Ksenia Se
AI & ML interests
Recent Activity
Organizations
Kseniase's activity
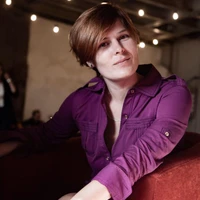
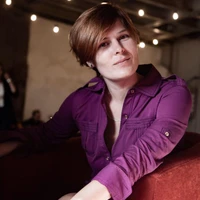
đ#87: Why DeepResearch Should Be Your New Hire
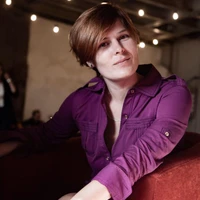
Other important RAG advancements:
SafeRAG (a benchmark) -> https://huggingface.co/papers/2501.18636
Establishes a security benchmark revealing how RAG systems are vulnerable to attacks like adversarial data injection, inter-context conflicts, and soft ad poisoning. Evaluates weaknesses in 14 RAG components, emphasizing the need for better filtering and security measures.Topic-FlipRAG: Adversarial Opinion Manipulation -> https://huggingface.co/papers/2502.01386
Demonstrates a two-stage adversarial attack that manipulates RAG-generated opinions on sensitive topics. Alters retrieval rankings and LLM reasoning to subtly flip the stance of generated answers, exposing the difficulty of mitigating semantic-level manipulation.Experiments with LLMs on RAG for Closed-Source Simulation Software -> https://huggingface.co/papers/2502.03916
Tests how RAG can support proprietary software by injecting relevant documentation dynamically. Shows that retrieval helps mitigate hallucinations in closed-source contexts, though some knowledge gaps remain, necessitating further improvements.Health-RAG -> https://huggingface.co/papers/2502.04666
Focuses on medical information retrieval by introducing a three-stage pipeline: retrieve, generate a reference summary (GenText), and re-rank based on factual alignment. Ensures accurate, evidence-backed health answers while mitigating misinformation risks.
Enhancing Health Information Retrieval with RAG by Prioritizing Topical Relevance and Factual Accuracy
Experiments with Large Language Models on Retrieval-Augmented Generation for Closed-Source Simulation Software
Improving Retrieval-Augmented Generation through Multi-Agent Reinforcement Learning
Topic-FlipRAG: Topic-Orientated Adversarial Opinion Manipulation Attacks to Retrieval-Augmented Generation Models
GFM-RAG: Graph Foundation Model for Retrieval Augmented Generation
Artificial Intelligence and Legal Analysis: Implications for Legal Education and the Profession
Limitations of Large Language Models in Clinical Problem-Solving Arising from Inflexible Reasoning
ParetoQ: Scaling Laws in Extremely Low-bit LLM Quantization
CoAT: Chain-of-Associated-Thoughts Framework for Enhancing Large Language Models Reasoning
Sundial: A Family of Highly Capable Time Series Foundation Models
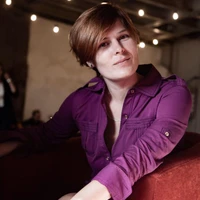
RAG techniques continuously evolve to enhance LLM response accuracy by retrieving relevant external data during generation. To keep up with current AI trends, new RAG types incorporate deep step-by-step reasoning, tree search, citations, multimodality and other effective techniques.
Here's a list of 8 latest RAG advancements:
1. DeepRAG -> DeepRAG: Thinking to Retrieval Step by Step for Large Language Models (2502.01142)
Models retrieval-augmented reasoning as a Markov Decision Process, enabling strategic retrieval. It dynamically decides when to retrieve external knowledge and when rely on parametric reasoning.
2. RealRAG -> RealRAG: Retrieval-augmented Realistic Image Generation via Self-reflective Contrastive Learning (2502.00848)
Enhances novel object generation by retrieving real-world images and using self-reflective contrastive learning to fill knowledge gap, improve realism and reduce distortions.
3. Chain-of-Retrieval Augmented Generation (CoRAG) -> Chain-of-Retrieval Augmented Generation (2501.14342)
Retrieves information step-by-step and adjusts it, also deciding how much compute power to use at test time. If needed it reformulates queries.
4. VideoRAG -> VideoRAG: Retrieval-Augmented Generation over Video Corpus (2501.05874)
Enables unlimited-length video processing, using dual-channel architecture that integrates graph-based textual grounding and multi-modal context encoding.
5. CFT-RAG ->Â CFT-RAG: An Entity Tree Based Retrieval Augmented Generation Algorithm With Cuckoo Filter (2501.15098)
A tree-RAG acceleration method uses an improved Cuckoo Filter to optimize entity localization, enabling faster retrieval.
6. Contextualized Graph RAG (CG-RAG) -> CG-RAG: Research Question Answering by Citation Graph Retrieval-Augmented LLMs (2501.15067)
Uses Lexical-Semantic Graph Retrieval (LeSeGR) to integrate sparse and dense signals within graph structure and capture citation relationships
7. GFM-RAG -> GFM-RAG: Graph Foundation Model for Retrieval Augmented Generation (2502.01113)
A graph foundation model that uses a graph neural network to refine query-knowledge connections
8. URAG -> URAG: Implementing a Unified Hybrid RAG for Precise Answers in University Admission Chatbots -- A Case Study at HCMUT (2501.16276)
A hybrid system combining rule-based and RAG methods to improve lightweight LLMs for educational chatbots
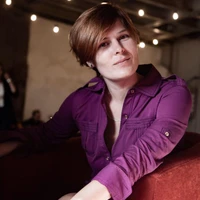
What is test-time compute and how to scale it?
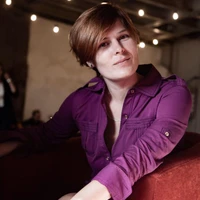
What is test-time compute and how to scale it?
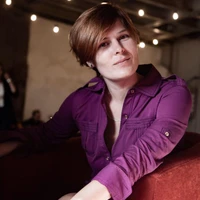
đ#86: Four Freedoms of truly open AI
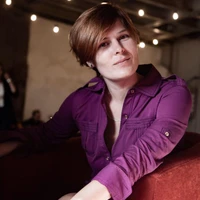