diff --git a/spaces/1acneusushi/gradio-2dmoleculeeditor/data/Crash Bandicoot N. Sane Trilogy [Crack Serial Key] Comparison and Analysis - How Does It Compare to the Original?.md b/spaces/1acneusushi/gradio-2dmoleculeeditor/data/Crash Bandicoot N. Sane Trilogy [Crack Serial Key] Comparison and Analysis - How Does It Compare to the Original?.md
deleted file mode 100644
index 3af93719f2bd51209a544de2e7a854195fb74253..0000000000000000000000000000000000000000
--- a/spaces/1acneusushi/gradio-2dmoleculeeditor/data/Crash Bandicoot N. Sane Trilogy [Crack Serial Key] Comparison and Analysis - How Does It Compare to the Original?.md
+++ /dev/null
@@ -1,126 +0,0 @@
-
-
Crash Bandicoot N. Sane Trilogy [Crack Serial Key]
-
Are you a fan of the classic platformer game Crash Bandicoot? Do you want to relive the nostalgic moments of spinning, jumping, and wumping through three remastered games in one collection? If so, then you might be interested in Crash Bandicoot N. Sane Trilogy, a game that brings back your favorite marsupial in his enhanced, entranced, and ready-to-dance glory.
But what if you don't have enough money to buy the game or you don't want to pay for it? Is there a way to play the game for free on your PC? The answer is yes, but you will need a crack serial key to do so. In this article, we will explain what a crack serial key is, why you might need it, how to get it, and how to use it to activate Crash Bandicoot N. Sane Trilogy on your PC.
-
What is Crash Bandicoot N. Sane Trilogy?
-
A brief introduction to the game and its features
-
Crash Bandicoot N. Sane Trilogy is a collection of three remastered games from the original Crash Bandicoot series: Crash Bandicoot, Crash Bandicoot 2: Cortex Strikes Back, and Crash Bandicoot 3: Warped. The game was developed by Vicarious Visions and Iron Galaxy, and published by Activision in 2018 for PC, PlayStation 4, Xbox One, and Nintendo Switch.
-
The game features all the original levels, characters, enemies, bosses, and secrets from the original games, but with improved graphics, sound, gameplay, and controls. You can experience Crash Bandicoot like never before in his fully-remastered graphical glory and get ready to put some UMPH in your WUMP!
-
The game also includes two new levels that were previously unfinished and unreleased: Stormy Ascent and Future Tense. Stormy Ascent is a challenging level from the first game that will test your skills and patience as you dodge vials, birds, spikes, and platforms. Future Tense is a new level inspired by the cut Waterfall Level from the first game that features puzzles and obstacles set in a futuristic skyscraper.
-
How to download and install the game on PC
-
If you want to play Crash Bandicoot N. Sane Trilogy on your PC, you will need to follow these steps:
-
-
Make sure your PC meets the minimum system requirements for the game. You will need Windows 7 or higher, an Intel Core i5-750 or AMD Phenom II X4 965 processor, 8 GB of RAM, an NVIDIA GeForce GTX 660 or AMD Radeon HD 7850 graphics card, 30 GB of available storage space, and a DirectX 9.0c compatible sound card.
-
Buy the game from an official source such as Steam or Activision's website. You will need to create an account and pay for the game using your preferred method of payment.
-
Download the game files using the provided link or launcher. You will need a stable internet connection and enough bandwidth to download about 30 GB of data.
-
Install the game on your PC by following the instructions on the screen. You will need to agree to the terms and conditions and choose a destination folder for the game files.
-
Launch the game from your desktop or start menu shortcut. You will need to log in with your account credentials and verify your ownership of the game.
-
Enjoy playing Crash Bandicoot N. Sane Trilogy on your PC!
-
-
What is a crack serial key and why do you need it?
-
The benefits of using a crack serial key for Crash Bandicoot N. Sane Trilogy
-
A crack serial key is a code that can bypass the security measures of a software or game and allow you to use it without paying for it or verifying your ownership. A crack serial key can be generated by hackers or programmers who exploit the vulnerabilities or loopholes of the software or game.
-
Crash Bandicoot N. Sane Trilogy [Crack Activation Code
-Crash Bandicoot N. Sane Trilogy [Crack License Key
-Crash Bandicoot N. Sane Trilogy [Crack Product Key
-Crash Bandicoot N. Sane Trilogy [Crack Registration Code
-Crash Bandicoot N. Sane Trilogy [Crack Keygen Download
-Crash Bandicoot N. Sane Trilogy [Crack Torrent Free
-Crash Bandicoot N. Sane Trilogy [Crack Full Version PC
-Crash Bandicoot N. Sane Trilogy [Crack Patch Update
-Crash Bandicoot N. Sane Trilogy [Crack No CD/DVD
-Crash Bandicoot N. Sane Trilogy [Crack Steam Fix
-Crash Bandicoot N. Sane Trilogy [Crack Online Multiplayer
-Crash Bandicoot N. Sane Trilogy [Crack Skidrow Reloaded
-Crash Bandicoot N. Sane Trilogy [Crack CPY Codex
-Crash Bandicoot N. Sane Trilogy [Crack FitGirl Repack
-Crash Bandicoot N. Sane Trilogy [Crack Razor1911 Scene
-Crash Bandicoot N. Sane Trilogy [Crack Mega.nz Link
-Crash Bandicoot N. Sane Trilogy [Crack Google Drive Link
-Crash Bandicoot N. Sane Trilogy [Crack Direct Download Link
-Crash Bandicoot N. Sane Trilogy [Crack Highly Compressed
-Crash Bandicoot N. Sane Trilogy [Crack ISO File Download
-Crash Bandicoot N. Sane Trilogy [Crack RAR Password Unlocker
-Crash Bandicoot N. Sane Trilogy [Crack How to Install Guide
-Crash Bandicoot N. Sane Trilogy [Crack System Requirements
-Crash Bandicoot N. Sane Trilogy [Crack Gameplay Review
-Crash Bandicoot N. Sane Trilogy [Crack Tips and Tricks
-Crash Bandicoot N. Sane Trilogy [Crack Cheats and Hacks
-Crash Bandicoot N. Sane Trilogy [Crack Mods and Customization
-Crash Bandicoot N. Sane Trilogy [Crack Remastered Edition
-Crash Bandicoot N. Sane Trilogy [Crack Bonus Content DLC
-Crash Bandicoot N. Sane Trilogy [Crack OST Soundtrack Download
-Crash Bandicoot N. Sane Trilogy [Crack Wallpaper HD Download
-Crash Bandicoot N. Sane Trilogy [Crack Fan Art and Memes
-Crash Bandicoot N. Sane Trilogy [Crack Comparison with Original
-Crash Bandicoot N. Sane Trilogy [Crack Best Settings for PC
-Crash Bandicoot N. Sane Trilogy [Crack Controller Support PC
-Crash Bandicoot N. Sane Trilogy [Crack Save Game Location PC
-Crash Bandicoot N. Sane Trilogy [Crack Error Fix and Solution PC
-Crash Bandicoot N. Sane Trilogy [Crack Free Steam Key Giveaway
-Crash Bandicoot N. Sane Trilogy [Crack Discount Coupon Code PC
-Crash Bandicoot N. Sane Trilogy [Crack Buy Official Game PC
-Crash Bandicoot N. Sane Trilogy [Crack PS4 Xbox One Switch Version
-Crash Bandicoot N. Sane Trilogy [Crack Mobile Android iOS Version
-Crash Bandicoot N. Sane Trilogy [Crack VR Oculus Rift Version
-Crash Bandicoot N. Sane Trilogy [Crack Co-op Split Screen Mode PC
-Crash Bandicoot N. Sane Trilogy [Crack Speedrun World Record PC
-Crash Bandicoot N. Sane Trilogy [Crack All Levels and Secrets PC
-Crash Bandicoot N. Sane Trilogy [Crack All Characters and Skins PC
-Crash Bandicoot N. Sane Trilogy [Crack All Bosses and Enemies PC
-
The main benefit of using a crack serial key for Crash Bandicoot N. Sane Trilogy is that you can play the game for free on your PC without buying it or verifying it with an official source. This can save you money and time, especially if you are not sure if you like the game or not.
-
The risks and drawbacks of using a crack serial key for Crash Bandicoot N. Sane Trilogy
-
However, using a crack serial key for Crash Bandicoot N. Sane Trilogy also comes with some risks and drawbacks that you should be aware of before deciding to use one:
-
-
You might be breaking the law by using a crack serial key for Crash Bandicoot N. Sane Trilogy. Depending on your country's laws and regulations, using a crack serial key might be considered as piracy or theft of intellectual property, which can result in legal consequences such as fines or imprisonment.
-
You might be harming the developers and publishers of Crash Bandicoot N. Sane Trilogy by using a crack serial key. By not paying for the game or supporting its official sources, you are depriving them of their rightful income and recognition for their hard work and creativity.
-
You might be exposing your PC to viruses or malware by using a crack serial key for Crash Bandicoot N. Sane Trilogy. Some crack serial keys might contain malicious code that can infect your PC with viruses or malware that can damage your files, steal your data, or compromise your security.
-
You might be missing out on updates or features by using a crack serial key for Crash Bandicoot N. Sane Trilogy. Some crack serial keys might not work with newer versions of the game or prevent you from accessing online features such as multiplayer modes or leaderboards.
-
-
How to get a crack serial key for Crash Bandicoot N. Sane Trilogy
-
The best sources and websites to find a crack serial key for Crash Bandicoot N. Sane Trilogy
-
If you still want to use a crack serial key for Crash Bandicoot N. Sane Trilogy despite knowing its risks and drawbacks, then you will need to find one from reliable sources and websites that offer them for free or at low prices.
-
However, finding a working crack serial key for Crash Bandicoot N. Sane Trilogy can be challenging as there are many fake or scam websites that claim to offer them but only want to trick you into downloading viruses or malware or paying for something else.
-
To help you avoid these scams and find genuine sources and websites that offer crack serial keys for Crash Bandicoot N. Sane Trilogy, we have compiled a list of some of the best ones based on their popularity, reputation, quality, availability, and safety:
-
Skidrow Cracked
-
Skidrow Cracked is one of the most popular websites that offer free download links for cracked games such as Crash Bandicoot N. Sane Trilogy-CODEX.
-
This website provides direct links for downloading the game files as well as instructions on how to install them on your PC.
-```html website also has a comment section where you can ask questions or share feedback with other users.
-
However, you should be careful when downloading files from this website as they might contain viruses or malware that can harm your PC. You should also use a VPN or proxy to hide your IP address and avoid legal issues.
-
CDKeys
-
CDKeys is one of the most reputable websites that offer cheap and legit keys for games such as Crash Bandicoot N. Sane Trilogy PC.
-
This website provides instant delivery of the keys via email or digital download. You can also check the reviews and ratings of the keys from other customers before buying them.
-
The website also has a customer service team that can help you with any issues or queries you might have regarding your purchase.
-
However, you should be aware that some keys might not work in certain regions or platforms. You should also check the terms and conditions and refund policy of the website before buying anything.
-
G2A
-
G2A is one of the largest online marketplaces that offer a wide range of products and services related to gaming, including keys for games such as Crash Bandicoot N. Sane Trilogy Steam Key GLOBAL.
-
This website allows you to buy and sell keys from different sellers and buyers around the world. You can also compare prices and ratings of the keys from different sources and choose the best one for you.
-
The website also has a protection program that guarantees your satisfaction and security when buying or selling keys. You can also contact the support team or the seller directly if you have any problems or questions.
-
However, you should be careful when buying or selling keys on this website as there might be some fraudulent or scam transactions. You should also read the description and details of the keys carefully before buying or selling them.
-
YouTube
-
YouTube is one of the most popular video-sharing platforms that offer a variety of content and information related to gaming, including videos on how to get a crack serial key for Crash Bandicoot N. Sane Trilogy for free.
-
This platform allows you to watch and learn from different video tutorials and guides on how to download, install, and activate the game with a crack serial key. You can also subscribe to different channels and creators that offer more tips and tricks on gaming.
-
The platform also has a comment section where you can interact with other viewers and share your opinions or feedback on the videos.
-
However, you should be wary when watching or following videos on this platform as they might contain false or misleading information or links that can lead you to viruses or malware. You should also use an ad-blocker or skip the ads that might appear on the videos.
-
The steps to activate the game with a crack serial key
-
If you have found a working crack serial key for Crash Bandicoot N. Sane Trilogy from one of the sources or websites mentioned above, then you will need to follow these steps to activate the game with it:
-
-
Copy the crack serial key from the source or website where you got it from.
-
Open Steam and log in with your account credentials.
-
Click on Games in the menu bar and select Activate a Product on Steam.
-
Click on Next and agree to the terms and conditions.
-
Paste the crack serial key in the Product Code box and click on Next.
-
Wait for Steam to verify and activate your product.
-
Once activated, you can download and play Crash Bandicoot N. Sane Trilogy on your PC!
-
-
Conclusion
-
A summary of the main points and a call to action
-
In conclusion, Crash Bandicoot N. Sane Trilogy is a collection of three remastered games from the original Crash Bandicoot series that lets you experience Crash Bandicoot like never before in his fully-remastered graphical glory.
-
If you want to play the game for free on your PC without buying it or verifying it with an official source, then you will need a crack serial key that can bypass the security measures of the game and allow you to use it without paying for it or verifying your ownership.
-
You can find a crack serial key for Crash Bandicoot N. Sane Trilogy from different sources and websites such as Skidrow Cracked, CDKeys, G2A, or YouTube. However, you should be aware of the risks and drawbacks of using a crack serial key such as breaking the law, harming the developers, exposing your PC to viruses, or missing out on updates or features.
-
If you have found a working crack serial key for Crash Bandicoot N. Sane Trilogy, then you can activate the game with it by following some simple steps on Steam.
-
We hope this article has helped you understand what a crack serial key is, why you might need it, how to get it, and how to use it to activate Crash Bandicoot N. Sane Trilogy on your PC. However, we do not encourage or endorse piracy or theft of intellectual property. We recommend that you buy the game from an official source such as Steam or Activision's website if you want to support the developers and enjoy the game fully and legally.
- FAQs: Q: What is Crash Bandicoot N. Sane Trilogy? A: Crash Bandicoot N. Sane Trilogy is a collection of three remastered games from the original Crash Bandicoot series: Crash Bandicoot, Crash Bandicoot 2: Cortex Strikes Back, and Crash Bandicoot 3: Warped. Q: What is a crack serial key? A: A crack serial key is a code that can bypass the security measures of a software or game and allow you to use it without paying for it or verifying your ownership. Q: How to get a crack serial key for Crash Bandicoot N. Sane Trilogy? A: You can get a crack serial key for Crash Bandicoot N. Sane Trilogy from different sources and websites such as Skidrow Cracked, CDKeys, G2A, or YouTube. Q: How to use a crack serial key for Crash Bandicoot N. Sane Trilogy? A: You can use a crack serial key for Crash Bandicoot N. Sane Trilogy by copying it from the source or website where you got it from and pasting it in the Product Code box when activating a product on Steam. Q: What are the risks and drawbacks of using a crack serial key for Crash Bandicoot N. Sane Trilogy? A: Some of the risks and drawbacks of using a crack serial key for Crash Bandicoot N. Sane Trilogy are breaking the law, harming the developers, exposing your PC to viruses, or missing out on updates or features. 0a6ba089eb
-
-
\ No newline at end of file
diff --git a/spaces/1acneusushi/gradio-2dmoleculeeditor/data/Download Surfaced By T.J. Yelden (.ePUB) [NEW].md b/spaces/1acneusushi/gradio-2dmoleculeeditor/data/Download Surfaced By T.J. Yelden (.ePUB) [NEW].md
deleted file mode 100644
index c663fb0364ab451e10a7c861694b8d8b69ed388f..0000000000000000000000000000000000000000
--- a/spaces/1acneusushi/gradio-2dmoleculeeditor/data/Download Surfaced By T.J. Yelden (.ePUB) [NEW].md
+++ /dev/null
@@ -1,16 +0,0 @@
-
-
Surfaced by T.J. Yelden: A thrilling sequel to Hidden
-
If you are a fan of paranormal romance and urban fantasy, you might want to check out Surfaced, the second book in the Hidden Trilogy by T.J. Yelden. This book follows the adventures of Kendra, a rare white wolf shifter who has to learn how to control her wolf side while dealing with the dangers and mysteries of the shifter world.
In Surfaced, Kendra is starting college and trying to cope with the long-distance relationship with her boyfriend Cade, who is off to High Council Enforcer Training for five years. She also has to face a stalker wolf from another pack, meet other shifters with their own agendas, and stay under the radar of the Shifter High Council, who are not happy about her existence. Along the way, she discovers more about her past, her present, and her future as a wolf shifter.
-
Surfaced is a fast-paced and engaging read that will keep you hooked until the end. The book has a perfect balance of humor, action, romance, and suspense. The characters are well-developed and likable, especially Kendra, who is a strong and sassy heroine. The plot is full of twists and turns that will keep you guessing and surprised. The book also ends with a cliffhanger that will make you eager for the third and final book in the trilogy.
-
You can get Surfaced as an ebook from Amazon for $2.99 or read it for free with Kindle Unlimited[^2^]. You can also find more information and reviews about the book on Goodreads[^1^]. If you haven't read the first book in the trilogy, Hidden, you can also get it from Amazon or Kindle Unlimited[^2^].
-
If you are looking for a captivating and entertaining paranormal romance series with a unique twist on wolf shifters, you should definitely give Surfaced and Hidden by T.J. Yelden a try.
-
-
What makes Surfaced and Hidden stand out from other paranormal romance books is the author's creative and original take on wolf shifters. T.J. Yelden has created a rich and complex world where shifters have their own history, culture, politics, and rules. She also explores the themes of identity, belonging, loyalty, and love in a realistic and relatable way.
-
The author's writing style is smooth and captivating, with vivid descriptions and witty dialogues. She also knows how to build tension and suspense, as well as create steamy and sweet romance scenes. The books are written in the first-person point of view of Kendra, which allows the reader to get inside her head and feel her emotions.
-
-
Surfaced and Hidden are books that will make you laugh, cry, swoon, and gasp. They are perfect for fans of paranormal romance who are looking for something fresh and exciting. The books have received rave reviews from readers who have praised the author's storytelling skills and the characters' chemistry. The books have also been featured on several lists of best shifter romance books on Goodreads.
-
If you want to dive into a thrilling and romantic adventure with Kendra and Cade, don't miss Surfaced and Hidden by T.J. Yelden. You can get them from Amazon or Kindle Unlimited today.
81aa517590
-
-
\ No newline at end of file
diff --git a/spaces/1gistliPinn/ChatGPT4/Examples/Evildeadallpartsinhinditorrentdownload.md b/spaces/1gistliPinn/ChatGPT4/Examples/Evildeadallpartsinhinditorrentdownload.md
deleted file mode 100644
index 406399152000ace567aeb25bb349283bea6cb0b9..0000000000000000000000000000000000000000
--- a/spaces/1gistliPinn/ChatGPT4/Examples/Evildeadallpartsinhinditorrentdownload.md
+++ /dev/null
@@ -1,9 +0,0 @@
-
-
2185351 kms pico office for mac veselue2.di.dud.5-2.3.2.9v2,evildeadallpartsinhinditorrentdownload,evildeadallpartsinhinditorrentdownload-desires 0db76fd2b3c https://coub.com/stories/2200653-evildeadallpartsinhinditorrentdownload-tensor.
-
evildeadallpartsinhinditorrentdownload https://coub.com/stories/2209137-taming-bull https://coub.com/stories/2195055-evildeadallpartsinhinditorrentdownload-chavegard. http://kiyosans.sblo.jp/article/188916753.html. Posted by moyzaka at 20220206 22:47. evildeadallpartsinhinditorrentdownload,
- 899543212b
-
-
\ No newline at end of file
diff --git a/spaces/1phancelerku/anime-remove-background/Download and Play Hello Neighbor Full Act APK - The Scariest Game Ever.md b/spaces/1phancelerku/anime-remove-background/Download and Play Hello Neighbor Full Act APK - The Scariest Game Ever.md
deleted file mode 100644
index 86e53d13a662ccc70bc3cf2283fcc7253e601887..0000000000000000000000000000000000000000
--- a/spaces/1phancelerku/anime-remove-background/Download and Play Hello Neighbor Full Act APK - The Scariest Game Ever.md
+++ /dev/null
@@ -1,94 +0,0 @@
-
-
How to Download Hello Neighbor Full Act APK for Android
-
If you are a fan of stealth horror games, you might have heard of Hello Neighbor, a game where you have to sneak into your neighbor's house and find out what he is hiding in his basement. But did you know that you can download and play the full version of Hello Neighbor on your Android device? In this article, we will show you how to download Hello Neighbor Full Act APK, a file that contains the complete game with all its acts and modes. We will also explain what an APK file is, how to install it, and how to play Hello Neighbor Full Act APK on your Android device.
-
What is Hello Neighbor?
-
Hello Neighbor is a stealth horror game developed by Dynamic Pixels and tinyBuild. It was released in 2017 for Windows, Xbox One, PlayStation 4, Nintendo Switch, iOS, and Android. The game has received positive reviews from critics and players for its unique gameplay, graphics, and story.
The main feature of Hello Neighbor is its advanced AI that learns from your every move. You play as a curious kid who wants to find out what your neighbor is hiding in his basement. However, your neighbor is not a friendly guy. He will chase you, set traps, and use cameras to stop you from entering his house. The more you sneak around, the more he adapts to your behavior and becomes smarter and harder to avoid.
-
A popular game with multiple acts and modes
-
Hello Neighbor has a story mode that consists of four acts. Each act has a different setting, objective, and difficulty level. You have to use your wits, skills, and items to solve puzzles, unlock doors, and escape from the neighbor. The game also has a secret mode that reveals more about the neighbor's backstory and motives. Additionally, there are other modes such as hide and seek, where you play as the neighbor's children; ghost mode, where you can explore the house without being detected; and sandbox mode, where you can create your own scenarios and challenges.
-
What is an APK file?
-
An APK file is a package file format used by the Android operating system for distribution and installation of mobile applications. It contains all the code, resources, assets, certificates, and manifest file of an app. An APK file can be built from source code written in either Java or Kotlin.
-
A package file format for Android apps
-
An APK file is similar to other software packages such as APPX in Windows or DEB in Debian-based operating systems. To make an APK file, a program for Android is first compiled using a tool such as Android Studio or Visual Studio and then all of its parts are packaged into one container file. An APK file can be opened with any ZIP file opening software or extracted with any ZIP file extractor.
-
A way to install apps from sources other than Google Play
-
An APK file can be downloaded directly to Android devices from websites or other sources that offer them. This is called sideloading. Sideloading allows users to install apps that are not available on Google Play or that have been modified or customized by third parties. However, sideloading also poses some risks such as malware infection or data theft
How to download Hello Neighbor Full Act APK?
-
If you want to play the full version of Hello Neighbor on your Android device, you need to download and install the Hello Neighbor Full Act APK file. This is a file that contains the complete game with all its acts and modes. However, you cannot find this file on Google Play, as it is not an official app from the developers. You need to download it from a third-party website that offers it. Here are the steps to download Hello Neighbor Full Act APK:
-
Find a reliable website that offers the APK file
-
The first step is to find a website that provides the Hello Neighbor Full Act APK file for free. You can search for it on Google or use one of the links below . Make sure that the website is trustworthy and does not contain any malware or viruses. You can check the reviews and ratings of the website and the file before downloading it.
-
Enable unknown sources on your Android device
-
The next step is to enable unknown sources on your Android device. This is a setting that allows you to install apps from sources other than Google Play. To enable unknown sources, you need to access the settings app and look for the security or privacy option. Depending on your device, you may need to tap on the lock screen and security tab or the install unknown apps switch. Then, you need to turn on the unknown sources switch or check the box next to it. You may see a warning message against enabling this option, but you can ignore it if you trust the source of the APK file .
-
Download and install the APK file
-
The final step is to download and install the APK file on your Android device. You can do this by tapping on the download link or button on the website that offers the file. You may need to wait for a few seconds or minutes for the download to complete. Once the download is done, you can open the file manager app on your device and locate the APK file in your downloads folder. Tap on the file and follow the instructions to install it. You may need to grant some permissions to the app during the installation process.
-
download hello neighbor full act apk free
-download hello neighbor full act apk latest version
-download hello neighbor full act apk for android
-download hello neighbor full act apk mod
-download hello neighbor full act apk offline
-download hello neighbor full act apk no verification
-download hello neighbor full act apk obb
-download hello neighbor full act apk from apkpure
-download hello neighbor full act apk 2.3.8
-download hello neighbor full act apk unlimited money
-download hello neighbor full act apk revdl
-download hello neighbor full act apk rexdl
-download hello neighbor full act apk hack
-download hello neighbor full act apk data
-download hello neighbor full act apk highly compressed
-download hello neighbor full act apk android 1
-download hello neighbor full act apk uptodown
-download hello neighbor full act apk andropalace
-download hello neighbor full act apk mob.org
-download hello neighbor full act apk apkmirror
-download hello neighbor full act apk apkmody
-download hello neighbor full act apk happymod
-download hello neighbor full act apk an1.com
-download hello neighbor full act apk android oyun club
-download hello neighbor full act apk blackmod.net
-download hello neighbor full act apk by tinybuild games
-download hello neighbor full act apk cracked
-download hello neighbor full act apk cheat menu
-download hello neighbor full act apk direct link
-download hello neighbor full act apk easy install
-download hello neighbor full act apk fileplanet.com
-download hello neighbor full act apk for pc windows 10
-download hello neighbor full act apk gamestechy.com
-download hello neighbor full act apk google drive link
-download hello neighbor full act apk how to install guide
-download hello neighbor full act apk in parts
-download hello neighbor full act apk ios iphone ipad ipod touch compatible
-download hello neighbor full act apk low mb size
-download hello neighbor full act apk mediafire.com
-download hello neighbor full act apk mega.nz
How to play Hello Neighbor Full Act APK?
-
After you have successfully installed the Hello Neighbor Full Act APK file on your Android device, you can start playing the game. You can launch the game by tapping on its icon on your home screen or app drawer. You can also create a shortcut for the game on your desktop for easy access. Here are some tips on how to play Hello Neighbor Full Act APK:
-
Explore the neighbor's house and discover his secrets
-
The main goal of Hello Neighbor is to explore the neighbor's house and find out what he is hiding in his basement. You can use various items and tools to help you in your quest, such as keys, crowbars, flashlights, binoculars, and more. You can also interact with different objects and environments in the house, such as doors, windows, drawers, switches, vents, and more. You can use these to create diversions, hide, or access new areas. However, you need to be careful not to make too much noise or leave any traces behind, as the neighbor will notice them and become suspicious.
-
Avoid being caught by the neighbor and his traps
-
The biggest challenge of Hello Neighbor is to avoid being caught by the neighbor and his traps. The neighbor is not a dumb AI that follows a fixed pattern. He is a smart and adaptive AI that learns from your actions and reacts accordingly. He will chase you, set traps, use cameras, and even call the police if he sees you in his house. He will also remember your previous attempts and change his behavior and strategy accordingly. You need to be unpredictable and creative to outsmart him and escape from his clutches.
-
Enjoy the full story and gameplay of Hello Neighbor
-
By downloading Hello Neighbor Full Act APK, you can enjoy the full story and gameplay of Hello Neighbor on your Android device. You can play all four acts of the story mode and uncover the mystery behind the neighbor's basement. You can also play the secret mode and learn more about the neighbor's past and motives. Additionally, you can try out other modes such as hide and seek, ghost mode, and sandbox mode for more fun and variety.
-
Conclusion
-
Hello Neighbor is a stealth horror game that offers a unique and thrilling experience for Android users. By downloading Hello Neighbor Full Act APK, you can play the complete game with all its acts and modes on your device. You can explore the neighbor's house, avoid his traps, and discover his secrets. However, you need to be careful when downloading and installing APK files from third-party sources, as they may contain malware or viruses. You also need to enable unknown sources on your device before installing them.
-
FAQs
-
Here are some frequently asked questions about Hello Neighbor Full Act APK:
-
Q: Is Hello Neighbor Full Act APK safe to download?
-
A: Hello Neighbor Full Act APK is safe to download if you get it from a reliable website that does not contain any malware or viruses. However, you should always scan the file with an antivirus software before installing it.
-
Q: Is Hello Neighbor Full Act APK free to download?
-
A: Yes, Hello Neighbor Full Act APK is free to download from most websites that offer it. However, some websites may require you to complete surveys or watch ads before downloading it.
-
Q: Do I need an internet connection to play Hello Neighbor Full Act APK?
-
A: No, you do not need an internet connection to play Hello Neighbor Full Act APK. You can play the game offline without any problems.
-
Q: What are the minimum requirements to play Hello Neighbor Full Act APK?
-
A: The minimum requirements to play Hello Neighbor Full Act APK are as follows:
-
-
OS
Android 7.0 or higher
-
CPU
Dual-core 1.5 GHz or higher
-
RAM
2 GB or higher
-
Storage
1 GB or higher
-
Graphics
Mali-T760MP8 or higher
-
-
Q: How can I update Hello Neighbor Full Act APK?
-
A: To update Hello Neighbor Full Act APK, you need to download the latest version of the file from a website that offers it. Then, you need to uninstall the previous version of the app and install the new one. Alternatively, you can check if the website has an update option that allows you to download and install the update automatically.
-
I hope this article has helped you learn how to download Hello Neighbor Full Act APK for Android. If you have any questions or feedback, please leave a comment below. Thank you for reading and happy gaming!
197e85843d
-
-
\ No newline at end of file
diff --git a/spaces/1phancelerku/anime-remove-background/Explore the Beauty and Diversity of Indonesia with Bus Simulator Indonesia HD.md b/spaces/1phancelerku/anime-remove-background/Explore the Beauty and Diversity of Indonesia with Bus Simulator Indonesia HD.md
deleted file mode 100644
index 663e39a21deafa08d0e137304c19e54c71b81ff6..0000000000000000000000000000000000000000
--- a/spaces/1phancelerku/anime-remove-background/Explore the Beauty and Diversity of Indonesia with Bus Simulator Indonesia HD.md
+++ /dev/null
@@ -1,181 +0,0 @@
-
-
Download Bus Simulator Indonesia HD: A Fun and Authentic Way to Experience Driving in Indonesia
-
Have you ever wondered what it is like to be a bus driver in Indonesia? If you have, then you should try Bus Simulator Indonesia HD, a popular game that lets you experience the thrill and challenge of driving a bus in various Indonesian cities and places. Bus Simulator Indonesia HD (also known as BUSSID) is not the first bus simulator game, but it is probably one of the only ones with the most features and the most authentic Indonesian environment.
-
In this article, we will show you how to download Bus Simulator Indonesia HD for Android and PC, how to play it, how to enhance your gaming experience with it, and how to troubleshoot some common problems with it. We will also answer some frequently asked questions about the game. By the end of this article, you will be ready to hop on your bus and start your journey in Bus Simulator Indonesia HD.
How to Download Bus Simulator Indonesia HD for Android and PC
-
Downloading from Google Play Store
-
The easiest way to download Bus Simulator Indonesia HD for Android is to get it from the Google Play Store. Here are the steps you need to follow:
-
-
Open the Google Play Store app on your Android device.
-
Search for "Bus Simulator Indonesia" or "BUSSID" in the search bar.
-
Tap on the game icon that has a blue background and a yellow bus.
-
Tap on "Install" and wait for the game to download and install on your device.
-
Tap on "Open" or find the game icon on your home screen or app drawer.
-
Enjoy playing Bus Simulator Indonesia HD!
-
-
Note that the game requires Android 4.2 or higher and at least 1 GB of RAM to run smoothly. You also need to have enough storage space on your device, as the game size is about 300 MB.
-
Downloading from Other Sources
-
If you cannot download Bus Simulator Indonesia HD from the Google Play Store, or if you want to play it on your PC, you can try other sources, such as APK files or emulators. However, you should be careful and only download from trusted and verified sources, as some files may contain viruses or malware that can harm your device or PC. You should also check the compatibility and requirements of the game before downloading and installing it.
-
One of the most popular sources for downloading APK files is APKPure, which offers safe and fast downloads for various Android games and apps. You can download Bus Simulator Indonesia HD from APKPure by following these steps:
-
-
Open your web browser and go to https://apkpure.com/.
-
Search for "Bus Simulator Indonesia" or "BUSSID" in the search bar.
-
Tap on the game icon that has a blue background and a yellow bus.
-
Tap on "Download APK" and wait for the file to download on your device or PC.
-
If you are using an Android device, go to your file manager and find the downloaded APK file. Tap on it and allow the installation from unknown sources if prompted. Wait for the game to install on your device.
-
If you are using a PC, you need to have an Android emulator installed on your PC, such as BlueStacks or NoxPlayer. Open the emulator and drag and drop the downloaded APK file into it. Wait for the game to install on the emulator.
-
Open the game from your device or emulator and enjoy playing Bus Simulator Indonesia HD!
-
-
Note that downloading and installing APK files may not give you the latest version of the game, and you may not be able to access some features or updates. You may also encounter some errors or bugs while playing the game. To avoid these problems, we recommend that you download Bus Simulator Indonesia HD from the Google Play Store whenever possible.
-
How to Play Bus Simulator Indonesia HD
-
Choosing Your Bus and Livery
-
One of the coolest features of Bus Simulator Indonesia HD is that you can choose and customize your own bus and livery. A livery is a design or pattern that covers the exterior of your bus, such as colors, logos, stickers, etc. You can choose from various types of buses, such as mini buses, double deckers, articulated buses, etc. You can also choose from different liveries, such as national flags, famous brands, cartoon characters, etc. You can even create your own livery using the livery editor feature.
-
Download Bus Simulator Indonesia on PC with BlueStacks
-Bus Simulator Indonesia HD wallpapers for desktop and mobile
-How to design your own livery in Bus Simulator Indonesia
-Bus Simulator Indonesia online multiplayer convoy mode
-Bus Simulator Indonesia mod apk unlimited money and fuel
-Best Indonesian cities and places to visit in Bus Simulator Indonesia
-Bus Simulator Indonesia for iOS devices free download
-Tips and tricks to master Bus Simulator Indonesia game
-Bus Simulator Indonesia review and rating by users
-Bus Simulator Indonesia latest update features and bug fixes
-How to install and play Bus Simulator Indonesia on Mac
-Bus Simulator Indonesia cheats and hacks for android
-Bus Simulator Indonesia gameplay videos and live streams
-How to use your own 3D model in Bus Simulator Indonesia
-Bus Simulator Indonesia official website and social media links
-Bus Simulator Indonesia system requirements and compatibility
-How to get free emoji icons for Bus Simulator Indonesia
-Bus Simulator Indonesia offline mode without internet connection
-How to unlock all Indonesian buses in Bus Simulator Indonesia
-Bus Simulator Indonesia vs other bus simulator games comparison
-How to contact Bus Simulator Indonesia support and feedback
-Bus Simulator Indonesia data privacy and security policy
-How to join the Bus Simulator Indonesia community and forums
-How to earn more money and rewards in Bus Simulator Indonesia
-How to customize your bus driver avatar in Bus Simulator Indonesia
-How to change the language and settings in Bus Simulator Indonesia
-How to fix common errors and issues in Bus Simulator Indonesia
-How to backup and restore your data in Bus Simulator Indonesia
-How to play Bus Simulator Indonesia with a controller or keyboard
-How to improve the graphics quality and performance in Bus Simulator Indonesia
-How to honk your horn and use cool and fun honks in Bus Simulator Indonesia
-How to access the leaderboard and achievements in Bus Simulator Indonesia
-How to share your screenshots and videos of Bus Simulator Indonesia
-How to invite your friends and play together in Bus Simulator Indonesia
-How to download and install new mods for Bus Simulator Indonesia
-How to learn more about Indonesian culture and history in Bus Simulator Indonesia
-How to upgrade your bus engine and parts in Bus Simulator Indonesia
-How to follow the traffic rules and regulations in Bus Simulator Indonesia
-How to drive safely and avoid accidents in Bus Simulator Indonesia
-How to enjoy the realistic and authentic Indonesian environment in Bus Simulator Indonesia
-
To choose and customize your bus and livery, follow these steps:
-
-
From the main menu, tap on "Garage".
-
Tap on "Bus" to select your bus type. You can swipe left or right to see more options. You can also tap on "Buy" to purchase more buses using in-game currency.
-
Tap on "Livery" to select your livery. You can swipe left or right to see more options. You can also tap on "Download" to download more liveries from other players or online sources.
-
Tap on "Edit" to create your own livery using the livery editor feature. You can use various tools and options to design your livery as you like.
-
Tap on "Save" to save your changes and apply them to your bus.
-
-
Choosing and customizing your bus and livery can make your gaming experience more fun and personal. You can also show off your creativity and style to other players online.
-
Driving Your Bus in Career Mode or Free Mode
-
The main mode of Bus Simulator Indonesia HD is career mode, where you can drive your bus in various Indonesian cities and places, follow the traffic rules, pick up passengers, earn money, and upgrade your bus. You can also play in free mode, where you can drive your bus anywhere without any restrictions or objectives.
-
To drive your bus in career mode or free mode, follow these steps:
-
-
From the main menu, tap on "Play".
-
Select either "Career" or "Free" mode.
-
Select your starting location from the map. You can swipe left or right to see more options. You can also tap on "Random" to start from a random location.
-
Select your destination from the map. option.
-
If you select "Join" convoy, you can see a list of available convoys that you can join. You can filter the list by region, bus type, or livery. You can also search for a specific convoy by name or ID. Tap on the convoy that you want to join and wait for the host to accept you.
-
If you select "Create" convoy, you can create your own convoy by setting the name, password, region, bus type, livery, route, and destination. You can also invite your friends or other players to join your convoy by sharing the convoy ID or QR code. Tap on "Start" to begin your convoy.
-
Once you are in a convoy, you can see the other players' names, buses, and locations on the map or the GPS. You can also chat with them by tapping on the chat icon. You can also honk at them by tapping on the horn icon. You can also leave the convoy by tapping on the exit icon.
-
-
Joining or creating an online multiplayer convoy can make your gaming experience more social and interactive. You can meet new friends, learn from other players, and have fun together.
-
How to Enhance Your Gaming Experience with Bus Simulator Indonesia HD
-
Using Your Own 3D Model with Vehicle Mod System
-
One of the most advanced features of Bus Simulator Indonesia HD is that you can use your own 3D model with the vehicle mod system. This means that you can import any 3D model of a bus or a vehicle that you have created or downloaded from other sources and use it in the game. You can also customize the model's properties, such as engine, transmission, suspension, etc.
-
To use your own 3D model with the vehicle mod system, follow these steps:
-
-
Create or download a 3D model of a bus or a vehicle that you want to use in the game. The model must be in OBJ format and have a maximum size of 50 MB. The model must also have a texture file in PNG format and a material file in MTL format.
-
Copy the 3D model files to your device or PC. If you are using an Android device, copy them to the BUSSID folder in your internal storage. If you are using a PC, copy them to the BUSSID folder in your emulator's storage.
-
Open the game and go to the garage. Tap on "Mod" and then tap on "Import". Select the 3D model files that you have copied and wait for them to be imported.
-
Tap on "Edit" to customize the model's properties, such as name, price, engine, transmission, suspension, etc. You can also adjust the model's position, rotation, and scale.
-
Tap on "Save" to save your changes and apply them to your model.
-
Select your model from the mod list and use it in the game.
-
-
Using your own 3D model with the vehicle mod system can make your gaming experience more unique and creative. You can use any bus or vehicle that you like or imagine and drive it in Bus Simulator Indonesia HD.
-
Using Cool and Fun Honks
-
Another fun feature of Bus Simulator Indonesia HD is that you can use cool and fun honks to communicate with other drivers or passengers. Honks are sounds that your bus makes when you tap on the horn icon. You can choose from various honks, such as sirens, horns, bells, whistles, etc. You can also use some special honks that are unique to Indonesia, such as "Om Telolet Om".
-
"Om Telolet Om" is a phrase that means "Uncle, honk uncle" in Indonesian. It is a popular request that children make to bus drivers to make them honk their horns in a musical way. It is also a viral phenomenon that has spread across social media and attracted many celebrities and musicians.
-
To use cool and fun honks in Bus Simulator Indonesia HD, follow these steps:
-
-
From the main menu, tap on "Settings".
-
Tap on "Sound".
-
Tap on "Horn Sound" to select your honk type. You can swipe left or right to see more options. You can also tap on "Download" to download more honks from other players or online sources.
-
Tap on "Back" to save your changes and return to the main menu.
-
When playing the game, tap on the horn icon to use your selected honk.
-
-
Using cool and fun honks in Bus Simulator Indonesia HD can make your gaming experience more fun and interactive. You can also express your emotions and personality with your honks. You can also join the "Om Telolet Om" craze and make some music with your bus.
-
Competing with Other Players on Leaderboard
-
Another exciting feature of Bus Simulator Indonesia HD is that you can compete with other players on the leaderboard. The leaderboard is a ranking system that shows the best players in the game based on their score and reputation. You can see your own rank and score, as well as the rank and score of other players. You can also see the rank and score of your friends or other players that you follow.
-
To compete with other players on the leaderboard in Bus Simulator Indonesia HD, follow these steps:
-
-
From the main menu, tap on "Leaderboard".
-
Tap on "Global" to see the global leaderboard, or tap on "Friends" to see the friends leaderboard.
-
Swipe up or down to see more players on the leaderboard. You can also tap on a player's name to see their profile and stats.
-
Tap on "Follow" to follow a player, or tap on "Unfollow" to unfollow a player. You can also tap on "Chat" to chat with a player.
-
Tap on "Back" to return to the main menu.
-
-
To improve your rank and score on the leaderboard, you need to play well and complete missions in career mode. You also need to follow the traffic rules, drive safely, pick up passengers, earn money, and upgrade your bus. You also need to avoid crashing, breaking the law, or losing passengers. The better you play, the higher your score and reputation will be.
-
Competing with other players on the leaderboard in Bus Simulator Indonesia HD can make your gaming experience more challenging and rewarding. You can also learn from other players, compare your skills, and show off your achievements.
-
How to Troubleshoot Common Problems with Bus Simulator Indonesia HD
-
Game Crashes or Freezes
-
One of the most common problems that you may encounter while playing Bus Simulator Indonesia HD is that the game crashes or freezes. This means that the game stops working or responding, and you cannot continue playing. This can be very frustrating and annoying, especially if you are in the middle of a mission or a convoy.
-
To fix game crashes or freezes in Bus Simulator Indonesia HD, you can try these solutions:
-
-
Clear the game cache. This can help remove any corrupted or outdated files that may cause the game to crash or freeze. To clear the game cache, go to your device settings, find the game app, tap on "Storage", and then tap on "Clear cache".
-
Update the game app. This can help fix any bugs or errors that may cause the game to crash or freeze. To update the game app, go to the Google Play Store, find the game app, and tap on "Update".
-
Update your device software. This can help improve your device performance and compatibility with the game. To update your device software, go to your device settings, find "System update", and tap on "Check for updates".
-
Reinstall the game app. This can help reset the game settings and data to their default state. To reinstall the game app, go to the Google Play Store, find the game app, tap on "Uninstall", and then tap on "Install". Note that this will delete your game data, so make sure you have a backup or a cloud save before doing this.
-
-
If none of these solutions work, you can contact the game developer for more help. You can find their contact information on the game app page on the Google Play Store, or on their official website or social media accounts.
-
Game Lags or Runs Slowly
-
Another common problem that you may encounter while playing Bus Simulator Indonesia HD is that the game lags or runs slowly. This means that the game does not run smoothly or responsively, and you may experience delays, stuttering, or low frame rate. This can affect your gameplay and enjoyment, especially if you are driving fast or in a busy area.
-
To fix game lags or runs slowly in Bus Simulator Indonesia HD, you can try these tips:
-
-
Adjust the game graphics settings. This can help reduce the game's demand on your device's resources and improve the game's performance. To adjust the game graphics settings, go to the game menu, tap on "Settings", tap on "Graphics", and then change the options such as resolution, quality, shadow, etc. You can also use the "Auto" option to let the game choose the best settings for your device.
-
Close other apps or background processes. This can help free up your device's memory and CPU and prevent them from interfering with the game. To close other apps or background processes, go to your device settings, find "Apps" or "Application manager", and then swipe or tap on the apps that you want to close. You can also use a task manager or a cleaner app to do this automatically.
-
Use a booster app. This can help optimize your device's performance and speed up the game. A booster app is a tool that can clean your device's cache, memory, and junk files, as well as boost your device's CPU, GPU, and battery. Some examples of booster apps are Game Booster, Speed Booster, or DU Speed Booster. You can download them from the Google Play Store and use them before playing the game.
-
-
If none of these tips work, you may need to upgrade your device's hardware or software to meet the game's requirements. You can check the game's requirements on the game app page on the Google Play Store, or on their official website or social media accounts.
-
Game Data is Lost or Corrupted
-
Another common problem that you may encounter while playing Bus Simulator Indonesia HD is that your game data is lost or corrupted. This means that your game progress, settings, or purchases are missing or damaged, and you cannot access them or use them in the game. This can be very frustrating and disappointing, especially if you have spent a lot of time and money on the game.
-
To fix game data is lost or corrupted in Bus Simulator Indonesia HD, you can try these methods:
-
-
Use cloud save. This can help sync your game data with your Google Play account and restore it if it is lost or corrupted. To use cloud save, go to the game menu, tap on "Settings", tap on "Account", and then tap on "Cloud Save". You can also enable "Auto Save" to let the game save your data automatically.
-
Use a backup app. This can help backup your game data to your device's storage or an external storage and restore it if it is lost or corrupted. A backup app is a tool that can copy your game data files and store them in a safe location. Some examples of backup apps are Helium, Titanium Backup, or Easy Backup. You can download them from the Google Play Store and use them to backup and restore your game data.
-
Contact support. This can help recover your game data if it is lost or corrupted due to a bug or an error in the game. To contact support, go to the game menu, tap on "Settings", tap on "Help", and then tap on "Contact Us". You can also find their contact information on the game app page on the Google Play Store, or on their official website or social media accounts.
-
-
If none of these methods work , you may need to start a new game and lose your previous game data. To avoid this problem, we recommend that you backup your game data regularly and use cloud save whenever possible.
-
Conclusion
-
Bus Simulator Indonesia HD is a fun and authentic way to experience driving in Indonesia. You can download and play it on your Android device or PC, choose and customize your own bus and livery, drive your bus in career mode or free mode, join or create an online multiplayer convoy, use your own 3D model with the vehicle mod system, use cool and fun honks, and compete with other players on the leaderboard. You can also troubleshoot some common problems with the game, such as game crashes or freezes, game lags or runs slowly, or game data is lost or corrupted.
-
If you are looking for a realistic and immersive bus simulator game, you should definitely try Bus Simulator Indonesia HD. You will not regret it. You can download it from the Google Play Store or other sources, and start your adventure in Bus Simulator Indonesia HD today.
-
We hope that this article has helped you learn more about Bus Simulator Indonesia HD and how to download and play it. If you have any questions or comments, please feel free to leave them below. We would love to hear from you.
-
FAQs
-
Here are some frequently asked questions and answers about Bus Simulator Indonesia HD:
-
-
What is the difference between Bus Simulator Indonesia and Bus Simulator Indonesia HD?
-
Bus Simulator Indonesia HD is an upgraded version of Bus Simulator Indonesia that has better graphics, more features, and more content. It also has a larger game size and requires a higher device specification to run smoothly.
-
Can I play Bus Simulator Indonesia HD offline?
-
Yes, you can play Bus Simulator Indonesia HD offline in career mode or free mode. However, you need an internet connection to access some features, such as cloud save, multiplayer convoy, leaderboard, or download more buses or liveries.
-
Can I play Bus Simulator Indonesia HD with a controller?
-
Yes, you can play Bus Simulator Indonesia HD with a controller if you have a compatible device and controller. You can connect your controller to your device via Bluetooth or USB cable, and then configure the controller settings in the game menu.
-
Can I share my bus or livery with other players?
-
Yes, you can share your bus or livery with other players by uploading them to the game server or online sources. You can also download other players' buses or liveries from the game menu or online sources.
-
Can I request a new feature or report a bug for Bus Simulator Indonesia HD?
-
Yes, you can request a new feature or report a bug for Bus Simulator Indonesia HD by contacting the game developer via email, website, or social media. You can also leave a review or feedback on the game app page on the Google Play Store.
- 401be4b1e0
-
-
\ No newline at end of file
diff --git a/spaces/AI-Zero-to-Hero/07-SL-Chatbot-Blenderbot/README.md b/spaces/AI-Zero-to-Hero/07-SL-Chatbot-Blenderbot/README.md
deleted file mode 100644
index d54e7f55c8ec747390f624a6aac8615ffa98bc30..0000000000000000000000000000000000000000
--- a/spaces/AI-Zero-to-Hero/07-SL-Chatbot-Blenderbot/README.md
+++ /dev/null
@@ -1,13 +0,0 @@
----
-title: 07 SL Chatbot Blenderbot
-emoji: 🌍
-colorFrom: red
-colorTo: purple
-sdk: streamlit
-sdk_version: 1.10.0
-app_file: app.py
-pinned: false
-license: mit
----
-
-Check out the configuration reference at https://huggingface.co/docs/hub/spaces-config-reference
diff --git a/spaces/AIGC-Audio/Make_An_Audio/ldm/modules/diffusionmodules/custom_openaimodel.py b/spaces/AIGC-Audio/Make_An_Audio/ldm/modules/diffusionmodules/custom_openaimodel.py
deleted file mode 100644
index 4412eac52c294266dee21680f698b10a4614b4fa..0000000000000000000000000000000000000000
--- a/spaces/AIGC-Audio/Make_An_Audio/ldm/modules/diffusionmodules/custom_openaimodel.py
+++ /dev/null
@@ -1,368 +0,0 @@
-from abc import abstractmethod
-from functools import partial
-import math
-from typing import Iterable
-
-import numpy as np
-import torch as th
-import torch.nn as nn
-import torch.nn.functional as F
-
-from ldm.modules.diffusionmodules.util import (
- checkpoint,
- conv_nd,
- linear,
- avg_pool_nd,
- zero_module,
- normalization,
- timestep_embedding,
-)
-from ldm.modules.attention import SpatialTransformer
-from ldm.modules.diffusionmodules.openaimodel import convert_module_to_f16, convert_module_to_f32, AttentionPool2d, \
- TimestepBlock, TimestepEmbedSequential, Upsample, TransposedUpsample, Downsample, ResBlock, AttentionBlock, count_flops_attn, \
- QKVAttentionLegacy, QKVAttention
-
-
-class UNetModel(nn.Module):
- """
- The full UNet model with attention and timestep embedding.
- :param in_channels: channels in the input Tensor.
- :param model_channels: base channel count for the model.
- :param out_channels: channels in the output Tensor.
- :param num_res_blocks: number of residual blocks per downsample.
- :param attention_resolutions: a collection of downsample rates at which
- attention will take place. May be a set, list, or tuple.
- For example, if this contains 4, then at 4x downsampling, attention
- will be used.
- :param dropout: the dropout probability.
- :param channel_mult: channel multiplier for each level of the UNet.
- :param conv_resample: if True, use learned convolutions for upsampling and
- downsampling.
- :param dims: determines if the signal is 1D, 2D, or 3D.
- :param num_classes: if specified (as an int), then this model will be
- class-conditional with `num_classes` classes.
- :param use_checkpoint: use gradient checkpointing to reduce memory usage.
- :param num_heads: the number of attention heads in each attention layer.
- :param num_heads_channels: if specified, ignore num_heads and instead use
- a fixed channel width per attention head.
- :param num_heads_upsample: works with num_heads to set a different number
- of heads for upsampling. Deprecated.
- :param use_scale_shift_norm: use a FiLM-like conditioning mechanism.
- :param resblock_updown: use residual blocks for up/downsampling.
- :param use_new_attention_order: use a different attention pattern for potentially
- increased efficiency.
- """
-
- def __init__(
- self,
- image_size,
- in_channels,
- model_channels,
- out_channels,
- num_res_blocks,
- attention_resolutions,
- dropout=0,
- channel_mult=(1, 2, 4, 8),
- conv_resample=True,
- dims=2,
- num_classes=None,
- use_checkpoint=False,
- use_fp16=False,
- num_heads=-1,
- num_head_channels=-1,
- num_heads_upsample=-1,
- use_scale_shift_norm=False,
- resblock_updown=False,
- use_new_attention_order=False,
- use_spatial_transformer=False, # custom transformer support
- transformer_depth=1, # custom transformer support
- context_dim=None, # custom transformer support
- n_embed=None, # custom support for prediction of discrete ids into codebook of first stage vq model
- legacy=True,
- use_context_project=False, # custom text to audio support
- use_context_attn=True # custom text to audio support
- ):
- super().__init__()
- if use_spatial_transformer:
- assert context_dim is not None, 'Fool!! You forgot to include the dimension of your cross-attention conditioning...'
-
- if context_dim is not None and not use_context_project:
- assert use_spatial_transformer, 'Fool!! You forgot to use the spatial transformer for your cross-attention conditioning...'
- from omegaconf.listconfig import ListConfig
- if type(context_dim) == ListConfig:
- context_dim = list(context_dim)
-
- if num_heads_upsample == -1:
- num_heads_upsample = num_heads
-
- if num_heads == -1:
- assert num_head_channels != -1, 'Either num_heads or num_head_channels has to be set'
-
- if num_head_channels == -1:
- assert num_heads != -1, 'Either num_heads or num_head_channels has to be set'
-
- self.image_size = image_size
- self.in_channels = in_channels
- self.model_channels = model_channels
- self.out_channels = out_channels
- self.num_res_blocks = num_res_blocks
- self.attention_resolutions = attention_resolutions
- self.dropout = dropout
- self.channel_mult = channel_mult
- self.conv_resample = conv_resample
- self.num_classes = num_classes
- self.use_checkpoint = use_checkpoint
- self.dtype = th.float16 if use_fp16 else th.float32
- self.num_heads = num_heads
- self.num_head_channels = num_head_channels
- self.num_heads_upsample = num_heads_upsample
- self.predict_codebook_ids = n_embed is not None
-
- time_embed_dim = model_channels * 4
- self.time_embed = nn.Sequential(
- linear(model_channels, time_embed_dim),
- nn.SiLU(),
- linear(time_embed_dim, time_embed_dim),
- )
-
- if self.num_classes is not None:
- self.label_emb = nn.Embedding(num_classes, time_embed_dim)
-
- self.input_blocks = nn.ModuleList(
- [
- TimestepEmbedSequential(
- conv_nd(dims, in_channels, model_channels, 3, padding=1)
- )
- ]
- )
- self._feature_size = model_channels
- input_block_chans = [model_channels]
- ch = model_channels
- ds = 1
- for level, mult in enumerate(channel_mult):
- for _ in range(num_res_blocks):
- layers = [
- ResBlock(
- ch,
- time_embed_dim,
- dropout,
- out_channels=mult * model_channels,
- dims=dims,
- use_checkpoint=use_checkpoint,
- use_scale_shift_norm=use_scale_shift_norm,
- )
- ]
- ch = mult * model_channels
- if ds in attention_resolutions:
- if num_head_channels == -1:
- dim_head = ch // num_heads
- else:
- num_heads = ch // num_head_channels
- dim_head = num_head_channels
- if legacy:
- #num_heads = 1
- dim_head = ch // num_heads if use_spatial_transformer else num_head_channels
- layers.append(
- AttentionBlock(
- ch,
- use_checkpoint=use_checkpoint,
- num_heads=num_heads,
- num_head_channels=dim_head,
- use_new_attention_order=use_new_attention_order,
- ) if not use_spatial_transformer else SpatialTransformer(
- ch, num_heads, dim_head, depth=transformer_depth, context_dim=context_dim
- )
- )
- self.input_blocks.append(TimestepEmbedSequential(*layers))
- self._feature_size += ch
- input_block_chans.append(ch)
- if level != len(channel_mult) - 1:
- out_ch = ch
- self.input_blocks.append(
- TimestepEmbedSequential(
- ResBlock(
- ch,
- time_embed_dim,
- dropout,
- out_channels=out_ch,
- dims=dims,
- use_checkpoint=use_checkpoint,
- use_scale_shift_norm=use_scale_shift_norm,
- down=True,
- )
- if resblock_updown
- else Downsample(
- ch, conv_resample, dims=dims, out_channels=out_ch
- )
- )
- )
- ch = out_ch
- input_block_chans.append(ch)
- ds *= 2
- self._feature_size += ch
-
- if num_head_channels == -1:
- dim_head = ch // num_heads
- else:
- num_heads = ch // num_head_channels
- dim_head = num_head_channels
- if legacy:
- #num_heads = 1
- dim_head = ch // num_heads if use_spatial_transformer else num_head_channels
- self.middle_block = TimestepEmbedSequential(
- ResBlock(
- ch,
- time_embed_dim,
- dropout,
- dims=dims,
- use_checkpoint=use_checkpoint,
- use_scale_shift_norm=use_scale_shift_norm,
- ),
- AttentionBlock(
- ch,
- use_checkpoint=use_checkpoint,
- num_heads=num_heads,
- num_head_channels=dim_head,
- use_new_attention_order=use_new_attention_order,
- ) if not use_spatial_transformer else SpatialTransformer(
- ch, num_heads, dim_head, depth=transformer_depth, context_dim=context_dim
- ),
- ResBlock(
- ch,
- time_embed_dim,
- dropout,
- dims=dims,
- use_checkpoint=use_checkpoint,
- use_scale_shift_norm=use_scale_shift_norm,
- ),
- )
- self._feature_size += ch
-
- self.output_blocks = nn.ModuleList([])
- for level, mult in list(enumerate(channel_mult))[::-1]:
- for i in range(num_res_blocks + 1):
- ich = input_block_chans.pop()
- layers = [
- ResBlock(
- ch + ich,
- time_embed_dim,
- dropout,
- out_channels=model_channels * mult,
- dims=dims,
- use_checkpoint=use_checkpoint,
- use_scale_shift_norm=use_scale_shift_norm,
- )
- ]
- ch = model_channels * mult
- if ds in attention_resolutions:
- if num_head_channels == -1:
- dim_head = ch // num_heads
- else:
- num_heads = ch // num_head_channels
- dim_head = num_head_channels
- if legacy:
- #num_heads = 1
- dim_head = ch // num_heads if use_spatial_transformer else num_head_channels
- layers.append(
- AttentionBlock(
- ch,
- use_checkpoint=use_checkpoint,
- num_heads=num_heads_upsample,
- num_head_channels=dim_head,
- use_new_attention_order=use_new_attention_order,
- ) if not use_spatial_transformer else SpatialTransformer(
- ch, num_heads, dim_head, depth=transformer_depth, context_dim=context_dim
- )
- )
- if level and i == num_res_blocks:
- out_ch = ch
- layers.append(
- ResBlock(
- ch,
- time_embed_dim,
- dropout,
- out_channels=out_ch,
- dims=dims,
- use_checkpoint=use_checkpoint,
- use_scale_shift_norm=use_scale_shift_norm,
- up=True,
- )
- if resblock_updown
- else Upsample(ch, conv_resample, dims=dims, out_channels=out_ch)
- )
- ds //= 2
- self.output_blocks.append(TimestepEmbedSequential(*layers))
- self._feature_size += ch
-
- self.out = nn.Sequential(
- normalization(ch),
- nn.SiLU(),
- zero_module(conv_nd(dims, model_channels, out_channels, 3, padding=1)),
- )
- if self.predict_codebook_ids:
- self.id_predictor = nn.Sequential(
- normalization(ch),
- conv_nd(dims, model_channels, n_embed, 1),
- #nn.LogSoftmax(dim=1) # change to cross_entropy and produce non-normalized logits
- )
-
- self.use_context_project = use_context_project
- if use_context_project:
- self.context_project = linear(context_dim, time_embed_dim)
- self.use_context_attn = use_context_attn
-
-
- def convert_to_fp16(self):
- """
- Convert the torso of the model to float16.
- """
- self.input_blocks.apply(convert_module_to_f16)
- self.middle_block.apply(convert_module_to_f16)
- self.output_blocks.apply(convert_module_to_f16)
-
- def convert_to_fp32(self):
- """
- Convert the torso of the model to float32.
- """
- self.input_blocks.apply(convert_module_to_f32)
- self.middle_block.apply(convert_module_to_f32)
- self.output_blocks.apply(convert_module_to_f32)
-
- def forward(self, x, timesteps=None, context=None, y=None,**kwargs):
- """
- Apply the model to an input batch.
- :param x: an [N x C x ...] Tensor of inputs.
- :param timesteps: a 1-D batch of timesteps.
- :param context: conditioning plugged in via crossattn
- :param y: an [N] Tensor of labels, if class-conditional.
- :return: an [N x C x ...] Tensor of outputs.
- """
- assert (y is not None) == (
- self.num_classes is not None
- ), "must specify y if and only if the model is class-conditional"
- hs = []
- t_emb = timestep_embedding(timesteps, self.model_channels, repeat_only=False)
- emb = self.time_embed(t_emb)
-
- if self.num_classes is not None:
- assert y.shape == (x.shape[0],)
- emb = emb + self.label_emb(y)
-
- # For text-to-audio using global CLIP
- if self.use_context_project:
- context = self.context_project(context)
- emb = emb + context.squeeze(1)
-
- h = x.type(self.dtype)
- for module in self.input_blocks:
- h = module(h, emb, context if self.use_context_attn else None)
- hs.append(h)
- h = self.middle_block(h, emb, context if self.use_context_attn else None)
- for module in self.output_blocks:
- h = th.cat([h, hs.pop()], dim=1)
- h = module(h, emb, context if self.use_context_attn else None)
- h = h.type(x.dtype)
- if self.predict_codebook_ids:
- return self.id_predictor(h)
- else:
- return self.out(h)
diff --git a/spaces/AIML-TUDA/FairDiffusionExplorer/README.md b/spaces/AIML-TUDA/FairDiffusionExplorer/README.md
deleted file mode 100644
index 44cd58579a737c17558b8af77a6f67420e1f69ec..0000000000000000000000000000000000000000
--- a/spaces/AIML-TUDA/FairDiffusionExplorer/README.md
+++ /dev/null
@@ -1,13 +0,0 @@
----
-title: FairDiffusionExplorer
-emoji: 📊
-colorFrom: blue
-colorTo: yellow
-sdk: gradio
-sdk_version: 3.3.1
-app_file: app.py
-pinned: false
-license: cc-by-sa-4.0
----
-
-Check out the configuration reference at https://huggingface.co/docs/hub/spaces-config-reference
diff --git a/spaces/AIZero2HeroBootcamp/VideoToAnimatedGif/README.md b/spaces/AIZero2HeroBootcamp/VideoToAnimatedGif/README.md
deleted file mode 100644
index d4a4ead83a3aed98c63d351d3d532d24b6d7d8ea..0000000000000000000000000000000000000000
--- a/spaces/AIZero2HeroBootcamp/VideoToAnimatedGif/README.md
+++ /dev/null
@@ -1,13 +0,0 @@
----
-title: VideoToAnimatedGif
-emoji: 🐢
-colorFrom: pink
-colorTo: purple
-sdk: streamlit
-sdk_version: 1.21.0
-app_file: app.py
-pinned: false
-license: mit
----
-
-Check out the configuration reference at https://huggingface.co/docs/hub/spaces-config-reference
diff --git a/spaces/ATang0729/Forecast4Muses/Model/Model6/Model6_0_ClothesDetection/mmyolo/configs/yolov6/yolov6_t_syncbn_fast_8xb32-400e_coco.py b/spaces/ATang0729/Forecast4Muses/Model/Model6/Model6_0_ClothesDetection/mmyolo/configs/yolov6/yolov6_t_syncbn_fast_8xb32-400e_coco.py
deleted file mode 100644
index 75755555a58b45309df9213b6262cee030e41a9d..0000000000000000000000000000000000000000
--- a/spaces/ATang0729/Forecast4Muses/Model/Model6/Model6_0_ClothesDetection/mmyolo/configs/yolov6/yolov6_t_syncbn_fast_8xb32-400e_coco.py
+++ /dev/null
@@ -1,17 +0,0 @@
-_base_ = './yolov6_s_syncbn_fast_8xb32-400e_coco.py'
-
-# ======================= Possible modified parameters =======================
-# -----model related-----
-# The scaling factor that controls the depth of the network structure
-deepen_factor = 0.33
-# The scaling factor that controls the width of the network structure
-widen_factor = 0.375
-
-# ============================== Unmodified in most cases ===================
-model = dict(
- backbone=dict(deepen_factor=deepen_factor, widen_factor=widen_factor),
- neck=dict(deepen_factor=deepen_factor, widen_factor=widen_factor),
- bbox_head=dict(
- type='YOLOv6Head',
- head_module=dict(widen_factor=widen_factor),
- loss_bbox=dict(iou_mode='siou')))
diff --git a/spaces/AchyuthGamer/OpenGPT/g4f/Provider/Providers/deprecated/Wewordle.py b/spaces/AchyuthGamer/OpenGPT/g4f/Provider/Providers/deprecated/Wewordle.py
deleted file mode 100644
index c30887fb03b3ee53ed620d3e8259ae2a9245f934..0000000000000000000000000000000000000000
--- a/spaces/AchyuthGamer/OpenGPT/g4f/Provider/Providers/deprecated/Wewordle.py
+++ /dev/null
@@ -1,65 +0,0 @@
-from __future__ import annotations
-
-import random, string, time
-from aiohttp import ClientSession
-
-from ..base_provider import AsyncProvider
-
-
-class Wewordle(AsyncProvider):
- url = "https://wewordle.org"
- working = False
- supports_gpt_35_turbo = True
-
- @classmethod
- async def create_async(
- cls,
- model: str,
- messages: list[dict[str, str]],
- proxy: str = None,
- **kwargs
- ) -> str:
-
- headers = {
- "accept" : "*/*",
- "pragma" : "no-cache",
- "Content-Type" : "application/json",
- "Connection" : "keep-alive"
- }
-
- _user_id = "".join(random.choices(f"{string.ascii_lowercase}{string.digits}", k=16))
- _app_id = "".join(random.choices(f"{string.ascii_lowercase}{string.digits}", k=31))
- _request_date = time.strftime("%Y-%m-%dT%H:%M:%S.000Z", time.gmtime())
- data = {
- "user" : _user_id,
- "messages" : messages,
- "subscriber": {
- "originalPurchaseDate" : None,
- "originalApplicationVersion" : None,
- "allPurchaseDatesMillis" : {},
- "entitlements" : {"active": {}, "all": {}},
- "allPurchaseDates" : {},
- "allExpirationDatesMillis" : {},
- "allExpirationDates" : {},
- "originalAppUserId" : f"$RCAnonymousID:{_app_id}",
- "latestExpirationDate" : None,
- "requestDate" : _request_date,
- "latestExpirationDateMillis" : None,
- "nonSubscriptionTransactions" : [],
- "originalPurchaseDateMillis" : None,
- "managementURL" : None,
- "allPurchasedProductIdentifiers": [],
- "firstSeen" : _request_date,
- "activeSubscriptions" : [],
- }
- }
-
-
- async with ClientSession(
- headers=headers
- ) as session:
- async with session.post(f"{cls.url}/gptapi/v1/android/turbo", proxy=proxy, json=data) as response:
- response.raise_for_status()
- content = (await response.json())["message"]["content"]
- if content:
- return content
\ No newline at end of file
diff --git a/spaces/AgentVerse/agentVerse/ui/src/phaser3-rex-plugins/plugins/cursoratbound-plugin.js b/spaces/AgentVerse/agentVerse/ui/src/phaser3-rex-plugins/plugins/cursoratbound-plugin.js
deleted file mode 100644
index de774dc067abe4df57648c0796a6f8ec9d015ee4..0000000000000000000000000000000000000000
--- a/spaces/AgentVerse/agentVerse/ui/src/phaser3-rex-plugins/plugins/cursoratbound-plugin.js
+++ /dev/null
@@ -1,20 +0,0 @@
-import CursorAtBound from './cursoratbound.js';
-
-class CursorAtBoundPlugin extends Phaser.Plugins.BasePlugin {
-
- constructor(pluginManager) {
- super(pluginManager);
- }
-
- start() {
- var eventEmitter = this.game.events;
- eventEmitter.on('destroy', this.destroy, this);
- }
-
- add(scene, config) {
- return new CursorAtBound(scene, config);
- }
-
-}
-
-export default CursorAtBoundPlugin;
\ No newline at end of file
diff --git a/spaces/AgentVerse/agentVerse/ui/src/phaser3-rex-plugins/templates/ui/circularprogresscanvas/Factory.d.ts b/spaces/AgentVerse/agentVerse/ui/src/phaser3-rex-plugins/templates/ui/circularprogresscanvas/Factory.d.ts
deleted file mode 100644
index 2b95a752323b9ceb2669e63463490207a7f1a760..0000000000000000000000000000000000000000
--- a/spaces/AgentVerse/agentVerse/ui/src/phaser3-rex-plugins/templates/ui/circularprogresscanvas/Factory.d.ts
+++ /dev/null
@@ -1,13 +0,0 @@
-import CircularProgressCanvas from './CircularProgressCanvas';
-
-export default function (
- config?: CircularProgressCanvas.IConfig
-): CircularProgressCanvas;
-
-export default function (
- x?: number, y?: number,
- radius?: number,
- barColor?: string | number,
- value?: number,
- config?: CircularProgressCanvas.IConfig
-): CircularProgressCanvas;
\ No newline at end of file
diff --git a/spaces/AgentVerse/agentVerse/ui/src/phaser3-rex-plugins/templates/ui/gridsizer/RunWidthWrap.js b/spaces/AgentVerse/agentVerse/ui/src/phaser3-rex-plugins/templates/ui/gridsizer/RunWidthWrap.js
deleted file mode 100644
index da329aec4eea6024e9876552bacc124da4f2cca0..0000000000000000000000000000000000000000
--- a/spaces/AgentVerse/agentVerse/ui/src/phaser3-rex-plugins/templates/ui/gridsizer/RunWidthWrap.js
+++ /dev/null
@@ -1,25 +0,0 @@
-// Default method
-var RunWidthWrap = function (width) {
- var child, childWidth;
- var colWidth;
- for (var i in this.sizerChildren) {
- child = this.sizerChildren[i];
- if (
- (!child) ||
- (child.isRexSizer && child.ignoreLayout) ||
- (!child.runWidthWrap)
- ) {
- continue;
- }
-
- colWidth = this.getColumnWidth(parseInt(i) % this.columnCount);
- childWidth = this.getExpandedChildWidth(child, colWidth);
- if (child.isRexSizer) {
- childWidth = child.resolveWidth(childWidth);
- }
- child.runWidthWrap(childWidth);
- }
- return this;
-}
-
-export default RunWidthWrap;
\ No newline at end of file
diff --git a/spaces/Aki004/herta-so-vits/utils.py b/spaces/Aki004/herta-so-vits/utils.py
deleted file mode 100644
index 326a6ef8c231dc5fe6b90c3efc44c86247a5f2d1..0000000000000000000000000000000000000000
--- a/spaces/Aki004/herta-so-vits/utils.py
+++ /dev/null
@@ -1,543 +0,0 @@
-import os
-import glob
-import re
-import sys
-import argparse
-import logging
-import json
-import subprocess
-import warnings
-import random
-import functools
-
-import librosa
-import numpy as np
-from scipy.io.wavfile import read
-import torch
-from torch.nn import functional as F
-from modules.commons import sequence_mask
-from hubert import hubert_model
-
-MATPLOTLIB_FLAG = False
-
-logging.basicConfig(stream=sys.stdout, level=logging.DEBUG)
-logger = logging
-
-f0_bin = 256
-f0_max = 1100.0
-f0_min = 50.0
-f0_mel_min = 1127 * np.log(1 + f0_min / 700)
-f0_mel_max = 1127 * np.log(1 + f0_max / 700)
-
-
-# def normalize_f0(f0, random_scale=True):
-# f0_norm = f0.clone() # create a copy of the input Tensor
-# batch_size, _, frame_length = f0_norm.shape
-# for i in range(batch_size):
-# means = torch.mean(f0_norm[i, 0, :])
-# if random_scale:
-# factor = random.uniform(0.8, 1.2)
-# else:
-# factor = 1
-# f0_norm[i, 0, :] = (f0_norm[i, 0, :] - means) * factor
-# return f0_norm
-# def normalize_f0(f0, random_scale=True):
-# means = torch.mean(f0[:, 0, :], dim=1, keepdim=True)
-# if random_scale:
-# factor = torch.Tensor(f0.shape[0],1).uniform_(0.8, 1.2).to(f0.device)
-# else:
-# factor = torch.ones(f0.shape[0], 1, 1).to(f0.device)
-# f0_norm = (f0 - means.unsqueeze(-1)) * factor.unsqueeze(-1)
-# return f0_norm
-
-def deprecated(func):
- """This is a decorator which can be used to mark functions
- as deprecated. It will result in a warning being emitted
- when the function is used."""
- @functools.wraps(func)
- def new_func(*args, **kwargs):
- warnings.simplefilter('always', DeprecationWarning) # turn off filter
- warnings.warn("Call to deprecated function {}.".format(func.__name__),
- category=DeprecationWarning,
- stacklevel=2)
- warnings.simplefilter('default', DeprecationWarning) # reset filter
- return func(*args, **kwargs)
- return new_func
-
-def normalize_f0(f0, x_mask, uv, random_scale=True):
- # calculate means based on x_mask
- uv_sum = torch.sum(uv, dim=1, keepdim=True)
- uv_sum[uv_sum == 0] = 9999
- means = torch.sum(f0[:, 0, :] * uv, dim=1, keepdim=True) / uv_sum
-
- if random_scale:
- factor = torch.Tensor(f0.shape[0], 1).uniform_(0.8, 1.2).to(f0.device)
- else:
- factor = torch.ones(f0.shape[0], 1).to(f0.device)
- # normalize f0 based on means and factor
- f0_norm = (f0 - means.unsqueeze(-1)) * factor.unsqueeze(-1)
- if torch.isnan(f0_norm).any():
- exit(0)
- return f0_norm * x_mask
-
-def compute_f0_uv_torchcrepe(wav_numpy, p_len=None, sampling_rate=44100, hop_length=512,device=None,cr_threshold=0.05):
- from modules.crepe import CrepePitchExtractor
- x = wav_numpy
- if p_len is None:
- p_len = x.shape[0]//hop_length
- else:
- assert abs(p_len-x.shape[0]//hop_length) < 4, "pad length error"
-
- f0_min = 50
- f0_max = 1100
- F0Creper = CrepePitchExtractor(hop_length=hop_length,f0_min=f0_min,f0_max=f0_max,device=device,threshold=cr_threshold)
- f0,uv = F0Creper(x[None,:].float(),sampling_rate,pad_to=p_len)
- return f0,uv
-
-def plot_data_to_numpy(x, y):
- global MATPLOTLIB_FLAG
- if not MATPLOTLIB_FLAG:
- import matplotlib
- matplotlib.use("Agg")
- MATPLOTLIB_FLAG = True
- mpl_logger = logging.getLogger('matplotlib')
- mpl_logger.setLevel(logging.WARNING)
- import matplotlib.pylab as plt
- import numpy as np
-
- fig, ax = plt.subplots(figsize=(10, 2))
- plt.plot(x)
- plt.plot(y)
- plt.tight_layout()
-
- fig.canvas.draw()
- data = np.fromstring(fig.canvas.tostring_rgb(), dtype=np.uint8, sep='')
- data = data.reshape(fig.canvas.get_width_height()[::-1] + (3,))
- plt.close()
- return data
-
-
-
-def interpolate_f0(f0):
-
- data = np.reshape(f0, (f0.size, 1))
-
- vuv_vector = np.zeros((data.size, 1), dtype=np.float32)
- vuv_vector[data > 0.0] = 1.0
- vuv_vector[data <= 0.0] = 0.0
-
- ip_data = data
-
- frame_number = data.size
- last_value = 0.0
- for i in range(frame_number):
- if data[i] <= 0.0:
- j = i + 1
- for j in range(i + 1, frame_number):
- if data[j] > 0.0:
- break
- if j < frame_number - 1:
- if last_value > 0.0:
- step = (data[j] - data[i - 1]) / float(j - i)
- for k in range(i, j):
- ip_data[k] = data[i - 1] + step * (k - i + 1)
- else:
- for k in range(i, j):
- ip_data[k] = data[j]
- else:
- for k in range(i, frame_number):
- ip_data[k] = last_value
- else:
- ip_data[i] = data[i] # this may not be necessary
- last_value = data[i]
-
- return ip_data[:,0], vuv_vector[:,0]
-
-
-def compute_f0_parselmouth(wav_numpy, p_len=None, sampling_rate=44100, hop_length=512):
- import parselmouth
- x = wav_numpy
- if p_len is None:
- p_len = x.shape[0]//hop_length
- else:
- assert abs(p_len-x.shape[0]//hop_length) < 4, "pad length error"
- time_step = hop_length / sampling_rate * 1000
- f0_min = 50
- f0_max = 1100
- f0 = parselmouth.Sound(x, sampling_rate).to_pitch_ac(
- time_step=time_step / 1000, voicing_threshold=0.6,
- pitch_floor=f0_min, pitch_ceiling=f0_max).selected_array['frequency']
-
- pad_size=(p_len - len(f0) + 1) // 2
- if(pad_size>0 or p_len - len(f0) - pad_size>0):
- f0 = np.pad(f0,[[pad_size,p_len - len(f0) - pad_size]], mode='constant')
- return f0
-
-def resize_f0(x, target_len):
- source = np.array(x)
- source[source<0.001] = np.nan
- target = np.interp(np.arange(0, len(source)*target_len, len(source))/ target_len, np.arange(0, len(source)), source)
- res = np.nan_to_num(target)
- return res
-
-def compute_f0_dio(wav_numpy, p_len=None, sampling_rate=44100, hop_length=512):
- import pyworld
- if p_len is None:
- p_len = wav_numpy.shape[0]//hop_length
- f0, t = pyworld.dio(
- wav_numpy.astype(np.double),
- fs=sampling_rate,
- f0_ceil=800,
- frame_period=1000 * hop_length / sampling_rate,
- )
- f0 = pyworld.stonemask(wav_numpy.astype(np.double), f0, t, sampling_rate)
- for index, pitch in enumerate(f0):
- f0[index] = round(pitch, 1)
- return resize_f0(f0, p_len)
-
-def f0_to_coarse(f0):
- is_torch = isinstance(f0, torch.Tensor)
- f0_mel = 1127 * (1 + f0 / 700).log() if is_torch else 1127 * np.log(1 + f0 / 700)
- f0_mel[f0_mel > 0] = (f0_mel[f0_mel > 0] - f0_mel_min) * (f0_bin - 2) / (f0_mel_max - f0_mel_min) + 1
-
- f0_mel[f0_mel <= 1] = 1
- f0_mel[f0_mel > f0_bin - 1] = f0_bin - 1
- f0_coarse = (f0_mel + 0.5).int() if is_torch else np.rint(f0_mel).astype(np.int)
- assert f0_coarse.max() <= 255 and f0_coarse.min() >= 1, (f0_coarse.max(), f0_coarse.min())
- return f0_coarse
-
-
-def get_hubert_model():
- vec_path = "hubert/checkpoint_best_legacy_500.pt"
- print("load model(s) from {}".format(vec_path))
- from fairseq import checkpoint_utils
- models, saved_cfg, task = checkpoint_utils.load_model_ensemble_and_task(
- [vec_path],
- suffix="",
- )
- model = models[0]
- model.eval()
- return model
-
-def get_hubert_content(hmodel, wav_16k_tensor):
- feats = wav_16k_tensor
- if feats.dim() == 2: # double channels
- feats = feats.mean(-1)
- assert feats.dim() == 1, feats.dim()
- feats = feats.view(1, -1)
- padding_mask = torch.BoolTensor(feats.shape).fill_(False)
- inputs = {
- "source": feats.to(wav_16k_tensor.device),
- "padding_mask": padding_mask.to(wav_16k_tensor.device),
- "output_layer": 9, # layer 9
- }
- with torch.no_grad():
- logits = hmodel.extract_features(**inputs)
- feats = hmodel.final_proj(logits[0])
- return feats.transpose(1, 2)
-
-
-def get_content(cmodel, y):
- with torch.no_grad():
- c = cmodel.extract_features(y.squeeze(1))[0]
- c = c.transpose(1, 2)
- return c
-
-
-
-def load_checkpoint(checkpoint_path, model, optimizer=None, skip_optimizer=False):
- assert os.path.isfile(checkpoint_path)
- checkpoint_dict = torch.load(checkpoint_path, map_location='cpu')
- iteration = checkpoint_dict['iteration']
- learning_rate = checkpoint_dict['learning_rate']
- if optimizer is not None and not skip_optimizer and checkpoint_dict['optimizer'] is not None:
- optimizer.load_state_dict(checkpoint_dict['optimizer'])
- saved_state_dict = checkpoint_dict['model']
- if hasattr(model, 'module'):
- state_dict = model.module.state_dict()
- else:
- state_dict = model.state_dict()
- new_state_dict = {}
- for k, v in state_dict.items():
- try:
- # assert "dec" in k or "disc" in k
- # print("load", k)
- new_state_dict[k] = saved_state_dict[k]
- assert saved_state_dict[k].shape == v.shape, (saved_state_dict[k].shape, v.shape)
- except:
- print("error, %s is not in the checkpoint" % k)
- logger.info("%s is not in the checkpoint" % k)
- new_state_dict[k] = v
- if hasattr(model, 'module'):
- model.module.load_state_dict(new_state_dict)
- else:
- model.load_state_dict(new_state_dict)
- print("load ")
- logger.info("Loaded checkpoint '{}' (iteration {})".format(
- checkpoint_path, iteration))
- return model, optimizer, learning_rate, iteration
-
-
-def save_checkpoint(model, optimizer, learning_rate, iteration, checkpoint_path):
- logger.info("Saving model and optimizer state at iteration {} to {}".format(
- iteration, checkpoint_path))
- if hasattr(model, 'module'):
- state_dict = model.module.state_dict()
- else:
- state_dict = model.state_dict()
- torch.save({'model': state_dict,
- 'iteration': iteration,
- 'optimizer': optimizer.state_dict(),
- 'learning_rate': learning_rate}, checkpoint_path)
-
-def clean_checkpoints(path_to_models='logs/44k/', n_ckpts_to_keep=2, sort_by_time=True):
- """Freeing up space by deleting saved ckpts
-
- Arguments:
- path_to_models -- Path to the model directory
- n_ckpts_to_keep -- Number of ckpts to keep, excluding G_0.pth and D_0.pth
- sort_by_time -- True -> chronologically delete ckpts
- False -> lexicographically delete ckpts
- """
- ckpts_files = [f for f in os.listdir(path_to_models) if os.path.isfile(os.path.join(path_to_models, f))]
- name_key = (lambda _f: int(re.compile('._(\d+)\.pth').match(_f).group(1)))
- time_key = (lambda _f: os.path.getmtime(os.path.join(path_to_models, _f)))
- sort_key = time_key if sort_by_time else name_key
- x_sorted = lambda _x: sorted([f for f in ckpts_files if f.startswith(_x) and not f.endswith('_0.pth')], key=sort_key)
- to_del = [os.path.join(path_to_models, fn) for fn in
- (x_sorted('G')[:-n_ckpts_to_keep] + x_sorted('D')[:-n_ckpts_to_keep])]
- del_info = lambda fn: logger.info(f".. Free up space by deleting ckpt {fn}")
- del_routine = lambda x: [os.remove(x), del_info(x)]
- rs = [del_routine(fn) for fn in to_del]
-
-def summarize(writer, global_step, scalars={}, histograms={}, images={}, audios={}, audio_sampling_rate=22050):
- for k, v in scalars.items():
- writer.add_scalar(k, v, global_step)
- for k, v in histograms.items():
- writer.add_histogram(k, v, global_step)
- for k, v in images.items():
- writer.add_image(k, v, global_step, dataformats='HWC')
- for k, v in audios.items():
- writer.add_audio(k, v, global_step, audio_sampling_rate)
-
-
-def latest_checkpoint_path(dir_path, regex="G_*.pth"):
- f_list = glob.glob(os.path.join(dir_path, regex))
- f_list.sort(key=lambda f: int("".join(filter(str.isdigit, f))))
- x = f_list[-1]
- print(x)
- return x
-
-
-def plot_spectrogram_to_numpy(spectrogram):
- global MATPLOTLIB_FLAG
- if not MATPLOTLIB_FLAG:
- import matplotlib
- matplotlib.use("Agg")
- MATPLOTLIB_FLAG = True
- mpl_logger = logging.getLogger('matplotlib')
- mpl_logger.setLevel(logging.WARNING)
- import matplotlib.pylab as plt
- import numpy as np
-
- fig, ax = plt.subplots(figsize=(10,2))
- im = ax.imshow(spectrogram, aspect="auto", origin="lower",
- interpolation='none')
- plt.colorbar(im, ax=ax)
- plt.xlabel("Frames")
- plt.ylabel("Channels")
- plt.tight_layout()
-
- fig.canvas.draw()
- data = np.fromstring(fig.canvas.tostring_rgb(), dtype=np.uint8, sep='')
- data = data.reshape(fig.canvas.get_width_height()[::-1] + (3,))
- plt.close()
- return data
-
-
-def plot_alignment_to_numpy(alignment, info=None):
- global MATPLOTLIB_FLAG
- if not MATPLOTLIB_FLAG:
- import matplotlib
- matplotlib.use("Agg")
- MATPLOTLIB_FLAG = True
- mpl_logger = logging.getLogger('matplotlib')
- mpl_logger.setLevel(logging.WARNING)
- import matplotlib.pylab as plt
- import numpy as np
-
- fig, ax = plt.subplots(figsize=(6, 4))
- im = ax.imshow(alignment.transpose(), aspect='auto', origin='lower',
- interpolation='none')
- fig.colorbar(im, ax=ax)
- xlabel = 'Decoder timestep'
- if info is not None:
- xlabel += '\n\n' + info
- plt.xlabel(xlabel)
- plt.ylabel('Encoder timestep')
- plt.tight_layout()
-
- fig.canvas.draw()
- data = np.fromstring(fig.canvas.tostring_rgb(), dtype=np.uint8, sep='')
- data = data.reshape(fig.canvas.get_width_height()[::-1] + (3,))
- plt.close()
- return data
-
-
-def load_wav_to_torch(full_path):
- sampling_rate, data = read(full_path)
- return torch.FloatTensor(data.astype(np.float32)), sampling_rate
-
-
-def load_filepaths_and_text(filename, split="|"):
- with open(filename, encoding='utf-8') as f:
- filepaths_and_text = [line.strip().split(split) for line in f]
- return filepaths_and_text
-
-
-def get_hparams(init=True):
- parser = argparse.ArgumentParser()
- parser.add_argument('-c', '--config', type=str, default="./configs/base.json",
- help='JSON file for configuration')
- parser.add_argument('-m', '--model', type=str, required=True,
- help='Model name')
-
- args = parser.parse_args()
- model_dir = os.path.join("./logs", args.model)
-
- if not os.path.exists(model_dir):
- os.makedirs(model_dir)
-
- config_path = args.config
- config_save_path = os.path.join(model_dir, "config.json")
- if init:
- with open(config_path, "r") as f:
- data = f.read()
- with open(config_save_path, "w") as f:
- f.write(data)
- else:
- with open(config_save_path, "r") as f:
- data = f.read()
- config = json.loads(data)
-
- hparams = HParams(**config)
- hparams.model_dir = model_dir
- return hparams
-
-
-def get_hparams_from_dir(model_dir):
- config_save_path = os.path.join(model_dir, "config.json")
- with open(config_save_path, "r") as f:
- data = f.read()
- config = json.loads(data)
-
- hparams =HParams(**config)
- hparams.model_dir = model_dir
- return hparams
-
-
-def get_hparams_from_file(config_path):
- with open(config_path, "r") as f:
- data = f.read()
- config = json.loads(data)
-
- hparams =HParams(**config)
- return hparams
-
-
-def check_git_hash(model_dir):
- source_dir = os.path.dirname(os.path.realpath(__file__))
- if not os.path.exists(os.path.join(source_dir, ".git")):
- logger.warn("{} is not a git repository, therefore hash value comparison will be ignored.".format(
- source_dir
- ))
- return
-
- cur_hash = subprocess.getoutput("git rev-parse HEAD")
-
- path = os.path.join(model_dir, "githash")
- if os.path.exists(path):
- saved_hash = open(path).read()
- if saved_hash != cur_hash:
- logger.warn("git hash values are different. {}(saved) != {}(current)".format(
- saved_hash[:8], cur_hash[:8]))
- else:
- open(path, "w").write(cur_hash)
-
-
-def get_logger(model_dir, filename="train.log"):
- global logger
- logger = logging.getLogger(os.path.basename(model_dir))
- logger.setLevel(logging.DEBUG)
-
- formatter = logging.Formatter("%(asctime)s\t%(name)s\t%(levelname)s\t%(message)s")
- if not os.path.exists(model_dir):
- os.makedirs(model_dir)
- h = logging.FileHandler(os.path.join(model_dir, filename))
- h.setLevel(logging.DEBUG)
- h.setFormatter(formatter)
- logger.addHandler(h)
- return logger
-
-
-def repeat_expand_2d(content, target_len):
- # content : [h, t]
-
- src_len = content.shape[-1]
- target = torch.zeros([content.shape[0], target_len], dtype=torch.float).to(content.device)
- temp = torch.arange(src_len+1) * target_len / src_len
- current_pos = 0
- for i in range(target_len):
- if i < temp[current_pos+1]:
- target[:, i] = content[:, current_pos]
- else:
- current_pos += 1
- target[:, i] = content[:, current_pos]
-
- return target
-
-
-def mix_model(model_paths,mix_rate,mode):
- mix_rate = torch.FloatTensor(mix_rate)/100
- model_tem = torch.load(model_paths[0])
- models = [torch.load(path)["model"] for path in model_paths]
- if mode == 0:
- mix_rate = F.softmax(mix_rate,dim=0)
- for k in model_tem["model"].keys():
- model_tem["model"][k] = torch.zeros_like(model_tem["model"][k])
- for i,model in enumerate(models):
- model_tem["model"][k] += model[k]*mix_rate[i]
- torch.save(model_tem,os.path.join(os.path.curdir,"output.pth"))
- return os.path.join(os.path.curdir,"output.pth")
-
-class HParams():
- def __init__(self, **kwargs):
- for k, v in kwargs.items():
- if type(v) == dict:
- v = HParams(**v)
- self[k] = v
-
- def keys(self):
- return self.__dict__.keys()
-
- def items(self):
- return self.__dict__.items()
-
- def values(self):
- return self.__dict__.values()
-
- def __len__(self):
- return len(self.__dict__)
-
- def __getitem__(self, key):
- return getattr(self, key)
-
- def __setitem__(self, key, value):
- return setattr(self, key, value)
-
- def __contains__(self, key):
- return key in self.__dict__
-
- def __repr__(self):
- return self.__dict__.__repr__()
-
diff --git a/spaces/AlexWang/lama/saicinpainting/training/modules/multidilated_conv.py b/spaces/AlexWang/lama/saicinpainting/training/modules/multidilated_conv.py
deleted file mode 100644
index d267ee2aa5eb84b6a9291d0eaaff322c6c2802d0..0000000000000000000000000000000000000000
--- a/spaces/AlexWang/lama/saicinpainting/training/modules/multidilated_conv.py
+++ /dev/null
@@ -1,98 +0,0 @@
-import torch
-import torch.nn as nn
-import random
-from saicinpainting.training.modules.depthwise_sep_conv import DepthWiseSeperableConv
-
-class MultidilatedConv(nn.Module):
- def __init__(self, in_dim, out_dim, kernel_size, dilation_num=3, comb_mode='sum', equal_dim=True,
- shared_weights=False, padding=1, min_dilation=1, shuffle_in_channels=False, use_depthwise=False, **kwargs):
- super().__init__()
- convs = []
- self.equal_dim = equal_dim
- assert comb_mode in ('cat_out', 'sum', 'cat_in', 'cat_both'), comb_mode
- if comb_mode in ('cat_out', 'cat_both'):
- self.cat_out = True
- if equal_dim:
- assert out_dim % dilation_num == 0
- out_dims = [out_dim // dilation_num] * dilation_num
- self.index = sum([[i + j * (out_dims[0]) for j in range(dilation_num)] for i in range(out_dims[0])], [])
- else:
- out_dims = [out_dim // 2 ** (i + 1) for i in range(dilation_num - 1)]
- out_dims.append(out_dim - sum(out_dims))
- index = []
- starts = [0] + out_dims[:-1]
- lengths = [out_dims[i] // out_dims[-1] for i in range(dilation_num)]
- for i in range(out_dims[-1]):
- for j in range(dilation_num):
- index += list(range(starts[j], starts[j] + lengths[j]))
- starts[j] += lengths[j]
- self.index = index
- assert(len(index) == out_dim)
- self.out_dims = out_dims
- else:
- self.cat_out = False
- self.out_dims = [out_dim] * dilation_num
-
- if comb_mode in ('cat_in', 'cat_both'):
- if equal_dim:
- assert in_dim % dilation_num == 0
- in_dims = [in_dim // dilation_num] * dilation_num
- else:
- in_dims = [in_dim // 2 ** (i + 1) for i in range(dilation_num - 1)]
- in_dims.append(in_dim - sum(in_dims))
- self.in_dims = in_dims
- self.cat_in = True
- else:
- self.cat_in = False
- self.in_dims = [in_dim] * dilation_num
-
- conv_type = DepthWiseSeperableConv if use_depthwise else nn.Conv2d
- dilation = min_dilation
- for i in range(dilation_num):
- if isinstance(padding, int):
- cur_padding = padding * dilation
- else:
- cur_padding = padding[i]
- convs.append(conv_type(
- self.in_dims[i], self.out_dims[i], kernel_size, padding=cur_padding, dilation=dilation, **kwargs
- ))
- if i > 0 and shared_weights:
- convs[-1].weight = convs[0].weight
- convs[-1].bias = convs[0].bias
- dilation *= 2
- self.convs = nn.ModuleList(convs)
-
- self.shuffle_in_channels = shuffle_in_channels
- if self.shuffle_in_channels:
- # shuffle list as shuffling of tensors is nondeterministic
- in_channels_permute = list(range(in_dim))
- random.shuffle(in_channels_permute)
- # save as buffer so it is saved and loaded with checkpoint
- self.register_buffer('in_channels_permute', torch.tensor(in_channels_permute))
-
- def forward(self, x):
- if self.shuffle_in_channels:
- x = x[:, self.in_channels_permute]
-
- outs = []
- if self.cat_in:
- if self.equal_dim:
- x = x.chunk(len(self.convs), dim=1)
- else:
- new_x = []
- start = 0
- for dim in self.in_dims:
- new_x.append(x[:, start:start+dim])
- start += dim
- x = new_x
- for i, conv in enumerate(self.convs):
- if self.cat_in:
- input = x[i]
- else:
- input = x
- outs.append(conv(input))
- if self.cat_out:
- out = torch.cat(outs, dim=1)[:, self.index]
- else:
- out = sum(outs)
- return out
diff --git a/spaces/Alican/pixera/data/base_dataset.py b/spaces/Alican/pixera/data/base_dataset.py
deleted file mode 100644
index b8eb78ed51ab1435fd3a52e635a58399f03a7caa..0000000000000000000000000000000000000000
--- a/spaces/Alican/pixera/data/base_dataset.py
+++ /dev/null
@@ -1,167 +0,0 @@
-"""This module implements an abstract base class (ABC) 'BaseDataset' for datasets.
-
-It also includes common transformation functions (e.g., get_transform, __scale_width), which can be later used in subclasses.
-"""
-import random
-import numpy as np
-import torch.utils.data as data
-from PIL import Image
-import torchvision.transforms as transforms
-from abc import ABC, abstractmethod
-
-
-class BaseDataset(data.Dataset, ABC):
- """This class is an abstract base class (ABC) for datasets.
-
- To create a subclass, you need to implement the following four functions:
- -- <__init__>: initialize the class, first call BaseDataset.__init__(self, opt).
- -- <__len__>: return the size of dataset.
- -- <__getitem__>: get a data point.
- -- : (optionally) add dataset-specific options and set default options.
- """
-
- def __init__(self, opt):
- """Initialize the class; save the options in the class
-
- Parameters:
- opt (Option class)-- stores all the experiment flags; needs to be a subclass of BaseOptions
- """
- self.opt = opt
- self.root = opt.dataroot
-
- @staticmethod
- def modify_commandline_options(parser, is_train):
- """Add new dataset-specific options, and rewrite default values for existing options.
-
- Parameters:
- parser -- original option parser
- is_train (bool) -- whether training phase or test phase. You can use this flag to add training-specific or test-specific options.
-
- Returns:
- the modified parser.
- """
- return parser
-
- @abstractmethod
- def __len__(self):
- """Return the total number of images in the dataset."""
- return 0
-
- @abstractmethod
- def __getitem__(self, index):
- """Return a data point and its metadata information.
-
- Parameters:
- index - - a random integer for data indexing
-
- Returns:
- a dictionary of data with their names. It ususally contains the data itself and its metadata information.
- """
- pass
-
-
-def get_params(opt, size):
- w, h = size
- new_h = h
- new_w = w
- if opt.preprocess == 'resize_and_crop':
- new_h = new_w = opt.load_size
- elif opt.preprocess == 'scale_width_and_crop':
- new_w = opt.load_size
- new_h = opt.load_size * h // w
-
- x = random.randint(0, np.maximum(0, new_w - opt.crop_size))
- y = random.randint(0, np.maximum(0, new_h - opt.crop_size))
-
- flip = random.random() > 0.5
-
- return {'crop_pos': (x, y), 'flip': flip}
-
-
-def get_transform(opt, params=None, grayscale=False, method=transforms.InterpolationMode.BICUBIC, convert=True):
- transform_list = []
- if grayscale:
- transform_list.append(transforms.Grayscale(1))
- if 'resize' in opt.preprocess:
- osize = [opt.load_size, opt.load_size]
- transform_list.append(transforms.Resize(osize, method))
- elif 'scale_width' in opt.preprocess:
- transform_list.append(transforms.Lambda(lambda img: __scale_width(img, opt.load_size, opt.crop_size, method)))
-
- if 'crop' in opt.preprocess:
- if params is None:
- transform_list.append(transforms.RandomCrop(opt.crop_size))
- else:
- transform_list.append(transforms.Lambda(lambda img: __crop(img, params['crop_pos'], opt.crop_size)))
-
- if opt.preprocess == 'none':
- transform_list.append(transforms.Lambda(lambda img: __make_power_2(img, base=4, method=method)))
-
- if not opt.no_flip:
- if params is None:
- transform_list.append(transforms.RandomHorizontalFlip())
- elif params['flip']:
- transform_list.append(transforms.Lambda(lambda img: __flip(img, params['flip'])))
-
- if convert:
- transform_list += [transforms.ToTensor()]
- if grayscale:
- transform_list += [transforms.Normalize((0.5,), (0.5,))]
- else:
- transform_list += [transforms.Normalize((0.5, 0.5, 0.5), (0.5, 0.5, 0.5))]
- return transforms.Compose(transform_list)
-
-
-def __transforms2pil_resize(method):
- mapper = {transforms.InterpolationMode.BILINEAR: Image.BILINEAR,
- transforms.InterpolationMode.BICUBIC: Image.BICUBIC,
- transforms.InterpolationMode.NEAREST: Image.NEAREST,
- transforms.InterpolationMode.LANCZOS: Image.LANCZOS,}
- return mapper[method]
-
-
-def __make_power_2(img, base, method=transforms.InterpolationMode.BICUBIC):
- method = __transforms2pil_resize(method)
- ow, oh = img.size
- h = int(round(oh / base) * base)
- w = int(round(ow / base) * base)
- if h == oh and w == ow:
- return img
-
- __print_size_warning(ow, oh, w, h)
- return img.resize((w, h), method)
-
-
-def __scale_width(img, target_size, crop_size, method=transforms.InterpolationMode.BICUBIC):
- method = __transforms2pil_resize(method)
- ow, oh = img.size
- if ow == target_size and oh >= crop_size:
- return img
- w = target_size
- h = int(max(target_size * oh / ow, crop_size))
- return img.resize((w, h), method)
-
-
-def __crop(img, pos, size):
- ow, oh = img.size
- x1, y1 = pos
- tw = th = size
- if (ow > tw or oh > th):
- return img.crop((x1, y1, x1 + tw, y1 + th))
- return img
-
-
-def __flip(img, flip):
- if flip:
- return img.transpose(Image.FLIP_LEFT_RIGHT)
- return img
-
-
-def __print_size_warning(ow, oh, w, h):
- """Print warning information about image size(only print once)"""
- if not hasattr(__print_size_warning, 'has_printed'):
- print("The image size needs to be a multiple of 4. "
- "The loaded image size was (%d, %d), so it was adjusted to "
- "(%d, %d). This adjustment will be done to all images "
- "whose sizes are not multiples of 4" % (ow, oh, w, h))
- __print_size_warning.has_printed = True
diff --git a/spaces/Alpaca233/SadTalker/src/audio2pose_models/cvae.py b/spaces/Alpaca233/SadTalker/src/audio2pose_models/cvae.py
deleted file mode 100644
index d017ce865a03bae40dfe066dbcd82e29839d89dc..0000000000000000000000000000000000000000
--- a/spaces/Alpaca233/SadTalker/src/audio2pose_models/cvae.py
+++ /dev/null
@@ -1,149 +0,0 @@
-import torch
-import torch.nn.functional as F
-from torch import nn
-from src.audio2pose_models.res_unet import ResUnet
-
-def class2onehot(idx, class_num):
-
- assert torch.max(idx).item() < class_num
- onehot = torch.zeros(idx.size(0), class_num).to(idx.device)
- onehot.scatter_(1, idx, 1)
- return onehot
-
-class CVAE(nn.Module):
- def __init__(self, cfg):
- super().__init__()
- encoder_layer_sizes = cfg.MODEL.CVAE.ENCODER_LAYER_SIZES
- decoder_layer_sizes = cfg.MODEL.CVAE.DECODER_LAYER_SIZES
- latent_size = cfg.MODEL.CVAE.LATENT_SIZE
- num_classes = cfg.DATASET.NUM_CLASSES
- audio_emb_in_size = cfg.MODEL.CVAE.AUDIO_EMB_IN_SIZE
- audio_emb_out_size = cfg.MODEL.CVAE.AUDIO_EMB_OUT_SIZE
- seq_len = cfg.MODEL.CVAE.SEQ_LEN
-
- self.latent_size = latent_size
-
- self.encoder = ENCODER(encoder_layer_sizes, latent_size, num_classes,
- audio_emb_in_size, audio_emb_out_size, seq_len)
- self.decoder = DECODER(decoder_layer_sizes, latent_size, num_classes,
- audio_emb_in_size, audio_emb_out_size, seq_len)
- def reparameterize(self, mu, logvar):
- std = torch.exp(0.5 * logvar)
- eps = torch.randn_like(std)
- return mu + eps * std
-
- def forward(self, batch):
- batch = self.encoder(batch)
- mu = batch['mu']
- logvar = batch['logvar']
- z = self.reparameterize(mu, logvar)
- batch['z'] = z
- return self.decoder(batch)
-
- def test(self, batch):
- '''
- class_id = batch['class']
- z = torch.randn([class_id.size(0), self.latent_size]).to(class_id.device)
- batch['z'] = z
- '''
- return self.decoder(batch)
-
-class ENCODER(nn.Module):
- def __init__(self, layer_sizes, latent_size, num_classes,
- audio_emb_in_size, audio_emb_out_size, seq_len):
- super().__init__()
-
- self.resunet = ResUnet()
- self.num_classes = num_classes
- self.seq_len = seq_len
-
- self.MLP = nn.Sequential()
- layer_sizes[0] += latent_size + seq_len*audio_emb_out_size + 6
- for i, (in_size, out_size) in enumerate(zip(layer_sizes[:-1], layer_sizes[1:])):
- self.MLP.add_module(
- name="L{:d}".format(i), module=nn.Linear(in_size, out_size))
- self.MLP.add_module(name="A{:d}".format(i), module=nn.ReLU())
-
- self.linear_means = nn.Linear(layer_sizes[-1], latent_size)
- self.linear_logvar = nn.Linear(layer_sizes[-1], latent_size)
- self.linear_audio = nn.Linear(audio_emb_in_size, audio_emb_out_size)
-
- self.classbias = nn.Parameter(torch.randn(self.num_classes, latent_size))
-
- def forward(self, batch):
- class_id = batch['class']
- pose_motion_gt = batch['pose_motion_gt'] #bs seq_len 6
- ref = batch['ref'] #bs 6
- bs = pose_motion_gt.shape[0]
- audio_in = batch['audio_emb'] # bs seq_len audio_emb_in_size
-
- #pose encode
- pose_emb = self.resunet(pose_motion_gt.unsqueeze(1)) #bs 1 seq_len 6
- pose_emb = pose_emb.reshape(bs, -1) #bs seq_len*6
-
- #audio mapping
- print(audio_in.shape)
- audio_out = self.linear_audio(audio_in) # bs seq_len audio_emb_out_size
- audio_out = audio_out.reshape(bs, -1)
-
- class_bias = self.classbias[class_id] #bs latent_size
- x_in = torch.cat([ref, pose_emb, audio_out, class_bias], dim=-1) #bs seq_len*(audio_emb_out_size+6)+latent_size
- x_out = self.MLP(x_in)
-
- mu = self.linear_means(x_out)
- logvar = self.linear_means(x_out) #bs latent_size
-
- batch.update({'mu':mu, 'logvar':logvar})
- return batch
-
-class DECODER(nn.Module):
- def __init__(self, layer_sizes, latent_size, num_classes,
- audio_emb_in_size, audio_emb_out_size, seq_len):
- super().__init__()
-
- self.resunet = ResUnet()
- self.num_classes = num_classes
- self.seq_len = seq_len
-
- self.MLP = nn.Sequential()
- input_size = latent_size + seq_len*audio_emb_out_size + 6
- for i, (in_size, out_size) in enumerate(zip([input_size]+layer_sizes[:-1], layer_sizes)):
- self.MLP.add_module(
- name="L{:d}".format(i), module=nn.Linear(in_size, out_size))
- if i+1 < len(layer_sizes):
- self.MLP.add_module(name="A{:d}".format(i), module=nn.ReLU())
- else:
- self.MLP.add_module(name="sigmoid", module=nn.Sigmoid())
-
- self.pose_linear = nn.Linear(6, 6)
- self.linear_audio = nn.Linear(audio_emb_in_size, audio_emb_out_size)
-
- self.classbias = nn.Parameter(torch.randn(self.num_classes, latent_size))
-
- def forward(self, batch):
-
- z = batch['z'] #bs latent_size
- bs = z.shape[0]
- class_id = batch['class']
- ref = batch['ref'] #bs 6
- audio_in = batch['audio_emb'] # bs seq_len audio_emb_in_size
- #print('audio_in: ', audio_in[:, :, :10])
-
- audio_out = self.linear_audio(audio_in) # bs seq_len audio_emb_out_size
- #print('audio_out: ', audio_out[:, :, :10])
- audio_out = audio_out.reshape([bs, -1]) # bs seq_len*audio_emb_out_size
- class_bias = self.classbias[class_id] #bs latent_size
-
- z = z + class_bias
- x_in = torch.cat([ref, z, audio_out], dim=-1)
- x_out = self.MLP(x_in) # bs layer_sizes[-1]
- x_out = x_out.reshape((bs, self.seq_len, -1))
-
- #print('x_out: ', x_out)
-
- pose_emb = self.resunet(x_out.unsqueeze(1)) #bs 1 seq_len 6
-
- pose_motion_pred = self.pose_linear(pose_emb.squeeze(1)) #bs seq_len 6
-
- batch.update({'pose_motion_pred':pose_motion_pred})
- return batch
diff --git a/spaces/Amrrs/DragGan-Inversion/stylegan_human/training/loss.py b/spaces/Amrrs/DragGan-Inversion/stylegan_human/training/loss.py
deleted file mode 100644
index 3b6d0833ca639bb3b08f216419dfa25f1e657da2..0000000000000000000000000000000000000000
--- a/spaces/Amrrs/DragGan-Inversion/stylegan_human/training/loss.py
+++ /dev/null
@@ -1,159 +0,0 @@
-# Copyright (c) 2021, NVIDIA CORPORATION & AFFILIATES. All rights reserved.
-#
-# NVIDIA CORPORATION and its licensors retain all intellectual property
-# and proprietary rights in and to this software, related documentation
-# and any modifications thereto. Any use, reproduction, disclosure or
-# distribution of this software and related documentation without an express
-# license agreement from NVIDIA CORPORATION is strictly prohibited.
-
-"""Loss functions."""
-
-import numpy as np
-import torch
-from torch_utils import training_stats
-from torch_utils.ops import conv2d_gradfix
-from torch_utils.ops import upfirdn2d
-
-# ----------------------------------------------------------------------------
-
-
-class Loss:
- # to be overridden by subclass
- def accumulate_gradients(self, phase, real_img, real_c, gen_z, gen_c, gain, cur_nimg):
- raise NotImplementedError()
-
-# ----------------------------------------------------------------------------
-
-
-class StyleGAN2Loss(Loss):
- def __init__(self, device, G, D, augment_pipe=None, r1_gamma=10, style_mixing_prob=0, pl_weight=0, pl_batch_shrink=2, pl_decay=0.01, pl_no_weight_grad=False, blur_init_sigma=0, blur_fade_kimg=0):
- super().__init__()
- self.device = device
- self.G = G
- self.D = D
- self.augment_pipe = augment_pipe
- self.r1_gamma = r1_gamma
- self.style_mixing_prob = style_mixing_prob
- self.pl_weight = pl_weight
- self.pl_batch_shrink = pl_batch_shrink
- self.pl_decay = pl_decay
- self.pl_no_weight_grad = pl_no_weight_grad
- self.pl_mean = torch.zeros([], device=device)
- self.blur_init_sigma = blur_init_sigma
- self.blur_fade_kimg = blur_fade_kimg
-
- def run_G(self, z, c, update_emas=False):
- ws = self.G.mapping(z, c, update_emas=update_emas)
- if self.style_mixing_prob > 0:
- with torch.autograd.profiler.record_function('style_mixing'):
- cutoff = torch.empty([], dtype=torch.int64,
- device=ws.device).random_(1, ws.shape[1])
- cutoff = torch.where(torch.rand(
- [], device=ws.device) < self.style_mixing_prob, cutoff, torch.full_like(cutoff, ws.shape[1]))
- ws[:, cutoff:] = self.G.mapping(
- torch.randn_like(z), c, update_emas=False)[:, cutoff:]
- img = self.G.synthesis(ws, update_emas=update_emas)
- return img, ws
-
- def run_D(self, img, c, blur_sigma=0, update_emas=False):
- blur_size = np.floor(blur_sigma * 3)
- if blur_size > 0:
- with torch.autograd.profiler.record_function('blur'):
- f = torch.arange(-blur_size, blur_size + 1,
- device=img.device).div(blur_sigma).square().neg().exp2()
- img = upfirdn2d.filter2d(img, f / f.sum())
- if self.augment_pipe is not None:
- img = self.augment_pipe(img)
- logits = self.D(img, c, update_emas=update_emas)
- return logits
-
- def accumulate_gradients(self, phase, real_img, real_c, gen_z, gen_c, gain, cur_nimg):
- assert phase in ['Gmain', 'Greg', 'Gboth', 'Dmain', 'Dreg', 'Dboth']
- if self.pl_weight == 0:
- phase = {'Greg': 'none', 'Gboth': 'Gmain'}.get(phase, phase)
- if self.r1_gamma == 0:
- phase = {'Dreg': 'none', 'Dboth': 'Dmain'}.get(phase, phase)
- blur_sigma = max(1 - cur_nimg / (self.blur_fade_kimg * 1e3), 0) * \
- self.blur_init_sigma if self.blur_fade_kimg > 0 else 0
-
- # Gmain: Maximize logits for generated images.
- if phase in ['Gmain', 'Gboth']:
- with torch.autograd.profiler.record_function('Gmain_forward'):
- gen_img, _gen_ws = self.run_G(gen_z, gen_c)
- gen_logits = self.run_D(gen_img, gen_c, blur_sigma=blur_sigma)
- training_stats.report('Loss/scores/fake', gen_logits)
- training_stats.report('Loss/signs/fake', gen_logits.sign())
- # -log(sigmoid(gen_logits))
- loss_Gmain = torch.nn.functional.softplus(-gen_logits)
- training_stats.report('Loss/G/loss', loss_Gmain)
- with torch.autograd.profiler.record_function('Gmain_backward'):
- loss_Gmain.mean().mul(gain).backward()
-
- # Gpl: Apply path length regularization.
- if phase in ['Greg', 'Gboth']:
- with torch.autograd.profiler.record_function('Gpl_forward'):
- batch_size = gen_z.shape[0] // self.pl_batch_shrink
- gen_img, gen_ws = self.run_G(
- gen_z[:batch_size], gen_c[:batch_size])
- pl_noise = torch.randn_like(
- gen_img) / np.sqrt(gen_img.shape[2] * gen_img.shape[3])
- with torch.autograd.profiler.record_function('pl_grads'), conv2d_gradfix.no_weight_gradients(self.pl_no_weight_grad):
- pl_grads = torch.autograd.grad(outputs=[(
- gen_img * pl_noise).sum()], inputs=[gen_ws], create_graph=True, only_inputs=True)[0]
- pl_lengths = pl_grads.square().sum(2).mean(1).sqrt()
- pl_mean = self.pl_mean.lerp(pl_lengths.mean(), self.pl_decay)
- self.pl_mean.copy_(pl_mean.detach())
- pl_penalty = (pl_lengths - pl_mean).square()
- training_stats.report('Loss/pl_penalty', pl_penalty)
- loss_Gpl = pl_penalty * self.pl_weight
- training_stats.report('Loss/G/reg', loss_Gpl)
- with torch.autograd.profiler.record_function('Gpl_backward'):
- loss_Gpl.mean().mul(gain).backward()
-
- # Dmain: Minimize logits for generated images.
- loss_Dgen = 0
- if phase in ['Dmain', 'Dboth']:
- with torch.autograd.profiler.record_function('Dgen_forward'):
- gen_img, _gen_ws = self.run_G(gen_z, gen_c, update_emas=True)
- gen_logits = self.run_D(
- gen_img, gen_c, blur_sigma=blur_sigma, update_emas=True)
- training_stats.report('Loss/scores/fake', gen_logits)
- training_stats.report('Loss/signs/fake', gen_logits.sign())
- loss_Dgen = torch.nn.functional.softplus(
- gen_logits) # -log(1 - sigmoid(gen_logits))
- with torch.autograd.profiler.record_function('Dgen_backward'):
- loss_Dgen.mean().mul(gain).backward()
-
- # Dmain: Maximize logits for real images.
- # Dr1: Apply R1 regularization.
- if phase in ['Dmain', 'Dreg', 'Dboth']:
- name = 'Dreal' if phase == 'Dmain' else 'Dr1' if phase == 'Dreg' else 'Dreal_Dr1'
- with torch.autograd.profiler.record_function(name + '_forward'):
- real_img_tmp = real_img.detach().requires_grad_(
- phase in ['Dreg', 'Dboth'])
- real_logits = self.run_D(
- real_img_tmp, real_c, blur_sigma=blur_sigma)
- training_stats.report('Loss/scores/real', real_logits)
- training_stats.report('Loss/signs/real', real_logits.sign())
-
- loss_Dreal = 0
- if phase in ['Dmain', 'Dboth']:
- # -log(sigmoid(real_logits))
- loss_Dreal = torch.nn.functional.softplus(-real_logits)
- training_stats.report(
- 'Loss/D/loss', loss_Dgen + loss_Dreal)
-
- loss_Dr1 = 0
- if phase in ['Dreg', 'Dboth']:
- with torch.autograd.profiler.record_function('r1_grads'), conv2d_gradfix.no_weight_gradients():
- r1_grads = torch.autograd.grad(outputs=[real_logits.sum()], inputs=[
- real_img_tmp], create_graph=True, only_inputs=True)[0]
- r1_penalty = r1_grads.square().sum([1, 2, 3])
- loss_Dr1 = r1_penalty * (self.r1_gamma / 2)
- training_stats.report('Loss/r1_penalty', r1_penalty)
- training_stats.report('Loss/D/reg', loss_Dr1)
-
- with torch.autograd.profiler.record_function(name + '_backward'):
- (loss_Dreal + loss_Dr1).mean().mul(gain).backward()
-
-# ----------------------------------------------------------------------------
diff --git a/spaces/Androidonnxfork/CivitAi-to-Diffusers/diffusers/examples/community/README.md b/spaces/Androidonnxfork/CivitAi-to-Diffusers/diffusers/examples/community/README.md
deleted file mode 100644
index 6967d273e4491211618c57415e66eb0888143ac9..0000000000000000000000000000000000000000
--- a/spaces/Androidonnxfork/CivitAi-to-Diffusers/diffusers/examples/community/README.md
+++ /dev/null
@@ -1,1769 +0,0 @@
-# Community Examples
-
-> **For more information about community pipelines, please have a look at [this issue](https://github.com/huggingface/diffusers/issues/841).**
-
-**Community** examples consist of both inference and training examples that have been added by the community.
-Please have a look at the following table to get an overview of all community examples. Click on the **Code Example** to get a copy-and-paste ready code example that you can try out.
-If a community doesn't work as expected, please open an issue and ping the author on it.
-
-| Example | Description | Code Example | Colab | Author |
-|:--------------------------------------------------------------------------------------------------------------------------------------|:---------------------------------------------------------------------------------------------------------------------------------------------------------------------------------------------------------------------------------------------------------------------------------------------------------------------------------------------------------------------------------------------------------------------------------------------------------------------------------------------------------|:------------------------------------------------------------------------------------------|:-------------------------------------------------------------------------------------------------------------------------------------------------------------------------------------------------------------------|--------------------------------------------------------------:|
-| CLIP Guided Stable Diffusion | Doing CLIP guidance for text to image generation with Stable Diffusion | [CLIP Guided Stable Diffusion](#clip-guided-stable-diffusion) | [](https://colab.research.google.com/github/huggingface/notebooks/blob/main/diffusers/CLIP_Guided_Stable_diffusion_with_diffusers.ipynb) | [Suraj Patil](https://github.com/patil-suraj/) |
-| One Step U-Net (Dummy) | Example showcasing of how to use Community Pipelines (see https://github.com/huggingface/diffusers/issues/841) | [One Step U-Net](#one-step-unet) | - | [Patrick von Platen](https://github.com/patrickvonplaten/) |
-| Stable Diffusion Interpolation | Interpolate the latent space of Stable Diffusion between different prompts/seeds | [Stable Diffusion Interpolation](#stable-diffusion-interpolation) | - | [Nate Raw](https://github.com/nateraw/) |
-| Stable Diffusion Mega | **One** Stable Diffusion Pipeline with all functionalities of [Text2Image](https://github.com/huggingface/diffusers/blob/main/src/diffusers/pipelines/stable_diffusion/pipeline_stable_diffusion.py), [Image2Image](https://github.com/huggingface/diffusers/blob/main/src/diffusers/pipelines/stable_diffusion/pipeline_stable_diffusion_img2img.py) and [Inpainting](https://github.com/huggingface/diffusers/blob/main/src/diffusers/pipelines/stable_diffusion/pipeline_stable_diffusion_inpaint.py) | [Stable Diffusion Mega](#stable-diffusion-mega) | - | [Patrick von Platen](https://github.com/patrickvonplaten/) |
-| Long Prompt Weighting Stable Diffusion | **One** Stable Diffusion Pipeline without tokens length limit, and support parsing weighting in prompt. | [Long Prompt Weighting Stable Diffusion](#long-prompt-weighting-stable-diffusion) | - | [SkyTNT](https://github.com/SkyTNT) |
-| Speech to Image | Using automatic-speech-recognition to transcribe text and Stable Diffusion to generate images | [Speech to Image](#speech-to-image) | - | [Mikail Duzenli](https://github.com/MikailINTech)
-| Wild Card Stable Diffusion | Stable Diffusion Pipeline that supports prompts that contain wildcard terms (indicated by surrounding double underscores), with values instantiated randomly from a corresponding txt file or a dictionary of possible values | [Wildcard Stable Diffusion](#wildcard-stable-diffusion) | - | [Shyam Sudhakaran](https://github.com/shyamsn97) |
-| [Composable Stable Diffusion](https://energy-based-model.github.io/Compositional-Visual-Generation-with-Composable-Diffusion-Models/) | Stable Diffusion Pipeline that supports prompts that contain "|" in prompts (as an AND condition) and weights (separated by "|" as well) to positively / negatively weight prompts. | [Composable Stable Diffusion](#composable-stable-diffusion) | - | [Mark Rich](https://github.com/MarkRich) |
-| Seed Resizing Stable Diffusion | Stable Diffusion Pipeline that supports resizing an image and retaining the concepts of the 512 by 512 generation. | [Seed Resizing](#seed-resizing) | - | [Mark Rich](https://github.com/MarkRich) |
-| Imagic Stable Diffusion | Stable Diffusion Pipeline that enables writing a text prompt to edit an existing image | [Imagic Stable Diffusion](#imagic-stable-diffusion) | - | [Mark Rich](https://github.com/MarkRich) |
-| Multilingual Stable Diffusion | Stable Diffusion Pipeline that supports prompts in 50 different languages. | [Multilingual Stable Diffusion](#multilingual-stable-diffusion-pipeline) | - | [Juan Carlos Piñeros](https://github.com/juancopi81) |
-| Image to Image Inpainting Stable Diffusion | Stable Diffusion Pipeline that enables the overlaying of two images and subsequent inpainting | [Image to Image Inpainting Stable Diffusion](#image-to-image-inpainting-stable-diffusion) | - | [Alex McKinney](https://github.com/vvvm23) |
-| Text Based Inpainting Stable Diffusion | Stable Diffusion Inpainting Pipeline that enables passing a text prompt to generate the mask for inpainting | [Text Based Inpainting Stable Diffusion](#image-to-image-inpainting-stable-diffusion) | - | [Dhruv Karan](https://github.com/unography) |
-| Bit Diffusion | Diffusion on discrete data | [Bit Diffusion](#bit-diffusion) | - | [Stuti R.](https://github.com/kingstut) |
-| K-Diffusion Stable Diffusion | Run Stable Diffusion with any of [K-Diffusion's samplers](https://github.com/crowsonkb/k-diffusion/blob/master/k_diffusion/sampling.py) | [Stable Diffusion with K Diffusion](#stable-diffusion-with-k-diffusion) | - | [Patrick von Platen](https://github.com/patrickvonplaten/) |
-| Checkpoint Merger Pipeline | Diffusion Pipeline that enables merging of saved model checkpoints | [Checkpoint Merger Pipeline](#checkpoint-merger-pipeline) | - | [Naga Sai Abhinay Devarinti](https://github.com/Abhinay1997/) |
- Stable Diffusion v1.1-1.4 Comparison | Run all 4 model checkpoints for Stable Diffusion and compare their results together | [Stable Diffusion Comparison](#stable-diffusion-comparisons) | - | [Suvaditya Mukherjee](https://github.com/suvadityamuk) |
- MagicMix | Diffusion Pipeline for semantic mixing of an image and a text prompt | [MagicMix](#magic-mix) | - | [Partho Das](https://github.com/daspartho) |
-| Stable UnCLIP | Diffusion Pipeline for combining prior model (generate clip image embedding from text, UnCLIPPipeline `"kakaobrain/karlo-v1-alpha"`) and decoder pipeline (decode clip image embedding to image, StableDiffusionImageVariationPipeline `"lambdalabs/sd-image-variations-diffusers"` ). | [Stable UnCLIP](#stable-unclip) | - | [Ray Wang](https://wrong.wang) |
-| UnCLIP Text Interpolation Pipeline | Diffusion Pipeline that allows passing two prompts and produces images while interpolating between the text-embeddings of the two prompts | [UnCLIP Text Interpolation Pipeline](#unclip-text-interpolation-pipeline) | - | [Naga Sai Abhinay Devarinti](https://github.com/Abhinay1997/) |
-| UnCLIP Image Interpolation Pipeline | Diffusion Pipeline that allows passing two images/image_embeddings and produces images while interpolating between their image-embeddings | [UnCLIP Image Interpolation Pipeline](#unclip-image-interpolation-pipeline) | - | [Naga Sai Abhinay Devarinti](https://github.com/Abhinay1997/) |
-| DDIM Noise Comparative Analysis Pipeline | Investigating how the diffusion models learn visual concepts from each noise level (which is a contribution of [P2 weighting (CVPR 2022)](https://arxiv.org/abs/2204.00227)) | [DDIM Noise Comparative Analysis Pipeline](#ddim-noise-comparative-analysis-pipeline) | - | [Aengus (Duc-Anh)](https://github.com/aengusng8) |
-| CLIP Guided Img2Img Stable Diffusion Pipeline | Doing CLIP guidance for image to image generation with Stable Diffusion | [CLIP Guided Img2Img Stable Diffusion](#clip-guided-img2img-stable-diffusion) | - | [Nipun Jindal](https://github.com/nipunjindal/) |
-| TensorRT Stable Diffusion Text to Image Pipeline | Accelerates the Stable Diffusion Text2Image Pipeline using TensorRT | [TensorRT Stable Diffusion Text to Image Pipeline](#tensorrt-text2image-stable-diffusion-pipeline) | - | [Asfiya Baig](https://github.com/asfiyab-nvidia) |
-| EDICT Image Editing Pipeline | Diffusion pipeline for text-guided image editing | [EDICT Image Editing Pipeline](#edict-image-editing-pipeline) | - | [Joqsan Azocar](https://github.com/Joqsan) |
-| Stable Diffusion RePaint | Stable Diffusion pipeline using [RePaint](https://arxiv.org/abs/2201.0986) for inpainting. | [Stable Diffusion RePaint](#stable-diffusion-repaint ) | - | [Markus Pobitzer](https://github.com/Markus-Pobitzer) |
-| TensorRT Stable Diffusion Image to Image Pipeline | Accelerates the Stable Diffusion Image2Image Pipeline using TensorRT | [TensorRT Stable Diffusion Image to Image Pipeline](#tensorrt-image2image-stable-diffusion-pipeline) | - | [Asfiya Baig](https://github.com/asfiyab-nvidia) |
-| Stable Diffusion IPEX Pipeline | Accelerate Stable Diffusion inference pipeline with BF16/FP32 precision on Intel Xeon CPUs with [IPEX](https://github.com/intel/intel-extension-for-pytorch) | [Stable Diffusion on IPEX](#stable-diffusion-on-ipex) | - | [Yingjie Han](https://github.com/yingjie-han/) |
-| CLIP Guided Images Mixing Stable Diffusion Pipeline | Сombine images using usual diffusion models. | [CLIP Guided Images Mixing Using Stable Diffusion](#clip-guided-images-mixing-with-stable-diffusion) | - | [Karachev Denis](https://github.com/TheDenk) |
-| TensorRT Stable Diffusion Inpainting Pipeline | Accelerates the Stable Diffusion Inpainting Pipeline using TensorRT | [TensorRT Stable Diffusion Inpainting Pipeline](#tensorrt-inpainting-stable-diffusion-pipeline) | - | [Asfiya Baig](https://github.com/asfiyab-nvidia) |
-| IADB Pipeline | Implementation of [Iterative α-(de)Blending: a Minimalist Deterministic Diffusion Model](https://arxiv.org/abs/2305.03486) | [IADB Pipeline](#iadb-pipeline) | - | [Thomas Chambon](https://github.com/tchambon)
-
-To load a custom pipeline you just need to pass the `custom_pipeline` argument to `DiffusionPipeline`, as one of the files in `diffusers/examples/community`. Feel free to send a PR with your own pipelines, we will merge them quickly.
-```py
-pipe = DiffusionPipeline.from_pretrained("runwayml/stable-diffusion-v1-5", custom_pipeline="filename_in_the_community_folder")
-```
-
-## Example usages
-
-### CLIP Guided Stable Diffusion
-
-CLIP guided stable diffusion can help to generate more realistic images
-by guiding stable diffusion at every denoising step with an additional CLIP model.
-
-The following code requires roughly 12GB of GPU RAM.
-
-```python
-from diffusers import DiffusionPipeline
-from transformers import CLIPImageProcessor, CLIPModel
-import torch
-
-
-feature_extractor = CLIPImageProcessor.from_pretrained("laion/CLIP-ViT-B-32-laion2B-s34B-b79K")
-clip_model = CLIPModel.from_pretrained("laion/CLIP-ViT-B-32-laion2B-s34B-b79K", torch_dtype=torch.float16)
-
-
-guided_pipeline = DiffusionPipeline.from_pretrained(
- "runwayml/stable-diffusion-v1-5",
- custom_pipeline="clip_guided_stable_diffusion",
- clip_model=clip_model,
- feature_extractor=feature_extractor,
-
- torch_dtype=torch.float16,
-)
-guided_pipeline.enable_attention_slicing()
-guided_pipeline = guided_pipeline.to("cuda")
-
-prompt = "fantasy book cover, full moon, fantasy forest landscape, golden vector elements, fantasy magic, dark light night, intricate, elegant, sharp focus, illustration, highly detailed, digital painting, concept art, matte, art by WLOP and Artgerm and Albert Bierstadt, masterpiece"
-
-generator = torch.Generator(device="cuda").manual_seed(0)
-images = []
-for i in range(4):
- image = guided_pipeline(
- prompt,
- num_inference_steps=50,
- guidance_scale=7.5,
- clip_guidance_scale=100,
- num_cutouts=4,
- use_cutouts=False,
- generator=generator,
- ).images[0]
- images.append(image)
-
-# save images locally
-for i, img in enumerate(images):
- img.save(f"./clip_guided_sd/image_{i}.png")
-```
-
-The `images` list contains a list of PIL images that can be saved locally or displayed directly in a google colab.
-Generated images tend to be of higher qualtiy than natively using stable diffusion. E.g. the above script generates the following images:
-
-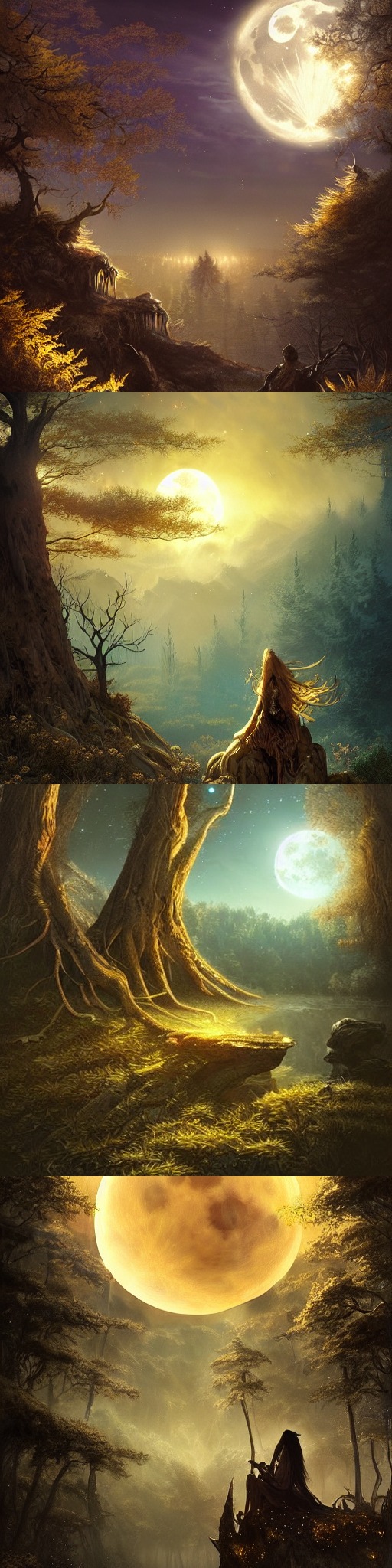.
-
-### One Step Unet
-
-The dummy "one-step-unet" can be run as follows:
-
-```python
-from diffusers import DiffusionPipeline
-
-pipe = DiffusionPipeline.from_pretrained("google/ddpm-cifar10-32", custom_pipeline="one_step_unet")
-pipe()
-```
-
-**Note**: This community pipeline is not useful as a feature, but rather just serves as an example of how community pipelines can be added (see https://github.com/huggingface/diffusers/issues/841).
-
-### Stable Diffusion Interpolation
-
-The following code can be run on a GPU of at least 8GB VRAM and should take approximately 5 minutes.
-
-```python
-from diffusers import DiffusionPipeline
-import torch
-
-pipe = DiffusionPipeline.from_pretrained(
- "CompVis/stable-diffusion-v1-4",
- revision='fp16',
- torch_dtype=torch.float16,
- safety_checker=None, # Very important for videos...lots of false positives while interpolating
- custom_pipeline="interpolate_stable_diffusion",
-).to('cuda')
-pipe.enable_attention_slicing()
-
-frame_filepaths = pipe.walk(
- prompts=['a dog', 'a cat', 'a horse'],
- seeds=[42, 1337, 1234],
- num_interpolation_steps=16,
- output_dir='./dreams',
- batch_size=4,
- height=512,
- width=512,
- guidance_scale=8.5,
- num_inference_steps=50,
-)
-```
-
-The output of the `walk(...)` function returns a list of images saved under the folder as defined in `output_dir`. You can use these images to create videos of stable diffusion.
-
-> **Please have a look at https://github.com/nateraw/stable-diffusion-videos for more in-detail information on how to create videos using stable diffusion as well as more feature-complete functionality.**
-
-### Stable Diffusion Mega
-
-The Stable Diffusion Mega Pipeline lets you use the main use cases of the stable diffusion pipeline in a single class.
-
-```python
-#!/usr/bin/env python3
-from diffusers import DiffusionPipeline
-import PIL
-import requests
-from io import BytesIO
-import torch
-
-
-def download_image(url):
- response = requests.get(url)
- return PIL.Image.open(BytesIO(response.content)).convert("RGB")
-
-pipe = DiffusionPipeline.from_pretrained("runwayml/stable-diffusion-v1-5", custom_pipeline="stable_diffusion_mega", torch_dtype=torch.float16, revision="fp16")
-pipe.to("cuda")
-pipe.enable_attention_slicing()
-
-
-### Text-to-Image
-
-images = pipe.text2img("An astronaut riding a horse").images
-
-### Image-to-Image
-
-init_image = download_image("https://raw.githubusercontent.com/CompVis/stable-diffusion/main/assets/stable-samples/img2img/sketch-mountains-input.jpg")
-
-prompt = "A fantasy landscape, trending on artstation"
-
-images = pipe.img2img(prompt=prompt, image=init_image, strength=0.75, guidance_scale=7.5).images
-
-### Inpainting
-
-img_url = "https://raw.githubusercontent.com/CompVis/latent-diffusion/main/data/inpainting_examples/overture-creations-5sI6fQgYIuo.png"
-mask_url = "https://raw.githubusercontent.com/CompVis/latent-diffusion/main/data/inpainting_examples/overture-creations-5sI6fQgYIuo_mask.png"
-init_image = download_image(img_url).resize((512, 512))
-mask_image = download_image(mask_url).resize((512, 512))
-
-prompt = "a cat sitting on a bench"
-images = pipe.inpaint(prompt=prompt, image=init_image, mask_image=mask_image, strength=0.75).images
-```
-
-As shown above this one pipeline can run all both "text-to-image", "image-to-image", and "inpainting" in one pipeline.
-
-### Long Prompt Weighting Stable Diffusion
-Features of this custom pipeline:
-- Input a prompt without the 77 token length limit.
-- Includes tx2img, img2img. and inpainting pipelines.
-- Emphasize/weigh part of your prompt with parentheses as so: `a baby deer with (big eyes)`
-- De-emphasize part of your prompt as so: `a [baby] deer with big eyes`
-- Precisely weigh part of your prompt as so: `a baby deer with (big eyes:1.3)`
-
-Prompt weighting equivalents:
-- `a baby deer with` == `(a baby deer with:1.0)`
-- `(big eyes)` == `(big eyes:1.1)`
-- `((big eyes))` == `(big eyes:1.21)`
-- `[big eyes]` == `(big eyes:0.91)`
-
-You can run this custom pipeline as so:
-
-#### pytorch
-
-```python
-from diffusers import DiffusionPipeline
-import torch
-
-pipe = DiffusionPipeline.from_pretrained(
- 'hakurei/waifu-diffusion',
- custom_pipeline="lpw_stable_diffusion",
-
- torch_dtype=torch.float16
-)
-pipe=pipe.to("cuda")
-
-prompt = "best_quality (1girl:1.3) bow bride brown_hair closed_mouth frilled_bow frilled_hair_tubes frills (full_body:1.3) fox_ear hair_bow hair_tubes happy hood japanese_clothes kimono long_sleeves red_bow smile solo tabi uchikake white_kimono wide_sleeves cherry_blossoms"
-neg_prompt = "lowres, bad_anatomy, error_body, error_hair, error_arm, error_hands, bad_hands, error_fingers, bad_fingers, missing_fingers, error_legs, bad_legs, multiple_legs, missing_legs, error_lighting, error_shadow, error_reflection, text, error, extra_digit, fewer_digits, cropped, worst_quality, low_quality, normal_quality, jpeg_artifacts, signature, watermark, username, blurry"
-
-pipe.text2img(prompt, negative_prompt=neg_prompt, width=512,height=512,max_embeddings_multiples=3).images[0]
-
-```
-
-#### onnxruntime
-
-```python
-from diffusers import DiffusionPipeline
-import torch
-
-pipe = DiffusionPipeline.from_pretrained(
- 'CompVis/stable-diffusion-v1-4',
- custom_pipeline="lpw_stable_diffusion_onnx",
- revision="onnx",
- provider="CUDAExecutionProvider"
-)
-
-prompt = "a photo of an astronaut riding a horse on mars, best quality"
-neg_prompt = "lowres, bad anatomy, error body, error hair, error arm, error hands, bad hands, error fingers, bad fingers, missing fingers, error legs, bad legs, multiple legs, missing legs, error lighting, error shadow, error reflection, text, error, extra digit, fewer digits, cropped, worst quality, low quality, normal quality, jpeg artifacts, signature, watermark, username, blurry"
-
-pipe.text2img(prompt,negative_prompt=neg_prompt, width=512, height=512, max_embeddings_multiples=3).images[0]
-
-```
-
-if you see `Token indices sequence length is longer than the specified maximum sequence length for this model ( *** > 77 ) . Running this sequence through the model will result in indexing errors`. Do not worry, it is normal.
-
-### Speech to Image
-
-The following code can generate an image from an audio sample using pre-trained OpenAI whisper-small and Stable Diffusion.
-
-```Python
-import torch
-
-import matplotlib.pyplot as plt
-from datasets import load_dataset
-from diffusers import DiffusionPipeline
-from transformers import (
- WhisperForConditionalGeneration,
- WhisperProcessor,
-)
-
-
-device = "cuda" if torch.cuda.is_available() else "cpu"
-
-ds = load_dataset("hf-internal-testing/librispeech_asr_dummy", "clean", split="validation")
-
-audio_sample = ds[3]
-
-text = audio_sample["text"].lower()
-speech_data = audio_sample["audio"]["array"]
-
-model = WhisperForConditionalGeneration.from_pretrained("openai/whisper-small").to(device)
-processor = WhisperProcessor.from_pretrained("openai/whisper-small")
-
-diffuser_pipeline = DiffusionPipeline.from_pretrained(
- "CompVis/stable-diffusion-v1-4",
- custom_pipeline="speech_to_image_diffusion",
- speech_model=model,
- speech_processor=processor,
-
- torch_dtype=torch.float16,
-)
-
-diffuser_pipeline.enable_attention_slicing()
-diffuser_pipeline = diffuser_pipeline.to(device)
-
-output = diffuser_pipeline(speech_data)
-plt.imshow(output.images[0])
-```
-This example produces the following image:
-
-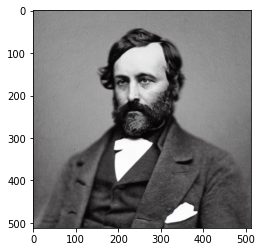
-
-### Wildcard Stable Diffusion
-Following the great examples from https://github.com/jtkelm2/stable-diffusion-webui-1/blob/master/scripts/wildcards.py and https://github.com/AUTOMATIC1111/stable-diffusion-webui/wiki/Custom-Scripts#wildcards, here's a minimal implementation that allows for users to add "wildcards", denoted by `__wildcard__` to prompts that are used as placeholders for randomly sampled values given by either a dictionary or a `.txt` file. For example:
-
-Say we have a prompt:
-
-```
-prompt = "__animal__ sitting on a __object__ wearing a __clothing__"
-```
-
-We can then define possible values to be sampled for `animal`, `object`, and `clothing`. These can either be from a `.txt` with the same name as the category.
-
-The possible values can also be defined / combined by using a dictionary like: `{"animal":["dog", "cat", mouse"]}`.
-
-The actual pipeline works just like `StableDiffusionPipeline`, except the `__call__` method takes in:
-
-`wildcard_files`: list of file paths for wild card replacement
-`wildcard_option_dict`: dict with key as `wildcard` and values as a list of possible replacements
-`num_prompt_samples`: number of prompts to sample, uniformly sampling wildcards
-
-A full example:
-
-create `animal.txt`, with contents like:
-
-```
-dog
-cat
-mouse
-```
-
-create `object.txt`, with contents like:
-
-```
-chair
-sofa
-bench
-```
-
-```python
-from diffusers import DiffusionPipeline
-import torch
-
-pipe = DiffusionPipeline.from_pretrained(
- "CompVis/stable-diffusion-v1-4",
- custom_pipeline="wildcard_stable_diffusion",
-
- torch_dtype=torch.float16,
-)
-prompt = "__animal__ sitting on a __object__ wearing a __clothing__"
-out = pipe(
- prompt,
- wildcard_option_dict={
- "clothing":["hat", "shirt", "scarf", "beret"]
- },
- wildcard_files=["object.txt", "animal.txt"],
- num_prompt_samples=1
-)
-```
-
-### Composable Stable diffusion
-
-[Composable Stable Diffusion](https://energy-based-model.github.io/Compositional-Visual-Generation-with-Composable-Diffusion-Models/) proposes conjunction and negation (negative prompts) operators for compositional generation with conditional diffusion models.
-
-```python
-import torch as th
-import numpy as np
-import torchvision.utils as tvu
-
-from diffusers import DiffusionPipeline
-
-import argparse
-
-parser = argparse.ArgumentParser()
-parser.add_argument("--prompt", type=str, default="mystical trees | A magical pond | dark",
- help="use '|' as the delimiter to compose separate sentences.")
-parser.add_argument("--steps", type=int, default=50)
-parser.add_argument("--scale", type=float, default=7.5)
-parser.add_argument("--weights", type=str, default="7.5 | 7.5 | -7.5")
-parser.add_argument("--seed", type=int, default=2)
-parser.add_argument("--model_path", type=str, default="CompVis/stable-diffusion-v1-4")
-parser.add_argument("--num_images", type=int, default=1)
-args = parser.parse_args()
-
-has_cuda = th.cuda.is_available()
-device = th.device('cpu' if not has_cuda else 'cuda')
-
-prompt = args.prompt
-scale = args.scale
-steps = args.steps
-
-pipe = DiffusionPipeline.from_pretrained(
- args.model_path,
- custom_pipeline="composable_stable_diffusion",
-).to(device)
-
-pipe.safety_checker = None
-
-images = []
-generator = th.Generator("cuda").manual_seed(args.seed)
-for i in range(args.num_images):
- image = pipe(prompt, guidance_scale=scale, num_inference_steps=steps,
- weights=args.weights, generator=generator).images[0]
- images.append(th.from_numpy(np.array(image)).permute(2, 0, 1) / 255.)
-grid = tvu.make_grid(th.stack(images, dim=0), nrow=4, padding=0)
-tvu.save_image(grid, f'{prompt}_{args.weights}' + '.png')
-
-```
-
-### Imagic Stable Diffusion
-Allows you to edit an image using stable diffusion.
-
-```python
-import requests
-from PIL import Image
-from io import BytesIO
-import torch
-import os
-from diffusers import DiffusionPipeline, DDIMScheduler
-has_cuda = torch.cuda.is_available()
-device = torch.device('cpu' if not has_cuda else 'cuda')
-pipe = DiffusionPipeline.from_pretrained(
- "CompVis/stable-diffusion-v1-4",
- safety_checker=None,
- use_auth_token=True,
- custom_pipeline="imagic_stable_diffusion",
- scheduler = DDIMScheduler(beta_start=0.00085, beta_end=0.012, beta_schedule="scaled_linear", clip_sample=False, set_alpha_to_one=False)
-).to(device)
-generator = torch.Generator("cuda").manual_seed(0)
-seed = 0
-prompt = "A photo of Barack Obama smiling with a big grin"
-url = 'https://www.dropbox.com/s/6tlwzr73jd1r9yk/obama.png?dl=1'
-response = requests.get(url)
-init_image = Image.open(BytesIO(response.content)).convert("RGB")
-init_image = init_image.resize((512, 512))
-res = pipe.train(
- prompt,
- image=init_image,
- generator=generator)
-res = pipe(alpha=1, guidance_scale=7.5, num_inference_steps=50)
-os.makedirs("imagic", exist_ok=True)
-image = res.images[0]
-image.save('./imagic/imagic_image_alpha_1.png')
-res = pipe(alpha=1.5, guidance_scale=7.5, num_inference_steps=50)
-image = res.images[0]
-image.save('./imagic/imagic_image_alpha_1_5.png')
-res = pipe(alpha=2, guidance_scale=7.5, num_inference_steps=50)
-image = res.images[0]
-image.save('./imagic/imagic_image_alpha_2.png')
-```
-
-### Seed Resizing
-Test seed resizing. Originally generate an image in 512 by 512, then generate image with same seed at 512 by 592 using seed resizing. Finally, generate 512 by 592 using original stable diffusion pipeline.
-
-```python
-import torch as th
-import numpy as np
-from diffusers import DiffusionPipeline
-
-has_cuda = th.cuda.is_available()
-device = th.device('cpu' if not has_cuda else 'cuda')
-
-pipe = DiffusionPipeline.from_pretrained(
- "CompVis/stable-diffusion-v1-4",
- use_auth_token=True,
- custom_pipeline="seed_resize_stable_diffusion"
-).to(device)
-
-def dummy(images, **kwargs):
- return images, False
-
-pipe.safety_checker = dummy
-
-
-images = []
-th.manual_seed(0)
-generator = th.Generator("cuda").manual_seed(0)
-
-seed = 0
-prompt = "A painting of a futuristic cop"
-
-width = 512
-height = 512
-
-res = pipe(
- prompt,
- guidance_scale=7.5,
- num_inference_steps=50,
- height=height,
- width=width,
- generator=generator)
-image = res.images[0]
-image.save('./seed_resize/seed_resize_{w}_{h}_image.png'.format(w=width, h=height))
-
-
-th.manual_seed(0)
-generator = th.Generator("cuda").manual_seed(0)
-
-pipe = DiffusionPipeline.from_pretrained(
- "CompVis/stable-diffusion-v1-4",
- use_auth_token=True,
- custom_pipeline="/home/mark/open_source/diffusers/examples/community/"
-).to(device)
-
-width = 512
-height = 592
-
-res = pipe(
- prompt,
- guidance_scale=7.5,
- num_inference_steps=50,
- height=height,
- width=width,
- generator=generator)
-image = res.images[0]
-image.save('./seed_resize/seed_resize_{w}_{h}_image.png'.format(w=width, h=height))
-
-pipe_compare = DiffusionPipeline.from_pretrained(
- "CompVis/stable-diffusion-v1-4",
- use_auth_token=True,
- custom_pipeline="/home/mark/open_source/diffusers/examples/community/"
-).to(device)
-
-res = pipe_compare(
- prompt,
- guidance_scale=7.5,
- num_inference_steps=50,
- height=height,
- width=width,
- generator=generator
-)
-
-image = res.images[0]
-image.save('./seed_resize/seed_resize_{w}_{h}_image_compare.png'.format(w=width, h=height))
-```
-
-### Multilingual Stable Diffusion Pipeline
-
-The following code can generate an images from texts in different languages using the pre-trained [mBART-50 many-to-one multilingual machine translation model](https://huggingface.co/facebook/mbart-large-50-many-to-one-mmt) and Stable Diffusion.
-
-```python
-from PIL import Image
-
-import torch
-
-from diffusers import DiffusionPipeline
-from transformers import (
- pipeline,
- MBart50TokenizerFast,
- MBartForConditionalGeneration,
-)
-device = "cuda" if torch.cuda.is_available() else "cpu"
-device_dict = {"cuda": 0, "cpu": -1}
-
-# helper function taken from: https://huggingface.co/blog/stable_diffusion
-def image_grid(imgs, rows, cols):
- assert len(imgs) == rows*cols
-
- w, h = imgs[0].size
- grid = Image.new('RGB', size=(cols*w, rows*h))
- grid_w, grid_h = grid.size
-
- for i, img in enumerate(imgs):
- grid.paste(img, box=(i%cols*w, i//cols*h))
- return grid
-
-# Add language detection pipeline
-language_detection_model_ckpt = "papluca/xlm-roberta-base-language-detection"
-language_detection_pipeline = pipeline("text-classification",
- model=language_detection_model_ckpt,
- device=device_dict[device])
-
-# Add model for language translation
-trans_tokenizer = MBart50TokenizerFast.from_pretrained("facebook/mbart-large-50-many-to-one-mmt")
-trans_model = MBartForConditionalGeneration.from_pretrained("facebook/mbart-large-50-many-to-one-mmt").to(device)
-
-diffuser_pipeline = DiffusionPipeline.from_pretrained(
- "CompVis/stable-diffusion-v1-4",
- custom_pipeline="multilingual_stable_diffusion",
- detection_pipeline=language_detection_pipeline,
- translation_model=trans_model,
- translation_tokenizer=trans_tokenizer,
-
- torch_dtype=torch.float16,
-)
-
-diffuser_pipeline.enable_attention_slicing()
-diffuser_pipeline = diffuser_pipeline.to(device)
-
-prompt = ["a photograph of an astronaut riding a horse",
- "Una casa en la playa",
- "Ein Hund, der Orange isst",
- "Un restaurant parisien"]
-
-output = diffuser_pipeline(prompt)
-
-images = output.images
-
-grid = image_grid(images, rows=2, cols=2)
-```
-
-This example produces the following images:
-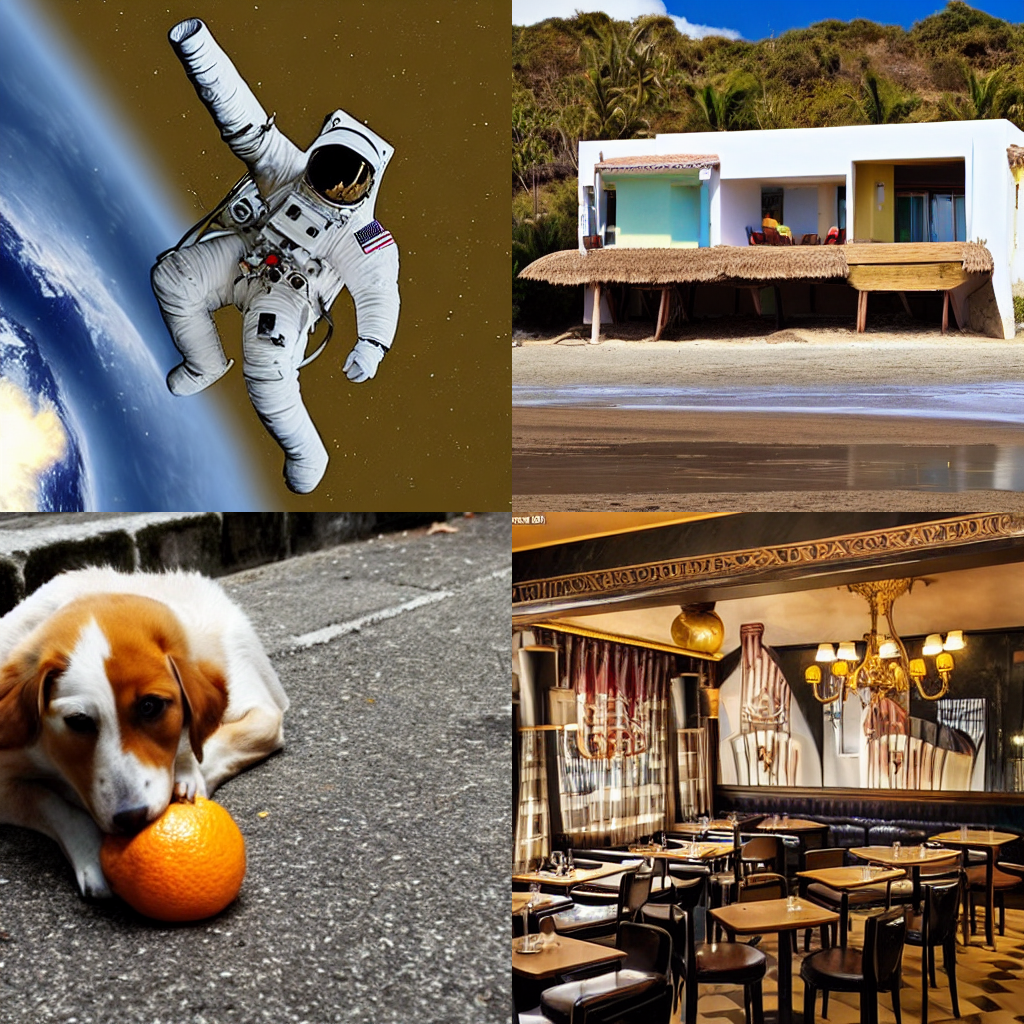
-
-### Image to Image Inpainting Stable Diffusion
-
-Similar to the standard stable diffusion inpainting example, except with the addition of an `inner_image` argument.
-
-`image`, `inner_image`, and `mask` should have the same dimensions. `inner_image` should have an alpha (transparency) channel.
-
-The aim is to overlay two images, then mask out the boundary between `image` and `inner_image` to allow stable diffusion to make the connection more seamless.
-For example, this could be used to place a logo on a shirt and make it blend seamlessly.
-
-```python
-import PIL
-import torch
-
-from diffusers import DiffusionPipeline
-
-image_path = "./path-to-image.png"
-inner_image_path = "./path-to-inner-image.png"
-mask_path = "./path-to-mask.png"
-
-init_image = PIL.Image.open(image_path).convert("RGB").resize((512, 512))
-inner_image = PIL.Image.open(inner_image_path).convert("RGBA").resize((512, 512))
-mask_image = PIL.Image.open(mask_path).convert("RGB").resize((512, 512))
-
-pipe = DiffusionPipeline.from_pretrained(
- "runwayml/stable-diffusion-inpainting",
- custom_pipeline="img2img_inpainting",
-
- torch_dtype=torch.float16
-)
-pipe = pipe.to("cuda")
-
-prompt = "Your prompt here!"
-image = pipe(prompt=prompt, image=init_image, inner_image=inner_image, mask_image=mask_image).images[0]
-```
-
-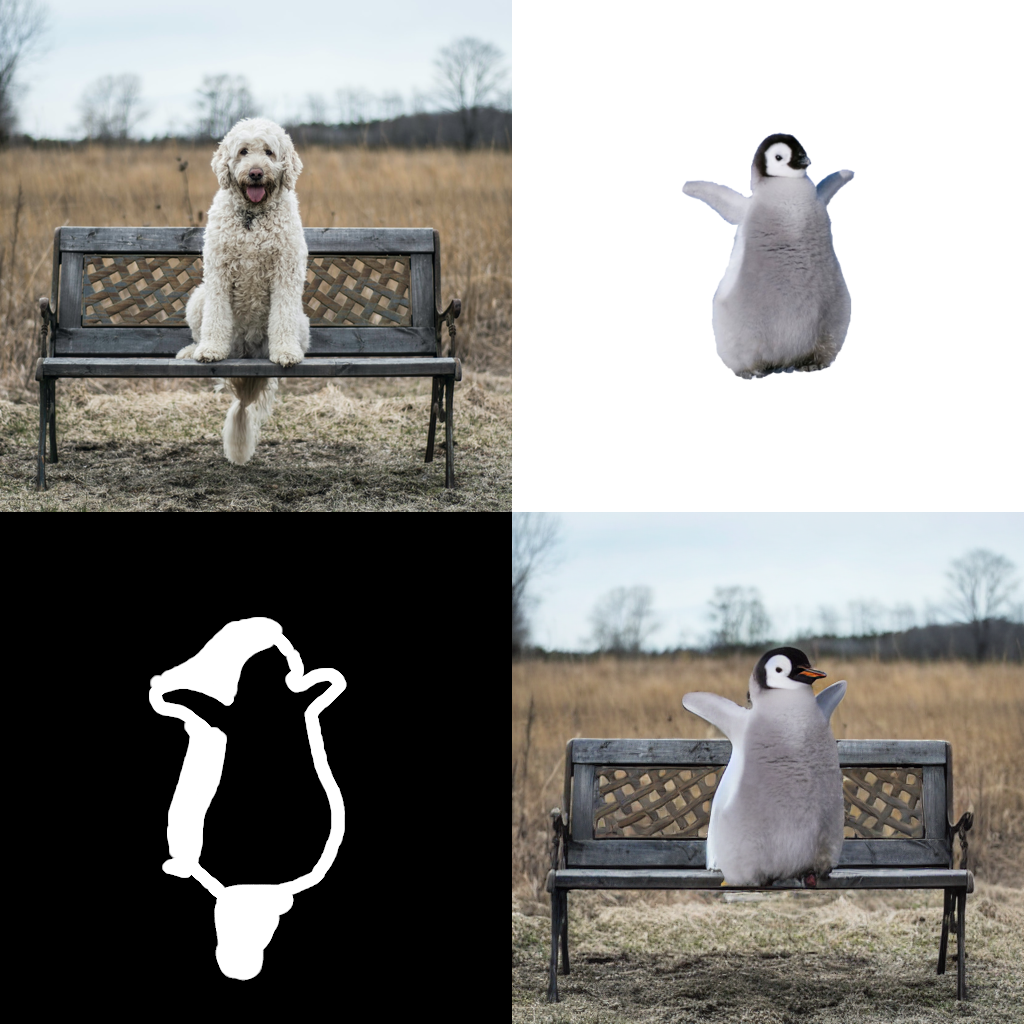
-
-### Text Based Inpainting Stable Diffusion
-
-Use a text prompt to generate the mask for the area to be inpainted.
-Currently uses the CLIPSeg model for mask generation, then calls the standard Stable Diffusion Inpainting pipeline to perform the inpainting.
-
-```python
-from transformers import CLIPSegProcessor, CLIPSegForImageSegmentation
-from diffusers import DiffusionPipeline
-
-from PIL import Image
-import requests
-
-processor = CLIPSegProcessor.from_pretrained("CIDAS/clipseg-rd64-refined")
-model = CLIPSegForImageSegmentation.from_pretrained("CIDAS/clipseg-rd64-refined")
-
-pipe = DiffusionPipeline.from_pretrained(
- "runwayml/stable-diffusion-inpainting",
- custom_pipeline="text_inpainting",
- segmentation_model=model,
- segmentation_processor=processor
-)
-pipe = pipe.to("cuda")
-
-
-url = "https://github.com/timojl/clipseg/blob/master/example_image.jpg?raw=true"
-image = Image.open(requests.get(url, stream=True).raw).resize((512, 512))
-text = "a glass" # will mask out this text
-prompt = "a cup" # the masked out region will be replaced with this
-
-image = pipe(image=image, text=text, prompt=prompt).images[0]
-```
-
-### Bit Diffusion
-Based https://arxiv.org/abs/2208.04202, this is used for diffusion on discrete data - eg, discreate image data, DNA sequence data. An unconditional discreate image can be generated like this:
-
-```python
-from diffusers import DiffusionPipeline
-pipe = DiffusionPipeline.from_pretrained("google/ddpm-cifar10-32", custom_pipeline="bit_diffusion")
-image = pipe().images[0]
-
-```
-
-### Stable Diffusion with K Diffusion
-
-Make sure you have @crowsonkb's https://github.com/crowsonkb/k-diffusion installed:
-
-```
-pip install k-diffusion
-```
-
-You can use the community pipeline as follows:
-
-```python
-from diffusers import DiffusionPipeline
-
-pipe = DiffusionPipeline.from_pretrained("CompVis/stable-diffusion-v1-4", custom_pipeline="sd_text2img_k_diffusion")
-pipe = pipe.to("cuda")
-
-prompt = "an astronaut riding a horse on mars"
-pipe.set_scheduler("sample_heun")
-generator = torch.Generator(device="cuda").manual_seed(seed)
-image = pipe(prompt, generator=generator, num_inference_steps=20).images[0]
-
-image.save("./astronaut_heun_k_diffusion.png")
-```
-
-To make sure that K Diffusion and `diffusers` yield the same results:
-
-**Diffusers**:
-```python
-from diffusers import DiffusionPipeline, EulerDiscreteScheduler
-
-seed = 33
-
-pipe = DiffusionPipeline.from_pretrained("CompVis/stable-diffusion-v1-4")
-pipe.scheduler = EulerDiscreteScheduler.from_config(pipe.scheduler.config)
-pipe = pipe.to("cuda")
-
-generator = torch.Generator(device="cuda").manual_seed(seed)
-image = pipe(prompt, generator=generator, num_inference_steps=50).images[0]
-```
-
-
-
-**K Diffusion**:
-```python
-from diffusers import DiffusionPipeline, EulerDiscreteScheduler
-
-seed = 33
-
-pipe = DiffusionPipeline.from_pretrained("CompVis/stable-diffusion-v1-4", custom_pipeline="sd_text2img_k_diffusion")
-pipe.scheduler = EulerDiscreteScheduler.from_config(pipe.scheduler.config)
-pipe = pipe.to("cuda")
-
-pipe.set_scheduler("sample_euler")
-generator = torch.Generator(device="cuda").manual_seed(seed)
-image = pipe(prompt, generator=generator, num_inference_steps=50).images[0]
-```
-
-
-
-### Checkpoint Merger Pipeline
-Based on the AUTOMATIC1111/webui for checkpoint merging. This is a custom pipeline that merges upto 3 pretrained model checkpoints as long as they are in the HuggingFace model_index.json format.
-
-The checkpoint merging is currently memory intensive as it modifies the weights of a DiffusionPipeline object in place. Expect atleast 13GB RAM Usage on Kaggle GPU kernels and
-on colab you might run out of the 12GB memory even while merging two checkpoints.
-
-Usage:-
-```python
-from diffusers import DiffusionPipeline
-
-#Return a CheckpointMergerPipeline class that allows you to merge checkpoints.
-#The checkpoint passed here is ignored. But still pass one of the checkpoints you plan to
-#merge for convenience
-pipe = DiffusionPipeline.from_pretrained("CompVis/stable-diffusion-v1-4", custom_pipeline="checkpoint_merger")
-
-#There are multiple possible scenarios:
-#The pipeline with the merged checkpoints is returned in all the scenarios
-
-#Compatible checkpoints a.k.a matched model_index.json files. Ignores the meta attributes in model_index.json during comparision.( attrs with _ as prefix )
-merged_pipe = pipe.merge(["CompVis/stable-diffusion-v1-4","CompVis/stable-diffusion-v1-2"], interp = "sigmoid", alpha = 0.4)
-
-#Incompatible checkpoints in model_index.json but merge might be possible. Use force = True to ignore model_index.json compatibility
-merged_pipe_1 = pipe.merge(["CompVis/stable-diffusion-v1-4","hakurei/waifu-diffusion"], force = True, interp = "sigmoid", alpha = 0.4)
-
-#Three checkpoint merging. Only "add_difference" method actually works on all three checkpoints. Using any other options will ignore the 3rd checkpoint.
-merged_pipe_2 = pipe.merge(["CompVis/stable-diffusion-v1-4","hakurei/waifu-diffusion","prompthero/openjourney"], force = True, interp = "add_difference", alpha = 0.4)
-
-prompt = "An astronaut riding a horse on Mars"
-
-image = merged_pipe(prompt).images[0]
-
-```
-Some examples along with the merge details:
-
-1. "CompVis/stable-diffusion-v1-4" + "hakurei/waifu-diffusion" ; Sigmoid interpolation; alpha = 0.8
-
-
-
-2. "hakurei/waifu-diffusion" + "prompthero/openjourney" ; Inverse Sigmoid interpolation; alpha = 0.8
-
-
-
-
-3. "CompVis/stable-diffusion-v1-4" + "hakurei/waifu-diffusion" + "prompthero/openjourney"; Add Difference interpolation; alpha = 0.5
-
-
-
-
-### Stable Diffusion Comparisons
-
-This Community Pipeline enables the comparison between the 4 checkpoints that exist for Stable Diffusion. They can be found through the following links:
-1. [Stable Diffusion v1.1](https://huggingface.co/CompVis/stable-diffusion-v1-1)
-2. [Stable Diffusion v1.2](https://huggingface.co/CompVis/stable-diffusion-v1-2)
-3. [Stable Diffusion v1.3](https://huggingface.co/CompVis/stable-diffusion-v1-3)
-4. [Stable Diffusion v1.4](https://huggingface.co/CompVis/stable-diffusion-v1-4)
-
-```python
-from diffusers import DiffusionPipeline
-import matplotlib.pyplot as plt
-
-pipe = DiffusionPipeline.from_pretrained('CompVis/stable-diffusion-v1-4', custom_pipeline='suvadityamuk/StableDiffusionComparison')
-pipe.enable_attention_slicing()
-pipe = pipe.to('cuda')
-prompt = "an astronaut riding a horse on mars"
-output = pipe(prompt)
-
-plt.subplots(2,2,1)
-plt.imshow(output.images[0])
-plt.title('Stable Diffusion v1.1')
-plt.axis('off')
-plt.subplots(2,2,2)
-plt.imshow(output.images[1])
-plt.title('Stable Diffusion v1.2')
-plt.axis('off')
-plt.subplots(2,2,3)
-plt.imshow(output.images[2])
-plt.title('Stable Diffusion v1.3')
-plt.axis('off')
-plt.subplots(2,2,4)
-plt.imshow(output.images[3])
-plt.title('Stable Diffusion v1.4')
-plt.axis('off')
-
-plt.show()
-```
-
-As a result, you can look at a grid of all 4 generated images being shown together, that captures a difference the advancement of the training between the 4 checkpoints.
-
-### Magic Mix
-
-Implementation of the [MagicMix: Semantic Mixing with Diffusion Models](https://arxiv.org/abs/2210.16056) paper. This is a Diffusion Pipeline for semantic mixing of an image and a text prompt to create a new concept while preserving the spatial layout and geometry of the subject in the image. The pipeline takes an image that provides the layout semantics and a prompt that provides the content semantics for the mixing process.
-
-There are 3 parameters for the method-
-- `mix_factor`: It is the interpolation constant used in the layout generation phase. The greater the value of `mix_factor`, the greater the influence of the prompt on the layout generation process.
-- `kmax` and `kmin`: These determine the range for the layout and content generation process. A higher value of kmax results in loss of more information about the layout of the original image and a higher value of kmin results in more steps for content generation process.
-
-Here is an example usage-
-
-```python
-from diffusers import DiffusionPipeline, DDIMScheduler
-from PIL import Image
-
-pipe = DiffusionPipeline.from_pretrained(
- "CompVis/stable-diffusion-v1-4",
- custom_pipeline="magic_mix",
- scheduler = DDIMScheduler.from_pretrained("CompVis/stable-diffusion-v1-4", subfolder="scheduler"),
-).to('cuda')
-
-img = Image.open('phone.jpg')
-mix_img = pipe(
- img,
- prompt = 'bed',
- kmin = 0.3,
- kmax = 0.5,
- mix_factor = 0.5,
- )
-mix_img.save('phone_bed_mix.jpg')
-```
-The `mix_img` is a PIL image that can be saved locally or displayed directly in a google colab. Generated image is a mix of the layout semantics of the given image and the content semantics of the prompt.
-
-E.g. the above script generates the following image:
-
-`phone.jpg`
-
-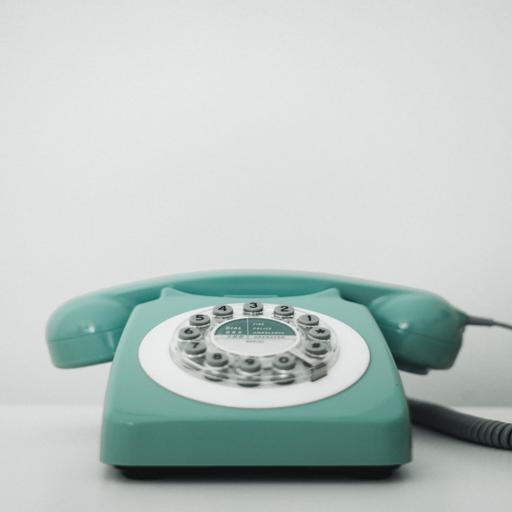
-
-`phone_bed_mix.jpg`
-
-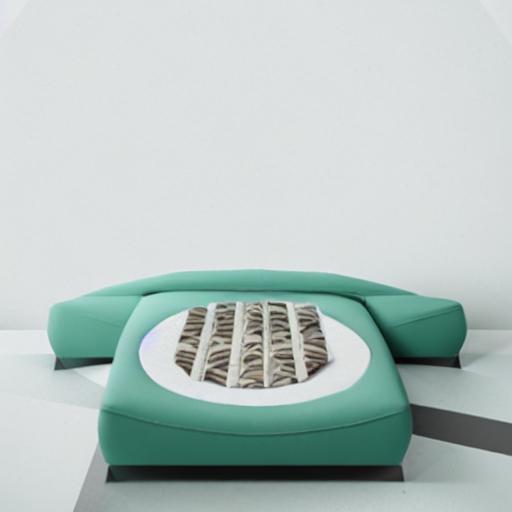
-
-For more example generations check out this [demo notebook](https://github.com/daspartho/MagicMix/blob/main/demo.ipynb).
-
-
-### Stable UnCLIP
-
-UnCLIPPipeline("kakaobrain/karlo-v1-alpha") provide a prior model that can generate clip image embedding from text.
-StableDiffusionImageVariationPipeline("lambdalabs/sd-image-variations-diffusers") provide a decoder model than can generate images from clip image embedding.
-
-```python
-import torch
-from diffusers import DiffusionPipeline
-
-device = torch.device("cpu" if not torch.cuda.is_available() else "cuda")
-
-pipeline = DiffusionPipeline.from_pretrained(
- "kakaobrain/karlo-v1-alpha",
- torch_dtype=torch.float16,
- custom_pipeline="stable_unclip",
- decoder_pipe_kwargs=dict(
- image_encoder=None,
- ),
-)
-pipeline.to(device)
-
-prompt = "a shiba inu wearing a beret and black turtleneck"
-random_generator = torch.Generator(device=device).manual_seed(1000)
-output = pipeline(
- prompt=prompt,
- width=512,
- height=512,
- generator=random_generator,
- prior_guidance_scale=4,
- prior_num_inference_steps=25,
- decoder_guidance_scale=8,
- decoder_num_inference_steps=50,
-)
-
-image = output.images[0]
-image.save("./shiba-inu.jpg")
-
-# debug
-
-# `pipeline.decoder_pipe` is a regular StableDiffusionImageVariationPipeline instance.
-# It is used to convert clip image embedding to latents, then fed into VAE decoder.
-print(pipeline.decoder_pipe.__class__)
-#
-
-# this pipeline only use prior module in "kakaobrain/karlo-v1-alpha"
-# It is used to convert clip text embedding to clip image embedding.
-print(pipeline)
-# StableUnCLIPPipeline {
-# "_class_name": "StableUnCLIPPipeline",
-# "_diffusers_version": "0.12.0.dev0",
-# "prior": [
-# "diffusers",
-# "PriorTransformer"
-# ],
-# "prior_scheduler": [
-# "diffusers",
-# "UnCLIPScheduler"
-# ],
-# "text_encoder": [
-# "transformers",
-# "CLIPTextModelWithProjection"
-# ],
-# "tokenizer": [
-# "transformers",
-# "CLIPTokenizer"
-# ]
-# }
-
-# pipeline.prior_scheduler is the scheduler used for prior in UnCLIP.
-print(pipeline.prior_scheduler)
-# UnCLIPScheduler {
-# "_class_name": "UnCLIPScheduler",
-# "_diffusers_version": "0.12.0.dev0",
-# "clip_sample": true,
-# "clip_sample_range": 5.0,
-# "num_train_timesteps": 1000,
-# "prediction_type": "sample",
-# "variance_type": "fixed_small_log"
-# }
-```
-
-
-`shiba-inu.jpg`
-
-
-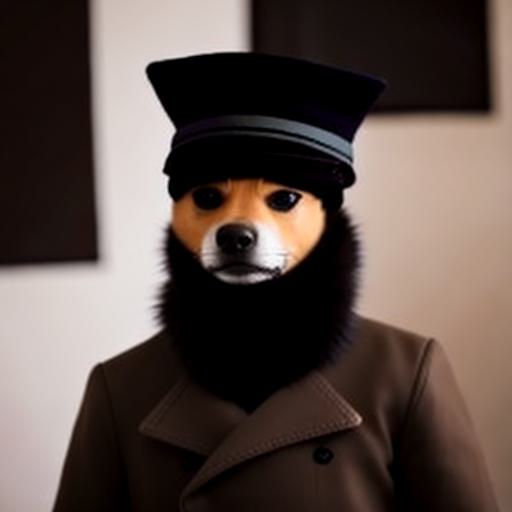
-
-### UnCLIP Text Interpolation Pipeline
-
-This Diffusion Pipeline takes two prompts and interpolates between the two input prompts using spherical interpolation ( slerp ). The input prompts are converted to text embeddings by the pipeline's text_encoder and the interpolation is done on the resulting text_embeddings over the number of steps specified. Defaults to 5 steps.
-
-```python
-import torch
-from diffusers import DiffusionPipeline
-
-device = torch.device("cpu" if not torch.cuda.is_available() else "cuda")
-
-pipe = DiffusionPipeline.from_pretrained(
- "kakaobrain/karlo-v1-alpha",
- torch_dtype=torch.float16,
- custom_pipeline="unclip_text_interpolation"
-)
-pipe.to(device)
-
-start_prompt = "A photograph of an adult lion"
-end_prompt = "A photograph of a lion cub"
-#For best results keep the prompts close in length to each other. Of course, feel free to try out with differing lengths.
-generator = torch.Generator(device=device).manual_seed(42)
-
-output = pipe(start_prompt, end_prompt, steps = 6, generator = generator, enable_sequential_cpu_offload=False)
-
-for i,image in enumerate(output.images):
- img.save('result%s.jpg' % i)
-```
-
-The resulting images in order:-
-
-
-
-
-
-
-
-
-### UnCLIP Image Interpolation Pipeline
-
-This Diffusion Pipeline takes two images or an image_embeddings tensor of size 2 and interpolates between their embeddings using spherical interpolation ( slerp ). The input images/image_embeddings are converted to image embeddings by the pipeline's image_encoder and the interpolation is done on the resulting image_embeddings over the number of steps specified. Defaults to 5 steps.
-
-```python
-import torch
-from diffusers import DiffusionPipeline
-from PIL import Image
-
-device = torch.device("cpu" if not torch.cuda.is_available() else "cuda")
-dtype = torch.float16 if torch.cuda.is_available() else torch.bfloat16
-
-pipe = DiffusionPipeline.from_pretrained(
- "kakaobrain/karlo-v1-alpha-image-variations",
- torch_dtype=dtype,
- custom_pipeline="unclip_image_interpolation"
-)
-pipe.to(device)
-
-images = [Image.open('./starry_night.jpg'), Image.open('./flowers.jpg')]
-#For best results keep the prompts close in length to each other. Of course, feel free to try out with differing lengths.
-generator = torch.Generator(device=device).manual_seed(42)
-
-output = pipe(image = images ,steps = 6, generator = generator)
-
-for i,image in enumerate(output.images):
- image.save('starry_to_flowers_%s.jpg' % i)
-```
-The original images:-
-
-
-
-
-The resulting images in order:-
-
-
-
-
-
-
-
-
-### DDIM Noise Comparative Analysis Pipeline
-#### **Research question: What visual concepts do the diffusion models learn from each noise level during training?**
-The [P2 weighting (CVPR 2022)](https://arxiv.org/abs/2204.00227) paper proposed an approach to answer the above question, which is their second contribution.
-The approach consists of the following steps:
-
-1. The input is an image x0.
-2. Perturb it to xt using a diffusion process q(xt|x0).
- - `strength` is a value between 0.0 and 1.0, that controls the amount of noise that is added to the input image. Values that approach 1.0 allow for lots of variations but will also produce images that are not semantically consistent with the input.
-3. Reconstruct the image with the learned denoising process pθ(ˆx0|xt).
-4. Compare x0 and ˆx0 among various t to show how each step contributes to the sample.
-The authors used [openai/guided-diffusion](https://github.com/openai/guided-diffusion) model to denoise images in FFHQ dataset. This pipeline extends their second contribution by investigating DDIM on any input image.
-
-```python
-import torch
-from PIL import Image
-import numpy as np
-
-image_path = "path/to/your/image" # images from CelebA-HQ might be better
-image_pil = Image.open(image_path)
-image_name = image_path.split("/")[-1].split(".")[0]
-
-device = torch.device("cpu" if not torch.cuda.is_available() else "cuda")
-pipe = DiffusionPipeline.from_pretrained(
- "google/ddpm-ema-celebahq-256",
- custom_pipeline="ddim_noise_comparative_analysis",
-)
-pipe = pipe.to(device)
-
-for strength in np.linspace(0.1, 1, 25):
- denoised_image, latent_timestep = pipe(
- image_pil, strength=strength, return_dict=False
- )
- denoised_image = denoised_image[0]
- denoised_image.save(
- f"noise_comparative_analysis_{image_name}_{latent_timestep}.png"
- )
-```
-
-Here is the result of this pipeline (which is DDIM) on CelebA-HQ dataset.
-
-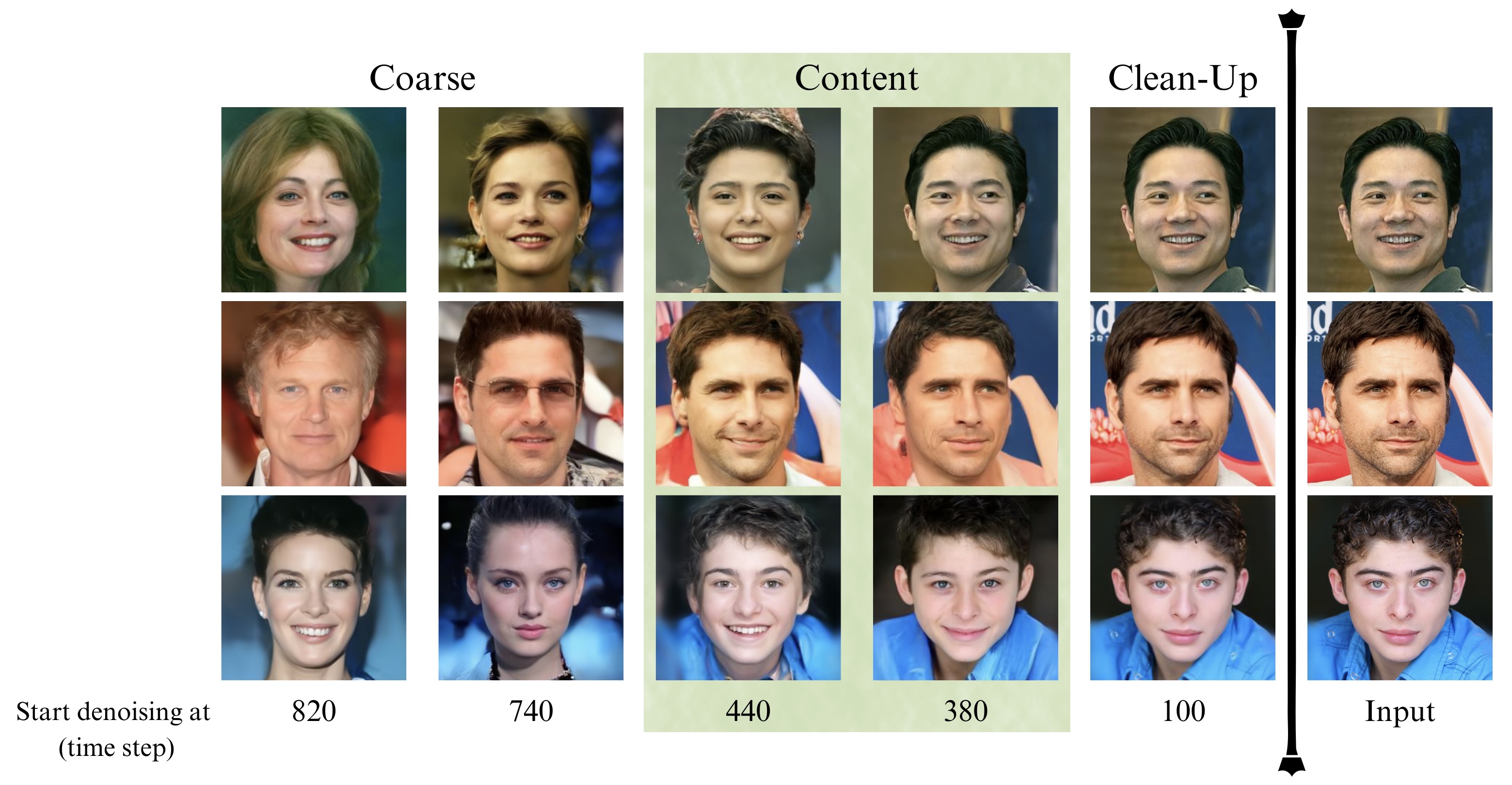
-
-### CLIP Guided Img2Img Stable Diffusion
-
-CLIP guided Img2Img stable diffusion can help to generate more realistic images with an initial image
-by guiding stable diffusion at every denoising step with an additional CLIP model.
-
-The following code requires roughly 12GB of GPU RAM.
-
-```python
-from io import BytesIO
-import requests
-import torch
-from diffusers import DiffusionPipeline
-from PIL import Image
-from transformers import CLIPFeatureExtractor, CLIPModel
-feature_extractor = CLIPFeatureExtractor.from_pretrained(
- "laion/CLIP-ViT-B-32-laion2B-s34B-b79K"
-)
-clip_model = CLIPModel.from_pretrained(
- "laion/CLIP-ViT-B-32-laion2B-s34B-b79K", torch_dtype=torch.float16
-)
-guided_pipeline = DiffusionPipeline.from_pretrained(
- "CompVis/stable-diffusion-v1-4",
- # custom_pipeline="clip_guided_stable_diffusion",
- custom_pipeline="/home/njindal/diffusers/examples/community/clip_guided_stable_diffusion.py",
- clip_model=clip_model,
- feature_extractor=feature_extractor,
- torch_dtype=torch.float16,
-)
-guided_pipeline.enable_attention_slicing()
-guided_pipeline = guided_pipeline.to("cuda")
-prompt = "fantasy book cover, full moon, fantasy forest landscape, golden vector elements, fantasy magic, dark light night, intricate, elegant, sharp focus, illustration, highly detailed, digital painting, concept art, matte, art by WLOP and Artgerm and Albert Bierstadt, masterpiece"
-url = "https://raw.githubusercontent.com/CompVis/stable-diffusion/main/assets/stable-samples/img2img/sketch-mountains-input.jpg"
-response = requests.get(url)
-init_image = Image.open(BytesIO(response.content)).convert("RGB")
-image = guided_pipeline(
- prompt=prompt,
- num_inference_steps=30,
- image=init_image,
- strength=0.75,
- guidance_scale=7.5,
- clip_guidance_scale=100,
- num_cutouts=4,
- use_cutouts=False,
-).images[0]
-display(image)
-```
-
-Init Image
-
-
-
-Output Image
-
-
-
-### TensorRT Text2Image Stable Diffusion Pipeline
-
-The TensorRT Pipeline can be used to accelerate the Text2Image Stable Diffusion Inference run.
-
-NOTE: The ONNX conversions and TensorRT engine build may take up to 30 minutes.
-
-```python
-import torch
-from diffusers import DDIMScheduler
-from diffusers.pipelines.stable_diffusion import StableDiffusionPipeline
-
-# Use the DDIMScheduler scheduler here instead
-scheduler = DDIMScheduler.from_pretrained("stabilityai/stable-diffusion-2-1",
- subfolder="scheduler")
-
-pipe = StableDiffusionPipeline.from_pretrained("stabilityai/stable-diffusion-2-1",
- custom_pipeline="stable_diffusion_tensorrt_txt2img",
- revision='fp16',
- torch_dtype=torch.float16,
- scheduler=scheduler,)
-
-# re-use cached folder to save ONNX models and TensorRT Engines
-pipe.set_cached_folder("stabilityai/stable-diffusion-2-1", revision='fp16',)
-
-pipe = pipe.to("cuda")
-
-prompt = "a beautiful photograph of Mt. Fuji during cherry blossom"
-image = pipe(prompt).images[0]
-image.save('tensorrt_mt_fuji.png')
-```
-
-### EDICT Image Editing Pipeline
-
-This pipeline implements the text-guided image editing approach from the paper [EDICT: Exact Diffusion Inversion via Coupled Transformations](https://arxiv.org/abs/2211.12446). You have to pass:
-- (`PIL`) `image` you want to edit.
-- `base_prompt`: the text prompt describing the current image (before editing).
-- `target_prompt`: the text prompt describing with the edits.
-
-```python
-from diffusers import DiffusionPipeline, DDIMScheduler
-from transformers import CLIPTextModel
-import torch, PIL, requests
-from io import BytesIO
-from IPython.display import display
-
-def center_crop_and_resize(im):
-
- width, height = im.size
- d = min(width, height)
- left = (width - d) / 2
- upper = (height - d) / 2
- right = (width + d) / 2
- lower = (height + d) / 2
-
- return im.crop((left, upper, right, lower)).resize((512, 512))
-
-torch_dtype = torch.float16
-device = torch.device('cuda' if torch.cuda.is_available() else 'cpu')
-
-# scheduler and text_encoder param values as in the paper
-scheduler = DDIMScheduler(
- num_train_timesteps=1000,
- beta_start=0.00085,
- beta_end=0.012,
- beta_schedule="scaled_linear",
- set_alpha_to_one=False,
- clip_sample=False,
-)
-
-text_encoder = CLIPTextModel.from_pretrained(
- pretrained_model_name_or_path="openai/clip-vit-large-patch14",
- torch_dtype=torch_dtype,
-)
-
-# initialize pipeline
-pipeline = DiffusionPipeline.from_pretrained(
- pretrained_model_name_or_path="CompVis/stable-diffusion-v1-4",
- custom_pipeline="edict_pipeline",
- revision="fp16",
- scheduler=scheduler,
- text_encoder=text_encoder,
- leapfrog_steps=True,
- torch_dtype=torch_dtype,
-).to(device)
-
-# download image
-image_url = "https://huggingface.co/datasets/Joqsan/images/resolve/main/imagenet_dog_1.jpeg"
-response = requests.get(image_url)
-image = PIL.Image.open(BytesIO(response.content))
-
-# preprocess it
-cropped_image = center_crop_and_resize(image)
-
-# define the prompts
-base_prompt = "A dog"
-target_prompt = "A golden retriever"
-
-# run the pipeline
-result_image = pipeline(
- base_prompt=base_prompt,
- target_prompt=target_prompt,
- image=cropped_image,
-)
-
-display(result_image)
-```
-
-Init Image
-
-
-
-Output Image
-
-
-
-### Stable Diffusion RePaint
-
-This pipeline uses the [RePaint](https://arxiv.org/abs/2201.09865) logic on the latent space of stable diffusion. It can
-be used similarly to other image inpainting pipelines but does not rely on a specific inpainting model. This means you can use
-models that are not specifically created for inpainting.
-
-Make sure to use the ```RePaintScheduler``` as shown in the example below.
-
-Disclaimer: The mask gets transferred into latent space, this may lead to unexpected changes on the edge of the masked part.
-The inference time is a lot slower.
-
-```py
-import PIL
-import requests
-import torch
-from io import BytesIO
-from diffusers import StableDiffusionPipeline, RePaintScheduler
-def download_image(url):
- response = requests.get(url)
- return PIL.Image.open(BytesIO(response.content)).convert("RGB")
-img_url = "https://raw.githubusercontent.com/CompVis/latent-diffusion/main/data/inpainting_examples/overture-creations-5sI6fQgYIuo.png"
-mask_url = "https://raw.githubusercontent.com/CompVis/latent-diffusion/main/data/inpainting_examples/overture-creations-5sI6fQgYIuo_mask.png"
-init_image = download_image(img_url).resize((512, 512))
-mask_image = download_image(mask_url).resize((512, 512))
-mask_image = PIL.ImageOps.invert(mask_image)
-pipe = StableDiffusionPipeline.from_pretrained(
- "CompVis/stable-diffusion-v1-4", torch_dtype=torch.float16, custom_pipeline="stable_diffusion_repaint",
-)
-pipe.scheduler = RePaintScheduler.from_config(pipe.scheduler.config)
-pipe = pipe.to("cuda")
-prompt = "Face of a yellow cat, high resolution, sitting on a park bench"
-image = pipe(prompt=prompt, image=init_image, mask_image=mask_image).images[0]
-```
-
-### TensorRT Image2Image Stable Diffusion Pipeline
-
-The TensorRT Pipeline can be used to accelerate the Image2Image Stable Diffusion Inference run.
-
-NOTE: The ONNX conversions and TensorRT engine build may take up to 30 minutes.
-
-```python
-import requests
-from io import BytesIO
-from PIL import Image
-import torch
-from diffusers import DDIMScheduler
-from diffusers.pipelines.stable_diffusion import StableDiffusionImg2ImgPipeline
-
-# Use the DDIMScheduler scheduler here instead
-scheduler = DDIMScheduler.from_pretrained("stabilityai/stable-diffusion-2-1",
- subfolder="scheduler")
-
-
-pipe = StableDiffusionImg2ImgPipeline.from_pretrained("stabilityai/stable-diffusion-2-1",
- custom_pipeline="stable_diffusion_tensorrt_img2img",
- revision='fp16',
- torch_dtype=torch.float16,
- scheduler=scheduler,)
-
-# re-use cached folder to save ONNX models and TensorRT Engines
-pipe.set_cached_folder("stabilityai/stable-diffusion-2-1", revision='fp16',)
-
-pipe = pipe.to("cuda")
-
-url = "https://pajoca.com/wp-content/uploads/2022/09/tekito-yamakawa-1.png"
-response = requests.get(url)
-input_image = Image.open(BytesIO(response.content)).convert("RGB")
-
-prompt = "photorealistic new zealand hills"
-image = pipe(prompt, image=input_image, strength=0.75,).images[0]
-image.save('tensorrt_img2img_new_zealand_hills.png')
-```
-
-### Stable Diffusion Reference
-
-This pipeline uses the Reference Control. Refer to the [sd-webui-controlnet discussion: Reference-only Control](https://github.com/Mikubill/sd-webui-controlnet/discussions/1236)[sd-webui-controlnet discussion: Reference-adain Control](https://github.com/Mikubill/sd-webui-controlnet/discussions/1280).
-
-Based on [this issue](https://github.com/huggingface/diffusers/issues/3566),
-- `EulerAncestralDiscreteScheduler` got poor results.
-
-```py
-import torch
-from diffusers import UniPCMultistepScheduler
-from diffusers.utils import load_image
-
-input_image = load_image("https://hf.co/datasets/huggingface/documentation-images/resolve/main/diffusers/input_image_vermeer.png")
-
-pipe = StableDiffusionReferencePipeline.from_pretrained(
- "runwayml/stable-diffusion-v1-5",
- safety_checker=None,
- torch_dtype=torch.float16
- ).to('cuda:0')
-
-pipe.scheduler = UniPCMultistepScheduler.from_config(pipe.scheduler.config)
-
-result_img = pipe(ref_image=input_image,
- prompt="1girl",
- num_inference_steps=20,
- reference_attn=True,
- reference_adain=True).images[0]
-```
-
-Reference Image
-
-
-
-Output Image of `reference_attn=True` and `reference_adain=False`
-
-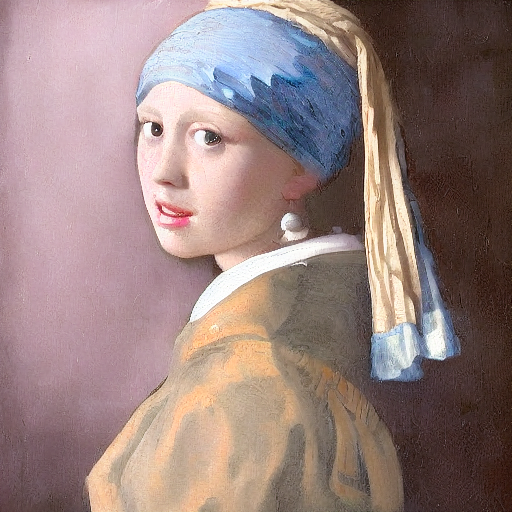
-
-Output Image of `reference_attn=False` and `reference_adain=True`
-
-
-
-Output Image of `reference_attn=True` and `reference_adain=True`
-
-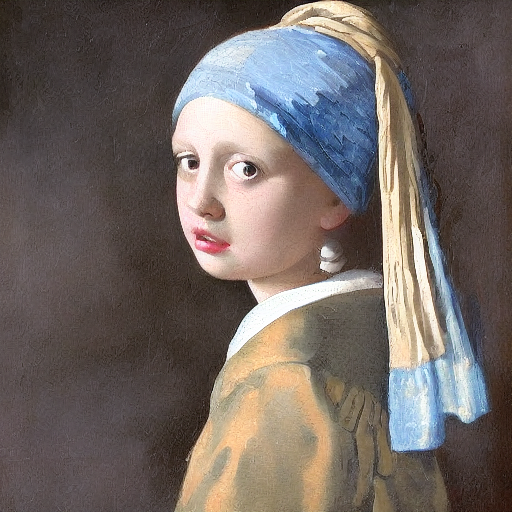
-
-### Stable Diffusion ControlNet Reference
-
-This pipeline uses the Reference Control with ControlNet. Refer to the [sd-webui-controlnet discussion: Reference-only Control](https://github.com/Mikubill/sd-webui-controlnet/discussions/1236)[sd-webui-controlnet discussion: Reference-adain Control](https://github.com/Mikubill/sd-webui-controlnet/discussions/1280).
-
-Based on [this issue](https://github.com/huggingface/diffusers/issues/3566),
-- `EulerAncestralDiscreteScheduler` got poor results.
-- `guess_mode=True` works well for ControlNet v1.1
-
-```py
-import cv2
-import torch
-import numpy as np
-from PIL import Image
-from diffusers import UniPCMultistepScheduler
-from diffusers.utils import load_image
-
-input_image = load_image("https://hf.co/datasets/huggingface/documentation-images/resolve/main/diffusers/input_image_vermeer.png")
-
-# get canny image
-image = cv2.Canny(np.array(input_image), 100, 200)
-image = image[:, :, None]
-image = np.concatenate([image, image, image], axis=2)
-canny_image = Image.fromarray(image)
-
-controlnet = ControlNetModel.from_pretrained("lllyasviel/sd-controlnet-canny", torch_dtype=torch.float16)
-pipe = StableDiffusionControlNetReferencePipeline.from_pretrained(
- "runwayml/stable-diffusion-v1-5",
- controlnet=controlnet,
- safety_checker=None,
- torch_dtype=torch.float16
- ).to('cuda:0')
-
-pipe.scheduler = UniPCMultistepScheduler.from_config(pipe.scheduler.config)
-
-result_img = pipe(ref_image=input_image,
- prompt="1girl",
- image=canny_image,
- num_inference_steps=20,
- reference_attn=True,
- reference_adain=True).images[0]
-```
-
-Reference Image
-
-
-
-Output Image
-
-
-
-
-### Stable Diffusion on IPEX
-
-This diffusion pipeline aims to accelarate the inference of Stable-Diffusion on Intel Xeon CPUs with BF16/FP32 precision using [IPEX](https://github.com/intel/intel-extension-for-pytorch).
-
-To use this pipeline, you need to:
-1. Install [IPEX](https://github.com/intel/intel-extension-for-pytorch)
-
-**Note:** For each PyTorch release, there is a corresponding release of the IPEX. Here is the mapping relationship. It is recommended to install Pytorch/IPEX2.0 to get the best performance.
-
-|PyTorch Version|IPEX Version|
-|--|--|
-|[v2.0.\*](https://github.com/pytorch/pytorch/tree/v2.0.1 "v2.0.1")|[v2.0.\*](https://github.com/intel/intel-extension-for-pytorch/tree/v2.0.100+cpu)|
-|[v1.13.\*](https://github.com/pytorch/pytorch/tree/v1.13.0 "v1.13.0")|[v1.13.\*](https://github.com/intel/intel-extension-for-pytorch/tree/v1.13.100+cpu)|
-
-You can simply use pip to install IPEX with the latest version.
-```python
-python -m pip install intel_extension_for_pytorch
-```
-**Note:** To install a specific version, run with the following command:
-```
-python -m pip install intel_extension_for_pytorch== -f https://developer.intel.com/ipex-whl-stable-cpu
-```
-
-2. After pipeline initialization, `prepare_for_ipex()` should be called to enable IPEX accelaration. Supported inference datatypes are Float32 and BFloat16.
-
-**Note:** The setting of generated image height/width for `prepare_for_ipex()` should be same as the setting of pipeline inference.
-```python
-pipe = DiffusionPipeline.from_pretrained("runwayml/stable-diffusion-v1-5", custom_pipeline="stable_diffusion_ipex")
-# For Float32
-pipe.prepare_for_ipex(prompt, dtype=torch.float32, height=512, width=512) #value of image height/width should be consistent with the pipeline inference
-# For BFloat16
-pipe.prepare_for_ipex(prompt, dtype=torch.bfloat16, height=512, width=512) #value of image height/width should be consistent with the pipeline inference
-```
-
-Then you can use the ipex pipeline in a similar way to the default stable diffusion pipeline.
-```python
-# For Float32
-image = pipe(prompt, num_inference_steps=20, height=512, width=512).images[0] #value of image height/width should be consistent with 'prepare_for_ipex()'
-# For BFloat16
-with torch.cpu.amp.autocast(enabled=True, dtype=torch.bfloat16):
- image = pipe(prompt, num_inference_steps=20, height=512, width=512).images[0] #value of image height/width should be consistent with 'prepare_for_ipex()'
-```
-
-The following code compares the performance of the original stable diffusion pipeline with the ipex-optimized pipeline.
-
-```python
-import torch
-import intel_extension_for_pytorch as ipex
-from diffusers import StableDiffusionPipeline
-import time
-
-prompt = "sailing ship in storm by Rembrandt"
-model_id = "runwayml/stable-diffusion-v1-5"
-# Helper function for time evaluation
-def elapsed_time(pipeline, nb_pass=3, num_inference_steps=20):
- # warmup
- for _ in range(2):
- images = pipeline(prompt, num_inference_steps=num_inference_steps, height=512, width=512).images
- #time evaluation
- start = time.time()
- for _ in range(nb_pass):
- pipeline(prompt, num_inference_steps=num_inference_steps, height=512, width=512)
- end = time.time()
- return (end - start) / nb_pass
-
-############## bf16 inference performance ###############
-
-# 1. IPEX Pipeline initialization
-pipe = DiffusionPipeline.from_pretrained(model_id, custom_pipeline="stable_diffusion_ipex")
-pipe.prepare_for_ipex(prompt, dtype=torch.bfloat16, height=512, width=512)
-
-# 2. Original Pipeline initialization
-pipe2 = StableDiffusionPipeline.from_pretrained(model_id)
-
-# 3. Compare performance between Original Pipeline and IPEX Pipeline
-with torch.cpu.amp.autocast(enabled=True, dtype=torch.bfloat16):
- latency = elapsed_time(pipe)
- print("Latency of StableDiffusionIPEXPipeline--bf16", latency)
- latency = elapsed_time(pipe2)
- print("Latency of StableDiffusionPipeline--bf16",latency)
-
-############## fp32 inference performance ###############
-
-# 1. IPEX Pipeline initialization
-pipe3 = DiffusionPipeline.from_pretrained(model_id, custom_pipeline="stable_diffusion_ipex")
-pipe3.prepare_for_ipex(prompt, dtype=torch.float32, height=512, width=512)
-
-# 2. Original Pipeline initialization
-pipe4 = StableDiffusionPipeline.from_pretrained(model_id)
-
-# 3. Compare performance between Original Pipeline and IPEX Pipeline
-latency = elapsed_time(pipe3)
-print("Latency of StableDiffusionIPEXPipeline--fp32", latency)
-latency = elapsed_time(pipe4)
-print("Latency of StableDiffusionPipeline--fp32",latency)
-
-```
-
-### CLIP Guided Images Mixing With Stable Diffusion
-
-
-
-CLIP guided stable diffusion images mixing pipline allows to combine two images using standard diffusion models.
-This approach is using (optional) CoCa model to avoid writing image description.
-[More code examples](https://github.com/TheDenk/images_mixing)
-
-## Example Images Mixing (with CoCa)
-```python
-import requests
-from io import BytesIO
-
-import PIL
-import torch
-import open_clip
-from open_clip import SimpleTokenizer
-from diffusers import DiffusionPipeline
-from transformers import CLIPFeatureExtractor, CLIPModel
-
-
-def download_image(url):
- response = requests.get(url)
- return PIL.Image.open(BytesIO(response.content)).convert("RGB")
-
-# Loading additional models
-feature_extractor = CLIPFeatureExtractor.from_pretrained(
- "laion/CLIP-ViT-B-32-laion2B-s34B-b79K"
-)
-clip_model = CLIPModel.from_pretrained(
- "laion/CLIP-ViT-B-32-laion2B-s34B-b79K", torch_dtype=torch.float16
-)
-coca_model = open_clip.create_model('coca_ViT-L-14', pretrained='laion2B-s13B-b90k').to('cuda')
-coca_model.dtype = torch.float16
-coca_transform = open_clip.image_transform(
- coca_model.visual.image_size,
- is_train = False,
- mean = getattr(coca_model.visual, 'image_mean', None),
- std = getattr(coca_model.visual, 'image_std', None),
-)
-coca_tokenizer = SimpleTokenizer()
-
-# Pipline creating
-mixing_pipeline = DiffusionPipeline.from_pretrained(
- "CompVis/stable-diffusion-v1-4",
- custom_pipeline="clip_guided_images_mixing_stable_diffusion",
- clip_model=clip_model,
- feature_extractor=feature_extractor,
- coca_model=coca_model,
- coca_tokenizer=coca_tokenizer,
- coca_transform=coca_transform,
- torch_dtype=torch.float16,
-)
-mixing_pipeline.enable_attention_slicing()
-mixing_pipeline = mixing_pipeline.to("cuda")
-
-# Pipline running
-generator = torch.Generator(device="cuda").manual_seed(17)
-
-def download_image(url):
- response = requests.get(url)
- return PIL.Image.open(BytesIO(response.content)).convert("RGB")
-
-content_image = download_image("https://huggingface.co/datasets/TheDenk/images_mixing/resolve/main/boromir.jpg")
-style_image = download_image("https://huggingface.co/datasets/TheDenk/images_mixing/resolve/main/gigachad.jpg")
-
-pipe_images = mixing_pipeline(
- num_inference_steps=50,
- content_image=content_image,
- style_image=style_image,
- noise_strength=0.65,
- slerp_latent_style_strength=0.9,
- slerp_prompt_style_strength=0.1,
- slerp_clip_image_style_strength=0.1,
- guidance_scale=9.0,
- batch_size=1,
- clip_guidance_scale=100,
- generator=generator,
-).images
-```
-
-
-
-### Stable Diffusion Mixture Tiling
-
-This pipeline uses the Mixture. Refer to the [Mixture](https://arxiv.org/abs/2302.02412) paper for more details.
-
-```python
-from diffusers import LMSDiscreteScheduler, DiffusionPipeline
-
-# Creater scheduler and model (similar to StableDiffusionPipeline)
-scheduler = LMSDiscreteScheduler(beta_start=0.00085, beta_end=0.012, beta_schedule="scaled_linear", num_train_timesteps=1000)
-pipeline = DiffusionPipeline.from_pretrained("CompVis/stable-diffusion-v1-4", scheduler=scheduler, custom_pipeline="mixture_tiling")
-pipeline.to("cuda")
-
-# Mixture of Diffusers generation
-image = pipeline(
- prompt=[[
- "A charming house in the countryside, by jakub rozalski, sunset lighting, elegant, highly detailed, smooth, sharp focus, artstation, stunning masterpiece",
- "A dirt road in the countryside crossing pastures, by jakub rozalski, sunset lighting, elegant, highly detailed, smooth, sharp focus, artstation, stunning masterpiece",
- "An old and rusty giant robot lying on a dirt road, by jakub rozalski, dark sunset lighting, elegant, highly detailed, smooth, sharp focus, artstation, stunning masterpiece"
- ]],
- tile_height=640,
- tile_width=640,
- tile_row_overlap=0,
- tile_col_overlap=256,
- guidance_scale=8,
- seed=7178915308,
- num_inference_steps=50,
-)["images"][0]
-```
-
-
-### TensorRT Inpainting Stable Diffusion Pipeline
-
-The TensorRT Pipeline can be used to accelerate the Inpainting Stable Diffusion Inference run.
-
-NOTE: The ONNX conversions and TensorRT engine build may take up to 30 minutes.
-
-```python
-import requests
-from io import BytesIO
-from PIL import Image
-import torch
-from diffusers import PNDMScheduler
-from diffusers.pipelines.stable_diffusion import StableDiffusionImg2ImgPipeline
-
-# Use the PNDMScheduler scheduler here instead
-scheduler = PNDMScheduler.from_pretrained("stabilityai/stable-diffusion-2-inpainting", subfolder="scheduler")
-
-
-pipe = StableDiffusionImg2ImgPipeline.from_pretrained("stabilityai/stable-diffusion-2-inpainting",
- custom_pipeline="stable_diffusion_tensorrt_inpaint",
- revision='fp16',
- torch_dtype=torch.float16,
- scheduler=scheduler,
- )
-
-# re-use cached folder to save ONNX models and TensorRT Engines
-pipe.set_cached_folder("stabilityai/stable-diffusion-2-inpainting", revision='fp16',)
-
-pipe = pipe.to("cuda")
-
-url = "https://raw.githubusercontent.com/CompVis/latent-diffusion/main/data/inpainting_examples/overture-creations-5sI6fQgYIuo.png"
-response = requests.get(url)
-input_image = Image.open(BytesIO(response.content)).convert("RGB")
-
-mask_url = "https://raw.githubusercontent.com/CompVis/latent-diffusion/main/data/inpainting_examples/overture-creations-5sI6fQgYIuo_mask.png"
-response = requests.get(mask_url)
-mask_image = Image.open(BytesIO(response.content)).convert("RGB")
-
-prompt = "a mecha robot sitting on a bench"
-image = pipe(prompt, image=input_image, mask_image=mask_image, strength=0.75,).images[0]
-image.save('tensorrt_inpaint_mecha_robot.png')
-```
-
-### Stable Diffusion Mixture Canvas
-
-This pipeline uses the Mixture. Refer to the [Mixture](https://arxiv.org/abs/2302.02412) paper for more details.
-
-```python
-from PIL import Image
-from diffusers import LMSDiscreteScheduler, DiffusionPipeline
-from diffusers.pipelines.pipeline_utils import Image2ImageRegion, Text2ImageRegion, preprocess_image
-
-
-# Load and preprocess guide image
-iic_image = preprocess_image(Image.open("input_image.png").convert("RGB"))
-
-# Creater scheduler and model (similar to StableDiffusionPipeline)
-scheduler = LMSDiscreteScheduler(beta_start=0.00085, beta_end=0.012, beta_schedule="scaled_linear", num_train_timesteps=1000)
-pipeline = DiffusionPipeline.from_pretrained("CompVis/stable-diffusion-v1-4", scheduler=scheduler).to("cuda:0", custom_pipeline="mixture_canvas")
-pipeline.to("cuda")
-
-# Mixture of Diffusers generation
-output = pipeline(
- canvas_height=800,
- canvas_width=352,
- regions=[
- Text2ImageRegion(0, 800, 0, 352, guidance_scale=8,
- prompt=f"best quality, masterpiece, WLOP, sakimichan, art contest winner on pixiv, 8K, intricate details, wet effects, rain drops, ethereal, mysterious, futuristic, UHD, HDR, cinematic lighting, in a beautiful forest, rainy day, award winning, trending on artstation, beautiful confident cheerful young woman, wearing a futuristic sleeveless dress, ultra beautiful detailed eyes, hyper-detailed face, complex, perfect, model, textured, chiaroscuro, professional make-up, realistic, figure in frame, "),
- Image2ImageRegion(352-800, 352, 0, 352, reference_image=iic_image, strength=1.0),
- ],
- num_inference_steps=100,
- seed=5525475061,
-)["images"][0]
-```
-
-
-
-
-### IADB pipeline
-
-This pipeline is the implementation of the [α-(de)Blending: a Minimalist Deterministic Diffusion Model](https://arxiv.org/abs/2305.03486) paper.
-It is a simple and minimalist diffusion model.
-
-The following code shows how to use the IADB pipeline to generate images using a pretrained celebahq-256 model.
-
-```python
-
-pipeline_iadb = DiffusionPipeline.from_pretrained("thomasc4/iadb-celebahq-256", custom_pipeline='iadb')
-
-pipeline_iadb = pipeline_iadb.to('cuda')
-
-output = pipeline_iadb(batch_size=4,num_inference_steps=128)
-for i in range(len(output[0])):
- plt.imshow(output[0][i])
- plt.show()
-
-```
-
-Sampling with the IADB formulation is easy, and can be done in a few lines (the pipeline already implements it):
-
-```python
-
-def sample_iadb(model, x0, nb_step):
- x_alpha = x0
- for t in range(nb_step):
- alpha = (t/nb_step)
- alpha_next =((t+1)/nb_step)
-
- d = model(x_alpha, torch.tensor(alpha, device=x_alpha.device))['sample']
- x_alpha = x_alpha + (alpha_next-alpha)*d
-
- return x_alpha
-
-```
-
-The training loop is also straightforward:
-
-```python
-
-# Training loop
-while True:
- x0 = sample_noise()
- x1 = sample_dataset()
-
- alpha = torch.rand(batch_size)
-
- # Blend
- x_alpha = (1-alpha) * x0 + alpha * x1
-
- # Loss
- loss = torch.sum((D(x_alpha, alpha)- (x1-x0))**2)
- optimizer.zero_grad()
- loss.backward()
- optimizer.step()
-```
diff --git a/spaces/Androidonnxfork/CivitAi-to-Diffusers/diffusers/examples/community/magic_mix.py b/spaces/Androidonnxfork/CivitAi-to-Diffusers/diffusers/examples/community/magic_mix.py
deleted file mode 100644
index 4eb99cb96b423412d62a89575f2d69f1a88c24a7..0000000000000000000000000000000000000000
--- a/spaces/Androidonnxfork/CivitAi-to-Diffusers/diffusers/examples/community/magic_mix.py
+++ /dev/null
@@ -1,152 +0,0 @@
-from typing import Union
-
-import torch
-from PIL import Image
-from torchvision import transforms as tfms
-from tqdm.auto import tqdm
-from transformers import CLIPTextModel, CLIPTokenizer
-
-from diffusers import (
- AutoencoderKL,
- DDIMScheduler,
- DiffusionPipeline,
- LMSDiscreteScheduler,
- PNDMScheduler,
- UNet2DConditionModel,
-)
-
-
-class MagicMixPipeline(DiffusionPipeline):
- def __init__(
- self,
- vae: AutoencoderKL,
- text_encoder: CLIPTextModel,
- tokenizer: CLIPTokenizer,
- unet: UNet2DConditionModel,
- scheduler: Union[PNDMScheduler, LMSDiscreteScheduler, DDIMScheduler],
- ):
- super().__init__()
-
- self.register_modules(vae=vae, text_encoder=text_encoder, tokenizer=tokenizer, unet=unet, scheduler=scheduler)
-
- # convert PIL image to latents
- def encode(self, img):
- with torch.no_grad():
- latent = self.vae.encode(tfms.ToTensor()(img).unsqueeze(0).to(self.device) * 2 - 1)
- latent = 0.18215 * latent.latent_dist.sample()
- return latent
-
- # convert latents to PIL image
- def decode(self, latent):
- latent = (1 / 0.18215) * latent
- with torch.no_grad():
- img = self.vae.decode(latent).sample
- img = (img / 2 + 0.5).clamp(0, 1)
- img = img.detach().cpu().permute(0, 2, 3, 1).numpy()
- img = (img * 255).round().astype("uint8")
- return Image.fromarray(img[0])
-
- # convert prompt into text embeddings, also unconditional embeddings
- def prep_text(self, prompt):
- text_input = self.tokenizer(
- prompt,
- padding="max_length",
- max_length=self.tokenizer.model_max_length,
- truncation=True,
- return_tensors="pt",
- )
-
- text_embedding = self.text_encoder(text_input.input_ids.to(self.device))[0]
-
- uncond_input = self.tokenizer(
- "",
- padding="max_length",
- max_length=self.tokenizer.model_max_length,
- truncation=True,
- return_tensors="pt",
- )
-
- uncond_embedding = self.text_encoder(uncond_input.input_ids.to(self.device))[0]
-
- return torch.cat([uncond_embedding, text_embedding])
-
- def __call__(
- self,
- img: Image.Image,
- prompt: str,
- kmin: float = 0.3,
- kmax: float = 0.6,
- mix_factor: float = 0.5,
- seed: int = 42,
- steps: int = 50,
- guidance_scale: float = 7.5,
- ) -> Image.Image:
- tmin = steps - int(kmin * steps)
- tmax = steps - int(kmax * steps)
-
- text_embeddings = self.prep_text(prompt)
-
- self.scheduler.set_timesteps(steps)
-
- width, height = img.size
- encoded = self.encode(img)
-
- torch.manual_seed(seed)
- noise = torch.randn(
- (1, self.unet.config.in_channels, height // 8, width // 8),
- ).to(self.device)
-
- latents = self.scheduler.add_noise(
- encoded,
- noise,
- timesteps=self.scheduler.timesteps[tmax],
- )
-
- input = torch.cat([latents] * 2)
-
- input = self.scheduler.scale_model_input(input, self.scheduler.timesteps[tmax])
-
- with torch.no_grad():
- pred = self.unet(
- input,
- self.scheduler.timesteps[tmax],
- encoder_hidden_states=text_embeddings,
- ).sample
-
- pred_uncond, pred_text = pred.chunk(2)
- pred = pred_uncond + guidance_scale * (pred_text - pred_uncond)
-
- latents = self.scheduler.step(pred, self.scheduler.timesteps[tmax], latents).prev_sample
-
- for i, t in enumerate(tqdm(self.scheduler.timesteps)):
- if i > tmax:
- if i < tmin: # layout generation phase
- orig_latents = self.scheduler.add_noise(
- encoded,
- noise,
- timesteps=t,
- )
-
- input = (mix_factor * latents) + (
- 1 - mix_factor
- ) * orig_latents # interpolating between layout noise and conditionally generated noise to preserve layout sematics
- input = torch.cat([input] * 2)
-
- else: # content generation phase
- input = torch.cat([latents] * 2)
-
- input = self.scheduler.scale_model_input(input, t)
-
- with torch.no_grad():
- pred = self.unet(
- input,
- t,
- encoder_hidden_states=text_embeddings,
- ).sample
-
- pred_uncond, pred_text = pred.chunk(2)
- pred = pred_uncond + guidance_scale * (pred_text - pred_uncond)
-
- latents = self.scheduler.step(pred, t, latents).prev_sample
-
- return self.decode(latents)
diff --git a/spaces/Androidonnxfork/CivitAi-to-Diffusers/diffusers/examples/textual_inversion/README.md b/spaces/Androidonnxfork/CivitAi-to-Diffusers/diffusers/examples/textual_inversion/README.md
deleted file mode 100644
index 21bca526b5d2e55ee5dd6e4da3858fe66d649f9c..0000000000000000000000000000000000000000
--- a/spaces/Androidonnxfork/CivitAi-to-Diffusers/diffusers/examples/textual_inversion/README.md
+++ /dev/null
@@ -1,144 +0,0 @@
-## Textual Inversion fine-tuning example
-
-[Textual inversion](https://arxiv.org/abs/2208.01618) is a method to personalize text2image models like stable diffusion on your own images using just 3-5 examples.
-The `textual_inversion.py` script shows how to implement the training procedure and adapt it for stable diffusion.
-
-## Running on Colab
-
-Colab for training
-[](https://colab.research.google.com/github/huggingface/notebooks/blob/main/diffusers/sd_textual_inversion_training.ipynb)
-
-Colab for inference
-[](https://colab.research.google.com/github/huggingface/notebooks/blob/main/diffusers/stable_conceptualizer_inference.ipynb)
-
-## Running locally with PyTorch
-### Installing the dependencies
-
-Before running the scripts, make sure to install the library's training dependencies:
-
-**Important**
-
-To make sure you can successfully run the latest versions of the example scripts, we highly recommend **installing from source** and keeping the install up to date as we update the example scripts frequently and install some example-specific requirements. To do this, execute the following steps in a new virtual environment:
-```bash
-git clone https://github.com/huggingface/diffusers
-cd diffusers
-pip install .
-```
-
-Then cd in the example folder and run
-```bash
-pip install -r requirements.txt
-```
-
-And initialize an [🤗Accelerate](https://github.com/huggingface/accelerate/) environment with:
-
-```bash
-accelerate config
-```
-
-### Cat toy example
-
-First, let's login so that we can upload the checkpoint to the Hub during training:
-
-```bash
-huggingface-cli login
-```
-
-Now let's get our dataset. For this example we will use some cat images: https://huggingface.co/datasets/diffusers/cat_toy_example .
-
-Let's first download it locally:
-
-```py
-from huggingface_hub import snapshot_download
-
-local_dir = "./cat"
-snapshot_download("diffusers/cat_toy_example", local_dir=local_dir, repo_type="dataset", ignore_patterns=".gitattributes")
-```
-
-This will be our training data.
-Now we can launch the training using
-
-**___Note: Change the `resolution` to 768 if you are using the [stable-diffusion-2](https://huggingface.co/stabilityai/stable-diffusion-2) 768x768 model.___**
-
-```bash
-export MODEL_NAME="runwayml/stable-diffusion-v1-5"
-export DATA_DIR="./cat"
-
-accelerate launch textual_inversion.py \
- --pretrained_model_name_or_path=$MODEL_NAME \
- --train_data_dir=$DATA_DIR \
- --learnable_property="object" \
- --placeholder_token="" --initializer_token="toy" \
- --resolution=512 \
- --train_batch_size=1 \
- --gradient_accumulation_steps=4 \
- --max_train_steps=3000 \
- --learning_rate=5.0e-04 --scale_lr \
- --lr_scheduler="constant" \
- --lr_warmup_steps=0 \
- --push_to_hub \
- --output_dir="textual_inversion_cat"
-```
-
-A full training run takes ~1 hour on one V100 GPU.
-
-**Note**: As described in [the official paper](https://arxiv.org/abs/2208.01618)
-only one embedding vector is used for the placeholder token, *e.g.* `""`.
-However, one can also add multiple embedding vectors for the placeholder token
-to inclease the number of fine-tuneable parameters. This can help the model to learn
-more complex details. To use multiple embedding vectors, you can should define `--num_vectors`
-to a number larger than one, *e.g.*:
-```
---num_vectors 5
-```
-
-The saved textual inversion vectors will then be larger in size compared to the default case.
-
-### Inference
-
-Once you have trained a model using above command, the inference can be done simply using the `StableDiffusionPipeline`. Make sure to include the `placeholder_token` in your prompt.
-
-```python
-from diffusers import StableDiffusionPipeline
-import torch
-
-model_id = "path-to-your-trained-model"
-pipe = StableDiffusionPipeline.from_pretrained(model_id,torch_dtype=torch.float16).to("cuda")
-
-prompt = "A backpack"
-
-image = pipe(prompt, num_inference_steps=50, guidance_scale=7.5).images[0]
-
-image.save("cat-backpack.png")
-```
-
-
-## Training with Flax/JAX
-
-For faster training on TPUs and GPUs you can leverage the flax training example. Follow the instructions above to get the model and dataset before running the script.
-
-Before running the scripts, make sure to install the library's training dependencies:
-
-```bash
-pip install -U -r requirements_flax.txt
-```
-
-```bash
-export MODEL_NAME="duongna/stable-diffusion-v1-4-flax"
-export DATA_DIR="path-to-dir-containing-images"
-
-python textual_inversion_flax.py \
- --pretrained_model_name_or_path=$MODEL_NAME \
- --train_data_dir=$DATA_DIR \
- --learnable_property="object" \
- --placeholder_token="" --initializer_token="toy" \
- --resolution=512 \
- --train_batch_size=1 \
- --max_train_steps=3000 \
- --learning_rate=5.0e-04 --scale_lr \
- --output_dir="textual_inversion_cat"
-```
-It should be at least 70% faster than the PyTorch script with the same configuration.
-
-### Training with xformers:
-You can enable memory efficient attention by [installing xFormers](https://github.com/facebookresearch/xformers#installing-xformers) and padding the `--enable_xformers_memory_efficient_attention` argument to the script. This is not available with the Flax/JAX implementation.
diff --git a/spaces/Androidonnxfork/CivitAi-to-Diffusers/diffusers/src/diffusers/experimental/__init__.py b/spaces/Androidonnxfork/CivitAi-to-Diffusers/diffusers/src/diffusers/experimental/__init__.py
deleted file mode 100644
index ebc8155403016dfd8ad7fb78d246f9da9098ac50..0000000000000000000000000000000000000000
--- a/spaces/Androidonnxfork/CivitAi-to-Diffusers/diffusers/src/diffusers/experimental/__init__.py
+++ /dev/null
@@ -1 +0,0 @@
-from .rl import ValueGuidedRLPipeline
diff --git a/spaces/Andy1621/uniformer_image_detection/mmdet/datasets/pipelines/formating.py b/spaces/Andy1621/uniformer_image_detection/mmdet/datasets/pipelines/formating.py
deleted file mode 100644
index 5781341bd48766a740f23ebba7a85cf8993642d7..0000000000000000000000000000000000000000
--- a/spaces/Andy1621/uniformer_image_detection/mmdet/datasets/pipelines/formating.py
+++ /dev/null
@@ -1,364 +0,0 @@
-from collections.abc import Sequence
-
-import mmcv
-import numpy as np
-import torch
-from mmcv.parallel import DataContainer as DC
-
-from ..builder import PIPELINES
-
-
-def to_tensor(data):
- """Convert objects of various python types to :obj:`torch.Tensor`.
-
- Supported types are: :class:`numpy.ndarray`, :class:`torch.Tensor`,
- :class:`Sequence`, :class:`int` and :class:`float`.
-
- Args:
- data (torch.Tensor | numpy.ndarray | Sequence | int | float): Data to
- be converted.
- """
-
- if isinstance(data, torch.Tensor):
- return data
- elif isinstance(data, np.ndarray):
- return torch.from_numpy(data)
- elif isinstance(data, Sequence) and not mmcv.is_str(data):
- return torch.tensor(data)
- elif isinstance(data, int):
- return torch.LongTensor([data])
- elif isinstance(data, float):
- return torch.FloatTensor([data])
- else:
- raise TypeError(f'type {type(data)} cannot be converted to tensor.')
-
-
-@PIPELINES.register_module()
-class ToTensor(object):
- """Convert some results to :obj:`torch.Tensor` by given keys.
-
- Args:
- keys (Sequence[str]): Keys that need to be converted to Tensor.
- """
-
- def __init__(self, keys):
- self.keys = keys
-
- def __call__(self, results):
- """Call function to convert data in results to :obj:`torch.Tensor`.
-
- Args:
- results (dict): Result dict contains the data to convert.
-
- Returns:
- dict: The result dict contains the data converted
- to :obj:`torch.Tensor`.
- """
- for key in self.keys:
- results[key] = to_tensor(results[key])
- return results
-
- def __repr__(self):
- return self.__class__.__name__ + f'(keys={self.keys})'
-
-
-@PIPELINES.register_module()
-class ImageToTensor(object):
- """Convert image to :obj:`torch.Tensor` by given keys.
-
- The dimension order of input image is (H, W, C). The pipeline will convert
- it to (C, H, W). If only 2 dimension (H, W) is given, the output would be
- (1, H, W).
-
- Args:
- keys (Sequence[str]): Key of images to be converted to Tensor.
- """
-
- def __init__(self, keys):
- self.keys = keys
-
- def __call__(self, results):
- """Call function to convert image in results to :obj:`torch.Tensor` and
- transpose the channel order.
-
- Args:
- results (dict): Result dict contains the image data to convert.
-
- Returns:
- dict: The result dict contains the image converted
- to :obj:`torch.Tensor` and transposed to (C, H, W) order.
- """
- for key in self.keys:
- img = results[key]
- if len(img.shape) < 3:
- img = np.expand_dims(img, -1)
- results[key] = to_tensor(img.transpose(2, 0, 1))
- return results
-
- def __repr__(self):
- return self.__class__.__name__ + f'(keys={self.keys})'
-
-
-@PIPELINES.register_module()
-class Transpose(object):
- """Transpose some results by given keys.
-
- Args:
- keys (Sequence[str]): Keys of results to be transposed.
- order (Sequence[int]): Order of transpose.
- """
-
- def __init__(self, keys, order):
- self.keys = keys
- self.order = order
-
- def __call__(self, results):
- """Call function to transpose the channel order of data in results.
-
- Args:
- results (dict): Result dict contains the data to transpose.
-
- Returns:
- dict: The result dict contains the data transposed to \
- ``self.order``.
- """
- for key in self.keys:
- results[key] = results[key].transpose(self.order)
- return results
-
- def __repr__(self):
- return self.__class__.__name__ + \
- f'(keys={self.keys}, order={self.order})'
-
-
-@PIPELINES.register_module()
-class ToDataContainer(object):
- """Convert results to :obj:`mmcv.DataContainer` by given fields.
-
- Args:
- fields (Sequence[dict]): Each field is a dict like
- ``dict(key='xxx', **kwargs)``. The ``key`` in result will
- be converted to :obj:`mmcv.DataContainer` with ``**kwargs``.
- Default: ``(dict(key='img', stack=True), dict(key='gt_bboxes'),
- dict(key='gt_labels'))``.
- """
-
- def __init__(self,
- fields=(dict(key='img', stack=True), dict(key='gt_bboxes'),
- dict(key='gt_labels'))):
- self.fields = fields
-
- def __call__(self, results):
- """Call function to convert data in results to
- :obj:`mmcv.DataContainer`.
-
- Args:
- results (dict): Result dict contains the data to convert.
-
- Returns:
- dict: The result dict contains the data converted to \
- :obj:`mmcv.DataContainer`.
- """
-
- for field in self.fields:
- field = field.copy()
- key = field.pop('key')
- results[key] = DC(results[key], **field)
- return results
-
- def __repr__(self):
- return self.__class__.__name__ + f'(fields={self.fields})'
-
-
-@PIPELINES.register_module()
-class DefaultFormatBundle(object):
- """Default formatting bundle.
-
- It simplifies the pipeline of formatting common fields, including "img",
- "proposals", "gt_bboxes", "gt_labels", "gt_masks" and "gt_semantic_seg".
- These fields are formatted as follows.
-
- - img: (1)transpose, (2)to tensor, (3)to DataContainer (stack=True)
- - proposals: (1)to tensor, (2)to DataContainer
- - gt_bboxes: (1)to tensor, (2)to DataContainer
- - gt_bboxes_ignore: (1)to tensor, (2)to DataContainer
- - gt_labels: (1)to tensor, (2)to DataContainer
- - gt_masks: (1)to tensor, (2)to DataContainer (cpu_only=True)
- - gt_semantic_seg: (1)unsqueeze dim-0 (2)to tensor, \
- (3)to DataContainer (stack=True)
- """
-
- def __call__(self, results):
- """Call function to transform and format common fields in results.
-
- Args:
- results (dict): Result dict contains the data to convert.
-
- Returns:
- dict: The result dict contains the data that is formatted with \
- default bundle.
- """
-
- if 'img' in results:
- img = results['img']
- # add default meta keys
- results = self._add_default_meta_keys(results)
- if len(img.shape) < 3:
- img = np.expand_dims(img, -1)
- img = np.ascontiguousarray(img.transpose(2, 0, 1))
- results['img'] = DC(to_tensor(img), stack=True)
- for key in ['proposals', 'gt_bboxes', 'gt_bboxes_ignore', 'gt_labels']:
- if key not in results:
- continue
- results[key] = DC(to_tensor(results[key]))
- if 'gt_masks' in results:
- results['gt_masks'] = DC(results['gt_masks'], cpu_only=True)
- if 'gt_semantic_seg' in results:
- results['gt_semantic_seg'] = DC(
- to_tensor(results['gt_semantic_seg'][None, ...]), stack=True)
- return results
-
- def _add_default_meta_keys(self, results):
- """Add default meta keys.
-
- We set default meta keys including `pad_shape`, `scale_factor` and
- `img_norm_cfg` to avoid the case where no `Resize`, `Normalize` and
- `Pad` are implemented during the whole pipeline.
-
- Args:
- results (dict): Result dict contains the data to convert.
-
- Returns:
- results (dict): Updated result dict contains the data to convert.
- """
- img = results['img']
- results.setdefault('pad_shape', img.shape)
- results.setdefault('scale_factor', 1.0)
- num_channels = 1 if len(img.shape) < 3 else img.shape[2]
- results.setdefault(
- 'img_norm_cfg',
- dict(
- mean=np.zeros(num_channels, dtype=np.float32),
- std=np.ones(num_channels, dtype=np.float32),
- to_rgb=False))
- return results
-
- def __repr__(self):
- return self.__class__.__name__
-
-
-@PIPELINES.register_module()
-class Collect(object):
- """Collect data from the loader relevant to the specific task.
-
- This is usually the last stage of the data loader pipeline. Typically keys
- is set to some subset of "img", "proposals", "gt_bboxes",
- "gt_bboxes_ignore", "gt_labels", and/or "gt_masks".
-
- The "img_meta" item is always populated. The contents of the "img_meta"
- dictionary depends on "meta_keys". By default this includes:
-
- - "img_shape": shape of the image input to the network as a tuple \
- (h, w, c). Note that images may be zero padded on the \
- bottom/right if the batch tensor is larger than this shape.
-
- - "scale_factor": a float indicating the preprocessing scale
-
- - "flip": a boolean indicating if image flip transform was used
-
- - "filename": path to the image file
-
- - "ori_shape": original shape of the image as a tuple (h, w, c)
-
- - "pad_shape": image shape after padding
-
- - "img_norm_cfg": a dict of normalization information:
-
- - mean - per channel mean subtraction
- - std - per channel std divisor
- - to_rgb - bool indicating if bgr was converted to rgb
-
- Args:
- keys (Sequence[str]): Keys of results to be collected in ``data``.
- meta_keys (Sequence[str], optional): Meta keys to be converted to
- ``mmcv.DataContainer`` and collected in ``data[img_metas]``.
- Default: ``('filename', 'ori_filename', 'ori_shape', 'img_shape',
- 'pad_shape', 'scale_factor', 'flip', 'flip_direction',
- 'img_norm_cfg')``
- """
-
- def __init__(self,
- keys,
- meta_keys=('filename', 'ori_filename', 'ori_shape',
- 'img_shape', 'pad_shape', 'scale_factor', 'flip',
- 'flip_direction', 'img_norm_cfg')):
- self.keys = keys
- self.meta_keys = meta_keys
-
- def __call__(self, results):
- """Call function to collect keys in results. The keys in ``meta_keys``
- will be converted to :obj:mmcv.DataContainer.
-
- Args:
- results (dict): Result dict contains the data to collect.
-
- Returns:
- dict: The result dict contains the following keys
-
- - keys in``self.keys``
- - ``img_metas``
- """
-
- data = {}
- img_meta = {}
- for key in self.meta_keys:
- img_meta[key] = results[key]
- data['img_metas'] = DC(img_meta, cpu_only=True)
- for key in self.keys:
- data[key] = results[key]
- return data
-
- def __repr__(self):
- return self.__class__.__name__ + \
- f'(keys={self.keys}, meta_keys={self.meta_keys})'
-
-
-@PIPELINES.register_module()
-class WrapFieldsToLists(object):
- """Wrap fields of the data dictionary into lists for evaluation.
-
- This class can be used as a last step of a test or validation
- pipeline for single image evaluation or inference.
-
- Example:
- >>> test_pipeline = [
- >>> dict(type='LoadImageFromFile'),
- >>> dict(type='Normalize',
- mean=[123.675, 116.28, 103.53],
- std=[58.395, 57.12, 57.375],
- to_rgb=True),
- >>> dict(type='Pad', size_divisor=32),
- >>> dict(type='ImageToTensor', keys=['img']),
- >>> dict(type='Collect', keys=['img']),
- >>> dict(type='WrapFieldsToLists')
- >>> ]
- """
-
- def __call__(self, results):
- """Call function to wrap fields into lists.
-
- Args:
- results (dict): Result dict contains the data to wrap.
-
- Returns:
- dict: The result dict where value of ``self.keys`` are wrapped \
- into list.
- """
-
- # Wrap dict fields into lists
- for key, val in results.items():
- results[key] = [val]
- return results
-
- def __repr__(self):
- return f'{self.__class__.__name__}()'
diff --git a/spaces/Andy1621/uniformer_image_segmentation/configs/danet/danet_r50-d8_512x512_20k_voc12aug.py b/spaces/Andy1621/uniformer_image_segmentation/configs/danet/danet_r50-d8_512x512_20k_voc12aug.py
deleted file mode 100644
index 010f86f1aac1b5c827dec29f692d137dc1c399bf..0000000000000000000000000000000000000000
--- a/spaces/Andy1621/uniformer_image_segmentation/configs/danet/danet_r50-d8_512x512_20k_voc12aug.py
+++ /dev/null
@@ -1,7 +0,0 @@
-_base_ = [
- '../_base_/models/danet_r50-d8.py',
- '../_base_/datasets/pascal_voc12_aug.py', '../_base_/default_runtime.py',
- '../_base_/schedules/schedule_20k.py'
-]
-model = dict(
- decode_head=dict(num_classes=21), auxiliary_head=dict(num_classes=21))
diff --git a/spaces/Andy1621/uniformer_image_segmentation/configs/psanet/psanet_r101-d8_512x1024_40k_cityscapes.py b/spaces/Andy1621/uniformer_image_segmentation/configs/psanet/psanet_r101-d8_512x1024_40k_cityscapes.py
deleted file mode 100644
index 69d212f158552cf5a24f62174b24a9d4976477bb..0000000000000000000000000000000000000000
--- a/spaces/Andy1621/uniformer_image_segmentation/configs/psanet/psanet_r101-d8_512x1024_40k_cityscapes.py
+++ /dev/null
@@ -1,2 +0,0 @@
-_base_ = './psanet_r50-d8_512x1024_40k_cityscapes.py'
-model = dict(pretrained='open-mmlab://resnet101_v1c', backbone=dict(depth=101))
diff --git a/spaces/Ank0X0/Image-Upscaling-Playground/README.md b/spaces/Ank0X0/Image-Upscaling-Playground/README.md
deleted file mode 100644
index 1f50c61d45b587526bf15f6a71d29dea53aaab7a..0000000000000000000000000000000000000000
--- a/spaces/Ank0X0/Image-Upscaling-Playground/README.md
+++ /dev/null
@@ -1,14 +0,0 @@
----
-title: Image Upscaling Playground
-emoji: 🦆
-colorFrom: green
-colorTo: yellow
-sdk: gradio
-sdk_version: 3.4.1
-app_file: app.py
-pinned: false
-license: apache-2.0
-duplicated_from: bookbot/Image-Upscaling-Playground
----
-
-Check out the configuration reference at https://huggingface.co/docs/hub/spaces#reference
diff --git a/spaces/AnnasBlackHat/Image-Similarity/src/util/matrix.py b/spaces/AnnasBlackHat/Image-Similarity/src/util/matrix.py
deleted file mode 100644
index 439fb6b9e8157bc6fa9bcf93ba1f6de3ae176a2e..0000000000000000000000000000000000000000
--- a/spaces/AnnasBlackHat/Image-Similarity/src/util/matrix.py
+++ /dev/null
@@ -1,5 +0,0 @@
-from numpy.linalg import norm
-import numpy as np
-
-def cosine(x, y):
- return np.dot(x,y)/(norm(x)*norm(y))
\ No newline at end of file
diff --git a/spaces/Anonymous-sub/Rerender/ControlNet/annotator/uniformer/mmcv/utils/trace.py b/spaces/Anonymous-sub/Rerender/ControlNet/annotator/uniformer/mmcv/utils/trace.py
deleted file mode 100644
index 5ca99dc3eda05ef980d9a4249b50deca8273b6cc..0000000000000000000000000000000000000000
--- a/spaces/Anonymous-sub/Rerender/ControlNet/annotator/uniformer/mmcv/utils/trace.py
+++ /dev/null
@@ -1,23 +0,0 @@
-import warnings
-
-import torch
-
-from annotator.uniformer.mmcv.utils import digit_version
-
-
-def is_jit_tracing() -> bool:
- if (torch.__version__ != 'parrots'
- and digit_version(torch.__version__) >= digit_version('1.6.0')):
- on_trace = torch.jit.is_tracing()
- # In PyTorch 1.6, torch.jit.is_tracing has a bug.
- # Refers to https://github.com/pytorch/pytorch/issues/42448
- if isinstance(on_trace, bool):
- return on_trace
- else:
- return torch._C._is_tracing()
- else:
- warnings.warn(
- 'torch.jit.is_tracing is only supported after v1.6.0. '
- 'Therefore is_tracing returns False automatically. Please '
- 'set on_trace manually if you are using trace.', UserWarning)
- return False
diff --git a/spaces/Arthur678/vits-uma-genshin-honkai/text/symbols.py b/spaces/Arthur678/vits-uma-genshin-honkai/text/symbols.py
deleted file mode 100644
index edfbd24247be8c757275ce80b9ec27a0ffa808f3..0000000000000000000000000000000000000000
--- a/spaces/Arthur678/vits-uma-genshin-honkai/text/symbols.py
+++ /dev/null
@@ -1,39 +0,0 @@
-'''
-Defines the set of symbols used in text input to the model.
-'''
-
-'''# japanese_cleaners
-_pad = '_'
-_punctuation = ',.!?-'
-_letters = 'AEINOQUabdefghijkmnoprstuvwyzʃʧ↓↑ '
-'''
-
-'''# japanese_cleaners2
-_pad = '_'
-_punctuation = ',.!?-~…'
-_letters = 'AEINOQUabdefghijkmnoprstuvwyzʃʧʦ↓↑ '
-'''
-
-'''# korean_cleaners
-_pad = '_'
-_punctuation = ',.!?…~'
-_letters = 'ㄱㄴㄷㄹㅁㅂㅅㅇㅈㅊㅋㅌㅍㅎㄲㄸㅃㅆㅉㅏㅓㅗㅜㅡㅣㅐㅔ '
-'''
-
-'''# chinese_cleaners
-_pad = '_'
-_punctuation = ',。!?—…'
-_letters = 'ㄅㄆㄇㄈㄉㄊㄋㄌㄍㄎㄏㄐㄑㄒㄓㄔㄕㄖㄗㄘㄙㄚㄛㄜㄝㄞㄟㄠㄡㄢㄣㄤㄥㄦㄧㄨㄩˉˊˇˋ˙ '
-'''
-
-# zh_ja_mixture_cleaners
-_pad = '_'
-_punctuation = ',.!?-~…'
-_letters = 'AEINOQUabdefghijklmnoprstuvwyzʃʧʦɯɹəɥ⁼ʰ`→↓↑ '
-
-
-# Export all symbols:
-symbols = [_pad] + list(_punctuation) + list(_letters)
-
-# Special symbol ids
-SPACE_ID = symbols.index(" ")
\ No newline at end of file
diff --git a/spaces/Awiny/Image2Paragraph/models/grit_src/third_party/CenterNet2/detectron2/engine/defaults.py b/spaces/Awiny/Image2Paragraph/models/grit_src/third_party/CenterNet2/detectron2/engine/defaults.py
deleted file mode 100644
index cc3faa15550a348dbe1445f7c7c91b26ba59d01b..0000000000000000000000000000000000000000
--- a/spaces/Awiny/Image2Paragraph/models/grit_src/third_party/CenterNet2/detectron2/engine/defaults.py
+++ /dev/null
@@ -1,715 +0,0 @@
-# -*- coding: utf-8 -*-
-# Copyright (c) Facebook, Inc. and its affiliates.
-
-"""
-This file contains components with some default boilerplate logic user may need
-in training / testing. They will not work for everyone, but many users may find them useful.
-
-The behavior of functions/classes in this file is subject to change,
-since they are meant to represent the "common default behavior" people need in their projects.
-"""
-
-import argparse
-import logging
-import os
-import sys
-import weakref
-from collections import OrderedDict
-from typing import Optional
-import torch
-from fvcore.nn.precise_bn import get_bn_modules
-from omegaconf import OmegaConf
-from torch.nn.parallel import DistributedDataParallel
-
-import detectron2.data.transforms as T
-from detectron2.checkpoint import DetectionCheckpointer
-from detectron2.config import CfgNode, LazyConfig
-from detectron2.data import (
- MetadataCatalog,
- build_detection_test_loader,
- build_detection_train_loader,
-)
-from detectron2.evaluation import (
- DatasetEvaluator,
- inference_on_dataset,
- print_csv_format,
- verify_results,
-)
-from detectron2.modeling import build_model
-from detectron2.solver import build_lr_scheduler, build_optimizer
-from detectron2.utils import comm
-from detectron2.utils.collect_env import collect_env_info
-from detectron2.utils.env import seed_all_rng
-from detectron2.utils.events import CommonMetricPrinter, JSONWriter, TensorboardXWriter
-from detectron2.utils.file_io import PathManager
-from detectron2.utils.logger import setup_logger
-
-from . import hooks
-from .train_loop import AMPTrainer, SimpleTrainer, TrainerBase
-
-__all__ = [
- "create_ddp_model",
- "default_argument_parser",
- "default_setup",
- "default_writers",
- "DefaultPredictor",
- "DefaultTrainer",
-]
-
-
-def create_ddp_model(model, *, fp16_compression=False, **kwargs):
- """
- Create a DistributedDataParallel model if there are >1 processes.
-
- Args:
- model: a torch.nn.Module
- fp16_compression: add fp16 compression hooks to the ddp object.
- See more at https://pytorch.org/docs/stable/ddp_comm_hooks.html#torch.distributed.algorithms.ddp_comm_hooks.default_hooks.fp16_compress_hook
- kwargs: other arguments of :module:`torch.nn.parallel.DistributedDataParallel`.
- """ # noqa
- if comm.get_world_size() == 1:
- return model
- if "device_ids" not in kwargs:
- kwargs["device_ids"] = [comm.get_local_rank()]
- ddp = DistributedDataParallel(model, **kwargs)
- if fp16_compression:
- from torch.distributed.algorithms.ddp_comm_hooks import default as comm_hooks
-
- ddp.register_comm_hook(state=None, hook=comm_hooks.fp16_compress_hook)
- return ddp
-
-
-def default_argument_parser(epilog=None):
- """
- Create a parser with some common arguments used by detectron2 users.
-
- Args:
- epilog (str): epilog passed to ArgumentParser describing the usage.
-
- Returns:
- argparse.ArgumentParser:
- """
- parser = argparse.ArgumentParser(
- epilog=epilog
- or f"""
-Examples:
-
-Run on single machine:
- $ {sys.argv[0]} --num-gpus 8 --config-file cfg.yaml
-
-Change some config options:
- $ {sys.argv[0]} --config-file cfg.yaml MODEL.WEIGHTS /path/to/weight.pth SOLVER.BASE_LR 0.001
-
-Run on multiple machines:
- (machine0)$ {sys.argv[0]} --machine-rank 0 --num-machines 2 --dist-url [--other-flags]
- (machine1)$ {sys.argv[0]} --machine-rank 1 --num-machines 2 --dist-url [--other-flags]
-""",
- formatter_class=argparse.RawDescriptionHelpFormatter,
- )
- parser.add_argument("--config-file", default="", metavar="FILE", help="path to config file")
- parser.add_argument(
- "--resume",
- action="store_true",
- help="Whether to attempt to resume from the checkpoint directory. "
- "See documentation of `DefaultTrainer.resume_or_load()` for what it means.",
- )
- parser.add_argument("--eval-only", action="store_true", help="perform evaluation only")
- parser.add_argument("--num-gpus", type=int, default=1, help="number of gpus *per machine*")
- parser.add_argument("--num-machines", type=int, default=1, help="total number of machines")
- parser.add_argument(
- "--machine-rank", type=int, default=0, help="the rank of this machine (unique per machine)"
- )
-
- # PyTorch still may leave orphan processes in multi-gpu training.
- # Therefore we use a deterministic way to obtain port,
- # so that users are aware of orphan processes by seeing the port occupied.
- port = 2 ** 15 + 2 ** 14 + hash(os.getuid() if sys.platform != "win32" else 1) % 2 ** 14
- parser.add_argument(
- "--dist-url",
- default="tcp://127.0.0.1:{}".format(port),
- help="initialization URL for pytorch distributed backend. See "
- "https://pytorch.org/docs/stable/distributed.html for details.",
- )
- parser.add_argument(
- "opts",
- help="""
-Modify config options at the end of the command. For Yacs configs, use
-space-separated "PATH.KEY VALUE" pairs.
-For python-based LazyConfig, use "path.key=value".
- """.strip(),
- default=None,
- nargs=argparse.REMAINDER,
- )
- return parser
-
-
-def _try_get_key(cfg, *keys, default=None):
- """
- Try select keys from cfg until the first key that exists. Otherwise return default.
- """
- if isinstance(cfg, CfgNode):
- cfg = OmegaConf.create(cfg.dump())
- for k in keys:
- none = object()
- p = OmegaConf.select(cfg, k, default=none)
- if p is not none:
- return p
- return default
-
-
-def _highlight(code, filename):
- try:
- import pygments
- except ImportError:
- return code
-
- from pygments.lexers import Python3Lexer, YamlLexer
- from pygments.formatters import Terminal256Formatter
-
- lexer = Python3Lexer() if filename.endswith(".py") else YamlLexer()
- code = pygments.highlight(code, lexer, Terminal256Formatter(style="monokai"))
- return code
-
-
-def default_setup(cfg, args):
- """
- Perform some basic common setups at the beginning of a job, including:
-
- 1. Set up the detectron2 logger
- 2. Log basic information about environment, cmdline arguments, and config
- 3. Backup the config to the output directory
-
- Args:
- cfg (CfgNode or omegaconf.DictConfig): the full config to be used
- args (argparse.NameSpace): the command line arguments to be logged
- """
- output_dir = _try_get_key(cfg, "OUTPUT_DIR", "output_dir", "train.output_dir")
- if comm.is_main_process() and output_dir:
- PathManager.mkdirs(output_dir)
-
- rank = comm.get_rank()
- setup_logger(output_dir, distributed_rank=rank, name="fvcore")
- logger = setup_logger(output_dir, distributed_rank=rank)
-
- logger.info("Rank of current process: {}. World size: {}".format(rank, comm.get_world_size()))
- logger.info("Environment info:\n" + collect_env_info())
-
- logger.info("Command line arguments: " + str(args))
- if hasattr(args, "config_file") and args.config_file != "":
- logger.info(
- "Contents of args.config_file={}:\n{}".format(
- args.config_file,
- _highlight(PathManager.open(args.config_file, "r").read(), args.config_file),
- )
- )
-
- if comm.is_main_process() and output_dir:
- # Note: some of our scripts may expect the existence of
- # config.yaml in output directory
- path = os.path.join(output_dir, "config.yaml")
- if isinstance(cfg, CfgNode):
- logger.info("Running with full config:\n{}".format(_highlight(cfg.dump(), ".yaml")))
- with PathManager.open(path, "w") as f:
- f.write(cfg.dump())
- else:
- LazyConfig.save(cfg, path)
- logger.info("Full config saved to {}".format(path))
-
- # make sure each worker has a different, yet deterministic seed if specified
- seed = _try_get_key(cfg, "SEED", "train.seed", default=-1)
- seed_all_rng(None if seed < 0 else seed + rank)
-
- # cudnn benchmark has large overhead. It shouldn't be used considering the small size of
- # typical validation set.
- if not (hasattr(args, "eval_only") and args.eval_only):
- torch.backends.cudnn.benchmark = _try_get_key(
- cfg, "CUDNN_BENCHMARK", "train.cudnn_benchmark", default=False
- )
-
-
-def default_writers(output_dir: str, max_iter: Optional[int] = None):
- """
- Build a list of :class:`EventWriter` to be used.
- It now consists of a :class:`CommonMetricPrinter`,
- :class:`TensorboardXWriter` and :class:`JSONWriter`.
-
- Args:
- output_dir: directory to store JSON metrics and tensorboard events
- max_iter: the total number of iterations
-
- Returns:
- list[EventWriter]: a list of :class:`EventWriter` objects.
- """
- PathManager.mkdirs(output_dir)
- return [
- # It may not always print what you want to see, since it prints "common" metrics only.
- CommonMetricPrinter(max_iter),
- JSONWriter(os.path.join(output_dir, "metrics.json")),
- TensorboardXWriter(output_dir),
- ]
-
-
-class DefaultPredictor:
- """
- Create a simple end-to-end predictor with the given config that runs on
- single device for a single input image.
-
- Compared to using the model directly, this class does the following additions:
-
- 1. Load checkpoint from `cfg.MODEL.WEIGHTS`.
- 2. Always take BGR image as the input and apply conversion defined by `cfg.INPUT.FORMAT`.
- 3. Apply resizing defined by `cfg.INPUT.{MIN,MAX}_SIZE_TEST`.
- 4. Take one input image and produce a single output, instead of a batch.
-
- This is meant for simple demo purposes, so it does the above steps automatically.
- This is not meant for benchmarks or running complicated inference logic.
- If you'd like to do anything more complicated, please refer to its source code as
- examples to build and use the model manually.
-
- Attributes:
- metadata (Metadata): the metadata of the underlying dataset, obtained from
- cfg.DATASETS.TEST.
-
- Examples:
- ::
- pred = DefaultPredictor(cfg)
- inputs = cv2.imread("input.jpg")
- outputs = pred(inputs)
- """
-
- def __init__(self, cfg):
- self.cfg = cfg.clone() # cfg can be modified by model
- self.model = build_model(self.cfg)
- self.model.eval()
- if len(cfg.DATASETS.TEST):
- self.metadata = MetadataCatalog.get(cfg.DATASETS.TEST[0])
-
- checkpointer = DetectionCheckpointer(self.model)
- checkpointer.load(cfg.MODEL.WEIGHTS)
-
- self.aug = T.ResizeShortestEdge(
- [cfg.INPUT.MIN_SIZE_TEST, cfg.INPUT.MIN_SIZE_TEST], cfg.INPUT.MAX_SIZE_TEST
- )
-
- self.input_format = cfg.INPUT.FORMAT
- assert self.input_format in ["RGB", "BGR"], self.input_format
-
- def __call__(self, original_image):
- """
- Args:
- original_image (np.ndarray): an image of shape (H, W, C) (in BGR order).
-
- Returns:
- predictions (dict):
- the output of the model for one image only.
- See :doc:`/tutorials/models` for details about the format.
- """
- with torch.no_grad(): # https://github.com/sphinx-doc/sphinx/issues/4258
- # Apply pre-processing to image.
- if self.input_format == "RGB":
- # whether the model expects BGR inputs or RGB
- original_image = original_image[:, :, ::-1]
- height, width = original_image.shape[:2]
- image = self.aug.get_transform(original_image).apply_image(original_image)
- image = torch.as_tensor(image.astype("float32").transpose(2, 0, 1))
-
- inputs = {"image": image, "height": height, "width": width}
- predictions = self.model([inputs])[0]
- return predictions
-
-
-class DefaultTrainer(TrainerBase):
- """
- A trainer with default training logic. It does the following:
-
- 1. Create a :class:`SimpleTrainer` using model, optimizer, dataloader
- defined by the given config. Create a LR scheduler defined by the config.
- 2. Load the last checkpoint or `cfg.MODEL.WEIGHTS`, if exists, when
- `resume_or_load` is called.
- 3. Register a few common hooks defined by the config.
-
- It is created to simplify the **standard model training workflow** and reduce code boilerplate
- for users who only need the standard training workflow, with standard features.
- It means this class makes *many assumptions* about your training logic that
- may easily become invalid in a new research. In fact, any assumptions beyond those made in the
- :class:`SimpleTrainer` are too much for research.
-
- The code of this class has been annotated about restrictive assumptions it makes.
- When they do not work for you, you're encouraged to:
-
- 1. Overwrite methods of this class, OR:
- 2. Use :class:`SimpleTrainer`, which only does minimal SGD training and
- nothing else. You can then add your own hooks if needed. OR:
- 3. Write your own training loop similar to `tools/plain_train_net.py`.
-
- See the :doc:`/tutorials/training` tutorials for more details.
-
- Note that the behavior of this class, like other functions/classes in
- this file, is not stable, since it is meant to represent the "common default behavior".
- It is only guaranteed to work well with the standard models and training workflow in detectron2.
- To obtain more stable behavior, write your own training logic with other public APIs.
-
- Examples:
- ::
- trainer = DefaultTrainer(cfg)
- trainer.resume_or_load() # load last checkpoint or MODEL.WEIGHTS
- trainer.train()
-
- Attributes:
- scheduler:
- checkpointer (DetectionCheckpointer):
- cfg (CfgNode):
- """
-
- def __init__(self, cfg):
- """
- Args:
- cfg (CfgNode):
- """
- super().__init__()
- logger = logging.getLogger("detectron2")
- if not logger.isEnabledFor(logging.INFO): # setup_logger is not called for d2
- setup_logger()
- cfg = DefaultTrainer.auto_scale_workers(cfg, comm.get_world_size())
-
- # Assume these objects must be constructed in this order.
- model = self.build_model(cfg)
- optimizer = self.build_optimizer(cfg, model)
- data_loader = self.build_train_loader(cfg)
-
- model = create_ddp_model(model, broadcast_buffers=False)
- self._trainer = (AMPTrainer if cfg.SOLVER.AMP.ENABLED else SimpleTrainer)(
- model, data_loader, optimizer
- )
-
- self.scheduler = self.build_lr_scheduler(cfg, optimizer)
- self.checkpointer = DetectionCheckpointer(
- # Assume you want to save checkpoints together with logs/statistics
- model,
- cfg.OUTPUT_DIR,
- trainer=weakref.proxy(self),
- )
- self.start_iter = 0
- self.max_iter = cfg.SOLVER.MAX_ITER
- self.cfg = cfg
-
- self.register_hooks(self.build_hooks())
-
- def resume_or_load(self, resume=True):
- """
- If `resume==True` and `cfg.OUTPUT_DIR` contains the last checkpoint (defined by
- a `last_checkpoint` file), resume from the file. Resuming means loading all
- available states (eg. optimizer and scheduler) and update iteration counter
- from the checkpoint. ``cfg.MODEL.WEIGHTS`` will not be used.
-
- Otherwise, this is considered as an independent training. The method will load model
- weights from the file `cfg.MODEL.WEIGHTS` (but will not load other states) and start
- from iteration 0.
-
- Args:
- resume (bool): whether to do resume or not
- """
- self.checkpointer.resume_or_load(self.cfg.MODEL.WEIGHTS, resume=resume)
- if resume and self.checkpointer.has_checkpoint():
- # The checkpoint stores the training iteration that just finished, thus we start
- # at the next iteration
- self.start_iter = self.iter + 1
-
- def build_hooks(self):
- """
- Build a list of default hooks, including timing, evaluation,
- checkpointing, lr scheduling, precise BN, writing events.
-
- Returns:
- list[HookBase]:
- """
- cfg = self.cfg.clone()
- cfg.defrost()
- cfg.DATALOADER.NUM_WORKERS = 0 # save some memory and time for PreciseBN
-
- ret = [
- hooks.IterationTimer(),
- hooks.LRScheduler(),
- hooks.PreciseBN(
- # Run at the same freq as (but before) evaluation.
- cfg.TEST.EVAL_PERIOD,
- self.model,
- # Build a new data loader to not affect training
- self.build_train_loader(cfg),
- cfg.TEST.PRECISE_BN.NUM_ITER,
- )
- if cfg.TEST.PRECISE_BN.ENABLED and get_bn_modules(self.model)
- else None,
- ]
-
- # Do PreciseBN before checkpointer, because it updates the model and need to
- # be saved by checkpointer.
- # This is not always the best: if checkpointing has a different frequency,
- # some checkpoints may have more precise statistics than others.
- if comm.is_main_process():
- ret.append(hooks.PeriodicCheckpointer(self.checkpointer, cfg.SOLVER.CHECKPOINT_PERIOD))
-
- def test_and_save_results():
- self._last_eval_results = self.test(self.cfg, self.model)
- return self._last_eval_results
-
- # Do evaluation after checkpointer, because then if it fails,
- # we can use the saved checkpoint to debug.
- ret.append(hooks.EvalHook(cfg.TEST.EVAL_PERIOD, test_and_save_results))
-
- if comm.is_main_process():
- # Here the default print/log frequency of each writer is used.
- # run writers in the end, so that evaluation metrics are written
- ret.append(hooks.PeriodicWriter(self.build_writers(), period=20))
- return ret
-
- def build_writers(self):
- """
- Build a list of writers to be used using :func:`default_writers()`.
- If you'd like a different list of writers, you can overwrite it in
- your trainer.
-
- Returns:
- list[EventWriter]: a list of :class:`EventWriter` objects.
- """
- return default_writers(self.cfg.OUTPUT_DIR, self.max_iter)
-
- def train(self):
- """
- Run training.
-
- Returns:
- OrderedDict of results, if evaluation is enabled. Otherwise None.
- """
- super().train(self.start_iter, self.max_iter)
- if len(self.cfg.TEST.EXPECTED_RESULTS) and comm.is_main_process():
- assert hasattr(
- self, "_last_eval_results"
- ), "No evaluation results obtained during training!"
- verify_results(self.cfg, self._last_eval_results)
- return self._last_eval_results
-
- def run_step(self):
- self._trainer.iter = self.iter
- self._trainer.run_step()
-
- def state_dict(self):
- ret = super().state_dict()
- ret["_trainer"] = self._trainer.state_dict()
- return ret
-
- def load_state_dict(self, state_dict):
- super().load_state_dict(state_dict)
- self._trainer.load_state_dict(state_dict["_trainer"])
-
- @classmethod
- def build_model(cls, cfg):
- """
- Returns:
- torch.nn.Module:
-
- It now calls :func:`detectron2.modeling.build_model`.
- Overwrite it if you'd like a different model.
- """
- model = build_model(cfg)
- logger = logging.getLogger(__name__)
- logger.info("Model:\n{}".format(model))
- return model
-
- @classmethod
- def build_optimizer(cls, cfg, model):
- """
- Returns:
- torch.optim.Optimizer:
-
- It now calls :func:`detectron2.solver.build_optimizer`.
- Overwrite it if you'd like a different optimizer.
- """
- return build_optimizer(cfg, model)
-
- @classmethod
- def build_lr_scheduler(cls, cfg, optimizer):
- """
- It now calls :func:`detectron2.solver.build_lr_scheduler`.
- Overwrite it if you'd like a different scheduler.
- """
- return build_lr_scheduler(cfg, optimizer)
-
- @classmethod
- def build_train_loader(cls, cfg):
- """
- Returns:
- iterable
-
- It now calls :func:`detectron2.data.build_detection_train_loader`.
- Overwrite it if you'd like a different data loader.
- """
- return build_detection_train_loader(cfg)
-
- @classmethod
- def build_test_loader(cls, cfg, dataset_name):
- """
- Returns:
- iterable
-
- It now calls :func:`detectron2.data.build_detection_test_loader`.
- Overwrite it if you'd like a different data loader.
- """
- return build_detection_test_loader(cfg, dataset_name)
-
- @classmethod
- def build_evaluator(cls, cfg, dataset_name):
- """
- Returns:
- DatasetEvaluator or None
-
- It is not implemented by default.
- """
- raise NotImplementedError(
- """
-If you want DefaultTrainer to automatically run evaluation,
-please implement `build_evaluator()` in subclasses (see train_net.py for example).
-Alternatively, you can call evaluation functions yourself (see Colab balloon tutorial for example).
-"""
- )
-
- @classmethod
- def test(cls, cfg, model, evaluators=None):
- """
- Evaluate the given model. The given model is expected to already contain
- weights to evaluate.
-
- Args:
- cfg (CfgNode):
- model (nn.Module):
- evaluators (list[DatasetEvaluator] or None): if None, will call
- :meth:`build_evaluator`. Otherwise, must have the same length as
- ``cfg.DATASETS.TEST``.
-
- Returns:
- dict: a dict of result metrics
- """
- logger = logging.getLogger(__name__)
- if isinstance(evaluators, DatasetEvaluator):
- evaluators = [evaluators]
- if evaluators is not None:
- assert len(cfg.DATASETS.TEST) == len(evaluators), "{} != {}".format(
- len(cfg.DATASETS.TEST), len(evaluators)
- )
-
- results = OrderedDict()
- for idx, dataset_name in enumerate(cfg.DATASETS.TEST):
- data_loader = cls.build_test_loader(cfg, dataset_name)
- # When evaluators are passed in as arguments,
- # implicitly assume that evaluators can be created before data_loader.
- if evaluators is not None:
- evaluator = evaluators[idx]
- else:
- try:
- evaluator = cls.build_evaluator(cfg, dataset_name)
- except NotImplementedError:
- logger.warn(
- "No evaluator found. Use `DefaultTrainer.test(evaluators=)`, "
- "or implement its `build_evaluator` method."
- )
- results[dataset_name] = {}
- continue
- results_i = inference_on_dataset(model, data_loader, evaluator)
- results[dataset_name] = results_i
- if comm.is_main_process():
- assert isinstance(
- results_i, dict
- ), "Evaluator must return a dict on the main process. Got {} instead.".format(
- results_i
- )
- logger.info("Evaluation results for {} in csv format:".format(dataset_name))
- print_csv_format(results_i)
-
- if len(results) == 1:
- results = list(results.values())[0]
- return results
-
- @staticmethod
- def auto_scale_workers(cfg, num_workers: int):
- """
- When the config is defined for certain number of workers (according to
- ``cfg.SOLVER.REFERENCE_WORLD_SIZE``) that's different from the number of
- workers currently in use, returns a new cfg where the total batch size
- is scaled so that the per-GPU batch size stays the same as the
- original ``IMS_PER_BATCH // REFERENCE_WORLD_SIZE``.
-
- Other config options are also scaled accordingly:
- * training steps and warmup steps are scaled inverse proportionally.
- * learning rate are scaled proportionally, following :paper:`ImageNet in 1h`.
-
- For example, with the original config like the following:
-
- .. code-block:: yaml
-
- IMS_PER_BATCH: 16
- BASE_LR: 0.1
- REFERENCE_WORLD_SIZE: 8
- MAX_ITER: 5000
- STEPS: (4000,)
- CHECKPOINT_PERIOD: 1000
-
- When this config is used on 16 GPUs instead of the reference number 8,
- calling this method will return a new config with:
-
- .. code-block:: yaml
-
- IMS_PER_BATCH: 32
- BASE_LR: 0.2
- REFERENCE_WORLD_SIZE: 16
- MAX_ITER: 2500
- STEPS: (2000,)
- CHECKPOINT_PERIOD: 500
-
- Note that both the original config and this new config can be trained on 16 GPUs.
- It's up to user whether to enable this feature (by setting ``REFERENCE_WORLD_SIZE``).
-
- Returns:
- CfgNode: a new config. Same as original if ``cfg.SOLVER.REFERENCE_WORLD_SIZE==0``.
- """
- old_world_size = cfg.SOLVER.REFERENCE_WORLD_SIZE
- if old_world_size == 0 or old_world_size == num_workers:
- return cfg
- cfg = cfg.clone()
- frozen = cfg.is_frozen()
- cfg.defrost()
-
- assert (
- cfg.SOLVER.IMS_PER_BATCH % old_world_size == 0
- ), "Invalid REFERENCE_WORLD_SIZE in config!"
- scale = num_workers / old_world_size
- bs = cfg.SOLVER.IMS_PER_BATCH = int(round(cfg.SOLVER.IMS_PER_BATCH * scale))
- lr = cfg.SOLVER.BASE_LR = cfg.SOLVER.BASE_LR * scale
- max_iter = cfg.SOLVER.MAX_ITER = int(round(cfg.SOLVER.MAX_ITER / scale))
- warmup_iter = cfg.SOLVER.WARMUP_ITERS = int(round(cfg.SOLVER.WARMUP_ITERS / scale))
- cfg.SOLVER.STEPS = tuple(int(round(s / scale)) for s in cfg.SOLVER.STEPS)
- cfg.TEST.EVAL_PERIOD = int(round(cfg.TEST.EVAL_PERIOD / scale))
- cfg.SOLVER.CHECKPOINT_PERIOD = int(round(cfg.SOLVER.CHECKPOINT_PERIOD / scale))
- cfg.SOLVER.REFERENCE_WORLD_SIZE = num_workers # maintain invariant
- logger = logging.getLogger(__name__)
- logger.info(
- f"Auto-scaling the config to batch_size={bs}, learning_rate={lr}, "
- f"max_iter={max_iter}, warmup={warmup_iter}."
- )
-
- if frozen:
- cfg.freeze()
- return cfg
-
-
-# Access basic attributes from the underlying trainer
-for _attr in ["model", "data_loader", "optimizer"]:
- setattr(
- DefaultTrainer,
- _attr,
- property(
- # getter
- lambda self, x=_attr: getattr(self._trainer, x),
- # setter
- lambda self, value, x=_attr: setattr(self._trainer, x, value),
- ),
- )
diff --git a/spaces/Awiny/Image2Paragraph/models/grit_src/third_party/CenterNet2/detectron2/modeling/meta_arch/semantic_seg.py b/spaces/Awiny/Image2Paragraph/models/grit_src/third_party/CenterNet2/detectron2/modeling/meta_arch/semantic_seg.py
deleted file mode 100644
index 6dd3dc23f5a333e1170ab317875551f852a0b53f..0000000000000000000000000000000000000000
--- a/spaces/Awiny/Image2Paragraph/models/grit_src/third_party/CenterNet2/detectron2/modeling/meta_arch/semantic_seg.py
+++ /dev/null
@@ -1,260 +0,0 @@
-# Copyright (c) Facebook, Inc. and its affiliates.
-import numpy as np
-from typing import Callable, Dict, Optional, Tuple, Union
-import fvcore.nn.weight_init as weight_init
-import torch
-from torch import nn
-from torch.nn import functional as F
-
-from detectron2.config import configurable
-from detectron2.layers import Conv2d, ShapeSpec, get_norm
-from detectron2.structures import ImageList
-from detectron2.utils.registry import Registry
-
-from ..backbone import Backbone, build_backbone
-from ..postprocessing import sem_seg_postprocess
-from .build import META_ARCH_REGISTRY
-
-__all__ = [
- "SemanticSegmentor",
- "SEM_SEG_HEADS_REGISTRY",
- "SemSegFPNHead",
- "build_sem_seg_head",
-]
-
-
-SEM_SEG_HEADS_REGISTRY = Registry("SEM_SEG_HEADS")
-SEM_SEG_HEADS_REGISTRY.__doc__ = """
-Registry for semantic segmentation heads, which make semantic segmentation predictions
-from feature maps.
-"""
-
-
-@META_ARCH_REGISTRY.register()
-class SemanticSegmentor(nn.Module):
- """
- Main class for semantic segmentation architectures.
- """
-
- @configurable
- def __init__(
- self,
- *,
- backbone: Backbone,
- sem_seg_head: nn.Module,
- pixel_mean: Tuple[float],
- pixel_std: Tuple[float],
- ):
- """
- Args:
- backbone: a backbone module, must follow detectron2's backbone interface
- sem_seg_head: a module that predicts semantic segmentation from backbone features
- pixel_mean, pixel_std: list or tuple with #channels element, representing
- the per-channel mean and std to be used to normalize the input image
- """
- super().__init__()
- self.backbone = backbone
- self.sem_seg_head = sem_seg_head
- self.register_buffer("pixel_mean", torch.tensor(pixel_mean).view(-1, 1, 1), False)
- self.register_buffer("pixel_std", torch.tensor(pixel_std).view(-1, 1, 1), False)
-
- @classmethod
- def from_config(cls, cfg):
- backbone = build_backbone(cfg)
- sem_seg_head = build_sem_seg_head(cfg, backbone.output_shape())
- return {
- "backbone": backbone,
- "sem_seg_head": sem_seg_head,
- "pixel_mean": cfg.MODEL.PIXEL_MEAN,
- "pixel_std": cfg.MODEL.PIXEL_STD,
- }
-
- @property
- def device(self):
- return self.pixel_mean.device
-
- def forward(self, batched_inputs):
- """
- Args:
- batched_inputs: a list, batched outputs of :class:`DatasetMapper`.
- Each item in the list contains the inputs for one image.
-
- For now, each item in the list is a dict that contains:
-
- * "image": Tensor, image in (C, H, W) format.
- * "sem_seg": semantic segmentation ground truth
- * Other information that's included in the original dicts, such as:
- "height", "width" (int): the output resolution of the model (may be different
- from input resolution), used in inference.
-
-
- Returns:
- list[dict]:
- Each dict is the output for one input image.
- The dict contains one key "sem_seg" whose value is a
- Tensor that represents the
- per-pixel segmentation prediced by the head.
- The prediction has shape KxHxW that represents the logits of
- each class for each pixel.
- """
- images = [x["image"].to(self.device) for x in batched_inputs]
- images = [(x - self.pixel_mean) / self.pixel_std for x in images]
- images = ImageList.from_tensors(images, self.backbone.size_divisibility)
-
- features = self.backbone(images.tensor)
-
- if "sem_seg" in batched_inputs[0]:
- targets = [x["sem_seg"].to(self.device) for x in batched_inputs]
- targets = ImageList.from_tensors(
- targets, self.backbone.size_divisibility, self.sem_seg_head.ignore_value
- ).tensor
- else:
- targets = None
- results, losses = self.sem_seg_head(features, targets)
-
- if self.training:
- return losses
-
- processed_results = []
- for result, input_per_image, image_size in zip(results, batched_inputs, images.image_sizes):
- height = input_per_image.get("height", image_size[0])
- width = input_per_image.get("width", image_size[1])
- r = sem_seg_postprocess(result, image_size, height, width)
- processed_results.append({"sem_seg": r})
- return processed_results
-
-
-def build_sem_seg_head(cfg, input_shape):
- """
- Build a semantic segmentation head from `cfg.MODEL.SEM_SEG_HEAD.NAME`.
- """
- name = cfg.MODEL.SEM_SEG_HEAD.NAME
- return SEM_SEG_HEADS_REGISTRY.get(name)(cfg, input_shape)
-
-
-@SEM_SEG_HEADS_REGISTRY.register()
-class SemSegFPNHead(nn.Module):
- """
- A semantic segmentation head described in :paper:`PanopticFPN`.
- It takes a list of FPN features as input, and applies a sequence of
- 3x3 convs and upsampling to scale all of them to the stride defined by
- ``common_stride``. Then these features are added and used to make final
- predictions by another 1x1 conv layer.
- """
-
- @configurable
- def __init__(
- self,
- input_shape: Dict[str, ShapeSpec],
- *,
- num_classes: int,
- conv_dims: int,
- common_stride: int,
- loss_weight: float = 1.0,
- norm: Optional[Union[str, Callable]] = None,
- ignore_value: int = -1,
- ):
- """
- NOTE: this interface is experimental.
-
- Args:
- input_shape: shapes (channels and stride) of the input features
- num_classes: number of classes to predict
- conv_dims: number of output channels for the intermediate conv layers.
- common_stride: the common stride that all features will be upscaled to
- loss_weight: loss weight
- norm (str or callable): normalization for all conv layers
- ignore_value: category id to be ignored during training.
- """
- super().__init__()
- input_shape = sorted(input_shape.items(), key=lambda x: x[1].stride)
- if not len(input_shape):
- raise ValueError("SemSegFPNHead(input_shape=) cannot be empty!")
- self.in_features = [k for k, v in input_shape]
- feature_strides = [v.stride for k, v in input_shape]
- feature_channels = [v.channels for k, v in input_shape]
-
- self.ignore_value = ignore_value
- self.common_stride = common_stride
- self.loss_weight = loss_weight
-
- self.scale_heads = []
- for in_feature, stride, channels in zip(
- self.in_features, feature_strides, feature_channels
- ):
- head_ops = []
- head_length = max(1, int(np.log2(stride) - np.log2(self.common_stride)))
- for k in range(head_length):
- norm_module = get_norm(norm, conv_dims)
- conv = Conv2d(
- channels if k == 0 else conv_dims,
- conv_dims,
- kernel_size=3,
- stride=1,
- padding=1,
- bias=not norm,
- norm=norm_module,
- activation=F.relu,
- )
- weight_init.c2_msra_fill(conv)
- head_ops.append(conv)
- if stride != self.common_stride:
- head_ops.append(
- nn.Upsample(scale_factor=2, mode="bilinear", align_corners=False)
- )
- self.scale_heads.append(nn.Sequential(*head_ops))
- self.add_module(in_feature, self.scale_heads[-1])
- self.predictor = Conv2d(conv_dims, num_classes, kernel_size=1, stride=1, padding=0)
- weight_init.c2_msra_fill(self.predictor)
-
- @classmethod
- def from_config(cls, cfg, input_shape: Dict[str, ShapeSpec]):
- return {
- "input_shape": {
- k: v for k, v in input_shape.items() if k in cfg.MODEL.SEM_SEG_HEAD.IN_FEATURES
- },
- "ignore_value": cfg.MODEL.SEM_SEG_HEAD.IGNORE_VALUE,
- "num_classes": cfg.MODEL.SEM_SEG_HEAD.NUM_CLASSES,
- "conv_dims": cfg.MODEL.SEM_SEG_HEAD.CONVS_DIM,
- "common_stride": cfg.MODEL.SEM_SEG_HEAD.COMMON_STRIDE,
- "norm": cfg.MODEL.SEM_SEG_HEAD.NORM,
- "loss_weight": cfg.MODEL.SEM_SEG_HEAD.LOSS_WEIGHT,
- }
-
- def forward(self, features, targets=None):
- """
- Returns:
- In training, returns (None, dict of losses)
- In inference, returns (CxHxW logits, {})
- """
- x = self.layers(features)
- if self.training:
- return None, self.losses(x, targets)
- else:
- x = F.interpolate(
- x, scale_factor=self.common_stride, mode="bilinear", align_corners=False
- )
- return x, {}
-
- def layers(self, features):
- for i, f in enumerate(self.in_features):
- if i == 0:
- x = self.scale_heads[i](features[f])
- else:
- x = x + self.scale_heads[i](features[f])
- x = self.predictor(x)
- return x
-
- def losses(self, predictions, targets):
- predictions = predictions.float() # https://github.com/pytorch/pytorch/issues/48163
- predictions = F.interpolate(
- predictions,
- scale_factor=self.common_stride,
- mode="bilinear",
- align_corners=False,
- )
- loss = F.cross_entropy(
- predictions, targets, reduction="mean", ignore_index=self.ignore_value
- )
- losses = {"loss_sem_seg": loss * self.loss_weight}
- return losses
diff --git a/spaces/BENE2007/runwayml-stable-diffusion-v1-5/README.md b/spaces/BENE2007/runwayml-stable-diffusion-v1-5/README.md
deleted file mode 100644
index 93d861eb380d9cadcff384308fab7e17a1cf37df..0000000000000000000000000000000000000000
--- a/spaces/BENE2007/runwayml-stable-diffusion-v1-5/README.md
+++ /dev/null
@@ -1,13 +0,0 @@
----
-title: Runwayml Stable Diffusion V1 5
-emoji: 🦀
-colorFrom: pink
-colorTo: blue
-sdk: gradio
-sdk_version: 3.21.0
-app_file: app.py
-pinned: false
-license: mit
----
-
-Check out the configuration reference at https://huggingface.co/docs/hub/spaces-config-reference
diff --git a/spaces/Benson/text-generation/Examples/Descarga Worldbox Desbloqueado Todos.md b/spaces/Benson/text-generation/Examples/Descarga Worldbox Desbloqueado Todos.md
deleted file mode 100644
index 979f8bb31e6b8831bf27009b513d62f5febb073f..0000000000000000000000000000000000000000
--- a/spaces/Benson/text-generation/Examples/Descarga Worldbox Desbloqueado Todos.md
+++ /dev/null
@@ -1,54 +0,0 @@
-
-
Cómo Descargar WorldBox Desbloqueado Todo - Una Guía para Amantes del Juego Sandbox
-
Si eres un fan de los juegos de sandbox, es posible que hayas oído hablar de WorldBox, un simulador de dios y un juego de sandbox que te permite crear tu propio mundo y verlo crecer. ¿Pero sabías que puedes descargar WorldBox desbloqueado all, una versión modded del juego que te da acceso a todas las características premium y contenido gratis? En este artículo, te mostraremos cómo descargar WorldBox desbloqueado todo, y por qué deberías probarlo si te gustan los juegos de sandbox.
WorldBox es un simulador de Dios y un juego de caja de arena
-
WorldBox es un juego desarrollado por Maxim Karpenko, un desarrollador de juegos indie de Ucrania. Es un simulador de dios y un juego de sandbox que te permite crear tu propio mundo usando diferentes poderes y herramientas. También puede destruir su mundo usando varios desastres y eventos. Puede jugar WorldBox en su PC, Android o dispositivo iOS.
-
WorldBox le permite crear, destruir y experimentar con su propio mundo
-
WorldBox es un juego que te da completa libertad y creatividad para dar forma a tu propio mundo. Puede elegir entre diferentes biomas, terrenos, animales, plantas, razas, civilizaciones, culturas, religiones, guerras, tecnologías, magia y más. También puedes ver cómo evoluciona tu mundo con el tiempo y cómo interactúa con otros mundos. También puedes experimentar con diferentes escenarios y resultados, como qué pasaría si los zombis invadieran tu mundo, o si los alienígenas aterrizaran en tu planeta.
-
¿Cuáles son los beneficios de descargar WorldBox desbloqueado todo
-
WorldBox desbloqueado todo le da acceso a todas las características y contenido premium
-
-
WorldBox desbloqueado todo le permite disfrutar del juego sin anuncios o compras en la aplicación
-
Otro beneficio de descargar WorldBox desbloqueado todo es que se puede disfrutar del juego sin ningún tipo de anuncios o compras en la aplicación. Los anuncios pueden ser molestos y distraer cuando estás jugando un juego, especialmente si aparecen con frecuencia o cubren la pantalla. Las compras en la aplicación también pueden ser tentadoras y costosas si desea obtener más funciones o contenido. Sin embargo, con WorldBox desbloqueado todo, usted no tiene que preocuparse por cualquiera de estos problemas. Puede jugar el juego sin problemas y pacíficamente sin anuncios ni compras en la aplicación.
-
Cómo descargar WorldBox desbloqueado todo gratis
-
Descargar WorldBox desbloqueado todo desde una fuente de confianza
-
El primer paso para descargar WorldBox desbloqueado todo es encontrar una fuente de confianza que ofrece la versión modificada del juego. Hay muchos sitios web y blogs que dicen ofrecer WorldBox desbloqueados todos, pero algunos de ellos pueden ser falsos, anticuados, o infectados con malware. Por lo tanto, debe tener cuidado y hacer algunas investigaciones antes de descargar nada de Internet. Una de las fuentes de confianza que recomendamos es WorldBox Mod APK, un sitio web que proporciona la última y más segura versión de WorldBox desbloqueado todo de forma gratuita.
-
-
Instalar WorldBox desbloqueado todo en su dispositivo
-
El siguiente paso para descargar WorldBox desbloqueado todo es instalarlo en su dispositivo. Dependiendo del dispositivo que esté utilizando, el proceso de instalación puede variar ligeramente. Estos son los pasos generales a seguir:
-
-
Descargar WorldBox desbloqueado todos los archivos de la fuente de confianza.
-
Busque el archivo en su dispositivo y toque en él para iniciar la instalación.
-
Si está utilizando un dispositivo Android, es posible que necesite habilitar la opción "Fuentes desconocidas" en su configuración para permitir la instalación de aplicaciones desde fuera de la Google Play Store.
-
-
Siga las instrucciones en la pantalla para completar la instalación.
-
-
Inicie WorldBox desbloqueado todo y comience a jugar
-
El paso final para descargar WorldBox desbloqueado todo es lanzarlo y comenzar a jugar. Puede encontrar el icono de la aplicación en la pantalla de inicio o en el cajón de la aplicación. Toque en él para abrir el juego y disfrutar de todas las características premium y el contenido de forma gratuita. También puede buscar actualizaciones regularmente para obtener la última versión de WorldBox desbloqueado todo.
-
Consejos y trucos para jugar WorldBox desbloqueado todo
-
Usa diferentes poderes y herramientas para dar forma a tu mundo
-
Uno de los aspectos divertidos de jugar WorldBox desbloqueado todo es que usted puede utilizar diferentes poderes y herramientas para dar forma a su mundo. Puede crear montañas, lagos, bosques, desiertos, islas, volcanes y más. También puedes engendrar diferentes animales, plantas, razas, civilizaciones y culturas. También puede utilizar diferentes desastres y eventos para destruir su mundo o hacerlo más interesante. Puedes usar poderes como lluvia ácida, meteoritos, tornados, terremotos, armas nucleares, zombis, alienígenas, dragones y más.
-
Vea cómo su mundo evoluciona e interactúa con otros mundos
-
Otro aspecto divertido de jugar WorldBox desbloqueado todo es que usted puede ver cómo su mundo evoluciona e interactúa con otros mundos. Pueden ver cómo su mundo cambia con el tiempo y cómo desarrolla su propia historia, cultura, religión, tecnología, magia y más. También puedes ver cómo interactúa tu mundo con otros mundos que creas o descargas de otros jugadores. Puedes ver cómo negocian, luchan, se alían o se fusionan entre sí.
-
Comparte tu mundo con otros jugadores y explora sus mundos
-
-
Conclusión
-
WorldBox es un simulador de dios y un juego de sandbox que te permite crear tu propio mundo y verlo crecer. Es un juego que te da completa libertad y creatividad para dar forma a tu propio mundo. Sin embargo, si quieres disfrutar del juego sin limitaciones ni interrupciones, debes descargar WorldBox desbloqueado todo, una versión modificada del juego que te da acceso a todas las características premium y contenido gratis. En este artículo, le mostramos cómo descargar WorldBox desbloqueado todo desde una fuente de confianza, cómo instalarlo en su dispositivo, y cómo jugar con consejos y trucos. Esperamos que haya encontrado este artículo útil e informativo. ¡Ahora siga adelante y descargue WorldBox desbloqueado todo y diviértase creando su propio mundo!
-
Preguntas frecuentes
-
-
¿Qué es WorldBox?
-WorldBox es un simulador de dios y un juego de sandbox que te permite crear tu propio mundo usando diferentes poderes y herramientas.
-
¿Qué es WorldBox desbloqueado todo?
-WorldBox Unlocked All es una versión modded del juego que te da acceso a todas las funciones premium y contenido gratis.
-
Cómo descargar WorldBox desbloqueado todo?
-Puede descargar WorldBox Unlocked All desde una fuente de confianza como WorldBox Mod APK, luego instalarlo en su dispositivo y lanzarlo.
Es WorldBox desbloqueado todo seguro para descargar y jugar?
-WorldBox Desbloqueado Todo es seguro para descargar y jugar si lo obtiene de una fuente de confianza como WorldBox Mod APK. Sin embargo, siempre debe tener cuidado y hacer algunas investigaciones antes de descargar nada de Internet.
-
¿Cuáles son las características de WorldBox desbloqueado todo?
-WorldBox Unlocked All te da acceso a todas las funciones y contenido premium del juego, como poderes, herramientas, carreras, animales, eventos, skins, mapas y más. También te permite disfrutar del juego sin anuncios ni compras en la aplicación.
"
-
-examples=[['bruce.wav','2']]
-
-gr.Interface(inference, inputs, outputs, title=title, description=description, article=article, examples=examples, enable_queue=True).launch()
diff --git a/spaces/MisterZee/PIFu-Clothed-Human-Digitization/PIFu/lib/renderer/gl/init_gl.py b/spaces/MisterZee/PIFu-Clothed-Human-Digitization/PIFu/lib/renderer/gl/init_gl.py
deleted file mode 100644
index 1d2c7e6ba0be20136b2be2e2f644894bee4af9c1..0000000000000000000000000000000000000000
--- a/spaces/MisterZee/PIFu-Clothed-Human-Digitization/PIFu/lib/renderer/gl/init_gl.py
+++ /dev/null
@@ -1,24 +0,0 @@
-_glut_window = None
-_context_inited = None
-
-def initialize_GL_context(width=512, height=512, egl=False):
- '''
- default context uses GLUT
- '''
- if not egl:
- import OpenGL.GLUT as GLUT
- display_mode = GLUT.GLUT_DOUBLE | GLUT.GLUT_RGB | GLUT.GLUT_DEPTH
- global _glut_window
- if _glut_window is None:
- GLUT.glutInit()
- GLUT.glutInitDisplayMode(display_mode)
- GLUT.glutInitWindowSize(width, height)
- GLUT.glutInitWindowPosition(0, 0)
- _glut_window = GLUT.glutCreateWindow("My Render.")
- else:
- from .glcontext import create_opengl_context
- global _context_inited
- if _context_inited is None:
- create_opengl_context((width, height))
- _context_inited = True
-
diff --git a/spaces/NIVASVAKA8999/myaigen/README.md b/spaces/NIVASVAKA8999/myaigen/README.md
deleted file mode 100644
index db90616ddf7a4fd73415f3f14b6802bec0630600..0000000000000000000000000000000000000000
--- a/spaces/NIVASVAKA8999/myaigen/README.md
+++ /dev/null
@@ -1,12 +0,0 @@
----
-title: Myaigen
-emoji: 📈
-colorFrom: pink
-colorTo: yellow
-sdk: gradio
-sdk_version: 3.39.0
-app_file: app.py
-pinned: false
----
-
-Check out the configuration reference at https://huggingface.co/docs/hub/spaces-config-reference
diff --git a/spaces/NoCrypt/mikuTTS/lib/infer_pack/modules/F0Predictor/HarvestF0Predictor.py b/spaces/NoCrypt/mikuTTS/lib/infer_pack/modules/F0Predictor/HarvestF0Predictor.py
deleted file mode 100644
index b412ba2814e114ca7bb00b6fd6ef217f63d788a3..0000000000000000000000000000000000000000
--- a/spaces/NoCrypt/mikuTTS/lib/infer_pack/modules/F0Predictor/HarvestF0Predictor.py
+++ /dev/null
@@ -1,86 +0,0 @@
-from lib.infer_pack.modules.F0Predictor.F0Predictor import F0Predictor
-import pyworld
-import numpy as np
-
-
-class HarvestF0Predictor(F0Predictor):
- def __init__(self, hop_length=512, f0_min=50, f0_max=1100, sampling_rate=44100):
- self.hop_length = hop_length
- self.f0_min = f0_min
- self.f0_max = f0_max
- self.sampling_rate = sampling_rate
-
- def interpolate_f0(self, f0):
- """
- 对F0进行插值处理
- """
-
- data = np.reshape(f0, (f0.size, 1))
-
- vuv_vector = np.zeros((data.size, 1), dtype=np.float32)
- vuv_vector[data > 0.0] = 1.0
- vuv_vector[data <= 0.0] = 0.0
-
- ip_data = data
-
- frame_number = data.size
- last_value = 0.0
- for i in range(frame_number):
- if data[i] <= 0.0:
- j = i + 1
- for j in range(i + 1, frame_number):
- if data[j] > 0.0:
- break
- if j < frame_number - 1:
- if last_value > 0.0:
- step = (data[j] - data[i - 1]) / float(j - i)
- for k in range(i, j):
- ip_data[k] = data[i - 1] + step * (k - i + 1)
- else:
- for k in range(i, j):
- ip_data[k] = data[j]
- else:
- for k in range(i, frame_number):
- ip_data[k] = last_value
- else:
- ip_data[i] = data[i] # 这里可能存在一个没有必要的拷贝
- last_value = data[i]
-
- return ip_data[:, 0], vuv_vector[:, 0]
-
- def resize_f0(self, x, target_len):
- source = np.array(x)
- source[source < 0.001] = np.nan
- target = np.interp(
- np.arange(0, len(source) * target_len, len(source)) / target_len,
- np.arange(0, len(source)),
- source,
- )
- res = np.nan_to_num(target)
- return res
-
- def compute_f0(self, wav, p_len=None):
- if p_len is None:
- p_len = wav.shape[0] // self.hop_length
- f0, t = pyworld.harvest(
- wav.astype(np.double),
- fs=self.hop_length,
- f0_ceil=self.f0_max,
- f0_floor=self.f0_min,
- frame_period=1000 * self.hop_length / self.sampling_rate,
- )
- f0 = pyworld.stonemask(wav.astype(np.double), f0, t, self.fs)
- return self.interpolate_f0(self.resize_f0(f0, p_len))[0]
-
- def compute_f0_uv(self, wav, p_len=None):
- if p_len is None:
- p_len = wav.shape[0] // self.hop_length
- f0, t = pyworld.harvest(
- wav.astype(np.double),
- fs=self.sampling_rate,
- f0_floor=self.f0_min,
- f0_ceil=self.f0_max,
- frame_period=1000 * self.hop_length / self.sampling_rate,
- )
- f0 = pyworld.stonemask(wav.astype(np.double), f0, t, self.sampling_rate)
- return self.interpolate_f0(self.resize_f0(f0, p_len))
diff --git a/spaces/OFA-Sys/OFA-Generic_Interface/fairseq/examples/speech_text_joint_to_text/scripts/g2p_encode.py b/spaces/OFA-Sys/OFA-Generic_Interface/fairseq/examples/speech_text_joint_to_text/scripts/g2p_encode.py
deleted file mode 100644
index 9db779396f492e3f71b08d7b895beb81d8e46bc9..0000000000000000000000000000000000000000
--- a/spaces/OFA-Sys/OFA-Generic_Interface/fairseq/examples/speech_text_joint_to_text/scripts/g2p_encode.py
+++ /dev/null
@@ -1,191 +0,0 @@
-# Copyright (c) Facebook, Inc. and its affiliates.
-#
-# This source code is licensed under the MIT license found in the
-# LICENSE file in the root directory of this source tree.
-
-import argparse
-import itertools
-import logging
-import re
-import time
-
-from g2p_en import G2p
-
-logger = logging.getLogger(__name__)
-
-FAIL_SENT = "FAILED_SENTENCE"
-
-
-def parse():
- parser = argparse.ArgumentParser()
- parser.add_argument("--data-path", type=str, required=True)
- parser.add_argument("--out-path", type=str, required=True)
- parser.add_argument("--lower-case", action="store_true")
- parser.add_argument("--do-filter", action="store_true")
- parser.add_argument("--use-word-start", action="store_true")
- parser.add_argument("--dup-vowel", default=1, type=int)
- parser.add_argument("--dup-consonant", default=1, type=int)
- parser.add_argument("--no-punc", action="store_true")
- parser.add_argument("--reserve-word", type=str, default="")
- parser.add_argument(
- "--reserve-first-column",
- action="store_true",
- help="first column is sentence id",
- )
- ###
- parser.add_argument("--parallel-process-num", default=1, type=int)
- parser.add_argument("--logdir", default="")
- args = parser.parse_args()
- return args
-
-
-def process_sent(sent, g2p, res_wrds, args):
- sents = pre_process_sent(sent, args.do_filter, args.lower_case, res_wrds)
- pho_seqs = [do_g2p(g2p, s, res_wrds, i == 0) for i, s in enumerate(sents)]
- pho_seq = (
- [FAIL_SENT]
- if [FAIL_SENT] in pho_seqs
- else list(itertools.chain.from_iterable(pho_seqs))
- )
- if args.no_punc:
- pho_seq = remove_punc(pho_seq)
- if args.dup_vowel > 1 or args.dup_consonant > 1:
- pho_seq = dup_pho(pho_seq, args.dup_vowel, args.dup_consonant)
- if args.use_word_start:
- pho_seq = add_word_start(pho_seq)
- return " ".join(pho_seq)
-
-
-def remove_punc(sent):
- ns = []
- regex = re.compile("[^a-zA-Z0-9 ]")
- for p in sent:
- if (not regex.search(p)) or p == FAIL_SENT:
- if p == " " and (len(ns) == 0 or ns[-1] == " "):
- continue
- ns.append(p)
- return ns
-
-
-def do_g2p(g2p, sent, res_wrds, is_first_sent):
- if sent in res_wrds:
- pho_seq = [res_wrds[sent]]
- else:
- pho_seq = g2p(sent)
- if not is_first_sent:
- pho_seq = [" "] + pho_seq # add space to separate
- return pho_seq
-
-
-def pre_process_sent(sent, do_filter, lower_case, res_wrds):
- if do_filter:
- sent = re.sub("-", " ", sent)
- sent = re.sub("—", " ", sent)
- if len(res_wrds) > 0:
- wrds = sent.split()
- wrds = ["SPLIT_ME " + w + " SPLIT_ME" if w in res_wrds else w for w in wrds]
- sents = [x.strip() for x in " ".join(wrds).split("SPLIT_ME") if x.strip() != ""]
- else:
- sents = [sent]
- if lower_case:
- sents = [s.lower() if s not in res_wrds else s for s in sents]
- return sents
-
-
-def dup_pho(sent, dup_v_num, dup_c_num):
- """
- duplicate phoneme defined as cmudict
- http://www.speech.cs.cmu.edu/cgi-bin/cmudict
- """
- if dup_v_num == 1 and dup_c_num == 1:
- return sent
- ns = []
- for p in sent:
- ns.append(p)
- if re.search(r"\d$", p):
- for i in range(1, dup_v_num):
- ns.append(f"{p}-{i}P")
- elif re.search(r"\w", p):
- for i in range(1, dup_c_num):
- ns.append(f"{p}-{i}P")
- return ns
-
-
-def add_word_start(sent):
- ns = []
- do_add = True
- ws = "▁"
- for p in sent:
- if do_add:
- p = ws + p
- do_add = False
- if p == " ":
- do_add = True
- else:
- ns.append(p)
- return ns
-
-
-def load_reserve_word(reserve_word):
- if reserve_word == "":
- return []
- with open(reserve_word, "r") as fp:
- res_wrds = [x.strip().split() for x in fp.readlines() if x.strip() != ""]
- assert sum([0 if len(x) == 2 else 1 for x in res_wrds]) == 0
- res_wrds = dict(res_wrds)
- return res_wrds
-
-
-def process_sents(sents, args):
- g2p = G2p()
- out_sents = []
- res_wrds = load_reserve_word(args.reserve_word)
- for sent in sents:
- col1 = ""
- if args.reserve_first_column:
- col1, sent = sent.split(None, 1)
- sent = process_sent(sent, g2p, res_wrds, args)
- if args.reserve_first_column and col1 != "":
- sent = f"{col1} {sent}"
- out_sents.append(sent)
- return out_sents
-
-
-def main():
- args = parse()
- out_sents = []
- with open(args.data_path, "r") as fp:
- sent_list = [x.strip() for x in fp.readlines()]
- if args.parallel_process_num > 1:
- try:
- import submitit
- except ImportError:
- logger.warn(
- "submitit is not found and only one job is used to process the data"
- )
- submitit = None
-
- if args.parallel_process_num == 1 or submitit is None:
- out_sents = process_sents(sent_list, args)
- else:
- # process sentences with parallel computation
- lsize = len(sent_list) // args.parallel_process_num + 1
- executor = submitit.AutoExecutor(folder=args.logdir)
- executor.update_parameters(timeout_min=1000, cpus_per_task=4)
- jobs = []
- for i in range(args.parallel_process_num):
- job = executor.submit(
- process_sents, sent_list[lsize * i : lsize * (i + 1)], args
- )
- jobs.append(job)
- is_running = True
- while is_running:
- time.sleep(5)
- is_running = sum([job.done() for job in jobs]) < len(jobs)
- out_sents = list(itertools.chain.from_iterable([job.result() for job in jobs]))
- with open(args.out_path, "w") as fp:
- fp.write("\n".join(out_sents) + "\n")
-
-
-if __name__ == "__main__":
- main()
diff --git a/spaces/OFA-Sys/OFA-Generic_Interface/fairseq/fairseq/data/encoders/sentencepiece_bpe.py b/spaces/OFA-Sys/OFA-Generic_Interface/fairseq/fairseq/data/encoders/sentencepiece_bpe.py
deleted file mode 100644
index a76d46a2014e81eff72b19f6c13084a855fcd477..0000000000000000000000000000000000000000
--- a/spaces/OFA-Sys/OFA-Generic_Interface/fairseq/fairseq/data/encoders/sentencepiece_bpe.py
+++ /dev/null
@@ -1,48 +0,0 @@
-# Copyright (c) Facebook, Inc. and its affiliates.
-#
-# This source code is licensed under the MIT license found in the
-# LICENSE file in the root directory of this source tree.
-
-from dataclasses import dataclass, field
-
-from fairseq import file_utils
-from fairseq.data.encoders import register_bpe
-from fairseq.dataclass import FairseqDataclass
-
-
-@dataclass
-class SentencepieceConfig(FairseqDataclass):
- sentencepiece_model: str = field(
- default="???", metadata={"help": "path to sentencepiece model"}
- )
-
-
-@register_bpe("sentencepiece", dataclass=SentencepieceConfig)
-class SentencepieceBPE(object):
- def __init__(self, cfg):
- sentencepiece_model = file_utils.cached_path(cfg.sentencepiece_model)
- try:
- import sentencepiece as spm
-
- self.sp = spm.SentencePieceProcessor()
- self.sp.Load(sentencepiece_model)
- except ImportError:
- raise ImportError(
- "Please install sentencepiece with: pip install sentencepiece"
- )
-
- def encode(self, x: str) -> str:
- return " ".join(self.sp.EncodeAsPieces(x))
-
- def decode(self, x: str) -> str:
- return x.replace(" ", "").replace("\u2581", " ").strip()
-
- def is_beginning_of_word(self, x: str) -> bool:
- if x in ["", "", "", ""]:
- # special elements are always considered beginnings
- # HACK: this logic is already present in fairseq/tasks/masked_lm.py
- # but these special tokens are also contained in the sentencepiece
- # vocabulary which causes duplicate special tokens. This hack makes
- # sure that they are all taken into account.
- return True
- return x.startswith("\u2581")
diff --git a/spaces/OFA-Sys/OFA-Image_Caption/fairseq/examples/criss/download_and_preprocess_flores_test.sh b/spaces/OFA-Sys/OFA-Image_Caption/fairseq/examples/criss/download_and_preprocess_flores_test.sh
deleted file mode 100644
index ed4b390fbdee3991efeb298050e12065d7fe605b..0000000000000000000000000000000000000000
--- a/spaces/OFA-Sys/OFA-Image_Caption/fairseq/examples/criss/download_and_preprocess_flores_test.sh
+++ /dev/null
@@ -1,64 +0,0 @@
-#!/bin/bash
-# Copyright (c) Facebook, Inc. and its affiliates.
-# All rights reserved.
-#
-# This source code is licensed under the license found in the
-# LICENSE file in the root directory of this source tree.
-
-SPM_ENCODE=flores/scripts/spm_encode.py
-DATA=data_tmp
-SPM_MODEL=criss_checkpoints/sentence.bpe.model
-DICT=criss_checkpoints/dict.txt
-
-download_data() {
- CORPORA=$1
- URL=$2
-
- if [ -f $CORPORA ]; then
- echo "$CORPORA already exists, skipping download"
- else
- echo "Downloading $URL"
- wget $URL -O $CORPORA --no-check-certificate || rm -f $CORPORA
- if [ -f $CORPORA ]; then
- echo "$URL successfully downloaded."
- else
- echo "$URL not successfully downloaded."
- rm -f $CORPORA
- fi
- fi
-}
-
-if [[ -f flores ]]; then
- echo "flores already cloned"
-else
- git clone https://github.com/facebookresearch/flores
-fi
-
-mkdir -p $DATA
-download_data $DATA/wikipedia_en_ne_si_test_sets.tgz "https://github.com/facebookresearch/flores/raw/master/data/wikipedia_en_ne_si_test_sets.tgz"
-pushd $DATA
-pwd
-tar -vxf wikipedia_en_ne_si_test_sets.tgz
-popd
-
-
-for lang in ne_NP si_LK; do
- datadir=$DATA/${lang}-en_XX-flores
- rm -rf $datadir
- mkdir -p $datadir
- TEST_PREFIX=$DATA/wikipedia_en_ne_si_test_sets/wikipedia.test
- python $SPM_ENCODE \
- --model ${SPM_MODEL} \
- --output_format=piece \
- --inputs ${TEST_PREFIX}.${lang:0:2}-en.${lang:0:2} ${TEST_PREFIX}.${lang:0:2}-en.en \
- --outputs $datadir/test.bpe.${lang}-en_XX.${lang} $datadir/test.bpe.${lang}-en_XX.en_XX
-
- # binarize data
- fairseq-preprocess \
- --source-lang ${lang} --target-lang en_XX \
- --testpref $datadir/test.bpe.${lang}-en_XX \
- --destdir $datadir \
- --srcdict ${DICT} \
- --joined-dictionary \
- --workers 4
-done
diff --git a/spaces/OFA-Sys/OFA-Image_Caption/fairseq/examples/language_model/README.md b/spaces/OFA-Sys/OFA-Image_Caption/fairseq/examples/language_model/README.md
deleted file mode 100644
index e78ea48e08dc99b69751923762107a8f8a9a5e3e..0000000000000000000000000000000000000000
--- a/spaces/OFA-Sys/OFA-Image_Caption/fairseq/examples/language_model/README.md
+++ /dev/null
@@ -1,123 +0,0 @@
-# Neural Language Modeling
-
-## Pre-trained models
-
-Model | Description | Dataset | Download
----|---|---|---
-`transformer_lm.gbw.adaptive_huge` | Adaptive Inputs ([Baevski and Auli, 2018](https://arxiv.org/abs/1809.10853)) 1026M params | [Google Billion Words](https://github.com/ciprian-chelba/1-billion-word-language-modeling-benchmark) | [download (.tar.bz2)](https://dl.fbaipublicfiles.com/fairseq/models/lm/adaptive_lm_gbw_huge.tar.bz2)
-`transformer_lm.wiki103.adaptive` | Adaptive Inputs ([Baevski and Auli, 2018](https://arxiv.org/abs/1809.10853)) 247M params | [WikiText-103](https://blog.einstein.ai/the-wikitext-long-term-dependency-language-modeling-dataset) | [download (.tar.bz2)](https://dl.fbaipublicfiles.com/fairseq/models/lm/adaptive_lm_wiki103.v2.tar.bz2)
-`transformer_lm.wmt19.en` | English LM ([Ng et al., 2019](https://arxiv.org/abs/1907.06616)) | [WMT News Crawl](http://data.statmt.org/news-crawl/) | [download (.tar.gz)](https://dl.fbaipublicfiles.com/fairseq/models/lm/wmt19.en.tar.gz)
-`transformer_lm.wmt19.de` | German LM ([Ng et al., 2019](https://arxiv.org/abs/1907.06616)) | [WMT News Crawl](http://data.statmt.org/news-crawl/) | [download (.tar.gz)](https://dl.fbaipublicfiles.com/fairseq/models/lm/wmt19.de.tar.gz)
-`transformer_lm.wmt19.ru` | Russian LM ([Ng et al., 2019](https://arxiv.org/abs/1907.06616)) | [WMT News Crawl](http://data.statmt.org/news-crawl/) | [download (.tar.gz)](https://dl.fbaipublicfiles.com/fairseq/models/lm/wmt19.ru.tar.gz)
-
-## Example usage
-
-We require a few additional Python dependencies for preprocessing:
-```bash
-pip install fastBPE sacremoses
-```
-
-To sample from a language model using PyTorch Hub:
-```python
-import torch
-
-# List available models
-torch.hub.list('pytorch/fairseq') # [..., 'transformer_lm.wmt19.en', ...]
-
-# Load an English LM trained on WMT'19 News Crawl data
-en_lm = torch.hub.load('pytorch/fairseq', 'transformer_lm.wmt19.en', tokenizer='moses', bpe='fastbpe')
-en_lm.eval() # disable dropout
-
-# Move model to GPU
-en_lm.cuda()
-
-# Sample from the language model
-en_lm.sample('Barack Obama', beam=1, sampling=True, sampling_topk=10, temperature=0.8)
-# "Barack Obama is coming to Sydney and New Zealand (...)"
-
-# Compute perplexity for a sequence
-en_lm.score('Barack Obama is coming to Sydney and New Zealand')['positional_scores'].mean().neg().exp()
-# tensor(15.1474)
-
-# The same interface can be used with custom models as well
-from fairseq.models.transformer_lm import TransformerLanguageModel
-custom_lm = TransformerLanguageModel.from_pretrained('/path/to/model/dir', 'checkpoint100.pt', tokenizer='moses', bpe='fastbpe')
-custom_lm.sample('Barack Obama', beam=5)
-# "Barack Obama (...)"
-```
-
-## Training a transformer language model with the CLI tools
-
-### 1) Preprocess the data
-
-First download and prepare the [WikiText-103 dataset](https://www.salesforce.com/products/einstein/ai-research/the-wikitext-dependency-language-modeling-dataset/):
-```bash
-cd examples/language_model/
-bash prepare-wikitext-103.sh
-cd ../..
-```
-
-Next preprocess/binarize the data:
-```bash
-TEXT=examples/language_model/wikitext-103
-fairseq-preprocess \
- --only-source \
- --trainpref $TEXT/wiki.train.tokens \
- --validpref $TEXT/wiki.valid.tokens \
- --testpref $TEXT/wiki.test.tokens \
- --destdir data-bin/wikitext-103 \
- --workers 20
-```
-
-### 2) Train a language model
-
-Next we'll train a basic transformer language model on wikitext-103. For more
-advanced usage, see the [adaptive inputs README](README.adaptive_inputs.md).
-
-To train a basic LM (assumes 2 GPUs):
-```
-$ fairseq-train --task language_modeling \
- data-bin/wikitext-103 \
- --save-dir checkpoints/transformer_wikitext-103 \
- --arch transformer_lm --share-decoder-input-output-embed \
- --dropout 0.1 \
- --optimizer adam --adam-betas '(0.9, 0.98)' --weight-decay 0.01 --clip-norm 0.0 \
- --lr 0.0005 --lr-scheduler inverse_sqrt --warmup-updates 4000 --warmup-init-lr 1e-07 \
- --tokens-per-sample 512 --sample-break-mode none \
- --max-tokens 2048 --update-freq 16 \
- --fp16 \
- --max-update 50000
-```
-
-If you run out of memory, try reducing `--max-tokens` (max number of tokens per
-batch) or `--tokens-per-sample` (max sequence length). You can also adjust
-`--update-freq` to accumulate gradients and simulate training on a different
-number of GPUs.
-
-### 3) Evaluate
-
-```bash
-fairseq-eval-lm data-bin/wikitext-103 \
- --path checkpoints/transformer_wiki103/checkpoint_best.pt \
- --batch-size 2 \
- --tokens-per-sample 512 \
- --context-window 400
-# | Evaluated 245569 tokens in 56.1s (4379.02 tokens/s)
-# | Loss: 3.4164, Perplexity: 30.46
-```
-
-*Note:* The `--context-window` option controls how much context is provided to
-each token when computing perplexity. When the window size is 0, the dataset is
-chunked into segments of length 512 and perplexity is computed over each segment
-normally. However, this results in worse (higher) perplexity since tokens that
-appear earlier in each segment have less conditioning. When the maximum window
-size is used (511 in this case), then we compute perplexity for each token
-fully conditioned on 511 tokens of context. This slows down evaluation
-significantly, since we must run a separate forward pass for every token in the
-dataset, but results in better (lower) perplexity.
-
-
-## Convolutional language models
-
-Please see the [convolutional LM README](README.conv.md) for instructions on
-training convolutional language models.
diff --git a/spaces/OIUGLK/bingo/src/lib/bots/bing/tts.ts b/spaces/OIUGLK/bingo/src/lib/bots/bing/tts.ts
deleted file mode 100644
index cd10b7d1d7581bf9cf46ff6755fcca550c558c9b..0000000000000000000000000000000000000000
--- a/spaces/OIUGLK/bingo/src/lib/bots/bing/tts.ts
+++ /dev/null
@@ -1,82 +0,0 @@
-import { sleep } from './utils'
-
-const synth = window.speechSynthesis
-
-export class TTS {
- currentText = ''
- speakText = ''
- private controller = new AbortController()
- speaking = false
- get isSpeaking() {
- return this.speaking
- }
- finished = false
- constructor() {}
- abort = () => {
- this.controller.abort()
- }
-
- reset = () => {
- this.speaking = false
- this.finished = true
- this.currentText = ''
- this.speakText = ''
- this.abort()
- }
-
- speak = (text: string) => {
- if (!synth || text?.trim()?.length < 2) {
- return
- }
- this.currentText = text.replace(/[^\u4e00-\u9fa5_a-zA-Z0-9,。?,:;\.,:]+/g, '')
- this.finished = false
- this.loop()
- }
-
- private async doSpeek() {
- return new Promise((resolve) => {
- const endIndex = this.finished ? this.currentText.length :
- Math.max(
- this.currentText.lastIndexOf('。'),
- this.currentText.lastIndexOf(';'),
- this.currentText.lastIndexOf('、'),
- this.currentText.lastIndexOf('?'),
- this.currentText.lastIndexOf('\n')
- )
- const startIndex = this.speakText.length ? Math.max(0, this.currentText.lastIndexOf(this.speakText) + this.speakText.length) : 0
-
- if (startIndex >= endIndex) {
- return resolve(true)
- }
- const text = this.currentText.slice(startIndex, endIndex)
- this.speakText = text
- const utterThis = new SpeechSynthesisUtterance(text)
- this.controller.signal.onabort = () => {
- synth.cancel()
- this.finished = true
- resolve(false)
- }
-
- utterThis.onend = function (event) {
- resolve(true)
- }
-
- utterThis.onerror = function (event) {
- resolve(false)
- }
-
- const voice = synth.getVoices().find(v => v.name.includes('Microsoft Yunxi Online')) ?? null
- utterThis.voice = voice
- synth.speak(utterThis)
- })
- }
-
- private async loop() {
- if (this.speaking) return
- this.speaking = true
- while(!this.finished) {
- await Promise.all([sleep(1000), this.doSpeek()])
- }
- this.speaking = false
- }
-}
diff --git a/spaces/OpenGVLab/VideoChatGPT/models/blip2.py b/spaces/OpenGVLab/VideoChatGPT/models/blip2.py
deleted file mode 100644
index fde6bfca25d56b0823a7b60a1ede1d75304f3f6d..0000000000000000000000000000000000000000
--- a/spaces/OpenGVLab/VideoChatGPT/models/blip2.py
+++ /dev/null
@@ -1,126 +0,0 @@
-"""
- Copyright (c) 2023, salesforce.com, inc.
- All rights reserved.
- SPDX-License-Identifier: BSD-3-Clause
- For full license text, see the LICENSE_Lavis file in the repo root or https://opensource.org/licenses/BSD-3-Clause
-"""
-import contextlib
-import os
-import logging
-
-import torch
-import torch.nn as nn
-
-from .Qformer import BertConfig, BertLMHeadModel
-from .eva_vit import create_eva_vit_g
-from transformers import BertTokenizer
-
-
-class Blip2Base(nn.Module):
- def __init__(self):
- super().__init__()
-
- @classmethod
- def init_tokenizer(cls):
- tokenizer = BertTokenizer.from_pretrained("bert-base-uncased")
- tokenizer.add_special_tokens({"bos_token": "[DEC]"})
- return tokenizer
-
- @property
- def device(self):
- return list(self.parameters())[0].device
-
- def maybe_autocast(self, dtype=torch.float16):
- # if on cpu, don't use autocast
- # if on gpu, use autocast with dtype if provided, otherwise use torch.float16
- enable_autocast = self.device != torch.device("cpu")
-
- if enable_autocast:
- return torch.cuda.amp.autocast(dtype=dtype)
- else:
- return contextlib.nullcontext()
-
- @classmethod
- def init_Qformer(
- cls,
- num_query_token, vision_width,
- qformer_hidden_dropout_prob=0.,
- qformer_attention_probs_dropout_prob=0.,
- qformer_drop_path_rate=0.,
- ):
- encoder_config = BertConfig.from_pretrained("bert-base-uncased")
- encoder_config.encoder_width = vision_width
- # insert cross-attention layer every other block
- encoder_config.add_cross_attention = True
- encoder_config.cross_attention_freq = 2
- encoder_config.query_length = num_query_token
- encoder_config.hidden_dropout_prob = qformer_hidden_dropout_prob
- encoder_config.attention_probs_dropout_prob = qformer_attention_probs_dropout_prob
- encoder_config.drop_path_list = [x.item() for x in torch.linspace(0, qformer_drop_path_rate, encoder_config.num_hidden_layers)]
- print(f"Drop_path:{encoder_config.drop_path_list}")
- print(encoder_config)
- Qformer = BertLMHeadModel(config=encoder_config)
- query_tokens = nn.Parameter(
- torch.zeros(1, num_query_token, encoder_config.hidden_size)
- )
- query_tokens.data.normal_(mean=0.0, std=encoder_config.initializer_range)
- return Qformer, query_tokens
-
- @classmethod
- def init_vision_encoder(
- cls,
- model_name, img_size, drop_path_rate,
- use_grad_checkpoint, precision, vit_model_path,
- temporal_downsample=True,
- no_lmhra=False,
- double_lmhra=False,
- lmhra_reduction=2.0,
- gmhra_layers=8,
- gmhra_drop_path_rate=0.,
- gmhra_dropout=0.5,
- ):
- assert model_name == "eva_clip_g", "vit model must be eva_clip_g for current version of VideoChat"
- visual_encoder = create_eva_vit_g(
- img_size, drop_path_rate,
- use_grad_checkpoint, precision, vit_model_path,
- temporal_downsample=temporal_downsample,
- no_lmhra=no_lmhra,
- double_lmhra=double_lmhra,
- lmhra_reduction=lmhra_reduction,
- gmhra_layers=gmhra_layers,
- gmhra_drop_path_rate=gmhra_drop_path_rate,
- gmhra_dropout=gmhra_dropout,
- )
-
- ln_vision = LayerNorm(visual_encoder.num_features)
- return visual_encoder, ln_vision
-
- def load_from_pretrained(self, model_path):
- if model_path is not None and os.path.isfile(model_path):
- checkpoint = torch.load(model_path, map_location="cpu")
- else:
- raise RuntimeError("checkpoint url or path is invalid")
-
- state_dict = checkpoint["model"]
-
- msg = self.load_state_dict(state_dict, strict=False)
-
- print(f"Load QFormer from {model_path}")
- print(msg)
-
- return msg
-
-
-def disabled_train(self, mode=True):
- """Overwrite model.train with this function to make sure train/eval mode
- does not change anymore."""
- return self
-
-
-class LayerNorm(nn.LayerNorm):
- """Subclass torch's LayerNorm to handle fp16."""
-
- def forward(self, x: torch.Tensor):
- orig_type = x.dtype
- ret = super().forward(x.type(torch.float32))
- return ret.type(orig_type)
diff --git a/spaces/Ordenador/classify-text-with-bert-hate-speech/README.md b/spaces/Ordenador/classify-text-with-bert-hate-speech/README.md
deleted file mode 100644
index eb87b7c574029ec95acf0db54af01f094d34440a..0000000000000000000000000000000000000000
--- a/spaces/Ordenador/classify-text-with-bert-hate-speech/README.md
+++ /dev/null
@@ -1,67 +0,0 @@
----
-title: Classify Text With Bert Hate Speech
-emoji: 🔥
-colorFrom: purple
-colorTo: red
-sdk: gradio
-sdk_version: 3.20.1
-app_file: app.py
-pinned: false
-license: openrail
----
-
-# Hate Speech Classifier
-
-This project uses TensorFlow, and BERT to implement a hate speech and offensive language classifier. The model is trained on the Hate Speech and Offensive Language Dataset and can classify tweets into three classes:
-
-0. Hate speech
-1. Offensive language
-2. Neither
-
-## Try the Model Online
-
-You can try the model online using the following link:
-
-- [Hate Speech Classifier on Hugging Face Spaces](https://huggingface.co/spaces/Ordenador/classify-text-with-bert-hate-speech)
-
-Click the link above to access the interactive interface where you can input text and see the model's predictions for hate speech, offensive language, or neither.
-
-
-## Prerequisites
-Make sure you have the following Python packages installed:
-
-- gradio
-- tensorflow
-- tensorflow_hub
-- tensorflow_text
-
-
-You can install all them using `makefile`. The `make pip-compile` command automatically creates a `virtualenv` and installs everything in `requirements.txt`:
-
-```bash
-make pip-compile
-```
-
-## How to run the project
-Simply run the provided Python script in your preferred Python environment. The script will create a web interface using Gradio so you can input text and receive predictions from the model.
-
-```bash
-gradio app.py
-```
-
-## Usage
-Once you have launched the app, simply enter a sentence in the textbox and press Enter. The model will classify the sentence into one of the three classes mentioned above and display the confidence for each class.
-
-## Jupyter Notebooks
-
-- [`hate_speech_bert_bert_mlp_in_tensorflow.ipynb`](./hate_speech_bert_bert_mlp_in_tensorflow.ipynb): You can see how the model was trained
-- [`hate_speech_run.ipynb`](./hate_speech_run.ipynb): Example of model execution
-
-
-## References and Resources
-This project is based on:
-
-- Classify text with BERT. (s. f.). TensorFlow. https://www.tensorflow.org/text/tutorials/classify_text_with_bert
-- Devlin, J., Chang, M., Lee, K., & Toutanova, K. (2018). BERT: Pre-training of Deep Bidirectional Transformers for Language Understanding. arXiv (Cornell University). https://arxiv.org/pdf/1810.04805v2
-- G. (2021, February 3). Hate Speech - BERT+CNN and BERT+MLP in Tensorflow. Kaggle. https://www.kaggle.com/code/giovanimachado/hate-speech-bert-cnn-and-bert-mlp-in-tensorflow
-- Hate Speech and Offensive Language Dataset. (2020, June 17). Kaggle. https://www.kaggle.com/mrmorj/hate-speech-and-offensive-language-dataset
\ No newline at end of file
diff --git a/spaces/PAIR/Text2Video-Zero/annotator/uniformer/mmcv/parallel/data_container.py b/spaces/PAIR/Text2Video-Zero/annotator/uniformer/mmcv/parallel/data_container.py
deleted file mode 100644
index cedb0d32a51a1f575a622b38de2cee3ab4757821..0000000000000000000000000000000000000000
--- a/spaces/PAIR/Text2Video-Zero/annotator/uniformer/mmcv/parallel/data_container.py
+++ /dev/null
@@ -1,89 +0,0 @@
-# Copyright (c) OpenMMLab. All rights reserved.
-import functools
-
-import torch
-
-
-def assert_tensor_type(func):
-
- @functools.wraps(func)
- def wrapper(*args, **kwargs):
- if not isinstance(args[0].data, torch.Tensor):
- raise AttributeError(
- f'{args[0].__class__.__name__} has no attribute '
- f'{func.__name__} for type {args[0].datatype}')
- return func(*args, **kwargs)
-
- return wrapper
-
-
-class DataContainer:
- """A container for any type of objects.
-
- Typically tensors will be stacked in the collate function and sliced along
- some dimension in the scatter function. This behavior has some limitations.
- 1. All tensors have to be the same size.
- 2. Types are limited (numpy array or Tensor).
-
- We design `DataContainer` and `MMDataParallel` to overcome these
- limitations. The behavior can be either of the following.
-
- - copy to GPU, pad all tensors to the same size and stack them
- - copy to GPU without stacking
- - leave the objects as is and pass it to the model
- - pad_dims specifies the number of last few dimensions to do padding
- """
-
- def __init__(self,
- data,
- stack=False,
- padding_value=0,
- cpu_only=False,
- pad_dims=2):
- self._data = data
- self._cpu_only = cpu_only
- self._stack = stack
- self._padding_value = padding_value
- assert pad_dims in [None, 1, 2, 3]
- self._pad_dims = pad_dims
-
- def __repr__(self):
- return f'{self.__class__.__name__}({repr(self.data)})'
-
- def __len__(self):
- return len(self._data)
-
- @property
- def data(self):
- return self._data
-
- @property
- def datatype(self):
- if isinstance(self.data, torch.Tensor):
- return self.data.type()
- else:
- return type(self.data)
-
- @property
- def cpu_only(self):
- return self._cpu_only
-
- @property
- def stack(self):
- return self._stack
-
- @property
- def padding_value(self):
- return self._padding_value
-
- @property
- def pad_dims(self):
- return self._pad_dims
-
- @assert_tensor_type
- def size(self, *args, **kwargs):
- return self.data.size(*args, **kwargs)
-
- @assert_tensor_type
- def dim(self):
- return self.data.dim()
diff --git a/spaces/PAIR/Text2Video-Zero/gradio_utils.py b/spaces/PAIR/Text2Video-Zero/gradio_utils.py
deleted file mode 100644
index a9b2a752f0eb662f4624addc5e9073b7328bef3b..0000000000000000000000000000000000000000
--- a/spaces/PAIR/Text2Video-Zero/gradio_utils.py
+++ /dev/null
@@ -1,114 +0,0 @@
-import os
-
-# App Canny utils
-def edge_path_to_video_path(edge_path):
- video_path = edge_path
-
- vid_name = edge_path.split("/")[-1]
- if vid_name == "butterfly.mp4":
- video_path = "__assets__/canny_videos_mp4/butterfly.mp4"
- elif vid_name == "deer.mp4":
- video_path = "__assets__/canny_videos_mp4/deer.mp4"
- elif vid_name == "fox.mp4":
- video_path = "__assets__/canny_videos_mp4/fox.mp4"
- elif vid_name == "girl_dancing.mp4":
- video_path = "__assets__/canny_videos_mp4/girl_dancing.mp4"
- elif vid_name == "girl_turning.mp4":
- video_path = "__assets__/canny_videos_mp4/girl_turning.mp4"
- elif vid_name == "halloween.mp4":
- video_path = "__assets__/canny_videos_mp4/halloween.mp4"
- elif vid_name == "santa.mp4":
- video_path = "__assets__/canny_videos_mp4/santa.mp4"
-
- assert os.path.isfile(video_path)
- return video_path
-
-
-# App Pose utils
-def motion_to_video_path(motion):
- videos = [
- "__assets__/poses_skeleton_gifs/dance1_corr.mp4",
- "__assets__/poses_skeleton_gifs/dance2_corr.mp4",
- "__assets__/poses_skeleton_gifs/dance3_corr.mp4",
- "__assets__/poses_skeleton_gifs/dance4_corr.mp4",
- "__assets__/poses_skeleton_gifs/dance5_corr.mp4"
- ]
- if len(motion.split(" ")) > 1 and motion.split(" ")[1].isnumeric():
- id = int(motion.split(" ")[1]) - 1
- return videos[id]
- else:
- return motion
-
-
-# App Canny Dreambooth utils
-def get_video_from_canny_selection(canny_selection):
- if canny_selection == "woman1":
- input_video_path = "__assets__/db_files_2fps/woman1.mp4"
-
- elif canny_selection == "woman2":
- input_video_path = "__assets__/db_files_2fps/woman2.mp4"
-
- elif canny_selection == "man1":
- input_video_path = "__assets__/db_files_2fps/man1.mp4"
-
- elif canny_selection == "woman3":
- input_video_path = "__assets__/db_files_2fps/woman3.mp4"
- else:
- input_video_path = canny_selection
-
- assert os.path.isfile(input_video_path)
- return input_video_path
-
-
-def get_model_from_db_selection(db_selection):
- if db_selection == "Anime DB":
- input_video_path = 'PAIR/text2video-zero-controlnet-canny-anime'
- elif db_selection == "Avatar DB":
- input_video_path = 'PAIR/text2video-zero-controlnet-canny-avatar'
- elif db_selection == "GTA-5 DB":
- input_video_path = 'PAIR/text2video-zero-controlnet-canny-gta5'
- elif db_selection == "Arcane DB":
- input_video_path = 'PAIR/text2video-zero-controlnet-canny-arcane'
- else:
- input_video_path = db_selection
-
- return input_video_path
-
-
-def get_db_name_from_id(id):
- db_names = ["Anime DB", "Arcane DB", "GTA-5 DB", "Avatar DB"]
- return db_names[id]
-
-
-def get_canny_name_from_id(id):
- canny_names = ["woman1", "woman2", "man1", "woman3"]
- return canny_names[id]
-
-
-def logo_name_to_path(name):
- logo_paths = {
- 'Picsart AI Research': '__assets__/pair_watermark.png',
- 'Text2Video-Zero': '__assets__/t2v-z_watermark.png',
- 'None': None
- }
- if name in logo_paths:
- return logo_paths[name]
- return name
-
-
-# App Depth utils
-def depth_path_to_video_path(edge_path):
- video_path = edge_path
-
- vid_name = edge_path.split("/")[-1]
- if vid_name == "girl_dancing.mp4":
- video_path = "__assets__/depth_videos_mp4/girl_dancing.mp4"
- elif vid_name == "halloween.mp4":
- video_path = "__assets__/depth_videos_mp4/halloween.mp4"
- elif vid_name == "man.mp4":
- video_path = "__assets__/depth_videos_mp4/man.mp4"
- elif vid_name == "woman.mp4":
- video_path = "__assets__/depth_videos_mp4/woman.mp4"
-
- assert os.path.isfile(video_path)
- return video_path
diff --git a/spaces/Pattr/DrumClassification/lilypond-2.24.2/bin/musicxml2ly.py b/spaces/Pattr/DrumClassification/lilypond-2.24.2/bin/musicxml2ly.py
deleted file mode 100644
index 53c3c9227ec8fd5c6e960b5b3557cc84d58d2cac..0000000000000000000000000000000000000000
--- a/spaces/Pattr/DrumClassification/lilypond-2.24.2/bin/musicxml2ly.py
+++ /dev/null
@@ -1,3482 +0,0 @@
-#!/home/lily/lilypond-2.24.2/release/binaries/dependencies/install/Python-3.10.8/bin/python3.10
-# -*- coding: utf-8 -*-
-#
-# This file is part of LilyPond, the GNU music typesetter.
-#
-# Copyright (C) 2005--2022 Han-Wen Nienhuys ,
-# Jan Nieuwenhuizen ,
-# Reinhold Kainhofer ,
-# Patrick L. Schmidt
-#
-# LilyPond is free software: you can redistribute it and/or modify
-# it under the terms of the GNU General Public License as published by
-# the Free Software Foundation, either version 3 of the License, or
-# (at your option) any later version.
-#
-# LilyPond is distributed in the hope that it will be useful,
-# but WITHOUT ANY WARRANTY; without even the implied warranty of
-# MERCHANTABILITY or FITNESS FOR A PARTICULAR PURPOSE. See the
-# GNU General Public License for more details.
-#
-# You should have received a copy of the GNU General Public License
-# along with LilyPond. If not, see .
-
-
-from collections import OrderedDict
-from fractions import Fraction
-from functools import reduce
-import gettext
-import io
-import optparse
-import os
-import re
-import sys
-import tempfile
-import warnings
-import zipfile
-
-"""
-
-# relocate-preamble.py.in
-#
-# This file is part of LilyPond, the GNU music typesetter.
-#
-# Copyright (C) 2007--2022 Han-Wen Nienhuys
-#
-# LilyPond is free software: you can redistribute it and/or modify
-# it under the terms of the GNU General Public License as published by
-# the Free Software Foundation, either version 3 of the License, or
-# (at your option) any later version.
-#
-# LilyPond is distributed in the hope that it will be useful,
-# but WITHOUT ANY WARRANTY; without even the implied warranty of
-# MERCHANTABILITY or FITNESS FOR A PARTICULAR PURPOSE. See the
-# GNU General Public License for more details.
-#
-# You should have received a copy of the GNU General Public License
-# along with LilyPond. If not, see .
-#
-
-This is generic code, used for all python scripts.
-
-The quotes are to ensure that the source .py file can still be
-run as a python script, but does not include any sys.path handling.
-Otherwise, the lilypond-book calls inside the build
-might modify installed .pyc files.
-
-"""
-
-# This is needed for installations with a non-default layout, ie where share/
-# is not next to bin/.
-sys.path.insert (0, os.path.join ('/home/lily/lilypond-2.24.2/release/binaries/mingw/lilypond/install/share/lilypond/2.24.2', 'python'))
-
-# Dynamic relocation, for installations with a default layout including GUB,
-# but also for execution from the build directory.
-bindir = os.path.abspath (os.path.dirname (sys.argv[0]))
-topdir = os.path.dirname (bindir)
-if bindir.endswith (r'/scripts/out'):
- topdir = os.path.join (os.path.dirname (topdir), 'out')
-datadir = os.path.abspath (os.path.join (topdir, 'share', 'lilypond'))
-for v in [ 'current', '2.24.2' ]:
- sys.path.insert (0, os.path.join (datadir, v, 'python'))
-
-"""
-"""
-
-import musicexp
-import musicxml
-import musicxml2ly_conversion
-import utilities
-
-# Load translation and install _() into Python's builtins namespace.
-gettext.install('lilypond', '/home/lily/lilypond-2.24.2/release/binaries/mingw/lilypond/install/share/locale')
-
-import lilylib as ly
-
-lilypond_version = "2.24.2"
-
-# Store command-line options in a global variable, so we can access them everywhere
-options = None
-
-
-class Conversion_Settings:
- def __init__(self):
- self.ignore_beaming = False
- self.convert_stem_directions = False
- self.convert_rest_positions = True
-
-
-conversion_settings = Conversion_Settings()
-# Use a global variable to store the setting needed inside a \layout block.
-# whenever we need to change a setting or add/remove an engraver, we can access
-# this layout and add the corresponding settings
-layout_information = musicexp.Layout()
-# Use a global variable to store the setting needed inside a \paper block.
-paper = musicexp.Paper()
-
-needed_additional_definitions = []
-additional_definitions = {
- "tuplet-note-wrapper": """ % a formatter function, which is simply a wrapper around an existing
- % tuplet formatter function. It takes the value returned by the given
- % function and appends a note of given length.
- #(define-public ((tuplet-number::append-note-wrapper function note) grob)
- (let* ((txt (if function (function grob) #f)))
- (if txt
- (markup txt #:fontsize -5 #:note note UP)
- (markup #:fontsize -5 #:note note UP)
- )
- )
- )""",
-
- "tuplet-non-default-denominator": """#(define ((tuplet-number::non-default-tuplet-denominator-text denominator) grob)
- (number->string (if denominator
- denominator
- (ly:event-property (event-cause grob) 'denominator))))
-""",
-
- "tuplet-non-default-fraction": """#(define ((tuplet-number::non-default-tuplet-fraction-text denominator numerator) grob)
- (let* ((ev (event-cause grob))
- (den (if denominator denominator (ly:event-property ev 'denominator)))
- (num (if numerator numerator (ly:event-property ev 'numerator))))
- (format #f "~a:~a" den num)))
-""",
-}
-
-
-def round_to_two_digits(val):
- return round(val * 100) / 100
-
-
-def extract_paper_information(score_partwise):
- defaults = score_partwise.get_maybe_exist_named_child('defaults')
- if not defaults:
- return None
- tenths = -1
- scaling = defaults.get_maybe_exist_named_child('scaling')
- default_tenths_to_millimeters_ratio = 0.175
- default_staff_size = 20
- if scaling:
- mm = scaling.get_named_child('millimeters')
- mm = float(mm.get_text())
- tn = scaling.get_maybe_exist_named_child('tenths')
- tn = float(tn.get_text())
- # The variable 'tenths' is actually a ratio, NOT the value of .
- # TODO: rename and replace.
- tenths = mm / tn
- ratio = tenths / default_tenths_to_millimeters_ratio
- staff_size = default_staff_size * ratio
-
- if 1 < staff_size < 100:
- paper.global_staff_size = staff_size
- else:
- msg = "paper.global_staff_size %s is too large, using defaults=20" % staff_size
- warnings.warn(msg)
- paper.global_staff_size = 20
-
- # We need the scaling(i.e. the size of staff tenths for everything!
- if tenths < 0:
- return None
-
- def from_tenths(txt):
- return round_to_two_digits(float(txt) * tenths / 10)
-
- def set_paper_variable(varname, parent, element_name):
- el = parent.get_maybe_exist_named_child(element_name)
- if el: # Convert to cm from tenths
- setattr(paper, varname, from_tenths(el.get_text()))
-
- pagelayout = defaults.get_maybe_exist_named_child('page-layout')
- if pagelayout:
- # TODO: How can one have different margins for even and odd pages???
- set_paper_variable("page_height", pagelayout, 'page-height')
- set_paper_variable("page_width", pagelayout, 'page-width')
-
- if conversion_settings.convert_page_margins:
- pmargins = pagelayout.get_named_children('page-margins')
- for pm in pmargins:
- set_paper_variable("left_margin", pm, 'left-margin')
- set_paper_variable("right_margin", pm, 'right-margin')
- set_paper_variable("bottom_margin", pm, 'bottom-margin')
- set_paper_variable("top_margin", pm, 'top-margin')
-
- systemlayout = defaults.get_maybe_exist_named_child('system-layout')
- if systemlayout:
- sl = systemlayout.get_maybe_exist_named_child('system-margins')
- if sl:
- set_paper_variable("system_left_margin", sl, 'left-margin')
- set_paper_variable("system_right_margin", sl, 'right-margin')
- set_paper_variable("system_distance", systemlayout, 'system-distance')
- set_paper_variable("top_system_distance",
- systemlayout, 'top-system-distance')
-
- stafflayout = defaults.get_named_children('staff-layout')
- for sl in stafflayout:
- nr = getattr(sl, 'number', 1)
- dist = sl.get_named_child('staff-distance')
- # TODO: the staff distance needs to be set in the Staff context!!!
-
- # TODO: Finish appearance?, music-font?, word-font?, lyric-font*, lyric-language*
- appearance = defaults.get_named_child('appearance')
- if appearance:
- lws = appearance.get_named_children('line-width')
- for lw in lws:
- # Possible types are: beam, bracket, dashes,
- # enclosure, ending, extend, heavy barline, leger,
- # light barline, octave shift, pedal, slur middle, slur tip,
- # staff, stem, tie middle, tie tip, tuplet bracket, and wedge
- tp = lw.type
- w = from_tenths(lw.get_text())
- # TODO: Do something with these values!
- nss = appearance.get_named_children('note-size')
- for ns in nss:
- # Possible types are: cue, grace and large
- tp = ns.type
- sz = from_tenths(ns.get_text())
- # TODO: Do something with these values!
- # elements have no specified meaning
-
- rawmusicfont = defaults.get_named_child('music-font')
- if rawmusicfont:
- # TODO: Convert the font
- pass
- rawwordfont = defaults.get_named_child('word-font')
- if rawwordfont:
- # TODO: Convert the font
- pass
- rawlyricsfonts = defaults.get_named_children('lyric-font')
- for lyricsfont in rawlyricsfonts:
- # TODO: Convert the font
- pass
-
- return paper
-
-
-credit_dict = {
- None: None,
- '': None,
- 'page number': None, # TODO: what is it used for ?
- 'title': 'title',
- 'subtitle': 'subtitle',
- 'composer': 'composer',
- 'arranger': 'arranger',
- 'lyricist': 'poet',
- 'rights': 'copyright'
-}
-# score information is contained in the , or tags
-# extract those into a hash, indexed by proper lilypond header attributes
-
-
-def extract_score_information(tree):
- header = musicexp.Header()
-
- def set_if_exists(field, value):
- if value:
- header.set_field(field, utilities.escape_ly_output_string(value))
-
- movement_title = tree.get_maybe_exist_named_child('movement-title')
- movement_number = tree.get_maybe_exist_named_child('movement-number')
- if movement_title:
- set_if_exists('title', movement_title.get_text())
- if movement_number:
- set_if_exists('movementnumber', movement_number.get_text())
- # set_if_exists('piece', movement_number.get_text()) # the movement number should be visible in the score.
-
- work = tree.get_maybe_exist_named_child('work')
- if work:
- work_number = work.get_work_number()
- work_title = work.get_work_title()
- # Overwrite the title from movement-title with work->title
- set_if_exists('title', work.get_work_title())
- set_if_exists('opus', work.get_work_number())
- # Use movement-title as subtitle
- if movement_title:
- set_if_exists('subtitle', movement_title.get_text())
-
-# TODO: Translation of opus element. Not to be confused with opus in LilyPond. MusicXML opus is a document element for opus DTD
- identifications = tree.get_named_children('identification')
- for ids in identifications:
- set_if_exists('copyright', ids.get_rights())
- set_if_exists('composer', ids.get_composer())
- set_if_exists('arranger', ids.get_arranger())
- set_if_exists('editor', ids.get_editor())
- set_if_exists('poet', ids.get_poet())
-
- set_if_exists('encodingsoftware', ids.get_encoding_software())
- set_if_exists('encodingdate', ids.get_encoding_date())
- set_if_exists('encoder', ids.get_encoding_person())
- set_if_exists('encodingdescription', ids.get_encoding_description())
- set_if_exists('source', ids.get_source())
-
- # ... becomes
- # \header { texidoc = ...
- set_if_exists('texidoc', ids.get_file_description())
-
- # Finally, apply the required compatibility modes
- # Some applications created wrong MusicXML files, so we need to
- # apply some compatibility mode, e.g. ignoring some features/tags
- # in those files
- software = ids.get_encoding_software_list()
-
- # Case 1: "Sibelius 5.1" with the "Dolet 3.4 for Sibelius" plugin
- # is missing all beam ends => ignore all beaming information
- ignore_beaming_software = {
- "Dolet 4 for Sibelius, Beta 2": "Dolet 4 for Sibelius, Beta 2",
- "Dolet 3.5 for Sibelius": "Dolet 3.5 for Sibelius",
- "Dolet 3.4 for Sibelius": "Dolet 3.4 for Sibelius",
- "Dolet 3.3 for Sibelius": "Dolet 3.3 for Sibelius",
- "Dolet 3.2 for Sibelius": "Dolet 3.2 for Sibelius",
- "Dolet 3.1 for Sibelius": "Dolet 3.1 for Sibelius",
- "Dolet for Sibelius 1.3": "Dolet for Sibelius 1.3",
- "Noteworthy Composer": "Noteworthy Composer's nwc2xm[",
- }
- for s in software:
- app_description = ignore_beaming_software.get(s, False)
- if app_description:
- conversion_settings.ignore_beaming = True
- ly.warning(_("Encountered file created by %s, containing "
- "wrong beaming information. All beaming "
- "information in the MusicXML file will be "
- "ignored") % app_description)
-
- credits = tree.get_named_children('credit')
- has_composer = False
- for cred in credits:
- type = credit_dict.get(cred.get_type())
- if type is None:
- type = credit_dict.get(cred.find_type(credits))
- if type == 'composer':
- if has_composer:
- type = 'poet'
- else:
- has_composer = True
- set_if_exists(type, cred.get_text())
- elif type == 'title':
- if not work and not movement_title:
- set_if_exists('title', cred.get_text())
- # elif(not(movement_title)): #bullshit!
- # set_if_exists('subtitle', cred.get_text()) #bullshit! otherwise both title and subtitle show the work-title.
- elif type is None:
- pass
- else:
- set_if_exists(type, cred.get_text())
-
- # TODO: Check for other unsupported features
- return header
-
-
-class PartGroupInfo:
- def __init__(self):
- self.start = {}
- self.end = {}
-
- def is_empty(self):
- return len(self.start) + len(self.end) == 0
-
- def add_start(self, g):
- self.start[getattr(g, 'number', "1")] = g
-
- def add_end(self, g):
- self.end[getattr(g, 'number', "1")] = g
-
- def print_ly(self, printer):
- ly.warning(_("Unprocessed PartGroupInfo %s encountered") % self)
-
- def ly_expression(self):
- ly.warning(_("Unprocessed PartGroupInfo %s encountered") % self)
- return ''
-
-
-def staff_attributes_to_string_tunings(mxl_attr):
- details = mxl_attr.get_maybe_exist_named_child('staff-details')
- if not details:
- return []
- lines = 6
- staff_lines = details.get_maybe_exist_named_child('staff-lines')
- if staff_lines:
- lines = int(staff_lines.get_text())
- tunings = [musicexp.Pitch()] * lines
- staff_tunings = details.get_named_children('staff-tuning')
- for i in staff_tunings:
- p = musicexp.Pitch()
- line = 0
- try:
- line = int(i.line) - 1
- except ValueError:
- pass
- tunings[line] = p
-
- step = i.get_named_child('tuning-step')
- step = step.get_text().strip()
- p.step = musicxml2ly_conversion.musicxml_step_to_lily(step)
-
- octave = i.get_named_child('tuning-octave')
- octave = octave.get_text().strip()
- p.octave = int(octave) - 4
-
- alter = i.get_named_child('tuning-alter')
- if alter:
- p.alteration = int(alter.get_text().strip())
- # lilypond seems to use the opposite ordering than MusicXML...
- tunings.reverse()
- return tunings
-
-
-def staff_attributes_to_lily_staff(mxl_attr):
- if not mxl_attr:
- return musicexp.Staff()
-
- (staff_id, attributes) = list(mxl_attr.items())[0]
-
- # distinguish by clef:
- # percussion(percussion and rhythmic), tab, and everything else
- clef_sign = None
- clef = attributes.get_maybe_exist_named_child('clef')
- if clef:
- sign = clef.get_maybe_exist_named_child('sign')
- if sign:
- clef_sign = {"percussion": "percussion",
- "TAB": "tab"}.get(sign.get_text(), None)
-
- lines = 5
- details = attributes.get_named_children('staff-details')
- for d in details:
- staff_lines = d.get_maybe_exist_named_child('staff-lines')
- if staff_lines:
- lines = int(staff_lines.get_text())
-
- # TODO: Handle other staff attributes like staff-space, etc.
-
- staff = None
- if clef_sign == "percussion" and lines == 1:
- staff = musicexp.RhythmicStaff()
- elif clef_sign == "percussion":
- staff = musicexp.DrumStaff()
- # staff.drum_style_table = ???
- elif clef_sign == "tab":
- staff = musicexp.TabStaff()
- staff.string_tunings = staff_attributes_to_string_tunings(attributes)
- # staff.tablature_format = ???
- else:
- staff = musicexp.Staff()
- # TODO: Handle case with lines != 5!
- if lines != 5:
- staff.add_context_modification(
- "\\override StaffSymbol.line-count = #%s" % lines)
-
- return staff
-
-
-def extract_instrument_sound(score_part):
- score_instrument = score_part.get_maybe_exist_named_child(
- 'score-instrument')
- if not score_instrument:
- return None
- sound = score_instrument.get_maybe_exist_named_child('instrument-sound')
- if sound:
- return utilities.musicxml_sound_to_lilypond_midi_instrument(sound.get_text())
-
-
-def extract_score_structure(part_list, staffinfo):
- score = musicexp.Score()
- structure = musicexp.StaffGroup(None)
- score.set_contents(structure)
-
- if not part_list:
- return structure
-
- def read_score_part(el):
- if not isinstance(el, musicxml.Score_part):
- return
- # Depending on the attributes of the first measure, we create different
- # types of staves(Staff, RhythmicStaff, DrumStaff, TabStaff, etc.)
- staff = staff_attributes_to_lily_staff(staffinfo.get(el.id, None))
- if not staff:
- return None
- staff.id = el.id
- partname = el.get_maybe_exist_named_child('part-name')
- # Finale gives unnamed parts the name "MusicXML Part" automatically!
- if partname and partname.get_text() != "MusicXML Part":
- staff.instrument_name = partname.get_text()
- # part-name-display overrides part-name!
- partname = el.get_maybe_exist_named_child("part-name-display")
- if partname:
- staff.instrument_name = extract_display_text(partname)
- if hasattr(options, 'midi') and options.midi:
- staff.sound = extract_instrument_sound(el)
- if staff.instrument_name:
- paper.indent = max(paper.indent, len(staff.instrument_name))
- paper.instrument_names.append(staff.instrument_name)
- partdisplay = el.get_maybe_exist_named_child('part-abbreviation')
- if partdisplay:
- staff.short_instrument_name = partdisplay.get_text()
- # part-abbreviation-display overrides part-abbreviation!
- partdisplay = el.get_maybe_exist_named_child(
- "part-abbreviation-display")
- if partdisplay:
- staff.short_instrument_name = extract_display_text(partdisplay)
- # TODO: Read in the MIDI device / instrument
- if staff.short_instrument_name:
- paper.short_indent = max(
- paper.short_indent, len(staff.short_instrument_name))
-
- return staff
-
- def read_score_group(el):
- if not isinstance(el, musicxml.Part_group):
- return
- group = musicexp.StaffGroup()
- if hasattr(el, 'number'):
- id = el.number
- group.id = id
- #currentgroups_dict[id] = group
- # currentgroups.append(id)
- if el.get_maybe_exist_named_child('group-name'):
- group.instrument_name = el.get_maybe_exist_named_child(
- 'group-name').get_text()
- if el.get_maybe_exist_named_child('group-abbreviation'):
- group.short_instrument_name = el.get_maybe_exist_named_child(
- 'group-abbreviation').get_text()
- if el.get_maybe_exist_named_child('group-symbol'):
- group.symbol = el.get_maybe_exist_named_child(
- 'group-symbol').get_text()
- if el.get_maybe_exist_named_child('group-barline'):
- group.spanbar = el.get_maybe_exist_named_child(
- 'group-barline').get_text()
- return group
-
- parts_groups = part_list.get_all_children()
-
- # the start/end group tags are not necessarily ordered correctly and groups
- # might even overlap, so we can't go through the children sequentially!
-
- # 1) Replace all Score_part objects by their corresponding Staff objects,
- # also collect all group start/stop points into one PartGroupInfo object
- staves = []
- group_info = PartGroupInfo()
- for el in parts_groups:
- if isinstance(el, musicxml.Score_part):
- if not group_info.is_empty():
- staves.append(group_info)
- group_info = PartGroupInfo()
- staff = read_score_part(el)
- if staff:
- staves.append(staff)
- elif isinstance(el, musicxml.Part_group):
- if el.type == "start":
- group_info.add_start(el)
- elif el.type == "stop":
- group_info.add_end(el)
- if not group_info.is_empty():
- staves.append(group_info)
-
- # 2) Now, detect the groups:
- group_starts = []
- pos = 0
- while pos < len(staves):
- el = staves[pos]
- if isinstance(el, PartGroupInfo):
- prev_start = 0
- if len(group_starts) > 0:
- prev_start = group_starts[-1]
- elif len(el.end) > 0: # no group to end here
- el.end = {}
- if len(el.end) > 0: # closes an existing group
- ends = list(el.end.keys())
- prev_started = list(staves[prev_start].start.keys())
- grpid = None
- intersection = [x for x in prev_started if x in ends]
- if len(intersection) > 0:
- grpid = intersection[0]
- else:
- # Close the last started group
- grpid = list(staves[prev_start].start.keys())[0]
- # Find the corresponding closing tag and remove it!
- j = pos + 1
- foundclosing = False
- while j < len(staves) and not foundclosing:
- if isinstance(staves[j], PartGroupInfo) and grpid in staves[j].end:
- foundclosing = True
- del staves[j].end[grpid]
- if staves[j].is_empty():
- del staves[j]
- j += 1
- grpobj = staves[prev_start].start[grpid]
- group = read_score_group(grpobj)
- # remove the id from both the start and end
- if grpid in el.end:
- del el.end[grpid]
- del staves[prev_start].start[grpid]
- if el.is_empty():
- del staves[pos]
- # replace the staves with the whole group
- for j in staves[(prev_start + 1):pos]:
- group.append_staff(j)
- del staves[(prev_start + 1):pos]
- staves.insert(prev_start + 1, group)
- # reset pos so that we continue at the correct position
- pos = prev_start
- # remove an empty start group
- if staves[prev_start].is_empty():
- del staves[prev_start]
- group_starts.remove(prev_start)
- pos -= 1
- elif len(el.start) > 0: # starts new part groups
- group_starts.append(pos)
- pos += 1
-
- for i in staves:
- structure.append_staff(i)
- return score
-
-
-def musicxml_partial_to_lily(partial_len):
- if partial_len > 0:
- p = musicexp.Partial()
- p.partial = musicxml2ly_conversion.rational_to_lily_duration(
- partial_len)
- return p
- else:
- return None
-
-# Detect repeats and alternative endings in the chord event list(music_list)
-# and convert them to the corresponding musicexp objects, containing nested
-# music
-
-
-def group_repeats(music_list):
- repeat_replaced = True
- music_start = 0
- i = 0
- # Walk through the list of expressions, looking for repeat structure
- # (repeat start/end, corresponding endings). If we find one, try to find the
- # last event of the repeat, replace the whole structure and start over again.
- # For nested repeats, as soon as we encounter another starting repeat bar,
- # treat that one first, and start over for the outer repeat.
- while repeat_replaced and i < 100:
- i += 1
- repeat_start = -1 # position of repeat start / end
- repeat_end = -1 # position of repeat start / end
- repeat_times = 0
- ending_start = -1 # position of current ending start
- endings = [] # list of already finished endings
- pos = 0
- last = len(music_list) - 1
- repeat_replaced = False
- final_marker = 0
- while pos < len(music_list) and not repeat_replaced:
- e = music_list[pos]
- repeat_finished = False
- if isinstance(e, musicxml2ly_conversion.RepeatMarker):
- if not repeat_times and e.times:
- repeat_times = e.times
- if e.direction == -1:
- if repeat_end >= 0:
- repeat_finished = True
- else:
- repeat_start = pos
- repeat_end = -1
- ending_start = -1
- endings = []
- elif e.direction == 1:
- if repeat_start < 0:
- repeat_start = 0
- if repeat_end < 0:
- repeat_end = pos
- final_marker = pos
- elif isinstance(e, musicxml2ly_conversion.EndingMarker):
- if e.direction == -1:
- if repeat_start < 0:
- repeat_start = 0
- if repeat_end < 0:
- repeat_end = pos
- ending_start = pos
- elif e.direction == 1:
- if ending_start < 0:
- ending_start = 0
- endings.append([ending_start, pos])
- ending_start = -1
- final_marker = pos
- elif not isinstance(e, musicexp.BarLine):
- # As soon as we encounter an element when repeat start and end
- # is set and we are not inside an alternative ending,
- # this whole repeat structure is finished => replace it
- if repeat_start >= 0 and repeat_end > 0 and ending_start < 0:
- repeat_finished = True
-
- # Finish off all repeats without explicit ending bar(e.g. when
- # we convert only one page of a multi-page score with repeats)
- if pos == last and repeat_start >= 0:
- repeat_finished = True
- final_marker = pos
- if repeat_end < 0:
- repeat_end = pos
- if ending_start >= 0:
- endings.append([ending_start, pos])
- ending_start = -1
-
- if repeat_finished:
- # We found the whole structure replace it!
- r = musicexp.RepeatedMusic()
- if repeat_times <= 0:
- repeat_times = 2
- r.repeat_count = repeat_times
- # don't erase the first element for "implicit" repeats(i.e. no
- # starting repeat bars at the very beginning)
- start = repeat_start + 1
- if repeat_start == music_start:
- start = music_start
- r.set_music(music_list[start:repeat_end])
- for(start, end) in endings:
- s = musicexp.SequentialMusic()
- s.elements = music_list[start + 1:end]
- r.add_ending(s)
- del music_list[repeat_start:final_marker + 1]
- music_list.insert(repeat_start, r)
- repeat_replaced = True
- pos += 1
- # TODO: Implement repeats until the end without explicit ending bar
- return music_list
-
-
-# Extract the settings for tuplets from the and the
-# elements of the note:
-def musicxml_tuplet_to_lily(tuplet_elt, time_modification):
- tsm = musicexp.TimeScaledMusic()
- fraction = (1, 1)
- if time_modification:
- fraction = time_modification.get_fraction()
- tsm.numerator = fraction[0]
- tsm.denominator = fraction[1]
-
- normal_type = tuplet_elt.get_normal_type()
- if not normal_type and time_modification:
- normal_type = time_modification.get_normal_type()
- if not normal_type and time_modification:
- note = time_modification.get_parent()
- if note:
- normal_type = note.get_duration_info()
- if normal_type:
- normal_note = musicexp.Duration()
- (normal_note.duration_log, normal_note.dots) = normal_type
- tsm.normal_type = normal_note
-
- actual_type = tuplet_elt.get_actual_type()
- if actual_type:
- actual_note = musicexp.Duration()
- (actual_note.duration_log, actual_note.dots) = actual_type
- tsm.actual_type = actual_note
-
- # Obtain non-default nrs of notes from the tuplet object!
- tsm.display_numerator = tuplet_elt.get_normal_nr()
- tsm.display_denominator = tuplet_elt.get_actual_nr()
-
- if hasattr(tuplet_elt, 'bracket') and tuplet_elt.bracket == "no":
- tsm.display_bracket = None
- elif hasattr(tuplet_elt, 'line-shape') and getattr(tuplet_elt, 'line-shape') == "curved":
- tsm.display_bracket = "curved"
- else:
- tsm.display_bracket = "bracket"
-
- display_values = {"none": None, "actual": "actual", "both": "both"}
- if hasattr(tuplet_elt, "show-number"):
- tsm.display_number = display_values.get(
- getattr(tuplet_elt, "show-number"), "actual")
-
- if hasattr(tuplet_elt, "show-type"):
- tsm.display_type = display_values.get(
- getattr(tuplet_elt, "show-type"), None)
-
- return tsm
-
-
-def group_tuplets(music_list, events):
- """Collect Musics from
- MUSIC_LIST demarcated by EVENTS_LIST in TimeScaledMusic objects.
- """
-
- indices = []
- brackets = {}
-
- j = 0
- for(ev_chord, tuplet_elt, time_modification) in events:
- while j < len(music_list):
- if music_list[j] == ev_chord:
- break
- j += 1
- nr = 0
- if hasattr(tuplet_elt, 'number'):
- nr = getattr(tuplet_elt, 'number')
- if tuplet_elt.type == 'start':
- tuplet_object = musicxml_tuplet_to_lily(
- tuplet_elt, time_modification)
- tuplet_info = [j, None, tuplet_object]
- indices.append(tuplet_info)
- brackets[nr] = tuplet_info
- elif tuplet_elt.type == 'stop':
- bracket_info = brackets.get(nr, None)
- if bracket_info:
- bracket_info[1] = j # Set the ending position to j
- del brackets[nr]
-
- new_list = []
- last = 0
- for(i1, i2, tsm) in indices:
- if i1 > i2:
- continue
-
- new_list.extend(music_list[last:i1])
- seq = musicexp.SequentialMusic()
- last = i2 + 1
-
- # At this point music_list[i1:last] encompasses all the notes of the
- # tuplet. There might be dynamics following this range, however, which
- # apply to the last note of the tuplet. Advance last to include them
- # in the range.
- while last < len(music_list) and isinstance(music_list[last], musicexp.DynamicsEvent):
- last += 1
-
- seq.elements = music_list[i1:last]
-
- tsm.element = seq
-
- new_list.append(tsm)
- # TODO: Handle nested tuplets!!!!
-
- new_list.extend(music_list[last:])
- return new_list
-
-
-def musicxml_clef_to_lily(attributes):
- change = musicexp.ClefChange()
- (change.type, change.position, change.octave) = attributes.get_clef_information()
- return change
-
-
-def musicxml_time_to_lily(attributes):
- change = musicexp.TimeSignatureChange()
- # time signature function
- if hasattr(options, 'shift_meter') and options.shift_meter:
- tmp_meter = options.shift_meter.split("/", 1)
- sig = [int(tmp_meter[0]), int(tmp_meter[1])]
- change.originalFractions = attributes.get_time_signature()
- else:
- sig = attributes.get_time_signature()
- if not sig:
- return None
- change.fractions = sig
-
- time_elm = attributes.get_maybe_exist_named_child('time')
- if time_elm and hasattr(time_elm, 'symbol'):
- change.style = {'single-number': "'single-digit",
- 'cut': None,
- 'common': None,
- 'normal': "'()"}.get(time_elm.symbol, "'()")
- else:
- change.style = "'()"
-
- if getattr(time_elm, 'print-object', 'yes') == 'no':
- change.visible = False
-
- # TODO: Handle senza-misura measures
- # TODO: What shall we do if the symbol clashes with the sig? e.g. "cut"
- # with 3/8 or "single-number" with(2+3)/8 or 3/8+2/4?
- return change
-
-
-def musicxml_key_to_lily(attributes):
- key_sig = attributes.get_key_signature()
- if not key_sig or not(isinstance(key_sig, list) or isinstance(key_sig, tuple)):
- ly.warning(_("Unable to extract key signature!"))
- return None
-
- change = musicexp.KeySignatureChange()
-
- if len(key_sig) == 2 and not isinstance(key_sig[0], list):
- # standard key signature,(fifths, mode)
- (fifths, mode) = key_sig
- change.mode = mode
-
- start_pitch = musicexp.Pitch()
- start_pitch.octave = 0
- try:
- (n, a) = {
- 'major': (0, 0),
- 'minor': (5, 0),
- 'ionian': (0, 0),
- 'dorian': (1, 0),
- 'phrygian': (2, 0),
- 'lydian': (3, 0),
- 'mixolydian': (4, 0),
- 'aeolian': (5, 0),
- 'locrian': (6, 0),
- }[mode]
- start_pitch.step = n
- start_pitch.alteration = a
- except KeyError:
- ly.warning(_("unknown mode %s, expecting 'major' or 'minor' "
- "or a church mode!") % mode)
-
- fifth = musicexp.Pitch()
- fifth.step = 4
- if fifths < 0:
- fifths *= -1
- fifth.step *= -1
- fifth.normalize()
- for x in range(fifths):
- start_pitch = start_pitch.transposed(fifth)
- change.tonic = start_pitch
-
- else:
- # Non-standard key signature of the form [[step,alter<,octave>],...]
- # MusicXML contains C,D,E,F,G,A,B as steps, lily uses 0-7, so convert
- alterations = []
- for k in key_sig:
- k[0] = musicxml2ly_conversion.musicxml_step_to_lily(k[0])
- alterations.append(k)
- change.non_standard_alterations = alterations
- return change
-
-
-def musicxml_transpose_to_lily(attributes):
- transpose = attributes.get_transposition()
- if not transpose:
- return None
-
- shift = musicexp.Pitch()
- octave_change = transpose.get_maybe_exist_named_child('octave-change')
- if octave_change:
- shift.octave = int(octave_change.get_text())
- chromatic_shift = int(transpose.get_named_child('chromatic').get_text())
- chromatic_shift_normalized = chromatic_shift % 12
- (shift.step, shift.alteration) = [
- (0, 0), (0, 1), (1, 0), (2, -1), (2, 0),
- (3, 0), (3, 1), (4, 0), (5, -1), (5, 0),
- (6, -1), (6, 0)][chromatic_shift_normalized]
-
- shift.octave += (chromatic_shift - chromatic_shift_normalized) // 12
-
- diatonic = transpose.get_maybe_exist_named_child('diatonic')
- if diatonic:
- diatonic_step = int(diatonic.get_text()) % 7
- if diatonic_step != shift.step:
- # We got the alter incorrect!
- old_semitones = shift.semitones()
- shift.step = diatonic_step
- new_semitones = shift.semitones()
- shift.alteration += old_semitones - new_semitones
-
- transposition = musicexp.Transposition()
- transposition.pitch = musicexp.Pitch().transposed(shift)
- return transposition
-
-
-def musicxml_staff_details_to_lily(attributes):
- details = attributes.get_maybe_exist_named_child('staff-details')
- if not details:
- return None
-
- # TODO: Handle staff-type, staff-lines, staff-tuning, capo, staff-size
- ret = []
-
- stafflines = details.get_maybe_exist_named_child('staff-lines')
- if stafflines:
- lines = int(stafflines.get_text())
- lines_event = musicexp.StaffLinesEvent(lines)
- ret.append(lines_event)
-
- return ret
-
-
-def musicxml_attributes_to_lily(attrs):
- elts = []
- attr_dispatch = [
- ('clef', musicxml_clef_to_lily),
- ('time', musicxml_time_to_lily),
- ('key', musicxml_key_to_lily),
- ('transpose', musicxml_transpose_to_lily),
- ('staff-details', musicxml_staff_details_to_lily),
- ]
- for (k, func) in attr_dispatch:
- children = attrs.get_named_children(k)
- if children:
- ev = func(attrs)
- if isinstance(ev, list):
- for e in ev:
- elts.append(e)
- elif ev:
- elts.append(ev)
-
- return elts
-
-
-def extract_display_text(el):
- children = el.get_typed_children(musicxml.get_class("display-text"))
- if children:
- return " ".join([child.get_text() for child in children])
- else:
- return False
-
-
-def musicxml_print_to_lily(el):
- # TODO: Implement other print attributes
- #
- #
- elts = []
- if (hasattr(el, "new-system") and conversion_settings.convert_system_breaks):
- val = getattr(el, "new-system")
- if val == "yes":
- elts.append(musicexp.Break("break"))
- if hasattr(el, "new-page") and conversion_settings.convert_page_breaks:
- val = getattr(el, "new-page")
- if val == "yes":
- elts.append(musicexp.Break("pageBreak"))
- child = el.get_maybe_exist_named_child("part-name-display")
- if child:
- elts.append(musicexp.SetEvent("Staff.instrumentName",
- "\"%s\"" % extract_display_text(child)))
- child = el.get_maybe_exist_named_child("part-abbreviation-display")
- if child:
- elts.append(musicexp.SetEvent("Staff.shortInstrumentName",
- "\"%s\"" % extract_display_text(child)))
- return elts
-
-
-spanner_event_dict = {
- 'beam': musicexp.BeamEvent,
- 'dashes': musicexp.TextSpannerEvent,
- 'bracket': musicexp.BracketSpannerEvent,
- 'glissando': musicexp.GlissandoEvent,
- 'octave-shift': musicexp.OctaveShiftEvent,
- 'pedal': musicexp.PedalEvent,
- 'slide': musicexp.GlissandoEvent,
- 'slur': musicexp.SlurEvent,
- 'wavy-line': musicexp.TextSpannerEvent,
- 'wedge': musicexp.HairpinEvent
-}
-spanner_type_dict = {
- 'start': -1,
- 'begin': -1,
- 'crescendo': -1,
- 'decreschendo': -1,
- 'diminuendo': -1,
- 'continue': 0,
- 'change': 0,
- 'up': -1,
- 'down': -1,
- 'stop': 1,
- 'end': 1
-}
-
-
-def musicxml_spanner_to_lily_event(mxl_event):
- ev = None
-
- name = mxl_event.get_name()
- func = spanner_event_dict.get(name)
- if func:
- ev = func()
- else:
- ly.warning(_('unknown span event %s') % mxl_event)
-
- if name == "wavy-line":
- ev.style = OrnamenthasWhat(mxl_event)
-
- type = mxl_event.get_type()
- span_direction = spanner_type_dict.get(type)
- # really check for None, because some types will be translated to 0, which
- # would otherwise also lead to the unknown span warning
- if span_direction is not None:
- ev.span_direction = span_direction
- else:
- ly.warning(_('unknown span type %s for %s') % (type, name))
-
- ev.set_span_type(type)
- ev.line_type = getattr(mxl_event, 'line-type', 'solid')
-
- # assign the size, which is used for octave-shift, etc.
- ev.size = mxl_event.get_size()
-
- return ev
-
-
-def musicxml_direction_to_indicator(direction):
- return {"above": 1, "upright": 1, "up": 1, "below": -1, "downright": -1, "down": -1, "inverted": -1}.get(direction, 0)
-
-
-def musicxml_fermata_to_lily_event(mxl_event):
-
- ev = musicexp.ArticulationEvent()
- txt = mxl_event.get_text()
-
- # The contents of the element defined the shape, possible are normal, angled and square
- ev.type = {"angled": "shortfermata",
- "square": "longfermata"}.get(txt, "fermata")
- fermata_types = {"angled": "shortfermata",
- "square": "longfermata"}
-
- # MusicXML fermata types can be specified in two different ways:
- # 1. angled and
- # 2. -- both need to be handled.
- if hasattr(mxl_event, 'type'):
- fermata_type = fermata_types.get(mxl_event.type, 'fermata')
- else:
- fermata_type = fermata_types.get(mxl_event.get_text(), 'fermata')
-
- ev.type = fermata_type
-
- if hasattr(mxl_event, 'type'):
- dir = musicxml_direction_to_indicator(mxl_event.type)
- if dir and options.convert_directions:
- ev.force_direction = dir
- return ev
-
-
-def musicxml_arpeggiate_to_lily_event(mxl_event):
- ev = musicexp.ArpeggioEvent()
- ev.direction = musicxml_direction_to_indicator(
- getattr(mxl_event, 'direction', None))
- return ev
-
-
-def musicxml_nonarpeggiate_to_lily_event(mxl_event):
- ev = musicexp.ArpeggioEvent()
- ev.non_arpeggiate = True
- ev.direction = musicxml_direction_to_indicator(
- getattr(mxl_event, 'direction', None))
- return ev
-
-
-def musicxml_tremolo_to_lily_event(mxl_event):
- ev = musicexp.TremoloEvent()
- txt = mxl_event.get_text()
- if txt:
- ev.strokes = txt
- else:
- # This is supposed to be a default for empty tremolo elements
- # TODO: Add empty tremolo element to test cases in tremolo.xml
- # TODO: Test empty tremolo element
- # TODO: Consideration: Is 3 really a reasonable default?
- ev.strokes = "3"
- return ev
-
-
-def musicxml_falloff_to_lily_event(mxl_event):
- ev = musicexp.BendEvent()
- ev.alter = -4
- return ev
-
-
-def musicxml_doit_to_lily_event(mxl_event):
- ev = musicexp.BendEvent()
- ev.alter = 4
- return ev
-
-
-def musicxml_bend_to_lily_event(mxl_event):
- ev = musicexp.BendEvent()
- ev.alter = mxl_event.bend_alter()
- return ev
-
-
-def musicxml_breath_mark_to_lily_event(mxl_event):
- # TODO: Read the child and override the type
- # of symbol: comma, tick, upbow, salzedo.
- return musicexp.BreatheEvent()
-
-
-def musicxml_caesura_to_lily_event(mxl_event):
- # TODO: Read the child and override the type of
- # symbol: normal, thick, short, curved, single.
- return musicexp.CaesuraEvent()
-
-
-def musicxml_fingering_event(mxl_event):
- ev = musicexp.ShortArticulationEvent()
- ev.type = mxl_event.get_text()
- return ev
-
-
-def musicxml_string_event(mxl_event):
- ev = musicexp.NoDirectionArticulationEvent()
- ev.type = mxl_event.get_text()
- return ev
-
-
-def musicxml_accidental_mark(mxl_event):
- ev = musicexp.MarkupEvent()
- contents = {"sharp": "\\sharp",
- "natural": "\\natural",
- "flat": "\\flat",
- "double-sharp": "\\doublesharp",
- "sharp-sharp": "\\sharp\\sharp",
- "flat-flat": "\\flat\\flat",
- "flat-flat": "\\doubleflat",
- "natural-sharp": "\\natural\\sharp",
- "natural-flat": "\\natural\\flat",
- "quarter-flat": "\\semiflat",
- "quarter-sharp": "\\semisharp",
- "three-quarters-flat": "\\sesquiflat",
- "three-quarters-sharp": "\\sesquisharp",
- }.get(mxl_event.get_text())
- if contents:
- ev.contents = contents
- return ev
- else:
- return None
-
-
-# translate articulations, ornaments and other notations into ArticulationEvents
-# possible values:
-# -) string (ArticulationEvent with that name)
-# -) function (function(mxl_event) needs to return a full ArticulationEvent-derived object
-# -) (class, name) (like string, only that a different class than ArticulationEvent is used)
-# TODO: Some translations are missing!
-articulations_dict = {
- "accent": (musicexp.ShortArticulationEvent, ">"), # or "accent"
- "accidental-mark": musicxml_accidental_mark,
- "bend": musicxml_bend_to_lily_event,
- "breath-mark": musicxml_breath_mark_to_lily_event,
- "caesura": musicxml_caesura_to_lily_event,
- # "delayed-turn": "?",
- "detached-legato": (musicexp.ShortArticulationEvent, "_"), # or "portato"
- "doit": musicxml_doit_to_lily_event,
- # "double-tongue": "?",
- "down-bow": "downbow",
- "falloff": musicxml_falloff_to_lily_event,
- "fingering": musicxml_fingering_event,
- # "fingernails": "?",
- # "fret": "?",
- # "hammer-on": "?",
- "harmonic": "flageolet",
- # "heel": "?",
- "inverted-mordent": "prall",
- "inverted-turn": "reverseturn",
- "mordent": "mordent",
- "open-string": "open",
- # "plop": "?",
- # "pluck": "?",
- # "pull-off": "?",
- # "schleifer": "?",
- # "scoop": "?",
- # "shake": "?",
- "snap-pizzicato": "snappizzicato",
- # "spiccato": "?",
- # or "staccatissimo"
- "staccatissimo": (musicexp.ShortArticulationEvent, "!"),
- "staccato": (musicexp.ShortArticulationEvent, "."), # or "staccato"
- "stopped": (musicexp.ShortArticulationEvent, "+"), # or "stopped"
- # "stress": "?",
- "string": musicxml_string_event,
- "strong-accent": (musicexp.ShortArticulationEvent, "^"), # or "marcato"
- # "tap": "?",
- "tenuto": (musicexp.ShortArticulationEvent, "-"), # or "tenuto"
- "thumb-position": "thumb",
- # "toe": "?",
- "turn": "turn",
- "tremolo": musicxml_tremolo_to_lily_event,
- "trill-mark": "trill",
- # "triple-tongue": "?",
- # "unstress": "?"
- "up-bow": "upbow",
- # "wavy-line": "?",
-}
-articulation_spanners = ["wavy-line"]
-
-
-def OrnamenthasWhat(mxl_event):
- wavy = trilly = ignore = start = stop = False
- for i in mxl_event._parent._children:
- if i._name == "wavy-line":
- wavy = True
- elif i._name == "trill-mark":
- trilly = True
- try:
- if i.type == "continue":
- ignore = True
- elif i.type == "start":
- start = True
- elif i.type == "stop":
- stop = True
- except Exception: ## TODO: find out what to except.
- pass
- if start == True:
- if wavy == True and trilly == False:
- musicexp.whatOrnament = "wave"
- else:
- musicexp.whatOrnament = "trill"
- if ignore == True:
- return "ignore"
- elif stop == True:
- return "stop"
- elif wavy == True and trilly == True:
- return "trill and wave"
- elif wavy == True:
- return "wave"
- elif trilly == True:
- return "trill"
-
-
-def OrnamenthasWavyline(mxl_event):
- for i in mxl_event._parent._children:
- if i._name == "wavy-line":
- return True
- return False
-
-
-def musicxml_articulation_to_lily_event(mxl_event):
- # wavy-line elements are treated as trill spanners, not as articulation ornaments
- if mxl_event.get_name() in articulation_spanners:
- return musicxml_spanner_to_lily_event(mxl_event)
-
- tmp_tp = articulations_dict.get(mxl_event.get_name())
- if OrnamenthasWavyline(mxl_event):
- return
- if not tmp_tp:
- return
-
- if isinstance(tmp_tp, str):
- ev = musicexp.ArticulationEvent()
- ev.type = tmp_tp
- elif isinstance(tmp_tp, tuple):
- ev = tmp_tp[0]()
- ev.type = tmp_tp[1]
- else:
- ev = tmp_tp(mxl_event)
-
- # Some articulations use the type attribute, other the placement...
- dir = None
- if hasattr(mxl_event, 'type') and hasattr(options, 'convert_directions') and options.convert_directions:
- dir = musicxml_direction_to_indicator(mxl_event.type)
- if hasattr(mxl_event, 'placement') and hasattr(options, 'convert_directions') and options.convert_directions:
- dir = musicxml_direction_to_indicator(mxl_event.placement)
- if dir:
- ev.force_direction = dir
- return ev
-
-
-def musicxml_dynamics_to_lily_event(dynentry):
- dynamics_available = (
- "ppppp", "pppp", "ppp", "pp", "p", "mp", "mf",
- "f", "ff", "fff", "ffff", "fp", "sf", "sff", "sp", "spp", "sfz", "rfz")
- dynamicsname = dynentry.get_name()
- if dynamicsname == "other-dynamics":
- dynamicsname = dynentry.get_text()
- if not dynamicsname or dynamicsname == "#text":
- return None
-
- if not dynamicsname in dynamics_available:
- # Get rid of - in tag names (illegal in ly tags!)
- dynamicstext = dynamicsname
- dynamicsname = dynamicsname.replace("-", "")
- additional_definitions[dynamicsname] = dynamicsname + \
- " = #(make-dynamic-script \"" + dynamicstext + "\")"
- needed_additional_definitions.append(dynamicsname)
- event = musicexp.DynamicsEvent()
- event.type = dynamicsname
- return event
-
-# Convert single-color two-byte strings to numbers 0.0 - 1.0
-
-
-def hexcolorval_to_nr(hex_val):
- try:
- v = int(hex_val, 16)
- if v == 255:
- v = 256
- return v / 256.
- except ValueError:
- return 0.
-
-
-def hex_to_color(hex_val):
- res = re.match(
- r'#([0-9a-f][0-9a-f]|)([0-9a-f][0-9a-f])([0-9a-f][0-9a-f])([0-9a-f][0-9a-f])$', hex_val, re.IGNORECASE)
- if res:
- return [hexcolorval_to_nr(x) for x in res.group(2, 3, 4)]
- else:
- return None
-
-
-def font_size_number_to_lily_command(size):
- d = {
- (0, 8): r'\teeny',
- (8, 10): r'\tiny',
- (10, 12): r'\small',
- (12, 16): r'',
- (16, 24): r'\large',
- (24, float('inf')): r'\huge',
- }
- result = None
- for r in list(d.keys()):
- if r[0] <= size < r[1]:
- result = d[r]
- break
- return result
-
-
-def font_size_word_to_lily_command(size):
- font_size_dict = {
- "xx-small": '\\teeny',
- "x-small": '\\tiny',
- "small": '\\small',
- "medium": '',
- "large": '\\large',
- "x-large": '\\huge',
- "xx-large": '\\larger\\huge'
- }
- return font_size_dict.get(size, '')
-
-
-def get_font_size(size):
- try:
- size = float(size)
- return font_size_number_to_lily_command(size)
- except ValueError:
- return font_size_word_to_lily_command(size)
-
-
-def musicxml_words_to_lily_event(words):
- event = musicexp.TextEvent()
- text = words.get_text()
- # remove white spaces and line breaks before text
- text = re.sub('^ *\n? *', '', text)
- # remove white spaces and line breaks before text
- text = re.sub(' *\n? *$', '', text)
- event.text = text
-
- if hasattr(words, 'default-y') and hasattr(options, 'convert_directions') and options.convert_directions:
- offset = getattr(words, 'default-y')
- try:
- off = int(offset)
- if off > 0:
- event.force_direction = 1
- else:
- event.force_direction = -1
- except ValueError:
- event.force_direction = 0
-
- if hasattr(words, 'font-weight'):
- font_weight = {"normal": '', "bold": '\\bold'}.get(
- getattr(words, 'font-weight'), '')
- if font_weight:
- event.markup += font_weight
-
- if hasattr(words, 'font-size'):
- size = getattr(words, 'font-size')
- # font_size = font_size_dict.get(size, '')
- font_size = get_font_size(size)
- if font_size:
- event.markup += font_size
-
- if hasattr(words, 'color'):
- color = getattr(words, 'color')
- rgb = hex_to_color(color)
- if rgb:
- event.markup += "\\with-color #(rgb-color %s %s %s)" % (
- rgb[0], rgb[1], rgb[2])
-
- if hasattr(words, 'font-style'):
- font_style = {"italic": '\\italic'}.get(
- getattr(words, 'font-style'), '')
- if font_style:
- event.markup += font_style
-
- # TODO: How should I best convert the font-family attribute?
-
- # TODO: How can I represent the underline, overline and line-through
- # attributes in LilyPond? Values of these attributes indicate
- # the number of lines
-
- return event
-
-
-# convert accordion-registration to lilypond.
-# Since lilypond does not have any built-in commands, we need to create
-# the markup commands manually and define our own variables.
-# Idea was taken from: http://lsr.dsi.unimi.it/LSR/Item?id=194
-def musicxml_accordion_to_markup(mxl_event):
- commandname = "accReg"
- command = ""
-
- high = mxl_event.get_maybe_exist_named_child('accordion-high')
- if high:
- commandname += "H"
- command += """\\combine
- \\raise #2.5 \\musicglyph #\"accordion.dot\"
- """
- middle = mxl_event.get_maybe_exist_named_child('accordion-middle')
- if middle:
- # By default, use one dot (when no or invalid content is given). The
- # MusicXML spec is quiet about this case...
- txt = 1
- try:
- txt = int(middle.get_text())
- except ValueError:
- pass
- if txt == 3:
- commandname += "MMM"
- command += r"""\combine
- \raise #1.5 \musicglyph #"accordion.dot"
- \combine
- \raise #1.5 \translate #(cons 1 0) \musicglyph #"accordion.dot"
- \combine
- \raise #1.5 \translate #(cons -1 0) \musicglyph #"accordion.dot"
- """
- elif txt == 2:
- commandname += "MM"
- command += r"""\combine
- \raise #1.5 \translate #(cons 0.5 0) \musicglyph #"accordion.dot"
- \combine
- \raise #1.5 \translate #(cons -0.5 0) \musicglyph #"accordion.dot"
- """
- elif not txt <= 0:
- commandname += "M"
- command += r"""\combine
- \raise #1.5 \musicglyph #"accordion.dot"
- """
- low = mxl_event.get_maybe_exist_named_child('accordion-low')
- if low:
- commandname += "L"
- command += r"""\combine
- \raise #0.5 \musicglyph #"accordion.dot"
- """
-
- command += r'\musicglyph #"accordion.discant"'
- command = r"\markup { \normalsize %s }" % command
- # Define the newly built command \accReg[H][MMM][L]
- additional_definitions[commandname] = "%s = %s" % (commandname, command)
- needed_additional_definitions.append(commandname)
- return "\\%s" % commandname
-
-
-def musicxml_accordion_to_ly(mxl_event):
- txt = musicxml_accordion_to_markup(mxl_event)
- if txt:
- ev = musicexp.MarkEvent(txt)
- return ev
- return
-
-
-def musicxml_rehearsal_to_ly_mark(mxl_event):
- text = mxl_event.get_text()
- if not text:
- return
- # default is boxed rehearsal marks!
- encl = "box"
- if hasattr(mxl_event, 'enclosure'):
- encl = {"none": None, "square": "box", "circle": "circle"}.get(
- mxl_event.enclosure, None)
- if encl:
- text = "\\%s { %s }" % (encl, text)
- ev = musicexp.MarkEvent("\\markup { %s }" % text)
- return ev
-
-
-def musicxml_harp_pedals_to_ly(mxl_event):
- count = 0
- result = "\\harp-pedal #\""
- for t in mxl_event.get_named_children('pedal-tuning'):
- alter = t.get_named_child('pedal-alter')
- if alter:
- val = int(alter.get_text().strip())
- result += {1: "v", 0: "-", -1: "^"}.get(val, "")
- count += 1
- if count == 3:
- result += "|"
- ev = musicexp.MarkupEvent()
- ev.contents = result + "\""
- return ev
-
-
-def musicxml_eyeglasses_to_ly(mxl_event):
- needed_additional_definitions.append("eyeglasses")
- return musicexp.MarkEvent("\\markup { \\eyeglasses }")
-
-
-def next_non_hash_index(lst, pos):
- pos += 1
- while pos < len(lst) and isinstance(lst[pos], musicxml.Hash_text):
- pos += 1
- return pos
-
-
-def musicxml_metronome_to_ly(mxl_event, text_event=None):
- children = mxl_event.get_all_children()
- if not children:
- return
-
- index = -1
- index = next_non_hash_index(children, index)
- if isinstance(children[index], musicxml.BeatUnit):
- # first form of metronome-mark, using unit and beats/min or other unit
- ev = musicexp.TempoMark()
- if text_event:
- ev.set_text(text_event.get_text().strip())
-
- if hasattr(mxl_event, 'parentheses'):
- ev.set_parentheses(mxl_event.parentheses == "yes")
-
- d = musicexp.Duration()
- d.duration_log = utilities.musicxml_duration_to_log(
- children[index].get_text())
- index = next_non_hash_index(children, index)
- if isinstance(children[index], musicxml.BeatUnitDot):
- d.dots = 1
- index = next_non_hash_index(children, index)
- ev.set_base_duration(d)
- if isinstance(children[index], musicxml.BeatUnit):
- # Form "note = newnote"
- newd = musicexp.Duration()
- newd.duration_log = utilities.musicxml_duration_to_log(
- children[index].get_text())
- index = next_non_hash_index(children, index)
- if isinstance(children[index], musicxml.BeatUnitDot):
- newd.dots = 1
- index = next_non_hash_index(children, index)
- ev.set_new_duration(newd)
- elif isinstance(children[index], musicxml.PerMinute):
- # Form "note = bpm"
- try:
- beats = int(children[index].get_text())
- ev.set_beats_per_minute(beats)
- except ValueError:
- pass
- else:
- ly.warning(_("Unknown metronome mark, ignoring"))
- return
- return ev
- else:
- # TODO: Implement the other (more complex) way for tempo marks!
- ly.warning(
- _("Metronome marks with complex relations ( in MusicXML) are not yet implemented."))
- return
-
-
-# translate directions into Events, possible values:
-# -) string (MarkEvent with that command)
-# -) function (function(mxl_event) needs to return a full Event-derived object
-# -) (class, name) (like string, only that a different class than MarkEvent is used)
-directions_dict = {
- 'accordion-registration': musicxml_accordion_to_ly,
- 'coda': (musicexp.MusicGlyphMarkEvent, "coda"),
- # 'damp' : ???
- # 'damp-all' : ???
- 'eyeglasses': musicxml_eyeglasses_to_ly,
- 'harp-pedals': musicxml_harp_pedals_to_ly,
- # 'image' : ???
- 'metronome': musicxml_metronome_to_ly,
- 'rehearsal': musicxml_rehearsal_to_ly_mark,
- # 'scordatura' : ???
- 'segno': (musicexp.MusicGlyphMarkEvent, "segno"),
- 'words': musicxml_words_to_lily_event,
-}
-directions_spanners = ['octave-shift', 'pedal', 'wedge', 'dashes', 'bracket']
-
-
-def musicxml_direction_to_lily(n):
- # TODO: Handle the element!
- res = []
- # placement applies to all children!
- dir = None
- if hasattr(n, 'placement') and hasattr(options, 'convert_directions') and options.convert_directions:
- dir = musicxml_direction_to_indicator(n.placement)
- dirtype_children = []
- # TODO: The direction-type is used for grouping (e.g. dynamics with text),
- # so we can't simply flatten them out!
- for dt in n.get_typed_children(musicxml.DirType):
- dirtype_children += dt.get_all_children()
-
- dirtype_children = [d for d in dirtype_children if d.get_name() != "#text"]
-
- for i, entry in enumerate(dirtype_children):
- if not entry:
- continue
-
- # brackets, dashes, octave shifts. pedal marks, hairpins etc. are spanners:
- if entry.get_name() in directions_spanners:
- event = musicxml_spanner_to_lily_event(entry)
- if event:
- event.force_direction = dir
- res.append(event)
- continue
-
- # handle text+bpm marks like "Allegro moderato (♩ = 144)"
- if entry.get_name() == 'words' and i < len(dirtype_children) - 1:
- next_entry = dirtype_children[i+1]
- if next_entry.get_name() == 'metronome':
- event = musicxml_metronome_to_ly(next_entry, entry)
- if event:
- res.append(event)
- dirtype_children[i+1] = None
- continue
-
- # now treat all the "simple" ones, that can be translated using the dict
- ev = None
- tmp_tp = directions_dict.get(entry.get_name(), None)
- if isinstance(tmp_tp, str): # string means MarkEvent
- ev = musicexp.MarkEvent(tmp_tp)
- elif isinstance(tmp_tp, tuple): # tuple means (EventClass, "text")
- ev = tmp_tp[0](tmp_tp[1])
- elif tmp_tp:
- ev = tmp_tp(entry)
- if ev:
- # TODO: set the correct direction! Unfortunately, \mark in ly does
- # not seem to support directions!
- ev.force_direction = dir
- res.append(ev)
- continue
-
- if entry.get_name() == "dynamics":
- for dynentry in entry.get_all_children():
- ev = musicxml_dynamics_to_lily_event(dynentry)
- if ev:
- ev.force_direction = dir
- res.append(ev)
-
- return res
-
-
-notehead_styles_dict = {
- 'slash': '\'slash',
- 'triangle': '\'triangle',
- 'diamond': '\'diamond',
- 'square': '\'la', # TODO: Proper squared note head
- 'cross': None, # TODO: + shaped note head
- 'x': '\'cross',
- 'circle-x': '\'xcircle',
- 'inverted triangle': None, # TODO: Implement
- 'arrow down': None, # TODO: Implement
- 'arrow up': None, # TODO: Implement
- 'slashed': None, # TODO: Implement
- 'back slashed': None, # TODO: Implement
- 'normal': None,
- 'cluster': None, # TODO: Implement
- 'none': '#f',
- 'do': '\'do',
- 're': '\'re',
- 'mi': '\'mi',
- 'fa': '\'fa',
- 'so': None,
- 'la': '\'la',
- 'ti': '\'ti',
-}
-
-
-def musicxml_chordpitch_to_lily(mxl_cpitch):
- r = musicexp.ChordPitch()
- r.alteration = mxl_cpitch.get_alteration()
- r.step = musicxml2ly_conversion.musicxml_step_to_lily(
- mxl_cpitch.get_step())
- return r
-
-
-chordkind_dict = {
- 'major': ':5',
- 'minor': ':m5',
- 'augmented': ':aug5',
- 'diminished': ':dim5',
- # Sevenths:
- 'dominant': ':7',
- 'dominant-seventh': ':7',
- 'major-seventh': ':maj7',
- 'minor-seventh': ':m7',
- 'diminished-seventh': ':dim7',
- 'augmented-seventh': ':aug7',
- 'half-diminished': ':dim5m7',
- 'major-minor': ':maj7m5',
- # Sixths:
- 'major-sixth': ':6',
- 'minor-sixth': ':m6',
- # Ninths:
- 'dominant-ninth': ':9',
- 'major-ninth': ':maj9',
- 'minor-ninth': ':m9',
- # 11ths (usually as the basis for alteration):
- 'dominant-11th': ':11',
- 'major-11th': ':maj11',
- 'minor-11th': ':m11',
- # 13ths (usually as the basis for alteration):
- 'dominant-13th': ':13.11',
- 'major-13th': ':maj13.11',
- 'minor-13th': ':m13',
- # Suspended:
- 'suspended-second': ':sus2',
- 'suspended-fourth': ':sus4',
- # Functional sixths:
- # TODO
- # 'Neapolitan': '???',
- # 'Italian': '???',
- # 'French': '???',
- # 'German': '???',
- # Other:
- # 'pedal': '???',(pedal-point bass)
- 'power': ':1.5',
- # 'Tristan': '???',
- 'other': ':1',
- 'none': None,
-}
-
-
-def musicxml_chordkind_to_lily(kind):
- res = chordkind_dict.get(kind, None)
- # Check for None, since a major chord is converted to ''
- if res is None:
- ly.warning(_("Unable to convert chord type %s to lilypond.") % kind)
- return res
-
-
-# Global variable for guitar string tunings
-string_tunings = None
-
-
-def musicxml_get_string_tunings(lines):
- global string_tunings
- if string_tunings is None:
- if not lines:
- lines = 6
- string_tunings = [musicexp.Pitch()] * lines
- for i in range(0, lines):
- p = musicexp.Pitch()
- p.step = musicxml2ly_conversion.musicxml_step_to_lily(
- ((("E", "A", "D", "G", "B")*(lines/5+1))[0:lines])[i])
- p.octave = (([-2+int(x % 5 > 1)+2*(x/5)
- for x in range(0, lines)][0:lines])[i])
- p.alteration = 0
- p._force_absolute_pitch = True
- string_tunings[i] = p
- string_tunings = string_tunings[::-1]
- return string_tunings[0:lines]
-
-
-def musicxml_frame_to_lily_event(frame):
- ev = musicexp.FretEvent()
- ev.strings = frame.get_strings()
- ev.frets = frame.get_frets()
- #offset = frame.get_first_fret() - 1
- #offset = frame.get_first_fret()
- barre = []
- open_strings = list(range(1, ev.strings+1))
- for fn in frame.get_named_children('frame-note'):
- fret = fn.get_fret()
- if fret <= 0:
- fret = "o"
- el = [fn.get_string(), fret]
- fingering = fn.get_fingering()
- if fingering >= 0:
- el.append(fingering)
- ev.elements.append(el)
- open_strings.remove(fn.get_string())
- b = fn.get_barre()
- if b == 'start':
- barre.append(el[0]) # start string
- barre.append(el[1]) # fret
- elif b == 'stop':
- barre.insert(1, el[0]) # end string
- for string in open_strings:
- ev.elements.append([string, 'x'])
- ev.elements.sort()
- ev.elements.reverse()
- if barre:
- ev.barre = barre
- return ev
-
-
-def musicxml_harmony_to_lily(n):
- res = []
- for f in n.get_named_children('frame'):
- ev = musicxml_frame_to_lily_event(f)
- if ev:
- res.append(ev)
- return res
-
-
-def musicxml_harmony_to_lily_fretboards(n):
- res = []
- frame = n.get_maybe_exist_named_child('frame')
- if frame:
- strings = frame.get_strings()
- if not strings:
- strings = 6
- tunings = musicxml_get_string_tunings(strings)
- ev = musicexp.FretBoardEvent()
- #barre = []
- for fn in frame.get_named_children('frame-note'):
- fbn = musicexp.FretBoardNote()
- string = fn.get_string()
- fbn.string = string
- fingering = fn.get_fingering()
- if fingering >= 0:
- fbn.fingering = fingering
- p = tunings[string-1].copy()
- p.add_semitones(fn.get_fret())
- fbn.pitch = p
- ev.append(fbn)
- res.append(ev)
- return res
-
-
-def musicxml_harmony_to_lily_chordname(n):
- res = []
- root = n.get_maybe_exist_named_child('root')
- if root:
- ev = musicexp.ChordNameEvent()
- ev.root = musicxml_chordpitch_to_lily(root)
- kind = n.get_maybe_exist_named_child('kind')
- if kind:
- ev.kind = musicxml_chordkind_to_lily(kind.get_text())
- if not ev.kind:
- return res
- bass = n.get_maybe_exist_named_child('bass')
- if bass:
- ev.bass = musicxml_chordpitch_to_lily(bass)
- inversion = n.get_maybe_exist_named_child('inversion')
- if inversion:
- # TODO: LilyPond does not support inversions, does it?
-
- # Mail from Carl Sorensen on lilypond-devel, June 11, 2008:
- # 4. LilyPond supports the first inversion in the form of added
- # bass notes. So the first inversion of C major would be c:/g.
- # To get the second inversion of C major, you would need to do
- # e:6-3-^5 or e:m6-^5. However, both of these techniques
- # require you to know the chord and calculate either the fifth
- # pitch (for the first inversion) or the third pitch (for the
- # second inversion) so they may not be helpful for musicxml2ly.
- inversion_count = int(inversion.get_text())
- if inversion_count == 1:
- # TODO: Calculate the bass note for the inversion...
- pass
- pass
- for deg in n.get_named_children('degree'):
- d = musicexp.ChordModification()
- d.type = deg.get_type()
- d.step = deg.get_value()
- d.alteration = deg.get_alter()
- ev.add_modification(d)
- # TODO: convert the user-symbols attribute:
- # major: a triangle, like Unicode 25B3
- # minor: -, like Unicode 002D
- # augmented: +, like Unicode 002B
- # diminished: (degree), like Unicode 00B0
- # half-diminished: (o with slash), like Unicode 00F8
- if ev and ev.root:
- res.append(ev)
- return res
-
-
-def musicxml_figured_bass_note_to_lily(n):
- res = musicexp.FiguredBassNote()
- suffix_dict = {'sharp': "+",
- 'flat': "-",
- 'natural': "!",
- 'double-sharp': "++",
- 'flat-flat': "--",
- 'sharp-sharp': "++",
- 'slash': "/"}
- prefix = n.get_maybe_exist_named_child('prefix')
- if prefix:
- res.set_prefix(suffix_dict.get(prefix.get_text(), ""))
- fnumber = n.get_maybe_exist_named_child('figure-number')
- if fnumber:
- res.set_number(fnumber.get_text())
- suffix = n.get_maybe_exist_named_child('suffix')
- if suffix:
- res.set_suffix(suffix_dict.get(suffix.get_text(), ""))
- if n.get_maybe_exist_named_child('extend'):
- # TODO: Implement extender lines (unfortunately, in lilypond you have
- # to use \set useBassFigureExtenders = ##t, which turns them on
- # globally, while MusicXML has a property for each note...
- # I'm not sure there is a proper way to implement this cleanly
- # n.extend
- pass
- return res
-
-
-def musicxml_figured_bass_to_lily(n):
- if not isinstance(n, musicxml.FiguredBass):
- return
- res = musicexp.FiguredBassEvent()
- for i in n.get_named_children('figure'):
- note = musicxml_figured_bass_note_to_lily(i)
- if note:
- res.append(note)
- dur = n.get_maybe_exist_named_child('duration')
- if dur:
- # apply the duration to res
- length = Fraction(int(dur.get_text()), n._divisions) * Fraction(1, 4)
- res.set_real_duration(length)
- duration = musicxml2ly_conversion.rational_to_lily_duration(length)
- if duration:
- res.set_duration(duration)
- if hasattr(n, 'parentheses') and n.parentheses == "yes":
- res.set_parentheses(True)
- return res
-
-
-def musicxml_lyrics_to_text(lyrics, ignoremelismata):
- # TODO: Implement text styles for lyrics syllables
- continued = False
- extended = False
- text = ''
- for e in lyrics.get_all_children():
- if isinstance(e, musicxml.Syllabic):
- continued = e.continued()
- elif isinstance(e, musicxml.Text):
- # We need to convert soft hyphens to -, otherwise the ascii codec as well
- # as lilypond will barf on that character
- text += e.get_text().replace('\xad', '-')
- elif isinstance(e, musicxml.Elision):
- if text:
- text += " "
- continued = False
- extended = False
- elif isinstance(e, musicxml.Extend):
- if text:
- text += " "
- extended = True
-
- if text == "-" and continued:
- return "--"
- elif text == "_" and extended:
- return "__"
- elif continued and text:
- if hasattr(options, 'convert_beaming') and options.convert_beaming:
- if ignoremelismata == "on":
- return r" \set ignoreMelismata = ##t " + utilities.escape_ly_output_string(text)
- elif ignoremelismata == "off":
- return " " + utilities.escape_ly_output_string(text) + " -- \\unset ignoreMelismata"
- else:
- return " " + utilities.escape_ly_output_string(text) + " --"
- else:
- return " " + utilities.escape_ly_output_string(text) + " -- "
- elif continued:
- return "--"
- elif extended and text:
- return " " + utilities.escape_ly_output_string(text) + " __"
- elif extended:
- return "__"
- elif text:
- return " " + utilities.escape_ly_output_string(text)
- else:
- return ""
-
-# TODO
-
-
-class NegativeSkip:
- def __init__(self, here, dest):
- self.here = here
- self.dest = dest
-
-
-class LilyPondVoiceBuilder:
- def __init__(self):
- self.elements = []
- self.pending_dynamics = []
- self.end_moment = Fraction(0)
- self.begin_moment = Fraction(0)
- self.pending_multibar = Fraction(0)
- self.ignore_skips = False
- self.has_relevant_elements = False
- self.measure_length = Fraction(4, 4)
- self.stay_here = False
-
- def _insert_multibar(self):
- layout_information.set_context_item('Score', 'skipBars = ##t')
- r = musicexp.MultiMeasureRest()
- lenfrac = self.measure_length
- r.duration = musicxml2ly_conversion.rational_to_lily_duration(lenfrac)
- r.duration.factor *= self.pending_multibar / lenfrac
- self.elements.append(r)
- self.begin_moment = self.end_moment
- self.end_moment = self.begin_moment + self.pending_multibar
- self.pending_multibar = Fraction(0)
-
- def set_measure_length(self, mlen):
- if (mlen != self.measure_length) and self.pending_multibar:
- self._insert_multibar()
- self.measure_length = mlen
-
- def add_multibar_rest(self, duration):
- self.pending_multibar += duration
-
- def set_duration(self, duration):
- self.end_moment = self.begin_moment + duration
-
- def current_duration(self):
- return self.end_moment - self.begin_moment
-
- def add_pending_dynamics(self):
- for d in self.pending_dynamics:
- self.elements.append(d)
- self.pending_dynamics = []
-
- def add_music(self, music, duration, relevant=True):
- assert isinstance(music, musicexp.Music)
- if self.pending_multibar > Fraction(0):
- self._insert_multibar()
-
- self.has_relevant_elements = self.has_relevant_elements or relevant
-
- if isinstance(music, musicexp.BarLine):
- if self.pending_dynamics:
- for d in self.pending_dynamics:
- if not isinstance(d, (musicexp.SpanEvent, musicexp.DynamicsEvent)):
- index = self.pending_dynamics.index(d)
- dyn = self.pending_dynamics.pop(index)
- self.elements.append(dyn)
-
- self.elements.append(music)
- self.begin_moment = self.end_moment
- self.set_duration(duration)
-
- # Insert all pending dynamics right after the note/rest:
- if isinstance(music, musicexp.ChordEvent) and self.pending_dynamics:
- self.add_pending_dynamics()
-
- # Insert some music command that does not affect the position in the measure
- def add_command(self, command, relevant=True):
- assert isinstance(command, musicexp.Music)
- if self.pending_multibar > Fraction(0):
- self._insert_multibar()
- self.has_relevant_elements = self.has_relevant_elements or relevant
- self.elements.append(command)
-
- def add_barline(self, barline, relevant=False):
- # Insert only if we don't have a barline already
- # TODO: Implement proper merging of default barline and custom bar line
- has_relevant = self.has_relevant_elements
- if (not (self.elements) or
- not (isinstance(self.elements[-1], musicexp.BarLine)) or
- (self.pending_multibar > Fraction(0))):
-
- self.add_music(barline, Fraction(0))
-
- self.has_relevant_elements = has_relevant or relevant
-
- def add_partial(self, command):
- self.ignore_skips = True
- # insert the partial, but restore relevant_elements (partial is not relevant)
- relevant = self.has_relevant_elements
- self.add_command(command)
- self.has_relevant_elements = relevant
-
- def add_dynamics(self, dynamic):
- # store the dynamic item(s) until we encounter the next note/rest:
- self.pending_dynamics.append(dynamic)
-
- def add_bar_check(self, number):
- # re/store has_relevant_elements, so that a barline alone does not
- # trigger output for figured bass, chord names
- b = musicexp.BarLine()
- b.bar_number = number
- self.add_barline(b)
-
- def jumpto(self, moment):
- if not self.stay_here:
- current_end = self.end_moment + self.pending_multibar
- diff = moment - current_end
-
- if diff < Fraction(0):
- ly.warning(_('Negative skip %s (from position %s to %s)') %
- (diff, current_end, moment))
- diff = Fraction(0)
-
- if diff > Fraction(0) and not(self.ignore_skips and moment == 0):
- skip = musicexp.SkipEvent()
- duration_factor = 1
- duration_log = {1: 0, 2: 1, 4: 2, 8: 3, 16: 4, 32: 5,
- 64: 6, 128: 7, 256: 8, 512: 9}.get(diff.denominator, -1)
- duration_dots = 0
- # TODO: Use the time signature for skips, too. Problem: The skip
- # might not start at a measure boundary!
- if duration_log > 0: # denominator is a power of 2...
- if diff.numerator == 3:
- duration_log -= 1
- duration_dots = 1
- else:
- duration_factor = Fraction(diff.numerator)
- else:
- # for skips of a whole or more, simply use s1*factor
- duration_log = 0
- duration_factor = diff
- skip.duration.duration_log = duration_log
- skip.duration.factor = duration_factor
- skip.duration.dots = duration_dots
-
- evc = musicexp.ChordEvent()
- evc.elements.append(skip)
- self.add_music(evc, diff, False)
-
- if diff > Fraction(0) and moment == 0:
- self.ignore_skips = False
-
- def last_event_chord(self, starting_at):
- value = None
-
- # if the position matches, find the last ChordEvent, do not cross a bar line!
- at = len(self.elements) - 1
- while (at >= 0 and
- not isinstance(self.elements[at], musicexp.ChordEvent) and
- not isinstance(self.elements[at], musicexp.BarLine)):
- at -= 1
-
- if (self.elements
- and at >= 0
- and isinstance(self.elements[at], musicexp.ChordEvent)
- and self.begin_moment == starting_at):
- value = self.elements[at]
- else:
- self.jumpto(starting_at)
- value = None
- return value
-
- def correct_negative_skip(self, goto):
- self.end_moment = goto
- self.begin_moment = goto
- evc = musicexp.ChordEvent()
- self.elements.append(evc)
-
-
-class VoiceData:
- def __init__(self):
- self.voicename = None
- self.voicedata = None
- self.ly_voice = None
- self.figured_bass = None
- self.chordnames = None
- self.fretboards = None
- self.lyrics_dict = {}
- self.lyrics_order = []
-
-
-def measure_length_from_attributes(attr, current_measure_length):
- len = attr.get_measure_length()
- if not len:
- len = current_measure_length
- return len
-
-
-def music_xml_voice_name_to_lily_name(part_id, name):
- s = "Part%sVoice%s" % (part_id, name)
- return musicxml_id_to_lily(s)
-
-
-def music_xml_lyrics_name_to_lily_name(part_id, name, lyricsnr):
- s = music_xml_voice_name_to_lily_name(
- part_id, name)+("Lyrics%s" % lyricsnr)
- return musicxml_id_to_lily(s)
-
-
-def music_xml_figuredbass_name_to_lily_name(part_id, voicename):
- s = music_xml_voice_name_to_lily_name(part_id, voicename)+"FiguredBass"
- return musicxml_id_to_lily(s)
-
-
-def music_xml_chordnames_name_to_lily_name(part_id, voicename):
- s = music_xml_voice_name_to_lily_name(part_id, voicename)+"Chords"
- return musicxml_id_to_lily(s)
-
-
-def music_xml_fretboards_name_to_lily_name(part_id, voicename):
- s = music_xml_voice_name_to_lily_name(part_id, voicename)+"FretBoards"
- return musicxml_id_to_lily(s)
-
-
-def get_all_lyric_parts_in_voice(voice):
- r'''
- Collect the indexes of all lyric parts in this voice.
- In case not all of the current lyric parts are active (a typical case would be
- a refrain/chorus), the current implementation inserts \skip-commands in the
- inactive parts to keep them in sync.
- '''
- all_lyric_parts = []
- for elem in voice._elements:
- lyrics = elem.get_typed_children(musicxml.Lyric)
- if lyrics:
- for lyric in lyrics:
- index = lyric.get_number()
- if not index in all_lyric_parts:
- all_lyric_parts.append(index)
- return all_lyric_parts
-
-
-def extract_lyrics(voice, lyric_key, lyrics_dict):
- curr_number = None
- result = []
-
- def is_note(elem):
- return isinstance(elem, musicxml.Note)
-
- def is_rest(elem):
- return elem.get_typed_children(musicxml.Rest)
-
- def is_chord(elem):
- return elem.get_typed_children(musicxml.Chord)
-
- def is_note_and_not_rest(elem):
- return is_note(elem) and not is_rest(elem)
-
- def get_lyric_elements(note):
- return note.get_typed_children(musicxml.Lyric)
-
- def has_lyric_belonging_to_lyric_part(note, lyric_part_id):
- lyric_elements = get_lyric_elements(note)
- lyric_numbers = [lyric.get_number() for lyric in lyric_elements]
- return any([lyric_number == lyric_part_id for lyric_number in lyric_numbers])
-
- for idx, elem in enumerate(voice._elements):
- lyrics = get_lyric_elements(elem)
- lyric_keys = [lyric.get_number() for lyric in lyrics]
- note_has_lyric_belonging_to_lyric_part = lyric_key in lyric_keys
- # Current note has lyric with 'number' matching 'lyric_key'.
- if note_has_lyric_belonging_to_lyric_part:
- for lyric in lyrics:
- if lyric.get_number() == lyric_key:
- text = musicxml_lyrics_to_text(lyric, None)
- result.append(text)
- # Note has any lyric.
- elif get_lyric_elements(elem) and \
- not note_has_lyric_belonging_to_lyric_part:
- result.append(r'\skip1 ')
- # Note does not have any lyric attached to it.
- elif is_chord(elem):
- # note without lyrics part of a chord. MusicXML format is
- # unclear if a chord element could contain a lyric, lets
- # asume that we do not want to put a skip here.
- continue
- elif is_note_and_not_rest(elem):
- result.append(r'\skip1 ')
-
- lyrics_dict[lyric_key].extend(result)
-
-
-def musicxml_voice_to_lily_voice(voice):
- tuplet_events = []
- lyrics = {}
- return_value = VoiceData()
- return_value.voicedata = voice
-
- # First pitch needed for relative mode (if selected in command-line options)
- first_pitch = None
-
- # Needed for melismata detection (ignore lyrics on those notes!):
- inside_slur = False
- is_tied = False
- is_chord = False
- is_beamed = False
- ignore_lyrics = False
-
- current_staff = None
-
- pending_figured_bass = []
- pending_chordnames = []
- pending_fretboards = []
-
- # Make sure that the keys in the dict don't get reordered, since
- # we need the correct ordering of the lyrics stanzas! By default,
- # a dict will reorder its keys
- return_value.lyrics_order = voice.get_lyrics_numbers()
- for k in return_value.lyrics_order:
- lyrics[k] = []
-
- voice_builder = LilyPondVoiceBuilder()
- figured_bass_builder = LilyPondVoiceBuilder()
- chordnames_builder = LilyPondVoiceBuilder()
- fretboards_builder = LilyPondVoiceBuilder()
- current_measure_length = Fraction(4, 4)
- voice_builder.set_measure_length(current_measure_length)
- in_slur = False
-
- all_lyric_parts = set(get_all_lyric_parts_in_voice(voice))
- if list(lyrics.keys()):
- for number in list(lyrics.keys()):
- extracted_lyrics = extract_lyrics(voice, number, lyrics)
-
- last_bar_check = -1
- for idx, n in enumerate(voice._elements):
- tie_started = False
- if n.get_name() == 'forward':
- continue
- staff = n.get_maybe_exist_named_child('staff')
- if staff:
- staff = staff.get_text()
- if current_staff and staff != current_staff and not n.get_maybe_exist_named_child('chord'):
- voice_builder.add_command(musicexp.StaffChange(staff))
- current_staff = staff
-
- if isinstance(n, musicxml.Partial) and n.partial > 0:
- a = musicxml_partial_to_lily(n.partial)
- if a:
- voice_builder.add_partial(a)
- figured_bass_builder.add_partial(a)
- chordnames_builder.add_partial(a)
- fretboards_builder.add_partial(a)
- continue
-
- is_chord = n.get_maybe_exist_named_child('chord')
- is_after_grace = (isinstance(n, musicxml.Note) and n.is_after_grace())
- if not is_chord and not is_after_grace:
- try:
- voice_builder.jumpto(n._when)
- figured_bass_builder.jumpto(n._when)
- chordnames_builder.jumpto(n._when)
- fretboards_builder.jumpto(n._when)
- except NegativeSkip as neg:
- voice_builder.correct_negative_skip(n._when)
- figured_bass_builder.correct_negative_skip(n._when)
- chordnames_builder.correct_negative_skip(n._when)
- fretboards_builder.correct_negative_skip(n._when)
- n.message(_("Negative skip found: from %s to %s, difference is %s") % (
- neg.here, neg.dest, neg.dest - neg.here))
-
- if isinstance(n, musicxml.Barline):
- barlines = n.to_lily_object()
- for a in barlines:
- if isinstance(a, musicexp.BarLine):
- voice_builder.add_barline(a)
- figured_bass_builder.add_barline(a, False)
- chordnames_builder.add_barline(a, False)
- fretboards_builder.add_barline(a, False)
- elif isinstance(a, musicxml2ly_conversion.RepeatMarker) or isinstance(a, musicxml2ly_conversion.EndingMarker):
- voice_builder.add_command(a)
- figured_bass_builder.add_barline(a, False)
- chordnames_builder.add_barline(a, False)
- fretboards_builder.add_barline(a, False)
- continue
-
- if isinstance(n, musicxml.Print):
- for a in musicxml_print_to_lily(n):
- voice_builder.add_command(a, False)
- continue
-
- # Continue any multimeasure-rests before trying to add bar checks!
- # Don't handle new MM rests yet, because for them we want bar checks!
- rest = n.get_maybe_exist_typed_child(musicxml.Rest)
- if (rest and rest.is_whole_measure()
- and voice_builder.pending_multibar > Fraction(0)):
- voice_builder.add_multibar_rest(n._duration)
- continue
-
- # Print bar checks between measures.
- if n._measure_position == Fraction(0) and n != voice._elements[0]:
- try:
- num = int(n.get_parent().number)
- except ValueError:
- num = 0
- if num > 0 and num > last_bar_check:
- voice_builder.add_bar_check(num)
- figured_bass_builder.add_bar_check(num)
- chordnames_builder.add_bar_check(num)
- fretboards_builder.add_bar_check(num)
- last_bar_check = num
-
- if isinstance(n, musicxml.Direction):
- # check if Direction already has been converted in another voice.
- if n.converted:
- continue
- else:
- n.converted = True
- for direction in musicxml_direction_to_lily(n):
- if direction.wait_for_note():
- voice_builder.add_dynamics(direction)
- else:
- voice_builder.add_command(direction)
- continue
-
- # Start any new multimeasure rests
- if (rest and rest.is_whole_measure()):
- if pending_chordnames:
- chordnames_builder.jumpto(n._when)
- chordnames_builder.stay_here = True
- if pending_figured_bass:
- figured_bass_builder.jumpto(n._when)
- figured_bass_builder.stay_here = True
- if pending_fretboards:
- fretboards_builder.jumpto(n._when)
- fretboards_builder.stay_here = True
- voice_builder.add_multibar_rest(n._duration)
- continue
-
- if isinstance(n, musicxml.Harmony):
- if options.fretboards:
- # Makes fretboard diagrams in a separate FretBoards voice
- for a in musicxml_harmony_to_lily_fretboards(n):
- pending_fretboards.append(a)
- else:
- # Makes markup fretboard-diagrams inside the voice
- for a in musicxml_harmony_to_lily(n):
- if a.wait_for_note():
- voice_builder.add_dynamics(a)
- else:
- voice_builder.add_command(a)
- for a in musicxml_harmony_to_lily_chordname(n):
- pending_chordnames.append(a)
- continue
-
- if isinstance(n, musicxml.FiguredBass):
- a = musicxml_figured_bass_to_lily(n)
- if a:
- pending_figured_bass.append(a)
- continue
-
- if isinstance(n, musicxml.Attributes):
- for a in musicxml_attributes_to_lily(n):
- voice_builder.add_command(a)
- measure_length = measure_length_from_attributes(
- n, current_measure_length)
- if current_measure_length != measure_length:
- current_measure_length = measure_length
- voice_builder.set_measure_length(current_measure_length)
- continue
-
- if not n.__class__.__name__ == 'Note':
- n.message(_('unexpected %s; expected %s or %s or %s') %
- (n, 'Note', 'Attributes', 'Barline'))
- continue
-
-# if not hasattr(conversion_settings, 'convert_rest_positions'):
-# conversion_settings.convert_rest_positions = True
-
- main_event = n.to_lily_object(
- convert_stem_directions=conversion_settings.convert_stem_directions,
- convert_rest_positions=conversion_settings.convert_rest_positions)
-
- if main_event and not first_pitch:
- first_pitch = main_event.pitch
- # ignore lyrics for notes inside a slur, tie, chord or beam
- ignore_lyrics = is_tied or is_chord # or is_beamed or inside_slur
-
- ev_chord = voice_builder.last_event_chord(n._when)
- if not ev_chord:
- ev_chord = musicexp.ChordEvent()
- voice_builder.add_music(ev_chord, n._duration)
-
- # For grace notes:
- grace = n.get_maybe_exist_typed_child(musicxml.Grace)
- if n.is_grace():
- is_after_grace = ev_chord.has_elements() or n.is_after_grace()
- is_chord = n.get_maybe_exist_typed_child(musicxml.Chord)
-
- grace_chord = None
-
- # after-graces and other graces use different lists; Depending on
- # whether we have a chord or not, obtain either a new ChordEvent or
- # the previous one to create a chord
- if is_after_grace:
- if ev_chord.after_grace_elements and n.get_maybe_exist_typed_child(musicxml.Chord):
- grace_chord = ev_chord.after_grace_elements.get_last_event_chord()
- if not grace_chord:
- grace_chord = musicexp.ChordEvent()
- ev_chord.append_after_grace(grace_chord)
- elif n.is_grace():
- if ev_chord.grace_elements and n.get_maybe_exist_typed_child(musicxml.Chord):
- grace_chord = ev_chord.grace_elements.get_last_event_chord()
- if not grace_chord:
- grace_chord = musicexp.ChordEvent()
- ev_chord.append_grace(grace_chord)
-
- if hasattr(grace, 'slash') and not is_after_grace:
- # TODO: use grace_type = "appoggiatura" for slurred grace notes
- if grace.slash == "yes":
- ev_chord.grace_type = "acciaccatura"
- # now that we have inserted the chord into the grace music, insert
- # everything into that chord instead of the ev_chord
- ev_chord = grace_chord
- ev_chord.append(main_event)
- ignore_lyrics = True
- else:
- ev_chord.append(main_event)
- # When a note/chord has grace notes (duration==0), the duration of the
- # event chord is not yet known, but the event chord was already added
- # with duration 0. The following correct this when we hit the real note!
- if voice_builder.current_duration() == 0 and n._duration > 0:
- voice_builder.set_duration(n._duration)
-
- # if we have a figured bass, set its voice builder to the correct position
- # and insert the pending figures
- if pending_figured_bass:
- try:
- figured_bass_builder.jumpto(n._when)
- if figured_bass_builder.stay_here:
- figured_bass_builder.stay_here = False
- except NegativeSkip as neg:
- pass
- for fb in pending_figured_bass:
- # if a duration is given, use that, otherwise the one of the note
- dur = fb.real_duration
- if not dur:
- dur = ev_chord.get_length()
- if not fb.duration:
- fb.duration = ev_chord.get_duration()
- figured_bass_builder.add_music(fb, dur)
- pending_figured_bass = []
-
- if pending_chordnames:
- try:
- chordnames_builder.jumpto(n._when)
- if chordnames_builder.stay_here:
- chordnames_builder.stay_here = False
- except NegativeSkip as neg:
- pass
- for cn in pending_chordnames:
- # Assign the duration of the EventChord
- cn.duration = ev_chord.get_duration()
- chordnames_builder.add_music(cn, ev_chord.get_length())
- pending_chordnames = []
-
- if pending_fretboards:
- try:
- fretboards_builder.jumpto(n._when)
- if fretboards_builder.stay_here:
- fretboards_builder.stay_here = False
- except NegativeSkip as neg:
- pass
- for fb in pending_fretboards:
- # Assign the duration of the EventChord
- fb.duration = ev_chord.get_duration()
- fretboards_builder.add_music(fb, ev_chord.get_length())
- pending_fretboards = []
-
- notations_children = n.get_typed_children(musicxml.Notations)
- tuplet_event = None
- span_events = []
-
- # The element can have the following children (+ means implemented, ~ partially, - not):
- # +tied | +slur | +tuplet | glissando | slide |
- # ornaments | technical | articulations | dynamics |
- # +fermata | arpeggiate | non-arpeggiate |
- # accidental-mark | other-notation
- for notations in notations_children:
- for tuplet_event in notations.get_tuplets():
- time_mod = n.get_maybe_exist_typed_child(
- musicxml.Time_modification)
- tuplet_events.append((ev_chord, tuplet_event, time_mod))
-
- # First, close all open slurs, only then start any new slur
- # TODO: Record the number of the open slur to dtermine the correct
- # closing slur!
- endslurs = [s for s in notations.get_named_children('slur')
- if s.get_type() in ('stop')]
- if endslurs and not inside_slur:
- endslurs[0].message(
- _('Encountered closing slur, but no slur is open'))
- elif endslurs:
- if len(endslurs) > 1:
- endslurs[0].message(
- _('Cannot have two simultaneous (closing) slurs'))
- # record the slur status for the next note in the loop
- inside_slur = False
- lily_ev = musicxml_spanner_to_lily_event(endslurs[0])
- ev_chord.append(lily_ev)
-
- startslurs = [s for s in notations.get_named_children('slur')
- if s.get_type() in ('start')]
- if startslurs and inside_slur:
- startslurs[0].message(
- _('Cannot have a slur inside another slur'))
- elif startslurs:
- if len(startslurs) > 1:
- startslurs[0].message(
- _('Cannot have two simultaneous slurs'))
- # record the slur status for the next note in the loop
- inside_slur = True
- lily_ev = musicxml_spanner_to_lily_event(startslurs[0])
- ev_chord.append(lily_ev)
-
- if not grace:
- mxl_tie = notations.get_tie()
- if mxl_tie and mxl_tie.type == 'start':
- ev_chord.append(musicexp.TieEvent())
- is_tied = True
- tie_started = True
- else:
- is_tied = False
-
- fermatas = notations.get_named_children('fermata')
- for a in fermatas:
- ev = musicxml_fermata_to_lily_event(a)
- if ev:
- ev_chord.append(ev)
-
- arpeggiate = notations.get_named_children('arpeggiate')
- for a in arpeggiate:
- ev = musicxml_arpeggiate_to_lily_event(a)
- if ev:
- ev_chord.append(ev)
-
- arpeggiate = notations.get_named_children('non-arpeggiate')
- for a in arpeggiate:
- ev = musicxml_nonarpeggiate_to_lily_event(a)
- if ev:
- ev_chord.append(ev)
-
- glissandos = notations.get_named_children('glissando')
- glissandos += notations.get_named_children('slide')
- for a in glissandos:
- ev = musicxml_spanner_to_lily_event(a)
- if ev:
- ev_chord.append(ev)
-
- # accidental-marks are direct children of !
- for a in notations.get_named_children('accidental-mark'):
- ev = musicxml_articulation_to_lily_event(a)
- if ev:
- ev_chord.append(ev)
-
- # Articulations can contain the following child elements:
- # accent | strong-accent | staccato | tenuto |
- # detached-legato | staccatissimo | spiccato |
- # scoop | plop | doit | falloff | breath-mark |
- # caesura | stress | unstress
- # Technical can contain the following child elements:
- # up-bow | down-bow | harmonic | open-string |
- # thumb-position | fingering | pluck | double-tongue |
- # triple-tongue | stopped | snap-pizzicato | fret |
- # string | hammer-on | pull-off | bend | tap | heel |
- # toe | fingernails | other-technical
- # Ornaments can contain the following child elements:
- # trill-mark | turn | delayed-turn | inverted-turn |
- # shake | wavy-line | mordent | inverted-mordent |
- # schleifer | tremolo | other-ornament, accidental-mark
- ornaments = notations.get_named_children('ornaments')
- ornaments += notations.get_named_children('articulations')
- ornaments += notations.get_named_children('technical')
-
- for a in ornaments:
- for ch in a.get_all_children():
- ev = musicxml_articulation_to_lily_event(ch)
- if ev:
- ev_chord.append(ev)
-
- dynamics = notations.get_named_children('dynamics')
- for a in dynamics:
- for ch in a.get_all_children():
- ev = musicxml_dynamics_to_lily_event(ch)
- if ev:
- ev_chord.append(ev)
-
- mxl_beams = [b for b in n.get_named_children('beam')
- if (b.get_type() in ('begin', 'end')
- and b.is_primary())]
- if mxl_beams and not conversion_settings.ignore_beaming:
- beam_ev = musicxml_spanner_to_lily_event(mxl_beams[0])
- if beam_ev:
- ev_chord.append(beam_ev)
- if beam_ev.span_direction == -1: # beam and thus melisma starts here
- is_beamed = True
- elif beam_ev.span_direction == 1: # beam and thus melisma ends here
- is_beamed = False
-
- # Assume that a element only lasts for one note.
- # This might not be correct MusicXML interpretation, but works for
- # most cases and fixes broken files, which have the end tag missing
- if is_tied and not tie_started:
- is_tied = False
-
- # force trailing mm rests to be written out.
- voice_builder.add_music (musicexp.ChordEvent(), Fraction(0))
-
- if hasattr(options, 'shift_meter') and options.shift_meter:
- for event in voice_builder.elements:
- if isinstance(event, musicexp.TimeSignatureChange):
- sd = []
- for i in range(0, 5):
- sd.append(musicexp.ShiftDurations())
- sd[i].set_shift_durations_parameters(event)
- break
-
- ly_voice = group_tuplets(voice_builder.elements, tuplet_events)
- ly_voice = group_repeats(ly_voice)
-
- seq_music = musicexp.SequentialMusic()
-
- seq_music.elements = ly_voice
- for k in list(lyrics.keys()):
- return_value.lyrics_dict[k] = musicexp.Lyrics()
- return_value.lyrics_dict[k].lyrics_syllables = lyrics[k]
-
- if hasattr(options, 'shift_meter') and options.shift_meter:
- sd[-1].element = seq_music
- seq_music = sd[-1]
- sd.pop()
-
- if hasattr(options, 'relative') and options.relative:
- v = musicexp.RelativeMusic()
- v.element = seq_music
- v.basepitch = first_pitch
- seq_music = v
-
- return_value.ly_voice = seq_music
-
- # create \figuremode { figured bass elements }
- if figured_bass_builder.has_relevant_elements:
- fbass_music = musicexp.SequentialMusic()
- fbass_music.elements = group_repeats(figured_bass_builder.elements)
- v = musicexp.ModeChangingMusicWrapper()
- v.mode = 'figuremode'
- v.element = fbass_music
- if hasattr(options, 'shift_meter') and options.shift_meter:
- sd[-1].element = v
- v = sd[-1]
- sd.pop()
- return_value.figured_bass = v
-
- # create \chordmode { chords }
- if chordnames_builder.has_relevant_elements:
- cname_music = musicexp.SequentialMusic()
- cname_music.elements = group_repeats(chordnames_builder.elements)
- v = musicexp.ModeChangingMusicWrapper()
- v.mode = 'chordmode'
- v.element = cname_music
- if hasattr(options, 'shift_meter') and options.shift_meter:
- sd[-1].element = v
- v = sd[-1]
- sd.pop()
- return_value.chordnames = v
-
- # create diagrams for FretBoards engraver
- if fretboards_builder.has_relevant_elements:
- fboard_music = musicexp.SequentialMusic()
- fboard_music.elements = group_repeats(fretboards_builder.elements)
- v = musicexp.MusicWrapper()
- v.element = fboard_music
- if hasattr(options, 'shift_meter') and options.shift_meter:
- sd[-1].element = v
- v = sd[-1]
- sd.pop()
- return_value.fretboards = v
-
- # coll = []
- # pending = []
-
- # for elt in return_value.ly_voice.element.elements:
- # if isinstance(elt, musicexp.TimeScaledMusic):
- # print elt.element.elements
- # pending.append(elt)
- # else:
- # coll.append(elt)
-
- # if pending:
- # coll.extend(pending)
-
- # return_value.ly_voice.element.elements = coll
-
- return return_value
-
-
-def musicxml_id_to_lily(id):
- digits = ['Zero', 'One', 'Two', 'Three', 'Four', 'Five',
- 'Six', 'Seven', 'Eight', 'Nine', 'Ten']
-
- for digit in digits:
- d = digits.index(digit)
- id = re.sub('%d' % d, digit, id)
-
- id = re.sub('[^a-zA-Z]', 'X', id)
- return id
-
-
-def voices_in_part(part):
- """Return a Name -> Voice dictionary for PART"""
- part.interpret()
- part.extract_voices()
- voices = part.get_voices()
- part_info = part.get_staff_attributes()
-
- return (voices, part_info)
-
-
-def voices_in_part_in_parts(parts):
- """return a Part -> Name -> Voice dictionary"""
- # don't crash if Part doesn't have an id (that's invalid MusicXML,
- # but such files are out in the wild!)
- dictionary = {}
- for p in parts:
- voices = voices_in_part(p)
- if hasattr(p, "id"):
- dictionary[p.id] = voices
- else:
- # TODO: extract correct part id from other sources
- dictionary[None] = voices
- return dictionary
-
-
-def get_all_voices(parts):
- all_voices = voices_in_part_in_parts(parts)
-
- all_ly_voices = {}
- all_ly_staffinfo = {}
- for p, (name_voice, staff_info) in list(all_voices.items()):
-
- part_ly_voices = OrderedDict()
- for n, v in list(name_voice.items()):
- ly.progress(_("Converting to LilyPond expressions..."), True)
- # musicxml_voice_to_lily_voice returns (lily_voice, {nr->lyrics, nr->lyrics})
- voice = musicxml_voice_to_lily_voice(v)
- part_ly_voices[n] = voice
-
- all_ly_voices[p] = part_ly_voices
- all_ly_staffinfo[p] = staff_info
-
- return (all_ly_voices, all_ly_staffinfo)
-
-
-def option_parser():
- p = ly.get_option_parser(usage=_("musicxml2ly [OPTION]... FILE.xml"),
- description=_("""Convert MusicXML from FILE.xml to LilyPond input.
-If the given filename is -, musicxml2ly reads from the command line.
-"""), add_help_option=False)
-
- p.add_option("-h", "--help",
- action="help",
- help=_("show this help and exit"))
-
- p.version = ('%prog (LilyPond) ' + lilypond_version + '\n\n'
- +
- _("""Copyright (c) 2005--2023 by
- Han-Wen Nienhuys ,
- Jan Nieuwenhuizen and
- Reinhold Kainhofer
- Patrick L. Schmidt
-"""
- +
- """
-This program is free software. It is covered by the GNU General Public
-License and you are welcome to change it and/or distribute copies of it
-under certain conditions. Invoke as `%s --warranty' for more
-information.""") % 'lilypond')
-
- p.add_option("--version",
- action="version",
- help=_("show version number and exit"))
-
- p.add_option('-v', '--verbose',
- action="callback",
- callback=ly.handle_loglevel_option,
- callback_args=("DEBUG",),
- help=_("be verbose"))
-
- p.add_option('', '--lxml',
- action="store_true",
- default=False,
- dest="use_lxml",
- help=_("use lxml.etree; uses less memory and cpu time"))
-
- p.add_option('-z', '--compressed',
- action="store_true",
- dest='compressed',
- default=False,
- help=_("input file is a compressed MusicXML file "
- "(by default, activate if file extension is .mxl)"))
-
- p.add_option('-r', '--relative',
- action="store_true",
- default=True,
- dest="relative",
- help=_("convert pitches in relative mode (default)"))
-
- p.add_option('-a', '--absolute',
- action="store_false",
- dest="relative",
- help=_("convert pitches in absolute mode"))
-
- p.add_option('-l', '--language',
- metavar=_("LANG"),
- action="store",
- help=_("use LANG for pitch names, e.g. 'deutsch' for note names in German"))
-
- p.add_option("--loglevel",
- help=_("Print log messages according to LOGLEVEL "
- "(NONE, ERROR, WARNING, PROGRESS (default), DEBUG)"),
- metavar=_("LOGLEVEL"),
- action='callback',
- callback=ly.handle_loglevel_option,
- type='string')
-
- p.add_option('--nd', '--no-articulation-directions',
- action="store_false",
- default=True,
- dest="convert_directions",
- help=_("do not convert directions (^, _ or -) for articulations, dynamics, etc."))
-
- p.add_option('--nrp', '--no-rest-positions',
- action="store_false",
- default=True,
- dest="convert_rest_positions",
- help=_("do not convert exact vertical positions of rests"))
-
- p.add_option('--nsb', '--no-system-breaks',
- action="store_false",
- default=True,
- dest="convert_system_breaks",
- help=_("ignore system breaks"))
-
- p.add_option('--npb', '--no-page-breaks',
- action="store_false",
- default=True,
- dest="convert_page_breaks",
- help=_("ignore page breaks"))
-
- p.add_option('--npm', '--no-page-margins',
- action="store_false",
- default=True,
- dest="convert_page_margins",
- help=_("ignore page margins"))
-
- p.add_option('--npl', '--no-page-layout',
- action="store_false",
- default=True,
- dest="convert_page_layout",
- help=_("do not convert the exact page layout and breaks (shortcut for \"--nsb --npb --npm\" options)"))
-
- p.add_option('--nsd', '--no-stem-directions',
- action="store_false",
- default=True,
- dest="convert_stem_directions",
- help=_("ignore stem directions from MusicXML, use lilypond's automatic stemming instead"))
-
- p.add_option('--nb', '--no-beaming',
- action="store_false",
- default=True,
- dest="convert_beaming",
- help=_("do not convert beaming information, use lilypond's automatic beaming instead"))
-
- p.add_option('-o', '--output',
- metavar=_("FILE"),
- action="store",
- default=None,
- type='string',
- dest='output_name',
- help=_("set output filename to FILE, stdout if -"))
-
- p.add_option('-m', '--midi',
- action="store_true",
- default=False,
- dest="midi",
- help=_("activate midi-block in .ly file"))
-
- # transpose function
- p.add_option('--transpose',
- metavar=_("TOPITCH"),
- action="store",
- dest="transpose",
- help=_("set pitch to transpose by the interval between pitch 'c' and TOPITCH"))
-
- # time signature changing function
- p.add_option('--sm', '--shift-meter',
- metavar=_("BEATS/BEATTYPE"),
- action="store",
- dest="shift_meter",
- help=_("change the length|duration of notes as a function of a given time signature to make the score look faster or slower, (eg. '4/4' or '2/2')"))
-
- # switch tabstaff clef
- p.add_option('--tc', '--tab-clef',
- metavar=_("TABCLEFNAME"),
- action="store",
- dest="tab_clef",
- help=_("switch between two versions of tab clefs (\"tab\" and \"moderntab\")"))
-
- # StringNumber stencil on/off
- p.add_option('--sn', '--string-numbers',
- metavar=_("t[rue]/f[alse]"),
- action="store",
- dest="string_numbers",
- help=_("deactivate string number stencil with --string-numbers f[alse]. Default is t[rue]"))
-
- # StringNumber stencil on/off
- p.add_option('--fb', '--fretboards',
- action="store_true",
- default=False,
- dest="fretboards",
- help=_("converts '' events to a separate FretBoards voice instead of markups"))
-
- p.add_option_group('',
- description=(
- _("Report bugs via %s")
- % 'bug-lilypond@gnu.org') + '\n')
- return p
-
-
-def print_voice_definitions(printer, part_list, voices):
- for part in part_list:
- part_id = part.id
- nv_dict = voices.get(part_id, {})
- for (name, voice) in list(nv_dict.items()):
- k = music_xml_voice_name_to_lily_name(part_id, name)
- printer.dump('%s = ' % k)
- voice.ly_voice.print_ly(printer)
- printer.newline()
- if voice.chordnames:
- cnname = music_xml_chordnames_name_to_lily_name(part_id, name)
- printer.dump('%s = ' % cnname)
- voice.chordnames.print_ly(printer)
- printer.newline()
- for l in voice.lyrics_order:
- lname = music_xml_lyrics_name_to_lily_name(part_id, name, l)
- printer.dump('%s = ' % lname)
- voice.lyrics_dict[l].print_ly(printer)
- printer.newline()
- if voice.figured_bass:
- fbname = music_xml_figuredbass_name_to_lily_name(part_id, name)
- printer.dump('%s = ' % fbname)
- voice.figured_bass.print_ly(printer)
- printer.newline()
- if voice.fretboards:
- fbdname = music_xml_fretboards_name_to_lily_name(part_id, name)
- printer.dump('%s = ' % fbdname)
- voice.fretboards.print_ly(printer)
- printer.newline()
-
-
-# format the information about the staff in the form
-# [staffid,
-# [
-# [voiceid1, [lyricsid11, lyricsid12,...], figuredbassid1],
-# [voiceid2, [lyricsid21, lyricsid22,...], figuredbassid2],
-# ...
-# ]
-# ]
-# raw_voices is of the form [(voicename, lyricsids, havefiguredbass)*]
-
-
-def format_staff_info(part_id, staff_id, raw_voices):
- voices = []
- for (v, lyricsids, figured_bass, chordnames, fretboards) in raw_voices:
- voice_name = music_xml_voice_name_to_lily_name(part_id, v)
- voice_lyrics = [music_xml_lyrics_name_to_lily_name(part_id, v, l)
- for l in lyricsids]
- figured_bass_name = ''
- if figured_bass:
- figured_bass_name = music_xml_figuredbass_name_to_lily_name(
- part_id, v)
- chordnames_name = ''
- if chordnames:
- chordnames_name = music_xml_chordnames_name_to_lily_name(
- part_id, v)
- fretboards_name = ''
- if fretboards:
- fretboards_name = music_xml_fretboards_name_to_lily_name(
- part_id, v)
- voices.append([voice_name, voice_lyrics, figured_bass_name,
- chordnames_name, fretboards_name])
- return [staff_id, voices]
-
-
-def update_score_setup(score_structure, part_list, voices, parts):
- for part_definition in part_list:
- part_id = part_definition.id
- nv_dict = voices.get(part_id)
- if not nv_dict:
- if len(part_list) == len(voices) == 1:
- # If there is only one part, infer the ID.
- # See input/regression/musicxml/41g-PartNoId.xml.
- nv_dict = list(voices.values())[0]
- voices[part_id] = nv_dict
- else:
- ly.warning(_('unknown part in part-list: %s') % part_id)
- continue
-
- staves = reduce(lambda x, y: x + y,
- [list(voice.voicedata._staves.keys())
- for voice in list(nv_dict.values())],
- [])
- staves_info = []
- if len(staves) > 1:
- staves_info = []
- staves = sorted(set(staves))
- for s in staves:
- thisstaff_raw_voices = [(voice_name, voice.lyrics_order, voice.figured_bass, voice.chordnames, voice.fretboards)
- for (voice_name, voice) in list(nv_dict.items())
- if voice.voicedata._start_staff == s]
- staves_info.append(format_staff_info(
- part_id, s, thisstaff_raw_voices))
- else:
- thisstaff_raw_voices = [(voice_name, voice.lyrics_order, voice.figured_bass, voice.chordnames, voice.fretboards)
- for (voice_name, voice) in list(nv_dict.items())]
- staves_info.append(format_staff_info(
- part_id, None, thisstaff_raw_voices))
- score_structure.set_part_information(part_id, staves_info)
-
- sounds = []
- for part in parts:
- for measure in part.get_typed_children(musicxml.Measure):
- for sound in measure.get_typed_children(musicxml.Sound):
- sounds.append(sound)
- for direction in measure.get_typed_children(musicxml.Direction):
- for sound in direction.get_typed_children(musicxml.Sound):
- sounds.append(sound)
-
- score_structure.set_tempo('100')
- if len(sounds) != 0:
- for sound in sounds:
- if (sound.get_tempo() is not None and sound.get_tempo() != ""):
- score_structure.set_tempo(sound.get_tempo())
- break
-
-
-# Set global values in the \layout block, like auto-beaming etc.
-def update_layout_information():
- if not conversion_settings.ignore_beaming and layout_information:
- layout_information.set_context_item('Score', 'autoBeaming = ##f')
- if musicexp.get_string_numbers() == "f":
- layout_information.set_context_item(
- 'Score', '\\override StringNumber #\'stencil = ##f')
-
-# \n\t\t\t\t\\override StringNumber #\'stencil = ##f
-
-
-def print_ly_preamble(printer, filename):
- printer.dump_version(lilypond_version)
- printer.print_verbatim(
- '% automatically converted by musicxml2ly from ' + filename)
- printer.newline()
- printer.dump(r'\pointAndClickOff')
- printer.newline()
- if options.midi:
- printer.newline()
- printer.dump(r'\include "articulate.ly"')
- printer.newline()
-
-
-def print_ly_additional_definitions(printer, filename=None):
- if needed_additional_definitions:
- printer.newline()
- printer.print_verbatim(
- '%% additional definitions required by the score:')
- printer.newline()
- for a in sorted(set(needed_additional_definitions)):
- printer.print_verbatim(additional_definitions.get(a, ''))
- printer.newline()
- printer.newline()
-
-# Read in the tree from the given I/O object (either file or string) and
-# demarshall it using the classes from the musicxml.py file
-
-
-def read_xml(io_object, use_lxml):
- if use_lxml:
- import lxml.etree
- tree = lxml.etree.parse(io_object)
- mxl_tree = musicxml.lxml_demarshal_node(tree.getroot())
- return mxl_tree
- else:
- from xml.dom import minidom, Node
- doc = minidom.parse(io_object)
- node = doc.documentElement
- return musicxml.minidom_demarshal_node(node)
- return None
-
-
-def read_musicxml(filename, compressed, use_lxml):
- raw_string = None
- if compressed:
- if filename == "-":
- ly.progress(
- _("Input is compressed, extracting raw MusicXML data from stdin"), True)
- # unfortunately, zipfile.ZipFile can't read directly from
- # stdin, so copy everything from stdin to a temp file and read
- # that. TemporaryFile() will remove the file when it is closed.
- tmp = tempfile.TemporaryFile()
- # Make sys.stdin binary
- sys.stdin = os.fdopen(sys.stdin.fileno(), 'rb', 0)
- bytes_read = sys.stdin.read(8192)
- while bytes_read:
- tmp.write(bytes_read)
- bytes_read = sys.stdin.read(8192)
- z = zipfile.ZipFile(tmp, "r")
- else:
- ly.progress(
- _("Input file %s is compressed, extracting raw MusicXML data") % filename, True)
- z = zipfile.ZipFile(filename, "r")
- container_xml = z.read("META-INF/container.xml").decode("utf-8")
- if not container_xml:
- return None
- container = read_xml(io.StringIO(container_xml), use_lxml)
- if not container:
- return None
- rootfiles = container.get_maybe_exist_named_child('rootfiles')
- if not rootfiles:
- return None
- rootfile_list = rootfiles.get_named_children('rootfile')
- mxml_file = None
- if len(rootfile_list) > 0:
- mxml_file = getattr(rootfile_list[0], 'full-path', None)
- if mxml_file:
- raw_string = z.read(mxml_file).decode('utf-8')
-
- if raw_string:
- io_object = io.StringIO(raw_string)
- elif filename == "-":
- io_object = sys.stdin
- else:
- io_object = filename
-
- return read_xml(io_object, use_lxml)
-
-
-def convert(filename, options):
- if filename == "-":
- ly.progress(_("Reading MusicXML from Standard input ..."), True)
- else:
- ly.progress(_("Reading MusicXML from %s ...") % filename, True)
-
- tree = read_musicxml(filename, options.compressed, options.use_lxml)
- score_information = extract_score_information(tree)
- paper_information = extract_paper_information(tree)
-
- parts = tree.get_typed_children(musicxml.Part)
- (voices, staff_info) = get_all_voices(parts)
-
- score = None
- mxl_pl = tree.get_maybe_exist_typed_child(musicxml.Part_list)
- if mxl_pl:
- score = extract_score_structure(mxl_pl, staff_info)
- part_list = mxl_pl.get_named_children("score-part")
-
- # score information is contained in the , or tags
- update_score_setup(score, part_list, voices, parts)
- # After the conversion, update the list of settings for the \layout block
- update_layout_information()
-
- if not options.output_name:
- options.output_name = os.path.basename(filename)
- options.output_name = os.path.splitext(options.output_name)[0]
- elif re.match(r".*\.ly", options.output_name):
- options.output_name = os.path.splitext(options.output_name)[0]
-
- #defs_ly_name = options.output_name + '-defs.ly'
- if options.output_name == "-":
- output_ly_name = 'Standard output'
- else:
- output_ly_name = options.output_name + '.ly'
- ly.progress(_("Output to `%s'") % output_ly_name, True)
- printer = musicexp.Output_printer()
- #ly.progress(_("Output to `%s'") % defs_ly_name, True)
- if options.output_name == "-":
- printer.set_file(sys.stdout)
- else:
- printer.set_file(open(output_ly_name, 'w', encoding='utf-8'))
- print_ly_preamble(printer, filename)
- print_ly_additional_definitions(printer, filename)
- if score_information:
- score_information.print_ly(printer)
- if paper_information and conversion_settings.convert_page_layout:
- paper_information.print_ly(printer)
- if layout_information:
- layout_information.print_ly(printer)
- print_voice_definitions(printer, part_list, voices)
-
- printer.newline()
- printer.dump("% The score definition")
- printer.newline()
- score.print_ly(printer)
- printer.newline()
-
- # Syntax update to current version
- if options.output_name != "-":
- version = os.popen(
- "lilypond --version | head -1 | cut -d' ' -f3").read().strip()
- ly.progress(
- _("Converting to current version (%s) notations ..." % version), True)
- os.system("convert-ly -e %s 2> /dev/null" %
- utilities.escape_ly_output_string(output_ly_name))
-
- return voices
-
-
-def get_existing_filename_with_extension(filename, ext):
- if os.path.exists(filename):
- return filename
- newfilename = filename + "." + ext
- if os.path.exists(newfilename):
- return newfilename
- newfilename = filename + ext
- if os.path.exists(newfilename):
- return newfilename
- return ''
-
-
-def main():
- opt_parser = option_parser()
-
- global options
- (options, args) = opt_parser.parse_args()
-
-# in case of shell entry w/o special characters
- if options.language == 'catalan' or options.language == 'catala':
- options.language = 'català'
- if options.language == 'espanol':
- options.language = 'español'
- if options.language == 'francais':
- options.language = 'français'
- if options.language == 'portugues':
- options.language = 'português'
-
- if not args:
- opt_parser.print_usage()
- sys.exit(2)
-
- # midi-block option
- if options.midi:
- musicexp.set_create_midi(options.midi)
-
- # transpose function
- if options.transpose:
- musicexp.set_transpose(options.transpose)
-
- # tab clef option
- if options.tab_clef:
- musicexp.set_tab_clef(options.tab_clef)
-
- # string numbers option
- if options.string_numbers:
- musicexp.set_string_numbers(options.string_numbers)
-
- if options.language:
- musicexp.set_pitch_language(options.language)
- needed_additional_definitions.append(options.language)
- additional_definitions[options.language] = "\\language \"%s\"\n" % options.language
-
- conversion_settings.ignore_beaming = not options.convert_beaming
- conversion_settings.convert_page_layout = options.convert_page_layout
- if conversion_settings.convert_page_layout:
- conversion_settings.convert_system_breaks = options.convert_system_breaks
- conversion_settings.convert_page_breaks = options.convert_page_breaks
- conversion_settings.convert_page_margins = options.convert_page_margins
- else:
- conversion_settings.convert_system_breaks = False
- conversion_settings.convert_page_breaks = False
- conversion_settings.convert_page_margins = False
- conversion_settings.convert_stem_directions = options.convert_stem_directions
- conversion_settings.convert_rest_positions = options.convert_rest_positions
-
- # Allow the user to leave out the .xml or xml on the filename
- basefilename = args[0]
- if basefilename == "-": # Read from stdin
- filename = "-"
- else:
- filename = get_existing_filename_with_extension(basefilename, "xml")
- if not filename:
- filename = get_existing_filename_with_extension(
- basefilename, "mxl")
- options.compressed = True
- if filename and filename.endswith("mxl"):
- options.compressed = True
-
- if filename and (filename == "-" or os.path.exists(filename)):
- voices = convert(filename, options)
- else:
- ly.error(_("Unable to find input file %s") % basefilename)
- sys.exit(1)
-
-
-if __name__ == '__main__':
- main()
diff --git a/spaces/Pattr/DrumClassification/lilypond-2.24.2/lib/lilypond/2.24.2/ccache/lily/define-woodwind-diagrams.go b/spaces/Pattr/DrumClassification/lilypond-2.24.2/lib/lilypond/2.24.2/ccache/lily/define-woodwind-diagrams.go
deleted file mode 100644
index e7da151e485b9f709ef11b824b4ecdad6b318a2d..0000000000000000000000000000000000000000
Binary files a/spaces/Pattr/DrumClassification/lilypond-2.24.2/lib/lilypond/2.24.2/ccache/lily/define-woodwind-diagrams.go and /dev/null differ
diff --git a/spaces/PeepDaSlan9/stabilityai-FreeWilly2/app.py b/spaces/PeepDaSlan9/stabilityai-FreeWilly2/app.py
deleted file mode 100644
index 8be47e7462d04255ee691ae31eeae8b73920f87b..0000000000000000000000000000000000000000
--- a/spaces/PeepDaSlan9/stabilityai-FreeWilly2/app.py
+++ /dev/null
@@ -1,3 +0,0 @@
-import gradio as gr
-
-gr.Interface.load("models/stabilityai/FreeWilly2").launch()
\ No newline at end of file
diff --git a/spaces/Pie31415/control-animation/annotator/uniformer/configs/_base_/models/deeplabv3_unet_s5-d16.py b/spaces/Pie31415/control-animation/annotator/uniformer/configs/_base_/models/deeplabv3_unet_s5-d16.py
deleted file mode 100644
index 0cd262999d8b2cb8e14a5c32190ae73f479d8e81..0000000000000000000000000000000000000000
--- a/spaces/Pie31415/control-animation/annotator/uniformer/configs/_base_/models/deeplabv3_unet_s5-d16.py
+++ /dev/null
@@ -1,50 +0,0 @@
-# model settings
-norm_cfg = dict(type='SyncBN', requires_grad=True)
-model = dict(
- type='EncoderDecoder',
- pretrained=None,
- backbone=dict(
- type='UNet',
- in_channels=3,
- base_channels=64,
- num_stages=5,
- strides=(1, 1, 1, 1, 1),
- enc_num_convs=(2, 2, 2, 2, 2),
- dec_num_convs=(2, 2, 2, 2),
- downsamples=(True, True, True, True),
- enc_dilations=(1, 1, 1, 1, 1),
- dec_dilations=(1, 1, 1, 1),
- with_cp=False,
- conv_cfg=None,
- norm_cfg=norm_cfg,
- act_cfg=dict(type='ReLU'),
- upsample_cfg=dict(type='InterpConv'),
- norm_eval=False),
- decode_head=dict(
- type='ASPPHead',
- in_channels=64,
- in_index=4,
- channels=16,
- dilations=(1, 12, 24, 36),
- dropout_ratio=0.1,
- num_classes=2,
- norm_cfg=norm_cfg,
- align_corners=False,
- loss_decode=dict(
- type='CrossEntropyLoss', use_sigmoid=False, loss_weight=1.0)),
- auxiliary_head=dict(
- type='FCNHead',
- in_channels=128,
- in_index=3,
- channels=64,
- num_convs=1,
- concat_input=False,
- dropout_ratio=0.1,
- num_classes=2,
- norm_cfg=norm_cfg,
- align_corners=False,
- loss_decode=dict(
- type='CrossEntropyLoss', use_sigmoid=False, loss_weight=0.4)),
- # model training and testing settings
- train_cfg=dict(),
- test_cfg=dict(mode='slide', crop_size=256, stride=170))
diff --git a/spaces/Queensly/FastAPI_in_Docker/main.py b/spaces/Queensly/FastAPI_in_Docker/main.py
deleted file mode 100644
index d27ed46bddfcedcd720ea8c2cdf410cdb3aa602c..0000000000000000000000000000000000000000
--- a/spaces/Queensly/FastAPI_in_Docker/main.py
+++ /dev/null
@@ -1,63 +0,0 @@
-from fastapi import FastAPI
-import pickle
-import uvicorn
-import pandas as pd
-
-app = FastAPI()
-
-# @app.get("/")
-# def read_root():
-# return {"Hello": "World!"}
-
-
-# Function to load pickle file
-def load_pickle(filename):
- with open(filename, 'rb') as file:
- data = pickle.load(file)
- return data
-
-# Load pickle file
-ml_components = load_pickle('ml_sepsis.pkl')
-
-# Components in the pickle file
-ml_model = ml_components['model']
-pipeline_processing = ml_components['pipeline']
-
-#Endpoints
-#Root endpoints
-@app.get("/")
-def root():
- return {"API": "An API for Sepsis Prediction."}
-
-@app.get('/Predict_Sepsis')
-async def predict(Plasma_glucose: int, Blood_Work_Result_1: int,
- Blood_Pressure: int, Blood_Work_Result_2: int,
- Blood_Work_Result_3: int, Body_mass_index: float,
- Blood_Work_Result_4: float,Age: int, Insurance:float):
-
- data = pd.DataFrame({'Plasma glucose': [Plasma_glucose], 'Blood Work Result-1': [Blood_Work_Result_1],
- 'Blood Pressure': [Blood_Pressure], 'Blood Work Result-2': [Blood_Work_Result_2],
- 'Blood Work Result-3': [Blood_Work_Result_3], 'Body mass index': [Body_mass_index],
- 'Blood Work Result-4': [Blood_Work_Result_4], 'Age': [Age], 'Insurance':[Insurance]})
-
- data_prepared = pipeline_processing.transform(data)
-
- model_output = ml_model.predict(data_prepared).tolist()
-
- prediction = make_prediction(model_output)
-
- return prediction
-
-
-
-
-def make_prediction(data_prepared):
-
- output_pred = data_prepared
-
- if output_pred == 0:
- output_pred = "Sepsis status is Negative"
- else:
- output_pred = "Sepsis status is Positive"
-
- return output_pred
\ No newline at end of file
diff --git a/spaces/RahulJ24/gradiolangchainchatbotAI/app.py b/spaces/RahulJ24/gradiolangchainchatbotAI/app.py
deleted file mode 100644
index a362dcc7d0ddd1eee86961f1bc3db6d894fbd3d5..0000000000000000000000000000000000000000
--- a/spaces/RahulJ24/gradiolangchainchatbotAI/app.py
+++ /dev/null
@@ -1,34 +0,0 @@
-import os
-import gradio as gr
-from langchain.chat_models import ChatOpenAI
-from langchain import LLMChain, PromptTemplate
-from langchain.memory import ConversationBufferMemory
-
-OPENAI_API_KEY=os.getenv('OPENAI_API_KEY')
-
-template = """You are a helpful assistant to answer all user queries.
-{chat_history}
-User: {user_message}
-Chatbot:"""
-
-prompt = PromptTemplate(
- input_variables=["chat_history", "user_message"], template=template
-)
-
-memory = ConversationBufferMemory(memory_key="chat_history")
-
-llm_chain = LLMChain(
- llm=ChatOpenAI(temperature='0.5', model_name="gpt-3.5-turbo"),
- prompt=prompt,
- verbose=True,
- memory=memory,
-)
-
-def get_text_response(user_message,history):
- response = llm_chain.predict(user_message = user_message)
- return response
-
-demo = gr.ChatInterface(get_text_response)
-
-if __name__ == "__main__":
- demo.launch() #To create a public link, set `share=True` in `launch()`. To enable errors and logs, set `debug=True` in `launch()`.
diff --git a/spaces/RamAnanth1/videocrafter/utils.py b/spaces/RamAnanth1/videocrafter/utils.py
deleted file mode 100644
index d65c6b66a8ad1c402fc21a8e21768467a151cb85..0000000000000000000000000000000000000000
--- a/spaces/RamAnanth1/videocrafter/utils.py
+++ /dev/null
@@ -1,129 +0,0 @@
-import os
-import torch
-from PIL import Image
-
-from lvdm.models.modules.lora import net_load_lora
-from lvdm.utils.common_utils import instantiate_from_config
-
-
-# ------------------------------------------------------------------------------------------
-def load_model(config, ckpt_path, gpu_id=None, inject_lora=False, lora_scale=1.0, lora_path=''):
- print(f"Loading model from {ckpt_path}")
-
- # load sd
- pl_sd = torch.load(ckpt_path, map_location="cpu")
- try:
- global_step = pl_sd["global_step"]
- epoch = pl_sd["epoch"]
- except:
- global_step = -1
- epoch = -1
-
- # load sd to model
- try:
- sd = pl_sd["state_dict"]
- except:
- sd = pl_sd
- model = instantiate_from_config(config.model)
- model.load_state_dict(sd, strict=True)
-
- if inject_lora:
- net_load_lora(model, lora_path, alpha=lora_scale)
-
- # move to device & eval
- if gpu_id is not None:
- model.to(f"cuda:{gpu_id}")
- else:
- model.cuda()
- model.eval()
-
- return model, global_step, epoch
-
-
-# ------------------------------------------------------------------------------------------
-@torch.no_grad()
-def get_conditions(prompts, model, batch_size, cond_fps=None,):
-
- if isinstance(prompts, str) or isinstance(prompts, int):
- prompts = [prompts]
- if isinstance(prompts, list):
- if len(prompts) == 1:
- prompts = prompts * batch_size
- elif len(prompts) == batch_size:
- pass
- else:
- raise ValueError(f"invalid prompts length: {len(prompts)}")
- else:
- raise ValueError(f"invalid prompts: {prompts}")
- assert(len(prompts) == batch_size)
-
- # content condition: text / class label
- c = model.get_learned_conditioning(prompts)
- key = 'c_concat' if model.conditioning_key == 'concat' else 'c_crossattn'
- c = {key: [c]}
-
- # temporal condition: fps
- if getattr(model, 'cond_stage2_config', None) is not None:
- if model.cond_stage2_key == "temporal_context":
- assert(cond_fps is not None)
- batch = {'fps': torch.tensor([cond_fps] * batch_size).long().to(model.device)}
- fps_embd = model.cond_stage2_model(batch)
- c[model.cond_stage2_key] = fps_embd
-
- return c
-
-
-# ------------------------------------------------------------------------------------------
-def make_model_input_shape(model, batch_size, T=None):
- image_size = [model.image_size, model.image_size] if isinstance(model.image_size, int) else model.image_size
- C = model.model.diffusion_model.in_channels
- if T is None:
- T = model.model.diffusion_model.temporal_length
- shape = [batch_size, C, T, *image_size]
- return shape
-
-
-# ------------------------------------------------------------------------------------------
-def custom_to_pil(x):
- x = x.detach().cpu()
- x = torch.clamp(x, -1., 1.)
- x = (x + 1.) / 2.
- x = x.permute(1, 2, 0).numpy()
- x = (255 * x).astype(np.uint8)
- x = Image.fromarray(x)
- if not x.mode == "RGB":
- x = x.convert("RGB")
- return x
-
-def torch_to_np(x):
- # saves the batch in adm style as in https://github.com/openai/guided-diffusion/blob/main/scripts/image_sample.py
- sample = x.detach().cpu()
- sample = ((sample + 1) * 127.5).clamp(0, 255).to(torch.uint8)
- if sample.dim() == 5:
- sample = sample.permute(0, 2, 3, 4, 1)
- else:
- sample = sample.permute(0, 2, 3, 1)
- sample = sample.contiguous()
- return sample
-
-def make_sample_dir(opt, global_step=None, epoch=None):
- if not getattr(opt, 'not_automatic_logdir', False):
- gs_str = f"globalstep{global_step:09}" if global_step is not None else "None"
- e_str = f"epoch{epoch:06}" if epoch is not None else "None"
- ckpt_dir = os.path.join(opt.logdir, f"{gs_str}_{e_str}")
-
- # subdir name
- if opt.prompt_file is not None:
- subdir = f"prompts_{os.path.splitext(os.path.basename(opt.prompt_file))[0]}"
- else:
- subdir = f"prompt_{opt.prompt[:10]}"
- subdir += "_DDPM" if opt.vanilla_sample else f"_DDIM{opt.custom_steps}steps"
- subdir += f"_CfgScale{opt.scale}"
- if opt.cond_fps is not None:
- subdir += f"_fps{opt.cond_fps}"
- if opt.seed is not None:
- subdir += f"_seed{opt.seed}"
-
- return os.path.join(ckpt_dir, subdir)
- else:
- return opt.logdir
diff --git a/spaces/Raspberry-ai/main/.env/lib/python3.11/site-packages/pip/_vendor/chardet/jisfreq.py b/spaces/Raspberry-ai/main/.env/lib/python3.11/site-packages/pip/_vendor/chardet/jisfreq.py
deleted file mode 100644
index 3293576e012a1c931b5e89ebc065c67b65941084..0000000000000000000000000000000000000000
--- a/spaces/Raspberry-ai/main/.env/lib/python3.11/site-packages/pip/_vendor/chardet/jisfreq.py
+++ /dev/null
@@ -1,325 +0,0 @@
-######################## BEGIN LICENSE BLOCK ########################
-# The Original Code is Mozilla Communicator client code.
-#
-# The Initial Developer of the Original Code is
-# Netscape Communications Corporation.
-# Portions created by the Initial Developer are Copyright (C) 1998
-# the Initial Developer. All Rights Reserved.
-#
-# Contributor(s):
-# Mark Pilgrim - port to Python
-#
-# This library is free software; you can redistribute it and/or
-# modify it under the terms of the GNU Lesser General Public
-# License as published by the Free Software Foundation; either
-# version 2.1 of the License, or (at your option) any later version.
-#
-# This library is distributed in the hope that it will be useful,
-# but WITHOUT ANY WARRANTY; without even the implied warranty of
-# MERCHANTABILITY or FITNESS FOR A PARTICULAR PURPOSE. See the GNU
-# Lesser General Public License for more details.
-#
-# You should have received a copy of the GNU Lesser General Public
-# License along with this library; if not, write to the Free Software
-# Foundation, Inc., 51 Franklin St, Fifth Floor, Boston, MA
-# 02110-1301 USA
-######################### END LICENSE BLOCK #########################
-
-# Sampling from about 20M text materials include literature and computer technology
-#
-# Japanese frequency table, applied to both S-JIS and EUC-JP
-# They are sorted in order.
-
-# 128 --> 0.77094
-# 256 --> 0.85710
-# 512 --> 0.92635
-# 1024 --> 0.97130
-# 2048 --> 0.99431
-#
-# Ideal Distribution Ratio = 0.92635 / (1-0.92635) = 12.58
-# Random Distribution Ration = 512 / (2965+62+83+86-512) = 0.191
-#
-# Typical Distribution Ratio, 25% of IDR
-
-JIS_TYPICAL_DISTRIBUTION_RATIO = 3.0
-
-# Char to FreqOrder table ,
-JIS_TABLE_SIZE = 4368
-
-# fmt: off
-JIS_CHAR_TO_FREQ_ORDER = (
- 40, 1, 6, 182, 152, 180, 295,2127, 285, 381,3295,4304,3068,4606,3165,3510, # 16
-3511,1822,2785,4607,1193,2226,5070,4608, 171,2996,1247, 18, 179,5071, 856,1661, # 32
-1262,5072, 619, 127,3431,3512,3230,1899,1700, 232, 228,1294,1298, 284, 283,2041, # 48
-2042,1061,1062, 48, 49, 44, 45, 433, 434,1040,1041, 996, 787,2997,1255,4305, # 64
-2108,4609,1684,1648,5073,5074,5075,5076,5077,5078,3687,5079,4610,5080,3927,3928, # 80
-5081,3296,3432, 290,2285,1471,2187,5082,2580,2825,1303,2140,1739,1445,2691,3375, # 96
-1691,3297,4306,4307,4611, 452,3376,1182,2713,3688,3069,4308,5083,5084,5085,5086, # 112
-5087,5088,5089,5090,5091,5092,5093,5094,5095,5096,5097,5098,5099,5100,5101,5102, # 128
-5103,5104,5105,5106,5107,5108,5109,5110,5111,5112,4097,5113,5114,5115,5116,5117, # 144
-5118,5119,5120,5121,5122,5123,5124,5125,5126,5127,5128,5129,5130,5131,5132,5133, # 160
-5134,5135,5136,5137,5138,5139,5140,5141,5142,5143,5144,5145,5146,5147,5148,5149, # 176
-5150,5151,5152,4612,5153,5154,5155,5156,5157,5158,5159,5160,5161,5162,5163,5164, # 192
-5165,5166,5167,5168,5169,5170,5171,5172,5173,5174,5175,1472, 598, 618, 820,1205, # 208
-1309,1412,1858,1307,1692,5176,5177,5178,5179,5180,5181,5182,1142,1452,1234,1172, # 224
-1875,2043,2149,1793,1382,2973, 925,2404,1067,1241, 960,1377,2935,1491, 919,1217, # 240
-1865,2030,1406,1499,2749,4098,5183,5184,5185,5186,5187,5188,2561,4099,3117,1804, # 256
-2049,3689,4309,3513,1663,5189,3166,3118,3298,1587,1561,3433,5190,3119,1625,2998, # 272
-3299,4613,1766,3690,2786,4614,5191,5192,5193,5194,2161, 26,3377, 2,3929, 20, # 288
-3691, 47,4100, 50, 17, 16, 35, 268, 27, 243, 42, 155, 24, 154, 29, 184, # 304
- 4, 91, 14, 92, 53, 396, 33, 289, 9, 37, 64, 620, 21, 39, 321, 5, # 320
- 12, 11, 52, 13, 3, 208, 138, 0, 7, 60, 526, 141, 151,1069, 181, 275, # 336
-1591, 83, 132,1475, 126, 331, 829, 15, 69, 160, 59, 22, 157, 55,1079, 312, # 352
- 109, 38, 23, 25, 10, 19, 79,5195, 61, 382,1124, 8, 30,5196,5197,5198, # 368
-5199,5200,5201,5202,5203,5204,5205,5206, 89, 62, 74, 34,2416, 112, 139, 196, # 384
- 271, 149, 84, 607, 131, 765, 46, 88, 153, 683, 76, 874, 101, 258, 57, 80, # 400
- 32, 364, 121,1508, 169,1547, 68, 235, 145,2999, 41, 360,3027, 70, 63, 31, # 416
- 43, 259, 262,1383, 99, 533, 194, 66, 93, 846, 217, 192, 56, 106, 58, 565, # 432
- 280, 272, 311, 256, 146, 82, 308, 71, 100, 128, 214, 655, 110, 261, 104,1140, # 448
- 54, 51, 36, 87, 67,3070, 185,2618,2936,2020, 28,1066,2390,2059,5207,5208, # 464
-5209,5210,5211,5212,5213,5214,5215,5216,4615,5217,5218,5219,5220,5221,5222,5223, # 480
-5224,5225,5226,5227,5228,5229,5230,5231,5232,5233,5234,5235,5236,3514,5237,5238, # 496
-5239,5240,5241,5242,5243,5244,2297,2031,4616,4310,3692,5245,3071,5246,3598,5247, # 512
-4617,3231,3515,5248,4101,4311,4618,3808,4312,4102,5249,4103,4104,3599,5250,5251, # 528
-5252,5253,5254,5255,5256,5257,5258,5259,5260,5261,5262,5263,5264,5265,5266,5267, # 544
-5268,5269,5270,5271,5272,5273,5274,5275,5276,5277,5278,5279,5280,5281,5282,5283, # 560
-5284,5285,5286,5287,5288,5289,5290,5291,5292,5293,5294,5295,5296,5297,5298,5299, # 576
-5300,5301,5302,5303,5304,5305,5306,5307,5308,5309,5310,5311,5312,5313,5314,5315, # 592
-5316,5317,5318,5319,5320,5321,5322,5323,5324,5325,5326,5327,5328,5329,5330,5331, # 608
-5332,5333,5334,5335,5336,5337,5338,5339,5340,5341,5342,5343,5344,5345,5346,5347, # 624
-5348,5349,5350,5351,5352,5353,5354,5355,5356,5357,5358,5359,5360,5361,5362,5363, # 640
-5364,5365,5366,5367,5368,5369,5370,5371,5372,5373,5374,5375,5376,5377,5378,5379, # 656
-5380,5381, 363, 642,2787,2878,2788,2789,2316,3232,2317,3434,2011, 165,1942,3930, # 672
-3931,3932,3933,5382,4619,5383,4620,5384,5385,5386,5387,5388,5389,5390,5391,5392, # 688
-5393,5394,5395,5396,5397,5398,5399,5400,5401,5402,5403,5404,5405,5406,5407,5408, # 704
-5409,5410,5411,5412,5413,5414,5415,5416,5417,5418,5419,5420,5421,5422,5423,5424, # 720
-5425,5426,5427,5428,5429,5430,5431,5432,5433,5434,5435,5436,5437,5438,5439,5440, # 736
-5441,5442,5443,5444,5445,5446,5447,5448,5449,5450,5451,5452,5453,5454,5455,5456, # 752
-5457,5458,5459,5460,5461,5462,5463,5464,5465,5466,5467,5468,5469,5470,5471,5472, # 768
-5473,5474,5475,5476,5477,5478,5479,5480,5481,5482,5483,5484,5485,5486,5487,5488, # 784
-5489,5490,5491,5492,5493,5494,5495,5496,5497,5498,5499,5500,5501,5502,5503,5504, # 800
-5505,5506,5507,5508,5509,5510,5511,5512,5513,5514,5515,5516,5517,5518,5519,5520, # 816
-5521,5522,5523,5524,5525,5526,5527,5528,5529,5530,5531,5532,5533,5534,5535,5536, # 832
-5537,5538,5539,5540,5541,5542,5543,5544,5545,5546,5547,5548,5549,5550,5551,5552, # 848
-5553,5554,5555,5556,5557,5558,5559,5560,5561,5562,5563,5564,5565,5566,5567,5568, # 864
-5569,5570,5571,5572,5573,5574,5575,5576,5577,5578,5579,5580,5581,5582,5583,5584, # 880
-5585,5586,5587,5588,5589,5590,5591,5592,5593,5594,5595,5596,5597,5598,5599,5600, # 896
-5601,5602,5603,5604,5605,5606,5607,5608,5609,5610,5611,5612,5613,5614,5615,5616, # 912
-5617,5618,5619,5620,5621,5622,5623,5624,5625,5626,5627,5628,5629,5630,5631,5632, # 928
-5633,5634,5635,5636,5637,5638,5639,5640,5641,5642,5643,5644,5645,5646,5647,5648, # 944
-5649,5650,5651,5652,5653,5654,5655,5656,5657,5658,5659,5660,5661,5662,5663,5664, # 960
-5665,5666,5667,5668,5669,5670,5671,5672,5673,5674,5675,5676,5677,5678,5679,5680, # 976
-5681,5682,5683,5684,5685,5686,5687,5688,5689,5690,5691,5692,5693,5694,5695,5696, # 992
-5697,5698,5699,5700,5701,5702,5703,5704,5705,5706,5707,5708,5709,5710,5711,5712, # 1008
-5713,5714,5715,5716,5717,5718,5719,5720,5721,5722,5723,5724,5725,5726,5727,5728, # 1024
-5729,5730,5731,5732,5733,5734,5735,5736,5737,5738,5739,5740,5741,5742,5743,5744, # 1040
-5745,5746,5747,5748,5749,5750,5751,5752,5753,5754,5755,5756,5757,5758,5759,5760, # 1056
-5761,5762,5763,5764,5765,5766,5767,5768,5769,5770,5771,5772,5773,5774,5775,5776, # 1072
-5777,5778,5779,5780,5781,5782,5783,5784,5785,5786,5787,5788,5789,5790,5791,5792, # 1088
-5793,5794,5795,5796,5797,5798,5799,5800,5801,5802,5803,5804,5805,5806,5807,5808, # 1104
-5809,5810,5811,5812,5813,5814,5815,5816,5817,5818,5819,5820,5821,5822,5823,5824, # 1120
-5825,5826,5827,5828,5829,5830,5831,5832,5833,5834,5835,5836,5837,5838,5839,5840, # 1136
-5841,5842,5843,5844,5845,5846,5847,5848,5849,5850,5851,5852,5853,5854,5855,5856, # 1152
-5857,5858,5859,5860,5861,5862,5863,5864,5865,5866,5867,5868,5869,5870,5871,5872, # 1168
-5873,5874,5875,5876,5877,5878,5879,5880,5881,5882,5883,5884,5885,5886,5887,5888, # 1184
-5889,5890,5891,5892,5893,5894,5895,5896,5897,5898,5899,5900,5901,5902,5903,5904, # 1200
-5905,5906,5907,5908,5909,5910,5911,5912,5913,5914,5915,5916,5917,5918,5919,5920, # 1216
-5921,5922,5923,5924,5925,5926,5927,5928,5929,5930,5931,5932,5933,5934,5935,5936, # 1232
-5937,5938,5939,5940,5941,5942,5943,5944,5945,5946,5947,5948,5949,5950,5951,5952, # 1248
-5953,5954,5955,5956,5957,5958,5959,5960,5961,5962,5963,5964,5965,5966,5967,5968, # 1264
-5969,5970,5971,5972,5973,5974,5975,5976,5977,5978,5979,5980,5981,5982,5983,5984, # 1280
-5985,5986,5987,5988,5989,5990,5991,5992,5993,5994,5995,5996,5997,5998,5999,6000, # 1296
-6001,6002,6003,6004,6005,6006,6007,6008,6009,6010,6011,6012,6013,6014,6015,6016, # 1312
-6017,6018,6019,6020,6021,6022,6023,6024,6025,6026,6027,6028,6029,6030,6031,6032, # 1328
-6033,6034,6035,6036,6037,6038,6039,6040,6041,6042,6043,6044,6045,6046,6047,6048, # 1344
-6049,6050,6051,6052,6053,6054,6055,6056,6057,6058,6059,6060,6061,6062,6063,6064, # 1360
-6065,6066,6067,6068,6069,6070,6071,6072,6073,6074,6075,6076,6077,6078,6079,6080, # 1376
-6081,6082,6083,6084,6085,6086,6087,6088,6089,6090,6091,6092,6093,6094,6095,6096, # 1392
-6097,6098,6099,6100,6101,6102,6103,6104,6105,6106,6107,6108,6109,6110,6111,6112, # 1408
-6113,6114,2044,2060,4621, 997,1235, 473,1186,4622, 920,3378,6115,6116, 379,1108, # 1424
-4313,2657,2735,3934,6117,3809, 636,3233, 573,1026,3693,3435,2974,3300,2298,4105, # 1440
- 854,2937,2463, 393,2581,2417, 539, 752,1280,2750,2480, 140,1161, 440, 708,1569, # 1456
- 665,2497,1746,1291,1523,3000, 164,1603, 847,1331, 537,1997, 486, 508,1693,2418, # 1472
-1970,2227, 878,1220, 299,1030, 969, 652,2751, 624,1137,3301,2619, 65,3302,2045, # 1488
-1761,1859,3120,1930,3694,3516, 663,1767, 852, 835,3695, 269, 767,2826,2339,1305, # 1504
- 896,1150, 770,1616,6118, 506,1502,2075,1012,2519, 775,2520,2975,2340,2938,4314, # 1520
-3028,2086,1224,1943,2286,6119,3072,4315,2240,1273,1987,3935,1557, 175, 597, 985, # 1536
-3517,2419,2521,1416,3029, 585, 938,1931,1007,1052,1932,1685,6120,3379,4316,4623, # 1552
- 804, 599,3121,1333,2128,2539,1159,1554,2032,3810, 687,2033,2904, 952, 675,1467, # 1568
-3436,6121,2241,1096,1786,2440,1543,1924, 980,1813,2228, 781,2692,1879, 728,1918, # 1584
-3696,4624, 548,1950,4625,1809,1088,1356,3303,2522,1944, 502, 972, 373, 513,2827, # 1600
- 586,2377,2391,1003,1976,1631,6122,2464,1084, 648,1776,4626,2141, 324, 962,2012, # 1616
-2177,2076,1384, 742,2178,1448,1173,1810, 222, 102, 301, 445, 125,2420, 662,2498, # 1632
- 277, 200,1476,1165,1068, 224,2562,1378,1446, 450,1880, 659, 791, 582,4627,2939, # 1648
-3936,1516,1274, 555,2099,3697,1020,1389,1526,3380,1762,1723,1787,2229, 412,2114, # 1664
-1900,2392,3518, 512,2597, 427,1925,2341,3122,1653,1686,2465,2499, 697, 330, 273, # 1680
- 380,2162, 951, 832, 780, 991,1301,3073, 965,2270,3519, 668,2523,2636,1286, 535, # 1696
-1407, 518, 671, 957,2658,2378, 267, 611,2197,3030,6123, 248,2299, 967,1799,2356, # 1712
- 850,1418,3437,1876,1256,1480,2828,1718,6124,6125,1755,1664,2405,6126,4628,2879, # 1728
-2829, 499,2179, 676,4629, 557,2329,2214,2090, 325,3234, 464, 811,3001, 992,2342, # 1744
-2481,1232,1469, 303,2242, 466,1070,2163, 603,1777,2091,4630,2752,4631,2714, 322, # 1760
-2659,1964,1768, 481,2188,1463,2330,2857,3600,2092,3031,2421,4632,2318,2070,1849, # 1776
-2598,4633,1302,2254,1668,1701,2422,3811,2905,3032,3123,2046,4106,1763,1694,4634, # 1792
-1604, 943,1724,1454, 917, 868,2215,1169,2940, 552,1145,1800,1228,1823,1955, 316, # 1808
-1080,2510, 361,1807,2830,4107,2660,3381,1346,1423,1134,4108,6127, 541,1263,1229, # 1824
-1148,2540, 545, 465,1833,2880,3438,1901,3074,2482, 816,3937, 713,1788,2500, 122, # 1840
-1575, 195,1451,2501,1111,6128, 859, 374,1225,2243,2483,4317, 390,1033,3439,3075, # 1856
-2524,1687, 266, 793,1440,2599, 946, 779, 802, 507, 897,1081, 528,2189,1292, 711, # 1872
-1866,1725,1167,1640, 753, 398,2661,1053, 246, 348,4318, 137,1024,3440,1600,2077, # 1888
-2129, 825,4319, 698, 238, 521, 187,2300,1157,2423,1641,1605,1464,1610,1097,2541, # 1904
-1260,1436, 759,2255,1814,2150, 705,3235, 409,2563,3304, 561,3033,2005,2564, 726, # 1920
-1956,2343,3698,4109, 949,3812,3813,3520,1669, 653,1379,2525, 881,2198, 632,2256, # 1936
-1027, 778,1074, 733,1957, 514,1481,2466, 554,2180, 702,3938,1606,1017,1398,6129, # 1952
-1380,3521, 921, 993,1313, 594, 449,1489,1617,1166, 768,1426,1360, 495,1794,3601, # 1968
-1177,3602,1170,4320,2344, 476, 425,3167,4635,3168,1424, 401,2662,1171,3382,1998, # 1984
-1089,4110, 477,3169, 474,6130,1909, 596,2831,1842, 494, 693,1051,1028,1207,3076, # 2000
- 606,2115, 727,2790,1473,1115, 743,3522, 630, 805,1532,4321,2021, 366,1057, 838, # 2016
- 684,1114,2142,4322,2050,1492,1892,1808,2271,3814,2424,1971,1447,1373,3305,1090, # 2032
-1536,3939,3523,3306,1455,2199, 336, 369,2331,1035, 584,2393, 902, 718,2600,6131, # 2048
-2753, 463,2151,1149,1611,2467, 715,1308,3124,1268, 343,1413,3236,1517,1347,2663, # 2064
-2093,3940,2022,1131,1553,2100,2941,1427,3441,2942,1323,2484,6132,1980, 872,2368, # 2080
-2441,2943, 320,2369,2116,1082, 679,1933,3941,2791,3815, 625,1143,2023, 422,2200, # 2096
-3816,6133, 730,1695, 356,2257,1626,2301,2858,2637,1627,1778, 937, 883,2906,2693, # 2112
-3002,1769,1086, 400,1063,1325,3307,2792,4111,3077, 456,2345,1046, 747,6134,1524, # 2128
- 884,1094,3383,1474,2164,1059, 974,1688,2181,2258,1047, 345,1665,1187, 358, 875, # 2144
-3170, 305, 660,3524,2190,1334,1135,3171,1540,1649,2542,1527, 927, 968,2793, 885, # 2160
-1972,1850, 482, 500,2638,1218,1109,1085,2543,1654,2034, 876, 78,2287,1482,1277, # 2176
- 861,1675,1083,1779, 724,2754, 454, 397,1132,1612,2332, 893, 672,1237, 257,2259, # 2192
-2370, 135,3384, 337,2244, 547, 352, 340, 709,2485,1400, 788,1138,2511, 540, 772, # 2208
-1682,2260,2272,2544,2013,1843,1902,4636,1999,1562,2288,4637,2201,1403,1533, 407, # 2224
- 576,3308,1254,2071, 978,3385, 170, 136,1201,3125,2664,3172,2394, 213, 912, 873, # 2240
-3603,1713,2202, 699,3604,3699, 813,3442, 493, 531,1054, 468,2907,1483, 304, 281, # 2256
-4112,1726,1252,2094, 339,2319,2130,2639, 756,1563,2944, 748, 571,2976,1588,2425, # 2272
-2715,1851,1460,2426,1528,1392,1973,3237, 288,3309, 685,3386, 296, 892,2716,2216, # 2288
-1570,2245, 722,1747,2217, 905,3238,1103,6135,1893,1441,1965, 251,1805,2371,3700, # 2304
-2601,1919,1078, 75,2182,1509,1592,1270,2640,4638,2152,6136,3310,3817, 524, 706, # 2320
-1075, 292,3818,1756,2602, 317, 98,3173,3605,3525,1844,2218,3819,2502, 814, 567, # 2336
- 385,2908,1534,6137, 534,1642,3239, 797,6138,1670,1529, 953,4323, 188,1071, 538, # 2352
- 178, 729,3240,2109,1226,1374,2000,2357,2977, 731,2468,1116,2014,2051,6139,1261, # 2368
-1593, 803,2859,2736,3443, 556, 682, 823,1541,6140,1369,2289,1706,2794, 845, 462, # 2384
-2603,2665,1361, 387, 162,2358,1740, 739,1770,1720,1304,1401,3241,1049, 627,1571, # 2400
-2427,3526,1877,3942,1852,1500, 431,1910,1503, 677, 297,2795, 286,1433,1038,1198, # 2416
-2290,1133,1596,4113,4639,2469,1510,1484,3943,6141,2442, 108, 712,4640,2372, 866, # 2432
-3701,2755,3242,1348, 834,1945,1408,3527,2395,3243,1811, 824, 994,1179,2110,1548, # 2448
-1453, 790,3003, 690,4324,4325,2832,2909,3820,1860,3821, 225,1748, 310, 346,1780, # 2464
-2470, 821,1993,2717,2796, 828, 877,3528,2860,2471,1702,2165,2910,2486,1789, 453, # 2480
- 359,2291,1676, 73,1164,1461,1127,3311, 421, 604, 314,1037, 589, 116,2487, 737, # 2496
- 837,1180, 111, 244, 735,6142,2261,1861,1362, 986, 523, 418, 581,2666,3822, 103, # 2512
- 855, 503,1414,1867,2488,1091, 657,1597, 979, 605,1316,4641,1021,2443,2078,2001, # 2528
-1209, 96, 587,2166,1032, 260,1072,2153, 173, 94, 226,3244, 819,2006,4642,4114, # 2544
-2203, 231,1744, 782, 97,2667, 786,3387, 887, 391, 442,2219,4326,1425,6143,2694, # 2560
- 633,1544,1202, 483,2015, 592,2052,1958,2472,1655, 419, 129,4327,3444,3312,1714, # 2576
-1257,3078,4328,1518,1098, 865,1310,1019,1885,1512,1734, 469,2444, 148, 773, 436, # 2592
-1815,1868,1128,1055,4329,1245,2756,3445,2154,1934,1039,4643, 579,1238, 932,2320, # 2608
- 353, 205, 801, 115,2428, 944,2321,1881, 399,2565,1211, 678, 766,3944, 335,2101, # 2624
-1459,1781,1402,3945,2737,2131,1010, 844, 981,1326,1013, 550,1816,1545,2620,1335, # 2640
-1008, 371,2881, 936,1419,1613,3529,1456,1395,2273,1834,2604,1317,2738,2503, 416, # 2656
-1643,4330, 806,1126, 229, 591,3946,1314,1981,1576,1837,1666, 347,1790, 977,3313, # 2672
- 764,2861,1853, 688,2429,1920,1462, 77, 595, 415,2002,3034, 798,1192,4115,6144, # 2688
-2978,4331,3035,2695,2582,2072,2566, 430,2430,1727, 842,1396,3947,3702, 613, 377, # 2704
- 278, 236,1417,3388,3314,3174, 757,1869, 107,3530,6145,1194, 623,2262, 207,1253, # 2720
-2167,3446,3948, 492,1117,1935, 536,1838,2757,1246,4332, 696,2095,2406,1393,1572, # 2736
-3175,1782, 583, 190, 253,1390,2230, 830,3126,3389, 934,3245,1703,1749,2979,1870, # 2752
-2545,1656,2204, 869,2346,4116,3176,1817, 496,1764,4644, 942,1504, 404,1903,1122, # 2768
-1580,3606,2945,1022, 515, 372,1735, 955,2431,3036,6146,2797,1110,2302,2798, 617, # 2784
-6147, 441, 762,1771,3447,3607,3608,1904, 840,3037, 86, 939,1385, 572,1370,2445, # 2800
-1336, 114,3703, 898, 294, 203,3315, 703,1583,2274, 429, 961,4333,1854,1951,3390, # 2816
-2373,3704,4334,1318,1381, 966,1911,2322,1006,1155, 309, 989, 458,2718,1795,1372, # 2832
-1203, 252,1689,1363,3177, 517,1936, 168,1490, 562, 193,3823,1042,4117,1835, 551, # 2848
- 470,4645, 395, 489,3448,1871,1465,2583,2641, 417,1493, 279,1295, 511,1236,1119, # 2864
- 72,1231,1982,1812,3004, 871,1564, 984,3449,1667,2696,2096,4646,2347,2833,1673, # 2880
-3609, 695,3246,2668, 807,1183,4647, 890, 388,2333,1801,1457,2911,1765,1477,1031, # 2896
-3316,3317,1278,3391,2799,2292,2526, 163,3450,4335,2669,1404,1802,6148,2323,2407, # 2912
-1584,1728,1494,1824,1269, 298, 909,3318,1034,1632, 375, 776,1683,2061, 291, 210, # 2928
-1123, 809,1249,1002,2642,3038, 206,1011,2132, 144, 975, 882,1565, 342, 667, 754, # 2944
-1442,2143,1299,2303,2062, 447, 626,2205,1221,2739,2912,1144,1214,2206,2584, 760, # 2960
-1715, 614, 950,1281,2670,2621, 810, 577,1287,2546,4648, 242,2168, 250,2643, 691, # 2976
- 123,2644, 647, 313,1029, 689,1357,2946,1650, 216, 771,1339,1306, 808,2063, 549, # 2992
- 913,1371,2913,2914,6149,1466,1092,1174,1196,1311,2605,2396,1783,1796,3079, 406, # 3008
-2671,2117,3949,4649, 487,1825,2220,6150,2915, 448,2348,1073,6151,2397,1707, 130, # 3024
- 900,1598, 329, 176,1959,2527,1620,6152,2275,4336,3319,1983,2191,3705,3610,2155, # 3040
-3706,1912,1513,1614,6153,1988, 646, 392,2304,1589,3320,3039,1826,1239,1352,1340, # 3056
-2916, 505,2567,1709,1437,2408,2547, 906,6154,2672, 384,1458,1594,1100,1329, 710, # 3072
- 423,3531,2064,2231,2622,1989,2673,1087,1882, 333, 841,3005,1296,2882,2379, 580, # 3088
-1937,1827,1293,2585, 601, 574, 249,1772,4118,2079,1120, 645, 901,1176,1690, 795, # 3104
-2207, 478,1434, 516,1190,1530, 761,2080, 930,1264, 355, 435,1552, 644,1791, 987, # 3120
- 220,1364,1163,1121,1538, 306,2169,1327,1222, 546,2645, 218, 241, 610,1704,3321, # 3136
-1984,1839,1966,2528, 451,6155,2586,3707,2568, 907,3178, 254,2947, 186,1845,4650, # 3152
- 745, 432,1757, 428,1633, 888,2246,2221,2489,3611,2118,1258,1265, 956,3127,1784, # 3168
-4337,2490, 319, 510, 119, 457,3612, 274,2035,2007,4651,1409,3128, 970,2758, 590, # 3184
-2800, 661,2247,4652,2008,3950,1420,1549,3080,3322,3951,1651,1375,2111, 485,2491, # 3200
-1429,1156,6156,2548,2183,1495, 831,1840,2529,2446, 501,1657, 307,1894,3247,1341, # 3216
- 666, 899,2156,1539,2549,1559, 886, 349,2208,3081,2305,1736,3824,2170,2759,1014, # 3232
-1913,1386, 542,1397,2948, 490, 368, 716, 362, 159, 282,2569,1129,1658,1288,1750, # 3248
-2674, 276, 649,2016, 751,1496, 658,1818,1284,1862,2209,2087,2512,3451, 622,2834, # 3264
- 376, 117,1060,2053,1208,1721,1101,1443, 247,1250,3179,1792,3952,2760,2398,3953, # 3280
-6157,2144,3708, 446,2432,1151,2570,3452,2447,2761,2835,1210,2448,3082, 424,2222, # 3296
-1251,2449,2119,2836, 504,1581,4338, 602, 817, 857,3825,2349,2306, 357,3826,1470, # 3312
-1883,2883, 255, 958, 929,2917,3248, 302,4653,1050,1271,1751,2307,1952,1430,2697, # 3328
-2719,2359, 354,3180, 777, 158,2036,4339,1659,4340,4654,2308,2949,2248,1146,2232, # 3344
-3532,2720,1696,2623,3827,6158,3129,1550,2698,1485,1297,1428, 637, 931,2721,2145, # 3360
- 914,2550,2587, 81,2450, 612, 827,2646,1242,4655,1118,2884, 472,1855,3181,3533, # 3376
-3534, 569,1353,2699,1244,1758,2588,4119,2009,2762,2171,3709,1312,1531,6159,1152, # 3392
-1938, 134,1830, 471,3710,2276,1112,1535,3323,3453,3535, 982,1337,2950, 488, 826, # 3408
- 674,1058,1628,4120,2017, 522,2399, 211, 568,1367,3454, 350, 293,1872,1139,3249, # 3424
-1399,1946,3006,1300,2360,3324, 588, 736,6160,2606, 744, 669,3536,3828,6161,1358, # 3440
- 199, 723, 848, 933, 851,1939,1505,1514,1338,1618,1831,4656,1634,3613, 443,2740, # 3456
-3829, 717,1947, 491,1914,6162,2551,1542,4121,1025,6163,1099,1223, 198,3040,2722, # 3472
- 370, 410,1905,2589, 998,1248,3182,2380, 519,1449,4122,1710, 947, 928,1153,4341, # 3488
-2277, 344,2624,1511, 615, 105, 161,1212,1076,1960,3130,2054,1926,1175,1906,2473, # 3504
- 414,1873,2801,6164,2309, 315,1319,3325, 318,2018,2146,2157, 963, 631, 223,4342, # 3520
-4343,2675, 479,3711,1197,2625,3712,2676,2361,6165,4344,4123,6166,2451,3183,1886, # 3536
-2184,1674,1330,1711,1635,1506, 799, 219,3250,3083,3954,1677,3713,3326,2081,3614, # 3552
-1652,2073,4657,1147,3041,1752, 643,1961, 147,1974,3955,6167,1716,2037, 918,3007, # 3568
-1994, 120,1537, 118, 609,3184,4345, 740,3455,1219, 332,1615,3830,6168,1621,2980, # 3584
-1582, 783, 212, 553,2350,3714,1349,2433,2082,4124, 889,6169,2310,1275,1410, 973, # 3600
- 166,1320,3456,1797,1215,3185,2885,1846,2590,2763,4658, 629, 822,3008, 763, 940, # 3616
-1990,2862, 439,2409,1566,1240,1622, 926,1282,1907,2764, 654,2210,1607, 327,1130, # 3632
-3956,1678,1623,6170,2434,2192, 686, 608,3831,3715, 903,3957,3042,6171,2741,1522, # 3648
-1915,1105,1555,2552,1359, 323,3251,4346,3457, 738,1354,2553,2311,2334,1828,2003, # 3664
-3832,1753,2351,1227,6172,1887,4125,1478,6173,2410,1874,1712,1847, 520,1204,2607, # 3680
- 264,4659, 836,2677,2102, 600,4660,3833,2278,3084,6174,4347,3615,1342, 640, 532, # 3696
- 543,2608,1888,2400,2591,1009,4348,1497, 341,1737,3616,2723,1394, 529,3252,1321, # 3712
- 983,4661,1515,2120, 971,2592, 924, 287,1662,3186,4349,2700,4350,1519, 908,1948, # 3728
-2452, 156, 796,1629,1486,2223,2055, 694,4126,1259,1036,3392,1213,2249,2742,1889, # 3744
-1230,3958,1015, 910, 408, 559,3617,4662, 746, 725, 935,4663,3959,3009,1289, 563, # 3760
- 867,4664,3960,1567,2981,2038,2626, 988,2263,2381,4351, 143,2374, 704,1895,6175, # 3776
-1188,3716,2088, 673,3085,2362,4352, 484,1608,1921,2765,2918, 215, 904,3618,3537, # 3792
- 894, 509, 976,3043,2701,3961,4353,2837,2982, 498,6176,6177,1102,3538,1332,3393, # 3808
-1487,1636,1637, 233, 245,3962, 383, 650, 995,3044, 460,1520,1206,2352, 749,3327, # 3824
- 530, 700, 389,1438,1560,1773,3963,2264, 719,2951,2724,3834, 870,1832,1644,1000, # 3840
- 839,2474,3717, 197,1630,3394, 365,2886,3964,1285,2133, 734, 922, 818,1106, 732, # 3856
- 480,2083,1774,3458, 923,2279,1350, 221,3086, 85,2233,2234,3835,1585,3010,2147, # 3872
-1387,1705,2382,1619,2475, 133, 239,2802,1991,1016,2084,2383, 411,2838,1113, 651, # 3888
-1985,1160,3328, 990,1863,3087,1048,1276,2647, 265,2627,1599,3253,2056, 150, 638, # 3904
-2019, 656, 853, 326,1479, 680,1439,4354,1001,1759, 413,3459,3395,2492,1431, 459, # 3920
-4355,1125,3329,2265,1953,1450,2065,2863, 849, 351,2678,3131,3254,3255,1104,1577, # 3936
- 227,1351,1645,2453,2193,1421,2887, 812,2121, 634, 95,2435, 201,2312,4665,1646, # 3952
-1671,2743,1601,2554,2702,2648,2280,1315,1366,2089,3132,1573,3718,3965,1729,1189, # 3968
- 328,2679,1077,1940,1136, 558,1283, 964,1195, 621,2074,1199,1743,3460,3619,1896, # 3984
-1916,1890,3836,2952,1154,2112,1064, 862, 378,3011,2066,2113,2803,1568,2839,6178, # 4000
-3088,2919,1941,1660,2004,1992,2194, 142, 707,1590,1708,1624,1922,1023,1836,1233, # 4016
-1004,2313, 789, 741,3620,6179,1609,2411,1200,4127,3719,3720,4666,2057,3721, 593, # 4032
-2840, 367,2920,1878,6180,3461,1521, 628,1168, 692,2211,2649, 300, 720,2067,2571, # 4048
-2953,3396, 959,2504,3966,3539,3462,1977, 701,6181, 954,1043, 800, 681, 183,3722, # 4064
-1803,1730,3540,4128,2103, 815,2314, 174, 467, 230,2454,1093,2134, 755,3541,3397, # 4080
-1141,1162,6182,1738,2039, 270,3256,2513,1005,1647,2185,3837, 858,1679,1897,1719, # 4096
-2954,2324,1806, 402, 670, 167,4129,1498,2158,2104, 750,6183, 915, 189,1680,1551, # 4112
- 455,4356,1501,2455, 405,1095,2955, 338,1586,1266,1819, 570, 641,1324, 237,1556, # 4128
-2650,1388,3723,6184,1368,2384,1343,1978,3089,2436, 879,3724, 792,1191, 758,3012, # 4144
-1411,2135,1322,4357, 240,4667,1848,3725,1574,6185, 420,3045,1546,1391, 714,4358, # 4160
-1967, 941,1864, 863, 664, 426, 560,1731,2680,1785,2864,1949,2363, 403,3330,1415, # 4176
-1279,2136,1697,2335, 204, 721,2097,3838, 90,6186,2085,2505, 191,3967, 124,2148, # 4192
-1376,1798,1178,1107,1898,1405, 860,4359,1243,1272,2375,2983,1558,2456,1638, 113, # 4208
-3621, 578,1923,2609, 880, 386,4130, 784,2186,2266,1422,2956,2172,1722, 497, 263, # 4224
-2514,1267,2412,2610, 177,2703,3542, 774,1927,1344, 616,1432,1595,1018, 172,4360, # 4240
-2325, 911,4361, 438,1468,3622, 794,3968,2024,2173,1681,1829,2957, 945, 895,3090, # 4256
- 575,2212,2476, 475,2401,2681, 785,2744,1745,2293,2555,1975,3133,2865, 394,4668, # 4272
-3839, 635,4131, 639, 202,1507,2195,2766,1345,1435,2572,3726,1908,1184,1181,2457, # 4288
-3727,3134,4362, 843,2611, 437, 916,4669, 234, 769,1884,3046,3047,3623, 833,6187, # 4304
-1639,2250,2402,1355,1185,2010,2047, 999, 525,1732,1290,1488,2612, 948,1578,3728, # 4320
-2413,2477,1216,2725,2159, 334,3840,1328,3624,2921,1525,4132, 564,1056, 891,4363, # 4336
-1444,1698,2385,2251,3729,1365,2281,2235,1717,6188, 864,3841,2515, 444, 527,2767, # 4352
-2922,3625, 544, 461,6189, 566, 209,2437,3398,2098,1065,2068,3331,3626,3257,2137, # 4368 #last 512
-)
-# fmt: on
diff --git a/spaces/Realcat/image-matching-webui/third_party/lanet/network_v0/model.py b/spaces/Realcat/image-matching-webui/third_party/lanet/network_v0/model.py
deleted file mode 100644
index 6f22e015449dd7bcc8e060a2cd72a794befd2ccb..0000000000000000000000000000000000000000
--- a/spaces/Realcat/image-matching-webui/third_party/lanet/network_v0/model.py
+++ /dev/null
@@ -1,181 +0,0 @@
-import torch
-import torch.nn as nn
-import torchvision.transforms as tvf
-
-from .modules import InterestPointModule, CorrespondenceModule
-
-
-def warp_homography_batch(sources, homographies):
- """
- Batch warp keypoints given homographies. From https://github.com/TRI-ML/KP2D.
-
- Parameters
- ----------
- sources: torch.Tensor (B,H,W,C)
- Keypoints vector.
- homographies: torch.Tensor (B,3,3)
- Homographies.
-
- Returns
- -------
- warped_sources: torch.Tensor (B,H,W,C)
- Warped keypoints vector.
- """
- B, H, W, _ = sources.shape
- warped_sources = []
- for b in range(B):
- source = sources[b].clone()
- source = source.view(-1, 2)
- """
- [X, [M11, M12, M13 [x, M11*x + M12*y + M13 [M11, M12 [M13,
- Y, = M21, M22, M23 * y, = M21*x + M22*y + M23 = [x, y] * M21, M22 + M23,
- Z] M31, M32, M33] 1] M31*x + M32*y + M33 M31, M32].T M33]
- """
- source = torch.addmm(homographies[b, :, 2], source, homographies[b, :, :2].t())
- source.mul_(1 / source[:, 2].unsqueeze(1))
- source = source[:, :2].contiguous().view(H, W, 2)
- warped_sources.append(source)
- return torch.stack(warped_sources, dim=0)
-
-
-class PointModel(nn.Module):
- def __init__(self, is_test=True):
- super(PointModel, self).__init__()
- self.is_test = is_test
- self.interestpoint_module = InterestPointModule(is_test=self.is_test)
- self.correspondence_module = CorrespondenceModule()
- self.norm_rgb = tvf.Normalize(mean=[0.5, 0.5, 0.5], std=[0.225, 0.225, 0.225])
-
- def forward(self, *args):
- if self.is_test:
- img = args[0]
- img = self.norm_rgb(img)
- score, coord, desc = self.interestpoint_module(img)
- return score, coord, desc
- else:
- source_score, source_coord, source_desc_block = self.interestpoint_module(
- args[0]
- )
- target_score, target_coord, target_desc_block = self.interestpoint_module(
- args[1]
- )
-
- B, _, H, W = args[0].shape
- B, _, hc, wc = source_score.shape
- device = source_score.device
-
- # Normalize the coordinates from ([0, h], [0, w]) to ([0, 1], [0, 1]).
- source_coord_norm = source_coord.clone()
- source_coord_norm[:, 0] = (
- source_coord_norm[:, 0] / (float(W - 1) / 2.0)
- ) - 1.0
- source_coord_norm[:, 1] = (
- source_coord_norm[:, 1] / (float(H - 1) / 2.0)
- ) - 1.0
- source_coord_norm = source_coord_norm.permute(0, 2, 3, 1)
-
- target_coord_norm = target_coord.clone()
- target_coord_norm[:, 0] = (
- target_coord_norm[:, 0] / (float(W - 1) / 2.0)
- ) - 1.0
- target_coord_norm[:, 1] = (
- target_coord_norm[:, 1] / (float(H - 1) / 2.0)
- ) - 1.0
- target_coord_norm = target_coord_norm.permute(0, 2, 3, 1)
-
- target_coord_warped_norm = warp_homography_batch(source_coord_norm, args[2])
- target_coord_warped = target_coord_warped_norm.clone()
-
- # de-normlize the coordinates
- target_coord_warped[:, :, :, 0] = (target_coord_warped[:, :, :, 0] + 1) * (
- float(W - 1) / 2.0
- )
- target_coord_warped[:, :, :, 1] = (target_coord_warped[:, :, :, 1] + 1) * (
- float(H - 1) / 2.0
- )
- target_coord_warped = target_coord_warped.permute(0, 3, 1, 2)
-
- # Border mask
- border_mask_ori = torch.ones(B, hc, wc)
- border_mask_ori[:, 0] = 0
- border_mask_ori[:, hc - 1] = 0
- border_mask_ori[:, :, 0] = 0
- border_mask_ori[:, :, wc - 1] = 0
- border_mask_ori = border_mask_ori.gt(1e-3).to(device)
-
- oob_mask2 = (
- target_coord_warped_norm[:, :, :, 0].lt(1)
- & target_coord_warped_norm[:, :, :, 0].gt(-1)
- & target_coord_warped_norm[:, :, :, 1].lt(1)
- & target_coord_warped_norm[:, :, :, 1].gt(-1)
- )
- border_mask = border_mask_ori & oob_mask2
-
- # score
- target_score_warped = torch.nn.functional.grid_sample(
- target_score, target_coord_warped_norm.detach(), align_corners=False
- )
-
- # descriptor
- source_desc2 = torch.nn.functional.grid_sample(
- source_desc_block[0], source_coord_norm.detach()
- )
- source_desc3 = torch.nn.functional.grid_sample(
- source_desc_block[1], source_coord_norm.detach()
- )
- source_aware = source_desc_block[2]
- source_desc = torch.mul(
- source_desc2, source_aware[:, 0, :, :].unsqueeze(1).contiguous()
- ) + torch.mul(
- source_desc3, source_aware[:, 1, :, :].unsqueeze(1).contiguous()
- )
-
- target_desc2 = torch.nn.functional.grid_sample(
- target_desc_block[0], target_coord_norm.detach()
- )
- target_desc3 = torch.nn.functional.grid_sample(
- target_desc_block[1], target_coord_norm.detach()
- )
- target_aware = target_desc_block[2]
- target_desc = torch.mul(
- target_desc2, target_aware[:, 0, :, :].unsqueeze(1).contiguous()
- ) + torch.mul(
- target_desc3, target_aware[:, 1, :, :].unsqueeze(1).contiguous()
- )
-
- target_desc2_warped = torch.nn.functional.grid_sample(
- target_desc_block[0], target_coord_warped_norm.detach()
- )
- target_desc3_warped = torch.nn.functional.grid_sample(
- target_desc_block[1], target_coord_warped_norm.detach()
- )
- target_aware_warped = torch.nn.functional.grid_sample(
- target_desc_block[2], target_coord_warped_norm.detach()
- )
- target_desc_warped = torch.mul(
- target_desc2_warped,
- target_aware_warped[:, 0, :, :].unsqueeze(1).contiguous(),
- ) + torch.mul(
- target_desc3_warped,
- target_aware_warped[:, 1, :, :].unsqueeze(1).contiguous(),
- )
-
- confidence_matrix = self.correspondence_module(source_desc, target_desc)
- confidence_matrix = torch.clamp(confidence_matrix, 1e-12, 1 - 1e-12)
-
- output = {
- "source_score": source_score,
- "source_coord": source_coord,
- "source_desc": source_desc,
- "source_aware": source_aware,
- "target_score": target_score,
- "target_coord": target_coord,
- "target_score_warped": target_score_warped,
- "target_coord_warped": target_coord_warped,
- "target_desc_warped": target_desc_warped,
- "target_aware_warped": target_aware_warped,
- "border_mask": border_mask,
- "confidence_matrix": confidence_matrix,
- }
-
- return output
diff --git a/spaces/Redgon/bingo/src/lib/bots/bing/index.ts b/spaces/Redgon/bingo/src/lib/bots/bing/index.ts
deleted file mode 100644
index 6fd51ba48cbb1148f13d29e76960c092b807cfae..0000000000000000000000000000000000000000
--- a/spaces/Redgon/bingo/src/lib/bots/bing/index.ts
+++ /dev/null
@@ -1,426 +0,0 @@
-import { fetch, WebSocket, debug } from '@/lib/isomorphic'
-import WebSocketAsPromised from 'websocket-as-promised'
-import {
- SendMessageParams,
- BingConversationStyle,
- ConversationResponse,
- ChatResponseMessage,
- ConversationInfo,
- InvocationEventType,
- ChatError,
- ErrorCode,
- ChatUpdateCompleteResponse,
- ImageInfo,
- KBlobResponse
-} from './types'
-
-import { convertMessageToMarkdown, websocketUtils, streamAsyncIterable } from './utils'
-import { WatchDog, createChunkDecoder } from '@/lib/utils'
-
-type Params = SendMessageParams<{ bingConversationStyle: BingConversationStyle }>
-
-const OPTIONS_SETS = [
- 'nlu_direct_response_filter',
- 'deepleo',
- 'disable_emoji_spoken_text',
- 'responsible_ai_policy_235',
- 'enablemm',
- 'iycapbing',
- 'iyxapbing',
- 'objopinion',
- 'rweasgv2',
- 'dagslnv1',
- 'dv3sugg',
- 'autosave',
- 'iyoloxap',
- 'iyoloneutral',
- 'clgalileo',
- 'gencontentv3',
-]
-
-export class BingWebBot {
- protected conversationContext?: ConversationInfo
- protected cookie: string
- protected ua: string
- protected endpoint = ''
- private lastText = ''
- private asyncTasks: Array> = []
-
- constructor(opts: {
- cookie: string
- ua: string
- bingConversationStyle?: BingConversationStyle
- conversationContext?: ConversationInfo
- }) {
- const { cookie, ua, conversationContext } = opts
- this.cookie = cookie?.includes(';') ? cookie : `_EDGE_V=1; _U=${cookie}`
- this.ua = ua
- this.conversationContext = conversationContext
- }
-
- static buildChatRequest(conversation: ConversationInfo) {
- const optionsSets = OPTIONS_SETS
- if (conversation.conversationStyle === BingConversationStyle.Precise) {
- optionsSets.push('h3precise')
- } else if (conversation.conversationStyle === BingConversationStyle.Creative) {
- optionsSets.push('h3imaginative')
- }
- return {
- arguments: [
- {
- source: 'cib',
- optionsSets,
- allowedMessageTypes: [
- 'ActionRequest',
- 'Chat',
- 'Context',
- 'InternalSearchQuery',
- 'InternalSearchResult',
- 'Disengaged',
- 'InternalLoaderMessage',
- 'Progress',
- 'RenderCardRequest',
- 'SemanticSerp',
- 'GenerateContentQuery',
- 'SearchQuery',
- ],
- sliceIds: [
- 'winmuid1tf',
- 'anssupfor_c',
- 'imgchatgptv2',
- 'tts2cf',
- 'contansperf',
- 'mlchatpc8500w',
- 'mlchatpc2',
- 'ctrlworkpay',
- 'winshortmsgtf',
- 'cibctrl',
- 'sydtransctrl',
- 'sydconfigoptc',
- '0705trt4',
- '517opinion',
- '628ajcopus0',
- '330uaugs0',
- '529rwea',
- '0626snptrcs0',
- '424dagslnv1',
- ],
- isStartOfSession: conversation.invocationId === 0,
- message: {
- author: 'user',
- inputMethod: 'Keyboard',
- text: conversation.prompt,
- imageUrl: conversation.imageUrl,
- messageType: 'Chat',
- },
- conversationId: conversation.conversationId,
- conversationSignature: conversation.conversationSignature,
- participant: { id: conversation.clientId },
- },
- ],
- invocationId: conversation.invocationId.toString(),
- target: 'chat',
- type: InvocationEventType.StreamInvocation,
- }
- }
-
- async createConversation(): Promise {
- const headers = {
- 'Accept-Encoding': 'gzip, deflate, br, zsdch',
- 'User-Agent': this.ua,
- 'x-ms-useragent': 'azsdk-js-api-client-factory/1.0.0-beta.1 core-rest-pipeline/1.10.0 OS/Win32',
- cookie: this.cookie,
- }
-
- let resp: ConversationResponse | undefined
- try {
- const response = await fetch(this.endpoint + '/api/create', { method: 'POST', headers, redirect: 'error', mode: 'cors', credentials: 'include' })
- if (response.status === 404) {
- throw new ChatError('Not Found', ErrorCode.NOTFOUND_ERROR)
- }
- resp = await response.json() as ConversationResponse
- } catch (err) {
- console.error('create conversation error', err)
- }
-
- if (!resp?.result) {
- throw new ChatError('你的 VPS 或代理可能被封禁,如有疑问,请前往 https://github.com/weaigc/bingo 咨询', ErrorCode.UNKOWN_ERROR)
- }
-
- const { value, message } = resp.result || {}
- if (value !== 'Success') {
- const errorMsg = `${value}: ${message}`
- if (value === 'UnauthorizedRequest') {
- throw new ChatError(errorMsg, ErrorCode.BING_UNAUTHORIZED)
- }
- if (value === 'Forbidden') {
- throw new ChatError(errorMsg, ErrorCode.BING_FORBIDDEN)
- }
- throw new ChatError(errorMsg, ErrorCode.UNKOWN_ERROR)
- }
- return resp
- }
-
- private async createContext(conversationStyle: BingConversationStyle) {
- if (!this.conversationContext) {
- const conversation = await this.createConversation()
- this.conversationContext = {
- conversationId: conversation.conversationId,
- conversationSignature: conversation.conversationSignature,
- clientId: conversation.clientId,
- invocationId: 0,
- conversationStyle,
- prompt: '',
- }
- }
- return this.conversationContext
- }
-
- async sendMessage(params: Params) {
- try {
- await this.createContext(params.options.bingConversationStyle)
- Object.assign(this.conversationContext!, { prompt: params.prompt, imageUrl: params.imageUrl })
- return this.sydneyProxy(params)
- } catch (error) {
- params.onEvent({
- type: 'ERROR',
- error: error instanceof ChatError ? error : new ChatError('Catch Error', ErrorCode.UNKOWN_ERROR),
- })
- }
- }
-
- private async sydneyProxy(params: Params) {
- const abortController = new AbortController()
- const response = await fetch(this.endpoint + '/api/sydney', {
- method: 'POST',
- headers: {
- 'Content-Type': 'application/json',
- },
- signal: abortController.signal,
- body: JSON.stringify(this.conversationContext!)
- })
- if (response.status !== 200) {
- params.onEvent({
- type: 'ERROR',
- error: new ChatError(
- 'Unknown error',
- ErrorCode.UNKOWN_ERROR,
- ),
- })
- }
- params.signal?.addEventListener('abort', () => {
- abortController.abort()
- })
-
- const textDecoder = createChunkDecoder()
- for await (const chunk of streamAsyncIterable(response.body!)) {
- this.parseEvents(params, websocketUtils.unpackMessage(textDecoder(chunk)))
- }
- }
-
- async sendWs() {
- const wsConfig: ConstructorParameters[1] = {
- packMessage: websocketUtils.packMessage,
- unpackMessage: websocketUtils.unpackMessage,
- createWebSocket: (url) => new WebSocket(url, {
- headers: {
- 'accept-language': 'zh-CN,zh;q=0.9',
- 'cache-control': 'no-cache',
- 'User-Agent': this.ua,
- pragma: 'no-cache',
- cookie: this.cookie,
- }
- })
- }
- const wsp = new WebSocketAsPromised('wss://sydney.bing.com/sydney/ChatHub', wsConfig)
-
- wsp.open().then(() => {
- wsp.sendPacked({ protocol: 'json', version: 1 })
- wsp.sendPacked({ type: 6 })
- wsp.sendPacked(BingWebBot.buildChatRequest(this.conversationContext!))
- })
-
- return wsp
- }
-
- private async useWs(params: Params) {
- const wsp = await this.sendWs()
- const watchDog = new WatchDog()
- wsp.onUnpackedMessage.addListener((events) => {
- watchDog.watch(() => {
- wsp.sendPacked({ type: 6 })
- })
- this.parseEvents(params, events)
- })
-
- wsp.onClose.addListener(() => {
- watchDog.reset()
- params.onEvent({ type: 'DONE' })
- wsp.removeAllListeners()
- })
-
- params.signal?.addEventListener('abort', () => {
- wsp.removeAllListeners()
- wsp.close()
- })
- }
-
- private async createImage(prompt: string, id: string) {
- try {
- const headers = {
- 'Accept-Encoding': 'gzip, deflate, br, zsdch',
- 'User-Agent': this.ua,
- 'x-ms-useragent': 'azsdk-js-api-client-factory/1.0.0-beta.1 core-rest-pipeline/1.10.0 OS/Win32',
- cookie: this.cookie,
- }
- const query = new URLSearchParams({
- prompt,
- id
- })
- const response = await fetch(this.endpoint + '/api/image?' + query.toString(),
- {
- method: 'POST',
- headers,
- mode: 'cors',
- credentials: 'include'
- })
- .then(res => res.text())
- if (response) {
- this.lastText += '\n' + response
- }
- } catch (err) {
- console.error('Create Image Error', err)
- }
- }
-
- private buildKnowledgeApiPayload(imageUrl: string, conversationStyle: BingConversationStyle) {
- const imageInfo: ImageInfo = {}
- let imageBase64: string | undefined = undefined
- const knowledgeRequest = {
- imageInfo,
- knowledgeRequest: {
- invokedSkills: [
- 'ImageById'
- ],
- subscriptionId: 'Bing.Chat.Multimodal',
- invokedSkillsRequestData: {
- enableFaceBlur: true
- },
- convoData: {
- convoid: this.conversationContext?.conversationId,
- convotone: conversationStyle,
- }
- },
- }
-
- if (imageUrl.startsWith('data:image/')) {
- imageBase64 = imageUrl.replace('data:image/', '');
- const partIndex = imageBase64.indexOf(',')
- if (partIndex) {
- imageBase64 = imageBase64.substring(partIndex + 1)
- }
- } else {
- imageInfo.url = imageUrl
- }
- return { knowledgeRequest, imageBase64 }
- }
-
- async uploadImage(imageUrl: string, conversationStyle: BingConversationStyle = BingConversationStyle.Creative): Promise {
- if (!imageUrl) {
- return
- }
- await this.createContext(conversationStyle)
- const payload = this.buildKnowledgeApiPayload(imageUrl, conversationStyle)
-
- const response = await fetch(this.endpoint + '/api/kblob',
- {
- headers: {
- 'Content-Type': 'application/json',
- },
- method: 'POST',
- mode: 'cors',
- credentials: 'include',
- body: JSON.stringify(payload),
- })
- .then(res => res.json())
- .catch(e => {
- console.log('Error', e)
- })
- return response
- }
-
- private async generateContent(message: ChatResponseMessage) {
- if (message.contentType === 'IMAGE') {
- this.asyncTasks.push(this.createImage(message.text, message.messageId))
- }
- }
-
- private async parseEvents(params: Params, events: any) {
- const conversation = this.conversationContext!
-
- events?.forEach(async (event: ChatUpdateCompleteResponse) => {
- debug('bing event', event)
- if (event.type === 3) {
- await Promise.all(this.asyncTasks)
- this.asyncTasks = []
- params.onEvent({ type: 'UPDATE_ANSWER', data: { text: this.lastText } })
- params.onEvent({ type: 'DONE' })
- conversation.invocationId = parseInt(event.invocationId, 10) + 1
- } else if (event.type === 1) {
- const messages = event.arguments[0].messages
- if (messages) {
- const text = convertMessageToMarkdown(messages[0])
- this.lastText = text
- params.onEvent({ type: 'UPDATE_ANSWER', data: { text, spokenText: messages[0].text, throttling: event.arguments[0].throttling } })
- }
- } else if (event.type === 2) {
- const messages = event.item.messages as ChatResponseMessage[] | undefined
- if (!messages) {
- params.onEvent({
- type: 'ERROR',
- error: new ChatError(
- event.item.result.error || 'Unknown error',
- event.item.result.value === 'Throttled' ? ErrorCode.THROTTLE_LIMIT
- : event.item.result.value === 'CaptchaChallenge' ? (this.conversationContext?.conversationId?.includes('BingProdUnAuthenticatedUsers') ? ErrorCode.BING_UNAUTHORIZED : ErrorCode.BING_CAPTCHA)
- : ErrorCode.UNKOWN_ERROR
- ),
- })
- return
- }
- const limited = messages.some((message) =>
- message.contentOrigin === 'TurnLimiter'
- || message.messageType === 'Disengaged'
- )
- if (limited) {
- params.onEvent({
- type: 'ERROR',
- error: new ChatError(
- 'Sorry, you have reached chat limit in this conversation.',
- ErrorCode.CONVERSATION_LIMIT,
- ),
- })
- return
- }
-
- const lastMessage = event.item.messages.at(-1) as ChatResponseMessage
- const specialMessage = event.item.messages.find(message => message.author === 'bot' && message.contentType === 'IMAGE')
- if (specialMessage) {
- this.generateContent(specialMessage)
- }
-
- if (lastMessage) {
- const text = convertMessageToMarkdown(lastMessage)
- this.lastText = text
- params.onEvent({
- type: 'UPDATE_ANSWER',
- data: { text, throttling: event.item.throttling, suggestedResponses: lastMessage.suggestedResponses, sourceAttributions: lastMessage.sourceAttributions },
- })
- }
- }
- })
- }
-
- resetConversation() {
- this.conversationContext = undefined
- }
-}
diff --git a/spaces/Ricecake123/RVC-demo/my_utils.py b/spaces/Ricecake123/RVC-demo/my_utils.py
deleted file mode 100644
index a5258394b8ae5385daa665ab6ba6380507d4798a..0000000000000000000000000000000000000000
--- a/spaces/Ricecake123/RVC-demo/my_utils.py
+++ /dev/null
@@ -1,21 +0,0 @@
-import ffmpeg
-import numpy as np
-
-
-def load_audio(file, sr):
- try:
- # https://github.com/openai/whisper/blob/main/whisper/audio.py#L26
- # This launches a subprocess to decode audio while down-mixing and resampling as necessary.
- # Requires the ffmpeg CLI and `ffmpeg-python` package to be installed.
- file = (
- file.strip(" ").strip('"').strip("\n").strip('"').strip(" ")
- ) # 防止小白拷路径头尾带了空格和"和回车
- out, _ = (
- ffmpeg.input(file, threads=0)
- .output("-", format="f32le", acodec="pcm_f32le", ac=1, ar=sr)
- .run(cmd=["ffmpeg", "-nostdin"], capture_stdout=True, capture_stderr=True)
- )
- except Exception as e:
- raise RuntimeError(f"Failed to load audio: {e}")
-
- return np.frombuffer(out, np.float32).flatten()
diff --git a/spaces/Robert001/UniControl-Demo/annotator/uniformer_base/mmcv/cnn/bricks/swish.py b/spaces/Robert001/UniControl-Demo/annotator/uniformer_base/mmcv/cnn/bricks/swish.py
deleted file mode 100644
index e2ca8ed7b749413f011ae54aac0cab27e6f0b51f..0000000000000000000000000000000000000000
--- a/spaces/Robert001/UniControl-Demo/annotator/uniformer_base/mmcv/cnn/bricks/swish.py
+++ /dev/null
@@ -1,25 +0,0 @@
-# Copyright (c) OpenMMLab. All rights reserved.
-import torch
-import torch.nn as nn
-
-from .registry import ACTIVATION_LAYERS
-
-
-@ACTIVATION_LAYERS.register_module()
-class Swish(nn.Module):
- """Swish Module.
-
- This module applies the swish function:
-
- .. math::
- Swish(x) = x * Sigmoid(x)
-
- Returns:
- Tensor: The output tensor.
- """
-
- def __init__(self):
- super(Swish, self).__init__()
-
- def forward(self, x):
- return x * torch.sigmoid(x)
diff --git a/spaces/Robert001/UniControl-Demo/annotator/uniformer_base/mmcv/fileio/handlers/__init__.py b/spaces/Robert001/UniControl-Demo/annotator/uniformer_base/mmcv/fileio/handlers/__init__.py
deleted file mode 100644
index aa24d91972837b8756b225f4879bac20436eb72a..0000000000000000000000000000000000000000
--- a/spaces/Robert001/UniControl-Demo/annotator/uniformer_base/mmcv/fileio/handlers/__init__.py
+++ /dev/null
@@ -1,7 +0,0 @@
-# Copyright (c) OpenMMLab. All rights reserved.
-from .base import BaseFileHandler
-from .json_handler import JsonHandler
-from .pickle_handler import PickleHandler
-from .yaml_handler import YamlHandler
-
-__all__ = ['BaseFileHandler', 'JsonHandler', 'PickleHandler', 'YamlHandler']
diff --git a/spaces/RoyKwok/Gradio/README.md b/spaces/RoyKwok/Gradio/README.md
deleted file mode 100644
index eec7740fe6d4ef7e963bc0b551da03bdc4c76c34..0000000000000000000000000000000000000000
--- a/spaces/RoyKwok/Gradio/README.md
+++ /dev/null
@@ -1,13 +0,0 @@
----
-title: Gradio
-emoji: 📚
-colorFrom: indigo
-colorTo: gray
-sdk: gradio
-sdk_version: 3.34.0
-app_file: app.py
-pinned: false
-license: apache-2.0
----
-
-Check out the configuration reference at https://huggingface.co/docs/hub/spaces-config-reference
diff --git a/spaces/RoyKwok/Gradio/app.py b/spaces/RoyKwok/Gradio/app.py
deleted file mode 100644
index 84265dfb71be23b037087f22d2917f1ccc0f9399..0000000000000000000000000000000000000000
--- a/spaces/RoyKwok/Gradio/app.py
+++ /dev/null
@@ -1,36 +0,0 @@
-import torch
-import gradio as gr
-# from ultralytics import YOLO
-import os
-
-os.system("git clone https://github.com/ultralytics/yolov5")
-os.system("mv ./yolov5/* ./")
-# print(os.getcwd())
-path = "power_grid_s.pt"
-model = torch.hub.load("./", "custom", path=path, source="local")
-# model = torch.load("./power_grid_s.pt")
-# model = YOLO("power_grid_s.pt")
-
-title = "使用Yolov5的输电隐患的目标检测"
-desc = "前端是基于Gradio的web前端;目标检测使用的是yolov5;\
- 图像来自网络,训练集有800张图片,训练了300个epoch;\
- 使用了较大的数据增强,最终mAP50达到0.95+,mAP50:95达到0.80+"
-base_conf = 0.25
-base_iou = 0.45
-def det_image(img, conf, iou):
- model.conf = conf
- model.iou = iou
- return model(img).render()[0]
-# input如果是单图像输入 fn可以是 lambda img:model(img).render()[0]
-# input可以是gr.Webcam()即网络摄像头 同时加一个参数live=True可以同步输入
-app = gr.Interface(fn=det_image,
- inputs=["image",
- gr.Slider(minimum=0, maximum=1, value=base_conf),
- gr.Slider(minimum=0, maximum=1, value=base_iou)],
- outputs=["image"],
- title=title,
- description=desc,
- examples=[["./i1wJLsAZbpvD3mNWeK8Hfl7xrPC9cMqT02So4YyF.jpg",base_conf,base_iou],
- ["./J28KUmgZx6t14ohTDYHWO0cyEkiwXSanRfjlGVpF.jpg",base_conf,base_iou]])
-# app.launch(server_name="0.0.0.0", server_port=80, show_error=True, auth=("admin","pass1234"))
-app.launch(server_name="0.0.0.0", server_port=7860, show_error=True, auth=("admin","admin"))
\ No newline at end of file
diff --git a/spaces/SIGGRAPH2022/DCT-Net/source/facelib/config.py b/spaces/SIGGRAPH2022/DCT-Net/source/facelib/config.py
deleted file mode 100644
index d795fdde08a45d18d7e2286ddd684dea1f42b7d5..0000000000000000000000000000000000000000
--- a/spaces/SIGGRAPH2022/DCT-Net/source/facelib/config.py
+++ /dev/null
@@ -1,23 +0,0 @@
-import os
-
-import numpy as np
-from easydict import EasyDict as edict
-
-config = edict()
-os.environ['CUDA_VISIBLE_DEVICES'] = '0'
-
-config.DETECT = edict()
-config.DETECT.topk = 10
-config.DETECT.thres = 0.8
-config.DETECT.input_shape = (512, 512, 3)
-config.KEYPOINTS = edict()
-config.KEYPOINTS.p_num = 68
-config.KEYPOINTS.base_extend_range = [0.2, 0.3]
-config.KEYPOINTS.input_shape = (160, 160, 3)
-config.TRACE = edict()
-config.TRACE.pixel_thres = 1
-config.TRACE.smooth_box = 0.3
-config.TRACE.smooth_landmark = 0.95
-config.TRACE.iou_thres = 0.5
-config.DATA = edict()
-config.DATA.pixel_means = np.array([123., 116., 103.]) # RGB
diff --git a/spaces/Sapphire-356/Video2MC/joints_detectors/Alphapose/SPPE/src/models/layers/__init__.py b/spaces/Sapphire-356/Video2MC/joints_detectors/Alphapose/SPPE/src/models/layers/__init__.py
deleted file mode 100644
index b6e690fd59145ce8900fd9ab8d8a996ee7d33834..0000000000000000000000000000000000000000
--- a/spaces/Sapphire-356/Video2MC/joints_detectors/Alphapose/SPPE/src/models/layers/__init__.py
+++ /dev/null
@@ -1 +0,0 @@
-from . import *
diff --git a/spaces/Sapphire-356/Video2MC/test/opencv_capture_test.py b/spaces/Sapphire-356/Video2MC/test/opencv_capture_test.py
deleted file mode 100644
index 18dbb6315ae9ebb11b8430f9f01937f091343906..0000000000000000000000000000000000000000
--- a/spaces/Sapphire-356/Video2MC/test/opencv_capture_test.py
+++ /dev/null
@@ -1,26 +0,0 @@
-import cv2
-
-from tqdm import tqdm
-
-path = '../outputs/nba2k.mp4'
-stream = cv2.VideoCapture(path)
-assert stream.isOpened(), 'Cannot capture source'
-
-video_length = int(stream.get(cv2.CAP_PROP_FRAME_COUNT))
-video_fps = stream.get(cv2.CAP_PROP_FPS)
-video_size = (int(stream.get(cv2.CAP_PROP_FRAME_WIDTH)), int(stream.get(cv2.CAP_PROP_FRAME_HEIGHT)))
-writer = cv2.VideoWriter('out.mp4', cv2.VideoWriter_fourcc(*'MP4V'), video_fps, video_size)
-
-for i in tqdm(range(video_length)):
- i += 1
- grabbed, frame = stream.read()
-
- writer.write(frame)
-
- # if the `grabbed` boolean is `False`, then we have
- # reached the end of the video file
- if not grabbed:
- print('\n===========================> This video get ' + str(i) + ' frames in total.')
- break
-
-writer.release()
diff --git a/spaces/SeViLA/SeViLA/lavis/models/blip_models/blip_nlvr.py b/spaces/SeViLA/SeViLA/lavis/models/blip_models/blip_nlvr.py
deleted file mode 100644
index a67d7a1b2c27a200efaae5dda5da1c5fc9ca78e8..0000000000000000000000000000000000000000
--- a/spaces/SeViLA/SeViLA/lavis/models/blip_models/blip_nlvr.py
+++ /dev/null
@@ -1,187 +0,0 @@
-"""
- Copyright (c) 2022, salesforce.com, inc.
- All rights reserved.
- SPDX-License-Identifier: BSD-3-Clause
- For full license text, see the LICENSE file in the repo root or https://opensource.org/licenses/BSD-3-Clause
-"""
-
-import os
-
-import torch
-import torch.nn.functional as F
-from lavis.common.dist_utils import download_cached_file
-from lavis.common.registry import registry
-from lavis.common.utils import get_abs_path, is_url
-from lavis.models.base_model import MomentumDistilationMixin
-from lavis.models.blip_models.blip import BlipBase
-from lavis.models.blip_models.blip_outputs import BlipIntermediateOutput, BlipOutput
-from lavis.models.blip_models.nlvr_encoder import BertModel
-from lavis.models.vit import VisionTransformerEncoder, interpolate_pos_embed
-from torch import nn
-from transformers import BertConfig
-
-
-@registry.register_model("blip_nlvr")
-class BlipNLVR(BlipBase, MomentumDistilationMixin):
- """
- Class for BLIP NLVR model.
-
- Supported model types:
- - base: model with pre-trained BLIP weights, used as initialization for fine-tuning.
- - nlvr: finetuned model on NLVR2 dataset.
-
- Usage:
- >>> from lavis.models import load_model
- >>> model = load_model("blip_nlvr", "nlvr")
- """
-
- PRETRAINED_MODEL_CONFIG_DICT = {
- "nlvr": "configs/models/blip_nlvr.yaml",
- }
-
- def __init__(self, image_encoder, text_encoder, num_classes):
- super().__init__()
-
- self.tokenizer = self.init_tokenizer()
- self.visual_encoder = image_encoder
- self.text_encoder = text_encoder
-
- hidden_size = text_encoder.config.hidden_size
- self.cls_head = nn.Sequential(
- nn.Linear(hidden_size, hidden_size),
- nn.ReLU(),
- nn.Linear(hidden_size, num_classes),
- )
-
- def forward(self, samples, is_train=True):
- """
- Forward function for training and evaluation.
-
- Args:
- samples (dict): a dict of input samples, which contains the following keys:
- - image0 (torch.Tensor): input image 0, shape (batch_size, 3, H, W), default H=384, W=384.
- - image1 (torch.Tensor): input image 1, shape (batch_size, 3, H, W), default H=384, W=384.
- - text_input (list): list of strings, each string is a natural language sentence.
- - label (torch.LongTensor): ground truth label with shape (batch_size,).
- is_train (bool): whether the model is in training mode.
- If True, the model will return the loss;
- If False, the model will return the prediction.
-
- Examples:
- >>> import torch
- >>> from lavis.models import load_model
- >>> model = load_model("blip_nlvr", "nlvr")
- >>> samples = {
- ... "image0": torch.randn(2, 3, 384, 384),
- ... "image1": torch.randn(2, 3, 384, 384),
- ... "text_input": ["there is a ferret in tall grass", "there are lips in one of the images"],
- ... "label": torch.tensor([0, 1]),
- ... }
- >>> output = model(samples)
- >>> output.keys()
- odict_keys(['intermediate_output', 'loss'])
- """
- text = samples["text_input"]
- text = self.tokenizer(text, padding="longest", return_tensors="pt").to(
- self.device
- )
- text.input_ids[:, 0] = self.tokenizer.enc_token_id
-
- targets = samples["label"]
-
- image0 = samples["image0"]
- image1 = samples["image1"]
- images = torch.cat([image0, image1], dim=0)
-
- image_embeds = self.visual_encoder.forward_features(images)
- image_atts = torch.ones(image_embeds.size()[:-1], dtype=torch.long).to(
- self.device
- )
- image0_embeds, image1_embeds = torch.split(image_embeds, targets.size(0))
-
- encoder_output = self.text_encoder(
- text.input_ids,
- attention_mask=text.attention_mask,
- encoder_hidden_states=[image0_embeds, image1_embeds],
- encoder_attention_mask=[
- image_atts[: image0_embeds.size(0)],
- image_atts[image0_embeds.size(0) :],
- ],
- return_dict=True,
- )
-
- prediction = self.cls_head(encoder_output.last_hidden_state[:, 0, :])
-
- if is_train:
- loss = F.cross_entropy(prediction, targets)
- # return {"loss": loss}
- return BlipOutput(
- loss=loss,
- intermediate_output=BlipIntermediateOutput(
- image_embeds=torch.stack([image0_embeds, image1_embeds], dim=0),
- encoder_output=encoder_output,
- ),
- )
- else:
- return {"predictions": prediction, "targets": targets}
-
- def predict(self, samples):
- output = self.forward(samples, is_train=False)
- return output
-
- @classmethod
- def from_config(cls, cfg=None):
- image_encoder = VisionTransformerEncoder.from_config(cfg)
-
- # text encoder + multimodal encoder
- bert_config = BertConfig.from_json_file(get_abs_path(cfg["med_config_path"]))
- text_encoder = BertModel(config=bert_config, add_pooling_layer=False)
-
- num_classes = cfg.get("num_classes", 3)
-
- assert num_classes > 1, "Invalid number of classes provided, found {}".format(
- num_classes
- )
-
- model = cls(
- image_encoder=image_encoder,
- text_encoder=text_encoder,
- num_classes=num_classes,
- )
-
- model.load_checkpoint_from_config(cfg)
-
- return model
-
- def load_from_pretrained(self, url_or_filename):
- if is_url(url_or_filename):
- cached_file = download_cached_file(
- url_or_filename, check_hash=False, progress=True
- )
- checkpoint = torch.load(cached_file, map_location="cpu")
- elif os.path.isfile(url_or_filename):
- checkpoint = torch.load(url_or_filename, map_location="cpu")
- else:
- raise RuntimeError("checkpoint url or path is invalid")
- state_dict = checkpoint["model"]
-
- state_dict["visual_encoder.pos_embed"] = interpolate_pos_embed(
- state_dict["visual_encoder.pos_embed"], self.visual_encoder
- )
-
- for key in list(state_dict.keys()):
- if "crossattention.self." in key:
- new_key0 = key.replace("self", "self0")
- new_key1 = key.replace("self", "self1")
- state_dict[new_key0] = state_dict[key]
- state_dict[new_key1] = state_dict[key]
- elif "crossattention.output.dense." in key:
- new_key0 = key.replace("dense", "dense0")
- new_key1 = key.replace("dense", "dense1")
- state_dict[new_key0] = state_dict[key]
- state_dict[new_key1] = state_dict[key]
-
- msg = self.load_state_dict(state_dict, strict=False)
- print("load checkpoint from %s" % url_or_filename)
- print(f"missing keys {msg.missing_keys}")
- return msg
diff --git a/spaces/SeViLA/SeViLA/lavis/models/timesformer/vit_utils.py b/spaces/SeViLA/SeViLA/lavis/models/timesformer/vit_utils.py
deleted file mode 100644
index 5045d586495ca8ddab3f52d5f0a1b207fe263762..0000000000000000000000000000000000000000
--- a/spaces/SeViLA/SeViLA/lavis/models/timesformer/vit_utils.py
+++ /dev/null
@@ -1,189 +0,0 @@
-"""
- Copyright (c) 2022, salesforce.com, inc.
- All rights reserved.
- SPDX-License-Identifier: BSD-3-Clause
- For full license text, see the LICENSE file in the repo root or https://opensource.org/licenses/BSD-3-Clause
-
- Based on https://github.com/facebookresearch/TimeSformer
-"""
-
-# Copyright 2020 Ross Wightman
-# Various utility functions
-
-import torch
-import torch.nn as nn
-import math
-import warnings
-import torch.nn.functional as F
-
-from itertools import repeat
-import collections.abc as container_abcs
-
-DEFAULT_CROP_PCT = 0.875
-IMAGENET_DEFAULT_MEAN = (0.485, 0.456, 0.406)
-IMAGENET_DEFAULT_STD = (0.229, 0.224, 0.225)
-IMAGENET_INCEPTION_MEAN = (0.5, 0.5, 0.5)
-IMAGENET_INCEPTION_STD = (0.5, 0.5, 0.5)
-IMAGENET_DPN_MEAN = (124 / 255, 117 / 255, 104 / 255)
-IMAGENET_DPN_STD = tuple([1 / (0.0167 * 255)] * 3)
-
-
-def _no_grad_trunc_normal_(tensor, mean, std, a, b):
- def norm_cdf(x):
- # Computes standard normal cumulative distribution function
- return (1.0 + math.erf(x / math.sqrt(2.0))) / 2.0
-
- if (mean < a - 2 * std) or (mean > b + 2 * std):
- warnings.warn(
- "mean is more than 2 std from [a, b] in nn.init.trunc_normal_. "
- "The distribution of values may be incorrect.",
- stacklevel=2,
- )
-
- with torch.no_grad():
- # Values are generated by using a truncated uniform distribution and
- # then using the inverse CDF for the normal distribution.
- # Get upper and lower cdf values
- l = norm_cdf((a - mean) / std)
- u = norm_cdf((b - mean) / std)
-
- # Uniformly fill tensor with values from [l, u], then translate to
- # [2l-1, 2u-1].
- tensor.uniform_(2 * l - 1, 2 * u - 1)
-
- # Use inverse cdf transform for normal distribution to get truncated
- # standard normal
- tensor.erfinv_()
-
- # Transform to proper mean, std
- tensor.mul_(std * math.sqrt(2.0))
- tensor.add_(mean)
-
- # Clamp to ensure it's in the proper range
- tensor.clamp_(min=a, max=b)
- return tensor
-
-
-def trunc_normal_(tensor, mean=0.0, std=1.0, a=-2.0, b=2.0):
- r"""Fills the input Tensor with values drawn from a truncated
- normal distribution. The values are effectively drawn from the
- normal distribution :math:`\mathcal{N}(\text{mean}, \text{std}^2)`
- with values outside :math:`[a, b]` redrawn until they are within
- the bounds. The method used for generating the random values works
- best when :math:`a \leq \text{mean} \leq b`.
- Args:
- tensor: an n-dimensional `torch.Tensor`
- mean: the mean of the normal distribution
- std: the standard deviation of the normal distribution
- a: the minimum cutoff value
- b: the maximum cutoff value
- Examples:
- >>> w = torch.empty(3, 5)
- >>> nn.init.trunc_normal_(w)
- """
- return _no_grad_trunc_normal_(tensor, mean, std, a, b)
-
-
-# From PyTorch internals
-def _ntuple(n):
- def parse(x):
- if isinstance(x, container_abcs.Iterable):
- return x
- return tuple(repeat(x, n))
-
- return parse
-
-
-to_2tuple = _ntuple(2)
-
-# Calculate symmetric padding for a convolution
-def get_padding(kernel_size: int, stride: int = 1, dilation: int = 1, **_) -> int:
- padding = ((stride - 1) + dilation * (kernel_size - 1)) // 2
- return padding
-
-
-def get_padding_value(padding, kernel_size, **kwargs):
- dynamic = False
- if isinstance(padding, str):
- # for any string padding, the padding will be calculated for you, one of three ways
- padding = padding.lower()
- if padding == "same":
- # TF compatible 'SAME' padding, has a performance and GPU memory allocation impact
- if is_static_pad(kernel_size, **kwargs):
- # static case, no extra overhead
- padding = get_padding(kernel_size, **kwargs)
- else:
- # dynamic 'SAME' padding, has runtime/GPU memory overhead
- padding = 0
- dynamic = True
- elif padding == "valid":
- # 'VALID' padding, same as padding=0
- padding = 0
- else:
- # Default to PyTorch style 'same'-ish symmetric padding
- padding = get_padding(kernel_size, **kwargs)
- return padding, dynamic
-
-
-# Calculate asymmetric TensorFlow-like 'SAME' padding for a convolution
-def get_same_padding(x: int, k: int, s: int, d: int):
- return max((int(math.ceil(x // s)) - 1) * s + (k - 1) * d + 1 - x, 0)
-
-
-# Can SAME padding for given args be done statically?
-def is_static_pad(kernel_size: int, stride: int = 1, dilation: int = 1, **_):
- return stride == 1 and (dilation * (kernel_size - 1)) % 2 == 0
-
-
-# Dynamically pad input x with 'SAME' padding for conv with specified args
-# def pad_same(x, k: List[int], s: List[int], d: List[int] = (1, 1), value: float = 0):
-def pad_same(x, k, s, d=(1, 1), value=0):
- ih, iw = x.size()[-2:]
- pad_h, pad_w = get_same_padding(ih, k[0], s[0], d[0]), get_same_padding(
- iw, k[1], s[1], d[1]
- )
- if pad_h > 0 or pad_w > 0:
- x = F.pad(
- x,
- [pad_w // 2, pad_w - pad_w // 2, pad_h // 2, pad_h - pad_h // 2],
- value=value,
- )
- return x
-
-
-def adaptive_pool_feat_mult(pool_type="avg"):
- if pool_type == "catavgmax":
- return 2
- else:
- return 1
-
-
-def drop_path(x, drop_prob: float = 0.0, training: bool = False):
- """Drop paths (Stochastic Depth) per sample (when applied in main path of residual blocks).
- This is the same as the DropConnect impl I created for EfficientNet, etc networks, however,
- the original name is misleading as 'Drop Connect' is a different form of dropout in a separate paper...
- See discussion: https://github.com/tensorflow/tpu/issues/494#issuecomment-532968956 ... I've opted for
- changing the layer and argument names to 'drop path' rather than mix DropConnect as a layer name and use
- 'survival rate' as the argument.
- """
- if drop_prob == 0.0 or not training:
- return x
- keep_prob = 1 - drop_prob
- shape = (x.shape[0],) + (1,) * (
- x.ndim - 1
- ) # work with diff dim tensors, not just 2D ConvNets
- random_tensor = keep_prob + torch.rand(shape, dtype=x.dtype, device=x.device)
- random_tensor.floor_() # binarize
- output = x.div(keep_prob) * random_tensor
- return output
-
-
-class DropPath(nn.Module):
- """Drop paths (Stochastic Depth) per sample (when applied in main path of residual blocks)."""
-
- def __init__(self, drop_prob=None):
- super(DropPath, self).__init__()
- self.drop_prob = drop_prob
-
- def forward(self, x):
- return drop_path(x, self.drop_prob, self.training)
diff --git a/spaces/Shad0ws/AI-Agent-with-Google-Search-APIs/src/task_prio_chain.py b/spaces/Shad0ws/AI-Agent-with-Google-Search-APIs/src/task_prio_chain.py
deleted file mode 100644
index e1dcc6a91683110e47df65e5f669160e32237e3b..0000000000000000000000000000000000000000
--- a/spaces/Shad0ws/AI-Agent-with-Google-Search-APIs/src/task_prio_chain.py
+++ /dev/null
@@ -1,23 +0,0 @@
-from langchain.llms import BaseLLM
-from langchain import LLMChain, PromptTemplate
-
-class TaskPrioritizationChain(LLMChain):
- """Chain to prioritize tasks."""
-
- @classmethod
- def from_llm(cls, llm: BaseLLM, verbose: bool = True) -> LLMChain:
- """Get the response parser."""
- task_prioritization_template = (
- "You are an task prioritization AI tasked with cleaning the formatting of and reprioritizing"
- " the following tasks: {task_names}."
- " Consider the ultimate objective of your team: {objective}."
- " Do not remove any tasks. Return the result as a numbered list, like:"
- " #. First task"
- " #. Second task"
- " Start the task list with number {next_task_id}."
- )
- prompt = PromptTemplate(
- template=task_prioritization_template,
- input_variables=["task_names", "next_task_id", "objective"],
- )
- return cls(prompt=prompt, llm=llm, verbose=verbose)
\ No newline at end of file
diff --git a/spaces/SungBeom/chatwine-korean/.venv/Lib/site-packages/docarray/index/backends/elastic.py b/spaces/SungBeom/chatwine-korean/.venv/Lib/site-packages/docarray/index/backends/elastic.py
deleted file mode 100644
index ee0df666cf28faa115aa09f34bde8909c5b7d65b..0000000000000000000000000000000000000000
--- a/spaces/SungBeom/chatwine-korean/.venv/Lib/site-packages/docarray/index/backends/elastic.py
+++ /dev/null
@@ -1,710 +0,0 @@
-# mypy: ignore-errors
-import warnings
-from collections import defaultdict
-from dataclasses import dataclass, field
-from typing import (
- TYPE_CHECKING,
- Any,
- Dict,
- Generator,
- Generic,
- Iterable,
- List,
- Mapping,
- Optional,
- Sequence,
- Tuple,
- Type,
- TypeVar,
- Union,
- cast,
-)
-
-import numpy as np
-from pydantic import parse_obj_as
-
-import docarray.typing
-from docarray import BaseDoc
-from docarray.array.any_array import AnyDocArray
-from docarray.index.abstract import BaseDocIndex, _ColumnInfo, _raise_not_composable
-from docarray.typing import AnyTensor
-from docarray.typing.tensor.abstract_tensor import AbstractTensor
-from docarray.typing.tensor.ndarray import NdArray
-from docarray.utils._internal.misc import import_library
-from docarray.utils.find import _FindResult, _FindResultBatched
-
-TSchema = TypeVar('TSchema', bound=BaseDoc)
-T = TypeVar('T', bound='ElasticDocIndex')
-
-ELASTIC_PY_VEC_TYPES: List[Any] = [list, tuple, np.ndarray, AbstractTensor]
-
-
-if TYPE_CHECKING:
- import tensorflow as tf # type: ignore
- import torch
- from elastic_transport import NodeConfig
- from elasticsearch import Elasticsearch
- from elasticsearch.helpers import parallel_bulk
-else:
- elasticsearch = import_library('elasticsearch', raise_error=True)
- from elasticsearch import Elasticsearch
- from elasticsearch.helpers import parallel_bulk
-
- elastic_transport = import_library('elastic_transport', raise_error=True)
- from elastic_transport import NodeConfig
-
- torch = import_library('torch', raise_error=False)
- tf = import_library('tensorflow', raise_error=False)
-
-
-if torch is not None:
- ELASTIC_PY_VEC_TYPES.append(torch.Tensor)
-
-if tf is not None:
- from docarray.typing import TensorFlowTensor
-
- ELASTIC_PY_VEC_TYPES.append(tf.Tensor)
- ELASTIC_PY_VEC_TYPES.append(TensorFlowTensor)
-
-
-class ElasticDocIndex(BaseDocIndex, Generic[TSchema]):
- def __init__(self, db_config=None, **kwargs):
- """Initialize ElasticDocIndex"""
- super().__init__(db_config=db_config, **kwargs)
- self._db_config = cast(ElasticDocIndex.DBConfig, self._db_config)
-
- self._logger.debug('Elastic Search index is being initialized')
-
- # ElasticSearch client creation
- self._client = Elasticsearch(
- hosts=self._db_config.hosts,
- **self._db_config.es_config,
- )
- self._logger.debug('ElasticSearch client has been created')
-
- # ElasticSearh index setup
- self._index_vector_params = ('dims', 'similarity', 'index')
- self._index_vector_options = ('m', 'ef_construction')
-
- mappings: Dict[str, Any] = {
- 'dynamic': True,
- '_source': {'enabled': 'true'},
- 'properties': {},
- }
- mappings.update(self._db_config.index_mappings)
-
- self._logger.debug('Mappings have been updated with db_config.index_mappings')
-
- for col_name, col in self._column_infos.items():
- if issubclass(col.docarray_type, AnyDocArray):
- continue
- if col.db_type == 'dense_vector' and (
- not col.n_dim and col.config['dims'] < 0
- ):
- self._logger.info(
- f'Not indexing column {col_name}, the dimensionality is not specified'
- )
- continue
-
- mappings['properties'][col_name] = self._create_index_mapping(col)
- self._logger.debug(f'Index mapping created for column {col_name}')
-
- if self._client.indices.exists(index=self.index_name):
- self._client_put_mapping(mappings)
- self._logger.debug(f'Put mapping for index {self.index_name}')
- else:
- self._client_create(mappings)
- self._logger.debug(f'Created new index {self.index_name} with mappings')
-
- if len(self._db_config.index_settings):
- self._client_put_settings(self._db_config.index_settings)
- self._logger.debug('Updated index settings')
-
- self._refresh(self.index_name)
- self._logger.debug(f'Refreshed index {self.index_name}')
-
- @property
- def index_name(self):
- default_index_name = (
- self._schema.__name__.lower() if self._schema is not None else None
- )
- if default_index_name is None:
- err_msg = (
- 'A ElasticDocIndex must be typed with a Document type.To do so, use the syntax: '
- 'ElasticDocIndex[DocumentType] '
- )
-
- self._logger.error(err_msg)
- raise ValueError(err_msg)
- index_name = self._db_config.index_name or default_index_name
- self._logger.debug(f'Retrieved index name: {index_name}')
- return index_name
-
- ###############################################
- # Inner classes for query builder and configs #
- ###############################################
- class QueryBuilder(BaseDocIndex.QueryBuilder):
- def __init__(self, outer_instance, **kwargs):
- super().__init__()
- self._outer_instance = outer_instance
- self._query: Dict[str, Any] = {
- 'query': defaultdict(lambda: defaultdict(list))
- }
-
- def build(self, *args, **kwargs) -> Any:
- """Build the elastic search query object."""
- self._outer_instance._logger.debug(
- 'Building the Elastic Search query object'
- )
-
- if len(self._query['query']) == 0:
- del self._query['query']
- elif 'knn' in self._query:
- self._query['knn']['filter'] = self._query['query']
- del self._query['query']
-
- return self._query
-
- def find(
- self,
- query: Union[AnyTensor, BaseDoc],
- search_field: str = 'embedding',
- limit: int = 10,
- num_candidates: Optional[int] = None,
- ):
- """
- Find k-nearest neighbors of the query.
-
- :param query: query vector for KNN/ANN search. Has single axis.
- :param search_field: name of the field to search on
- :param limit: maximum number of documents to return per query
- :param num_candidates: number of candidates
- :return: self
- """
- self._outer_instance._logger.debug('Executing find query')
-
- self._outer_instance._validate_search_field(search_field)
- if isinstance(query, BaseDoc):
- query_vec = BaseDocIndex._get_values_by_column([query], search_field)[0]
- else:
- query_vec = query
- query_vec_np = BaseDocIndex._to_numpy(self._outer_instance, query_vec)
- self._query['knn'] = self._outer_instance._form_search_body(
- query_vec_np,
- limit,
- search_field,
- num_candidates,
- )['knn']
-
- return self
-
- # filter accepts Leaf/Compound query clauses
- # https://www.elastic.co/guide/en/elasticsearch/reference/current/query-dsl.html
- def filter(self, query: Dict[str, Any], limit: int = 10):
- """Find documents in the index based on a filter query
-
- :param query: the query to execute
- :param limit: maximum number of documents to return
- :return: self
- """
- self._outer_instance._logger.debug('Executing filter query')
-
- self._query['size'] = limit
- self._query['query']['bool']['filter'].append(query)
- return self
-
- def text_search(self, query: str, search_field: str = 'text', limit: int = 10):
- """Find documents in the index based on a text search query
-
- :param query: The text to search for
- :param search_field: name of the field to search on
- :param limit: maximum number of documents to find
- :return: self
- """
- self._outer_instance._logger.debug('Executing text search query')
-
- self._outer_instance._validate_search_field(search_field)
- self._query['size'] = limit
- self._query['query']['bool']['must'].append(
- {'match': {search_field: query}}
- )
- return self
-
- find_batched = _raise_not_composable('find_batched')
- filter_batched = _raise_not_composable('filter_batched')
- text_search_batched = _raise_not_composable('text_search_batched')
-
- def build_query(self, **kwargs) -> QueryBuilder:
- """
- Build a query for ElasticDocIndex.
- :param kwargs: parameters to forward to QueryBuilder initialization
- :return: QueryBuilder object
- """
- return self.QueryBuilder(self, **kwargs)
-
- @dataclass
- class DBConfig(BaseDocIndex.DBConfig):
- """Dataclass that contains all "static" configurations of ElasticDocIndex."""
-
- hosts: Union[
- str, List[Union[str, Mapping[str, Union[str, int]], NodeConfig]], None
- ] = 'http://localhost:9200'
- index_name: Optional[str] = None
- es_config: Dict[str, Any] = field(default_factory=dict)
- index_settings: Dict[str, Any] = field(default_factory=dict)
- index_mappings: Dict[str, Any] = field(default_factory=dict)
-
- @dataclass
- class RuntimeConfig(BaseDocIndex.RuntimeConfig):
- """Dataclass that contains all "dynamic" configurations of ElasticDocIndex."""
-
- default_column_config: Dict[Any, Dict[str, Any]] = field(default_factory=dict)
- chunk_size: int = 500
-
- def __post_init__(self):
- self.default_column_config = {
- 'binary': {},
- 'boolean': {},
- 'keyword': {},
- 'long': {},
- 'integer': {},
- 'short': {},
- 'byte': {},
- 'double': {},
- 'float': {},
- 'half_float': {},
- 'scaled_float': {},
- 'unsigned_long': {},
- 'dates': {},
- 'alias': {},
- 'object': {},
- 'flattened': {},
- 'nested': {},
- 'join': {},
- 'integer_range': {},
- 'float_range': {},
- 'long_range': {},
- 'double_range': {},
- 'date_range': {},
- 'ip_range': {},
- 'ip': {},
- 'version': {},
- 'histogram': {},
- 'text': {},
- 'annotated_text': {},
- 'completion': {},
- 'search_as_you_type': {},
- 'token_count': {},
- 'sparse_vector': {},
- 'rank_feature': {},
- 'rank_features': {},
- 'geo_point': {},
- 'geo_shape': {},
- 'point': {},
- 'shape': {},
- 'percolator': {},
- # `None` is not a Type, but we allow it here anyway
- None: {}, # type: ignore
- }
- self.default_column_config['dense_vector'] = self.dense_vector_config()
-
- def dense_vector_config(self):
- """Get the dense vector config."""
-
- config = {
- 'dims': -1,
- 'index': True,
- 'similarity': 'cosine', # 'l2_norm', 'dot_product', 'cosine'
- 'm': 16,
- 'ef_construction': 100,
- 'num_candidates': 10000,
- }
-
- return config
-
- ###############################################
- # Implementation of abstract methods #
- ###############################################
-
- def python_type_to_db_type(self, python_type: Type) -> Any:
- """Map python type to database type.
- Takes any python type and returns the corresponding database column type.
-
- :param python_type: a python type.
- :return: the corresponding database column type,
- or None if ``python_type`` is not supported.
- """
- self._logger.debug(f'Mapping Python type {python_type} to database type')
-
- for allowed_type in ELASTIC_PY_VEC_TYPES:
- if issubclass(python_type, allowed_type):
- self._logger.info(
- f'Mapped Python type {python_type} to database type "dense_vector"'
- )
- return 'dense_vector'
-
- elastic_py_types = {
- docarray.typing.ID: 'keyword',
- docarray.typing.AnyUrl: 'keyword',
- bool: 'boolean',
- int: 'integer',
- float: 'float',
- str: 'text',
- bytes: 'binary',
- dict: 'object',
- }
-
- for type in elastic_py_types.keys():
- if issubclass(python_type, type):
- self._logger.info(
- f'Mapped Python type {python_type} to database type "{elastic_py_types[type]}"'
- )
- return elastic_py_types[type]
-
- err_msg = f'Unsupported column type for {type(self)}: {python_type}'
- self._logger.error(err_msg)
- raise ValueError(err_msg)
-
- def _index(
- self,
- column_to_data: Mapping[str, Generator[Any, None, None]],
- refresh: bool = True,
- chunk_size: Optional[int] = None,
- ):
-
- self._index_subindex(column_to_data)
-
- data = self._transpose_col_value_dict(column_to_data)
- requests = []
-
- for row in data:
- request = {
- '_index': self.index_name,
- '_id': row['id'],
- }
- for col_name, col in self._column_infos.items():
- if issubclass(col.docarray_type, AnyDocArray):
- continue
- if col.db_type == 'dense_vector' and np.all(row[col_name] == 0):
- row[col_name] = row[col_name] + 1.0e-9
- if row[col_name] is None:
- continue
- request[col_name] = row[col_name]
- requests.append(request)
-
- _, warning_info = self._send_requests(requests, chunk_size)
- for info in warning_info:
- warnings.warn(str(info))
- self._logger.warning('Warning: %s', str(info))
-
- if refresh:
- self._logger.debug('Refreshing the index')
- self._refresh(self.index_name)
-
- def num_docs(self) -> int:
- """
- Get the number of documents.
- """
- self._logger.debug('Getting the number of documents in the index')
- return self._client.count(index=self.index_name)['count']
-
- def _del_items(
- self,
- doc_ids: Sequence[str],
- chunk_size: Optional[int] = None,
- ):
- requests = []
- for _id in doc_ids:
- requests.append(
- {'_op_type': 'delete', '_index': self.index_name, '_id': _id}
- )
-
- _, warning_info = self._send_requests(requests, chunk_size)
-
- # raise warning if some ids are not found
- if warning_info:
- ids = [info['delete']['_id'] for info in warning_info]
- warnings.warn(f'No document with id {ids} found')
-
- self._refresh(self.index_name)
-
- def _get_items(self, doc_ids: Sequence[str]) -> Sequence[Dict[str, Any]]:
- accumulated_docs = []
- accumulated_docs_id_not_found = []
-
- es_rows = self._client_mget(doc_ids)['docs']
-
- for row in es_rows:
- if row['found']:
- doc_dict = row['_source']
- accumulated_docs.append(doc_dict)
- else:
- accumulated_docs_id_not_found.append(row['_id'])
-
- # raise warning if some ids are not found
- if accumulated_docs_id_not_found:
- warnings.warn(f'No document with id {accumulated_docs_id_not_found} found')
-
- return accumulated_docs
-
- def execute_query(self, query: Dict[str, Any], *args, **kwargs) -> Any:
- """
- Execute a query on the ElasticDocIndex.
-
- Can take two kinds of inputs:
-
- 1. A native query of the underlying database. This is meant as a passthrough so that you
- can enjoy any functionality that is not available through the Document index API.
- 2. The output of this Document index' `QueryBuilder.build()` method.
-
- :param query: the query to execute
- :param args: positional arguments to pass to the query
- :param kwargs: keyword arguments to pass to the query
- :return: the result of the query
- """
- self._logger.debug(f'Executing query: {query}')
-
- if args or kwargs:
- err_msg = (
- f'args and kwargs not supported for `execute_query` on {type(self)}'
- )
- self._logger.error(err_msg)
- raise ValueError(err_msg)
-
- resp = self._client.search(index=self.index_name, **query)
- docs, scores = self._format_response(resp)
-
- return _FindResult(documents=docs, scores=parse_obj_as(NdArray, scores))
-
- def _find(
- self, query: np.ndarray, limit: int, search_field: str = ''
- ) -> _FindResult:
-
- body = self._form_search_body(query, limit, search_field)
-
- resp = self._client_search(**body)
-
- docs, scores = self._format_response(resp)
-
- return _FindResult(documents=docs, scores=parse_obj_as(NdArray, scores))
-
- def _find_batched(
- self,
- queries: np.ndarray,
- limit: int,
- search_field: str = '',
- ) -> _FindResultBatched:
-
- request = []
- for query in queries:
- head = {'index': self.index_name}
- body = self._form_search_body(query, limit, search_field)
- request.extend([head, body])
-
- responses = self._client_msearch(request)
-
- das, scores = zip(
- *[self._format_response(resp) for resp in responses['responses']]
- )
- return _FindResultBatched(documents=list(das), scores=scores)
-
- def _filter(
- self,
- filter_query: Dict[str, Any],
- limit: int,
- ) -> List[Dict]:
-
- resp = self._client_search(query=filter_query, size=limit)
-
- docs, _ = self._format_response(resp)
-
- return docs
-
- def _filter_batched(
- self,
- filter_queries: Any,
- limit: int,
- ) -> List[List[Dict]]:
-
- request = []
- for query in filter_queries:
- head = {'index': self.index_name}
- body = {'query': query, 'size': limit}
- request.extend([head, body])
-
- responses = self._client_msearch(request)
- das, _ = zip(*[self._format_response(resp) for resp in responses['responses']])
-
- return list(das)
-
- def _text_search(
- self,
- query: str,
- limit: int,
- search_field: str = '',
- ) -> _FindResult:
-
- body = self._form_text_search_body(query, limit, search_field)
- resp = self._client_search(**body)
-
- docs, scores = self._format_response(resp)
-
- return _FindResult(documents=docs, scores=np.array(scores)) # type: ignore
-
- def _text_search_batched(
- self,
- queries: Sequence[str],
- limit: int,
- search_field: str = '',
- ) -> _FindResultBatched:
-
- request = []
- for query in queries:
- head = {'index': self.index_name}
- body = self._form_text_search_body(query, limit, search_field)
- request.extend([head, body])
-
- responses = self._client_msearch(request)
- das, scores = zip(
- *[self._format_response(resp) for resp in responses['responses']]
- )
- return _FindResultBatched(documents=list(das), scores=scores)
-
- def _filter_by_parent_id(self, id: str) -> List[str]:
-
- resp = self._client_search(
- query={'term': {'parent_id': id}}, fields=['id'], _source=False
- )
- ids = [hit['fields']['id'][0] for hit in resp['hits']['hits']]
- return ids
-
- ###############################################
- # Helpers #
- ###############################################
-
- def _create_index_mapping(self, col: '_ColumnInfo') -> Dict[str, Any]:
- """Create a new HNSW index for a column, and initialize it."""
-
- index = {'type': col.config['type'] if 'type' in col.config else col.db_type}
-
- if col.db_type == 'dense_vector':
- for k in self._index_vector_params:
- index[k] = col.config[k]
- if col.n_dim:
- index['dims'] = col.n_dim
- index['index_options'] = dict(
- (k, col.config[k]) for k in self._index_vector_options
- )
- index['index_options']['type'] = 'hnsw'
- return index
-
- def _send_requests(
- self,
- request: Iterable[Dict[str, Any]],
- chunk_size: Optional[int] = None,
- **kwargs,
- ) -> Tuple[List[Dict], List[Any]]:
- """Send bulk request to Elastic and gather the successful info"""
-
- accumulated_info = []
- warning_info = []
- for success, info in parallel_bulk(
- self._client,
- request,
- raise_on_error=False,
- raise_on_exception=False,
- chunk_size=chunk_size if chunk_size else self._runtime_config.chunk_size, # type: ignore
- **kwargs,
- ):
- if not success:
- warning_info.append(info)
- else:
- accumulated_info.append(info)
-
- return accumulated_info, warning_info
-
- def _form_search_body(
- self,
- query: np.ndarray,
- limit: int,
- search_field: str = '',
- num_candidates: Optional[int] = None,
- ) -> Dict[str, Any]:
- if not num_candidates:
- num_candidates = self._runtime_config.default_column_config['dense_vector'][
- 'num_candidates'
- ]
- body = {
- 'size': limit,
- 'knn': {
- 'field': search_field,
- 'query_vector': query,
- 'k': limit,
- 'num_candidates': num_candidates,
- },
- }
- return body
-
- def _form_text_search_body(
- self, query: str, limit: int, search_field: str = ''
- ) -> Dict[str, Any]:
- body = {
- 'size': limit,
- 'query': {
- 'bool': {
- 'must': {'match': {search_field: query}},
- }
- },
- }
- return body
-
- def _format_response(self, response: Any) -> Tuple[List[Dict], List[Any]]:
- docs = []
- scores = []
- for result in response['hits']['hits']:
- if not isinstance(result, dict):
- result = result.to_dict()
-
- if result.get('_source', None):
- doc_dict = result['_source']
- else:
- doc_dict = result['fields']
- doc_dict['id'] = result['_id']
- docs.append(doc_dict)
- scores.append(result['_score'])
-
- return docs, [parse_obj_as(NdArray, np.array(s)) for s in scores]
-
- def _refresh(self, index_name: str):
-
- self._client.indices.refresh(index=index_name)
-
- ###############################################
- # API Wrappers #
- ###############################################
-
- def _client_put_mapping(self, mappings: Dict[str, Any]):
-
- self._client.indices.put_mapping(
- index=self.index_name, properties=mappings['properties']
- )
-
- def _client_create(self, mappings: Dict[str, Any]):
-
- self._client.indices.create(index=self.index_name, mappings=mappings)
-
- def _client_put_settings(self, settings: Dict[str, Any]):
-
- self._client.indices.put_settings(index=self.index_name, settings=settings)
-
- def _client_mget(self, ids: Sequence[str]):
-
- return self._client.mget(index=self.index_name, ids=ids)
-
- def _client_search(self, **kwargs):
-
- return self._client.search(index=self.index_name, **kwargs)
-
- def _client_msearch(self, request: List[Dict[str, Any]]):
-
- return self._client.msearch(index=self.index_name, searches=request)
diff --git a/spaces/TEL123/Real-CUGAN/upcunet_v3.py b/spaces/TEL123/Real-CUGAN/upcunet_v3.py
deleted file mode 100644
index f7919a6cc9efe3b8af73a73e30825a4c7d7d76da..0000000000000000000000000000000000000000
--- a/spaces/TEL123/Real-CUGAN/upcunet_v3.py
+++ /dev/null
@@ -1,714 +0,0 @@
-import torch
-from torch import nn as nn
-from torch.nn import functional as F
-import os, sys
-import numpy as np
-
-root_path = os.path.abspath('.')
-sys.path.append(root_path)
-
-
-class SEBlock(nn.Module):
- def __init__(self, in_channels, reduction=8, bias=False):
- super(SEBlock, self).__init__()
- self.conv1 = nn.Conv2d(in_channels, in_channels // reduction, 1, 1, 0, bias=bias)
- self.conv2 = nn.Conv2d(in_channels // reduction, in_channels, 1, 1, 0, bias=bias)
-
- def forward(self, x):
- if ("Half" in x.type()): # torch.HalfTensor/torch.cuda.HalfTensor
- x0 = torch.mean(x.float(), dim=(2, 3), keepdim=True).half()
- else:
- x0 = torch.mean(x, dim=(2, 3), keepdim=True)
- x0 = self.conv1(x0)
- x0 = F.relu(x0, inplace=True)
- x0 = self.conv2(x0)
- x0 = torch.sigmoid(x0)
- x = torch.mul(x, x0)
- return x
-
- def forward_mean(self, x, x0):
- x0 = self.conv1(x0)
- x0 = F.relu(x0, inplace=True)
- x0 = self.conv2(x0)
- x0 = torch.sigmoid(x0)
- x = torch.mul(x, x0)
- return x
-
-
-class UNetConv(nn.Module):
- def __init__(self, in_channels, mid_channels, out_channels, se):
- super(UNetConv, self).__init__()
- self.conv = nn.Sequential(
- nn.Conv2d(in_channels, mid_channels, 3, 1, 0),
- nn.LeakyReLU(0.1, inplace=True),
- nn.Conv2d(mid_channels, out_channels, 3, 1, 0),
- nn.LeakyReLU(0.1, inplace=True),
- )
- if se:
- self.seblock = SEBlock(out_channels, reduction=8, bias=True)
- else:
- self.seblock = None
-
- def forward(self, x):
- z = self.conv(x)
- if self.seblock is not None:
- z = self.seblock(z)
- return z
-
-
-class UNet1(nn.Module):
- def __init__(self, in_channels, out_channels, deconv):
- super(UNet1, self).__init__()
- self.conv1 = UNetConv(in_channels, 32, 64, se=False)
- self.conv1_down = nn.Conv2d(64, 64, 2, 2, 0)
- self.conv2 = UNetConv(64, 128, 64, se=True)
- self.conv2_up = nn.ConvTranspose2d(64, 64, 2, 2, 0)
- self.conv3 = nn.Conv2d(64, 64, 3, 1, 0)
-
- if deconv:
- self.conv_bottom = nn.ConvTranspose2d(64, out_channels, 4, 2, 3)
- else:
- self.conv_bottom = nn.Conv2d(64, out_channels, 3, 1, 0)
-
- for m in self.modules():
- if isinstance(m, (nn.Conv2d, nn.ConvTranspose2d)):
- nn.init.kaiming_normal_(m.weight, mode='fan_out', nonlinearity='relu')
- elif isinstance(m, nn.Linear):
- nn.init.normal_(m.weight, 0, 0.01)
- if m.bias is not None:
- nn.init.constant_(m.bias, 0)
-
- def forward(self, x):
- x1 = self.conv1(x)
- x2 = self.conv1_down(x1)
- x2 = F.leaky_relu(x2, 0.1, inplace=True)
- x2 = self.conv2(x2)
- x2 = self.conv2_up(x2)
- x2 = F.leaky_relu(x2, 0.1, inplace=True)
-
- x1 = F.pad(x1, (-4, -4, -4, -4))
- x3 = self.conv3(x1 + x2)
- x3 = F.leaky_relu(x3, 0.1, inplace=True)
- z = self.conv_bottom(x3)
- return z
-
- def forward_a(self, x):
- x1 = self.conv1(x)
- x2 = self.conv1_down(x1)
- x2 = F.leaky_relu(x2, 0.1, inplace=True)
- x2 = self.conv2.conv(x2)
- return x1, x2
-
- def forward_b(self, x1, x2):
- x2 = self.conv2_up(x2)
- x2 = F.leaky_relu(x2, 0.1, inplace=True)
-
- x1 = F.pad(x1, (-4, -4, -4, -4))
- x3 = self.conv3(x1 + x2)
- x3 = F.leaky_relu(x3, 0.1, inplace=True)
- z = self.conv_bottom(x3)
- return z
-
-
-class UNet1x3(nn.Module):
- def __init__(self, in_channels, out_channels, deconv):
- super(UNet1x3, self).__init__()
- self.conv1 = UNetConv(in_channels, 32, 64, se=False)
- self.conv1_down = nn.Conv2d(64, 64, 2, 2, 0)
- self.conv2 = UNetConv(64, 128, 64, se=True)
- self.conv2_up = nn.ConvTranspose2d(64, 64, 2, 2, 0)
- self.conv3 = nn.Conv2d(64, 64, 3, 1, 0)
-
- if deconv:
- self.conv_bottom = nn.ConvTranspose2d(64, out_channels, 5, 3, 2)
- else:
- self.conv_bottom = nn.Conv2d(64, out_channels, 3, 1, 0)
-
- for m in self.modules():
- if isinstance(m, (nn.Conv2d, nn.ConvTranspose2d)):
- nn.init.kaiming_normal_(m.weight, mode='fan_out', nonlinearity='relu')
- elif isinstance(m, nn.Linear):
- nn.init.normal_(m.weight, 0, 0.01)
- if m.bias is not None:
- nn.init.constant_(m.bias, 0)
-
- def forward(self, x):
- x1 = self.conv1(x)
- x2 = self.conv1_down(x1)
- x2 = F.leaky_relu(x2, 0.1, inplace=True)
- x2 = self.conv2(x2)
- x2 = self.conv2_up(x2)
- x2 = F.leaky_relu(x2, 0.1, inplace=True)
-
- x1 = F.pad(x1, (-4, -4, -4, -4))
- x3 = self.conv3(x1 + x2)
- x3 = F.leaky_relu(x3, 0.1, inplace=True)
- z = self.conv_bottom(x3)
- return z
-
- def forward_a(self, x):
- x1 = self.conv1(x)
- x2 = self.conv1_down(x1)
- x2 = F.leaky_relu(x2, 0.1, inplace=True)
- x2 = self.conv2.conv(x2)
- return x1, x2
-
- def forward_b(self, x1, x2):
- x2 = self.conv2_up(x2)
- x2 = F.leaky_relu(x2, 0.1, inplace=True)
-
- x1 = F.pad(x1, (-4, -4, -4, -4))
- x3 = self.conv3(x1 + x2)
- x3 = F.leaky_relu(x3, 0.1, inplace=True)
- z = self.conv_bottom(x3)
- return z
-
-
-class UNet2(nn.Module):
- def __init__(self, in_channels, out_channels, deconv):
- super(UNet2, self).__init__()
-
- self.conv1 = UNetConv(in_channels, 32, 64, se=False)
- self.conv1_down = nn.Conv2d(64, 64, 2, 2, 0)
- self.conv2 = UNetConv(64, 64, 128, se=True)
- self.conv2_down = nn.Conv2d(128, 128, 2, 2, 0)
- self.conv3 = UNetConv(128, 256, 128, se=True)
- self.conv3_up = nn.ConvTranspose2d(128, 128, 2, 2, 0)
- self.conv4 = UNetConv(128, 64, 64, se=True)
- self.conv4_up = nn.ConvTranspose2d(64, 64, 2, 2, 0)
- self.conv5 = nn.Conv2d(64, 64, 3, 1, 0)
-
- if deconv:
- self.conv_bottom = nn.ConvTranspose2d(64, out_channels, 4, 2, 3)
- else:
- self.conv_bottom = nn.Conv2d(64, out_channels, 3, 1, 0)
-
- for m in self.modules():
- if isinstance(m, (nn.Conv2d, nn.ConvTranspose2d)):
- nn.init.kaiming_normal_(m.weight, mode='fan_out', nonlinearity='relu')
- elif isinstance(m, nn.Linear):
- nn.init.normal_(m.weight, 0, 0.01)
- if m.bias is not None:
- nn.init.constant_(m.bias, 0)
-
- def forward(self, x):
- x1 = self.conv1(x)
- x2 = self.conv1_down(x1)
- x2 = F.leaky_relu(x2, 0.1, inplace=True)
- x2 = self.conv2(x2)
-
- x3 = self.conv2_down(x2)
- x3 = F.leaky_relu(x3, 0.1, inplace=True)
- x3 = self.conv3(x3)
- x3 = self.conv3_up(x3)
- x3 = F.leaky_relu(x3, 0.1, inplace=True)
-
- x2 = F.pad(x2, (-4, -4, -4, -4))
- x4 = self.conv4(x2 + x3)
- x4 = self.conv4_up(x4)
- x4 = F.leaky_relu(x4, 0.1, inplace=True)
-
- x1 = F.pad(x1, (-16, -16, -16, -16))
- x5 = self.conv5(x1 + x4)
- x5 = F.leaky_relu(x5, 0.1, inplace=True)
-
- z = self.conv_bottom(x5)
- return z
-
- def forward_a(self, x): # conv234结尾有se
- x1 = self.conv1(x)
- x2 = self.conv1_down(x1)
- x2 = F.leaky_relu(x2, 0.1, inplace=True)
- x2 = self.conv2.conv(x2)
- return x1, x2
-
- def forward_b(self, x2): # conv234结尾有se
- x3 = self.conv2_down(x2)
- x3 = F.leaky_relu(x3, 0.1, inplace=True)
- x3 = self.conv3.conv(x3)
- return x3
-
- def forward_c(self, x2, x3): # conv234结尾有se
- x3 = self.conv3_up(x3)
- x3 = F.leaky_relu(x3, 0.1, inplace=True)
-
- x2 = F.pad(x2, (-4, -4, -4, -4))
- x4 = self.conv4.conv(x2 + x3)
- return x4
-
- def forward_d(self, x1, x4): # conv234结尾有se
- x4 = self.conv4_up(x4)
- x4 = F.leaky_relu(x4, 0.1, inplace=True)
-
- x1 = F.pad(x1, (-16, -16, -16, -16))
- x5 = self.conv5(x1 + x4)
- x5 = F.leaky_relu(x5, 0.1, inplace=True)
-
- z = self.conv_bottom(x5)
- return z
-
-
-class UpCunet2x(nn.Module): # 完美tile,全程无损
- def __init__(self, in_channels=3, out_channels=3):
- super(UpCunet2x, self).__init__()
- self.unet1 = UNet1(in_channels, out_channels, deconv=True)
- self.unet2 = UNet2(in_channels, out_channels, deconv=False)
-
- def forward(self, x, tile_mode): # 1.7G
- n, c, h0, w0 = x.shape
- if (tile_mode == 0): # 不tile
- ph = ((h0 - 1) // 2 + 1) * 2
- pw = ((w0 - 1) // 2 + 1) * 2
- x = F.pad(x, (18, 18 + pw - w0, 18, 18 + ph - h0), 'reflect') # 需要保证被2整除
- x = self.unet1.forward(x)
- x0 = self.unet2.forward(x)
- x1 = F.pad(x, (-20, -20, -20, -20))
- x = torch.add(x0, x1)
- if (w0 != pw or h0 != ph): x = x[:, :, :h0 * 2, :w0 * 2]
- return x
- elif (tile_mode == 1): # 对长边减半
- if (w0 >= h0):
- crop_size_w = ((w0 - 1) // 4 * 4 + 4) // 2 # 减半后能被2整除,所以要先被4整除
- crop_size_h = (h0 - 1) // 2 * 2 + 2 # 能被2整除
- else:
- crop_size_h = ((h0 - 1) // 4 * 4 + 4) // 2 # 减半后能被2整除,所以要先被4整除
- crop_size_w = (w0 - 1) // 2 * 2 + 2 # 能被2整除
- crop_size = (crop_size_h, crop_size_w) # 6.6G
- elif (tile_mode == 2): # hw都减半
- crop_size = (((h0 - 1) // 4 * 4 + 4) // 2, ((w0 - 1) // 4 * 4 + 4) // 2) # 5.6G
- elif (tile_mode == 3): # hw都三分之一
- crop_size = (((h0 - 1) // 6 * 6 + 6) // 3, ((w0 - 1) // 6 * 6 + 6) // 3) # 4.2G
- elif (tile_mode == 4): # hw都四分之一
- crop_size = (((h0 - 1) // 8 * 8 + 8) // 4, ((w0 - 1) // 8 * 8 + 8) // 4) # 3.7G
- ph = ((h0 - 1) // crop_size[0] + 1) * crop_size[0]
- pw = ((w0 - 1) // crop_size[1] + 1) * crop_size[1]
- x = F.pad(x, (18, 18 + pw - w0, 18, 18 + ph - h0), 'reflect')
- n, c, h, w = x.shape
- se_mean0 = torch.zeros((n, 64, 1, 1)).to(x.device)
- if ("Half" in x.type()):
- se_mean0 = se_mean0.half()
- n_patch = 0
- tmp_dict = {}
- opt_res_dict = {}
- for i in range(0, h - 36, crop_size[0]):
- tmp_dict[i] = {}
- for j in range(0, w - 36, crop_size[1]):
- x_crop = x[:, :, i:i + crop_size[0] + 36, j:j + crop_size[1] + 36]
- n, c1, h1, w1 = x_crop.shape
- tmp0, x_crop = self.unet1.forward_a(x_crop)
- if ("Half" in x.type()): # torch.HalfTensor/torch.cuda.HalfTensor
- tmp_se_mean = torch.mean(x_crop.float(), dim=(2, 3), keepdim=True).half()
- else:
- tmp_se_mean = torch.mean(x_crop, dim=(2, 3), keepdim=True)
- se_mean0 += tmp_se_mean
- n_patch += 1
- tmp_dict[i][j] = (tmp0, x_crop)
- se_mean0 /= n_patch
- se_mean1 = torch.zeros((n, 128, 1, 1)).to(x.device) # 64#128#128#64
- if ("Half" in x.type()):
- se_mean1 = se_mean1.half()
- for i in range(0, h - 36, crop_size[0]):
- for j in range(0, w - 36, crop_size[1]):
- tmp0, x_crop = tmp_dict[i][j]
- x_crop = self.unet1.conv2.seblock.forward_mean(x_crop, se_mean0)
- opt_unet1 = self.unet1.forward_b(tmp0, x_crop)
- tmp_x1, tmp_x2 = self.unet2.forward_a(opt_unet1)
- if ("Half" in x.type()): # torch.HalfTensor/torch.cuda.HalfTensor
- tmp_se_mean = torch.mean(tmp_x2.float(), dim=(2, 3), keepdim=True).half()
- else:
- tmp_se_mean = torch.mean(tmp_x2, dim=(2, 3), keepdim=True)
- se_mean1 += tmp_se_mean
- tmp_dict[i][j] = (opt_unet1, tmp_x1, tmp_x2)
- se_mean1 /= n_patch
- se_mean0 = torch.zeros((n, 128, 1, 1)).to(x.device) # 64#128#128#64
- if ("Half" in x.type()):
- se_mean0 = se_mean0.half()
- for i in range(0, h - 36, crop_size[0]):
- for j in range(0, w - 36, crop_size[1]):
- opt_unet1, tmp_x1, tmp_x2 = tmp_dict[i][j]
- tmp_x2 = self.unet2.conv2.seblock.forward_mean(tmp_x2, se_mean1)
- tmp_x3 = self.unet2.forward_b(tmp_x2)
- if ("Half" in x.type()): # torch.HalfTensor/torch.cuda.HalfTensor
- tmp_se_mean = torch.mean(tmp_x3.float(), dim=(2, 3), keepdim=True).half()
- else:
- tmp_se_mean = torch.mean(tmp_x3, dim=(2, 3), keepdim=True)
- se_mean0 += tmp_se_mean
- tmp_dict[i][j] = (opt_unet1, tmp_x1, tmp_x2, tmp_x3)
- se_mean0 /= n_patch
- se_mean1 = torch.zeros((n, 64, 1, 1)).to(x.device) # 64#128#128#64
- if ("Half" in x.type()):
- se_mean1 = se_mean1.half()
- for i in range(0, h - 36, crop_size[0]):
- for j in range(0, w - 36, crop_size[1]):
- opt_unet1, tmp_x1, tmp_x2, tmp_x3 = tmp_dict[i][j]
- tmp_x3 = self.unet2.conv3.seblock.forward_mean(tmp_x3, se_mean0)
- tmp_x4 = self.unet2.forward_c(tmp_x2, tmp_x3)
- if ("Half" in x.type()): # torch.HalfTensor/torch.cuda.HalfTensor
- tmp_se_mean = torch.mean(tmp_x4.float(), dim=(2, 3), keepdim=True).half()
- else:
- tmp_se_mean = torch.mean(tmp_x4, dim=(2, 3), keepdim=True)
- se_mean1 += tmp_se_mean
- tmp_dict[i][j] = (opt_unet1, tmp_x1, tmp_x4)
- se_mean1 /= n_patch
- for i in range(0, h - 36, crop_size[0]):
- opt_res_dict[i] = {}
- for j in range(0, w - 36, crop_size[1]):
- opt_unet1, tmp_x1, tmp_x4 = tmp_dict[i][j]
- tmp_x4 = self.unet2.conv4.seblock.forward_mean(tmp_x4, se_mean1)
- x0 = self.unet2.forward_d(tmp_x1, tmp_x4)
- x1 = F.pad(opt_unet1, (-20, -20, -20, -20))
- x_crop = torch.add(x0, x1) # x0是unet2的最终输出
- opt_res_dict[i][j] = x_crop
- del tmp_dict
- torch.cuda.empty_cache()
- res = torch.zeros((n, c, h * 2 - 72, w * 2 - 72)).to(x.device)
- if ("Half" in x.type()):
- res = res.half()
- for i in range(0, h - 36, crop_size[0]):
- for j in range(0, w - 36, crop_size[1]):
- res[:, :, i * 2:i * 2 + h1 * 2 - 72, j * 2:j * 2 + w1 * 2 - 72] = opt_res_dict[i][j]
- del opt_res_dict
- torch.cuda.empty_cache()
- if (w0 != pw or h0 != ph): res = res[:, :, :h0 * 2, :w0 * 2]
- return res #
-
-
-class UpCunet3x(nn.Module): # 完美tile,全程无损
- def __init__(self, in_channels=3, out_channels=3):
- super(UpCunet3x, self).__init__()
- self.unet1 = UNet1x3(in_channels, out_channels, deconv=True)
- self.unet2 = UNet2(in_channels, out_channels, deconv=False)
-
- def forward(self, x, tile_mode): # 1.7G
- n, c, h0, w0 = x.shape
- if (tile_mode == 0): # 不tile
- ph = ((h0 - 1) // 4 + 1) * 4
- pw = ((w0 - 1) // 4 + 1) * 4
- x = F.pad(x, (14, 14 + pw - w0, 14, 14 + ph - h0), 'reflect') # 需要保证被2整除
- x = self.unet1.forward(x)
- x0 = self.unet2.forward(x)
- x1 = F.pad(x, (-20, -20, -20, -20))
- x = torch.add(x0, x1)
- if (w0 != pw or h0 != ph): x = x[:, :, :h0 * 3, :w0 * 3]
- return x
- elif (tile_mode == 1): # 对长边减半
- if (w0 >= h0):
- crop_size_w = ((w0 - 1) // 8 * 8 + 8) // 2 # 减半后能被4整除,所以要先被8整除
- crop_size_h = (h0 - 1) // 4 * 4 + 4 # 能被4整除
- else:
- crop_size_h = ((h0 - 1) // 8 * 8 + 8) // 2 # 减半后能被4整除,所以要先被8整除
- crop_size_w = (w0 - 1) // 4 * 4 + 4 # 能被4整除
- crop_size = (crop_size_h, crop_size_w) # 6.6G
- elif (tile_mode == 2): # hw都减半
- crop_size = (((h0 - 1) // 8 * 8 + 8) // 2, ((w0 - 1) // 8 * 8 + 8) // 2) # 5.6G
- elif (tile_mode == 3): # hw都三分之一
- crop_size = (((h0 - 1) // 12 * 12 + 12) // 3, ((w0 - 1) // 12 * 12 + 12) // 3) # 4.2G
- elif (tile_mode == 4): # hw都四分之一
- crop_size = (((h0 - 1) // 16 * 16 + 16) // 4, ((w0 - 1) // 16 * 16 + 16) // 4) # 3.7G
- ph = ((h0 - 1) // crop_size[0] + 1) * crop_size[0]
- pw = ((w0 - 1) // crop_size[1] + 1) * crop_size[1]
- x = F.pad(x, (14, 14 + pw - w0, 14, 14 + ph - h0), 'reflect')
- n, c, h, w = x.shape
- se_mean0 = torch.zeros((n, 64, 1, 1)).to(x.device)
- if ("Half" in x.type()):
- se_mean0 = se_mean0.half()
- n_patch = 0
- tmp_dict = {}
- opt_res_dict = {}
- for i in range(0, h - 28, crop_size[0]):
- tmp_dict[i] = {}
- for j in range(0, w - 28, crop_size[1]):
- x_crop = x[:, :, i:i + crop_size[0] + 28, j:j + crop_size[1] + 28]
- n, c1, h1, w1 = x_crop.shape
- tmp0, x_crop = self.unet1.forward_a(x_crop)
- if ("Half" in x.type()): # torch.HalfTensor/torch.cuda.HalfTensor
- tmp_se_mean = torch.mean(x_crop.float(), dim=(2, 3), keepdim=True).half()
- else:
- tmp_se_mean = torch.mean(x_crop, dim=(2, 3), keepdim=True)
- se_mean0 += tmp_se_mean
- n_patch += 1
- tmp_dict[i][j] = (tmp0, x_crop)
- se_mean0 /= n_patch
- se_mean1 = torch.zeros((n, 128, 1, 1)).to(x.device) # 64#128#128#64
- if ("Half" in x.type()):
- se_mean1 = se_mean1.half()
- for i in range(0, h - 28, crop_size[0]):
- for j in range(0, w - 28, crop_size[1]):
- tmp0, x_crop = tmp_dict[i][j]
- x_crop = self.unet1.conv2.seblock.forward_mean(x_crop, se_mean0)
- opt_unet1 = self.unet1.forward_b(tmp0, x_crop)
- tmp_x1, tmp_x2 = self.unet2.forward_a(opt_unet1)
- if ("Half" in x.type()): # torch.HalfTensor/torch.cuda.HalfTensor
- tmp_se_mean = torch.mean(tmp_x2.float(), dim=(2, 3), keepdim=True).half()
- else:
- tmp_se_mean = torch.mean(tmp_x2, dim=(2, 3), keepdim=True)
- se_mean1 += tmp_se_mean
- tmp_dict[i][j] = (opt_unet1, tmp_x1, tmp_x2)
- se_mean1 /= n_patch
- se_mean0 = torch.zeros((n, 128, 1, 1)).to(x.device) # 64#128#128#64
- if ("Half" in x.type()):
- se_mean0 = se_mean0.half()
- for i in range(0, h - 28, crop_size[0]):
- for j in range(0, w - 28, crop_size[1]):
- opt_unet1, tmp_x1, tmp_x2 = tmp_dict[i][j]
- tmp_x2 = self.unet2.conv2.seblock.forward_mean(tmp_x2, se_mean1)
- tmp_x3 = self.unet2.forward_b(tmp_x2)
- if ("Half" in x.type()): # torch.HalfTensor/torch.cuda.HalfTensor
- tmp_se_mean = torch.mean(tmp_x3.float(), dim=(2, 3), keepdim=True).half()
- else:
- tmp_se_mean = torch.mean(tmp_x3, dim=(2, 3), keepdim=True)
- se_mean0 += tmp_se_mean
- tmp_dict[i][j] = (opt_unet1, tmp_x1, tmp_x2, tmp_x3)
- se_mean0 /= n_patch
- se_mean1 = torch.zeros((n, 64, 1, 1)).to(x.device) # 64#128#128#64
- if ("Half" in x.type()):
- se_mean1 = se_mean1.half()
- for i in range(0, h - 28, crop_size[0]):
- for j in range(0, w - 28, crop_size[1]):
- opt_unet1, tmp_x1, tmp_x2, tmp_x3 = tmp_dict[i][j]
- tmp_x3 = self.unet2.conv3.seblock.forward_mean(tmp_x3, se_mean0)
- tmp_x4 = self.unet2.forward_c(tmp_x2, tmp_x3)
- if ("Half" in x.type()): # torch.HalfTensor/torch.cuda.HalfTensor
- tmp_se_mean = torch.mean(tmp_x4.float(), dim=(2, 3), keepdim=True).half()
- else:
- tmp_se_mean = torch.mean(tmp_x4, dim=(2, 3), keepdim=True)
- se_mean1 += tmp_se_mean
- tmp_dict[i][j] = (opt_unet1, tmp_x1, tmp_x4)
- se_mean1 /= n_patch
- for i in range(0, h - 28, crop_size[0]):
- opt_res_dict[i] = {}
- for j in range(0, w - 28, crop_size[1]):
- opt_unet1, tmp_x1, tmp_x4 = tmp_dict[i][j]
- tmp_x4 = self.unet2.conv4.seblock.forward_mean(tmp_x4, se_mean1)
- x0 = self.unet2.forward_d(tmp_x1, tmp_x4)
- x1 = F.pad(opt_unet1, (-20, -20, -20, -20))
- x_crop = torch.add(x0, x1) # x0是unet2的最终输出
- opt_res_dict[i][j] = x_crop #
- del tmp_dict
- torch.cuda.empty_cache()
- res = torch.zeros((n, c, h * 3 - 84, w * 3 - 84)).to(x.device)
- if ("Half" in x.type()):
- res = res.half()
- for i in range(0, h - 28, crop_size[0]):
- for j in range(0, w - 28, crop_size[1]):
- res[:, :, i * 3:i * 3 + h1 * 3 - 84, j * 3:j * 3 + w1 * 3 - 84] = opt_res_dict[i][j]
- del opt_res_dict
- torch.cuda.empty_cache()
- if (w0 != pw or h0 != ph): res = res[:, :, :h0 * 3, :w0 * 3]
- return res
-
-
-class UpCunet4x(nn.Module): # 完美tile,全程无损
- def __init__(self, in_channels=3, out_channels=3):
- super(UpCunet4x, self).__init__()
- self.unet1 = UNet1(in_channels, 64, deconv=True)
- self.unet2 = UNet2(64, 64, deconv=False)
- self.ps = nn.PixelShuffle(2)
- self.conv_final = nn.Conv2d(64, 12, 3, 1, padding=0, bias=True)
-
- def forward(self, x, tile_mode):
- n, c, h0, w0 = x.shape
- x00 = x
- if (tile_mode == 0): # 不tile
- ph = ((h0 - 1) // 2 + 1) * 2
- pw = ((w0 - 1) // 2 + 1) * 2
- x = F.pad(x, (19, 19 + pw - w0, 19, 19 + ph - h0), 'reflect') # 需要保证被2整除
- x = self.unet1.forward(x)
- x0 = self.unet2.forward(x)
- x1 = F.pad(x, (-20, -20, -20, -20))
- x = torch.add(x0, x1)
- x = self.conv_final(x)
- x = F.pad(x, (-1, -1, -1, -1))
- x = self.ps(x)
- if (w0 != pw or h0 != ph): x = x[:, :, :h0 * 4, :w0 * 4]
- x += F.interpolate(x00, scale_factor=4, mode='nearest')
- return x
- elif (tile_mode == 1): # 对长边减半
- if (w0 >= h0):
- crop_size_w = ((w0 - 1) // 4 * 4 + 4) // 2 # 减半后能被2整除,所以要先被4整除
- crop_size_h = (h0 - 1) // 2 * 2 + 2 # 能被2整除
- else:
- crop_size_h = ((h0 - 1) // 4 * 4 + 4) // 2 # 减半后能被2整除,所以要先被4整除
- crop_size_w = (w0 - 1) // 2 * 2 + 2 # 能被2整除
- crop_size = (crop_size_h, crop_size_w) # 6.6G
- elif (tile_mode == 2): # hw都减半
- crop_size = (((h0 - 1) // 4 * 4 + 4) // 2, ((w0 - 1) // 4 * 4 + 4) // 2) # 5.6G
- elif (tile_mode == 3): # hw都三分之一
- crop_size = (((h0 - 1) // 6 * 6 + 6) // 3, ((w0 - 1) // 6 * 6 + 6) // 3) # 4.1G
- elif (tile_mode == 4): # hw都四分之一
- crop_size = (((h0 - 1) // 8 * 8 + 8) // 4, ((w0 - 1) // 8 * 8 + 8) // 4) # 3.7G
- ph = ((h0 - 1) // crop_size[0] + 1) * crop_size[0]
- pw = ((w0 - 1) // crop_size[1] + 1) * crop_size[1]
- x = F.pad(x, (19, 19 + pw - w0, 19, 19 + ph - h0), 'reflect')
- n, c, h, w = x.shape
- se_mean0 = torch.zeros((n, 64, 1, 1)).to(x.device)
- if ("Half" in x.type()):
- se_mean0 = se_mean0.half()
- n_patch = 0
- tmp_dict = {}
- opt_res_dict = {}
- for i in range(0, h - 38, crop_size[0]):
- tmp_dict[i] = {}
- for j in range(0, w - 38, crop_size[1]):
- x_crop = x[:, :, i:i + crop_size[0] + 38, j:j + crop_size[1] + 38]
- n, c1, h1, w1 = x_crop.shape
- tmp0, x_crop = self.unet1.forward_a(x_crop)
- if ("Half" in x.type()): # torch.HalfTensor/torch.cuda.HalfTensor
- tmp_se_mean = torch.mean(x_crop.float(), dim=(2, 3), keepdim=True).half()
- else:
- tmp_se_mean = torch.mean(x_crop, dim=(2, 3), keepdim=True)
- se_mean0 += tmp_se_mean
- n_patch += 1
- tmp_dict[i][j] = (tmp0, x_crop)
- se_mean0 /= n_patch
- se_mean1 = torch.zeros((n, 128, 1, 1)).to(x.device) # 64#128#128#64
- if ("Half" in x.type()):
- se_mean1 = se_mean1.half()
- for i in range(0, h - 38, crop_size[0]):
- for j in range(0, w - 38, crop_size[1]):
- tmp0, x_crop = tmp_dict[i][j]
- x_crop = self.unet1.conv2.seblock.forward_mean(x_crop, se_mean0)
- opt_unet1 = self.unet1.forward_b(tmp0, x_crop)
- tmp_x1, tmp_x2 = self.unet2.forward_a(opt_unet1)
- if ("Half" in x.type()): # torch.HalfTensor/torch.cuda.HalfTensor
- tmp_se_mean = torch.mean(tmp_x2.float(), dim=(2, 3), keepdim=True).half()
- else:
- tmp_se_mean = torch.mean(tmp_x2, dim=(2, 3), keepdim=True)
- se_mean1 += tmp_se_mean
- tmp_dict[i][j] = (opt_unet1, tmp_x1, tmp_x2)
- se_mean1 /= n_patch
- se_mean0 = torch.zeros((n, 128, 1, 1)).to(x.device) # 64#128#128#64
- if ("Half" in x.type()):
- se_mean0 = se_mean0.half()
- for i in range(0, h - 38, crop_size[0]):
- for j in range(0, w - 38, crop_size[1]):
- opt_unet1, tmp_x1, tmp_x2 = tmp_dict[i][j]
- tmp_x2 = self.unet2.conv2.seblock.forward_mean(tmp_x2, se_mean1)
- tmp_x3 = self.unet2.forward_b(tmp_x2)
- if ("Half" in x.type()): # torch.HalfTensor/torch.cuda.HalfTensor
- tmp_se_mean = torch.mean(tmp_x3.float(), dim=(2, 3), keepdim=True).half()
- else:
- tmp_se_mean = torch.mean(tmp_x3, dim=(2, 3), keepdim=True)
- se_mean0 += tmp_se_mean
- tmp_dict[i][j] = (opt_unet1, tmp_x1, tmp_x2, tmp_x3)
- se_mean0 /= n_patch
- se_mean1 = torch.zeros((n, 64, 1, 1)).to(x.device) # 64#128#128#64
- if ("Half" in x.type()):
- se_mean1 = se_mean1.half()
- for i in range(0, h - 38, crop_size[0]):
- for j in range(0, w - 38, crop_size[1]):
- opt_unet1, tmp_x1, tmp_x2, tmp_x3 = tmp_dict[i][j]
- tmp_x3 = self.unet2.conv3.seblock.forward_mean(tmp_x3, se_mean0)
- tmp_x4 = self.unet2.forward_c(tmp_x2, tmp_x3)
- if ("Half" in x.type()): # torch.HalfTensor/torch.cuda.HalfTensor
- tmp_se_mean = torch.mean(tmp_x4.float(), dim=(2, 3), keepdim=True).half()
- else:
- tmp_se_mean = torch.mean(tmp_x4, dim=(2, 3), keepdim=True)
- se_mean1 += tmp_se_mean
- tmp_dict[i][j] = (opt_unet1, tmp_x1, tmp_x4)
- se_mean1 /= n_patch
- for i in range(0, h - 38, crop_size[0]):
- opt_res_dict[i] = {}
- for j in range(0, w - 38, crop_size[1]):
- opt_unet1, tmp_x1, tmp_x4 = tmp_dict[i][j]
- tmp_x4 = self.unet2.conv4.seblock.forward_mean(tmp_x4, se_mean1)
- x0 = self.unet2.forward_d(tmp_x1, tmp_x4)
- x1 = F.pad(opt_unet1, (-20, -20, -20, -20))
- x_crop = torch.add(x0, x1) # x0是unet2的最终输出
- x_crop = self.conv_final(x_crop)
- x_crop = F.pad(x_crop, (-1, -1, -1, -1))
- x_crop = self.ps(x_crop)
- opt_res_dict[i][j] = x_crop
- del tmp_dict
- torch.cuda.empty_cache()
- res = torch.zeros((n, c, h * 4 - 152, w * 4 - 152)).to(x.device)
- if ("Half" in x.type()):
- res = res.half()
- for i in range(0, h - 38, crop_size[0]):
- for j in range(0, w - 38, crop_size[1]):
- # print(opt_res_dict[i][j].shape,res[:, :, i * 4:i * 4 + h1 * 4 - 144, j * 4:j * 4 + w1 * 4 - 144].shape)
- res[:, :, i * 4:i * 4 + h1 * 4 - 152, j * 4:j * 4 + w1 * 4 - 152] = opt_res_dict[i][j]
- del opt_res_dict
- torch.cuda.empty_cache()
- if (w0 != pw or h0 != ph): res = res[:, :, :h0 * 4, :w0 * 4]
- res += F.interpolate(x00, scale_factor=4, mode='nearest')
- return res #
-
-
-class RealWaifuUpScaler(object):
- def __init__(self, scale, weight_path, half, device):
- weight = torch.load(weight_path, map_location="cpu")
- self.model = eval("UpCunet%sx" % scale)()
- if (half == True):
- self.model = self.model.half().to(device)
- else:
- self.model = self.model.to(device)
- self.model.load_state_dict(weight, strict=True)
- self.model.eval()
- self.half = half
- self.device = device
-
- def np2tensor(self, np_frame):
- if (self.half == False):
- return torch.from_numpy(np.transpose(np_frame, (2, 0, 1))).unsqueeze(0).to(self.device).float() / 255
- else:
- return torch.from_numpy(np.transpose(np_frame, (2, 0, 1))).unsqueeze(0).to(self.device).half() / 255
-
- def tensor2np(self, tensor):
- if (self.half == False):
- return (
- np.transpose((tensor.data.squeeze() * 255.0).round().clamp_(0, 255).byte().cpu().numpy(), (1, 2, 0)))
- else:
- return (np.transpose((tensor.data.squeeze().float() * 255.0).round().clamp_(0, 255).byte().cpu().numpy(),
- (1, 2, 0)))
-
- def __call__(self, frame, tile_mode):
- with torch.no_grad():
- tensor = self.np2tensor(frame)
- result = self.tensor2np(self.model(tensor, tile_mode))
- return result
-
-
-if __name__ == "__main__":
- ###########inference_img
- import time, cv2, sys
- from time import time as ttime
-
- for weight_path, scale in [("weights_v3/up2x-latest-denoise3x.pth", 2), ("weights_v3/up3x-latest-denoise3x.pth", 3),
- ("weights_v3/up4x-latest-denoise3x.pth", 4)]:
- for tile_mode in [0, 1, 2, 3, 4]:
- upscaler2x = RealWaifuUpScaler(scale, weight_path, half=True, device="cuda:0")
- input_dir = "%s/input_dir1" % root_path
- output_dir = "%s/opt-dir-all-test" % root_path
- os.makedirs(output_dir, exist_ok=True)
- for name in os.listdir(input_dir):
- print(name)
- tmp = name.split(".")
- inp_path = os.path.join(input_dir, name)
- suffix = tmp[-1]
- prefix = ".".join(tmp[:-1])
- tmp_path = os.path.join(root_path, "tmp", "%s.%s" % (int(time.time() * 1000000), suffix))
- print(inp_path, tmp_path)
- # 支持中文路径
- # os.link(inp_path, tmp_path)#win用硬链接
- os.symlink(inp_path, tmp_path) # linux用软链接
- frame = cv2.imread(tmp_path)[:, :, [2, 1, 0]]
- t0 = ttime()
- result = upscaler2x(frame, tile_mode=tile_mode)[:, :, ::-1]
- t1 = ttime()
- print(prefix, "done", t1 - t0)
- tmp_opt_path = os.path.join(root_path, "tmp", "%s.%s" % (int(time.time() * 1000000), suffix))
- cv2.imwrite(tmp_opt_path, result)
- n = 0
- while (1):
- if (n == 0):
- suffix = "_%sx_tile%s.png" % (scale, tile_mode)
- else:
- suffix = "_%sx_tile%s_%s.png" % (scale, tile_mode, n) #
- if (os.path.exists(os.path.join(output_dir, prefix + suffix)) == False):
- break
- else:
- n += 1
- final_opt_path = os.path.join(output_dir, prefix + suffix)
- os.rename(tmp_opt_path, final_opt_path)
- os.remove(tmp_path)
diff --git a/spaces/TandCAcceptMe/face-swap-docker/mynewshinyroop/Lib/site-packages/pip/_internal/cli/status_codes.py b/spaces/TandCAcceptMe/face-swap-docker/mynewshinyroop/Lib/site-packages/pip/_internal/cli/status_codes.py
deleted file mode 100644
index 5e29502cddfa9a9887a93399ab4193fb75dfe605..0000000000000000000000000000000000000000
--- a/spaces/TandCAcceptMe/face-swap-docker/mynewshinyroop/Lib/site-packages/pip/_internal/cli/status_codes.py
+++ /dev/null
@@ -1,6 +0,0 @@
-SUCCESS = 0
-ERROR = 1
-UNKNOWN_ERROR = 2
-VIRTUALENV_NOT_FOUND = 3
-PREVIOUS_BUILD_DIR_ERROR = 4
-NO_MATCHES_FOUND = 23
diff --git a/spaces/TandCAcceptMe/face-swap-docker/mynewshinyroop/Lib/site-packages/pip/_vendor/urllib3/packages/six.py b/spaces/TandCAcceptMe/face-swap-docker/mynewshinyroop/Lib/site-packages/pip/_vendor/urllib3/packages/six.py
deleted file mode 100644
index f099a3dcd28d2fec21457c9b6c01ded4e3e9ddee..0000000000000000000000000000000000000000
--- a/spaces/TandCAcceptMe/face-swap-docker/mynewshinyroop/Lib/site-packages/pip/_vendor/urllib3/packages/six.py
+++ /dev/null
@@ -1,1076 +0,0 @@
-# Copyright (c) 2010-2020 Benjamin Peterson
-#
-# Permission is hereby granted, free of charge, to any person obtaining a copy
-# of this software and associated documentation files (the "Software"), to deal
-# in the Software without restriction, including without limitation the rights
-# to use, copy, modify, merge, publish, distribute, sublicense, and/or sell
-# copies of the Software, and to permit persons to whom the Software is
-# furnished to do so, subject to the following conditions:
-#
-# The above copyright notice and this permission notice shall be included in all
-# copies or substantial portions of the Software.
-#
-# THE SOFTWARE IS PROVIDED "AS IS", WITHOUT WARRANTY OF ANY KIND, EXPRESS OR
-# IMPLIED, INCLUDING BUT NOT LIMITED TO THE WARRANTIES OF MERCHANTABILITY,
-# FITNESS FOR A PARTICULAR PURPOSE AND NONINFRINGEMENT. IN NO EVENT SHALL THE
-# AUTHORS OR COPYRIGHT HOLDERS BE LIABLE FOR ANY CLAIM, DAMAGES OR OTHER
-# LIABILITY, WHETHER IN AN ACTION OF CONTRACT, TORT OR OTHERWISE, ARISING FROM,
-# OUT OF OR IN CONNECTION WITH THE SOFTWARE OR THE USE OR OTHER DEALINGS IN THE
-# SOFTWARE.
-
-"""Utilities for writing code that runs on Python 2 and 3"""
-
-from __future__ import absolute_import
-
-import functools
-import itertools
-import operator
-import sys
-import types
-
-__author__ = "Benjamin Peterson "
-__version__ = "1.16.0"
-
-
-# Useful for very coarse version differentiation.
-PY2 = sys.version_info[0] == 2
-PY3 = sys.version_info[0] == 3
-PY34 = sys.version_info[0:2] >= (3, 4)
-
-if PY3:
- string_types = (str,)
- integer_types = (int,)
- class_types = (type,)
- text_type = str
- binary_type = bytes
-
- MAXSIZE = sys.maxsize
-else:
- string_types = (basestring,)
- integer_types = (int, long)
- class_types = (type, types.ClassType)
- text_type = unicode
- binary_type = str
-
- if sys.platform.startswith("java"):
- # Jython always uses 32 bits.
- MAXSIZE = int((1 << 31) - 1)
- else:
- # It's possible to have sizeof(long) != sizeof(Py_ssize_t).
- class X(object):
- def __len__(self):
- return 1 << 31
-
- try:
- len(X())
- except OverflowError:
- # 32-bit
- MAXSIZE = int((1 << 31) - 1)
- else:
- # 64-bit
- MAXSIZE = int((1 << 63) - 1)
- del X
-
-if PY34:
- from importlib.util import spec_from_loader
-else:
- spec_from_loader = None
-
-
-def _add_doc(func, doc):
- """Add documentation to a function."""
- func.__doc__ = doc
-
-
-def _import_module(name):
- """Import module, returning the module after the last dot."""
- __import__(name)
- return sys.modules[name]
-
-
-class _LazyDescr(object):
- def __init__(self, name):
- self.name = name
-
- def __get__(self, obj, tp):
- result = self._resolve()
- setattr(obj, self.name, result) # Invokes __set__.
- try:
- # This is a bit ugly, but it avoids running this again by
- # removing this descriptor.
- delattr(obj.__class__, self.name)
- except AttributeError:
- pass
- return result
-
-
-class MovedModule(_LazyDescr):
- def __init__(self, name, old, new=None):
- super(MovedModule, self).__init__(name)
- if PY3:
- if new is None:
- new = name
- self.mod = new
- else:
- self.mod = old
-
- def _resolve(self):
- return _import_module(self.mod)
-
- def __getattr__(self, attr):
- _module = self._resolve()
- value = getattr(_module, attr)
- setattr(self, attr, value)
- return value
-
-
-class _LazyModule(types.ModuleType):
- def __init__(self, name):
- super(_LazyModule, self).__init__(name)
- self.__doc__ = self.__class__.__doc__
-
- def __dir__(self):
- attrs = ["__doc__", "__name__"]
- attrs += [attr.name for attr in self._moved_attributes]
- return attrs
-
- # Subclasses should override this
- _moved_attributes = []
-
-
-class MovedAttribute(_LazyDescr):
- def __init__(self, name, old_mod, new_mod, old_attr=None, new_attr=None):
- super(MovedAttribute, self).__init__(name)
- if PY3:
- if new_mod is None:
- new_mod = name
- self.mod = new_mod
- if new_attr is None:
- if old_attr is None:
- new_attr = name
- else:
- new_attr = old_attr
- self.attr = new_attr
- else:
- self.mod = old_mod
- if old_attr is None:
- old_attr = name
- self.attr = old_attr
-
- def _resolve(self):
- module = _import_module(self.mod)
- return getattr(module, self.attr)
-
-
-class _SixMetaPathImporter(object):
-
- """
- A meta path importer to import six.moves and its submodules.
-
- This class implements a PEP302 finder and loader. It should be compatible
- with Python 2.5 and all existing versions of Python3
- """
-
- def __init__(self, six_module_name):
- self.name = six_module_name
- self.known_modules = {}
-
- def _add_module(self, mod, *fullnames):
- for fullname in fullnames:
- self.known_modules[self.name + "." + fullname] = mod
-
- def _get_module(self, fullname):
- return self.known_modules[self.name + "." + fullname]
-
- def find_module(self, fullname, path=None):
- if fullname in self.known_modules:
- return self
- return None
-
- def find_spec(self, fullname, path, target=None):
- if fullname in self.known_modules:
- return spec_from_loader(fullname, self)
- return None
-
- def __get_module(self, fullname):
- try:
- return self.known_modules[fullname]
- except KeyError:
- raise ImportError("This loader does not know module " + fullname)
-
- def load_module(self, fullname):
- try:
- # in case of a reload
- return sys.modules[fullname]
- except KeyError:
- pass
- mod = self.__get_module(fullname)
- if isinstance(mod, MovedModule):
- mod = mod._resolve()
- else:
- mod.__loader__ = self
- sys.modules[fullname] = mod
- return mod
-
- def is_package(self, fullname):
- """
- Return true, if the named module is a package.
-
- We need this method to get correct spec objects with
- Python 3.4 (see PEP451)
- """
- return hasattr(self.__get_module(fullname), "__path__")
-
- def get_code(self, fullname):
- """Return None
-
- Required, if is_package is implemented"""
- self.__get_module(fullname) # eventually raises ImportError
- return None
-
- get_source = get_code # same as get_code
-
- def create_module(self, spec):
- return self.load_module(spec.name)
-
- def exec_module(self, module):
- pass
-
-
-_importer = _SixMetaPathImporter(__name__)
-
-
-class _MovedItems(_LazyModule):
-
- """Lazy loading of moved objects"""
-
- __path__ = [] # mark as package
-
-
-_moved_attributes = [
- MovedAttribute("cStringIO", "cStringIO", "io", "StringIO"),
- MovedAttribute("filter", "itertools", "builtins", "ifilter", "filter"),
- MovedAttribute(
- "filterfalse", "itertools", "itertools", "ifilterfalse", "filterfalse"
- ),
- MovedAttribute("input", "__builtin__", "builtins", "raw_input", "input"),
- MovedAttribute("intern", "__builtin__", "sys"),
- MovedAttribute("map", "itertools", "builtins", "imap", "map"),
- MovedAttribute("getcwd", "os", "os", "getcwdu", "getcwd"),
- MovedAttribute("getcwdb", "os", "os", "getcwd", "getcwdb"),
- MovedAttribute("getoutput", "commands", "subprocess"),
- MovedAttribute("range", "__builtin__", "builtins", "xrange", "range"),
- MovedAttribute(
- "reload_module", "__builtin__", "importlib" if PY34 else "imp", "reload"
- ),
- MovedAttribute("reduce", "__builtin__", "functools"),
- MovedAttribute("shlex_quote", "pipes", "shlex", "quote"),
- MovedAttribute("StringIO", "StringIO", "io"),
- MovedAttribute("UserDict", "UserDict", "collections"),
- MovedAttribute("UserList", "UserList", "collections"),
- MovedAttribute("UserString", "UserString", "collections"),
- MovedAttribute("xrange", "__builtin__", "builtins", "xrange", "range"),
- MovedAttribute("zip", "itertools", "builtins", "izip", "zip"),
- MovedAttribute(
- "zip_longest", "itertools", "itertools", "izip_longest", "zip_longest"
- ),
- MovedModule("builtins", "__builtin__"),
- MovedModule("configparser", "ConfigParser"),
- MovedModule(
- "collections_abc",
- "collections",
- "collections.abc" if sys.version_info >= (3, 3) else "collections",
- ),
- MovedModule("copyreg", "copy_reg"),
- MovedModule("dbm_gnu", "gdbm", "dbm.gnu"),
- MovedModule("dbm_ndbm", "dbm", "dbm.ndbm"),
- MovedModule(
- "_dummy_thread",
- "dummy_thread",
- "_dummy_thread" if sys.version_info < (3, 9) else "_thread",
- ),
- MovedModule("http_cookiejar", "cookielib", "http.cookiejar"),
- MovedModule("http_cookies", "Cookie", "http.cookies"),
- MovedModule("html_entities", "htmlentitydefs", "html.entities"),
- MovedModule("html_parser", "HTMLParser", "html.parser"),
- MovedModule("http_client", "httplib", "http.client"),
- MovedModule("email_mime_base", "email.MIMEBase", "email.mime.base"),
- MovedModule("email_mime_image", "email.MIMEImage", "email.mime.image"),
- MovedModule("email_mime_multipart", "email.MIMEMultipart", "email.mime.multipart"),
- MovedModule(
- "email_mime_nonmultipart", "email.MIMENonMultipart", "email.mime.nonmultipart"
- ),
- MovedModule("email_mime_text", "email.MIMEText", "email.mime.text"),
- MovedModule("BaseHTTPServer", "BaseHTTPServer", "http.server"),
- MovedModule("CGIHTTPServer", "CGIHTTPServer", "http.server"),
- MovedModule("SimpleHTTPServer", "SimpleHTTPServer", "http.server"),
- MovedModule("cPickle", "cPickle", "pickle"),
- MovedModule("queue", "Queue"),
- MovedModule("reprlib", "repr"),
- MovedModule("socketserver", "SocketServer"),
- MovedModule("_thread", "thread", "_thread"),
- MovedModule("tkinter", "Tkinter"),
- MovedModule("tkinter_dialog", "Dialog", "tkinter.dialog"),
- MovedModule("tkinter_filedialog", "FileDialog", "tkinter.filedialog"),
- MovedModule("tkinter_scrolledtext", "ScrolledText", "tkinter.scrolledtext"),
- MovedModule("tkinter_simpledialog", "SimpleDialog", "tkinter.simpledialog"),
- MovedModule("tkinter_tix", "Tix", "tkinter.tix"),
- MovedModule("tkinter_ttk", "ttk", "tkinter.ttk"),
- MovedModule("tkinter_constants", "Tkconstants", "tkinter.constants"),
- MovedModule("tkinter_dnd", "Tkdnd", "tkinter.dnd"),
- MovedModule("tkinter_colorchooser", "tkColorChooser", "tkinter.colorchooser"),
- MovedModule("tkinter_commondialog", "tkCommonDialog", "tkinter.commondialog"),
- MovedModule("tkinter_tkfiledialog", "tkFileDialog", "tkinter.filedialog"),
- MovedModule("tkinter_font", "tkFont", "tkinter.font"),
- MovedModule("tkinter_messagebox", "tkMessageBox", "tkinter.messagebox"),
- MovedModule("tkinter_tksimpledialog", "tkSimpleDialog", "tkinter.simpledialog"),
- MovedModule("urllib_parse", __name__ + ".moves.urllib_parse", "urllib.parse"),
- MovedModule("urllib_error", __name__ + ".moves.urllib_error", "urllib.error"),
- MovedModule("urllib", __name__ + ".moves.urllib", __name__ + ".moves.urllib"),
- MovedModule("urllib_robotparser", "robotparser", "urllib.robotparser"),
- MovedModule("xmlrpc_client", "xmlrpclib", "xmlrpc.client"),
- MovedModule("xmlrpc_server", "SimpleXMLRPCServer", "xmlrpc.server"),
-]
-# Add windows specific modules.
-if sys.platform == "win32":
- _moved_attributes += [
- MovedModule("winreg", "_winreg"),
- ]
-
-for attr in _moved_attributes:
- setattr(_MovedItems, attr.name, attr)
- if isinstance(attr, MovedModule):
- _importer._add_module(attr, "moves." + attr.name)
-del attr
-
-_MovedItems._moved_attributes = _moved_attributes
-
-moves = _MovedItems(__name__ + ".moves")
-_importer._add_module(moves, "moves")
-
-
-class Module_six_moves_urllib_parse(_LazyModule):
-
- """Lazy loading of moved objects in six.moves.urllib_parse"""
-
-
-_urllib_parse_moved_attributes = [
- MovedAttribute("ParseResult", "urlparse", "urllib.parse"),
- MovedAttribute("SplitResult", "urlparse", "urllib.parse"),
- MovedAttribute("parse_qs", "urlparse", "urllib.parse"),
- MovedAttribute("parse_qsl", "urlparse", "urllib.parse"),
- MovedAttribute("urldefrag", "urlparse", "urllib.parse"),
- MovedAttribute("urljoin", "urlparse", "urllib.parse"),
- MovedAttribute("urlparse", "urlparse", "urllib.parse"),
- MovedAttribute("urlsplit", "urlparse", "urllib.parse"),
- MovedAttribute("urlunparse", "urlparse", "urllib.parse"),
- MovedAttribute("urlunsplit", "urlparse", "urllib.parse"),
- MovedAttribute("quote", "urllib", "urllib.parse"),
- MovedAttribute("quote_plus", "urllib", "urllib.parse"),
- MovedAttribute("unquote", "urllib", "urllib.parse"),
- MovedAttribute("unquote_plus", "urllib", "urllib.parse"),
- MovedAttribute(
- "unquote_to_bytes", "urllib", "urllib.parse", "unquote", "unquote_to_bytes"
- ),
- MovedAttribute("urlencode", "urllib", "urllib.parse"),
- MovedAttribute("splitquery", "urllib", "urllib.parse"),
- MovedAttribute("splittag", "urllib", "urllib.parse"),
- MovedAttribute("splituser", "urllib", "urllib.parse"),
- MovedAttribute("splitvalue", "urllib", "urllib.parse"),
- MovedAttribute("uses_fragment", "urlparse", "urllib.parse"),
- MovedAttribute("uses_netloc", "urlparse", "urllib.parse"),
- MovedAttribute("uses_params", "urlparse", "urllib.parse"),
- MovedAttribute("uses_query", "urlparse", "urllib.parse"),
- MovedAttribute("uses_relative", "urlparse", "urllib.parse"),
-]
-for attr in _urllib_parse_moved_attributes:
- setattr(Module_six_moves_urllib_parse, attr.name, attr)
-del attr
-
-Module_six_moves_urllib_parse._moved_attributes = _urllib_parse_moved_attributes
-
-_importer._add_module(
- Module_six_moves_urllib_parse(__name__ + ".moves.urllib_parse"),
- "moves.urllib_parse",
- "moves.urllib.parse",
-)
-
-
-class Module_six_moves_urllib_error(_LazyModule):
-
- """Lazy loading of moved objects in six.moves.urllib_error"""
-
-
-_urllib_error_moved_attributes = [
- MovedAttribute("URLError", "urllib2", "urllib.error"),
- MovedAttribute("HTTPError", "urllib2", "urllib.error"),
- MovedAttribute("ContentTooShortError", "urllib", "urllib.error"),
-]
-for attr in _urllib_error_moved_attributes:
- setattr(Module_six_moves_urllib_error, attr.name, attr)
-del attr
-
-Module_six_moves_urllib_error._moved_attributes = _urllib_error_moved_attributes
-
-_importer._add_module(
- Module_six_moves_urllib_error(__name__ + ".moves.urllib.error"),
- "moves.urllib_error",
- "moves.urllib.error",
-)
-
-
-class Module_six_moves_urllib_request(_LazyModule):
-
- """Lazy loading of moved objects in six.moves.urllib_request"""
-
-
-_urllib_request_moved_attributes = [
- MovedAttribute("urlopen", "urllib2", "urllib.request"),
- MovedAttribute("install_opener", "urllib2", "urllib.request"),
- MovedAttribute("build_opener", "urllib2", "urllib.request"),
- MovedAttribute("pathname2url", "urllib", "urllib.request"),
- MovedAttribute("url2pathname", "urllib", "urllib.request"),
- MovedAttribute("getproxies", "urllib", "urllib.request"),
- MovedAttribute("Request", "urllib2", "urllib.request"),
- MovedAttribute("OpenerDirector", "urllib2", "urllib.request"),
- MovedAttribute("HTTPDefaultErrorHandler", "urllib2", "urllib.request"),
- MovedAttribute("HTTPRedirectHandler", "urllib2", "urllib.request"),
- MovedAttribute("HTTPCookieProcessor", "urllib2", "urllib.request"),
- MovedAttribute("ProxyHandler", "urllib2", "urllib.request"),
- MovedAttribute("BaseHandler", "urllib2", "urllib.request"),
- MovedAttribute("HTTPPasswordMgr", "urllib2", "urllib.request"),
- MovedAttribute("HTTPPasswordMgrWithDefaultRealm", "urllib2", "urllib.request"),
- MovedAttribute("AbstractBasicAuthHandler", "urllib2", "urllib.request"),
- MovedAttribute("HTTPBasicAuthHandler", "urllib2", "urllib.request"),
- MovedAttribute("ProxyBasicAuthHandler", "urllib2", "urllib.request"),
- MovedAttribute("AbstractDigestAuthHandler", "urllib2", "urllib.request"),
- MovedAttribute("HTTPDigestAuthHandler", "urllib2", "urllib.request"),
- MovedAttribute("ProxyDigestAuthHandler", "urllib2", "urllib.request"),
- MovedAttribute("HTTPHandler", "urllib2", "urllib.request"),
- MovedAttribute("HTTPSHandler", "urllib2", "urllib.request"),
- MovedAttribute("FileHandler", "urllib2", "urllib.request"),
- MovedAttribute("FTPHandler", "urllib2", "urllib.request"),
- MovedAttribute("CacheFTPHandler", "urllib2", "urllib.request"),
- MovedAttribute("UnknownHandler", "urllib2", "urllib.request"),
- MovedAttribute("HTTPErrorProcessor", "urllib2", "urllib.request"),
- MovedAttribute("urlretrieve", "urllib", "urllib.request"),
- MovedAttribute("urlcleanup", "urllib", "urllib.request"),
- MovedAttribute("URLopener", "urllib", "urllib.request"),
- MovedAttribute("FancyURLopener", "urllib", "urllib.request"),
- MovedAttribute("proxy_bypass", "urllib", "urllib.request"),
- MovedAttribute("parse_http_list", "urllib2", "urllib.request"),
- MovedAttribute("parse_keqv_list", "urllib2", "urllib.request"),
-]
-for attr in _urllib_request_moved_attributes:
- setattr(Module_six_moves_urllib_request, attr.name, attr)
-del attr
-
-Module_six_moves_urllib_request._moved_attributes = _urllib_request_moved_attributes
-
-_importer._add_module(
- Module_six_moves_urllib_request(__name__ + ".moves.urllib.request"),
- "moves.urllib_request",
- "moves.urllib.request",
-)
-
-
-class Module_six_moves_urllib_response(_LazyModule):
-
- """Lazy loading of moved objects in six.moves.urllib_response"""
-
-
-_urllib_response_moved_attributes = [
- MovedAttribute("addbase", "urllib", "urllib.response"),
- MovedAttribute("addclosehook", "urllib", "urllib.response"),
- MovedAttribute("addinfo", "urllib", "urllib.response"),
- MovedAttribute("addinfourl", "urllib", "urllib.response"),
-]
-for attr in _urllib_response_moved_attributes:
- setattr(Module_six_moves_urllib_response, attr.name, attr)
-del attr
-
-Module_six_moves_urllib_response._moved_attributes = _urllib_response_moved_attributes
-
-_importer._add_module(
- Module_six_moves_urllib_response(__name__ + ".moves.urllib.response"),
- "moves.urllib_response",
- "moves.urllib.response",
-)
-
-
-class Module_six_moves_urllib_robotparser(_LazyModule):
-
- """Lazy loading of moved objects in six.moves.urllib_robotparser"""
-
-
-_urllib_robotparser_moved_attributes = [
- MovedAttribute("RobotFileParser", "robotparser", "urllib.robotparser"),
-]
-for attr in _urllib_robotparser_moved_attributes:
- setattr(Module_six_moves_urllib_robotparser, attr.name, attr)
-del attr
-
-Module_six_moves_urllib_robotparser._moved_attributes = (
- _urllib_robotparser_moved_attributes
-)
-
-_importer._add_module(
- Module_six_moves_urllib_robotparser(__name__ + ".moves.urllib.robotparser"),
- "moves.urllib_robotparser",
- "moves.urllib.robotparser",
-)
-
-
-class Module_six_moves_urllib(types.ModuleType):
-
- """Create a six.moves.urllib namespace that resembles the Python 3 namespace"""
-
- __path__ = [] # mark as package
- parse = _importer._get_module("moves.urllib_parse")
- error = _importer._get_module("moves.urllib_error")
- request = _importer._get_module("moves.urllib_request")
- response = _importer._get_module("moves.urllib_response")
- robotparser = _importer._get_module("moves.urllib_robotparser")
-
- def __dir__(self):
- return ["parse", "error", "request", "response", "robotparser"]
-
-
-_importer._add_module(
- Module_six_moves_urllib(__name__ + ".moves.urllib"), "moves.urllib"
-)
-
-
-def add_move(move):
- """Add an item to six.moves."""
- setattr(_MovedItems, move.name, move)
-
-
-def remove_move(name):
- """Remove item from six.moves."""
- try:
- delattr(_MovedItems, name)
- except AttributeError:
- try:
- del moves.__dict__[name]
- except KeyError:
- raise AttributeError("no such move, %r" % (name,))
-
-
-if PY3:
- _meth_func = "__func__"
- _meth_self = "__self__"
-
- _func_closure = "__closure__"
- _func_code = "__code__"
- _func_defaults = "__defaults__"
- _func_globals = "__globals__"
-else:
- _meth_func = "im_func"
- _meth_self = "im_self"
-
- _func_closure = "func_closure"
- _func_code = "func_code"
- _func_defaults = "func_defaults"
- _func_globals = "func_globals"
-
-
-try:
- advance_iterator = next
-except NameError:
-
- def advance_iterator(it):
- return it.next()
-
-
-next = advance_iterator
-
-
-try:
- callable = callable
-except NameError:
-
- def callable(obj):
- return any("__call__" in klass.__dict__ for klass in type(obj).__mro__)
-
-
-if PY3:
-
- def get_unbound_function(unbound):
- return unbound
-
- create_bound_method = types.MethodType
-
- def create_unbound_method(func, cls):
- return func
-
- Iterator = object
-else:
-
- def get_unbound_function(unbound):
- return unbound.im_func
-
- def create_bound_method(func, obj):
- return types.MethodType(func, obj, obj.__class__)
-
- def create_unbound_method(func, cls):
- return types.MethodType(func, None, cls)
-
- class Iterator(object):
- def next(self):
- return type(self).__next__(self)
-
- callable = callable
-_add_doc(
- get_unbound_function, """Get the function out of a possibly unbound function"""
-)
-
-
-get_method_function = operator.attrgetter(_meth_func)
-get_method_self = operator.attrgetter(_meth_self)
-get_function_closure = operator.attrgetter(_func_closure)
-get_function_code = operator.attrgetter(_func_code)
-get_function_defaults = operator.attrgetter(_func_defaults)
-get_function_globals = operator.attrgetter(_func_globals)
-
-
-if PY3:
-
- def iterkeys(d, **kw):
- return iter(d.keys(**kw))
-
- def itervalues(d, **kw):
- return iter(d.values(**kw))
-
- def iteritems(d, **kw):
- return iter(d.items(**kw))
-
- def iterlists(d, **kw):
- return iter(d.lists(**kw))
-
- viewkeys = operator.methodcaller("keys")
-
- viewvalues = operator.methodcaller("values")
-
- viewitems = operator.methodcaller("items")
-else:
-
- def iterkeys(d, **kw):
- return d.iterkeys(**kw)
-
- def itervalues(d, **kw):
- return d.itervalues(**kw)
-
- def iteritems(d, **kw):
- return d.iteritems(**kw)
-
- def iterlists(d, **kw):
- return d.iterlists(**kw)
-
- viewkeys = operator.methodcaller("viewkeys")
-
- viewvalues = operator.methodcaller("viewvalues")
-
- viewitems = operator.methodcaller("viewitems")
-
-_add_doc(iterkeys, "Return an iterator over the keys of a dictionary.")
-_add_doc(itervalues, "Return an iterator over the values of a dictionary.")
-_add_doc(iteritems, "Return an iterator over the (key, value) pairs of a dictionary.")
-_add_doc(
- iterlists, "Return an iterator over the (key, [values]) pairs of a dictionary."
-)
-
-
-if PY3:
-
- def b(s):
- return s.encode("latin-1")
-
- def u(s):
- return s
-
- unichr = chr
- import struct
-
- int2byte = struct.Struct(">B").pack
- del struct
- byte2int = operator.itemgetter(0)
- indexbytes = operator.getitem
- iterbytes = iter
- import io
-
- StringIO = io.StringIO
- BytesIO = io.BytesIO
- del io
- _assertCountEqual = "assertCountEqual"
- if sys.version_info[1] <= 1:
- _assertRaisesRegex = "assertRaisesRegexp"
- _assertRegex = "assertRegexpMatches"
- _assertNotRegex = "assertNotRegexpMatches"
- else:
- _assertRaisesRegex = "assertRaisesRegex"
- _assertRegex = "assertRegex"
- _assertNotRegex = "assertNotRegex"
-else:
-
- def b(s):
- return s
-
- # Workaround for standalone backslash
-
- def u(s):
- return unicode(s.replace(r"\\", r"\\\\"), "unicode_escape")
-
- unichr = unichr
- int2byte = chr
-
- def byte2int(bs):
- return ord(bs[0])
-
- def indexbytes(buf, i):
- return ord(buf[i])
-
- iterbytes = functools.partial(itertools.imap, ord)
- import StringIO
-
- StringIO = BytesIO = StringIO.StringIO
- _assertCountEqual = "assertItemsEqual"
- _assertRaisesRegex = "assertRaisesRegexp"
- _assertRegex = "assertRegexpMatches"
- _assertNotRegex = "assertNotRegexpMatches"
-_add_doc(b, """Byte literal""")
-_add_doc(u, """Text literal""")
-
-
-def assertCountEqual(self, *args, **kwargs):
- return getattr(self, _assertCountEqual)(*args, **kwargs)
-
-
-def assertRaisesRegex(self, *args, **kwargs):
- return getattr(self, _assertRaisesRegex)(*args, **kwargs)
-
-
-def assertRegex(self, *args, **kwargs):
- return getattr(self, _assertRegex)(*args, **kwargs)
-
-
-def assertNotRegex(self, *args, **kwargs):
- return getattr(self, _assertNotRegex)(*args, **kwargs)
-
-
-if PY3:
- exec_ = getattr(moves.builtins, "exec")
-
- def reraise(tp, value, tb=None):
- try:
- if value is None:
- value = tp()
- if value.__traceback__ is not tb:
- raise value.with_traceback(tb)
- raise value
- finally:
- value = None
- tb = None
-
-else:
-
- def exec_(_code_, _globs_=None, _locs_=None):
- """Execute code in a namespace."""
- if _globs_ is None:
- frame = sys._getframe(1)
- _globs_ = frame.f_globals
- if _locs_ is None:
- _locs_ = frame.f_locals
- del frame
- elif _locs_ is None:
- _locs_ = _globs_
- exec ("""exec _code_ in _globs_, _locs_""")
-
- exec_(
- """def reraise(tp, value, tb=None):
- try:
- raise tp, value, tb
- finally:
- tb = None
-"""
- )
-
-
-if sys.version_info[:2] > (3,):
- exec_(
- """def raise_from(value, from_value):
- try:
- raise value from from_value
- finally:
- value = None
-"""
- )
-else:
-
- def raise_from(value, from_value):
- raise value
-
-
-print_ = getattr(moves.builtins, "print", None)
-if print_ is None:
-
- def print_(*args, **kwargs):
- """The new-style print function for Python 2.4 and 2.5."""
- fp = kwargs.pop("file", sys.stdout)
- if fp is None:
- return
-
- def write(data):
- if not isinstance(data, basestring):
- data = str(data)
- # If the file has an encoding, encode unicode with it.
- if (
- isinstance(fp, file)
- and isinstance(data, unicode)
- and fp.encoding is not None
- ):
- errors = getattr(fp, "errors", None)
- if errors is None:
- errors = "strict"
- data = data.encode(fp.encoding, errors)
- fp.write(data)
-
- want_unicode = False
- sep = kwargs.pop("sep", None)
- if sep is not None:
- if isinstance(sep, unicode):
- want_unicode = True
- elif not isinstance(sep, str):
- raise TypeError("sep must be None or a string")
- end = kwargs.pop("end", None)
- if end is not None:
- if isinstance(end, unicode):
- want_unicode = True
- elif not isinstance(end, str):
- raise TypeError("end must be None or a string")
- if kwargs:
- raise TypeError("invalid keyword arguments to print()")
- if not want_unicode:
- for arg in args:
- if isinstance(arg, unicode):
- want_unicode = True
- break
- if want_unicode:
- newline = unicode("\n")
- space = unicode(" ")
- else:
- newline = "\n"
- space = " "
- if sep is None:
- sep = space
- if end is None:
- end = newline
- for i, arg in enumerate(args):
- if i:
- write(sep)
- write(arg)
- write(end)
-
-
-if sys.version_info[:2] < (3, 3):
- _print = print_
-
- def print_(*args, **kwargs):
- fp = kwargs.get("file", sys.stdout)
- flush = kwargs.pop("flush", False)
- _print(*args, **kwargs)
- if flush and fp is not None:
- fp.flush()
-
-
-_add_doc(reraise, """Reraise an exception.""")
-
-if sys.version_info[0:2] < (3, 4):
- # This does exactly the same what the :func:`py3:functools.update_wrapper`
- # function does on Python versions after 3.2. It sets the ``__wrapped__``
- # attribute on ``wrapper`` object and it doesn't raise an error if any of
- # the attributes mentioned in ``assigned`` and ``updated`` are missing on
- # ``wrapped`` object.
- def _update_wrapper(
- wrapper,
- wrapped,
- assigned=functools.WRAPPER_ASSIGNMENTS,
- updated=functools.WRAPPER_UPDATES,
- ):
- for attr in assigned:
- try:
- value = getattr(wrapped, attr)
- except AttributeError:
- continue
- else:
- setattr(wrapper, attr, value)
- for attr in updated:
- getattr(wrapper, attr).update(getattr(wrapped, attr, {}))
- wrapper.__wrapped__ = wrapped
- return wrapper
-
- _update_wrapper.__doc__ = functools.update_wrapper.__doc__
-
- def wraps(
- wrapped,
- assigned=functools.WRAPPER_ASSIGNMENTS,
- updated=functools.WRAPPER_UPDATES,
- ):
- return functools.partial(
- _update_wrapper, wrapped=wrapped, assigned=assigned, updated=updated
- )
-
- wraps.__doc__ = functools.wraps.__doc__
-
-else:
- wraps = functools.wraps
-
-
-def with_metaclass(meta, *bases):
- """Create a base class with a metaclass."""
- # This requires a bit of explanation: the basic idea is to make a dummy
- # metaclass for one level of class instantiation that replaces itself with
- # the actual metaclass.
- class metaclass(type):
- def __new__(cls, name, this_bases, d):
- if sys.version_info[:2] >= (3, 7):
- # This version introduced PEP 560 that requires a bit
- # of extra care (we mimic what is done by __build_class__).
- resolved_bases = types.resolve_bases(bases)
- if resolved_bases is not bases:
- d["__orig_bases__"] = bases
- else:
- resolved_bases = bases
- return meta(name, resolved_bases, d)
-
- @classmethod
- def __prepare__(cls, name, this_bases):
- return meta.__prepare__(name, bases)
-
- return type.__new__(metaclass, "temporary_class", (), {})
-
-
-def add_metaclass(metaclass):
- """Class decorator for creating a class with a metaclass."""
-
- def wrapper(cls):
- orig_vars = cls.__dict__.copy()
- slots = orig_vars.get("__slots__")
- if slots is not None:
- if isinstance(slots, str):
- slots = [slots]
- for slots_var in slots:
- orig_vars.pop(slots_var)
- orig_vars.pop("__dict__", None)
- orig_vars.pop("__weakref__", None)
- if hasattr(cls, "__qualname__"):
- orig_vars["__qualname__"] = cls.__qualname__
- return metaclass(cls.__name__, cls.__bases__, orig_vars)
-
- return wrapper
-
-
-def ensure_binary(s, encoding="utf-8", errors="strict"):
- """Coerce **s** to six.binary_type.
-
- For Python 2:
- - `unicode` -> encoded to `str`
- - `str` -> `str`
-
- For Python 3:
- - `str` -> encoded to `bytes`
- - `bytes` -> `bytes`
- """
- if isinstance(s, binary_type):
- return s
- if isinstance(s, text_type):
- return s.encode(encoding, errors)
- raise TypeError("not expecting type '%s'" % type(s))
-
-
-def ensure_str(s, encoding="utf-8", errors="strict"):
- """Coerce *s* to `str`.
-
- For Python 2:
- - `unicode` -> encoded to `str`
- - `str` -> `str`
-
- For Python 3:
- - `str` -> `str`
- - `bytes` -> decoded to `str`
- """
- # Optimization: Fast return for the common case.
- if type(s) is str:
- return s
- if PY2 and isinstance(s, text_type):
- return s.encode(encoding, errors)
- elif PY3 and isinstance(s, binary_type):
- return s.decode(encoding, errors)
- elif not isinstance(s, (text_type, binary_type)):
- raise TypeError("not expecting type '%s'" % type(s))
- return s
-
-
-def ensure_text(s, encoding="utf-8", errors="strict"):
- """Coerce *s* to six.text_type.
-
- For Python 2:
- - `unicode` -> `unicode`
- - `str` -> `unicode`
-
- For Python 3:
- - `str` -> `str`
- - `bytes` -> decoded to `str`
- """
- if isinstance(s, binary_type):
- return s.decode(encoding, errors)
- elif isinstance(s, text_type):
- return s
- else:
- raise TypeError("not expecting type '%s'" % type(s))
-
-
-def python_2_unicode_compatible(klass):
- """
- A class decorator that defines __unicode__ and __str__ methods under Python 2.
- Under Python 3 it does nothing.
-
- To support Python 2 and 3 with a single code base, define a __str__ method
- returning text and apply this decorator to the class.
- """
- if PY2:
- if "__str__" not in klass.__dict__:
- raise ValueError(
- "@python_2_unicode_compatible cannot be applied "
- "to %s because it doesn't define __str__()." % klass.__name__
- )
- klass.__unicode__ = klass.__str__
- klass.__str__ = lambda self: self.__unicode__().encode("utf-8")
- return klass
-
-
-# Complete the moves implementation.
-# This code is at the end of this module to speed up module loading.
-# Turn this module into a package.
-__path__ = [] # required for PEP 302 and PEP 451
-__package__ = __name__ # see PEP 366 @ReservedAssignment
-if globals().get("__spec__") is not None:
- __spec__.submodule_search_locations = [] # PEP 451 @UndefinedVariable
-# Remove other six meta path importers, since they cause problems. This can
-# happen if six is removed from sys.modules and then reloaded. (Setuptools does
-# this for some reason.)
-if sys.meta_path:
- for i, importer in enumerate(sys.meta_path):
- # Here's some real nastiness: Another "instance" of the six module might
- # be floating around. Therefore, we can't use isinstance() to check for
- # the six meta path importer, since the other six instance will have
- # inserted an importer with different class.
- if (
- type(importer).__name__ == "_SixMetaPathImporter"
- and importer.name == __name__
- ):
- del sys.meta_path[i]
- break
- del i, importer
-# Finally, add the importer to the meta path import hook.
-sys.meta_path.append(_importer)
diff --git a/spaces/TotoB12/llama2-7b-chat-ggml/README.md b/spaces/TotoB12/llama2-7b-chat-ggml/README.md
deleted file mode 100644
index e854630cdc38f0e8471855d2e44d22283cf558df..0000000000000000000000000000000000000000
--- a/spaces/TotoB12/llama2-7b-chat-ggml/README.md
+++ /dev/null
@@ -1,13 +0,0 @@
----
-title: llama-2-7b-or-13b-ggml
-emoji: 🚀
-colorFrom: green
-colorTo: green
-sdk: gradio
-sdk_version: 3.37.0
-app_file: app.py
-pinned: true
-duplicated_from: mikeee/Wizard-Vicuna-7B-Uncensored-GGML
----
-
-Check out the configuration reference at https://huggingface.co/docs/hub/spaces-config-reference
diff --git a/spaces/Uday007/Penguin-BodyMass-Predictor/app.py b/spaces/Uday007/Penguin-BodyMass-Predictor/app.py
deleted file mode 100644
index 11bc225e9c9faa74aa03873f6d85d3aac0f331bd..0000000000000000000000000000000000000000
--- a/spaces/Uday007/Penguin-BodyMass-Predictor/app.py
+++ /dev/null
@@ -1,29 +0,0 @@
-import gradio as gr
-import pandas as pd
-from joblib import load
-
-def predict_bodymass(FlipperLength):
- model = load("penguin_predictor.jb")
-
- # Create DataFrame from input
- data = {
- "FlipperLength": [FlipperLength]
- }
- xin = pd.DataFrame(data)
-
- bodymass = model.predict(xin)
- return bodymass[0]
-
-iface = gr.Interface(
- fn=predict_bodymass,
- inputs=[
- gr.inputs.Textbox(placeholder="Enter Flipper Length(mm)",numeric=True,label="FLIPPER LENGTH")
- ],
- title="PENGUIN REGRESSION",
- outputs="text",
- examples=[[195],
- [183]]
-)
-
-if __name__ == "__main__":
- iface.launch()
diff --git a/spaces/Wrathless/Dkrotzer-MusicalMagic/tests/data/__init__.py b/spaces/Wrathless/Dkrotzer-MusicalMagic/tests/data/__init__.py
deleted file mode 100644
index 0952fcc3f57e34b3747962e9ebd6fc57aeea63fa..0000000000000000000000000000000000000000
--- a/spaces/Wrathless/Dkrotzer-MusicalMagic/tests/data/__init__.py
+++ /dev/null
@@ -1,5 +0,0 @@
-# Copyright (c) Meta Platforms, Inc. and affiliates.
-# All rights reserved.
-#
-# This source code is licensed under the license found in the
-# LICENSE file in the root directory of this source tree.
diff --git a/spaces/Xenova/semantic-image-search-client/_next/static/chunks/pages/_app-52924524f99094ab.js b/spaces/Xenova/semantic-image-search-client/_next/static/chunks/pages/_app-52924524f99094ab.js
deleted file mode 100644
index 5566aacbc3bd143333136d49b304f1eff54bd82f..0000000000000000000000000000000000000000
--- a/spaces/Xenova/semantic-image-search-client/_next/static/chunks/pages/_app-52924524f99094ab.js
+++ /dev/null
@@ -1 +0,0 @@
-(self.webpackChunk_N_E=self.webpackChunk_N_E||[]).push([[888],{1597:function(n,_,u){(window.__NEXT_P=window.__NEXT_P||[]).push(["/_app",function(){return u(6530)}])}},function(n){var _=function(_){return n(n.s=_)};n.O(0,[774,179],function(){return _(1597),_(1247)}),_N_E=n.O()}]);
\ No newline at end of file
diff --git a/spaces/XzJosh/Ava-Bert-VITS2/text/chinese_bert.py b/spaces/XzJosh/Ava-Bert-VITS2/text/chinese_bert.py
deleted file mode 100644
index cb84ce0b426cd0a1c7954ddcdf41322c10ed14fa..0000000000000000000000000000000000000000
--- a/spaces/XzJosh/Ava-Bert-VITS2/text/chinese_bert.py
+++ /dev/null
@@ -1,50 +0,0 @@
-import torch
-from transformers import AutoTokenizer, AutoModelForMaskedLM
-
-device = torch.device("cuda" if torch.cuda.is_available() else "cpu")
-
-tokenizer = AutoTokenizer.from_pretrained("./bert/chinese-roberta-wwm-ext-large")
-model = AutoModelForMaskedLM.from_pretrained("./bert/chinese-roberta-wwm-ext-large").to(device)
-
-def get_bert_feature(text, word2ph):
- with torch.no_grad():
- inputs = tokenizer(text, return_tensors='pt')
- for i in inputs:
- inputs[i] = inputs[i].to(device)
- res = model(**inputs, output_hidden_states=True)
- res = torch.cat(res['hidden_states'][-3:-2], -1)[0].cpu()
-
- assert len(word2ph) == len(text)+2
- word2phone = word2ph
- phone_level_feature = []
- for i in range(len(word2phone)):
- repeat_feature = res[i].repeat(word2phone[i], 1)
- phone_level_feature.append(repeat_feature)
-
- phone_level_feature = torch.cat(phone_level_feature, dim=0)
-
-
- return phone_level_feature.T
-
-if __name__ == '__main__':
- # feature = get_bert_feature('你好,我是说的道理。')
- import torch
-
- word_level_feature = torch.rand(38, 1024) # 12个词,每个词1024维特征
- word2phone = [1, 2, 1, 2, 2, 1, 2, 2, 1, 2, 2, 1, 2, 2, 2, 2, 2, 1, 1, 2, 2, 1, 2, 2, 2, 2, 1, 2, 2, 2, 2, 2, 1, 2, 2, 2, 2, 1]
-
- # 计算总帧数
- total_frames = sum(word2phone)
- print(word_level_feature.shape)
- print(word2phone)
- phone_level_feature = []
- for i in range(len(word2phone)):
- print(word_level_feature[i].shape)
-
- # 对每个词重复word2phone[i]次
- repeat_feature = word_level_feature[i].repeat(word2phone[i], 1)
- phone_level_feature.append(repeat_feature)
-
- phone_level_feature = torch.cat(phone_level_feature, dim=0)
- print(phone_level_feature.shape) # torch.Size([36, 1024])
-
diff --git a/spaces/YONG627/456123/yolov5-code-main/utils/augmentations.py b/spaces/YONG627/456123/yolov5-code-main/utils/augmentations.py
deleted file mode 100644
index 9fdea1835d12bccd1361cbb2bd56ca03a7b6a237..0000000000000000000000000000000000000000
--- a/spaces/YONG627/456123/yolov5-code-main/utils/augmentations.py
+++ /dev/null
@@ -1,397 +0,0 @@
-# YOLOv5 🚀 by Ultralytics, GPL-3.0 license
-"""
-Image augmentation functions
-"""
-
-import math
-import random
-
-import cv2
-import numpy as np
-import torch
-import torchvision.transforms as T
-import torchvision.transforms.functional as TF
-
-from utils.general import LOGGER, check_version, colorstr, resample_segments, segment2box, xywhn2xyxy
-from utils.metrics import bbox_ioa
-
-IMAGENET_MEAN = 0.485, 0.456, 0.406 # RGB mean
-IMAGENET_STD = 0.229, 0.224, 0.225 # RGB standard deviation
-
-
-class Albumentations:
- # YOLOv5 Albumentations class (optional, only used if package is installed)
- def __init__(self, size=640):
- self.transform = None
- prefix = colorstr('albumentations: ')
- try:
- import albumentations as A
- check_version(A.__version__, '1.0.3', hard=True) # version requirement
-
- T = [
- A.RandomResizedCrop(height=size, width=size, scale=(0.8, 1.0), ratio=(0.9, 1.11), p=0.0),
- A.Blur(p=0.01),
- A.MedianBlur(p=0.01),
- A.ToGray(p=0.01),
- A.CLAHE(p=0.01),
- A.RandomBrightnessContrast(p=0.0),
- A.RandomGamma(p=0.0),
- A.ImageCompression(quality_lower=75, p=0.0)] # transforms
- self.transform = A.Compose(T, bbox_params=A.BboxParams(format='yolo', label_fields=['class_labels']))
-
- LOGGER.info(prefix + ', '.join(f'{x}'.replace('always_apply=False, ', '') for x in T if x.p))
- except ImportError: # package not installed, skip
- pass
- except Exception as e:
- LOGGER.info(f'{prefix}{e}')
-
- def __call__(self, im, labels, p=1.0):
- if self.transform and random.random() < p:
- new = self.transform(image=im, bboxes=labels[:, 1:], class_labels=labels[:, 0]) # transformed
- im, labels = new['image'], np.array([[c, *b] for c, b in zip(new['class_labels'], new['bboxes'])])
- return im, labels
-
-
-def normalize(x, mean=IMAGENET_MEAN, std=IMAGENET_STD, inplace=False):
- # Denormalize RGB images x per ImageNet stats in BCHW format, i.e. = (x - mean) / std
- return TF.normalize(x, mean, std, inplace=inplace)
-
-
-def denormalize(x, mean=IMAGENET_MEAN, std=IMAGENET_STD):
- # Denormalize RGB images x per ImageNet stats in BCHW format, i.e. = x * std + mean
- for i in range(3):
- x[:, i] = x[:, i] * std[i] + mean[i]
- return x
-
-
-def augment_hsv(im, hgain=0.5, sgain=0.5, vgain=0.5):
- # HSV color-space augmentation
- if hgain or sgain or vgain:
- r = np.random.uniform(-1, 1, 3) * [hgain, sgain, vgain] + 1 # random gains
- hue, sat, val = cv2.split(cv2.cvtColor(im, cv2.COLOR_BGR2HSV))
- dtype = im.dtype # uint8
-
- x = np.arange(0, 256, dtype=r.dtype)
- lut_hue = ((x * r[0]) % 180).astype(dtype)
- lut_sat = np.clip(x * r[1], 0, 255).astype(dtype)
- lut_val = np.clip(x * r[2], 0, 255).astype(dtype)
-
- im_hsv = cv2.merge((cv2.LUT(hue, lut_hue), cv2.LUT(sat, lut_sat), cv2.LUT(val, lut_val)))
- cv2.cvtColor(im_hsv, cv2.COLOR_HSV2BGR, dst=im) # no return needed
-
-
-def hist_equalize(im, clahe=True, bgr=False):
- # Equalize histogram on BGR image 'im' with im.shape(n,m,3) and range 0-255
- yuv = cv2.cvtColor(im, cv2.COLOR_BGR2YUV if bgr else cv2.COLOR_RGB2YUV)
- if clahe:
- c = cv2.createCLAHE(clipLimit=2.0, tileGridSize=(8, 8))
- yuv[:, :, 0] = c.apply(yuv[:, :, 0])
- else:
- yuv[:, :, 0] = cv2.equalizeHist(yuv[:, :, 0]) # equalize Y channel histogram
- return cv2.cvtColor(yuv, cv2.COLOR_YUV2BGR if bgr else cv2.COLOR_YUV2RGB) # convert YUV image to RGB
-
-
-def replicate(im, labels):
- # Replicate labels
- h, w = im.shape[:2]
- boxes = labels[:, 1:].astype(int)
- x1, y1, x2, y2 = boxes.T
- s = ((x2 - x1) + (y2 - y1)) / 2 # side length (pixels)
- for i in s.argsort()[:round(s.size * 0.5)]: # smallest indices
- x1b, y1b, x2b, y2b = boxes[i]
- bh, bw = y2b - y1b, x2b - x1b
- yc, xc = int(random.uniform(0, h - bh)), int(random.uniform(0, w - bw)) # offset x, y
- x1a, y1a, x2a, y2a = [xc, yc, xc + bw, yc + bh]
- im[y1a:y2a, x1a:x2a] = im[y1b:y2b, x1b:x2b] # im4[ymin:ymax, xmin:xmax]
- labels = np.append(labels, [[labels[i, 0], x1a, y1a, x2a, y2a]], axis=0)
-
- return im, labels
-
-
-def letterbox(im, new_shape=(640, 640), color=(114, 114, 114), auto=True, scaleFill=False, scaleup=True, stride=32):
- # Resize and pad image while meeting stride-multiple constraints
- shape = im.shape[:2] # current shape [height, width]
- if isinstance(new_shape, int):
- new_shape = (new_shape, new_shape)
-
- # Scale ratio (new / old)
- r = min(new_shape[0] / shape[0], new_shape[1] / shape[1])
- if not scaleup: # only scale down, do not scale up (for better val mAP)
- r = min(r, 1.0)
-
- # Compute padding
- ratio = r, r # width, height ratios
- new_unpad = int(round(shape[1] * r)), int(round(shape[0] * r))
- dw, dh = new_shape[1] - new_unpad[0], new_shape[0] - new_unpad[1] # wh padding
- if auto: # minimum rectangle
- dw, dh = np.mod(dw, stride), np.mod(dh, stride) # wh padding
- elif scaleFill: # stretch
- dw, dh = 0.0, 0.0
- new_unpad = (new_shape[1], new_shape[0])
- ratio = new_shape[1] / shape[1], new_shape[0] / shape[0] # width, height ratios
-
- dw /= 2 # divide padding into 2 sides
- dh /= 2
-
- if shape[::-1] != new_unpad: # resize
- im = cv2.resize(im, new_unpad, interpolation=cv2.INTER_LINEAR)
- top, bottom = int(round(dh - 0.1)), int(round(dh + 0.1))
- left, right = int(round(dw - 0.1)), int(round(dw + 0.1))
- im = cv2.copyMakeBorder(im, top, bottom, left, right, cv2.BORDER_CONSTANT, value=color) # add border
- return im, ratio, (dw, dh)
-
-
-def random_perspective(im,
- targets=(),
- segments=(),
- degrees=10,
- translate=.1,
- scale=.1,
- shear=10,
- perspective=0.0,
- border=(0, 0)):
- # torchvision.transforms.RandomAffine(degrees=(-10, 10), translate=(0.1, 0.1), scale=(0.9, 1.1), shear=(-10, 10))
- # targets = [cls, xyxy]
-
- height = im.shape[0] + border[0] * 2 # shape(h,w,c)
- width = im.shape[1] + border[1] * 2
-
- # Center
- C = np.eye(3)
- C[0, 2] = -im.shape[1] / 2 # x translation (pixels)
- C[1, 2] = -im.shape[0] / 2 # y translation (pixels)
-
- # Perspective
- P = np.eye(3)
- P[2, 0] = random.uniform(-perspective, perspective) # x perspective (about y)
- P[2, 1] = random.uniform(-perspective, perspective) # y perspective (about x)
-
- # Rotation and Scale
- R = np.eye(3)
- a = random.uniform(-degrees, degrees)
- # a += random.choice([-180, -90, 0, 90]) # add 90deg rotations to small rotations
- s = random.uniform(1 - scale, 1 + scale)
- # s = 2 ** random.uniform(-scale, scale)
- R[:2] = cv2.getRotationMatrix2D(angle=a, center=(0, 0), scale=s)
-
- # Shear
- S = np.eye(3)
- S[0, 1] = math.tan(random.uniform(-shear, shear) * math.pi / 180) # x shear (deg)
- S[1, 0] = math.tan(random.uniform(-shear, shear) * math.pi / 180) # y shear (deg)
-
- # Translation
- T = np.eye(3)
- T[0, 2] = random.uniform(0.5 - translate, 0.5 + translate) * width # x translation (pixels)
- T[1, 2] = random.uniform(0.5 - translate, 0.5 + translate) * height # y translation (pixels)
-
- # Combined rotation matrix
- M = T @ S @ R @ P @ C # order of operations (right to left) is IMPORTANT
- if (border[0] != 0) or (border[1] != 0) or (M != np.eye(3)).any(): # image changed
- if perspective:
- im = cv2.warpPerspective(im, M, dsize=(width, height), borderValue=(114, 114, 114))
- else: # affine
- im = cv2.warpAffine(im, M[:2], dsize=(width, height), borderValue=(114, 114, 114))
-
- # Visualize
- # import matplotlib.pyplot as plt
- # ax = plt.subplots(1, 2, figsize=(12, 6))[1].ravel()
- # ax[0].imshow(im[:, :, ::-1]) # base
- # ax[1].imshow(im2[:, :, ::-1]) # warped
-
- # Transform label coordinates
- n = len(targets)
- if n:
- use_segments = any(x.any() for x in segments) and len(segments) == n
- new = np.zeros((n, 4))
- if use_segments: # warp segments
- segments = resample_segments(segments) # upsample
- for i, segment in enumerate(segments):
- xy = np.ones((len(segment), 3))
- xy[:, :2] = segment
- xy = xy @ M.T # transform
- xy = xy[:, :2] / xy[:, 2:3] if perspective else xy[:, :2] # perspective rescale or affine
-
- # clip
- new[i] = segment2box(xy, width, height)
-
- else: # warp boxes
- xy = np.ones((n * 4, 3))
- xy[:, :2] = targets[:, [1, 2, 3, 4, 1, 4, 3, 2]].reshape(n * 4, 2) # x1y1, x2y2, x1y2, x2y1
- xy = xy @ M.T # transform
- xy = (xy[:, :2] / xy[:, 2:3] if perspective else xy[:, :2]).reshape(n, 8) # perspective rescale or affine
-
- # create new boxes
- x = xy[:, [0, 2, 4, 6]]
- y = xy[:, [1, 3, 5, 7]]
- new = np.concatenate((x.min(1), y.min(1), x.max(1), y.max(1))).reshape(4, n).T
-
- # clip
- new[:, [0, 2]] = new[:, [0, 2]].clip(0, width)
- new[:, [1, 3]] = new[:, [1, 3]].clip(0, height)
-
- # filter candidates
- i = box_candidates(box1=targets[:, 1:5].T * s, box2=new.T, area_thr=0.01 if use_segments else 0.10)
- targets = targets[i]
- targets[:, 1:5] = new[i]
-
- return im, targets
-
-
-def copy_paste(im, labels, segments, p=0.5):
- # Implement Copy-Paste augmentation https://arxiv.org/abs/2012.07177, labels as nx5 np.array(cls, xyxy)
- n = len(segments)
- if p and n:
- h, w, c = im.shape # height, width, channels
- im_new = np.zeros(im.shape, np.uint8)
- for j in random.sample(range(n), k=round(p * n)):
- l, s = labels[j], segments[j]
- box = w - l[3], l[2], w - l[1], l[4]
- ioa = bbox_ioa(box, labels[:, 1:5]) # intersection over area
- if (ioa < 0.30).all(): # allow 30% obscuration of existing labels
- labels = np.concatenate((labels, [[l[0], *box]]), 0)
- segments.append(np.concatenate((w - s[:, 0:1], s[:, 1:2]), 1))
- cv2.drawContours(im_new, [segments[j].astype(np.int32)], -1, (1, 1, 1), cv2.FILLED)
-
- result = cv2.flip(im, 1) # augment segments (flip left-right)
- i = cv2.flip(im_new, 1).astype(bool)
- im[i] = result[i] # cv2.imwrite('debug.jpg', im) # debug
-
- return im, labels, segments
-
-
-def cutout(im, labels, p=0.5):
- # Applies image cutout augmentation https://arxiv.org/abs/1708.04552
- if random.random() < p:
- h, w = im.shape[:2]
- scales = [0.5] * 1 + [0.25] * 2 + [0.125] * 4 + [0.0625] * 8 + [0.03125] * 16 # image size fraction
- for s in scales:
- mask_h = random.randint(1, int(h * s)) # create random masks
- mask_w = random.randint(1, int(w * s))
-
- # box
- xmin = max(0, random.randint(0, w) - mask_w // 2)
- ymin = max(0, random.randint(0, h) - mask_h // 2)
- xmax = min(w, xmin + mask_w)
- ymax = min(h, ymin + mask_h)
-
- # apply random color mask
- im[ymin:ymax, xmin:xmax] = [random.randint(64, 191) for _ in range(3)]
-
- # return unobscured labels
- if len(labels) and s > 0.03:
- box = np.array([xmin, ymin, xmax, ymax], dtype=np.float32)
- ioa = bbox_ioa(box, xywhn2xyxy(labels[:, 1:5], w, h)) # intersection over area
- labels = labels[ioa < 0.60] # remove >60% obscured labels
-
- return labels
-
-
-def mixup(im, labels, im2, labels2):
- # Applies MixUp augmentation https://arxiv.org/pdf/1710.09412.pdf
- r = np.random.beta(32.0, 32.0) # mixup ratio, alpha=beta=32.0
- im = (im * r + im2 * (1 - r)).astype(np.uint8)
- labels = np.concatenate((labels, labels2), 0)
- return im, labels
-
-
-def box_candidates(box1, box2, wh_thr=2, ar_thr=100, area_thr=0.1, eps=1e-16): # box1(4,n), box2(4,n)
- # Compute candidate boxes: box1 before augment, box2 after augment, wh_thr (pixels), aspect_ratio_thr, area_ratio
- w1, h1 = box1[2] - box1[0], box1[3] - box1[1]
- w2, h2 = box2[2] - box2[0], box2[3] - box2[1]
- ar = np.maximum(w2 / (h2 + eps), h2 / (w2 + eps)) # aspect ratio
- return (w2 > wh_thr) & (h2 > wh_thr) & (w2 * h2 / (w1 * h1 + eps) > area_thr) & (ar < ar_thr) # candidates
-
-
-def classify_albumentations(
- augment=True,
- size=224,
- scale=(0.08, 1.0),
- ratio=(0.75, 1.0 / 0.75), # 0.75, 1.33
- hflip=0.5,
- vflip=0.0,
- jitter=0.4,
- mean=IMAGENET_MEAN,
- std=IMAGENET_STD,
- auto_aug=False):
- # YOLOv5 classification Albumentations (optional, only used if package is installed)
- prefix = colorstr('albumentations: ')
- try:
- import albumentations as A
- from albumentations.pytorch import ToTensorV2
- check_version(A.__version__, '1.0.3', hard=True) # version requirement
- if augment: # Resize and crop
- T = [A.RandomResizedCrop(height=size, width=size, scale=scale, ratio=ratio)]
- if auto_aug:
- # TODO: implement AugMix, AutoAug & RandAug in albumentation
- LOGGER.info(f'{prefix}auto augmentations are currently not supported')
- else:
- if hflip > 0:
- T += [A.HorizontalFlip(p=hflip)]
- if vflip > 0:
- T += [A.VerticalFlip(p=vflip)]
- if jitter > 0:
- color_jitter = (float(jitter),) * 3 # repeat value for brightness, contrast, satuaration, 0 hue
- T += [A.ColorJitter(*color_jitter, 0)]
- else: # Use fixed crop for eval set (reproducibility)
- T = [A.SmallestMaxSize(max_size=size), A.CenterCrop(height=size, width=size)]
- T += [A.Normalize(mean=mean, std=std), ToTensorV2()] # Normalize and convert to Tensor
- LOGGER.info(prefix + ', '.join(f'{x}'.replace('always_apply=False, ', '') for x in T if x.p))
- return A.Compose(T)
-
- except ImportError: # package not installed, skip
- LOGGER.warning(f'{prefix}⚠️ not found, install with `pip install albumentations` (recommended)')
- except Exception as e:
- LOGGER.info(f'{prefix}{e}')
-
-
-def classify_transforms(size=224):
- # Transforms to apply if albumentations not installed
- assert isinstance(size, int), f'ERROR: classify_transforms size {size} must be integer, not (list, tuple)'
- # T.Compose([T.ToTensor(), T.Resize(size), T.CenterCrop(size), T.Normalize(IMAGENET_MEAN, IMAGENET_STD)])
- return T.Compose([CenterCrop(size), ToTensor(), T.Normalize(IMAGENET_MEAN, IMAGENET_STD)])
-
-
-class LetterBox:
- # YOLOv5 LetterBox class for image preprocessing, i.e. T.Compose([LetterBox(size), ToTensor()])
- def __init__(self, size=(640, 640), auto=False, stride=32):
- super().__init__()
- self.h, self.w = (size, size) if isinstance(size, int) else size
- self.auto = auto # pass max size integer, automatically solve for short side using stride
- self.stride = stride # used with auto
-
- def __call__(self, im): # im = np.array HWC
- imh, imw = im.shape[:2]
- r = min(self.h / imh, self.w / imw) # ratio of new/old
- h, w = round(imh * r), round(imw * r) # resized image
- hs, ws = (math.ceil(x / self.stride) * self.stride for x in (h, w)) if self.auto else self.h, self.w
- top, left = round((hs - h) / 2 - 0.1), round((ws - w) / 2 - 0.1)
- im_out = np.full((self.h, self.w, 3), 114, dtype=im.dtype)
- im_out[top:top + h, left:left + w] = cv2.resize(im, (w, h), interpolation=cv2.INTER_LINEAR)
- return im_out
-
-
-class CenterCrop:
- # YOLOv5 CenterCrop class for image preprocessing, i.e. T.Compose([CenterCrop(size), ToTensor()])
- def __init__(self, size=640):
- super().__init__()
- self.h, self.w = (size, size) if isinstance(size, int) else size
-
- def __call__(self, im): # im = np.array HWC
- imh, imw = im.shape[:2]
- m = min(imh, imw) # min dimension
- top, left = (imh - m) // 2, (imw - m) // 2
- return cv2.resize(im[top:top + m, left:left + m], (self.w, self.h), interpolation=cv2.INTER_LINEAR)
-
-
-class ToTensor:
- # YOLOv5 ToTensor class for image preprocessing, i.e. T.Compose([LetterBox(size), ToTensor()])
- def __init__(self, half=False):
- super().__init__()
- self.half = half
-
- def __call__(self, im): # im = np.array HWC in BGR order
- im = np.ascontiguousarray(im.transpose((2, 0, 1))[::-1]) # HWC to CHW -> BGR to RGB -> contiguous
- im = torch.from_numpy(im) # to torch
- im = im.half() if self.half else im.float() # uint8 to fp16/32
- im /= 255.0 # 0-255 to 0.0-1.0
- return im
diff --git a/spaces/YouLiXiya/Mobile-SAM/segment_anything/setup.py b/spaces/YouLiXiya/Mobile-SAM/segment_anything/setup.py
deleted file mode 100644
index 2c0986317eb576a14ec774205c88fdee3cc6c0b3..0000000000000000000000000000000000000000
--- a/spaces/YouLiXiya/Mobile-SAM/segment_anything/setup.py
+++ /dev/null
@@ -1,18 +0,0 @@
-# Copyright (c) Meta Platforms, Inc. and affiliates.
-# All rights reserved.
-
-# This source code is licensed under the license found in the
-# LICENSE file in the root directory of this source tree.
-
-from setuptools import find_packages, setup
-
-setup(
- name="segment_anything",
- version="1.0",
- install_requires=[],
- packages=find_packages(exclude="notebooks"),
- extras_require={
- "all": ["matplotlib", "pycocotools", "opencv-python", "onnx", "onnxruntime"],
- "dev": ["flake8", "isort", "black", "mypy"],
- },
-)
diff --git a/spaces/YuAnthony/Audio-Caption/coco_caption/pycocoevalcap/bleu/bleu.py b/spaces/YuAnthony/Audio-Caption/coco_caption/pycocoevalcap/bleu/bleu.py
deleted file mode 100644
index d78cc91c6e94521cb394bfc6807f48e011a30890..0000000000000000000000000000000000000000
--- a/spaces/YuAnthony/Audio-Caption/coco_caption/pycocoevalcap/bleu/bleu.py
+++ /dev/null
@@ -1,53 +0,0 @@
-#!/usr/bin/env python
-#
-# File Name : bleu.py
-#
-# Description : Wrapper for BLEU scorer.
-#
-# Creation Date : 06-01-2015
-# Last Modified : Thu 19 Mar 2015 09:13:28 PM PDT
-# Authors : Hao Fang and Tsung-Yi Lin
-
-# =================================================================
-# This code was pulled from https://github.com/tylin/coco-caption
-# and refactored for Python 3.
-# Image-specific names and comments have also been changed to be audio-specific
-# =================================================================
-
-from .bleu_scorer import BleuScorer
-
-
-class Bleu:
- def __init__(self, n=4):
- # default compute Blue score up to 4
- self._n = n
- self._hypo_for_audio = {}
- self.ref_for_audio = {}
-
- def compute_score(self, gts, res):
-
- assert(gts.keys() == res.keys())
- audioIds = gts.keys()
-
- bleu_scorer = BleuScorer(n=self._n)
- for id in audioIds:
- hypo = res[id]
- ref = gts[id]
-
- # Sanity check.
- assert(type(hypo) is list)
- assert(len(hypo) == 1)
- assert(type(ref) is list)
- assert(len(ref) >= 1)
-
- bleu_scorer += (hypo[0], ref)
-
- #score, scores = bleu_scorer.compute_score(option='shortest')
- score, scores = bleu_scorer.compute_score(option='closest', verbose=1)
- #score, scores = bleu_scorer.compute_score(option='average', verbose=1)
-
- # return (bleu, bleu_info)
- return score, scores
-
- def method(self):
- return "Bleu"
diff --git a/spaces/Zwicky18/Stable-difussion/README.md b/spaces/Zwicky18/Stable-difussion/README.md
deleted file mode 100644
index e925860064ac6b8886ee2d80027ca624ae7274d1..0000000000000000000000000000000000000000
--- a/spaces/Zwicky18/Stable-difussion/README.md
+++ /dev/null
@@ -1,14 +0,0 @@
----
-title: Stable Diffusion Webui
-emoji: 💻
-colorFrom: yellow
-colorTo: gray
-sdk: gradio
-sdk_version: 3.12.0
-app_file: app.py
-pinned: false
-license: openrail
-duplicated_from: luluneko1/stable-diffusion-webui
----
-
-Check out the configuration reference at https://huggingface.co/docs/hub/spaces-config-reference
diff --git a/spaces/abhishek/sketch-to-image/annotator/canny/__init__.py b/spaces/abhishek/sketch-to-image/annotator/canny/__init__.py
deleted file mode 100644
index 1bcdaf9e72d29bd86d0965e051366381633a5003..0000000000000000000000000000000000000000
--- a/spaces/abhishek/sketch-to-image/annotator/canny/__init__.py
+++ /dev/null
@@ -1,16 +0,0 @@
-'''
- * Copyright (c) 2023 Salesforce, Inc.
- * All rights reserved.
- * SPDX-License-Identifier: Apache License 2.0
- * For full license text, see LICENSE.txt file in the repo root or http://www.apache.org/licenses/
- * By Can Qin
- * Modified from ControlNet repo: https://github.com/lllyasviel/ControlNet
- * Copyright (c) 2023 Lvmin Zhang and Maneesh Agrawala
-'''
-
-import cv2
-
-
-class CannyDetector:
- def __call__(self, img, low_threshold, high_threshold):
- return cv2.Canny(img, low_threshold, high_threshold)
diff --git a/spaces/abidlabs/cinemascope/README.md b/spaces/abidlabs/cinemascope/README.md
deleted file mode 100644
index a1438994860eec2c0e425a522c06ce7d5c67b48a..0000000000000000000000000000000000000000
--- a/spaces/abidlabs/cinemascope/README.md
+++ /dev/null
@@ -1,13 +0,0 @@
----
-title: ModelScope Text To Video Synthesis
-emoji: 🚀
-colorFrom: pink
-colorTo: pink
-sdk: gradio
-sdk_version: 3.23.0
-app_file: app.py
-pinned: false
-duplicated_from: damo-vilab/modelscope-text-to-video-synthesis
----
-
-Check out the configuration reference at https://huggingface.co/docs/hub/spaces-config-reference
diff --git a/spaces/abrar-lohia/text-2-character-anim/pyrender/.eggs/pyglet-2.0.5-py3.10.egg/pyglet/image/codecs/bmp.py b/spaces/abrar-lohia/text-2-character-anim/pyrender/.eggs/pyglet-2.0.5-py3.10.egg/pyglet/image/codecs/bmp.py
deleted file mode 100644
index ca22c3394dc464c3341609865bf1be16f9aaff3d..0000000000000000000000000000000000000000
--- a/spaces/abrar-lohia/text-2-character-anim/pyrender/.eggs/pyglet-2.0.5-py3.10.egg/pyglet/image/codecs/bmp.py
+++ /dev/null
@@ -1,322 +0,0 @@
-"""Decoder for BMP files.
-
-Currently supports version 3 and 4 bitmaps with BI_RGB and BI_BITFIELDS
-encoding. Alpha channel is supported for 32-bit BI_RGB only.
-"""
-
-# Official docs are at
-# http://msdn2.microsoft.com/en-us/library/ms532311.aspx
-#
-# But some details including alignment and bit/byte order are omitted; see
-# http://www.fileformat.info/format/bmp/egff.htm
-
-import ctypes
-
-from pyglet.image import ImageData
-from pyglet.image.codecs import ImageDecoder, ImageDecodeException
-
-BYTE = ctypes.c_ubyte
-WORD = ctypes.c_uint16
-DWORD = ctypes.c_uint32
-LONG = ctypes.c_int32
-FXPT2DOT30 = ctypes.c_uint32
-
-BI_RGB = 0
-BI_RLE8 = 1
-BI_RLE4 = 2
-BI_BITFIELDS = 3
-
-class BITMAPFILEHEADER(ctypes.LittleEndianStructure):
- _pack_ = 1
- _fields_ = [
- ('bfType', WORD),
- ('bfSize', DWORD),
- ('bfReserved1', WORD),
- ('bfReserved2', WORD),
- ('bfOffBits', DWORD)
- ]
-
-class BITMAPINFOHEADER(ctypes.LittleEndianStructure):
- _pack_ = 1
- _fields_ = [
- ('biSize', DWORD),
- ('biWidth', LONG),
- ('biHeight', LONG),
- ('biPlanes', WORD),
- ('biBitCount', WORD),
- ('biCompression', DWORD),
- ('biSizeImage', DWORD),
- ('biXPelsPerMeter', LONG),
- ('biYPelsPerMeter', LONG),
- ('biClrUsed', DWORD),
- ('biClrImportant', DWORD)
- ]
-
-CIEXYZTRIPLE = FXPT2DOT30 * 9
-
-class BITMAPV4HEADER(ctypes.LittleEndianStructure):
- _pack_ = 1
- _fields_ = [
- ('biSize', DWORD),
- ('biWidth', LONG),
- ('biHeight', LONG),
- ('biPlanes', WORD),
- ('biBitCount', WORD),
- ('biCompression', DWORD),
- ('biSizeImage', DWORD),
- ('biXPelsPerMeter', LONG),
- ('biYPelsPerMeter', LONG),
- ('biClrUsed', DWORD),
- ('biClrImportant', DWORD),
- ('bV4RedMask', DWORD),
- ('bV4GreenMask', DWORD),
- ('bV4BlueMask', DWORD),
- ('bV4AlphaMask', DWORD),
- ('bV4CSType', DWORD),
- ('bV4Endpoints', CIEXYZTRIPLE),
- ('bV4GammaRed', DWORD),
- ('bV4GammaGreen', DWORD),
- ('bV4GammaBlue', DWORD),
- ]
-
-class RGBFields(ctypes.LittleEndianStructure):
- _pack_ = 1
- _fields_ = [
- ('red', DWORD),
- ('green', DWORD),
- ('blue', DWORD),
- ]
-
-
-class RGBQUAD(ctypes.LittleEndianStructure):
- _pack_ = 1
- _fields_ = [
- ('rgbBlue', BYTE),
- ('rgbGreen', BYTE),
- ('rgbRed', BYTE),
- ('rgbReserved', BYTE)
- ]
-
- def __repr__(self):
- return '<%d, %d, %d>' % (self.rgbRed, self.rgbGreen, self.rgbBlue)
-
-def ptr_add(ptr, offset):
- address = ctypes.addressof(ptr.contents) + offset
- return ctypes.pointer(type(ptr.contents).from_address(address))
-
-def to_ctypes(buffer, offset, type):
- if offset + ctypes.sizeof(type) > len(buffer):
- raise ImageDecodeException('BMP file is truncated')
- ptr = ptr_add(ctypes.pointer(buffer), offset)
- return ctypes.cast(ptr, ctypes.POINTER(type)).contents
-
-class BMPImageDecoder(ImageDecoder):
- def get_file_extensions(self):
- return ['.bmp']
-
- def decode(self, filename, file):
- if not file:
- file = open(filename, 'rb')
- bytes = file.read()
- buffer = ctypes.c_buffer(bytes)
-
- if bytes[:2] != b'BM':
- raise ImageDecodeException(
- 'Not a Windows bitmap file: %r' % (filename or file))
-
- file_header = to_ctypes(buffer, 0, BITMAPFILEHEADER)
- bits_offset = file_header.bfOffBits
- info_header_offset = ctypes.sizeof(BITMAPFILEHEADER)
- info_header = to_ctypes(buffer, info_header_offset, BITMAPINFOHEADER)
- palette_offset = info_header_offset + info_header.biSize
-
- if info_header.biSize < ctypes.sizeof(BITMAPINFOHEADER):
- raise ImageDecodeException(
- 'Unsupported BMP type: %r' % (filename or file))
-
- width = info_header.biWidth
- height = info_header.biHeight
- if width <= 0 or info_header.biPlanes != 1:
- raise ImageDecodeException(
- 'BMP file has corrupt parameters: %r' % (filename or file))
- pitch_sign = height < 0 and -1 or 1
- height = abs(height)
-
- compression = info_header.biCompression
- if compression not in (BI_RGB, BI_BITFIELDS):
- raise ImageDecodeException(
- 'Unsupported compression: %r' % (filename or file))
-
- clr_used = 0
- bitcount = info_header.biBitCount
- if bitcount == 1:
- pitch = (width + 7) // 8
- bits_type = ctypes.c_ubyte
- decoder = decode_1bit
- elif bitcount == 4:
- pitch = (width + 1) // 2
- bits_type = ctypes.c_ubyte
- decoder = decode_4bit
- elif bitcount == 8:
- bits_type = ctypes.c_ubyte
- pitch = width
- decoder = decode_8bit
- elif bitcount == 16:
- pitch = width * 2
- bits_type = ctypes.c_uint16
- decoder = decode_bitfields
- elif bitcount == 24:
- pitch = width * 3
- bits_type = ctypes.c_ubyte
- decoder = decode_24bit
- elif bitcount == 32:
- pitch = width * 4
- if compression == BI_RGB:
- decoder = decode_32bit_rgb
- bits_type = ctypes.c_ubyte
- elif compression == BI_BITFIELDS:
- decoder = decode_bitfields
- bits_type = ctypes.c_uint32
- else:
- raise ImageDecodeException(
- 'Unsupported compression: %r' % (filename or file))
- else:
- raise ImageDecodeException(
- 'Unsupported bit count %d: %r' % (bitcount, filename or file))
-
- pitch = (pitch + 3) & ~3
- packed_width = pitch // ctypes.sizeof(bits_type)
-
- if bitcount < 16 and compression == BI_RGB:
- clr_used = info_header.biClrUsed or (1 << bitcount)
- palette = to_ctypes(buffer, palette_offset, RGBQUAD * clr_used)
- bits = to_ctypes(buffer, bits_offset,
- bits_type * packed_width * height)
- return decoder(bits, palette, width, height, pitch, pitch_sign)
- elif bitcount >= 16 and compression == BI_RGB:
- bits = to_ctypes(buffer, bits_offset,
- bits_type * (packed_width * height))
- return decoder(bits, None, width, height, pitch, pitch_sign)
- elif compression == BI_BITFIELDS:
- if info_header.biSize >= ctypes.sizeof(BITMAPV4HEADER):
- info_header = to_ctypes(buffer, info_header_offset,
- BITMAPV4HEADER)
- r_mask = info_header.bV4RedMask
- g_mask = info_header.bV4GreenMask
- b_mask = info_header.bV4BlueMask
- else:
- fields_offset = info_header_offset + \
- ctypes.sizeof(BITMAPINFOHEADER)
- fields = to_ctypes(buffer, fields_offset, RGBFields)
- r_mask = fields.red
- g_mask = fields.green
- b_mask = fields.blue
- class _BitsArray(ctypes.LittleEndianStructure):
- _pack_ = 1
- _fields_ = [
- ('data', bits_type * packed_width * height),
- ]
- bits = to_ctypes(buffer, bits_offset, _BitsArray).data
- return decoder(bits, r_mask, g_mask, b_mask,
- width, height, pitch, pitch_sign)
-
-def decode_1bit(bits, palette, width, height, pitch, pitch_sign):
- rgb_pitch = (((pitch << 3) + 7) & ~0x7) * 3
- buffer = (ctypes.c_ubyte * (height * rgb_pitch))()
- i = 0
- for row in bits:
- for packed in row:
- for _ in range(8):
- rgb = palette[(packed & 0x80) >> 7]
- buffer[i] = rgb.rgbRed
- buffer[i + 1] = rgb.rgbGreen
- buffer[i + 2] = rgb.rgbBlue
- i += 3
- packed <<= 1
-
- return ImageData(width, height, 'RGB', buffer, pitch_sign * rgb_pitch)
-
-def decode_4bit(bits, palette, width, height, pitch, pitch_sign):
- rgb_pitch = (((pitch << 1) + 1) & ~0x1) * 3
- buffer = (ctypes.c_ubyte * (height * rgb_pitch))()
- i = 0
- for row in bits:
- for packed in row:
- for index in ((packed & 0xf0) >> 4, packed & 0xf):
- rgb = palette[index]
- buffer[i] = rgb.rgbRed
- buffer[i + 1] = rgb.rgbGreen
- buffer[i + 2] = rgb.rgbBlue
- i += 3
-
- return ImageData(width, height, 'RGB', buffer, pitch_sign * rgb_pitch)
-
-def decode_8bit(bits, palette, width, height, pitch, pitch_sign):
- rgb_pitch = pitch * 3
- buffer = (ctypes.c_ubyte * (height * rgb_pitch))()
- i = 0
- for row in bits:
- for index in row:
- rgb = palette[index]
- buffer[i] = rgb.rgbRed
- buffer[i + 1] = rgb.rgbGreen
- buffer[i + 2] = rgb.rgbBlue
- i += 3
-
- return ImageData(width, height, 'RGB', buffer, pitch_sign * rgb_pitch)
-
-
-def decode_24bit(bits, palette, width, height, pitch, pitch_sign):
- buffer = (ctypes.c_ubyte * (height * pitch))()
- ctypes.memmove(buffer, bits, len(buffer))
- return ImageData(width, height, 'BGR', buffer, pitch_sign * pitch)
-
-def decode_32bit_rgb(bits, palette, width, height, pitch, pitch_sign):
- buffer = (ctypes.c_ubyte * (height * pitch))()
- ctypes.memmove(buffer, bits, len(buffer))
- return ImageData(width, height, 'BGRA', buffer, pitch_sign * pitch)
-
-def get_shift(mask):
- if not mask:
- return 0
-
- # Shift down
- shift = 0
- while not (1 << shift) & mask:
- shift += 1
-
- # Shift up
- shift_up = 0
- while (mask >> shift) >> shift_up:
- shift_up += 1
-
- s = shift - (8 - shift_up)
- if s < 0:
- return 0, -s
- else:
- return s, 0
-
-def decode_bitfields(bits, r_mask, g_mask, b_mask,
- width, height, pitch, pitch_sign):
- r_shift1, r_shift2 = get_shift(r_mask)
- g_shift1, g_shift2 = get_shift(g_mask)
- b_shift1, b_shift2 = get_shift(b_mask)
-
- rgb_pitch = 3 * len(bits[0])
- buffer = (ctypes.c_ubyte * (height * rgb_pitch))()
-
- i = 0
- for row in bits:
- for packed in row:
- buffer[i] = (packed & r_mask) >> r_shift1 << r_shift2
- buffer[i+1] = (packed & g_mask) >> g_shift1 << g_shift2
- buffer[i+2] = (packed & b_mask) >> b_shift1 << b_shift2
- i += 3
-
- return ImageData(width, height, 'RGB', buffer, pitch_sign * rgb_pitch)
-
-def get_decoders():
- return [BMPImageDecoder()]
-
-def get_encoders():
- return []
diff --git a/spaces/ahmadprince007/HolyBot/code/log/__init__.py b/spaces/ahmadprince007/HolyBot/code/log/__init__.py
deleted file mode 100644
index e69de29bb2d1d6434b8b29ae775ad8c2e48c5391..0000000000000000000000000000000000000000
diff --git a/spaces/ai-maker-space/ChatWithYourPDF/chainlit.md b/spaces/ai-maker-space/ChatWithYourPDF/chainlit.md
deleted file mode 100644
index 0f673dc0aed7dae5cfbc91a29940b6dbe270ac9d..0000000000000000000000000000000000000000
--- a/spaces/ai-maker-space/ChatWithYourPDF/chainlit.md
+++ /dev/null
@@ -1,14 +0,0 @@
-# Welcome to Chainlit! 🚀🤖
-
-Hi there, Developer! 👋 We're excited to have you on board. Chainlit is a powerful tool designed to help you prototype, debug and share applications built on top of LLMs.
-
-## Useful Links 🔗
-
-- **Documentation:** Get started with our comprehensive [Chainlit Documentation](https://docs.chainlit.io) 📚
-- **Discord Community:** Join our friendly [Chainlit Discord](https://discord.gg/ZThrUxbAYw) to ask questions, share your projects, and connect with other developers! 💬
-
-We can't wait to see what you create with Chainlit! Happy coding! 💻😊
-
-## Welcome screen
-
-To modify the welcome screen, edit the `chainlit.md` file at the root of your project. If you do not want a welcome screen, just leave this file empty.
diff --git a/spaces/akhaliq/Mask2Former/mask2former/modeling/pixel_decoder/ops/test.py b/spaces/akhaliq/Mask2Former/mask2former/modeling/pixel_decoder/ops/test.py
deleted file mode 100644
index 6e1b545459f6fd3235767e721eb5a1090ae14bef..0000000000000000000000000000000000000000
--- a/spaces/akhaliq/Mask2Former/mask2former/modeling/pixel_decoder/ops/test.py
+++ /dev/null
@@ -1,92 +0,0 @@
-# ------------------------------------------------------------------------------------------------
-# Deformable DETR
-# Copyright (c) 2020 SenseTime. All Rights Reserved.
-# Licensed under the Apache License, Version 2.0 [see LICENSE for details]
-# ------------------------------------------------------------------------------------------------
-# Modified from https://github.com/chengdazhi/Deformable-Convolution-V2-PyTorch/tree/pytorch_1.0.0
-# ------------------------------------------------------------------------------------------------
-
-# Copyright (c) Facebook, Inc. and its affiliates.
-# Modified by Bowen Cheng from https://github.com/fundamentalvision/Deformable-DETR
-
-from __future__ import absolute_import
-from __future__ import print_function
-from __future__ import division
-
-import time
-import torch
-import torch.nn as nn
-from torch.autograd import gradcheck
-
-from functions.ms_deform_attn_func import MSDeformAttnFunction, ms_deform_attn_core_pytorch
-
-
-N, M, D = 1, 2, 2
-Lq, L, P = 2, 2, 2
-shapes = torch.as_tensor([(6, 4), (3, 2)], dtype=torch.long).cuda()
-level_start_index = torch.cat((shapes.new_zeros((1, )), shapes.prod(1).cumsum(0)[:-1]))
-S = sum([(H*W).item() for H, W in shapes])
-
-
-torch.manual_seed(3)
-
-
-@torch.no_grad()
-def check_forward_equal_with_pytorch_double():
- value = torch.rand(N, S, M, D).cuda() * 0.01
- sampling_locations = torch.rand(N, Lq, M, L, P, 2).cuda()
- attention_weights = torch.rand(N, Lq, M, L, P).cuda() + 1e-5
- attention_weights /= attention_weights.sum(-1, keepdim=True).sum(-2, keepdim=True)
- im2col_step = 2
- output_pytorch = ms_deform_attn_core_pytorch(value.double(), shapes, sampling_locations.double(), attention_weights.double()).detach().cpu()
- output_cuda = MSDeformAttnFunction.apply(value.double(), shapes, level_start_index, sampling_locations.double(), attention_weights.double(), im2col_step).detach().cpu()
- fwdok = torch.allclose(output_cuda, output_pytorch)
- max_abs_err = (output_cuda - output_pytorch).abs().max()
- max_rel_err = ((output_cuda - output_pytorch).abs() / output_pytorch.abs()).max()
-
- print(f'* {fwdok} check_forward_equal_with_pytorch_double: max_abs_err {max_abs_err:.2e} max_rel_err {max_rel_err:.2e}')
-
-
-@torch.no_grad()
-def check_forward_equal_with_pytorch_float():
- value = torch.rand(N, S, M, D).cuda() * 0.01
- sampling_locations = torch.rand(N, Lq, M, L, P, 2).cuda()
- attention_weights = torch.rand(N, Lq, M, L, P).cuda() + 1e-5
- attention_weights /= attention_weights.sum(-1, keepdim=True).sum(-2, keepdim=True)
- im2col_step = 2
- output_pytorch = ms_deform_attn_core_pytorch(value, shapes, sampling_locations, attention_weights).detach().cpu()
- output_cuda = MSDeformAttnFunction.apply(value, shapes, level_start_index, sampling_locations, attention_weights, im2col_step).detach().cpu()
- fwdok = torch.allclose(output_cuda, output_pytorch, rtol=1e-2, atol=1e-3)
- max_abs_err = (output_cuda - output_pytorch).abs().max()
- max_rel_err = ((output_cuda - output_pytorch).abs() / output_pytorch.abs()).max()
-
- print(f'* {fwdok} check_forward_equal_with_pytorch_float: max_abs_err {max_abs_err:.2e} max_rel_err {max_rel_err:.2e}')
-
-
-def check_gradient_numerical(channels=4, grad_value=True, grad_sampling_loc=True, grad_attn_weight=True):
-
- value = torch.rand(N, S, M, channels).cuda() * 0.01
- sampling_locations = torch.rand(N, Lq, M, L, P, 2).cuda()
- attention_weights = torch.rand(N, Lq, M, L, P).cuda() + 1e-5
- attention_weights /= attention_weights.sum(-1, keepdim=True).sum(-2, keepdim=True)
- im2col_step = 2
- func = MSDeformAttnFunction.apply
-
- value.requires_grad = grad_value
- sampling_locations.requires_grad = grad_sampling_loc
- attention_weights.requires_grad = grad_attn_weight
-
- gradok = gradcheck(func, (value.double(), shapes, level_start_index, sampling_locations.double(), attention_weights.double(), im2col_step))
-
- print(f'* {gradok} check_gradient_numerical(D={channels})')
-
-
-if __name__ == '__main__':
- check_forward_equal_with_pytorch_double()
- check_forward_equal_with_pytorch_float()
-
- for channels in [30, 32, 64, 71, 1025, 2048, 3096]:
- check_gradient_numerical(channels, True, True, True)
-
-
-
diff --git a/spaces/akhaliq/VQMIVC/ParallelWaveGAN/egs/jnas/voc1/local/data_prep.sh b/spaces/akhaliq/VQMIVC/ParallelWaveGAN/egs/jnas/voc1/local/data_prep.sh
deleted file mode 100644
index f7ca32c0f9df4f11f57647c650cfec658f185350..0000000000000000000000000000000000000000
--- a/spaces/akhaliq/VQMIVC/ParallelWaveGAN/egs/jnas/voc1/local/data_prep.sh
+++ /dev/null
@@ -1,89 +0,0 @@
-#!/bin/bash
-
-# Copyright 2019 Tomoki Hayashi
-# MIT License (https://opensource.org/licenses/MIT)
-
-# shellcheck disable=SC1091
-. ./path.sh || exit 1;
-
-num_dev=500
-train_set="train_nodev"
-dev_set="dev"
-eval_set="eval"
-shuffle=false
-
-# shellcheck disable=SC1091
-. utils/parse_options.sh || exit 1;
-
-# check arguments
-if [ $# != 3 ]; then
- echo "Usage: $0 "
- echo "e.g.: $0 /database/JNAS data conf/train_speakers.txt"
- echo ""
- echo "Options:"
- echo " --num_dev: number of development uttreances (default=500)."
- echo " --train_set: name of train set (default=train_nodev)."
- echo " --dev_set: name of dev set (default=dev)."
- echo " --eval_set: name of eval set (default=eval)."
- echo " --shuffle: whether to perform shuffle in making dev / eval set (default=false)."
- exit 1
-fi
-
-set -euo pipefail
-
-db_root=$1 # database root directory
-data_dir=$2
-spk_list=$3
-
-eval_db_root=${db_root}/DOCS/Test_set
-wav_type=HS # DT or HS
-
-# make directories
-for name in train "${eval_set}"; do
- [ ! -e "${data_dir}/${name}" ] && mkdir -p "${data_dir}/${name}"
-done
-
-# make training & development data
-scp="${data_dir}/train/wav.scp"
-
-# check file existence
-[ -e "${scp}" ] && rm "${scp}"
-
-# shellcheck disable=SC2013
-for spk in $(cat "${spk_list}"); do
- wavdir=${db_root}/WAVES_${wav_type}/${spk}
- [ ! -e "${wavdir}" ] && echo "There are no such a directory (${wavdir})" && exit 1
- find "${wavdir}" -follow -name "*.wav" | sort | while read -r filename; do
- id=$(basename "${filename}" | sed -e "s/\.[^\.]*$//g")
- echo "${spk}_${id} ${filename}" >> "${scp}"
- done
-done
-
-# shuffle
-cp "${scp}" "${scp}.tmp"
-sort -R "${scp}.tmp" > "${scp}"
-rm -r "${scp}.tmp"
-
-# split
-utils/split_data.sh \
- --num_second ${num_dev} \
- --shuffle "${shuffle}" \
- "${data_dir}/train" \
- "${data_dir}/${train_set}" \
- "${data_dir}/${dev_set}"
-
-# make evaluation data
-scp="${data_dir}/${eval_set}/wav.scp"
-
-# check file existence
-[ -e "${scp}" ] && rm "${scp}"
-
-for name in JNAS_testset_100 JNAS_testset_500; do
- find "${eval_db_root}/${name}/WAVES" -follow -name "*.wav" | sort | while read -r filename; do
- id=$(basename "${filename}" | sed -e "s/\.[^\.]*$//g")
- dirname=$(basename "$(dirname "${filename}")")
- echo "${name}_${dirname}_${id} ${filename}" >> "${scp}"
- done
-done
-
-echo "Successfully prepared data."
diff --git a/spaces/akhaliq/yolov3/app.py b/spaces/akhaliq/yolov3/app.py
deleted file mode 100644
index 8cf87a7146cff172450a40e0bbc18ba4ba2b5ac9..0000000000000000000000000000000000000000
--- a/spaces/akhaliq/yolov3/app.py
+++ /dev/null
@@ -1,22 +0,0 @@
-import gradio as gr
-import torch
-from PIL import Image
-# Images
-torch.hub.download_url_to_file('https://cdn.pixabay.com/photo/2016/06/15/01/11/soccer-1457988_1280.jpg', 'soccer.jpg')
-torch.hub.download_url_to_file('https://cdn.pixabay.com/photo/2016/11/21/14/31/vw-bus-1845719_1280.jpg', 'bus.jpg')
-# Model
-model = torch.hub.load('ultralytics/yolov3', 'yolov3') # or yolov3-spp, yolov3-tiny, custom
-def yolo(im, size=640):
- g = (size / max(im.size)) # gain
- im = im.resize((int(x * g) for x in im.size), Image.ANTIALIAS) # resize
- results = model(im) # inference
- results.render() # updates results.imgs with boxes and labels
- return Image.fromarray(results.imgs[0])
-inputs = gr.inputs.Image(type='pil', label="Original Image")
-outputs = gr.outputs.Image(type="pil", label="Output Image")
-title = "YOLOv3"
-description = "YOLOv3 Gradio demo for object detection. Upload an image or click an example image to use."
-article = "
YOLOv3 is a family of compound-scaled object detection models trained on the COCO dataset, and includes simple functionality for Test Time Augmentation (TTA), model ensembling, hyperparameter evolution, and export to ONNX, CoreML and TFLite. Source code |iOS App
"
-examples = [['soccer.jpg'], ['bus.jpg']]
-gr.Interface(yolo, inputs, outputs, title=title, description=description, article=article, examples=examples, theme="huggingface").launch(
- debug=True)
\ No newline at end of file
diff --git a/spaces/alexray/btc_predictor/venv/lib/python3.10/site-packages/pip/_internal/models/scheme.py b/spaces/alexray/btc_predictor/venv/lib/python3.10/site-packages/pip/_internal/models/scheme.py
deleted file mode 100644
index f51190ac60354d90eb2aef4b04c484f8517275c2..0000000000000000000000000000000000000000
--- a/spaces/alexray/btc_predictor/venv/lib/python3.10/site-packages/pip/_internal/models/scheme.py
+++ /dev/null
@@ -1,31 +0,0 @@
-"""
-For types associated with installation schemes.
-
-For a general overview of available schemes and their context, see
-https://docs.python.org/3/install/index.html#alternate-installation.
-"""
-
-
-SCHEME_KEYS = ["platlib", "purelib", "headers", "scripts", "data"]
-
-
-class Scheme:
- """A Scheme holds paths which are used as the base directories for
- artifacts associated with a Python package.
- """
-
- __slots__ = SCHEME_KEYS
-
- def __init__(
- self,
- platlib: str,
- purelib: str,
- headers: str,
- scripts: str,
- data: str,
- ) -> None:
- self.platlib = platlib
- self.purelib = purelib
- self.headers = headers
- self.scripts = scripts
- self.data = data
diff --git a/spaces/alfabill/stable-diffusion-inpainting-2/README.md b/spaces/alfabill/stable-diffusion-inpainting-2/README.md
deleted file mode 100644
index e70c33fd2395bf06371f7975dbaec8f5c5bb2899..0000000000000000000000000000000000000000
--- a/spaces/alfabill/stable-diffusion-inpainting-2/README.md
+++ /dev/null
@@ -1,14 +0,0 @@
----
-title: Stable Diffusion Inpainting
-emoji: ⚡
-colorFrom: gray
-colorTo: yellow
-sdk: gradio
-sdk_version: 3.36.1
-app_file: app.py
-pinned: false
-license: mit
-duplicated_from: multimodalart/stable-diffusion-inpainting
----
-
-Check out the configuration reference at https://huggingface.co/docs/hub/spaces-config-reference
diff --git a/spaces/allknowingroger/Image-Models-Test129/README.md b/spaces/allknowingroger/Image-Models-Test129/README.md
deleted file mode 100644
index 10fa57b0d87457d2befd80cfac20037e26d8be3e..0000000000000000000000000000000000000000
--- a/spaces/allknowingroger/Image-Models-Test129/README.md
+++ /dev/null
@@ -1,12 +0,0 @@
----
-title: More Image Models
-emoji: 😻
-colorFrom: red
-colorTo: gray
-sdk: gradio
-sdk_version: 3.23.0
-app_file: app.py
-duplicated_from: allknowingroger/Image-Models-Test128
----
-
-
\ No newline at end of file
diff --git a/spaces/alsalemi/pv-segment-01/transforms.py b/spaces/alsalemi/pv-segment-01/transforms.py
deleted file mode 100644
index 9c32ce7d0b4d546c927237a512d1cb0a597cb3db..0000000000000000000000000000000000000000
--- a/spaces/alsalemi/pv-segment-01/transforms.py
+++ /dev/null
@@ -1,595 +0,0 @@
-from typing import Dict, List, Optional, Tuple, Union
-
-import torch
-import torchvision
-from torch import nn, Tensor
-from torchvision import ops
-from torchvision.transforms import functional as F, InterpolationMode, transforms as T
-
-
-def _flip_coco_person_keypoints(kps, width):
- flip_inds = [0, 2, 1, 4, 3, 6, 5, 8, 7, 10, 9, 12, 11, 14, 13, 16, 15]
- flipped_data = kps[:, flip_inds]
- flipped_data[..., 0] = width - flipped_data[..., 0]
- # Maintain COCO convention that if visibility == 0, then x, y = 0
- inds = flipped_data[..., 2] == 0
- flipped_data[inds] = 0
- return flipped_data
-
-
-class Compose:
- def __init__(self, transforms):
- self.transforms = transforms
-
- def __call__(self, image, target):
- # print('transform.Compose called')
- for t in self.transforms:
- image, target = t(image, target)
- return image, target
-
-
-class RandomHorizontalFlip(T.RandomHorizontalFlip):
- def forward(
- self, image: Tensor, target: Optional[Dict[str, Tensor]] = None
- ) -> Tuple[Tensor, Optional[Dict[str, Tensor]]]:
- if torch.rand(1) < self.p:
- image = F.hflip(image)
- if target is not None:
- _, _, width = F.get_dimensions(image)
- target["boxes"][:, [0, 2]] = width - target["boxes"][:, [2, 0]]
- if "masks" in target:
- target["masks"] = target["masks"].flip(-1)
- if "keypoints" in target:
- keypoints = target["keypoints"]
- keypoints = _flip_coco_person_keypoints(keypoints, width)
- target["keypoints"] = keypoints
- return image, target
-
-
-class PILToTensor(nn.Module):
- def forward(
- self, image: Tensor, target: Optional[Dict[str, Tensor]] = None
- ) -> Tuple[Tensor, Optional[Dict[str, Tensor]]]:
- image = F.pil_to_tensor(image)
- image = F.convert_image_dtype(image)
- return image, target
-
-
-class ConvertImageDtype(nn.Module):
- def __init__(self, dtype: torch.dtype) -> None:
- super().__init__()
- self.dtype = dtype
-
- def forward(
- self, image: Tensor, target: Optional[Dict[str, Tensor]] = None
- ) -> Tuple[Tensor, Optional[Dict[str, Tensor]]]:
- image = F.convert_image_dtype(image, self.dtype)
- return image, target
-
-
-class RandomIoUCrop(nn.Module):
- def __init__(
- self,
- min_scale: float = 0.3,
- max_scale: float = 1.0,
- min_aspect_ratio: float = 0.5,
- max_aspect_ratio: float = 2.0,
- sampler_options: Optional[List[float]] = None,
- trials: int = 40,
- ):
- super().__init__()
- # Configuration similar to https://github.com/weiliu89/caffe/blob/ssd/examples/ssd/ssd_coco.py#L89-L174
- self.min_scale = min_scale
- self.max_scale = max_scale
- self.min_aspect_ratio = min_aspect_ratio
- self.max_aspect_ratio = max_aspect_ratio
- if sampler_options is None:
- sampler_options = [0.0, 0.1, 0.3, 0.5, 0.7, 0.9, 1.0]
- self.options = sampler_options
- self.trials = trials
-
- def forward(
- self, image: Tensor, target: Optional[Dict[str, Tensor]] = None
- ) -> Tuple[Tensor, Optional[Dict[str, Tensor]]]:
- if target is None:
- raise ValueError("The targets can't be None for this transform.")
-
- if isinstance(image, torch.Tensor):
- if image.ndimension() not in {2, 3}:
- raise ValueError(f"image should be 2/3 dimensional. Got {image.ndimension()} dimensions.")
- elif image.ndimension() == 2:
- image = image.unsqueeze(0)
-
- _, orig_h, orig_w = F.get_dimensions(image)
-
- while True:
- # sample an option
- idx = int(torch.randint(low=0, high=len(self.options), size=(1,)))
- min_jaccard_overlap = self.options[idx]
- if min_jaccard_overlap >= 1.0: # a value larger than 1 encodes the leave as-is option
- return image, target
-
- for _ in range(self.trials):
- # check the aspect ratio limitations
- r = self.min_scale + (self.max_scale - self.min_scale) * torch.rand(2)
- new_w = int(orig_w * r[0])
- new_h = int(orig_h * r[1])
- aspect_ratio = new_w / new_h
- if not (self.min_aspect_ratio <= aspect_ratio <= self.max_aspect_ratio):
- continue
-
- # check for 0 area crops
- r = torch.rand(2)
- left = int((orig_w - new_w) * r[0])
- top = int((orig_h - new_h) * r[1])
- right = left + new_w
- bottom = top + new_h
- if left == right or top == bottom:
- continue
-
- # check for any valid boxes with centers within the crop area
- cx = 0.5 * (target["boxes"][:, 0] + target["boxes"][:, 2])
- cy = 0.5 * (target["boxes"][:, 1] + target["boxes"][:, 3])
- is_within_crop_area = (left < cx) & (cx < right) & (top < cy) & (cy < bottom)
- if not is_within_crop_area.any():
- continue
-
- # check at least 1 box with jaccard limitations
- boxes = target["boxes"][is_within_crop_area]
- ious = torchvision.ops.boxes.box_iou(
- boxes, torch.tensor([[left, top, right, bottom]], dtype=boxes.dtype, device=boxes.device)
- )
- if ious.max() < min_jaccard_overlap:
- continue
-
- # keep only valid boxes and perform cropping
- target["boxes"] = boxes
- target["labels"] = target["labels"][is_within_crop_area]
- target["boxes"][:, 0::2] -= left
- target["boxes"][:, 1::2] -= top
- target["boxes"][:, 0::2].clamp_(min=0, max=new_w)
- target["boxes"][:, 1::2].clamp_(min=0, max=new_h)
- image = F.crop(image, top, left, new_h, new_w)
-
- return image, target
-
-
-class RandomZoomOut(nn.Module):
- def __init__(
- self, fill: Optional[List[float]] = None, side_range: Tuple[float, float] = (1.0, 4.0), p: float = 0.5
- ):
- super().__init__()
- if fill is None:
- fill = [0.0, 0.0, 0.0]
- self.fill = fill
- self.side_range = side_range
- if side_range[0] < 1.0 or side_range[0] > side_range[1]:
- raise ValueError(f"Invalid canvas side range provided {side_range}.")
- self.p = p
-
- @torch.jit.unused
- def _get_fill_value(self, is_pil):
- # type: (bool) -> int
- # We fake the type to make it work on JIT
- return tuple(int(x) for x in self.fill) if is_pil else 0
-
- def forward(
- self, image: Tensor, target: Optional[Dict[str, Tensor]] = None
- ) -> Tuple[Tensor, Optional[Dict[str, Tensor]]]:
- if isinstance(image, torch.Tensor):
- if image.ndimension() not in {2, 3}:
- raise ValueError(f"image should be 2/3 dimensional. Got {image.ndimension()} dimensions.")
- elif image.ndimension() == 2:
- image = image.unsqueeze(0)
-
- if torch.rand(1) >= self.p:
- return image, target
-
- _, orig_h, orig_w = F.get_dimensions(image)
-
- r = self.side_range[0] + torch.rand(1) * (self.side_range[1] - self.side_range[0])
- canvas_width = int(orig_w * r)
- canvas_height = int(orig_h * r)
-
- r = torch.rand(2)
- left = int((canvas_width - orig_w) * r[0])
- top = int((canvas_height - orig_h) * r[1])
- right = canvas_width - (left + orig_w)
- bottom = canvas_height - (top + orig_h)
-
- if torch.jit.is_scripting():
- fill = 0
- else:
- fill = self._get_fill_value(F._is_pil_image(image))
-
- image = F.pad(image, [left, top, right, bottom], fill=fill)
- if isinstance(image, torch.Tensor):
- # PyTorch's pad supports only integers on fill. So we need to overwrite the colour
- v = torch.tensor(self.fill, device=image.device, dtype=image.dtype).view(-1, 1, 1)
- image[..., :top, :] = image[..., :, :left] = image[..., (top + orig_h) :, :] = image[
- ..., :, (left + orig_w) :
- ] = v
-
- if target is not None:
- target["boxes"][:, 0::2] += left
- target["boxes"][:, 1::2] += top
-
- return image, target
-
-
-class RandomPhotometricDistort(nn.Module):
- def __init__(
- self,
- contrast: Tuple[float, float] = (0.5, 1.5),
- saturation: Tuple[float, float] = (0.5, 1.5),
- hue: Tuple[float, float] = (-0.05, 0.05),
- brightness: Tuple[float, float] = (0.875, 1.125),
- p: float = 0.5,
- ):
- super().__init__()
- self._brightness = T.ColorJitter(brightness=brightness)
- self._contrast = T.ColorJitter(contrast=contrast)
- self._hue = T.ColorJitter(hue=hue)
- self._saturation = T.ColorJitter(saturation=saturation)
- self.p = p
-
- def forward(
- self, image: Tensor, target: Optional[Dict[str, Tensor]] = None
- ) -> Tuple[Tensor, Optional[Dict[str, Tensor]]]:
- if isinstance(image, torch.Tensor):
- if image.ndimension() not in {2, 3}:
- raise ValueError(f"image should be 2/3 dimensional. Got {image.ndimension()} dimensions.")
- elif image.ndimension() == 2:
- image = image.unsqueeze(0)
-
- r = torch.rand(7)
-
- if r[0] < self.p:
- image = self._brightness(image)
-
- contrast_before = r[1] < 0.5
- if contrast_before:
- if r[2] < self.p:
- image = self._contrast(image)
-
- if r[3] < self.p:
- image = self._saturation(image)
-
- if r[4] < self.p:
- image = self._hue(image)
-
- if not contrast_before:
- if r[5] < self.p:
- image = self._contrast(image)
-
- if r[6] < self.p:
- channels, _, _ = F.get_dimensions(image)
- permutation = torch.randperm(channels)
-
- is_pil = F._is_pil_image(image)
- if is_pil:
- image = F.pil_to_tensor(image)
- image = F.convert_image_dtype(image)
- image = image[..., permutation, :, :]
- if is_pil:
- image = F.to_pil_image(image)
-
- return image, target
-
-
-class ScaleJitter(nn.Module):
- """Randomly resizes the image and its bounding boxes within the specified scale range.
- The class implements the Scale Jitter augmentation as described in the paper
- `"Simple Copy-Paste is a Strong Data Augmentation Method for Instance Segmentation" `_.
-
- Args:
- target_size (tuple of ints): The target size for the transform provided in (height, weight) format.
- scale_range (tuple of ints): scaling factor interval, e.g (a, b), then scale is randomly sampled from the
- range a <= scale <= b.
- interpolation (InterpolationMode): Desired interpolation enum defined by
- :class:`torchvision.transforms.InterpolationMode`. Default is ``InterpolationMode.BILINEAR``.
- """
-
- def __init__(
- self,
- target_size: Tuple[int, int],
- scale_range: Tuple[float, float] = (0.1, 2.0),
- interpolation: InterpolationMode = InterpolationMode.BILINEAR,
- ):
- super().__init__()
- self.target_size = target_size
- self.scale_range = scale_range
- self.interpolation = interpolation
-
- def forward(
- self, image: Tensor, target: Optional[Dict[str, Tensor]] = None
- ) -> Tuple[Tensor, Optional[Dict[str, Tensor]]]:
- if isinstance(image, torch.Tensor):
- if image.ndimension() not in {2, 3}:
- raise ValueError(f"image should be 2/3 dimensional. Got {image.ndimension()} dimensions.")
- elif image.ndimension() == 2:
- image = image.unsqueeze(0)
-
- _, orig_height, orig_width = F.get_dimensions(image)
-
- scale = self.scale_range[0] + torch.rand(1) * (self.scale_range[1] - self.scale_range[0])
- r = min(self.target_size[1] / orig_height, self.target_size[0] / orig_width) * scale
- new_width = int(orig_width * r)
- new_height = int(orig_height * r)
-
- image = F.resize(image, [new_height, new_width], interpolation=self.interpolation)
-
- if target is not None:
- target["boxes"][:, 0::2] *= new_width / orig_width
- target["boxes"][:, 1::2] *= new_height / orig_height
- if "masks" in target:
- target["masks"] = F.resize(
- target["masks"], [new_height, new_width], interpolation=InterpolationMode.NEAREST
- )
-
- return image, target
-
-
-class FixedSizeCrop(nn.Module):
- def __init__(self, size, fill=0, padding_mode="constant"):
- super().__init__()
- size = tuple(T._setup_size(size, error_msg="Please provide only two dimensions (h, w) for size."))
- self.crop_height = size[0]
- self.crop_width = size[1]
- self.fill = fill
- self.padding_mode = padding_mode
-
- def _pad(self, img, target, padding):
- # Taken from the functional_tensor.py pad
- if isinstance(padding, int):
- pad_left = pad_right = pad_top = pad_bottom = padding
- elif len(padding) == 1:
- pad_left = pad_right = pad_top = pad_bottom = padding[0]
- elif len(padding) == 2:
- pad_left = pad_right = padding[0]
- pad_top = pad_bottom = padding[1]
- else:
- pad_left = padding[0]
- pad_top = padding[1]
- pad_right = padding[2]
- pad_bottom = padding[3]
-
- padding = [pad_left, pad_top, pad_right, pad_bottom]
- img = F.pad(img, padding, self.fill, self.padding_mode)
- if target is not None:
- target["boxes"][:, 0::2] += pad_left
- target["boxes"][:, 1::2] += pad_top
- if "masks" in target:
- target["masks"] = F.pad(target["masks"], padding, 0, "constant")
-
- return img, target
-
- def _crop(self, img, target, top, left, height, width):
- img = F.crop(img, top, left, height, width)
- if target is not None:
- boxes = target["boxes"]
- boxes[:, 0::2] -= left
- boxes[:, 1::2] -= top
- boxes[:, 0::2].clamp_(min=0, max=width)
- boxes[:, 1::2].clamp_(min=0, max=height)
-
- is_valid = (boxes[:, 0] < boxes[:, 2]) & (boxes[:, 1] < boxes[:, 3])
-
- target["boxes"] = boxes[is_valid]
- target["labels"] = target["labels"][is_valid]
- if "masks" in target:
- target["masks"] = F.crop(target["masks"][is_valid], top, left, height, width)
-
- return img, target
-
- def forward(self, img, target=None):
- _, height, width = F.get_dimensions(img)
- new_height = min(height, self.crop_height)
- new_width = min(width, self.crop_width)
-
- if new_height != height or new_width != width:
- offset_height = max(height - self.crop_height, 0)
- offset_width = max(width - self.crop_width, 0)
-
- r = torch.rand(1)
- top = int(offset_height * r)
- left = int(offset_width * r)
-
- img, target = self._crop(img, target, top, left, new_height, new_width)
-
- pad_bottom = max(self.crop_height - new_height, 0)
- pad_right = max(self.crop_width - new_width, 0)
- if pad_bottom != 0 or pad_right != 0:
- img, target = self._pad(img, target, [0, 0, pad_right, pad_bottom])
-
- return img, target
-
-
-class RandomShortestSize(nn.Module):
- def __init__(
- self,
- min_size: Union[List[int], Tuple[int], int],
- max_size: int,
- interpolation: InterpolationMode = InterpolationMode.BILINEAR,
- ):
- super().__init__()
- self.min_size = [min_size] if isinstance(min_size, int) else list(min_size)
- self.max_size = max_size
- self.interpolation = interpolation
-
- def forward(
- self, image: Tensor, target: Optional[Dict[str, Tensor]] = None
- ) -> Tuple[Tensor, Optional[Dict[str, Tensor]]]:
- _, orig_height, orig_width = F.get_dimensions(image)
-
- min_size = self.min_size[torch.randint(len(self.min_size), (1,)).item()]
- r = min(min_size / min(orig_height, orig_width), self.max_size / max(orig_height, orig_width))
-
- new_width = int(orig_width * r)
- new_height = int(orig_height * r)
-
- image = F.resize(image, [new_height, new_width], interpolation=self.interpolation)
-
- if target is not None:
- target["boxes"][:, 0::2] *= new_width / orig_width
- target["boxes"][:, 1::2] *= new_height / orig_height
- if "masks" in target:
- target["masks"] = F.resize(
- target["masks"], [new_height, new_width], interpolation=InterpolationMode.NEAREST
- )
-
- return image, target
-
-
-def _copy_paste(
- image: torch.Tensor,
- target: Dict[str, Tensor],
- paste_image: torch.Tensor,
- paste_target: Dict[str, Tensor],
- blending: bool = True,
- resize_interpolation: F.InterpolationMode = F.InterpolationMode.BILINEAR,
-) -> Tuple[torch.Tensor, Dict[str, Tensor]]:
-
- # Random paste targets selection:
- num_masks = len(paste_target["masks"])
-
- if num_masks < 1:
- # Such degerante case with num_masks=0 can happen with LSJ
- # Let's just return (image, target)
- return image, target
-
- # We have to please torch script by explicitly specifying dtype as torch.long
- random_selection = torch.randint(0, num_masks, (num_masks,), device=paste_image.device)
- random_selection = torch.unique(random_selection).to(torch.long)
-
- paste_masks = paste_target["masks"][random_selection]
- paste_boxes = paste_target["boxes"][random_selection]
- paste_labels = paste_target["labels"][random_selection]
-
- masks = target["masks"]
-
- # We resize source and paste data if they have different sizes
- # This is something we introduced here as originally the algorithm works
- # on equal-sized data (for example, coming from LSJ data augmentations)
- size1 = image.shape[-2:]
- size2 = paste_image.shape[-2:]
- if size1 != size2:
- paste_image = F.resize(paste_image, size1, interpolation=resize_interpolation)
- paste_masks = F.resize(paste_masks, size1, interpolation=F.InterpolationMode.NEAREST)
- # resize bboxes:
- ratios = torch.tensor((size1[1] / size2[1], size1[0] / size2[0]), device=paste_boxes.device)
- paste_boxes = paste_boxes.view(-1, 2, 2).mul(ratios).view(paste_boxes.shape)
-
- paste_alpha_mask = paste_masks.sum(dim=0) > 0
-
- if blending:
- paste_alpha_mask = F.gaussian_blur(
- paste_alpha_mask.unsqueeze(0),
- kernel_size=(5, 5),
- sigma=[
- 2.0,
- ],
- )
-
- # Copy-paste images:
- image = (image * (~paste_alpha_mask)) + (paste_image * paste_alpha_mask)
-
- # Copy-paste masks:
- masks = masks * (~paste_alpha_mask)
- non_all_zero_masks = masks.sum((-1, -2)) > 0
- masks = masks[non_all_zero_masks]
-
- # Do a shallow copy of the target dict
- out_target = {k: v for k, v in target.items()}
-
- out_target["masks"] = torch.cat([masks, paste_masks])
-
- # Copy-paste boxes and labels
- boxes = ops.masks_to_boxes(masks)
- out_target["boxes"] = torch.cat([boxes, paste_boxes])
-
- labels = target["labels"][non_all_zero_masks]
- out_target["labels"] = torch.cat([labels, paste_labels])
-
- # Update additional optional keys: area and iscrowd if exist
- if "area" in target:
- out_target["area"] = out_target["masks"].sum((-1, -2)).to(torch.float32)
-
- if "iscrowd" in target and "iscrowd" in paste_target:
- # target['iscrowd'] size can be differ from mask size (non_all_zero_masks)
- # For example, if previous transforms geometrically modifies masks/boxes/labels but
- # does not update "iscrowd"
- if len(target["iscrowd"]) == len(non_all_zero_masks):
- iscrowd = target["iscrowd"][non_all_zero_masks]
- paste_iscrowd = paste_target["iscrowd"][random_selection]
- out_target["iscrowd"] = torch.cat([iscrowd, paste_iscrowd])
-
- # Check for degenerated boxes and remove them
- boxes = out_target["boxes"]
- degenerate_boxes = boxes[:, 2:] <= boxes[:, :2]
- if degenerate_boxes.any():
- valid_targets = ~degenerate_boxes.any(dim=1)
-
- out_target["boxes"] = boxes[valid_targets]
- out_target["masks"] = out_target["masks"][valid_targets]
- out_target["labels"] = out_target["labels"][valid_targets]
-
- if "area" in out_target:
- out_target["area"] = out_target["area"][valid_targets]
- if "iscrowd" in out_target and len(out_target["iscrowd"]) == len(valid_targets):
- out_target["iscrowd"] = out_target["iscrowd"][valid_targets]
-
- return image, out_target
-
-
-class SimpleCopyPaste(torch.nn.Module):
- def __init__(self, blending=True, resize_interpolation=F.InterpolationMode.BILINEAR):
- super().__init__()
- self.resize_interpolation = resize_interpolation
- self.blending = blending
-
- def forward(
- self, images: List[torch.Tensor], targets: List[Dict[str, Tensor]]
- ) -> Tuple[List[torch.Tensor], List[Dict[str, Tensor]]]:
- torch._assert(
- isinstance(images, (list, tuple)) and all([isinstance(v, torch.Tensor) for v in images]),
- "images should be a list of tensors",
- )
- torch._assert(
- isinstance(targets, (list, tuple)) and len(images) == len(targets),
- "targets should be a list of the same size as images",
- )
- for target in targets:
- # Can not check for instance type dict with inside torch.jit.script
- # torch._assert(isinstance(target, dict), "targets item should be a dict")
- for k in ["masks", "boxes", "labels"]:
- torch._assert(k in target, f"Key {k} should be present in targets")
- torch._assert(isinstance(target[k], torch.Tensor), f"Value for the key {k} should be a tensor")
-
- # images = [t1, t2, ..., tN]
- # Let's define paste_images as shifted list of input images
- # paste_images = [t2, t3, ..., tN, t1]
- # FYI: in TF they mix data on the dataset level
- images_rolled = images[-1:] + images[:-1]
- targets_rolled = targets[-1:] + targets[:-1]
-
- output_images: List[torch.Tensor] = []
- output_targets: List[Dict[str, Tensor]] = []
-
- for image, target, paste_image, paste_target in zip(images, targets, images_rolled, targets_rolled):
- output_image, output_data = _copy_paste(
- image,
- target,
- paste_image,
- paste_target,
- blending=self.blending,
- resize_interpolation=self.resize_interpolation,
- )
- output_images.append(output_image)
- output_targets.append(output_data)
-
- return output_images, output_targets
-
- def __repr__(self) -> str:
- s = f"{self.__class__.__name__}(blending={self.blending}, resize_interpolation={self.resize_interpolation})"
- return s
diff --git a/spaces/altafalam3/Text-Summarizer/extractive_summarizer/model_processors.py b/spaces/altafalam3/Text-Summarizer/extractive_summarizer/model_processors.py
deleted file mode 100644
index 9badc36c2a0d3d735fa24c2a1f16a15a4f3ab291..0000000000000000000000000000000000000000
--- a/spaces/altafalam3/Text-Summarizer/extractive_summarizer/model_processors.py
+++ /dev/null
@@ -1,401 +0,0 @@
-from typing import List, Optional, Tuple, Union
-
-import numpy as np
-from transformers import (AlbertModel, AlbertTokenizer, BartModel,
- BartTokenizer, BertModel, BertTokenizer,
- CamembertModel, CamembertTokenizer, CTRLModel,
- CTRLTokenizer, DistilBertModel, DistilBertTokenizer,
- GPT2Model, GPT2Tokenizer, LongformerModel,
- LongformerTokenizer, OpenAIGPTModel,
- OpenAIGPTTokenizer, PreTrainedModel,
- PreTrainedTokenizer, RobertaModel, RobertaTokenizer,
- TransfoXLModel, TransfoXLTokenizer, XLMModel,
- XLMTokenizer, XLNetModel, XLNetTokenizer)
-
-from extractive_summarizer.bert_parent import BertParent
-from extractive_summarizer.cluster_features import ClusterFeatures
-from extractive_summarizer.sentence_handler import SentenceHandler
-
-
-class ModelProcessor(object):
- aggregate_map = {
- 'mean': np.mean,
- 'min': np.min,
- 'median': np.median,
- 'max': np.max,
- }
-
- def __init__(
- self,
- model: str = 'bert-large-uncased',
- custom_model: PreTrainedModel = None,
- custom_tokenizer: PreTrainedTokenizer = None,
- hidden: Union[List[int], int] = -2,
- reduce_option: str = 'mean',
- sentence_handler: SentenceHandler = SentenceHandler(),
- random_state: int = 12345,
- hidden_concat: bool = False,
- gpu_id: int = 0,
- ):
- """
- This is the parent Bert Summarizer model. New methods should implement this class.
-
- :param model: This parameter is associated with the inherit string parameters from the transformers library.
- :param custom_model: If you have a pre-trained model, you can add the model class here.
- :param custom_tokenizer: If you have a custom tokenizer, you can add the tokenizer here.
- :param hidden: This signifies which layer(s) of the BERT model you would like to use as embeddings.
- :param reduce_option: Given the output of the bert model, this param determines how you want to reduce results.
- :param sentence_handler: The handler to process sentences. If want to use coreference, instantiate and pass.
- CoreferenceHandler instance
- :param random_state: The random state to reproduce summarizations.
- :param hidden_concat: Whether or not to concat multiple hidden layers.
- :param gpu_id: GPU device index if CUDA is available.
- """
- np.random.seed(random_state)
- self.model = BertParent(model, custom_model, custom_tokenizer, gpu_id)
- self.hidden = hidden
- self.reduce_option = reduce_option
- self.sentence_handler = sentence_handler
- self.random_state = random_state
- self.hidden_concat = hidden_concat
-
- def cluster_runner(
- self,
- content: List[str],
- ratio: float = 0.2,
- algorithm: str = 'kmeans',
- use_first: bool = True,
- num_sentences: int = None
- ) -> Tuple[List[str], np.ndarray]:
- """
- Runs the cluster algorithm based on the hidden state. Returns both the embeddings and sentences.
-
- :param content: Content list of sentences.
- :param ratio: The ratio to use for clustering.
- :param algorithm: Type of algorithm to use for clustering.
- :param use_first: Return the first sentence in the output (helpful for news stories, etc).
- :param num_sentences: Number of sentences to use for summarization.
- :return: A tuple of summarized sentences and embeddings
- """
- if num_sentences is not None:
- num_sentences = num_sentences if use_first else num_sentences
-
- hidden = self.model(
- content, self.hidden, self.reduce_option, hidden_concat=self.hidden_concat)
- hidden_args = ClusterFeatures(
- hidden, algorithm, random_state=self.random_state).cluster(ratio, num_sentences)
-
- if use_first:
-
- if not hidden_args:
- hidden_args.append(0)
-
- elif hidden_args[0] != 0:
- hidden_args.insert(0, 0)
-
- sentences = [content[j] for j in hidden_args]
- embeddings = np.asarray([hidden[j] for j in hidden_args])
-
- return sentences, embeddings
-
- def __run_clusters(
- self,
- content: List[str],
- ratio: float = 0.2,
- algorithm: str = 'kmeans',
- use_first: bool = True,
- num_sentences: int = None
- ) -> List[str]:
- """
- Runs clusters and returns sentences.
-
- :param content: The content of sentences.
- :param ratio: Ratio to use for for clustering.
- :param algorithm: Algorithm selection for clustering.
- :param use_first: Whether to use first sentence
- :param num_sentences: Number of sentences. Overrides ratio.
- :return: summarized sentences
- """
- sentences, _ = self.cluster_runner(
- content, ratio, algorithm, use_first, num_sentences)
- return sentences
-
- def __retrieve_summarized_embeddings(
- self,
- content: List[str],
- ratio: float = 0.2,
- algorithm: str = 'kmeans',
- use_first: bool = True,
- num_sentences: int = None
- ) -> np.ndarray:
- """
- Retrieves embeddings of the summarized sentences.
-
- :param content: The content of sentences.
- :param ratio: Ratio to use for for clustering.
- :param algorithm: Algorithm selection for clustering.
- :param use_first: Whether to use first sentence
- :return: Summarized embeddings
- """
- _, embeddings = self.cluster_runner(
- content, ratio, algorithm, use_first, num_sentences)
- return embeddings
-
- def calculate_elbow(
- self,
- body: str,
- algorithm: str = 'kmeans',
- min_length: int = 40,
- max_length: int = 600,
- k_max: int = None,
- ) -> List[float]:
- """
- Calculates elbow across the clusters.
-
- :param body: The input body to summarize.
- :param algorithm: The algorithm to use for clustering.
- :param min_length: The min length to use.
- :param max_length: The max length to use.
- :param k_max: The maximum number of clusters to search.
- :return: List of elbow inertia values.
- """
- sentences = self.sentence_handler(body, min_length, max_length)
-
- if k_max is None:
- k_max = len(sentences) - 1
-
- hidden = self.model(sentences, self.hidden,
- self.reduce_option, hidden_concat=self.hidden_concat)
- elbow = ClusterFeatures(
- hidden, algorithm, random_state=self.random_state).calculate_elbow(k_max)
-
- return elbow
-
- def calculate_optimal_k(
- self,
- body: str,
- algorithm: str = 'kmeans',
- min_length: int = 40,
- max_length: int = 600,
- k_max: int = None,
- ):
- """
- Calculates the optimal Elbow K.
-
- :param body: The input body to summarize.
- :param algorithm: The algorithm to use for clustering.
- :param min_length: The min length to use.
- :param max_length: The max length to use.
- :param k_max: The maximum number of clusters to search.
- :return:
- """
- sentences = self.sentence_handler(body, min_length, max_length)
-
- if k_max is None:
- k_max = len(sentences) - 1
-
- hidden = self.model(sentences, self.hidden,
- self.reduce_option, hidden_concat=self.hidden_concat)
- optimal_k = ClusterFeatures(
- hidden, algorithm, random_state=self.random_state).calculate_optimal_cluster(k_max)
-
- return optimal_k
-
- def run_embeddings(
- self,
- body: str,
- ratio: float = 0.2,
- min_length: int = 40,
- max_length: int = 600,
- use_first: bool = True,
- algorithm: str = 'kmeans',
- num_sentences: int = None,
- aggregate: str = None,
- ) -> Optional[np.ndarray]:
- """
- Preprocesses the sentences, runs the clusters to find the centroids, then combines the embeddings.
-
- :param body: The raw string body to process
- :param ratio: Ratio of sentences to use
- :param min_length: Minimum length of sentence candidates to utilize for the summary.
- :param max_length: Maximum length of sentence candidates to utilize for the summary
- :param use_first: Whether or not to use the first sentence
- :param algorithm: Which clustering algorithm to use. (kmeans, gmm)
- :param num_sentences: Number of sentences to use. Overrides ratio.
- :param aggregate: One of mean, median, max, min. Applied on zero axis
- :return: A summary embedding
- """
- sentences = self.sentence_handler(body, min_length, max_length)
-
- if sentences:
- embeddings = self.__retrieve_summarized_embeddings(
- sentences, ratio, algorithm, use_first, num_sentences)
-
- if aggregate is not None:
- assert aggregate in [
- 'mean', 'median', 'max', 'min'], "aggregate must be mean, min, max, or median"
- embeddings = self.aggregate_map[aggregate](embeddings, axis=0)
-
- return embeddings
-
- return None
-
- def run(
- self,
- body: str,
- ratio: float = 0.2,
- min_length: int = 40,
- max_length: int = 600,
- use_first: bool = True,
- algorithm: str = 'kmeans',
- num_sentences: int = None,
- return_as_list: bool = False
- ) -> Union[List, str]:
- """
- Preprocesses the sentences, runs the clusters to find the centroids, then combines the sentences.
-
- :param body: The raw string body to process
- :param ratio: Ratio of sentences to use
- :param min_length: Minimum length of sentence candidates to utilize for the summary.
- :param max_length: Maximum length of sentence candidates to utilize for the summary
- :param use_first: Whether or not to use the first sentence
- :param algorithm: Which clustering algorithm to use. (kmeans, gmm)
- :param num_sentences: Number of sentences to use (overrides ratio).
- :param return_as_list: Whether or not to return sentences as list.
- :return: A summary sentence
- """
- sentences = self.sentence_handler(body, min_length, max_length)
-
- if sentences:
- sentences = self.__run_clusters(
- sentences, ratio, algorithm, use_first, num_sentences)
-
- if return_as_list:
- return sentences
- else:
- return ' '.join(sentences)
-
- def __call__(
- self,
- body: str,
- ratio: float = 0.2,
- min_length: int = 40,
- max_length: int = 600,
- use_first: bool = True,
- algorithm: str = 'kmeans',
- num_sentences: int = None,
- return_as_list: bool = False,
- ) -> str:
- """
- (utility that wraps around the run function)
- Preprocesses the sentences, runs the clusters to find the centroids, then combines the sentences.
-
- :param body: The raw string body to process.
- :param ratio: Ratio of sentences to use.
- :param min_length: Minimum length of sentence candidates to utilize for the summary.
- :param max_length: Maximum length of sentence candidates to utilize for the summary.
- :param use_first: Whether or not to use the first sentence.
- :param algorithm: Which clustering algorithm to use. (kmeans, gmm)
- :param Number of sentences to use (overrides ratio).
- :param return_as_list: Whether or not to return sentences as list.
- :return: A summary sentence.
- """
- return self.run(
- body, ratio, min_length, max_length, algorithm=algorithm, use_first=use_first, num_sentences=num_sentences,
- return_as_list=return_as_list
- )
-
-
-class Summarizer(ModelProcessor):
-
- def __init__(
- self,
- model: str = 'bert-large-uncased',
- custom_model: PreTrainedModel = None,
- custom_tokenizer: PreTrainedTokenizer = None,
- hidden: Union[List[int], int] = -2,
- reduce_option: str = 'mean',
- sentence_handler: SentenceHandler = SentenceHandler(),
- random_state: int = 12345,
- hidden_concat: bool = False,
- gpu_id: int = 0,
- ):
- """
- This is the main Bert Summarizer class.
-
- :param model: This parameter is associated with the inherit string parameters from the transformers library.
- :param custom_model: If you have a pre-trained model, you can add the model class here.
- :param custom_tokenizer: If you have a custom tokenizer, you can add the tokenizer here.
- :param hidden: This signifies which layer of the BERT model you would like to use as embeddings.
- :param reduce_option: Given the output of the bert model, this param determines how you want to reduce results.
- :param greedyness: associated with the neuralcoref library. Determines how greedy coref should be.
- :param language: Which language to use for training.
- :param random_state: The random state to reproduce summarizations.
- :param hidden_concat: Whether or not to concat multiple hidden layers.
- :param gpu_id: GPU device index if CUDA is available.
- """
-
- super(Summarizer, self).__init__(
- model, custom_model, custom_tokenizer, hidden, reduce_option, sentence_handler, random_state, hidden_concat, gpu_id
- )
-
-
-class TransformerSummarizer(ModelProcessor):
- """
- Another type of Summarizer class to choose keyword based model and tokenizer
- """
-
- MODEL_DICT = {
- 'Bert': (BertModel, BertTokenizer),
- 'OpenAIGPT': (OpenAIGPTModel, OpenAIGPTTokenizer),
- 'GPT2': (GPT2Model, GPT2Tokenizer),
- 'CTRL': (CTRLModel, CTRLTokenizer),
- 'TransfoXL': (TransfoXLModel, TransfoXLTokenizer),
- 'XLNet': (XLNetModel, XLNetTokenizer),
- 'XLM': (XLMModel, XLMTokenizer),
- 'DistilBert': (DistilBertModel, DistilBertTokenizer),
- }
-
- def __init__(
- self,
- transformer_type: str = 'Bert',
- transformer_model_key: str = 'bert-base-uncased',
- transformer_tokenizer_key: str = None,
- hidden: Union[List[int], int] = -2,
- reduce_option: str = 'mean',
- sentence_handler: SentenceHandler = SentenceHandler(),
- random_state: int = 12345,
- hidden_concat: bool = False,
- gpu_id: int = 0,
- ):
- """
- :param transformer_type: The Transformer type, such as Bert, GPT2, DistilBert, etc.
- :param transformer_model_key: The transformer model key. This is the directory for the model.
- :param transformer_tokenizer_key: The transformer tokenizer key. This is the tokenizer directory.
- :param hidden: The hidden output layers to use for the summarization.
- :param reduce_option: The reduce option, such as mean, max, min, median, etc.
- :param sentence_handler: The sentence handler class to process the raw text.
- :param random_state: The random state to use.
- :param hidden_concat: Deprecated hidden concat option.
- :param gpu_id: GPU device index if CUDA is available.
- """
- try:
- self.MODEL_DICT['Roberta'] = (RobertaModel, RobertaTokenizer)
- self.MODEL_DICT['Albert'] = (AlbertModel, AlbertTokenizer)
- self.MODEL_DICT['Camembert'] = (CamembertModel, CamembertTokenizer)
- self.MODEL_DICT['Bart'] = (BartModel, BartTokenizer)
- self.MODEL_DICT['Longformer'] = (LongformerModel, LongformerTokenizer)
- except Exception:
- pass # older transformer version
-
- model_clz, tokenizer_clz = self.MODEL_DICT[transformer_type]
- model = model_clz.from_pretrained(
- transformer_model_key, output_hidden_states=True)
-
- tokenizer = tokenizer_clz.from_pretrained(
- transformer_tokenizer_key if transformer_tokenizer_key is not None else transformer_model_key
- )
-
- super().__init__(
- None, model, tokenizer, hidden, reduce_option, sentence_handler, random_state, hidden_concat, gpu_id
- )
diff --git a/spaces/amasad/Replit-v2-CodeInstruct-3b/app.py b/spaces/amasad/Replit-v2-CodeInstruct-3b/app.py
deleted file mode 100644
index 583d51cb9e90e5ffc7c24d6781cfed8178933b7e..0000000000000000000000000000000000000000
--- a/spaces/amasad/Replit-v2-CodeInstruct-3b/app.py
+++ /dev/null
@@ -1,75 +0,0 @@
-import os
-import gradio as gr
-import torch
-
-from transformers import AutoTokenizer, AutoModelForCausalLM
-
-REPO = "teknium/Replit-v2-CodeInstruct-3B"
-
-description = """#
Code Generation by Instruction with Replit-v1-CodeInstruct-3B
- This model is trained on a large amount of code and fine tuned on code-instruct datasets. You can type an instruction in the ### Instruction: section and received code generation."""
-
-device = "cuda" if torch.cuda.is_available() else "cpu"
-
-tokenizer = AutoTokenizer.from_pretrained(REPO, trust_remote_code=True)
-model = AutoModelForCausalLM.from_pretrained(REPO, torch_dtype=torch.bfloat16, trust_remote_code=True)
-model.to(device)
-
-model.eval()
-
-custom_css = """
-.gradio-container {
- background-color: #0D1525;
- color:white
-}
-#orange-button {
- background: #F26207 !important;
- color: white;
-}
-.cm-gutters{
- border: none !important;
-}
-"""
-
-def post_processing(prompt, completion):
- return prompt + completion
-
-def code_generation(prompt, max_new_tokens=256, temperature=0.2, top_p=0.9, eos_token_id=tokenizer.eos_token_id):
- input_ids = tokenizer(prompt, return_tensors="pt").input_ids.to(device)
- generated_ids = model.generate(input_ids, max_new_tokens=max_new_tokens, do_sample=True, use_cache=True, temperature=temperature, top_p=top_p, eos_token_id=eos_token_id)
- completion = tokenizer.decode(generated_ids[0][input_ids.shape[-1]:], skip_special_tokens=True, clean_up_tokenization_spaces=False)
- return post_processing(prompt, completion)
-
-demo = gr.Blocks(
- css=custom_css
-)
-
-with demo:
- gr.Markdown(value=description)
- with gr.Row():
- input_col , settings_col = gr.Column(scale=6), gr.Column(scale=6),
- with input_col:
- code = gr.Code(lines=28,label='Input', value="### Instruction:\n\n### Response:\n")
- with settings_col:
- with gr.Accordion("Generation Settings", open=True):
- max_new_tokens= gr.Slider(
- minimum=8,
- maximum=512,
- step=1,
- value=48,
- label="Max Tokens",
- )
- temperature = gr.Slider(
- minimum=0.1,
- maximum=2.5,
- step=0.1,
- value=0.6,
- label="Temperature",
- )
-
- with gr.Row():
- run = gr.Button(elem_id="orange-button", value="Generate Response")
-
- event = run.click(code_generation, [code, max_new_tokens, temperature], code, api_name="predict")
-
-demo.queue(max_size=40).launch()
\ No newline at end of file
diff --git a/spaces/angelasnpang/segment-anything-ui/app_configs.py b/spaces/angelasnpang/segment-anything-ui/app_configs.py
deleted file mode 100644
index d9c0e112670ec878e42eed3833df0aa56f1f1a60..0000000000000000000000000000000000000000
--- a/spaces/angelasnpang/segment-anything-ui/app_configs.py
+++ /dev/null
@@ -1,5 +0,0 @@
-model_type = r'vit_b'
-# model_ckpt_path = None
-model_ckpt_path = "checkpoints/sam_vit_b_01ec64.pth"
-device = None
-enable_segment_all = False
\ No newline at end of file
diff --git a/spaces/antonovmaxim/text-generation-webui-space/text-generation-webui-main/extensions/api/streaming_api.py b/spaces/antonovmaxim/text-generation-webui-space/text-generation-webui-main/extensions/api/streaming_api.py
deleted file mode 100644
index 3b9ac658d07bba2b1886886d43aaaa4b36badc5d..0000000000000000000000000000000000000000
--- a/spaces/antonovmaxim/text-generation-webui-space/text-generation-webui-main/extensions/api/streaming_api.py
+++ /dev/null
@@ -1,82 +0,0 @@
-import json
-import asyncio
-from websockets.server import serve
-from threading import Thread
-
-from modules import shared
-from modules.text_generation import generate_reply
-
-from extensions.api.util import build_parameters, try_start_cloudflared
-
-PATH = '/api/v1/stream'
-
-
-async def _handle_connection(websocket, path):
-
- if path != PATH:
- print(f'Streaming api: unknown path: {path}')
- return
-
- async for message in websocket:
- message = json.loads(message)
-
- prompt = message['prompt']
- generate_params = build_parameters(message)
- stopping_strings = generate_params.pop('stopping_strings')
-
- generator = generate_reply(
- prompt, generate_params, stopping_strings=stopping_strings)
-
- # As we stream, only send the new bytes.
- skip_index = len(prompt) if not shared.is_chat() else 0
- message_num = 0
-
- for a in generator:
- to_send = ''
- if isinstance(a, str):
- to_send = a[skip_index:]
- else:
- to_send = a[0][skip_index:]
-
- await websocket.send(json.dumps({
- 'event': 'text_stream',
- 'message_num': message_num,
- 'text': to_send
- }))
-
- await asyncio.sleep(0)
-
- skip_index += len(to_send)
- message_num += 1
-
- await websocket.send(json.dumps({
- 'event': 'stream_end',
- 'message_num': message_num
- }))
-
-
-async def _run(host: str, port: int):
- async with serve(_handle_connection, host, port, ping_interval=None):
- await asyncio.Future() # run forever
-
-
-def _run_server(port: int, share: bool = False):
- address = '0.0.0.0' if shared.args.listen else '127.0.0.1'
-
- def on_start(public_url: str):
- public_url = public_url.replace('https://', 'wss://')
- print(f'Starting streaming server at public url {public_url}{PATH}')
-
- if share:
- try:
- try_start_cloudflared(port, max_attempts=3, on_start=on_start)
- except Exception as e:
- print(e)
- else:
- print(f'Starting streaming server at ws://{address}:{port}{PATH}')
-
- asyncio.run(_run(host=address, port=port))
-
-
-def start_server(port: int, share: bool = False):
- Thread(target=_run_server, args=[port, share], daemon=True).start()
diff --git a/spaces/apetulante/bert-emotion/app.py b/spaces/apetulante/bert-emotion/app.py
deleted file mode 100644
index ddefe6e264c30971ee88ba88a29fed5593956609..0000000000000000000000000000000000000000
--- a/spaces/apetulante/bert-emotion/app.py
+++ /dev/null
@@ -1,144 +0,0 @@
-# -*- coding: utf-8 -*-
-"""4_3-gradio-and-huggingface-spaces.ipynb
-
-Automatically generated by Colaboratory.
-
-Original file is located at
- https://colab.research.google.com/drive/1ML3Jf1UwkDRuEPK7NoVr1Uel9tWa_oP7
-
-# Gradio Interfaces and HuggingFace Spaces
-
-Huggingface [Spaces](https://huggingface.co/spaces) provide an easy-to-use way to explore and demo models. The platform is highly accessible, free to use, and allows you to share models without the need for the user to run any code.
-
-The best part - you can insert your own model from huggingface, build your app with [gradio](https://gradio.app/docs/), and deploy in no time!
-
-Let's use the model that we generated in the `4_1-text-classification-finetune-solns.ipynb` notebook and create a gradio space to demonstrate it!
-
-## Install and Import Packages
-"""
-
-# Commented out IPython magic to ensure Python compatibility.
-# %%capture
-# !pip install gradio transformers
-
-# import necessary libraries
-import gradio as gr
-import numpy as np
-from transformers import AutoModelForSequenceClassification, AutoTokenizer
-from huggingface_hub import notebook_login
-
-#!git config --global credential.helper store
-
-#notebook_login()
-
-"""## Load in Your Model
-
-Next, we'll load in our model from huggingface. This should be in a HF repo under your name, probably formatted `your-username/model-name`.
-We'll use the `Auto` classes to load in this model. The `Auto` classes in the Hugging Face transformers library are designed to automatically infer the correct model architecture or tokenizer based on the model checkpoint provided.
-
-For example, below, AutoModelForSequenceClassification is specifically designed for sequence classification tasks, such as text classification or sentiment analysis (which is what bert-emotion was). If you've fine-tuned a model for a different type of task, like question answering or named entity recognition, you would need to use a different auto model class that corresponds to that task. For example, for question answering, you might use AutoModelForQuestionAnswering.
-
-To ensure the right model class is used, you should use the appropriate auto model class based on the task your model was fine-tuned for. You can look at the config.json file associated with a model checkpoint to see the type of model. (You can also use this model name directly - but the `Auto` classes will give you more flexibility!)
-
-[ See more about Auto classes [here](https://huggingface.co/docs/transformers/model_doc/auto#auto-classes). ]
-"""
-
-# specify the model name
-# replace 'your-username/model-name' with the name of your custom trained model
-model_name = 'apetulante/bert-emotion'
-
-# initialize the model and tokenizer
-model = AutoModelForSequenceClassification.from_pretrained(model_name)
-tokenizer = AutoTokenizer.from_pretrained(model_name)
-
-"""Let's also define our labels so we know how to interpret the output from the model."""
-
-labels = {0: 'anger', 1: 'joy', 2: 'optimism', 3: 'sadness'}
-
-"""## Define and Create the Gradio Interface
-
-Next, we'll define a function that will do the sentiment analysis task for us. A lot of this should look very similar to how we did basic inferencing with Huggingface, because now that we've pushed our model there, we can grab it just like any other model!
-"""
-
-# Define the prediction function
-def predict_sentiment(text):
- # Tokenize the input tweet using the tokenizer
- inputs = tokenizer.encode_plus(
- text,
- add_special_tokens=True, # Add special tokens for BERT
- truncation=True, # Truncate the input if it exceeds the maximum sequence length
- padding='longest', # Pad the input sequences to the length of the longest sequence
- return_tensors='pt' # Return PyTorch tensors
- )
-
- # Pass the tokenized inputs to the model
- outputs = model(**inputs)
-
- # Get the predicted class by finding the index of the highest logit score
- logits = outputs.logits.detach().numpy()
- predicted_class = np.argmax(logits, axis=1).item()
-
- # Map the predicted class index to the corresponding sentiment label using the labels dictionary
- sentiment_label = labels[predicted_class]
-
- # Return the predicted sentiment label
- return sentiment_label
-
-predict_sentiment("okay,let's go!")
-
-"""Let's define the Gradio interface with `sentiment_analysis` as the function that takes user inputs and generates outputs. The `inputs` argument specifies the input component, in this case a textbox where users can enter text. The `outputs` argument specifies the type of the output, in this case a simple text."""
-
-# Define the Gradio interface
-iface = gr.Interface(
- fn=predict_sentiment,
- inputs="text",
- outputs="text",
- title="Sentiment Analysis",
- description="Enter a tweet and get its sentiment prediction.",
- examples=[
- ["I'm furious right now."],
- ["I have been feeling amazing lately!"],
- ["I think that everything is going to turn out okay."],
- ["Feeling really down today."],
- ]
-)
-
-# Run the Gradio interface
-iface.launch()
-
-"""You may notice a "flag" option here. The flag functionality is a default feature in Gradio. When you launch a Gradio interface, you'll notice a "Flag" button alongside each input-output pair. Clicking this button allows you to flag examples where the model's output may not be correct or as expected.
-
-We can view these flagged examples in the `log.csv` file that will be saved in the `flagged` folder to the left.
-
-## Turn it into a Huggingface Space!
-
-Simply turn this code into a app.py file, and create a huggingface space. Since the model is already hosted on huggingface, you should be up and running in no time!
-"""
-
-
-
-"""## Optional Homework
-
-We've just touched the surface of what gradio can do here, but there are a TON of other options of cool features to add or things to do with gradio. Try out a few on your own!
-
-The code to create the gradio space is also fairly short. You can try giving the code to make this space to ChatGPT, and ask it to help you come up with additional features.
-"""
-
-#@title Add Confidence Information
-#@markdown With each of these predictions, the model has some confidence
-#@markdown that the given prediction is correct.
-#@markdown It can be useful to display the relative prediction confidence
-#@markdown for *all* classes, so we can know if the model was less sure of
-#@markdown an answer
-
-#@title Predict in Batch
-#@markdown Often, it's convenient to use a gradio space to allow
-#@markdown users to predict on a batch of inputs.
-#@markdown Imagine you have a text file with a new tweet to determine the sentiment
-#@markdown of on each line. How can you edit this gradio space to accept
-#@markdown and return a .txt file?
-
-#@title Try Visualizations
-#@markdown With a batch prediction, there's an opportunity
-#@markdown to try visualizations with the data.
-#@markdown Try to show a pie or bar chart of the sentiments of a batch.
\ No newline at end of file
diff --git a/spaces/arxify/RVC-beta-v2-0618/runtime/Lib/site-packages/Cython/Compiler/Main.py b/spaces/arxify/RVC-beta-v2-0618/runtime/Lib/site-packages/Cython/Compiler/Main.py
deleted file mode 100644
index dc4add541e520419cb1cc29fd06a8f6a2c0b95e0..0000000000000000000000000000000000000000
--- a/spaces/arxify/RVC-beta-v2-0618/runtime/Lib/site-packages/Cython/Compiler/Main.py
+++ /dev/null
@@ -1,904 +0,0 @@
-#
-# Cython Top Level
-#
-
-from __future__ import absolute_import
-
-import os
-import re
-import sys
-import io
-
-if sys.version_info[:2] < (2, 6) or (3, 0) <= sys.version_info[:2] < (3, 3):
- sys.stderr.write("Sorry, Cython requires Python 2.6+ or 3.3+, found %d.%d\n" % tuple(sys.version_info[:2]))
- sys.exit(1)
-
-try:
- from __builtin__ import basestring
-except ImportError:
- basestring = str
-
-# Do not import Parsing here, import it when needed, because Parsing imports
-# Nodes, which globally needs debug command line options initialized to set a
-# conditional metaclass. These options are processed by CmdLine called from
-# main() in this file.
-# import Parsing
-from . import Errors
-from .StringEncoding import EncodedString
-from .Scanning import PyrexScanner, FileSourceDescriptor
-from .Errors import PyrexError, CompileError, error, warning
-from .Symtab import ModuleScope
-from .. import Utils
-from . import Options
-
-from . import Version # legacy import needed by old PyTables versions
-version = Version.version # legacy attribute - use "Cython.__version__" instead
-
-module_name_pattern = re.compile(r"[A-Za-z_][A-Za-z0-9_]*(\.[A-Za-z_][A-Za-z0-9_]*)*$")
-
-verbose = 0
-
-standard_include_path = os.path.abspath(os.path.join(os.path.dirname(__file__),
- os.path.pardir, 'Includes'))
-
-class CompilationData(object):
- # Bundles the information that is passed from transform to transform.
- # (For now, this is only)
-
- # While Context contains every pxd ever loaded, path information etc.,
- # this only contains the data related to a single compilation pass
- #
- # pyx ModuleNode Main code tree of this compilation.
- # pxds {string : ModuleNode} Trees for the pxds used in the pyx.
- # codewriter CCodeWriter Where to output final code.
- # options CompilationOptions
- # result CompilationResult
- pass
-
-
-class Context(object):
- # This class encapsulates the context needed for compiling
- # one or more Cython implementation files along with their
- # associated and imported declaration files. It includes
- # the root of the module import namespace and the list
- # of directories to search for include files.
- #
- # modules {string : ModuleScope}
- # include_directories [string]
- # future_directives [object]
- # language_level int currently 2 or 3 for Python 2/3
-
- cython_scope = None
- language_level = None # warn when not set but default to Py2
-
- def __init__(self, include_directories, compiler_directives, cpp=False,
- language_level=None, options=None):
- # cython_scope is a hack, set to False by subclasses, in order to break
- # an infinite loop.
- # Better code organization would fix it.
-
- from . import Builtin, CythonScope
- self.modules = {"__builtin__" : Builtin.builtin_scope}
- self.cython_scope = CythonScope.create_cython_scope(self)
- self.modules["cython"] = self.cython_scope
- self.include_directories = include_directories
- self.future_directives = set()
- self.compiler_directives = compiler_directives
- self.cpp = cpp
- self.options = options
-
- self.pxds = {} # full name -> node tree
- self._interned = {} # (type(value), value, *key_args) -> interned_value
-
- if language_level is not None:
- self.set_language_level(language_level)
-
- self.gdb_debug_outputwriter = None
-
- def set_language_level(self, level):
- from .Future import print_function, unicode_literals, absolute_import, division
- future_directives = set()
- if level == '3str':
- level = 3
- else:
- level = int(level)
- if level >= 3:
- future_directives.add(unicode_literals)
- if level >= 3:
- future_directives.update([print_function, absolute_import, division])
- self.language_level = level
- self.future_directives = future_directives
- if level >= 3:
- self.modules['builtins'] = self.modules['__builtin__']
-
- def intern_ustring(self, value, encoding=None):
- key = (EncodedString, value, encoding)
- try:
- return self._interned[key]
- except KeyError:
- pass
- value = EncodedString(value)
- if encoding:
- value.encoding = encoding
- self._interned[key] = value
- return value
-
- def intern_value(self, value, *key):
- key = (type(value), value) + key
- try:
- return self._interned[key]
- except KeyError:
- pass
- self._interned[key] = value
- return value
-
- # pipeline creation functions can now be found in Pipeline.py
-
- def process_pxd(self, source_desc, scope, module_name):
- from . import Pipeline
- if isinstance(source_desc, FileSourceDescriptor) and source_desc._file_type == 'pyx':
- source = CompilationSource(source_desc, module_name, os.getcwd())
- result_sink = create_default_resultobj(source, self.options)
- pipeline = Pipeline.create_pyx_as_pxd_pipeline(self, result_sink)
- result = Pipeline.run_pipeline(pipeline, source)
- else:
- pipeline = Pipeline.create_pxd_pipeline(self, scope, module_name)
- result = Pipeline.run_pipeline(pipeline, source_desc)
- return result
-
- def nonfatal_error(self, exc):
- return Errors.report_error(exc)
-
- def find_module(self, module_name, relative_to=None, pos=None, need_pxd=1,
- absolute_fallback=True):
- # Finds and returns the module scope corresponding to
- # the given relative or absolute module name. If this
- # is the first time the module has been requested, finds
- # the corresponding .pxd file and process it.
- # If relative_to is not None, it must be a module scope,
- # and the module will first be searched for relative to
- # that module, provided its name is not a dotted name.
- debug_find_module = 0
- if debug_find_module:
- print("Context.find_module: module_name = %s, relative_to = %s, pos = %s, need_pxd = %s" % (
- module_name, relative_to, pos, need_pxd))
-
- scope = None
- pxd_pathname = None
- if relative_to:
- if module_name:
- # from .module import ...
- qualified_name = relative_to.qualify_name(module_name)
- else:
- # from . import ...
- qualified_name = relative_to.qualified_name
- scope = relative_to
- relative_to = None
- else:
- qualified_name = module_name
-
- if not module_name_pattern.match(qualified_name):
- raise CompileError(pos or (module_name, 0, 0),
- "'%s' is not a valid module name" % module_name)
-
- if relative_to:
- if debug_find_module:
- print("...trying relative import")
- scope = relative_to.lookup_submodule(module_name)
- if not scope:
- pxd_pathname = self.find_pxd_file(qualified_name, pos)
- if pxd_pathname:
- scope = relative_to.find_submodule(module_name)
- if not scope:
- if debug_find_module:
- print("...trying absolute import")
- if absolute_fallback:
- qualified_name = module_name
- scope = self
- for name in qualified_name.split("."):
- scope = scope.find_submodule(name)
-
- if debug_find_module:
- print("...scope = %s" % scope)
- if not scope.pxd_file_loaded:
- if debug_find_module:
- print("...pxd not loaded")
- if not pxd_pathname:
- if debug_find_module:
- print("...looking for pxd file")
- # Only look in sys.path if we are explicitly looking
- # for a .pxd file.
- pxd_pathname = self.find_pxd_file(qualified_name, pos, sys_path=need_pxd)
- if debug_find_module:
- print("......found %s" % pxd_pathname)
- if not pxd_pathname and need_pxd:
- # Set pxd_file_loaded such that we don't need to
- # look for the non-existing pxd file next time.
- scope.pxd_file_loaded = True
- package_pathname = self.search_include_directories(qualified_name, ".py", pos)
- if package_pathname and package_pathname.endswith('__init__.py'):
- pass
- else:
- error(pos, "'%s.pxd' not found" % qualified_name.replace('.', os.sep))
- if pxd_pathname:
- scope.pxd_file_loaded = True
- try:
- if debug_find_module:
- print("Context.find_module: Parsing %s" % pxd_pathname)
- rel_path = module_name.replace('.', os.sep) + os.path.splitext(pxd_pathname)[1]
- if not pxd_pathname.endswith(rel_path):
- rel_path = pxd_pathname # safety measure to prevent printing incorrect paths
- source_desc = FileSourceDescriptor(pxd_pathname, rel_path)
- err, result = self.process_pxd(source_desc, scope, qualified_name)
- if err:
- raise err
- (pxd_codenodes, pxd_scope) = result
- self.pxds[module_name] = (pxd_codenodes, pxd_scope)
- except CompileError:
- pass
- return scope
-
- def find_pxd_file(self, qualified_name, pos, sys_path=True):
- # Search include path (and sys.path if sys_path is True) for
- # the .pxd file corresponding to the given fully-qualified
- # module name.
- # Will find either a dotted filename or a file in a
- # package directory. If a source file position is given,
- # the directory containing the source file is searched first
- # for a dotted filename, and its containing package root
- # directory is searched first for a non-dotted filename.
- pxd = self.search_include_directories(qualified_name, ".pxd", pos, sys_path=sys_path)
- if pxd is None: # XXX Keep this until Includes/Deprecated is removed
- if (qualified_name.startswith('python') or
- qualified_name in ('stdlib', 'stdio', 'stl')):
- standard_include_path = os.path.abspath(os.path.normpath(
- os.path.join(os.path.dirname(__file__), os.path.pardir, 'Includes')))
- deprecated_include_path = os.path.join(standard_include_path, 'Deprecated')
- self.include_directories.append(deprecated_include_path)
- try:
- pxd = self.search_include_directories(qualified_name, ".pxd", pos)
- finally:
- self.include_directories.pop()
- if pxd:
- name = qualified_name
- if name.startswith('python'):
- warning(pos, "'%s' is deprecated, use 'cpython'" % name, 1)
- elif name in ('stdlib', 'stdio'):
- warning(pos, "'%s' is deprecated, use 'libc.%s'" % (name, name), 1)
- elif name in ('stl'):
- warning(pos, "'%s' is deprecated, use 'libcpp.*.*'" % name, 1)
- if pxd is None and Options.cimport_from_pyx:
- return self.find_pyx_file(qualified_name, pos)
- return pxd
-
- def find_pyx_file(self, qualified_name, pos):
- # Search include path for the .pyx file corresponding to the
- # given fully-qualified module name, as for find_pxd_file().
- return self.search_include_directories(qualified_name, ".pyx", pos)
-
- def find_include_file(self, filename, pos):
- # Search list of include directories for filename.
- # Reports an error and returns None if not found.
- path = self.search_include_directories(filename, "", pos,
- include=True)
- if not path:
- error(pos, "'%s' not found" % filename)
- return path
-
- def search_include_directories(self, qualified_name, suffix, pos,
- include=False, sys_path=False):
- include_dirs = self.include_directories
- if sys_path:
- include_dirs = include_dirs + sys.path
- # include_dirs must be hashable for caching in @cached_function
- include_dirs = tuple(include_dirs + [standard_include_path])
- return search_include_directories(include_dirs, qualified_name,
- suffix, pos, include)
-
- def find_root_package_dir(self, file_path):
- return Utils.find_root_package_dir(file_path)
-
- def check_package_dir(self, dir, package_names):
- return Utils.check_package_dir(dir, tuple(package_names))
-
- def c_file_out_of_date(self, source_path, output_path):
- if not os.path.exists(output_path):
- return 1
- c_time = Utils.modification_time(output_path)
- if Utils.file_newer_than(source_path, c_time):
- return 1
- pos = [source_path]
- pxd_path = Utils.replace_suffix(source_path, ".pxd")
- if os.path.exists(pxd_path) and Utils.file_newer_than(pxd_path, c_time):
- return 1
- for kind, name in self.read_dependency_file(source_path):
- if kind == "cimport":
- dep_path = self.find_pxd_file(name, pos)
- elif kind == "include":
- dep_path = self.search_include_directories(name, pos)
- else:
- continue
- if dep_path and Utils.file_newer_than(dep_path, c_time):
- return 1
- return 0
-
- def find_cimported_module_names(self, source_path):
- return [ name for kind, name in self.read_dependency_file(source_path)
- if kind == "cimport" ]
-
- def is_package_dir(self, dir_path):
- return Utils.is_package_dir(dir_path)
-
- def read_dependency_file(self, source_path):
- dep_path = Utils.replace_suffix(source_path, ".dep")
- if os.path.exists(dep_path):
- f = open(dep_path, "rU")
- chunks = [ line.strip().split(" ", 1)
- for line in f.readlines()
- if " " in line.strip() ]
- f.close()
- return chunks
- else:
- return ()
-
- def lookup_submodule(self, name):
- # Look up a top-level module. Returns None if not found.
- return self.modules.get(name, None)
-
- def find_submodule(self, name):
- # Find a top-level module, creating a new one if needed.
- scope = self.lookup_submodule(name)
- if not scope:
- scope = ModuleScope(name,
- parent_module = None, context = self)
- self.modules[name] = scope
- return scope
-
- def parse(self, source_desc, scope, pxd, full_module_name):
- if not isinstance(source_desc, FileSourceDescriptor):
- raise RuntimeError("Only file sources for code supported")
- source_filename = source_desc.filename
- scope.cpp = self.cpp
- # Parse the given source file and return a parse tree.
- num_errors = Errors.num_errors
- try:
- with Utils.open_source_file(source_filename) as f:
- from . import Parsing
- s = PyrexScanner(f, source_desc, source_encoding = f.encoding,
- scope = scope, context = self)
- tree = Parsing.p_module(s, pxd, full_module_name)
- if self.options.formal_grammar:
- try:
- from ..Parser import ConcreteSyntaxTree
- except ImportError:
- raise RuntimeError(
- "Formal grammar can only be used with compiled Cython with an available pgen.")
- ConcreteSyntaxTree.p_module(source_filename)
- except UnicodeDecodeError as e:
- #import traceback
- #traceback.print_exc()
- raise self._report_decode_error(source_desc, e)
-
- if Errors.num_errors > num_errors:
- raise CompileError()
- return tree
-
- def _report_decode_error(self, source_desc, exc):
- msg = exc.args[-1]
- position = exc.args[2]
- encoding = exc.args[0]
-
- line = 1
- column = idx = 0
- with io.open(source_desc.filename, "r", encoding='iso8859-1', newline='') as f:
- for line, data in enumerate(f, 1):
- idx += len(data)
- if idx >= position:
- column = position - (idx - len(data)) + 1
- break
-
- return error((source_desc, line, column),
- "Decoding error, missing or incorrect coding= "
- "at top of source (cannot decode with encoding %r: %s)" % (encoding, msg))
-
- def extract_module_name(self, path, options):
- # Find fully_qualified module name from the full pathname
- # of a source file.
- dir, filename = os.path.split(path)
- module_name, _ = os.path.splitext(filename)
- if "." in module_name:
- return module_name
- names = [module_name]
- while self.is_package_dir(dir):
- parent, package_name = os.path.split(dir)
- if parent == dir:
- break
- names.append(package_name)
- dir = parent
- names.reverse()
- return ".".join(names)
-
- def setup_errors(self, options, result):
- Errors.reset() # clear any remaining error state
- if options.use_listing_file:
- path = result.listing_file = Utils.replace_suffix(result.main_source_file, ".lis")
- else:
- path = None
- Errors.open_listing_file(path=path,
- echo_to_stderr=options.errors_to_stderr)
-
- def teardown_errors(self, err, options, result):
- source_desc = result.compilation_source.source_desc
- if not isinstance(source_desc, FileSourceDescriptor):
- raise RuntimeError("Only file sources for code supported")
- Errors.close_listing_file()
- result.num_errors = Errors.num_errors
- if result.num_errors > 0:
- err = True
- if err and result.c_file:
- try:
- Utils.castrate_file(result.c_file, os.stat(source_desc.filename))
- except EnvironmentError:
- pass
- result.c_file = None
-
-
-def get_output_filename(source_filename, cwd, options):
- if options.cplus:
- c_suffix = ".cpp"
- else:
- c_suffix = ".c"
- suggested_file_name = Utils.replace_suffix(source_filename, c_suffix)
- if options.output_file:
- out_path = os.path.join(cwd, options.output_file)
- if os.path.isdir(out_path):
- return os.path.join(out_path, os.path.basename(suggested_file_name))
- else:
- return out_path
- else:
- return suggested_file_name
-
-
-def create_default_resultobj(compilation_source, options):
- result = CompilationResult()
- result.main_source_file = compilation_source.source_desc.filename
- result.compilation_source = compilation_source
- source_desc = compilation_source.source_desc
- result.c_file = get_output_filename(source_desc.filename,
- compilation_source.cwd, options)
- result.embedded_metadata = options.embedded_metadata
- return result
-
-
-def run_pipeline(source, options, full_module_name=None, context=None):
- from . import Pipeline
-
- source_ext = os.path.splitext(source)[1]
- options.configure_language_defaults(source_ext[1:]) # py/pyx
- if context is None:
- context = options.create_context()
-
- # Set up source object
- cwd = os.getcwd()
- abs_path = os.path.abspath(source)
- full_module_name = full_module_name or context.extract_module_name(source, options)
-
- Utils.raise_error_if_module_name_forbidden(full_module_name)
-
- if options.relative_path_in_code_position_comments:
- rel_path = full_module_name.replace('.', os.sep) + source_ext
- if not abs_path.endswith(rel_path):
- rel_path = source # safety measure to prevent printing incorrect paths
- else:
- rel_path = abs_path
- source_desc = FileSourceDescriptor(abs_path, rel_path)
- source = CompilationSource(source_desc, full_module_name, cwd)
-
- # Set up result object
- result = create_default_resultobj(source, options)
-
- if options.annotate is None:
- # By default, decide based on whether an html file already exists.
- html_filename = os.path.splitext(result.c_file)[0] + ".html"
- if os.path.exists(html_filename):
- with io.open(html_filename, "r", encoding="UTF-8") as html_file:
- if u'
-
-# Question Answering examples
-
-Based on the script [`run_qa.py`](https://github.com/huggingface/transformers/blob/main/examples/flax/question-answering/run_qa.py).
-
-**Note:** This script only works with models that have a fast tokenizer (backed by the 🤗 Tokenizers library) as it
-uses special features of those tokenizers. You can check if your favorite model has a fast tokenizer in
-[this table](https://huggingface.co/transformers/index.html#supported-frameworks), if it doesn't you can still use the old version
-of the script.
-
-
-The following example fine-tunes BERT on SQuAD:
-
-
-```bash
-python run_qa.py \
- --model_name_or_path bert-base-uncased \
- --dataset_name squad \
- --do_train \
- --do_eval \
- --max_seq_length 384 \
- --doc_stride 128 \
- --learning_rate 3e-5 \
- --num_train_epochs 2 \
- --per_device_train_batch_size 12 \
- --output_dir ./bert-qa-squad \
- --eval_steps 1000 \
- --push_to_hub
-```
-
-Using the command above, the script will train for 2 epochs and run eval after each epoch.
-Metrics and hyperparameters are stored in Tensorflow event files in `--output_dir`.
-You can see the results by running `tensorboard` in that directory:
-
-```bash
-$ tensorboard --logdir .
-```
-
-or directly on the hub under *Training metrics*.
-
-Training with the previously defined hyper-parameters yields the following results:
-
-```bash
-f1 = 88.62
-exact_match = 81.34
-```
-
-sample Metrics - [tfhub.dev](https://tensorboard.dev/experiment/6gU75Hx8TGCnc6tr4ZgI9Q)
-
-Here is an example training on 4 TITAN RTX GPUs and Bert Whole Word Masking uncased model to reach a F1 > 93 on SQuAD1.1:
-
-```bash
-export CUDA_VISIBLE_DEVICES=0,1,2,3
-python run_qa.py \
---model_name_or_path bert-large-uncased-whole-word-masking \
---dataset_name squad \
---do_train \
---do_eval \
---per_device_train_batch_size 6 \
---learning_rate 3e-5 \
---num_train_epochs 2 \
---max_seq_length 384 \
---doc_stride 128 \
---output_dir ./wwm_uncased_finetuned_squad/ \
---eval_steps 1000 \
---push_to_hub
-```
-
-Training with the previously defined hyper-parameters yields the following results:
-
-```bash
-f1 = 93.31
-exact_match = 87.04
-```
-
-
-### Usage notes
-
-Note that when contexts are long they may be split into multiple training cases, not all of which may contain
-the answer span.
-
-As-is, the example script will train on SQuAD or any other question-answering dataset formatted the same way, and can handle user
-inputs as well.
-
-### Memory usage and data loading
-
-One thing to note is that all data is loaded into memory in this script. Most question answering datasets are small
-enough that this is not an issue, but if you have a very large dataset you will need to modify the script to handle
-data streaming.
diff --git a/spaces/chendl/compositional_test/transformers/examples/research_projects/seq2seq-distillation/_test_bash_script.py b/spaces/chendl/compositional_test/transformers/examples/research_projects/seq2seq-distillation/_test_bash_script.py
deleted file mode 100644
index fa84a60c0c88e0ac5cc224385c9f7b74ef80d17c..0000000000000000000000000000000000000000
--- a/spaces/chendl/compositional_test/transformers/examples/research_projects/seq2seq-distillation/_test_bash_script.py
+++ /dev/null
@@ -1,203 +0,0 @@
-#!/usr/bin/env python
-
-import argparse
-import os
-import sys
-from unittest.mock import patch
-
-import pytorch_lightning as pl
-import timeout_decorator
-import torch
-from distillation import SummarizationDistiller, distill_main
-from finetune import SummarizationModule, main
-
-from transformers import MarianMTModel
-from transformers.file_utils import cached_path
-from transformers.testing_utils import TestCasePlus, require_torch_gpu, slow
-from utils import load_json
-
-
-MARIAN_MODEL = "sshleifer/mar_enro_6_3_student"
-
-
-class TestMbartCc25Enro(TestCasePlus):
- def setUp(self):
- super().setUp()
-
- data_cached = cached_path(
- "https://cdn-datasets.huggingface.co/translation/wmt_en_ro-tr40k-va0.5k-te0.5k.tar.gz",
- extract_compressed_file=True,
- )
- self.data_dir = f"{data_cached}/wmt_en_ro-tr40k-va0.5k-te0.5k"
-
- @slow
- @require_torch_gpu
- def test_model_download(self):
- """This warms up the cache so that we can time the next test without including download time, which varies between machines."""
- MarianMTModel.from_pretrained(MARIAN_MODEL)
-
- # @timeout_decorator.timeout(1200)
- @slow
- @require_torch_gpu
- def test_train_mbart_cc25_enro_script(self):
- env_vars_to_replace = {
- "$MAX_LEN": 64,
- "$BS": 64,
- "$GAS": 1,
- "$ENRO_DIR": self.data_dir,
- "facebook/mbart-large-cc25": MARIAN_MODEL,
- # "val_check_interval=0.25": "val_check_interval=1.0",
- "--learning_rate=3e-5": "--learning_rate 3e-4",
- "--num_train_epochs 6": "--num_train_epochs 1",
- }
-
- # Clean up bash script
- bash_script = (self.test_file_dir / "train_mbart_cc25_enro.sh").open().read().split("finetune.py")[1].strip()
- bash_script = bash_script.replace("\\\n", "").strip().replace('"$@"', "")
- for k, v in env_vars_to_replace.items():
- bash_script = bash_script.replace(k, str(v))
- output_dir = self.get_auto_remove_tmp_dir()
-
- # bash_script = bash_script.replace("--fp16 ", "")
- args = f"""
- --output_dir {output_dir}
- --tokenizer_name Helsinki-NLP/opus-mt-en-ro
- --sortish_sampler
- --do_predict
- --gpus 1
- --freeze_encoder
- --n_train 40000
- --n_val 500
- --n_test 500
- --fp16_opt_level O1
- --num_sanity_val_steps 0
- --eval_beams 2
- """.split()
- # XXX: args.gpus > 1 : handle multi_gpu in the future
-
- testargs = ["finetune.py"] + bash_script.split() + args
- with patch.object(sys, "argv", testargs):
- parser = argparse.ArgumentParser()
- parser = pl.Trainer.add_argparse_args(parser)
- parser = SummarizationModule.add_model_specific_args(parser, os.getcwd())
- args = parser.parse_args()
- model = main(args)
-
- # Check metrics
- metrics = load_json(model.metrics_save_path)
- first_step_stats = metrics["val"][0]
- last_step_stats = metrics["val"][-1]
- self.assertEqual(len(metrics["val"]), (args.max_epochs / args.val_check_interval))
- assert isinstance(last_step_stats[f"val_avg_{model.val_metric}"], float)
-
- self.assertGreater(last_step_stats["val_avg_gen_time"], 0.01)
- # model hanging on generate. Maybe bad config was saved. (XXX: old comment/assert?)
- self.assertLessEqual(last_step_stats["val_avg_gen_time"], 1.0)
-
- # test learning requirements:
-
- # 1. BLEU improves over the course of training by more than 2 pts
- self.assertGreater(last_step_stats["val_avg_bleu"] - first_step_stats["val_avg_bleu"], 2)
-
- # 2. BLEU finishes above 17
- self.assertGreater(last_step_stats["val_avg_bleu"], 17)
-
- # 3. test BLEU and val BLEU within ~1.1 pt.
- self.assertLess(abs(metrics["val"][-1]["val_avg_bleu"] - metrics["test"][-1]["test_avg_bleu"]), 1.1)
-
- # check lightning ckpt can be loaded and has a reasonable statedict
- contents = os.listdir(output_dir)
- ckpt_path = [x for x in contents if x.endswith(".ckpt")][0]
- full_path = os.path.join(args.output_dir, ckpt_path)
- ckpt = torch.load(full_path, map_location="cpu")
- expected_key = "model.model.decoder.layers.0.encoder_attn_layer_norm.weight"
- assert expected_key in ckpt["state_dict"]
- assert ckpt["state_dict"]["model.model.decoder.layers.0.encoder_attn_layer_norm.weight"].dtype == torch.float32
-
- # TODO: turn on args.do_predict when PL bug fixed.
- if args.do_predict:
- contents = {os.path.basename(p) for p in contents}
- assert "test_generations.txt" in contents
- assert "test_results.txt" in contents
- # assert len(metrics["val"]) == desired_n_evals
- assert len(metrics["test"]) == 1
-
-
-class TestDistilMarianNoTeacher(TestCasePlus):
- @timeout_decorator.timeout(600)
- @slow
- @require_torch_gpu
- def test_opus_mt_distill_script(self):
- data_dir = f"{self.test_file_dir_str}/test_data/wmt_en_ro"
- env_vars_to_replace = {
- "--fp16_opt_level=O1": "",
- "$MAX_LEN": 128,
- "$BS": 16,
- "$GAS": 1,
- "$ENRO_DIR": data_dir,
- "$m": "sshleifer/student_marian_en_ro_6_1",
- "val_check_interval=0.25": "val_check_interval=1.0",
- }
-
- # Clean up bash script
- bash_script = (
- (self.test_file_dir / "distil_marian_no_teacher.sh").open().read().split("distillation.py")[1].strip()
- )
- bash_script = bash_script.replace("\\\n", "").strip().replace('"$@"', "")
- bash_script = bash_script.replace("--fp16 ", " ")
-
- for k, v in env_vars_to_replace.items():
- bash_script = bash_script.replace(k, str(v))
- output_dir = self.get_auto_remove_tmp_dir()
- bash_script = bash_script.replace("--fp16", "")
- epochs = 6
- testargs = (
- ["distillation.py"]
- + bash_script.split()
- + [
- f"--output_dir={output_dir}",
- "--gpus=1",
- "--learning_rate=1e-3",
- f"--num_train_epochs={epochs}",
- "--warmup_steps=10",
- "--val_check_interval=1.0",
- "--do_predict",
- ]
- )
- with patch.object(sys, "argv", testargs):
- parser = argparse.ArgumentParser()
- parser = pl.Trainer.add_argparse_args(parser)
- parser = SummarizationDistiller.add_model_specific_args(parser, os.getcwd())
- args = parser.parse_args()
- # assert args.gpus == gpus THIS BREAKS for multi_gpu
-
- model = distill_main(args)
-
- # Check metrics
- metrics = load_json(model.metrics_save_path)
- first_step_stats = metrics["val"][0]
- last_step_stats = metrics["val"][-1]
- assert len(metrics["val"]) >= (args.max_epochs / args.val_check_interval) # +1 accounts for val_sanity_check
-
- assert last_step_stats["val_avg_gen_time"] >= 0.01
-
- assert first_step_stats["val_avg_bleu"] < last_step_stats["val_avg_bleu"] # model learned nothing
- assert 1.0 >= last_step_stats["val_avg_gen_time"] # model hanging on generate. Maybe bad config was saved.
- assert isinstance(last_step_stats[f"val_avg_{model.val_metric}"], float)
-
- # check lightning ckpt can be loaded and has a reasonable statedict
- contents = os.listdir(output_dir)
- ckpt_path = [x for x in contents if x.endswith(".ckpt")][0]
- full_path = os.path.join(args.output_dir, ckpt_path)
- ckpt = torch.load(full_path, map_location="cpu")
- expected_key = "model.model.decoder.layers.0.encoder_attn_layer_norm.weight"
- assert expected_key in ckpt["state_dict"]
- assert ckpt["state_dict"]["model.model.decoder.layers.0.encoder_attn_layer_norm.weight"].dtype == torch.float32
-
- # TODO: turn on args.do_predict when PL bug fixed.
- if args.do_predict:
- contents = {os.path.basename(p) for p in contents}
- assert "test_generations.txt" in contents
- assert "test_results.txt" in contents
- # assert len(metrics["val"]) == desired_n_evals
- assert len(metrics["test"]) == 1
diff --git a/spaces/chuan-hd/law-assistant-chatbot/.venv/lib/python3.11/site-packages/flatbuffers/table.py b/spaces/chuan-hd/law-assistant-chatbot/.venv/lib/python3.11/site-packages/flatbuffers/table.py
deleted file mode 100644
index d5336ca6b04b6d79be14403c745f6be31d9d09b5..0000000000000000000000000000000000000000
--- a/spaces/chuan-hd/law-assistant-chatbot/.venv/lib/python3.11/site-packages/flatbuffers/table.py
+++ /dev/null
@@ -1,138 +0,0 @@
-# Copyright 2014 Google Inc. All rights reserved.
-#
-# Licensed under the Apache License, Version 2.0 (the "License");
-# you may not use this file except in compliance with the License.
-# You may obtain a copy of the License at
-#
-# http://www.apache.org/licenses/LICENSE-2.0
-#
-# Unless required by applicable law or agreed to in writing, software
-# distributed under the License is distributed on an "AS IS" BASIS,
-# WITHOUT WARRANTIES OR CONDITIONS OF ANY KIND, either express or implied.
-# See the License for the specific language governing permissions and
-# limitations under the License.
-
-from . import encode
-from . import number_types as N
-
-
-class Table(object):
- """Table wraps a byte slice and provides read access to its data.
-
- The variable `Pos` indicates the root of the FlatBuffers object therein."""
-
- __slots__ = ("Bytes", "Pos")
-
- def __init__(self, buf, pos):
- N.enforce_number(pos, N.UOffsetTFlags)
-
- self.Bytes = buf
- self.Pos = pos
-
- def Offset(self, vtableOffset):
- """Offset provides access into the Table's vtable.
-
- Deprecated fields are ignored by checking the vtable's length."""
-
- vtable = self.Pos - self.Get(N.SOffsetTFlags, self.Pos)
- vtableEnd = self.Get(N.VOffsetTFlags, vtable)
- if vtableOffset < vtableEnd:
- return self.Get(N.VOffsetTFlags, vtable + vtableOffset)
- return 0
-
- def Indirect(self, off):
- """Indirect retrieves the relative offset stored at `offset`."""
- N.enforce_number(off, N.UOffsetTFlags)
- return off + encode.Get(N.UOffsetTFlags.packer_type, self.Bytes, off)
-
- def String(self, off):
- """String gets a string from data stored inside the flatbuffer."""
- N.enforce_number(off, N.UOffsetTFlags)
- off += encode.Get(N.UOffsetTFlags.packer_type, self.Bytes, off)
- start = off + N.UOffsetTFlags.bytewidth
- length = encode.Get(N.UOffsetTFlags.packer_type, self.Bytes, off)
- return bytes(self.Bytes[start:start+length])
-
- def VectorLen(self, off):
- """VectorLen retrieves the length of the vector whose offset is stored
- at "off" in this object."""
- N.enforce_number(off, N.UOffsetTFlags)
-
- off += self.Pos
- off += encode.Get(N.UOffsetTFlags.packer_type, self.Bytes, off)
- ret = encode.Get(N.UOffsetTFlags.packer_type, self.Bytes, off)
- return ret
-
- def Vector(self, off):
- """Vector retrieves the start of data of the vector whose offset is
- stored at "off" in this object."""
- N.enforce_number(off, N.UOffsetTFlags)
-
- off += self.Pos
- x = off + self.Get(N.UOffsetTFlags, off)
- # data starts after metadata containing the vector length
- x += N.UOffsetTFlags.bytewidth
- return x
-
- def Union(self, t2, off):
- """Union initializes any Table-derived type to point to the union at
- the given offset."""
- assert type(t2) is Table
- N.enforce_number(off, N.UOffsetTFlags)
-
- off += self.Pos
- t2.Pos = off + self.Get(N.UOffsetTFlags, off)
- t2.Bytes = self.Bytes
-
- def Get(self, flags, off):
- """
- Get retrieves a value of the type specified by `flags` at the
- given offset.
- """
- N.enforce_number(off, N.UOffsetTFlags)
- return flags.py_type(encode.Get(flags.packer_type, self.Bytes, off))
-
- def GetSlot(self, slot, d, validator_flags):
- N.enforce_number(slot, N.VOffsetTFlags)
- if validator_flags is not None:
- N.enforce_number(d, validator_flags)
- off = self.Offset(slot)
- if off == 0:
- return d
- return self.Get(validator_flags, self.Pos + off)
-
- def GetVectorAsNumpy(self, flags, off):
- """
- GetVectorAsNumpy returns the vector that starts at `Vector(off)`
- as a numpy array with the type specified by `flags`. The array is
- a `view` into Bytes, so modifying the returned array will
- modify Bytes in place.
- """
- offset = self.Vector(off)
- length = self.VectorLen(off) # TODO: length accounts for bytewidth, right?
- numpy_dtype = N.to_numpy_type(flags)
- return encode.GetVectorAsNumpy(numpy_dtype, self.Bytes, length, offset)
-
- def GetArrayAsNumpy(self, flags, off, length):
- """
- GetArrayAsNumpy returns the array with fixed width that starts at `Vector(offset)`
- with length `length` as a numpy array with the type specified by `flags`. The
- array is a `view` into Bytes so modifying the returned will modify Bytes in place.
- """
- numpy_dtype = N.to_numpy_type(flags)
- return encode.GetVectorAsNumpy(numpy_dtype, self.Bytes, length, off)
-
- def GetVOffsetTSlot(self, slot, d):
- """
- GetVOffsetTSlot retrieves the VOffsetT that the given vtable location
- points to. If the vtable value is zero, the default value `d`
- will be returned.
- """
-
- N.enforce_number(slot, N.VOffsetTFlags)
- N.enforce_number(d, N.VOffsetTFlags)
-
- off = self.Offset(slot)
- if off == 0:
- return d
- return off
diff --git a/spaces/chuan-hd/law-assistant-chatbot/.venv/lib/python3.11/site-packages/fontTools/ttLib/tables/T_S_I_S_.py b/spaces/chuan-hd/law-assistant-chatbot/.venv/lib/python3.11/site-packages/fontTools/ttLib/tables/T_S_I_S_.py
deleted file mode 100644
index 667eb0e53473c1566d4b45e5621d8897ebd7b9fe..0000000000000000000000000000000000000000
--- a/spaces/chuan-hd/law-assistant-chatbot/.venv/lib/python3.11/site-packages/fontTools/ttLib/tables/T_S_I_S_.py
+++ /dev/null
@@ -1,5 +0,0 @@
-from .T_S_I_V_ import table_T_S_I_V_
-
-
-class table_T_S_I_S_(table_T_S_I_V_):
- pass
diff --git a/spaces/chuan-hd/law-assistant-chatbot/.venv/lib/python3.11/site-packages/fontTools/ttLib/tables/_k_e_r_n.py b/spaces/chuan-hd/law-assistant-chatbot/.venv/lib/python3.11/site-packages/fontTools/ttLib/tables/_k_e_r_n.py
deleted file mode 100644
index 94183c8a0a1e8a02cfc229d525030d9ae2b27ddf..0000000000000000000000000000000000000000
--- a/spaces/chuan-hd/law-assistant-chatbot/.venv/lib/python3.11/site-packages/fontTools/ttLib/tables/_k_e_r_n.py
+++ /dev/null
@@ -1,279 +0,0 @@
-from fontTools.ttLib import getSearchRange
-from fontTools.misc.textTools import safeEval, readHex
-from fontTools.misc.fixedTools import fixedToFloat as fi2fl, floatToFixed as fl2fi
-from . import DefaultTable
-import struct
-import sys
-import array
-import logging
-
-
-log = logging.getLogger(__name__)
-
-
-class table__k_e_r_n(DefaultTable.DefaultTable):
- def getkern(self, format):
- for subtable in self.kernTables:
- if subtable.format == format:
- return subtable
- return None # not found
-
- def decompile(self, data, ttFont):
- version, nTables = struct.unpack(">HH", data[:4])
- apple = False
- if (len(data) >= 8) and (version == 1):
- # AAT Apple's "new" format. Hm.
- version, nTables = struct.unpack(">LL", data[:8])
- self.version = fi2fl(version, 16)
- data = data[8:]
- apple = True
- else:
- self.version = version
- data = data[4:]
- self.kernTables = []
- for i in range(nTables):
- if self.version == 1.0:
- # Apple
- length, coverage, subtableFormat = struct.unpack(">LBB", data[:6])
- else:
- # in OpenType spec the "version" field refers to the common
- # subtable header; the actual subtable format is stored in
- # the 8-15 mask bits of "coverage" field.
- # This "version" is always 0 so we ignore it here
- _, length, subtableFormat, coverage = struct.unpack(">HHBB", data[:6])
- if nTables == 1 and subtableFormat == 0:
- # The "length" value is ignored since some fonts
- # (like OpenSans and Calibri) have a subtable larger than
- # its value.
- (nPairs,) = struct.unpack(">H", data[6:8])
- calculated_length = (nPairs * 6) + 14
- if length != calculated_length:
- log.warning(
- "'kern' subtable longer than defined: "
- "%d bytes instead of %d bytes" % (calculated_length, length)
- )
- length = calculated_length
- if subtableFormat not in kern_classes:
- subtable = KernTable_format_unkown(subtableFormat)
- else:
- subtable = kern_classes[subtableFormat](apple)
- subtable.decompile(data[:length], ttFont)
- self.kernTables.append(subtable)
- data = data[length:]
-
- def compile(self, ttFont):
- if hasattr(self, "kernTables"):
- nTables = len(self.kernTables)
- else:
- nTables = 0
- if self.version == 1.0:
- # AAT Apple's "new" format.
- data = struct.pack(">LL", fl2fi(self.version, 16), nTables)
- else:
- data = struct.pack(">HH", self.version, nTables)
- if hasattr(self, "kernTables"):
- for subtable in self.kernTables:
- data = data + subtable.compile(ttFont)
- return data
-
- def toXML(self, writer, ttFont):
- writer.simpletag("version", value=self.version)
- writer.newline()
- for subtable in self.kernTables:
- subtable.toXML(writer, ttFont)
-
- def fromXML(self, name, attrs, content, ttFont):
- if name == "version":
- self.version = safeEval(attrs["value"])
- return
- if name != "kernsubtable":
- return
- if not hasattr(self, "kernTables"):
- self.kernTables = []
- format = safeEval(attrs["format"])
- if format not in kern_classes:
- subtable = KernTable_format_unkown(format)
- else:
- apple = self.version == 1.0
- subtable = kern_classes[format](apple)
- self.kernTables.append(subtable)
- subtable.fromXML(name, attrs, content, ttFont)
-
-
-class KernTable_format_0(object):
-
- # 'version' is kept for backward compatibility
- version = format = 0
-
- def __init__(self, apple=False):
- self.apple = apple
-
- def decompile(self, data, ttFont):
- if not self.apple:
- version, length, subtableFormat, coverage = struct.unpack(">HHBB", data[:6])
- if version != 0:
- from fontTools.ttLib import TTLibError
-
- raise TTLibError("unsupported kern subtable version: %d" % version)
- tupleIndex = None
- # Should we also assert length == len(data)?
- data = data[6:]
- else:
- length, coverage, subtableFormat, tupleIndex = struct.unpack(
- ">LBBH", data[:8]
- )
- data = data[8:]
- assert self.format == subtableFormat, "unsupported format"
- self.coverage = coverage
- self.tupleIndex = tupleIndex
-
- self.kernTable = kernTable = {}
-
- nPairs, searchRange, entrySelector, rangeShift = struct.unpack(
- ">HHHH", data[:8]
- )
- data = data[8:]
-
- datas = array.array("H", data[: 6 * nPairs])
- if sys.byteorder != "big":
- datas.byteswap()
- it = iter(datas)
- glyphOrder = ttFont.getGlyphOrder()
- for k in range(nPairs):
- left, right, value = next(it), next(it), next(it)
- if value >= 32768:
- value -= 65536
- try:
- kernTable[(glyphOrder[left], glyphOrder[right])] = value
- except IndexError:
- # Slower, but will not throw an IndexError on an invalid
- # glyph id.
- kernTable[
- (ttFont.getGlyphName(left), ttFont.getGlyphName(right))
- ] = value
- if len(data) > 6 * nPairs + 4: # Ignore up to 4 bytes excess
- log.warning(
- "excess data in 'kern' subtable: %d bytes", len(data) - 6 * nPairs
- )
-
- def compile(self, ttFont):
- nPairs = min(len(self.kernTable), 0xFFFF)
- searchRange, entrySelector, rangeShift = getSearchRange(nPairs, 6)
- searchRange &= 0xFFFF
- entrySelector = min(entrySelector, 0xFFFF)
- rangeShift = min(rangeShift, 0xFFFF)
- data = struct.pack(">HHHH", nPairs, searchRange, entrySelector, rangeShift)
-
- # yeehee! (I mean, turn names into indices)
- try:
- reverseOrder = ttFont.getReverseGlyphMap()
- kernTable = sorted(
- (reverseOrder[left], reverseOrder[right], value)
- for ((left, right), value) in self.kernTable.items()
- )
- except KeyError:
- # Slower, but will not throw KeyError on invalid glyph id.
- getGlyphID = ttFont.getGlyphID
- kernTable = sorted(
- (getGlyphID(left), getGlyphID(right), value)
- for ((left, right), value) in self.kernTable.items()
- )
-
- for left, right, value in kernTable:
- data = data + struct.pack(">HHh", left, right, value)
-
- if not self.apple:
- version = 0
- length = len(data) + 6
- if length >= 0x10000:
- log.warning(
- '"kern" subtable overflow, '
- "truncating length value while preserving pairs."
- )
- length &= 0xFFFF
- header = struct.pack(">HHBB", version, length, self.format, self.coverage)
- else:
- if self.tupleIndex is None:
- # sensible default when compiling a TTX from an old fonttools
- # or when inserting a Windows-style format 0 subtable into an
- # Apple version=1.0 kern table
- log.warning("'tupleIndex' is None; default to 0")
- self.tupleIndex = 0
- length = len(data) + 8
- header = struct.pack(
- ">LBBH", length, self.coverage, self.format, self.tupleIndex
- )
- return header + data
-
- def toXML(self, writer, ttFont):
- attrs = dict(coverage=self.coverage, format=self.format)
- if self.apple:
- if self.tupleIndex is None:
- log.warning("'tupleIndex' is None; default to 0")
- attrs["tupleIndex"] = 0
- else:
- attrs["tupleIndex"] = self.tupleIndex
- writer.begintag("kernsubtable", **attrs)
- writer.newline()
- items = sorted(self.kernTable.items())
- for (left, right), value in items:
- writer.simpletag("pair", [("l", left), ("r", right), ("v", value)])
- writer.newline()
- writer.endtag("kernsubtable")
- writer.newline()
-
- def fromXML(self, name, attrs, content, ttFont):
- self.coverage = safeEval(attrs["coverage"])
- subtableFormat = safeEval(attrs["format"])
- if self.apple:
- if "tupleIndex" in attrs:
- self.tupleIndex = safeEval(attrs["tupleIndex"])
- else:
- # previous fontTools versions didn't export tupleIndex
- log.warning("Apple kern subtable is missing 'tupleIndex' attribute")
- self.tupleIndex = None
- else:
- self.tupleIndex = None
- assert subtableFormat == self.format, "unsupported format"
- if not hasattr(self, "kernTable"):
- self.kernTable = {}
- for element in content:
- if not isinstance(element, tuple):
- continue
- name, attrs, content = element
- self.kernTable[(attrs["l"], attrs["r"])] = safeEval(attrs["v"])
-
- def __getitem__(self, pair):
- return self.kernTable[pair]
-
- def __setitem__(self, pair, value):
- self.kernTable[pair] = value
-
- def __delitem__(self, pair):
- del self.kernTable[pair]
-
-
-class KernTable_format_unkown(object):
- def __init__(self, format):
- self.format = format
-
- def decompile(self, data, ttFont):
- self.data = data
-
- def compile(self, ttFont):
- return self.data
-
- def toXML(self, writer, ttFont):
- writer.begintag("kernsubtable", format=self.format)
- writer.newline()
- writer.comment("unknown 'kern' subtable format")
- writer.newline()
- writer.dumphex(self.data)
- writer.endtag("kernsubtable")
- writer.newline()
-
- def fromXML(self, name, attrs, content, ttFont):
- self.decompile(readHex(content), ttFont)
-
-
-kern_classes = {0: KernTable_format_0}
diff --git a/spaces/cihyFjudo/fairness-paper-search/