Spaces:
Running
Running
File size: 6,581 Bytes
1999a98 |
1 2 3 4 5 6 7 8 9 10 11 12 13 14 15 16 17 18 19 20 21 22 23 24 25 26 27 28 29 30 31 32 33 34 35 36 37 38 39 40 41 42 43 44 45 46 47 48 49 50 51 52 53 54 55 56 57 58 59 60 61 62 63 64 65 66 67 68 69 70 71 72 73 74 75 76 77 78 79 80 81 82 83 84 85 86 87 88 89 90 91 92 93 94 95 96 97 98 99 100 101 102 103 104 105 106 107 108 109 110 111 112 113 114 115 116 117 118 119 120 121 122 123 124 125 126 127 128 129 130 131 132 133 134 135 136 137 138 139 140 141 142 143 144 145 146 147 148 149 150 151 152 153 154 155 156 157 158 159 160 161 162 163 164 165 166 167 168 169 170 171 172 173 174 175 176 177 178 179 180 181 182 183 184 185 186 187 188 189 190 191 192 193 194 195 196 197 198 199 200 201 202 203 204 205 206 207 208 209 210 211 212 213 |
# YOLOv8-ONNXRuntime-Rust for All the Key YOLO Tasks
This repository provides a Rust demo for performing YOLOv8 tasks like `Classification`, `Segmentation`, `Detection`, `Pose Detection` and `OBB` using ONNXRuntime.
## Recently Updated
- Add YOLOv8-OBB demo
- Update ONNXRuntime to 1.19.x
Newly updated YOLOv8 example code is located in [this repository](https://github.com/jamjamjon/usls/tree/main/examples/yolo)
## Features
- Support `Classification`, `Segmentation`, `Detection`, `Pose(Keypoints)-Detection`, `OBB` tasks.
- Support `FP16` & `FP32` ONNX models.
- Support `CPU`, `CUDA` and `TensorRT` execution provider to accelerate computation.
- Support dynamic input shapes(`batch`, `width`, `height`).
## Installation
### 1. Install Rust
Please follow the Rust official installation. (https://www.rust-lang.org/tools/install)
### 2. ONNXRuntime Linking
- #### For detailed setup instructions, refer to the [ORT documentation](https://ort.pyke.io/setup/linking).
- #### For Linux or macOS Users:
- Download the ONNX Runtime package from the [Releases page](https://github.com/microsoft/onnxruntime/releases).
- Set up the library path by exporting the `ORT_DYLIB_PATH` environment variable:
```shell
export ORT_DYLIB_PATH=/path/to/onnxruntime/lib/libonnxruntime.so.1.19.0
```
### 3. \[Optional\] Install CUDA & CuDNN & TensorRT
- CUDA execution provider requires CUDA v11.6+.
- TensorRT execution provider requires CUDA v11.4+ and TensorRT v8.4+.
## Get Started
### 1. Export the YOLOv8 ONNX Models
```bash
pip install -U ultralytics
# export onnx model with dynamic shapes
yolo export model=yolov8m.pt format=onnx simplify dynamic
yolo export model=yolov8m-cls.pt format=onnx simplify dynamic
yolo export model=yolov8m-pose.pt format=onnx simplify dynamic
yolo export model=yolov8m-seg.pt format=onnx simplify dynamic
# export onnx model with constant shapes
yolo export model=yolov8m.pt format=onnx simplify
yolo export model=yolov8m-cls.pt format=onnx simplify
yolo export model=yolov8m-pose.pt format=onnx simplify
yolo export model=yolov8m-seg.pt format=onnx simplify
```
### 2. Run Inference
It will perform inference with the ONNX model on the source image.
```bash
cargo run --release -- --model <MODEL> --source <SOURCE>
```
Set `--cuda` to use CUDA execution provider to speed up inference.
```bash
cargo run --release -- --cuda --model <MODEL> --source <SOURCE>
```
Set `--trt` to use TensorRT execution provider, and you can set `--fp16` at the same time to use TensorRT FP16 engine.
```bash
cargo run --release -- --trt --fp16 --model <MODEL> --source <SOURCE>
```
Set `--device_id` to select which device to run. When you have only one GPU, and you set `device_id` to 1 will not cause program panic, the `ort` would automatically fall back to `CPU` EP.
```bash
cargo run --release -- --cuda --device_id 0 --model <MODEL> --source <SOURCE>
```
Set `--batch` to do multi-batch-size inference.
If you're using `--trt`, you can also set `--batch-min` and `--batch-max` to explicitly specify min/max/opt batch for dynamic batch input.(https://onnxruntime.ai/docs/execution-providers/TensorRT-ExecutionProvider.html#explicit-shape-range-for-dynamic-shape-input).(Note that the ONNX model should be exported with dynamic shapes.)
```bash
cargo run --release -- --cuda --batch 2 --model <MODEL> --source <SOURCE>
```
Set `--height` and `--width` to do dynamic image size inference. (Note that the ONNX model should be exported with dynamic shapes.)
```bash
cargo run --release -- --cuda --width 480 --height 640 --model <MODEL> --source <SOURCE>
```
Set `--profile` to check time consumed in each stage.(Note that the model usually needs to take 1~3 times dry run to warmup. Make sure to run enough times to evaluate the result.)
```bash
cargo run --release -- --trt --fp16 --profile --model <MODEL> --source <SOURCE>
```
Results: (yolov8m.onnx, batch=1, 3 times, trt, fp16, RTX 3060Ti)
```bash
==> 0
[Model Preprocess]: 12.75788ms
[ORT H2D]: 237.118µs
[ORT Inference]: 507.895469ms
[ORT D2H]: 191.655µs
[Model Inference]: 508.34589ms
[Model Postprocess]: 1.061122ms
==> 1
[Model Preprocess]: 13.658655ms
[ORT H2D]: 209.975µs
[ORT Inference]: 5.12372ms
[ORT D2H]: 182.389µs
[Model Inference]: 5.530022ms
[Model Postprocess]: 1.04851ms
==> 2
[Model Preprocess]: 12.475332ms
[ORT H2D]: 246.127µs
[ORT Inference]: 5.048432ms
[ORT D2H]: 187.117µs
[Model Inference]: 5.493119ms
[Model Postprocess]: 1.040906ms
```
And also:
`--conf`: confidence threshold \[default: 0.3\]
`--iou`: iou threshold in NMS \[default: 0.45\]
`--kconf`: confidence threshold of keypoint \[default: 0.55\]
`--plot`: plot inference result with random RGB color and save
you can check out all CLI arguments by:
```bash
git clone https://github.com/ultralytics/ultralytics
cd ultralytics/examples/YOLOv8-ONNXRuntime-Rust
cargo run --release -- --help
```
## Examples
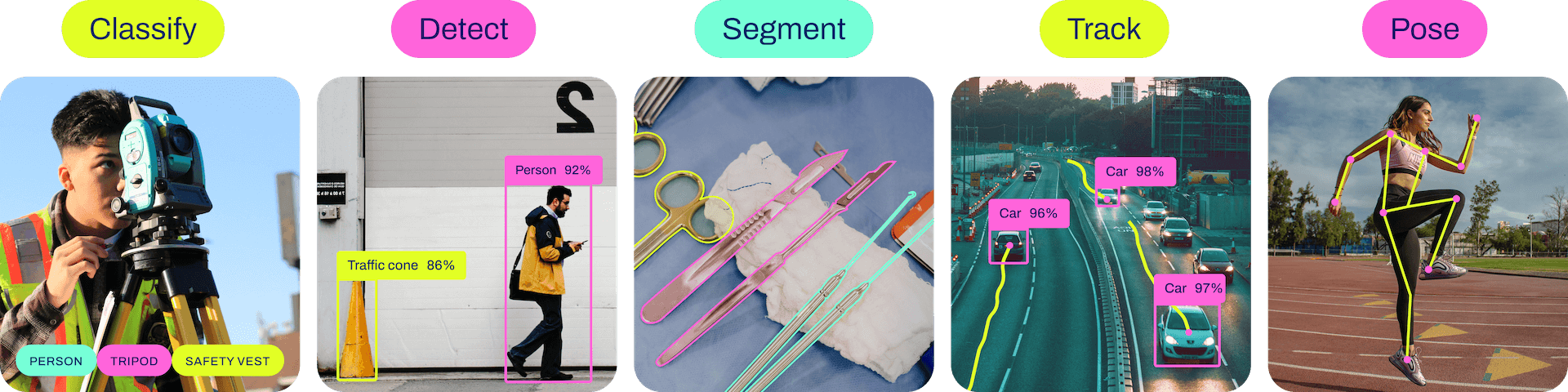
### Classification
Running dynamic shape ONNX model on `CPU` with image size `--height 224 --width 224`. Saving plotted image in `runs` directory.
```bash
cargo run --release -- --model ../assets/weights/yolov8m-cls-dyn.onnx --source ../assets/images/dog.jpg --height 224 --width 224 --plot --profile
```
You will see result like:
```bash
Summary:
> Task: Classify (Ultralytics 8.0.217)
> EP: Cpu
> Dtype: Float32
> Batch: 1 (Dynamic), Height: 224 (Dynamic), Width: 224 (Dynamic)
> nc: 1000 nk: 0, nm: 0, conf: 0.3, kconf: 0.55, iou: 0.45
[Model Preprocess]: 16.363477ms
[ORT H2D]: 50.722µs
[ORT Inference]: 16.295808ms
[ORT D2H]: 8.37µs
[Model Inference]: 16.367046ms
[Model Postprocess]: 3.527µs
[
YOLOResult {
Probs(top5): Some([(208, 0.6950566), (209, 0.13823675), (178, 0.04849795), (215, 0.019029364), (212, 0.016506357)]),
Bboxes: None,
Keypoints: None,
Masks: None,
},
]
```
### Object Detection
Using `CUDA` EP and dynamic image size `--height 640 --width 480`
```bash
cargo run --release -- --cuda --model ../assets/weights/yolov8m-dynamic.onnx --source ../assets/images/bus.jpg --plot --height 640 --width 480
```
### Pose Detection
using `TensorRT` EP
```bash
cargo run --release -- --trt --model ../assets/weights/yolov8m-pose.onnx --source ../assets/images/bus.jpg --plot
```
### Instance Segmentation
using `TensorRT` EP and FP16 model `--fp16`
```bash
cargo run --release -- --trt --fp16 --model ../assets/weights/yolov8m-seg.onnx --source ../assets/images/0172.jpg --plot
```
|