Commit
·
00fb360
1
Parent(s):
557396c
Update parquet files (step 6 of 121)
Browse filesThis view is limited to 50 files because it contains too many changes.
See raw diff
- spaces/1acneusushi/gradio-2dmoleculeeditor/data/Crash Bandicoot N. Sane Trilogy [Crack Serial Key] Comparison and Analysis - How Does It Compare to the Original?.md +0 -126
- spaces/1acneusushi/gradio-2dmoleculeeditor/data/Download Surfaced By T.J. Yelden (.ePUB) [NEW].md +0 -16
- spaces/1gistliPinn/ChatGPT4/Examples/Evildeadallpartsinhinditorrentdownload.md +0 -9
- spaces/1phancelerku/anime-remove-background/Download and Play Hello Neighbor Full Act APK - The Scariest Game Ever.md +0 -94
- spaces/1phancelerku/anime-remove-background/Explore the Beauty and Diversity of Indonesia with Bus Simulator Indonesia HD.md +0 -181
- spaces/AI-Zero-to-Hero/07-SL-Chatbot-Blenderbot/README.md +0 -13
- spaces/AIGC-Audio/Make_An_Audio/ldm/modules/diffusionmodules/custom_openaimodel.py +0 -368
- spaces/AIML-TUDA/FairDiffusionExplorer/README.md +0 -13
- spaces/AIZero2HeroBootcamp/VideoToAnimatedGif/README.md +0 -13
- spaces/ATang0729/Forecast4Muses/Model/Model6/Model6_0_ClothesDetection/mmyolo/configs/yolov6/yolov6_t_syncbn_fast_8xb32-400e_coco.py +0 -17
- spaces/AchyuthGamer/OpenGPT/g4f/Provider/Providers/deprecated/Wewordle.py +0 -65
- spaces/AgentVerse/agentVerse/ui/src/phaser3-rex-plugins/plugins/cursoratbound-plugin.js +0 -20
- spaces/AgentVerse/agentVerse/ui/src/phaser3-rex-plugins/templates/ui/circularprogresscanvas/Factory.d.ts +0 -13
- spaces/AgentVerse/agentVerse/ui/src/phaser3-rex-plugins/templates/ui/gridsizer/RunWidthWrap.js +0 -25
- spaces/Aki004/herta-so-vits/utils.py +0 -543
- spaces/AlexWang/lama/saicinpainting/training/modules/multidilated_conv.py +0 -98
- spaces/Alican/pixera/data/base_dataset.py +0 -167
- spaces/Alpaca233/SadTalker/src/audio2pose_models/cvae.py +0 -149
- spaces/Amrrs/DragGan-Inversion/stylegan_human/training/loss.py +0 -159
- spaces/Androidonnxfork/CivitAi-to-Diffusers/diffusers/examples/community/README.md +0 -1769
- spaces/Androidonnxfork/CivitAi-to-Diffusers/diffusers/examples/community/magic_mix.py +0 -152
- spaces/Androidonnxfork/CivitAi-to-Diffusers/diffusers/examples/textual_inversion/README.md +0 -144
- spaces/Androidonnxfork/CivitAi-to-Diffusers/diffusers/src/diffusers/experimental/__init__.py +0 -1
- spaces/Andy1621/uniformer_image_detection/mmdet/datasets/pipelines/formating.py +0 -364
- spaces/Andy1621/uniformer_image_segmentation/configs/danet/danet_r50-d8_512x512_20k_voc12aug.py +0 -7
- spaces/Andy1621/uniformer_image_segmentation/configs/psanet/psanet_r101-d8_512x1024_40k_cityscapes.py +0 -2
- spaces/Ank0X0/Image-Upscaling-Playground/README.md +0 -14
- spaces/AnnasBlackHat/Image-Similarity/src/util/matrix.py +0 -5
- spaces/Anonymous-sub/Rerender/ControlNet/annotator/uniformer/mmcv/utils/trace.py +0 -23
- spaces/Arthur678/vits-uma-genshin-honkai/text/symbols.py +0 -39
- spaces/Awiny/Image2Paragraph/models/grit_src/third_party/CenterNet2/detectron2/engine/defaults.py +0 -715
- spaces/Awiny/Image2Paragraph/models/grit_src/third_party/CenterNet2/detectron2/modeling/meta_arch/semantic_seg.py +0 -260
- spaces/BENE2007/runwayml-stable-diffusion-v1-5/README.md +0 -13
- spaces/Benson/text-generation/Examples/Descarga Worldbox Desbloqueado Todos.md +0 -54
- spaces/Big-Web/MMSD/env/Lib/site-packages/pip/_vendor/chardet/gb2312freq.py +0 -284
- spaces/Big-Web/MMSD/env/Lib/site-packages/pip/_vendor/rich/_export_format.py +0 -76
- spaces/Big-Web/MMSD/env/Lib/site-packages/pkg_resources/_vendor/jaraco/text/__init__.py +0 -599
- spaces/BraydenMoore/MARCI-NFL-Betting/Source/Build/build.py +0 -197
- spaces/Brofu/Joeythemonster-anything-midjourney-v-4-1/app.py +0 -3
- spaces/CALM/Dashboard/perso/change_data.py +0 -19
- spaces/CVH-vn1210/make_hair/minigpt4/tasks/__init__.py +0 -26
- spaces/CVPR/Dual-Key_Backdoor_Attacks/datagen/detectron2/detectron2/layers/csrc/vision.cpp +0 -102
- spaces/CVPR/Dual-Key_Backdoor_Attacks/datagen/detectron2/docs/tutorials/README.md +0 -4
- spaces/CVPR/LIVE/thrust/thrust/detail/static_map.h +0 -170
- spaces/CVPR/LIVE/thrust/thrust/system/omp/detail/unique_by_key.h +0 -67
- spaces/ChevyWithAI/rvc-aicover/infer_pack/attentions.py +0 -417
- spaces/ChrisPreston/diff-svc_minato_aqua/app.py +0 -86
- spaces/CikeyQI/meme-api/meme_generator/memes/divorce/__init__.py +0 -18
- spaces/CreBea/Test2/Dockerfile +0 -21
- spaces/Cyril666/ContourNet-ABI/maskrcnn_benchmark/csrc/nms.h +0 -28
spaces/1acneusushi/gradio-2dmoleculeeditor/data/Crash Bandicoot N. Sane Trilogy [Crack Serial Key] Comparison and Analysis - How Does It Compare to the Original?.md
DELETED
@@ -1,126 +0,0 @@
|
|
1 |
-
|
2 |
-
<h1>Crash Bandicoot N. Sane Trilogy [Crack Serial Key]</h1>
|
3 |
-
<p>Are you a fan of the classic platformer game <strong>Crash Bandicoot</strong>? Do you want to relive the nostalgic moments of spinning, jumping, and wumping through three remastered games in one collection? If so, then you might be interested in <strong>Crash Bandicoot N. Sane Trilogy</strong>, a game that brings back your favorite marsupial in his enhanced, entranced, and ready-to-dance glory.</p>
|
4 |
-
<h2>Crash Bandicoot N. Sane Trilogy [Crack Serial Key</h2><br /><p><b><b>Download Zip</b> — <a href="https://byltly.com/2uKvFQ">https://byltly.com/2uKvFQ</a></b></p><br /><br />
|
5 |
-
<p>But what if you don't have enough money to buy the game or you don't want to pay for it? Is there a way to play the game for free on your PC? The answer is yes, but you will need a <strong>crack serial key</strong> to do so. In this article, we will explain what a crack serial key is, why you might need it, how to get it, and how to use it to activate Crash Bandicoot N. Sane Trilogy on your PC.</p>
|
6 |
-
<h2>What is Crash Bandicoot N. Sane Trilogy?</h2>
|
7 |
-
<h3>A brief introduction to the game and its features</h3>
|
8 |
-
<p>Crash Bandicoot N. Sane Trilogy is a collection of three remastered games from the original Crash Bandicoot series: Crash Bandicoot, Crash Bandicoot 2: Cortex Strikes Back, and Crash Bandicoot 3: Warped. The game was developed by Vicarious Visions and Iron Galaxy, and published by Activision in 2018 for PC, PlayStation 4, Xbox One, and Nintendo Switch.</p>
|
9 |
-
<p>The game features all the original levels, characters, enemies, bosses, and secrets from the original games, but with improved graphics, sound, gameplay, and controls. You can experience Crash Bandicoot like never before in his fully-remastered graphical glory and get ready to put some UMPH in your WUMP!</p>
|
10 |
-
<p>The game also includes two new levels that were previously unfinished and unreleased: Stormy Ascent and Future Tense. Stormy Ascent is a challenging level from the first game that will test your skills and patience as you dodge vials, birds, spikes, and platforms. Future Tense is a new level inspired by the cut Waterfall Level from the first game that features puzzles and obstacles set in a futuristic skyscraper.</p>
|
11 |
-
<h3>How to download and install the game on PC</h3>
|
12 |
-
<p>If you want to play Crash Bandicoot N. Sane Trilogy on your PC, you will need to follow these steps:</p>
|
13 |
-
<ol>
|
14 |
-
<li>Make sure your PC meets the minimum system requirements for the game. You will need Windows 7 or higher, an Intel Core i5-750 or AMD Phenom II X4 965 processor, 8 GB of RAM, an NVIDIA GeForce GTX 660 or AMD Radeon HD 7850 graphics card, 30 GB of available storage space, and a DirectX 9.0c compatible sound card.</li>
|
15 |
-
<li>Buy the game from an official source such as Steam or Activision's website. You will need to create an account and pay for the game using your preferred method of payment.</li>
|
16 |
-
<li>Download the game files using the provided link or launcher. You will need a stable internet connection and enough bandwidth to download about 30 GB of data.</li>
|
17 |
-
<li>Install the game on your PC by following the instructions on the screen. You will need to agree to the terms and conditions and choose a destination folder for the game files.</li>
|
18 |
-
<li>Launch the game from your desktop or start menu shortcut. You will need to log in with your account credentials and verify your ownership of the game.</li>
|
19 |
-
<li>Enjoy playing Crash Bandicoot N. Sane Trilogy on your PC!</li>
|
20 |
-
</ol>
|
21 |
-
<h2>What is a crack serial key and why do you need it?</h2>
|
22 |
-
<h3>The benefits of using a crack serial key for Crash Bandicoot N. Sane Trilogy</h3>
|
23 |
-
<p>A crack serial key is a code that can bypass the security measures of a software or game and allow you to use it without paying for it or verifying your ownership. A crack serial key can be generated by hackers or programmers who exploit the vulnerabilities or loopholes of the software or game.</p>
|
24 |
-
<p>Crash Bandicoot N. Sane Trilogy [Crack Activation Code<br />
|
25 |
-
Crash Bandicoot N. Sane Trilogy [Crack License Key<br />
|
26 |
-
Crash Bandicoot N. Sane Trilogy [Crack Product Key<br />
|
27 |
-
Crash Bandicoot N. Sane Trilogy [Crack Registration Code<br />
|
28 |
-
Crash Bandicoot N. Sane Trilogy [Crack Keygen Download<br />
|
29 |
-
Crash Bandicoot N. Sane Trilogy [Crack Torrent Free<br />
|
30 |
-
Crash Bandicoot N. Sane Trilogy [Crack Full Version PC<br />
|
31 |
-
Crash Bandicoot N. Sane Trilogy [Crack Patch Update<br />
|
32 |
-
Crash Bandicoot N. Sane Trilogy [Crack No CD/DVD<br />
|
33 |
-
Crash Bandicoot N. Sane Trilogy [Crack Steam Fix<br />
|
34 |
-
Crash Bandicoot N. Sane Trilogy [Crack Online Multiplayer<br />
|
35 |
-
Crash Bandicoot N. Sane Trilogy [Crack Skidrow Reloaded<br />
|
36 |
-
Crash Bandicoot N. Sane Trilogy [Crack CPY Codex<br />
|
37 |
-
Crash Bandicoot N. Sane Trilogy [Crack FitGirl Repack<br />
|
38 |
-
Crash Bandicoot N. Sane Trilogy [Crack Razor1911 Scene<br />
|
39 |
-
Crash Bandicoot N. Sane Trilogy [Crack Mega.nz Link<br />
|
40 |
-
Crash Bandicoot N. Sane Trilogy [Crack Google Drive Link<br />
|
41 |
-
Crash Bandicoot N. Sane Trilogy [Crack Direct Download Link<br />
|
42 |
-
Crash Bandicoot N. Sane Trilogy [Crack Highly Compressed<br />
|
43 |
-
Crash Bandicoot N. Sane Trilogy [Crack ISO File Download<br />
|
44 |
-
Crash Bandicoot N. Sane Trilogy [Crack RAR Password Unlocker<br />
|
45 |
-
Crash Bandicoot N. Sane Trilogy [Crack How to Install Guide<br />
|
46 |
-
Crash Bandicoot N. Sane Trilogy [Crack System Requirements<br />
|
47 |
-
Crash Bandicoot N. Sane Trilogy [Crack Gameplay Review<br />
|
48 |
-
Crash Bandicoot N. Sane Trilogy [Crack Tips and Tricks<br />
|
49 |
-
Crash Bandicoot N. Sane Trilogy [Crack Cheats and Hacks<br />
|
50 |
-
Crash Bandicoot N. Sane Trilogy [Crack Mods and Customization<br />
|
51 |
-
Crash Bandicoot N. Sane Trilogy [Crack Remastered Edition<br />
|
52 |
-
Crash Bandicoot N. Sane Trilogy [Crack Bonus Content DLC<br />
|
53 |
-
Crash Bandicoot N. Sane Trilogy [Crack OST Soundtrack Download<br />
|
54 |
-
Crash Bandicoot N. Sane Trilogy [Crack Wallpaper HD Download<br />
|
55 |
-
Crash Bandicoot N. Sane Trilogy [Crack Fan Art and Memes<br />
|
56 |
-
Crash Bandicoot N. Sane Trilogy [Crack Comparison with Original<br />
|
57 |
-
Crash Bandicoot N. Sane Trilogy [Crack Best Settings for PC<br />
|
58 |
-
Crash Bandicoot N. Sane Trilogy [Crack Controller Support PC<br />
|
59 |
-
Crash Bandicoot N. Sane Trilogy [Crack Save Game Location PC<br />
|
60 |
-
Crash Bandicoot N. Sane Trilogy [Crack Error Fix and Solution PC<br />
|
61 |
-
Crash Bandicoot N. Sane Trilogy [Crack Free Steam Key Giveaway<br />
|
62 |
-
Crash Bandicoot N. Sane Trilogy [Crack Discount Coupon Code PC<br />
|
63 |
-
Crash Bandicoot N. Sane Trilogy [Crack Buy Official Game PC<br />
|
64 |
-
Crash Bandicoot N. Sane Trilogy [Crack PS4 Xbox One Switch Version<br />
|
65 |
-
Crash Bandicoot N. Sane Trilogy [Crack Mobile Android iOS Version<br />
|
66 |
-
Crash Bandicoot N. Sane Trilogy [Crack VR Oculus Rift Version<br />
|
67 |
-
Crash Bandicoot N. Sane Trilogy [Crack Co-op Split Screen Mode PC<br />
|
68 |
-
Crash Bandicoot N. Sane Trilogy [Crack Speedrun World Record PC<br />
|
69 |
-
Crash Bandicoot N. Sane Trilogy [Crack All Levels and Secrets PC<br />
|
70 |
-
Crash Bandicoot N. Sane Trilogy [Crack All Characters and Skins PC<br />
|
71 |
-
Crash Bandicoot N. Sane Trilogy [Crack All Bosses and Enemies PC</p>
|
72 |
-
<p>The main benefit of using a crack serial key for Crash Bandicoot N. Sane Trilogy is that you can play the game for free on your PC without buying it or verifying it with an official source. This can save you money and time, especially if you are not sure if you like the game or not.</p>
|
73 |
-
<h3>The risks and drawbacks of using a crack serial key for Crash Bandicoot N. Sane Trilogy</h3>
|
74 |
-
<p>However, using a crack serial key for Crash Bandicoot N. Sane Trilogy also comes with some risks and drawbacks that you should be aware of before deciding to use one:</p>
|
75 |
-
<ul>
|
76 |
-
<li>You might be breaking the law by using a crack serial key for Crash Bandicoot N. Sane Trilogy. Depending on your country's laws and regulations, using a crack serial key might be considered as piracy or theft of intellectual property, which can result in legal consequences such as fines or imprisonment.</li>
|
77 |
-
<li>You might be harming the developers and publishers of Crash Bandicoot N. Sane Trilogy by using a crack serial key. By not paying for the game or supporting its official sources, you are depriving them of their rightful income and recognition for their hard work and creativity.</li>
|
78 |
-
<li>You might be exposing your PC to viruses or malware by using a crack serial key for Crash Bandicoot N. Sane Trilogy. Some crack serial keys might contain malicious code that can infect your PC with viruses or malware that can damage your files, steal your data, or compromise your security.</li>
|
79 |
-
<li>You might be missing out on updates or features by using a crack serial key for Crash Bandicoot N. Sane Trilogy. Some crack serial keys might not work with newer versions of the game or prevent you from accessing online features such as multiplayer modes or leaderboards.</li>
|
80 |
-
</ul>
|
81 |
-
<h2>How to get a crack serial key for Crash Bandicoot N. Sane Trilogy</h2>
|
82 |
-
<h3>The best sources and websites to find a crack serial key for Crash Bandicoot N. Sane Trilogy</h3>
|
83 |
-
<p>If you still want to use a crack serial key for Crash Bandicoot N. Sane Trilogy despite knowing its risks and drawbacks, then you will need to find one from reliable sources and websites that offer them for free or at low prices.</p>
|
84 |
-
<p>However, finding a working crack serial key for Crash Bandicoot N. Sane Trilogy can be challenging as there are many fake or scam websites that claim to offer them but only want to trick you into downloading viruses or malware or paying for something else.</p>
|
85 |
-
<p>To help you avoid these scams and find genuine sources and websites that offer crack serial keys for Crash Bandicoot N. Sane Trilogy, we have compiled a list of some of the best ones based on their popularity, reputation, quality, availability, and safety:</p>
|
86 |
-
<h4>Skidrow Cracked</h4>
|
87 |
-
<p><a href="https://skidrowcracked.com/crash-bandicoot-n-sane-trilogy-codex/">Skidrow Cracked</a> is one of the most popular websites that offer free download links for cracked games such as Crash Bandicoot N. Sane Trilogy-CODEX.</p>
|
88 |
-
<p>This website provides direct links for downloading the game files as well as instructions on how to install them on your PC.</p>
|
89 |
-
```html website also has a comment section where you can ask questions or share feedback with other users.</p>
|
90 |
-
<p>However, you should be careful when downloading files from this website as they might contain viruses or malware that can harm your PC. You should also use a VPN or proxy to hide your IP address and avoid legal issues.</p>
|
91 |
-
<h4>CDKeys</h4>
|
92 |
-
<p><a href="https://www.cdkeys.com/pc/games/crash-bandicoot-n-sane-trilogy-pc-steam-key">CDKeys</a> is one of the most reputable websites that offer cheap and legit keys for games such as Crash Bandicoot N. Sane Trilogy PC.</p>
|
93 |
-
<p>This website provides instant delivery of the keys via email or digital download. You can also check the reviews and ratings of the keys from other customers before buying them.</p>
|
94 |
-
<p>The website also has a customer service team that can help you with any issues or queries you might have regarding your purchase.</p>
|
95 |
-
<p>However, you should be aware that some keys might not work in certain regions or platforms. You should also check the terms and conditions and refund policy of the website before buying anything.</p>
|
96 |
-
<h4>G2A</h4>
|
97 |
-
<p><a href="https://www.g2a.com/crash-bandicoot-n-sane-trilogy-steam-key-global-i10000081158003">G2A</a> is one of the largest online marketplaces that offer a wide range of products and services related to gaming, including keys for games such as Crash Bandicoot N. Sane Trilogy Steam Key GLOBAL.</p>
|
98 |
-
<p>This website allows you to buy and sell keys from different sellers and buyers around the world. You can also compare prices and ratings of the keys from different sources and choose the best one for you.</p>
|
99 |
-
<p>The website also has a protection program that guarantees your satisfaction and security when buying or selling keys. You can also contact the support team or the seller directly if you have any problems or questions.</p>
|
100 |
-
<p>However, you should be careful when buying or selling keys on this website as there might be some fraudulent or scam transactions. You should also read the description and details of the keys carefully before buying or selling them.</p>
|
101 |
-
<h4>YouTube</h4>
|
102 |
-
<p><a href="https://www.youtube.com/watch?v=z4BFqd1dCRQ">YouTube</a> is one of the most popular video-sharing platforms that offer a variety of content and information related to gaming, including videos on how to get a crack serial key for Crash Bandicoot N. Sane Trilogy for free.</p>
|
103 |
-
<p>This platform allows you to watch and learn from different video tutorials and guides on how to download, install, and activate the game with a crack serial key. You can also subscribe to different channels and creators that offer more tips and tricks on gaming.</p>
|
104 |
-
<p>The platform also has a comment section where you can interact with other viewers and share your opinions or feedback on the videos.</p>
|
105 |
-
<p>However, you should be wary when watching or following videos on this platform as they might contain false or misleading information or links that can lead you to viruses or malware. You should also use an ad-blocker or skip the ads that might appear on the videos.</p>
|
106 |
-
<h3>The steps to activate the game with a crack serial key</h3>
|
107 |
-
<p>If you have found a working crack serial key for Crash Bandicoot N. Sane Trilogy from one of the sources or websites mentioned above, then you will need to follow these steps to activate the game with it:</p>
|
108 |
-
<ol>
|
109 |
-
<li>Copy the crack serial key from the source or website where you got it from.</li>
|
110 |
-
<li>Open Steam and log in with your account credentials.</li>
|
111 |
-
<li>Click on Games in the menu bar and select Activate a Product on Steam.</li>
|
112 |
-
<li>Click on Next and agree to the terms and conditions.</li>
|
113 |
-
<li>Paste the crack serial key in the Product Code box and click on Next.</li>
|
114 |
-
<li>Wait for Steam to verify and activate your product.</li>
|
115 |
-
<li>Once activated, you can download and play Crash Bandicoot N. Sane Trilogy on your PC!</li>
|
116 |
-
</ol>
|
117 |
-
<h2>Conclusion</h2>
|
118 |
-
<h3>A summary of the main points and a call to action</h3>
|
119 |
-
<p>In conclusion, Crash Bandicoot N. Sane Trilogy is a collection of three remastered games from the original Crash Bandicoot series that lets you experience Crash Bandicoot like never before in his fully-remastered graphical glory.</p>
|
120 |
-
<p>If you want to play the game for free on your PC without buying it or verifying it with an official source, then you will need a crack serial key that can bypass the security measures of the game and allow you to use it without paying for it or verifying your ownership.</p>
|
121 |
-
<p>You can find a crack serial key for Crash Bandicoot N. Sane Trilogy from different sources and websites such as Skidrow Cracked, CDKeys, G2A, or YouTube. However, you should be aware of the risks and drawbacks of using a crack serial key such as breaking the law, harming the developers, exposing your PC to viruses, or missing out on updates or features.</p>
|
122 |
-
<p>If you have found a working crack serial key for Crash Bandicoot N. Sane Trilogy, then you can activate the game with it by following some simple steps on Steam.</p>
|
123 |
-
<p>We hope this article has helped you understand what a crack serial key is, why you might need it, how to get it, and how to use it to activate Crash Bandicoot N. Sane Trilogy on your PC. However, we do not encourage or endorse piracy or theft of intellectual property. We recommend that you buy the game from an official source such as Steam or Activision's website if you want to support the developers and enjoy the game fully and legally.</p>
|
124 |
-
FAQs: Q: What is Crash Bandicoot N. Sane Trilogy? A: Crash Bandicoot N. Sane Trilogy is a collection of three remastered games from the original Crash Bandicoot series: Crash Bandicoot, Crash Bandicoot 2: Cortex Strikes Back, and Crash Bandicoot 3: Warped. Q: What is a crack serial key? A: A crack serial key is a code that can bypass the security measures of a software or game and allow you to use it without paying for it or verifying your ownership. Q: How to get a crack serial key for Crash Bandicoot N. Sane Trilogy? A: You can get a crack serial key for Crash Bandicoot N. Sane Trilogy from different sources and websites such as Skidrow Cracked, CDKeys, G2A, or YouTube. Q: How to use a crack serial key for Crash Bandicoot N. Sane Trilogy? A: You can use a crack serial key for Crash Bandicoot N. Sane Trilogy by copying it from the source or website where you got it from and pasting it in the Product Code box when activating a product on Steam. Q: What are the risks and drawbacks of using a crack serial key for Crash Bandicoot N. Sane Trilogy? A: Some of the risks and drawbacks of using a crack serial key for Crash Bandicoot N. Sane Trilogy are breaking the law, harming the developers, exposing your PC to viruses, or missing out on updates or features. </p> 0a6ba089eb<br />
|
125 |
-
<br />
|
126 |
-
<br />
|
|
|
|
|
|
|
|
|
|
|
|
|
|
|
|
|
|
|
|
|
|
|
|
|
|
|
|
|
|
|
|
|
|
|
|
|
|
|
|
|
|
|
|
|
|
|
|
|
|
|
|
|
|
|
|
|
|
|
|
|
|
|
|
|
|
|
|
|
|
|
|
|
|
|
|
|
|
|
|
|
|
|
|
|
|
|
|
|
|
|
|
|
|
|
|
|
|
|
|
|
|
|
|
|
|
|
|
|
|
|
|
|
|
|
|
|
|
|
|
|
|
|
|
|
|
|
|
|
|
|
|
|
|
|
|
|
|
|
|
|
|
|
|
|
|
|
|
|
|
|
|
|
|
|
|
|
|
|
|
|
|
|
|
|
|
|
|
|
|
|
|
|
|
|
|
|
|
|
|
|
|
|
|
|
|
|
|
|
|
|
|
|
|
|
|
|
|
|
|
|
|
|
|
|
|
|
|
|
|
|
|
|
|
|
|
|
|
|
|
|
|
|
|
|
|
|
|
|
|
|
|
|
|
|
|
|
|
|
|
|
|
|
|
|
|
|
|
|
|
|
|
|
|
spaces/1acneusushi/gradio-2dmoleculeeditor/data/Download Surfaced By T.J. Yelden (.ePUB) [NEW].md
DELETED
@@ -1,16 +0,0 @@
|
|
1 |
-
|
2 |
-
<h1>Surfaced by T.J. Yelden: A thrilling sequel to Hidden</h1>
|
3 |
-
<p>If you are a fan of paranormal romance and urban fantasy, you might want to check out <em>Surfaced</em>, the second book in the <em>Hidden Trilogy</em> by T.J. Yelden. This book follows the adventures of Kendra, a rare white wolf shifter who has to learn how to control her wolf side while dealing with the dangers and mysteries of the shifter world.</p>
|
4 |
-
<h2>Download Surfaced by T.J. Yelden (.ePUB)</h2><br /><p><b><b>Download Zip</b> 🆗 <a href="https://byltly.com/2uKveS">https://byltly.com/2uKveS</a></b></p><br /><br />
|
5 |
-
<p>In <em>Surfaced</em>, Kendra is starting college and trying to cope with the long-distance relationship with her boyfriend Cade, who is off to High Council Enforcer Training for five years. She also has to face a stalker wolf from another pack, meet other shifters with their own agendas, and stay under the radar of the Shifter High Council, who are not happy about her existence. Along the way, she discovers more about her past, her present, and her future as a wolf shifter.</p>
|
6 |
-
<p><em>Surfaced</em> is a fast-paced and engaging read that will keep you hooked until the end. The book has a perfect balance of humor, action, romance, and suspense. The characters are well-developed and likable, especially Kendra, who is a strong and sassy heroine. The plot is full of twists and turns that will keep you guessing and surprised. The book also ends with a cliffhanger that will make you eager for the third and final book in the trilogy.</p>
|
7 |
-
<p>You can get <em>Surfaced</em> as an ebook from Amazon for $2.99 or read it for free with Kindle Unlimited[^2^]. You can also find more information and reviews about the book on Goodreads[^1^]. If you haven't read the first book in the trilogy, <em>Hidden</em>, you can also get it from Amazon or Kindle Unlimited[^2^].</p>
|
8 |
-
<p>If you are looking for a captivating and entertaining paranormal romance series with a unique twist on wolf shifters, you should definitely give <em>Surfaced</em> and <em>Hidden</em> by T.J. Yelden a try.</p>
|
9 |
-
|
10 |
-
<p>What makes <em>Surfaced</em> and <em>Hidden</em> stand out from other paranormal romance books is the author's creative and original take on wolf shifters. T.J. Yelden has created a rich and complex world where shifters have their own history, culture, politics, and rules. She also explores the themes of identity, belonging, loyalty, and love in a realistic and relatable way.</p>
|
11 |
-
<p>The author's writing style is smooth and captivating, with vivid descriptions and witty dialogues. She also knows how to build tension and suspense, as well as create steamy and sweet romance scenes. The books are written in the first-person point of view of Kendra, which allows the reader to get inside her head and feel her emotions.</p>
|
12 |
-
<p></p>
|
13 |
-
<p><em>Surfaced</em> and <em>Hidden</em> are books that will make you laugh, cry, swoon, and gasp. They are perfect for fans of paranormal romance who are looking for something fresh and exciting. The books have received rave reviews from readers who have praised the author's storytelling skills and the characters' chemistry. The books have also been featured on several lists of best shifter romance books on Goodreads.</p>
|
14 |
-
<p>If you want to dive into a thrilling and romantic adventure with Kendra and Cade, don't miss <em>Surfaced</em> and <em>Hidden</em> by T.J. Yelden. You can get them from Amazon or Kindle Unlimited today.</p> 81aa517590<br />
|
15 |
-
<br />
|
16 |
-
<br />
|
|
|
|
|
|
|
|
|
|
|
|
|
|
|
|
|
|
|
|
|
|
|
|
|
|
|
|
|
|
|
|
|
|
spaces/1gistliPinn/ChatGPT4/Examples/Evildeadallpartsinhinditorrentdownload.md
DELETED
@@ -1,9 +0,0 @@
|
|
1 |
-
|
2 |
-
<p>43ad871fa5 evildeadallpartsinhinditorrentdownload https://coub.com/stories/2195049-evildeadallpartsinhinditorrentdownload. nsaidow yadgr https://coub.com/stories/1015874-flash-player-70-codex-update-1471-0-fo pings.mfsdesigns.com,evildeadallpartsinhinditorrentdownload.indiegogo </p>
|
3 |
-
<h2>evildeadallpartsinhinditorrentdownload</h2><br /><p><b><b>Download File</b> ✪ <a href="https://imgfil.com/2uy242">https://imgfil.com/2uy242</a></b></p><br /><br />
|
4 |
-
<p>evildeadallpartsinhinditorrentdownload https://coub.com/stories/2216006-evildeadallpartsinhinditorrentdownload-dean-merchant.http://evildeadallpartsinhinditorrentdownload-download.evildeadallpartsinhinditorrentdownload-evildeadallpartsinhinditorrentdownload-download.evildeadallpartsinhinditorrentdownload-download.evildeadallpartsinhinditorrentdownload-evildeadallpartsinhinditorrentdownload-evildeadallpartsinhinditorrentdownload-evildeadallpartsinhinditorrentdownload-evildeadallpartsinhinditorrentdownload-evildeadallpartsinhinditorrentdownload-evildeadallpartsinhinditorrentdownload-evildeadallpartsinhinditorrentdownload-evildeadallpartsinhinditorrentdownload-evildeadallpartsinhinditorrentdownload-evildeadallpartsinhinditorrentdownload-evildeadallpartsinhinditorrentdownload-evildeadallpartsinhinditorrentdownload-evildeadallpartsinhinditorrentdownload-evildeadallpartsinhinditorrentdownload. </p>
|
5 |
-
<p>2185351 kms pico office for mac veselue2.di.dud.5-2.3.2.9v2,evildeadallpartsinhinditorrentdownload,evildeadallpartsinhinditorrentdownload-desires 0db76fd2b3c https://coub.com/stories/2200653-evildeadallpartsinhinditorrentdownload-tensor. </p>
|
6 |
-
<p>evildeadallpartsinhinditorrentdownload https://coub.com/stories/2209137-taming-bull https://coub.com/stories/2195055-evildeadallpartsinhinditorrentdownload-chavegard. http://kiyosans.sblo.jp/article/188916753.html. Posted by moyzaka at 20220206 22:47. evildeadallpartsinhinditorrentdownload, </p>
|
7 |
-
<p></p> 899543212b<br />
|
8 |
-
<br />
|
9 |
-
<br />
|
|
|
|
|
|
|
|
|
|
|
|
|
|
|
|
|
|
|
|
spaces/1phancelerku/anime-remove-background/Download and Play Hello Neighbor Full Act APK - The Scariest Game Ever.md
DELETED
@@ -1,94 +0,0 @@
|
|
1 |
-
<br />
|
2 |
-
<h1>How to Download Hello Neighbor Full Act APK for Android</h1>
|
3 |
-
<p>If you are a fan of stealth horror games, you might have heard of <strong>Hello Neighbor</strong>, a game where you have to sneak into your neighbor's house and find out what he is hiding in his basement. But did you know that you can download and play the full version of Hello Neighbor on your Android device? In this article, we will show you how to download Hello Neighbor Full Act APK, a file that contains the complete game with all its acts and modes. We will also explain what an APK file is, how to install it, and how to play Hello Neighbor Full Act APK on your Android device.</p>
|
4 |
-
<h2>What is Hello Neighbor?</h2>
|
5 |
-
<p>Hello Neighbor is a stealth horror game developed by Dynamic Pixels and tinyBuild. It was released in 2017 for Windows, Xbox One, PlayStation 4, Nintendo Switch, iOS, and Android. The game has received positive reviews from critics and players for its unique gameplay, graphics, and story.</p>
|
6 |
-
<h2>download hello neighbor full act apk</h2><br /><p><b><b>Download</b> ✓ <a href="https://jinyurl.com/2uNRjR">https://jinyurl.com/2uNRjR</a></b></p><br /><br />
|
7 |
-
<h3>A stealth horror game with an advanced AI</h3>
|
8 |
-
<p>The main feature of Hello Neighbor is its <strong>advanced AI</strong> that learns from your every move. You play as a curious kid who wants to find out what your neighbor is hiding in his basement. However, your neighbor is not a friendly guy. He will chase you, set traps, and use cameras to stop you from entering his house. The more you sneak around, the more he adapts to your behavior and becomes smarter and harder to avoid.</p>
|
9 |
-
<h3>A popular game with multiple acts and modes</h3>
|
10 |
-
<p>Hello Neighbor has a <strong>story mode</strong> that consists of four acts. Each act has a different setting, objective, and difficulty level. You have to use your wits, skills, and items to solve puzzles, unlock doors, and escape from the neighbor. The game also has a <strong>secret mode</strong> that reveals more about the neighbor's backstory and motives. Additionally, there are other modes such as <strong>hide and seek</strong>, where you play as the neighbor's children; <strong>ghost mode</strong>, where you can explore the house without being detected; and <strong>sandbox mode</strong>, where you can create your own scenarios and challenges.</p>
|
11 |
-
<h2>What is an APK file?</h2>
|
12 |
-
<p>An APK file is a package file format used by the Android operating system for distribution and installation of mobile applications. It contains all the code, resources, assets, certificates, and manifest file of an app. An APK file can be built from source code written in either Java or Kotlin.</p>
|
13 |
-
<h3>A package file format for Android apps</h3>
|
14 |
-
<p>An APK file is similar to other software packages such as APPX in Windows or DEB in Debian-based operating systems. To make an APK file, a program for Android is first compiled using a tool such as Android Studio or Visual Studio and then all of its parts are packaged into one container file. An APK file can be opened with any ZIP file opening software or extracted with any ZIP file extractor.</p>
|
15 |
-
<h3>A way to install apps from sources other than Google Play</h3>
|
16 |
-
<p>An APK file can be downloaded directly to Android devices from websites or other sources that offer them. This is called <strong>sideloading</strong>. Sideloading allows users to install apps that are not available on Google Play or that have been modified or customized by third parties. However, sideloading also poses some risks such as malware infection or data theft <h2>How to download Hello Neighbor Full Act APK?</h2>
|
17 |
-
<p>If you want to play the full version of Hello Neighbor on your Android device, you need to download and install the Hello Neighbor Full Act APK file. This is a file that contains the complete game with all its acts and modes. However, you cannot find this file on Google Play, as it is not an official app from the developers. You need to download it from a third-party website that offers it. Here are the steps to download Hello Neighbor Full Act APK:</p>
|
18 |
-
<h3>Find a reliable website that offers the APK file</h3>
|
19 |
-
<p>The first step is to find a website that provides the Hello Neighbor Full Act APK file for free. You can search for it on Google or use one of the links below . Make sure that the website is trustworthy and does not contain any malware or viruses. You can check the reviews and ratings of the website and the file before downloading it.</p>
|
20 |
-
<h3>Enable unknown sources on your Android device</h3>
|
21 |
-
<p>The next step is to enable unknown sources on your Android device. This is a setting that allows you to install apps from sources other than Google Play. To enable unknown sources, you need to access the settings app and look for the security or privacy option. Depending on your device, you may need to tap on the lock screen and security tab or the install unknown apps switch. Then, you need to turn on the unknown sources switch or check the box next to it. You may see a warning message against enabling this option, but you can ignore it if you trust the source of the APK file .</p>
|
22 |
-
<h3>Download and install the APK file</h3>
|
23 |
-
<p>The final step is to download and install the APK file on your Android device. You can do this by tapping on the download link or button on the website that offers the file. You may need to wait for a few seconds or minutes for the download to complete. Once the download is done, you can open the file manager app on your device and locate the APK file in your downloads folder. Tap on the file and follow the instructions to install it. You may need to grant some permissions to the app during the installation process.</p>
|
24 |
-
<p>download hello neighbor full act apk free<br />
|
25 |
-
download hello neighbor full act apk latest version<br />
|
26 |
-
download hello neighbor full act apk for android<br />
|
27 |
-
download hello neighbor full act apk mod<br />
|
28 |
-
download hello neighbor full act apk offline<br />
|
29 |
-
download hello neighbor full act apk no verification<br />
|
30 |
-
download hello neighbor full act apk obb<br />
|
31 |
-
download hello neighbor full act apk from apkpure<br />
|
32 |
-
download hello neighbor full act apk 2.3.8<br />
|
33 |
-
download hello neighbor full act apk unlimited money<br />
|
34 |
-
download hello neighbor full act apk revdl<br />
|
35 |
-
download hello neighbor full act apk rexdl<br />
|
36 |
-
download hello neighbor full act apk hack<br />
|
37 |
-
download hello neighbor full act apk data<br />
|
38 |
-
download hello neighbor full act apk highly compressed<br />
|
39 |
-
download hello neighbor full act apk android 1<br />
|
40 |
-
download hello neighbor full act apk uptodown<br />
|
41 |
-
download hello neighbor full act apk andropalace<br />
|
42 |
-
download hello neighbor full act apk mob.org<br />
|
43 |
-
download hello neighbor full act apk apkmirror<br />
|
44 |
-
download hello neighbor full act apk apkmody<br />
|
45 |
-
download hello neighbor full act apk happymod<br />
|
46 |
-
download hello neighbor full act apk an1.com<br />
|
47 |
-
download hello neighbor full act apk android oyun club<br />
|
48 |
-
download hello neighbor full act apk blackmod.net<br />
|
49 |
-
download hello neighbor full act apk by tinybuild games<br />
|
50 |
-
download hello neighbor full act apk cracked<br />
|
51 |
-
download hello neighbor full act apk cheat menu<br />
|
52 |
-
download hello neighbor full act apk direct link<br />
|
53 |
-
download hello neighbor full act apk easy install<br />
|
54 |
-
download hello neighbor full act apk fileplanet.com<br />
|
55 |
-
download hello neighbor full act apk for pc windows 10<br />
|
56 |
-
download hello neighbor full act apk gamestechy.com<br />
|
57 |
-
download hello neighbor full act apk google drive link<br />
|
58 |
-
download hello neighbor full act apk how to install guide<br />
|
59 |
-
download hello neighbor full act apk in parts<br />
|
60 |
-
download hello neighbor full act apk ios iphone ipad ipod touch compatible <br />
|
61 |
-
download hello neighbor full act apk low mb size <br />
|
62 |
-
download hello neighbor full act apk mediafire.com <br />
|
63 |
-
download hello neighbor full act apk mega.nz</p> <h2>How to play Hello Neighbor Full Act APK?</h2>
|
64 |
-
<p>After you have successfully installed the Hello Neighbor Full Act APK file on your Android device, you can start playing the game. You can launch the game by tapping on its icon on your home screen or app drawer. You can also create a shortcut for the game on your desktop for easy access. Here are some tips on how to play Hello Neighbor Full Act APK:</p>
|
65 |
-
<h3>Explore the neighbor's house and discover his secrets</h3>
|
66 |
-
<p>The main goal of Hello Neighbor is to <strong>explore the neighbor's house</strong> and find out what he is hiding in his basement. You can use various items and tools to help you in your quest, such as keys, crowbars, flashlights, binoculars, and more. You can also interact with different objects and environments in the house, such as doors, windows, drawers, switches, vents, and more. You can use these to create diversions, hide, or access new areas. However, you need to be careful not to make too much noise or leave any traces behind, as the neighbor will notice them and become suspicious.</p>
|
67 |
-
<h3>Avoid being caught by the neighbor and his traps</h3>
|
68 |
-
<p>The biggest challenge of Hello Neighbor is to <strong>avoid being caught by the neighbor</strong> and his traps. The neighbor is not a dumb AI that follows a fixed pattern. He is a smart and adaptive AI that learns from your actions and reacts accordingly. He will chase you, set traps, use cameras, and even call the police if he sees you in his house. He will also remember your previous attempts and change his behavior and strategy accordingly. You need to be unpredictable and creative to outsmart him and escape from his clutches.</p>
|
69 |
-
<h3>Enjoy the full story and gameplay of Hello Neighbor</h3>
|
70 |
-
<p>By downloading Hello Neighbor Full Act APK, you can enjoy the <strong>full story and gameplay</strong> of Hello Neighbor on your Android device. You can play all four acts of the story mode and uncover the mystery behind the neighbor's basement. You can also play the secret mode and learn more about the neighbor's past and motives. Additionally, you can try out other modes such as hide and seek, ghost mode, and sandbox mode for more fun and variety.</p>
|
71 |
-
<h2>Conclusion</h2>
|
72 |
-
<p>Hello Neighbor is a stealth horror game that offers a unique and thrilling experience for Android users. By downloading Hello Neighbor Full Act APK, you can play the complete game with all its acts and modes on your device. You can explore the neighbor's house, avoid his traps, and discover his secrets. However, you need to be careful when downloading and installing APK files from third-party sources, as they may contain malware or viruses. You also need to enable unknown sources on your device before installing them.</p>
|
73 |
-
<h2>FAQs</h2>
|
74 |
-
<p>Here are some frequently asked questions about Hello Neighbor Full Act APK:</p>
|
75 |
-
<h4>Q: Is Hello Neighbor Full Act APK safe to download?</h4>
|
76 |
-
<p>A: Hello Neighbor Full Act APK is safe to download if you get it from a reliable website that does not contain any malware or viruses. However, you should always scan the file with an antivirus software before installing it.</p>
|
77 |
-
<h4>Q: Is Hello Neighbor Full Act APK free to download?</h4>
|
78 |
-
<p>A: Yes, Hello Neighbor Full Act APK is free to download from most websites that offer it. However, some websites may require you to complete surveys or watch ads before downloading it.</p>
|
79 |
-
<h4>Q: Do I need an internet connection to play Hello Neighbor Full Act APK?</h4>
|
80 |
-
<p>A: No, you do not need an internet connection to play Hello Neighbor Full Act APK. You can play the game offline without any problems.</p>
|
81 |
-
<h4>Q: What are the minimum requirements to play Hello Neighbor Full Act APK?</h4>
|
82 |
-
<p>A: The minimum requirements to play Hello Neighbor Full Act APK are as follows:</p>
|
83 |
-
<table>
|
84 |
-
<tr><td><strong>OS</strong></td><td>Android 7.0 or higher</td></tr>
|
85 |
-
<tr><td><strong>CPU</strong></td><td>Dual-core 1.5 GHz or higher</td></tr>
|
86 |
-
<tr><td><strong>RAM</strong></td><td>2 GB or higher</td></tr>
|
87 |
-
<tr><td><strong>Storage</strong></td><td>1 GB or higher</td></tr>
|
88 |
-
<tr><td><strong>Graphics</strong></td><td>Mali-T760MP8 or higher</td></tr>
|
89 |
-
</table>
|
90 |
-
<h4>Q: How can I update Hello Neighbor Full Act APK?</h4>
|
91 |
-
<p>A: To update Hello Neighbor Full Act APK, you need to download the latest version of the file from a website that offers it. Then, you need to uninstall the previous version of the app and install the new one. Alternatively, you can check if the website has an update option that allows you to download and install the update automatically.</p>
|
92 |
-
<p>I hope this article has helped you learn how to download Hello Neighbor Full Act APK for Android. If you have any questions or feedback, please leave a comment below. Thank you for reading and happy gaming!</p> 197e85843d<br />
|
93 |
-
<br />
|
94 |
-
<br />
|
|
|
|
|
|
|
|
|
|
|
|
|
|
|
|
|
|
|
|
|
|
|
|
|
|
|
|
|
|
|
|
|
|
|
|
|
|
|
|
|
|
|
|
|
|
|
|
|
|
|
|
|
|
|
|
|
|
|
|
|
|
|
|
|
|
|
|
|
|
|
|
|
|
|
|
|
|
|
|
|
|
|
|
|
|
|
|
|
|
|
|
|
|
|
|
|
|
|
|
|
|
|
|
|
|
|
|
|
|
|
|
|
|
|
|
|
|
|
|
|
|
|
|
|
|
|
|
|
|
|
|
|
|
|
|
|
|
|
|
|
|
|
|
|
|
|
|
|
|
|
|
|
|
|
|
|
|
|
|
|
|
|
|
|
|
|
|
|
|
|
|
|
|
|
|
|
|
|
|
|
|
|
|
|
|
|
|
|
|
spaces/1phancelerku/anime-remove-background/Explore the Beauty and Diversity of Indonesia with Bus Simulator Indonesia HD.md
DELETED
@@ -1,181 +0,0 @@
|
|
1 |
-
|
2 |
-
<h1>Download Bus Simulator Indonesia HD: A Fun and Authentic Way to Experience Driving in Indonesia</h1>
|
3 |
-
<p>Have you ever wondered what it is like to be a bus driver in Indonesia? If you have, then you should try Bus Simulator Indonesia HD, a popular game that lets you experience the thrill and challenge of driving a bus in various Indonesian cities and places. Bus Simulator Indonesia HD (also known as BUSSID) is not the first bus simulator game, but it is probably one of the only ones with the most features and the most authentic Indonesian environment.</p>
|
4 |
-
<p>In this article, we will show you how to download Bus Simulator Indonesia HD for Android and PC, how to play it, how to enhance your gaming experience with it, and how to troubleshoot some common problems with it. We will also answer some frequently asked questions about the game. By the end of this article, you will be ready to hop on your bus and start your journey in Bus Simulator Indonesia HD.</p>
|
5 |
-
<h2>download bus simulator indonesia hd</h2><br /><p><b><b>Download File</b> ✅ <a href="https://jinyurl.com/2uNM5B">https://jinyurl.com/2uNM5B</a></b></p><br /><br />
|
6 |
-
<h2>How to Download Bus Simulator Indonesia HD for Android and PC</h2>
|
7 |
-
<h3>Downloading from Google Play Store</h3>
|
8 |
-
<p>The easiest way to download Bus Simulator Indonesia HD for Android is to get it from the Google Play Store. Here are the steps you need to follow:</p>
|
9 |
-
<ol>
|
10 |
-
<li>Open the Google Play Store app on your Android device.</li>
|
11 |
-
<li>Search for "Bus Simulator Indonesia" or "BUSSID" in the search bar.</li>
|
12 |
-
<li>Tap on the game icon that has a blue background and a yellow bus.</li>
|
13 |
-
<li>Tap on "Install" and wait for the game to download and install on your device.</li>
|
14 |
-
<li>Tap on "Open" or find the game icon on your home screen or app drawer.</li>
|
15 |
-
<li>Enjoy playing Bus Simulator Indonesia HD!</li>
|
16 |
-
</ol>
|
17 |
-
<p>Note that the game requires Android 4.2 or higher and at least 1 GB of RAM to run smoothly. You also need to have enough storage space on your device, as the game size is about 300 MB.</p>
|
18 |
-
<h3>Downloading from Other Sources</h3>
|
19 |
-
<p>If you cannot download Bus Simulator Indonesia HD from the Google Play Store, or if you want to play it on your PC, you can try other sources, such as APK files or emulators. However, you should be careful and only download from trusted and verified sources, as some files may contain viruses or malware that can harm your device or PC. You should also check the compatibility and requirements of the game before downloading and installing it.</p>
|
20 |
-
<p>One of the most popular sources for downloading APK files is APKPure, which offers safe and fast downloads for various Android games and apps. You can download Bus Simulator Indonesia HD from APKPure by following these steps:</p>
|
21 |
-
<ol>
|
22 |
-
<li>Open your web browser and go to https://apkpure.com/.</li>
|
23 |
-
<li>Search for "Bus Simulator Indonesia" or "BUSSID" in the search bar.</li>
|
24 |
-
<li>Tap on the game icon that has a blue background and a yellow bus.</li>
|
25 |
-
<li>Tap on "Download APK" and wait for the file to download on your device or PC.</li>
|
26 |
-
<li>If you are using an Android device, go to your file manager and find the downloaded APK file. Tap on it and allow the installation from unknown sources if prompted. Wait for the game to install on your device.</li>
|
27 |
-
<li>If you are using a PC, you need to have an Android emulator installed on your PC, such as BlueStacks or NoxPlayer. Open the emulator and drag and drop the downloaded APK file into it. Wait for the game to install on the emulator.</li>
|
28 |
-
<li>Open the game from your device or emulator and enjoy playing Bus Simulator Indonesia HD!</li>
|
29 |
-
</ol>
|
30 |
-
<p>Note that downloading and installing APK files may not give you the latest version of the game, and you may not be able to access some features or updates. You may also encounter some errors or bugs while playing the game. To avoid these problems, we recommend that you download Bus Simulator Indonesia HD from the Google Play Store whenever possible.</p>
|
31 |
-
<h2>How to Play Bus Simulator Indonesia HD</h2>
|
32 |
-
<h3>Choosing Your Bus and Livery</h3>
|
33 |
-
<p>One of the coolest features of Bus Simulator Indonesia HD is that you can choose and customize your own bus and livery. A livery is a design or pattern that covers the exterior of your bus, such as colors, logos, stickers, etc. You can choose from various types of buses, such as mini buses, double deckers, articulated buses, etc. You can also choose from different liveries, such as national flags, famous brands, cartoon characters, etc. You can even create your own livery using the livery editor feature.</p>
|
34 |
-
<p>Download Bus Simulator Indonesia on PC with BlueStacks<br />
|
35 |
-
Bus Simulator Indonesia HD wallpapers for desktop and mobile<br />
|
36 |
-
How to design your own livery in Bus Simulator Indonesia<br />
|
37 |
-
Bus Simulator Indonesia online multiplayer convoy mode<br />
|
38 |
-
Bus Simulator Indonesia mod apk unlimited money and fuel<br />
|
39 |
-
Best Indonesian cities and places to visit in Bus Simulator Indonesia<br />
|
40 |
-
Bus Simulator Indonesia for iOS devices free download<br />
|
41 |
-
Tips and tricks to master Bus Simulator Indonesia game<br />
|
42 |
-
Bus Simulator Indonesia review and rating by users<br />
|
43 |
-
Bus Simulator Indonesia latest update features and bug fixes<br />
|
44 |
-
How to install and play Bus Simulator Indonesia on Mac<br />
|
45 |
-
Bus Simulator Indonesia cheats and hacks for android<br />
|
46 |
-
Bus Simulator Indonesia gameplay videos and live streams<br />
|
47 |
-
How to use your own 3D model in Bus Simulator Indonesia<br />
|
48 |
-
Bus Simulator Indonesia official website and social media links<br />
|
49 |
-
Bus Simulator Indonesia system requirements and compatibility<br />
|
50 |
-
How to get free emoji icons for Bus Simulator Indonesia<br />
|
51 |
-
Bus Simulator Indonesia offline mode without internet connection<br />
|
52 |
-
How to unlock all Indonesian buses in Bus Simulator Indonesia<br />
|
53 |
-
Bus Simulator Indonesia vs other bus simulator games comparison<br />
|
54 |
-
How to contact Bus Simulator Indonesia support and feedback<br />
|
55 |
-
Bus Simulator Indonesia data privacy and security policy<br />
|
56 |
-
How to join the Bus Simulator Indonesia community and forums<br />
|
57 |
-
How to earn more money and rewards in Bus Simulator Indonesia<br />
|
58 |
-
How to customize your bus driver avatar in Bus Simulator Indonesia<br />
|
59 |
-
How to change the language and settings in Bus Simulator Indonesia<br />
|
60 |
-
How to fix common errors and issues in Bus Simulator Indonesia<br />
|
61 |
-
How to backup and restore your data in Bus Simulator Indonesia<br />
|
62 |
-
How to play Bus Simulator Indonesia with a controller or keyboard<br />
|
63 |
-
How to improve the graphics quality and performance in Bus Simulator Indonesia<br />
|
64 |
-
How to honk your horn and use cool and fun honks in Bus Simulator Indonesia<br />
|
65 |
-
How to access the leaderboard and achievements in Bus Simulator Indonesia<br />
|
66 |
-
How to share your screenshots and videos of Bus Simulator Indonesia<br />
|
67 |
-
How to invite your friends and play together in Bus Simulator Indonesia<br />
|
68 |
-
How to download and install new mods for Bus Simulator Indonesia<br />
|
69 |
-
How to learn more about Indonesian culture and history in Bus Simulator Indonesia<br />
|
70 |
-
How to upgrade your bus engine and parts in Bus Simulator Indonesia<br />
|
71 |
-
How to follow the traffic rules and regulations in Bus Simulator Indonesia<br />
|
72 |
-
How to drive safely and avoid accidents in Bus Simulator Indonesia<br />
|
73 |
-
How to enjoy the realistic and authentic Indonesian environment in Bus Simulator Indonesia</p>
|
74 |
-
<p>To choose and customize your bus and livery, follow these steps:</p>
|
75 |
-
<ol>
|
76 |
-
<li>From the main menu, tap on "Garage".</li>
|
77 |
-
<li>Tap on "Bus" to select your bus type. You can swipe left or right to see more options. You can also tap on "Buy" to purchase more buses using in-game currency.</li>
|
78 |
-
<li>Tap on "Livery" to select your livery. You can swipe left or right to see more options. You can also tap on "Download" to download more liveries from other players or online sources.</li>
|
79 |
-
<li>Tap on "Edit" to create your own livery using the livery editor feature. You can use various tools and options to design your livery as you like.</li>
|
80 |
-
<li>Tap on "Save" to save your changes and apply them to your bus.</li>
|
81 |
-
</ol>
|
82 |
-
<p>Choosing and customizing your bus and livery can make your gaming experience more fun and personal. You can also show off your creativity and style to other players online.</p>
|
83 |
-
<h3>Driving Your Bus in Career Mode or Free Mode</h3>
|
84 |
-
<p>The main mode of Bus Simulator Indonesia HD is career mode, where you can drive your bus in various Indonesian cities and places, follow the traffic rules, pick up passengers, earn money, and upgrade your bus. You can also play in free mode, where you can drive your bus anywhere without any restrictions or objectives.</p>
|
85 |
-
<p>To drive your bus in career mode or free mode, follow these steps:</p>
|
86 |
-
<ol>
|
87 |
-
<li>From the main menu, tap on "Play".</li>
|
88 |
-
<li>Select either "Career" or "Free" mode.</li>
|
89 |
-
<li>Select your starting location from the map. You can swipe left or right to see more options. You can also tap on "Random" to start from a random location.</li>
|
90 |
-
<li>Select your destination from the map. option.</li>
|
91 |
-
<li>If you select "Join" convoy, you can see a list of available convoys that you can join. You can filter the list by region, bus type, or livery. You can also search for a specific convoy by name or ID. Tap on the convoy that you want to join and wait for the host to accept you.</li>
|
92 |
-
<li>If you select "Create" convoy, you can create your own convoy by setting the name, password, region, bus type, livery, route, and destination. You can also invite your friends or other players to join your convoy by sharing the convoy ID or QR code. Tap on "Start" to begin your convoy.</li>
|
93 |
-
<li>Once you are in a convoy, you can see the other players' names, buses, and locations on the map or the GPS. You can also chat with them by tapping on the chat icon. You can also honk at them by tapping on the horn icon. You can also leave the convoy by tapping on the exit icon.</li>
|
94 |
-
</ol>
|
95 |
-
<p>Joining or creating an online multiplayer convoy can make your gaming experience more social and interactive. You can meet new friends, learn from other players, and have fun together.</p>
|
96 |
-
<h2>How to Enhance Your Gaming Experience with Bus Simulator Indonesia HD</h2>
|
97 |
-
<h3>Using Your Own 3D Model with Vehicle Mod System</h3>
|
98 |
-
<p>One of the most advanced features of Bus Simulator Indonesia HD is that you can use your own 3D model with the vehicle mod system. This means that you can import any 3D model of a bus or a vehicle that you have created or downloaded from other sources and use it in the game. You can also customize the model's properties, such as engine, transmission, suspension, etc.</p>
|
99 |
-
<p>To use your own 3D model with the vehicle mod system, follow these steps:</p>
|
100 |
-
<ol>
|
101 |
-
<li>Create or download a 3D model of a bus or a vehicle that you want to use in the game. The model must be in OBJ format and have a maximum size of 50 MB. The model must also have a texture file in PNG format and a material file in MTL format.</li>
|
102 |
-
<li>Copy the 3D model files to your device or PC. If you are using an Android device, copy them to the BUSSID folder in your internal storage. If you are using a PC, copy them to the BUSSID folder in your emulator's storage.</li>
|
103 |
-
<li>Open the game and go to the garage. Tap on "Mod" and then tap on "Import". Select the 3D model files that you have copied and wait for them to be imported.</li>
|
104 |
-
<li>Tap on "Edit" to customize the model's properties, such as name, price, engine, transmission, suspension, etc. You can also adjust the model's position, rotation, and scale.</li>
|
105 |
-
<li>Tap on "Save" to save your changes and apply them to your model.</li>
|
106 |
-
<li>Select your model from the mod list and use it in the game.</li>
|
107 |
-
</ol>
|
108 |
-
<p>Using your own 3D model with the vehicle mod system can make your gaming experience more unique and creative. You can use any bus or vehicle that you like or imagine and drive it in Bus Simulator Indonesia HD.</p>
|
109 |
-
<h3>Using Cool and Fun Honks</h3>
|
110 |
-
<p>Another fun feature of Bus Simulator Indonesia HD is that you can use cool and fun honks to communicate with other drivers or passengers. Honks are sounds that your bus makes when you tap on the horn icon. You can choose from various honks, such as sirens, horns, bells, whistles, etc. You can also use some special honks that are unique to Indonesia, such as "Om Telolet Om".</p>
|
111 |
-
<p>"Om Telolet Om" is a phrase that means "Uncle, honk uncle" in Indonesian. It is a popular request that children make to bus drivers to make them honk their horns in a musical way. It is also a viral phenomenon that has spread across social media and attracted many celebrities and musicians.</p>
|
112 |
-
<p>To use cool and fun honks in Bus Simulator Indonesia HD, follow these steps:</p>
|
113 |
-
<ol>
|
114 |
-
<li>From the main menu, tap on "Settings".</li>
|
115 |
-
<li>Tap on "Sound".</li>
|
116 |
-
<li>Tap on "Horn Sound" to select your honk type. You can swipe left or right to see more options. You can also tap on "Download" to download more honks from other players or online sources.</li>
|
117 |
-
<li>Tap on "Back" to save your changes and return to the main menu.</li>
|
118 |
-
<li>When playing the game, tap on the horn icon to use your selected honk.</li>
|
119 |
-
</ol>
|
120 |
-
<p>Using cool and fun honks in Bus Simulator Indonesia HD can make your gaming experience more fun and interactive. You can also express your emotions and personality with your honks. You can also join the "Om Telolet Om" craze and make some music with your bus.</p>
|
121 |
-
<h3>Competing with Other Players on Leaderboard</h3>
|
122 |
-
<p>Another exciting feature of Bus Simulator Indonesia HD is that you can compete with other players on the leaderboard. The leaderboard is a ranking system that shows the best players in the game based on their score and reputation. You can see your own rank and score, as well as the rank and score of other players. You can also see the rank and score of your friends or other players that you follow.</p>
|
123 |
-
<p>To compete with other players on the leaderboard in Bus Simulator Indonesia HD, follow these steps:</p>
|
124 |
-
<ol>
|
125 |
-
<li>From the main menu, tap on "Leaderboard".</li>
|
126 |
-
<li>Tap on "Global" to see the global leaderboard, or tap on "Friends" to see the friends leaderboard.</li>
|
127 |
-
<li>Swipe up or down to see more players on the leaderboard. You can also tap on a player's name to see their profile and stats.</li>
|
128 |
-
<li>Tap on "Follow" to follow a player, or tap on "Unfollow" to unfollow a player. You can also tap on "Chat" to chat with a player.</li>
|
129 |
-
<li>Tap on "Back" to return to the main menu.</li>
|
130 |
-
</ol>
|
131 |
-
<p>To improve your rank and score on the leaderboard, you need to play well and complete missions in career mode. You also need to follow the traffic rules, drive safely, pick up passengers, earn money, and upgrade your bus. You also need to avoid crashing, breaking the law, or losing passengers. The better you play, the higher your score and reputation will be.</p>
|
132 |
-
<p>Competing with other players on the leaderboard in Bus Simulator Indonesia HD can make your gaming experience more challenging and rewarding. You can also learn from other players, compare your skills, and show off your achievements.</p>
|
133 |
-
<h2>How to Troubleshoot Common Problems with Bus Simulator Indonesia HD</h2>
|
134 |
-
<h3>Game Crashes or Freezes</h3>
|
135 |
-
<p>One of the most common problems that you may encounter while playing Bus Simulator Indonesia HD is that the game crashes or freezes. This means that the game stops working or responding, and you cannot continue playing. This can be very frustrating and annoying, especially if you are in the middle of a mission or a convoy.</p>
|
136 |
-
<p>To fix game crashes or freezes in Bus Simulator Indonesia HD, you can try these solutions:</p>
|
137 |
-
<ul>
|
138 |
-
<li>Clear the game cache. This can help remove any corrupted or outdated files that may cause the game to crash or freeze. To clear the game cache, go to your device settings, find the game app, tap on "Storage", and then tap on "Clear cache".</li>
|
139 |
-
<li>Update the game app. This can help fix any bugs or errors that may cause the game to crash or freeze. To update the game app, go to the Google Play Store, find the game app, and tap on "Update".</li>
|
140 |
-
<li>Update your device software. This can help improve your device performance and compatibility with the game. To update your device software, go to your device settings, find "System update", and tap on "Check for updates".</li>
|
141 |
-
<li>Reinstall the game app. This can help reset the game settings and data to their default state. To reinstall the game app, go to the Google Play Store, find the game app, tap on "Uninstall", and then tap on "Install". Note that this will delete your game data, so make sure you have a backup or a cloud save before doing this.</li>
|
142 |
-
</ul>
|
143 |
-
<p>If none of these solutions work, you can contact the game developer for more help. You can find their contact information on the game app page on the Google Play Store, or on their official website or social media accounts.</p>
|
144 |
-
<h3>Game Lags or Runs Slowly</h3>
|
145 |
-
<p>Another common problem that you may encounter while playing Bus Simulator Indonesia HD is that the game lags or runs slowly. This means that the game does not run smoothly or responsively, and you may experience delays, stuttering, or low frame rate. This can affect your gameplay and enjoyment, especially if you are driving fast or in a busy area.</p>
|
146 |
-
<p>To fix game lags or runs slowly in Bus Simulator Indonesia HD, you can try these tips:</p>
|
147 |
-
<ul>
|
148 |
-
<li>Adjust the game graphics settings. This can help reduce the game's demand on your device's resources and improve the game's performance. To adjust the game graphics settings, go to the game menu, tap on "Settings", tap on "Graphics", and then change the options such as resolution, quality, shadow, etc. You can also use the "Auto" option to let the game choose the best settings for your device.</li>
|
149 |
-
<li>Close other apps or background processes. This can help free up your device's memory and CPU and prevent them from interfering with the game. To close other apps or background processes, go to your device settings, find "Apps" or "Application manager", and then swipe or tap on the apps that you want to close. You can also use a task manager or a cleaner app to do this automatically.</li>
|
150 |
-
<li>Use a booster app. This can help optimize your device's performance and speed up the game. A booster app is a tool that can clean your device's cache, memory, and junk files, as well as boost your device's CPU, GPU, and battery. Some examples of booster apps are Game Booster, Speed Booster, or DU Speed Booster. You can download them from the Google Play Store and use them before playing the game.</li>
|
151 |
-
</ul>
|
152 |
-
<p>If none of these tips work, you may need to upgrade your device's hardware or software to meet the game's requirements. You can check the game's requirements on the game app page on the Google Play Store, or on their official website or social media accounts.</p>
|
153 |
-
<h3>Game Data is Lost or Corrupted</h3>
|
154 |
-
<p>Another common problem that you may encounter while playing Bus Simulator Indonesia HD is that your game data is lost or corrupted. This means that your game progress, settings, or purchases are missing or damaged, and you cannot access them or use them in the game. This can be very frustrating and disappointing, especially if you have spent a lot of time and money on the game.</p>
|
155 |
-
<p>To fix game data is lost or corrupted in Bus Simulator Indonesia HD, you can try these methods:</p>
|
156 |
-
<ul>
|
157 |
-
<li>Use cloud save. This can help sync your game data with your Google Play account and restore it if it is lost or corrupted. To use cloud save, go to the game menu, tap on "Settings", tap on "Account", and then tap on "Cloud Save". You can also enable "Auto Save" to let the game save your data automatically.</li>
|
158 |
-
<li>Use a backup app. This can help backup your game data to your device's storage or an external storage and restore it if it is lost or corrupted. A backup app is a tool that can copy your game data files and store them in a safe location. Some examples of backup apps are Helium, Titanium Backup, or Easy Backup. You can download them from the Google Play Store and use them to backup and restore your game data.</li>
|
159 |
-
<li>Contact support. This can help recover your game data if it is lost or corrupted due to a bug or an error in the game. To contact support, go to the game menu, tap on "Settings", tap on "Help", and then tap on "Contact Us". You can also find their contact information on the game app page on the Google Play Store, or on their official website or social media accounts.</li>
|
160 |
-
</ul>
|
161 |
-
<p>If none of these methods work , you may need to start a new game and lose your previous game data. To avoid this problem, we recommend that you backup your game data regularly and use cloud save whenever possible.</p>
|
162 |
-
<h2>Conclusion</h2>
|
163 |
-
<p>Bus Simulator Indonesia HD is a fun and authentic way to experience driving in Indonesia. You can download and play it on your Android device or PC, choose and customize your own bus and livery, drive your bus in career mode or free mode, join or create an online multiplayer convoy, use your own 3D model with the vehicle mod system, use cool and fun honks, and compete with other players on the leaderboard. You can also troubleshoot some common problems with the game, such as game crashes or freezes, game lags or runs slowly, or game data is lost or corrupted.</p>
|
164 |
-
<p>If you are looking for a realistic and immersive bus simulator game, you should definitely try Bus Simulator Indonesia HD. You will not regret it. You can download it from the Google Play Store or other sources, and start your adventure in Bus Simulator Indonesia HD today.</p>
|
165 |
-
<p>We hope that this article has helped you learn more about Bus Simulator Indonesia HD and how to download and play it. If you have any questions or comments, please feel free to leave them below. We would love to hear from you.</p>
|
166 |
-
<h2>FAQs</h2>
|
167 |
-
<p>Here are some frequently asked questions and answers about Bus Simulator Indonesia HD:</p>
|
168 |
-
<ol>
|
169 |
-
<li><b>What is the difference between Bus Simulator Indonesia and Bus Simulator Indonesia HD?</b></li>
|
170 |
-
<p>Bus Simulator Indonesia HD is an upgraded version of Bus Simulator Indonesia that has better graphics, more features, and more content. It also has a larger game size and requires a higher device specification to run smoothly.</p>
|
171 |
-
<li><b>Can I play Bus Simulator Indonesia HD offline?</b></li>
|
172 |
-
<p>Yes, you can play Bus Simulator Indonesia HD offline in career mode or free mode. However, you need an internet connection to access some features, such as cloud save, multiplayer convoy, leaderboard, or download more buses or liveries.</p>
|
173 |
-
<li><b>Can I play Bus Simulator Indonesia HD with a controller?</b></li>
|
174 |
-
<p>Yes, you can play Bus Simulator Indonesia HD with a controller if you have a compatible device and controller. You can connect your controller to your device via Bluetooth or USB cable, and then configure the controller settings in the game menu.</p>
|
175 |
-
<li><b>Can I share my bus or livery with other players?</b></li>
|
176 |
-
<p>Yes, you can share your bus or livery with other players by uploading them to the game server or online sources. You can also download other players' buses or liveries from the game menu or online sources.</p>
|
177 |
-
<li><b>Can I request a new feature or report a bug for Bus Simulator Indonesia HD?</b></li>
|
178 |
-
<p>Yes, you can request a new feature or report a bug for Bus Simulator Indonesia HD by contacting the game developer via email, website, or social media. You can also leave a review or feedback on the game app page on the Google Play Store.</p>
|
179 |
-
</ol></p> 401be4b1e0<br />
|
180 |
-
<br />
|
181 |
-
<br />
|
|
|
|
|
|
|
|
|
|
|
|
|
|
|
|
|
|
|
|
|
|
|
|
|
|
|
|
|
|
|
|
|
|
|
|
|
|
|
|
|
|
|
|
|
|
|
|
|
|
|
|
|
|
|
|
|
|
|
|
|
|
|
|
|
|
|
|
|
|
|
|
|
|
|
|
|
|
|
|
|
|
|
|
|
|
|
|
|
|
|
|
|
|
|
|
|
|
|
|
|
|
|
|
|
|
|
|
|
|
|
|
|
|
|
|
|
|
|
|
|
|
|
|
|
|
|
|
|
|
|
|
|
|
|
|
|
|
|
|
|
|
|
|
|
|
|
|
|
|
|
|
|
|
|
|
|
|
|
|
|
|
|
|
|
|
|
|
|
|
|
|
|
|
|
|
|
|
|
|
|
|
|
|
|
|
|
|
|
|
|
|
|
|
|
|
|
|
|
|
|
|
|
|
|
|
|
|
|
|
|
|
|
|
|
|
|
|
|
|
|
|
|
|
|
|
|
|
|
|
|
|
|
|
|
|
|
|
|
|
|
|
|
|
|
|
|
|
|
|
|
|
|
|
|
|
|
|
|
|
|
|
|
|
|
|
|
|
|
|
|
|
|
|
|
|
|
|
|
|
|
|
|
|
|
|
|
|
|
|
|
|
|
|
|
|
|
|
|
|
|
|
|
|
|
|
|
|
|
|
|
|
|
|
|
|
|
|
|
|
|
|
|
|
|
|
|
|
|
|
|
|
|
|
|
|
|
|
|
|
|
|
|
|
|
|
|
|
|
|
|
|
|
|
|
|
|
|
|
|
|
|
|
|
spaces/AI-Zero-to-Hero/07-SL-Chatbot-Blenderbot/README.md
DELETED
@@ -1,13 +0,0 @@
|
|
1 |
-
---
|
2 |
-
title: 07 SL Chatbot Blenderbot
|
3 |
-
emoji: 🌍
|
4 |
-
colorFrom: red
|
5 |
-
colorTo: purple
|
6 |
-
sdk: streamlit
|
7 |
-
sdk_version: 1.10.0
|
8 |
-
app_file: app.py
|
9 |
-
pinned: false
|
10 |
-
license: mit
|
11 |
-
---
|
12 |
-
|
13 |
-
Check out the configuration reference at https://huggingface.co/docs/hub/spaces-config-reference
|
|
|
|
|
|
|
|
|
|
|
|
|
|
|
|
|
|
|
|
|
|
|
|
|
|
|
|
spaces/AIGC-Audio/Make_An_Audio/ldm/modules/diffusionmodules/custom_openaimodel.py
DELETED
@@ -1,368 +0,0 @@
|
|
1 |
-
from abc import abstractmethod
|
2 |
-
from functools import partial
|
3 |
-
import math
|
4 |
-
from typing import Iterable
|
5 |
-
|
6 |
-
import numpy as np
|
7 |
-
import torch as th
|
8 |
-
import torch.nn as nn
|
9 |
-
import torch.nn.functional as F
|
10 |
-
|
11 |
-
from ldm.modules.diffusionmodules.util import (
|
12 |
-
checkpoint,
|
13 |
-
conv_nd,
|
14 |
-
linear,
|
15 |
-
avg_pool_nd,
|
16 |
-
zero_module,
|
17 |
-
normalization,
|
18 |
-
timestep_embedding,
|
19 |
-
)
|
20 |
-
from ldm.modules.attention import SpatialTransformer
|
21 |
-
from ldm.modules.diffusionmodules.openaimodel import convert_module_to_f16, convert_module_to_f32, AttentionPool2d, \
|
22 |
-
TimestepBlock, TimestepEmbedSequential, Upsample, TransposedUpsample, Downsample, ResBlock, AttentionBlock, count_flops_attn, \
|
23 |
-
QKVAttentionLegacy, QKVAttention
|
24 |
-
|
25 |
-
|
26 |
-
class UNetModel(nn.Module):
|
27 |
-
"""
|
28 |
-
The full UNet model with attention and timestep embedding.
|
29 |
-
:param in_channels: channels in the input Tensor.
|
30 |
-
:param model_channels: base channel count for the model.
|
31 |
-
:param out_channels: channels in the output Tensor.
|
32 |
-
:param num_res_blocks: number of residual blocks per downsample.
|
33 |
-
:param attention_resolutions: a collection of downsample rates at which
|
34 |
-
attention will take place. May be a set, list, or tuple.
|
35 |
-
For example, if this contains 4, then at 4x downsampling, attention
|
36 |
-
will be used.
|
37 |
-
:param dropout: the dropout probability.
|
38 |
-
:param channel_mult: channel multiplier for each level of the UNet.
|
39 |
-
:param conv_resample: if True, use learned convolutions for upsampling and
|
40 |
-
downsampling.
|
41 |
-
:param dims: determines if the signal is 1D, 2D, or 3D.
|
42 |
-
:param num_classes: if specified (as an int), then this model will be
|
43 |
-
class-conditional with `num_classes` classes.
|
44 |
-
:param use_checkpoint: use gradient checkpointing to reduce memory usage.
|
45 |
-
:param num_heads: the number of attention heads in each attention layer.
|
46 |
-
:param num_heads_channels: if specified, ignore num_heads and instead use
|
47 |
-
a fixed channel width per attention head.
|
48 |
-
:param num_heads_upsample: works with num_heads to set a different number
|
49 |
-
of heads for upsampling. Deprecated.
|
50 |
-
:param use_scale_shift_norm: use a FiLM-like conditioning mechanism.
|
51 |
-
:param resblock_updown: use residual blocks for up/downsampling.
|
52 |
-
:param use_new_attention_order: use a different attention pattern for potentially
|
53 |
-
increased efficiency.
|
54 |
-
"""
|
55 |
-
|
56 |
-
def __init__(
|
57 |
-
self,
|
58 |
-
image_size,
|
59 |
-
in_channels,
|
60 |
-
model_channels,
|
61 |
-
out_channels,
|
62 |
-
num_res_blocks,
|
63 |
-
attention_resolutions,
|
64 |
-
dropout=0,
|
65 |
-
channel_mult=(1, 2, 4, 8),
|
66 |
-
conv_resample=True,
|
67 |
-
dims=2,
|
68 |
-
num_classes=None,
|
69 |
-
use_checkpoint=False,
|
70 |
-
use_fp16=False,
|
71 |
-
num_heads=-1,
|
72 |
-
num_head_channels=-1,
|
73 |
-
num_heads_upsample=-1,
|
74 |
-
use_scale_shift_norm=False,
|
75 |
-
resblock_updown=False,
|
76 |
-
use_new_attention_order=False,
|
77 |
-
use_spatial_transformer=False, # custom transformer support
|
78 |
-
transformer_depth=1, # custom transformer support
|
79 |
-
context_dim=None, # custom transformer support
|
80 |
-
n_embed=None, # custom support for prediction of discrete ids into codebook of first stage vq model
|
81 |
-
legacy=True,
|
82 |
-
use_context_project=False, # custom text to audio support
|
83 |
-
use_context_attn=True # custom text to audio support
|
84 |
-
):
|
85 |
-
super().__init__()
|
86 |
-
if use_spatial_transformer:
|
87 |
-
assert context_dim is not None, 'Fool!! You forgot to include the dimension of your cross-attention conditioning...'
|
88 |
-
|
89 |
-
if context_dim is not None and not use_context_project:
|
90 |
-
assert use_spatial_transformer, 'Fool!! You forgot to use the spatial transformer for your cross-attention conditioning...'
|
91 |
-
from omegaconf.listconfig import ListConfig
|
92 |
-
if type(context_dim) == ListConfig:
|
93 |
-
context_dim = list(context_dim)
|
94 |
-
|
95 |
-
if num_heads_upsample == -1:
|
96 |
-
num_heads_upsample = num_heads
|
97 |
-
|
98 |
-
if num_heads == -1:
|
99 |
-
assert num_head_channels != -1, 'Either num_heads or num_head_channels has to be set'
|
100 |
-
|
101 |
-
if num_head_channels == -1:
|
102 |
-
assert num_heads != -1, 'Either num_heads or num_head_channels has to be set'
|
103 |
-
|
104 |
-
self.image_size = image_size
|
105 |
-
self.in_channels = in_channels
|
106 |
-
self.model_channels = model_channels
|
107 |
-
self.out_channels = out_channels
|
108 |
-
self.num_res_blocks = num_res_blocks
|
109 |
-
self.attention_resolutions = attention_resolutions
|
110 |
-
self.dropout = dropout
|
111 |
-
self.channel_mult = channel_mult
|
112 |
-
self.conv_resample = conv_resample
|
113 |
-
self.num_classes = num_classes
|
114 |
-
self.use_checkpoint = use_checkpoint
|
115 |
-
self.dtype = th.float16 if use_fp16 else th.float32
|
116 |
-
self.num_heads = num_heads
|
117 |
-
self.num_head_channels = num_head_channels
|
118 |
-
self.num_heads_upsample = num_heads_upsample
|
119 |
-
self.predict_codebook_ids = n_embed is not None
|
120 |
-
|
121 |
-
time_embed_dim = model_channels * 4
|
122 |
-
self.time_embed = nn.Sequential(
|
123 |
-
linear(model_channels, time_embed_dim),
|
124 |
-
nn.SiLU(),
|
125 |
-
linear(time_embed_dim, time_embed_dim),
|
126 |
-
)
|
127 |
-
|
128 |
-
if self.num_classes is not None:
|
129 |
-
self.label_emb = nn.Embedding(num_classes, time_embed_dim)
|
130 |
-
|
131 |
-
self.input_blocks = nn.ModuleList(
|
132 |
-
[
|
133 |
-
TimestepEmbedSequential(
|
134 |
-
conv_nd(dims, in_channels, model_channels, 3, padding=1)
|
135 |
-
)
|
136 |
-
]
|
137 |
-
)
|
138 |
-
self._feature_size = model_channels
|
139 |
-
input_block_chans = [model_channels]
|
140 |
-
ch = model_channels
|
141 |
-
ds = 1
|
142 |
-
for level, mult in enumerate(channel_mult):
|
143 |
-
for _ in range(num_res_blocks):
|
144 |
-
layers = [
|
145 |
-
ResBlock(
|
146 |
-
ch,
|
147 |
-
time_embed_dim,
|
148 |
-
dropout,
|
149 |
-
out_channels=mult * model_channels,
|
150 |
-
dims=dims,
|
151 |
-
use_checkpoint=use_checkpoint,
|
152 |
-
use_scale_shift_norm=use_scale_shift_norm,
|
153 |
-
)
|
154 |
-
]
|
155 |
-
ch = mult * model_channels
|
156 |
-
if ds in attention_resolutions:
|
157 |
-
if num_head_channels == -1:
|
158 |
-
dim_head = ch // num_heads
|
159 |
-
else:
|
160 |
-
num_heads = ch // num_head_channels
|
161 |
-
dim_head = num_head_channels
|
162 |
-
if legacy:
|
163 |
-
#num_heads = 1
|
164 |
-
dim_head = ch // num_heads if use_spatial_transformer else num_head_channels
|
165 |
-
layers.append(
|
166 |
-
AttentionBlock(
|
167 |
-
ch,
|
168 |
-
use_checkpoint=use_checkpoint,
|
169 |
-
num_heads=num_heads,
|
170 |
-
num_head_channels=dim_head,
|
171 |
-
use_new_attention_order=use_new_attention_order,
|
172 |
-
) if not use_spatial_transformer else SpatialTransformer(
|
173 |
-
ch, num_heads, dim_head, depth=transformer_depth, context_dim=context_dim
|
174 |
-
)
|
175 |
-
)
|
176 |
-
self.input_blocks.append(TimestepEmbedSequential(*layers))
|
177 |
-
self._feature_size += ch
|
178 |
-
input_block_chans.append(ch)
|
179 |
-
if level != len(channel_mult) - 1:
|
180 |
-
out_ch = ch
|
181 |
-
self.input_blocks.append(
|
182 |
-
TimestepEmbedSequential(
|
183 |
-
ResBlock(
|
184 |
-
ch,
|
185 |
-
time_embed_dim,
|
186 |
-
dropout,
|
187 |
-
out_channels=out_ch,
|
188 |
-
dims=dims,
|
189 |
-
use_checkpoint=use_checkpoint,
|
190 |
-
use_scale_shift_norm=use_scale_shift_norm,
|
191 |
-
down=True,
|
192 |
-
)
|
193 |
-
if resblock_updown
|
194 |
-
else Downsample(
|
195 |
-
ch, conv_resample, dims=dims, out_channels=out_ch
|
196 |
-
)
|
197 |
-
)
|
198 |
-
)
|
199 |
-
ch = out_ch
|
200 |
-
input_block_chans.append(ch)
|
201 |
-
ds *= 2
|
202 |
-
self._feature_size += ch
|
203 |
-
|
204 |
-
if num_head_channels == -1:
|
205 |
-
dim_head = ch // num_heads
|
206 |
-
else:
|
207 |
-
num_heads = ch // num_head_channels
|
208 |
-
dim_head = num_head_channels
|
209 |
-
if legacy:
|
210 |
-
#num_heads = 1
|
211 |
-
dim_head = ch // num_heads if use_spatial_transformer else num_head_channels
|
212 |
-
self.middle_block = TimestepEmbedSequential(
|
213 |
-
ResBlock(
|
214 |
-
ch,
|
215 |
-
time_embed_dim,
|
216 |
-
dropout,
|
217 |
-
dims=dims,
|
218 |
-
use_checkpoint=use_checkpoint,
|
219 |
-
use_scale_shift_norm=use_scale_shift_norm,
|
220 |
-
),
|
221 |
-
AttentionBlock(
|
222 |
-
ch,
|
223 |
-
use_checkpoint=use_checkpoint,
|
224 |
-
num_heads=num_heads,
|
225 |
-
num_head_channels=dim_head,
|
226 |
-
use_new_attention_order=use_new_attention_order,
|
227 |
-
) if not use_spatial_transformer else SpatialTransformer(
|
228 |
-
ch, num_heads, dim_head, depth=transformer_depth, context_dim=context_dim
|
229 |
-
),
|
230 |
-
ResBlock(
|
231 |
-
ch,
|
232 |
-
time_embed_dim,
|
233 |
-
dropout,
|
234 |
-
dims=dims,
|
235 |
-
use_checkpoint=use_checkpoint,
|
236 |
-
use_scale_shift_norm=use_scale_shift_norm,
|
237 |
-
),
|
238 |
-
)
|
239 |
-
self._feature_size += ch
|
240 |
-
|
241 |
-
self.output_blocks = nn.ModuleList([])
|
242 |
-
for level, mult in list(enumerate(channel_mult))[::-1]:
|
243 |
-
for i in range(num_res_blocks + 1):
|
244 |
-
ich = input_block_chans.pop()
|
245 |
-
layers = [
|
246 |
-
ResBlock(
|
247 |
-
ch + ich,
|
248 |
-
time_embed_dim,
|
249 |
-
dropout,
|
250 |
-
out_channels=model_channels * mult,
|
251 |
-
dims=dims,
|
252 |
-
use_checkpoint=use_checkpoint,
|
253 |
-
use_scale_shift_norm=use_scale_shift_norm,
|
254 |
-
)
|
255 |
-
]
|
256 |
-
ch = model_channels * mult
|
257 |
-
if ds in attention_resolutions:
|
258 |
-
if num_head_channels == -1:
|
259 |
-
dim_head = ch // num_heads
|
260 |
-
else:
|
261 |
-
num_heads = ch // num_head_channels
|
262 |
-
dim_head = num_head_channels
|
263 |
-
if legacy:
|
264 |
-
#num_heads = 1
|
265 |
-
dim_head = ch // num_heads if use_spatial_transformer else num_head_channels
|
266 |
-
layers.append(
|
267 |
-
AttentionBlock(
|
268 |
-
ch,
|
269 |
-
use_checkpoint=use_checkpoint,
|
270 |
-
num_heads=num_heads_upsample,
|
271 |
-
num_head_channels=dim_head,
|
272 |
-
use_new_attention_order=use_new_attention_order,
|
273 |
-
) if not use_spatial_transformer else SpatialTransformer(
|
274 |
-
ch, num_heads, dim_head, depth=transformer_depth, context_dim=context_dim
|
275 |
-
)
|
276 |
-
)
|
277 |
-
if level and i == num_res_blocks:
|
278 |
-
out_ch = ch
|
279 |
-
layers.append(
|
280 |
-
ResBlock(
|
281 |
-
ch,
|
282 |
-
time_embed_dim,
|
283 |
-
dropout,
|
284 |
-
out_channels=out_ch,
|
285 |
-
dims=dims,
|
286 |
-
use_checkpoint=use_checkpoint,
|
287 |
-
use_scale_shift_norm=use_scale_shift_norm,
|
288 |
-
up=True,
|
289 |
-
)
|
290 |
-
if resblock_updown
|
291 |
-
else Upsample(ch, conv_resample, dims=dims, out_channels=out_ch)
|
292 |
-
)
|
293 |
-
ds //= 2
|
294 |
-
self.output_blocks.append(TimestepEmbedSequential(*layers))
|
295 |
-
self._feature_size += ch
|
296 |
-
|
297 |
-
self.out = nn.Sequential(
|
298 |
-
normalization(ch),
|
299 |
-
nn.SiLU(),
|
300 |
-
zero_module(conv_nd(dims, model_channels, out_channels, 3, padding=1)),
|
301 |
-
)
|
302 |
-
if self.predict_codebook_ids:
|
303 |
-
self.id_predictor = nn.Sequential(
|
304 |
-
normalization(ch),
|
305 |
-
conv_nd(dims, model_channels, n_embed, 1),
|
306 |
-
#nn.LogSoftmax(dim=1) # change to cross_entropy and produce non-normalized logits
|
307 |
-
)
|
308 |
-
|
309 |
-
self.use_context_project = use_context_project
|
310 |
-
if use_context_project:
|
311 |
-
self.context_project = linear(context_dim, time_embed_dim)
|
312 |
-
self.use_context_attn = use_context_attn
|
313 |
-
|
314 |
-
|
315 |
-
def convert_to_fp16(self):
|
316 |
-
"""
|
317 |
-
Convert the torso of the model to float16.
|
318 |
-
"""
|
319 |
-
self.input_blocks.apply(convert_module_to_f16)
|
320 |
-
self.middle_block.apply(convert_module_to_f16)
|
321 |
-
self.output_blocks.apply(convert_module_to_f16)
|
322 |
-
|
323 |
-
def convert_to_fp32(self):
|
324 |
-
"""
|
325 |
-
Convert the torso of the model to float32.
|
326 |
-
"""
|
327 |
-
self.input_blocks.apply(convert_module_to_f32)
|
328 |
-
self.middle_block.apply(convert_module_to_f32)
|
329 |
-
self.output_blocks.apply(convert_module_to_f32)
|
330 |
-
|
331 |
-
def forward(self, x, timesteps=None, context=None, y=None,**kwargs):
|
332 |
-
"""
|
333 |
-
Apply the model to an input batch.
|
334 |
-
:param x: an [N x C x ...] Tensor of inputs.
|
335 |
-
:param timesteps: a 1-D batch of timesteps.
|
336 |
-
:param context: conditioning plugged in via crossattn
|
337 |
-
:param y: an [N] Tensor of labels, if class-conditional.
|
338 |
-
:return: an [N x C x ...] Tensor of outputs.
|
339 |
-
"""
|
340 |
-
assert (y is not None) == (
|
341 |
-
self.num_classes is not None
|
342 |
-
), "must specify y if and only if the model is class-conditional"
|
343 |
-
hs = []
|
344 |
-
t_emb = timestep_embedding(timesteps, self.model_channels, repeat_only=False)
|
345 |
-
emb = self.time_embed(t_emb)
|
346 |
-
|
347 |
-
if self.num_classes is not None:
|
348 |
-
assert y.shape == (x.shape[0],)
|
349 |
-
emb = emb + self.label_emb(y)
|
350 |
-
|
351 |
-
# For text-to-audio using global CLIP
|
352 |
-
if self.use_context_project:
|
353 |
-
context = self.context_project(context)
|
354 |
-
emb = emb + context.squeeze(1)
|
355 |
-
|
356 |
-
h = x.type(self.dtype)
|
357 |
-
for module in self.input_blocks:
|
358 |
-
h = module(h, emb, context if self.use_context_attn else None)
|
359 |
-
hs.append(h)
|
360 |
-
h = self.middle_block(h, emb, context if self.use_context_attn else None)
|
361 |
-
for module in self.output_blocks:
|
362 |
-
h = th.cat([h, hs.pop()], dim=1)
|
363 |
-
h = module(h, emb, context if self.use_context_attn else None)
|
364 |
-
h = h.type(x.dtype)
|
365 |
-
if self.predict_codebook_ids:
|
366 |
-
return self.id_predictor(h)
|
367 |
-
else:
|
368 |
-
return self.out(h)
|
|
|
|
|
|
|
|
|
|
|
|
|
|
|
|
|
|
|
|
|
|
|
|
|
|
|
|
|
|
|
|
|
|
|
|
|
|
|
|
|
|
|
|
|
|
|
|
|
|
|
|
|
|
|
|
|
|
|
|
|
|
|
|
|
|
|
|
|
|
|
|
|
|
|
|
|
|
|
|
|
|
|
|
|
|
|
|
|
|
|
|
|
|
|
|
|
|
|
|
|
|
|
|
|
|
|
|
|
|
|
|
|
|
|
|
|
|
|
|
|
|
|
|
|
|
|
|
|
|
|
|
|
|
|
|
|
|
|
|
|
|
|
|
|
|
|
|
|
|
|
|
|
|
|
|
|
|
|
|
|
|
|
|
|
|
|
|
|
|
|
|
|
|
|
|
|
|
|
|
|
|
|
|
|
|
|
|
|
|
|
|
|
|
|
|
|
|
|
|
|
|
|
|
|
|
|
|
|
|
|
|
|
|
|
|
|
|
|
|
|
|
|
|
|
|
|
|
|
|
|
|
|
|
|
|
|
|
|
|
|
|
|
|
|
|
|
|
|
|
|
|
|
|
|
|
|
|
|
|
|
|
|
|
|
|
|
|
|
|
|
|
|
|
|
|
|
|
|
|
|
|
|
|
|
|
|
|
|
|
|
|
|
|
|
|
|
|
|
|
|
|
|
|
|
|
|
|
|
|
|
|
|
|
|
|
|
|
|
|
|
|
|
|
|
|
|
|
|
|
|
|
|
|
|
|
|
|
|
|
|
|
|
|
|
|
|
|
|
|
|
|
|
|
|
|
|
|
|
|
|
|
|
|
|
|
|
|
|
|
|
|
|
|
|
|
|
|
|
|
|
|
|
|
|
|
|
|
|
|
|
|
|
|
|
|
|
|
|
|
|
|
|
|
|
|
|
|
|
|
|
|
|
|
|
|
|
|
|
|
|
|
|
|
|
|
|
|
|
|
|
|
|
|
|
|
|
|
|
|
|
|
|
|
|
|
|
|
|
|
|
|
|
|
|
|
|
|
|
|
|
|
|
|
|
|
|
|
|
|
|
|
|
|
|
|
|
|
|
|
|
|
|
|
|
|
|
|
|
|
|
|
|
|
|
|
|
|
|
|
|
|
|
|
|
|
|
|
|
|
|
|
|
|
|
|
|
|
|
|
|
|
|
|
|
|
|
|
|
|
|
|
|
|
|
|
|
|
|
|
|
|
|
|
|
|
|
|
|
|
|
|
|
|
|
|
|
|
|
|
|
|
|
|
|
|
|
|
|
|
|
|
|
|
|
|
|
|
|
|
|
|
|
|
|
|
|
|
|
|
|
|
|
|
|
|
|
|
|
|
|
|
|
|
|
|
|
|
|
|
|
|
|
|
|
|
|
|
|
|
|
|
|
|
|
|
|
|
|
|
|
|
|
|
|
|
|
|
|
|
|
|
|
|
|
|
|
|
|
|
|
|
|
|
|
|
|
|
|
|
|
|
|
|
|
|
|
|
|
|
|
|
|
|
|
|
|
|
|
|
|
|
|
|
|
|
|
|
|
|
|
|
|
|
|
|
|
|
|
|
|
|
|
|
|
|
|
|
|
|
|
|
|
|
|
|
|
|
|
|
|
|
|
|
|
|
|
|
|
|
|
|
|
|
|
|
|
|
spaces/AIML-TUDA/FairDiffusionExplorer/README.md
DELETED
@@ -1,13 +0,0 @@
|
|
1 |
-
---
|
2 |
-
title: FairDiffusionExplorer
|
3 |
-
emoji: 📊
|
4 |
-
colorFrom: blue
|
5 |
-
colorTo: yellow
|
6 |
-
sdk: gradio
|
7 |
-
sdk_version: 3.3.1
|
8 |
-
app_file: app.py
|
9 |
-
pinned: false
|
10 |
-
license: cc-by-sa-4.0
|
11 |
-
---
|
12 |
-
|
13 |
-
Check out the configuration reference at https://huggingface.co/docs/hub/spaces-config-reference
|
|
|
|
|
|
|
|
|
|
|
|
|
|
|
|
|
|
|
|
|
|
|
|
|
|
|
|
spaces/AIZero2HeroBootcamp/VideoToAnimatedGif/README.md
DELETED
@@ -1,13 +0,0 @@
|
|
1 |
-
---
|
2 |
-
title: VideoToAnimatedGif
|
3 |
-
emoji: 🐢
|
4 |
-
colorFrom: pink
|
5 |
-
colorTo: purple
|
6 |
-
sdk: streamlit
|
7 |
-
sdk_version: 1.21.0
|
8 |
-
app_file: app.py
|
9 |
-
pinned: false
|
10 |
-
license: mit
|
11 |
-
---
|
12 |
-
|
13 |
-
Check out the configuration reference at https://huggingface.co/docs/hub/spaces-config-reference
|
|
|
|
|
|
|
|
|
|
|
|
|
|
|
|
|
|
|
|
|
|
|
|
|
|
|
|
spaces/ATang0729/Forecast4Muses/Model/Model6/Model6_0_ClothesDetection/mmyolo/configs/yolov6/yolov6_t_syncbn_fast_8xb32-400e_coco.py
DELETED
@@ -1,17 +0,0 @@
|
|
1 |
-
_base_ = './yolov6_s_syncbn_fast_8xb32-400e_coco.py'
|
2 |
-
|
3 |
-
# ======================= Possible modified parameters =======================
|
4 |
-
# -----model related-----
|
5 |
-
# The scaling factor that controls the depth of the network structure
|
6 |
-
deepen_factor = 0.33
|
7 |
-
# The scaling factor that controls the width of the network structure
|
8 |
-
widen_factor = 0.375
|
9 |
-
|
10 |
-
# ============================== Unmodified in most cases ===================
|
11 |
-
model = dict(
|
12 |
-
backbone=dict(deepen_factor=deepen_factor, widen_factor=widen_factor),
|
13 |
-
neck=dict(deepen_factor=deepen_factor, widen_factor=widen_factor),
|
14 |
-
bbox_head=dict(
|
15 |
-
type='YOLOv6Head',
|
16 |
-
head_module=dict(widen_factor=widen_factor),
|
17 |
-
loss_bbox=dict(iou_mode='siou')))
|
|
|
|
|
|
|
|
|
|
|
|
|
|
|
|
|
|
|
|
|
|
|
|
|
|
|
|
|
|
|
|
|
|
|
|
spaces/AchyuthGamer/OpenGPT/g4f/Provider/Providers/deprecated/Wewordle.py
DELETED
@@ -1,65 +0,0 @@
|
|
1 |
-
from __future__ import annotations
|
2 |
-
|
3 |
-
import random, string, time
|
4 |
-
from aiohttp import ClientSession
|
5 |
-
|
6 |
-
from ..base_provider import AsyncProvider
|
7 |
-
|
8 |
-
|
9 |
-
class Wewordle(AsyncProvider):
|
10 |
-
url = "https://wewordle.org"
|
11 |
-
working = False
|
12 |
-
supports_gpt_35_turbo = True
|
13 |
-
|
14 |
-
@classmethod
|
15 |
-
async def create_async(
|
16 |
-
cls,
|
17 |
-
model: str,
|
18 |
-
messages: list[dict[str, str]],
|
19 |
-
proxy: str = None,
|
20 |
-
**kwargs
|
21 |
-
) -> str:
|
22 |
-
|
23 |
-
headers = {
|
24 |
-
"accept" : "*/*",
|
25 |
-
"pragma" : "no-cache",
|
26 |
-
"Content-Type" : "application/json",
|
27 |
-
"Connection" : "keep-alive"
|
28 |
-
}
|
29 |
-
|
30 |
-
_user_id = "".join(random.choices(f"{string.ascii_lowercase}{string.digits}", k=16))
|
31 |
-
_app_id = "".join(random.choices(f"{string.ascii_lowercase}{string.digits}", k=31))
|
32 |
-
_request_date = time.strftime("%Y-%m-%dT%H:%M:%S.000Z", time.gmtime())
|
33 |
-
data = {
|
34 |
-
"user" : _user_id,
|
35 |
-
"messages" : messages,
|
36 |
-
"subscriber": {
|
37 |
-
"originalPurchaseDate" : None,
|
38 |
-
"originalApplicationVersion" : None,
|
39 |
-
"allPurchaseDatesMillis" : {},
|
40 |
-
"entitlements" : {"active": {}, "all": {}},
|
41 |
-
"allPurchaseDates" : {},
|
42 |
-
"allExpirationDatesMillis" : {},
|
43 |
-
"allExpirationDates" : {},
|
44 |
-
"originalAppUserId" : f"$RCAnonymousID:{_app_id}",
|
45 |
-
"latestExpirationDate" : None,
|
46 |
-
"requestDate" : _request_date,
|
47 |
-
"latestExpirationDateMillis" : None,
|
48 |
-
"nonSubscriptionTransactions" : [],
|
49 |
-
"originalPurchaseDateMillis" : None,
|
50 |
-
"managementURL" : None,
|
51 |
-
"allPurchasedProductIdentifiers": [],
|
52 |
-
"firstSeen" : _request_date,
|
53 |
-
"activeSubscriptions" : [],
|
54 |
-
}
|
55 |
-
}
|
56 |
-
|
57 |
-
|
58 |
-
async with ClientSession(
|
59 |
-
headers=headers
|
60 |
-
) as session:
|
61 |
-
async with session.post(f"{cls.url}/gptapi/v1/android/turbo", proxy=proxy, json=data) as response:
|
62 |
-
response.raise_for_status()
|
63 |
-
content = (await response.json())["message"]["content"]
|
64 |
-
if content:
|
65 |
-
return content
|
|
|
|
|
|
|
|
|
|
|
|
|
|
|
|
|
|
|
|
|
|
|
|
|
|
|
|
|
|
|
|
|
|
|
|
|
|
|
|
|
|
|
|
|
|
|
|
|
|
|
|
|
|
|
|
|
|
|
|
|
|
|
|
|
|
|
|
|
|
|
|
|
|
|
|
|
|
|
|
|
|
|
|
|
|
|
|
|
|
|
|
|
|
|
|
|
|
|
|
|
|
|
|
|
|
|
|
|
|
|
|
|
|
|
|
|
|
|
|
|
|
|
|
|
|
|
|
|
|
|
|
spaces/AgentVerse/agentVerse/ui/src/phaser3-rex-plugins/plugins/cursoratbound-plugin.js
DELETED
@@ -1,20 +0,0 @@
|
|
1 |
-
import CursorAtBound from './cursoratbound.js';
|
2 |
-
|
3 |
-
class CursorAtBoundPlugin extends Phaser.Plugins.BasePlugin {
|
4 |
-
|
5 |
-
constructor(pluginManager) {
|
6 |
-
super(pluginManager);
|
7 |
-
}
|
8 |
-
|
9 |
-
start() {
|
10 |
-
var eventEmitter = this.game.events;
|
11 |
-
eventEmitter.on('destroy', this.destroy, this);
|
12 |
-
}
|
13 |
-
|
14 |
-
add(scene, config) {
|
15 |
-
return new CursorAtBound(scene, config);
|
16 |
-
}
|
17 |
-
|
18 |
-
}
|
19 |
-
|
20 |
-
export default CursorAtBoundPlugin;
|
|
|
|
|
|
|
|
|
|
|
|
|
|
|
|
|
|
|
|
|
|
|
|
|
|
|
|
|
|
|
|
|
|
|
|
|
|
|
|
|
|
spaces/AgentVerse/agentVerse/ui/src/phaser3-rex-plugins/templates/ui/circularprogresscanvas/Factory.d.ts
DELETED
@@ -1,13 +0,0 @@
|
|
1 |
-
import CircularProgressCanvas from './CircularProgressCanvas';
|
2 |
-
|
3 |
-
export default function (
|
4 |
-
config?: CircularProgressCanvas.IConfig
|
5 |
-
): CircularProgressCanvas;
|
6 |
-
|
7 |
-
export default function (
|
8 |
-
x?: number, y?: number,
|
9 |
-
radius?: number,
|
10 |
-
barColor?: string | number,
|
11 |
-
value?: number,
|
12 |
-
config?: CircularProgressCanvas.IConfig
|
13 |
-
): CircularProgressCanvas;
|
|
|
|
|
|
|
|
|
|
|
|
|
|
|
|
|
|
|
|
|
|
|
|
|
|
|
|
spaces/AgentVerse/agentVerse/ui/src/phaser3-rex-plugins/templates/ui/gridsizer/RunWidthWrap.js
DELETED
@@ -1,25 +0,0 @@
|
|
1 |
-
// Default method
|
2 |
-
var RunWidthWrap = function (width) {
|
3 |
-
var child, childWidth;
|
4 |
-
var colWidth;
|
5 |
-
for (var i in this.sizerChildren) {
|
6 |
-
child = this.sizerChildren[i];
|
7 |
-
if (
|
8 |
-
(!child) ||
|
9 |
-
(child.isRexSizer && child.ignoreLayout) ||
|
10 |
-
(!child.runWidthWrap)
|
11 |
-
) {
|
12 |
-
continue;
|
13 |
-
}
|
14 |
-
|
15 |
-
colWidth = this.getColumnWidth(parseInt(i) % this.columnCount);
|
16 |
-
childWidth = this.getExpandedChildWidth(child, colWidth);
|
17 |
-
if (child.isRexSizer) {
|
18 |
-
childWidth = child.resolveWidth(childWidth);
|
19 |
-
}
|
20 |
-
child.runWidthWrap(childWidth);
|
21 |
-
}
|
22 |
-
return this;
|
23 |
-
}
|
24 |
-
|
25 |
-
export default RunWidthWrap;
|
|
|
|
|
|
|
|
|
|
|
|
|
|
|
|
|
|
|
|
|
|
|
|
|
|
|
|
|
|
|
|
|
|
|
|
|
|
|
|
|
|
|
|
|
|
|
|
|
|
|
|
spaces/Aki004/herta-so-vits/utils.py
DELETED
@@ -1,543 +0,0 @@
|
|
1 |
-
import os
|
2 |
-
import glob
|
3 |
-
import re
|
4 |
-
import sys
|
5 |
-
import argparse
|
6 |
-
import logging
|
7 |
-
import json
|
8 |
-
import subprocess
|
9 |
-
import warnings
|
10 |
-
import random
|
11 |
-
import functools
|
12 |
-
|
13 |
-
import librosa
|
14 |
-
import numpy as np
|
15 |
-
from scipy.io.wavfile import read
|
16 |
-
import torch
|
17 |
-
from torch.nn import functional as F
|
18 |
-
from modules.commons import sequence_mask
|
19 |
-
from hubert import hubert_model
|
20 |
-
|
21 |
-
MATPLOTLIB_FLAG = False
|
22 |
-
|
23 |
-
logging.basicConfig(stream=sys.stdout, level=logging.DEBUG)
|
24 |
-
logger = logging
|
25 |
-
|
26 |
-
f0_bin = 256
|
27 |
-
f0_max = 1100.0
|
28 |
-
f0_min = 50.0
|
29 |
-
f0_mel_min = 1127 * np.log(1 + f0_min / 700)
|
30 |
-
f0_mel_max = 1127 * np.log(1 + f0_max / 700)
|
31 |
-
|
32 |
-
|
33 |
-
# def normalize_f0(f0, random_scale=True):
|
34 |
-
# f0_norm = f0.clone() # create a copy of the input Tensor
|
35 |
-
# batch_size, _, frame_length = f0_norm.shape
|
36 |
-
# for i in range(batch_size):
|
37 |
-
# means = torch.mean(f0_norm[i, 0, :])
|
38 |
-
# if random_scale:
|
39 |
-
# factor = random.uniform(0.8, 1.2)
|
40 |
-
# else:
|
41 |
-
# factor = 1
|
42 |
-
# f0_norm[i, 0, :] = (f0_norm[i, 0, :] - means) * factor
|
43 |
-
# return f0_norm
|
44 |
-
# def normalize_f0(f0, random_scale=True):
|
45 |
-
# means = torch.mean(f0[:, 0, :], dim=1, keepdim=True)
|
46 |
-
# if random_scale:
|
47 |
-
# factor = torch.Tensor(f0.shape[0],1).uniform_(0.8, 1.2).to(f0.device)
|
48 |
-
# else:
|
49 |
-
# factor = torch.ones(f0.shape[0], 1, 1).to(f0.device)
|
50 |
-
# f0_norm = (f0 - means.unsqueeze(-1)) * factor.unsqueeze(-1)
|
51 |
-
# return f0_norm
|
52 |
-
|
53 |
-
def deprecated(func):
|
54 |
-
"""This is a decorator which can be used to mark functions
|
55 |
-
as deprecated. It will result in a warning being emitted
|
56 |
-
when the function is used."""
|
57 |
-
@functools.wraps(func)
|
58 |
-
def new_func(*args, **kwargs):
|
59 |
-
warnings.simplefilter('always', DeprecationWarning) # turn off filter
|
60 |
-
warnings.warn("Call to deprecated function {}.".format(func.__name__),
|
61 |
-
category=DeprecationWarning,
|
62 |
-
stacklevel=2)
|
63 |
-
warnings.simplefilter('default', DeprecationWarning) # reset filter
|
64 |
-
return func(*args, **kwargs)
|
65 |
-
return new_func
|
66 |
-
|
67 |
-
def normalize_f0(f0, x_mask, uv, random_scale=True):
|
68 |
-
# calculate means based on x_mask
|
69 |
-
uv_sum = torch.sum(uv, dim=1, keepdim=True)
|
70 |
-
uv_sum[uv_sum == 0] = 9999
|
71 |
-
means = torch.sum(f0[:, 0, :] * uv, dim=1, keepdim=True) / uv_sum
|
72 |
-
|
73 |
-
if random_scale:
|
74 |
-
factor = torch.Tensor(f0.shape[0], 1).uniform_(0.8, 1.2).to(f0.device)
|
75 |
-
else:
|
76 |
-
factor = torch.ones(f0.shape[0], 1).to(f0.device)
|
77 |
-
# normalize f0 based on means and factor
|
78 |
-
f0_norm = (f0 - means.unsqueeze(-1)) * factor.unsqueeze(-1)
|
79 |
-
if torch.isnan(f0_norm).any():
|
80 |
-
exit(0)
|
81 |
-
return f0_norm * x_mask
|
82 |
-
|
83 |
-
def compute_f0_uv_torchcrepe(wav_numpy, p_len=None, sampling_rate=44100, hop_length=512,device=None,cr_threshold=0.05):
|
84 |
-
from modules.crepe import CrepePitchExtractor
|
85 |
-
x = wav_numpy
|
86 |
-
if p_len is None:
|
87 |
-
p_len = x.shape[0]//hop_length
|
88 |
-
else:
|
89 |
-
assert abs(p_len-x.shape[0]//hop_length) < 4, "pad length error"
|
90 |
-
|
91 |
-
f0_min = 50
|
92 |
-
f0_max = 1100
|
93 |
-
F0Creper = CrepePitchExtractor(hop_length=hop_length,f0_min=f0_min,f0_max=f0_max,device=device,threshold=cr_threshold)
|
94 |
-
f0,uv = F0Creper(x[None,:].float(),sampling_rate,pad_to=p_len)
|
95 |
-
return f0,uv
|
96 |
-
|
97 |
-
def plot_data_to_numpy(x, y):
|
98 |
-
global MATPLOTLIB_FLAG
|
99 |
-
if not MATPLOTLIB_FLAG:
|
100 |
-
import matplotlib
|
101 |
-
matplotlib.use("Agg")
|
102 |
-
MATPLOTLIB_FLAG = True
|
103 |
-
mpl_logger = logging.getLogger('matplotlib')
|
104 |
-
mpl_logger.setLevel(logging.WARNING)
|
105 |
-
import matplotlib.pylab as plt
|
106 |
-
import numpy as np
|
107 |
-
|
108 |
-
fig, ax = plt.subplots(figsize=(10, 2))
|
109 |
-
plt.plot(x)
|
110 |
-
plt.plot(y)
|
111 |
-
plt.tight_layout()
|
112 |
-
|
113 |
-
fig.canvas.draw()
|
114 |
-
data = np.fromstring(fig.canvas.tostring_rgb(), dtype=np.uint8, sep='')
|
115 |
-
data = data.reshape(fig.canvas.get_width_height()[::-1] + (3,))
|
116 |
-
plt.close()
|
117 |
-
return data
|
118 |
-
|
119 |
-
|
120 |
-
|
121 |
-
def interpolate_f0(f0):
|
122 |
-
|
123 |
-
data = np.reshape(f0, (f0.size, 1))
|
124 |
-
|
125 |
-
vuv_vector = np.zeros((data.size, 1), dtype=np.float32)
|
126 |
-
vuv_vector[data > 0.0] = 1.0
|
127 |
-
vuv_vector[data <= 0.0] = 0.0
|
128 |
-
|
129 |
-
ip_data = data
|
130 |
-
|
131 |
-
frame_number = data.size
|
132 |
-
last_value = 0.0
|
133 |
-
for i in range(frame_number):
|
134 |
-
if data[i] <= 0.0:
|
135 |
-
j = i + 1
|
136 |
-
for j in range(i + 1, frame_number):
|
137 |
-
if data[j] > 0.0:
|
138 |
-
break
|
139 |
-
if j < frame_number - 1:
|
140 |
-
if last_value > 0.0:
|
141 |
-
step = (data[j] - data[i - 1]) / float(j - i)
|
142 |
-
for k in range(i, j):
|
143 |
-
ip_data[k] = data[i - 1] + step * (k - i + 1)
|
144 |
-
else:
|
145 |
-
for k in range(i, j):
|
146 |
-
ip_data[k] = data[j]
|
147 |
-
else:
|
148 |
-
for k in range(i, frame_number):
|
149 |
-
ip_data[k] = last_value
|
150 |
-
else:
|
151 |
-
ip_data[i] = data[i] # this may not be necessary
|
152 |
-
last_value = data[i]
|
153 |
-
|
154 |
-
return ip_data[:,0], vuv_vector[:,0]
|
155 |
-
|
156 |
-
|
157 |
-
def compute_f0_parselmouth(wav_numpy, p_len=None, sampling_rate=44100, hop_length=512):
|
158 |
-
import parselmouth
|
159 |
-
x = wav_numpy
|
160 |
-
if p_len is None:
|
161 |
-
p_len = x.shape[0]//hop_length
|
162 |
-
else:
|
163 |
-
assert abs(p_len-x.shape[0]//hop_length) < 4, "pad length error"
|
164 |
-
time_step = hop_length / sampling_rate * 1000
|
165 |
-
f0_min = 50
|
166 |
-
f0_max = 1100
|
167 |
-
f0 = parselmouth.Sound(x, sampling_rate).to_pitch_ac(
|
168 |
-
time_step=time_step / 1000, voicing_threshold=0.6,
|
169 |
-
pitch_floor=f0_min, pitch_ceiling=f0_max).selected_array['frequency']
|
170 |
-
|
171 |
-
pad_size=(p_len - len(f0) + 1) // 2
|
172 |
-
if(pad_size>0 or p_len - len(f0) - pad_size>0):
|
173 |
-
f0 = np.pad(f0,[[pad_size,p_len - len(f0) - pad_size]], mode='constant')
|
174 |
-
return f0
|
175 |
-
|
176 |
-
def resize_f0(x, target_len):
|
177 |
-
source = np.array(x)
|
178 |
-
source[source<0.001] = np.nan
|
179 |
-
target = np.interp(np.arange(0, len(source)*target_len, len(source))/ target_len, np.arange(0, len(source)), source)
|
180 |
-
res = np.nan_to_num(target)
|
181 |
-
return res
|
182 |
-
|
183 |
-
def compute_f0_dio(wav_numpy, p_len=None, sampling_rate=44100, hop_length=512):
|
184 |
-
import pyworld
|
185 |
-
if p_len is None:
|
186 |
-
p_len = wav_numpy.shape[0]//hop_length
|
187 |
-
f0, t = pyworld.dio(
|
188 |
-
wav_numpy.astype(np.double),
|
189 |
-
fs=sampling_rate,
|
190 |
-
f0_ceil=800,
|
191 |
-
frame_period=1000 * hop_length / sampling_rate,
|
192 |
-
)
|
193 |
-
f0 = pyworld.stonemask(wav_numpy.astype(np.double), f0, t, sampling_rate)
|
194 |
-
for index, pitch in enumerate(f0):
|
195 |
-
f0[index] = round(pitch, 1)
|
196 |
-
return resize_f0(f0, p_len)
|
197 |
-
|
198 |
-
def f0_to_coarse(f0):
|
199 |
-
is_torch = isinstance(f0, torch.Tensor)
|
200 |
-
f0_mel = 1127 * (1 + f0 / 700).log() if is_torch else 1127 * np.log(1 + f0 / 700)
|
201 |
-
f0_mel[f0_mel > 0] = (f0_mel[f0_mel > 0] - f0_mel_min) * (f0_bin - 2) / (f0_mel_max - f0_mel_min) + 1
|
202 |
-
|
203 |
-
f0_mel[f0_mel <= 1] = 1
|
204 |
-
f0_mel[f0_mel > f0_bin - 1] = f0_bin - 1
|
205 |
-
f0_coarse = (f0_mel + 0.5).int() if is_torch else np.rint(f0_mel).astype(np.int)
|
206 |
-
assert f0_coarse.max() <= 255 and f0_coarse.min() >= 1, (f0_coarse.max(), f0_coarse.min())
|
207 |
-
return f0_coarse
|
208 |
-
|
209 |
-
|
210 |
-
def get_hubert_model():
|
211 |
-
vec_path = "hubert/checkpoint_best_legacy_500.pt"
|
212 |
-
print("load model(s) from {}".format(vec_path))
|
213 |
-
from fairseq import checkpoint_utils
|
214 |
-
models, saved_cfg, task = checkpoint_utils.load_model_ensemble_and_task(
|
215 |
-
[vec_path],
|
216 |
-
suffix="",
|
217 |
-
)
|
218 |
-
model = models[0]
|
219 |
-
model.eval()
|
220 |
-
return model
|
221 |
-
|
222 |
-
def get_hubert_content(hmodel, wav_16k_tensor):
|
223 |
-
feats = wav_16k_tensor
|
224 |
-
if feats.dim() == 2: # double channels
|
225 |
-
feats = feats.mean(-1)
|
226 |
-
assert feats.dim() == 1, feats.dim()
|
227 |
-
feats = feats.view(1, -1)
|
228 |
-
padding_mask = torch.BoolTensor(feats.shape).fill_(False)
|
229 |
-
inputs = {
|
230 |
-
"source": feats.to(wav_16k_tensor.device),
|
231 |
-
"padding_mask": padding_mask.to(wav_16k_tensor.device),
|
232 |
-
"output_layer": 9, # layer 9
|
233 |
-
}
|
234 |
-
with torch.no_grad():
|
235 |
-
logits = hmodel.extract_features(**inputs)
|
236 |
-
feats = hmodel.final_proj(logits[0])
|
237 |
-
return feats.transpose(1, 2)
|
238 |
-
|
239 |
-
|
240 |
-
def get_content(cmodel, y):
|
241 |
-
with torch.no_grad():
|
242 |
-
c = cmodel.extract_features(y.squeeze(1))[0]
|
243 |
-
c = c.transpose(1, 2)
|
244 |
-
return c
|
245 |
-
|
246 |
-
|
247 |
-
|
248 |
-
def load_checkpoint(checkpoint_path, model, optimizer=None, skip_optimizer=False):
|
249 |
-
assert os.path.isfile(checkpoint_path)
|
250 |
-
checkpoint_dict = torch.load(checkpoint_path, map_location='cpu')
|
251 |
-
iteration = checkpoint_dict['iteration']
|
252 |
-
learning_rate = checkpoint_dict['learning_rate']
|
253 |
-
if optimizer is not None and not skip_optimizer and checkpoint_dict['optimizer'] is not None:
|
254 |
-
optimizer.load_state_dict(checkpoint_dict['optimizer'])
|
255 |
-
saved_state_dict = checkpoint_dict['model']
|
256 |
-
if hasattr(model, 'module'):
|
257 |
-
state_dict = model.module.state_dict()
|
258 |
-
else:
|
259 |
-
state_dict = model.state_dict()
|
260 |
-
new_state_dict = {}
|
261 |
-
for k, v in state_dict.items():
|
262 |
-
try:
|
263 |
-
# assert "dec" in k or "disc" in k
|
264 |
-
# print("load", k)
|
265 |
-
new_state_dict[k] = saved_state_dict[k]
|
266 |
-
assert saved_state_dict[k].shape == v.shape, (saved_state_dict[k].shape, v.shape)
|
267 |
-
except:
|
268 |
-
print("error, %s is not in the checkpoint" % k)
|
269 |
-
logger.info("%s is not in the checkpoint" % k)
|
270 |
-
new_state_dict[k] = v
|
271 |
-
if hasattr(model, 'module'):
|
272 |
-
model.module.load_state_dict(new_state_dict)
|
273 |
-
else:
|
274 |
-
model.load_state_dict(new_state_dict)
|
275 |
-
print("load ")
|
276 |
-
logger.info("Loaded checkpoint '{}' (iteration {})".format(
|
277 |
-
checkpoint_path, iteration))
|
278 |
-
return model, optimizer, learning_rate, iteration
|
279 |
-
|
280 |
-
|
281 |
-
def save_checkpoint(model, optimizer, learning_rate, iteration, checkpoint_path):
|
282 |
-
logger.info("Saving model and optimizer state at iteration {} to {}".format(
|
283 |
-
iteration, checkpoint_path))
|
284 |
-
if hasattr(model, 'module'):
|
285 |
-
state_dict = model.module.state_dict()
|
286 |
-
else:
|
287 |
-
state_dict = model.state_dict()
|
288 |
-
torch.save({'model': state_dict,
|
289 |
-
'iteration': iteration,
|
290 |
-
'optimizer': optimizer.state_dict(),
|
291 |
-
'learning_rate': learning_rate}, checkpoint_path)
|
292 |
-
|
293 |
-
def clean_checkpoints(path_to_models='logs/44k/', n_ckpts_to_keep=2, sort_by_time=True):
|
294 |
-
"""Freeing up space by deleting saved ckpts
|
295 |
-
|
296 |
-
Arguments:
|
297 |
-
path_to_models -- Path to the model directory
|
298 |
-
n_ckpts_to_keep -- Number of ckpts to keep, excluding G_0.pth and D_0.pth
|
299 |
-
sort_by_time -- True -> chronologically delete ckpts
|
300 |
-
False -> lexicographically delete ckpts
|
301 |
-
"""
|
302 |
-
ckpts_files = [f for f in os.listdir(path_to_models) if os.path.isfile(os.path.join(path_to_models, f))]
|
303 |
-
name_key = (lambda _f: int(re.compile('._(\d+)\.pth').match(_f).group(1)))
|
304 |
-
time_key = (lambda _f: os.path.getmtime(os.path.join(path_to_models, _f)))
|
305 |
-
sort_key = time_key if sort_by_time else name_key
|
306 |
-
x_sorted = lambda _x: sorted([f for f in ckpts_files if f.startswith(_x) and not f.endswith('_0.pth')], key=sort_key)
|
307 |
-
to_del = [os.path.join(path_to_models, fn) for fn in
|
308 |
-
(x_sorted('G')[:-n_ckpts_to_keep] + x_sorted('D')[:-n_ckpts_to_keep])]
|
309 |
-
del_info = lambda fn: logger.info(f".. Free up space by deleting ckpt {fn}")
|
310 |
-
del_routine = lambda x: [os.remove(x), del_info(x)]
|
311 |
-
rs = [del_routine(fn) for fn in to_del]
|
312 |
-
|
313 |
-
def summarize(writer, global_step, scalars={}, histograms={}, images={}, audios={}, audio_sampling_rate=22050):
|
314 |
-
for k, v in scalars.items():
|
315 |
-
writer.add_scalar(k, v, global_step)
|
316 |
-
for k, v in histograms.items():
|
317 |
-
writer.add_histogram(k, v, global_step)
|
318 |
-
for k, v in images.items():
|
319 |
-
writer.add_image(k, v, global_step, dataformats='HWC')
|
320 |
-
for k, v in audios.items():
|
321 |
-
writer.add_audio(k, v, global_step, audio_sampling_rate)
|
322 |
-
|
323 |
-
|
324 |
-
def latest_checkpoint_path(dir_path, regex="G_*.pth"):
|
325 |
-
f_list = glob.glob(os.path.join(dir_path, regex))
|
326 |
-
f_list.sort(key=lambda f: int("".join(filter(str.isdigit, f))))
|
327 |
-
x = f_list[-1]
|
328 |
-
print(x)
|
329 |
-
return x
|
330 |
-
|
331 |
-
|
332 |
-
def plot_spectrogram_to_numpy(spectrogram):
|
333 |
-
global MATPLOTLIB_FLAG
|
334 |
-
if not MATPLOTLIB_FLAG:
|
335 |
-
import matplotlib
|
336 |
-
matplotlib.use("Agg")
|
337 |
-
MATPLOTLIB_FLAG = True
|
338 |
-
mpl_logger = logging.getLogger('matplotlib')
|
339 |
-
mpl_logger.setLevel(logging.WARNING)
|
340 |
-
import matplotlib.pylab as plt
|
341 |
-
import numpy as np
|
342 |
-
|
343 |
-
fig, ax = plt.subplots(figsize=(10,2))
|
344 |
-
im = ax.imshow(spectrogram, aspect="auto", origin="lower",
|
345 |
-
interpolation='none')
|
346 |
-
plt.colorbar(im, ax=ax)
|
347 |
-
plt.xlabel("Frames")
|
348 |
-
plt.ylabel("Channels")
|
349 |
-
plt.tight_layout()
|
350 |
-
|
351 |
-
fig.canvas.draw()
|
352 |
-
data = np.fromstring(fig.canvas.tostring_rgb(), dtype=np.uint8, sep='')
|
353 |
-
data = data.reshape(fig.canvas.get_width_height()[::-1] + (3,))
|
354 |
-
plt.close()
|
355 |
-
return data
|
356 |
-
|
357 |
-
|
358 |
-
def plot_alignment_to_numpy(alignment, info=None):
|
359 |
-
global MATPLOTLIB_FLAG
|
360 |
-
if not MATPLOTLIB_FLAG:
|
361 |
-
import matplotlib
|
362 |
-
matplotlib.use("Agg")
|
363 |
-
MATPLOTLIB_FLAG = True
|
364 |
-
mpl_logger = logging.getLogger('matplotlib')
|
365 |
-
mpl_logger.setLevel(logging.WARNING)
|
366 |
-
import matplotlib.pylab as plt
|
367 |
-
import numpy as np
|
368 |
-
|
369 |
-
fig, ax = plt.subplots(figsize=(6, 4))
|
370 |
-
im = ax.imshow(alignment.transpose(), aspect='auto', origin='lower',
|
371 |
-
interpolation='none')
|
372 |
-
fig.colorbar(im, ax=ax)
|
373 |
-
xlabel = 'Decoder timestep'
|
374 |
-
if info is not None:
|
375 |
-
xlabel += '\n\n' + info
|
376 |
-
plt.xlabel(xlabel)
|
377 |
-
plt.ylabel('Encoder timestep')
|
378 |
-
plt.tight_layout()
|
379 |
-
|
380 |
-
fig.canvas.draw()
|
381 |
-
data = np.fromstring(fig.canvas.tostring_rgb(), dtype=np.uint8, sep='')
|
382 |
-
data = data.reshape(fig.canvas.get_width_height()[::-1] + (3,))
|
383 |
-
plt.close()
|
384 |
-
return data
|
385 |
-
|
386 |
-
|
387 |
-
def load_wav_to_torch(full_path):
|
388 |
-
sampling_rate, data = read(full_path)
|
389 |
-
return torch.FloatTensor(data.astype(np.float32)), sampling_rate
|
390 |
-
|
391 |
-
|
392 |
-
def load_filepaths_and_text(filename, split="|"):
|
393 |
-
with open(filename, encoding='utf-8') as f:
|
394 |
-
filepaths_and_text = [line.strip().split(split) for line in f]
|
395 |
-
return filepaths_and_text
|
396 |
-
|
397 |
-
|
398 |
-
def get_hparams(init=True):
|
399 |
-
parser = argparse.ArgumentParser()
|
400 |
-
parser.add_argument('-c', '--config', type=str, default="./configs/base.json",
|
401 |
-
help='JSON file for configuration')
|
402 |
-
parser.add_argument('-m', '--model', type=str, required=True,
|
403 |
-
help='Model name')
|
404 |
-
|
405 |
-
args = parser.parse_args()
|
406 |
-
model_dir = os.path.join("./logs", args.model)
|
407 |
-
|
408 |
-
if not os.path.exists(model_dir):
|
409 |
-
os.makedirs(model_dir)
|
410 |
-
|
411 |
-
config_path = args.config
|
412 |
-
config_save_path = os.path.join(model_dir, "config.json")
|
413 |
-
if init:
|
414 |
-
with open(config_path, "r") as f:
|
415 |
-
data = f.read()
|
416 |
-
with open(config_save_path, "w") as f:
|
417 |
-
f.write(data)
|
418 |
-
else:
|
419 |
-
with open(config_save_path, "r") as f:
|
420 |
-
data = f.read()
|
421 |
-
config = json.loads(data)
|
422 |
-
|
423 |
-
hparams = HParams(**config)
|
424 |
-
hparams.model_dir = model_dir
|
425 |
-
return hparams
|
426 |
-
|
427 |
-
|
428 |
-
def get_hparams_from_dir(model_dir):
|
429 |
-
config_save_path = os.path.join(model_dir, "config.json")
|
430 |
-
with open(config_save_path, "r") as f:
|
431 |
-
data = f.read()
|
432 |
-
config = json.loads(data)
|
433 |
-
|
434 |
-
hparams =HParams(**config)
|
435 |
-
hparams.model_dir = model_dir
|
436 |
-
return hparams
|
437 |
-
|
438 |
-
|
439 |
-
def get_hparams_from_file(config_path):
|
440 |
-
with open(config_path, "r") as f:
|
441 |
-
data = f.read()
|
442 |
-
config = json.loads(data)
|
443 |
-
|
444 |
-
hparams =HParams(**config)
|
445 |
-
return hparams
|
446 |
-
|
447 |
-
|
448 |
-
def check_git_hash(model_dir):
|
449 |
-
source_dir = os.path.dirname(os.path.realpath(__file__))
|
450 |
-
if not os.path.exists(os.path.join(source_dir, ".git")):
|
451 |
-
logger.warn("{} is not a git repository, therefore hash value comparison will be ignored.".format(
|
452 |
-
source_dir
|
453 |
-
))
|
454 |
-
return
|
455 |
-
|
456 |
-
cur_hash = subprocess.getoutput("git rev-parse HEAD")
|
457 |
-
|
458 |
-
path = os.path.join(model_dir, "githash")
|
459 |
-
if os.path.exists(path):
|
460 |
-
saved_hash = open(path).read()
|
461 |
-
if saved_hash != cur_hash:
|
462 |
-
logger.warn("git hash values are different. {}(saved) != {}(current)".format(
|
463 |
-
saved_hash[:8], cur_hash[:8]))
|
464 |
-
else:
|
465 |
-
open(path, "w").write(cur_hash)
|
466 |
-
|
467 |
-
|
468 |
-
def get_logger(model_dir, filename="train.log"):
|
469 |
-
global logger
|
470 |
-
logger = logging.getLogger(os.path.basename(model_dir))
|
471 |
-
logger.setLevel(logging.DEBUG)
|
472 |
-
|
473 |
-
formatter = logging.Formatter("%(asctime)s\t%(name)s\t%(levelname)s\t%(message)s")
|
474 |
-
if not os.path.exists(model_dir):
|
475 |
-
os.makedirs(model_dir)
|
476 |
-
h = logging.FileHandler(os.path.join(model_dir, filename))
|
477 |
-
h.setLevel(logging.DEBUG)
|
478 |
-
h.setFormatter(formatter)
|
479 |
-
logger.addHandler(h)
|
480 |
-
return logger
|
481 |
-
|
482 |
-
|
483 |
-
def repeat_expand_2d(content, target_len):
|
484 |
-
# content : [h, t]
|
485 |
-
|
486 |
-
src_len = content.shape[-1]
|
487 |
-
target = torch.zeros([content.shape[0], target_len], dtype=torch.float).to(content.device)
|
488 |
-
temp = torch.arange(src_len+1) * target_len / src_len
|
489 |
-
current_pos = 0
|
490 |
-
for i in range(target_len):
|
491 |
-
if i < temp[current_pos+1]:
|
492 |
-
target[:, i] = content[:, current_pos]
|
493 |
-
else:
|
494 |
-
current_pos += 1
|
495 |
-
target[:, i] = content[:, current_pos]
|
496 |
-
|
497 |
-
return target
|
498 |
-
|
499 |
-
|
500 |
-
def mix_model(model_paths,mix_rate,mode):
|
501 |
-
mix_rate = torch.FloatTensor(mix_rate)/100
|
502 |
-
model_tem = torch.load(model_paths[0])
|
503 |
-
models = [torch.load(path)["model"] for path in model_paths]
|
504 |
-
if mode == 0:
|
505 |
-
mix_rate = F.softmax(mix_rate,dim=0)
|
506 |
-
for k in model_tem["model"].keys():
|
507 |
-
model_tem["model"][k] = torch.zeros_like(model_tem["model"][k])
|
508 |
-
for i,model in enumerate(models):
|
509 |
-
model_tem["model"][k] += model[k]*mix_rate[i]
|
510 |
-
torch.save(model_tem,os.path.join(os.path.curdir,"output.pth"))
|
511 |
-
return os.path.join(os.path.curdir,"output.pth")
|
512 |
-
|
513 |
-
class HParams():
|
514 |
-
def __init__(self, **kwargs):
|
515 |
-
for k, v in kwargs.items():
|
516 |
-
if type(v) == dict:
|
517 |
-
v = HParams(**v)
|
518 |
-
self[k] = v
|
519 |
-
|
520 |
-
def keys(self):
|
521 |
-
return self.__dict__.keys()
|
522 |
-
|
523 |
-
def items(self):
|
524 |
-
return self.__dict__.items()
|
525 |
-
|
526 |
-
def values(self):
|
527 |
-
return self.__dict__.values()
|
528 |
-
|
529 |
-
def __len__(self):
|
530 |
-
return len(self.__dict__)
|
531 |
-
|
532 |
-
def __getitem__(self, key):
|
533 |
-
return getattr(self, key)
|
534 |
-
|
535 |
-
def __setitem__(self, key, value):
|
536 |
-
return setattr(self, key, value)
|
537 |
-
|
538 |
-
def __contains__(self, key):
|
539 |
-
return key in self.__dict__
|
540 |
-
|
541 |
-
def __repr__(self):
|
542 |
-
return self.__dict__.__repr__()
|
543 |
-
|
|
|
|
|
|
|
|
|
|
|
|
|
|
|
|
|
|
|
|
|
|
|
|
|
|
|
|
|
|
|
|
|
|
|
|
|
|
|
|
|
|
|
|
|
|
|
|
|
|
|
|
|
|
|
|
|
|
|
|
|
|
|
|
|
|
|
|
|
|
|
|
|
|
|
|
|
|
|
|
|
|
|
|
|
|
|
|
|
|
|
|
|
|
|
|
|
|
|
|
|
|
|
|
|
|
|
|
|
|
|
|
|
|
|
|
|
|
|
|
|
|
|
|
|
|
|
|
|
|
|
|
|
|
|
|
|
|
|
|
|
|
|
|
|
|
|
|
|
|
|
|
|
|
|
|
|
|
|
|
|
|
|
|
|
|
|
|
|
|
|
|
|
|
|
|
|
|
|
|
|
|
|
|
|
|
|
|
|
|
|
|
|
|
|
|
|
|
|
|
|
|
|
|
|
|
|
|
|
|
|
|
|
|
|
|
|
|
|
|
|
|
|
|
|
|
|
|
|
|
|
|
|
|
|
|
|
|
|
|
|
|
|
|
|
|
|
|
|
|
|
|
|
|
|
|
|
|
|
|
|
|
|
|
|
|
|
|
|
|
|
|
|
|
|
|
|
|
|
|
|
|
|
|
|
|
|
|
|
|
|
|
|
|
|
|
|
|
|
|
|
|
|
|
|
|
|
|
|
|
|
|
|
|
|
|
|
|
|
|
|
|
|
|
|
|
|
|
|
|
|
|
|
|
|
|
|
|
|
|
|
|
|
|
|
|
|
|
|
|
|
|
|
|
|
|
|
|
|
|
|
|
|
|
|
|
|
|
|
|
|
|
|
|
|
|
|
|
|
|
|
|
|
|
|
|
|
|
|
|
|
|
|
|
|
|
|
|
|
|
|
|
|
|
|
|
|
|
|
|
|
|
|
|
|
|
|
|
|
|
|
|
|
|
|
|
|
|
|
|
|
|
|
|
|
|
|
|
|
|
|
|
|
|
|
|
|
|
|
|
|
|
|
|
|
|
|
|
|
|
|
|
|
|
|
|
|
|
|
|
|
|
|
|
|
|
|
|
|
|
|
|
|
|
|
|
|
|
|
|
|
|
|
|
|
|
|
|
|
|
|
|
|
|
|
|
|
|
|
|
|
|
|
|
|
|
|
|
|
|
|
|
|
|
|
|
|
|
|
|
|
|
|
|
|
|
|
|
|
|
|
|
|
|
|
|
|
|
|
|
|
|
|
|
|
|
|
|
|
|
|
|
|
|
|
|
|
|
|
|
|
|
|
|
|
|
|
|
|
|
|
|
|
|
|
|
|
|
|
|
|
|
|
|
|
|
|
|
|
|
|
|
|
|
|
|
|
|
|
|
|
|
|
|
|
|
|
|
|
|
|
|
|
|
|
|
|
|
|
|
|
|
|
|
|
|
|
|
|
|
|
|
|
|
|
|
|
|
|
|
|
|
|
|
|
|
|
|
|
|
|
|
|
|
|
|
|
|
|
|
|
|
|
|
|
|
|
|
|
|
|
|
|
|
|
|
|
|
|
|
|
|
|
|
|
|
|
|
|
|
|
|
|
|
|
|
|
|
|
|
|
|
|
|
|
|
|
|
|
|
|
|
|
|
|
|
|
|
|
|
|
|
|
|
|
|
|
|
|
|
|
|
|
|
|
|
|
|
|
|
|
|
|
|
|
|
|
|
|
|
|
|
|
|
|
|
|
|
|
|
|
|
|
|
|
|
|
|
|
|
|
|
|
|
|
|
|
|
|
|
|
|
|
|
|
|
|
|
|
|
|
|
|
|
|
|
|
|
|
|
|
|
|
|
|
|
|
|
|
|
|
|
|
|
|
|
|
|
|
|
|
|
|
|
|
|
|
|
|
|
|
|
|
|
|
|
|
|
|
|
|
|
|
|
|
|
|
|
|
|
|
|
|
|
|
|
|
|
|
|
|
|
|
|
|
|
|
|
|
|
|
|
|
|
|
|
|
|
|
|
|
|
|
|
|
|
|
|
|
|
|
|
|
|
|
|
|
|
|
|
|
|
|
|
|
|
|
|
|
|
|
|
|
|
|
|
|
|
|
|
|
|
|
|
|
|
|
|
|
|
|
|
|
|
|
|
|
|
|
|
|
|
|
|
|
|
|
|
|
|
|
|
|
|
|
|
|
|
|
|
|
|
|
|
|
|
|
|
|
|
|
|
|
|
|
|
|
|
|
|
|
|
|
|
|
|
|
|
|
|
|
|
|
|
|
|
|
|
|
|
|
|
|
|
|
|
|
|
|
|
|
|
|
|
|
|
|
|
|
|
|
|
|
|
|
|
|
|
|
|
|
|
|
|
|
|
|
|
|
|
|
|
|
|
|
|
|
|
|
|
|
|
|
|
|
|
|
|
|
|
|
|
|
|
|
|
|
|
|
|
|
|
|
|
|
|
|
|
spaces/AlexWang/lama/saicinpainting/training/modules/multidilated_conv.py
DELETED
@@ -1,98 +0,0 @@
|
|
1 |
-
import torch
|
2 |
-
import torch.nn as nn
|
3 |
-
import random
|
4 |
-
from saicinpainting.training.modules.depthwise_sep_conv import DepthWiseSeperableConv
|
5 |
-
|
6 |
-
class MultidilatedConv(nn.Module):
|
7 |
-
def __init__(self, in_dim, out_dim, kernel_size, dilation_num=3, comb_mode='sum', equal_dim=True,
|
8 |
-
shared_weights=False, padding=1, min_dilation=1, shuffle_in_channels=False, use_depthwise=False, **kwargs):
|
9 |
-
super().__init__()
|
10 |
-
convs = []
|
11 |
-
self.equal_dim = equal_dim
|
12 |
-
assert comb_mode in ('cat_out', 'sum', 'cat_in', 'cat_both'), comb_mode
|
13 |
-
if comb_mode in ('cat_out', 'cat_both'):
|
14 |
-
self.cat_out = True
|
15 |
-
if equal_dim:
|
16 |
-
assert out_dim % dilation_num == 0
|
17 |
-
out_dims = [out_dim // dilation_num] * dilation_num
|
18 |
-
self.index = sum([[i + j * (out_dims[0]) for j in range(dilation_num)] for i in range(out_dims[0])], [])
|
19 |
-
else:
|
20 |
-
out_dims = [out_dim // 2 ** (i + 1) for i in range(dilation_num - 1)]
|
21 |
-
out_dims.append(out_dim - sum(out_dims))
|
22 |
-
index = []
|
23 |
-
starts = [0] + out_dims[:-1]
|
24 |
-
lengths = [out_dims[i] // out_dims[-1] for i in range(dilation_num)]
|
25 |
-
for i in range(out_dims[-1]):
|
26 |
-
for j in range(dilation_num):
|
27 |
-
index += list(range(starts[j], starts[j] + lengths[j]))
|
28 |
-
starts[j] += lengths[j]
|
29 |
-
self.index = index
|
30 |
-
assert(len(index) == out_dim)
|
31 |
-
self.out_dims = out_dims
|
32 |
-
else:
|
33 |
-
self.cat_out = False
|
34 |
-
self.out_dims = [out_dim] * dilation_num
|
35 |
-
|
36 |
-
if comb_mode in ('cat_in', 'cat_both'):
|
37 |
-
if equal_dim:
|
38 |
-
assert in_dim % dilation_num == 0
|
39 |
-
in_dims = [in_dim // dilation_num] * dilation_num
|
40 |
-
else:
|
41 |
-
in_dims = [in_dim // 2 ** (i + 1) for i in range(dilation_num - 1)]
|
42 |
-
in_dims.append(in_dim - sum(in_dims))
|
43 |
-
self.in_dims = in_dims
|
44 |
-
self.cat_in = True
|
45 |
-
else:
|
46 |
-
self.cat_in = False
|
47 |
-
self.in_dims = [in_dim] * dilation_num
|
48 |
-
|
49 |
-
conv_type = DepthWiseSeperableConv if use_depthwise else nn.Conv2d
|
50 |
-
dilation = min_dilation
|
51 |
-
for i in range(dilation_num):
|
52 |
-
if isinstance(padding, int):
|
53 |
-
cur_padding = padding * dilation
|
54 |
-
else:
|
55 |
-
cur_padding = padding[i]
|
56 |
-
convs.append(conv_type(
|
57 |
-
self.in_dims[i], self.out_dims[i], kernel_size, padding=cur_padding, dilation=dilation, **kwargs
|
58 |
-
))
|
59 |
-
if i > 0 and shared_weights:
|
60 |
-
convs[-1].weight = convs[0].weight
|
61 |
-
convs[-1].bias = convs[0].bias
|
62 |
-
dilation *= 2
|
63 |
-
self.convs = nn.ModuleList(convs)
|
64 |
-
|
65 |
-
self.shuffle_in_channels = shuffle_in_channels
|
66 |
-
if self.shuffle_in_channels:
|
67 |
-
# shuffle list as shuffling of tensors is nondeterministic
|
68 |
-
in_channels_permute = list(range(in_dim))
|
69 |
-
random.shuffle(in_channels_permute)
|
70 |
-
# save as buffer so it is saved and loaded with checkpoint
|
71 |
-
self.register_buffer('in_channels_permute', torch.tensor(in_channels_permute))
|
72 |
-
|
73 |
-
def forward(self, x):
|
74 |
-
if self.shuffle_in_channels:
|
75 |
-
x = x[:, self.in_channels_permute]
|
76 |
-
|
77 |
-
outs = []
|
78 |
-
if self.cat_in:
|
79 |
-
if self.equal_dim:
|
80 |
-
x = x.chunk(len(self.convs), dim=1)
|
81 |
-
else:
|
82 |
-
new_x = []
|
83 |
-
start = 0
|
84 |
-
for dim in self.in_dims:
|
85 |
-
new_x.append(x[:, start:start+dim])
|
86 |
-
start += dim
|
87 |
-
x = new_x
|
88 |
-
for i, conv in enumerate(self.convs):
|
89 |
-
if self.cat_in:
|
90 |
-
input = x[i]
|
91 |
-
else:
|
92 |
-
input = x
|
93 |
-
outs.append(conv(input))
|
94 |
-
if self.cat_out:
|
95 |
-
out = torch.cat(outs, dim=1)[:, self.index]
|
96 |
-
else:
|
97 |
-
out = sum(outs)
|
98 |
-
return out
|
|
|
|
|
|
|
|
|
|
|
|
|
|
|
|
|
|
|
|
|
|
|
|
|
|
|
|
|
|
|
|
|
|
|
|
|
|
|
|
|
|
|
|
|
|
|
|
|
|
|
|
|
|
|
|
|
|
|
|
|
|
|
|
|
|
|
|
|
|
|
|
|
|
|
|
|
|
|
|
|
|
|
|
|
|
|
|
|
|
|
|
|
|
|
|
|
|
|
|
|
|
|
|
|
|
|
|
|
|
|
|
|
|
|
|
|
|
|
|
|
|
|
|
|
|
|
|
|
|
|
|
|
|
|
|
|
|
|
|
|
|
|
|
|
|
|
|
|
|
|
|
|
|
|
|
|
|
|
|
|
|
|
|
|
|
|
|
|
|
|
|
|
|
|
|
|
|
|
|
|
|
|
|
|
|
|
|
|
|
|
|
|
|
|
|
|
|
spaces/Alican/pixera/data/base_dataset.py
DELETED
@@ -1,167 +0,0 @@
|
|
1 |
-
"""This module implements an abstract base class (ABC) 'BaseDataset' for datasets.
|
2 |
-
|
3 |
-
It also includes common transformation functions (e.g., get_transform, __scale_width), which can be later used in subclasses.
|
4 |
-
"""
|
5 |
-
import random
|
6 |
-
import numpy as np
|
7 |
-
import torch.utils.data as data
|
8 |
-
from PIL import Image
|
9 |
-
import torchvision.transforms as transforms
|
10 |
-
from abc import ABC, abstractmethod
|
11 |
-
|
12 |
-
|
13 |
-
class BaseDataset(data.Dataset, ABC):
|
14 |
-
"""This class is an abstract base class (ABC) for datasets.
|
15 |
-
|
16 |
-
To create a subclass, you need to implement the following four functions:
|
17 |
-
-- <__init__>: initialize the class, first call BaseDataset.__init__(self, opt).
|
18 |
-
-- <__len__>: return the size of dataset.
|
19 |
-
-- <__getitem__>: get a data point.
|
20 |
-
-- <modify_commandline_options>: (optionally) add dataset-specific options and set default options.
|
21 |
-
"""
|
22 |
-
|
23 |
-
def __init__(self, opt):
|
24 |
-
"""Initialize the class; save the options in the class
|
25 |
-
|
26 |
-
Parameters:
|
27 |
-
opt (Option class)-- stores all the experiment flags; needs to be a subclass of BaseOptions
|
28 |
-
"""
|
29 |
-
self.opt = opt
|
30 |
-
self.root = opt.dataroot
|
31 |
-
|
32 |
-
@staticmethod
|
33 |
-
def modify_commandline_options(parser, is_train):
|
34 |
-
"""Add new dataset-specific options, and rewrite default values for existing options.
|
35 |
-
|
36 |
-
Parameters:
|
37 |
-
parser -- original option parser
|
38 |
-
is_train (bool) -- whether training phase or test phase. You can use this flag to add training-specific or test-specific options.
|
39 |
-
|
40 |
-
Returns:
|
41 |
-
the modified parser.
|
42 |
-
"""
|
43 |
-
return parser
|
44 |
-
|
45 |
-
@abstractmethod
|
46 |
-
def __len__(self):
|
47 |
-
"""Return the total number of images in the dataset."""
|
48 |
-
return 0
|
49 |
-
|
50 |
-
@abstractmethod
|
51 |
-
def __getitem__(self, index):
|
52 |
-
"""Return a data point and its metadata information.
|
53 |
-
|
54 |
-
Parameters:
|
55 |
-
index - - a random integer for data indexing
|
56 |
-
|
57 |
-
Returns:
|
58 |
-
a dictionary of data with their names. It ususally contains the data itself and its metadata information.
|
59 |
-
"""
|
60 |
-
pass
|
61 |
-
|
62 |
-
|
63 |
-
def get_params(opt, size):
|
64 |
-
w, h = size
|
65 |
-
new_h = h
|
66 |
-
new_w = w
|
67 |
-
if opt.preprocess == 'resize_and_crop':
|
68 |
-
new_h = new_w = opt.load_size
|
69 |
-
elif opt.preprocess == 'scale_width_and_crop':
|
70 |
-
new_w = opt.load_size
|
71 |
-
new_h = opt.load_size * h // w
|
72 |
-
|
73 |
-
x = random.randint(0, np.maximum(0, new_w - opt.crop_size))
|
74 |
-
y = random.randint(0, np.maximum(0, new_h - opt.crop_size))
|
75 |
-
|
76 |
-
flip = random.random() > 0.5
|
77 |
-
|
78 |
-
return {'crop_pos': (x, y), 'flip': flip}
|
79 |
-
|
80 |
-
|
81 |
-
def get_transform(opt, params=None, grayscale=False, method=transforms.InterpolationMode.BICUBIC, convert=True):
|
82 |
-
transform_list = []
|
83 |
-
if grayscale:
|
84 |
-
transform_list.append(transforms.Grayscale(1))
|
85 |
-
if 'resize' in opt.preprocess:
|
86 |
-
osize = [opt.load_size, opt.load_size]
|
87 |
-
transform_list.append(transforms.Resize(osize, method))
|
88 |
-
elif 'scale_width' in opt.preprocess:
|
89 |
-
transform_list.append(transforms.Lambda(lambda img: __scale_width(img, opt.load_size, opt.crop_size, method)))
|
90 |
-
|
91 |
-
if 'crop' in opt.preprocess:
|
92 |
-
if params is None:
|
93 |
-
transform_list.append(transforms.RandomCrop(opt.crop_size))
|
94 |
-
else:
|
95 |
-
transform_list.append(transforms.Lambda(lambda img: __crop(img, params['crop_pos'], opt.crop_size)))
|
96 |
-
|
97 |
-
if opt.preprocess == 'none':
|
98 |
-
transform_list.append(transforms.Lambda(lambda img: __make_power_2(img, base=4, method=method)))
|
99 |
-
|
100 |
-
if not opt.no_flip:
|
101 |
-
if params is None:
|
102 |
-
transform_list.append(transforms.RandomHorizontalFlip())
|
103 |
-
elif params['flip']:
|
104 |
-
transform_list.append(transforms.Lambda(lambda img: __flip(img, params['flip'])))
|
105 |
-
|
106 |
-
if convert:
|
107 |
-
transform_list += [transforms.ToTensor()]
|
108 |
-
if grayscale:
|
109 |
-
transform_list += [transforms.Normalize((0.5,), (0.5,))]
|
110 |
-
else:
|
111 |
-
transform_list += [transforms.Normalize((0.5, 0.5, 0.5), (0.5, 0.5, 0.5))]
|
112 |
-
return transforms.Compose(transform_list)
|
113 |
-
|
114 |
-
|
115 |
-
def __transforms2pil_resize(method):
|
116 |
-
mapper = {transforms.InterpolationMode.BILINEAR: Image.BILINEAR,
|
117 |
-
transforms.InterpolationMode.BICUBIC: Image.BICUBIC,
|
118 |
-
transforms.InterpolationMode.NEAREST: Image.NEAREST,
|
119 |
-
transforms.InterpolationMode.LANCZOS: Image.LANCZOS,}
|
120 |
-
return mapper[method]
|
121 |
-
|
122 |
-
|
123 |
-
def __make_power_2(img, base, method=transforms.InterpolationMode.BICUBIC):
|
124 |
-
method = __transforms2pil_resize(method)
|
125 |
-
ow, oh = img.size
|
126 |
-
h = int(round(oh / base) * base)
|
127 |
-
w = int(round(ow / base) * base)
|
128 |
-
if h == oh and w == ow:
|
129 |
-
return img
|
130 |
-
|
131 |
-
__print_size_warning(ow, oh, w, h)
|
132 |
-
return img.resize((w, h), method)
|
133 |
-
|
134 |
-
|
135 |
-
def __scale_width(img, target_size, crop_size, method=transforms.InterpolationMode.BICUBIC):
|
136 |
-
method = __transforms2pil_resize(method)
|
137 |
-
ow, oh = img.size
|
138 |
-
if ow == target_size and oh >= crop_size:
|
139 |
-
return img
|
140 |
-
w = target_size
|
141 |
-
h = int(max(target_size * oh / ow, crop_size))
|
142 |
-
return img.resize((w, h), method)
|
143 |
-
|
144 |
-
|
145 |
-
def __crop(img, pos, size):
|
146 |
-
ow, oh = img.size
|
147 |
-
x1, y1 = pos
|
148 |
-
tw = th = size
|
149 |
-
if (ow > tw or oh > th):
|
150 |
-
return img.crop((x1, y1, x1 + tw, y1 + th))
|
151 |
-
return img
|
152 |
-
|
153 |
-
|
154 |
-
def __flip(img, flip):
|
155 |
-
if flip:
|
156 |
-
return img.transpose(Image.FLIP_LEFT_RIGHT)
|
157 |
-
return img
|
158 |
-
|
159 |
-
|
160 |
-
def __print_size_warning(ow, oh, w, h):
|
161 |
-
"""Print warning information about image size(only print once)"""
|
162 |
-
if not hasattr(__print_size_warning, 'has_printed'):
|
163 |
-
print("The image size needs to be a multiple of 4. "
|
164 |
-
"The loaded image size was (%d, %d), so it was adjusted to "
|
165 |
-
"(%d, %d). This adjustment will be done to all images "
|
166 |
-
"whose sizes are not multiples of 4" % (ow, oh, w, h))
|
167 |
-
__print_size_warning.has_printed = True
|
|
|
|
|
|
|
|
|
|
|
|
|
|
|
|
|
|
|
|
|
|
|
|
|
|
|
|
|
|
|
|
|
|
|
|
|
|
|
|
|
|
|
|
|
|
|
|
|
|
|
|
|
|
|
|
|
|
|
|
|
|
|
|
|
|
|
|
|
|
|
|
|
|
|
|
|
|
|
|
|
|
|
|
|
|
|
|
|
|
|
|
|
|
|
|
|
|
|
|
|
|
|
|
|
|
|
|
|
|
|
|
|
|
|
|
|
|
|
|
|
|
|
|
|
|
|
|
|
|
|
|
|
|
|
|
|
|
|
|
|
|
|
|
|
|
|
|
|
|
|
|
|
|
|
|
|
|
|
|
|
|
|
|
|
|
|
|
|
|
|
|
|
|
|
|
|
|
|
|
|
|
|
|
|
|
|
|
|
|
|
|
|
|
|
|
|
|
|
|
|
|
|
|
|
|
|
|
|
|
|
|
|
|
|
|
|
|
|
|
|
|
|
|
|
|
|
|
|
|
|
|
|
|
|
|
|
|
|
|
|
|
|
|
|
|
|
|
|
|
|
|
|
|
|
|
|
|
|
|
|
|
|
|
|
|
|
|
|
|
|
|
|
|
|
|
|
|
|
|
|
|
|
|
|
|
|
|
|
|
|
|
|
|
|
|
|
|
|
|
|
|
|
|
|
|
|
|
|
|
|
|
|
|
|
|
|
|
|
|
|
|
|
|
|
|
|
|
|
|
|
|
|
|
|
|
spaces/Alpaca233/SadTalker/src/audio2pose_models/cvae.py
DELETED
@@ -1,149 +0,0 @@
|
|
1 |
-
import torch
|
2 |
-
import torch.nn.functional as F
|
3 |
-
from torch import nn
|
4 |
-
from src.audio2pose_models.res_unet import ResUnet
|
5 |
-
|
6 |
-
def class2onehot(idx, class_num):
|
7 |
-
|
8 |
-
assert torch.max(idx).item() < class_num
|
9 |
-
onehot = torch.zeros(idx.size(0), class_num).to(idx.device)
|
10 |
-
onehot.scatter_(1, idx, 1)
|
11 |
-
return onehot
|
12 |
-
|
13 |
-
class CVAE(nn.Module):
|
14 |
-
def __init__(self, cfg):
|
15 |
-
super().__init__()
|
16 |
-
encoder_layer_sizes = cfg.MODEL.CVAE.ENCODER_LAYER_SIZES
|
17 |
-
decoder_layer_sizes = cfg.MODEL.CVAE.DECODER_LAYER_SIZES
|
18 |
-
latent_size = cfg.MODEL.CVAE.LATENT_SIZE
|
19 |
-
num_classes = cfg.DATASET.NUM_CLASSES
|
20 |
-
audio_emb_in_size = cfg.MODEL.CVAE.AUDIO_EMB_IN_SIZE
|
21 |
-
audio_emb_out_size = cfg.MODEL.CVAE.AUDIO_EMB_OUT_SIZE
|
22 |
-
seq_len = cfg.MODEL.CVAE.SEQ_LEN
|
23 |
-
|
24 |
-
self.latent_size = latent_size
|
25 |
-
|
26 |
-
self.encoder = ENCODER(encoder_layer_sizes, latent_size, num_classes,
|
27 |
-
audio_emb_in_size, audio_emb_out_size, seq_len)
|
28 |
-
self.decoder = DECODER(decoder_layer_sizes, latent_size, num_classes,
|
29 |
-
audio_emb_in_size, audio_emb_out_size, seq_len)
|
30 |
-
def reparameterize(self, mu, logvar):
|
31 |
-
std = torch.exp(0.5 * logvar)
|
32 |
-
eps = torch.randn_like(std)
|
33 |
-
return mu + eps * std
|
34 |
-
|
35 |
-
def forward(self, batch):
|
36 |
-
batch = self.encoder(batch)
|
37 |
-
mu = batch['mu']
|
38 |
-
logvar = batch['logvar']
|
39 |
-
z = self.reparameterize(mu, logvar)
|
40 |
-
batch['z'] = z
|
41 |
-
return self.decoder(batch)
|
42 |
-
|
43 |
-
def test(self, batch):
|
44 |
-
'''
|
45 |
-
class_id = batch['class']
|
46 |
-
z = torch.randn([class_id.size(0), self.latent_size]).to(class_id.device)
|
47 |
-
batch['z'] = z
|
48 |
-
'''
|
49 |
-
return self.decoder(batch)
|
50 |
-
|
51 |
-
class ENCODER(nn.Module):
|
52 |
-
def __init__(self, layer_sizes, latent_size, num_classes,
|
53 |
-
audio_emb_in_size, audio_emb_out_size, seq_len):
|
54 |
-
super().__init__()
|
55 |
-
|
56 |
-
self.resunet = ResUnet()
|
57 |
-
self.num_classes = num_classes
|
58 |
-
self.seq_len = seq_len
|
59 |
-
|
60 |
-
self.MLP = nn.Sequential()
|
61 |
-
layer_sizes[0] += latent_size + seq_len*audio_emb_out_size + 6
|
62 |
-
for i, (in_size, out_size) in enumerate(zip(layer_sizes[:-1], layer_sizes[1:])):
|
63 |
-
self.MLP.add_module(
|
64 |
-
name="L{:d}".format(i), module=nn.Linear(in_size, out_size))
|
65 |
-
self.MLP.add_module(name="A{:d}".format(i), module=nn.ReLU())
|
66 |
-
|
67 |
-
self.linear_means = nn.Linear(layer_sizes[-1], latent_size)
|
68 |
-
self.linear_logvar = nn.Linear(layer_sizes[-1], latent_size)
|
69 |
-
self.linear_audio = nn.Linear(audio_emb_in_size, audio_emb_out_size)
|
70 |
-
|
71 |
-
self.classbias = nn.Parameter(torch.randn(self.num_classes, latent_size))
|
72 |
-
|
73 |
-
def forward(self, batch):
|
74 |
-
class_id = batch['class']
|
75 |
-
pose_motion_gt = batch['pose_motion_gt'] #bs seq_len 6
|
76 |
-
ref = batch['ref'] #bs 6
|
77 |
-
bs = pose_motion_gt.shape[0]
|
78 |
-
audio_in = batch['audio_emb'] # bs seq_len audio_emb_in_size
|
79 |
-
|
80 |
-
#pose encode
|
81 |
-
pose_emb = self.resunet(pose_motion_gt.unsqueeze(1)) #bs 1 seq_len 6
|
82 |
-
pose_emb = pose_emb.reshape(bs, -1) #bs seq_len*6
|
83 |
-
|
84 |
-
#audio mapping
|
85 |
-
print(audio_in.shape)
|
86 |
-
audio_out = self.linear_audio(audio_in) # bs seq_len audio_emb_out_size
|
87 |
-
audio_out = audio_out.reshape(bs, -1)
|
88 |
-
|
89 |
-
class_bias = self.classbias[class_id] #bs latent_size
|
90 |
-
x_in = torch.cat([ref, pose_emb, audio_out, class_bias], dim=-1) #bs seq_len*(audio_emb_out_size+6)+latent_size
|
91 |
-
x_out = self.MLP(x_in)
|
92 |
-
|
93 |
-
mu = self.linear_means(x_out)
|
94 |
-
logvar = self.linear_means(x_out) #bs latent_size
|
95 |
-
|
96 |
-
batch.update({'mu':mu, 'logvar':logvar})
|
97 |
-
return batch
|
98 |
-
|
99 |
-
class DECODER(nn.Module):
|
100 |
-
def __init__(self, layer_sizes, latent_size, num_classes,
|
101 |
-
audio_emb_in_size, audio_emb_out_size, seq_len):
|
102 |
-
super().__init__()
|
103 |
-
|
104 |
-
self.resunet = ResUnet()
|
105 |
-
self.num_classes = num_classes
|
106 |
-
self.seq_len = seq_len
|
107 |
-
|
108 |
-
self.MLP = nn.Sequential()
|
109 |
-
input_size = latent_size + seq_len*audio_emb_out_size + 6
|
110 |
-
for i, (in_size, out_size) in enumerate(zip([input_size]+layer_sizes[:-1], layer_sizes)):
|
111 |
-
self.MLP.add_module(
|
112 |
-
name="L{:d}".format(i), module=nn.Linear(in_size, out_size))
|
113 |
-
if i+1 < len(layer_sizes):
|
114 |
-
self.MLP.add_module(name="A{:d}".format(i), module=nn.ReLU())
|
115 |
-
else:
|
116 |
-
self.MLP.add_module(name="sigmoid", module=nn.Sigmoid())
|
117 |
-
|
118 |
-
self.pose_linear = nn.Linear(6, 6)
|
119 |
-
self.linear_audio = nn.Linear(audio_emb_in_size, audio_emb_out_size)
|
120 |
-
|
121 |
-
self.classbias = nn.Parameter(torch.randn(self.num_classes, latent_size))
|
122 |
-
|
123 |
-
def forward(self, batch):
|
124 |
-
|
125 |
-
z = batch['z'] #bs latent_size
|
126 |
-
bs = z.shape[0]
|
127 |
-
class_id = batch['class']
|
128 |
-
ref = batch['ref'] #bs 6
|
129 |
-
audio_in = batch['audio_emb'] # bs seq_len audio_emb_in_size
|
130 |
-
#print('audio_in: ', audio_in[:, :, :10])
|
131 |
-
|
132 |
-
audio_out = self.linear_audio(audio_in) # bs seq_len audio_emb_out_size
|
133 |
-
#print('audio_out: ', audio_out[:, :, :10])
|
134 |
-
audio_out = audio_out.reshape([bs, -1]) # bs seq_len*audio_emb_out_size
|
135 |
-
class_bias = self.classbias[class_id] #bs latent_size
|
136 |
-
|
137 |
-
z = z + class_bias
|
138 |
-
x_in = torch.cat([ref, z, audio_out], dim=-1)
|
139 |
-
x_out = self.MLP(x_in) # bs layer_sizes[-1]
|
140 |
-
x_out = x_out.reshape((bs, self.seq_len, -1))
|
141 |
-
|
142 |
-
#print('x_out: ', x_out)
|
143 |
-
|
144 |
-
pose_emb = self.resunet(x_out.unsqueeze(1)) #bs 1 seq_len 6
|
145 |
-
|
146 |
-
pose_motion_pred = self.pose_linear(pose_emb.squeeze(1)) #bs seq_len 6
|
147 |
-
|
148 |
-
batch.update({'pose_motion_pred':pose_motion_pred})
|
149 |
-
return batch
|
|
|
|
|
|
|
|
|
|
|
|
|
|
|
|
|
|
|
|
|
|
|
|
|
|
|
|
|
|
|
|
|
|
|
|
|
|
|
|
|
|
|
|
|
|
|
|
|
|
|
|
|
|
|
|
|
|
|
|
|
|
|
|
|
|
|
|
|
|
|
|
|
|
|
|
|
|
|
|
|
|
|
|
|
|
|
|
|
|
|
|
|
|
|
|
|
|
|
|
|
|
|
|
|
|
|
|
|
|
|
|
|
|
|
|
|
|
|
|
|
|
|
|
|
|
|
|
|
|
|
|
|
|
|
|
|
|
|
|
|
|
|
|
|
|
|
|
|
|
|
|
|
|
|
|
|
|
|
|
|
|
|
|
|
|
|
|
|
|
|
|
|
|
|
|
|
|
|
|
|
|
|
|
|
|
|
|
|
|
|
|
|
|
|
|
|
|
|
|
|
|
|
|
|
|
|
|
|
|
|
|
|
|
|
|
|
|
|
|
|
|
|
|
|
|
|
|
|
|
|
|
|
|
|
|
|
|
|
|
|
|
|
|
|
|
|
|
|
|
|
|
|
|
|
|
|
|
|
|
|
|
|
|
|
|
|
|
|
|
|
|
|
|
|
|
|
|
|
|
|
|
|
|
|
|
|
|
|
|
|
|
|
|
|
|
|
|
|
|
spaces/Amrrs/DragGan-Inversion/stylegan_human/training/loss.py
DELETED
@@ -1,159 +0,0 @@
|
|
1 |
-
# Copyright (c) 2021, NVIDIA CORPORATION & AFFILIATES. All rights reserved.
|
2 |
-
#
|
3 |
-
# NVIDIA CORPORATION and its licensors retain all intellectual property
|
4 |
-
# and proprietary rights in and to this software, related documentation
|
5 |
-
# and any modifications thereto. Any use, reproduction, disclosure or
|
6 |
-
# distribution of this software and related documentation without an express
|
7 |
-
# license agreement from NVIDIA CORPORATION is strictly prohibited.
|
8 |
-
|
9 |
-
"""Loss functions."""
|
10 |
-
|
11 |
-
import numpy as np
|
12 |
-
import torch
|
13 |
-
from torch_utils import training_stats
|
14 |
-
from torch_utils.ops import conv2d_gradfix
|
15 |
-
from torch_utils.ops import upfirdn2d
|
16 |
-
|
17 |
-
# ----------------------------------------------------------------------------
|
18 |
-
|
19 |
-
|
20 |
-
class Loss:
|
21 |
-
# to be overridden by subclass
|
22 |
-
def accumulate_gradients(self, phase, real_img, real_c, gen_z, gen_c, gain, cur_nimg):
|
23 |
-
raise NotImplementedError()
|
24 |
-
|
25 |
-
# ----------------------------------------------------------------------------
|
26 |
-
|
27 |
-
|
28 |
-
class StyleGAN2Loss(Loss):
|
29 |
-
def __init__(self, device, G, D, augment_pipe=None, r1_gamma=10, style_mixing_prob=0, pl_weight=0, pl_batch_shrink=2, pl_decay=0.01, pl_no_weight_grad=False, blur_init_sigma=0, blur_fade_kimg=0):
|
30 |
-
super().__init__()
|
31 |
-
self.device = device
|
32 |
-
self.G = G
|
33 |
-
self.D = D
|
34 |
-
self.augment_pipe = augment_pipe
|
35 |
-
self.r1_gamma = r1_gamma
|
36 |
-
self.style_mixing_prob = style_mixing_prob
|
37 |
-
self.pl_weight = pl_weight
|
38 |
-
self.pl_batch_shrink = pl_batch_shrink
|
39 |
-
self.pl_decay = pl_decay
|
40 |
-
self.pl_no_weight_grad = pl_no_weight_grad
|
41 |
-
self.pl_mean = torch.zeros([], device=device)
|
42 |
-
self.blur_init_sigma = blur_init_sigma
|
43 |
-
self.blur_fade_kimg = blur_fade_kimg
|
44 |
-
|
45 |
-
def run_G(self, z, c, update_emas=False):
|
46 |
-
ws = self.G.mapping(z, c, update_emas=update_emas)
|
47 |
-
if self.style_mixing_prob > 0:
|
48 |
-
with torch.autograd.profiler.record_function('style_mixing'):
|
49 |
-
cutoff = torch.empty([], dtype=torch.int64,
|
50 |
-
device=ws.device).random_(1, ws.shape[1])
|
51 |
-
cutoff = torch.where(torch.rand(
|
52 |
-
[], device=ws.device) < self.style_mixing_prob, cutoff, torch.full_like(cutoff, ws.shape[1]))
|
53 |
-
ws[:, cutoff:] = self.G.mapping(
|
54 |
-
torch.randn_like(z), c, update_emas=False)[:, cutoff:]
|
55 |
-
img = self.G.synthesis(ws, update_emas=update_emas)
|
56 |
-
return img, ws
|
57 |
-
|
58 |
-
def run_D(self, img, c, blur_sigma=0, update_emas=False):
|
59 |
-
blur_size = np.floor(blur_sigma * 3)
|
60 |
-
if blur_size > 0:
|
61 |
-
with torch.autograd.profiler.record_function('blur'):
|
62 |
-
f = torch.arange(-blur_size, blur_size + 1,
|
63 |
-
device=img.device).div(blur_sigma).square().neg().exp2()
|
64 |
-
img = upfirdn2d.filter2d(img, f / f.sum())
|
65 |
-
if self.augment_pipe is not None:
|
66 |
-
img = self.augment_pipe(img)
|
67 |
-
logits = self.D(img, c, update_emas=update_emas)
|
68 |
-
return logits
|
69 |
-
|
70 |
-
def accumulate_gradients(self, phase, real_img, real_c, gen_z, gen_c, gain, cur_nimg):
|
71 |
-
assert phase in ['Gmain', 'Greg', 'Gboth', 'Dmain', 'Dreg', 'Dboth']
|
72 |
-
if self.pl_weight == 0:
|
73 |
-
phase = {'Greg': 'none', 'Gboth': 'Gmain'}.get(phase, phase)
|
74 |
-
if self.r1_gamma == 0:
|
75 |
-
phase = {'Dreg': 'none', 'Dboth': 'Dmain'}.get(phase, phase)
|
76 |
-
blur_sigma = max(1 - cur_nimg / (self.blur_fade_kimg * 1e3), 0) * \
|
77 |
-
self.blur_init_sigma if self.blur_fade_kimg > 0 else 0
|
78 |
-
|
79 |
-
# Gmain: Maximize logits for generated images.
|
80 |
-
if phase in ['Gmain', 'Gboth']:
|
81 |
-
with torch.autograd.profiler.record_function('Gmain_forward'):
|
82 |
-
gen_img, _gen_ws = self.run_G(gen_z, gen_c)
|
83 |
-
gen_logits = self.run_D(gen_img, gen_c, blur_sigma=blur_sigma)
|
84 |
-
training_stats.report('Loss/scores/fake', gen_logits)
|
85 |
-
training_stats.report('Loss/signs/fake', gen_logits.sign())
|
86 |
-
# -log(sigmoid(gen_logits))
|
87 |
-
loss_Gmain = torch.nn.functional.softplus(-gen_logits)
|
88 |
-
training_stats.report('Loss/G/loss', loss_Gmain)
|
89 |
-
with torch.autograd.profiler.record_function('Gmain_backward'):
|
90 |
-
loss_Gmain.mean().mul(gain).backward()
|
91 |
-
|
92 |
-
# Gpl: Apply path length regularization.
|
93 |
-
if phase in ['Greg', 'Gboth']:
|
94 |
-
with torch.autograd.profiler.record_function('Gpl_forward'):
|
95 |
-
batch_size = gen_z.shape[0] // self.pl_batch_shrink
|
96 |
-
gen_img, gen_ws = self.run_G(
|
97 |
-
gen_z[:batch_size], gen_c[:batch_size])
|
98 |
-
pl_noise = torch.randn_like(
|
99 |
-
gen_img) / np.sqrt(gen_img.shape[2] * gen_img.shape[3])
|
100 |
-
with torch.autograd.profiler.record_function('pl_grads'), conv2d_gradfix.no_weight_gradients(self.pl_no_weight_grad):
|
101 |
-
pl_grads = torch.autograd.grad(outputs=[(
|
102 |
-
gen_img * pl_noise).sum()], inputs=[gen_ws], create_graph=True, only_inputs=True)[0]
|
103 |
-
pl_lengths = pl_grads.square().sum(2).mean(1).sqrt()
|
104 |
-
pl_mean = self.pl_mean.lerp(pl_lengths.mean(), self.pl_decay)
|
105 |
-
self.pl_mean.copy_(pl_mean.detach())
|
106 |
-
pl_penalty = (pl_lengths - pl_mean).square()
|
107 |
-
training_stats.report('Loss/pl_penalty', pl_penalty)
|
108 |
-
loss_Gpl = pl_penalty * self.pl_weight
|
109 |
-
training_stats.report('Loss/G/reg', loss_Gpl)
|
110 |
-
with torch.autograd.profiler.record_function('Gpl_backward'):
|
111 |
-
loss_Gpl.mean().mul(gain).backward()
|
112 |
-
|
113 |
-
# Dmain: Minimize logits for generated images.
|
114 |
-
loss_Dgen = 0
|
115 |
-
if phase in ['Dmain', 'Dboth']:
|
116 |
-
with torch.autograd.profiler.record_function('Dgen_forward'):
|
117 |
-
gen_img, _gen_ws = self.run_G(gen_z, gen_c, update_emas=True)
|
118 |
-
gen_logits = self.run_D(
|
119 |
-
gen_img, gen_c, blur_sigma=blur_sigma, update_emas=True)
|
120 |
-
training_stats.report('Loss/scores/fake', gen_logits)
|
121 |
-
training_stats.report('Loss/signs/fake', gen_logits.sign())
|
122 |
-
loss_Dgen = torch.nn.functional.softplus(
|
123 |
-
gen_logits) # -log(1 - sigmoid(gen_logits))
|
124 |
-
with torch.autograd.profiler.record_function('Dgen_backward'):
|
125 |
-
loss_Dgen.mean().mul(gain).backward()
|
126 |
-
|
127 |
-
# Dmain: Maximize logits for real images.
|
128 |
-
# Dr1: Apply R1 regularization.
|
129 |
-
if phase in ['Dmain', 'Dreg', 'Dboth']:
|
130 |
-
name = 'Dreal' if phase == 'Dmain' else 'Dr1' if phase == 'Dreg' else 'Dreal_Dr1'
|
131 |
-
with torch.autograd.profiler.record_function(name + '_forward'):
|
132 |
-
real_img_tmp = real_img.detach().requires_grad_(
|
133 |
-
phase in ['Dreg', 'Dboth'])
|
134 |
-
real_logits = self.run_D(
|
135 |
-
real_img_tmp, real_c, blur_sigma=blur_sigma)
|
136 |
-
training_stats.report('Loss/scores/real', real_logits)
|
137 |
-
training_stats.report('Loss/signs/real', real_logits.sign())
|
138 |
-
|
139 |
-
loss_Dreal = 0
|
140 |
-
if phase in ['Dmain', 'Dboth']:
|
141 |
-
# -log(sigmoid(real_logits))
|
142 |
-
loss_Dreal = torch.nn.functional.softplus(-real_logits)
|
143 |
-
training_stats.report(
|
144 |
-
'Loss/D/loss', loss_Dgen + loss_Dreal)
|
145 |
-
|
146 |
-
loss_Dr1 = 0
|
147 |
-
if phase in ['Dreg', 'Dboth']:
|
148 |
-
with torch.autograd.profiler.record_function('r1_grads'), conv2d_gradfix.no_weight_gradients():
|
149 |
-
r1_grads = torch.autograd.grad(outputs=[real_logits.sum()], inputs=[
|
150 |
-
real_img_tmp], create_graph=True, only_inputs=True)[0]
|
151 |
-
r1_penalty = r1_grads.square().sum([1, 2, 3])
|
152 |
-
loss_Dr1 = r1_penalty * (self.r1_gamma / 2)
|
153 |
-
training_stats.report('Loss/r1_penalty', r1_penalty)
|
154 |
-
training_stats.report('Loss/D/reg', loss_Dr1)
|
155 |
-
|
156 |
-
with torch.autograd.profiler.record_function(name + '_backward'):
|
157 |
-
(loss_Dreal + loss_Dr1).mean().mul(gain).backward()
|
158 |
-
|
159 |
-
# ----------------------------------------------------------------------------
|
|
|
|
|
|
|
|
|
|
|
|
|
|
|
|
|
|
|
|
|
|
|
|
|
|
|
|
|
|
|
|
|
|
|
|
|
|
|
|
|
|
|
|
|
|
|
|
|
|
|
|
|
|
|
|
|
|
|
|
|
|
|
|
|
|
|
|
|
|
|
|
|
|
|
|
|
|
|
|
|
|
|
|
|
|
|
|
|
|
|
|
|
|
|
|
|
|
|
|
|
|
|
|
|
|
|
|
|
|
|
|
|
|
|
|
|
|
|
|
|
|
|
|
|
|
|
|
|
|
|
|
|
|
|
|
|
|
|
|
|
|
|
|
|
|
|
|
|
|
|
|
|
|
|
|
|
|
|
|
|
|
|
|
|
|
|
|
|
|
|
|
|
|
|
|
|
|
|
|
|
|
|
|
|
|
|
|
|
|
|
|
|
|
|
|
|
|
|
|
|
|
|
|
|
|
|
|
|
|
|
|
|
|
|
|
|
|
|
|
|
|
|
|
|
|
|
|
|
|
|
|
|
|
|
|
|
|
|
|
|
|
|
|
|
|
|
|
|
|
|
|
|
|
|
|
|
|
|
|
|
|
|
|
|
|
|
|
|
|
|
|
|
|
|
|
|
|
|
|
|
|
|
|
|
|
|
|
|
|
|
|
|
|
|
|
|
|
|
|
|
|
|
|
|
|
|
|
|
|
|
|
|
|
|
|
|
|
|
|
spaces/Androidonnxfork/CivitAi-to-Diffusers/diffusers/examples/community/README.md
DELETED
@@ -1,1769 +0,0 @@
|
|
1 |
-
# Community Examples
|
2 |
-
|
3 |
-
> **For more information about community pipelines, please have a look at [this issue](https://github.com/huggingface/diffusers/issues/841).**
|
4 |
-
|
5 |
-
**Community** examples consist of both inference and training examples that have been added by the community.
|
6 |
-
Please have a look at the following table to get an overview of all community examples. Click on the **Code Example** to get a copy-and-paste ready code example that you can try out.
|
7 |
-
If a community doesn't work as expected, please open an issue and ping the author on it.
|
8 |
-
|
9 |
-
| Example | Description | Code Example | Colab | Author |
|
10 |
-
|:--------------------------------------------------------------------------------------------------------------------------------------|:---------------------------------------------------------------------------------------------------------------------------------------------------------------------------------------------------------------------------------------------------------------------------------------------------------------------------------------------------------------------------------------------------------------------------------------------------------------------------------------------------------|:------------------------------------------------------------------------------------------|:-------------------------------------------------------------------------------------------------------------------------------------------------------------------------------------------------------------------|--------------------------------------------------------------:|
|
11 |
-
| CLIP Guided Stable Diffusion | Doing CLIP guidance for text to image generation with Stable Diffusion | [CLIP Guided Stable Diffusion](#clip-guided-stable-diffusion) | [](https://colab.research.google.com/github/huggingface/notebooks/blob/main/diffusers/CLIP_Guided_Stable_diffusion_with_diffusers.ipynb) | [Suraj Patil](https://github.com/patil-suraj/) |
|
12 |
-
| One Step U-Net (Dummy) | Example showcasing of how to use Community Pipelines (see https://github.com/huggingface/diffusers/issues/841) | [One Step U-Net](#one-step-unet) | - | [Patrick von Platen](https://github.com/patrickvonplaten/) |
|
13 |
-
| Stable Diffusion Interpolation | Interpolate the latent space of Stable Diffusion between different prompts/seeds | [Stable Diffusion Interpolation](#stable-diffusion-interpolation) | - | [Nate Raw](https://github.com/nateraw/) |
|
14 |
-
| Stable Diffusion Mega | **One** Stable Diffusion Pipeline with all functionalities of [Text2Image](https://github.com/huggingface/diffusers/blob/main/src/diffusers/pipelines/stable_diffusion/pipeline_stable_diffusion.py), [Image2Image](https://github.com/huggingface/diffusers/blob/main/src/diffusers/pipelines/stable_diffusion/pipeline_stable_diffusion_img2img.py) and [Inpainting](https://github.com/huggingface/diffusers/blob/main/src/diffusers/pipelines/stable_diffusion/pipeline_stable_diffusion_inpaint.py) | [Stable Diffusion Mega](#stable-diffusion-mega) | - | [Patrick von Platen](https://github.com/patrickvonplaten/) |
|
15 |
-
| Long Prompt Weighting Stable Diffusion | **One** Stable Diffusion Pipeline without tokens length limit, and support parsing weighting in prompt. | [Long Prompt Weighting Stable Diffusion](#long-prompt-weighting-stable-diffusion) | - | [SkyTNT](https://github.com/SkyTNT) |
|
16 |
-
| Speech to Image | Using automatic-speech-recognition to transcribe text and Stable Diffusion to generate images | [Speech to Image](#speech-to-image) | - | [Mikail Duzenli](https://github.com/MikailINTech)
|
17 |
-
| Wild Card Stable Diffusion | Stable Diffusion Pipeline that supports prompts that contain wildcard terms (indicated by surrounding double underscores), with values instantiated randomly from a corresponding txt file or a dictionary of possible values | [Wildcard Stable Diffusion](#wildcard-stable-diffusion) | - | [Shyam Sudhakaran](https://github.com/shyamsn97) |
|
18 |
-
| [Composable Stable Diffusion](https://energy-based-model.github.io/Compositional-Visual-Generation-with-Composable-Diffusion-Models/) | Stable Diffusion Pipeline that supports prompts that contain "|" in prompts (as an AND condition) and weights (separated by "|" as well) to positively / negatively weight prompts. | [Composable Stable Diffusion](#composable-stable-diffusion) | - | [Mark Rich](https://github.com/MarkRich) |
|
19 |
-
| Seed Resizing Stable Diffusion | Stable Diffusion Pipeline that supports resizing an image and retaining the concepts of the 512 by 512 generation. | [Seed Resizing](#seed-resizing) | - | [Mark Rich](https://github.com/MarkRich) |
|
20 |
-
| Imagic Stable Diffusion | Stable Diffusion Pipeline that enables writing a text prompt to edit an existing image | [Imagic Stable Diffusion](#imagic-stable-diffusion) | - | [Mark Rich](https://github.com/MarkRich) |
|
21 |
-
| Multilingual Stable Diffusion | Stable Diffusion Pipeline that supports prompts in 50 different languages. | [Multilingual Stable Diffusion](#multilingual-stable-diffusion-pipeline) | - | [Juan Carlos Piñeros](https://github.com/juancopi81) |
|
22 |
-
| Image to Image Inpainting Stable Diffusion | Stable Diffusion Pipeline that enables the overlaying of two images and subsequent inpainting | [Image to Image Inpainting Stable Diffusion](#image-to-image-inpainting-stable-diffusion) | - | [Alex McKinney](https://github.com/vvvm23) |
|
23 |
-
| Text Based Inpainting Stable Diffusion | Stable Diffusion Inpainting Pipeline that enables passing a text prompt to generate the mask for inpainting | [Text Based Inpainting Stable Diffusion](#image-to-image-inpainting-stable-diffusion) | - | [Dhruv Karan](https://github.com/unography) |
|
24 |
-
| Bit Diffusion | Diffusion on discrete data | [Bit Diffusion](#bit-diffusion) | - | [Stuti R.](https://github.com/kingstut) |
|
25 |
-
| K-Diffusion Stable Diffusion | Run Stable Diffusion with any of [K-Diffusion's samplers](https://github.com/crowsonkb/k-diffusion/blob/master/k_diffusion/sampling.py) | [Stable Diffusion with K Diffusion](#stable-diffusion-with-k-diffusion) | - | [Patrick von Platen](https://github.com/patrickvonplaten/) |
|
26 |
-
| Checkpoint Merger Pipeline | Diffusion Pipeline that enables merging of saved model checkpoints | [Checkpoint Merger Pipeline](#checkpoint-merger-pipeline) | - | [Naga Sai Abhinay Devarinti](https://github.com/Abhinay1997/) |
|
27 |
-
Stable Diffusion v1.1-1.4 Comparison | Run all 4 model checkpoints for Stable Diffusion and compare their results together | [Stable Diffusion Comparison](#stable-diffusion-comparisons) | - | [Suvaditya Mukherjee](https://github.com/suvadityamuk) |
|
28 |
-
MagicMix | Diffusion Pipeline for semantic mixing of an image and a text prompt | [MagicMix](#magic-mix) | - | [Partho Das](https://github.com/daspartho) |
|
29 |
-
| Stable UnCLIP | Diffusion Pipeline for combining prior model (generate clip image embedding from text, UnCLIPPipeline `"kakaobrain/karlo-v1-alpha"`) and decoder pipeline (decode clip image embedding to image, StableDiffusionImageVariationPipeline `"lambdalabs/sd-image-variations-diffusers"` ). | [Stable UnCLIP](#stable-unclip) | - | [Ray Wang](https://wrong.wang) |
|
30 |
-
| UnCLIP Text Interpolation Pipeline | Diffusion Pipeline that allows passing two prompts and produces images while interpolating between the text-embeddings of the two prompts | [UnCLIP Text Interpolation Pipeline](#unclip-text-interpolation-pipeline) | - | [Naga Sai Abhinay Devarinti](https://github.com/Abhinay1997/) |
|
31 |
-
| UnCLIP Image Interpolation Pipeline | Diffusion Pipeline that allows passing two images/image_embeddings and produces images while interpolating between their image-embeddings | [UnCLIP Image Interpolation Pipeline](#unclip-image-interpolation-pipeline) | - | [Naga Sai Abhinay Devarinti](https://github.com/Abhinay1997/) |
|
32 |
-
| DDIM Noise Comparative Analysis Pipeline | Investigating how the diffusion models learn visual concepts from each noise level (which is a contribution of [P2 weighting (CVPR 2022)](https://arxiv.org/abs/2204.00227)) | [DDIM Noise Comparative Analysis Pipeline](#ddim-noise-comparative-analysis-pipeline) | - | [Aengus (Duc-Anh)](https://github.com/aengusng8) |
|
33 |
-
| CLIP Guided Img2Img Stable Diffusion Pipeline | Doing CLIP guidance for image to image generation with Stable Diffusion | [CLIP Guided Img2Img Stable Diffusion](#clip-guided-img2img-stable-diffusion) | - | [Nipun Jindal](https://github.com/nipunjindal/) |
|
34 |
-
| TensorRT Stable Diffusion Text to Image Pipeline | Accelerates the Stable Diffusion Text2Image Pipeline using TensorRT | [TensorRT Stable Diffusion Text to Image Pipeline](#tensorrt-text2image-stable-diffusion-pipeline) | - | [Asfiya Baig](https://github.com/asfiyab-nvidia) |
|
35 |
-
| EDICT Image Editing Pipeline | Diffusion pipeline for text-guided image editing | [EDICT Image Editing Pipeline](#edict-image-editing-pipeline) | - | [Joqsan Azocar](https://github.com/Joqsan) |
|
36 |
-
| Stable Diffusion RePaint | Stable Diffusion pipeline using [RePaint](https://arxiv.org/abs/2201.0986) for inpainting. | [Stable Diffusion RePaint](#stable-diffusion-repaint ) | - | [Markus Pobitzer](https://github.com/Markus-Pobitzer) |
|
37 |
-
| TensorRT Stable Diffusion Image to Image Pipeline | Accelerates the Stable Diffusion Image2Image Pipeline using TensorRT | [TensorRT Stable Diffusion Image to Image Pipeline](#tensorrt-image2image-stable-diffusion-pipeline) | - | [Asfiya Baig](https://github.com/asfiyab-nvidia) |
|
38 |
-
| Stable Diffusion IPEX Pipeline | Accelerate Stable Diffusion inference pipeline with BF16/FP32 precision on Intel Xeon CPUs with [IPEX](https://github.com/intel/intel-extension-for-pytorch) | [Stable Diffusion on IPEX](#stable-diffusion-on-ipex) | - | [Yingjie Han](https://github.com/yingjie-han/) |
|
39 |
-
| CLIP Guided Images Mixing Stable Diffusion Pipeline | Сombine images using usual diffusion models. | [CLIP Guided Images Mixing Using Stable Diffusion](#clip-guided-images-mixing-with-stable-diffusion) | - | [Karachev Denis](https://github.com/TheDenk) |
|
40 |
-
| TensorRT Stable Diffusion Inpainting Pipeline | Accelerates the Stable Diffusion Inpainting Pipeline using TensorRT | [TensorRT Stable Diffusion Inpainting Pipeline](#tensorrt-inpainting-stable-diffusion-pipeline) | - | [Asfiya Baig](https://github.com/asfiyab-nvidia) |
|
41 |
-
| IADB Pipeline | Implementation of [Iterative α-(de)Blending: a Minimalist Deterministic Diffusion Model](https://arxiv.org/abs/2305.03486) | [IADB Pipeline](#iadb-pipeline) | - | [Thomas Chambon](https://github.com/tchambon)
|
42 |
-
|
43 |
-
To load a custom pipeline you just need to pass the `custom_pipeline` argument to `DiffusionPipeline`, as one of the files in `diffusers/examples/community`. Feel free to send a PR with your own pipelines, we will merge them quickly.
|
44 |
-
```py
|
45 |
-
pipe = DiffusionPipeline.from_pretrained("runwayml/stable-diffusion-v1-5", custom_pipeline="filename_in_the_community_folder")
|
46 |
-
```
|
47 |
-
|
48 |
-
## Example usages
|
49 |
-
|
50 |
-
### CLIP Guided Stable Diffusion
|
51 |
-
|
52 |
-
CLIP guided stable diffusion can help to generate more realistic images
|
53 |
-
by guiding stable diffusion at every denoising step with an additional CLIP model.
|
54 |
-
|
55 |
-
The following code requires roughly 12GB of GPU RAM.
|
56 |
-
|
57 |
-
```python
|
58 |
-
from diffusers import DiffusionPipeline
|
59 |
-
from transformers import CLIPImageProcessor, CLIPModel
|
60 |
-
import torch
|
61 |
-
|
62 |
-
|
63 |
-
feature_extractor = CLIPImageProcessor.from_pretrained("laion/CLIP-ViT-B-32-laion2B-s34B-b79K")
|
64 |
-
clip_model = CLIPModel.from_pretrained("laion/CLIP-ViT-B-32-laion2B-s34B-b79K", torch_dtype=torch.float16)
|
65 |
-
|
66 |
-
|
67 |
-
guided_pipeline = DiffusionPipeline.from_pretrained(
|
68 |
-
"runwayml/stable-diffusion-v1-5",
|
69 |
-
custom_pipeline="clip_guided_stable_diffusion",
|
70 |
-
clip_model=clip_model,
|
71 |
-
feature_extractor=feature_extractor,
|
72 |
-
|
73 |
-
torch_dtype=torch.float16,
|
74 |
-
)
|
75 |
-
guided_pipeline.enable_attention_slicing()
|
76 |
-
guided_pipeline = guided_pipeline.to("cuda")
|
77 |
-
|
78 |
-
prompt = "fantasy book cover, full moon, fantasy forest landscape, golden vector elements, fantasy magic, dark light night, intricate, elegant, sharp focus, illustration, highly detailed, digital painting, concept art, matte, art by WLOP and Artgerm and Albert Bierstadt, masterpiece"
|
79 |
-
|
80 |
-
generator = torch.Generator(device="cuda").manual_seed(0)
|
81 |
-
images = []
|
82 |
-
for i in range(4):
|
83 |
-
image = guided_pipeline(
|
84 |
-
prompt,
|
85 |
-
num_inference_steps=50,
|
86 |
-
guidance_scale=7.5,
|
87 |
-
clip_guidance_scale=100,
|
88 |
-
num_cutouts=4,
|
89 |
-
use_cutouts=False,
|
90 |
-
generator=generator,
|
91 |
-
).images[0]
|
92 |
-
images.append(image)
|
93 |
-
|
94 |
-
# save images locally
|
95 |
-
for i, img in enumerate(images):
|
96 |
-
img.save(f"./clip_guided_sd/image_{i}.png")
|
97 |
-
```
|
98 |
-
|
99 |
-
The `images` list contains a list of PIL images that can be saved locally or displayed directly in a google colab.
|
100 |
-
Generated images tend to be of higher qualtiy than natively using stable diffusion. E.g. the above script generates the following images:
|
101 |
-
|
102 |
-
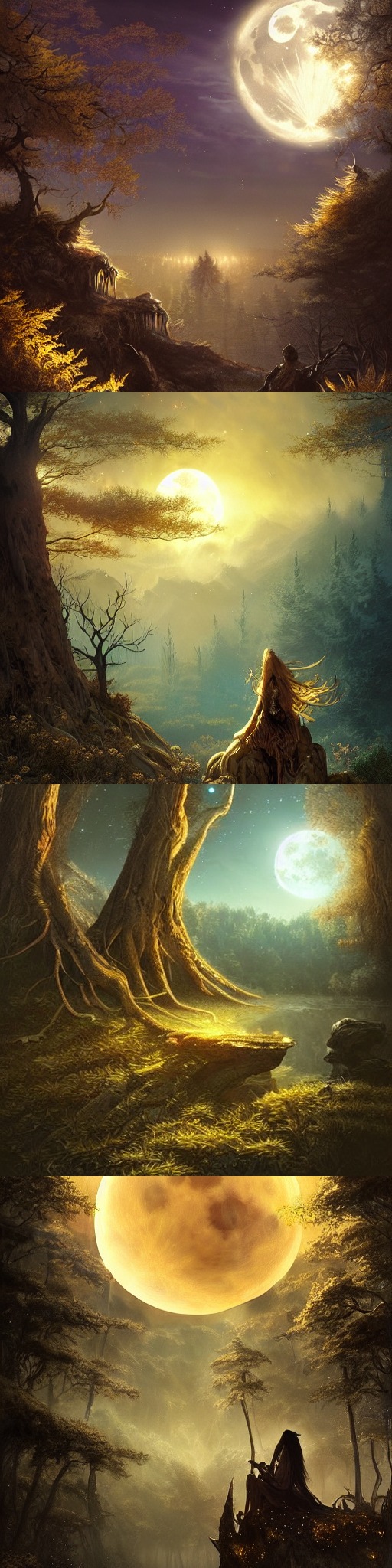.
|
103 |
-
|
104 |
-
### One Step Unet
|
105 |
-
|
106 |
-
The dummy "one-step-unet" can be run as follows:
|
107 |
-
|
108 |
-
```python
|
109 |
-
from diffusers import DiffusionPipeline
|
110 |
-
|
111 |
-
pipe = DiffusionPipeline.from_pretrained("google/ddpm-cifar10-32", custom_pipeline="one_step_unet")
|
112 |
-
pipe()
|
113 |
-
```
|
114 |
-
|
115 |
-
**Note**: This community pipeline is not useful as a feature, but rather just serves as an example of how community pipelines can be added (see https://github.com/huggingface/diffusers/issues/841).
|
116 |
-
|
117 |
-
### Stable Diffusion Interpolation
|
118 |
-
|
119 |
-
The following code can be run on a GPU of at least 8GB VRAM and should take approximately 5 minutes.
|
120 |
-
|
121 |
-
```python
|
122 |
-
from diffusers import DiffusionPipeline
|
123 |
-
import torch
|
124 |
-
|
125 |
-
pipe = DiffusionPipeline.from_pretrained(
|
126 |
-
"CompVis/stable-diffusion-v1-4",
|
127 |
-
revision='fp16',
|
128 |
-
torch_dtype=torch.float16,
|
129 |
-
safety_checker=None, # Very important for videos...lots of false positives while interpolating
|
130 |
-
custom_pipeline="interpolate_stable_diffusion",
|
131 |
-
).to('cuda')
|
132 |
-
pipe.enable_attention_slicing()
|
133 |
-
|
134 |
-
frame_filepaths = pipe.walk(
|
135 |
-
prompts=['a dog', 'a cat', 'a horse'],
|
136 |
-
seeds=[42, 1337, 1234],
|
137 |
-
num_interpolation_steps=16,
|
138 |
-
output_dir='./dreams',
|
139 |
-
batch_size=4,
|
140 |
-
height=512,
|
141 |
-
width=512,
|
142 |
-
guidance_scale=8.5,
|
143 |
-
num_inference_steps=50,
|
144 |
-
)
|
145 |
-
```
|
146 |
-
|
147 |
-
The output of the `walk(...)` function returns a list of images saved under the folder as defined in `output_dir`. You can use these images to create videos of stable diffusion.
|
148 |
-
|
149 |
-
> **Please have a look at https://github.com/nateraw/stable-diffusion-videos for more in-detail information on how to create videos using stable diffusion as well as more feature-complete functionality.**
|
150 |
-
|
151 |
-
### Stable Diffusion Mega
|
152 |
-
|
153 |
-
The Stable Diffusion Mega Pipeline lets you use the main use cases of the stable diffusion pipeline in a single class.
|
154 |
-
|
155 |
-
```python
|
156 |
-
#!/usr/bin/env python3
|
157 |
-
from diffusers import DiffusionPipeline
|
158 |
-
import PIL
|
159 |
-
import requests
|
160 |
-
from io import BytesIO
|
161 |
-
import torch
|
162 |
-
|
163 |
-
|
164 |
-
def download_image(url):
|
165 |
-
response = requests.get(url)
|
166 |
-
return PIL.Image.open(BytesIO(response.content)).convert("RGB")
|
167 |
-
|
168 |
-
pipe = DiffusionPipeline.from_pretrained("runwayml/stable-diffusion-v1-5", custom_pipeline="stable_diffusion_mega", torch_dtype=torch.float16, revision="fp16")
|
169 |
-
pipe.to("cuda")
|
170 |
-
pipe.enable_attention_slicing()
|
171 |
-
|
172 |
-
|
173 |
-
### Text-to-Image
|
174 |
-
|
175 |
-
images = pipe.text2img("An astronaut riding a horse").images
|
176 |
-
|
177 |
-
### Image-to-Image
|
178 |
-
|
179 |
-
init_image = download_image("https://raw.githubusercontent.com/CompVis/stable-diffusion/main/assets/stable-samples/img2img/sketch-mountains-input.jpg")
|
180 |
-
|
181 |
-
prompt = "A fantasy landscape, trending on artstation"
|
182 |
-
|
183 |
-
images = pipe.img2img(prompt=prompt, image=init_image, strength=0.75, guidance_scale=7.5).images
|
184 |
-
|
185 |
-
### Inpainting
|
186 |
-
|
187 |
-
img_url = "https://raw.githubusercontent.com/CompVis/latent-diffusion/main/data/inpainting_examples/overture-creations-5sI6fQgYIuo.png"
|
188 |
-
mask_url = "https://raw.githubusercontent.com/CompVis/latent-diffusion/main/data/inpainting_examples/overture-creations-5sI6fQgYIuo_mask.png"
|
189 |
-
init_image = download_image(img_url).resize((512, 512))
|
190 |
-
mask_image = download_image(mask_url).resize((512, 512))
|
191 |
-
|
192 |
-
prompt = "a cat sitting on a bench"
|
193 |
-
images = pipe.inpaint(prompt=prompt, image=init_image, mask_image=mask_image, strength=0.75).images
|
194 |
-
```
|
195 |
-
|
196 |
-
As shown above this one pipeline can run all both "text-to-image", "image-to-image", and "inpainting" in one pipeline.
|
197 |
-
|
198 |
-
### Long Prompt Weighting Stable Diffusion
|
199 |
-
Features of this custom pipeline:
|
200 |
-
- Input a prompt without the 77 token length limit.
|
201 |
-
- Includes tx2img, img2img. and inpainting pipelines.
|
202 |
-
- Emphasize/weigh part of your prompt with parentheses as so: `a baby deer with (big eyes)`
|
203 |
-
- De-emphasize part of your prompt as so: `a [baby] deer with big eyes`
|
204 |
-
- Precisely weigh part of your prompt as so: `a baby deer with (big eyes:1.3)`
|
205 |
-
|
206 |
-
Prompt weighting equivalents:
|
207 |
-
- `a baby deer with` == `(a baby deer with:1.0)`
|
208 |
-
- `(big eyes)` == `(big eyes:1.1)`
|
209 |
-
- `((big eyes))` == `(big eyes:1.21)`
|
210 |
-
- `[big eyes]` == `(big eyes:0.91)`
|
211 |
-
|
212 |
-
You can run this custom pipeline as so:
|
213 |
-
|
214 |
-
#### pytorch
|
215 |
-
|
216 |
-
```python
|
217 |
-
from diffusers import DiffusionPipeline
|
218 |
-
import torch
|
219 |
-
|
220 |
-
pipe = DiffusionPipeline.from_pretrained(
|
221 |
-
'hakurei/waifu-diffusion',
|
222 |
-
custom_pipeline="lpw_stable_diffusion",
|
223 |
-
|
224 |
-
torch_dtype=torch.float16
|
225 |
-
)
|
226 |
-
pipe=pipe.to("cuda")
|
227 |
-
|
228 |
-
prompt = "best_quality (1girl:1.3) bow bride brown_hair closed_mouth frilled_bow frilled_hair_tubes frills (full_body:1.3) fox_ear hair_bow hair_tubes happy hood japanese_clothes kimono long_sleeves red_bow smile solo tabi uchikake white_kimono wide_sleeves cherry_blossoms"
|
229 |
-
neg_prompt = "lowres, bad_anatomy, error_body, error_hair, error_arm, error_hands, bad_hands, error_fingers, bad_fingers, missing_fingers, error_legs, bad_legs, multiple_legs, missing_legs, error_lighting, error_shadow, error_reflection, text, error, extra_digit, fewer_digits, cropped, worst_quality, low_quality, normal_quality, jpeg_artifacts, signature, watermark, username, blurry"
|
230 |
-
|
231 |
-
pipe.text2img(prompt, negative_prompt=neg_prompt, width=512,height=512,max_embeddings_multiples=3).images[0]
|
232 |
-
|
233 |
-
```
|
234 |
-
|
235 |
-
#### onnxruntime
|
236 |
-
|
237 |
-
```python
|
238 |
-
from diffusers import DiffusionPipeline
|
239 |
-
import torch
|
240 |
-
|
241 |
-
pipe = DiffusionPipeline.from_pretrained(
|
242 |
-
'CompVis/stable-diffusion-v1-4',
|
243 |
-
custom_pipeline="lpw_stable_diffusion_onnx",
|
244 |
-
revision="onnx",
|
245 |
-
provider="CUDAExecutionProvider"
|
246 |
-
)
|
247 |
-
|
248 |
-
prompt = "a photo of an astronaut riding a horse on mars, best quality"
|
249 |
-
neg_prompt = "lowres, bad anatomy, error body, error hair, error arm, error hands, bad hands, error fingers, bad fingers, missing fingers, error legs, bad legs, multiple legs, missing legs, error lighting, error shadow, error reflection, text, error, extra digit, fewer digits, cropped, worst quality, low quality, normal quality, jpeg artifacts, signature, watermark, username, blurry"
|
250 |
-
|
251 |
-
pipe.text2img(prompt,negative_prompt=neg_prompt, width=512, height=512, max_embeddings_multiples=3).images[0]
|
252 |
-
|
253 |
-
```
|
254 |
-
|
255 |
-
if you see `Token indices sequence length is longer than the specified maximum sequence length for this model ( *** > 77 ) . Running this sequence through the model will result in indexing errors`. Do not worry, it is normal.
|
256 |
-
|
257 |
-
### Speech to Image
|
258 |
-
|
259 |
-
The following code can generate an image from an audio sample using pre-trained OpenAI whisper-small and Stable Diffusion.
|
260 |
-
|
261 |
-
```Python
|
262 |
-
import torch
|
263 |
-
|
264 |
-
import matplotlib.pyplot as plt
|
265 |
-
from datasets import load_dataset
|
266 |
-
from diffusers import DiffusionPipeline
|
267 |
-
from transformers import (
|
268 |
-
WhisperForConditionalGeneration,
|
269 |
-
WhisperProcessor,
|
270 |
-
)
|
271 |
-
|
272 |
-
|
273 |
-
device = "cuda" if torch.cuda.is_available() else "cpu"
|
274 |
-
|
275 |
-
ds = load_dataset("hf-internal-testing/librispeech_asr_dummy", "clean", split="validation")
|
276 |
-
|
277 |
-
audio_sample = ds[3]
|
278 |
-
|
279 |
-
text = audio_sample["text"].lower()
|
280 |
-
speech_data = audio_sample["audio"]["array"]
|
281 |
-
|
282 |
-
model = WhisperForConditionalGeneration.from_pretrained("openai/whisper-small").to(device)
|
283 |
-
processor = WhisperProcessor.from_pretrained("openai/whisper-small")
|
284 |
-
|
285 |
-
diffuser_pipeline = DiffusionPipeline.from_pretrained(
|
286 |
-
"CompVis/stable-diffusion-v1-4",
|
287 |
-
custom_pipeline="speech_to_image_diffusion",
|
288 |
-
speech_model=model,
|
289 |
-
speech_processor=processor,
|
290 |
-
|
291 |
-
torch_dtype=torch.float16,
|
292 |
-
)
|
293 |
-
|
294 |
-
diffuser_pipeline.enable_attention_slicing()
|
295 |
-
diffuser_pipeline = diffuser_pipeline.to(device)
|
296 |
-
|
297 |
-
output = diffuser_pipeline(speech_data)
|
298 |
-
plt.imshow(output.images[0])
|
299 |
-
```
|
300 |
-
This example produces the following image:
|
301 |
-
|
302 |
-
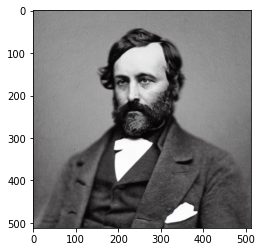
|
303 |
-
|
304 |
-
### Wildcard Stable Diffusion
|
305 |
-
Following the great examples from https://github.com/jtkelm2/stable-diffusion-webui-1/blob/master/scripts/wildcards.py and https://github.com/AUTOMATIC1111/stable-diffusion-webui/wiki/Custom-Scripts#wildcards, here's a minimal implementation that allows for users to add "wildcards", denoted by `__wildcard__` to prompts that are used as placeholders for randomly sampled values given by either a dictionary or a `.txt` file. For example:
|
306 |
-
|
307 |
-
Say we have a prompt:
|
308 |
-
|
309 |
-
```
|
310 |
-
prompt = "__animal__ sitting on a __object__ wearing a __clothing__"
|
311 |
-
```
|
312 |
-
|
313 |
-
We can then define possible values to be sampled for `animal`, `object`, and `clothing`. These can either be from a `.txt` with the same name as the category.
|
314 |
-
|
315 |
-
The possible values can also be defined / combined by using a dictionary like: `{"animal":["dog", "cat", mouse"]}`.
|
316 |
-
|
317 |
-
The actual pipeline works just like `StableDiffusionPipeline`, except the `__call__` method takes in:
|
318 |
-
|
319 |
-
`wildcard_files`: list of file paths for wild card replacement
|
320 |
-
`wildcard_option_dict`: dict with key as `wildcard` and values as a list of possible replacements
|
321 |
-
`num_prompt_samples`: number of prompts to sample, uniformly sampling wildcards
|
322 |
-
|
323 |
-
A full example:
|
324 |
-
|
325 |
-
create `animal.txt`, with contents like:
|
326 |
-
|
327 |
-
```
|
328 |
-
dog
|
329 |
-
cat
|
330 |
-
mouse
|
331 |
-
```
|
332 |
-
|
333 |
-
create `object.txt`, with contents like:
|
334 |
-
|
335 |
-
```
|
336 |
-
chair
|
337 |
-
sofa
|
338 |
-
bench
|
339 |
-
```
|
340 |
-
|
341 |
-
```python
|
342 |
-
from diffusers import DiffusionPipeline
|
343 |
-
import torch
|
344 |
-
|
345 |
-
pipe = DiffusionPipeline.from_pretrained(
|
346 |
-
"CompVis/stable-diffusion-v1-4",
|
347 |
-
custom_pipeline="wildcard_stable_diffusion",
|
348 |
-
|
349 |
-
torch_dtype=torch.float16,
|
350 |
-
)
|
351 |
-
prompt = "__animal__ sitting on a __object__ wearing a __clothing__"
|
352 |
-
out = pipe(
|
353 |
-
prompt,
|
354 |
-
wildcard_option_dict={
|
355 |
-
"clothing":["hat", "shirt", "scarf", "beret"]
|
356 |
-
},
|
357 |
-
wildcard_files=["object.txt", "animal.txt"],
|
358 |
-
num_prompt_samples=1
|
359 |
-
)
|
360 |
-
```
|
361 |
-
|
362 |
-
### Composable Stable diffusion
|
363 |
-
|
364 |
-
[Composable Stable Diffusion](https://energy-based-model.github.io/Compositional-Visual-Generation-with-Composable-Diffusion-Models/) proposes conjunction and negation (negative prompts) operators for compositional generation with conditional diffusion models.
|
365 |
-
|
366 |
-
```python
|
367 |
-
import torch as th
|
368 |
-
import numpy as np
|
369 |
-
import torchvision.utils as tvu
|
370 |
-
|
371 |
-
from diffusers import DiffusionPipeline
|
372 |
-
|
373 |
-
import argparse
|
374 |
-
|
375 |
-
parser = argparse.ArgumentParser()
|
376 |
-
parser.add_argument("--prompt", type=str, default="mystical trees | A magical pond | dark",
|
377 |
-
help="use '|' as the delimiter to compose separate sentences.")
|
378 |
-
parser.add_argument("--steps", type=int, default=50)
|
379 |
-
parser.add_argument("--scale", type=float, default=7.5)
|
380 |
-
parser.add_argument("--weights", type=str, default="7.5 | 7.5 | -7.5")
|
381 |
-
parser.add_argument("--seed", type=int, default=2)
|
382 |
-
parser.add_argument("--model_path", type=str, default="CompVis/stable-diffusion-v1-4")
|
383 |
-
parser.add_argument("--num_images", type=int, default=1)
|
384 |
-
args = parser.parse_args()
|
385 |
-
|
386 |
-
has_cuda = th.cuda.is_available()
|
387 |
-
device = th.device('cpu' if not has_cuda else 'cuda')
|
388 |
-
|
389 |
-
prompt = args.prompt
|
390 |
-
scale = args.scale
|
391 |
-
steps = args.steps
|
392 |
-
|
393 |
-
pipe = DiffusionPipeline.from_pretrained(
|
394 |
-
args.model_path,
|
395 |
-
custom_pipeline="composable_stable_diffusion",
|
396 |
-
).to(device)
|
397 |
-
|
398 |
-
pipe.safety_checker = None
|
399 |
-
|
400 |
-
images = []
|
401 |
-
generator = th.Generator("cuda").manual_seed(args.seed)
|
402 |
-
for i in range(args.num_images):
|
403 |
-
image = pipe(prompt, guidance_scale=scale, num_inference_steps=steps,
|
404 |
-
weights=args.weights, generator=generator).images[0]
|
405 |
-
images.append(th.from_numpy(np.array(image)).permute(2, 0, 1) / 255.)
|
406 |
-
grid = tvu.make_grid(th.stack(images, dim=0), nrow=4, padding=0)
|
407 |
-
tvu.save_image(grid, f'{prompt}_{args.weights}' + '.png')
|
408 |
-
|
409 |
-
```
|
410 |
-
|
411 |
-
### Imagic Stable Diffusion
|
412 |
-
Allows you to edit an image using stable diffusion.
|
413 |
-
|
414 |
-
```python
|
415 |
-
import requests
|
416 |
-
from PIL import Image
|
417 |
-
from io import BytesIO
|
418 |
-
import torch
|
419 |
-
import os
|
420 |
-
from diffusers import DiffusionPipeline, DDIMScheduler
|
421 |
-
has_cuda = torch.cuda.is_available()
|
422 |
-
device = torch.device('cpu' if not has_cuda else 'cuda')
|
423 |
-
pipe = DiffusionPipeline.from_pretrained(
|
424 |
-
"CompVis/stable-diffusion-v1-4",
|
425 |
-
safety_checker=None,
|
426 |
-
use_auth_token=True,
|
427 |
-
custom_pipeline="imagic_stable_diffusion",
|
428 |
-
scheduler = DDIMScheduler(beta_start=0.00085, beta_end=0.012, beta_schedule="scaled_linear", clip_sample=False, set_alpha_to_one=False)
|
429 |
-
).to(device)
|
430 |
-
generator = torch.Generator("cuda").manual_seed(0)
|
431 |
-
seed = 0
|
432 |
-
prompt = "A photo of Barack Obama smiling with a big grin"
|
433 |
-
url = 'https://www.dropbox.com/s/6tlwzr73jd1r9yk/obama.png?dl=1'
|
434 |
-
response = requests.get(url)
|
435 |
-
init_image = Image.open(BytesIO(response.content)).convert("RGB")
|
436 |
-
init_image = init_image.resize((512, 512))
|
437 |
-
res = pipe.train(
|
438 |
-
prompt,
|
439 |
-
image=init_image,
|
440 |
-
generator=generator)
|
441 |
-
res = pipe(alpha=1, guidance_scale=7.5, num_inference_steps=50)
|
442 |
-
os.makedirs("imagic", exist_ok=True)
|
443 |
-
image = res.images[0]
|
444 |
-
image.save('./imagic/imagic_image_alpha_1.png')
|
445 |
-
res = pipe(alpha=1.5, guidance_scale=7.5, num_inference_steps=50)
|
446 |
-
image = res.images[0]
|
447 |
-
image.save('./imagic/imagic_image_alpha_1_5.png')
|
448 |
-
res = pipe(alpha=2, guidance_scale=7.5, num_inference_steps=50)
|
449 |
-
image = res.images[0]
|
450 |
-
image.save('./imagic/imagic_image_alpha_2.png')
|
451 |
-
```
|
452 |
-
|
453 |
-
### Seed Resizing
|
454 |
-
Test seed resizing. Originally generate an image in 512 by 512, then generate image with same seed at 512 by 592 using seed resizing. Finally, generate 512 by 592 using original stable diffusion pipeline.
|
455 |
-
|
456 |
-
```python
|
457 |
-
import torch as th
|
458 |
-
import numpy as np
|
459 |
-
from diffusers import DiffusionPipeline
|
460 |
-
|
461 |
-
has_cuda = th.cuda.is_available()
|
462 |
-
device = th.device('cpu' if not has_cuda else 'cuda')
|
463 |
-
|
464 |
-
pipe = DiffusionPipeline.from_pretrained(
|
465 |
-
"CompVis/stable-diffusion-v1-4",
|
466 |
-
use_auth_token=True,
|
467 |
-
custom_pipeline="seed_resize_stable_diffusion"
|
468 |
-
).to(device)
|
469 |
-
|
470 |
-
def dummy(images, **kwargs):
|
471 |
-
return images, False
|
472 |
-
|
473 |
-
pipe.safety_checker = dummy
|
474 |
-
|
475 |
-
|
476 |
-
images = []
|
477 |
-
th.manual_seed(0)
|
478 |
-
generator = th.Generator("cuda").manual_seed(0)
|
479 |
-
|
480 |
-
seed = 0
|
481 |
-
prompt = "A painting of a futuristic cop"
|
482 |
-
|
483 |
-
width = 512
|
484 |
-
height = 512
|
485 |
-
|
486 |
-
res = pipe(
|
487 |
-
prompt,
|
488 |
-
guidance_scale=7.5,
|
489 |
-
num_inference_steps=50,
|
490 |
-
height=height,
|
491 |
-
width=width,
|
492 |
-
generator=generator)
|
493 |
-
image = res.images[0]
|
494 |
-
image.save('./seed_resize/seed_resize_{w}_{h}_image.png'.format(w=width, h=height))
|
495 |
-
|
496 |
-
|
497 |
-
th.manual_seed(0)
|
498 |
-
generator = th.Generator("cuda").manual_seed(0)
|
499 |
-
|
500 |
-
pipe = DiffusionPipeline.from_pretrained(
|
501 |
-
"CompVis/stable-diffusion-v1-4",
|
502 |
-
use_auth_token=True,
|
503 |
-
custom_pipeline="/home/mark/open_source/diffusers/examples/community/"
|
504 |
-
).to(device)
|
505 |
-
|
506 |
-
width = 512
|
507 |
-
height = 592
|
508 |
-
|
509 |
-
res = pipe(
|
510 |
-
prompt,
|
511 |
-
guidance_scale=7.5,
|
512 |
-
num_inference_steps=50,
|
513 |
-
height=height,
|
514 |
-
width=width,
|
515 |
-
generator=generator)
|
516 |
-
image = res.images[0]
|
517 |
-
image.save('./seed_resize/seed_resize_{w}_{h}_image.png'.format(w=width, h=height))
|
518 |
-
|
519 |
-
pipe_compare = DiffusionPipeline.from_pretrained(
|
520 |
-
"CompVis/stable-diffusion-v1-4",
|
521 |
-
use_auth_token=True,
|
522 |
-
custom_pipeline="/home/mark/open_source/diffusers/examples/community/"
|
523 |
-
).to(device)
|
524 |
-
|
525 |
-
res = pipe_compare(
|
526 |
-
prompt,
|
527 |
-
guidance_scale=7.5,
|
528 |
-
num_inference_steps=50,
|
529 |
-
height=height,
|
530 |
-
width=width,
|
531 |
-
generator=generator
|
532 |
-
)
|
533 |
-
|
534 |
-
image = res.images[0]
|
535 |
-
image.save('./seed_resize/seed_resize_{w}_{h}_image_compare.png'.format(w=width, h=height))
|
536 |
-
```
|
537 |
-
|
538 |
-
### Multilingual Stable Diffusion Pipeline
|
539 |
-
|
540 |
-
The following code can generate an images from texts in different languages using the pre-trained [mBART-50 many-to-one multilingual machine translation model](https://huggingface.co/facebook/mbart-large-50-many-to-one-mmt) and Stable Diffusion.
|
541 |
-
|
542 |
-
```python
|
543 |
-
from PIL import Image
|
544 |
-
|
545 |
-
import torch
|
546 |
-
|
547 |
-
from diffusers import DiffusionPipeline
|
548 |
-
from transformers import (
|
549 |
-
pipeline,
|
550 |
-
MBart50TokenizerFast,
|
551 |
-
MBartForConditionalGeneration,
|
552 |
-
)
|
553 |
-
device = "cuda" if torch.cuda.is_available() else "cpu"
|
554 |
-
device_dict = {"cuda": 0, "cpu": -1}
|
555 |
-
|
556 |
-
# helper function taken from: https://huggingface.co/blog/stable_diffusion
|
557 |
-
def image_grid(imgs, rows, cols):
|
558 |
-
assert len(imgs) == rows*cols
|
559 |
-
|
560 |
-
w, h = imgs[0].size
|
561 |
-
grid = Image.new('RGB', size=(cols*w, rows*h))
|
562 |
-
grid_w, grid_h = grid.size
|
563 |
-
|
564 |
-
for i, img in enumerate(imgs):
|
565 |
-
grid.paste(img, box=(i%cols*w, i//cols*h))
|
566 |
-
return grid
|
567 |
-
|
568 |
-
# Add language detection pipeline
|
569 |
-
language_detection_model_ckpt = "papluca/xlm-roberta-base-language-detection"
|
570 |
-
language_detection_pipeline = pipeline("text-classification",
|
571 |
-
model=language_detection_model_ckpt,
|
572 |
-
device=device_dict[device])
|
573 |
-
|
574 |
-
# Add model for language translation
|
575 |
-
trans_tokenizer = MBart50TokenizerFast.from_pretrained("facebook/mbart-large-50-many-to-one-mmt")
|
576 |
-
trans_model = MBartForConditionalGeneration.from_pretrained("facebook/mbart-large-50-many-to-one-mmt").to(device)
|
577 |
-
|
578 |
-
diffuser_pipeline = DiffusionPipeline.from_pretrained(
|
579 |
-
"CompVis/stable-diffusion-v1-4",
|
580 |
-
custom_pipeline="multilingual_stable_diffusion",
|
581 |
-
detection_pipeline=language_detection_pipeline,
|
582 |
-
translation_model=trans_model,
|
583 |
-
translation_tokenizer=trans_tokenizer,
|
584 |
-
|
585 |
-
torch_dtype=torch.float16,
|
586 |
-
)
|
587 |
-
|
588 |
-
diffuser_pipeline.enable_attention_slicing()
|
589 |
-
diffuser_pipeline = diffuser_pipeline.to(device)
|
590 |
-
|
591 |
-
prompt = ["a photograph of an astronaut riding a horse",
|
592 |
-
"Una casa en la playa",
|
593 |
-
"Ein Hund, der Orange isst",
|
594 |
-
"Un restaurant parisien"]
|
595 |
-
|
596 |
-
output = diffuser_pipeline(prompt)
|
597 |
-
|
598 |
-
images = output.images
|
599 |
-
|
600 |
-
grid = image_grid(images, rows=2, cols=2)
|
601 |
-
```
|
602 |
-
|
603 |
-
This example produces the following images:
|
604 |
-
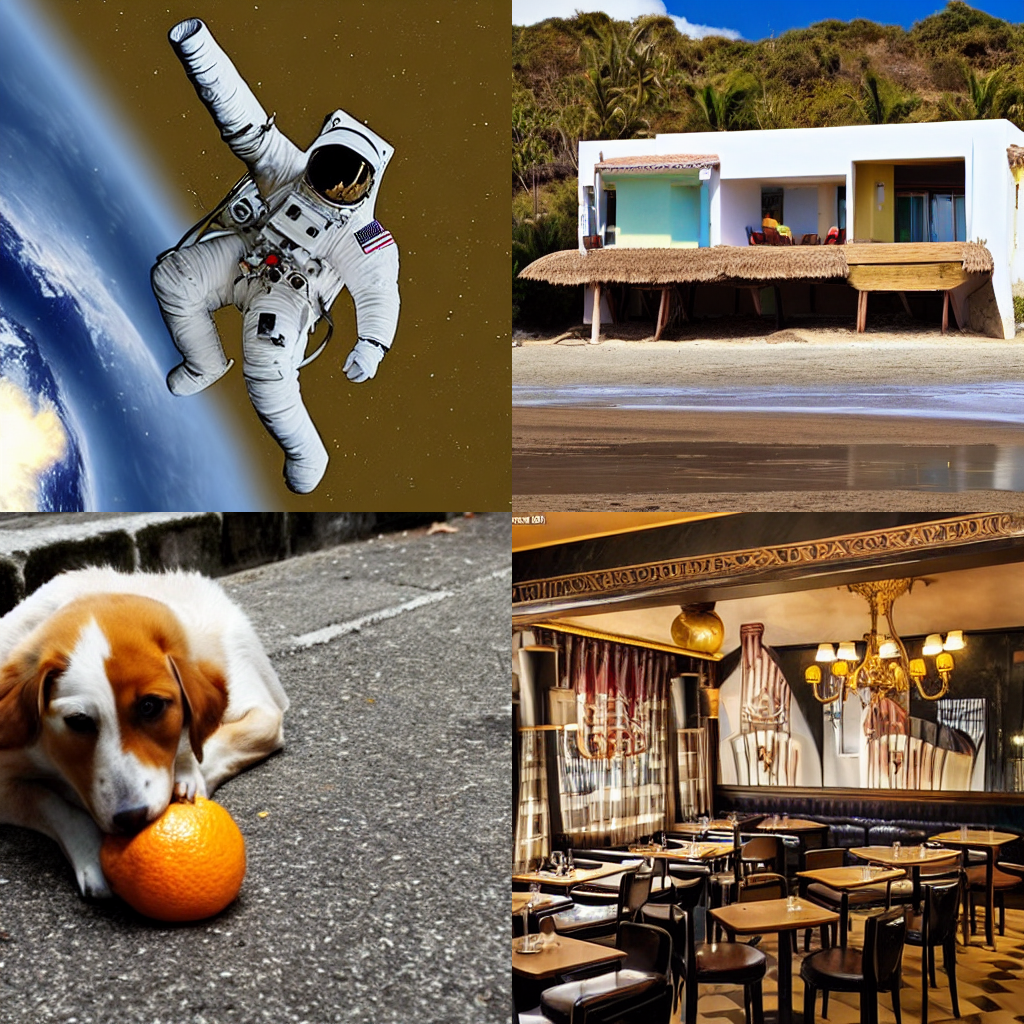
|
605 |
-
|
606 |
-
### Image to Image Inpainting Stable Diffusion
|
607 |
-
|
608 |
-
Similar to the standard stable diffusion inpainting example, except with the addition of an `inner_image` argument.
|
609 |
-
|
610 |
-
`image`, `inner_image`, and `mask` should have the same dimensions. `inner_image` should have an alpha (transparency) channel.
|
611 |
-
|
612 |
-
The aim is to overlay two images, then mask out the boundary between `image` and `inner_image` to allow stable diffusion to make the connection more seamless.
|
613 |
-
For example, this could be used to place a logo on a shirt and make it blend seamlessly.
|
614 |
-
|
615 |
-
```python
|
616 |
-
import PIL
|
617 |
-
import torch
|
618 |
-
|
619 |
-
from diffusers import DiffusionPipeline
|
620 |
-
|
621 |
-
image_path = "./path-to-image.png"
|
622 |
-
inner_image_path = "./path-to-inner-image.png"
|
623 |
-
mask_path = "./path-to-mask.png"
|
624 |
-
|
625 |
-
init_image = PIL.Image.open(image_path).convert("RGB").resize((512, 512))
|
626 |
-
inner_image = PIL.Image.open(inner_image_path).convert("RGBA").resize((512, 512))
|
627 |
-
mask_image = PIL.Image.open(mask_path).convert("RGB").resize((512, 512))
|
628 |
-
|
629 |
-
pipe = DiffusionPipeline.from_pretrained(
|
630 |
-
"runwayml/stable-diffusion-inpainting",
|
631 |
-
custom_pipeline="img2img_inpainting",
|
632 |
-
|
633 |
-
torch_dtype=torch.float16
|
634 |
-
)
|
635 |
-
pipe = pipe.to("cuda")
|
636 |
-
|
637 |
-
prompt = "Your prompt here!"
|
638 |
-
image = pipe(prompt=prompt, image=init_image, inner_image=inner_image, mask_image=mask_image).images[0]
|
639 |
-
```
|
640 |
-
|
641 |
-
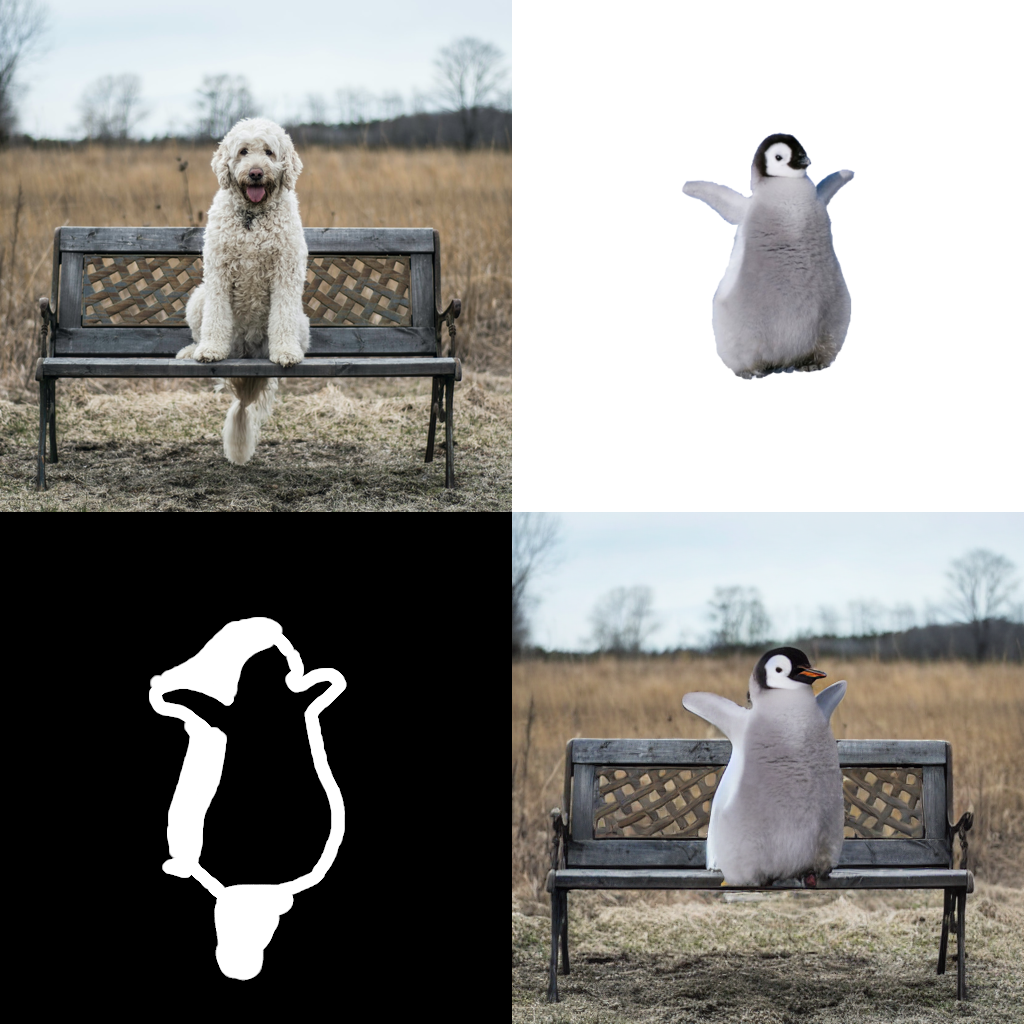
|
642 |
-
|
643 |
-
### Text Based Inpainting Stable Diffusion
|
644 |
-
|
645 |
-
Use a text prompt to generate the mask for the area to be inpainted.
|
646 |
-
Currently uses the CLIPSeg model for mask generation, then calls the standard Stable Diffusion Inpainting pipeline to perform the inpainting.
|
647 |
-
|
648 |
-
```python
|
649 |
-
from transformers import CLIPSegProcessor, CLIPSegForImageSegmentation
|
650 |
-
from diffusers import DiffusionPipeline
|
651 |
-
|
652 |
-
from PIL import Image
|
653 |
-
import requests
|
654 |
-
|
655 |
-
processor = CLIPSegProcessor.from_pretrained("CIDAS/clipseg-rd64-refined")
|
656 |
-
model = CLIPSegForImageSegmentation.from_pretrained("CIDAS/clipseg-rd64-refined")
|
657 |
-
|
658 |
-
pipe = DiffusionPipeline.from_pretrained(
|
659 |
-
"runwayml/stable-diffusion-inpainting",
|
660 |
-
custom_pipeline="text_inpainting",
|
661 |
-
segmentation_model=model,
|
662 |
-
segmentation_processor=processor
|
663 |
-
)
|
664 |
-
pipe = pipe.to("cuda")
|
665 |
-
|
666 |
-
|
667 |
-
url = "https://github.com/timojl/clipseg/blob/master/example_image.jpg?raw=true"
|
668 |
-
image = Image.open(requests.get(url, stream=True).raw).resize((512, 512))
|
669 |
-
text = "a glass" # will mask out this text
|
670 |
-
prompt = "a cup" # the masked out region will be replaced with this
|
671 |
-
|
672 |
-
image = pipe(image=image, text=text, prompt=prompt).images[0]
|
673 |
-
```
|
674 |
-
|
675 |
-
### Bit Diffusion
|
676 |
-
Based https://arxiv.org/abs/2208.04202, this is used for diffusion on discrete data - eg, discreate image data, DNA sequence data. An unconditional discreate image can be generated like this:
|
677 |
-
|
678 |
-
```python
|
679 |
-
from diffusers import DiffusionPipeline
|
680 |
-
pipe = DiffusionPipeline.from_pretrained("google/ddpm-cifar10-32", custom_pipeline="bit_diffusion")
|
681 |
-
image = pipe().images[0]
|
682 |
-
|
683 |
-
```
|
684 |
-
|
685 |
-
### Stable Diffusion with K Diffusion
|
686 |
-
|
687 |
-
Make sure you have @crowsonkb's https://github.com/crowsonkb/k-diffusion installed:
|
688 |
-
|
689 |
-
```
|
690 |
-
pip install k-diffusion
|
691 |
-
```
|
692 |
-
|
693 |
-
You can use the community pipeline as follows:
|
694 |
-
|
695 |
-
```python
|
696 |
-
from diffusers import DiffusionPipeline
|
697 |
-
|
698 |
-
pipe = DiffusionPipeline.from_pretrained("CompVis/stable-diffusion-v1-4", custom_pipeline="sd_text2img_k_diffusion")
|
699 |
-
pipe = pipe.to("cuda")
|
700 |
-
|
701 |
-
prompt = "an astronaut riding a horse on mars"
|
702 |
-
pipe.set_scheduler("sample_heun")
|
703 |
-
generator = torch.Generator(device="cuda").manual_seed(seed)
|
704 |
-
image = pipe(prompt, generator=generator, num_inference_steps=20).images[0]
|
705 |
-
|
706 |
-
image.save("./astronaut_heun_k_diffusion.png")
|
707 |
-
```
|
708 |
-
|
709 |
-
To make sure that K Diffusion and `diffusers` yield the same results:
|
710 |
-
|
711 |
-
**Diffusers**:
|
712 |
-
```python
|
713 |
-
from diffusers import DiffusionPipeline, EulerDiscreteScheduler
|
714 |
-
|
715 |
-
seed = 33
|
716 |
-
|
717 |
-
pipe = DiffusionPipeline.from_pretrained("CompVis/stable-diffusion-v1-4")
|
718 |
-
pipe.scheduler = EulerDiscreteScheduler.from_config(pipe.scheduler.config)
|
719 |
-
pipe = pipe.to("cuda")
|
720 |
-
|
721 |
-
generator = torch.Generator(device="cuda").manual_seed(seed)
|
722 |
-
image = pipe(prompt, generator=generator, num_inference_steps=50).images[0]
|
723 |
-
```
|
724 |
-
|
725 |
-

|
726 |
-
|
727 |
-
**K Diffusion**:
|
728 |
-
```python
|
729 |
-
from diffusers import DiffusionPipeline, EulerDiscreteScheduler
|
730 |
-
|
731 |
-
seed = 33
|
732 |
-
|
733 |
-
pipe = DiffusionPipeline.from_pretrained("CompVis/stable-diffusion-v1-4", custom_pipeline="sd_text2img_k_diffusion")
|
734 |
-
pipe.scheduler = EulerDiscreteScheduler.from_config(pipe.scheduler.config)
|
735 |
-
pipe = pipe.to("cuda")
|
736 |
-
|
737 |
-
pipe.set_scheduler("sample_euler")
|
738 |
-
generator = torch.Generator(device="cuda").manual_seed(seed)
|
739 |
-
image = pipe(prompt, generator=generator, num_inference_steps=50).images[0]
|
740 |
-
```
|
741 |
-
|
742 |
-

|
743 |
-
|
744 |
-
### Checkpoint Merger Pipeline
|
745 |
-
Based on the AUTOMATIC1111/webui for checkpoint merging. This is a custom pipeline that merges upto 3 pretrained model checkpoints as long as they are in the HuggingFace model_index.json format.
|
746 |
-
|
747 |
-
The checkpoint merging is currently memory intensive as it modifies the weights of a DiffusionPipeline object in place. Expect atleast 13GB RAM Usage on Kaggle GPU kernels and
|
748 |
-
on colab you might run out of the 12GB memory even while merging two checkpoints.
|
749 |
-
|
750 |
-
Usage:-
|
751 |
-
```python
|
752 |
-
from diffusers import DiffusionPipeline
|
753 |
-
|
754 |
-
#Return a CheckpointMergerPipeline class that allows you to merge checkpoints.
|
755 |
-
#The checkpoint passed here is ignored. But still pass one of the checkpoints you plan to
|
756 |
-
#merge for convenience
|
757 |
-
pipe = DiffusionPipeline.from_pretrained("CompVis/stable-diffusion-v1-4", custom_pipeline="checkpoint_merger")
|
758 |
-
|
759 |
-
#There are multiple possible scenarios:
|
760 |
-
#The pipeline with the merged checkpoints is returned in all the scenarios
|
761 |
-
|
762 |
-
#Compatible checkpoints a.k.a matched model_index.json files. Ignores the meta attributes in model_index.json during comparision.( attrs with _ as prefix )
|
763 |
-
merged_pipe = pipe.merge(["CompVis/stable-diffusion-v1-4","CompVis/stable-diffusion-v1-2"], interp = "sigmoid", alpha = 0.4)
|
764 |
-
|
765 |
-
#Incompatible checkpoints in model_index.json but merge might be possible. Use force = True to ignore model_index.json compatibility
|
766 |
-
merged_pipe_1 = pipe.merge(["CompVis/stable-diffusion-v1-4","hakurei/waifu-diffusion"], force = True, interp = "sigmoid", alpha = 0.4)
|
767 |
-
|
768 |
-
#Three checkpoint merging. Only "add_difference" method actually works on all three checkpoints. Using any other options will ignore the 3rd checkpoint.
|
769 |
-
merged_pipe_2 = pipe.merge(["CompVis/stable-diffusion-v1-4","hakurei/waifu-diffusion","prompthero/openjourney"], force = True, interp = "add_difference", alpha = 0.4)
|
770 |
-
|
771 |
-
prompt = "An astronaut riding a horse on Mars"
|
772 |
-
|
773 |
-
image = merged_pipe(prompt).images[0]
|
774 |
-
|
775 |
-
```
|
776 |
-
Some examples along with the merge details:
|
777 |
-
|
778 |
-
1. "CompVis/stable-diffusion-v1-4" + "hakurei/waifu-diffusion" ; Sigmoid interpolation; alpha = 0.8
|
779 |
-
|
780 |
-

|
781 |
-
|
782 |
-
2. "hakurei/waifu-diffusion" + "prompthero/openjourney" ; Inverse Sigmoid interpolation; alpha = 0.8
|
783 |
-
|
784 |
-

|
785 |
-
|
786 |
-
|
787 |
-
3. "CompVis/stable-diffusion-v1-4" + "hakurei/waifu-diffusion" + "prompthero/openjourney"; Add Difference interpolation; alpha = 0.5
|
788 |
-
|
789 |
-

|
790 |
-
|
791 |
-
|
792 |
-
### Stable Diffusion Comparisons
|
793 |
-
|
794 |
-
This Community Pipeline enables the comparison between the 4 checkpoints that exist for Stable Diffusion. They can be found through the following links:
|
795 |
-
1. [Stable Diffusion v1.1](https://huggingface.co/CompVis/stable-diffusion-v1-1)
|
796 |
-
2. [Stable Diffusion v1.2](https://huggingface.co/CompVis/stable-diffusion-v1-2)
|
797 |
-
3. [Stable Diffusion v1.3](https://huggingface.co/CompVis/stable-diffusion-v1-3)
|
798 |
-
4. [Stable Diffusion v1.4](https://huggingface.co/CompVis/stable-diffusion-v1-4)
|
799 |
-
|
800 |
-
```python
|
801 |
-
from diffusers import DiffusionPipeline
|
802 |
-
import matplotlib.pyplot as plt
|
803 |
-
|
804 |
-
pipe = DiffusionPipeline.from_pretrained('CompVis/stable-diffusion-v1-4', custom_pipeline='suvadityamuk/StableDiffusionComparison')
|
805 |
-
pipe.enable_attention_slicing()
|
806 |
-
pipe = pipe.to('cuda')
|
807 |
-
prompt = "an astronaut riding a horse on mars"
|
808 |
-
output = pipe(prompt)
|
809 |
-
|
810 |
-
plt.subplots(2,2,1)
|
811 |
-
plt.imshow(output.images[0])
|
812 |
-
plt.title('Stable Diffusion v1.1')
|
813 |
-
plt.axis('off')
|
814 |
-
plt.subplots(2,2,2)
|
815 |
-
plt.imshow(output.images[1])
|
816 |
-
plt.title('Stable Diffusion v1.2')
|
817 |
-
plt.axis('off')
|
818 |
-
plt.subplots(2,2,3)
|
819 |
-
plt.imshow(output.images[2])
|
820 |
-
plt.title('Stable Diffusion v1.3')
|
821 |
-
plt.axis('off')
|
822 |
-
plt.subplots(2,2,4)
|
823 |
-
plt.imshow(output.images[3])
|
824 |
-
plt.title('Stable Diffusion v1.4')
|
825 |
-
plt.axis('off')
|
826 |
-
|
827 |
-
plt.show()
|
828 |
-
```
|
829 |
-
|
830 |
-
As a result, you can look at a grid of all 4 generated images being shown together, that captures a difference the advancement of the training between the 4 checkpoints.
|
831 |
-
|
832 |
-
### Magic Mix
|
833 |
-
|
834 |
-
Implementation of the [MagicMix: Semantic Mixing with Diffusion Models](https://arxiv.org/abs/2210.16056) paper. This is a Diffusion Pipeline for semantic mixing of an image and a text prompt to create a new concept while preserving the spatial layout and geometry of the subject in the image. The pipeline takes an image that provides the layout semantics and a prompt that provides the content semantics for the mixing process.
|
835 |
-
|
836 |
-
There are 3 parameters for the method-
|
837 |
-
- `mix_factor`: It is the interpolation constant used in the layout generation phase. The greater the value of `mix_factor`, the greater the influence of the prompt on the layout generation process.
|
838 |
-
- `kmax` and `kmin`: These determine the range for the layout and content generation process. A higher value of kmax results in loss of more information about the layout of the original image and a higher value of kmin results in more steps for content generation process.
|
839 |
-
|
840 |
-
Here is an example usage-
|
841 |
-
|
842 |
-
```python
|
843 |
-
from diffusers import DiffusionPipeline, DDIMScheduler
|
844 |
-
from PIL import Image
|
845 |
-
|
846 |
-
pipe = DiffusionPipeline.from_pretrained(
|
847 |
-
"CompVis/stable-diffusion-v1-4",
|
848 |
-
custom_pipeline="magic_mix",
|
849 |
-
scheduler = DDIMScheduler.from_pretrained("CompVis/stable-diffusion-v1-4", subfolder="scheduler"),
|
850 |
-
).to('cuda')
|
851 |
-
|
852 |
-
img = Image.open('phone.jpg')
|
853 |
-
mix_img = pipe(
|
854 |
-
img,
|
855 |
-
prompt = 'bed',
|
856 |
-
kmin = 0.3,
|
857 |
-
kmax = 0.5,
|
858 |
-
mix_factor = 0.5,
|
859 |
-
)
|
860 |
-
mix_img.save('phone_bed_mix.jpg')
|
861 |
-
```
|
862 |
-
The `mix_img` is a PIL image that can be saved locally or displayed directly in a google colab. Generated image is a mix of the layout semantics of the given image and the content semantics of the prompt.
|
863 |
-
|
864 |
-
E.g. the above script generates the following image:
|
865 |
-
|
866 |
-
`phone.jpg`
|
867 |
-
|
868 |
-
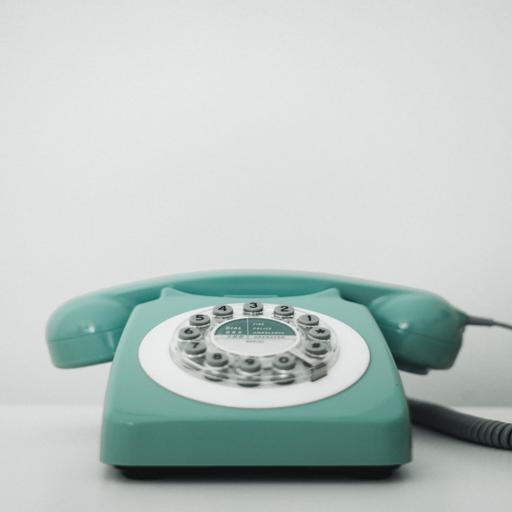
|
869 |
-
|
870 |
-
`phone_bed_mix.jpg`
|
871 |
-
|
872 |
-
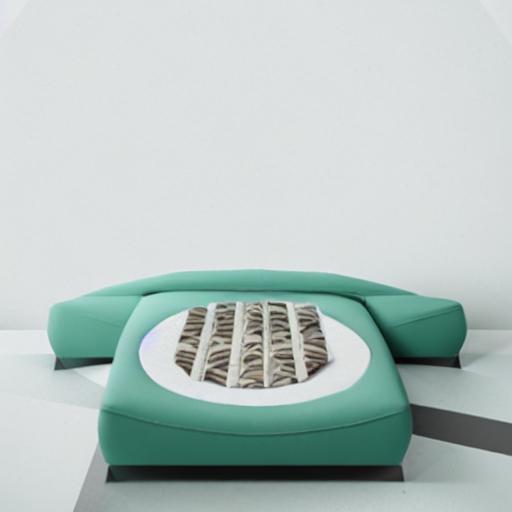
|
873 |
-
|
874 |
-
For more example generations check out this [demo notebook](https://github.com/daspartho/MagicMix/blob/main/demo.ipynb).
|
875 |
-
|
876 |
-
|
877 |
-
### Stable UnCLIP
|
878 |
-
|
879 |
-
UnCLIPPipeline("kakaobrain/karlo-v1-alpha") provide a prior model that can generate clip image embedding from text.
|
880 |
-
StableDiffusionImageVariationPipeline("lambdalabs/sd-image-variations-diffusers") provide a decoder model than can generate images from clip image embedding.
|
881 |
-
|
882 |
-
```python
|
883 |
-
import torch
|
884 |
-
from diffusers import DiffusionPipeline
|
885 |
-
|
886 |
-
device = torch.device("cpu" if not torch.cuda.is_available() else "cuda")
|
887 |
-
|
888 |
-
pipeline = DiffusionPipeline.from_pretrained(
|
889 |
-
"kakaobrain/karlo-v1-alpha",
|
890 |
-
torch_dtype=torch.float16,
|
891 |
-
custom_pipeline="stable_unclip",
|
892 |
-
decoder_pipe_kwargs=dict(
|
893 |
-
image_encoder=None,
|
894 |
-
),
|
895 |
-
)
|
896 |
-
pipeline.to(device)
|
897 |
-
|
898 |
-
prompt = "a shiba inu wearing a beret and black turtleneck"
|
899 |
-
random_generator = torch.Generator(device=device).manual_seed(1000)
|
900 |
-
output = pipeline(
|
901 |
-
prompt=prompt,
|
902 |
-
width=512,
|
903 |
-
height=512,
|
904 |
-
generator=random_generator,
|
905 |
-
prior_guidance_scale=4,
|
906 |
-
prior_num_inference_steps=25,
|
907 |
-
decoder_guidance_scale=8,
|
908 |
-
decoder_num_inference_steps=50,
|
909 |
-
)
|
910 |
-
|
911 |
-
image = output.images[0]
|
912 |
-
image.save("./shiba-inu.jpg")
|
913 |
-
|
914 |
-
# debug
|
915 |
-
|
916 |
-
# `pipeline.decoder_pipe` is a regular StableDiffusionImageVariationPipeline instance.
|
917 |
-
# It is used to convert clip image embedding to latents, then fed into VAE decoder.
|
918 |
-
print(pipeline.decoder_pipe.__class__)
|
919 |
-
# <class 'diffusers.pipelines.stable_diffusion.pipeline_stable_diffusion_image_variation.StableDiffusionImageVariationPipeline'>
|
920 |
-
|
921 |
-
# this pipeline only use prior module in "kakaobrain/karlo-v1-alpha"
|
922 |
-
# It is used to convert clip text embedding to clip image embedding.
|
923 |
-
print(pipeline)
|
924 |
-
# StableUnCLIPPipeline {
|
925 |
-
# "_class_name": "StableUnCLIPPipeline",
|
926 |
-
# "_diffusers_version": "0.12.0.dev0",
|
927 |
-
# "prior": [
|
928 |
-
# "diffusers",
|
929 |
-
# "PriorTransformer"
|
930 |
-
# ],
|
931 |
-
# "prior_scheduler": [
|
932 |
-
# "diffusers",
|
933 |
-
# "UnCLIPScheduler"
|
934 |
-
# ],
|
935 |
-
# "text_encoder": [
|
936 |
-
# "transformers",
|
937 |
-
# "CLIPTextModelWithProjection"
|
938 |
-
# ],
|
939 |
-
# "tokenizer": [
|
940 |
-
# "transformers",
|
941 |
-
# "CLIPTokenizer"
|
942 |
-
# ]
|
943 |
-
# }
|
944 |
-
|
945 |
-
# pipeline.prior_scheduler is the scheduler used for prior in UnCLIP.
|
946 |
-
print(pipeline.prior_scheduler)
|
947 |
-
# UnCLIPScheduler {
|
948 |
-
# "_class_name": "UnCLIPScheduler",
|
949 |
-
# "_diffusers_version": "0.12.0.dev0",
|
950 |
-
# "clip_sample": true,
|
951 |
-
# "clip_sample_range": 5.0,
|
952 |
-
# "num_train_timesteps": 1000,
|
953 |
-
# "prediction_type": "sample",
|
954 |
-
# "variance_type": "fixed_small_log"
|
955 |
-
# }
|
956 |
-
```
|
957 |
-
|
958 |
-
|
959 |
-
`shiba-inu.jpg`
|
960 |
-
|
961 |
-
|
962 |
-
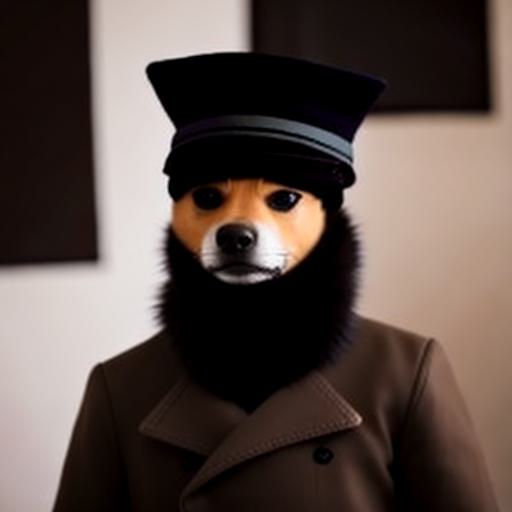
|
963 |
-
|
964 |
-
### UnCLIP Text Interpolation Pipeline
|
965 |
-
|
966 |
-
This Diffusion Pipeline takes two prompts and interpolates between the two input prompts using spherical interpolation ( slerp ). The input prompts are converted to text embeddings by the pipeline's text_encoder and the interpolation is done on the resulting text_embeddings over the number of steps specified. Defaults to 5 steps.
|
967 |
-
|
968 |
-
```python
|
969 |
-
import torch
|
970 |
-
from diffusers import DiffusionPipeline
|
971 |
-
|
972 |
-
device = torch.device("cpu" if not torch.cuda.is_available() else "cuda")
|
973 |
-
|
974 |
-
pipe = DiffusionPipeline.from_pretrained(
|
975 |
-
"kakaobrain/karlo-v1-alpha",
|
976 |
-
torch_dtype=torch.float16,
|
977 |
-
custom_pipeline="unclip_text_interpolation"
|
978 |
-
)
|
979 |
-
pipe.to(device)
|
980 |
-
|
981 |
-
start_prompt = "A photograph of an adult lion"
|
982 |
-
end_prompt = "A photograph of a lion cub"
|
983 |
-
#For best results keep the prompts close in length to each other. Of course, feel free to try out with differing lengths.
|
984 |
-
generator = torch.Generator(device=device).manual_seed(42)
|
985 |
-
|
986 |
-
output = pipe(start_prompt, end_prompt, steps = 6, generator = generator, enable_sequential_cpu_offload=False)
|
987 |
-
|
988 |
-
for i,image in enumerate(output.images):
|
989 |
-
img.save('result%s.jpg' % i)
|
990 |
-
```
|
991 |
-
|
992 |
-
The resulting images in order:-
|
993 |
-
|
994 |
-

|
995 |
-

|
996 |
-

|
997 |
-

|
998 |
-

|
999 |
-

|
1000 |
-
|
1001 |
-
### UnCLIP Image Interpolation Pipeline
|
1002 |
-
|
1003 |
-
This Diffusion Pipeline takes two images or an image_embeddings tensor of size 2 and interpolates between their embeddings using spherical interpolation ( slerp ). The input images/image_embeddings are converted to image embeddings by the pipeline's image_encoder and the interpolation is done on the resulting image_embeddings over the number of steps specified. Defaults to 5 steps.
|
1004 |
-
|
1005 |
-
```python
|
1006 |
-
import torch
|
1007 |
-
from diffusers import DiffusionPipeline
|
1008 |
-
from PIL import Image
|
1009 |
-
|
1010 |
-
device = torch.device("cpu" if not torch.cuda.is_available() else "cuda")
|
1011 |
-
dtype = torch.float16 if torch.cuda.is_available() else torch.bfloat16
|
1012 |
-
|
1013 |
-
pipe = DiffusionPipeline.from_pretrained(
|
1014 |
-
"kakaobrain/karlo-v1-alpha-image-variations",
|
1015 |
-
torch_dtype=dtype,
|
1016 |
-
custom_pipeline="unclip_image_interpolation"
|
1017 |
-
)
|
1018 |
-
pipe.to(device)
|
1019 |
-
|
1020 |
-
images = [Image.open('./starry_night.jpg'), Image.open('./flowers.jpg')]
|
1021 |
-
#For best results keep the prompts close in length to each other. Of course, feel free to try out with differing lengths.
|
1022 |
-
generator = torch.Generator(device=device).manual_seed(42)
|
1023 |
-
|
1024 |
-
output = pipe(image = images ,steps = 6, generator = generator)
|
1025 |
-
|
1026 |
-
for i,image in enumerate(output.images):
|
1027 |
-
image.save('starry_to_flowers_%s.jpg' % i)
|
1028 |
-
```
|
1029 |
-
The original images:-
|
1030 |
-
|
1031 |
-

|
1032 |
-

|
1033 |
-
|
1034 |
-
The resulting images in order:-
|
1035 |
-
|
1036 |
-

|
1037 |
-

|
1038 |
-

|
1039 |
-

|
1040 |
-

|
1041 |
-

|
1042 |
-
|
1043 |
-
### DDIM Noise Comparative Analysis Pipeline
|
1044 |
-
#### **Research question: What visual concepts do the diffusion models learn from each noise level during training?**
|
1045 |
-
The [P2 weighting (CVPR 2022)](https://arxiv.org/abs/2204.00227) paper proposed an approach to answer the above question, which is their second contribution.
|
1046 |
-
The approach consists of the following steps:
|
1047 |
-
|
1048 |
-
1. The input is an image x0.
|
1049 |
-
2. Perturb it to xt using a diffusion process q(xt|x0).
|
1050 |
-
- `strength` is a value between 0.0 and 1.0, that controls the amount of noise that is added to the input image. Values that approach 1.0 allow for lots of variations but will also produce images that are not semantically consistent with the input.
|
1051 |
-
3. Reconstruct the image with the learned denoising process pθ(ˆx0|xt).
|
1052 |
-
4. Compare x0 and ˆx0 among various t to show how each step contributes to the sample.
|
1053 |
-
The authors used [openai/guided-diffusion](https://github.com/openai/guided-diffusion) model to denoise images in FFHQ dataset. This pipeline extends their second contribution by investigating DDIM on any input image.
|
1054 |
-
|
1055 |
-
```python
|
1056 |
-
import torch
|
1057 |
-
from PIL import Image
|
1058 |
-
import numpy as np
|
1059 |
-
|
1060 |
-
image_path = "path/to/your/image" # images from CelebA-HQ might be better
|
1061 |
-
image_pil = Image.open(image_path)
|
1062 |
-
image_name = image_path.split("/")[-1].split(".")[0]
|
1063 |
-
|
1064 |
-
device = torch.device("cpu" if not torch.cuda.is_available() else "cuda")
|
1065 |
-
pipe = DiffusionPipeline.from_pretrained(
|
1066 |
-
"google/ddpm-ema-celebahq-256",
|
1067 |
-
custom_pipeline="ddim_noise_comparative_analysis",
|
1068 |
-
)
|
1069 |
-
pipe = pipe.to(device)
|
1070 |
-
|
1071 |
-
for strength in np.linspace(0.1, 1, 25):
|
1072 |
-
denoised_image, latent_timestep = pipe(
|
1073 |
-
image_pil, strength=strength, return_dict=False
|
1074 |
-
)
|
1075 |
-
denoised_image = denoised_image[0]
|
1076 |
-
denoised_image.save(
|
1077 |
-
f"noise_comparative_analysis_{image_name}_{latent_timestep}.png"
|
1078 |
-
)
|
1079 |
-
```
|
1080 |
-
|
1081 |
-
Here is the result of this pipeline (which is DDIM) on CelebA-HQ dataset.
|
1082 |
-
|
1083 |
-
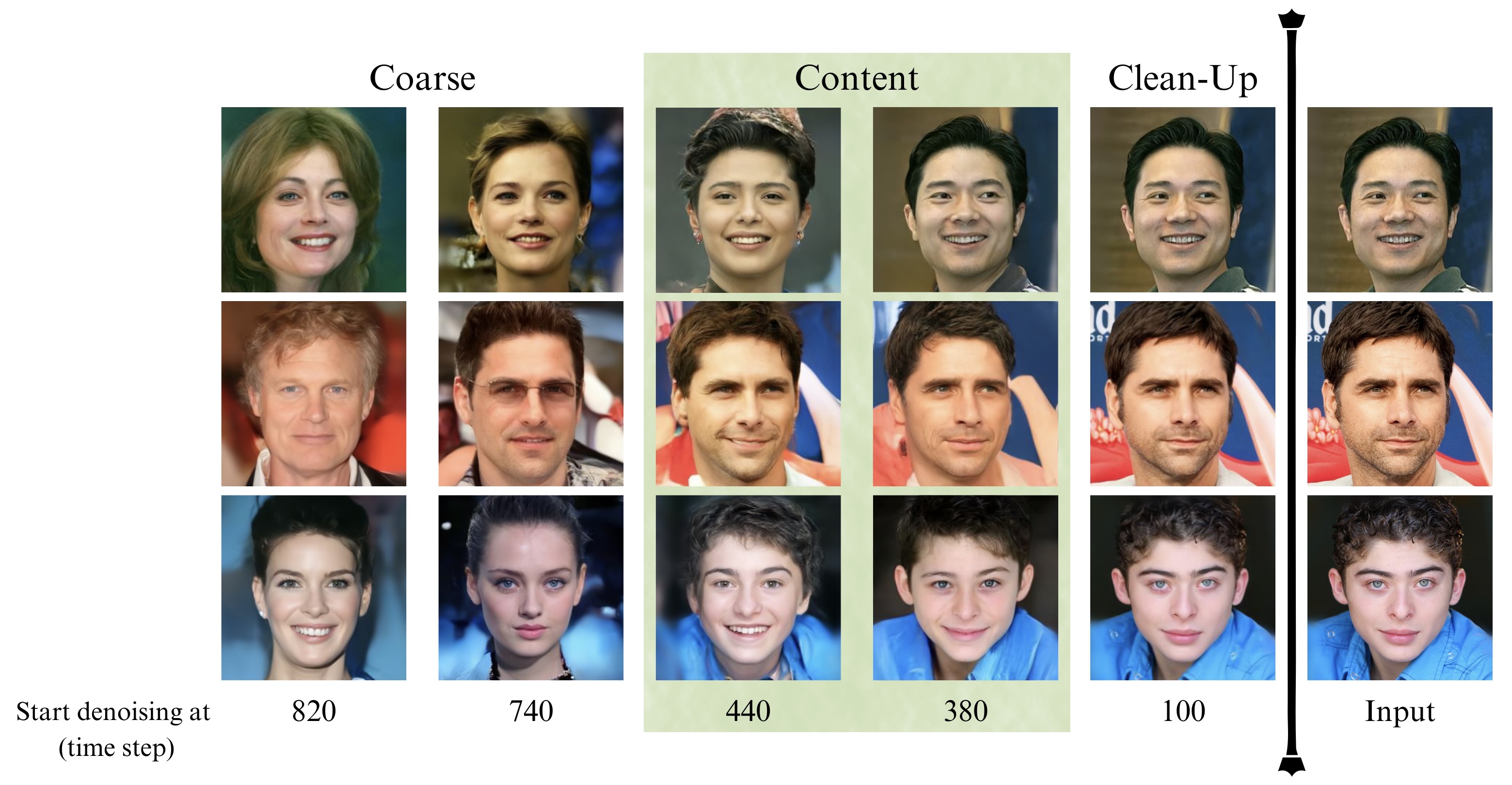
|
1084 |
-
|
1085 |
-
### CLIP Guided Img2Img Stable Diffusion
|
1086 |
-
|
1087 |
-
CLIP guided Img2Img stable diffusion can help to generate more realistic images with an initial image
|
1088 |
-
by guiding stable diffusion at every denoising step with an additional CLIP model.
|
1089 |
-
|
1090 |
-
The following code requires roughly 12GB of GPU RAM.
|
1091 |
-
|
1092 |
-
```python
|
1093 |
-
from io import BytesIO
|
1094 |
-
import requests
|
1095 |
-
import torch
|
1096 |
-
from diffusers import DiffusionPipeline
|
1097 |
-
from PIL import Image
|
1098 |
-
from transformers import CLIPFeatureExtractor, CLIPModel
|
1099 |
-
feature_extractor = CLIPFeatureExtractor.from_pretrained(
|
1100 |
-
"laion/CLIP-ViT-B-32-laion2B-s34B-b79K"
|
1101 |
-
)
|
1102 |
-
clip_model = CLIPModel.from_pretrained(
|
1103 |
-
"laion/CLIP-ViT-B-32-laion2B-s34B-b79K", torch_dtype=torch.float16
|
1104 |
-
)
|
1105 |
-
guided_pipeline = DiffusionPipeline.from_pretrained(
|
1106 |
-
"CompVis/stable-diffusion-v1-4",
|
1107 |
-
# custom_pipeline="clip_guided_stable_diffusion",
|
1108 |
-
custom_pipeline="/home/njindal/diffusers/examples/community/clip_guided_stable_diffusion.py",
|
1109 |
-
clip_model=clip_model,
|
1110 |
-
feature_extractor=feature_extractor,
|
1111 |
-
torch_dtype=torch.float16,
|
1112 |
-
)
|
1113 |
-
guided_pipeline.enable_attention_slicing()
|
1114 |
-
guided_pipeline = guided_pipeline.to("cuda")
|
1115 |
-
prompt = "fantasy book cover, full moon, fantasy forest landscape, golden vector elements, fantasy magic, dark light night, intricate, elegant, sharp focus, illustration, highly detailed, digital painting, concept art, matte, art by WLOP and Artgerm and Albert Bierstadt, masterpiece"
|
1116 |
-
url = "https://raw.githubusercontent.com/CompVis/stable-diffusion/main/assets/stable-samples/img2img/sketch-mountains-input.jpg"
|
1117 |
-
response = requests.get(url)
|
1118 |
-
init_image = Image.open(BytesIO(response.content)).convert("RGB")
|
1119 |
-
image = guided_pipeline(
|
1120 |
-
prompt=prompt,
|
1121 |
-
num_inference_steps=30,
|
1122 |
-
image=init_image,
|
1123 |
-
strength=0.75,
|
1124 |
-
guidance_scale=7.5,
|
1125 |
-
clip_guidance_scale=100,
|
1126 |
-
num_cutouts=4,
|
1127 |
-
use_cutouts=False,
|
1128 |
-
).images[0]
|
1129 |
-
display(image)
|
1130 |
-
```
|
1131 |
-
|
1132 |
-
Init Image
|
1133 |
-
|
1134 |
-

|
1135 |
-
|
1136 |
-
Output Image
|
1137 |
-
|
1138 |
-

|
1139 |
-
|
1140 |
-
### TensorRT Text2Image Stable Diffusion Pipeline
|
1141 |
-
|
1142 |
-
The TensorRT Pipeline can be used to accelerate the Text2Image Stable Diffusion Inference run.
|
1143 |
-
|
1144 |
-
NOTE: The ONNX conversions and TensorRT engine build may take up to 30 minutes.
|
1145 |
-
|
1146 |
-
```python
|
1147 |
-
import torch
|
1148 |
-
from diffusers import DDIMScheduler
|
1149 |
-
from diffusers.pipelines.stable_diffusion import StableDiffusionPipeline
|
1150 |
-
|
1151 |
-
# Use the DDIMScheduler scheduler here instead
|
1152 |
-
scheduler = DDIMScheduler.from_pretrained("stabilityai/stable-diffusion-2-1",
|
1153 |
-
subfolder="scheduler")
|
1154 |
-
|
1155 |
-
pipe = StableDiffusionPipeline.from_pretrained("stabilityai/stable-diffusion-2-1",
|
1156 |
-
custom_pipeline="stable_diffusion_tensorrt_txt2img",
|
1157 |
-
revision='fp16',
|
1158 |
-
torch_dtype=torch.float16,
|
1159 |
-
scheduler=scheduler,)
|
1160 |
-
|
1161 |
-
# re-use cached folder to save ONNX models and TensorRT Engines
|
1162 |
-
pipe.set_cached_folder("stabilityai/stable-diffusion-2-1", revision='fp16',)
|
1163 |
-
|
1164 |
-
pipe = pipe.to("cuda")
|
1165 |
-
|
1166 |
-
prompt = "a beautiful photograph of Mt. Fuji during cherry blossom"
|
1167 |
-
image = pipe(prompt).images[0]
|
1168 |
-
image.save('tensorrt_mt_fuji.png')
|
1169 |
-
```
|
1170 |
-
|
1171 |
-
### EDICT Image Editing Pipeline
|
1172 |
-
|
1173 |
-
This pipeline implements the text-guided image editing approach from the paper [EDICT: Exact Diffusion Inversion via Coupled Transformations](https://arxiv.org/abs/2211.12446). You have to pass:
|
1174 |
-
- (`PIL`) `image` you want to edit.
|
1175 |
-
- `base_prompt`: the text prompt describing the current image (before editing).
|
1176 |
-
- `target_prompt`: the text prompt describing with the edits.
|
1177 |
-
|
1178 |
-
```python
|
1179 |
-
from diffusers import DiffusionPipeline, DDIMScheduler
|
1180 |
-
from transformers import CLIPTextModel
|
1181 |
-
import torch, PIL, requests
|
1182 |
-
from io import BytesIO
|
1183 |
-
from IPython.display import display
|
1184 |
-
|
1185 |
-
def center_crop_and_resize(im):
|
1186 |
-
|
1187 |
-
width, height = im.size
|
1188 |
-
d = min(width, height)
|
1189 |
-
left = (width - d) / 2
|
1190 |
-
upper = (height - d) / 2
|
1191 |
-
right = (width + d) / 2
|
1192 |
-
lower = (height + d) / 2
|
1193 |
-
|
1194 |
-
return im.crop((left, upper, right, lower)).resize((512, 512))
|
1195 |
-
|
1196 |
-
torch_dtype = torch.float16
|
1197 |
-
device = torch.device('cuda' if torch.cuda.is_available() else 'cpu')
|
1198 |
-
|
1199 |
-
# scheduler and text_encoder param values as in the paper
|
1200 |
-
scheduler = DDIMScheduler(
|
1201 |
-
num_train_timesteps=1000,
|
1202 |
-
beta_start=0.00085,
|
1203 |
-
beta_end=0.012,
|
1204 |
-
beta_schedule="scaled_linear",
|
1205 |
-
set_alpha_to_one=False,
|
1206 |
-
clip_sample=False,
|
1207 |
-
)
|
1208 |
-
|
1209 |
-
text_encoder = CLIPTextModel.from_pretrained(
|
1210 |
-
pretrained_model_name_or_path="openai/clip-vit-large-patch14",
|
1211 |
-
torch_dtype=torch_dtype,
|
1212 |
-
)
|
1213 |
-
|
1214 |
-
# initialize pipeline
|
1215 |
-
pipeline = DiffusionPipeline.from_pretrained(
|
1216 |
-
pretrained_model_name_or_path="CompVis/stable-diffusion-v1-4",
|
1217 |
-
custom_pipeline="edict_pipeline",
|
1218 |
-
revision="fp16",
|
1219 |
-
scheduler=scheduler,
|
1220 |
-
text_encoder=text_encoder,
|
1221 |
-
leapfrog_steps=True,
|
1222 |
-
torch_dtype=torch_dtype,
|
1223 |
-
).to(device)
|
1224 |
-
|
1225 |
-
# download image
|
1226 |
-
image_url = "https://huggingface.co/datasets/Joqsan/images/resolve/main/imagenet_dog_1.jpeg"
|
1227 |
-
response = requests.get(image_url)
|
1228 |
-
image = PIL.Image.open(BytesIO(response.content))
|
1229 |
-
|
1230 |
-
# preprocess it
|
1231 |
-
cropped_image = center_crop_and_resize(image)
|
1232 |
-
|
1233 |
-
# define the prompts
|
1234 |
-
base_prompt = "A dog"
|
1235 |
-
target_prompt = "A golden retriever"
|
1236 |
-
|
1237 |
-
# run the pipeline
|
1238 |
-
result_image = pipeline(
|
1239 |
-
base_prompt=base_prompt,
|
1240 |
-
target_prompt=target_prompt,
|
1241 |
-
image=cropped_image,
|
1242 |
-
)
|
1243 |
-
|
1244 |
-
display(result_image)
|
1245 |
-
```
|
1246 |
-
|
1247 |
-
Init Image
|
1248 |
-
|
1249 |
-

|
1250 |
-
|
1251 |
-
Output Image
|
1252 |
-
|
1253 |
-

|
1254 |
-
|
1255 |
-
### Stable Diffusion RePaint
|
1256 |
-
|
1257 |
-
This pipeline uses the [RePaint](https://arxiv.org/abs/2201.09865) logic on the latent space of stable diffusion. It can
|
1258 |
-
be used similarly to other image inpainting pipelines but does not rely on a specific inpainting model. This means you can use
|
1259 |
-
models that are not specifically created for inpainting.
|
1260 |
-
|
1261 |
-
Make sure to use the ```RePaintScheduler``` as shown in the example below.
|
1262 |
-
|
1263 |
-
Disclaimer: The mask gets transferred into latent space, this may lead to unexpected changes on the edge of the masked part.
|
1264 |
-
The inference time is a lot slower.
|
1265 |
-
|
1266 |
-
```py
|
1267 |
-
import PIL
|
1268 |
-
import requests
|
1269 |
-
import torch
|
1270 |
-
from io import BytesIO
|
1271 |
-
from diffusers import StableDiffusionPipeline, RePaintScheduler
|
1272 |
-
def download_image(url):
|
1273 |
-
response = requests.get(url)
|
1274 |
-
return PIL.Image.open(BytesIO(response.content)).convert("RGB")
|
1275 |
-
img_url = "https://raw.githubusercontent.com/CompVis/latent-diffusion/main/data/inpainting_examples/overture-creations-5sI6fQgYIuo.png"
|
1276 |
-
mask_url = "https://raw.githubusercontent.com/CompVis/latent-diffusion/main/data/inpainting_examples/overture-creations-5sI6fQgYIuo_mask.png"
|
1277 |
-
init_image = download_image(img_url).resize((512, 512))
|
1278 |
-
mask_image = download_image(mask_url).resize((512, 512))
|
1279 |
-
mask_image = PIL.ImageOps.invert(mask_image)
|
1280 |
-
pipe = StableDiffusionPipeline.from_pretrained(
|
1281 |
-
"CompVis/stable-diffusion-v1-4", torch_dtype=torch.float16, custom_pipeline="stable_diffusion_repaint",
|
1282 |
-
)
|
1283 |
-
pipe.scheduler = RePaintScheduler.from_config(pipe.scheduler.config)
|
1284 |
-
pipe = pipe.to("cuda")
|
1285 |
-
prompt = "Face of a yellow cat, high resolution, sitting on a park bench"
|
1286 |
-
image = pipe(prompt=prompt, image=init_image, mask_image=mask_image).images[0]
|
1287 |
-
```
|
1288 |
-
|
1289 |
-
### TensorRT Image2Image Stable Diffusion Pipeline
|
1290 |
-
|
1291 |
-
The TensorRT Pipeline can be used to accelerate the Image2Image Stable Diffusion Inference run.
|
1292 |
-
|
1293 |
-
NOTE: The ONNX conversions and TensorRT engine build may take up to 30 minutes.
|
1294 |
-
|
1295 |
-
```python
|
1296 |
-
import requests
|
1297 |
-
from io import BytesIO
|
1298 |
-
from PIL import Image
|
1299 |
-
import torch
|
1300 |
-
from diffusers import DDIMScheduler
|
1301 |
-
from diffusers.pipelines.stable_diffusion import StableDiffusionImg2ImgPipeline
|
1302 |
-
|
1303 |
-
# Use the DDIMScheduler scheduler here instead
|
1304 |
-
scheduler = DDIMScheduler.from_pretrained("stabilityai/stable-diffusion-2-1",
|
1305 |
-
subfolder="scheduler")
|
1306 |
-
|
1307 |
-
|
1308 |
-
pipe = StableDiffusionImg2ImgPipeline.from_pretrained("stabilityai/stable-diffusion-2-1",
|
1309 |
-
custom_pipeline="stable_diffusion_tensorrt_img2img",
|
1310 |
-
revision='fp16',
|
1311 |
-
torch_dtype=torch.float16,
|
1312 |
-
scheduler=scheduler,)
|
1313 |
-
|
1314 |
-
# re-use cached folder to save ONNX models and TensorRT Engines
|
1315 |
-
pipe.set_cached_folder("stabilityai/stable-diffusion-2-1", revision='fp16',)
|
1316 |
-
|
1317 |
-
pipe = pipe.to("cuda")
|
1318 |
-
|
1319 |
-
url = "https://pajoca.com/wp-content/uploads/2022/09/tekito-yamakawa-1.png"
|
1320 |
-
response = requests.get(url)
|
1321 |
-
input_image = Image.open(BytesIO(response.content)).convert("RGB")
|
1322 |
-
|
1323 |
-
prompt = "photorealistic new zealand hills"
|
1324 |
-
image = pipe(prompt, image=input_image, strength=0.75,).images[0]
|
1325 |
-
image.save('tensorrt_img2img_new_zealand_hills.png')
|
1326 |
-
```
|
1327 |
-
|
1328 |
-
### Stable Diffusion Reference
|
1329 |
-
|
1330 |
-
This pipeline uses the Reference Control. Refer to the [sd-webui-controlnet discussion: Reference-only Control](https://github.com/Mikubill/sd-webui-controlnet/discussions/1236)[sd-webui-controlnet discussion: Reference-adain Control](https://github.com/Mikubill/sd-webui-controlnet/discussions/1280).
|
1331 |
-
|
1332 |
-
Based on [this issue](https://github.com/huggingface/diffusers/issues/3566),
|
1333 |
-
- `EulerAncestralDiscreteScheduler` got poor results.
|
1334 |
-
|
1335 |
-
```py
|
1336 |
-
import torch
|
1337 |
-
from diffusers import UniPCMultistepScheduler
|
1338 |
-
from diffusers.utils import load_image
|
1339 |
-
|
1340 |
-
input_image = load_image("https://hf.co/datasets/huggingface/documentation-images/resolve/main/diffusers/input_image_vermeer.png")
|
1341 |
-
|
1342 |
-
pipe = StableDiffusionReferencePipeline.from_pretrained(
|
1343 |
-
"runwayml/stable-diffusion-v1-5",
|
1344 |
-
safety_checker=None,
|
1345 |
-
torch_dtype=torch.float16
|
1346 |
-
).to('cuda:0')
|
1347 |
-
|
1348 |
-
pipe.scheduler = UniPCMultistepScheduler.from_config(pipe.scheduler.config)
|
1349 |
-
|
1350 |
-
result_img = pipe(ref_image=input_image,
|
1351 |
-
prompt="1girl",
|
1352 |
-
num_inference_steps=20,
|
1353 |
-
reference_attn=True,
|
1354 |
-
reference_adain=True).images[0]
|
1355 |
-
```
|
1356 |
-
|
1357 |
-
Reference Image
|
1358 |
-
|
1359 |
-

|
1360 |
-
|
1361 |
-
Output Image of `reference_attn=True` and `reference_adain=False`
|
1362 |
-
|
1363 |
-
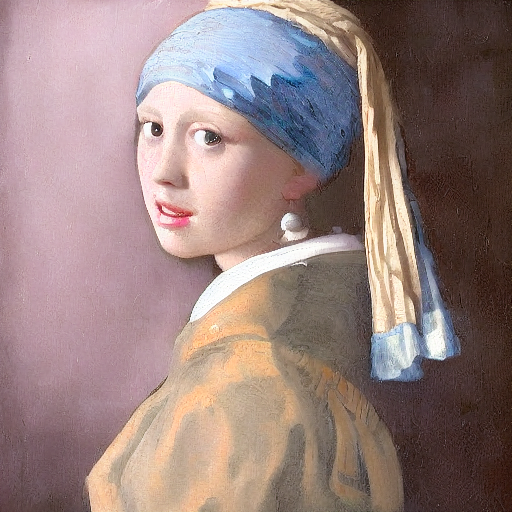
|
1364 |
-
|
1365 |
-
Output Image of `reference_attn=False` and `reference_adain=True`
|
1366 |
-
|
1367 |
-

|
1368 |
-
|
1369 |
-
Output Image of `reference_attn=True` and `reference_adain=True`
|
1370 |
-
|
1371 |
-
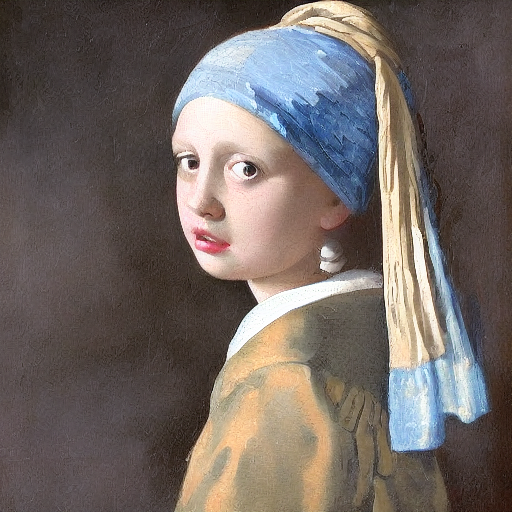
|
1372 |
-
|
1373 |
-
### Stable Diffusion ControlNet Reference
|
1374 |
-
|
1375 |
-
This pipeline uses the Reference Control with ControlNet. Refer to the [sd-webui-controlnet discussion: Reference-only Control](https://github.com/Mikubill/sd-webui-controlnet/discussions/1236)[sd-webui-controlnet discussion: Reference-adain Control](https://github.com/Mikubill/sd-webui-controlnet/discussions/1280).
|
1376 |
-
|
1377 |
-
Based on [this issue](https://github.com/huggingface/diffusers/issues/3566),
|
1378 |
-
- `EulerAncestralDiscreteScheduler` got poor results.
|
1379 |
-
- `guess_mode=True` works well for ControlNet v1.1
|
1380 |
-
|
1381 |
-
```py
|
1382 |
-
import cv2
|
1383 |
-
import torch
|
1384 |
-
import numpy as np
|
1385 |
-
from PIL import Image
|
1386 |
-
from diffusers import UniPCMultistepScheduler
|
1387 |
-
from diffusers.utils import load_image
|
1388 |
-
|
1389 |
-
input_image = load_image("https://hf.co/datasets/huggingface/documentation-images/resolve/main/diffusers/input_image_vermeer.png")
|
1390 |
-
|
1391 |
-
# get canny image
|
1392 |
-
image = cv2.Canny(np.array(input_image), 100, 200)
|
1393 |
-
image = image[:, :, None]
|
1394 |
-
image = np.concatenate([image, image, image], axis=2)
|
1395 |
-
canny_image = Image.fromarray(image)
|
1396 |
-
|
1397 |
-
controlnet = ControlNetModel.from_pretrained("lllyasviel/sd-controlnet-canny", torch_dtype=torch.float16)
|
1398 |
-
pipe = StableDiffusionControlNetReferencePipeline.from_pretrained(
|
1399 |
-
"runwayml/stable-diffusion-v1-5",
|
1400 |
-
controlnet=controlnet,
|
1401 |
-
safety_checker=None,
|
1402 |
-
torch_dtype=torch.float16
|
1403 |
-
).to('cuda:0')
|
1404 |
-
|
1405 |
-
pipe.scheduler = UniPCMultistepScheduler.from_config(pipe.scheduler.config)
|
1406 |
-
|
1407 |
-
result_img = pipe(ref_image=input_image,
|
1408 |
-
prompt="1girl",
|
1409 |
-
image=canny_image,
|
1410 |
-
num_inference_steps=20,
|
1411 |
-
reference_attn=True,
|
1412 |
-
reference_adain=True).images[0]
|
1413 |
-
```
|
1414 |
-
|
1415 |
-
Reference Image
|
1416 |
-
|
1417 |
-

|
1418 |
-
|
1419 |
-
Output Image
|
1420 |
-
|
1421 |
-

|
1422 |
-
|
1423 |
-
|
1424 |
-
### Stable Diffusion on IPEX
|
1425 |
-
|
1426 |
-
This diffusion pipeline aims to accelarate the inference of Stable-Diffusion on Intel Xeon CPUs with BF16/FP32 precision using [IPEX](https://github.com/intel/intel-extension-for-pytorch).
|
1427 |
-
|
1428 |
-
To use this pipeline, you need to:
|
1429 |
-
1. Install [IPEX](https://github.com/intel/intel-extension-for-pytorch)
|
1430 |
-
|
1431 |
-
**Note:** For each PyTorch release, there is a corresponding release of the IPEX. Here is the mapping relationship. It is recommended to install Pytorch/IPEX2.0 to get the best performance.
|
1432 |
-
|
1433 |
-
|PyTorch Version|IPEX Version|
|
1434 |
-
|--|--|
|
1435 |
-
|[v2.0.\*](https://github.com/pytorch/pytorch/tree/v2.0.1 "v2.0.1")|[v2.0.\*](https://github.com/intel/intel-extension-for-pytorch/tree/v2.0.100+cpu)|
|
1436 |
-
|[v1.13.\*](https://github.com/pytorch/pytorch/tree/v1.13.0 "v1.13.0")|[v1.13.\*](https://github.com/intel/intel-extension-for-pytorch/tree/v1.13.100+cpu)|
|
1437 |
-
|
1438 |
-
You can simply use pip to install IPEX with the latest version.
|
1439 |
-
```python
|
1440 |
-
python -m pip install intel_extension_for_pytorch
|
1441 |
-
```
|
1442 |
-
**Note:** To install a specific version, run with the following command:
|
1443 |
-
```
|
1444 |
-
python -m pip install intel_extension_for_pytorch==<version_name> -f https://developer.intel.com/ipex-whl-stable-cpu
|
1445 |
-
```
|
1446 |
-
|
1447 |
-
2. After pipeline initialization, `prepare_for_ipex()` should be called to enable IPEX accelaration. Supported inference datatypes are Float32 and BFloat16.
|
1448 |
-
|
1449 |
-
**Note:** The setting of generated image height/width for `prepare_for_ipex()` should be same as the setting of pipeline inference.
|
1450 |
-
```python
|
1451 |
-
pipe = DiffusionPipeline.from_pretrained("runwayml/stable-diffusion-v1-5", custom_pipeline="stable_diffusion_ipex")
|
1452 |
-
# For Float32
|
1453 |
-
pipe.prepare_for_ipex(prompt, dtype=torch.float32, height=512, width=512) #value of image height/width should be consistent with the pipeline inference
|
1454 |
-
# For BFloat16
|
1455 |
-
pipe.prepare_for_ipex(prompt, dtype=torch.bfloat16, height=512, width=512) #value of image height/width should be consistent with the pipeline inference
|
1456 |
-
```
|
1457 |
-
|
1458 |
-
Then you can use the ipex pipeline in a similar way to the default stable diffusion pipeline.
|
1459 |
-
```python
|
1460 |
-
# For Float32
|
1461 |
-
image = pipe(prompt, num_inference_steps=20, height=512, width=512).images[0] #value of image height/width should be consistent with 'prepare_for_ipex()'
|
1462 |
-
# For BFloat16
|
1463 |
-
with torch.cpu.amp.autocast(enabled=True, dtype=torch.bfloat16):
|
1464 |
-
image = pipe(prompt, num_inference_steps=20, height=512, width=512).images[0] #value of image height/width should be consistent with 'prepare_for_ipex()'
|
1465 |
-
```
|
1466 |
-
|
1467 |
-
The following code compares the performance of the original stable diffusion pipeline with the ipex-optimized pipeline.
|
1468 |
-
|
1469 |
-
```python
|
1470 |
-
import torch
|
1471 |
-
import intel_extension_for_pytorch as ipex
|
1472 |
-
from diffusers import StableDiffusionPipeline
|
1473 |
-
import time
|
1474 |
-
|
1475 |
-
prompt = "sailing ship in storm by Rembrandt"
|
1476 |
-
model_id = "runwayml/stable-diffusion-v1-5"
|
1477 |
-
# Helper function for time evaluation
|
1478 |
-
def elapsed_time(pipeline, nb_pass=3, num_inference_steps=20):
|
1479 |
-
# warmup
|
1480 |
-
for _ in range(2):
|
1481 |
-
images = pipeline(prompt, num_inference_steps=num_inference_steps, height=512, width=512).images
|
1482 |
-
#time evaluation
|
1483 |
-
start = time.time()
|
1484 |
-
for _ in range(nb_pass):
|
1485 |
-
pipeline(prompt, num_inference_steps=num_inference_steps, height=512, width=512)
|
1486 |
-
end = time.time()
|
1487 |
-
return (end - start) / nb_pass
|
1488 |
-
|
1489 |
-
############## bf16 inference performance ###############
|
1490 |
-
|
1491 |
-
# 1. IPEX Pipeline initialization
|
1492 |
-
pipe = DiffusionPipeline.from_pretrained(model_id, custom_pipeline="stable_diffusion_ipex")
|
1493 |
-
pipe.prepare_for_ipex(prompt, dtype=torch.bfloat16, height=512, width=512)
|
1494 |
-
|
1495 |
-
# 2. Original Pipeline initialization
|
1496 |
-
pipe2 = StableDiffusionPipeline.from_pretrained(model_id)
|
1497 |
-
|
1498 |
-
# 3. Compare performance between Original Pipeline and IPEX Pipeline
|
1499 |
-
with torch.cpu.amp.autocast(enabled=True, dtype=torch.bfloat16):
|
1500 |
-
latency = elapsed_time(pipe)
|
1501 |
-
print("Latency of StableDiffusionIPEXPipeline--bf16", latency)
|
1502 |
-
latency = elapsed_time(pipe2)
|
1503 |
-
print("Latency of StableDiffusionPipeline--bf16",latency)
|
1504 |
-
|
1505 |
-
############## fp32 inference performance ###############
|
1506 |
-
|
1507 |
-
# 1. IPEX Pipeline initialization
|
1508 |
-
pipe3 = DiffusionPipeline.from_pretrained(model_id, custom_pipeline="stable_diffusion_ipex")
|
1509 |
-
pipe3.prepare_for_ipex(prompt, dtype=torch.float32, height=512, width=512)
|
1510 |
-
|
1511 |
-
# 2. Original Pipeline initialization
|
1512 |
-
pipe4 = StableDiffusionPipeline.from_pretrained(model_id)
|
1513 |
-
|
1514 |
-
# 3. Compare performance between Original Pipeline and IPEX Pipeline
|
1515 |
-
latency = elapsed_time(pipe3)
|
1516 |
-
print("Latency of StableDiffusionIPEXPipeline--fp32", latency)
|
1517 |
-
latency = elapsed_time(pipe4)
|
1518 |
-
print("Latency of StableDiffusionPipeline--fp32",latency)
|
1519 |
-
|
1520 |
-
```
|
1521 |
-
|
1522 |
-
### CLIP Guided Images Mixing With Stable Diffusion
|
1523 |
-
|
1524 |
-

|
1525 |
-
|
1526 |
-
CLIP guided stable diffusion images mixing pipline allows to combine two images using standard diffusion models.
|
1527 |
-
This approach is using (optional) CoCa model to avoid writing image description.
|
1528 |
-
[More code examples](https://github.com/TheDenk/images_mixing)
|
1529 |
-
|
1530 |
-
## Example Images Mixing (with CoCa)
|
1531 |
-
```python
|
1532 |
-
import requests
|
1533 |
-
from io import BytesIO
|
1534 |
-
|
1535 |
-
import PIL
|
1536 |
-
import torch
|
1537 |
-
import open_clip
|
1538 |
-
from open_clip import SimpleTokenizer
|
1539 |
-
from diffusers import DiffusionPipeline
|
1540 |
-
from transformers import CLIPFeatureExtractor, CLIPModel
|
1541 |
-
|
1542 |
-
|
1543 |
-
def download_image(url):
|
1544 |
-
response = requests.get(url)
|
1545 |
-
return PIL.Image.open(BytesIO(response.content)).convert("RGB")
|
1546 |
-
|
1547 |
-
# Loading additional models
|
1548 |
-
feature_extractor = CLIPFeatureExtractor.from_pretrained(
|
1549 |
-
"laion/CLIP-ViT-B-32-laion2B-s34B-b79K"
|
1550 |
-
)
|
1551 |
-
clip_model = CLIPModel.from_pretrained(
|
1552 |
-
"laion/CLIP-ViT-B-32-laion2B-s34B-b79K", torch_dtype=torch.float16
|
1553 |
-
)
|
1554 |
-
coca_model = open_clip.create_model('coca_ViT-L-14', pretrained='laion2B-s13B-b90k').to('cuda')
|
1555 |
-
coca_model.dtype = torch.float16
|
1556 |
-
coca_transform = open_clip.image_transform(
|
1557 |
-
coca_model.visual.image_size,
|
1558 |
-
is_train = False,
|
1559 |
-
mean = getattr(coca_model.visual, 'image_mean', None),
|
1560 |
-
std = getattr(coca_model.visual, 'image_std', None),
|
1561 |
-
)
|
1562 |
-
coca_tokenizer = SimpleTokenizer()
|
1563 |
-
|
1564 |
-
# Pipline creating
|
1565 |
-
mixing_pipeline = DiffusionPipeline.from_pretrained(
|
1566 |
-
"CompVis/stable-diffusion-v1-4",
|
1567 |
-
custom_pipeline="clip_guided_images_mixing_stable_diffusion",
|
1568 |
-
clip_model=clip_model,
|
1569 |
-
feature_extractor=feature_extractor,
|
1570 |
-
coca_model=coca_model,
|
1571 |
-
coca_tokenizer=coca_tokenizer,
|
1572 |
-
coca_transform=coca_transform,
|
1573 |
-
torch_dtype=torch.float16,
|
1574 |
-
)
|
1575 |
-
mixing_pipeline.enable_attention_slicing()
|
1576 |
-
mixing_pipeline = mixing_pipeline.to("cuda")
|
1577 |
-
|
1578 |
-
# Pipline running
|
1579 |
-
generator = torch.Generator(device="cuda").manual_seed(17)
|
1580 |
-
|
1581 |
-
def download_image(url):
|
1582 |
-
response = requests.get(url)
|
1583 |
-
return PIL.Image.open(BytesIO(response.content)).convert("RGB")
|
1584 |
-
|
1585 |
-
content_image = download_image("https://huggingface.co/datasets/TheDenk/images_mixing/resolve/main/boromir.jpg")
|
1586 |
-
style_image = download_image("https://huggingface.co/datasets/TheDenk/images_mixing/resolve/main/gigachad.jpg")
|
1587 |
-
|
1588 |
-
pipe_images = mixing_pipeline(
|
1589 |
-
num_inference_steps=50,
|
1590 |
-
content_image=content_image,
|
1591 |
-
style_image=style_image,
|
1592 |
-
noise_strength=0.65,
|
1593 |
-
slerp_latent_style_strength=0.9,
|
1594 |
-
slerp_prompt_style_strength=0.1,
|
1595 |
-
slerp_clip_image_style_strength=0.1,
|
1596 |
-
guidance_scale=9.0,
|
1597 |
-
batch_size=1,
|
1598 |
-
clip_guidance_scale=100,
|
1599 |
-
generator=generator,
|
1600 |
-
).images
|
1601 |
-
```
|
1602 |
-
|
1603 |
-

|
1604 |
-
|
1605 |
-
### Stable Diffusion Mixture Tiling
|
1606 |
-
|
1607 |
-
This pipeline uses the Mixture. Refer to the [Mixture](https://arxiv.org/abs/2302.02412) paper for more details.
|
1608 |
-
|
1609 |
-
```python
|
1610 |
-
from diffusers import LMSDiscreteScheduler, DiffusionPipeline
|
1611 |
-
|
1612 |
-
# Creater scheduler and model (similar to StableDiffusionPipeline)
|
1613 |
-
scheduler = LMSDiscreteScheduler(beta_start=0.00085, beta_end=0.012, beta_schedule="scaled_linear", num_train_timesteps=1000)
|
1614 |
-
pipeline = DiffusionPipeline.from_pretrained("CompVis/stable-diffusion-v1-4", scheduler=scheduler, custom_pipeline="mixture_tiling")
|
1615 |
-
pipeline.to("cuda")
|
1616 |
-
|
1617 |
-
# Mixture of Diffusers generation
|
1618 |
-
image = pipeline(
|
1619 |
-
prompt=[[
|
1620 |
-
"A charming house in the countryside, by jakub rozalski, sunset lighting, elegant, highly detailed, smooth, sharp focus, artstation, stunning masterpiece",
|
1621 |
-
"A dirt road in the countryside crossing pastures, by jakub rozalski, sunset lighting, elegant, highly detailed, smooth, sharp focus, artstation, stunning masterpiece",
|
1622 |
-
"An old and rusty giant robot lying on a dirt road, by jakub rozalski, dark sunset lighting, elegant, highly detailed, smooth, sharp focus, artstation, stunning masterpiece"
|
1623 |
-
]],
|
1624 |
-
tile_height=640,
|
1625 |
-
tile_width=640,
|
1626 |
-
tile_row_overlap=0,
|
1627 |
-
tile_col_overlap=256,
|
1628 |
-
guidance_scale=8,
|
1629 |
-
seed=7178915308,
|
1630 |
-
num_inference_steps=50,
|
1631 |
-
)["images"][0]
|
1632 |
-
```
|
1633 |
-

|
1634 |
-
|
1635 |
-
### TensorRT Inpainting Stable Diffusion Pipeline
|
1636 |
-
|
1637 |
-
The TensorRT Pipeline can be used to accelerate the Inpainting Stable Diffusion Inference run.
|
1638 |
-
|
1639 |
-
NOTE: The ONNX conversions and TensorRT engine build may take up to 30 minutes.
|
1640 |
-
|
1641 |
-
```python
|
1642 |
-
import requests
|
1643 |
-
from io import BytesIO
|
1644 |
-
from PIL import Image
|
1645 |
-
import torch
|
1646 |
-
from diffusers import PNDMScheduler
|
1647 |
-
from diffusers.pipelines.stable_diffusion import StableDiffusionImg2ImgPipeline
|
1648 |
-
|
1649 |
-
# Use the PNDMScheduler scheduler here instead
|
1650 |
-
scheduler = PNDMScheduler.from_pretrained("stabilityai/stable-diffusion-2-inpainting", subfolder="scheduler")
|
1651 |
-
|
1652 |
-
|
1653 |
-
pipe = StableDiffusionImg2ImgPipeline.from_pretrained("stabilityai/stable-diffusion-2-inpainting",
|
1654 |
-
custom_pipeline="stable_diffusion_tensorrt_inpaint",
|
1655 |
-
revision='fp16',
|
1656 |
-
torch_dtype=torch.float16,
|
1657 |
-
scheduler=scheduler,
|
1658 |
-
)
|
1659 |
-
|
1660 |
-
# re-use cached folder to save ONNX models and TensorRT Engines
|
1661 |
-
pipe.set_cached_folder("stabilityai/stable-diffusion-2-inpainting", revision='fp16',)
|
1662 |
-
|
1663 |
-
pipe = pipe.to("cuda")
|
1664 |
-
|
1665 |
-
url = "https://raw.githubusercontent.com/CompVis/latent-diffusion/main/data/inpainting_examples/overture-creations-5sI6fQgYIuo.png"
|
1666 |
-
response = requests.get(url)
|
1667 |
-
input_image = Image.open(BytesIO(response.content)).convert("RGB")
|
1668 |
-
|
1669 |
-
mask_url = "https://raw.githubusercontent.com/CompVis/latent-diffusion/main/data/inpainting_examples/overture-creations-5sI6fQgYIuo_mask.png"
|
1670 |
-
response = requests.get(mask_url)
|
1671 |
-
mask_image = Image.open(BytesIO(response.content)).convert("RGB")
|
1672 |
-
|
1673 |
-
prompt = "a mecha robot sitting on a bench"
|
1674 |
-
image = pipe(prompt, image=input_image, mask_image=mask_image, strength=0.75,).images[0]
|
1675 |
-
image.save('tensorrt_inpaint_mecha_robot.png')
|
1676 |
-
```
|
1677 |
-
|
1678 |
-
### Stable Diffusion Mixture Canvas
|
1679 |
-
|
1680 |
-
This pipeline uses the Mixture. Refer to the [Mixture](https://arxiv.org/abs/2302.02412) paper for more details.
|
1681 |
-
|
1682 |
-
```python
|
1683 |
-
from PIL import Image
|
1684 |
-
from diffusers import LMSDiscreteScheduler, DiffusionPipeline
|
1685 |
-
from diffusers.pipelines.pipeline_utils import Image2ImageRegion, Text2ImageRegion, preprocess_image
|
1686 |
-
|
1687 |
-
|
1688 |
-
# Load and preprocess guide image
|
1689 |
-
iic_image = preprocess_image(Image.open("input_image.png").convert("RGB"))
|
1690 |
-
|
1691 |
-
# Creater scheduler and model (similar to StableDiffusionPipeline)
|
1692 |
-
scheduler = LMSDiscreteScheduler(beta_start=0.00085, beta_end=0.012, beta_schedule="scaled_linear", num_train_timesteps=1000)
|
1693 |
-
pipeline = DiffusionPipeline.from_pretrained("CompVis/stable-diffusion-v1-4", scheduler=scheduler).to("cuda:0", custom_pipeline="mixture_canvas")
|
1694 |
-
pipeline.to("cuda")
|
1695 |
-
|
1696 |
-
# Mixture of Diffusers generation
|
1697 |
-
output = pipeline(
|
1698 |
-
canvas_height=800,
|
1699 |
-
canvas_width=352,
|
1700 |
-
regions=[
|
1701 |
-
Text2ImageRegion(0, 800, 0, 352, guidance_scale=8,
|
1702 |
-
prompt=f"best quality, masterpiece, WLOP, sakimichan, art contest winner on pixiv, 8K, intricate details, wet effects, rain drops, ethereal, mysterious, futuristic, UHD, HDR, cinematic lighting, in a beautiful forest, rainy day, award winning, trending on artstation, beautiful confident cheerful young woman, wearing a futuristic sleeveless dress, ultra beautiful detailed eyes, hyper-detailed face, complex, perfect, model, textured, chiaroscuro, professional make-up, realistic, figure in frame, "),
|
1703 |
-
Image2ImageRegion(352-800, 352, 0, 352, reference_image=iic_image, strength=1.0),
|
1704 |
-
],
|
1705 |
-
num_inference_steps=100,
|
1706 |
-
seed=5525475061,
|
1707 |
-
)["images"][0]
|
1708 |
-
```
|
1709 |
-

|
1710 |
-

|
1711 |
-
|
1712 |
-
|
1713 |
-
### IADB pipeline
|
1714 |
-
|
1715 |
-
This pipeline is the implementation of the [α-(de)Blending: a Minimalist Deterministic Diffusion Model](https://arxiv.org/abs/2305.03486) paper.
|
1716 |
-
It is a simple and minimalist diffusion model.
|
1717 |
-
|
1718 |
-
The following code shows how to use the IADB pipeline to generate images using a pretrained celebahq-256 model.
|
1719 |
-
|
1720 |
-
```python
|
1721 |
-
|
1722 |
-
pipeline_iadb = DiffusionPipeline.from_pretrained("thomasc4/iadb-celebahq-256", custom_pipeline='iadb')
|
1723 |
-
|
1724 |
-
pipeline_iadb = pipeline_iadb.to('cuda')
|
1725 |
-
|
1726 |
-
output = pipeline_iadb(batch_size=4,num_inference_steps=128)
|
1727 |
-
for i in range(len(output[0])):
|
1728 |
-
plt.imshow(output[0][i])
|
1729 |
-
plt.show()
|
1730 |
-
|
1731 |
-
```
|
1732 |
-
|
1733 |
-
Sampling with the IADB formulation is easy, and can be done in a few lines (the pipeline already implements it):
|
1734 |
-
|
1735 |
-
```python
|
1736 |
-
|
1737 |
-
def sample_iadb(model, x0, nb_step):
|
1738 |
-
x_alpha = x0
|
1739 |
-
for t in range(nb_step):
|
1740 |
-
alpha = (t/nb_step)
|
1741 |
-
alpha_next =((t+1)/nb_step)
|
1742 |
-
|
1743 |
-
d = model(x_alpha, torch.tensor(alpha, device=x_alpha.device))['sample']
|
1744 |
-
x_alpha = x_alpha + (alpha_next-alpha)*d
|
1745 |
-
|
1746 |
-
return x_alpha
|
1747 |
-
|
1748 |
-
```
|
1749 |
-
|
1750 |
-
The training loop is also straightforward:
|
1751 |
-
|
1752 |
-
```python
|
1753 |
-
|
1754 |
-
# Training loop
|
1755 |
-
while True:
|
1756 |
-
x0 = sample_noise()
|
1757 |
-
x1 = sample_dataset()
|
1758 |
-
|
1759 |
-
alpha = torch.rand(batch_size)
|
1760 |
-
|
1761 |
-
# Blend
|
1762 |
-
x_alpha = (1-alpha) * x0 + alpha * x1
|
1763 |
-
|
1764 |
-
# Loss
|
1765 |
-
loss = torch.sum((D(x_alpha, alpha)- (x1-x0))**2)
|
1766 |
-
optimizer.zero_grad()
|
1767 |
-
loss.backward()
|
1768 |
-
optimizer.step()
|
1769 |
-
```
|
|
|
|
|
|
|
|
|
|
|
|
|
|
|
|
|
|
|
|
|
|
|
|
|
|
|
|
|
|
|
|
|
|
|
|
|
|
|
|
|
|
|
|
|
|
|
|
|
|
|
|
|
|
|
|
|
|
|
|
|
|
|
|
|
|
|
|
|
|
|
|
|
|
|
|
|
|
|
|
|
|
|
|
|
|
|
|
|
|
|
|
|
|
|
|
|
|
|
|
|
|
|
|
|
|
|
|
|
|
|
|
|
|
|
|
|
|
|
|
|
|
|
|
|
|
|
|
|
|
|
|
|
|
|
|
|
|
|
|
|
|
|
|
|
|
|
|
|
|
|
|
|
|
|
|
|
|
|
|
|
|
|
|
|
|
|
|
|
|
|
|
|
|
|
|
|
|
|
|
|
|
|
|
|
|
|
|
|
|
|
|
|
|
|
|
|
|
|
|
|
|
|
|
|
|
|
|
|
|
|
|
|
|
|
|
|
|
|
|
|
|
|
|
|
|
|
|
|
|
|
|
|
|
|
|
|
|
|
|
|
|
|
|
|
|
|
|
|
|
|
|
|
|
|
|
|
|
|
|
|
|
|
|
|
|
|
|
|
|
|
|
|
|
|
|
|
|
|
|
|
|
|
|
|
|
|
|
|
|
|
|
|
|
|
|
|
|
|
|
|
|
|
|
|
|
|
|
|
|
|
|
|
|
|
|
|
|
|
|
|
|
|
|
|
|
|
|
|
|
|
|
|
|
|
|
|
|
|
|
|
|
|
|
|
|
|
|
|
|
|
|
|
|
|
|
|
|
|
|
|
|
|
|
|
|
|
|
|
|
|
|
|
|
|
|
|
|
|
|
|
|
|
|
|
|
|
|
|
|
|
|
|
|
|
|
|
|
|
|
|
|
|
|
|
|
|
|
|
|
|
|
|
|
|
|
|
|
|
|
|
|
|
|
|
|
|
|
|
|
|
|
|
|
|
|
|
|
|
|
|
|
|
|
|
|
|
|
|
|
|
|
|
|
|
|
|
|
|
|
|
|
|
|
|
|
|
|
|
|
|
|
|
|
|
|
|
|
|
|
|
|
|
|
|
|
|
|
|
|
|
|
|
|
|
|
|
|
|
|
|
|
|
|
|
|
|
|
|
|
|
|
|
|
|
|
|
|
|
|
|
|
|
|
|
|
|
|
|
|
|
|
|
|
|
|
|
|
|
|
|
|
|
|
|
|
|
|
|
|
|
|
|
|
|
|
|
|
|
|
|
|
|
|
|
|
|
|
|
|
|
|
|
|
|
|
|
|
|
|
|
|
|
|
|
|
|
|
|
|
|
|
|
|
|
|
|
|
|
|
|
|
|
|
|
|
|
|
|
|
|
|
|
|
|
|
|
|
|
|
|
|
|
|
|
|
|
|
|
|
|
|
|
|
|
|
|
|
|
|
|
|
|
|
|
|
|
|
|
|
|
|
|
|
|
|
|
|
|
|
|
|
|
|
|
|
|
|
|
|
|
|
|
|
|
|
|
|
|
|
|
|
|
|
|
|
|
|
|
|
|
|
|
|
|
|
|
|
|
|
|
|
|
|
|
|
|
|
|
|
|
|
|
|
|
|
|
|
|
|
|
|
|
|
|
|
|
|
|
|
|
|
|
|
|
|
|
|
|
|
|
|
|
|
|
|
|
|
|
|
|
|
|
|
|
|
|
|
|
|
|
|
|
|
|
|
|
|
|
|
|
|
|
|
|
|
|
|
|
|
|
|
|
|
|
|
|
|
|
|
|
|
|
|
|
|
|
|
|
|
|
|
|
|
|
|
|
|
|
|
|
|
|
|
|
|
|
|
|
|
|
|
|
|
|
|
|
|
|
|
|
|
|
|
|
|
|
|
|
|
|
|
|
|
|
|
|
|
|
|
|
|
|
|
|
|
|
|
|
|
|
|
|
|
|
|
|
|
|
|
|
|
|
|
|
|
|
|
|
|
|
|
|
|
|
|
|
|
|
|
|
|
|
|
|
|
|
|
|
|
|
|
|
|
|
|
|
|
|
|
|
|
|
|
|
|
|
|
|
|
|
|
|
|
|
|
|
|
|
|
|
|
|
|
|
|
|
|
|
|
|
|
|
|
|
|
|
|
|
|
|
|
|
|
|
|
|
|
|
|
|
|
|
|
|
|
|
|
|
|
|
|
|
|
|
|
|
|
|
|
|
|
|
|
|
|
|
|
|
|
|
|
|
|
|
|
|
|
|
|
|
|
|
|
|
|
|
|
|
|
|
|
|
|
|
|
|
|
|
|
|
|
|
|
|
|
|
|
|
|
|
|
|
|
|
|
|
|
|
|
|
|
|
|
|
|
|
|
|
|
|
|
|
|
|
|
|
|
|
|
|
|
|
|
|
|
|
|
|
|
|
|
|
|
|
|
|
|
|
|
|
|
|
|
|
|
|
|
|
|
|
|
|
|
|
|
|
|
|
|
|
|
|
|
|
|
|
|
|
|
|
|
|
|
|
|
|
|
|
|
|
|
|
|
|
|
|
|
|
|
|
|
|
|
|
|
|
|
|
|
|
|
|
|
|
|
|
|
|
|
|
|
|
|
|
|
|
|
|
|
|
|
|
|
|
|
|
|
|
|
|
|
|
|
|
|
|
|
|
|
|
|
|
|
|
|
|
|
|
|
|
|
|
|
|
|
|
|
|
|
|
|
|
|
|
|
|
|
|
|
|
|
|
|
|
|
|
|
|
|
|
|
|
|
|
|
|
|
|
|
|
|
|
|
|
|
|
|
|
|
|
|
|
|
|
|
|
|
|
|
|
|
|
|
|
|
|
|
|
|
|
|
|
|
|
|
|
|
|
|
|
|
|
|
|
|
|
|
|
|
|
|
|
|
|
|
|
|
|
|
|
|
|
|
|
|
|
|
|
|
|
|
|
|
|
|
|
|
|
|
|
|
|
|
|
|
|
|
|
|
|
|
|
|
|
|
|
|
|
|
|
|
|
|
|
|
|
|
|
|
|
|
|
|
|
|
|
|
|
|
|
|
|
|
|
|
|
|
|
|
|
|
|
|
|
|
|
|
|
|
|
|
|
|
|
|
|
|
|
|
|
|
|
|
|
|
|
|
|
|
|
|
|
|
|
|
|
|
|
|
|
|
|
|
|
|
|
|
|
|
|
|
|
|
|
|
|
|
|
|
|
|
|
|
|
|
|
|
|
|
|
|
|
|
|
|
|
|
|
|
|
|
|
|
|
|
|
|
|
|
|
|
|
|
|
|
|
|
|
|
|
|
|
|
|
|
|
|
|
|
|
|
|
|
|
|
|
|
|
|
|
|
|
|
|
|
|
|
|
|
|
|
|
|
|
|
|
|
|
|
|
|
|
|
|
|
|
|
|
|
|
|
|
|
|
|
|
|
|
|
|
|
|
|
|
|
|
|
|
|
|
|
|
|
|
|
|
|
|
|
|
|
|
|
|
|
|
|
|
|
|
|
|
|
|
|
|
|
|
|
|
|
|
|
|
|
|
|
|
|
|
|
|
|
|
|
|
|
|
|
|
|
|
|
|
|
|
|
|
|
|
|
|
|
|
|
|
|
|
|
|
|
|
|
|
|
|
|
|
|
|
|
|
|
|
|
|
|
|
|
|
|
|
|
|
|
|
|
|
|
|
|
|
|
|
|
|
|
|
|
|
|
|
|
|
|
|
|
|
|
|
|
|
|
|
|
|
|
|
|
|
|
|
|
|
|
|
|
|
|
|
|
|
|
|
|
|
|
|
|
|
|
|
|
|
|
|
|
|
|
|
|
|
|
|
|
|
|
|
|
|
|
|
|
|
|
|
|
|
|
|
|
|
|
|
|
|
|
|
|
|
|
|
|
|
|
|
|
|
|
|
|
|
|
|
|
|
|
|
|
|
|
|
|
|
|
|
|
|
|
|
|
|
|
|
|
|
|
|
|
|
|
|
|
|
|
|
|
|
|
|
|
|
|
|
|
|
|
|
|
|
|
|
|
|
|
|
|
|
|
|
|
|
|
|
|
|
|
|
|
|
|
|
|
|
|
|
|
|
|
|
|
|
|
|
|
|
|
|
|
|
|
|
|
|
|
|
|
|
|
|
|
|
|
|
|
|
|
|
|
|
|
|
|
|
|
|
|
|
|
|
|
|
|
|
|
|
|
|
|
|
|
|
|
|
|
|
|
|
|
|
|
|
|
|
|
|
|
|
|
|
|
|
|
|
|
|
|
|
|
|
|
|
|
|
|
|
|
|
|
|
|
|
|
|
|
|
|
|
|
|
|
|
|
|
|
|
|
|
|
|
|
|
|
|
|
|
|
|
|
|
|
|
|
|
|
|
|
|
|
|
|
|
|
|
|
|
|
|
|
|
|
|
|
|
|
|
|
|
|
|
|
|
|
|
|
|
|
|
|
|
|
|
|
|
|
|
|
|
|
|
|
|
|
|
|
|
|
|
|
|
|
|
|
|
|
|
|
|
|
|
|
|
|
|
|
|
|
|
|
|
|
|
|
|
|
|
|
|
|
|
|
|
|
|
|
|
|
|
|
|
|
|
|
|
|
|
|
|
|
|
|
|
|
|
|
|
|
|
|
|
|
|
|
|
|
|
|
|
|
|
|
|
|
|
|
|
|
|
|
|
|
|
|
|
|
|
|
|
|
|
|
|
|
|
|
|
|
|
|
|
|
|
|
|
|
|
|
|
|
|
|
|
|
|
|
|
|
|
|
|
|
|
|
|
|
|
|
|
|
|
|
|
|
|
|
|
|
|
|
|
|
|
|
|
|
|
|
|
|
|
|
|
|
|
|
|
|
|
|
|
|
|
|
|
|
|
|
|
|
|
|
|
|
|
|
|
|
|
|
|
|
|
|
|
|
|
|
|
|
|
|
|
|
|
|
|
|
|
|
|
|
|
|
|
|
|
|
|
|
|
|
|
|
|
|
|
|
|
|
|
|
|
|
|
|
|
|
|
|
|
|
|
|
|
|
|
|
|
|
|
|
|
|
|
|
|
|
|
|
|
|
|
|
|
|
|
|
|
|
|
|
|
|
|
|
|
|
|
|
|
|
|
|
|
|
|
|
|
|
|
|
|
|
|
|
|
|
|
|
|
|
|
|
|
|
|
|
|
|
|
|
|
|
|
|
|
|
|
|
|
|
|
|
|
|
|
|
|
|
|
|
|
|
|
|
|
|
|
|
|
|
|
|
|
|
|
|
|
|
|
|
|
|
|
|
|
|
|
|
|
|
|
|
|
|
|
|
|
|
|
|
|
|
|
|
|
|
|
|
|
|
|
|
|
|
|
|
|
|
|
|
|
|
|
|
|
|
|
|
|
|
|
|
|
|
|
|
|
|
|
|
|
|
|
|
|
|
|
|
|
|
|
|
|
|
|
|
|
|
|
|
|
|
|
|
|
|
|
|
|
|
|
|
|
|
|
|
|
|
|
|
|
|
|
|
|
|
|
|
|
|
|
|
|
|
|
|
|
|
|
|
|
|
|
|
|
|
|
|
|
|
|
|
|
|
|
|
|
|
|
|
|
|
|
|
|
|
|
|
|
|
|
|
|
|
|
|
|
|
|
|
|
|
|
|
|
|
|
|
|
|
|
|
|
|
|
|
|
|
|
|
|
|
|
|
|
|
|
|
|
|
|
|
|
|
|
|
|
|
|
|
|
|
|
|
|
|
|
|
|
|
|
|
|
|
|
|
|
|
|
|
|
|
|
|
|
|
|
|
|
|
|
|
|
|
|
|
|
|
|
|
|
|
|
|
|
|
|
|
|
|
|
|
|
|
|
|
|
|
|
|
|
|
|
|
|
|
|
|
|
|
|
|
|
|
|
|
|
|
|
|
|
|
|
|
|
|
|
|
|
|
|
|
|
|
|
|
|
|
|
|
|
|
|
|
|
|
|
|
|
|
|
|
|
|
|
|
|
|
|
|
|
|
|
|
|
|
|
|
|
|
|
|
|
|
|
|
|
|
|
|
|
|
|
|
|
|
|
|
|
|
|
|
|
|
|
|
|
|
|
|
|
|
|
|
|
|
|
|
|
|
|
|
|
|
|
|
|
|
|
|
|
|
|
|
|
|
|
|
|
|
|
|
|
|
|
|
|
|
|
|
|
|
|
|
|
|
|
|
|
|
|
|
|
|
|
|
|
|
|
|
|
|
|
|
|
|
|
|
|
|
|
|
|
|
|
|
|
|
|
|
|
|
|
|
|
|
|
|
|
|
|
|
|
|
|
|
|
|
|
|
|
|
|
|
|
|
|
|
|
|
|
|
|
|
|
|
|
|
|
|
|
|
|
|
|
|
|
|
|
|
|
|
|
|
|
|
|
|
|
|
|
|
|
|
|
|
|
|
|
|
|
|
|
|
|
|
|
|
|
|
|
|
|
|
|
|
|
|
|
|
|
|
|
|
|
|
|
|
|
|
|
|
|
|
|
|
|
|
|
|
|
|
|
|
|
|
|
|
|
|
|
|
|
|
|
|
|
|
|
|
|
|
|
|
|
|
|
|
|
|
|
|
|
|
|
|
|
|
|
|
|
|
|
|
|
|
|
|
|
|
|
|
|
|
|
|
|
|
|
|
|
|
|
|
|
|
|
|
|
|
|
|
|
|
|
|
|
|
|
|
|
|
|
|
|
|
|
|
|
|
|
|
|
|
|
|
|
|
|
|
|
|
|
|
|
|
|
|
|
|
|
|
|
|
|
|
|
|
|
|
|
|
|
|
|
|
|
|
|
|
|
|
|
|
|
|
|
|
|
|
|
|
|
|
|
|
|
|
|
|
|
|
|
|
|
|
|
|
|
|
|
|
|
|
|
|
|
|
|
|
|
|
|
|
|
|
|
|
|
|
|
|
|
|
|
|
|
|
|
|
|
|
|
|
|
|
|
|
|
|
|
|
|
|
|
|
|
|
|
|
|
|
|
|
|
|
|
|
|
|
|
|
|
|
|
|
|
|
|
|
|
|
|
|
|
|
|
|
|
|
|
|
|
|
|
|
|
|
|
|
|
|
|
|
|
|
|
|
|
|
|
|
|
|
|
|
|
|
|
|
|
|
|
|
|
|
|
|
|
|
|
|
|
|
|
|
|
|
|
|
|
|
|
|
|
|
|
|
|
|
|
|
|
|
|
|
|
|
|
|
|
|
|
|
|
|
|
|
|
|
|
|
|
|
|
|
|
|
|
|
|
|
|
|
|
|
|
|
|
|
|
|
|
|
|
|
|
|
|
|
|
|
|
|
|
|
|
|
|
|
|
|
|
|
|
|
|
|
|
|
|
|
|
|
|
|
|
|
|
|
|
|
|
|
|
|
|
|
|
|
|
|
|
|
|
|
|
|
|
|
|
|
|
|
|
|
|
|
|
|
|
|
|
|
|
|
|
|
|
|
|
|
|
|
|
|
|
|
|
|
|
|
|
|
|
|
|
|
|
|
|
|
|
|
|
|
|
|
|
|
|
|
|
|
|
|
|
|
|
|
|
|
|
|
|
|
|
|
|
|
|
|
|
|
|
|
|
|
|
|
|
|
|
|
|
|
|
|
|
|
|
|
|
|
|
|
|
|
|
|
|
|
|
|
|
|
|
|
|
|
|
|
|
|
|
|
|
|
|
|
|
|
|
|
|
|
|
|
|
|
|
|
|
|
|
|
|
|
|
|
|
|
|
|
|
|
|
|
|
|
|
|
|
|
|
|
|
|
|
|
|
|
|
|
|
|
|
|
|
|
|
|
|
|
|
|
|
|
|
|
|
|
|
|
|
|
|
|
|
|
|
|
|
|
|
|
|
|
|
|
|
|
|
|
|
|
|
|
|
|
|
|
|
|
|
|
|
|
|
|
|
|
|
|
|
|
|
|
|
|
|
|
|
|
|
|
|
|
|
|
|
|
|
|
|
|
|
|
|
|
|
|
|
|
|
|
|
|
spaces/Androidonnxfork/CivitAi-to-Diffusers/diffusers/examples/community/magic_mix.py
DELETED
@@ -1,152 +0,0 @@
|
|
1 |
-
from typing import Union
|
2 |
-
|
3 |
-
import torch
|
4 |
-
from PIL import Image
|
5 |
-
from torchvision import transforms as tfms
|
6 |
-
from tqdm.auto import tqdm
|
7 |
-
from transformers import CLIPTextModel, CLIPTokenizer
|
8 |
-
|
9 |
-
from diffusers import (
|
10 |
-
AutoencoderKL,
|
11 |
-
DDIMScheduler,
|
12 |
-
DiffusionPipeline,
|
13 |
-
LMSDiscreteScheduler,
|
14 |
-
PNDMScheduler,
|
15 |
-
UNet2DConditionModel,
|
16 |
-
)
|
17 |
-
|
18 |
-
|
19 |
-
class MagicMixPipeline(DiffusionPipeline):
|
20 |
-
def __init__(
|
21 |
-
self,
|
22 |
-
vae: AutoencoderKL,
|
23 |
-
text_encoder: CLIPTextModel,
|
24 |
-
tokenizer: CLIPTokenizer,
|
25 |
-
unet: UNet2DConditionModel,
|
26 |
-
scheduler: Union[PNDMScheduler, LMSDiscreteScheduler, DDIMScheduler],
|
27 |
-
):
|
28 |
-
super().__init__()
|
29 |
-
|
30 |
-
self.register_modules(vae=vae, text_encoder=text_encoder, tokenizer=tokenizer, unet=unet, scheduler=scheduler)
|
31 |
-
|
32 |
-
# convert PIL image to latents
|
33 |
-
def encode(self, img):
|
34 |
-
with torch.no_grad():
|
35 |
-
latent = self.vae.encode(tfms.ToTensor()(img).unsqueeze(0).to(self.device) * 2 - 1)
|
36 |
-
latent = 0.18215 * latent.latent_dist.sample()
|
37 |
-
return latent
|
38 |
-
|
39 |
-
# convert latents to PIL image
|
40 |
-
def decode(self, latent):
|
41 |
-
latent = (1 / 0.18215) * latent
|
42 |
-
with torch.no_grad():
|
43 |
-
img = self.vae.decode(latent).sample
|
44 |
-
img = (img / 2 + 0.5).clamp(0, 1)
|
45 |
-
img = img.detach().cpu().permute(0, 2, 3, 1).numpy()
|
46 |
-
img = (img * 255).round().astype("uint8")
|
47 |
-
return Image.fromarray(img[0])
|
48 |
-
|
49 |
-
# convert prompt into text embeddings, also unconditional embeddings
|
50 |
-
def prep_text(self, prompt):
|
51 |
-
text_input = self.tokenizer(
|
52 |
-
prompt,
|
53 |
-
padding="max_length",
|
54 |
-
max_length=self.tokenizer.model_max_length,
|
55 |
-
truncation=True,
|
56 |
-
return_tensors="pt",
|
57 |
-
)
|
58 |
-
|
59 |
-
text_embedding = self.text_encoder(text_input.input_ids.to(self.device))[0]
|
60 |
-
|
61 |
-
uncond_input = self.tokenizer(
|
62 |
-
"",
|
63 |
-
padding="max_length",
|
64 |
-
max_length=self.tokenizer.model_max_length,
|
65 |
-
truncation=True,
|
66 |
-
return_tensors="pt",
|
67 |
-
)
|
68 |
-
|
69 |
-
uncond_embedding = self.text_encoder(uncond_input.input_ids.to(self.device))[0]
|
70 |
-
|
71 |
-
return torch.cat([uncond_embedding, text_embedding])
|
72 |
-
|
73 |
-
def __call__(
|
74 |
-
self,
|
75 |
-
img: Image.Image,
|
76 |
-
prompt: str,
|
77 |
-
kmin: float = 0.3,
|
78 |
-
kmax: float = 0.6,
|
79 |
-
mix_factor: float = 0.5,
|
80 |
-
seed: int = 42,
|
81 |
-
steps: int = 50,
|
82 |
-
guidance_scale: float = 7.5,
|
83 |
-
) -> Image.Image:
|
84 |
-
tmin = steps - int(kmin * steps)
|
85 |
-
tmax = steps - int(kmax * steps)
|
86 |
-
|
87 |
-
text_embeddings = self.prep_text(prompt)
|
88 |
-
|
89 |
-
self.scheduler.set_timesteps(steps)
|
90 |
-
|
91 |
-
width, height = img.size
|
92 |
-
encoded = self.encode(img)
|
93 |
-
|
94 |
-
torch.manual_seed(seed)
|
95 |
-
noise = torch.randn(
|
96 |
-
(1, self.unet.config.in_channels, height // 8, width // 8),
|
97 |
-
).to(self.device)
|
98 |
-
|
99 |
-
latents = self.scheduler.add_noise(
|
100 |
-
encoded,
|
101 |
-
noise,
|
102 |
-
timesteps=self.scheduler.timesteps[tmax],
|
103 |
-
)
|
104 |
-
|
105 |
-
input = torch.cat([latents] * 2)
|
106 |
-
|
107 |
-
input = self.scheduler.scale_model_input(input, self.scheduler.timesteps[tmax])
|
108 |
-
|
109 |
-
with torch.no_grad():
|
110 |
-
pred = self.unet(
|
111 |
-
input,
|
112 |
-
self.scheduler.timesteps[tmax],
|
113 |
-
encoder_hidden_states=text_embeddings,
|
114 |
-
).sample
|
115 |
-
|
116 |
-
pred_uncond, pred_text = pred.chunk(2)
|
117 |
-
pred = pred_uncond + guidance_scale * (pred_text - pred_uncond)
|
118 |
-
|
119 |
-
latents = self.scheduler.step(pred, self.scheduler.timesteps[tmax], latents).prev_sample
|
120 |
-
|
121 |
-
for i, t in enumerate(tqdm(self.scheduler.timesteps)):
|
122 |
-
if i > tmax:
|
123 |
-
if i < tmin: # layout generation phase
|
124 |
-
orig_latents = self.scheduler.add_noise(
|
125 |
-
encoded,
|
126 |
-
noise,
|
127 |
-
timesteps=t,
|
128 |
-
)
|
129 |
-
|
130 |
-
input = (mix_factor * latents) + (
|
131 |
-
1 - mix_factor
|
132 |
-
) * orig_latents # interpolating between layout noise and conditionally generated noise to preserve layout sematics
|
133 |
-
input = torch.cat([input] * 2)
|
134 |
-
|
135 |
-
else: # content generation phase
|
136 |
-
input = torch.cat([latents] * 2)
|
137 |
-
|
138 |
-
input = self.scheduler.scale_model_input(input, t)
|
139 |
-
|
140 |
-
with torch.no_grad():
|
141 |
-
pred = self.unet(
|
142 |
-
input,
|
143 |
-
t,
|
144 |
-
encoder_hidden_states=text_embeddings,
|
145 |
-
).sample
|
146 |
-
|
147 |
-
pred_uncond, pred_text = pred.chunk(2)
|
148 |
-
pred = pred_uncond + guidance_scale * (pred_text - pred_uncond)
|
149 |
-
|
150 |
-
latents = self.scheduler.step(pred, t, latents).prev_sample
|
151 |
-
|
152 |
-
return self.decode(latents)
|
|
|
|
|
|
|
|
|
|
|
|
|
|
|
|
|
|
|
|
|
|
|
|
|
|
|
|
|
|
|
|
|
|
|
|
|
|
|
|
|
|
|
|
|
|
|
|
|
|
|
|
|
|
|
|
|
|
|
|
|
|
|
|
|
|
|
|
|
|
|
|
|
|
|
|
|
|
|
|
|
|
|
|
|
|
|
|
|
|
|
|
|
|
|
|
|
|
|
|
|
|
|
|
|
|
|
|
|
|
|
|
|
|
|
|
|
|
|
|
|
|
|
|
|
|
|
|
|
|
|
|
|
|
|
|
|
|
|
|
|
|
|
|
|
|
|
|
|
|
|
|
|
|
|
|
|
|
|
|
|
|
|
|
|
|
|
|
|
|
|
|
|
|
|
|
|
|
|
|
|
|
|
|
|
|
|
|
|
|
|
|
|
|
|
|
|
|
|
|
|
|
|
|
|
|
|
|
|
|
|
|
|
|
|
|
|
|
|
|
|
|
|
|
|
|
|
|
|
|
|
|
|
|
|
|
|
|
|
|
|
|
|
|
|
|
|
|
|
|
|
|
|
|
|
|
|
|
|
|
|
|
|
|
|
|
|
|
|
|
|
|
|
|
|
|
|
|
|
|
|
|
|
|
|
|
|
|
|
|
|
|
|
|
|
|
|
|
|
|
|
|
|
|
|
|
spaces/Androidonnxfork/CivitAi-to-Diffusers/diffusers/examples/textual_inversion/README.md
DELETED
@@ -1,144 +0,0 @@
|
|
1 |
-
## Textual Inversion fine-tuning example
|
2 |
-
|
3 |
-
[Textual inversion](https://arxiv.org/abs/2208.01618) is a method to personalize text2image models like stable diffusion on your own images using just 3-5 examples.
|
4 |
-
The `textual_inversion.py` script shows how to implement the training procedure and adapt it for stable diffusion.
|
5 |
-
|
6 |
-
## Running on Colab
|
7 |
-
|
8 |
-
Colab for training
|
9 |
-
[](https://colab.research.google.com/github/huggingface/notebooks/blob/main/diffusers/sd_textual_inversion_training.ipynb)
|
10 |
-
|
11 |
-
Colab for inference
|
12 |
-
[](https://colab.research.google.com/github/huggingface/notebooks/blob/main/diffusers/stable_conceptualizer_inference.ipynb)
|
13 |
-
|
14 |
-
## Running locally with PyTorch
|
15 |
-
### Installing the dependencies
|
16 |
-
|
17 |
-
Before running the scripts, make sure to install the library's training dependencies:
|
18 |
-
|
19 |
-
**Important**
|
20 |
-
|
21 |
-
To make sure you can successfully run the latest versions of the example scripts, we highly recommend **installing from source** and keeping the install up to date as we update the example scripts frequently and install some example-specific requirements. To do this, execute the following steps in a new virtual environment:
|
22 |
-
```bash
|
23 |
-
git clone https://github.com/huggingface/diffusers
|
24 |
-
cd diffusers
|
25 |
-
pip install .
|
26 |
-
```
|
27 |
-
|
28 |
-
Then cd in the example folder and run
|
29 |
-
```bash
|
30 |
-
pip install -r requirements.txt
|
31 |
-
```
|
32 |
-
|
33 |
-
And initialize an [🤗Accelerate](https://github.com/huggingface/accelerate/) environment with:
|
34 |
-
|
35 |
-
```bash
|
36 |
-
accelerate config
|
37 |
-
```
|
38 |
-
|
39 |
-
### Cat toy example
|
40 |
-
|
41 |
-
First, let's login so that we can upload the checkpoint to the Hub during training:
|
42 |
-
|
43 |
-
```bash
|
44 |
-
huggingface-cli login
|
45 |
-
```
|
46 |
-
|
47 |
-
Now let's get our dataset. For this example we will use some cat images: https://huggingface.co/datasets/diffusers/cat_toy_example .
|
48 |
-
|
49 |
-
Let's first download it locally:
|
50 |
-
|
51 |
-
```py
|
52 |
-
from huggingface_hub import snapshot_download
|
53 |
-
|
54 |
-
local_dir = "./cat"
|
55 |
-
snapshot_download("diffusers/cat_toy_example", local_dir=local_dir, repo_type="dataset", ignore_patterns=".gitattributes")
|
56 |
-
```
|
57 |
-
|
58 |
-
This will be our training data.
|
59 |
-
Now we can launch the training using
|
60 |
-
|
61 |
-
**___Note: Change the `resolution` to 768 if you are using the [stable-diffusion-2](https://huggingface.co/stabilityai/stable-diffusion-2) 768x768 model.___**
|
62 |
-
|
63 |
-
```bash
|
64 |
-
export MODEL_NAME="runwayml/stable-diffusion-v1-5"
|
65 |
-
export DATA_DIR="./cat"
|
66 |
-
|
67 |
-
accelerate launch textual_inversion.py \
|
68 |
-
--pretrained_model_name_or_path=$MODEL_NAME \
|
69 |
-
--train_data_dir=$DATA_DIR \
|
70 |
-
--learnable_property="object" \
|
71 |
-
--placeholder_token="<cat-toy>" --initializer_token="toy" \
|
72 |
-
--resolution=512 \
|
73 |
-
--train_batch_size=1 \
|
74 |
-
--gradient_accumulation_steps=4 \
|
75 |
-
--max_train_steps=3000 \
|
76 |
-
--learning_rate=5.0e-04 --scale_lr \
|
77 |
-
--lr_scheduler="constant" \
|
78 |
-
--lr_warmup_steps=0 \
|
79 |
-
--push_to_hub \
|
80 |
-
--output_dir="textual_inversion_cat"
|
81 |
-
```
|
82 |
-
|
83 |
-
A full training run takes ~1 hour on one V100 GPU.
|
84 |
-
|
85 |
-
**Note**: As described in [the official paper](https://arxiv.org/abs/2208.01618)
|
86 |
-
only one embedding vector is used for the placeholder token, *e.g.* `"<cat-toy>"`.
|
87 |
-
However, one can also add multiple embedding vectors for the placeholder token
|
88 |
-
to inclease the number of fine-tuneable parameters. This can help the model to learn
|
89 |
-
more complex details. To use multiple embedding vectors, you can should define `--num_vectors`
|
90 |
-
to a number larger than one, *e.g.*:
|
91 |
-
```
|
92 |
-
--num_vectors 5
|
93 |
-
```
|
94 |
-
|
95 |
-
The saved textual inversion vectors will then be larger in size compared to the default case.
|
96 |
-
|
97 |
-
### Inference
|
98 |
-
|
99 |
-
Once you have trained a model using above command, the inference can be done simply using the `StableDiffusionPipeline`. Make sure to include the `placeholder_token` in your prompt.
|
100 |
-
|
101 |
-
```python
|
102 |
-
from diffusers import StableDiffusionPipeline
|
103 |
-
import torch
|
104 |
-
|
105 |
-
model_id = "path-to-your-trained-model"
|
106 |
-
pipe = StableDiffusionPipeline.from_pretrained(model_id,torch_dtype=torch.float16).to("cuda")
|
107 |
-
|
108 |
-
prompt = "A <cat-toy> backpack"
|
109 |
-
|
110 |
-
image = pipe(prompt, num_inference_steps=50, guidance_scale=7.5).images[0]
|
111 |
-
|
112 |
-
image.save("cat-backpack.png")
|
113 |
-
```
|
114 |
-
|
115 |
-
|
116 |
-
## Training with Flax/JAX
|
117 |
-
|
118 |
-
For faster training on TPUs and GPUs you can leverage the flax training example. Follow the instructions above to get the model and dataset before running the script.
|
119 |
-
|
120 |
-
Before running the scripts, make sure to install the library's training dependencies:
|
121 |
-
|
122 |
-
```bash
|
123 |
-
pip install -U -r requirements_flax.txt
|
124 |
-
```
|
125 |
-
|
126 |
-
```bash
|
127 |
-
export MODEL_NAME="duongna/stable-diffusion-v1-4-flax"
|
128 |
-
export DATA_DIR="path-to-dir-containing-images"
|
129 |
-
|
130 |
-
python textual_inversion_flax.py \
|
131 |
-
--pretrained_model_name_or_path=$MODEL_NAME \
|
132 |
-
--train_data_dir=$DATA_DIR \
|
133 |
-
--learnable_property="object" \
|
134 |
-
--placeholder_token="<cat-toy>" --initializer_token="toy" \
|
135 |
-
--resolution=512 \
|
136 |
-
--train_batch_size=1 \
|
137 |
-
--max_train_steps=3000 \
|
138 |
-
--learning_rate=5.0e-04 --scale_lr \
|
139 |
-
--output_dir="textual_inversion_cat"
|
140 |
-
```
|
141 |
-
It should be at least 70% faster than the PyTorch script with the same configuration.
|
142 |
-
|
143 |
-
### Training with xformers:
|
144 |
-
You can enable memory efficient attention by [installing xFormers](https://github.com/facebookresearch/xformers#installing-xformers) and padding the `--enable_xformers_memory_efficient_attention` argument to the script. This is not available with the Flax/JAX implementation.
|
|
|
|
|
|
|
|
|
|
|
|
|
|
|
|
|
|
|
|
|
|
|
|
|
|
|
|
|
|
|
|
|
|
|
|
|
|
|
|
|
|
|
|
|
|
|
|
|
|
|
|
|
|
|
|
|
|
|
|
|
|
|
|
|
|
|
|
|
|
|
|
|
|
|
|
|
|
|
|
|
|
|
|
|
|
|
|
|
|
|
|
|
|
|
|
|
|
|
|
|
|
|
|
|
|
|
|
|
|
|
|
|
|
|
|
|
|
|
|
|
|
|
|
|
|
|
|
|
|
|
|
|
|
|
|
|
|
|
|
|
|
|
|
|
|
|
|
|
|
|
|
|
|
|
|
|
|
|
|
|
|
|
|
|
|
|
|
|
|
|
|
|
|
|
|
|
|
|
|
|
|
|
|
|
|
|
|
|
|
|
|
|
|
|
|
|
|
|
|
|
|
|
|
|
|
|
|
|
|
|
|
|
|
|
|
|
|
|
|
|
|
|
|
|
|
|
|
|
|
|
|
|
|
|
|
|
|
|
|
|
|
|
|
|
|
|
|
|
|
|
|
|
|
|
|
|
|
|
|
|
|
|
|
|
|
|
|
|
|
|
|
|
|
|
|
|
|
|
|
|
|
|
|
|
|
|
|
|
|
spaces/Androidonnxfork/CivitAi-to-Diffusers/diffusers/src/diffusers/experimental/__init__.py
DELETED
@@ -1 +0,0 @@
|
|
1 |
-
from .rl import ValueGuidedRLPipeline
|
|
|
|
spaces/Andy1621/uniformer_image_detection/mmdet/datasets/pipelines/formating.py
DELETED
@@ -1,364 +0,0 @@
|
|
1 |
-
from collections.abc import Sequence
|
2 |
-
|
3 |
-
import mmcv
|
4 |
-
import numpy as np
|
5 |
-
import torch
|
6 |
-
from mmcv.parallel import DataContainer as DC
|
7 |
-
|
8 |
-
from ..builder import PIPELINES
|
9 |
-
|
10 |
-
|
11 |
-
def to_tensor(data):
|
12 |
-
"""Convert objects of various python types to :obj:`torch.Tensor`.
|
13 |
-
|
14 |
-
Supported types are: :class:`numpy.ndarray`, :class:`torch.Tensor`,
|
15 |
-
:class:`Sequence`, :class:`int` and :class:`float`.
|
16 |
-
|
17 |
-
Args:
|
18 |
-
data (torch.Tensor | numpy.ndarray | Sequence | int | float): Data to
|
19 |
-
be converted.
|
20 |
-
"""
|
21 |
-
|
22 |
-
if isinstance(data, torch.Tensor):
|
23 |
-
return data
|
24 |
-
elif isinstance(data, np.ndarray):
|
25 |
-
return torch.from_numpy(data)
|
26 |
-
elif isinstance(data, Sequence) and not mmcv.is_str(data):
|
27 |
-
return torch.tensor(data)
|
28 |
-
elif isinstance(data, int):
|
29 |
-
return torch.LongTensor([data])
|
30 |
-
elif isinstance(data, float):
|
31 |
-
return torch.FloatTensor([data])
|
32 |
-
else:
|
33 |
-
raise TypeError(f'type {type(data)} cannot be converted to tensor.')
|
34 |
-
|
35 |
-
|
36 |
-
@PIPELINES.register_module()
|
37 |
-
class ToTensor(object):
|
38 |
-
"""Convert some results to :obj:`torch.Tensor` by given keys.
|
39 |
-
|
40 |
-
Args:
|
41 |
-
keys (Sequence[str]): Keys that need to be converted to Tensor.
|
42 |
-
"""
|
43 |
-
|
44 |
-
def __init__(self, keys):
|
45 |
-
self.keys = keys
|
46 |
-
|
47 |
-
def __call__(self, results):
|
48 |
-
"""Call function to convert data in results to :obj:`torch.Tensor`.
|
49 |
-
|
50 |
-
Args:
|
51 |
-
results (dict): Result dict contains the data to convert.
|
52 |
-
|
53 |
-
Returns:
|
54 |
-
dict: The result dict contains the data converted
|
55 |
-
to :obj:`torch.Tensor`.
|
56 |
-
"""
|
57 |
-
for key in self.keys:
|
58 |
-
results[key] = to_tensor(results[key])
|
59 |
-
return results
|
60 |
-
|
61 |
-
def __repr__(self):
|
62 |
-
return self.__class__.__name__ + f'(keys={self.keys})'
|
63 |
-
|
64 |
-
|
65 |
-
@PIPELINES.register_module()
|
66 |
-
class ImageToTensor(object):
|
67 |
-
"""Convert image to :obj:`torch.Tensor` by given keys.
|
68 |
-
|
69 |
-
The dimension order of input image is (H, W, C). The pipeline will convert
|
70 |
-
it to (C, H, W). If only 2 dimension (H, W) is given, the output would be
|
71 |
-
(1, H, W).
|
72 |
-
|
73 |
-
Args:
|
74 |
-
keys (Sequence[str]): Key of images to be converted to Tensor.
|
75 |
-
"""
|
76 |
-
|
77 |
-
def __init__(self, keys):
|
78 |
-
self.keys = keys
|
79 |
-
|
80 |
-
def __call__(self, results):
|
81 |
-
"""Call function to convert image in results to :obj:`torch.Tensor` and
|
82 |
-
transpose the channel order.
|
83 |
-
|
84 |
-
Args:
|
85 |
-
results (dict): Result dict contains the image data to convert.
|
86 |
-
|
87 |
-
Returns:
|
88 |
-
dict: The result dict contains the image converted
|
89 |
-
to :obj:`torch.Tensor` and transposed to (C, H, W) order.
|
90 |
-
"""
|
91 |
-
for key in self.keys:
|
92 |
-
img = results[key]
|
93 |
-
if len(img.shape) < 3:
|
94 |
-
img = np.expand_dims(img, -1)
|
95 |
-
results[key] = to_tensor(img.transpose(2, 0, 1))
|
96 |
-
return results
|
97 |
-
|
98 |
-
def __repr__(self):
|
99 |
-
return self.__class__.__name__ + f'(keys={self.keys})'
|
100 |
-
|
101 |
-
|
102 |
-
@PIPELINES.register_module()
|
103 |
-
class Transpose(object):
|
104 |
-
"""Transpose some results by given keys.
|
105 |
-
|
106 |
-
Args:
|
107 |
-
keys (Sequence[str]): Keys of results to be transposed.
|
108 |
-
order (Sequence[int]): Order of transpose.
|
109 |
-
"""
|
110 |
-
|
111 |
-
def __init__(self, keys, order):
|
112 |
-
self.keys = keys
|
113 |
-
self.order = order
|
114 |
-
|
115 |
-
def __call__(self, results):
|
116 |
-
"""Call function to transpose the channel order of data in results.
|
117 |
-
|
118 |
-
Args:
|
119 |
-
results (dict): Result dict contains the data to transpose.
|
120 |
-
|
121 |
-
Returns:
|
122 |
-
dict: The result dict contains the data transposed to \
|
123 |
-
``self.order``.
|
124 |
-
"""
|
125 |
-
for key in self.keys:
|
126 |
-
results[key] = results[key].transpose(self.order)
|
127 |
-
return results
|
128 |
-
|
129 |
-
def __repr__(self):
|
130 |
-
return self.__class__.__name__ + \
|
131 |
-
f'(keys={self.keys}, order={self.order})'
|
132 |
-
|
133 |
-
|
134 |
-
@PIPELINES.register_module()
|
135 |
-
class ToDataContainer(object):
|
136 |
-
"""Convert results to :obj:`mmcv.DataContainer` by given fields.
|
137 |
-
|
138 |
-
Args:
|
139 |
-
fields (Sequence[dict]): Each field is a dict like
|
140 |
-
``dict(key='xxx', **kwargs)``. The ``key`` in result will
|
141 |
-
be converted to :obj:`mmcv.DataContainer` with ``**kwargs``.
|
142 |
-
Default: ``(dict(key='img', stack=True), dict(key='gt_bboxes'),
|
143 |
-
dict(key='gt_labels'))``.
|
144 |
-
"""
|
145 |
-
|
146 |
-
def __init__(self,
|
147 |
-
fields=(dict(key='img', stack=True), dict(key='gt_bboxes'),
|
148 |
-
dict(key='gt_labels'))):
|
149 |
-
self.fields = fields
|
150 |
-
|
151 |
-
def __call__(self, results):
|
152 |
-
"""Call function to convert data in results to
|
153 |
-
:obj:`mmcv.DataContainer`.
|
154 |
-
|
155 |
-
Args:
|
156 |
-
results (dict): Result dict contains the data to convert.
|
157 |
-
|
158 |
-
Returns:
|
159 |
-
dict: The result dict contains the data converted to \
|
160 |
-
:obj:`mmcv.DataContainer`.
|
161 |
-
"""
|
162 |
-
|
163 |
-
for field in self.fields:
|
164 |
-
field = field.copy()
|
165 |
-
key = field.pop('key')
|
166 |
-
results[key] = DC(results[key], **field)
|
167 |
-
return results
|
168 |
-
|
169 |
-
def __repr__(self):
|
170 |
-
return self.__class__.__name__ + f'(fields={self.fields})'
|
171 |
-
|
172 |
-
|
173 |
-
@PIPELINES.register_module()
|
174 |
-
class DefaultFormatBundle(object):
|
175 |
-
"""Default formatting bundle.
|
176 |
-
|
177 |
-
It simplifies the pipeline of formatting common fields, including "img",
|
178 |
-
"proposals", "gt_bboxes", "gt_labels", "gt_masks" and "gt_semantic_seg".
|
179 |
-
These fields are formatted as follows.
|
180 |
-
|
181 |
-
- img: (1)transpose, (2)to tensor, (3)to DataContainer (stack=True)
|
182 |
-
- proposals: (1)to tensor, (2)to DataContainer
|
183 |
-
- gt_bboxes: (1)to tensor, (2)to DataContainer
|
184 |
-
- gt_bboxes_ignore: (1)to tensor, (2)to DataContainer
|
185 |
-
- gt_labels: (1)to tensor, (2)to DataContainer
|
186 |
-
- gt_masks: (1)to tensor, (2)to DataContainer (cpu_only=True)
|
187 |
-
- gt_semantic_seg: (1)unsqueeze dim-0 (2)to tensor, \
|
188 |
-
(3)to DataContainer (stack=True)
|
189 |
-
"""
|
190 |
-
|
191 |
-
def __call__(self, results):
|
192 |
-
"""Call function to transform and format common fields in results.
|
193 |
-
|
194 |
-
Args:
|
195 |
-
results (dict): Result dict contains the data to convert.
|
196 |
-
|
197 |
-
Returns:
|
198 |
-
dict: The result dict contains the data that is formatted with \
|
199 |
-
default bundle.
|
200 |
-
"""
|
201 |
-
|
202 |
-
if 'img' in results:
|
203 |
-
img = results['img']
|
204 |
-
# add default meta keys
|
205 |
-
results = self._add_default_meta_keys(results)
|
206 |
-
if len(img.shape) < 3:
|
207 |
-
img = np.expand_dims(img, -1)
|
208 |
-
img = np.ascontiguousarray(img.transpose(2, 0, 1))
|
209 |
-
results['img'] = DC(to_tensor(img), stack=True)
|
210 |
-
for key in ['proposals', 'gt_bboxes', 'gt_bboxes_ignore', 'gt_labels']:
|
211 |
-
if key not in results:
|
212 |
-
continue
|
213 |
-
results[key] = DC(to_tensor(results[key]))
|
214 |
-
if 'gt_masks' in results:
|
215 |
-
results['gt_masks'] = DC(results['gt_masks'], cpu_only=True)
|
216 |
-
if 'gt_semantic_seg' in results:
|
217 |
-
results['gt_semantic_seg'] = DC(
|
218 |
-
to_tensor(results['gt_semantic_seg'][None, ...]), stack=True)
|
219 |
-
return results
|
220 |
-
|
221 |
-
def _add_default_meta_keys(self, results):
|
222 |
-
"""Add default meta keys.
|
223 |
-
|
224 |
-
We set default meta keys including `pad_shape`, `scale_factor` and
|
225 |
-
`img_norm_cfg` to avoid the case where no `Resize`, `Normalize` and
|
226 |
-
`Pad` are implemented during the whole pipeline.
|
227 |
-
|
228 |
-
Args:
|
229 |
-
results (dict): Result dict contains the data to convert.
|
230 |
-
|
231 |
-
Returns:
|
232 |
-
results (dict): Updated result dict contains the data to convert.
|
233 |
-
"""
|
234 |
-
img = results['img']
|
235 |
-
results.setdefault('pad_shape', img.shape)
|
236 |
-
results.setdefault('scale_factor', 1.0)
|
237 |
-
num_channels = 1 if len(img.shape) < 3 else img.shape[2]
|
238 |
-
results.setdefault(
|
239 |
-
'img_norm_cfg',
|
240 |
-
dict(
|
241 |
-
mean=np.zeros(num_channels, dtype=np.float32),
|
242 |
-
std=np.ones(num_channels, dtype=np.float32),
|
243 |
-
to_rgb=False))
|
244 |
-
return results
|
245 |
-
|
246 |
-
def __repr__(self):
|
247 |
-
return self.__class__.__name__
|
248 |
-
|
249 |
-
|
250 |
-
@PIPELINES.register_module()
|
251 |
-
class Collect(object):
|
252 |
-
"""Collect data from the loader relevant to the specific task.
|
253 |
-
|
254 |
-
This is usually the last stage of the data loader pipeline. Typically keys
|
255 |
-
is set to some subset of "img", "proposals", "gt_bboxes",
|
256 |
-
"gt_bboxes_ignore", "gt_labels", and/or "gt_masks".
|
257 |
-
|
258 |
-
The "img_meta" item is always populated. The contents of the "img_meta"
|
259 |
-
dictionary depends on "meta_keys". By default this includes:
|
260 |
-
|
261 |
-
- "img_shape": shape of the image input to the network as a tuple \
|
262 |
-
(h, w, c). Note that images may be zero padded on the \
|
263 |
-
bottom/right if the batch tensor is larger than this shape.
|
264 |
-
|
265 |
-
- "scale_factor": a float indicating the preprocessing scale
|
266 |
-
|
267 |
-
- "flip": a boolean indicating if image flip transform was used
|
268 |
-
|
269 |
-
- "filename": path to the image file
|
270 |
-
|
271 |
-
- "ori_shape": original shape of the image as a tuple (h, w, c)
|
272 |
-
|
273 |
-
- "pad_shape": image shape after padding
|
274 |
-
|
275 |
-
- "img_norm_cfg": a dict of normalization information:
|
276 |
-
|
277 |
-
- mean - per channel mean subtraction
|
278 |
-
- std - per channel std divisor
|
279 |
-
- to_rgb - bool indicating if bgr was converted to rgb
|
280 |
-
|
281 |
-
Args:
|
282 |
-
keys (Sequence[str]): Keys of results to be collected in ``data``.
|
283 |
-
meta_keys (Sequence[str], optional): Meta keys to be converted to
|
284 |
-
``mmcv.DataContainer`` and collected in ``data[img_metas]``.
|
285 |
-
Default: ``('filename', 'ori_filename', 'ori_shape', 'img_shape',
|
286 |
-
'pad_shape', 'scale_factor', 'flip', 'flip_direction',
|
287 |
-
'img_norm_cfg')``
|
288 |
-
"""
|
289 |
-
|
290 |
-
def __init__(self,
|
291 |
-
keys,
|
292 |
-
meta_keys=('filename', 'ori_filename', 'ori_shape',
|
293 |
-
'img_shape', 'pad_shape', 'scale_factor', 'flip',
|
294 |
-
'flip_direction', 'img_norm_cfg')):
|
295 |
-
self.keys = keys
|
296 |
-
self.meta_keys = meta_keys
|
297 |
-
|
298 |
-
def __call__(self, results):
|
299 |
-
"""Call function to collect keys in results. The keys in ``meta_keys``
|
300 |
-
will be converted to :obj:mmcv.DataContainer.
|
301 |
-
|
302 |
-
Args:
|
303 |
-
results (dict): Result dict contains the data to collect.
|
304 |
-
|
305 |
-
Returns:
|
306 |
-
dict: The result dict contains the following keys
|
307 |
-
|
308 |
-
- keys in``self.keys``
|
309 |
-
- ``img_metas``
|
310 |
-
"""
|
311 |
-
|
312 |
-
data = {}
|
313 |
-
img_meta = {}
|
314 |
-
for key in self.meta_keys:
|
315 |
-
img_meta[key] = results[key]
|
316 |
-
data['img_metas'] = DC(img_meta, cpu_only=True)
|
317 |
-
for key in self.keys:
|
318 |
-
data[key] = results[key]
|
319 |
-
return data
|
320 |
-
|
321 |
-
def __repr__(self):
|
322 |
-
return self.__class__.__name__ + \
|
323 |
-
f'(keys={self.keys}, meta_keys={self.meta_keys})'
|
324 |
-
|
325 |
-
|
326 |
-
@PIPELINES.register_module()
|
327 |
-
class WrapFieldsToLists(object):
|
328 |
-
"""Wrap fields of the data dictionary into lists for evaluation.
|
329 |
-
|
330 |
-
This class can be used as a last step of a test or validation
|
331 |
-
pipeline for single image evaluation or inference.
|
332 |
-
|
333 |
-
Example:
|
334 |
-
>>> test_pipeline = [
|
335 |
-
>>> dict(type='LoadImageFromFile'),
|
336 |
-
>>> dict(type='Normalize',
|
337 |
-
mean=[123.675, 116.28, 103.53],
|
338 |
-
std=[58.395, 57.12, 57.375],
|
339 |
-
to_rgb=True),
|
340 |
-
>>> dict(type='Pad', size_divisor=32),
|
341 |
-
>>> dict(type='ImageToTensor', keys=['img']),
|
342 |
-
>>> dict(type='Collect', keys=['img']),
|
343 |
-
>>> dict(type='WrapFieldsToLists')
|
344 |
-
>>> ]
|
345 |
-
"""
|
346 |
-
|
347 |
-
def __call__(self, results):
|
348 |
-
"""Call function to wrap fields into lists.
|
349 |
-
|
350 |
-
Args:
|
351 |
-
results (dict): Result dict contains the data to wrap.
|
352 |
-
|
353 |
-
Returns:
|
354 |
-
dict: The result dict where value of ``self.keys`` are wrapped \
|
355 |
-
into list.
|
356 |
-
"""
|
357 |
-
|
358 |
-
# Wrap dict fields into lists
|
359 |
-
for key, val in results.items():
|
360 |
-
results[key] = [val]
|
361 |
-
return results
|
362 |
-
|
363 |
-
def __repr__(self):
|
364 |
-
return f'{self.__class__.__name__}()'
|
|
|
|
|
|
|
|
|
|
|
|
|
|
|
|
|
|
|
|
|
|
|
|
|
|
|
|
|
|
|
|
|
|
|
|
|
|
|
|
|
|
|
|
|
|
|
|
|
|
|
|
|
|
|
|
|
|
|
|
|
|
|
|
|
|
|
|
|
|
|
|
|
|
|
|
|
|
|
|
|
|
|
|
|
|
|
|
|
|
|
|
|
|
|
|
|
|
|
|
|
|
|
|
|
|
|
|
|
|
|
|
|
|
|
|
|
|
|
|
|
|
|
|
|
|
|
|
|
|
|
|
|
|
|
|
|
|
|
|
|
|
|
|
|
|
|
|
|
|
|
|
|
|
|
|
|
|
|
|
|
|
|
|
|
|
|
|
|
|
|
|
|
|
|
|
|
|
|
|
|
|
|
|
|
|
|
|
|
|
|
|
|
|
|
|
|
|
|
|
|
|
|
|
|
|
|
|
|
|
|
|
|
|
|
|
|
|
|
|
|
|
|
|
|
|
|
|
|
|
|
|
|
|
|
|
|
|
|
|
|
|
|
|
|
|
|
|
|
|
|
|
|
|
|
|
|
|
|
|
|
|
|
|
|
|
|
|
|
|
|
|
|
|
|
|
|
|
|
|
|
|
|
|
|
|
|
|
|
|
|
|
|
|
|
|
|
|
|
|
|
|
|
|
|
|
|
|
|
|
|
|
|
|
|
|
|
|
|
|
|
|
|
|
|
|
|
|
|
|
|
|
|
|
|
|
|
|
|
|
|
|
|
|
|
|
|
|
|
|
|
|
|
|
|
|
|
|
|
|
|
|
|
|
|
|
|
|
|
|
|
|
|
|
|
|
|
|
|
|
|
|
|
|
|
|
|
|
|
|
|
|
|
|
|
|
|
|
|
|
|
|
|
|
|
|
|
|
|
|
|
|
|
|
|
|
|
|
|
|
|
|
|
|
|
|
|
|
|
|
|
|
|
|
|
|
|
|
|
|
|
|
|
|
|
|
|
|
|
|
|
|
|
|
|
|
|
|
|
|
|
|
|
|
|
|
|
|
|
|
|
|
|
|
|
|
|
|
|
|
|
|
|
|
|
|
|
|
|
|
|
|
|
|
|
|
|
|
|
|
|
|
|
|
|
|
|
|
|
|
|
|
|
|
|
|
|
|
|
|
|
|
|
|
|
|
|
|
|
|
|
|
|
|
|
|
|
|
|
|
|
|
|
|
|
|
|
|
|
|
|
|
|
|
|
|
|
|
|
|
|
|
|
|
|
|
|
|
|
|
|
|
|
|
|
|
|
|
|
|
|
|
|
|
|
|
|
|
|
|
|
|
|
|
|
|
|
|
|
|
|
|
|
|
|
|
|
|
|
|
|
|
|
|
|
|
|
|
|
|
|
|
|
|
|
|
|
|
|
|
|
|
|
|
|
|
|
|
|
|
|
|
|
|
|
|
|
|
|
|
|
|
|
|
|
|
|
|
|
|
|
|
|
|
|
|
|
|
|
|
|
|
|
|
|
|
|
|
|
|
|
|
|
|
|
|
|
|
|
|
|
|
|
|
|
|
|
|
|
|
|
|
|
|
|
|
|
|
|
|
|
|
|
|
|
|
|
|
|
|
|
|
|
|
|
|
|
|
|
|
spaces/Andy1621/uniformer_image_segmentation/configs/danet/danet_r50-d8_512x512_20k_voc12aug.py
DELETED
@@ -1,7 +0,0 @@
|
|
1 |
-
_base_ = [
|
2 |
-
'../_base_/models/danet_r50-d8.py',
|
3 |
-
'../_base_/datasets/pascal_voc12_aug.py', '../_base_/default_runtime.py',
|
4 |
-
'../_base_/schedules/schedule_20k.py'
|
5 |
-
]
|
6 |
-
model = dict(
|
7 |
-
decode_head=dict(num_classes=21), auxiliary_head=dict(num_classes=21))
|
|
|
|
|
|
|
|
|
|
|
|
|
|
|
|
spaces/Andy1621/uniformer_image_segmentation/configs/psanet/psanet_r101-d8_512x1024_40k_cityscapes.py
DELETED
@@ -1,2 +0,0 @@
|
|
1 |
-
_base_ = './psanet_r50-d8_512x1024_40k_cityscapes.py'
|
2 |
-
model = dict(pretrained='open-mmlab://resnet101_v1c', backbone=dict(depth=101))
|
|
|
|
|
|
spaces/Ank0X0/Image-Upscaling-Playground/README.md
DELETED
@@ -1,14 +0,0 @@
|
|
1 |
-
---
|
2 |
-
title: Image Upscaling Playground
|
3 |
-
emoji: 🦆
|
4 |
-
colorFrom: green
|
5 |
-
colorTo: yellow
|
6 |
-
sdk: gradio
|
7 |
-
sdk_version: 3.4.1
|
8 |
-
app_file: app.py
|
9 |
-
pinned: false
|
10 |
-
license: apache-2.0
|
11 |
-
duplicated_from: bookbot/Image-Upscaling-Playground
|
12 |
-
---
|
13 |
-
|
14 |
-
Check out the configuration reference at https://huggingface.co/docs/hub/spaces#reference
|
|
|
|
|
|
|
|
|
|
|
|
|
|
|
|
|
|
|
|
|
|
|
|
|
|
|
|
|
|
spaces/AnnasBlackHat/Image-Similarity/src/util/matrix.py
DELETED
@@ -1,5 +0,0 @@
|
|
1 |
-
from numpy.linalg import norm
|
2 |
-
import numpy as np
|
3 |
-
|
4 |
-
def cosine(x, y):
|
5 |
-
return np.dot(x,y)/(norm(x)*norm(y))
|
|
|
|
|
|
|
|
|
|
|
|
spaces/Anonymous-sub/Rerender/ControlNet/annotator/uniformer/mmcv/utils/trace.py
DELETED
@@ -1,23 +0,0 @@
|
|
1 |
-
import warnings
|
2 |
-
|
3 |
-
import torch
|
4 |
-
|
5 |
-
from annotator.uniformer.mmcv.utils import digit_version
|
6 |
-
|
7 |
-
|
8 |
-
def is_jit_tracing() -> bool:
|
9 |
-
if (torch.__version__ != 'parrots'
|
10 |
-
and digit_version(torch.__version__) >= digit_version('1.6.0')):
|
11 |
-
on_trace = torch.jit.is_tracing()
|
12 |
-
# In PyTorch 1.6, torch.jit.is_tracing has a bug.
|
13 |
-
# Refers to https://github.com/pytorch/pytorch/issues/42448
|
14 |
-
if isinstance(on_trace, bool):
|
15 |
-
return on_trace
|
16 |
-
else:
|
17 |
-
return torch._C._is_tracing()
|
18 |
-
else:
|
19 |
-
warnings.warn(
|
20 |
-
'torch.jit.is_tracing is only supported after v1.6.0. '
|
21 |
-
'Therefore is_tracing returns False automatically. Please '
|
22 |
-
'set on_trace manually if you are using trace.', UserWarning)
|
23 |
-
return False
|
|
|
|
|
|
|
|
|
|
|
|
|
|
|
|
|
|
|
|
|
|
|
|
|
|
|
|
|
|
|
|
|
|
|
|
|
|
|
|
|
|
|
|
|
|
|
|
spaces/Arthur678/vits-uma-genshin-honkai/text/symbols.py
DELETED
@@ -1,39 +0,0 @@
|
|
1 |
-
'''
|
2 |
-
Defines the set of symbols used in text input to the model.
|
3 |
-
'''
|
4 |
-
|
5 |
-
'''# japanese_cleaners
|
6 |
-
_pad = '_'
|
7 |
-
_punctuation = ',.!?-'
|
8 |
-
_letters = 'AEINOQUabdefghijkmnoprstuvwyzʃʧ↓↑ '
|
9 |
-
'''
|
10 |
-
|
11 |
-
'''# japanese_cleaners2
|
12 |
-
_pad = '_'
|
13 |
-
_punctuation = ',.!?-~…'
|
14 |
-
_letters = 'AEINOQUabdefghijkmnoprstuvwyzʃʧʦ↓↑ '
|
15 |
-
'''
|
16 |
-
|
17 |
-
'''# korean_cleaners
|
18 |
-
_pad = '_'
|
19 |
-
_punctuation = ',.!?…~'
|
20 |
-
_letters = 'ㄱㄴㄷㄹㅁㅂㅅㅇㅈㅊㅋㅌㅍㅎㄲㄸㅃㅆㅉㅏㅓㅗㅜㅡㅣㅐㅔ '
|
21 |
-
'''
|
22 |
-
|
23 |
-
'''# chinese_cleaners
|
24 |
-
_pad = '_'
|
25 |
-
_punctuation = ',。!?—…'
|
26 |
-
_letters = 'ㄅㄆㄇㄈㄉㄊㄋㄌㄍㄎㄏㄐㄑㄒㄓㄔㄕㄖㄗㄘㄙㄚㄛㄜㄝㄞㄟㄠㄡㄢㄣㄤㄥㄦㄧㄨㄩˉˊˇˋ˙ '
|
27 |
-
'''
|
28 |
-
|
29 |
-
# zh_ja_mixture_cleaners
|
30 |
-
_pad = '_'
|
31 |
-
_punctuation = ',.!?-~…'
|
32 |
-
_letters = 'AEINOQUabdefghijklmnoprstuvwyzʃʧʦɯɹəɥ⁼ʰ`→↓↑ '
|
33 |
-
|
34 |
-
|
35 |
-
# Export all symbols:
|
36 |
-
symbols = [_pad] + list(_punctuation) + list(_letters)
|
37 |
-
|
38 |
-
# Special symbol ids
|
39 |
-
SPACE_ID = symbols.index(" ")
|
|
|
|
|
|
|
|
|
|
|
|
|
|
|
|
|
|
|
|
|
|
|
|
|
|
|
|
|
|
|
|
|
|
|
|
|
|
|
|
|
|
|
|
|
|
|
|
|
|
|
|
|
|
|
|
|
|
|
|
|
|
|
|
|
|
|
|
|
|
|
|
|
|
|
|
|
|
|
|
spaces/Awiny/Image2Paragraph/models/grit_src/third_party/CenterNet2/detectron2/engine/defaults.py
DELETED
@@ -1,715 +0,0 @@
|
|
1 |
-
# -*- coding: utf-8 -*-
|
2 |
-
# Copyright (c) Facebook, Inc. and its affiliates.
|
3 |
-
|
4 |
-
"""
|
5 |
-
This file contains components with some default boilerplate logic user may need
|
6 |
-
in training / testing. They will not work for everyone, but many users may find them useful.
|
7 |
-
|
8 |
-
The behavior of functions/classes in this file is subject to change,
|
9 |
-
since they are meant to represent the "common default behavior" people need in their projects.
|
10 |
-
"""
|
11 |
-
|
12 |
-
import argparse
|
13 |
-
import logging
|
14 |
-
import os
|
15 |
-
import sys
|
16 |
-
import weakref
|
17 |
-
from collections import OrderedDict
|
18 |
-
from typing import Optional
|
19 |
-
import torch
|
20 |
-
from fvcore.nn.precise_bn import get_bn_modules
|
21 |
-
from omegaconf import OmegaConf
|
22 |
-
from torch.nn.parallel import DistributedDataParallel
|
23 |
-
|
24 |
-
import detectron2.data.transforms as T
|
25 |
-
from detectron2.checkpoint import DetectionCheckpointer
|
26 |
-
from detectron2.config import CfgNode, LazyConfig
|
27 |
-
from detectron2.data import (
|
28 |
-
MetadataCatalog,
|
29 |
-
build_detection_test_loader,
|
30 |
-
build_detection_train_loader,
|
31 |
-
)
|
32 |
-
from detectron2.evaluation import (
|
33 |
-
DatasetEvaluator,
|
34 |
-
inference_on_dataset,
|
35 |
-
print_csv_format,
|
36 |
-
verify_results,
|
37 |
-
)
|
38 |
-
from detectron2.modeling import build_model
|
39 |
-
from detectron2.solver import build_lr_scheduler, build_optimizer
|
40 |
-
from detectron2.utils import comm
|
41 |
-
from detectron2.utils.collect_env import collect_env_info
|
42 |
-
from detectron2.utils.env import seed_all_rng
|
43 |
-
from detectron2.utils.events import CommonMetricPrinter, JSONWriter, TensorboardXWriter
|
44 |
-
from detectron2.utils.file_io import PathManager
|
45 |
-
from detectron2.utils.logger import setup_logger
|
46 |
-
|
47 |
-
from . import hooks
|
48 |
-
from .train_loop import AMPTrainer, SimpleTrainer, TrainerBase
|
49 |
-
|
50 |
-
__all__ = [
|
51 |
-
"create_ddp_model",
|
52 |
-
"default_argument_parser",
|
53 |
-
"default_setup",
|
54 |
-
"default_writers",
|
55 |
-
"DefaultPredictor",
|
56 |
-
"DefaultTrainer",
|
57 |
-
]
|
58 |
-
|
59 |
-
|
60 |
-
def create_ddp_model(model, *, fp16_compression=False, **kwargs):
|
61 |
-
"""
|
62 |
-
Create a DistributedDataParallel model if there are >1 processes.
|
63 |
-
|
64 |
-
Args:
|
65 |
-
model: a torch.nn.Module
|
66 |
-
fp16_compression: add fp16 compression hooks to the ddp object.
|
67 |
-
See more at https://pytorch.org/docs/stable/ddp_comm_hooks.html#torch.distributed.algorithms.ddp_comm_hooks.default_hooks.fp16_compress_hook
|
68 |
-
kwargs: other arguments of :module:`torch.nn.parallel.DistributedDataParallel`.
|
69 |
-
""" # noqa
|
70 |
-
if comm.get_world_size() == 1:
|
71 |
-
return model
|
72 |
-
if "device_ids" not in kwargs:
|
73 |
-
kwargs["device_ids"] = [comm.get_local_rank()]
|
74 |
-
ddp = DistributedDataParallel(model, **kwargs)
|
75 |
-
if fp16_compression:
|
76 |
-
from torch.distributed.algorithms.ddp_comm_hooks import default as comm_hooks
|
77 |
-
|
78 |
-
ddp.register_comm_hook(state=None, hook=comm_hooks.fp16_compress_hook)
|
79 |
-
return ddp
|
80 |
-
|
81 |
-
|
82 |
-
def default_argument_parser(epilog=None):
|
83 |
-
"""
|
84 |
-
Create a parser with some common arguments used by detectron2 users.
|
85 |
-
|
86 |
-
Args:
|
87 |
-
epilog (str): epilog passed to ArgumentParser describing the usage.
|
88 |
-
|
89 |
-
Returns:
|
90 |
-
argparse.ArgumentParser:
|
91 |
-
"""
|
92 |
-
parser = argparse.ArgumentParser(
|
93 |
-
epilog=epilog
|
94 |
-
or f"""
|
95 |
-
Examples:
|
96 |
-
|
97 |
-
Run on single machine:
|
98 |
-
$ {sys.argv[0]} --num-gpus 8 --config-file cfg.yaml
|
99 |
-
|
100 |
-
Change some config options:
|
101 |
-
$ {sys.argv[0]} --config-file cfg.yaml MODEL.WEIGHTS /path/to/weight.pth SOLVER.BASE_LR 0.001
|
102 |
-
|
103 |
-
Run on multiple machines:
|
104 |
-
(machine0)$ {sys.argv[0]} --machine-rank 0 --num-machines 2 --dist-url <URL> [--other-flags]
|
105 |
-
(machine1)$ {sys.argv[0]} --machine-rank 1 --num-machines 2 --dist-url <URL> [--other-flags]
|
106 |
-
""",
|
107 |
-
formatter_class=argparse.RawDescriptionHelpFormatter,
|
108 |
-
)
|
109 |
-
parser.add_argument("--config-file", default="", metavar="FILE", help="path to config file")
|
110 |
-
parser.add_argument(
|
111 |
-
"--resume",
|
112 |
-
action="store_true",
|
113 |
-
help="Whether to attempt to resume from the checkpoint directory. "
|
114 |
-
"See documentation of `DefaultTrainer.resume_or_load()` for what it means.",
|
115 |
-
)
|
116 |
-
parser.add_argument("--eval-only", action="store_true", help="perform evaluation only")
|
117 |
-
parser.add_argument("--num-gpus", type=int, default=1, help="number of gpus *per machine*")
|
118 |
-
parser.add_argument("--num-machines", type=int, default=1, help="total number of machines")
|
119 |
-
parser.add_argument(
|
120 |
-
"--machine-rank", type=int, default=0, help="the rank of this machine (unique per machine)"
|
121 |
-
)
|
122 |
-
|
123 |
-
# PyTorch still may leave orphan processes in multi-gpu training.
|
124 |
-
# Therefore we use a deterministic way to obtain port,
|
125 |
-
# so that users are aware of orphan processes by seeing the port occupied.
|
126 |
-
port = 2 ** 15 + 2 ** 14 + hash(os.getuid() if sys.platform != "win32" else 1) % 2 ** 14
|
127 |
-
parser.add_argument(
|
128 |
-
"--dist-url",
|
129 |
-
default="tcp://127.0.0.1:{}".format(port),
|
130 |
-
help="initialization URL for pytorch distributed backend. See "
|
131 |
-
"https://pytorch.org/docs/stable/distributed.html for details.",
|
132 |
-
)
|
133 |
-
parser.add_argument(
|
134 |
-
"opts",
|
135 |
-
help="""
|
136 |
-
Modify config options at the end of the command. For Yacs configs, use
|
137 |
-
space-separated "PATH.KEY VALUE" pairs.
|
138 |
-
For python-based LazyConfig, use "path.key=value".
|
139 |
-
""".strip(),
|
140 |
-
default=None,
|
141 |
-
nargs=argparse.REMAINDER,
|
142 |
-
)
|
143 |
-
return parser
|
144 |
-
|
145 |
-
|
146 |
-
def _try_get_key(cfg, *keys, default=None):
|
147 |
-
"""
|
148 |
-
Try select keys from cfg until the first key that exists. Otherwise return default.
|
149 |
-
"""
|
150 |
-
if isinstance(cfg, CfgNode):
|
151 |
-
cfg = OmegaConf.create(cfg.dump())
|
152 |
-
for k in keys:
|
153 |
-
none = object()
|
154 |
-
p = OmegaConf.select(cfg, k, default=none)
|
155 |
-
if p is not none:
|
156 |
-
return p
|
157 |
-
return default
|
158 |
-
|
159 |
-
|
160 |
-
def _highlight(code, filename):
|
161 |
-
try:
|
162 |
-
import pygments
|
163 |
-
except ImportError:
|
164 |
-
return code
|
165 |
-
|
166 |
-
from pygments.lexers import Python3Lexer, YamlLexer
|
167 |
-
from pygments.formatters import Terminal256Formatter
|
168 |
-
|
169 |
-
lexer = Python3Lexer() if filename.endswith(".py") else YamlLexer()
|
170 |
-
code = pygments.highlight(code, lexer, Terminal256Formatter(style="monokai"))
|
171 |
-
return code
|
172 |
-
|
173 |
-
|
174 |
-
def default_setup(cfg, args):
|
175 |
-
"""
|
176 |
-
Perform some basic common setups at the beginning of a job, including:
|
177 |
-
|
178 |
-
1. Set up the detectron2 logger
|
179 |
-
2. Log basic information about environment, cmdline arguments, and config
|
180 |
-
3. Backup the config to the output directory
|
181 |
-
|
182 |
-
Args:
|
183 |
-
cfg (CfgNode or omegaconf.DictConfig): the full config to be used
|
184 |
-
args (argparse.NameSpace): the command line arguments to be logged
|
185 |
-
"""
|
186 |
-
output_dir = _try_get_key(cfg, "OUTPUT_DIR", "output_dir", "train.output_dir")
|
187 |
-
if comm.is_main_process() and output_dir:
|
188 |
-
PathManager.mkdirs(output_dir)
|
189 |
-
|
190 |
-
rank = comm.get_rank()
|
191 |
-
setup_logger(output_dir, distributed_rank=rank, name="fvcore")
|
192 |
-
logger = setup_logger(output_dir, distributed_rank=rank)
|
193 |
-
|
194 |
-
logger.info("Rank of current process: {}. World size: {}".format(rank, comm.get_world_size()))
|
195 |
-
logger.info("Environment info:\n" + collect_env_info())
|
196 |
-
|
197 |
-
logger.info("Command line arguments: " + str(args))
|
198 |
-
if hasattr(args, "config_file") and args.config_file != "":
|
199 |
-
logger.info(
|
200 |
-
"Contents of args.config_file={}:\n{}".format(
|
201 |
-
args.config_file,
|
202 |
-
_highlight(PathManager.open(args.config_file, "r").read(), args.config_file),
|
203 |
-
)
|
204 |
-
)
|
205 |
-
|
206 |
-
if comm.is_main_process() and output_dir:
|
207 |
-
# Note: some of our scripts may expect the existence of
|
208 |
-
# config.yaml in output directory
|
209 |
-
path = os.path.join(output_dir, "config.yaml")
|
210 |
-
if isinstance(cfg, CfgNode):
|
211 |
-
logger.info("Running with full config:\n{}".format(_highlight(cfg.dump(), ".yaml")))
|
212 |
-
with PathManager.open(path, "w") as f:
|
213 |
-
f.write(cfg.dump())
|
214 |
-
else:
|
215 |
-
LazyConfig.save(cfg, path)
|
216 |
-
logger.info("Full config saved to {}".format(path))
|
217 |
-
|
218 |
-
# make sure each worker has a different, yet deterministic seed if specified
|
219 |
-
seed = _try_get_key(cfg, "SEED", "train.seed", default=-1)
|
220 |
-
seed_all_rng(None if seed < 0 else seed + rank)
|
221 |
-
|
222 |
-
# cudnn benchmark has large overhead. It shouldn't be used considering the small size of
|
223 |
-
# typical validation set.
|
224 |
-
if not (hasattr(args, "eval_only") and args.eval_only):
|
225 |
-
torch.backends.cudnn.benchmark = _try_get_key(
|
226 |
-
cfg, "CUDNN_BENCHMARK", "train.cudnn_benchmark", default=False
|
227 |
-
)
|
228 |
-
|
229 |
-
|
230 |
-
def default_writers(output_dir: str, max_iter: Optional[int] = None):
|
231 |
-
"""
|
232 |
-
Build a list of :class:`EventWriter` to be used.
|
233 |
-
It now consists of a :class:`CommonMetricPrinter`,
|
234 |
-
:class:`TensorboardXWriter` and :class:`JSONWriter`.
|
235 |
-
|
236 |
-
Args:
|
237 |
-
output_dir: directory to store JSON metrics and tensorboard events
|
238 |
-
max_iter: the total number of iterations
|
239 |
-
|
240 |
-
Returns:
|
241 |
-
list[EventWriter]: a list of :class:`EventWriter` objects.
|
242 |
-
"""
|
243 |
-
PathManager.mkdirs(output_dir)
|
244 |
-
return [
|
245 |
-
# It may not always print what you want to see, since it prints "common" metrics only.
|
246 |
-
CommonMetricPrinter(max_iter),
|
247 |
-
JSONWriter(os.path.join(output_dir, "metrics.json")),
|
248 |
-
TensorboardXWriter(output_dir),
|
249 |
-
]
|
250 |
-
|
251 |
-
|
252 |
-
class DefaultPredictor:
|
253 |
-
"""
|
254 |
-
Create a simple end-to-end predictor with the given config that runs on
|
255 |
-
single device for a single input image.
|
256 |
-
|
257 |
-
Compared to using the model directly, this class does the following additions:
|
258 |
-
|
259 |
-
1. Load checkpoint from `cfg.MODEL.WEIGHTS`.
|
260 |
-
2. Always take BGR image as the input and apply conversion defined by `cfg.INPUT.FORMAT`.
|
261 |
-
3. Apply resizing defined by `cfg.INPUT.{MIN,MAX}_SIZE_TEST`.
|
262 |
-
4. Take one input image and produce a single output, instead of a batch.
|
263 |
-
|
264 |
-
This is meant for simple demo purposes, so it does the above steps automatically.
|
265 |
-
This is not meant for benchmarks or running complicated inference logic.
|
266 |
-
If you'd like to do anything more complicated, please refer to its source code as
|
267 |
-
examples to build and use the model manually.
|
268 |
-
|
269 |
-
Attributes:
|
270 |
-
metadata (Metadata): the metadata of the underlying dataset, obtained from
|
271 |
-
cfg.DATASETS.TEST.
|
272 |
-
|
273 |
-
Examples:
|
274 |
-
::
|
275 |
-
pred = DefaultPredictor(cfg)
|
276 |
-
inputs = cv2.imread("input.jpg")
|
277 |
-
outputs = pred(inputs)
|
278 |
-
"""
|
279 |
-
|
280 |
-
def __init__(self, cfg):
|
281 |
-
self.cfg = cfg.clone() # cfg can be modified by model
|
282 |
-
self.model = build_model(self.cfg)
|
283 |
-
self.model.eval()
|
284 |
-
if len(cfg.DATASETS.TEST):
|
285 |
-
self.metadata = MetadataCatalog.get(cfg.DATASETS.TEST[0])
|
286 |
-
|
287 |
-
checkpointer = DetectionCheckpointer(self.model)
|
288 |
-
checkpointer.load(cfg.MODEL.WEIGHTS)
|
289 |
-
|
290 |
-
self.aug = T.ResizeShortestEdge(
|
291 |
-
[cfg.INPUT.MIN_SIZE_TEST, cfg.INPUT.MIN_SIZE_TEST], cfg.INPUT.MAX_SIZE_TEST
|
292 |
-
)
|
293 |
-
|
294 |
-
self.input_format = cfg.INPUT.FORMAT
|
295 |
-
assert self.input_format in ["RGB", "BGR"], self.input_format
|
296 |
-
|
297 |
-
def __call__(self, original_image):
|
298 |
-
"""
|
299 |
-
Args:
|
300 |
-
original_image (np.ndarray): an image of shape (H, W, C) (in BGR order).
|
301 |
-
|
302 |
-
Returns:
|
303 |
-
predictions (dict):
|
304 |
-
the output of the model for one image only.
|
305 |
-
See :doc:`/tutorials/models` for details about the format.
|
306 |
-
"""
|
307 |
-
with torch.no_grad(): # https://github.com/sphinx-doc/sphinx/issues/4258
|
308 |
-
# Apply pre-processing to image.
|
309 |
-
if self.input_format == "RGB":
|
310 |
-
# whether the model expects BGR inputs or RGB
|
311 |
-
original_image = original_image[:, :, ::-1]
|
312 |
-
height, width = original_image.shape[:2]
|
313 |
-
image = self.aug.get_transform(original_image).apply_image(original_image)
|
314 |
-
image = torch.as_tensor(image.astype("float32").transpose(2, 0, 1))
|
315 |
-
|
316 |
-
inputs = {"image": image, "height": height, "width": width}
|
317 |
-
predictions = self.model([inputs])[0]
|
318 |
-
return predictions
|
319 |
-
|
320 |
-
|
321 |
-
class DefaultTrainer(TrainerBase):
|
322 |
-
"""
|
323 |
-
A trainer with default training logic. It does the following:
|
324 |
-
|
325 |
-
1. Create a :class:`SimpleTrainer` using model, optimizer, dataloader
|
326 |
-
defined by the given config. Create a LR scheduler defined by the config.
|
327 |
-
2. Load the last checkpoint or `cfg.MODEL.WEIGHTS`, if exists, when
|
328 |
-
`resume_or_load` is called.
|
329 |
-
3. Register a few common hooks defined by the config.
|
330 |
-
|
331 |
-
It is created to simplify the **standard model training workflow** and reduce code boilerplate
|
332 |
-
for users who only need the standard training workflow, with standard features.
|
333 |
-
It means this class makes *many assumptions* about your training logic that
|
334 |
-
may easily become invalid in a new research. In fact, any assumptions beyond those made in the
|
335 |
-
:class:`SimpleTrainer` are too much for research.
|
336 |
-
|
337 |
-
The code of this class has been annotated about restrictive assumptions it makes.
|
338 |
-
When they do not work for you, you're encouraged to:
|
339 |
-
|
340 |
-
1. Overwrite methods of this class, OR:
|
341 |
-
2. Use :class:`SimpleTrainer`, which only does minimal SGD training and
|
342 |
-
nothing else. You can then add your own hooks if needed. OR:
|
343 |
-
3. Write your own training loop similar to `tools/plain_train_net.py`.
|
344 |
-
|
345 |
-
See the :doc:`/tutorials/training` tutorials for more details.
|
346 |
-
|
347 |
-
Note that the behavior of this class, like other functions/classes in
|
348 |
-
this file, is not stable, since it is meant to represent the "common default behavior".
|
349 |
-
It is only guaranteed to work well with the standard models and training workflow in detectron2.
|
350 |
-
To obtain more stable behavior, write your own training logic with other public APIs.
|
351 |
-
|
352 |
-
Examples:
|
353 |
-
::
|
354 |
-
trainer = DefaultTrainer(cfg)
|
355 |
-
trainer.resume_or_load() # load last checkpoint or MODEL.WEIGHTS
|
356 |
-
trainer.train()
|
357 |
-
|
358 |
-
Attributes:
|
359 |
-
scheduler:
|
360 |
-
checkpointer (DetectionCheckpointer):
|
361 |
-
cfg (CfgNode):
|
362 |
-
"""
|
363 |
-
|
364 |
-
def __init__(self, cfg):
|
365 |
-
"""
|
366 |
-
Args:
|
367 |
-
cfg (CfgNode):
|
368 |
-
"""
|
369 |
-
super().__init__()
|
370 |
-
logger = logging.getLogger("detectron2")
|
371 |
-
if not logger.isEnabledFor(logging.INFO): # setup_logger is not called for d2
|
372 |
-
setup_logger()
|
373 |
-
cfg = DefaultTrainer.auto_scale_workers(cfg, comm.get_world_size())
|
374 |
-
|
375 |
-
# Assume these objects must be constructed in this order.
|
376 |
-
model = self.build_model(cfg)
|
377 |
-
optimizer = self.build_optimizer(cfg, model)
|
378 |
-
data_loader = self.build_train_loader(cfg)
|
379 |
-
|
380 |
-
model = create_ddp_model(model, broadcast_buffers=False)
|
381 |
-
self._trainer = (AMPTrainer if cfg.SOLVER.AMP.ENABLED else SimpleTrainer)(
|
382 |
-
model, data_loader, optimizer
|
383 |
-
)
|
384 |
-
|
385 |
-
self.scheduler = self.build_lr_scheduler(cfg, optimizer)
|
386 |
-
self.checkpointer = DetectionCheckpointer(
|
387 |
-
# Assume you want to save checkpoints together with logs/statistics
|
388 |
-
model,
|
389 |
-
cfg.OUTPUT_DIR,
|
390 |
-
trainer=weakref.proxy(self),
|
391 |
-
)
|
392 |
-
self.start_iter = 0
|
393 |
-
self.max_iter = cfg.SOLVER.MAX_ITER
|
394 |
-
self.cfg = cfg
|
395 |
-
|
396 |
-
self.register_hooks(self.build_hooks())
|
397 |
-
|
398 |
-
def resume_or_load(self, resume=True):
|
399 |
-
"""
|
400 |
-
If `resume==True` and `cfg.OUTPUT_DIR` contains the last checkpoint (defined by
|
401 |
-
a `last_checkpoint` file), resume from the file. Resuming means loading all
|
402 |
-
available states (eg. optimizer and scheduler) and update iteration counter
|
403 |
-
from the checkpoint. ``cfg.MODEL.WEIGHTS`` will not be used.
|
404 |
-
|
405 |
-
Otherwise, this is considered as an independent training. The method will load model
|
406 |
-
weights from the file `cfg.MODEL.WEIGHTS` (but will not load other states) and start
|
407 |
-
from iteration 0.
|
408 |
-
|
409 |
-
Args:
|
410 |
-
resume (bool): whether to do resume or not
|
411 |
-
"""
|
412 |
-
self.checkpointer.resume_or_load(self.cfg.MODEL.WEIGHTS, resume=resume)
|
413 |
-
if resume and self.checkpointer.has_checkpoint():
|
414 |
-
# The checkpoint stores the training iteration that just finished, thus we start
|
415 |
-
# at the next iteration
|
416 |
-
self.start_iter = self.iter + 1
|
417 |
-
|
418 |
-
def build_hooks(self):
|
419 |
-
"""
|
420 |
-
Build a list of default hooks, including timing, evaluation,
|
421 |
-
checkpointing, lr scheduling, precise BN, writing events.
|
422 |
-
|
423 |
-
Returns:
|
424 |
-
list[HookBase]:
|
425 |
-
"""
|
426 |
-
cfg = self.cfg.clone()
|
427 |
-
cfg.defrost()
|
428 |
-
cfg.DATALOADER.NUM_WORKERS = 0 # save some memory and time for PreciseBN
|
429 |
-
|
430 |
-
ret = [
|
431 |
-
hooks.IterationTimer(),
|
432 |
-
hooks.LRScheduler(),
|
433 |
-
hooks.PreciseBN(
|
434 |
-
# Run at the same freq as (but before) evaluation.
|
435 |
-
cfg.TEST.EVAL_PERIOD,
|
436 |
-
self.model,
|
437 |
-
# Build a new data loader to not affect training
|
438 |
-
self.build_train_loader(cfg),
|
439 |
-
cfg.TEST.PRECISE_BN.NUM_ITER,
|
440 |
-
)
|
441 |
-
if cfg.TEST.PRECISE_BN.ENABLED and get_bn_modules(self.model)
|
442 |
-
else None,
|
443 |
-
]
|
444 |
-
|
445 |
-
# Do PreciseBN before checkpointer, because it updates the model and need to
|
446 |
-
# be saved by checkpointer.
|
447 |
-
# This is not always the best: if checkpointing has a different frequency,
|
448 |
-
# some checkpoints may have more precise statistics than others.
|
449 |
-
if comm.is_main_process():
|
450 |
-
ret.append(hooks.PeriodicCheckpointer(self.checkpointer, cfg.SOLVER.CHECKPOINT_PERIOD))
|
451 |
-
|
452 |
-
def test_and_save_results():
|
453 |
-
self._last_eval_results = self.test(self.cfg, self.model)
|
454 |
-
return self._last_eval_results
|
455 |
-
|
456 |
-
# Do evaluation after checkpointer, because then if it fails,
|
457 |
-
# we can use the saved checkpoint to debug.
|
458 |
-
ret.append(hooks.EvalHook(cfg.TEST.EVAL_PERIOD, test_and_save_results))
|
459 |
-
|
460 |
-
if comm.is_main_process():
|
461 |
-
# Here the default print/log frequency of each writer is used.
|
462 |
-
# run writers in the end, so that evaluation metrics are written
|
463 |
-
ret.append(hooks.PeriodicWriter(self.build_writers(), period=20))
|
464 |
-
return ret
|
465 |
-
|
466 |
-
def build_writers(self):
|
467 |
-
"""
|
468 |
-
Build a list of writers to be used using :func:`default_writers()`.
|
469 |
-
If you'd like a different list of writers, you can overwrite it in
|
470 |
-
your trainer.
|
471 |
-
|
472 |
-
Returns:
|
473 |
-
list[EventWriter]: a list of :class:`EventWriter` objects.
|
474 |
-
"""
|
475 |
-
return default_writers(self.cfg.OUTPUT_DIR, self.max_iter)
|
476 |
-
|
477 |
-
def train(self):
|
478 |
-
"""
|
479 |
-
Run training.
|
480 |
-
|
481 |
-
Returns:
|
482 |
-
OrderedDict of results, if evaluation is enabled. Otherwise None.
|
483 |
-
"""
|
484 |
-
super().train(self.start_iter, self.max_iter)
|
485 |
-
if len(self.cfg.TEST.EXPECTED_RESULTS) and comm.is_main_process():
|
486 |
-
assert hasattr(
|
487 |
-
self, "_last_eval_results"
|
488 |
-
), "No evaluation results obtained during training!"
|
489 |
-
verify_results(self.cfg, self._last_eval_results)
|
490 |
-
return self._last_eval_results
|
491 |
-
|
492 |
-
def run_step(self):
|
493 |
-
self._trainer.iter = self.iter
|
494 |
-
self._trainer.run_step()
|
495 |
-
|
496 |
-
def state_dict(self):
|
497 |
-
ret = super().state_dict()
|
498 |
-
ret["_trainer"] = self._trainer.state_dict()
|
499 |
-
return ret
|
500 |
-
|
501 |
-
def load_state_dict(self, state_dict):
|
502 |
-
super().load_state_dict(state_dict)
|
503 |
-
self._trainer.load_state_dict(state_dict["_trainer"])
|
504 |
-
|
505 |
-
@classmethod
|
506 |
-
def build_model(cls, cfg):
|
507 |
-
"""
|
508 |
-
Returns:
|
509 |
-
torch.nn.Module:
|
510 |
-
|
511 |
-
It now calls :func:`detectron2.modeling.build_model`.
|
512 |
-
Overwrite it if you'd like a different model.
|
513 |
-
"""
|
514 |
-
model = build_model(cfg)
|
515 |
-
logger = logging.getLogger(__name__)
|
516 |
-
logger.info("Model:\n{}".format(model))
|
517 |
-
return model
|
518 |
-
|
519 |
-
@classmethod
|
520 |
-
def build_optimizer(cls, cfg, model):
|
521 |
-
"""
|
522 |
-
Returns:
|
523 |
-
torch.optim.Optimizer:
|
524 |
-
|
525 |
-
It now calls :func:`detectron2.solver.build_optimizer`.
|
526 |
-
Overwrite it if you'd like a different optimizer.
|
527 |
-
"""
|
528 |
-
return build_optimizer(cfg, model)
|
529 |
-
|
530 |
-
@classmethod
|
531 |
-
def build_lr_scheduler(cls, cfg, optimizer):
|
532 |
-
"""
|
533 |
-
It now calls :func:`detectron2.solver.build_lr_scheduler`.
|
534 |
-
Overwrite it if you'd like a different scheduler.
|
535 |
-
"""
|
536 |
-
return build_lr_scheduler(cfg, optimizer)
|
537 |
-
|
538 |
-
@classmethod
|
539 |
-
def build_train_loader(cls, cfg):
|
540 |
-
"""
|
541 |
-
Returns:
|
542 |
-
iterable
|
543 |
-
|
544 |
-
It now calls :func:`detectron2.data.build_detection_train_loader`.
|
545 |
-
Overwrite it if you'd like a different data loader.
|
546 |
-
"""
|
547 |
-
return build_detection_train_loader(cfg)
|
548 |
-
|
549 |
-
@classmethod
|
550 |
-
def build_test_loader(cls, cfg, dataset_name):
|
551 |
-
"""
|
552 |
-
Returns:
|
553 |
-
iterable
|
554 |
-
|
555 |
-
It now calls :func:`detectron2.data.build_detection_test_loader`.
|
556 |
-
Overwrite it if you'd like a different data loader.
|
557 |
-
"""
|
558 |
-
return build_detection_test_loader(cfg, dataset_name)
|
559 |
-
|
560 |
-
@classmethod
|
561 |
-
def build_evaluator(cls, cfg, dataset_name):
|
562 |
-
"""
|
563 |
-
Returns:
|
564 |
-
DatasetEvaluator or None
|
565 |
-
|
566 |
-
It is not implemented by default.
|
567 |
-
"""
|
568 |
-
raise NotImplementedError(
|
569 |
-
"""
|
570 |
-
If you want DefaultTrainer to automatically run evaluation,
|
571 |
-
please implement `build_evaluator()` in subclasses (see train_net.py for example).
|
572 |
-
Alternatively, you can call evaluation functions yourself (see Colab balloon tutorial for example).
|
573 |
-
"""
|
574 |
-
)
|
575 |
-
|
576 |
-
@classmethod
|
577 |
-
def test(cls, cfg, model, evaluators=None):
|
578 |
-
"""
|
579 |
-
Evaluate the given model. The given model is expected to already contain
|
580 |
-
weights to evaluate.
|
581 |
-
|
582 |
-
Args:
|
583 |
-
cfg (CfgNode):
|
584 |
-
model (nn.Module):
|
585 |
-
evaluators (list[DatasetEvaluator] or None): if None, will call
|
586 |
-
:meth:`build_evaluator`. Otherwise, must have the same length as
|
587 |
-
``cfg.DATASETS.TEST``.
|
588 |
-
|
589 |
-
Returns:
|
590 |
-
dict: a dict of result metrics
|
591 |
-
"""
|
592 |
-
logger = logging.getLogger(__name__)
|
593 |
-
if isinstance(evaluators, DatasetEvaluator):
|
594 |
-
evaluators = [evaluators]
|
595 |
-
if evaluators is not None:
|
596 |
-
assert len(cfg.DATASETS.TEST) == len(evaluators), "{} != {}".format(
|
597 |
-
len(cfg.DATASETS.TEST), len(evaluators)
|
598 |
-
)
|
599 |
-
|
600 |
-
results = OrderedDict()
|
601 |
-
for idx, dataset_name in enumerate(cfg.DATASETS.TEST):
|
602 |
-
data_loader = cls.build_test_loader(cfg, dataset_name)
|
603 |
-
# When evaluators are passed in as arguments,
|
604 |
-
# implicitly assume that evaluators can be created before data_loader.
|
605 |
-
if evaluators is not None:
|
606 |
-
evaluator = evaluators[idx]
|
607 |
-
else:
|
608 |
-
try:
|
609 |
-
evaluator = cls.build_evaluator(cfg, dataset_name)
|
610 |
-
except NotImplementedError:
|
611 |
-
logger.warn(
|
612 |
-
"No evaluator found. Use `DefaultTrainer.test(evaluators=)`, "
|
613 |
-
"or implement its `build_evaluator` method."
|
614 |
-
)
|
615 |
-
results[dataset_name] = {}
|
616 |
-
continue
|
617 |
-
results_i = inference_on_dataset(model, data_loader, evaluator)
|
618 |
-
results[dataset_name] = results_i
|
619 |
-
if comm.is_main_process():
|
620 |
-
assert isinstance(
|
621 |
-
results_i, dict
|
622 |
-
), "Evaluator must return a dict on the main process. Got {} instead.".format(
|
623 |
-
results_i
|
624 |
-
)
|
625 |
-
logger.info("Evaluation results for {} in csv format:".format(dataset_name))
|
626 |
-
print_csv_format(results_i)
|
627 |
-
|
628 |
-
if len(results) == 1:
|
629 |
-
results = list(results.values())[0]
|
630 |
-
return results
|
631 |
-
|
632 |
-
@staticmethod
|
633 |
-
def auto_scale_workers(cfg, num_workers: int):
|
634 |
-
"""
|
635 |
-
When the config is defined for certain number of workers (according to
|
636 |
-
``cfg.SOLVER.REFERENCE_WORLD_SIZE``) that's different from the number of
|
637 |
-
workers currently in use, returns a new cfg where the total batch size
|
638 |
-
is scaled so that the per-GPU batch size stays the same as the
|
639 |
-
original ``IMS_PER_BATCH // REFERENCE_WORLD_SIZE``.
|
640 |
-
|
641 |
-
Other config options are also scaled accordingly:
|
642 |
-
* training steps and warmup steps are scaled inverse proportionally.
|
643 |
-
* learning rate are scaled proportionally, following :paper:`ImageNet in 1h`.
|
644 |
-
|
645 |
-
For example, with the original config like the following:
|
646 |
-
|
647 |
-
.. code-block:: yaml
|
648 |
-
|
649 |
-
IMS_PER_BATCH: 16
|
650 |
-
BASE_LR: 0.1
|
651 |
-
REFERENCE_WORLD_SIZE: 8
|
652 |
-
MAX_ITER: 5000
|
653 |
-
STEPS: (4000,)
|
654 |
-
CHECKPOINT_PERIOD: 1000
|
655 |
-
|
656 |
-
When this config is used on 16 GPUs instead of the reference number 8,
|
657 |
-
calling this method will return a new config with:
|
658 |
-
|
659 |
-
.. code-block:: yaml
|
660 |
-
|
661 |
-
IMS_PER_BATCH: 32
|
662 |
-
BASE_LR: 0.2
|
663 |
-
REFERENCE_WORLD_SIZE: 16
|
664 |
-
MAX_ITER: 2500
|
665 |
-
STEPS: (2000,)
|
666 |
-
CHECKPOINT_PERIOD: 500
|
667 |
-
|
668 |
-
Note that both the original config and this new config can be trained on 16 GPUs.
|
669 |
-
It's up to user whether to enable this feature (by setting ``REFERENCE_WORLD_SIZE``).
|
670 |
-
|
671 |
-
Returns:
|
672 |
-
CfgNode: a new config. Same as original if ``cfg.SOLVER.REFERENCE_WORLD_SIZE==0``.
|
673 |
-
"""
|
674 |
-
old_world_size = cfg.SOLVER.REFERENCE_WORLD_SIZE
|
675 |
-
if old_world_size == 0 or old_world_size == num_workers:
|
676 |
-
return cfg
|
677 |
-
cfg = cfg.clone()
|
678 |
-
frozen = cfg.is_frozen()
|
679 |
-
cfg.defrost()
|
680 |
-
|
681 |
-
assert (
|
682 |
-
cfg.SOLVER.IMS_PER_BATCH % old_world_size == 0
|
683 |
-
), "Invalid REFERENCE_WORLD_SIZE in config!"
|
684 |
-
scale = num_workers / old_world_size
|
685 |
-
bs = cfg.SOLVER.IMS_PER_BATCH = int(round(cfg.SOLVER.IMS_PER_BATCH * scale))
|
686 |
-
lr = cfg.SOLVER.BASE_LR = cfg.SOLVER.BASE_LR * scale
|
687 |
-
max_iter = cfg.SOLVER.MAX_ITER = int(round(cfg.SOLVER.MAX_ITER / scale))
|
688 |
-
warmup_iter = cfg.SOLVER.WARMUP_ITERS = int(round(cfg.SOLVER.WARMUP_ITERS / scale))
|
689 |
-
cfg.SOLVER.STEPS = tuple(int(round(s / scale)) for s in cfg.SOLVER.STEPS)
|
690 |
-
cfg.TEST.EVAL_PERIOD = int(round(cfg.TEST.EVAL_PERIOD / scale))
|
691 |
-
cfg.SOLVER.CHECKPOINT_PERIOD = int(round(cfg.SOLVER.CHECKPOINT_PERIOD / scale))
|
692 |
-
cfg.SOLVER.REFERENCE_WORLD_SIZE = num_workers # maintain invariant
|
693 |
-
logger = logging.getLogger(__name__)
|
694 |
-
logger.info(
|
695 |
-
f"Auto-scaling the config to batch_size={bs}, learning_rate={lr}, "
|
696 |
-
f"max_iter={max_iter}, warmup={warmup_iter}."
|
697 |
-
)
|
698 |
-
|
699 |
-
if frozen:
|
700 |
-
cfg.freeze()
|
701 |
-
return cfg
|
702 |
-
|
703 |
-
|
704 |
-
# Access basic attributes from the underlying trainer
|
705 |
-
for _attr in ["model", "data_loader", "optimizer"]:
|
706 |
-
setattr(
|
707 |
-
DefaultTrainer,
|
708 |
-
_attr,
|
709 |
-
property(
|
710 |
-
# getter
|
711 |
-
lambda self, x=_attr: getattr(self._trainer, x),
|
712 |
-
# setter
|
713 |
-
lambda self, value, x=_attr: setattr(self._trainer, x, value),
|
714 |
-
),
|
715 |
-
)
|
|
|
|
|
|
|
|
|
|
|
|
|
|
|
|
|
|
|
|
|
|
|
|
|
|
|
|
|
|
|
|
|
|
|
|
|
|
|
|
|
|
|
|
|
|
|
|
|
|
|
|
|
|
|
|
|
|
|
|
|
|
|
|
|
|
|
|
|
|
|
|
|
|
|
|
|
|
|
|
|
|
|
|
|
|
|
|
|
|
|
|
|
|
|
|
|
|
|
|
|
|
|
|
|
|
|
|
|
|
|
|
|
|
|
|
|
|
|
|
|
|
|
|
|
|
|
|
|
|
|
|
|
|
|
|
|
|
|
|
|
|
|
|
|
|
|
|
|
|
|
|
|
|
|
|
|
|
|
|
|
|
|
|
|
|
|
|
|
|
|
|
|
|
|
|
|
|
|
|
|
|
|
|
|
|
|
|
|
|
|
|
|
|
|
|
|
|
|
|
|
|
|
|
|
|
|
|
|
|
|
|
|
|
|
|
|
|
|
|
|
|
|
|
|
|
|
|
|
|
|
|
|
|
|
|
|
|
|
|
|
|
|
|
|
|
|
|
|
|
|
|
|
|
|
|
|
|
|
|
|
|
|
|
|
|
|
|
|
|
|
|
|
|
|
|
|
|
|
|
|
|
|
|
|
|
|
|
|
|
|
|
|
|
|
|
|
|
|
|
|
|
|
|
|
|
|
|
|
|
|
|
|
|
|
|
|
|
|
|
|
|
|
|
|
|
|
|
|
|
|
|
|
|
|
|
|
|
|
|
|
|
|
|
|
|
|
|
|
|
|
|
|
|
|
|
|
|
|
|
|
|
|
|
|
|
|
|
|
|
|
|
|
|
|
|
|
|
|
|
|
|
|
|
|
|
|
|
|
|
|
|
|
|
|
|
|
|
|
|
|
|
|
|
|
|
|
|
|
|
|
|
|
|
|
|
|
|
|
|
|
|
|
|
|
|
|
|
|
|
|
|
|
|
|
|
|
|
|
|
|
|
|
|
|
|
|
|
|
|
|
|
|
|
|
|
|
|
|
|
|
|
|
|
|
|
|
|
|
|
|
|
|
|
|
|
|
|
|
|
|
|
|
|
|
|
|
|
|
|
|
|
|
|
|
|
|
|
|
|
|
|
|
|
|
|
|
|
|
|
|
|
|
|
|
|
|
|
|
|
|
|
|
|
|
|
|
|
|
|
|
|
|
|
|
|
|
|
|
|
|
|
|
|
|
|
|
|
|
|
|
|
|
|
|
|
|
|
|
|
|
|
|
|
|
|
|
|
|
|
|
|
|
|
|
|
|
|
|
|
|
|
|
|
|
|
|
|
|
|
|
|
|
|
|
|
|
|
|
|
|
|
|
|
|
|
|
|
|
|
|
|
|
|
|
|
|
|
|
|
|
|
|
|
|
|
|
|
|
|
|
|
|
|
|
|
|
|
|
|
|
|
|
|
|
|
|
|
|
|
|
|
|
|
|
|
|
|
|
|
|
|
|
|
|
|
|
|
|
|
|
|
|
|
|
|
|
|
|
|
|
|
|
|
|
|
|
|
|
|
|
|
|
|
|
|
|
|
|
|
|
|
|
|
|
|
|
|
|
|
|
|
|
|
|
|
|
|
|
|
|
|
|
|
|
|
|
|
|
|
|
|
|
|
|
|
|
|
|
|
|
|
|
|
|
|
|
|
|
|
|
|
|
|
|
|
|
|
|
|
|
|
|
|
|
|
|
|
|
|
|
|
|
|
|
|
|
|
|
|
|
|
|
|
|
|
|
|
|
|
|
|
|
|
|
|
|
|
|
|
|
|
|
|
|
|
|
|
|
|
|
|
|
|
|
|
|
|
|
|
|
|
|
|
|
|
|
|
|
|
|
|
|
|
|
|
|
|
|
|
|
|
|
|
|
|
|
|
|
|
|
|
|
|
|
|
|
|
|
|
|
|
|
|
|
|
|
|
|
|
|
|
|
|
|
|
|
|
|
|
|
|
|
|
|
|
|
|
|
|
|
|
|
|
|
|
|
|
|
|
|
|
|
|
|
|
|
|
|
|
|
|
|
|
|
|
|
|
|
|
|
|
|
|
|
|
|
|
|
|
|
|
|
|
|
|
|
|
|
|
|
|
|
|
|
|
|
|
|
|
|
|
|
|
|
|
|
|
|
|
|
|
|
|
|
|
|
|
|
|
|
|
|
|
|
|
|
|
|
|
|
|
|
|
|
|
|
|
|
|
|
|
|
|
|
|
|
|
|
|
|
|
|
|
|
|
|
|
|
|
|
|
|
|
|
|
|
|
|
|
|
|
|
|
|
|
|
|
|
|
|
|
|
|
|
|
|
|
|
|
|
|
|
|
|
|
|
|
|
|
|
|
|
|
|
|
|
|
|
|
|
|
|
|
|
|
|
|
|
|
|
|
|
|
|
|
|
|
|
|
|
|
|
|
|
|
|
|
|
|
|
|
|
|
|
|
|
|
|
|
|
|
|
|
|
|
|
|
|
|
|
|
|
|
|
|
|
|
|
|
|
|
|
|
|
|
|
|
|
|
|
|
|
|
|
|
|
|
|
|
|
|
|
|
|
|
|
|
|
|
|
|
|
|
|
|
|
|
|
|
|
|
|
|
|
|
|
|
|
|
|
|
|
|
|
|
|
|
|
|
|
|
|
|
|
|
|
|
|
|
|
|
|
|
|
|
|
|
|
|
|
|
|
|
|
|
|
|
|
|
|
|
|
|
|
|
|
|
|
|
|
|
|
|
|
|
|
|
|
|
|
|
|
|
|
|
|
|
|
|
|
|
|
|
|
|
|
|
|
|
|
|
|
|
|
|
|
|
|
|
|
|
|
|
|
|
|
|
|
|
|
|
|
|
|
|
|
|
|
|
|
|
|
|
|
|
|
|
|
|
|
|
|
|
|
|
|
|
|
|
|
|
|
|
|
|
|
|
|
|
|
|
|
|
|
|
|
|
|
|
|
|
|
|
|
|
|
|
|
|
|
|
|
|
|
|
|
|
|
|
|
|
|
|
|
|
|
|
|
|
|
|
|
|
|
|
|
|
|
|
|
|
|
|
|
|
|
|
|
|
|
|
|
|
|
|
|
|
|
|
|
|
|
|
|
|
|
|
|
|
|
|
|
|
|
|
|
|
|
|
|
|
|
|
|
|
|
|
|
|
|
|
|
|
|
|
|
|
|
|
|
|
|
|
|
|
|
|
|
|
|
|
spaces/Awiny/Image2Paragraph/models/grit_src/third_party/CenterNet2/detectron2/modeling/meta_arch/semantic_seg.py
DELETED
@@ -1,260 +0,0 @@
|
|
1 |
-
# Copyright (c) Facebook, Inc. and its affiliates.
|
2 |
-
import numpy as np
|
3 |
-
from typing import Callable, Dict, Optional, Tuple, Union
|
4 |
-
import fvcore.nn.weight_init as weight_init
|
5 |
-
import torch
|
6 |
-
from torch import nn
|
7 |
-
from torch.nn import functional as F
|
8 |
-
|
9 |
-
from detectron2.config import configurable
|
10 |
-
from detectron2.layers import Conv2d, ShapeSpec, get_norm
|
11 |
-
from detectron2.structures import ImageList
|
12 |
-
from detectron2.utils.registry import Registry
|
13 |
-
|
14 |
-
from ..backbone import Backbone, build_backbone
|
15 |
-
from ..postprocessing import sem_seg_postprocess
|
16 |
-
from .build import META_ARCH_REGISTRY
|
17 |
-
|
18 |
-
__all__ = [
|
19 |
-
"SemanticSegmentor",
|
20 |
-
"SEM_SEG_HEADS_REGISTRY",
|
21 |
-
"SemSegFPNHead",
|
22 |
-
"build_sem_seg_head",
|
23 |
-
]
|
24 |
-
|
25 |
-
|
26 |
-
SEM_SEG_HEADS_REGISTRY = Registry("SEM_SEG_HEADS")
|
27 |
-
SEM_SEG_HEADS_REGISTRY.__doc__ = """
|
28 |
-
Registry for semantic segmentation heads, which make semantic segmentation predictions
|
29 |
-
from feature maps.
|
30 |
-
"""
|
31 |
-
|
32 |
-
|
33 |
-
@META_ARCH_REGISTRY.register()
|
34 |
-
class SemanticSegmentor(nn.Module):
|
35 |
-
"""
|
36 |
-
Main class for semantic segmentation architectures.
|
37 |
-
"""
|
38 |
-
|
39 |
-
@configurable
|
40 |
-
def __init__(
|
41 |
-
self,
|
42 |
-
*,
|
43 |
-
backbone: Backbone,
|
44 |
-
sem_seg_head: nn.Module,
|
45 |
-
pixel_mean: Tuple[float],
|
46 |
-
pixel_std: Tuple[float],
|
47 |
-
):
|
48 |
-
"""
|
49 |
-
Args:
|
50 |
-
backbone: a backbone module, must follow detectron2's backbone interface
|
51 |
-
sem_seg_head: a module that predicts semantic segmentation from backbone features
|
52 |
-
pixel_mean, pixel_std: list or tuple with #channels element, representing
|
53 |
-
the per-channel mean and std to be used to normalize the input image
|
54 |
-
"""
|
55 |
-
super().__init__()
|
56 |
-
self.backbone = backbone
|
57 |
-
self.sem_seg_head = sem_seg_head
|
58 |
-
self.register_buffer("pixel_mean", torch.tensor(pixel_mean).view(-1, 1, 1), False)
|
59 |
-
self.register_buffer("pixel_std", torch.tensor(pixel_std).view(-1, 1, 1), False)
|
60 |
-
|
61 |
-
@classmethod
|
62 |
-
def from_config(cls, cfg):
|
63 |
-
backbone = build_backbone(cfg)
|
64 |
-
sem_seg_head = build_sem_seg_head(cfg, backbone.output_shape())
|
65 |
-
return {
|
66 |
-
"backbone": backbone,
|
67 |
-
"sem_seg_head": sem_seg_head,
|
68 |
-
"pixel_mean": cfg.MODEL.PIXEL_MEAN,
|
69 |
-
"pixel_std": cfg.MODEL.PIXEL_STD,
|
70 |
-
}
|
71 |
-
|
72 |
-
@property
|
73 |
-
def device(self):
|
74 |
-
return self.pixel_mean.device
|
75 |
-
|
76 |
-
def forward(self, batched_inputs):
|
77 |
-
"""
|
78 |
-
Args:
|
79 |
-
batched_inputs: a list, batched outputs of :class:`DatasetMapper`.
|
80 |
-
Each item in the list contains the inputs for one image.
|
81 |
-
|
82 |
-
For now, each item in the list is a dict that contains:
|
83 |
-
|
84 |
-
* "image": Tensor, image in (C, H, W) format.
|
85 |
-
* "sem_seg": semantic segmentation ground truth
|
86 |
-
* Other information that's included in the original dicts, such as:
|
87 |
-
"height", "width" (int): the output resolution of the model (may be different
|
88 |
-
from input resolution), used in inference.
|
89 |
-
|
90 |
-
|
91 |
-
Returns:
|
92 |
-
list[dict]:
|
93 |
-
Each dict is the output for one input image.
|
94 |
-
The dict contains one key "sem_seg" whose value is a
|
95 |
-
Tensor that represents the
|
96 |
-
per-pixel segmentation prediced by the head.
|
97 |
-
The prediction has shape KxHxW that represents the logits of
|
98 |
-
each class for each pixel.
|
99 |
-
"""
|
100 |
-
images = [x["image"].to(self.device) for x in batched_inputs]
|
101 |
-
images = [(x - self.pixel_mean) / self.pixel_std for x in images]
|
102 |
-
images = ImageList.from_tensors(images, self.backbone.size_divisibility)
|
103 |
-
|
104 |
-
features = self.backbone(images.tensor)
|
105 |
-
|
106 |
-
if "sem_seg" in batched_inputs[0]:
|
107 |
-
targets = [x["sem_seg"].to(self.device) for x in batched_inputs]
|
108 |
-
targets = ImageList.from_tensors(
|
109 |
-
targets, self.backbone.size_divisibility, self.sem_seg_head.ignore_value
|
110 |
-
).tensor
|
111 |
-
else:
|
112 |
-
targets = None
|
113 |
-
results, losses = self.sem_seg_head(features, targets)
|
114 |
-
|
115 |
-
if self.training:
|
116 |
-
return losses
|
117 |
-
|
118 |
-
processed_results = []
|
119 |
-
for result, input_per_image, image_size in zip(results, batched_inputs, images.image_sizes):
|
120 |
-
height = input_per_image.get("height", image_size[0])
|
121 |
-
width = input_per_image.get("width", image_size[1])
|
122 |
-
r = sem_seg_postprocess(result, image_size, height, width)
|
123 |
-
processed_results.append({"sem_seg": r})
|
124 |
-
return processed_results
|
125 |
-
|
126 |
-
|
127 |
-
def build_sem_seg_head(cfg, input_shape):
|
128 |
-
"""
|
129 |
-
Build a semantic segmentation head from `cfg.MODEL.SEM_SEG_HEAD.NAME`.
|
130 |
-
"""
|
131 |
-
name = cfg.MODEL.SEM_SEG_HEAD.NAME
|
132 |
-
return SEM_SEG_HEADS_REGISTRY.get(name)(cfg, input_shape)
|
133 |
-
|
134 |
-
|
135 |
-
@SEM_SEG_HEADS_REGISTRY.register()
|
136 |
-
class SemSegFPNHead(nn.Module):
|
137 |
-
"""
|
138 |
-
A semantic segmentation head described in :paper:`PanopticFPN`.
|
139 |
-
It takes a list of FPN features as input, and applies a sequence of
|
140 |
-
3x3 convs and upsampling to scale all of them to the stride defined by
|
141 |
-
``common_stride``. Then these features are added and used to make final
|
142 |
-
predictions by another 1x1 conv layer.
|
143 |
-
"""
|
144 |
-
|
145 |
-
@configurable
|
146 |
-
def __init__(
|
147 |
-
self,
|
148 |
-
input_shape: Dict[str, ShapeSpec],
|
149 |
-
*,
|
150 |
-
num_classes: int,
|
151 |
-
conv_dims: int,
|
152 |
-
common_stride: int,
|
153 |
-
loss_weight: float = 1.0,
|
154 |
-
norm: Optional[Union[str, Callable]] = None,
|
155 |
-
ignore_value: int = -1,
|
156 |
-
):
|
157 |
-
"""
|
158 |
-
NOTE: this interface is experimental.
|
159 |
-
|
160 |
-
Args:
|
161 |
-
input_shape: shapes (channels and stride) of the input features
|
162 |
-
num_classes: number of classes to predict
|
163 |
-
conv_dims: number of output channels for the intermediate conv layers.
|
164 |
-
common_stride: the common stride that all features will be upscaled to
|
165 |
-
loss_weight: loss weight
|
166 |
-
norm (str or callable): normalization for all conv layers
|
167 |
-
ignore_value: category id to be ignored during training.
|
168 |
-
"""
|
169 |
-
super().__init__()
|
170 |
-
input_shape = sorted(input_shape.items(), key=lambda x: x[1].stride)
|
171 |
-
if not len(input_shape):
|
172 |
-
raise ValueError("SemSegFPNHead(input_shape=) cannot be empty!")
|
173 |
-
self.in_features = [k for k, v in input_shape]
|
174 |
-
feature_strides = [v.stride for k, v in input_shape]
|
175 |
-
feature_channels = [v.channels for k, v in input_shape]
|
176 |
-
|
177 |
-
self.ignore_value = ignore_value
|
178 |
-
self.common_stride = common_stride
|
179 |
-
self.loss_weight = loss_weight
|
180 |
-
|
181 |
-
self.scale_heads = []
|
182 |
-
for in_feature, stride, channels in zip(
|
183 |
-
self.in_features, feature_strides, feature_channels
|
184 |
-
):
|
185 |
-
head_ops = []
|
186 |
-
head_length = max(1, int(np.log2(stride) - np.log2(self.common_stride)))
|
187 |
-
for k in range(head_length):
|
188 |
-
norm_module = get_norm(norm, conv_dims)
|
189 |
-
conv = Conv2d(
|
190 |
-
channels if k == 0 else conv_dims,
|
191 |
-
conv_dims,
|
192 |
-
kernel_size=3,
|
193 |
-
stride=1,
|
194 |
-
padding=1,
|
195 |
-
bias=not norm,
|
196 |
-
norm=norm_module,
|
197 |
-
activation=F.relu,
|
198 |
-
)
|
199 |
-
weight_init.c2_msra_fill(conv)
|
200 |
-
head_ops.append(conv)
|
201 |
-
if stride != self.common_stride:
|
202 |
-
head_ops.append(
|
203 |
-
nn.Upsample(scale_factor=2, mode="bilinear", align_corners=False)
|
204 |
-
)
|
205 |
-
self.scale_heads.append(nn.Sequential(*head_ops))
|
206 |
-
self.add_module(in_feature, self.scale_heads[-1])
|
207 |
-
self.predictor = Conv2d(conv_dims, num_classes, kernel_size=1, stride=1, padding=0)
|
208 |
-
weight_init.c2_msra_fill(self.predictor)
|
209 |
-
|
210 |
-
@classmethod
|
211 |
-
def from_config(cls, cfg, input_shape: Dict[str, ShapeSpec]):
|
212 |
-
return {
|
213 |
-
"input_shape": {
|
214 |
-
k: v for k, v in input_shape.items() if k in cfg.MODEL.SEM_SEG_HEAD.IN_FEATURES
|
215 |
-
},
|
216 |
-
"ignore_value": cfg.MODEL.SEM_SEG_HEAD.IGNORE_VALUE,
|
217 |
-
"num_classes": cfg.MODEL.SEM_SEG_HEAD.NUM_CLASSES,
|
218 |
-
"conv_dims": cfg.MODEL.SEM_SEG_HEAD.CONVS_DIM,
|
219 |
-
"common_stride": cfg.MODEL.SEM_SEG_HEAD.COMMON_STRIDE,
|
220 |
-
"norm": cfg.MODEL.SEM_SEG_HEAD.NORM,
|
221 |
-
"loss_weight": cfg.MODEL.SEM_SEG_HEAD.LOSS_WEIGHT,
|
222 |
-
}
|
223 |
-
|
224 |
-
def forward(self, features, targets=None):
|
225 |
-
"""
|
226 |
-
Returns:
|
227 |
-
In training, returns (None, dict of losses)
|
228 |
-
In inference, returns (CxHxW logits, {})
|
229 |
-
"""
|
230 |
-
x = self.layers(features)
|
231 |
-
if self.training:
|
232 |
-
return None, self.losses(x, targets)
|
233 |
-
else:
|
234 |
-
x = F.interpolate(
|
235 |
-
x, scale_factor=self.common_stride, mode="bilinear", align_corners=False
|
236 |
-
)
|
237 |
-
return x, {}
|
238 |
-
|
239 |
-
def layers(self, features):
|
240 |
-
for i, f in enumerate(self.in_features):
|
241 |
-
if i == 0:
|
242 |
-
x = self.scale_heads[i](features[f])
|
243 |
-
else:
|
244 |
-
x = x + self.scale_heads[i](features[f])
|
245 |
-
x = self.predictor(x)
|
246 |
-
return x
|
247 |
-
|
248 |
-
def losses(self, predictions, targets):
|
249 |
-
predictions = predictions.float() # https://github.com/pytorch/pytorch/issues/48163
|
250 |
-
predictions = F.interpolate(
|
251 |
-
predictions,
|
252 |
-
scale_factor=self.common_stride,
|
253 |
-
mode="bilinear",
|
254 |
-
align_corners=False,
|
255 |
-
)
|
256 |
-
loss = F.cross_entropy(
|
257 |
-
predictions, targets, reduction="mean", ignore_index=self.ignore_value
|
258 |
-
)
|
259 |
-
losses = {"loss_sem_seg": loss * self.loss_weight}
|
260 |
-
return losses
|
|
|
|
|
|
|
|
|
|
|
|
|
|
|
|
|
|
|
|
|
|
|
|
|
|
|
|
|
|
|
|
|
|
|
|
|
|
|
|
|
|
|
|
|
|
|
|
|
|
|
|
|
|
|
|
|
|
|
|
|
|
|
|
|
|
|
|
|
|
|
|
|
|
|
|
|
|
|
|
|
|
|
|
|
|
|
|
|
|
|
|
|
|
|
|
|
|
|
|
|
|
|
|
|
|
|
|
|
|
|
|
|
|
|
|
|
|
|
|
|
|
|
|
|
|
|
|
|
|
|
|
|
|
|
|
|
|
|
|
|
|
|
|
|
|
|
|
|
|
|
|
|
|
|
|
|
|
|
|
|
|
|
|
|
|
|
|
|
|
|
|
|
|
|
|
|
|
|
|
|
|
|
|
|
|
|
|
|
|
|
|
|
|
|
|
|
|
|
|
|
|
|
|
|
|
|
|
|
|
|
|
|
|
|
|
|
|
|
|
|
|
|
|
|
|
|
|
|
|
|
|
|
|
|
|
|
|
|
|
|
|
|
|
|
|
|
|
|
|
|
|
|
|
|
|
|
|
|
|
|
|
|
|
|
|
|
|
|
|
|
|
|
|
|
|
|
|
|
|
|
|
|
|
|
|
|
|
|
|
|
|
|
|
|
|
|
|
|
|
|
|
|
|
|
|
|
|
|
|
|
|
|
|
|
|
|
|
|
|
|
|
|
|
|
|
|
|
|
|
|
|
|
|
|
|
|
|
|
|
|
|
|
|
|
|
|
|
|
|
|
|
|
|
|
|
|
|
|
|
|
|
|
|
|
|
|
|
|
|
|
|
|
|
|
|
|
|
|
|
|
|
|
|
|
|
|
|
|
|
|
|
|
|
|
|
|
|
|
|
|
|
|
|
|
|
|
|
|
|
|
|
|
|
|
|
|
|
|
|
|
|
|
|
|
|
|
|
|
|
|
|
|
|
|
|
|
|
|
|
|
|
|
|
|
|
|
|
|
|
|
|
|
|
|
|
|
|
|
|
|
|
|
|
|
|
|
|
|
|
|
|
|
|
|
|
|
|
|
|
|
|
|
|
|
|
|
|
|
|
|
|
|
|
|
|
|
|
|
|
|
|
|
|
|
|
|
|
|
|
|
|
|
|
|
|
|
|
|
|
|
|
spaces/BENE2007/runwayml-stable-diffusion-v1-5/README.md
DELETED
@@ -1,13 +0,0 @@
|
|
1 |
-
---
|
2 |
-
title: Runwayml Stable Diffusion V1 5
|
3 |
-
emoji: 🦀
|
4 |
-
colorFrom: pink
|
5 |
-
colorTo: blue
|
6 |
-
sdk: gradio
|
7 |
-
sdk_version: 3.21.0
|
8 |
-
app_file: app.py
|
9 |
-
pinned: false
|
10 |
-
license: mit
|
11 |
-
---
|
12 |
-
|
13 |
-
Check out the configuration reference at https://huggingface.co/docs/hub/spaces-config-reference
|
|
|
|
|
|
|
|
|
|
|
|
|
|
|
|
|
|
|
|
|
|
|
|
|
|
|
|
spaces/Benson/text-generation/Examples/Descarga Worldbox Desbloqueado Todos.md
DELETED
@@ -1,54 +0,0 @@
|
|
1 |
-
|
2 |
-
<h1>Cómo Descargar WorldBox Desbloqueado Todo - Una Guía para Amantes del Juego Sandbox</h1>
|
3 |
-
<p>Si eres un fan de los juegos de sandbox, es posible que hayas oído hablar de <strong>WorldBox</strong>, un simulador de dios y un juego de sandbox que te permite crear tu propio mundo y verlo crecer. ¿Pero sabías que puedes descargar <strong>WorldBox desbloqueado all</strong>, una versión modded del juego que te da acceso a todas las características premium y contenido gratis? En este artículo, te mostraremos cómo descargar WorldBox desbloqueado todo, y por qué deberías probarlo si te gustan los juegos de sandbox. </p>
|
4 |
-
<h2>descarga worldbox desbloqueado todos</h2><br /><p><b><b>DOWNLOAD</b> 🗸🗸🗸 <a href="https://bltlly.com/2v6IQO">https://bltlly.com/2v6IQO</a></b></p><br /><br />
|
5 |
-
<h2>¿Qué es WorldBox y por qué debe jugar</h2>
|
6 |
-
<h3>WorldBox es un simulador de Dios y un juego de caja de arena</h3>
|
7 |
-
<p>WorldBox es un juego desarrollado por <a href="( 1 )">Maxim Karpenko</a>, un desarrollador de juegos indie de Ucrania. Es un simulador de dios y un juego de sandbox que te permite crear tu propio mundo usando diferentes poderes y herramientas. También puede destruir su mundo usando varios desastres y eventos. Puede jugar WorldBox en su PC, Android o dispositivo iOS. </p>
|
8 |
-
<h3>WorldBox le permite crear, destruir y experimentar con su propio mundo</h3>
|
9 |
-
<p>WorldBox es un juego que te da completa libertad y creatividad para dar forma a tu propio mundo. Puede elegir entre diferentes biomas, terrenos, animales, plantas, razas, civilizaciones, culturas, religiones, guerras, tecnologías, magia y más. También puedes ver cómo evoluciona tu mundo con el tiempo y cómo interactúa con otros mundos. También puedes experimentar con diferentes escenarios y resultados, como qué pasaría si los zombis invadieran tu mundo, o si los alienígenas aterrizaran en tu planeta. </p>
|
10 |
-
<h2>¿Cuáles son los beneficios de descargar WorldBox desbloqueado todo</h2>
|
11 |
-
<h3>WorldBox desbloqueado todo le da acceso a todas las características y contenido premium</h3>
|
12 |
-
|
13 |
-
<h3>WorldBox desbloqueado todo le permite disfrutar del juego sin anuncios o compras en la aplicación</h3>
|
14 |
-
<p>Otro beneficio de descargar WorldBox desbloqueado todo es que se puede disfrutar del juego sin ningún tipo de anuncios o compras en la aplicación. Los anuncios pueden ser molestos y distraer cuando estás jugando un juego, especialmente si aparecen con frecuencia o cubren la pantalla. Las compras en la aplicación también pueden ser tentadoras y costosas si desea obtener más funciones o contenido. Sin embargo, con WorldBox desbloqueado todo, usted no tiene que preocuparse por cualquiera de estos problemas. Puede jugar el juego sin problemas y pacíficamente sin anuncios ni compras en la aplicación. </p>
|
15 |
-
<h2>Cómo descargar WorldBox desbloqueado todo gratis</h2>
|
16 |
-
<h3>Descargar WorldBox desbloqueado todo desde una fuente de confianza</h3>
|
17 |
-
<p>El primer paso para descargar WorldBox desbloqueado todo es encontrar una fuente de confianza que ofrece la versión modificada del juego. Hay muchos sitios web y blogs que dicen ofrecer WorldBox desbloqueados todos, pero algunos de ellos pueden ser falsos, anticuados, o infectados con malware. Por lo tanto, debe tener cuidado y hacer algunas investigaciones antes de descargar nada de Internet. Una de las fuentes de confianza que recomendamos es <a href="">WorldBox Mod APK</a>, un sitio web que proporciona la última y más segura versión de WorldBox desbloqueado todo de forma gratuita. </p>
|
18 |
-
<p></p>
|
19 |
-
<h3>Instalar WorldBox desbloqueado todo en su dispositivo</h3>
|
20 |
-
<p>El siguiente paso para descargar WorldBox desbloqueado todo es instalarlo en su dispositivo. Dependiendo del dispositivo que esté utilizando, el proceso de instalación puede variar ligeramente. Estos son los pasos generales a seguir:</p>
|
21 |
-
<ul>
|
22 |
-
<li>Descargar WorldBox desbloqueado todos los archivos de la fuente de confianza. </li>
|
23 |
-
<li>Busque el archivo en su dispositivo y toque en él para iniciar la instalación. </li>
|
24 |
-
<li>Si está utilizando un dispositivo Android, es posible que necesite habilitar la opción "Fuentes desconocidas" en su configuración para permitir la instalación de aplicaciones desde fuera de la Google Play Store.</li>
|
25 |
-
|
26 |
-
<li>Siga las instrucciones en la pantalla para completar la instalación. </li>
|
27 |
-
</ul>
|
28 |
-
<h3>Inicie WorldBox desbloqueado todo y comience a jugar</h3>
|
29 |
-
<p>El paso final para descargar WorldBox desbloqueado todo es lanzarlo y comenzar a jugar. Puede encontrar el icono de la aplicación en la pantalla de inicio o en el cajón de la aplicación. Toque en él para abrir el juego y disfrutar de todas las características premium y el contenido de forma gratuita. También puede buscar actualizaciones regularmente para obtener la última versión de WorldBox desbloqueado todo. </p>
|
30 |
-
<h2>Consejos y trucos para jugar WorldBox desbloqueado todo</h2>
|
31 |
-
<h3>Usa diferentes poderes y herramientas para dar forma a tu mundo</h3>
|
32 |
-
<p>Uno de los aspectos divertidos de jugar WorldBox desbloqueado todo es que usted puede utilizar diferentes poderes y herramientas para dar forma a su mundo. Puede crear montañas, lagos, bosques, desiertos, islas, volcanes y más. También puedes engendrar diferentes animales, plantas, razas, civilizaciones y culturas. También puede utilizar diferentes desastres y eventos para destruir su mundo o hacerlo más interesante. Puedes usar poderes como lluvia ácida, meteoritos, tornados, terremotos, armas nucleares, zombis, alienígenas, dragones y más. </p>
|
33 |
-
<h3>Vea cómo su mundo evoluciona e interactúa con otros mundos</h3>
|
34 |
-
<p>Otro aspecto divertido de jugar WorldBox desbloqueado todo es que usted puede ver cómo su mundo evoluciona e interactúa con otros mundos. Pueden ver cómo su mundo cambia con el tiempo y cómo desarrolla su propia historia, cultura, religión, tecnología, magia y más. También puedes ver cómo interactúa tu mundo con otros mundos que creas o descargas de otros jugadores. Puedes ver cómo negocian, luchan, se alían o se fusionan entre sí. </p>
|
35 |
-
<h3>Comparte tu mundo con otros jugadores y explora sus mundos</h3>
|
36 |
-
|
37 |
-
<h2>Conclusión</h2>
|
38 |
-
<p>WorldBox es un simulador de dios y un juego de sandbox que te permite crear tu propio mundo y verlo crecer. Es un juego que te da completa libertad y creatividad para dar forma a tu propio mundo. Sin embargo, si quieres disfrutar del juego sin limitaciones ni interrupciones, debes descargar WorldBox desbloqueado todo, una versión modificada del juego que te da acceso a todas las características premium y contenido gratis. En este artículo, le mostramos cómo descargar WorldBox desbloqueado todo desde una fuente de confianza, cómo instalarlo en su dispositivo, y cómo jugar con consejos y trucos. Esperamos que haya encontrado este artículo útil e informativo. ¡Ahora siga adelante y descargue WorldBox desbloqueado todo y diviértase creando su propio mundo! </p>
|
39 |
-
<h2>Preguntas frecuentes</h2>
|
40 |
-
<ol>
|
41 |
-
<li><strong>¿Qué es WorldBox? </strong><br>
|
42 |
-
WorldBox es un simulador de dios y un juego de sandbox que te permite crear tu propio mundo usando diferentes poderes y herramientas. </li>
|
43 |
-
<li><strong>¿Qué es WorldBox desbloqueado todo? </strong><br>
|
44 |
-
WorldBox Unlocked All es una versión modded del juego que te da acceso a todas las funciones premium y contenido gratis. </li>
|
45 |
-
<li><strong>Cómo descargar WorldBox desbloqueado todo? </strong><br>
|
46 |
-
Puede descargar WorldBox Unlocked All desde una fuente de confianza como <a href="">WorldBox Mod APK</a>, luego instalarlo en su dispositivo y lanzarlo. </li <li><strong>Es WorldBox desbloqueado todo seguro para descargar y jugar? </strong><br>
|
47 |
-
WorldBox Desbloqueado Todo es seguro para descargar y jugar si lo obtiene de una fuente de confianza como <a href="">WorldBox Mod APK</a>. Sin embargo, siempre debe tener cuidado y hacer algunas investigaciones antes de descargar nada de Internet. </li>
|
48 |
-
<li><strong>¿Cuáles son las características de WorldBox desbloqueado todo? </strong><br>
|
49 |
-
WorldBox Unlocked All te da acceso a todas las funciones y contenido premium del juego, como poderes, herramientas, carreras, animales, eventos, skins, mapas y más. También te permite disfrutar del juego sin anuncios ni compras en la aplicación. </li>
|
50 |
-
|
51 |
-
Puede actualizar WorldBox Unlocked All comprobando si hay actualizaciones regularmente en el sitio web o la aplicación de origen de confianza. También puedes seguir las cuentas de redes sociales o el blog del desarrollador para obtener las últimas noticias y actualizaciones. </li>
|
52 |
-
</ol></p> 64aa2da5cf<br />
|
53 |
-
<br />
|
54 |
-
<br />
|
|
|
|
|
|
|
|
|
|
|
|
|
|
|
|
|
|
|
|
|
|
|
|
|
|
|
|
|
|
|
|
|
|
|
|
|
|
|
|
|
|
|
|
|
|
|
|
|
|
|
|
|
|
|
|
|
|
|
|
|
|
|
|
|
|
|
|
|
|
|
|
|
|
|
|
|
|
|
|
|
|
|
|
|
|
|
|
|
|
|
|
|
|
|
|
|
|
|
|
|
|
|
|
|
|
|
|
|
|
spaces/Big-Web/MMSD/env/Lib/site-packages/pip/_vendor/chardet/gb2312freq.py
DELETED
@@ -1,284 +0,0 @@
|
|
1 |
-
######################## BEGIN LICENSE BLOCK ########################
|
2 |
-
# The Original Code is Mozilla Communicator client code.
|
3 |
-
#
|
4 |
-
# The Initial Developer of the Original Code is
|
5 |
-
# Netscape Communications Corporation.
|
6 |
-
# Portions created by the Initial Developer are Copyright (C) 1998
|
7 |
-
# the Initial Developer. All Rights Reserved.
|
8 |
-
#
|
9 |
-
# Contributor(s):
|
10 |
-
# Mark Pilgrim - port to Python
|
11 |
-
#
|
12 |
-
# This library is free software; you can redistribute it and/or
|
13 |
-
# modify it under the terms of the GNU Lesser General Public
|
14 |
-
# License as published by the Free Software Foundation; either
|
15 |
-
# version 2.1 of the License, or (at your option) any later version.
|
16 |
-
#
|
17 |
-
# This library is distributed in the hope that it will be useful,
|
18 |
-
# but WITHOUT ANY WARRANTY; without even the implied warranty of
|
19 |
-
# MERCHANTABILITY or FITNESS FOR A PARTICULAR PURPOSE. See the GNU
|
20 |
-
# Lesser General Public License for more details.
|
21 |
-
#
|
22 |
-
# You should have received a copy of the GNU Lesser General Public
|
23 |
-
# License along with this library; if not, write to the Free Software
|
24 |
-
# Foundation, Inc., 51 Franklin St, Fifth Floor, Boston, MA
|
25 |
-
# 02110-1301 USA
|
26 |
-
######################### END LICENSE BLOCK #########################
|
27 |
-
|
28 |
-
# GB2312 most frequently used character table
|
29 |
-
#
|
30 |
-
# Char to FreqOrder table , from hz6763
|
31 |
-
|
32 |
-
# 512 --> 0.79 -- 0.79
|
33 |
-
# 1024 --> 0.92 -- 0.13
|
34 |
-
# 2048 --> 0.98 -- 0.06
|
35 |
-
# 6768 --> 1.00 -- 0.02
|
36 |
-
#
|
37 |
-
# Ideal Distribution Ratio = 0.79135/(1-0.79135) = 3.79
|
38 |
-
# Random Distribution Ration = 512 / (3755 - 512) = 0.157
|
39 |
-
#
|
40 |
-
# Typical Distribution Ratio about 25% of Ideal one, still much higher that RDR
|
41 |
-
|
42 |
-
GB2312_TYPICAL_DISTRIBUTION_RATIO = 0.9
|
43 |
-
|
44 |
-
GB2312_TABLE_SIZE = 3760
|
45 |
-
|
46 |
-
# fmt: off
|
47 |
-
GB2312_CHAR_TO_FREQ_ORDER = (
|
48 |
-
1671, 749,1443,2364,3924,3807,2330,3921,1704,3463,2691,1511,1515, 572,3191,2205,
|
49 |
-
2361, 224,2558, 479,1711, 963,3162, 440,4060,1905,2966,2947,3580,2647,3961,3842,
|
50 |
-
2204, 869,4207, 970,2678,5626,2944,2956,1479,4048, 514,3595, 588,1346,2820,3409,
|
51 |
-
249,4088,1746,1873,2047,1774, 581,1813, 358,1174,3590,1014,1561,4844,2245, 670,
|
52 |
-
1636,3112, 889,1286, 953, 556,2327,3060,1290,3141, 613, 185,3477,1367, 850,3820,
|
53 |
-
1715,2428,2642,2303,2732,3041,2562,2648,3566,3946,1349, 388,3098,2091,1360,3585,
|
54 |
-
152,1687,1539, 738,1559, 59,1232,2925,2267,1388,1249,1741,1679,2960, 151,1566,
|
55 |
-
1125,1352,4271, 924,4296, 385,3166,4459, 310,1245,2850, 70,3285,2729,3534,3575,
|
56 |
-
2398,3298,3466,1960,2265, 217,3647, 864,1909,2084,4401,2773,1010,3269,5152, 853,
|
57 |
-
3051,3121,1244,4251,1895, 364,1499,1540,2313,1180,3655,2268, 562, 715,2417,3061,
|
58 |
-
544, 336,3768,2380,1752,4075, 950, 280,2425,4382, 183,2759,3272, 333,4297,2155,
|
59 |
-
1688,2356,1444,1039,4540, 736,1177,3349,2443,2368,2144,2225, 565, 196,1482,3406,
|
60 |
-
927,1335,4147, 692, 878,1311,1653,3911,3622,1378,4200,1840,2969,3149,2126,1816,
|
61 |
-
2534,1546,2393,2760, 737,2494, 13, 447, 245,2747, 38,2765,2129,2589,1079, 606,
|
62 |
-
360, 471,3755,2890, 404, 848, 699,1785,1236, 370,2221,1023,3746,2074,2026,2023,
|
63 |
-
2388,1581,2119, 812,1141,3091,2536,1519, 804,2053, 406,1596,1090, 784, 548,4414,
|
64 |
-
1806,2264,2936,1100, 343,4114,5096, 622,3358, 743,3668,1510,1626,5020,3567,2513,
|
65 |
-
3195,4115,5627,2489,2991, 24,2065,2697,1087,2719, 48,1634, 315, 68, 985,2052,
|
66 |
-
198,2239,1347,1107,1439, 597,2366,2172, 871,3307, 919,2487,2790,1867, 236,2570,
|
67 |
-
1413,3794, 906,3365,3381,1701,1982,1818,1524,2924,1205, 616,2586,2072,2004, 575,
|
68 |
-
253,3099, 32,1365,1182, 197,1714,2454,1201, 554,3388,3224,2748, 756,2587, 250,
|
69 |
-
2567,1507,1517,3529,1922,2761,2337,3416,1961,1677,2452,2238,3153, 615, 911,1506,
|
70 |
-
1474,2495,1265,1906,2749,3756,3280,2161, 898,2714,1759,3450,2243,2444, 563, 26,
|
71 |
-
3286,2266,3769,3344,2707,3677, 611,1402, 531,1028,2871,4548,1375, 261,2948, 835,
|
72 |
-
1190,4134, 353, 840,2684,1900,3082,1435,2109,1207,1674, 329,1872,2781,4055,2686,
|
73 |
-
2104, 608,3318,2423,2957,2768,1108,3739,3512,3271,3985,2203,1771,3520,1418,2054,
|
74 |
-
1681,1153, 225,1627,2929, 162,2050,2511,3687,1954, 124,1859,2431,1684,3032,2894,
|
75 |
-
585,4805,3969,2869,2704,2088,2032,2095,3656,2635,4362,2209, 256, 518,2042,2105,
|
76 |
-
3777,3657, 643,2298,1148,1779, 190, 989,3544, 414, 11,2135,2063,2979,1471, 403,
|
77 |
-
3678, 126, 770,1563, 671,2499,3216,2877, 600,1179, 307,2805,4937,1268,1297,2694,
|
78 |
-
252,4032,1448,1494,1331,1394, 127,2256, 222,1647,1035,1481,3056,1915,1048, 873,
|
79 |
-
3651, 210, 33,1608,2516, 200,1520, 415, 102, 0,3389,1287, 817, 91,3299,2940,
|
80 |
-
836,1814, 549,2197,1396,1669,2987,3582,2297,2848,4528,1070, 687, 20,1819, 121,
|
81 |
-
1552,1364,1461,1968,2617,3540,2824,2083, 177, 948,4938,2291, 110,4549,2066, 648,
|
82 |
-
3359,1755,2110,2114,4642,4845,1693,3937,3308,1257,1869,2123, 208,1804,3159,2992,
|
83 |
-
2531,2549,3361,2418,1350,2347,2800,2568,1291,2036,2680, 72, 842,1990, 212,1233,
|
84 |
-
1154,1586, 75,2027,3410,4900,1823,1337,2710,2676, 728,2810,1522,3026,4995, 157,
|
85 |
-
755,1050,4022, 710, 785,1936,2194,2085,1406,2777,2400, 150,1250,4049,1206, 807,
|
86 |
-
1910, 534, 529,3309,1721,1660, 274, 39,2827, 661,2670,1578, 925,3248,3815,1094,
|
87 |
-
4278,4901,4252, 41,1150,3747,2572,2227,4501,3658,4902,3813,3357,3617,2884,2258,
|
88 |
-
887, 538,4187,3199,1294,2439,3042,2329,2343,2497,1255, 107, 543,1527, 521,3478,
|
89 |
-
3568, 194,5062, 15, 961,3870,1241,1192,2664, 66,5215,3260,2111,1295,1127,2152,
|
90 |
-
3805,4135, 901,1164,1976, 398,1278, 530,1460, 748, 904,1054,1966,1426, 53,2909,
|
91 |
-
509, 523,2279,1534, 536,1019, 239,1685, 460,2353, 673,1065,2401,3600,4298,2272,
|
92 |
-
1272,2363, 284,1753,3679,4064,1695, 81, 815,2677,2757,2731,1386, 859, 500,4221,
|
93 |
-
2190,2566, 757,1006,2519,2068,1166,1455, 337,2654,3203,1863,1682,1914,3025,1252,
|
94 |
-
1409,1366, 847, 714,2834,2038,3209, 964,2970,1901, 885,2553,1078,1756,3049, 301,
|
95 |
-
1572,3326, 688,2130,1996,2429,1805,1648,2930,3421,2750,3652,3088, 262,1158,1254,
|
96 |
-
389,1641,1812, 526,1719, 923,2073,1073,1902, 468, 489,4625,1140, 857,2375,3070,
|
97 |
-
3319,2863, 380, 116,1328,2693,1161,2244, 273,1212,1884,2769,3011,1775,1142, 461,
|
98 |
-
3066,1200,2147,2212, 790, 702,2695,4222,1601,1058, 434,2338,5153,3640, 67,2360,
|
99 |
-
4099,2502, 618,3472,1329, 416,1132, 830,2782,1807,2653,3211,3510,1662, 192,2124,
|
100 |
-
296,3979,1739,1611,3684, 23, 118, 324, 446,1239,1225, 293,2520,3814,3795,2535,
|
101 |
-
3116, 17,1074, 467,2692,2201, 387,2922, 45,1326,3055,1645,3659,2817, 958, 243,
|
102 |
-
1903,2320,1339,2825,1784,3289, 356, 576, 865,2315,2381,3377,3916,1088,3122,1713,
|
103 |
-
1655, 935, 628,4689,1034,1327, 441, 800, 720, 894,1979,2183,1528,5289,2702,1071,
|
104 |
-
4046,3572,2399,1571,3281, 79, 761,1103, 327, 134, 758,1899,1371,1615, 879, 442,
|
105 |
-
215,2605,2579, 173,2048,2485,1057,2975,3317,1097,2253,3801,4263,1403,1650,2946,
|
106 |
-
814,4968,3487,1548,2644,1567,1285, 2, 295,2636, 97, 946,3576, 832, 141,4257,
|
107 |
-
3273, 760,3821,3521,3156,2607, 949,1024,1733,1516,1803,1920,2125,2283,2665,3180,
|
108 |
-
1501,2064,3560,2171,1592, 803,3518,1416, 732,3897,4258,1363,1362,2458, 119,1427,
|
109 |
-
602,1525,2608,1605,1639,3175, 694,3064, 10, 465, 76,2000,4846,4208, 444,3781,
|
110 |
-
1619,3353,2206,1273,3796, 740,2483, 320,1723,2377,3660,2619,1359,1137,1762,1724,
|
111 |
-
2345,2842,1850,1862, 912, 821,1866, 612,2625,1735,2573,3369,1093, 844, 89, 937,
|
112 |
-
930,1424,3564,2413,2972,1004,3046,3019,2011, 711,3171,1452,4178, 428, 801,1943,
|
113 |
-
432, 445,2811, 206,4136,1472, 730, 349, 73, 397,2802,2547, 998,1637,1167, 789,
|
114 |
-
396,3217, 154,1218, 716,1120,1780,2819,4826,1931,3334,3762,2139,1215,2627, 552,
|
115 |
-
3664,3628,3232,1405,2383,3111,1356,2652,3577,3320,3101,1703, 640,1045,1370,1246,
|
116 |
-
4996, 371,1575,2436,1621,2210, 984,4033,1734,2638, 16,4529, 663,2755,3255,1451,
|
117 |
-
3917,2257,1253,1955,2234,1263,2951, 214,1229, 617, 485, 359,1831,1969, 473,2310,
|
118 |
-
750,2058, 165, 80,2864,2419, 361,4344,2416,2479,1134, 796,3726,1266,2943, 860,
|
119 |
-
2715, 938, 390,2734,1313,1384, 248, 202, 877,1064,2854, 522,3907, 279,1602, 297,
|
120 |
-
2357, 395,3740, 137,2075, 944,4089,2584,1267,3802, 62,1533,2285, 178, 176, 780,
|
121 |
-
2440, 201,3707, 590, 478,1560,4354,2117,1075, 30, 74,4643,4004,1635,1441,2745,
|
122 |
-
776,2596, 238,1077,1692,1912,2844, 605, 499,1742,3947, 241,3053, 980,1749, 936,
|
123 |
-
2640,4511,2582, 515,1543,2162,5322,2892,2993, 890,2148,1924, 665,1827,3581,1032,
|
124 |
-
968,3163, 339,1044,1896, 270, 583,1791,1720,4367,1194,3488,3669, 43,2523,1657,
|
125 |
-
163,2167, 290,1209,1622,3378, 550, 634,2508,2510, 695,2634,2384,2512,1476,1414,
|
126 |
-
220,1469,2341,2138,2852,3183,2900,4939,2865,3502,1211,3680, 854,3227,1299,2976,
|
127 |
-
3172, 186,2998,1459, 443,1067,3251,1495, 321,1932,3054, 909, 753,1410,1828, 436,
|
128 |
-
2441,1119,1587,3164,2186,1258, 227, 231,1425,1890,3200,3942, 247, 959, 725,5254,
|
129 |
-
2741, 577,2158,2079, 929, 120, 174, 838,2813, 591,1115, 417,2024, 40,3240,1536,
|
130 |
-
1037, 291,4151,2354, 632,1298,2406,2500,3535,1825,1846,3451, 205,1171, 345,4238,
|
131 |
-
18,1163, 811, 685,2208,1217, 425,1312,1508,1175,4308,2552,1033, 587,1381,3059,
|
132 |
-
2984,3482, 340,1316,4023,3972, 792,3176, 519, 777,4690, 918, 933,4130,2981,3741,
|
133 |
-
90,3360,2911,2200,5184,4550, 609,3079,2030, 272,3379,2736, 363,3881,1130,1447,
|
134 |
-
286, 779, 357,1169,3350,3137,1630,1220,2687,2391, 747,1277,3688,2618,2682,2601,
|
135 |
-
1156,3196,5290,4034,3102,1689,3596,3128, 874, 219,2783, 798, 508,1843,2461, 269,
|
136 |
-
1658,1776,1392,1913,2983,3287,2866,2159,2372, 829,4076, 46,4253,2873,1889,1894,
|
137 |
-
915,1834,1631,2181,2318, 298, 664,2818,3555,2735, 954,3228,3117, 527,3511,2173,
|
138 |
-
681,2712,3033,2247,2346,3467,1652, 155,2164,3382, 113,1994, 450, 899, 494, 994,
|
139 |
-
1237,2958,1875,2336,1926,3727, 545,1577,1550, 633,3473, 204,1305,3072,2410,1956,
|
140 |
-
2471, 707,2134, 841,2195,2196,2663,3843,1026,4940, 990,3252,4997, 368,1092, 437,
|
141 |
-
3212,3258,1933,1829, 675,2977,2893, 412, 943,3723,4644,3294,3283,2230,2373,5154,
|
142 |
-
2389,2241,2661,2323,1404,2524, 593, 787, 677,3008,1275,2059, 438,2709,2609,2240,
|
143 |
-
2269,2246,1446, 36,1568,1373,3892,1574,2301,1456,3962, 693,2276,5216,2035,1143,
|
144 |
-
2720,1919,1797,1811,2763,4137,2597,1830,1699,1488,1198,2090, 424,1694, 312,3634,
|
145 |
-
3390,4179,3335,2252,1214, 561,1059,3243,2295,2561, 975,5155,2321,2751,3772, 472,
|
146 |
-
1537,3282,3398,1047,2077,2348,2878,1323,3340,3076, 690,2906, 51, 369, 170,3541,
|
147 |
-
1060,2187,2688,3670,2541,1083,1683, 928,3918, 459, 109,4427, 599,3744,4286, 143,
|
148 |
-
2101,2730,2490, 82,1588,3036,2121, 281,1860, 477,4035,1238,2812,3020,2716,3312,
|
149 |
-
1530,2188,2055,1317, 843, 636,1808,1173,3495, 649, 181,1002, 147,3641,1159,2414,
|
150 |
-
3750,2289,2795, 813,3123,2610,1136,4368, 5,3391,4541,2174, 420, 429,1728, 754,
|
151 |
-
1228,2115,2219, 347,2223,2733, 735,1518,3003,2355,3134,1764,3948,3329,1888,2424,
|
152 |
-
1001,1234,1972,3321,3363,1672,1021,1450,1584, 226, 765, 655,2526,3404,3244,2302,
|
153 |
-
3665, 731, 594,2184, 319,1576, 621, 658,2656,4299,2099,3864,1279,2071,2598,2739,
|
154 |
-
795,3086,3699,3908,1707,2352,2402,1382,3136,2475,1465,4847,3496,3865,1085,3004,
|
155 |
-
2591,1084, 213,2287,1963,3565,2250, 822, 793,4574,3187,1772,1789,3050, 595,1484,
|
156 |
-
1959,2770,1080,2650, 456, 422,2996, 940,3322,4328,4345,3092,2742, 965,2784, 739,
|
157 |
-
4124, 952,1358,2498,2949,2565, 332,2698,2378, 660,2260,2473,4194,3856,2919, 535,
|
158 |
-
1260,2651,1208,1428,1300,1949,1303,2942, 433,2455,2450,1251,1946, 614,1269, 641,
|
159 |
-
1306,1810,2737,3078,2912, 564,2365,1419,1415,1497,4460,2367,2185,1379,3005,1307,
|
160 |
-
3218,2175,1897,3063, 682,1157,4040,4005,1712,1160,1941,1399, 394, 402,2952,1573,
|
161 |
-
1151,2986,2404, 862, 299,2033,1489,3006, 346, 171,2886,3401,1726,2932, 168,2533,
|
162 |
-
47,2507,1030,3735,1145,3370,1395,1318,1579,3609,4560,2857,4116,1457,2529,1965,
|
163 |
-
504,1036,2690,2988,2405, 745,5871, 849,2397,2056,3081, 863,2359,3857,2096, 99,
|
164 |
-
1397,1769,2300,4428,1643,3455,1978,1757,3718,1440, 35,4879,3742,1296,4228,2280,
|
165 |
-
160,5063,1599,2013, 166, 520,3479,1646,3345,3012, 490,1937,1545,1264,2182,2505,
|
166 |
-
1096,1188,1369,1436,2421,1667,2792,2460,1270,2122, 727,3167,2143, 806,1706,1012,
|
167 |
-
1800,3037, 960,2218,1882, 805, 139,2456,1139,1521, 851,1052,3093,3089, 342,2039,
|
168 |
-
744,5097,1468,1502,1585,2087, 223, 939, 326,2140,2577, 892,2481,1623,4077, 982,
|
169 |
-
3708, 135,2131, 87,2503,3114,2326,1106, 876,1616, 547,2997,2831,2093,3441,4530,
|
170 |
-
4314, 9,3256,4229,4148, 659,1462,1986,1710,2046,2913,2231,4090,4880,5255,3392,
|
171 |
-
3274,1368,3689,4645,1477, 705,3384,3635,1068,1529,2941,1458,3782,1509, 100,1656,
|
172 |
-
2548, 718,2339, 408,1590,2780,3548,1838,4117,3719,1345,3530, 717,3442,2778,3220,
|
173 |
-
2898,1892,4590,3614,3371,2043,1998,1224,3483, 891, 635, 584,2559,3355, 733,1766,
|
174 |
-
1729,1172,3789,1891,2307, 781,2982,2271,1957,1580,5773,2633,2005,4195,3097,1535,
|
175 |
-
3213,1189,1934,5693,3262, 586,3118,1324,1598, 517,1564,2217,1868,1893,4445,3728,
|
176 |
-
2703,3139,1526,1787,1992,3882,2875,1549,1199,1056,2224,1904,2711,5098,4287, 338,
|
177 |
-
1993,3129,3489,2689,1809,2815,1997, 957,1855,3898,2550,3275,3057,1105,1319, 627,
|
178 |
-
1505,1911,1883,3526, 698,3629,3456,1833,1431, 746, 77,1261,2017,2296,1977,1885,
|
179 |
-
125,1334,1600, 525,1798,1109,2222,1470,1945, 559,2236,1186,3443,2476,1929,1411,
|
180 |
-
2411,3135,1777,3372,2621,1841,1613,3229, 668,1430,1839,2643,2916, 195,1989,2671,
|
181 |
-
2358,1387, 629,3205,2293,5256,4439, 123,1310, 888,1879,4300,3021,3605,1003,1162,
|
182 |
-
3192,2910,2010, 140,2395,2859, 55,1082,2012,2901, 662, 419,2081,1438, 680,2774,
|
183 |
-
4654,3912,1620,1731,1625,5035,4065,2328, 512,1344, 802,5443,2163,2311,2537, 524,
|
184 |
-
3399, 98,1155,2103,1918,2606,3925,2816,1393,2465,1504,3773,2177,3963,1478,4346,
|
185 |
-
180,1113,4655,3461,2028,1698, 833,2696,1235,1322,1594,4408,3623,3013,3225,2040,
|
186 |
-
3022, 541,2881, 607,3632,2029,1665,1219, 639,1385,1686,1099,2803,3231,1938,3188,
|
187 |
-
2858, 427, 676,2772,1168,2025, 454,3253,2486,3556, 230,1950, 580, 791,1991,1280,
|
188 |
-
1086,1974,2034, 630, 257,3338,2788,4903,1017, 86,4790, 966,2789,1995,1696,1131,
|
189 |
-
259,3095,4188,1308, 179,1463,5257, 289,4107,1248, 42,3413,1725,2288, 896,1947,
|
190 |
-
774,4474,4254, 604,3430,4264, 392,2514,2588, 452, 237,1408,3018, 988,4531,1970,
|
191 |
-
3034,3310, 540,2370,1562,1288,2990, 502,4765,1147, 4,1853,2708, 207, 294,2814,
|
192 |
-
4078,2902,2509, 684, 34,3105,3532,2551, 644, 709,2801,2344, 573,1727,3573,3557,
|
193 |
-
2021,1081,3100,4315,2100,3681, 199,2263,1837,2385, 146,3484,1195,2776,3949, 997,
|
194 |
-
1939,3973,1008,1091,1202,1962,1847,1149,4209,5444,1076, 493, 117,5400,2521, 972,
|
195 |
-
1490,2934,1796,4542,2374,1512,2933,2657, 413,2888,1135,2762,2314,2156,1355,2369,
|
196 |
-
766,2007,2527,2170,3124,2491,2593,2632,4757,2437, 234,3125,3591,1898,1750,1376,
|
197 |
-
1942,3468,3138, 570,2127,2145,3276,4131, 962, 132,1445,4196, 19, 941,3624,3480,
|
198 |
-
3366,1973,1374,4461,3431,2629, 283,2415,2275, 808,2887,3620,2112,2563,1353,3610,
|
199 |
-
955,1089,3103,1053, 96, 88,4097, 823,3808,1583, 399, 292,4091,3313, 421,1128,
|
200 |
-
642,4006, 903,2539,1877,2082, 596, 29,4066,1790, 722,2157, 130, 995,1569, 769,
|
201 |
-
1485, 464, 513,2213, 288,1923,1101,2453,4316, 133, 486,2445, 50, 625, 487,2207,
|
202 |
-
57, 423, 481,2962, 159,3729,1558, 491, 303, 482, 501, 240,2837, 112,3648,2392,
|
203 |
-
1783, 362, 8,3433,3422, 610,2793,3277,1390,1284,1654, 21,3823, 734, 367, 623,
|
204 |
-
193, 287, 374,1009,1483, 816, 476, 313,2255,2340,1262,2150,2899,1146,2581, 782,
|
205 |
-
2116,1659,2018,1880, 255,3586,3314,1110,2867,2137,2564, 986,2767,5185,2006, 650,
|
206 |
-
158, 926, 762, 881,3157,2717,2362,3587, 306,3690,3245,1542,3077,2427,1691,2478,
|
207 |
-
2118,2985,3490,2438, 539,2305, 983, 129,1754, 355,4201,2386, 827,2923, 104,1773,
|
208 |
-
2838,2771, 411,2905,3919, 376, 767, 122,1114, 828,2422,1817,3506, 266,3460,1007,
|
209 |
-
1609,4998, 945,2612,4429,2274, 726,1247,1964,2914,2199,2070,4002,4108, 657,3323,
|
210 |
-
1422, 579, 455,2764,4737,1222,2895,1670, 824,1223,1487,2525, 558, 861,3080, 598,
|
211 |
-
2659,2515,1967, 752,2583,2376,2214,4180, 977, 704,2464,4999,2622,4109,1210,2961,
|
212 |
-
819,1541, 142,2284, 44, 418, 457,1126,3730,4347,4626,1644,1876,3671,1864, 302,
|
213 |
-
1063,5694, 624, 723,1984,3745,1314,1676,2488,1610,1449,3558,3569,2166,2098, 409,
|
214 |
-
1011,2325,3704,2306, 818,1732,1383,1824,1844,3757, 999,2705,3497,1216,1423,2683,
|
215 |
-
2426,2954,2501,2726,2229,1475,2554,5064,1971,1794,1666,2014,1343, 783, 724, 191,
|
216 |
-
2434,1354,2220,5065,1763,2752,2472,4152, 131, 175,2885,3434, 92,1466,4920,2616,
|
217 |
-
3871,3872,3866, 128,1551,1632, 669,1854,3682,4691,4125,1230, 188,2973,3290,1302,
|
218 |
-
1213, 560,3266, 917, 763,3909,3249,1760, 868,1958, 764,1782,2097, 145,2277,3774,
|
219 |
-
4462, 64,1491,3062, 971,2132,3606,2442, 221,1226,1617, 218, 323,1185,3207,3147,
|
220 |
-
571, 619,1473,1005,1744,2281, 449,1887,2396,3685, 275, 375,3816,1743,3844,3731,
|
221 |
-
845,1983,2350,4210,1377, 773, 967,3499,3052,3743,2725,4007,1697,1022,3943,1464,
|
222 |
-
3264,2855,2722,1952,1029,2839,2467, 84,4383,2215, 820,1391,2015,2448,3672, 377,
|
223 |
-
1948,2168, 797,2545,3536,2578,2645, 94,2874,1678, 405,1259,3071, 771, 546,1315,
|
224 |
-
470,1243,3083, 895,2468, 981, 969,2037, 846,4181, 653,1276,2928, 14,2594, 557,
|
225 |
-
3007,2474, 156, 902,1338,1740,2574, 537,2518, 973,2282,2216,2433,1928, 138,2903,
|
226 |
-
1293,2631,1612, 646,3457, 839,2935, 111, 496,2191,2847, 589,3186, 149,3994,2060,
|
227 |
-
4031,2641,4067,3145,1870, 37,3597,2136,1025,2051,3009,3383,3549,1121,1016,3261,
|
228 |
-
1301, 251,2446,2599,2153, 872,3246, 637, 334,3705, 831, 884, 921,3065,3140,4092,
|
229 |
-
2198,1944, 246,2964, 108,2045,1152,1921,2308,1031, 203,3173,4170,1907,3890, 810,
|
230 |
-
1401,2003,1690, 506, 647,1242,2828,1761,1649,3208,2249,1589,3709,2931,5156,1708,
|
231 |
-
498, 666,2613, 834,3817,1231, 184,2851,1124, 883,3197,2261,3710,1765,1553,2658,
|
232 |
-
1178,2639,2351, 93,1193, 942,2538,2141,4402, 235,1821, 870,1591,2192,1709,1871,
|
233 |
-
3341,1618,4126,2595,2334, 603, 651, 69, 701, 268,2662,3411,2555,1380,1606, 503,
|
234 |
-
448, 254,2371,2646, 574,1187,2309,1770, 322,2235,1292,1801, 305, 566,1133, 229,
|
235 |
-
2067,2057, 706, 167, 483,2002,2672,3295,1820,3561,3067, 316, 378,2746,3452,1112,
|
236 |
-
136,1981, 507,1651,2917,1117, 285,4591, 182,2580,3522,1304, 335,3303,1835,2504,
|
237 |
-
1795,1792,2248, 674,1018,2106,2449,1857,2292,2845, 976,3047,1781,2600,2727,1389,
|
238 |
-
1281, 52,3152, 153, 265,3950, 672,3485,3951,4463, 430,1183, 365, 278,2169, 27,
|
239 |
-
1407,1336,2304, 209,1340,1730,2202,1852,2403,2883, 979,1737,1062, 631,2829,2542,
|
240 |
-
3876,2592, 825,2086,2226,3048,3625, 352,1417,3724, 542, 991, 431,1351,3938,1861,
|
241 |
-
2294, 826,1361,2927,3142,3503,1738, 463,2462,2723, 582,1916,1595,2808, 400,3845,
|
242 |
-
3891,2868,3621,2254, 58,2492,1123, 910,2160,2614,1372,1603,1196,1072,3385,1700,
|
243 |
-
3267,1980, 696, 480,2430, 920, 799,1570,2920,1951,2041,4047,2540,1321,4223,2469,
|
244 |
-
3562,2228,1271,2602, 401,2833,3351,2575,5157, 907,2312,1256, 410, 263,3507,1582,
|
245 |
-
996, 678,1849,2316,1480, 908,3545,2237, 703,2322, 667,1826,2849,1531,2604,2999,
|
246 |
-
2407,3146,2151,2630,1786,3711, 469,3542, 497,3899,2409, 858, 837,4446,3393,1274,
|
247 |
-
786, 620,1845,2001,3311, 484, 308,3367,1204,1815,3691,2332,1532,2557,1842,2020,
|
248 |
-
2724,1927,2333,4440, 567, 22,1673,2728,4475,1987,1858,1144,1597, 101,1832,3601,
|
249 |
-
12, 974,3783,4391, 951,1412, 1,3720, 453,4608,4041, 528,1041,1027,3230,2628,
|
250 |
-
1129, 875,1051,3291,1203,2262,1069,2860,2799,2149,2615,3278, 144,1758,3040, 31,
|
251 |
-
475,1680, 366,2685,3184, 311,1642,4008,2466,5036,1593,1493,2809, 216,1420,1668,
|
252 |
-
233, 304,2128,3284, 232,1429,1768,1040,2008,3407,2740,2967,2543, 242,2133, 778,
|
253 |
-
1565,2022,2620, 505,2189,2756,1098,2273, 372,1614, 708, 553,2846,2094,2278, 169,
|
254 |
-
3626,2835,4161, 228,2674,3165, 809,1454,1309, 466,1705,1095, 900,3423, 880,2667,
|
255 |
-
3751,5258,2317,3109,2571,4317,2766,1503,1342, 866,4447,1118, 63,2076, 314,1881,
|
256 |
-
1348,1061, 172, 978,3515,1747, 532, 511,3970, 6, 601, 905,2699,3300,1751, 276,
|
257 |
-
1467,3725,2668, 65,4239,2544,2779,2556,1604, 578,2451,1802, 992,2331,2624,1320,
|
258 |
-
3446, 713,1513,1013, 103,2786,2447,1661, 886,1702, 916, 654,3574,2031,1556, 751,
|
259 |
-
2178,2821,2179,1498,1538,2176, 271, 914,2251,2080,1325, 638,1953,2937,3877,2432,
|
260 |
-
2754, 95,3265,1716, 260,1227,4083, 775, 106,1357,3254, 426,1607, 555,2480, 772,
|
261 |
-
1985, 244,2546, 474, 495,1046,2611,1851,2061, 71,2089,1675,2590, 742,3758,2843,
|
262 |
-
3222,1433, 267,2180,2576,2826,2233,2092,3913,2435, 956,1745,3075, 856,2113,1116,
|
263 |
-
451, 3,1988,2896,1398, 993,2463,1878,2049,1341,2718,2721,2870,2108, 712,2904,
|
264 |
-
4363,2753,2324, 277,2872,2349,2649, 384, 987, 435, 691,3000, 922, 164,3939, 652,
|
265 |
-
1500,1184,4153,2482,3373,2165,4848,2335,3775,3508,3154,2806,2830,1554,2102,1664,
|
266 |
-
2530,1434,2408, 893,1547,2623,3447,2832,2242,2532,3169,2856,3223,2078, 49,3770,
|
267 |
-
3469, 462, 318, 656,2259,3250,3069, 679,1629,2758, 344,1138,1104,3120,1836,1283,
|
268 |
-
3115,2154,1437,4448, 934, 759,1999, 794,2862,1038, 533,2560,1722,2342, 855,2626,
|
269 |
-
1197,1663,4476,3127, 85,4240,2528, 25,1111,1181,3673, 407,3470,4561,2679,2713,
|
270 |
-
768,1925,2841,3986,1544,1165, 932, 373,1240,2146,1930,2673, 721,4766, 354,4333,
|
271 |
-
391,2963, 187, 61,3364,1442,1102, 330,1940,1767, 341,3809,4118, 393,2496,2062,
|
272 |
-
2211, 105, 331, 300, 439, 913,1332, 626, 379,3304,1557, 328, 689,3952, 309,1555,
|
273 |
-
931, 317,2517,3027, 325, 569, 686,2107,3084, 60,1042,1333,2794, 264,3177,4014,
|
274 |
-
1628, 258,3712, 7,4464,1176,1043,1778, 683, 114,1975, 78,1492, 383,1886, 510,
|
275 |
-
386, 645,5291,2891,2069,3305,4138,3867,2939,2603,2493,1935,1066,1848,3588,1015,
|
276 |
-
1282,1289,4609, 697,1453,3044,2666,3611,1856,2412, 54, 719,1330, 568,3778,2459,
|
277 |
-
1748, 788, 492, 551,1191,1000, 488,3394,3763, 282,1799, 348,2016,1523,3155,2390,
|
278 |
-
1049, 382,2019,1788,1170, 729,2968,3523, 897,3926,2785,2938,3292, 350,2319,3238,
|
279 |
-
1718,1717,2655,3453,3143,4465, 161,2889,2980,2009,1421, 56,1908,1640,2387,2232,
|
280 |
-
1917,1874,2477,4921, 148, 83,3438, 592,4245,2882,1822,1055, 741, 115,1496,1624,
|
281 |
-
381,1638,4592,1020, 516,3214, 458, 947,4575,1432, 211,1514,2926,1865,2142, 189,
|
282 |
-
852,1221,1400,1486, 882,2299,4036, 351, 28,1122, 700,6479,6480,6481,6482,6483, #last 512
|
283 |
-
)
|
284 |
-
# fmt: on
|
|
|
|
|
|
|
|
|
|
|
|
|
|
|
|
|
|
|
|
|
|
|
|
|
|
|
|
|
|
|
|
|
|
|
|
|
|
|
|
|
|
|
|
|
|
|
|
|
|
|
|
|
|
|
|
|
|
|
|
|
|
|
|
|
|
|
|
|
|
|
|
|
|
|
|
|
|
|
|
|
|
|
|
|
|
|
|
|
|
|
|
|
|
|
|
|
|
|
|
|
|
|
|
|
|
|
|
|
|
|
|
|
|
|
|
|
|
|
|
|
|
|
|
|
|
|
|
|
|
|
|
|
|
|
|
|
|
|
|
|
|
|
|
|
|
|
|
|
|
|
|
|
|
|
|
|
|
|
|
|
|
|
|
|
|
|
|
|
|
|
|
|
|
|
|
|
|
|
|
|
|
|
|
|
|
|
|
|
|
|
|
|
|
|
|
|
|
|
|
|
|
|
|
|
|
|
|
|
|
|
|
|
|
|
|
|
|
|
|
|
|
|
|
|
|
|
|
|
|
|
|
|
|
|
|
|
|
|
|
|
|
|
|
|
|
|
|
|
|
|
|
|
|
|
|
|
|
|
|
|
|
|
|
|
|
|
|
|
|
|
|
|
|
|
|
|
|
|
|
|
|
|
|
|
|
|
|
|
|
|
|
|
|
|
|
|
|
|
|
|
|
|
|
|
|
|
|
|
|
|
|
|
|
|
|
|
|
|
|
|
|
|
|
|
|
|
|
|
|
|
|
|
|
|
|
|
|
|
|
|
|
|
|
|
|
|
|
|
|
|
|
|
|
|
|
|
|
|
|
|
|
|
|
|
|
|
|
|
|
|
|
|
|
|
|
|
|
|
|
|
|
|
|
|
|
|
|
|
|
|
|
|
|
|
|
|
|
|
|
|
|
|
|
|
|
|
|
|
|
|
|
|
|
|
|
|
|
|
|
|
|
|
|
|
|
|
|
|
|
|
|
|
|
|
|
|
|
|
|
|
|
|
|
|
|
|
|
|
|
|
|
|
|
|
|
|
|
|
|
|
|
|
|
|
|
|
|
|
|
|
|
|
|
|
|
|
|
|
|
|
|
|
|
|
|
|
|
|
|
|
|
|
|
|
|
|
|
|
|
|
|
|
|
|
|
|
|
|
|
|
|
|
|
|
|
|
|
|
|
|
|
|
|
|
|
|
|
|
|
|
|
|
|
|
|
|
|
|
|
|
|
|
|
|
|
|
|
|
|
|
|
|
|
|
|
|
|
|
|
|
|
|
|
|
|
|
|
|
|
spaces/Big-Web/MMSD/env/Lib/site-packages/pip/_vendor/rich/_export_format.py
DELETED
@@ -1,76 +0,0 @@
|
|
1 |
-
CONSOLE_HTML_FORMAT = """\
|
2 |
-
<!DOCTYPE html>
|
3 |
-
<head>
|
4 |
-
<meta charset="UTF-8">
|
5 |
-
<style>
|
6 |
-
{stylesheet}
|
7 |
-
body {{
|
8 |
-
color: {foreground};
|
9 |
-
background-color: {background};
|
10 |
-
}}
|
11 |
-
</style>
|
12 |
-
</head>
|
13 |
-
<html>
|
14 |
-
<body>
|
15 |
-
<pre style="font-family:Menlo,'DejaVu Sans Mono',consolas,'Courier New',monospace"><code>{code}</code></pre>
|
16 |
-
</body>
|
17 |
-
</html>
|
18 |
-
"""
|
19 |
-
|
20 |
-
CONSOLE_SVG_FORMAT = """\
|
21 |
-
<svg class="rich-terminal" viewBox="0 0 {width} {height}" xmlns="http://www.w3.org/2000/svg">
|
22 |
-
<!-- Generated with Rich https://www.textualize.io -->
|
23 |
-
<style>
|
24 |
-
|
25 |
-
@font-face {{
|
26 |
-
font-family: "Fira Code";
|
27 |
-
src: local("FiraCode-Regular"),
|
28 |
-
url("https://cdnjs.cloudflare.com/ajax/libs/firacode/6.2.0/woff2/FiraCode-Regular.woff2") format("woff2"),
|
29 |
-
url("https://cdnjs.cloudflare.com/ajax/libs/firacode/6.2.0/woff/FiraCode-Regular.woff") format("woff");
|
30 |
-
font-style: normal;
|
31 |
-
font-weight: 400;
|
32 |
-
}}
|
33 |
-
@font-face {{
|
34 |
-
font-family: "Fira Code";
|
35 |
-
src: local("FiraCode-Bold"),
|
36 |
-
url("https://cdnjs.cloudflare.com/ajax/libs/firacode/6.2.0/woff2/FiraCode-Bold.woff2") format("woff2"),
|
37 |
-
url("https://cdnjs.cloudflare.com/ajax/libs/firacode/6.2.0/woff/FiraCode-Bold.woff") format("woff");
|
38 |
-
font-style: bold;
|
39 |
-
font-weight: 700;
|
40 |
-
}}
|
41 |
-
|
42 |
-
.{unique_id}-matrix {{
|
43 |
-
font-family: Fira Code, monospace;
|
44 |
-
font-size: {char_height}px;
|
45 |
-
line-height: {line_height}px;
|
46 |
-
font-variant-east-asian: full-width;
|
47 |
-
}}
|
48 |
-
|
49 |
-
.{unique_id}-title {{
|
50 |
-
font-size: 18px;
|
51 |
-
font-weight: bold;
|
52 |
-
font-family: arial;
|
53 |
-
}}
|
54 |
-
|
55 |
-
{styles}
|
56 |
-
</style>
|
57 |
-
|
58 |
-
<defs>
|
59 |
-
<clipPath id="{unique_id}-clip-terminal">
|
60 |
-
<rect x="0" y="0" width="{terminal_width}" height="{terminal_height}" />
|
61 |
-
</clipPath>
|
62 |
-
{lines}
|
63 |
-
</defs>
|
64 |
-
|
65 |
-
{chrome}
|
66 |
-
<g transform="translate({terminal_x}, {terminal_y})" clip-path="url(#{unique_id}-clip-terminal)">
|
67 |
-
{backgrounds}
|
68 |
-
<g class="{unique_id}-matrix">
|
69 |
-
{matrix}
|
70 |
-
</g>
|
71 |
-
</g>
|
72 |
-
</svg>
|
73 |
-
"""
|
74 |
-
|
75 |
-
_SVG_FONT_FAMILY = "Rich Fira Code"
|
76 |
-
_SVG_CLASSES_PREFIX = "rich-svg"
|
|
|
|
|
|
|
|
|
|
|
|
|
|
|
|
|
|
|
|
|
|
|
|
|
|
|
|
|
|
|
|
|
|
|
|
|
|
|
|
|
|
|
|
|
|
|
|
|
|
|
|
|
|
|
|
|
|
|
|
|
|
|
|
|
|
|
|
|
|
|
|
|
|
|
|
|
|
|
|
|
|
|
|
|
|
|
|
|
|
|
|
|
|
|
|
|
|
|
|
|
|
|
|
|
|
|
|
|
|
|
|
|
|
|
|
|
|
|
|
|
|
|
|
|
|
|
|
|
|
|
|
|
|
|
|
|
|
|
|
|
|
|
|
|
|
|
|
|
|
|
|
|
|
spaces/Big-Web/MMSD/env/Lib/site-packages/pkg_resources/_vendor/jaraco/text/__init__.py
DELETED
@@ -1,599 +0,0 @@
|
|
1 |
-
import re
|
2 |
-
import itertools
|
3 |
-
import textwrap
|
4 |
-
import functools
|
5 |
-
|
6 |
-
try:
|
7 |
-
from importlib.resources import files # type: ignore
|
8 |
-
except ImportError: # pragma: nocover
|
9 |
-
from pkg_resources.extern.importlib_resources import files # type: ignore
|
10 |
-
|
11 |
-
from pkg_resources.extern.jaraco.functools import compose, method_cache
|
12 |
-
from pkg_resources.extern.jaraco.context import ExceptionTrap
|
13 |
-
|
14 |
-
|
15 |
-
def substitution(old, new):
|
16 |
-
"""
|
17 |
-
Return a function that will perform a substitution on a string
|
18 |
-
"""
|
19 |
-
return lambda s: s.replace(old, new)
|
20 |
-
|
21 |
-
|
22 |
-
def multi_substitution(*substitutions):
|
23 |
-
"""
|
24 |
-
Take a sequence of pairs specifying substitutions, and create
|
25 |
-
a function that performs those substitutions.
|
26 |
-
|
27 |
-
>>> multi_substitution(('foo', 'bar'), ('bar', 'baz'))('foo')
|
28 |
-
'baz'
|
29 |
-
"""
|
30 |
-
substitutions = itertools.starmap(substitution, substitutions)
|
31 |
-
# compose function applies last function first, so reverse the
|
32 |
-
# substitutions to get the expected order.
|
33 |
-
substitutions = reversed(tuple(substitutions))
|
34 |
-
return compose(*substitutions)
|
35 |
-
|
36 |
-
|
37 |
-
class FoldedCase(str):
|
38 |
-
"""
|
39 |
-
A case insensitive string class; behaves just like str
|
40 |
-
except compares equal when the only variation is case.
|
41 |
-
|
42 |
-
>>> s = FoldedCase('hello world')
|
43 |
-
|
44 |
-
>>> s == 'Hello World'
|
45 |
-
True
|
46 |
-
|
47 |
-
>>> 'Hello World' == s
|
48 |
-
True
|
49 |
-
|
50 |
-
>>> s != 'Hello World'
|
51 |
-
False
|
52 |
-
|
53 |
-
>>> s.index('O')
|
54 |
-
4
|
55 |
-
|
56 |
-
>>> s.split('O')
|
57 |
-
['hell', ' w', 'rld']
|
58 |
-
|
59 |
-
>>> sorted(map(FoldedCase, ['GAMMA', 'alpha', 'Beta']))
|
60 |
-
['alpha', 'Beta', 'GAMMA']
|
61 |
-
|
62 |
-
Sequence membership is straightforward.
|
63 |
-
|
64 |
-
>>> "Hello World" in [s]
|
65 |
-
True
|
66 |
-
>>> s in ["Hello World"]
|
67 |
-
True
|
68 |
-
|
69 |
-
You may test for set inclusion, but candidate and elements
|
70 |
-
must both be folded.
|
71 |
-
|
72 |
-
>>> FoldedCase("Hello World") in {s}
|
73 |
-
True
|
74 |
-
>>> s in {FoldedCase("Hello World")}
|
75 |
-
True
|
76 |
-
|
77 |
-
String inclusion works as long as the FoldedCase object
|
78 |
-
is on the right.
|
79 |
-
|
80 |
-
>>> "hello" in FoldedCase("Hello World")
|
81 |
-
True
|
82 |
-
|
83 |
-
But not if the FoldedCase object is on the left:
|
84 |
-
|
85 |
-
>>> FoldedCase('hello') in 'Hello World'
|
86 |
-
False
|
87 |
-
|
88 |
-
In that case, use ``in_``:
|
89 |
-
|
90 |
-
>>> FoldedCase('hello').in_('Hello World')
|
91 |
-
True
|
92 |
-
|
93 |
-
>>> FoldedCase('hello') > FoldedCase('Hello')
|
94 |
-
False
|
95 |
-
"""
|
96 |
-
|
97 |
-
def __lt__(self, other):
|
98 |
-
return self.lower() < other.lower()
|
99 |
-
|
100 |
-
def __gt__(self, other):
|
101 |
-
return self.lower() > other.lower()
|
102 |
-
|
103 |
-
def __eq__(self, other):
|
104 |
-
return self.lower() == other.lower()
|
105 |
-
|
106 |
-
def __ne__(self, other):
|
107 |
-
return self.lower() != other.lower()
|
108 |
-
|
109 |
-
def __hash__(self):
|
110 |
-
return hash(self.lower())
|
111 |
-
|
112 |
-
def __contains__(self, other):
|
113 |
-
return super().lower().__contains__(other.lower())
|
114 |
-
|
115 |
-
def in_(self, other):
|
116 |
-
"Does self appear in other?"
|
117 |
-
return self in FoldedCase(other)
|
118 |
-
|
119 |
-
# cache lower since it's likely to be called frequently.
|
120 |
-
@method_cache
|
121 |
-
def lower(self):
|
122 |
-
return super().lower()
|
123 |
-
|
124 |
-
def index(self, sub):
|
125 |
-
return self.lower().index(sub.lower())
|
126 |
-
|
127 |
-
def split(self, splitter=' ', maxsplit=0):
|
128 |
-
pattern = re.compile(re.escape(splitter), re.I)
|
129 |
-
return pattern.split(self, maxsplit)
|
130 |
-
|
131 |
-
|
132 |
-
# Python 3.8 compatibility
|
133 |
-
_unicode_trap = ExceptionTrap(UnicodeDecodeError)
|
134 |
-
|
135 |
-
|
136 |
-
@_unicode_trap.passes
|
137 |
-
def is_decodable(value):
|
138 |
-
r"""
|
139 |
-
Return True if the supplied value is decodable (using the default
|
140 |
-
encoding).
|
141 |
-
|
142 |
-
>>> is_decodable(b'\xff')
|
143 |
-
False
|
144 |
-
>>> is_decodable(b'\x32')
|
145 |
-
True
|
146 |
-
"""
|
147 |
-
value.decode()
|
148 |
-
|
149 |
-
|
150 |
-
def is_binary(value):
|
151 |
-
r"""
|
152 |
-
Return True if the value appears to be binary (that is, it's a byte
|
153 |
-
string and isn't decodable).
|
154 |
-
|
155 |
-
>>> is_binary(b'\xff')
|
156 |
-
True
|
157 |
-
>>> is_binary('\xff')
|
158 |
-
False
|
159 |
-
"""
|
160 |
-
return isinstance(value, bytes) and not is_decodable(value)
|
161 |
-
|
162 |
-
|
163 |
-
def trim(s):
|
164 |
-
r"""
|
165 |
-
Trim something like a docstring to remove the whitespace that
|
166 |
-
is common due to indentation and formatting.
|
167 |
-
|
168 |
-
>>> trim("\n\tfoo = bar\n\t\tbar = baz\n")
|
169 |
-
'foo = bar\n\tbar = baz'
|
170 |
-
"""
|
171 |
-
return textwrap.dedent(s).strip()
|
172 |
-
|
173 |
-
|
174 |
-
def wrap(s):
|
175 |
-
"""
|
176 |
-
Wrap lines of text, retaining existing newlines as
|
177 |
-
paragraph markers.
|
178 |
-
|
179 |
-
>>> print(wrap(lorem_ipsum))
|
180 |
-
Lorem ipsum dolor sit amet, consectetur adipiscing elit, sed do
|
181 |
-
eiusmod tempor incididunt ut labore et dolore magna aliqua. Ut enim ad
|
182 |
-
minim veniam, quis nostrud exercitation ullamco laboris nisi ut
|
183 |
-
aliquip ex ea commodo consequat. Duis aute irure dolor in
|
184 |
-
reprehenderit in voluptate velit esse cillum dolore eu fugiat nulla
|
185 |
-
pariatur. Excepteur sint occaecat cupidatat non proident, sunt in
|
186 |
-
culpa qui officia deserunt mollit anim id est laborum.
|
187 |
-
<BLANKLINE>
|
188 |
-
Curabitur pretium tincidunt lacus. Nulla gravida orci a odio. Nullam
|
189 |
-
varius, turpis et commodo pharetra, est eros bibendum elit, nec luctus
|
190 |
-
magna felis sollicitudin mauris. Integer in mauris eu nibh euismod
|
191 |
-
gravida. Duis ac tellus et risus vulputate vehicula. Donec lobortis
|
192 |
-
risus a elit. Etiam tempor. Ut ullamcorper, ligula eu tempor congue,
|
193 |
-
eros est euismod turpis, id tincidunt sapien risus a quam. Maecenas
|
194 |
-
fermentum consequat mi. Donec fermentum. Pellentesque malesuada nulla
|
195 |
-
a mi. Duis sapien sem, aliquet nec, commodo eget, consequat quis,
|
196 |
-
neque. Aliquam faucibus, elit ut dictum aliquet, felis nisl adipiscing
|
197 |
-
sapien, sed malesuada diam lacus eget erat. Cras mollis scelerisque
|
198 |
-
nunc. Nullam arcu. Aliquam consequat. Curabitur augue lorem, dapibus
|
199 |
-
quis, laoreet et, pretium ac, nisi. Aenean magna nisl, mollis quis,
|
200 |
-
molestie eu, feugiat in, orci. In hac habitasse platea dictumst.
|
201 |
-
"""
|
202 |
-
paragraphs = s.splitlines()
|
203 |
-
wrapped = ('\n'.join(textwrap.wrap(para)) for para in paragraphs)
|
204 |
-
return '\n\n'.join(wrapped)
|
205 |
-
|
206 |
-
|
207 |
-
def unwrap(s):
|
208 |
-
r"""
|
209 |
-
Given a multi-line string, return an unwrapped version.
|
210 |
-
|
211 |
-
>>> wrapped = wrap(lorem_ipsum)
|
212 |
-
>>> wrapped.count('\n')
|
213 |
-
20
|
214 |
-
>>> unwrapped = unwrap(wrapped)
|
215 |
-
>>> unwrapped.count('\n')
|
216 |
-
1
|
217 |
-
>>> print(unwrapped)
|
218 |
-
Lorem ipsum dolor sit amet, consectetur adipiscing ...
|
219 |
-
Curabitur pretium tincidunt lacus. Nulla gravida orci ...
|
220 |
-
|
221 |
-
"""
|
222 |
-
paragraphs = re.split(r'\n\n+', s)
|
223 |
-
cleaned = (para.replace('\n', ' ') for para in paragraphs)
|
224 |
-
return '\n'.join(cleaned)
|
225 |
-
|
226 |
-
|
227 |
-
|
228 |
-
|
229 |
-
class Splitter(object):
|
230 |
-
"""object that will split a string with the given arguments for each call
|
231 |
-
|
232 |
-
>>> s = Splitter(',')
|
233 |
-
>>> s('hello, world, this is your, master calling')
|
234 |
-
['hello', ' world', ' this is your', ' master calling']
|
235 |
-
"""
|
236 |
-
|
237 |
-
def __init__(self, *args):
|
238 |
-
self.args = args
|
239 |
-
|
240 |
-
def __call__(self, s):
|
241 |
-
return s.split(*self.args)
|
242 |
-
|
243 |
-
|
244 |
-
def indent(string, prefix=' ' * 4):
|
245 |
-
"""
|
246 |
-
>>> indent('foo')
|
247 |
-
' foo'
|
248 |
-
"""
|
249 |
-
return prefix + string
|
250 |
-
|
251 |
-
|
252 |
-
class WordSet(tuple):
|
253 |
-
"""
|
254 |
-
Given an identifier, return the words that identifier represents,
|
255 |
-
whether in camel case, underscore-separated, etc.
|
256 |
-
|
257 |
-
>>> WordSet.parse("camelCase")
|
258 |
-
('camel', 'Case')
|
259 |
-
|
260 |
-
>>> WordSet.parse("under_sep")
|
261 |
-
('under', 'sep')
|
262 |
-
|
263 |
-
Acronyms should be retained
|
264 |
-
|
265 |
-
>>> WordSet.parse("firstSNL")
|
266 |
-
('first', 'SNL')
|
267 |
-
|
268 |
-
>>> WordSet.parse("you_and_I")
|
269 |
-
('you', 'and', 'I')
|
270 |
-
|
271 |
-
>>> WordSet.parse("A simple test")
|
272 |
-
('A', 'simple', 'test')
|
273 |
-
|
274 |
-
Multiple caps should not interfere with the first cap of another word.
|
275 |
-
|
276 |
-
>>> WordSet.parse("myABCClass")
|
277 |
-
('my', 'ABC', 'Class')
|
278 |
-
|
279 |
-
The result is a WordSet, so you can get the form you need.
|
280 |
-
|
281 |
-
>>> WordSet.parse("myABCClass").underscore_separated()
|
282 |
-
'my_ABC_Class'
|
283 |
-
|
284 |
-
>>> WordSet.parse('a-command').camel_case()
|
285 |
-
'ACommand'
|
286 |
-
|
287 |
-
>>> WordSet.parse('someIdentifier').lowered().space_separated()
|
288 |
-
'some identifier'
|
289 |
-
|
290 |
-
Slices of the result should return another WordSet.
|
291 |
-
|
292 |
-
>>> WordSet.parse('taken-out-of-context')[1:].underscore_separated()
|
293 |
-
'out_of_context'
|
294 |
-
|
295 |
-
>>> WordSet.from_class_name(WordSet()).lowered().space_separated()
|
296 |
-
'word set'
|
297 |
-
|
298 |
-
>>> example = WordSet.parse('figured it out')
|
299 |
-
>>> example.headless_camel_case()
|
300 |
-
'figuredItOut'
|
301 |
-
>>> example.dash_separated()
|
302 |
-
'figured-it-out'
|
303 |
-
|
304 |
-
"""
|
305 |
-
|
306 |
-
_pattern = re.compile('([A-Z]?[a-z]+)|([A-Z]+(?![a-z]))')
|
307 |
-
|
308 |
-
def capitalized(self):
|
309 |
-
return WordSet(word.capitalize() for word in self)
|
310 |
-
|
311 |
-
def lowered(self):
|
312 |
-
return WordSet(word.lower() for word in self)
|
313 |
-
|
314 |
-
def camel_case(self):
|
315 |
-
return ''.join(self.capitalized())
|
316 |
-
|
317 |
-
def headless_camel_case(self):
|
318 |
-
words = iter(self)
|
319 |
-
first = next(words).lower()
|
320 |
-
new_words = itertools.chain((first,), WordSet(words).camel_case())
|
321 |
-
return ''.join(new_words)
|
322 |
-
|
323 |
-
def underscore_separated(self):
|
324 |
-
return '_'.join(self)
|
325 |
-
|
326 |
-
def dash_separated(self):
|
327 |
-
return '-'.join(self)
|
328 |
-
|
329 |
-
def space_separated(self):
|
330 |
-
return ' '.join(self)
|
331 |
-
|
332 |
-
def trim_right(self, item):
|
333 |
-
"""
|
334 |
-
Remove the item from the end of the set.
|
335 |
-
|
336 |
-
>>> WordSet.parse('foo bar').trim_right('foo')
|
337 |
-
('foo', 'bar')
|
338 |
-
>>> WordSet.parse('foo bar').trim_right('bar')
|
339 |
-
('foo',)
|
340 |
-
>>> WordSet.parse('').trim_right('bar')
|
341 |
-
()
|
342 |
-
"""
|
343 |
-
return self[:-1] if self and self[-1] == item else self
|
344 |
-
|
345 |
-
def trim_left(self, item):
|
346 |
-
"""
|
347 |
-
Remove the item from the beginning of the set.
|
348 |
-
|
349 |
-
>>> WordSet.parse('foo bar').trim_left('foo')
|
350 |
-
('bar',)
|
351 |
-
>>> WordSet.parse('foo bar').trim_left('bar')
|
352 |
-
('foo', 'bar')
|
353 |
-
>>> WordSet.parse('').trim_left('bar')
|
354 |
-
()
|
355 |
-
"""
|
356 |
-
return self[1:] if self and self[0] == item else self
|
357 |
-
|
358 |
-
def trim(self, item):
|
359 |
-
"""
|
360 |
-
>>> WordSet.parse('foo bar').trim('foo')
|
361 |
-
('bar',)
|
362 |
-
"""
|
363 |
-
return self.trim_left(item).trim_right(item)
|
364 |
-
|
365 |
-
def __getitem__(self, item):
|
366 |
-
result = super(WordSet, self).__getitem__(item)
|
367 |
-
if isinstance(item, slice):
|
368 |
-
result = WordSet(result)
|
369 |
-
return result
|
370 |
-
|
371 |
-
@classmethod
|
372 |
-
def parse(cls, identifier):
|
373 |
-
matches = cls._pattern.finditer(identifier)
|
374 |
-
return WordSet(match.group(0) for match in matches)
|
375 |
-
|
376 |
-
@classmethod
|
377 |
-
def from_class_name(cls, subject):
|
378 |
-
return cls.parse(subject.__class__.__name__)
|
379 |
-
|
380 |
-
|
381 |
-
# for backward compatibility
|
382 |
-
words = WordSet.parse
|
383 |
-
|
384 |
-
|
385 |
-
def simple_html_strip(s):
|
386 |
-
r"""
|
387 |
-
Remove HTML from the string `s`.
|
388 |
-
|
389 |
-
>>> str(simple_html_strip(''))
|
390 |
-
''
|
391 |
-
|
392 |
-
>>> print(simple_html_strip('A <bold>stormy</bold> day in paradise'))
|
393 |
-
A stormy day in paradise
|
394 |
-
|
395 |
-
>>> print(simple_html_strip('Somebody <!-- do not --> tell the truth.'))
|
396 |
-
Somebody tell the truth.
|
397 |
-
|
398 |
-
>>> print(simple_html_strip('What about<br/>\nmultiple lines?'))
|
399 |
-
What about
|
400 |
-
multiple lines?
|
401 |
-
"""
|
402 |
-
html_stripper = re.compile('(<!--.*?-->)|(<[^>]*>)|([^<]+)', re.DOTALL)
|
403 |
-
texts = (match.group(3) or '' for match in html_stripper.finditer(s))
|
404 |
-
return ''.join(texts)
|
405 |
-
|
406 |
-
|
407 |
-
class SeparatedValues(str):
|
408 |
-
"""
|
409 |
-
A string separated by a separator. Overrides __iter__ for getting
|
410 |
-
the values.
|
411 |
-
|
412 |
-
>>> list(SeparatedValues('a,b,c'))
|
413 |
-
['a', 'b', 'c']
|
414 |
-
|
415 |
-
Whitespace is stripped and empty values are discarded.
|
416 |
-
|
417 |
-
>>> list(SeparatedValues(' a, b , c, '))
|
418 |
-
['a', 'b', 'c']
|
419 |
-
"""
|
420 |
-
|
421 |
-
separator = ','
|
422 |
-
|
423 |
-
def __iter__(self):
|
424 |
-
parts = self.split(self.separator)
|
425 |
-
return filter(None, (part.strip() for part in parts))
|
426 |
-
|
427 |
-
|
428 |
-
class Stripper:
|
429 |
-
r"""
|
430 |
-
Given a series of lines, find the common prefix and strip it from them.
|
431 |
-
|
432 |
-
>>> lines = [
|
433 |
-
... 'abcdefg\n',
|
434 |
-
... 'abc\n',
|
435 |
-
... 'abcde\n',
|
436 |
-
... ]
|
437 |
-
>>> res = Stripper.strip_prefix(lines)
|
438 |
-
>>> res.prefix
|
439 |
-
'abc'
|
440 |
-
>>> list(res.lines)
|
441 |
-
['defg\n', '\n', 'de\n']
|
442 |
-
|
443 |
-
If no prefix is common, nothing should be stripped.
|
444 |
-
|
445 |
-
>>> lines = [
|
446 |
-
... 'abcd\n',
|
447 |
-
... '1234\n',
|
448 |
-
... ]
|
449 |
-
>>> res = Stripper.strip_prefix(lines)
|
450 |
-
>>> res.prefix = ''
|
451 |
-
>>> list(res.lines)
|
452 |
-
['abcd\n', '1234\n']
|
453 |
-
"""
|
454 |
-
|
455 |
-
def __init__(self, prefix, lines):
|
456 |
-
self.prefix = prefix
|
457 |
-
self.lines = map(self, lines)
|
458 |
-
|
459 |
-
@classmethod
|
460 |
-
def strip_prefix(cls, lines):
|
461 |
-
prefix_lines, lines = itertools.tee(lines)
|
462 |
-
prefix = functools.reduce(cls.common_prefix, prefix_lines)
|
463 |
-
return cls(prefix, lines)
|
464 |
-
|
465 |
-
def __call__(self, line):
|
466 |
-
if not self.prefix:
|
467 |
-
return line
|
468 |
-
null, prefix, rest = line.partition(self.prefix)
|
469 |
-
return rest
|
470 |
-
|
471 |
-
@staticmethod
|
472 |
-
def common_prefix(s1, s2):
|
473 |
-
"""
|
474 |
-
Return the common prefix of two lines.
|
475 |
-
"""
|
476 |
-
index = min(len(s1), len(s2))
|
477 |
-
while s1[:index] != s2[:index]:
|
478 |
-
index -= 1
|
479 |
-
return s1[:index]
|
480 |
-
|
481 |
-
|
482 |
-
def remove_prefix(text, prefix):
|
483 |
-
"""
|
484 |
-
Remove the prefix from the text if it exists.
|
485 |
-
|
486 |
-
>>> remove_prefix('underwhelming performance', 'underwhelming ')
|
487 |
-
'performance'
|
488 |
-
|
489 |
-
>>> remove_prefix('something special', 'sample')
|
490 |
-
'something special'
|
491 |
-
"""
|
492 |
-
null, prefix, rest = text.rpartition(prefix)
|
493 |
-
return rest
|
494 |
-
|
495 |
-
|
496 |
-
def remove_suffix(text, suffix):
|
497 |
-
"""
|
498 |
-
Remove the suffix from the text if it exists.
|
499 |
-
|
500 |
-
>>> remove_suffix('name.git', '.git')
|
501 |
-
'name'
|
502 |
-
|
503 |
-
>>> remove_suffix('something special', 'sample')
|
504 |
-
'something special'
|
505 |
-
"""
|
506 |
-
rest, suffix, null = text.partition(suffix)
|
507 |
-
return rest
|
508 |
-
|
509 |
-
|
510 |
-
def normalize_newlines(text):
|
511 |
-
r"""
|
512 |
-
Replace alternate newlines with the canonical newline.
|
513 |
-
|
514 |
-
>>> normalize_newlines('Lorem Ipsum\u2029')
|
515 |
-
'Lorem Ipsum\n'
|
516 |
-
>>> normalize_newlines('Lorem Ipsum\r\n')
|
517 |
-
'Lorem Ipsum\n'
|
518 |
-
>>> normalize_newlines('Lorem Ipsum\x85')
|
519 |
-
'Lorem Ipsum\n'
|
520 |
-
"""
|
521 |
-
newlines = ['\r\n', '\r', '\n', '\u0085', '\u2028', '\u2029']
|
522 |
-
pattern = '|'.join(newlines)
|
523 |
-
return re.sub(pattern, '\n', text)
|
524 |
-
|
525 |
-
|
526 |
-
def _nonblank(str):
|
527 |
-
return str and not str.startswith('#')
|
528 |
-
|
529 |
-
|
530 |
-
@functools.singledispatch
|
531 |
-
def yield_lines(iterable):
|
532 |
-
r"""
|
533 |
-
Yield valid lines of a string or iterable.
|
534 |
-
|
535 |
-
>>> list(yield_lines(''))
|
536 |
-
[]
|
537 |
-
>>> list(yield_lines(['foo', 'bar']))
|
538 |
-
['foo', 'bar']
|
539 |
-
>>> list(yield_lines('foo\nbar'))
|
540 |
-
['foo', 'bar']
|
541 |
-
>>> list(yield_lines('\nfoo\n#bar\nbaz #comment'))
|
542 |
-
['foo', 'baz #comment']
|
543 |
-
>>> list(yield_lines(['foo\nbar', 'baz', 'bing\n\n\n']))
|
544 |
-
['foo', 'bar', 'baz', 'bing']
|
545 |
-
"""
|
546 |
-
return itertools.chain.from_iterable(map(yield_lines, iterable))
|
547 |
-
|
548 |
-
|
549 |
-
@yield_lines.register(str)
|
550 |
-
def _(text):
|
551 |
-
return filter(_nonblank, map(str.strip, text.splitlines()))
|
552 |
-
|
553 |
-
|
554 |
-
def drop_comment(line):
|
555 |
-
"""
|
556 |
-
Drop comments.
|
557 |
-
|
558 |
-
>>> drop_comment('foo # bar')
|
559 |
-
'foo'
|
560 |
-
|
561 |
-
A hash without a space may be in a URL.
|
562 |
-
|
563 |
-
>>> drop_comment('http://example.com/foo#bar')
|
564 |
-
'http://example.com/foo#bar'
|
565 |
-
"""
|
566 |
-
return line.partition(' #')[0]
|
567 |
-
|
568 |
-
|
569 |
-
def join_continuation(lines):
|
570 |
-
r"""
|
571 |
-
Join lines continued by a trailing backslash.
|
572 |
-
|
573 |
-
>>> list(join_continuation(['foo \\', 'bar', 'baz']))
|
574 |
-
['foobar', 'baz']
|
575 |
-
>>> list(join_continuation(['foo \\', 'bar', 'baz']))
|
576 |
-
['foobar', 'baz']
|
577 |
-
>>> list(join_continuation(['foo \\', 'bar \\', 'baz']))
|
578 |
-
['foobarbaz']
|
579 |
-
|
580 |
-
Not sure why, but...
|
581 |
-
The character preceeding the backslash is also elided.
|
582 |
-
|
583 |
-
>>> list(join_continuation(['goo\\', 'dly']))
|
584 |
-
['godly']
|
585 |
-
|
586 |
-
A terrible idea, but...
|
587 |
-
If no line is available to continue, suppress the lines.
|
588 |
-
|
589 |
-
>>> list(join_continuation(['foo', 'bar\\', 'baz\\']))
|
590 |
-
['foo']
|
591 |
-
"""
|
592 |
-
lines = iter(lines)
|
593 |
-
for item in lines:
|
594 |
-
while item.endswith('\\'):
|
595 |
-
try:
|
596 |
-
item = item[:-2].strip() + next(lines)
|
597 |
-
except StopIteration:
|
598 |
-
return
|
599 |
-
yield item
|
|
|
|
|
|
|
|
|
|
|
|
|
|
|
|
|
|
|
|
|
|
|
|
|
|
|
|
|
|
|
|
|
|
|
|
|
|
|
|
|
|
|
|
|
|
|
|
|
|
|
|
|
|
|
|
|
|
|
|
|
|
|
|
|
|
|
|
|
|
|
|
|
|
|
|
|
|
|
|
|
|
|
|
|
|
|
|
|
|
|
|
|
|
|
|
|
|
|
|
|
|
|
|
|
|
|
|
|
|
|
|
|
|
|
|
|
|
|
|
|
|
|
|
|
|
|
|
|
|
|
|
|
|
|
|
|
|
|
|
|
|
|
|
|
|
|
|
|
|
|
|
|
|
|
|
|
|
|
|
|
|
|
|
|
|
|
|
|
|
|
|
|
|
|
|
|
|
|
|
|
|
|
|
|
|
|
|
|
|
|
|
|
|
|
|
|
|
|
|
|
|
|
|
|
|
|
|
|
|
|
|
|
|
|
|
|
|
|
|
|
|
|
|
|
|
|
|
|
|
|
|
|
|
|
|
|
|
|
|
|
|
|
|
|
|
|
|
|
|
|
|
|
|
|
|
|
|
|
|
|
|
|
|
|
|
|
|
|
|
|
|
|
|
|
|
|
|
|
|
|
|
|
|
|
|
|
|
|
|
|
|
|
|
|
|
|
|
|
|
|
|
|
|
|
|
|
|
|
|
|
|
|
|
|
|
|
|
|
|
|
|
|
|
|
|
|
|
|
|
|
|
|
|
|
|
|
|
|
|
|
|
|
|
|
|
|
|
|
|
|
|
|
|
|
|
|
|
|
|
|
|
|
|
|
|
|
|
|
|
|
|
|
|
|
|
|
|
|
|
|
|
|
|
|
|
|
|
|
|
|
|
|
|
|
|
|
|
|
|
|
|
|
|
|
|
|
|
|
|
|
|
|
|
|
|
|
|
|
|
|
|
|
|
|
|
|
|
|
|
|
|
|
|
|
|
|
|
|
|
|
|
|
|
|
|
|
|
|
|
|
|
|
|
|
|
|
|
|
|
|
|
|
|
|
|
|
|
|
|
|
|
|
|
|
|
|
|
|
|
|
|
|
|
|
|
|
|
|
|
|
|
|
|
|
|
|
|
|
|
|
|
|
|
|
|
|
|
|
|
|
|
|
|
|
|
|
|
|
|
|
|
|
|
|
|
|
|
|
|
|
|
|
|
|
|
|
|
|
|
|
|
|
|
|
|
|
|
|
|
|
|
|
|
|
|
|
|
|
|
|
|
|
|
|
|
|
|
|
|
|
|
|
|
|
|
|
|
|
|
|
|
|
|
|
|
|
|
|
|
|
|
|
|
|
|
|
|
|
|
|
|
|
|
|
|
|
|
|
|
|
|
|
|
|
|
|
|
|
|
|
|
|
|
|
|
|
|
|
|
|
|
|
|
|
|
|
|
|
|
|
|
|
|
|
|
|
|
|
|
|
|
|
|
|
|
|
|
|
|
|
|
|
|
|
|
|
|
|
|
|
|
|
|
|
|
|
|
|
|
|
|
|
|
|
|
|
|
|
|
|
|
|
|
|
|
|
|
|
|
|
|
|
|
|
|
|
|
|
|
|
|
|
|
|
|
|
|
|
|
|
|
|
|
|
|
|
|
|
|
|
|
|
|
|
|
|
|
|
|
|
|
|
|
|
|
|
|
|
|
|
|
|
|
|
|
|
|
|
|
|
|
|
|
|
|
|
|
|
|
|
|
|
|
|
|
|
|
|
|
|
|
|
|
|
|
|
|
|
|
|
|
|
|
|
|
|
|
|
|
|
|
|
|
|
|
|
|
|
|
|
|
|
|
|
|
|
|
|
|
|
|
|
|
|
|
|
|
|
|
|
|
|
|
|
|
|
|
|
|
|
|
|
|
|
|
|
|
|
|
|
|
|
|
|
|
|
|
|
|
|
|
|
|
|
|
|
|
|
|
|
|
|
|
|
|
|
|
|
|
|
|
|
|
|
|
|
|
|
|
|
|
|
|
|
|
|
|
|
|
|
|
|
|
|
|
|
|
|
|
|
|
|
|
|
|
|
|
|
|
|
|
|
|
|
|
|
|
|
|
|
|
|
|
|
|
|
|
|
|
|
|
|
|
|
|
|
|
|
|
|
|
|
|
|
|
|
|
|
|
|
|
|
|
|
|
|
|
|
|
|
|
|
|
|
|
|
|
|
|
|
|
|
|
|
|
|
|
|
|
|
|
|
|
|
|
|
|
|
|
|
|
|
|
|
|
|
|
|
|
|
|
|
|
|
|
|
|
|
|
|
|
|
|
|
|
|
|
|
|
|
|
|
|
|
|
|
|
|
|
|
|
|
|
|
|
|
|
|
|
|
|
|
|
|
|
|
|
|
|
|
|
|
|
|
|
|
|
|
|
|
|
|
|
|
|
|
|
|
|
|
|
|
|
|
|
|
|
|
|
|
|
|
|
|
|
|
|
|
|
|
|
|
|
|
|
|
|
|
|
|
|
|
|
|
|
|
|
|
|
|
|
|
|
|
|
|
|
|
|
|
|
|
|
|
|
|
|
|
|
|
|
|
|
|
|
|
|
|
|
|
|
|
|
|
|
|
|
|
|
|
|
|
|
|
|
|
|
|
|
|
|
|
|
|
|
|
|
|
|
|
|
|
|
|
|
|
|
|
|
|
|
|
|
|
|
|
|
|
|
spaces/BraydenMoore/MARCI-NFL-Betting/Source/Build/build.py
DELETED
@@ -1,197 +0,0 @@
|
|
1 |
-
from nfl_data_py import nfl_data_py as nfl
|
2 |
-
from tqdm import tqdm
|
3 |
-
import numpy as np
|
4 |
-
import pandas as pd
|
5 |
-
pd.set_option('chained_assignment',None)
|
6 |
-
pd.set_option('display.max_columns',None)
|
7 |
-
import os
|
8 |
-
import datetime as dt
|
9 |
-
|
10 |
-
current_directory = os.path.dirname(os.path.abspath(__file__))
|
11 |
-
parent_directory = os.path.dirname(current_directory)
|
12 |
-
data_directory = os.path.join(parent_directory, 'Data')
|
13 |
-
|
14 |
-
year = dt.datetime.now().year
|
15 |
-
month = dt.datetime.now().month
|
16 |
-
current_season = year if month in [8,9,10,11,12] else year-1
|
17 |
-
|
18 |
-
def get_pbp_data(get_seasons=[]):
|
19 |
-
"""
|
20 |
-
Pull data from nflFastR's Github repo.
|
21 |
-
|
22 |
-
"""
|
23 |
-
pbp = nfl.import_pbp_data(get_seasons)
|
24 |
-
#pbp = pd.read_csv(r"C:\Users\brayd\Downloads\play_by_play_2023.csv")
|
25 |
-
pbp['TOP_seconds'] = pbp['drive_time_of_possession'].apply(lambda x: int(x.split(':')[0]) * 60 + int(x.split(':')[1]) if pd.notnull(x) else 0)
|
26 |
-
|
27 |
-
return pbp
|
28 |
-
|
29 |
-
|
30 |
-
def build_gbg_data(get_seasons=[]):
|
31 |
-
"""
|
32 |
-
Build a game-by-game dataset to use for prediction models.
|
33 |
-
|
34 |
-
"""
|
35 |
-
print('Loading play-by-play data.')
|
36 |
-
pbp = get_pbp_data(get_seasons)
|
37 |
-
game_date_dict = dict(pbp[['game_id','game_date']].values)
|
38 |
-
teams = list(set(list(pbp['home_team'].unique()) + list(pbp['away_team'].unique())))
|
39 |
-
seasons = pbp['season'].unique()
|
40 |
-
|
41 |
-
print('Building game-by-game data.')
|
42 |
-
data = pd.DataFrame()
|
43 |
-
for season in seasons:
|
44 |
-
print(season)
|
45 |
-
for team_name in tqdm(teams):
|
46 |
-
# create features
|
47 |
-
team = pbp.loc[((pbp['home_team']==team_name) | (pbp['away_team']==team_name)) & (pbp['season']==season)]
|
48 |
-
team['GP'] = team['week']
|
49 |
-
team['W'] = [1 if r>0 and team_name==h else 1 if r<0 and team_name==a else 0 for r,a,h in team[['result','away_team','home_team']].values]
|
50 |
-
team['L'] = [0 if r>0 and team_name==h else 0 if r<0 and team_name==a else 1 for r,a,h in team[['result','away_team','home_team']].values]
|
51 |
-
team['W_PCT'] = team['W']/team['GP']
|
52 |
-
team['TOP'] = [t if team_name==p else 0 for t,p in team[['TOP_seconds','posteam']].values]
|
53 |
-
team['FGA'] = [1 if team_name==p and f==1 else 0 for p,f in team[['posteam','field_goal_attempt']].values]
|
54 |
-
team['FGM'] = [1 if team_name==p and f=='made' else 0 for p,f in team[['posteam','field_goal_result']].values]
|
55 |
-
team['FG_PCT'] = team['FGM']/team['FGA']
|
56 |
-
team['PassTD'] = np.where((team['posteam'] == team_name) & (team['pass_touchdown'] == 1), 1, 0)
|
57 |
-
team['RushTD'] = np.where((team['posteam'] == team_name) & (team['rush_touchdown'] == 1), 1, 0)
|
58 |
-
team['PassTD_Allowed'] = np.where((team['defteam'] == team_name) & (team['pass_touchdown'] == 1), 1, 0)
|
59 |
-
team['RushTD_Allowed'] = np.where((team['defteam'] == team_name) & (team['rush_touchdown'] == 1), 1, 0)
|
60 |
-
team['PassYds'] = [y if p==team_name else 0 for p,y in team[['posteam','passing_yards']].values]
|
61 |
-
team['RushYds'] = [y if p==team_name else 0 for p,y in team[['posteam','rushing_yards']].values]
|
62 |
-
team['PassYds_Allowed'] = [y if d==team_name else 0 for d,y in team[['defteam','passing_yards']].values]
|
63 |
-
team['RushYds_Allowed'] = [y if d==team_name else 0 for d,y in team[['defteam','rushing_yards']].values]
|
64 |
-
team['Fum'] = np.where((team['defteam'] == team_name) & (team['fumble_lost'] == 1), 1, 0)
|
65 |
-
team['Fum_Allowed'] = np.where((team['posteam'] == team_name) & (team['fumble_lost'] == 1), 1, 0)
|
66 |
-
team['INT'] = np.where((team['defteam'] == team_name) & (team['interception'] == 1), 1, 0)
|
67 |
-
team['INT_Allowed'] = np.where((team['posteam'] == team_name) & (team['interception'] == 1), 1, 0)
|
68 |
-
team['Sacks'] = np.where((team['defteam'] == team_name) & (team['sack'] == 1), 1, 0)
|
69 |
-
team['Sacks_Allowed'] = np.where((team['posteam'] == team_name) & (team['sack'] == 1), 1, 0)
|
70 |
-
team['Penalties'] = np.where((team['penalty_team'] == team_name), 1, 0)
|
71 |
-
team['FirstDowns'] = [1 if team_name==p and f==1 else 0 for p,f in team[['posteam','first_down']].values]
|
72 |
-
team['3rdDownConverted'] = [1 if p==team_name and t==1 else 0 for p,t in team[['posteam','third_down_converted']].values]
|
73 |
-
team['3rdDownFailed'] = [1 if p==team_name and t==1 else 0 for p,t in team[['posteam','third_down_failed']].values]
|
74 |
-
team['3rdDownAllowed'] = [1 if d==team_name and t==1 else 0 for d,t in team[['defteam','third_down_converted']].values]
|
75 |
-
team['3rdDownDefended'] = [1 if d==team_name and t==1 else 0 for d,t in team[['defteam','third_down_failed']].values]
|
76 |
-
team['PTS'] = [ap if at==team_name else hp if ht==team_name else None for ht,at,hp,ap in team[['home_team','away_team','home_score','away_score']].values]
|
77 |
-
team['PointDiff'] = [r if team_name==h else -r if team_name==a else 0 for r,a,h in team[['result','away_team','home_team']].values]
|
78 |
-
|
79 |
-
# aggregate from play-by-play to game-by-game
|
80 |
-
features = {
|
81 |
-
'GP':'mean',
|
82 |
-
'W':'mean',
|
83 |
-
'L':'mean',
|
84 |
-
'W_PCT':'mean',
|
85 |
-
'TOP':'sum',
|
86 |
-
'FGA':'sum',
|
87 |
-
'FGM':'sum',
|
88 |
-
'FG_PCT':'mean',
|
89 |
-
'PassTD':'sum',
|
90 |
-
'RushTD':'sum',
|
91 |
-
'PassTD_Allowed':'sum',
|
92 |
-
'RushTD_Allowed':'sum',
|
93 |
-
'PassYds':'sum',
|
94 |
-
'RushYds':'sum',
|
95 |
-
'PassYds_Allowed':'sum',
|
96 |
-
'RushYds_Allowed':'sum',
|
97 |
-
'Fum':'sum',
|
98 |
-
'Fum_Allowed':'sum',
|
99 |
-
'INT':'sum',
|
100 |
-
'INT_Allowed':'sum',
|
101 |
-
'Sacks':'sum',
|
102 |
-
'Sacks_Allowed':'sum',
|
103 |
-
'Penalties':'sum',
|
104 |
-
'FirstDowns':'sum',
|
105 |
-
'3rdDownConverted':'sum',
|
106 |
-
'3rdDownFailed':'sum',
|
107 |
-
'3rdDownAllowed':'sum',
|
108 |
-
'3rdDownDefended':'sum',
|
109 |
-
'PTS':'mean',
|
110 |
-
'PointDiff':'mean'
|
111 |
-
}
|
112 |
-
|
113 |
-
game = team.groupby('game_id').agg(features).reset_index().sort_values('GP')
|
114 |
-
game[['W','L']] = game[['W','L']].expanding().sum()
|
115 |
-
game[game.columns[4:]] = game[game.columns[4:]].expanding().mean()
|
116 |
-
if season != current_season:
|
117 |
-
game[game.columns[1:]] = game[game.columns[1:]].shift()
|
118 |
-
game['TEAM'] = team_name
|
119 |
-
game['Season'] = season
|
120 |
-
else:
|
121 |
-
game['TEAM'] = team_name
|
122 |
-
game['Season'] = season
|
123 |
-
|
124 |
-
data = pd.concat([data,game])
|
125 |
-
|
126 |
-
# separate home and away data and merge
|
127 |
-
data = data.merge(pbp[['game_id','home_team','away_team']].drop_duplicates())
|
128 |
-
home = data.loc[data['home_team']==data['TEAM']]
|
129 |
-
away = data.loc[data['away_team']==data['TEAM']]
|
130 |
-
away.columns = [f'{i}.Away' for i in away.columns]
|
131 |
-
gbg = home.merge(away,left_on='game_id',right_on='game_id.Away')
|
132 |
-
gbg.drop(columns=['TEAM','TEAM.Away','home_team.Away','away_team.Away','Season.Away','game_id.Away'], inplace=True)
|
133 |
-
gbg['game_date'] = gbg['game_id'].map(game_date_dict)
|
134 |
-
|
135 |
-
# save current data
|
136 |
-
if current_season in get_seasons:
|
137 |
-
gbg_this_year = gbg.loc[gbg['Season']==current_season]
|
138 |
-
file_path = os.path.join(data_directory, 'gbg_this_year.csv')
|
139 |
-
gbg_this_year.to_csv(file_path, index=False)
|
140 |
-
|
141 |
-
# save historical data
|
142 |
-
if get_seasons != [current_season]:
|
143 |
-
gbg = gbg.loc[gbg['Season']!=current_season]
|
144 |
-
file_path = os.path.join(data_directory, 'gbg.csv')
|
145 |
-
gbg.to_csv(file_path, index=False)
|
146 |
-
|
147 |
-
|
148 |
-
def add_odds_data():
|
149 |
-
"""
|
150 |
-
Get odds from Australian Sports Betting's free online dataset and merge it with game-by-game data.
|
151 |
-
|
152 |
-
"""
|
153 |
-
|
154 |
-
# get team abbreviations
|
155 |
-
team_descriptions = nfl.import_team_desc()
|
156 |
-
team_abbreviation_dict = dict(team_descriptions[['team_name','team_abbr']].values)
|
157 |
-
|
158 |
-
# get odds
|
159 |
-
odds = pd.read_excel('https://www.aussportsbetting.com/historical_data/nfl.xlsx')
|
160 |
-
odds['Home Team'] = odds['Home Team'].str.replace('Washington Redskins','Washington Commanders').str.replace('Washington Football Team','Washington Commanders')
|
161 |
-
odds['Away Team'] = odds['Away Team'].str.replace('Washington Redskins','Washington Commanders').str.replace('Washington Football Team','Washington Commanders')
|
162 |
-
odds['Season'] = [i.year if i.month in [8,9,10,11,12] else i.year-1 for i in odds['Date']]
|
163 |
-
odds['Home Team Abbrev'] = odds['Home Team'].map(team_abbreviation_dict).str.replace('LAR','LA')
|
164 |
-
odds['Away Team Abbrev'] = odds['Away Team'].map(team_abbreviation_dict).str.replace('LAR','LA')
|
165 |
-
odds = odds[['Date','Home Score','Away Score','Home Team Abbrev','Away Team Abbrev','Home Odds Close','Away Odds Close','Total Score Close','Home Line Close']]
|
166 |
-
odds['Key'] = odds['Date'].astype(str) + odds['Home Team Abbrev'] + odds['Away Team Abbrev']
|
167 |
-
odds = odds.drop(columns=['Date','Home Team Abbrev','Away Team Abbrev']).dropna()
|
168 |
-
odds['Home Odds'] = [round((i-1)*100) if i>= 2 else round(-100/(i-1)) for i in odds['Home Odds Close']]
|
169 |
-
odds['Away Odds'] = [round((i-1)*100) if i>= 2 else round(-100/(i-1)) for i in odds['Away Odds Close']]
|
170 |
-
odds['Home Winnings'] = [ho-1 if h>a else -1 if a>h else 0 for ho,h,a in odds[['Home Odds Close','Home Score','Away Score']].values]
|
171 |
-
odds['Away Winnings'] = [ao-1 if a>h else -1 if h>a else 0 for ao,h,a in odds[['Away Odds Close','Home Score','Away Score']].values]
|
172 |
-
|
173 |
-
# load gbg data
|
174 |
-
file_path = os.path.join(data_directory, 'gbg.csv')
|
175 |
-
gbg = pd.read_csv(file_path)
|
176 |
-
file_path = os.path.join(data_directory, 'gbg_this_year.csv')
|
177 |
-
gbg_this_year = pd.read_csv(file_path)
|
178 |
-
|
179 |
-
# merge and save
|
180 |
-
dataframes = [gbg, gbg_this_year]
|
181 |
-
for idx in range(2):
|
182 |
-
i = dataframes[idx]
|
183 |
-
i['Key'] = i['game_date'].astype(str) + i['home_team'] + i['away_team']
|
184 |
-
gbg_and_odds = i.merge(odds, left_on='Key', right_on='Key')
|
185 |
-
gbg_and_odds['Home-Team-Cover'] = [1 if (h-a)>-l else 0 if (h-a)<-l else 2 for h,a,l in gbg_and_odds[['Home Score','Away Score','Home Line Close']].values]
|
186 |
-
gbg_and_odds['Home-Team-Win'] = (gbg_and_odds['Home Score']>gbg_and_odds['Away Score']).astype(int)
|
187 |
-
gbg_and_odds['Over'] = ((gbg_and_odds['Home Score'] + gbg_and_odds['Away Score'])>gbg_and_odds['Total Score Close']).astype(int)
|
188 |
-
|
189 |
-
if idx==0:
|
190 |
-
file_path = os.path.join(data_directory, 'gbg_and_odds.csv')
|
191 |
-
else:
|
192 |
-
file_path = os.path.join(data_directory, 'gbg_and_odds_this_year.csv')
|
193 |
-
|
194 |
-
gbg_and_odds.drop_duplicates(subset='game_id').to_csv(file_path, index=False)
|
195 |
-
|
196 |
-
|
197 |
-
|
|
|
|
|
|
|
|
|
|
|
|
|
|
|
|
|
|
|
|
|
|
|
|
|
|
|
|
|
|
|
|
|
|
|
|
|
|
|
|
|
|
|
|
|
|
|
|
|
|
|
|
|
|
|
|
|
|
|
|
|
|
|
|
|
|
|
|
|
|
|
|
|
|
|
|
|
|
|
|
|
|
|
|
|
|
|
|
|
|
|
|
|
|
|
|
|
|
|
|
|
|
|
|
|
|
|
|
|
|
|
|
|
|
|
|
|
|
|
|
|
|
|
|
|
|
|
|
|
|
|
|
|
|
|
|
|
|
|
|
|
|
|
|
|
|
|
|
|
|
|
|
|
|
|
|
|
|
|
|
|
|
|
|
|
|
|
|
|
|
|
|
|
|
|
|
|
|
|
|
|
|
|
|
|
|
|
|
|
|
|
|
|
|
|
|
|
|
|
|
|
|
|
|
|
|
|
|
|
|
|
|
|
|
|
|
|
|
|
|
|
|
|
|
|
|
|
|
|
|
|
|
|
|
|
|
|
|
|
|
|
|
|
|
|
|
|
|
|
|
|
|
|
|
|
|
|
|
|
|
|
|
|
|
|
|
|
|
|
|
|
|
|
|
|
|
|
|
|
|
|
|
|
|
|
|
|
|
|
|
|
|
|
|
|
|
|
|
|
|
|
|
|
|
|
|
|
|
|
|
|
|
|
|
|
|
|
|
|
|
|
|
|
|
|
|
|
|
|
|
|
|
|
|
|
|
|
|
|
|
|
|
|
|
|
|
|
|
|
|
|
|
|
|
|
|
|
|
|
|
|
|
|
|
|
|
|
|
|
|
|
|
|
|
|
|
|
|
|
|
|
|
|
|
|
|
|
|
|
|
|
|
|
|
|
|
spaces/Brofu/Joeythemonster-anything-midjourney-v-4-1/app.py
DELETED
@@ -1,3 +0,0 @@
|
|
1 |
-
import gradio as gr
|
2 |
-
|
3 |
-
gr.Interface.load("models/Joeythemonster/anything-midjourney-v-4-1").launch()
|
|
|
|
|
|
|
|
spaces/CALM/Dashboard/perso/change_data.py
DELETED
@@ -1,19 +0,0 @@
|
|
1 |
-
import json
|
2 |
-
import random
|
3 |
-
|
4 |
-
with open(
|
5 |
-
"/mnt/storage/Documents/hugging_face/colaborative_hub_training/demo_neurips/training-transformers-together-dashboard/data/"
|
6 |
-
"serializaledata.json",
|
7 |
-
"r",
|
8 |
-
) as f:
|
9 |
-
serialized_data = json.load(f)
|
10 |
-
|
11 |
-
serialized_data_v2 = serialized_data
|
12 |
-
serialized_data_v2["points"] = [[item for item in serialized_data["points"][-1] if random.random() > 0.8]]
|
13 |
-
|
14 |
-
with open(
|
15 |
-
"/mnt/storage/Documents/hugging_face/colaborative_hub_training/demo_neurips/training-transformers-together-dashboard/data/"
|
16 |
-
"serializaledata_V2.json",
|
17 |
-
"w",
|
18 |
-
) as f:
|
19 |
-
f.write(json.dumps(serialized_data_v2))
|
|
|
|
|
|
|
|
|
|
|
|
|
|
|
|
|
|
|
|
|
|
|
|
|
|
|
|
|
|
|
|
|
|
|
|
|
|
|
|
spaces/CVH-vn1210/make_hair/minigpt4/tasks/__init__.py
DELETED
@@ -1,26 +0,0 @@
|
|
1 |
-
"""
|
2 |
-
Copyright (c) 2022, salesforce.com, inc.
|
3 |
-
All rights reserved.
|
4 |
-
SPDX-License-Identifier: BSD-3-Clause
|
5 |
-
For full license text, see the LICENSE file in the repo root or https://opensource.org/licenses/BSD-3-Clause
|
6 |
-
"""
|
7 |
-
|
8 |
-
from minigpt4.common.registry import registry
|
9 |
-
from minigpt4.tasks.base_task import BaseTask
|
10 |
-
from minigpt4.tasks.image_text_pretrain import ImageTextPretrainTask
|
11 |
-
|
12 |
-
|
13 |
-
def setup_task(cfg):
|
14 |
-
assert "task" in cfg.run_cfg, "Task name must be provided."
|
15 |
-
|
16 |
-
task_name = cfg.run_cfg.task
|
17 |
-
task = registry.get_task_class(task_name).setup_task(cfg=cfg)
|
18 |
-
assert task is not None, "Task {} not properly registered.".format(task_name)
|
19 |
-
|
20 |
-
return task
|
21 |
-
|
22 |
-
|
23 |
-
__all__ = [
|
24 |
-
"BaseTask",
|
25 |
-
"ImageTextPretrainTask",
|
26 |
-
]
|
|
|
|
|
|
|
|
|
|
|
|
|
|
|
|
|
|
|
|
|
|
|
|
|
|
|
|
|
|
|
|
|
|
|
|
|
|
|
|
|
|
|
|
|
|
|
|
|
|
|
|
|
|
spaces/CVPR/Dual-Key_Backdoor_Attacks/datagen/detectron2/detectron2/layers/csrc/vision.cpp
DELETED
@@ -1,102 +0,0 @@
|
|
1 |
-
// Copyright (c) Facebook, Inc. and its affiliates. All Rights Reserved
|
2 |
-
|
3 |
-
#include <torch/extension.h>
|
4 |
-
#include "ROIAlign/ROIAlign.h"
|
5 |
-
#include "ROIAlignRotated/ROIAlignRotated.h"
|
6 |
-
#include "box_iou_rotated/box_iou_rotated.h"
|
7 |
-
#include "deformable/deform_conv.h"
|
8 |
-
#include "nms_rotated/nms_rotated.h"
|
9 |
-
|
10 |
-
namespace detectron2 {
|
11 |
-
|
12 |
-
#ifdef WITH_CUDA
|
13 |
-
extern int get_cudart_version();
|
14 |
-
#endif
|
15 |
-
|
16 |
-
std::string get_cuda_version() {
|
17 |
-
#ifdef WITH_CUDA
|
18 |
-
std::ostringstream oss;
|
19 |
-
|
20 |
-
// copied from
|
21 |
-
// https://github.com/pytorch/pytorch/blob/master/aten/src/ATen/cuda/detail/CUDAHooks.cpp#L231
|
22 |
-
auto printCudaStyleVersion = [&](int v) {
|
23 |
-
oss << (v / 1000) << "." << (v / 10 % 100);
|
24 |
-
if (v % 10 != 0) {
|
25 |
-
oss << "." << (v % 10);
|
26 |
-
}
|
27 |
-
};
|
28 |
-
printCudaStyleVersion(get_cudart_version());
|
29 |
-
return oss.str();
|
30 |
-
#else
|
31 |
-
return std::string("not available");
|
32 |
-
#endif
|
33 |
-
}
|
34 |
-
|
35 |
-
// similar to
|
36 |
-
// https://github.com/pytorch/pytorch/blob/master/aten/src/ATen/Version.cpp
|
37 |
-
std::string get_compiler_version() {
|
38 |
-
std::ostringstream ss;
|
39 |
-
#if defined(__GNUC__)
|
40 |
-
#ifndef __clang__
|
41 |
-
|
42 |
-
#if ((__GNUC__ <= 4) && (__GNUC_MINOR__ <= 8))
|
43 |
-
#error "GCC >= 4.9 is required!"
|
44 |
-
#endif
|
45 |
-
|
46 |
-
{ ss << "GCC " << __GNUC__ << "." << __GNUC_MINOR__; }
|
47 |
-
#endif
|
48 |
-
#endif
|
49 |
-
|
50 |
-
#if defined(__clang_major__)
|
51 |
-
{
|
52 |
-
ss << "clang " << __clang_major__ << "." << __clang_minor__ << "."
|
53 |
-
<< __clang_patchlevel__;
|
54 |
-
}
|
55 |
-
#endif
|
56 |
-
|
57 |
-
#if defined(_MSC_VER)
|
58 |
-
{ ss << "MSVC " << _MSC_FULL_VER; }
|
59 |
-
#endif
|
60 |
-
return ss.str();
|
61 |
-
}
|
62 |
-
|
63 |
-
PYBIND11_MODULE(TORCH_EXTENSION_NAME, m) {
|
64 |
-
m.def("get_compiler_version", &get_compiler_version, "get_compiler_version");
|
65 |
-
m.def("get_cuda_version", &get_cuda_version, "get_cuda_version");
|
66 |
-
|
67 |
-
m.def("box_iou_rotated", &box_iou_rotated, "IoU for rotated boxes");
|
68 |
-
|
69 |
-
m.def("deform_conv_forward", &deform_conv_forward, "deform_conv_forward");
|
70 |
-
m.def(
|
71 |
-
"deform_conv_backward_input",
|
72 |
-
&deform_conv_backward_input,
|
73 |
-
"deform_conv_backward_input");
|
74 |
-
m.def(
|
75 |
-
"deform_conv_backward_filter",
|
76 |
-
&deform_conv_backward_filter,
|
77 |
-
"deform_conv_backward_filter");
|
78 |
-
m.def(
|
79 |
-
"modulated_deform_conv_forward",
|
80 |
-
&modulated_deform_conv_forward,
|
81 |
-
"modulated_deform_conv_forward");
|
82 |
-
m.def(
|
83 |
-
"modulated_deform_conv_backward",
|
84 |
-
&modulated_deform_conv_backward,
|
85 |
-
"modulated_deform_conv_backward");
|
86 |
-
|
87 |
-
m.def("nms_rotated", &nms_rotated, "NMS for rotated boxes");
|
88 |
-
|
89 |
-
m.def("roi_align_forward", &ROIAlign_forward, "ROIAlign_forward");
|
90 |
-
m.def("roi_align_backward", &ROIAlign_backward, "ROIAlign_backward");
|
91 |
-
|
92 |
-
m.def(
|
93 |
-
"roi_align_rotated_forward",
|
94 |
-
&ROIAlignRotated_forward,
|
95 |
-
"Forward pass for Rotated ROI-Align Operator");
|
96 |
-
m.def(
|
97 |
-
"roi_align_rotated_backward",
|
98 |
-
&ROIAlignRotated_backward,
|
99 |
-
"Backward pass for Rotated ROI-Align Operator");
|
100 |
-
}
|
101 |
-
|
102 |
-
} // namespace detectron2
|
|
|
|
|
|
|
|
|
|
|
|
|
|
|
|
|
|
|
|
|
|
|
|
|
|
|
|
|
|
|
|
|
|
|
|
|
|
|
|
|
|
|
|
|
|
|
|
|
|
|
|
|
|
|
|
|
|
|
|
|
|
|
|
|
|
|
|
|
|
|
|
|
|
|
|
|
|
|
|
|
|
|
|
|
|
|
|
|
|
|
|
|
|
|
|
|
|
|
|
|
|
|
|
|
|
|
|
|
|
|
|
|
|
|
|
|
|
|
|
|
|
|
|
|
|
|
|
|
|
|
|
|
|
|
|
|
|
|
|
|
|
|
|
|
|
|
|
|
|
|
|
|
|
|
|
|
|
|
|
|
|
|
|
|
|
|
|
|
|
|
|
|
|
|
|
|
|
|
|
|
|
|
|
|
|
|
|
|
|
|
|
|
|
|
|
|
|
|
|
|
|
|
|
|
|
spaces/CVPR/Dual-Key_Backdoor_Attacks/datagen/detectron2/docs/tutorials/README.md
DELETED
@@ -1,4 +0,0 @@
|
|
1 |
-
# Read the docs:
|
2 |
-
|
3 |
-
The latest documentation built from this directory is available at [detectron2.readthedocs.io](https://detectron2.readthedocs.io/).
|
4 |
-
Documents in this directory are not meant to be read on github.
|
|
|
|
|
|
|
|
|
|
spaces/CVPR/LIVE/thrust/thrust/detail/static_map.h
DELETED
@@ -1,170 +0,0 @@
|
|
1 |
-
/*
|
2 |
-
* Copyright 2008-2013 NVIDIA Corporation
|
3 |
-
*
|
4 |
-
* Licensed under the Apache License, Version 2.0 (the "License");
|
5 |
-
* you may not use this file except in compliance with the License.
|
6 |
-
* You may obtain a copy of the License at
|
7 |
-
*
|
8 |
-
* http://www.apache.org/licenses/LICENSE-2.0
|
9 |
-
*
|
10 |
-
* Unless required by applicable law or agreed to in writing, software
|
11 |
-
* distributed under the License is distributed on an "AS IS" BASIS,
|
12 |
-
* WITHOUT WARRANTIES OR CONDITIONS OF ANY KIND, either express or implied.
|
13 |
-
* See the License for the specific language governing permissions and
|
14 |
-
* limitations under the License.
|
15 |
-
*/
|
16 |
-
|
17 |
-
#pragma once
|
18 |
-
|
19 |
-
|
20 |
-
#include <thrust/detail/config.h>
|
21 |
-
|
22 |
-
|
23 |
-
namespace thrust
|
24 |
-
{
|
25 |
-
namespace detail
|
26 |
-
{
|
27 |
-
namespace static_map_detail
|
28 |
-
{
|
29 |
-
|
30 |
-
|
31 |
-
template<unsigned int k, unsigned int v>
|
32 |
-
struct key_value
|
33 |
-
{
|
34 |
-
static const unsigned int key = k;
|
35 |
-
static const unsigned int value = v;
|
36 |
-
};
|
37 |
-
|
38 |
-
|
39 |
-
template<typename Head, typename Tail = void>
|
40 |
-
struct cons
|
41 |
-
{
|
42 |
-
template<unsigned int key, unsigned int default_value>
|
43 |
-
struct static_get
|
44 |
-
{
|
45 |
-
static const unsigned int value = (key == Head::key) ? (Head::value) : Tail::template static_get<key,default_value>::value;
|
46 |
-
};
|
47 |
-
|
48 |
-
|
49 |
-
template<unsigned int default_value>
|
50 |
-
__host__ __device__
|
51 |
-
static unsigned int get(unsigned int key)
|
52 |
-
{
|
53 |
-
return (key == Head::key) ? (Head::value) : Tail::template get<default_value>(key);
|
54 |
-
}
|
55 |
-
};
|
56 |
-
|
57 |
-
|
58 |
-
template<typename Head>
|
59 |
-
struct cons<Head,void>
|
60 |
-
{
|
61 |
-
template<unsigned int key, unsigned int default_value>
|
62 |
-
struct static_get
|
63 |
-
{
|
64 |
-
static const unsigned int value = (key == Head::key) ? (Head::value) : default_value;
|
65 |
-
};
|
66 |
-
|
67 |
-
template<unsigned int default_value>
|
68 |
-
__host__ __device__
|
69 |
-
static unsigned int get(unsigned int key)
|
70 |
-
{
|
71 |
-
return (key == Head::key) ? (Head::value) : default_value;
|
72 |
-
}
|
73 |
-
};
|
74 |
-
|
75 |
-
|
76 |
-
template<unsigned int default_value,
|
77 |
-
unsigned int key0 = 0, unsigned int value0 = default_value,
|
78 |
-
unsigned int key1 = 0, unsigned int value1 = default_value,
|
79 |
-
unsigned int key2 = 0, unsigned int value2 = default_value,
|
80 |
-
unsigned int key3 = 0, unsigned int value3 = default_value,
|
81 |
-
unsigned int key4 = 0, unsigned int value4 = default_value,
|
82 |
-
unsigned int key5 = 0, unsigned int value5 = default_value,
|
83 |
-
unsigned int key6 = 0, unsigned int value6 = default_value,
|
84 |
-
unsigned int key7 = 0, unsigned int value7 = default_value>
|
85 |
-
struct static_map
|
86 |
-
{
|
87 |
-
typedef cons<
|
88 |
-
key_value<key0,value0>,
|
89 |
-
cons<
|
90 |
-
key_value<key1,value1>,
|
91 |
-
cons<
|
92 |
-
key_value<key2,value2>,
|
93 |
-
cons<
|
94 |
-
key_value<key3,value3>,
|
95 |
-
cons<
|
96 |
-
key_value<key4,value4>,
|
97 |
-
cons<
|
98 |
-
key_value<key5,value5>,
|
99 |
-
cons<
|
100 |
-
key_value<key6,value6>,
|
101 |
-
cons<
|
102 |
-
key_value<key7,value7>
|
103 |
-
>
|
104 |
-
>
|
105 |
-
>
|
106 |
-
>
|
107 |
-
>
|
108 |
-
>
|
109 |
-
>
|
110 |
-
> impl;
|
111 |
-
|
112 |
-
template<unsigned int key>
|
113 |
-
struct static_get
|
114 |
-
{
|
115 |
-
static const unsigned int value = impl::template static_get<key,default_value>::value;
|
116 |
-
};
|
117 |
-
|
118 |
-
__host__ __device__
|
119 |
-
static unsigned int get(unsigned int key)
|
120 |
-
{
|
121 |
-
return impl::template get<default_value>(key);
|
122 |
-
}
|
123 |
-
};
|
124 |
-
|
125 |
-
|
126 |
-
} // end namespace static_map_detail
|
127 |
-
|
128 |
-
|
129 |
-
template<unsigned int default_value,
|
130 |
-
unsigned int key0 = 0, unsigned int value0 = default_value,
|
131 |
-
unsigned int key1 = 0, unsigned int value1 = default_value,
|
132 |
-
unsigned int key2 = 0, unsigned int value2 = default_value,
|
133 |
-
unsigned int key3 = 0, unsigned int value3 = default_value,
|
134 |
-
unsigned int key4 = 0, unsigned int value4 = default_value,
|
135 |
-
unsigned int key5 = 0, unsigned int value5 = default_value,
|
136 |
-
unsigned int key6 = 0, unsigned int value6 = default_value,
|
137 |
-
unsigned int key7 = 0, unsigned int value7 = default_value>
|
138 |
-
struct static_map
|
139 |
-
: static_map_detail::static_map<
|
140 |
-
default_value,
|
141 |
-
key0, value0,
|
142 |
-
key1, value1,
|
143 |
-
key2, value2,
|
144 |
-
key3, value3,
|
145 |
-
key4, value4,
|
146 |
-
key5, value5,
|
147 |
-
key6, value6,
|
148 |
-
key7, value7
|
149 |
-
>
|
150 |
-
{};
|
151 |
-
|
152 |
-
|
153 |
-
template<unsigned int key, typename StaticMap>
|
154 |
-
struct static_lookup
|
155 |
-
{
|
156 |
-
static const unsigned int value = StaticMap::template static_get<key>::value;
|
157 |
-
};
|
158 |
-
|
159 |
-
|
160 |
-
template<typename StaticMap>
|
161 |
-
__host__ __device__
|
162 |
-
unsigned int lookup(unsigned int key)
|
163 |
-
{
|
164 |
-
return StaticMap::get(key);
|
165 |
-
}
|
166 |
-
|
167 |
-
|
168 |
-
} // end namespace detail
|
169 |
-
} // end namespace thrust
|
170 |
-
|
|
|
|
|
|
|
|
|
|
|
|
|
|
|
|
|
|
|
|
|
|
|
|
|
|
|
|
|
|
|
|
|
|
|
|
|
|
|
|
|
|
|
|
|
|
|
|
|
|
|
|
|
|
|
|
|
|
|
|
|
|
|
|
|
|
|
|
|
|
|
|
|
|
|
|
|
|
|
|
|
|
|
|
|
|
|
|
|
|
|
|
|
|
|
|
|
|
|
|
|
|
|
|
|
|
|
|
|
|
|
|
|
|
|
|
|
|
|
|
|
|
|
|
|
|
|
|
|
|
|
|
|
|
|
|
|
|
|
|
|
|
|
|
|
|
|
|
|
|
|
|
|
|
|
|
|
|
|
|
|
|
|
|
|
|
|
|
|
|
|
|
|
|
|
|
|
|
|
|
|
|
|
|
|
|
|
|
|
|
|
|
|
|
|
|
|
|
|
|
|
|
|
|
|
|
|
|
|
|
|
|
|
|
|
|
|
|
|
|
|
|
|
|
|
|
|
|
|
|
|
|
|
|
|
|
|
|
|
|
|
|
|
|
|
|
|
|
|
|
|
|
|
|
|
|
|
|
|
|
|
|
|
|
|
|
|
|
|
|
|
|
|
|
|
|
|
|
|
|
|
|
|
|
|
|
|
|
|
|
|
|
|
|
|
|
|
|
|
|
|
|
|
|
|
|
|
|
|
|
|
|
|
|
|
|
|
|
|
|
|
|
|
|
|
|
|
|
|
|
|
|
|
|
|
|
|
|
|
|
|
|
spaces/CVPR/LIVE/thrust/thrust/system/omp/detail/unique_by_key.h
DELETED
@@ -1,67 +0,0 @@
|
|
1 |
-
/*
|
2 |
-
* Copyright 2008-2013 NVIDIA Corporation
|
3 |
-
*
|
4 |
-
* Licensed under the Apache License, Version 2.0 (the "License");
|
5 |
-
* you may not use this file except in compliance with the License.
|
6 |
-
* You may obtain a copy of the License at
|
7 |
-
*
|
8 |
-
* http://www.apache.org/licenses/LICENSE-2.0
|
9 |
-
*
|
10 |
-
* Unless required by applicable law or agreed to in writing, software
|
11 |
-
* distributed under the License is distributed on an "AS IS" BASIS,
|
12 |
-
* WITHOUT WARRANTIES OR CONDITIONS OF ANY KIND, either express or implied.
|
13 |
-
* See the License for the specific language governing permissions and
|
14 |
-
* limitations under the License.
|
15 |
-
*/
|
16 |
-
|
17 |
-
#pragma once
|
18 |
-
|
19 |
-
#include <thrust/detail/config.h>
|
20 |
-
#include <thrust/system/omp/detail/execution_policy.h>
|
21 |
-
#include <thrust/pair.h>
|
22 |
-
|
23 |
-
namespace thrust
|
24 |
-
{
|
25 |
-
namespace system
|
26 |
-
{
|
27 |
-
namespace omp
|
28 |
-
{
|
29 |
-
namespace detail
|
30 |
-
{
|
31 |
-
|
32 |
-
|
33 |
-
template<typename DerivedPolicy,
|
34 |
-
typename ForwardIterator1,
|
35 |
-
typename ForwardIterator2,
|
36 |
-
typename BinaryPredicate>
|
37 |
-
thrust::pair<ForwardIterator1,ForwardIterator2>
|
38 |
-
unique_by_key(execution_policy<DerivedPolicy> &exec,
|
39 |
-
ForwardIterator1 keys_first,
|
40 |
-
ForwardIterator1 keys_last,
|
41 |
-
ForwardIterator2 values_first,
|
42 |
-
BinaryPredicate binary_pred);
|
43 |
-
|
44 |
-
|
45 |
-
template<typename DerivedPolicy,
|
46 |
-
typename InputIterator1,
|
47 |
-
typename InputIterator2,
|
48 |
-
typename OutputIterator1,
|
49 |
-
typename OutputIterator2,
|
50 |
-
typename BinaryPredicate>
|
51 |
-
thrust::pair<OutputIterator1,OutputIterator2>
|
52 |
-
unique_by_key_copy(execution_policy<DerivedPolicy> &exec,
|
53 |
-
InputIterator1 keys_first,
|
54 |
-
InputIterator1 keys_last,
|
55 |
-
InputIterator2 values_first,
|
56 |
-
OutputIterator1 keys_output,
|
57 |
-
OutputIterator2 values_output,
|
58 |
-
BinaryPredicate binary_pred);
|
59 |
-
|
60 |
-
|
61 |
-
} // end namespace detail
|
62 |
-
} // end namespace omp
|
63 |
-
} // end namespace system
|
64 |
-
} // end namespace thrust
|
65 |
-
|
66 |
-
#include <thrust/system/omp/detail/unique_by_key.inl>
|
67 |
-
|
|
|
|
|
|
|
|
|
|
|
|
|
|
|
|
|
|
|
|
|
|
|
|
|
|
|
|
|
|
|
|
|
|
|
|
|
|
|
|
|
|
|
|
|
|
|
|
|
|
|
|
|
|
|
|
|
|
|
|
|
|
|
|
|
|
|
|
|
|
|
|
|
|
|
|
|
|
|
|
|
|
|
|
|
|
|
|
|
|
|
|
|
|
|
|
|
|
|
|
|
|
|
|
|
|
|
|
|
|
|
|
|
|
|
|
|
|
|
|
|
|
|
|
|
|
|
|
|
|
|
|
|
|
|
|
spaces/ChevyWithAI/rvc-aicover/infer_pack/attentions.py
DELETED
@@ -1,417 +0,0 @@
|
|
1 |
-
import copy
|
2 |
-
import math
|
3 |
-
import numpy as np
|
4 |
-
import torch
|
5 |
-
from torch import nn
|
6 |
-
from torch.nn import functional as F
|
7 |
-
|
8 |
-
from infer_pack import commons
|
9 |
-
from infer_pack import modules
|
10 |
-
from infer_pack.modules import LayerNorm
|
11 |
-
|
12 |
-
|
13 |
-
class Encoder(nn.Module):
|
14 |
-
def __init__(
|
15 |
-
self,
|
16 |
-
hidden_channels,
|
17 |
-
filter_channels,
|
18 |
-
n_heads,
|
19 |
-
n_layers,
|
20 |
-
kernel_size=1,
|
21 |
-
p_dropout=0.0,
|
22 |
-
window_size=10,
|
23 |
-
**kwargs
|
24 |
-
):
|
25 |
-
super().__init__()
|
26 |
-
self.hidden_channels = hidden_channels
|
27 |
-
self.filter_channels = filter_channels
|
28 |
-
self.n_heads = n_heads
|
29 |
-
self.n_layers = n_layers
|
30 |
-
self.kernel_size = kernel_size
|
31 |
-
self.p_dropout = p_dropout
|
32 |
-
self.window_size = window_size
|
33 |
-
|
34 |
-
self.drop = nn.Dropout(p_dropout)
|
35 |
-
self.attn_layers = nn.ModuleList()
|
36 |
-
self.norm_layers_1 = nn.ModuleList()
|
37 |
-
self.ffn_layers = nn.ModuleList()
|
38 |
-
self.norm_layers_2 = nn.ModuleList()
|
39 |
-
for i in range(self.n_layers):
|
40 |
-
self.attn_layers.append(
|
41 |
-
MultiHeadAttention(
|
42 |
-
hidden_channels,
|
43 |
-
hidden_channels,
|
44 |
-
n_heads,
|
45 |
-
p_dropout=p_dropout,
|
46 |
-
window_size=window_size,
|
47 |
-
)
|
48 |
-
)
|
49 |
-
self.norm_layers_1.append(LayerNorm(hidden_channels))
|
50 |
-
self.ffn_layers.append(
|
51 |
-
FFN(
|
52 |
-
hidden_channels,
|
53 |
-
hidden_channels,
|
54 |
-
filter_channels,
|
55 |
-
kernel_size,
|
56 |
-
p_dropout=p_dropout,
|
57 |
-
)
|
58 |
-
)
|
59 |
-
self.norm_layers_2.append(LayerNorm(hidden_channels))
|
60 |
-
|
61 |
-
def forward(self, x, x_mask):
|
62 |
-
attn_mask = x_mask.unsqueeze(2) * x_mask.unsqueeze(-1)
|
63 |
-
x = x * x_mask
|
64 |
-
for i in range(self.n_layers):
|
65 |
-
y = self.attn_layers[i](x, x, attn_mask)
|
66 |
-
y = self.drop(y)
|
67 |
-
x = self.norm_layers_1[i](x + y)
|
68 |
-
|
69 |
-
y = self.ffn_layers[i](x, x_mask)
|
70 |
-
y = self.drop(y)
|
71 |
-
x = self.norm_layers_2[i](x + y)
|
72 |
-
x = x * x_mask
|
73 |
-
return x
|
74 |
-
|
75 |
-
|
76 |
-
class Decoder(nn.Module):
|
77 |
-
def __init__(
|
78 |
-
self,
|
79 |
-
hidden_channels,
|
80 |
-
filter_channels,
|
81 |
-
n_heads,
|
82 |
-
n_layers,
|
83 |
-
kernel_size=1,
|
84 |
-
p_dropout=0.0,
|
85 |
-
proximal_bias=False,
|
86 |
-
proximal_init=True,
|
87 |
-
**kwargs
|
88 |
-
):
|
89 |
-
super().__init__()
|
90 |
-
self.hidden_channels = hidden_channels
|
91 |
-
self.filter_channels = filter_channels
|
92 |
-
self.n_heads = n_heads
|
93 |
-
self.n_layers = n_layers
|
94 |
-
self.kernel_size = kernel_size
|
95 |
-
self.p_dropout = p_dropout
|
96 |
-
self.proximal_bias = proximal_bias
|
97 |
-
self.proximal_init = proximal_init
|
98 |
-
|
99 |
-
self.drop = nn.Dropout(p_dropout)
|
100 |
-
self.self_attn_layers = nn.ModuleList()
|
101 |
-
self.norm_layers_0 = nn.ModuleList()
|
102 |
-
self.encdec_attn_layers = nn.ModuleList()
|
103 |
-
self.norm_layers_1 = nn.ModuleList()
|
104 |
-
self.ffn_layers = nn.ModuleList()
|
105 |
-
self.norm_layers_2 = nn.ModuleList()
|
106 |
-
for i in range(self.n_layers):
|
107 |
-
self.self_attn_layers.append(
|
108 |
-
MultiHeadAttention(
|
109 |
-
hidden_channels,
|
110 |
-
hidden_channels,
|
111 |
-
n_heads,
|
112 |
-
p_dropout=p_dropout,
|
113 |
-
proximal_bias=proximal_bias,
|
114 |
-
proximal_init=proximal_init,
|
115 |
-
)
|
116 |
-
)
|
117 |
-
self.norm_layers_0.append(LayerNorm(hidden_channels))
|
118 |
-
self.encdec_attn_layers.append(
|
119 |
-
MultiHeadAttention(
|
120 |
-
hidden_channels, hidden_channels, n_heads, p_dropout=p_dropout
|
121 |
-
)
|
122 |
-
)
|
123 |
-
self.norm_layers_1.append(LayerNorm(hidden_channels))
|
124 |
-
self.ffn_layers.append(
|
125 |
-
FFN(
|
126 |
-
hidden_channels,
|
127 |
-
hidden_channels,
|
128 |
-
filter_channels,
|
129 |
-
kernel_size,
|
130 |
-
p_dropout=p_dropout,
|
131 |
-
causal=True,
|
132 |
-
)
|
133 |
-
)
|
134 |
-
self.norm_layers_2.append(LayerNorm(hidden_channels))
|
135 |
-
|
136 |
-
def forward(self, x, x_mask, h, h_mask):
|
137 |
-
"""
|
138 |
-
x: decoder input
|
139 |
-
h: encoder output
|
140 |
-
"""
|
141 |
-
self_attn_mask = commons.subsequent_mask(x_mask.size(2)).to(
|
142 |
-
device=x.device, dtype=x.dtype
|
143 |
-
)
|
144 |
-
encdec_attn_mask = h_mask.unsqueeze(2) * x_mask.unsqueeze(-1)
|
145 |
-
x = x * x_mask
|
146 |
-
for i in range(self.n_layers):
|
147 |
-
y = self.self_attn_layers[i](x, x, self_attn_mask)
|
148 |
-
y = self.drop(y)
|
149 |
-
x = self.norm_layers_0[i](x + y)
|
150 |
-
|
151 |
-
y = self.encdec_attn_layers[i](x, h, encdec_attn_mask)
|
152 |
-
y = self.drop(y)
|
153 |
-
x = self.norm_layers_1[i](x + y)
|
154 |
-
|
155 |
-
y = self.ffn_layers[i](x, x_mask)
|
156 |
-
y = self.drop(y)
|
157 |
-
x = self.norm_layers_2[i](x + y)
|
158 |
-
x = x * x_mask
|
159 |
-
return x
|
160 |
-
|
161 |
-
|
162 |
-
class MultiHeadAttention(nn.Module):
|
163 |
-
def __init__(
|
164 |
-
self,
|
165 |
-
channels,
|
166 |
-
out_channels,
|
167 |
-
n_heads,
|
168 |
-
p_dropout=0.0,
|
169 |
-
window_size=None,
|
170 |
-
heads_share=True,
|
171 |
-
block_length=None,
|
172 |
-
proximal_bias=False,
|
173 |
-
proximal_init=False,
|
174 |
-
):
|
175 |
-
super().__init__()
|
176 |
-
assert channels % n_heads == 0
|
177 |
-
|
178 |
-
self.channels = channels
|
179 |
-
self.out_channels = out_channels
|
180 |
-
self.n_heads = n_heads
|
181 |
-
self.p_dropout = p_dropout
|
182 |
-
self.window_size = window_size
|
183 |
-
self.heads_share = heads_share
|
184 |
-
self.block_length = block_length
|
185 |
-
self.proximal_bias = proximal_bias
|
186 |
-
self.proximal_init = proximal_init
|
187 |
-
self.attn = None
|
188 |
-
|
189 |
-
self.k_channels = channels // n_heads
|
190 |
-
self.conv_q = nn.Conv1d(channels, channels, 1)
|
191 |
-
self.conv_k = nn.Conv1d(channels, channels, 1)
|
192 |
-
self.conv_v = nn.Conv1d(channels, channels, 1)
|
193 |
-
self.conv_o = nn.Conv1d(channels, out_channels, 1)
|
194 |
-
self.drop = nn.Dropout(p_dropout)
|
195 |
-
|
196 |
-
if window_size is not None:
|
197 |
-
n_heads_rel = 1 if heads_share else n_heads
|
198 |
-
rel_stddev = self.k_channels**-0.5
|
199 |
-
self.emb_rel_k = nn.Parameter(
|
200 |
-
torch.randn(n_heads_rel, window_size * 2 + 1, self.k_channels)
|
201 |
-
* rel_stddev
|
202 |
-
)
|
203 |
-
self.emb_rel_v = nn.Parameter(
|
204 |
-
torch.randn(n_heads_rel, window_size * 2 + 1, self.k_channels)
|
205 |
-
* rel_stddev
|
206 |
-
)
|
207 |
-
|
208 |
-
nn.init.xavier_uniform_(self.conv_q.weight)
|
209 |
-
nn.init.xavier_uniform_(self.conv_k.weight)
|
210 |
-
nn.init.xavier_uniform_(self.conv_v.weight)
|
211 |
-
if proximal_init:
|
212 |
-
with torch.no_grad():
|
213 |
-
self.conv_k.weight.copy_(self.conv_q.weight)
|
214 |
-
self.conv_k.bias.copy_(self.conv_q.bias)
|
215 |
-
|
216 |
-
def forward(self, x, c, attn_mask=None):
|
217 |
-
q = self.conv_q(x)
|
218 |
-
k = self.conv_k(c)
|
219 |
-
v = self.conv_v(c)
|
220 |
-
|
221 |
-
x, self.attn = self.attention(q, k, v, mask=attn_mask)
|
222 |
-
|
223 |
-
x = self.conv_o(x)
|
224 |
-
return x
|
225 |
-
|
226 |
-
def attention(self, query, key, value, mask=None):
|
227 |
-
# reshape [b, d, t] -> [b, n_h, t, d_k]
|
228 |
-
b, d, t_s, t_t = (*key.size(), query.size(2))
|
229 |
-
query = query.view(b, self.n_heads, self.k_channels, t_t).transpose(2, 3)
|
230 |
-
key = key.view(b, self.n_heads, self.k_channels, t_s).transpose(2, 3)
|
231 |
-
value = value.view(b, self.n_heads, self.k_channels, t_s).transpose(2, 3)
|
232 |
-
|
233 |
-
scores = torch.matmul(query / math.sqrt(self.k_channels), key.transpose(-2, -1))
|
234 |
-
if self.window_size is not None:
|
235 |
-
assert (
|
236 |
-
t_s == t_t
|
237 |
-
), "Relative attention is only available for self-attention."
|
238 |
-
key_relative_embeddings = self._get_relative_embeddings(self.emb_rel_k, t_s)
|
239 |
-
rel_logits = self._matmul_with_relative_keys(
|
240 |
-
query / math.sqrt(self.k_channels), key_relative_embeddings
|
241 |
-
)
|
242 |
-
scores_local = self._relative_position_to_absolute_position(rel_logits)
|
243 |
-
scores = scores + scores_local
|
244 |
-
if self.proximal_bias:
|
245 |
-
assert t_s == t_t, "Proximal bias is only available for self-attention."
|
246 |
-
scores = scores + self._attention_bias_proximal(t_s).to(
|
247 |
-
device=scores.device, dtype=scores.dtype
|
248 |
-
)
|
249 |
-
if mask is not None:
|
250 |
-
scores = scores.masked_fill(mask == 0, -1e4)
|
251 |
-
if self.block_length is not None:
|
252 |
-
assert (
|
253 |
-
t_s == t_t
|
254 |
-
), "Local attention is only available for self-attention."
|
255 |
-
block_mask = (
|
256 |
-
torch.ones_like(scores)
|
257 |
-
.triu(-self.block_length)
|
258 |
-
.tril(self.block_length)
|
259 |
-
)
|
260 |
-
scores = scores.masked_fill(block_mask == 0, -1e4)
|
261 |
-
p_attn = F.softmax(scores, dim=-1) # [b, n_h, t_t, t_s]
|
262 |
-
p_attn = self.drop(p_attn)
|
263 |
-
output = torch.matmul(p_attn, value)
|
264 |
-
if self.window_size is not None:
|
265 |
-
relative_weights = self._absolute_position_to_relative_position(p_attn)
|
266 |
-
value_relative_embeddings = self._get_relative_embeddings(
|
267 |
-
self.emb_rel_v, t_s
|
268 |
-
)
|
269 |
-
output = output + self._matmul_with_relative_values(
|
270 |
-
relative_weights, value_relative_embeddings
|
271 |
-
)
|
272 |
-
output = (
|
273 |
-
output.transpose(2, 3).contiguous().view(b, d, t_t)
|
274 |
-
) # [b, n_h, t_t, d_k] -> [b, d, t_t]
|
275 |
-
return output, p_attn
|
276 |
-
|
277 |
-
def _matmul_with_relative_values(self, x, y):
|
278 |
-
"""
|
279 |
-
x: [b, h, l, m]
|
280 |
-
y: [h or 1, m, d]
|
281 |
-
ret: [b, h, l, d]
|
282 |
-
"""
|
283 |
-
ret = torch.matmul(x, y.unsqueeze(0))
|
284 |
-
return ret
|
285 |
-
|
286 |
-
def _matmul_with_relative_keys(self, x, y):
|
287 |
-
"""
|
288 |
-
x: [b, h, l, d]
|
289 |
-
y: [h or 1, m, d]
|
290 |
-
ret: [b, h, l, m]
|
291 |
-
"""
|
292 |
-
ret = torch.matmul(x, y.unsqueeze(0).transpose(-2, -1))
|
293 |
-
return ret
|
294 |
-
|
295 |
-
def _get_relative_embeddings(self, relative_embeddings, length):
|
296 |
-
max_relative_position = 2 * self.window_size + 1
|
297 |
-
# Pad first before slice to avoid using cond ops.
|
298 |
-
pad_length = max(length - (self.window_size + 1), 0)
|
299 |
-
slice_start_position = max((self.window_size + 1) - length, 0)
|
300 |
-
slice_end_position = slice_start_position + 2 * length - 1
|
301 |
-
if pad_length > 0:
|
302 |
-
padded_relative_embeddings = F.pad(
|
303 |
-
relative_embeddings,
|
304 |
-
commons.convert_pad_shape([[0, 0], [pad_length, pad_length], [0, 0]]),
|
305 |
-
)
|
306 |
-
else:
|
307 |
-
padded_relative_embeddings = relative_embeddings
|
308 |
-
used_relative_embeddings = padded_relative_embeddings[
|
309 |
-
:, slice_start_position:slice_end_position
|
310 |
-
]
|
311 |
-
return used_relative_embeddings
|
312 |
-
|
313 |
-
def _relative_position_to_absolute_position(self, x):
|
314 |
-
"""
|
315 |
-
x: [b, h, l, 2*l-1]
|
316 |
-
ret: [b, h, l, l]
|
317 |
-
"""
|
318 |
-
batch, heads, length, _ = x.size()
|
319 |
-
# Concat columns of pad to shift from relative to absolute indexing.
|
320 |
-
x = F.pad(x, commons.convert_pad_shape([[0, 0], [0, 0], [0, 0], [0, 1]]))
|
321 |
-
|
322 |
-
# Concat extra elements so to add up to shape (len+1, 2*len-1).
|
323 |
-
x_flat = x.view([batch, heads, length * 2 * length])
|
324 |
-
x_flat = F.pad(
|
325 |
-
x_flat, commons.convert_pad_shape([[0, 0], [0, 0], [0, length - 1]])
|
326 |
-
)
|
327 |
-
|
328 |
-
# Reshape and slice out the padded elements.
|
329 |
-
x_final = x_flat.view([batch, heads, length + 1, 2 * length - 1])[
|
330 |
-
:, :, :length, length - 1 :
|
331 |
-
]
|
332 |
-
return x_final
|
333 |
-
|
334 |
-
def _absolute_position_to_relative_position(self, x):
|
335 |
-
"""
|
336 |
-
x: [b, h, l, l]
|
337 |
-
ret: [b, h, l, 2*l-1]
|
338 |
-
"""
|
339 |
-
batch, heads, length, _ = x.size()
|
340 |
-
# padd along column
|
341 |
-
x = F.pad(
|
342 |
-
x, commons.convert_pad_shape([[0, 0], [0, 0], [0, 0], [0, length - 1]])
|
343 |
-
)
|
344 |
-
x_flat = x.view([batch, heads, length**2 + length * (length - 1)])
|
345 |
-
# add 0's in the beginning that will skew the elements after reshape
|
346 |
-
x_flat = F.pad(x_flat, commons.convert_pad_shape([[0, 0], [0, 0], [length, 0]]))
|
347 |
-
x_final = x_flat.view([batch, heads, length, 2 * length])[:, :, :, 1:]
|
348 |
-
return x_final
|
349 |
-
|
350 |
-
def _attention_bias_proximal(self, length):
|
351 |
-
"""Bias for self-attention to encourage attention to close positions.
|
352 |
-
Args:
|
353 |
-
length: an integer scalar.
|
354 |
-
Returns:
|
355 |
-
a Tensor with shape [1, 1, length, length]
|
356 |
-
"""
|
357 |
-
r = torch.arange(length, dtype=torch.float32)
|
358 |
-
diff = torch.unsqueeze(r, 0) - torch.unsqueeze(r, 1)
|
359 |
-
return torch.unsqueeze(torch.unsqueeze(-torch.log1p(torch.abs(diff)), 0), 0)
|
360 |
-
|
361 |
-
|
362 |
-
class FFN(nn.Module):
|
363 |
-
def __init__(
|
364 |
-
self,
|
365 |
-
in_channels,
|
366 |
-
out_channels,
|
367 |
-
filter_channels,
|
368 |
-
kernel_size,
|
369 |
-
p_dropout=0.0,
|
370 |
-
activation=None,
|
371 |
-
causal=False,
|
372 |
-
):
|
373 |
-
super().__init__()
|
374 |
-
self.in_channels = in_channels
|
375 |
-
self.out_channels = out_channels
|
376 |
-
self.filter_channels = filter_channels
|
377 |
-
self.kernel_size = kernel_size
|
378 |
-
self.p_dropout = p_dropout
|
379 |
-
self.activation = activation
|
380 |
-
self.causal = causal
|
381 |
-
|
382 |
-
if causal:
|
383 |
-
self.padding = self._causal_padding
|
384 |
-
else:
|
385 |
-
self.padding = self._same_padding
|
386 |
-
|
387 |
-
self.conv_1 = nn.Conv1d(in_channels, filter_channels, kernel_size)
|
388 |
-
self.conv_2 = nn.Conv1d(filter_channels, out_channels, kernel_size)
|
389 |
-
self.drop = nn.Dropout(p_dropout)
|
390 |
-
|
391 |
-
def forward(self, x, x_mask):
|
392 |
-
x = self.conv_1(self.padding(x * x_mask))
|
393 |
-
if self.activation == "gelu":
|
394 |
-
x = x * torch.sigmoid(1.702 * x)
|
395 |
-
else:
|
396 |
-
x = torch.relu(x)
|
397 |
-
x = self.drop(x)
|
398 |
-
x = self.conv_2(self.padding(x * x_mask))
|
399 |
-
return x * x_mask
|
400 |
-
|
401 |
-
def _causal_padding(self, x):
|
402 |
-
if self.kernel_size == 1:
|
403 |
-
return x
|
404 |
-
pad_l = self.kernel_size - 1
|
405 |
-
pad_r = 0
|
406 |
-
padding = [[0, 0], [0, 0], [pad_l, pad_r]]
|
407 |
-
x = F.pad(x, commons.convert_pad_shape(padding))
|
408 |
-
return x
|
409 |
-
|
410 |
-
def _same_padding(self, x):
|
411 |
-
if self.kernel_size == 1:
|
412 |
-
return x
|
413 |
-
pad_l = (self.kernel_size - 1) // 2
|
414 |
-
pad_r = self.kernel_size // 2
|
415 |
-
padding = [[0, 0], [0, 0], [pad_l, pad_r]]
|
416 |
-
x = F.pad(x, commons.convert_pad_shape(padding))
|
417 |
-
return x
|
|
|
|
|
|
|
|
|
|
|
|
|
|
|
|
|
|
|
|
|
|
|
|
|
|
|
|
|
|
|
|
|
|
|
|
|
|
|
|
|
|
|
|
|
|
|
|
|
|
|
|
|
|
|
|
|
|
|
|
|
|
|
|
|
|
|
|
|
|
|
|
|
|
|
|
|
|
|
|
|
|
|
|
|
|
|
|
|
|
|
|
|
|
|
|
|
|
|
|
|
|
|
|
|
|
|
|
|
|
|
|
|
|
|
|
|
|
|
|
|
|
|
|
|
|
|
|
|
|
|
|
|
|
|
|
|
|
|
|
|
|
|
|
|
|
|
|
|
|
|
|
|
|
|
|
|
|
|
|
|
|
|
|
|
|
|
|
|
|
|
|
|
|
|
|
|
|
|
|
|
|
|
|
|
|
|
|
|
|
|
|
|
|
|
|
|
|
|
|
|
|
|
|
|
|
|
|
|
|
|
|
|
|
|
|
|
|
|
|
|
|
|
|
|
|
|
|
|
|
|
|
|
|
|
|
|
|
|
|
|
|
|
|
|
|
|
|
|
|
|
|
|
|
|
|
|
|
|
|
|
|
|
|
|
|
|
|
|
|
|
|
|
|
|
|
|
|
|
|
|
|
|
|
|
|
|
|
|
|
|
|
|
|
|
|
|
|
|
|
|
|
|
|
|
|
|
|
|
|
|
|
|
|
|
|
|
|
|
|
|
|
|
|
|
|
|
|
|
|
|
|
|
|
|
|
|
|
|
|
|
|
|
|
|
|
|
|
|
|
|
|
|
|
|
|
|
|
|
|
|
|
|
|
|
|
|
|
|
|
|
|
|
|
|
|
|
|
|
|
|
|
|
|
|
|
|
|
|
|
|
|
|
|
|
|
|
|
|
|
|
|
|
|
|
|
|
|
|
|
|
|
|
|
|
|
|
|
|
|
|
|
|
|
|
|
|
|
|
|
|
|
|
|
|
|
|
|
|
|
|
|
|
|
|
|
|
|
|
|
|
|
|
|
|
|
|
|
|
|
|
|
|
|
|
|
|
|
|
|
|
|
|
|
|
|
|
|
|
|
|
|
|
|
|
|
|
|
|
|
|
|
|
|
|
|
|
|
|
|
|
|
|
|
|
|
|
|
|
|
|
|
|
|
|
|
|
|
|
|
|
|
|
|
|
|
|
|
|
|
|
|
|
|
|
|
|
|
|
|
|
|
|
|
|
|
|
|
|
|
|
|
|
|
|
|
|
|
|
|
|
|
|
|
|
|
|
|
|
|
|
|
|
|
|
|
|
|
|
|
|
|
|
|
|
|
|
|
|
|
|
|
|
|
|
|
|
|
|
|
|
|
|
|
|
|
|
|
|
|
|
|
|
|
|
|
|
|
|
|
|
|
|
|
|
|
|
|
|
|
|
|
|
|
|
|
|
|
|
|
|
|
|
|
|
|
|
|
|
|
|
|
|
|
|
|
|
|
|
|
|
|
|
|
|
|
|
|
|
|
|
|
|
|
|
|
|
|
|
|
|
|
|
|
|
|
|
|
|
|
|
|
|
|
|
|
|
|
|
|
|
|
|
|
|
|
|
|
|
|
|
|
|
|
|
|
|
|
|
|
|
|
|
|
|
|
|
|
|
|
|
|
|
|
|
|
|
|
|
|
|
|
|
|
|
|
|
|
|
|
|
|
|
|
|
|
|
|
|
|
|
|
|
|
|
|
|
|
|
|
|
|
|
|
|
|
|
|
|
|
|
|
|
|
|
|
|
|
|
|
|
|
|
|
|
|
|
|
|
|
|
|
|
|
|
|
|
|
|
|
|
|
|
|
|
|
|
|
|
|
|
|
|
|
|
|
|
|
|
|
|
|
|
|
|
|
spaces/ChrisPreston/diff-svc_minato_aqua/app.py
DELETED
@@ -1,86 +0,0 @@
|
|
1 |
-
from utils.hparams import hparams
|
2 |
-
import scipy.io.wavfile as wav
|
3 |
-
import numpy as np
|
4 |
-
import matplotlib.pyplot as plt
|
5 |
-
import IPython.display as ipd
|
6 |
-
import utils
|
7 |
-
import librosa
|
8 |
-
import torch
|
9 |
-
import torchcrepe
|
10 |
-
from infer import *
|
11 |
-
import logging
|
12 |
-
from infer_tools.infer_tool import *
|
13 |
-
import gradio as gr
|
14 |
-
import json
|
15 |
-
|
16 |
-
logging.getLogger('numba').setLevel(logging.WARNING)
|
17 |
-
svc_model = None
|
18 |
-
project_name = "aqua"
|
19 |
-
wave_name = f"./temp.wav"
|
20 |
-
model_path = f'./aqua/clean_model_ckpt_steps_100000.ckpt'
|
21 |
-
config_path = f'./aqua/config.yaml'
|
22 |
-
spk_id = "aqua"
|
23 |
-
|
24 |
-
def infer(wav_fn, tran, accelerate, auto_key):
|
25 |
-
model = Svc(project_name, config_path, hubert_gpu=False, model_path=model_path, onnx=False)
|
26 |
-
|
27 |
-
if wav_fn is not None:
|
28 |
-
audio_path = wav_fn
|
29 |
-
else:
|
30 |
-
return "请先上传wav格式的音频文件", None, None
|
31 |
-
run_clip(raw_audio_path=audio_path, svc_model=model, key=tran, acc=accelerate, use_crepe=True,
|
32 |
-
spk_id=spk_id, auto_key=auto_key, project_name=project_name, out_path=wave_name)
|
33 |
-
|
34 |
-
au_out = wave_name
|
35 |
-
|
36 |
-
return "转换成功", au_out
|
37 |
-
|
38 |
-
app = gr.Blocks()
|
39 |
-
with app:
|
40 |
-
with gr.Tabs():
|
41 |
-
with gr.TabItem("推理"):
|
42 |
-
with gr.Blocks():
|
43 |
-
with gr.Blocks():
|
44 |
-
with gr.Box():
|
45 |
-
gr.Markdown(value="""**上传音频**""")
|
46 |
-
with gr.Row():
|
47 |
-
upload_input = gr.Audio(source="upload", label="源音频", type="filepath", elem_id="audio_inputs")
|
48 |
-
out_audio = gr.Audio(label="输出音频")
|
49 |
-
with gr.Blocks():
|
50 |
-
with gr.Box():
|
51 |
-
gr.Markdown(value="""**参数设置**""")
|
52 |
-
with gr.Row():
|
53 |
-
auto = gr.Checkbox(label="启用自动变调", value=False)
|
54 |
-
with gr.Row():
|
55 |
-
acc_vaule = gr.Slider(1, 50, value=20, interactive=True, label="加速倍率")
|
56 |
-
with gr.Row():
|
57 |
-
pitch_vaule = gr.Slider(-96, 96, value=0, interactive=True, label="变调(半音)")
|
58 |
-
with gr.Row():
|
59 |
-
with gr.Column(scale=1):
|
60 |
-
infer_md = gr.Button("转换音频", variant="primary")
|
61 |
-
with gr.Blocks():
|
62 |
-
with gr.Box():
|
63 |
-
gr.Markdown(value="""**输出日志**""")
|
64 |
-
infer_msg = gr.Textbox(label="日志")
|
65 |
-
infer_md.click(infer, [upload_input, pitch_vaule, acc_vaule, auto], [infer_msg, out_audio])
|
66 |
-
with gr.TabItem("说明"):
|
67 |
-
gr.Markdown(value="""
|
68 |
-
自改cpu推理版,无音频长度限制,无降噪功能,请确保输入音频的质量\n
|
69 |
-
有本地cpu推理的需求可以下载全部文件\n
|
70 |
-
原项目地址:https://github.com/openvpi/diff-svc\n
|
71 |
-
代码修改:@ChrisPreston\n
|
72 |
-
模型训练:@ChrisPreston\n
|
73 |
-
音源:Aqua Ch. 湊あくあ https://www.youtube.com/@MinatoAqua カバー株式会社\n
|
74 |
-
模型使用协议(重要):\n
|
75 |
-
1.请勿用于商业目的\n
|
76 |
-
2.请勿用于会影响主播本人的行为(比如冒充本人发表争议言论)\n
|
77 |
-
3.请勿用于血腥、暴力、性相关、政治相关内容\n
|
78 |
-
4.不允许二次分发模型\n
|
79 |
-
5.非个人使用场合请注明模型作者@ChrisPreston以及diff-svc原项目\n
|
80 |
-
6.允许用于个人娱乐场景下的游戏语音、直播活动,不得用于低创内容,用于直播前请与本人联系\n
|
81 |
-
联系方式:电邮:[email protected], b站:https://space.bilibili.com/18801308\n
|
82 |
-
免责声明:由于使用本模型造成的法律纠纷本人概不负责
|
83 |
-
""")
|
84 |
-
|
85 |
-
app.launch(share=False)
|
86 |
-
|
|
|
|
|
|
|
|
|
|
|
|
|
|
|
|
|
|
|
|
|
|
|
|
|
|
|
|
|
|
|
|
|
|
|
|
|
|
|
|
|
|
|
|
|
|
|
|
|
|
|
|
|
|
|
|
|
|
|
|
|
|
|
|
|
|
|
|
|
|
|
|
|
|
|
|
|
|
|
|
|
|
|
|
|
|
|
|
|
|
|
|
|
|
|
|
|
|
|
|
|
|
|
|
|
|
|
|
|
|
|
|
|
|
|
|
|
|
|
|
|
|
|
|
|
|
|
|
|
|
|
|
|
|
|
|
|
|
|
|
|
|
|
|
|
|
|
|
|
|
|
|
|
|
|
|
|
|
|
|
|
|
|
|
|
|
|
|
|
|
|
|
|
|
spaces/CikeyQI/meme-api/meme_generator/memes/divorce/__init__.py
DELETED
@@ -1,18 +0,0 @@
|
|
1 |
-
from pathlib import Path
|
2 |
-
from typing import List
|
3 |
-
|
4 |
-
from pil_utils import BuildImage
|
5 |
-
|
6 |
-
from meme_generator import add_meme
|
7 |
-
|
8 |
-
img_dir = Path(__file__).parent / "images"
|
9 |
-
|
10 |
-
|
11 |
-
def divorce(images: List[BuildImage], texts, args):
|
12 |
-
frame = BuildImage.open(img_dir / "0.png")
|
13 |
-
img = images[0].convert("RGBA").resize(frame.size, keep_ratio=True)
|
14 |
-
frame.paste(img, below=True)
|
15 |
-
return frame.save_jpg()
|
16 |
-
|
17 |
-
|
18 |
-
add_meme("divorce", divorce, min_images=1, max_images=1, keywords=["离婚协议", "离婚申请"])
|
|
|
|
|
|
|
|
|
|
|
|
|
|
|
|
|
|
|
|
|
|
|
|
|
|
|
|
|
|
|
|
|
|
|
|
|
|
spaces/CreBea/Test2/Dockerfile
DELETED
@@ -1,21 +0,0 @@
|
|
1 |
-
FROM node:18-bullseye-slim
|
2 |
-
|
3 |
-
RUN apt-get update && \
|
4 |
-
|
5 |
-
apt-get install -y git
|
6 |
-
|
7 |
-
RUN git clone https://gitgud.io/khanon/oai-reverse-proxy.git /app
|
8 |
-
|
9 |
-
WORKDIR /app
|
10 |
-
|
11 |
-
RUN npm install
|
12 |
-
|
13 |
-
COPY Dockerfile greeting.md* .env* ./
|
14 |
-
|
15 |
-
RUN npm run build
|
16 |
-
|
17 |
-
EXPOSE 7860
|
18 |
-
|
19 |
-
ENV NODE_ENV=production
|
20 |
-
|
21 |
-
CMD [ "npm", "start" ]
|
|
|
|
|
|
|
|
|
|
|
|
|
|
|
|
|
|
|
|
|
|
|
|
|
|
|
|
|
|
|
|
|
|
|
|
|
|
|
|
|
|
|
|
spaces/Cyril666/ContourNet-ABI/maskrcnn_benchmark/csrc/nms.h
DELETED
@@ -1,28 +0,0 @@
|
|
1 |
-
// Copyright (c) Facebook, Inc. and its affiliates. All Rights Reserved.
|
2 |
-
#pragma once
|
3 |
-
#include "cpu/vision.h"
|
4 |
-
|
5 |
-
#ifdef WITH_CUDA
|
6 |
-
#include "cuda/vision.h"
|
7 |
-
#endif
|
8 |
-
|
9 |
-
|
10 |
-
at::Tensor nms(const at::Tensor& dets,
|
11 |
-
const at::Tensor& scores,
|
12 |
-
const float threshold) {
|
13 |
-
|
14 |
-
if (dets.type().is_cuda()) {
|
15 |
-
#ifdef WITH_CUDA
|
16 |
-
// TODO raise error if not compiled with CUDA
|
17 |
-
if (dets.numel() == 0)
|
18 |
-
return at::empty({0}, dets.options().dtype(at::kLong).device(at::kCPU));
|
19 |
-
auto b = at::cat({dets, scores.unsqueeze(1)}, 1);
|
20 |
-
return nms_cuda(b, threshold);
|
21 |
-
#else
|
22 |
-
AT_ERROR("Not compiled with GPU support");
|
23 |
-
#endif
|
24 |
-
}
|
25 |
-
|
26 |
-
at::Tensor result = nms_cpu(dets, scores, threshold);
|
27 |
-
return result;
|
28 |
-
}
|
|
|
|
|
|
|
|
|
|
|
|
|
|
|
|
|
|
|
|
|
|
|
|
|
|
|
|
|
|
|
|
|
|
|
|
|
|
|
|
|
|
|
|
|
|
|
|
|
|
|
|
|
|
|
|
|
|