artist is None:
- patch_artist = mpl.rcParams['boxplot.patchartist']
- if meanline is None:
- meanline = mpl.rcParams['boxplot.meanline']
- if showmeans is None:
- showmeans = mpl.rcParams['boxplot.showmeans']
- if showcaps is None:
- showcaps = mpl.rcParams['boxplot.showcaps']
- if showbox is None:
- showbox = mpl.rcParams['boxplot.showbox']
- if showfliers is None:
- showfliers = mpl.rcParams['boxplot.showfliers']
-
- if boxprops is None:
- boxprops = {}
- if whiskerprops is None:
- whiskerprops = {}
- if capprops is None:
- capprops = {}
- if medianprops is None:
- medianprops = {}
- if meanprops is None:
- meanprops = {}
- if flierprops is None:
- flierprops = {}
-
- if patch_artist:
- boxprops['linestyle'] = 'solid' # Not consistent with bxp.
- if 'color' in boxprops:
- boxprops['edgecolor'] = boxprops.pop('color')
-
- # if non-default sym value, put it into the flier dictionary
- # the logic for providing the default symbol ('b+') now lives
- # in bxp in the initial value of flierkw
- # handle all of the *sym* related logic here so we only have to pass
- # on the flierprops dict.
- if sym is not None:
- # no-flier case, which should really be done with
- # 'showfliers=False' but none-the-less deal with it to keep back
- # compatibility
- if sym == '':
- # blow away existing dict and make one for invisible markers
- flierprops = dict(linestyle='none', marker='', color='none')
- # turn the fliers off just to be safe
- showfliers = False
- # now process the symbol string
- else:
- # process the symbol string
- # discarded linestyle
- _, marker, color = _process_plot_format(sym)
- # if we have a marker, use it
- if marker is not None:
- flierprops['marker'] = marker
- # if we have a color, use it
- if color is not None:
- # assume that if color is passed in the user want
- # filled symbol, if the users want more control use
- # flierprops
- flierprops['color'] = color
- flierprops['markerfacecolor'] = color
- flierprops['markeredgecolor'] = color
-
- # replace medians if necessary:
- if usermedians is not None:
- if (len(np.ravel(usermedians)) != len(bxpstats) or
- np.shape(usermedians)[0] != len(bxpstats)):
- raise ValueError(
- "'usermedians' and 'x' have different lengths")
- else:
- # reassign medians as necessary
- for stats, med in zip(bxpstats, usermedians):
- if med is not None:
- stats['med'] = med
-
- if conf_intervals is not None:
- if len(conf_intervals) != len(bxpstats):
- raise ValueError(
- "'conf_intervals' and 'x' have different lengths")
- else:
- for stats, ci in zip(bxpstats, conf_intervals):
- if ci is not None:
- if len(ci) != 2:
- raise ValueError('each confidence interval must '
- 'have two values')
- else:
- if ci[0] is not None:
- stats['cilo'] = ci[0]
- if ci[1] is not None:
- stats['cihi'] = ci[1]
-
- artists = self.bxp(bxpstats, positions=positions, widths=widths,
- vert=vert, patch_artist=patch_artist,
- shownotches=notch, showmeans=showmeans,
- showcaps=showcaps, showbox=showbox,
- boxprops=boxprops, flierprops=flierprops,
- medianprops=medianprops, meanprops=meanprops,
- meanline=meanline, showfliers=showfliers,
- capprops=capprops, whiskerprops=whiskerprops,
- manage_ticks=manage_ticks, zorder=zorder,
- capwidths=capwidths)
- return artists
-
- def bxp(self, bxpstats, positions=None, widths=None, vert=True,
- patch_artist=False, shownotches=False, showmeans=False,
- showcaps=True, showbox=True, showfliers=True,
- boxprops=None, whiskerprops=None, flierprops=None,
- medianprops=None, capprops=None, meanprops=None,
- meanline=False, manage_ticks=True, zorder=None,
- capwidths=None):
- """
- Drawing function for box and whisker plots.
-
- Make a box and whisker plot for each column of *x* or each
- vector in sequence *x*. The box extends from the lower to
- upper quartile values of the data, with a line at the median.
- The whiskers extend from the box to show the range of the
- data. Flier points are those past the end of the whiskers.
-
- Parameters
- ----------
- bxpstats : list of dicts
- A list of dictionaries containing stats for each boxplot.
- Required keys are:
-
- - ``med``: Median (scalar).
- - ``q1``, ``q3``: First & third quartiles (scalars).
- - ``whislo``, ``whishi``: Lower & upper whisker positions (scalars).
-
- Optional keys are:
-
- - ``mean``: Mean (scalar). Needed if ``showmeans=True``.
- - ``fliers``: Data beyond the whiskers (array-like).
- Needed if ``showfliers=True``.
- - ``cilo``, ``cihi``: Lower & upper confidence intervals
- about the median. Needed if ``shownotches=True``.
- - ``label``: Name of the dataset (str). If available,
- this will be used a tick label for the boxplot
-
- positions : array-like, default: [1, 2, ..., n]
- The positions of the boxes. The ticks and limits
- are automatically set to match the positions.
-
- widths : float or array-like, default: None
- The widths of the boxes. The default is
- ``clip(0.15*(distance between extreme positions), 0.15, 0.5)``.
-
- capwidths : float or array-like, default: None
- Either a scalar or a vector and sets the width of each cap.
- The default is ``0.5*(with of the box)``, see *widths*.
-
- vert : bool, default: True
- If `True` (default), makes the boxes vertical.
- If `False`, makes horizontal boxes.
-
- patch_artist : bool, default: False
- If `False` produces boxes with the `.Line2D` artist.
- If `True` produces boxes with the `~matplotlib.patches.Patch` artist.
-
- shownotches, showmeans, showcaps, showbox, showfliers : bool
- Whether to draw the CI notches, the mean value (both default to
- False), the caps, the box, and the fliers (all three default to
- True).
-
- boxprops, whiskerprops, capprops, flierprops, medianprops, meanprops :\
- dict, optional
- Artist properties for the boxes, whiskers, caps, fliers, medians, and
- means.
-
- meanline : bool, default: False
- If `True` (and *showmeans* is `True`), will try to render the mean
- as a line spanning the full width of the box according to
- *meanprops*. Not recommended if *shownotches* is also True.
- Otherwise, means will be shown as points.
-
- manage_ticks : bool, default: True
- If True, the tick locations and labels will be adjusted to match the
- boxplot positions.
-
- zorder : float, default: ``Line2D.zorder = 2``
- The zorder of the resulting boxplot.
-
- Returns
- -------
- dict
- A dictionary mapping each component of the boxplot to a list
- of the `.Line2D` instances created. That dictionary has the
- following keys (assuming vertical boxplots):
-
- - ``boxes``: main bodies of the boxplot showing the quartiles, and
- the median's confidence intervals if enabled.
- - ``medians``: horizontal lines at the median of each box.
- - ``whiskers``: vertical lines up to the last non-outlier data.
- - ``caps``: horizontal lines at the ends of the whiskers.
- - ``fliers``: points representing data beyond the whiskers (fliers).
- - ``means``: points or lines representing the means.
-
- Examples
- --------
- .. plot:: gallery/statistics/bxp.py
- """
-
- # lists of artists to be output
- whiskers = []
- caps = []
- boxes = []
- medians = []
- means = []
- fliers = []
-
- # empty list of xticklabels
- datalabels = []
-
- # Use default zorder if none specified
- if zorder is None:
- zorder = mlines.Line2D.zorder
-
- zdelta = 0.1
-
- def merge_kw_rc(subkey, explicit, zdelta=0, usemarker=True):
- d = {k.split('.')[-1]: v for k, v in mpl.rcParams.items()
- if k.startswith(f'boxplot.{subkey}props')}
- d['zorder'] = zorder + zdelta
- if not usemarker:
- d['marker'] = ''
- d.update(cbook.normalize_kwargs(explicit, mlines.Line2D))
- return d
-
- box_kw = {
- 'linestyle': mpl.rcParams['boxplot.boxprops.linestyle'],
- 'linewidth': mpl.rcParams['boxplot.boxprops.linewidth'],
- 'edgecolor': mpl.rcParams['boxplot.boxprops.color'],
- 'facecolor': ('white' if mpl.rcParams['_internal.classic_mode']
- else mpl.rcParams['patch.facecolor']),
- 'zorder': zorder,
- **cbook.normalize_kwargs(boxprops, mpatches.PathPatch)
- } if patch_artist else merge_kw_rc('box', boxprops, usemarker=False)
- whisker_kw = merge_kw_rc('whisker', whiskerprops, usemarker=False)
- cap_kw = merge_kw_rc('cap', capprops, usemarker=False)
- flier_kw = merge_kw_rc('flier', flierprops)
- median_kw = merge_kw_rc('median', medianprops, zdelta, usemarker=False)
- mean_kw = merge_kw_rc('mean', meanprops, zdelta)
- removed_prop = 'marker' if meanline else 'linestyle'
- # Only remove the property if it's not set explicitly as a parameter.
- if meanprops is None or removed_prop not in meanprops:
- mean_kw[removed_prop] = ''
-
- # vertical or horizontal plot?
- maybe_swap = slice(None) if vert else slice(None, None, -1)
-
- def do_plot(xs, ys, **kwargs):
- return self.plot(*[xs, ys][maybe_swap], **kwargs)[0]
-
- def do_patch(xs, ys, **kwargs):
- path = mpath.Path._create_closed(
- np.column_stack([xs, ys][maybe_swap]))
- patch = mpatches.PathPatch(path, **kwargs)
- self.add_artist(patch)
- return patch
-
- # input validation
- N = len(bxpstats)
- datashape_message = ("List of boxplot statistics and `{0}` "
- "values must have same the length")
- # check position
- if positions is None:
- positions = list(range(1, N + 1))
- elif len(positions) != N:
- raise ValueError(datashape_message.format("positions"))
-
- positions = np.array(positions)
- if len(positions) > 0 and not isinstance(positions[0], Number):
- raise TypeError("positions should be an iterable of numbers")
-
- # width
- if widths is None:
- widths = [np.clip(0.15 * np.ptp(positions), 0.15, 0.5)] * N
- elif np.isscalar(widths):
- widths = [widths] * N
- elif len(widths) != N:
- raise ValueError(datashape_message.format("widths"))
-
- # capwidth
- if capwidths is None:
- capwidths = 0.5 * np.array(widths)
- elif np.isscalar(capwidths):
- capwidths = [capwidths] * N
- elif len(capwidths) != N:
- raise ValueError(datashape_message.format("capwidths"))
-
- for pos, width, stats, capwidth in zip(positions, widths, bxpstats,
- capwidths):
- # try to find a new label
- datalabels.append(stats.get('label', pos))
-
- # whisker coords
- whis_x = [pos, pos]
- whislo_y = [stats['q1'], stats['whislo']]
- whishi_y = [stats['q3'], stats['whishi']]
- # cap coords
- cap_left = pos - capwidth * 0.5
- cap_right = pos + capwidth * 0.5
- cap_x = [cap_left, cap_right]
- cap_lo = np.full(2, stats['whislo'])
- cap_hi = np.full(2, stats['whishi'])
- # box and median coords
- box_left = pos - width * 0.5
- box_right = pos + width * 0.5
- med_y = [stats['med'], stats['med']]
- # notched boxes
- if shownotches:
- notch_left = pos - width * 0.25
- notch_right = pos + width * 0.25
- box_x = [box_left, box_right, box_right, notch_right,
- box_right, box_right, box_left, box_left, notch_left,
- box_left, box_left]
- box_y = [stats['q1'], stats['q1'], stats['cilo'],
- stats['med'], stats['cihi'], stats['q3'],
- stats['q3'], stats['cihi'], stats['med'],
- stats['cilo'], stats['q1']]
- med_x = [notch_left, notch_right]
- # plain boxes
- else:
- box_x = [box_left, box_right, box_right, box_left, box_left]
- box_y = [stats['q1'], stats['q1'], stats['q3'], stats['q3'],
- stats['q1']]
- med_x = [box_left, box_right]
-
- # maybe draw the box
- if showbox:
- do_box = do_patch if patch_artist else do_plot
- boxes.append(do_box(box_x, box_y, **box_kw))
- # draw the whiskers
- whiskers.append(do_plot(whis_x, whislo_y, **whisker_kw))
- whiskers.append(do_plot(whis_x, whishi_y, **whisker_kw))
- # maybe draw the caps
- if showcaps:
- caps.append(do_plot(cap_x, cap_lo, **cap_kw))
- caps.append(do_plot(cap_x, cap_hi, **cap_kw))
- # draw the medians
- medians.append(do_plot(med_x, med_y, **median_kw))
- # maybe draw the means
- if showmeans:
- if meanline:
- means.append(do_plot(
- [box_left, box_right], [stats['mean'], stats['mean']],
- **mean_kw
- ))
- else:
- means.append(do_plot([pos], [stats['mean']], **mean_kw))
- # maybe draw the fliers
- if showfliers:
- flier_x = np.full(len(stats['fliers']), pos, dtype=np.float64)
- flier_y = stats['fliers']
- fliers.append(do_plot(flier_x, flier_y, **flier_kw))
-
- if manage_ticks:
- axis_name = "x" if vert else "y"
- interval = getattr(self.dataLim, f"interval{axis_name}")
- axis = getattr(self, f"{axis_name}axis")
- positions = axis.convert_units(positions)
- # The 0.5 additional padding ensures reasonable-looking boxes
- # even when drawing a single box. We set the sticky edge to
- # prevent margins expansion, in order to match old behavior (back
- # when separate calls to boxplot() would completely reset the axis
- # limits regardless of what was drawn before). The sticky edges
- # are attached to the median lines, as they are always present.
- interval[:] = (min(interval[0], min(positions) - .5),
- max(interval[1], max(positions) + .5))
- for median, position in zip(medians, positions):
- getattr(median.sticky_edges, axis_name).extend(
- [position - .5, position + .5])
- # Modified from Axis.set_ticks and Axis.set_ticklabels.
- locator = axis.get_major_locator()
- if not isinstance(axis.get_major_locator(),
- mticker.FixedLocator):
- locator = mticker.FixedLocator([])
- axis.set_major_locator(locator)
- locator.locs = np.array([*locator.locs, *positions])
- formatter = axis.get_major_formatter()
- if not isinstance(axis.get_major_formatter(),
- mticker.FixedFormatter):
- formatter = mticker.FixedFormatter([])
- axis.set_major_formatter(formatter)
- formatter.seq = [*formatter.seq, *datalabels]
-
- self._request_autoscale_view()
-
- return dict(whiskers=whiskers, caps=caps, boxes=boxes,
- medians=medians, fliers=fliers, means=means)
-
- @staticmethod
- def _parse_scatter_color_args(c, edgecolors, kwargs, xsize,
- get_next_color_func):
- """
- Helper function to process color related arguments of `.Axes.scatter`.
-
- Argument precedence for facecolors:
-
- - c (if not None)
- - kwargs['facecolor']
- - kwargs['facecolors']
- - kwargs['color'] (==kwcolor)
- - 'b' if in classic mode else the result of ``get_next_color_func()``
-
- Argument precedence for edgecolors:
-
- - kwargs['edgecolor']
- - edgecolors (is an explicit kw argument in scatter())
- - kwargs['color'] (==kwcolor)
- - 'face' if not in classic mode else None
-
- Parameters
- ----------
- c : color or sequence or sequence of color or None
- See argument description of `.Axes.scatter`.
- edgecolors : color or sequence of color or {'face', 'none'} or None
- See argument description of `.Axes.scatter`.
- kwargs : dict
- Additional kwargs. If these keys exist, we pop and process them:
- 'facecolors', 'facecolor', 'edgecolor', 'color'
- Note: The dict is modified by this function.
- xsize : int
- The size of the x and y arrays passed to `.Axes.scatter`.
- get_next_color_func : callable
- A callable that returns a color. This color is used as facecolor
- if no other color is provided.
-
- Note, that this is a function rather than a fixed color value to
- support conditional evaluation of the next color. As of the
- current implementation obtaining the next color from the
- property cycle advances the cycle. This must only happen if we
- actually use the color, which will only be decided within this
- method.
-
- Returns
- -------
- c
- The input *c* if it was not *None*, else a color derived from the
- other inputs or defaults.
- colors : array(N, 4) or None
- The facecolors as RGBA values, or *None* if a colormap is used.
- edgecolors
- The edgecolor.
-
- """
- facecolors = kwargs.pop('facecolors', None)
- facecolors = kwargs.pop('facecolor', facecolors)
- edgecolors = kwargs.pop('edgecolor', edgecolors)
-
- kwcolor = kwargs.pop('color', None)
-
- if kwcolor is not None and c is not None:
- raise ValueError("Supply a 'c' argument or a 'color'"
- " kwarg but not both; they differ but"
- " their functionalities overlap.")
-
- if kwcolor is not None:
- try:
- mcolors.to_rgba_array(kwcolor)
- except ValueError as err:
- raise ValueError(
- "'color' kwarg must be a color or sequence of color "
- "specs. For a sequence of values to be color-mapped, use "
- "the 'c' argument instead.") from err
- if edgecolors is None:
- edgecolors = kwcolor
- if facecolors is None:
- facecolors = kwcolor
-
- if edgecolors is None and not mpl.rcParams['_internal.classic_mode']:
- edgecolors = mpl.rcParams['scatter.edgecolors']
-
- c_was_none = c is None
- if c is None:
- c = (facecolors if facecolors is not None
- else "b" if mpl.rcParams['_internal.classic_mode']
- else get_next_color_func())
- c_is_string_or_strings = (
- isinstance(c, str)
- or (np.iterable(c) and len(c) > 0
- and isinstance(cbook._safe_first_finite(c), str)))
-
- def invalid_shape_exception(csize, xsize):
- return ValueError(
- f"'c' argument has {csize} elements, which is inconsistent "
- f"with 'x' and 'y' with size {xsize}.")
-
- c_is_mapped = False # Unless proven otherwise below.
- valid_shape = True # Unless proven otherwise below.
- if not c_was_none and kwcolor is None and not c_is_string_or_strings:
- try: # First, does 'c' look suitable for value-mapping?
- c = np.asanyarray(c, dtype=float)
- except ValueError:
- pass # Failed to convert to float array; must be color specs.
- else:
- # handle the documented special case of a 2D array with 1
- # row which as RGB(A) to broadcast.
- if c.shape == (1, 4) or c.shape == (1, 3):
- c_is_mapped = False
- if c.size != xsize:
- valid_shape = False
- # If c can be either mapped values or an RGB(A) color, prefer
- # the former if shapes match, the latter otherwise.
- elif c.size == xsize:
- c = c.ravel()
- c_is_mapped = True
- else: # Wrong size; it must not be intended for mapping.
- if c.shape in ((3,), (4,)):
- _api.warn_external(
- "*c* argument looks like a single numeric RGB or "
- "RGBA sequence, which should be avoided as value-"
- "mapping will have precedence in case its length "
- "matches with *x* & *y*. Please use the *color* "
- "keyword-argument or provide a 2D array "
- "with a single row if you intend to specify "
- "the same RGB or RGBA value for all points.")
- valid_shape = False
- if not c_is_mapped:
- try: # Is 'c' acceptable as PathCollection facecolors?
- colors = mcolors.to_rgba_array(c)
- except (TypeError, ValueError) as err:
- if "RGBA values should be within 0-1 range" in str(err):
- raise
- else:
- if not valid_shape:
- raise invalid_shape_exception(c.size, xsize) from err
- # Both the mapping *and* the RGBA conversion failed: pretty
- # severe failure => one may appreciate a verbose feedback.
- raise ValueError(
- f"'c' argument must be a color, a sequence of colors, "
- f"or a sequence of numbers, not {c!r}") from err
- else:
- if len(colors) not in (0, 1, xsize):
- # NB: remember that a single color is also acceptable.
- # Besides *colors* will be an empty array if c == 'none'.
- raise invalid_shape_exception(len(colors), xsize)
- else:
- colors = None # use cmap, norm after collection is created
- return c, colors, edgecolors
-
- @_preprocess_data(replace_names=["x", "y", "s", "linewidths",
- "edgecolors", "c", "facecolor",
- "facecolors", "color"],
- label_namer="y")
- @_docstring.interpd
- def scatter(self, x, y, s=None, c=None, marker=None, cmap=None, norm=None,
- vmin=None, vmax=None, alpha=None, linewidths=None, *,
- edgecolors=None, plotnonfinite=False, **kwargs):
- """
- A scatter plot of *y* vs. *x* with varying marker size and/or color.
-
- Parameters
- ----------
- x, y : float or array-like, shape (n, )
- The data positions.
-
- s : float or array-like, shape (n, ), optional
- The marker size in points**2 (typographic points are 1/72 in.).
- Default is ``rcParams['lines.markersize'] ** 2``.
-
- c : array-like or list of colors or color, optional
- The marker colors. Possible values:
-
- - A scalar or sequence of n numbers to be mapped to colors using
- *cmap* and *norm*.
- - A 2D array in which the rows are RGB or RGBA.
- - A sequence of colors of length n.
- - A single color format string.
-
- Note that *c* should not be a single numeric RGB or RGBA sequence
- because that is indistinguishable from an array of values to be
- colormapped. If you want to specify the same RGB or RGBA value for
- all points, use a 2D array with a single row. Otherwise,
- value-matching will have precedence in case of a size matching with
- *x* and *y*.
-
- If you wish to specify a single color for all points
- prefer the *color* keyword argument.
-
- Defaults to `None`. In that case the marker color is determined
- by the value of *color*, *facecolor* or *facecolors*. In case
- those are not specified or `None`, the marker color is determined
- by the next color of the ``Axes``' current "shape and fill" color
- cycle. This cycle defaults to :rc:`axes.prop_cycle`.
-
- marker : `~.markers.MarkerStyle`, default: :rc:`scatter.marker`
- The marker style. *marker* can be either an instance of the class
- or the text shorthand for a particular marker.
- See :mod:`matplotlib.markers` for more information about marker
- styles.
-
- %(cmap_doc)s
-
- This parameter is ignored if *c* is RGB(A).
-
- %(norm_doc)s
-
- This parameter is ignored if *c* is RGB(A).
-
- %(vmin_vmax_doc)s
-
- This parameter is ignored if *c* is RGB(A).
-
- alpha : float, default: None
- The alpha blending value, between 0 (transparent) and 1 (opaque).
-
- linewidths : float or array-like, default: :rc:`lines.linewidth`
- The linewidth of the marker edges. Note: The default *edgecolors*
- is 'face'. You may want to change this as well.
-
- edgecolors : {'face', 'none', *None*} or color or sequence of color, \
-default: :rc:`scatter.edgecolors`
- The edge color of the marker. Possible values:
-
- - 'face': The edge color will always be the same as the face color.
- - 'none': No patch boundary will be drawn.
- - A color or sequence of colors.
-
- For non-filled markers, *edgecolors* is ignored. Instead, the color
- is determined like with 'face', i.e. from *c*, *colors*, or
- *facecolors*.
-
- plotnonfinite : bool, default: False
- Whether to plot points with nonfinite *c* (i.e. ``inf``, ``-inf``
- or ``nan``). If ``True`` the points are drawn with the *bad*
- colormap color (see `.Colormap.set_bad`).
-
- Returns
- -------
- `~matplotlib.collections.PathCollection`
-
- Other Parameters
- ----------------
- data : indexable object, optional
- DATA_PARAMETER_PLACEHOLDER
- **kwargs : `~matplotlib.collections.Collection` properties
-
- See Also
- --------
- plot : To plot scatter plots when markers are identical in size and
- color.
-
- Notes
- -----
- * The `.plot` function will be faster for scatterplots where markers
- don't vary in size or color.
-
- * Any or all of *x*, *y*, *s*, and *c* may be masked arrays, in which
- case all masks will be combined and only unmasked points will be
- plotted.
-
- * Fundamentally, scatter works with 1D arrays; *x*, *y*, *s*, and *c*
- may be input as N-D arrays, but within scatter they will be
- flattened. The exception is *c*, which will be flattened only if its
- size matches the size of *x* and *y*.
-
- """
- # Process **kwargs to handle aliases, conflicts with explicit kwargs:
- x, y = self._process_unit_info([("x", x), ("y", y)], kwargs)
- # np.ma.ravel yields an ndarray, not a masked array,
- # unless its argument is a masked array.
- x = np.ma.ravel(x)
- y = np.ma.ravel(y)
- if x.size != y.size:
- raise ValueError("x and y must be the same size")
-
- if s is None:
- s = (20 if mpl.rcParams['_internal.classic_mode'] else
- mpl.rcParams['lines.markersize'] ** 2.0)
- s = np.ma.ravel(s)
- if (len(s) not in (1, x.size) or
- (not np.issubdtype(s.dtype, np.floating) and
- not np.issubdtype(s.dtype, np.integer))):
- raise ValueError(
- "s must be a scalar, "
- "or float array-like with the same size as x and y")
-
- # get the original edgecolor the user passed before we normalize
- orig_edgecolor = edgecolors
- if edgecolors is None:
- orig_edgecolor = kwargs.get('edgecolor', None)
- c, colors, edgecolors = \
- self._parse_scatter_color_args(
- c, edgecolors, kwargs, x.size,
- get_next_color_func=self._get_patches_for_fill.get_next_color)
-
- if plotnonfinite and colors is None:
- c = np.ma.masked_invalid(c)
- x, y, s, edgecolors, linewidths = \
- cbook._combine_masks(x, y, s, edgecolors, linewidths)
- else:
- x, y, s, c, colors, edgecolors, linewidths = \
- cbook._combine_masks(
- x, y, s, c, colors, edgecolors, linewidths)
- # Unmask edgecolors if it was actually a single RGB or RGBA.
- if (x.size in (3, 4)
- and np.ma.is_masked(edgecolors)
- and not np.ma.is_masked(orig_edgecolor)):
- edgecolors = edgecolors.data
-
- scales = s # Renamed for readability below.
-
- # load default marker from rcParams
- if marker is None:
- marker = mpl.rcParams['scatter.marker']
-
- if isinstance(marker, mmarkers.MarkerStyle):
- marker_obj = marker
- else:
- marker_obj = mmarkers.MarkerStyle(marker)
-
- path = marker_obj.get_path().transformed(
- marker_obj.get_transform())
- if not marker_obj.is_filled():
- if orig_edgecolor is not None:
- _api.warn_external(
- f"You passed a edgecolor/edgecolors ({orig_edgecolor!r}) "
- f"for an unfilled marker ({marker!r}). Matplotlib is "
- "ignoring the edgecolor in favor of the facecolor. This "
- "behavior may change in the future."
- )
- # We need to handle markers that can not be filled (like
- # '+' and 'x') differently than markers that can be
- # filled, but have their fillstyle set to 'none'. This is
- # to get:
- #
- # - respecting the fillestyle if set
- # - maintaining back-compatibility for querying the facecolor of
- # the un-fillable markers.
- #
- # While not an ideal situation, but is better than the
- # alternatives.
- if marker_obj.get_fillstyle() == 'none':
- # promote the facecolor to be the edgecolor
- edgecolors = colors
- # set the facecolor to 'none' (at the last chance) because
- # we can not fill a path if the facecolor is non-null
- # (which is defendable at the renderer level).
- colors = 'none'
- else:
- # if we are not nulling the face color we can do this
- # simpler
- edgecolors = 'face'
-
- if linewidths is None:
- linewidths = mpl.rcParams['lines.linewidth']
- elif np.iterable(linewidths):
- linewidths = [
- lw if lw is not None else mpl.rcParams['lines.linewidth']
- for lw in linewidths]
-
- offsets = np.ma.column_stack([x, y])
-
- collection = mcoll.PathCollection(
- (path,), scales,
- facecolors=colors,
- edgecolors=edgecolors,
- linewidths=linewidths,
- offsets=offsets,
- offset_transform=kwargs.pop('transform', self.transData),
- alpha=alpha,
- )
- collection.set_transform(mtransforms.IdentityTransform())
- if colors is None:
- collection.set_array(c)
- collection.set_cmap(cmap)
- collection.set_norm(norm)
- collection._scale_norm(norm, vmin, vmax)
- else:
- extra_kwargs = {
- 'cmap': cmap, 'norm': norm, 'vmin': vmin, 'vmax': vmax
- }
- extra_keys = [k for k, v in extra_kwargs.items() if v is not None]
- if any(extra_keys):
- keys_str = ", ".join(f"'{k}'" for k in extra_keys)
- _api.warn_external(
- "No data for colormapping provided via 'c'. "
- f"Parameters {keys_str} will be ignored")
- collection._internal_update(kwargs)
-
- # Classic mode only:
- # ensure there are margins to allow for the
- # finite size of the symbols. In v2.x, margins
- # are present by default, so we disable this
- # scatter-specific override.
- if mpl.rcParams['_internal.classic_mode']:
- if self._xmargin < 0.05 and x.size > 0:
- self.set_xmargin(0.05)
- if self._ymargin < 0.05 and x.size > 0:
- self.set_ymargin(0.05)
-
- self.add_collection(collection)
- self._request_autoscale_view()
-
- return collection
-
- @_preprocess_data(replace_names=["x", "y", "C"], label_namer="y")
- @_docstring.dedent_interpd
- def hexbin(self, x, y, C=None, gridsize=100, bins=None,
- xscale='linear', yscale='linear', extent=None,
- cmap=None, norm=None, vmin=None, vmax=None,
- alpha=None, linewidths=None, edgecolors='face',
- reduce_C_function=np.mean, mincnt=None, marginals=False,
- **kwargs):
- """
- Make a 2D hexagonal binning plot of points *x*, *y*.
-
- If *C* is *None*, the value of the hexagon is determined by the number
- of points in the hexagon. Otherwise, *C* specifies values at the
- coordinate (x[i], y[i]). For each hexagon, these values are reduced
- using *reduce_C_function*.
-
- Parameters
- ----------
- x, y : array-like
- The data positions. *x* and *y* must be of the same length.
-
- C : array-like, optional
- If given, these values are accumulated in the bins. Otherwise,
- every point has a value of 1. Must be of the same length as *x*
- and *y*.
-
- gridsize : int or (int, int), default: 100
- If a single int, the number of hexagons in the *x*-direction.
- The number of hexagons in the *y*-direction is chosen such that
- the hexagons are approximately regular.
-
- Alternatively, if a tuple (*nx*, *ny*), the number of hexagons
- in the *x*-direction and the *y*-direction. In the
- *y*-direction, counting is done along vertically aligned
- hexagons, not along the zig-zag chains of hexagons; see the
- following illustration.
-
- .. plot::
-
- import numpy
- import matplotlib.pyplot as plt
-
- np.random.seed(19680801)
- n= 300
- x = np.random.standard_normal(n)
- y = np.random.standard_normal(n)
-
- fig, ax = plt.subplots(figsize=(4, 4))
- h = ax.hexbin(x, y, gridsize=(5, 3))
- hx, hy = h.get_offsets().T
- ax.plot(hx[24::3], hy[24::3], 'ro-')
- ax.plot(hx[-3:], hy[-3:], 'ro-')
- ax.set_title('gridsize=(5, 3)')
- ax.axis('off')
-
- To get approximately regular hexagons, choose
- :math:`n_x = \\sqrt{3}\\,n_y`.
-
- bins : 'log' or int or sequence, default: None
- Discretization of the hexagon values.
-
- - If *None*, no binning is applied; the color of each hexagon
- directly corresponds to its count value.
- - If 'log', use a logarithmic scale for the colormap.
- Internally, :math:`log_{10}(i+1)` is used to determine the
- hexagon color. This is equivalent to ``norm=LogNorm()``.
- - If an integer, divide the counts in the specified number
- of bins, and color the hexagons accordingly.
- - If a sequence of values, the values of the lower bound of
- the bins to be used.
-
- xscale : {'linear', 'log'}, default: 'linear'
- Use a linear or log10 scale on the horizontal axis.
-
- yscale : {'linear', 'log'}, default: 'linear'
- Use a linear or log10 scale on the vertical axis.
-
- mincnt : int > 0, default: *None*
- If not *None*, only display cells with more than *mincnt*
- number of points in the cell.
-
- marginals : bool, default: *False*
- If marginals is *True*, plot the marginal density as
- colormapped rectangles along the bottom of the x-axis and
- left of the y-axis.
-
- extent : 4-tuple of float, default: *None*
- The limits of the bins (xmin, xmax, ymin, ymax).
- The default assigns the limits based on
- *gridsize*, *x*, *y*, *xscale* and *yscale*.
-
- If *xscale* or *yscale* is set to 'log', the limits are
- expected to be the exponent for a power of 10. E.g. for
- x-limits of 1 and 50 in 'linear' scale and y-limits
- of 10 and 1000 in 'log' scale, enter (1, 50, 1, 3).
-
- Returns
- -------
- `~matplotlib.collections.PolyCollection`
- A `.PolyCollection` defining the hexagonal bins.
-
- - `.PolyCollection.get_offsets` contains a Mx2 array containing
- the x, y positions of the M hexagon centers.
- - `.PolyCollection.get_array` contains the values of the M
- hexagons.
-
- If *marginals* is *True*, horizontal
- bar and vertical bar (both PolyCollections) will be attached
- to the return collection as attributes *hbar* and *vbar*.
-
- Other Parameters
- ----------------
- %(cmap_doc)s
-
- %(norm_doc)s
-
- %(vmin_vmax_doc)s
-
- alpha : float between 0 and 1, optional
- The alpha blending value, between 0 (transparent) and 1 (opaque).
-
- linewidths : float, default: *None*
- If *None*, defaults to 1.0.
-
- edgecolors : {'face', 'none', *None*} or color, default: 'face'
- The color of the hexagon edges. Possible values are:
-
- - 'face': Draw the edges in the same color as the fill color.
- - 'none': No edges are drawn. This can sometimes lead to unsightly
- unpainted pixels between the hexagons.
- - *None*: Draw outlines in the default color.
- - An explicit color.
-
- reduce_C_function : callable, default: `numpy.mean`
- The function to aggregate *C* within the bins. It is ignored if
- *C* is not given. This must have the signature::
-
- def reduce_C_function(C: array) -> float
-
- Commonly used functions are:
-
- - `numpy.mean`: average of the points
- - `numpy.sum`: integral of the point values
- - `numpy.amax`: value taken from the largest point
-
- data : indexable object, optional
- DATA_PARAMETER_PLACEHOLDER
-
- **kwargs : `~matplotlib.collections.PolyCollection` properties
- All other keyword arguments are passed on to `.PolyCollection`:
-
- %(PolyCollection:kwdoc)s
-
- See Also
- --------
- hist2d : 2D histogram rectangular bins
- """
- self._process_unit_info([("x", x), ("y", y)], kwargs, convert=False)
-
- x, y, C = cbook.delete_masked_points(x, y, C)
-
- # Set the size of the hexagon grid
- if np.iterable(gridsize):
- nx, ny = gridsize
- else:
- nx = gridsize
- ny = int(nx / math.sqrt(3))
- # Count the number of data in each hexagon
- x = np.asarray(x, float)
- y = np.asarray(y, float)
-
- # Will be log()'d if necessary, and then rescaled.
- tx = x
- ty = y
-
- if xscale == 'log':
- if np.any(x <= 0.0):
- raise ValueError("x contains non-positive values, so can not "
- "be log-scaled")
- tx = np.log10(tx)
- if yscale == 'log':
- if np.any(y <= 0.0):
- raise ValueError("y contains non-positive values, so can not "
- "be log-scaled")
- ty = np.log10(ty)
- if extent is not None:
- xmin, xmax, ymin, ymax = extent
- else:
- xmin, xmax = (tx.min(), tx.max()) if len(x) else (0, 1)
- ymin, ymax = (ty.min(), ty.max()) if len(y) else (0, 1)
-
- # to avoid issues with singular data, expand the min/max pairs
- xmin, xmax = mtransforms.nonsingular(xmin, xmax, expander=0.1)
- ymin, ymax = mtransforms.nonsingular(ymin, ymax, expander=0.1)
-
- nx1 = nx + 1
- ny1 = ny + 1
- nx2 = nx
- ny2 = ny
- n = nx1 * ny1 + nx2 * ny2
-
- # In the x-direction, the hexagons exactly cover the region from
- # xmin to xmax. Need some padding to avoid roundoff errors.
- padding = 1.e-9 * (xmax - xmin)
- xmin -= padding
- xmax += padding
- sx = (xmax - xmin) / nx
- sy = (ymax - ymin) / ny
- # Positions in hexagon index coordinates.
- ix = (tx - xmin) / sx
- iy = (ty - ymin) / sy
- ix1 = np.round(ix).astype(int)
- iy1 = np.round(iy).astype(int)
- ix2 = np.floor(ix).astype(int)
- iy2 = np.floor(iy).astype(int)
- # flat indices, plus one so that out-of-range points go to position 0.
- i1 = np.where((0 <= ix1) & (ix1 < nx1) & (0 <= iy1) & (iy1 < ny1),
- ix1 * ny1 + iy1 + 1, 0)
- i2 = np.where((0 <= ix2) & (ix2 < nx2) & (0 <= iy2) & (iy2 < ny2),
- ix2 * ny2 + iy2 + 1, 0)
-
- d1 = (ix - ix1) ** 2 + 3.0 * (iy - iy1) ** 2
- d2 = (ix - ix2 - 0.5) ** 2 + 3.0 * (iy - iy2 - 0.5) ** 2
- bdist = (d1 < d2)
-
- if C is None: # [1:] drops out-of-range points.
- counts1 = np.bincount(i1[bdist], minlength=1 + nx1 * ny1)[1:]
- counts2 = np.bincount(i2[~bdist], minlength=1 + nx2 * ny2)[1:]
- accum = np.concatenate([counts1, counts2]).astype(float)
- if mincnt is not None:
- accum[accum < mincnt] = np.nan
- C = np.ones(len(x))
- else:
- # store the C values in a list per hexagon index
- Cs_at_i1 = [[] for _ in range(1 + nx1 * ny1)]
- Cs_at_i2 = [[] for _ in range(1 + nx2 * ny2)]
- for i in range(len(x)):
- if bdist[i]:
- Cs_at_i1[i1[i]].append(C[i])
- else:
- Cs_at_i2[i2[i]].append(C[i])
- if mincnt is None:
- mincnt = 0
- accum = np.array(
- [reduce_C_function(acc) if len(acc) > mincnt else np.nan
- for Cs_at_i in [Cs_at_i1, Cs_at_i2]
- for acc in Cs_at_i[1:]], # [1:] drops out-of-range points.
- float)
-
- good_idxs = ~np.isnan(accum)
-
- offsets = np.zeros((n, 2), float)
- offsets[:nx1 * ny1, 0] = np.repeat(np.arange(nx1), ny1)
- offsets[:nx1 * ny1, 1] = np.tile(np.arange(ny1), nx1)
- offsets[nx1 * ny1:, 0] = np.repeat(np.arange(nx2) + 0.5, ny2)
- offsets[nx1 * ny1:, 1] = np.tile(np.arange(ny2), nx2) + 0.5
- offsets[:, 0] *= sx
- offsets[:, 1] *= sy
- offsets[:, 0] += xmin
- offsets[:, 1] += ymin
- # remove accumulation bins with no data
- offsets = offsets[good_idxs, :]
- accum = accum[good_idxs]
-
- polygon = [sx, sy / 3] * np.array(
- [[.5, -.5], [.5, .5], [0., 1.], [-.5, .5], [-.5, -.5], [0., -1.]])
-
- if linewidths is None:
- linewidths = [1.0]
-
- if xscale == 'log' or yscale == 'log':
- polygons = np.expand_dims(polygon, 0) + np.expand_dims(offsets, 1)
- if xscale == 'log':
- polygons[:, :, 0] = 10.0 ** polygons[:, :, 0]
- xmin = 10.0 ** xmin
- xmax = 10.0 ** xmax
- self.set_xscale(xscale)
- if yscale == 'log':
- polygons[:, :, 1] = 10.0 ** polygons[:, :, 1]
- ymin = 10.0 ** ymin
- ymax = 10.0 ** ymax
- self.set_yscale(yscale)
- collection = mcoll.PolyCollection(
- polygons,
- edgecolors=edgecolors,
- linewidths=linewidths,
- )
- else:
- collection = mcoll.PolyCollection(
- [polygon],
- edgecolors=edgecolors,
- linewidths=linewidths,
- offsets=offsets,
- offset_transform=mtransforms.AffineDeltaTransform(
- self.transData),
- )
-
- # Set normalizer if bins is 'log'
- if bins == 'log':
- if norm is not None:
- _api.warn_external("Only one of 'bins' and 'norm' arguments "
- f"can be supplied, ignoring bins={bins}")
- else:
- norm = mcolors.LogNorm(vmin=vmin, vmax=vmax)
- vmin = vmax = None
- bins = None
-
- # autoscale the norm with current accum values if it hasn't been set
- if norm is not None:
- if norm.vmin is None and norm.vmax is None:
- norm.autoscale(accum)
-
- if bins is not None:
- if not np.iterable(bins):
- minimum, maximum = min(accum), max(accum)
- bins -= 1 # one less edge than bins
- bins = minimum + (maximum - minimum) * np.arange(bins) / bins
- bins = np.sort(bins)
- accum = bins.searchsorted(accum)
-
- collection.set_array(accum)
- collection.set_cmap(cmap)
- collection.set_norm(norm)
- collection.set_alpha(alpha)
- collection._internal_update(kwargs)
- collection._scale_norm(norm, vmin, vmax)
-
- corners = ((xmin, ymin), (xmax, ymax))
- self.update_datalim(corners)
- self._request_autoscale_view(tight=True)
-
- # add the collection last
- self.add_collection(collection, autolim=False)
- if not marginals:
- return collection
-
- # Process marginals
- bars = []
- for zname, z, zmin, zmax, zscale, nbins in [
- ("x", x, xmin, xmax, xscale, nx),
- ("y", y, ymin, ymax, yscale, 2 * ny),
- ]:
-
- if zscale == "log":
- bin_edges = np.geomspace(zmin, zmax, nbins + 1)
- else:
- bin_edges = np.linspace(zmin, zmax, nbins + 1)
-
- verts = np.empty((nbins, 4, 2))
- verts[:, 0, 0] = verts[:, 1, 0] = bin_edges[:-1]
- verts[:, 2, 0] = verts[:, 3, 0] = bin_edges[1:]
- verts[:, 0, 1] = verts[:, 3, 1] = .00
- verts[:, 1, 1] = verts[:, 2, 1] = .05
- if zname == "y":
- verts = verts[:, :, ::-1] # Swap x and y.
-
- # Sort z-values into bins defined by bin_edges.
- bin_idxs = np.searchsorted(bin_edges, z) - 1
- values = np.empty(nbins)
- for i in range(nbins):
- # Get C-values for each bin, and compute bin value with
- # reduce_C_function.
- ci = C[bin_idxs == i]
- values[i] = reduce_C_function(ci) if len(ci) > 0 else np.nan
-
- mask = ~np.isnan(values)
- verts = verts[mask]
- values = values[mask]
-
- trans = getattr(self, f"get_{zname}axis_transform")(which="grid")
- bar = mcoll.PolyCollection(
- verts, transform=trans, edgecolors="face")
- bar.set_array(values)
- bar.set_cmap(cmap)
- bar.set_norm(norm)
- bar.set_alpha(alpha)
- bar._internal_update(kwargs)
- bars.append(self.add_collection(bar, autolim=False))
-
- collection.hbar, collection.vbar = bars
-
- def on_changed(collection):
- collection.hbar.set_cmap(collection.get_cmap())
- collection.hbar.set_cmap(collection.get_cmap())
- collection.vbar.set_clim(collection.get_clim())
- collection.vbar.set_clim(collection.get_clim())
-
- collection.callbacks.connect('changed', on_changed)
-
- return collection
-
- @_docstring.dedent_interpd
- def arrow(self, x, y, dx, dy, **kwargs):
- """
- Add an arrow to the Axes.
-
- This draws an arrow from ``(x, y)`` to ``(x+dx, y+dy)``.
-
- Parameters
- ----------
- %(FancyArrow)s
-
- Returns
- -------
- `.FancyArrow`
- The created `.FancyArrow` object.
-
- Notes
- -----
- The resulting arrow is affected by the Axes aspect ratio and limits.
- This may produce an arrow whose head is not square with its stem. To
- create an arrow whose head is square with its stem,
- use :meth:`annotate` for example:
-
- >>> ax.annotate("", xy=(0.5, 0.5), xytext=(0, 0),
- ... arrowprops=dict(arrowstyle="->"))
-
- """
- # Strip away units for the underlying patch since units
- # do not make sense to most patch-like code
- x = self.convert_xunits(x)
- y = self.convert_yunits(y)
- dx = self.convert_xunits(dx)
- dy = self.convert_yunits(dy)
-
- a = mpatches.FancyArrow(x, y, dx, dy, **kwargs)
- self.add_patch(a)
- self._request_autoscale_view()
- return a
-
- @_docstring.copy(mquiver.QuiverKey.__init__)
- def quiverkey(self, Q, X, Y, U, label, **kwargs):
- qk = mquiver.QuiverKey(Q, X, Y, U, label, **kwargs)
- self.add_artist(qk)
- return qk
-
- # Handle units for x and y, if they've been passed
- def _quiver_units(self, args, kwargs):
- if len(args) > 3:
- x, y = args[0:2]
- x, y = self._process_unit_info([("x", x), ("y", y)], kwargs)
- return (x, y) + args[2:]
- return args
-
- # args can be a combination of X, Y, U, V, C and all should be replaced
- @_preprocess_data()
- @_docstring.dedent_interpd
- def quiver(self, *args, **kwargs):
- """%(quiver_doc)s"""
- # Make sure units are handled for x and y values
- args = self._quiver_units(args, kwargs)
- q = mquiver.Quiver(self, *args, **kwargs)
- self.add_collection(q, autolim=True)
- self._request_autoscale_view()
- return q
-
- # args can be some combination of X, Y, U, V, C and all should be replaced
- @_preprocess_data()
- @_docstring.dedent_interpd
- def barbs(self, *args, **kwargs):
- """%(barbs_doc)s"""
- # Make sure units are handled for x and y values
- args = self._quiver_units(args, kwargs)
- b = mquiver.Barbs(self, *args, **kwargs)
- self.add_collection(b, autolim=True)
- self._request_autoscale_view()
- return b
-
- # Uses a custom implementation of data-kwarg handling in
- # _process_plot_var_args.
- def fill(self, *args, data=None, **kwargs):
- """
- Plot filled polygons.
-
- Parameters
- ----------
- *args : sequence of x, y, [color]
- Each polygon is defined by the lists of *x* and *y* positions of
- its nodes, optionally followed by a *color* specifier. See
- :mod:`matplotlib.colors` for supported color specifiers. The
- standard color cycle is used for polygons without a color
- specifier.
-
- You can plot multiple polygons by providing multiple *x*, *y*,
- *[color]* groups.
-
- For example, each of the following is legal::
-
- ax.fill(x, y) # a polygon with default color
- ax.fill(x, y, "b") # a blue polygon
- ax.fill(x, y, x2, y2) # two polygons
- ax.fill(x, y, "b", x2, y2, "r") # a blue and a red polygon
-
- data : indexable object, optional
- An object with labelled data. If given, provide the label names to
- plot in *x* and *y*, e.g.::
-
- ax.fill("time", "signal",
- data={"time": [0, 1, 2], "signal": [0, 1, 0]})
-
- Returns
- -------
- list of `~matplotlib.patches.Polygon`
-
- Other Parameters
- ----------------
- **kwargs : `~matplotlib.patches.Polygon` properties
-
- Notes
- -----
- Use :meth:`fill_between` if you would like to fill the region between
- two curves.
- """
- # For compatibility(!), get aliases from Line2D rather than Patch.
- kwargs = cbook.normalize_kwargs(kwargs, mlines.Line2D)
- # _get_patches_for_fill returns a generator, convert it to a list.
- patches = [*self._get_patches_for_fill(*args, data=data, **kwargs)]
- for poly in patches:
- self.add_patch(poly)
- self._request_autoscale_view()
- return patches
-
- def _fill_between_x_or_y(
- self, ind_dir, ind, dep1, dep2=0, *,
- where=None, interpolate=False, step=None, **kwargs):
- # Common implementation between fill_between (*ind_dir*="x") and
- # fill_betweenx (*ind_dir*="y"). *ind* is the independent variable,
- # *dep* the dependent variable. The docstring below is interpolated
- # to generate both methods' docstrings.
- """
- Fill the area between two {dir} curves.
-
- The curves are defined by the points (*{ind}*, *{dep}1*) and (*{ind}*,
- *{dep}2*). This creates one or multiple polygons describing the filled
- area.
-
- You may exclude some {dir} sections from filling using *where*.
-
- By default, the edges connect the given points directly. Use *step*
- if the filling should be a step function, i.e. constant in between
- *{ind}*.
-
- Parameters
- ----------
- {ind} : array (length N)
- The {ind} coordinates of the nodes defining the curves.
-
- {dep}1 : array (length N) or scalar
- The {dep} coordinates of the nodes defining the first curve.
-
- {dep}2 : array (length N) or scalar, default: 0
- The {dep} coordinates of the nodes defining the second curve.
-
- where : array of bool (length N), optional
- Define *where* to exclude some {dir} regions from being filled.
- The filled regions are defined by the coordinates ``{ind}[where]``.
- More precisely, fill between ``{ind}[i]`` and ``{ind}[i+1]`` if
- ``where[i] and where[i+1]``. Note that this definition implies
- that an isolated *True* value between two *False* values in *where*
- will not result in filling. Both sides of the *True* position
- remain unfilled due to the adjacent *False* values.
-
- interpolate : bool, default: False
- This option is only relevant if *where* is used and the two curves
- are crossing each other.
-
- Semantically, *where* is often used for *{dep}1* > *{dep}2* or
- similar. By default, the nodes of the polygon defining the filled
- region will only be placed at the positions in the *{ind}* array.
- Such a polygon cannot describe the above semantics close to the
- intersection. The {ind}-sections containing the intersection are
- simply clipped.
-
- Setting *interpolate* to *True* will calculate the actual
- intersection point and extend the filled region up to this point.
-
- step : {{'pre', 'post', 'mid'}}, optional
- Define *step* if the filling should be a step function,
- i.e. constant in between *{ind}*. The value determines where the
- step will occur:
-
- - 'pre': The y value is continued constantly to the left from
- every *x* position, i.e. the interval ``(x[i-1], x[i]]`` has the
- value ``y[i]``.
- - 'post': The y value is continued constantly to the right from
- every *x* position, i.e. the interval ``[x[i], x[i+1])`` has the
- value ``y[i]``.
- - 'mid': Steps occur half-way between the *x* positions.
-
- Returns
- -------
- `.PolyCollection`
- A `.PolyCollection` containing the plotted polygons.
-
- Other Parameters
- ----------------
- data : indexable object, optional
- DATA_PARAMETER_PLACEHOLDER
-
- **kwargs
- All other keyword arguments are passed on to `.PolyCollection`.
- They control the `.Polygon` properties:
-
- %(PolyCollection:kwdoc)s
-
- See Also
- --------
- fill_between : Fill between two sets of y-values.
- fill_betweenx : Fill between two sets of x-values.
- """
-
- dep_dir = {"x": "y", "y": "x"}[ind_dir]
-
- if not mpl.rcParams["_internal.classic_mode"]:
- kwargs = cbook.normalize_kwargs(kwargs, mcoll.Collection)
- if not any(c in kwargs for c in ("color", "facecolor")):
- kwargs["facecolor"] = \
- self._get_patches_for_fill.get_next_color()
-
- # Handle united data, such as dates
- ind, dep1, dep2 = map(
- ma.masked_invalid, self._process_unit_info(
- [(ind_dir, ind), (dep_dir, dep1), (dep_dir, dep2)], kwargs))
-
- for name, array in [
- (ind_dir, ind), (f"{dep_dir}1", dep1), (f"{dep_dir}2", dep2)]:
- if array.ndim > 1:
- raise ValueError(f"{name!r} is not 1-dimensional")
-
- if where is None:
- where = True
- else:
- where = np.asarray(where, dtype=bool)
- if where.size != ind.size:
- raise ValueError(f"where size ({where.size}) does not match "
- f"{ind_dir} size ({ind.size})")
- where = where & ~functools.reduce(
- np.logical_or, map(np.ma.getmaskarray, [ind, dep1, dep2]))
-
- ind, dep1, dep2 = np.broadcast_arrays(
- np.atleast_1d(ind), dep1, dep2, subok=True)
-
- polys = []
- for idx0, idx1 in cbook.contiguous_regions(where):
- indslice = ind[idx0:idx1]
- dep1slice = dep1[idx0:idx1]
- dep2slice = dep2[idx0:idx1]
- if step is not None:
- step_func = cbook.STEP_LOOKUP_MAP["steps-" + step]
- indslice, dep1slice, dep2slice = \
- step_func(indslice, dep1slice, dep2slice)
-
- if not len(indslice):
- continue
-
- N = len(indslice)
- pts = np.zeros((2 * N + 2, 2))
-
- if interpolate:
- def get_interp_point(idx):
- im1 = max(idx - 1, 0)
- ind_values = ind[im1:idx+1]
- diff_values = dep1[im1:idx+1] - dep2[im1:idx+1]
- dep1_values = dep1[im1:idx+1]
-
- if len(diff_values) == 2:
- if np.ma.is_masked(diff_values[1]):
- return ind[im1], dep1[im1]
- elif np.ma.is_masked(diff_values[0]):
- return ind[idx], dep1[idx]
-
- diff_order = diff_values.argsort()
- diff_root_ind = np.interp(
- 0, diff_values[diff_order], ind_values[diff_order])
- ind_order = ind_values.argsort()
- diff_root_dep = np.interp(
- diff_root_ind,
- ind_values[ind_order], dep1_values[ind_order])
- return diff_root_ind, diff_root_dep
-
- start = get_interp_point(idx0)
- end = get_interp_point(idx1)
- else:
- # Handle scalar dep2 (e.g. 0): the fill should go all
- # the way down to 0 even if none of the dep1 sample points do.
- start = indslice[0], dep2slice[0]
- end = indslice[-1], dep2slice[-1]
-
- pts[0] = start
- pts[N + 1] = end
-
- pts[1:N+1, 0] = indslice
- pts[1:N+1, 1] = dep1slice
- pts[N+2:, 0] = indslice[::-1]
- pts[N+2:, 1] = dep2slice[::-1]
-
- if ind_dir == "y":
- pts = pts[:, ::-1]
-
- polys.append(pts)
-
- collection = mcoll.PolyCollection(polys, **kwargs)
-
- # now update the datalim and autoscale
- pts = np.row_stack([np.column_stack([ind[where], dep1[where]]),
- np.column_stack([ind[where], dep2[where]])])
- if ind_dir == "y":
- pts = pts[:, ::-1]
- self.update_datalim(pts, updatex=True, updatey=True)
- self.add_collection(collection, autolim=False)
- self._request_autoscale_view()
- return collection
-
- def fill_between(self, x, y1, y2=0, where=None, interpolate=False,
- step=None, **kwargs):
- return self._fill_between_x_or_y(
- "x", x, y1, y2,
- where=where, interpolate=interpolate, step=step, **kwargs)
-
- if _fill_between_x_or_y.__doc__:
- fill_between.__doc__ = _fill_between_x_or_y.__doc__.format(
- dir="horizontal", ind="x", dep="y"
- )
- fill_between = _preprocess_data(
- _docstring.dedent_interpd(fill_between),
- replace_names=["x", "y1", "y2", "where"])
-
- def fill_betweenx(self, y, x1, x2=0, where=None,
- step=None, interpolate=False, **kwargs):
- return self._fill_between_x_or_y(
- "y", y, x1, x2,
- where=where, interpolate=interpolate, step=step, **kwargs)
-
- if _fill_between_x_or_y.__doc__:
- fill_betweenx.__doc__ = _fill_between_x_or_y.__doc__.format(
- dir="vertical", ind="y", dep="x"
- )
- fill_betweenx = _preprocess_data(
- _docstring.dedent_interpd(fill_betweenx),
- replace_names=["y", "x1", "x2", "where"])
-
- #### plotting z(x, y): imshow, pcolor and relatives, contour
-
- @_preprocess_data()
- @_docstring.interpd
- def imshow(self, X, cmap=None, norm=None, *, aspect=None,
- interpolation=None, alpha=None,
- vmin=None, vmax=None, origin=None, extent=None,
- interpolation_stage=None, filternorm=True, filterrad=4.0,
- resample=None, url=None, **kwargs):
- """
- Display data as an image, i.e., on a 2D regular raster.
-
- The input may either be actual RGB(A) data, or 2D scalar data, which
- will be rendered as a pseudocolor image. For displaying a grayscale
- image set up the colormapping using the parameters
- ``cmap='gray', vmin=0, vmax=255``.
-
- The number of pixels used to render an image is set by the Axes size
- and the *dpi* of the figure. This can lead to aliasing artifacts when
- the image is resampled because the displayed image size will usually
- not match the size of *X* (see
- :doc:`/gallery/images_contours_and_fields/image_antialiasing`).
- The resampling can be controlled via the *interpolation* parameter
- and/or :rc:`image.interpolation`.
-
- Parameters
- ----------
- X : array-like or PIL image
- The image data. Supported array shapes are:
-
- - (M, N): an image with scalar data. The values are mapped to
- colors using normalization and a colormap. See parameters *norm*,
- *cmap*, *vmin*, *vmax*.
- - (M, N, 3): an image with RGB values (0-1 float or 0-255 int).
- - (M, N, 4): an image with RGBA values (0-1 float or 0-255 int),
- i.e. including transparency.
-
- The first two dimensions (M, N) define the rows and columns of
- the image.
-
- Out-of-range RGB(A) values are clipped.
-
- %(cmap_doc)s
-
- This parameter is ignored if *X* is RGB(A).
-
- %(norm_doc)s
-
- This parameter is ignored if *X* is RGB(A).
-
- %(vmin_vmax_doc)s
-
- This parameter is ignored if *X* is RGB(A).
-
- aspect : {'equal', 'auto'} or float, default: :rc:`image.aspect`
- The aspect ratio of the Axes. This parameter is particularly
- relevant for images since it determines whether data pixels are
- square.
-
- This parameter is a shortcut for explicitly calling
- `.Axes.set_aspect`. See there for further details.
-
- - 'equal': Ensures an aspect ratio of 1. Pixels will be square
- (unless pixel sizes are explicitly made non-square in data
- coordinates using *extent*).
- - 'auto': The Axes is kept fixed and the aspect is adjusted so
- that the data fit in the Axes. In general, this will result in
- non-square pixels.
-
- interpolation : str, default: :rc:`image.interpolation`
- The interpolation method used.
-
- Supported values are 'none', 'antialiased', 'nearest', 'bilinear',
- 'bicubic', 'spline16', 'spline36', 'hanning', 'hamming', 'hermite',
- 'kaiser', 'quadric', 'catrom', 'gaussian', 'bessel', 'mitchell',
- 'sinc', 'lanczos', 'blackman'.
-
- The data *X* is resampled to the pixel size of the image on the
- figure canvas, using the interpolation method to either up- or
- downsample the data.
-
- If *interpolation* is 'none', then for the ps, pdf, and svg
- backends no down- or upsampling occurs, and the image data is
- passed to the backend as a native image. Note that different ps,
- pdf, and svg viewers may display these raw pixels differently. On
- other backends, 'none' is the same as 'nearest'.
-
- If *interpolation* is the default 'antialiased', then 'nearest'
- interpolation is used if the image is upsampled by more than a
- factor of three (i.e. the number of display pixels is at least
- three times the size of the data array). If the upsampling rate is
- smaller than 3, or the image is downsampled, then 'hanning'
- interpolation is used to act as an anti-aliasing filter, unless the
- image happens to be upsampled by exactly a factor of two or one.
-
- See
- :doc:`/gallery/images_contours_and_fields/interpolation_methods`
- for an overview of the supported interpolation methods, and
- :doc:`/gallery/images_contours_and_fields/image_antialiasing` for
- a discussion of image antialiasing.
-
- Some interpolation methods require an additional radius parameter,
- which can be set by *filterrad*. Additionally, the antigrain image
- resize filter is controlled by the parameter *filternorm*.
-
- interpolation_stage : {'data', 'rgba'}, default: 'data'
- If 'data', interpolation
- is carried out on the data provided by the user. If 'rgba', the
- interpolation is carried out after the colormapping has been
- applied (visual interpolation).
-
- alpha : float or array-like, optional
- The alpha blending value, between 0 (transparent) and 1 (opaque).
- If *alpha* is an array, the alpha blending values are applied pixel
- by pixel, and *alpha* must have the same shape as *X*.
-
- origin : {'upper', 'lower'}, default: :rc:`image.origin`
- Place the [0, 0] index of the array in the upper left or lower
- left corner of the Axes. The convention (the default) 'upper' is
- typically used for matrices and images.
-
- Note that the vertical axis points upward for 'lower'
- but downward for 'upper'.
-
- See the :doc:`/tutorials/intermediate/imshow_extent` tutorial for
- examples and a more detailed description.
-
- extent : floats (left, right, bottom, top), optional
- The bounding box in data coordinates that the image will fill.
- These values may be unitful and match the units of the Axes.
- The image is stretched individually along x and y to fill the box.
-
- The default extent is determined by the following conditions.
- Pixels have unit size in data coordinates. Their centers are on
- integer coordinates, and their center coordinates range from 0 to
- columns-1 horizontally and from 0 to rows-1 vertically.
-
- Note that the direction of the vertical axis and thus the default
- values for top and bottom depend on *origin*:
-
- - For ``origin == 'upper'`` the default is
- ``(-0.5, numcols-0.5, numrows-0.5, -0.5)``.
- - For ``origin == 'lower'`` the default is
- ``(-0.5, numcols-0.5, -0.5, numrows-0.5)``.
-
- See the :doc:`/tutorials/intermediate/imshow_extent` tutorial for
- examples and a more detailed description.
-
- filternorm : bool, default: True
- A parameter for the antigrain image resize filter (see the
- antigrain documentation). If *filternorm* is set, the filter
- normalizes integer values and corrects the rounding errors. It
- doesn't do anything with the source floating point values, it
- corrects only integers according to the rule of 1.0 which means
- that any sum of pixel weights must be equal to 1.0. So, the
- filter function must produce a graph of the proper shape.
-
- filterrad : float > 0, default: 4.0
- The filter radius for filters that have a radius parameter, i.e.
- when interpolation is one of: 'sinc', 'lanczos' or 'blackman'.
-
- resample : bool, default: :rc:`image.resample`
- When *True*, use a full resampling method. When *False*, only
- resample when the output image is larger than the input image.
-
- url : str, optional
- Set the url of the created `.AxesImage`. See `.Artist.set_url`.
-
- Returns
- -------
- `~matplotlib.image.AxesImage`
-
- Other Parameters
- ----------------
- data : indexable object, optional
- DATA_PARAMETER_PLACEHOLDER
-
- **kwargs : `~matplotlib.artist.Artist` properties
- These parameters are passed on to the constructor of the
- `.AxesImage` artist.
-
- See Also
- --------
- matshow : Plot a matrix or an array as an image.
-
- Notes
- -----
- Unless *extent* is used, pixel centers will be located at integer
- coordinates. In other words: the origin will coincide with the center
- of pixel (0, 0).
-
- There are two common representations for RGB images with an alpha
- channel:
-
- - Straight (unassociated) alpha: R, G, and B channels represent the
- color of the pixel, disregarding its opacity.
- - Premultiplied (associated) alpha: R, G, and B channels represent
- the color of the pixel, adjusted for its opacity by multiplication.
-
- `~matplotlib.pyplot.imshow` expects RGB images adopting the straight
- (unassociated) alpha representation.
- """
- if aspect is None:
- aspect = mpl.rcParams['image.aspect']
- self.set_aspect(aspect)
- im = mimage.AxesImage(self, cmap=cmap, norm=norm,
- interpolation=interpolation, origin=origin,
- extent=extent, filternorm=filternorm,
- filterrad=filterrad, resample=resample,
- interpolation_stage=interpolation_stage,
- **kwargs)
-
- im.set_data(X)
- im.set_alpha(alpha)
- if im.get_clip_path() is None:
- # image does not already have clipping set, clip to axes patch
- im.set_clip_path(self.patch)
- im._scale_norm(norm, vmin, vmax)
- im.set_url(url)
-
- # update ax.dataLim, and, if autoscaling, set viewLim
- # to tightly fit the image, regardless of dataLim.
- im.set_extent(im.get_extent())
-
- self.add_image(im)
- return im
-
- def _pcolorargs(self, funcname, *args, shading='auto', **kwargs):
- # - create X and Y if not present;
- # - reshape X and Y as needed if they are 1-D;
- # - check for proper sizes based on `shading` kwarg;
- # - reset shading if shading='auto' to flat or nearest
- # depending on size;
-
- _valid_shading = ['gouraud', 'nearest', 'flat', 'auto']
- try:
- _api.check_in_list(_valid_shading, shading=shading)
- except ValueError:
- _api.warn_external(f"shading value '{shading}' not in list of "
- f"valid values {_valid_shading}. Setting "
- "shading='auto'.")
- shading = 'auto'
-
- if len(args) == 1:
- C = np.asanyarray(args[0])
- nrows, ncols = C.shape[:2]
- if shading in ['gouraud', 'nearest']:
- X, Y = np.meshgrid(np.arange(ncols), np.arange(nrows))
- else:
- X, Y = np.meshgrid(np.arange(ncols + 1), np.arange(nrows + 1))
- shading = 'flat'
- C = cbook.safe_masked_invalid(C, copy=True)
- return X, Y, C, shading
-
- if len(args) == 3:
- # Check x and y for bad data...
- C = np.asanyarray(args[2])
- # unit conversion allows e.g. datetime objects as axis values
- X, Y = args[:2]
- X, Y = self._process_unit_info([("x", X), ("y", Y)], kwargs)
- X, Y = [cbook.safe_masked_invalid(a, copy=True) for a in [X, Y]]
-
- if funcname == 'pcolormesh':
- if np.ma.is_masked(X) or np.ma.is_masked(Y):
- raise ValueError(
- 'x and y arguments to pcolormesh cannot have '
- 'non-finite values or be of type '
- 'numpy.ma.core.MaskedArray with masked values')
- # safe_masked_invalid() returns an ndarray for dtypes other
- # than floating point.
- if isinstance(X, np.ma.core.MaskedArray):
- X = X.data # strip mask as downstream doesn't like it...
- if isinstance(Y, np.ma.core.MaskedArray):
- Y = Y.data
- nrows, ncols = C.shape[:2]
- else:
- raise _api.nargs_error(funcname, takes="1 or 3", given=len(args))
-
- Nx = X.shape[-1]
- Ny = Y.shape[0]
- if X.ndim != 2 or X.shape[0] == 1:
- x = X.reshape(1, Nx)
- X = x.repeat(Ny, axis=0)
- if Y.ndim != 2 or Y.shape[1] == 1:
- y = Y.reshape(Ny, 1)
- Y = y.repeat(Nx, axis=1)
- if X.shape != Y.shape:
- raise TypeError(f'Incompatible X, Y inputs to {funcname}; '
- f'see help({funcname})')
-
- if shading == 'auto':
- if ncols == Nx and nrows == Ny:
- shading = 'nearest'
- else:
- shading = 'flat'
-
- if shading == 'flat':
- if (Nx, Ny) != (ncols + 1, nrows + 1):
- raise TypeError(f"Dimensions of C {C.shape} should"
- f" be one smaller than X({Nx}) and Y({Ny})"
- f" while using shading='flat'"
- f" see help({funcname})")
- else: # ['nearest', 'gouraud']:
- if (Nx, Ny) != (ncols, nrows):
- raise TypeError('Dimensions of C %s are incompatible with'
- ' X (%d) and/or Y (%d); see help(%s)' % (
- C.shape, Nx, Ny, funcname))
- if shading == 'nearest':
- # grid is specified at the center, so define corners
- # at the midpoints between the grid centers and then use the
- # flat algorithm.
- def _interp_grid(X):
- # helper for below
- if np.shape(X)[1] > 1:
- dX = np.diff(X, axis=1)/2.
- if not (np.all(dX >= 0) or np.all(dX <= 0)):
- _api.warn_external(
- f"The input coordinates to {funcname} are "
- "interpreted as cell centers, but are not "
- "monotonically increasing or decreasing. "
- "This may lead to incorrectly calculated cell "
- "edges, in which case, please supply "
- f"explicit cell edges to {funcname}.")
- X = np.hstack((X[:, [0]] - dX[:, [0]],
- X[:, :-1] + dX,
- X[:, [-1]] + dX[:, [-1]]))
- else:
- # This is just degenerate, but we can't reliably guess
- # a dX if there is just one value.
- X = np.hstack((X, X))
- return X
-
- if ncols == Nx:
- X = _interp_grid(X)
- Y = _interp_grid(Y)
- if nrows == Ny:
- X = _interp_grid(X.T).T
- Y = _interp_grid(Y.T).T
- shading = 'flat'
-
- C = cbook.safe_masked_invalid(C, copy=True)
- return X, Y, C, shading
-
- @_preprocess_data()
- @_docstring.dedent_interpd
- def pcolor(self, *args, shading=None, alpha=None, norm=None, cmap=None,
- vmin=None, vmax=None, **kwargs):
- r"""
- Create a pseudocolor plot with a non-regular rectangular grid.
-
- Call signature::
-
- pcolor([X, Y,] C, **kwargs)
-
- *X* and *Y* can be used to specify the corners of the quadrilaterals.
-
- .. hint::
-
- ``pcolor()`` can be very slow for large arrays. In most
- cases you should use the similar but much faster
- `~.Axes.pcolormesh` instead. See
- :ref:`Differences between pcolor() and pcolormesh()
- ` for a discussion of the
- differences.
-
- Parameters
- ----------
- C : 2D array-like
- The color-mapped values. Color-mapping is controlled by *cmap*,
- *norm*, *vmin*, and *vmax*.
-
- X, Y : array-like, optional
- The coordinates of the corners of quadrilaterals of a pcolormesh::
-
- (X[i+1, j], Y[i+1, j]) (X[i+1, j+1], Y[i+1, j+1])
- ●╶───╴●
- │ │
- ●╶───╴●
- (X[i, j], Y[i, j]) (X[i, j+1], Y[i, j+1])
-
- Note that the column index corresponds to the x-coordinate, and
- the row index corresponds to y. For details, see the
- :ref:`Notes ` section below.
-
- If ``shading='flat'`` the dimensions of *X* and *Y* should be one
- greater than those of *C*, and the quadrilateral is colored due
- to the value at ``C[i, j]``. If *X*, *Y* and *C* have equal
- dimensions, a warning will be raised and the last row and column
- of *C* will be ignored.
-
- If ``shading='nearest'``, the dimensions of *X* and *Y* should be
- the same as those of *C* (if not, a ValueError will be raised). The
- color ``C[i, j]`` will be centered on ``(X[i, j], Y[i, j])``.
-
- If *X* and/or *Y* are 1-D arrays or column vectors they will be
- expanded as needed into the appropriate 2D arrays, making a
- rectangular grid.
-
- shading : {'flat', 'nearest', 'auto'}, default: :rc:`pcolor.shading`
- The fill style for the quadrilateral. Possible values:
-
- - 'flat': A solid color is used for each quad. The color of the
- quad (i, j), (i+1, j), (i, j+1), (i+1, j+1) is given by
- ``C[i, j]``. The dimensions of *X* and *Y* should be
- one greater than those of *C*; if they are the same as *C*,
- then a deprecation warning is raised, and the last row
- and column of *C* are dropped.
- - 'nearest': Each grid point will have a color centered on it,
- extending halfway between the adjacent grid centers. The
- dimensions of *X* and *Y* must be the same as *C*.
- - 'auto': Choose 'flat' if dimensions of *X* and *Y* are one
- larger than *C*. Choose 'nearest' if dimensions are the same.
-
- See :doc:`/gallery/images_contours_and_fields/pcolormesh_grids`
- for more description.
-
- %(cmap_doc)s
-
- %(norm_doc)s
-
- %(vmin_vmax_doc)s
-
- edgecolors : {'none', None, 'face', color, color sequence}, optional
- The color of the edges. Defaults to 'none'. Possible values:
-
- - 'none' or '': No edge.
- - *None*: :rc:`patch.edgecolor` will be used. Note that currently
- :rc:`patch.force_edgecolor` has to be True for this to work.
- - 'face': Use the adjacent face color.
- - A color or sequence of colors will set the edge color.
-
- The singular form *edgecolor* works as an alias.
-
- alpha : float, default: None
- The alpha blending value of the face color, between 0 (transparent)
- and 1 (opaque). Note: The edgecolor is currently not affected by
- this.
-
- snap : bool, default: False
- Whether to snap the mesh to pixel boundaries.
-
- Returns
- -------
- `matplotlib.collections.Collection`
-
- Other Parameters
- ----------------
- antialiaseds : bool, default: False
- The default *antialiaseds* is False if the default
- *edgecolors*\ ="none" is used. This eliminates artificial lines
- at patch boundaries, and works regardless of the value of alpha.
- If *edgecolors* is not "none", then the default *antialiaseds*
- is taken from :rc:`patch.antialiased`.
- Stroking the edges may be preferred if *alpha* is 1, but will
- cause artifacts otherwise.
-
- data : indexable object, optional
- DATA_PARAMETER_PLACEHOLDER
-
- **kwargs
- Additionally, the following arguments are allowed. They are passed
- along to the `~matplotlib.collections.PolyCollection` constructor:
-
- %(PolyCollection:kwdoc)s
-
- See Also
- --------
- pcolormesh : for an explanation of the differences between
- pcolor and pcolormesh.
- imshow : If *X* and *Y* are each equidistant, `~.Axes.imshow` can be a
- faster alternative.
-
- Notes
- -----
- **Masked arrays**
-
- *X*, *Y* and *C* may be masked arrays. If either ``C[i, j]``, or one
- of the vertices surrounding ``C[i, j]`` (*X* or *Y* at
- ``[i, j], [i+1, j], [i, j+1], [i+1, j+1]``) is masked, nothing is
- plotted.
-
- .. _axes-pcolor-grid-orientation:
-
- **Grid orientation**
-
- The grid orientation follows the standard matrix convention: An array
- *C* with shape (nrows, ncolumns) is plotted with the column number as
- *X* and the row number as *Y*.
- """
-
- if shading is None:
- shading = mpl.rcParams['pcolor.shading']
- shading = shading.lower()
- X, Y, C, shading = self._pcolorargs('pcolor', *args, shading=shading,
- kwargs=kwargs)
- Ny, Nx = X.shape
-
- # convert to MA, if necessary.
- C = ma.asarray(C)
- X = ma.asarray(X)
- Y = ma.asarray(Y)
-
- mask = ma.getmaskarray(X) + ma.getmaskarray(Y)
- xymask = (mask[0:-1, 0:-1] + mask[1:, 1:] +
- mask[0:-1, 1:] + mask[1:, 0:-1])
- # don't plot if C or any of the surrounding vertices are masked.
- mask = ma.getmaskarray(C) + xymask
-
- unmask = ~mask
- X1 = ma.filled(X[:-1, :-1])[unmask]
- Y1 = ma.filled(Y[:-1, :-1])[unmask]
- X2 = ma.filled(X[1:, :-1])[unmask]
- Y2 = ma.filled(Y[1:, :-1])[unmask]
- X3 = ma.filled(X[1:, 1:])[unmask]
- Y3 = ma.filled(Y[1:, 1:])[unmask]
- X4 = ma.filled(X[:-1, 1:])[unmask]
- Y4 = ma.filled(Y[:-1, 1:])[unmask]
- npoly = len(X1)
-
- xy = np.stack([X1, Y1, X2, Y2, X3, Y3, X4, Y4, X1, Y1], axis=-1)
- verts = xy.reshape((npoly, 5, 2))
-
- C = ma.filled(C[:Ny - 1, :Nx - 1])[unmask]
-
- linewidths = (0.25,)
- if 'linewidth' in kwargs:
- kwargs['linewidths'] = kwargs.pop('linewidth')
- kwargs.setdefault('linewidths', linewidths)
-
- if 'edgecolor' in kwargs:
- kwargs['edgecolors'] = kwargs.pop('edgecolor')
- ec = kwargs.setdefault('edgecolors', 'none')
-
- # aa setting will default via collections to patch.antialiased
- # unless the boundary is not stroked, in which case the
- # default will be False; with unstroked boundaries, aa
- # makes artifacts that are often disturbing.
- if 'antialiased' in kwargs:
- kwargs['antialiaseds'] = kwargs.pop('antialiased')
- if 'antialiaseds' not in kwargs and cbook._str_lower_equal(ec, "none"):
- kwargs['antialiaseds'] = False
-
- kwargs.setdefault('snap', False)
-
- collection = mcoll.PolyCollection(
- verts, array=C, cmap=cmap, norm=norm, alpha=alpha, **kwargs)
- collection._scale_norm(norm, vmin, vmax)
-
- x = X.compressed()
- y = Y.compressed()
-
- # Transform from native to data coordinates?
- t = collection._transform
- if (not isinstance(t, mtransforms.Transform) and
- hasattr(t, '_as_mpl_transform')):
- t = t._as_mpl_transform(self.axes)
-
- if t and any(t.contains_branch_seperately(self.transData)):
- trans_to_data = t - self.transData
- pts = np.vstack([x, y]).T.astype(float)
- transformed_pts = trans_to_data.transform(pts)
- x = transformed_pts[..., 0]
- y = transformed_pts[..., 1]
-
- self.add_collection(collection, autolim=False)
-
- minx = np.min(x)
- maxx = np.max(x)
- miny = np.min(y)
- maxy = np.max(y)
- collection.sticky_edges.x[:] = [minx, maxx]
- collection.sticky_edges.y[:] = [miny, maxy]
- corners = (minx, miny), (maxx, maxy)
- self.update_datalim(corners)
- self._request_autoscale_view()
- return collection
-
- @_preprocess_data()
- @_docstring.dedent_interpd
- def pcolormesh(self, *args, alpha=None, norm=None, cmap=None, vmin=None,
- vmax=None, shading=None, antialiased=False, **kwargs):
- """
- Create a pseudocolor plot with a non-regular rectangular grid.
-
- Call signature::
-
- pcolormesh([X, Y,] C, **kwargs)
-
- *X* and *Y* can be used to specify the corners of the quadrilaterals.
-
- .. hint::
-
- `~.Axes.pcolormesh` is similar to `~.Axes.pcolor`. It is much faster
- and preferred in most cases. For a detailed discussion on the
- differences see :ref:`Differences between pcolor() and pcolormesh()
- `.
-
- Parameters
- ----------
- C : array-like
- The mesh data. Supported array shapes are:
-
- - (M, N) or M*N: a mesh with scalar data. The values are mapped to
- colors using normalization and a colormap. See parameters *norm*,
- *cmap*, *vmin*, *vmax*.
- - (M, N, 3): an image with RGB values (0-1 float or 0-255 int).
- - (M, N, 4): an image with RGBA values (0-1 float or 0-255 int),
- i.e. including transparency.
-
- The first two dimensions (M, N) define the rows and columns of
- the mesh data.
-
- X, Y : array-like, optional
- The coordinates of the corners of quadrilaterals of a pcolormesh::
-
- (X[i+1, j], Y[i+1, j]) (X[i+1, j+1], Y[i+1, j+1])
- ●╶───╴●
- │ │
- ●╶───╴●
- (X[i, j], Y[i, j]) (X[i, j+1], Y[i, j+1])
-
- Note that the column index corresponds to the x-coordinate, and
- the row index corresponds to y. For details, see the
- :ref:`Notes ` section below.
-
- If ``shading='flat'`` the dimensions of *X* and *Y* should be one
- greater than those of *C*, and the quadrilateral is colored due
- to the value at ``C[i, j]``. If *X*, *Y* and *C* have equal
- dimensions, a warning will be raised and the last row and column
- of *C* will be ignored.
-
- If ``shading='nearest'`` or ``'gouraud'``, the dimensions of *X*
- and *Y* should be the same as those of *C* (if not, a ValueError
- will be raised). For ``'nearest'`` the color ``C[i, j]`` is
- centered on ``(X[i, j], Y[i, j])``. For ``'gouraud'``, a smooth
- interpolation is caried out between the quadrilateral corners.
-
- If *X* and/or *Y* are 1-D arrays or column vectors they will be
- expanded as needed into the appropriate 2D arrays, making a
- rectangular grid.
-
- %(cmap_doc)s
-
- %(norm_doc)s
-
- %(vmin_vmax_doc)s
-
- edgecolors : {'none', None, 'face', color, color sequence}, optional
- The color of the edges. Defaults to 'none'. Possible values:
-
- - 'none' or '': No edge.
- - *None*: :rc:`patch.edgecolor` will be used. Note that currently
- :rc:`patch.force_edgecolor` has to be True for this to work.
- - 'face': Use the adjacent face color.
- - A color or sequence of colors will set the edge color.
-
- The singular form *edgecolor* works as an alias.
-
- alpha : float, default: None
- The alpha blending value, between 0 (transparent) and 1 (opaque).
-
- shading : {'flat', 'nearest', 'gouraud', 'auto'}, optional
- The fill style for the quadrilateral; defaults to
- :rc:`pcolor.shading`. Possible values:
-
- - 'flat': A solid color is used for each quad. The color of the
- quad (i, j), (i+1, j), (i, j+1), (i+1, j+1) is given by
- ``C[i, j]``. The dimensions of *X* and *Y* should be
- one greater than those of *C*; if they are the same as *C*,
- then a deprecation warning is raised, and the last row
- and column of *C* are dropped.
- - 'nearest': Each grid point will have a color centered on it,
- extending halfway between the adjacent grid centers. The
- dimensions of *X* and *Y* must be the same as *C*.
- - 'gouraud': Each quad will be Gouraud shaded: The color of the
- corners (i', j') are given by ``C[i', j']``. The color values of
- the area in between is interpolated from the corner values.
- The dimensions of *X* and *Y* must be the same as *C*. When
- Gouraud shading is used, *edgecolors* is ignored.
- - 'auto': Choose 'flat' if dimensions of *X* and *Y* are one
- larger than *C*. Choose 'nearest' if dimensions are the same.
-
- See :doc:`/gallery/images_contours_and_fields/pcolormesh_grids`
- for more description.
-
- snap : bool, default: False
- Whether to snap the mesh to pixel boundaries.
-
- rasterized : bool, optional
- Rasterize the pcolormesh when drawing vector graphics. This can
- speed up rendering and produce smaller files for large data sets.
- See also :doc:`/gallery/misc/rasterization_demo`.
-
- Returns
- -------
- `matplotlib.collections.QuadMesh`
-
- Other Parameters
- ----------------
- data : indexable object, optional
- DATA_PARAMETER_PLACEHOLDER
-
- **kwargs
- Additionally, the following arguments are allowed. They are passed
- along to the `~matplotlib.collections.QuadMesh` constructor:
-
- %(QuadMesh:kwdoc)s
-
- See Also
- --------
- pcolor : An alternative implementation with slightly different
- features. For a detailed discussion on the differences see
- :ref:`Differences between pcolor() and pcolormesh()
- `.
- imshow : If *X* and *Y* are each equidistant, `~.Axes.imshow` can be a
- faster alternative.
-
- Notes
- -----
- **Masked arrays**
-
- *C* may be a masked array. If ``C[i, j]`` is masked, the corresponding
- quadrilateral will be transparent. Masking of *X* and *Y* is not
- supported. Use `~.Axes.pcolor` if you need this functionality.
-
- .. _axes-pcolormesh-grid-orientation:
-
- **Grid orientation**
-
- The grid orientation follows the standard matrix convention: An array
- *C* with shape (nrows, ncolumns) is plotted with the column number as
- *X* and the row number as *Y*.
-
- .. _differences-pcolor-pcolormesh:
-
- **Differences between pcolor() and pcolormesh()**
-
- Both methods are used to create a pseudocolor plot of a 2D array
- using quadrilaterals.
-
- The main difference lies in the created object and internal data
- handling:
- While `~.Axes.pcolor` returns a `.PolyCollection`, `~.Axes.pcolormesh`
- returns a `.QuadMesh`. The latter is more specialized for the given
- purpose and thus is faster. It should almost always be preferred.
-
- There is also a slight difference in the handling of masked arrays.
- Both `~.Axes.pcolor` and `~.Axes.pcolormesh` support masked arrays
- for *C*. However, only `~.Axes.pcolor` supports masked arrays for *X*
- and *Y*. The reason lies in the internal handling of the masked values.
- `~.Axes.pcolor` leaves out the respective polygons from the
- PolyCollection. `~.Axes.pcolormesh` sets the facecolor of the masked
- elements to transparent. You can see the difference when using
- edgecolors. While all edges are drawn irrespective of masking in a
- QuadMesh, the edge between two adjacent masked quadrilaterals in
- `~.Axes.pcolor` is not drawn as the corresponding polygons do not
- exist in the PolyCollection.
-
- Another difference is the support of Gouraud shading in
- `~.Axes.pcolormesh`, which is not available with `~.Axes.pcolor`.
-
- """
- if shading is None:
- shading = mpl.rcParams['pcolor.shading']
- shading = shading.lower()
- kwargs.setdefault('edgecolors', 'none')
-
- X, Y, C, shading = self._pcolorargs('pcolormesh', *args,
- shading=shading, kwargs=kwargs)
- coords = np.stack([X, Y], axis=-1)
- # convert to one dimensional array, except for 3D RGB(A) arrays
- if C.ndim != 3:
- C = C.ravel()
-
- kwargs.setdefault('snap', mpl.rcParams['pcolormesh.snap'])
-
- collection = mcoll.QuadMesh(
- coords, antialiased=antialiased, shading=shading,
- array=C, cmap=cmap, norm=norm, alpha=alpha, **kwargs)
- collection._scale_norm(norm, vmin, vmax)
-
- coords = coords.reshape(-1, 2) # flatten the grid structure; keep x, y
-
- # Transform from native to data coordinates?
- t = collection._transform
- if (not isinstance(t, mtransforms.Transform) and
- hasattr(t, '_as_mpl_transform')):
- t = t._as_mpl_transform(self.axes)
-
- if t and any(t.contains_branch_seperately(self.transData)):
- trans_to_data = t - self.transData
- coords = trans_to_data.transform(coords)
-
- self.add_collection(collection, autolim=False)
-
- minx, miny = np.min(coords, axis=0)
- maxx, maxy = np.max(coords, axis=0)
- collection.sticky_edges.x[:] = [minx, maxx]
- collection.sticky_edges.y[:] = [miny, maxy]
- corners = (minx, miny), (maxx, maxy)
- self.update_datalim(corners)
- self._request_autoscale_view()
- return collection
-
- @_preprocess_data()
- @_docstring.dedent_interpd
- def pcolorfast(self, *args, alpha=None, norm=None, cmap=None, vmin=None,
- vmax=None, **kwargs):
- """
- Create a pseudocolor plot with a non-regular rectangular grid.
-
- Call signature::
-
- ax.pcolorfast([X, Y], C, /, **kwargs)
-
- This method is similar to `~.Axes.pcolor` and `~.Axes.pcolormesh`.
- It's designed to provide the fastest pcolor-type plotting with the
- Agg backend. To achieve this, it uses different algorithms internally
- depending on the complexity of the input grid (regular rectangular,
- non-regular rectangular or arbitrary quadrilateral).
-
- .. warning::
-
- This method is experimental. Compared to `~.Axes.pcolor` or
- `~.Axes.pcolormesh` it has some limitations:
-
- - It supports only flat shading (no outlines)
- - It lacks support for log scaling of the axes.
- - It does not have a pyplot wrapper.
-
- Parameters
- ----------
- C : array-like
- The image data. Supported array shapes are:
-
- - (M, N): an image with scalar data. Color-mapping is controlled
- by *cmap*, *norm*, *vmin*, and *vmax*.
- - (M, N, 3): an image with RGB values (0-1 float or 0-255 int).
- - (M, N, 4): an image with RGBA values (0-1 float or 0-255 int),
- i.e. including transparency.
-
- The first two dimensions (M, N) define the rows and columns of
- the image.
-
- This parameter can only be passed positionally.
-
- X, Y : tuple or array-like, default: ``(0, N)``, ``(0, M)``
- *X* and *Y* are used to specify the coordinates of the
- quadrilaterals. There are different ways to do this:
-
- - Use tuples ``X=(xmin, xmax)`` and ``Y=(ymin, ymax)`` to define
- a *uniform rectangular grid*.
-
- The tuples define the outer edges of the grid. All individual
- quadrilaterals will be of the same size. This is the fastest
- version.
-
- - Use 1D arrays *X*, *Y* to specify a *non-uniform rectangular
- grid*.
-
- In this case *X* and *Y* have to be monotonic 1D arrays of length
- *N+1* and *M+1*, specifying the x and y boundaries of the cells.
-
- The speed is intermediate. Note: The grid is checked, and if
- found to be uniform the fast version is used.
-
- - Use 2D arrays *X*, *Y* if you need an *arbitrary quadrilateral
- grid* (i.e. if the quadrilaterals are not rectangular).
-
- In this case *X* and *Y* are 2D arrays with shape (M + 1, N + 1),
- specifying the x and y coordinates of the corners of the colored
- quadrilaterals.
-
- This is the most general, but the slowest to render. It may
- produce faster and more compact output using ps, pdf, and
- svg backends, however.
-
- These arguments can only be passed positionally.
-
- %(cmap_doc)s
-
- This parameter is ignored if *C* is RGB(A).
-
- %(norm_doc)s
-
- This parameter is ignored if *C* is RGB(A).
-
- %(vmin_vmax_doc)s
-
- This parameter is ignored if *C* is RGB(A).
-
- alpha : float, default: None
- The alpha blending value, between 0 (transparent) and 1 (opaque).
-
- snap : bool, default: False
- Whether to snap the mesh to pixel boundaries.
-
- Returns
- -------
- `.AxesImage` or `.PcolorImage` or `.QuadMesh`
- The return type depends on the type of grid:
-
- - `.AxesImage` for a regular rectangular grid.
- - `.PcolorImage` for a non-regular rectangular grid.
- - `.QuadMesh` for a non-rectangular grid.
-
- Other Parameters
- ----------------
- data : indexable object, optional
- DATA_PARAMETER_PLACEHOLDER
-
- **kwargs
- Supported additional parameters depend on the type of grid.
- See return types of *image* for further description.
- """
-
- C = args[-1]
- nr, nc = np.shape(C)[:2]
- if len(args) == 1:
- style = "image"
- x = [0, nc]
- y = [0, nr]
- elif len(args) == 3:
- x, y = args[:2]
- x = np.asarray(x)
- y = np.asarray(y)
- if x.ndim == 1 and y.ndim == 1:
- if x.size == 2 and y.size == 2:
- style = "image"
- else:
- dx = np.diff(x)
- dy = np.diff(y)
- if (np.ptp(dx) < 0.01 * abs(dx.mean()) and
- np.ptp(dy) < 0.01 * abs(dy.mean())):
- style = "image"
- else:
- style = "pcolorimage"
- elif x.ndim == 2 and y.ndim == 2:
- style = "quadmesh"
- else:
- raise TypeError("arguments do not match valid signatures")
- else:
- raise TypeError("need 1 argument or 3 arguments")
-
- if style == "quadmesh":
- # data point in each cell is value at lower left corner
- coords = np.stack([x, y], axis=-1)
- if np.ndim(C) not in {2, 3}:
- raise ValueError("C must be 2D or 3D")
- collection = mcoll.QuadMesh(
- coords, array=C,
- alpha=alpha, cmap=cmap, norm=norm,
- antialiased=False, edgecolors="none")
- self.add_collection(collection, autolim=False)
- xl, xr, yb, yt = x.min(), x.max(), y.min(), y.max()
- ret = collection
-
- else: # It's one of the two image styles.
- extent = xl, xr, yb, yt = x[0], x[-1], y[0], y[-1]
- if style == "image":
- im = mimage.AxesImage(
- self, cmap=cmap, norm=norm,
- data=C, alpha=alpha, extent=extent,
- interpolation='nearest', origin='lower',
- **kwargs)
- elif style == "pcolorimage":
- im = mimage.PcolorImage(
- self, x, y, C,
- cmap=cmap, norm=norm, alpha=alpha, extent=extent,
- **kwargs)
- self.add_image(im)
- ret = im
-
- if np.ndim(C) == 2: # C.ndim == 3 is RGB(A) so doesn't need scaling.
- ret._scale_norm(norm, vmin, vmax)
-
- if ret.get_clip_path() is None:
- # image does not already have clipping set, clip to axes patch
- ret.set_clip_path(self.patch)
-
- ret.sticky_edges.x[:] = [xl, xr]
- ret.sticky_edges.y[:] = [yb, yt]
- self.update_datalim(np.array([[xl, yb], [xr, yt]]))
- self._request_autoscale_view(tight=True)
- return ret
-
- @_preprocess_data()
- @_docstring.dedent_interpd
- def contour(self, *args, **kwargs):
- """
- Plot contour lines.
-
- Call signature::
-
- contour([X, Y,] Z, [levels], **kwargs)
- %(contour_doc)s
- """
- kwargs['filled'] = False
- contours = mcontour.QuadContourSet(self, *args, **kwargs)
- self._request_autoscale_view()
- return contours
-
- @_preprocess_data()
- @_docstring.dedent_interpd
- def contourf(self, *args, **kwargs):
- """
- Plot filled contours.
-
- Call signature::
-
- contourf([X, Y,] Z, [levels], **kwargs)
- %(contour_doc)s
- """
- kwargs['filled'] = True
- contours = mcontour.QuadContourSet(self, *args, **kwargs)
- self._request_autoscale_view()
- return contours
-
- def clabel(self, CS, levels=None, **kwargs):
- """
- Label a contour plot.
-
- Adds labels to line contours in given `.ContourSet`.
-
- Parameters
- ----------
- CS : `.ContourSet` instance
- Line contours to label.
-
- levels : array-like, optional
- A list of level values, that should be labeled. The list must be
- a subset of ``CS.levels``. If not given, all levels are labeled.
-
- **kwargs
- All other parameters are documented in `~.ContourLabeler.clabel`.
- """
- return CS.clabel(levels, **kwargs)
-
- #### Data analysis
-
- @_preprocess_data(replace_names=["x", 'weights'], label_namer="x")
- def hist(self, x, bins=None, range=None, density=False, weights=None,
- cumulative=False, bottom=None, histtype='bar', align='mid',
- orientation='vertical', rwidth=None, log=False,
- color=None, label=None, stacked=False, **kwargs):
- """
- Compute and plot a histogram.
-
- This method uses `numpy.histogram` to bin the data in *x* and count the
- number of values in each bin, then draws the distribution either as a
- `.BarContainer` or `.Polygon`. The *bins*, *range*, *density*, and
- *weights* parameters are forwarded to `numpy.histogram`.
-
- If the data has already been binned and counted, use `~.bar` or
- `~.stairs` to plot the distribution::
-
- counts, bins = np.histogram(x)
- plt.stairs(counts, bins)
-
- Alternatively, plot pre-computed bins and counts using ``hist()`` by
- treating each bin as a single point with a weight equal to its count::
-
- plt.hist(bins[:-1], bins, weights=counts)
-
- The data input *x* can be a singular array, a list of datasets of
- potentially different lengths ([*x0*, *x1*, ...]), or a 2D ndarray in
- which each column is a dataset. Note that the ndarray form is
- transposed relative to the list form. If the input is an array, then
- the return value is a tuple (*n*, *bins*, *patches*); if the input is a
- sequence of arrays, then the return value is a tuple
- ([*n0*, *n1*, ...], *bins*, [*patches0*, *patches1*, ...]).
-
- Masked arrays are not supported.
-
- Parameters
- ----------
- x : (n,) array or sequence of (n,) arrays
- Input values, this takes either a single array or a sequence of
- arrays which are not required to be of the same length.
-
- bins : int or sequence or str, default: :rc:`hist.bins`
- If *bins* is an integer, it defines the number of equal-width bins
- in the range.
-
- If *bins* is a sequence, it defines the bin edges, including the
- left edge of the first bin and the right edge of the last bin;
- in this case, bins may be unequally spaced. All but the last
- (righthand-most) bin is half-open. In other words, if *bins* is::
-
- [1, 2, 3, 4]
-
- then the first bin is ``[1, 2)`` (including 1, but excluding 2) and
- the second ``[2, 3)``. The last bin, however, is ``[3, 4]``, which
- *includes* 4.
-
- If *bins* is a string, it is one of the binning strategies
- supported by `numpy.histogram_bin_edges`: 'auto', 'fd', 'doane',
- 'scott', 'stone', 'rice', 'sturges', or 'sqrt'.
-
- range : tuple or None, default: None
- The lower and upper range of the bins. Lower and upper outliers
- are ignored. If not provided, *range* is ``(x.min(), x.max())``.
- Range has no effect if *bins* is a sequence.
-
- If *bins* is a sequence or *range* is specified, autoscaling
- is based on the specified bin range instead of the
- range of x.
-
- density : bool, default: False
- If ``True``, draw and return a probability density: each bin
- will display the bin's raw count divided by the total number of
- counts *and the bin width*
- (``density = counts / (sum(counts) * np.diff(bins))``),
- so that the area under the histogram integrates to 1
- (``np.sum(density * np.diff(bins)) == 1``).
-
- If *stacked* is also ``True``, the sum of the histograms is
- normalized to 1.
-
- weights : (n,) array-like or None, default: None
- An array of weights, of the same shape as *x*. Each value in
- *x* only contributes its associated weight towards the bin count
- (instead of 1). If *density* is ``True``, the weights are
- normalized, so that the integral of the density over the range
- remains 1.
-
- cumulative : bool or -1, default: False
- If ``True``, then a histogram is computed where each bin gives the
- counts in that bin plus all bins for smaller values. The last bin
- gives the total number of datapoints.
-
- If *density* is also ``True`` then the histogram is normalized such
- that the last bin equals 1.
-
- If *cumulative* is a number less than 0 (e.g., -1), the direction
- of accumulation is reversed. In this case, if *density* is also
- ``True``, then the histogram is normalized such that the first bin
- equals 1.
-
- bottom : array-like, scalar, or None, default: None
- Location of the bottom of each bin, i.e. bins are drawn from
- ``bottom`` to ``bottom + hist(x, bins)`` If a scalar, the bottom
- of each bin is shifted by the same amount. If an array, each bin
- is shifted independently and the length of bottom must match the
- number of bins. If None, defaults to 0.
-
- histtype : {'bar', 'barstacked', 'step', 'stepfilled'}, default: 'bar'
- The type of histogram to draw.
-
- - 'bar' is a traditional bar-type histogram. If multiple data
- are given the bars are arranged side by side.
- - 'barstacked' is a bar-type histogram where multiple
- data are stacked on top of each other.
- - 'step' generates a lineplot that is by default unfilled.
- - 'stepfilled' generates a lineplot that is by default filled.
-
- align : {'left', 'mid', 'right'}, default: 'mid'
- The horizontal alignment of the histogram bars.
-
- - 'left': bars are centered on the left bin edges.
- - 'mid': bars are centered between the bin edges.
- - 'right': bars are centered on the right bin edges.
-
- orientation : {'vertical', 'horizontal'}, default: 'vertical'
- If 'horizontal', `~.Axes.barh` will be used for bar-type histograms
- and the *bottom* kwarg will be the left edges.
-
- rwidth : float or None, default: None
- The relative width of the bars as a fraction of the bin width. If
- ``None``, automatically compute the width.
-
- Ignored if *histtype* is 'step' or 'stepfilled'.
-
- log : bool, default: False
- If ``True``, the histogram axis will be set to a log scale.
-
- color : color or array-like of colors or None, default: None
- Color or sequence of colors, one per dataset. Default (``None``)
- uses the standard line color sequence.
-
- label : str or None, default: None
- String, or sequence of strings to match multiple datasets. Bar
- charts yield multiple patches per dataset, but only the first gets
- the label, so that `~.Axes.legend` will work as expected.
-
- stacked : bool, default: False
- If ``True``, multiple data are stacked on top of each other If
- ``False`` multiple data are arranged side by side if histtype is
- 'bar' or on top of each other if histtype is 'step'
-
- Returns
- -------
- n : array or list of arrays
- The values of the histogram bins. See *density* and *weights* for a
- description of the possible semantics. If input *x* is an array,
- then this is an array of length *nbins*. If input is a sequence of
- arrays ``[data1, data2, ...]``, then this is a list of arrays with
- the values of the histograms for each of the arrays in the same
- order. The dtype of the array *n* (or of its element arrays) will
- always be float even if no weighting or normalization is used.
-
- bins : array
- The edges of the bins. Length nbins + 1 (nbins left edges and right
- edge of last bin). Always a single array even when multiple data
- sets are passed in.
-
- patches : `.BarContainer` or list of a single `.Polygon` or list of \
-such objects
- Container of individual artists used to create the histogram
- or list of such containers if there are multiple input datasets.
-
- Other Parameters
- ----------------
- data : indexable object, optional
- DATA_PARAMETER_PLACEHOLDER
-
- **kwargs
- `~matplotlib.patches.Patch` properties
-
- See Also
- --------
- hist2d : 2D histogram with rectangular bins
- hexbin : 2D histogram with hexagonal bins
- stairs : Plot a pre-computed histogram
- bar : Plot a pre-computed histogram
-
- Notes
- -----
- For large numbers of bins (>1000), plotting can be significantly
- accelerated by using `~.Axes.stairs` to plot a pre-computed histogram
- (``plt.stairs(*np.histogram(data))``), or by setting *histtype* to
- 'step' or 'stepfilled' rather than 'bar' or 'barstacked'.
- """
- # Avoid shadowing the builtin.
- bin_range = range
- from builtins import range
-
- if np.isscalar(x):
- x = [x]
-
- if bins is None:
- bins = mpl.rcParams['hist.bins']
-
- # Validate string inputs here to avoid cluttering subsequent code.
- _api.check_in_list(['bar', 'barstacked', 'step', 'stepfilled'],
- histtype=histtype)
- _api.check_in_list(['left', 'mid', 'right'], align=align)
- _api.check_in_list(['horizontal', 'vertical'], orientation=orientation)
-
- if histtype == 'barstacked' and not stacked:
- stacked = True
-
- # Massage 'x' for processing.
- x = cbook._reshape_2D(x, 'x')
- nx = len(x) # number of datasets
-
- # Process unit information. _process_unit_info sets the unit and
- # converts the first dataset; then we convert each following dataset
- # one at a time.
- if orientation == "vertical":
- convert_units = self.convert_xunits
- x = [*self._process_unit_info([("x", x[0])], kwargs),
- *map(convert_units, x[1:])]
- else: # horizontal
- convert_units = self.convert_yunits
- x = [*self._process_unit_info([("y", x[0])], kwargs),
- *map(convert_units, x[1:])]
-
- if bin_range is not None:
- bin_range = convert_units(bin_range)
-
- if not cbook.is_scalar_or_string(bins):
- bins = convert_units(bins)
-
- # We need to do to 'weights' what was done to 'x'
- if weights is not None:
- w = cbook._reshape_2D(weights, 'weights')
- else:
- w = [None] * nx
-
- if len(w) != nx:
- raise ValueError('weights should have the same shape as x')
-
- input_empty = True
- for xi, wi in zip(x, w):
- len_xi = len(xi)
- if wi is not None and len(wi) != len_xi:
- raise ValueError('weights should have the same shape as x')
- if len_xi:
- input_empty = False
-
- if color is None:
- colors = [self._get_lines.get_next_color() for i in range(nx)]
- else:
- colors = mcolors.to_rgba_array(color)
- if len(colors) != nx:
- raise ValueError(f"The 'color' keyword argument must have one "
- f"color per dataset, but {nx} datasets and "
- f"{len(colors)} colors were provided")
-
- hist_kwargs = dict()
-
- # if the bin_range is not given, compute without nan numpy
- # does not do this for us when guessing the range (but will
- # happily ignore nans when computing the histogram).
- if bin_range is None:
- xmin = np.inf
- xmax = -np.inf
- for xi in x:
- if len(xi):
- # python's min/max ignore nan,
- # np.minnan returns nan for all nan input
- xmin = min(xmin, np.nanmin(xi))
- xmax = max(xmax, np.nanmax(xi))
- if xmin <= xmax: # Only happens if we have seen a finite value.
- bin_range = (xmin, xmax)
-
- # If bins are not specified either explicitly or via range,
- # we need to figure out the range required for all datasets,
- # and supply that to np.histogram.
- if not input_empty and len(x) > 1:
- if weights is not None:
- _w = np.concatenate(w)
- else:
- _w = None
- bins = np.histogram_bin_edges(
- np.concatenate(x), bins, bin_range, _w)
- else:
- hist_kwargs['range'] = bin_range
-
- density = bool(density)
- if density and not stacked:
- hist_kwargs['density'] = density
-
- # List to store all the top coordinates of the histograms
- tops = [] # Will have shape (n_datasets, n_bins).
- # Loop through datasets
- for i in range(nx):
- # this will automatically overwrite bins,
- # so that each histogram uses the same bins
- m, bins = np.histogram(x[i], bins, weights=w[i], **hist_kwargs)
- tops.append(m)
- tops = np.array(tops, float) # causes problems later if it's an int
- bins = np.array(bins, float) # causes problems if float16
- if stacked:
- tops = tops.cumsum(axis=0)
- # If a stacked density plot, normalize so the area of all the
- # stacked histograms together is 1
- if density:
- tops = (tops / np.diff(bins)) / tops[-1].sum()
- if cumulative:
- slc = slice(None)
- if isinstance(cumulative, Number) and cumulative < 0:
- slc = slice(None, None, -1)
- if density:
- tops = (tops * np.diff(bins))[:, slc].cumsum(axis=1)[:, slc]
- else:
- tops = tops[:, slc].cumsum(axis=1)[:, slc]
-
- patches = []
-
- if histtype.startswith('bar'):
-
- totwidth = np.diff(bins)
-
- if rwidth is not None:
- dr = np.clip(rwidth, 0, 1)
- elif (len(tops) > 1 and
- ((not stacked) or mpl.rcParams['_internal.classic_mode'])):
- dr = 0.8
- else:
- dr = 1.0
-
- if histtype == 'bar' and not stacked:
- width = dr * totwidth / nx
- dw = width
- boffset = -0.5 * dr * totwidth * (1 - 1 / nx)
- elif histtype == 'barstacked' or stacked:
- width = dr * totwidth
- boffset, dw = 0.0, 0.0
-
- if align == 'mid':
- boffset += 0.5 * totwidth
- elif align == 'right':
- boffset += totwidth
-
- if orientation == 'horizontal':
- _barfunc = self.barh
- bottom_kwarg = 'left'
- else: # orientation == 'vertical'
- _barfunc = self.bar
- bottom_kwarg = 'bottom'
-
- for top, color in zip(tops, colors):
- if bottom is None:
- bottom = np.zeros(len(top))
- if stacked:
- height = top - bottom
- else:
- height = top
- bars = _barfunc(bins[:-1]+boffset, height, width,
- align='center', log=log,
- color=color, **{bottom_kwarg: bottom})
- patches.append(bars)
- if stacked:
- bottom = top
- boffset += dw
- # Remove stickies from all bars but the lowest ones, as otherwise
- # margin expansion would be unable to cross the stickies in the
- # middle of the bars.
- for bars in patches[1:]:
- for patch in bars:
- patch.sticky_edges.x[:] = patch.sticky_edges.y[:] = []
-
- elif histtype.startswith('step'):
- # these define the perimeter of the polygon
- x = np.zeros(4 * len(bins) - 3)
- y = np.zeros(4 * len(bins) - 3)
-
- x[0:2*len(bins)-1:2], x[1:2*len(bins)-1:2] = bins, bins[:-1]
- x[2*len(bins)-1:] = x[1:2*len(bins)-1][::-1]
-
- if bottom is None:
- bottom = 0
-
- y[1:2*len(bins)-1:2] = y[2:2*len(bins):2] = bottom
- y[2*len(bins)-1:] = y[1:2*len(bins)-1][::-1]
-
- if log:
- if orientation == 'horizontal':
- self.set_xscale('log', nonpositive='clip')
- else: # orientation == 'vertical'
- self.set_yscale('log', nonpositive='clip')
-
- if align == 'left':
- x -= 0.5*(bins[1]-bins[0])
- elif align == 'right':
- x += 0.5*(bins[1]-bins[0])
-
- # If fill kwarg is set, it will be passed to the patch collection,
- # overriding this
- fill = (histtype == 'stepfilled')
-
- xvals, yvals = [], []
- for top in tops:
- if stacked:
- # top of the previous polygon becomes the bottom
- y[2*len(bins)-1:] = y[1:2*len(bins)-1][::-1]
- # set the top of this polygon
- y[1:2*len(bins)-1:2] = y[2:2*len(bins):2] = top + bottom
-
- # The starting point of the polygon has not yet been
- # updated. So far only the endpoint was adjusted. This
- # assignment closes the polygon. The redundant endpoint is
- # later discarded (for step and stepfilled).
- y[0] = y[-1]
-
- if orientation == 'horizontal':
- xvals.append(y.copy())
- yvals.append(x.copy())
- else:
- xvals.append(x.copy())
- yvals.append(y.copy())
-
- # stepfill is closed, step is not
- split = -1 if fill else 2 * len(bins)
- # add patches in reverse order so that when stacking,
- # items lower in the stack are plotted on top of
- # items higher in the stack
- for x, y, color in reversed(list(zip(xvals, yvals, colors))):
- patches.append(self.fill(
- x[:split], y[:split],
- closed=True if fill else None,
- facecolor=color,
- edgecolor=None if fill else color,
- fill=fill if fill else None,
- zorder=None if fill else mlines.Line2D.zorder))
- for patch_list in patches:
- for patch in patch_list:
- if orientation == 'vertical':
- patch.sticky_edges.y.append(0)
- elif orientation == 'horizontal':
- patch.sticky_edges.x.append(0)
-
- # we return patches, so put it back in the expected order
- patches.reverse()
-
- # If None, make all labels None (via zip_longest below); otherwise,
- # cast each element to str, but keep a single str as it.
- labels = [] if label is None else np.atleast_1d(np.asarray(label, str))
- for patch, lbl in itertools.zip_longest(patches, labels):
- if patch:
- p = patch[0]
- p._internal_update(kwargs)
- if lbl is not None:
- p.set_label(lbl)
- for p in patch[1:]:
- p._internal_update(kwargs)
- p.set_label('_nolegend_')
-
- if nx == 1:
- return tops[0], bins, patches[0]
- else:
- patch_type = ("BarContainer" if histtype.startswith("bar")
- else "list[Polygon]")
- return tops, bins, cbook.silent_list(patch_type, patches)
-
- @_preprocess_data()
- def stairs(self, values, edges=None, *,
- orientation='vertical', baseline=0, fill=False, **kwargs):
- """
- A stepwise constant function as a line with bounding edges
- or a filled plot.
-
- Parameters
- ----------
- values : array-like
- The step heights.
-
- edges : array-like
- The edge positions, with ``len(edges) == len(vals) + 1``,
- between which the curve takes on vals values.
-
- orientation : {'vertical', 'horizontal'}, default: 'vertical'
- The direction of the steps. Vertical means that *values* are along
- the y-axis, and edges are along the x-axis.
-
- baseline : float, array-like or None, default: 0
- The bottom value of the bounding edges or when
- ``fill=True``, position of lower edge. If *fill* is
- True or an array is passed to *baseline*, a closed
- path is drawn.
-
- fill : bool, default: False
- Whether the area under the step curve should be filled.
-
- Returns
- -------
- StepPatch : `~matplotlib.patches.StepPatch`
-
- Other Parameters
- ----------------
- data : indexable object, optional
- DATA_PARAMETER_PLACEHOLDER
-
- **kwargs
- `~matplotlib.patches.StepPatch` properties
-
- """
-
- if 'color' in kwargs:
- _color = kwargs.pop('color')
- else:
- _color = self._get_lines.get_next_color()
- if fill:
- kwargs.setdefault('linewidth', 0)
- kwargs.setdefault('facecolor', _color)
- else:
- kwargs.setdefault('edgecolor', _color)
-
- if edges is None:
- edges = np.arange(len(values) + 1)
-
- edges, values, baseline = self._process_unit_info(
- [("x", edges), ("y", values), ("y", baseline)], kwargs)
-
- patch = mpatches.StepPatch(values,
- edges,
- baseline=baseline,
- orientation=orientation,
- fill=fill,
- **kwargs)
- self.add_patch(patch)
- if baseline is None:
- baseline = 0
- if orientation == 'vertical':
- patch.sticky_edges.y.append(np.min(baseline))
- self.update_datalim([(edges[0], np.min(baseline))])
- else:
- patch.sticky_edges.x.append(np.min(baseline))
- self.update_datalim([(np.min(baseline), edges[0])])
- self._request_autoscale_view()
- return patch
-
- @_preprocess_data(replace_names=["x", "y", "weights"])
- @_docstring.dedent_interpd
- def hist2d(self, x, y, bins=10, range=None, density=False, weights=None,
- cmin=None, cmax=None, **kwargs):
- """
- Make a 2D histogram plot.
-
- Parameters
- ----------
- x, y : array-like, shape (n, )
- Input values
-
- bins : None or int or [int, int] or array-like or [array, array]
-
- The bin specification:
-
- - If int, the number of bins for the two dimensions
- (nx=ny=bins).
- - If ``[int, int]``, the number of bins in each dimension
- (nx, ny = bins).
- - If array-like, the bin edges for the two dimensions
- (x_edges=y_edges=bins).
- - If ``[array, array]``, the bin edges in each dimension
- (x_edges, y_edges = bins).
-
- The default value is 10.
-
- range : array-like shape(2, 2), optional
- The leftmost and rightmost edges of the bins along each dimension
- (if not specified explicitly in the bins parameters): ``[[xmin,
- xmax], [ymin, ymax]]``. All values outside of this range will be
- considered outliers and not tallied in the histogram.
-
- density : bool, default: False
- Normalize histogram. See the documentation for the *density*
- parameter of `~.Axes.hist` for more details.
-
- weights : array-like, shape (n, ), optional
- An array of values w_i weighing each sample (x_i, y_i).
-
- cmin, cmax : float, default: None
- All bins that has count less than *cmin* or more than *cmax* will
- not be displayed (set to NaN before passing to imshow) and these
- count values in the return value count histogram will also be set
- to nan upon return.
-
- Returns
- -------
- h : 2D array
- The bi-dimensional histogram of samples x and y. Values in x are
- histogrammed along the first dimension and values in y are
- histogrammed along the second dimension.
- xedges : 1D array
- The bin edges along the x-axis.
- yedges : 1D array
- The bin edges along the y-axis.
- image : `~.matplotlib.collections.QuadMesh`
-
- Other Parameters
- ----------------
- %(cmap_doc)s
-
- %(norm_doc)s
-
- %(vmin_vmax_doc)s
-
- alpha : ``0 <= scalar <= 1`` or ``None``, optional
- The alpha blending value.
-
- data : indexable object, optional
- DATA_PARAMETER_PLACEHOLDER
-
- **kwargs
- Additional parameters are passed along to the
- `~.Axes.pcolormesh` method and `~matplotlib.collections.QuadMesh`
- constructor.
-
- See Also
- --------
- hist : 1D histogram plotting
- hexbin : 2D histogram with hexagonal bins
-
- Notes
- -----
- - Currently ``hist2d`` calculates its own axis limits, and any limits
- previously set are ignored.
- - Rendering the histogram with a logarithmic color scale is
- accomplished by passing a `.colors.LogNorm` instance to the *norm*
- keyword argument. Likewise, power-law normalization (similar
- in effect to gamma correction) can be accomplished with
- `.colors.PowerNorm`.
- """
-
- h, xedges, yedges = np.histogram2d(x, y, bins=bins, range=range,
- density=density, weights=weights)
-
- if cmin is not None:
- h[h < cmin] = None
- if cmax is not None:
- h[h > cmax] = None
-
- pc = self.pcolormesh(xedges, yedges, h.T, **kwargs)
- self.set_xlim(xedges[0], xedges[-1])
- self.set_ylim(yedges[0], yedges[-1])
-
- return h, xedges, yedges, pc
-
- @_preprocess_data(replace_names=["x"])
- @_docstring.dedent_interpd
- def psd(self, x, NFFT=None, Fs=None, Fc=None, detrend=None,
- window=None, noverlap=None, pad_to=None,
- sides=None, scale_by_freq=None, return_line=None, **kwargs):
- r"""
- Plot the power spectral density.
-
- The power spectral density :math:`P_{xx}` by Welch's average
- periodogram method. The vector *x* is divided into *NFFT* length
- segments. Each segment is detrended by function *detrend* and
- windowed by function *window*. *noverlap* gives the length of
- the overlap between segments. The :math:`|\mathrm{fft}(i)|^2`
- of each segment :math:`i` are averaged to compute :math:`P_{xx}`,
- with a scaling to correct for power loss due to windowing.
-
- If len(*x*) < *NFFT*, it will be zero padded to *NFFT*.
-
- Parameters
- ----------
- x : 1-D array or sequence
- Array or sequence containing the data
-
- %(Spectral)s
-
- %(PSD)s
-
- noverlap : int, default: 0 (no overlap)
- The number of points of overlap between segments.
-
- Fc : int, default: 0
- The center frequency of *x*, which offsets the x extents of the
- plot to reflect the frequency range used when a signal is acquired
- and then filtered and downsampled to baseband.
-
- return_line : bool, default: False
- Whether to include the line object plotted in the returned values.
-
- Returns
- -------
- Pxx : 1-D array
- The values for the power spectrum :math:`P_{xx}` before scaling
- (real valued).
-
- freqs : 1-D array
- The frequencies corresponding to the elements in *Pxx*.
-
- line : `~matplotlib.lines.Line2D`
- The line created by this function.
- Only returned if *return_line* is True.
-
- Other Parameters
- ----------------
- data : indexable object, optional
- DATA_PARAMETER_PLACEHOLDER
-
- **kwargs
- Keyword arguments control the `.Line2D` properties:
-
- %(Line2D:kwdoc)s
-
- See Also
- --------
- specgram
- Differs in the default overlap; in not returning the mean of the
- segment periodograms; in returning the times of the segments; and
- in plotting a colormap instead of a line.
- magnitude_spectrum
- Plots the magnitude spectrum.
- csd
- Plots the spectral density between two signals.
-
- Notes
- -----
- For plotting, the power is plotted as
- :math:`10\log_{10}(P_{xx})` for decibels, though *Pxx* itself
- is returned.
-
- References
- ----------
- Bendat & Piersol -- Random Data: Analysis and Measurement Procedures,
- John Wiley & Sons (1986)
- """
- if Fc is None:
- Fc = 0
-
- pxx, freqs = mlab.psd(x=x, NFFT=NFFT, Fs=Fs, detrend=detrend,
- window=window, noverlap=noverlap, pad_to=pad_to,
- sides=sides, scale_by_freq=scale_by_freq)
- freqs += Fc
-
- if scale_by_freq in (None, True):
- psd_units = 'dB/Hz'
- else:
- psd_units = 'dB'
-
- line = self.plot(freqs, 10 * np.log10(pxx), **kwargs)
- self.set_xlabel('Frequency')
- self.set_ylabel('Power Spectral Density (%s)' % psd_units)
- self.grid(True)
-
- vmin, vmax = self.get_ybound()
- step = max(10 * int(np.log10(vmax - vmin)), 1)
- ticks = np.arange(math.floor(vmin), math.ceil(vmax) + 1, step)
- self.set_yticks(ticks)
-
- if return_line is None or not return_line:
- return pxx, freqs
- else:
- return pxx, freqs, line
-
- @_preprocess_data(replace_names=["x", "y"], label_namer="y")
- @_docstring.dedent_interpd
- def csd(self, x, y, NFFT=None, Fs=None, Fc=None, detrend=None,
- window=None, noverlap=None, pad_to=None,
- sides=None, scale_by_freq=None, return_line=None, **kwargs):
- r"""
- Plot the cross-spectral density.
-
- The cross spectral density :math:`P_{xy}` by Welch's average
- periodogram method. The vectors *x* and *y* are divided into
- *NFFT* length segments. Each segment is detrended by function
- *detrend* and windowed by function *window*. *noverlap* gives
- the length of the overlap between segments. The product of
- the direct FFTs of *x* and *y* are averaged over each segment
- to compute :math:`P_{xy}`, with a scaling to correct for power
- loss due to windowing.
-
- If len(*x*) < *NFFT* or len(*y*) < *NFFT*, they will be zero
- padded to *NFFT*.
-
- Parameters
- ----------
- x, y : 1-D arrays or sequences
- Arrays or sequences containing the data.
-
- %(Spectral)s
-
- %(PSD)s
-
- noverlap : int, default: 0 (no overlap)
- The number of points of overlap between segments.
-
- Fc : int, default: 0
- The center frequency of *x*, which offsets the x extents of the
- plot to reflect the frequency range used when a signal is acquired
- and then filtered and downsampled to baseband.
-
- return_line : bool, default: False
- Whether to include the line object plotted in the returned values.
-
- Returns
- -------
- Pxy : 1-D array
- The values for the cross spectrum :math:`P_{xy}` before scaling
- (complex valued).
-
- freqs : 1-D array
- The frequencies corresponding to the elements in *Pxy*.
-
- line : `~matplotlib.lines.Line2D`
- The line created by this function.
- Only returned if *return_line* is True.
-
- Other Parameters
- ----------------
- data : indexable object, optional
- DATA_PARAMETER_PLACEHOLDER
-
- **kwargs
- Keyword arguments control the `.Line2D` properties:
-
- %(Line2D:kwdoc)s
-
- See Also
- --------
- psd : is equivalent to setting ``y = x``.
-
- Notes
- -----
- For plotting, the power is plotted as
- :math:`10 \log_{10}(P_{xy})` for decibels, though :math:`P_{xy}` itself
- is returned.
-
- References
- ----------
- Bendat & Piersol -- Random Data: Analysis and Measurement Procedures,
- John Wiley & Sons (1986)
- """
- if Fc is None:
- Fc = 0
-
- pxy, freqs = mlab.csd(x=x, y=y, NFFT=NFFT, Fs=Fs, detrend=detrend,
- window=window, noverlap=noverlap, pad_to=pad_to,
- sides=sides, scale_by_freq=scale_by_freq)
- # pxy is complex
- freqs += Fc
-
- line = self.plot(freqs, 10 * np.log10(np.abs(pxy)), **kwargs)
- self.set_xlabel('Frequency')
- self.set_ylabel('Cross Spectrum Magnitude (dB)')
- self.grid(True)
-
- vmin, vmax = self.get_ybound()
- step = max(10 * int(np.log10(vmax - vmin)), 1)
- ticks = np.arange(math.floor(vmin), math.ceil(vmax) + 1, step)
- self.set_yticks(ticks)
-
- if return_line is None or not return_line:
- return pxy, freqs
- else:
- return pxy, freqs, line
-
- @_preprocess_data(replace_names=["x"])
- @_docstring.dedent_interpd
- def magnitude_spectrum(self, x, Fs=None, Fc=None, window=None,
- pad_to=None, sides=None, scale=None,
- **kwargs):
- """
- Plot the magnitude spectrum.
-
- Compute the magnitude spectrum of *x*. Data is padded to a
- length of *pad_to* and the windowing function *window* is applied to
- the signal.
-
- Parameters
- ----------
- x : 1-D array or sequence
- Array or sequence containing the data.
-
- %(Spectral)s
-
- %(Single_Spectrum)s
-
- scale : {'default', 'linear', 'dB'}
- The scaling of the values in the *spec*. 'linear' is no scaling.
- 'dB' returns the values in dB scale, i.e., the dB amplitude
- (20 * log10). 'default' is 'linear'.
-
- Fc : int, default: 0
- The center frequency of *x*, which offsets the x extents of the
- plot to reflect the frequency range used when a signal is acquired
- and then filtered and downsampled to baseband.
-
- Returns
- -------
- spectrum : 1-D array
- The values for the magnitude spectrum before scaling (real valued).
-
- freqs : 1-D array
- The frequencies corresponding to the elements in *spectrum*.
-
- line : `~matplotlib.lines.Line2D`
- The line created by this function.
-
- Other Parameters
- ----------------
- data : indexable object, optional
- DATA_PARAMETER_PLACEHOLDER
-
- **kwargs
- Keyword arguments control the `.Line2D` properties:
-
- %(Line2D:kwdoc)s
-
- See Also
- --------
- psd
- Plots the power spectral density.
- angle_spectrum
- Plots the angles of the corresponding frequencies.
- phase_spectrum
- Plots the phase (unwrapped angle) of the corresponding frequencies.
- specgram
- Can plot the magnitude spectrum of segments within the signal in a
- colormap.
- """
- if Fc is None:
- Fc = 0
-
- spec, freqs = mlab.magnitude_spectrum(x=x, Fs=Fs, window=window,
- pad_to=pad_to, sides=sides)
- freqs += Fc
-
- yunits = _api.check_getitem(
- {None: 'energy', 'default': 'energy', 'linear': 'energy',
- 'dB': 'dB'},
- scale=scale)
- if yunits == 'energy':
- Z = spec
- else: # yunits == 'dB'
- Z = 20. * np.log10(spec)
-
- line, = self.plot(freqs, Z, **kwargs)
- self.set_xlabel('Frequency')
- self.set_ylabel('Magnitude (%s)' % yunits)
-
- return spec, freqs, line
-
- @_preprocess_data(replace_names=["x"])
- @_docstring.dedent_interpd
- def angle_spectrum(self, x, Fs=None, Fc=None, window=None,
- pad_to=None, sides=None, **kwargs):
- """
- Plot the angle spectrum.
-
- Compute the angle spectrum (wrapped phase spectrum) of *x*.
- Data is padded to a length of *pad_to* and the windowing function
- *window* is applied to the signal.
-
- Parameters
- ----------
- x : 1-D array or sequence
- Array or sequence containing the data.
-
- %(Spectral)s
-
- %(Single_Spectrum)s
-
- Fc : int, default: 0
- The center frequency of *x*, which offsets the x extents of the
- plot to reflect the frequency range used when a signal is acquired
- and then filtered and downsampled to baseband.
-
- Returns
- -------
- spectrum : 1-D array
- The values for the angle spectrum in radians (real valued).
-
- freqs : 1-D array
- The frequencies corresponding to the elements in *spectrum*.
-
- line : `~matplotlib.lines.Line2D`
- The line created by this function.
-
- Other Parameters
- ----------------
- data : indexable object, optional
- DATA_PARAMETER_PLACEHOLDER
-
- **kwargs
- Keyword arguments control the `.Line2D` properties:
-
- %(Line2D:kwdoc)s
-
- See Also
- --------
- magnitude_spectrum
- Plots the magnitudes of the corresponding frequencies.
- phase_spectrum
- Plots the unwrapped version of this function.
- specgram
- Can plot the angle spectrum of segments within the signal in a
- colormap.
- """
- if Fc is None:
- Fc = 0
-
- spec, freqs = mlab.angle_spectrum(x=x, Fs=Fs, window=window,
- pad_to=pad_to, sides=sides)
- freqs += Fc
-
- lines = self.plot(freqs, spec, **kwargs)
- self.set_xlabel('Frequency')
- self.set_ylabel('Angle (radians)')
-
- return spec, freqs, lines[0]
-
- @_preprocess_data(replace_names=["x"])
- @_docstring.dedent_interpd
- def phase_spectrum(self, x, Fs=None, Fc=None, window=None,
- pad_to=None, sides=None, **kwargs):
- """
- Plot the phase spectrum.
-
- Compute the phase spectrum (unwrapped angle spectrum) of *x*.
- Data is padded to a length of *pad_to* and the windowing function
- *window* is applied to the signal.
-
- Parameters
- ----------
- x : 1-D array or sequence
- Array or sequence containing the data
-
- %(Spectral)s
-
- %(Single_Spectrum)s
-
- Fc : int, default: 0
- The center frequency of *x*, which offsets the x extents of the
- plot to reflect the frequency range used when a signal is acquired
- and then filtered and downsampled to baseband.
-
- Returns
- -------
- spectrum : 1-D array
- The values for the phase spectrum in radians (real valued).
-
- freqs : 1-D array
- The frequencies corresponding to the elements in *spectrum*.
-
- line : `~matplotlib.lines.Line2D`
- The line created by this function.
-
- Other Parameters
- ----------------
- data : indexable object, optional
- DATA_PARAMETER_PLACEHOLDER
-
- **kwargs
- Keyword arguments control the `.Line2D` properties:
-
- %(Line2D:kwdoc)s
-
- See Also
- --------
- magnitude_spectrum
- Plots the magnitudes of the corresponding frequencies.
- angle_spectrum
- Plots the wrapped version of this function.
- specgram
- Can plot the phase spectrum of segments within the signal in a
- colormap.
- """
- if Fc is None:
- Fc = 0
-
- spec, freqs = mlab.phase_spectrum(x=x, Fs=Fs, window=window,
- pad_to=pad_to, sides=sides)
- freqs += Fc
-
- lines = self.plot(freqs, spec, **kwargs)
- self.set_xlabel('Frequency')
- self.set_ylabel('Phase (radians)')
-
- return spec, freqs, lines[0]
-
- @_preprocess_data(replace_names=["x", "y"])
- @_docstring.dedent_interpd
- def cohere(self, x, y, NFFT=256, Fs=2, Fc=0, detrend=mlab.detrend_none,
- window=mlab.window_hanning, noverlap=0, pad_to=None,
- sides='default', scale_by_freq=None, **kwargs):
- r"""
- Plot the coherence between *x* and *y*.
-
- Coherence is the normalized cross spectral density:
-
- .. math::
-
- C_{xy} = \frac{|P_{xy}|^2}{P_{xx}P_{yy}}
-
- Parameters
- ----------
- %(Spectral)s
-
- %(PSD)s
-
- noverlap : int, default: 0 (no overlap)
- The number of points of overlap between blocks.
-
- Fc : int, default: 0
- The center frequency of *x*, which offsets the x extents of the
- plot to reflect the frequency range used when a signal is acquired
- and then filtered and downsampled to baseband.
-
- Returns
- -------
- Cxy : 1-D array
- The coherence vector.
-
- freqs : 1-D array
- The frequencies for the elements in *Cxy*.
-
- Other Parameters
- ----------------
- data : indexable object, optional
- DATA_PARAMETER_PLACEHOLDER
-
- **kwargs
- Keyword arguments control the `.Line2D` properties:
-
- %(Line2D:kwdoc)s
-
- References
- ----------
- Bendat & Piersol -- Random Data: Analysis and Measurement Procedures,
- John Wiley & Sons (1986)
- """
- cxy, freqs = mlab.cohere(x=x, y=y, NFFT=NFFT, Fs=Fs, detrend=detrend,
- window=window, noverlap=noverlap,
- scale_by_freq=scale_by_freq, sides=sides,
- pad_to=pad_to)
- freqs += Fc
-
- self.plot(freqs, cxy, **kwargs)
- self.set_xlabel('Frequency')
- self.set_ylabel('Coherence')
- self.grid(True)
-
- return cxy, freqs
-
- @_preprocess_data(replace_names=["x"])
- @_docstring.dedent_interpd
- def specgram(self, x, NFFT=None, Fs=None, Fc=None, detrend=None,
- window=None, noverlap=None,
- cmap=None, xextent=None, pad_to=None, sides=None,
- scale_by_freq=None, mode=None, scale=None,
- vmin=None, vmax=None, **kwargs):
- """
- Plot a spectrogram.
-
- Compute and plot a spectrogram of data in *x*. Data are split into
- *NFFT* length segments and the spectrum of each section is
- computed. The windowing function *window* is applied to each
- segment, and the amount of overlap of each segment is
- specified with *noverlap*. The spectrogram is plotted as a colormap
- (using imshow).
-
- Parameters
- ----------
- x : 1-D array or sequence
- Array or sequence containing the data.
-
- %(Spectral)s
-
- %(PSD)s
-
- mode : {'default', 'psd', 'magnitude', 'angle', 'phase'}
- What sort of spectrum to use. Default is 'psd', which takes the
- power spectral density. 'magnitude' returns the magnitude
- spectrum. 'angle' returns the phase spectrum without unwrapping.
- 'phase' returns the phase spectrum with unwrapping.
-
- noverlap : int, default: 128
- The number of points of overlap between blocks.
-
- scale : {'default', 'linear', 'dB'}
- The scaling of the values in the *spec*. 'linear' is no scaling.
- 'dB' returns the values in dB scale. When *mode* is 'psd',
- this is dB power (10 * log10). Otherwise, this is dB amplitude
- (20 * log10). 'default' is 'dB' if *mode* is 'psd' or
- 'magnitude' and 'linear' otherwise. This must be 'linear'
- if *mode* is 'angle' or 'phase'.
-
- Fc : int, default: 0
- The center frequency of *x*, which offsets the x extents of the
- plot to reflect the frequency range used when a signal is acquired
- and then filtered and downsampled to baseband.
-
- cmap : `.Colormap`, default: :rc:`image.cmap`
-
- xextent : *None* or (xmin, xmax)
- The image extent along the x-axis. The default sets *xmin* to the
- left border of the first bin (*spectrum* column) and *xmax* to the
- right border of the last bin. Note that for *noverlap>0* the width
- of the bins is smaller than those of the segments.
-
- data : indexable object, optional
- DATA_PARAMETER_PLACEHOLDER
-
- **kwargs
- Additional keyword arguments are passed on to `~.axes.Axes.imshow`
- which makes the specgram image. The origin keyword argument
- is not supported.
-
- Returns
- -------
- spectrum : 2D array
- Columns are the periodograms of successive segments.
-
- freqs : 1-D array
- The frequencies corresponding to the rows in *spectrum*.
-
- t : 1-D array
- The times corresponding to midpoints of segments (i.e., the columns
- in *spectrum*).
-
- im : `.AxesImage`
- The image created by imshow containing the spectrogram.
-
- See Also
- --------
- psd
- Differs in the default overlap; in returning the mean of the
- segment periodograms; in not returning times; and in generating a
- line plot instead of colormap.
- magnitude_spectrum
- A single spectrum, similar to having a single segment when *mode*
- is 'magnitude'. Plots a line instead of a colormap.
- angle_spectrum
- A single spectrum, similar to having a single segment when *mode*
- is 'angle'. Plots a line instead of a colormap.
- phase_spectrum
- A single spectrum, similar to having a single segment when *mode*
- is 'phase'. Plots a line instead of a colormap.
-
- Notes
- -----
- The parameters *detrend* and *scale_by_freq* do only apply when *mode*
- is set to 'psd'.
- """
- if NFFT is None:
- NFFT = 256 # same default as in mlab.specgram()
- if Fc is None:
- Fc = 0 # same default as in mlab._spectral_helper()
- if noverlap is None:
- noverlap = 128 # same default as in mlab.specgram()
- if Fs is None:
- Fs = 2 # same default as in mlab._spectral_helper()
-
- if mode == 'complex':
- raise ValueError('Cannot plot a complex specgram')
-
- if scale is None or scale == 'default':
- if mode in ['angle', 'phase']:
- scale = 'linear'
- else:
- scale = 'dB'
- elif mode in ['angle', 'phase'] and scale == 'dB':
- raise ValueError('Cannot use dB scale with angle or phase mode')
-
- spec, freqs, t = mlab.specgram(x=x, NFFT=NFFT, Fs=Fs,
- detrend=detrend, window=window,
- noverlap=noverlap, pad_to=pad_to,
- sides=sides,
- scale_by_freq=scale_by_freq,
- mode=mode)
-
- if scale == 'linear':
- Z = spec
- elif scale == 'dB':
- if mode is None or mode == 'default' or mode == 'psd':
- Z = 10. * np.log10(spec)
- else:
- Z = 20. * np.log10(spec)
- else:
- raise ValueError(f'Unknown scale {scale!r}')
-
- Z = np.flipud(Z)
-
- if xextent is None:
- # padding is needed for first and last segment:
- pad_xextent = (NFFT-noverlap) / Fs / 2
- xextent = np.min(t) - pad_xextent, np.max(t) + pad_xextent
- xmin, xmax = xextent
- freqs += Fc
- extent = xmin, xmax, freqs[0], freqs[-1]
-
- if 'origin' in kwargs:
- raise _api.kwarg_error("specgram", "origin")
-
- im = self.imshow(Z, cmap, extent=extent, vmin=vmin, vmax=vmax,
- origin='upper', **kwargs)
- self.axis('auto')
-
- return spec, freqs, t, im
-
- @_docstring.dedent_interpd
- def spy(self, Z, precision=0, marker=None, markersize=None,
- aspect='equal', origin="upper", **kwargs):
- """
- Plot the sparsity pattern of a 2D array.
-
- This visualizes the non-zero values of the array.
-
- Two plotting styles are available: image and marker. Both
- are available for full arrays, but only the marker style
- works for `scipy.sparse.spmatrix` instances.
-
- **Image style**
-
- If *marker* and *markersize* are *None*, `~.Axes.imshow` is used. Any
- extra remaining keyword arguments are passed to this method.
-
- **Marker style**
-
- If *Z* is a `scipy.sparse.spmatrix` or *marker* or *markersize* are
- *None*, a `.Line2D` object will be returned with the value of marker
- determining the marker type, and any remaining keyword arguments
- passed to `~.Axes.plot`.
-
- Parameters
- ----------
- Z : (M, N) array-like
- The array to be plotted.
-
- precision : float or 'present', default: 0
- If *precision* is 0, any non-zero value will be plotted. Otherwise,
- values of :math:`|Z| > precision` will be plotted.
-
- For `scipy.sparse.spmatrix` instances, you can also
- pass 'present'. In this case any value present in the array
- will be plotted, even if it is identically zero.
-
- aspect : {'equal', 'auto', None} or float, default: 'equal'
- The aspect ratio of the Axes. This parameter is particularly
- relevant for images since it determines whether data pixels are
- square.
-
- This parameter is a shortcut for explicitly calling
- `.Axes.set_aspect`. See there for further details.
-
- - 'equal': Ensures an aspect ratio of 1. Pixels will be square.
- - 'auto': The Axes is kept fixed and the aspect is adjusted so
- that the data fit in the Axes. In general, this will result in
- non-square pixels.
- - *None*: Use :rc:`image.aspect`.
-
- origin : {'upper', 'lower'}, default: :rc:`image.origin`
- Place the [0, 0] index of the array in the upper left or lower left
- corner of the Axes. The convention 'upper' is typically used for
- matrices and images.
-
- Returns
- -------
- `~matplotlib.image.AxesImage` or `.Line2D`
- The return type depends on the plotting style (see above).
-
- Other Parameters
- ----------------
- **kwargs
- The supported additional parameters depend on the plotting style.
-
- For the image style, you can pass the following additional
- parameters of `~.Axes.imshow`:
-
- - *cmap*
- - *alpha*
- - *url*
- - any `.Artist` properties (passed on to the `.AxesImage`)
-
- For the marker style, you can pass any `.Line2D` property except
- for *linestyle*:
-
- %(Line2D:kwdoc)s
- """
- if marker is None and markersize is None and hasattr(Z, 'tocoo'):
- marker = 's'
- _api.check_in_list(["upper", "lower"], origin=origin)
- if marker is None and markersize is None:
- Z = np.asarray(Z)
- mask = np.abs(Z) > precision
-
- if 'cmap' not in kwargs:
- kwargs['cmap'] = mcolors.ListedColormap(['w', 'k'],
- name='binary')
- if 'interpolation' in kwargs:
- raise _api.kwarg_error("spy", "interpolation")
- if 'norm' not in kwargs:
- kwargs['norm'] = mcolors.NoNorm()
- ret = self.imshow(mask, interpolation='nearest',
- aspect=aspect, origin=origin,
- **kwargs)
- else:
- if hasattr(Z, 'tocoo'):
- c = Z.tocoo()
- if precision == 'present':
- y = c.row
- x = c.col
- else:
- nonzero = np.abs(c.data) > precision
- y = c.row[nonzero]
- x = c.col[nonzero]
- else:
- Z = np.asarray(Z)
- nonzero = np.abs(Z) > precision
- y, x = np.nonzero(nonzero)
- if marker is None:
- marker = 's'
- if markersize is None:
- markersize = 10
- if 'linestyle' in kwargs:
- raise _api.kwarg_error("spy", "linestyle")
- ret = mlines.Line2D(
- x, y, linestyle='None', marker=marker, markersize=markersize,
- **kwargs)
- self.add_line(ret)
- nr, nc = Z.shape
- self.set_xlim(-0.5, nc - 0.5)
- if origin == "upper":
- self.set_ylim(nr - 0.5, -0.5)
- else:
- self.set_ylim(-0.5, nr - 0.5)
- self.set_aspect(aspect)
- self.title.set_y(1.05)
- if origin == "upper":
- self.xaxis.tick_top()
- else: # lower
- self.xaxis.tick_bottom()
- self.xaxis.set_ticks_position('both')
- self.xaxis.set_major_locator(
- mticker.MaxNLocator(nbins=9, steps=[1, 2, 5, 10], integer=True))
- self.yaxis.set_major_locator(
- mticker.MaxNLocator(nbins=9, steps=[1, 2, 5, 10], integer=True))
- return ret
-
- def matshow(self, Z, **kwargs):
- """
- Plot the values of a 2D matrix or array as color-coded image.
-
- The matrix will be shown the way it would be printed, with the first
- row at the top. Row and column numbering is zero-based.
-
- Parameters
- ----------
- Z : (M, N) array-like
- The matrix to be displayed.
-
- Returns
- -------
- `~matplotlib.image.AxesImage`
-
- Other Parameters
- ----------------
- **kwargs : `~matplotlib.axes.Axes.imshow` arguments
-
- See Also
- --------
- imshow : More general function to plot data on a 2D regular raster.
-
- Notes
- -----
- This is just a convenience function wrapping `.imshow` to set useful
- defaults for displaying a matrix. In particular:
-
- - Set ``origin='upper'``.
- - Set ``interpolation='nearest'``.
- - Set ``aspect='equal'``.
- - Ticks are placed to the left and above.
- - Ticks are formatted to show integer indices.
-
- """
- Z = np.asanyarray(Z)
- kw = {'origin': 'upper',
- 'interpolation': 'nearest',
- 'aspect': 'equal', # (already the imshow default)
- **kwargs}
- im = self.imshow(Z, **kw)
- self.title.set_y(1.05)
- self.xaxis.tick_top()
- self.xaxis.set_ticks_position('both')
- self.xaxis.set_major_locator(
- mticker.MaxNLocator(nbins=9, steps=[1, 2, 5, 10], integer=True))
- self.yaxis.set_major_locator(
- mticker.MaxNLocator(nbins=9, steps=[1, 2, 5, 10], integer=True))
- return im
-
- @_preprocess_data(replace_names=["dataset"])
- def violinplot(self, dataset, positions=None, vert=True, widths=0.5,
- showmeans=False, showextrema=True, showmedians=False,
- quantiles=None, points=100, bw_method=None):
- """
- Make a violin plot.
-
- Make a violin plot for each column of *dataset* or each vector in
- sequence *dataset*. Each filled area extends to represent the
- entire data range, with optional lines at the mean, the median,
- the minimum, the maximum, and user-specified quantiles.
-
- Parameters
- ----------
- dataset : Array or a sequence of vectors.
- The input data.
-
- positions : array-like, default: [1, 2, ..., n]
- The positions of the violins. The ticks and limits are
- automatically set to match the positions.
-
- vert : bool, default: True.
- If true, creates a vertical violin plot.
- Otherwise, creates a horizontal violin plot.
-
- widths : array-like, default: 0.5
- Either a scalar or a vector that sets the maximal width of
- each violin. The default is 0.5, which uses about half of the
- available horizontal space.
-
- showmeans : bool, default: False
- If `True`, will toggle rendering of the means.
-
- showextrema : bool, default: True
- If `True`, will toggle rendering of the extrema.
-
- showmedians : bool, default: False
- If `True`, will toggle rendering of the medians.
-
- quantiles : array-like, default: None
- If not None, set a list of floats in interval [0, 1] for each violin,
- which stands for the quantiles that will be rendered for that
- violin.
-
- points : int, default: 100
- Defines the number of points to evaluate each of the
- gaussian kernel density estimations at.
-
- bw_method : str, scalar or callable, optional
- The method used to calculate the estimator bandwidth. This can be
- 'scott', 'silverman', a scalar constant or a callable. If a
- scalar, this will be used directly as `kde.factor`. If a
- callable, it should take a `matplotlib.mlab.GaussianKDE` instance as
- its only parameter and return a scalar. If None (default), 'scott'
- is used.
-
- data : indexable object, optional
- DATA_PARAMETER_PLACEHOLDER
-
- Returns
- -------
- dict
- A dictionary mapping each component of the violinplot to a
- list of the corresponding collection instances created. The
- dictionary has the following keys:
-
- - ``bodies``: A list of the `~.collections.PolyCollection`
- instances containing the filled area of each violin.
-
- - ``cmeans``: A `~.collections.LineCollection` instance that marks
- the mean values of each of the violin's distribution.
-
- - ``cmins``: A `~.collections.LineCollection` instance that marks
- the bottom of each violin's distribution.
-
- - ``cmaxes``: A `~.collections.LineCollection` instance that marks
- the top of each violin's distribution.
-
- - ``cbars``: A `~.collections.LineCollection` instance that marks
- the centers of each violin's distribution.
-
- - ``cmedians``: A `~.collections.LineCollection` instance that
- marks the median values of each of the violin's distribution.
-
- - ``cquantiles``: A `~.collections.LineCollection` instance created
- to identify the quantile values of each of the violin's
- distribution.
-
- """
-
- def _kde_method(X, coords):
- # Unpack in case of e.g. Pandas or xarray object
- X = cbook._unpack_to_numpy(X)
- # fallback gracefully if the vector contains only one value
- if np.all(X[0] == X):
- return (X[0] == coords).astype(float)
- kde = mlab.GaussianKDE(X, bw_method)
- return kde.evaluate(coords)
-
- vpstats = cbook.violin_stats(dataset, _kde_method, points=points,
- quantiles=quantiles)
- return self.violin(vpstats, positions=positions, vert=vert,
- widths=widths, showmeans=showmeans,
- showextrema=showextrema, showmedians=showmedians)
-
- def violin(self, vpstats, positions=None, vert=True, widths=0.5,
- showmeans=False, showextrema=True, showmedians=False):
- """
- Drawing function for violin plots.
-
- Draw a violin plot for each column of *vpstats*. Each filled area
- extends to represent the entire data range, with optional lines at the
- mean, the median, the minimum, the maximum, and the quantiles values.
-
- Parameters
- ----------
- vpstats : list of dicts
- A list of dictionaries containing stats for each violin plot.
- Required keys are:
-
- - ``coords``: A list of scalars containing the coordinates that
- the violin's kernel density estimate were evaluated at.
-
- - ``vals``: A list of scalars containing the values of the
- kernel density estimate at each of the coordinates given
- in *coords*.
-
- - ``mean``: The mean value for this violin's dataset.
-
- - ``median``: The median value for this violin's dataset.
-
- - ``min``: The minimum value for this violin's dataset.
-
- - ``max``: The maximum value for this violin's dataset.
-
- Optional keys are:
-
- - ``quantiles``: A list of scalars containing the quantile values
- for this violin's dataset.
-
- positions : array-like, default: [1, 2, ..., n]
- The positions of the violins. The ticks and limits are
- automatically set to match the positions.
-
- vert : bool, default: True.
- If true, plots the violins vertically.
- Otherwise, plots the violins horizontally.
-
- widths : array-like, default: 0.5
- Either a scalar or a vector that sets the maximal width of
- each violin. The default is 0.5, which uses about half of the
- available horizontal space.
-
- showmeans : bool, default: False
- If true, will toggle rendering of the means.
-
- showextrema : bool, default: True
- If true, will toggle rendering of the extrema.
-
- showmedians : bool, default: False
- If true, will toggle rendering of the medians.
-
- Returns
- -------
- dict
- A dictionary mapping each component of the violinplot to a
- list of the corresponding collection instances created. The
- dictionary has the following keys:
-
- - ``bodies``: A list of the `~.collections.PolyCollection`
- instances containing the filled area of each violin.
-
- - ``cmeans``: A `~.collections.LineCollection` instance that marks
- the mean values of each of the violin's distribution.
-
- - ``cmins``: A `~.collections.LineCollection` instance that marks
- the bottom of each violin's distribution.
-
- - ``cmaxes``: A `~.collections.LineCollection` instance that marks
- the top of each violin's distribution.
-
- - ``cbars``: A `~.collections.LineCollection` instance that marks
- the centers of each violin's distribution.
-
- - ``cmedians``: A `~.collections.LineCollection` instance that
- marks the median values of each of the violin's distribution.
-
- - ``cquantiles``: A `~.collections.LineCollection` instance created
- to identify the quantiles values of each of the violin's
- distribution.
- """
-
- # Statistical quantities to be plotted on the violins
- means = []
- mins = []
- maxes = []
- medians = []
- quantiles = []
-
- qlens = [] # Number of quantiles in each dataset.
-
- artists = {} # Collections to be returned
-
- N = len(vpstats)
- datashape_message = ("List of violinplot statistics and `{0}` "
- "values must have the same length")
-
- # Validate positions
- if positions is None:
- positions = range(1, N + 1)
- elif len(positions) != N:
- raise ValueError(datashape_message.format("positions"))
-
- # Validate widths
- if np.isscalar(widths):
- widths = [widths] * N
- elif len(widths) != N:
- raise ValueError(datashape_message.format("widths"))
-
- # Calculate ranges for statistics lines (shape (2, N)).
- line_ends = [[-0.25], [0.25]] * np.array(widths) + positions
-
- # Colors.
- if mpl.rcParams['_internal.classic_mode']:
- fillcolor = 'y'
- linecolor = 'r'
- else:
- fillcolor = linecolor = self._get_lines.get_next_color()
-
- # Check whether we are rendering vertically or horizontally
- if vert:
- fill = self.fill_betweenx
- perp_lines = functools.partial(self.hlines, colors=linecolor)
- par_lines = functools.partial(self.vlines, colors=linecolor)
- else:
- fill = self.fill_between
- perp_lines = functools.partial(self.vlines, colors=linecolor)
- par_lines = functools.partial(self.hlines, colors=linecolor)
-
- # Render violins
- bodies = []
- for stats, pos, width in zip(vpstats, positions, widths):
- # The 0.5 factor reflects the fact that we plot from v-p to v+p.
- vals = np.array(stats['vals'])
- vals = 0.5 * width * vals / vals.max()
- bodies += [fill(stats['coords'], -vals + pos, vals + pos,
- facecolor=fillcolor, alpha=0.3)]
- means.append(stats['mean'])
- mins.append(stats['min'])
- maxes.append(stats['max'])
- medians.append(stats['median'])
- q = stats.get('quantiles') # a list of floats, or None
- if q is None:
- q = []
- quantiles.extend(q)
- qlens.append(len(q))
- artists['bodies'] = bodies
-
- if showmeans: # Render means
- artists['cmeans'] = perp_lines(means, *line_ends)
- if showextrema: # Render extrema
- artists['cmaxes'] = perp_lines(maxes, *line_ends)
- artists['cmins'] = perp_lines(mins, *line_ends)
- artists['cbars'] = par_lines(positions, mins, maxes)
- if showmedians: # Render medians
- artists['cmedians'] = perp_lines(medians, *line_ends)
- if quantiles: # Render quantiles: each width is repeated qlen times.
- artists['cquantiles'] = perp_lines(
- quantiles, *np.repeat(line_ends, qlens, axis=1))
-
- return artists
-
- # Methods that are entirely implemented in other modules.
-
- table = mtable.table
-
- # args can be either Y or y1, y2, ... and all should be replaced
- stackplot = _preprocess_data()(mstack.stackplot)
-
- streamplot = _preprocess_data(
- replace_names=["x", "y", "u", "v", "start_points"])(mstream.streamplot)
-
- tricontour = mtri.tricontour
- tricontourf = mtri.tricontourf
- tripcolor = mtri.tripcolor
- triplot = mtri.triplot
-
- def _get_aspect_ratio(self):
- """
- Convenience method to calculate the aspect ratio of the axes in
- the display coordinate system.
- """
- figure_size = self.get_figure().get_size_inches()
- ll, ur = self.get_position() * figure_size
- width, height = ur - ll
- return height / (width * self.get_data_ratio())
diff --git a/spaces/declare-lab/tango/diffusers/src/diffusers/pipelines/paint_by_example/image_encoder.py b/spaces/declare-lab/tango/diffusers/src/diffusers/pipelines/paint_by_example/image_encoder.py
deleted file mode 100644
index 831489eefed167264c8fd8f57e1ed59610ebb858..0000000000000000000000000000000000000000
--- a/spaces/declare-lab/tango/diffusers/src/diffusers/pipelines/paint_by_example/image_encoder.py
+++ /dev/null
@@ -1,67 +0,0 @@
-# Copyright 2023 The HuggingFace Team. All rights reserved.
-#
-# Licensed under the Apache License, Version 2.0 (the "License");
-# you may not use this file except in compliance with the License.
-# You may obtain a copy of the License at
-#
-# http://www.apache.org/licenses/LICENSE-2.0
-#
-# Unless required by applicable law or agreed to in writing, software
-# distributed under the License is distributed on an "AS IS" BASIS,
-# WITHOUT WARRANTIES OR CONDITIONS OF ANY KIND, either express or implied.
-# See the License for the specific language governing permissions and
-# limitations under the License.
-import torch
-from torch import nn
-from transformers import CLIPPreTrainedModel, CLIPVisionModel
-
-from ...models.attention import BasicTransformerBlock
-from ...utils import logging
-
-
-logger = logging.get_logger(__name__) # pylint: disable=invalid-name
-
-
-class PaintByExampleImageEncoder(CLIPPreTrainedModel):
- def __init__(self, config, proj_size=768):
- super().__init__(config)
- self.proj_size = proj_size
-
- self.model = CLIPVisionModel(config)
- self.mapper = PaintByExampleMapper(config)
- self.final_layer_norm = nn.LayerNorm(config.hidden_size)
- self.proj_out = nn.Linear(config.hidden_size, self.proj_size)
-
- # uncondition for scaling
- self.uncond_vector = nn.Parameter(torch.randn((1, 1, self.proj_size)))
-
- def forward(self, pixel_values, return_uncond_vector=False):
- clip_output = self.model(pixel_values=pixel_values)
- latent_states = clip_output.pooler_output
- latent_states = self.mapper(latent_states[:, None])
- latent_states = self.final_layer_norm(latent_states)
- latent_states = self.proj_out(latent_states)
- if return_uncond_vector:
- return latent_states, self.uncond_vector
-
- return latent_states
-
-
-class PaintByExampleMapper(nn.Module):
- def __init__(self, config):
- super().__init__()
- num_layers = (config.num_hidden_layers + 1) // 5
- hid_size = config.hidden_size
- num_heads = 1
- self.blocks = nn.ModuleList(
- [
- BasicTransformerBlock(hid_size, num_heads, hid_size, activation_fn="gelu", attention_bias=True)
- for _ in range(num_layers)
- ]
- )
-
- def forward(self, hidden_states):
- for block in self.blocks:
- hidden_states = block(hidden_states)
-
- return hidden_states
diff --git a/spaces/deelerb/3dselfie/PIFu/lib/model/ConvPIFuNet.py b/spaces/deelerb/3dselfie/PIFu/lib/model/ConvPIFuNet.py
deleted file mode 100644
index 1d43d262aa237d03db0cf329b4d199061ee6a006..0000000000000000000000000000000000000000
--- a/spaces/deelerb/3dselfie/PIFu/lib/model/ConvPIFuNet.py
+++ /dev/null
@@ -1,99 +0,0 @@
-import torch
-import torch.nn as nn
-import torch.nn.functional as F
-from .BasePIFuNet import BasePIFuNet
-from .SurfaceClassifier import SurfaceClassifier
-from .DepthNormalizer import DepthNormalizer
-from .ConvFilters import *
-from ..net_util import init_net
-
-class ConvPIFuNet(BasePIFuNet):
- '''
- Conv Piximp network is the standard 3-phase network that we will use.
- The image filter is a pure multi-layer convolutional network,
- while during feature extraction phase all features in the pyramid at the projected location
- will be aggregated.
- It does the following:
- 1. Compute image feature pyramids and store it in self.im_feat_list
- 2. Calculate calibration and indexing on each of the feat, and append them together
- 3. Classification.
- '''
-
- def __init__(self,
- opt,
- projection_mode='orthogonal',
- error_term=nn.MSELoss(),
- ):
- super(ConvPIFuNet, self).__init__(
- projection_mode=projection_mode,
- error_term=error_term)
-
- self.name = 'convpifu'
-
- self.opt = opt
- self.num_views = self.opt.num_views
-
- self.image_filter = self.define_imagefilter(opt)
-
- self.surface_classifier = SurfaceClassifier(
- filter_channels=self.opt.mlp_dim,
- num_views=self.opt.num_views,
- no_residual=self.opt.no_residual,
- last_op=nn.Sigmoid())
-
- self.normalizer = DepthNormalizer(opt)
-
- # This is a list of [B x Feat_i x H x W] features
- self.im_feat_list = []
-
- init_net(self)
-
- def define_imagefilter(self, opt):
- net = None
- if opt.netIMF == 'multiconv':
- net = MultiConv(opt.enc_dim)
- elif 'resnet' in opt.netIMF:
- net = ResNet(model=opt.netIMF)
- elif opt.netIMF == 'vgg16':
- net = Vgg16()
- else:
- raise NotImplementedError('model name [%s] is not recognized' % opt.imf_type)
-
- return net
-
- def filter(self, images):
- '''
- Filter the input images
- store all intermediate features.
- :param images: [B, C, H, W] input images
- '''
- self.im_feat_list = self.image_filter(images)
-
- def query(self, points, calibs, transforms=None, labels=None):
- '''
- Given 3D points, query the network predictions for each point.
- Image features should be pre-computed before this call.
- store all intermediate features.
- query() function may behave differently during training/testing.
- :param points: [B, 3, N] world space coordinates of points
- :param calibs: [B, 3, 4] calibration matrices for each image
- :param transforms: Optional [B, 2, 3] image space coordinate transforms
- :param labels: Optional [B, Res, N] gt labeling
- :return: [B, Res, N] predictions for each point
- '''
- if labels is not None:
- self.labels = labels
-
- xyz = self.projection(points, calibs, transforms)
- xy = xyz[:, :2, :]
- z = xyz[:, 2:3, :]
-
- z_feat = self.normalizer(z)
-
- # This is a list of [B, Feat_i, N] features
- point_local_feat_list = [self.index(im_feat, xy) for im_feat in self.im_feat_list]
- point_local_feat_list.append(z_feat)
- # [B, Feat_all, N]
- point_local_feat = torch.cat(point_local_feat_list, 1)
-
- self.preds = self.surface_classifier(point_local_feat)
diff --git a/spaces/deeplearning/audioldm-text-to-audio-generation/audioldm/latent_diffusion/openaimodel.py b/spaces/deeplearning/audioldm-text-to-audio-generation/audioldm/latent_diffusion/openaimodel.py
deleted file mode 100644
index 831d7aafb36bba16888e4389153979a6c13639f5..0000000000000000000000000000000000000000
--- a/spaces/deeplearning/audioldm-text-to-audio-generation/audioldm/latent_diffusion/openaimodel.py
+++ /dev/null
@@ -1,1069 +0,0 @@
-from abc import abstractmethod
-import math
-
-import numpy as np
-import torch as th
-import torch.nn as nn
-import torch.nn.functional as F
-
-from audioldm.latent_diffusion.util import (
- checkpoint,
- conv_nd,
- linear,
- avg_pool_nd,
- zero_module,
- normalization,
- timestep_embedding,
-)
-from audioldm.latent_diffusion.attention import SpatialTransformer
-
-
-# dummy replace
-def convert_module_to_f16(x):
- pass
-
-
-def convert_module_to_f32(x):
- pass
-
-
-## go
-class AttentionPool2d(nn.Module):
- """
- Adapted from CLIP: https://github.com/openai/CLIP/blob/main/clip/model.py
- """
-
- def __init__(
- self,
- spacial_dim: int,
- embed_dim: int,
- num_heads_channels: int,
- output_dim: int = None,
- ):
- super().__init__()
- self.positional_embedding = nn.Parameter(
- th.randn(embed_dim, spacial_dim**2 + 1) / embed_dim**0.5
- )
- self.qkv_proj = conv_nd(1, embed_dim, 3 * embed_dim, 1)
- self.c_proj = conv_nd(1, embed_dim, output_dim or embed_dim, 1)
- self.num_heads = embed_dim // num_heads_channels
- self.attention = QKVAttention(self.num_heads)
-
- def forward(self, x):
- b, c, *_spatial = x.shape
- x = x.reshape(b, c, -1).contiguous() # NC(HW)
- x = th.cat([x.mean(dim=-1, keepdim=True), x], dim=-1) # NC(HW+1)
- x = x + self.positional_embedding[None, :, :].to(x.dtype) # NC(HW+1)
- x = self.qkv_proj(x)
- x = self.attention(x)
- x = self.c_proj(x)
- return x[:, :, 0]
-
-
-class TimestepBlock(nn.Module):
- """
- Any module where forward() takes timestep embeddings as a second argument.
- """
-
- @abstractmethod
- def forward(self, x, emb):
- """
- Apply the module to `x` given `emb` timestep embeddings.
- """
-
-
-class TimestepEmbedSequential(nn.Sequential, TimestepBlock):
- """
- A sequential module that passes timestep embeddings to the children that
- support it as an extra input.
- """
-
- def forward(self, x, emb, context=None):
- for layer in self:
- if isinstance(layer, TimestepBlock):
- x = layer(x, emb)
- elif isinstance(layer, SpatialTransformer):
- x = layer(x, context)
- else:
- x = layer(x)
- return x
-
-
-class Upsample(nn.Module):
- """
- An upsampling layer with an optional convolution.
- :param channels: channels in the inputs and outputs.
- :param use_conv: a bool determining if a convolution is applied.
- :param dims: determines if the signal is 1D, 2D, or 3D. If 3D, then
- upsampling occurs in the inner-two dimensions.
- """
-
- def __init__(self, channels, use_conv, dims=2, out_channels=None, padding=1):
- super().__init__()
- self.channels = channels
- self.out_channels = out_channels or channels
- self.use_conv = use_conv
- self.dims = dims
- if use_conv:
- self.conv = conv_nd(
- dims, self.channels, self.out_channels, 3, padding=padding
- )
-
- def forward(self, x):
- assert x.shape[1] == self.channels
- if self.dims == 3:
- x = F.interpolate(
- x, (x.shape[2], x.shape[3] * 2, x.shape[4] * 2), mode="nearest"
- )
- else:
- x = F.interpolate(x, scale_factor=2, mode="nearest")
- if self.use_conv:
- x = self.conv(x)
- return x
-
-
-class TransposedUpsample(nn.Module):
- "Learned 2x upsampling without padding"
-
- def __init__(self, channels, out_channels=None, ks=5):
- super().__init__()
- self.channels = channels
- self.out_channels = out_channels or channels
-
- self.up = nn.ConvTranspose2d(
- self.channels, self.out_channels, kernel_size=ks, stride=2
- )
-
- def forward(self, x):
- return self.up(x)
-
-
-class Downsample(nn.Module):
- """
- A downsampling layer with an optional convolution.
- :param channels: channels in the inputs and outputs.
- :param use_conv: a bool determining if a convolution is applied.
- :param dims: determines if the signal is 1D, 2D, or 3D. If 3D, then
- downsampling occurs in the inner-two dimensions.
- """
-
- def __init__(self, channels, use_conv, dims=2, out_channels=None, padding=1):
- super().__init__()
- self.channels = channels
- self.out_channels = out_channels or channels
- self.use_conv = use_conv
- self.dims = dims
- stride = 2 if dims != 3 else (1, 2, 2)
- if use_conv:
- self.op = conv_nd(
- dims,
- self.channels,
- self.out_channels,
- 3,
- stride=stride,
- padding=padding,
- )
- else:
- assert self.channels == self.out_channels
- self.op = avg_pool_nd(dims, kernel_size=stride, stride=stride)
-
- def forward(self, x):
- assert x.shape[1] == self.channels
- return self.op(x)
-
-
-class ResBlock(TimestepBlock):
- """
- A residual block that can optionally change the number of channels.
- :param channels: the number of input channels.
- :param emb_channels: the number of timestep embedding channels.
- :param dropout: the rate of dropout.
- :param out_channels: if specified, the number of out channels.
- :param use_conv: if True and out_channels is specified, use a spatial
- convolution instead of a smaller 1x1 convolution to change the
- channels in the skip connection.
- :param dims: determines if the signal is 1D, 2D, or 3D.
- :param use_checkpoint: if True, use gradient checkpointing on this module.
- :param up: if True, use this block for upsampling.
- :param down: if True, use this block for downsampling.
- """
-
- def __init__(
- self,
- channels,
- emb_channels,
- dropout,
- out_channels=None,
- use_conv=False,
- use_scale_shift_norm=False,
- dims=2,
- use_checkpoint=False,
- up=False,
- down=False,
- ):
- super().__init__()
- self.channels = channels
- self.emb_channels = emb_channels
- self.dropout = dropout
- self.out_channels = out_channels or channels
- self.use_conv = use_conv
- self.use_checkpoint = use_checkpoint
- self.use_scale_shift_norm = use_scale_shift_norm
-
- self.in_layers = nn.Sequential(
- normalization(channels),
- nn.SiLU(),
- conv_nd(dims, channels, self.out_channels, 3, padding=1),
- )
-
- self.updown = up or down
-
- if up:
- self.h_upd = Upsample(channels, False, dims)
- self.x_upd = Upsample(channels, False, dims)
- elif down:
- self.h_upd = Downsample(channels, False, dims)
- self.x_upd = Downsample(channels, False, dims)
- else:
- self.h_upd = self.x_upd = nn.Identity()
-
- self.emb_layers = nn.Sequential(
- nn.SiLU(),
- linear(
- emb_channels,
- 2 * self.out_channels if use_scale_shift_norm else self.out_channels,
- ),
- )
- self.out_layers = nn.Sequential(
- normalization(self.out_channels),
- nn.SiLU(),
- nn.Dropout(p=dropout),
- zero_module(
- conv_nd(dims, self.out_channels, self.out_channels, 3, padding=1)
- ),
- )
-
- if self.out_channels == channels:
- self.skip_connection = nn.Identity()
- elif use_conv:
- self.skip_connection = conv_nd(
- dims, channels, self.out_channels, 3, padding=1
- )
- else:
- self.skip_connection = conv_nd(dims, channels, self.out_channels, 1)
-
- def forward(self, x, emb):
- """
- Apply the block to a Tensor, conditioned on a timestep embedding.
- :param x: an [N x C x ...] Tensor of features.
- :param emb: an [N x emb_channels] Tensor of timestep embeddings.
- :return: an [N x C x ...] Tensor of outputs.
- """
- return checkpoint(
- self._forward, (x, emb), self.parameters(), self.use_checkpoint
- )
-
- def _forward(self, x, emb):
- if self.updown:
- in_rest, in_conv = self.in_layers[:-1], self.in_layers[-1]
- h = in_rest(x)
- h = self.h_upd(h)
- x = self.x_upd(x)
- h = in_conv(h)
- else:
- h = self.in_layers(x)
- emb_out = self.emb_layers(emb).type(h.dtype)
- while len(emb_out.shape) < len(h.shape):
- emb_out = emb_out[..., None]
- if self.use_scale_shift_norm:
- out_norm, out_rest = self.out_layers[0], self.out_layers[1:]
- scale, shift = th.chunk(emb_out, 2, dim=1)
- h = out_norm(h) * (1 + scale) + shift
- h = out_rest(h)
- else:
- h = h + emb_out
- h = self.out_layers(h)
- return self.skip_connection(x) + h
-
-
-class AttentionBlock(nn.Module):
- """
- An attention block that allows spatial positions to attend to each other.
- Originally ported from here, but adapted to the N-d case.
- https://github.com/hojonathanho/diffusion/blob/1e0dceb3b3495bbe19116a5e1b3596cd0706c543/diffusion_tf/models/unet.py#L66.
- """
-
- def __init__(
- self,
- channels,
- num_heads=1,
- num_head_channels=-1,
- use_checkpoint=False,
- use_new_attention_order=False,
- ):
- super().__init__()
- self.channels = channels
- if num_head_channels == -1:
- self.num_heads = num_heads
- else:
- assert (
- channels % num_head_channels == 0
- ), f"q,k,v channels {channels} is not divisible by num_head_channels {num_head_channels}"
- self.num_heads = channels // num_head_channels
- self.use_checkpoint = use_checkpoint
- self.norm = normalization(channels)
- self.qkv = conv_nd(1, channels, channels * 3, 1)
- if use_new_attention_order:
- # split qkv before split heads
- self.attention = QKVAttention(self.num_heads)
- else:
- # split heads before split qkv
- self.attention = QKVAttentionLegacy(self.num_heads)
-
- self.proj_out = zero_module(conv_nd(1, channels, channels, 1))
-
- def forward(self, x):
- return checkpoint(
- self._forward, (x,), self.parameters(), True
- ) # TODO: check checkpoint usage, is True # TODO: fix the .half call!!!
- # return pt_checkpoint(self._forward, x) # pytorch
-
- def _forward(self, x):
- b, c, *spatial = x.shape
- x = x.reshape(b, c, -1).contiguous()
- qkv = self.qkv(self.norm(x)).contiguous()
- h = self.attention(qkv).contiguous()
- h = self.proj_out(h).contiguous()
- return (x + h).reshape(b, c, *spatial).contiguous()
-
-
-def count_flops_attn(model, _x, y):
- """
- A counter for the `thop` package to count the operations in an
- attention operation.
- Meant to be used like:
- macs, params = thop.profile(
- model,
- inputs=(inputs, timestamps),
- custom_ops={QKVAttention: QKVAttention.count_flops},
- )
- """
- b, c, *spatial = y[0].shape
- num_spatial = int(np.prod(spatial))
- # We perform two matmuls with the same number of ops.
- # The first computes the weight matrix, the second computes
- # the combination of the value vectors.
- matmul_ops = 2 * b * (num_spatial**2) * c
- model.total_ops += th.DoubleTensor([matmul_ops])
-
-
-class QKVAttentionLegacy(nn.Module):
- """
- A module which performs QKV attention. Matches legacy QKVAttention + input/ouput heads shaping
- """
-
- def __init__(self, n_heads):
- super().__init__()
- self.n_heads = n_heads
-
- def forward(self, qkv):
- """
- Apply QKV attention.
- :param qkv: an [N x (H * 3 * C) x T] tensor of Qs, Ks, and Vs.
- :return: an [N x (H * C) x T] tensor after attention.
- """
- bs, width, length = qkv.shape
- assert width % (3 * self.n_heads) == 0
- ch = width // (3 * self.n_heads)
- q, k, v = (
- qkv.reshape(bs * self.n_heads, ch * 3, length).contiguous().split(ch, dim=1)
- )
- scale = 1 / math.sqrt(math.sqrt(ch))
- weight = th.einsum(
- "bct,bcs->bts", q * scale, k * scale
- ) # More stable with f16 than dividing afterwards
- weight = th.softmax(weight.float(), dim=-1).type(weight.dtype)
- a = th.einsum("bts,bcs->bct", weight, v)
- return a.reshape(bs, -1, length).contiguous()
-
- @staticmethod
- def count_flops(model, _x, y):
- return count_flops_attn(model, _x, y)
-
-
-class QKVAttention(nn.Module):
- """
- A module which performs QKV attention and splits in a different order.
- """
-
- def __init__(self, n_heads):
- super().__init__()
- self.n_heads = n_heads
-
- def forward(self, qkv):
- """
- Apply QKV attention.
- :param qkv: an [N x (3 * H * C) x T] tensor of Qs, Ks, and Vs.
- :return: an [N x (H * C) x T] tensor after attention.
- """
- bs, width, length = qkv.shape
- assert width % (3 * self.n_heads) == 0
- ch = width // (3 * self.n_heads)
- q, k, v = qkv.chunk(3, dim=1)
- scale = 1 / math.sqrt(math.sqrt(ch))
- weight = th.einsum(
- "bct,bcs->bts",
- (q * scale).view(bs * self.n_heads, ch, length),
- (k * scale).view(bs * self.n_heads, ch, length),
- ) # More stable with f16 than dividing afterwards
- weight = th.softmax(weight.float(), dim=-1).type(weight.dtype)
- a = th.einsum(
- "bts,bcs->bct",
- weight,
- v.reshape(bs * self.n_heads, ch, length).contiguous(),
- )
- return a.reshape(bs, -1, length).contiguous()
-
- @staticmethod
- def count_flops(model, _x, y):
- return count_flops_attn(model, _x, y)
-
-
-class UNetModel(nn.Module):
- """
- The full UNet model with attention and timestep embedding.
- :param in_channels: channels in the input Tensor.
- :param model_channels: base channel count for the model.
- :param out_channels: channels in the output Tensor.
- :param num_res_blocks: number of residual blocks per downsample.
- :param attention_resolutions: a collection of downsample rates at which
- attention will take place. May be a set, list, or tuple.
- For example, if this contains 4, then at 4x downsampling, attention
- will be used.
- :param dropout: the dropout probability.
- :param channel_mult: channel multiplier for each level of the UNet.
- :param conv_resample: if True, use learned convolutions for upsampling and
- downsampling.
- :param dims: determines if the signal is 1D, 2D, or 3D.
- :param num_classes: if specified (as an int), then this model will be
- class-conditional with `num_classes` classes.
- :param use_checkpoint: use gradient checkpointing to reduce memory usage.
- :param num_heads: the number of attention heads in each attention layer.
- :param num_heads_channels: if specified, ignore num_heads and instead use
- a fixed channel width per attention head.
- :param num_heads_upsample: works with num_heads to set a different number
- of heads for upsampling. Deprecated.
- :param use_scale_shift_norm: use a FiLM-like conditioning mechanism.
- :param resblock_updown: use residual blocks for up/downsampling.
- :param use_new_attention_order: use a different attention pattern for potentially
- increased efficiency.
- """
-
- def __init__(
- self,
- image_size,
- in_channels,
- model_channels,
- out_channels,
- num_res_blocks,
- attention_resolutions,
- dropout=0,
- channel_mult=(1, 2, 4, 8),
- conv_resample=True,
- dims=2,
- num_classes=None,
- extra_film_condition_dim=None,
- use_checkpoint=False,
- use_fp16=False,
- num_heads=-1,
- num_head_channels=-1,
- num_heads_upsample=-1,
- use_scale_shift_norm=False,
- extra_film_use_concat=False, # If true, concatenate extrafilm condition with time embedding, else addition
- resblock_updown=False,
- use_new_attention_order=False,
- use_spatial_transformer=False, # custom transformer support
- transformer_depth=1, # custom transformer support
- context_dim=None, # custom transformer support
- n_embed=None, # custom support for prediction of discrete ids into codebook of first stage vq model
- legacy=True,
- ):
- super().__init__()
- if num_heads_upsample == -1:
- num_heads_upsample = num_heads
-
- if num_heads == -1:
- assert (
- num_head_channels != -1
- ), "Either num_heads or num_head_channels has to be set"
-
- if num_head_channels == -1:
- assert (
- num_heads != -1
- ), "Either num_heads or num_head_channels has to be set"
-
- self.image_size = image_size
- self.in_channels = in_channels
- self.model_channels = model_channels
- self.out_channels = out_channels
- self.num_res_blocks = num_res_blocks
- self.attention_resolutions = attention_resolutions
- self.dropout = dropout
- self.channel_mult = channel_mult
- self.conv_resample = conv_resample
- self.num_classes = num_classes
- self.extra_film_condition_dim = extra_film_condition_dim
- self.use_checkpoint = use_checkpoint
- self.dtype = th.float16 if use_fp16 else th.float32
- self.num_heads = num_heads
- self.num_head_channels = num_head_channels
- self.num_heads_upsample = num_heads_upsample
- self.predict_codebook_ids = n_embed is not None
- self.extra_film_use_concat = extra_film_use_concat
- time_embed_dim = model_channels * 4
- self.time_embed = nn.Sequential(
- linear(model_channels, time_embed_dim),
- nn.SiLU(),
- linear(time_embed_dim, time_embed_dim),
- )
-
- assert not (
- self.num_classes is not None and self.extra_film_condition_dim is not None
- ), "As for the condition of theh UNet model, you can only set using class label or an extra embedding vector (such as from CLAP). You cannot set both num_classes and extra_film_condition_dim."
-
- if self.num_classes is not None:
- self.label_emb = nn.Embedding(num_classes, time_embed_dim)
-
- self.use_extra_film_by_concat = (
- self.extra_film_condition_dim is not None and self.extra_film_use_concat
- )
- self.use_extra_film_by_addition = (
- self.extra_film_condition_dim is not None and not self.extra_film_use_concat
- )
-
- if self.extra_film_condition_dim is not None:
- self.film_emb = nn.Linear(self.extra_film_condition_dim, time_embed_dim)
- # print("+ Use extra condition on UNet channel using Film. Extra condition dimension is %s. " % self.extra_film_condition_dim)
- # if(self.use_extra_film_by_concat):
- # print("\t By concatenation with time embedding")
- # elif(self.use_extra_film_by_concat):
- # print("\t By addition with time embedding")
-
- if use_spatial_transformer and (
- self.use_extra_film_by_concat or self.use_extra_film_by_addition
- ):
- # print("+ Spatial transformer will only be used as self-attention. Because you have choose to use film as your global condition.")
- spatial_transformer_no_context = True
- else:
- spatial_transformer_no_context = False
-
- if use_spatial_transformer and not spatial_transformer_no_context:
- assert (
- context_dim is not None
- ), "Fool!! You forgot to include the dimension of your cross-attention conditioning..."
-
- if context_dim is not None and not spatial_transformer_no_context:
- assert (
- use_spatial_transformer
- ), "Fool!! You forgot to use the spatial transformer for your cross-attention conditioning..."
- from omegaconf.listconfig import ListConfig
-
- if type(context_dim) == ListConfig:
- context_dim = list(context_dim)
-
- self.input_blocks = nn.ModuleList(
- [
- TimestepEmbedSequential(
- conv_nd(dims, in_channels, model_channels, 3, padding=1)
- )
- ]
- )
- self._feature_size = model_channels
- input_block_chans = [model_channels]
- ch = model_channels
- ds = 1
- for level, mult in enumerate(channel_mult):
- for _ in range(num_res_blocks):
- layers = [
- ResBlock(
- ch,
- time_embed_dim
- if (not self.use_extra_film_by_concat)
- else time_embed_dim * 2,
- dropout,
- out_channels=mult * model_channels,
- dims=dims,
- use_checkpoint=use_checkpoint,
- use_scale_shift_norm=use_scale_shift_norm,
- )
- ]
- ch = mult * model_channels
- if ds in attention_resolutions:
- if num_head_channels == -1:
- dim_head = ch // num_heads
- else:
- num_heads = ch // num_head_channels
- dim_head = num_head_channels
- if legacy:
- dim_head = (
- ch // num_heads
- if use_spatial_transformer
- else num_head_channels
- )
- layers.append(
- AttentionBlock(
- ch,
- use_checkpoint=use_checkpoint,
- num_heads=num_heads,
- num_head_channels=dim_head,
- use_new_attention_order=use_new_attention_order,
- )
- if not use_spatial_transformer
- else SpatialTransformer(
- ch,
- num_heads,
- dim_head,
- depth=transformer_depth,
- context_dim=context_dim,
- no_context=spatial_transformer_no_context,
- )
- )
- self.input_blocks.append(TimestepEmbedSequential(*layers))
- self._feature_size += ch
- input_block_chans.append(ch)
- if level != len(channel_mult) - 1:
- out_ch = ch
- self.input_blocks.append(
- TimestepEmbedSequential(
- ResBlock(
- ch,
- time_embed_dim
- if (not self.use_extra_film_by_concat)
- else time_embed_dim * 2,
- dropout,
- out_channels=out_ch,
- dims=dims,
- use_checkpoint=use_checkpoint,
- use_scale_shift_norm=use_scale_shift_norm,
- down=True,
- )
- if resblock_updown
- else Downsample(
- ch, conv_resample, dims=dims, out_channels=out_ch
- )
- )
- )
- ch = out_ch
- input_block_chans.append(ch)
- ds *= 2
- self._feature_size += ch
-
- if num_head_channels == -1:
- dim_head = ch // num_heads
- else:
- num_heads = ch // num_head_channels
- dim_head = num_head_channels
- if legacy:
- # num_heads = 1
- dim_head = ch // num_heads if use_spatial_transformer else num_head_channels
- self.middle_block = TimestepEmbedSequential(
- ResBlock(
- ch,
- time_embed_dim
- if (not self.use_extra_film_by_concat)
- else time_embed_dim * 2,
- dropout,
- dims=dims,
- use_checkpoint=use_checkpoint,
- use_scale_shift_norm=use_scale_shift_norm,
- ),
- AttentionBlock(
- ch,
- use_checkpoint=use_checkpoint,
- num_heads=num_heads,
- num_head_channels=dim_head,
- use_new_attention_order=use_new_attention_order,
- )
- if not use_spatial_transformer
- else SpatialTransformer(
- ch,
- num_heads,
- dim_head,
- depth=transformer_depth,
- context_dim=context_dim,
- no_context=spatial_transformer_no_context,
- ),
- ResBlock(
- ch,
- time_embed_dim
- if (not self.use_extra_film_by_concat)
- else time_embed_dim * 2,
- dropout,
- dims=dims,
- use_checkpoint=use_checkpoint,
- use_scale_shift_norm=use_scale_shift_norm,
- ),
- )
- self._feature_size += ch
-
- self.output_blocks = nn.ModuleList([])
- for level, mult in list(enumerate(channel_mult))[::-1]:
- for i in range(num_res_blocks + 1):
- ich = input_block_chans.pop()
- layers = [
- ResBlock(
- ch + ich,
- time_embed_dim
- if (not self.use_extra_film_by_concat)
- else time_embed_dim * 2,
- dropout,
- out_channels=model_channels * mult,
- dims=dims,
- use_checkpoint=use_checkpoint,
- use_scale_shift_norm=use_scale_shift_norm,
- )
- ]
- ch = model_channels * mult
- if ds in attention_resolutions:
- if num_head_channels == -1:
- dim_head = ch // num_heads
- else:
- num_heads = ch // num_head_channels
- dim_head = num_head_channels
- if legacy:
- # num_heads = 1
- dim_head = (
- ch // num_heads
- if use_spatial_transformer
- else num_head_channels
- )
- layers.append(
- AttentionBlock(
- ch,
- use_checkpoint=use_checkpoint,
- num_heads=num_heads_upsample,
- num_head_channels=dim_head,
- use_new_attention_order=use_new_attention_order,
- )
- if not use_spatial_transformer
- else SpatialTransformer(
- ch,
- num_heads,
- dim_head,
- depth=transformer_depth,
- context_dim=context_dim,
- no_context=spatial_transformer_no_context,
- )
- )
- if level and i == num_res_blocks:
- out_ch = ch
- layers.append(
- ResBlock(
- ch,
- time_embed_dim
- if (not self.use_extra_film_by_concat)
- else time_embed_dim * 2,
- dropout,
- out_channels=out_ch,
- dims=dims,
- use_checkpoint=use_checkpoint,
- use_scale_shift_norm=use_scale_shift_norm,
- up=True,
- )
- if resblock_updown
- else Upsample(ch, conv_resample, dims=dims, out_channels=out_ch)
- )
- ds //= 2
- self.output_blocks.append(TimestepEmbedSequential(*layers))
- self._feature_size += ch
-
- self.out = nn.Sequential(
- normalization(ch),
- nn.SiLU(),
- zero_module(conv_nd(dims, model_channels, out_channels, 3, padding=1)),
- )
- if self.predict_codebook_ids:
- self.id_predictor = nn.Sequential(
- normalization(ch),
- conv_nd(dims, model_channels, n_embed, 1),
- # nn.LogSoftmax(dim=1) # change to cross_entropy and produce non-normalized logits
- )
-
- self.shape_reported = False
-
- def convert_to_fp16(self):
- """
- Convert the torso of the model to float16.
- """
- self.input_blocks.apply(convert_module_to_f16)
- self.middle_block.apply(convert_module_to_f16)
- self.output_blocks.apply(convert_module_to_f16)
-
- def convert_to_fp32(self):
- """
- Convert the torso of the model to float32.
- """
- self.input_blocks.apply(convert_module_to_f32)
- self.middle_block.apply(convert_module_to_f32)
- self.output_blocks.apply(convert_module_to_f32)
-
- def forward(self, x, timesteps=None, context=None, y=None, **kwargs):
- """
- Apply the model to an input batch.
- :param x: an [N x C x ...] Tensor of inputs.
- :param timesteps: a 1-D batch of timesteps.
- :param context: conditioning plugged in via crossattn
- :param y: an [N] Tensor of labels, if class-conditional. an [N, extra_film_condition_dim] Tensor if film-embed conditional
- :return: an [N x C x ...] Tensor of outputs.
- """
- if not self.shape_reported:
- # print("The shape of UNet input is", x.size())
- self.shape_reported = True
-
- assert (y is not None) == (
- self.num_classes is not None or self.extra_film_condition_dim is not None
- ), "must specify y if and only if the model is class-conditional or film embedding conditional"
- hs = []
- t_emb = timestep_embedding(timesteps, self.model_channels, repeat_only=False)
- emb = self.time_embed(t_emb)
-
- if self.num_classes is not None:
- assert y.shape == (x.shape[0],)
- emb = emb + self.label_emb(y)
-
- if self.use_extra_film_by_addition:
- emb = emb + self.film_emb(y)
- elif self.use_extra_film_by_concat:
- emb = th.cat([emb, self.film_emb(y)], dim=-1)
-
- h = x.type(self.dtype)
- for module in self.input_blocks:
- h = module(h, emb, context)
- hs.append(h)
- h = self.middle_block(h, emb, context)
- for module in self.output_blocks:
- h = th.cat([h, hs.pop()], dim=1)
- h = module(h, emb, context)
- h = h.type(x.dtype)
- if self.predict_codebook_ids:
- return self.id_predictor(h)
- else:
- return self.out(h)
-
-
-class EncoderUNetModel(nn.Module):
- """
- The half UNet model with attention and timestep embedding.
- For usage, see UNet.
- """
-
- def __init__(
- self,
- image_size,
- in_channels,
- model_channels,
- out_channels,
- num_res_blocks,
- attention_resolutions,
- dropout=0,
- channel_mult=(1, 2, 4, 8),
- conv_resample=True,
- dims=2,
- use_checkpoint=False,
- use_fp16=False,
- num_heads=1,
- num_head_channels=-1,
- num_heads_upsample=-1,
- use_scale_shift_norm=False,
- resblock_updown=False,
- use_new_attention_order=False,
- pool="adaptive",
- *args,
- **kwargs,
- ):
- super().__init__()
-
- if num_heads_upsample == -1:
- num_heads_upsample = num_heads
-
- self.in_channels = in_channels
- self.model_channels = model_channels
- self.out_channels = out_channels
- self.num_res_blocks = num_res_blocks
- self.attention_resolutions = attention_resolutions
- self.dropout = dropout
- self.channel_mult = channel_mult
- self.conv_resample = conv_resample
- self.use_checkpoint = use_checkpoint
- self.dtype = th.float16 if use_fp16 else th.float32
- self.num_heads = num_heads
- self.num_head_channels = num_head_channels
- self.num_heads_upsample = num_heads_upsample
-
- time_embed_dim = model_channels * 4
- self.time_embed = nn.Sequential(
- linear(model_channels, time_embed_dim),
- nn.SiLU(),
- linear(time_embed_dim, time_embed_dim),
- )
-
- self.input_blocks = nn.ModuleList(
- [
- TimestepEmbedSequential(
- conv_nd(dims, in_channels, model_channels, 3, padding=1)
- )
- ]
- )
- self._feature_size = model_channels
- input_block_chans = [model_channels]
- ch = model_channels
- ds = 1
- for level, mult in enumerate(channel_mult):
- for _ in range(num_res_blocks):
- layers = [
- ResBlock(
- ch,
- time_embed_dim,
- dropout,
- out_channels=mult * model_channels,
- dims=dims,
- use_checkpoint=use_checkpoint,
- use_scale_shift_norm=use_scale_shift_norm,
- )
- ]
- ch = mult * model_channels
- if ds in attention_resolutions:
- layers.append(
- AttentionBlock(
- ch,
- use_checkpoint=use_checkpoint,
- num_heads=num_heads,
- num_head_channels=num_head_channels,
- use_new_attention_order=use_new_attention_order,
- )
- )
- self.input_blocks.append(TimestepEmbedSequential(*layers))
- self._feature_size += ch
- input_block_chans.append(ch)
- if level != len(channel_mult) - 1:
- out_ch = ch
- self.input_blocks.append(
- TimestepEmbedSequential(
- ResBlock(
- ch,
- time_embed_dim,
- dropout,
- out_channels=out_ch,
- dims=dims,
- use_checkpoint=use_checkpoint,
- use_scale_shift_norm=use_scale_shift_norm,
- down=True,
- )
- if resblock_updown
- else Downsample(
- ch, conv_resample, dims=dims, out_channels=out_ch
- )
- )
- )
- ch = out_ch
- input_block_chans.append(ch)
- ds *= 2
- self._feature_size += ch
-
- self.middle_block = TimestepEmbedSequential(
- ResBlock(
- ch,
- time_embed_dim,
- dropout,
- dims=dims,
- use_checkpoint=use_checkpoint,
- use_scale_shift_norm=use_scale_shift_norm,
- ),
- AttentionBlock(
- ch,
- use_checkpoint=use_checkpoint,
- num_heads=num_heads,
- num_head_channels=num_head_channels,
- use_new_attention_order=use_new_attention_order,
- ),
- ResBlock(
- ch,
- time_embed_dim,
- dropout,
- dims=dims,
- use_checkpoint=use_checkpoint,
- use_scale_shift_norm=use_scale_shift_norm,
- ),
- )
- self._feature_size += ch
- self.pool = pool
- if pool == "adaptive":
- self.out = nn.Sequential(
- normalization(ch),
- nn.SiLU(),
- nn.AdaptiveAvgPool2d((1, 1)),
- zero_module(conv_nd(dims, ch, out_channels, 1)),
- nn.Flatten(),
- )
- elif pool == "attention":
- assert num_head_channels != -1
- self.out = nn.Sequential(
- normalization(ch),
- nn.SiLU(),
- AttentionPool2d(
- (image_size // ds), ch, num_head_channels, out_channels
- ),
- )
- elif pool == "spatial":
- self.out = nn.Sequential(
- nn.Linear(self._feature_size, 2048),
- nn.ReLU(),
- nn.Linear(2048, self.out_channels),
- )
- elif pool == "spatial_v2":
- self.out = nn.Sequential(
- nn.Linear(self._feature_size, 2048),
- normalization(2048),
- nn.SiLU(),
- nn.Linear(2048, self.out_channels),
- )
- else:
- raise NotImplementedError(f"Unexpected {pool} pooling")
-
- def convert_to_fp16(self):
- """
- Convert the torso of the model to float16.
- """
- self.input_blocks.apply(convert_module_to_f16)
- self.middle_block.apply(convert_module_to_f16)
-
- def convert_to_fp32(self):
- """
- Convert the torso of the model to float32.
- """
- self.input_blocks.apply(convert_module_to_f32)
- self.middle_block.apply(convert_module_to_f32)
-
- def forward(self, x, timesteps):
- """
- Apply the model to an input batch.
- :param x: an [N x C x ...] Tensor of inputs.
- :param timesteps: a 1-D batch of timesteps.
- :return: an [N x K] Tensor of outputs.
- """
- emb = self.time_embed(timestep_embedding(timesteps, self.model_channels))
-
- results = []
- h = x.type(self.dtype)
- for module in self.input_blocks:
- h = module(h, emb)
- if self.pool.startswith("spatial"):
- results.append(h.type(x.dtype).mean(dim=(2, 3)))
- h = self.middle_block(h, emb)
- if self.pool.startswith("spatial"):
- results.append(h.type(x.dtype).mean(dim=(2, 3)))
- h = th.cat(results, axis=-1)
- return self.out(h)
- else:
- h = h.type(x.dtype)
- return self.out(h)
diff --git a/spaces/deepwisdom/MetaGPT/metagpt/prompts/structure_action.py b/spaces/deepwisdom/MetaGPT/metagpt/prompts/structure_action.py
deleted file mode 100644
index 97c57cf249556cfc2af8f534bbd4fe8284d6a683..0000000000000000000000000000000000000000
--- a/spaces/deepwisdom/MetaGPT/metagpt/prompts/structure_action.py
+++ /dev/null
@@ -1,22 +0,0 @@
-#!/usr/bin/env python
-# -*- coding: utf-8 -*-
-"""
-@Time : 2023/5/30 10:12
-@Author : alexanderwu
-@File : structure_action.py
-"""
-
-ACTION_SYSTEM = """SYSTEM:
-You serve as an assistant that helps me play Minecraft.
-I will give you a sentence. Please convert this sentence into one or several actions according to the following instructions.
-Each action should be a tuple of four items, written in the form (’verb’, ’object’, ’tools’, ’materials’)
-’verb’ is the verb of this action.
-’object’ refers to the target object of the action.
-’tools’ specifies the tools required for the action.
-’material’ specifies the materials required for the action.
-If some of the items are not required, set them to be ’None’.
-"""
-
-ACTION_USER = """USER:
-The sentence is {sentence}. Generate the action tuple according to the requirements.
-"""
diff --git a/spaces/derina/BartSummarizer/README.md b/spaces/derina/BartSummarizer/README.md
deleted file mode 100644
index 175a7da038f05ad0b4775429f77dbdd4c12f62cb..0000000000000000000000000000000000000000
--- a/spaces/derina/BartSummarizer/README.md
+++ /dev/null
@@ -1,13 +0,0 @@
----
-title: OpenAISummarizer
-emoji: 👁
-colorFrom: gray
-colorTo: yellow
-sdk: gradio
-sdk_version: 3.28.1
-app_file: app.py
-pinned: false
-license: bsd
----
-
-Check out the configuration reference at https://huggingface.co/docs/hub/spaces-config-reference
diff --git a/spaces/digitalxingtong/Miiu-Bert-Vits2/text/chinese.py b/spaces/digitalxingtong/Miiu-Bert-Vits2/text/chinese.py
deleted file mode 100644
index 276753880b73de2e8889dcb2101cd98c09e0710b..0000000000000000000000000000000000000000
--- a/spaces/digitalxingtong/Miiu-Bert-Vits2/text/chinese.py
+++ /dev/null
@@ -1,193 +0,0 @@
-import os
-import re
-
-import cn2an
-from pypinyin import lazy_pinyin, Style
-
-from text import symbols
-from text.symbols import punctuation
-from text.tone_sandhi import ToneSandhi
-
-current_file_path = os.path.dirname(__file__)
-pinyin_to_symbol_map = {line.split("\t")[0]: line.strip().split("\t")[1] for line in
- open(os.path.join(current_file_path, 'opencpop-strict.txt')).readlines()}
-
-import jieba.posseg as psg
-
-
-rep_map = {
- ':': ',',
- ';': ',',
- ',': ',',
- '。': '.',
- '!': '!',
- '?': '?',
- '\n': '.',
- "·": ",",
- '、': ",",
- '...': '…',
- '$': '.',
- '“': "'",
- '”': "'",
- '‘': "'",
- '’': "'",
- '(': "'",
- ')': "'",
- '(': "'",
- ')': "'",
- '《': "'",
- '》': "'",
- '【': "'",
- '】': "'",
- '[': "'",
- ']': "'",
- '—': "-",
- '~': "-",
- '~': "-",
- '「': "'",
- '」': "'",
-
-}
-
-tone_modifier = ToneSandhi()
-
-def replace_punctuation(text):
- text = text.replace("嗯", "恩").replace("呣","母")
- pattern = re.compile('|'.join(re.escape(p) for p in rep_map.keys()))
-
- replaced_text = pattern.sub(lambda x: rep_map[x.group()], text)
-
- replaced_text = re.sub(r'[^\u4e00-\u9fa5'+"".join(punctuation)+r']+', '', replaced_text)
-
- return replaced_text
-
-def g2p(text):
- pattern = r'(?<=[{0}])\s*'.format(''.join(punctuation))
- sentences = [i for i in re.split(pattern, text) if i.strip()!='']
- phones, tones, word2ph = _g2p(sentences)
- assert sum(word2ph) == len(phones)
- assert len(word2ph) == len(text) #Sometimes it will crash,you can add a try-catch.
- phones = ['_'] + phones + ["_"]
- tones = [0] + tones + [0]
- word2ph = [1] + word2ph + [1]
- return phones, tones, word2ph
-
-
-def _get_initials_finals(word):
- initials = []
- finals = []
- orig_initials = lazy_pinyin(
- word, neutral_tone_with_five=True, style=Style.INITIALS)
- orig_finals = lazy_pinyin(
- word, neutral_tone_with_five=True, style=Style.FINALS_TONE3)
- for c, v in zip(orig_initials, orig_finals):
- initials.append(c)
- finals.append(v)
- return initials, finals
-
-
-def _g2p(segments):
- phones_list = []
- tones_list = []
- word2ph = []
- for seg in segments:
- pinyins = []
- # Replace all English words in the sentence
- seg = re.sub('[a-zA-Z]+', '', seg)
- seg_cut = psg.lcut(seg)
- initials = []
- finals = []
- seg_cut = tone_modifier.pre_merge_for_modify(seg_cut)
- for word, pos in seg_cut:
- if pos == 'eng':
- continue
- sub_initials, sub_finals = _get_initials_finals(word)
- sub_finals = tone_modifier.modified_tone(word, pos,
- sub_finals)
- initials.append(sub_initials)
- finals.append(sub_finals)
-
- # assert len(sub_initials) == len(sub_finals) == len(word)
- initials = sum(initials, [])
- finals = sum(finals, [])
- #
- for c, v in zip(initials, finals):
- raw_pinyin = c+v
- # NOTE: post process for pypinyin outputs
- # we discriminate i, ii and iii
- if c == v:
- assert c in punctuation
- phone = [c]
- tone = '0'
- word2ph.append(1)
- else:
- v_without_tone = v[:-1]
- tone = v[-1]
-
- pinyin = c+v_without_tone
- assert tone in '12345'
-
- if c:
- # 多音节
- v_rep_map = {
- "uei": 'ui',
- 'iou': 'iu',
- 'uen': 'un',
- }
- if v_without_tone in v_rep_map.keys():
- pinyin = c+v_rep_map[v_without_tone]
- else:
- # 单音节
- pinyin_rep_map = {
- 'ing': 'ying',
- 'i': 'yi',
- 'in': 'yin',
- 'u': 'wu',
- }
- if pinyin in pinyin_rep_map.keys():
- pinyin = pinyin_rep_map[pinyin]
- else:
- single_rep_map = {
- 'v': 'yu',
- 'e': 'e',
- 'i': 'y',
- 'u': 'w',
- }
- if pinyin[0] in single_rep_map.keys():
- pinyin = single_rep_map[pinyin[0]]+pinyin[1:]
-
- assert pinyin in pinyin_to_symbol_map.keys(), (pinyin, seg, raw_pinyin)
- phone = pinyin_to_symbol_map[pinyin].split(' ')
- word2ph.append(len(phone))
-
- phones_list += phone
- tones_list += [int(tone)] * len(phone)
- return phones_list, tones_list, word2ph
-
-
-
-def text_normalize(text):
- numbers = re.findall(r'\d+(?:\.?\d+)?', text)
- for number in numbers:
- text = text.replace(number, cn2an.an2cn(number), 1)
- text = replace_punctuation(text)
- return text
-
-def get_bert_feature(text, word2ph):
- from text import chinese_bert
- return chinese_bert.get_bert_feature(text, word2ph)
-
-if __name__ == '__main__':
- from text.chinese_bert import get_bert_feature
- text = "啊!但是《原神》是由,米哈\游自主, [研发]的一款全.新开放世界.冒险游戏"
- text = text_normalize(text)
- print(text)
- phones, tones, word2ph = g2p(text)
- bert = get_bert_feature(text, word2ph)
-
- print(phones, tones, word2ph, bert.shape)
-
-
-# # 示例用法
-# text = "这是一个示例文本:,你好!这是一个测试...."
-# print(g2p_paddle(text)) # 输出: 这是一个示例文本你好这是一个测试
diff --git a/spaces/digitalxingtong/Taffy-Bert-VITS2/monotonic_align/setup.py b/spaces/digitalxingtong/Taffy-Bert-VITS2/monotonic_align/setup.py
deleted file mode 100644
index 30c224807a70faa9df9c9eb75f8e80c8c867b16b..0000000000000000000000000000000000000000
--- a/spaces/digitalxingtong/Taffy-Bert-VITS2/monotonic_align/setup.py
+++ /dev/null
@@ -1,9 +0,0 @@
-from distutils.core import setup
-from Cython.Build import cythonize
-import numpy
-
-setup(
- name = 'monotonic_align',
- ext_modules = cythonize("core.pyx"),
- include_dirs=[numpy.get_include()]
-)
diff --git a/spaces/digitalxingtong/Xingtong-All-in-One/README.md b/spaces/digitalxingtong/Xingtong-All-in-One/README.md
deleted file mode 100644
index 4171b70798d66b8e0a4b8319ad2c8c9dc582510f..0000000000000000000000000000000000000000
--- a/spaces/digitalxingtong/Xingtong-All-in-One/README.md
+++ /dev/null
@@ -1,13 +0,0 @@
----
-title: Xingtong All In One
-emoji: 🌖
-colorFrom: gray
-colorTo: green
-sdk: streamlit
-sdk_version: 1.27.2
-app_file: app.py
-pinned: false
-license: mit
----
-
-Check out the configuration reference at https://huggingface.co/docs/hub/spaces-config-reference
diff --git a/spaces/dineshreddy/WALT/configs/_base_/models/mask_rcnn_swin_fpn.py b/spaces/dineshreddy/WALT/configs/_base_/models/mask_rcnn_swin_fpn.py
deleted file mode 100644
index e3d42197f4646cd9ecafac2095d3f8e079f0a729..0000000000000000000000000000000000000000
--- a/spaces/dineshreddy/WALT/configs/_base_/models/mask_rcnn_swin_fpn.py
+++ /dev/null
@@ -1,127 +0,0 @@
-# model settings
-model = dict(
- type='MaskRCNN',
- pretrained=None,
- backbone=dict(
- type='SwinTransformer',
- embed_dim=96,
- depths=[2, 2, 6, 2],
- num_heads=[3, 6, 12, 24],
- window_size=7,
- mlp_ratio=4.,
- qkv_bias=True,
- qk_scale=None,
- drop_rate=0.,
- attn_drop_rate=0.,
- drop_path_rate=0.2,
- ape=False,
- patch_norm=True,
- out_indices=(0, 1, 2, 3),
- use_checkpoint=False),
- neck=dict(
- type='FPN',
- in_channels=[96, 192, 384, 768],
- out_channels=256,
- num_outs=5),
- rpn_head=dict(
- type='RPNHead',
- in_channels=256,
- feat_channels=256,
- anchor_generator=dict(
- type='AnchorGenerator',
- scales=[8],
- ratios=[0.5, 1.0, 2.0],
- strides=[4, 8, 16, 32, 64]),
- bbox_coder=dict(
- type='DeltaXYWHBBoxCoder',
- target_means=[.0, .0, .0, .0],
- target_stds=[1.0, 1.0, 1.0, 1.0]),
- loss_cls=dict(
- type='CrossEntropyLoss', use_sigmoid=True, loss_weight=1.0),
- loss_bbox=dict(type='L1Loss', loss_weight=1.0)),
- roi_head=dict(
- type='StandardRoIHead',
- bbox_roi_extractor=dict(
- type='SingleRoIExtractor',
- roi_layer=dict(type='RoIAlign', output_size=7, sampling_ratio=0),
- out_channels=256,
- featmap_strides=[4, 8, 16, 32]),
- bbox_head=dict(
- type='Shared2FCBBoxHead',
- in_channels=256,
- fc_out_channels=1024,
- roi_feat_size=7,
- num_classes=80,
- bbox_coder=dict(
- type='DeltaXYWHBBoxCoder',
- target_means=[0., 0., 0., 0.],
- target_stds=[0.1, 0.1, 0.2, 0.2]),
- reg_class_agnostic=False,
- loss_cls=dict(
- type='CrossEntropyLoss', use_sigmoid=False, loss_weight=1.0),
- loss_bbox=dict(type='L1Loss', loss_weight=1.0)),
- mask_roi_extractor=dict(
- type='SingleRoIExtractor',
- roi_layer=dict(type='RoIAlign', output_size=14, sampling_ratio=0),
- out_channels=256,
- featmap_strides=[4, 8, 16, 32]),
- mask_head=dict(
- type='FCNMaskHead',
- num_convs=4,
- in_channels=256,
- conv_out_channels=256,
- num_classes=80,
- loss_mask=dict(
- type='CrossEntropyLoss', use_mask=True, loss_weight=1.0))),
- # model training and testing settings
- train_cfg=dict(
- rpn=dict(
- assigner=dict(
- type='MaxIoUAssigner',
- pos_iou_thr=0.7,
- neg_iou_thr=0.3,
- min_pos_iou=0.3,
- match_low_quality=True,
- ignore_iof_thr=-1),
- sampler=dict(
- type='RandomSampler',
- num=256,
- pos_fraction=0.5,
- neg_pos_ub=-1,
- add_gt_as_proposals=False),
- allowed_border=-1,
- pos_weight=-1,
- debug=False),
- rpn_proposal=dict(
- nms_pre=2000,
- max_per_img=1000,
- nms=dict(type='nms', iou_threshold=0.7),
- min_bbox_size=0),
- rcnn=dict(
- assigner=dict(
- type='MaxIoUAssigner',
- pos_iou_thr=0.5,
- neg_iou_thr=0.5,
- min_pos_iou=0.5,
- match_low_quality=True,
- ignore_iof_thr=-1),
- sampler=dict(
- type='RandomSampler',
- num=512,
- pos_fraction=0.25,
- neg_pos_ub=-1,
- add_gt_as_proposals=True),
- mask_size=28,
- pos_weight=-1,
- debug=False)),
- test_cfg=dict(
- rpn=dict(
- nms_pre=1000,
- max_per_img=1000,
- nms=dict(type='nms', iou_threshold=0.7),
- min_bbox_size=0),
- rcnn=dict(
- score_thr=0.05,
- nms=dict(type='nms', iou_threshold=0.5),
- max_per_img=100,
- mask_thr_binary=0.5)))
diff --git a/spaces/dinhminh20521597/OCR_DEMO/configs/textdet/dbnet/README.md b/spaces/dinhminh20521597/OCR_DEMO/configs/textdet/dbnet/README.md
deleted file mode 100644
index d2007c72ec2b45e70d30c6edea128b7e0be2baca..0000000000000000000000000000000000000000
--- a/spaces/dinhminh20521597/OCR_DEMO/configs/textdet/dbnet/README.md
+++ /dev/null
@@ -1,33 +0,0 @@
-# DBNet
-
-> [Real-time Scene Text Detection with Differentiable Binarization](https://arxiv.org/abs/1911.08947)
-
-
-
-## Abstract
-
-Recently, segmentation-based methods are quite popular in scene text detection, as the segmentation results can more accurately describe scene text of various shapes such as curve text. However, the post-processing of binarization is essential for segmentation-based detection, which converts probability maps produced by a segmentation method into bounding boxes/regions of text. In this paper, we propose a module named Differentiable Binarization (DB), which can perform the binarization process in a segmentation network. Optimized along with a DB module, a segmentation network can adaptively set the thresholds for binarization, which not only simplifies the post-processing but also enhances the performance of text detection. Based on a simple segmentation network, we validate the performance improvements of DB on five benchmark datasets, which consistently achieves state-of-the-art results, in terms of both detection accuracy and speed. In particular, with a light-weight backbone, the performance improvements by DB are significant so that we can look for an ideal tradeoff between detection accuracy and efficiency. Specifically, with a backbone of ResNet-18, our detector achieves an F-measure of 82.8, running at 62 FPS, on the MSRA-TD500 dataset.
-
-
"
-
-examples = [
- """la seguente SENTENZA sul ricorso 24817-2015 proposto da: ANDREA FORMISANO, elettivamente domiciliato in ROMA VIA S. TOMMASO D'AQUINO 7, presso lo studio dell'avvocato CARLO BORELLO, che lo rappresenta e difende giusta delega in calce; - ricorrente - contro SOGET SPA, CAMERA DI COMMERCIO DI PESCARA; - intimati - avverso la sentenza n. 169/2012 della COMM.TRIB.REG.SEZ.DIST. di PESCARA, depositata il 13/03/2012; udita la relazione della causa svolta nella pubblica udienza del 04/04/2018 dal Consigliere Dott. MILENA BALSAMO; udito il P.M. in persona del Sostituto Procuratore Generale Dott. GIOVANNI GIACALONE che ha concluso per l'inammissibilità in subordine rigetto del ricorso.""",
- """la seguente SENTENZA sul ricorso 17668-2016 proposto da: C.B.H. CITTA DI BARI HOSPITAL S.P.A., in persona del legale rappresentante pro tempore, elettivamente domiciliata in ROMA, LUNGOTEVERE DEI MELLINI 10, presso lo studio dell'avvocato CRISTIANO MARINESE, rappresentata e difesa dagli avvocati GIUSEPPE LUIGI 2022 POLITO, FRANCESCO ANTONUCCI; 51 - ricorrente - contro I.N.P.S. - ISTITUTO NAZIONALE PREVIDENZA SOCIALE, in persona del legale rappresentante pro tempore, elettivamente domiciliato in ROMA, VIA CESARE BECCARIA 29, presso l'Avvocatura Centrale dell'Istituto, rappresentato e difeso dagli avvocati ANTONINO SGROI, CARLA D'ALOISIO, ESTER ADA SCIPLINO, EMANUELE DE ROSE, LELIO MARITATO, GIUSEPPE MATANO; - controricorrente - nonchè contro EQUITALIA SERVIZI DI RISCOSIONE S.P.A. già EQUITALIA SUD S.P.A. agente della riscossione della provincia di Bari; - intimata - avverso la sentenza n. 2696/2015 della CORTE D'APPELLO di BARI, depositata il 13/01/2016 R.G.N. 1439/2013; udita la relazione della causa svolta nella pubblica udienza del 12/01/2022 dal Consigliere Dott. DANIELA CALAFIORE; udito il P.M. in persona del Sostituto Procuratore Generale Dott. STEFANO VISONA' che ha concluso per il rigetto del ricorso; udito l'avvocato ANTONINO SGROI. R.g. n. 17668/2016""",
- """4. SENTENZA sul ricorso 4005-2012 proposto da: BANCA NAZIONALE DEL LAVORO S.P.A. C.E. 09339391006, in persona del legale rappresentante pro tempore, elettivamente domiciliata in ROMA, VIA PO 25/B, presso lo studio degli avvocati ROBERTO PESSI, FRANCESCO GIAMMARIA, che la rappresentano e difendono, giusta procura speciale notarile in atti; 2015 - ricorrente - 4680 contro CAMPAGNOLI ALESSANDRO MARIA C.F. CMPLSN59L29G388P; 4 - intimato - Nonché da: CAMPAGNOLI ALESSANDRO MARIA C.E. CMPLSN59L29G388P, domiciliato in ROMA PIAZZA CAVOUR, presso LA CANCELLERIA DELLA CORTE SUPREMA DI CASSAZIONE, rappresentato e difeso dall'avvocato FABRIZIA MAURICI, giusta procura speciale notarile in atti; - controricorrente e ricorrente incidentale - contro BANCA NAZIONALE DEL LAVORO S.P.A. C.E. 09339391006, in persona del legale rappresentante pro tempore, elettivamente domiciliata in ROMA, VIA PO 25/B, presso lo studio degli avvocati ROBERTO FESSI, FRANCESCO GIAMMARIA, che la rappresentano e difendono, giusta procura speciale notarile in atti; - controricorrente al ricorso incidentale - avverso la sentenza n. 1091/2011 della CORTE D'APPELLO di MILANO, depositata il 28/10/2011 R.G.N. 537/2008; udita la relazione della causa svolta nella pubblica udienza del 02/12/2015 dal Consigliere Dott. UMBERTO BERRINO; udito l'Avvocato SERRANI TIZIANA per delega verbale FESSI ROBERTO; udito l'Avvocato MAURICI FABRIZIA (per procura speciale notarile); udito il P.M. in persona del Sostituto Procuratore Generale Dott. RITA SANLORENZO che ha concluso per il rigetto del ricorso principale e del ricorso incidentale.""",
- #"""SENTENZA sul ricorso 11948-2014 proposto da: VENTURA VINCENZO C.F. VNTVCN47T08A841S, già elettivamente domiciliato in ROMA, VIA VALLISNERI 11, presso lo studio dell'avvocato PAOLO PACIFICI, che lo rappresenta e difende unitamente all'avvocato DIEGO TOSI, giusta delega in atti e da ultimo domiciliato 2015 presso LA CANCELLERIA DELLA CORTE SUPREMA DI CASSAZIONE; 4525 - ricorrente - contro k RAI RADIOTELEVISIONE ITALIANA S.P.A. C.F. 06382641006, in persona del legale rappresentante pro tempore, elettivamente domiciliata in ROMA, VIA P.L. DA PALESTRINA 47, presso lo studio dell'avvocato RINALDO GEREMIA, rappresentata e difesa dall'avvocato NATALIA FERRO, giusta delega in atti; - controri corrente nonchè contro I.N.A.I.L - ISTITUTO NAZIONALE PER L'ASSICURAZIONE CONTRO GLI INFORTUNI SUL LAVORO C.F. 01165400589, in persona del legale rappresentante pro tempore, elettivamente domiciliato in ROMA, VIA IV NOVEMBRE 144, presso lo studio degli avvocati LUCIANA ROMEO, LETIZIA CRIPPA, che lo rappresentano e difendono giusta delega in atti; - controricorrente - avverso la sentenza n. 1423/2013 della CORTE D'APPELLO di TORINO, depositata il 03/02/2014 R.G.N. 275/2013; udita la relazione della causa svolta nella pubblica udienza del 25/11/2015 dal Consigliere Dott. NICOLA DE MARINIS; AVV, udito l'Avvocato OTTOLINI TERESA per delega', ROMEO LUCIANA; udito l'Avvocato GEREMIA RINALDO per delega'-eFERRO NATALIA; udito il P.M. in persona del Sostituto Procuratore Generale Dott. RENATO FINOCCHI GHERSI che ha concluso per ESTINZIONE PER RINUNCIA. ... , z , I ? F""",
-
-]
-
-model_name = "fabiod20/italian-legal-ner"
-model = AutoModelForTokenClassification.from_pretrained(model_name, use_auth_token=os.environ['token'])
-tokenizer = AutoTokenizer.from_pretrained(model_name, use_auth_token=os.environ['token'])
-
-ner_pipe = pipeline("ner", model=model, tokenizer=tokenizer)
-
-nlp = spacy.load("it_core_news_sm")
-nlp.disable_pipes("ner")
-
-def ner(input_text):
- entities = ner_pipe(input_text, aggregation_strategy="first")
-
- doc = nlp(input_text)
-
- potential_entities = []
-
- for entity in entities:
- start = entity["start"]
- end = entity["end"]
- label = entity["entity_group"]
-
- ent = doc.char_span(start, end, label=label)
- if ent != None:
- doc.ents += (ent,)
- else:
- potential_entities.append(entity)
-
- potential_entities.append({"entity_group": "NONE", "start": -1, "end": -1})
-
- start = potential_entities[0]["start"]
- end = potential_entities[0]["end"]
- label = potential_entities[0]["entity_group"]
-
- for item in potential_entities:
- if item["entity_group"] == label and item["start"] == end:
- end = item["end"]
- continue
- else:
- if item["start"] != start:
- ent = doc.char_span(start, end, label=label)
- doc.ents += (ent,)
-
- start = item["start"]
- end = item["end"]
- label = item["entity_group"]
-
- colors = {
- "RIC": "#ff5e5e",
- "RCR": "#ff9999",
- "CTR": "#ffd699",
- "DOM": "#c3a1c9",
- "AVV": "#80c5c5",
- "CNS": "#ff9500",
- "PMI": "#0ea5e9",
- "CDA": "#84b351",
- "SNT": "#ffff5e",
- }
- options = {"ents": colors.keys(), "colors": colors}
-
- output = displacy.render(doc, style="ent", options=options)
- return output
-
-interface = gr.Interface(
- title=title,
- description=description,
- article=article,
- allow_screenshot=False,
- allow_flagging=False,
- fn=ner,
- inputs=gr.inputs.Textbox(placeholder="Insert an Italian judgments (you can click on an example below)", lines=10),
- outputs=gr.outputs.HTML(),
- examples=examples
- )
-
-interface.launch()
\ No newline at end of file
diff --git a/spaces/facebook/StyleNeRF/training/dataset.py b/spaces/facebook/StyleNeRF/training/dataset.py
deleted file mode 100644
index 0df9031f874cb4ee5ba1a5c6ea016991bbbbd749..0000000000000000000000000000000000000000
--- a/spaces/facebook/StyleNeRF/training/dataset.py
+++ /dev/null
@@ -1,275 +0,0 @@
-# Copyright (c) Facebook, Inc. and its affiliates. All Rights Reserved
-
-# Copyright (c) 2021, NVIDIA CORPORATION. All rights reserved.
-#
-# NVIDIA CORPORATION and its licensors retain all intellectual property
-# and proprietary rights in and to this software, related documentation
-# and any modifications thereto. Any use, reproduction, disclosure or
-# distribution of this software and related documentation without an express
-# license agreement from NVIDIA CORPORATION is strictly prohibited.
-
-from curses import raw
-import os
-from urllib import response
-import numpy as np
-import zipfile
-import PIL.Image
-import cv2
-import json
-import torch
-import dnnlib
-
-try:
- import pyspng
-except ImportError:
- pyspng = None
-
-#----------------------------------------------------------------------------
-
-class Dataset(torch.utils.data.Dataset):
- def __init__(self,
- name, # Name of the dataset.
- raw_shape, # Shape of the raw image data (NCHW).
- max_size = None, # Artificially limit the size of the dataset. None = no limit. Applied before xflip.
- use_labels = False, # Enable conditioning labels? False = label dimension is zero.
- xflip = False, # Artificially double the size of the dataset via x-flips. Applied after max_size.
- random_seed = 0, # Random seed to use when applying max_size.
- ):
- self._name = name
- self._raw_shape = list(raw_shape)
- self._use_labels = use_labels
- self._raw_labels = None
- self._label_shape = None
-
- # Apply max_size.
- self._raw_idx = np.arange(self._raw_shape[0], dtype=np.int64)
- if (max_size is not None) and (self._raw_idx.size > max_size):
- np.random.RandomState(random_seed).shuffle(self._raw_idx)
- self._raw_idx = np.sort(self._raw_idx[:max_size])
-
- # Apply xflip.
- self.xflip = xflip
- self._xflip = np.zeros(self._raw_idx.size, dtype=np.uint8)
- if xflip:
- self._raw_idx = np.tile(self._raw_idx, 2)
- self._xflip = np.concatenate([self._xflip, np.ones_like(self._xflip)])
-
- def _get_raw_labels(self):
- if self._raw_labels is None:
- self._raw_labels = self._load_raw_labels() if self._use_labels else None
- if self._raw_labels is None:
- self._raw_labels = np.zeros([self._raw_shape[0], 0], dtype=np.float32)
- assert isinstance(self._raw_labels, np.ndarray)
- assert self._raw_labels.shape[0] == self._raw_shape[0]
- assert self._raw_labels.dtype in [np.float32, np.int64]
- if self._raw_labels.dtype == np.int64:
- assert self._raw_labels.ndim == 1
- assert np.all(self._raw_labels >= 0)
- return self._raw_labels
-
- def close(self): # to be overridden by subclass
- pass
-
- def _load_raw_image(self, raw_idx): # to be overridden by subclass
- raise NotImplementedError
-
- def _load_raw_labels(self): # to be overridden by subclass
- raise NotImplementedError
-
- def __getstate__(self):
- return dict(self.__dict__, _raw_labels=None)
-
- def __del__(self):
- try:
- self.close()
- except:
- pass
-
- def __len__(self):
- return self._raw_idx.size
-
- def __getitem__(self, idx):
- image = self._load_raw_image(self._raw_idx[idx])
- assert isinstance(image, np.ndarray)
- assert list(image.shape) == self.image_shape
- assert image.dtype == np.uint8
- if self._xflip[idx]:
- assert image.ndim == 3 # CHW
- image = image[:, :, ::-1]
- return image.copy(), self.get_label(idx), idx
-
- def get_label(self, idx):
- label = self._get_raw_labels()[self._raw_idx[idx]]
- if label.dtype == np.int64:
- onehot = np.zeros(self.label_shape, dtype=np.float32)
- onehot[label] = 1
- label = onehot
- return label.copy()
-
- def get_details(self, idx):
- d = dnnlib.EasyDict()
- d.raw_idx = int(self._raw_idx[idx])
- d.xflip = (int(self._xflip[idx]) != 0)
- d.raw_label = self._get_raw_labels()[d.raw_idx].copy()
- return d
-
- @property
- def name(self):
- return self._name
-
- @property
- def image_shape(self):
- return list(self._raw_shape[1:])
-
- @property
- def num_channels(self):
- assert len(self.image_shape) == 3 # CHW
- return self.image_shape[0]
-
- @property
- def resolution(self):
- assert len(self.image_shape) == 3 # CHW
- assert self.image_shape[1] == self.image_shape[2]
- return self.image_shape[1]
-
- @property
- def label_shape(self):
- if self._label_shape is None:
- raw_labels = self._get_raw_labels()
- if raw_labels.dtype == np.int64:
- self._label_shape = [int(np.max(raw_labels)) + 1]
- else:
- self._label_shape = raw_labels.shape[1:]
- return list(self._label_shape)
-
- @property
- def label_dim(self):
- assert len(self.label_shape) == 1
- return self.label_shape[0]
-
- @property
- def has_labels(self):
- return any(x != 0 for x in self.label_shape)
-
- @property
- def has_onehot_labels(self):
- return self._get_raw_labels().dtype == np.int64
-
-#----------------------------------------------------------------------------
-
-class ImageFolderDataset(Dataset):
- def __init__(self,
- path, # Path to directory or zip.
- resolution = None, # Ensure specific resolution, None = highest available.
- **super_kwargs, # Additional arguments for the Dataset base class.
- ):
- self._path = path
- self._zipfile = None
-
- if os.path.isdir(self._path):
- self._type = 'dir'
- self._all_fnames = {os.path.relpath(os.path.join(root, fname), start=self._path) for root, _dirs, files in os.walk(self._path) for fname in files}
- elif self._file_ext(self._path) == '.zip':
- self._type = 'zip'
- self._all_fnames = set(self._get_zipfile().namelist())
- else:
- raise IOError('Path must point to a directory or zip')
-
- PIL.Image.init()
- self._image_fnames = sorted(fname for fname in self._all_fnames if self._file_ext(fname) in PIL.Image.EXTENSION)
- if len(self._image_fnames) == 0:
- raise IOError('No image files found in the specified path')
-
- name = os.path.splitext(os.path.basename(self._path))[0]
- raw_shape = [len(self._image_fnames)] + list(self._load_raw_image(0).shape)
- if resolution is not None:
- raw_shape[2] = raw_shape[3] = resolution
- # if resolution is not None and (raw_shape[2] != resolution or raw_shape[3] != resolution):
- # raise IOError('Image files do not match the specified resolution')
- super().__init__(name=name, raw_shape=raw_shape, **super_kwargs)
-
- @staticmethod
- def _file_ext(fname):
- return os.path.splitext(fname)[1].lower()
-
- def _get_zipfile(self):
- assert self._type == 'zip'
- if self._zipfile is None:
- self._zipfile = zipfile.ZipFile(self._path)
- return self._zipfile
-
- def _open_file(self, fname):
- if self._type == 'dir':
- return open(os.path.join(self._path, fname), 'rb')
- if self._type == 'zip':
- return self._get_zipfile().open(fname, 'r')
- return None
-
- def close(self):
- try:
- if self._zipfile is not None:
- self._zipfile.close()
- finally:
- self._zipfile = None
-
- def __getstate__(self):
- return dict(super().__getstate__(), _zipfile=None)
-
- def _load_raw_image(self, raw_idx):
- fname = self._image_fnames[raw_idx]
- with self._open_file(fname) as f:
- if pyspng is not None and self._file_ext(fname) == '.png':
- image = pyspng.load(f.read())
- else:
- image = np.array(PIL.Image.open(f))
- if image.ndim == 2:
- image = image[:, :, np.newaxis] # HW => HWC
- if hasattr(self, '_raw_shape') and image.shape[-1] != self.resolution: # resize input image
- image = cv2.resize(image, (self.resolution, self.resolution), interpolation=cv2.INTER_AREA)
- image = image.transpose(2, 0, 1) # HWC => CHW
- return image
-
- def _load_raw_labels(self):
- fname = 'dataset.json'
- if fname not in self._all_fnames:
- return None
- with self._open_file(fname) as f:
- labels = json.load(f)['labels']
- if labels is None:
- return None
- labels = dict(labels)
- labels = [labels[fname.replace('\\', '/')] for fname in self._image_fnames]
- labels = np.array(labels)
- labels = labels.astype({1: np.int64, 2: np.float32}[labels.ndim])
- return labels
-
- def get_dali_dataloader(self, batch_size, world_size, rank, gpu): # TODO
- from nvidia.dali import pipeline_def, Pipeline
- import nvidia.dali.fn as fn
- import nvidia.dali.types as types
- from nvidia.dali.plugin.pytorch import DALIGenericIterator
-
- @pipeline_def
- def pipeline():
- jpegs, _ = fn.readers.file(
- file_root=self._path,
- files=list(self._all_fnames),
- random_shuffle=True,
- shard_id=rank,
- num_shards=world_size,
- name='reader')
- images = fn.decoders.image(jpegs, device='mixed')
- mirror = fn.random.coin_flip(probability=0.5) if self.xflip else False
- images = fn.crop_mirror_normalize(
- images.gpu(), output_layout="CHW", dtype=types.UINT8, mirror=mirror)
- labels = np.zeros([1, 0], dtype=np.float32)
- return images, labels
-
- dali_pipe = pipeline(batch_size=batch_size//world_size, num_threads=2, device_id=gpu)
- dali_pipe.build()
- training_set_iterator = DALIGenericIterator([dali_pipe], ['img', 'label'])
- for data in training_set_iterator:
- yield data[0]['img'], data[0]['label']
-
-#----------------------------------------------------------------------------
-
diff --git a/spaces/falterWliame/Face_Mask_Detection/Elit Egitim Seti Almanca.md b/spaces/falterWliame/Face_Mask_Detection/Elit Egitim Seti Almanca.md
deleted file mode 100644
index 13e4207260ed956641feb5a0ec4140a574d41273..0000000000000000000000000000000000000000
--- a/spaces/falterWliame/Face_Mask_Detection/Elit Egitim Seti Almanca.md
+++ /dev/null
@@ -1,6 +0,0 @@
-
Marvel Contest of Champions adalah salah satu game fighting terbaik yang bisa kamu mainkan di smartphone kamu. Game ini menawarkan aksi pertarungan yang seru dan spektakuler dengan karakter-karakter favorit kamu dari Marvel Universe. Apakah kamu ingin tahu cara download marvel contest of champions di perangkat kamu? Simak artikel ini sampai habis untuk mengetahui caranya.
Marvel Contest of Champions adalah game fighting yang dirilis oleh Kabam Games, Inc. pada tahun 2014. Game ini menghadirkan lebih dari 200 pahlawan dan penjahat dari Marvel Comics yang bisa kamu kumpulkan, tingkatkan, dan bawa ke pertempuran. Kamu bisa memilih karakter seperti Spider-Man, Iron Man, Wolverine, Captain America, Deadpool, Thanos, dan banyak lagi.
-
Game ini memiliki mode cerita yang menarik dan penuh tantangan, di mana kamu harus menghadapi musuh-musuh kuat seperti Kang the Conqueror, Thanos, dan The Collector. Kamu juga bisa bermain bersama teman-teman kamu dalam mode aliansi, di mana kamu bisa berkolaborasi, berstrategi, dan berkompetisi dengan aliansi lain dari seluruh dunia. Selain itu, game ini juga memiliki mode arena, incursions, battlegrounds, dan event-event spesial yang bisa kamu ikuti untuk mendapatkan hadiah-hadiah menarik.
-
The benefits of playing Marvel Contest of Champions
-
Marvel Contest of Champions bukan hanya sekedar game fighting biasa. Game ini juga memiliki banyak manfaat yang bisa kamu rasakan saat bermain, seperti:
-
cara download marvel contest of champions di android
-cara download marvel contest of champions mod apk
-cara download marvel contest of champions di pc
-cara download marvel contest of champions di iphone
-cara download marvel contest of champions tanpa wifi
-cara download marvel contest of champions versi terbaru
-cara download marvel contest of champions offline
-cara download marvel contest of champions dengan cepat
-cara download marvel contest of champions dari play store
-cara download marvel contest of champions gratis
-cara install marvel contest of champions di android
-cara install marvel contest of champions mod apk
-cara install marvel contest of champions di pc
-cara install marvel contest of champions di iphone
-cara install marvel contest of champions tanpa wifi
-cara install marvel contest of champions versi terbaru
-cara install marvel contest of champions offline
-cara install marvel contest of champions dengan cepat
-cara install marvel contest of champions dari play store
-cara install marvel contest of champions gratis
-cara main marvel contest of champions di android
-cara main marvel contest of champions mod apk
-cara main marvel contest of champions di pc
-cara main marvel contest of champions di iphone
-cara main marvel contest of champions tanpa wifi
-cara main marvel contest of champions versi terbaru
-cara main marvel contest of champions offline
-cara main marvel contest of champions dengan cepat
-cara main marvel contest of champions dari play store
-cara main marvel contest of champions gratis
-tips dan trik bermain marvel contest of champions di android
-tips dan trik bermain marvel contest of champions mod apk
-tips dan trik bermain marvel contest of champions di pc
-tips dan trik bermain marvel contest of champions di iphone
-tips dan trik bermain marvel contest of champions tanpa wifi
-tips dan trik bermain marvel contest of champions versi terbaru
-tips dan trik bermain marvel contest of champions offline
-tips dan trik bermain marvel contest of champions dengan cepat
-tips dan trik bermain marvel contest of champions dari play store
-tips dan trik bermain marvel contest of champions gratis
-
-
Meningkatkan keterampilan berpikir kritis dan strategis kamu. Kamu harus memilih tim yang tepat, memanfaatkan bonus sinergi, dan mengatur serangan dan pertahanan kamu dengan cerdas untuk mengalahkan lawan-lawan kamu.
-
Mengasah refleks dan koordinasi mata-tangan kamu. Kamu harus menguasai kontrol yang responsif dan intuitif untuk melakukan gerakan-gerakan dasar, serangan khusus, blok, esquive, dan parry dengan tepat dan cepat.
-
Menambah pengetahuan dan apresiasi kamu terhadap Marvel Universe. Kamu bisa melihat karakter-karakter Marvel dari sudut pandang yang berbeda, mengetahui latar belakang dan hubungan mereka, serta menikmati grafis dan suara yang berkualitas.
-
Bersenang-senang dan bersosialisasi dengan pemain lain. Kamu bisa bermain bersama teman-teman kamu atau bertemu dengan pemain baru dari seluruh dunia. Kamu bisa berbagi tips, saran, pengalaman, dan dukungan dengan mereka melalui fitur chat dan forum yang tersedia.
-
-
How to download Marvel Contest of Champions on Android devices?
-
The steps to download the game from Google Play Store
-
Untuk bisa bermain Marvel Contest of Champions di perangkat Android kamu, kamu harus mengunduh game ini dari Google Play Store. Berikut adalah langkah-langkahnya:
-
-
Buka aplikasi Google Play Store di perangkat kamu.
-
Ketik "Marvel Contest of Champions" di kolom pencarian dan tekan tombol cari.
-
Pilih game Marvel Contest of Champions dari daftar hasil pencarian dan tekan tombol instal.
-
Tunggu proses unduhan dan instalasi selesai. Pastikan kamu memiliki koneksi internet yang stabil dan cukup ruang penyimpanan di perangkat kamu.
-
Setelah instalasi selesai, tekan tombol buka untuk memulai game.
-
-
The requirements and permissions for installing the game
-
Sebelum kamu mengunduh dan memainkan Marvel Contest of Champions di perangkat Android kamu, kamu harus memenuhi beberapa persyaratan dan izin berikut:
-
-
Perangkat kamu harus memiliki sistem operasi Android versi 6.0 (Marshmallow) atau lebih tinggi.
-
Perangkat kamu harus memiliki RAM minimal 1 GB dan ruang penyimpanan minimal 2 GB.
-
Perangkat kamu harus mendukung OpenGL ES 3.0 atau lebih tinggi.
-
Kamu harus memberikan izin akses ke kamera, mikrofon, lokasi, media, dan kontak perangkat kamu saat pertama kali membuka game.
-
Kamu harus terhubung ke internet saat bermain game, baik melalui Wi-Fi atau data seluler.
-
-
How to download Marvel Contest of Champions on iOS devices?
-
The steps to download the game from App Store
-
Untuk bisa bermain Marvel Contest of Champions di perangkat iOS kamu, kamu harus mengunduh game ini dari App Store. Berikut adalah langkah-langkahnya:
-
-
Buka aplikasi App Store di perangkat kamu.
-
Ketik "Marvel Contest of Champions" di kolom pencarian dan tekan tombol cari.
-
Pilih game Marvel Contest of Champions dari daftar hasil pencarian dan tekan tombol unduh.
-
Masukkan kata sandi ID Apple kamu atau gunakan Face ID atau Touch ID jika diminta.
-
Tunggu proses unduhan dan instalasi selesai. Pastikan kamu memiliki koneksi internet yang stabil dan cukup ruang penyimpanan di perangkat kamu.
-
Setelah instalasi selesai, tekan tombol buka untuk memulai game.
-
-
The requirements and permissions for installing the game
-
Sebelum kamu mengunduh dan memainkan Marvel Contest of Champions di perangkat iOS kamu, kamu harus memenuhi beberapa persyaratan dan izin berikut:
-
-
Perangkat kamu harus memiliki sistem operasi iOS versi 10.0 atau lebih tinggi.
-
Perangkat kamu harus kompatibel dengan iPhone 5S atau lebih baru, iPad Air atau lebih baru, iPad mini 2 atau lebih baru, atau iPod touch (generasi ke-6) atau lebih baru.
-
Perangkat kamu harus memiliki ruang penyimpanan minimal 2 GB.
-
Kamu harus memberikan izin akses ke kamera, mikrofon, lokasi, media, dan kontak perangkat kamu saat pertama kali membuka game.
-
Kamu harus terhubung ke internet saat bermain game, baik melalui Wi-Fi atau data seluler.
-
-
How to play Marvel Contest of Champions?
The basics of the gameplay and the controls
-
Marvel Contest of Champions adalah game fighting yang mudah dipelajari tapi sulit dikuasai. Kamu harus mengontrol karakter kamu dengan menyentuh dan menggeser layar perangkat kamu. Berikut adalah beberapa gerakan dasar yang bisa kamu lakukan:
-
-
Tap layar di sebelah kanan untuk melakukan serangan ringan. Kamu bisa melakukan serangan combo dengan mengetuk layar beberapa kali.
-
Swipe layar di sebelah kanan untuk melakukan serangan berat. Serangan ini lebih kuat tapi lebih lambat dan bisa diblok oleh lawan.
-
Tap layar di sebelah kiri untuk melakukan blok. Blok bisa mengurangi kerusakan yang kamu terima dari serangan lawan.
-
Swipe layar di sebelah kiri untuk melakukan esquive. Esquive bisa menghindari serangan lawan sepenuhnya, tapi membutuhkan waktu yang tepat.
-
Swipe layar di sebelah kanan dan tahan untuk melakukan parry. Parry bisa memblok serangan lawan dan membuat mereka terpukul, memberi kamu kesempatan untuk menyerang balik.
-
Tap ikon serangan khusus di bagian bawah layar untuk melakukan serangan khusus. Serangan khusus adalah serangan yang sangat kuat dan unik untuk setiap karakter. Kamu bisa mengisi meter serangan khusus dengan melakukan serangan normal atau menerima kerusakan.
-
-
The tips and tricks to master the game and win battles
-
Marvel Contest of Champions adalah game yang menguji keterampilan dan pengetahuan kamu tentang karakter-karakter Marvel. Berikut adalah beberapa tips dan trik yang bisa kamu gunakan untuk meningkatkan kemampuan kamu dan memenangkan pertempuran:
-
-
Pilih tim yang sesuai dengan gaya bermain kamu. Setiap karakter memiliki kelas, atribut, kekuatan, kelemahan, dan bonus sinergi yang berbeda-beda. Kamu harus mempelajari karakter-karakter yang kamu miliki dan memilih tim yang seimbang dan efektif.
-
Tingkatkan karakter-karakter kamu secara rutin. Kamu bisa menggunakan item-item seperti ISO-8, catalyst, gold, dan signature stone untuk meningkatkan level, rank, tier, dan signature ability karakter-karakter kamu. Karakter-karakter yang lebih kuat akan membantu kamu menghadapi lawan-lawan yang lebih sulit.
-
Gunakan strategi yang tepat untuk setiap lawan. Kamu harus memperhatikan kelas, kekuatan, kelemahan, dan pola serangan lawan-lawan kamu. Kamu harus menyesuaikan gerakan-gerakan kamu dengan situasi dan kondisi pertempuran. Kamu juga harus memanfaatkan item-item seperti potion, revive, boost, dan synergy team untuk mendapatkan keuntungan.
-
Bergabunglah dengan aliansi yang aktif dan komunikatif. Aliansi adalah kelompok pemain yang bisa berkolaborasi, berstrategi, dan berkompetisi bersama. Bergabung dengan aliansi akan memberi kamu akses ke fitur-fitur seperti alliance quest, alliance war, alliance help, alliance chat, dan alliance store. Kamu juga bisa mendapatkan hadiah-hadiah berharga dari aliansi kamu.
-
Jadilah pemain yang sportif dan sopan. Marvel Contest of Champions adalah game yang menyenangkan dan menghibur, tapi juga menantang dan kompetitif. Kamu harus menghormati pemain lain, baik teman maupun lawan. Kamu harus mengikuti aturan-aturan yang berlaku dan tidak melakukan kecurangan atau penyalahgunaan. Kamu juga harus memberikan feedback yang konstruktif dan positif kepada pengembang game.
-
-
Conclusion
-
A summary of the main points and a call to action
-
Marvel Contest of Champions adalah game fighting yang wajib kamu coba jika kamu adalah penggemar Marvel Comics. Game ini menawarkan gameplay yang seru dan spektakuler dengan karakter-karakter Marvel yang beragam dan menarik. Kamu bisa mengunduh game ini secara gratis di perangkat Android atau iOS kamu dengan mengikuti langkah-langkah yang sudah kami jelaskan di atas. Kamu juga bisa belajar cara bermain game ini dengan mudah dan cepat dengan mengikuti tips dan trik yang sudah kami berikan di atas. Jadi, tunggu apa lagi? Segera download dan mainkan Marvel Contest of Champions sekarang juga dan rasakan sensasi menjadi juara di kontes Marvel. Selamat bermain!
-
FAQs
-
Q1. Is Marvel Contest of Champions free to play?
-
A1. Yes, Marvel Contest of Champions is free to play. You can download and play the game without spending any money. However, the game also offers some optional in-app purchases that can enhance your gaming experience. You can buy items such as crystals, units, bundles, and subscriptions with real money. You can also disable the in-app purchases feature in your device settings if you want.
-
Q2. What are the best champions in Marvel Contest of Champions?
-
A2. There is no definitive answer to this question, as the best champions may vary depending on your preferences, play style, and game mode. However, some of the most popular and powerful champions in the game are Doctor Doom, Ghost, Corvus Glaive, Quake, Nick Fury, Captain America (Infinity War), Archangel, and Hyperion. You can also check the online tier lists and rankings to see the opinions of other players and experts.
-
Q3. How can I get more crystals and units in Marvel Contest of Champions?
-
A3. Crystals and units are two of the most valuable resources in Marvel Contest of Champions. You can use them to unlock new champions, upgrade your existing ones, and buy various items. There are several ways to get more crystals and units in the game, such as:
-
-
Completing quests and events. You can earn different types of crystals and units by finishing the story mode, alliance quests, alliance wars, arena battles, incursions, battlegrounds, and special events.
-
Claiming daily and weekly rewards. You can get free crystals and units by logging in to the game every day and every week.
-
Opening free crystals. You can get free crystals every four hours and every 24 hours by tapping the crystal icon on the home screen.
-
Joining an alliance. You can get alliance crystals and units by participating in alliance activities and helping your alliance members.
-
Spending real money. You can buy crystals and units with real money by tapping the store icon on the home screen.
-
-
Q4. How can I join an alliance in Marvel Contest of Champions?
-
A4. Joining an alliance is one of the best ways to enjoy Marvel Contest of Champions. You can join an alliance by following these steps:
-
-
Tap the alliance icon on the home screen.
-
Tap the join or create alliance button.
-
Choose whether you want to join an existing alliance or create your own alliance.
-
If you want to join an existing alliance, you can browse the list of recommended alliances or search for a specific alliance by name or tag.
-
If you want to create your own alliance, you can choose a name, a tag, a description, a logo, and a language for your alliance.
-
Tap the join or create button to confirm your choice.
-
-
Q5. How can I contact the support team of Marvel Contest of Champions?
-
A5. If you have any questions, issues, or feedback regarding Marvel Contest of Champions, you can contact the support team by following these steps:
-
-
Tap the gear icon on the home screen to open the settings menu.
-
Tap the support button to open the support page.
-
Choose whether you want to visit the help center or submit a ticket.
-
If you want to visit the help center, you can browse the articles and FAQs that may answer your queries.
-
If you want to submit a ticket, you can fill out a form with your details and your message.
-
Tap the send button to submit your ticket.
- 401be4b1e0
-
-
\ No newline at end of file
diff --git a/spaces/feregVcuzo/sanity-test-midi/checkpoint/Download Real Football APK and Join Millions of Fans Worldwide.md b/spaces/feregVcuzo/sanity-test-midi/checkpoint/Download Real Football APK and Join Millions of Fans Worldwide.md
deleted file mode 100644
index 99a7733d88eb12e09e6134c2e9ca2e1c44844a38..0000000000000000000000000000000000000000
--- a/spaces/feregVcuzo/sanity-test-midi/checkpoint/Download Real Football APK and Join Millions of Fans Worldwide.md
+++ /dev/null
@@ -1,127 +0,0 @@
-
-
Real Football Download APK: A Guide for Soccer Fans
-
If you are a soccer fan, you might have heard of Real Football, a mobile game developed and published by Gameloft. Real Football is a realistic and immersive soccer simulation game that lets you experience soccer both on and off the pitch. You can build your dream team, upgrade your facilities, challenge other players online, and enjoy stunning 3D graphics and animations. In this article, we will tell you everything you need to know about Real Football download apk, including its features, modes, tips, reviews, and FAQs.
-
What are the main features of Real Football game?
-
Real Football game has many features that make it one of the best soccer games on mobile devices. Here are some of them:
3D stadiums: You can play in realistic 3D stadiums where polished shadows, detailed textures, and spectators all come together to provide an exciting atmosphere.
-
Multiple camera views: You can enjoy multiple camera views during cutscenes and set pieces for a richer broadcast and first-person sensation.
-
Improved opponents and positioning: You can face smarter players who make for a more realistic and challenging experience.
-
Dream team: You can build your dream team by recruiting star players through the lottery. You can also enhance your players' abilities by acquiring skill items through the lottery and matches.
-
Team facilities: You can upgrade your team facilities including Stadiums, Hospitals, Physiotherapy Centers and a Youth Camp.
-
PvP World Arena mode: You can challenge other players in asynchronous PvP World Arena mode and climb the leaderboards.
-
-
What are the different game modes available in Real Football?
-
Real Football game offers various game modes to suit your preferences and skills. Here are some of them:
-
-
Career mode: You can play as a manager and lead your team to glory in various tournaments and leagues. You can also customize your team name, logo, jersey, and players.
-
Friendly mode: You can play a quick match against any team of your choice. You can also adjust the difficulty level, match duration, weather, and other settings.
-
Cup mode: You can participate in various cup competitions such as the World Cup, the European Championship, the Copa America, and more. You can also create your own custom cup with your own rules and teams.
-
Training mode: You can practice your skills and tactics in various training drills such as dribbling, passing, shooting, defending, and more.
-
-
How to play Real Football better and win more matches?
-
If you want to improve your performance and win more matches in Real Football game, here are some tips that might help you:
-
-
Use the right controls: You can choose between two types of controls: virtual buttons or gestures. Virtual buttons are more precise and responsive, while gestures are more intuitive and fluid. You can also customize the size and position of the buttons according to your preference.
-
Use the right tactics: You can choose between different formations, strategies, and styles for your team. You can also adjust the roles and positions of your players according to their strengths and weaknesses. For example, you can use a 4-4-2 formation with a defensive style for a balanced approach, or a 4-3-3 formation with an attacking style for a more aggressive approach.
-
Use the right skills: You can use various skills to outsmart your opponents and create chances. For example, you can use sprint to run faster, dribble to evade defenders, pass to find teammates, shoot to score goals, tackle to dispossess opponents, slide to block shots, switch to change players, and more.
-
Use the right items: You can use various items to enhance your players' abilities and skills. For example, you can use boots to increase speed, gloves to improve handling, kits to boost stamina, balls to improve shooting, and more. You can also use skill items to perform special moves such as curve shots, bicycle kicks, long passes, and more.
-
-
What are some of the user reviews of Real Football game?
-
Real Football game has received mostly positive reviews from users who have downloaded and played it. Here are some of the user reviews from Google Play Store:
-
-
-
User
-
Rating
-
Review
-
-
-
John Smith
-
5 stars
-
This game is awesome. The graphics are amazing and the gameplay is smooth and realistic. I love the different modes and the online features. I recommend this game to all soccer fans.
-
-
-
Jane Doe
-
4 stars
-
I like this game a lot. It has a lot of features and options to customize your team and players. The only thing I don't like is that it takes too long to load sometimes and it crashes occasionally. Please fix these issues.
-
-
-
Bob Lee
-
3 stars
-
This game is good but not great. It has some nice graphics and animations but the controls are not very responsive and the AI is not very smart. It also has some bugs and glitches that need to be fixed.
-
-
-
Alice Cooper
-
2 stars
-
This game is disappointing. It has poor graphics and sound quality and the gameplay is boring and repetitive. It also has a lot of ads and in-app purchases that ruin the experience. I don't recommend this game.
-
-
-
Tom Cruise
-
1 star
-
This game is terrible. It doesn't work at all on my device. It always freezes and crashes and I can't even play it. It also has a lot of viruses and malware that damage my device. I hate this game.
-
-
-
Conclusion: Why download Real Football apk?
-
In conclusion, Real Football download apk is a great option for soccer fans who want to enjoy a realistic and immersive soccer simulation game on their mobile devices. Real Football game has many features, modes, tips, and reviews that make it one of the best soccer games on the market. You can download Real Football apk from various sources such as Google Play Store, APKPure, APKMirror, and more. However, you should always be careful and check the authenticity and security of the apk file before downloading it. You should also make sure that your device meets the minimum requirements for running the game smoothly.
-
If you are ready to download Real Football apk and start playing, click on the link below and follow the instructions:
Frequently Asked Questions (FAQs) about Real Football game
-
Here are some of the most common questions that users have about Real Football game:
-
Q: How much space does Real Football game require on my device?
-
A: Real Football game requires about 500 MB of free space on your device.
-
real football apk free download
-download real football 2023 apk
-real football mod apk download
-real football game download apk
-real football 2022 apk download
-download real football offline apk
-real football 2021 apk download
-real football hack apk download
-real football 2020 apk download
-download real football 2019 apk
-real football 2018 apk download
-real football unlimited money apk download
-real football 2017 apk download
-real football latest version apk download
-real football 2016 apk download
-real football 2015 apk download
-real football old version apk download
-real football 2014 apk download
-real football 2013 apk download
-real football 2012 apk download
-real football 2011 apk download
-real football 2010 apk download
-real football 2009 apk download
-real football 2008 apk download
-real football 2007 apk download
-gameloft real football apk download
-real soccer (football) apk download
-real world soccer league: football worldcup 2021 apk download
-dream league soccer - classic (real soccer) apk download
-ultimate soccer - football (real soccer) apk download
-soccer star 2021 top leagues: play the best soccer game (real soccer) apk download
-score! hero (real soccer) apk download
-pes club manager (real soccer) apk download
-fifa mobile soccer (real soccer) apk download
-pes 2021 pro evolution soccer (real soccer) apk download
-fifa 16 ultimate team (real soccer) apk download
-pes 2012 pro evolution soccer (real soccer) apk download
-fifa 14 by ea sports™ (real soccer) apk download
-pes 2011 pro evolution soccer (real soccer) apk download
-fifa 12 by ea sports™ (real soccer) apk download
-pes 2010 pro evolution soccer (real soccer) apk download
-fifa 10 by ea sports™ (real soccer) apk download
-pes 2009 pro evolution soccer (real soccer) apk download
-fifa 09 by ea sports™ (real soccer) apk download
-pes 2008 pro evolution soccer (real soccer) apk download
-
Q: What are the minimum requirements for running Real Football game on my device?
-
A: Real Football game requires Android 4.1 or higher and at least 1 GB of RAM.
-
Q: How can I update Real Football game to the latest version?
-
A: You can update Real Football game by downloading the latest apk file from the same source that you downloaded it from or by checking for updates in the game settings.
-
Q: How can I contact the developers of Real Football game for feedback or support?
-
A: You can contact the developers of Real Football game by sending an email to support@gameloft.com or by visiting their official website at www.gameloft.com.
-
Q: How can I play Real Football game offline?
-
A: You can play Real Football game offline by turning off your internet connection before launching the game. However, you will not be able to access some features such as online matches, leaderboards, achievements, etc.
401be4b1e0
-
-
\ No newline at end of file
diff --git a/spaces/feregVcuzo/sanity-test-midi/checkpoint/Download True Love For Her APK and Find Your Soulmate in this Dating Simulation.md b/spaces/feregVcuzo/sanity-test-midi/checkpoint/Download True Love For Her APK and Find Your Soulmate in this Dating Simulation.md
deleted file mode 100644
index 8cdfb789417f74cc0fbfbcacb68edb1cdfb92388..0000000000000000000000000000000000000000
--- a/spaces/feregVcuzo/sanity-test-midi/checkpoint/Download True Love For Her APK and Find Your Soulmate in this Dating Simulation.md
+++ /dev/null
@@ -1,101 +0,0 @@
-
-
True Love for Her APK Download: A Romantic Game for Android Users
-
Are you looking for a romantic game that will keep you hooked for hours? Do you want to experience a thrilling story of love, passion, and madness? If yes, then you should try True Love for Her APK, a fan-made game based on the popular Yandere Simulator. In this game, you will play as Ayano Aishi, a girl who is obsessed with her crush, Taro Yamada. You will do anything to make him yours, even if it means eliminating your rivals in the most brutal ways. But be careful, your actions will have consequences and affect the outcome of the game. Read on to find out more about this game and how to download it on your Android device.
True Love for Her is a game created by Ayano-Dev, a fan of Yandere Simulator, a stealth action game that revolves around a yandere girl who stalks and kills her love interest's admirers. True Love for Her is inspired by Yandere Simulator, but it has its own original story, characters, and features. Here are some of the aspects of True Love for Her that make it an interesting game to play.
-
A fan-made game based on Yandere Simulator
-
True Love for Her is not an official game by YandereDev, the developer of Yandere Simulator. It is a fan-made game that uses some of the assets and mechanics from Yandere Simulator, but it also adds new elements and twists to the original game. For example, True Love for Her has different rivals, locations, events, and endings than Yandere Simulator. It also has more romance and drama than the original game. True Love for Her is a tribute to Yandere Simulator, but it is also a unique game that stands on its own.
-
A story of obsession, jealousy, and murder
-
True Love for Her follows the story of Ayano Aishi, a girl who suffers from a condition that makes her unable to feel emotions. She only feels alive when she is near her crush, Taro Yamada, whom she calls Senpai. She believes that he is her true love and that they are destined to be together. However, she faces many obstacles in her way, such as other girls who are interested in Senpai. She decides to eliminate them one by one using various methods, such as poisoning, kidnapping, blackmailing, or stabbing. She also has to deal with other threats, such as the police, the school council, or Senpai himself. Will she be able to win Senpai's heart without getting caught or losing her sanity?
-
A game with multiple endings and choices
-
True Love for Her is not a linear game that has only one outcome. It is a game that has multiple endings and choices that affect the story and the gameplay. Depending on your actions and decisions, you can get different results and consequences. For example, you can choose to be stealthy or aggressive when eliminating your rivals. You can also choose to be friendly or hostile when interacting with other characters. You can also choose to confess your love to Senpai or keep it a secret until the end. Each choice will have an impact on how Senpai and others perceive you and how the game ends. There are many possible endings in True Love for Her, ranging from happy to tragic, from romantic to horrific. You can replay the game multiple times to see different outcomes and discover new secrets.
-
true love for her android game
-true love for her yandere simulator fan game
-true love for her apk latest version
-true love for her apk combo download
-true love for her new update youtube
-true love for her pc and android test build
-true love for her ayano dev game
-true love for her discord server link
-true love for her download page link
-true love for her mobile app game
-true love for her romantic quotes
-true love for her love calculator
-true love for her dating chat flirt
-true love for her real love test
-true love for her eharmony app
-true love for her delicious gamehouse
-true love for her bloom dating app
-true love for her hey love adam texting game
-true love for her quotes and sayings
-true love for her messages sms
-true love for her tester viralappspro
-true love for her smart apps pro
-true love for her happy verse entertainment
-true love for her kode makers app
-true love for her share and enjoy app
-true love for her peafowl apps social
-true love for her style photo studio apps
-true love for her zaran dev social
-true love for her piapps social
-true love for her gv apps entertainment
-true love for her manjul saini lifestyle
-true love for her winkle studio education
-true love for her lv apps studio entertainment
-true love for her only tools entertainment
-true love for her weloveapps dating
-true love for her nutnut simulation
-true love for her zeekoapps entertainment
-true love for her mobilplug dating
-true love for her deep messages gv apps
-true love for her solar core wikipedia
-true love for her montana sun fact sheet
-true love for her cornell sun layers
-true love for her nasa sun fact sheet
-true love for her yahoo nuclear fusion breakthrough
-true love for her the sun holy grail fusion experiments
-true love for her new scientist korean nuclear fusion reactor
-true love for her the i cricket world cup
-true love for her ndtv shubman gill
-true love for her indian express ind vs aus 3rd odi
-
How to download and install True Love for Her APK?
-
If you are interested in playing True Love for Her, you will need to download and install the APK file on your Android device. APK stands for Android Package Kit, and it is a file format that allows you to install applications that are not available on the Google Play Store. Here are the steps that you need to follow to download and install True Love for Her APK on your device.
-
Download the APK file from the official website
-
The first step is to download the APK file from the official website of True Love for Her. You can visit the website by clicking [here]. On the website, you will find a download button that will direct you to a secure link where you can download the APK file. The file size is about 200 MB, so make sure you have enough space on your device and a stable internet connection.
-
Enable unknown sources on your device settings
-
The second step is to enable unknown sources on your device settings. This will allow you to install applications that are not from the Google Play Store. To do this, go to your device settings and look for the security or privacy option. Then, find the unknown sources option and toggle it on. You may see a warning message that says installing apps from unknown sources may harm your device, but don't worry, True Love for Her APK is safe and virus-free.
-
Install the APK file and enjoy the game
-
The third and final step is to install the APK file and enjoy the game. To do this, locate the downloaded APK file on your device storage and tap on it. You may see a pop-up message that asks for your permission to install the app, just tap on install and wait for the process to finish. Once the installation is done, you can open the app and start playing True Love for Her. Have fun!
-
What are the features of True Love for Her APK?
-
True Love for Her APK is not just a simple game that you can play on your Android device. It is a game that has many features that make it more enjoyable and immersive. Here are some of the features that you can expect from True Love for Her APK.
-
High-quality graphics and sound effects
-
True Love for Her APK has high-quality graphics and sound effects that create a realistic and captivating atmosphere. The game has detailed and colorful graphics that show the characters, the environments, and the actions in a clear and vivid way. The game also has sound effects that match the mood and tone of the game, such as romantic music, creepy noises, or dramatic sounds. The game also has voice acting for some of the characters, which adds more personality and emotion to them.
-
Interactive gameplay and dialogue options
-
True Love for Her APK has interactive gameplay and dialogue options that make you feel like you are part of the story. The game has gameplay mechanics that allow you to control Ayano's actions, such as walking, running, crouching, attacking, or interacting with objects. The game also has dialogue options that allow you to choose what Ayano says or does in certain situations, such as talking to Senpai, confronting rivals, or making decisions. The game also has mini-games that test your skills and reflexes, such as stealth mode, combat mode, or puzzle mode.
-
Different modes and difficulty levels
-
True Love for Her APK has different modes and difficulty levels that offer different challenges and experiences. The game has two main modes: story mode and sandbox mode. Story mode is where you follow Ayano's story and try to get one of the endings. Sandbox mode is where you can explore the school and do whatever you want without any restrictions or consequences. The game also has three difficulty levels: easy, normal, and hard. Each difficulty level affects how easy or hard it is to eliminate rivals, avoid detection, or complete tasks.
-
Customizable characters and outfits
-
True Love for Her APK has customizable characters and outfits that allow you to personalize your appearance and style. The game has a character creator feature that allows you to change Ayano's hair color, eye color, skin tone, facial features, or accessories. The game also has an outfit selector feature that allows you to change Ayano's clothes, shoes, or accessories. You can choose from various outfits that suit different occasions, such as school uniform, casual wear, formal wear, or cosplay.
-
What are the pros and cons of True Love for Her APK?
-
True Love for Her APK is a game that has many pros and cons that you should consider before playing it. Here are some of the advantages and disadvantages of True Love for Her APK.
-
Pros: Free, fun, and addictive game
-
One of the pros of True Love for Her APK is that it is a free, fun, and addictive game that you can enjoy on your Android device. You don't have to pay anything to download or play the game, and you can access all the features and content without any limitations or ads. The game is also fun and addictive, as it offers a captivating story, engaging gameplay, and multiple endings that will keep you hooked for hours. You will never get bored of playing True Love for Her APK, as there is always something new to discover or try.
-
Cons: Mature content, violence, and bugs
-
One of the cons of True Love for Her APK is that it has mature content, violence, and bugs that may not be suitable for everyone. The game has mature content that involves themes such as obsession, jealousy, murder, suicide, and gore. The game also has violence that shows graphic scenes of blood, torture, and death. The game also has bugs that may cause crashes, glitches, or errors. The game is not recommended for children or sensitive people, and it may require parental guidance or discretion.
-
Conclusion
-
True Love for Her APK is a romantic game for Android users that is based on Yandere Simulator. It is a game that tells the story of Ayano Aishi, a girl who is obsessed with her crush, Taro Yamada. She will do anything to make him hers, even if it means killing her rivals in the most brutal ways. The game has multiple endings and choices that affect the story and the gameplay. The game also has many features that make it more enjoyable and immersive, such as high-quality graphics and sound effects, interactive gameplay and dialogue options, different modes and difficulty levels, and customizable characters and outfits. The game also has pros and cons that you should consider before playing it, such as being free, fun, and addictive, but also having mature content, violence, and bugs. If you are looking for a romantic game that will keep you hooked for hours, you should try True Love for Her APK.
-
FAQs
-
Here are some of the frequently asked questions about True Love for Her APK.
-
Q: Is True Love for Her APK safe to download?
-
A: Yes, True Love for Her APK is safe to download from the official website. It does not contain any viruses or malware that can harm your device or data. However, you should always be careful when downloading apps from unknown sources and scan them with an antivirus before installing them.
-
Q: Is True Love for Her APK compatible with my device?
-
A: True Love for Her APK is compatible with most Android devices that have Android 4.4 or higher. However, some devices may not support the game or run it smoothly due to different specifications or performance issues. You can check the compatibility of your device by visiting the official website or contacting the developer.
-
Q: How can I update True Love for Her APK?
-
A: You can update True Love for Her APK by visiting the official website and downloading the latest version of the APK file. You can also follow the developer on social media or join their Discord server to get notified about new updates or features.
-
Q: How can I contact the developer of True Love for Her APK?
-
A: You can contact the developer of True Love for Her APK by visiting their website or social media accounts. You can also join their Discord server or email them at ayano.dev@gmail.com. You can give them feedback, suggestions, bug reports, or fan art.
-
Q: Where can I find more information about True Love for Her APK?
-
A: You can find more information about True Love for Her APK by visiting their website or social media accounts. You can also watch gameplay videos or reviews on YouTube or read articles or blogs on the internet.
401be4b1e0
-
-
\ No newline at end of file
diff --git a/spaces/fffiloni/audioldm-text-to-audio-generation-copy/audioldm/clap/training/zero_shot.py b/spaces/fffiloni/audioldm-text-to-audio-generation-copy/audioldm/clap/training/zero_shot.py
deleted file mode 100644
index 28b8fccc1af17fc69002857a7f529ac041c374f2..0000000000000000000000000000000000000000
--- a/spaces/fffiloni/audioldm-text-to-audio-generation-copy/audioldm/clap/training/zero_shot.py
+++ /dev/null
@@ -1,95 +0,0 @@
-# NOTE: This script is currently not supported for CLAP.
-import logging
-from contextlib import suppress
-
-import torch
-import torch.nn.functional as F
-from tqdm import tqdm
-
-from open_clip import tokenize
-from .imagenet_zeroshot_data import imagenet_classnames, openai_imagenet_template
-
-
-def zero_shot_classifier(model, classnames, templates, args):
- with torch.no_grad():
- zeroshot_weights = []
- for classname in tqdm(classnames):
- texts = [template(classname) for template in templates] # format with class
- texts = tokenize(texts).to(args.device) # tokenize
- if args.distributed and not args.horovod:
- class_embeddings = model.module.encode_text(texts)
- else:
- class_embeddings = model.encode_text(texts)
- class_embedding = F.normalize(class_embeddings, dim=-1).mean(dim=0)
- class_embedding /= class_embedding.norm()
- zeroshot_weights.append(class_embedding)
- zeroshot_weights = torch.stack(zeroshot_weights, dim=1).to(args.device)
- return zeroshot_weights
-
-
-def accuracy(output, target, topk=(1,)):
- pred = output.topk(max(topk), 1, True, True)[1].t()
- correct = pred.eq(target.view(1, -1).expand_as(pred))
- return [
- float(correct[:k].reshape(-1).float().sum(0, keepdim=True).cpu().numpy())
- for k in topk
- ]
-
-
-def run(model, classifier, dataloader, args):
- autocast = torch.cuda.amp.autocast if args.precision == "amp" else suppress
- with torch.no_grad():
- top1, top5, n = 0.0, 0.0, 0.0
- for images, target in tqdm(dataloader, unit_scale=args.batch_size):
- images = images.to(args.device)
- target = target.to(args.device)
-
- with autocast():
- # predict
- if args.distributed and not args.horovod:
- image_features = model.module.encode_image(images)
- else:
- image_features = model.encode_image(images)
- image_features = F.normalize(image_features, dim=-1)
- logits = 100.0 * image_features @ classifier
-
- # measure accuracy
- acc1, acc5 = accuracy(logits, target, topk=(1, 5))
- top1 += acc1
- top5 += acc5
- n += images.size(0)
-
- top1 = top1 / n
- top5 = top5 / n
- return top1, top5
-
-
-def zero_shot_eval(model, data, epoch, args):
- if "imagenet-val" not in data and "imagenet-v2" not in data:
- return {}
- if args.zeroshot_frequency == 0:
- return {}
- if (epoch % args.zeroshot_frequency) != 0 and epoch != args.epochs:
- return {}
-
- logging.info("Starting zero-shot imagenet.")
-
- logging.info("Building zero-shot classifier")
- classifier = zero_shot_classifier(
- model, imagenet_classnames, openai_imagenet_template, args
- )
-
- logging.info("Using classifier")
- results = {}
- if "imagenet-val" in data:
- top1, top5 = run(model, classifier, data["imagenet-val"].dataloader, args)
- results["imagenet-zeroshot-val-top1"] = top1
- results["imagenet-zeroshot-val-top5"] = top5
- if "imagenet-v2" in data:
- top1, top5 = run(model, classifier, data["imagenet-v2"].dataloader, args)
- results["imagenetv2-zeroshot-val-top1"] = top1
- results["imagenetv2-zeroshot-val-top5"] = top5
-
- logging.info("Finished zero-shot imagenet.")
-
- return results
diff --git a/spaces/fffiloni/controlnet-animation-doodle/node_modules/@types/node/ts4.8/http2.d.ts b/spaces/fffiloni/controlnet-animation-doodle/node_modules/@types/node/ts4.8/http2.d.ts
deleted file mode 100644
index 0e3682609f32c1783ba84ea2331f7197526a1cc9..0000000000000000000000000000000000000000
--- a/spaces/fffiloni/controlnet-animation-doodle/node_modules/@types/node/ts4.8/http2.d.ts
+++ /dev/null
@@ -1,2134 +0,0 @@
-/**
- * The `http2` module provides an implementation of the [HTTP/2](https://tools.ietf.org/html/rfc7540) protocol. It
- * can be accessed using:
- *
- * ```js
- * const http2 = require('http2');
- * ```
- * @since v8.4.0
- * @see [source](https://github.com/nodejs/node/blob/v18.0.0/lib/http2.js)
- */
-declare module 'http2' {
- import EventEmitter = require('node:events');
- import * as fs from 'node:fs';
- import * as net from 'node:net';
- import * as stream from 'node:stream';
- import * as tls from 'node:tls';
- import * as url from 'node:url';
- import { IncomingHttpHeaders as Http1IncomingHttpHeaders, OutgoingHttpHeaders, IncomingMessage, ServerResponse } from 'node:http';
- export { OutgoingHttpHeaders } from 'node:http';
- export interface IncomingHttpStatusHeader {
- ':status'?: number | undefined;
- }
- export interface IncomingHttpHeaders extends Http1IncomingHttpHeaders {
- ':path'?: string | undefined;
- ':method'?: string | undefined;
- ':authority'?: string | undefined;
- ':scheme'?: string | undefined;
- }
- // Http2Stream
- export interface StreamPriorityOptions {
- exclusive?: boolean | undefined;
- parent?: number | undefined;
- weight?: number | undefined;
- silent?: boolean | undefined;
- }
- export interface StreamState {
- localWindowSize?: number | undefined;
- state?: number | undefined;
- localClose?: number | undefined;
- remoteClose?: number | undefined;
- sumDependencyWeight?: number | undefined;
- weight?: number | undefined;
- }
- export interface ServerStreamResponseOptions {
- endStream?: boolean | undefined;
- waitForTrailers?: boolean | undefined;
- }
- export interface StatOptions {
- offset: number;
- length: number;
- }
- export interface ServerStreamFileResponseOptions {
- statCheck?(stats: fs.Stats, headers: OutgoingHttpHeaders, statOptions: StatOptions): void | boolean;
- waitForTrailers?: boolean | undefined;
- offset?: number | undefined;
- length?: number | undefined;
- }
- export interface ServerStreamFileResponseOptionsWithError extends ServerStreamFileResponseOptions {
- onError?(err: NodeJS.ErrnoException): void;
- }
- export interface Http2Stream extends stream.Duplex {
- /**
- * Set to `true` if the `Http2Stream` instance was aborted abnormally. When set,
- * the `'aborted'` event will have been emitted.
- * @since v8.4.0
- */
- readonly aborted: boolean;
- /**
- * This property shows the number of characters currently buffered to be written.
- * See `net.Socket.bufferSize` for details.
- * @since v11.2.0, v10.16.0
- */
- readonly bufferSize: number;
- /**
- * Set to `true` if the `Http2Stream` instance has been closed.
- * @since v9.4.0
- */
- readonly closed: boolean;
- /**
- * Set to `true` if the `Http2Stream` instance has been destroyed and is no longer
- * usable.
- * @since v8.4.0
- */
- readonly destroyed: boolean;
- /**
- * Set to `true` if the `END_STREAM` flag was set in the request or response
- * HEADERS frame received, indicating that no additional data should be received
- * and the readable side of the `Http2Stream` will be closed.
- * @since v10.11.0
- */
- readonly endAfterHeaders: boolean;
- /**
- * The numeric stream identifier of this `Http2Stream` instance. Set to `undefined`if the stream identifier has not yet been assigned.
- * @since v8.4.0
- */
- readonly id?: number | undefined;
- /**
- * Set to `true` if the `Http2Stream` instance has not yet been assigned a
- * numeric stream identifier.
- * @since v9.4.0
- */
- readonly pending: boolean;
- /**
- * Set to the `RST_STREAM` `error code` reported when the `Http2Stream` is
- * destroyed after either receiving an `RST_STREAM` frame from the connected peer,
- * calling `http2stream.close()`, or `http2stream.destroy()`. Will be`undefined` if the `Http2Stream` has not been closed.
- * @since v8.4.0
- */
- readonly rstCode: number;
- /**
- * An object containing the outbound headers sent for this `Http2Stream`.
- * @since v9.5.0
- */
- readonly sentHeaders: OutgoingHttpHeaders;
- /**
- * An array of objects containing the outbound informational (additional) headers
- * sent for this `Http2Stream`.
- * @since v9.5.0
- */
- readonly sentInfoHeaders?: OutgoingHttpHeaders[] | undefined;
- /**
- * An object containing the outbound trailers sent for this `HttpStream`.
- * @since v9.5.0
- */
- readonly sentTrailers?: OutgoingHttpHeaders | undefined;
- /**
- * A reference to the `Http2Session` instance that owns this `Http2Stream`. The
- * value will be `undefined` after the `Http2Stream` instance is destroyed.
- * @since v8.4.0
- */
- readonly session: Http2Session;
- /**
- * Provides miscellaneous information about the current state of the`Http2Stream`.
- *
- * A current state of this `Http2Stream`.
- * @since v8.4.0
- */
- readonly state: StreamState;
- /**
- * Closes the `Http2Stream` instance by sending an `RST_STREAM` frame to the
- * connected HTTP/2 peer.
- * @since v8.4.0
- * @param [code=http2.constants.NGHTTP2_NO_ERROR] Unsigned 32-bit integer identifying the error code.
- * @param callback An optional function registered to listen for the `'close'` event.
- */
- close(code?: number, callback?: () => void): void;
- /**
- * Updates the priority for this `Http2Stream` instance.
- * @since v8.4.0
- */
- priority(options: StreamPriorityOptions): void;
- /**
- * ```js
- * const http2 = require('http2');
- * const client = http2.connect('http://example.org:8000');
- * const { NGHTTP2_CANCEL } = http2.constants;
- * const req = client.request({ ':path': '/' });
- *
- * // Cancel the stream if there's no activity after 5 seconds
- * req.setTimeout(5000, () => req.close(NGHTTP2_CANCEL));
- * ```
- * @since v8.4.0
- */
- setTimeout(msecs: number, callback?: () => void): void;
- /**
- * Sends a trailing `HEADERS` frame to the connected HTTP/2 peer. This method
- * will cause the `Http2Stream` to be immediately closed and must only be
- * called after the `'wantTrailers'` event has been emitted. When sending a
- * request or sending a response, the `options.waitForTrailers` option must be set
- * in order to keep the `Http2Stream` open after the final `DATA` frame so that
- * trailers can be sent.
- *
- * ```js
- * const http2 = require('http2');
- * const server = http2.createServer();
- * server.on('stream', (stream) => {
- * stream.respond(undefined, { waitForTrailers: true });
- * stream.on('wantTrailers', () => {
- * stream.sendTrailers({ xyz: 'abc' });
- * });
- * stream.end('Hello World');
- * });
- * ```
- *
- * The HTTP/1 specification forbids trailers from containing HTTP/2 pseudo-header
- * fields (e.g. `':method'`, `':path'`, etc).
- * @since v10.0.0
- */
- sendTrailers(headers: OutgoingHttpHeaders): void;
- addListener(event: 'aborted', listener: () => void): this;
- addListener(event: 'close', listener: () => void): this;
- addListener(event: 'data', listener: (chunk: Buffer | string) => void): this;
- addListener(event: 'drain', listener: () => void): this;
- addListener(event: 'end', listener: () => void): this;
- addListener(event: 'error', listener: (err: Error) => void): this;
- addListener(event: 'finish', listener: () => void): this;
- addListener(event: 'frameError', listener: (frameType: number, errorCode: number) => void): this;
- addListener(event: 'pipe', listener: (src: stream.Readable) => void): this;
- addListener(event: 'unpipe', listener: (src: stream.Readable) => void): this;
- addListener(event: 'streamClosed', listener: (code: number) => void): this;
- addListener(event: 'timeout', listener: () => void): this;
- addListener(event: 'trailers', listener: (trailers: IncomingHttpHeaders, flags: number) => void): this;
- addListener(event: 'wantTrailers', listener: () => void): this;
- addListener(event: string | symbol, listener: (...args: any[]) => void): this;
- emit(event: 'aborted'): boolean;
- emit(event: 'close'): boolean;
- emit(event: 'data', chunk: Buffer | string): boolean;
- emit(event: 'drain'): boolean;
- emit(event: 'end'): boolean;
- emit(event: 'error', err: Error): boolean;
- emit(event: 'finish'): boolean;
- emit(event: 'frameError', frameType: number, errorCode: number): boolean;
- emit(event: 'pipe', src: stream.Readable): boolean;
- emit(event: 'unpipe', src: stream.Readable): boolean;
- emit(event: 'streamClosed', code: number): boolean;
- emit(event: 'timeout'): boolean;
- emit(event: 'trailers', trailers: IncomingHttpHeaders, flags: number): boolean;
- emit(event: 'wantTrailers'): boolean;
- emit(event: string | symbol, ...args: any[]): boolean;
- on(event: 'aborted', listener: () => void): this;
- on(event: 'close', listener: () => void): this;
- on(event: 'data', listener: (chunk: Buffer | string) => void): this;
- on(event: 'drain', listener: () => void): this;
- on(event: 'end', listener: () => void): this;
- on(event: 'error', listener: (err: Error) => void): this;
- on(event: 'finish', listener: () => void): this;
- on(event: 'frameError', listener: (frameType: number, errorCode: number) => void): this;
- on(event: 'pipe', listener: (src: stream.Readable) => void): this;
- on(event: 'unpipe', listener: (src: stream.Readable) => void): this;
- on(event: 'streamClosed', listener: (code: number) => void): this;
- on(event: 'timeout', listener: () => void): this;
- on(event: 'trailers', listener: (trailers: IncomingHttpHeaders, flags: number) => void): this;
- on(event: 'wantTrailers', listener: () => void): this;
- on(event: string | symbol, listener: (...args: any[]) => void): this;
- once(event: 'aborted', listener: () => void): this;
- once(event: 'close', listener: () => void): this;
- once(event: 'data', listener: (chunk: Buffer | string) => void): this;
- once(event: 'drain', listener: () => void): this;
- once(event: 'end', listener: () => void): this;
- once(event: 'error', listener: (err: Error) => void): this;
- once(event: 'finish', listener: () => void): this;
- once(event: 'frameError', listener: (frameType: number, errorCode: number) => void): this;
- once(event: 'pipe', listener: (src: stream.Readable) => void): this;
- once(event: 'unpipe', listener: (src: stream.Readable) => void): this;
- once(event: 'streamClosed', listener: (code: number) => void): this;
- once(event: 'timeout', listener: () => void): this;
- once(event: 'trailers', listener: (trailers: IncomingHttpHeaders, flags: number) => void): this;
- once(event: 'wantTrailers', listener: () => void): this;
- once(event: string | symbol, listener: (...args: any[]) => void): this;
- prependListener(event: 'aborted', listener: () => void): this;
- prependListener(event: 'close', listener: () => void): this;
- prependListener(event: 'data', listener: (chunk: Buffer | string) => void): this;
- prependListener(event: 'drain', listener: () => void): this;
- prependListener(event: 'end', listener: () => void): this;
- prependListener(event: 'error', listener: (err: Error) => void): this;
- prependListener(event: 'finish', listener: () => void): this;
- prependListener(event: 'frameError', listener: (frameType: number, errorCode: number) => void): this;
- prependListener(event: 'pipe', listener: (src: stream.Readable) => void): this;
- prependListener(event: 'unpipe', listener: (src: stream.Readable) => void): this;
- prependListener(event: 'streamClosed', listener: (code: number) => void): this;
- prependListener(event: 'timeout', listener: () => void): this;
- prependListener(event: 'trailers', listener: (trailers: IncomingHttpHeaders, flags: number) => void): this;
- prependListener(event: 'wantTrailers', listener: () => void): this;
- prependListener(event: string | symbol, listener: (...args: any[]) => void): this;
- prependOnceListener(event: 'aborted', listener: () => void): this;
- prependOnceListener(event: 'close', listener: () => void): this;
- prependOnceListener(event: 'data', listener: (chunk: Buffer | string) => void): this;
- prependOnceListener(event: 'drain', listener: () => void): this;
- prependOnceListener(event: 'end', listener: () => void): this;
- prependOnceListener(event: 'error', listener: (err: Error) => void): this;
- prependOnceListener(event: 'finish', listener: () => void): this;
- prependOnceListener(event: 'frameError', listener: (frameType: number, errorCode: number) => void): this;
- prependOnceListener(event: 'pipe', listener: (src: stream.Readable) => void): this;
- prependOnceListener(event: 'unpipe', listener: (src: stream.Readable) => void): this;
- prependOnceListener(event: 'streamClosed', listener: (code: number) => void): this;
- prependOnceListener(event: 'timeout', listener: () => void): this;
- prependOnceListener(event: 'trailers', listener: (trailers: IncomingHttpHeaders, flags: number) => void): this;
- prependOnceListener(event: 'wantTrailers', listener: () => void): this;
- prependOnceListener(event: string | symbol, listener: (...args: any[]) => void): this;
- }
- export interface ClientHttp2Stream extends Http2Stream {
- addListener(event: 'continue', listener: () => {}): this;
- addListener(event: 'headers', listener: (headers: IncomingHttpHeaders & IncomingHttpStatusHeader, flags: number) => void): this;
- addListener(event: 'push', listener: (headers: IncomingHttpHeaders, flags: number) => void): this;
- addListener(event: 'response', listener: (headers: IncomingHttpHeaders & IncomingHttpStatusHeader, flags: number) => void): this;
- addListener(event: string | symbol, listener: (...args: any[]) => void): this;
- emit(event: 'continue'): boolean;
- emit(event: 'headers', headers: IncomingHttpHeaders & IncomingHttpStatusHeader, flags: number): boolean;
- emit(event: 'push', headers: IncomingHttpHeaders, flags: number): boolean;
- emit(event: 'response', headers: IncomingHttpHeaders & IncomingHttpStatusHeader, flags: number): boolean;
- emit(event: string | symbol, ...args: any[]): boolean;
- on(event: 'continue', listener: () => {}): this;
- on(event: 'headers', listener: (headers: IncomingHttpHeaders & IncomingHttpStatusHeader, flags: number) => void): this;
- on(event: 'push', listener: (headers: IncomingHttpHeaders, flags: number) => void): this;
- on(event: 'response', listener: (headers: IncomingHttpHeaders & IncomingHttpStatusHeader, flags: number) => void): this;
- on(event: string | symbol, listener: (...args: any[]) => void): this;
- once(event: 'continue', listener: () => {}): this;
- once(event: 'headers', listener: (headers: IncomingHttpHeaders & IncomingHttpStatusHeader, flags: number) => void): this;
- once(event: 'push', listener: (headers: IncomingHttpHeaders, flags: number) => void): this;
- once(event: 'response', listener: (headers: IncomingHttpHeaders & IncomingHttpStatusHeader, flags: number) => void): this;
- once(event: string | symbol, listener: (...args: any[]) => void): this;
- prependListener(event: 'continue', listener: () => {}): this;
- prependListener(event: 'headers', listener: (headers: IncomingHttpHeaders & IncomingHttpStatusHeader, flags: number) => void): this;
- prependListener(event: 'push', listener: (headers: IncomingHttpHeaders, flags: number) => void): this;
- prependListener(event: 'response', listener: (headers: IncomingHttpHeaders & IncomingHttpStatusHeader, flags: number) => void): this;
- prependListener(event: string | symbol, listener: (...args: any[]) => void): this;
- prependOnceListener(event: 'continue', listener: () => {}): this;
- prependOnceListener(event: 'headers', listener: (headers: IncomingHttpHeaders & IncomingHttpStatusHeader, flags: number) => void): this;
- prependOnceListener(event: 'push', listener: (headers: IncomingHttpHeaders, flags: number) => void): this;
- prependOnceListener(event: 'response', listener: (headers: IncomingHttpHeaders & IncomingHttpStatusHeader, flags: number) => void): this;
- prependOnceListener(event: string | symbol, listener: (...args: any[]) => void): this;
- }
- export interface ServerHttp2Stream extends Http2Stream {
- /**
- * True if headers were sent, false otherwise (read-only).
- * @since v8.4.0
- */
- readonly headersSent: boolean;
- /**
- * Read-only property mapped to the `SETTINGS_ENABLE_PUSH` flag of the remote
- * client's most recent `SETTINGS` frame. Will be `true` if the remote peer
- * accepts push streams, `false` otherwise. Settings are the same for every`Http2Stream` in the same `Http2Session`.
- * @since v8.4.0
- */
- readonly pushAllowed: boolean;
- /**
- * Sends an additional informational `HEADERS` frame to the connected HTTP/2 peer.
- * @since v8.4.0
- */
- additionalHeaders(headers: OutgoingHttpHeaders): void;
- /**
- * Initiates a push stream. The callback is invoked with the new `Http2Stream`instance created for the push stream passed as the second argument, or an`Error` passed as the first argument.
- *
- * ```js
- * const http2 = require('http2');
- * const server = http2.createServer();
- * server.on('stream', (stream) => {
- * stream.respond({ ':status': 200 });
- * stream.pushStream({ ':path': '/' }, (err, pushStream, headers) => {
- * if (err) throw err;
- * pushStream.respond({ ':status': 200 });
- * pushStream.end('some pushed data');
- * });
- * stream.end('some data');
- * });
- * ```
- *
- * Setting the weight of a push stream is not allowed in the `HEADERS` frame. Pass
- * a `weight` value to `http2stream.priority` with the `silent` option set to`true` to enable server-side bandwidth balancing between concurrent streams.
- *
- * Calling `http2stream.pushStream()` from within a pushed stream is not permitted
- * and will throw an error.
- * @since v8.4.0
- * @param callback Callback that is called once the push stream has been initiated.
- */
- pushStream(headers: OutgoingHttpHeaders, callback?: (err: Error | null, pushStream: ServerHttp2Stream, headers: OutgoingHttpHeaders) => void): void;
- pushStream(headers: OutgoingHttpHeaders, options?: StreamPriorityOptions, callback?: (err: Error | null, pushStream: ServerHttp2Stream, headers: OutgoingHttpHeaders) => void): void;
- /**
- * ```js
- * const http2 = require('http2');
- * const server = http2.createServer();
- * server.on('stream', (stream) => {
- * stream.respond({ ':status': 200 });
- * stream.end('some data');
- * });
- * ```
- *
- * When the `options.waitForTrailers` option is set, the `'wantTrailers'` event
- * will be emitted immediately after queuing the last chunk of payload data to be
- * sent. The `http2stream.sendTrailers()` method can then be used to sent trailing
- * header fields to the peer.
- *
- * When `options.waitForTrailers` is set, the `Http2Stream` will not automatically
- * close when the final `DATA` frame is transmitted. User code must call either`http2stream.sendTrailers()` or `http2stream.close()` to close the`Http2Stream`.
- *
- * ```js
- * const http2 = require('http2');
- * const server = http2.createServer();
- * server.on('stream', (stream) => {
- * stream.respond({ ':status': 200 }, { waitForTrailers: true });
- * stream.on('wantTrailers', () => {
- * stream.sendTrailers({ ABC: 'some value to send' });
- * });
- * stream.end('some data');
- * });
- * ```
- * @since v8.4.0
- */
- respond(headers?: OutgoingHttpHeaders, options?: ServerStreamResponseOptions): void;
- /**
- * Initiates a response whose data is read from the given file descriptor. No
- * validation is performed on the given file descriptor. If an error occurs while
- * attempting to read data using the file descriptor, the `Http2Stream` will be
- * closed using an `RST_STREAM` frame using the standard `INTERNAL_ERROR` code.
- *
- * When used, the `Http2Stream` object's `Duplex` interface will be closed
- * automatically.
- *
- * ```js
- * const http2 = require('http2');
- * const fs = require('fs');
- *
- * const server = http2.createServer();
- * server.on('stream', (stream) => {
- * const fd = fs.openSync('/some/file', 'r');
- *
- * const stat = fs.fstatSync(fd);
- * const headers = {
- * 'content-length': stat.size,
- * 'last-modified': stat.mtime.toUTCString(),
- * 'content-type': 'text/plain; charset=utf-8'
- * };
- * stream.respondWithFD(fd, headers);
- * stream.on('close', () => fs.closeSync(fd));
- * });
- * ```
- *
- * The optional `options.statCheck` function may be specified to give user code
- * an opportunity to set additional content headers based on the `fs.Stat` details
- * of the given fd. If the `statCheck` function is provided, the`http2stream.respondWithFD()` method will perform an `fs.fstat()` call to
- * collect details on the provided file descriptor.
- *
- * The `offset` and `length` options may be used to limit the response to a
- * specific range subset. This can be used, for instance, to support HTTP Range
- * requests.
- *
- * The file descriptor or `FileHandle` is not closed when the stream is closed,
- * so it will need to be closed manually once it is no longer needed.
- * Using the same file descriptor concurrently for multiple streams
- * is not supported and may result in data loss. Re-using a file descriptor
- * after a stream has finished is supported.
- *
- * When the `options.waitForTrailers` option is set, the `'wantTrailers'` event
- * will be emitted immediately after queuing the last chunk of payload data to be
- * sent. The `http2stream.sendTrailers()` method can then be used to sent trailing
- * header fields to the peer.
- *
- * When `options.waitForTrailers` is set, the `Http2Stream` will not automatically
- * close when the final `DATA` frame is transmitted. User code _must_ call either`http2stream.sendTrailers()` or `http2stream.close()` to close the`Http2Stream`.
- *
- * ```js
- * const http2 = require('http2');
- * const fs = require('fs');
- *
- * const server = http2.createServer();
- * server.on('stream', (stream) => {
- * const fd = fs.openSync('/some/file', 'r');
- *
- * const stat = fs.fstatSync(fd);
- * const headers = {
- * 'content-length': stat.size,
- * 'last-modified': stat.mtime.toUTCString(),
- * 'content-type': 'text/plain; charset=utf-8'
- * };
- * stream.respondWithFD(fd, headers, { waitForTrailers: true });
- * stream.on('wantTrailers', () => {
- * stream.sendTrailers({ ABC: 'some value to send' });
- * });
- *
- * stream.on('close', () => fs.closeSync(fd));
- * });
- * ```
- * @since v8.4.0
- * @param fd A readable file descriptor.
- */
- respondWithFD(fd: number | fs.promises.FileHandle, headers?: OutgoingHttpHeaders, options?: ServerStreamFileResponseOptions): void;
- /**
- * Sends a regular file as the response. The `path` must specify a regular file
- * or an `'error'` event will be emitted on the `Http2Stream` object.
- *
- * When used, the `Http2Stream` object's `Duplex` interface will be closed
- * automatically.
- *
- * The optional `options.statCheck` function may be specified to give user code
- * an opportunity to set additional content headers based on the `fs.Stat` details
- * of the given file:
- *
- * If an error occurs while attempting to read the file data, the `Http2Stream`will be closed using an `RST_STREAM` frame using the standard `INTERNAL_ERROR`code. If the `onError` callback is
- * defined, then it will be called. Otherwise
- * the stream will be destroyed.
- *
- * Example using a file path:
- *
- * ```js
- * const http2 = require('http2');
- * const server = http2.createServer();
- * server.on('stream', (stream) => {
- * function statCheck(stat, headers) {
- * headers['last-modified'] = stat.mtime.toUTCString();
- * }
- *
- * function onError(err) {
- * // stream.respond() can throw if the stream has been destroyed by
- * // the other side.
- * try {
- * if (err.code === 'ENOENT') {
- * stream.respond({ ':status': 404 });
- * } else {
- * stream.respond({ ':status': 500 });
- * }
- * } catch (err) {
- * // Perform actual error handling.
- * console.log(err);
- * }
- * stream.end();
- * }
- *
- * stream.respondWithFile('/some/file',
- * { 'content-type': 'text/plain; charset=utf-8' },
- * { statCheck, onError });
- * });
- * ```
- *
- * The `options.statCheck` function may also be used to cancel the send operation
- * by returning `false`. For instance, a conditional request may check the stat
- * results to determine if the file has been modified to return an appropriate`304` response:
- *
- * ```js
- * const http2 = require('http2');
- * const server = http2.createServer();
- * server.on('stream', (stream) => {
- * function statCheck(stat, headers) {
- * // Check the stat here...
- * stream.respond({ ':status': 304 });
- * return false; // Cancel the send operation
- * }
- * stream.respondWithFile('/some/file',
- * { 'content-type': 'text/plain; charset=utf-8' },
- * { statCheck });
- * });
- * ```
- *
- * The `content-length` header field will be automatically set.
- *
- * The `offset` and `length` options may be used to limit the response to a
- * specific range subset. This can be used, for instance, to support HTTP Range
- * requests.
- *
- * The `options.onError` function may also be used to handle all the errors
- * that could happen before the delivery of the file is initiated. The
- * default behavior is to destroy the stream.
- *
- * When the `options.waitForTrailers` option is set, the `'wantTrailers'` event
- * will be emitted immediately after queuing the last chunk of payload data to be
- * sent. The `http2stream.sendTrailers()` method can then be used to sent trailing
- * header fields to the peer.
- *
- * When `options.waitForTrailers` is set, the `Http2Stream` will not automatically
- * close when the final `DATA` frame is transmitted. User code must call either`http2stream.sendTrailers()` or `http2stream.close()` to close the`Http2Stream`.
- *
- * ```js
- * const http2 = require('http2');
- * const server = http2.createServer();
- * server.on('stream', (stream) => {
- * stream.respondWithFile('/some/file',
- * { 'content-type': 'text/plain; charset=utf-8' },
- * { waitForTrailers: true });
- * stream.on('wantTrailers', () => {
- * stream.sendTrailers({ ABC: 'some value to send' });
- * });
- * });
- * ```
- * @since v8.4.0
- */
- respondWithFile(path: string, headers?: OutgoingHttpHeaders, options?: ServerStreamFileResponseOptionsWithError): void;
- }
- // Http2Session
- export interface Settings {
- headerTableSize?: number | undefined;
- enablePush?: boolean | undefined;
- initialWindowSize?: number | undefined;
- maxFrameSize?: number | undefined;
- maxConcurrentStreams?: number | undefined;
- maxHeaderListSize?: number | undefined;
- enableConnectProtocol?: boolean | undefined;
- }
- export interface ClientSessionRequestOptions {
- endStream?: boolean | undefined;
- exclusive?: boolean | undefined;
- parent?: number | undefined;
- weight?: number | undefined;
- waitForTrailers?: boolean | undefined;
- signal?: AbortSignal | undefined;
- }
- export interface SessionState {
- effectiveLocalWindowSize?: number | undefined;
- effectiveRecvDataLength?: number | undefined;
- nextStreamID?: number | undefined;
- localWindowSize?: number | undefined;
- lastProcStreamID?: number | undefined;
- remoteWindowSize?: number | undefined;
- outboundQueueSize?: number | undefined;
- deflateDynamicTableSize?: number | undefined;
- inflateDynamicTableSize?: number | undefined;
- }
- export interface Http2Session extends EventEmitter {
- /**
- * Value will be `undefined` if the `Http2Session` is not yet connected to a
- * socket, `h2c` if the `Http2Session` is not connected to a `TLSSocket`, or
- * will return the value of the connected `TLSSocket`'s own `alpnProtocol`property.
- * @since v9.4.0
- */
- readonly alpnProtocol?: string | undefined;
- /**
- * Will be `true` if this `Http2Session` instance has been closed, otherwise`false`.
- * @since v9.4.0
- */
- readonly closed: boolean;
- /**
- * Will be `true` if this `Http2Session` instance is still connecting, will be set
- * to `false` before emitting `connect` event and/or calling the `http2.connect`callback.
- * @since v10.0.0
- */
- readonly connecting: boolean;
- /**
- * Will be `true` if this `Http2Session` instance has been destroyed and must no
- * longer be used, otherwise `false`.
- * @since v8.4.0
- */
- readonly destroyed: boolean;
- /**
- * Value is `undefined` if the `Http2Session` session socket has not yet been
- * connected, `true` if the `Http2Session` is connected with a `TLSSocket`,
- * and `false` if the `Http2Session` is connected to any other kind of socket
- * or stream.
- * @since v9.4.0
- */
- readonly encrypted?: boolean | undefined;
- /**
- * A prototype-less object describing the current local settings of this`Http2Session`. The local settings are local to _this_`Http2Session` instance.
- * @since v8.4.0
- */
- readonly localSettings: Settings;
- /**
- * If the `Http2Session` is connected to a `TLSSocket`, the `originSet` property
- * will return an `Array` of origins for which the `Http2Session` may be
- * considered authoritative.
- *
- * The `originSet` property is only available when using a secure TLS connection.
- * @since v9.4.0
- */
- readonly originSet?: string[] | undefined;
- /**
- * Indicates whether the `Http2Session` is currently waiting for acknowledgment of
- * a sent `SETTINGS` frame. Will be `true` after calling the`http2session.settings()` method. Will be `false` once all sent `SETTINGS`frames have been acknowledged.
- * @since v8.4.0
- */
- readonly pendingSettingsAck: boolean;
- /**
- * A prototype-less object describing the current remote settings of this`Http2Session`. The remote settings are set by the _connected_ HTTP/2 peer.
- * @since v8.4.0
- */
- readonly remoteSettings: Settings;
- /**
- * Returns a `Proxy` object that acts as a `net.Socket` (or `tls.TLSSocket`) but
- * limits available methods to ones safe to use with HTTP/2.
- *
- * `destroy`, `emit`, `end`, `pause`, `read`, `resume`, and `write` will throw
- * an error with code `ERR_HTTP2_NO_SOCKET_MANIPULATION`. See `Http2Session and Sockets` for more information.
- *
- * `setTimeout` method will be called on this `Http2Session`.
- *
- * All other interactions will be routed directly to the socket.
- * @since v8.4.0
- */
- readonly socket: net.Socket | tls.TLSSocket;
- /**
- * Provides miscellaneous information about the current state of the`Http2Session`.
- *
- * An object describing the current status of this `Http2Session`.
- * @since v8.4.0
- */
- readonly state: SessionState;
- /**
- * The `http2session.type` will be equal to`http2.constants.NGHTTP2_SESSION_SERVER` if this `Http2Session` instance is a
- * server, and `http2.constants.NGHTTP2_SESSION_CLIENT` if the instance is a
- * client.
- * @since v8.4.0
- */
- readonly type: number;
- /**
- * Gracefully closes the `Http2Session`, allowing any existing streams to
- * complete on their own and preventing new `Http2Stream` instances from being
- * created. Once closed, `http2session.destroy()`_might_ be called if there
- * are no open `Http2Stream` instances.
- *
- * If specified, the `callback` function is registered as a handler for the`'close'` event.
- * @since v9.4.0
- */
- close(callback?: () => void): void;
- /**
- * Immediately terminates the `Http2Session` and the associated `net.Socket` or`tls.TLSSocket`.
- *
- * Once destroyed, the `Http2Session` will emit the `'close'` event. If `error`is not undefined, an `'error'` event will be emitted immediately before the`'close'` event.
- *
- * If there are any remaining open `Http2Streams` associated with the`Http2Session`, those will also be destroyed.
- * @since v8.4.0
- * @param error An `Error` object if the `Http2Session` is being destroyed due to an error.
- * @param code The HTTP/2 error code to send in the final `GOAWAY` frame. If unspecified, and `error` is not undefined, the default is `INTERNAL_ERROR`, otherwise defaults to `NO_ERROR`.
- */
- destroy(error?: Error, code?: number): void;
- /**
- * Transmits a `GOAWAY` frame to the connected peer _without_ shutting down the`Http2Session`.
- * @since v9.4.0
- * @param code An HTTP/2 error code
- * @param lastStreamID The numeric ID of the last processed `Http2Stream`
- * @param opaqueData A `TypedArray` or `DataView` instance containing additional data to be carried within the `GOAWAY` frame.
- */
- goaway(code?: number, lastStreamID?: number, opaqueData?: NodeJS.ArrayBufferView): void;
- /**
- * Sends a `PING` frame to the connected HTTP/2 peer. A `callback` function must
- * be provided. The method will return `true` if the `PING` was sent, `false`otherwise.
- *
- * The maximum number of outstanding (unacknowledged) pings is determined by the`maxOutstandingPings` configuration option. The default maximum is 10.
- *
- * If provided, the `payload` must be a `Buffer`, `TypedArray`, or `DataView`containing 8 bytes of data that will be transmitted with the `PING` and
- * returned with the ping acknowledgment.
- *
- * The callback will be invoked with three arguments: an error argument that will
- * be `null` if the `PING` was successfully acknowledged, a `duration` argument
- * that reports the number of milliseconds elapsed since the ping was sent and the
- * acknowledgment was received, and a `Buffer` containing the 8-byte `PING`payload.
- *
- * ```js
- * session.ping(Buffer.from('abcdefgh'), (err, duration, payload) => {
- * if (!err) {
- * console.log(`Ping acknowledged in ${duration} milliseconds`);
- * console.log(`With payload '${payload.toString()}'`);
- * }
- * });
- * ```
- *
- * If the `payload` argument is not specified, the default payload will be the
- * 64-bit timestamp (little endian) marking the start of the `PING` duration.
- * @since v8.9.3
- * @param payload Optional ping payload.
- */
- ping(callback: (err: Error | null, duration: number, payload: Buffer) => void): boolean;
- ping(payload: NodeJS.ArrayBufferView, callback: (err: Error | null, duration: number, payload: Buffer) => void): boolean;
- /**
- * Calls `ref()` on this `Http2Session`instance's underlying `net.Socket`.
- * @since v9.4.0
- */
- ref(): void;
- /**
- * Sets the local endpoint's window size.
- * The `windowSize` is the total window size to set, not
- * the delta.
- *
- * ```js
- * const http2 = require('http2');
- *
- * const server = http2.createServer();
- * const expectedWindowSize = 2 ** 20;
- * server.on('connect', (session) => {
- *
- * // Set local window size to be 2 ** 20
- * session.setLocalWindowSize(expectedWindowSize);
- * });
- * ```
- * @since v15.3.0, v14.18.0
- */
- setLocalWindowSize(windowSize: number): void;
- /**
- * Used to set a callback function that is called when there is no activity on
- * the `Http2Session` after `msecs` milliseconds. The given `callback` is
- * registered as a listener on the `'timeout'` event.
- * @since v8.4.0
- */
- setTimeout(msecs: number, callback?: () => void): void;
- /**
- * Updates the current local settings for this `Http2Session` and sends a new`SETTINGS` frame to the connected HTTP/2 peer.
- *
- * Once called, the `http2session.pendingSettingsAck` property will be `true`while the session is waiting for the remote peer to acknowledge the new
- * settings.
- *
- * The new settings will not become effective until the `SETTINGS` acknowledgment
- * is received and the `'localSettings'` event is emitted. It is possible to send
- * multiple `SETTINGS` frames while acknowledgment is still pending.
- * @since v8.4.0
- * @param callback Callback that is called once the session is connected or right away if the session is already connected.
- */
- settings(settings: Settings, callback?: (err: Error | null, settings: Settings, duration: number) => void): void;
- /**
- * Calls `unref()` on this `Http2Session`instance's underlying `net.Socket`.
- * @since v9.4.0
- */
- unref(): void;
- addListener(event: 'close', listener: () => void): this;
- addListener(event: 'error', listener: (err: Error) => void): this;
- addListener(event: 'frameError', listener: (frameType: number, errorCode: number, streamID: number) => void): this;
- addListener(event: 'goaway', listener: (errorCode: number, lastStreamID: number, opaqueData: Buffer) => void): this;
- addListener(event: 'localSettings', listener: (settings: Settings) => void): this;
- addListener(event: 'ping', listener: () => void): this;
- addListener(event: 'remoteSettings', listener: (settings: Settings) => void): this;
- addListener(event: 'timeout', listener: () => void): this;
- addListener(event: string | symbol, listener: (...args: any[]) => void): this;
- emit(event: 'close'): boolean;
- emit(event: 'error', err: Error): boolean;
- emit(event: 'frameError', frameType: number, errorCode: number, streamID: number): boolean;
- emit(event: 'goaway', errorCode: number, lastStreamID: number, opaqueData: Buffer): boolean;
- emit(event: 'localSettings', settings: Settings): boolean;
- emit(event: 'ping'): boolean;
- emit(event: 'remoteSettings', settings: Settings): boolean;
- emit(event: 'timeout'): boolean;
- emit(event: string | symbol, ...args: any[]): boolean;
- on(event: 'close', listener: () => void): this;
- on(event: 'error', listener: (err: Error) => void): this;
- on(event: 'frameError', listener: (frameType: number, errorCode: number, streamID: number) => void): this;
- on(event: 'goaway', listener: (errorCode: number, lastStreamID: number, opaqueData: Buffer) => void): this;
- on(event: 'localSettings', listener: (settings: Settings) => void): this;
- on(event: 'ping', listener: () => void): this;
- on(event: 'remoteSettings', listener: (settings: Settings) => void): this;
- on(event: 'timeout', listener: () => void): this;
- on(event: string | symbol, listener: (...args: any[]) => void): this;
- once(event: 'close', listener: () => void): this;
- once(event: 'error', listener: (err: Error) => void): this;
- once(event: 'frameError', listener: (frameType: number, errorCode: number, streamID: number) => void): this;
- once(event: 'goaway', listener: (errorCode: number, lastStreamID: number, opaqueData: Buffer) => void): this;
- once(event: 'localSettings', listener: (settings: Settings) => void): this;
- once(event: 'ping', listener: () => void): this;
- once(event: 'remoteSettings', listener: (settings: Settings) => void): this;
- once(event: 'timeout', listener: () => void): this;
- once(event: string | symbol, listener: (...args: any[]) => void): this;
- prependListener(event: 'close', listener: () => void): this;
- prependListener(event: 'error', listener: (err: Error) => void): this;
- prependListener(event: 'frameError', listener: (frameType: number, errorCode: number, streamID: number) => void): this;
- prependListener(event: 'goaway', listener: (errorCode: number, lastStreamID: number, opaqueData: Buffer) => void): this;
- prependListener(event: 'localSettings', listener: (settings: Settings) => void): this;
- prependListener(event: 'ping', listener: () => void): this;
- prependListener(event: 'remoteSettings', listener: (settings: Settings) => void): this;
- prependListener(event: 'timeout', listener: () => void): this;
- prependListener(event: string | symbol, listener: (...args: any[]) => void): this;
- prependOnceListener(event: 'close', listener: () => void): this;
- prependOnceListener(event: 'error', listener: (err: Error) => void): this;
- prependOnceListener(event: 'frameError', listener: (frameType: number, errorCode: number, streamID: number) => void): this;
- prependOnceListener(event: 'goaway', listener: (errorCode: number, lastStreamID: number, opaqueData: Buffer) => void): this;
- prependOnceListener(event: 'localSettings', listener: (settings: Settings) => void): this;
- prependOnceListener(event: 'ping', listener: () => void): this;
- prependOnceListener(event: 'remoteSettings', listener: (settings: Settings) => void): this;
- prependOnceListener(event: 'timeout', listener: () => void): this;
- prependOnceListener(event: string | symbol, listener: (...args: any[]) => void): this;
- }
- export interface ClientHttp2Session extends Http2Session {
- /**
- * For HTTP/2 Client `Http2Session` instances only, the `http2session.request()`creates and returns an `Http2Stream` instance that can be used to send an
- * HTTP/2 request to the connected server.
- *
- * When a `ClientHttp2Session` is first created, the socket may not yet be
- * connected. if `clienthttp2session.request()` is called during this time, the
- * actual request will be deferred until the socket is ready to go.
- * If the `session` is closed before the actual request be executed, an`ERR_HTTP2_GOAWAY_SESSION` is thrown.
- *
- * This method is only available if `http2session.type` is equal to`http2.constants.NGHTTP2_SESSION_CLIENT`.
- *
- * ```js
- * const http2 = require('http2');
- * const clientSession = http2.connect('https://localhost:1234');
- * const {
- * HTTP2_HEADER_PATH,
- * HTTP2_HEADER_STATUS
- * } = http2.constants;
- *
- * const req = clientSession.request({ [HTTP2_HEADER_PATH]: '/' });
- * req.on('response', (headers) => {
- * console.log(headers[HTTP2_HEADER_STATUS]);
- * req.on('data', (chunk) => { // .. });
- * req.on('end', () => { // .. });
- * });
- * ```
- *
- * When the `options.waitForTrailers` option is set, the `'wantTrailers'` event
- * is emitted immediately after queuing the last chunk of payload data to be sent.
- * The `http2stream.sendTrailers()` method can then be called to send trailing
- * headers to the peer.
- *
- * When `options.waitForTrailers` is set, the `Http2Stream` will not automatically
- * close when the final `DATA` frame is transmitted. User code must call either`http2stream.sendTrailers()` or `http2stream.close()` to close the`Http2Stream`.
- *
- * When `options.signal` is set with an `AbortSignal` and then `abort` on the
- * corresponding `AbortController` is called, the request will emit an `'error'`event with an `AbortError` error.
- *
- * The `:method` and `:path` pseudo-headers are not specified within `headers`,
- * they respectively default to:
- *
- * * `:method` \= `'GET'`
- * * `:path` \= `/`
- * @since v8.4.0
- */
- request(headers?: OutgoingHttpHeaders, options?: ClientSessionRequestOptions): ClientHttp2Stream;
- addListener(event: 'altsvc', listener: (alt: string, origin: string, stream: number) => void): this;
- addListener(event: 'origin', listener: (origins: string[]) => void): this;
- addListener(event: 'connect', listener: (session: ClientHttp2Session, socket: net.Socket | tls.TLSSocket) => void): this;
- addListener(event: 'stream', listener: (stream: ClientHttp2Stream, headers: IncomingHttpHeaders & IncomingHttpStatusHeader, flags: number) => void): this;
- addListener(event: string | symbol, listener: (...args: any[]) => void): this;
- emit(event: 'altsvc', alt: string, origin: string, stream: number): boolean;
- emit(event: 'origin', origins: ReadonlyArray): boolean;
- emit(event: 'connect', session: ClientHttp2Session, socket: net.Socket | tls.TLSSocket): boolean;
- emit(event: 'stream', stream: ClientHttp2Stream, headers: IncomingHttpHeaders & IncomingHttpStatusHeader, flags: number): boolean;
- emit(event: string | symbol, ...args: any[]): boolean;
- on(event: 'altsvc', listener: (alt: string, origin: string, stream: number) => void): this;
- on(event: 'origin', listener: (origins: string[]) => void): this;
- on(event: 'connect', listener: (session: ClientHttp2Session, socket: net.Socket | tls.TLSSocket) => void): this;
- on(event: 'stream', listener: (stream: ClientHttp2Stream, headers: IncomingHttpHeaders & IncomingHttpStatusHeader, flags: number) => void): this;
- on(event: string | symbol, listener: (...args: any[]) => void): this;
- once(event: 'altsvc', listener: (alt: string, origin: string, stream: number) => void): this;
- once(event: 'origin', listener: (origins: string[]) => void): this;
- once(event: 'connect', listener: (session: ClientHttp2Session, socket: net.Socket | tls.TLSSocket) => void): this;
- once(event: 'stream', listener: (stream: ClientHttp2Stream, headers: IncomingHttpHeaders & IncomingHttpStatusHeader, flags: number) => void): this;
- once(event: string | symbol, listener: (...args: any[]) => void): this;
- prependListener(event: 'altsvc', listener: (alt: string, origin: string, stream: number) => void): this;
- prependListener(event: 'origin', listener: (origins: string[]) => void): this;
- prependListener(event: 'connect', listener: (session: ClientHttp2Session, socket: net.Socket | tls.TLSSocket) => void): this;
- prependListener(event: 'stream', listener: (stream: ClientHttp2Stream, headers: IncomingHttpHeaders & IncomingHttpStatusHeader, flags: number) => void): this;
- prependListener(event: string | symbol, listener: (...args: any[]) => void): this;
- prependOnceListener(event: 'altsvc', listener: (alt: string, origin: string, stream: number) => void): this;
- prependOnceListener(event: 'origin', listener: (origins: string[]) => void): this;
- prependOnceListener(event: 'connect', listener: (session: ClientHttp2Session, socket: net.Socket | tls.TLSSocket) => void): this;
- prependOnceListener(event: 'stream', listener: (stream: ClientHttp2Stream, headers: IncomingHttpHeaders & IncomingHttpStatusHeader, flags: number) => void): this;
- prependOnceListener(event: string | symbol, listener: (...args: any[]) => void): this;
- }
- export interface AlternativeServiceOptions {
- origin: number | string | url.URL;
- }
- export interface ServerHttp2Session extends Http2Session {
- readonly server: Http2Server | Http2SecureServer;
- /**
- * Submits an `ALTSVC` frame (as defined by [RFC 7838](https://tools.ietf.org/html/rfc7838)) to the connected client.
- *
- * ```js
- * const http2 = require('http2');
- *
- * const server = http2.createServer();
- * server.on('session', (session) => {
- * // Set altsvc for origin https://example.org:80
- * session.altsvc('h2=":8000"', 'https://example.org:80');
- * });
- *
- * server.on('stream', (stream) => {
- * // Set altsvc for a specific stream
- * stream.session.altsvc('h2=":8000"', stream.id);
- * });
- * ```
- *
- * Sending an `ALTSVC` frame with a specific stream ID indicates that the alternate
- * service is associated with the origin of the given `Http2Stream`.
- *
- * The `alt` and origin string _must_ contain only ASCII bytes and are
- * strictly interpreted as a sequence of ASCII bytes. The special value `'clear'`may be passed to clear any previously set alternative service for a given
- * domain.
- *
- * When a string is passed for the `originOrStream` argument, it will be parsed as
- * a URL and the origin will be derived. For instance, the origin for the
- * HTTP URL `'https://example.org/foo/bar'` is the ASCII string`'https://example.org'`. An error will be thrown if either the given string
- * cannot be parsed as a URL or if a valid origin cannot be derived.
- *
- * A `URL` object, or any object with an `origin` property, may be passed as`originOrStream`, in which case the value of the `origin` property will be
- * used. The value of the `origin` property _must_ be a properly serialized
- * ASCII origin.
- * @since v9.4.0
- * @param alt A description of the alternative service configuration as defined by `RFC 7838`.
- * @param originOrStream Either a URL string specifying the origin (or an `Object` with an `origin` property) or the numeric identifier of an active `Http2Stream` as given by the
- * `http2stream.id` property.
- */
- altsvc(alt: string, originOrStream: number | string | url.URL | AlternativeServiceOptions): void;
- /**
- * Submits an `ORIGIN` frame (as defined by [RFC 8336](https://tools.ietf.org/html/rfc8336)) to the connected client
- * to advertise the set of origins for which the server is capable of providing
- * authoritative responses.
- *
- * ```js
- * const http2 = require('http2');
- * const options = getSecureOptionsSomehow();
- * const server = http2.createSecureServer(options);
- * server.on('stream', (stream) => {
- * stream.respond();
- * stream.end('ok');
- * });
- * server.on('session', (session) => {
- * session.origin('https://example.com', 'https://example.org');
- * });
- * ```
- *
- * When a string is passed as an `origin`, it will be parsed as a URL and the
- * origin will be derived. For instance, the origin for the HTTP URL`'https://example.org/foo/bar'` is the ASCII string`'https://example.org'`. An error will be thrown if either the given
- * string
- * cannot be parsed as a URL or if a valid origin cannot be derived.
- *
- * A `URL` object, or any object with an `origin` property, may be passed as
- * an `origin`, in which case the value of the `origin` property will be
- * used. The value of the `origin` property _must_ be a properly serialized
- * ASCII origin.
- *
- * Alternatively, the `origins` option may be used when creating a new HTTP/2
- * server using the `http2.createSecureServer()` method:
- *
- * ```js
- * const http2 = require('http2');
- * const options = getSecureOptionsSomehow();
- * options.origins = ['https://example.com', 'https://example.org'];
- * const server = http2.createSecureServer(options);
- * server.on('stream', (stream) => {
- * stream.respond();
- * stream.end('ok');
- * });
- * ```
- * @since v10.12.0
- * @param origins One or more URL Strings passed as separate arguments.
- */
- origin(
- ...origins: Array<
- | string
- | url.URL
- | {
- origin: string;
- }
- >
- ): void;
- addListener(event: 'connect', listener: (session: ServerHttp2Session, socket: net.Socket | tls.TLSSocket) => void): this;
- addListener(event: 'stream', listener: (stream: ServerHttp2Stream, headers: IncomingHttpHeaders, flags: number) => void): this;
- addListener(event: string | symbol, listener: (...args: any[]) => void): this;
- emit(event: 'connect', session: ServerHttp2Session, socket: net.Socket | tls.TLSSocket): boolean;
- emit(event: 'stream', stream: ServerHttp2Stream, headers: IncomingHttpHeaders, flags: number): boolean;
- emit(event: string | symbol, ...args: any[]): boolean;
- on(event: 'connect', listener: (session: ServerHttp2Session, socket: net.Socket | tls.TLSSocket) => void): this;
- on(event: 'stream', listener: (stream: ServerHttp2Stream, headers: IncomingHttpHeaders, flags: number) => void): this;
- on(event: string | symbol, listener: (...args: any[]) => void): this;
- once(event: 'connect', listener: (session: ServerHttp2Session, socket: net.Socket | tls.TLSSocket) => void): this;
- once(event: 'stream', listener: (stream: ServerHttp2Stream, headers: IncomingHttpHeaders, flags: number) => void): this;
- once(event: string | symbol, listener: (...args: any[]) => void): this;
- prependListener(event: 'connect', listener: (session: ServerHttp2Session, socket: net.Socket | tls.TLSSocket) => void): this;
- prependListener(event: 'stream', listener: (stream: ServerHttp2Stream, headers: IncomingHttpHeaders, flags: number) => void): this;
- prependListener(event: string | symbol, listener: (...args: any[]) => void): this;
- prependOnceListener(event: 'connect', listener: (session: ServerHttp2Session, socket: net.Socket | tls.TLSSocket) => void): this;
- prependOnceListener(event: 'stream', listener: (stream: ServerHttp2Stream, headers: IncomingHttpHeaders, flags: number) => void): this;
- prependOnceListener(event: string | symbol, listener: (...args: any[]) => void): this;
- }
- // Http2Server
- export interface SessionOptions {
- maxDeflateDynamicTableSize?: number | undefined;
- maxSessionMemory?: number | undefined;
- maxHeaderListPairs?: number | undefined;
- maxOutstandingPings?: number | undefined;
- maxSendHeaderBlockLength?: number | undefined;
- paddingStrategy?: number | undefined;
- peerMaxConcurrentStreams?: number | undefined;
- settings?: Settings | undefined;
- /**
- * Specifies a timeout in milliseconds that
- * a server should wait when an [`'unknownProtocol'`][] is emitted. If the
- * socket has not been destroyed by that time the server will destroy it.
- * @default 100000
- */
- unknownProtocolTimeout?: number | undefined;
- selectPadding?(frameLen: number, maxFrameLen: number): number;
- createConnection?(authority: url.URL, option: SessionOptions): stream.Duplex;
- }
- export interface ClientSessionOptions extends SessionOptions {
- maxReservedRemoteStreams?: number | undefined;
- createConnection?: ((authority: url.URL, option: SessionOptions) => stream.Duplex) | undefined;
- protocol?: 'http:' | 'https:' | undefined;
- }
- export interface ServerSessionOptions extends SessionOptions {
- Http1IncomingMessage?: typeof IncomingMessage | undefined;
- Http1ServerResponse?: typeof ServerResponse | undefined;
- Http2ServerRequest?: typeof Http2ServerRequest | undefined;
- Http2ServerResponse?: typeof Http2ServerResponse | undefined;
- }
- export interface SecureClientSessionOptions extends ClientSessionOptions, tls.ConnectionOptions {}
- export interface SecureServerSessionOptions extends ServerSessionOptions, tls.TlsOptions {}
- export interface ServerOptions extends ServerSessionOptions {}
- export interface SecureServerOptions extends SecureServerSessionOptions {
- allowHTTP1?: boolean | undefined;
- origins?: string[] | undefined;
- }
- interface HTTP2ServerCommon {
- setTimeout(msec?: number, callback?: () => void): this;
- /**
- * Throws ERR_HTTP2_INVALID_SETTING_VALUE for invalid settings values.
- * Throws ERR_INVALID_ARG_TYPE for invalid settings argument.
- */
- updateSettings(settings: Settings): void;
- }
- export interface Http2Server extends net.Server, HTTP2ServerCommon {
- addListener(event: 'checkContinue', listener: (request: Http2ServerRequest, response: Http2ServerResponse) => void): this;
- addListener(event: 'request', listener: (request: Http2ServerRequest, response: Http2ServerResponse) => void): this;
- addListener(event: 'session', listener: (session: ServerHttp2Session) => void): this;
- addListener(event: 'sessionError', listener: (err: Error) => void): this;
- addListener(event: 'stream', listener: (stream: ServerHttp2Stream, headers: IncomingHttpHeaders, flags: number) => void): this;
- addListener(event: 'timeout', listener: () => void): this;
- addListener(event: string | symbol, listener: (...args: any[]) => void): this;
- emit(event: 'checkContinue', request: Http2ServerRequest, response: Http2ServerResponse): boolean;
- emit(event: 'request', request: Http2ServerRequest, response: Http2ServerResponse): boolean;
- emit(event: 'session', session: ServerHttp2Session): boolean;
- emit(event: 'sessionError', err: Error): boolean;
- emit(event: 'stream', stream: ServerHttp2Stream, headers: IncomingHttpHeaders, flags: number): boolean;
- emit(event: 'timeout'): boolean;
- emit(event: string | symbol, ...args: any[]): boolean;
- on(event: 'checkContinue', listener: (request: Http2ServerRequest, response: Http2ServerResponse) => void): this;
- on(event: 'request', listener: (request: Http2ServerRequest, response: Http2ServerResponse) => void): this;
- on(event: 'session', listener: (session: ServerHttp2Session) => void): this;
- on(event: 'sessionError', listener: (err: Error) => void): this;
- on(event: 'stream', listener: (stream: ServerHttp2Stream, headers: IncomingHttpHeaders, flags: number) => void): this;
- on(event: 'timeout', listener: () => void): this;
- on(event: string | symbol, listener: (...args: any[]) => void): this;
- once(event: 'checkContinue', listener: (request: Http2ServerRequest, response: Http2ServerResponse) => void): this;
- once(event: 'request', listener: (request: Http2ServerRequest, response: Http2ServerResponse) => void): this;
- once(event: 'session', listener: (session: ServerHttp2Session) => void): this;
- once(event: 'sessionError', listener: (err: Error) => void): this;
- once(event: 'stream', listener: (stream: ServerHttp2Stream, headers: IncomingHttpHeaders, flags: number) => void): this;
- once(event: 'timeout', listener: () => void): this;
- once(event: string | symbol, listener: (...args: any[]) => void): this;
- prependListener(event: 'checkContinue', listener: (request: Http2ServerRequest, response: Http2ServerResponse) => void): this;
- prependListener(event: 'request', listener: (request: Http2ServerRequest, response: Http2ServerResponse) => void): this;
- prependListener(event: 'session', listener: (session: ServerHttp2Session) => void): this;
- prependListener(event: 'sessionError', listener: (err: Error) => void): this;
- prependListener(event: 'stream', listener: (stream: ServerHttp2Stream, headers: IncomingHttpHeaders, flags: number) => void): this;
- prependListener(event: 'timeout', listener: () => void): this;
- prependListener(event: string | symbol, listener: (...args: any[]) => void): this;
- prependOnceListener(event: 'checkContinue', listener: (request: Http2ServerRequest, response: Http2ServerResponse) => void): this;
- prependOnceListener(event: 'request', listener: (request: Http2ServerRequest, response: Http2ServerResponse) => void): this;
- prependOnceListener(event: 'session', listener: (session: ServerHttp2Session) => void): this;
- prependOnceListener(event: 'sessionError', listener: (err: Error) => void): this;
- prependOnceListener(event: 'stream', listener: (stream: ServerHttp2Stream, headers: IncomingHttpHeaders, flags: number) => void): this;
- prependOnceListener(event: 'timeout', listener: () => void): this;
- prependOnceListener(event: string | symbol, listener: (...args: any[]) => void): this;
- }
- export interface Http2SecureServer extends tls.Server, HTTP2ServerCommon {
- addListener(event: 'checkContinue', listener: (request: Http2ServerRequest, response: Http2ServerResponse) => void): this;
- addListener(event: 'request', listener: (request: Http2ServerRequest, response: Http2ServerResponse) => void): this;
- addListener(event: 'session', listener: (session: ServerHttp2Session) => void): this;
- addListener(event: 'sessionError', listener: (err: Error) => void): this;
- addListener(event: 'stream', listener: (stream: ServerHttp2Stream, headers: IncomingHttpHeaders, flags: number) => void): this;
- addListener(event: 'timeout', listener: () => void): this;
- addListener(event: 'unknownProtocol', listener: (socket: tls.TLSSocket) => void): this;
- addListener(event: string | symbol, listener: (...args: any[]) => void): this;
- emit(event: 'checkContinue', request: Http2ServerRequest, response: Http2ServerResponse): boolean;
- emit(event: 'request', request: Http2ServerRequest, response: Http2ServerResponse): boolean;
- emit(event: 'session', session: ServerHttp2Session): boolean;
- emit(event: 'sessionError', err: Error): boolean;
- emit(event: 'stream', stream: ServerHttp2Stream, headers: IncomingHttpHeaders, flags: number): boolean;
- emit(event: 'timeout'): boolean;
- emit(event: 'unknownProtocol', socket: tls.TLSSocket): boolean;
- emit(event: string | symbol, ...args: any[]): boolean;
- on(event: 'checkContinue', listener: (request: Http2ServerRequest, response: Http2ServerResponse) => void): this;
- on(event: 'request', listener: (request: Http2ServerRequest, response: Http2ServerResponse) => void): this;
- on(event: 'session', listener: (session: ServerHttp2Session) => void): this;
- on(event: 'sessionError', listener: (err: Error) => void): this;
- on(event: 'stream', listener: (stream: ServerHttp2Stream, headers: IncomingHttpHeaders, flags: number) => void): this;
- on(event: 'timeout', listener: () => void): this;
- on(event: 'unknownProtocol', listener: (socket: tls.TLSSocket) => void): this;
- on(event: string | symbol, listener: (...args: any[]) => void): this;
- once(event: 'checkContinue', listener: (request: Http2ServerRequest, response: Http2ServerResponse) => void): this;
- once(event: 'request', listener: (request: Http2ServerRequest, response: Http2ServerResponse) => void): this;
- once(event: 'session', listener: (session: ServerHttp2Session) => void): this;
- once(event: 'sessionError', listener: (err: Error) => void): this;
- once(event: 'stream', listener: (stream: ServerHttp2Stream, headers: IncomingHttpHeaders, flags: number) => void): this;
- once(event: 'timeout', listener: () => void): this;
- once(event: 'unknownProtocol', listener: (socket: tls.TLSSocket) => void): this;
- once(event: string | symbol, listener: (...args: any[]) => void): this;
- prependListener(event: 'checkContinue', listener: (request: Http2ServerRequest, response: Http2ServerResponse) => void): this;
- prependListener(event: 'request', listener: (request: Http2ServerRequest, response: Http2ServerResponse) => void): this;
- prependListener(event: 'session', listener: (session: ServerHttp2Session) => void): this;
- prependListener(event: 'sessionError', listener: (err: Error) => void): this;
- prependListener(event: 'stream', listener: (stream: ServerHttp2Stream, headers: IncomingHttpHeaders, flags: number) => void): this;
- prependListener(event: 'timeout', listener: () => void): this;
- prependListener(event: 'unknownProtocol', listener: (socket: tls.TLSSocket) => void): this;
- prependListener(event: string | symbol, listener: (...args: any[]) => void): this;
- prependOnceListener(event: 'checkContinue', listener: (request: Http2ServerRequest, response: Http2ServerResponse) => void): this;
- prependOnceListener(event: 'request', listener: (request: Http2ServerRequest, response: Http2ServerResponse) => void): this;
- prependOnceListener(event: 'session', listener: (session: ServerHttp2Session) => void): this;
- prependOnceListener(event: 'sessionError', listener: (err: Error) => void): this;
- prependOnceListener(event: 'stream', listener: (stream: ServerHttp2Stream, headers: IncomingHttpHeaders, flags: number) => void): this;
- prependOnceListener(event: 'timeout', listener: () => void): this;
- prependOnceListener(event: 'unknownProtocol', listener: (socket: tls.TLSSocket) => void): this;
- prependOnceListener(event: string | symbol, listener: (...args: any[]) => void): this;
- }
- /**
- * A `Http2ServerRequest` object is created by {@link Server} or {@link SecureServer} and passed as the first argument to the `'request'` event. It may be used to access a request status,
- * headers, and
- * data.
- * @since v8.4.0
- */
- export class Http2ServerRequest extends stream.Readable {
- constructor(stream: ServerHttp2Stream, headers: IncomingHttpHeaders, options: stream.ReadableOptions, rawHeaders: ReadonlyArray);
- /**
- * The `request.aborted` property will be `true` if the request has
- * been aborted.
- * @since v10.1.0
- */
- readonly aborted: boolean;
- /**
- * The request authority pseudo header field. Because HTTP/2 allows requests
- * to set either `:authority` or `host`, this value is derived from`req.headers[':authority']` if present. Otherwise, it is derived from`req.headers['host']`.
- * @since v8.4.0
- */
- readonly authority: string;
- /**
- * See `request.socket`.
- * @since v8.4.0
- * @deprecated Since v13.0.0 - Use `socket`.
- */
- readonly connection: net.Socket | tls.TLSSocket;
- /**
- * The `request.complete` property will be `true` if the request has
- * been completed, aborted, or destroyed.
- * @since v12.10.0
- */
- readonly complete: boolean;
- /**
- * The request/response headers object.
- *
- * Key-value pairs of header names and values. Header names are lower-cased.
- *
- * ```js
- * // Prints something like:
- * //
- * // { 'user-agent': 'curl/7.22.0',
- * // host: '127.0.0.1:8000',
- * // accept: '*' }
- * console.log(request.headers);
- * ```
- *
- * See `HTTP/2 Headers Object`.
- *
- * In HTTP/2, the request path, host name, protocol, and method are represented as
- * special headers prefixed with the `:` character (e.g. `':path'`). These special
- * headers will be included in the `request.headers` object. Care must be taken not
- * to inadvertently modify these special headers or errors may occur. For instance,
- * removing all headers from the request will cause errors to occur:
- *
- * ```js
- * removeAllHeaders(request.headers);
- * assert(request.url); // Fails because the :path header has been removed
- * ```
- * @since v8.4.0
- */
- readonly headers: IncomingHttpHeaders;
- /**
- * In case of server request, the HTTP version sent by the client. In the case of
- * client response, the HTTP version of the connected-to server. Returns`'2.0'`.
- *
- * Also `message.httpVersionMajor` is the first integer and`message.httpVersionMinor` is the second.
- * @since v8.4.0
- */
- readonly httpVersion: string;
- readonly httpVersionMinor: number;
- readonly httpVersionMajor: number;
- /**
- * The request method as a string. Read-only. Examples: `'GET'`, `'DELETE'`.
- * @since v8.4.0
- */
- readonly method: string;
- /**
- * The raw request/response headers list exactly as they were received.
- *
- * The keys and values are in the same list. It is _not_ a
- * list of tuples. So, the even-numbered offsets are key values, and the
- * odd-numbered offsets are the associated values.
- *
- * Header names are not lowercased, and duplicates are not merged.
- *
- * ```js
- * // Prints something like:
- * //
- * // [ 'user-agent',
- * // 'this is invalid because there can be only one',
- * // 'User-Agent',
- * // 'curl/7.22.0',
- * // 'Host',
- * // '127.0.0.1:8000',
- * // 'ACCEPT',
- * // '*' ]
- * console.log(request.rawHeaders);
- * ```
- * @since v8.4.0
- */
- readonly rawHeaders: string[];
- /**
- * The raw request/response trailer keys and values exactly as they were
- * received. Only populated at the `'end'` event.
- * @since v8.4.0
- */
- readonly rawTrailers: string[];
- /**
- * The request scheme pseudo header field indicating the scheme
- * portion of the target URL.
- * @since v8.4.0
- */
- readonly scheme: string;
- /**
- * Returns a `Proxy` object that acts as a `net.Socket` (or `tls.TLSSocket`) but
- * applies getters, setters, and methods based on HTTP/2 logic.
- *
- * `destroyed`, `readable`, and `writable` properties will be retrieved from and
- * set on `request.stream`.
- *
- * `destroy`, `emit`, `end`, `on` and `once` methods will be called on`request.stream`.
- *
- * `setTimeout` method will be called on `request.stream.session`.
- *
- * `pause`, `read`, `resume`, and `write` will throw an error with code`ERR_HTTP2_NO_SOCKET_MANIPULATION`. See `Http2Session and Sockets` for
- * more information.
- *
- * All other interactions will be routed directly to the socket. With TLS support,
- * use `request.socket.getPeerCertificate()` to obtain the client's
- * authentication details.
- * @since v8.4.0
- */
- readonly socket: net.Socket | tls.TLSSocket;
- /**
- * The `Http2Stream` object backing the request.
- * @since v8.4.0
- */
- readonly stream: ServerHttp2Stream;
- /**
- * The request/response trailers object. Only populated at the `'end'` event.
- * @since v8.4.0
- */
- readonly trailers: IncomingHttpHeaders;
- /**
- * Request URL string. This contains only the URL that is present in the actual
- * HTTP request. If the request is:
- *
- * ```http
- * GET /status?name=ryan HTTP/1.1
- * Accept: text/plain
- * ```
- *
- * Then `request.url` will be:
- *
- * ```js
- * '/status?name=ryan'
- * ```
- *
- * To parse the url into its parts, `new URL()` can be used:
- *
- * ```console
- * $ node
- * > new URL('/status?name=ryan', 'http://example.com')
- * URL {
- * href: 'http://example.com/status?name=ryan',
- * origin: 'http://example.com',
- * protocol: 'http:',
- * username: '',
- * password: '',
- * host: 'example.com',
- * hostname: 'example.com',
- * port: '',
- * pathname: '/status',
- * search: '?name=ryan',
- * searchParams: URLSearchParams { 'name' => 'ryan' },
- * hash: ''
- * }
- * ```
- * @since v8.4.0
- */
- url: string;
- /**
- * Sets the `Http2Stream`'s timeout value to `msecs`. If a callback is
- * provided, then it is added as a listener on the `'timeout'` event on
- * the response object.
- *
- * If no `'timeout'` listener is added to the request, the response, or
- * the server, then `Http2Stream` s are destroyed when they time out. If a
- * handler is assigned to the request, the response, or the server's `'timeout'`events, timed out sockets must be handled explicitly.
- * @since v8.4.0
- */
- setTimeout(msecs: number, callback?: () => void): void;
- read(size?: number): Buffer | string | null;
- addListener(event: 'aborted', listener: (hadError: boolean, code: number) => void): this;
- addListener(event: 'close', listener: () => void): this;
- addListener(event: 'data', listener: (chunk: Buffer | string) => void): this;
- addListener(event: 'end', listener: () => void): this;
- addListener(event: 'readable', listener: () => void): this;
- addListener(event: 'error', listener: (err: Error) => void): this;
- addListener(event: string | symbol, listener: (...args: any[]) => void): this;
- emit(event: 'aborted', hadError: boolean, code: number): boolean;
- emit(event: 'close'): boolean;
- emit(event: 'data', chunk: Buffer | string): boolean;
- emit(event: 'end'): boolean;
- emit(event: 'readable'): boolean;
- emit(event: 'error', err: Error): boolean;
- emit(event: string | symbol, ...args: any[]): boolean;
- on(event: 'aborted', listener: (hadError: boolean, code: number) => void): this;
- on(event: 'close', listener: () => void): this;
- on(event: 'data', listener: (chunk: Buffer | string) => void): this;
- on(event: 'end', listener: () => void): this;
- on(event: 'readable', listener: () => void): this;
- on(event: 'error', listener: (err: Error) => void): this;
- on(event: string | symbol, listener: (...args: any[]) => void): this;
- once(event: 'aborted', listener: (hadError: boolean, code: number) => void): this;
- once(event: 'close', listener: () => void): this;
- once(event: 'data', listener: (chunk: Buffer | string) => void): this;
- once(event: 'end', listener: () => void): this;
- once(event: 'readable', listener: () => void): this;
- once(event: 'error', listener: (err: Error) => void): this;
- once(event: string | symbol, listener: (...args: any[]) => void): this;
- prependListener(event: 'aborted', listener: (hadError: boolean, code: number) => void): this;
- prependListener(event: 'close', listener: () => void): this;
- prependListener(event: 'data', listener: (chunk: Buffer | string) => void): this;
- prependListener(event: 'end', listener: () => void): this;
- prependListener(event: 'readable', listener: () => void): this;
- prependListener(event: 'error', listener: (err: Error) => void): this;
- prependListener(event: string | symbol, listener: (...args: any[]) => void): this;
- prependOnceListener(event: 'aborted', listener: (hadError: boolean, code: number) => void): this;
- prependOnceListener(event: 'close', listener: () => void): this;
- prependOnceListener(event: 'data', listener: (chunk: Buffer | string) => void): this;
- prependOnceListener(event: 'end', listener: () => void): this;
- prependOnceListener(event: 'readable', listener: () => void): this;
- prependOnceListener(event: 'error', listener: (err: Error) => void): this;
- prependOnceListener(event: string | symbol, listener: (...args: any[]) => void): this;
- }
- /**
- * This object is created internally by an HTTP server, not by the user. It is
- * passed as the second parameter to the `'request'` event.
- * @since v8.4.0
- */
- export class Http2ServerResponse extends stream.Writable {
- constructor(stream: ServerHttp2Stream);
- /**
- * See `response.socket`.
- * @since v8.4.0
- * @deprecated Since v13.0.0 - Use `socket`.
- */
- readonly connection: net.Socket | tls.TLSSocket;
- /**
- * Boolean value that indicates whether the response has completed. Starts
- * as `false`. After `response.end()` executes, the value will be `true`.
- * @since v8.4.0
- * @deprecated Since v13.4.0,v12.16.0 - Use `writableEnded`.
- */
- readonly finished: boolean;
- /**
- * True if headers were sent, false otherwise (read-only).
- * @since v8.4.0
- */
- readonly headersSent: boolean;
- /**
- * A reference to the original HTTP2 request object.
- * @since v15.7.0
- */
- readonly req: Http2ServerRequest;
- /**
- * Returns a `Proxy` object that acts as a `net.Socket` (or `tls.TLSSocket`) but
- * applies getters, setters, and methods based on HTTP/2 logic.
- *
- * `destroyed`, `readable`, and `writable` properties will be retrieved from and
- * set on `response.stream`.
- *
- * `destroy`, `emit`, `end`, `on` and `once` methods will be called on`response.stream`.
- *
- * `setTimeout` method will be called on `response.stream.session`.
- *
- * `pause`, `read`, `resume`, and `write` will throw an error with code`ERR_HTTP2_NO_SOCKET_MANIPULATION`. See `Http2Session and Sockets` for
- * more information.
- *
- * All other interactions will be routed directly to the socket.
- *
- * ```js
- * const http2 = require('http2');
- * const server = http2.createServer((req, res) => {
- * const ip = req.socket.remoteAddress;
- * const port = req.socket.remotePort;
- * res.end(`Your IP address is ${ip} and your source port is ${port}.`);
- * }).listen(3000);
- * ```
- * @since v8.4.0
- */
- readonly socket: net.Socket | tls.TLSSocket;
- /**
- * The `Http2Stream` object backing the response.
- * @since v8.4.0
- */
- readonly stream: ServerHttp2Stream;
- /**
- * When true, the Date header will be automatically generated and sent in
- * the response if it is not already present in the headers. Defaults to true.
- *
- * This should only be disabled for testing; HTTP requires the Date header
- * in responses.
- * @since v8.4.0
- */
- sendDate: boolean;
- /**
- * When using implicit headers (not calling `response.writeHead()` explicitly),
- * this property controls the status code that will be sent to the client when
- * the headers get flushed.
- *
- * ```js
- * response.statusCode = 404;
- * ```
- *
- * After response header was sent to the client, this property indicates the
- * status code which was sent out.
- * @since v8.4.0
- */
- statusCode: number;
- /**
- * Status message is not supported by HTTP/2 (RFC 7540 8.1.2.4). It returns
- * an empty string.
- * @since v8.4.0
- */
- statusMessage: '';
- /**
- * This method adds HTTP trailing headers (a header but at the end of the
- * message) to the response.
- *
- * Attempting to set a header field name or value that contains invalid characters
- * will result in a `TypeError` being thrown.
- * @since v8.4.0
- */
- addTrailers(trailers: OutgoingHttpHeaders): void;
- /**
- * This method signals to the server that all of the response headers and body
- * have been sent; that server should consider this message complete.
- * The method, `response.end()`, MUST be called on each response.
- *
- * If `data` is specified, it is equivalent to calling `response.write(data, encoding)` followed by `response.end(callback)`.
- *
- * If `callback` is specified, it will be called when the response stream
- * is finished.
- * @since v8.4.0
- */
- end(callback?: () => void): this;
- end(data: string | Uint8Array, callback?: () => void): this;
- end(data: string | Uint8Array, encoding: BufferEncoding, callback?: () => void): this;
- /**
- * Reads out a header that has already been queued but not sent to the client.
- * The name is case-insensitive.
- *
- * ```js
- * const contentType = response.getHeader('content-type');
- * ```
- * @since v8.4.0
- */
- getHeader(name: string): string;
- /**
- * Returns an array containing the unique names of the current outgoing headers.
- * All header names are lowercase.
- *
- * ```js
- * response.setHeader('Foo', 'bar');
- * response.setHeader('Set-Cookie', ['foo=bar', 'bar=baz']);
- *
- * const headerNames = response.getHeaderNames();
- * // headerNames === ['foo', 'set-cookie']
- * ```
- * @since v8.4.0
- */
- getHeaderNames(): string[];
- /**
- * Returns a shallow copy of the current outgoing headers. Since a shallow copy
- * is used, array values may be mutated without additional calls to various
- * header-related http module methods. The keys of the returned object are the
- * header names and the values are the respective header values. All header names
- * are lowercase.
- *
- * The object returned by the `response.getHeaders()` method _does not_prototypically inherit from the JavaScript `Object`. This means that typical`Object` methods such as `obj.toString()`,
- * `obj.hasOwnProperty()`, and others
- * are not defined and _will not work_.
- *
- * ```js
- * response.setHeader('Foo', 'bar');
- * response.setHeader('Set-Cookie', ['foo=bar', 'bar=baz']);
- *
- * const headers = response.getHeaders();
- * // headers === { foo: 'bar', 'set-cookie': ['foo=bar', 'bar=baz'] }
- * ```
- * @since v8.4.0
- */
- getHeaders(): OutgoingHttpHeaders;
- /**
- * Returns `true` if the header identified by `name` is currently set in the
- * outgoing headers. The header name matching is case-insensitive.
- *
- * ```js
- * const hasContentType = response.hasHeader('content-type');
- * ```
- * @since v8.4.0
- */
- hasHeader(name: string): boolean;
- /**
- * Removes a header that has been queued for implicit sending.
- *
- * ```js
- * response.removeHeader('Content-Encoding');
- * ```
- * @since v8.4.0
- */
- removeHeader(name: string): void;
- /**
- * Sets a single header value for implicit headers. If this header already exists
- * in the to-be-sent headers, its value will be replaced. Use an array of strings
- * here to send multiple headers with the same name.
- *
- * ```js
- * response.setHeader('Content-Type', 'text/html; charset=utf-8');
- * ```
- *
- * or
- *
- * ```js
- * response.setHeader('Set-Cookie', ['type=ninja', 'language=javascript']);
- * ```
- *
- * Attempting to set a header field name or value that contains invalid characters
- * will result in a `TypeError` being thrown.
- *
- * When headers have been set with `response.setHeader()`, they will be merged
- * with any headers passed to `response.writeHead()`, with the headers passed
- * to `response.writeHead()` given precedence.
- *
- * ```js
- * // Returns content-type = text/plain
- * const server = http2.createServer((req, res) => {
- * res.setHeader('Content-Type', 'text/html; charset=utf-8');
- * res.setHeader('X-Foo', 'bar');
- * res.writeHead(200, { 'Content-Type': 'text/plain; charset=utf-8' });
- * res.end('ok');
- * });
- * ```
- * @since v8.4.0
- */
- setHeader(name: string, value: number | string | ReadonlyArray): void;
- /**
- * Sets the `Http2Stream`'s timeout value to `msecs`. If a callback is
- * provided, then it is added as a listener on the `'timeout'` event on
- * the response object.
- *
- * If no `'timeout'` listener is added to the request, the response, or
- * the server, then `Http2Stream` s are destroyed when they time out. If a
- * handler is assigned to the request, the response, or the server's `'timeout'`events, timed out sockets must be handled explicitly.
- * @since v8.4.0
- */
- setTimeout(msecs: number, callback?: () => void): void;
- /**
- * If this method is called and `response.writeHead()` has not been called,
- * it will switch to implicit header mode and flush the implicit headers.
- *
- * This sends a chunk of the response body. This method may
- * be called multiple times to provide successive parts of the body.
- *
- * In the `http` module, the response body is omitted when the
- * request is a HEAD request. Similarly, the `204` and `304` responses _must not_ include a message body.
- *
- * `chunk` can be a string or a buffer. If `chunk` is a string,
- * the second parameter specifies how to encode it into a byte stream.
- * By default the `encoding` is `'utf8'`. `callback` will be called when this chunk
- * of data is flushed.
- *
- * This is the raw HTTP body and has nothing to do with higher-level multi-part
- * body encodings that may be used.
- *
- * The first time `response.write()` is called, it will send the buffered
- * header information and the first chunk of the body to the client. The second
- * time `response.write()` is called, Node.js assumes data will be streamed,
- * and sends the new data separately. That is, the response is buffered up to the
- * first chunk of the body.
- *
- * Returns `true` if the entire data was flushed successfully to the kernel
- * buffer. Returns `false` if all or part of the data was queued in user memory.`'drain'` will be emitted when the buffer is free again.
- * @since v8.4.0
- */
- write(chunk: string | Uint8Array, callback?: (err: Error) => void): boolean;
- write(chunk: string | Uint8Array, encoding: BufferEncoding, callback?: (err: Error) => void): boolean;
- /**
- * Sends a status `100 Continue` to the client, indicating that the request body
- * should be sent. See the `'checkContinue'` event on `Http2Server` and`Http2SecureServer`.
- * @since v8.4.0
- */
- writeContinue(): void;
- /**
- * Sends a status `103 Early Hints` to the client with a Link header,
- * indicating that the user agent can preload/preconnect the linked resources.
- * The `hints` is an object containing the values of headers to be sent with
- * early hints message.
- *
- * Example:
- *
- * ```js
- * const earlyHintsLink = '; rel=preload; as=style';
- * response.writeEarlyHints({
- * 'link': earlyHintsLink,
- * });
- *
- * const earlyHintsLinks = [
- * '; rel=preload; as=style',
- * '; rel=preload; as=script',
- * ];
- * response.writeEarlyHints({
- * 'link': earlyHintsLinks,
- * 'x-trace-id': 'id for diagnostics'
- * });
- * ```
- *
- * @since v18.11.0
- * @param hints An object containing the values of headers
- */
- writeEarlyHints(hints: Record): void;
- /**
- * Sends a response header to the request. The status code is a 3-digit HTTP
- * status code, like `404`. The last argument, `headers`, are the response headers.
- *
- * Returns a reference to the `Http2ServerResponse`, so that calls can be chained.
- *
- * For compatibility with `HTTP/1`, a human-readable `statusMessage` may be
- * passed as the second argument. However, because the `statusMessage` has no
- * meaning within HTTP/2, the argument will have no effect and a process warning
- * will be emitted.
- *
- * ```js
- * const body = 'hello world';
- * response.writeHead(200, {
- * 'Content-Length': Buffer.byteLength(body),
- * 'Content-Type': 'text/plain; charset=utf-8',
- * });
- * ```
- *
- * `Content-Length` is given in bytes not characters. The`Buffer.byteLength()` API may be used to determine the number of bytes in a
- * given encoding. On outbound messages, Node.js does not check if Content-Length
- * and the length of the body being transmitted are equal or not. However, when
- * receiving messages, Node.js will automatically reject messages when the`Content-Length` does not match the actual payload size.
- *
- * This method may be called at most one time on a message before `response.end()` is called.
- *
- * If `response.write()` or `response.end()` are called before calling
- * this, the implicit/mutable headers will be calculated and call this function.
- *
- * When headers have been set with `response.setHeader()`, they will be merged
- * with any headers passed to `response.writeHead()`, with the headers passed
- * to `response.writeHead()` given precedence.
- *
- * ```js
- * // Returns content-type = text/plain
- * const server = http2.createServer((req, res) => {
- * res.setHeader('Content-Type', 'text/html; charset=utf-8');
- * res.setHeader('X-Foo', 'bar');
- * res.writeHead(200, { 'Content-Type': 'text/plain; charset=utf-8' });
- * res.end('ok');
- * });
- * ```
- *
- * Attempting to set a header field name or value that contains invalid characters
- * will result in a `TypeError` being thrown.
- * @since v8.4.0
- */
- writeHead(statusCode: number, headers?: OutgoingHttpHeaders): this;
- writeHead(statusCode: number, statusMessage: string, headers?: OutgoingHttpHeaders): this;
- /**
- * Call `http2stream.pushStream()` with the given headers, and wrap the
- * given `Http2Stream` on a newly created `Http2ServerResponse` as the callback
- * parameter if successful. When `Http2ServerRequest` is closed, the callback is
- * called with an error `ERR_HTTP2_INVALID_STREAM`.
- * @since v8.4.0
- * @param headers An object describing the headers
- * @param callback Called once `http2stream.pushStream()` is finished, or either when the attempt to create the pushed `Http2Stream` has failed or has been rejected, or the state of
- * `Http2ServerRequest` is closed prior to calling the `http2stream.pushStream()` method
- */
- createPushResponse(headers: OutgoingHttpHeaders, callback: (err: Error | null, res: Http2ServerResponse) => void): void;
- addListener(event: 'close', listener: () => void): this;
- addListener(event: 'drain', listener: () => void): this;
- addListener(event: 'error', listener: (error: Error) => void): this;
- addListener(event: 'finish', listener: () => void): this;
- addListener(event: 'pipe', listener: (src: stream.Readable) => void): this;
- addListener(event: 'unpipe', listener: (src: stream.Readable) => void): this;
- addListener(event: string | symbol, listener: (...args: any[]) => void): this;
- emit(event: 'close'): boolean;
- emit(event: 'drain'): boolean;
- emit(event: 'error', error: Error): boolean;
- emit(event: 'finish'): boolean;
- emit(event: 'pipe', src: stream.Readable): boolean;
- emit(event: 'unpipe', src: stream.Readable): boolean;
- emit(event: string | symbol, ...args: any[]): boolean;
- on(event: 'close', listener: () => void): this;
- on(event: 'drain', listener: () => void): this;
- on(event: 'error', listener: (error: Error) => void): this;
- on(event: 'finish', listener: () => void): this;
- on(event: 'pipe', listener: (src: stream.Readable) => void): this;
- on(event: 'unpipe', listener: (src: stream.Readable) => void): this;
- on(event: string | symbol, listener: (...args: any[]) => void): this;
- once(event: 'close', listener: () => void): this;
- once(event: 'drain', listener: () => void): this;
- once(event: 'error', listener: (error: Error) => void): this;
- once(event: 'finish', listener: () => void): this;
- once(event: 'pipe', listener: (src: stream.Readable) => void): this;
- once(event: 'unpipe', listener: (src: stream.Readable) => void): this;
- once(event: string | symbol, listener: (...args: any[]) => void): this;
- prependListener(event: 'close', listener: () => void): this;
- prependListener(event: 'drain', listener: () => void): this;
- prependListener(event: 'error', listener: (error: Error) => void): this;
- prependListener(event: 'finish', listener: () => void): this;
- prependListener(event: 'pipe', listener: (src: stream.Readable) => void): this;
- prependListener(event: 'unpipe', listener: (src: stream.Readable) => void): this;
- prependListener(event: string | symbol, listener: (...args: any[]) => void): this;
- prependOnceListener(event: 'close', listener: () => void): this;
- prependOnceListener(event: 'drain', listener: () => void): this;
- prependOnceListener(event: 'error', listener: (error: Error) => void): this;
- prependOnceListener(event: 'finish', listener: () => void): this;
- prependOnceListener(event: 'pipe', listener: (src: stream.Readable) => void): this;
- prependOnceListener(event: 'unpipe', listener: (src: stream.Readable) => void): this;
- prependOnceListener(event: string | symbol, listener: (...args: any[]) => void): this;
- }
- export namespace constants {
- const NGHTTP2_SESSION_SERVER: number;
- const NGHTTP2_SESSION_CLIENT: number;
- const NGHTTP2_STREAM_STATE_IDLE: number;
- const NGHTTP2_STREAM_STATE_OPEN: number;
- const NGHTTP2_STREAM_STATE_RESERVED_LOCAL: number;
- const NGHTTP2_STREAM_STATE_RESERVED_REMOTE: number;
- const NGHTTP2_STREAM_STATE_HALF_CLOSED_LOCAL: number;
- const NGHTTP2_STREAM_STATE_HALF_CLOSED_REMOTE: number;
- const NGHTTP2_STREAM_STATE_CLOSED: number;
- const NGHTTP2_NO_ERROR: number;
- const NGHTTP2_PROTOCOL_ERROR: number;
- const NGHTTP2_INTERNAL_ERROR: number;
- const NGHTTP2_FLOW_CONTROL_ERROR: number;
- const NGHTTP2_SETTINGS_TIMEOUT: number;
- const NGHTTP2_STREAM_CLOSED: number;
- const NGHTTP2_FRAME_SIZE_ERROR: number;
- const NGHTTP2_REFUSED_STREAM: number;
- const NGHTTP2_CANCEL: number;
- const NGHTTP2_COMPRESSION_ERROR: number;
- const NGHTTP2_CONNECT_ERROR: number;
- const NGHTTP2_ENHANCE_YOUR_CALM: number;
- const NGHTTP2_INADEQUATE_SECURITY: number;
- const NGHTTP2_HTTP_1_1_REQUIRED: number;
- const NGHTTP2_ERR_FRAME_SIZE_ERROR: number;
- const NGHTTP2_FLAG_NONE: number;
- const NGHTTP2_FLAG_END_STREAM: number;
- const NGHTTP2_FLAG_END_HEADERS: number;
- const NGHTTP2_FLAG_ACK: number;
- const NGHTTP2_FLAG_PADDED: number;
- const NGHTTP2_FLAG_PRIORITY: number;
- const DEFAULT_SETTINGS_HEADER_TABLE_SIZE: number;
- const DEFAULT_SETTINGS_ENABLE_PUSH: number;
- const DEFAULT_SETTINGS_INITIAL_WINDOW_SIZE: number;
- const DEFAULT_SETTINGS_MAX_FRAME_SIZE: number;
- const MAX_MAX_FRAME_SIZE: number;
- const MIN_MAX_FRAME_SIZE: number;
- const MAX_INITIAL_WINDOW_SIZE: number;
- const NGHTTP2_DEFAULT_WEIGHT: number;
- const NGHTTP2_SETTINGS_HEADER_TABLE_SIZE: number;
- const NGHTTP2_SETTINGS_ENABLE_PUSH: number;
- const NGHTTP2_SETTINGS_MAX_CONCURRENT_STREAMS: number;
- const NGHTTP2_SETTINGS_INITIAL_WINDOW_SIZE: number;
- const NGHTTP2_SETTINGS_MAX_FRAME_SIZE: number;
- const NGHTTP2_SETTINGS_MAX_HEADER_LIST_SIZE: number;
- const PADDING_STRATEGY_NONE: number;
- const PADDING_STRATEGY_MAX: number;
- const PADDING_STRATEGY_CALLBACK: number;
- const HTTP2_HEADER_STATUS: string;
- const HTTP2_HEADER_METHOD: string;
- const HTTP2_HEADER_AUTHORITY: string;
- const HTTP2_HEADER_SCHEME: string;
- const HTTP2_HEADER_PATH: string;
- const HTTP2_HEADER_ACCEPT_CHARSET: string;
- const HTTP2_HEADER_ACCEPT_ENCODING: string;
- const HTTP2_HEADER_ACCEPT_LANGUAGE: string;
- const HTTP2_HEADER_ACCEPT_RANGES: string;
- const HTTP2_HEADER_ACCEPT: string;
- const HTTP2_HEADER_ACCESS_CONTROL_ALLOW_ORIGIN: string;
- const HTTP2_HEADER_AGE: string;
- const HTTP2_HEADER_ALLOW: string;
- const HTTP2_HEADER_AUTHORIZATION: string;
- const HTTP2_HEADER_CACHE_CONTROL: string;
- const HTTP2_HEADER_CONNECTION: string;
- const HTTP2_HEADER_CONTENT_DISPOSITION: string;
- const HTTP2_HEADER_CONTENT_ENCODING: string;
- const HTTP2_HEADER_CONTENT_LANGUAGE: string;
- const HTTP2_HEADER_CONTENT_LENGTH: string;
- const HTTP2_HEADER_CONTENT_LOCATION: string;
- const HTTP2_HEADER_CONTENT_MD5: string;
- const HTTP2_HEADER_CONTENT_RANGE: string;
- const HTTP2_HEADER_CONTENT_TYPE: string;
- const HTTP2_HEADER_COOKIE: string;
- const HTTP2_HEADER_DATE: string;
- const HTTP2_HEADER_ETAG: string;
- const HTTP2_HEADER_EXPECT: string;
- const HTTP2_HEADER_EXPIRES: string;
- const HTTP2_HEADER_FROM: string;
- const HTTP2_HEADER_HOST: string;
- const HTTP2_HEADER_IF_MATCH: string;
- const HTTP2_HEADER_IF_MODIFIED_SINCE: string;
- const HTTP2_HEADER_IF_NONE_MATCH: string;
- const HTTP2_HEADER_IF_RANGE: string;
- const HTTP2_HEADER_IF_UNMODIFIED_SINCE: string;
- const HTTP2_HEADER_LAST_MODIFIED: string;
- const HTTP2_HEADER_LINK: string;
- const HTTP2_HEADER_LOCATION: string;
- const HTTP2_HEADER_MAX_FORWARDS: string;
- const HTTP2_HEADER_PREFER: string;
- const HTTP2_HEADER_PROXY_AUTHENTICATE: string;
- const HTTP2_HEADER_PROXY_AUTHORIZATION: string;
- const HTTP2_HEADER_RANGE: string;
- const HTTP2_HEADER_REFERER: string;
- const HTTP2_HEADER_REFRESH: string;
- const HTTP2_HEADER_RETRY_AFTER: string;
- const HTTP2_HEADER_SERVER: string;
- const HTTP2_HEADER_SET_COOKIE: string;
- const HTTP2_HEADER_STRICT_TRANSPORT_SECURITY: string;
- const HTTP2_HEADER_TRANSFER_ENCODING: string;
- const HTTP2_HEADER_TE: string;
- const HTTP2_HEADER_UPGRADE: string;
- const HTTP2_HEADER_USER_AGENT: string;
- const HTTP2_HEADER_VARY: string;
- const HTTP2_HEADER_VIA: string;
- const HTTP2_HEADER_WWW_AUTHENTICATE: string;
- const HTTP2_HEADER_HTTP2_SETTINGS: string;
- const HTTP2_HEADER_KEEP_ALIVE: string;
- const HTTP2_HEADER_PROXY_CONNECTION: string;
- const HTTP2_METHOD_ACL: string;
- const HTTP2_METHOD_BASELINE_CONTROL: string;
- const HTTP2_METHOD_BIND: string;
- const HTTP2_METHOD_CHECKIN: string;
- const HTTP2_METHOD_CHECKOUT: string;
- const HTTP2_METHOD_CONNECT: string;
- const HTTP2_METHOD_COPY: string;
- const HTTP2_METHOD_DELETE: string;
- const HTTP2_METHOD_GET: string;
- const HTTP2_METHOD_HEAD: string;
- const HTTP2_METHOD_LABEL: string;
- const HTTP2_METHOD_LINK: string;
- const HTTP2_METHOD_LOCK: string;
- const HTTP2_METHOD_MERGE: string;
- const HTTP2_METHOD_MKACTIVITY: string;
- const HTTP2_METHOD_MKCALENDAR: string;
- const HTTP2_METHOD_MKCOL: string;
- const HTTP2_METHOD_MKREDIRECTREF: string;
- const HTTP2_METHOD_MKWORKSPACE: string;
- const HTTP2_METHOD_MOVE: string;
- const HTTP2_METHOD_OPTIONS: string;
- const HTTP2_METHOD_ORDERPATCH: string;
- const HTTP2_METHOD_PATCH: string;
- const HTTP2_METHOD_POST: string;
- const HTTP2_METHOD_PRI: string;
- const HTTP2_METHOD_PROPFIND: string;
- const HTTP2_METHOD_PROPPATCH: string;
- const HTTP2_METHOD_PUT: string;
- const HTTP2_METHOD_REBIND: string;
- const HTTP2_METHOD_REPORT: string;
- const HTTP2_METHOD_SEARCH: string;
- const HTTP2_METHOD_TRACE: string;
- const HTTP2_METHOD_UNBIND: string;
- const HTTP2_METHOD_UNCHECKOUT: string;
- const HTTP2_METHOD_UNLINK: string;
- const HTTP2_METHOD_UNLOCK: string;
- const HTTP2_METHOD_UPDATE: string;
- const HTTP2_METHOD_UPDATEREDIRECTREF: string;
- const HTTP2_METHOD_VERSION_CONTROL: string;
- const HTTP_STATUS_CONTINUE: number;
- const HTTP_STATUS_SWITCHING_PROTOCOLS: number;
- const HTTP_STATUS_PROCESSING: number;
- const HTTP_STATUS_OK: number;
- const HTTP_STATUS_CREATED: number;
- const HTTP_STATUS_ACCEPTED: number;
- const HTTP_STATUS_NON_AUTHORITATIVE_INFORMATION: number;
- const HTTP_STATUS_NO_CONTENT: number;
- const HTTP_STATUS_RESET_CONTENT: number;
- const HTTP_STATUS_PARTIAL_CONTENT: number;
- const HTTP_STATUS_MULTI_STATUS: number;
- const HTTP_STATUS_ALREADY_REPORTED: number;
- const HTTP_STATUS_IM_USED: number;
- const HTTP_STATUS_MULTIPLE_CHOICES: number;
- const HTTP_STATUS_MOVED_PERMANENTLY: number;
- const HTTP_STATUS_FOUND: number;
- const HTTP_STATUS_SEE_OTHER: number;
- const HTTP_STATUS_NOT_MODIFIED: number;
- const HTTP_STATUS_USE_PROXY: number;
- const HTTP_STATUS_TEMPORARY_REDIRECT: number;
- const HTTP_STATUS_PERMANENT_REDIRECT: number;
- const HTTP_STATUS_BAD_REQUEST: number;
- const HTTP_STATUS_UNAUTHORIZED: number;
- const HTTP_STATUS_PAYMENT_REQUIRED: number;
- const HTTP_STATUS_FORBIDDEN: number;
- const HTTP_STATUS_NOT_FOUND: number;
- const HTTP_STATUS_METHOD_NOT_ALLOWED: number;
- const HTTP_STATUS_NOT_ACCEPTABLE: number;
- const HTTP_STATUS_PROXY_AUTHENTICATION_REQUIRED: number;
- const HTTP_STATUS_REQUEST_TIMEOUT: number;
- const HTTP_STATUS_CONFLICT: number;
- const HTTP_STATUS_GONE: number;
- const HTTP_STATUS_LENGTH_REQUIRED: number;
- const HTTP_STATUS_PRECONDITION_FAILED: number;
- const HTTP_STATUS_PAYLOAD_TOO_LARGE: number;
- const HTTP_STATUS_URI_TOO_LONG: number;
- const HTTP_STATUS_UNSUPPORTED_MEDIA_TYPE: number;
- const HTTP_STATUS_RANGE_NOT_SATISFIABLE: number;
- const HTTP_STATUS_EXPECTATION_FAILED: number;
- const HTTP_STATUS_TEAPOT: number;
- const HTTP_STATUS_MISDIRECTED_REQUEST: number;
- const HTTP_STATUS_UNPROCESSABLE_ENTITY: number;
- const HTTP_STATUS_LOCKED: number;
- const HTTP_STATUS_FAILED_DEPENDENCY: number;
- const HTTP_STATUS_UNORDERED_COLLECTION: number;
- const HTTP_STATUS_UPGRADE_REQUIRED: number;
- const HTTP_STATUS_PRECONDITION_REQUIRED: number;
- const HTTP_STATUS_TOO_MANY_REQUESTS: number;
- const HTTP_STATUS_REQUEST_HEADER_FIELDS_TOO_LARGE: number;
- const HTTP_STATUS_UNAVAILABLE_FOR_LEGAL_REASONS: number;
- const HTTP_STATUS_INTERNAL_SERVER_ERROR: number;
- const HTTP_STATUS_NOT_IMPLEMENTED: number;
- const HTTP_STATUS_BAD_GATEWAY: number;
- const HTTP_STATUS_SERVICE_UNAVAILABLE: number;
- const HTTP_STATUS_GATEWAY_TIMEOUT: number;
- const HTTP_STATUS_HTTP_VERSION_NOT_SUPPORTED: number;
- const HTTP_STATUS_VARIANT_ALSO_NEGOTIATES: number;
- const HTTP_STATUS_INSUFFICIENT_STORAGE: number;
- const HTTP_STATUS_LOOP_DETECTED: number;
- const HTTP_STATUS_BANDWIDTH_LIMIT_EXCEEDED: number;
- const HTTP_STATUS_NOT_EXTENDED: number;
- const HTTP_STATUS_NETWORK_AUTHENTICATION_REQUIRED: number;
- }
- /**
- * This symbol can be set as a property on the HTTP/2 headers object with
- * an array value in order to provide a list of headers considered sensitive.
- */
- export const sensitiveHeaders: symbol;
- /**
- * Returns an object containing the default settings for an `Http2Session`instance. This method returns a new object instance every time it is called
- * so instances returned may be safely modified for use.
- * @since v8.4.0
- */
- export function getDefaultSettings(): Settings;
- /**
- * Returns a `Buffer` instance containing serialized representation of the given
- * HTTP/2 settings as specified in the [HTTP/2](https://tools.ietf.org/html/rfc7540) specification. This is intended
- * for use with the `HTTP2-Settings` header field.
- *
- * ```js
- * const http2 = require('http2');
- *
- * const packed = http2.getPackedSettings({ enablePush: false });
- *
- * console.log(packed.toString('base64'));
- * // Prints: AAIAAAAA
- * ```
- * @since v8.4.0
- */
- export function getPackedSettings(settings: Settings): Buffer;
- /**
- * Returns a `HTTP/2 Settings Object` containing the deserialized settings from
- * the given `Buffer` as generated by `http2.getPackedSettings()`.
- * @since v8.4.0
- * @param buf The packed settings.
- */
- export function getUnpackedSettings(buf: Uint8Array): Settings;
- /**
- * Returns a `net.Server` instance that creates and manages `Http2Session`instances.
- *
- * Since there are no browsers known that support [unencrypted HTTP/2](https://http2.github.io/faq/#does-http2-require-encryption), the use of {@link createSecureServer} is necessary when
- * communicating
- * with browser clients.
- *
- * ```js
- * const http2 = require('http2');
- *
- * // Create an unencrypted HTTP/2 server.
- * // Since there are no browsers known that support
- * // unencrypted HTTP/2, the use of `http2.createSecureServer()`
- * // is necessary when communicating with browser clients.
- * const server = http2.createServer();
- *
- * server.on('stream', (stream, headers) => {
- * stream.respond({
- * 'content-type': 'text/html; charset=utf-8',
- * ':status': 200
- * });
- * stream.end('
');
- * });
- *
- * server.listen(80);
- * ```
- * @since v8.4.0
- * @param onRequestHandler See `Compatibility API`
- */
- export function createSecureServer(onRequestHandler?: (request: Http2ServerRequest, response: Http2ServerResponse) => void): Http2SecureServer;
- export function createSecureServer(options: SecureServerOptions, onRequestHandler?: (request: Http2ServerRequest, response: Http2ServerResponse) => void): Http2SecureServer;
- /**
- * Returns a `ClientHttp2Session` instance.
- *
- * ```js
- * const http2 = require('http2');
- * const client = http2.connect('https://localhost:1234');
- *
- * // Use the client
- *
- * client.close();
- * ```
- * @since v8.4.0
- * @param authority The remote HTTP/2 server to connect to. This must be in the form of a minimal, valid URL with the `http://` or `https://` prefix, host name, and IP port (if a non-default port
- * is used). Userinfo (user ID and password), path, querystring, and fragment details in the URL will be ignored.
- * @param listener Will be registered as a one-time listener of the {@link 'connect'} event.
- */
- export function connect(authority: string | url.URL, listener: (session: ClientHttp2Session, socket: net.Socket | tls.TLSSocket) => void): ClientHttp2Session;
- export function connect(
- authority: string | url.URL,
- options?: ClientSessionOptions | SecureClientSessionOptions,
- listener?: (session: ClientHttp2Session, socket: net.Socket | tls.TLSSocket) => void
- ): ClientHttp2Session;
-}
-declare module 'node:http2' {
- export * from 'http2';
-}
diff --git a/spaces/fffiloni/controlnet-animation-doodle/node_modules/object-inspect/test-core-js.js b/spaces/fffiloni/controlnet-animation-doodle/node_modules/object-inspect/test-core-js.js
deleted file mode 100644
index e53c40022533f691fd17d623cd24a8ecb5a82669..0000000000000000000000000000000000000000
--- a/spaces/fffiloni/controlnet-animation-doodle/node_modules/object-inspect/test-core-js.js
+++ /dev/null
@@ -1,26 +0,0 @@
-'use strict';
-
-require('core-js');
-
-var inspect = require('./');
-var test = require('tape');
-
-test('Maps', function (t) {
- t.equal(inspect(new Map([[1, 2]])), 'Map (1) {1 => 2}');
- t.end();
-});
-
-test('WeakMaps', function (t) {
- t.equal(inspect(new WeakMap([[{}, 2]])), 'WeakMap { ? }');
- t.end();
-});
-
-test('Sets', function (t) {
- t.equal(inspect(new Set([[1, 2]])), 'Set (1) {[ 1, 2 ]}');
- t.end();
-});
-
-test('WeakSets', function (t) {
- t.equal(inspect(new WeakSet([[1, 2]])), 'WeakSet { ? }');
- t.end();
-});
diff --git a/spaces/fishaudio/fish-diffusion/configs/Kiritan.py b/spaces/fishaudio/fish-diffusion/configs/Kiritan.py
deleted file mode 100644
index adbcc11bdf74cac263ee428f2f84a62a6aff9aef..0000000000000000000000000000000000000000
--- a/spaces/fishaudio/fish-diffusion/configs/Kiritan.py
+++ /dev/null
@@ -1,39 +0,0 @@
-_base_ = [
- "./_base_/archs/hifi_svc.py",
-]
-
-speaker_mapping = {'kiritan': 0,}
-
-model = dict(
- type="HiFiSVC",
- speaker_encoder=dict(
- input_size=len(speaker_mapping),
- ),
-)
-
-preprocessing = dict(
- text_features_extractor=dict(
- type="ContentVec",
- ),
- pitch_extractor=dict(
- type="ParselMouthPitchExtractor",
- keep_zeros=False,
- f0_min=40.0,
- f0_max=1600.0,
- ),
- energy_extractor=dict(
- type="RMSEnergyExtractor",
- ),
- augmentations=[
- dict(
- type="RandomPitchShifting",
- key_shifts=[-5., 5.],
- probability=1.5,
- ),
- dict(
- type="RandomTimeStretching",
- factors=[0.8, 1.2],
- probability=0.75,
- )
- ],
-)
\ No newline at end of file
diff --git a/spaces/flax-community/koclip/text2patch.py b/spaces/flax-community/koclip/text2patch.py
deleted file mode 100644
index 907b155f70bab08ccaa2d812717aa1688533ae29..0000000000000000000000000000000000000000
--- a/spaces/flax-community/koclip/text2patch.py
+++ /dev/null
@@ -1,93 +0,0 @@
-import os
-
-import jax
-import jax.numpy as jnp
-import numpy as np
-import requests
-import streamlit as st
-from PIL import Image
-
-from utils import load_model
-
-
-def split_image(im, num_rows=3, num_cols=3):
- im = np.array(im)
- row_size = im.shape[0] // num_rows
- col_size = im.shape[1] // num_cols
- tiles = [
- im[row : row + row_size, col : col + col_size]
- for row in range(0, num_rows * row_size, row_size)
- for col in range(0, num_cols * col_size, col_size)
- ]
- return tiles
-
-
-def app(model_name):
- model, processor = load_model(f"koclip/{model_name}")
-
- st.title("Patch-based Relevance Ranking")
- st.markdown(
- """
- Given a piece of text, the CLIP model finds the part of an image that best explains the text.
- To try it out, you can
-
- 1. Upload an image
- 2. Explain a part of the image in text
-
- which will yield the most relevant image tile from a grid of the image. You can specify how
- granular you want to be with your search by specifying the number of rows and columns that
- make up the image grid.
-
- ---
- """
- )
-
- query1 = st.text_input(
- "Enter a URL to an image...",
- value="https://img.sbs.co.kr/newimg/news/20200823/201463830_1280.jpg",
- )
- query2 = st.file_uploader("or upload an image...", type=["jpg", "jpeg", "png"])
- captions = st.text_input(
- "Enter a prompt to query the image.",
- value="이건 서울의 경복궁 사진이다.",
- )
-
- col1, col2 = st.beta_columns(2)
- with col1:
- num_rows = st.slider(
- "Number of rows", min_value=1, max_value=5, value=3, step=1
- )
- with col2:
- num_cols = st.slider(
- "Number of columns", min_value=1, max_value=5, value=3, step=1
- )
-
- if st.button("질문 (Query)"):
- if not any([query1, query2]):
- st.error("Please upload an image or paste an image URL.")
- else:
- st.markdown("""---""")
- with st.spinner("Computing..."):
- image_data = (
- query2
- if query2 is not None
- else requests.get(query1, stream=True).raw
- )
- image = Image.open(image_data)
- st.image(image)
-
- images = split_image(image, num_rows, num_cols)
-
- inputs = processor(
- text=captions, images=images, return_tensors="jax", padding=True
- )
- inputs["pixel_values"] = jnp.transpose(
- inputs["pixel_values"], axes=[0, 2, 3, 1]
- )
- outputs = model(**inputs)
- probs = jax.nn.softmax(outputs.logits_per_image, axis=0)
- for idx, prob in sorted(
- enumerate(probs), key=lambda x: x[1], reverse=True
- ):
- st.text(f"Score: {prob[0]:.3f}")
- st.image(images[idx])
diff --git a/spaces/flowers-team/SocialAISchool/data_analysis_neurips.py b/spaces/flowers-team/SocialAISchool/data_analysis_neurips.py
deleted file mode 100644
index 3b413df8effc9c15fa8b27f00d8a9a27a99c3994..0000000000000000000000000000000000000000
--- a/spaces/flowers-team/SocialAISchool/data_analysis_neurips.py
+++ /dev/null
@@ -1,570 +0,0 @@
-#!/usr/bin/env python
-import seaborn
-import numpy as np
-import os
-from collections import OrderedDict
-import pandas as pd
-import matplotlib.pyplot as plt
-import sys
-from termcolor import cprint
-
-# Load data
-
-# Global vars for tracking and labeling data at load time.
-exp_idx = 0
-label_parser_dict = None
-
-smooth_factor = 10
-leg_size = 30
-
-subsample_step = 1
-load_subsample_step = 50
-
-default_colors = ["blue","orange","green","magenta", "brown", "red",'black',"grey",u'#ff7f0e',
- "cyan", "pink",'purple', u'#1f77b4',
- "darkorchid","sienna","lightpink", "indigo","mediumseagreen",'aqua',
- 'deeppink','silver','khaki','goldenrod','y','y','y','y','y','y','y','y','y','y','y','y' ] + ['y']*50
-
-def get_all_runs(logdir, load_subsample_step=1):
- """
- Recursively look through logdir for output files produced by
- Assumes that any file "progress.txt" is a valid hit.
- """
- global exp_idx
- global units
- datasets = []
- for root, _, files in os.walk(logdir):
- if 'log.csv' in files:
- run_name = root[8:]
- exp_name = None
-
- # try to load a config file containing hyperparameters
- config = None
- try:
- config_path = open(os.path.join(root,'config.json'))
- config = json.load(config_path)
- if 'exp_name' in config:
- exp_name = config['exp_name']
- except:
- print('No file named config.json')
-
- exp_idx += 1
-
- # load progress data
- try:
- print(os.path.join(root,'log.csv'))
- exp_data = pd.read_csv(os.path.join(root,'log.csv'))
- except:
- raise ValueError("CSV {} faulty".format(os.path.join(root, 'log.csv')))
-
- exp_data = exp_data[::load_subsample_step]
- data_dict = exp_data.to_dict("list")
-
- data_dict['config'] = config
- nb_epochs = len(data_dict['frames'])
- print('{} -> {}'.format(run_name, nb_epochs))
-
-
- datasets.append(data_dict)
-
- return datasets
-
-def get_datasets(rootdir, load_only="", load_subsample_step=1, ignore_pattern="ignore"):
- _, models_list, _ = next(os.walk(rootdir))
- print(models_list)
- for dir_name in models_list.copy():
- # add "ignore" in a directory name to avoid loading its content
- if ignore_pattern in dir_name or load_only not in dir_name:
- models_list.remove(dir_name)
- for expe_name in list(labels.keys()):
- if expe_name not in models_list:
- del labels[expe_name]
-
- # setting per-model type colors
- for i,m_name in enumerate(models_list):
- for m_type, m_color in per_model_colors.items():
- if m_type in m_name:
- colors[m_name] = m_color
- print("extracting data for {}...".format(m_name))
- m_id = m_name
- models_saves[m_id] = OrderedDict()
- models_saves[m_id]['data'] = get_all_runs(rootdir+m_name, load_subsample_step=load_subsample_step)
- print("done")
- if m_name not in labels:
- labels[m_name] = m_name
-
- """
- retrieve all experiences located in "data to vizu" folder
- """
-labels = OrderedDict()
-per_model_colors = OrderedDict()
-# per_model_colors = OrderedDict([('ALP-GMM',u'#1f77b4'),
-# ('hmn','pink'),
-# ('ADR','black')])
-
-# LOAD DATA
-models_saves = OrderedDict()
-colors = OrderedDict()
-
-static_lines = {}
-# get_datasets("storage/",load_only="RERUN_WizardGuide")
-# get_datasets("storage/",load_only="RERUN_WizardTwoGuides")
-try:
- figure_id = eval(sys.argv[1])
-except:
- figure_id = sys.argv[1]
-
-print("fig:", figure_id)
-if figure_id == 0:
- # train change
- env_type = "No_NPC_environment"
- fig_type = "train"
-
- get_datasets("storage/", "RERUN_WizardGuide_lang64_mm", load_subsample_step=load_subsample_step)
- get_datasets("storage/", "RERUN_WizardGuide_lang64_deaf_no_explo", load_subsample_step=load_subsample_step)
- get_datasets("storage/", "RERUN_WizardGuide_lang64_no_explo", load_subsample_step=load_subsample_step)
- get_datasets("storage/", "RERUN_WizardGuide_lang64_curr_dial", load_subsample_step=load_subsample_step)
- top_n = 16
-elif figure_id == 1:
- # arch change
- env_type = "No_NPC_environment"
- fig_type = "arch"
-
- get_datasets("storage/", "RERUN_WizardGuide_lang64_mm", load_subsample_step=load_subsample_step)
- get_datasets("storage/", "RERUN_WizardGuide_lang64_bow", load_subsample_step=load_subsample_step)
- get_datasets("storage/", "RERUN_WizardGuide_lang64_no_mem", load_subsample_step=load_subsample_step)
- get_datasets("storage/", "RERUN_WizardGuide_lang64_bigru", load_subsample_step=load_subsample_step)
- get_datasets("storage/", "RERUN_WizardGuide_lang64_attgru", load_subsample_step=load_subsample_step)
- top_n = 16
-elif figure_id == 2:
- # train change FULL
- env_type = "FULL_environment"
- fig_type = "train"
-
- get_datasets("storage/", "RERUN_WizardTwoGuides_lang64_mm", load_subsample_step=load_subsample_step)
- get_datasets("storage/", "RERUN_WizardTwoGuides_lang64_deaf_no_explo", load_subsample_step=load_subsample_step)
- get_datasets("storage/", "RERUN_WizardTwoGuides_lang64_no_explo", load_subsample_step=load_subsample_step)
- get_datasets("storage/", "RERUN_WizardTwoGuides_lang64_curr_dial", load_subsample_step=load_subsample_step)
- top_n = 16
-elif figure_id == 3:
- # arch change FULL
- env_type = "FULL_environment"
- fig_type = "arch"
-
- get_datasets("storage/", "RERUN_WizardTwoGuides_lang64_mm", load_subsample_step=load_subsample_step)
- get_datasets("storage/", "RERUN_WizardTwoGuides_lang64_bow", load_subsample_step=load_subsample_step)
- get_datasets("storage/", "RERUN_WizardTwoGuides_lang64_no_mem", load_subsample_step=load_subsample_step)
- get_datasets("storage/", "RERUN_WizardTwoGuides_lang64_bigru", load_subsample_step=load_subsample_step)
- get_datasets("storage/", "RERUN_WizardTwoGuides_lang64_attgru", load_subsample_step=load_subsample_step)
- top_n = 16
-elif str(figure_id) == "ShowMe":
-
- get_datasets("storage/", "20-05_NeurIPS_ShowMe_ABL_CEB", load_subsample_step=load_subsample_step, ignore_pattern="tanh_0.3")
- get_datasets("storage/", "20-05_NeurIPS_ShowMe_NO_BONUS_ABL", load_subsample_step=load_subsample_step)
- get_datasets("storage/", "20-05_NeurIPS_ShowMe_CEB", load_subsample_step=load_subsample_step, ignore_pattern="tanh_0.3")
- get_datasets("storage/", "20-05_NeurIPS_ShowMe_NO_BONUS_env", load_subsample_step=load_subsample_step)
-
- label_parser_dict = {
- "20-05_NeurIPS_ShowMe_ABL_CEB" : "ShowMe_exp_bonus_no_social_skills_required",
- "20-05_NeurIPS_ShowMe_NO_BONUS_ABL" : "ShowMe_no_bonus_no_social_skills_required",
- "20-05_NeurIPS_ShowMe_CEB" : "ShowMe_exp_bonus",
- "20-05_NeurIPS_ShowMe_NO_BONUS_env" : "ShowMe_no_bonus",
- }
-
- env_type = str(figure_id)
-
- fig_type = "test"
- top_n = 16
-
-elif str(figure_id) == "Help":
-
- # env_type = "Bobo"
- # get_datasets("storage/", "Bobo")
- get_datasets("storage/", "24-05_NeurIPS_Help", load_subsample_step=load_subsample_step, ignore_pattern="ABL")
- # get_datasets("storage/", "26-05_NeurIPS_gpu_Help_NoSocial_NO_BONUS_ABL", load_subsample_step=load_subsample_step)
- get_datasets("storage/", "26-05_NeurIPS_gpu_Help_NoSocial_NO_BONUS_env", load_subsample_step=load_subsample_step)
-
- label_parser_dict = {
- "Help_NO_BONUS_env": "PPO",
- "Help_BONUS_env": "PPO+Explo",
- # "Help_NO_BONUS_ABL_env": "ExiterRole_no_bonus_no_NPC",
- # "Help_BONUS_ABL_env": "ExiterRole_bonus_no_NPC",
- "26-05_NeurIPS_gpu_Help_NoSocial_NO_BONUS_env": "Unsocial PPO",
- # "26-05_NeurIPS_gpu_Help_NoSocial_NO_BONUS_ABL": "ExiterRole_Insocial_ABL"
- }
-
- static_lines = {
- "PPO (helper)": (0.12, 0.05, "#1f77b4"),
- "PPO+Explo (helper)": (0.11, 0.04, "indianred"),
- # "Help_exp_bonus": (0.11525, 0.04916 , default_colors[2]),
- # "HelperRole_ABL_no_exp_bonus": (0.022375, 0.01848, default_colors[3]),
- "Unsocial PPO (helper)": (0.15, 0.06, "grey"),
- # "HelperRole_ABL_Insocial": (0.01775, 0.010544, default_colors[4]),
- }
-
- env_type = str(figure_id)
-
- fig_type = "test"
- top_n = 16
-
-elif str(figure_id) == "TalkItOut":
- print("You mean Polite")
- exit()
-
-elif str(figure_id) == "TalkItOutPolite":
- # env_type = "TalkItOut"
- # get_datasets("storage/", "ORIENT_env_MiniGrid-TalkItOut")
-
- # env_type = "GuideThief"
- # get_datasets("storage/", "GuideThief")
-
- # env_type = "Bobo"
- # get_datasets("storage/", "Bobo")
- get_datasets("storage/", "20-05_NeurIPS_TalkItOutPolite", load_subsample_step=load_subsample_step)
- # get_datasets("storage/", "21-05_NeurIPS_small_bonus_TalkItOutPolite")
- get_datasets("storage/", "26-05_NeurIPS_gpu_TalkItOutPolite_NoSocial_NO_BONUS_env", load_subsample_step=load_subsample_step)
- get_datasets("storage/", "26-05_NeurIPS_gpu_TalkItOutPolite_NoSocial_NO_BONUS_NoLiar", load_subsample_step=load_subsample_step)
-
- label_parser_dict = {
- "TalkItOutPolite_NO_BONUS_env": "PPO",
- "TalkItOutPolite_e": "PPO+Explo",
- "TalkItOutPolite_NO_BONUS_NoLiar": "PPO (no liar)",
- "TalkItOutPolite_NoLiar_e": "PPO+Explo (no liar)",
- "26-05_NeurIPS_gpu_TalkItOutPolite_NoSocial_NO_BONUS_env": "Unsocial PPO",
- "26-05_NeurIPS_gpu_TalkItOutPolite_NoSocial_NO_BONUS_NoLiar": "Unsocial PPO (no liar)",
- }
-
-
- env_type = str(figure_id)
-
- fig_type = "test"
- top_n = 16
-
-elif str(figure_id) == "DiverseExit":
- get_datasets("storage/", "24-05_NeurIPS_DiverseExit", load_subsample_step=load_subsample_step)
- get_datasets("storage/", "26-05_NeurIPS_gpu_DiverseExit", load_subsample_step=load_subsample_step)
-
- label_parser_dict = {
- "DiverseExit_NO_BONUS": "No_bonus",
- "DiverseExit_BONUS": "BOnus",
- "gpu_DiverseExit_NoSocial": "No_social",
- }
-
- env_type = str(figure_id)
-
- fig_type = "test"
- top_n = 16
-
-else:
- get_datasets("storage/", str(figure_id), load_subsample_step=load_subsample_step)
-
- env_type = str(figure_id)
-
- fig_type = "test"
- top_n = 8
-
-#### get_datasets("storage/", "RERUN_WizardGuide_lang64_nameless")
-#### get_datasets("storage/", "RERUN_WizardTwoGuides_lang64_nameless")
-
-
-if per_model_colors: # order runs for legend order as in per_models_colors, with corresponding colors
- ordered_labels = OrderedDict()
- for teacher_type in per_model_colors.keys():
- for k,v in labels.items():
- if teacher_type in k:
- ordered_labels[k] = v
- labels = ordered_labels
-else:
- print('not using per_model_color')
- for k in models_saves.keys():
- labels[k] = k
-
-def plot_with_shade(subplot_nb, ax,x,y,err,color,shade_color,label,
- y_min=None,y_max=None, legend=False, leg_size=30, leg_loc='best', title=None,
- ylim=[0,100], xlim=[0,40], leg_args={}, leg_linewidth=13.0, linewidth=10.0, ticksize=20,
- zorder=None, xlabel='perf',ylabel='env steps'):
- #plt.rcParams.update({'font.size': 15})
- ax.locator_params(axis='x', nbins=4)
- ax.locator_params(axis='y', nbins=3)
- ax.tick_params(axis='both', which='major', labelsize=ticksize)
- ax.plot(x,y, color=color, label=label,linewidth=linewidth,zorder=zorder)
- ax.fill_between(x,y-err,y+err,color=shade_color,alpha=0.2)
- if legend:
- leg = ax.legend(loc=leg_loc, **leg_args) #34
- for legobj in leg.legendHandles:
- legobj.set_linewidth(leg_linewidth)
- ax.set_xlabel(xlabel, fontsize=30)
- if subplot_nb == 0:
- ax.set_ylabel(ylabel, fontsize=30,labelpad=-4)
- ax.set_xlim(xmin=xlim[0],xmax=xlim[1])
- ax.set_ylim(bottom=ylim[0],top=ylim[1])
- if title:
- ax.set_title(title, fontsize=22)
-# Plot utils
-def plot_with_shade_grg(subplot_nb, ax,x,y,err,color,shade_color,label,
- y_min=None,y_max=None, legend=False, leg_size=30, leg_loc='best', title=None,
- ylim=[0,100], xlim=[0,40], leg_args={}, leg_linewidth=13.0, linewidth=10.0, ticksize=20,
- zorder=None, xlabel='perf',ylabel='env steps', linestyle="-"):
- #plt.rcParams.update({'font.size': 15})
- ax.locator_params(axis='x', nbins=4)
- ax.locator_params(axis='y', nbins=3)
- ax.tick_params(axis='both', which='major', labelsize=ticksize)
-
-
- ax.plot(x, y, color=color, label=label,linewidth=linewidth,zorder=zorder, linestyle=linestyle)
- ax.fill_between(x, y-err, y+err,color=shade_color,alpha=0.2)
- if legend:
- leg = ax.legend(loc=leg_loc, **leg_args) #34
- for legobj in leg.legendHandles:
- legobj.set_linewidth(leg_linewidth)
- ax.set_xlabel(xlabel, fontsize=30)
- if subplot_nb == 0:
- ax.set_ylabel(ylabel, fontsize=30, labelpad=-4)
- ax.set_xlim(xmin=xlim[0],xmax=xlim[1])
- ax.set_ylim(bottom=ylim[0],top=ylim[1])
- if title:
- ax.set_title(title, fontsize=22)
-
-
-# Metric plot
-metric = 'bin_extrinsic_return_mean'
-# metric = 'mission_string_observed_mean'
-# metric = 'extrinsic_return_mean'
-# metric = 'extrinsic_return_max'
-# metric = "rreturn_mean"
-# metric = 'rreturn_max'
-# metric = 'FPS'
-
-f, ax = plt.subplots(1, 1, figsize=(10.0, 6.0))
-ax = [ax]
-max_y = -np.inf
-min_y = np.inf
-# hardcoded
-min_y, max_y = 0.0, 1.0
-max_steps = 0
-exclude_patterns = []
-include_patterns = []
-
-
-def label_parser(label, figure_id, label_parser_dict=None):
- if label_parser_dict:
- if sum([1 for k, v in label_parser_dict.items() if k in label]) != 1:
- if label in label_parser_dict:
- # see if there is an exact match
- return label_parser_dict[label]
- else:
- print("ERROR multiple curves match a lable and there is no exact match")
- print(label)
- exit()
-
- for k, v in label_parser_dict.items():
- if k in label: return v
-
- else:
- # return label.split("_env_")[1]
- if figure_id not in [1,2,3,4]:
- return label
- else:
- label_parser_dict = {
- "RERUN_WizardGuide_lang64_no_explo": "MH-BabyAI",
- "RERUN_WizardTwoGuides_lang64_no_explo": "MH-BabyAI",
-
- "RERUN_WizardGuide_lang64_mm_baby_short_rec_env": "MH-BabyAI-ExpBonus",
- "RERUN_WizardTwoGuides_lang64_mm_baby_short_rec_env": "MH-BabyAI-ExpBonus",
-
- "RERUN_WizardGuide_lang64_deaf_no_explo": "Deaf-MH-BabyAI",
- "RERUN_WizardTwoGuides_lang64_deaf_no_explo": "Deaf-MH-BabyAI",
-
- "RERUN_WizardGuide_lang64_bow": "MH-BabyAI-ExpBonus-BOW",
- "RERUN_WizardTwoGuides_lang64_bow": "MH-BabyAI-ExpBonus-BOW",
-
- "RERUN_WizardGuide_lang64_no_mem": "MH-BabyAI-ExpBonus-no-mem",
- "RERUN_WizardTwoGuides_lang64_no_mem": "MH-BabyAI-ExpBonus-no-mem",
-
- "RERUN_WizardGuide_lang64_bigru": "MH-BabyAI-ExpBonus-bigru",
- "RERUN_WizardTwoGuides_lang64_bigru": "MH-BabyAI-ExpBonus-bigru",
-
- "RERUN_WizardGuide_lang64_attgru": "MH-BabyAI-ExpBonus-attgru",
- "RERUN_WizardTwoGuides_lang64_attgru": "MH-BabyAI-ExpBonus-attgru",
-
- "RERUN_WizardGuide_lang64_curr_dial": "MH-BabyAI-ExpBonus-current-dialogue",
- "RERUN_WizardTwoGuides_lang64_curr_dial": "MH-BabyAI-ExpBonus-current-dialogue",
-
- "RERUN_WizardTwoGuides_lang64_mm_baby_short_rec_100M": "MH-BabyAI-ExpBonus-100M"
- }
- if sum([1 for k, v in label_parser_dict.items() if k in label]) != 1:
- print("ERROR multiple curves match a lable")
- print(label)
- exit()
-
- for k, v in label_parser_dict.items():
- if k in label: return v
-
- return label
-
-per_seed=False
-
-for i, m_id in enumerate(models_saves.keys()):
- #excluding some experiments
- if any([ex_pat in m_id for ex_pat in exclude_patterns]):
- continue
- if len(include_patterns) > 0:
- if not any([in_pat in m_id for in_pat in include_patterns]):
- continue
- runs_data = models_saves[m_id]['data']
- ys = []
-
- # DIRTY FIX FOR FAULTY LOGGING
- print("m_id:", m_id)
- if runs_data[0]['frames'][1] == 'frames':
- runs_data[0]['frames'] = list(filter(('frames').__ne__, runs_data[0]['frames']))
- ###########################################
-
-
- # determine minimal run length across seeds
- minimum = sorted([len(run['frames']) for run in runs_data if len(run['frames'])])[-top_n]
- min_len = np.min([len(run['frames']) for run in runs_data if len(run['frames']) >= minimum])
-
-# min_len = np.min([len(run['frames']) for run in runs_data if len(run['frames']) > 10])
-
-
- print("min_len:", min_len)
-
- #compute env steps (x axis)
- longest_id = np.argmax([len(rd['frames']) for rd in runs_data])
- steps = np.array(runs_data[longest_id]['frames'], dtype=np.int) / 1000000
- steps = steps[:min_len]
- for run in runs_data:
- data = run[metric]
- # DIRTY FIX FOR FAULTY LOGGING (headers in data)
- if data[1] == metric:
- data = np.array(list(filter((metric).__ne__, data)), dtype=np.float16)
- ###########################################
- if len(data) >= min_len:
- if len(data) > min_len:
- print("run has too many {} datapoints ({}). Discarding {}".format(m_id, len(data),
- len(data)-min_len))
- data = data[0:min_len]
- ys.append(data)
- ys_same_len = ys # RUNS MUST HAVE SAME LEN
-
- # computes stats
- n_seeds = len(ys_same_len)
- sems = np.std(ys_same_len,axis=0)/np.sqrt(len(ys_same_len)) # sem
- stds = np.std(ys_same_len,axis=0) # std
- means = np.mean(ys_same_len,axis=0)
- color = default_colors[i]
-
- # per-metric adjusments
- ylabel=metric
- if metric == 'bin_extrinsic_return_mean':
- ylabel = "success rate"
- if metric == 'duration':
- ylabel = "time (hours)"
- means = means / 3600
- sems = sems / 3600
- stds = stds / 3600
-
- #plot x y bounds
- curr_max_y = np.max(means)
- curr_min_y = np.min(means)
- curr_max_steps = np.max(steps)
- if curr_max_y > max_y:
- max_y = curr_max_y
- if curr_min_y < min_y:
- min_y = curr_min_y
- if curr_max_steps > max_steps:
- max_steps = curr_max_steps
-
- if subsample_step:
- steps = steps[0::subsample_step]
- means = means[0::subsample_step]
- stds = stds[0::subsample_step]
- sems = sems[0::subsample_step]
- ys_same_len = [y[0::subsample_step] for y in ys_same_len]
-
- # display seeds separtely
- if per_seed:
- for s_i, seed_ys in enumerate(ys_same_len):
- seed_c = default_colors[i+s_i]
- label = m_id#+"(s:{})".format(s_i)
- plot_with_shade(0, ax[0], steps, seed_ys, stds*0, seed_c, seed_c, label,
- legend=False, xlim=[0, max_steps], ylim=[min_y, max_y],
- leg_size=leg_size, xlabel="env steps (millions)", ylabel=ylabel, smooth_factor=smooth_factor,
- )
- else:
- label = label_parser(m_id, figure_id, label_parser_dict=label_parser_dict)
- label = label #+"({})".format(n_seeds)
-
-
- def smooth(x_, n=50):
- if type(x_) == list:
- x_ = np.array(x_)
- return np.array([x_[max(i - n, 0):i + 1].mean() for i in range(len(x_))])
- if smooth_factor:
- means = smooth(means,smooth_factor)
- stds = smooth(stds,smooth_factor)
- x_lim = 30
- if figure_id == "TalkItOutPolite":
- leg_args = {
- 'ncol': 1,
- 'columnspacing': 1.0,
- 'handlelength': 1.0,
- 'frameon': False,
- # 'bbox_to_anchor': (0.00, 0.23, 0.10, .102),
- 'bbox_to_anchor': (0.55, 0.35, 0.10, .102),
- 'labelspacing': 0.2,
- 'fontsize': 27
- }
- elif figure_id == "Help":
- leg_args = {
- 'ncol': 1,
- 'columnspacing': 1.0,
- 'handlelength': 1.0,
- 'frameon': False,
- # 'bbox_to_anchor': (0.00, 0.23, 0.10, .102),
- 'bbox_to_anchor': (0.39, 0.20, 0.10, .102),
- 'labelspacing': 0.2,
- 'fontsize': 27
- }
- else:
- leg_args = {}
-
- color_code = dict([
- ('PPO+Explo', 'indianred'),
- ('PPO', "#1f77b4"),
- ('Unsocial PPO', "grey"),
- ('PPO (no liar)', "#043252"),
- ('PPO+Explo (no liar)', "darkred"),
- ('Unsocial PPO (no liar)', "black"),
- ('PPO+Explo (helper)', 'indianred'),
- ('PPO (helper)', "#1f77b4"),
- ('Unsocial PPO (helper)', "grey")]
- )
- color = color_code.get(label, np.random.choice(default_colors))
- print("C:",color)
- plot_with_shade_grg(
- 0, ax[0], steps, means, stds, color, color, label,
- legend=True,
- xlim=[0, steps[-1] if not x_lim else x_lim],
- ylim=[0, 1.0], xlabel="env steps (millions)", ylabel=ylabel, title=None,
- leg_args =leg_args)
- #
- # plot_with_shade(0, ax[0], steps, means, stds, color, color,label,
- # legend=True, xlim=[0, max_steps], ylim=[min_y, max_y],
- # leg_size=leg_size, xlabel="Env steps (millions)", ylabel=ylabel, linewidth=5.0, smooth_factor=smooth_factor)
-
-
-for label, (mean, std, color) in static_lines.items():
- plot_with_shade_grg(
- 0, ax[0], steps, np.array([mean]*len(steps)), np.array([std]*len(steps)), color, color, label,
- legend=True,
- xlim=[0, max_steps],
- ylim=[0, 1.0],
- xlabel="env steps (millions)", ylabel=ylabel, linestyle=":",
- leg_args=leg_args)
-
-plt.tight_layout()
-f.savefig('graphics/{}_results.svg'.format(str(figure_id)))
-f.savefig('graphics/{}_results.png'.format(str(figure_id)))
-plt.show()
\ No newline at end of file
diff --git a/spaces/flowers-team/SocialAISchool/torch-ac/torch_ac/utils/penv.py b/spaces/flowers-team/SocialAISchool/torch-ac/torch_ac/utils/penv.py
deleted file mode 100644
index e92891cb2138265e8b8135f1fc444529aefde0e5..0000000000000000000000000000000000000000
--- a/spaces/flowers-team/SocialAISchool/torch-ac/torch_ac/utils/penv.py
+++ /dev/null
@@ -1,74 +0,0 @@
-from multiprocessing import Process, Pipe
-import gym
-
-def worker(conn, env):
- while True:
- cmd, data = conn.recv()
- if cmd == "step":
- obs, reward, done, info = env.step(data)
- if done:
- obs = env.reset()
- conn.send((obs, reward, done, info))
- elif cmd == "set_curriculum_parameters":
- env.set_curriculum_parameters(data)
- conn.send(None)
- elif cmd == "reset":
- obs = env.reset()
- conn.send(obs)
- elif cmd == "get_mission":
- ks = env.get_mission()
- conn.send(ks)
- else:
- raise NotImplementedError
-
-class ParallelEnv(gym.Env):
- """A concurrent execution of environments in multiple processes."""
-
- def __init__(self, envs):
- assert len(envs) >= 1, "No environment given."
-
- self.envs = envs
- self.observation_space = self.envs[0].observation_space
- self.action_space = self.envs[0].action_space
-
- if hasattr(self.envs[0], "curriculum"):
- self.curriculum = self.envs[0].curriculum
-
- self.locals = []
- for env in self.envs[1:]:
- local, remote = Pipe()
- self.locals.append(local)
- p = Process(target=worker, args=(remote, env))
- p.daemon = True
- p.start()
- remote.close()
-
- def broadcast_curriculum_parameters(self, data):
- # broadcast curriculum_data to every worker
- for local in self.locals:
- local.send(("set_curriculum_parameters", data))
- results = [self.envs[0].set_curriculum_parameters(data)] + [local.recv() for local in self.locals]
-
- def get_mission(self):
- for local in self.locals:
- local.send(("get_mission", None))
- results = [self.envs[0].get_mission()] + [local.recv() for local in self.locals]
- return results
-
- def reset(self):
- for local in self.locals:
- local.send(("reset", None))
- results = [self.envs[0].reset()] + [local.recv() for local in self.locals]
- return results
-
- def step(self, actions):
- for local, action in zip(self.locals, actions[1:]):
- local.send(("step", action))
- obs, reward, done, info = self.envs[0].step(actions[0])
- if done:
- obs = self.envs[0].reset()
- results = zip(*[(obs, reward, done, info)] + [local.recv() for local in self.locals])
- return results
-
- def render(self):
- raise NotImplementedError
\ No newline at end of file
diff --git a/spaces/georgefen/Face-Landmark-ControlNet/annotator/uniformer/configs/_base_/models/deeplabv3_unet_s5-d16.py b/spaces/georgefen/Face-Landmark-ControlNet/annotator/uniformer/configs/_base_/models/deeplabv3_unet_s5-d16.py
deleted file mode 100644
index 0cd262999d8b2cb8e14a5c32190ae73f479d8e81..0000000000000000000000000000000000000000
--- a/spaces/georgefen/Face-Landmark-ControlNet/annotator/uniformer/configs/_base_/models/deeplabv3_unet_s5-d16.py
+++ /dev/null
@@ -1,50 +0,0 @@
-# model settings
-norm_cfg = dict(type='SyncBN', requires_grad=True)
-model = dict(
- type='EncoderDecoder',
- pretrained=None,
- backbone=dict(
- type='UNet',
- in_channels=3,
- base_channels=64,
- num_stages=5,
- strides=(1, 1, 1, 1, 1),
- enc_num_convs=(2, 2, 2, 2, 2),
- dec_num_convs=(2, 2, 2, 2),
- downsamples=(True, True, True, True),
- enc_dilations=(1, 1, 1, 1, 1),
- dec_dilations=(1, 1, 1, 1),
- with_cp=False,
- conv_cfg=None,
- norm_cfg=norm_cfg,
- act_cfg=dict(type='ReLU'),
- upsample_cfg=dict(type='InterpConv'),
- norm_eval=False),
- decode_head=dict(
- type='ASPPHead',
- in_channels=64,
- in_index=4,
- channels=16,
- dilations=(1, 12, 24, 36),
- dropout_ratio=0.1,
- num_classes=2,
- norm_cfg=norm_cfg,
- align_corners=False,
- loss_decode=dict(
- type='CrossEntropyLoss', use_sigmoid=False, loss_weight=1.0)),
- auxiliary_head=dict(
- type='FCNHead',
- in_channels=128,
- in_index=3,
- channels=64,
- num_convs=1,
- concat_input=False,
- dropout_ratio=0.1,
- num_classes=2,
- norm_cfg=norm_cfg,
- align_corners=False,
- loss_decode=dict(
- type='CrossEntropyLoss', use_sigmoid=False, loss_weight=0.4)),
- # model training and testing settings
- train_cfg=dict(),
- test_cfg=dict(mode='slide', crop_size=256, stride=170))
diff --git a/spaces/georgefen/Face-Landmark-ControlNet/annotator/uniformer/mmcv/image/__init__.py b/spaces/georgefen/Face-Landmark-ControlNet/annotator/uniformer/mmcv/image/__init__.py
deleted file mode 100644
index d0051d609d3de4e7562e3fe638335c66617c4d91..0000000000000000000000000000000000000000
--- a/spaces/georgefen/Face-Landmark-ControlNet/annotator/uniformer/mmcv/image/__init__.py
+++ /dev/null
@@ -1,28 +0,0 @@
-# Copyright (c) OpenMMLab. All rights reserved.
-from .colorspace import (bgr2gray, bgr2hls, bgr2hsv, bgr2rgb, bgr2ycbcr,
- gray2bgr, gray2rgb, hls2bgr, hsv2bgr, imconvert,
- rgb2bgr, rgb2gray, rgb2ycbcr, ycbcr2bgr, ycbcr2rgb)
-from .geometric import (cutout, imcrop, imflip, imflip_, impad,
- impad_to_multiple, imrescale, imresize, imresize_like,
- imresize_to_multiple, imrotate, imshear, imtranslate,
- rescale_size)
-from .io import imfrombytes, imread, imwrite, supported_backends, use_backend
-from .misc import tensor2imgs
-from .photometric import (adjust_brightness, adjust_color, adjust_contrast,
- adjust_lighting, adjust_sharpness, auto_contrast,
- clahe, imdenormalize, imequalize, iminvert,
- imnormalize, imnormalize_, lut_transform, posterize,
- solarize)
-
-__all__ = [
- 'bgr2gray', 'bgr2hls', 'bgr2hsv', 'bgr2rgb', 'gray2bgr', 'gray2rgb',
- 'hls2bgr', 'hsv2bgr', 'imconvert', 'rgb2bgr', 'rgb2gray', 'imrescale',
- 'imresize', 'imresize_like', 'imresize_to_multiple', 'rescale_size',
- 'imcrop', 'imflip', 'imflip_', 'impad', 'impad_to_multiple', 'imrotate',
- 'imfrombytes', 'imread', 'imwrite', 'supported_backends', 'use_backend',
- 'imdenormalize', 'imnormalize', 'imnormalize_', 'iminvert', 'posterize',
- 'solarize', 'rgb2ycbcr', 'bgr2ycbcr', 'ycbcr2rgb', 'ycbcr2bgr',
- 'tensor2imgs', 'imshear', 'imtranslate', 'adjust_color', 'imequalize',
- 'adjust_brightness', 'adjust_contrast', 'lut_transform', 'clahe',
- 'adjust_sharpness', 'auto_contrast', 'cutout', 'adjust_lighting'
-]
diff --git a/spaces/georgefen/Face-Landmark-ControlNet/annotator/uniformer/mmcv/image/colorspace.py b/spaces/georgefen/Face-Landmark-ControlNet/annotator/uniformer/mmcv/image/colorspace.py
deleted file mode 100644
index 814533952fdfda23d67cb6a3073692d8c1156add..0000000000000000000000000000000000000000
--- a/spaces/georgefen/Face-Landmark-ControlNet/annotator/uniformer/mmcv/image/colorspace.py
+++ /dev/null
@@ -1,306 +0,0 @@
-# Copyright (c) OpenMMLab. All rights reserved.
-import cv2
-import numpy as np
-
-
-def imconvert(img, src, dst):
- """Convert an image from the src colorspace to dst colorspace.
-
- Args:
- img (ndarray): The input image.
- src (str): The source colorspace, e.g., 'rgb', 'hsv'.
- dst (str): The destination colorspace, e.g., 'rgb', 'hsv'.
-
- Returns:
- ndarray: The converted image.
- """
- code = getattr(cv2, f'COLOR_{src.upper()}2{dst.upper()}')
- out_img = cv2.cvtColor(img, code)
- return out_img
-
-
-def bgr2gray(img, keepdim=False):
- """Convert a BGR image to grayscale image.
-
- Args:
- img (ndarray): The input image.
- keepdim (bool): If False (by default), then return the grayscale image
- with 2 dims, otherwise 3 dims.
-
- Returns:
- ndarray: The converted grayscale image.
- """
- out_img = cv2.cvtColor(img, cv2.COLOR_BGR2GRAY)
- if keepdim:
- out_img = out_img[..., None]
- return out_img
-
-
-def rgb2gray(img, keepdim=False):
- """Convert a RGB image to grayscale image.
-
- Args:
- img (ndarray): The input image.
- keepdim (bool): If False (by default), then return the grayscale image
- with 2 dims, otherwise 3 dims.
-
- Returns:
- ndarray: The converted grayscale image.
- """
- out_img = cv2.cvtColor(img, cv2.COLOR_RGB2GRAY)
- if keepdim:
- out_img = out_img[..., None]
- return out_img
-
-
-def gray2bgr(img):
- """Convert a grayscale image to BGR image.
-
- Args:
- img (ndarray): The input image.
-
- Returns:
- ndarray: The converted BGR image.
- """
- img = img[..., None] if img.ndim == 2 else img
- out_img = cv2.cvtColor(img, cv2.COLOR_GRAY2BGR)
- return out_img
-
-
-def gray2rgb(img):
- """Convert a grayscale image to RGB image.
-
- Args:
- img (ndarray): The input image.
-
- Returns:
- ndarray: The converted RGB image.
- """
- img = img[..., None] if img.ndim == 2 else img
- out_img = cv2.cvtColor(img, cv2.COLOR_GRAY2RGB)
- return out_img
-
-
-def _convert_input_type_range(img):
- """Convert the type and range of the input image.
-
- It converts the input image to np.float32 type and range of [0, 1].
- It is mainly used for pre-processing the input image in colorspace
- conversion functions such as rgb2ycbcr and ycbcr2rgb.
-
- Args:
- img (ndarray): The input image. It accepts:
- 1. np.uint8 type with range [0, 255];
- 2. np.float32 type with range [0, 1].
-
- Returns:
- (ndarray): The converted image with type of np.float32 and range of
- [0, 1].
- """
- img_type = img.dtype
- img = img.astype(np.float32)
- if img_type == np.float32:
- pass
- elif img_type == np.uint8:
- img /= 255.
- else:
- raise TypeError('The img type should be np.float32 or np.uint8, '
- f'but got {img_type}')
- return img
-
-
-def _convert_output_type_range(img, dst_type):
- """Convert the type and range of the image according to dst_type.
-
- It converts the image to desired type and range. If `dst_type` is np.uint8,
- images will be converted to np.uint8 type with range [0, 255]. If
- `dst_type` is np.float32, it converts the image to np.float32 type with
- range [0, 1].
- It is mainly used for post-processing images in colorspace conversion
- functions such as rgb2ycbcr and ycbcr2rgb.
-
- Args:
- img (ndarray): The image to be converted with np.float32 type and
- range [0, 255].
- dst_type (np.uint8 | np.float32): If dst_type is np.uint8, it
- converts the image to np.uint8 type with range [0, 255]. If
- dst_type is np.float32, it converts the image to np.float32 type
- with range [0, 1].
-
- Returns:
- (ndarray): The converted image with desired type and range.
- """
- if dst_type not in (np.uint8, np.float32):
- raise TypeError('The dst_type should be np.float32 or np.uint8, '
- f'but got {dst_type}')
- if dst_type == np.uint8:
- img = img.round()
- else:
- img /= 255.
- return img.astype(dst_type)
-
-
-def rgb2ycbcr(img, y_only=False):
- """Convert a RGB image to YCbCr image.
-
- This function produces the same results as Matlab's `rgb2ycbcr` function.
- It implements the ITU-R BT.601 conversion for standard-definition
- television. See more details in
- https://en.wikipedia.org/wiki/YCbCr#ITU-R_BT.601_conversion.
-
- It differs from a similar function in cv2.cvtColor: `RGB <-> YCrCb`.
- In OpenCV, it implements a JPEG conversion. See more details in
- https://en.wikipedia.org/wiki/YCbCr#JPEG_conversion.
-
- Args:
- img (ndarray): The input image. It accepts:
- 1. np.uint8 type with range [0, 255];
- 2. np.float32 type with range [0, 1].
- y_only (bool): Whether to only return Y channel. Default: False.
-
- Returns:
- ndarray: The converted YCbCr image. The output image has the same type
- and range as input image.
- """
- img_type = img.dtype
- img = _convert_input_type_range(img)
- if y_only:
- out_img = np.dot(img, [65.481, 128.553, 24.966]) + 16.0
- else:
- out_img = np.matmul(
- img, [[65.481, -37.797, 112.0], [128.553, -74.203, -93.786],
- [24.966, 112.0, -18.214]]) + [16, 128, 128]
- out_img = _convert_output_type_range(out_img, img_type)
- return out_img
-
-
-def bgr2ycbcr(img, y_only=False):
- """Convert a BGR image to YCbCr image.
-
- The bgr version of rgb2ycbcr.
- It implements the ITU-R BT.601 conversion for standard-definition
- television. See more details in
- https://en.wikipedia.org/wiki/YCbCr#ITU-R_BT.601_conversion.
-
- It differs from a similar function in cv2.cvtColor: `BGR <-> YCrCb`.
- In OpenCV, it implements a JPEG conversion. See more details in
- https://en.wikipedia.org/wiki/YCbCr#JPEG_conversion.
-
- Args:
- img (ndarray): The input image. It accepts:
- 1. np.uint8 type with range [0, 255];
- 2. np.float32 type with range [0, 1].
- y_only (bool): Whether to only return Y channel. Default: False.
-
- Returns:
- ndarray: The converted YCbCr image. The output image has the same type
- and range as input image.
- """
- img_type = img.dtype
- img = _convert_input_type_range(img)
- if y_only:
- out_img = np.dot(img, [24.966, 128.553, 65.481]) + 16.0
- else:
- out_img = np.matmul(
- img, [[24.966, 112.0, -18.214], [128.553, -74.203, -93.786],
- [65.481, -37.797, 112.0]]) + [16, 128, 128]
- out_img = _convert_output_type_range(out_img, img_type)
- return out_img
-
-
-def ycbcr2rgb(img):
- """Convert a YCbCr image to RGB image.
-
- This function produces the same results as Matlab's ycbcr2rgb function.
- It implements the ITU-R BT.601 conversion for standard-definition
- television. See more details in
- https://en.wikipedia.org/wiki/YCbCr#ITU-R_BT.601_conversion.
-
- It differs from a similar function in cv2.cvtColor: `YCrCb <-> RGB`.
- In OpenCV, it implements a JPEG conversion. See more details in
- https://en.wikipedia.org/wiki/YCbCr#JPEG_conversion.
-
- Args:
- img (ndarray): The input image. It accepts:
- 1. np.uint8 type with range [0, 255];
- 2. np.float32 type with range [0, 1].
-
- Returns:
- ndarray: The converted RGB image. The output image has the same type
- and range as input image.
- """
- img_type = img.dtype
- img = _convert_input_type_range(img) * 255
- out_img = np.matmul(img, [[0.00456621, 0.00456621, 0.00456621],
- [0, -0.00153632, 0.00791071],
- [0.00625893, -0.00318811, 0]]) * 255.0 + [
- -222.921, 135.576, -276.836
- ]
- out_img = _convert_output_type_range(out_img, img_type)
- return out_img
-
-
-def ycbcr2bgr(img):
- """Convert a YCbCr image to BGR image.
-
- The bgr version of ycbcr2rgb.
- It implements the ITU-R BT.601 conversion for standard-definition
- television. See more details in
- https://en.wikipedia.org/wiki/YCbCr#ITU-R_BT.601_conversion.
-
- It differs from a similar function in cv2.cvtColor: `YCrCb <-> BGR`.
- In OpenCV, it implements a JPEG conversion. See more details in
- https://en.wikipedia.org/wiki/YCbCr#JPEG_conversion.
-
- Args:
- img (ndarray): The input image. It accepts:
- 1. np.uint8 type with range [0, 255];
- 2. np.float32 type with range [0, 1].
-
- Returns:
- ndarray: The converted BGR image. The output image has the same type
- and range as input image.
- """
- img_type = img.dtype
- img = _convert_input_type_range(img) * 255
- out_img = np.matmul(img, [[0.00456621, 0.00456621, 0.00456621],
- [0.00791071, -0.00153632, 0],
- [0, -0.00318811, 0.00625893]]) * 255.0 + [
- -276.836, 135.576, -222.921
- ]
- out_img = _convert_output_type_range(out_img, img_type)
- return out_img
-
-
-def convert_color_factory(src, dst):
-
- code = getattr(cv2, f'COLOR_{src.upper()}2{dst.upper()}')
-
- def convert_color(img):
- out_img = cv2.cvtColor(img, code)
- return out_img
-
- convert_color.__doc__ = f"""Convert a {src.upper()} image to {dst.upper()}
- image.
-
- Args:
- img (ndarray or str): The input image.
-
- Returns:
- ndarray: The converted {dst.upper()} image.
- """
-
- return convert_color
-
-
-bgr2rgb = convert_color_factory('bgr', 'rgb')
-
-rgb2bgr = convert_color_factory('rgb', 'bgr')
-
-bgr2hsv = convert_color_factory('bgr', 'hsv')
-
-hsv2bgr = convert_color_factory('hsv', 'bgr')
-
-bgr2hls = convert_color_factory('bgr', 'hls')
-
-hls2bgr = convert_color_factory('hls', 'bgr')
diff --git a/spaces/georgefen/Face-Landmark-ControlNet/annotator/uniformer/mmcv/ops/upfirdn2d.py b/spaces/georgefen/Face-Landmark-ControlNet/annotator/uniformer/mmcv/ops/upfirdn2d.py
deleted file mode 100644
index c8bb2c3c949eed38a6465ed369fa881538dca010..0000000000000000000000000000000000000000
--- a/spaces/georgefen/Face-Landmark-ControlNet/annotator/uniformer/mmcv/ops/upfirdn2d.py
+++ /dev/null
@@ -1,330 +0,0 @@
-# modified from https://github.com/rosinality/stylegan2-pytorch/blob/master/op/upfirdn2d.py # noqa:E501
-
-# Copyright (c) 2021, NVIDIA Corporation. All rights reserved.
-# NVIDIA Source Code License for StyleGAN2 with Adaptive Discriminator
-# Augmentation (ADA)
-# =======================================================================
-
-# 1. Definitions
-
-# "Licensor" means any person or entity that distributes its Work.
-
-# "Software" means the original work of authorship made available under
-# this License.
-
-# "Work" means the Software and any additions to or derivative works of
-# the Software that are made available under this License.
-
-# The terms "reproduce," "reproduction," "derivative works," and
-# "distribution" have the meaning as provided under U.S. copyright law;
-# provided, however, that for the purposes of this License, derivative
-# works shall not include works that remain separable from, or merely
-# link (or bind by name) to the interfaces of, the Work.
-
-# Works, including the Software, are "made available" under this License
-# by including in or with the Work either (a) a copyright notice
-# referencing the applicability of this License to the Work, or (b) a
-# copy of this License.
-
-# 2. License Grants
-
-# 2.1 Copyright Grant. Subject to the terms and conditions of this
-# License, each Licensor grants to you a perpetual, worldwide,
-# non-exclusive, royalty-free, copyright license to reproduce,
-# prepare derivative works of, publicly display, publicly perform,
-# sublicense and distribute its Work and any resulting derivative
-# works in any form.
-
-# 3. Limitations
-
-# 3.1 Redistribution. You may reproduce or distribute the Work only
-# if (a) you do so under this License, (b) you include a complete
-# copy of this License with your distribution, and (c) you retain
-# without modification any copyright, patent, trademark, or
-# attribution notices that are present in the Work.
-
-# 3.2 Derivative Works. You may specify that additional or different
-# terms apply to the use, reproduction, and distribution of your
-# derivative works of the Work ("Your Terms") only if (a) Your Terms
-# provide that the use limitation in Section 3.3 applies to your
-# derivative works, and (b) you identify the specific derivative
-# works that are subject to Your Terms. Notwithstanding Your Terms,
-# this License (including the redistribution requirements in Section
-# 3.1) will continue to apply to the Work itself.
-
-# 3.3 Use Limitation. The Work and any derivative works thereof only
-# may be used or intended for use non-commercially. Notwithstanding
-# the foregoing, NVIDIA and its affiliates may use the Work and any
-# derivative works commercially. As used herein, "non-commercially"
-# means for research or evaluation purposes only.
-
-# 3.4 Patent Claims. If you bring or threaten to bring a patent claim
-# against any Licensor (including any claim, cross-claim or
-# counterclaim in a lawsuit) to enforce any patents that you allege
-# are infringed by any Work, then your rights under this License from
-# such Licensor (including the grant in Section 2.1) will terminate
-# immediately.
-
-# 3.5 Trademarks. This License does not grant any rights to use any
-# Licensor’s or its affiliates’ names, logos, or trademarks, except
-# as necessary to reproduce the notices described in this License.
-
-# 3.6 Termination. If you violate any term of this License, then your
-# rights under this License (including the grant in Section 2.1) will
-# terminate immediately.
-
-# 4. Disclaimer of Warranty.
-
-# THE WORK IS PROVIDED "AS IS" WITHOUT WARRANTIES OR CONDITIONS OF ANY
-# KIND, EITHER EXPRESS OR IMPLIED, INCLUDING WARRANTIES OR CONDITIONS OF
-# MERCHANTABILITY, FITNESS FOR A PARTICULAR PURPOSE, TITLE OR
-# NON-INFRINGEMENT. YOU BEAR THE RISK OF UNDERTAKING ANY ACTIVITIES UNDER
-# THIS LICENSE.
-
-# 5. Limitation of Liability.
-
-# EXCEPT AS PROHIBITED BY APPLICABLE LAW, IN NO EVENT AND UNDER NO LEGAL
-# THEORY, WHETHER IN TORT (INCLUDING NEGLIGENCE), CONTRACT, OR OTHERWISE
-# SHALL ANY LICENSOR BE LIABLE TO YOU FOR DAMAGES, INCLUDING ANY DIRECT,
-# INDIRECT, SPECIAL, INCIDENTAL, OR CONSEQUENTIAL DAMAGES ARISING OUT OF
-# OR RELATED TO THIS LICENSE, THE USE OR INABILITY TO USE THE WORK
-# (INCLUDING BUT NOT LIMITED TO LOSS OF GOODWILL, BUSINESS INTERRUPTION,
-# LOST PROFITS OR DATA, COMPUTER FAILURE OR MALFUNCTION, OR ANY OTHER
-# COMMERCIAL DAMAGES OR LOSSES), EVEN IF THE LICENSOR HAS BEEN ADVISED OF
-# THE POSSIBILITY OF SUCH DAMAGES.
-
-# =======================================================================
-
-import torch
-from torch.autograd import Function
-from torch.nn import functional as F
-
-from annotator.uniformer.mmcv.utils import to_2tuple
-from ..utils import ext_loader
-
-upfirdn2d_ext = ext_loader.load_ext('_ext', ['upfirdn2d'])
-
-
-class UpFirDn2dBackward(Function):
-
- @staticmethod
- def forward(ctx, grad_output, kernel, grad_kernel, up, down, pad, g_pad,
- in_size, out_size):
-
- up_x, up_y = up
- down_x, down_y = down
- g_pad_x0, g_pad_x1, g_pad_y0, g_pad_y1 = g_pad
-
- grad_output = grad_output.reshape(-1, out_size[0], out_size[1], 1)
-
- grad_input = upfirdn2d_ext.upfirdn2d(
- grad_output,
- grad_kernel,
- up_x=down_x,
- up_y=down_y,
- down_x=up_x,
- down_y=up_y,
- pad_x0=g_pad_x0,
- pad_x1=g_pad_x1,
- pad_y0=g_pad_y0,
- pad_y1=g_pad_y1)
- grad_input = grad_input.view(in_size[0], in_size[1], in_size[2],
- in_size[3])
-
- ctx.save_for_backward(kernel)
-
- pad_x0, pad_x1, pad_y0, pad_y1 = pad
-
- ctx.up_x = up_x
- ctx.up_y = up_y
- ctx.down_x = down_x
- ctx.down_y = down_y
- ctx.pad_x0 = pad_x0
- ctx.pad_x1 = pad_x1
- ctx.pad_y0 = pad_y0
- ctx.pad_y1 = pad_y1
- ctx.in_size = in_size
- ctx.out_size = out_size
-
- return grad_input
-
- @staticmethod
- def backward(ctx, gradgrad_input):
- kernel, = ctx.saved_tensors
-
- gradgrad_input = gradgrad_input.reshape(-1, ctx.in_size[2],
- ctx.in_size[3], 1)
-
- gradgrad_out = upfirdn2d_ext.upfirdn2d(
- gradgrad_input,
- kernel,
- up_x=ctx.up_x,
- up_y=ctx.up_y,
- down_x=ctx.down_x,
- down_y=ctx.down_y,
- pad_x0=ctx.pad_x0,
- pad_x1=ctx.pad_x1,
- pad_y0=ctx.pad_y0,
- pad_y1=ctx.pad_y1)
- # gradgrad_out = gradgrad_out.view(ctx.in_size[0], ctx.out_size[0],
- # ctx.out_size[1], ctx.in_size[3])
- gradgrad_out = gradgrad_out.view(ctx.in_size[0], ctx.in_size[1],
- ctx.out_size[0], ctx.out_size[1])
-
- return gradgrad_out, None, None, None, None, None, None, None, None
-
-
-class UpFirDn2d(Function):
-
- @staticmethod
- def forward(ctx, input, kernel, up, down, pad):
- up_x, up_y = up
- down_x, down_y = down
- pad_x0, pad_x1, pad_y0, pad_y1 = pad
-
- kernel_h, kernel_w = kernel.shape
- batch, channel, in_h, in_w = input.shape
- ctx.in_size = input.shape
-
- input = input.reshape(-1, in_h, in_w, 1)
-
- ctx.save_for_backward(kernel, torch.flip(kernel, [0, 1]))
-
- out_h = (in_h * up_y + pad_y0 + pad_y1 - kernel_h) // down_y + 1
- out_w = (in_w * up_x + pad_x0 + pad_x1 - kernel_w) // down_x + 1
- ctx.out_size = (out_h, out_w)
-
- ctx.up = (up_x, up_y)
- ctx.down = (down_x, down_y)
- ctx.pad = (pad_x0, pad_x1, pad_y0, pad_y1)
-
- g_pad_x0 = kernel_w - pad_x0 - 1
- g_pad_y0 = kernel_h - pad_y0 - 1
- g_pad_x1 = in_w * up_x - out_w * down_x + pad_x0 - up_x + 1
- g_pad_y1 = in_h * up_y - out_h * down_y + pad_y0 - up_y + 1
-
- ctx.g_pad = (g_pad_x0, g_pad_x1, g_pad_y0, g_pad_y1)
-
- out = upfirdn2d_ext.upfirdn2d(
- input,
- kernel,
- up_x=up_x,
- up_y=up_y,
- down_x=down_x,
- down_y=down_y,
- pad_x0=pad_x0,
- pad_x1=pad_x1,
- pad_y0=pad_y0,
- pad_y1=pad_y1)
- # out = out.view(major, out_h, out_w, minor)
- out = out.view(-1, channel, out_h, out_w)
-
- return out
-
- @staticmethod
- def backward(ctx, grad_output):
- kernel, grad_kernel = ctx.saved_tensors
-
- grad_input = UpFirDn2dBackward.apply(
- grad_output,
- kernel,
- grad_kernel,
- ctx.up,
- ctx.down,
- ctx.pad,
- ctx.g_pad,
- ctx.in_size,
- ctx.out_size,
- )
-
- return grad_input, None, None, None, None
-
-
-def upfirdn2d(input, kernel, up=1, down=1, pad=(0, 0)):
- """UpFRIDn for 2d features.
-
- UpFIRDn is short for upsample, apply FIR filter and downsample. More
- details can be found in:
- https://www.mathworks.com/help/signal/ref/upfirdn.html
-
- Args:
- input (Tensor): Tensor with shape of (n, c, h, w).
- kernel (Tensor): Filter kernel.
- up (int | tuple[int], optional): Upsampling factor. If given a number,
- we will use this factor for the both height and width side.
- Defaults to 1.
- down (int | tuple[int], optional): Downsampling factor. If given a
- number, we will use this factor for the both height and width side.
- Defaults to 1.
- pad (tuple[int], optional): Padding for tensors, (x_pad, y_pad) or
- (x_pad_0, x_pad_1, y_pad_0, y_pad_1). Defaults to (0, 0).
-
- Returns:
- Tensor: Tensor after UpFIRDn.
- """
- if input.device.type == 'cpu':
- if len(pad) == 2:
- pad = (pad[0], pad[1], pad[0], pad[1])
-
- up = to_2tuple(up)
-
- down = to_2tuple(down)
-
- out = upfirdn2d_native(input, kernel, up[0], up[1], down[0], down[1],
- pad[0], pad[1], pad[2], pad[3])
- else:
- _up = to_2tuple(up)
-
- _down = to_2tuple(down)
-
- if len(pad) == 4:
- _pad = pad
- elif len(pad) == 2:
- _pad = (pad[0], pad[1], pad[0], pad[1])
-
- out = UpFirDn2d.apply(input, kernel, _up, _down, _pad)
-
- return out
-
-
-def upfirdn2d_native(input, kernel, up_x, up_y, down_x, down_y, pad_x0, pad_x1,
- pad_y0, pad_y1):
- _, channel, in_h, in_w = input.shape
- input = input.reshape(-1, in_h, in_w, 1)
-
- _, in_h, in_w, minor = input.shape
- kernel_h, kernel_w = kernel.shape
-
- out = input.view(-1, in_h, 1, in_w, 1, minor)
- out = F.pad(out, [0, 0, 0, up_x - 1, 0, 0, 0, up_y - 1])
- out = out.view(-1, in_h * up_y, in_w * up_x, minor)
-
- out = F.pad(
- out,
- [0, 0,
- max(pad_x0, 0),
- max(pad_x1, 0),
- max(pad_y0, 0),
- max(pad_y1, 0)])
- out = out[:,
- max(-pad_y0, 0):out.shape[1] - max(-pad_y1, 0),
- max(-pad_x0, 0):out.shape[2] - max(-pad_x1, 0), :, ]
-
- out = out.permute(0, 3, 1, 2)
- out = out.reshape(
- [-1, 1, in_h * up_y + pad_y0 + pad_y1, in_w * up_x + pad_x0 + pad_x1])
- w = torch.flip(kernel, [0, 1]).view(1, 1, kernel_h, kernel_w)
- out = F.conv2d(out, w)
- out = out.reshape(
- -1,
- minor,
- in_h * up_y + pad_y0 + pad_y1 - kernel_h + 1,
- in_w * up_x + pad_x0 + pad_x1 - kernel_w + 1,
- )
- out = out.permute(0, 2, 3, 1)
- out = out[:, ::down_y, ::down_x, :]
-
- out_h = (in_h * up_y + pad_y0 + pad_y1 - kernel_h) // down_y + 1
- out_w = (in_w * up_x + pad_x0 + pad_x1 - kernel_w) // down_x + 1
-
- return out.view(-1, channel, out_h, out_w)
diff --git a/spaces/godot-demo/godot-3d-voxel/README.md b/spaces/godot-demo/godot-3d-voxel/README.md
deleted file mode 100644
index b4eca11d82ddbafa831284bd2684a7cc37992db9..0000000000000000000000000000000000000000
--- a/spaces/godot-demo/godot-3d-voxel/README.md
+++ /dev/null
@@ -1,10 +0,0 @@
----
-title: Godot 3d Voxel
-emoji: 🌍
-colorFrom: red
-colorTo: blue
-sdk: static
-pinned: false
----
-
-Check out the configuration reference at https://huggingface.co/docs/hub/spaces#reference
diff --git a/spaces/gradio/HuBERT/fairseq/modules/unfold.py b/spaces/gradio/HuBERT/fairseq/modules/unfold.py
deleted file mode 100644
index 138272f1ef4f673b29e36aed4531106f7ce95968..0000000000000000000000000000000000000000
--- a/spaces/gradio/HuBERT/fairseq/modules/unfold.py
+++ /dev/null
@@ -1,19 +0,0 @@
-# Copyright (c) Facebook, Inc. and its affiliates.
-#
-# This source code is licensed under the MIT license found in the
-# LICENSE file in the root directory of this source tree.
-
-import torch.nn.functional as F
-
-
-def unfold1d(x, kernel_size, padding_l, pad_value=0):
- """unfold T x B x C to T x B x C x K"""
- if kernel_size > 1:
- T, B, C = x.size()
- x = F.pad(
- x, (0, 0, 0, 0, padding_l, kernel_size - 1 - padding_l), value=pad_value
- )
- x = x.as_strided((T, B, C, kernel_size), (B * C, C, 1, B * C))
- else:
- x = x.unsqueeze(3)
- return x
diff --git a/spaces/gradio/HuBERT/tests/test_concat_dataset.py b/spaces/gradio/HuBERT/tests/test_concat_dataset.py
deleted file mode 100644
index d94aeffd481a2e107eb5747e41d76435b3f3dc8a..0000000000000000000000000000000000000000
--- a/spaces/gradio/HuBERT/tests/test_concat_dataset.py
+++ /dev/null
@@ -1,58 +0,0 @@
-# Copyright (c) Facebook, Inc. and its affiliates.
-#
-# This source code is licensed under the MIT license found in the
-# LICENSE file in the root directory of this source tree.
-
-import unittest
-
-import torch
-from fairseq.data import LanguagePairDataset, TokenBlockDataset
-from fairseq.data.concat_dataset import ConcatDataset
-from tests.test_train import mock_dict
-
-
-class TestConcatDataset(unittest.TestCase):
- def setUp(self):
- d = mock_dict()
- tokens_1 = torch.LongTensor([1]).view(1, -1)
- tokens_ds1 = TokenBlockDataset(
- tokens_1,
- sizes=[tokens_1.size(-1)],
- block_size=1,
- pad=0,
- eos=1,
- include_targets=False,
- )
- self.dataset_1 = LanguagePairDataset(
- tokens_ds1, tokens_ds1.sizes, d, shuffle=False
- )
- tokens_2 = torch.LongTensor([2]).view(1, -1)
- tokens_ds2 = TokenBlockDataset(
- tokens_2,
- sizes=[tokens_2.size(-1)],
- block_size=1,
- pad=0,
- eos=1,
- include_targets=False,
- )
- self.dataset_2 = LanguagePairDataset(
- tokens_ds2, tokens_ds2.sizes, d, shuffle=False
- )
-
- def test_concat_dataset_basics(self):
- d = ConcatDataset([self.dataset_1, self.dataset_2])
- assert len(d) == 2
- assert d[0]["source"][0] == 1
- assert d[1]["source"][0] == 2
-
- d = ConcatDataset([self.dataset_1, self.dataset_2], sample_ratios=[1, 2])
- assert len(d) == 3
- assert d[0]["source"][0] == 1
- assert d[1]["source"][0] == 2
- assert d[2]["source"][0] == 2
-
- d = ConcatDataset([self.dataset_1, self.dataset_2], sample_ratios=[2, 1])
- assert len(d) == 3
- assert d[0]["source"][0] == 1
- assert d[1]["source"][0] == 1
- assert d[2]["source"][0] == 2
diff --git a/spaces/gradio/longformer/tvm/ndarray.py b/spaces/gradio/longformer/tvm/ndarray.py
deleted file mode 100644
index 9a00f78eb77fa6f591396caffd8e1b430a11d37b..0000000000000000000000000000000000000000
--- a/spaces/gradio/longformer/tvm/ndarray.py
+++ /dev/null
@@ -1,232 +0,0 @@
-# Licensed to the Apache Software Foundation (ASF) under one
-# or more contributor license agreements. See the NOTICE file
-# distributed with this work for additional information
-# regarding copyright ownership. The ASF licenses this file
-# to you under the Apache License, Version 2.0 (the
-# "License"); you may not use this file except in compliance
-# with the License. You may obtain a copy of the License at
-#
-# http://www.apache.org/licenses/LICENSE-2.0
-#
-# Unless required by applicable law or agreed to in writing,
-# software distributed under the License is distributed on an
-# "AS IS" BASIS, WITHOUT WARRANTIES OR CONDITIONS OF ANY
-# KIND, either express or implied. See the License for the
-# specific language governing permissions and limitations
-# under the License.
-"""TVM Runtime NDArray API.
-
-tvm.ndarray provides a minimum runtime array API to test
-the correctness of the program.
-"""
-# pylint: disable=invalid-name,unused-import
-from __future__ import absolute_import as _abs
-import numpy as _np
-
-from ._ffi.ndarray import TVMContext, TVMType, NDArrayBase
-from ._ffi.ndarray import context, empty, from_dlpack
-from ._ffi.ndarray import _set_class_ndarray
-from ._ffi.ndarray import register_extension, free_extension_handle
-
-class NDArray(NDArrayBase):
- """Lightweight NDArray class of TVM runtime.
-
- Strictly this is only an Array Container (a buffer object)
- No arthimetic operations are defined.
- All operations are performed by TVM functions.
-
- The goal is not to re-build yet another array library.
- Instead, this is a minimal data structure to demonstrate
- how can we use TVM in existing project which might have their own array containers.
- """
-
-
-def cpu(dev_id=0):
- """Construct a CPU device
-
- Parameters
- ----------
- dev_id : int, optional
- The integer device id
-
- Returns
- -------
- ctx : TVMContext
- The created context
- """
- return TVMContext(1, dev_id)
-
-
-def gpu(dev_id=0):
- """Construct a CPU device
-
- Parameters
- ----------
- dev_id : int, optional
- The integer device id
-
- Returns
- -------
- ctx : TVMContext
- The created context
- """
- return TVMContext(2, dev_id)
-
-def rocm(dev_id=0):
- """Construct a ROCM device
-
- Parameters
- ----------
- dev_id : int, optional
- The integer device id
-
- Returns
- -------
- ctx : TVMContext
- The created context
- """
- return TVMContext(10, dev_id)
-
-
-def opencl(dev_id=0):
- """Construct a OpenCL device
-
- Parameters
- ----------
- dev_id : int, optional
- The integer device id
-
- Returns
- -------
- ctx : TVMContext
- The created context
- """
- return TVMContext(4, dev_id)
-
-
-def metal(dev_id=0):
- """Construct a metal device
-
- Parameters
- ----------
- dev_id : int, optional
- The integer device id
-
- Returns
- -------
- ctx : TVMContext
- The created context
- """
- return TVMContext(8, dev_id)
-
-
-def vpi(dev_id=0):
- """Construct a VPI simulated device
-
- Parameters
- ----------
- dev_id : int, optional
- The integer device id
-
- Returns
- -------
- ctx : TVMContext
- The created context
- """
- return TVMContext(9, dev_id)
-
-
-def vulkan(dev_id=0):
- """Construct a Vulkan device
-
- Parameters
- ----------
- dev_id : int, optional
- The integer device id
-
- Returns
- -------
- ctx : TVMContext
- The created context
- """
- return TVMContext(7, dev_id)
-
-
-def opengl(dev_id=0):
- """Construct a OpenGL device
-
- Parameters
- ----------
- dev_id : int, optional
- The integer device id
-
- Returns
- -------
- ctx : TVMContext
- The created context
- """
- return TVMContext(11, dev_id)
-
-
-def ext_dev(dev_id=0):
- """Construct a extension device
-
- Parameters
- ----------
- dev_id : int, optional
- The integer device id
-
- Returns
- -------
- ctx : TVMContext
- The created context
-
- Note
- ----
- This API is reserved for quick testing of new
- device by plugin device API as ext_dev.
- """
- return TVMContext(12, dev_id)
-
-
-def micro_dev(dev_id=0):
- """Construct a micro device
-
- Parameters
- ----------
- dev_id : int, optional
- The integer device id
-
- Returns
- -------
- ctx : TVMContext
- The created context
- """
- return TVMContext(13, dev_id)
-
-
-cl = opencl
-mtl = metal
-
-
-def array(arr, ctx=cpu(0)):
- """Create an array from source arr.
-
- Parameters
- ----------
- arr : numpy.ndarray
- The array to be copied from
-
- ctx : TVMContext, optional
- The device context to create the array
-
- Returns
- -------
- ret : NDArray
- The created array
- """
- if not isinstance(arr, (_np.ndarray, NDArray)):
- arr = _np.array(arr)
- return empty(arr.shape, arr.dtype, ctx).copyfrom(arr)
-
-_set_class_ndarray(NDArray)
diff --git a/spaces/h2oai/wave-tour/examples/textbox.py b/spaces/h2oai/wave-tour/examples/textbox.py
deleted file mode 100644
index 39c29bdee321e568ee2811b5c1e198351362ea3f..0000000000000000000000000000000000000000
--- a/spaces/h2oai/wave-tour/examples/textbox.py
+++ /dev/null
@@ -1,45 +0,0 @@
-# Form / Textbox
-# Use a #textbox to allow users to provide text inputs.
-# #form
-# ---
-from h2o_wave import main, app, Q, ui
-
-
-@app('/demo')
-async def serve(q: Q):
- if q.args.show_inputs:
- q.page['example'].items = [
- ui.text(f'textbox={q.args.textbox}'),
- ui.text(f'textbox_disabled={q.args.textbox_disabled}'),
- ui.text(f'textbox_readonly={q.args.textbox_readonly}'),
- ui.text(f'textbox_required={q.args.textbox_required}'),
- ui.text(f'textbox_error={q.args.textbox_error}'),
- ui.text(f'textbox_mask={q.args.textbox_mask}'),
- ui.text(f'textbox_icon={q.args.textbox_icon}'),
- ui.text(f'textbox_prefix={q.args.textbox_prefix}'),
- ui.text(f'textbox_suffix={q.args.textbox_suffix}'),
- ui.text(f'textbox_placeholder={q.args.textbox_placeholder}'),
- ui.text(f'textbox_disabled_placeholder={q.args.textbox_disabled_placeholder}'),
- ui.text(f'textbox_multiline={q.args.textbox_multiline}'),
- ui.text(f'textbox_spellcheck_disabled={q.args.textbox_spellcheck_disabled}'),
- ui.button(name='show_form', label='Back', primary=True),
- ]
- else:
- q.page['example'] = ui.form_card(box='1 1 -1 -1', items=[
- ui.textbox(name='textbox', label='Standard'),
- ui.textbox(name='textbox_disabled', label='Disabled', value='I am disabled', disabled=True),
- ui.textbox(name='textbox_readonly', label='Read-only', value='I am read-only', readonly=True),
- ui.textbox(name='textbox_required', label='Required', required=True),
- ui.textbox(name='textbox_error', label='With error message', error='I have an error'),
- ui.textbox(name='textbox_mask', label='With input mask', mask='(999) 999 - 9999'),
- ui.textbox(name='textbox_icon', label='With icon', icon='Calendar'),
- ui.textbox(name='textbox_prefix', label='With prefix', prefix='http://'),
- ui.textbox(name='textbox_suffix', label='With suffix', suffix='@h2o.ai'),
- ui.textbox(name='textbox_placeholder', label='With placeholder', placeholder='I need some input'),
- ui.textbox(name='textbox_disabled_placeholder', label='Disabled with placeholder', disabled=True,
- placeholder='I am disabled'),
- ui.textbox(name='textbox_multiline', label='Multiline textarea', multiline=True),
- ui.textbox(name='textbox_spellcheck_disabled', label='Spellcheck disabled', spellcheck=False),
- ui.button(name='show_inputs', label='Submit', primary=True),
- ])
- await q.page.save()
diff --git a/spaces/hackathon-pln-es/DemoAcosoTwitter/README.md b/spaces/hackathon-pln-es/DemoAcosoTwitter/README.md
deleted file mode 100644
index 16cb44f4fadf3e5e277ad472540cee6e58616eb9..0000000000000000000000000000000000000000
--- a/spaces/hackathon-pln-es/DemoAcosoTwitter/README.md
+++ /dev/null
@@ -1,23 +0,0 @@
----
-title: Demo-Acoso-Twitter
-emoji: 👁️🗨️💻
-colorFrom: blue
-colorTo: green
-sdk: gradio
-sdk_version: 2.8.14
-app_file: app.py
-pinned: false
-license: apache-2.0
-models : hackathon-pln-es/Detect-Acoso-Twitter-Es
-datasets: hackathon-pln-es/Dataset-Acoso-Twitter-Es
----
-
-Check out the configuration reference at https://huggingface.co/docs/hub/spaces#reference
-# UNL: Universidad Nacional de Loja
-
-## Miembros del equipo:
-- Anderson Quizhpe
-- Luis Negrón
-- David Pacheco
-- Bryan Requenes
-- Paul Pasaca
\ No newline at end of file
diff --git a/spaces/hamacojr/SAM-CAT-Seg/cat_seg/data/datasets/register_coco_stuff.py b/spaces/hamacojr/SAM-CAT-Seg/cat_seg/data/datasets/register_coco_stuff.py
deleted file mode 100644
index 35c823dee37b1657dc61d1f5beab8c0ecaa98855..0000000000000000000000000000000000000000
--- a/spaces/hamacojr/SAM-CAT-Seg/cat_seg/data/datasets/register_coco_stuff.py
+++ /dev/null
@@ -1,216 +0,0 @@
-import os
-
-from detectron2.data import DatasetCatalog, MetadataCatalog
-from detectron2.data.datasets import load_sem_seg
-
-COCO_CATEGORIES = [
- {"color": [220, 20, 60], "isthing": 1, "id": 1, "name": "person"},
- {"color": [119, 11, 32], "isthing": 1, "id": 2, "name": "bicycle"},
- {"color": [0, 0, 142], "isthing": 1, "id": 3, "name": "car"},
- {"color": [0, 0, 230], "isthing": 1, "id": 4, "name": "motorcycle"},
- {"color": [106, 0, 228], "isthing": 1, "id": 5, "name": "airplane"},
- {"color": [0, 60, 100], "isthing": 1, "id": 6, "name": "bus"},
- {"color": [0, 80, 100], "isthing": 1, "id": 7, "name": "train"},
- {"color": [0, 0, 70], "isthing": 1, "id": 8, "name": "truck"},
- {"color": [0, 0, 192], "isthing": 1, "id": 9, "name": "boat"},
- {"color": [250, 170, 30], "isthing": 1, "id": 10, "name": "traffic light"},
- {"color": [100, 170, 30], "isthing": 1, "id": 11, "name": "fire hydrant"},
- {"color": [220, 220, 0], "isthing": 1, "id": 13, "name": "stop sign"},
- {"color": [175, 116, 175], "isthing": 1, "id": 14, "name": "parking meter"},
- {"color": [250, 0, 30], "isthing": 1, "id": 15, "name": "bench"},
- {"color": [165, 42, 42], "isthing": 1, "id": 16, "name": "bird"},
- {"color": [255, 77, 255], "isthing": 1, "id": 17, "name": "cat"},
- {"color": [0, 226, 252], "isthing": 1, "id": 18, "name": "dog"},
- {"color": [182, 182, 255], "isthing": 1, "id": 19, "name": "horse"},
- {"color": [0, 82, 0], "isthing": 1, "id": 20, "name": "sheep"},
- {"color": [120, 166, 157], "isthing": 1, "id": 21, "name": "cow"},
- {"color": [110, 76, 0], "isthing": 1, "id": 22, "name": "elephant"},
- {"color": [174, 57, 255], "isthing": 1, "id": 23, "name": "bear"},
- {"color": [199, 100, 0], "isthing": 1, "id": 24, "name": "zebra"},
- {"color": [72, 0, 118], "isthing": 1, "id": 25, "name": "giraffe"},
- {"color": [255, 179, 240], "isthing": 1, "id": 27, "name": "backpack"},
- {"color": [0, 125, 92], "isthing": 1, "id": 28, "name": "umbrella"},
- {"color": [209, 0, 151], "isthing": 1, "id": 31, "name": "handbag"},
- {"color": [188, 208, 182], "isthing": 1, "id": 32, "name": "tie"},
- {"color": [0, 220, 176], "isthing": 1, "id": 33, "name": "suitcase"},
- {"color": [255, 99, 164], "isthing": 1, "id": 34, "name": "frisbee"},
- {"color": [92, 0, 73], "isthing": 1, "id": 35, "name": "skis"},
- {"color": [133, 129, 255], "isthing": 1, "id": 36, "name": "snowboard"},
- {"color": [78, 180, 255], "isthing": 1, "id": 37, "name": "sports ball"},
- {"color": [0, 228, 0], "isthing": 1, "id": 38, "name": "kite"},
- {"color": [174, 255, 243], "isthing": 1, "id": 39, "name": "baseball bat"},
- {"color": [45, 89, 255], "isthing": 1, "id": 40, "name": "baseball glove"},
- {"color": [134, 134, 103], "isthing": 1, "id": 41, "name": "skateboard"},
- {"color": [145, 148, 174], "isthing": 1, "id": 42, "name": "surfboard"},
- {"color": [255, 208, 186], "isthing": 1, "id": 43, "name": "tennis racket"},
- {"color": [197, 226, 255], "isthing": 1, "id": 44, "name": "bottle"},
- {"color": [171, 134, 1], "isthing": 1, "id": 46, "name": "wine glass"},
- {"color": [109, 63, 54], "isthing": 1, "id": 47, "name": "cup"},
- {"color": [207, 138, 255], "isthing": 1, "id": 48, "name": "fork"},
- {"color": [151, 0, 95], "isthing": 1, "id": 49, "name": "knife"},
- {"color": [9, 80, 61], "isthing": 1, "id": 50, "name": "spoon"},
- {"color": [84, 105, 51], "isthing": 1, "id": 51, "name": "bowl"},
- {"color": [74, 65, 105], "isthing": 1, "id": 52, "name": "banana"},
- {"color": [166, 196, 102], "isthing": 1, "id": 53, "name": "apple"},
- {"color": [208, 195, 210], "isthing": 1, "id": 54, "name": "sandwich"},
- {"color": [255, 109, 65], "isthing": 1, "id": 55, "name": "orange"},
- {"color": [0, 143, 149], "isthing": 1, "id": 56, "name": "broccoli"},
- {"color": [179, 0, 194], "isthing": 1, "id": 57, "name": "carrot"},
- {"color": [209, 99, 106], "isthing": 1, "id": 58, "name": "hot dog"},
- {"color": [5, 121, 0], "isthing": 1, "id": 59, "name": "pizza"},
- {"color": [227, 255, 205], "isthing": 1, "id": 60, "name": "donut"},
- {"color": [147, 186, 208], "isthing": 1, "id": 61, "name": "cake"},
- {"color": [153, 69, 1], "isthing": 1, "id": 62, "name": "chair"},
- {"color": [3, 95, 161], "isthing": 1, "id": 63, "name": "couch"},
- {"color": [163, 255, 0], "isthing": 1, "id": 64, "name": "potted plant"},
- {"color": [119, 0, 170], "isthing": 1, "id": 65, "name": "bed"},
- {"color": [0, 182, 199], "isthing": 1, "id": 67, "name": "dining table"},
- {"color": [0, 165, 120], "isthing": 1, "id": 70, "name": "toilet"},
- {"color": [183, 130, 88], "isthing": 1, "id": 72, "name": "tv"},
- {"color": [95, 32, 0], "isthing": 1, "id": 73, "name": "laptop"},
- {"color": [130, 114, 135], "isthing": 1, "id": 74, "name": "mouse"},
- {"color": [110, 129, 133], "isthing": 1, "id": 75, "name": "remote"},
- {"color": [166, 74, 118], "isthing": 1, "id": 76, "name": "keyboard"},
- {"color": [219, 142, 185], "isthing": 1, "id": 77, "name": "cell phone"},
- {"color": [79, 210, 114], "isthing": 1, "id": 78, "name": "microwave"},
- {"color": [178, 90, 62], "isthing": 1, "id": 79, "name": "oven"},
- {"color": [65, 70, 15], "isthing": 1, "id": 80, "name": "toaster"},
- {"color": [127, 167, 115], "isthing": 1, "id": 81, "name": "sink"},
- {"color": [59, 105, 106], "isthing": 1, "id": 82, "name": "refrigerator"},
- {"color": [142, 108, 45], "isthing": 1, "id": 84, "name": "book"},
- {"color": [196, 172, 0], "isthing": 1, "id": 85, "name": "clock"},
- {"color": [95, 54, 80], "isthing": 1, "id": 86, "name": "vase"},
- {"color": [128, 76, 255], "isthing": 1, "id": 87, "name": "scissors"},
- {"color": [201, 57, 1], "isthing": 1, "id": 88, "name": "teddy bear"},
- {"color": [246, 0, 122], "isthing": 1, "id": 89, "name": "hair drier"},
- {"color": [191, 162, 208], "isthing": 1, "id": 90, "name": "toothbrush"},
- {"id": 92, "name": "banner", "supercategory": "textile"},
- {"id": 93, "name": "blanket", "supercategory": "textile"},
- {"id": 94, "name": "branch", "supercategory": "plant"},
- {"id": 95, "name": "bridge", "supercategory": "building"},
- {"id": 96, "name": "building-other", "supercategory": "building"},
- {"id": 97, "name": "bush", "supercategory": "plant"},
- {"id": 98, "name": "cabinet", "supercategory": "furniture-stuff"},
- {"id": 99, "name": "cage", "supercategory": "structural"},
- {"id": 100, "name": "cardboard", "supercategory": "raw-material"},
- {"id": 101, "name": "carpet", "supercategory": "floor"},
- {"id": 102, "name": "ceiling-other", "supercategory": "ceiling"},
- {"id": 103, "name": "ceiling-tile", "supercategory": "ceiling"},
- {"id": 104, "name": "cloth", "supercategory": "textile"},
- {"id": 105, "name": "clothes", "supercategory": "textile"},
- {"id": 106, "name": "clouds", "supercategory": "sky"},
- {"id": 107, "name": "counter", "supercategory": "furniture-stuff"},
- {"id": 108, "name": "cupboard", "supercategory": "furniture-stuff"},
- {"id": 109, "name": "curtain", "supercategory": "textile"},
- {"id": 110, "name": "desk-stuff", "supercategory": "furniture-stuff"},
- {"id": 111, "name": "dirt", "supercategory": "ground"},
- {"id": 112, "name": "door-stuff", "supercategory": "furniture-stuff"},
- {"id": 113, "name": "fence", "supercategory": "structural"},
- {"id": 114, "name": "floor-marble", "supercategory": "floor"},
- {"id": 115, "name": "floor-other", "supercategory": "floor"},
- {"id": 116, "name": "floor-stone", "supercategory": "floor"},
- {"id": 117, "name": "floor-tile", "supercategory": "floor"},
- {"id": 118, "name": "floor-wood", "supercategory": "floor"},
- {"id": 119, "name": "flower", "supercategory": "plant"},
- {"id": 120, "name": "fog", "supercategory": "water"},
- {"id": 121, "name": "food-other", "supercategory": "food-stuff"},
- {"id": 122, "name": "fruit", "supercategory": "food-stuff"},
- {"id": 123, "name": "furniture-other", "supercategory": "furniture-stuff"},
- {"id": 124, "name": "grass", "supercategory": "plant"},
- {"id": 125, "name": "gravel", "supercategory": "ground"},
- {"id": 126, "name": "ground-other", "supercategory": "ground"},
- {"id": 127, "name": "hill", "supercategory": "solid"},
- {"id": 128, "name": "house", "supercategory": "building"},
- {"id": 129, "name": "leaves", "supercategory": "plant"},
- {"id": 130, "name": "light", "supercategory": "furniture-stuff"},
- {"id": 131, "name": "mat", "supercategory": "textile"},
- {"id": 132, "name": "metal", "supercategory": "raw-material"},
- {"id": 133, "name": "mirror-stuff", "supercategory": "furniture-stuff"},
- {"id": 134, "name": "moss", "supercategory": "plant"},
- {"id": 135, "name": "mountain", "supercategory": "solid"},
- {"id": 136, "name": "mud", "supercategory": "ground"},
- {"id": 137, "name": "napkin", "supercategory": "textile"},
- {"id": 138, "name": "net", "supercategory": "structural"},
- {"id": 139, "name": "paper", "supercategory": "raw-material"},
- {"id": 140, "name": "pavement", "supercategory": "ground"},
- {"id": 141, "name": "pillow", "supercategory": "textile"},
- {"id": 142, "name": "plant-other", "supercategory": "plant"},
- {"id": 143, "name": "plastic", "supercategory": "raw-material"},
- {"id": 144, "name": "platform", "supercategory": "ground"},
- {"id": 145, "name": "playingfield", "supercategory": "ground"},
- {"id": 146, "name": "railing", "supercategory": "structural"},
- {"id": 147, "name": "railroad", "supercategory": "ground"},
- {"id": 148, "name": "river", "supercategory": "water"},
- {"id": 149, "name": "road", "supercategory": "ground"},
- {"id": 150, "name": "rock", "supercategory": "solid"},
- {"id": 151, "name": "roof", "supercategory": "building"},
- {"id": 152, "name": "rug", "supercategory": "textile"},
- {"id": 153, "name": "salad", "supercategory": "food-stuff"},
- {"id": 154, "name": "sand", "supercategory": "ground"},
- {"id": 155, "name": "sea", "supercategory": "water"},
- {"id": 156, "name": "shelf", "supercategory": "furniture-stuff"},
- {"id": 157, "name": "sky-other", "supercategory": "sky"},
- {"id": 158, "name": "skyscraper", "supercategory": "building"},
- {"id": 159, "name": "snow", "supercategory": "ground"},
- {"id": 160, "name": "solid-other", "supercategory": "solid"},
- {"id": 161, "name": "stairs", "supercategory": "furniture-stuff"},
- {"id": 162, "name": "stone", "supercategory": "solid"},
- {"id": 163, "name": "straw", "supercategory": "plant"},
- {"id": 164, "name": "structural-other", "supercategory": "structural"},
- {"id": 165, "name": "table", "supercategory": "furniture-stuff"},
- {"id": 166, "name": "tent", "supercategory": "building"},
- {"id": 167, "name": "textile-other", "supercategory": "textile"},
- {"id": 168, "name": "towel", "supercategory": "textile"},
- {"id": 169, "name": "tree", "supercategory": "plant"},
- {"id": 170, "name": "vegetable", "supercategory": "food-stuff"},
- {"id": 171, "name": "wall-brick", "supercategory": "wall"},
- {"id": 172, "name": "wall-concrete", "supercategory": "wall"},
- {"id": 173, "name": "wall-other", "supercategory": "wall"},
- {"id": 174, "name": "wall-panel", "supercategory": "wall"},
- {"id": 175, "name": "wall-stone", "supercategory": "wall"},
- {"id": 176, "name": "wall-tile", "supercategory": "wall"},
- {"id": 177, "name": "wall-wood", "supercategory": "wall"},
- {"id": 178, "name": "water-other", "supercategory": "water"},
- {"id": 179, "name": "waterdrops", "supercategory": "water"},
- {"id": 180, "name": "window-blind", "supercategory": "window"},
- {"id": 181, "name": "window-other", "supercategory": "window"},
- {"id": 182, "name": "wood", "supercategory": "solid"},
-]
-
-
-def _get_coco_stuff_meta():
- stuff_ids = [k["id"] for k in COCO_CATEGORIES]
- assert len(stuff_ids) == 171, len(stuff_ids)
-
- stuff_dataset_id_to_contiguous_id = {k: i for i, k in enumerate(stuff_ids)}
- stuff_classes = [k["name"] for k in COCO_CATEGORIES]
-
- ret = {
- "stuff_dataset_id_to_contiguous_id": stuff_dataset_id_to_contiguous_id,
- "stuff_classes": stuff_classes,
- }
- return ret
-
-def register_all_coco_stuff_10k(root):
- root = os.path.join(root, "coco-stuff")
- meta = _get_coco_stuff_meta()
- for name, image_dirname, sem_seg_dirname in [
- ("train", "images/train2017", "annotations_detectron2/train2017"),
- ("test", "images/val2017", "annotations_detectron2/val2017"),
- ]:
- image_dir = os.path.join(root, image_dirname)
- gt_dir = os.path.join(root, sem_seg_dirname)
- name = f"coco_2017_{name}_stuff_all_sem_seg"
- DatasetCatalog.register(
- name, lambda x=image_dir, y=gt_dir: load_sem_seg(y, x, gt_ext="png", image_ext="jpg")
- )
- MetadataCatalog.get(name).set(
- image_root=image_dir,
- sem_seg_root=gt_dir,
- evaluator_type="sem_seg",
- ignore_label=255,
- **meta,
- )
-
-_root = os.getenv("DETECTRON2_DATASETS", "datasets")
-register_all_coco_stuff_10k(_root)
diff --git a/spaces/hamzapehlivan/StyleRes/models/torch_utils/ops/conv2d_gradfix.py b/spaces/hamzapehlivan/StyleRes/models/torch_utils/ops/conv2d_gradfix.py
deleted file mode 100644
index 388778fa971d7bc5c64b5fd6c0e5492863ee1c5f..0000000000000000000000000000000000000000
--- a/spaces/hamzapehlivan/StyleRes/models/torch_utils/ops/conv2d_gradfix.py
+++ /dev/null
@@ -1,198 +0,0 @@
-# Copyright (c) 2021, NVIDIA CORPORATION & AFFILIATES. All rights reserved.
-#
-# NVIDIA CORPORATION and its licensors retain all intellectual property
-# and proprietary rights in and to this software, related documentation
-# and any modifications thereto. Any use, reproduction, disclosure or
-# distribution of this software and related documentation without an express
-# license agreement from NVIDIA CORPORATION is strictly prohibited.
-
-"""Custom replacement for `torch.nn.functional.conv2d` that supports
-arbitrarily high order gradients with zero performance penalty."""
-
-import contextlib
-import torch
-
-# pylint: disable=redefined-builtin
-# pylint: disable=arguments-differ
-# pylint: disable=protected-access
-
-#----------------------------------------------------------------------------
-
-enabled = False # Enable the custom op by setting this to true.
-weight_gradients_disabled = False # Forcefully disable computation of gradients with respect to the weights.
-
-@contextlib.contextmanager
-def no_weight_gradients(disable=True):
- global weight_gradients_disabled
- old = weight_gradients_disabled
- if disable:
- weight_gradients_disabled = True
- yield
- weight_gradients_disabled = old
-
-#----------------------------------------------------------------------------
-
-def conv2d(input, weight, bias=None, stride=1, padding=0, dilation=1, groups=1):
- if _should_use_custom_op(input):
- return _conv2d_gradfix(transpose=False, weight_shape=weight.shape, stride=stride, padding=padding, output_padding=0, dilation=dilation, groups=groups).apply(input, weight, bias)
- return torch.nn.functional.conv2d(input=input, weight=weight, bias=bias, stride=stride, padding=padding, dilation=dilation, groups=groups)
-
-def conv_transpose2d(input, weight, bias=None, stride=1, padding=0, output_padding=0, groups=1, dilation=1):
- if _should_use_custom_op(input):
- return _conv2d_gradfix(transpose=True, weight_shape=weight.shape, stride=stride, padding=padding, output_padding=output_padding, groups=groups, dilation=dilation).apply(input, weight, bias)
- return torch.nn.functional.conv_transpose2d(input=input, weight=weight, bias=bias, stride=stride, padding=padding, output_padding=output_padding, groups=groups, dilation=dilation)
-
-#----------------------------------------------------------------------------
-
-def _should_use_custom_op(input):
- assert isinstance(input, torch.Tensor)
- if (not enabled) or (not torch.backends.cudnn.enabled):
- return False
- if input.device.type != 'cuda':
- return False
- return True
-
-def _tuple_of_ints(xs, ndim):
- xs = tuple(xs) if isinstance(xs, (tuple, list)) else (xs,) * ndim
- assert len(xs) == ndim
- assert all(isinstance(x, int) for x in xs)
- return xs
-
-#----------------------------------------------------------------------------
-
-_conv2d_gradfix_cache = dict()
-_null_tensor = torch.empty([0])
-
-def _conv2d_gradfix(transpose, weight_shape, stride, padding, output_padding, dilation, groups):
- # Parse arguments.
- ndim = 2
- weight_shape = tuple(weight_shape)
- stride = _tuple_of_ints(stride, ndim)
- padding = _tuple_of_ints(padding, ndim)
- output_padding = _tuple_of_ints(output_padding, ndim)
- dilation = _tuple_of_ints(dilation, ndim)
-
- # Lookup from cache.
- key = (transpose, weight_shape, stride, padding, output_padding, dilation, groups)
- if key in _conv2d_gradfix_cache:
- return _conv2d_gradfix_cache[key]
-
- # Validate arguments.
- assert groups >= 1
- assert len(weight_shape) == ndim + 2
- assert all(stride[i] >= 1 for i in range(ndim))
- assert all(padding[i] >= 0 for i in range(ndim))
- assert all(dilation[i] >= 0 for i in range(ndim))
- if not transpose:
- assert all(output_padding[i] == 0 for i in range(ndim))
- else: # transpose
- assert all(0 <= output_padding[i] < max(stride[i], dilation[i]) for i in range(ndim))
-
- # Helpers.
- common_kwargs = dict(stride=stride, padding=padding, dilation=dilation, groups=groups)
- def calc_output_padding(input_shape, output_shape):
- if transpose:
- return [0, 0]
- return [
- input_shape[i + 2]
- - (output_shape[i + 2] - 1) * stride[i]
- - (1 - 2 * padding[i])
- - dilation[i] * (weight_shape[i + 2] - 1)
- for i in range(ndim)
- ]
-
- # Forward & backward.
- class Conv2d(torch.autograd.Function):
- @staticmethod
- def forward(ctx, input, weight, bias):
- assert weight.shape == weight_shape
- ctx.save_for_backward(
- input if weight.requires_grad else _null_tensor,
- weight if input.requires_grad else _null_tensor,
- )
- ctx.input_shape = input.shape
-
- # Simple 1x1 convolution => cuBLAS (only on Volta, not on Ampere).
- if weight_shape[2:] == stride == dilation == (1, 1) and padding == (0, 0) and torch.cuda.get_device_capability(input.device) < (8, 0):
- a = weight.reshape(groups, weight_shape[0] // groups, weight_shape[1])
- b = input.reshape(input.shape[0], groups, input.shape[1] // groups, -1)
- c = (a.transpose(1, 2) if transpose else a) @ b.permute(1, 2, 0, 3).flatten(2)
- c = c.reshape(-1, input.shape[0], *input.shape[2:]).transpose(0, 1)
- c = c if bias is None else c + bias.unsqueeze(0).unsqueeze(2).unsqueeze(3)
- return c.contiguous(memory_format=(torch.channels_last if input.stride(1) == 1 else torch.contiguous_format))
-
- # General case => cuDNN.
- if transpose:
- return torch.nn.functional.conv_transpose2d(input=input, weight=weight, bias=bias, output_padding=output_padding, **common_kwargs)
- return torch.nn.functional.conv2d(input=input, weight=weight, bias=bias, **common_kwargs)
-
- @staticmethod
- def backward(ctx, grad_output):
- input, weight = ctx.saved_tensors
- input_shape = ctx.input_shape
- grad_input = None
- grad_weight = None
- grad_bias = None
-
- if ctx.needs_input_grad[0]:
- p = calc_output_padding(input_shape=input_shape, output_shape=grad_output.shape)
- op = _conv2d_gradfix(transpose=(not transpose), weight_shape=weight_shape, output_padding=p, **common_kwargs)
- grad_input = op.apply(grad_output, weight, None)
- assert grad_input.shape == input_shape
-
- if ctx.needs_input_grad[1] and not weight_gradients_disabled:
- grad_weight = Conv2dGradWeight.apply(grad_output, input)
- assert grad_weight.shape == weight_shape
-
- if ctx.needs_input_grad[2]:
- grad_bias = grad_output.sum([0, 2, 3])
-
- return grad_input, grad_weight, grad_bias
-
- # Gradient with respect to the weights.
- class Conv2dGradWeight(torch.autograd.Function):
- @staticmethod
- def forward(ctx, grad_output, input):
- ctx.save_for_backward(
- grad_output if input.requires_grad else _null_tensor,
- input if grad_output.requires_grad else _null_tensor,
- )
- ctx.grad_output_shape = grad_output.shape
- ctx.input_shape = input.shape
-
- # Simple 1x1 convolution => cuBLAS (on both Volta and Ampere).
- if weight_shape[2:] == stride == dilation == (1, 1) and padding == (0, 0):
- a = grad_output.reshape(grad_output.shape[0], groups, grad_output.shape[1] // groups, -1).permute(1, 2, 0, 3).flatten(2)
- b = input.reshape(input.shape[0], groups, input.shape[1] // groups, -1).permute(1, 2, 0, 3).flatten(2)
- c = (b @ a.transpose(1, 2) if transpose else a @ b.transpose(1, 2)).reshape(weight_shape)
- return c.contiguous(memory_format=(torch.channels_last if input.stride(1) == 1 else torch.contiguous_format))
-
- # General case => cuDNN.
- name = 'aten::cudnn_convolution_transpose_backward_weight' if transpose else 'aten::cudnn_convolution_backward_weight'
- flags = [torch.backends.cudnn.benchmark, torch.backends.cudnn.deterministic, torch.backends.cudnn.allow_tf32]
- return torch._C._jit_get_operation(name)(weight_shape, grad_output, input, padding, stride, dilation, groups, *flags)
-
- @staticmethod
- def backward(ctx, grad2_grad_weight):
- grad_output, input = ctx.saved_tensors
- grad_output_shape = ctx.grad_output_shape
- input_shape = ctx.input_shape
- grad2_grad_output = None
- grad2_input = None
-
- if ctx.needs_input_grad[0]:
- grad2_grad_output = Conv2d.apply(input, grad2_grad_weight, None)
- assert grad2_grad_output.shape == grad_output_shape
-
- if ctx.needs_input_grad[1]:
- p = calc_output_padding(input_shape=input_shape, output_shape=grad_output_shape)
- op = _conv2d_gradfix(transpose=(not transpose), weight_shape=weight_shape, output_padding=p, **common_kwargs)
- grad2_input = op.apply(grad_output, grad2_grad_weight, None)
- assert grad2_input.shape == input_shape
-
- return grad2_grad_output, grad2_input
-
- _conv2d_gradfix_cache[key] = Conv2d
- return Conv2d
-
-#----------------------------------------------------------------------------
diff --git a/spaces/hca97/Mosquito-Detection/my_models/torch_hub_cache/yolov5/utils/activations.py b/spaces/hca97/Mosquito-Detection/my_models/torch_hub_cache/yolov5/utils/activations.py
deleted file mode 100644
index e4d4bbde5ec8610a5ff13fe2ef2281721c14ca1a..0000000000000000000000000000000000000000
--- a/spaces/hca97/Mosquito-Detection/my_models/torch_hub_cache/yolov5/utils/activations.py
+++ /dev/null
@@ -1,103 +0,0 @@
-# YOLOv5 🚀 by Ultralytics, AGPL-3.0 license
-"""
-Activation functions
-"""
-
-import torch
-import torch.nn as nn
-import torch.nn.functional as F
-
-
-class SiLU(nn.Module):
- # SiLU activation https://arxiv.org/pdf/1606.08415.pdf
- @staticmethod
- def forward(x):
- return x * torch.sigmoid(x)
-
-
-class Hardswish(nn.Module):
- # Hard-SiLU activation
- @staticmethod
- def forward(x):
- # return x * F.hardsigmoid(x) # for TorchScript and CoreML
- return x * F.hardtanh(x + 3, 0.0, 6.0) / 6.0 # for TorchScript, CoreML and ONNX
-
-
-class Mish(nn.Module):
- # Mish activation https://github.com/digantamisra98/Mish
- @staticmethod
- def forward(x):
- return x * F.softplus(x).tanh()
-
-
-class MemoryEfficientMish(nn.Module):
- # Mish activation memory-efficient
- class F(torch.autograd.Function):
-
- @staticmethod
- def forward(ctx, x):
- ctx.save_for_backward(x)
- return x.mul(torch.tanh(F.softplus(x))) # x * tanh(ln(1 + exp(x)))
-
- @staticmethod
- def backward(ctx, grad_output):
- x = ctx.saved_tensors[0]
- sx = torch.sigmoid(x)
- fx = F.softplus(x).tanh()
- return grad_output * (fx + x * sx * (1 - fx * fx))
-
- def forward(self, x):
- return self.F.apply(x)
-
-
-class FReLU(nn.Module):
- # FReLU activation https://arxiv.org/abs/2007.11824
- def __init__(self, c1, k=3): # ch_in, kernel
- super().__init__()
- self.conv = nn.Conv2d(c1, c1, k, 1, 1, groups=c1, bias=False)
- self.bn = nn.BatchNorm2d(c1)
-
- def forward(self, x):
- return torch.max(x, self.bn(self.conv(x)))
-
-
-class AconC(nn.Module):
- r""" ACON activation (activate or not)
- AconC: (p1*x-p2*x) * sigmoid(beta*(p1*x-p2*x)) + p2*x, beta is a learnable parameter
- according to "Activate or Not: Learning Customized Activation" .
- """
-
- def __init__(self, c1):
- super().__init__()
- self.p1 = nn.Parameter(torch.randn(1, c1, 1, 1))
- self.p2 = nn.Parameter(torch.randn(1, c1, 1, 1))
- self.beta = nn.Parameter(torch.ones(1, c1, 1, 1))
-
- def forward(self, x):
- dpx = (self.p1 - self.p2) * x
- return dpx * torch.sigmoid(self.beta * dpx) + self.p2 * x
-
-
-class MetaAconC(nn.Module):
- r""" ACON activation (activate or not)
- MetaAconC: (p1*x-p2*x) * sigmoid(beta*(p1*x-p2*x)) + p2*x, beta is generated by a small network
- according to "Activate or Not: Learning Customized Activation" .
- """
-
- def __init__(self, c1, k=1, s=1, r=16): # ch_in, kernel, stride, r
- super().__init__()
- c2 = max(r, c1 // r)
- self.p1 = nn.Parameter(torch.randn(1, c1, 1, 1))
- self.p2 = nn.Parameter(torch.randn(1, c1, 1, 1))
- self.fc1 = nn.Conv2d(c1, c2, k, s, bias=True)
- self.fc2 = nn.Conv2d(c2, c1, k, s, bias=True)
- # self.bn1 = nn.BatchNorm2d(c2)
- # self.bn2 = nn.BatchNorm2d(c1)
-
- def forward(self, x):
- y = x.mean(dim=2, keepdims=True).mean(dim=3, keepdims=True)
- # batch-size 1 bug/instabilities https://github.com/ultralytics/yolov5/issues/2891
- # beta = torch.sigmoid(self.bn2(self.fc2(self.bn1(self.fc1(y))))) # bug/unstable
- beta = torch.sigmoid(self.fc2(self.fc1(y))) # bug patch BN layers removed
- dpx = (self.p1 - self.p2) * x
- return dpx * torch.sigmoid(beta * dpx) + self.p2 * x
diff --git a/spaces/hebert2099/MusicGen/tests/modules/test_transformer.py b/spaces/hebert2099/MusicGen/tests/modules/test_transformer.py
deleted file mode 100644
index 8c9953d9e8f139db7b8ce3063e3d5a78d2f5d088..0000000000000000000000000000000000000000
--- a/spaces/hebert2099/MusicGen/tests/modules/test_transformer.py
+++ /dev/null
@@ -1,247 +0,0 @@
-# Copyright (c) Meta Platforms, Inc. and affiliates.
-# All rights reserved.
-#
-# This source code is licensed under the license found in the
-# LICENSE file in the root directory of this source tree.
-
-from itertools import product
-
-import pytest
-import torch
-
-from audiocraft.modules.transformer import StreamingMultiheadAttention, StreamingTransformer
-
-
-def test_transformer_causal_streaming():
- torch.manual_seed(1234)
-
- for context, custom in product([None, 10], [False, True]):
- # Test that causality and receptive fields are properly handled.
- # looking at the gradients
- tr = StreamingTransformer(
- 16, 4, 1 if context else 2,
- causal=True, past_context=context, custom=custom,
- dropout=0.)
- steps = 20
- for k in [0, 10, 15, 19]:
- x = torch.randn(4, steps, 16, requires_grad=True)
- y = tr(x)
- y[:, k].abs().sum().backward()
- if k + 1 < steps:
- assert torch.allclose(x.grad[:, k + 1:], torch.tensor(0.)), x.grad[:, k + 1:].norm()
- assert not torch.allclose(x.grad[:, :k + 1], torch.tensor(0.)), x.grad[:, :k + 1].norm()
- if context is not None and k > context:
- limit = k - context - 1
- assert torch.allclose(x.grad[:, :limit],
- torch.tensor(0.)), x.grad[:, :limit].norm()
-
- # Now check that streaming gives the same result at batch eval.
- x = torch.randn(4, steps, 16)
- y = tr(x)
- ys = []
- with tr.streaming():
- for k in range(steps):
- chunk = x[:, k:k + 1, :]
- ys.append(tr(chunk))
- y_stream = torch.cat(ys, dim=1)
- delta = torch.norm(y_stream - y) / torch.norm(y)
- assert delta < 1e-6, delta
-
-
-def test_transformer_vs_pytorch():
- torch.manual_seed(1234)
- # Check that in the non causal setting, we get the same result as
- # PyTorch Transformer encoder.
- for custom in [False, True]:
- tr = StreamingTransformer(
- 16, 4, 2,
- causal=False, custom=custom, dropout=0., positional_scale=0.)
- layer = torch.nn.TransformerEncoderLayer(16, 4, dropout=0., batch_first=True)
- tr_ref = torch.nn.TransformerEncoder(layer, 2)
- tr.load_state_dict(tr_ref.state_dict())
-
- x = torch.randn(4, 20, 16)
- y = tr(x)
- y2 = tr_ref(x)
- delta = torch.norm(y2 - y) / torch.norm(y)
- assert delta < 1e-6, delta
-
-
-def test_streaming_api():
- tr = StreamingTransformer(16, 4, 2, causal=True, dropout=0.)
- tr.eval()
- steps = 12
- x = torch.randn(1, steps, 16)
-
- with torch.no_grad():
- with tr.streaming():
- _ = tr(x[:, :1])
- state = {k: v.clone() for k, v in tr.get_streaming_state().items()}
- y = tr(x[:, 1:2])
- tr.set_streaming_state(state)
- y2 = tr(x[:, 1:2])
- assert torch.allclose(y, y2), (y - y2).norm()
- assert tr.flush() is None
-
-
-def test_memory_efficient():
- torch.manual_seed(1234)
- tr = StreamingTransformer(
- 16, 4, 2, custom=True, dropout=0., layer_scale=0.1)
- tr_mem_efficient = StreamingTransformer(
- 16, 4, 2, dropout=0., memory_efficient=True, layer_scale=0.1)
- tr_mem_efficient.load_state_dict(tr.state_dict())
- tr.eval()
- steps = 12
- x = torch.randn(3, steps, 16)
-
- with torch.no_grad():
- y = tr(x)
- y2 = tr_mem_efficient(x)
- assert torch.allclose(y, y2), (y - y2).norm()
-
-
-def test_attention_as_float32():
- torch.manual_seed(1234)
- cases = [
- {'custom': True},
- {'custom': False},
- ]
- for case in cases:
- tr = StreamingTransformer(16, 4, 2, dropout=0., dtype=torch.bfloat16, **case)
- tr_float32 = StreamingTransformer(
- 16, 4, 2, dropout=0., attention_as_float32=True, dtype=torch.bfloat16, **case)
- if not case['custom']:
- # we are not using autocast here because it doesn't really
- # work as expected on CPU, so we have to manually cast the weights of the MHA.
- for layer in tr_float32.layers:
- layer.self_attn.mha.to(torch.float32)
- tr_float32.load_state_dict(tr.state_dict())
- steps = 12
- x = torch.randn(3, steps, 16, dtype=torch.bfloat16)
-
- with torch.no_grad():
- y = tr(x)
- y2 = tr_float32(x)
- assert not torch.allclose(y, y2), (y - y2).norm()
-
-
-@torch.no_grad()
-def test_streaming_memory_efficient():
- torch.manual_seed(1234)
- tr = StreamingTransformer(16, 4, 2, causal=True, dropout=0., custom=True)
- tr_mem_efficient = StreamingTransformer(
- 16, 4, 2, dropout=0., memory_efficient=True, causal=True)
- tr.load_state_dict(tr_mem_efficient.state_dict())
- tr.eval()
- tr_mem_efficient.eval()
- steps = 12
- x = torch.randn(3, steps, 16)
-
- ref = tr(x)
-
- with tr_mem_efficient.streaming():
- outs = []
- # frame_sizes = [2] + [1] * (steps - 2)
- frame_sizes = [1] * steps
-
- for frame_size in frame_sizes:
- frame = x[:, :frame_size]
- x = x[:, frame_size:]
- outs.append(tr_mem_efficient(frame))
-
- out = torch.cat(outs, dim=1)
- delta = torch.norm(out - ref) / torch.norm(out)
- assert delta < 1e-6, delta
-
-
-def test_cross_attention():
- torch.manual_seed(1234)
- for norm_first in [True, False]:
- m = StreamingTransformer(
- 16, 4, 2, cross_attention=False, norm_first=norm_first, dropout=0., custom=True)
- m_cross = StreamingTransformer(
- 16, 4, 2, cross_attention=True, norm_first=norm_first, dropout=0., custom=True)
- m_cross.load_state_dict(m.state_dict(), strict=False)
- x = torch.randn(2, 5, 16)
- cross_x = torch.randn(2, 3, 16)
- y_ref = m(x)
- y_cross_zero = m_cross(x, cross_attention_src=0 * cross_x)
- # With norm_first, the two should be exactly yhe same,
- # but with norm_first=False, we get 2 normalization in a row
- # and the epsilon value leads to a tiny change.
- atol = 0. if norm_first else 1e-6
- print((y_ref - y_cross_zero).norm() / y_ref.norm())
- assert torch.allclose(y_ref, y_cross_zero, atol=atol)
-
- # We now expect a difference even with a generous atol of 1e-2.
- y_cross = m_cross(x, cross_attention_src=cross_x)
- assert not torch.allclose(y_cross, y_cross_zero, atol=1e-2)
-
- with pytest.raises(AssertionError):
- _ = m_cross(x)
- _ = m(x, cross_attention_src=cross_x)
-
-
-def test_cross_attention_compat():
- torch.manual_seed(1234)
- num_heads = 2
- dim = num_heads * 64
- with pytest.raises(AssertionError):
- StreamingMultiheadAttention(dim, num_heads, causal=True, cross_attention=True)
-
- cross_attn = StreamingMultiheadAttention(
- dim, num_heads, dropout=0, cross_attention=True, custom=True)
- ref_attn = torch.nn.MultiheadAttention(dim, num_heads, dropout=0, batch_first=True)
-
- # We can load the regular attention state dict
- # so we have compat when loading old checkpoints.
- cross_attn.load_state_dict(ref_attn.state_dict())
-
- queries = torch.randn(3, 7, dim)
- keys = torch.randn(3, 9, dim)
- values = torch.randn(3, 9, dim)
-
- y = cross_attn(queries, keys, values)[0]
- y_ref = ref_attn(queries, keys, values)[0]
- assert torch.allclose(y, y_ref, atol=1e-7)
-
- # Now let's check that streaming is working properly.
- with cross_attn.streaming():
- ys = []
- for step in range(queries.shape[1]):
- ys.append(cross_attn(queries[:, step: step + 1], keys, values)[0])
- y_streaming = torch.cat(ys, dim=1)
- assert torch.allclose(y_streaming, y, atol=1e-7)
-
-
-def test_repeat_kv():
- torch.manual_seed(1234)
- num_heads = 8
- kv_repeat = 4
- dim = num_heads * 64
- with pytest.raises(AssertionError):
- mha = StreamingMultiheadAttention(
- dim, num_heads, causal=True, kv_repeat=kv_repeat, cross_attention=True)
- mha = StreamingMultiheadAttention(
- dim, num_heads, causal=True, kv_repeat=kv_repeat)
- mha = StreamingMultiheadAttention(
- dim, num_heads, causal=True, kv_repeat=kv_repeat, custom=True)
- x = torch.randn(4, 18, dim)
- y = mha(x, x, x)[0]
- assert x.shape == y.shape
-
-
-def test_qk_layer_norm():
- torch.manual_seed(1234)
- tr = StreamingTransformer(
- 16, 4, 2, custom=True, dropout=0., qk_layer_norm=True, bias_attn=False)
- steps = 12
- x = torch.randn(3, steps, 16)
- y = tr(x)
-
- tr = StreamingTransformer(
- 16, 4, 2, custom=True, dropout=0., qk_layer_norm=True, cross_attention=True)
- z = torch.randn(3, 21, 16)
- y = tr(x, cross_attention_src=z)
- assert y.shape == x.shape
diff --git a/spaces/hhhyrhe/vits-uma-genshin-honkai/Docker/vits.sh b/spaces/hhhyrhe/vits-uma-genshin-honkai/Docker/vits.sh
deleted file mode 100644
index 2b87f26eda96d3800b73b4a21b210c78888a2299..0000000000000000000000000000000000000000
--- a/spaces/hhhyrhe/vits-uma-genshin-honkai/Docker/vits.sh
+++ /dev/null
@@ -1,20 +0,0 @@
-#!/bin/bash
-run() {
- echo -e "\033[32m已完成初始化,启动服务...\033[0m"
- python3 /app/vits-uma-genshin-honkai/app.py
-}
-install() {
- echo -e "\033[33m正在初始化:安装依赖....\033[0m"
- pip install -r /app/vits-uma-genshin-honkai/requirements.txt -i https://mirrors.ustc.edu.cn/pypi/web/simple
- echo -e "\033[33m正在下载模型....\033[0m"
- rm -f /app/vits-uma-genshin-honkai/model/G_953000.pth
- wget -O /app/vits-uma-genshin-honkai/model/G_953000.pth https://huggingface.co/spaces/ikechan8370/vits-uma-genshin-honkai/resolve/main/model/G_953000.pth
- echo -e "\033[32m初始化完成!\033[0m"
- run
-}
-
-if [ ! -f "/app/vits-uma-genshin-honkai/model/G_953000.pth" ] || [ "$(stat -c%s "/app/vits-uma-genshin-honkai/model/G_953000.pth")" -lt 10000 ]; then
- install
-else
- run
-fi
diff --git a/spaces/hizkifw/clipbooru/README.md b/spaces/hizkifw/clipbooru/README.md
deleted file mode 100644
index c672e2e480cf8c6e63b01add53a78c8d432942f8..0000000000000000000000000000000000000000
--- a/spaces/hizkifw/clipbooru/README.md
+++ /dev/null
@@ -1,12 +0,0 @@
----
-title: Clipbooru
-emoji: 🌍
-colorFrom: indigo
-colorTo: gray
-sdk: gradio
-sdk_version: 3.9
-app_file: app.py
-pinned: false
----
-
-Check out the configuration reference at https://huggingface.co/docs/hub/spaces-config-reference
diff --git a/spaces/ho11laqe/nnUNet_calvingfront_detection/scripts_new/run_glacier_zone_1.sh b/spaces/ho11laqe/nnUNet_calvingfront_detection/scripts_new/run_glacier_zone_1.sh
deleted file mode 100644
index ab33a894696f61a6a952c870f1c586c870d8429e..0000000000000000000000000000000000000000
--- a/spaces/ho11laqe/nnUNet_calvingfront_detection/scripts_new/run_glacier_zone_1.sh
+++ /dev/null
@@ -1,17 +0,0 @@
-#!/bin/bash -l
-#SBATCH --nodes=1 --gres=gpu:1 --time=24:00:00
-#SBATCH --job-name=Task502_glacier_zone_1
-
-export data_raw="/home/woody/iwi5/iwi5039h/data_raw"
-export nnUNet_raw_data_base="/home/woody/iwi5/iwi5039h/nnUNet_data/nnUNet_raw_data_base/"
-export nnUNet_preprocessed="/home/woody/iwi5/iwi5039h/nnUNet_data/nnUNet_preprocessed/"
-export RESULTS_FOLDER="/home/woody/iwi5/iwi5039h/nnUNet_data/RESULTS_FOLDER"
-
-cd nnunet_glacer
-pwd
-conda activate nnunet
-
-python3 nnunet/run/run_training.py 2d nnUNetTrainerV2 502 1 --disable_postprocessing_on_folds
-python3 nnunet/inference/predict_simple.py -i $nnUNet_raw_data_base/nnUNet_raw_data/Task502_Glacier_zone/imagesTs -o $RESULTS_FOLDER/test_predictions/Task502_Glacier_zone/fold_1 -t 502 -m 2d -f 1
-python3 nnunet/dataset_conversion/Task502_Glacier_reverse.py -i $RESULTS_FOLDER/test_predictions/Task502_Glacier_zone/fold_1
-python3 ./evaluate_nnUNet.py --predictions $RESULTS_FOLDER/test_predictions/Task502_Glacier_zone/fold_1/pngs --labels_fronts $data_raw/fronts/test --labels_zones $data_raw/zones/test --sar_images $data_raw/sar_images/test
diff --git a/spaces/hongaik/hc_text_classification/.ipynb_checkpoints/app-checkpoint.py b/spaces/hongaik/hc_text_classification/.ipynb_checkpoints/app-checkpoint.py
deleted file mode 100644
index a063cd369a469888e50c7f06db4f18abf0890d74..0000000000000000000000000000000000000000
--- a/spaces/hongaik/hc_text_classification/.ipynb_checkpoints/app-checkpoint.py
+++ /dev/null
@@ -1,59 +0,0 @@
-import streamlit as st
-import plotly.express as px
-from plotly.subplots import make_subplots
-from utils import *
-
-########## Title for the Web App ##########
-st.title("Text Classification for HC")
-
-########## Create Input field ##########
-feedback = st.text_input('Type your text here', 'Customer suggested that the customer service needs to be improved and the response time needs to be improved.')
-
-if st.button('Click for predictions!'):
- with st.spinner('Generating predictions...'):
-
- topics_prob, sentiment_prob, touchpoint_prob = get_single_prediction(feedback)
-
- bar_topic = px.bar(topics_prob, x='probability', y='topic')
-
- bar_touchpoint = px.bar(touchpoint_prob, x='probability', y='touchpoint')
-
- pie = px.pie(sentiment_prob,
- values='probability',
- names='sentiment',
- color_discrete_map={'positive':'rgb(0, 204, 0)',
- 'negative':'rgb(215, 11, 11)'
- },
- color='sentiment'
- )
-
- st.plotly_chart(bar_topic, use_container_width=True)
- st.plotly_chart(bar_touchpoint, use_container_width=True)
- st.plotly_chart(pie, use_container_width=True)
-
-st.write("\n")
-st.subheader('Or... Upload a csv file if you have a file instead.')
-st.write("\n")
-
-st.download_button(
- label="Download sample file here",
- data=sample_file,
- file_name='sample_data.csv',
- mime='text/csv',
- )
-
-uploaded_file = st.file_uploader("Please upload a csv file with only 1 column of texts.")
-
-if uploaded_file is not None:
-
- with st.spinner('Generating predictions...'):
- results = get_multiple_predictions(uploaded_file)
-
- st.download_button(
- label="Download results as CSV",
- data=results,
- file_name='results.csv',
- mime='text/csv',
- )
-
-
\ No newline at end of file
diff --git a/spaces/hyxue/HiFiFace-inference-demo/Deep3DFaceRecon_pytorch/models/arcface_torch/configs/ms1mv2_mbf.py b/spaces/hyxue/HiFiFace-inference-demo/Deep3DFaceRecon_pytorch/models/arcface_torch/configs/ms1mv2_mbf.py
deleted file mode 100644
index 098afd8d2d6ca353d0b02281d02ac54e584f8281..0000000000000000000000000000000000000000
--- a/spaces/hyxue/HiFiFace-inference-demo/Deep3DFaceRecon_pytorch/models/arcface_torch/configs/ms1mv2_mbf.py
+++ /dev/null
@@ -1,27 +0,0 @@
-from easydict import EasyDict as edict
-
-# make training faster
-# our RAM is 256G
-# mount -t tmpfs -o size=140G tmpfs /train_tmp
-
-config = edict()
-config.margin_list = (1.0, 0.5, 0.0)
-config.network = "mbf"
-config.resume = False
-config.output = None
-config.embedding_size = 512
-config.sample_rate = 1.0
-config.fp16 = True
-config.momentum = 0.9
-config.weight_decay = 1e-4
-config.batch_size = 128
-config.lr = 0.1
-config.verbose = 2000
-config.dali = False
-
-config.rec = "/train_tmp/faces_emore"
-config.num_classes = 85742
-config.num_image = 5822653
-config.num_epoch = 40
-config.warmup_epoch = 0
-config.val_targets = ["lfw", "cfp_fp", "agedb_30"]
diff --git a/spaces/hzy123/bingo/src/components/chat-scroll-anchor.tsx b/spaces/hzy123/bingo/src/components/chat-scroll-anchor.tsx
deleted file mode 100644
index ac809f4486a48e134cb69314c3d0dae5e68d614e..0000000000000000000000000000000000000000
--- a/spaces/hzy123/bingo/src/components/chat-scroll-anchor.tsx
+++ /dev/null
@@ -1,29 +0,0 @@
-'use client'
-
-import * as React from 'react'
-import { useInView } from 'react-intersection-observer'
-
-import { useAtBottom } from '@/lib/hooks/use-at-bottom'
-
-interface ChatScrollAnchorProps {
- trackVisibility?: boolean
-}
-
-export function ChatScrollAnchor({ trackVisibility }: ChatScrollAnchorProps) {
- const isAtBottom = useAtBottom()
- const { ref, entry, inView } = useInView({
- trackVisibility,
- delay: 100,
- rootMargin: '0px 0px -150px 0px'
- })
-
- React.useEffect(() => {
- if (isAtBottom && trackVisibility && !inView) {
- entry?.target.scrollIntoView({
- block: 'start'
- })
- }
- }, [inView, entry, isAtBottom, trackVisibility])
-
- return
-}
diff --git a/spaces/inamXcontru/PoeticTTS/4K Stogram 2.7.2.1795 With Crack Full Download The Ultimate Solution for Instagram Marketing.md b/spaces/inamXcontru/PoeticTTS/4K Stogram 2.7.2.1795 With Crack Full Download The Ultimate Solution for Instagram Marketing.md
deleted file mode 100644
index 102e15669ab5d12f6e22b8cd1a1a8879019d1fa8..0000000000000000000000000000000000000000
--- a/spaces/inamXcontru/PoeticTTS/4K Stogram 2.7.2.1795 With Crack Full Download The Ultimate Solution for Instagram Marketing.md
+++ /dev/null
@@ -1,5 +0,0 @@
-
-
4K Stogram 3.3.3 Crack is a special tool which is equipped with the features for the viewing and then downloading of the videos, photos, stories and different audio tracks from Instagram. There is no restriction on the status of the account. You can download these all contents from the public accounts and the private accounts. For performing actions, it provides you with a lot of features. With just simple clicks, you can download videos, photos and various backup on Instagram.This program is a complete set of features and is very simple to use. With this program, you can save your important Instagram data which you can import or export anytime and anywhere without any kind of problem. You can back up all of the data which is completely secure. While you make a comparison with all other social software, you will find this one is the worlds best tool. With this application support, you can perform a lot of functions with the media of your Instagram.
-
-Student Solutions Guide for Ebbing / Gammon's General Chemistry, 10th. ISBN-13: 9781111989415. The Student Solutions Guide features designed solutions for everyone. Tutorial.
-Solving problems in chemistry.
-Grade 9
-To the textbook of Rudzitis G.E., Feldman F.F.
-Student Solutions Guide for General Chemistry, 10th edition.
-ISBN: 9781119498544.
-Description: The Student Solutions Guide for General Chemistry, 10th edition is an electronic version of the study guide that collects problem-solving materials from the fields of General Chemistry and Environmental Chemistry. 8a78ff9644
-
-
-
diff --git a/spaces/inplisQlawa/anything-midjourney-v4-1/Mathematicallogicdiscretemathematicsbytremblaymanoharpdffree [PORTABLE]125.md b/spaces/inplisQlawa/anything-midjourney-v4-1/Mathematicallogicdiscretemathematicsbytremblaymanoharpdffree [PORTABLE]125.md
deleted file mode 100644
index 3456799f29ac4c05ac8c8c43d523fe1b8d34ffaf..0000000000000000000000000000000000000000
--- a/spaces/inplisQlawa/anything-midjourney-v4-1/Mathematicallogicdiscretemathematicsbytremblaymanoharpdffree [PORTABLE]125.md
+++ /dev/null
@@ -1,9 +0,0 @@
-
-
-None for this reason, so as not to give you the opportunity to use it, we
-For this reason, not to give you the opportunity to use it, we
-. Online store (hereinafter referred to as the site) - a store that sells goods via the Internet.
-. For this reason, not to give you the opportunity to use it, we 8a78ff9644
-
-
-
diff --git a/spaces/inreVtussa/clothingai/Examples/Biblia Nacar Colunga Comentada Pdfl TOP.md b/spaces/inreVtussa/clothingai/Examples/Biblia Nacar Colunga Comentada Pdfl TOP.md
deleted file mode 100644
index acdd8f35016517e9612473acc668d4d8a399658b..0000000000000000000000000000000000000000
--- a/spaces/inreVtussa/clothingai/Examples/Biblia Nacar Colunga Comentada Pdfl TOP.md
+++ /dev/null
@@ -1,6 +0,0 @@
-
-
-August 18, 2016 - Sagrada BIBI-de-Okhar-Colung. Date added: 2016-08-18 23:57:04. FOLDOUTCOUNT: 0. ID: SagradabiblianAnacarcolunga1944. I had a feeling that I was looking at the picture Salvador Dali. In the park next to the main square. I walked alone, so no one could take pictures there. All people passed down the street and around the cathedral. At the time when I looked at the cathedral, I saw only him, and not people who were there. I felt in the surrealistic world. I was in the world drawn by Salvador Dali. It was a surreal landscape. I liked what I see: a cathedral that looked old and ancient. 8a78ff9644
-
-
-
diff --git a/spaces/isabel/mental-health-project/reader.py b/spaces/isabel/mental-health-project/reader.py
deleted file mode 100644
index 2089f121665bf06f1c4d8a54d78df7b435b01ae9..0000000000000000000000000000000000000000
--- a/spaces/isabel/mental-health-project/reader.py
+++ /dev/null
@@ -1,161 +0,0 @@
-import os
-from yattag import Doc
-## --------------------------------- ###
-### reading: info.txt ###
-### -------------------------------- ###
-# placeholders in case info.txt does not exist
-def get_article(acc, most_imp_feat):
- filename = "info.txt"
- placeholder = "please create an info.txt to customize this text"
- note = "**Note that model accuracy is based on the uploaded data.csv and reflects how well the AI model can give correct recommendations for that dataset. An accuracy of 50% means that half of the model's predictions for that dataset were accurate. Model accuracy and most important feature can be helpful for understanding how the model works, but should not be considered absolute facts about the real world."
-
- title = bkgd = data_collection = priv_cons = bias_cons = img_src = membs = description = placeholder
- # check if info.txt is present
- if os.path.isfile(filename):
- # open info.txt in read mode
- info = open(filename, "r")
-
- # read each line to a string
- description = "An AI project created by " + info.readline()
- title = info.readline()
- bkgd = info.readline()
- data_collection = info.readline()
- priv_cons = info.readline()
- bias_cons = info.readline()
- img_src = info.readline()
- membs = info.readline()
-
- # close file
- info.close()
-
- # use yattag library to generate html
- doc, tag, text, line = Doc().ttl()
- # create html based on info.txt
- with tag('div'):
- with tag('div', klass='box model-container'):
- with tag('div', klass='spacer'):
- with tag('div', klass='box model-div'):
- line('h2', "Model Accuracy", klass='acc')
- line('p', acc)
- with tag('div', klass='box model-div'):
- line('h2', "Most Important Feature", klass='feat')
- line('p', most_imp_feat)
- with tag('div', klass='spacer'):
- line('p', note)
- with tag('div', klass='box'):
- line('h2', 'Problem Statement and Research Summary', klass='prj')
- line('p', bkgd)
- with tag('div', klass='box'):
- line('h2', 'Data Collection Plan', klass='data')
- line('p', data_collection)
- with tag('div', klass='box'):
- line('h2', 'Ethical Considerations (Data Privacy and Bias)', klass='ethics')
- with tag('ul'):
- line('li', priv_cons)
- line('li', bias_cons)
- with tag('div', klass='box'):
- line('h2', 'Our Team', klass='team')
- line('p', membs)
- doc.stag('img', src=img_src)
-
- css = '''
- .box {
- border: 2px solid black;
- text-align: center;
- margin: 10px;
- padding: 5%;
- }
- ul {
- display: inline-block;
- text-align: left;
- }
- img {
- display: block;
- margin: auto;
- }
- .description {
- text-align: center;
- }
- .panel_button {
- display: block !important;
- width: 100% !important;
- background-color: #00EACD !important;
- color: #000;
- transition: all .2s ease-out 0s !important;
- box-shadow: 0 10px #00AEAB !important;
- border-radius: 10px !important;
- }
- .panel_button:hover {
- box-shadow: 0 5px #00AEAB;
- transform: translateY(5px);
- }
- .submit {
- color: black !important;
- }
- .selected {
- background-color: #656bd6 !important;
- }
- .radio_item {
- border-radius: 10px;
- padding-left: 10px !important;
- padding-right: 10px !important;
- }
- .radio_item:hover {
- color: #656bd6 !important;
- }
- .title {
- background-image: url(https://media.giphy.com/media/26BROrSHlmyzzHf3i/giphy.gif);
- background-size: cover;
- color: transparent;
- -moz-background-clip: text;
- -webkit-background-clip: text;
- text-transform: uppercase;
- font-size: 60px;
- line-height: .75;
- margin: 10px 0;
- }
- .panel_header {
- color: black !important;
- }
- input {
- background-color: #efeffa !important;
- }
- .acc, .feat {
- background-color: #FF3399 !important
- }
- .prj {
- background-color: #FFCE3B !important;
- }
- .data {
- background-color: #ED6800 !important;
- }
- .ethics {
- background-color: #3EE6F9 !important;
- }
- .team {
- background-color: #9581EF !important;
- }
- .model-container {
- display: flex;
- flex-direction: column;
- justify-content: center;
- }
- .spacer {
- display: flex;
- justify-content: center;
- }
- .model-div {
- width: 45%;
- }
- @media screen and (max-width: 700px) {
- .model-container {
- flex-wrap: wrap;
- }
- }
- '''
- return {
- 'article': doc.getvalue(),
- 'css': css,
- 'title': title,
- 'description': description,
- }
\ No newline at end of file
diff --git a/spaces/ismot/8testi1/LICENSE.md b/spaces/ismot/8testi1/LICENSE.md
deleted file mode 100644
index f288702d2fa16d3cdf0035b15a9fcbc552cd88e7..0000000000000000000000000000000000000000
--- a/spaces/ismot/8testi1/LICENSE.md
+++ /dev/null
@@ -1,674 +0,0 @@
- GNU GENERAL PUBLIC LICENSE
- Version 3, 29 June 2007
-
- Copyright (C) 2007 Free Software Foundation, Inc.
- Everyone is permitted to copy and distribute verbatim copies
- of this license document, but changing it is not allowed.
-
- Preamble
-
- The GNU General Public License is a free, copyleft license for
-software and other kinds of works.
-
- The licenses for most software and other practical works are designed
-to take away your freedom to share and change the works. By contrast,
-the GNU General Public License is intended to guarantee your freedom to
-share and change all versions of a program--to make sure it remains free
-software for all its users. We, the Free Software Foundation, use the
-GNU General Public License for most of our software; it applies also to
-any other work released this way by its authors. You can apply it to
-your programs, too.
-
- When we speak of free software, we are referring to freedom, not
-price. Our General Public Licenses are designed to make sure that you
-have the freedom to distribute copies of free software (and charge for
-them if you wish), that you receive source code or can get it if you
-want it, that you can change the software or use pieces of it in new
-free programs, and that you know you can do these things.
-
- To protect your rights, we need to prevent others from denying you
-these rights or asking you to surrender the rights. Therefore, you have
-certain responsibilities if you distribute copies of the software, or if
-you modify it: responsibilities to respect the freedom of others.
-
- For example, if you distribute copies of such a program, whether
-gratis or for a fee, you must pass on to the recipients the same
-freedoms that you received. You must make sure that they, too, receive
-or can get the source code. And you must show them these terms so they
-know their rights.
-
- Developers that use the GNU GPL protect your rights with two steps:
-(1) assert copyright on the software, and (2) offer you this License
-giving you legal permission to copy, distribute and/or modify it.
-
- For the developers' and authors' protection, the GPL clearly explains
-that there is no warranty for this free software. For both users' and
-authors' sake, the GPL requires that modified versions be marked as
-changed, so that their problems will not be attributed erroneously to
-authors of previous versions.
-
- Some devices are designed to deny users access to install or run
-modified versions of the software inside them, although the manufacturer
-can do so. This is fundamentally incompatible with the aim of
-protecting users' freedom to change the software. The systematic
-pattern of such abuse occurs in the area of products for individuals to
-use, which is precisely where it is most unacceptable. Therefore, we
-have designed this version of the GPL to prohibit the practice for those
-products. If such problems arise substantially in other domains, we
-stand ready to extend this provision to those domains in future versions
-of the GPL, as needed to protect the freedom of users.
-
- Finally, every program is threatened constantly by software patents.
-States should not allow patents to restrict development and use of
-software on general-purpose computers, but in those that do, we wish to
-avoid the special danger that patents applied to a free program could
-make it effectively proprietary. To prevent this, the GPL assures that
-patents cannot be used to render the program non-free.
-
- The precise terms and conditions for copying, distribution and
-modification follow.
-
- TERMS AND CONDITIONS
-
- 0. Definitions.
-
- "This License" refers to version 3 of the GNU General Public License.
-
- "Copyright" also means copyright-like laws that apply to other kinds of
-works, such as semiconductor masks.
-
- "The Program" refers to any copyrightable work licensed under this
-License. Each licensee is addressed as "you". "Licensees" and
-"recipients" may be individuals or organizations.
-
- To "modify" a work means to copy from or adapt all or part of the work
-in a fashion requiring copyright permission, other than the making of an
-exact copy. The resulting work is called a "modified version" of the
-earlier work or a work "based on" the earlier work.
-
- A "covered work" means either the unmodified Program or a work based
-on the Program.
-
- To "propagate" a work means to do anything with it that, without
-permission, would make you directly or secondarily liable for
-infringement under applicable copyright law, except executing it on a
-computer or modifying a private copy. Propagation includes copying,
-distribution (with or without modification), making available to the
-public, and in some countries other activities as well.
-
- To "convey" a work means any kind of propagation that enables other
-parties to make or receive copies. Mere interaction with a user through
-a computer network, with no transfer of a copy, is not conveying.
-
- An interactive user interface displays "Appropriate Legal Notices"
-to the extent that it includes a convenient and prominently visible
-feature that (1) displays an appropriate copyright notice, and (2)
-tells the user that there is no warranty for the work (except to the
-extent that warranties are provided), that licensees may convey the
-work under this License, and how to view a copy of this License. If
-the interface presents a list of user commands or options, such as a
-menu, a prominent item in the list meets this criterion.
-
- 1. Source Code.
-
- The "source code" for a work means the preferred form of the work
-for making modifications to it. "Object code" means any non-source
-form of a work.
-
- A "Standard Interface" means an interface that either is an official
-standard defined by a recognized standards body, or, in the case of
-interfaces specified for a particular programming language, one that
-is widely used among developers working in that language.
-
- The "System Libraries" of an executable work include anything, other
-than the work as a whole, that (a) is included in the normal form of
-packaging a Major Component, but which is not part of that Major
-Component, and (b) serves only to enable use of the work with that
-Major Component, or to implement a Standard Interface for which an
-implementation is available to the public in source code form. A
-"Major Component", in this context, means a major essential component
-(kernel, window system, and so on) of the specific operating system
-(if any) on which the executable work runs, or a compiler used to
-produce the work, or an object code interpreter used to run it.
-
- The "Corresponding Source" for a work in object code form means all
-the source code needed to generate, install, and (for an executable
-work) run the object code and to modify the work, including scripts to
-control those activities. However, it does not include the work's
-System Libraries, or general-purpose tools or generally available free
-programs which are used unmodified in performing those activities but
-which are not part of the work. For example, Corresponding Source
-includes interface definition files associated with source files for
-the work, and the source code for shared libraries and dynamically
-linked subprograms that the work is specifically designed to require,
-such as by intimate data communication or control flow between those
-subprograms and other parts of the work.
-
- The Corresponding Source need not include anything that users
-can regenerate automatically from other parts of the Corresponding
-Source.
-
- The Corresponding Source for a work in source code form is that
-same work.
-
- 2. Basic Permissions.
-
- All rights granted under this License are granted for the term of
-copyright on the Program, and are irrevocable provided the stated
-conditions are met. This License explicitly affirms your unlimited
-permission to run the unmodified Program. The output from running a
-covered work is covered by this License only if the output, given its
-content, constitutes a covered work. This License acknowledges your
-rights of fair use or other equivalent, as provided by copyright law.
-
- You may make, run and propagate covered works that you do not
-convey, without conditions so long as your license otherwise remains
-in force. You may convey covered works to others for the sole purpose
-of having them make modifications exclusively for you, or provide you
-with facilities for running those works, provided that you comply with
-the terms of this License in conveying all material for which you do
-not control copyright. Those thus making or running the covered works
-for you must do so exclusively on your behalf, under your direction
-and control, on terms that prohibit them from making any copies of
-your copyrighted material outside their relationship with you.
-
- Conveying under any other circumstances is permitted solely under
-the conditions stated below. Sublicensing is not allowed; section 10
-makes it unnecessary.
-
- 3. Protecting Users' Legal Rights From Anti-Circumvention Law.
-
- No covered work shall be deemed part of an effective technological
-measure under any applicable law fulfilling obligations under article
-11 of the WIPO copyright treaty adopted on 20 December 1996, or
-similar laws prohibiting or restricting circumvention of such
-measures.
-
- When you convey a covered work, you waive any legal power to forbid
-circumvention of technological measures to the extent such circumvention
-is effected by exercising rights under this License with respect to
-the covered work, and you disclaim any intention to limit operation or
-modification of the work as a means of enforcing, against the work's
-users, your or third parties' legal rights to forbid circumvention of
-technological measures.
-
- 4. Conveying Verbatim Copies.
-
- You may convey verbatim copies of the Program's source code as you
-receive it, in any medium, provided that you conspicuously and
-appropriately publish on each copy an appropriate copyright notice;
-keep intact all notices stating that this License and any
-non-permissive terms added in accord with section 7 apply to the code;
-keep intact all notices of the absence of any warranty; and give all
-recipients a copy of this License along with the Program.
-
- You may charge any price or no price for each copy that you convey,
-and you may offer support or warranty protection for a fee.
-
- 5. Conveying Modified Source Versions.
-
- You may convey a work based on the Program, or the modifications to
-produce it from the Program, in the form of source code under the
-terms of section 4, provided that you also meet all of these conditions:
-
- a) The work must carry prominent notices stating that you modified
- it, and giving a relevant date.
-
- b) The work must carry prominent notices stating that it is
- released under this License and any conditions added under section
- 7. This requirement modifies the requirement in section 4 to
- "keep intact all notices".
-
- c) You must license the entire work, as a whole, under this
- License to anyone who comes into possession of a copy. This
- License will therefore apply, along with any applicable section 7
- additional terms, to the whole of the work, and all its parts,
- regardless of how they are packaged. This License gives no
- permission to license the work in any other way, but it does not
- invalidate such permission if you have separately received it.
-
- d) If the work has interactive user interfaces, each must display
- Appropriate Legal Notices; however, if the Program has interactive
- interfaces that do not display Appropriate Legal Notices, your
- work need not make them do so.
-
- A compilation of a covered work with other separate and independent
-works, which are not by their nature extensions of the covered work,
-and which are not combined with it such as to form a larger program,
-in or on a volume of a storage or distribution medium, is called an
-"aggregate" if the compilation and its resulting copyright are not
-used to limit the access or legal rights of the compilation's users
-beyond what the individual works permit. Inclusion of a covered work
-in an aggregate does not cause this License to apply to the other
-parts of the aggregate.
-
- 6. Conveying Non-Source Forms.
-
- You may convey a covered work in object code form under the terms
-of sections 4 and 5, provided that you also convey the
-machine-readable Corresponding Source under the terms of this License,
-in one of these ways:
-
- a) Convey the object code in, or embodied in, a physical product
- (including a physical distribution medium), accompanied by the
- Corresponding Source fixed on a durable physical medium
- customarily used for software interchange.
-
- b) Convey the object code in, or embodied in, a physical product
- (including a physical distribution medium), accompanied by a
- written offer, valid for at least three years and valid for as
- long as you offer spare parts or customer support for that product
- model, to give anyone who possesses the object code either (1) a
- copy of the Corresponding Source for all the software in the
- product that is covered by this License, on a durable physical
- medium customarily used for software interchange, for a price no
- more than your reasonable cost of physically performing this
- conveying of source, or (2) access to copy the
- Corresponding Source from a network server at no charge.
-
- c) Convey individual copies of the object code with a copy of the
- written offer to provide the Corresponding Source. This
- alternative is allowed only occasionally and noncommercially, and
- only if you received the object code with such an offer, in accord
- with subsection 6b.
-
- d) Convey the object code by offering access from a designated
- place (gratis or for a charge), and offer equivalent access to the
- Corresponding Source in the same way through the same place at no
- further charge. You need not require recipients to copy the
- Corresponding Source along with the object code. If the place to
- copy the object code is a network server, the Corresponding Source
- may be on a different server (operated by you or a third party)
- that supports equivalent copying facilities, provided you maintain
- clear directions next to the object code saying where to find the
- Corresponding Source. Regardless of what server hosts the
- Corresponding Source, you remain obligated to ensure that it is
- available for as long as needed to satisfy these requirements.
-
- e) Convey the object code using peer-to-peer transmission, provided
- you inform other peers where the object code and Corresponding
- Source of the work are being offered to the general public at no
- charge under subsection 6d.
-
- A separable portion of the object code, whose source code is excluded
-from the Corresponding Source as a System Library, need not be
-included in conveying the object code work.
-
- A "User Product" is either (1) a "consumer product", which means any
-tangible personal property which is normally used for personal, family,
-or household purposes, or (2) anything designed or sold for incorporation
-into a dwelling. In determining whether a product is a consumer product,
-doubtful cases shall be resolved in favor of coverage. For a particular
-product received by a particular user, "normally used" refers to a
-typical or common use of that class of product, regardless of the status
-of the particular user or of the way in which the particular user
-actually uses, or expects or is expected to use, the product. A product
-is a consumer product regardless of whether the product has substantial
-commercial, industrial or non-consumer uses, unless such uses represent
-the only significant mode of use of the product.
-
- "Installation Information" for a User Product means any methods,
-procedures, authorization keys, or other information required to install
-and execute modified versions of a covered work in that User Product from
-a modified version of its Corresponding Source. The information must
-suffice to ensure that the continued functioning of the modified object
-code is in no case prevented or interfered with solely because
-modification has been made.
-
- If you convey an object code work under this section in, or with, or
-specifically for use in, a User Product, and the conveying occurs as
-part of a transaction in which the right of possession and use of the
-User Product is transferred to the recipient in perpetuity or for a
-fixed term (regardless of how the transaction is characterized), the
-Corresponding Source conveyed under this section must be accompanied
-by the Installation Information. But this requirement does not apply
-if neither you nor any third party retains the ability to install
-modified object code on the User Product (for example, the work has
-been installed in ROM).
-
- The requirement to provide Installation Information does not include a
-requirement to continue to provide support service, warranty, or updates
-for a work that has been modified or installed by the recipient, or for
-the User Product in which it has been modified or installed. Access to a
-network may be denied when the modification itself materially and
-adversely affects the operation of the network or violates the rules and
-protocols for communication across the network.
-
- Corresponding Source conveyed, and Installation Information provided,
-in accord with this section must be in a format that is publicly
-documented (and with an implementation available to the public in
-source code form), and must require no special password or key for
-unpacking, reading or copying.
-
- 7. Additional Terms.
-
- "Additional permissions" are terms that supplement the terms of this
-License by making exceptions from one or more of its conditions.
-Additional permissions that are applicable to the entire Program shall
-be treated as though they were included in this License, to the extent
-that they are valid under applicable law. If additional permissions
-apply only to part of the Program, that part may be used separately
-under those permissions, but the entire Program remains governed by
-this License without regard to the additional permissions.
-
- When you convey a copy of a covered work, you may at your option
-remove any additional permissions from that copy, or from any part of
-it. (Additional permissions may be written to require their own
-removal in certain cases when you modify the work.) You may place
-additional permissions on material, added by you to a covered work,
-for which you have or can give appropriate copyright permission.
-
- Notwithstanding any other provision of this License, for material you
-add to a covered work, you may (if authorized by the copyright holders of
-that material) supplement the terms of this License with terms:
-
- a) Disclaiming warranty or limiting liability differently from the
- terms of sections 15 and 16 of this License; or
-
- b) Requiring preservation of specified reasonable legal notices or
- author attributions in that material or in the Appropriate Legal
- Notices displayed by works containing it; or
-
- c) Prohibiting misrepresentation of the origin of that material, or
- requiring that modified versions of such material be marked in
- reasonable ways as different from the original version; or
-
- d) Limiting the use for publicity purposes of names of licensors or
- authors of the material; or
-
- e) Declining to grant rights under trademark law for use of some
- trade names, trademarks, or service marks; or
-
- f) Requiring indemnification of licensors and authors of that
- material by anyone who conveys the material (or modified versions of
- it) with contractual assumptions of liability to the recipient, for
- any liability that these contractual assumptions directly impose on
- those licensors and authors.
-
- All other non-permissive additional terms are considered "further
-restrictions" within the meaning of section 10. If the Program as you
-received it, or any part of it, contains a notice stating that it is
-governed by this License along with a term that is a further
-restriction, you may remove that term. If a license document contains
-a further restriction but permits relicensing or conveying under this
-License, you may add to a covered work material governed by the terms
-of that license document, provided that the further restriction does
-not survive such relicensing or conveying.
-
- If you add terms to a covered work in accord with this section, you
-must place, in the relevant source files, a statement of the
-additional terms that apply to those files, or a notice indicating
-where to find the applicable terms.
-
- Additional terms, permissive or non-permissive, may be stated in the
-form of a separately written license, or stated as exceptions;
-the above requirements apply either way.
-
- 8. Termination.
-
- You may not propagate or modify a covered work except as expressly
-provided under this License. Any attempt otherwise to propagate or
-modify it is void, and will automatically terminate your rights under
-this License (including any patent licenses granted under the third
-paragraph of section 11).
-
- However, if you cease all violation of this License, then your
-license from a particular copyright holder is reinstated (a)
-provisionally, unless and until the copyright holder explicitly and
-finally terminates your license, and (b) permanently, if the copyright
-holder fails to notify you of the violation by some reasonable means
-prior to 60 days after the cessation.
-
- Moreover, your license from a particular copyright holder is
-reinstated permanently if the copyright holder notifies you of the
-violation by some reasonable means, this is the first time you have
-received notice of violation of this License (for any work) from that
-copyright holder, and you cure the violation prior to 30 days after
-your receipt of the notice.
-
- Termination of your rights under this section does not terminate the
-licenses of parties who have received copies or rights from you under
-this License. If your rights have been terminated and not permanently
-reinstated, you do not qualify to receive new licenses for the same
-material under section 10.
-
- 9. Acceptance Not Required for Having Copies.
-
- You are not required to accept this License in order to receive or
-run a copy of the Program. Ancillary propagation of a covered work
-occurring solely as a consequence of using peer-to-peer transmission
-to receive a copy likewise does not require acceptance. However,
-nothing other than this License grants you permission to propagate or
-modify any covered work. These actions infringe copyright if you do
-not accept this License. Therefore, by modifying or propagating a
-covered work, you indicate your acceptance of this License to do so.
-
- 10. Automatic Licensing of Downstream Recipients.
-
- Each time you convey a covered work, the recipient automatically
-receives a license from the original licensors, to run, modify and
-propagate that work, subject to this License. You are not responsible
-for enforcing compliance by third parties with this License.
-
- An "entity transaction" is a transaction transferring control of an
-organization, or substantially all assets of one, or subdividing an
-organization, or merging organizations. If propagation of a covered
-work results from an entity transaction, each party to that
-transaction who receives a copy of the work also receives whatever
-licenses to the work the party's predecessor in interest had or could
-give under the previous paragraph, plus a right to possession of the
-Corresponding Source of the work from the predecessor in interest, if
-the predecessor has it or can get it with reasonable efforts.
-
- You may not impose any further restrictions on the exercise of the
-rights granted or affirmed under this License. For example, you may
-not impose a license fee, royalty, or other charge for exercise of
-rights granted under this License, and you may not initiate litigation
-(including a cross-claim or counterclaim in a lawsuit) alleging that
-any patent claim is infringed by making, using, selling, offering for
-sale, or importing the Program or any portion of it.
-
- 11. Patents.
-
- A "contributor" is a copyright holder who authorizes use under this
-License of the Program or a work on which the Program is based. The
-work thus licensed is called the contributor's "contributor version".
-
- A contributor's "essential patent claims" are all patent claims
-owned or controlled by the contributor, whether already acquired or
-hereafter acquired, that would be infringed by some manner, permitted
-by this License, of making, using, or selling its contributor version,
-but do not include claims that would be infringed only as a
-consequence of further modification of the contributor version. For
-purposes of this definition, "control" includes the right to grant
-patent sublicenses in a manner consistent with the requirements of
-this License.
-
- Each contributor grants you a non-exclusive, worldwide, royalty-free
-patent license under the contributor's essential patent claims, to
-make, use, sell, offer for sale, import and otherwise run, modify and
-propagate the contents of its contributor version.
-
- In the following three paragraphs, a "patent license" is any express
-agreement or commitment, however denominated, not to enforce a patent
-(such as an express permission to practice a patent or covenant not to
-sue for patent infringement). To "grant" such a patent license to a
-party means to make such an agreement or commitment not to enforce a
-patent against the party.
-
- If you convey a covered work, knowingly relying on a patent license,
-and the Corresponding Source of the work is not available for anyone
-to copy, free of charge and under the terms of this License, through a
-publicly available network server or other readily accessible means,
-then you must either (1) cause the Corresponding Source to be so
-available, or (2) arrange to deprive yourself of the benefit of the
-patent license for this particular work, or (3) arrange, in a manner
-consistent with the requirements of this License, to extend the patent
-license to downstream recipients. "Knowingly relying" means you have
-actual knowledge that, but for the patent license, your conveying the
-covered work in a country, or your recipient's use of the covered work
-in a country, would infringe one or more identifiable patents in that
-country that you have reason to believe are valid.
-
- If, pursuant to or in connection with a single transaction or
-arrangement, you convey, or propagate by procuring conveyance of, a
-covered work, and grant a patent license to some of the parties
-receiving the covered work authorizing them to use, propagate, modify
-or convey a specific copy of the covered work, then the patent license
-you grant is automatically extended to all recipients of the covered
-work and works based on it.
-
- A patent license is "discriminatory" if it does not include within
-the scope of its coverage, prohibits the exercise of, or is
-conditioned on the non-exercise of one or more of the rights that are
-specifically granted under this License. You may not convey a covered
-work if you are a party to an arrangement with a third party that is
-in the business of distributing software, under which you make payment
-to the third party based on the extent of your activity of conveying
-the work, and under which the third party grants, to any of the
-parties who would receive the covered work from you, a discriminatory
-patent license (a) in connection with copies of the covered work
-conveyed by you (or copies made from those copies), or (b) primarily
-for and in connection with specific products or compilations that
-contain the covered work, unless you entered into that arrangement,
-or that patent license was granted, prior to 28 March 2007.
-
- Nothing in this License shall be construed as excluding or limiting
-any implied license or other defenses to infringement that may
-otherwise be available to you under applicable patent law.
-
- 12. No Surrender of Others' Freedom.
-
- If conditions are imposed on you (whether by court order, agreement or
-otherwise) that contradict the conditions of this License, they do not
-excuse you from the conditions of this License. If you cannot convey a
-covered work so as to satisfy simultaneously your obligations under this
-License and any other pertinent obligations, then as a consequence you may
-not convey it at all. For example, if you agree to terms that obligate you
-to collect a royalty for further conveying from those to whom you convey
-the Program, the only way you could satisfy both those terms and this
-License would be to refrain entirely from conveying the Program.
-
- 13. Use with the GNU Affero General Public License.
-
- Notwithstanding any other provision of this License, you have
-permission to link or combine any covered work with a work licensed
-under version 3 of the GNU Affero General Public License into a single
-combined work, and to convey the resulting work. The terms of this
-License will continue to apply to the part which is the covered work,
-but the special requirements of the GNU Affero General Public License,
-section 13, concerning interaction through a network will apply to the
-combination as such.
-
- 14. Revised Versions of this License.
-
- The Free Software Foundation may publish revised and/or new versions of
-the GNU General Public License from time to time. Such new versions will
-be similar in spirit to the present version, but may differ in detail to
-address new problems or concerns.
-
- Each version is given a distinguishing version number. If the
-Program specifies that a certain numbered version of the GNU General
-Public License "or any later version" applies to it, you have the
-option of following the terms and conditions either of that numbered
-version or of any later version published by the Free Software
-Foundation. If the Program does not specify a version number of the
-GNU General Public License, you may choose any version ever published
-by the Free Software Foundation.
-
- If the Program specifies that a proxy can decide which future
-versions of the GNU General Public License can be used, that proxy's
-public statement of acceptance of a version permanently authorizes you
-to choose that version for the Program.
-
- Later license versions may give you additional or different
-permissions. However, no additional obligations are imposed on any
-author or copyright holder as a result of your choosing to follow a
-later version.
-
- 15. Disclaimer of Warranty.
-
- THERE IS NO WARRANTY FOR THE PROGRAM, TO THE EXTENT PERMITTED BY
-APPLICABLE LAW. EXCEPT WHEN OTHERWISE STATED IN WRITING THE COPYRIGHT
-HOLDERS AND/OR OTHER PARTIES PROVIDE THE PROGRAM "AS IS" WITHOUT WARRANTY
-OF ANY KIND, EITHER EXPRESSED OR IMPLIED, INCLUDING, BUT NOT LIMITED TO,
-THE IMPLIED WARRANTIES OF MERCHANTABILITY AND FITNESS FOR A PARTICULAR
-PURPOSE. THE ENTIRE RISK AS TO THE QUALITY AND PERFORMANCE OF THE PROGRAM
-IS WITH YOU. SHOULD THE PROGRAM PROVE DEFECTIVE, YOU ASSUME THE COST OF
-ALL NECESSARY SERVICING, REPAIR OR CORRECTION.
-
- 16. Limitation of Liability.
-
- IN NO EVENT UNLESS REQUIRED BY APPLICABLE LAW OR AGREED TO IN WRITING
-WILL ANY COPYRIGHT HOLDER, OR ANY OTHER PARTY WHO MODIFIES AND/OR CONVEYS
-THE PROGRAM AS PERMITTED ABOVE, BE LIABLE TO YOU FOR DAMAGES, INCLUDING ANY
-GENERAL, SPECIAL, INCIDENTAL OR CONSEQUENTIAL DAMAGES ARISING OUT OF THE
-USE OR INABILITY TO USE THE PROGRAM (INCLUDING BUT NOT LIMITED TO LOSS OF
-DATA OR DATA BEING RENDERED INACCURATE OR LOSSES SUSTAINED BY YOU OR THIRD
-PARTIES OR A FAILURE OF THE PROGRAM TO OPERATE WITH ANY OTHER PROGRAMS),
-EVEN IF SUCH HOLDER OR OTHER PARTY HAS BEEN ADVISED OF THE POSSIBILITY OF
-SUCH DAMAGES.
-
- 17. Interpretation of Sections 15 and 16.
-
- If the disclaimer of warranty and limitation of liability provided
-above cannot be given local legal effect according to their terms,
-reviewing courts shall apply local law that most closely approximates
-an absolute waiver of all civil liability in connection with the
-Program, unless a warranty or assumption of liability accompanies a
-copy of the Program in return for a fee.
-
- END OF TERMS AND CONDITIONS
-
- How to Apply These Terms to Your New Programs
-
- If you develop a new program, and you want it to be of the greatest
-possible use to the public, the best way to achieve this is to make it
-free software which everyone can redistribute and change under these terms.
-
- To do so, attach the following notices to the program. It is safest
-to attach them to the start of each source file to most effectively
-state the exclusion of warranty; and each file should have at least
-the "copyright" line and a pointer to where the full notice is found.
-
-
- Copyright (C)
-
- This program is free software: you can redistribute it and/or modify
- it under the terms of the GNU General Public License as published by
- the Free Software Foundation, either version 3 of the License, or
- (at your option) any later version.
-
- This program is distributed in the hope that it will be useful,
- but WITHOUT ANY WARRANTY; without even the implied warranty of
- MERCHANTABILITY or FITNESS FOR A PARTICULAR PURPOSE. See the
- GNU General Public License for more details.
-
- You should have received a copy of the GNU General Public License
- along with this program. If not, see .
-
-Also add information on how to contact you by electronic and paper mail.
-
- If the program does terminal interaction, make it output a short
-notice like this when it starts in an interactive mode:
-
- Copyright (C)
- This program comes with ABSOLUTELY NO WARRANTY; for details type `show w'.
- This is free software, and you are welcome to redistribute it
- under certain conditions; type `show c' for details.
-
-The hypothetical commands `show w' and `show c' should show the appropriate
-parts of the General Public License. Of course, your program's commands
-might be different; for a GUI interface, you would use an "about box".
-
- You should also get your employer (if you work as a programmer) or school,
-if any, to sign a "copyright disclaimer" for the program, if necessary.
-For more information on this, and how to apply and follow the GNU GPL, see
-.
-
- The GNU General Public License does not permit incorporating your program
-into proprietary programs. If your program is a subroutine library, you
-may consider it more useful to permit linking proprietary applications with
-the library. If this is what you want to do, use the GNU Lesser General
-Public License instead of this License. But first, please read
-.
diff --git a/spaces/ivanmeyer/dreamlike-photoreal-2.0/README.md b/spaces/ivanmeyer/dreamlike-photoreal-2.0/README.md
deleted file mode 100644
index a70a7b6bfda1bdeb1d5d103e33a80e6780b24740..0000000000000000000000000000000000000000
--- a/spaces/ivanmeyer/dreamlike-photoreal-2.0/README.md
+++ /dev/null
@@ -1,13 +0,0 @@
----
-title: Dreamlike Photoreal 2.0
-emoji: 📉
-colorFrom: pink
-colorTo: pink
-sdk: gradio
-sdk_version: 3.15.0
-app_file: app.py
-pinned: false
-duplicated_from: akhaliq/dreamlike-photoreal-2.0
----
-
-Check out the configuration reference at https://huggingface.co/docs/hub/spaces-config-reference
diff --git a/spaces/izumi-lab/llama-13b-japanese-lora-v0-1ep/Makefile b/spaces/izumi-lab/llama-13b-japanese-lora-v0-1ep/Makefile
deleted file mode 100644
index 4f458021aed1d71e5ce346617b3b02d29985b5af..0000000000000000000000000000000000000000
--- a/spaces/izumi-lab/llama-13b-japanese-lora-v0-1ep/Makefile
+++ /dev/null
@@ -1,35 +0,0 @@
-
-RUN := poetry run
-
-.PHONY: check
-check: lint mypy
-
-.PHONY: lint
-lint: lint-black lint-isort lint-flake8
-
-.PHONY: lint-black
-lint-black:
- $(RUN) black --check --diff --quiet .
-
-.PHONY: lint-isort
-lint-isort:
- $(RUN) isort --check --quiet .
-
-.PHONY: lint-flake8
-lint-flake8:
- $(RUN) pflake8 .
-
-.PHONY: mypy
-mypy:
- $(RUN) mypy .
-
-.PHONY: format
-format: format-black format-isort
-
-.PHONY: format-black
-format-black:
- $(RUN) black --quiet .
-
-.PHONY: format-isort
-format-isort:
- $(RUN) isort --quiet .
diff --git a/spaces/jackli888/stable-diffusion-webui/modules/extensions.py b/spaces/jackli888/stable-diffusion-webui/modules/extensions.py
deleted file mode 100644
index 1be7509685e5c11a6f0e44cd39d11613c8ba3e9f..0000000000000000000000000000000000000000
--- a/spaces/jackli888/stable-diffusion-webui/modules/extensions.py
+++ /dev/null
@@ -1,107 +0,0 @@
-import os
-import sys
-import traceback
-
-import time
-import git
-
-from modules import paths, shared
-
-extensions = []
-extensions_dir = os.path.join(paths.data_path, "extensions")
-extensions_builtin_dir = os.path.join(paths.script_path, "extensions-builtin")
-
-if not os.path.exists(extensions_dir):
- os.makedirs(extensions_dir)
-
-def active():
- return [x for x in extensions if x.enabled]
-
-
-class Extension:
- def __init__(self, name, path, enabled=True, is_builtin=False):
- self.name = name
- self.path = path
- self.enabled = enabled
- self.status = ''
- self.can_update = False
- self.is_builtin = is_builtin
- self.version = ''
-
- repo = None
- try:
- if os.path.exists(os.path.join(path, ".git")):
- repo = git.Repo(path)
- except Exception:
- print(f"Error reading github repository info from {path}:", file=sys.stderr)
- print(traceback.format_exc(), file=sys.stderr)
-
- if repo is None or repo.bare:
- self.remote = None
- else:
- try:
- self.remote = next(repo.remote().urls, None)
- self.status = 'unknown'
- head = repo.head.commit
- ts = time.asctime(time.gmtime(repo.head.commit.committed_date))
- self.version = f'{head.hexsha[:8]} ({ts})'
-
- except Exception:
- self.remote = None
-
- def list_files(self, subdir, extension):
- from modules import scripts
-
- dirpath = os.path.join(self.path, subdir)
- if not os.path.isdir(dirpath):
- return []
-
- res = []
- for filename in sorted(os.listdir(dirpath)):
- res.append(scripts.ScriptFile(self.path, filename, os.path.join(dirpath, filename)))
-
- res = [x for x in res if os.path.splitext(x.path)[1].lower() == extension and os.path.isfile(x.path)]
-
- return res
-
- def check_updates(self):
- repo = git.Repo(self.path)
- for fetch in repo.remote().fetch("--dry-run"):
- if fetch.flags != fetch.HEAD_UPTODATE:
- self.can_update = True
- self.status = "behind"
- return
-
- self.can_update = False
- self.status = "latest"
-
- def fetch_and_reset_hard(self):
- repo = git.Repo(self.path)
- # Fix: `error: Your local changes to the following files would be overwritten by merge`,
- # because WSL2 Docker set 755 file permissions instead of 644, this results to the error.
- repo.git.fetch('--all')
- repo.git.reset('--hard', 'origin')
-
-
-def list_extensions():
- extensions.clear()
-
- if not os.path.isdir(extensions_dir):
- return
-
- paths = []
- for dirname in [extensions_dir, extensions_builtin_dir]:
- if not os.path.isdir(dirname):
- return
-
- for extension_dirname in sorted(os.listdir(dirname)):
- path = os.path.join(dirname, extension_dirname)
- if not os.path.isdir(path):
- continue
-
- paths.append((extension_dirname, path, dirname == extensions_builtin_dir))
-
- for dirname, path, is_builtin in paths:
- extension = Extension(name=dirname, path=path, enabled=dirname not in shared.opts.disabled_extensions, is_builtin=is_builtin)
- extensions.append(extension)
-
diff --git a/spaces/jdczlx/ChatGPT-chuanhu/modules/presets.py b/spaces/jdczlx/ChatGPT-chuanhu/modules/presets.py
deleted file mode 100644
index fcfb53e73e9c5217d312e1a53a7b82c3dbbc82d5..0000000000000000000000000000000000000000
--- a/spaces/jdczlx/ChatGPT-chuanhu/modules/presets.py
+++ /dev/null
@@ -1,165 +0,0 @@
-# -*- coding:utf-8 -*-
-import gradio as gr
-
-# ChatGPT 设置
-initial_prompt = "You are a helpful assistant."
-API_URL = "https://api.openai.com/v1/chat/completions"
-BALANCE_API_URL="https://api.openai.com/dashboard/billing/credit_grants"
-HISTORY_DIR = "history"
-TEMPLATES_DIR = "templates"
-
-# 错误信息
-standard_error_msg = "☹️发生了错误:" # 错误信息的标准前缀
-error_retrieve_prompt = "请检查网络连接,或者API-Key是否有效。" # 获取对话时发生错误
-connection_timeout_prompt = "连接超时,无法获取对话。" # 连接超时
-read_timeout_prompt = "读取超时,无法获取对话。" # 读取超时
-proxy_error_prompt = "代理错误,无法获取对话。" # 代理错误
-ssl_error_prompt = "SSL错误,无法获取对话。" # SSL 错误
-no_apikey_msg = "API key长度不是51位,请检查是否输入正确。" # API key 长度不足 51 位
-no_input_msg = "请输入对话内容。" # 未输入对话内容
-
-max_token_streaming = 3500 # 流式对话时的最大 token 数
-timeout_streaming = 10 # 流式对话时的超时时间
-max_token_all = 3500 # 非流式对话时的最大 token 数
-timeout_all = 200 # 非流式对话时的超时时间
-enable_streaming_option = True # 是否启用选择选择是否实时显示回答的勾选框
-HIDE_MY_KEY = False # 如果你想在UI中隐藏你的 API 密钥,将此值设置为 True
-CONCURRENT_COUNT = 100 # 允许同时使用的用户数量
-
-SIM_K = 5
-INDEX_QUERY_TEMPRATURE = 1.0
-
-title = """
-"""
-
-summarize_prompt = "你是谁?我们刚才聊了什么?" # 总结对话时的 prompt
-
-MODELS = [
- "gpt-3.5-turbo",
- "gpt-3.5-turbo-0301",
- "gpt-4",
- "gpt-4-0314",
- "gpt-4-32k",
- "gpt-4-32k-0314",
-] # 可选的模型
-
-REPLY_LANGUAGES = [
- "中文",
- "English",
- "日本語",
- "Español",
- "Français",
- "Deutsch",
- "跟随问题语言(不稳定)"
-]
-
-
-WEBSEARCH_PTOMPT_TEMPLATE = """\
-Web search results:
-
-{web_results}
-Current date: {current_date}
-
-Instructions: Using the provided web search results, write a comprehensive reply to the given query. Make sure to cite results using [[number](URL)] notation after the reference. If the provided search results refer to multiple subjects with the same name, write separate answers for each subject.
-Query: {query}
-Reply in {reply_language}
-"""
-
-PROMPT_TEMPLATE = """\
-Context information is below.
----------------------
-{context_str}
----------------------
-Current date: {current_date}.
-Using the provided context information, write a comprehensive reply to the given query.
-Make sure to cite results using [number] notation after the reference.
-If the provided context information refer to multiple subjects with the same name, write separate answers for each subject.
-Use prior knowledge only if the given context didn't provide enough information.
-Answer the question: {query_str}
-Reply in {reply_language}
-"""
-
-REFINE_TEMPLATE = """\
-The original question is as follows: {query_str}
-We have provided an existing answer: {existing_answer}
-We have the opportunity to refine the existing answer
-(only if needed) with some more context below.
-------------
-{context_msg}
-------------
-Given the new context, refine the original answer to better
-Reply in {reply_language}
-If the context isn't useful, return the original answer.
-"""
-
-ALREADY_CONVERTED_MARK = ""
-
-small_and_beautiful_theme = gr.themes.Soft(
- primary_hue=gr.themes.Color(
- c50="#02C160",
- c100="rgba(2, 193, 96, 0.2)",
- c200="#02C160",
- c300="rgba(2, 193, 96, 0.32)",
- c400="rgba(2, 193, 96, 0.32)",
- c500="rgba(2, 193, 96, 1.0)",
- c600="rgba(2, 193, 96, 1.0)",
- c700="rgba(2, 193, 96, 0.32)",
- c800="rgba(2, 193, 96, 0.32)",
- c900="#02C160",
- c950="#02C160",
- ),
- secondary_hue=gr.themes.Color(
- c50="#576b95",
- c100="#576b95",
- c200="#576b95",
- c300="#576b95",
- c400="#576b95",
- c500="#576b95",
- c600="#576b95",
- c700="#576b95",
- c800="#576b95",
- c900="#576b95",
- c950="#576b95",
- ),
- neutral_hue=gr.themes.Color(
- name="gray",
- c50="#f9fafb",
- c100="#f3f4f6",
- c200="#e5e7eb",
- c300="#d1d5db",
- c400="#B2B2B2",
- c500="#808080",
- c600="#636363",
- c700="#515151",
- c800="#393939",
- c900="#272727",
- c950="#171717",
- ),
- radius_size=gr.themes.sizes.radius_sm,
- ).set(
- button_primary_background_fill="#06AE56",
- button_primary_background_fill_dark="#06AE56",
- button_primary_background_fill_hover="#07C863",
- button_primary_border_color="#06AE56",
- button_primary_border_color_dark="#06AE56",
- button_primary_text_color="#FFFFFF",
- button_primary_text_color_dark="#FFFFFF",
- button_secondary_background_fill="#F2F2F2",
- button_secondary_background_fill_dark="#2B2B2B",
- button_secondary_text_color="#393939",
- button_secondary_text_color_dark="#FFFFFF",
- # background_fill_primary="#F7F7F7",
- # background_fill_primary_dark="#1F1F1F",
- block_title_text_color="*primary_500",
- block_title_background_fill="*primary_100",
- input_background_fill="#F6F6F6",
- )
diff --git a/spaces/jennysun/jwsun-multisubject-render-model/gligen/ldm/modules/diffusionmodules/positionnet.py b/spaces/jennysun/jwsun-multisubject-render-model/gligen/ldm/modules/diffusionmodules/positionnet.py
deleted file mode 100644
index 8cfa9bf3a43964b1e1669fec71d2d32356356e70..0000000000000000000000000000000000000000
--- a/spaces/jennysun/jwsun-multisubject-render-model/gligen/ldm/modules/diffusionmodules/positionnet.py
+++ /dev/null
@@ -1,50 +0,0 @@
-import torch
-import torch.nn as nn
-from ldm.modules.attention import BasicTransformerBlock
-from ldm.modules.diffusionmodules.util import checkpoint, FourierEmbedder
-import torch.nn.functional as F
-
-
-
-class PositionNet(nn.Module):
- def __init__(self, positive_len, out_dim, fourier_freqs=8):
- super().__init__()
- self.positive_len = positive_len
- self.out_dim = out_dim
-
- self.fourier_embedder = FourierEmbedder(num_freqs=fourier_freqs)
- self.position_dim = fourier_freqs*2*4 # 2 is sin&cos, 4 is xyxy
-
- self.linears = nn.Sequential(
- nn.Linear( self.positive_len + self.position_dim, 512),
- nn.SiLU(),
- nn.Linear( 512, 512),
- nn.SiLU(),
- nn.Linear(512, out_dim),
- )
-
- self.null_positive_feature = torch.nn.Parameter(torch.zeros([self.positive_len]))
- self.null_position_feature = torch.nn.Parameter(torch.zeros([self.position_dim]))
-
-
- def forward(self, boxes, masks, positive_embeddings):
- B, N, _ = boxes.shape
- masks = masks.unsqueeze(-1)
-
- # embedding position (it may includes padding as placeholder)
- xyxy_embedding = self.fourier_embedder(boxes) # B*N*4 --> B*N*C
-
- # learnable null embedding
- positive_null = self.null_positive_feature.view(1,1,-1)
- xyxy_null = self.null_position_feature.view(1,1,-1)
-
- # replace padding with learnable null embedding
- positive_embeddings = positive_embeddings*masks + (1-masks)*positive_null
- xyxy_embedding = xyxy_embedding*masks + (1-masks)*xyxy_null
-
- objs = self.linears( torch.cat([positive_embeddings, xyxy_embedding], dim=-1) )
- assert objs.shape == torch.Size([B,N,self.out_dim])
- return objs
-
-
-
diff --git a/spaces/jeonsworld/whisper-medium-ko/README.md b/spaces/jeonsworld/whisper-medium-ko/README.md
deleted file mode 100644
index 22bc36ebcecfb16cec2c86ce05d4efc16fb06c90..0000000000000000000000000000000000000000
--- a/spaces/jeonsworld/whisper-medium-ko/README.md
+++ /dev/null
@@ -1,10 +0,0 @@
----
-title: whisper-medium-ko
-emoji: 📉
-colorFrom: pink
-colorTo: yellow
-sdk: gradio
-sdk_version: 3.3.1
-app_file: app.py
-pinned: false
----
\ No newline at end of file
diff --git a/spaces/jhwen/bingo/tests/parse.ts b/spaces/jhwen/bingo/tests/parse.ts
deleted file mode 100644
index 92940fe6315f1d7cb2b267ba5e5a7e26460a1de3..0000000000000000000000000000000000000000
--- a/spaces/jhwen/bingo/tests/parse.ts
+++ /dev/null
@@ -1,13 +0,0 @@
-import { promises as fs } from 'fs'
-import { join } from 'path'
-import { parseHeadersFromCurl } from '@/lib/utils'
-
-(async () => {
- const content = await fs.readFile(join(__dirname, './fixtures/curl.txt'), 'utf-8')
- const headers = parseHeadersFromCurl(content)
- console.log(headers)
-
- const cmdContent = await fs.readFile(join(__dirname, './fixtures/cmd.txt'), 'utf-8')
- const cmdHeaders = parseHeadersFromCurl(cmdContent)
- console.log(cmdHeaders)
-})()
diff --git a/spaces/jie1/succ1/DLKcat/DeeplearningApproach/Code/model/run_model.py b/spaces/jie1/succ1/DLKcat/DeeplearningApproach/Code/model/run_model.py
deleted file mode 100644
index 9d3abbb2fa471b9406094e4d33b0a9ec3817395c..0000000000000000000000000000000000000000
--- a/spaces/jie1/succ1/DLKcat/DeeplearningApproach/Code/model/run_model.py
+++ /dev/null
@@ -1,254 +0,0 @@
-#!/usr/bin/python
-# coding: utf-8
-
-# Author: LE YUAN
-# Date: 2020-10-23
-
-import pickle
-import sys
-import timeit
-import math
-import numpy as np
-import torch
-import torch.nn as nn
-import torch.nn.functional as F
-import torch.optim as optim
-from sklearn.metrics import mean_squared_error,r2_score
-
-
-class KcatPrediction(nn.Module):
- def __init__(self):
- super(KcatPrediction, self).__init__()
- self.embed_fingerprint = nn.Embedding(n_fingerprint, dim)
- self.embed_word = nn.Embedding(n_word, dim)
- self.W_gnn = nn.ModuleList([nn.Linear(dim, dim)
- for _ in range(layer_gnn)])
- self.W_cnn = nn.ModuleList([nn.Conv2d(
- in_channels=1, out_channels=1, kernel_size=2*window+1,
- stride=1, padding=window) for _ in range(layer_cnn)])
- self.W_attention = nn.Linear(dim, dim)
- self.W_out = nn.ModuleList([nn.Linear(2*dim, 2*dim)
- for _ in range(layer_output)])
- # self.W_interaction = nn.Linear(2*dim, 2)
- self.W_interaction = nn.Linear(2*dim, 1)
-
- def gnn(self, xs, A, layer):
- for i in range(layer):
- hs = torch.relu(self.W_gnn[i](xs))
- xs = xs + torch.matmul(A, hs)
- # return torch.unsqueeze(torch.sum(xs, 0), 0)
- return torch.unsqueeze(torch.mean(xs, 0), 0)
-
- def attention_cnn(self, x, xs, layer):
- """The attention mechanism is applied to the last layer of CNN."""
-
- xs = torch.unsqueeze(torch.unsqueeze(xs, 0), 0)
- for i in range(layer):
- xs = torch.relu(self.W_cnn[i](xs))
- xs = torch.squeeze(torch.squeeze(xs, 0), 0)
-
- h = torch.relu(self.W_attention(x))
- hs = torch.relu(self.W_attention(xs))
- weights = torch.tanh(F.linear(h, hs))
- ys = torch.t(weights) * hs
-
- # return torch.unsqueeze(torch.sum(ys, 0), 0)
- return torch.unsqueeze(torch.mean(ys, 0), 0)
-
- def forward(self, inputs):
-
- fingerprints, adjacency, words = inputs
-
- """Compound vector with GNN."""
- fingerprint_vectors = self.embed_fingerprint(fingerprints)
- compound_vector = self.gnn(fingerprint_vectors, adjacency, layer_gnn)
-
- """Protein vector with attention-CNN."""
- word_vectors = self.embed_word(words)
- protein_vector = self.attention_cnn(compound_vector,
- word_vectors, layer_cnn)
-
- """Concatenate the above two vectors and output the interaction."""
- cat_vector = torch.cat((compound_vector, protein_vector), 1)
- for j in range(layer_output):
- cat_vector = torch.relu(self.W_out[j](cat_vector))
- interaction = self.W_interaction(cat_vector)
- # print(interaction)
-
- return interaction
-
- def __call__(self, data, train=True):
-
- inputs, correct_interaction = data[:-1], data[-1]
- predicted_interaction = self.forward(inputs)
- # print(predicted_interaction)
-
- if train:
- loss = F.mse_loss(predicted_interaction, correct_interaction)
- correct_values = correct_interaction.to('cpu').data.numpy()
- predicted_values = predicted_interaction.to('cpu').data.numpy()[0]
- return loss, correct_values, predicted_values
- else:
- correct_values = correct_interaction.to('cpu').data.numpy()
- predicted_values = predicted_interaction.to('cpu').data.numpy()[0]
- # correct_values = np.concatenate(correct_values)
- # predicted_values = np.concatenate(predicted_values)
- # ys = F.softmax(predicted_interaction, 1).to('cpu').data.numpy()
- # predicted_values = list(map(lambda x: np.argmax(x), ys))
- # print(correct_values)
- # print(predicted_values)
- # predicted_scores = list(map(lambda x: x[1], ys))
- return correct_values, predicted_values
-
-
-class Trainer(object):
- def __init__(self, model):
- self.model = model
- self.optimizer = optim.Adam(self.model.parameters(),
- lr=lr, weight_decay=weight_decay)
-
- def train(self, dataset):
- np.random.shuffle(dataset)
- N = len(dataset)
- loss_total = 0
- trainCorrect, trainPredict = [], []
- for data in dataset:
- loss, correct_values, predicted_values = self.model(data)
- self.optimizer.zero_grad()
- loss.backward()
- self.optimizer.step()
- loss_total += loss.to('cpu').data.numpy()
-
- correct_values = math.log10(math.pow(2,correct_values))
- predicted_values = math.log10(math.pow(2,predicted_values))
- trainCorrect.append(correct_values)
- trainPredict.append(predicted_values)
- rmse_train = np.sqrt(mean_squared_error(trainCorrect,trainPredict))
- r2_train = r2_score(trainCorrect,trainPredict)
- return loss_total, rmse_train, r2_train
-
-
-class Tester(object):
- def __init__(self, model):
- self.model = model
-
- def test(self, dataset):
- N = len(dataset)
- SAE = 0 # sum absolute error.
- testY, testPredict = [], []
- for data in dataset :
- (correct_values, predicted_values) = self.model(data, train=False)
- correct_values = math.log10(math.pow(2,correct_values))
- predicted_values = math.log10(math.pow(2,predicted_values))
- SAE += np.abs(predicted_values-correct_values)
- # SAE += sum(np.abs(predicted_values-correct_values))
- testY.append(correct_values)
- testPredict.append(predicted_values)
- MAE = SAE / N # mean absolute error.
- rmse = np.sqrt(mean_squared_error(testY,testPredict))
- r2 = r2_score(testY,testPredict)
- return MAE, rmse, r2
-
- def save_MAEs(self, MAEs, filename):
- with open(filename, 'a') as f:
- f.write('\t'.join(map(str, MAEs)) + '\n')
-
- def save_model(self, model, filename):
- torch.save(model.state_dict(), filename)
-
-def load_tensor(file_name, dtype):
- return [dtype(d).to(device) for d in np.load(file_name + '.npy', allow_pickle=True)]
-
-
-def load_pickle(file_name):
- with open(file_name, 'rb') as f:
- return pickle.load(f)
-
-def shuffle_dataset(dataset, seed):
- np.random.seed(seed)
- np.random.shuffle(dataset)
- return dataset
-
-def split_dataset(dataset, ratio):
- n = int(ratio * len(dataset))
- dataset_1, dataset_2 = dataset[:n], dataset[n:]
- return dataset_1, dataset_2
-
-
-if __name__ == "__main__":
-
- """Hyperparameters."""
- (DATASET, radius, ngram, dim, layer_gnn, window, layer_cnn, layer_output,
- lr, lr_decay, decay_interval, weight_decay, iteration,
- setting) = sys.argv[1:]
- (dim, layer_gnn, window, layer_cnn, layer_output, decay_interval,
- iteration) = map(int, [dim, layer_gnn, window, layer_cnn, layer_output,
- decay_interval, iteration])
- lr, lr_decay, weight_decay = map(float, [lr, lr_decay, weight_decay])
-
- # print(type(radius))
-
- """CPU or GPU."""
- if torch.cuda.is_available():
- device = torch.device('cuda')
- print('The code uses GPU...')
- else:
- device = torch.device('cpu')
- print('The code uses CPU!!!')
-
- """Load preprocessed data."""
- dir_input = ('../../Data/input/')
- compounds = load_tensor(dir_input + 'compounds', torch.LongTensor)
- adjacencies = load_tensor(dir_input + 'adjacencies', torch.FloatTensor)
- proteins = load_tensor(dir_input + 'proteins', torch.LongTensor)
- interactions = load_tensor(dir_input + 'regression', torch.FloatTensor)
- fingerprint_dict = load_pickle(dir_input + 'fingerprint_dict.pickle')
- word_dict = load_pickle(dir_input + 'sequence_dict.pickle')
- n_fingerprint = len(fingerprint_dict)
- n_word = len(word_dict)
- # print(n_fingerprint) # 3958
- # print(n_word) # 8542
- # 394 and 474 when radius=1 and ngram=2
-
- """Create a dataset and split it into train/dev/test."""
- dataset = list(zip(compounds, adjacencies, proteins, interactions))
- dataset = shuffle_dataset(dataset, 1234)
- dataset_train, dataset_ = split_dataset(dataset, 0.8)
- dataset_dev, dataset_test = split_dataset(dataset_, 0.5)
-
- """Set a model."""
- torch.manual_seed(1234)
- model = KcatPrediction().to(device)
- trainer = Trainer(model)
- tester = Tester(model)
-
- """Output files."""
- file_MAEs = '../../Data/Results/output/MAEs--' + setting + '.txt'
- file_model = '../../Data/Results/output/' + setting
- MAEs = ('Epoch\tTime(sec)\tRMSE_train\tR2_train\tMAE_dev\tMAE_test\tRMSE_dev\tRMSE_test\tR2_dev\tR2_test')
- with open(file_MAEs, 'w') as f:
- f.write(MAEs + '\n')
-
- """Start training."""
- print('Training...')
- print(MAEs)
- start = timeit.default_timer()
-
- for epoch in range(1, iteration+1):
-
- if epoch % decay_interval == 0:
- trainer.optimizer.param_groups[0]['lr'] *= lr_decay
-
- loss_train, rmse_train, r2_train = trainer.train(dataset_train)
- MAE_dev, RMSE_dev, R2_dev = tester.test(dataset_dev)
- MAE_test, RMSE_test, R2_test = tester.test(dataset_test)
-
- end = timeit.default_timer()
- time = end - start
-
- MAEs = [epoch, time, rmse_train, r2_train, MAE_dev,
- MAE_test, RMSE_dev, RMSE_test, R2_dev, R2_test]
- tester.save_MAEs(MAEs, file_MAEs)
- tester.save_model(model, file_model)
-
- print('\t'.join(map(str, MAEs)))
diff --git a/spaces/jinlinyi/PerspectiveFields/README.md b/spaces/jinlinyi/PerspectiveFields/README.md
deleted file mode 100644
index 321a23de483401ffaec2babdd075daf2ba51afb5..0000000000000000000000000000000000000000
--- a/spaces/jinlinyi/PerspectiveFields/README.md
+++ /dev/null
@@ -1,13 +0,0 @@
----
-title: PerspectiveFields
-emoji: 🏃
-colorFrom: gray
-colorTo: indigo
-sdk: gradio
-sdk_version: 3.29.0
-app_file: app.py
-pinned: false
-license: mit
----
-
-Check out the configuration reference at https://huggingface.co/docs/hub/spaces-config-reference
diff --git a/spaces/joaopdrm/Emotion_Analisys/app.py b/spaces/joaopdrm/Emotion_Analisys/app.py
deleted file mode 100644
index 4e2c90cad231ca07c85f85cabdec7bfe2c98978b..0000000000000000000000000000000000000000
--- a/spaces/joaopdrm/Emotion_Analisys/app.py
+++ /dev/null
@@ -1,46 +0,0 @@
-import gradio as gr
-from transformers import AutoModelForSequenceClassification, AutoTokenizer, pipeline
-
-
-class Emotionclass:
- def __init__(self, model: str):
- self.model = AutoModelForSequenceClassification.from_pretrained(model)
- self.tokenizer = AutoTokenizer.from_pretrained(model)
- self.pipeline = pipeline(
- "text-classification",
- model=self.model,
- tokenizer=self.tokenizer,
- return_all_scores=True,
- )
-
- def predict(self, input: str):
- output = self.pipeline(input)[0]
- result = {
- "sad": output[0]["score"],
- "joy": output[1]["score"],
- "love": output[2]["score"],
- "anger": output[3]["score"],
- "fear": output[4]["score"],
- "surprise": output[5]["score"],
- }
- return result
-
-
-def main():
- model = Emotionclass("bhadresh-savani/bert-base-uncased-emotion")
- iface = gr.Interface(
- fn=model.predict,
- inputs=gr.inputs.Textbox(
- lines=3,
- placeholder="type here",
- label="Input",
- ),
- outputs="label",
- title="Sentiment Classification",
- )
-
- iface.launch()
-
-
-if __name__ == "__main__":
- main()
\ No newline at end of file
diff --git a/spaces/joaopereirajp/livvieChatBot/venv/lib/python3.9/site-packages/aiohttp/pytest_plugin.py b/spaces/joaopereirajp/livvieChatBot/venv/lib/python3.9/site-packages/aiohttp/pytest_plugin.py
deleted file mode 100644
index dd9a9f617901ef2c2fa7c1b4ceb5dd92ecbfd5de..0000000000000000000000000000000000000000
--- a/spaces/joaopereirajp/livvieChatBot/venv/lib/python3.9/site-packages/aiohttp/pytest_plugin.py
+++ /dev/null
@@ -1,391 +0,0 @@
-import asyncio
-import contextlib
-import warnings
-from collections.abc import Callable
-from typing import Any, Awaitable, Callable, Dict, Generator, Optional, Union
-
-import pytest
-
-from aiohttp.helpers import PY_37, isasyncgenfunction
-from aiohttp.web import Application
-
-from .test_utils import (
- BaseTestServer,
- RawTestServer,
- TestClient,
- TestServer,
- loop_context,
- setup_test_loop,
- teardown_test_loop,
- unused_port as _unused_port,
-)
-
-try:
- import uvloop
-except ImportError: # pragma: no cover
- uvloop = None
-
-try:
- import tokio
-except ImportError: # pragma: no cover
- tokio = None
-
-AiohttpClient = Callable[[Union[Application, BaseTestServer]], Awaitable[TestClient]]
-
-
-def pytest_addoption(parser): # type: ignore[no-untyped-def]
- parser.addoption(
- "--aiohttp-fast",
- action="store_true",
- default=False,
- help="run tests faster by disabling extra checks",
- )
- parser.addoption(
- "--aiohttp-loop",
- action="store",
- default="pyloop",
- help="run tests with specific loop: pyloop, uvloop, tokio or all",
- )
- parser.addoption(
- "--aiohttp-enable-loop-debug",
- action="store_true",
- default=False,
- help="enable event loop debug mode",
- )
-
-
-def pytest_fixture_setup(fixturedef): # type: ignore[no-untyped-def]
- """Set up pytest fixture.
-
- Allow fixtures to be coroutines. Run coroutine fixtures in an event loop.
- """
- func = fixturedef.func
-
- if isasyncgenfunction(func):
- # async generator fixture
- is_async_gen = True
- elif asyncio.iscoroutinefunction(func):
- # regular async fixture
- is_async_gen = False
- else:
- # not an async fixture, nothing to do
- return
-
- strip_request = False
- if "request" not in fixturedef.argnames:
- fixturedef.argnames += ("request",)
- strip_request = True
-
- def wrapper(*args, **kwargs): # type: ignore[no-untyped-def]
- request = kwargs["request"]
- if strip_request:
- del kwargs["request"]
-
- # if neither the fixture nor the test use the 'loop' fixture,
- # 'getfixturevalue' will fail because the test is not parameterized
- # (this can be removed someday if 'loop' is no longer parameterized)
- if "loop" not in request.fixturenames:
- raise Exception(
- "Asynchronous fixtures must depend on the 'loop' fixture or "
- "be used in tests depending from it."
- )
-
- _loop = request.getfixturevalue("loop")
-
- if is_async_gen:
- # for async generators, we need to advance the generator once,
- # then advance it again in a finalizer
- gen = func(*args, **kwargs)
-
- def finalizer(): # type: ignore[no-untyped-def]
- try:
- return _loop.run_until_complete(gen.__anext__())
- except StopAsyncIteration:
- pass
-
- request.addfinalizer(finalizer)
- return _loop.run_until_complete(gen.__anext__())
- else:
- return _loop.run_until_complete(func(*args, **kwargs))
-
- fixturedef.func = wrapper
-
-
-@pytest.fixture
-def fast(request): # type: ignore[no-untyped-def]
- """--fast config option"""
- return request.config.getoption("--aiohttp-fast")
-
-
-@pytest.fixture
-def loop_debug(request): # type: ignore[no-untyped-def]
- """--enable-loop-debug config option"""
- return request.config.getoption("--aiohttp-enable-loop-debug")
-
-
-@contextlib.contextmanager
-def _runtime_warning_context(): # type: ignore[no-untyped-def]
- """Context manager which checks for RuntimeWarnings.
-
- This exists specifically to
- avoid "coroutine 'X' was never awaited" warnings being missed.
-
- If RuntimeWarnings occur in the context a RuntimeError is raised.
- """
- with warnings.catch_warnings(record=True) as _warnings:
- yield
- rw = [
- "{w.filename}:{w.lineno}:{w.message}".format(w=w)
- for w in _warnings
- if w.category == RuntimeWarning
- ]
- if rw:
- raise RuntimeError(
- "{} Runtime Warning{},\n{}".format(
- len(rw), "" if len(rw) == 1 else "s", "\n".join(rw)
- )
- )
-
-
-@contextlib.contextmanager
-def _passthrough_loop_context(loop, fast=False): # type: ignore[no-untyped-def]
- """Passthrough loop context.
-
- Sets up and tears down a loop unless one is passed in via the loop
- argument when it's passed straight through.
- """
- if loop:
- # loop already exists, pass it straight through
- yield loop
- else:
- # this shadows loop_context's standard behavior
- loop = setup_test_loop()
- yield loop
- teardown_test_loop(loop, fast=fast)
-
-
-def pytest_pycollect_makeitem(collector, name, obj): # type: ignore[no-untyped-def]
- """Fix pytest collecting for coroutines."""
- if collector.funcnamefilter(name) and asyncio.iscoroutinefunction(obj):
- return list(collector._genfunctions(name, obj))
-
-
-def pytest_pyfunc_call(pyfuncitem): # type: ignore[no-untyped-def]
- """Run coroutines in an event loop instead of a normal function call."""
- fast = pyfuncitem.config.getoption("--aiohttp-fast")
- if asyncio.iscoroutinefunction(pyfuncitem.function):
- existing_loop = pyfuncitem.funcargs.get(
- "proactor_loop"
- ) or pyfuncitem.funcargs.get("loop", None)
- with _runtime_warning_context():
- with _passthrough_loop_context(existing_loop, fast=fast) as _loop:
- testargs = {
- arg: pyfuncitem.funcargs[arg]
- for arg in pyfuncitem._fixtureinfo.argnames
- }
- _loop.run_until_complete(pyfuncitem.obj(**testargs))
-
- return True
-
-
-def pytest_generate_tests(metafunc): # type: ignore[no-untyped-def]
- if "loop_factory" not in metafunc.fixturenames:
- return
-
- loops = metafunc.config.option.aiohttp_loop
- avail_factories = {"pyloop": asyncio.DefaultEventLoopPolicy}
-
- if uvloop is not None: # pragma: no cover
- avail_factories["uvloop"] = uvloop.EventLoopPolicy
-
- if tokio is not None: # pragma: no cover
- avail_factories["tokio"] = tokio.EventLoopPolicy
-
- if loops == "all":
- loops = "pyloop,uvloop?,tokio?"
-
- factories = {} # type: ignore[var-annotated]
- for name in loops.split(","):
- required = not name.endswith("?")
- name = name.strip(" ?")
- if name not in avail_factories: # pragma: no cover
- if required:
- raise ValueError(
- "Unknown loop '%s', available loops: %s"
- % (name, list(factories.keys()))
- )
- else:
- continue
- factories[name] = avail_factories[name]
- metafunc.parametrize(
- "loop_factory", list(factories.values()), ids=list(factories.keys())
- )
-
-
-@pytest.fixture
-def loop(loop_factory, fast, loop_debug): # type: ignore[no-untyped-def]
- """Return an instance of the event loop."""
- policy = loop_factory()
- asyncio.set_event_loop_policy(policy)
- with loop_context(fast=fast) as _loop:
- if loop_debug:
- _loop.set_debug(True) # pragma: no cover
- asyncio.set_event_loop(_loop)
- yield _loop
-
-
-@pytest.fixture
-def proactor_loop(): # type: ignore[no-untyped-def]
- if not PY_37:
- policy = asyncio.get_event_loop_policy()
- policy._loop_factory = asyncio.ProactorEventLoop # type: ignore[attr-defined]
- else:
- policy = asyncio.WindowsProactorEventLoopPolicy() # type: ignore[attr-defined]
- asyncio.set_event_loop_policy(policy)
-
- with loop_context(policy.new_event_loop) as _loop:
- asyncio.set_event_loop(_loop)
- yield _loop
-
-
-@pytest.fixture
-def unused_port(aiohttp_unused_port): # type: ignore[no-untyped-def] # pragma: no cover
- warnings.warn(
- "Deprecated, use aiohttp_unused_port fixture instead",
- DeprecationWarning,
- stacklevel=2,
- )
- return aiohttp_unused_port
-
-
-@pytest.fixture
-def aiohttp_unused_port(): # type: ignore[no-untyped-def]
- """Return a port that is unused on the current host."""
- return _unused_port
-
-
-@pytest.fixture
-def aiohttp_server(loop): # type: ignore[no-untyped-def]
- """Factory to create a TestServer instance, given an app.
-
- aiohttp_server(app, **kwargs)
- """
- servers = []
-
- async def go(app, *, port=None, **kwargs): # type: ignore[no-untyped-def]
- server = TestServer(app, port=port)
- await server.start_server(loop=loop, **kwargs)
- servers.append(server)
- return server
-
- yield go
-
- async def finalize() -> None:
- while servers:
- await servers.pop().close()
-
- loop.run_until_complete(finalize())
-
-
-@pytest.fixture
-def test_server(aiohttp_server): # type: ignore[no-untyped-def] # pragma: no cover
- warnings.warn(
- "Deprecated, use aiohttp_server fixture instead",
- DeprecationWarning,
- stacklevel=2,
- )
- return aiohttp_server
-
-
-@pytest.fixture
-def aiohttp_raw_server(loop): # type: ignore[no-untyped-def]
- """Factory to create a RawTestServer instance, given a web handler.
-
- aiohttp_raw_server(handler, **kwargs)
- """
- servers = []
-
- async def go(handler, *, port=None, **kwargs): # type: ignore[no-untyped-def]
- server = RawTestServer(handler, port=port)
- await server.start_server(loop=loop, **kwargs)
- servers.append(server)
- return server
-
- yield go
-
- async def finalize() -> None:
- while servers:
- await servers.pop().close()
-
- loop.run_until_complete(finalize())
-
-
-@pytest.fixture
-def raw_test_server( # type: ignore[no-untyped-def] # pragma: no cover
- aiohttp_raw_server,
-):
- warnings.warn(
- "Deprecated, use aiohttp_raw_server fixture instead",
- DeprecationWarning,
- stacklevel=2,
- )
- return aiohttp_raw_server
-
-
-@pytest.fixture
-def aiohttp_client(
- loop: asyncio.AbstractEventLoop,
-) -> Generator[AiohttpClient, None, None]:
- """Factory to create a TestClient instance.
-
- aiohttp_client(app, **kwargs)
- aiohttp_client(server, **kwargs)
- aiohttp_client(raw_server, **kwargs)
- """
- clients = []
-
- async def go(
- __param: Union[Application, BaseTestServer],
- *args: Any,
- server_kwargs: Optional[Dict[str, Any]] = None,
- **kwargs: Any
- ) -> TestClient:
-
- if isinstance(__param, Callable) and not isinstance( # type: ignore[arg-type]
- __param, (Application, BaseTestServer)
- ):
- __param = __param(loop, *args, **kwargs)
- kwargs = {}
- else:
- assert not args, "args should be empty"
-
- if isinstance(__param, Application):
- server_kwargs = server_kwargs or {}
- server = TestServer(__param, loop=loop, **server_kwargs)
- client = TestClient(server, loop=loop, **kwargs)
- elif isinstance(__param, BaseTestServer):
- client = TestClient(__param, loop=loop, **kwargs)
- else:
- raise ValueError("Unknown argument type: %r" % type(__param))
-
- await client.start_server()
- clients.append(client)
- return client
-
- yield go
-
- async def finalize() -> None:
- while clients:
- await clients.pop().close()
-
- loop.run_until_complete(finalize())
-
-
-@pytest.fixture
-def test_client(aiohttp_client): # type: ignore[no-untyped-def] # pragma: no cover
- warnings.warn(
- "Deprecated, use aiohttp_client fixture instead",
- DeprecationWarning,
- stacklevel=2,
- )
- return aiohttp_client
diff --git a/spaces/joaopereirajp/livvieChatBot/venv/lib/python3.9/site-packages/altair/jupyter/__init__.py b/spaces/joaopereirajp/livvieChatBot/venv/lib/python3.9/site-packages/altair/jupyter/__init__.py
deleted file mode 100644
index 651ab11e4cf8de15370bbf02efd36315c1d27e82..0000000000000000000000000000000000000000
--- a/spaces/joaopereirajp/livvieChatBot/venv/lib/python3.9/site-packages/altair/jupyter/__init__.py
+++ /dev/null
@@ -1,20 +0,0 @@
-try:
- import anywidget # noqa: F401
-except ImportError:
- # When anywidget isn't available, create stand-in JupyterChart class
- # that raises an informative import error on construction. This
- # way we can make JupyterChart available in the altair namespace
- # when anywidget is not installed
- class JupyterChart:
- def __init__(self, *args, **kwargs):
- raise ImportError(
- "The Altair JupyterChart requires the anywidget \n"
- "Python package which may be installed using pip with\n"
- " pip install anywidget\n"
- "or using conda with\n"
- " conda install -c conda-forge anywidget\n"
- "Afterwards, you will need to restart your Python kernel."
- )
-
-else:
- from .jupyter_chart import JupyterChart # noqa: F401
diff --git a/spaces/joaopereirajp/livvieChatBot/venv/lib/python3.9/site-packages/fontTools/misc/bezierTools.py b/spaces/joaopereirajp/livvieChatBot/venv/lib/python3.9/site-packages/fontTools/misc/bezierTools.py
deleted file mode 100644
index 7772a4bf8588d2723f2435c7a2ba56ce47a71cf1..0000000000000000000000000000000000000000
--- a/spaces/joaopereirajp/livvieChatBot/venv/lib/python3.9/site-packages/fontTools/misc/bezierTools.py
+++ /dev/null
@@ -1,1474 +0,0 @@
-# -*- coding: utf-8 -*-
-"""fontTools.misc.bezierTools.py -- tools for working with Bezier path segments.
-"""
-
-from fontTools.misc.arrayTools import calcBounds, sectRect, rectArea
-from fontTools.misc.transform import Identity
-import math
-from collections import namedtuple
-
-try:
- import cython
-
- COMPILED = cython.compiled
-except (AttributeError, ImportError):
- # if cython not installed, use mock module with no-op decorators and types
- from fontTools.misc import cython
-
- COMPILED = False
-
-
-Intersection = namedtuple("Intersection", ["pt", "t1", "t2"])
-
-
-__all__ = [
- "approximateCubicArcLength",
- "approximateCubicArcLengthC",
- "approximateQuadraticArcLength",
- "approximateQuadraticArcLengthC",
- "calcCubicArcLength",
- "calcCubicArcLengthC",
- "calcQuadraticArcLength",
- "calcQuadraticArcLengthC",
- "calcCubicBounds",
- "calcQuadraticBounds",
- "splitLine",
- "splitQuadratic",
- "splitCubic",
- "splitQuadraticAtT",
- "splitCubicAtT",
- "splitCubicAtTC",
- "splitCubicIntoTwoAtTC",
- "solveQuadratic",
- "solveCubic",
- "quadraticPointAtT",
- "cubicPointAtT",
- "cubicPointAtTC",
- "linePointAtT",
- "segmentPointAtT",
- "lineLineIntersections",
- "curveLineIntersections",
- "curveCurveIntersections",
- "segmentSegmentIntersections",
-]
-
-
-def calcCubicArcLength(pt1, pt2, pt3, pt4, tolerance=0.005):
- """Calculates the arc length for a cubic Bezier segment.
-
- Whereas :func:`approximateCubicArcLength` approximates the length, this
- function calculates it by "measuring", recursively dividing the curve
- until the divided segments are shorter than ``tolerance``.
-
- Args:
- pt1,pt2,pt3,pt4: Control points of the Bezier as 2D tuples.
- tolerance: Controls the precision of the calcuation.
-
- Returns:
- Arc length value.
- """
- return calcCubicArcLengthC(
- complex(*pt1), complex(*pt2), complex(*pt3), complex(*pt4), tolerance
- )
-
-
-def _split_cubic_into_two(p0, p1, p2, p3):
- mid = (p0 + 3 * (p1 + p2) + p3) * 0.125
- deriv3 = (p3 + p2 - p1 - p0) * 0.125
- return (
- (p0, (p0 + p1) * 0.5, mid - deriv3, mid),
- (mid, mid + deriv3, (p2 + p3) * 0.5, p3),
- )
-
-
-@cython.returns(cython.double)
-@cython.locals(
- p0=cython.complex,
- p1=cython.complex,
- p2=cython.complex,
- p3=cython.complex,
-)
-@cython.locals(mult=cython.double, arch=cython.double, box=cython.double)
-def _calcCubicArcLengthCRecurse(mult, p0, p1, p2, p3):
- arch = abs(p0 - p3)
- box = abs(p0 - p1) + abs(p1 - p2) + abs(p2 - p3)
- if arch * mult >= box:
- return (arch + box) * 0.5
- else:
- one, two = _split_cubic_into_two(p0, p1, p2, p3)
- return _calcCubicArcLengthCRecurse(mult, *one) + _calcCubicArcLengthCRecurse(
- mult, *two
- )
-
-
-@cython.returns(cython.double)
-@cython.locals(
- pt1=cython.complex,
- pt2=cython.complex,
- pt3=cython.complex,
- pt4=cython.complex,
-)
-@cython.locals(
- tolerance=cython.double,
- mult=cython.double,
-)
-def calcCubicArcLengthC(pt1, pt2, pt3, pt4, tolerance=0.005):
- """Calculates the arc length for a cubic Bezier segment.
-
- Args:
- pt1,pt2,pt3,pt4: Control points of the Bezier as complex numbers.
- tolerance: Controls the precision of the calcuation.
-
- Returns:
- Arc length value.
- """
- mult = 1.0 + 1.5 * tolerance # The 1.5 is a empirical hack; no math
- return _calcCubicArcLengthCRecurse(mult, pt1, pt2, pt3, pt4)
-
-
-epsilonDigits = 6
-epsilon = 1e-10
-
-
-@cython.cfunc
-@cython.inline
-@cython.returns(cython.double)
-@cython.locals(v1=cython.complex, v2=cython.complex)
-def _dot(v1, v2):
- return (v1 * v2.conjugate()).real
-
-
-@cython.cfunc
-@cython.inline
-@cython.returns(cython.double)
-@cython.locals(x=cython.complex)
-def _intSecAtan(x):
- # In : sympy.integrate(sp.sec(sp.atan(x)))
- # Out: x*sqrt(x**2 + 1)/2 + asinh(x)/2
- return x * math.sqrt(x**2 + 1) / 2 + math.asinh(x) / 2
-
-
-def calcQuadraticArcLength(pt1, pt2, pt3):
- """Calculates the arc length for a quadratic Bezier segment.
-
- Args:
- pt1: Start point of the Bezier as 2D tuple.
- pt2: Handle point of the Bezier as 2D tuple.
- pt3: End point of the Bezier as 2D tuple.
-
- Returns:
- Arc length value.
-
- Example::
-
- >>> calcQuadraticArcLength((0, 0), (0, 0), (0, 0)) # empty segment
- 0.0
- >>> calcQuadraticArcLength((0, 0), (50, 0), (80, 0)) # collinear points
- 80.0
- >>> calcQuadraticArcLength((0, 0), (0, 50), (0, 80)) # collinear points vertical
- 80.0
- >>> calcQuadraticArcLength((0, 0), (50, 20), (100, 40)) # collinear points
- 107.70329614269008
- >>> calcQuadraticArcLength((0, 0), (0, 100), (100, 0))
- 154.02976155645263
- >>> calcQuadraticArcLength((0, 0), (0, 50), (100, 0))
- 120.21581243984076
- >>> calcQuadraticArcLength((0, 0), (50, -10), (80, 50))
- 102.53273816445825
- >>> calcQuadraticArcLength((0, 0), (40, 0), (-40, 0)) # collinear points, control point outside
- 66.66666666666667
- >>> calcQuadraticArcLength((0, 0), (40, 0), (0, 0)) # collinear points, looping back
- 40.0
- """
- return calcQuadraticArcLengthC(complex(*pt1), complex(*pt2), complex(*pt3))
-
-
-@cython.returns(cython.double)
-@cython.locals(
- pt1=cython.complex,
- pt2=cython.complex,
- pt3=cython.complex,
- d0=cython.complex,
- d1=cython.complex,
- d=cython.complex,
- n=cython.complex,
-)
-@cython.locals(
- scale=cython.double,
- origDist=cython.double,
- a=cython.double,
- b=cython.double,
- x0=cython.double,
- x1=cython.double,
- Len=cython.double,
-)
-def calcQuadraticArcLengthC(pt1, pt2, pt3):
- """Calculates the arc length for a quadratic Bezier segment.
-
- Args:
- pt1: Start point of the Bezier as a complex number.
- pt2: Handle point of the Bezier as a complex number.
- pt3: End point of the Bezier as a complex number.
-
- Returns:
- Arc length value.
- """
- # Analytical solution to the length of a quadratic bezier.
- # Documentation: https://github.com/fonttools/fonttools/issues/3055
- d0 = pt2 - pt1
- d1 = pt3 - pt2
- d = d1 - d0
- n = d * 1j
- scale = abs(n)
- if scale == 0.0:
- return abs(pt3 - pt1)
- origDist = _dot(n, d0)
- if abs(origDist) < epsilon:
- if _dot(d0, d1) >= 0:
- return abs(pt3 - pt1)
- a, b = abs(d0), abs(d1)
- return (a * a + b * b) / (a + b)
- x0 = _dot(d, d0) / origDist
- x1 = _dot(d, d1) / origDist
- Len = abs(2 * (_intSecAtan(x1) - _intSecAtan(x0)) * origDist / (scale * (x1 - x0)))
- return Len
-
-
-def approximateQuadraticArcLength(pt1, pt2, pt3):
- """Calculates the arc length for a quadratic Bezier segment.
-
- Uses Gauss-Legendre quadrature for a branch-free approximation.
- See :func:`calcQuadraticArcLength` for a slower but more accurate result.
-
- Args:
- pt1: Start point of the Bezier as 2D tuple.
- pt2: Handle point of the Bezier as 2D tuple.
- pt3: End point of the Bezier as 2D tuple.
-
- Returns:
- Approximate arc length value.
- """
- return approximateQuadraticArcLengthC(complex(*pt1), complex(*pt2), complex(*pt3))
-
-
-@cython.returns(cython.double)
-@cython.locals(
- pt1=cython.complex,
- pt2=cython.complex,
- pt3=cython.complex,
-)
-@cython.locals(
- v0=cython.double,
- v1=cython.double,
- v2=cython.double,
-)
-def approximateQuadraticArcLengthC(pt1, pt2, pt3):
- """Calculates the arc length for a quadratic Bezier segment.
-
- Uses Gauss-Legendre quadrature for a branch-free approximation.
- See :func:`calcQuadraticArcLength` for a slower but more accurate result.
-
- Args:
- pt1: Start point of the Bezier as a complex number.
- pt2: Handle point of the Bezier as a complex number.
- pt3: End point of the Bezier as a complex number.
-
- Returns:
- Approximate arc length value.
- """
- # This, essentially, approximates the length-of-derivative function
- # to be integrated with the best-matching fifth-degree polynomial
- # approximation of it.
- #
- # https://en.wikipedia.org/wiki/Gaussian_quadrature#Gauss.E2.80.93Legendre_quadrature
-
- # abs(BezierCurveC[2].diff(t).subs({t:T})) for T in sorted(.5, .5±sqrt(3/5)/2),
- # weighted 5/18, 8/18, 5/18 respectively.
- v0 = abs(
- -0.492943519233745 * pt1 + 0.430331482911935 * pt2 + 0.0626120363218102 * pt3
- )
- v1 = abs(pt3 - pt1) * 0.4444444444444444
- v2 = abs(
- -0.0626120363218102 * pt1 - 0.430331482911935 * pt2 + 0.492943519233745 * pt3
- )
-
- return v0 + v1 + v2
-
-
-def calcQuadraticBounds(pt1, pt2, pt3):
- """Calculates the bounding rectangle for a quadratic Bezier segment.
-
- Args:
- pt1: Start point of the Bezier as a 2D tuple.
- pt2: Handle point of the Bezier as a 2D tuple.
- pt3: End point of the Bezier as a 2D tuple.
-
- Returns:
- A four-item tuple representing the bounding rectangle ``(xMin, yMin, xMax, yMax)``.
-
- Example::
-
- >>> calcQuadraticBounds((0, 0), (50, 100), (100, 0))
- (0, 0, 100, 50.0)
- >>> calcQuadraticBounds((0, 0), (100, 0), (100, 100))
- (0.0, 0.0, 100, 100)
- """
- (ax, ay), (bx, by), (cx, cy) = calcQuadraticParameters(pt1, pt2, pt3)
- ax2 = ax * 2.0
- ay2 = ay * 2.0
- roots = []
- if ax2 != 0:
- roots.append(-bx / ax2)
- if ay2 != 0:
- roots.append(-by / ay2)
- points = [
- (ax * t * t + bx * t + cx, ay * t * t + by * t + cy)
- for t in roots
- if 0 <= t < 1
- ] + [pt1, pt3]
- return calcBounds(points)
-
-
-def approximateCubicArcLength(pt1, pt2, pt3, pt4):
- """Approximates the arc length for a cubic Bezier segment.
-
- Uses Gauss-Lobatto quadrature with n=5 points to approximate arc length.
- See :func:`calcCubicArcLength` for a slower but more accurate result.
-
- Args:
- pt1,pt2,pt3,pt4: Control points of the Bezier as 2D tuples.
-
- Returns:
- Arc length value.
-
- Example::
-
- >>> approximateCubicArcLength((0, 0), (25, 100), (75, 100), (100, 0))
- 190.04332968932817
- >>> approximateCubicArcLength((0, 0), (50, 0), (100, 50), (100, 100))
- 154.8852074945903
- >>> approximateCubicArcLength((0, 0), (50, 0), (100, 0), (150, 0)) # line; exact result should be 150.
- 149.99999999999991
- >>> approximateCubicArcLength((0, 0), (50, 0), (100, 0), (-50, 0)) # cusp; exact result should be 150.
- 136.9267662156362
- >>> approximateCubicArcLength((0, 0), (50, 0), (100, -50), (-50, 0)) # cusp
- 154.80848416537057
- """
- return approximateCubicArcLengthC(
- complex(*pt1), complex(*pt2), complex(*pt3), complex(*pt4)
- )
-
-
-@cython.returns(cython.double)
-@cython.locals(
- pt1=cython.complex,
- pt2=cython.complex,
- pt3=cython.complex,
- pt4=cython.complex,
-)
-@cython.locals(
- v0=cython.double,
- v1=cython.double,
- v2=cython.double,
- v3=cython.double,
- v4=cython.double,
-)
-def approximateCubicArcLengthC(pt1, pt2, pt3, pt4):
- """Approximates the arc length for a cubic Bezier segment.
-
- Args:
- pt1,pt2,pt3,pt4: Control points of the Bezier as complex numbers.
-
- Returns:
- Arc length value.
- """
- # This, essentially, approximates the length-of-derivative function
- # to be integrated with the best-matching seventh-degree polynomial
- # approximation of it.
- #
- # https://en.wikipedia.org/wiki/Gaussian_quadrature#Gauss.E2.80.93Lobatto_rules
-
- # abs(BezierCurveC[3].diff(t).subs({t:T})) for T in sorted(0, .5±(3/7)**.5/2, .5, 1),
- # weighted 1/20, 49/180, 32/90, 49/180, 1/20 respectively.
- v0 = abs(pt2 - pt1) * 0.15
- v1 = abs(
- -0.558983582205757 * pt1
- + 0.325650248872424 * pt2
- + 0.208983582205757 * pt3
- + 0.024349751127576 * pt4
- )
- v2 = abs(pt4 - pt1 + pt3 - pt2) * 0.26666666666666666
- v3 = abs(
- -0.024349751127576 * pt1
- - 0.208983582205757 * pt2
- - 0.325650248872424 * pt3
- + 0.558983582205757 * pt4
- )
- v4 = abs(pt4 - pt3) * 0.15
-
- return v0 + v1 + v2 + v3 + v4
-
-
-def calcCubicBounds(pt1, pt2, pt3, pt4):
- """Calculates the bounding rectangle for a quadratic Bezier segment.
-
- Args:
- pt1,pt2,pt3,pt4: Control points of the Bezier as 2D tuples.
-
- Returns:
- A four-item tuple representing the bounding rectangle ``(xMin, yMin, xMax, yMax)``.
-
- Example::
-
- >>> calcCubicBounds((0, 0), (25, 100), (75, 100), (100, 0))
- (0, 0, 100, 75.0)
- >>> calcCubicBounds((0, 0), (50, 0), (100, 50), (100, 100))
- (0.0, 0.0, 100, 100)
- >>> print("%f %f %f %f" % calcCubicBounds((50, 0), (0, 100), (100, 100), (50, 0)))
- 35.566243 0.000000 64.433757 75.000000
- """
- (ax, ay), (bx, by), (cx, cy), (dx, dy) = calcCubicParameters(pt1, pt2, pt3, pt4)
- # calc first derivative
- ax3 = ax * 3.0
- ay3 = ay * 3.0
- bx2 = bx * 2.0
- by2 = by * 2.0
- xRoots = [t for t in solveQuadratic(ax3, bx2, cx) if 0 <= t < 1]
- yRoots = [t for t in solveQuadratic(ay3, by2, cy) if 0 <= t < 1]
- roots = xRoots + yRoots
-
- points = [
- (
- ax * t * t * t + bx * t * t + cx * t + dx,
- ay * t * t * t + by * t * t + cy * t + dy,
- )
- for t in roots
- ] + [pt1, pt4]
- return calcBounds(points)
-
-
-def splitLine(pt1, pt2, where, isHorizontal):
- """Split a line at a given coordinate.
-
- Args:
- pt1: Start point of line as 2D tuple.
- pt2: End point of line as 2D tuple.
- where: Position at which to split the line.
- isHorizontal: Direction of the ray splitting the line. If true,
- ``where`` is interpreted as a Y coordinate; if false, then
- ``where`` is interpreted as an X coordinate.
-
- Returns:
- A list of two line segments (each line segment being two 2D tuples)
- if the line was successfully split, or a list containing the original
- line.
-
- Example::
-
- >>> printSegments(splitLine((0, 0), (100, 100), 50, True))
- ((0, 0), (50, 50))
- ((50, 50), (100, 100))
- >>> printSegments(splitLine((0, 0), (100, 100), 100, True))
- ((0, 0), (100, 100))
- >>> printSegments(splitLine((0, 0), (100, 100), 0, True))
- ((0, 0), (0, 0))
- ((0, 0), (100, 100))
- >>> printSegments(splitLine((0, 0), (100, 100), 0, False))
- ((0, 0), (0, 0))
- ((0, 0), (100, 100))
- >>> printSegments(splitLine((100, 0), (0, 0), 50, False))
- ((100, 0), (50, 0))
- ((50, 0), (0, 0))
- >>> printSegments(splitLine((0, 100), (0, 0), 50, True))
- ((0, 100), (0, 50))
- ((0, 50), (0, 0))
- """
- pt1x, pt1y = pt1
- pt2x, pt2y = pt2
-
- ax = pt2x - pt1x
- ay = pt2y - pt1y
-
- bx = pt1x
- by = pt1y
-
- a = (ax, ay)[isHorizontal]
-
- if a == 0:
- return [(pt1, pt2)]
- t = (where - (bx, by)[isHorizontal]) / a
- if 0 <= t < 1:
- midPt = ax * t + bx, ay * t + by
- return [(pt1, midPt), (midPt, pt2)]
- else:
- return [(pt1, pt2)]
-
-
-def splitQuadratic(pt1, pt2, pt3, where, isHorizontal):
- """Split a quadratic Bezier curve at a given coordinate.
-
- Args:
- pt1,pt2,pt3: Control points of the Bezier as 2D tuples.
- where: Position at which to split the curve.
- isHorizontal: Direction of the ray splitting the curve. If true,
- ``where`` is interpreted as a Y coordinate; if false, then
- ``where`` is interpreted as an X coordinate.
-
- Returns:
- A list of two curve segments (each curve segment being three 2D tuples)
- if the curve was successfully split, or a list containing the original
- curve.
-
- Example::
-
- >>> printSegments(splitQuadratic((0, 0), (50, 100), (100, 0), 150, False))
- ((0, 0), (50, 100), (100, 0))
- >>> printSegments(splitQuadratic((0, 0), (50, 100), (100, 0), 50, False))
- ((0, 0), (25, 50), (50, 50))
- ((50, 50), (75, 50), (100, 0))
- >>> printSegments(splitQuadratic((0, 0), (50, 100), (100, 0), 25, False))
- ((0, 0), (12.5, 25), (25, 37.5))
- ((25, 37.5), (62.5, 75), (100, 0))
- >>> printSegments(splitQuadratic((0, 0), (50, 100), (100, 0), 25, True))
- ((0, 0), (7.32233, 14.6447), (14.6447, 25))
- ((14.6447, 25), (50, 75), (85.3553, 25))
- ((85.3553, 25), (92.6777, 14.6447), (100, -7.10543e-15))
- >>> # XXX I'm not at all sure if the following behavior is desirable:
- >>> printSegments(splitQuadratic((0, 0), (50, 100), (100, 0), 50, True))
- ((0, 0), (25, 50), (50, 50))
- ((50, 50), (50, 50), (50, 50))
- ((50, 50), (75, 50), (100, 0))
- """
- a, b, c = calcQuadraticParameters(pt1, pt2, pt3)
- solutions = solveQuadratic(
- a[isHorizontal], b[isHorizontal], c[isHorizontal] - where
- )
- solutions = sorted(t for t in solutions if 0 <= t < 1)
- if not solutions:
- return [(pt1, pt2, pt3)]
- return _splitQuadraticAtT(a, b, c, *solutions)
-
-
-def splitCubic(pt1, pt2, pt3, pt4, where, isHorizontal):
- """Split a cubic Bezier curve at a given coordinate.
-
- Args:
- pt1,pt2,pt3,pt4: Control points of the Bezier as 2D tuples.
- where: Position at which to split the curve.
- isHorizontal: Direction of the ray splitting the curve. If true,
- ``where`` is interpreted as a Y coordinate; if false, then
- ``where`` is interpreted as an X coordinate.
-
- Returns:
- A list of two curve segments (each curve segment being four 2D tuples)
- if the curve was successfully split, or a list containing the original
- curve.
-
- Example::
-
- >>> printSegments(splitCubic((0, 0), (25, 100), (75, 100), (100, 0), 150, False))
- ((0, 0), (25, 100), (75, 100), (100, 0))
- >>> printSegments(splitCubic((0, 0), (25, 100), (75, 100), (100, 0), 50, False))
- ((0, 0), (12.5, 50), (31.25, 75), (50, 75))
- ((50, 75), (68.75, 75), (87.5, 50), (100, 0))
- >>> printSegments(splitCubic((0, 0), (25, 100), (75, 100), (100, 0), 25, True))
- ((0, 0), (2.29379, 9.17517), (4.79804, 17.5085), (7.47414, 25))
- ((7.47414, 25), (31.2886, 91.6667), (68.7114, 91.6667), (92.5259, 25))
- ((92.5259, 25), (95.202, 17.5085), (97.7062, 9.17517), (100, 1.77636e-15))
- """
- a, b, c, d = calcCubicParameters(pt1, pt2, pt3, pt4)
- solutions = solveCubic(
- a[isHorizontal], b[isHorizontal], c[isHorizontal], d[isHorizontal] - where
- )
- solutions = sorted(t for t in solutions if 0 <= t < 1)
- if not solutions:
- return [(pt1, pt2, pt3, pt4)]
- return _splitCubicAtT(a, b, c, d, *solutions)
-
-
-def splitQuadraticAtT(pt1, pt2, pt3, *ts):
- """Split a quadratic Bezier curve at one or more values of t.
-
- Args:
- pt1,pt2,pt3: Control points of the Bezier as 2D tuples.
- *ts: Positions at which to split the curve.
-
- Returns:
- A list of curve segments (each curve segment being three 2D tuples).
-
- Examples::
-
- >>> printSegments(splitQuadraticAtT((0, 0), (50, 100), (100, 0), 0.5))
- ((0, 0), (25, 50), (50, 50))
- ((50, 50), (75, 50), (100, 0))
- >>> printSegments(splitQuadraticAtT((0, 0), (50, 100), (100, 0), 0.5, 0.75))
- ((0, 0), (25, 50), (50, 50))
- ((50, 50), (62.5, 50), (75, 37.5))
- ((75, 37.5), (87.5, 25), (100, 0))
- """
- a, b, c = calcQuadraticParameters(pt1, pt2, pt3)
- return _splitQuadraticAtT(a, b, c, *ts)
-
-
-def splitCubicAtT(pt1, pt2, pt3, pt4, *ts):
- """Split a cubic Bezier curve at one or more values of t.
-
- Args:
- pt1,pt2,pt3,pt4: Control points of the Bezier as 2D tuples.
- *ts: Positions at which to split the curve.
-
- Returns:
- A list of curve segments (each curve segment being four 2D tuples).
-
- Examples::
-
- >>> printSegments(splitCubicAtT((0, 0), (25, 100), (75, 100), (100, 0), 0.5))
- ((0, 0), (12.5, 50), (31.25, 75), (50, 75))
- ((50, 75), (68.75, 75), (87.5, 50), (100, 0))
- >>> printSegments(splitCubicAtT((0, 0), (25, 100), (75, 100), (100, 0), 0.5, 0.75))
- ((0, 0), (12.5, 50), (31.25, 75), (50, 75))
- ((50, 75), (59.375, 75), (68.75, 68.75), (77.3438, 56.25))
- ((77.3438, 56.25), (85.9375, 43.75), (93.75, 25), (100, 0))
- """
- a, b, c, d = calcCubicParameters(pt1, pt2, pt3, pt4)
- return _splitCubicAtT(a, b, c, d, *ts)
-
-
-@cython.locals(
- pt1=cython.complex,
- pt2=cython.complex,
- pt3=cython.complex,
- pt4=cython.complex,
- a=cython.complex,
- b=cython.complex,
- c=cython.complex,
- d=cython.complex,
-)
-def splitCubicAtTC(pt1, pt2, pt3, pt4, *ts):
- """Split a cubic Bezier curve at one or more values of t.
-
- Args:
- pt1,pt2,pt3,pt4: Control points of the Bezier as complex numbers..
- *ts: Positions at which to split the curve.
-
- Yields:
- Curve segments (each curve segment being four complex numbers).
- """
- a, b, c, d = calcCubicParametersC(pt1, pt2, pt3, pt4)
- yield from _splitCubicAtTC(a, b, c, d, *ts)
-
-
-@cython.returns(cython.complex)
-@cython.locals(
- t=cython.double,
- pt1=cython.complex,
- pt2=cython.complex,
- pt3=cython.complex,
- pt4=cython.complex,
- pointAtT=cython.complex,
- off1=cython.complex,
- off2=cython.complex,
-)
-@cython.locals(
- t2=cython.double, _1_t=cython.double, _1_t_2=cython.double, _2_t_1_t=cython.double
-)
-def splitCubicIntoTwoAtTC(pt1, pt2, pt3, pt4, t):
- """Split a cubic Bezier curve at t.
-
- Args:
- pt1,pt2,pt3,pt4: Control points of the Bezier as complex numbers.
- t: Position at which to split the curve.
-
- Returns:
- A tuple of two curve segments (each curve segment being four complex numbers).
- """
- t2 = t * t
- _1_t = 1 - t
- _1_t_2 = _1_t * _1_t
- _2_t_1_t = 2 * t * _1_t
- pointAtT = (
- _1_t_2 * _1_t * pt1 + 3 * (_1_t_2 * t * pt2 + _1_t * t2 * pt3) + t2 * t * pt4
- )
- off1 = _1_t_2 * pt1 + _2_t_1_t * pt2 + t2 * pt3
- off2 = _1_t_2 * pt2 + _2_t_1_t * pt3 + t2 * pt4
-
- pt2 = pt1 + (pt2 - pt1) * t
- pt3 = pt4 + (pt3 - pt4) * _1_t
-
- return ((pt1, pt2, off1, pointAtT), (pointAtT, off2, pt3, pt4))
-
-
-def _splitQuadraticAtT(a, b, c, *ts):
- ts = list(ts)
- segments = []
- ts.insert(0, 0.0)
- ts.append(1.0)
- ax, ay = a
- bx, by = b
- cx, cy = c
- for i in range(len(ts) - 1):
- t1 = ts[i]
- t2 = ts[i + 1]
- delta = t2 - t1
- # calc new a, b and c
- delta_2 = delta * delta
- a1x = ax * delta_2
- a1y = ay * delta_2
- b1x = (2 * ax * t1 + bx) * delta
- b1y = (2 * ay * t1 + by) * delta
- t1_2 = t1 * t1
- c1x = ax * t1_2 + bx * t1 + cx
- c1y = ay * t1_2 + by * t1 + cy
-
- pt1, pt2, pt3 = calcQuadraticPoints((a1x, a1y), (b1x, b1y), (c1x, c1y))
- segments.append((pt1, pt2, pt3))
- return segments
-
-
-def _splitCubicAtT(a, b, c, d, *ts):
- ts = list(ts)
- ts.insert(0, 0.0)
- ts.append(1.0)
- segments = []
- ax, ay = a
- bx, by = b
- cx, cy = c
- dx, dy = d
- for i in range(len(ts) - 1):
- t1 = ts[i]
- t2 = ts[i + 1]
- delta = t2 - t1
-
- delta_2 = delta * delta
- delta_3 = delta * delta_2
- t1_2 = t1 * t1
- t1_3 = t1 * t1_2
-
- # calc new a, b, c and d
- a1x = ax * delta_3
- a1y = ay * delta_3
- b1x = (3 * ax * t1 + bx) * delta_2
- b1y = (3 * ay * t1 + by) * delta_2
- c1x = (2 * bx * t1 + cx + 3 * ax * t1_2) * delta
- c1y = (2 * by * t1 + cy + 3 * ay * t1_2) * delta
- d1x = ax * t1_3 + bx * t1_2 + cx * t1 + dx
- d1y = ay * t1_3 + by * t1_2 + cy * t1 + dy
- pt1, pt2, pt3, pt4 = calcCubicPoints(
- (a1x, a1y), (b1x, b1y), (c1x, c1y), (d1x, d1y)
- )
- segments.append((pt1, pt2, pt3, pt4))
- return segments
-
-
-@cython.locals(
- a=cython.complex,
- b=cython.complex,
- c=cython.complex,
- d=cython.complex,
- t1=cython.double,
- t2=cython.double,
- delta=cython.double,
- delta_2=cython.double,
- delta_3=cython.double,
- a1=cython.complex,
- b1=cython.complex,
- c1=cython.complex,
- d1=cython.complex,
-)
-def _splitCubicAtTC(a, b, c, d, *ts):
- ts = list(ts)
- ts.insert(0, 0.0)
- ts.append(1.0)
- for i in range(len(ts) - 1):
- t1 = ts[i]
- t2 = ts[i + 1]
- delta = t2 - t1
-
- delta_2 = delta * delta
- delta_3 = delta * delta_2
- t1_2 = t1 * t1
- t1_3 = t1 * t1_2
-
- # calc new a, b, c and d
- a1 = a * delta_3
- b1 = (3 * a * t1 + b) * delta_2
- c1 = (2 * b * t1 + c + 3 * a * t1_2) * delta
- d1 = a * t1_3 + b * t1_2 + c * t1 + d
- pt1, pt2, pt3, pt4 = calcCubicPointsC(a1, b1, c1, d1)
- yield (pt1, pt2, pt3, pt4)
-
-
-#
-# Equation solvers.
-#
-
-from math import sqrt, acos, cos, pi
-
-
-def solveQuadratic(a, b, c, sqrt=sqrt):
- """Solve a quadratic equation.
-
- Solves *a*x*x + b*x + c = 0* where a, b and c are real.
-
- Args:
- a: coefficient of *x²*
- b: coefficient of *x*
- c: constant term
-
- Returns:
- A list of roots. Note that the returned list is neither guaranteed to
- be sorted nor to contain unique values!
- """
- if abs(a) < epsilon:
- if abs(b) < epsilon:
- # We have a non-equation; therefore, we have no valid solution
- roots = []
- else:
- # We have a linear equation with 1 root.
- roots = [-c / b]
- else:
- # We have a true quadratic equation. Apply the quadratic formula to find two roots.
- DD = b * b - 4.0 * a * c
- if DD >= 0.0:
- rDD = sqrt(DD)
- roots = [(-b + rDD) / 2.0 / a, (-b - rDD) / 2.0 / a]
- else:
- # complex roots, ignore
- roots = []
- return roots
-
-
-def solveCubic(a, b, c, d):
- """Solve a cubic equation.
-
- Solves *a*x*x*x + b*x*x + c*x + d = 0* where a, b, c and d are real.
-
- Args:
- a: coefficient of *x³*
- b: coefficient of *x²*
- c: coefficient of *x*
- d: constant term
-
- Returns:
- A list of roots. Note that the returned list is neither guaranteed to
- be sorted nor to contain unique values!
-
- Examples::
-
- >>> solveCubic(1, 1, -6, 0)
- [-3.0, -0.0, 2.0]
- >>> solveCubic(-10.0, -9.0, 48.0, -29.0)
- [-2.9, 1.0, 1.0]
- >>> solveCubic(-9.875, -9.0, 47.625, -28.75)
- [-2.911392, 1.0, 1.0]
- >>> solveCubic(1.0, -4.5, 6.75, -3.375)
- [1.5, 1.5, 1.5]
- >>> solveCubic(-12.0, 18.0, -9.0, 1.50023651123)
- [0.5, 0.5, 0.5]
- >>> solveCubic(
- ... 9.0, 0.0, 0.0, -7.62939453125e-05
- ... ) == [-0.0, -0.0, -0.0]
- True
- """
- #
- # adapted from:
- # CUBIC.C - Solve a cubic polynomial
- # public domain by Ross Cottrell
- # found at: http://www.strangecreations.com/library/snippets/Cubic.C
- #
- if abs(a) < epsilon:
- # don't just test for zero; for very small values of 'a' solveCubic()
- # returns unreliable results, so we fall back to quad.
- return solveQuadratic(b, c, d)
- a = float(a)
- a1 = b / a
- a2 = c / a
- a3 = d / a
-
- Q = (a1 * a1 - 3.0 * a2) / 9.0
- R = (2.0 * a1 * a1 * a1 - 9.0 * a1 * a2 + 27.0 * a3) / 54.0
-
- R2 = R * R
- Q3 = Q * Q * Q
- R2 = 0 if R2 < epsilon else R2
- Q3 = 0 if abs(Q3) < epsilon else Q3
-
- R2_Q3 = R2 - Q3
-
- if R2 == 0.0 and Q3 == 0.0:
- x = round(-a1 / 3.0, epsilonDigits)
- return [x, x, x]
- elif R2_Q3 <= epsilon * 0.5:
- # The epsilon * .5 above ensures that Q3 is not zero.
- theta = acos(max(min(R / sqrt(Q3), 1.0), -1.0))
- rQ2 = -2.0 * sqrt(Q)
- a1_3 = a1 / 3.0
- x0 = rQ2 * cos(theta / 3.0) - a1_3
- x1 = rQ2 * cos((theta + 2.0 * pi) / 3.0) - a1_3
- x2 = rQ2 * cos((theta + 4.0 * pi) / 3.0) - a1_3
- x0, x1, x2 = sorted([x0, x1, x2])
- # Merge roots that are close-enough
- if x1 - x0 < epsilon and x2 - x1 < epsilon:
- x0 = x1 = x2 = round((x0 + x1 + x2) / 3.0, epsilonDigits)
- elif x1 - x0 < epsilon:
- x0 = x1 = round((x0 + x1) / 2.0, epsilonDigits)
- x2 = round(x2, epsilonDigits)
- elif x2 - x1 < epsilon:
- x0 = round(x0, epsilonDigits)
- x1 = x2 = round((x1 + x2) / 2.0, epsilonDigits)
- else:
- x0 = round(x0, epsilonDigits)
- x1 = round(x1, epsilonDigits)
- x2 = round(x2, epsilonDigits)
- return [x0, x1, x2]
- else:
- x = pow(sqrt(R2_Q3) + abs(R), 1 / 3.0)
- x = x + Q / x
- if R >= 0.0:
- x = -x
- x = round(x - a1 / 3.0, epsilonDigits)
- return [x]
-
-
-#
-# Conversion routines for points to parameters and vice versa
-#
-
-
-def calcQuadraticParameters(pt1, pt2, pt3):
- x2, y2 = pt2
- x3, y3 = pt3
- cx, cy = pt1
- bx = (x2 - cx) * 2.0
- by = (y2 - cy) * 2.0
- ax = x3 - cx - bx
- ay = y3 - cy - by
- return (ax, ay), (bx, by), (cx, cy)
-
-
-def calcCubicParameters(pt1, pt2, pt3, pt4):
- x2, y2 = pt2
- x3, y3 = pt3
- x4, y4 = pt4
- dx, dy = pt1
- cx = (x2 - dx) * 3.0
- cy = (y2 - dy) * 3.0
- bx = (x3 - x2) * 3.0 - cx
- by = (y3 - y2) * 3.0 - cy
- ax = x4 - dx - cx - bx
- ay = y4 - dy - cy - by
- return (ax, ay), (bx, by), (cx, cy), (dx, dy)
-
-
-@cython.cfunc
-@cython.inline
-@cython.locals(
- pt1=cython.complex,
- pt2=cython.complex,
- pt3=cython.complex,
- pt4=cython.complex,
- a=cython.complex,
- b=cython.complex,
- c=cython.complex,
-)
-def calcCubicParametersC(pt1, pt2, pt3, pt4):
- c = (pt2 - pt1) * 3.0
- b = (pt3 - pt2) * 3.0 - c
- a = pt4 - pt1 - c - b
- return (a, b, c, pt1)
-
-
-def calcQuadraticPoints(a, b, c):
- ax, ay = a
- bx, by = b
- cx, cy = c
- x1 = cx
- y1 = cy
- x2 = (bx * 0.5) + cx
- y2 = (by * 0.5) + cy
- x3 = ax + bx + cx
- y3 = ay + by + cy
- return (x1, y1), (x2, y2), (x3, y3)
-
-
-def calcCubicPoints(a, b, c, d):
- ax, ay = a
- bx, by = b
- cx, cy = c
- dx, dy = d
- x1 = dx
- y1 = dy
- x2 = (cx / 3.0) + dx
- y2 = (cy / 3.0) + dy
- x3 = (bx + cx) / 3.0 + x2
- y3 = (by + cy) / 3.0 + y2
- x4 = ax + dx + cx + bx
- y4 = ay + dy + cy + by
- return (x1, y1), (x2, y2), (x3, y3), (x4, y4)
-
-
-@cython.cfunc
-@cython.inline
-@cython.locals(
- a=cython.complex,
- b=cython.complex,
- c=cython.complex,
- d=cython.complex,
- p2=cython.complex,
- p3=cython.complex,
- p4=cython.complex,
-)
-def calcCubicPointsC(a, b, c, d):
- p2 = c * (1 / 3) + d
- p3 = (b + c) * (1 / 3) + p2
- p4 = a + b + c + d
- return (d, p2, p3, p4)
-
-
-#
-# Point at time
-#
-
-
-def linePointAtT(pt1, pt2, t):
- """Finds the point at time `t` on a line.
-
- Args:
- pt1, pt2: Coordinates of the line as 2D tuples.
- t: The time along the line.
-
- Returns:
- A 2D tuple with the coordinates of the point.
- """
- return ((pt1[0] * (1 - t) + pt2[0] * t), (pt1[1] * (1 - t) + pt2[1] * t))
-
-
-def quadraticPointAtT(pt1, pt2, pt3, t):
- """Finds the point at time `t` on a quadratic curve.
-
- Args:
- pt1, pt2, pt3: Coordinates of the curve as 2D tuples.
- t: The time along the curve.
-
- Returns:
- A 2D tuple with the coordinates of the point.
- """
- x = (1 - t) * (1 - t) * pt1[0] + 2 * (1 - t) * t * pt2[0] + t * t * pt3[0]
- y = (1 - t) * (1 - t) * pt1[1] + 2 * (1 - t) * t * pt2[1] + t * t * pt3[1]
- return (x, y)
-
-
-def cubicPointAtT(pt1, pt2, pt3, pt4, t):
- """Finds the point at time `t` on a cubic curve.
-
- Args:
- pt1, pt2, pt3, pt4: Coordinates of the curve as 2D tuples.
- t: The time along the curve.
-
- Returns:
- A 2D tuple with the coordinates of the point.
- """
- t2 = t * t
- _1_t = 1 - t
- _1_t_2 = _1_t * _1_t
- x = (
- _1_t_2 * _1_t * pt1[0]
- + 3 * (_1_t_2 * t * pt2[0] + _1_t * t2 * pt3[0])
- + t2 * t * pt4[0]
- )
- y = (
- _1_t_2 * _1_t * pt1[1]
- + 3 * (_1_t_2 * t * pt2[1] + _1_t * t2 * pt3[1])
- + t2 * t * pt4[1]
- )
- return (x, y)
-
-
-@cython.returns(cython.complex)
-@cython.locals(
- t=cython.double,
- pt1=cython.complex,
- pt2=cython.complex,
- pt3=cython.complex,
- pt4=cython.complex,
-)
-@cython.locals(t2=cython.double, _1_t=cython.double, _1_t_2=cython.double)
-def cubicPointAtTC(pt1, pt2, pt3, pt4, t):
- """Finds the point at time `t` on a cubic curve.
-
- Args:
- pt1, pt2, pt3, pt4: Coordinates of the curve as complex numbers.
- t: The time along the curve.
-
- Returns:
- A complex number with the coordinates of the point.
- """
- t2 = t * t
- _1_t = 1 - t
- _1_t_2 = _1_t * _1_t
- return _1_t_2 * _1_t * pt1 + 3 * (_1_t_2 * t * pt2 + _1_t * t2 * pt3) + t2 * t * pt4
-
-
-def segmentPointAtT(seg, t):
- if len(seg) == 2:
- return linePointAtT(*seg, t)
- elif len(seg) == 3:
- return quadraticPointAtT(*seg, t)
- elif len(seg) == 4:
- return cubicPointAtT(*seg, t)
- raise ValueError("Unknown curve degree")
-
-
-#
-# Intersection finders
-#
-
-
-def _line_t_of_pt(s, e, pt):
- sx, sy = s
- ex, ey = e
- px, py = pt
- if abs(sx - ex) < epsilon and abs(sy - ey) < epsilon:
- # Line is a point!
- return -1
- # Use the largest
- if abs(sx - ex) > abs(sy - ey):
- return (px - sx) / (ex - sx)
- else:
- return (py - sy) / (ey - sy)
-
-
-def _both_points_are_on_same_side_of_origin(a, b, origin):
- xDiff = (a[0] - origin[0]) * (b[0] - origin[0])
- yDiff = (a[1] - origin[1]) * (b[1] - origin[1])
- return not (xDiff <= 0.0 and yDiff <= 0.0)
-
-
-def lineLineIntersections(s1, e1, s2, e2):
- """Finds intersections between two line segments.
-
- Args:
- s1, e1: Coordinates of the first line as 2D tuples.
- s2, e2: Coordinates of the second line as 2D tuples.
-
- Returns:
- A list of ``Intersection`` objects, each object having ``pt``, ``t1``
- and ``t2`` attributes containing the intersection point, time on first
- segment and time on second segment respectively.
-
- Examples::
-
- >>> a = lineLineIntersections( (310,389), (453, 222), (289, 251), (447, 367))
- >>> len(a)
- 1
- >>> intersection = a[0]
- >>> intersection.pt
- (374.44882952482897, 313.73458370177315)
- >>> (intersection.t1, intersection.t2)
- (0.45069111555824465, 0.5408153767394238)
- """
- s1x, s1y = s1
- e1x, e1y = e1
- s2x, s2y = s2
- e2x, e2y = e2
- if (
- math.isclose(s2x, e2x) and math.isclose(s1x, e1x) and not math.isclose(s1x, s2x)
- ): # Parallel vertical
- return []
- if (
- math.isclose(s2y, e2y) and math.isclose(s1y, e1y) and not math.isclose(s1y, s2y)
- ): # Parallel horizontal
- return []
- if math.isclose(s2x, e2x) and math.isclose(s2y, e2y): # Line segment is tiny
- return []
- if math.isclose(s1x, e1x) and math.isclose(s1y, e1y): # Line segment is tiny
- return []
- if math.isclose(e1x, s1x):
- x = s1x
- slope34 = (e2y - s2y) / (e2x - s2x)
- y = slope34 * (x - s2x) + s2y
- pt = (x, y)
- return [
- Intersection(
- pt=pt, t1=_line_t_of_pt(s1, e1, pt), t2=_line_t_of_pt(s2, e2, pt)
- )
- ]
- if math.isclose(s2x, e2x):
- x = s2x
- slope12 = (e1y - s1y) / (e1x - s1x)
- y = slope12 * (x - s1x) + s1y
- pt = (x, y)
- return [
- Intersection(
- pt=pt, t1=_line_t_of_pt(s1, e1, pt), t2=_line_t_of_pt(s2, e2, pt)
- )
- ]
-
- slope12 = (e1y - s1y) / (e1x - s1x)
- slope34 = (e2y - s2y) / (e2x - s2x)
- if math.isclose(slope12, slope34):
- return []
- x = (slope12 * s1x - s1y - slope34 * s2x + s2y) / (slope12 - slope34)
- y = slope12 * (x - s1x) + s1y
- pt = (x, y)
- if _both_points_are_on_same_side_of_origin(
- pt, e1, s1
- ) and _both_points_are_on_same_side_of_origin(pt, s2, e2):
- return [
- Intersection(
- pt=pt, t1=_line_t_of_pt(s1, e1, pt), t2=_line_t_of_pt(s2, e2, pt)
- )
- ]
- return []
-
-
-def _alignment_transformation(segment):
- # Returns a transformation which aligns a segment horizontally at the
- # origin. Apply this transformation to curves and root-find to find
- # intersections with the segment.
- start = segment[0]
- end = segment[-1]
- angle = math.atan2(end[1] - start[1], end[0] - start[0])
- return Identity.rotate(-angle).translate(-start[0], -start[1])
-
-
-def _curve_line_intersections_t(curve, line):
- aligned_curve = _alignment_transformation(line).transformPoints(curve)
- if len(curve) == 3:
- a, b, c = calcQuadraticParameters(*aligned_curve)
- intersections = solveQuadratic(a[1], b[1], c[1])
- elif len(curve) == 4:
- a, b, c, d = calcCubicParameters(*aligned_curve)
- intersections = solveCubic(a[1], b[1], c[1], d[1])
- else:
- raise ValueError("Unknown curve degree")
- return sorted(i for i in intersections if 0.0 <= i <= 1)
-
-
-def curveLineIntersections(curve, line):
- """Finds intersections between a curve and a line.
-
- Args:
- curve: List of coordinates of the curve segment as 2D tuples.
- line: List of coordinates of the line segment as 2D tuples.
-
- Returns:
- A list of ``Intersection`` objects, each object having ``pt``, ``t1``
- and ``t2`` attributes containing the intersection point, time on first
- segment and time on second segment respectively.
-
- Examples::
- >>> curve = [ (100, 240), (30, 60), (210, 230), (160, 30) ]
- >>> line = [ (25, 260), (230, 20) ]
- >>> intersections = curveLineIntersections(curve, line)
- >>> len(intersections)
- 3
- >>> intersections[0].pt
- (84.9000930760723, 189.87306176459828)
- """
- if len(curve) == 3:
- pointFinder = quadraticPointAtT
- elif len(curve) == 4:
- pointFinder = cubicPointAtT
- else:
- raise ValueError("Unknown curve degree")
- intersections = []
- for t in _curve_line_intersections_t(curve, line):
- pt = pointFinder(*curve, t)
- # Back-project the point onto the line, to avoid problems with
- # numerical accuracy in the case of vertical and horizontal lines
- line_t = _line_t_of_pt(*line, pt)
- pt = linePointAtT(*line, line_t)
- intersections.append(Intersection(pt=pt, t1=t, t2=line_t))
- return intersections
-
-
-def _curve_bounds(c):
- if len(c) == 3:
- return calcQuadraticBounds(*c)
- elif len(c) == 4:
- return calcCubicBounds(*c)
- raise ValueError("Unknown curve degree")
-
-
-def _split_segment_at_t(c, t):
- if len(c) == 2:
- s, e = c
- midpoint = linePointAtT(s, e, t)
- return [(s, midpoint), (midpoint, e)]
- if len(c) == 3:
- return splitQuadraticAtT(*c, t)
- elif len(c) == 4:
- return splitCubicAtT(*c, t)
- raise ValueError("Unknown curve degree")
-
-
-def _curve_curve_intersections_t(
- curve1, curve2, precision=1e-3, range1=None, range2=None
-):
- bounds1 = _curve_bounds(curve1)
- bounds2 = _curve_bounds(curve2)
-
- if not range1:
- range1 = (0.0, 1.0)
- if not range2:
- range2 = (0.0, 1.0)
-
- # If bounds don't intersect, go home
- intersects, _ = sectRect(bounds1, bounds2)
- if not intersects:
- return []
-
- def midpoint(r):
- return 0.5 * (r[0] + r[1])
-
- # If they do overlap but they're tiny, approximate
- if rectArea(bounds1) < precision and rectArea(bounds2) < precision:
- return [(midpoint(range1), midpoint(range2))]
-
- c11, c12 = _split_segment_at_t(curve1, 0.5)
- c11_range = (range1[0], midpoint(range1))
- c12_range = (midpoint(range1), range1[1])
-
- c21, c22 = _split_segment_at_t(curve2, 0.5)
- c21_range = (range2[0], midpoint(range2))
- c22_range = (midpoint(range2), range2[1])
-
- found = []
- found.extend(
- _curve_curve_intersections_t(
- c11, c21, precision, range1=c11_range, range2=c21_range
- )
- )
- found.extend(
- _curve_curve_intersections_t(
- c12, c21, precision, range1=c12_range, range2=c21_range
- )
- )
- found.extend(
- _curve_curve_intersections_t(
- c11, c22, precision, range1=c11_range, range2=c22_range
- )
- )
- found.extend(
- _curve_curve_intersections_t(
- c12, c22, precision, range1=c12_range, range2=c22_range
- )
- )
-
- unique_key = lambda ts: (int(ts[0] / precision), int(ts[1] / precision))
- seen = set()
- unique_values = []
-
- for ts in found:
- key = unique_key(ts)
- if key in seen:
- continue
- seen.add(key)
- unique_values.append(ts)
-
- return unique_values
-
-
-def curveCurveIntersections(curve1, curve2):
- """Finds intersections between a curve and a curve.
-
- Args:
- curve1: List of coordinates of the first curve segment as 2D tuples.
- curve2: List of coordinates of the second curve segment as 2D tuples.
-
- Returns:
- A list of ``Intersection`` objects, each object having ``pt``, ``t1``
- and ``t2`` attributes containing the intersection point, time on first
- segment and time on second segment respectively.
-
- Examples::
- >>> curve1 = [ (10,100), (90,30), (40,140), (220,220) ]
- >>> curve2 = [ (5,150), (180,20), (80,250), (210,190) ]
- >>> intersections = curveCurveIntersections(curve1, curve2)
- >>> len(intersections)
- 3
- >>> intersections[0].pt
- (81.7831487395506, 109.88904552375288)
- """
- intersection_ts = _curve_curve_intersections_t(curve1, curve2)
- return [
- Intersection(pt=segmentPointAtT(curve1, ts[0]), t1=ts[0], t2=ts[1])
- for ts in intersection_ts
- ]
-
-
-def segmentSegmentIntersections(seg1, seg2):
- """Finds intersections between two segments.
-
- Args:
- seg1: List of coordinates of the first segment as 2D tuples.
- seg2: List of coordinates of the second segment as 2D tuples.
-
- Returns:
- A list of ``Intersection`` objects, each object having ``pt``, ``t1``
- and ``t2`` attributes containing the intersection point, time on first
- segment and time on second segment respectively.
-
- Examples::
- >>> curve1 = [ (10,100), (90,30), (40,140), (220,220) ]
- >>> curve2 = [ (5,150), (180,20), (80,250), (210,190) ]
- >>> intersections = segmentSegmentIntersections(curve1, curve2)
- >>> len(intersections)
- 3
- >>> intersections[0].pt
- (81.7831487395506, 109.88904552375288)
- >>> curve3 = [ (100, 240), (30, 60), (210, 230), (160, 30) ]
- >>> line = [ (25, 260), (230, 20) ]
- >>> intersections = segmentSegmentIntersections(curve3, line)
- >>> len(intersections)
- 3
- >>> intersections[0].pt
- (84.9000930760723, 189.87306176459828)
-
- """
- # Arrange by degree
- swapped = False
- if len(seg2) > len(seg1):
- seg2, seg1 = seg1, seg2
- swapped = True
- if len(seg1) > 2:
- if len(seg2) > 2:
- intersections = curveCurveIntersections(seg1, seg2)
- else:
- intersections = curveLineIntersections(seg1, seg2)
- elif len(seg1) == 2 and len(seg2) == 2:
- intersections = lineLineIntersections(*seg1, *seg2)
- else:
- raise ValueError("Couldn't work out which intersection function to use")
- if not swapped:
- return intersections
- return [Intersection(pt=i.pt, t1=i.t2, t2=i.t1) for i in intersections]
-
-
-def _segmentrepr(obj):
- """
- >>> _segmentrepr([1, [2, 3], [], [[2, [3, 4], [0.1, 2.2]]]])
- '(1, (2, 3), (), ((2, (3, 4), (0.1, 2.2))))'
- """
- try:
- it = iter(obj)
- except TypeError:
- return "%g" % obj
- else:
- return "(%s)" % ", ".join(_segmentrepr(x) for x in it)
-
-
-def printSegments(segments):
- """Helper for the doctests, displaying each segment in a list of
- segments on a single line as a tuple.
- """
- for segment in segments:
- print(_segmentrepr(segment))
-
-
-if __name__ == "__main__":
- import sys
- import doctest
-
- sys.exit(doctest.testmod().failed)
diff --git a/spaces/jonatanklosko/chai/assets/js/hooks/messages.js b/spaces/jonatanklosko/chai/assets/js/hooks/messages.js
deleted file mode 100644
index 1289a4559e09ec6d774004baa393be83fbaaa914..0000000000000000000000000000000000000000
--- a/spaces/jonatanklosko/chai/assets/js/hooks/messages.js
+++ /dev/null
@@ -1,15 +0,0 @@
-const Messages = {
- mounted() {
- this.scroll();
- },
-
- updated() {
- this.scroll();
- },
-
- scroll() {
- this.el.scrollTop = this.el.scrollHeight;
- },
-};
-
-export default Messages;
diff --git a/spaces/jordonpeter01/ai-comic-factory/src/lib/fonts.ts b/spaces/jordonpeter01/ai-comic-factory/src/lib/fonts.ts
deleted file mode 100644
index 7498aa46bc21fe19cc1b878ee928f9d55c31f927..0000000000000000000000000000000000000000
--- a/spaces/jordonpeter01/ai-comic-factory/src/lib/fonts.ts
+++ /dev/null
@@ -1,119 +0,0 @@
-import {
- Indie_Flower,
- The_Girl_Next_Door,
-
-} from "next/font/google"
-import localFont from "next/font/local"
-
-export const indieflower = Indie_Flower({
- subsets: ["latin"],
- weight: "400",
- variable: "--font-indieflower",
-})
-
-export const thegirlnextdoor = The_Girl_Next_Door({
- subsets: ["latin"],
- weight: "400",
- variable: "--font-the-girl-next-door",
-})
-
-export const komika = localFont({
- src: "../fonts/Komika-Hand/Komika-Hand.woff2",
- variable: "--font-komika"
-})
-
-export const actionman = localFont({
- src: "../fonts/Action-Man/Action-Man.woff2",
- variable: "--font-action-man"
-})
-
-export const karantula = localFont({
- src: "../fonts/Karantula/Karantula.woff2",
- variable: "--font-karantula"
-})
-
-export const manoskope = localFont({
- src: "../fonts/Manoskope/MANOSKOPE-Bold.woff2",
- variable: "--font-manoskope"
-})
-
-export const paeteround = localFont({
- src: "../fonts/Paete-Round/Paete-Round.woff2",
- variable: "--font-paete-round"
-})
-
-export const qarmic = localFont({
- src: "../fonts/Qarmic-Sans/Qarmic-Sans-Abridged.woff2",
- variable: "--font-qarmic-sans"
-})
-
-export const archrival = localFont({
- src: "../fonts/SF-Arch-Rival/SF-Arch-Rival.woff2",
- variable: "--font-sf-arch-rival"
-})
-
-export const cartoonist = localFont({
- src: "../fonts/SF-Cartoonist-Hand/SF-Cartoonist-Hand.woff2",
- variable: "--font-sf-cartoonist-hand"
-})
-
-export const toontime = localFont({
- src: "../fonts/SF-Toontime/SF-Toontime.woff2",
- variable: "--font-sf-toontime"
-})
-
-export const vtc = localFont({
- src: "../fonts/VTC-Letterer-Pro/VTC-Letterer-Pro.woff2",
- variable: "--font-vtc-letterer-pro"
-})
-
-
-export const digitalstrip = localFont({
- src: "../fonts/DigitalStripBB/DigitalStripBB_Reg.woff2",
- variable: "--font-digital-strip-bb"
-})
-
-// https://nextjs.org/docs/pages/building-your-application/optimizing/fonts
-// If loading a variable font, you don"t need to specify the font weight
-export const fonts = {
- indieflower,
- thegirlnextdoor,
- // komika,
- actionman,
- karantula,
- manoskope,
- // paeteround,
- // qarmic,
- // archrival,
- // cartoonist,
- // toontime,
- // vtc,
- digitalstrip
-}
-
-// https://nextjs.org/docs/pages/building-your-application/optimizing/fonts
-// If loading a variable font, you don"t need to specify the font weight
-export const fontList = Object.keys(fonts)
-
-export type FontName = keyof typeof fonts
-
-export const defaultFont = "cartoonist" as FontName
-
-export const classNames = Object.values(fonts).map(font => font.className)
-
-export const className = classNames.join(" ")
-
-export type FontClass =
- | "font-indieflower"
- | "font-thegirlnextdoor"
- | "font-komika"
- | "font-actionman"
- | "font-karantula"
- | "font-manoskope"
- | "font-paeteround"
- | "font-qarmic"
- | "font-archrival"
- | "font-cartoonist"
- | "font-toontime"
- | "font-vtc"
- | "font-digitalstrip"
diff --git a/spaces/jw2yang/unicl-img-recog-demo/model/text_encoder/__init__.py b/spaces/jw2yang/unicl-img-recog-demo/model/text_encoder/__init__.py
deleted file mode 100644
index e09753c06e7cd77d8df3bee03b04ae9f85ce80bb..0000000000000000000000000000000000000000
--- a/spaces/jw2yang/unicl-img-recog-demo/model/text_encoder/__init__.py
+++ /dev/null
@@ -1,9 +0,0 @@
-from __future__ import absolute_import
-from __future__ import division
-from __future__ import print_function
-
-from .build import build_lang_encoder as build_text_encoder
-from .build import build_tokenizer
-
-from .transformer import *
-from .hf_model import *
diff --git a/spaces/jyseo/3DFuse/my/utils/event.py b/spaces/jyseo/3DFuse/my/utils/event.py
deleted file mode 100644
index 741ab144fef51eef800dc7a03208059675ee8860..0000000000000000000000000000000000000000
--- a/spaces/jyseo/3DFuse/my/utils/event.py
+++ /dev/null
@@ -1,143 +0,0 @@
-# design inspiration from detectron2
-from pathlib import Path
-import json
-import os
-from contextlib import contextmanager
-from .ticker import IntervalTicker
-
-
-_CURRENT_STORAGE_STACK = []
-
-
-def get_event_storage():
- """
- Returns:
- The :class:`EventStorage` object that's currently being used.
- Throws an error if no :class:`EventStorage` is currently enabled.
- """
- assert len(
- _CURRENT_STORAGE_STACK
- ), "get_event_storage() has to be called inside a 'with EventStorage(...)' context!"
- return _CURRENT_STORAGE_STACK[-1]
-
-
-def read_lined_json(fname):
- with Path(fname).open('r') as f:
- for line in f:
- item = json.loads(line)
- yield item
-
-
-def read_stats(dirname, key):
- if dirname is None or not (fname := Path(dirname) / "history.json").is_file():
- return [], []
- stats = read_lined_json(fname)
- stats = list(filter(lambda x: key in x, stats))
- xs = [e['iter'] for e in stats]
- ys = [e[key] for e in stats]
- return xs, ys
-
-
-class EventStorage():
- def __init__(self, output_dir="./hotdog", start_iter=0, flush_period=60):
- self.iter = start_iter
- self.ticker = IntervalTicker(flush_period)
- self.history = []
- self._current_prefix = ""
- self._init_curr_buffer_()
-
- self.output_dir = output_dir
- self.writable = False
-
- def _open(self):
- if self.writable:
- output_dir = Path(self.output_dir)
- if not output_dir.is_dir():
- output_dir.mkdir(parents=True, exist_ok=True)
- json_fname = output_dir / 'history.json'
-
- self._file_handle = json_fname.open('a', encoding='utf8')
- self.output_dir = output_dir # make sure it's a path object
-
- def _init_curr_buffer_(self):
- self.curr_buffer = {'iter': self.iter}
-
- def step(self, flush=False):
- self.history.append(self.curr_buffer)
-
- on_flush_period = self.ticker.tick()
- if flush or on_flush_period:
- self.flush_history()
-
- self.iter += 1
- self._init_curr_buffer_()
-
- def flush_history(self):
- if self.writable:
- for item in self.history:
- line = json.dumps(item, sort_keys=True, ensure_ascii=False) + "\n"
- self._file_handle.write(line)
- self._file_handle.flush()
- self.history = []
-
- def full_key(self, key):
- assert isinstance(key, str)
- name = self._current_prefix + key
- return name
-
- def put(self, key, val):
- key = self.full_key(key)
- assert isinstance(val, (int, float, str))
- if isinstance(val, float):
- val = round(val, 3)
- self.curr_buffer[key] = val
-
- def put_scalars(self, **kwargs):
- for k, v in kwargs.items():
- self.put(k, v)
-
- def put_artifact(self, key, ext,p, save_func):
- if not self.writable:
- return
- p=p.replace(" ","_")
- os.makedirs(self.output_dir / key, exist_ok=True)
- fname = (self.output_dir / key / f"step_{self.iter}_{p}").with_suffix(ext)
- fname = str(fname)
-
- # must be called inside so that
- # 1. the func is not executed if the metric is not writable
- # 2. the key is only inserted if the func succeeds
- save_func(fname)
- self.put(key, fname)
- return fname
-
- def close(self):
- self.flush_history()
- if self.writable:
- self._file_handle.close()
-
- def get_last(self):
- if len(self.history) > 0:
- last = self.history[-1]
- return last
-
- def __enter__(self):
- if len(_CURRENT_STORAGE_STACK) > 0:
- parent = _CURRENT_STORAGE_STACK[-1]
- root, dirname = parent.output_dir, self.output_dir
- if root is not None and dirname is not None:
- child_dir = parent.output_dir / f"{self.output_dir}_{parent.iter}"
- self.output_dir = child_dir
- parent.put(str(dirname), str(child_dir))
-
- if self.output_dir is not None:
- self.writable = True
- self._open()
-
- _CURRENT_STORAGE_STACK.append(self)
- return self
-
- def __exit__(self, exc_type, exc_val, exc_tb):
- assert _CURRENT_STORAGE_STACK[-1] == self
- _CURRENT_STORAGE_STACK.pop()
- self.close()
diff --git a/spaces/kangvcar/RealChar/client/web/src/index.js b/spaces/kangvcar/RealChar/client/web/src/index.js
deleted file mode 100644
index d563c0fb10ba0e42724b21286eb546ee4e5734fc..0000000000000000000000000000000000000000
--- a/spaces/kangvcar/RealChar/client/web/src/index.js
+++ /dev/null
@@ -1,17 +0,0 @@
-import React from 'react';
-import ReactDOM from 'react-dom/client';
-import './index.css';
-import App from './App';
-import reportWebVitals from './reportWebVitals';
-
-const root = ReactDOM.createRoot(document.getElementById('root'));
-root.render(
-
-
-
-);
-
-// If you want to start measuring performance in your app, pass a function
-// to log results (for example: reportWebVitals(console.log))
-// or send to an analytics endpoint. Learn more: https://bit.ly/CRA-vitals
-reportWebVitals();
diff --git a/spaces/karay/diar_speech/player.html b/spaces/karay/diar_speech/player.html
deleted file mode 100644
index a267066b4d794c70661838a617c36ecfc59c54cb..0000000000000000000000000000000000000000
--- a/spaces/karay/diar_speech/player.html
+++ /dev/null
@@ -1,274 +0,0 @@
-
-
-
-Speakers
-
-
-
-
-
-
-
-
-
-
-
-
-
-
-
-
00:00 / 00:00
-
-
-
-
-
-
-
\ No newline at end of file
diff --git a/spaces/kastan/ai-teaching-assistant-beta/gpu_memory_utils.py b/spaces/kastan/ai-teaching-assistant-beta/gpu_memory_utils.py
deleted file mode 100644
index 573713a52280fd8cb828600dab3faa20fc2696d7..0000000000000000000000000000000000000000
--- a/spaces/kastan/ai-teaching-assistant-beta/gpu_memory_utils.py
+++ /dev/null
@@ -1,57 +0,0 @@
-import GPUtil # pip install gputil
-
-
-def get_gpu_ids_with_sufficient_memory(memory_requirement_GB):
- '''
- Returns the MINIMAL SET of GPU IDs that, combined, have at least `memory_requirement` MB of free memory.
- You will need to use all returned GPU IDs to get the desired memory requirement.
- It returns lower IDs first [0, 1, ...]
-
- If `memory_requirement` is 0, returns all available GPUs.
- If `memory_requirement` is not available, returns an empty list.
- '''
- memory_requirement_MB = float(memory_requirement_GB * 1024)
- GPUs = sorted(GPUtil.getGPUs(), key=lambda x: x.memoryFree, reverse=True)
- total_memory = sum(gpu.memoryFree for gpu in GPUs)
- if memory_requirement_MB > total_memory:
- return []
- GPU_IDs = []
- for gpu in GPUs:
- if memory_requirement_MB <= 0:
- break
- GPU_IDs.append(gpu.id)
- memory_requirement_MB -= gpu.memoryFree
- return GPU_IDs
-
-
-def get_device_with_most_free_memory():
- '''
- Returns the GPU ID of the GPU with the most free memory.
- '''
- GPUs = GPUtil.getGPUs()
- return sorted(GPUs, key=lambda x: x.memoryFree, reverse=True)[0].id
-
-
-def get_free_memory_dict(leave_extra_memory_unused_GiB: float = 2, leave_extra_memory_unused_gpu0_GiB: float = 3):
- '''
- Returns a dictionary of GPU IDs and their free memory, in MiB.
- Compatible with huggingface Accelerate formatting: `max_memory=get_free_memory_dict()`
-
- Accelerate seems to use more memory than we give it, so we default to telling Accelerate we have 2 GiB less than we actually do.
-
- Example output:
- {0: '24753MiB', 1: '26223MiB', 2: '25603MiB', 3: '9044MiB'}
- '''
- GPUs = GPUtil.getGPUs()
- memory_map = {gpu.id: int(round(gpu.memoryFree)) for gpu in GPUs}
- if leave_extra_memory_unused_GiB > 0:
- for device_id, memory_MiB in memory_map.items():
- memory_map[device_id] = memory_MiB - (leave_extra_memory_unused_GiB * 1024)
- if leave_extra_memory_unused_gpu0_GiB > 0 and 0 in memory_map:
- memory_map[0] = memory_map[0] - (leave_extra_memory_unused_gpu0_GiB * 1024)
-
- # format to Accelerate's liking
- for device_id, memory_MiB in memory_map.items():
- memory_map[device_id] = f"{int(round(memory_MiB))}MiB"
-
- return memory_map
diff --git a/spaces/kdrkdrkdr/ShirokoTTS/text/cleaners.py b/spaces/kdrkdrkdr/ShirokoTTS/text/cleaners.py
deleted file mode 100644
index e48d53fed89e6e163bc4285dc24682cc3efcb56a..0000000000000000000000000000000000000000
--- a/spaces/kdrkdrkdr/ShirokoTTS/text/cleaners.py
+++ /dev/null
@@ -1 +0,0 @@
-from ptml2ja import ml2ja_ipa
\ No newline at end of file
diff --git a/spaces/kdrkdrkdr/YuukaTTS/export_model.py b/spaces/kdrkdrkdr/YuukaTTS/export_model.py
deleted file mode 100644
index 98a49835df5a7a2486e76ddf94fbbb4444b52203..0000000000000000000000000000000000000000
--- a/spaces/kdrkdrkdr/YuukaTTS/export_model.py
+++ /dev/null
@@ -1,13 +0,0 @@
-import torch
-
-if __name__ == '__main__':
- model_path = "saved_model/11/model.pth"
- output_path = "saved_model/11/model1.pth"
- checkpoint_dict = torch.load(model_path, map_location='cpu')
- checkpoint_dict_new = {}
- for k, v in checkpoint_dict.items():
- if k == "optimizer":
- print("remove optimizer")
- continue
- checkpoint_dict_new[k] = v
- torch.save(checkpoint_dict_new, output_path)
diff --git a/spaces/kevinwang676/ChatGLM2-SadTalker-VC/src/face3d/options/train_options.py b/spaces/kevinwang676/ChatGLM2-SadTalker-VC/src/face3d/options/train_options.py
deleted file mode 100644
index 1337bfdd5f372b5c686a91b394a2aadbe5741f44..0000000000000000000000000000000000000000
--- a/spaces/kevinwang676/ChatGLM2-SadTalker-VC/src/face3d/options/train_options.py
+++ /dev/null
@@ -1,53 +0,0 @@
-"""This script contains the training options for Deep3DFaceRecon_pytorch
-"""
-
-from .base_options import BaseOptions
-from util import util
-
-class TrainOptions(BaseOptions):
- """This class includes training options.
-
- It also includes shared options defined in BaseOptions.
- """
-
- def initialize(self, parser):
- parser = BaseOptions.initialize(self, parser)
- # dataset parameters
- # for train
- parser.add_argument('--data_root', type=str, default='./', help='dataset root')
- parser.add_argument('--flist', type=str, default='datalist/train/masks.txt', help='list of mask names of training set')
- parser.add_argument('--batch_size', type=int, default=32)
- parser.add_argument('--dataset_mode', type=str, default='flist', help='chooses how datasets are loaded. [None | flist]')
- parser.add_argument('--serial_batches', action='store_true', help='if true, takes images in order to make batches, otherwise takes them randomly')
- parser.add_argument('--num_threads', default=4, type=int, help='# threads for loading data')
- parser.add_argument('--max_dataset_size', type=int, default=float("inf"), help='Maximum number of samples allowed per dataset. If the dataset directory contains more than max_dataset_size, only a subset is loaded.')
- parser.add_argument('--preprocess', type=str, default='shift_scale_rot_flip', help='scaling and cropping of images at load time [shift_scale_rot_flip | shift_scale | shift | shift_rot_flip ]')
- parser.add_argument('--use_aug', type=util.str2bool, nargs='?', const=True, default=True, help='whether use data augmentation')
-
- # for val
- parser.add_argument('--flist_val', type=str, default='datalist/val/masks.txt', help='list of mask names of val set')
- parser.add_argument('--batch_size_val', type=int, default=32)
-
-
- # visualization parameters
- parser.add_argument('--display_freq', type=int, default=1000, help='frequency of showing training results on screen')
- parser.add_argument('--print_freq', type=int, default=100, help='frequency of showing training results on console')
-
- # network saving and loading parameters
- parser.add_argument('--save_latest_freq', type=int, default=5000, help='frequency of saving the latest results')
- parser.add_argument('--save_epoch_freq', type=int, default=1, help='frequency of saving checkpoints at the end of epochs')
- parser.add_argument('--evaluation_freq', type=int, default=5000, help='evaluation freq')
- parser.add_argument('--save_by_iter', action='store_true', help='whether saves model by iteration')
- parser.add_argument('--continue_train', action='store_true', help='continue training: load the latest model')
- parser.add_argument('--epoch_count', type=int, default=1, help='the starting epoch count, we save the model by , +, ...')
- parser.add_argument('--phase', type=str, default='train', help='train, val, test, etc')
- parser.add_argument('--pretrained_name', type=str, default=None, help='resume training from another checkpoint')
-
- # training parameters
- parser.add_argument('--n_epochs', type=int, default=20, help='number of epochs with the initial learning rate')
- parser.add_argument('--lr', type=float, default=0.0001, help='initial learning rate for adam')
- parser.add_argument('--lr_policy', type=str, default='step', help='learning rate policy. [linear | step | plateau | cosine]')
- parser.add_argument('--lr_decay_epochs', type=int, default=10, help='multiply by a gamma every lr_decay_epochs epoches')
-
- self.isTrain = True
- return parser
diff --git a/spaces/kevinwang676/ChatGLM2-SadTalker/src/facerender/sync_batchnorm/replicate.py b/spaces/kevinwang676/ChatGLM2-SadTalker/src/facerender/sync_batchnorm/replicate.py
deleted file mode 100644
index b71c7b8ed51a1d6c55b1f753bdd8d90bad79bd06..0000000000000000000000000000000000000000
--- a/spaces/kevinwang676/ChatGLM2-SadTalker/src/facerender/sync_batchnorm/replicate.py
+++ /dev/null
@@ -1,94 +0,0 @@
-# -*- coding: utf-8 -*-
-# File : replicate.py
-# Author : Jiayuan Mao
-# Email : maojiayuan@gmail.com
-# Date : 27/01/2018
-#
-# This file is part of Synchronized-BatchNorm-PyTorch.
-# https://github.com/vacancy/Synchronized-BatchNorm-PyTorch
-# Distributed under MIT License.
-
-import functools
-
-from torch.nn.parallel.data_parallel import DataParallel
-
-__all__ = [
- 'CallbackContext',
- 'execute_replication_callbacks',
- 'DataParallelWithCallback',
- 'patch_replication_callback'
-]
-
-
-class CallbackContext(object):
- pass
-
-
-def execute_replication_callbacks(modules):
- """
- Execute an replication callback `__data_parallel_replicate__` on each module created by original replication.
-
- The callback will be invoked with arguments `__data_parallel_replicate__(ctx, copy_id)`
-
- Note that, as all modules are isomorphism, we assign each sub-module with a context
- (shared among multiple copies of this module on different devices).
- Through this context, different copies can share some information.
-
- We guarantee that the callback on the master copy (the first copy) will be called ahead of calling the callback
- of any slave copies.
- """
- master_copy = modules[0]
- nr_modules = len(list(master_copy.modules()))
- ctxs = [CallbackContext() for _ in range(nr_modules)]
-
- for i, module in enumerate(modules):
- for j, m in enumerate(module.modules()):
- if hasattr(m, '__data_parallel_replicate__'):
- m.__data_parallel_replicate__(ctxs[j], i)
-
-
-class DataParallelWithCallback(DataParallel):
- """
- Data Parallel with a replication callback.
-
- An replication callback `__data_parallel_replicate__` of each module will be invoked after being created by
- original `replicate` function.
- The callback will be invoked with arguments `__data_parallel_replicate__(ctx, copy_id)`
-
- Examples:
- > sync_bn = SynchronizedBatchNorm1d(10, eps=1e-5, affine=False)
- > sync_bn = DataParallelWithCallback(sync_bn, device_ids=[0, 1])
- # sync_bn.__data_parallel_replicate__ will be invoked.
- """
-
- def replicate(self, module, device_ids):
- modules = super(DataParallelWithCallback, self).replicate(module, device_ids)
- execute_replication_callbacks(modules)
- return modules
-
-
-def patch_replication_callback(data_parallel):
- """
- Monkey-patch an existing `DataParallel` object. Add the replication callback.
- Useful when you have customized `DataParallel` implementation.
-
- Examples:
- > sync_bn = SynchronizedBatchNorm1d(10, eps=1e-5, affine=False)
- > sync_bn = DataParallel(sync_bn, device_ids=[0, 1])
- > patch_replication_callback(sync_bn)
- # this is equivalent to
- > sync_bn = SynchronizedBatchNorm1d(10, eps=1e-5, affine=False)
- > sync_bn = DataParallelWithCallback(sync_bn, device_ids=[0, 1])
- """
-
- assert isinstance(data_parallel, DataParallel)
-
- old_replicate = data_parallel.replicate
-
- @functools.wraps(old_replicate)
- def new_replicate(module, device_ids):
- modules = old_replicate(module, device_ids)
- execute_replication_callbacks(modules)
- return modules
-
- data_parallel.replicate = new_replicate
diff --git a/spaces/kevinwang676/ChatGLM2-VC-SadTalker/src/face3d/models/arcface_torch/utils/__init__.py b/spaces/kevinwang676/ChatGLM2-VC-SadTalker/src/face3d/models/arcface_torch/utils/__init__.py
deleted file mode 100644
index e69de29bb2d1d6434b8b29ae775ad8c2e48c5391..0000000000000000000000000000000000000000
diff --git a/spaces/kevinwang676/FreeVC-en/speaker_encoder/voice_encoder.py b/spaces/kevinwang676/FreeVC-en/speaker_encoder/voice_encoder.py
deleted file mode 100644
index 88cdee2de76b72db58c5dd19a888597e0fe12fbb..0000000000000000000000000000000000000000
--- a/spaces/kevinwang676/FreeVC-en/speaker_encoder/voice_encoder.py
+++ /dev/null
@@ -1,173 +0,0 @@
-from speaker_encoder.hparams import *
-from speaker_encoder import audio
-from pathlib import Path
-from typing import Union, List
-from torch import nn
-from time import perf_counter as timer
-import numpy as np
-import torch
-
-
-class SpeakerEncoder(nn.Module):
- def __init__(self, weights_fpath, device: Union[str, torch.device]=None, verbose=True):
- """
- :param device: either a torch device or the name of a torch device (e.g. "cpu", "cuda").
- If None, defaults to cuda if it is available on your machine, otherwise the model will
- run on cpu. Outputs are always returned on the cpu, as numpy arrays.
- """
- super().__init__()
-
- # Define the network
- self.lstm = nn.LSTM(mel_n_channels, model_hidden_size, model_num_layers, batch_first=True)
- self.linear = nn.Linear(model_hidden_size, model_embedding_size)
- self.relu = nn.ReLU()
-
- # Get the target device
- if device is None:
- device = torch.device("cuda" if torch.cuda.is_available() else "cpu")
- elif isinstance(device, str):
- device = torch.device(device)
- self.device = device
-
- # Load the pretrained model'speaker weights
- # weights_fpath = Path(__file__).resolve().parent.joinpath("pretrained.pt")
- # if not weights_fpath.exists():
- # raise Exception("Couldn't find the voice encoder pretrained model at %s." %
- # weights_fpath)
-
- start = timer()
- checkpoint = torch.load(weights_fpath, map_location="cpu")
-
- self.load_state_dict(checkpoint["model_state"], strict=False)
- self.to(device)
-
- if verbose:
- print("Loaded the voice encoder model on %s in %.2f seconds." %
- (device.type, timer() - start))
-
- def forward(self, mels: torch.FloatTensor):
- """
- Computes the embeddings of a batch of utterance spectrograms.
- :param mels: a batch of mel spectrograms of same duration as a float32 tensor of shape
- (batch_size, n_frames, n_channels)
- :return: the embeddings as a float 32 tensor of shape (batch_size, embedding_size).
- Embeddings are positive and L2-normed, thus they lay in the range [0, 1].
- """
- # Pass the input through the LSTM layers and retrieve the final hidden state of the last
- # layer. Apply a cutoff to 0 for negative values and L2 normalize the embeddings.
- _, (hidden, _) = self.lstm(mels)
- embeds_raw = self.relu(self.linear(hidden[-1]))
- return embeds_raw / torch.norm(embeds_raw, dim=1, keepdim=True)
-
- @staticmethod
- def compute_partial_slices(n_samples: int, rate, min_coverage):
- """
- Computes where to split an utterance waveform and its corresponding mel spectrogram to
- obtain partial utterances of each. Both the waveform and the
- mel spectrogram slices are returned, so as to make each partial utterance waveform
- correspond to its spectrogram.
-
- The returned ranges may be indexing further than the length of the waveform. It is
- recommended that you pad the waveform with zeros up to wav_slices[-1].stop.
-
- :param n_samples: the number of samples in the waveform
- :param rate: how many partial utterances should occur per second. Partial utterances must
- cover the span of the entire utterance, thus the rate should not be lower than the inverse
- of the duration of a partial utterance. By default, partial utterances are 1.6s long and
- the minimum rate is thus 0.625.
- :param min_coverage: when reaching the last partial utterance, it may or may not have
- enough frames. If at least of are present,
- then the last partial utterance will be considered by zero-padding the audio. Otherwise,
- it will be discarded. If there aren't enough frames for one partial utterance,
- this parameter is ignored so that the function always returns at least one slice.
- :return: the waveform slices and mel spectrogram slices as lists of array slices. Index
- respectively the waveform and the mel spectrogram with these slices to obtain the partial
- utterances.
- """
- assert 0 < min_coverage <= 1
-
- # Compute how many frames separate two partial utterances
- samples_per_frame = int((sampling_rate * mel_window_step / 1000))
- n_frames = int(np.ceil((n_samples + 1) / samples_per_frame))
- frame_step = int(np.round((sampling_rate / rate) / samples_per_frame))
- assert 0 < frame_step, "The rate is too high"
- assert frame_step <= partials_n_frames, "The rate is too low, it should be %f at least" % \
- (sampling_rate / (samples_per_frame * partials_n_frames))
-
- # Compute the slices
- wav_slices, mel_slices = [], []
- steps = max(1, n_frames - partials_n_frames + frame_step + 1)
- for i in range(0, steps, frame_step):
- mel_range = np.array([i, i + partials_n_frames])
- wav_range = mel_range * samples_per_frame
- mel_slices.append(slice(*mel_range))
- wav_slices.append(slice(*wav_range))
-
- # Evaluate whether extra padding is warranted or not
- last_wav_range = wav_slices[-1]
- coverage = (n_samples - last_wav_range.start) / (last_wav_range.stop - last_wav_range.start)
- if coverage < min_coverage and len(mel_slices) > 1:
- mel_slices = mel_slices[:-1]
- wav_slices = wav_slices[:-1]
-
- return wav_slices, mel_slices
-
- def embed_utterance(self, wav: np.ndarray, return_partials=False, rate=1.3, min_coverage=0.75):
- """
- Computes an embedding for a single utterance. The utterance is divided in partial
- utterances and an embedding is computed for each. The complete utterance embedding is the
- L2-normed average embedding of the partial utterances.
-
- TODO: independent batched version of this function
-
- :param wav: a preprocessed utterance waveform as a numpy array of float32
- :param return_partials: if True, the partial embeddings will also be returned along with
- the wav slices corresponding to each partial utterance.
- :param rate: how many partial utterances should occur per second. Partial utterances must
- cover the span of the entire utterance, thus the rate should not be lower than the inverse
- of the duration of a partial utterance. By default, partial utterances are 1.6s long and
- the minimum rate is thus 0.625.
- :param min_coverage: when reaching the last partial utterance, it may or may not have
- enough frames. If at least of are present,
- then the last partial utterance will be considered by zero-padding the audio. Otherwise,
- it will be discarded. If there aren't enough frames for one partial utterance,
- this parameter is ignored so that the function always returns at least one slice.
- :return: the embedding as a numpy array of float32 of shape (model_embedding_size,). If
- is True, the partial utterances as a numpy array of float32 of shape
- (n_partials, model_embedding_size) and the wav partials as a list of slices will also be
- returned.
- """
- # Compute where to split the utterance into partials and pad the waveform with zeros if
- # the partial utterances cover a larger range.
- wav_slices, mel_slices = self.compute_partial_slices(len(wav), rate, min_coverage)
- max_wave_length = wav_slices[-1].stop
- if max_wave_length >= len(wav):
- wav = np.pad(wav, (0, max_wave_length - len(wav)), "constant")
-
- # Split the utterance into partials and forward them through the model
- mel = audio.wav_to_mel_spectrogram(wav)
- mels = np.array([mel[s] for s in mel_slices])
- with torch.no_grad():
- mels = torch.from_numpy(mels).to(self.device)
- partial_embeds = self(mels).cpu().numpy()
-
- # Compute the utterance embedding from the partial embeddings
- raw_embed = np.mean(partial_embeds, axis=0)
- embed = raw_embed / np.linalg.norm(raw_embed, 2)
-
- if return_partials:
- return embed, partial_embeds, wav_slices
- return embed
-
- def embed_speaker(self, wavs: List[np.ndarray], **kwargs):
- """
- Compute the embedding of a collection of wavs (presumably from the same speaker) by
- averaging their embedding and L2-normalizing it.
-
- :param wavs: list of wavs a numpy arrays of float32.
- :param kwargs: extra arguments to embed_utterance()
- :return: the embedding as a numpy array of float32 of shape (model_embedding_size,).
- """
- raw_embed = np.mean([self.embed_utterance(wav, return_partials=False, **kwargs) \
- for wav in wavs], axis=0)
- return raw_embed / np.linalg.norm(raw_embed, 2)
\ No newline at end of file
diff --git a/spaces/kevinwang676/voice-conversion-yourtts/parseinput.py b/spaces/kevinwang676/voice-conversion-yourtts/parseinput.py
deleted file mode 100644
index 990a4edbabc9b81e275e203d654cda6ba8561ac4..0000000000000000000000000000000000000000
--- a/spaces/kevinwang676/voice-conversion-yourtts/parseinput.py
+++ /dev/null
@@ -1,128 +0,0 @@
-import re
-import xml.etree.ElementTree as ET
-from xml.sax import saxutils
-#import nltk
-
-# Chunked generation originally from https://github.com/serp-ai/bark-with-voice-clone
-def split_and_recombine_text(text, desired_length=100, max_length=150):
- # return nltk.sent_tokenize(text)
-
- # from https://github.com/neonbjb/tortoise-tts
- """Split text it into chunks of a desired length trying to keep sentences intact."""
- # normalize text, remove redundant whitespace and convert non-ascii quotes to ascii
- text = re.sub(r"\n\n+", "\n", text)
- text = re.sub(r"\s+", " ", text)
- text = re.sub(r"[“”]", '"', text)
-
- rv = []
- in_quote = False
- current = ""
- split_pos = []
- pos = -1
- end_pos = len(text) - 1
-
- def seek(delta):
- nonlocal pos, in_quote, current
- is_neg = delta < 0
- for _ in range(abs(delta)):
- if is_neg:
- pos -= 1
- current = current[:-1]
- else:
- pos += 1
- current += text[pos]
- if text[pos] == '"':
- in_quote = not in_quote
- return text[pos]
-
- def peek(delta):
- p = pos + delta
- return text[p] if p < end_pos and p >= 0 else ""
-
- def commit():
- nonlocal rv, current, split_pos
- rv.append(current)
- current = ""
- split_pos = []
-
- while pos < end_pos:
- c = seek(1)
- # do we need to force a split?
- if len(current) >= max_length:
- if len(split_pos) > 0 and len(current) > (desired_length / 2):
- # we have at least one sentence and we are over half the desired length, seek back to the last split
- d = pos - split_pos[-1]
- seek(-d)
- else:
- # no full sentences, seek back until we are not in the middle of a word and split there
- while c not in "!?.,\n " and pos > 0 and len(current) > desired_length:
- c = seek(-1)
- commit()
- # check for sentence boundaries
- elif not in_quote and (c in "!?]\n" or (c == "." and peek(1) in "\n ")):
- # seek forward if we have consecutive boundary markers but still within the max length
- while (
- pos < len(text) - 1 and len(current) < max_length and peek(1) in "!?.]"
- ):
- c = seek(1)
- split_pos.append(pos)
- if len(current) >= desired_length:
- commit()
- # treat end of quote as a boundary if its followed by a space or newline
- elif in_quote and peek(1) == '"' and peek(2) in "\n ":
- seek(2)
- split_pos.append(pos)
- rv.append(current)
-
- # clean up, remove lines with only whitespace or punctuation
- rv = [s.strip() for s in rv]
- rv = [s for s in rv if len(s) > 0 and not re.match(r"^[\s\.,;:!?]*$", s)]
-
- return rv
-
-def is_ssml(value):
- try:
- ET.fromstring(value)
- except ET.ParseError:
- return False
- return True
-
-def build_ssml(rawtext, selected_voice):
- texts = rawtext.split("\n")
- joinedparts = ""
- for textpart in texts:
- textpart = textpart.strip()
- if len(textpart) < 1:
- continue
- joinedparts = joinedparts + f"\n{saxutils.escape(textpart)}"
- ssml = f"""
-
- {joinedparts}
-
- """
- return ssml
-
-def create_clips_from_ssml(ssmlinput):
- # Parse the XML
- tree = ET.ElementTree(ET.fromstring(ssmlinput))
- root = tree.getroot()
-
- # Create an empty list
- voice_list = []
-
- # Loop through all voice tags
- for voice in root.iter('{http://www.w3.org/2001/10/synthesis}voice'):
- # Extract the voice name attribute and the content text
- voice_name = voice.attrib['name']
- voice_content = voice.text.strip() if voice.text else ''
- if(len(voice_content) > 0):
- parts = split_and_recombine_text(voice_content)
- for p in parts:
- if(len(p) > 1):
- # add to tuple list
- voice_list.append((voice_name, p))
- return voice_list
diff --git a/spaces/koajoel/PolyFormer/fairseq/examples/noisychannel/rerank_options.py b/spaces/koajoel/PolyFormer/fairseq/examples/noisychannel/rerank_options.py
deleted file mode 100644
index de91939e6635bdf33c9dc330116be07d9e8be6a2..0000000000000000000000000000000000000000
--- a/spaces/koajoel/PolyFormer/fairseq/examples/noisychannel/rerank_options.py
+++ /dev/null
@@ -1,149 +0,0 @@
-# Copyright (c) Facebook, Inc. and its affiliates.
-#
-# This source code is licensed under the MIT license found in the
-# LICENSE file in the root directory of this source tree.
-
-from fairseq import options
-
-
-def get_reranking_parser(default_task="translation"):
- parser = options.get_parser("Generation and reranking", default_task)
- add_reranking_args(parser)
- return parser
-
-
-def get_tuning_parser(default_task="translation"):
- parser = options.get_parser("Reranking tuning", default_task)
- add_reranking_args(parser)
- add_tuning_args(parser)
- return parser
-
-
-def add_reranking_args(parser):
- group = parser.add_argument_group("Reranking")
- # fmt: off
- group.add_argument('--score-model1', '-s1', type=str, metavar='FILE', required=True,
- help='path to first model or ensemble of models for rescoring')
- group.add_argument('--score-model2', '-s2', type=str, metavar='FILE', required=False,
- help='path to second model or ensemble of models for rescoring')
- group.add_argument('--num-rescore', '-n', type=int, metavar='N', default=10,
- help='the number of candidate hypothesis to rescore')
- group.add_argument('-bz', '--batch-size', type=int, metavar='N', default=128,
- help='batch size for generating the nbest list')
- group.add_argument('--gen-subset', default='test', metavar='SET', choices=['test', 'train', 'valid'],
- help='data subset to generate (train, valid, test)')
- group.add_argument('--gen-model', default=None, metavar='FILE',
- help='the model to generate translations')
- group.add_argument('-b1', '--backwards1', action='store_true',
- help='whether or not the first model group is backwards')
- group.add_argument('-b2', '--backwards2', action='store_true',
- help='whether or not the second model group is backwards')
- group.add_argument('-a', '--weight1', default=1, nargs='+', type=float,
- help='the weight(s) of the first model')
- group.add_argument('-b', '--weight2', default=1, nargs='+', type=float,
- help='the weight(s) of the second model, or the gen model if using nbest from interactive.py')
- group.add_argument('-c', '--weight3', default=1, nargs='+', type=float,
- help='the weight(s) of the third model')
-
- # lm arguments
- group.add_argument('-lm', '--language-model', default=None, metavar='FILE',
- help='language model for target language to rescore translations')
- group.add_argument('--lm-dict', default=None, metavar='FILE',
- help='the dict of the language model for the target language')
- group.add_argument('--lm-name', default=None,
- help='the name of the language model for the target language')
- group.add_argument('--lm-bpe-code', default=None, metavar='FILE',
- help='the bpe code for the language model for the target language')
- group.add_argument('--data-dir-name', default=None,
- help='name of data directory')
- group.add_argument('--lenpen', default=1, nargs='+', type=float,
- help='length penalty: <1.0 favors shorter, >1.0 favors longer sentences')
- group.add_argument('--score-dict-dir', default=None,
- help='the directory with dictionaries for the scoring models')
- group.add_argument('--right-to-left1', action='store_true',
- help='whether the first model group is a right to left model')
- group.add_argument('--right-to-left2', action='store_true',
- help='whether the second model group is a right to left model')
- group.add_argument('--post-process', '--remove-bpe', default='@@ ',
- help='the bpe symbol, used for the bitext and LM')
- group.add_argument('--prefix-len', default=None, type=int,
- help='the length of the target prefix to use in rescoring (in terms of words wo bpe)')
- group.add_argument('--sampling', action='store_true',
- help='use sampling instead of beam search for generating n best list')
- group.add_argument('--diff-bpe', action='store_true',
- help='bpe for rescoring and nbest list not the same')
- group.add_argument('--rescore-bpe-code', default=None,
- help='bpe code for rescoring models')
- group.add_argument('--nbest-list', default=None,
- help='use predefined nbest list in interactive.py format')
- group.add_argument('--write-hypos', default=None,
- help='filename prefix to write hypos to')
- group.add_argument('--ref-translation', default=None,
- help='reference translation to use with nbest list from interactive.py')
- group.add_argument('--backwards-score-dict-dir', default=None,
- help='the directory with dictionaries for the backwards model,'
- 'if None then it is assumed the fw and backwards models share dictionaries')
-
- # extra scaling args
- group.add_argument('--gen-model-name', default=None,
- help='the name of the models that generated the nbest list')
- group.add_argument('--model1-name', default=None,
- help='the name of the set for model1 group ')
- group.add_argument('--model2-name', default=None,
- help='the name of the set for model2 group')
- group.add_argument('--shard-id', default=0, type=int,
- help='the id of the shard to generate')
- group.add_argument('--num-shards', default=1, type=int,
- help='the number of shards to generate across')
- group.add_argument('--all-shards', action='store_true',
- help='use all shards')
- group.add_argument('--target-prefix-frac', default=None, type=float,
- help='the fraction of the target prefix to use in rescoring (in terms of words wo bpe)')
- group.add_argument('--source-prefix-frac', default=None, type=float,
- help='the fraction of the source prefix to use in rescoring (in terms of words wo bpe)')
- group.add_argument('--normalize', action='store_true',
- help='whether to normalize by src and target len')
- # fmt: on
- return group
-
-
-def add_tuning_args(parser):
- group = parser.add_argument_group("Tuning")
-
- group.add_argument(
- "--lower-bound",
- default=[-0.7],
- nargs="+",
- type=float,
- help="lower bound of search space",
- )
- group.add_argument(
- "--upper-bound",
- default=[3],
- nargs="+",
- type=float,
- help="upper bound of search space",
- )
- group.add_argument(
- "--tune-param",
- default=["lenpen"],
- nargs="+",
- choices=["lenpen", "weight1", "weight2", "weight3"],
- help="the parameter(s) to tune",
- )
- group.add_argument(
- "--tune-subset",
- default="valid",
- choices=["valid", "test", "train"],
- help="the subset to tune on ",
- )
- group.add_argument(
- "--num-trials",
- default=1000,
- type=int,
- help="number of trials to do for random search",
- )
- group.add_argument(
- "--share-weights", action="store_true", help="share weight2 and weight 3"
- )
- return group
diff --git a/spaces/kobayashi123/bingo/README.md b/spaces/kobayashi123/bingo/README.md
deleted file mode 100644
index 5d6936218874c647b5d22e13ad4be7edb8936f92..0000000000000000000000000000000000000000
--- a/spaces/kobayashi123/bingo/README.md
+++ /dev/null
@@ -1,28 +0,0 @@
----
-title: bingo
-emoji: 😊
-colorFrom: red
-colorTo: red
-sdk: docker
-license: mit
-duplicated_from: hf4all/bingo
----
-
-
-
-# Bingo
-
-Bingo,一个让你呼吸顺畅 New Bing。
-
-高度还原 New Bing 网页版的主要操作,国内可用,兼容绝大多数微软 Bing AI 的功能,可自行部署使用。
-
-
-
-[](https://hub.docker.com/repository/docker/weaigc/bingo/)
-[](https://hub.docker.com/repository/docker/weaigc/bingo/)
-[](https://github.com/weaigc/bingo/blob/main/license)
-
-问题反馈请前往 https://github.com/weaigc/bingo/issues
-
-
-
diff --git a/spaces/ky2k/Toxicity_Classifier_POC/.venv/lib/python3.9/site-packages/jinja2/constants.py b/spaces/ky2k/Toxicity_Classifier_POC/.venv/lib/python3.9/site-packages/jinja2/constants.py
deleted file mode 100644
index 41a1c23b0a7fe134b1f662545876eb65b31b071e..0000000000000000000000000000000000000000
--- a/spaces/ky2k/Toxicity_Classifier_POC/.venv/lib/python3.9/site-packages/jinja2/constants.py
+++ /dev/null
@@ -1,20 +0,0 @@
-#: list of lorem ipsum words used by the lipsum() helper function
-LOREM_IPSUM_WORDS = """\
-a ac accumsan ad adipiscing aenean aliquam aliquet amet ante aptent arcu at
-auctor augue bibendum blandit class commodo condimentum congue consectetuer
-consequat conubia convallis cras cubilia cum curabitur curae cursus dapibus
-diam dictum dictumst dignissim dis dolor donec dui duis egestas eget eleifend
-elementum elit enim erat eros est et etiam eu euismod facilisi facilisis fames
-faucibus felis fermentum feugiat fringilla fusce gravida habitant habitasse hac
-hendrerit hymenaeos iaculis id imperdiet in inceptos integer interdum ipsum
-justo lacinia lacus laoreet lectus leo libero ligula litora lobortis lorem
-luctus maecenas magna magnis malesuada massa mattis mauris metus mi molestie
-mollis montes morbi mus nam nascetur natoque nec neque netus nibh nisi nisl non
-nonummy nostra nulla nullam nunc odio orci ornare parturient pede pellentesque
-penatibus per pharetra phasellus placerat platea porta porttitor posuere
-potenti praesent pretium primis proin pulvinar purus quam quis quisque rhoncus
-ridiculus risus rutrum sagittis sapien scelerisque sed sem semper senectus sit
-sociis sociosqu sodales sollicitudin suscipit suspendisse taciti tellus tempor
-tempus tincidunt torquent tortor tristique turpis ullamcorper ultrices
-ultricies urna ut varius vehicula vel velit venenatis vestibulum vitae vivamus
-viverra volutpat vulputate"""
diff --git a/spaces/ky2k/summarize_text/app.py b/spaces/ky2k/summarize_text/app.py
deleted file mode 100644
index fb58c3c2cb3991a2a582482a2628f92aa8f971ee..0000000000000000000000000000000000000000
--- a/spaces/ky2k/summarize_text/app.py
+++ /dev/null
@@ -1,47 +0,0 @@
-import gradio as gr
-from summarizer import TransformerSummarizer, Summarizer
-
-title = "Summarizer"
-description = """
-This is a demo of a text summarization NN - based on GPT-2, XLNet, BERT,
-works with English, Ukrainian, and Russian (and a few other languages too, these are SOTA NN after all).
-"""
-
-NN_OPTIONS_LIST = ["mean", "max", "min", "median"]
-NN_LIST = ["GPT-2", "XLNet", "BERT"]
-
-
-def start_fn(article_input: str, reduce_option="mean", model_type='GPT-2') -> str:
- """
- GPT-2 based solution, input full text, output summarized text
- :param model_type:
- :param reduce_option:
- :param article_input:
- :return summarized article_output:
- """
- if model_type == "GPT-2":
- GPT2_model = TransformerSummarizer(transformer_type="GPT2", transformer_model_key="gpt2-medium",
- reduce_option=reduce_option)
- full = ''.join(GPT2_model(article_input, min_length=60))
- return full
- elif model_type == "XLNet":
- XLNet_model = TransformerSummarizer(transformer_type="XLNet", transformer_model_key="xlnet-base-cased",
- reduce_option=reduce_option)
- full = ''.join(XLNet_model(article_input, min_length=60))
- return full
-
- elif model_type == "BERT":
- BERT_model = Summarizer(reduce_option=reduce_option)
- full = ''.join(BERT_model(article_input, min_length=60))
- return full
-
-
-face = gr.Interface(fn=start_fn,
- inputs=[gr.inputs.Textbox(lines=2, placeholder="Paste article here.", label='Input Article'),
- gr.inputs.Dropdown(NN_OPTIONS_LIST, label="Summarize mode"),
- gr.inputs.Dropdown(NN_LIST, label="Selected NN")],
- outputs=gr.inputs.Textbox(lines=2, placeholder="Summarized article here.", label='Summarized '
- 'Article'),
- title=title,
- description=description, )
-face.launch(server_name="0.0.0.0", share=True)
diff --git a/spaces/kyleebrooks/VectorDatabaseCreate/app.py b/spaces/kyleebrooks/VectorDatabaseCreate/app.py
deleted file mode 100644
index a003267dbeb7d95a35f53d84fbb4ef023b8ac807..0000000000000000000000000000000000000000
--- a/spaces/kyleebrooks/VectorDatabaseCreate/app.py
+++ /dev/null
@@ -1,233 +0,0 @@
-from llama_index import SimpleDirectoryReader, Prompt, LLMPredictor, GPTVectorStoreIndex, VectorStoreIndex, PromptHelper, ServiceContext, load_index_from_storage, StorageContext
-from llama_index.node_parser import SimpleNodeParser
-from llama_index.data_structs import Node
-from langchain.chat_models import ChatOpenAI
-from huggingface_hub import whoami
-from huggingface_hub import HfApi
-from huggingface_hub import login
-import os
-import openai
-import tiktoken
-import shutil
-import gradio as gr
-
-
-
-#if you have OpenAI API key as a string, enable the below
-openai.api_key = ""
-os.environ["OPENAI_API_KEY"] = ''
-large_document=""
-api=HfApi()
-model_type=""
-messages = []
-Chat_message = []
-chat_history=[]
-custom_chat_history=[]
-max_input_size = 4096
-num_outputs = 512
-chunk_size_limit = 600
-chunk_overlap_ratio = .1
-
-
-prompt_helper = PromptHelper(max_input_size, num_outputs, chunk_overlap_ratio, chunk_size_limit)
-
-store = './storage'
-#store = 'kyleebrooks/Data/storage'
-
-max_response_tokens = 1000
-token_limit= 4097
-
-template = (
- "This Chatbot is helpful, accurate, and will use the context below for answering all questions. This Chatbot will not answer questions not included in the context provided \n"
- "---------------------\n"
- "{context_str}"
- "\n---------------------\n"
- "Given this information, please answer the question by providing a detailed summary and provide accurate citations for all referenced areas at the end of each response. {query_str}\n"
-)
-qa_template = Prompt(template)
-
-def upload_file (index, input_file):
- login(token="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")
- json_list=["docstore.json", "graph_store.json", "index_store.json", "vector_store.json"]
- os.mkdir("/tmp/gradio/json")
- index.storage_context.persist(persist_dir="/tmp/gradio/json")
- for i in json_list:
- print(i)
- api.upload_file(
- path_or_fileobj="/tmp/gradio/json/"+i,
- #path_or_fileobj=i.name,
- path_in_repo="storage/"+i,
- repo_id="kyleebrooks/VectorDatabaseCreate",
- repo_type="space" # dataset
- )
-
-#loads openai key
-def load_api_key (api_key):
- os.environ["OPENAI_API_KEY"] = str(api_key)
- openai.api_key = str(api_key)
-
-#identifies the current number of tokens used for the conversation
-def num_tokens_from_messages(messages, model_type):
- encoding = tiktoken.encoding_for_model(model_type)
- num_tokens = 0
- for message in messages:
- num_tokens += 4 # every message follows {role/name}\n{content}\n
- for key, value in message.items():
- num_tokens += len(encoding.encode(value))
- if key == "name": # if there's a name, the role is omitted
- num_tokens += -1 # role is always required and always 1 token
- num_tokens += 2 # every reply is primed with assistant
- print(num_tokens)
- return num_tokens
-
-#constructs the index and saves to a subfolder
-def construct_index(create_index, input_file, model_type, save_index):
- if create_index == "Yes":
- login(token="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")
- source=input_file[0].name
- suffix = source.rsplit("/", 1)[1]
- prefix = source.rsplit("/", 2)[0]
- directories=[]
- print(prefix+" This is the Prefix")
- for i in input_file:
- directories.append(i.name)
- print(i.name)
- response="constructing index"
- print('Constructing index')
- # load in the documents from the docs subfolder
- llm_predictor = LLMPredictor(llm=ChatOpenAI(temperature=0.1, model_name=model_type, max_tokens=num_outputs))
- service_context = ServiceContext.from_defaults(llm_predictor=llm_predictor, prompt_helper=prompt_helper)
- docs = SimpleDirectoryReader(input_files=directories, filename_as_id=True).load_data()
- #Large_document=str(docs)
- #node_parser = SimpleNodeParser.from_defaults(chunk_size=1024, chunk_overlap=20)
- # Use the Node Parser to get nodes from the document
- #nodes = node_parser.get_nodes_from_documents([large_document], show_progress=False)
- # Each node in the 'nodes' list will contain a smaller chunk of the text file
-
- #index = GPTVectorStoreIndex.from_documents(nodes, service_context=service_context)
- index = GPTVectorStoreIndex.from_documents(docs, service_context=service_context)
- #index = VectorStoreIndex.from_documents(docs, service_context=service_context)
- index.set_index_id('vector_index')
- # Stores json files in a subfolder
- if save_index=="Yes":
- upload_file(index, input_file)
- index_status="Index constructed and saved, allow time for loading"
- else:
- index_status="Index constructed but not saved for future use"
- index.storage_context.persist(persist_dir=store)
- # Clears out temporary files
- shutil.rmtree(prefix)
- response=index_status
- return response
- else:
- response= "You did not select Yes to load a new index."
-
- return response
-
-
-#resets the conversation
-def generate_restart(prompt, model_type):
-
- messages.clear()
- messages.append({"role":"system", "content": "Tell the user that this conversation has been reset due to the discussion size reaching maximum size, and to please start by asking a new question."})
- storage_context = StorageContext.from_defaults(persist_dir=store)
- llm_predictor = LLMPredictor(llm=ChatOpenAI(temperature=0.1, model_name=model_type, max_tokens=num_outputs))
- service_context = ServiceContext.from_defaults(llm_predictor=llm_predictor, prompt_helper=prompt_helper)
- #index = load_index_from_storage(storage_context)
- index = load_index_from_storage(
- StorageContext.from_defaults(persist_dir=store),
- service_context=service_context,
- )
- #query_engine = index.as_query_engine(text_qa_template=qa_template)
- chat_engine = index.as_chat_engine(text_qa_template=qa_template)
- string_message=str(messages)
- #response = query_engine.query(string_message)
- response = chat_engine.chat(messages)
- messages.clear()
- messages.append({"role":"system", "content": "This Chatbot is helpful, accurate, and provides all relevnt information from the Treasury Financial Manual (TFM) when responding. This Chatbot always provides accurate citations from the TFM."})
- messages.append({"role":"user","content": ""})
- messages.append({"role":"assistant","content": ""})
- print("restert initiated")
- print(messages)
- return response.response
-
-#generates the ChatGPT call
-def generate_response(prompt, model_type):
-
- messages.append({"role": "user", "content": prompt})
- storage_context = StorageContext.from_defaults(persist_dir=store)
- llm_predictor = LLMPredictor(llm=ChatOpenAI(temperature=0.1, model_name=model_type, max_tokens=num_outputs))
- service_context = ServiceContext.from_defaults(llm=ChatOpenAI(temperature=0., model_name=model_type))
- #service_context = ServiceContext.from_defaults(llm_predictor=llm_predictor, prompt_helper=prompt_helper)
- index = load_index_from_storage(
- StorageContext.from_defaults(persist_dir=store),
- service_context=service_context,
- )
- #chat_engine = index.as_chat_engine(verbose=True, chat_history=chat_history, text_qa_template=qa_template, chat_mode='condense_question')
- query_engine = index.as_query_engine(text_qa_template=qa_template)
- string_message=str(messages)
- response = query_engine.query(prompt)
- #response = chat_engine.chat(prompt, chat_history)
- string_response=str(response)
- messages.append({"role": "assistant", "content":string_response})
- num_tokens_from_messages(messages, model_type)
- print(messages)
- print("below is history")
- print(chat_history)
-
- return ('MIL Custom Index Chatbot: '+response.response)
-
-
-def my_chatbot(input, history, model_type):
- history = history or []
- if num_tokens_from_messages(messages, model_type)<(int(token_limit)-int(max_response_tokens)):
- output = generate_response(input, model_type)
- history.append((input, output))
- return history, history
- else:
- history.clear()
- output = generate_restart(input, model_type)
- history.append((input, output))
- prompt=input
- return prompt, prompt
-
-def index_chatbot(input_text):
- if not hasattr(chatbot, 'index'):
- storage_context = StorageContext.from_defaults(persist_dir=store)
- index = load_index_from_storage(storage_context)
- query_engine = chatbot.index.as_query_engine(text_qa_template=QA_TEMPLATE)
- response = chatbot.query_engine.query(input_text)
- return response.response
-
-
-with gr.Blocks() as demo:
-
- gr.Markdown("""
MIL Custom Vector Index Chatbot
""")
- gr.Image(value="logo.PNG", width=200, height=150, interactive=False, show_share_button=False)
- api_key = gr.Textbox(type='password', label="Enter the API key", width=250)
- input_file = gr.Files()
- #load_btn.click(in_to_out,input_file,output_file)
- with gr.Row().style(equal_height=True):
- create_index = gr.Radio(["Yes", "No"], label = "index creation", info="Would you like to create a new index?", value="No")
- model_type = gr.Radio(["gpt-3.5-turbo", "gpt-4"], label = "Model_Type", info="Would you like to create a new index?", value="gpt-3.5-turbo")
- save_index = gr.Radio(["Yes", "No"], label = "Save Index", info="Would you like to save the index for future use?", value="No")
- output = gr.Textbox(
- label="Output",
- info="",
- lines=1
- )
- submit_index = gr.Button("Create Index")
- submit_index.click(load_api_key, [api_key])
- chatbot = gr.Chatbot()
- state = gr.State()
- text = gr.Textbox(label="Input", info="", lines=2, placeholder="Hello. Ask me a question about the indexed content. Please approach each question as if it is a new question, my memory is limited in this model.")
- submit = gr.Button("SEND")
- submit.click(load_api_key, [api_key])
- submit.click(my_chatbot, inputs=[text, state, model_type], outputs=[chatbot, state])
- submit_index.click(construct_index, [create_index, input_file, model_type, save_index], output, show_progress=True)
-
-
-demo.launch(share = False)
-
-
-
diff --git a/spaces/lcipolina/Print_Gallery/README.md b/spaces/lcipolina/Print_Gallery/README.md
deleted file mode 100644
index 39cf16214b7935f26f605cc9b8f0cf3b418657c0..0000000000000000000000000000000000000000
--- a/spaces/lcipolina/Print_Gallery/README.md
+++ /dev/null
@@ -1,13 +0,0 @@
----
-title: Print_Gallery
-emoji: 😻
-colorFrom: gray
-colorTo: indigo
-sdk: gradio
-sdk_version: 2.8.14
-app_file: app.py
-pinned: false
-license: afl-3.0
----
-
-Check out the configuration reference at https://huggingface.co/docs/hub/spaces#reference
diff --git a/spaces/legoandmars/glide-inpainting/glide_text2im/xf.py b/spaces/legoandmars/glide-inpainting/glide_text2im/xf.py
deleted file mode 100644
index 5dfff440b489f3cc3c62450dc28c2f35f692dd94..0000000000000000000000000000000000000000
--- a/spaces/legoandmars/glide-inpainting/glide_text2im/xf.py
+++ /dev/null
@@ -1,130 +0,0 @@
-"""
-Transformer implementation adapted from CLIP ViT:
-https://github.com/openai/CLIP/blob/4c0275784d6d9da97ca1f47eaaee31de1867da91/clip/model.py
-"""
-
-import math
-
-import torch as th
-import torch.nn as nn
-
-
-def convert_module_to_f16(l):
- """
- Convert primitive modules to float16.
- """
- if isinstance(l, (nn.Linear, nn.Conv2d, nn.ConvTranspose2d)):
- l.weight.data = l.weight.data.half()
- if l.bias is not None:
- l.bias.data = l.bias.data.half()
-
-
-class LayerNorm(nn.LayerNorm):
- """
- Implementation that supports fp16 inputs but fp32 gains/biases.
- """
-
- def forward(self, x: th.Tensor):
- return super().forward(x.float()).to(x.dtype)
-
-
-class MultiheadAttention(nn.Module):
- def __init__(self, n_ctx, width, heads):
- super().__init__()
- self.n_ctx = n_ctx
- self.width = width
- self.heads = heads
- self.c_qkv = nn.Linear(width, width * 3)
- self.c_proj = nn.Linear(width, width)
- self.attention = QKVMultiheadAttention(heads, n_ctx)
-
- def forward(self, x):
- x = self.c_qkv(x)
- x = self.attention(x)
- x = self.c_proj(x)
- return x
-
-
-class MLP(nn.Module):
- def __init__(self, width):
- super().__init__()
- self.width = width
- self.c_fc = nn.Linear(width, width * 4)
- self.c_proj = nn.Linear(width * 4, width)
- self.gelu = nn.GELU()
-
- def forward(self, x):
- return self.c_proj(self.gelu(self.c_fc(x)))
-
-
-class QKVMultiheadAttention(nn.Module):
- def __init__(self, n_heads: int, n_ctx: int):
- super().__init__()
- self.n_heads = n_heads
- self.n_ctx = n_ctx
-
- def forward(self, qkv):
- bs, n_ctx, width = qkv.shape
- attn_ch = width // self.n_heads // 3
- scale = 1 / math.sqrt(math.sqrt(attn_ch))
- qkv = qkv.view(bs, n_ctx, self.n_heads, -1)
- q, k, v = th.split(qkv, attn_ch, dim=-1)
- weight = th.einsum(
- "bthc,bshc->bhts", q * scale, k * scale
- ) # More stable with f16 than dividing afterwards
- wdtype = weight.dtype
- weight = th.softmax(weight.float(), dim=-1).type(wdtype)
- return th.einsum("bhts,bshc->bthc", weight, v).reshape(bs, n_ctx, -1)
-
-
-class ResidualAttentionBlock(nn.Module):
- def __init__(
- self,
- n_ctx: int,
- width: int,
- heads: int,
- ):
- super().__init__()
-
- self.attn = MultiheadAttention(
- n_ctx,
- width,
- heads,
- )
- self.ln_1 = LayerNorm(width)
- self.mlp = MLP(width)
- self.ln_2 = LayerNorm(width)
-
- def forward(self, x: th.Tensor):
- x = x + self.attn(self.ln_1(x))
- x = x + self.mlp(self.ln_2(x))
- return x
-
-
-class Transformer(nn.Module):
- def __init__(
- self,
- n_ctx: int,
- width: int,
- layers: int,
- heads: int,
- ):
- super().__init__()
- self.n_ctx = n_ctx
- self.width = width
- self.layers = layers
- self.resblocks = nn.ModuleList(
- [
- ResidualAttentionBlock(
- n_ctx,
- width,
- heads,
- )
- for _ in range(layers)
- ]
- )
-
- def forward(self, x: th.Tensor):
- for block in self.resblocks:
- x = block(x)
- return x
diff --git a/spaces/librarian-bot/webhook_metadata_reviewer/README.md b/spaces/librarian-bot/webhook_metadata_reviewer/README.md
deleted file mode 100644
index d4a21b80bbb7eb653339abd00d89f87982008bbb..0000000000000000000000000000000000000000
--- a/spaces/librarian-bot/webhook_metadata_reviewer/README.md
+++ /dev/null
@@ -1,11 +0,0 @@
----
-title: Automatic metadata review bot
-emoji: 🧐
-colorFrom: blue
-colorTo: pink
-sdk: docker
-pinned: false
-duplicated_from: davanstrien/webhook_metadata_reviewer
----
-
-Check out the configuration reference at https://huggingface.co/docs/hub/spaces-config-reference
diff --git a/spaces/liimefruit/RVCollection/config.py b/spaces/liimefruit/RVCollection/config.py
deleted file mode 100644
index 03275af5912b923cf6e74f7de743fde92eacf2ad..0000000000000000000000000000000000000000
--- a/spaces/liimefruit/RVCollection/config.py
+++ /dev/null
@@ -1,105 +0,0 @@
-import argparse
-import torch
-from multiprocessing import cpu_count
-
-class Config:
- def __init__(self):
- self.device = "cuda:0"
- self.is_half = True
- self.n_cpu = 0
- self.gpu_name = None
- self.gpu_mem = None
- (
- self.python_cmd,
- self.listen_port,
- self.colab,
- self.noparallel,
- self.noautoopen,
- self.api
- ) = self.arg_parse()
- self.x_pad, self.x_query, self.x_center, self.x_max = self.device_config()
-
- @staticmethod
- def arg_parse() -> tuple:
- parser = argparse.ArgumentParser()
- parser.add_argument("--port", type=int, default=7865, help="Listen port")
- parser.add_argument(
- "--pycmd", type=str, default="python", help="Python command"
- )
- parser.add_argument("--colab", action="store_true", help="Launch in colab")
- parser.add_argument(
- "--noparallel", action="store_true", help="Disable parallel processing"
- )
- parser.add_argument(
- "--noautoopen",
- action="store_true",
- help="Do not open in browser automatically",
- )
- parser.add_argument("--api", action="store_true", help="Launch with api")
- cmd_opts = parser.parse_args()
-
- cmd_opts.port = cmd_opts.port if 0 <= cmd_opts.port <= 65535 else 7865
-
- return (
- cmd_opts.pycmd,
- cmd_opts.port,
- cmd_opts.colab,
- cmd_opts.noparallel,
- cmd_opts.noautoopen,
- cmd_opts.api
- )
-
- def device_config(self) -> tuple:
- if torch.cuda.is_available():
- i_device = int(self.device.split(":")[-1])
- self.gpu_name = torch.cuda.get_device_name(i_device)
- if (
- ("16" in self.gpu_name and "V100" not in self.gpu_name.upper())
- or "P40" in self.gpu_name.upper()
- or "1060" in self.gpu_name
- or "1070" in self.gpu_name
- or "1080" in self.gpu_name
- ):
- print("16系/10系显卡和P40强制单精度")
- self.is_half = False
- else:
- self.gpu_name = None
- self.gpu_mem = int(
- torch.cuda.get_device_properties(i_device).total_memory
- / 1024
- / 1024
- / 1024
- + 0.4
- )
- elif torch.backends.mps.is_available():
- print("没有发现支持的N卡, 使用MPS进行推理")
- self.device = "mps"
- self.is_half = False
- else:
- print("没有发现支持的N卡, 使用CPU进行推理")
- self.device = "cpu"
- self.is_half = False
-
- if self.n_cpu == 0:
- self.n_cpu = cpu_count()
-
- if self.is_half:
- # 6G显存配置
- x_pad = 3
- x_query = 10
- x_center = 60
- x_max = 65
- else:
- # 5G显存配置
- x_pad = 1
- x_query = 6
- x_center = 38
- x_max = 41
-
- if self.gpu_mem != None and self.gpu_mem <= 4:
- x_pad = 1
- x_query = 5
- x_center = 30
- x_max = 32
-
- return x_pad, x_query, x_center, x_max
\ No newline at end of file
diff --git a/spaces/lincquiQcaudo/Top-20-Diffusion/Antares Auto Tune 8 Mac Crack Torrent.md b/spaces/lincquiQcaudo/Top-20-Diffusion/Antares Auto Tune 8 Mac Crack Torrent.md
deleted file mode 100644
index 3a9795dc0ae474fbf198a5a3f823ab3498151616..0000000000000000000000000000000000000000
--- a/spaces/lincquiQcaudo/Top-20-Diffusion/Antares Auto Tune 8 Mac Crack Torrent.md
+++ /dev/null
@@ -1,6 +0,0 @@
-
-
-Antares Auto-Tune 8 Torrent Incl Patch + Full Version Setup Antares Auto-Tune Crack – is available here to download. The Audio industry is ... 4d29de3e1b
-
-
-
diff --git a/spaces/lincquiQcaudo/Top-20-Diffusion/Doraemon Story Of Seasons Update 1.0.2 PLAZA FitGirl.md b/spaces/lincquiQcaudo/Top-20-Diffusion/Doraemon Story Of Seasons Update 1.0.2 PLAZA FitGirl.md
deleted file mode 100644
index 20f9e9f27787bf3c1c5908d0db76678f4365ce2a..0000000000000000000000000000000000000000
--- a/spaces/lincquiQcaudo/Top-20-Diffusion/Doraemon Story Of Seasons Update 1.0.2 PLAZA FitGirl.md
+++ /dev/null
@@ -1,6 +0,0 @@
-
Doraemon Story of Seasons Update 1.0.2 PLAZA, FitGirl
-
-For Doraemon: Story of Seasons on the Nintendo Switch, a GameFAQs message board topic titled "Version 1.0.2 - Patch Notes?".. Download ... 4d29de3e1b
-
-
-
diff --git a/spaces/lincquiQcaudo/Top-20-Diffusion/Dragon Age Inquisition Patch V.1.11 24 TOP.md b/spaces/lincquiQcaudo/Top-20-Diffusion/Dragon Age Inquisition Patch V.1.11 24 TOP.md
deleted file mode 100644
index 7038050d368cc5870dc6ffad46f7ff9924720edf..0000000000000000000000000000000000000000
--- a/spaces/lincquiQcaudo/Top-20-Diffusion/Dragon Age Inquisition Patch V.1.11 24 TOP.md
+++ /dev/null
@@ -1,106 +0,0 @@
-
-
Dragon Age: Inquisition Patch v.1.11 24 - A Comprehensive Overview
-
Dragon Age: Inquisition is one of the most popular and critically acclaimed role-playing games of the last decade. It offers a vast and immersive world, a rich and diverse story, and a dynamic and engaging gameplay. However, like any complex game, it also has its share of bugs, glitches, and issues that may affect the player's experience.
That's why the developers at BioWare have been constantly working on improving and updating the game with patches that fix various problems, add new features, and enhance performance. The latest patch for Dragon Age: Inquisition is v.1.11 24, which was released on January 27th, 2023 for Windows 10 users.
-
In this article, we will give you a comprehensive overview of what this patch does, how to install it, and what are the pros and cons of using it. We will also answer some frequently asked questions and provide some tips and tricks for getting the most out of Dragon Age: Inquisition Patch v.1.11 24.
-
What Does Dragon Age: Inquisition Patch v.1.11 24 Do?
-
Dragon Age: Inquisition Patch v.1.11 24 is a major update that brings several improvements and fixes to the game. Here are some of the main changes that this patch introduces:
-
-
It adds SplitCam Video Driver and SplitCam Audio Driver for Windows 10 users, which allow them to use their webcam with multiple applications at the same time and add effects to their video stream.
-
It updates the codecs for better video and audio quality and compatibility.
-
It fixes the incorrect log for the Desktop custom mode.
-
It fixes the issue where some programs did not detect SplitCam Video Driver in Windows 10.
-
It fixes the sound lagging issue in Windows 10 1703.
-
It fixes the crash that occurred at the start if the player selected a video source.
-
-
How to Install Dragon Age: Inquisition Patch v.1.11 24?
-
Dragon Age: Inquisition Patch v.1.11 24 is compatible with Windows 10 (both 32-bit and 64-bit versions) and it is totally free without any restrictions or hidden payments. You can download it from SteamDB, which provides curated patch notes for Dragon Age: Inquisition on Steam: https://steamdb.info/app/1222690/patchnotes/
-
The installation process is simple and straightforward. Just follow these steps:
-
-
Run Steam and launch Dragon Age: Inquisition from your library.
-
Steam will automatically download and install the patch for you.
-
Wait for the installation to finish and restart the game.
-
-
What are the Pros and Cons of Dragon Age: Inquisition Patch v.1.11 24?
-
Dragon Age: Inquisition Patch v.1.11 24 is a great update that brings many benefits and few drawbacks to the game. Here are some of them:
-
-
-
Pros
Cons
-
It improves the video and audio quality and compatibility of the game.
It may not work with some webcam models or applications.
-
It adds new features and effects for webcam users.
It may cause some lag or delay in video stream.
-
It fixes various bugs and issues that affected the gameplay.
It may consume some CPU resources or disk space.
-
It enhances the performance and stability of the game.
It may have some bugs or errors in some features.
-
-
Frequently Asked Questions
-
If you have any questions or issues with Dragon Age: Inquisition Patch v.1.11 24, you can check the FAQs section on BioWare Blog, which provides official patch notes for Dragon Age: Inquisition: https://blog.bioware.com/dragon-age-inquisition-patch-notes/ Here are some of the common FAQs:
-
-
Q: How can I check which patch/version I'm using of Dragon Age: Inquisition?
-
A: You can check your patch/version by following these steps:
-
-
Navigate to C:\Program Files (x86)\Origin Games\Dragon Age Inquisition\Update\Patch\package.mft
-
Open it with any ASCII text editor.
-
The version number is displayed at the top of the file.
-
-
Q: How can I uninstall Dragon Age: Inquisition Patch v.1.11 24?
-
A: You can uninstall Dragon Age: Inquisition Patch v.1.11 24 by following these steps:
-
-
Close Dragon Age: Inquisition and any other application that uses your webcam.
-
Navigate to C:\Program Files (x86)\Origin Games\Dragon Age Inquisition\Update\Patch\
-
Delete package.mft file.
-
Delete SplitCam folder if present.
-
Delete SplitCamAudio folder if present.
-
Delete SplitCamVideo folder if present.
-
-
-
How to Use Dragon Age: Inquisition Patch v.1.11 24?
-
Dragon Age: Inquisition Patch v.1.11 24 is easy and intuitive to use. Here are some basic steps to get you started:
-
-
Launch Dragon Age: Inquisition from Steam and select your saved game.
-
To use your webcam with multiple applications and add effects to your video stream, click on the "SplitCam" icon on the top right corner of the game screen.
-
To select the video source you want to use, click on the drop-down menu at the top left corner of the SplitCam window.
-
To add effects to your video stream, click on the "Effects" tab at the bottom left corner of the SplitCam window and choose from the categories on the left panel.
-
To zoom your video stream, use the slider at the bottom right corner of the SplitCam window or press Ctrl + mouse wheel.
-
To stream video to a livestream website or record it to Youtube, click on the "Stream" tab at the bottom left corner of the SplitCam window and select the platform you want to use from the list on the left panel.
-
To mix audio sources in one audio stream, click on the "Audio" tab at the bottom left corner of the SplitCam window and select the sources you want to use from the list on the left panel.
-
-
What are the Best Practices for Using Dragon Age: Inquisition Patch v.1.11 24?
-
To get the most out of Dragon Age: Inquisition Patch v.1.11 24 and enjoy a smooth and high-quality video chat experience, you can follow these best practices:
-
-
Make sure your webcam driver and SplitCam software are updated to the latest version.
-
Close any unnecessary programs or processes that may interfere with SplitCam or consume CPU resources.
-
Adjust the settings of SplitCam according to your preferences and needs, such as resolution, frame rate, brightness, contrast, saturation, etc.
-
Choose the effects and masks that suit your video chat purpose and mood, and don't overuse them.
-
Test your video stream before broadcasting it to a livestream website or recording it to Youtube.
-
Have fun and be creative with SplitCam!
-
-
Where to Get More Information About Dragon Age: Inquisition Patch v.1.11 24?
-
If you want to learn more about Dragon Age: Inquisition Patch v.1.11 24, you can visit the following sources:
-
-
The official website: https://www.ea.com/games/dragon-age/dragon-age-inquisition Here you can find the latest news, updates, media, and community content for Dragon Age: Inquisition.
-
The official blog: https://blog.bioware.com/category/dragon-age/ Here you can read new articles and insights from the developers and writers of Dragon Age: Inquisition.
-
The official forum: https://answers.ea.com/t5/Dragon-Age-Inquisition/bd-p/Dragon-Age-Inquisition Here you can join the discussion with other players and get help from the support team.
-
The official social media: https://www.facebook.com/DragonAge/ https://twitter.com/dragonage Here you can follow Dragon Age: Inquisition on Facebook and Twitter and get the latest updates and interact with the community.
-
-
What are the Tips and Tricks for Playing Dragon Age: Inquisition Patch v.1.11 24?
-
Dragon Age: Inquisition Patch v.1.11 24 is a fun and immersive game that offers a lot of options and possibilities for the player. Here are some tips and tricks to help you enjoy the game even more:
-
-
Explore the world and collect resources. Dragon Age: Inquisition has a huge and beautiful world that is full of secrets, quests, and loot. You can use your resources to craft weapons, armor, potions, and upgrades for your equipment and your base.
-
Manage your party and your relationships. Dragon Age: Inquisition has a diverse and interesting cast of characters that you can recruit, interact with, and romance. You can choose who to bring with you on your missions, who to talk to, who to support, and who to romance. Your choices will affect your relationships with them and their loyalty to you.
-
Customize your character and your playstyle. Dragon Age: Inquisition allows you to create your own character from four different races (human, elf, dwarf, or qunari) and three different classes (warrior, rogue, or mage). You can also choose from various specializations that give you unique abilities and skills. You can also customize your appearance, your gear, your skills, and your tactics.
-
Play online with other players. Dragon Age: Inquisition has a multiplayer mode that lets you team up with up to three other players and take on various missions and challenges. You can choose from different characters with different abilities and roles, earn rewards, and unlock new content.
-
-
What are the Reviews for Dragon Age: Inquisition Patch v.1.11 24?
-
Dragon Age: Inquisition Patch v.1.11 24 has received mostly positive reviews from users and critics alike. Here are some of the comments from various sources:
-
-
"Dragon Age: Inquisition Patch v.1.11 24 is a great update that improves the game in many ways. I love the new SplitCam feature that lets me use my webcam with multiple applications and add effects to my video stream. The game also runs smoother and looks better than before." - User review on Steam
-
"Dragon Age: Inquisition Patch v.1.11 24 is a must-have for any fan of the game. It fixes many bugs and issues that plagued the game since launch, and adds new features and enhancements that make the game more enjoyable and immersive. The SplitCam feature is especially cool and fun to use." - Editor review on IGN
-
"Dragon Age: Inquisition Patch v.1.11 24 is a welcome update that brings a lot of improvements and fixes to the game. The SplitCam feature is a nice addition that allows you to use your webcam with multiple applications and add effects to your video stream. The game also looks and performs better than ever." - Review on GameSpot
-
-
Conclusion
-
Dragon Age: Inquisition Patch v.1.11 24 is a powerful and comprehensive update that enhances the game in various ways. It adds SplitCam Video Driver and SplitCam Audio Driver for Windows 10 users, which allow them to use their webcam with multiple applications at the same time and add effects to their video stream. It also updates the codecs for better video and audio quality and compatibility, fixes various bugs and issues, and improves the performance and stability of the game.
-
If you are looking for a free and versatile update for Dragon Age: Inquisition, you should definitely give Dragon Age: Inquisition Patch v.1.11 24 a try.
-
Dragon Age: Inquisition Patch v.1.11 24 is a powerful and comprehensive update that enhances the game in various ways. It adds SplitCam Video Driver and SplitCam Audio Driver for Windows 10 users, which allow them to use their webcam with multiple applications at the same time and add effects to their video stream. It also updates the codecs for better video and audio quality and compatibility, fixes various bugs and issues, and improves the performance and stability of the game.
-
If you are looking for a free and versatile update for Dragon Age: Inquisition, you should definitely give Dragon Age: Inquisition Patch v.1.11 24 a try.
3cee63e6c2
-
-
\ No newline at end of file
diff --git a/spaces/lincquiQcaudo/Top-20-Diffusion/HACK SONY Vegas Pro 13.0 Build 373 (x64) RePack By D!akov NEW!.md b/spaces/lincquiQcaudo/Top-20-Diffusion/HACK SONY Vegas Pro 13.0 Build 373 (x64) RePack By D!akov NEW!.md
deleted file mode 100644
index 04067382a96ec8edbcbf6b22378183f8155de814..0000000000000000000000000000000000000000
--- a/spaces/lincquiQcaudo/Top-20-Diffusion/HACK SONY Vegas Pro 13.0 Build 373 (x64) RePack By D!akov NEW!.md
+++ /dev/null
@@ -1,5 +0,0 @@
-
-
84c4c6b66f GiliSoft Smart wifi USB KNetwok 2.2.0.0 (MAC/PC) with Patch.rar COFFEE PAD - TV Hacked REPACK - 4.6.2.1 FULL Version Osmera Torrent 1.3.6.2 The Ranger Best of Pdf - KriptoVirus 2 Training Files.zip THIS IS A FULLY PATCHED FILE! ALL FREE KEYS ARE LISTED BELOW I. I will not be held responsible for any viruses to your computer, hard drive, or other media. I can assure you that this is the real thing and that it has been fully tested. http://www.expressvpn.net/ This is the brand new premium VPN service that offers users a feeling of anonymity when they are online. I have seen both free and paid VPN services, and I must say that ExpressVPN is one of the finest VPN services I have ever used. It offers strong online security features, such as 256-bit SSL encryption on all servers, scrupulous customer service, and three platforms for all your devices. This is the best VPN service I have tried because it runs perfectly on both Windows and Mac. ExpressVPN is definitely the choice VPN service for all those who are looking for safe online browsing experience. Now you can easily enjoy the free ExpressVPN services if you want. I am a big fan of ExpressVPN because their customer service is simply impeccable. I have never experienced any problems or technical issues when using ExpressVPN, and the customer support staff is always very helpful, responsive, and professional. It is totally worth its money. The website is easy to use and it is never complicated to access the information you need. ExpressVPN is a bit pricey, but considering the vast number of users who use it, I would say it is a good investment. It offers 3 platforms for the three of their most popular devices: Android, iOS, and Windows. I’ve tried all three platforms, and I must say that Android is a dream! You can control everything through the 3D interface, which is free from unwanted ads and clutter. The basic functions of Android are easily manageable, so you have no problem navigating around the website. I prefer that the app is able to manage Android settings for my Wi-Fi connection settings. As far as online browsing experience using ExpressVPN is concerned, I must say that Android is not the best out of the three platforms. iOS is the only iOS compatible app that we can control. The touchscreen Android mobile, IOS, iOS 9.2.1 Theme Torrent for greek Note- Added and removed files [ 30 ] Iso Crack.rar [31] Full Version Rar Torrent. Full Windows!BETTER!! full free 1080p deudacop UPC Reference Model Of Paas Pdf 2017 PRO 24 Crack With Licence Key Free Download 2019.
-
HACK SONY Vegas Pro 13.0 Build 373 (x64) RePack By D!akov
899543212b
-
-
\ No newline at end of file
diff --git a/spaces/lllqqq/so-vits-svc-models-pcr/diffusion/dpm_solver_pytorch.py b/spaces/lllqqq/so-vits-svc-models-pcr/diffusion/dpm_solver_pytorch.py
deleted file mode 100644
index dee5e280661b61e0a99038ce0bd240db51344ead..0000000000000000000000000000000000000000
--- a/spaces/lllqqq/so-vits-svc-models-pcr/diffusion/dpm_solver_pytorch.py
+++ /dev/null
@@ -1,1201 +0,0 @@
-import math
-
-import torch
-
-
-class NoiseScheduleVP:
- def __init__(
- self,
- schedule='discrete',
- betas=None,
- alphas_cumprod=None,
- continuous_beta_0=0.1,
- continuous_beta_1=20.,
- ):
- """Create a wrapper class for the forward SDE (VP type).
-
- ***
- Update: We support discrete-time diffusion models by implementing a picewise linear interpolation for log_alpha_t.
- We recommend to use schedule='discrete' for the discrete-time diffusion models, especially for high-resolution images.
- ***
-
- The forward SDE ensures that the condition distribution q_{t|0}(x_t | x_0) = N ( alpha_t * x_0, sigma_t^2 * I ).
- We further define lambda_t = log(alpha_t) - log(sigma_t), which is the half-logSNR (described in the DPM-Solver paper).
- Therefore, we implement the functions for computing alpha_t, sigma_t and lambda_t. For t in [0, T], we have:
-
- log_alpha_t = self.marginal_log_mean_coeff(t)
- sigma_t = self.marginal_std(t)
- lambda_t = self.marginal_lambda(t)
-
- Moreover, as lambda(t) is an invertible function, we also support its inverse function:
-
- t = self.inverse_lambda(lambda_t)
-
- ===============================================================
-
- We support both discrete-time DPMs (trained on n = 0, 1, ..., N-1) and continuous-time DPMs (trained on t in [t_0, T]).
-
- 1. For discrete-time DPMs:
-
- For discrete-time DPMs trained on n = 0, 1, ..., N-1, we convert the discrete steps to continuous time steps by:
- t_i = (i + 1) / N
- e.g. for N = 1000, we have t_0 = 1e-3 and T = t_{N-1} = 1.
- We solve the corresponding diffusion ODE from time T = 1 to time t_0 = 1e-3.
-
- Args:
- betas: A `torch.Tensor`. The beta array for the discrete-time DPM. (See the original DDPM paper for details)
- alphas_cumprod: A `torch.Tensor`. The cumprod alphas for the discrete-time DPM. (See the original DDPM paper for details)
-
- Note that we always have alphas_cumprod = cumprod(betas). Therefore, we only need to set one of `betas` and `alphas_cumprod`.
-
- **Important**: Please pay special attention for the args for `alphas_cumprod`:
- The `alphas_cumprod` is the \hat{alpha_n} arrays in the notations of DDPM. Specifically, DDPMs assume that
- q_{t_n | 0}(x_{t_n} | x_0) = N ( \sqrt{\hat{alpha_n}} * x_0, (1 - \hat{alpha_n}) * I ).
- Therefore, the notation \hat{alpha_n} is different from the notation alpha_t in DPM-Solver. In fact, we have
- alpha_{t_n} = \sqrt{\hat{alpha_n}},
- and
- log(alpha_{t_n}) = 0.5 * log(\hat{alpha_n}).
-
-
- 2. For continuous-time DPMs:
-
- We support two types of VPSDEs: linear (DDPM) and cosine (improved-DDPM). The hyperparameters for the noise
- schedule are the default settings in DDPM and improved-DDPM:
-
- Args:
- beta_min: A `float` number. The smallest beta for the linear schedule.
- beta_max: A `float` number. The largest beta for the linear schedule.
- cosine_s: A `float` number. The hyperparameter in the cosine schedule.
- cosine_beta_max: A `float` number. The hyperparameter in the cosine schedule.
- T: A `float` number. The ending time of the forward process.
-
- ===============================================================
-
- Args:
- schedule: A `str`. The noise schedule of the forward SDE. 'discrete' for discrete-time DPMs,
- 'linear' or 'cosine' for continuous-time DPMs.
- Returns:
- A wrapper object of the forward SDE (VP type).
-
- ===============================================================
-
- Example:
-
- # For discrete-time DPMs, given betas (the beta array for n = 0, 1, ..., N - 1):
- >>> ns = NoiseScheduleVP('discrete', betas=betas)
-
- # For discrete-time DPMs, given alphas_cumprod (the \hat{alpha_n} array for n = 0, 1, ..., N - 1):
- >>> ns = NoiseScheduleVP('discrete', alphas_cumprod=alphas_cumprod)
-
- # For continuous-time DPMs (VPSDE), linear schedule:
- >>> ns = NoiseScheduleVP('linear', continuous_beta_0=0.1, continuous_beta_1=20.)
-
- """
-
- if schedule not in ['discrete', 'linear', 'cosine']:
- raise ValueError(
- "Unsupported noise schedule {}. The schedule needs to be 'discrete' or 'linear' or 'cosine'".format(
- schedule))
-
- self.schedule = schedule
- if schedule == 'discrete':
- if betas is not None:
- log_alphas = 0.5 * torch.log(1 - betas).cumsum(dim=0)
- else:
- assert alphas_cumprod is not None
- log_alphas = 0.5 * torch.log(alphas_cumprod)
- self.total_N = len(log_alphas)
- self.T = 1.
- self.t_array = torch.linspace(0., 1., self.total_N + 1)[1:].reshape((1, -1))
- self.log_alpha_array = log_alphas.reshape((1, -1,))
- else:
- self.total_N = 1000
- self.beta_0 = continuous_beta_0
- self.beta_1 = continuous_beta_1
- self.cosine_s = 0.008
- self.cosine_beta_max = 999.
- self.cosine_t_max = math.atan(self.cosine_beta_max * (1. + self.cosine_s) / math.pi) * 2. * (
- 1. + self.cosine_s) / math.pi - self.cosine_s
- self.cosine_log_alpha_0 = math.log(math.cos(self.cosine_s / (1. + self.cosine_s) * math.pi / 2.))
- self.schedule = schedule
- if schedule == 'cosine':
- # For the cosine schedule, T = 1 will have numerical issues. So we manually set the ending time T.
- # Note that T = 0.9946 may be not the optimal setting. However, we find it works well.
- self.T = 0.9946
- else:
- self.T = 1.
-
- def marginal_log_mean_coeff(self, t):
- """
- Compute log(alpha_t) of a given continuous-time label t in [0, T].
- """
- if self.schedule == 'discrete':
- return interpolate_fn(t.reshape((-1, 1)), self.t_array.to(t.device),
- self.log_alpha_array.to(t.device)).reshape((-1))
- elif self.schedule == 'linear':
- return -0.25 * t ** 2 * (self.beta_1 - self.beta_0) - 0.5 * t * self.beta_0
- elif self.schedule == 'cosine':
- log_alpha_fn = lambda s: torch.log(torch.cos((s + self.cosine_s) / (1. + self.cosine_s) * math.pi / 2.))
- log_alpha_t = log_alpha_fn(t) - self.cosine_log_alpha_0
- return log_alpha_t
-
- def marginal_alpha(self, t):
- """
- Compute alpha_t of a given continuous-time label t in [0, T].
- """
- return torch.exp(self.marginal_log_mean_coeff(t))
-
- def marginal_std(self, t):
- """
- Compute sigma_t of a given continuous-time label t in [0, T].
- """
- return torch.sqrt(1. - torch.exp(2. * self.marginal_log_mean_coeff(t)))
-
- def marginal_lambda(self, t):
- """
- Compute lambda_t = log(alpha_t) - log(sigma_t) of a given continuous-time label t in [0, T].
- """
- log_mean_coeff = self.marginal_log_mean_coeff(t)
- log_std = 0.5 * torch.log(1. - torch.exp(2. * log_mean_coeff))
- return log_mean_coeff - log_std
-
- def inverse_lambda(self, lamb):
- """
- Compute the continuous-time label t in [0, T] of a given half-logSNR lambda_t.
- """
- if self.schedule == 'linear':
- tmp = 2. * (self.beta_1 - self.beta_0) * torch.logaddexp(-2. * lamb, torch.zeros((1,)).to(lamb))
- Delta = self.beta_0 ** 2 + tmp
- return tmp / (torch.sqrt(Delta) + self.beta_0) / (self.beta_1 - self.beta_0)
- elif self.schedule == 'discrete':
- log_alpha = -0.5 * torch.logaddexp(torch.zeros((1,)).to(lamb.device), -2. * lamb)
- t = interpolate_fn(log_alpha.reshape((-1, 1)), torch.flip(self.log_alpha_array.to(lamb.device), [1]),
- torch.flip(self.t_array.to(lamb.device), [1]))
- return t.reshape((-1,))
- else:
- log_alpha = -0.5 * torch.logaddexp(-2. * lamb, torch.zeros((1,)).to(lamb))
- t_fn = lambda log_alpha_t: torch.arccos(torch.exp(log_alpha_t + self.cosine_log_alpha_0)) * 2. * (
- 1. + self.cosine_s) / math.pi - self.cosine_s
- t = t_fn(log_alpha)
- return t
-
-
-def model_wrapper(
- model,
- noise_schedule,
- model_type="noise",
- model_kwargs={},
- guidance_type="uncond",
- condition=None,
- unconditional_condition=None,
- guidance_scale=1.,
- classifier_fn=None,
- classifier_kwargs={},
-):
- """Create a wrapper function for the noise prediction model.
-
- DPM-Solver needs to solve the continuous-time diffusion ODEs. For DPMs trained on discrete-time labels, we need to
- firstly wrap the model function to a noise prediction model that accepts the continuous time as the input.
-
- We support four types of the diffusion model by setting `model_type`:
-
- 1. "noise": noise prediction model. (Trained by predicting noise).
-
- 2. "x_start": data prediction model. (Trained by predicting the data x_0 at time 0).
-
- 3. "v": velocity prediction model. (Trained by predicting the velocity).
- The "v" prediction is derivation detailed in Appendix D of [1], and is used in Imagen-Video [2].
-
- [1] Salimans, Tim, and Jonathan Ho. "Progressive distillation for fast sampling of diffusion models."
- arXiv preprint arXiv:2202.00512 (2022).
- [2] Ho, Jonathan, et al. "Imagen Video: High Definition Video Generation with Diffusion Models."
- arXiv preprint arXiv:2210.02303 (2022).
-
- 4. "score": marginal score function. (Trained by denoising score matching).
- Note that the score function and the noise prediction model follows a simple relationship:
- ```
- noise(x_t, t) = -sigma_t * score(x_t, t)
- ```
-
- We support three types of guided sampling by DPMs by setting `guidance_type`:
- 1. "uncond": unconditional sampling by DPMs.
- The input `model` has the following format:
- ``
- model(x, t_input, **model_kwargs) -> noise | x_start | v | score
- ``
-
- 2. "classifier": classifier guidance sampling [3] by DPMs and another classifier.
- The input `model` has the following format:
- ``
- model(x, t_input, **model_kwargs) -> noise | x_start | v | score
- ``
-
- The input `classifier_fn` has the following format:
- ``
- classifier_fn(x, t_input, cond, **classifier_kwargs) -> logits(x, t_input, cond)
- ``
-
- [3] P. Dhariwal and A. Q. Nichol, "Diffusion models beat GANs on image synthesis,"
- in Advances in Neural Information Processing Systems, vol. 34, 2021, pp. 8780-8794.
-
- 3. "classifier-free": classifier-free guidance sampling by conditional DPMs.
- The input `model` has the following format:
- ``
- model(x, t_input, cond, **model_kwargs) -> noise | x_start | v | score
- ``
- And if cond == `unconditional_condition`, the model output is the unconditional DPM output.
-
- [4] Ho, Jonathan, and Tim Salimans. "Classifier-free diffusion guidance."
- arXiv preprint arXiv:2207.12598 (2022).
-
-
- The `t_input` is the time label of the model, which may be discrete-time labels (i.e. 0 to 999)
- or continuous-time labels (i.e. epsilon to T).
-
- We wrap the model function to accept only `x` and `t_continuous` as inputs, and outputs the predicted noise:
- ``
- def model_fn(x, t_continuous) -> noise:
- t_input = get_model_input_time(t_continuous)
- return noise_pred(model, x, t_input, **model_kwargs)
- ``
- where `t_continuous` is the continuous time labels (i.e. epsilon to T). And we use `model_fn` for DPM-Solver.
-
- ===============================================================
-
- Args:
- model: A diffusion model with the corresponding format described above.
- noise_schedule: A noise schedule object, such as NoiseScheduleVP.
- model_type: A `str`. The parameterization type of the diffusion model.
- "noise" or "x_start" or "v" or "score".
- model_kwargs: A `dict`. A dict for the other inputs of the model function.
- guidance_type: A `str`. The type of the guidance for sampling.
- "uncond" or "classifier" or "classifier-free".
- condition: A pytorch tensor. The condition for the guided sampling.
- Only used for "classifier" or "classifier-free" guidance type.
- unconditional_condition: A pytorch tensor. The condition for the unconditional sampling.
- Only used for "classifier-free" guidance type.
- guidance_scale: A `float`. The scale for the guided sampling.
- classifier_fn: A classifier function. Only used for the classifier guidance.
- classifier_kwargs: A `dict`. A dict for the other inputs of the classifier function.
- Returns:
- A noise prediction model that accepts the noised data and the continuous time as the inputs.
- """
-
- def get_model_input_time(t_continuous):
- """
- Convert the continuous-time `t_continuous` (in [epsilon, T]) to the model input time.
- For discrete-time DPMs, we convert `t_continuous` in [1 / N, 1] to `t_input` in [0, 1000 * (N - 1) / N].
- For continuous-time DPMs, we just use `t_continuous`.
- """
- if noise_schedule.schedule == 'discrete':
- return (t_continuous - 1. / noise_schedule.total_N) * noise_schedule.total_N
- else:
- return t_continuous
-
- def noise_pred_fn(x, t_continuous, cond=None):
- if t_continuous.reshape((-1,)).shape[0] == 1:
- t_continuous = t_continuous.expand((x.shape[0]))
- t_input = get_model_input_time(t_continuous)
- if cond is None:
- output = model(x, t_input, **model_kwargs)
- else:
- output = model(x, t_input, cond, **model_kwargs)
- if model_type == "noise":
- return output
- elif model_type == "x_start":
- alpha_t, sigma_t = noise_schedule.marginal_alpha(t_continuous), noise_schedule.marginal_std(t_continuous)
- dims = x.dim()
- return (x - expand_dims(alpha_t, dims) * output) / expand_dims(sigma_t, dims)
- elif model_type == "v":
- alpha_t, sigma_t = noise_schedule.marginal_alpha(t_continuous), noise_schedule.marginal_std(t_continuous)
- dims = x.dim()
- return expand_dims(alpha_t, dims) * output + expand_dims(sigma_t, dims) * x
- elif model_type == "score":
- sigma_t = noise_schedule.marginal_std(t_continuous)
- dims = x.dim()
- return -expand_dims(sigma_t, dims) * output
-
- def cond_grad_fn(x, t_input):
- """
- Compute the gradient of the classifier, i.e. nabla_{x} log p_t(cond | x_t).
- """
- with torch.enable_grad():
- x_in = x.detach().requires_grad_(True)
- log_prob = classifier_fn(x_in, t_input, condition, **classifier_kwargs)
- return torch.autograd.grad(log_prob.sum(), x_in)[0]
-
- def model_fn(x, t_continuous):
- """
- The noise predicition model function that is used for DPM-Solver.
- """
- if t_continuous.reshape((-1,)).shape[0] == 1:
- t_continuous = t_continuous.expand((x.shape[0]))
- if guidance_type == "uncond":
- return noise_pred_fn(x, t_continuous)
- elif guidance_type == "classifier":
- assert classifier_fn is not None
- t_input = get_model_input_time(t_continuous)
- cond_grad = cond_grad_fn(x, t_input)
- sigma_t = noise_schedule.marginal_std(t_continuous)
- noise = noise_pred_fn(x, t_continuous)
- return noise - guidance_scale * expand_dims(sigma_t, dims=cond_grad.dim()) * cond_grad
- elif guidance_type == "classifier-free":
- if guidance_scale == 1. or unconditional_condition is None:
- return noise_pred_fn(x, t_continuous, cond=condition)
- else:
- x_in = torch.cat([x] * 2)
- t_in = torch.cat([t_continuous] * 2)
- c_in = torch.cat([unconditional_condition, condition])
- noise_uncond, noise = noise_pred_fn(x_in, t_in, cond=c_in).chunk(2)
- return noise_uncond + guidance_scale * (noise - noise_uncond)
-
- assert model_type in ["noise", "x_start", "v"]
- assert guidance_type in ["uncond", "classifier", "classifier-free"]
- return model_fn
-
-
-class DPM_Solver:
- def __init__(self, model_fn, noise_schedule, predict_x0=False, thresholding=False, max_val=1.):
- """Construct a DPM-Solver.
-
- We support both the noise prediction model ("predicting epsilon") and the data prediction model ("predicting x0").
- If `predict_x0` is False, we use the solver for the noise prediction model (DPM-Solver).
- If `predict_x0` is True, we use the solver for the data prediction model (DPM-Solver++).
- In such case, we further support the "dynamic thresholding" in [1] when `thresholding` is True.
- The "dynamic thresholding" can greatly improve the sample quality for pixel-space DPMs with large guidance scales.
-
- Args:
- model_fn: A noise prediction model function which accepts the continuous-time input (t in [epsilon, T]):
- ``
- def model_fn(x, t_continuous):
- return noise
- ``
- noise_schedule: A noise schedule object, such as NoiseScheduleVP.
- predict_x0: A `bool`. If true, use the data prediction model; else, use the noise prediction model.
- thresholding: A `bool`. Valid when `predict_x0` is True. Whether to use the "dynamic thresholding" in [1].
- max_val: A `float`. Valid when both `predict_x0` and `thresholding` are True. The max value for thresholding.
-
- [1] Chitwan Saharia, William Chan, Saurabh Saxena, Lala Li, Jay Whang, Emily Denton, Seyed Kamyar Seyed Ghasemipour, Burcu Karagol Ayan, S Sara Mahdavi, Rapha Gontijo Lopes, et al. Photorealistic text-to-image diffusion models with deep language understanding. arXiv preprint arXiv:2205.11487, 2022b.
- """
- self.model = model_fn
- self.noise_schedule = noise_schedule
- self.predict_x0 = predict_x0
- self.thresholding = thresholding
- self.max_val = max_val
-
- def noise_prediction_fn(self, x, t):
- """
- Return the noise prediction model.
- """
- return self.model(x, t)
-
- def data_prediction_fn(self, x, t):
- """
- Return the data prediction model (with thresholding).
- """
- noise = self.noise_prediction_fn(x, t)
- dims = x.dim()
- alpha_t, sigma_t = self.noise_schedule.marginal_alpha(t), self.noise_schedule.marginal_std(t)
- x0 = (x - expand_dims(sigma_t, dims) * noise) / expand_dims(alpha_t, dims)
- if self.thresholding:
- p = 0.995 # A hyperparameter in the paper of "Imagen" [1].
- s = torch.quantile(torch.abs(x0).reshape((x0.shape[0], -1)), p, dim=1)
- s = expand_dims(torch.maximum(s, self.max_val * torch.ones_like(s).to(s.device)), dims)
- x0 = torch.clamp(x0, -s, s) / s
- return x0
-
- def model_fn(self, x, t):
- """
- Convert the model to the noise prediction model or the data prediction model.
- """
- if self.predict_x0:
- return self.data_prediction_fn(x, t)
- else:
- return self.noise_prediction_fn(x, t)
-
- def get_time_steps(self, skip_type, t_T, t_0, N, device):
- """Compute the intermediate time steps for sampling.
-
- Args:
- skip_type: A `str`. The type for the spacing of the time steps. We support three types:
- - 'logSNR': uniform logSNR for the time steps.
- - 'time_uniform': uniform time for the time steps. (**Recommended for high-resolutional data**.)
- - 'time_quadratic': quadratic time for the time steps. (Used in DDIM for low-resolutional data.)
- t_T: A `float`. The starting time of the sampling (default is T).
- t_0: A `float`. The ending time of the sampling (default is epsilon).
- N: A `int`. The total number of the spacing of the time steps.
- device: A torch device.
- Returns:
- A pytorch tensor of the time steps, with the shape (N + 1,).
- """
- if skip_type == 'logSNR':
- lambda_T = self.noise_schedule.marginal_lambda(torch.tensor(t_T).to(device))
- lambda_0 = self.noise_schedule.marginal_lambda(torch.tensor(t_0).to(device))
- logSNR_steps = torch.linspace(lambda_T.cpu().item(), lambda_0.cpu().item(), N + 1).to(device)
- return self.noise_schedule.inverse_lambda(logSNR_steps)
- elif skip_type == 'time_uniform':
- return torch.linspace(t_T, t_0, N + 1).to(device)
- elif skip_type == 'time_quadratic':
- t_order = 2
- t = torch.linspace(t_T ** (1. / t_order), t_0 ** (1. / t_order), N + 1).pow(t_order).to(device)
- return t
- else:
- raise ValueError(
- "Unsupported skip_type {}, need to be 'logSNR' or 'time_uniform' or 'time_quadratic'".format(skip_type))
-
- def get_orders_and_timesteps_for_singlestep_solver(self, steps, order, skip_type, t_T, t_0, device):
- """
- Get the order of each step for sampling by the singlestep DPM-Solver.
-
- We combine both DPM-Solver-1,2,3 to use all the function evaluations, which is named as "DPM-Solver-fast".
- Given a fixed number of function evaluations by `steps`, the sampling procedure by DPM-Solver-fast is:
- - If order == 1:
- We take `steps` of DPM-Solver-1 (i.e. DDIM).
- - If order == 2:
- - Denote K = (steps // 2). We take K or (K + 1) intermediate time steps for sampling.
- - If steps % 2 == 0, we use K steps of DPM-Solver-2.
- - If steps % 2 == 1, we use K steps of DPM-Solver-2 and 1 step of DPM-Solver-1.
- - If order == 3:
- - Denote K = (steps // 3 + 1). We take K intermediate time steps for sampling.
- - If steps % 3 == 0, we use (K - 2) steps of DPM-Solver-3, and 1 step of DPM-Solver-2 and 1 step of DPM-Solver-1.
- - If steps % 3 == 1, we use (K - 1) steps of DPM-Solver-3 and 1 step of DPM-Solver-1.
- - If steps % 3 == 2, we use (K - 1) steps of DPM-Solver-3 and 1 step of DPM-Solver-2.
-
- ============================================
- Args:
- order: A `int`. The max order for the solver (2 or 3).
- steps: A `int`. The total number of function evaluations (NFE).
- skip_type: A `str`. The type for the spacing of the time steps. We support three types:
- - 'logSNR': uniform logSNR for the time steps.
- - 'time_uniform': uniform time for the time steps. (**Recommended for high-resolutional data**.)
- - 'time_quadratic': quadratic time for the time steps. (Used in DDIM for low-resolutional data.)
- t_T: A `float`. The starting time of the sampling (default is T).
- t_0: A `float`. The ending time of the sampling (default is epsilon).
- device: A torch device.
- Returns:
- orders: A list of the solver order of each step.
- """
- if order == 3:
- K = steps // 3 + 1
- if steps % 3 == 0:
- orders = [3, ] * (K - 2) + [2, 1]
- elif steps % 3 == 1:
- orders = [3, ] * (K - 1) + [1]
- else:
- orders = [3, ] * (K - 1) + [2]
- elif order == 2:
- if steps % 2 == 0:
- K = steps // 2
- orders = [2, ] * K
- else:
- K = steps // 2 + 1
- orders = [2, ] * (K - 1) + [1]
- elif order == 1:
- K = 1
- orders = [1, ] * steps
- else:
- raise ValueError("'order' must be '1' or '2' or '3'.")
- if skip_type == 'logSNR':
- # To reproduce the results in DPM-Solver paper
- timesteps_outer = self.get_time_steps(skip_type, t_T, t_0, K, device)
- else:
- timesteps_outer = self.get_time_steps(skip_type, t_T, t_0, steps, device)[
- torch.cumsum(torch.tensor([0, ] + orders), dim=0).to(device)]
- return timesteps_outer, orders
-
- def denoise_fn(self, x, s):
- """
- Denoise at the final step, which is equivalent to solve the ODE from lambda_s to infty by first-order discretization.
- """
- return self.data_prediction_fn(x, s)
-
- def dpm_solver_first_update(self, x, s, t, model_s=None, return_intermediate=False):
- """
- DPM-Solver-1 (equivalent to DDIM) from time `s` to time `t`.
-
- Args:
- x: A pytorch tensor. The initial value at time `s`.
- s: A pytorch tensor. The starting time, with the shape (x.shape[0],).
- t: A pytorch tensor. The ending time, with the shape (x.shape[0],).
- model_s: A pytorch tensor. The model function evaluated at time `s`.
- If `model_s` is None, we evaluate the model by `x` and `s`; otherwise we directly use it.
- return_intermediate: A `bool`. If true, also return the model value at time `s`.
- Returns:
- x_t: A pytorch tensor. The approximated solution at time `t`.
- """
- ns = self.noise_schedule
- dims = x.dim()
- lambda_s, lambda_t = ns.marginal_lambda(s), ns.marginal_lambda(t)
- h = lambda_t - lambda_s
- log_alpha_s, log_alpha_t = ns.marginal_log_mean_coeff(s), ns.marginal_log_mean_coeff(t)
- sigma_s, sigma_t = ns.marginal_std(s), ns.marginal_std(t)
- alpha_t = torch.exp(log_alpha_t)
-
- if self.predict_x0:
- phi_1 = torch.expm1(-h)
- if model_s is None:
- model_s = self.model_fn(x, s)
- x_t = (
- expand_dims(sigma_t / sigma_s, dims) * x
- - expand_dims(alpha_t * phi_1, dims) * model_s
- )
- if return_intermediate:
- return x_t, {'model_s': model_s}
- else:
- return x_t
- else:
- phi_1 = torch.expm1(h)
- if model_s is None:
- model_s = self.model_fn(x, s)
- x_t = (
- expand_dims(torch.exp(log_alpha_t - log_alpha_s), dims) * x
- - expand_dims(sigma_t * phi_1, dims) * model_s
- )
- if return_intermediate:
- return x_t, {'model_s': model_s}
- else:
- return x_t
-
- def singlestep_dpm_solver_second_update(self, x, s, t, r1=0.5, model_s=None, return_intermediate=False,
- solver_type='dpm_solver'):
- """
- Singlestep solver DPM-Solver-2 from time `s` to time `t`.
-
- Args:
- x: A pytorch tensor. The initial value at time `s`.
- s: A pytorch tensor. The starting time, with the shape (x.shape[0],).
- t: A pytorch tensor. The ending time, with the shape (x.shape[0],).
- r1: A `float`. The hyperparameter of the second-order solver.
- model_s: A pytorch tensor. The model function evaluated at time `s`.
- If `model_s` is None, we evaluate the model by `x` and `s`; otherwise we directly use it.
- return_intermediate: A `bool`. If true, also return the model value at time `s` and `s1` (the intermediate time).
- solver_type: either 'dpm_solver' or 'taylor'. The type for the high-order solvers.
- The type slightly impacts the performance. We recommend to use 'dpm_solver' type.
- Returns:
- x_t: A pytorch tensor. The approximated solution at time `t`.
- """
- if solver_type not in ['dpm_solver', 'taylor']:
- raise ValueError("'solver_type' must be either 'dpm_solver' or 'taylor', got {}".format(solver_type))
- if r1 is None:
- r1 = 0.5
- ns = self.noise_schedule
- dims = x.dim()
- lambda_s, lambda_t = ns.marginal_lambda(s), ns.marginal_lambda(t)
- h = lambda_t - lambda_s
- lambda_s1 = lambda_s + r1 * h
- s1 = ns.inverse_lambda(lambda_s1)
- log_alpha_s, log_alpha_s1, log_alpha_t = ns.marginal_log_mean_coeff(s), ns.marginal_log_mean_coeff(
- s1), ns.marginal_log_mean_coeff(t)
- sigma_s, sigma_s1, sigma_t = ns.marginal_std(s), ns.marginal_std(s1), ns.marginal_std(t)
- alpha_s1, alpha_t = torch.exp(log_alpha_s1), torch.exp(log_alpha_t)
-
- if self.predict_x0:
- phi_11 = torch.expm1(-r1 * h)
- phi_1 = torch.expm1(-h)
-
- if model_s is None:
- model_s = self.model_fn(x, s)
- x_s1 = (
- expand_dims(sigma_s1 / sigma_s, dims) * x
- - expand_dims(alpha_s1 * phi_11, dims) * model_s
- )
- model_s1 = self.model_fn(x_s1, s1)
- if solver_type == 'dpm_solver':
- x_t = (
- expand_dims(sigma_t / sigma_s, dims) * x
- - expand_dims(alpha_t * phi_1, dims) * model_s
- - (0.5 / r1) * expand_dims(alpha_t * phi_1, dims) * (model_s1 - model_s)
- )
- elif solver_type == 'taylor':
- x_t = (
- expand_dims(sigma_t / sigma_s, dims) * x
- - expand_dims(alpha_t * phi_1, dims) * model_s
- + (1. / r1) * expand_dims(alpha_t * ((torch.exp(-h) - 1.) / h + 1.), dims) * (
- model_s1 - model_s)
- )
- else:
- phi_11 = torch.expm1(r1 * h)
- phi_1 = torch.expm1(h)
-
- if model_s is None:
- model_s = self.model_fn(x, s)
- x_s1 = (
- expand_dims(torch.exp(log_alpha_s1 - log_alpha_s), dims) * x
- - expand_dims(sigma_s1 * phi_11, dims) * model_s
- )
- model_s1 = self.model_fn(x_s1, s1)
- if solver_type == 'dpm_solver':
- x_t = (
- expand_dims(torch.exp(log_alpha_t - log_alpha_s), dims) * x
- - expand_dims(sigma_t * phi_1, dims) * model_s
- - (0.5 / r1) * expand_dims(sigma_t * phi_1, dims) * (model_s1 - model_s)
- )
- elif solver_type == 'taylor':
- x_t = (
- expand_dims(torch.exp(log_alpha_t - log_alpha_s), dims) * x
- - expand_dims(sigma_t * phi_1, dims) * model_s
- - (1. / r1) * expand_dims(sigma_t * ((torch.exp(h) - 1.) / h - 1.), dims) * (model_s1 - model_s)
- )
- if return_intermediate:
- return x_t, {'model_s': model_s, 'model_s1': model_s1}
- else:
- return x_t
-
- def singlestep_dpm_solver_third_update(self, x, s, t, r1=1. / 3., r2=2. / 3., model_s=None, model_s1=None,
- return_intermediate=False, solver_type='dpm_solver'):
- """
- Singlestep solver DPM-Solver-3 from time `s` to time `t`.
-
- Args:
- x: A pytorch tensor. The initial value at time `s`.
- s: A pytorch tensor. The starting time, with the shape (x.shape[0],).
- t: A pytorch tensor. The ending time, with the shape (x.shape[0],).
- r1: A `float`. The hyperparameter of the third-order solver.
- r2: A `float`. The hyperparameter of the third-order solver.
- model_s: A pytorch tensor. The model function evaluated at time `s`.
- If `model_s` is None, we evaluate the model by `x` and `s`; otherwise we directly use it.
- model_s1: A pytorch tensor. The model function evaluated at time `s1` (the intermediate time given by `r1`).
- If `model_s1` is None, we evaluate the model at `s1`; otherwise we directly use it.
- return_intermediate: A `bool`. If true, also return the model value at time `s`, `s1` and `s2` (the intermediate times).
- solver_type: either 'dpm_solver' or 'taylor'. The type for the high-order solvers.
- The type slightly impacts the performance. We recommend to use 'dpm_solver' type.
- Returns:
- x_t: A pytorch tensor. The approximated solution at time `t`.
- """
- if solver_type not in ['dpm_solver', 'taylor']:
- raise ValueError("'solver_type' must be either 'dpm_solver' or 'taylor', got {}".format(solver_type))
- if r1 is None:
- r1 = 1. / 3.
- if r2 is None:
- r2 = 2. / 3.
- ns = self.noise_schedule
- dims = x.dim()
- lambda_s, lambda_t = ns.marginal_lambda(s), ns.marginal_lambda(t)
- h = lambda_t - lambda_s
- lambda_s1 = lambda_s + r1 * h
- lambda_s2 = lambda_s + r2 * h
- s1 = ns.inverse_lambda(lambda_s1)
- s2 = ns.inverse_lambda(lambda_s2)
- log_alpha_s, log_alpha_s1, log_alpha_s2, log_alpha_t = ns.marginal_log_mean_coeff(
- s), ns.marginal_log_mean_coeff(s1), ns.marginal_log_mean_coeff(s2), ns.marginal_log_mean_coeff(t)
- sigma_s, sigma_s1, sigma_s2, sigma_t = ns.marginal_std(s), ns.marginal_std(s1), ns.marginal_std(
- s2), ns.marginal_std(t)
- alpha_s1, alpha_s2, alpha_t = torch.exp(log_alpha_s1), torch.exp(log_alpha_s2), torch.exp(log_alpha_t)
-
- if self.predict_x0:
- phi_11 = torch.expm1(-r1 * h)
- phi_12 = torch.expm1(-r2 * h)
- phi_1 = torch.expm1(-h)
- phi_22 = torch.expm1(-r2 * h) / (r2 * h) + 1.
- phi_2 = phi_1 / h + 1.
- phi_3 = phi_2 / h - 0.5
-
- if model_s is None:
- model_s = self.model_fn(x, s)
- if model_s1 is None:
- x_s1 = (
- expand_dims(sigma_s1 / sigma_s, dims) * x
- - expand_dims(alpha_s1 * phi_11, dims) * model_s
- )
- model_s1 = self.model_fn(x_s1, s1)
- x_s2 = (
- expand_dims(sigma_s2 / sigma_s, dims) * x
- - expand_dims(alpha_s2 * phi_12, dims) * model_s
- + r2 / r1 * expand_dims(alpha_s2 * phi_22, dims) * (model_s1 - model_s)
- )
- model_s2 = self.model_fn(x_s2, s2)
- if solver_type == 'dpm_solver':
- x_t = (
- expand_dims(sigma_t / sigma_s, dims) * x
- - expand_dims(alpha_t * phi_1, dims) * model_s
- + (1. / r2) * expand_dims(alpha_t * phi_2, dims) * (model_s2 - model_s)
- )
- elif solver_type == 'taylor':
- D1_0 = (1. / r1) * (model_s1 - model_s)
- D1_1 = (1. / r2) * (model_s2 - model_s)
- D1 = (r2 * D1_0 - r1 * D1_1) / (r2 - r1)
- D2 = 2. * (D1_1 - D1_0) / (r2 - r1)
- x_t = (
- expand_dims(sigma_t / sigma_s, dims) * x
- - expand_dims(alpha_t * phi_1, dims) * model_s
- + expand_dims(alpha_t * phi_2, dims) * D1
- - expand_dims(alpha_t * phi_3, dims) * D2
- )
- else:
- phi_11 = torch.expm1(r1 * h)
- phi_12 = torch.expm1(r2 * h)
- phi_1 = torch.expm1(h)
- phi_22 = torch.expm1(r2 * h) / (r2 * h) - 1.
- phi_2 = phi_1 / h - 1.
- phi_3 = phi_2 / h - 0.5
-
- if model_s is None:
- model_s = self.model_fn(x, s)
- if model_s1 is None:
- x_s1 = (
- expand_dims(torch.exp(log_alpha_s1 - log_alpha_s), dims) * x
- - expand_dims(sigma_s1 * phi_11, dims) * model_s
- )
- model_s1 = self.model_fn(x_s1, s1)
- x_s2 = (
- expand_dims(torch.exp(log_alpha_s2 - log_alpha_s), dims) * x
- - expand_dims(sigma_s2 * phi_12, dims) * model_s
- - r2 / r1 * expand_dims(sigma_s2 * phi_22, dims) * (model_s1 - model_s)
- )
- model_s2 = self.model_fn(x_s2, s2)
- if solver_type == 'dpm_solver':
- x_t = (
- expand_dims(torch.exp(log_alpha_t - log_alpha_s), dims) * x
- - expand_dims(sigma_t * phi_1, dims) * model_s
- - (1. / r2) * expand_dims(sigma_t * phi_2, dims) * (model_s2 - model_s)
- )
- elif solver_type == 'taylor':
- D1_0 = (1. / r1) * (model_s1 - model_s)
- D1_1 = (1. / r2) * (model_s2 - model_s)
- D1 = (r2 * D1_0 - r1 * D1_1) / (r2 - r1)
- D2 = 2. * (D1_1 - D1_0) / (r2 - r1)
- x_t = (
- expand_dims(torch.exp(log_alpha_t - log_alpha_s), dims) * x
- - expand_dims(sigma_t * phi_1, dims) * model_s
- - expand_dims(sigma_t * phi_2, dims) * D1
- - expand_dims(sigma_t * phi_3, dims) * D2
- )
-
- if return_intermediate:
- return x_t, {'model_s': model_s, 'model_s1': model_s1, 'model_s2': model_s2}
- else:
- return x_t
-
- def multistep_dpm_solver_second_update(self, x, model_prev_list, t_prev_list, t, solver_type="dpm_solver"):
- """
- Multistep solver DPM-Solver-2 from time `t_prev_list[-1]` to time `t`.
-
- Args:
- x: A pytorch tensor. The initial value at time `s`.
- model_prev_list: A list of pytorch tensor. The previous computed model values.
- t_prev_list: A list of pytorch tensor. The previous times, each time has the shape (x.shape[0],)
- t: A pytorch tensor. The ending time, with the shape (x.shape[0],).
- solver_type: either 'dpm_solver' or 'taylor'. The type for the high-order solvers.
- The type slightly impacts the performance. We recommend to use 'dpm_solver' type.
- Returns:
- x_t: A pytorch tensor. The approximated solution at time `t`.
- """
- if solver_type not in ['dpm_solver', 'taylor']:
- raise ValueError("'solver_type' must be either 'dpm_solver' or 'taylor', got {}".format(solver_type))
- ns = self.noise_schedule
- dims = x.dim()
- model_prev_1, model_prev_0 = model_prev_list
- t_prev_1, t_prev_0 = t_prev_list
- lambda_prev_1, lambda_prev_0, lambda_t = ns.marginal_lambda(t_prev_1), ns.marginal_lambda(
- t_prev_0), ns.marginal_lambda(t)
- log_alpha_prev_0, log_alpha_t = ns.marginal_log_mean_coeff(t_prev_0), ns.marginal_log_mean_coeff(t)
- sigma_prev_0, sigma_t = ns.marginal_std(t_prev_0), ns.marginal_std(t)
- alpha_t = torch.exp(log_alpha_t)
-
- h_0 = lambda_prev_0 - lambda_prev_1
- h = lambda_t - lambda_prev_0
- r0 = h_0 / h
- D1_0 = expand_dims(1. / r0, dims) * (model_prev_0 - model_prev_1)
- if self.predict_x0:
- if solver_type == 'dpm_solver':
- x_t = (
- expand_dims(sigma_t / sigma_prev_0, dims) * x
- - expand_dims(alpha_t * (torch.exp(-h) - 1.), dims) * model_prev_0
- - 0.5 * expand_dims(alpha_t * (torch.exp(-h) - 1.), dims) * D1_0
- )
- elif solver_type == 'taylor':
- x_t = (
- expand_dims(sigma_t / sigma_prev_0, dims) * x
- - expand_dims(alpha_t * (torch.exp(-h) - 1.), dims) * model_prev_0
- + expand_dims(alpha_t * ((torch.exp(-h) - 1.) / h + 1.), dims) * D1_0
- )
- else:
- if solver_type == 'dpm_solver':
- x_t = (
- expand_dims(torch.exp(log_alpha_t - log_alpha_prev_0), dims) * x
- - expand_dims(sigma_t * (torch.exp(h) - 1.), dims) * model_prev_0
- - 0.5 * expand_dims(sigma_t * (torch.exp(h) - 1.), dims) * D1_0
- )
- elif solver_type == 'taylor':
- x_t = (
- expand_dims(torch.exp(log_alpha_t - log_alpha_prev_0), dims) * x
- - expand_dims(sigma_t * (torch.exp(h) - 1.), dims) * model_prev_0
- - expand_dims(sigma_t * ((torch.exp(h) - 1.) / h - 1.), dims) * D1_0
- )
- return x_t
-
- def multistep_dpm_solver_third_update(self, x, model_prev_list, t_prev_list, t, solver_type='dpm_solver'):
- """
- Multistep solver DPM-Solver-3 from time `t_prev_list[-1]` to time `t`.
-
- Args:
- x: A pytorch tensor. The initial value at time `s`.
- model_prev_list: A list of pytorch tensor. The previous computed model values.
- t_prev_list: A list of pytorch tensor. The previous times, each time has the shape (x.shape[0],)
- t: A pytorch tensor. The ending time, with the shape (x.shape[0],).
- solver_type: either 'dpm_solver' or 'taylor'. The type for the high-order solvers.
- The type slightly impacts the performance. We recommend to use 'dpm_solver' type.
- Returns:
- x_t: A pytorch tensor. The approximated solution at time `t`.
- """
- ns = self.noise_schedule
- dims = x.dim()
- model_prev_2, model_prev_1, model_prev_0 = model_prev_list
- t_prev_2, t_prev_1, t_prev_0 = t_prev_list
- lambda_prev_2, lambda_prev_1, lambda_prev_0, lambda_t = ns.marginal_lambda(t_prev_2), ns.marginal_lambda(
- t_prev_1), ns.marginal_lambda(t_prev_0), ns.marginal_lambda(t)
- log_alpha_prev_0, log_alpha_t = ns.marginal_log_mean_coeff(t_prev_0), ns.marginal_log_mean_coeff(t)
- sigma_prev_0, sigma_t = ns.marginal_std(t_prev_0), ns.marginal_std(t)
- alpha_t = torch.exp(log_alpha_t)
-
- h_1 = lambda_prev_1 - lambda_prev_2
- h_0 = lambda_prev_0 - lambda_prev_1
- h = lambda_t - lambda_prev_0
- r0, r1 = h_0 / h, h_1 / h
- D1_0 = expand_dims(1. / r0, dims) * (model_prev_0 - model_prev_1)
- D1_1 = expand_dims(1. / r1, dims) * (model_prev_1 - model_prev_2)
- D1 = D1_0 + expand_dims(r0 / (r0 + r1), dims) * (D1_0 - D1_1)
- D2 = expand_dims(1. / (r0 + r1), dims) * (D1_0 - D1_1)
- if self.predict_x0:
- x_t = (
- expand_dims(sigma_t / sigma_prev_0, dims) * x
- - expand_dims(alpha_t * (torch.exp(-h) - 1.), dims) * model_prev_0
- + expand_dims(alpha_t * ((torch.exp(-h) - 1.) / h + 1.), dims) * D1
- - expand_dims(alpha_t * ((torch.exp(-h) - 1. + h) / h ** 2 - 0.5), dims) * D2
- )
- else:
- x_t = (
- expand_dims(torch.exp(log_alpha_t - log_alpha_prev_0), dims) * x
- - expand_dims(sigma_t * (torch.exp(h) - 1.), dims) * model_prev_0
- - expand_dims(sigma_t * ((torch.exp(h) - 1.) / h - 1.), dims) * D1
- - expand_dims(sigma_t * ((torch.exp(h) - 1. - h) / h ** 2 - 0.5), dims) * D2
- )
- return x_t
-
- def singlestep_dpm_solver_update(self, x, s, t, order, return_intermediate=False, solver_type='dpm_solver', r1=None,
- r2=None):
- """
- Singlestep DPM-Solver with the order `order` from time `s` to time `t`.
-
- Args:
- x: A pytorch tensor. The initial value at time `s`.
- s: A pytorch tensor. The starting time, with the shape (x.shape[0],).
- t: A pytorch tensor. The ending time, with the shape (x.shape[0],).
- order: A `int`. The order of DPM-Solver. We only support order == 1 or 2 or 3.
- return_intermediate: A `bool`. If true, also return the model value at time `s`, `s1` and `s2` (the intermediate times).
- solver_type: either 'dpm_solver' or 'taylor'. The type for the high-order solvers.
- The type slightly impacts the performance. We recommend to use 'dpm_solver' type.
- r1: A `float`. The hyperparameter of the second-order or third-order solver.
- r2: A `float`. The hyperparameter of the third-order solver.
- Returns:
- x_t: A pytorch tensor. The approximated solution at time `t`.
- """
- if order == 1:
- return self.dpm_solver_first_update(x, s, t, return_intermediate=return_intermediate)
- elif order == 2:
- return self.singlestep_dpm_solver_second_update(x, s, t, return_intermediate=return_intermediate,
- solver_type=solver_type, r1=r1)
- elif order == 3:
- return self.singlestep_dpm_solver_third_update(x, s, t, return_intermediate=return_intermediate,
- solver_type=solver_type, r1=r1, r2=r2)
- else:
- raise ValueError("Solver order must be 1 or 2 or 3, got {}".format(order))
-
- def multistep_dpm_solver_update(self, x, model_prev_list, t_prev_list, t, order, solver_type='dpm_solver'):
- """
- Multistep DPM-Solver with the order `order` from time `t_prev_list[-1]` to time `t`.
-
- Args:
- x: A pytorch tensor. The initial value at time `s`.
- model_prev_list: A list of pytorch tensor. The previous computed model values.
- t_prev_list: A list of pytorch tensor. The previous times, each time has the shape (x.shape[0],)
- t: A pytorch tensor. The ending time, with the shape (x.shape[0],).
- order: A `int`. The order of DPM-Solver. We only support order == 1 or 2 or 3.
- solver_type: either 'dpm_solver' or 'taylor'. The type for the high-order solvers.
- The type slightly impacts the performance. We recommend to use 'dpm_solver' type.
- Returns:
- x_t: A pytorch tensor. The approximated solution at time `t`.
- """
- if order == 1:
- return self.dpm_solver_first_update(x, t_prev_list[-1], t, model_s=model_prev_list[-1])
- elif order == 2:
- return self.multistep_dpm_solver_second_update(x, model_prev_list, t_prev_list, t, solver_type=solver_type)
- elif order == 3:
- return self.multistep_dpm_solver_third_update(x, model_prev_list, t_prev_list, t, solver_type=solver_type)
- else:
- raise ValueError("Solver order must be 1 or 2 or 3, got {}".format(order))
-
- def dpm_solver_adaptive(self, x, order, t_T, t_0, h_init=0.05, atol=0.0078, rtol=0.05, theta=0.9, t_err=1e-5,
- solver_type='dpm_solver'):
- """
- The adaptive step size solver based on singlestep DPM-Solver.
-
- Args:
- x: A pytorch tensor. The initial value at time `t_T`.
- order: A `int`. The (higher) order of the solver. We only support order == 2 or 3.
- t_T: A `float`. The starting time of the sampling (default is T).
- t_0: A `float`. The ending time of the sampling (default is epsilon).
- h_init: A `float`. The initial step size (for logSNR).
- atol: A `float`. The absolute tolerance of the solver. For image data, the default setting is 0.0078, followed [1].
- rtol: A `float`. The relative tolerance of the solver. The default setting is 0.05.
- theta: A `float`. The safety hyperparameter for adapting the step size. The default setting is 0.9, followed [1].
- t_err: A `float`. The tolerance for the time. We solve the diffusion ODE until the absolute error between the
- current time and `t_0` is less than `t_err`. The default setting is 1e-5.
- solver_type: either 'dpm_solver' or 'taylor'. The type for the high-order solvers.
- The type slightly impacts the performance. We recommend to use 'dpm_solver' type.
- Returns:
- x_0: A pytorch tensor. The approximated solution at time `t_0`.
-
- [1] A. Jolicoeur-Martineau, K. Li, R. Piché-Taillefer, T. Kachman, and I. Mitliagkas, "Gotta go fast when generating data with score-based models," arXiv preprint arXiv:2105.14080, 2021.
- """
- ns = self.noise_schedule
- s = t_T * torch.ones((x.shape[0],)).to(x)
- lambda_s = ns.marginal_lambda(s)
- lambda_0 = ns.marginal_lambda(t_0 * torch.ones_like(s).to(x))
- h = h_init * torch.ones_like(s).to(x)
- x_prev = x
- nfe = 0
- if order == 2:
- r1 = 0.5
- lower_update = lambda x, s, t: self.dpm_solver_first_update(x, s, t, return_intermediate=True)
- higher_update = lambda x, s, t, **kwargs: self.singlestep_dpm_solver_second_update(x, s, t, r1=r1,
- solver_type=solver_type,
- **kwargs)
- elif order == 3:
- r1, r2 = 1. / 3., 2. / 3.
- lower_update = lambda x, s, t: self.singlestep_dpm_solver_second_update(x, s, t, r1=r1,
- return_intermediate=True,
- solver_type=solver_type)
- higher_update = lambda x, s, t, **kwargs: self.singlestep_dpm_solver_third_update(x, s, t, r1=r1, r2=r2,
- solver_type=solver_type,
- **kwargs)
- else:
- raise ValueError("For adaptive step size solver, order must be 2 or 3, got {}".format(order))
- while torch.abs((s - t_0)).mean() > t_err:
- t = ns.inverse_lambda(lambda_s + h)
- x_lower, lower_noise_kwargs = lower_update(x, s, t)
- x_higher = higher_update(x, s, t, **lower_noise_kwargs)
- delta = torch.max(torch.ones_like(x).to(x) * atol, rtol * torch.max(torch.abs(x_lower), torch.abs(x_prev)))
- norm_fn = lambda v: torch.sqrt(torch.square(v.reshape((v.shape[0], -1))).mean(dim=-1, keepdim=True))
- E = norm_fn((x_higher - x_lower) / delta).max()
- if torch.all(E <= 1.):
- x = x_higher
- s = t
- x_prev = x_lower
- lambda_s = ns.marginal_lambda(s)
- h = torch.min(theta * h * torch.float_power(E, -1. / order).float(), lambda_0 - lambda_s)
- nfe += order
- print('adaptive solver nfe', nfe)
- return x
-
- def sample(self, x, steps=20, t_start=None, t_end=None, order=3, skip_type='time_uniform',
- method='singlestep', denoise=False, solver_type='dpm_solver', atol=0.0078,
- rtol=0.05,
- ):
- """
- Compute the sample at time `t_end` by DPM-Solver, given the initial `x` at time `t_start`.
-
- =====================================================
-
- We support the following algorithms for both noise prediction model and data prediction model:
- - 'singlestep':
- Singlestep DPM-Solver (i.e. "DPM-Solver-fast" in the paper), which combines different orders of singlestep DPM-Solver.
- We combine all the singlestep solvers with order <= `order` to use up all the function evaluations (steps).
- The total number of function evaluations (NFE) == `steps`.
- Given a fixed NFE == `steps`, the sampling procedure is:
- - If `order` == 1:
- - Denote K = steps. We use K steps of DPM-Solver-1 (i.e. DDIM).
- - If `order` == 2:
- - Denote K = (steps // 2) + (steps % 2). We take K intermediate time steps for sampling.
- - If steps % 2 == 0, we use K steps of singlestep DPM-Solver-2.
- - If steps % 2 == 1, we use (K - 1) steps of singlestep DPM-Solver-2 and 1 step of DPM-Solver-1.
- - If `order` == 3:
- - Denote K = (steps // 3 + 1). We take K intermediate time steps for sampling.
- - If steps % 3 == 0, we use (K - 2) steps of singlestep DPM-Solver-3, and 1 step of singlestep DPM-Solver-2 and 1 step of DPM-Solver-1.
- - If steps % 3 == 1, we use (K - 1) steps of singlestep DPM-Solver-3 and 1 step of DPM-Solver-1.
- - If steps % 3 == 2, we use (K - 1) steps of singlestep DPM-Solver-3 and 1 step of singlestep DPM-Solver-2.
- - 'multistep':
- Multistep DPM-Solver with the order of `order`. The total number of function evaluations (NFE) == `steps`.
- We initialize the first `order` values by lower order multistep solvers.
- Given a fixed NFE == `steps`, the sampling procedure is:
- Denote K = steps.
- - If `order` == 1:
- - We use K steps of DPM-Solver-1 (i.e. DDIM).
- - If `order` == 2:
- - We firstly use 1 step of DPM-Solver-1, then use (K - 1) step of multistep DPM-Solver-2.
- - If `order` == 3:
- - We firstly use 1 step of DPM-Solver-1, then 1 step of multistep DPM-Solver-2, then (K - 2) step of multistep DPM-Solver-3.
- - 'singlestep_fixed':
- Fixed order singlestep DPM-Solver (i.e. DPM-Solver-1 or singlestep DPM-Solver-2 or singlestep DPM-Solver-3).
- We use singlestep DPM-Solver-`order` for `order`=1 or 2 or 3, with total [`steps` // `order`] * `order` NFE.
- - 'adaptive':
- Adaptive step size DPM-Solver (i.e. "DPM-Solver-12" and "DPM-Solver-23" in the paper).
- We ignore `steps` and use adaptive step size DPM-Solver with a higher order of `order`.
- You can adjust the absolute tolerance `atol` and the relative tolerance `rtol` to balance the computatation costs
- (NFE) and the sample quality.
- - If `order` == 2, we use DPM-Solver-12 which combines DPM-Solver-1 and singlestep DPM-Solver-2.
- - If `order` == 3, we use DPM-Solver-23 which combines singlestep DPM-Solver-2 and singlestep DPM-Solver-3.
-
- =====================================================
-
- Some advices for choosing the algorithm:
- - For **unconditional sampling** or **guided sampling with small guidance scale** by DPMs:
- Use singlestep DPM-Solver ("DPM-Solver-fast" in the paper) with `order = 3`.
- e.g.
- >>> dpm_solver = DPM_Solver(model_fn, noise_schedule, predict_x0=False)
- >>> x_sample = dpm_solver.sample(x, steps=steps, t_start=t_start, t_end=t_end, order=3,
- skip_type='time_uniform', method='singlestep')
- - For **guided sampling with large guidance scale** by DPMs:
- Use multistep DPM-Solver with `predict_x0 = True` and `order = 2`.
- e.g.
- >>> dpm_solver = DPM_Solver(model_fn, noise_schedule, predict_x0=True)
- >>> x_sample = dpm_solver.sample(x, steps=steps, t_start=t_start, t_end=t_end, order=2,
- skip_type='time_uniform', method='multistep')
-
- We support three types of `skip_type`:
- - 'logSNR': uniform logSNR for the time steps. **Recommended for low-resolutional images**
- - 'time_uniform': uniform time for the time steps. **Recommended for high-resolutional images**.
- - 'time_quadratic': quadratic time for the time steps.
-
- =====================================================
- Args:
- x: A pytorch tensor. The initial value at time `t_start`
- e.g. if `t_start` == T, then `x` is a sample from the standard normal distribution.
- steps: A `int`. The total number of function evaluations (NFE).
- t_start: A `float`. The starting time of the sampling.
- If `T` is None, we use self.noise_schedule.T (default is 1.0).
- t_end: A `float`. The ending time of the sampling.
- If `t_end` is None, we use 1. / self.noise_schedule.total_N.
- e.g. if total_N == 1000, we have `t_end` == 1e-3.
- For discrete-time DPMs:
- - We recommend `t_end` == 1. / self.noise_schedule.total_N.
- For continuous-time DPMs:
- - We recommend `t_end` == 1e-3 when `steps` <= 15; and `t_end` == 1e-4 when `steps` > 15.
- order: A `int`. The order of DPM-Solver.
- skip_type: A `str`. The type for the spacing of the time steps. 'time_uniform' or 'logSNR' or 'time_quadratic'.
- method: A `str`. The method for sampling. 'singlestep' or 'multistep' or 'singlestep_fixed' or 'adaptive'.
- denoise: A `bool`. Whether to denoise at the final step. Default is False.
- If `denoise` is True, the total NFE is (`steps` + 1).
- solver_type: A `str`. The taylor expansion type for the solver. `dpm_solver` or `taylor`. We recommend `dpm_solver`.
- atol: A `float`. The absolute tolerance of the adaptive step size solver. Valid when `method` == 'adaptive'.
- rtol: A `float`. The relative tolerance of the adaptive step size solver. Valid when `method` == 'adaptive'.
- Returns:
- x_end: A pytorch tensor. The approximated solution at time `t_end`.
-
- """
- t_0 = 1. / self.noise_schedule.total_N if t_end is None else t_end
- t_T = self.noise_schedule.T if t_start is None else t_start
- device = x.device
- if method == 'adaptive':
- with torch.no_grad():
- x = self.dpm_solver_adaptive(x, order=order, t_T=t_T, t_0=t_0, atol=atol, rtol=rtol,
- solver_type=solver_type)
- elif method == 'multistep':
- assert steps >= order
- timesteps = self.get_time_steps(skip_type=skip_type, t_T=t_T, t_0=t_0, N=steps, device=device)
- assert timesteps.shape[0] - 1 == steps
- with torch.no_grad():
- vec_t = timesteps[0].expand((x.shape[0]))
- model_prev_list = [self.model_fn(x, vec_t)]
- t_prev_list = [vec_t]
- # Init the first `order` values by lower order multistep DPM-Solver.
- for init_order in range(1, order):
- vec_t = timesteps[init_order].expand(x.shape[0])
- x = self.multistep_dpm_solver_update(x, model_prev_list, t_prev_list, vec_t, init_order,
- solver_type=solver_type)
- model_prev_list.append(self.model_fn(x, vec_t))
- t_prev_list.append(vec_t)
- # Compute the remaining values by `order`-th order multistep DPM-Solver.
- for step in range(order, steps + 1):
- vec_t = timesteps[step].expand(x.shape[0])
- x = self.multistep_dpm_solver_update(x, model_prev_list, t_prev_list, vec_t, order,
- solver_type=solver_type)
- for i in range(order - 1):
- t_prev_list[i] = t_prev_list[i + 1]
- model_prev_list[i] = model_prev_list[i + 1]
- t_prev_list[-1] = vec_t
- # We do not need to evaluate the final model value.
- if step < steps:
- model_prev_list[-1] = self.model_fn(x, vec_t)
- elif method in ['singlestep', 'singlestep_fixed']:
- if method == 'singlestep':
- timesteps_outer, orders = self.get_orders_and_timesteps_for_singlestep_solver(steps=steps, order=order,
- skip_type=skip_type,
- t_T=t_T, t_0=t_0,
- device=device)
- elif method == 'singlestep_fixed':
- K = steps // order
- orders = [order, ] * K
- timesteps_outer = self.get_time_steps(skip_type=skip_type, t_T=t_T, t_0=t_0, N=K, device=device)
- for i, order in enumerate(orders):
- t_T_inner, t_0_inner = timesteps_outer[i], timesteps_outer[i + 1]
- timesteps_inner = self.get_time_steps(skip_type=skip_type, t_T=t_T_inner.item(), t_0=t_0_inner.item(),
- N=order, device=device)
- lambda_inner = self.noise_schedule.marginal_lambda(timesteps_inner)
- vec_s, vec_t = t_T_inner.repeat(x.shape[0]), t_0_inner.repeat(x.shape[0])
- h = lambda_inner[-1] - lambda_inner[0]
- r1 = None if order <= 1 else (lambda_inner[1] - lambda_inner[0]) / h
- r2 = None if order <= 2 else (lambda_inner[2] - lambda_inner[0]) / h
- x = self.singlestep_dpm_solver_update(x, vec_s, vec_t, order, solver_type=solver_type, r1=r1, r2=r2)
- if denoise:
- x = self.denoise_fn(x, torch.ones((x.shape[0],)).to(device) * t_0)
- return x
-
-
-#############################################################
-# other utility functions
-#############################################################
-
-def interpolate_fn(x, xp, yp):
- """
- A piecewise linear function y = f(x), using xp and yp as keypoints.
- We implement f(x) in a differentiable way (i.e. applicable for autograd).
- The function f(x) is well-defined for all x-axis. (For x beyond the bounds of xp, we use the outmost points of xp to define the linear function.)
-
- Args:
- x: PyTorch tensor with shape [N, C], where N is the batch size, C is the number of channels (we use C = 1 for DPM-Solver).
- xp: PyTorch tensor with shape [C, K], where K is the number of keypoints.
- yp: PyTorch tensor with shape [C, K].
- Returns:
- The function values f(x), with shape [N, C].
- """
- N, K = x.shape[0], xp.shape[1]
- all_x = torch.cat([x.unsqueeze(2), xp.unsqueeze(0).repeat((N, 1, 1))], dim=2)
- sorted_all_x, x_indices = torch.sort(all_x, dim=2)
- x_idx = torch.argmin(x_indices, dim=2)
- cand_start_idx = x_idx - 1
- start_idx = torch.where(
- torch.eq(x_idx, 0),
- torch.tensor(1, device=x.device),
- torch.where(
- torch.eq(x_idx, K), torch.tensor(K - 2, device=x.device), cand_start_idx,
- ),
- )
- end_idx = torch.where(torch.eq(start_idx, cand_start_idx), start_idx + 2, start_idx + 1)
- start_x = torch.gather(sorted_all_x, dim=2, index=start_idx.unsqueeze(2)).squeeze(2)
- end_x = torch.gather(sorted_all_x, dim=2, index=end_idx.unsqueeze(2)).squeeze(2)
- start_idx2 = torch.where(
- torch.eq(x_idx, 0),
- torch.tensor(0, device=x.device),
- torch.where(
- torch.eq(x_idx, K), torch.tensor(K - 2, device=x.device), cand_start_idx,
- ),
- )
- y_positions_expanded = yp.unsqueeze(0).expand(N, -1, -1)
- start_y = torch.gather(y_positions_expanded, dim=2, index=start_idx2.unsqueeze(2)).squeeze(2)
- end_y = torch.gather(y_positions_expanded, dim=2, index=(start_idx2 + 1).unsqueeze(2)).squeeze(2)
- cand = start_y + (x - start_x) * (end_y - start_y) / (end_x - start_x)
- return cand
-
-
-def expand_dims(v, dims):
- """
- Expand the tensor `v` to the dim `dims`.
-
- Args:
- `v`: a PyTorch tensor with shape [N].
- `dim`: a `int`.
- Returns:
- a PyTorch tensor with shape [N, 1, 1, ..., 1] and the total dimension is `dims`.
- """
- return v[(...,) + (None,) * (dims - 1)]
diff --git a/spaces/lnyan/stablediffusion-infinity/PyPatchMatch/csrc/inpaint.cpp b/spaces/lnyan/stablediffusion-infinity/PyPatchMatch/csrc/inpaint.cpp
deleted file mode 100644
index de1f4b0c8bc74a2d4daf712827a903cc1385a2a7..0000000000000000000000000000000000000000
--- a/spaces/lnyan/stablediffusion-infinity/PyPatchMatch/csrc/inpaint.cpp
+++ /dev/null
@@ -1,234 +0,0 @@
-#include
-#include
-#include
-#include
-#include
-
-#include "inpaint.h"
-
-namespace {
- static std::vector kDistance2Similarity;
-
- void init_kDistance2Similarity() {
- double base[11] = {1.0, 0.99, 0.96, 0.83, 0.38, 0.11, 0.02, 0.005, 0.0006, 0.0001, 0};
- int length = (PatchDistanceMetric::kDistanceScale + 1);
- kDistance2Similarity.resize(length);
- for (int i = 0; i < length; ++i) {
- double t = (double) i / length;
- int j = (int) (100 * t);
- int k = j + 1;
- double vj = (j < 11) ? base[j] : 0;
- double vk = (k < 11) ? base[k] : 0;
- kDistance2Similarity[i] = vj + (100 * t - j) * (vk - vj);
- }
- }
-
-
- inline void _weighted_copy(const MaskedImage &source, int ys, int xs, cv::Mat &target, int yt, int xt, double weight) {
- if (source.is_masked(ys, xs)) return;
- if (source.is_globally_masked(ys, xs)) return;
-
- auto source_ptr = source.get_image(ys, xs);
- auto target_ptr = target.ptr(yt, xt);
-
-#pragma unroll
- for (int c = 0; c < 3; ++c)
- target_ptr[c] += static_cast(source_ptr[c]) * weight;
- target_ptr[3] += weight;
- }
-}
-
-/**
- * This algorithme uses a version proposed by Xavier Philippeau.
- */
-
-Inpainting::Inpainting(cv::Mat image, cv::Mat mask, const PatchDistanceMetric *metric)
- : m_initial(image, mask), m_distance_metric(metric), m_pyramid(), m_source2target(), m_target2source() {
- _initialize_pyramid();
-}
-
-Inpainting::Inpainting(cv::Mat image, cv::Mat mask, cv::Mat global_mask, const PatchDistanceMetric *metric)
- : m_initial(image, mask, global_mask), m_distance_metric(metric), m_pyramid(), m_source2target(), m_target2source() {
- _initialize_pyramid();
-}
-
-void Inpainting::_initialize_pyramid() {
- auto source = m_initial;
- m_pyramid.push_back(source);
- while (source.size().height > m_distance_metric->patch_size() && source.size().width > m_distance_metric->patch_size()) {
- source = source.downsample();
- m_pyramid.push_back(source);
- }
-
- if (kDistance2Similarity.size() == 0) {
- init_kDistance2Similarity();
- }
-}
-
-cv::Mat Inpainting::run(bool verbose, bool verbose_visualize, unsigned int random_seed) {
- srand(random_seed);
- const int nr_levels = m_pyramid.size();
-
- MaskedImage source, target;
- for (int level = nr_levels - 1; level >= 0; --level) {
- if (verbose) std::cerr << "Inpainting level: " << level << std::endl;
-
- source = m_pyramid[level];
-
- if (level == nr_levels - 1) {
- target = source.clone();
- target.clear_mask();
- m_source2target = NearestNeighborField(source, target, m_distance_metric);
- m_target2source = NearestNeighborField(target, source, m_distance_metric);
- } else {
- m_source2target = NearestNeighborField(source, target, m_distance_metric, m_source2target);
- m_target2source = NearestNeighborField(target, source, m_distance_metric, m_target2source);
- }
-
- if (verbose) std::cerr << "Initialization done." << std::endl;
-
- if (verbose_visualize) {
- auto visualize_size = m_initial.size();
- cv::Mat source_visualize(visualize_size, m_initial.image().type());
- cv::resize(source.image(), source_visualize, visualize_size);
- cv::imshow("Source", source_visualize);
- cv::Mat target_visualize(visualize_size, m_initial.image().type());
- cv::resize(target.image(), target_visualize, visualize_size);
- cv::imshow("Target", target_visualize);
- cv::waitKey(0);
- }
-
- target = _expectation_maximization(source, target, level, verbose);
- }
-
- return target.image();
-}
-
-// EM-Like algorithm (see "PatchMatch" - page 6).
-// Returns a double sized target image (unless level = 0).
-MaskedImage Inpainting::_expectation_maximization(MaskedImage source, MaskedImage target, int level, bool verbose) {
- const int nr_iters_em = 1 + 2 * level;
- const int nr_iters_nnf = static_cast(std::min(7, 1 + level));
- const int patch_size = m_distance_metric->patch_size();
-
- MaskedImage new_source, new_target;
-
- for (int iter_em = 0; iter_em < nr_iters_em; ++iter_em) {
- if (iter_em != 0) {
- m_source2target.set_target(new_target);
- m_target2source.set_source(new_target);
- target = new_target;
- }
-
- if (verbose) std::cerr << "EM Iteration: " << iter_em << std::endl;
-
- auto size = source.size();
- for (int i = 0; i < size.height; ++i) {
- for (int j = 0; j < size.width; ++j) {
- if (!source.contains_mask(i, j, patch_size)) {
- m_source2target.set_identity(i, j);
- m_target2source.set_identity(i, j);
- }
- }
- }
- if (verbose) std::cerr << " NNF minimization started." << std::endl;
- m_source2target.minimize(nr_iters_nnf);
- m_target2source.minimize(nr_iters_nnf);
- if (verbose) std::cerr << " NNF minimization finished." << std::endl;
-
- // Instead of upsizing the final target, we build the last target from the next level source image.
- // Thus, the final target is less blurry (see "Space-Time Video Completion" - page 5).
- bool upscaled = false;
- if (level >= 1 && iter_em == nr_iters_em - 1) {
- new_source = m_pyramid[level - 1];
- new_target = target.upsample(new_source.size().width, new_source.size().height, m_pyramid[level - 1].global_mask());
- upscaled = true;
- } else {
- new_source = m_pyramid[level];
- new_target = target.clone();
- }
-
- auto vote = cv::Mat(new_target.size(), CV_64FC4);
- vote.setTo(cv::Scalar::all(0));
-
- // Votes for best patch from NNF Source->Target (completeness) and Target->Source (coherence).
- _expectation_step(m_source2target, 1, vote, new_source, upscaled);
- if (verbose) std::cerr << " Expectation source to target finished." << std::endl;
- _expectation_step(m_target2source, 0, vote, new_source, upscaled);
- if (verbose) std::cerr << " Expectation target to source finished." << std::endl;
-
- // Compile votes and update pixel values.
- _maximization_step(new_target, vote);
- if (verbose) std::cerr << " Minimization step finished." << std::endl;
- }
-
- return new_target;
-}
-
-// Expectation step: vote for best estimations of each pixel.
-void Inpainting::_expectation_step(
- const NearestNeighborField &nnf, bool source2target,
- cv::Mat &vote, const MaskedImage &source, bool upscaled
-) {
- auto source_size = nnf.source_size();
- auto target_size = nnf.target_size();
- const int patch_size = m_distance_metric->patch_size();
-
- for (int i = 0; i < source_size.height; ++i) {
- for (int j = 0; j < source_size.width; ++j) {
- if (nnf.source().is_globally_masked(i, j)) continue;
- int yp = nnf.at(i, j, 0), xp = nnf.at(i, j, 1), dp = nnf.at(i, j, 2);
- double w = kDistance2Similarity[dp];
-
- for (int di = -patch_size; di <= patch_size; ++di) {
- for (int dj = -patch_size; dj <= patch_size; ++dj) {
- int ys = i + di, xs = j + dj, yt = yp + di, xt = xp + dj;
- if (!(ys >= 0 && ys < source_size.height && xs >= 0 && xs < source_size.width)) continue;
- if (nnf.source().is_globally_masked(ys, xs)) continue;
- if (!(yt >= 0 && yt < target_size.height && xt >= 0 && xt < target_size.width)) continue;
- if (nnf.target().is_globally_masked(yt, xt)) continue;
-
- if (!source2target) {
- std::swap(ys, yt);
- std::swap(xs, xt);
- }
-
- if (upscaled) {
- for (int uy = 0; uy < 2; ++uy) {
- for (int ux = 0; ux < 2; ++ux) {
- _weighted_copy(source, 2 * ys + uy, 2 * xs + ux, vote, 2 * yt + uy, 2 * xt + ux, w);
- }
- }
- } else {
- _weighted_copy(source, ys, xs, vote, yt, xt, w);
- }
- }
- }
- }
- }
-}
-
-// Maximization Step: maximum likelihood of target pixel.
-void Inpainting::_maximization_step(MaskedImage &target, const cv::Mat &vote) {
- auto target_size = target.size();
- for (int i = 0; i < target_size.height; ++i) {
- for (int j = 0; j < target_size.width; ++j) {
- const double *source_ptr = vote.ptr(i, j);
- unsigned char *target_ptr = target.get_mutable_image(i, j);
-
- if (target.is_globally_masked(i, j)) {
- continue;
- }
-
- if (source_ptr[3] > 0) {
- unsigned char r = cv::saturate_cast(source_ptr[0] / source_ptr[3]);
- unsigned char g = cv::saturate_cast(source_ptr[1] / source_ptr[3]);
- unsigned char b = cv::saturate_cast(source_ptr[2] / source_ptr[3]);
- target_ptr[0] = r, target_ptr[1] = g, target_ptr[2] = b;
- } else {
- target.set_mask(i, j, 0);
- }
- }
- }
-}
-
diff --git a/spaces/lvwerra/license/README.md b/spaces/lvwerra/license/README.md
deleted file mode 100644
index 9371e023f138523c78b8bf3c4d42c1535d322354..0000000000000000000000000000000000000000
--- a/spaces/lvwerra/license/README.md
+++ /dev/null
@@ -1,12 +0,0 @@
----
-title: License
-emoji: ⚖️
-colorFrom: red
-colorTo: indigo
-sdk: streamlit
-sdk_version: 1.9.0
-app_file: app.py
-pinned: false
----
-
-Check out the configuration reference at https://huggingface.co/docs/hub/spaces#reference
diff --git a/spaces/lwchen/CodeFormer/CodeFormer/basicsr/ops/dcn/deform_conv.py b/spaces/lwchen/CodeFormer/CodeFormer/basicsr/ops/dcn/deform_conv.py
deleted file mode 100644
index 734154f9ed9447d585eae7df6886acb136f8a3cf..0000000000000000000000000000000000000000
--- a/spaces/lwchen/CodeFormer/CodeFormer/basicsr/ops/dcn/deform_conv.py
+++ /dev/null
@@ -1,377 +0,0 @@
-import math
-import torch
-from torch import nn as nn
-from torch.autograd import Function
-from torch.autograd.function import once_differentiable
-from torch.nn import functional as F
-from torch.nn.modules.utils import _pair, _single
-
-try:
- from . import deform_conv_ext
-except ImportError:
- import os
- BASICSR_JIT = os.getenv('BASICSR_JIT')
- if BASICSR_JIT == 'True':
- from torch.utils.cpp_extension import load
- module_path = os.path.dirname(__file__)
- deform_conv_ext = load(
- 'deform_conv',
- sources=[
- os.path.join(module_path, 'src', 'deform_conv_ext.cpp'),
- os.path.join(module_path, 'src', 'deform_conv_cuda.cpp'),
- os.path.join(module_path, 'src', 'deform_conv_cuda_kernel.cu'),
- ],
- )
-
-
-class DeformConvFunction(Function):
-
- @staticmethod
- def forward(ctx,
- input,
- offset,
- weight,
- stride=1,
- padding=0,
- dilation=1,
- groups=1,
- deformable_groups=1,
- im2col_step=64):
- if input is not None and input.dim() != 4:
- raise ValueError(f'Expected 4D tensor as input, got {input.dim()}' 'D tensor instead.')
- ctx.stride = _pair(stride)
- ctx.padding = _pair(padding)
- ctx.dilation = _pair(dilation)
- ctx.groups = groups
- ctx.deformable_groups = deformable_groups
- ctx.im2col_step = im2col_step
-
- ctx.save_for_backward(input, offset, weight)
-
- output = input.new_empty(DeformConvFunction._output_size(input, weight, ctx.padding, ctx.dilation, ctx.stride))
-
- ctx.bufs_ = [input.new_empty(0), input.new_empty(0)] # columns, ones
-
- if not input.is_cuda:
- raise NotImplementedError
- else:
- cur_im2col_step = min(ctx.im2col_step, input.shape[0])
- assert (input.shape[0] % cur_im2col_step) == 0, 'im2col step must divide batchsize'
- deform_conv_ext.deform_conv_forward(input, weight,
- offset, output, ctx.bufs_[0], ctx.bufs_[1], weight.size(3),
- weight.size(2), ctx.stride[1], ctx.stride[0], ctx.padding[1],
- ctx.padding[0], ctx.dilation[1], ctx.dilation[0], ctx.groups,
- ctx.deformable_groups, cur_im2col_step)
- return output
-
- @staticmethod
- @once_differentiable
- def backward(ctx, grad_output):
- input, offset, weight = ctx.saved_tensors
-
- grad_input = grad_offset = grad_weight = None
-
- if not grad_output.is_cuda:
- raise NotImplementedError
- else:
- cur_im2col_step = min(ctx.im2col_step, input.shape[0])
- assert (input.shape[0] % cur_im2col_step) == 0, 'im2col step must divide batchsize'
-
- if ctx.needs_input_grad[0] or ctx.needs_input_grad[1]:
- grad_input = torch.zeros_like(input)
- grad_offset = torch.zeros_like(offset)
- deform_conv_ext.deform_conv_backward_input(input, offset, grad_output, grad_input,
- grad_offset, weight, ctx.bufs_[0], weight.size(3),
- weight.size(2), ctx.stride[1], ctx.stride[0], ctx.padding[1],
- ctx.padding[0], ctx.dilation[1], ctx.dilation[0], ctx.groups,
- ctx.deformable_groups, cur_im2col_step)
-
- if ctx.needs_input_grad[2]:
- grad_weight = torch.zeros_like(weight)
- deform_conv_ext.deform_conv_backward_parameters(input, offset, grad_output, grad_weight,
- ctx.bufs_[0], ctx.bufs_[1], weight.size(3),
- weight.size(2), ctx.stride[1], ctx.stride[0],
- ctx.padding[1], ctx.padding[0], ctx.dilation[1],
- ctx.dilation[0], ctx.groups, ctx.deformable_groups, 1,
- cur_im2col_step)
-
- return (grad_input, grad_offset, grad_weight, None, None, None, None, None)
-
- @staticmethod
- def _output_size(input, weight, padding, dilation, stride):
- channels = weight.size(0)
- output_size = (input.size(0), channels)
- for d in range(input.dim() - 2):
- in_size = input.size(d + 2)
- pad = padding[d]
- kernel = dilation[d] * (weight.size(d + 2) - 1) + 1
- stride_ = stride[d]
- output_size += ((in_size + (2 * pad) - kernel) // stride_ + 1, )
- if not all(map(lambda s: s > 0, output_size)):
- raise ValueError('convolution input is too small (output would be ' f'{"x".join(map(str, output_size))})')
- return output_size
-
-
-class ModulatedDeformConvFunction(Function):
-
- @staticmethod
- def forward(ctx,
- input,
- offset,
- mask,
- weight,
- bias=None,
- stride=1,
- padding=0,
- dilation=1,
- groups=1,
- deformable_groups=1):
- ctx.stride = stride
- ctx.padding = padding
- ctx.dilation = dilation
- ctx.groups = groups
- ctx.deformable_groups = deformable_groups
- ctx.with_bias = bias is not None
- if not ctx.with_bias:
- bias = input.new_empty(1) # fake tensor
- if not input.is_cuda:
- raise NotImplementedError
- if weight.requires_grad or mask.requires_grad or offset.requires_grad \
- or input.requires_grad:
- ctx.save_for_backward(input, offset, mask, weight, bias)
- output = input.new_empty(ModulatedDeformConvFunction._infer_shape(ctx, input, weight))
- ctx._bufs = [input.new_empty(0), input.new_empty(0)]
- deform_conv_ext.modulated_deform_conv_forward(input, weight, bias, ctx._bufs[0], offset, mask, output,
- ctx._bufs[1], weight.shape[2], weight.shape[3], ctx.stride,
- ctx.stride, ctx.padding, ctx.padding, ctx.dilation, ctx.dilation,
- ctx.groups, ctx.deformable_groups, ctx.with_bias)
- return output
-
- @staticmethod
- @once_differentiable
- def backward(ctx, grad_output):
- if not grad_output.is_cuda:
- raise NotImplementedError
- input, offset, mask, weight, bias = ctx.saved_tensors
- grad_input = torch.zeros_like(input)
- grad_offset = torch.zeros_like(offset)
- grad_mask = torch.zeros_like(mask)
- grad_weight = torch.zeros_like(weight)
- grad_bias = torch.zeros_like(bias)
- deform_conv_ext.modulated_deform_conv_backward(input, weight, bias, ctx._bufs[0], offset, mask, ctx._bufs[1],
- grad_input, grad_weight, grad_bias, grad_offset, grad_mask,
- grad_output, weight.shape[2], weight.shape[3], ctx.stride,
- ctx.stride, ctx.padding, ctx.padding, ctx.dilation, ctx.dilation,
- ctx.groups, ctx.deformable_groups, ctx.with_bias)
- if not ctx.with_bias:
- grad_bias = None
-
- return (grad_input, grad_offset, grad_mask, grad_weight, grad_bias, None, None, None, None, None)
-
- @staticmethod
- def _infer_shape(ctx, input, weight):
- n = input.size(0)
- channels_out = weight.size(0)
- height, width = input.shape[2:4]
- kernel_h, kernel_w = weight.shape[2:4]
- height_out = (height + 2 * ctx.padding - (ctx.dilation * (kernel_h - 1) + 1)) // ctx.stride + 1
- width_out = (width + 2 * ctx.padding - (ctx.dilation * (kernel_w - 1) + 1)) // ctx.stride + 1
- return n, channels_out, height_out, width_out
-
-
-deform_conv = DeformConvFunction.apply
-modulated_deform_conv = ModulatedDeformConvFunction.apply
-
-
-class DeformConv(nn.Module):
-
- def __init__(self,
- in_channels,
- out_channels,
- kernel_size,
- stride=1,
- padding=0,
- dilation=1,
- groups=1,
- deformable_groups=1,
- bias=False):
- super(DeformConv, self).__init__()
-
- assert not bias
- assert in_channels % groups == 0, \
- f'in_channels {in_channels} is not divisible by groups {groups}'
- assert out_channels % groups == 0, \
- f'out_channels {out_channels} is not divisible ' \
- f'by groups {groups}'
-
- self.in_channels = in_channels
- self.out_channels = out_channels
- self.kernel_size = _pair(kernel_size)
- self.stride = _pair(stride)
- self.padding = _pair(padding)
- self.dilation = _pair(dilation)
- self.groups = groups
- self.deformable_groups = deformable_groups
- # enable compatibility with nn.Conv2d
- self.transposed = False
- self.output_padding = _single(0)
-
- self.weight = nn.Parameter(torch.Tensor(out_channels, in_channels // self.groups, *self.kernel_size))
-
- self.reset_parameters()
-
- def reset_parameters(self):
- n = self.in_channels
- for k in self.kernel_size:
- n *= k
- stdv = 1. / math.sqrt(n)
- self.weight.data.uniform_(-stdv, stdv)
-
- def forward(self, x, offset):
- # To fix an assert error in deform_conv_cuda.cpp:128
- # input image is smaller than kernel
- input_pad = (x.size(2) < self.kernel_size[0] or x.size(3) < self.kernel_size[1])
- if input_pad:
- pad_h = max(self.kernel_size[0] - x.size(2), 0)
- pad_w = max(self.kernel_size[1] - x.size(3), 0)
- x = F.pad(x, (0, pad_w, 0, pad_h), 'constant', 0).contiguous()
- offset = F.pad(offset, (0, pad_w, 0, pad_h), 'constant', 0).contiguous()
- out = deform_conv(x, offset, self.weight, self.stride, self.padding, self.dilation, self.groups,
- self.deformable_groups)
- if input_pad:
- out = out[:, :, :out.size(2) - pad_h, :out.size(3) - pad_w].contiguous()
- return out
-
-
-class DeformConvPack(DeformConv):
- """A Deformable Conv Encapsulation that acts as normal Conv layers.
-
- Args:
- in_channels (int): Same as nn.Conv2d.
- out_channels (int): Same as nn.Conv2d.
- kernel_size (int or tuple[int]): Same as nn.Conv2d.
- stride (int or tuple[int]): Same as nn.Conv2d.
- padding (int or tuple[int]): Same as nn.Conv2d.
- dilation (int or tuple[int]): Same as nn.Conv2d.
- groups (int): Same as nn.Conv2d.
- bias (bool or str): If specified as `auto`, it will be decided by the
- norm_cfg. Bias will be set as True if norm_cfg is None, otherwise
- False.
- """
-
- _version = 2
-
- def __init__(self, *args, **kwargs):
- super(DeformConvPack, self).__init__(*args, **kwargs)
-
- self.conv_offset = nn.Conv2d(
- self.in_channels,
- self.deformable_groups * 2 * self.kernel_size[0] * self.kernel_size[1],
- kernel_size=self.kernel_size,
- stride=_pair(self.stride),
- padding=_pair(self.padding),
- dilation=_pair(self.dilation),
- bias=True)
- self.init_offset()
-
- def init_offset(self):
- self.conv_offset.weight.data.zero_()
- self.conv_offset.bias.data.zero_()
-
- def forward(self, x):
- offset = self.conv_offset(x)
- return deform_conv(x, offset, self.weight, self.stride, self.padding, self.dilation, self.groups,
- self.deformable_groups)
-
-
-class ModulatedDeformConv(nn.Module):
-
- def __init__(self,
- in_channels,
- out_channels,
- kernel_size,
- stride=1,
- padding=0,
- dilation=1,
- groups=1,
- deformable_groups=1,
- bias=True):
- super(ModulatedDeformConv, self).__init__()
- self.in_channels = in_channels
- self.out_channels = out_channels
- self.kernel_size = _pair(kernel_size)
- self.stride = stride
- self.padding = padding
- self.dilation = dilation
- self.groups = groups
- self.deformable_groups = deformable_groups
- self.with_bias = bias
- # enable compatibility with nn.Conv2d
- self.transposed = False
- self.output_padding = _single(0)
-
- self.weight = nn.Parameter(torch.Tensor(out_channels, in_channels // groups, *self.kernel_size))
- if bias:
- self.bias = nn.Parameter(torch.Tensor(out_channels))
- else:
- self.register_parameter('bias', None)
- self.init_weights()
-
- def init_weights(self):
- n = self.in_channels
- for k in self.kernel_size:
- n *= k
- stdv = 1. / math.sqrt(n)
- self.weight.data.uniform_(-stdv, stdv)
- if self.bias is not None:
- self.bias.data.zero_()
-
- def forward(self, x, offset, mask):
- return modulated_deform_conv(x, offset, mask, self.weight, self.bias, self.stride, self.padding, self.dilation,
- self.groups, self.deformable_groups)
-
-
-class ModulatedDeformConvPack(ModulatedDeformConv):
- """A ModulatedDeformable Conv Encapsulation that acts as normal Conv layers.
-
- Args:
- in_channels (int): Same as nn.Conv2d.
- out_channels (int): Same as nn.Conv2d.
- kernel_size (int or tuple[int]): Same as nn.Conv2d.
- stride (int or tuple[int]): Same as nn.Conv2d.
- padding (int or tuple[int]): Same as nn.Conv2d.
- dilation (int or tuple[int]): Same as nn.Conv2d.
- groups (int): Same as nn.Conv2d.
- bias (bool or str): If specified as `auto`, it will be decided by the
- norm_cfg. Bias will be set as True if norm_cfg is None, otherwise
- False.
- """
-
- _version = 2
-
- def __init__(self, *args, **kwargs):
- super(ModulatedDeformConvPack, self).__init__(*args, **kwargs)
-
- self.conv_offset = nn.Conv2d(
- self.in_channels,
- self.deformable_groups * 3 * self.kernel_size[0] * self.kernel_size[1],
- kernel_size=self.kernel_size,
- stride=_pair(self.stride),
- padding=_pair(self.padding),
- dilation=_pair(self.dilation),
- bias=True)
- self.init_weights()
-
- def init_weights(self):
- super(ModulatedDeformConvPack, self).init_weights()
- if hasattr(self, 'conv_offset'):
- self.conv_offset.weight.data.zero_()
- self.conv_offset.bias.data.zero_()
-
- def forward(self, x):
- out = self.conv_offset(x)
- o1, o2, mask = torch.chunk(out, 3, dim=1)
- offset = torch.cat((o1, o2), dim=1)
- mask = torch.sigmoid(mask)
- return modulated_deform_conv(x, offset, mask, self.weight, self.bias, self.stride, self.padding, self.dilation,
- self.groups, self.deformable_groups)
diff --git a/spaces/ma-xu/LIVE/thrust/thrust/detail/config/host_device.h b/spaces/ma-xu/LIVE/thrust/thrust/detail/config/host_device.h
deleted file mode 100644
index 5540f91260d807bfb2ef06064767aeaccea2fc1a..0000000000000000000000000000000000000000
--- a/spaces/ma-xu/LIVE/thrust/thrust/detail/config/host_device.h
+++ /dev/null
@@ -1,44 +0,0 @@
-/*
- * Copyright 2008-2013 NVIDIA Corporation
- *
- * Licensed under the Apache License, Version 2.0 (the "License");
- * you may not use this file except in compliance with the License.
- * You may obtain a copy of the License at
- *
- * http://www.apache.org/licenses/LICENSE-2.0
- *
- * Unless required by applicable law or agreed to in writing, software
- * distributed under the License is distributed on an "AS IS" BASIS,
- * WITHOUT WARRANTIES OR CONDITIONS OF ANY KIND, either express or implied.
- * See the License for the specific language governing permissions and
- * limitations under the License.
- */
-
-/*! \file host_device.h
- * \brief Defines __host__ and __device__
- */
-
-#pragma once
-
-#include
-
-// since nvcc defines __host__ and __device__ for us,
-// and only nvcc knows what to do with __host__ and __device__,
-// define them to be the empty string for other compilers
-
-#if THRUST_DEVICE_COMPILER != THRUST_DEVICE_COMPILER_NVCC
-
-// since __host__ & __device__ might have already be defined, only
-// #define them if not defined already
-// XXX this will break if the client does #include later
-
-#ifndef __host__
-#define __host__
-#endif // __host__
-
-#ifndef __device__
-#define __device__
-#endif // __device__
-
-#endif
-
diff --git a/spaces/ma-xu/LIVE/thrust/thrust/system/tbb/detail/for_each.h b/spaces/ma-xu/LIVE/thrust/thrust/system/tbb/detail/for_each.h
deleted file mode 100644
index dfe5329b84ed273e60dacab576a559e351d26c42..0000000000000000000000000000000000000000
--- a/spaces/ma-xu/LIVE/thrust/thrust/system/tbb/detail/for_each.h
+++ /dev/null
@@ -1,54 +0,0 @@
-/*
- * Copyright 2008-2013 NVIDIA Corporation
- *
- * Licensed under the Apache License, Version 2.0 (the "License");
- * you may not use this file except in compliance with the License.
- * You may obtain a copy of the License at
- *
- * http://www.apache.org/licenses/LICENSE-2.0
- *
- * Unless required by applicable law or agreed to in writing, software
- * distributed under the License is distributed on an "AS IS" BASIS,
- * WITHOUT WARRANTIES OR CONDITIONS OF ANY KIND, either express or implied.
- * See the License for the specific language governing permissions and
- * limitations under the License.
- */
-
-#pragma once
-
-#include
-#include
-
-namespace thrust
-{
-namespace system
-{
-namespace tbb
-{
-namespace detail
-{
-
-template
- RandomAccessIterator for_each(execution_policy &exec,
- RandomAccessIterator first,
- RandomAccessIterator last,
- UnaryFunction f);
-
-template
- RandomAccessIterator for_each_n(execution_policy &exec,
- RandomAccessIterator first,
- Size n,
- UnaryFunction f);
-
-} // end namespace detail
-} // end namespace tbb
-} // end namespace system
-} // end namespace thrust
-
-#include
-
diff --git a/spaces/maminghui/ChatGPT/overwrites.py b/spaces/maminghui/ChatGPT/overwrites.py
deleted file mode 100644
index 436fcf46b5807ca045e77ac762039ba0ffc16f6d..0000000000000000000000000000000000000000
--- a/spaces/maminghui/ChatGPT/overwrites.py
+++ /dev/null
@@ -1,38 +0,0 @@
-from __future__ import annotations
-import logging
-
-from llama_index import Prompt
-from typing import List, Tuple
-import mdtex2html
-
-from presets import *
-from llama_func import *
-
-
-def compact_text_chunks(self, prompt: Prompt, text_chunks: List[str]) -> List[str]:
- logging.debug("Compacting text chunks...🚀🚀🚀")
- combined_str = [c.strip() for c in text_chunks if c.strip()]
- combined_str = [f"[{index+1}] {c}" for index, c in enumerate(combined_str)]
- combined_str = "\n\n".join(combined_str)
- # resplit based on self.max_chunk_overlap
- text_splitter = self.get_text_splitter_given_prompt(prompt, 1, padding=1)
- return text_splitter.split_text(combined_str)
-
-
-def postprocess(
- self, y: List[Tuple[str | None, str | None]]
-) -> List[Tuple[str | None, str | None]]:
- """
- Parameters:
- y: List of tuples representing the message and response pairs. Each message and response should be a string, which may be in Markdown format.
- Returns:
- List of tuples representing the message and response. Each message and response will be a string of HTML.
- """
- if y is None or y == []:
- return []
- tag_regex = re.compile(r"^<\w+>[^<]+\w+>")
- if tag_regex.search(y[-1][1]):
- y[-1] = (y[-1][0].replace("\n", " "), y[-1][1])
- else:
- y[-1] = (y[-1][0].replace("\n", " "), convert_mdtext(y[-1][1]))
- return y
diff --git a/spaces/manavisrani07/gradio-lipsync-wav2lip/basicsr/ops/__init__.py b/spaces/manavisrani07/gradio-lipsync-wav2lip/basicsr/ops/__init__.py
deleted file mode 100644
index e69de29bb2d1d6434b8b29ae775ad8c2e48c5391..0000000000000000000000000000000000000000
diff --git a/spaces/marioboy/neil-breen/vocoder_train.py b/spaces/marioboy/neil-breen/vocoder_train.py
deleted file mode 100644
index d712ffa3e6c92a091aa18dc90f0027f46940e400..0000000000000000000000000000000000000000
--- a/spaces/marioboy/neil-breen/vocoder_train.py
+++ /dev/null
@@ -1,56 +0,0 @@
-from utils.argutils import print_args
-from vocoder.train import train
-from pathlib import Path
-import argparse
-
-
-if __name__ == "__main__":
- parser = argparse.ArgumentParser(
- description="Trains the vocoder from the synthesizer audios and the GTA synthesized mels, "
- "or ground truth mels.",
- formatter_class=argparse.ArgumentDefaultsHelpFormatter
- )
-
- parser.add_argument("run_id", type=str, help= \
- "Name for this model instance. If a model state from the same run ID was previously "
- "saved, the training will restart from there. Pass -f to overwrite saved states and "
- "restart from scratch.")
- parser.add_argument("datasets_root", type=str, help= \
- "Path to the directory containing your SV2TTS directory. Specifying --syn_dir or --voc_dir "
- "will take priority over this argument.")
- parser.add_argument("--syn_dir", type=str, default=argparse.SUPPRESS, help= \
- "Path to the synthesizer directory that contains the ground truth mel spectrograms, "
- "the wavs and the embeds. Defaults to /SV2TTS/synthesizer/.")
- parser.add_argument("--voc_dir", type=str, default=argparse.SUPPRESS, help= \
- "Path to the vocoder directory that contains the GTA synthesized mel spectrograms. "
- "Defaults to /SV2TTS/vocoder/. Unused if --ground_truth is passed.")
- parser.add_argument("-m", "--models_dir", type=str, default="vocoder/saved_models/", help=\
- "Path to the directory that will contain the saved model weights, as well as backups "
- "of those weights and wavs generated during training.")
- parser.add_argument("-g", "--ground_truth", action="store_true", help= \
- "Train on ground truth spectrograms (/SV2TTS/synthesizer/mels).")
- parser.add_argument("-s", "--save_every", type=int, default=1000, help= \
- "Number of steps between updates of the model on the disk. Set to 0 to never save the "
- "model.")
- parser.add_argument("-b", "--backup_every", type=int, default=25000, help= \
- "Number of steps between backups of the model. Set to 0 to never make backups of the "
- "model.")
- parser.add_argument("-f", "--force_restart", action="store_true", help= \
- "Do not load any saved model and restart from scratch.")
- args = parser.parse_args()
-
- # Process the arguments
- if not hasattr(args, "syn_dir"):
- args.syn_dir = Path(args.datasets_root, "SV2TTS", "synthesizer")
- args.syn_dir = Path(args.syn_dir)
- if not hasattr(args, "voc_dir"):
- args.voc_dir = Path(args.datasets_root, "SV2TTS", "vocoder")
- args.voc_dir = Path(args.voc_dir)
- del args.datasets_root
- args.models_dir = Path(args.models_dir)
- args.models_dir.mkdir(exist_ok=True)
-
- # Run the training
- print_args(args, parser)
- train(**vars(args))
-
\ No newline at end of file
diff --git a/spaces/matthoffner/starchat-ui/components/Settings/Key.tsx b/spaces/matthoffner/starchat-ui/components/Settings/Key.tsx
deleted file mode 100644
index fe056e9d9e0d0827d44b1cf82bf2c0dac1deccae..0000000000000000000000000000000000000000
--- a/spaces/matthoffner/starchat-ui/components/Settings/Key.tsx
+++ /dev/null
@@ -1,15 +0,0 @@
-import { IconCheck, IconKey, IconX } from '@tabler/icons-react';
-import { FC, KeyboardEvent, useEffect, useRef, useState } from 'react';
-
-import { useTranslation } from 'next-i18next';
-
-import { SidebarButton } from '../Sidebar/SidebarButton';
-
-interface Props {
- apiKey: string;
- onApiKeyChange: (apiKey: string) => void;
-}
-
-export const Key: FC = ({ apiKey, onApiKeyChange }) => {
- return null;
-};
diff --git a/spaces/meaqua33/White-box-Cartoonization/app.py b/spaces/meaqua33/White-box-Cartoonization/app.py
deleted file mode 100644
index c55ced56bd87a85f59d1c8ef84b7eca87422720f..0000000000000000000000000000000000000000
--- a/spaces/meaqua33/White-box-Cartoonization/app.py
+++ /dev/null
@@ -1,108 +0,0 @@
-#!/usr/bin/env python
-
-from __future__ import annotations
-import argparse
-import functools
-import os
-import pathlib
-import sys
-from typing import Callable
-import uuid
-
-import gradio as gr
-import huggingface_hub
-import numpy as np
-import PIL.Image
-
-from io import BytesIO
-from wbc.cartoonize import Cartoonize
-
-ORIGINAL_REPO_URL = 'https://github.com/SystemErrorWang/White-box-Cartoonization'
-TITLE = 'SystemErrorWang/White-box-Cartoonization'
-DESCRIPTION = f"""This is a demo for {ORIGINAL_REPO_URL}.
-
-"""
-ARTICLE = """
-
-"""
-
-SAFEHASH = [x for x in "0123456789-abcdefghijklmnopqrstuvwxyz_ABCDEFGHIJKLMNOPQRSTUVWXYZ"]
-def compress_UUID():
- '''
- 根据http://www.ietf.org/rfc/rfc1738.txt,由uuid编码扩bai大字符域生成du串
- 包括:[0-9a-zA-Z\-_]共64个
- 长度:(32-2)/3*2=20
- 备注:可在地球上人zhi人都用,使用100年不重复(2^120)
- :return:String
- '''
- row = str(uuid.uuid4()).replace('-', '')
- safe_code = ''
- for i in range(10):
- enbin = "%012d" % int(bin(int(row[i * 3] + row[i * 3 + 1] + row[i * 3 + 2], 16))[2:], 10)
- safe_code += (SAFEHASH[int(enbin[0:6], 2)] + SAFEHASH[int(enbin[6:12], 2)])
- safe_code = safe_code.replace('-', '')
- return safe_code
-
-
-def parse_args() -> argparse.Namespace:
- parser = argparse.ArgumentParser()
- parser.add_argument('--device', type=str, default='cpu')
- parser.add_argument('--theme', type=str)
- parser.add_argument('--live', action='store_true')
- parser.add_argument('--share', action='store_true')
- parser.add_argument('--port', type=int)
- parser.add_argument('--disable-queue',
- dest='enable_queue',
- action='store_false')
- parser.add_argument('--allow-flagging', type=str, default='never')
- parser.add_argument('--allow-screenshot', action='store_true')
- return parser.parse_args()
-
-def run(
- image,
- cartoonize : Cartoonize
-) -> tuple[PIL.Image.Image]:
-
- out_path = compress_UUID()+'.png'
- cartoonize.run_sigle(image.name, out_path)
-
- return PIL.Image.open(out_path)
-
-
-def main():
- gr.close_all()
-
- args = parse_args()
-
- cartoonize = Cartoonize(os.path.join(os.path.dirname(os.path.abspath(__file__)),'wbc/saved_models/'))
-
- func = functools.partial(run, cartoonize=cartoonize)
- func = functools.update_wrapper(func, run)
-
- gr.Interface(
- func,
- [
- gr.inputs.Image(type='file', label='Input Image'),
- ],
- [
- gr.outputs.Image(
- type='pil',
- label='Result'),
- ],
- # examples=examples,
- theme=args.theme,
- title=TITLE,
- description=DESCRIPTION,
- article=ARTICLE,
- allow_screenshot=args.allow_screenshot,
- allow_flagging=args.allow_flagging,
- live=args.live,
- ).launch(
- enable_queue=args.enable_queue,
- server_port=args.port,
- share=args.share,
- )
-
-
-if __name__ == '__main__':
- main()
diff --git a/spaces/merve/KerasBERTv1/README.md b/spaces/merve/KerasBERTv1/README.md
deleted file mode 100644
index 6f78e92386f9e0aa355a0b41839a9724f91ee79e..0000000000000000000000000000000000000000
--- a/spaces/merve/KerasBERTv1/README.md
+++ /dev/null
@@ -1,37 +0,0 @@
----
-title: KerasBERTv1
-emoji: ❤️
-colorFrom: green
-colorTo: pink
-sdk: gradio
-app_file: app.py
-pinned: false
----
-
-# Configuration
-
-`title`: _string_
-Display title for the Space
-
-`emoji`: _string_
-Space emoji (emoji-only character allowed)
-
-`colorFrom`: _string_
-Color for Thumbnail gradient (red, yellow, green, blue, indigo, purple, pink, gray)
-
-`colorTo`: _string_
-Color for Thumbnail gradient (red, yellow, green, blue, indigo, purple, pink, gray)
-
-`sdk`: _string_
-Can be either `gradio` or `streamlit`
-
-`sdk_version` : _string_
-Only applicable for `streamlit` SDK.
-See [doc](https://hf.co/docs/hub/spaces) for more info on supported versions.
-
-`app_file`: _string_
-Path to your main application file (which contains either `gradio` or `streamlit` Python code).
-Path is relative to the root of the repository.
-
-`pinned`: _boolean_
-Whether the Space stays on top of your list.
diff --git a/spaces/merve/fill-in-the-blank/source/_posts/2020-09-27-diversity-metrics.md b/spaces/merve/fill-in-the-blank/source/_posts/2020-09-27-diversity-metrics.md
deleted file mode 100644
index 4c84423fe9a6f8566a0b7182bc378feec97d9654..0000000000000000000000000000000000000000
--- a/spaces/merve/fill-in-the-blank/source/_posts/2020-09-27-diversity-metrics.md
+++ /dev/null
@@ -1,100 +0,0 @@
----
-template: post.html
-title: Measuring Diversity
-titlex: Diversity and Inclusion Metrics
-summary: Search results that reflect historic inequities can amplify stereotypes and perpetuate under-representation. Carefully measuring diversity in data sets can help.
-shareimg: https://pair.withgoogle.com/explorables/images/measuring-diversity.png
-permalink: /measuring-diversity/
-date: 2021-03-01
----
-
-
-
-Search, ranking and recommendation systems can help find useful documents in large datasets. However, these datasets reflect the biases of the society in which they were created and the systems risk re-entrenching those biases. For example, if someone who is not a white man searches for "CEO pictures" and sees a [page of white men](https://www.nytimes.com/interactive/2018/04/24/upshot/women-and-men-named-john.html), they may feel that only white men can be CEOs, further perpetuating lack of representation at companies' executive levels.
-
-Using the careful quantification outlined in a recent paper, [Diversity and Inclusion Metrics in Subset Selection](https://arxiv.org/pdf/2002.03256.pdf), we can quantify biases and push these systems to return a wider range of results.
-
-The mathematics of all this is a little easier to follow with abstract shapes. Let's take a look at some of them:
-
-
-
-Suppose we want to return about 30% green boxes to reflect the distribution of some larger universe of shapes. Try clicking on the shapes below to select some of them — can you find a better subset to return?
-
-
-
-Another diversity metric we care about is the percentage of dots... how close to 35% dots can you get?
-
-
-
-If we can only return a single subset, how should we consider multiple diversity metrics? Sometimes it isn't possible to reduce the difference of every metric to zero. One natural approach: find the selection with the **lowest mean difference** across all the metrics to get as close as possible to all the targets.
-
-In other circumstances, like picking a panel of speakers, avoiding badly representing any single category might be more important. This can be done by finding the subset with the **lowest max difference**. Try minimizing both below:
-
-
-
-Notice that minimizing the mean results in a different subset than minimizing the max; how else might using one over the other change the results?
-
-### Ranking Measures
-
-We can pull out more detail by showing how the mean difference and maximum difference rank lots of sets. Below, there are 20 sets of 10 shapes sorted by the two measures. Try adjusting the target slider on the left to see how the rankings change; each set's percentage of green, dots and small shapes are shown in the small histograms.
-
-
-
-At the extremes, the choice of measure can have a big impact: if we want to try and return all green results, we can shift the green target up to 100%. With this target, the minimum difference basically sorts the sets by the number of green items and uses the other targets as a tiebreaker. In contrast, sorting by the mean difference balances the green target more with the dot and small targets.
-
-
-
-Beyond mean and max differences, there are more ways to combine diversity metrics, like taking the cross of two metrics to account for [intersectionality](https://en.wikipedia.org/wiki/Intersectionality). The absolute value of the difference in target and actual percentages can also be quantified in other ways — you might want to penalize undershooting more than overshooting, for example. It's important to keep in mind what exactly you're trying to maximize and the dataset that you're operating on.
-
-### Which Measure is Best?
-
-In a vacuum, all of these ranking methods are defensible. Picking one requires knowledge of the dataset and broader societal context.
-
-For example, the doctors on the left have more variance along the shirt color attribute, but they're less diverse by gender than the doctors on the right. With the shirt color and gender targets we've picked, the two subsets have the same mean and max differences However, in most applications, it's more important to have a representative sample of socially relevant characteristics, like gender, rather than something less salient, like clothing color.
-
-
-
-Just selecting a diverse sample isn't sufficient either. [Diversity and Inclusion Metrics in Subset Selection](https://arxiv.org/pdf/2002.03256.pdf) introduces a way of measuring "inclusion" - how well does the searcher feel represented in the results?
-
-Below, we have gender diversity, without inclusion for women, in the “construction worker” image domain. Masculine-presenting individuals are shown in realistic, modern construction worker situations, while feminine-presenting individuals and other gender presentations are depicted as historic nostalgia, toys, clipart, or passive.
-
-
-
-The context of the query and the searcher also plays in the quality of search results. A search for "work clothing" that shows a mixed palette of colors for men's clothing and only pink women's clothing might make the searcher feel that women need to appear stereotypically feminine in a professional setting. But the same set of women's clothes might be appropriate to show for a "pink women work clothes" search or if the searcher had previously expressed a preference for pink.
-
-We saw how a small switch from mean to max made a huge difference in what abstract shapes are returned – and how things can get even more complex when socially salient characteristics are layered in. Defaults and small decisions can encode our priorities and values; intentionally thinking about how diversity and inclusion are being measured and which characteristics are emphasized is a step towards designing more equitable systems.
-
-### More Reading
-
-The [Diversity and Inclusion Metrics](https://arxiv.org/pdf/2002.03256.pdf) paper has a [Colab](https://colab.research.google.com/github/PAIR-code/ai-explorables/blob/master/source/measuring-diversity/diversity-and-inclusion.ipynb) with a detailed desciption of the metrics, additional visualizations and a reference Python implementation.
-
-The difficulties of [measuring fairness](https://pair.withgoogle.com/explorables/measuring-fairness/) in general have been well studied; subset selection is still an active area of research. [Fairness of Exposure in Rankings](https://www.cs.cornell.edu/~tj/publications/singh_joachims_18a.pdf) proposes a ranking algorithm that incorporates fairness constraints. [Toward creating a fairer ranking in search engine results](https://www.ilab.cs.rutgers.edu/~rg522/publication/gao-2020-ipm/gao-2020-ipm.pdf) measures diversity bias in actual search results.
-
-Inferring user preferences is also tricky; you can checkout ways to design for user feedback and control over queries in the [People + AI Guidebook](https://pair.withgoogle.com/chapter/feedback-controls/).
-
-### Credits
-
-Adam Pearce, Dylan Baker, Ellen Jiang, Meg Mitchell\* and Timnit Gebru\* // March 2021
-
-*Work done while at Google
-
-Thanks to Alex Hanna, Carey Radebaugh, Emily Denton, Fernanda Viégas, James Wexler, Jess Holbrook, Ludovic Peran, Martin Wattenberg, Michael Terry, Yannick Assogba and Zan Armstrong for their help with this piece.
-
-
-
More Explorables
-
-
-
-
-
-
-
-
-
-
-
-
-
-
-
\ No newline at end of file
diff --git a/spaces/merve/fill-in-the-blank/source/fill-in-the-blank/style.css b/spaces/merve/fill-in-the-blank/source/fill-in-the-blank/style.css
deleted file mode 100644
index 726984190483443c3da0905eae281514eccc7487..0000000000000000000000000000000000000000
--- a/spaces/merve/fill-in-the-blank/source/fill-in-the-blank/style.css
+++ /dev/null
@@ -1,737 +0,0 @@
-@media (max-width: 1100px){
- body{
- /*overflow-x: hidden;*/
- }
-}
-
-
-.tooltip {
- top: -1000px;
- position: absolute;
- padding: 10px;
- background: rgba(255, 255, 255, .8);
- border: 0px solid lightgray;
-
- width: 300px;
- font-size: 14px;
- line-height: 1.4em;
- background: rgba(0, 0, 0, .8);
- color: #fff;
- pointer-events: all !important;
-}
-.tooltip a{
- color: #fff !important;
-}
-.tooltip:hover{
-/* opacity: 1;
- pointer-events: all !important;
-*/}
-
-.tooltip-hidden{
- opacity: 0;
- transition: all .3s;
- transition-delay: .2s;
- pointer-events: none !important;
-}
-
-@media (max-width: 590px){
- .footend{
- margin-left: 0px;
- width: 10px;
- }
-
-
- div.tooltip{
- transition: all 0s !important;
- transition-delay: 0s !important;
-
- display: none;
- position: fixed;
- bottom: -1px;
- width: calc(100%);
- left: -1px !important;
- right: -1px !important;
- top: auto !important;
- width: auto !important;
- }
-}
-
-svg{
- overflow: visible;
-}
-
-.domain{
- display: none;
-}
-
-.tick{
- display: none;
-}
-
-.bg-tick{
- stroke: #eee;
-}
-
-text{
- pointer-events: none;
- /*fill: #fff;*/
- text-shadow: 0 1px 0 #fff, 1px 0 0 #fff, 0 -1px 0 #fff, -1px 0 0 #fff;
-}
-
-.pair{
- width: 820px;
- /*height: 550px;*/
- margin: 0px auto;
- margin-top: 25px !important
-}
-
-.nurse-name-zari-cda{
- margin-bottom: 35px;
-}
-
-.pair > div{
- display: inline-block;
- vertical-align: top;
-}
-
-.pair .graph{
- width: 500px;
-}
-
-.pair .options{
- width: 250px;
- padding-right: 20px;
-}
-
-.pair .warning{
- width: 250px;
- /*border: 1px solid orange;*/
- /*background: #fff9e4;*/
- /*padding: 10px;*/
- margin-top: 15px;
- padding-left: 0px;
- font-size: 14px;
- line-height: 1.25em;
- opacity: 0;
- transition: all .2s;
-}
-
-.pair .reset{
- width: 58px;
- /*border: 1px solid orange;*/
- /*background: #fff9e4;*/
- /*padding: 10px;*/
- margin-top: 15px;
- font-size: 14px;
- line-height: 1.25em;
- opacity: 0;
- transition: opacity .2s;
- cursor: pointer;
- user-select: none;
- outline: 1px solid #ccc;
- padding: 5px;
-
-}
-.pair .reset span{
- position: relative;
- top: -1px;
- padding-right: 4px;
- padding-left: 1px;
- /*font-size: ;*/
-}
-
-.pair .reset:hover{
- background: #eee;
- color: #000;
- outline: 1px solid #000;
-}
-
-.options > *{
- margin-right: 10px;
-}
-
-.options b{
- display: block;
- margin-bottom: 5px;
- margin-top: 10px;
-}
-
-
-
-
-.flex-row{
- width: 100%;
- display: flex;
- justify-content: space-between;
- column-gap: 10px
-}
-
-.flex-row > *{
- flex-grow: 1;
- margin-right: 0px !important;
-}
-
-.options > *{
- margin-right: 0px;
-}
-
-.pair textarea{
- width: 100%;
-}
-
-.flex-row-textarea{
- display: block;
-}
-
-@media (max-width: 820px){
- .pair{
- width: 100%;
- height: auto;
- max-width: 500px;
- margin: 0px auto;
- }
-
- .flex-row{
- margin-bottom: -10px;
- }
-
- .flex-row-textarea{
- display: flex;
- margin-bottom: 10px;
- }
-
-
- .pair .options{
- width: auto;
- padding-right: 0px;
- }
-
- .warning{
- display: none !important;
- }
-
- .reset{
- display: none !important;
- }
-
- .pair .graph{
- width: 100%;
- }
-
- .annotations{
- display: none;
- }
-}
-
-
-
-.pair.difference{
- width: 1000px;
- margin-left: 0px;
-}
-
-.pair.difference .pair-container{
-}
-
-.pair .options.wide{
- width: 100%;
- margin-bottom: 20px;
-}
-.pair .options.wide > div{
- display: inline-block;
-}
-
-.options.wide .option-type .button{
- width: 78px !important;
-}
-
-.options.wide .option-model .button{
- width: 40px !important;
-}
-
-.options.wide .update.button{
- width: 80px !important;
-}
-
-textarea{
- font-family: 'Roboto', Helvetica, sans-serif;
- font-weight: 300;
- line-height: 1.55em;
- font-size: 16px;
- font-weight: bold;
- border: 1px #ccc solid;
- resize: none;
-}
-
-.button.update{
- /*height: 20px;*/
- /*position: relative;*/
- /*top: -30px;*/
- /*margin-bottom: -10px;*/
- /*vertical-align: center;*/
- margin-top: 25px;
- width: 252px;
- text-align: center;
- font-weight: 500;
-}
-.button{
- display: inline-block;
- outline: 1px solid #ccc;
- padding: 5px;
- margin-top: 10px;
- margin-right: 10px;
- position: relative;
- top: -12px;
- cursor: pointer;
- user-select: none;
-}
-
-@media (hover: hover) and (pointer: fine) {
- .button:hover{
- outline-color: #000;
- }
-}
-
-@media screen and (-webkit-min-device-pixel-ratio:0) and @media (max-width: 900px) {
- select,
- textarea,
- input {
- font-size: 16px !important;
- }
-
- textarea{
- height: 80px !important;
- }
-}
-
-
-.button.active{
- background: #eee;
- color: #000;
- /*font-weight: 500;*/
-}
-
-
-.button.loading i{
- opacity: 1;
-}
-
-.button.loading{
- pointer-events: none;
- /*opacity: .6;*/
-}
-.p-button{
- /*position: relative;*/
- /*top: -3px;*/
- /*line-height: 10px;*/
- /*line-height: */
- display: inline-block;
- margin-right: 15px;
-}
-.p-button-link{
- text-decoration: underline;
- cursor: pointer;
- padding-right: 10px;
-}
-.interesting-pair-alts .p-button-link{
- display: block;
- text-decoration: none;
-}
-.interesting-pair-alts .p-button-link div{
- padding-left: 10px;
- padding-right: 10px;
- padding-top: 5px;
- padding-bottom: 5px;
- outline: 1px solid #ccc;
- margin-top: 5px;
- margin-bottom: 5px;
- margin-left: 10px;
-
-}
-.difference-difference-alts .p-button-link:hover div{
- outline: 1px solid #000;
-}
-
-.difference-difference-alts .p-button-link{
- display: block;
- text-decoration: none;
-}
-.difference-difference-alts .p-button-link div{
- padding-left: 10px;
- padding-right: 10px;
- padding-top: 5px;
- padding-bottom: 5px;
- outline: 1px solid #ccc;
- margin-top: 5px;
- margin-bottom: 5px;
- margin-left: 10px;
-
-}
-.difference-difference-alts .p-button-link:hover div{
- outline: 1px solid #000;
-}
-
-
-.wide .flex-row{
- width: 220px;
-}
-
-.wide > *{
- margin-right: 40px;
-}
-
-.wide textarea{
- position: relative;
- top: 12px;
-}
-
-
-@media (max-width: 1100px){
- .pair-container-overflow{
- overflow-x: scroll;
- width: 100% !important;
- }
-
- .pair.difference{
- width: auto;
- max-width: 2000px;
- }
-
- .pair.difference .options{
- margin: 0px auto;
- margin-left: max(50vh - 500px, 0px);
- width: min(500px, 100%);
- }
-
-}
-
-.pair-container{
- width: 1000px;
-}
-
-
-
-
-
-.checkbox{
- display: inline-block;
- position: relative;
- top: -10px;
- margin-left: 10px;
-
-}
-
-circle:hover{
- stroke: blue;
-}
-
-
-
-.hover text{
- fill: #000;
- font-weight: 300;
- /*stroke-width: 2px;*/
- /*text-shadow: 0 2px 0 #000, 2px 0 0 #000, 0 -2px 0 #000, -2px 0 0 #000;*/
-}
-
-#graph > div{
- display: inline-block;
-}
-
-text.tiny{
- font-size: 9px;
- font-family: monospace;
- /*fill: #555;*/
-}
-
-
-
-
-
-svg{
- overflow: visible;
-}
-
-
-input{
- font-family: monospace;
- width: 900px;
- overflow: hidden;
- background-color: rgba(0,0,0,0);
- border: 0px;
-}
-
-textarea{
- font-family: monospace;
- font-size: 14px;
-}
-
-/* Hide scrollbar for Chrome, Safari and Opera */
-.top-sents::-webkit-scrollbar {
- /*display: none;*/
-}
-
-/* Hide scrollbar for IE, Edge and Firefox */
-.top-sents {
- -ms-overflow-style: none; /* IE and Edge */
- scrollbar-width: none; /* Firefox */
-}
-
-.sent{
- margin-top: -15px;
-}
-
-
-
-.post-summary{
- display: none;
-}
-
-
-.token-container{
- text-align: center;
- line-height: 2em;
-}
-
-.token{
- display: inline-block;
- padding: 5px;
- margin: 10px;
- margin-top: 0px;
- margin-bottom: 0px;
- font-size: 20px;
- font-family: monospace;
- outline: 1px solid #ccc;
- color: #000;
- cursor: pointer;
- background: #fff;
- border: 0px;
-}
-
-.token:hover, .token.active{
- outline: 1px solid #000;
-}
-
-
-.xy-only, .rotate-only{
- opacity: 0;
- transition: all .2s;
-}
-
-.annotations{
- transition: opacity .2s;
-}
-
-.is-xy .xy-only{
- opacity: 1 !important;
-}
-.is-rotate .rotate-only{
- opacity: 1 !important;
-}
-
-.hamlet{
- min-height: 304px;
- margin-bottom: 20px;
-}
-
-.hamlet-edit .button{
- color: #ccc;
- pointer-events: none;
-}
-.hamlet-edit.changed .button{
- color: #000;
- pointer-events: all;
-}
-
-@media (max-width: 500px){
- .hamlet-edit .button{
- display: block;
- text-align: center;
- top: 0px !important;
- margin: 0px auto !important;
- margin-top: 5px !important;
- width: 100%;
- }
-}
-
-
-
-.pair .update{
- color: #ccc;
- pointer-events: none;
-}
-.pair.changed .update{
- color: #000;
- pointer-events: all;
-}
-
-
-
-
-.difference-difference-list{
- display: none;
-}
-
-.pair-container{
- width: 900px;
-}
-.pair-container > div{
- display: inline-block;
-}
-
-
-.difference-difference textarea{
- height: 52px;
-}
-
-.not-is-color-by .y-axis-label text, .not-is-color-by .sent-1 text, .not-is-color-by .x-axis-label{
- fill: #444 !important;
-}
-
-.is-color-by .y-axis-label text, .is-color-by .sent-1 text, .is-color-by .x-axis-label{
- font-weight: 400;
- /*text-decoration: underline;*/
-}
-
-
-
-.time-token.active path{
- stroke: #f0f;
- opacity: 1;
-}
-.time-token.active text{
- fill: #f0f !important;
- opacity: 1 !important;
- font-size: 14px;
-}
-
-
-.token{
-
-}
-
-.gender-over-time{
- width: 1100px;
- margin: 0px auto;
- font-size: 14px;
- margin-left: -91px;
-}
-
-.gender-over-time .tick{
- display: block;
-}
-
-.gender-over-time .axis{
- opacity: .7;
-}
-
-.gender-over-time .sentence{
- /*position: relative;*/
- width: 32%;
-}
-
-.gender-over-time .sentence .sentence-title{
- right: 42px;
- position: relative;
- text-align: right;
- font-family: monospace;
-
-}
-.gender-over-time .sentence.is-bear .sentence-title{
- /*text-align: center;*/
- right: 115px;
-}
-
-.gender-over-time .g-caption{
- line-height: 18px;
- margin-bottom: 30px;
- margin-top: 5px;
- width: 290px;
- font-size: 13px;
- left: 365px;
- position: relative;
-}
-
-@media (max-width: 1100px){
- .gender-over-time{
- width: 100%;
- margin-left: 0px;
- max-width: 500px;
- margin: 0px auto;
- }
-
- .gender-over-time .sentence{
- width: 100% !important;
- margin-bottom: 20px;
- }
-
- .gender-over-time .g-caption{
- left: 0px;
- width: 100%;
- }
-}
-
-.time-token text{
- font-family: monospace;
- pointer-events: all !important;
- cursor: default;
-}
-
-
-
-img[src*="img/wiki-years.png"] {
- width: 300px;
-}
-
-
-#more-explorables{
- margin-top: 100px;
-}
-
-
-
-
-/*html{
- font-smooth: never;
- -webkit-font-smoothing: none;
- background: transparent;
-}
-
-path{
- display: none;
-}*/
-
-
-button {
- display: inline-block;
- border: none;
- margin: 0;
- text-decoration: none;
- background: #fff;
- color: #ffffff;
- font-size: 1em;
- cursor: pointer;
- text-align: center;
- -webkit-appearance: none;
- -moz-appearance: none;
- font-family : inherit;
-
-}
-
-button:active {
- transform: scale(0.99);
-}
-
-
-info{
- font-weight: 300;
- font-size: 12px;
- line-height: 0em;
- position: relative;
- left: 7px;
- top: -1px;
- cursor: default;
-}
-info:hover{
- font-weight: 600;
-}
\ No newline at end of file
diff --git a/spaces/merve/hidden-bias/server-side/fill-in-the-blank/py/model_bert_large.py b/spaces/merve/hidden-bias/server-side/fill-in-the-blank/py/model_bert_large.py
deleted file mode 100644
index 6ddb175a7158944305a2a8d9f99948ef41f7ec1a..0000000000000000000000000000000000000000
--- a/spaces/merve/hidden-bias/server-side/fill-in-the-blank/py/model_bert_large.py
+++ /dev/null
@@ -1,124 +0,0 @@
-import torch
-import json
-import numpy as np
-
-from transformers import (BertForMaskedLM, BertTokenizer)
-
-modelpath = 'bert-large-uncased-whole-word-masking/'
-tokenizer = BertTokenizer.from_pretrained(modelpath)
-model = BertForMaskedLM.from_pretrained(modelpath)
-model.eval()
-
-id_of_mask = 103
-
-def get_embeddings(sentence):
- with torch.no_grad():
- processed_sentence = '' + sentence + ''
- tokenized = tokenizer.encode(processed_sentence)
- input_ids = torch.tensor(tokenized).unsqueeze(0) # Batch size 1
- outputs = model(input_ids)
- index_of_mask = tokenized.index(id_of_mask)
-
- # batch, tokens, vocab_size
- prediction_scores = outputs[0]
-
- return prediction_scores[0][index_of_mask].cpu().numpy().tolist()
-
-
-def get_embedding_group(tokens):
- print(tokens)
-
- mutated = []
- for i, v in enumerate(tokens):
- array = tokens.copy()
- array[i] = id_of_mask
- mutated.append(array)
-
- print('Running model')
- output = model(torch.tensor(mutated))[0]
-
- print('Converting to list')
- array = output.detach().numpy().tolist()
-
- print('Constructing out array')
- # only grab mask embedding
- # can probaby do this in torch? not sure how
- out = []
- for i, v in enumerate(array):
- out.append(v[i])
-
- return out
-
-def get_embedding_group_top(tokens):
- sents = get_embedding_group(tokens)
- out = []
-
- print('get_embedding_group done')
-
- for sent_i, sent in enumerate(sents):
- all_tokens = []
-
- for i, v in enumerate(sent):
- all_tokens.append({'i': i, 'v': float(v)})
-
- all_tokens.sort(key=lambda d: d['v'], reverse=True)
-
- topTokens = all_tokens[:90]
-
- sum = np.sum(np.exp(sent))
- for i, token in enumerate(topTokens):
- token['p'] = float(np.exp(token['v'])/sum)
-
- out.append(all_tokens[:90])
-
- return out
-
-
-# Runs one token at a time to stay under memory limit
-def get_embedding_group_low_mem(tokens):
- print(tokens)
-
- out = []
- for index_of_mask, v in enumerate(tokens):
- array = tokens.copy()
- array[index_of_mask] = id_of_mask
-
- input_ids = torch.tensor(array).unsqueeze(0)
- prediction_scores = model(input_ids)[0]
-
- out.append(prediction_scores[0][index_of_mask].detach().numpy())
-
- return out
-
-def get_embedding_group_top_low_mem(tokens):
- sents = get_embedding_group_low_mem(tokens)
- out = []
-
- print('get_embedding_group done')
-
- for sent_i, sent in enumerate(sents):
- all_tokens = []
-
- for i, v in enumerate(sent):
- all_tokens.append({'i': i, 'v': float(v)})
-
- all_tokens.sort(key=lambda d: d['v'], reverse=True)
-
- topTokens = all_tokens[:90]
-
- sum = np.sum(np.exp(sent))
- for i, token in enumerate(topTokens):
- token['p'] = float(np.exp(token['v'])/sum)
-
- out.append(all_tokens[:90])
-
- return out
-
-
-import os
-import shutil
-
-# Free up memory
-if os.environ.get('REMOVE_WEIGHTS') == 'TRUE':
- print('removing bert-large-uncased-whole-word-masking from filesystem')
- shutil.rmtree('bert-large-uncased-whole-word-masking', ignore_errors=True)
diff --git a/spaces/merve/measuring-fairness/server-side/fill-in-the-blank/py/main.py b/spaces/merve/measuring-fairness/server-side/fill-in-the-blank/py/main.py
deleted file mode 100644
index 2ac15bda96de733df52cd7730895ae18baf20529..0000000000000000000000000000000000000000
--- a/spaces/merve/measuring-fairness/server-side/fill-in-the-blank/py/main.py
+++ /dev/null
@@ -1,59 +0,0 @@
-import os
-import json
-import shutil
-
-from flask import Flask, request
-from flask_cors import CORS
-
-import model_bert_large
-import model_bert_zari_cda
-
-app = Flask(__name__)
-CORS(app)
-
-
-@app.route('/')
-def hello_world():
- name = os.environ.get('NAME', 'Test')
- print('[Hello]')
- return 'Hello {}!'.format(name)
-
-
-@app.route('/embed_test')
-def embed_test():
- sentence = 'The dog went to the [MASK].'
- print('[TEST] ', sentence)
- return json.dumps(model_bert_large.get_embeddings(sentence))
-
-
-@app.route('/embed', methods=['POST'])
-def embed():
- data = json.loads(request.data)
- sentence = data['sentence']
- print('[BASE] ' + sentence)
- return json.dumps(model_bert_large.get_embeddings(sentence))
-
-@app.route('/embed_zari_cda', methods=['POST'])
-def embed_zari_cda():
- data = json.loads(request.data)
- sentence = data['sentence']
- print('[ZARI] ' + sentence)
- return json.dumps(model_bert_zari_cda.get_embeddings(sentence))
-
-
-@app.route('/embed_group_top', methods=['POST'])
-def embed_group_top():
- data = json.loads(request.data)
- tokens = data['tokens']
- return json.dumps(model_bert_large.get_embedding_group_top(tokens))
-
-@app.route('/get_embedding_group_top_low_mem', methods=['POST'])
-def embed_group():
- data = json.loads(request.data)
- tokens = data['tokens']
- return json.dumps(model_bert_large.get_embedding_group(tokens))
-
-if __name__ == '__main__':
- app.run(debug=True, host='0.0.0.0', port=int(os.environ.get('PORT', 5004)))
-
-
diff --git a/spaces/metricspace/OcTra/df_local/model.py b/spaces/metricspace/OcTra/df_local/model.py
deleted file mode 100644
index d8802766131c82536a511ab1a65c52bff0801edc..0000000000000000000000000000000000000000
--- a/spaces/metricspace/OcTra/df_local/model.py
+++ /dev/null
@@ -1,24 +0,0 @@
-from importlib import import_module
-
-import torch
-from loguru import logger
-
-from df_local.config import DfParams, config
-
-
-class ModelParams(DfParams):
- def __init__(self):
- self.__model = config("MODEL", default="deepfilternet", section="train")
- self.__params = getattr(import_module("df_local." + self.__model), "ModelParams")()
-
- def __getattr__(self, attr: str):
- return getattr(self.__params, attr)
-
-
-def init_model(*args, **kwargs):
- """Initialize the model specified in the config."""
- model = config("MODEL", default="deepfilternet", section="train")
- logger.info(f"Initializing model `{model}`")
- model = getattr(import_module("df_local." + model), "init_model")(*args, **kwargs)
- model.to(memory_format=torch.channels_last)
- return model
diff --git a/spaces/mfrashad/ClothingGAN/models/stylegan2/stylegan2-pytorch/inception.py b/spaces/mfrashad/ClothingGAN/models/stylegan2/stylegan2-pytorch/inception.py
deleted file mode 100644
index f3afed8123e595f65c1333dea7151e653a836e2b..0000000000000000000000000000000000000000
--- a/spaces/mfrashad/ClothingGAN/models/stylegan2/stylegan2-pytorch/inception.py
+++ /dev/null
@@ -1,310 +0,0 @@
-import torch
-import torch.nn as nn
-import torch.nn.functional as F
-from torchvision import models
-
-try:
- from torchvision.models.utils import load_state_dict_from_url
-except ImportError:
- from torch.utils.model_zoo import load_url as load_state_dict_from_url
-
-# Inception weights ported to Pytorch from
-# http://download.tensorflow.org/models/image/imagenet/inception-2015-12-05.tgz
-FID_WEIGHTS_URL = 'https://github.com/mseitzer/pytorch-fid/releases/download/fid_weights/pt_inception-2015-12-05-6726825d.pth'
-
-
-class InceptionV3(nn.Module):
- """Pretrained InceptionV3 network returning feature maps"""
-
- # Index of default block of inception to return,
- # corresponds to output of final average pooling
- DEFAULT_BLOCK_INDEX = 3
-
- # Maps feature dimensionality to their output blocks indices
- BLOCK_INDEX_BY_DIM = {
- 64: 0, # First max pooling features
- 192: 1, # Second max pooling featurs
- 768: 2, # Pre-aux classifier features
- 2048: 3 # Final average pooling features
- }
-
- def __init__(self,
- output_blocks=[DEFAULT_BLOCK_INDEX],
- resize_input=True,
- normalize_input=True,
- requires_grad=False,
- use_fid_inception=True):
- """Build pretrained InceptionV3
-
- Parameters
- ----------
- output_blocks : list of int
- Indices of blocks to return features of. Possible values are:
- - 0: corresponds to output of first max pooling
- - 1: corresponds to output of second max pooling
- - 2: corresponds to output which is fed to aux classifier
- - 3: corresponds to output of final average pooling
- resize_input : bool
- If true, bilinearly resizes input to width and height 299 before
- feeding input to model. As the network without fully connected
- layers is fully convolutional, it should be able to handle inputs
- of arbitrary size, so resizing might not be strictly needed
- normalize_input : bool
- If true, scales the input from range (0, 1) to the range the
- pretrained Inception network expects, namely (-1, 1)
- requires_grad : bool
- If true, parameters of the model require gradients. Possibly useful
- for finetuning the network
- use_fid_inception : bool
- If true, uses the pretrained Inception model used in Tensorflow's
- FID implementation. If false, uses the pretrained Inception model
- available in torchvision. The FID Inception model has different
- weights and a slightly different structure from torchvision's
- Inception model. If you want to compute FID scores, you are
- strongly advised to set this parameter to true to get comparable
- results.
- """
- super(InceptionV3, self).__init__()
-
- self.resize_input = resize_input
- self.normalize_input = normalize_input
- self.output_blocks = sorted(output_blocks)
- self.last_needed_block = max(output_blocks)
-
- assert self.last_needed_block <= 3, \
- 'Last possible output block index is 3'
-
- self.blocks = nn.ModuleList()
-
- if use_fid_inception:
- inception = fid_inception_v3()
- else:
- inception = models.inception_v3(pretrained=True)
-
- # Block 0: input to maxpool1
- block0 = [
- inception.Conv2d_1a_3x3,
- inception.Conv2d_2a_3x3,
- inception.Conv2d_2b_3x3,
- nn.MaxPool2d(kernel_size=3, stride=2)
- ]
- self.blocks.append(nn.Sequential(*block0))
-
- # Block 1: maxpool1 to maxpool2
- if self.last_needed_block >= 1:
- block1 = [
- inception.Conv2d_3b_1x1,
- inception.Conv2d_4a_3x3,
- nn.MaxPool2d(kernel_size=3, stride=2)
- ]
- self.blocks.append(nn.Sequential(*block1))
-
- # Block 2: maxpool2 to aux classifier
- if self.last_needed_block >= 2:
- block2 = [
- inception.Mixed_5b,
- inception.Mixed_5c,
- inception.Mixed_5d,
- inception.Mixed_6a,
- inception.Mixed_6b,
- inception.Mixed_6c,
- inception.Mixed_6d,
- inception.Mixed_6e,
- ]
- self.blocks.append(nn.Sequential(*block2))
-
- # Block 3: aux classifier to final avgpool
- if self.last_needed_block >= 3:
- block3 = [
- inception.Mixed_7a,
- inception.Mixed_7b,
- inception.Mixed_7c,
- nn.AdaptiveAvgPool2d(output_size=(1, 1))
- ]
- self.blocks.append(nn.Sequential(*block3))
-
- for param in self.parameters():
- param.requires_grad = requires_grad
-
- def forward(self, inp):
- """Get Inception feature maps
-
- Parameters
- ----------
- inp : torch.autograd.Variable
- Input tensor of shape Bx3xHxW. Values are expected to be in
- range (0, 1)
-
- Returns
- -------
- List of torch.autograd.Variable, corresponding to the selected output
- block, sorted ascending by index
- """
- outp = []
- x = inp
-
- if self.resize_input:
- x = F.interpolate(x,
- size=(299, 299),
- mode='bilinear',
- align_corners=False)
-
- if self.normalize_input:
- x = 2 * x - 1 # Scale from range (0, 1) to range (-1, 1)
-
- for idx, block in enumerate(self.blocks):
- x = block(x)
- if idx in self.output_blocks:
- outp.append(x)
-
- if idx == self.last_needed_block:
- break
-
- return outp
-
-
-def fid_inception_v3():
- """Build pretrained Inception model for FID computation
-
- The Inception model for FID computation uses a different set of weights
- and has a slightly different structure than torchvision's Inception.
-
- This method first constructs torchvision's Inception and then patches the
- necessary parts that are different in the FID Inception model.
- """
- inception = models.inception_v3(num_classes=1008,
- aux_logits=False,
- pretrained=False)
- inception.Mixed_5b = FIDInceptionA(192, pool_features=32)
- inception.Mixed_5c = FIDInceptionA(256, pool_features=64)
- inception.Mixed_5d = FIDInceptionA(288, pool_features=64)
- inception.Mixed_6b = FIDInceptionC(768, channels_7x7=128)
- inception.Mixed_6c = FIDInceptionC(768, channels_7x7=160)
- inception.Mixed_6d = FIDInceptionC(768, channels_7x7=160)
- inception.Mixed_6e = FIDInceptionC(768, channels_7x7=192)
- inception.Mixed_7b = FIDInceptionE_1(1280)
- inception.Mixed_7c = FIDInceptionE_2(2048)
-
- state_dict = load_state_dict_from_url(FID_WEIGHTS_URL, progress=True)
- inception.load_state_dict(state_dict)
- return inception
-
-
-class FIDInceptionA(models.inception.InceptionA):
- """InceptionA block patched for FID computation"""
- def __init__(self, in_channels, pool_features):
- super(FIDInceptionA, self).__init__(in_channels, pool_features)
-
- def forward(self, x):
- branch1x1 = self.branch1x1(x)
-
- branch5x5 = self.branch5x5_1(x)
- branch5x5 = self.branch5x5_2(branch5x5)
-
- branch3x3dbl = self.branch3x3dbl_1(x)
- branch3x3dbl = self.branch3x3dbl_2(branch3x3dbl)
- branch3x3dbl = self.branch3x3dbl_3(branch3x3dbl)
-
- # Patch: Tensorflow's average pool does not use the padded zero's in
- # its average calculation
- branch_pool = F.avg_pool2d(x, kernel_size=3, stride=1, padding=1,
- count_include_pad=False)
- branch_pool = self.branch_pool(branch_pool)
-
- outputs = [branch1x1, branch5x5, branch3x3dbl, branch_pool]
- return torch.cat(outputs, 1)
-
-
-class FIDInceptionC(models.inception.InceptionC):
- """InceptionC block patched for FID computation"""
- def __init__(self, in_channels, channels_7x7):
- super(FIDInceptionC, self).__init__(in_channels, channels_7x7)
-
- def forward(self, x):
- branch1x1 = self.branch1x1(x)
-
- branch7x7 = self.branch7x7_1(x)
- branch7x7 = self.branch7x7_2(branch7x7)
- branch7x7 = self.branch7x7_3(branch7x7)
-
- branch7x7dbl = self.branch7x7dbl_1(x)
- branch7x7dbl = self.branch7x7dbl_2(branch7x7dbl)
- branch7x7dbl = self.branch7x7dbl_3(branch7x7dbl)
- branch7x7dbl = self.branch7x7dbl_4(branch7x7dbl)
- branch7x7dbl = self.branch7x7dbl_5(branch7x7dbl)
-
- # Patch: Tensorflow's average pool does not use the padded zero's in
- # its average calculation
- branch_pool = F.avg_pool2d(x, kernel_size=3, stride=1, padding=1,
- count_include_pad=False)
- branch_pool = self.branch_pool(branch_pool)
-
- outputs = [branch1x1, branch7x7, branch7x7dbl, branch_pool]
- return torch.cat(outputs, 1)
-
-
-class FIDInceptionE_1(models.inception.InceptionE):
- """First InceptionE block patched for FID computation"""
- def __init__(self, in_channels):
- super(FIDInceptionE_1, self).__init__(in_channels)
-
- def forward(self, x):
- branch1x1 = self.branch1x1(x)
-
- branch3x3 = self.branch3x3_1(x)
- branch3x3 = [
- self.branch3x3_2a(branch3x3),
- self.branch3x3_2b(branch3x3),
- ]
- branch3x3 = torch.cat(branch3x3, 1)
-
- branch3x3dbl = self.branch3x3dbl_1(x)
- branch3x3dbl = self.branch3x3dbl_2(branch3x3dbl)
- branch3x3dbl = [
- self.branch3x3dbl_3a(branch3x3dbl),
- self.branch3x3dbl_3b(branch3x3dbl),
- ]
- branch3x3dbl = torch.cat(branch3x3dbl, 1)
-
- # Patch: Tensorflow's average pool does not use the padded zero's in
- # its average calculation
- branch_pool = F.avg_pool2d(x, kernel_size=3, stride=1, padding=1,
- count_include_pad=False)
- branch_pool = self.branch_pool(branch_pool)
-
- outputs = [branch1x1, branch3x3, branch3x3dbl, branch_pool]
- return torch.cat(outputs, 1)
-
-
-class FIDInceptionE_2(models.inception.InceptionE):
- """Second InceptionE block patched for FID computation"""
- def __init__(self, in_channels):
- super(FIDInceptionE_2, self).__init__(in_channels)
-
- def forward(self, x):
- branch1x1 = self.branch1x1(x)
-
- branch3x3 = self.branch3x3_1(x)
- branch3x3 = [
- self.branch3x3_2a(branch3x3),
- self.branch3x3_2b(branch3x3),
- ]
- branch3x3 = torch.cat(branch3x3, 1)
-
- branch3x3dbl = self.branch3x3dbl_1(x)
- branch3x3dbl = self.branch3x3dbl_2(branch3x3dbl)
- branch3x3dbl = [
- self.branch3x3dbl_3a(branch3x3dbl),
- self.branch3x3dbl_3b(branch3x3dbl),
- ]
- branch3x3dbl = torch.cat(branch3x3dbl, 1)
-
- # Patch: The FID Inception model uses max pooling instead of average
- # pooling. This is likely an error in this specific Inception
- # implementation, as other Inception models use average pooling here
- # (which matches the description in the paper).
- branch_pool = F.max_pool2d(x, kernel_size=3, stride=1, padding=1)
- branch_pool = self.branch_pool(branch_pool)
-
- outputs = [branch1x1, branch3x3, branch3x3dbl, branch_pool]
- return torch.cat(outputs, 1)
diff --git a/spaces/mfrashad/ClothingGAN/netdissect/dissection.py b/spaces/mfrashad/ClothingGAN/netdissect/dissection.py
deleted file mode 100644
index 6eef0dfd0b8804e45eb878aca68e72f8c6493474..0000000000000000000000000000000000000000
--- a/spaces/mfrashad/ClothingGAN/netdissect/dissection.py
+++ /dev/null
@@ -1,1617 +0,0 @@
-'''
-To run dissection:
-
-1. Load up the convolutional model you wish to dissect, and wrap it in
- an InstrumentedModel; then call imodel.retain_layers([layernames,..])
- to instrument the layers of interest.
-2. Load the segmentation dataset using the BrodenDataset class;
- use the transform_image argument to normalize images to be
- suitable for the model, or the size argument to truncate the dataset.
-3. Choose a directory in which to write the output, and call
- dissect(outdir, model, dataset).
-
-Example:
-
- from dissect import InstrumentedModel, dissect
- from broden import BrodenDataset
-
- model = InstrumentedModel(load_my_model())
- model.eval()
- model.cuda()
- model.retain_layers(['conv1', 'conv2', 'conv3', 'conv4', 'conv5'])
- bds = BrodenDataset('dataset/broden1_227',
- transform_image=transforms.Compose([
- transforms.ToTensor(),
- transforms.Normalize(IMAGE_MEAN, IMAGE_STDEV)]),
- size=1000)
- dissect('result/dissect', model, bds,
- examples_per_unit=10)
-'''
-
-import torch, numpy, os, re, json, shutil, types, tempfile, torchvision
-# import warnings
-# warnings.simplefilter('error', UserWarning)
-from PIL import Image
-from xml.etree import ElementTree as et
-from collections import OrderedDict, defaultdict
-from .progress import verbose_progress, default_progress, print_progress
-from .progress import desc_progress
-from .runningstats import RunningQuantile, RunningTopK
-from .runningstats import RunningCrossCovariance, RunningConditionalQuantile
-from .sampler import FixedSubsetSampler
-from .actviz import activation_visualization
-from .segviz import segment_visualization, high_contrast
-from .workerpool import WorkerBase, WorkerPool
-from .segmenter import UnifiedParsingSegmenter
-
-def dissect(outdir, model, dataset,
- segrunner=None,
- train_dataset=None,
- model_segmenter=None,
- quantile_threshold=0.005,
- iou_threshold=0.05,
- iqr_threshold=0.01,
- examples_per_unit=100,
- batch_size=100,
- num_workers=24,
- seg_batch_size=5,
- make_images=True,
- make_labels=True,
- make_maxiou=False,
- make_covariance=False,
- make_report=True,
- make_row_images=True,
- make_single_images=False,
- rank_all_labels=False,
- netname=None,
- meta=None,
- merge=None,
- settings=None,
- ):
- '''
- Runs net dissection in-memory, using pytorch, and saves visualizations
- and metadata into outdir.
- '''
- assert not model.training, 'Run model.eval() before dissection'
- if netname is None:
- netname = type(model).__name__
- if segrunner is None:
- segrunner = ClassifierSegRunner(dataset)
- if train_dataset is None:
- train_dataset = dataset
- make_iqr = (quantile_threshold == 'iqr')
- with torch.no_grad():
- device = next(model.parameters()).device
- levels = None
- labelnames, catnames = None, None
- maxioudata, iqrdata = None, None
- labeldata = None
- iqrdata, cov = None, None
-
- labelnames, catnames = segrunner.get_label_and_category_names()
- label_category = [catnames.index(c) if c in catnames else 0
- for l, c in labelnames]
-
- # First, always collect qunatiles and topk information.
- segloader = torch.utils.data.DataLoader(dataset,
- batch_size=batch_size, num_workers=num_workers,
- pin_memory=(device.type == 'cuda'))
- quantiles, topk = collect_quantiles_and_topk(outdir, model,
- segloader, segrunner, k=examples_per_unit)
-
- # Thresholds can be automatically chosen by maximizing iqr
- if make_iqr:
- # Get thresholds based on an IQR optimization
- segloader = torch.utils.data.DataLoader(train_dataset,
- batch_size=1, num_workers=num_workers,
- pin_memory=(device.type == 'cuda'))
- iqrdata = collect_iqr(outdir, model, segloader, segrunner)
- max_iqr, full_iqr_levels = iqrdata[:2]
- max_iqr_agreement = iqrdata[4]
- # qualified_iqr[max_iqr_quantile[layer] > 0.5] = 0
- levels = {layer: full_iqr_levels[layer][
- max_iqr[layer].max(0)[1],
- torch.arange(max_iqr[layer].shape[1])].to(device)
- for layer in full_iqr_levels}
- else:
- levels = {k: qc.quantiles([1.0 - quantile_threshold])[:,0]
- for k, qc in quantiles.items()}
-
- quantiledata = (topk, quantiles, levels, quantile_threshold)
-
- if make_images:
- segloader = torch.utils.data.DataLoader(dataset,
- batch_size=batch_size, num_workers=num_workers,
- pin_memory=(device.type == 'cuda'))
- generate_images(outdir, model, dataset, topk, levels, segrunner,
- row_length=examples_per_unit, batch_size=seg_batch_size,
- row_images=make_row_images,
- single_images=make_single_images,
- num_workers=num_workers)
-
- if make_maxiou:
- assert train_dataset, "Need training dataset for maxiou."
- segloader = torch.utils.data.DataLoader(train_dataset,
- batch_size=1, num_workers=num_workers,
- pin_memory=(device.type == 'cuda'))
- maxioudata = collect_maxiou(outdir, model, segloader,
- segrunner)
-
- if make_labels:
- segloader = torch.utils.data.DataLoader(dataset,
- batch_size=1, num_workers=num_workers,
- pin_memory=(device.type == 'cuda'))
- iou_scores, iqr_scores, tcs, lcs, ccs, ics = (
- collect_bincounts(outdir, model, segloader,
- levels, segrunner))
- labeldata = (iou_scores, iqr_scores, lcs, ccs, ics, iou_threshold,
- iqr_threshold)
-
- if make_covariance:
- segloader = torch.utils.data.DataLoader(dataset,
- batch_size=seg_batch_size,
- num_workers=num_workers,
- pin_memory=(device.type == 'cuda'))
- cov = collect_covariance(outdir, model, segloader, segrunner)
-
- if make_report:
- generate_report(outdir,
- quantiledata=quantiledata,
- labelnames=labelnames,
- catnames=catnames,
- labeldata=labeldata,
- maxioudata=maxioudata,
- iqrdata=iqrdata,
- covariancedata=cov,
- rank_all_labels=rank_all_labels,
- netname=netname,
- meta=meta,
- mergedata=merge,
- settings=settings)
-
- return quantiledata, labeldata
-
-def generate_report(outdir, quantiledata, labelnames=None, catnames=None,
- labeldata=None, maxioudata=None, iqrdata=None, covariancedata=None,
- rank_all_labels=False, netname='Model', meta=None, settings=None,
- mergedata=None):
- '''
- Creates dissection.json reports and summary bargraph.svg files in the
- specified output directory, and copies a dissection.html interface
- to go along with it.
- '''
- all_layers = []
- # Current source code directory, for html to copy.
- srcdir = os.path.realpath(
- os.path.join(os.getcwd(), os.path.dirname(__file__)))
- # Unpack arguments
- topk, quantiles, levels, quantile_threshold = quantiledata
- top_record = dict(
- netname=netname,
- meta=meta,
- default_ranking='unit',
- quantile_threshold=quantile_threshold)
- if settings is not None:
- top_record['settings'] = settings
- if labeldata is not None:
- iou_scores, iqr_scores, lcs, ccs, ics, iou_threshold, iqr_threshold = (
- labeldata)
- catorder = {'object': -7, 'scene': -6, 'part': -5,
- 'piece': -4,
- 'material': -3, 'texture': -2, 'color': -1}
- for i, cat in enumerate(c for c in catnames if c not in catorder):
- catorder[cat] = i
- catnumber = {n: i for i, n in enumerate(catnames)}
- catnumber['-'] = 0
- top_record['default_ranking'] = 'label'
- top_record['iou_threshold'] = iou_threshold
- top_record['iqr_threshold'] = iqr_threshold
- labelnumber = dict((name[0], num)
- for num, name in enumerate(labelnames))
- # Make a segmentation color dictionary
- segcolors = {}
- for i, name in enumerate(labelnames):
- key = ','.join(str(s) for s in high_contrast[i % len(high_contrast)])
- if key in segcolors:
- segcolors[key] += '/' + name[0]
- else:
- segcolors[key] = name[0]
- top_record['segcolors'] = segcolors
- for layer in topk.keys():
- units, rankings = [], []
- record = dict(layer=layer, units=units, rankings=rankings)
- # For every unit, we always have basic visualization information.
- topa, topi = topk[layer].result()
- lev = levels[layer]
- for u in range(len(topa)):
- units.append(dict(
- unit=u,
- interp=True,
- level=lev[u].item(),
- top=[dict(imgnum=i.item(), maxact=a.item())
- for i, a in zip(topi[u], topa[u])],
- ))
- rankings.append(dict(name="unit", score=list([
- u for u in range(len(topa))])))
- # TODO: consider including stats and ranking based on quantiles,
- # variance, connectedness here.
-
- # if we have labeldata, then every unit also gets a bunch of other info
- if labeldata is not None:
- lscore, qscore, cc, ic = [dat[layer]
- for dat in [iou_scores, iqr_scores, ccs, ics]]
- if iqrdata is not None:
- # If we have IQR thresholds, assign labels based on that
- max_iqr, max_iqr_level = iqrdata[:2]
- best_label = max_iqr[layer].max(0)[1]
- best_score = lscore[best_label, torch.arange(lscore.shape[1])]
- best_qscore = qscore[best_label, torch.arange(lscore.shape[1])]
- else:
- # Otherwise, assign labels based on max iou
- best_score, best_label = lscore.max(0)
- best_qscore = qscore[best_label, torch.arange(qscore.shape[1])]
- record['iou_threshold'] = iou_threshold,
- for u, urec in enumerate(units):
- score, qscore, label = (
- best_score[u], best_qscore[u], best_label[u])
- urec.update(dict(
- iou=score.item(),
- iou_iqr=qscore.item(),
- lc=lcs[label].item(),
- cc=cc[catnumber[labelnames[label][1]], u].item(),
- ic=ic[label, u].item(),
- interp=(qscore.item() > iqr_threshold and
- score.item() > iou_threshold),
- iou_labelnum=label.item(),
- iou_label=labelnames[label.item()][0],
- iou_cat=labelnames[label.item()][1],
- ))
- if maxioudata is not None:
- max_iou, max_iou_level, max_iou_quantile = maxioudata
- qualified_iou = max_iou[layer].clone()
- # qualified_iou[max_iou_quantile[layer] > 0.75] = 0
- best_score, best_label = qualified_iou.max(0)
- for u, urec in enumerate(units):
- urec.update(dict(
- maxiou=best_score[u].item(),
- maxiou_label=labelnames[best_label[u].item()][0],
- maxiou_cat=labelnames[best_label[u].item()][1],
- maxiou_level=max_iou_level[layer][best_label[u], u].item(),
- maxiou_quantile=max_iou_quantile[layer][
- best_label[u], u].item()))
- if iqrdata is not None:
- [max_iqr, max_iqr_level, max_iqr_quantile,
- max_iqr_iou, max_iqr_agreement] = iqrdata
- qualified_iqr = max_iqr[layer].clone()
- qualified_iqr[max_iqr_quantile[layer] > 0.5] = 0
- best_score, best_label = qualified_iqr.max(0)
- for u, urec in enumerate(units):
- urec.update(dict(
- iqr=best_score[u].item(),
- iqr_label=labelnames[best_label[u].item()][0],
- iqr_cat=labelnames[best_label[u].item()][1],
- iqr_level=max_iqr_level[layer][best_label[u], u].item(),
- iqr_quantile=max_iqr_quantile[layer][
- best_label[u], u].item(),
- iqr_iou=max_iqr_iou[layer][best_label[u], u].item()
- ))
- if covariancedata is not None:
- score = covariancedata[layer].correlation()
- best_score, best_label = score.max(1)
- for u, urec in enumerate(units):
- urec.update(dict(
- cor=best_score[u].item(),
- cor_label=labelnames[best_label[u].item()][0],
- cor_cat=labelnames[best_label[u].item()][1]
- ))
- if mergedata is not None:
- # Final step: if the user passed any data to merge into the
- # units, merge them now. This can be used, for example, to
- # indiate that a unit is not interpretable based on some
- # outside analysis of unit statistics.
- for lrec in mergedata.get('layers', []):
- if lrec['layer'] == layer:
- break
- else:
- lrec = None
- for u, urec in enumerate(lrec.get('units', []) if lrec else []):
- units[u].update(urec)
- # After populating per-unit info, populate per-layer ranking info
- if labeldata is not None:
- # Collect all labeled units
- labelunits = defaultdict(list)
- all_labelunits = defaultdict(list)
- for u, urec in enumerate(units):
- if urec['interp']:
- labelunits[urec['iou_labelnum']].append(u)
- all_labelunits[urec['iou_labelnum']].append(u)
- # Sort all units in order with most popular label first.
- label_ordering = sorted(units,
- # Sort by:
- key=lambda r: (-1 if r['interp'] else 0, # interpretable
- -len(labelunits[r['iou_labelnum']]), # label freq, score
- -max([units[u]['iou']
- for u in labelunits[r['iou_labelnum']]], default=0),
- r['iou_labelnum'], # label
- -r['iou'])) # unit score
- # Add label and iou ranking.
- rankings.append(dict(name="label", score=(numpy.argsort(list(
- ur['unit'] for ur in label_ordering))).tolist()))
- rankings.append(dict(name="max iou", metric="iou", score=list(
- -ur['iou'] for ur in units)))
- # Add ranking for top labels
- # for labelnum in [n for n in sorted(
- # all_labelunits.keys(), key=lambda x:
- # -len(all_labelunits[x])) if len(all_labelunits[n])]:
- # label = labelnames[labelnum][0]
- # rankings.append(dict(name="%s-iou" % label,
- # concept=label, metric='iou',
- # score=(-lscore[labelnum, :]).tolist()))
- # Collate labels by category then frequency.
- record['labels'] = [dict(
- label=labelnames[label][0],
- labelnum=label,
- units=labelunits[label],
- cat=labelnames[label][1])
- for label in (sorted(labelunits.keys(),
- # Sort by:
- key=lambda l: (catorder.get( # category
- labelnames[l][1], 0),
- -len(labelunits[l]), # label freq
- -max([units[u]['iou'] for u in labelunits[l]],
- default=0) # score
- ))) if len(labelunits[label])]
- # Total number of interpretable units.
- record['interpretable'] = sum(len(group['units'])
- for group in record['labels'])
- # Make a bargraph of labels
- os.makedirs(os.path.join(outdir, safe_dir_name(layer)),
- exist_ok=True)
- catgroups = OrderedDict()
- for _, cat in sorted([(v, k) for k, v in catorder.items()]):
- catgroups[cat] = []
- for rec in record['labels']:
- if rec['cat'] not in catgroups:
- catgroups[rec['cat']] = []
- catgroups[rec['cat']].append(rec['label'])
- make_svg_bargraph(
- [rec['label'] for rec in record['labels']],
- [len(rec['units']) for rec in record['labels']],
- [(cat, len(group)) for cat, group in catgroups.items()],
- filename=os.path.join(outdir, safe_dir_name(layer),
- 'bargraph.svg'))
- # Only show the bargraph if it is non-empty.
- if len(record['labels']):
- record['bargraph'] = 'bargraph.svg'
- if maxioudata is not None:
- rankings.append(dict(name="max maxiou", metric="maxiou", score=list(
- -ur['maxiou'] for ur in units)))
- if iqrdata is not None:
- rankings.append(dict(name="max iqr", metric="iqr", score=list(
- -ur['iqr'] for ur in units)))
- if covariancedata is not None:
- rankings.append(dict(name="max cor", metric="cor", score=list(
- -ur['cor'] for ur in units)))
-
- all_layers.append(record)
- # Now add the same rankings to every layer...
- all_labels = None
- if rank_all_labels:
- all_labels = [name for name, cat in labelnames]
- if labeldata is not None:
- # Count layers+quadrants with a given label, and sort by freq
- counted_labels = defaultdict(int)
- for label in [
- re.sub(r'-(?:t|b|l|r|tl|tr|bl|br)$', '', unitrec['iou_label'])
- for record in all_layers for unitrec in record['units']]:
- counted_labels[label] += 1
- if all_labels is None:
- all_labels = [label for count, label in sorted((-v, k)
- for k, v in counted_labels.items())]
- for record in all_layers:
- layer = record['layer']
- for label in all_labels:
- labelnum = labelnumber[label]
- record['rankings'].append(dict(name="%s-iou" % label,
- concept=label, metric='iou',
- score=(-iou_scores[layer][labelnum, :]).tolist()))
-
- if maxioudata is not None:
- if all_labels is None:
- counted_labels = defaultdict(int)
- for label in [
- re.sub(r'-(?:t|b|l|r|tl|tr|bl|br)$', '',
- unitrec['maxiou_label'])
- for record in all_layers for unitrec in record['units']]:
- counted_labels[label] += 1
- all_labels = [label for count, label in sorted((-v, k)
- for k, v in counted_labels.items())]
- qualified_iou = max_iou[layer].clone()
- qualified_iou[max_iou_quantile[layer] > 0.5] = 0
- for record in all_layers:
- layer = record['layer']
- for label in all_labels:
- labelnum = labelnumber[label]
- record['rankings'].append(dict(name="%s-maxiou" % label,
- concept=label, metric='maxiou',
- score=(-qualified_iou[labelnum, :]).tolist()))
-
- if iqrdata is not None:
- if all_labels is None:
- counted_labels = defaultdict(int)
- for label in [
- re.sub(r'-(?:t|b|l|r|tl|tr|bl|br)$', '',
- unitrec['iqr_label'])
- for record in all_layers for unitrec in record['units']]:
- counted_labels[label] += 1
- all_labels = [label for count, label in sorted((-v, k)
- for k, v in counted_labels.items())]
- # qualified_iqr[max_iqr_quantile[layer] > 0.5] = 0
- for record in all_layers:
- layer = record['layer']
- qualified_iqr = max_iqr[layer].clone()
- for label in all_labels:
- labelnum = labelnumber[label]
- record['rankings'].append(dict(name="%s-iqr" % label,
- concept=label, metric='iqr',
- score=(-qualified_iqr[labelnum, :]).tolist()))
-
- if covariancedata is not None:
- if all_labels is None:
- counted_labels = defaultdict(int)
- for label in [
- re.sub(r'-(?:t|b|l|r|tl|tr|bl|br)$', '',
- unitrec['cor_label'])
- for record in all_layers for unitrec in record['units']]:
- counted_labels[label] += 1
- all_labels = [label for count, label in sorted((-v, k)
- for k, v in counted_labels.items())]
- for record in all_layers:
- layer = record['layer']
- score = covariancedata[layer].correlation()
- for label in all_labels:
- labelnum = labelnumber[label]
- record['rankings'].append(dict(name="%s-cor" % label,
- concept=label, metric='cor',
- score=(-score[:, labelnum]).tolist()))
-
- for record in all_layers:
- layer = record['layer']
- # Dump per-layer json inside per-layer directory
- record['dirname'] = '.'
- with open(os.path.join(outdir, safe_dir_name(layer), 'dissect.json'),
- 'w') as jsonfile:
- top_record['layers'] = [record]
- json.dump(top_record, jsonfile, indent=1)
- # Copy the per-layer html
- shutil.copy(os.path.join(srcdir, 'dissect.html'),
- os.path.join(outdir, safe_dir_name(layer), 'dissect.html'))
- record['dirname'] = safe_dir_name(layer)
-
- # Dump all-layer json in parent directory
- with open(os.path.join(outdir, 'dissect.json'), 'w') as jsonfile:
- top_record['layers'] = all_layers
- json.dump(top_record, jsonfile, indent=1)
- # Copy the all-layer html
- shutil.copy(os.path.join(srcdir, 'dissect.html'),
- os.path.join(outdir, 'dissect.html'))
- shutil.copy(os.path.join(srcdir, 'edit.html'),
- os.path.join(outdir, 'edit.html'))
-
-
-def generate_images(outdir, model, dataset, topk, levels,
- segrunner, row_length=None, gap_pixels=5,
- row_images=True, single_images=False, prefix='',
- batch_size=100, num_workers=24):
- '''
- Creates an image strip file for every unit of every retained layer
- of the model, in the format [outdir]/[layername]/[unitnum]-top.jpg.
- Assumes that the indexes of topk refer to the indexes of dataset.
- Limits each strip to the top row_length images.
- '''
- progress = default_progress()
- needed_images = {}
- if row_images is False:
- row_length = 1
- # Pass 1: needed_images lists all images that are topk for some unit.
- for layer in topk:
- topresult = topk[layer].result()[1].cpu()
- for unit, row in enumerate(topresult):
- for rank, imgnum in enumerate(row[:row_length]):
- imgnum = imgnum.item()
- if imgnum not in needed_images:
- needed_images[imgnum] = []
- needed_images[imgnum].append((layer, unit, rank))
- levels = {k: v.cpu().numpy() for k, v in levels.items()}
- row_length = len(row[:row_length])
- needed_sample = FixedSubsetSampler(sorted(needed_images.keys()))
- device = next(model.parameters()).device
- segloader = torch.utils.data.DataLoader(dataset,
- batch_size=batch_size, num_workers=num_workers,
- pin_memory=(device.type == 'cuda'),
- sampler=needed_sample)
- vizgrid, maskgrid, origrid, seggrid = [{} for _ in range(4)]
- # Pass 2: populate vizgrid with visualizations of top units.
- pool = None
- for i, batch in enumerate(
- progress(segloader, desc='Making images')):
- # Reverse transformation to get the image in byte form.
- seg, _, byte_im, _ = segrunner.run_and_segment_batch(batch, model,
- want_rgb=True)
- torch_features = model.retained_features()
- scale_offset = getattr(model, 'scale_offset', None)
- if pool is None:
- # Distribute the work across processes: create shared mmaps.
- for layer, tf in torch_features.items():
- [vizgrid[layer], maskgrid[layer], origrid[layer],
- seggrid[layer]] = [
- create_temp_mmap_grid((tf.shape[1],
- byte_im.shape[1], row_length,
- byte_im.shape[2] + gap_pixels, depth),
- dtype='uint8',
- fill=255)
- for depth in [3, 4, 3, 3]]
- # Pass those mmaps to worker processes.
- pool = WorkerPool(worker=VisualizeImageWorker,
- memmap_grid_info=[
- {layer: (g.filename, g.shape, g.dtype)
- for layer, g in grid.items()}
- for grid in [vizgrid, maskgrid, origrid, seggrid]])
- byte_im = byte_im.cpu().numpy()
- numpy_seg = seg.cpu().numpy()
- features = {}
- for index in range(len(byte_im)):
- imgnum = needed_sample.samples[index + i*segloader.batch_size]
- for layer, unit, rank in needed_images[imgnum]:
- if layer not in features:
- features[layer] = torch_features[layer].cpu().numpy()
- pool.add(layer, unit, rank,
- byte_im[index],
- features[layer][index, unit],
- levels[layer][unit],
- scale_offset[layer] if scale_offset else None,
- numpy_seg[index])
- pool.join()
- # Pass 3: save image strips as [outdir]/[layer]/[unitnum]-[top/orig].jpg
- pool = WorkerPool(worker=SaveImageWorker)
- for layer, vg in progress(vizgrid.items(), desc='Saving images'):
- os.makedirs(os.path.join(outdir, safe_dir_name(layer),
- prefix + 'image'), exist_ok=True)
- if single_images:
- os.makedirs(os.path.join(outdir, safe_dir_name(layer),
- prefix + 's-image'), exist_ok=True)
- og, sg, mg = origrid[layer], seggrid[layer], maskgrid[layer]
- for unit in progress(range(len(vg)), desc='Units'):
- for suffix, grid in [('top.jpg', vg), ('orig.jpg', og),
- ('seg.png', sg), ('mask.png', mg)]:
- strip = grid[unit].reshape(
- (grid.shape[1], grid.shape[2] * grid.shape[3],
- grid.shape[4]))
- if row_images:
- filename = os.path.join(outdir, safe_dir_name(layer),
- prefix + 'image', '%d-%s' % (unit, suffix))
- pool.add(strip[:,:-gap_pixels,:].copy(), filename)
- # Image.fromarray(strip[:,:-gap_pixels,:]).save(filename,
- # optimize=True, quality=80)
- if single_images:
- single_filename = os.path.join(outdir, safe_dir_name(layer),
- prefix + 's-image', '%d-%s' % (unit, suffix))
- pool.add(strip[:,:strip.shape[1] // row_length
- - gap_pixels,:].copy(), single_filename)
- # Image.fromarray(strip[:,:strip.shape[1] // row_length
- # - gap_pixels,:]).save(single_filename,
- # optimize=True, quality=80)
- pool.join()
- # Delete the shared memory map files
- clear_global_shared_files([g.filename
- for grid in [vizgrid, maskgrid, origrid, seggrid]
- for g in grid.values()])
-
-global_shared_files = {}
-def create_temp_mmap_grid(shape, dtype, fill):
- dtype = numpy.dtype(dtype)
- filename = os.path.join(tempfile.mkdtemp(), 'temp-%s-%s.mmap' %
- ('x'.join('%d' % s for s in shape), dtype.name))
- fid = open(filename, mode='w+b')
- original = numpy.memmap(fid, dtype=dtype, mode='w+', shape=shape)
- original.fid = fid
- original[...] = fill
- global_shared_files[filename] = original
- return original
-
-def shared_temp_mmap_grid(filename, shape, dtype):
- if filename not in global_shared_files:
- global_shared_files[filename] = numpy.memmap(
- filename, dtype=dtype, mode='r+', shape=shape)
- return global_shared_files[filename]
-
-def clear_global_shared_files(filenames):
- for fn in filenames:
- if fn in global_shared_files:
- del global_shared_files[fn]
- try:
- os.unlink(fn)
- except OSError:
- pass
-
-class VisualizeImageWorker(WorkerBase):
- def setup(self, memmap_grid_info):
- self.vizgrid, self.maskgrid, self.origrid, self.seggrid = [
- {layer: shared_temp_mmap_grid(*info)
- for layer, info in grid.items()}
- for grid in memmap_grid_info]
- def work(self, layer, unit, rank,
- byte_im, acts, level, scale_offset, seg):
- self.origrid[layer][unit,:,rank,:byte_im.shape[0],:] = byte_im
- [self.vizgrid[layer][unit,:,rank,:byte_im.shape[0],:],
- self.maskgrid[layer][unit,:,rank,:byte_im.shape[0],:]] = (
- activation_visualization(
- byte_im,
- acts,
- level,
- scale_offset=scale_offset,
- return_mask=True))
- self.seggrid[layer][unit,:,rank,:byte_im.shape[0],:] = (
- segment_visualization(seg, byte_im.shape[0:2]))
-
-class SaveImageWorker(WorkerBase):
- def work(self, data, filename):
- Image.fromarray(data).save(filename, optimize=True, quality=80)
-
-def score_tally_stats(label_category, tc, truth, cc, ic):
- pred = cc[label_category]
- total = tc[label_category][:, None]
- truth = truth[:, None]
- epsilon = 1e-20 # avoid division-by-zero
- union = pred + truth - ic
- iou = ic.double() / (union.double() + epsilon)
- arr = torch.empty(size=(2, 2) + ic.shape, dtype=ic.dtype, device=ic.device)
- arr[0, 0] = ic
- arr[0, 1] = pred - ic
- arr[1, 0] = truth - ic
- arr[1, 1] = total - union
- arr = arr.double() / total.double()
- mi = mutual_information(arr)
- je = joint_entropy(arr)
- iqr = mi / je
- iqr[torch.isnan(iqr)] = 0 # Zero out any 0/0
- return iou, iqr
-
-def collect_quantiles_and_topk(outdir, model, segloader,
- segrunner, k=100, resolution=1024):
- '''
- Collects (estimated) quantile information and (exact) sorted top-K lists
- for every channel in the retained layers of the model. Returns
- a map of quantiles (one RunningQuantile for each layer) along with
- a map of topk (one RunningTopK for each layer).
- '''
- device = next(model.parameters()).device
- features = model.retained_features()
- cached_quantiles = {
- layer: load_quantile_if_present(os.path.join(outdir,
- safe_dir_name(layer)), 'quantiles.npz',
- device=torch.device('cpu'))
- for layer in features }
- cached_topks = {
- layer: load_topk_if_present(os.path.join(outdir,
- safe_dir_name(layer)), 'topk.npz',
- device=torch.device('cpu'))
- for layer in features }
- if (all(value is not None for value in cached_quantiles.values()) and
- all(value is not None for value in cached_topks.values())):
- return cached_quantiles, cached_topks
-
- layer_batch_size = 8
- all_layers = list(features.keys())
- layer_batches = [all_layers[i:i+layer_batch_size]
- for i in range(0, len(all_layers), layer_batch_size)]
-
- quantiles, topks = {}, {}
- progress = default_progress()
- for layer_batch in layer_batches:
- for i, batch in enumerate(progress(segloader, desc='Quantiles')):
- # We don't actually care about the model output.
- model(batch[0].to(device))
- features = model.retained_features()
- # We care about the retained values
- for key in layer_batch:
- value = features[key]
- if topks.get(key, None) is None:
- topks[key] = RunningTopK(k)
- if quantiles.get(key, None) is None:
- quantiles[key] = RunningQuantile(resolution=resolution)
- topvalue = value
- if len(value.shape) > 2:
- topvalue, _ = value.view(*(value.shape[:2] + (-1,))).max(2)
- # Put the channel index last.
- value = value.permute(
- (0,) + tuple(range(2, len(value.shape))) + (1,)
- ).contiguous().view(-1, value.shape[1])
- quantiles[key].add(value)
- topks[key].add(topvalue)
- # Save GPU memory
- for key in layer_batch:
- quantiles[key].to_(torch.device('cpu'))
- topks[key].to_(torch.device('cpu'))
- for layer in quantiles:
- save_state_dict(quantiles[layer],
- os.path.join(outdir, safe_dir_name(layer), 'quantiles.npz'))
- save_state_dict(topks[layer],
- os.path.join(outdir, safe_dir_name(layer), 'topk.npz'))
- return quantiles, topks
-
-def collect_bincounts(outdir, model, segloader, levels, segrunner):
- '''
- Returns label_counts, category_activation_counts, and intersection_counts,
- across the data set, counting the pixels of intersection between upsampled,
- thresholded model featuremaps, with segmentation classes in the segloader.
-
- label_counts (independent of model): pixels across the data set that
- are labeled with the given label.
- category_activation_counts (one per layer): for each feature channel,
- pixels across the dataset where the channel exceeds the level
- threshold. There is one count per category: activations only
- contribute to the categories for which any category labels are
- present on the images.
- intersection_counts (one per layer): for each feature channel and
- label, pixels across the dataset where the channel exceeds
- the level, and the labeled segmentation class is also present.
-
- This is a performance-sensitive function. Best performance is
- achieved with a counting scheme which assumes a segloader with
- batch_size 1.
- '''
- # Load cached data if present
- (iou_scores, iqr_scores,
- total_counts, label_counts, category_activation_counts,
- intersection_counts) = {}, {}, None, None, {}, {}
- found_all = True
- for layer in model.retained_features():
- filename = os.path.join(outdir, safe_dir_name(layer), 'bincounts.npz')
- if os.path.isfile(filename):
- data = numpy.load(filename)
- iou_scores[layer] = torch.from_numpy(data['iou_scores'])
- iqr_scores[layer] = torch.from_numpy(data['iqr_scores'])
- total_counts = torch.from_numpy(data['total_counts'])
- label_counts = torch.from_numpy(data['label_counts'])
- category_activation_counts[layer] = torch.from_numpy(
- data['category_activation_counts'])
- intersection_counts[layer] = torch.from_numpy(
- data['intersection_counts'])
- else:
- found_all = False
- if found_all:
- return (iou_scores, iqr_scores,
- total_counts, label_counts, category_activation_counts,
- intersection_counts)
-
- device = next(model.parameters()).device
- labelcat, categories = segrunner.get_label_and_category_names()
- label_category = [categories.index(c) if c in categories else 0
- for l, c in labelcat]
- num_labels, num_categories = (len(n) for n in [labelcat, categories])
-
- # One-hot vector of category for each label
- labelcat = torch.zeros(num_labels, num_categories,
- dtype=torch.long, device=device)
- labelcat.scatter_(1, torch.from_numpy(numpy.array(label_category,
- dtype='int64')).to(device)[:,None], 1)
- # Running bincounts
- # activation_counts = {}
- assert segloader.batch_size == 1 # category_activation_counts needs this.
- category_activation_counts = {}
- intersection_counts = {}
- label_counts = torch.zeros(num_labels, dtype=torch.long, device=device)
- total_counts = torch.zeros(num_categories, dtype=torch.long, device=device)
- progress = default_progress()
- scale_offset_map = getattr(model, 'scale_offset', None)
- upsample_grids = {}
- # total_batch_categories = torch.zeros(
- # labelcat.shape[1], dtype=torch.long, device=device)
- for i, batch in enumerate(progress(segloader, desc='Bincounts')):
- seg, batch_label_counts, _, imshape = segrunner.run_and_segment_batch(
- batch, model, want_bincount=True, want_rgb=True)
- bc = batch_label_counts.cpu()
- batch_label_counts = batch_label_counts.to(device)
- seg = seg.to(device)
- features = model.retained_features()
- # Accumulate bincounts and identify nonzeros
- label_counts += batch_label_counts[0]
- batch_labels = bc[0].nonzero()[:,0]
- batch_categories = labelcat[batch_labels].max(0)[0]
- total_counts += batch_categories * (
- seg.shape[0] * seg.shape[2] * seg.shape[3])
- for key, value in features.items():
- if key not in upsample_grids:
- upsample_grids[key] = upsample_grid(value.shape[2:],
- seg.shape[2:], imshape,
- scale_offset=scale_offset_map.get(key, None)
- if scale_offset_map is not None else None,
- dtype=value.dtype, device=value.device)
- upsampled = torch.nn.functional.grid_sample(value,
- upsample_grids[key], padding_mode='border')
- amask = (upsampled > levels[key][None,:,None,None].to(
- upsampled.device))
- ac = amask.int().view(amask.shape[1], -1).sum(1)
- # if key not in activation_counts:
- # activation_counts[key] = ac
- # else:
- # activation_counts[key] += ac
- # The fastest approach: sum over each label separately!
- for label in batch_labels.tolist():
- if label == 0:
- continue # ignore the background label
- imask = amask * ((seg == label).max(dim=1, keepdim=True)[0])
- ic = imask.int().view(imask.shape[1], -1).sum(1)
- if key not in intersection_counts:
- intersection_counts[key] = torch.zeros(num_labels,
- amask.shape[1], dtype=torch.long, device=device)
- intersection_counts[key][label] += ic
- # Count activations within images that have category labels.
- # Note: This only makes sense with batch-size one
- # total_batch_categories += batch_categories
- cc = batch_categories[:,None] * ac[None,:]
- if key not in category_activation_counts:
- category_activation_counts[key] = cc
- else:
- category_activation_counts[key] += cc
- iou_scores = {}
- iqr_scores = {}
- for k in intersection_counts:
- iou_scores[k], iqr_scores[k] = score_tally_stats(
- label_category, total_counts, label_counts,
- category_activation_counts[k], intersection_counts[k])
- for k in intersection_counts:
- numpy.savez(os.path.join(outdir, safe_dir_name(k), 'bincounts.npz'),
- iou_scores=iou_scores[k].cpu().numpy(),
- iqr_scores=iqr_scores[k].cpu().numpy(),
- total_counts=total_counts.cpu().numpy(),
- label_counts=label_counts.cpu().numpy(),
- category_activation_counts=category_activation_counts[k]
- .cpu().numpy(),
- intersection_counts=intersection_counts[k].cpu().numpy(),
- levels=levels[k].cpu().numpy())
- return (iou_scores, iqr_scores,
- total_counts, label_counts, category_activation_counts,
- intersection_counts)
-
-def collect_cond_quantiles(outdir, model, segloader, segrunner):
- '''
- Returns maxiou and maxiou_level across the data set, one per layer.
-
- This is a performance-sensitive function. Best performance is
- achieved with a counting scheme which assumes a segloader with
- batch_size 1.
- '''
- device = next(model.parameters()).device
- cached_cond_quantiles = {
- layer: load_conditional_quantile_if_present(os.path.join(outdir,
- safe_dir_name(layer)), 'cond_quantiles.npz') # on cpu
- for layer in model.retained_features() }
- label_fracs = load_npy_if_present(outdir, 'label_fracs.npy', 'cpu')
- if label_fracs is not None and all(
- value is not None for value in cached_cond_quantiles.values()):
- return cached_cond_quantiles, label_fracs
-
- labelcat, categories = segrunner.get_label_and_category_names()
- label_category = [categories.index(c) if c in categories else 0
- for l, c in labelcat]
- num_labels, num_categories = (len(n) for n in [labelcat, categories])
-
- # One-hot vector of category for each label
- labelcat = torch.zeros(num_labels, num_categories,
- dtype=torch.long, device=device)
- labelcat.scatter_(1, torch.from_numpy(numpy.array(label_category,
- dtype='int64')).to(device)[:,None], 1)
- # Running maxiou
- assert segloader.batch_size == 1 # category_activation_counts needs this.
- conditional_quantiles = {}
- label_counts = torch.zeros(num_labels, dtype=torch.long, device=device)
- pixel_count = 0
- progress = default_progress()
- scale_offset_map = getattr(model, 'scale_offset', None)
- upsample_grids = {}
- common_conditions = set()
- if label_fracs is None or label_fracs is 0:
- for i, batch in enumerate(progress(segloader, desc='label fracs')):
- seg, batch_label_counts, im, _ = segrunner.run_and_segment_batch(
- batch, model, want_bincount=True, want_rgb=True)
- batch_label_counts = batch_label_counts.to(device)
- features = model.retained_features()
- # Accumulate bincounts and identify nonzeros
- label_counts += batch_label_counts[0]
- pixel_count += seg.shape[2] * seg.shape[3]
- label_fracs = (label_counts.cpu().float() / pixel_count)[:, None, None]
- numpy.save(os.path.join(outdir, 'label_fracs.npy'), label_fracs)
-
- skip_threshold = 1e-4
- skip_labels = set(i.item()
- for i in (label_fracs.view(-1) < skip_threshold).nonzero().view(-1))
-
- for layer in progress(model.retained_features().keys(), desc='CQ layers'):
- if cached_cond_quantiles.get(layer, None) is not None:
- conditional_quantiles[layer] = cached_cond_quantiles[layer]
- continue
-
- for i, batch in enumerate(progress(segloader, desc='Condquant')):
- seg, batch_label_counts, _, imshape = (
- segrunner.run_and_segment_batch(
- batch, model, want_bincount=True, want_rgb=True))
- bc = batch_label_counts.cpu()
- batch_label_counts = batch_label_counts.to(device)
- features = model.retained_features()
- # Accumulate bincounts and identify nonzeros
- label_counts += batch_label_counts[0]
- pixel_count += seg.shape[2] * seg.shape[3]
- batch_labels = bc[0].nonzero()[:,0]
- batch_categories = labelcat[batch_labels].max(0)[0]
- cpu_seg = None
- value = features[layer]
- if layer not in upsample_grids:
- upsample_grids[layer] = upsample_grid(value.shape[2:],
- seg.shape[2:], imshape,
- scale_offset=scale_offset_map.get(layer, None)
- if scale_offset_map is not None else None,
- dtype=value.dtype, device=value.device)
- if layer not in conditional_quantiles:
- conditional_quantiles[layer] = RunningConditionalQuantile(
- resolution=2048)
- upsampled = torch.nn.functional.grid_sample(value,
- upsample_grids[layer], padding_mode='border').view(
- value.shape[1], -1)
- conditional_quantiles[layer].add(('all',), upsampled.t())
- cpu_upsampled = None
- for label in batch_labels.tolist():
- if label in skip_labels:
- continue
- label_key = ('label', label)
- if label_key in common_conditions:
- imask = (seg == label).max(dim=1)[0].view(-1)
- intersected = upsampled[:, imask]
- conditional_quantiles[layer].add(('label', label),
- intersected.t())
- else:
- if cpu_seg is None:
- cpu_seg = seg.cpu()
- if cpu_upsampled is None:
- cpu_upsampled = upsampled.cpu()
- imask = (cpu_seg == label).max(dim=1)[0].view(-1)
- intersected = cpu_upsampled[:, imask]
- conditional_quantiles[layer].add(('label', label),
- intersected.t())
- if num_categories > 1:
- for cat in batch_categories.nonzero()[:,0]:
- conditional_quantiles[layer].add(('cat', cat.item()),
- upsampled.t())
- # Move the most common conditions to the GPU.
- if i and not i & (i - 1): # if i is a power of 2:
- cq = conditional_quantiles[layer]
- common_conditions = set(cq.most_common_conditions(64))
- cq.to_('cpu', [k for k in cq.running_quantiles.keys()
- if k not in common_conditions])
- # When a layer is done, get it off the GPU
- conditional_quantiles[layer].to_('cpu')
-
- label_fracs = (label_counts.cpu().float() / pixel_count)[:, None, None]
-
- for cq in conditional_quantiles.values():
- cq.to_('cpu')
-
- for layer in conditional_quantiles:
- save_state_dict(conditional_quantiles[layer],
- os.path.join(outdir, safe_dir_name(layer), 'cond_quantiles.npz'))
- numpy.save(os.path.join(outdir, 'label_fracs.npy'), label_fracs)
-
- return conditional_quantiles, label_fracs
-
-
-def collect_maxiou(outdir, model, segloader, segrunner):
- '''
- Returns maxiou and maxiou_level across the data set, one per layer.
-
- This is a performance-sensitive function. Best performance is
- achieved with a counting scheme which assumes a segloader with
- batch_size 1.
- '''
- device = next(model.parameters()).device
- conditional_quantiles, label_fracs = collect_cond_quantiles(
- outdir, model, segloader, segrunner)
-
- labelcat, categories = segrunner.get_label_and_category_names()
- label_category = [categories.index(c) if c in categories else 0
- for l, c in labelcat]
- num_labels, num_categories = (len(n) for n in [labelcat, categories])
-
- label_list = [('label', i) for i in range(num_labels)]
- category_list = [('all',)] if num_categories <= 1 else (
- [('cat', i) for i in range(num_categories)])
- max_iou, max_iou_level, max_iou_quantile = {}, {}, {}
- fracs = torch.logspace(-3, 0, 100)
- progress = default_progress()
- for layer, cq in progress(conditional_quantiles.items(), desc='Maxiou'):
- levels = cq.conditional(('all',)).quantiles(1 - fracs)
- denoms = 1 - cq.collected_normalize(category_list, levels)
- isects = (1 - cq.collected_normalize(label_list, levels)) * label_fracs
- unions = label_fracs + denoms[label_category, :, :] - isects
- iou = isects / unions
- # TODO: erase any for which threshold is bad
- max_iou[layer], level_bucket = iou.max(2)
- max_iou_level[layer] = levels[
- torch.arange(levels.shape[0])[None,:], level_bucket]
- max_iou_quantile[layer] = fracs[level_bucket]
- for layer in model.retained_features():
- numpy.savez(os.path.join(outdir, safe_dir_name(layer), 'max_iou.npz'),
- max_iou=max_iou[layer].cpu().numpy(),
- max_iou_level=max_iou_level[layer].cpu().numpy(),
- max_iou_quantile=max_iou_quantile[layer].cpu().numpy())
- return (max_iou, max_iou_level, max_iou_quantile)
-
-def collect_iqr(outdir, model, segloader, segrunner):
- '''
- Returns iqr and iqr_level.
-
- This is a performance-sensitive function. Best performance is
- achieved with a counting scheme which assumes a segloader with
- batch_size 1.
- '''
- max_iqr, max_iqr_level, max_iqr_quantile, max_iqr_iou = {}, {}, {}, {}
- max_iqr_agreement = {}
- found_all = True
- for layer in model.retained_features():
- filename = os.path.join(outdir, safe_dir_name(layer), 'iqr.npz')
- if os.path.isfile(filename):
- data = numpy.load(filename)
- max_iqr[layer] = torch.from_numpy(data['max_iqr'])
- max_iqr_level[layer] = torch.from_numpy(data['max_iqr_level'])
- max_iqr_quantile[layer] = torch.from_numpy(data['max_iqr_quantile'])
- max_iqr_iou[layer] = torch.from_numpy(data['max_iqr_iou'])
- max_iqr_agreement[layer] = torch.from_numpy(
- data['max_iqr_agreement'])
- else:
- found_all = False
- if found_all:
- return (max_iqr, max_iqr_level, max_iqr_quantile, max_iqr_iou,
- max_iqr_agreement)
-
-
- device = next(model.parameters()).device
- conditional_quantiles, label_fracs = collect_cond_quantiles(
- outdir, model, segloader, segrunner)
-
- labelcat, categories = segrunner.get_label_and_category_names()
- label_category = [categories.index(c) if c in categories else 0
- for l, c in labelcat]
- num_labels, num_categories = (len(n) for n in [labelcat, categories])
-
- label_list = [('label', i) for i in range(num_labels)]
- category_list = [('all',)] if num_categories <= 1 else (
- [('cat', i) for i in range(num_categories)])
- full_mi, full_je, full_iqr = {}, {}, {}
- fracs = torch.logspace(-3, 0, 100)
- progress = default_progress()
- for layer, cq in progress(conditional_quantiles.items(), desc='IQR'):
- levels = cq.conditional(('all',)).quantiles(1 - fracs)
- truth = label_fracs.to(device)
- preds = (1 - cq.collected_normalize(category_list, levels)
- )[label_category, :, :].to(device)
- cond_isects = 1 - cq.collected_normalize(label_list, levels).to(device)
- isects = cond_isects * truth
- unions = truth + preds - isects
- arr = torch.empty(size=(2, 2) + isects.shape, dtype=isects.dtype,
- device=device)
- arr[0, 0] = isects
- arr[0, 1] = preds - isects
- arr[1, 0] = truth - isects
- arr[1, 1] = 1 - unions
- arr.clamp_(0, 1)
- mi = mutual_information(arr)
- mi[:,:,-1] = 0 # at the 1.0 quantile should be no MI.
- # Don't trust mi when less than label_frac is less than 1e-3,
- # because our samples are too small.
- mi[label_fracs.view(-1) < 1e-3, :, :] = 0
- je = joint_entropy(arr)
- iqr = mi / je
- iqr[torch.isnan(iqr)] = 0 # Zero out any 0/0
- full_mi[layer] = mi.cpu()
- full_je[layer] = je.cpu()
- full_iqr[layer] = iqr.cpu()
- del mi, je
- agreement = isects + arr[1, 1]
- # When optimizing, maximize only over those pairs where the
- # unit is positively correlated with the label, and where the
- # threshold level is positive
- positive_iqr = iqr
- positive_iqr[agreement <= 0.8] = 0
- positive_iqr[(levels <= 0.0)[None, :, :].expand(positive_iqr.shape)] = 0
- # TODO: erase any for which threshold is bad
- maxiqr, level_bucket = positive_iqr.max(2)
- max_iqr[layer] = maxiqr.cpu()
- max_iqr_level[layer] = levels.to(device)[
- torch.arange(levels.shape[0])[None,:], level_bucket].cpu()
- max_iqr_quantile[layer] = fracs.to(device)[level_bucket].cpu()
- max_iqr_agreement[layer] = agreement[
- torch.arange(agreement.shape[0])[:, None],
- torch.arange(agreement.shape[1])[None, :],
- level_bucket].cpu()
-
- # Compute the iou that goes with each maximized iqr
- matching_iou = (isects[
- torch.arange(isects.shape[0])[:, None],
- torch.arange(isects.shape[1])[None, :],
- level_bucket] /
- unions[
- torch.arange(unions.shape[0])[:, None],
- torch.arange(unions.shape[1])[None, :],
- level_bucket])
- matching_iou[torch.isnan(matching_iou)] = 0
- max_iqr_iou[layer] = matching_iou.cpu()
- for layer in model.retained_features():
- numpy.savez(os.path.join(outdir, safe_dir_name(layer), 'iqr.npz'),
- max_iqr=max_iqr[layer].cpu().numpy(),
- max_iqr_level=max_iqr_level[layer].cpu().numpy(),
- max_iqr_quantile=max_iqr_quantile[layer].cpu().numpy(),
- max_iqr_iou=max_iqr_iou[layer].cpu().numpy(),
- max_iqr_agreement=max_iqr_agreement[layer].cpu().numpy(),
- full_mi=full_mi[layer].cpu().numpy(),
- full_je=full_je[layer].cpu().numpy(),
- full_iqr=full_iqr[layer].cpu().numpy())
- return (max_iqr, max_iqr_level, max_iqr_quantile, max_iqr_iou,
- max_iqr_agreement)
-
-def mutual_information(arr):
- total = 0
- for j in range(arr.shape[0]):
- for k in range(arr.shape[1]):
- joint = arr[j,k]
- ind = arr[j,:].sum(dim=0) * arr[:,k].sum(dim=0)
- term = joint * (joint / ind).log()
- term[torch.isnan(term)] = 0
- total += term
- return total.clamp_(0)
-
-def joint_entropy(arr):
- total = 0
- for j in range(arr.shape[0]):
- for k in range(arr.shape[1]):
- joint = arr[j,k]
- term = joint * joint.log()
- term[torch.isnan(term)] = 0
- total += term
- return (-total).clamp_(0)
-
-def information_quality_ratio(arr):
- iqr = mutual_information(arr) / joint_entropy(arr)
- iqr[torch.isnan(iqr)] = 0
- return iqr
-
-def collect_covariance(outdir, model, segloader, segrunner):
- '''
- Returns label_mean, label_variance, unit_mean, unit_variance,
- and cross_covariance across the data set.
-
- label_mean, label_variance (independent of model):
- treating the label as a one-hot, each label's mean and variance.
- unit_mean, unit_variance (one per layer): for each feature channel,
- the mean and variance of the activations in that channel.
- cross_covariance (one per layer): the cross covariance between the
- labels and the units in the layer.
- '''
- device = next(model.parameters()).device
- cached_covariance = {
- layer: load_covariance_if_present(os.path.join(outdir,
- safe_dir_name(layer)), 'covariance.npz', device=device)
- for layer in model.retained_features() }
- if all(value is not None for value in cached_covariance.values()):
- return cached_covariance
- labelcat, categories = segrunner.get_label_and_category_names()
- label_category = [categories.index(c) if c in categories else 0
- for l, c in labelcat]
- num_labels, num_categories = (len(n) for n in [labelcat, categories])
-
- # Running covariance
- cov = {}
- progress = default_progress()
- scale_offset_map = getattr(model, 'scale_offset', None)
- upsample_grids = {}
- for i, batch in enumerate(progress(segloader, desc='Covariance')):
- seg, _, _, imshape = segrunner.run_and_segment_batch(batch, model,
- want_rgb=True)
- features = model.retained_features()
- ohfeats = multilabel_onehot(seg, num_labels, ignore_index=0)
- # Accumulate bincounts and identify nonzeros
- for key, value in features.items():
- if key not in upsample_grids:
- upsample_grids[key] = upsample_grid(value.shape[2:],
- seg.shape[2:], imshape,
- scale_offset=scale_offset_map.get(key, None)
- if scale_offset_map is not None else None,
- dtype=value.dtype, device=value.device)
- upsampled = torch.nn.functional.grid_sample(value,
- upsample_grids[key].expand(
- (value.shape[0],) + upsample_grids[key].shape[1:]),
- padding_mode='border')
- if key not in cov:
- cov[key] = RunningCrossCovariance()
- cov[key].add(upsampled, ohfeats)
- for layer in cov:
- save_state_dict(cov[layer],
- os.path.join(outdir, safe_dir_name(layer), 'covariance.npz'))
- return cov
-
-def multilabel_onehot(labels, num_labels, dtype=None, ignore_index=None):
- '''
- Converts a multilabel tensor into a onehot tensor.
-
- The input labels is a tensor of shape (samples, multilabels, y, x).
- The output is a tensor of shape (samples, num_labels, y, x).
- If ignore_index is specified, labels with that index are ignored.
- Each x in labels should be 0 <= x < num_labels, or x == ignore_index.
- '''
- assert ignore_index is None or ignore_index <= 0
- if dtype is None:
- dtype = torch.float
- device = labels.device
- chans = num_labels + (-ignore_index if ignore_index else 0)
- outshape = (labels.shape[0], chans) + labels.shape[2:]
- result = torch.zeros(outshape, device=device, dtype=dtype)
- if ignore_index and ignore_index < 0:
- labels = labels + (-ignore_index)
- result.scatter_(1, labels, 1)
- if ignore_index and ignore_index < 0:
- result = result[:, -ignore_index:]
- elif ignore_index is not None:
- result[:, ignore_index] = 0
- return result
-
-def load_npy_if_present(outdir, filename, device):
- filepath = os.path.join(outdir, filename)
- if os.path.isfile(filepath):
- data = numpy.load(filepath)
- return torch.from_numpy(data).to(device)
- return 0
-
-def load_npz_if_present(outdir, filename, varnames, device):
- filepath = os.path.join(outdir, filename)
- if os.path.isfile(filepath):
- data = numpy.load(filepath)
- numpy_result = [data[n] for n in varnames]
- return tuple(torch.from_numpy(data).to(device) for data in numpy_result)
- return None
-
-def load_quantile_if_present(outdir, filename, device):
- filepath = os.path.join(outdir, filename)
- if os.path.isfile(filepath):
- data = numpy.load(filepath)
- result = RunningQuantile(state=data)
- result.to_(device)
- return result
- return None
-
-def load_conditional_quantile_if_present(outdir, filename):
- filepath = os.path.join(outdir, filename)
- if os.path.isfile(filepath):
- data = numpy.load(filepath)
- result = RunningConditionalQuantile(state=data)
- return result
- return None
-
-def load_topk_if_present(outdir, filename, device):
- filepath = os.path.join(outdir, filename)
- if os.path.isfile(filepath):
- data = numpy.load(filepath)
- result = RunningTopK(state=data)
- result.to_(device)
- return result
- return None
-
-def load_covariance_if_present(outdir, filename, device):
- filepath = os.path.join(outdir, filename)
- if os.path.isfile(filepath):
- data = numpy.load(filepath)
- result = RunningCrossCovariance(state=data)
- result.to_(device)
- return result
- return None
-
-def save_state_dict(obj, filepath):
- dirname = os.path.dirname(filepath)
- os.makedirs(dirname, exist_ok=True)
- dic = obj.state_dict()
- numpy.savez(filepath, **dic)
-
-def upsample_grid(data_shape, target_shape, input_shape=None,
- scale_offset=None, dtype=torch.float, device=None):
- '''Prepares a grid to use with grid_sample to upsample a batch of
- features in data_shape to the target_shape. Can use scale_offset
- and input_shape to center the grid in a nondefault way: scale_offset
- maps feature pixels to input_shape pixels, and it is assumed that
- the target_shape is a uniform downsampling of input_shape.'''
- # Default is that nothing is resized.
- if target_shape is None:
- target_shape = data_shape
- # Make a default scale_offset to fill the image if there isn't one
- if scale_offset is None:
- scale = tuple(float(ts) / ds
- for ts, ds in zip(target_shape, data_shape))
- offset = tuple(0.5 * s - 0.5 for s in scale)
- else:
- scale, offset = (v for v in zip(*scale_offset))
- # Handle downsampling for different input vs target shape.
- if input_shape is not None:
- scale = tuple(s * (ts - 1) / (ns - 1)
- for s, ns, ts in zip(scale, input_shape, target_shape))
- offset = tuple(o * (ts - 1) / (ns - 1)
- for o, ns, ts in zip(offset, input_shape, target_shape))
- # Pytorch needs target coordinates in terms of source coordinates [-1..1]
- ty, tx = (((torch.arange(ts, dtype=dtype, device=device) - o)
- * (2 / (s * (ss - 1))) - 1)
- for ts, ss, s, o, in zip(target_shape, data_shape, scale, offset))
- # Whoa, note that grid_sample reverses the order y, x -> x, y.
- grid = torch.stack(
- (tx[None,:].expand(target_shape), ty[:,None].expand(target_shape)),2
- )[None,:,:,:].expand((1, target_shape[0], target_shape[1], 2))
- return grid
-
-def safe_dir_name(filename):
- keepcharacters = (' ','.','_','-')
- return ''.join(c
- for c in filename if c.isalnum() or c in keepcharacters).rstrip()
-
-bargraph_palette = [
- ('#4B4CBF', '#B6B6F2'),
- ('#55B05B', '#B6F2BA'),
- ('#50BDAC', '#A5E5DB'),
- ('#81C679', '#C0FF9B'),
- ('#F0883B', '#F2CFB6'),
- ('#D4CF24', '#F2F1B6'),
- ('#D92E2B', '#F2B6B6'),
- ('#AB6BC6', '#CFAAFF'),
-]
-
-def make_svg_bargraph(labels, heights, categories,
- barheight=100, barwidth=12, show_labels=True, filename=None):
- # if len(labels) == 0:
- # return # Nothing to do
- unitheight = float(barheight) / max(max(heights, default=1), 1)
- textheight = barheight if show_labels else 0
- labelsize = float(barwidth)
- gap = float(barwidth) / 4
- textsize = barwidth + gap
- rollup = max(heights, default=1)
- textmargin = float(labelsize) * 2 / 3
- leftmargin = 32
- rightmargin = 8
- svgwidth = len(heights) * (barwidth + gap) + 2 * leftmargin + rightmargin
- svgheight = barheight + textheight
-
- # create an SVG XML element
- svg = et.Element('svg', width=str(svgwidth), height=str(svgheight),
- version='1.1', xmlns='http://www.w3.org/2000/svg')
-
- # Draw the bar graph
- basey = svgheight - textheight
- x = leftmargin
- # Add units scale on left
- if len(heights):
- for h in [1, (max(heights) + 1) // 2, max(heights)]:
- et.SubElement(svg, 'text', x='0', y='0',
- style=('font-family:sans-serif;font-size:%dpx;' +
- 'text-anchor:end;alignment-baseline:hanging;' +
- 'transform:translate(%dpx, %dpx);') %
- (textsize, x - gap, basey - h * unitheight)).text = str(h)
- et.SubElement(svg, 'text', x='0', y='0',
- style=('font-family:sans-serif;font-size:%dpx;' +
- 'text-anchor:middle;' +
- 'transform:translate(%dpx, %dpx) rotate(-90deg)') %
- (textsize, x - gap - textsize, basey - h * unitheight / 2)
- ).text = 'units'
- # Draw big category background rectangles
- for catindex, (cat, catcount) in enumerate(categories):
- if not catcount:
- continue
- et.SubElement(svg, 'rect', x=str(x), y=str(basey - rollup * unitheight),
- width=(str((barwidth + gap) * catcount - gap)),
- height = str(rollup*unitheight),
- fill=bargraph_palette[catindex % len(bargraph_palette)][1])
- x += (barwidth + gap) * catcount
- # Draw small bars as well as 45degree text labels
- x = leftmargin
- catindex = -1
- catcount = 0
- for label, height in zip(labels, heights):
- while not catcount and catindex <= len(categories):
- catindex += 1
- catcount = categories[catindex][1]
- color = bargraph_palette[catindex % len(bargraph_palette)][0]
- et.SubElement(svg, 'rect', x=str(x), y=str(basey-(height * unitheight)),
- width=str(barwidth), height=str(height * unitheight),
- fill=color)
- x += barwidth
- if show_labels:
- et.SubElement(svg, 'text', x='0', y='0',
- style=('font-family:sans-serif;font-size:%dpx;text-anchor:end;'+
- 'transform:translate(%dpx, %dpx) rotate(-45deg);') %
- (labelsize, x, basey + textmargin)).text = readable(label)
- x += gap
- catcount -= 1
- # Text labels for each category
- x = leftmargin
- for cat, catcount in categories:
- if not catcount:
- continue
- et.SubElement(svg, 'text', x='0', y='0',
- style=('font-family:sans-serif;font-size:%dpx;text-anchor:end;'+
- 'transform:translate(%dpx, %dpx) rotate(-90deg);') %
- (textsize, x + (barwidth + gap) * catcount - gap,
- basey - rollup * unitheight + gap)).text = '%d %s' % (
- catcount, readable(cat + ('s' if catcount != 1 else '')))
- x += (barwidth + gap) * catcount
- # Output - this is the bare svg.
- result = et.tostring(svg)
- if filename:
- f = open(filename, 'wb')
- # When writing to a file a special header is needed.
- f.write(''.join([
- '\n',
- '\n']
- ).encode('utf-8'))
- f.write(result)
- f.close()
- return result
-
-readable_replacements = [(re.compile(r[0]), r[1]) for r in [
- (r'-[sc]$', ''),
- (r'_', ' '),
- ]]
-
-def readable(label):
- for pattern, subst in readable_replacements:
- label= re.sub(pattern, subst, label)
- return label
-
-def reverse_normalize_from_transform(transform):
- '''
- Crawl around the transforms attached to a dataset looking for a
- Normalize transform, and return it a corresponding ReverseNormalize,
- or None if no normalization is found.
- '''
- if isinstance(transform, torchvision.transforms.Normalize):
- return ReverseNormalize(transform.mean, transform.std)
- t = getattr(transform, 'transform', None)
- if t is not None:
- return reverse_normalize_from_transform(t)
- transforms = getattr(transform, 'transforms', None)
- if transforms is not None:
- for t in reversed(transforms):
- result = reverse_normalize_from_transform(t)
- if result is not None:
- return result
- return None
-
-class ReverseNormalize:
- '''
- Applies the reverse of torchvision.transforms.Normalize.
- '''
- def __init__(self, mean, stdev):
- mean = numpy.array(mean)
- stdev = numpy.array(stdev)
- self.mean = torch.from_numpy(mean)[None,:,None,None].float()
- self.stdev = torch.from_numpy(stdev)[None,:,None,None].float()
- def __call__(self, data):
- device = data.device
- return data.mul(self.stdev.to(device)).add_(self.mean.to(device))
-
-class ImageOnlySegRunner:
- def __init__(self, dataset, recover_image=None):
- if recover_image is None:
- recover_image = reverse_normalize_from_transform(dataset)
- self.recover_image = recover_image
- self.dataset = dataset
- def get_label_and_category_names(self):
- return [('-', '-')], ['-']
- def run_and_segment_batch(self, batch, model,
- want_bincount=False, want_rgb=False):
- [im] = batch
- device = next(model.parameters()).device
- if want_rgb:
- rgb = self.recover_image(im.clone()
- ).permute(0, 2, 3, 1).mul_(255).clamp(0, 255).byte()
- else:
- rgb = None
- # Stubs for seg and bc
- seg = torch.zeros(im.shape[0], 1, 1, 1, dtype=torch.long)
- bc = torch.ones(im.shape[0], 1, dtype=torch.long)
- # Run the model.
- model(im.to(device))
- return seg, bc, rgb, im.shape[2:]
-
-class ClassifierSegRunner:
- def __init__(self, dataset, recover_image=None):
- # The dataset contains explicit segmentations
- if recover_image is None:
- recover_image = reverse_normalize_from_transform(dataset)
- self.recover_image = recover_image
- self.dataset = dataset
- def get_label_and_category_names(self):
- catnames = self.dataset.categories
- label_and_cat_names = [(readable(label),
- catnames[self.dataset.label_category[i]])
- for i, label in enumerate(self.dataset.labels)]
- return label_and_cat_names, catnames
- def run_and_segment_batch(self, batch, model,
- want_bincount=False, want_rgb=False):
- '''
- Runs the dissected model on one batch of the dataset, and
- returns a multilabel semantic segmentation for the data.
- Given a batch of size (n, c, y, x) the segmentation should
- be a (long integer) tensor of size (n, d, y//r, x//r) where
- d is the maximum number of simultaneous labels given to a pixel,
- and where r is some (optional) resolution reduction factor.
- In the segmentation returned, the label `0` is reserved for
- the background "no-label".
-
- In addition to the segmentation, bc, rgb, and shape are returned
- where bc is a per-image bincount counting returned label pixels,
- rgb is a viewable (n, y, x, rgb) byte image tensor for the data
- for visualizations (reversing normalizations, for example), and
- shape is the (y, x) size of the data. If want_bincount or
- want_rgb are False, those return values may be None.
- '''
- im, seg, bc = batch
- device = next(model.parameters()).device
- if want_rgb:
- rgb = self.recover_image(im.clone()
- ).permute(0, 2, 3, 1).mul_(255).clamp(0, 255).byte()
- else:
- rgb = None
- # Run the model.
- model(im.to(device))
- return seg, bc, rgb, im.shape[2:]
-
-class GeneratorSegRunner:
- def __init__(self, segmenter):
- # The segmentations are given by an algorithm
- if segmenter is None:
- segmenter = UnifiedParsingSegmenter(segsizes=[256], segdiv='quad')
- self.segmenter = segmenter
- self.num_classes = len(segmenter.get_label_and_category_names()[0])
- def get_label_and_category_names(self):
- return self.segmenter.get_label_and_category_names()
- def run_and_segment_batch(self, batch, model,
- want_bincount=False, want_rgb=False):
- '''
- Runs the dissected model on one batch of the dataset, and
- returns a multilabel semantic segmentation for the data.
- Given a batch of size (n, c, y, x) the segmentation should
- be a (long integer) tensor of size (n, d, y//r, x//r) where
- d is the maximum number of simultaneous labels given to a pixel,
- and where r is some (optional) resolution reduction factor.
- In the segmentation returned, the label `0` is reserved for
- the background "no-label".
-
- In addition to the segmentation, bc, rgb, and shape are returned
- where bc is a per-image bincount counting returned label pixels,
- rgb is a viewable (n, y, x, rgb) byte image tensor for the data
- for visualizations (reversing normalizations, for example), and
- shape is the (y, x) size of the data. If want_bincount or
- want_rgb are False, those return values may be None.
- '''
- device = next(model.parameters()).device
- z_batch = batch[0]
- tensor_images = model(z_batch.to(device))
- seg = self.segmenter.segment_batch(tensor_images, downsample=2)
- if want_bincount:
- index = torch.arange(z_batch.shape[0],
- dtype=torch.long, device=device)
- bc = (seg + index[:, None, None, None] * self.num_classes).view(-1
- ).bincount(minlength=z_batch.shape[0] * self.num_classes)
- bc = bc.view(z_batch.shape[0], self.num_classes)
- else:
- bc = None
- if want_rgb:
- images = ((tensor_images + 1) / 2 * 255)
- rgb = images.permute(0, 2, 3, 1).clamp(0, 255).byte()
- else:
- rgb = None
- return seg, bc, rgb, tensor_images.shape[2:]
diff --git a/spaces/mikeee/radiobee-aligner/radiobee/app.py b/spaces/mikeee/radiobee-aligner/radiobee/app.py
deleted file mode 100644
index 8db39bd7832424dbf98733a76b14e401b40a604f..0000000000000000000000000000000000000000
--- a/spaces/mikeee/radiobee-aligner/radiobee/app.py
+++ /dev/null
@@ -1,38 +0,0 @@
-"""Talk to spaces VM via subprocess.check_output."""
-# pylint: disable=unused-variable, invalid-name
-
-# import httpx
-import subprocess as sp
-from shlex import split
-import gradio as gr
-
-
-def greet(command):
- """Probe vm."""
- try:
- out = sp.check_output(split(command), encoding="utf8")
- except Exception as e:
- out = str(e)
- # return "Hello " + name + "!!"
- if not (out and out.strip()):
- out = "No output, that's all we know."
- return out
-
-
-iface = gr.Interface(
- fn=greet,
- inputs="text",
- outputs="text",
- examples=[
- "cat /proc/version",
- "free # show free memory",
- "uname -m",
- "df -h .",
- "cat /proc/cpuinfo",
- ],
- title="probe the system",
- description="talk to the system via subprocess.check_output ",
-)
-
-# iface.launch(share=True, debug=True)
-iface.launch(debug=True)
diff --git a/spaces/mikeee/radiobee-dev/tests/test_shuffle_sents.py b/spaces/mikeee/radiobee-dev/tests/test_shuffle_sents.py
deleted file mode 100644
index 2c09d0253786f05946d007b64df43a30cd1fc032..0000000000000000000000000000000000000000
--- a/spaces/mikeee/radiobee-dev/tests/test_shuffle_sents.py
+++ /dev/null
@@ -1,136 +0,0 @@
-"""Test shuffle_sents.
-
- eps: float = 6
- min_samples: int = 4
- tf_type: str = "linear"
- idf_type: Optional[str] = None
- dl_type: Optional[str] = None
- norm: Optional[str] = None
- lang1: Optional[str] = "en"
- lang2: Optional[str] = "zh"
-"""
-from radiobee.seg_text import seg_text
-from radiobee.shuffle_sents import shuffle_sents
-from radiobee.align_sents import align_sents
-
-text1 = """`Wretched inmates!' I ejaculated mentally, `you deserve perpetual isolation from your species for your churlish inhospitality. At least, I would not keep my doors barred in the day time. I don't care--I will get in!' So resolved, I grasped the latch and shook it vehemently. Vinegar-faced Joseph projected his head from a round window of the barn."""
-text2 = """“被囚禁的囚犯!”我在精神上被射精,“你应该永远与你的物种隔绝,因为你这种粗鲁的病态。至少,我白天不会锁门,我不在乎,我进去了!”我决心如此,我抓住了门锁,狠狠地摇了一下。醋脸的约瑟夫从谷仓的圆窗朝他的头照射。"""
-text3 = """"Elende Insassen! ejakulierte ich im Geiste, "ihr verdient die ewige Isolation von eurer Spezies für eure rüpelhafte Ungastlichkeit. Zumindest würde ich meine Türen tagsüber nicht verriegeln. Das ist mir egal - ich werde reinkommen!' So entschlossen, ergriff ich die Klinke und rüttelte heftig daran. Der essiggesichtige Joseph streckte seinen Kopf aus einem runden Fenster der Scheune."""
-
-
-def test_shuffle_sents_en_zh():
- """Test shuffle_sents_en_zh."""
- sents_en = seg_text(text1)
- sents_zh = seg_text(text2)
-
- lang1 = "en"
- lang2 = "zh"
-
- pairs = shuffle_sents(sents_en, sents_zh)
- pairs_ = shuffle_sents(sents_en, sents_zh, lang1=lang1, lang2=lang2)
-
- # pairs[3] == ('', "I don't care--I will get in!'", '')
- assert pairs == pairs_
-
- # assert not pairs[3][0]
- # after swapping
- assert not pairs[3][1]
-
-
-def test_shuffle_sents_en_de():
- """Test shuffle_sents_en_de."""
- sents_en = seg_text(text1)
- sents_de = seg_text(text3)
-
- lang1 = "en"
- lang2 = "de"
-
- pairs = shuffle_sents(sents_en, sents_de)
- pairs_ = shuffle_sents(sents_en, sents_de, lang1=lang1, lang2=lang2)
-
- assert pairs == pairs_
-
- #
- # assert not pairs[3][0]
- _ = """In [218]: pairs[:2]
- Out[218]:
- [["`Wretched inmates!'", '', ''],
- ['I ejaculated mentally, `you deserve perpetual isolation from your species for your churlish inhospitality.',
- '"Elende Insassen! ejakulierte ich im Geiste, "ihr verdient die ewige Isolation von eurer Spezies für eure rüpelhafte Ungastlichkeit.',
- 0.62]]
- """
- assert not pairs[0][1]
- assert "mentally" in str(pairs[1]) and "Elende" in str(pairs[1])
-
- # [elm[2] for elm in pairs]
- # ['', 0.62, 0.72, 0.74, 0.68, 0.79]
- if isinstance(pairs[1][2], float):
- assert pairs[1][2] > 0.6
- if isinstance(pairs[2][2], float):
- assert pairs[2][2] > 0.7
- if isinstance(pairs[3][2], float):
- assert pairs[3][2] > 0.7
- if isinstance(pairs[4][2], float):
- assert pairs[4][2] > 0.6
- if isinstance(pairs[5][2], float):
- assert pairs[5][2] > 0.7
-
-
-_ = """
-In [232]: shuffle_sents.cmat.round(2)
-Out[232]:
-array([[ 0.27, 0.62, 0.07, 0.11, 0.02, 0.02],
- [ 0.03, 0.09, 0.72, 0.18, 0.07, -0.07],
- [ 0.19, 0.07, 0.16, 0.74, -0.01, -0.02],
- [-0.02, 0.18, 0.16, 0.06, 0.68, -0.04],
- [ 0.02, 0.07, 0.04, -0.04, 0.02, 0.79]], dtype=float32)
-pairs[1]
-sents_en[1], sents_de[0], shuffle_sents.cmat[0, 1]
-['I ejaculated mentally, `you deserve perpetual isolation from your species for your churlish inhospitality.',
- '"Elende Insassen! ejakulierte ich im Geiste, "ihr verdient die ewige Isolation von eurer Spezies für eure rüpelhafte Ungastlichkeit.',
- 0.62]
-
-pairs[2]
-sents_en[2], sents_de[1], shuffle_sents.cmat[1, 2].round(2)
-Out[244]:
-('At least, I would not keep my doors barred in the day time.',
- 'Zumindest würde ich meine Türen tagsüber nicht verriegeln.',
- 0.72)
-...
-
-import mtplotlib
-import matplotlib.pyplot as plt
-import seaborn as sns
-
-sns.set()
-set_style("darkgrind")
-plt.ion()
-
-ali = shuffle_sents(sents_en, sents_de)
-sns.heatmap(shuffle_sents.cmat, cmap="viridis_r").invert_yaxis()
-ax = plt.gca()
-ax.set_xlabel(shuffle_sents.lang1)
-ax.set_ylabel(shuffle_sents.lang2)
-
-ali == [["`Wretched inmates!'", '', ''],
- ['I ejaculated mentally, `you deserve perpetual isolation from your species for your churlish inhospitality.',
- '"Elende Insassen! ejakulierte ich im Geiste, "ihr verdient die ewige Isolation von eurer Spezies für eure rüpelhafte Ungastlichkeit.',
- 0.62],
- ['At least, I would not keep my doors barred in the day time.',
- 'Zumindest würde ich meine Türen tagsüber nicht verriegeln.',
- 0.72],
- ["I don't care--I will get in!'",
- "Das ist mir egal - ich werde reinkommen!'",
- 0.74],
- ['So resolved, I grasped the latch and shook it vehemently.',
- 'So entschlossen, ergriff ich die Klinke und rüttelte heftig daran.',
- 0.68],
- ['Vinegar-faced Joseph projected his head from a round window of the barn.',
- 'Der essiggesichtige Joseph streckte seinen Kopf aus einem runden Fenster der Scheune.',
- 0.79]]
-
-res1 = align_sents(sents_en, sents_de)
-ali = shuffle_sents(sents_en, sents_de)
-for idx in range(1, 6):
- assert res1[idx] == tuple(ali[idx][:2])
-"""
diff --git a/spaces/miracle01/white-emotion-recognition/app.py b/spaces/miracle01/white-emotion-recognition/app.py
deleted file mode 100644
index 26f18be352ae39a6eca77faee4fb7f2a5f54f65b..0000000000000000000000000000000000000000
--- a/spaces/miracle01/white-emotion-recognition/app.py
+++ /dev/null
@@ -1,3 +0,0 @@
-import gradio as gr
-
-gr.Interface.load("models/tahiyacy/emotion-recognition").launch()
\ No newline at end of file
diff --git a/spaces/mithril-security/blind_chat/.svelte-kit/types/src/routes/settings/$types.d.ts b/spaces/mithril-security/blind_chat/.svelte-kit/types/src/routes/settings/$types.d.ts
deleted file mode 100644
index 11802b80d201eeb689785235bcb7a8a567da64f3..0000000000000000000000000000000000000000
--- a/spaces/mithril-security/blind_chat/.svelte-kit/types/src/routes/settings/$types.d.ts
+++ /dev/null
@@ -1,28 +0,0 @@
-import type * as Kit from '@sveltejs/kit';
-
-type Expand = T extends infer O ? { [K in keyof O]: O[K] } : never;
-type RouteParams = { }
-type RouteId = '/settings';
-type MaybeWithVoid = {} extends T ? T | void : T;
-export type RequiredKeys = { [K in keyof T]-?: {} extends { [P in K]: T[K] } ? never : K; }[keyof T];
-type OutputDataShape = MaybeWithVoid> & Partial> & Record>
-type EnsureDefined = T extends null | undefined ? {} : T;
-type OptionalUnion, A extends keyof U = U extends U ? keyof U : never> = U extends unknown ? { [P in Exclude]?: never } & U : never;
-export type Snapshot = Kit.Snapshot;
-type PageServerParentData = EnsureDefined;
-type PageParentData = EnsureDefined;
-
-export type PageServerLoad = OutputDataShape> = Kit.ServerLoad;
-export type PageServerLoadEvent = Parameters[0];
-type ExcludeActionFailure = T extends Kit.ActionFailure ? never : T extends void ? never : T;
-type ActionsSuccess any>> = { [Key in keyof T]: ExcludeActionFailure>>; }[keyof T];
-type ExtractActionFailure = T extends Kit.ActionFailure ? X extends void ? never : X : never;
-type ActionsFailure any>> = { [Key in keyof T]: Exclude>>, void>; }[keyof T];
-type ActionsExport = typeof import('../../../../../src/routes/settings/+page.server.js').actions
-export type SubmitFunction = Kit.SubmitFunction>, Expand>>
-export type ActionData = Expand> | null;
-export type PageServerData = null;
-export type PageData = Expand;
-export type Action | void = Record | void> = Kit.Action
-export type Actions | void = Record | void> = Kit.Actions
-export type RequestEvent = Kit.RequestEvent;
\ No newline at end of file
diff --git a/spaces/mnauf/detect-bees/val.py b/spaces/mnauf/detect-bees/val.py
deleted file mode 100644
index 127acf8100297f6a15e9008ea3eb674550d743b3..0000000000000000000000000000000000000000
--- a/spaces/mnauf/detect-bees/val.py
+++ /dev/null
@@ -1,406 +0,0 @@
-# YOLOv5 🚀 by Ultralytics, GPL-3.0 license
-"""
-Validate a trained YOLOv5 detection model on a detection dataset
-
-Usage:
- $ python val.py --weights yolov5s.pt --data coco128.yaml --img 640
-
-Usage - formats:
- $ python val.py --weights yolov5s.pt # PyTorch
- yolov5s.torchscript # TorchScript
- yolov5s.onnx # ONNX Runtime or OpenCV DNN with --dnn
- yolov5s_openvino_model # OpenVINO
- yolov5s.engine # TensorRT
- yolov5s.mlmodel # CoreML (macOS-only)
- yolov5s_saved_model # TensorFlow SavedModel
- yolov5s.pb # TensorFlow GraphDef
- yolov5s.tflite # TensorFlow Lite
- yolov5s_edgetpu.tflite # TensorFlow Edge TPU
- yolov5s_paddle_model # PaddlePaddle
-"""
-
-import argparse
-import json
-import os
-import sys
-from pathlib import Path
-
-import numpy as np
-import torch
-from tqdm import tqdm
-
-FILE = Path(__file__).resolve()
-ROOT = FILE.parents[0] # YOLOv5 root directory
-if str(ROOT) not in sys.path:
- sys.path.append(str(ROOT)) # add ROOT to PATH
-ROOT = Path(os.path.relpath(ROOT, Path.cwd())) # relative
-
-from models.common import DetectMultiBackend
-from utils.callbacks import Callbacks
-from utils.dataloaders import create_dataloader
-from utils.general import (LOGGER, Profile, check_dataset, check_img_size, check_requirements, check_yaml,
- coco80_to_coco91_class, colorstr, increment_path, non_max_suppression, print_args,
- scale_boxes, xywh2xyxy, xyxy2xywh)
-from utils.metrics import ConfusionMatrix, ap_per_class, box_iou
-from utils.plots import output_to_target, plot_images, plot_val_study
-from utils.torch_utils import select_device, smart_inference_mode
-
-
-def save_one_txt(predn, save_conf, shape, file):
- # Save one txt result
- gn = torch.tensor(shape)[[1, 0, 1, 0]] # normalization gain whwh
- for *xyxy, conf, cls in predn.tolist():
- xywh = (xyxy2xywh(torch.tensor(xyxy).view(1, 4)) / gn).view(-1).tolist() # normalized xywh
- line = (cls, *xywh, conf) if save_conf else (cls, *xywh) # label format
- with open(file, 'a') as f:
- f.write(('%g ' * len(line)).rstrip() % line + '\n')
-
-
-def save_one_json(predn, jdict, path, class_map):
- # Save one JSON result {"image_id": 42, "category_id": 18, "bbox": [258.15, 41.29, 348.26, 243.78], "score": 0.236}
- image_id = int(path.stem) if path.stem.isnumeric() else path.stem
- box = xyxy2xywh(predn[:, :4]) # xywh
- box[:, :2] -= box[:, 2:] / 2 # xy center to top-left corner
- for p, b in zip(predn.tolist(), box.tolist()):
- jdict.append({
- 'image_id': image_id,
- 'category_id': class_map[int(p[5])],
- 'bbox': [round(x, 3) for x in b],
- 'score': round(p[4], 5)})
-
-
-def process_batch(detections, labels, iouv):
- """
- Return correct prediction matrix
- Arguments:
- detections (array[N, 6]), x1, y1, x2, y2, conf, class
- labels (array[M, 5]), class, x1, y1, x2, y2
- Returns:
- correct (array[N, 10]), for 10 IoU levels
- """
- correct = np.zeros((detections.shape[0], iouv.shape[0])).astype(bool)
- iou = box_iou(labels[:, 1:], detections[:, :4])
- correct_class = labels[:, 0:1] == detections[:, 5]
- for i in range(len(iouv)):
- x = torch.where((iou >= iouv[i]) & correct_class) # IoU > threshold and classes match
- if x[0].shape[0]:
- matches = torch.cat((torch.stack(x, 1), iou[x[0], x[1]][:, None]), 1).cpu().numpy() # [label, detect, iou]
- if x[0].shape[0] > 1:
- matches = matches[matches[:, 2].argsort()[::-1]]
- matches = matches[np.unique(matches[:, 1], return_index=True)[1]]
- # matches = matches[matches[:, 2].argsort()[::-1]]
- matches = matches[np.unique(matches[:, 0], return_index=True)[1]]
- correct[matches[:, 1].astype(int), i] = True
- return torch.tensor(correct, dtype=torch.bool, device=iouv.device)
-
-
-@smart_inference_mode()
-def run(
- data,
- weights=None, # model.pt path(s)
- batch_size=32, # batch size
- imgsz=640, # inference size (pixels)
- conf_thres=0.001, # confidence threshold
- iou_thres=0.6, # NMS IoU threshold
- max_det=300, # maximum detections per image
- task='val', # train, val, test, speed or study
- device='', # cuda device, i.e. 0 or 0,1,2,3 or cpu
- workers=8, # max dataloader workers (per RANK in DDP mode)
- single_cls=False, # treat as single-class dataset
- augment=False, # augmented inference
- verbose=False, # verbose output
- save_txt=False, # save results to *.txt
- save_hybrid=False, # save label+prediction hybrid results to *.txt
- save_conf=False, # save confidences in --save-txt labels
- save_json=False, # save a COCO-JSON results file
- project=ROOT / 'runs/val', # save to project/name
- name='exp', # save to project/name
- exist_ok=False, # existing project/name ok, do not increment
- half=True, # use FP16 half-precision inference
- dnn=False, # use OpenCV DNN for ONNX inference
- model=None,
- dataloader=None,
- save_dir=Path(''),
- plots=True,
- callbacks=Callbacks(),
- compute_loss=None,
-):
- # Initialize/load model and set device
- training = model is not None
- if training: # called by train.py
- device, pt, jit, engine = next(model.parameters()).device, True, False, False # get model device, PyTorch model
- half &= device.type != 'cpu' # half precision only supported on CUDA
- model.half() if half else model.float()
- else: # called directly
- device = select_device(device, batch_size=batch_size)
-
- # Directories
- save_dir = increment_path(Path(project) / name, exist_ok=exist_ok) # increment run
- (save_dir / 'labels' if save_txt else save_dir).mkdir(parents=True, exist_ok=True) # make dir
-
- # Load model
- model = DetectMultiBackend(weights, device=device, dnn=dnn, data=data, fp16=half)
- stride, pt, jit, engine = model.stride, model.pt, model.jit, model.engine
- imgsz = check_img_size(imgsz, s=stride) # check image size
- half = model.fp16 # FP16 supported on limited backends with CUDA
- if engine:
- batch_size = model.batch_size
- else:
- device = model.device
- if not (pt or jit):
- batch_size = 1 # export.py models default to batch-size 1
- LOGGER.info(f'Forcing --batch-size 1 square inference (1,3,{imgsz},{imgsz}) for non-PyTorch models')
-
- # Data
- data = check_dataset(data) # check
-
- # Configure
- model.eval()
- cuda = device.type != 'cpu'
- is_coco = isinstance(data.get('val'), str) and data['val'].endswith(f'coco{os.sep}val2017.txt') # COCO dataset
- nc = 1 if single_cls else int(data['nc']) # number of classes
- iouv = torch.linspace(0.5, 0.95, 10, device=device) # iou vector for mAP@0.5:0.95
- niou = iouv.numel()
-
- # Dataloader
- if not training:
- if pt and not single_cls: # check --weights are trained on --data
- ncm = model.model.nc
- assert ncm == nc, f'{weights} ({ncm} classes) trained on different --data than what you passed ({nc} ' \
- f'classes). Pass correct combination of --weights and --data that are trained together.'
- model.warmup(imgsz=(1 if pt else batch_size, 3, imgsz, imgsz)) # warmup
- pad, rect = (0.0, False) if task == 'speed' else (0.5, pt) # square inference for benchmarks
- task = task if task in ('train', 'val', 'test') else 'val' # path to train/val/test images
- dataloader = create_dataloader(data[task],
- imgsz,
- batch_size,
- stride,
- single_cls,
- pad=pad,
- rect=rect,
- workers=workers,
- prefix=colorstr(f'{task}: '))[0]
-
- seen = 0
- confusion_matrix = ConfusionMatrix(nc=nc)
- names = model.names if hasattr(model, 'names') else model.module.names # get class names
- if isinstance(names, (list, tuple)): # old format
- names = dict(enumerate(names))
- class_map = coco80_to_coco91_class() if is_coco else list(range(1000))
- s = ('%22s' + '%11s' * 6) % ('Class', 'Images', 'Instances', 'P', 'R', 'mAP50', 'mAP50-95')
- tp, fp, p, r, f1, mp, mr, map50, ap50, map = 0.0, 0.0, 0.0, 0.0, 0.0, 0.0, 0.0, 0.0, 0.0, 0.0
- dt = Profile(), Profile(), Profile() # profiling times
- loss = torch.zeros(3, device=device)
- jdict, stats, ap, ap_class = [], [], [], []
- callbacks.run('on_val_start')
- pbar = tqdm(dataloader, desc=s, bar_format='{l_bar}{bar:10}{r_bar}{bar:-10b}') # progress bar
- for batch_i, (im, targets, paths, shapes) in enumerate(pbar):
- callbacks.run('on_val_batch_start')
- with dt[0]:
- if cuda:
- im = im.to(device, non_blocking=True)
- targets = targets.to(device)
- im = im.half() if half else im.float() # uint8 to fp16/32
- im /= 255 # 0 - 255 to 0.0 - 1.0
- nb, _, height, width = im.shape # batch size, channels, height, width
-
- # Inference
- with dt[1]:
- preds, train_out = model(im) if compute_loss else (model(im, augment=augment), None)
-
- # Loss
- if compute_loss:
- loss += compute_loss(train_out, targets)[1] # box, obj, cls
-
- # NMS
- targets[:, 2:] *= torch.tensor((width, height, width, height), device=device) # to pixels
- lb = [targets[targets[:, 0] == i, 1:] for i in range(nb)] if save_hybrid else [] # for autolabelling
- with dt[2]:
- preds = non_max_suppression(preds,
- conf_thres,
- iou_thres,
- labels=lb,
- multi_label=True,
- agnostic=single_cls,
- max_det=max_det)
-
- # Metrics
- for si, pred in enumerate(preds):
- labels = targets[targets[:, 0] == si, 1:]
- nl, npr = labels.shape[0], pred.shape[0] # number of labels, predictions
- path, shape = Path(paths[si]), shapes[si][0]
- correct = torch.zeros(npr, niou, dtype=torch.bool, device=device) # init
- seen += 1
-
- if npr == 0:
- if nl:
- stats.append((correct, *torch.zeros((2, 0), device=device), labels[:, 0]))
- if plots:
- confusion_matrix.process_batch(detections=None, labels=labels[:, 0])
- continue
-
- # Predictions
- if single_cls:
- pred[:, 5] = 0
- predn = pred.clone()
- scale_boxes(im[si].shape[1:], predn[:, :4], shape, shapes[si][1]) # native-space pred
-
- # Evaluate
- if nl:
- tbox = xywh2xyxy(labels[:, 1:5]) # target boxes
- scale_boxes(im[si].shape[1:], tbox, shape, shapes[si][1]) # native-space labels
- labelsn = torch.cat((labels[:, 0:1], tbox), 1) # native-space labels
- correct = process_batch(predn, labelsn, iouv)
- if plots:
- confusion_matrix.process_batch(predn, labelsn)
- stats.append((correct, pred[:, 4], pred[:, 5], labels[:, 0])) # (correct, conf, pcls, tcls)
-
- # Save/log
- if save_txt:
- save_one_txt(predn, save_conf, shape, file=save_dir / 'labels' / f'{path.stem}.txt')
- if save_json:
- save_one_json(predn, jdict, path, class_map) # append to COCO-JSON dictionary
- callbacks.run('on_val_image_end', pred, predn, path, names, im[si])
-
- # Plot images
- if plots and batch_i < 3:
- plot_images(im, targets, paths, save_dir / f'val_batch{batch_i}_labels.jpg', names) # labels
- plot_images(im, output_to_target(preds), paths, save_dir / f'val_batch{batch_i}_pred.jpg', names) # pred
-
- callbacks.run('on_val_batch_end', batch_i, im, targets, paths, shapes, preds)
-
- # Compute metrics
- stats = [torch.cat(x, 0).cpu().numpy() for x in zip(*stats)] # to numpy
- if len(stats) and stats[0].any():
- tp, fp, p, r, f1, ap, ap_class = ap_per_class(*stats, plot=plots, save_dir=save_dir, names=names)
- ap50, ap = ap[:, 0], ap.mean(1) # AP@0.5, AP@0.5:0.95
- mp, mr, map50, map = p.mean(), r.mean(), ap50.mean(), ap.mean()
- nt = np.bincount(stats[3].astype(int), minlength=nc) # number of targets per class
-
- # Print results
- pf = '%22s' + '%11i' * 2 + '%11.3g' * 4 # print format
- LOGGER.info(pf % ('all', seen, nt.sum(), mp, mr, map50, map))
- if nt.sum() == 0:
- LOGGER.warning(f'WARNING ⚠️ no labels found in {task} set, can not compute metrics without labels')
-
- # Print results per class
- if (verbose or (nc < 50 and not training)) and nc > 1 and len(stats):
- for i, c in enumerate(ap_class):
- LOGGER.info(pf % (names[c], seen, nt[c], p[i], r[i], ap50[i], ap[i]))
-
- # Print speeds
- t = tuple(x.t / seen * 1E3 for x in dt) # speeds per image
- if not training:
- shape = (batch_size, 3, imgsz, imgsz)
- LOGGER.info(f'Speed: %.1fms pre-process, %.1fms inference, %.1fms NMS per image at shape {shape}' % t)
-
- # Plots
- if plots:
- confusion_matrix.plot(save_dir=save_dir, names=list(names.values()))
- callbacks.run('on_val_end', nt, tp, fp, p, r, f1, ap, ap50, ap_class, confusion_matrix)
-
- # Save JSON
- if save_json and len(jdict):
- w = Path(weights[0] if isinstance(weights, list) else weights).stem if weights is not None else '' # weights
- anno_json = str(Path(data.get('path', '../coco')) / 'annotations/instances_val2017.json') # annotations json
- pred_json = str(save_dir / f"{w}_predictions.json") # predictions json
- LOGGER.info(f'\nEvaluating pycocotools mAP... saving {pred_json}...')
- with open(pred_json, 'w') as f:
- json.dump(jdict, f)
-
- try: # https://github.com/cocodataset/cocoapi/blob/master/PythonAPI/pycocoEvalDemo.ipynb
- check_requirements('pycocotools')
- from pycocotools.coco import COCO
- from pycocotools.cocoeval import COCOeval
-
- anno = COCO(anno_json) # init annotations api
- pred = anno.loadRes(pred_json) # init predictions api
- eval = COCOeval(anno, pred, 'bbox')
- if is_coco:
- eval.params.imgIds = [int(Path(x).stem) for x in dataloader.dataset.im_files] # image IDs to evaluate
- eval.evaluate()
- eval.accumulate()
- eval.summarize()
- map, map50 = eval.stats[:2] # update results (mAP@0.5:0.95, mAP@0.5)
- except Exception as e:
- LOGGER.info(f'pycocotools unable to run: {e}')
-
- # Return results
- model.float() # for training
- if not training:
- s = f"\n{len(list(save_dir.glob('labels/*.txt')))} labels saved to {save_dir / 'labels'}" if save_txt else ''
- LOGGER.info(f"Results saved to {colorstr('bold', save_dir)}{s}")
- maps = np.zeros(nc) + map
- for i, c in enumerate(ap_class):
- maps[c] = ap[i]
- return (mp, mr, map50, map, *(loss.cpu() / len(dataloader)).tolist()), maps, t
-
-
-def parse_opt():
- parser = argparse.ArgumentParser()
- parser.add_argument('--data', type=str, default=ROOT / 'data/coco128.yaml', help='dataset.yaml path')
- parser.add_argument('--weights', nargs='+', type=str, default=ROOT / 'yolov5s.pt', help='model path(s)')
- parser.add_argument('--batch-size', type=int, default=32, help='batch size')
- parser.add_argument('--imgsz', '--img', '--img-size', type=int, default=640, help='inference size (pixels)')
- parser.add_argument('--conf-thres', type=float, default=0.001, help='confidence threshold')
- parser.add_argument('--iou-thres', type=float, default=0.6, help='NMS IoU threshold')
- parser.add_argument('--max-det', type=int, default=300, help='maximum detections per image')
- parser.add_argument('--task', default='val', help='train, val, test, speed or study')
- parser.add_argument('--device', default='', help='cuda device, i.e. 0 or 0,1,2,3 or cpu')
- parser.add_argument('--workers', type=int, default=8, help='max dataloader workers (per RANK in DDP mode)')
- parser.add_argument('--single-cls', action='store_true', help='treat as single-class dataset')
- parser.add_argument('--augment', action='store_true', help='augmented inference')
- parser.add_argument('--verbose', action='store_true', help='report mAP by class')
- parser.add_argument('--save-txt', action='store_true', help='save results to *.txt')
- parser.add_argument('--save-hybrid', action='store_true', help='save label+prediction hybrid results to *.txt')
- parser.add_argument('--save-conf', action='store_true', help='save confidences in --save-txt labels')
- parser.add_argument('--save-json', action='store_true', help='save a COCO-JSON results file')
- parser.add_argument('--project', default=ROOT / 'runs/val', help='save to project/name')
- parser.add_argument('--name', default='exp', help='save to project/name')
- parser.add_argument('--exist-ok', action='store_true', help='existing project/name ok, do not increment')
- parser.add_argument('--half', action='store_true', help='use FP16 half-precision inference')
- parser.add_argument('--dnn', action='store_true', help='use OpenCV DNN for ONNX inference')
- opt = parser.parse_args()
- opt.data = check_yaml(opt.data) # check YAML
- opt.save_json |= opt.data.endswith('coco.yaml')
- opt.save_txt |= opt.save_hybrid
- print_args(vars(opt))
- return opt
-
-
-def main(opt):
- check_requirements(exclude=('tensorboard', 'thop'))
-
- if opt.task in ('train', 'val', 'test'): # run normally
- if opt.conf_thres > 0.001: # https://github.com/ultralytics/yolov5/issues/1466
- LOGGER.info(f'WARNING ⚠️ confidence threshold {opt.conf_thres} > 0.001 produces invalid results')
- if opt.save_hybrid:
- LOGGER.info('WARNING ⚠️ --save-hybrid will return high mAP from hybrid labels, not from predictions alone')
- run(**vars(opt))
-
- else:
- weights = opt.weights if isinstance(opt.weights, list) else [opt.weights]
- opt.half = True # FP16 for fastest results
- if opt.task == 'speed': # speed benchmarks
- # python val.py --task speed --data coco.yaml --batch 1 --weights yolov5n.pt yolov5s.pt...
- opt.conf_thres, opt.iou_thres, opt.save_json = 0.25, 0.45, False
- for opt.weights in weights:
- run(**vars(opt), plots=False)
-
- elif opt.task == 'study': # speed vs mAP benchmarks
- # python val.py --task study --data coco.yaml --iou 0.7 --weights yolov5n.pt yolov5s.pt...
- for opt.weights in weights:
- f = f'study_{Path(opt.data).stem}_{Path(opt.weights).stem}.txt' # filename to save to
- x, y = list(range(256, 1536 + 128, 128)), [] # x axis (image sizes), y axis
- for opt.imgsz in x: # img-size
- LOGGER.info(f'\nRunning {f} --imgsz {opt.imgsz}...')
- r, _, t = run(**vars(opt), plots=False)
- y.append(r + t) # results and times
- np.savetxt(f, y, fmt='%10.4g') # save
- os.system('zip -r study.zip study_*.txt')
- plot_val_study(x=x) # plot
-
-
-if __name__ == "__main__":
- opt = parse_opt()
- main(opt)
diff --git a/spaces/mshukor/UnIVAL/fairseq/fairseq/optim/lr_scheduler/manual_lr_scheduler.py b/spaces/mshukor/UnIVAL/fairseq/fairseq/optim/lr_scheduler/manual_lr_scheduler.py
deleted file mode 100644
index 0269a1e2853854745e23b07931294f37b67d0295..0000000000000000000000000000000000000000
--- a/spaces/mshukor/UnIVAL/fairseq/fairseq/optim/lr_scheduler/manual_lr_scheduler.py
+++ /dev/null
@@ -1,110 +0,0 @@
-# Copyright (c) Facebook, Inc. and its affiliates.
-#
-# This source code is licensed under the MIT license found in the
-# LICENSE file in the root directory of this source tree.
-
-from . import LegacyFairseqLRScheduler, register_lr_scheduler
-import logging
-import ast
-
-logger = logging.getLogger(__name__)
-logger.setLevel(logging.WARNING)
-
-
-@register_lr_scheduler("manual")
-class ManualSchedule(LegacyFairseqLRScheduler):
- """Decay the LR on a manual schedule."""
-
- def __init__(self, args, optimizer):
- super().__init__(args, optimizer)
-
- self.epoch2lr = self.parse_manuallr_args(args.epoch2lr)
- self.update2lr = self.parse_manuallr_args(args.update2lr)
- logger.info("@@@ ManualSchedule epoch2lr={}".format(self.epoch2lr))
- logger.info("@@@ ManualSchedule update2lr={}".format(self.update2lr))
-
- if 1 in self.epoch2lr:
- self.lr = self.epoch2lr[1]
- elif 1 in self.update2lr:
- self.lr = self.update2lr[1]
- else:
- self.lr = args.lr[0]
- self.optimizer.set_lr(self.lr) # Set the beginning of the epoch.
-
- def parse_manuallr_args(self, lr_args_str):
- lr_dict = ast.literal_eval(lr_args_str.replace(' ', ''))
- if not isinstance(lr_dict, dict):
- raise ValueError("epoch2lr/update2lr must be abel to evaluated to a dict")
-
- lr_args = {}
- logger.info("@@@ after parsing input dictionary lr_dict = {}".format(lr_dict))
- for key, val in lr_dict.items():
- if "," in key:
- for k in key.split(","):
- lr_args[int(k)] = float(val)
- elif "-" in key:
- s = int(key.split("-")[0])
- e = int(key.split("-")[1])
- for k in range(s, e + 1, 1):
- lr_args[k] = float(val)
- else:
- lr_args[int(key)] = float(val)
-
- return lr_args
-
- @staticmethod
- def add_args(parser):
- """Add arguments to the parser for this LR scheduler."""
- # fmt: off
- parser.add_argument(
- "--epoch2lr",
- type=str,
- metavar="DICT",
- default="{}",
- help="a dictionary used to set lr for each epoch manually",
- )
- parser.add_argument(
- "--update2lr",
- type=str,
- metavar="DICT",
- default="{}",
- help="a dictionary used to set lr for each update manually",
- )
- # fmt: on
-
- def state_dict(self):
- return {"lr": self.lr}
-
- def load_state_dict(self, state_dict):
- if "lr" in state_dict:
- self.lr = state_dict["lr"]
-
- def get_next_lr(self, epoch):
- manual_keys = [k for k in self.epoch2lr if k <= epoch]
- if manual_keys:
- manual_lr = self.epoch2lr[max(manual_keys)]
- else:
- logger.warning("@@@ epoch={} does not exist in manual lr input. epoch2lr={}...".format(
- epoch, list(self.epoch2lr.items())[:min(10, len(self.epoch2lr.keys())-1)]
- ))
- manual_lr = self.optimizer.get_lr()
- return manual_lr
-
- def step_begin_epoch(self, epoch):
- """Update the learning rate at the beginning of the given epoch."""
- self.lr = self.get_next_lr(epoch)
- self.optimizer.set_lr(self.lr)
- return self.optimizer.get_lr()
-
- def step_update(self, num_updates):
- """Update the learning rate after each update."""
- manual_keys = [k for k in self.update2lr if k <= num_updates]
- if manual_keys:
- manual_lr = self.update2lr[max(manual_keys)]
- else:
- logger.warning("epoch={} does not exist in manual lr input update2lr={}...".format(
- num_updates, list(self.update2lr.items())[:min(10, len(self.update2lr.keys())-1)]))
- manual_lr = self.optimizer.get_lr()
-
- self.optimizer.set_lr(manual_lr)
- return self.optimizer.get_lr()
diff --git a/spaces/mshukor/UnIVAL/fairseq/fairseq/optim/shard.py b/spaces/mshukor/UnIVAL/fairseq/fairseq/optim/shard.py
deleted file mode 100644
index 9d7f2eb9e5de6086fe2435d432bde7521ebb8155..0000000000000000000000000000000000000000
--- a/spaces/mshukor/UnIVAL/fairseq/fairseq/optim/shard.py
+++ /dev/null
@@ -1,58 +0,0 @@
-# Copyright (c) Facebook, Inc. and its affiliates.
-#
-# This source code is licensed under the MIT license found in the
-# LICENSE file in the root directory of this source tree.
-
-from typing import Any, Dict
-
-from fairseq.distributed import utils
-
-
-try:
- from fairscale.optim import OSS
-
- _has_fairscale = True
-except ImportError:
- _has_fairscale = False
-
-
-def shard_(optimizer, group):
- if not _has_fairscale:
- raise ImportError(
- "\n\nPlease install the fairscale package:" "\n\n pip install fairscale"
- )
-
- class FairseqOSS(OSS):
- @property
- def disable_mem_eff_fp16_loading_hack(self):
- return True
-
- def __getattr__(self, name):
- if name.startswith("supports") and hasattr(self.optim, name):
- return getattr(self.optim, name)
- raise AttributeError(
- "'FairseqOSS' object has no attribute {0!r}".format(name)
- )
-
- def broadcast_global_state_dict(
- self, state_dict: Dict[str, Any]
- ) -> Dict[str, Any]:
- """
- Broadcasts the entire state_dict to all other ranks
- each rank is responsible to load their own partition of data
- """
- return utils.broadcast_object(
- state_dict,
- src_rank=0,
- group=self.group,
- )
-
- torch_optimizer = optimizer.optimizer
- optim_cls = type(torch_optimizer)
-
- optimizer.optimizer = FairseqOSS(
- torch_optimizer.param_groups,
- optim_cls,
- group=group,
- **optimizer.optimizer_config
- )
diff --git a/spaces/mueller-franzes/medfusion-app/medical_diffusion/utils/train_utils.py b/spaces/mueller-franzes/medfusion-app/medical_diffusion/utils/train_utils.py
deleted file mode 100644
index dbbc73701c6afe3043fb437761c78ca8f4805cc6..0000000000000000000000000000000000000000
--- a/spaces/mueller-franzes/medfusion-app/medical_diffusion/utils/train_utils.py
+++ /dev/null
@@ -1,88 +0,0 @@
-import copy
-import torch
-import torch.nn as nn
-
-class EMAModel(nn.Module):
- # See: https://github.com/huggingface/diffusers/blob/3100bc967084964480628ae61210b7eaa7436f1d/src/diffusers/training_utils.py#L42
- """
- Exponential Moving Average of models weights
- """
-
- def __init__(
- self,
- model,
- update_after_step=0,
- inv_gamma=1.0,
- power=2 / 3,
- min_value=0.0,
- max_value=0.9999,
- ):
- super().__init__()
- """
- @crowsonkb's notes on EMA Warmup:
- If gamma=1 and power=1, implements a simple average. gamma=1, power=2/3 are good values for models you plan
- to train for a million or more steps (reaches decay factor 0.999 at 31.6K steps, 0.9999 at 1M steps),
- gamma=1, power=3/4 for models you plan to train for less (reaches decay factor 0.999 at 10K steps, 0.9999
- at 215.4k steps).
- Args:
- inv_gamma (float): Inverse multiplicative factor of EMA warmup. Default: 1.
- power (float): Exponential factor of EMA warmup. Default: 2/3.
- min_value (float): The minimum EMA decay rate. Default: 0.
- """
-
- self.averaged_model = copy.deepcopy(model).eval()
- self.averaged_model.requires_grad_(False)
-
- self.update_after_step = update_after_step
- self.inv_gamma = inv_gamma
- self.power = power
- self.min_value = min_value
- self.max_value = max_value
-
- self.averaged_model = self.averaged_model #.to(device=model.device)
-
- self.decay = 0.0
- self.optimization_step = 0
-
- def get_decay(self, optimization_step):
- """
- Compute the decay factor for the exponential moving average.
- """
- step = max(0, optimization_step - self.update_after_step - 1)
- value = 1 - (1 + step / self.inv_gamma) ** -self.power
-
- if step <= 0:
- return 0.0
-
- return max(self.min_value, min(value, self.max_value))
-
- @torch.no_grad()
- def step(self, new_model):
- ema_state_dict = {}
- ema_params = self.averaged_model.state_dict()
-
- self.decay = self.get_decay(self.optimization_step)
-
- for key, param in new_model.named_parameters():
- if isinstance(param, dict):
- continue
- try:
- ema_param = ema_params[key]
- except KeyError:
- ema_param = param.float().clone() if param.ndim == 1 else copy.deepcopy(param)
- ema_params[key] = ema_param
-
- if not param.requires_grad:
- ema_params[key].copy_(param.to(dtype=ema_param.dtype).data)
- ema_param = ema_params[key]
- else:
- ema_param.mul_(self.decay)
- ema_param.add_(param.data.to(dtype=ema_param.dtype), alpha=1 - self.decay)
-
- ema_state_dict[key] = ema_param
-
- for key, param in new_model.named_buffers():
- ema_state_dict[key] = param
-
- self.averaged_model.load_state_dict(ema_state_dict, strict=False)
- self.optimization_step += 1
\ No newline at end of file
diff --git a/spaces/ner4archives/ner4archives-NEL-vizualizer-app/README.md b/spaces/ner4archives/ner4archives-NEL-vizualizer-app/README.md
deleted file mode 100644
index f62ccdfb761f2d0882da304421d4b05d6bef1c7f..0000000000000000000000000000000000000000
--- a/spaces/ner4archives/ner4archives-NEL-vizualizer-app/README.md
+++ /dev/null
@@ -1,16 +0,0 @@
----
-title: NER4Archives Visualizer App
-emoji: 📜
-colorFrom: indigo
-colorTo: gray
-sdk: streamlit
-sdk_version: 1.10.0
-app_file: app.py
-pinned: false
-
-
----
-
-Check out the configuration reference at https://huggingface.co/docs/hub/spaces-config-reference
-
-
diff --git a/spaces/netiMophi/DreamlikeArt-Diffusion-1.0/3ds Emulator V1.1.7 Bios 14 VERIFIED.md b/spaces/netiMophi/DreamlikeArt-Diffusion-1.0/3ds Emulator V1.1.7 Bios 14 VERIFIED.md
deleted file mode 100644
index 60dab103aa6e5c199611a6bd3536faec7f316fa3..0000000000000000000000000000000000000000
--- a/spaces/netiMophi/DreamlikeArt-Diffusion-1.0/3ds Emulator V1.1.7 Bios 14 VERIFIED.md
+++ /dev/null
@@ -1,61 +0,0 @@
-
-
An emulator is a program that mimics the functions of another device or system. A 3ds emulator is an emulator that mimics the functions of a Nintendo 3DS, which is a handheld gaming console that can display stereoscopic 3D effects without the need for special glasses.
-
A 3ds emulator allows you to play Nintendo 3DS games on your PC, Android, or iOS devices, as if you were playing them on a real console. You can enjoy the same graphics, sound, and gameplay as on a real device, but with some added benefits, such as saving and loading states, customizing controls, and enhancing performance.
-
What is a bios file?
-
A bios file is a file that contains the basic input/output system (BIOS) of a device or system. The BIOS is a firmware that controls the booting process, hardware configuration, and communication between different components of a device or system.
-
A bios file is essential for running an emulator, as it provides the information and instructions that the emulator needs to mimic the functions of the device or system that it emulates. Without a bios file, an emulator cannot run properly or at all.
-
What is the version 1.1.7 of the 3ds emulator?
-
The version number of an emulator indicates the updates and improvements that have been made to it over time. The version 1.1.7 of the 3ds emulator is one of the latest versions that has been released by its developers.
-
The version 1.1.7 of the 3ds emulator claims to have fixed some bugs and glitches, improved compatibility and performance, added new features and options, and enhanced user interface and experience.
-
Why do you need 3ds Emulator V1.1.7 Bios 14?
-
Now that you know what this software is, you might wonder why you need it. Here are some reasons why you might want to use this software.
-
The benefits of using a 3ds emulator
-
Using a 3ds emulator has many benefits, such as: - You can play Nintendo 3DS games on your PC, Android, or iOS devices, without having to buy a real console or game cartridges. - You can save and load your game progress anytime and anywhere, without worrying about losing data or battery life. - You can customize your controls, screen size, resolution, sound, and other settings to suit your preferences and device specifications. - You can enhance the graphics, speed, and performance of the games, by using filters, shaders, cheats, and other options. - You can access a large library of games and roms, by downloading them from various sources online.
The features of the version 1.1.7 of the 3ds emulator
-
The version 1.1.7 of the 3ds emulator has many features that make it one of the best and most popular emulators available. Some of these features are:
-
- - It supports all Nintendo 3DS games, including the latest releases and updates. - It has a high compatibility rate, meaning that most games run smoothly and without errors. - It has a fast and stable performance, meaning that the games run at full speed and without lag or crashes. - It has a user-friendly and intuitive interface, meaning that the emulator is easy to use and navigate. - It has a multi-language support, meaning that the emulator can be used in different languages, such as English, Spanish, French, German, Italian, Japanese, Chinese, and more. - It has a multiplayer mode, meaning that you can play online with other players using the same emulator or different devices.
The compatibility of the bios file with the emulator
-
The bios file is compatible with the version 1.1.7 of the 3ds emulator, meaning that it works well with it and does not cause any problems or conflicts. The bios file is also compatible with other versions of the 3ds emulator, as well as other emulators that use the same bios file.
-
The bios file is also compatible with different devices and operating systems, such as Windows, Mac OS X, Linux, Android, iOS, and more. The bios file is also compatible with different processors and architectures, such as x86, x64, ARM, and more.
-
How to download and install 3ds Emulator V1.1.7 Bios 14?
-
Now that you know why you need this software, you might want to know how to get it. Here are some steps to download and install this software.
-
The sources of the emulator and the bios file
-
The first step is to find reliable and safe sources for downloading the emulator and the bios file. There are many websites that offer these files for free or for a fee, but not all of them are trustworthy or legitimate.
-
Some websites may contain malware or viruses that can harm your device or steal your personal information. Some websites may also provide fake or outdated files that do not work or cause errors.
-
To avoid these risks, you should only download from reputable and verified sources that have positive reviews and feedback from other users. Some examples of such sources are:
- - The official website of the emulator: [https://www.3dsemulator.org/] - The official website of the bios file: [https://www.bios-files.com/] - The official website of Nintendo: [https://www.nintendo.com/]
The steps to download and install the emulator and the bios file
-
The second step is to follow these steps to download and install the emulator and the bios file:
- - Go to one of the sources mentioned above and find the download link for the emulator and the bios file. - Click on the download link and save the files to your device. - Extract the files from their compressed format using a program such as WinRAR or 7-Zip. - Open the folder where you extracted the files and find the executable file for the emulator (usually named 3dsemulator.exe). - Double-click on the executable file to launch the emulator. - Go to File > Open Bios File and browse to the folder where you extracted the bios file (usually named bios.bin). - Select the bios file and click Open. - Wait for a few seconds until you see a message saying "Bios Loaded Successfully". - Congratulations! You have successfully installed the emulator and the bios file.
The tips to avoid scams and viruses
-
The third step is to follow these tips to avoid scams and viruses when downloading and installing this software:
- - Always scan your files with an antivirus program before opening them. - Always read the terms and conditions before agreeing to anything. - Always check the file size and format before downloading them. - Always backup your data and settings before installing anything. - Always be careful of pop-ups, ads, and links that ask you to download or install something. - Always research the source and the file before downloading or installing them.
How to use 3ds Emulator V1.1.7 Bios 14?
-
Now that you have downloaded and installed this software, you might want to know how to use it. Here are some steps to use this software.
-
The requirements for running the emulator
-
The first step is to make sure that your device meets the minimum requirements for running the emulator. These are:
- - A device with a processor of at least 1 GHz and a RAM of at least 512 MB. - A device with an operating system of Windows XP or higher, Mac OS X 10.6 or higher, Linux, Android 4.0 or higher, or iOS 7.0 or higher. - A device with a graphics card that supports OpenGL ES 2.0 or higher. - A device with a sound card that supports DirectSound or OpenAL. - A device with a storage space of at least 100 MB for the emulator and the bios file, and more for the games and roms.
The settings and options of the emulator
-
The second step is to adjust the settings and options of the emulator to optimize your gaming experience. These are:
- - Go to Options > Emulation Settings and choose the emulation mode that suits your device and game. You can choose between Hardware, Software, and Hybrid modes, depending on the performance and compatibility of your device and game. - Go to Options > Graphics Settings and choose the graphics settings that suit your device and game. You can adjust the screen size, resolution, aspect ratio, filter, shader, anti-aliasing, anisotropic filtering, and more, depending on the quality and speed of your device and game. - Go to Options > Sound Settings and choose the sound settings that suit your device and game. You can adjust the volume, frequency, latency, reverb, interpolation, and more, depending on the clarity and realism of your device and game. - Go to Options > Control Settings and choose the control settings that suit your device and game. You can customize the keyboard, mouse, touch screen, joystick, or gamepad controls, depending on the convenience and accuracy of your device and game.
The games and roms that you can play with the emulator
-
The third step is to find and play the games and roms that you want to play with the emulator. These are:
- - Go to one of the sources mentioned above or any other source that offers Nintendo 3DS games and roms for free or for a fee. - Download the games and roms that you want to play to your device. - Extract the games and roms from their compressed format using a program such as WinRAR or 7-Zip. - Open the folder where you extracted the games and roms and find the file for the game or rom (usually named .3ds or .cia). - Double-click on the file to launch the game or rom with the emulator. - Enjoy playing your favorite Nintendo 3DS games on your PC, Android, or iOS devices.
Conclusion
-
In conclusion, 3ds Emulator V1.1.7 Bios 14 is a software that allows you to play Nintendo 3DS games on your PC, Android, or iOS devices. It has many benefits, features, and options that make it one of the best emulators available. It is also easy to download, install, and use.
-
If you are looking for a way to enjoy Nintendo 3DS games without having to buy a real console or game cartridges, you should definitely try this software. You will not regret it.
-
FAQs
-
Here are some frequently asked questions about this software:
-
Q: Is this software legal?
-
A: This software is legal as long as you own a copy of the original Nintendo 3DS console and games that you want to play with it. However, downloading games and roms from unauthorized sources may be illegal in some countries. You should check your local laws before doing so.
-
Q: Is this software safe?
-
A: This software is safe as long as you download it from reputable and verified sources that do not contain malware or viruses. You should also scan your files with an antivirus program before opening them.
-
Q: Is this software free?
-
A: This software is free as long as you download it from official sources that do not charge any fees. However, some sources may require you to complete surveys or offers before downloading them. You should be careful of these sources as they may contain scams or viruses. You should only download from sources that you trust and that have positive reviews and feedback from other users.
-
Q: How can I update this software?
-
A: You can update this software by visiting the official website of the emulator or the bios file and downloading the latest version available. You should also check for updates regularly to enjoy the new features and improvements that the developers make.
-
Q: How can I contact the developers of this software?
-
A: You can contact the developers of this software by visiting their official website or their social media pages and sending them a message or a comment. You can also report any bugs or issues that you encounter or suggest any ideas or feedback that you have.
-
Q: How can I support the developers of this software?
-
A: You can support the developers of this software by donating to them via their official website or their social media pages. You can also support them by sharing their software with your friends and family, rating and reviewing their software, and joining their community.
b2dd77e56b
-
-
\ No newline at end of file
diff --git a/spaces/netiMophi/DreamlikeArt-Diffusion-1.0/Blaze And Blade Eternal Quest [1998 PC Full ISO] (CRS) DRM Free [NEW].md b/spaces/netiMophi/DreamlikeArt-Diffusion-1.0/Blaze And Blade Eternal Quest [1998 PC Full ISO] (CRS) DRM Free [NEW].md
deleted file mode 100644
index 53420b6c39530d218ad2f51a80507b962bf4eb49..0000000000000000000000000000000000000000
--- a/spaces/netiMophi/DreamlikeArt-Diffusion-1.0/Blaze And Blade Eternal Quest [1998 PC Full ISO] (CRS) DRM Free [NEW].md
+++ /dev/null
@@ -1,17 +0,0 @@
-
-
Blaze And Blade: Eternal Quest - A Classic Action RPG for PC
-
Blaze And Blade: Eternal Quest is a multiplayer action role-playing game that was released in 1998 for the PlayStation and Microsoft Windows. It is the first game in the Blaze And Blade series, which also includes Blaze And Blade Busters, a Japan-only sequel.
-
The game allows players to create their own characters from eight different classes, such as fighter, mage, thief, or priest, and choose their gender, appearance, and personality. The game supports up to four players in co-op mode, using either a MultiTap or a cable link for the PlayStation version, or a LAN connection for the PC version.
-
Blaze And Blade: Eternal Quest [1998 PC, Full ISO] (CRS) DRM Free
The game's story revolves around a group of adventurers who discover an ancient lithograph that is said to grant great power to those who can collect the magical gems that fit into it. The adventurers explore various dungeons and locations in the Forbidden Land, formerly known as Foresia, and face enemies, traps, and puzzles along the way.
-
The game features a real-time combat system that allows players to switch between characters and use different skills and items. The game also has a unique character growth system that depends on the actions and choices of the players, rather than fixed levels and stats. For example, a character's strength can increase by using heavy weapons or carrying heavy items, while their intelligence can increase by using magic or solving puzzles.
-
Blaze And Blade: Eternal Quest is a game that offers a lot of freedom and customization for RPG fans who enjoy exploring and experimenting. The game has a retro charm and a quirky sense of humor that make it stand out from other games of its genre. The game is also DRM-free, meaning that it does not require any activation or online connection to play.
-
If you are looking for a classic action RPG that you can play with your friends or by yourself, you might want to check out Blaze And Blade: Eternal Quest. You can download the full ISO file from CRS (Classic Retro Software), a website that specializes in preserving and distributing old PC games. You will need an emulator or a virtual machine to run the game on modern systems.
-
Blaze And Blade: Eternal Quest is a game that deserves more recognition and appreciation for its originality and fun factor. It is a hidden gem that you should not miss if you love action RPGs.
-
-
Blaze And Blade: Eternal Quest has a colorful and detailed graphics style that creates a vivid and immersive world. The game's soundtrack is composed by Ken Kojima, who also worked on other T&E Soft games such as Hydlide and Daikoukai Jidai. The music is catchy and atmospheric, and fits well with the game's mood and setting.
-
-
The game's difficulty level can be adjusted by the players, who can choose to play on easy, normal, or hard mode. The game also has a permadeath option, which means that if a character dies, they are gone forever and cannot be revived. This adds an extra challenge and risk to the game, as well as a sense of realism and consequence.
-
Blaze And Blade: Eternal Quest is a game that can provide hours of entertainment and replay value, as each playthrough can be different depending on the characters, choices, and actions of the players. The game also has a lot of secrets and hidden content that can be discovered by exploring and experimenting. The game is a true gem that deserves more attention and appreciation from RPG fans.
7b8c122e87
-
-
\ No newline at end of file
diff --git a/spaces/netiMophi/DreamlikeArt-Diffusion-1.0/Corel Photoimpact X3 Activation Code Serial Number ((TOP)).md b/spaces/netiMophi/DreamlikeArt-Diffusion-1.0/Corel Photoimpact X3 Activation Code Serial Number ((TOP)).md
deleted file mode 100644
index 9035b9eca06c0a4b984e9147f0e48f25b883808c..0000000000000000000000000000000000000000
--- a/spaces/netiMophi/DreamlikeArt-Diffusion-1.0/Corel Photoimpact X3 Activation Code Serial Number ((TOP)).md
+++ /dev/null
@@ -1,95 +0,0 @@
-
-
Corel PhotoImpact X3 Activation Code Serial Number: How to Get It and Why You Need It
-
If you are looking for a powerful and easy-to-use photo editing software that combines inspiring photo projects and amazing digital art, you might want to check out Corel PhotoImpact X3. This software can help you make digital photography and image creativity fun, fast, and easy. But before you can enjoy all the features and benefits of Corel PhotoImpact X3, you need to activate it with a serial number and an activation code. In this article, we will explain what these terms mean, how to get them, how to activate your software, what to do if you lose or forget them, and what are some alternatives and competitors of Corel PhotoImpact X3. We will also share some reviews and ratings of Corel PhotoImpact X3 from real users.
-
What is Corel PhotoImpact X3?
-
Corel PhotoImpact X3 is a graphic design software that enables users to view, edit, and manage images using a drag-and-drop interface, effects, filters galleries, and more. It was originally developed by Ulead Systems, but later acquired by Corel Corporation in 2006. Corel PhotoImpact X3 was released in 2008 as the 13th version of the software, and it is still available for purchase from the official website or other online platforms.
-
corel photoimpact x3 activation code serial number
Corel PhotoImpact X3 offers a range of features and benefits for photo editing enthusiasts, such as:
-
-
ExpressFix: A handy mode that provides automated enhancements and easy-to-understand options for quickly fixing exposure, color, composition, noise, red-eye, straightening, cropping, and more.
-
Corel MediaOne Plus: A digital media management suite that allows users to import, tag, sort, organize, search, share, and create slideshows from their photos and videos.
-
EasyPalette: A library of over 800 objects and 2,500 customizable effects that users can drag-and-drop to apply to their images.
-
SmartGuide: A feature that shows step-by-step directions on-screen for completing various photo-editing, web design, video, and DVD menu tasks.
-
Welcome Screen: A feature that lets users jump directly to browsing photos, photo editing, or creating photo projects.
-
RAW File Support: A feature that supports a greater number of camera models and provides brighter previews, improved performance, and easier editing of RAW images.
-
Share Button: A feature that offers easy wizards to create fun photo projects and gifts using over 200 customizable templates.
-
-
System requirements and compatibility of Corel PhotoImpact X3
-
To run Corel PhotoImpact X3 smoothly on your computer, you need to meet the following minimum system requirements:
-
-
Windows XP SP2 Home Edition/Professional (32-bit), Windows Vista (32-bit or 64-bit editions), Windows 7 (32-bit or 64-bit editions), Windows 8, Windows 10
Intel Pentium III, AMD Athlon 800 or above CPU
512 MB RAM (for Windows XP), 1 GB RAM (for Windows Vista and Windows 7)
750 MB available hard disk space
1024 x 768 resolution, 16-bit color display or higher
CD-ROM drive
Internet connection required for online activation and web services
-
-
Corel PhotoImpact X3 is compatible with the following file formats:
-
-
-
Image
-
Video
-
Audio
-
-
-
BMP, CLP, CUR, DCS, DCX, EPS, FAX, FPX, GIF, ICO, IFF, IMG, JP2, JPC, JPG, MAC, MSP, PBM, PCD*, PCT, PCX, PDF*, PEF*, PGM, PIC, PNG, PPM, PSD, PSPImage, PXR, RAS, SCI, SCT, SHG, TGA, TIF/TIFF*, UFO*, UFP*, WBM and WBMP. RAW file support for over 250 camera models including the following file extensions: 3FR*, ARW*, BAY*, CR2*, CRW*, CS1*, DCR*, DNG*, ERF*, FFF*, HDR*, K25*, KDC*, MDC*, MRW*, NEF*, NRW*, ORF*, PEF*, RAF*, RAW*, SR2*, SRF* and X3F*.
-
ASF (MPEG-4), AVI (MPEG-4), DAT (MPEG-1), MOV (MPEG-4), MPEG-1 and MPEG-2
-
MIDI and WAV
-
-
-
What is a serial number and an activation code?
-
A serial number and an activation code are two types of codes that are required to activate Corel PhotoImpact X3. They are different from each other in terms of their purpose and format.
-
The difference between a serial number and an activation code
-
A serial number is a unique alphanumeric code that identifies your copy of Corel PhotoImpact X3. It is usually composed of 18 digits divided into six groups of three digits each. For example: XXX-XXX-XXX-XXX-XXX-XXX. A serial number is provided to you when you purchase Corel PhotoImpact X3 from the official website or other authorized resellers. You need to enter your serial number during the installation process of Corel PhotoImpact X3.
-
-
An activation code is a one-time use code that verifies that your copy of Corel PhotoImpact X3 is genuine and not pirated. It is usually composed of 16 digits divided into four groups of four digits each. For example: XXXX-XXXX-XXXX-XXXX. An activation code is generated by Corel after you enter your serial number and some personal information online or by phone. You need to enter your activation code after the installation process of Corel PhotoImpact X3.
-
How to find your serial number and activation code
-
If you purchased Corel PhotoImpact X3 from the official website or other online platforms, you can find your serial number in the confirmation email that was sent to you after your purchase. You can also find your serial number in your Corel account if you registered your product online.
-
If you purchased Corel PhotoImpact X3 from a physical store or received it as a gift, you can find your serial number on the back of the CD case or on the sticker inside the DVD box.
-
To get your activation code, you need to follow these steps:
-
-
Launch Corel PhotoImpact X3 and click on Activate Now.
-
Select Activate Online or Activate by Phone.
-
If you choose Activate Online, you need to enter your serial number and some personal information such as your name and email address. Then click on Submit. You will receive your activation code on the screen and in your email.
-
If you choose Activate by Phone, you need to call the toll-free number that is displayed on the screen and provide your serial number and some personal information. You will receive your activation code from the customer service representative.
-
Enter your activation code in the corresponding field and click on Finish.
-
-
How to activate Corel PhotoImpact X3 with your serial number and layers. GIMP is suitable for users who want a free and flexible photo editing software that can handle various tasks. However, GIMP is also less user-friendly, intuitive, and stable than Corel PhotoImpact X3.
-
Reviews and ratings of Corel PhotoImpact X3
-
Corel PhotoImpact X3 has received mixed reviews and ratings from users and critics. Some users praised its ease of use, versatility, and affordability, while others criticized its outdated interface, limited support, and lack of updates. Here are some examples of reviews and ratings of Corel PhotoImpact X3 from different sources:
-
Pros and cons of Corel PhotoImpact X3
-
According to Software Advice, a website that provides reviews and ratings of various software, Corel PhotoImpact X3 has the following pros and cons:
-
-
-
Pros
-
Cons
-
-
-
- Easy to learn and use - Offers a lot of features and effects for photo editing - Has a good balance between power and simplicity - Has a low price compared to other photo editing software - Includes Corel MediaOne Plus for managing photos and videos
-
- Has an outdated and cluttered interface - Does not support some newer file formats and camera models - Does not receive regular updates or bug fixes - Has limited customer support and online resources - Lacks some advanced tools and options for professional photo editing
-
-
-
User feedback and testimonials of Corel PhotoImpact X3
-
According to Amazon, a website that sells and reviews various products, Corel PhotoImpact X3 has an average rating of 4.1 out of 5 stars based on 111 customer reviews. Here are some examples of user feedback and testimonials of Corel PhotoImpact X3 from Amazon:
-
-
"I have been using PhotoImpact for years and love it. It is easy to use and has many features that I use regularly. I especially like the ExpressFix mode that allows me to quickly fix common problems with my photos. I also like the Share button that lets me create fun photo projects and gifts. I would recommend this software to anyone who wants a simple but powerful photo editing software." - 5 stars
-
"I bought this software because I needed a photo editing software that could handle RAW files from my camera. However, I was disappointed to find out that it does not support my camera model. I contacted Corel customer support but they were not helpful at all. They told me to wait for an update that might or might not come. I feel like I wasted my money on this software." - 1 star
-
"I have been using PhotoImpact for a long time and I still like it. It is not as fancy or complicated as Photoshop, but it does what I need it to do. It is easy to use and has a lot of options for editing photos. It also works well with other Corel products such as PaintShop Pro and VideoStudio. I think it is a great value for the money." - 4 stars
-
"I bought this software because I wanted to try something new for photo editing. However, I regret my decision because this software is very outdated and buggy. It crashes frequently, freezes my computer, and corrupts my files. It also has a very poor interface that is hard to navigate and understand. It does not have many features or effects that other photo editing software have. I do not recommend this software to anyone." - 2 stars
-
-
Conclusion
-
Corel PhotoImpact X3 is a graphic design software that can help you edit, enhance, and create amazing images with ease. It has a lot of features and benefits that make it suitable for photo editing enthusiasts who want a simple but powerful software. However, it also has some drawbacks such as an outdated interface, limited support, and lack of updates that make it less appealing for professional photographers who want a more advanced and updated software.
-
If you want to use Corel PhotoImpact X3, you need to activate it with a serial number and an activation code that you can get from your purchase confirmation email or from Corel customer support. You can activate your software online or offline by following the instructions on the screen.
-
If you lose or forget your serial number or activation code, you can contact Corel customer support or use a third-party software or website to retrieve them. However, you should be careful about the security risks or the terms of service violations that might occur.
-
If you are not satisfied with Corel PhotoImpact X3 or want to try other photo editing software, you can consider some alternatives and competitors such as Adobe Photoshop or GIMP that offer more features, effects, and updates for photo editing. However, they also have their own pros and cons that you should weigh before making a decision.
-
We hope this article has helped you understand more about Corel PhotoImpact X3 activation code serial number and how to get it and why you need it. If you have any questions or feedback, please feel free to leave a comment below.
-
FAQs
-
Here are some frequently asked questions and answers about Corel PhotoImpact X3 activation code serial number:
-
Q: How much does Corel PhotoImpact X3 cost?
-
A: Corel PhotoImpact X3 costs $29.99 USD for a one-time purchase from the official website or other online platforms. You can also get a free trial version for 30 days from the official website.
-
Q: Can I use Corel PhotoImpact X3 on multiple computers?
-
A: Yes, you can use Corel PhotoImpact X3 on up to three computers with the same serial number and activation code. However, you cannot use the software on more than one computer at the same time.
-
Q: Can I transfer Corel PhotoImpact X3 to another computer?
-
A: Yes, you can transfer Corel PhotoImpact X3 to another computer by uninstalling it from the old computer and installing it on the new computer. You need to enter your serial number and activation code again on the new computer.
-
Q: Can I upgrade Corel PhotoImpact X3 to a newer version?
-
A: No, Corel PhotoImpact X3 is the latest and final version of the software. There are no updates or upgrades available for Corel PhotoImpact X3.
-
Q: Is Corel PhotoImpact X3 compatible with Windows 10?
-
A: Yes, Corel PhotoImpact X3 is compatible with Windows 10. However, some users have reported some issues or errors when using the software on Windows 10. You can try to run the software in compatibility mode or as an administrator to solve these problems.
b2dd77e56b
-
-
\ No newline at end of file
diff --git a/spaces/netiMophi/DreamlikeArt-Diffusion-1.0/Mainconcept Aac Encoder V1.0.6 Serial 30.md b/spaces/netiMophi/DreamlikeArt-Diffusion-1.0/Mainconcept Aac Encoder V1.0.6 Serial 30.md
deleted file mode 100644
index 44d943cd2d39a1535f767855ddb6138a016bd866..0000000000000000000000000000000000000000
--- a/spaces/netiMophi/DreamlikeArt-Diffusion-1.0/Mainconcept Aac Encoder V1.0.6 Serial 30.md
+++ /dev/null
@@ -1,162 +0,0 @@
-
-
MainConcept AAC Encoder v1.0.6 Serial 30: A Review
-
If you are looking for a professional and reliable audio encoding software, you might have heard of MainConcept AAC Encoder v1.0.6 Serial 30. This software is designed to enrich Adobe products with state-of-the-art codec solutions, especially for the Adobe Flash Media Live Encoder 2.5 that only comes with Nellymoser or MP3 audio encoding as standard.
In this article, we will review MainConcept AAC Encoder v1.0.6 Serial 30, and show you what it is, how to install and activate it, how to use it, and what are its pros and cons.
-
What is MainConcept AAC Encoder?
-
MainConcept AAC Encoder is a plug-in that offers professional AAC encoding within the Adobe Flash Media Live Encoder 2.5. It supports AAC (MPEG-4 AAC & HE Audio), which is the emerging future audio standard that might replace existing ones, such as MP3.
-
What is AAC?
-
AAC stands for Advanced Audio Coding, which is a lossy audio compression format that provides better sound quality and efficiency than MP3. It is widely supported by popular devices such as Apple iPod, Sony PSP, Sony PS3, Nintendo Wii, various cell phones, etc.
-
AAC has different versions, such as Low Complexity (LC), High-Efficiency (HE) v1 and v2, and Extended High-Efficiency (xHE). The HE versions use Spectral Band Replication (SBR) and Parametric Stereo (PS) techniques to enhance the audio quality at low bit rates. The xHE version uses Unified Speech and Audio Coding (USAC) to improve the speech and music quality at very low bit rates.
-
What are the features of MainConcept AAC Encoder?
-
MainConcept AAC Encoder has the following features:
-
-
Fully compliant to ISO/IEC 14496-3 (MPEG-4 AAC) and ISO/IEC 13818-7 (MPEG-2 AAC) audio streams specification
-
Encodes PCM audio streams to MPEG-2 / MPEG-4 Low Complexity, HE AAC v1 as SBR, and HE AAC v2 as Parametric Stereo audio streams
-
Supports common output formats like RAW (no header), ADTS (Audio Data Transport Stream header), and LOAS/LATM (used for multiplexing into MPEG-2 streams)
-
Supports different channel layouts from mono, stereo, 5.1 up to 7.1
-
Supports different sampling rates from 8 kHz up to 96 kHz
-
Supports different bit rates from 8 kbit/s up to 320 kbit/s
-
Supports different profiles such as LC, HE, and HEv2
-
Supports different modes such as CBR (Constant Bit Rate), VBR (Variable Bit Rate), and ABR (Average Bit Rate)
-
Supports different quality levels from 0 (lowest) to 5 (highest)
-
Supports metadata such as title, artist, album, genre, etc
-
Supports gapless encoding for seamless playback of consecutive tracks
-
-
What are the benefits of using MainConcept AAC Encoder?
-
MainConcept AAC Encoder has the following benefits:
-
-
-
It provides high-quality audio encoding for Adobe Flash Media Live Encoder 2.5, which only supports Nellymoser or MP3 audio encoding by default
-
It allows you to stream audio files in AAC format, which is compatible with most popular devices and platforms
-
It enables you to save bandwidth and storage space by using efficient compression techniques such as SBR and PS
-
It gives you flexibility and control over the encoding parameters such as bit rate, mode, quality, etc
-
It supports various input and output formats such as RAW, ADTS, and LOAS/LATM
-
It supports various channel layouts and sampling rates for different audio scenarios
-
It supports metadata and gapless encoding for better user experience
-
-
How to install and activate MainConcept AAC Encoder v1.0.6 Serial 30?
-
In order to install and activate MainConcept AAC Encoder v1.0.6 Serial 30, you need to follow these steps:
-
How to download MainConcept AAC Encoder v1.0.6?
-
You can download MainConcept AAC Encoder v1.0.6 from the official website of MainConcept. You need to register an account and provide some basic information before you can access the download link. You will also receive an email with the serial number for activation.
-
How to install MainConcept AAC Encoder v1.0.6?
-
After you download the installer file, you need to run it and follow the instructions on the screen. You will be asked to accept the license agreement, choose the installation folder, and select the components to install. You can choose to install the plug-in for Adobe Flash Media Live Encoder 2.5 or Adobe Premiere Pro CS4/CS5/CS6/CC.
-
The installation process will take a few minutes, and you will see a confirmation message when it is done.
-
How to activate MainConcept AAC Encoder v1.0.6 with Serial 30?
-
To activate MainConcept AAC Encoder v1.0.6 with Serial 30, you need to launch Adobe Flash Media Live Encoder 2.5 or Adobe Premiere Pro CS4/CS5/CS6/CC, depending on which component you installed. You will see a dialog box asking you to enter the serial number that you received by email.
-
You need to enter the serial number exactly as it is shown in the email, including the dashes and spaces. Then, click on Activate Online button to complete the activation process. You will see a message saying that your product has been successfully activated.
-
If you have any problems with the activation process, you can contact the customer support of MainConcept.
How to use MainConcept AAC Encoder v1.0.6 Serial 30?
-
Once you have installed and activated MainConcept AAC Encoder v1.0.6 Serial 30, you can start using it to encode and stream audio files in AAC format. Here are some tips on how to use it:
-
How to encode audio files with MainConcept AAC Encoder v1.0.6?
-
To encode audio files with MainConcept AAC Encoder v1.0.6, you need to use Adobe Flash Media Live Encoder 2.5 or Adobe Premiere Pro CS4/CS5/CS6/CC, depending on which component you installed.
-
If you use Adobe Flash Media Live Encoder 2.5, you need to do the following steps:
-
-
Launch Adobe Flash Media Live Encoder 2.5 and select the input source for your audio file
-
Click on the Audio tab and select MainConcept AAC Encoder from the Format drop-down menu
-
Click on the Settings button to open the MainConcept AAC Encoder Settings dialog box
-
Select the output format, channel layout, sampling rate, bit rate, mode, quality, profile, and metadata for your audio file
-
Click on OK to save the settings and close the dialog box
-
Click on Start to begin the encoding process
-
Click on Stop to end the encoding process
-
-
If you use Adobe Premiere Pro CS4/CS5/CS6/CC, you need to do the following steps:
-
-
Launch Adobe Premiere Pro CS4/CS5/CS6/CC and import your audio file into the project panel
-
Drag and drop your audio file into the timeline and edit it as you wish
-
Select File > Export > Media to open the Export Settings dialog box
-
Select MainConcept AAC Encoder from the Format drop-down menu
-
Select the output format, channel layout, sampling rate, bit rate, mode, quality, profile, and metadata for your audio file
-
Click on Export to begin the encoding process
-
-
How to configure the encoding settings with MainConcept AAC Encoder v1.0.6?
-
To configure the encoding settings with MainConcept AAC Encoder v1.0.6, you need to open the MainConcept AAC Encoder Settings dialog box by clicking on the Settings button in Adobe Flash Media Live Encoder 2.5 or Adobe Premiere Pro CS4/CS5/CS6/CC.
-
In this dialog box, you can adjust the following parameters:
-
-
Parameter
Description
-
Output Format
The output format of the encoded audio file. You can choose from RAW (no header), ADTS (Audio Data Transport Stream header), or LOAS/LATM (used for multiplexing into MPEG-2 streams).
-
Channel Layout
The channel layout of the encoded audio file. You can choose from mono, stereo, 5.1 up to 7.1.
-
Sampling Rate
The sampling rate of the encoded audio file. You can choose from 8 kHz up to 96 kHz.
-
Bit Rate
The bit rate of the encoded audio file. You can choose from 8 kbit/s up to 320 kbit/s.
-
Mode
The mode of the encoded audio file. You can choose from CBR (Constant Bit Rate), VBR (Variable Bit Rate), or ABR (Average Bit Rate).
-
Quality
The quality level of the encoded audio file. You can choose from 0 (lowest) to 5 (highest).
-
Profile
The profile of the encoded audio file. You can choose from LC (Low Complexity), HE (High-Efficiency), or HEv2 (High-Efficiency version 2).
-
Metadata
The metadata of the encoded audio file. You can enter information such as title, artist, album, genre, etc.
-
-
How to stream audio files with MainConcept AAC Encoder v1.0.6?
-
To stream audio files with MainConcept AAC Encoder v1.0.6, you need to use Adobe Flash Media Live Encoder 2.5 or Adobe Premiere Pro CS4/CS5/CS 6/CC, depending on which component you installed. If you use Adobe Flash Media Live Encoder 2.5, you need to do the following steps:
-
Launch Adobe Flash Media Live Encoder 2.5 and select the input source for your audio file
-
Click on the Audio tab and select MainConcept AAC Encoder from the Format drop-down menu
-
Click on the Settings button to open the MainConcept AAC Encoder Settings dialog box and configure the encoding settings as you wish
-
Click on OK to save the settings and close the dialog box
-
Click on the Output tab and select Stream to Flash Media Server from the Output Type drop-down menu
-
Enter the URL, username, and password of your Flash Media Server in the corresponding fields
-
Click on Connect to connect to your Flash Media Server
-
Enter the stream name and select the stream type for your audio file
-
Click on Start to begin streaming your audio file
-
Click on Stop to end streaming your audio file
-
- If you use Adobe Premiere Pro CS4/CS5/CS6/CC, you need to do the following steps:
-
Launch Adobe Premiere Pro CS4/CS5/CS6/CC and import your audio file into the project panel
-
Drag and drop your audio file into the timeline and edit it as you wish
-
Select File > Export > Media to open the Export Settings dialog box
-
Select MainConcept AAC Encoder from the Format drop-down menu and configure the encoding settings as you wish
-
Select Publish > Adobe Flash Media Server from the left panel and check the box next to it
-
Enter the URL, username, and password of your Flash Media Server in the corresponding fields
-
Enter the stream name and select the stream type for your audio file
-
Click on Queue to add your audio file to the Adobe Media Encoder queue
-
Launch Adobe Media Encoder and click on Start Queue to begin streaming your audio file
-
Click on Stop Queue to end streaming your audio file
-
-
What are the pros and cons of MainConcept AAC Encoder v1.0.6 Serial 30?
-
MainConcept AAC Encoder v1.0.6 Serial 30 is a powerful and versatile audio encoding software, but it also has some drawbacks. Here are some of the pros and cons of using it:
-
Pros of MainConcept AAC Encoder v1.0.6 Serial 30
-
-
It provides high-quality audio encoding for Adobe Flash Media Live Encoder 2.5, which only supports Nellymoser or MP3 audio encoding by default
-
It allows you to stream audio files in AAC format, which is compatible with most popular devices and platforms
-
It enables you to save bandwidth and storage space by using efficient compression techniques such as SBR and PS
-
It gives you flexibility and control over the encoding parameters such as bit rate, mode, quality, etc.
-
It supports various input and output formats such as RAW, ADTS, and LOAS/LATM
-
It supports various channel layouts and sampling rates for different audio scenarios
-
It supports metadata and gapless encoding for better user experience
-
-
Cons of MainConcept AAC Encoder v1.0.6 Serial 30
-
-
It requires a serial number for activation, which might be lost or stolen by hackers or malware
-
It is not compatible with other versions of Adobe Flash Media Live Encoder or Adobe Premiere Pro than 2.5 or CS4/CS5/CS6/CC respectively
-
It does not support xHE-AAC profile, which is the latest version of AAC that offers better speech and music quality at very low bit rates
-
It does not support Dolby Digital Plus or Dolby Atmos formats, which are advanced surround sound formats that offer immersive audio experience
-
It might have some compatibility issues with some devices or platforms that do not support AAC format or certain profiles or modes of AAC format
-
-
Conclusion
-
MainConcept AAC Encoder v1.0.6 Serial 30 is a plug-in that offers professional AAC encoding within the Adobe Flash Media Live Encoder 2.5 or Adobe Premiere Pro CS4/CS5/CS6/CC. It supports AAC (MPEG-4 AAC & HE Audio), which is the emerging future audio standard that might replace existing ones, such as MP3.
-
MainConcept AAC Encoder v1.0.6 Serial 30 has many features and benefits, such as high-quality audio encoding, compatibility with popular devices and platforms, bandwidth and storage saving, flexibility and control over encoding parameters, various input and output formats, metadata and gapless encoding support, etc.
-
However, MainConcept AAC Encoder v1.0.6 Serial 30 also has some drawbacks, such as requiring a serial number for activation, not being compatible with other versions of Adobe Flash Media Live Encoder or Adobe Premiere Pro, not supporting xHE-AAC profile or Dolby Digital Plus or Dolby Atmos formats, and having some compatibility issues with some devices or platforms that do not support AAC format or certain profiles or modes of AAC format.
-
Therefore, MainConcept AAC Encoder v1.0.6 Serial 30 is a great choice for professional and reliable audio encoding software, but it also has some limitations that you should be aware of before using it.
-
FAQs
-
Here are some frequently asked questions about MainConcept AAC Encoder v1.0.6 Serial 30:
-
-
Q: Where can I get MainConcept AAC Encoder v1.0.6 Serial 30?
-
A: You can get MainConcept AAC Encoder v1.0.6 Serial 30 from the official website of MainConcept. You need to register an account and provide some basic information before you can access the download link. You will also receive an email with the serial number for activation.
-
Q: How much does MainConcept AAC Encoder v1.0.6 Serial 30 cost?
-
A: MainConcept AAC Encoder v1.0.6 Serial 30 costs $180 for a single user license. You can also get a free trial version for 30 days from the official website of MainConcept.
-
Q: What are the system requirements for MainConcept AAC Encoder v1.0.6 Serial 30?
-
A: The system requirements for MainConcept AAC Encoder v1.0.6 Serial 30 are as follows:
-
-
Operating System: Windows XP/Vista/7/8/10
-
Processor: Pentium IV or higher
-
Memory: 512 MB RAM or higher
-
Disk Space: 100 MB free disk space or higher
-
Software: Adobe Flash Media Live Encoder 2.5 or Adobe Premiere Pro CS4/CS5/CS6/CC
-
-
Q: How can I contact the customer support of MainConcept?
-
A: You can contact the customer support of MainConcept by filling out the online form on their website, sending an email to support@mainconcept.com, or calling +49 (0)2408-9383-0.
-
Q: What are some alternatives to MainConcept AAC Encoder v1.0.6 Serial 30?
-
A: Some alternatives to MainConcept AAC Encoder v1.0.6 Serial 30 are as follows:
-
-
Fraunhofer FDK AAC Codec Library for Android: A software library that provides high-quality encoding and decoding of AAC audio on Android devices.
-
Nero AAC Codec: A freeware tool that allows you to convert WAV files to AAC files and vice versa.
-
Foobar2000: A free and advanced audio player that supports various audio formats, including AAC.
-
- b2dd77e56b
-
-
\ No newline at end of file
diff --git a/spaces/nikitaPDL2023/assignment4/detectron2/detectron2/layers/csrc/nms_rotated/nms_rotated.h b/spaces/nikitaPDL2023/assignment4/detectron2/detectron2/layers/csrc/nms_rotated/nms_rotated.h
deleted file mode 100644
index 12aca388e47b12dafd20999f2991a9d42f4b904b..0000000000000000000000000000000000000000
--- a/spaces/nikitaPDL2023/assignment4/detectron2/detectron2/layers/csrc/nms_rotated/nms_rotated.h
+++ /dev/null
@@ -1,39 +0,0 @@
-// Copyright (c) Facebook, Inc. and its affiliates.
-#pragma once
-#include
-
-namespace detectron2 {
-
-at::Tensor nms_rotated_cpu(
- const at::Tensor& dets,
- const at::Tensor& scores,
- const double iou_threshold);
-
-#if defined(WITH_CUDA) || defined(WITH_HIP)
-at::Tensor nms_rotated_cuda(
- const at::Tensor& dets,
- const at::Tensor& scores,
- const double iou_threshold);
-#endif
-
-// Interface for Python
-// inline is needed to prevent multiple function definitions when this header is
-// included by different cpps
-inline at::Tensor nms_rotated(
- const at::Tensor& dets,
- const at::Tensor& scores,
- const double iou_threshold) {
- assert(dets.device().is_cuda() == scores.device().is_cuda());
- if (dets.device().is_cuda()) {
-#if defined(WITH_CUDA) || defined(WITH_HIP)
- return nms_rotated_cuda(
- dets.contiguous(), scores.contiguous(), iou_threshold);
-#else
- AT_ERROR("Detectron2 is not compiled with GPU support!");
-#endif
- }
-
- return nms_rotated_cpu(dets.contiguous(), scores.contiguous(), iou_threshold);
-}
-
-} // namespace detectron2
diff --git a/spaces/nikitaPDL2023/assignment4/detectron2/detectron2/utils/collect_env.py b/spaces/nikitaPDL2023/assignment4/detectron2/detectron2/utils/collect_env.py
deleted file mode 100644
index 2846d7a56c3efbdec5ccc5a9c4890ff47cff9512..0000000000000000000000000000000000000000
--- a/spaces/nikitaPDL2023/assignment4/detectron2/detectron2/utils/collect_env.py
+++ /dev/null
@@ -1,246 +0,0 @@
-# Copyright (c) Facebook, Inc. and its affiliates.
-import importlib
-import numpy as np
-import os
-import re
-import subprocess
-import sys
-from collections import defaultdict
-import PIL
-import torch
-import torchvision
-from tabulate import tabulate
-
-__all__ = ["collect_env_info"]
-
-
-def collect_torch_env():
- try:
- import torch.__config__
-
- return torch.__config__.show()
- except ImportError:
- # compatible with older versions of pytorch
- from torch.utils.collect_env import get_pretty_env_info
-
- return get_pretty_env_info()
-
-
-def get_env_module():
- var_name = "DETECTRON2_ENV_MODULE"
- return var_name, os.environ.get(var_name, "")
-
-
-def detect_compute_compatibility(CUDA_HOME, so_file):
- try:
- cuobjdump = os.path.join(CUDA_HOME, "bin", "cuobjdump")
- if os.path.isfile(cuobjdump):
- output = subprocess.check_output(
- "'{}' --list-elf '{}'".format(cuobjdump, so_file), shell=True
- )
- output = output.decode("utf-8").strip().split("\n")
- arch = []
- for line in output:
- line = re.findall(r"\.sm_([0-9]*)\.", line)[0]
- arch.append(".".join(line))
- arch = sorted(set(arch))
- return ", ".join(arch)
- else:
- return so_file + "; cannot find cuobjdump"
- except Exception:
- # unhandled failure
- return so_file
-
-
-def collect_env_info():
- has_gpu = torch.cuda.is_available() # true for both CUDA & ROCM
- torch_version = torch.__version__
-
- # NOTE that CUDA_HOME/ROCM_HOME could be None even when CUDA runtime libs are functional
- from torch.utils.cpp_extension import CUDA_HOME, ROCM_HOME
-
- has_rocm = False
- if (getattr(torch.version, "hip", None) is not None) and (ROCM_HOME is not None):
- has_rocm = True
- has_cuda = has_gpu and (not has_rocm)
-
- data = []
- data.append(("sys.platform", sys.platform)) # check-template.yml depends on it
- data.append(("Python", sys.version.replace("\n", "")))
- data.append(("numpy", np.__version__))
-
- try:
- import detectron2 # noqa
-
- data.append(
- ("detectron2", detectron2.__version__ + " @" + os.path.dirname(detectron2.__file__))
- )
- except ImportError:
- data.append(("detectron2", "failed to import"))
- except AttributeError:
- data.append(("detectron2", "imported a wrong installation"))
-
- try:
- import detectron2._C as _C
- except ImportError as e:
- data.append(("detectron2._C", f"not built correctly: {e}"))
-
- # print system compilers when extension fails to build
- if sys.platform != "win32": # don't know what to do for windows
- try:
- # this is how torch/utils/cpp_extensions.py choose compiler
- cxx = os.environ.get("CXX", "c++")
- cxx = subprocess.check_output("'{}' --version".format(cxx), shell=True)
- cxx = cxx.decode("utf-8").strip().split("\n")[0]
- except subprocess.SubprocessError:
- cxx = "Not found"
- data.append(("Compiler ($CXX)", cxx))
-
- if has_cuda and CUDA_HOME is not None:
- try:
- nvcc = os.path.join(CUDA_HOME, "bin", "nvcc")
- nvcc = subprocess.check_output("'{}' -V".format(nvcc), shell=True)
- nvcc = nvcc.decode("utf-8").strip().split("\n")[-1]
- except subprocess.SubprocessError:
- nvcc = "Not found"
- data.append(("CUDA compiler", nvcc))
- if has_cuda and sys.platform != "win32":
- try:
- so_file = importlib.util.find_spec("detectron2._C").origin
- except (ImportError, AttributeError):
- pass
- else:
- data.append(
- ("detectron2 arch flags", detect_compute_compatibility(CUDA_HOME, so_file))
- )
- else:
- # print compilers that are used to build extension
- data.append(("Compiler", _C.get_compiler_version()))
- data.append(("CUDA compiler", _C.get_cuda_version())) # cuda or hip
- if has_cuda and getattr(_C, "has_cuda", lambda: True)():
- data.append(
- ("detectron2 arch flags", detect_compute_compatibility(CUDA_HOME, _C.__file__))
- )
-
- data.append(get_env_module())
- data.append(("PyTorch", torch_version + " @" + os.path.dirname(torch.__file__)))
- data.append(("PyTorch debug build", torch.version.debug))
- try:
- data.append(("torch._C._GLIBCXX_USE_CXX11_ABI", torch._C._GLIBCXX_USE_CXX11_ABI))
- except Exception:
- pass
-
- if not has_gpu:
- has_gpu_text = "No: torch.cuda.is_available() == False"
- else:
- has_gpu_text = "Yes"
- data.append(("GPU available", has_gpu_text))
- if has_gpu:
- devices = defaultdict(list)
- for k in range(torch.cuda.device_count()):
- cap = ".".join((str(x) for x in torch.cuda.get_device_capability(k)))
- name = torch.cuda.get_device_name(k) + f" (arch={cap})"
- devices[name].append(str(k))
- for name, devids in devices.items():
- data.append(("GPU " + ",".join(devids), name))
-
- if has_rocm:
- msg = " - invalid!" if not (ROCM_HOME and os.path.isdir(ROCM_HOME)) else ""
- data.append(("ROCM_HOME", str(ROCM_HOME) + msg))
- else:
- try:
- from torch.utils.collect_env import get_nvidia_driver_version, run as _run
-
- data.append(("Driver version", get_nvidia_driver_version(_run)))
- except Exception:
- pass
- msg = " - invalid!" if not (CUDA_HOME and os.path.isdir(CUDA_HOME)) else ""
- data.append(("CUDA_HOME", str(CUDA_HOME) + msg))
-
- cuda_arch_list = os.environ.get("TORCH_CUDA_ARCH_LIST", None)
- if cuda_arch_list:
- data.append(("TORCH_CUDA_ARCH_LIST", cuda_arch_list))
- data.append(("Pillow", PIL.__version__))
-
- try:
- data.append(
- (
- "torchvision",
- str(torchvision.__version__) + " @" + os.path.dirname(torchvision.__file__),
- )
- )
- if has_cuda:
- try:
- torchvision_C = importlib.util.find_spec("torchvision._C").origin
- msg = detect_compute_compatibility(CUDA_HOME, torchvision_C)
- data.append(("torchvision arch flags", msg))
- except (ImportError, AttributeError):
- data.append(("torchvision._C", "Not found"))
- except AttributeError:
- data.append(("torchvision", "unknown"))
-
- try:
- import fvcore
-
- data.append(("fvcore", fvcore.__version__))
- except (ImportError, AttributeError):
- pass
-
- try:
- import iopath
-
- data.append(("iopath", iopath.__version__))
- except (ImportError, AttributeError):
- pass
-
- try:
- import cv2
-
- data.append(("cv2", cv2.__version__))
- except (ImportError, AttributeError):
- data.append(("cv2", "Not found"))
- env_str = tabulate(data) + "\n"
- env_str += collect_torch_env()
- return env_str
-
-
-def test_nccl_ops():
- num_gpu = torch.cuda.device_count()
- if os.access("/tmp", os.W_OK):
- import torch.multiprocessing as mp
-
- dist_url = "file:///tmp/nccl_tmp_file"
- print("Testing NCCL connectivity ... this should not hang.")
- mp.spawn(_test_nccl_worker, nprocs=num_gpu, args=(num_gpu, dist_url), daemon=False)
- print("NCCL succeeded.")
-
-
-def _test_nccl_worker(rank, num_gpu, dist_url):
- import torch.distributed as dist
-
- dist.init_process_group(backend="NCCL", init_method=dist_url, rank=rank, world_size=num_gpu)
- dist.barrier(device_ids=[rank])
-
-
-if __name__ == "__main__":
- try:
- from detectron2.utils.collect_env import collect_env_info as f
-
- print(f())
- except ImportError:
- print(collect_env_info())
-
- if torch.cuda.is_available():
- num_gpu = torch.cuda.device_count()
- for k in range(num_gpu):
- device = f"cuda:{k}"
- try:
- x = torch.tensor([1, 2.0], dtype=torch.float32)
- x = x.to(device)
- except Exception as e:
- print(
- f"Unable to copy tensor to device={device}: {e}. "
- "Your CUDA environment is broken."
- )
- if num_gpu > 1:
- test_nccl_ops()
diff --git a/spaces/niro-private/chatCSV/files.py b/spaces/niro-private/chatCSV/files.py
deleted file mode 100644
index 061ae907ec528e1f4fead599877eec57642a2859..0000000000000000000000000000000000000000
--- a/spaces/niro-private/chatCSV/files.py
+++ /dev/null
@@ -1,191 +0,0 @@
-import os
-from typing import (
- Any,
- Union,
-)
-import zipfile
-import streamlit as st
-from streamlit.runtime.uploaded_file_manager import (
- UploadedFile,
- UploadedFileRec,
- UploadedFileManager,
-)
-from streamlit.runtime.scriptrunner import get_script_run_ctx
-from supabase.client import Client
-from langchain.vectorstores.supabase import SupabaseVectorStore
-from components_keys import ComponentsKeys
-from loaders.audio import process_audio
-from loaders.txt import process_txt
-from loaders.csv import process_csv
-from loaders.markdown import process_markdown
-from loaders.pdf import process_pdf
-from loaders.html import (
- create_html_file,
- delete_tempfile,
- get_html,
- process_html,
-)
-from loaders.powerpoint import process_powerpoint
-from loaders.docx import process_docx
-from utils import compute_sha1_from_content
-
-
-ctx = get_script_run_ctx()
-manager = UploadedFileManager()
-file_processors = {
- ".txt": process_txt,
- ".csv": process_csv,
- ".md": process_markdown,
- ".markdown": process_markdown,
- ".m4a": process_audio,
- ".mp3": process_audio,
- ".webm": process_audio,
- ".mp4": process_audio,
- ".mpga": process_audio,
- ".wav": process_audio,
- ".mpeg": process_audio,
- ".pdf": process_pdf,
- ".html": process_html,
- ".pptx": process_powerpoint,
- ".docx": process_docx
-}
-
-def file_uploader(supabase, vector_store):
- # Omit zip file support if the `st.secrets.self_hosted` != "true" because
- # a zip file can consist of multiple files so the limit on 1 file uploaded
- # at a time in the demo can be circumvented.
- accepted_file_extensions = list(file_processors.keys())
- accept_multiple_files = st.secrets.self_hosted == "true"
- if accept_multiple_files:
- accepted_file_extensions += [".zip"]
-
- files = st.file_uploader(
- "**Upload a file**",
- accept_multiple_files=accept_multiple_files,
- type=accepted_file_extensions,
- key=ComponentsKeys.FILE_UPLOADER,
- )
- if st.secrets.self_hosted == "false":
- st.markdown("**In demo mode, the max file size is 1MB**")
- if st.button("Add to Database"):
- # Single file upload
- if isinstance(files, UploadedFile):
- filter_file(files, supabase, vector_store)
- # Multiple files upload
- elif isinstance(files, list):
- for file in files:
- filter_file(file, supabase, vector_store)
-
-def file_already_exists(supabase, file):
- file_sha1 = compute_sha1_from_content(file.getvalue())
- response = supabase.table("documents").select("id").eq("metadata->>file_sha1", file_sha1).execute()
- return len(response.data) > 0
-
-def file_to_uploaded_file(file: Any) -> Union[None, UploadedFile]:
- """Convert a file to a streamlit `UploadedFile` object.
-
- This allows us to unzip files and treat them the same way
- streamlit treats files uploaded through the file uploader.
-
- Parameters
- ---------
- file : Any
- The file. Can be any file supported by this app.
-
- Returns
- -------
- Union[None, UploadedFile]
- The file converted to a streamlit `UploadedFile` object.
- Returns `None` if the script context cannot be grabbed.
- """
-
- if ctx is None:
- print("script context not found, skipping uploading file:", file.name)
- return
-
- file_extension = os.path.splitext(file.name)[-1]
- file_name = file.name
- file_data = file.read()
- # The file manager will automatically assign an ID so pass `None`
- # Reference: https://github.com/streamlit/streamlit/blob/9a6ce804b7977bdc1f18906d1672c45f9a9b3398/lib/streamlit/runtime/uploaded_file_manager.py#LL98C6-L98C6
- uploaded_file_rec = UploadedFileRec(None, file_name, file_extension, file_data)
- uploaded_file_rec = manager.add_file(
- ctx.session_id,
- ComponentsKeys.FILE_UPLOADER,
- uploaded_file_rec,
- )
- return UploadedFile(uploaded_file_rec)
-
-def filter_zip_file(
- file: UploadedFile,
- supabase: Client,
- vector_store: SupabaseVectorStore,
-) -> None:
- """Unzip the zip file then filter each unzipped file.
-
- Parameters
- ----------
- file : UploadedFile
- The uploaded file from the file uploader.
- supabase : Client
- The supabase client.
- vector_store : SupabaseVectorStore
- The vector store in the database.
- """
-
- with zipfile.ZipFile(file, "r") as z:
- unzipped_files = z.namelist()
- for unzipped_file in unzipped_files:
- with z.open(unzipped_file, "r") as f:
- filter_file(f, supabase, vector_store)
-
-def filter_file(file, supabase, vector_store):
- # Streamlit file uploads are of type `UploadedFile` which has the
- # necessary methods and attributes for this app to work.
- if not isinstance(file, UploadedFile):
- file = file_to_uploaded_file(file)
-
- file_extension = os.path.splitext(file.name)[-1]
- if file_extension == ".zip":
- filter_zip_file(file, supabase, vector_store)
- return True
-
- if file_already_exists(supabase, file):
- st.write(f"😎 {file.name} is already in the database.")
- return False
-
- if file.size < 1:
- st.write(f"💨 {file.name} is empty.")
- return False
-
- if file_extension in file_processors:
- if st.secrets.self_hosted == "false":
- file_processors[file_extension](vector_store, file, stats_db=supabase)
- else:
- file_processors[file_extension](vector_store, file, stats_db=None)
- st.write(f"✅ {file.name} ")
- return True
-
- st.write(f"❌ {file.name} is not a valid file type.")
- return False
-
-def url_uploader(supabase, vector_store):
- url = st.text_area("**Add an url**",placeholder="vanti.ai")
- button = st.button("Add the URL to the database")
-
- if button:
- if not st.session_state["overused"]:
- html = get_html(url)
- if html:
- st.write(f"Getting content ... {url} ")
- try:
- file, temp_file_path = create_html_file(url, html)
- except UnicodeEncodeError as e:
- st.write(f"❌ Error encoding character: {e}")
- file, temp_file_path = create_html_file(url, html)
- ret = filter_file(file, supabase, vector_store)
- delete_tempfile(temp_file_path, url, ret)
- else:
- st.write(f"❌ Failed to access to {url} .")
- else:
- st.write("You have reached your daily limit. Please come back later or self host the solution.")
\ No newline at end of file
diff --git a/spaces/nomic-ai/BelleGroup_train_1M_CN/index.html b/spaces/nomic-ai/BelleGroup_train_1M_CN/index.html
deleted file mode 100644
index 221dc1b90167be35e486b264c5a56cf1cd1dd3f3..0000000000000000000000000000000000000000
--- a/spaces/nomic-ai/BelleGroup_train_1M_CN/index.html
+++ /dev/null
@@ -1,42 +0,0 @@
-
-
-
- BelleGroup/train_1M_CN
-
-
-
-
-
-
-
-
-
-
\ No newline at end of file
diff --git a/spaces/nomic-ai/nomic-ai_gpt4all-j-prompt-generations/index.html b/spaces/nomic-ai/nomic-ai_gpt4all-j-prompt-generations/index.html
deleted file mode 100644
index e3588b476f4862a682a483659f3408fd7dd928e7..0000000000000000000000000000000000000000
--- a/spaces/nomic-ai/nomic-ai_gpt4all-j-prompt-generations/index.html
+++ /dev/null
@@ -1,42 +0,0 @@
-
-
-
- nomic-ai/gpt4all-j-prompt-generations
-
-
-
-
-
-
-
-
-
-
\ No newline at end of file
diff --git a/spaces/nomic-ai/wikiann/style.css b/spaces/nomic-ai/wikiann/style.css
deleted file mode 100644
index 114adf441e9032febb46bc056b2a8bb651075f0d..0000000000000000000000000000000000000000
--- a/spaces/nomic-ai/wikiann/style.css
+++ /dev/null
@@ -1,28 +0,0 @@
-body {
- padding: 2rem;
- font-family: -apple-system, BlinkMacSystemFont, "Arial", sans-serif;
-}
-
-h1 {
- font-size: 16px;
- margin-top: 0;
-}
-
-p {
- color: rgb(107, 114, 128);
- font-size: 15px;
- margin-bottom: 10px;
- margin-top: 5px;
-}
-
-.card {
- max-width: 620px;
- margin: 0 auto;
- padding: 16px;
- border: 1px solid lightgray;
- border-radius: 16px;
-}
-
-.card p:last-child {
- margin-bottom: 0;
-}
diff --git a/spaces/ntt123/vietnam-male-voice-wavegru-tts/sparse_matmul/vector/cachealignedvector_test.cc b/spaces/ntt123/vietnam-male-voice-wavegru-tts/sparse_matmul/vector/cachealignedvector_test.cc
deleted file mode 100644
index 245c64d7dc3de69e5e37c4445c9ce4c599b28ab0..0000000000000000000000000000000000000000
--- a/spaces/ntt123/vietnam-male-voice-wavegru-tts/sparse_matmul/vector/cachealignedvector_test.cc
+++ /dev/null
@@ -1,405 +0,0 @@
-// Copyright 2021 Google LLC
-//
-// Licensed under the Apache License, Version 2.0 (the "License");
-// you may not use this file except in compliance with the License.
-// You may obtain a copy of the License at
-//
-// http://www.apache.org/licenses/LICENSE-2.0
-//
-// Unless required by applicable law or agreed to in writing, software
-// distributed under the License is distributed on an "AS IS" BASIS,
-// WITHOUT WARRANTIES OR CONDITIONS OF ANY KIND, either express or implied.
-// See the License for the specific language governing permissions and
-// limitations under the License.
-
-#include "sparse_matmul/vector/cache_aligned_vector.h"
-
-#if defined __aarch64__
-#include
-#endif
-
-#include
-
-#include
-#include
-#include
-#include
-#include
-
-#include "gmock/gmock.h"
-#include "gtest/gtest.h"
-#include "sparse_matmul/numerics/test_utils.h"
-#include "sparse_matmul/os/coop_threads.h"
-
-namespace csrblocksparse {
-
-const float kExpRelTolerance = .03f; // 3% relative
-#ifdef SIGMOID_AS_TANH
-const float kSigmoidRelTolerance = .09f; // 9.0% relative
-const float kSigmoidAbsTolerance = .003f;
-#else
-const float kSigmoidRelTolerance = .031f; // 3.1% relative
-const float kSigmoidAbsTolerance = .006f;
-#endif
-const float kTanhRelTolerance = .014f; // 1.4% relative
-const float kTanhAbsTolerance = .00525f;
-
-TEST(Transcendentals, CacheAlignedVectorExp) {
- const int kTestSize = 1 << 16;
- CacheAlignedVector values(kTestSize);
- values.FillRandom();
- CacheAlignedVector values_ref = values;
-
- values.Exp();
- for (int i = 0; i < kTestSize; ++i) {
- float exact_val = std::exp(values_ref[i]);
- float rel_diff = RelDiff(exact_val, values[i]);
-
- EXPECT_LT(rel_diff, kExpRelTolerance)
- << exact_val << " " << values[i] << " " << values_ref[i];
- }
-}
-
-TEST(Transcendentals, CacheAlignedVectorSigmoid) {
- const int kTestSize = 1 << 16;
- CacheAlignedVector values(kTestSize);
- values.FillRandom();
- CacheAlignedVector values_ref = values;
-
- values.Sigmoid();
- for (int i = 0; i < kTestSize; ++i) {
- float exact_val = 1. / (1. + std::exp(-values_ref[i]));
- float rel_diff = RelDiff(exact_val, values[i]);
-
- EXPECT_LT(rel_diff, kSigmoidRelTolerance)
- << exact_val << " " << values[i] << " " << values_ref[i];
- EXPECT_NEAR(values[i], exact_val, kSigmoidAbsTolerance) << values_ref[i];
- }
-}
-
-TEST(Transcendentals, CacheAlignedVectorTanh) {
- const int kTestSize = 1 << 16;
- CacheAlignedVector values(kTestSize);
- values.FillRandom();
- CacheAlignedVector values_ref = values;
-
- values.Tanh();
- for (int i = 0; i < kTestSize; ++i) {
- float exact_val = std::tanh(values_ref[i]);
- float rel_diff = RelDiff(exact_val, values[i]);
-
- EXPECT_LT(rel_diff, kTanhRelTolerance)
- << exact_val << " " << values[i] << " " << values_ref[i];
- EXPECT_NEAR(values[i], exact_val, kTanhAbsTolerance) << values_ref[i];
- }
-}
-
-// Uniformly sample logits and check that the resulting sample choices are
-// also (nearly) uniformly distributed.
-TEST(Sampling, Random) {
- const int kSize = 256;
-
- CacheAlignedVector logits(kSize);
- logits.FillZero();
-
- double histogram[kSize] = {};
-
- const int kIterations = 10000;
- for (int i = 0; i < kIterations; ++i) {
- histogram[logits.Sample()]++;
- }
-
- for (int i = 0; i < kSize; ++i) {
- // .002 is an empirical bound
- EXPECT_GT(histogram[i] / kIterations, 1. / kSize - .002f);
- EXPECT_LT(histogram[i] / kIterations, 1. / kSize + .002f);
- }
-}
-
-// Put (nearly) all the probability mass on one bin and make sure only that bin
-// is chosen.
-TEST(Sampling, FixedDistribution) {
- const int kSize = 256;
-
- CacheAlignedVector logits(kSize);
-
- int histogram[kSize] = {};
-
- const int kIterations = 1000;
- const int kIndex = 3;
- const int kAllProbabilityMass = 10;
- const int kNoProbabilityMass = -10;
- for (int i = 0; i < kIterations; ++i) {
- for (int i = 1; i <= kSize; ++i) {
- logits.data()[i - 1] =
- i == (kIndex + 1) ? kAllProbabilityMass : kNoProbabilityMass;
- }
-
- histogram[logits.Sample()]++;
- }
-
- EXPECT_EQ(histogram[kIndex], 1000);
-}
-
-// Put (nearly) all the probability mass on one bin outside the target range,
-// and make sure that bin is not chosen.
-TEST(ScalarSample, ThreadedMasked) {
- const int kSize = 256;
- const int mindex = 2;
- const int maxdex = 3;
- const int kNumThreads = 4;
- const int kIterations = 1000;
- const int kIndex = 3;
- const int kMostProbabilityMass = 3;
- const int kLittleProbabilityMass = -3;
-
- CacheAlignedVector logits(kSize);
- std::vector> tmp_vectors;
- std::vector generators(kNumThreads);
-
- for (int i = 0; i < kNumThreads; ++i) {
- tmp_vectors.emplace_back(kSize);
- }
-
- for (int i = 0; i < kSize; ++i) {
- logits.data()[i] =
- (i + 1) == (kIndex + 1) ? kMostProbabilityMass : kLittleProbabilityMass;
- }
-
- std::vector> histograms;
- for (int i = 0; i < kNumThreads; ++i) {
- histograms.emplace_back(kSize);
- }
-
- auto f = [&](csrblocksparse::SpinBarrier* /*barrier*/, int tid) {
- for (int i = 0; i < kIterations; ++i) {
- histograms[tid][logits.ScalarSample(
- 1.f, &generators[tid], &tmp_vectors[tid], 0, mindex, maxdex)]++;
- }
- };
-
- csrblocksparse::LaunchOnThreadsWithBarrier(kNumThreads, f);
-
- // Every thread should generate the exact same set of samples.
- for (int i = 0; i < kSize; ++i) {
- int val = histograms[0][i];
- for (int tid = 1; tid < kNumThreads; ++tid) {
- EXPECT_EQ(val, histograms[tid][i]);
- }
- }
-
- // The most probable sample should be the only one we're sampling.
- for (int tid = 0; tid < kNumThreads; ++tid) {
- EXPECT_EQ(std::distance(histograms[tid].begin(),
- std::max_element(histograms[tid].begin(),
- histograms[tid].end())),
- mindex);
- }
-}
-
-TEST(Sampling, Threaded) {
- const int kSize = 256;
- const int kNumThreads = 4;
- const int kIterations = 1000;
- const int kIndex = 3;
- const int kMostProbabilityMass = 3;
- const int kLittleProbabilityMass = -3;
-
- CacheAlignedVector logits(kSize);
- std::vector> tmp_vectors;
- std::vector generators(kNumThreads);
-
- for (int i = 0; i < kNumThreads; ++i) {
- tmp_vectors.emplace_back(kSize);
- }
-
- for (int i = 0; i < kSize; ++i) {
- logits.data()[i] =
- (i + 1) == (kIndex + 1) ? kMostProbabilityMass : kLittleProbabilityMass;
- }
-
- std::vector> histograms;
- for (int i = 0; i < kNumThreads; ++i) {
- histograms.emplace_back(kSize);
- }
-
- auto f = [&](csrblocksparse::SpinBarrier* /*barrier*/, int tid) {
- for (int i = 0; i < kIterations; ++i) {
- histograms[tid]
- [logits.Sample(1.f, &generators[tid], &tmp_vectors[tid])]++;
- }
- };
-
- csrblocksparse::LaunchOnThreadsWithBarrier(kNumThreads, f);
-
- // Every thread should generate the exact same set of samples.
- for (int i = 0; i < kSize; ++i) {
- int val = histograms[0][i];
- for (int tid = 1; tid < kNumThreads; ++tid) {
- EXPECT_EQ(val, histograms[tid][i]);
- }
- }
-
- // The most probable sample should be the one with the most probability mass.
- for (int tid = 0; tid < kNumThreads; ++tid) {
- EXPECT_EQ(std::distance(histograms[tid].begin(),
- std::max_element(histograms[tid].begin(),
- histograms[tid].end())),
- kIndex);
- }
-}
-
-void CreateVectorHelper(
- csrblocksparse::FatCacheAlignedVector* fat_vector, int cols,
- int rows, std::unique_ptr>* view) {
- *view = absl::make_unique>(*fat_vector,
- cols, rows);
-}
-
-void CreateVectorHelper(
- csrblocksparse::FatCacheAlignedVector* fat_vector, int cols,
- int rows, std::unique_ptr>* view) {
- *view = absl::make_unique>(
- fat_vector, cols, rows);
-}
-
-csrblocksparse::FatCacheAlignedVector CreateFatAlignedVector(int rows,
- int cols) {
- csrblocksparse::FatCacheAlignedVector fat_vector(rows, cols);
- // Usage intent of FatCacheAlignedVector is that they are COLUMN MAJOR.
- float v = 0;
- for (int c = 0; c < cols; ++c) {
- for (int r = 0; r < rows; ++r) {
- fat_vector.data()[c * rows + r] = v++;
- }
- }
-
- return fat_vector;
-}
-
-template
-void TestFatVectorView() {
- const int kRows = 6;
- const int kCols = 6;
- auto fat_vector = CreateFatAlignedVector(kRows, kCols);
-
- std::unique_ptr top;
- CreateVectorHelper(&fat_vector, 0, kRows / 2, &top);
- std::unique_ptr bottom;
- CreateVectorHelper(&fat_vector, kRows / 2, kRows / 2, &bottom);
-
- EXPECT_EQ(top->cols(), kCols);
- EXPECT_EQ(bottom->cols(), kCols);
- EXPECT_EQ(top->rows(), kRows / 2);
- EXPECT_EQ(bottom->rows(), kRows / 2);
- EXPECT_EQ(top->col_stride(), kRows);
- EXPECT_EQ(bottom->col_stride(), kRows);
-
- for (int c = 0; c < kCols; ++c) {
- for (int r = 0; r < kRows; ++r) {
- if (r < kRows / 2) {
- EXPECT_EQ(fat_vector[c * kRows + r],
- top->data()[c * top->col_stride() + r]);
- } else {
- EXPECT_EQ(fat_vector[c * kRows + r],
- bottom->data()[c * top->col_stride() + r - kRows / 2]);
- }
- }
- }
-}
-
-TEST(FatVector, View) {
- TestFatVectorView>();
-}
-TEST(FatVector, MutableView) {
- TestFatVectorView>();
-}
-
-TEST(FatVector, SliceMutableView) {
- const int kRows = 6;
- const int kCols = 3;
- auto fat_vector = CreateFatAlignedVector(kRows, kCols);
-
- int c = 1;
- csrblocksparse::MutableVectorView slice = fat_vector.slice(c);
- for (int r = 0; r < kRows; ++r) {
- EXPECT_EQ(slice[r], c * kRows + r);
- }
-}
-
-TEST(FatVector, SliceConstView) {
- const int kRows = 6;
- const int kCols = 3;
- auto fat_vector = CreateFatAlignedVector(kRows, kCols);
-
- int c = 1;
- csrblocksparse::VectorView const_slice;
- {
- // Take a VectorView from a non-const slice.
- const_slice = fat_vector.slice(c);
- for (int r = 0; r < kRows; ++r) {
- EXPECT_EQ(const_slice[r], c * kRows + r);
- }
- }
-
- {
- // Take a VectorView from a const slice.
- const auto& const_fat_vector = fat_vector;
- const_slice = const_fat_vector.slice(c);
- for (int r = 0; r < kRows; ++r) {
- EXPECT_EQ(const_slice[r], c * kRows + r);
- }
- }
-}
-
-TEST(View, FromMutableToConst) {
- const int kRows = 6;
- const int kCols = 3;
- auto fat_vector = CreateFatAlignedVector(kRows, kCols);
- csrblocksparse::MutableVectorView slice = fat_vector.slice(0);
-
- csrblocksparse::VectorView const_slice(slice);
- for (int r = 0; r < kRows; ++r) {
- EXPECT_EQ(const_slice[r], r);
- }
-}
-
-TEST(View, CopyTest) {
- const int kRows = 6;
- const int kCols = 3;
- auto fat_vector = CreateFatAlignedVector(kRows, kCols);
- csrblocksparse::MutableVectorView slice = fat_vector.slice(0);
- csrblocksparse::MutableVectorView slice2(slice);
-
- for (int r = 0; r < kRows; ++r) {
- EXPECT_EQ(slice2[r], r);
- }
-}
-
-TEST(Vector, CopyNull) {
- // Check that we can copy a vector with a null generator without segfault.
- CacheAlignedVector foo((CacheAlignedVector()));
- // This is here to prevent foo from being optimized out.
- CHECK_EQ(foo.size(), 0);
- CacheAlignedVector foo_bar = CacheAlignedVector();
- CHECK_EQ(foo_bar.size(), 0);
-}
-
-TEST(Vector, FromRawPointer) {
- std::vector input;
- for (int i = 0; i < 5; ++i) {
- input.push_back(i * 2);
- }
-
- // Calls first constructor.
- CacheAlignedVector foo(input.data(), 5);
- CHECK_EQ(foo.size(), 5);
- EXPECT_THAT(input, testing::ElementsAreArray(foo.data(), 5));
-
- // Calls the second constructor.
- CacheAlignedVector foo2(input.data(), 5);
- CHECK_EQ(foo2.size(), 5);
- EXPECT_THAT(input, testing::ElementsAreArray(foo2.data(), 5));
-}
-
-} // namespace csrblocksparse
diff --git a/spaces/ohmyteeth/seo-tools/README.md b/spaces/ohmyteeth/seo-tools/README.md
deleted file mode 100644
index 2ad62afaf453e2fb7327030ba6562a72a1eeab61..0000000000000000000000000000000000000000
--- a/spaces/ohmyteeth/seo-tools/README.md
+++ /dev/null
@@ -1,13 +0,0 @@
----
-title: SEO Tools
-emoji: 🚀
-colorFrom: gray
-colorTo: gray
-sdk: gradio
-sdk_version: 3.28.0
-app_file: app.py
-pinned: false
-license: apache-2.0
----
-
-Check out the configuration reference at https://huggingface.co/docs/hub/spaces-config-reference
\ No newline at end of file
diff --git a/spaces/owaiskha9654/Yolo-v7/app.py b/spaces/owaiskha9654/Yolo-v7/app.py
deleted file mode 100644
index 572009c8ca945a46e72781c847213b9d7e40c044..0000000000000000000000000000000000000000
--- a/spaces/owaiskha9654/Yolo-v7/app.py
+++ /dev/null
@@ -1,49 +0,0 @@
-import torch
-import gradio as gr
-from huggingface_hub import hf_hub_download
-from PIL import Image
-
-REPO_ID = "owaiskha9654/Yolov7_Custom_Object_Detection"
-FILENAME = "best.pt"
-
-
-yolov7_custom_weights = hf_hub_download(repo_id=REPO_ID, filename=FILENAME)
-
-model = torch.hub.load('Owaiskhan9654/yolov7-1:main',model='custom', path_or_model=yolov7_custom_weights, force_reload=True) # My Github repository https://github.com/Owaiskhan9654
-
-def object_detection(im, size=416):
- results = model(im)
- results.render()
- return Image.fromarray(results.imgs[0])
-
-title = "Yolov7 Custom"
-
-image = gr.inputs.Image(shape=(416, 416), image_mode="RGB", source="upload", label="Upload Image", optional=False)
-outputs = gr.outputs.Image(type="pil", label="Output Image")
-
-Custom_description="
🚗Car and 👦Person Detection"
-css = ".output-image, .input-image {height: 50rem !important; width: 100% !important;}"
-css = ".image-preview {height: auto !important;}"
-
-gr.Interface(
- fn=object_detection,
- inputs=image,
- outputs=outputs,
- title=Top_Title,
- description=Custom_description,
- article=Footer,
- examples=[["car-person-2.jpg"], ["car-person-2.jpg"]]).launch()
\ No newline at end of file
diff --git a/spaces/pablodawson/ldm3d-inpainting/diffuserslocal/docs/source/en/api/pipelines/repaint.md b/spaces/pablodawson/ldm3d-inpainting/diffuserslocal/docs/source/en/api/pipelines/repaint.md
deleted file mode 100644
index 9529893c354b160c4c4ded38dc5a2410693afefb..0000000000000000000000000000000000000000
--- a/spaces/pablodawson/ldm3d-inpainting/diffuserslocal/docs/source/en/api/pipelines/repaint.md
+++ /dev/null
@@ -1,37 +0,0 @@
-
-
-# RePaint
-
-[RePaint: Inpainting using Denoising Diffusion Probabilistic Models](https://huggingface.co/papers/2201.09865) is by Andreas Lugmayr, Martin Danelljan, Andres Romero, Fisher Yu, Radu Timofte, Luc Van Gool.
-
-The abstract from the paper is:
-
-*Free-form inpainting is the task of adding new content to an image in the regions specified by an arbitrary binary mask. Most existing approaches train for a certain distribution of masks, which limits their generalization capabilities to unseen mask types. Furthermore, training with pixel-wise and perceptual losses often leads to simple textural extensions towards the missing areas instead of semantically meaningful generation. In this work, we propose RePaint: A Denoising Diffusion Probabilistic Model (DDPM) based inpainting approach that is applicable to even extreme masks. We employ a pretrained unconditional DDPM as the generative prior. To condition the generation process, we only alter the reverse diffusion iterations by sampling the unmasked regions using the given image information. Since this technique does not modify or condition the original DDPM network itself, the model produces high-quality and diverse output images for any inpainting form. We validate our method for both faces and general-purpose image inpainting using standard and extreme masks.
-RePaint outperforms state-of-the-art Autoregressive, and GAN approaches for at least five out of six mask distributions.*
-
-The original codebase can be found at [andreas128/RePaint](https://github.com/andreas128/RePaint).
-
-
-
-Make sure to check out the Schedulers [guide](/using-diffusers/schedulers) to learn how to explore the tradeoff between scheduler speed and quality, and see the [reuse components across pipelines](/using-diffusers/loading#reuse-components-across-pipelines) section to learn how to efficiently load the same components into multiple pipelines.
-
-
-
-
-## RePaintPipeline
-[[autodoc]] RePaintPipeline
- - all
- - __call__
-
-## ImagePipelineOutput
-[[autodoc]] pipelines.ImagePipelineOutput
diff --git a/spaces/pablodawson/ldm3d-inpainting/diffuserslocal/examples/t2i_adapter/README_sdxl.md b/spaces/pablodawson/ldm3d-inpainting/diffuserslocal/examples/t2i_adapter/README_sdxl.md
deleted file mode 100644
index 03053c85d8a53564d5361c8c050e73238e65da03..0000000000000000000000000000000000000000
--- a/spaces/pablodawson/ldm3d-inpainting/diffuserslocal/examples/t2i_adapter/README_sdxl.md
+++ /dev/null
@@ -1,131 +0,0 @@
-# T2I-Adapter training example for Stable Diffusion XL (SDXL)
-
-The `train_t2i_adapter_sdxl.py` script shows how to implement the [T2I-Adapter training procedure](https://hf.co/papers/2302.08453) for [Stable Diffusion XL](https://huggingface.co/papers/2307.01952).
-
-## Running locally with PyTorch
-
-### Installing the dependencies
-
-Before running the scripts, make sure to install the library's training dependencies:
-
-**Important**
-
-To make sure you can successfully run the latest versions of the example scripts, we highly recommend **installing from source** and keeping the install up to date as we update the example scripts frequently and install some example-specific requirements. To do this, execute the following steps in a new virtual environment:
-
-```bash
-git clone https://github.com/huggingface/diffusers
-cd diffusers
-pip install -e .
-```
-
-Then cd in the `examples/t2i_adapter` folder and run
-```bash
-pip install -r requirements_sdxl.txt
-```
-
-And initialize an [🤗Accelerate](https://github.com/huggingface/accelerate/) environment with:
-
-```bash
-accelerate config
-```
-
-Or for a default accelerate configuration without answering questions about your environment
-
-```bash
-accelerate config default
-```
-
-Or if your environment doesn't support an interactive shell (e.g., a notebook)
-
-```python
-from accelerate.utils import write_basic_config
-write_basic_config()
-```
-
-When running `accelerate config`, if we specify torch compile mode to True there can be dramatic speedups.
-
-## Circle filling dataset
-
-The original dataset is hosted in the [ControlNet repo](https://huggingface.co/lllyasviel/ControlNet/blob/main/training/fill50k.zip). We re-uploaded it to be compatible with `datasets` [here](https://huggingface.co/datasets/fusing/fill50k). Note that `datasets` handles dataloading within the training script.
-
-## Training
-
-Our training examples use two test conditioning images. They can be downloaded by running
-
-```sh
-wget https://huggingface.co/datasets/huggingface/documentation-images/resolve/main/diffusers/controlnet_training/conditioning_image_1.png
-
-wget https://huggingface.co/datasets/huggingface/documentation-images/resolve/main/diffusers/controlnet_training/conditioning_image_2.png
-```
-
-Then run `huggingface-cli login` to log into your Hugging Face account. This is needed to be able to push the trained T2IAdapter parameters to Hugging Face Hub.
-
-```bash
-export MODEL_DIR="stabilityai/stable-diffusion-xl-base-1.0"
-export OUTPUT_DIR="path to save model"
-
-accelerate launch train_t2i_adapter_sdxl.py \
- --pretrained_model_name_or_path=$MODEL_DIR \
- --output_dir=$OUTPUT_DIR \
- --dataset_name=fusing/fill50k \
- --mixed_precision="fp16" \
- --resolution=1024 \
- --learning_rate=1e-5 \
- --max_train_steps=15000 \
- --validation_image "./conditioning_image_1.png" "./conditioning_image_2.png" \
- --validation_prompt "red circle with blue background" "cyan circle with brown floral background" \
- --validation_steps=100 \
- --train_batch_size=1 \
- --gradient_accumulation_steps=4 \
- --report_to="wandb" \
- --seed=42 \
- --push_to_hub
-```
-
-To better track our training experiments, we're using the following flags in the command above:
-
-* `report_to="wandb` will ensure the training runs are tracked on Weights and Biases. To use it, be sure to install `wandb` with `pip install wandb`.
-* `validation_image`, `validation_prompt`, and `validation_steps` to allow the script to do a few validation inference runs. This allows us to qualitatively check if the training is progressing as expected.
-
-Our experiments were conducted on a single 40GB A100 GPU.
-
-### Inference
-
-Once training is done, we can perform inference like so:
-
-```python
-from diffusers import StableDiffusionXLAdapterPipeline, T2IAdapter, EulerAncestralDiscreteSchedulerTest
-from diffusers.utils import load_image
-import torch
-
-base_model_path = "stabilityai/stable-diffusion-xl-base-1.0"
-adapter_path = "path to adapter"
-
-adapter = T2IAdapter.from_pretrained(adapter_path, torch_dtype=torch.float16)
-pipe = StableDiffusionXLAdapterPipeline.from_pretrained(
- base_model_path, adapter=adapter, torch_dtype=torch.float16
-)
-
-# speed up diffusion process with faster scheduler and memory optimization
-pipe.scheduler = EulerAncestralDiscreteSchedulerTest.from_config(pipe.scheduler.config)
-# remove following line if xformers is not installed or when using Torch 2.0.
-pipe.enable_xformers_memory_efficient_attention()
-# memory optimization.
-pipe.enable_model_cpu_offload()
-
-control_image = load_image("./conditioning_image_1.png")
-prompt = "pale golden rod circle with old lace background"
-
-# generate image
-generator = torch.manual_seed(0)
-image = pipe(
- prompt, num_inference_steps=20, generator=generator, image=control_image
-).images[0]
-image.save("./output.png")
-```
-
-## Notes
-
-### Specifying a better VAE
-
-SDXL's VAE is known to suffer from numerical instability issues. This is why we also expose a CLI argument namely `--pretrained_vae_model_name_or_path` that lets you specify the location of a better VAE (such as [this one](https://huggingface.co/madebyollin/sdxl-vae-fp16-fix)).
diff --git a/spaces/pierreguillou/tesseract-ocr-pt/app.py b/spaces/pierreguillou/tesseract-ocr-pt/app.py
deleted file mode 100644
index 2d8af11fc6d28f21ae155dc79a8cbd07deb8309f..0000000000000000000000000000000000000000
--- a/spaces/pierreguillou/tesseract-ocr-pt/app.py
+++ /dev/null
@@ -1,46 +0,0 @@
-import os
-import gradio as gr
-import re
-
-print(os.popen(f'cat /etc/debian_version').read())
-print(os.popen(f'cat /etc/issue').read())
-print(os.popen(f'apt search tesseract').read())
-
-# choices = os.popen('tesseract --list-langs').read().split('\n')[1:-1]
-
-def correction(text):
- # replace 3 lines break (\n\n\n) or more by 2 lines break
- text = text.replace('\n \n','\n\n')
- text = re.sub(r'\n(\n+)', '\n\n', text).strip()
-
- # delete \n at the end of a line
- text = re.sub(r'(?Tesseract documentation | Github Repo"
-#examples = [['eurotext.png', ['eng']], ['tesseract_sample.png', ['jpn', 'eng']], ['chi.jpg', ['HanS', 'HanT']]]
-examples = [['exemple.png']]
-allow_flagging = "never"
-live = True
-
-gr.Interface(
- inference,
- #[gr.inputs.Image(type="filepath", label="Input"), gr.inputs.CheckboxGroup(choices, type="value", default=['eng'], label='language')],
- gr.Image(type="filepath", label="Input"),
- "text",
- title=title,
- description=description,
- article=article,
- examples=examples,
- allow_flagging=allow_flagging,
- live=live
-).launch(debug=False, enable_queue=True)
\ No newline at end of file
diff --git a/spaces/pknez/face-swap-docker/mynewshinyroop/Lib/site-packages/pip/_vendor/pyparsing/exceptions.py b/spaces/pknez/face-swap-docker/mynewshinyroop/Lib/site-packages/pip/_vendor/pyparsing/exceptions.py
deleted file mode 100644
index 12219f124aeca6d3d7edd2621071f100c7ecd90a..0000000000000000000000000000000000000000
--- a/spaces/pknez/face-swap-docker/mynewshinyroop/Lib/site-packages/pip/_vendor/pyparsing/exceptions.py
+++ /dev/null
@@ -1,299 +0,0 @@
-# exceptions.py
-
-import re
-import sys
-import typing
-
-from .util import (
- col,
- line,
- lineno,
- _collapse_string_to_ranges,
- replaced_by_pep8,
-)
-from .unicode import pyparsing_unicode as ppu
-
-
-class ExceptionWordUnicode(ppu.Latin1, ppu.LatinA, ppu.LatinB, ppu.Greek, ppu.Cyrillic):
- pass
-
-
-_extract_alphanums = _collapse_string_to_ranges(ExceptionWordUnicode.alphanums)
-_exception_word_extractor = re.compile("([" + _extract_alphanums + "]{1,16})|.")
-
-
-class ParseBaseException(Exception):
- """base exception class for all parsing runtime exceptions"""
-
- loc: int
- msg: str
- pstr: str
- parser_element: typing.Any # "ParserElement"
- args: typing.Tuple[str, int, typing.Optional[str]]
-
- __slots__ = (
- "loc",
- "msg",
- "pstr",
- "parser_element",
- "args",
- )
-
- # Performance tuning: we construct a *lot* of these, so keep this
- # constructor as small and fast as possible
- def __init__(
- self,
- pstr: str,
- loc: int = 0,
- msg: typing.Optional[str] = None,
- elem=None,
- ):
- self.loc = loc
- if msg is None:
- self.msg = pstr
- self.pstr = ""
- else:
- self.msg = msg
- self.pstr = pstr
- self.parser_element = elem
- self.args = (pstr, loc, msg)
-
- @staticmethod
- def explain_exception(exc, depth=16):
- """
- Method to take an exception and translate the Python internal traceback into a list
- of the pyparsing expressions that caused the exception to be raised.
-
- Parameters:
-
- - exc - exception raised during parsing (need not be a ParseException, in support
- of Python exceptions that might be raised in a parse action)
- - depth (default=16) - number of levels back in the stack trace to list expression
- and function names; if None, the full stack trace names will be listed; if 0, only
- the failing input line, marker, and exception string will be shown
-
- Returns a multi-line string listing the ParserElements and/or function names in the
- exception's stack trace.
- """
- import inspect
- from .core import ParserElement
-
- if depth is None:
- depth = sys.getrecursionlimit()
- ret = []
- if isinstance(exc, ParseBaseException):
- ret.append(exc.line)
- ret.append(" " * (exc.column - 1) + "^")
- ret.append(f"{type(exc).__name__}: {exc}")
-
- if depth > 0:
- callers = inspect.getinnerframes(exc.__traceback__, context=depth)
- seen = set()
- for i, ff in enumerate(callers[-depth:]):
- frm = ff[0]
-
- f_self = frm.f_locals.get("self", None)
- if isinstance(f_self, ParserElement):
- if not frm.f_code.co_name.startswith(
- ("parseImpl", "_parseNoCache")
- ):
- continue
- if id(f_self) in seen:
- continue
- seen.add(id(f_self))
-
- self_type = type(f_self)
- ret.append(
- f"{self_type.__module__}.{self_type.__name__} - {f_self}"
- )
-
- elif f_self is not None:
- self_type = type(f_self)
- ret.append(f"{self_type.__module__}.{self_type.__name__}")
-
- else:
- code = frm.f_code
- if code.co_name in ("wrapper", ""):
- continue
-
- ret.append(code.co_name)
-
- depth -= 1
- if not depth:
- break
-
- return "\n".join(ret)
-
- @classmethod
- def _from_exception(cls, pe):
- """
- internal factory method to simplify creating one type of ParseException
- from another - avoids having __init__ signature conflicts among subclasses
- """
- return cls(pe.pstr, pe.loc, pe.msg, pe.parser_element)
-
- @property
- def line(self) -> str:
- """
- Return the line of text where the exception occurred.
- """
- return line(self.loc, self.pstr)
-
- @property
- def lineno(self) -> int:
- """
- Return the 1-based line number of text where the exception occurred.
- """
- return lineno(self.loc, self.pstr)
-
- @property
- def col(self) -> int:
- """
- Return the 1-based column on the line of text where the exception occurred.
- """
- return col(self.loc, self.pstr)
-
- @property
- def column(self) -> int:
- """
- Return the 1-based column on the line of text where the exception occurred.
- """
- return col(self.loc, self.pstr)
-
- # pre-PEP8 compatibility
- @property
- def parserElement(self):
- return self.parser_element
-
- @parserElement.setter
- def parserElement(self, elem):
- self.parser_element = elem
-
- def __str__(self) -> str:
- if self.pstr:
- if self.loc >= len(self.pstr):
- foundstr = ", found end of text"
- else:
- # pull out next word at error location
- found_match = _exception_word_extractor.match(self.pstr, self.loc)
- if found_match is not None:
- found = found_match.group(0)
- else:
- found = self.pstr[self.loc : self.loc + 1]
- foundstr = (", found %r" % found).replace(r"\\", "\\")
- else:
- foundstr = ""
- return f"{self.msg}{foundstr} (at char {self.loc}), (line:{self.lineno}, col:{self.column})"
-
- def __repr__(self):
- return str(self)
-
- def mark_input_line(
- self, marker_string: typing.Optional[str] = None, *, markerString: str = ">!<"
- ) -> str:
- """
- Extracts the exception line from the input string, and marks
- the location of the exception with a special symbol.
- """
- markerString = marker_string if marker_string is not None else markerString
- line_str = self.line
- line_column = self.column - 1
- if markerString:
- line_str = "".join(
- (line_str[:line_column], markerString, line_str[line_column:])
- )
- return line_str.strip()
-
- def explain(self, depth=16) -> str:
- """
- Method to translate the Python internal traceback into a list
- of the pyparsing expressions that caused the exception to be raised.
-
- Parameters:
-
- - depth (default=16) - number of levels back in the stack trace to list expression
- and function names; if None, the full stack trace names will be listed; if 0, only
- the failing input line, marker, and exception string will be shown
-
- Returns a multi-line string listing the ParserElements and/or function names in the
- exception's stack trace.
-
- Example::
-
- expr = pp.Word(pp.nums) * 3
- try:
- expr.parse_string("123 456 A789")
- except pp.ParseException as pe:
- print(pe.explain(depth=0))
-
- prints::
-
- 123 456 A789
- ^
- ParseException: Expected W:(0-9), found 'A' (at char 8), (line:1, col:9)
-
- Note: the diagnostic output will include string representations of the expressions
- that failed to parse. These representations will be more helpful if you use `set_name` to
- give identifiable names to your expressions. Otherwise they will use the default string
- forms, which may be cryptic to read.
-
- Note: pyparsing's default truncation of exception tracebacks may also truncate the
- stack of expressions that are displayed in the ``explain`` output. To get the full listing
- of parser expressions, you may have to set ``ParserElement.verbose_stacktrace = True``
- """
- return self.explain_exception(self, depth)
-
- # fmt: off
- @replaced_by_pep8(mark_input_line)
- def markInputline(self): ...
- # fmt: on
-
-
-class ParseException(ParseBaseException):
- """
- Exception thrown when a parse expression doesn't match the input string
-
- Example::
-
- try:
- Word(nums).set_name("integer").parse_string("ABC")
- except ParseException as pe:
- print(pe)
- print("column: {}".format(pe.column))
-
- prints::
-
- Expected integer (at char 0), (line:1, col:1)
- column: 1
-
- """
-
-
-class ParseFatalException(ParseBaseException):
- """
- User-throwable exception thrown when inconsistent parse content
- is found; stops all parsing immediately
- """
-
-
-class ParseSyntaxException(ParseFatalException):
- """
- Just like :class:`ParseFatalException`, but thrown internally
- when an :class:`ErrorStop` ('-' operator) indicates
- that parsing is to stop immediately because an unbacktrackable
- syntax error has been found.
- """
-
-
-class RecursiveGrammarException(Exception):
- """
- Exception thrown by :class:`ParserElement.validate` if the
- grammar could be left-recursive; parser may need to enable
- left recursion using :class:`ParserElement.enable_left_recursion`
- """
-
- def __init__(self, parseElementList):
- self.parseElementTrace = parseElementList
-
- def __str__(self) -> str:
- return f"RecursiveGrammarException: {self.parseElementTrace}"
diff --git a/spaces/platzi/platzi-curso-streamlit-segmentacion-imagenes/app.py b/spaces/platzi/platzi-curso-streamlit-segmentacion-imagenes/app.py
deleted file mode 100644
index d80e0f51365480c5d6a14e1daf0b695226675b27..0000000000000000000000000000000000000000
--- a/spaces/platzi/platzi-curso-streamlit-segmentacion-imagenes/app.py
+++ /dev/null
@@ -1,108 +0,0 @@
-import streamlit as st
-from PIL import Image
-import numpy as np
-import cv2
-from huggingface_hub import from_pretrained_keras
-
-st.header("Segmentación de dientes con rayos X")
-
-st.markdown(
- """
-
-Hola estudiantes de Platzi 🚀. Este modelo usa UNet para segmentar imágenes
-de dientes en rayos X. Se utila un modelo de Keras importado con la función
-`huggingface_hub.from_pretrained_keras`. Recuerda que el Hub de Hugging Face está integrado
-con muchas librerías como Keras, scikit-learn, fastai y otras.
-
-El modelo fue creado por [SerdarHelli](https://huggingface.co/SerdarHelli/Segmentation-of-Teeth-in-Panoramic-X-ray-Image-Using-U-Net).
-
-"""
-)
-
-## Seleccionamos y cargamos el modelo
-model_id = "SerdarHelli/Segmentation-of-Teeth-in-Panoramic-X-ray-Image-Using-U-Net"
-model = from_pretrained_keras(model_id)
-
-## Permitimos a la usuaria cargar una imagen
-archivo_imagen = st.file_uploader("Sube aquí tu imagen.", type=["png", "jpg", "jpeg"])
-
-## Si una imagen tiene más de un canal entonces se convierte a escala de grises (1 canal)
-def convertir_one_channel(img):
- if len(img.shape) > 2:
- img = cv2.cvtColor(img, cv2.COLOR_BGR2GRAY)
- return img
- else:
- return img
-
-
-def convertir_rgb(img):
- if len(img.shape) == 2:
- img = cv2.cvtColor(img, cv2.COLOR_GRAY2RGB)
- return img
- else:
- return img
-
-
-## Manipularemos la interfaz para que podamos usar imágenes ejemplo
-## Si el usuario da click en un ejemplo entonces el modelo correrá con él
-ejemplos = ["dientes_1.png", "dientes_2.png", "dientes_3.png"]
-
-## Creamos tres columnas; en cada una estará una imagen ejemplo
-col1, col2, col3 = st.columns(3)
-with col1:
- ## Se carga la imagen y se muestra en la interfaz
- ex = Image.open(ejemplos[0])
- st.image(ex, width=200)
- ## Si oprime el botón entonces usaremos ese ejemplo en el modelo
- if st.button("Corre este ejemplo 1"):
- archivo_imagen = ejemplos[0]
-
-with col2:
- ex1 = Image.open(ejemplos[1])
- st.image(ex1, width=200)
- if st.button("Corre este ejemplo 2"):
- archivo_imagen = ejemplos[1]
-
-with col3:
- ex2 = Image.open(ejemplos[2])
- st.image(ex2, width=200)
- if st.button("Corre este ejemplo 3"):
- archivo_imagen = ejemplos[2]
-
-## Si tenemos una imagen para ingresar en el modelo entonces
-## la procesamos e ingresamos al modelo
-if archivo_imagen is not None:
- ## Cargamos la imagen con PIL, la mostramos y la convertimos a un array de NumPy
- img = Image.open(archivo_imagen)
- st.image(img, width=850)
- img = np.asarray(img)
-
- ## Procesamos la imagen para ingresarla al modelo
- img_cv = convertir_one_channel(img)
- img_cv = cv2.resize(img_cv, (512, 512), interpolation=cv2.INTER_LANCZOS4)
- img_cv = np.float32(img_cv / 255)
- img_cv = np.reshape(img_cv, (1, 512, 512, 1))
-
- ## Ingresamos el array de NumPy al modelo
- predicted = model.predict(img_cv)
- predicted = predicted[0]
-
- ## Regresamos la imagen a su forma original y agregamos las máscaras de la segmentación
- predicted = cv2.resize(
- predicted, (img.shape[1], img.shape[0]), interpolation=cv2.INTER_LANCZOS4
- )
- mask = np.uint8(predicted * 255) #
- _, mask = cv2.threshold(
- mask, thresh=0, maxval=255, type=cv2.THRESH_BINARY + cv2.THRESH_OTSU
- )
- kernel = np.ones((5, 5), dtype=np.float32)
- mask = cv2.morphologyEx(mask, cv2.MORPH_OPEN, kernel, iterations=1)
- mask = cv2.morphologyEx(mask, cv2.MORPH_CLOSE, kernel, iterations=1)
- cnts, hieararch = cv2.findContours(mask, cv2.RETR_TREE, cv2.CHAIN_APPROX_SIMPLE)
- output = cv2.drawContours(convertir_one_channel(img), cnts, -1, (255, 0, 0), 3)
-
- ## Si obtuvimos exitosamente un resultadod entonces lo mostramos en la interfaz
- if output is not None:
- st.subheader("Segmentación:")
- st.write(output.shape)
- st.image(output, width=850)
diff --git a/spaces/pojitha/sinhala_hate_speech/README.md b/spaces/pojitha/sinhala_hate_speech/README.md
deleted file mode 100644
index f5df92ac2886e20c0ddc5fd371611a427427417b..0000000000000000000000000000000000000000
--- a/spaces/pojitha/sinhala_hate_speech/README.md
+++ /dev/null
@@ -1,12 +0,0 @@
----
-title: Sinhala Hate Speech
-emoji: 📚
-colorFrom: purple
-colorTo: green
-sdk: streamlit
-sdk_version: 1.17.0
-app_file: app.py
-pinned: false
----
-
-Check out the configuration reference at https://huggingface.co/docs/hub/spaces-config-reference
diff --git a/spaces/prerna9811/Chord/portaudio/bindings/java/jportaudio/src/com/portaudio/DeviceInfo.java b/spaces/prerna9811/Chord/portaudio/bindings/java/jportaudio/src/com/portaudio/DeviceInfo.java
deleted file mode 100644
index 1c4682ec50732882a9d657c26d5b6ab19990691a..0000000000000000000000000000000000000000
--- a/spaces/prerna9811/Chord/portaudio/bindings/java/jportaudio/src/com/portaudio/DeviceInfo.java
+++ /dev/null
@@ -1,65 +0,0 @@
-/*
- * Portable Audio I/O Library
- * Java Binding for PortAudio
- *
- * Based on the Open Source API proposed by Ross Bencina
- * Copyright (c) 2008 Ross Bencina
- *
- * Permission is hereby granted, free of charge, to any person obtaining
- * a copy of this software and associated documentation files
- * (the "Software"), to deal in the Software without restriction,
- * including without limitation the rights to use, copy, modify, merge,
- * publish, distribute, sublicense, and/or sell copies of the Software,
- * and to permit persons to whom the Software is furnished to do so,
- * subject to the following conditions:
- *
- * The above copyright notice and this permission notice shall be
- * included in all copies or substantial portions of the Software.
- *
- * THE SOFTWARE IS PROVIDED "AS IS", WITHOUT WARRANTY OF ANY KIND,
- * EXPRESS OR IMPLIED, INCLUDING BUT NOT LIMITED TO THE WARRANTIES OF
- * MERCHANTABILITY, FITNESS FOR A PARTICULAR PURPOSE AND NONINFRINGEMENT.
- * IN NO EVENT SHALL THE AUTHORS OR COPYRIGHT HOLDERS BE LIABLE FOR
- * ANY CLAIM, DAMAGES OR OTHER LIABILITY, WHETHER IN AN ACTION OF
- * CONTRACT, TORT OR OTHERWISE, ARISING FROM, OUT OF OR IN CONNECTION
- * WITH THE SOFTWARE OR THE USE OR OTHER DEALINGS IN THE SOFTWARE.
- */
-
-/*
- * The text above constitutes the entire PortAudio license; however,
- * the PortAudio community also makes the following non-binding requests:
- *
- * Any person wishing to distribute modifications to the Software is
- * requested to send the modifications to the original developer so that
- * they can be incorporated into the canonical version. It is also
- * requested that these non-binding requests be included along with the
- * license above.
- */
-
-/** @file
- @ingroup bindings_java
-
- @brief Information about a JPortAudio device.
-*/
-package com.portaudio;
-
-/**
- * Equivalent to PaDeviceInfo
- * @see PortAudio
- * @see HostApiInfo
- * @author Phil Burk
- *
- */
-public class DeviceInfo
-{
- public int version;
- public String name;
- public int hostApi;
- public int maxInputChannels;
- public int maxOutputChannels;
- public double defaultLowInputLatency;
- public double defaultHighInputLatency;
- public double defaultLowOutputLatency;
- public double defaultHighOutputLatency;
- public double defaultSampleRate;
-}
diff --git a/spaces/prithvihehe/TheBotFather/app.py b/spaces/prithvihehe/TheBotFather/app.py
deleted file mode 100644
index fe63dcfe56c0524b4ace27f39afba71f2d29f727..0000000000000000000000000000000000000000
--- a/spaces/prithvihehe/TheBotFather/app.py
+++ /dev/null
@@ -1,42 +0,0 @@
-import openai
-import gradio
-
-
-openai.api_key = "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"
-
-messages = [{"role": "system", "content": "You are Vito Corleone from the Godfather, act wise and help people who come to you, and also speak like him"}]
-
-def MyChatGPT(user_input):
- messages.append({"role": "user", "content": user_input})
- response = openai.ChatCompletion.create(
- model = "gpt-3.5-turbo",
- messages = messages
- )
- reply = response["choices"][0]["message"]["content"]
- messages.append({"role": "assistant", "content": reply})
- return reply
-
-def chatbot(input, history = []):
- output = MyChatGPT(input)
- avatar_url = "https://mcdn.wallpapersafari.com/medium/43/60/bFauO9.jpg"
- message_with_avatar = f'
{output}
'
- history.append((input, message_with_avatar))
- return history, history
-
-
-demo = gradio.Interface(fn=chatbot, inputs = ["text", 'state'], outputs = ["chatbot",'state'], title = "TheBotFather")
-
-css = """
-body {
- background-image: url('https://c4.wallpaperflare.com/wallpaper/484/369/194/movies-the-godfather-al-pacino-wallpaper-preview.jpg');
- background-size: cover;
- opacity: 0.9;
-}
-.gradio-input-wrapper, .gradio-output-wrapper {
- background-color: rgba(255, 255, 255, 0.95) !important;
-
-}
-"""
-demo.css = css
-
-demo.launch()
diff --git a/spaces/profayle/TerrapinTalk/myenv/lib/python3.9/site-packages/altair/utils/core.py b/spaces/profayle/TerrapinTalk/myenv/lib/python3.9/site-packages/altair/utils/core.py
deleted file mode 100644
index f365ce96235d5ee633ee08ba0de14d3dacc3efe3..0000000000000000000000000000000000000000
--- a/spaces/profayle/TerrapinTalk/myenv/lib/python3.9/site-packages/altair/utils/core.py
+++ /dev/null
@@ -1,843 +0,0 @@
-"""
-Utility routines
-"""
-from collections.abc import Mapping, MutableMapping
-from copy import deepcopy
-import json
-import itertools
-import re
-import sys
-import traceback
-import warnings
-from typing import (
- Callable,
- TypeVar,
- Any,
- Union,
- Dict,
- Optional,
- Tuple,
- Sequence,
- Type,
- cast,
-)
-from types import ModuleType
-
-import jsonschema
-import pandas as pd
-import numpy as np
-from pandas.api.types import infer_dtype
-
-from altair.utils.schemapi import SchemaBase
-from altair.utils._dfi_types import Column, DtypeKind, DataFrame as DfiDataFrame
-
-if sys.version_info >= (3, 10):
- from typing import ParamSpec
-else:
- from typing_extensions import ParamSpec
-
-from typing import Literal, Protocol, TYPE_CHECKING
-
-if TYPE_CHECKING:
- from pandas.core.interchange.dataframe_protocol import Column as PandasColumn
-
-_V = TypeVar("_V")
-_P = ParamSpec("_P")
-
-
-class _DataFrameLike(Protocol):
- def __dataframe__(self, *args, **kwargs) -> DfiDataFrame:
- ...
-
-
-TYPECODE_MAP = {
- "ordinal": "O",
- "nominal": "N",
- "quantitative": "Q",
- "temporal": "T",
- "geojson": "G",
-}
-
-INV_TYPECODE_MAP = {v: k for k, v in TYPECODE_MAP.items()}
-
-
-# aggregates from vega-lite version 4.6.0
-AGGREGATES = [
- "argmax",
- "argmin",
- "average",
- "count",
- "distinct",
- "max",
- "mean",
- "median",
- "min",
- "missing",
- "product",
- "q1",
- "q3",
- "ci0",
- "ci1",
- "stderr",
- "stdev",
- "stdevp",
- "sum",
- "valid",
- "values",
- "variance",
- "variancep",
-]
-
-# window aggregates from vega-lite version 4.6.0
-WINDOW_AGGREGATES = [
- "row_number",
- "rank",
- "dense_rank",
- "percent_rank",
- "cume_dist",
- "ntile",
- "lag",
- "lead",
- "first_value",
- "last_value",
- "nth_value",
-]
-
-# timeUnits from vega-lite version 4.17.0
-TIMEUNITS = [
- "year",
- "quarter",
- "month",
- "week",
- "day",
- "dayofyear",
- "date",
- "hours",
- "minutes",
- "seconds",
- "milliseconds",
- "yearquarter",
- "yearquartermonth",
- "yearmonth",
- "yearmonthdate",
- "yearmonthdatehours",
- "yearmonthdatehoursminutes",
- "yearmonthdatehoursminutesseconds",
- "yearweek",
- "yearweekday",
- "yearweekdayhours",
- "yearweekdayhoursminutes",
- "yearweekdayhoursminutesseconds",
- "yeardayofyear",
- "quartermonth",
- "monthdate",
- "monthdatehours",
- "monthdatehoursminutes",
- "monthdatehoursminutesseconds",
- "weekday",
- "weeksdayhours",
- "weekdayhoursminutes",
- "weekdayhoursminutesseconds",
- "dayhours",
- "dayhoursminutes",
- "dayhoursminutesseconds",
- "hoursminutes",
- "hoursminutesseconds",
- "minutesseconds",
- "secondsmilliseconds",
- "utcyear",
- "utcquarter",
- "utcmonth",
- "utcweek",
- "utcday",
- "utcdayofyear",
- "utcdate",
- "utchours",
- "utcminutes",
- "utcseconds",
- "utcmilliseconds",
- "utcyearquarter",
- "utcyearquartermonth",
- "utcyearmonth",
- "utcyearmonthdate",
- "utcyearmonthdatehours",
- "utcyearmonthdatehoursminutes",
- "utcyearmonthdatehoursminutesseconds",
- "utcyearweek",
- "utcyearweekday",
- "utcyearweekdayhours",
- "utcyearweekdayhoursminutes",
- "utcyearweekdayhoursminutesseconds",
- "utcyeardayofyear",
- "utcquartermonth",
- "utcmonthdate",
- "utcmonthdatehours",
- "utcmonthdatehoursminutes",
- "utcmonthdatehoursminutesseconds",
- "utcweekday",
- "utcweeksdayhours",
- "utcweekdayhoursminutes",
- "utcweekdayhoursminutesseconds",
- "utcdayhours",
- "utcdayhoursminutes",
- "utcdayhoursminutesseconds",
- "utchoursminutes",
- "utchoursminutesseconds",
- "utcminutesseconds",
- "utcsecondsmilliseconds",
-]
-
-
-_InferredVegaLiteType = Literal["ordinal", "nominal", "quantitative", "temporal"]
-
-
-def infer_vegalite_type(
- data: object,
-) -> Union[_InferredVegaLiteType, Tuple[_InferredVegaLiteType, list]]:
- """
- From an array-like input, infer the correct vega typecode
- ('ordinal', 'nominal', 'quantitative', or 'temporal')
-
- Parameters
- ----------
- data: object
- """
- typ = infer_dtype(data, skipna=False)
-
- if typ in [
- "floating",
- "mixed-integer-float",
- "integer",
- "mixed-integer",
- "complex",
- ]:
- return "quantitative"
- elif typ == "categorical" and hasattr(data, "cat") and data.cat.ordered:
- return ("ordinal", data.cat.categories.tolist())
- elif typ in ["string", "bytes", "categorical", "boolean", "mixed", "unicode"]:
- return "nominal"
- elif typ in [
- "datetime",
- "datetime64",
- "timedelta",
- "timedelta64",
- "date",
- "time",
- "period",
- ]:
- return "temporal"
- else:
- warnings.warn(
- "I don't know how to infer vegalite type from '{}'. "
- "Defaulting to nominal.".format(typ),
- stacklevel=1,
- )
- return "nominal"
-
-
-def merge_props_geom(feat: dict) -> dict:
- """
- Merge properties with geometry
- * Overwrites 'type' and 'geometry' entries if existing
- """
-
- geom = {k: feat[k] for k in ("type", "geometry")}
- try:
- feat["properties"].update(geom)
- props_geom = feat["properties"]
- except (AttributeError, KeyError):
- # AttributeError when 'properties' equals None
- # KeyError when 'properties' is non-existing
- props_geom = geom
-
- return props_geom
-
-
-def sanitize_geo_interface(geo: MutableMapping) -> dict:
- """Santize a geo_interface to prepare it for serialization.
-
- * Make a copy
- * Convert type array or _Array to list
- * Convert tuples to lists (using json.loads/dumps)
- * Merge properties with geometry
- """
-
- geo = deepcopy(geo)
-
- # convert type _Array or array to list
- for key in geo.keys():
- if str(type(geo[key]).__name__).startswith(("_Array", "array")):
- geo[key] = geo[key].tolist()
-
- # convert (nested) tuples to lists
- geo_dct: dict = json.loads(json.dumps(geo))
-
- # sanitize features
- if geo_dct["type"] == "FeatureCollection":
- geo_dct = geo_dct["features"]
- if len(geo_dct) > 0:
- for idx, feat in enumerate(geo_dct):
- geo_dct[idx] = merge_props_geom(feat)
- elif geo_dct["type"] == "Feature":
- geo_dct = merge_props_geom(geo_dct)
- else:
- geo_dct = {"type": "Feature", "geometry": geo_dct}
-
- return geo_dct
-
-
-def numpy_is_subtype(dtype: Any, subtype: Any) -> bool:
- try:
- return np.issubdtype(dtype, subtype)
- except (NotImplementedError, TypeError):
- return False
-
-
-def sanitize_dataframe(df: pd.DataFrame) -> pd.DataFrame: # noqa: C901
- """Sanitize a DataFrame to prepare it for serialization.
-
- * Make a copy
- * Convert RangeIndex columns to strings
- * Raise ValueError if column names are not strings
- * Raise ValueError if it has a hierarchical index.
- * Convert categoricals to strings.
- * Convert np.bool_ dtypes to Python bool objects
- * Convert np.int dtypes to Python int objects
- * Convert floats to objects and replace NaNs/infs with None.
- * Convert DateTime dtypes into appropriate string representations
- * Convert Nullable integers to objects and replace NaN with None
- * Convert Nullable boolean to objects and replace NaN with None
- * convert dedicated string column to objects and replace NaN with None
- * Raise a ValueError for TimeDelta dtypes
- """
- df = df.copy()
-
- if isinstance(df.columns, pd.RangeIndex):
- df.columns = df.columns.astype(str)
-
- for col_name in df.columns:
- if not isinstance(col_name, str):
- raise ValueError(
- "Dataframe contains invalid column name: {0!r}. "
- "Column names must be strings".format(col_name)
- )
-
- if isinstance(df.index, pd.MultiIndex):
- raise ValueError("Hierarchical indices not supported")
- if isinstance(df.columns, pd.MultiIndex):
- raise ValueError("Hierarchical indices not supported")
-
- def to_list_if_array(val):
- if isinstance(val, np.ndarray):
- return val.tolist()
- else:
- return val
-
- for dtype_item in df.dtypes.items():
- # We know that the column names are strings from the isinstance check
- # further above but mypy thinks it is of type Hashable and therefore does not
- # let us assign it to the col_name variable which is already of type str.
- col_name = cast(str, dtype_item[0])
- dtype = dtype_item[1]
- dtype_name = str(dtype)
- if dtype_name == "category":
- # Work around bug in to_json for categorical types in older versions
- # of pandas as they do not properly convert NaN values to null in to_json.
- # We can probably remove this part once we require Pandas >= 1.0
- col = df[col_name].astype(object)
- df[col_name] = col.where(col.notnull(), None)
- elif dtype_name == "string":
- # dedicated string datatype (since 1.0)
- # https://pandas.pydata.org/pandas-docs/version/1.0.0/whatsnew/v1.0.0.html#dedicated-string-data-type
- col = df[col_name].astype(object)
- df[col_name] = col.where(col.notnull(), None)
- elif dtype_name == "bool":
- # convert numpy bools to objects; np.bool is not JSON serializable
- df[col_name] = df[col_name].astype(object)
- elif dtype_name == "boolean":
- # dedicated boolean datatype (since 1.0)
- # https://pandas.io/docs/user_guide/boolean.html
- col = df[col_name].astype(object)
- df[col_name] = col.where(col.notnull(), None)
- elif dtype_name.startswith("datetime") or dtype_name.startswith("timestamp"):
- # Convert datetimes to strings. This needs to be a full ISO string
- # with time, which is why we cannot use ``col.astype(str)``.
- # This is because Javascript parses date-only times in UTC, but
- # parses full ISO-8601 dates as local time, and dates in Vega and
- # Vega-Lite are displayed in local time by default.
- # (see https://github.com/altair-viz/altair/issues/1027)
- df[col_name] = (
- df[col_name].apply(lambda x: x.isoformat()).replace("NaT", "")
- )
- elif dtype_name.startswith("timedelta"):
- raise ValueError(
- 'Field "{col_name}" has type "{dtype}" which is '
- "not supported by Altair. Please convert to "
- "either a timestamp or a numerical value."
- "".format(col_name=col_name, dtype=dtype)
- )
- elif dtype_name.startswith("geometry"):
- # geopandas >=0.6.1 uses the dtype geometry. Continue here
- # otherwise it will give an error on np.issubdtype(dtype, np.integer)
- continue
- elif dtype_name in {
- "Int8",
- "Int16",
- "Int32",
- "Int64",
- "UInt8",
- "UInt16",
- "UInt32",
- "UInt64",
- "Float32",
- "Float64",
- }: # nullable integer datatypes (since 24.0) and nullable float datatypes (since 1.2.0)
- # https://pandas.pydata.org/pandas-docs/version/0.25/whatsnew/v0.24.0.html#optional-integer-na-support
- col = df[col_name].astype(object)
- df[col_name] = col.where(col.notnull(), None)
- elif numpy_is_subtype(dtype, np.integer):
- # convert integers to objects; np.int is not JSON serializable
- df[col_name] = df[col_name].astype(object)
- elif numpy_is_subtype(dtype, np.floating):
- # For floats, convert to Python float: np.float is not JSON serializable
- # Also convert NaN/inf values to null, as they are not JSON serializable
- col = df[col_name]
- bad_values = col.isnull() | np.isinf(col)
- df[col_name] = col.astype(object).where(~bad_values, None)
- elif dtype == object:
- # Convert numpy arrays saved as objects to lists
- # Arrays are not JSON serializable
- col = df[col_name].astype(object).apply(to_list_if_array)
- df[col_name] = col.where(col.notnull(), None)
- return df
-
-
-def sanitize_arrow_table(pa_table):
- """Sanitize arrow table for JSON serialization"""
- import pyarrow as pa
- import pyarrow.compute as pc
-
- arrays = []
- schema = pa_table.schema
- for name in schema.names:
- array = pa_table[name]
- dtype = schema.field(name).type
- if str(dtype).startswith("timestamp"):
- arrays.append(pc.strftime(array))
- elif str(dtype).startswith("duration"):
- raise ValueError(
- 'Field "{col_name}" has type "{dtype}" which is '
- "not supported by Altair. Please convert to "
- "either a timestamp or a numerical value."
- "".format(col_name=name, dtype=dtype)
- )
- else:
- arrays.append(array)
-
- return pa.Table.from_arrays(arrays, names=schema.names)
-
-
-def parse_shorthand(
- shorthand: Union[Dict[str, Any], str],
- data: Optional[Union[pd.DataFrame, _DataFrameLike]] = None,
- parse_aggregates: bool = True,
- parse_window_ops: bool = False,
- parse_timeunits: bool = True,
- parse_types: bool = True,
-) -> Dict[str, Any]:
- """General tool to parse shorthand values
-
- These are of the form:
-
- - "col_name"
- - "col_name:O"
- - "average(col_name)"
- - "average(col_name):O"
-
- Optionally, a dataframe may be supplied, from which the type
- will be inferred if not specified in the shorthand.
-
- Parameters
- ----------
- shorthand : dict or string
- The shorthand representation to be parsed
- data : DataFrame, optional
- If specified and of type DataFrame, then use these values to infer the
- column type if not provided by the shorthand.
- parse_aggregates : boolean
- If True (default), then parse aggregate functions within the shorthand.
- parse_window_ops : boolean
- If True then parse window operations within the shorthand (default:False)
- parse_timeunits : boolean
- If True (default), then parse timeUnits from within the shorthand
- parse_types : boolean
- If True (default), then parse typecodes within the shorthand
-
- Returns
- -------
- attrs : dict
- a dictionary of attributes extracted from the shorthand
-
- Examples
- --------
- >>> data = pd.DataFrame({'foo': ['A', 'B', 'A', 'B'],
- ... 'bar': [1, 2, 3, 4]})
-
- >>> parse_shorthand('name') == {'field': 'name'}
- True
-
- >>> parse_shorthand('name:Q') == {'field': 'name', 'type': 'quantitative'}
- True
-
- >>> parse_shorthand('average(col)') == {'aggregate': 'average', 'field': 'col'}
- True
-
- >>> parse_shorthand('foo:O') == {'field': 'foo', 'type': 'ordinal'}
- True
-
- >>> parse_shorthand('min(foo):Q') == {'aggregate': 'min', 'field': 'foo', 'type': 'quantitative'}
- True
-
- >>> parse_shorthand('month(col)') == {'field': 'col', 'timeUnit': 'month', 'type': 'temporal'}
- True
-
- >>> parse_shorthand('year(col):O') == {'field': 'col', 'timeUnit': 'year', 'type': 'ordinal'}
- True
-
- >>> parse_shorthand('foo', data) == {'field': 'foo', 'type': 'nominal'}
- True
-
- >>> parse_shorthand('bar', data) == {'field': 'bar', 'type': 'quantitative'}
- True
-
- >>> parse_shorthand('bar:O', data) == {'field': 'bar', 'type': 'ordinal'}
- True
-
- >>> parse_shorthand('sum(bar)', data) == {'aggregate': 'sum', 'field': 'bar', 'type': 'quantitative'}
- True
-
- >>> parse_shorthand('count()', data) == {'aggregate': 'count', 'type': 'quantitative'}
- True
- """
- from altair.utils._importers import pyarrow_available
-
- if not shorthand:
- return {}
-
- valid_typecodes = list(TYPECODE_MAP) + list(INV_TYPECODE_MAP)
-
- units = {
- "field": "(?P.*)",
- "type": "(?P{})".format("|".join(valid_typecodes)),
- "agg_count": "(?Pcount)",
- "op_count": "(?Pcount)",
- "aggregate": "(?P{})".format("|".join(AGGREGATES)),
- "window_op": "(?P{})".format("|".join(AGGREGATES + WINDOW_AGGREGATES)),
- "timeUnit": "(?P{})".format("|".join(TIMEUNITS)),
- }
-
- patterns = []
-
- if parse_aggregates:
- patterns.extend([r"{agg_count}\(\)"])
- patterns.extend([r"{aggregate}\({field}\)"])
- if parse_window_ops:
- patterns.extend([r"{op_count}\(\)"])
- patterns.extend([r"{window_op}\({field}\)"])
- if parse_timeunits:
- patterns.extend([r"{timeUnit}\({field}\)"])
-
- patterns.extend([r"{field}"])
-
- if parse_types:
- patterns = list(itertools.chain(*((p + ":{type}", p) for p in patterns)))
-
- regexps = (
- re.compile(r"\A" + p.format(**units) + r"\Z", re.DOTALL) for p in patterns
- )
-
- # find matches depending on valid fields passed
- if isinstance(shorthand, dict):
- attrs = shorthand
- else:
- attrs = next(
- exp.match(shorthand).groupdict() # type: ignore[union-attr]
- for exp in regexps
- if exp.match(shorthand) is not None
- )
-
- # Handle short form of the type expression
- if "type" in attrs:
- attrs["type"] = INV_TYPECODE_MAP.get(attrs["type"], attrs["type"])
-
- # counts are quantitative by default
- if attrs == {"aggregate": "count"}:
- attrs["type"] = "quantitative"
-
- # times are temporal by default
- if "timeUnit" in attrs and "type" not in attrs:
- attrs["type"] = "temporal"
-
- # if data is specified and type is not, infer type from data
- if "type" not in attrs:
- if pyarrow_available() and data is not None and hasattr(data, "__dataframe__"):
- dfi = data.__dataframe__()
- if "field" in attrs:
- unescaped_field = attrs["field"].replace("\\", "")
- if unescaped_field in dfi.column_names():
- column = dfi.get_column_by_name(unescaped_field)
- try:
- attrs["type"] = infer_vegalite_type_for_dfi_column(column)
- except (NotImplementedError, AttributeError, ValueError):
- # Fall back to pandas-based inference.
- # Note: The AttributeError catch is a workaround for
- # https://github.com/pandas-dev/pandas/issues/55332
- if isinstance(data, pd.DataFrame):
- attrs["type"] = infer_vegalite_type(data[unescaped_field])
- else:
- raise
-
- if isinstance(attrs["type"], tuple):
- attrs["sort"] = attrs["type"][1]
- attrs["type"] = attrs["type"][0]
- elif isinstance(data, pd.DataFrame):
- # Fallback if pyarrow is not installed or if pandas is older than 1.5
- #
- # Remove escape sequences so that types can be inferred for columns with special characters
- if "field" in attrs and attrs["field"].replace("\\", "") in data.columns:
- attrs["type"] = infer_vegalite_type(
- data[attrs["field"].replace("\\", "")]
- )
- # ordered categorical dataframe columns return the type and sort order as a tuple
- if isinstance(attrs["type"], tuple):
- attrs["sort"] = attrs["type"][1]
- attrs["type"] = attrs["type"][0]
-
- # If an unescaped colon is still present, it's often due to an incorrect data type specification
- # but could also be due to using a column name with ":" in it.
- if (
- "field" in attrs
- and ":" in attrs["field"]
- and attrs["field"][attrs["field"].rfind(":") - 1] != "\\"
- ):
- raise ValueError(
- '"{}" '.format(attrs["field"].split(":")[-1])
- + "is not one of the valid encoding data types: {}.".format(
- ", ".join(TYPECODE_MAP.values())
- )
- + "\nFor more details, see https://altair-viz.github.io/user_guide/encodings/index.html#encoding-data-types. "
- + "If you are trying to use a column name that contains a colon, "
- + 'prefix it with a backslash; for example "column\\:name" instead of "column:name".'
- )
- return attrs
-
-
-def infer_vegalite_type_for_dfi_column(
- column: Union[Column, "PandasColumn"],
-) -> Union[_InferredVegaLiteType, Tuple[_InferredVegaLiteType, list]]:
- from pyarrow.interchange.from_dataframe import column_to_array
-
- try:
- kind = column.dtype[0]
- except NotImplementedError as e:
- # Edge case hack:
- # dtype access fails for pandas column with datetime64[ns, UTC] type,
- # but all we need to know is that its temporal, so check the
- # error message for the presence of datetime64.
- #
- # See https://github.com/pandas-dev/pandas/issues/54239
- if "datetime64" in e.args[0] or "timestamp" in e.args[0]:
- return "temporal"
- raise e
-
- if (
- kind == DtypeKind.CATEGORICAL
- and column.describe_categorical["is_ordered"]
- and column.describe_categorical["categories"] is not None
- ):
- # Treat ordered categorical column as Vega-Lite ordinal
- categories_column = column.describe_categorical["categories"]
- categories_array = column_to_array(categories_column)
- return "ordinal", categories_array.to_pylist()
- if kind in (DtypeKind.STRING, DtypeKind.CATEGORICAL, DtypeKind.BOOL):
- return "nominal"
- elif kind in (DtypeKind.INT, DtypeKind.UINT, DtypeKind.FLOAT):
- return "quantitative"
- elif kind == DtypeKind.DATETIME:
- return "temporal"
- else:
- raise ValueError(f"Unexpected DtypeKind: {kind}")
-
-
-def use_signature(Obj: Callable[_P, Any]):
- """Apply call signature and documentation of Obj to the decorated method"""
-
- def decorate(f: Callable[..., _V]) -> Callable[_P, _V]:
- # call-signature of f is exposed via __wrapped__.
- # we want it to mimic Obj.__init__
- f.__wrapped__ = Obj.__init__ # type: ignore
- f._uses_signature = Obj # type: ignore
-
- # Supplement the docstring of f with information from Obj
- if Obj.__doc__:
- # Patch in a reference to the class this docstring is copied from,
- # to generate a hyperlink.
- doclines = Obj.__doc__.splitlines()
- doclines[0] = f"Refer to :class:`{Obj.__name__}`"
-
- if f.__doc__:
- doc = f.__doc__ + "\n".join(doclines[1:])
- else:
- doc = "\n".join(doclines)
- try:
- f.__doc__ = doc
- except AttributeError:
- # __doc__ is not modifiable for classes in Python < 3.3
- pass
-
- return f
-
- return decorate
-
-
-def update_nested(
- original: MutableMapping, update: Mapping, copy: bool = False
-) -> MutableMapping:
- """Update nested dictionaries
-
- Parameters
- ----------
- original : MutableMapping
- the original (nested) dictionary, which will be updated in-place
- update : Mapping
- the nested dictionary of updates
- copy : bool, default False
- if True, then copy the original dictionary rather than modifying it
-
- Returns
- -------
- original : MutableMapping
- a reference to the (modified) original dict
-
- Examples
- --------
- >>> original = {'x': {'b': 2, 'c': 4}}
- >>> update = {'x': {'b': 5, 'd': 6}, 'y': 40}
- >>> update_nested(original, update) # doctest: +SKIP
- {'x': {'b': 5, 'c': 4, 'd': 6}, 'y': 40}
- >>> original # doctest: +SKIP
- {'x': {'b': 5, 'c': 4, 'd': 6}, 'y': 40}
- """
- if copy:
- original = deepcopy(original)
- for key, val in update.items():
- if isinstance(val, Mapping):
- orig_val = original.get(key, {})
- if isinstance(orig_val, MutableMapping):
- original[key] = update_nested(orig_val, val)
- else:
- original[key] = val
- else:
- original[key] = val
- return original
-
-
-def display_traceback(in_ipython: bool = True):
- exc_info = sys.exc_info()
-
- if in_ipython:
- from IPython.core.getipython import get_ipython
-
- ip = get_ipython()
- else:
- ip = None
-
- if ip is not None:
- ip.showtraceback(exc_info)
- else:
- traceback.print_exception(*exc_info)
-
-
-def infer_encoding_types(args: Sequence, kwargs: MutableMapping, channels: ModuleType):
- """Infer typed keyword arguments for args and kwargs
-
- Parameters
- ----------
- args : Sequence
- Sequence of function args
- kwargs : MutableMapping
- Dict of function kwargs
- channels : ModuleType
- The module containing all altair encoding channel classes.
-
- Returns
- -------
- kwargs : dict
- All args and kwargs in a single dict, with keys and types
- based on the channels mapping.
- """
- # Construct a dictionary of channel type to encoding name
- # TODO: cache this somehow?
- channel_objs = (getattr(channels, name) for name in dir(channels))
- channel_objs = (
- c for c in channel_objs if isinstance(c, type) and issubclass(c, SchemaBase)
- )
- channel_to_name: Dict[Type[SchemaBase], str] = {
- c: c._encoding_name for c in channel_objs
- }
- name_to_channel: Dict[str, Dict[str, Type[SchemaBase]]] = {}
- for chan, name in channel_to_name.items():
- chans = name_to_channel.setdefault(name, {})
- if chan.__name__.endswith("Datum"):
- key = "datum"
- elif chan.__name__.endswith("Value"):
- key = "value"
- else:
- key = "field"
- chans[key] = chan
-
- # First use the mapping to convert args to kwargs based on their types.
- for arg in args:
- if isinstance(arg, (list, tuple)) and len(arg) > 0:
- type_ = type(arg[0])
- else:
- type_ = type(arg)
-
- encoding = channel_to_name.get(type_, None)
- if encoding is None:
- raise NotImplementedError("positional of type {}" "".format(type_))
- if encoding in kwargs:
- raise ValueError("encoding {} specified twice.".format(encoding))
- kwargs[encoding] = arg
-
- def _wrap_in_channel_class(obj, encoding):
- if isinstance(obj, SchemaBase):
- return obj
-
- if isinstance(obj, str):
- obj = {"shorthand": obj}
-
- if isinstance(obj, (list, tuple)):
- return [_wrap_in_channel_class(subobj, encoding) for subobj in obj]
-
- if encoding not in name_to_channel:
- warnings.warn(
- "Unrecognized encoding channel '{}'".format(encoding), stacklevel=1
- )
- return obj
-
- classes = name_to_channel[encoding]
- cls = classes["value"] if "value" in obj else classes["field"]
-
- try:
- # Don't force validation here; some objects won't be valid until
- # they're created in the context of a chart.
- return cls.from_dict(obj, validate=False)
- except jsonschema.ValidationError:
- # our attempts at finding the correct class have failed
- return obj
-
- return {
- encoding: _wrap_in_channel_class(obj, encoding)
- for encoding, obj in kwargs.items()
- }
diff --git a/spaces/profayle/TerrapinTalk/myenv/lib/python3.9/site-packages/anyio/__init__.py b/spaces/profayle/TerrapinTalk/myenv/lib/python3.9/site-packages/anyio/__init__.py
deleted file mode 100644
index 29fb3561e4f2dc9d3a764e756439c0dea2c9897a..0000000000000000000000000000000000000000
--- a/spaces/profayle/TerrapinTalk/myenv/lib/python3.9/site-packages/anyio/__init__.py
+++ /dev/null
@@ -1,169 +0,0 @@
-from __future__ import annotations
-
-__all__ = (
- "maybe_async",
- "maybe_async_cm",
- "run",
- "sleep",
- "sleep_forever",
- "sleep_until",
- "current_time",
- "get_all_backends",
- "get_cancelled_exc_class",
- "BrokenResourceError",
- "BrokenWorkerProcess",
- "BusyResourceError",
- "ClosedResourceError",
- "DelimiterNotFound",
- "EndOfStream",
- "ExceptionGroup",
- "IncompleteRead",
- "TypedAttributeLookupError",
- "WouldBlock",
- "AsyncFile",
- "Path",
- "open_file",
- "wrap_file",
- "aclose_forcefully",
- "open_signal_receiver",
- "connect_tcp",
- "connect_unix",
- "create_tcp_listener",
- "create_unix_listener",
- "create_udp_socket",
- "create_connected_udp_socket",
- "getaddrinfo",
- "getnameinfo",
- "wait_socket_readable",
- "wait_socket_writable",
- "create_memory_object_stream",
- "run_process",
- "open_process",
- "create_lock",
- "CapacityLimiter",
- "CapacityLimiterStatistics",
- "Condition",
- "ConditionStatistics",
- "Event",
- "EventStatistics",
- "Lock",
- "LockStatistics",
- "Semaphore",
- "SemaphoreStatistics",
- "create_condition",
- "create_event",
- "create_semaphore",
- "create_capacity_limiter",
- "open_cancel_scope",
- "fail_after",
- "move_on_after",
- "current_effective_deadline",
- "TASK_STATUS_IGNORED",
- "CancelScope",
- "create_task_group",
- "TaskInfo",
- "get_current_task",
- "get_running_tasks",
- "wait_all_tasks_blocked",
- "run_sync_in_worker_thread",
- "run_async_from_thread",
- "run_sync_from_thread",
- "current_default_worker_thread_limiter",
- "create_blocking_portal",
- "start_blocking_portal",
- "typed_attribute",
- "TypedAttributeSet",
- "TypedAttributeProvider",
-)
-
-from typing import Any
-
-from ._core._compat import maybe_async, maybe_async_cm
-from ._core._eventloop import (
- current_time,
- get_all_backends,
- get_cancelled_exc_class,
- run,
- sleep,
- sleep_forever,
- sleep_until,
-)
-from ._core._exceptions import (
- BrokenResourceError,
- BrokenWorkerProcess,
- BusyResourceError,
- ClosedResourceError,
- DelimiterNotFound,
- EndOfStream,
- ExceptionGroup,
- IncompleteRead,
- TypedAttributeLookupError,
- WouldBlock,
-)
-from ._core._fileio import AsyncFile, Path, open_file, wrap_file
-from ._core._resources import aclose_forcefully
-from ._core._signals import open_signal_receiver
-from ._core._sockets import (
- connect_tcp,
- connect_unix,
- create_connected_udp_socket,
- create_tcp_listener,
- create_udp_socket,
- create_unix_listener,
- getaddrinfo,
- getnameinfo,
- wait_socket_readable,
- wait_socket_writable,
-)
-from ._core._streams import create_memory_object_stream
-from ._core._subprocesses import open_process, run_process
-from ._core._synchronization import (
- CapacityLimiter,
- CapacityLimiterStatistics,
- Condition,
- ConditionStatistics,
- Event,
- EventStatistics,
- Lock,
- LockStatistics,
- Semaphore,
- SemaphoreStatistics,
- create_capacity_limiter,
- create_condition,
- create_event,
- create_lock,
- create_semaphore,
-)
-from ._core._tasks import (
- TASK_STATUS_IGNORED,
- CancelScope,
- create_task_group,
- current_effective_deadline,
- fail_after,
- move_on_after,
- open_cancel_scope,
-)
-from ._core._testing import (
- TaskInfo,
- get_current_task,
- get_running_tasks,
- wait_all_tasks_blocked,
-)
-from ._core._typedattr import TypedAttributeProvider, TypedAttributeSet, typed_attribute
-
-# Re-exported here, for backwards compatibility
-# isort: off
-from .to_thread import current_default_worker_thread_limiter, run_sync_in_worker_thread
-from .from_thread import (
- create_blocking_portal,
- run_async_from_thread,
- run_sync_from_thread,
- start_blocking_portal,
-)
-
-# Re-export imports so they look like they live directly in this package
-key: str
-value: Any
-for key, value in list(locals().items()):
- if getattr(value, "__module__", "").startswith("anyio."):
- value.__module__ = __name__
diff --git a/spaces/profayle/TerrapinTalk/myenv/lib/python3.9/site-packages/click/_compat.py b/spaces/profayle/TerrapinTalk/myenv/lib/python3.9/site-packages/click/_compat.py
deleted file mode 100644
index 23f8866598b4b4eb836b9d9b210ebd395fd0c557..0000000000000000000000000000000000000000
--- a/spaces/profayle/TerrapinTalk/myenv/lib/python3.9/site-packages/click/_compat.py
+++ /dev/null
@@ -1,623 +0,0 @@
-import codecs
-import io
-import os
-import re
-import sys
-import typing as t
-from weakref import WeakKeyDictionary
-
-CYGWIN = sys.platform.startswith("cygwin")
-WIN = sys.platform.startswith("win")
-auto_wrap_for_ansi: t.Optional[t.Callable[[t.TextIO], t.TextIO]] = None
-_ansi_re = re.compile(r"\033\[[;?0-9]*[a-zA-Z]")
-
-
-def _make_text_stream(
- stream: t.BinaryIO,
- encoding: t.Optional[str],
- errors: t.Optional[str],
- force_readable: bool = False,
- force_writable: bool = False,
-) -> t.TextIO:
- if encoding is None:
- encoding = get_best_encoding(stream)
- if errors is None:
- errors = "replace"
- return _NonClosingTextIOWrapper(
- stream,
- encoding,
- errors,
- line_buffering=True,
- force_readable=force_readable,
- force_writable=force_writable,
- )
-
-
-def is_ascii_encoding(encoding: str) -> bool:
- """Checks if a given encoding is ascii."""
- try:
- return codecs.lookup(encoding).name == "ascii"
- except LookupError:
- return False
-
-
-def get_best_encoding(stream: t.IO[t.Any]) -> str:
- """Returns the default stream encoding if not found."""
- rv = getattr(stream, "encoding", None) or sys.getdefaultencoding()
- if is_ascii_encoding(rv):
- return "utf-8"
- return rv
-
-
-class _NonClosingTextIOWrapper(io.TextIOWrapper):
- def __init__(
- self,
- stream: t.BinaryIO,
- encoding: t.Optional[str],
- errors: t.Optional[str],
- force_readable: bool = False,
- force_writable: bool = False,
- **extra: t.Any,
- ) -> None:
- self._stream = stream = t.cast(
- t.BinaryIO, _FixupStream(stream, force_readable, force_writable)
- )
- super().__init__(stream, encoding, errors, **extra)
-
- def __del__(self) -> None:
- try:
- self.detach()
- except Exception:
- pass
-
- def isatty(self) -> bool:
- # https://bitbucket.org/pypy/pypy/issue/1803
- return self._stream.isatty()
-
-
-class _FixupStream:
- """The new io interface needs more from streams than streams
- traditionally implement. As such, this fix-up code is necessary in
- some circumstances.
-
- The forcing of readable and writable flags are there because some tools
- put badly patched objects on sys (one such offender are certain version
- of jupyter notebook).
- """
-
- def __init__(
- self,
- stream: t.BinaryIO,
- force_readable: bool = False,
- force_writable: bool = False,
- ):
- self._stream = stream
- self._force_readable = force_readable
- self._force_writable = force_writable
-
- def __getattr__(self, name: str) -> t.Any:
- return getattr(self._stream, name)
-
- def read1(self, size: int) -> bytes:
- f = getattr(self._stream, "read1", None)
-
- if f is not None:
- return t.cast(bytes, f(size))
-
- return self._stream.read(size)
-
- def readable(self) -> bool:
- if self._force_readable:
- return True
- x = getattr(self._stream, "readable", None)
- if x is not None:
- return t.cast(bool, x())
- try:
- self._stream.read(0)
- except Exception:
- return False
- return True
-
- def writable(self) -> bool:
- if self._force_writable:
- return True
- x = getattr(self._stream, "writable", None)
- if x is not None:
- return t.cast(bool, x())
- try:
- self._stream.write("") # type: ignore
- except Exception:
- try:
- self._stream.write(b"")
- except Exception:
- return False
- return True
-
- def seekable(self) -> bool:
- x = getattr(self._stream, "seekable", None)
- if x is not None:
- return t.cast(bool, x())
- try:
- self._stream.seek(self._stream.tell())
- except Exception:
- return False
- return True
-
-
-def _is_binary_reader(stream: t.IO[t.Any], default: bool = False) -> bool:
- try:
- return isinstance(stream.read(0), bytes)
- except Exception:
- return default
- # This happens in some cases where the stream was already
- # closed. In this case, we assume the default.
-
-
-def _is_binary_writer(stream: t.IO[t.Any], default: bool = False) -> bool:
- try:
- stream.write(b"")
- except Exception:
- try:
- stream.write("")
- return False
- except Exception:
- pass
- return default
- return True
-
-
-def _find_binary_reader(stream: t.IO[t.Any]) -> t.Optional[t.BinaryIO]:
- # We need to figure out if the given stream is already binary.
- # This can happen because the official docs recommend detaching
- # the streams to get binary streams. Some code might do this, so
- # we need to deal with this case explicitly.
- if _is_binary_reader(stream, False):
- return t.cast(t.BinaryIO, stream)
-
- buf = getattr(stream, "buffer", None)
-
- # Same situation here; this time we assume that the buffer is
- # actually binary in case it's closed.
- if buf is not None and _is_binary_reader(buf, True):
- return t.cast(t.BinaryIO, buf)
-
- return None
-
-
-def _find_binary_writer(stream: t.IO[t.Any]) -> t.Optional[t.BinaryIO]:
- # We need to figure out if the given stream is already binary.
- # This can happen because the official docs recommend detaching
- # the streams to get binary streams. Some code might do this, so
- # we need to deal with this case explicitly.
- if _is_binary_writer(stream, False):
- return t.cast(t.BinaryIO, stream)
-
- buf = getattr(stream, "buffer", None)
-
- # Same situation here; this time we assume that the buffer is
- # actually binary in case it's closed.
- if buf is not None and _is_binary_writer(buf, True):
- return t.cast(t.BinaryIO, buf)
-
- return None
-
-
-def _stream_is_misconfigured(stream: t.TextIO) -> bool:
- """A stream is misconfigured if its encoding is ASCII."""
- # If the stream does not have an encoding set, we assume it's set
- # to ASCII. This appears to happen in certain unittest
- # environments. It's not quite clear what the correct behavior is
- # but this at least will force Click to recover somehow.
- return is_ascii_encoding(getattr(stream, "encoding", None) or "ascii")
-
-
-def _is_compat_stream_attr(stream: t.TextIO, attr: str, value: t.Optional[str]) -> bool:
- """A stream attribute is compatible if it is equal to the
- desired value or the desired value is unset and the attribute
- has a value.
- """
- stream_value = getattr(stream, attr, None)
- return stream_value == value or (value is None and stream_value is not None)
-
-
-def _is_compatible_text_stream(
- stream: t.TextIO, encoding: t.Optional[str], errors: t.Optional[str]
-) -> bool:
- """Check if a stream's encoding and errors attributes are
- compatible with the desired values.
- """
- return _is_compat_stream_attr(
- stream, "encoding", encoding
- ) and _is_compat_stream_attr(stream, "errors", errors)
-
-
-def _force_correct_text_stream(
- text_stream: t.IO[t.Any],
- encoding: t.Optional[str],
- errors: t.Optional[str],
- is_binary: t.Callable[[t.IO[t.Any], bool], bool],
- find_binary: t.Callable[[t.IO[t.Any]], t.Optional[t.BinaryIO]],
- force_readable: bool = False,
- force_writable: bool = False,
-) -> t.TextIO:
- if is_binary(text_stream, False):
- binary_reader = t.cast(t.BinaryIO, text_stream)
- else:
- text_stream = t.cast(t.TextIO, text_stream)
- # If the stream looks compatible, and won't default to a
- # misconfigured ascii encoding, return it as-is.
- if _is_compatible_text_stream(text_stream, encoding, errors) and not (
- encoding is None and _stream_is_misconfigured(text_stream)
- ):
- return text_stream
-
- # Otherwise, get the underlying binary reader.
- possible_binary_reader = find_binary(text_stream)
-
- # If that's not possible, silently use the original reader
- # and get mojibake instead of exceptions.
- if possible_binary_reader is None:
- return text_stream
-
- binary_reader = possible_binary_reader
-
- # Default errors to replace instead of strict in order to get
- # something that works.
- if errors is None:
- errors = "replace"
-
- # Wrap the binary stream in a text stream with the correct
- # encoding parameters.
- return _make_text_stream(
- binary_reader,
- encoding,
- errors,
- force_readable=force_readable,
- force_writable=force_writable,
- )
-
-
-def _force_correct_text_reader(
- text_reader: t.IO[t.Any],
- encoding: t.Optional[str],
- errors: t.Optional[str],
- force_readable: bool = False,
-) -> t.TextIO:
- return _force_correct_text_stream(
- text_reader,
- encoding,
- errors,
- _is_binary_reader,
- _find_binary_reader,
- force_readable=force_readable,
- )
-
-
-def _force_correct_text_writer(
- text_writer: t.IO[t.Any],
- encoding: t.Optional[str],
- errors: t.Optional[str],
- force_writable: bool = False,
-) -> t.TextIO:
- return _force_correct_text_stream(
- text_writer,
- encoding,
- errors,
- _is_binary_writer,
- _find_binary_writer,
- force_writable=force_writable,
- )
-
-
-def get_binary_stdin() -> t.BinaryIO:
- reader = _find_binary_reader(sys.stdin)
- if reader is None:
- raise RuntimeError("Was not able to determine binary stream for sys.stdin.")
- return reader
-
-
-def get_binary_stdout() -> t.BinaryIO:
- writer = _find_binary_writer(sys.stdout)
- if writer is None:
- raise RuntimeError("Was not able to determine binary stream for sys.stdout.")
- return writer
-
-
-def get_binary_stderr() -> t.BinaryIO:
- writer = _find_binary_writer(sys.stderr)
- if writer is None:
- raise RuntimeError("Was not able to determine binary stream for sys.stderr.")
- return writer
-
-
-def get_text_stdin(
- encoding: t.Optional[str] = None, errors: t.Optional[str] = None
-) -> t.TextIO:
- rv = _get_windows_console_stream(sys.stdin, encoding, errors)
- if rv is not None:
- return rv
- return _force_correct_text_reader(sys.stdin, encoding, errors, force_readable=True)
-
-
-def get_text_stdout(
- encoding: t.Optional[str] = None, errors: t.Optional[str] = None
-) -> t.TextIO:
- rv = _get_windows_console_stream(sys.stdout, encoding, errors)
- if rv is not None:
- return rv
- return _force_correct_text_writer(sys.stdout, encoding, errors, force_writable=True)
-
-
-def get_text_stderr(
- encoding: t.Optional[str] = None, errors: t.Optional[str] = None
-) -> t.TextIO:
- rv = _get_windows_console_stream(sys.stderr, encoding, errors)
- if rv is not None:
- return rv
- return _force_correct_text_writer(sys.stderr, encoding, errors, force_writable=True)
-
-
-def _wrap_io_open(
- file: t.Union[str, "os.PathLike[str]", int],
- mode: str,
- encoding: t.Optional[str],
- errors: t.Optional[str],
-) -> t.IO[t.Any]:
- """Handles not passing ``encoding`` and ``errors`` in binary mode."""
- if "b" in mode:
- return open(file, mode)
-
- return open(file, mode, encoding=encoding, errors=errors)
-
-
-def open_stream(
- filename: "t.Union[str, os.PathLike[str]]",
- mode: str = "r",
- encoding: t.Optional[str] = None,
- errors: t.Optional[str] = "strict",
- atomic: bool = False,
-) -> t.Tuple[t.IO[t.Any], bool]:
- binary = "b" in mode
- filename = os.fspath(filename)
-
- # Standard streams first. These are simple because they ignore the
- # atomic flag. Use fsdecode to handle Path("-").
- if os.fsdecode(filename) == "-":
- if any(m in mode for m in ["w", "a", "x"]):
- if binary:
- return get_binary_stdout(), False
- return get_text_stdout(encoding=encoding, errors=errors), False
- if binary:
- return get_binary_stdin(), False
- return get_text_stdin(encoding=encoding, errors=errors), False
-
- # Non-atomic writes directly go out through the regular open functions.
- if not atomic:
- return _wrap_io_open(filename, mode, encoding, errors), True
-
- # Some usability stuff for atomic writes
- if "a" in mode:
- raise ValueError(
- "Appending to an existing file is not supported, because that"
- " would involve an expensive `copy`-operation to a temporary"
- " file. Open the file in normal `w`-mode and copy explicitly"
- " if that's what you're after."
- )
- if "x" in mode:
- raise ValueError("Use the `overwrite`-parameter instead.")
- if "w" not in mode:
- raise ValueError("Atomic writes only make sense with `w`-mode.")
-
- # Atomic writes are more complicated. They work by opening a file
- # as a proxy in the same folder and then using the fdopen
- # functionality to wrap it in a Python file. Then we wrap it in an
- # atomic file that moves the file over on close.
- import errno
- import random
-
- try:
- perm: t.Optional[int] = os.stat(filename).st_mode
- except OSError:
- perm = None
-
- flags = os.O_RDWR | os.O_CREAT | os.O_EXCL
-
- if binary:
- flags |= getattr(os, "O_BINARY", 0)
-
- while True:
- tmp_filename = os.path.join(
- os.path.dirname(filename),
- f".__atomic-write{random.randrange(1 << 32):08x}",
- )
- try:
- fd = os.open(tmp_filename, flags, 0o666 if perm is None else perm)
- break
- except OSError as e:
- if e.errno == errno.EEXIST or (
- os.name == "nt"
- and e.errno == errno.EACCES
- and os.path.isdir(e.filename)
- and os.access(e.filename, os.W_OK)
- ):
- continue
- raise
-
- if perm is not None:
- os.chmod(tmp_filename, perm) # in case perm includes bits in umask
-
- f = _wrap_io_open(fd, mode, encoding, errors)
- af = _AtomicFile(f, tmp_filename, os.path.realpath(filename))
- return t.cast(t.IO[t.Any], af), True
-
-
-class _AtomicFile:
- def __init__(self, f: t.IO[t.Any], tmp_filename: str, real_filename: str) -> None:
- self._f = f
- self._tmp_filename = tmp_filename
- self._real_filename = real_filename
- self.closed = False
-
- @property
- def name(self) -> str:
- return self._real_filename
-
- def close(self, delete: bool = False) -> None:
- if self.closed:
- return
- self._f.close()
- os.replace(self._tmp_filename, self._real_filename)
- self.closed = True
-
- def __getattr__(self, name: str) -> t.Any:
- return getattr(self._f, name)
-
- def __enter__(self) -> "_AtomicFile":
- return self
-
- def __exit__(self, exc_type: t.Optional[t.Type[BaseException]], *_: t.Any) -> None:
- self.close(delete=exc_type is not None)
-
- def __repr__(self) -> str:
- return repr(self._f)
-
-
-def strip_ansi(value: str) -> str:
- return _ansi_re.sub("", value)
-
-
-def _is_jupyter_kernel_output(stream: t.IO[t.Any]) -> bool:
- while isinstance(stream, (_FixupStream, _NonClosingTextIOWrapper)):
- stream = stream._stream
-
- return stream.__class__.__module__.startswith("ipykernel.")
-
-
-def should_strip_ansi(
- stream: t.Optional[t.IO[t.Any]] = None, color: t.Optional[bool] = None
-) -> bool:
- if color is None:
- if stream is None:
- stream = sys.stdin
- return not isatty(stream) and not _is_jupyter_kernel_output(stream)
- return not color
-
-
-# On Windows, wrap the output streams with colorama to support ANSI
-# color codes.
-# NOTE: double check is needed so mypy does not analyze this on Linux
-if sys.platform.startswith("win") and WIN:
- from ._winconsole import _get_windows_console_stream
-
- def _get_argv_encoding() -> str:
- import locale
-
- return locale.getpreferredencoding()
-
- _ansi_stream_wrappers: t.MutableMapping[t.TextIO, t.TextIO] = WeakKeyDictionary()
-
- def auto_wrap_for_ansi( # noqa: F811
- stream: t.TextIO, color: t.Optional[bool] = None
- ) -> t.TextIO:
- """Support ANSI color and style codes on Windows by wrapping a
- stream with colorama.
- """
- try:
- cached = _ansi_stream_wrappers.get(stream)
- except Exception:
- cached = None
-
- if cached is not None:
- return cached
-
- import colorama
-
- strip = should_strip_ansi(stream, color)
- ansi_wrapper = colorama.AnsiToWin32(stream, strip=strip)
- rv = t.cast(t.TextIO, ansi_wrapper.stream)
- _write = rv.write
-
- def _safe_write(s):
- try:
- return _write(s)
- except BaseException:
- ansi_wrapper.reset_all()
- raise
-
- rv.write = _safe_write
-
- try:
- _ansi_stream_wrappers[stream] = rv
- except Exception:
- pass
-
- return rv
-
-else:
-
- def _get_argv_encoding() -> str:
- return getattr(sys.stdin, "encoding", None) or sys.getfilesystemencoding()
-
- def _get_windows_console_stream(
- f: t.TextIO, encoding: t.Optional[str], errors: t.Optional[str]
- ) -> t.Optional[t.TextIO]:
- return None
-
-
-def term_len(x: str) -> int:
- return len(strip_ansi(x))
-
-
-def isatty(stream: t.IO[t.Any]) -> bool:
- try:
- return stream.isatty()
- except Exception:
- return False
-
-
-def _make_cached_stream_func(
- src_func: t.Callable[[], t.Optional[t.TextIO]],
- wrapper_func: t.Callable[[], t.TextIO],
-) -> t.Callable[[], t.Optional[t.TextIO]]:
- cache: t.MutableMapping[t.TextIO, t.TextIO] = WeakKeyDictionary()
-
- def func() -> t.Optional[t.TextIO]:
- stream = src_func()
-
- if stream is None:
- return None
-
- try:
- rv = cache.get(stream)
- except Exception:
- rv = None
- if rv is not None:
- return rv
- rv = wrapper_func()
- try:
- cache[stream] = rv
- except Exception:
- pass
- return rv
-
- return func
-
-
-_default_text_stdin = _make_cached_stream_func(lambda: sys.stdin, get_text_stdin)
-_default_text_stdout = _make_cached_stream_func(lambda: sys.stdout, get_text_stdout)
-_default_text_stderr = _make_cached_stream_func(lambda: sys.stderr, get_text_stderr)
-
-
-binary_streams: t.Mapping[str, t.Callable[[], t.BinaryIO]] = {
- "stdin": get_binary_stdin,
- "stdout": get_binary_stdout,
- "stderr": get_binary_stderr,
-}
-
-text_streams: t.Mapping[
- str, t.Callable[[t.Optional[str], t.Optional[str]], t.TextIO]
-] = {
- "stdin": get_text_stdin,
- "stdout": get_text_stdout,
- "stderr": get_text_stderr,
-}
diff --git a/spaces/profayle/TerrapinTalk/myenv/lib/python3.9/site-packages/fontTools/ttLib/tables/T_S_I_D_.py b/spaces/profayle/TerrapinTalk/myenv/lib/python3.9/site-packages/fontTools/ttLib/tables/T_S_I_D_.py
deleted file mode 100644
index 536ff2f98a0abb8b27fe6da44199534a32fd0c3e..0000000000000000000000000000000000000000
--- a/spaces/profayle/TerrapinTalk/myenv/lib/python3.9/site-packages/fontTools/ttLib/tables/T_S_I_D_.py
+++ /dev/null
@@ -1,5 +0,0 @@
-from .T_S_I_V_ import table_T_S_I_V_
-
-
-class table_T_S_I_D_(table_T_S_I_V_):
- pass
diff --git a/spaces/profayle/TerrapinTalk/myenv/lib/python3.9/site-packages/matplotlib/backends/backend_gtk4.py b/spaces/profayle/TerrapinTalk/myenv/lib/python3.9/site-packages/matplotlib/backends/backend_gtk4.py
deleted file mode 100644
index cb4006048d5536b08acc264a5e5766209ca085ef..0000000000000000000000000000000000000000
--- a/spaces/profayle/TerrapinTalk/myenv/lib/python3.9/site-packages/matplotlib/backends/backend_gtk4.py
+++ /dev/null
@@ -1,606 +0,0 @@
-import functools
-import io
-import os
-
-import matplotlib as mpl
-from matplotlib import _api, backend_tools, cbook
-from matplotlib.backend_bases import (
- ToolContainerBase, KeyEvent, LocationEvent, MouseEvent, ResizeEvent,
- CloseEvent)
-
-try:
- import gi
-except ImportError as err:
- raise ImportError("The GTK4 backends require PyGObject") from err
-
-try:
- # :raises ValueError: If module/version is already loaded, already
- # required, or unavailable.
- gi.require_version("Gtk", "4.0")
-except ValueError as e:
- # in this case we want to re-raise as ImportError so the
- # auto-backend selection logic correctly skips.
- raise ImportError(e) from e
-
-from gi.repository import Gio, GLib, Gtk, Gdk, GdkPixbuf
-from . import _backend_gtk
-from ._backend_gtk import ( # noqa: F401 # pylint: disable=W0611
- _BackendGTK, _FigureCanvasGTK, _FigureManagerGTK, _NavigationToolbar2GTK,
- TimerGTK as TimerGTK4,
-)
-
-
-class FigureCanvasGTK4(_FigureCanvasGTK, Gtk.DrawingArea):
- required_interactive_framework = "gtk4"
- supports_blit = False
- manager_class = _api.classproperty(lambda cls: FigureManagerGTK4)
- _context_is_scaled = False
-
- def __init__(self, figure=None):
- super().__init__(figure=figure)
-
- self.set_hexpand(True)
- self.set_vexpand(True)
-
- self._idle_draw_id = 0
- self._rubberband_rect = None
-
- self.set_draw_func(self._draw_func)
- self.connect('resize', self.resize_event)
- self.connect('notify::scale-factor', self._update_device_pixel_ratio)
-
- click = Gtk.GestureClick()
- click.set_button(0) # All buttons.
- click.connect('pressed', self.button_press_event)
- click.connect('released', self.button_release_event)
- self.add_controller(click)
-
- key = Gtk.EventControllerKey()
- key.connect('key-pressed', self.key_press_event)
- key.connect('key-released', self.key_release_event)
- self.add_controller(key)
-
- motion = Gtk.EventControllerMotion()
- motion.connect('motion', self.motion_notify_event)
- motion.connect('enter', self.enter_notify_event)
- motion.connect('leave', self.leave_notify_event)
- self.add_controller(motion)
-
- scroll = Gtk.EventControllerScroll.new(
- Gtk.EventControllerScrollFlags.VERTICAL)
- scroll.connect('scroll', self.scroll_event)
- self.add_controller(scroll)
-
- self.set_focusable(True)
-
- css = Gtk.CssProvider()
- style = '.matplotlib-canvas { background-color: white; }'
- if Gtk.check_version(4, 9, 3) is None:
- css.load_from_data(style, -1)
- else:
- css.load_from_data(style.encode('utf-8'))
- style_ctx = self.get_style_context()
- style_ctx.add_provider(css, Gtk.STYLE_PROVIDER_PRIORITY_APPLICATION)
- style_ctx.add_class("matplotlib-canvas")
-
- def destroy(self):
- CloseEvent("close_event", self)._process()
-
- def set_cursor(self, cursor):
- # docstring inherited
- self.set_cursor_from_name(_backend_gtk.mpl_to_gtk_cursor_name(cursor))
-
- def _mpl_coords(self, xy=None):
- """
- Convert the *xy* position of a GTK event, or of the current cursor
- position if *xy* is None, to Matplotlib coordinates.
-
- GTK use logical pixels, but the figure is scaled to physical pixels for
- rendering. Transform to physical pixels so that all of the down-stream
- transforms work as expected.
-
- Also, the origin is different and needs to be corrected.
- """
- if xy is None:
- surface = self.get_native().get_surface()
- is_over, x, y, mask = surface.get_device_position(
- self.get_display().get_default_seat().get_pointer())
- else:
- x, y = xy
- x = x * self.device_pixel_ratio
- # flip y so y=0 is bottom of canvas
- y = self.figure.bbox.height - y * self.device_pixel_ratio
- return x, y
-
- def scroll_event(self, controller, dx, dy):
- MouseEvent(
- "scroll_event", self, *self._mpl_coords(), step=dy,
- modifiers=self._mpl_modifiers(controller),
- )._process()
- return True
-
- def button_press_event(self, controller, n_press, x, y):
- MouseEvent(
- "button_press_event", self, *self._mpl_coords((x, y)),
- controller.get_current_button(),
- modifiers=self._mpl_modifiers(controller),
- )._process()
- self.grab_focus()
-
- def button_release_event(self, controller, n_press, x, y):
- MouseEvent(
- "button_release_event", self, *self._mpl_coords((x, y)),
- controller.get_current_button(),
- modifiers=self._mpl_modifiers(controller),
- )._process()
-
- def key_press_event(self, controller, keyval, keycode, state):
- KeyEvent(
- "key_press_event", self, self._get_key(keyval, keycode, state),
- *self._mpl_coords(),
- )._process()
- return True
-
- def key_release_event(self, controller, keyval, keycode, state):
- KeyEvent(
- "key_release_event", self, self._get_key(keyval, keycode, state),
- *self._mpl_coords(),
- )._process()
- return True
-
- def motion_notify_event(self, controller, x, y):
- MouseEvent(
- "motion_notify_event", self, *self._mpl_coords((x, y)),
- modifiers=self._mpl_modifiers(controller),
- )._process()
-
- def enter_notify_event(self, controller, x, y):
- LocationEvent(
- "figure_enter_event", self, *self._mpl_coords((x, y)),
- modifiers=self._mpl_modifiers(),
- )._process()
-
- def leave_notify_event(self, controller):
- LocationEvent(
- "figure_leave_event", self, *self._mpl_coords(),
- modifiers=self._mpl_modifiers(),
- )._process()
-
- def resize_event(self, area, width, height):
- self._update_device_pixel_ratio()
- dpi = self.figure.dpi
- winch = width * self.device_pixel_ratio / dpi
- hinch = height * self.device_pixel_ratio / dpi
- self.figure.set_size_inches(winch, hinch, forward=False)
- ResizeEvent("resize_event", self)._process()
- self.draw_idle()
-
- def _mpl_modifiers(self, controller=None):
- if controller is None:
- surface = self.get_native().get_surface()
- is_over, x, y, event_state = surface.get_device_position(
- self.get_display().get_default_seat().get_pointer())
- else:
- event_state = controller.get_current_event_state()
- mod_table = [
- ("ctrl", Gdk.ModifierType.CONTROL_MASK),
- ("alt", Gdk.ModifierType.ALT_MASK),
- ("shift", Gdk.ModifierType.SHIFT_MASK),
- ("super", Gdk.ModifierType.SUPER_MASK),
- ]
- return [name for name, mask in mod_table if event_state & mask]
-
- def _get_key(self, keyval, keycode, state):
- unikey = chr(Gdk.keyval_to_unicode(keyval))
- key = cbook._unikey_or_keysym_to_mplkey(
- unikey,
- Gdk.keyval_name(keyval))
- modifiers = [
- ("ctrl", Gdk.ModifierType.CONTROL_MASK, "control"),
- ("alt", Gdk.ModifierType.ALT_MASK, "alt"),
- ("shift", Gdk.ModifierType.SHIFT_MASK, "shift"),
- ("super", Gdk.ModifierType.SUPER_MASK, "super"),
- ]
- mods = [
- mod for mod, mask, mod_key in modifiers
- if (mod_key != key and state & mask
- and not (mod == "shift" and unikey.isprintable()))]
- return "+".join([*mods, key])
-
- def _update_device_pixel_ratio(self, *args, **kwargs):
- # We need to be careful in cases with mixed resolution displays if
- # device_pixel_ratio changes.
- if self._set_device_pixel_ratio(self.get_scale_factor()):
- self.draw()
-
- def _draw_rubberband(self, rect):
- self._rubberband_rect = rect
- # TODO: Only update the rubberband area.
- self.queue_draw()
-
- def _draw_func(self, drawing_area, ctx, width, height):
- self.on_draw_event(self, ctx)
- self._post_draw(self, ctx)
-
- def _post_draw(self, widget, ctx):
- if self._rubberband_rect is None:
- return
-
- lw = 1
- dash = 3
- if not self._context_is_scaled:
- x0, y0, w, h = (dim / self.device_pixel_ratio
- for dim in self._rubberband_rect)
- else:
- x0, y0, w, h = self._rubberband_rect
- lw *= self.device_pixel_ratio
- dash *= self.device_pixel_ratio
- x1 = x0 + w
- y1 = y0 + h
-
- # Draw the lines from x0, y0 towards x1, y1 so that the
- # dashes don't "jump" when moving the zoom box.
- ctx.move_to(x0, y0)
- ctx.line_to(x0, y1)
- ctx.move_to(x0, y0)
- ctx.line_to(x1, y0)
- ctx.move_to(x0, y1)
- ctx.line_to(x1, y1)
- ctx.move_to(x1, y0)
- ctx.line_to(x1, y1)
-
- ctx.set_antialias(1)
- ctx.set_line_width(lw)
- ctx.set_dash((dash, dash), 0)
- ctx.set_source_rgb(0, 0, 0)
- ctx.stroke_preserve()
-
- ctx.set_dash((dash, dash), dash)
- ctx.set_source_rgb(1, 1, 1)
- ctx.stroke()
-
- def on_draw_event(self, widget, ctx):
- # to be overwritten by GTK4Agg or GTK4Cairo
- pass
-
- def draw(self):
- # docstring inherited
- if self.is_drawable():
- self.queue_draw()
-
- def draw_idle(self):
- # docstring inherited
- if self._idle_draw_id != 0:
- return
- def idle_draw(*args):
- try:
- self.draw()
- finally:
- self._idle_draw_id = 0
- return False
- self._idle_draw_id = GLib.idle_add(idle_draw)
-
- def flush_events(self):
- # docstring inherited
- context = GLib.MainContext.default()
- while context.pending():
- context.iteration(True)
-
-
-class NavigationToolbar2GTK4(_NavigationToolbar2GTK, Gtk.Box):
- def __init__(self, canvas):
- Gtk.Box.__init__(self)
-
- self.add_css_class('toolbar')
-
- self._gtk_ids = {}
- for text, tooltip_text, image_file, callback in self.toolitems:
- if text is None:
- self.append(Gtk.Separator())
- continue
- image = Gtk.Image.new_from_gicon(
- Gio.Icon.new_for_string(
- str(cbook._get_data_path('images',
- f'{image_file}-symbolic.svg'))))
- self._gtk_ids[text] = button = (
- Gtk.ToggleButton() if callback in ['zoom', 'pan'] else
- Gtk.Button())
- button.set_child(image)
- button.add_css_class('flat')
- button.add_css_class('image-button')
- # Save the handler id, so that we can block it as needed.
- button._signal_handler = button.connect(
- 'clicked', getattr(self, callback))
- button.set_tooltip_text(tooltip_text)
- self.append(button)
-
- # This filler item ensures the toolbar is always at least two text
- # lines high. Otherwise the canvas gets redrawn as the mouse hovers
- # over images because those use two-line messages which resize the
- # toolbar.
- label = Gtk.Label()
- label.set_markup(
- '\N{NO-BREAK SPACE}\n\N{NO-BREAK SPACE}')
- label.set_hexpand(True) # Push real message to the right.
- self.append(label)
-
- self.message = Gtk.Label()
- self.message.set_justify(Gtk.Justification.RIGHT)
- self.append(self.message)
-
- _NavigationToolbar2GTK.__init__(self, canvas)
-
- def save_figure(self, *args):
- dialog = Gtk.FileChooserNative(
- title='Save the figure',
- transient_for=self.canvas.get_root(),
- action=Gtk.FileChooserAction.SAVE,
- modal=True)
- self._save_dialog = dialog # Must keep a reference.
-
- ff = Gtk.FileFilter()
- ff.set_name('All files')
- ff.add_pattern('*')
- dialog.add_filter(ff)
- dialog.set_filter(ff)
-
- formats = []
- default_format = None
- for i, (name, fmts) in enumerate(
- self.canvas.get_supported_filetypes_grouped().items()):
- ff = Gtk.FileFilter()
- ff.set_name(name)
- for fmt in fmts:
- ff.add_pattern(f'*.{fmt}')
- dialog.add_filter(ff)
- formats.append(name)
- if self.canvas.get_default_filetype() in fmts:
- default_format = i
- # Setting the choice doesn't always work, so make sure the default
- # format is first.
- formats = [formats[default_format], *formats[:default_format],
- *formats[default_format+1:]]
- dialog.add_choice('format', 'File format', formats, formats)
- dialog.set_choice('format', formats[default_format])
-
- dialog.set_current_folder(Gio.File.new_for_path(
- os.path.expanduser(mpl.rcParams['savefig.directory'])))
- dialog.set_current_name(self.canvas.get_default_filename())
-
- @functools.partial(dialog.connect, 'response')
- def on_response(dialog, response):
- file = dialog.get_file()
- fmt = dialog.get_choice('format')
- fmt = self.canvas.get_supported_filetypes_grouped()[fmt][0]
- dialog.destroy()
- self._save_dialog = None
- if response != Gtk.ResponseType.ACCEPT:
- return
- # Save dir for next time, unless empty str (which means use cwd).
- if mpl.rcParams['savefig.directory']:
- parent = file.get_parent()
- mpl.rcParams['savefig.directory'] = parent.get_path()
- try:
- self.canvas.figure.savefig(file.get_path(), format=fmt)
- except Exception as e:
- msg = Gtk.MessageDialog(
- transient_for=self.canvas.get_root(),
- message_type=Gtk.MessageType.ERROR,
- buttons=Gtk.ButtonsType.OK, modal=True,
- text=str(e))
- msg.show()
-
- dialog.show()
-
-
-class ToolbarGTK4(ToolContainerBase, Gtk.Box):
- _icon_extension = '-symbolic.svg'
-
- def __init__(self, toolmanager):
- ToolContainerBase.__init__(self, toolmanager)
- Gtk.Box.__init__(self)
- self.set_property('orientation', Gtk.Orientation.HORIZONTAL)
-
- # Tool items are created later, but must appear before the message.
- self._tool_box = Gtk.Box()
- self.append(self._tool_box)
- self._groups = {}
- self._toolitems = {}
-
- # This filler item ensures the toolbar is always at least two text
- # lines high. Otherwise the canvas gets redrawn as the mouse hovers
- # over images because those use two-line messages which resize the
- # toolbar.
- label = Gtk.Label()
- label.set_markup(
- '\N{NO-BREAK SPACE}\n\N{NO-BREAK SPACE}')
- label.set_hexpand(True) # Push real message to the right.
- self.append(label)
-
- self._message = Gtk.Label()
- self._message.set_justify(Gtk.Justification.RIGHT)
- self.append(self._message)
-
- def add_toolitem(self, name, group, position, image_file, description,
- toggle):
- if toggle:
- button = Gtk.ToggleButton()
- else:
- button = Gtk.Button()
- button.set_label(name)
- button.add_css_class('flat')
-
- if image_file is not None:
- image = Gtk.Image.new_from_gicon(
- Gio.Icon.new_for_string(image_file))
- button.set_child(image)
- button.add_css_class('image-button')
-
- if position is None:
- position = -1
-
- self._add_button(button, group, position)
- signal = button.connect('clicked', self._call_tool, name)
- button.set_tooltip_text(description)
- self._toolitems.setdefault(name, [])
- self._toolitems[name].append((button, signal))
-
- def _find_child_at_position(self, group, position):
- children = [None]
- child = self._groups[group].get_first_child()
- while child is not None:
- children.append(child)
- child = child.get_next_sibling()
- return children[position]
-
- def _add_button(self, button, group, position):
- if group not in self._groups:
- if self._groups:
- self._add_separator()
- group_box = Gtk.Box()
- self._tool_box.append(group_box)
- self._groups[group] = group_box
- self._groups[group].insert_child_after(
- button, self._find_child_at_position(group, position))
-
- def _call_tool(self, btn, name):
- self.trigger_tool(name)
-
- def toggle_toolitem(self, name, toggled):
- if name not in self._toolitems:
- return
- for toolitem, signal in self._toolitems[name]:
- toolitem.handler_block(signal)
- toolitem.set_active(toggled)
- toolitem.handler_unblock(signal)
-
- def remove_toolitem(self, name):
- if name not in self._toolitems:
- self.toolmanager.message_event(f'{name} not in toolbar', self)
- return
-
- for group in self._groups:
- for toolitem, _signal in self._toolitems[name]:
- if toolitem in self._groups[group]:
- self._groups[group].remove(toolitem)
- del self._toolitems[name]
-
- def _add_separator(self):
- sep = Gtk.Separator()
- sep.set_property("orientation", Gtk.Orientation.VERTICAL)
- self._tool_box.append(sep)
-
- def set_message(self, s):
- self._message.set_label(s)
-
-
-@backend_tools._register_tool_class(FigureCanvasGTK4)
-class SaveFigureGTK4(backend_tools.SaveFigureBase):
- def trigger(self, *args, **kwargs):
- NavigationToolbar2GTK4.save_figure(
- self._make_classic_style_pseudo_toolbar())
-
-
-@backend_tools._register_tool_class(FigureCanvasGTK4)
-class HelpGTK4(backend_tools.ToolHelpBase):
- def _normalize_shortcut(self, key):
- """
- Convert Matplotlib key presses to GTK+ accelerator identifiers.
-
- Related to `FigureCanvasGTK4._get_key`.
- """
- special = {
- 'backspace': 'BackSpace',
- 'pagedown': 'Page_Down',
- 'pageup': 'Page_Up',
- 'scroll_lock': 'Scroll_Lock',
- }
-
- parts = key.split('+')
- mods = ['<' + mod + '>' for mod in parts[:-1]]
- key = parts[-1]
-
- if key in special:
- key = special[key]
- elif len(key) > 1:
- key = key.capitalize()
- elif key.isupper():
- mods += ['']
-
- return ''.join(mods) + key
-
- def _is_valid_shortcut(self, key):
- """
- Check for a valid shortcut to be displayed.
-
- - GTK will never send 'cmd+' (see `FigureCanvasGTK4._get_key`).
- - The shortcut window only shows keyboard shortcuts, not mouse buttons.
- """
- return 'cmd+' not in key and not key.startswith('MouseButton.')
-
- def trigger(self, *args):
- section = Gtk.ShortcutsSection()
-
- for name, tool in sorted(self.toolmanager.tools.items()):
- if not tool.description:
- continue
-
- # Putting everything in a separate group allows GTK to
- # automatically split them into separate columns/pages, which is
- # useful because we have lots of shortcuts, some with many keys
- # that are very wide.
- group = Gtk.ShortcutsGroup()
- section.append(group)
- # A hack to remove the title since we have no group naming.
- child = group.get_first_child()
- while child is not None:
- child.set_visible(False)
- child = child.get_next_sibling()
-
- shortcut = Gtk.ShortcutsShortcut(
- accelerator=' '.join(
- self._normalize_shortcut(key)
- for key in self.toolmanager.get_tool_keymap(name)
- if self._is_valid_shortcut(key)),
- title=tool.name,
- subtitle=tool.description)
- group.append(shortcut)
-
- window = Gtk.ShortcutsWindow(
- title='Help',
- modal=True,
- transient_for=self._figure.canvas.get_root())
- window.set_child(section)
-
- window.show()
-
-
-@backend_tools._register_tool_class(FigureCanvasGTK4)
-class ToolCopyToClipboardGTK4(backend_tools.ToolCopyToClipboardBase):
- def trigger(self, *args, **kwargs):
- with io.BytesIO() as f:
- self.canvas.print_rgba(f)
- w, h = self.canvas.get_width_height()
- pb = GdkPixbuf.Pixbuf.new_from_data(f.getbuffer(),
- GdkPixbuf.Colorspace.RGB, True,
- 8, w, h, w*4)
- clipboard = self.canvas.get_clipboard()
- clipboard.set(pb)
-
-
-backend_tools._register_tool_class(
- FigureCanvasGTK4, _backend_gtk.ConfigureSubplotsGTK)
-backend_tools._register_tool_class(
- FigureCanvasGTK4, _backend_gtk.RubberbandGTK)
-Toolbar = ToolbarGTK4
-
-
-class FigureManagerGTK4(_FigureManagerGTK):
- _toolbar2_class = NavigationToolbar2GTK4
- _toolmanager_toolbar_class = ToolbarGTK4
-
-
-@_BackendGTK.export
-class _BackendGTK4(_BackendGTK):
- FigureCanvas = FigureCanvasGTK4
- FigureManager = FigureManagerGTK4
diff --git a/spaces/profayle/TerrapinTalk/myenv/lib/python3.9/site-packages/numpy/random/__init__.py b/spaces/profayle/TerrapinTalk/myenv/lib/python3.9/site-packages/numpy/random/__init__.py
deleted file mode 100644
index 2e8f99fe3045b9c2b691a8ece67d0f06d9d73b08..0000000000000000000000000000000000000000
--- a/spaces/profayle/TerrapinTalk/myenv/lib/python3.9/site-packages/numpy/random/__init__.py
+++ /dev/null
@@ -1,215 +0,0 @@
-"""
-========================
-Random Number Generation
-========================
-
-Use ``default_rng()`` to create a `Generator` and call its methods.
-
-=============== =========================================================
-Generator
---------------- ---------------------------------------------------------
-Generator Class implementing all of the random number distributions
-default_rng Default constructor for ``Generator``
-=============== =========================================================
-
-============================================= ===
-BitGenerator Streams that work with Generator
---------------------------------------------- ---
-MT19937
-PCG64
-PCG64DXSM
-Philox
-SFC64
-============================================= ===
-
-============================================= ===
-Getting entropy to initialize a BitGenerator
---------------------------------------------- ---
-SeedSequence
-============================================= ===
-
-
-Legacy
-------
-
-For backwards compatibility with previous versions of numpy before 1.17, the
-various aliases to the global `RandomState` methods are left alone and do not
-use the new `Generator` API.
-
-==================== =========================================================
-Utility functions
--------------------- ---------------------------------------------------------
-random Uniformly distributed floats over ``[0, 1)``
-bytes Uniformly distributed random bytes.
-permutation Randomly permute a sequence / generate a random sequence.
-shuffle Randomly permute a sequence in place.
-choice Random sample from 1-D array.
-==================== =========================================================
-
-==================== =========================================================
-Compatibility
-functions - removed
-in the new API
--------------------- ---------------------------------------------------------
-rand Uniformly distributed values.
-randn Normally distributed values.
-ranf Uniformly distributed floating point numbers.
-random_integers Uniformly distributed integers in a given range.
- (deprecated, use ``integers(..., closed=True)`` instead)
-random_sample Alias for `random_sample`
-randint Uniformly distributed integers in a given range
-seed Seed the legacy random number generator.
-==================== =========================================================
-
-==================== =========================================================
-Univariate
-distributions
--------------------- ---------------------------------------------------------
-beta Beta distribution over ``[0, 1]``.
-binomial Binomial distribution.
-chisquare :math:`\\chi^2` distribution.
-exponential Exponential distribution.
-f F (Fisher-Snedecor) distribution.
-gamma Gamma distribution.
-geometric Geometric distribution.
-gumbel Gumbel distribution.
-hypergeometric Hypergeometric distribution.
-laplace Laplace distribution.
-logistic Logistic distribution.
-lognormal Log-normal distribution.
-logseries Logarithmic series distribution.
-negative_binomial Negative binomial distribution.
-noncentral_chisquare Non-central chi-square distribution.
-noncentral_f Non-central F distribution.
-normal Normal / Gaussian distribution.
-pareto Pareto distribution.
-poisson Poisson distribution.
-power Power distribution.
-rayleigh Rayleigh distribution.
-triangular Triangular distribution.
-uniform Uniform distribution.
-vonmises Von Mises circular distribution.
-wald Wald (inverse Gaussian) distribution.
-weibull Weibull distribution.
-zipf Zipf's distribution over ranked data.
-==================== =========================================================
-
-==================== ==========================================================
-Multivariate
-distributions
--------------------- ----------------------------------------------------------
-dirichlet Multivariate generalization of Beta distribution.
-multinomial Multivariate generalization of the binomial distribution.
-multivariate_normal Multivariate generalization of the normal distribution.
-==================== ==========================================================
-
-==================== =========================================================
-Standard
-distributions
--------------------- ---------------------------------------------------------
-standard_cauchy Standard Cauchy-Lorentz distribution.
-standard_exponential Standard exponential distribution.
-standard_gamma Standard Gamma distribution.
-standard_normal Standard normal distribution.
-standard_t Standard Student's t-distribution.
-==================== =========================================================
-
-==================== =========================================================
-Internal functions
--------------------- ---------------------------------------------------------
-get_state Get tuple representing internal state of generator.
-set_state Set state of generator.
-==================== =========================================================
-
-
-"""
-__all__ = [
- 'beta',
- 'binomial',
- 'bytes',
- 'chisquare',
- 'choice',
- 'dirichlet',
- 'exponential',
- 'f',
- 'gamma',
- 'geometric',
- 'get_state',
- 'gumbel',
- 'hypergeometric',
- 'laplace',
- 'logistic',
- 'lognormal',
- 'logseries',
- 'multinomial',
- 'multivariate_normal',
- 'negative_binomial',
- 'noncentral_chisquare',
- 'noncentral_f',
- 'normal',
- 'pareto',
- 'permutation',
- 'poisson',
- 'power',
- 'rand',
- 'randint',
- 'randn',
- 'random',
- 'random_integers',
- 'random_sample',
- 'ranf',
- 'rayleigh',
- 'sample',
- 'seed',
- 'set_state',
- 'shuffle',
- 'standard_cauchy',
- 'standard_exponential',
- 'standard_gamma',
- 'standard_normal',
- 'standard_t',
- 'triangular',
- 'uniform',
- 'vonmises',
- 'wald',
- 'weibull',
- 'zipf',
-]
-
-# add these for module-freeze analysis (like PyInstaller)
-from . import _pickle
-from . import _common
-from . import _bounded_integers
-
-from ._generator import Generator, default_rng
-from .bit_generator import SeedSequence, BitGenerator
-from ._mt19937 import MT19937
-from ._pcg64 import PCG64, PCG64DXSM
-from ._philox import Philox
-from ._sfc64 import SFC64
-from .mtrand import *
-
-__all__ += ['Generator', 'RandomState', 'SeedSequence', 'MT19937',
- 'Philox', 'PCG64', 'PCG64DXSM', 'SFC64', 'default_rng',
- 'BitGenerator']
-
-
-def __RandomState_ctor():
- """Return a RandomState instance.
-
- This function exists solely to assist (un)pickling.
-
- Note that the state of the RandomState returned here is irrelevant, as this
- function's entire purpose is to return a newly allocated RandomState whose
- state pickle can set. Consequently the RandomState returned by this function
- is a freshly allocated copy with a seed=0.
-
- See https://github.com/numpy/numpy/issues/4763 for a detailed discussion
-
- """
- return RandomState(seed=0)
-
-
-from numpy._pytesttester import PytestTester
-test = PytestTester(__name__)
-del PytestTester
diff --git a/spaces/profayle/TerrapinTalk/myenv/lib/python3.9/site-packages/pandas/core/arrays/base.py b/spaces/profayle/TerrapinTalk/myenv/lib/python3.9/site-packages/pandas/core/arrays/base.py
deleted file mode 100644
index bfd6ae361e1e8fdf0526a754476903b2274f5d7c..0000000000000000000000000000000000000000
--- a/spaces/profayle/TerrapinTalk/myenv/lib/python3.9/site-packages/pandas/core/arrays/base.py
+++ /dev/null
@@ -1,2451 +0,0 @@
-"""
-An interface for extending pandas with custom arrays.
-
-.. warning::
-
- This is an experimental API and subject to breaking changes
- without warning.
-"""
-from __future__ import annotations
-
-import operator
-from typing import (
- TYPE_CHECKING,
- Any,
- Callable,
- ClassVar,
- Literal,
- cast,
- overload,
-)
-import warnings
-
-import numpy as np
-
-from pandas._libs import (
- algos as libalgos,
- lib,
-)
-from pandas.compat import set_function_name
-from pandas.compat.numpy import function as nv
-from pandas.errors import AbstractMethodError
-from pandas.util._decorators import (
- Appender,
- Substitution,
- cache_readonly,
-)
-from pandas.util._exceptions import find_stack_level
-from pandas.util._validators import (
- validate_bool_kwarg,
- validate_fillna_kwargs,
- validate_insert_loc,
-)
-
-from pandas.core.dtypes.cast import maybe_cast_pointwise_result
-from pandas.core.dtypes.common import (
- is_list_like,
- is_scalar,
- pandas_dtype,
-)
-from pandas.core.dtypes.dtypes import ExtensionDtype
-from pandas.core.dtypes.generic import (
- ABCDataFrame,
- ABCIndex,
- ABCSeries,
-)
-from pandas.core.dtypes.missing import isna
-
-from pandas.core import (
- arraylike,
- missing,
- roperator,
-)
-from pandas.core.algorithms import (
- factorize_array,
- isin,
- map_array,
- mode,
- rank,
- unique,
-)
-from pandas.core.array_algos.quantile import quantile_with_mask
-from pandas.core.sorting import (
- nargminmax,
- nargsort,
-)
-
-if TYPE_CHECKING:
- from collections.abc import (
- Iterator,
- Sequence,
- )
-
- from pandas._typing import (
- ArrayLike,
- AstypeArg,
- AxisInt,
- Dtype,
- FillnaOptions,
- InterpolateOptions,
- NumpySorter,
- NumpyValueArrayLike,
- PositionalIndexer,
- ScalarIndexer,
- Self,
- SequenceIndexer,
- Shape,
- SortKind,
- TakeIndexer,
- npt,
- )
-
- from pandas import Index
-
-_extension_array_shared_docs: dict[str, str] = {}
-
-
-class ExtensionArray:
- """
- Abstract base class for custom 1-D array types.
-
- pandas will recognize instances of this class as proper arrays
- with a custom type and will not attempt to coerce them to objects. They
- may be stored directly inside a :class:`DataFrame` or :class:`Series`.
-
- Attributes
- ----------
- dtype
- nbytes
- ndim
- shape
-
- Methods
- -------
- argsort
- astype
- copy
- dropna
- factorize
- fillna
- equals
- insert
- interpolate
- isin
- isna
- ravel
- repeat
- searchsorted
- shift
- take
- tolist
- unique
- view
- _accumulate
- _concat_same_type
- _formatter
- _from_factorized
- _from_sequence
- _from_sequence_of_strings
- _hash_pandas_object
- _pad_or_backfill
- _reduce
- _values_for_argsort
- _values_for_factorize
-
- Notes
- -----
- The interface includes the following abstract methods that must be
- implemented by subclasses:
-
- * _from_sequence
- * _from_factorized
- * __getitem__
- * __len__
- * __eq__
- * dtype
- * nbytes
- * isna
- * take
- * copy
- * _concat_same_type
- * interpolate
-
- A default repr displaying the type, (truncated) data, length,
- and dtype is provided. It can be customized or replaced by
- by overriding:
-
- * __repr__ : A default repr for the ExtensionArray.
- * _formatter : Print scalars inside a Series or DataFrame.
-
- Some methods require casting the ExtensionArray to an ndarray of Python
- objects with ``self.astype(object)``, which may be expensive. When
- performance is a concern, we highly recommend overriding the following
- methods:
-
- * fillna
- * _pad_or_backfill
- * dropna
- * unique
- * factorize / _values_for_factorize
- * argsort, argmax, argmin / _values_for_argsort
- * searchsorted
- * map
-
- The remaining methods implemented on this class should be performant,
- as they only compose abstract methods. Still, a more efficient
- implementation may be available, and these methods can be overridden.
-
- One can implement methods to handle array accumulations or reductions.
-
- * _accumulate
- * _reduce
-
- One can implement methods to handle parsing from strings that will be used
- in methods such as ``pandas.io.parsers.read_csv``.
-
- * _from_sequence_of_strings
-
- This class does not inherit from 'abc.ABCMeta' for performance reasons.
- Methods and properties required by the interface raise
- ``pandas.errors.AbstractMethodError`` and no ``register`` method is
- provided for registering virtual subclasses.
-
- ExtensionArrays are limited to 1 dimension.
-
- They may be backed by none, one, or many NumPy arrays. For example,
- ``pandas.Categorical`` is an extension array backed by two arrays,
- one for codes and one for categories. An array of IPv6 address may
- be backed by a NumPy structured array with two fields, one for the
- lower 64 bits and one for the upper 64 bits. Or they may be backed
- by some other storage type, like Python lists. Pandas makes no
- assumptions on how the data are stored, just that it can be converted
- to a NumPy array.
- The ExtensionArray interface does not impose any rules on how this data
- is stored. However, currently, the backing data cannot be stored in
- attributes called ``.values`` or ``._values`` to ensure full compatibility
- with pandas internals. But other names as ``.data``, ``._data``,
- ``._items``, ... can be freely used.
-
- If implementing NumPy's ``__array_ufunc__`` interface, pandas expects
- that
-
- 1. You defer by returning ``NotImplemented`` when any Series are present
- in `inputs`. Pandas will extract the arrays and call the ufunc again.
- 2. You define a ``_HANDLED_TYPES`` tuple as an attribute on the class.
- Pandas inspect this to determine whether the ufunc is valid for the
- types present.
-
- See :ref:`extending.extension.ufunc` for more.
-
- By default, ExtensionArrays are not hashable. Immutable subclasses may
- override this behavior.
-
- Examples
- --------
- Please see the following:
-
- https://github.com/pandas-dev/pandas/blob/main/pandas/tests/extension/list/array.py
- """
-
- # '_typ' is for pandas.core.dtypes.generic.ABCExtensionArray.
- # Don't override this.
- _typ = "extension"
-
- # similar to __array_priority__, positions ExtensionArray after Index,
- # Series, and DataFrame. EA subclasses may override to choose which EA
- # subclass takes priority. If overriding, the value should always be
- # strictly less than 2000 to be below Index.__pandas_priority__.
- __pandas_priority__ = 1000
-
- # ------------------------------------------------------------------------
- # Constructors
- # ------------------------------------------------------------------------
-
- @classmethod
- def _from_sequence(cls, scalars, *, dtype: Dtype | None = None, copy: bool = False):
- """
- Construct a new ExtensionArray from a sequence of scalars.
-
- Parameters
- ----------
- scalars : Sequence
- Each element will be an instance of the scalar type for this
- array, ``cls.dtype.type`` or be converted into this type in this method.
- dtype : dtype, optional
- Construct for this particular dtype. This should be a Dtype
- compatible with the ExtensionArray.
- copy : bool, default False
- If True, copy the underlying data.
-
- Returns
- -------
- ExtensionArray
-
- Examples
- --------
- >>> pd.arrays.IntegerArray._from_sequence([4, 5])
-
- [4, 5]
- Length: 2, dtype: Int64
- """
- raise AbstractMethodError(cls)
-
- @classmethod
- def _from_sequence_of_strings(
- cls, strings, *, dtype: Dtype | None = None, copy: bool = False
- ):
- """
- Construct a new ExtensionArray from a sequence of strings.
-
- Parameters
- ----------
- strings : Sequence
- Each element will be an instance of the scalar type for this
- array, ``cls.dtype.type``.
- dtype : dtype, optional
- Construct for this particular dtype. This should be a Dtype
- compatible with the ExtensionArray.
- copy : bool, default False
- If True, copy the underlying data.
-
- Returns
- -------
- ExtensionArray
-
- Examples
- --------
- >>> pd.arrays.IntegerArray._from_sequence_of_strings(["1", "2", "3"])
-
- [1, 2, 3]
- Length: 3, dtype: Int64
- """
- raise AbstractMethodError(cls)
-
- @classmethod
- def _from_factorized(cls, values, original):
- """
- Reconstruct an ExtensionArray after factorization.
-
- Parameters
- ----------
- values : ndarray
- An integer ndarray with the factorized values.
- original : ExtensionArray
- The original ExtensionArray that factorize was called on.
-
- See Also
- --------
- factorize : Top-level factorize method that dispatches here.
- ExtensionArray.factorize : Encode the extension array as an enumerated type.
-
- Examples
- --------
- >>> interv_arr = pd.arrays.IntervalArray([pd.Interval(0, 1),
- ... pd.Interval(1, 5), pd.Interval(1, 5)])
- >>> codes, uniques = pd.factorize(interv_arr)
- >>> pd.arrays.IntervalArray._from_factorized(uniques, interv_arr)
-
- [(0, 1], (1, 5]]
- Length: 2, dtype: interval[int64, right]
- """
- raise AbstractMethodError(cls)
-
- # ------------------------------------------------------------------------
- # Must be a Sequence
- # ------------------------------------------------------------------------
- @overload
- def __getitem__(self, item: ScalarIndexer) -> Any:
- ...
-
- @overload
- def __getitem__(self, item: SequenceIndexer) -> Self:
- ...
-
- def __getitem__(self, item: PositionalIndexer) -> Self | Any:
- """
- Select a subset of self.
-
- Parameters
- ----------
- item : int, slice, or ndarray
- * int: The position in 'self' to get.
-
- * slice: A slice object, where 'start', 'stop', and 'step' are
- integers or None
-
- * ndarray: A 1-d boolean NumPy ndarray the same length as 'self'
-
- * list[int]: A list of int
-
- Returns
- -------
- item : scalar or ExtensionArray
-
- Notes
- -----
- For scalar ``item``, return a scalar value suitable for the array's
- type. This should be an instance of ``self.dtype.type``.
-
- For slice ``key``, return an instance of ``ExtensionArray``, even
- if the slice is length 0 or 1.
-
- For a boolean mask, return an instance of ``ExtensionArray``, filtered
- to the values where ``item`` is True.
- """
- raise AbstractMethodError(self)
-
- def __setitem__(self, key, value) -> None:
- """
- Set one or more values inplace.
-
- This method is not required to satisfy the pandas extension array
- interface.
-
- Parameters
- ----------
- key : int, ndarray, or slice
- When called from, e.g. ``Series.__setitem__``, ``key`` will be
- one of
-
- * scalar int
- * ndarray of integers.
- * boolean ndarray
- * slice object
-
- value : ExtensionDtype.type, Sequence[ExtensionDtype.type], or object
- value or values to be set of ``key``.
-
- Returns
- -------
- None
- """
- # Some notes to the ExtensionArray implementor who may have ended up
- # here. While this method is not required for the interface, if you
- # *do* choose to implement __setitem__, then some semantics should be
- # observed:
- #
- # * Setting multiple values : ExtensionArrays should support setting
- # multiple values at once, 'key' will be a sequence of integers and
- # 'value' will be a same-length sequence.
- #
- # * Broadcasting : For a sequence 'key' and a scalar 'value',
- # each position in 'key' should be set to 'value'.
- #
- # * Coercion : Most users will expect basic coercion to work. For
- # example, a string like '2018-01-01' is coerced to a datetime
- # when setting on a datetime64ns array. In general, if the
- # __init__ method coerces that value, then so should __setitem__
- # Note, also, that Series/DataFrame.where internally use __setitem__
- # on a copy of the data.
- raise NotImplementedError(f"{type(self)} does not implement __setitem__.")
-
- def __len__(self) -> int:
- """
- Length of this array
-
- Returns
- -------
- length : int
- """
- raise AbstractMethodError(self)
-
- def __iter__(self) -> Iterator[Any]:
- """
- Iterate over elements of the array.
- """
- # This needs to be implemented so that pandas recognizes extension
- # arrays as list-like. The default implementation makes successive
- # calls to ``__getitem__``, which may be slower than necessary.
- for i in range(len(self)):
- yield self[i]
-
- def __contains__(self, item: object) -> bool | np.bool_:
- """
- Return for `item in self`.
- """
- # GH37867
- # comparisons of any item to pd.NA always return pd.NA, so e.g. "a" in [pd.NA]
- # would raise a TypeError. The implementation below works around that.
- if is_scalar(item) and isna(item):
- if not self._can_hold_na:
- return False
- elif item is self.dtype.na_value or isinstance(item, self.dtype.type):
- return self._hasna
- else:
- return False
- else:
- # error: Item "ExtensionArray" of "Union[ExtensionArray, ndarray]" has no
- # attribute "any"
- return (item == self).any() # type: ignore[union-attr]
-
- # error: Signature of "__eq__" incompatible with supertype "object"
- def __eq__(self, other: Any) -> ArrayLike: # type: ignore[override]
- """
- Return for `self == other` (element-wise equality).
- """
- # Implementer note: this should return a boolean numpy ndarray or
- # a boolean ExtensionArray.
- # When `other` is one of Series, Index, or DataFrame, this method should
- # return NotImplemented (to ensure that those objects are responsible for
- # first unpacking the arrays, and then dispatch the operation to the
- # underlying arrays)
- raise AbstractMethodError(self)
-
- # error: Signature of "__ne__" incompatible with supertype "object"
- def __ne__(self, other: Any) -> ArrayLike: # type: ignore[override]
- """
- Return for `self != other` (element-wise in-equality).
- """
- return ~(self == other)
-
- def to_numpy(
- self,
- dtype: npt.DTypeLike | None = None,
- copy: bool = False,
- na_value: object = lib.no_default,
- ) -> np.ndarray:
- """
- Convert to a NumPy ndarray.
-
- This is similar to :meth:`numpy.asarray`, but may provide additional control
- over how the conversion is done.
-
- Parameters
- ----------
- dtype : str or numpy.dtype, optional
- The dtype to pass to :meth:`numpy.asarray`.
- copy : bool, default False
- Whether to ensure that the returned value is a not a view on
- another array. Note that ``copy=False`` does not *ensure* that
- ``to_numpy()`` is no-copy. Rather, ``copy=True`` ensure that
- a copy is made, even if not strictly necessary.
- na_value : Any, optional
- The value to use for missing values. The default value depends
- on `dtype` and the type of the array.
-
- Returns
- -------
- numpy.ndarray
- """
- result = np.asarray(self, dtype=dtype)
- if copy or na_value is not lib.no_default:
- result = result.copy()
- if na_value is not lib.no_default:
- result[self.isna()] = na_value
- return result
-
- # ------------------------------------------------------------------------
- # Required attributes
- # ------------------------------------------------------------------------
-
- @property
- def dtype(self) -> ExtensionDtype:
- """
- An instance of ExtensionDtype.
-
- Examples
- --------
- >>> pd.array([1, 2, 3]).dtype
- Int64Dtype()
- """
- raise AbstractMethodError(self)
-
- @property
- def shape(self) -> Shape:
- """
- Return a tuple of the array dimensions.
-
- Examples
- --------
- >>> arr = pd.array([1, 2, 3])
- >>> arr.shape
- (3,)
- """
- return (len(self),)
-
- @property
- def size(self) -> int:
- """
- The number of elements in the array.
- """
- # error: Incompatible return value type (got "signedinteger[_64Bit]",
- # expected "int") [return-value]
- return np.prod(self.shape) # type: ignore[return-value]
-
- @property
- def ndim(self) -> int:
- """
- Extension Arrays are only allowed to be 1-dimensional.
-
- Examples
- --------
- >>> arr = pd.array([1, 2, 3])
- >>> arr.ndim
- 1
- """
- return 1
-
- @property
- def nbytes(self) -> int:
- """
- The number of bytes needed to store this object in memory.
-
- Examples
- --------
- >>> pd.array([1, 2, 3]).nbytes
- 27
- """
- # If this is expensive to compute, return an approximate lower bound
- # on the number of bytes needed.
- raise AbstractMethodError(self)
-
- # ------------------------------------------------------------------------
- # Additional Methods
- # ------------------------------------------------------------------------
-
- @overload
- def astype(self, dtype: npt.DTypeLike, copy: bool = ...) -> np.ndarray:
- ...
-
- @overload
- def astype(self, dtype: ExtensionDtype, copy: bool = ...) -> ExtensionArray:
- ...
-
- @overload
- def astype(self, dtype: AstypeArg, copy: bool = ...) -> ArrayLike:
- ...
-
- def astype(self, dtype: AstypeArg, copy: bool = True) -> ArrayLike:
- """
- Cast to a NumPy array or ExtensionArray with 'dtype'.
-
- Parameters
- ----------
- dtype : str or dtype
- Typecode or data-type to which the array is cast.
- copy : bool, default True
- Whether to copy the data, even if not necessary. If False,
- a copy is made only if the old dtype does not match the
- new dtype.
-
- Returns
- -------
- np.ndarray or pandas.api.extensions.ExtensionArray
- An ``ExtensionArray`` if ``dtype`` is ``ExtensionDtype``,
- otherwise a Numpy ndarray with ``dtype`` for its dtype.
-
- Examples
- --------
- >>> arr = pd.array([1, 2, 3])
- >>> arr
-
- [1, 2, 3]
- Length: 3, dtype: Int64
-
- Casting to another ``ExtensionDtype`` returns an ``ExtensionArray``:
-
- >>> arr1 = arr.astype('Float64')
- >>> arr1
-
- [1.0, 2.0, 3.0]
- Length: 3, dtype: Float64
- >>> arr1.dtype
- Float64Dtype()
-
- Otherwise, we will get a Numpy ndarray:
-
- >>> arr2 = arr.astype('float64')
- >>> arr2
- array([1., 2., 3.])
- >>> arr2.dtype
- dtype('float64')
- """
- dtype = pandas_dtype(dtype)
- if dtype == self.dtype:
- if not copy:
- return self
- else:
- return self.copy()
-
- if isinstance(dtype, ExtensionDtype):
- cls = dtype.construct_array_type()
- return cls._from_sequence(self, dtype=dtype, copy=copy)
-
- elif lib.is_np_dtype(dtype, "M"):
- from pandas.core.arrays import DatetimeArray
-
- return DatetimeArray._from_sequence(self, dtype=dtype, copy=copy)
-
- elif lib.is_np_dtype(dtype, "m"):
- from pandas.core.arrays import TimedeltaArray
-
- return TimedeltaArray._from_sequence(self, dtype=dtype, copy=copy)
-
- return np.array(self, dtype=dtype, copy=copy)
-
- def isna(self) -> np.ndarray | ExtensionArraySupportsAnyAll:
- """
- A 1-D array indicating if each value is missing.
-
- Returns
- -------
- numpy.ndarray or pandas.api.extensions.ExtensionArray
- In most cases, this should return a NumPy ndarray. For
- exceptional cases like ``SparseArray``, where returning
- an ndarray would be expensive, an ExtensionArray may be
- returned.
-
- Notes
- -----
- If returning an ExtensionArray, then
-
- * ``na_values._is_boolean`` should be True
- * `na_values` should implement :func:`ExtensionArray._reduce`
- * ``na_values.any`` and ``na_values.all`` should be implemented
-
- Examples
- --------
- >>> arr = pd.array([1, 2, np.nan, np.nan])
- >>> arr.isna()
- array([False, False, True, True])
- """
- raise AbstractMethodError(self)
-
- @property
- def _hasna(self) -> bool:
- # GH#22680
- """
- Equivalent to `self.isna().any()`.
-
- Some ExtensionArray subclasses may be able to optimize this check.
- """
- return bool(self.isna().any())
-
- def _values_for_argsort(self) -> np.ndarray:
- """
- Return values for sorting.
-
- Returns
- -------
- ndarray
- The transformed values should maintain the ordering between values
- within the array.
-
- See Also
- --------
- ExtensionArray.argsort : Return the indices that would sort this array.
-
- Notes
- -----
- The caller is responsible for *not* modifying these values in-place, so
- it is safe for implementors to give views on ``self``.
-
- Functions that use this (e.g. ``ExtensionArray.argsort``) should ignore
- entries with missing values in the original array (according to
- ``self.isna()``). This means that the corresponding entries in the returned
- array don't need to be modified to sort correctly.
-
- Examples
- --------
- In most cases, this is the underlying Numpy array of the ``ExtensionArray``:
-
- >>> arr = pd.array([1, 2, 3])
- >>> arr._values_for_argsort()
- array([1, 2, 3])
- """
- # Note: this is used in `ExtensionArray.argsort/argmin/argmax`.
- return np.array(self)
-
- def argsort(
- self,
- *,
- ascending: bool = True,
- kind: SortKind = "quicksort",
- na_position: str = "last",
- **kwargs,
- ) -> np.ndarray:
- """
- Return the indices that would sort this array.
-
- Parameters
- ----------
- ascending : bool, default True
- Whether the indices should result in an ascending
- or descending sort.
- kind : {'quicksort', 'mergesort', 'heapsort', 'stable'}, optional
- Sorting algorithm.
- na_position : {'first', 'last'}, default 'last'
- If ``'first'``, put ``NaN`` values at the beginning.
- If ``'last'``, put ``NaN`` values at the end.
- *args, **kwargs:
- Passed through to :func:`numpy.argsort`.
-
- Returns
- -------
- np.ndarray[np.intp]
- Array of indices that sort ``self``. If NaN values are contained,
- NaN values are placed at the end.
-
- See Also
- --------
- numpy.argsort : Sorting implementation used internally.
-
- Examples
- --------
- >>> arr = pd.array([3, 1, 2, 5, 4])
- >>> arr.argsort()
- array([1, 2, 0, 4, 3])
- """
- # Implementor note: You have two places to override the behavior of
- # argsort.
- # 1. _values_for_argsort : construct the values passed to np.argsort
- # 2. argsort : total control over sorting. In case of overriding this,
- # it is recommended to also override argmax/argmin
- ascending = nv.validate_argsort_with_ascending(ascending, (), kwargs)
-
- values = self._values_for_argsort()
- return nargsort(
- values,
- kind=kind,
- ascending=ascending,
- na_position=na_position,
- mask=np.asarray(self.isna()),
- )
-
- def argmin(self, skipna: bool = True) -> int:
- """
- Return the index of minimum value.
-
- In case of multiple occurrences of the minimum value, the index
- corresponding to the first occurrence is returned.
-
- Parameters
- ----------
- skipna : bool, default True
-
- Returns
- -------
- int
-
- See Also
- --------
- ExtensionArray.argmax : Return the index of the maximum value.
-
- Examples
- --------
- >>> arr = pd.array([3, 1, 2, 5, 4])
- >>> arr.argmin()
- 1
- """
- # Implementor note: You have two places to override the behavior of
- # argmin.
- # 1. _values_for_argsort : construct the values used in nargminmax
- # 2. argmin itself : total control over sorting.
- validate_bool_kwarg(skipna, "skipna")
- if not skipna and self._hasna:
- raise NotImplementedError
- return nargminmax(self, "argmin")
-
- def argmax(self, skipna: bool = True) -> int:
- """
- Return the index of maximum value.
-
- In case of multiple occurrences of the maximum value, the index
- corresponding to the first occurrence is returned.
-
- Parameters
- ----------
- skipna : bool, default True
-
- Returns
- -------
- int
-
- See Also
- --------
- ExtensionArray.argmin : Return the index of the minimum value.
-
- Examples
- --------
- >>> arr = pd.array([3, 1, 2, 5, 4])
- >>> arr.argmax()
- 3
- """
- # Implementor note: You have two places to override the behavior of
- # argmax.
- # 1. _values_for_argsort : construct the values used in nargminmax
- # 2. argmax itself : total control over sorting.
- validate_bool_kwarg(skipna, "skipna")
- if not skipna and self._hasna:
- raise NotImplementedError
- return nargminmax(self, "argmax")
-
- def interpolate(
- self,
- *,
- method: InterpolateOptions,
- axis: int,
- index: Index,
- limit,
- limit_direction,
- limit_area,
- copy: bool,
- **kwargs,
- ) -> Self:
- """
- See DataFrame.interpolate.__doc__.
-
- Examples
- --------
- >>> arr = pd.arrays.NumpyExtensionArray(np.array([0, 1, np.nan, 3]))
- >>> arr.interpolate(method="linear",
- ... limit=3,
- ... limit_direction="forward",
- ... index=pd.Index([1, 2, 3, 4]),
- ... fill_value=1,
- ... copy=False,
- ... axis=0,
- ... limit_area="inside"
- ... )
-
- [0.0, 1.0, 2.0, 3.0]
- Length: 4, dtype: float64
- """
- # NB: we return type(self) even if copy=False
- raise NotImplementedError(
- f"{type(self).__name__} does not implement interpolate"
- )
-
- def _pad_or_backfill(
- self, *, method: FillnaOptions, limit: int | None = None, copy: bool = True
- ) -> Self:
- """
- Pad or backfill values, used by Series/DataFrame ffill and bfill.
-
- Parameters
- ----------
- method : {'backfill', 'bfill', 'pad', 'ffill'}
- Method to use for filling holes in reindexed Series:
-
- * pad / ffill: propagate last valid observation forward to next valid.
- * backfill / bfill: use NEXT valid observation to fill gap.
-
- limit : int, default None
- This is the maximum number of consecutive
- NaN values to forward/backward fill. In other words, if there is
- a gap with more than this number of consecutive NaNs, it will only
- be partially filled. If method is not specified, this is the
- maximum number of entries along the entire axis where NaNs will be
- filled.
-
- copy : bool, default True
- Whether to make a copy of the data before filling. If False, then
- the original should be modified and no new memory should be allocated.
- For ExtensionArray subclasses that cannot do this, it is at the
- author's discretion whether to ignore "copy=False" or to raise.
- The base class implementation ignores the keyword if any NAs are
- present.
-
- Returns
- -------
- Same type as self
-
- Examples
- --------
- >>> arr = pd.array([np.nan, np.nan, 2, 3, np.nan, np.nan])
- >>> arr._pad_or_backfill(method="backfill", limit=1)
-
- [, 2, 2, 3, , ]
- Length: 6, dtype: Int64
- """
-
- # If a 3rd-party EA has implemented this functionality in fillna,
- # we warn that they need to implement _pad_or_backfill instead.
- if (
- type(self).fillna is not ExtensionArray.fillna
- and type(self)._pad_or_backfill is ExtensionArray._pad_or_backfill
- ):
- # Check for _pad_or_backfill here allows us to call
- # super()._pad_or_backfill without getting this warning
- warnings.warn(
- "ExtensionArray.fillna 'method' keyword is deprecated. "
- "In a future version. arr._pad_or_backfill will be called "
- "instead. 3rd-party ExtensionArray authors need to implement "
- "_pad_or_backfill.",
- DeprecationWarning,
- stacklevel=find_stack_level(),
- )
- return self.fillna(method=method, limit=limit)
-
- mask = self.isna()
-
- if mask.any():
- # NB: the base class does not respect the "copy" keyword
- meth = missing.clean_fill_method(method)
-
- npmask = np.asarray(mask)
- if meth == "pad":
- indexer = libalgos.get_fill_indexer(npmask, limit=limit)
- return self.take(indexer, allow_fill=True)
- else:
- # i.e. meth == "backfill"
- indexer = libalgos.get_fill_indexer(npmask[::-1], limit=limit)[::-1]
- return self[::-1].take(indexer, allow_fill=True)
-
- else:
- if not copy:
- return self
- new_values = self.copy()
- return new_values
-
- def fillna(
- self,
- value: object | ArrayLike | None = None,
- method: FillnaOptions | None = None,
- limit: int | None = None,
- copy: bool = True,
- ) -> Self:
- """
- Fill NA/NaN values using the specified method.
-
- Parameters
- ----------
- value : scalar, array-like
- If a scalar value is passed it is used to fill all missing values.
- Alternatively, an array-like "value" can be given. It's expected
- that the array-like have the same length as 'self'.
- method : {'backfill', 'bfill', 'pad', 'ffill', None}, default None
- Method to use for filling holes in reindexed Series:
-
- * pad / ffill: propagate last valid observation forward to next valid.
- * backfill / bfill: use NEXT valid observation to fill gap.
-
- .. deprecated:: 2.1.0
-
- limit : int, default None
- If method is specified, this is the maximum number of consecutive
- NaN values to forward/backward fill. In other words, if there is
- a gap with more than this number of consecutive NaNs, it will only
- be partially filled. If method is not specified, this is the
- maximum number of entries along the entire axis where NaNs will be
- filled.
-
- .. deprecated:: 2.1.0
-
- copy : bool, default True
- Whether to make a copy of the data before filling. If False, then
- the original should be modified and no new memory should be allocated.
- For ExtensionArray subclasses that cannot do this, it is at the
- author's discretion whether to ignore "copy=False" or to raise.
- The base class implementation ignores the keyword in pad/backfill
- cases.
-
- Returns
- -------
- ExtensionArray
- With NA/NaN filled.
-
- Examples
- --------
- >>> arr = pd.array([np.nan, np.nan, 2, 3, np.nan, np.nan])
- >>> arr.fillna(0)
-
- [0, 0, 2, 3, 0, 0]
- Length: 6, dtype: Int64
- """
- if method is not None:
- warnings.warn(
- f"The 'method' keyword in {type(self).__name__}.fillna is "
- "deprecated and will be removed in a future version.",
- FutureWarning,
- stacklevel=find_stack_level(),
- )
-
- value, method = validate_fillna_kwargs(value, method)
-
- mask = self.isna()
- # error: Argument 2 to "check_value_size" has incompatible type
- # "ExtensionArray"; expected "ndarray"
- value = missing.check_value_size(
- value, mask, len(self) # type: ignore[arg-type]
- )
-
- if mask.any():
- if method is not None:
- meth = missing.clean_fill_method(method)
-
- npmask = np.asarray(mask)
- if meth == "pad":
- indexer = libalgos.get_fill_indexer(npmask, limit=limit)
- return self.take(indexer, allow_fill=True)
- else:
- # i.e. meth == "backfill"
- indexer = libalgos.get_fill_indexer(npmask[::-1], limit=limit)[::-1]
- return self[::-1].take(indexer, allow_fill=True)
- else:
- # fill with value
- if not copy:
- new_values = self[:]
- else:
- new_values = self.copy()
- new_values[mask] = value
- else:
- if not copy:
- new_values = self[:]
- else:
- new_values = self.copy()
- return new_values
-
- def dropna(self) -> Self:
- """
- Return ExtensionArray without NA values.
-
- Returns
- -------
-
- Examples
- --------
- >>> pd.array([1, 2, np.nan]).dropna()
-
- [1, 2]
- Length: 2, dtype: Int64
- """
- # error: Unsupported operand type for ~ ("ExtensionArray")
- return self[~self.isna()] # type: ignore[operator]
-
- def shift(self, periods: int = 1, fill_value: object = None) -> ExtensionArray:
- """
- Shift values by desired number.
-
- Newly introduced missing values are filled with
- ``self.dtype.na_value``.
-
- Parameters
- ----------
- periods : int, default 1
- The number of periods to shift. Negative values are allowed
- for shifting backwards.
-
- fill_value : object, optional
- The scalar value to use for newly introduced missing values.
- The default is ``self.dtype.na_value``.
-
- Returns
- -------
- ExtensionArray
- Shifted.
-
- Notes
- -----
- If ``self`` is empty or ``periods`` is 0, a copy of ``self`` is
- returned.
-
- If ``periods > len(self)``, then an array of size
- len(self) is returned, with all values filled with
- ``self.dtype.na_value``.
-
- For 2-dimensional ExtensionArrays, we are always shifting along axis=0.
-
- Examples
- --------
- >>> arr = pd.array([1, 2, 3])
- >>> arr.shift(2)
-
- [, , 1]
- Length: 3, dtype: Int64
- """
- # Note: this implementation assumes that `self.dtype.na_value` can be
- # stored in an instance of your ExtensionArray with `self.dtype`.
- if not len(self) or periods == 0:
- return self.copy()
-
- if isna(fill_value):
- fill_value = self.dtype.na_value
-
- empty = self._from_sequence(
- [fill_value] * min(abs(periods), len(self)), dtype=self.dtype
- )
- if periods > 0:
- a = empty
- b = self[:-periods]
- else:
- a = self[abs(periods) :]
- b = empty
- return self._concat_same_type([a, b])
-
- def unique(self) -> Self:
- """
- Compute the ExtensionArray of unique values.
-
- Returns
- -------
- pandas.api.extensions.ExtensionArray
-
- Examples
- --------
- >>> arr = pd.array([1, 2, 3, 1, 2, 3])
- >>> arr.unique()
-
- [1, 2, 3]
- Length: 3, dtype: Int64
- """
- uniques = unique(self.astype(object))
- return self._from_sequence(uniques, dtype=self.dtype)
-
- def searchsorted(
- self,
- value: NumpyValueArrayLike | ExtensionArray,
- side: Literal["left", "right"] = "left",
- sorter: NumpySorter | None = None,
- ) -> npt.NDArray[np.intp] | np.intp:
- """
- Find indices where elements should be inserted to maintain order.
-
- Find the indices into a sorted array `self` (a) such that, if the
- corresponding elements in `value` were inserted before the indices,
- the order of `self` would be preserved.
-
- Assuming that `self` is sorted:
-
- ====== ================================
- `side` returned index `i` satisfies
- ====== ================================
- left ``self[i-1] < value <= self[i]``
- right ``self[i-1] <= value < self[i]``
- ====== ================================
-
- Parameters
- ----------
- value : array-like, list or scalar
- Value(s) to insert into `self`.
- side : {'left', 'right'}, optional
- If 'left', the index of the first suitable location found is given.
- If 'right', return the last such index. If there is no suitable
- index, return either 0 or N (where N is the length of `self`).
- sorter : 1-D array-like, optional
- Optional array of integer indices that sort array a into ascending
- order. They are typically the result of argsort.
-
- Returns
- -------
- array of ints or int
- If value is array-like, array of insertion points.
- If value is scalar, a single integer.
-
- See Also
- --------
- numpy.searchsorted : Similar method from NumPy.
-
- Examples
- --------
- >>> arr = pd.array([1, 2, 3, 5])
- >>> arr.searchsorted([4])
- array([3])
- """
- # Note: the base tests provided by pandas only test the basics.
- # We do not test
- # 1. Values outside the range of the `data_for_sorting` fixture
- # 2. Values between the values in the `data_for_sorting` fixture
- # 3. Missing values.
- arr = self.astype(object)
- if isinstance(value, ExtensionArray):
- value = value.astype(object)
- return arr.searchsorted(value, side=side, sorter=sorter)
-
- def equals(self, other: object) -> bool:
- """
- Return if another array is equivalent to this array.
-
- Equivalent means that both arrays have the same shape and dtype, and
- all values compare equal. Missing values in the same location are
- considered equal (in contrast with normal equality).
-
- Parameters
- ----------
- other : ExtensionArray
- Array to compare to this Array.
-
- Returns
- -------
- boolean
- Whether the arrays are equivalent.
-
- Examples
- --------
- >>> arr1 = pd.array([1, 2, np.nan])
- >>> arr2 = pd.array([1, 2, np.nan])
- >>> arr1.equals(arr2)
- True
- """
- if type(self) != type(other):
- return False
- other = cast(ExtensionArray, other)
- if self.dtype != other.dtype:
- return False
- elif len(self) != len(other):
- return False
- else:
- equal_values = self == other
- if isinstance(equal_values, ExtensionArray):
- # boolean array with NA -> fill with False
- equal_values = equal_values.fillna(False)
- # error: Unsupported left operand type for & ("ExtensionArray")
- equal_na = self.isna() & other.isna() # type: ignore[operator]
- return bool((equal_values | equal_na).all())
-
- def isin(self, values) -> npt.NDArray[np.bool_]:
- """
- Pointwise comparison for set containment in the given values.
-
- Roughly equivalent to `np.array([x in values for x in self])`
-
- Parameters
- ----------
- values : Sequence
-
- Returns
- -------
- np.ndarray[bool]
-
- Examples
- --------
- >>> arr = pd.array([1, 2, 3])
- >>> arr.isin([1])
-
- [True, False, False]
- Length: 3, dtype: boolean
- """
- return isin(np.asarray(self), values)
-
- def _values_for_factorize(self) -> tuple[np.ndarray, Any]:
- """
- Return an array and missing value suitable for factorization.
-
- Returns
- -------
- values : ndarray
- An array suitable for factorization. This should maintain order
- and be a supported dtype (Float64, Int64, UInt64, String, Object).
- By default, the extension array is cast to object dtype.
- na_value : object
- The value in `values` to consider missing. This will be treated
- as NA in the factorization routines, so it will be coded as
- `-1` and not included in `uniques`. By default,
- ``np.nan`` is used.
-
- Notes
- -----
- The values returned by this method are also used in
- :func:`pandas.util.hash_pandas_object`. If needed, this can be
- overridden in the ``self._hash_pandas_object()`` method.
-
- Examples
- --------
- >>> pd.array([1, 2, 3])._values_for_factorize()
- (array([1, 2, 3], dtype=object), nan)
- """
- return self.astype(object), np.nan
-
- def factorize(
- self,
- use_na_sentinel: bool = True,
- ) -> tuple[np.ndarray, ExtensionArray]:
- """
- Encode the extension array as an enumerated type.
-
- Parameters
- ----------
- use_na_sentinel : bool, default True
- If True, the sentinel -1 will be used for NaN values. If False,
- NaN values will be encoded as non-negative integers and will not drop the
- NaN from the uniques of the values.
-
- .. versionadded:: 1.5.0
-
- Returns
- -------
- codes : ndarray
- An integer NumPy array that's an indexer into the original
- ExtensionArray.
- uniques : ExtensionArray
- An ExtensionArray containing the unique values of `self`.
-
- .. note::
-
- uniques will *not* contain an entry for the NA value of
- the ExtensionArray if there are any missing values present
- in `self`.
-
- See Also
- --------
- factorize : Top-level factorize method that dispatches here.
-
- Notes
- -----
- :meth:`pandas.factorize` offers a `sort` keyword as well.
-
- Examples
- --------
- >>> idx1 = pd.PeriodIndex(["2014-01", "2014-01", "2014-02", "2014-02",
- ... "2014-03", "2014-03"], freq="M")
- >>> arr, idx = idx1.factorize()
- >>> arr
- array([0, 0, 1, 1, 2, 2])
- >>> idx
- PeriodIndex(['2014-01', '2014-02', '2014-03'], dtype='period[M]')
- """
- # Implementer note: There are two ways to override the behavior of
- # pandas.factorize
- # 1. _values_for_factorize and _from_factorize.
- # Specify the values passed to pandas' internal factorization
- # routines, and how to convert from those values back to the
- # original ExtensionArray.
- # 2. ExtensionArray.factorize.
- # Complete control over factorization.
- arr, na_value = self._values_for_factorize()
-
- codes, uniques = factorize_array(
- arr, use_na_sentinel=use_na_sentinel, na_value=na_value
- )
-
- uniques_ea = self._from_factorized(uniques, self)
- return codes, uniques_ea
-
- _extension_array_shared_docs[
- "repeat"
- ] = """
- Repeat elements of a %(klass)s.
-
- Returns a new %(klass)s where each element of the current %(klass)s
- is repeated consecutively a given number of times.
-
- Parameters
- ----------
- repeats : int or array of ints
- The number of repetitions for each element. This should be a
- non-negative integer. Repeating 0 times will return an empty
- %(klass)s.
- axis : None
- Must be ``None``. Has no effect but is accepted for compatibility
- with numpy.
-
- Returns
- -------
- %(klass)s
- Newly created %(klass)s with repeated elements.
-
- See Also
- --------
- Series.repeat : Equivalent function for Series.
- Index.repeat : Equivalent function for Index.
- numpy.repeat : Similar method for :class:`numpy.ndarray`.
- ExtensionArray.take : Take arbitrary positions.
-
- Examples
- --------
- >>> cat = pd.Categorical(['a', 'b', 'c'])
- >>> cat
- ['a', 'b', 'c']
- Categories (3, object): ['a', 'b', 'c']
- >>> cat.repeat(2)
- ['a', 'a', 'b', 'b', 'c', 'c']
- Categories (3, object): ['a', 'b', 'c']
- >>> cat.repeat([1, 2, 3])
- ['a', 'b', 'b', 'c', 'c', 'c']
- Categories (3, object): ['a', 'b', 'c']
- """
-
- @Substitution(klass="ExtensionArray")
- @Appender(_extension_array_shared_docs["repeat"])
- def repeat(self, repeats: int | Sequence[int], axis: AxisInt | None = None) -> Self:
- nv.validate_repeat((), {"axis": axis})
- ind = np.arange(len(self)).repeat(repeats)
- return self.take(ind)
-
- # ------------------------------------------------------------------------
- # Indexing methods
- # ------------------------------------------------------------------------
-
- def take(
- self,
- indices: TakeIndexer,
- *,
- allow_fill: bool = False,
- fill_value: Any = None,
- ) -> Self:
- """
- Take elements from an array.
-
- Parameters
- ----------
- indices : sequence of int or one-dimensional np.ndarray of int
- Indices to be taken.
- allow_fill : bool, default False
- How to handle negative values in `indices`.
-
- * False: negative values in `indices` indicate positional indices
- from the right (the default). This is similar to
- :func:`numpy.take`.
-
- * True: negative values in `indices` indicate
- missing values. These values are set to `fill_value`. Any other
- other negative values raise a ``ValueError``.
-
- fill_value : any, optional
- Fill value to use for NA-indices when `allow_fill` is True.
- This may be ``None``, in which case the default NA value for
- the type, ``self.dtype.na_value``, is used.
-
- For many ExtensionArrays, there will be two representations of
- `fill_value`: a user-facing "boxed" scalar, and a low-level
- physical NA value. `fill_value` should be the user-facing version,
- and the implementation should handle translating that to the
- physical version for processing the take if necessary.
-
- Returns
- -------
- ExtensionArray
-
- Raises
- ------
- IndexError
- When the indices are out of bounds for the array.
- ValueError
- When `indices` contains negative values other than ``-1``
- and `allow_fill` is True.
-
- See Also
- --------
- numpy.take : Take elements from an array along an axis.
- api.extensions.take : Take elements from an array.
-
- Notes
- -----
- ExtensionArray.take is called by ``Series.__getitem__``, ``.loc``,
- ``iloc``, when `indices` is a sequence of values. Additionally,
- it's called by :meth:`Series.reindex`, or any other method
- that causes realignment, with a `fill_value`.
-
- Examples
- --------
- Here's an example implementation, which relies on casting the
- extension array to object dtype. This uses the helper method
- :func:`pandas.api.extensions.take`.
-
- .. code-block:: python
-
- def take(self, indices, allow_fill=False, fill_value=None):
- from pandas.core.algorithms import take
-
- # If the ExtensionArray is backed by an ndarray, then
- # just pass that here instead of coercing to object.
- data = self.astype(object)
-
- if allow_fill and fill_value is None:
- fill_value = self.dtype.na_value
-
- # fill value should always be translated from the scalar
- # type for the array, to the physical storage type for
- # the data, before passing to take.
-
- result = take(data, indices, fill_value=fill_value,
- allow_fill=allow_fill)
- return self._from_sequence(result, dtype=self.dtype)
- """
- # Implementer note: The `fill_value` parameter should be a user-facing
- # value, an instance of self.dtype.type. When passed `fill_value=None`,
- # the default of `self.dtype.na_value` should be used.
- # This may differ from the physical storage type your ExtensionArray
- # uses. In this case, your implementation is responsible for casting
- # the user-facing type to the storage type, before using
- # pandas.api.extensions.take
- raise AbstractMethodError(self)
-
- def copy(self) -> Self:
- """
- Return a copy of the array.
-
- Returns
- -------
- ExtensionArray
-
- Examples
- --------
- >>> arr = pd.array([1, 2, 3])
- >>> arr2 = arr.copy()
- >>> arr[0] = 2
- >>> arr2
-
- [1, 2, 3]
- Length: 3, dtype: Int64
- """
- raise AbstractMethodError(self)
-
- def view(self, dtype: Dtype | None = None) -> ArrayLike:
- """
- Return a view on the array.
-
- Parameters
- ----------
- dtype : str, np.dtype, or ExtensionDtype, optional
- Default None.
-
- Returns
- -------
- ExtensionArray or np.ndarray
- A view on the :class:`ExtensionArray`'s data.
-
- Examples
- --------
- This gives view on the underlying data of an ``ExtensionArray`` and is not a
- copy. Modifications on either the view or the original ``ExtensionArray``
- will be reflectd on the underlying data:
-
- >>> arr = pd.array([1, 2, 3])
- >>> arr2 = arr.view()
- >>> arr[0] = 2
- >>> arr2
-
- [2, 2, 3]
- Length: 3, dtype: Int64
- """
- # NB:
- # - This must return a *new* object referencing the same data, not self.
- # - The only case that *must* be implemented is with dtype=None,
- # giving a view with the same dtype as self.
- if dtype is not None:
- raise NotImplementedError(dtype)
- return self[:]
-
- # ------------------------------------------------------------------------
- # Printing
- # ------------------------------------------------------------------------
-
- def __repr__(self) -> str:
- if self.ndim > 1:
- return self._repr_2d()
-
- from pandas.io.formats.printing import format_object_summary
-
- # the short repr has no trailing newline, while the truncated
- # repr does. So we include a newline in our template, and strip
- # any trailing newlines from format_object_summary
- data = format_object_summary(
- self, self._formatter(), indent_for_name=False
- ).rstrip(", \n")
- class_name = f"<{type(self).__name__}>\n"
- return f"{class_name}{data}\nLength: {len(self)}, dtype: {self.dtype}"
-
- def _repr_2d(self) -> str:
- from pandas.io.formats.printing import format_object_summary
-
- # the short repr has no trailing newline, while the truncated
- # repr does. So we include a newline in our template, and strip
- # any trailing newlines from format_object_summary
- lines = [
- format_object_summary(x, self._formatter(), indent_for_name=False).rstrip(
- ", \n"
- )
- for x in self
- ]
- data = ",\n".join(lines)
- class_name = f"<{type(self).__name__}>"
- return f"{class_name}\n[\n{data}\n]\nShape: {self.shape}, dtype: {self.dtype}"
-
- def _formatter(self, boxed: bool = False) -> Callable[[Any], str | None]:
- """
- Formatting function for scalar values.
-
- This is used in the default '__repr__'. The returned formatting
- function receives instances of your scalar type.
-
- Parameters
- ----------
- boxed : bool, default False
- An indicated for whether or not your array is being printed
- within a Series, DataFrame, or Index (True), or just by
- itself (False). This may be useful if you want scalar values
- to appear differently within a Series versus on its own (e.g.
- quoted or not).
-
- Returns
- -------
- Callable[[Any], str]
- A callable that gets instances of the scalar type and
- returns a string. By default, :func:`repr` is used
- when ``boxed=False`` and :func:`str` is used when
- ``boxed=True``.
-
- Examples
- --------
- >>> class MyExtensionArray(pd.arrays.NumpyExtensionArray):
- ... def _formatter(self, boxed=False):
- ... return lambda x: '*' + str(x) + '*' if boxed else repr(x) + '*'
- >>> MyExtensionArray(np.array([1, 2, 3, 4]))
-
- [1*, 2*, 3*, 4*]
- Length: 4, dtype: int64
- """
- if boxed:
- return str
- return repr
-
- # ------------------------------------------------------------------------
- # Reshaping
- # ------------------------------------------------------------------------
-
- def transpose(self, *axes: int) -> ExtensionArray:
- """
- Return a transposed view on this array.
-
- Because ExtensionArrays are always 1D, this is a no-op. It is included
- for compatibility with np.ndarray.
- """
- return self[:]
-
- @property
- def T(self) -> ExtensionArray:
- return self.transpose()
-
- def ravel(self, order: Literal["C", "F", "A", "K"] | None = "C") -> ExtensionArray:
- """
- Return a flattened view on this array.
-
- Parameters
- ----------
- order : {None, 'C', 'F', 'A', 'K'}, default 'C'
-
- Returns
- -------
- ExtensionArray
-
- Notes
- -----
- - Because ExtensionArrays are 1D-only, this is a no-op.
- - The "order" argument is ignored, is for compatibility with NumPy.
-
- Examples
- --------
- >>> pd.array([1, 2, 3]).ravel()
-
- [1, 2, 3]
- Length: 3, dtype: Int64
- """
- return self
-
- @classmethod
- def _concat_same_type(cls, to_concat: Sequence[Self]) -> Self:
- """
- Concatenate multiple array of this dtype.
-
- Parameters
- ----------
- to_concat : sequence of this type
-
- Returns
- -------
- ExtensionArray
-
- Examples
- --------
- >>> arr1 = pd.array([1, 2, 3])
- >>> arr2 = pd.array([4, 5, 6])
- >>> pd.arrays.IntegerArray._concat_same_type([arr1, arr2])
-
- [1, 2, 3, 4, 5, 6]
- Length: 6, dtype: Int64
- """
- # Implementer note: this method will only be called with a sequence of
- # ExtensionArrays of this class and with the same dtype as self. This
- # should allow "easy" concatenation (no upcasting needed), and result
- # in a new ExtensionArray of the same dtype.
- # Note: this strict behaviour is only guaranteed starting with pandas 1.1
- raise AbstractMethodError(cls)
-
- # The _can_hold_na attribute is set to True so that pandas internals
- # will use the ExtensionDtype.na_value as the NA value in operations
- # such as take(), reindex(), shift(), etc. In addition, those results
- # will then be of the ExtensionArray subclass rather than an array
- # of objects
- @cache_readonly
- def _can_hold_na(self) -> bool:
- return self.dtype._can_hold_na
-
- def _accumulate(
- self, name: str, *, skipna: bool = True, **kwargs
- ) -> ExtensionArray:
- """
- Return an ExtensionArray performing an accumulation operation.
-
- The underlying data type might change.
-
- Parameters
- ----------
- name : str
- Name of the function, supported values are:
- - cummin
- - cummax
- - cumsum
- - cumprod
- skipna : bool, default True
- If True, skip NA values.
- **kwargs
- Additional keyword arguments passed to the accumulation function.
- Currently, there is no supported kwarg.
-
- Returns
- -------
- array
-
- Raises
- ------
- NotImplementedError : subclass does not define accumulations
-
- Examples
- --------
- >>> arr = pd.array([1, 2, 3])
- >>> arr._accumulate(name='cumsum')
-
- [1, 3, 6]
- Length: 3, dtype: Int64
- """
- raise NotImplementedError(f"cannot perform {name} with type {self.dtype}")
-
- def _reduce(
- self, name: str, *, skipna: bool = True, keepdims: bool = False, **kwargs
- ):
- """
- Return a scalar result of performing the reduction operation.
-
- Parameters
- ----------
- name : str
- Name of the function, supported values are:
- { any, all, min, max, sum, mean, median, prod,
- std, var, sem, kurt, skew }.
- skipna : bool, default True
- If True, skip NaN values.
- keepdims : bool, default False
- If False, a scalar is returned.
- If True, the result has dimension with size one along the reduced axis.
-
- .. versionadded:: 2.1
-
- This parameter is not required in the _reduce signature to keep backward
- compatibility, but will become required in the future. If the parameter
- is not found in the method signature, a FutureWarning will be emitted.
- **kwargs
- Additional keyword arguments passed to the reduction function.
- Currently, `ddof` is the only supported kwarg.
-
- Returns
- -------
- scalar
-
- Raises
- ------
- TypeError : subclass does not define reductions
-
- Examples
- --------
- >>> pd.array([1, 2, 3])._reduce("min")
- 1
- """
- meth = getattr(self, name, None)
- if meth is None:
- raise TypeError(
- f"'{type(self).__name__}' with dtype {self.dtype} "
- f"does not support reduction '{name}'"
- )
- result = meth(skipna=skipna, **kwargs)
- if keepdims:
- result = np.array([result])
-
- return result
-
- # https://github.com/python/typeshed/issues/2148#issuecomment-520783318
- # Incompatible types in assignment (expression has type "None", base class
- # "object" defined the type as "Callable[[object], int]")
- __hash__: ClassVar[None] # type: ignore[assignment]
-
- # ------------------------------------------------------------------------
- # Non-Optimized Default Methods; in the case of the private methods here,
- # these are not guaranteed to be stable across pandas versions.
-
- def _values_for_json(self) -> np.ndarray:
- """
- Specify how to render our entries in to_json.
-
- Notes
- -----
- The dtype on the returned ndarray is not restricted, but for non-native
- types that are not specifically handled in objToJSON.c, to_json is
- liable to raise. In these cases, it may be safer to return an ndarray
- of strings.
- """
- return np.asarray(self)
-
- def _hash_pandas_object(
- self, *, encoding: str, hash_key: str, categorize: bool
- ) -> npt.NDArray[np.uint64]:
- """
- Hook for hash_pandas_object.
-
- Default is to use the values returned by _values_for_factorize.
-
- Parameters
- ----------
- encoding : str
- Encoding for data & key when strings.
- hash_key : str
- Hash_key for string key to encode.
- categorize : bool
- Whether to first categorize object arrays before hashing. This is more
- efficient when the array contains duplicate values.
-
- Returns
- -------
- np.ndarray[uint64]
-
- Examples
- --------
- >>> pd.array([1, 2])._hash_pandas_object(encoding='utf-8',
- ... hash_key="1000000000000000",
- ... categorize=False
- ... )
- array([11381023671546835630, 4641644667904626417], dtype=uint64)
- """
- from pandas.core.util.hashing import hash_array
-
- values, _ = self._values_for_factorize()
- return hash_array(
- values, encoding=encoding, hash_key=hash_key, categorize=categorize
- )
-
- def tolist(self) -> list:
- """
- Return a list of the values.
-
- These are each a scalar type, which is a Python scalar
- (for str, int, float) or a pandas scalar
- (for Timestamp/Timedelta/Interval/Period)
-
- Returns
- -------
- list
-
- Examples
- --------
- >>> arr = pd.array([1, 2, 3])
- >>> arr.tolist()
- [1, 2, 3]
- """
- if self.ndim > 1:
- return [x.tolist() for x in self]
- return list(self)
-
- def delete(self, loc: PositionalIndexer) -> Self:
- indexer = np.delete(np.arange(len(self)), loc)
- return self.take(indexer)
-
- def insert(self, loc: int, item) -> Self:
- """
- Insert an item at the given position.
-
- Parameters
- ----------
- loc : int
- item : scalar-like
-
- Returns
- -------
- same type as self
-
- Notes
- -----
- This method should be both type and dtype-preserving. If the item
- cannot be held in an array of this type/dtype, either ValueError or
- TypeError should be raised.
-
- The default implementation relies on _from_sequence to raise on invalid
- items.
-
- Examples
- --------
- >>> arr = pd.array([1, 2, 3])
- >>> arr.insert(2, -1)
-
- [1, 2, -1, 3]
- Length: 4, dtype: Int64
- """
- loc = validate_insert_loc(loc, len(self))
-
- item_arr = type(self)._from_sequence([item], dtype=self.dtype)
-
- return type(self)._concat_same_type([self[:loc], item_arr, self[loc:]])
-
- def _putmask(self, mask: npt.NDArray[np.bool_], value) -> None:
- """
- Analogue to np.putmask(self, mask, value)
-
- Parameters
- ----------
- mask : np.ndarray[bool]
- value : scalar or listlike
- If listlike, must be arraylike with same length as self.
-
- Returns
- -------
- None
-
- Notes
- -----
- Unlike np.putmask, we do not repeat listlike values with mismatched length.
- 'value' should either be a scalar or an arraylike with the same length
- as self.
- """
- if is_list_like(value):
- val = value[mask]
- else:
- val = value
-
- self[mask] = val
-
- def _where(self, mask: npt.NDArray[np.bool_], value) -> Self:
- """
- Analogue to np.where(mask, self, value)
-
- Parameters
- ----------
- mask : np.ndarray[bool]
- value : scalar or listlike
-
- Returns
- -------
- same type as self
- """
- result = self.copy()
-
- if is_list_like(value):
- val = value[~mask]
- else:
- val = value
-
- result[~mask] = val
- return result
-
- def _fill_mask_inplace(
- self, method: str, limit: int | None, mask: npt.NDArray[np.bool_]
- ) -> None:
- """
- Replace values in locations specified by 'mask' using pad or backfill.
-
- See also
- --------
- ExtensionArray.fillna
- """
- func = missing.get_fill_func(method)
- npvalues = self.astype(object)
- # NB: if we don't copy mask here, it may be altered inplace, which
- # would mess up the `self[mask] = ...` below.
- func(npvalues, limit=limit, mask=mask.copy())
- new_values = self._from_sequence(npvalues, dtype=self.dtype)
- self[mask] = new_values[mask]
-
- def _rank(
- self,
- *,
- axis: AxisInt = 0,
- method: str = "average",
- na_option: str = "keep",
- ascending: bool = True,
- pct: bool = False,
- ):
- """
- See Series.rank.__doc__.
- """
- if axis != 0:
- raise NotImplementedError
-
- return rank(
- self._values_for_argsort(),
- axis=axis,
- method=method,
- na_option=na_option,
- ascending=ascending,
- pct=pct,
- )
-
- @classmethod
- def _empty(cls, shape: Shape, dtype: ExtensionDtype):
- """
- Create an ExtensionArray with the given shape and dtype.
-
- See also
- --------
- ExtensionDtype.empty
- ExtensionDtype.empty is the 'official' public version of this API.
- """
- # Implementer note: while ExtensionDtype.empty is the public way to
- # call this method, it is still required to implement this `_empty`
- # method as well (it is called internally in pandas)
- obj = cls._from_sequence([], dtype=dtype)
-
- taker = np.broadcast_to(np.intp(-1), shape)
- result = obj.take(taker, allow_fill=True)
- if not isinstance(result, cls) or dtype != result.dtype:
- raise NotImplementedError(
- f"Default 'empty' implementation is invalid for dtype='{dtype}'"
- )
- return result
-
- def _quantile(self, qs: npt.NDArray[np.float64], interpolation: str) -> Self:
- """
- Compute the quantiles of self for each quantile in `qs`.
-
- Parameters
- ----------
- qs : np.ndarray[float64]
- interpolation: str
-
- Returns
- -------
- same type as self
- """
- mask = np.asarray(self.isna())
- arr = np.asarray(self)
- fill_value = np.nan
-
- res_values = quantile_with_mask(arr, mask, fill_value, qs, interpolation)
- return type(self)._from_sequence(res_values)
-
- def _mode(self, dropna: bool = True) -> Self:
- """
- Returns the mode(s) of the ExtensionArray.
-
- Always returns `ExtensionArray` even if only one value.
-
- Parameters
- ----------
- dropna : bool, default True
- Don't consider counts of NA values.
-
- Returns
- -------
- same type as self
- Sorted, if possible.
- """
- # error: Incompatible return value type (got "Union[ExtensionArray,
- # ndarray[Any, Any]]", expected "Self")
- return mode(self, dropna=dropna) # type: ignore[return-value]
-
- def __array_ufunc__(self, ufunc: np.ufunc, method: str, *inputs, **kwargs):
- if any(
- isinstance(other, (ABCSeries, ABCIndex, ABCDataFrame)) for other in inputs
- ):
- return NotImplemented
-
- result = arraylike.maybe_dispatch_ufunc_to_dunder_op(
- self, ufunc, method, *inputs, **kwargs
- )
- if result is not NotImplemented:
- return result
-
- if "out" in kwargs:
- return arraylike.dispatch_ufunc_with_out(
- self, ufunc, method, *inputs, **kwargs
- )
-
- if method == "reduce":
- result = arraylike.dispatch_reduction_ufunc(
- self, ufunc, method, *inputs, **kwargs
- )
- if result is not NotImplemented:
- return result
-
- return arraylike.default_array_ufunc(self, ufunc, method, *inputs, **kwargs)
-
- def map(self, mapper, na_action=None):
- """
- Map values using an input mapping or function.
-
- Parameters
- ----------
- mapper : function, dict, or Series
- Mapping correspondence.
- na_action : {None, 'ignore'}, default None
- If 'ignore', propagate NA values, without passing them to the
- mapping correspondence. If 'ignore' is not supported, a
- ``NotImplementedError`` should be raised.
-
- Returns
- -------
- Union[ndarray, Index, ExtensionArray]
- The output of the mapping function applied to the array.
- If the function returns a tuple with more than one element
- a MultiIndex will be returned.
- """
- return map_array(self, mapper, na_action=na_action)
-
- # ------------------------------------------------------------------------
- # GroupBy Methods
-
- def _groupby_op(
- self,
- *,
- how: str,
- has_dropped_na: bool,
- min_count: int,
- ngroups: int,
- ids: npt.NDArray[np.intp],
- **kwargs,
- ) -> ArrayLike:
- """
- Dispatch GroupBy reduction or transformation operation.
-
- This is an *experimental* API to allow ExtensionArray authors to implement
- reductions and transformations. The API is subject to change.
-
- Parameters
- ----------
- how : {'any', 'all', 'sum', 'prod', 'min', 'max', 'mean', 'median',
- 'median', 'var', 'std', 'sem', 'nth', 'last', 'ohlc',
- 'cumprod', 'cumsum', 'cummin', 'cummax', 'rank'}
- has_dropped_na : bool
- min_count : int
- ngroups : int
- ids : np.ndarray[np.intp]
- ids[i] gives the integer label for the group that self[i] belongs to.
- **kwargs : operation-specific
- 'any', 'all' -> ['skipna']
- 'var', 'std', 'sem' -> ['ddof']
- 'cumprod', 'cumsum', 'cummin', 'cummax' -> ['skipna']
- 'rank' -> ['ties_method', 'ascending', 'na_option', 'pct']
-
- Returns
- -------
- np.ndarray or ExtensionArray
- """
- from pandas.core.arrays.string_ import StringDtype
- from pandas.core.groupby.ops import WrappedCythonOp
-
- kind = WrappedCythonOp.get_kind_from_how(how)
- op = WrappedCythonOp(how=how, kind=kind, has_dropped_na=has_dropped_na)
-
- # GH#43682
- if isinstance(self.dtype, StringDtype):
- # StringArray
- npvalues = self.to_numpy(object, na_value=np.nan)
- else:
- raise NotImplementedError(
- f"function is not implemented for this dtype: {self.dtype}"
- )
-
- res_values = op._cython_op_ndim_compat(
- npvalues,
- min_count=min_count,
- ngroups=ngroups,
- comp_ids=ids,
- mask=None,
- **kwargs,
- )
-
- if op.how in op.cast_blocklist:
- # i.e. how in ["rank"], since other cast_blocklist methods don't go
- # through cython_operation
- return res_values
-
- if isinstance(self.dtype, StringDtype):
- dtype = self.dtype
- string_array_cls = dtype.construct_array_type()
- return string_array_cls._from_sequence(res_values, dtype=dtype)
-
- else:
- raise NotImplementedError
-
-
-class ExtensionArraySupportsAnyAll(ExtensionArray):
- def any(self, *, skipna: bool = True) -> bool:
- raise AbstractMethodError(self)
-
- def all(self, *, skipna: bool = True) -> bool:
- raise AbstractMethodError(self)
-
-
-class ExtensionOpsMixin:
- """
- A base class for linking the operators to their dunder names.
-
- .. note::
-
- You may want to set ``__array_priority__`` if you want your
- implementation to be called when involved in binary operations
- with NumPy arrays.
- """
-
- @classmethod
- def _create_arithmetic_method(cls, op):
- raise AbstractMethodError(cls)
-
- @classmethod
- def _add_arithmetic_ops(cls) -> None:
- setattr(cls, "__add__", cls._create_arithmetic_method(operator.add))
- setattr(cls, "__radd__", cls._create_arithmetic_method(roperator.radd))
- setattr(cls, "__sub__", cls._create_arithmetic_method(operator.sub))
- setattr(cls, "__rsub__", cls._create_arithmetic_method(roperator.rsub))
- setattr(cls, "__mul__", cls._create_arithmetic_method(operator.mul))
- setattr(cls, "__rmul__", cls._create_arithmetic_method(roperator.rmul))
- setattr(cls, "__pow__", cls._create_arithmetic_method(operator.pow))
- setattr(cls, "__rpow__", cls._create_arithmetic_method(roperator.rpow))
- setattr(cls, "__mod__", cls._create_arithmetic_method(operator.mod))
- setattr(cls, "__rmod__", cls._create_arithmetic_method(roperator.rmod))
- setattr(cls, "__floordiv__", cls._create_arithmetic_method(operator.floordiv))
- setattr(
- cls, "__rfloordiv__", cls._create_arithmetic_method(roperator.rfloordiv)
- )
- setattr(cls, "__truediv__", cls._create_arithmetic_method(operator.truediv))
- setattr(cls, "__rtruediv__", cls._create_arithmetic_method(roperator.rtruediv))
- setattr(cls, "__divmod__", cls._create_arithmetic_method(divmod))
- setattr(cls, "__rdivmod__", cls._create_arithmetic_method(roperator.rdivmod))
-
- @classmethod
- def _create_comparison_method(cls, op):
- raise AbstractMethodError(cls)
-
- @classmethod
- def _add_comparison_ops(cls) -> None:
- setattr(cls, "__eq__", cls._create_comparison_method(operator.eq))
- setattr(cls, "__ne__", cls._create_comparison_method(operator.ne))
- setattr(cls, "__lt__", cls._create_comparison_method(operator.lt))
- setattr(cls, "__gt__", cls._create_comparison_method(operator.gt))
- setattr(cls, "__le__", cls._create_comparison_method(operator.le))
- setattr(cls, "__ge__", cls._create_comparison_method(operator.ge))
-
- @classmethod
- def _create_logical_method(cls, op):
- raise AbstractMethodError(cls)
-
- @classmethod
- def _add_logical_ops(cls) -> None:
- setattr(cls, "__and__", cls._create_logical_method(operator.and_))
- setattr(cls, "__rand__", cls._create_logical_method(roperator.rand_))
- setattr(cls, "__or__", cls._create_logical_method(operator.or_))
- setattr(cls, "__ror__", cls._create_logical_method(roperator.ror_))
- setattr(cls, "__xor__", cls._create_logical_method(operator.xor))
- setattr(cls, "__rxor__", cls._create_logical_method(roperator.rxor))
-
-
-class ExtensionScalarOpsMixin(ExtensionOpsMixin):
- """
- A mixin for defining ops on an ExtensionArray.
-
- It is assumed that the underlying scalar objects have the operators
- already defined.
-
- Notes
- -----
- If you have defined a subclass MyExtensionArray(ExtensionArray), then
- use MyExtensionArray(ExtensionArray, ExtensionScalarOpsMixin) to
- get the arithmetic operators. After the definition of MyExtensionArray,
- insert the lines
-
- MyExtensionArray._add_arithmetic_ops()
- MyExtensionArray._add_comparison_ops()
-
- to link the operators to your class.
-
- .. note::
-
- You may want to set ``__array_priority__`` if you want your
- implementation to be called when involved in binary operations
- with NumPy arrays.
- """
-
- @classmethod
- def _create_method(cls, op, coerce_to_dtype: bool = True, result_dtype=None):
- """
- A class method that returns a method that will correspond to an
- operator for an ExtensionArray subclass, by dispatching to the
- relevant operator defined on the individual elements of the
- ExtensionArray.
-
- Parameters
- ----------
- op : function
- An operator that takes arguments op(a, b)
- coerce_to_dtype : bool, default True
- boolean indicating whether to attempt to convert
- the result to the underlying ExtensionArray dtype.
- If it's not possible to create a new ExtensionArray with the
- values, an ndarray is returned instead.
-
- Returns
- -------
- Callable[[Any, Any], Union[ndarray, ExtensionArray]]
- A method that can be bound to a class. When used, the method
- receives the two arguments, one of which is the instance of
- this class, and should return an ExtensionArray or an ndarray.
-
- Returning an ndarray may be necessary when the result of the
- `op` cannot be stored in the ExtensionArray. The dtype of the
- ndarray uses NumPy's normal inference rules.
-
- Examples
- --------
- Given an ExtensionArray subclass called MyExtensionArray, use
-
- __add__ = cls._create_method(operator.add)
-
- in the class definition of MyExtensionArray to create the operator
- for addition, that will be based on the operator implementation
- of the underlying elements of the ExtensionArray
- """
-
- def _binop(self, other):
- def convert_values(param):
- if isinstance(param, ExtensionArray) or is_list_like(param):
- ovalues = param
- else: # Assume its an object
- ovalues = [param] * len(self)
- return ovalues
-
- if isinstance(other, (ABCSeries, ABCIndex, ABCDataFrame)):
- # rely on pandas to unbox and dispatch to us
- return NotImplemented
-
- lvalues = self
- rvalues = convert_values(other)
-
- # If the operator is not defined for the underlying objects,
- # a TypeError should be raised
- res = [op(a, b) for (a, b) in zip(lvalues, rvalues)]
-
- def _maybe_convert(arr):
- if coerce_to_dtype:
- # https://github.com/pandas-dev/pandas/issues/22850
- # We catch all regular exceptions here, and fall back
- # to an ndarray.
- res = maybe_cast_pointwise_result(arr, self.dtype, same_dtype=False)
- if not isinstance(res, type(self)):
- # exception raised in _from_sequence; ensure we have ndarray
- res = np.asarray(arr)
- else:
- res = np.asarray(arr, dtype=result_dtype)
- return res
-
- if op.__name__ in {"divmod", "rdivmod"}:
- a, b = zip(*res)
- return _maybe_convert(a), _maybe_convert(b)
-
- return _maybe_convert(res)
-
- op_name = f"__{op.__name__}__"
- return set_function_name(_binop, op_name, cls)
-
- @classmethod
- def _create_arithmetic_method(cls, op):
- return cls._create_method(op)
-
- @classmethod
- def _create_comparison_method(cls, op):
- return cls._create_method(op, coerce_to_dtype=False, result_dtype=bool)
diff --git a/spaces/profayle/TerrapinTalk/myenv/lib/python3.9/site-packages/pandas/core/groupby/ops.py b/spaces/profayle/TerrapinTalk/myenv/lib/python3.9/site-packages/pandas/core/groupby/ops.py
deleted file mode 100644
index 3c4a22d0094062730eee561cc63cf8356505930a..0000000000000000000000000000000000000000
--- a/spaces/profayle/TerrapinTalk/myenv/lib/python3.9/site-packages/pandas/core/groupby/ops.py
+++ /dev/null
@@ -1,1197 +0,0 @@
-"""
-Provide classes to perform the groupby aggregate operations.
-
-These are not exposed to the user and provide implementations of the grouping
-operations, primarily in cython. These classes (BaseGrouper and BinGrouper)
-are contained *in* the SeriesGroupBy and DataFrameGroupBy objects.
-"""
-from __future__ import annotations
-
-import collections
-import functools
-from typing import (
- TYPE_CHECKING,
- Callable,
- Generic,
- final,
-)
-
-import numpy as np
-
-from pandas._libs import (
- NaT,
- lib,
-)
-import pandas._libs.groupby as libgroupby
-from pandas._typing import (
- ArrayLike,
- AxisInt,
- NDFrameT,
- Shape,
- npt,
-)
-from pandas.errors import AbstractMethodError
-from pandas.util._decorators import cache_readonly
-
-from pandas.core.dtypes.cast import (
- maybe_cast_pointwise_result,
- maybe_downcast_to_dtype,
-)
-from pandas.core.dtypes.common import (
- ensure_float64,
- ensure_int64,
- ensure_platform_int,
- ensure_uint64,
- is_1d_only_ea_dtype,
-)
-from pandas.core.dtypes.missing import (
- isna,
- maybe_fill,
-)
-
-from pandas.core.frame import DataFrame
-from pandas.core.groupby import grouper
-from pandas.core.indexes.api import (
- CategoricalIndex,
- Index,
- MultiIndex,
- ensure_index,
-)
-from pandas.core.series import Series
-from pandas.core.sorting import (
- compress_group_index,
- decons_obs_group_ids,
- get_flattened_list,
- get_group_index,
- get_group_index_sorter,
- get_indexer_dict,
-)
-
-if TYPE_CHECKING:
- from collections.abc import (
- Hashable,
- Iterator,
- Sequence,
- )
-
- from pandas.core.generic import NDFrame
-
-
-def check_result_array(obj, dtype):
- # Our operation is supposed to be an aggregation/reduction. If
- # it returns an ndarray, this likely means an invalid operation has
- # been passed. See test_apply_without_aggregation, test_agg_must_agg
- if isinstance(obj, np.ndarray):
- if dtype != object:
- # If it is object dtype, the function can be a reduction/aggregation
- # and still return an ndarray e.g. test_agg_over_numpy_arrays
- raise ValueError("Must produce aggregated value")
-
-
-def extract_result(res):
- """
- Extract the result object, it might be a 0-dim ndarray
- or a len-1 0-dim, or a scalar
- """
- if hasattr(res, "_values"):
- # Preserve EA
- res = res._values
- if res.ndim == 1 and len(res) == 1:
- # see test_agg_lambda_with_timezone, test_resampler_grouper.py::test_apply
- res = res[0]
- return res
-
-
-class WrappedCythonOp:
- """
- Dispatch logic for functions defined in _libs.groupby
-
- Parameters
- ----------
- kind: str
- Whether the operation is an aggregate or transform.
- how: str
- Operation name, e.g. "mean".
- has_dropped_na: bool
- True precisely when dropna=True and the grouper contains a null value.
- """
-
- # Functions for which we do _not_ attempt to cast the cython result
- # back to the original dtype.
- cast_blocklist = frozenset(
- ["any", "all", "rank", "count", "size", "idxmin", "idxmax"]
- )
-
- def __init__(self, kind: str, how: str, has_dropped_na: bool) -> None:
- self.kind = kind
- self.how = how
- self.has_dropped_na = has_dropped_na
-
- _CYTHON_FUNCTIONS: dict[str, dict] = {
- "aggregate": {
- "any": functools.partial(libgroupby.group_any_all, val_test="any"),
- "all": functools.partial(libgroupby.group_any_all, val_test="all"),
- "sum": "group_sum",
- "prod": "group_prod",
- "min": "group_min",
- "max": "group_max",
- "mean": "group_mean",
- "median": "group_median_float64",
- "var": "group_var",
- "std": functools.partial(libgroupby.group_var, name="std"),
- "sem": functools.partial(libgroupby.group_var, name="sem"),
- "skew": "group_skew",
- "first": "group_nth",
- "last": "group_last",
- "ohlc": "group_ohlc",
- },
- "transform": {
- "cumprod": "group_cumprod",
- "cumsum": "group_cumsum",
- "cummin": "group_cummin",
- "cummax": "group_cummax",
- "rank": "group_rank",
- },
- }
-
- _cython_arity = {"ohlc": 4} # OHLC
-
- @classmethod
- def get_kind_from_how(cls, how: str) -> str:
- if how in cls._CYTHON_FUNCTIONS["aggregate"]:
- return "aggregate"
- return "transform"
-
- # Note: we make this a classmethod and pass kind+how so that caching
- # works at the class level and not the instance level
- @classmethod
- @functools.cache
- def _get_cython_function(
- cls, kind: str, how: str, dtype: np.dtype, is_numeric: bool
- ):
- dtype_str = dtype.name
- ftype = cls._CYTHON_FUNCTIONS[kind][how]
-
- # see if there is a fused-type version of function
- # only valid for numeric
- if callable(ftype):
- f = ftype
- else:
- f = getattr(libgroupby, ftype)
- if is_numeric:
- return f
- elif dtype == np.dtype(object):
- if how in ["median", "cumprod"]:
- # no fused types -> no __signatures__
- raise NotImplementedError(
- f"function is not implemented for this dtype: "
- f"[how->{how},dtype->{dtype_str}]"
- )
- elif how in ["std", "sem"]:
- # We have a partial object that does not have __signatures__
- return f
- elif how == "skew":
- # _get_cython_vals will convert to float64
- pass
- elif "object" not in f.__signatures__:
- # raise NotImplementedError here rather than TypeError later
- raise NotImplementedError(
- f"function is not implemented for this dtype: "
- f"[how->{how},dtype->{dtype_str}]"
- )
- return f
- else:
- raise NotImplementedError(
- "This should not be reached. Please report a bug at "
- "github.com/pandas-dev/pandas/",
- dtype,
- )
-
- def _get_cython_vals(self, values: np.ndarray) -> np.ndarray:
- """
- Cast numeric dtypes to float64 for functions that only support that.
-
- Parameters
- ----------
- values : np.ndarray
-
- Returns
- -------
- values : np.ndarray
- """
- how = self.how
-
- if how in ["median", "std", "sem", "skew"]:
- # median only has a float64 implementation
- # We should only get here with is_numeric, as non-numeric cases
- # should raise in _get_cython_function
- values = ensure_float64(values)
-
- elif values.dtype.kind in "iu":
- if how in ["var", "mean"] or (
- self.kind == "transform" and self.has_dropped_na
- ):
- # has_dropped_na check need for test_null_group_str_transformer
- # result may still include NaN, so we have to cast
- values = ensure_float64(values)
-
- elif how in ["sum", "ohlc", "prod", "cumsum", "cumprod"]:
- # Avoid overflow during group op
- if values.dtype.kind == "i":
- values = ensure_int64(values)
- else:
- values = ensure_uint64(values)
-
- return values
-
- def _get_output_shape(self, ngroups: int, values: np.ndarray) -> Shape:
- how = self.how
- kind = self.kind
-
- arity = self._cython_arity.get(how, 1)
-
- out_shape: Shape
- if how == "ohlc":
- out_shape = (ngroups, arity)
- elif arity > 1:
- raise NotImplementedError(
- "arity of more than 1 is not supported for the 'how' argument"
- )
- elif kind == "transform":
- out_shape = values.shape
- else:
- out_shape = (ngroups,) + values.shape[1:]
- return out_shape
-
- def _get_out_dtype(self, dtype: np.dtype) -> np.dtype:
- how = self.how
-
- if how == "rank":
- out_dtype = "float64"
- else:
- if dtype.kind in "iufcb":
- out_dtype = f"{dtype.kind}{dtype.itemsize}"
- else:
- out_dtype = "object"
- return np.dtype(out_dtype)
-
- def _get_result_dtype(self, dtype: np.dtype) -> np.dtype:
- """
- Get the desired dtype of a result based on the
- input dtype and how it was computed.
-
- Parameters
- ----------
- dtype : np.dtype
-
- Returns
- -------
- np.dtype
- The desired dtype of the result.
- """
- how = self.how
-
- if how in ["sum", "cumsum", "sum", "prod", "cumprod"]:
- if dtype == np.dtype(bool):
- return np.dtype(np.int64)
- elif how in ["mean", "median", "var", "std", "sem"]:
- if dtype.kind in "fc":
- return dtype
- elif dtype.kind in "iub":
- return np.dtype(np.float64)
- return dtype
-
- @final
- def _cython_op_ndim_compat(
- self,
- values: np.ndarray,
- *,
- min_count: int,
- ngroups: int,
- comp_ids: np.ndarray,
- mask: npt.NDArray[np.bool_] | None = None,
- result_mask: npt.NDArray[np.bool_] | None = None,
- **kwargs,
- ) -> np.ndarray:
- if values.ndim == 1:
- # expand to 2d, dispatch, then squeeze if appropriate
- values2d = values[None, :]
- if mask is not None:
- mask = mask[None, :]
- if result_mask is not None:
- result_mask = result_mask[None, :]
- res = self._call_cython_op(
- values2d,
- min_count=min_count,
- ngroups=ngroups,
- comp_ids=comp_ids,
- mask=mask,
- result_mask=result_mask,
- **kwargs,
- )
- if res.shape[0] == 1:
- return res[0]
-
- # otherwise we have OHLC
- return res.T
-
- return self._call_cython_op(
- values,
- min_count=min_count,
- ngroups=ngroups,
- comp_ids=comp_ids,
- mask=mask,
- result_mask=result_mask,
- **kwargs,
- )
-
- @final
- def _call_cython_op(
- self,
- values: np.ndarray, # np.ndarray[ndim=2]
- *,
- min_count: int,
- ngroups: int,
- comp_ids: np.ndarray,
- mask: npt.NDArray[np.bool_] | None,
- result_mask: npt.NDArray[np.bool_] | None,
- **kwargs,
- ) -> np.ndarray: # np.ndarray[ndim=2]
- orig_values = values
-
- dtype = values.dtype
- is_numeric = dtype.kind in "iufcb"
-
- is_datetimelike = dtype.kind in "mM"
-
- if is_datetimelike:
- values = values.view("int64")
- is_numeric = True
- elif dtype.kind == "b":
- values = values.view("uint8")
- if values.dtype == "float16":
- values = values.astype(np.float32)
-
- if self.how in ["any", "all"]:
- if mask is None:
- mask = isna(values)
- if dtype == object:
- if kwargs["skipna"]:
- # GH#37501: don't raise on pd.NA when skipna=True
- if mask.any():
- # mask on original values computed separately
- values = values.copy()
- values[mask] = True
- values = values.astype(bool, copy=False).view(np.int8)
- is_numeric = True
-
- values = values.T
- if mask is not None:
- mask = mask.T
- if result_mask is not None:
- result_mask = result_mask.T
-
- out_shape = self._get_output_shape(ngroups, values)
- func = self._get_cython_function(self.kind, self.how, values.dtype, is_numeric)
- values = self._get_cython_vals(values)
- out_dtype = self._get_out_dtype(values.dtype)
-
- result = maybe_fill(np.empty(out_shape, dtype=out_dtype))
- if self.kind == "aggregate":
- counts = np.zeros(ngroups, dtype=np.int64)
- if self.how in ["min", "max", "mean", "last", "first", "sum"]:
- func(
- out=result,
- counts=counts,
- values=values,
- labels=comp_ids,
- min_count=min_count,
- mask=mask,
- result_mask=result_mask,
- is_datetimelike=is_datetimelike,
- )
- elif self.how in ["sem", "std", "var", "ohlc", "prod", "median"]:
- if self.how in ["std", "sem"]:
- kwargs["is_datetimelike"] = is_datetimelike
- func(
- result,
- counts,
- values,
- comp_ids,
- min_count=min_count,
- mask=mask,
- result_mask=result_mask,
- **kwargs,
- )
- elif self.how in ["any", "all"]:
- func(
- out=result,
- values=values,
- labels=comp_ids,
- mask=mask,
- result_mask=result_mask,
- **kwargs,
- )
- result = result.astype(bool, copy=False)
- elif self.how in ["skew"]:
- func(
- out=result,
- counts=counts,
- values=values,
- labels=comp_ids,
- mask=mask,
- result_mask=result_mask,
- **kwargs,
- )
- if dtype == object:
- result = result.astype(object)
-
- else:
- raise NotImplementedError(f"{self.how} is not implemented")
- else:
- # TODO: min_count
- if self.how != "rank":
- # TODO: should rank take result_mask?
- kwargs["result_mask"] = result_mask
- func(
- out=result,
- values=values,
- labels=comp_ids,
- ngroups=ngroups,
- is_datetimelike=is_datetimelike,
- mask=mask,
- **kwargs,
- )
-
- if self.kind == "aggregate":
- # i.e. counts is defined. Locations where count None:
- if values.ndim > 2:
- raise NotImplementedError("number of dimensions is currently limited to 2")
- if values.ndim == 2:
- assert axis == 1, axis
- elif not is_1d_only_ea_dtype(values.dtype):
- # Note: it is *not* the case that axis is always 0 for 1-dim values,
- # as we can have 1D ExtensionArrays that we need to treat as 2D
- assert axis == 0
-
- @final
- def cython_operation(
- self,
- *,
- values: ArrayLike,
- axis: AxisInt,
- min_count: int = -1,
- comp_ids: np.ndarray,
- ngroups: int,
- **kwargs,
- ) -> ArrayLike:
- """
- Call our cython function, with appropriate pre- and post- processing.
- """
- self._validate_axis(axis, values)
-
- if not isinstance(values, np.ndarray):
- # i.e. ExtensionArray
- return values._groupby_op(
- how=self.how,
- has_dropped_na=self.has_dropped_na,
- min_count=min_count,
- ngroups=ngroups,
- ids=comp_ids,
- **kwargs,
- )
-
- return self._cython_op_ndim_compat(
- values,
- min_count=min_count,
- ngroups=ngroups,
- comp_ids=comp_ids,
- mask=None,
- **kwargs,
- )
-
-
-class BaseGrouper:
- """
- This is an internal Grouper class, which actually holds
- the generated groups
-
- Parameters
- ----------
- axis : Index
- groupings : Sequence[Grouping]
- all the grouping instances to handle in this grouper
- for example for grouper list to groupby, need to pass the list
- sort : bool, default True
- whether this grouper will give sorted result or not
-
- """
-
- axis: Index
-
- def __init__(
- self,
- axis: Index,
- groupings: Sequence[grouper.Grouping],
- sort: bool = True,
- dropna: bool = True,
- ) -> None:
- assert isinstance(axis, Index), axis
-
- self.axis = axis
- self._groupings: list[grouper.Grouping] = list(groupings)
- self._sort = sort
- self.dropna = dropna
-
- @property
- def groupings(self) -> list[grouper.Grouping]:
- return self._groupings
-
- @property
- def shape(self) -> Shape:
- return tuple(ping.ngroups for ping in self.groupings)
-
- def __iter__(self) -> Iterator[Hashable]:
- return iter(self.indices)
-
- @property
- def nkeys(self) -> int:
- return len(self.groupings)
-
- def get_iterator(
- self, data: NDFrameT, axis: AxisInt = 0
- ) -> Iterator[tuple[Hashable, NDFrameT]]:
- """
- Groupby iterator
-
- Returns
- -------
- Generator yielding sequence of (name, subsetted object)
- for each group
- """
- splitter = self._get_splitter(data, axis=axis)
- keys = self.group_keys_seq
- yield from zip(keys, splitter)
-
- @final
- def _get_splitter(self, data: NDFrame, axis: AxisInt = 0) -> DataSplitter:
- """
- Returns
- -------
- Generator yielding subsetted objects
- """
- ids, _, ngroups = self.group_info
- return _get_splitter(
- data,
- ids,
- ngroups,
- sorted_ids=self._sorted_ids,
- sort_idx=self._sort_idx,
- axis=axis,
- )
-
- @final
- @cache_readonly
- def group_keys_seq(self):
- if len(self.groupings) == 1:
- return self.levels[0]
- else:
- ids, _, ngroups = self.group_info
-
- # provide "flattened" iterator for multi-group setting
- return get_flattened_list(ids, ngroups, self.levels, self.codes)
-
- @cache_readonly
- def indices(self) -> dict[Hashable, npt.NDArray[np.intp]]:
- """dict {group name -> group indices}"""
- if len(self.groupings) == 1 and isinstance(self.result_index, CategoricalIndex):
- # This shows unused categories in indices GH#38642
- return self.groupings[0].indices
- codes_list = [ping.codes for ping in self.groupings]
- keys = [ping.group_index for ping in self.groupings]
- return get_indexer_dict(codes_list, keys)
-
- @final
- def result_ilocs(self) -> npt.NDArray[np.intp]:
- """
- Get the original integer locations of result_index in the input.
- """
- # Original indices are where group_index would go via sorting.
- # But when dropna is true, we need to remove null values while accounting for
- # any gaps that then occur because of them.
- group_index = get_group_index(
- self.codes, self.shape, sort=self._sort, xnull=True
- )
- group_index, _ = compress_group_index(group_index, sort=self._sort)
-
- if self.has_dropped_na:
- mask = np.where(group_index >= 0)
- # Count how many gaps are caused by previous null values for each position
- null_gaps = np.cumsum(group_index == -1)[mask]
- group_index = group_index[mask]
-
- result = get_group_index_sorter(group_index, self.ngroups)
-
- if self.has_dropped_na:
- # Shift by the number of prior null gaps
- result += np.take(null_gaps, result)
-
- return result
-
- @final
- @property
- def codes(self) -> list[npt.NDArray[np.signedinteger]]:
- return [ping.codes for ping in self.groupings]
-
- @property
- def levels(self) -> list[Index]:
- return [ping.group_index for ping in self.groupings]
-
- @property
- def names(self) -> list[Hashable]:
- return [ping.name for ping in self.groupings]
-
- @final
- def size(self) -> Series:
- """
- Compute group sizes.
- """
- ids, _, ngroups = self.group_info
- out: np.ndarray | list
- if ngroups:
- out = np.bincount(ids[ids != -1], minlength=ngroups)
- else:
- out = []
- return Series(out, index=self.result_index, dtype="int64")
-
- @cache_readonly
- def groups(self) -> dict[Hashable, np.ndarray]:
- """dict {group name -> group labels}"""
- if len(self.groupings) == 1:
- return self.groupings[0].groups
- else:
- to_groupby = []
- for ping in self.groupings:
- gv = ping.grouping_vector
- if not isinstance(gv, BaseGrouper):
- to_groupby.append(gv)
- else:
- to_groupby.append(gv.groupings[0].grouping_vector)
- index = MultiIndex.from_arrays(to_groupby)
- return self.axis.groupby(index)
-
- @final
- @cache_readonly
- def is_monotonic(self) -> bool:
- # return if my group orderings are monotonic
- return Index(self.group_info[0]).is_monotonic_increasing
-
- @final
- @cache_readonly
- def has_dropped_na(self) -> bool:
- """
- Whether grouper has null value(s) that are dropped.
- """
- return bool((self.group_info[0] < 0).any())
-
- @cache_readonly
- def group_info(self) -> tuple[npt.NDArray[np.intp], npt.NDArray[np.intp], int]:
- comp_ids, obs_group_ids = self._get_compressed_codes()
-
- ngroups = len(obs_group_ids)
- comp_ids = ensure_platform_int(comp_ids)
-
- return comp_ids, obs_group_ids, ngroups
-
- @cache_readonly
- def codes_info(self) -> npt.NDArray[np.intp]:
- # return the codes of items in original grouped axis
- ids, _, _ = self.group_info
- return ids
-
- @final
- def _get_compressed_codes(
- self,
- ) -> tuple[npt.NDArray[np.signedinteger], npt.NDArray[np.intp]]:
- # The first returned ndarray may have any signed integer dtype
- if len(self.groupings) > 1:
- group_index = get_group_index(self.codes, self.shape, sort=True, xnull=True)
- return compress_group_index(group_index, sort=self._sort)
- # FIXME: compress_group_index's second return value is int64, not intp
-
- ping = self.groupings[0]
- return ping.codes, np.arange(len(ping.group_index), dtype=np.intp)
-
- @final
- @cache_readonly
- def ngroups(self) -> int:
- return len(self.result_index)
-
- @property
- def reconstructed_codes(self) -> list[npt.NDArray[np.intp]]:
- codes = self.codes
- ids, obs_ids, _ = self.group_info
- return decons_obs_group_ids(ids, obs_ids, self.shape, codes, xnull=True)
-
- @cache_readonly
- def result_index(self) -> Index:
- if len(self.groupings) == 1:
- return self.groupings[0].result_index.rename(self.names[0])
-
- codes = self.reconstructed_codes
- levels = [ping.result_index for ping in self.groupings]
- return MultiIndex(
- levels=levels, codes=codes, verify_integrity=False, names=self.names
- )
-
- @final
- def get_group_levels(self) -> list[ArrayLike]:
- # Note: only called from _insert_inaxis_grouper, which
- # is only called for BaseGrouper, never for BinGrouper
- if len(self.groupings) == 1:
- return [self.groupings[0].group_arraylike]
-
- name_list = []
- for ping, codes in zip(self.groupings, self.reconstructed_codes):
- codes = ensure_platform_int(codes)
- levels = ping.group_arraylike.take(codes)
-
- name_list.append(levels)
-
- return name_list
-
- # ------------------------------------------------------------
- # Aggregation functions
-
- @final
- def _cython_operation(
- self,
- kind: str,
- values,
- how: str,
- axis: AxisInt,
- min_count: int = -1,
- **kwargs,
- ) -> ArrayLike:
- """
- Returns the values of a cython operation.
- """
- assert kind in ["transform", "aggregate"]
-
- cy_op = WrappedCythonOp(kind=kind, how=how, has_dropped_na=self.has_dropped_na)
-
- ids, _, _ = self.group_info
- ngroups = self.ngroups
- return cy_op.cython_operation(
- values=values,
- axis=axis,
- min_count=min_count,
- comp_ids=ids,
- ngroups=ngroups,
- **kwargs,
- )
-
- @final
- def agg_series(
- self, obj: Series, func: Callable, preserve_dtype: bool = False
- ) -> ArrayLike:
- """
- Parameters
- ----------
- obj : Series
- func : function taking a Series and returning a scalar-like
- preserve_dtype : bool
- Whether the aggregation is known to be dtype-preserving.
-
- Returns
- -------
- np.ndarray or ExtensionArray
- """
- # test_groupby_empty_with_category gets here with self.ngroups == 0
- # and len(obj) > 0
-
- if len(obj) > 0 and not isinstance(obj._values, np.ndarray):
- # we can preserve a little bit more aggressively with EA dtype
- # because maybe_cast_pointwise_result will do a try/except
- # with _from_sequence. NB we are assuming here that _from_sequence
- # is sufficiently strict that it casts appropriately.
- preserve_dtype = True
-
- result = self._aggregate_series_pure_python(obj, func)
-
- npvalues = lib.maybe_convert_objects(result, try_float=False)
- if preserve_dtype:
- out = maybe_cast_pointwise_result(npvalues, obj.dtype, numeric_only=True)
- else:
- out = npvalues
- return out
-
- @final
- def _aggregate_series_pure_python(
- self, obj: Series, func: Callable
- ) -> npt.NDArray[np.object_]:
- _, _, ngroups = self.group_info
-
- result = np.empty(ngroups, dtype="O")
- initialized = False
-
- splitter = self._get_splitter(obj, axis=0)
-
- for i, group in enumerate(splitter):
- res = func(group)
- res = extract_result(res)
-
- if not initialized:
- # We only do this validation on the first iteration
- check_result_array(res, group.dtype)
- initialized = True
-
- result[i] = res
-
- return result
-
- @final
- def apply_groupwise(
- self, f: Callable, data: DataFrame | Series, axis: AxisInt = 0
- ) -> tuple[list, bool]:
- mutated = False
- splitter = self._get_splitter(data, axis=axis)
- group_keys = self.group_keys_seq
- result_values = []
-
- # This calls DataSplitter.__iter__
- zipped = zip(group_keys, splitter)
-
- for key, group in zipped:
- # Pinning name is needed for
- # test_group_apply_once_per_group,
- # test_inconsistent_return_type, test_set_group_name,
- # test_group_name_available_in_inference_pass,
- # test_groupby_multi_timezone
- object.__setattr__(group, "name", key)
-
- # group might be modified
- group_axes = group.axes
- res = f(group)
- if not mutated and not _is_indexed_like(res, group_axes, axis):
- mutated = True
- result_values.append(res)
- # getattr pattern for __name__ is needed for functools.partial objects
- if len(group_keys) == 0 and getattr(f, "__name__", None) in [
- "skew",
- "sum",
- "prod",
- ]:
- # If group_keys is empty, then no function calls have been made,
- # so we will not have raised even if this is an invalid dtype.
- # So do one dummy call here to raise appropriate TypeError.
- f(data.iloc[:0])
-
- return result_values, mutated
-
- # ------------------------------------------------------------
- # Methods for sorting subsets of our GroupBy's object
-
- @final
- @cache_readonly
- def _sort_idx(self) -> npt.NDArray[np.intp]:
- # Counting sort indexer
- ids, _, ngroups = self.group_info
- return get_group_index_sorter(ids, ngroups)
-
- @final
- @cache_readonly
- def _sorted_ids(self) -> npt.NDArray[np.intp]:
- ids, _, _ = self.group_info
- return ids.take(self._sort_idx)
-
-
-class BinGrouper(BaseGrouper):
- """
- This is an internal Grouper class
-
- Parameters
- ----------
- bins : the split index of binlabels to group the item of axis
- binlabels : the label list
- indexer : np.ndarray[np.intp], optional
- the indexer created by Grouper
- some groupers (TimeGrouper) will sort its axis and its
- group_info is also sorted, so need the indexer to reorder
-
- Examples
- --------
- bins: [2, 4, 6, 8, 10]
- binlabels: DatetimeIndex(['2005-01-01', '2005-01-03',
- '2005-01-05', '2005-01-07', '2005-01-09'],
- dtype='datetime64[ns]', freq='2D')
-
- the group_info, which contains the label of each item in grouped
- axis, the index of label in label list, group number, is
-
- (array([0, 0, 1, 1, 2, 2, 3, 3, 4, 4]), array([0, 1, 2, 3, 4]), 5)
-
- means that, the grouped axis has 10 items, can be grouped into 5
- labels, the first and second items belong to the first label, the
- third and forth items belong to the second label, and so on
-
- """
-
- bins: npt.NDArray[np.int64]
- binlabels: Index
-
- def __init__(
- self,
- bins,
- binlabels,
- indexer=None,
- ) -> None:
- self.bins = ensure_int64(bins)
- self.binlabels = ensure_index(binlabels)
- self.indexer = indexer
-
- # These lengths must match, otherwise we could call agg_series
- # with empty self.bins, which would raise later.
- assert len(self.binlabels) == len(self.bins)
-
- @cache_readonly
- def groups(self):
- """dict {group name -> group labels}"""
- # this is mainly for compat
- # GH 3881
- result = {
- key: value
- for key, value in zip(self.binlabels, self.bins)
- if key is not NaT
- }
- return result
-
- def __iter__(self) -> Iterator[Hashable]:
- return iter(self.groupings[0].grouping_vector)
-
- @property
- def nkeys(self) -> int:
- # still matches len(self.groupings), but we can hard-code
- return 1
-
- @cache_readonly
- def codes_info(self) -> npt.NDArray[np.intp]:
- # return the codes of items in original grouped axis
- ids, _, _ = self.group_info
- if self.indexer is not None:
- sorter = np.lexsort((ids, self.indexer))
- ids = ids[sorter]
- return ids
-
- def get_iterator(self, data: NDFrame, axis: AxisInt = 0):
- """
- Groupby iterator
-
- Returns
- -------
- Generator yielding sequence of (name, subsetted object)
- for each group
- """
- if axis == 0:
- slicer = lambda start, edge: data.iloc[start:edge]
- else:
- slicer = lambda start, edge: data.iloc[:, start:edge]
-
- length = len(data.axes[axis])
-
- start = 0
- for edge, label in zip(self.bins, self.binlabels):
- if label is not NaT:
- yield label, slicer(start, edge)
- start = edge
-
- if start < length:
- yield self.binlabels[-1], slicer(start, None)
-
- @cache_readonly
- def indices(self):
- indices = collections.defaultdict(list)
-
- i = 0
- for label, bin in zip(self.binlabels, self.bins):
- if i < bin:
- if label is not NaT:
- indices[label] = list(range(i, bin))
- i = bin
- return indices
-
- @cache_readonly
- def group_info(self) -> tuple[npt.NDArray[np.intp], npt.NDArray[np.intp], int]:
- ngroups = self.ngroups
- obs_group_ids = np.arange(ngroups, dtype=np.intp)
- rep = np.diff(np.r_[0, self.bins])
-
- rep = ensure_platform_int(rep)
- if ngroups == len(self.bins):
- comp_ids = np.repeat(np.arange(ngroups), rep)
- else:
- comp_ids = np.repeat(np.r_[-1, np.arange(ngroups)], rep)
-
- return (
- ensure_platform_int(comp_ids),
- obs_group_ids,
- ngroups,
- )
-
- @cache_readonly
- def reconstructed_codes(self) -> list[np.ndarray]:
- # get unique result indices, and prepend 0 as groupby starts from the first
- return [np.r_[0, np.flatnonzero(self.bins[1:] != self.bins[:-1]) + 1]]
-
- @cache_readonly
- def result_index(self) -> Index:
- if len(self.binlabels) != 0 and isna(self.binlabels[0]):
- return self.binlabels[1:]
-
- return self.binlabels
-
- @property
- def levels(self) -> list[Index]:
- return [self.binlabels]
-
- @property
- def names(self) -> list[Hashable]:
- return [self.binlabels.name]
-
- @property
- def groupings(self) -> list[grouper.Grouping]:
- lev = self.binlabels
- codes = self.group_info[0]
- labels = lev.take(codes)
- ping = grouper.Grouping(
- labels, labels, in_axis=False, level=None, uniques=lev._values
- )
- return [ping]
-
-
-def _is_indexed_like(obj, axes, axis: AxisInt) -> bool:
- if isinstance(obj, Series):
- if len(axes) > 1:
- return False
- return obj.axes[axis].equals(axes[axis])
- elif isinstance(obj, DataFrame):
- return obj.axes[axis].equals(axes[axis])
-
- return False
-
-
-# ----------------------------------------------------------------------
-# Splitting / application
-
-
-class DataSplitter(Generic[NDFrameT]):
- def __init__(
- self,
- data: NDFrameT,
- labels: npt.NDArray[np.intp],
- ngroups: int,
- *,
- sort_idx: npt.NDArray[np.intp],
- sorted_ids: npt.NDArray[np.intp],
- axis: AxisInt = 0,
- ) -> None:
- self.data = data
- self.labels = ensure_platform_int(labels) # _should_ already be np.intp
- self.ngroups = ngroups
-
- self._slabels = sorted_ids
- self._sort_idx = sort_idx
-
- self.axis = axis
- assert isinstance(axis, int), axis
-
- def __iter__(self) -> Iterator:
- sdata = self._sorted_data
-
- if self.ngroups == 0:
- # we are inside a generator, rather than raise StopIteration
- # we merely return signal the end
- return
-
- starts, ends = lib.generate_slices(self._slabels, self.ngroups)
-
- for start, end in zip(starts, ends):
- yield self._chop(sdata, slice(start, end))
-
- @cache_readonly
- def _sorted_data(self) -> NDFrameT:
- return self.data.take(self._sort_idx, axis=self.axis)
-
- def _chop(self, sdata, slice_obj: slice) -> NDFrame:
- raise AbstractMethodError(self)
-
-
-class SeriesSplitter(DataSplitter):
- def _chop(self, sdata: Series, slice_obj: slice) -> Series:
- # fastpath equivalent to `sdata.iloc[slice_obj]`
- mgr = sdata._mgr.get_slice(slice_obj)
- ser = sdata._constructor_from_mgr(mgr, axes=mgr.axes)
- ser._name = sdata.name
- return ser.__finalize__(sdata, method="groupby")
-
-
-class FrameSplitter(DataSplitter):
- def _chop(self, sdata: DataFrame, slice_obj: slice) -> DataFrame:
- # Fastpath equivalent to:
- # if self.axis == 0:
- # return sdata.iloc[slice_obj]
- # else:
- # return sdata.iloc[:, slice_obj]
- mgr = sdata._mgr.get_slice(slice_obj, axis=1 - self.axis)
- df = sdata._constructor_from_mgr(mgr, axes=mgr.axes)
- return df.__finalize__(sdata, method="groupby")
-
-
-def _get_splitter(
- data: NDFrame,
- labels: npt.NDArray[np.intp],
- ngroups: int,
- *,
- sort_idx: npt.NDArray[np.intp],
- sorted_ids: npt.NDArray[np.intp],
- axis: AxisInt = 0,
-) -> DataSplitter:
- if isinstance(data, Series):
- klass: type[DataSplitter] = SeriesSplitter
- else:
- # i.e. DataFrame
- klass = FrameSplitter
-
- return klass(
- data, labels, ngroups, sort_idx=sort_idx, sorted_ids=sorted_ids, axis=axis
- )
diff --git a/spaces/profayle/TerrapinTalk/myenv/lib/python3.9/site-packages/pandas/tests/dtypes/__init__.py b/spaces/profayle/TerrapinTalk/myenv/lib/python3.9/site-packages/pandas/tests/dtypes/__init__.py
deleted file mode 100644
index e69de29bb2d1d6434b8b29ae775ad8c2e48c5391..0000000000000000000000000000000000000000
diff --git a/spaces/profayle/TerrapinTalk/myenv/lib/python3.9/site-packages/pandas/tests/frame/methods/test_rename_axis.py b/spaces/profayle/TerrapinTalk/myenv/lib/python3.9/site-packages/pandas/tests/frame/methods/test_rename_axis.py
deleted file mode 100644
index dd4a77c6509b8de7eb767bb44238004399c159a4..0000000000000000000000000000000000000000
--- a/spaces/profayle/TerrapinTalk/myenv/lib/python3.9/site-packages/pandas/tests/frame/methods/test_rename_axis.py
+++ /dev/null
@@ -1,111 +0,0 @@
-import numpy as np
-import pytest
-
-from pandas import (
- DataFrame,
- Index,
- MultiIndex,
-)
-import pandas._testing as tm
-
-
-class TestDataFrameRenameAxis:
- def test_rename_axis_inplace(self, float_frame):
- # GH#15704
- expected = float_frame.rename_axis("foo")
- result = float_frame.copy()
- return_value = no_return = result.rename_axis("foo", inplace=True)
- assert return_value is None
-
- assert no_return is None
- tm.assert_frame_equal(result, expected)
-
- expected = float_frame.rename_axis("bar", axis=1)
- result = float_frame.copy()
- return_value = no_return = result.rename_axis("bar", axis=1, inplace=True)
- assert return_value is None
-
- assert no_return is None
- tm.assert_frame_equal(result, expected)
-
- def test_rename_axis_raises(self):
- # GH#17833
- df = DataFrame({"A": [1, 2], "B": [1, 2]})
- with pytest.raises(ValueError, match="Use `.rename`"):
- df.rename_axis(id, axis=0)
-
- with pytest.raises(ValueError, match="Use `.rename`"):
- df.rename_axis({0: 10, 1: 20}, axis=0)
-
- with pytest.raises(ValueError, match="Use `.rename`"):
- df.rename_axis(id, axis=1)
-
- with pytest.raises(ValueError, match="Use `.rename`"):
- df["A"].rename_axis(id)
-
- def test_rename_axis_mapper(self):
- # GH#19978
- mi = MultiIndex.from_product([["a", "b", "c"], [1, 2]], names=["ll", "nn"])
- df = DataFrame(
- {"x": list(range(len(mi))), "y": [i * 10 for i in range(len(mi))]}, index=mi
- )
-
- # Test for rename of the Index object of columns
- result = df.rename_axis("cols", axis=1)
- tm.assert_index_equal(result.columns, Index(["x", "y"], name="cols"))
-
- # Test for rename of the Index object of columns using dict
- result = result.rename_axis(columns={"cols": "new"}, axis=1)
- tm.assert_index_equal(result.columns, Index(["x", "y"], name="new"))
-
- # Test for renaming index using dict
- result = df.rename_axis(index={"ll": "foo"})
- assert result.index.names == ["foo", "nn"]
-
- # Test for renaming index using a function
- result = df.rename_axis(index=str.upper, axis=0)
- assert result.index.names == ["LL", "NN"]
-
- # Test for renaming index providing complete list
- result = df.rename_axis(index=["foo", "goo"])
- assert result.index.names == ["foo", "goo"]
-
- # Test for changing index and columns at same time
- sdf = df.reset_index().set_index("nn").drop(columns=["ll", "y"])
- result = sdf.rename_axis(index="foo", columns="meh")
- assert result.index.name == "foo"
- assert result.columns.name == "meh"
-
- # Test different error cases
- with pytest.raises(TypeError, match="Must pass"):
- df.rename_axis(index="wrong")
-
- with pytest.raises(ValueError, match="Length of names"):
- df.rename_axis(index=["wrong"])
-
- with pytest.raises(TypeError, match="bogus"):
- df.rename_axis(bogus=None)
-
- @pytest.mark.parametrize(
- "kwargs, rename_index, rename_columns",
- [
- ({"mapper": None, "axis": 0}, True, False),
- ({"mapper": None, "axis": 1}, False, True),
- ({"index": None}, True, False),
- ({"columns": None}, False, True),
- ({"index": None, "columns": None}, True, True),
- ({}, False, False),
- ],
- )
- def test_rename_axis_none(self, kwargs, rename_index, rename_columns):
- # GH 25034
- index = Index(list("abc"), name="foo")
- columns = Index(["col1", "col2"], name="bar")
- data = np.arange(6).reshape(3, 2)
- df = DataFrame(data, index, columns)
-
- result = df.rename_axis(**kwargs)
- expected_index = index.rename(None) if rename_index else index
- expected_columns = columns.rename(None) if rename_columns else columns
- expected = DataFrame(data, expected_index, expected_columns)
- tm.assert_frame_equal(result, expected)
diff --git a/spaces/profayle/TerrapinTalk/myenv/lib/python3.9/site-packages/pandas/tests/groupby/__init__.py b/spaces/profayle/TerrapinTalk/myenv/lib/python3.9/site-packages/pandas/tests/groupby/__init__.py
deleted file mode 100644
index 446d9da4377712b073d76dac7672dcf1de00cf04..0000000000000000000000000000000000000000
--- a/spaces/profayle/TerrapinTalk/myenv/lib/python3.9/site-packages/pandas/tests/groupby/__init__.py
+++ /dev/null
@@ -1,25 +0,0 @@
-def get_groupby_method_args(name, obj):
- """
- Get required arguments for a groupby method.
-
- When parametrizing a test over groupby methods (e.g. "sum", "mean", "fillna"),
- it is often the case that arguments are required for certain methods.
-
- Parameters
- ----------
- name: str
- Name of the method.
- obj: Series or DataFrame
- pandas object that is being grouped.
-
- Returns
- -------
- A tuple of required arguments for the method.
- """
- if name in ("nth", "fillna", "take"):
- return (0,)
- if name == "quantile":
- return (0.5,)
- if name == "corrwith":
- return (obj,)
- return ()
diff --git a/spaces/profayle/TerrapinTalk/myenv/lib/python3.9/site-packages/pandas/tests/indexes/categorical/test_indexing.py b/spaces/profayle/TerrapinTalk/myenv/lib/python3.9/site-packages/pandas/tests/indexes/categorical/test_indexing.py
deleted file mode 100644
index 49eb79da616e7603b70ee3189e9004dd51fb33e7..0000000000000000000000000000000000000000
--- a/spaces/profayle/TerrapinTalk/myenv/lib/python3.9/site-packages/pandas/tests/indexes/categorical/test_indexing.py
+++ /dev/null
@@ -1,420 +0,0 @@
-import numpy as np
-import pytest
-
-from pandas.errors import InvalidIndexError
-
-import pandas as pd
-from pandas import (
- CategoricalIndex,
- Index,
- IntervalIndex,
- Timestamp,
-)
-import pandas._testing as tm
-
-
-class TestTake:
- def test_take_fill_value(self):
- # GH 12631
-
- # numeric category
- idx = CategoricalIndex([1, 2, 3], name="xxx")
- result = idx.take(np.array([1, 0, -1]))
- expected = CategoricalIndex([2, 1, 3], name="xxx")
- tm.assert_index_equal(result, expected)
- tm.assert_categorical_equal(result.values, expected.values)
-
- # fill_value
- result = idx.take(np.array([1, 0, -1]), fill_value=True)
- expected = CategoricalIndex([2, 1, np.nan], categories=[1, 2, 3], name="xxx")
- tm.assert_index_equal(result, expected)
- tm.assert_categorical_equal(result.values, expected.values)
-
- # allow_fill=False
- result = idx.take(np.array([1, 0, -1]), allow_fill=False, fill_value=True)
- expected = CategoricalIndex([2, 1, 3], name="xxx")
- tm.assert_index_equal(result, expected)
- tm.assert_categorical_equal(result.values, expected.values)
-
- # object category
- idx = CategoricalIndex(
- list("CBA"), categories=list("ABC"), ordered=True, name="xxx"
- )
- result = idx.take(np.array([1, 0, -1]))
- expected = CategoricalIndex(
- list("BCA"), categories=list("ABC"), ordered=True, name="xxx"
- )
- tm.assert_index_equal(result, expected)
- tm.assert_categorical_equal(result.values, expected.values)
-
- # fill_value
- result = idx.take(np.array([1, 0, -1]), fill_value=True)
- expected = CategoricalIndex(
- ["B", "C", np.nan], categories=list("ABC"), ordered=True, name="xxx"
- )
- tm.assert_index_equal(result, expected)
- tm.assert_categorical_equal(result.values, expected.values)
-
- # allow_fill=False
- result = idx.take(np.array([1, 0, -1]), allow_fill=False, fill_value=True)
- expected = CategoricalIndex(
- list("BCA"), categories=list("ABC"), ordered=True, name="xxx"
- )
- tm.assert_index_equal(result, expected)
- tm.assert_categorical_equal(result.values, expected.values)
-
- msg = (
- "When allow_fill=True and fill_value is not None, "
- "all indices must be >= -1"
- )
- with pytest.raises(ValueError, match=msg):
- idx.take(np.array([1, 0, -2]), fill_value=True)
- with pytest.raises(ValueError, match=msg):
- idx.take(np.array([1, 0, -5]), fill_value=True)
-
- msg = "index -5 is out of bounds for (axis 0 with )?size 3"
- with pytest.raises(IndexError, match=msg):
- idx.take(np.array([1, -5]))
-
- def test_take_fill_value_datetime(self):
- # datetime category
- idx = pd.DatetimeIndex(["2011-01-01", "2011-02-01", "2011-03-01"], name="xxx")
- idx = CategoricalIndex(idx)
- result = idx.take(np.array([1, 0, -1]))
- expected = pd.DatetimeIndex(
- ["2011-02-01", "2011-01-01", "2011-03-01"], name="xxx"
- )
- expected = CategoricalIndex(expected)
- tm.assert_index_equal(result, expected)
-
- # fill_value
- result = idx.take(np.array([1, 0, -1]), fill_value=True)
- expected = pd.DatetimeIndex(["2011-02-01", "2011-01-01", "NaT"], name="xxx")
- exp_cats = pd.DatetimeIndex(["2011-01-01", "2011-02-01", "2011-03-01"])
- expected = CategoricalIndex(expected, categories=exp_cats)
- tm.assert_index_equal(result, expected)
-
- # allow_fill=False
- result = idx.take(np.array([1, 0, -1]), allow_fill=False, fill_value=True)
- expected = pd.DatetimeIndex(
- ["2011-02-01", "2011-01-01", "2011-03-01"], name="xxx"
- )
- expected = CategoricalIndex(expected)
- tm.assert_index_equal(result, expected)
-
- msg = (
- "When allow_fill=True and fill_value is not None, "
- "all indices must be >= -1"
- )
- with pytest.raises(ValueError, match=msg):
- idx.take(np.array([1, 0, -2]), fill_value=True)
- with pytest.raises(ValueError, match=msg):
- idx.take(np.array([1, 0, -5]), fill_value=True)
-
- msg = "index -5 is out of bounds for (axis 0 with )?size 3"
- with pytest.raises(IndexError, match=msg):
- idx.take(np.array([1, -5]))
-
- def test_take_invalid_kwargs(self):
- idx = CategoricalIndex([1, 2, 3], name="foo")
- indices = [1, 0, -1]
-
- msg = r"take\(\) got an unexpected keyword argument 'foo'"
- with pytest.raises(TypeError, match=msg):
- idx.take(indices, foo=2)
-
- msg = "the 'out' parameter is not supported"
- with pytest.raises(ValueError, match=msg):
- idx.take(indices, out=indices)
-
- msg = "the 'mode' parameter is not supported"
- with pytest.raises(ValueError, match=msg):
- idx.take(indices, mode="clip")
-
-
-class TestGetLoc:
- def test_get_loc(self):
- # GH 12531
- cidx1 = CategoricalIndex(list("abcde"), categories=list("edabc"))
- idx1 = Index(list("abcde"))
- assert cidx1.get_loc("a") == idx1.get_loc("a")
- assert cidx1.get_loc("e") == idx1.get_loc("e")
-
- for i in [cidx1, idx1]:
- with pytest.raises(KeyError, match="'NOT-EXIST'"):
- i.get_loc("NOT-EXIST")
-
- # non-unique
- cidx2 = CategoricalIndex(list("aacded"), categories=list("edabc"))
- idx2 = Index(list("aacded"))
-
- # results in bool array
- res = cidx2.get_loc("d")
- tm.assert_numpy_array_equal(res, idx2.get_loc("d"))
- tm.assert_numpy_array_equal(
- res, np.array([False, False, False, True, False, True])
- )
- # unique element results in scalar
- res = cidx2.get_loc("e")
- assert res == idx2.get_loc("e")
- assert res == 4
-
- for i in [cidx2, idx2]:
- with pytest.raises(KeyError, match="'NOT-EXIST'"):
- i.get_loc("NOT-EXIST")
-
- # non-unique, sliceable
- cidx3 = CategoricalIndex(list("aabbb"), categories=list("abc"))
- idx3 = Index(list("aabbb"))
-
- # results in slice
- res = cidx3.get_loc("a")
- assert res == idx3.get_loc("a")
- assert res == slice(0, 2, None)
-
- res = cidx3.get_loc("b")
- assert res == idx3.get_loc("b")
- assert res == slice(2, 5, None)
-
- for i in [cidx3, idx3]:
- with pytest.raises(KeyError, match="'c'"):
- i.get_loc("c")
-
- def test_get_loc_unique(self):
- cidx = CategoricalIndex(list("abc"))
- result = cidx.get_loc("b")
- assert result == 1
-
- def test_get_loc_monotonic_nonunique(self):
- cidx = CategoricalIndex(list("abbc"))
- result = cidx.get_loc("b")
- expected = slice(1, 3, None)
- assert result == expected
-
- def test_get_loc_nonmonotonic_nonunique(self):
- cidx = CategoricalIndex(list("abcb"))
- result = cidx.get_loc("b")
- expected = np.array([False, True, False, True], dtype=bool)
- tm.assert_numpy_array_equal(result, expected)
-
- def test_get_loc_nan(self):
- # GH#41933
- ci = CategoricalIndex(["A", "B", np.nan])
- res = ci.get_loc(np.nan)
-
- assert res == 2
-
-
-class TestGetIndexer:
- def test_get_indexer_base(self):
- # Determined by cat ordering.
- idx = CategoricalIndex(list("cab"), categories=list("cab"))
- expected = np.arange(len(idx), dtype=np.intp)
-
- actual = idx.get_indexer(idx)
- tm.assert_numpy_array_equal(expected, actual)
-
- with pytest.raises(ValueError, match="Invalid fill method"):
- idx.get_indexer(idx, method="invalid")
-
- def test_get_indexer_requires_unique(self):
- ci = CategoricalIndex(list("aabbca"), categories=list("cab"), ordered=False)
- oidx = Index(np.array(ci))
-
- msg = "Reindexing only valid with uniquely valued Index objects"
-
- for n in [1, 2, 5, len(ci)]:
- finder = oidx[np.random.default_rng(2).integers(0, len(ci), size=n)]
-
- with pytest.raises(InvalidIndexError, match=msg):
- ci.get_indexer(finder)
-
- # see gh-17323
- #
- # Even when indexer is equal to the
- # members in the index, we should
- # respect duplicates instead of taking
- # the fast-track path.
- for finder in [list("aabbca"), list("aababca")]:
- with pytest.raises(InvalidIndexError, match=msg):
- ci.get_indexer(finder)
-
- def test_get_indexer_non_unique(self):
- idx1 = CategoricalIndex(list("aabcde"), categories=list("edabc"))
- idx2 = CategoricalIndex(list("abf"))
-
- for indexer in [idx2, list("abf"), Index(list("abf"))]:
- msg = "Reindexing only valid with uniquely valued Index objects"
- with pytest.raises(InvalidIndexError, match=msg):
- idx1.get_indexer(indexer)
-
- r1, _ = idx1.get_indexer_non_unique(indexer)
- expected = np.array([0, 1, 2, -1], dtype=np.intp)
- tm.assert_almost_equal(r1, expected)
-
- def test_get_indexer_method(self):
- idx1 = CategoricalIndex(list("aabcde"), categories=list("edabc"))
- idx2 = CategoricalIndex(list("abf"))
-
- msg = "method pad not yet implemented for CategoricalIndex"
- with pytest.raises(NotImplementedError, match=msg):
- idx2.get_indexer(idx1, method="pad")
- msg = "method backfill not yet implemented for CategoricalIndex"
- with pytest.raises(NotImplementedError, match=msg):
- idx2.get_indexer(idx1, method="backfill")
-
- msg = "method nearest not yet implemented for CategoricalIndex"
- with pytest.raises(NotImplementedError, match=msg):
- idx2.get_indexer(idx1, method="nearest")
-
- def test_get_indexer_array(self):
- arr = np.array(
- [Timestamp("1999-12-31 00:00:00"), Timestamp("2000-12-31 00:00:00")],
- dtype=object,
- )
- cats = [Timestamp("1999-12-31 00:00:00"), Timestamp("2000-12-31 00:00:00")]
- ci = CategoricalIndex(cats, categories=cats, ordered=False, dtype="category")
- result = ci.get_indexer(arr)
- expected = np.array([0, 1], dtype="intp")
- tm.assert_numpy_array_equal(result, expected)
-
- def test_get_indexer_same_categories_same_order(self):
- ci = CategoricalIndex(["a", "b"], categories=["a", "b"])
-
- result = ci.get_indexer(CategoricalIndex(["b", "b"], categories=["a", "b"]))
- expected = np.array([1, 1], dtype="intp")
- tm.assert_numpy_array_equal(result, expected)
-
- def test_get_indexer_same_categories_different_order(self):
- # https://github.com/pandas-dev/pandas/issues/19551
- ci = CategoricalIndex(["a", "b"], categories=["a", "b"])
-
- result = ci.get_indexer(CategoricalIndex(["b", "b"], categories=["b", "a"]))
- expected = np.array([1, 1], dtype="intp")
- tm.assert_numpy_array_equal(result, expected)
-
- def test_get_indexer_nans_in_index_and_target(self):
- # GH 45361
- ci = CategoricalIndex([1, 2, np.nan, 3])
- other1 = [2, 3, 4, np.nan]
- res1 = ci.get_indexer(other1)
- expected1 = np.array([1, 3, -1, 2], dtype=np.intp)
- tm.assert_numpy_array_equal(res1, expected1)
- other2 = [1, 4, 2, 3]
- res2 = ci.get_indexer(other2)
- expected2 = np.array([0, -1, 1, 3], dtype=np.intp)
- tm.assert_numpy_array_equal(res2, expected2)
-
-
-class TestWhere:
- def test_where(self, listlike_box):
- klass = listlike_box
-
- i = CategoricalIndex(list("aabbca"), categories=list("cab"), ordered=False)
- cond = [True] * len(i)
- expected = i
- result = i.where(klass(cond))
- tm.assert_index_equal(result, expected)
-
- cond = [False] + [True] * (len(i) - 1)
- expected = CategoricalIndex([np.nan] + i[1:].tolist(), categories=i.categories)
- result = i.where(klass(cond))
- tm.assert_index_equal(result, expected)
-
- def test_where_non_categories(self):
- ci = CategoricalIndex(["a", "b", "c", "d"])
- mask = np.array([True, False, True, False])
-
- result = ci.where(mask, 2)
- expected = Index(["a", 2, "c", 2], dtype=object)
- tm.assert_index_equal(result, expected)
-
- msg = "Cannot setitem on a Categorical with a new category"
- with pytest.raises(TypeError, match=msg):
- # Test the Categorical method directly
- ci._data._where(mask, 2)
-
-
-class TestContains:
- def test_contains(self):
- ci = CategoricalIndex(list("aabbca"), categories=list("cabdef"), ordered=False)
-
- assert "a" in ci
- assert "z" not in ci
- assert "e" not in ci
- assert np.nan not in ci
-
- # assert codes NOT in index
- assert 0 not in ci
- assert 1 not in ci
-
- def test_contains_nan(self):
- ci = CategoricalIndex(list("aabbca") + [np.nan], categories=list("cabdef"))
- assert np.nan in ci
-
- @pytest.mark.parametrize("unwrap", [True, False])
- def test_contains_na_dtype(self, unwrap):
- dti = pd.date_range("2016-01-01", periods=100).insert(0, pd.NaT)
- pi = dti.to_period("D")
- tdi = dti - dti[-1]
- ci = CategoricalIndex(dti)
-
- obj = ci
- if unwrap:
- obj = ci._data
-
- assert np.nan in obj
- assert None in obj
- assert pd.NaT in obj
- assert np.datetime64("NaT") in obj
- assert np.timedelta64("NaT") not in obj
-
- obj2 = CategoricalIndex(tdi)
- if unwrap:
- obj2 = obj2._data
-
- assert np.nan in obj2
- assert None in obj2
- assert pd.NaT in obj2
- assert np.datetime64("NaT") not in obj2
- assert np.timedelta64("NaT") in obj2
-
- obj3 = CategoricalIndex(pi)
- if unwrap:
- obj3 = obj3._data
-
- assert np.nan in obj3
- assert None in obj3
- assert pd.NaT in obj3
- assert np.datetime64("NaT") not in obj3
- assert np.timedelta64("NaT") not in obj3
-
- @pytest.mark.parametrize(
- "item, expected",
- [
- (pd.Interval(0, 1), True),
- (1.5, True),
- (pd.Interval(0.5, 1.5), False),
- ("a", False),
- (Timestamp(1), False),
- (pd.Timedelta(1), False),
- ],
- ids=str,
- )
- def test_contains_interval(self, item, expected):
- # GH 23705
- ci = CategoricalIndex(IntervalIndex.from_breaks(range(3)))
- result = item in ci
- assert result is expected
-
- def test_contains_list(self):
- # GH#21729
- idx = CategoricalIndex([1, 2, 3])
-
- assert "a" not in idx
-
- with pytest.raises(TypeError, match="unhashable type"):
- ["a"] in idx
-
- with pytest.raises(TypeError, match="unhashable type"):
- ["a", "b"] in idx
diff --git a/spaces/profayle/TerrapinTalk/myenv/lib/python3.9/site-packages/pandas/tests/tslibs/test_np_datetime.py b/spaces/profayle/TerrapinTalk/myenv/lib/python3.9/site-packages/pandas/tests/tslibs/test_np_datetime.py
deleted file mode 100644
index 02edf1a09387766d71097ea0baedc2640cfb824b..0000000000000000000000000000000000000000
--- a/spaces/profayle/TerrapinTalk/myenv/lib/python3.9/site-packages/pandas/tests/tslibs/test_np_datetime.py
+++ /dev/null
@@ -1,222 +0,0 @@
-import numpy as np
-import pytest
-
-from pandas._libs.tslibs.dtypes import NpyDatetimeUnit
-from pandas._libs.tslibs.np_datetime import (
- OutOfBoundsDatetime,
- OutOfBoundsTimedelta,
- astype_overflowsafe,
- is_unitless,
- py_get_unit_from_dtype,
- py_td64_to_tdstruct,
-)
-
-import pandas._testing as tm
-
-
-def test_is_unitless():
- dtype = np.dtype("M8[ns]")
- assert not is_unitless(dtype)
-
- dtype = np.dtype("datetime64")
- assert is_unitless(dtype)
-
- dtype = np.dtype("m8[ns]")
- assert not is_unitless(dtype)
-
- dtype = np.dtype("timedelta64")
- assert is_unitless(dtype)
-
- msg = "dtype must be datetime64 or timedelta64"
- with pytest.raises(ValueError, match=msg):
- is_unitless(np.dtype(np.int64))
-
- msg = "Argument 'dtype' has incorrect type"
- with pytest.raises(TypeError, match=msg):
- is_unitless("foo")
-
-
-def test_get_unit_from_dtype():
- # datetime64
- assert py_get_unit_from_dtype(np.dtype("M8[Y]")) == NpyDatetimeUnit.NPY_FR_Y.value
- assert py_get_unit_from_dtype(np.dtype("M8[M]")) == NpyDatetimeUnit.NPY_FR_M.value
- assert py_get_unit_from_dtype(np.dtype("M8[W]")) == NpyDatetimeUnit.NPY_FR_W.value
- # B has been deprecated and removed -> no 3
- assert py_get_unit_from_dtype(np.dtype("M8[D]")) == NpyDatetimeUnit.NPY_FR_D.value
- assert py_get_unit_from_dtype(np.dtype("M8[h]")) == NpyDatetimeUnit.NPY_FR_h.value
- assert py_get_unit_from_dtype(np.dtype("M8[m]")) == NpyDatetimeUnit.NPY_FR_m.value
- assert py_get_unit_from_dtype(np.dtype("M8[s]")) == NpyDatetimeUnit.NPY_FR_s.value
- assert py_get_unit_from_dtype(np.dtype("M8[ms]")) == NpyDatetimeUnit.NPY_FR_ms.value
- assert py_get_unit_from_dtype(np.dtype("M8[us]")) == NpyDatetimeUnit.NPY_FR_us.value
- assert py_get_unit_from_dtype(np.dtype("M8[ns]")) == NpyDatetimeUnit.NPY_FR_ns.value
- assert py_get_unit_from_dtype(np.dtype("M8[ps]")) == NpyDatetimeUnit.NPY_FR_ps.value
- assert py_get_unit_from_dtype(np.dtype("M8[fs]")) == NpyDatetimeUnit.NPY_FR_fs.value
- assert py_get_unit_from_dtype(np.dtype("M8[as]")) == NpyDatetimeUnit.NPY_FR_as.value
-
- # timedelta64
- assert py_get_unit_from_dtype(np.dtype("m8[Y]")) == NpyDatetimeUnit.NPY_FR_Y.value
- assert py_get_unit_from_dtype(np.dtype("m8[M]")) == NpyDatetimeUnit.NPY_FR_M.value
- assert py_get_unit_from_dtype(np.dtype("m8[W]")) == NpyDatetimeUnit.NPY_FR_W.value
- # B has been deprecated and removed -> no 3
- assert py_get_unit_from_dtype(np.dtype("m8[D]")) == NpyDatetimeUnit.NPY_FR_D.value
- assert py_get_unit_from_dtype(np.dtype("m8[h]")) == NpyDatetimeUnit.NPY_FR_h.value
- assert py_get_unit_from_dtype(np.dtype("m8[m]")) == NpyDatetimeUnit.NPY_FR_m.value
- assert py_get_unit_from_dtype(np.dtype("m8[s]")) == NpyDatetimeUnit.NPY_FR_s.value
- assert py_get_unit_from_dtype(np.dtype("m8[ms]")) == NpyDatetimeUnit.NPY_FR_ms.value
- assert py_get_unit_from_dtype(np.dtype("m8[us]")) == NpyDatetimeUnit.NPY_FR_us.value
- assert py_get_unit_from_dtype(np.dtype("m8[ns]")) == NpyDatetimeUnit.NPY_FR_ns.value
- assert py_get_unit_from_dtype(np.dtype("m8[ps]")) == NpyDatetimeUnit.NPY_FR_ps.value
- assert py_get_unit_from_dtype(np.dtype("m8[fs]")) == NpyDatetimeUnit.NPY_FR_fs.value
- assert py_get_unit_from_dtype(np.dtype("m8[as]")) == NpyDatetimeUnit.NPY_FR_as.value
-
-
-def test_td64_to_tdstruct():
- val = 12454636234 # arbitrary value
-
- res1 = py_td64_to_tdstruct(val, NpyDatetimeUnit.NPY_FR_ns.value)
- exp1 = {
- "days": 0,
- "hrs": 0,
- "min": 0,
- "sec": 12,
- "ms": 454,
- "us": 636,
- "ns": 234,
- "seconds": 12,
- "microseconds": 454636,
- "nanoseconds": 234,
- }
- assert res1 == exp1
-
- res2 = py_td64_to_tdstruct(val, NpyDatetimeUnit.NPY_FR_us.value)
- exp2 = {
- "days": 0,
- "hrs": 3,
- "min": 27,
- "sec": 34,
- "ms": 636,
- "us": 234,
- "ns": 0,
- "seconds": 12454,
- "microseconds": 636234,
- "nanoseconds": 0,
- }
- assert res2 == exp2
-
- res3 = py_td64_to_tdstruct(val, NpyDatetimeUnit.NPY_FR_ms.value)
- exp3 = {
- "days": 144,
- "hrs": 3,
- "min": 37,
- "sec": 16,
- "ms": 234,
- "us": 0,
- "ns": 0,
- "seconds": 13036,
- "microseconds": 234000,
- "nanoseconds": 0,
- }
- assert res3 == exp3
-
- # Note this out of bounds for nanosecond Timedelta
- res4 = py_td64_to_tdstruct(val, NpyDatetimeUnit.NPY_FR_s.value)
- exp4 = {
- "days": 144150,
- "hrs": 21,
- "min": 10,
- "sec": 34,
- "ms": 0,
- "us": 0,
- "ns": 0,
- "seconds": 76234,
- "microseconds": 0,
- "nanoseconds": 0,
- }
- assert res4 == exp4
-
-
-class TestAstypeOverflowSafe:
- def test_pass_non_dt64_array(self):
- # check that we raise, not segfault
- arr = np.arange(5)
- dtype = np.dtype("M8[ns]")
-
- msg = (
- "astype_overflowsafe values.dtype and dtype must be either "
- "both-datetime64 or both-timedelta64"
- )
- with pytest.raises(TypeError, match=msg):
- astype_overflowsafe(arr, dtype, copy=True)
-
- with pytest.raises(TypeError, match=msg):
- astype_overflowsafe(arr, dtype, copy=False)
-
- def test_pass_non_dt64_dtype(self):
- # check that we raise, not segfault
- arr = np.arange(5, dtype="i8").view("M8[D]")
- dtype = np.dtype("m8[ns]")
-
- msg = (
- "astype_overflowsafe values.dtype and dtype must be either "
- "both-datetime64 or both-timedelta64"
- )
- with pytest.raises(TypeError, match=msg):
- astype_overflowsafe(arr, dtype, copy=True)
-
- with pytest.raises(TypeError, match=msg):
- astype_overflowsafe(arr, dtype, copy=False)
-
- def test_astype_overflowsafe_dt64(self):
- dtype = np.dtype("M8[ns]")
-
- dt = np.datetime64("2262-04-05", "D")
- arr = dt + np.arange(10, dtype="m8[D]")
-
- # arr.astype silently overflows, so this
- wrong = arr.astype(dtype)
- roundtrip = wrong.astype(arr.dtype)
- assert not (wrong == roundtrip).all()
-
- msg = "Out of bounds nanosecond timestamp"
- with pytest.raises(OutOfBoundsDatetime, match=msg):
- astype_overflowsafe(arr, dtype)
-
- # But converting to microseconds is fine, and we match numpy's results.
- dtype2 = np.dtype("M8[us]")
- result = astype_overflowsafe(arr, dtype2)
- expected = arr.astype(dtype2)
- tm.assert_numpy_array_equal(result, expected)
-
- def test_astype_overflowsafe_td64(self):
- dtype = np.dtype("m8[ns]")
-
- dt = np.datetime64("2262-04-05", "D")
- arr = dt + np.arange(10, dtype="m8[D]")
- arr = arr.view("m8[D]")
-
- # arr.astype silently overflows, so this
- wrong = arr.astype(dtype)
- roundtrip = wrong.astype(arr.dtype)
- assert not (wrong == roundtrip).all()
-
- msg = r"Cannot convert 106752 days to timedelta64\[ns\] without overflow"
- with pytest.raises(OutOfBoundsTimedelta, match=msg):
- astype_overflowsafe(arr, dtype)
-
- # But converting to microseconds is fine, and we match numpy's results.
- dtype2 = np.dtype("m8[us]")
- result = astype_overflowsafe(arr, dtype2)
- expected = arr.astype(dtype2)
- tm.assert_numpy_array_equal(result, expected)
-
- def test_astype_overflowsafe_disallow_rounding(self):
- arr = np.array([-1500, 1500], dtype="M8[ns]")
- dtype = np.dtype("M8[us]")
-
- msg = "Cannot losslessly cast '-1500 ns' to us"
- with pytest.raises(ValueError, match=msg):
- astype_overflowsafe(arr, dtype, round_ok=False)
-
- result = astype_overflowsafe(arr, dtype, round_ok=True)
- expected = arr.astype(dtype)
- tm.assert_numpy_array_equal(result, expected)
diff --git a/spaces/profayle/TerrapinTalk/myenv/lib/python3.9/site-packages/pygments/lexers/diff.py b/spaces/profayle/TerrapinTalk/myenv/lib/python3.9/site-packages/pygments/lexers/diff.py
deleted file mode 100644
index 0ab85bfbf32b307f0e7a99058847d941cb35e911..0000000000000000000000000000000000000000
--- a/spaces/profayle/TerrapinTalk/myenv/lib/python3.9/site-packages/pygments/lexers/diff.py
+++ /dev/null
@@ -1,168 +0,0 @@
-"""
- pygments.lexers.diff
- ~~~~~~~~~~~~~~~~~~~~
-
- Lexers for diff/patch formats.
-
- :copyright: Copyright 2006-2023 by the Pygments team, see AUTHORS.
- :license: BSD, see LICENSE for details.
-"""
-
-import re
-
-from pygments.lexer import RegexLexer, include, bygroups
-from pygments.token import Text, Comment, Operator, Keyword, Name, Generic, \
- Literal, Whitespace
-
-__all__ = ['DiffLexer', 'DarcsPatchLexer', 'WDiffLexer']
-
-
-class DiffLexer(RegexLexer):
- """
- Lexer for unified or context-style diffs or patches.
- """
-
- name = 'Diff'
- aliases = ['diff', 'udiff']
- filenames = ['*.diff', '*.patch']
- mimetypes = ['text/x-diff', 'text/x-patch']
-
- tokens = {
- 'root': [
- (r'( )(.*)(\n)', bygroups(Whitespace, Text, Whitespace)),
- (r'(!.*|---)(\n)', bygroups(Generic.Strong, Whitespace)),
- (r'((?:< |-).*)(\n)', bygroups(Generic.Deleted, Whitespace)),
- (r'((?:> |\+).*)(\n)', bygroups(Generic.Inserted, Whitespace)),
- (
- r'(@.*|\d(?:,\d+)?(?:a|c|d)\d+(?:,\d+)?)(\n)',
- bygroups(Generic.Subheading, Whitespace),
- ),
- (r'((?:[Ii]ndex|diff).*)(\n)', bygroups(Generic.Heading, Whitespace)),
- (r'(=.*)(\n)', bygroups(Generic.Heading, Whitespace)),
- (r'(.*)(\n)', bygroups(Text, Whitespace)),
- ]
- }
-
- def analyse_text(text):
- if text[:7] == 'Index: ':
- return True
- if text[:5] == 'diff ':
- return True
- if text[:4] == '--- ':
- return 0.9
-
-
-class DarcsPatchLexer(RegexLexer):
- """
- DarcsPatchLexer is a lexer for the various versions of the darcs patch
- format. Examples of this format are derived by commands such as
- ``darcs annotate --patch`` and ``darcs send``.
-
- .. versionadded:: 0.10
- """
-
- name = 'Darcs Patch'
- aliases = ['dpatch']
- filenames = ['*.dpatch', '*.darcspatch']
-
- DPATCH_KEYWORDS = ('hunk', 'addfile', 'adddir', 'rmfile', 'rmdir', 'move',
- 'replace')
-
- tokens = {
- 'root': [
- (r'<', Operator),
- (r'>', Operator),
- (r'\{', Operator),
- (r'\}', Operator),
- (r'(\[)((?:TAG )?)(.*)(\n)(.*)(\*\*)(\d+)(\s?)(\])',
- bygroups(Operator, Keyword, Name, Whitespace, Name, Operator,
- Literal.Date, Whitespace, Operator)),
- (r'(\[)((?:TAG )?)(.*)(\n)(.*)(\*\*)(\d+)(\s?)',
- bygroups(Operator, Keyword, Name, Whitespace, Name, Operator,
- Literal.Date, Whitespace), 'comment'),
- (r'New patches:', Generic.Heading),
- (r'Context:', Generic.Heading),
- (r'Patch bundle hash:', Generic.Heading),
- (r'(\s*)(%s)(.*)(\n)' % '|'.join(DPATCH_KEYWORDS),
- bygroups(Whitespace, Keyword, Text, Whitespace)),
- (r'\+', Generic.Inserted, "insert"),
- (r'-', Generic.Deleted, "delete"),
- (r'(.*)(\n)', bygroups(Text, Whitespace)),
- ],
- 'comment': [
- (r'[^\]].*\n', Comment),
- (r'\]', Operator, "#pop"),
- ],
- 'specialText': [ # darcs add [_CODE_] special operators for clarity
- (r'\n', Whitespace, "#pop"), # line-based
- (r'\[_[^_]*_]', Operator),
- ],
- 'insert': [
- include('specialText'),
- (r'\[', Generic.Inserted),
- (r'[^\n\[]+', Generic.Inserted),
- ],
- 'delete': [
- include('specialText'),
- (r'\[', Generic.Deleted),
- (r'[^\n\[]+', Generic.Deleted),
- ],
- }
-
-
-class WDiffLexer(RegexLexer):
- """
- A wdiff lexer.
-
- Note that:
-
- * It only works with normal output (without options like ``-l``).
- * If the target files contain "[-", "-]", "{+", or "+}",
- especially they are unbalanced, the lexer will get confused.
-
- .. versionadded:: 2.2
- """
-
- name = 'WDiff'
- url = 'https://www.gnu.org/software/wdiff/'
- aliases = ['wdiff']
- filenames = ['*.wdiff']
- mimetypes = []
-
- flags = re.MULTILINE | re.DOTALL
-
- # We can only assume "[-" after "[-" before "-]" is `nested`,
- # for instance wdiff to wdiff outputs. We have no way to
- # distinct these marker is of wdiff output from original text.
-
- ins_op = r"\{\+"
- ins_cl = r"\+\}"
- del_op = r"\[\-"
- del_cl = r"\-\]"
- normal = r'[^{}[\]+-]+' # for performance
- tokens = {
- 'root': [
- (ins_op, Generic.Inserted, 'inserted'),
- (del_op, Generic.Deleted, 'deleted'),
- (normal, Text),
- (r'.', Text),
- ],
- 'inserted': [
- (ins_op, Generic.Inserted, '#push'),
- (del_op, Generic.Inserted, '#push'),
- (del_cl, Generic.Inserted, '#pop'),
-
- (ins_cl, Generic.Inserted, '#pop'),
- (normal, Generic.Inserted),
- (r'.', Generic.Inserted),
- ],
- 'deleted': [
- (del_op, Generic.Deleted, '#push'),
- (ins_op, Generic.Deleted, '#push'),
- (ins_cl, Generic.Deleted, '#pop'),
-
- (del_cl, Generic.Deleted, '#pop'),
- (normal, Generic.Deleted),
- (r'.', Generic.Deleted),
- ],
- }
diff --git a/spaces/profayle/TerrapinTalk/myenv/lib/python3.9/site-packages/setuptools/dep_util.py b/spaces/profayle/TerrapinTalk/myenv/lib/python3.9/site-packages/setuptools/dep_util.py
deleted file mode 100644
index 521eb716a5ebbcbc2c59654c4e71c3f0ff1abf26..0000000000000000000000000000000000000000
--- a/spaces/profayle/TerrapinTalk/myenv/lib/python3.9/site-packages/setuptools/dep_util.py
+++ /dev/null
@@ -1,25 +0,0 @@
-from distutils.dep_util import newer_group
-
-
-# yes, this is was almost entirely copy-pasted from
-# 'newer_pairwise()', this is just another convenience
-# function.
-def newer_pairwise_group(sources_groups, targets):
- """Walk both arguments in parallel, testing if each source group is newer
- than its corresponding target. Returns a pair of lists (sources_groups,
- targets) where sources is newer than target, according to the semantics
- of 'newer_group()'.
- """
- if len(sources_groups) != len(targets):
- raise ValueError(
- "'sources_group' and 'targets' must be the same length")
-
- # build a pair of lists (sources_groups, targets) where source is newer
- n_sources = []
- n_targets = []
- for i in range(len(sources_groups)):
- if newer_group(sources_groups[i], targets[i]):
- n_sources.append(sources_groups[i])
- n_targets.append(targets[i])
-
- return n_sources, n_targets
diff --git a/spaces/quidiaMuxgu/Expedit-SAM/Adminpaq 2012 Activador Crack PATCHED.md b/spaces/quidiaMuxgu/Expedit-SAM/Adminpaq 2012 Activador Crack PATCHED.md
deleted file mode 100644
index 27b4302a9ac333c229e5f3059603052bbd54edcd..0000000000000000000000000000000000000000
--- a/spaces/quidiaMuxgu/Expedit-SAM/Adminpaq 2012 Activador Crack PATCHED.md
+++ /dev/null
@@ -1,11 +0,0 @@
-
-
-Adminpaq 2012 activador crack. DOWNLOAD: activador adminpaq 2012, activador adminpaq 2a1358a15e. Related links:. Name: Activation AdminPE Program developer: AdminPAQ Year: 2012 Platform: Windows XP/Vista/7 Interface language: Russian Tablet: not required.
-Activation instructions: Copy the Activator AdminPE.exe file to the Windows folder and run as Administrator.
-In the "Activation Status" window, click "Activate".
-After that, in the main window of the "Activation Log" program, an inscription about the successful activation of AdminPE will appear.
-Download Adminpaq 2012 activador crack.
-AdminPAQ AdminPE crack, AdminPAQ AdminPE 8a78ff9644
-
-
-
diff --git a/spaces/quidiaMuxgu/Expedit-SAM/Bioquimica De Richard A Harvey 5ta Edicion Pdf Gratis.md b/spaces/quidiaMuxgu/Expedit-SAM/Bioquimica De Richard A Harvey 5ta Edicion Pdf Gratis.md
deleted file mode 100644
index e06bcf05d70f7d2b85f7b53fdd16e1bfc6b130be..0000000000000000000000000000000000000000
--- a/spaces/quidiaMuxgu/Expedit-SAM/Bioquimica De Richard A Harvey 5ta Edicion Pdf Gratis.md
+++ /dev/null
@@ -1,22 +0,0 @@
-
-
BioquÃmica de Richard A. Harvey y Denise R. Ferrier: una obra de referencia para estudiantes y profesionales
Entre los libros de texto de bioquÃmica más utilizados y reconocidos se encuentra el de Richard A. Harvey y Denise R. Ferrier, que ha llegado a su quinta edición en español. Se trata de una obra que combina rigor cientÃfico, claridad expositiva y un enfoque didáctico que facilita el aprendizaje.
-
El libro se divide en cuatro secciones que abarcan los principales temas de la bioquÃmica: estructura y función de las proteÃnas, metabolismo intermedio, metabolismo de los lÃpidos y metabolismo del nitrógeno. Cada sección se compone de varias unidades que presentan los conceptos clave, los mecanismos moleculares y las aplicaciones clÃnicas de cada tema.
-
El libro cuenta con numerosos recursos pedagógicos que ayudan al estudiante a consolidar sus conocimientos y a evaluar su progreso. Entre ellos se destacan:
-
-
Recuadros con información clÃnica y casos de estudio que relacionan la bioquÃmica con la medicina.
-
Ilustraciones a todo color que facilitan la comprensión de las estructuras y las reacciones quÃmicas.
-
Preguntas al final de cada unidad que permiten repasar y autoevaluarse.
-
Resúmenes al final de cada sección que sintetizan las ideas más importantes.
BioquÃmica de Richard A. Harvey y Denise R. Ferrier es un libro imprescindible para los estudiantes y profesionales de ciencias de la salud que quieran adquirir una base sólida y actualizada de bioquÃmica.
-
-
Fuente: Adaptado de los resultados web [^1^] [^2^] [^3^] [^4^]
A continuación se presentan algunos párrafos adicionales que podrÃan formar parte del artÃculo:
-
-damage inc. pacific squadron wwii pc - All Latest Cheats Codes Free Games, Pc ... Download full free pc games, highly compressed and torrent games for this ... 4d29de3e1b
-
-
-
diff --git a/spaces/quidiaMuxgu/Expedit-SAM/Evil Dead Movie In Tamil Free Download ((NEW)).md b/spaces/quidiaMuxgu/Expedit-SAM/Evil Dead Movie In Tamil Free Download ((NEW)).md
deleted file mode 100644
index 9cb6dd4a187e2653356c059d22258f3ccfcc8496..0000000000000000000000000000000000000000
--- a/spaces/quidiaMuxgu/Expedit-SAM/Evil Dead Movie In Tamil Free Download ((NEW)).md
+++ /dev/null
@@ -1,6 +0,0 @@
-
-
- d5da3c52bf
-
-
-
diff --git a/spaces/qwieug123467/Linaqruf-anything-v3.0/README.md b/spaces/qwieug123467/Linaqruf-anything-v3.0/README.md
deleted file mode 100644
index c5f56337e16c1edb91a62dd61575eb359cdbcf92..0000000000000000000000000000000000000000
--- a/spaces/qwieug123467/Linaqruf-anything-v3.0/README.md
+++ /dev/null
@@ -1,12 +0,0 @@
----
-title: Linaqruf Anything V3.0
-emoji: 👀
-colorFrom: green
-colorTo: yellow
-sdk: gradio
-sdk_version: 3.13.2
-app_file: app.py
-pinned: false
----
-
-Check out the configuration reference at https://huggingface.co/docs/hub/spaces-config-reference
diff --git a/spaces/raedeXanto/academic-chatgpt-beta/Astral Neverwinter Bot Cracked ArMa The Best Way to Level Up and Dominate Neverwinter Online.md b/spaces/raedeXanto/academic-chatgpt-beta/Astral Neverwinter Bot Cracked ArMa The Best Way to Level Up and Dominate Neverwinter Online.md
deleted file mode 100644
index fcb2d471f547ec0bbca8e107fdf1b8088934c9e8..0000000000000000000000000000000000000000
--- a/spaces/raedeXanto/academic-chatgpt-beta/Astral Neverwinter Bot Cracked ArMa The Best Way to Level Up and Dominate Neverwinter Online.md
+++ /dev/null
@@ -1,178 +0,0 @@
-
-
Astral Neverwinter Bot Cracked ArMa: How to Get It and Use It
-
If you are a fan of Neverwinter Online, you might have heard of Astral Neverwinter Bot, a powerful tool that automates various tasks in the game. But did you know that there is a way to get it for free, thanks to a crack by ArMa? In this article, we will tell you everything you need to know about Astral Neverwinter Bot Cracked ArMa, including what it is, how to get it, and how to use it. Read on and discover how you can take your gaming experience to the next level!
Astral Neverwinter Bot is a software that allows you to automate various aspects of Neverwinter Online, such as questing, farming, crafting, fishing, refining, and more. It can also perform complex actions such as combat rotations, looting, selling, repairing, and using potions. With Astral Neverwinter Bot, you can save time and effort while enjoying the game at your own pace.
-
Features and Benefits of Astral Neverwinter Bot
-
Some of the features and benefits of Astral Neverwinter Bot are:
-
-
It supports all classes and races in the game.
-
It has a user-friendly interface that allows you to customize your settings and preferences.
-
It has a smart pathfinding system that avoids obstacles and enemies.
-
It has a built-in anti-afk system that prevents you from being kicked out of the game.
-
It has a stealth mode that hides your botting activity from other players and GMs.
-
It has a premium mode that unlocks additional features such as PvP botting, dungeon botting, profession botting, and more.
-
-
With Astral Neverwinter Bot, you can enjoy the game without having to worry about grinding, leveling, or farming. You can also earn more astral diamonds, gold, items, and rewards while playing. You can even use it to boost your friends or guild members in the game.
-
How to Download and Install Astral Neverwinter Bot
-
To download and install Astral Neverwinter Bot, you need to follow these steps:
Log in to your account and go to the download page.
-
Download the latest version of Astral Neverwinter Bot for your operating system (Windows or Linux).
-
Extract the zip file to a folder of your choice.
-
Run the launcher.exe file as administrator.
-
Enter your username and password and click on login.
-
Select your server and click on start.
-
Astral Neverwinter Bot will launch and connect to your game client.
-
-
Congratulations! You have successfully downloaded and installed Astral Neverwinter Bot. Now you can start using it in the game.
-
Astral Neverwinter Bot free download cracked version
-How to use Astral Neverwinter Bot crack ArMa
-Astral Neverwinter Bot cracked by ArMa features
-Astral Neverwinter Bot ArMa crack tutorial
-Astral Neverwinter Bot crack ArMa update
-Download Astral Neverwinter Bot cracked ArMa 2023
-Astral Neverwinter Bot crack ArMa license key
-Astral Neverwinter Bot cracked ArMa review
-Astral Neverwinter Bot crack ArMa reddit
-Astral Neverwinter Bot cracked ArMa forum
-Astral Neverwinter Bot crack ArMa discord
-Astral Neverwinter Bot cracked ArMa youtube
-Astral Neverwinter Bot crack ArMa gameplay
-Astral Neverwinter Bot cracked ArMa settings
-Astral Neverwinter Bot crack ArMa support
-Astral Neverwinter Bot cracked ArMa guide
-Astral Neverwinter Bot crack ArMa tips and tricks
-Astral Neverwinter Bot cracked ArMa best settings
-Astral Neverwinter Bot crack ArMa safe to use
-Astral Neverwinter Bot cracked ArMa virus free
-Astral Neverwinter Bot crack ArMa working 2023
-Astral Neverwinter Bot cracked ArMa no survey
-Astral Neverwinter Bot crack ArMa direct link
-Astral Neverwinter Bot cracked ArMa mega.nz
-Astral Neverwinter Bot crack ArMa mediafire
-Astral Neverwinter Bot cracked ArMa google drive
-Astral Neverwinter Bot crack ArMa dropbox
-Astral Neverwinter Bot cracked ArMa torrent
-Astral Neverwinter Bot crack ArMa magnet link
-Astral Neverwinter Bot cracked ArMa rar password
-Astral Neverwinter Bot crack ArMa zip file
-Astral Neverwinter Bot cracked ArMa installer
-Astral Neverwinter Bot crack ArMa setup.exe
-Astral Neverwinter Bot cracked ArMa patch notes
-Astral Neverwinter Bot crack ArMa changelog
-Astral Neverwinter Bot cracked ArMa latest version
-Astral Neverwinter Bot crack ArMa compatible windows 10
-Astral Neverwinter Bot cracked ArMa for mac os x
-Astral Neverwinter Bot crack ArMa for linux ubuntu
-Astral Neverwinter Bot cracked ArMa for android apk
-Astral Neverwinter Bot crack ArMa for ios iphone ipad ipod touch
-Astral Neverwinter Bot cracked ArMa for xbox one ps4 switch
-Astral Neverwinter Bot crack ArMa for steam origin uplay epic games
-Astral Neverwinter Bot cracked ArMa for never winter online mmorpg
-Astral Neverwinter Bot crack ArMa for never winter nights enhanced edition
-Astral Neverwinter Bot cracked ArMa for dungeons and dragons dnd
-Astral Neverwinter Bot crack ArMa for forgotten realms campaign setting
-Astral Neverwinter Bot cracked ArMa for sword coast adventurer's guide
-Astral Neverwinter Bot crack ArMa for baldur's gate 3 bg3
-Astral Neverwinter Bot cracked by arma for astraldynamics.com
-
What is ArMa?
-
ArMa is a hacker who specializes in cracking various bots and cheats for online games. He is known for his skills and generosity in sharing his cracks with the gaming community. He has cracked many popular bots such as WoW Glider, Honorbuddy, Demonbuddy, Rebornbuddy, Exiledbot, Pokefarmer, and more. He has also cracked some cheats such as Aimbot, Wallhack, ESP, Speedhack, No Recoil, No Spread, and more.
-
How ArMa Cracked Astral Neverwinter Bot
-
ArMa cracked Astral Neverwinter Bot by reverse engineering its code and bypassing its protection mechanisms. He managed to find and exploit several vulnerabilities in the bot's encryption, authentication, licensing, and anti-debugging systems. He also modified some of the bot's functions to improve its performance and stability. He then released his crack for free on his website at https://arma-project.ru/.
-
How to Get ArMa's Crack for Astral Neverwinter Bot
-
To get ArMa's crack for Astral Neverwinter Bot, you need to follow these steps:
Log in to your account and go to the download page.
-
Download the latest version of ArMa's crack for Astral Neverwinter Bot.
-
Extract the zip file to the same folder where you installed Astral Neverwinter Bot.
-
Replace the original launcher.exe file with the cracked one.
-
Run the cracked launcher.exe file as administrator.
-
You will see a message saying "Cracked by ArMa" on the login screen.
-
You can now use any username and password to log in.
-
-
Congratulations! You have successfully obtained ArMa's crack for Astral Neverwinter Bot. Now you can use it for free without having to pay for a subscription or a premium mode.
-
How to Use Astral Neverwinter Bot Cracked ArMa
-
To use Astral Neverwinter Bot Cracked ArMa, you need to follow these steps:
-
How to Configure and Run Astral Neverwinter Bot
-
-
After logging in with the cracked launcher.exe file, you will see the main interface of Astral Neverwinter Bot.
-
Select your character from the drop-down menu on the top left corner.
-
Select your profile from the drop-down menu on the top right corner. A profile is a set of settings that determines how your bot will behave in the game. You can choose from predefined profiles or create your own custom ones.
-
If you want to create or edit a profile, click on the profile editor button on the bottom right corner. You will see a new window where you can adjust various parameters such as movement speed, combat strategy, looting options, inventory management options, etc. You can also add or remove tasks from your profile such as quests, farming locations, crafting recipes, fishing spots, refining methods, etc. You can save your profile by clicking on the save button on the top left corner. You can load your profile by clicking on the load button on the top right corner. You can close the profile editor by clicking on the X button on the top right corner.
-
If you want to use a predefined profile, you can browse through the available ones by clicking on the browse button on the bottom left corner. You will see a new window where you can search for profiles by name, category, rating, or author. You can also sort them by date, popularity, or relevance. You can download a profile by clicking on the download button next to it. You can rate a profile by clicking on the stars next to it. You can close the browse window by clicking on the X button on the top right corner.
-
After selecting or creating your profile, you can start the bot by clicking on the start button on the bottom center. You will see a message saying "Bot started" on the status bar. You can stop the bot by clicking on the stop button next to it. You will see a message saying "Bot stopped" on the status bar. You can pause the bot by clicking on the pause button next to it. You will see a message saying "Bot paused" on the status bar. You can resume the bot by clicking on the resume button next to it. You will see Bot resumed" on the status bar.
-
You can also control the bot using hotkeys. The default hotkeys are F1 for start, F2 for stop, F3 for pause, and F4 for resume. You can change the hotkeys by clicking on the settings button on the top right corner. You will see a new window where you can assign different keys for different functions. You can close the settings window by clicking on the X button on the top right corner.
-
-
That's it! You have successfully configured and run Astral Neverwinter Bot. Now you can sit back and relax while the bot does all the work for you.
-
How to Avoid Detection and Bans from Neverwinter Online
-
While using Astral Neverwinter Bot Cracked ArMa, you need to be careful and avoid detection and bans from Neverwinter Online. Here are some tips and tricks to help you stay safe:
-
-
Do not use the bot for too long or too often. Take breaks and play manually from time to time.
-
Do not use the bot in crowded or public areas. Choose secluded or hidden spots for your botting activities.
-
Do not use the bot in PvP or dungeons. These modes require human interaction and coordination, and using a bot will make you stand out and attract attention.
-
Do not use the bot with unrealistic settings or profiles. For example, do not set your movement speed too high, do not use combat skills that are not available for your class or level, do not loot items that are not appropriate for your character, etc.
-
Do not brag or advertise about using the bot in chat or forums. Keep a low profile and do not draw attention to yourself.
-
Do not share your account or your bot with anyone else. This will increase the risk of getting reported or banned.
-
Do not use the same username and password for your game account and your bot account. Use different and unique credentials for each one.
-
Do not use outdated or cracked versions of the bot. Always update to the latest version of Astral Neverwinter Bot Cracked ArMa from ArMa's website.
-
-
By following these tips and tricks, you can reduce the chances of getting detected and banned from Neverwinter Online while using Astral Neverwinter Bot Cracked ArMa.
-
Tips and Tricks for Using Astral Neverwinter Bot Cracked ArMa
-
Besides avoiding detection and bans, there are some other tips and tricks that can help you get the most out of Astral Neverwinter Bot Cracked ArMa. Here are some of them:
-
-
You can use multiple instances of the bot on different computers or virtual machines. This way, you can run multiple characters at the same time and increase your productivity and efficiency.
-
You can use a VPN or a proxy to hide your IP address and location from Neverwinter Online. This way, you can avoid IP bans and geo-restrictions.
-
You can use a sandbox or a virtual machine to isolate your bot from your main system. This way, you can protect your computer from viruses, malware, or spyware that might come with the bot or the crack.
-
You can use a backup tool to save your settings and profiles. This way, you can restore them in case of data loss or corruption.
-
You can use a forum or a community to get support and feedback from other users of Astral Neverwinter Bot Cracked ArMa. You can also share your own experiences and tips with them.
-
-
By using these tips and tricks, you can enhance your experience and performance while using Astral Neverwinter Bot Cracked ArMa.
-
Conclusion
-
Summary of the Main Points
-
In this article, we have covered everything you need to know about Astral Neverwinter Bot Cracked ArMa, including:
-
-
What is Astral Neverwinter Bot and what are its features and benefits?
-
What is ArMa and how did he crack Astral Neverwinter Bot?
-
How to get ArMa's crack for Astral Neverwinter Bot?
-
How to configure and run Astral Neverwinter Bot?
-
How to avoid detection and bans from Neverwinter Online?
-
Tips and tricks for using Astral Neverwinter Bot Cracked ArMa.
-
-
We hope that this article has been informative and helpful for you. If you have any questions or comments, feel free to leave them below.
-
Call to Action for the Readers
-
If you are interested in trying out Astral Neverwinter Bot Cracked ArMa, we have good news for you. You can download it for free from ArMa's website at https://arma-project.ru/. All you need is an account and a valid email address. You can also check out his other cracks for various bots and cheats for online games.
-
However, we must warn you that using bots and cheats in online games is against their terms of service and may result in account suspension or termination. Therefore, we advise you to use them at your own risk and discretion. We are not responsible for any consequences that may arise from using them.
-
If you are looking for a legit and safe way to play Neverwinter Online without bots or cheats, we recommend you to check out our partner site at https://www.mmorpg.com/neverwinter. There you can find guides, reviews, news, videos, forums, and more about this amazing game. You can also join their community of players who share your passion and enthusiasm for Neverwinter Online.
-
So what are you waiting for? Go ahead and download Astral Neverwinter Bot Cracked ArMa today and enjoy the game like never before! Or visit our partner site at https://www.mmorpg.com/neverwinter and discover everything there is to know about Neverwinter Online!
-
FAQs
-
Here are some frequently asked questions about Astral Neverwinter Bot Cracked ArMa:
-
-
What is Neverwinter Online?
-
Neverwinter Online is a free-to-play massively multiplayer online role-playing game (MMORPG) based on the Dungeons & Dragons fantasy franchise. It was developed by Cryptic Studios and published by Perfect World Entertainment in 2013. It is available for Windows, PlayStation 4, and Xbox One platforms. It features an immersive story, dynamic combat, customizable characters, rich lore, and a vibrant community. It has received positive reviews and awards from critics and players alike. It has over 18 million registered users as of 2019. You can learn more about it at https://www.arcgames.com/en/games/neverwinter.
-
Is Astral Neverwinter Bot legal?
-
No, Astral Neverwinter Bot is not legal. It is a third-party software that violates the terms of service of Neverwinter Online. Using it may result in account suspension or termination. Therefore, we advise you to use it at your own risk and discretion. We are not responsible for any consequences that may arise from using it.
-
Is ArMa's crack safe?
-
We cannot guarantee that ArMa's crack is safe. It may contain viruses, malware, or spyware that could harm your computer or compromise your personal information. Therefore, we advise you to use it at your own risk and discretion. We recommend you to use a sandbox or a virtual machine to isolate it from your main system. We also recommend you to use a VPN or a proxy to hide your IP address and location from Neverwinter Online. We are not responsible for any consequences that may arise from using it.
-
How do I update Astral Neverwinter Bot Cracked ArMa?
-
To update Astral Neverwinter Bot Cracked ArMa, you need to visit ArMa's website at https://arma-project.ru/. There you can find the latest version of his crack for Astral Neverwinter Bot. You need to download and install it over your existing one. You also need to check the official website of Astral Neverwinter Bot at https://www.neverwinter-bot.com/. There you can find the latest version of the bot itself. You need to download and install it over your existing one. You need to make sure that both versions are compatible with each other and with current version of Neverwinter Online. You need to update both the bot and the crack regularly to avoid errors and issues.
-
How do I contact ArMa or Astral Neverwinter Bot?
-
To contact ArMa, you can visit his website at https://arma-project.ru/. There you can find his email address, his Discord server, his Telegram channel, and his VK group. You can also leave a comment on his blog or forum posts. He is usually friendly and helpful, but he may not respond to every message or request.
-
To contact Astral Neverwinter Bot, you can visit their website at https://www.neverwinter-bot.com/. There you can find their email address, their Discord server, their Facebook page, and their Twitter account. You can also leave a comment on their blog or forum posts. They are usually professional and supportive, but they may not tolerate or assist users of cracked versions of their bot.
-
Where can I find more information or support for Astral Neverwinter Bot Cracked ArMa?
-
If you need more information or support for Astral Neverwinter Bot Cracked ArMa, you can try the following sources:
-
-
You can read the documentation and the FAQ on the official website of Astral Neverwinter Bot at https://www.neverwinter-bot.com/. There you can find detailed instructions and explanations on how to use the bot and its features.
-
You can watch the videos and tutorials on the official YouTube channel of Astral Neverwinter Bot at https://www.youtube.com/channel/UC0yQ6Z7J4vY0Q6Z7J4vY0Q. There you can see the bot in action and learn some tips and tricks on how to optimize it.
-
You can join the community and the discussion on the official forum of Astral Neverwinter Bot at https://www.neverwinter-bot.com/forums/. There you can interact with other users and developers of the bot and share your feedback and suggestions.
-
You can also join the community and the discussion on ArMa's website at https://arma-project.ru/. There you can interact with other users and fans of ArMa's cracks and share your experiences and problems.
-
-
These sources may provide you with some useful information or support for Astral Neverwinter Bot Cracked ArMa. However, they may not cover everything or answer all your questions. Therefore, you may need to do some research or experimentation on your own to find out more.
- 0a6ba089eb
-
-
\ No newline at end of file
diff --git a/spaces/raedeXanto/academic-chatgpt-beta/Download Ebook Purpose Driven Life Bahasa Indonesia Inggris The Bestselling Book that Changed Millions of Lives.md b/spaces/raedeXanto/academic-chatgpt-beta/Download Ebook Purpose Driven Life Bahasa Indonesia Inggris The Bestselling Book that Changed Millions of Lives.md
deleted file mode 100644
index ee84ef3105a5e12b71314e71c9102ac0b3dedb09..0000000000000000000000000000000000000000
--- a/spaces/raedeXanto/academic-chatgpt-beta/Download Ebook Purpose Driven Life Bahasa Indonesia Inggris The Bestselling Book that Changed Millions of Lives.md
+++ /dev/null
@@ -1,179 +0,0 @@
-
-
Download Ebook Purpose Driven Life Bahasa Indonesia Inggris
-
Have you ever wondered what your purpose in life is? Do you feel like you are living a meaningless and aimless existence? If you answered yes to these questions, then you might want to read Purpose Driven Life, a bestselling book by Rick Warren that has transformed millions of lives around the world. In this article, we will tell you everything you need to know about this book and how you can download it as an ebook in both Indonesian and English languages.
-
download ebook purpose driven life bahasa indonesia inggris
Purpose Driven Life is a Christian devotional book that was published in 2002 by Rick Warren, a pastor and founder of Saddleback Church in California. The book is based on Warren's 40-day spiritual journey program that he developed for his congregation. The book has sold over 50 million copies worldwide and has been translated into more than 80 languages.
-
A brief introduction to the book and its author
-
Rick Warren is one of the most influential pastors and authors in the world. He has been named as one of the "100 Most Influential People in the World" by Time magazine and one of the "15 World Leaders Who Mattered Most in 2004" by Newsweek. He is also a global strategist, philanthropist, and humanitarian who has initiated various projects to fight poverty, disease, illiteracy, and injustice.
-
Warren wrote Purpose Driven Life as a response to his own personal crisis. He said that he was feeling empty and restless despite his success and achievements. He realized that he needed to find his true purpose in life, not just his goals and ambitions. He decided to share his insights and discoveries with others who might be going through the same struggle.
-
Download buku rohani kristen purpose driven life bahasa indonesia
-Cara download ebook hidup yang digerakkan oleh tujuan Rick Warren
-Tempat download pdf purpose driven life bahasa indonesia gratis
-Review buku the purpose driven life what on earth am I here for
-Beli buku purpose driven life bahasa indonesia online
-Download ebook purpose driven life bahasa inggris pdf
-Baca online buku purpose driven life bahasa indonesia
-Sinopsis buku purpose driven life Rick Warren bahasa indonesia
-Download ebook perjalanan spiritual pribadi 40 hari purpose driven life
-Jual buku purpose driven life bahasa indonesia murah
-Download ebook lima tujuan Allah bagi hidup manusia purpose driven life
-Resensi buku purpose driven life bahasa indonesia
-Download ebook purpose driven life bahasa indonesia epub
-Buku purpose driven life pdf bahasa indonesia docplayer
-Download ebook hidup dengan tujuan Rick Warren bahasa indonesia
-Ebook the purpose driven life in bahasa indonesia guide to serving God
-Download ebook purpose driven life bahasa indonesia inggris sway
-Ebook purpose driven life bahasa indonesia terjemahan
-Download ebook purpose driven life bahasa indonesia full version
-Ebook purpose driven life bahasa indonesia best seller
-Download ebook mengenal tujuan hidup kita purpose driven life
-Ebook purpose driven life bahasa indonesia dan inggris bilingual
-Download ebook purpose driven life bahasa indonesia lengkap
-Ebook purpose driven life bahasa indonesia hardcover
-Download ebook purpose driven life bahasa indonesia softcover
-Ebook hidup yang digerakkan oleh tujuan Rick Warren pdf
-Download ebook the purpose driven life what on earth am I here for by Rick Warren
-Ebook hidup yang digerakkan oleh tujuan Rick Warren epub
-Download ebook the purpose driven life what on earth am I here for by Rick Warren pdf gratis
-Ebook hidup yang digerakkan oleh tujuan Rick Warren online
-Download ebook the purpose driven life what on earth am I here for by Rick Warren epub gratis
-Ebook hidup yang digerakkan oleh tujuan Rick Warren docplayer
-Download ebook the purpose driven life what on earth am I here for by Rick Warren sway
-Ebook hidup yang digerakkan oleh tujuan Rick Warren terjemahan
-Download ebook the purpose driven life what on earth am I here for by Rick Warren bilingual
-Ebook hidup yang digerakkan oleh tujuan Rick Warren full version
-Download ebook the purpose driven life what on earth am I here for by Rick Warren best seller
-Ebook hidup yang digerakkan oleh tujuan Rick Warren hardcover
-Download ebook the purpose driven life what on earth am I here for by Rick Warren softcover
-Ebook perjalanan spiritual pribadi 40 hari purpose driven life pdf
-Download ebook perjalanan spiritual pribadi 40 hari purpose driven life epub
-Ebook perjalanan spiritual pribadi 40 hari purpose driven life online
-Download ebook perjalanan spiritual pribadi 40 hari purpose driven life gratis
-Ebook perjalanan spiritual pribadi 40 hari purpose driven life docplayer
-Download ebook perjalanan spiritual pribadi 40 hari purpose driven life sway
-Ebook perjalanan spiritual pribadi 40 hari purpose driven life terjemahan
-Download ebook perjalanan spiritual pribadi 40 hari purpose driven life bilingual
-Ebook perjalanan spiritual pribadi 40 hari purpose driven life full version
-Download ebook perjalanan spiritual pribadi 40 hari purpose driven life best seller
-
The main themes and messages of the book
-
The book is divided into 40 chapters, each corresponding to a day of the program. The chapters are grouped into six sections that cover the following topics:
-
-
What on Earth Am I Here For?
-
Purpose #1: You Were Planned for God's Pleasure
-
Purpose #2: You Were Formed for God's Family
-
Purpose #3: You Were Created to Become Like Christ
-
Purpose #4: You Were Shaped for Serving God
-
Purpose #5: You Were Made for a Mission
-
-
The book teaches that God has a specific plan and purpose for each person's life, and that finding and fulfilling that purpose is the key to happiness and fulfillment. The book also emphasizes that life is not about oneself, but about God and others. The book challenges readers to surrender their lives to God, worship Him, join His family, grow in His likeness, serve Him, and share His love with others.
-
How the book can help you find your purpose and live a fulfilling life
-
Purpose Driven Life can help you find your purpose and live a fulfilling life by:
-
-
Giving you a clear vision of God's plan and will for your life
-
Helping you discover your unique gifts, talents, passions, and personality
-
Guiding you to align your goals and actions with God's purposes
-
Inspiring you to live a life of worship, fellowship, discipleship, ministry, and evangelism
-
Motivating you to make a positive difference in the world with your skills and resources
-
Encouraging you to trust God's promises and power in every situation
-
Providing you with practical tools and tips to apply the principles in your daily life
-
-
Why Download Ebook Purpose Driven Life Bahasa Indonesia Inggris?
-
If you are interested in reading Purpose Driven Life, you might want to consider downloading it as an ebook in both Indonesian and English languages. There are many benefits of reading ebooks over physical books, such as:
-
The benefits of reading ebooks over physical books
-
Some of the benefits of reading ebooks over physical books are:
-
-
Ebooks are more convenient and accessible. You can download them instantly from anywhere with an internet connection. You can also store thousands of ebooks on your device without taking up much space.
-
Ebooks are more affordable and eco-friendly. You can save money by buying ebooks at lower prices or even getting them for free from some sources. You can also reduce paper waste and environmental impact by reading ebooks instead of printed books.
-
Ebooks are more customizable and interactive. You can adjust the font size, brightness, color, orientation, etc. according to your preference. You can also use features like bookmarks, highlights, notes, dictionary, search, etc. to enhance your reading experience. You can also access multimedia content like audio, video, images, links, etc. that might be embedded in some ebooks.
Click on "Register" or "Login" if you already have an account.
-
Fill in the required information and verify your email address.
-
Go back to the ebook page and click on "Download Ebook".
-
Select the ebook format (PDF or EPUB) and click on "Download".
-
Save the ebook file on your device.
-
-
-
A comparison of the quality and features of different ebook formats and versions
-
You might be wondering which ebook format and version is best for you. There are two main types of ebook formats: PDF and EPUB. Each has its own advantages and disadvantages. Here is a comparison of them:
-
-
-
Ebook Format
-
Description
-
Pros
-
Cons
-
-
-
PDF
-
This is a fixed-layout format that preserves the original design and layout of the book. It is compatible with most devices and apps.
-
- Easy to print and share - Good for books with complex graphics and tables - Supports multimedia content like audio and video
-
- Not very flexible and adaptable - Difficult to adjust font size, color, etc. - May not fit well on small screens - Does not support features like bookmarks, highlights, notes, etc.
-
-
-
EPUB
-
This is a reflowable format that adapts to the screen size and orientation of the device. It is compatible with most devices and apps except Kindle.
-
- Flexible and adaptable - Easy to adjust font size, color, etc. - Fits well on any screen size - Supports features like bookmarks, highlights, notes, etc.
-
- Not easy to print and share - Not good for books with complex graphics and tables - Does not support multimedia content like audio and video
-
A list of tips and tricks to enhance your reading experience and comprehension
-
Now that you have downloaded Purpose Driven Life as an ebook in both Indonesian and English languages, you might want to make the most out of your reading experience and comprehension. Here are some tips and tricks that can help you:
-
-
Set a reading schedule and stick to it. The book is designed to be read in 40 days, one chapter per day. You can follow this plan or create your own based on your availability and preference.
-
Read the book in both languages alternately or simultaneously. You can read one chapter in Indonesian and then the same chapter in English, or vice versa. You can also read both versions side by side or on different devices.
-
Use a dictionary or translator app to look up unfamiliar words or phrases. You can also use online tools like Google Translate or DeepL to translate whole sentences or paragraphs.
-
Take notes and write summaries of each chapter. You can use a notebook, a word processor, or an app like Evernote to record your thoughts and reflections on each chapter. You can also write summaries of each chapter in both languages to practice your writing skills.
-
Discuss the book with others who are reading it or have read it. You can join online forums, groups, or communities where you can share your insights and questions with other readers. You can also find a reading partner or a mentor who can help you understand and apply the book better.
-
-
Conclusion
-
In conclusion, Purpose Driven Life is a book that can help you discover and fulfill your God-given purpose in life. It is a book that has changed millions of lives around the world and can change yours too. You can download it as an ebook in both Indonesian and English languages from various sources and platforms for free or at a low cost. You can also use different ebook formats and versions to suit your preferences and needs. You can also follow some tips and tricks to enhance your reading experience and comprehension. We hope that this article has been helpful and informative for you. We encourage you to download Purpose Driven Life as an ebook in both Indonesian and English languages today and start your journey of finding your purpose.
-
A call to action for the readers to download the ebook and start their journey of finding their purpose
-
If you are ready to download Purpose Driven Life as an ebook in both Indonesian and English languages, you can click on any of the links below to get started:
If you are not sure which source or platform to choose, you can refer to our comparison table above to see the pros and cons of each option.
-
If you have already downloaded Purpose Driven Life as an ebook in both Indonesian and English languages, you can follow our step-by-step guide above to learn how to download it from different websites and apps.
-
If you have not yet started reading Purpose Driven Life, you can follow our reading schedule and tips above to make the most out of your reading experience and comprehension.
-
Whatever stage you are in, we hope that you will enjoy reading Purpose Driven Life as an ebook in both Indonesian and English languages and that it will help you find your purpose and live a fulfilling life.
-
FAQs
-
Here are some frequently asked questions and answers about Purpose Driven Life and how to download it as an ebook in both Indonesian and English languages:
-
-
What is the difference between Purpose Driven Life and The Purpose Driven Church?
-
Purpose Driven Life is a book for individuals who want to find their personal purpose in life. The Purpose Driven Church is a book for pastors and church leaders who want to build healthy and effective churches based on God's purposes.
-
Is Purpose Driven Life a Bible study or a devotional?
-
Purpose Driven Life is both a Bible study and a devotional. It is a Bible study because it is based on the teachings and principles of the Bible. It is a devotional because it helps readers to apply the Bible to their daily lives and to grow closer to God.
-
Can I read Purpose Driven Life without being a Christian?
-
Yes, you can read Purpose Driven Life without being a Christian. The book is written for anyone who wants to find their purpose in life, regardless of their religious background or beliefs. However, the book does present a Christian perspective on life and purpose, and it invites readers to consider accepting Jesus Christ as their Lord and Savior.
-
Can I read Purpose Driven Life more than once?
-
Yes, you can read Purpose Driven Life more than once. In fact, the author recommends that you read it at least once every year. He says that each time you read it, you will discover new insights and applications that will help you grow in your purpose.
-
Can I share Purpose Driven Life with others?
-
Yes, you can share Purpose Driven Life with others. You can share your ebook with your friends or family members who have compatible devices or apps. You can also share your thoughts and reflections on the book with others through social media, blogs, podcasts, etc. You can also join or start a small group or a class where you can discuss the book with others who are reading it or have read it.
-
- 0a6ba089eb
-
-
\ No newline at end of file
diff --git a/spaces/raedeXanto/academic-chatgpt-beta/Download Mr Bechara 2 Movie 1080p.md b/spaces/raedeXanto/academic-chatgpt-beta/Download Mr Bechara 2 Movie 1080p.md
deleted file mode 100644
index 5ace71f7e0882b503980f65f4324c99bbb311be3..0000000000000000000000000000000000000000
--- a/spaces/raedeXanto/academic-chatgpt-beta/Download Mr Bechara 2 Movie 1080p.md
+++ /dev/null
@@ -1,24 +0,0 @@
-
-
Download Mr Bechara 2 Movie 1080p: A Sequel to the 1996 Romantic Comedy
-
-
If you are a fan of the 1996 Hindi-language romantic comedy film Mr. Bechara, starring Anil Kapoor, Sridevi and Nagarjuna Akkineni, you will be delighted to know that a sequel is in the works. Mr. Bechara 2 is expected to release in 2023, and will feature the same lead actors reprising their roles as Anand Verma, Asha/Anita and Ajay.
In case you are not familiar with the plot of Mr. Bechara, here is a brief summary: Anand Verma is a shy widower and a single father to his infant son. He admits a woman into the hospital who has lost her memory due to an accident. The doctor names her Asha and makes her believe that she is married to Anand and has a child. Anand reluctantly agrees to take care of her until she recovers from amnesia. However, he soon falls in love with her, while she also gets attached to him and his son. But on the day of their wedding, Asha regains her memory and realizes that she is actually Anita, and Ajay is her lover. Anand sacrifices his happiness and reunites Anita with Ajay, but Anita realizes that she loves Anand more and returns to him.
-
-
Mr. Bechara 2 will continue the story of Anand and Anita, who are now happily married and have a daughter. Ajay also moves on with his life and finds a new partner. But their lives take a dramatic turn when Anita's brother, who was presumed dead in the accident that caused her amnesia, returns to claim his share of their family property. He also has a grudge against Anand and Ajay, and plots to ruin their lives. Will Anand and Anita be able to overcome this new challenge? Will Ajay be able to help them? Will there be more twists and turns in their love story?
-
-
To find out the answers, you will have to wait for Mr. Bechara 2 to release in theatres. But if you can't wait that long, you can download Mr. Bechara 2 movie 1080p from our website. We have the best quality and fastest download speed for all your Bollywood movie needs. Just click on the link below and enjoy Mr. Bechara 2 movie 1080p on your device.
Mr. Bechara 2 is directed by K. Bhagyaraj, who also directed the original film. He has written the screenplay and the story for the sequel, based on his own Tamil film Veetla Visheshanga (1994), which was the source material for Mr. Bechara. The music for Mr. Bechara 2 is composed by Anand Milind, who also composed the songs for the first film. The lyrics are written by Sameer.
-
-
The film has been shot in various locations in India and abroad, including Mumbai, Goa, Ooty, London and Switzerland. The film features some of the original cast members from Mr. Bechara, such as Anupam Kher as Dr. Dayanand, Shakti Kapoor as Mr. Natwarlal 'Romeo', Tiku Talsania as Inspector V.P. Chaturvedi and Shammi as the caretaker. The film also introduces some new characters, such as Anita's brother played by Abhimanyu Singh, Ajay's partner played by Heera Rajgopal and Anand's daughter played by Baby Akshay.
-
-
Mr. Bechara 2 promises to be a fun-filled and heartwarming comedy that will make you laugh and cry. The film has some hilarious scenes, such as Anand trying to impress Anita's brother with his fake wealth, Ajay getting into trouble with Romeo's gang, Anita and Ajay competing in a dance contest and Dr. Dayanand using his crazy methods to help Anand and Anita. The film also has some emotional moments, such as Anita's brother revealing his true intentions, Anand and Anita facing a life-threatening situation, Ajay sacrificing his love for Anita and Anand and Anita renewing their vows.
-
-
If you loved Mr. Bechara, you will surely love Mr. Bechara 2. And if you haven't seen Mr. Bechara, you can still enjoy Mr. Bechara 2, as it is a standalone story that does not require any prior knowledge of the first film. So don't miss this opportunity to watch Mr. Bechara 2 movie 1080p on your device. Just download it from our website and have a great time.
81aa517590
-
-
\ No newline at end of file
diff --git "a/spaces/rainy3/chatgpt_academic/crazy_functions/\344\273\243\347\240\201\351\207\215\345\206\231\344\270\272\345\205\250\350\213\261\346\226\207_\345\244\232\347\272\277\347\250\213.py" "b/spaces/rainy3/chatgpt_academic/crazy_functions/\344\273\243\347\240\201\351\207\215\345\206\231\344\270\272\345\205\250\350\213\261\346\226\207_\345\244\232\347\272\277\347\250\213.py"
deleted file mode 100644
index f74704aec2730fc8e9198a6c79ef45a43346a261..0000000000000000000000000000000000000000
--- "a/spaces/rainy3/chatgpt_academic/crazy_functions/\344\273\243\347\240\201\351\207\215\345\206\231\344\270\272\345\205\250\350\213\261\346\226\207_\345\244\232\347\272\277\347\250\213.py"
+++ /dev/null
@@ -1,139 +0,0 @@
-import threading
-from request_llm.bridge_chatgpt import predict_no_ui_long_connection
-from toolbox import update_ui
-from toolbox import CatchException, write_results_to_file, report_execption
-from .crazy_utils import breakdown_txt_to_satisfy_token_limit
-
-def extract_code_block_carefully(txt):
- splitted = txt.split('```')
- n_code_block_seg = len(splitted) - 1
- if n_code_block_seg <= 1: return txt
- # 剩下的情况都开头除去 ``` 结尾除去一次 ```
- txt_out = '```'.join(splitted[1:-1])
- return txt_out
-
-
-
-def break_txt_into_half_at_some_linebreak(txt):
- lines = txt.split('\n')
- n_lines = len(lines)
- pre = lines[:(n_lines//2)]
- post = lines[(n_lines//2):]
- return "\n".join(pre), "\n".join(post)
-
-
-@CatchException
-def 全项目切换英文(txt, llm_kwargs, plugin_kwargs, chatbot, history, sys_prompt, web_port):
- # 第1步:清空历史,以免输入溢出
- history = []
-
- # 第2步:尝试导入依赖,如果缺少依赖,则给出安装建议
- try:
- import tiktoken
- except:
- report_execption(chatbot, history,
- a = f"解析项目: {txt}",
- b = f"导入软件依赖失败。使用该模块需要额外依赖,安装方法```pip install --upgrade tiktoken```。")
- yield from update_ui(chatbot=chatbot, history=history) # 刷新界面
- return
-
- # 第3步:集合文件
- import time, glob, os, shutil, re
- os.makedirs('gpt_log/generated_english_version', exist_ok=True)
- os.makedirs('gpt_log/generated_english_version/crazy_functions', exist_ok=True)
- file_manifest = [f for f in glob.glob('./*.py') if ('test_project' not in f) and ('gpt_log' not in f)] + \
- [f for f in glob.glob('./crazy_functions/*.py') if ('test_project' not in f) and ('gpt_log' not in f)]
- # file_manifest = ['./toolbox.py']
- i_say_show_user_buffer = []
-
- # 第4步:随便显示点什么防止卡顿的感觉
- for index, fp in enumerate(file_manifest):
- # if 'test_project' in fp: continue
- with open(fp, 'r', encoding='utf-8', errors='replace') as f:
- file_content = f.read()
- i_say_show_user =f'[{index}/{len(file_manifest)}] 接下来请将以下代码中包含的所有中文转化为英文,只输出转化后的英文代码,请用代码块输出代码: {os.path.abspath(fp)}'
- i_say_show_user_buffer.append(i_say_show_user)
- chatbot.append((i_say_show_user, "[Local Message] 等待多线程操作,中间过程不予显示."))
- yield from update_ui(chatbot=chatbot, history=history) # 刷新界面
-
-
- # 第5步:Token限制下的截断与处理
- MAX_TOKEN = 3000
- import tiktoken
- from toolbox import get_conf
- enc = tiktoken.encoding_for_model(*get_conf('LLM_MODEL'))
- def get_token_fn(txt): return len(enc.encode(txt))
-
-
- # 第6步:任务函数
- mutable_return = [None for _ in file_manifest]
- observe_window = [[""] for _ in file_manifest]
- def thread_worker(fp,index):
- if index > 10:
- time.sleep(60)
- print('Openai 限制免费用户每分钟20次请求,降低请求频率中。')
- with open(fp, 'r', encoding='utf-8', errors='replace') as f:
- file_content = f.read()
- i_say_template = lambda fp, file_content: f'接下来请将以下代码中包含的所有中文转化为英文,只输出代码,文件名是{fp},文件代码是 ```{file_content}```'
- try:
- gpt_say = ""
- # 分解代码文件
- file_content_breakdown = breakdown_txt_to_satisfy_token_limit(file_content, get_token_fn, MAX_TOKEN)
- for file_content_partial in file_content_breakdown:
- i_say = i_say_template(fp, file_content_partial)
- # # ** gpt request **
- gpt_say_partial = predict_no_ui_long_connection(inputs=i_say, llm_kwargs=llm_kwargs, history=[], sys_prompt=sys_prompt, observe_window=observe_window[index])
- gpt_say_partial = extract_code_block_carefully(gpt_say_partial)
- gpt_say += gpt_say_partial
- mutable_return[index] = gpt_say
- except ConnectionAbortedError as token_exceed_err:
- print('至少一个线程任务Token溢出而失败', e)
- except Exception as e:
- print('至少一个线程任务意外失败', e)
-
- # 第7步:所有线程同时开始执行任务函数
- handles = [threading.Thread(target=thread_worker, args=(fp,index)) for index, fp in enumerate(file_manifest)]
- for h in handles:
- h.daemon = True
- h.start()
- chatbot.append(('开始了吗?', f'多线程操作已经开始'))
- yield from update_ui(chatbot=chatbot, history=history) # 刷新界面
-
- # 第8步:循环轮询各个线程是否执行完毕
- cnt = 0
- while True:
- cnt += 1
- time.sleep(0.2)
- th_alive = [h.is_alive() for h in handles]
- if not any(th_alive): break
- # 更好的UI视觉效果
- observe_win = []
- for thread_index, alive in enumerate(th_alive):
- observe_win.append("[ ..."+observe_window[thread_index][0][-60:].replace('\n','').replace('```','...').replace(' ','.').replace(' ','.....').replace('$','.')+"... ]")
- stat = [f'执行中: {obs}\n\n' if alive else '已完成\n\n' for alive, obs in zip(th_alive, observe_win)]
- stat_str = ''.join(stat)
- chatbot[-1] = (chatbot[-1][0], f'多线程操作已经开始,完成情况: \n\n{stat_str}' + ''.join(['.']*(cnt%10+1)))
- yield from update_ui(chatbot=chatbot, history=history) # 刷新界面
-
- # 第9步:把结果写入文件
- for index, h in enumerate(handles):
- h.join() # 这里其实不需要join了,肯定已经都结束了
- fp = file_manifest[index]
- gpt_say = mutable_return[index]
- i_say_show_user = i_say_show_user_buffer[index]
-
- where_to_relocate = f'gpt_log/generated_english_version/{fp}'
- if gpt_say is not None:
- with open(where_to_relocate, 'w+', encoding='utf-8') as f:
- f.write(gpt_say)
- else: # 失败
- shutil.copyfile(file_manifest[index], where_to_relocate)
- chatbot.append((i_say_show_user, f'[Local Message] 已完成{os.path.abspath(fp)}的转化,\n\n存入{os.path.abspath(where_to_relocate)}'))
- history.append(i_say_show_user); history.append(gpt_say)
- yield from update_ui(chatbot=chatbot, history=history) # 刷新界面
- time.sleep(1)
-
- # 第10步:备份一个文件
- res = write_results_to_file(history)
- chatbot.append(("生成一份任务执行报告", res))
- yield from update_ui(chatbot=chatbot, history=history) # 刷新界面
diff --git a/spaces/rayan-saleh/whisper2notion/server/node_modules/@types/node/diagnostics_channel.d.ts b/spaces/rayan-saleh/whisper2notion/server/node_modules/@types/node/diagnostics_channel.d.ts
deleted file mode 100644
index 3dcaa035a56d95e3e6bcfb39246f8b4bb6348ba7..0000000000000000000000000000000000000000
--- a/spaces/rayan-saleh/whisper2notion/server/node_modules/@types/node/diagnostics_channel.d.ts
+++ /dev/null
@@ -1,153 +0,0 @@
-/**
- * The `diagnostics_channel` module provides an API to create named channels
- * to report arbitrary message data for diagnostics purposes.
- *
- * It can be accessed using:
- *
- * ```js
- * import diagnostics_channel from 'diagnostics_channel';
- * ```
- *
- * It is intended that a module writer wanting to report diagnostics messages
- * will create one or many top-level channels to report messages through.
- * Channels may also be acquired at runtime but it is not encouraged
- * due to the additional overhead of doing so. Channels may be exported for
- * convenience, but as long as the name is known it can be acquired anywhere.
- *
- * If you intend for your module to produce diagnostics data for others to
- * consume it is recommended that you include documentation of what named
- * channels are used along with the shape of the message data. Channel names
- * should generally include the module name to avoid collisions with data from
- * other modules.
- * @experimental
- * @see [source](https://github.com/nodejs/node/blob/v18.0.0/lib/diagnostics_channel.js)
- */
-declare module 'diagnostics_channel' {
- /**
- * Check if there are active subscribers to the named channel. This is helpful if
- * the message you want to send might be expensive to prepare.
- *
- * This API is optional but helpful when trying to publish messages from very
- * performance-sensitive code.
- *
- * ```js
- * import diagnostics_channel from 'diagnostics_channel';
- *
- * if (diagnostics_channel.hasSubscribers('my-channel')) {
- * // There are subscribers, prepare and publish message
- * }
- * ```
- * @since v15.1.0, v14.17.0
- * @param name The channel name
- * @return If there are active subscribers
- */
- function hasSubscribers(name: string | symbol): boolean;
- /**
- * This is the primary entry-point for anyone wanting to interact with a named
- * channel. It produces a channel object which is optimized to reduce overhead at
- * publish time as much as possible.
- *
- * ```js
- * import diagnostics_channel from 'diagnostics_channel';
- *
- * const channel = diagnostics_channel.channel('my-channel');
- * ```
- * @since v15.1.0, v14.17.0
- * @param name The channel name
- * @return The named channel object
- */
- function channel(name: string | symbol): Channel;
- type ChannelListener = (message: unknown, name: string | symbol) => void;
- /**
- * The class `Channel` represents an individual named channel within the data
- * pipeline. It is use to track subscribers and to publish messages when there
- * are subscribers present. It exists as a separate object to avoid channel
- * lookups at publish time, enabling very fast publish speeds and allowing
- * for heavy use while incurring very minimal cost. Channels are created with {@link channel}, constructing a channel directly
- * with `new Channel(name)` is not supported.
- * @since v15.1.0, v14.17.0
- */
- class Channel {
- readonly name: string | symbol;
- /**
- * Check if there are active subscribers to this channel. This is helpful if
- * the message you want to send might be expensive to prepare.
- *
- * This API is optional but helpful when trying to publish messages from very
- * performance-sensitive code.
- *
- * ```js
- * import diagnostics_channel from 'diagnostics_channel';
- *
- * const channel = diagnostics_channel.channel('my-channel');
- *
- * if (channel.hasSubscribers) {
- * // There are subscribers, prepare and publish message
- * }
- * ```
- * @since v15.1.0, v14.17.0
- */
- readonly hasSubscribers: boolean;
- private constructor(name: string | symbol);
- /**
- * Publish a message to any subscribers to the channel. This will
- * trigger message handlers synchronously so they will execute within
- * the same context.
- *
- * ```js
- * import diagnostics_channel from 'diagnostics_channel';
- *
- * const channel = diagnostics_channel.channel('my-channel');
- *
- * channel.publish({
- * some: 'message'
- * });
- * ```
- * @since v15.1.0, v14.17.0
- * @param message The message to send to the channel subscribers
- */
- publish(message: unknown): void;
- /**
- * Register a message handler to subscribe to this channel. This message handler
- * will be run synchronously whenever a message is published to the channel. Any
- * errors thrown in the message handler will trigger an `'uncaughtException'`.
- *
- * ```js
- * import diagnostics_channel from 'diagnostics_channel';
- *
- * const channel = diagnostics_channel.channel('my-channel');
- *
- * channel.subscribe((message, name) => {
- * // Received data
- * });
- * ```
- * @since v15.1.0, v14.17.0
- * @param onMessage The handler to receive channel messages
- */
- subscribe(onMessage: ChannelListener): void;
- /**
- * Remove a message handler previously registered to this channel with `channel.subscribe(onMessage)`.
- *
- * ```js
- * import diagnostics_channel from 'diagnostics_channel';
- *
- * const channel = diagnostics_channel.channel('my-channel');
- *
- * function onMessage(message, name) {
- * // Received data
- * }
- *
- * channel.subscribe(onMessage);
- *
- * channel.unsubscribe(onMessage);
- * ```
- * @since v15.1.0, v14.17.0
- * @param onMessage The previous subscribed handler to remove
- * @return `true` if the handler was found, `false` otherwise.
- */
- unsubscribe(onMessage: ChannelListener): void;
- }
-}
-declare module 'node:diagnostics_channel' {
- export * from 'diagnostics_channel';
-}
diff --git a/spaces/rayan-saleh/whisper2notion/server/node_modules/@types/node/ts4.8/inspector.d.ts b/spaces/rayan-saleh/whisper2notion/server/node_modules/@types/node/ts4.8/inspector.d.ts
deleted file mode 100644
index eba0b55d8bca0ef10cbf24922fb899b67c35f3a9..0000000000000000000000000000000000000000
--- a/spaces/rayan-saleh/whisper2notion/server/node_modules/@types/node/ts4.8/inspector.d.ts
+++ /dev/null
@@ -1,2741 +0,0 @@
-// eslint-disable-next-line dt-header
-// Type definitions for inspector
-
-// These definitions are auto-generated.
-// Please see https://github.com/DefinitelyTyped/DefinitelyTyped/pull/19330
-// for more information.
-
-// tslint:disable:max-line-length
-
-/**
- * The `inspector` module provides an API for interacting with the V8 inspector.
- *
- * It can be accessed using:
- *
- * ```js
- * const inspector = require('inspector');
- * ```
- * @see [source](https://github.com/nodejs/node/blob/v18.0.0/lib/inspector.js)
- */
-declare module 'inspector' {
- import EventEmitter = require('node:events');
- interface InspectorNotification {
- method: string;
- params: T;
- }
- namespace Schema {
- /**
- * Description of the protocol domain.
- */
- interface Domain {
- /**
- * Domain name.
- */
- name: string;
- /**
- * Domain version.
- */
- version: string;
- }
- interface GetDomainsReturnType {
- /**
- * List of supported domains.
- */
- domains: Domain[];
- }
- }
- namespace Runtime {
- /**
- * Unique script identifier.
- */
- type ScriptId = string;
- /**
- * Unique object identifier.
- */
- type RemoteObjectId = string;
- /**
- * Primitive value which cannot be JSON-stringified.
- */
- type UnserializableValue = string;
- /**
- * Mirror object referencing original JavaScript object.
- */
- interface RemoteObject {
- /**
- * Object type.
- */
- type: string;
- /**
- * Object subtype hint. Specified for object type values only.
- */
- subtype?: string | undefined;
- /**
- * Object class (constructor) name. Specified for object type values only.
- */
- className?: string | undefined;
- /**
- * Remote object value in case of primitive values or JSON values (if it was requested).
- */
- value?: any;
- /**
- * Primitive value which can not be JSON-stringified does not have value, but gets this property.
- */
- unserializableValue?: UnserializableValue | undefined;
- /**
- * String representation of the object.
- */
- description?: string | undefined;
- /**
- * Unique object identifier (for non-primitive values).
- */
- objectId?: RemoteObjectId | undefined;
- /**
- * Preview containing abbreviated property values. Specified for object type values only.
- * @experimental
- */
- preview?: ObjectPreview | undefined;
- /**
- * @experimental
- */
- customPreview?: CustomPreview | undefined;
- }
- /**
- * @experimental
- */
- interface CustomPreview {
- header: string;
- hasBody: boolean;
- formatterObjectId: RemoteObjectId;
- bindRemoteObjectFunctionId: RemoteObjectId;
- configObjectId?: RemoteObjectId | undefined;
- }
- /**
- * Object containing abbreviated remote object value.
- * @experimental
- */
- interface ObjectPreview {
- /**
- * Object type.
- */
- type: string;
- /**
- * Object subtype hint. Specified for object type values only.
- */
- subtype?: string | undefined;
- /**
- * String representation of the object.
- */
- description?: string | undefined;
- /**
- * True iff some of the properties or entries of the original object did not fit.
- */
- overflow: boolean;
- /**
- * List of the properties.
- */
- properties: PropertyPreview[];
- /**
- * List of the entries. Specified for map and set subtype values only.
- */
- entries?: EntryPreview[] | undefined;
- }
- /**
- * @experimental
- */
- interface PropertyPreview {
- /**
- * Property name.
- */
- name: string;
- /**
- * Object type. Accessor means that the property itself is an accessor property.
- */
- type: string;
- /**
- * User-friendly property value string.
- */
- value?: string | undefined;
- /**
- * Nested value preview.
- */
- valuePreview?: ObjectPreview | undefined;
- /**
- * Object subtype hint. Specified for object type values only.
- */
- subtype?: string | undefined;
- }
- /**
- * @experimental
- */
- interface EntryPreview {
- /**
- * Preview of the key. Specified for map-like collection entries.
- */
- key?: ObjectPreview | undefined;
- /**
- * Preview of the value.
- */
- value: ObjectPreview;
- }
- /**
- * Object property descriptor.
- */
- interface PropertyDescriptor {
- /**
- * Property name or symbol description.
- */
- name: string;
- /**
- * The value associated with the property.
- */
- value?: RemoteObject | undefined;
- /**
- * True if the value associated with the property may be changed (data descriptors only).
- */
- writable?: boolean | undefined;
- /**
- * A function which serves as a getter for the property, or undefined if there is no getter (accessor descriptors only).
- */
- get?: RemoteObject | undefined;
- /**
- * A function which serves as a setter for the property, or undefined if there is no setter (accessor descriptors only).
- */
- set?: RemoteObject | undefined;
- /**
- * True if the type of this property descriptor may be changed and if the property may be deleted from the corresponding object.
- */
- configurable: boolean;
- /**
- * True if this property shows up during enumeration of the properties on the corresponding object.
- */
- enumerable: boolean;
- /**
- * True if the result was thrown during the evaluation.
- */
- wasThrown?: boolean | undefined;
- /**
- * True if the property is owned for the object.
- */
- isOwn?: boolean | undefined;
- /**
- * Property symbol object, if the property is of the symbol type.
- */
- symbol?: RemoteObject | undefined;
- }
- /**
- * Object internal property descriptor. This property isn't normally visible in JavaScript code.
- */
- interface InternalPropertyDescriptor {
- /**
- * Conventional property name.
- */
- name: string;
- /**
- * The value associated with the property.
- */
- value?: RemoteObject | undefined;
- }
- /**
- * Represents function call argument. Either remote object id objectId, primitive value, unserializable primitive value or neither of (for undefined) them should be specified.
- */
- interface CallArgument {
- /**
- * Primitive value or serializable javascript object.
- */
- value?: any;
- /**
- * Primitive value which can not be JSON-stringified.
- */
- unserializableValue?: UnserializableValue | undefined;
- /**
- * Remote object handle.
- */
- objectId?: RemoteObjectId | undefined;
- }
- /**
- * Id of an execution context.
- */
- type ExecutionContextId = number;
- /**
- * Description of an isolated world.
- */
- interface ExecutionContextDescription {
- /**
- * Unique id of the execution context. It can be used to specify in which execution context script evaluation should be performed.
- */
- id: ExecutionContextId;
- /**
- * Execution context origin.
- */
- origin: string;
- /**
- * Human readable name describing given context.
- */
- name: string;
- /**
- * Embedder-specific auxiliary data.
- */
- auxData?: {} | undefined;
- }
- /**
- * Detailed information about exception (or error) that was thrown during script compilation or execution.
- */
- interface ExceptionDetails {
- /**
- * Exception id.
- */
- exceptionId: number;
- /**
- * Exception text, which should be used together with exception object when available.
- */
- text: string;
- /**
- * Line number of the exception location (0-based).
- */
- lineNumber: number;
- /**
- * Column number of the exception location (0-based).
- */
- columnNumber: number;
- /**
- * Script ID of the exception location.
- */
- scriptId?: ScriptId | undefined;
- /**
- * URL of the exception location, to be used when the script was not reported.
- */
- url?: string | undefined;
- /**
- * JavaScript stack trace if available.
- */
- stackTrace?: StackTrace | undefined;
- /**
- * Exception object if available.
- */
- exception?: RemoteObject | undefined;
- /**
- * Identifier of the context where exception happened.
- */
- executionContextId?: ExecutionContextId | undefined;
- }
- /**
- * Number of milliseconds since epoch.
- */
- type Timestamp = number;
- /**
- * Stack entry for runtime errors and assertions.
- */
- interface CallFrame {
- /**
- * JavaScript function name.
- */
- functionName: string;
- /**
- * JavaScript script id.
- */
- scriptId: ScriptId;
- /**
- * JavaScript script name or url.
- */
- url: string;
- /**
- * JavaScript script line number (0-based).
- */
- lineNumber: number;
- /**
- * JavaScript script column number (0-based).
- */
- columnNumber: number;
- }
- /**
- * Call frames for assertions or error messages.
- */
- interface StackTrace {
- /**
- * String label of this stack trace. For async traces this may be a name of the function that initiated the async call.
- */
- description?: string | undefined;
- /**
- * JavaScript function name.
- */
- callFrames: CallFrame[];
- /**
- * Asynchronous JavaScript stack trace that preceded this stack, if available.
- */
- parent?: StackTrace | undefined;
- /**
- * Asynchronous JavaScript stack trace that preceded this stack, if available.
- * @experimental
- */
- parentId?: StackTraceId | undefined;
- }
- /**
- * Unique identifier of current debugger.
- * @experimental
- */
- type UniqueDebuggerId = string;
- /**
- * If debuggerId is set stack trace comes from another debugger and can be resolved there. This allows to track cross-debugger calls. See Runtime.StackTrace and Debugger.paused for usages.
- * @experimental
- */
- interface StackTraceId {
- id: string;
- debuggerId?: UniqueDebuggerId | undefined;
- }
- interface EvaluateParameterType {
- /**
- * Expression to evaluate.
- */
- expression: string;
- /**
- * Symbolic group name that can be used to release multiple objects.
- */
- objectGroup?: string | undefined;
- /**
- * Determines whether Command Line API should be available during the evaluation.
- */
- includeCommandLineAPI?: boolean | undefined;
- /**
- * In silent mode exceptions thrown during evaluation are not reported and do not pause execution. Overrides setPauseOnException state.
- */
- silent?: boolean | undefined;
- /**
- * Specifies in which execution context to perform evaluation. If the parameter is omitted the evaluation will be performed in the context of the inspected page.
- */
- contextId?: ExecutionContextId | undefined;
- /**
- * Whether the result is expected to be a JSON object that should be sent by value.
- */
- returnByValue?: boolean | undefined;
- /**
- * Whether preview should be generated for the result.
- * @experimental
- */
- generatePreview?: boolean | undefined;
- /**
- * Whether execution should be treated as initiated by user in the UI.
- */
- userGesture?: boolean | undefined;
- /**
- * Whether execution should await for resulting value and return once awaited promise is resolved.
- */
- awaitPromise?: boolean | undefined;
- }
- interface AwaitPromiseParameterType {
- /**
- * Identifier of the promise.
- */
- promiseObjectId: RemoteObjectId;
- /**
- * Whether the result is expected to be a JSON object that should be sent by value.
- */
- returnByValue?: boolean | undefined;
- /**
- * Whether preview should be generated for the result.
- */
- generatePreview?: boolean | undefined;
- }
- interface CallFunctionOnParameterType {
- /**
- * Declaration of the function to call.
- */
- functionDeclaration: string;
- /**
- * Identifier of the object to call function on. Either objectId or executionContextId should be specified.
- */
- objectId?: RemoteObjectId | undefined;
- /**
- * Call arguments. All call arguments must belong to the same JavaScript world as the target object.
- */
- arguments?: CallArgument[] | undefined;
- /**
- * In silent mode exceptions thrown during evaluation are not reported and do not pause execution. Overrides setPauseOnException state.
- */
- silent?: boolean | undefined;
- /**
- * Whether the result is expected to be a JSON object which should be sent by value.
- */
- returnByValue?: boolean | undefined;
- /**
- * Whether preview should be generated for the result.
- * @experimental
- */
- generatePreview?: boolean | undefined;
- /**
- * Whether execution should be treated as initiated by user in the UI.
- */
- userGesture?: boolean | undefined;
- /**
- * Whether execution should await for resulting value and return once awaited promise is resolved.
- */
- awaitPromise?: boolean | undefined;
- /**
- * Specifies execution context which global object will be used to call function on. Either executionContextId or objectId should be specified.
- */
- executionContextId?: ExecutionContextId | undefined;
- /**
- * Symbolic group name that can be used to release multiple objects. If objectGroup is not specified and objectId is, objectGroup will be inherited from object.
- */
- objectGroup?: string | undefined;
- }
- interface GetPropertiesParameterType {
- /**
- * Identifier of the object to return properties for.
- */
- objectId: RemoteObjectId;
- /**
- * If true, returns properties belonging only to the element itself, not to its prototype chain.
- */
- ownProperties?: boolean | undefined;
- /**
- * If true, returns accessor properties (with getter/setter) only; internal properties are not returned either.
- * @experimental
- */
- accessorPropertiesOnly?: boolean | undefined;
- /**
- * Whether preview should be generated for the results.
- * @experimental
- */
- generatePreview?: boolean | undefined;
- }
- interface ReleaseObjectParameterType {
- /**
- * Identifier of the object to release.
- */
- objectId: RemoteObjectId;
- }
- interface ReleaseObjectGroupParameterType {
- /**
- * Symbolic object group name.
- */
- objectGroup: string;
- }
- interface SetCustomObjectFormatterEnabledParameterType {
- enabled: boolean;
- }
- interface CompileScriptParameterType {
- /**
- * Expression to compile.
- */
- expression: string;
- /**
- * Source url to be set for the script.
- */
- sourceURL: string;
- /**
- * Specifies whether the compiled script should be persisted.
- */
- persistScript: boolean;
- /**
- * Specifies in which execution context to perform script run. If the parameter is omitted the evaluation will be performed in the context of the inspected page.
- */
- executionContextId?: ExecutionContextId | undefined;
- }
- interface RunScriptParameterType {
- /**
- * Id of the script to run.
- */
- scriptId: ScriptId;
- /**
- * Specifies in which execution context to perform script run. If the parameter is omitted the evaluation will be performed in the context of the inspected page.
- */
- executionContextId?: ExecutionContextId | undefined;
- /**
- * Symbolic group name that can be used to release multiple objects.
- */
- objectGroup?: string | undefined;
- /**
- * In silent mode exceptions thrown during evaluation are not reported and do not pause execution. Overrides setPauseOnException state.
- */
- silent?: boolean | undefined;
- /**
- * Determines whether Command Line API should be available during the evaluation.
- */
- includeCommandLineAPI?: boolean | undefined;
- /**
- * Whether the result is expected to be a JSON object which should be sent by value.
- */
- returnByValue?: boolean | undefined;
- /**
- * Whether preview should be generated for the result.
- */
- generatePreview?: boolean | undefined;
- /**
- * Whether execution should await for resulting value and return once awaited promise is resolved.
- */
- awaitPromise?: boolean | undefined;
- }
- interface QueryObjectsParameterType {
- /**
- * Identifier of the prototype to return objects for.
- */
- prototypeObjectId: RemoteObjectId;
- }
- interface GlobalLexicalScopeNamesParameterType {
- /**
- * Specifies in which execution context to lookup global scope variables.
- */
- executionContextId?: ExecutionContextId | undefined;
- }
- interface EvaluateReturnType {
- /**
- * Evaluation result.
- */
- result: RemoteObject;
- /**
- * Exception details.
- */
- exceptionDetails?: ExceptionDetails | undefined;
- }
- interface AwaitPromiseReturnType {
- /**
- * Promise result. Will contain rejected value if promise was rejected.
- */
- result: RemoteObject;
- /**
- * Exception details if stack strace is available.
- */
- exceptionDetails?: ExceptionDetails | undefined;
- }
- interface CallFunctionOnReturnType {
- /**
- * Call result.
- */
- result: RemoteObject;
- /**
- * Exception details.
- */
- exceptionDetails?: ExceptionDetails | undefined;
- }
- interface GetPropertiesReturnType {
- /**
- * Object properties.
- */
- result: PropertyDescriptor[];
- /**
- * Internal object properties (only of the element itself).
- */
- internalProperties?: InternalPropertyDescriptor[] | undefined;
- /**
- * Exception details.
- */
- exceptionDetails?: ExceptionDetails | undefined;
- }
- interface CompileScriptReturnType {
- /**
- * Id of the script.
- */
- scriptId?: ScriptId | undefined;
- /**
- * Exception details.
- */
- exceptionDetails?: ExceptionDetails | undefined;
- }
- interface RunScriptReturnType {
- /**
- * Run result.
- */
- result: RemoteObject;
- /**
- * Exception details.
- */
- exceptionDetails?: ExceptionDetails | undefined;
- }
- interface QueryObjectsReturnType {
- /**
- * Array with objects.
- */
- objects: RemoteObject;
- }
- interface GlobalLexicalScopeNamesReturnType {
- names: string[];
- }
- interface ExecutionContextCreatedEventDataType {
- /**
- * A newly created execution context.
- */
- context: ExecutionContextDescription;
- }
- interface ExecutionContextDestroyedEventDataType {
- /**
- * Id of the destroyed context
- */
- executionContextId: ExecutionContextId;
- }
- interface ExceptionThrownEventDataType {
- /**
- * Timestamp of the exception.
- */
- timestamp: Timestamp;
- exceptionDetails: ExceptionDetails;
- }
- interface ExceptionRevokedEventDataType {
- /**
- * Reason describing why exception was revoked.
- */
- reason: string;
- /**
- * The id of revoked exception, as reported in exceptionThrown.
- */
- exceptionId: number;
- }
- interface ConsoleAPICalledEventDataType {
- /**
- * Type of the call.
- */
- type: string;
- /**
- * Call arguments.
- */
- args: RemoteObject[];
- /**
- * Identifier of the context where the call was made.
- */
- executionContextId: ExecutionContextId;
- /**
- * Call timestamp.
- */
- timestamp: Timestamp;
- /**
- * Stack trace captured when the call was made.
- */
- stackTrace?: StackTrace | undefined;
- /**
- * Console context descriptor for calls on non-default console context (not console.*): 'anonymous#unique-logger-id' for call on unnamed context, 'name#unique-logger-id' for call on named context.
- * @experimental
- */
- context?: string | undefined;
- }
- interface InspectRequestedEventDataType {
- object: RemoteObject;
- hints: {};
- }
- }
- namespace Debugger {
- /**
- * Breakpoint identifier.
- */
- type BreakpointId = string;
- /**
- * Call frame identifier.
- */
- type CallFrameId = string;
- /**
- * Location in the source code.
- */
- interface Location {
- /**
- * Script identifier as reported in the Debugger.scriptParsed.
- */
- scriptId: Runtime.ScriptId;
- /**
- * Line number in the script (0-based).
- */
- lineNumber: number;
- /**
- * Column number in the script (0-based).
- */
- columnNumber?: number | undefined;
- }
- /**
- * Location in the source code.
- * @experimental
- */
- interface ScriptPosition {
- lineNumber: number;
- columnNumber: number;
- }
- /**
- * JavaScript call frame. Array of call frames form the call stack.
- */
- interface CallFrame {
- /**
- * Call frame identifier. This identifier is only valid while the virtual machine is paused.
- */
- callFrameId: CallFrameId;
- /**
- * Name of the JavaScript function called on this call frame.
- */
- functionName: string;
- /**
- * Location in the source code.
- */
- functionLocation?: Location | undefined;
- /**
- * Location in the source code.
- */
- location: Location;
- /**
- * JavaScript script name or url.
- */
- url: string;
- /**
- * Scope chain for this call frame.
- */
- scopeChain: Scope[];
- /**
- * this object for this call frame.
- */
- this: Runtime.RemoteObject;
- /**
- * The value being returned, if the function is at return point.
- */
- returnValue?: Runtime.RemoteObject | undefined;
- }
- /**
- * Scope description.
- */
- interface Scope {
- /**
- * Scope type.
- */
- type: string;
- /**
- * Object representing the scope. For global and with scopes it represents the actual object; for the rest of the scopes, it is artificial transient object enumerating scope variables as its properties.
- */
- object: Runtime.RemoteObject;
- name?: string | undefined;
- /**
- * Location in the source code where scope starts
- */
- startLocation?: Location | undefined;
- /**
- * Location in the source code where scope ends
- */
- endLocation?: Location | undefined;
- }
- /**
- * Search match for resource.
- */
- interface SearchMatch {
- /**
- * Line number in resource content.
- */
- lineNumber: number;
- /**
- * Line with match content.
- */
- lineContent: string;
- }
- interface BreakLocation {
- /**
- * Script identifier as reported in the Debugger.scriptParsed.
- */
- scriptId: Runtime.ScriptId;
- /**
- * Line number in the script (0-based).
- */
- lineNumber: number;
- /**
- * Column number in the script (0-based).
- */
- columnNumber?: number | undefined;
- type?: string | undefined;
- }
- interface SetBreakpointsActiveParameterType {
- /**
- * New value for breakpoints active state.
- */
- active: boolean;
- }
- interface SetSkipAllPausesParameterType {
- /**
- * New value for skip pauses state.
- */
- skip: boolean;
- }
- interface SetBreakpointByUrlParameterType {
- /**
- * Line number to set breakpoint at.
- */
- lineNumber: number;
- /**
- * URL of the resources to set breakpoint on.
- */
- url?: string | undefined;
- /**
- * Regex pattern for the URLs of the resources to set breakpoints on. Either url or urlRegex must be specified.
- */
- urlRegex?: string | undefined;
- /**
- * Script hash of the resources to set breakpoint on.
- */
- scriptHash?: string | undefined;
- /**
- * Offset in the line to set breakpoint at.
- */
- columnNumber?: number | undefined;
- /**
- * Expression to use as a breakpoint condition. When specified, debugger will only stop on the breakpoint if this expression evaluates to true.
- */
- condition?: string | undefined;
- }
- interface SetBreakpointParameterType {
- /**
- * Location to set breakpoint in.
- */
- location: Location;
- /**
- * Expression to use as a breakpoint condition. When specified, debugger will only stop on the breakpoint if this expression evaluates to true.
- */
- condition?: string | undefined;
- }
- interface RemoveBreakpointParameterType {
- breakpointId: BreakpointId;
- }
- interface GetPossibleBreakpointsParameterType {
- /**
- * Start of range to search possible breakpoint locations in.
- */
- start: Location;
- /**
- * End of range to search possible breakpoint locations in (excluding). When not specified, end of scripts is used as end of range.
- */
- end?: Location | undefined;
- /**
- * Only consider locations which are in the same (non-nested) function as start.
- */
- restrictToFunction?: boolean | undefined;
- }
- interface ContinueToLocationParameterType {
- /**
- * Location to continue to.
- */
- location: Location;
- targetCallFrames?: string | undefined;
- }
- interface PauseOnAsyncCallParameterType {
- /**
- * Debugger will pause when async call with given stack trace is started.
- */
- parentStackTraceId: Runtime.StackTraceId;
- }
- interface StepIntoParameterType {
- /**
- * Debugger will issue additional Debugger.paused notification if any async task is scheduled before next pause.
- * @experimental
- */
- breakOnAsyncCall?: boolean | undefined;
- }
- interface GetStackTraceParameterType {
- stackTraceId: Runtime.StackTraceId;
- }
- interface SearchInContentParameterType {
- /**
- * Id of the script to search in.
- */
- scriptId: Runtime.ScriptId;
- /**
- * String to search for.
- */
- query: string;
- /**
- * If true, search is case sensitive.
- */
- caseSensitive?: boolean | undefined;
- /**
- * If true, treats string parameter as regex.
- */
- isRegex?: boolean | undefined;
- }
- interface SetScriptSourceParameterType {
- /**
- * Id of the script to edit.
- */
- scriptId: Runtime.ScriptId;
- /**
- * New content of the script.
- */
- scriptSource: string;
- /**
- * If true the change will not actually be applied. Dry run may be used to get result description without actually modifying the code.
- */
- dryRun?: boolean | undefined;
- }
- interface RestartFrameParameterType {
- /**
- * Call frame identifier to evaluate on.
- */
- callFrameId: CallFrameId;
- }
- interface GetScriptSourceParameterType {
- /**
- * Id of the script to get source for.
- */
- scriptId: Runtime.ScriptId;
- }
- interface SetPauseOnExceptionsParameterType {
- /**
- * Pause on exceptions mode.
- */
- state: string;
- }
- interface EvaluateOnCallFrameParameterType {
- /**
- * Call frame identifier to evaluate on.
- */
- callFrameId: CallFrameId;
- /**
- * Expression to evaluate.
- */
- expression: string;
- /**
- * String object group name to put result into (allows rapid releasing resulting object handles using releaseObjectGroup).
- */
- objectGroup?: string | undefined;
- /**
- * Specifies whether command line API should be available to the evaluated expression, defaults to false.
- */
- includeCommandLineAPI?: boolean | undefined;
- /**
- * In silent mode exceptions thrown during evaluation are not reported and do not pause execution. Overrides setPauseOnException state.
- */
- silent?: boolean | undefined;
- /**
- * Whether the result is expected to be a JSON object that should be sent by value.
- */
- returnByValue?: boolean | undefined;
- /**
- * Whether preview should be generated for the result.
- * @experimental
- */
- generatePreview?: boolean | undefined;
- /**
- * Whether to throw an exception if side effect cannot be ruled out during evaluation.
- */
- throwOnSideEffect?: boolean | undefined;
- }
- interface SetVariableValueParameterType {
- /**
- * 0-based number of scope as was listed in scope chain. Only 'local', 'closure' and 'catch' scope types are allowed. Other scopes could be manipulated manually.
- */
- scopeNumber: number;
- /**
- * Variable name.
- */
- variableName: string;
- /**
- * New variable value.
- */
- newValue: Runtime.CallArgument;
- /**
- * Id of callframe that holds variable.
- */
- callFrameId: CallFrameId;
- }
- interface SetReturnValueParameterType {
- /**
- * New return value.
- */
- newValue: Runtime.CallArgument;
- }
- interface SetAsyncCallStackDepthParameterType {
- /**
- * Maximum depth of async call stacks. Setting to 0 will effectively disable collecting async call stacks (default).
- */
- maxDepth: number;
- }
- interface SetBlackboxPatternsParameterType {
- /**
- * Array of regexps that will be used to check script url for blackbox state.
- */
- patterns: string[];
- }
- interface SetBlackboxedRangesParameterType {
- /**
- * Id of the script.
- */
- scriptId: Runtime.ScriptId;
- positions: ScriptPosition[];
- }
- interface EnableReturnType {
- /**
- * Unique identifier of the debugger.
- * @experimental
- */
- debuggerId: Runtime.UniqueDebuggerId;
- }
- interface SetBreakpointByUrlReturnType {
- /**
- * Id of the created breakpoint for further reference.
- */
- breakpointId: BreakpointId;
- /**
- * List of the locations this breakpoint resolved into upon addition.
- */
- locations: Location[];
- }
- interface SetBreakpointReturnType {
- /**
- * Id of the created breakpoint for further reference.
- */
- breakpointId: BreakpointId;
- /**
- * Location this breakpoint resolved into.
- */
- actualLocation: Location;
- }
- interface GetPossibleBreakpointsReturnType {
- /**
- * List of the possible breakpoint locations.
- */
- locations: BreakLocation[];
- }
- interface GetStackTraceReturnType {
- stackTrace: Runtime.StackTrace;
- }
- interface SearchInContentReturnType {
- /**
- * List of search matches.
- */
- result: SearchMatch[];
- }
- interface SetScriptSourceReturnType {
- /**
- * New stack trace in case editing has happened while VM was stopped.
- */
- callFrames?: CallFrame[] | undefined;
- /**
- * Whether current call stack was modified after applying the changes.
- */
- stackChanged?: boolean | undefined;
- /**
- * Async stack trace, if any.
- */
- asyncStackTrace?: Runtime.StackTrace | undefined;
- /**
- * Async stack trace, if any.
- * @experimental
- */
- asyncStackTraceId?: Runtime.StackTraceId | undefined;
- /**
- * Exception details if any.
- */
- exceptionDetails?: Runtime.ExceptionDetails | undefined;
- }
- interface RestartFrameReturnType {
- /**
- * New stack trace.
- */
- callFrames: CallFrame[];
- /**
- * Async stack trace, if any.
- */
- asyncStackTrace?: Runtime.StackTrace | undefined;
- /**
- * Async stack trace, if any.
- * @experimental
- */
- asyncStackTraceId?: Runtime.StackTraceId | undefined;
- }
- interface GetScriptSourceReturnType {
- /**
- * Script source.
- */
- scriptSource: string;
- }
- interface EvaluateOnCallFrameReturnType {
- /**
- * Object wrapper for the evaluation result.
- */
- result: Runtime.RemoteObject;
- /**
- * Exception details.
- */
- exceptionDetails?: Runtime.ExceptionDetails | undefined;
- }
- interface ScriptParsedEventDataType {
- /**
- * Identifier of the script parsed.
- */
- scriptId: Runtime.ScriptId;
- /**
- * URL or name of the script parsed (if any).
- */
- url: string;
- /**
- * Line offset of the script within the resource with given URL (for script tags).
- */
- startLine: number;
- /**
- * Column offset of the script within the resource with given URL.
- */
- startColumn: number;
- /**
- * Last line of the script.
- */
- endLine: number;
- /**
- * Length of the last line of the script.
- */
- endColumn: number;
- /**
- * Specifies script creation context.
- */
- executionContextId: Runtime.ExecutionContextId;
- /**
- * Content hash of the script.
- */
- hash: string;
- /**
- * Embedder-specific auxiliary data.
- */
- executionContextAuxData?: {} | undefined;
- /**
- * True, if this script is generated as a result of the live edit operation.
- * @experimental
- */
- isLiveEdit?: boolean | undefined;
- /**
- * URL of source map associated with script (if any).
- */
- sourceMapURL?: string | undefined;
- /**
- * True, if this script has sourceURL.
- */
- hasSourceURL?: boolean | undefined;
- /**
- * True, if this script is ES6 module.
- */
- isModule?: boolean | undefined;
- /**
- * This script length.
- */
- length?: number | undefined;
- /**
- * JavaScript top stack frame of where the script parsed event was triggered if available.
- * @experimental
- */
- stackTrace?: Runtime.StackTrace | undefined;
- }
- interface ScriptFailedToParseEventDataType {
- /**
- * Identifier of the script parsed.
- */
- scriptId: Runtime.ScriptId;
- /**
- * URL or name of the script parsed (if any).
- */
- url: string;
- /**
- * Line offset of the script within the resource with given URL (for script tags).
- */
- startLine: number;
- /**
- * Column offset of the script within the resource with given URL.
- */
- startColumn: number;
- /**
- * Last line of the script.
- */
- endLine: number;
- /**
- * Length of the last line of the script.
- */
- endColumn: number;
- /**
- * Specifies script creation context.
- */
- executionContextId: Runtime.ExecutionContextId;
- /**
- * Content hash of the script.
- */
- hash: string;
- /**
- * Embedder-specific auxiliary data.
- */
- executionContextAuxData?: {} | undefined;
- /**
- * URL of source map associated with script (if any).
- */
- sourceMapURL?: string | undefined;
- /**
- * True, if this script has sourceURL.
- */
- hasSourceURL?: boolean | undefined;
- /**
- * True, if this script is ES6 module.
- */
- isModule?: boolean | undefined;
- /**
- * This script length.
- */
- length?: number | undefined;
- /**
- * JavaScript top stack frame of where the script parsed event was triggered if available.
- * @experimental
- */
- stackTrace?: Runtime.StackTrace | undefined;
- }
- interface BreakpointResolvedEventDataType {
- /**
- * Breakpoint unique identifier.
- */
- breakpointId: BreakpointId;
- /**
- * Actual breakpoint location.
- */
- location: Location;
- }
- interface PausedEventDataType {
- /**
- * Call stack the virtual machine stopped on.
- */
- callFrames: CallFrame[];
- /**
- * Pause reason.
- */
- reason: string;
- /**
- * Object containing break-specific auxiliary properties.
- */
- data?: {} | undefined;
- /**
- * Hit breakpoints IDs
- */
- hitBreakpoints?: string[] | undefined;
- /**
- * Async stack trace, if any.
- */
- asyncStackTrace?: Runtime.StackTrace | undefined;
- /**
- * Async stack trace, if any.
- * @experimental
- */
- asyncStackTraceId?: Runtime.StackTraceId | undefined;
- /**
- * Just scheduled async call will have this stack trace as parent stack during async execution. This field is available only after Debugger.stepInto call with breakOnAsynCall flag.
- * @experimental
- */
- asyncCallStackTraceId?: Runtime.StackTraceId | undefined;
- }
- }
- namespace Console {
- /**
- * Console message.
- */
- interface ConsoleMessage {
- /**
- * Message source.
- */
- source: string;
- /**
- * Message severity.
- */
- level: string;
- /**
- * Message text.
- */
- text: string;
- /**
- * URL of the message origin.
- */
- url?: string | undefined;
- /**
- * Line number in the resource that generated this message (1-based).
- */
- line?: number | undefined;
- /**
- * Column number in the resource that generated this message (1-based).
- */
- column?: number | undefined;
- }
- interface MessageAddedEventDataType {
- /**
- * Console message that has been added.
- */
- message: ConsoleMessage;
- }
- }
- namespace Profiler {
- /**
- * Profile node. Holds callsite information, execution statistics and child nodes.
- */
- interface ProfileNode {
- /**
- * Unique id of the node.
- */
- id: number;
- /**
- * Function location.
- */
- callFrame: Runtime.CallFrame;
- /**
- * Number of samples where this node was on top of the call stack.
- */
- hitCount?: number | undefined;
- /**
- * Child node ids.
- */
- children?: number[] | undefined;
- /**
- * The reason of being not optimized. The function may be deoptimized or marked as don't optimize.
- */
- deoptReason?: string | undefined;
- /**
- * An array of source position ticks.
- */
- positionTicks?: PositionTickInfo[] | undefined;
- }
- /**
- * Profile.
- */
- interface Profile {
- /**
- * The list of profile nodes. First item is the root node.
- */
- nodes: ProfileNode[];
- /**
- * Profiling start timestamp in microseconds.
- */
- startTime: number;
- /**
- * Profiling end timestamp in microseconds.
- */
- endTime: number;
- /**
- * Ids of samples top nodes.
- */
- samples?: number[] | undefined;
- /**
- * Time intervals between adjacent samples in microseconds. The first delta is relative to the profile startTime.
- */
- timeDeltas?: number[] | undefined;
- }
- /**
- * Specifies a number of samples attributed to a certain source position.
- */
- interface PositionTickInfo {
- /**
- * Source line number (1-based).
- */
- line: number;
- /**
- * Number of samples attributed to the source line.
- */
- ticks: number;
- }
- /**
- * Coverage data for a source range.
- */
- interface CoverageRange {
- /**
- * JavaScript script source offset for the range start.
- */
- startOffset: number;
- /**
- * JavaScript script source offset for the range end.
- */
- endOffset: number;
- /**
- * Collected execution count of the source range.
- */
- count: number;
- }
- /**
- * Coverage data for a JavaScript function.
- */
- interface FunctionCoverage {
- /**
- * JavaScript function name.
- */
- functionName: string;
- /**
- * Source ranges inside the function with coverage data.
- */
- ranges: CoverageRange[];
- /**
- * Whether coverage data for this function has block granularity.
- */
- isBlockCoverage: boolean;
- }
- /**
- * Coverage data for a JavaScript script.
- */
- interface ScriptCoverage {
- /**
- * JavaScript script id.
- */
- scriptId: Runtime.ScriptId;
- /**
- * JavaScript script name or url.
- */
- url: string;
- /**
- * Functions contained in the script that has coverage data.
- */
- functions: FunctionCoverage[];
- }
- /**
- * Describes a type collected during runtime.
- * @experimental
- */
- interface TypeObject {
- /**
- * Name of a type collected with type profiling.
- */
- name: string;
- }
- /**
- * Source offset and types for a parameter or return value.
- * @experimental
- */
- interface TypeProfileEntry {
- /**
- * Source offset of the parameter or end of function for return values.
- */
- offset: number;
- /**
- * The types for this parameter or return value.
- */
- types: TypeObject[];
- }
- /**
- * Type profile data collected during runtime for a JavaScript script.
- * @experimental
- */
- interface ScriptTypeProfile {
- /**
- * JavaScript script id.
- */
- scriptId: Runtime.ScriptId;
- /**
- * JavaScript script name or url.
- */
- url: string;
- /**
- * Type profile entries for parameters and return values of the functions in the script.
- */
- entries: TypeProfileEntry[];
- }
- interface SetSamplingIntervalParameterType {
- /**
- * New sampling interval in microseconds.
- */
- interval: number;
- }
- interface StartPreciseCoverageParameterType {
- /**
- * Collect accurate call counts beyond simple 'covered' or 'not covered'.
- */
- callCount?: boolean | undefined;
- /**
- * Collect block-based coverage.
- */
- detailed?: boolean | undefined;
- }
- interface StopReturnType {
- /**
- * Recorded profile.
- */
- profile: Profile;
- }
- interface TakePreciseCoverageReturnType {
- /**
- * Coverage data for the current isolate.
- */
- result: ScriptCoverage[];
- }
- interface GetBestEffortCoverageReturnType {
- /**
- * Coverage data for the current isolate.
- */
- result: ScriptCoverage[];
- }
- interface TakeTypeProfileReturnType {
- /**
- * Type profile for all scripts since startTypeProfile() was turned on.
- */
- result: ScriptTypeProfile[];
- }
- interface ConsoleProfileStartedEventDataType {
- id: string;
- /**
- * Location of console.profile().
- */
- location: Debugger.Location;
- /**
- * Profile title passed as an argument to console.profile().
- */
- title?: string | undefined;
- }
- interface ConsoleProfileFinishedEventDataType {
- id: string;
- /**
- * Location of console.profileEnd().
- */
- location: Debugger.Location;
- profile: Profile;
- /**
- * Profile title passed as an argument to console.profile().
- */
- title?: string | undefined;
- }
- }
- namespace HeapProfiler {
- /**
- * Heap snapshot object id.
- */
- type HeapSnapshotObjectId = string;
- /**
- * Sampling Heap Profile node. Holds callsite information, allocation statistics and child nodes.
- */
- interface SamplingHeapProfileNode {
- /**
- * Function location.
- */
- callFrame: Runtime.CallFrame;
- /**
- * Allocations size in bytes for the node excluding children.
- */
- selfSize: number;
- /**
- * Child nodes.
- */
- children: SamplingHeapProfileNode[];
- }
- /**
- * Profile.
- */
- interface SamplingHeapProfile {
- head: SamplingHeapProfileNode;
- }
- interface StartTrackingHeapObjectsParameterType {
- trackAllocations?: boolean | undefined;
- }
- interface StopTrackingHeapObjectsParameterType {
- /**
- * If true 'reportHeapSnapshotProgress' events will be generated while snapshot is being taken when the tracking is stopped.
- */
- reportProgress?: boolean | undefined;
- }
- interface TakeHeapSnapshotParameterType {
- /**
- * If true 'reportHeapSnapshotProgress' events will be generated while snapshot is being taken.
- */
- reportProgress?: boolean | undefined;
- }
- interface GetObjectByHeapObjectIdParameterType {
- objectId: HeapSnapshotObjectId;
- /**
- * Symbolic group name that can be used to release multiple objects.
- */
- objectGroup?: string | undefined;
- }
- interface AddInspectedHeapObjectParameterType {
- /**
- * Heap snapshot object id to be accessible by means of $x command line API.
- */
- heapObjectId: HeapSnapshotObjectId;
- }
- interface GetHeapObjectIdParameterType {
- /**
- * Identifier of the object to get heap object id for.
- */
- objectId: Runtime.RemoteObjectId;
- }
- interface StartSamplingParameterType {
- /**
- * Average sample interval in bytes. Poisson distribution is used for the intervals. The default value is 32768 bytes.
- */
- samplingInterval?: number | undefined;
- }
- interface GetObjectByHeapObjectIdReturnType {
- /**
- * Evaluation result.
- */
- result: Runtime.RemoteObject;
- }
- interface GetHeapObjectIdReturnType {
- /**
- * Id of the heap snapshot object corresponding to the passed remote object id.
- */
- heapSnapshotObjectId: HeapSnapshotObjectId;
- }
- interface StopSamplingReturnType {
- /**
- * Recorded sampling heap profile.
- */
- profile: SamplingHeapProfile;
- }
- interface GetSamplingProfileReturnType {
- /**
- * Return the sampling profile being collected.
- */
- profile: SamplingHeapProfile;
- }
- interface AddHeapSnapshotChunkEventDataType {
- chunk: string;
- }
- interface ReportHeapSnapshotProgressEventDataType {
- done: number;
- total: number;
- finished?: boolean | undefined;
- }
- interface LastSeenObjectIdEventDataType {
- lastSeenObjectId: number;
- timestamp: number;
- }
- interface HeapStatsUpdateEventDataType {
- /**
- * An array of triplets. Each triplet describes a fragment. The first integer is the fragment index, the second integer is a total count of objects for the fragment, the third integer is a total size of the objects for the fragment.
- */
- statsUpdate: number[];
- }
- }
- namespace NodeTracing {
- interface TraceConfig {
- /**
- * Controls how the trace buffer stores data.
- */
- recordMode?: string | undefined;
- /**
- * Included category filters.
- */
- includedCategories: string[];
- }
- interface StartParameterType {
- traceConfig: TraceConfig;
- }
- interface GetCategoriesReturnType {
- /**
- * A list of supported tracing categories.
- */
- categories: string[];
- }
- interface DataCollectedEventDataType {
- value: Array<{}>;
- }
- }
- namespace NodeWorker {
- type WorkerID = string;
- /**
- * Unique identifier of attached debugging session.
- */
- type SessionID = string;
- interface WorkerInfo {
- workerId: WorkerID;
- type: string;
- title: string;
- url: string;
- }
- interface SendMessageToWorkerParameterType {
- message: string;
- /**
- * Identifier of the session.
- */
- sessionId: SessionID;
- }
- interface EnableParameterType {
- /**
- * Whether to new workers should be paused until the frontend sends `Runtime.runIfWaitingForDebugger`
- * message to run them.
- */
- waitForDebuggerOnStart: boolean;
- }
- interface DetachParameterType {
- sessionId: SessionID;
- }
- interface AttachedToWorkerEventDataType {
- /**
- * Identifier assigned to the session used to send/receive messages.
- */
- sessionId: SessionID;
- workerInfo: WorkerInfo;
- waitingForDebugger: boolean;
- }
- interface DetachedFromWorkerEventDataType {
- /**
- * Detached session identifier.
- */
- sessionId: SessionID;
- }
- interface ReceivedMessageFromWorkerEventDataType {
- /**
- * Identifier of a session which sends a message.
- */
- sessionId: SessionID;
- message: string;
- }
- }
- namespace NodeRuntime {
- interface NotifyWhenWaitingForDisconnectParameterType {
- enabled: boolean;
- }
- }
- /**
- * The `inspector.Session` is used for dispatching messages to the V8 inspector
- * back-end and receiving message responses and notifications.
- */
- class Session extends EventEmitter {
- /**
- * Create a new instance of the inspector.Session class.
- * The inspector session needs to be connected through session.connect() before the messages can be dispatched to the inspector backend.
- */
- constructor();
- /**
- * Connects a session to the inspector back-end.
- * @since v8.0.0
- */
- connect(): void;
- /**
- * Immediately close the session. All pending message callbacks will be called
- * with an error. `session.connect()` will need to be called to be able to send
- * messages again. Reconnected session will lose all inspector state, such as
- * enabled agents or configured breakpoints.
- * @since v8.0.0
- */
- disconnect(): void;
- /**
- * Posts a message to the inspector back-end. `callback` will be notified when
- * a response is received. `callback` is a function that accepts two optional
- * arguments: error and message-specific result.
- *
- * ```js
- * session.post('Runtime.evaluate', { expression: '2 + 2' },
- * (error, { result }) => console.log(result));
- * // Output: { type: 'number', value: 4, description: '4' }
- * ```
- *
- * The latest version of the V8 inspector protocol is published on the [Chrome DevTools Protocol Viewer](https://chromedevtools.github.io/devtools-protocol/v8/).
- *
- * Node.js inspector supports all the Chrome DevTools Protocol domains declared
- * by V8\. Chrome DevTools Protocol domain provides an interface for interacting
- * with one of the runtime agents used to inspect the application state and listen
- * to the run-time events.
- *
- * ## Example usage
- *
- * Apart from the debugger, various V8 Profilers are available through the DevTools
- * protocol.
- * @since v8.0.0
- */
- post(method: string, params?: {}, callback?: (err: Error | null, params?: {}) => void): void;
- post(method: string, callback?: (err: Error | null, params?: {}) => void): void;
- /**
- * Returns supported domains.
- */
- post(method: 'Schema.getDomains', callback?: (err: Error | null, params: Schema.GetDomainsReturnType) => void): void;
- /**
- * Evaluates expression on global object.
- */
- post(method: 'Runtime.evaluate', params?: Runtime.EvaluateParameterType, callback?: (err: Error | null, params: Runtime.EvaluateReturnType) => void): void;
- post(method: 'Runtime.evaluate', callback?: (err: Error | null, params: Runtime.EvaluateReturnType) => void): void;
- /**
- * Add handler to promise with given promise object id.
- */
- post(method: 'Runtime.awaitPromise', params?: Runtime.AwaitPromiseParameterType, callback?: (err: Error | null, params: Runtime.AwaitPromiseReturnType) => void): void;
- post(method: 'Runtime.awaitPromise', callback?: (err: Error | null, params: Runtime.AwaitPromiseReturnType) => void): void;
- /**
- * Calls function with given declaration on the given object. Object group of the result is inherited from the target object.
- */
- post(method: 'Runtime.callFunctionOn', params?: Runtime.CallFunctionOnParameterType, callback?: (err: Error | null, params: Runtime.CallFunctionOnReturnType) => void): void;
- post(method: 'Runtime.callFunctionOn', callback?: (err: Error | null, params: Runtime.CallFunctionOnReturnType) => void): void;
- /**
- * Returns properties of a given object. Object group of the result is inherited from the target object.
- */
- post(method: 'Runtime.getProperties', params?: Runtime.GetPropertiesParameterType, callback?: (err: Error | null, params: Runtime.GetPropertiesReturnType) => void): void;
- post(method: 'Runtime.getProperties', callback?: (err: Error | null, params: Runtime.GetPropertiesReturnType) => void): void;
- /**
- * Releases remote object with given id.
- */
- post(method: 'Runtime.releaseObject', params?: Runtime.ReleaseObjectParameterType, callback?: (err: Error | null) => void): void;
- post(method: 'Runtime.releaseObject', callback?: (err: Error | null) => void): void;
- /**
- * Releases all remote objects that belong to a given group.
- */
- post(method: 'Runtime.releaseObjectGroup', params?: Runtime.ReleaseObjectGroupParameterType, callback?: (err: Error | null) => void): void;
- post(method: 'Runtime.releaseObjectGroup', callback?: (err: Error | null) => void): void;
- /**
- * Tells inspected instance to run if it was waiting for debugger to attach.
- */
- post(method: 'Runtime.runIfWaitingForDebugger', callback?: (err: Error | null) => void): void;
- /**
- * Enables reporting of execution contexts creation by means of executionContextCreated event. When the reporting gets enabled the event will be sent immediately for each existing execution context.
- */
- post(method: 'Runtime.enable', callback?: (err: Error | null) => void): void;
- /**
- * Disables reporting of execution contexts creation.
- */
- post(method: 'Runtime.disable', callback?: (err: Error | null) => void): void;
- /**
- * Discards collected exceptions and console API calls.
- */
- post(method: 'Runtime.discardConsoleEntries', callback?: (err: Error | null) => void): void;
- /**
- * @experimental
- */
- post(method: 'Runtime.setCustomObjectFormatterEnabled', params?: Runtime.SetCustomObjectFormatterEnabledParameterType, callback?: (err: Error | null) => void): void;
- post(method: 'Runtime.setCustomObjectFormatterEnabled', callback?: (err: Error | null) => void): void;
- /**
- * Compiles expression.
- */
- post(method: 'Runtime.compileScript', params?: Runtime.CompileScriptParameterType, callback?: (err: Error | null, params: Runtime.CompileScriptReturnType) => void): void;
- post(method: 'Runtime.compileScript', callback?: (err: Error | null, params: Runtime.CompileScriptReturnType) => void): void;
- /**
- * Runs script with given id in a given context.
- */
- post(method: 'Runtime.runScript', params?: Runtime.RunScriptParameterType, callback?: (err: Error | null, params: Runtime.RunScriptReturnType) => void): void;
- post(method: 'Runtime.runScript', callback?: (err: Error | null, params: Runtime.RunScriptReturnType) => void): void;
- post(method: 'Runtime.queryObjects', params?: Runtime.QueryObjectsParameterType, callback?: (err: Error | null, params: Runtime.QueryObjectsReturnType) => void): void;
- post(method: 'Runtime.queryObjects', callback?: (err: Error | null, params: Runtime.QueryObjectsReturnType) => void): void;
- /**
- * Returns all let, const and class variables from global scope.
- */
- post(
- method: 'Runtime.globalLexicalScopeNames',
- params?: Runtime.GlobalLexicalScopeNamesParameterType,
- callback?: (err: Error | null, params: Runtime.GlobalLexicalScopeNamesReturnType) => void
- ): void;
- post(method: 'Runtime.globalLexicalScopeNames', callback?: (err: Error | null, params: Runtime.GlobalLexicalScopeNamesReturnType) => void): void;
- /**
- * Enables debugger for the given page. Clients should not assume that the debugging has been enabled until the result for this command is received.
- */
- post(method: 'Debugger.enable', callback?: (err: Error | null, params: Debugger.EnableReturnType) => void): void;
- /**
- * Disables debugger for given page.
- */
- post(method: 'Debugger.disable', callback?: (err: Error | null) => void): void;
- /**
- * Activates / deactivates all breakpoints on the page.
- */
- post(method: 'Debugger.setBreakpointsActive', params?: Debugger.SetBreakpointsActiveParameterType, callback?: (err: Error | null) => void): void;
- post(method: 'Debugger.setBreakpointsActive', callback?: (err: Error | null) => void): void;
- /**
- * Makes page not interrupt on any pauses (breakpoint, exception, dom exception etc).
- */
- post(method: 'Debugger.setSkipAllPauses', params?: Debugger.SetSkipAllPausesParameterType, callback?: (err: Error | null) => void): void;
- post(method: 'Debugger.setSkipAllPauses', callback?: (err: Error | null) => void): void;
- /**
- * Sets JavaScript breakpoint at given location specified either by URL or URL regex. Once this command is issued, all existing parsed scripts will have breakpoints resolved and returned in locations property. Further matching script parsing will result in subsequent breakpointResolved events issued. This logical breakpoint will survive page reloads.
- */
- post(method: 'Debugger.setBreakpointByUrl', params?: Debugger.SetBreakpointByUrlParameterType, callback?: (err: Error | null, params: Debugger.SetBreakpointByUrlReturnType) => void): void;
- post(method: 'Debugger.setBreakpointByUrl', callback?: (err: Error | null, params: Debugger.SetBreakpointByUrlReturnType) => void): void;
- /**
- * Sets JavaScript breakpoint at a given location.
- */
- post(method: 'Debugger.setBreakpoint', params?: Debugger.SetBreakpointParameterType, callback?: (err: Error | null, params: Debugger.SetBreakpointReturnType) => void): void;
- post(method: 'Debugger.setBreakpoint', callback?: (err: Error | null, params: Debugger.SetBreakpointReturnType) => void): void;
- /**
- * Removes JavaScript breakpoint.
- */
- post(method: 'Debugger.removeBreakpoint', params?: Debugger.RemoveBreakpointParameterType, callback?: (err: Error | null) => void): void;
- post(method: 'Debugger.removeBreakpoint', callback?: (err: Error | null) => void): void;
- /**
- * Returns possible locations for breakpoint. scriptId in start and end range locations should be the same.
- */
- post(
- method: 'Debugger.getPossibleBreakpoints',
- params?: Debugger.GetPossibleBreakpointsParameterType,
- callback?: (err: Error | null, params: Debugger.GetPossibleBreakpointsReturnType) => void
- ): void;
- post(method: 'Debugger.getPossibleBreakpoints', callback?: (err: Error | null, params: Debugger.GetPossibleBreakpointsReturnType) => void): void;
- /**
- * Continues execution until specific location is reached.
- */
- post(method: 'Debugger.continueToLocation', params?: Debugger.ContinueToLocationParameterType, callback?: (err: Error | null) => void): void;
- post(method: 'Debugger.continueToLocation', callback?: (err: Error | null) => void): void;
- /**
- * @experimental
- */
- post(method: 'Debugger.pauseOnAsyncCall', params?: Debugger.PauseOnAsyncCallParameterType, callback?: (err: Error | null) => void): void;
- post(method: 'Debugger.pauseOnAsyncCall', callback?: (err: Error | null) => void): void;
- /**
- * Steps over the statement.
- */
- post(method: 'Debugger.stepOver', callback?: (err: Error | null) => void): void;
- /**
- * Steps into the function call.
- */
- post(method: 'Debugger.stepInto', params?: Debugger.StepIntoParameterType, callback?: (err: Error | null) => void): void;
- post(method: 'Debugger.stepInto', callback?: (err: Error | null) => void): void;
- /**
- * Steps out of the function call.
- */
- post(method: 'Debugger.stepOut', callback?: (err: Error | null) => void): void;
- /**
- * Stops on the next JavaScript statement.
- */
- post(method: 'Debugger.pause', callback?: (err: Error | null) => void): void;
- /**
- * This method is deprecated - use Debugger.stepInto with breakOnAsyncCall and Debugger.pauseOnAsyncTask instead. Steps into next scheduled async task if any is scheduled before next pause. Returns success when async task is actually scheduled, returns error if no task were scheduled or another scheduleStepIntoAsync was called.
- * @experimental
- */
- post(method: 'Debugger.scheduleStepIntoAsync', callback?: (err: Error | null) => void): void;
- /**
- * Resumes JavaScript execution.
- */
- post(method: 'Debugger.resume', callback?: (err: Error | null) => void): void;
- /**
- * Returns stack trace with given stackTraceId.
- * @experimental
- */
- post(method: 'Debugger.getStackTrace', params?: Debugger.GetStackTraceParameterType, callback?: (err: Error | null, params: Debugger.GetStackTraceReturnType) => void): void;
- post(method: 'Debugger.getStackTrace', callback?: (err: Error | null, params: Debugger.GetStackTraceReturnType) => void): void;
- /**
- * Searches for given string in script content.
- */
- post(method: 'Debugger.searchInContent', params?: Debugger.SearchInContentParameterType, callback?: (err: Error | null, params: Debugger.SearchInContentReturnType) => void): void;
- post(method: 'Debugger.searchInContent', callback?: (err: Error | null, params: Debugger.SearchInContentReturnType) => void): void;
- /**
- * Edits JavaScript source live.
- */
- post(method: 'Debugger.setScriptSource', params?: Debugger.SetScriptSourceParameterType, callback?: (err: Error | null, params: Debugger.SetScriptSourceReturnType) => void): void;
- post(method: 'Debugger.setScriptSource', callback?: (err: Error | null, params: Debugger.SetScriptSourceReturnType) => void): void;
- /**
- * Restarts particular call frame from the beginning.
- */
- post(method: 'Debugger.restartFrame', params?: Debugger.RestartFrameParameterType, callback?: (err: Error | null, params: Debugger.RestartFrameReturnType) => void): void;
- post(method: 'Debugger.restartFrame', callback?: (err: Error | null, params: Debugger.RestartFrameReturnType) => void): void;
- /**
- * Returns source for the script with given id.
- */
- post(method: 'Debugger.getScriptSource', params?: Debugger.GetScriptSourceParameterType, callback?: (err: Error | null, params: Debugger.GetScriptSourceReturnType) => void): void;
- post(method: 'Debugger.getScriptSource', callback?: (err: Error | null, params: Debugger.GetScriptSourceReturnType) => void): void;
- /**
- * Defines pause on exceptions state. Can be set to stop on all exceptions, uncaught exceptions or no exceptions. Initial pause on exceptions state is none.
- */
- post(method: 'Debugger.setPauseOnExceptions', params?: Debugger.SetPauseOnExceptionsParameterType, callback?: (err: Error | null) => void): void;
- post(method: 'Debugger.setPauseOnExceptions', callback?: (err: Error | null) => void): void;
- /**
- * Evaluates expression on a given call frame.
- */
- post(method: 'Debugger.evaluateOnCallFrame', params?: Debugger.EvaluateOnCallFrameParameterType, callback?: (err: Error | null, params: Debugger.EvaluateOnCallFrameReturnType) => void): void;
- post(method: 'Debugger.evaluateOnCallFrame', callback?: (err: Error | null, params: Debugger.EvaluateOnCallFrameReturnType) => void): void;
- /**
- * Changes value of variable in a callframe. Object-based scopes are not supported and must be mutated manually.
- */
- post(method: 'Debugger.setVariableValue', params?: Debugger.SetVariableValueParameterType, callback?: (err: Error | null) => void): void;
- post(method: 'Debugger.setVariableValue', callback?: (err: Error | null) => void): void;
- /**
- * Changes return value in top frame. Available only at return break position.
- * @experimental
- */
- post(method: 'Debugger.setReturnValue', params?: Debugger.SetReturnValueParameterType, callback?: (err: Error | null) => void): void;
- post(method: 'Debugger.setReturnValue', callback?: (err: Error | null) => void): void;
- /**
- * Enables or disables async call stacks tracking.
- */
- post(method: 'Debugger.setAsyncCallStackDepth', params?: Debugger.SetAsyncCallStackDepthParameterType, callback?: (err: Error | null) => void): void;
- post(method: 'Debugger.setAsyncCallStackDepth', callback?: (err: Error | null) => void): void;
- /**
- * Replace previous blackbox patterns with passed ones. Forces backend to skip stepping/pausing in scripts with url matching one of the patterns. VM will try to leave blackboxed script by performing 'step in' several times, finally resorting to 'step out' if unsuccessful.
- * @experimental
- */
- post(method: 'Debugger.setBlackboxPatterns', params?: Debugger.SetBlackboxPatternsParameterType, callback?: (err: Error | null) => void): void;
- post(method: 'Debugger.setBlackboxPatterns', callback?: (err: Error | null) => void): void;
- /**
- * Makes backend skip steps in the script in blackboxed ranges. VM will try leave blacklisted scripts by performing 'step in' several times, finally resorting to 'step out' if unsuccessful. Positions array contains positions where blackbox state is changed. First interval isn't blackboxed. Array should be sorted.
- * @experimental
- */
- post(method: 'Debugger.setBlackboxedRanges', params?: Debugger.SetBlackboxedRangesParameterType, callback?: (err: Error | null) => void): void;
- post(method: 'Debugger.setBlackboxedRanges', callback?: (err: Error | null) => void): void;
- /**
- * Enables console domain, sends the messages collected so far to the client by means of the messageAdded notification.
- */
- post(method: 'Console.enable', callback?: (err: Error | null) => void): void;
- /**
- * Disables console domain, prevents further console messages from being reported to the client.
- */
- post(method: 'Console.disable', callback?: (err: Error | null) => void): void;
- /**
- * Does nothing.
- */
- post(method: 'Console.clearMessages', callback?: (err: Error | null) => void): void;
- post(method: 'Profiler.enable', callback?: (err: Error | null) => void): void;
- post(method: 'Profiler.disable', callback?: (err: Error | null) => void): void;
- /**
- * Changes CPU profiler sampling interval. Must be called before CPU profiles recording started.
- */
- post(method: 'Profiler.setSamplingInterval', params?: Profiler.SetSamplingIntervalParameterType, callback?: (err: Error | null) => void): void;
- post(method: 'Profiler.setSamplingInterval', callback?: (err: Error | null) => void): void;
- post(method: 'Profiler.start', callback?: (err: Error | null) => void): void;
- post(method: 'Profiler.stop', callback?: (err: Error | null, params: Profiler.StopReturnType) => void): void;
- /**
- * Enable precise code coverage. Coverage data for JavaScript executed before enabling precise code coverage may be incomplete. Enabling prevents running optimized code and resets execution counters.
- */
- post(method: 'Profiler.startPreciseCoverage', params?: Profiler.StartPreciseCoverageParameterType, callback?: (err: Error | null) => void): void;
- post(method: 'Profiler.startPreciseCoverage', callback?: (err: Error | null) => void): void;
- /**
- * Disable precise code coverage. Disabling releases unnecessary execution count records and allows executing optimized code.
- */
- post(method: 'Profiler.stopPreciseCoverage', callback?: (err: Error | null) => void): void;
- /**
- * Collect coverage data for the current isolate, and resets execution counters. Precise code coverage needs to have started.
- */
- post(method: 'Profiler.takePreciseCoverage', callback?: (err: Error | null, params: Profiler.TakePreciseCoverageReturnType) => void): void;
- /**
- * Collect coverage data for the current isolate. The coverage data may be incomplete due to garbage collection.
- */
- post(method: 'Profiler.getBestEffortCoverage', callback?: (err: Error | null, params: Profiler.GetBestEffortCoverageReturnType) => void): void;
- /**
- * Enable type profile.
- * @experimental
- */
- post(method: 'Profiler.startTypeProfile', callback?: (err: Error | null) => void): void;
- /**
- * Disable type profile. Disabling releases type profile data collected so far.
- * @experimental
- */
- post(method: 'Profiler.stopTypeProfile', callback?: (err: Error | null) => void): void;
- /**
- * Collect type profile.
- * @experimental
- */
- post(method: 'Profiler.takeTypeProfile', callback?: (err: Error | null, params: Profiler.TakeTypeProfileReturnType) => void): void;
- post(method: 'HeapProfiler.enable', callback?: (err: Error | null) => void): void;
- post(method: 'HeapProfiler.disable', callback?: (err: Error | null) => void): void;
- post(method: 'HeapProfiler.startTrackingHeapObjects', params?: HeapProfiler.StartTrackingHeapObjectsParameterType, callback?: (err: Error | null) => void): void;
- post(method: 'HeapProfiler.startTrackingHeapObjects', callback?: (err: Error | null) => void): void;
- post(method: 'HeapProfiler.stopTrackingHeapObjects', params?: HeapProfiler.StopTrackingHeapObjectsParameterType, callback?: (err: Error | null) => void): void;
- post(method: 'HeapProfiler.stopTrackingHeapObjects', callback?: (err: Error | null) => void): void;
- post(method: 'HeapProfiler.takeHeapSnapshot', params?: HeapProfiler.TakeHeapSnapshotParameterType, callback?: (err: Error | null) => void): void;
- post(method: 'HeapProfiler.takeHeapSnapshot', callback?: (err: Error | null) => void): void;
- post(method: 'HeapProfiler.collectGarbage', callback?: (err: Error | null) => void): void;
- post(
- method: 'HeapProfiler.getObjectByHeapObjectId',
- params?: HeapProfiler.GetObjectByHeapObjectIdParameterType,
- callback?: (err: Error | null, params: HeapProfiler.GetObjectByHeapObjectIdReturnType) => void
- ): void;
- post(method: 'HeapProfiler.getObjectByHeapObjectId', callback?: (err: Error | null, params: HeapProfiler.GetObjectByHeapObjectIdReturnType) => void): void;
- /**
- * Enables console to refer to the node with given id via $x (see Command Line API for more details $x functions).
- */
- post(method: 'HeapProfiler.addInspectedHeapObject', params?: HeapProfiler.AddInspectedHeapObjectParameterType, callback?: (err: Error | null) => void): void;
- post(method: 'HeapProfiler.addInspectedHeapObject', callback?: (err: Error | null) => void): void;
- post(method: 'HeapProfiler.getHeapObjectId', params?: HeapProfiler.GetHeapObjectIdParameterType, callback?: (err: Error | null, params: HeapProfiler.GetHeapObjectIdReturnType) => void): void;
- post(method: 'HeapProfiler.getHeapObjectId', callback?: (err: Error | null, params: HeapProfiler.GetHeapObjectIdReturnType) => void): void;
- post(method: 'HeapProfiler.startSampling', params?: HeapProfiler.StartSamplingParameterType, callback?: (err: Error | null) => void): void;
- post(method: 'HeapProfiler.startSampling', callback?: (err: Error | null) => void): void;
- post(method: 'HeapProfiler.stopSampling', callback?: (err: Error | null, params: HeapProfiler.StopSamplingReturnType) => void): void;
- post(method: 'HeapProfiler.getSamplingProfile', callback?: (err: Error | null, params: HeapProfiler.GetSamplingProfileReturnType) => void): void;
- /**
- * Gets supported tracing categories.
- */
- post(method: 'NodeTracing.getCategories', callback?: (err: Error | null, params: NodeTracing.GetCategoriesReturnType) => void): void;
- /**
- * Start trace events collection.
- */
- post(method: 'NodeTracing.start', params?: NodeTracing.StartParameterType, callback?: (err: Error | null) => void): void;
- post(method: 'NodeTracing.start', callback?: (err: Error | null) => void): void;
- /**
- * Stop trace events collection. Remaining collected events will be sent as a sequence of
- * dataCollected events followed by tracingComplete event.
- */
- post(method: 'NodeTracing.stop', callback?: (err: Error | null) => void): void;
- /**
- * Sends protocol message over session with given id.
- */
- post(method: 'NodeWorker.sendMessageToWorker', params?: NodeWorker.SendMessageToWorkerParameterType, callback?: (err: Error | null) => void): void;
- post(method: 'NodeWorker.sendMessageToWorker', callback?: (err: Error | null) => void): void;
- /**
- * Instructs the inspector to attach to running workers. Will also attach to new workers
- * as they start
- */
- post(method: 'NodeWorker.enable', params?: NodeWorker.EnableParameterType, callback?: (err: Error | null) => void): void;
- post(method: 'NodeWorker.enable', callback?: (err: Error | null) => void): void;
- /**
- * Detaches from all running workers and disables attaching to new workers as they are started.
- */
- post(method: 'NodeWorker.disable', callback?: (err: Error | null) => void): void;
- /**
- * Detached from the worker with given sessionId.
- */
- post(method: 'NodeWorker.detach', params?: NodeWorker.DetachParameterType, callback?: (err: Error | null) => void): void;
- post(method: 'NodeWorker.detach', callback?: (err: Error | null) => void): void;
- /**
- * Enable the `NodeRuntime.waitingForDisconnect`.
- */
- post(method: 'NodeRuntime.notifyWhenWaitingForDisconnect', params?: NodeRuntime.NotifyWhenWaitingForDisconnectParameterType, callback?: (err: Error | null) => void): void;
- post(method: 'NodeRuntime.notifyWhenWaitingForDisconnect', callback?: (err: Error | null) => void): void;
- // Events
- addListener(event: string, listener: (...args: any[]) => void): this;
- /**
- * Emitted when any notification from the V8 Inspector is received.
- */
- addListener(event: 'inspectorNotification', listener: (message: InspectorNotification<{}>) => void): this;
- /**
- * Issued when new execution context is created.
- */
- addListener(event: 'Runtime.executionContextCreated', listener: (message: InspectorNotification) => void): this;
- /**
- * Issued when execution context is destroyed.
- */
- addListener(event: 'Runtime.executionContextDestroyed', listener: (message: InspectorNotification) => void): this;
- /**
- * Issued when all executionContexts were cleared in browser
- */
- addListener(event: 'Runtime.executionContextsCleared', listener: () => void): this;
- /**
- * Issued when exception was thrown and unhandled.
- */
- addListener(event: 'Runtime.exceptionThrown', listener: (message: InspectorNotification) => void): this;
- /**
- * Issued when unhandled exception was revoked.
- */
- addListener(event: 'Runtime.exceptionRevoked', listener: (message: InspectorNotification) => void): this;
- /**
- * Issued when console API was called.
- */
- addListener(event: 'Runtime.consoleAPICalled', listener: (message: InspectorNotification) => void): this;
- /**
- * Issued when object should be inspected (for example, as a result of inspect() command line API call).
- */
- addListener(event: 'Runtime.inspectRequested', listener: (message: InspectorNotification) => void): this;
- /**
- * Fired when virtual machine parses script. This event is also fired for all known and uncollected scripts upon enabling debugger.
- */
- addListener(event: 'Debugger.scriptParsed', listener: (message: InspectorNotification) => void): this;
- /**
- * Fired when virtual machine fails to parse the script.
- */
- addListener(event: 'Debugger.scriptFailedToParse', listener: (message: InspectorNotification) => void): this;
- /**
- * Fired when breakpoint is resolved to an actual script and location.
- */
- addListener(event: 'Debugger.breakpointResolved', listener: (message: InspectorNotification) => void): this;
- /**
- * Fired when the virtual machine stopped on breakpoint or exception or any other stop criteria.
- */
- addListener(event: 'Debugger.paused', listener: (message: InspectorNotification) => void): this;
- /**
- * Fired when the virtual machine resumed execution.
- */
- addListener(event: 'Debugger.resumed', listener: () => void): this;
- /**
- * Issued when new console message is added.
- */
- addListener(event: 'Console.messageAdded', listener: (message: InspectorNotification) => void): this;
- /**
- * Sent when new profile recording is started using console.profile() call.
- */
- addListener(event: 'Profiler.consoleProfileStarted', listener: (message: InspectorNotification) => void): this;
- addListener(event: 'Profiler.consoleProfileFinished', listener: (message: InspectorNotification) => void): this;
- addListener(event: 'HeapProfiler.addHeapSnapshotChunk', listener: (message: InspectorNotification) => void): this;
- addListener(event: 'HeapProfiler.resetProfiles', listener: () => void): this;
- addListener(event: 'HeapProfiler.reportHeapSnapshotProgress', listener: (message: InspectorNotification) => void): this;
- /**
- * If heap objects tracking has been started then backend regularly sends a current value for last seen object id and corresponding timestamp. If the were changes in the heap since last event then one or more heapStatsUpdate events will be sent before a new lastSeenObjectId event.
- */
- addListener(event: 'HeapProfiler.lastSeenObjectId', listener: (message: InspectorNotification) => void): this;
- /**
- * If heap objects tracking has been started then backend may send update for one or more fragments
- */
- addListener(event: 'HeapProfiler.heapStatsUpdate', listener: (message: InspectorNotification) => void): this;
- /**
- * Contains an bucket of collected trace events.
- */
- addListener(event: 'NodeTracing.dataCollected', listener: (message: InspectorNotification) => void): this;
- /**
- * Signals that tracing is stopped and there is no trace buffers pending flush, all data were
- * delivered via dataCollected events.
- */
- addListener(event: 'NodeTracing.tracingComplete', listener: () => void): this;
- /**
- * Issued when attached to a worker.
- */
- addListener(event: 'NodeWorker.attachedToWorker', listener: (message: InspectorNotification) => void): this;
- /**
- * Issued when detached from the worker.
- */
- addListener(event: 'NodeWorker.detachedFromWorker', listener: (message: InspectorNotification) => void): this;
- /**
- * Notifies about a new protocol message received from the session
- * (session ID is provided in attachedToWorker notification).
- */
- addListener(event: 'NodeWorker.receivedMessageFromWorker', listener: (message: InspectorNotification) => void): this;
- /**
- * This event is fired instead of `Runtime.executionContextDestroyed` when
- * enabled.
- * It is fired when the Node process finished all code execution and is
- * waiting for all frontends to disconnect.
- */
- addListener(event: 'NodeRuntime.waitingForDisconnect', listener: () => void): this;
- emit(event: string | symbol, ...args: any[]): boolean;
- emit(event: 'inspectorNotification', message: InspectorNotification<{}>): boolean;
- emit(event: 'Runtime.executionContextCreated', message: InspectorNotification): boolean;
- emit(event: 'Runtime.executionContextDestroyed', message: InspectorNotification): boolean;
- emit(event: 'Runtime.executionContextsCleared'): boolean;
- emit(event: 'Runtime.exceptionThrown', message: InspectorNotification): boolean;
- emit(event: 'Runtime.exceptionRevoked', message: InspectorNotification): boolean;
- emit(event: 'Runtime.consoleAPICalled', message: InspectorNotification): boolean;
- emit(event: 'Runtime.inspectRequested', message: InspectorNotification): boolean;
- emit(event: 'Debugger.scriptParsed', message: InspectorNotification): boolean;
- emit(event: 'Debugger.scriptFailedToParse', message: InspectorNotification): boolean;
- emit(event: 'Debugger.breakpointResolved', message: InspectorNotification): boolean;
- emit(event: 'Debugger.paused', message: InspectorNotification): boolean;
- emit(event: 'Debugger.resumed'): boolean;
- emit(event: 'Console.messageAdded', message: InspectorNotification): boolean;
- emit(event: 'Profiler.consoleProfileStarted', message: InspectorNotification): boolean;
- emit(event: 'Profiler.consoleProfileFinished', message: InspectorNotification): boolean;
- emit(event: 'HeapProfiler.addHeapSnapshotChunk', message: InspectorNotification): boolean;
- emit(event: 'HeapProfiler.resetProfiles'): boolean;
- emit(event: 'HeapProfiler.reportHeapSnapshotProgress', message: InspectorNotification): boolean;
- emit(event: 'HeapProfiler.lastSeenObjectId', message: InspectorNotification): boolean;
- emit(event: 'HeapProfiler.heapStatsUpdate', message: InspectorNotification): boolean;
- emit(event: 'NodeTracing.dataCollected', message: InspectorNotification): boolean;
- emit(event: 'NodeTracing.tracingComplete'): boolean;
- emit(event: 'NodeWorker.attachedToWorker', message: InspectorNotification): boolean;
- emit(event: 'NodeWorker.detachedFromWorker', message: InspectorNotification): boolean;
- emit(event: 'NodeWorker.receivedMessageFromWorker', message: InspectorNotification): boolean;
- emit(event: 'NodeRuntime.waitingForDisconnect'): boolean;
- on(event: string, listener: (...args: any[]) => void): this;
- /**
- * Emitted when any notification from the V8 Inspector is received.
- */
- on(event: 'inspectorNotification', listener: (message: InspectorNotification<{}>) => void): this;
- /**
- * Issued when new execution context is created.
- */
- on(event: 'Runtime.executionContextCreated', listener: (message: InspectorNotification) => void): this;
- /**
- * Issued when execution context is destroyed.
- */
- on(event: 'Runtime.executionContextDestroyed', listener: (message: InspectorNotification) => void): this;
- /**
- * Issued when all executionContexts were cleared in browser
- */
- on(event: 'Runtime.executionContextsCleared', listener: () => void): this;
- /**
- * Issued when exception was thrown and unhandled.
- */
- on(event: 'Runtime.exceptionThrown', listener: (message: InspectorNotification