Spaces:
Running
Running
File size: 11,559 Bytes
cfd3735 |
1 2 3 4 5 6 7 8 9 10 11 12 13 14 15 16 17 18 19 20 21 22 23 24 25 26 27 28 29 30 31 32 33 34 35 36 37 38 39 40 41 42 43 44 45 46 47 48 49 50 51 52 53 54 55 56 57 58 59 60 61 62 63 64 65 66 67 68 69 70 71 72 73 74 75 76 77 78 79 80 81 82 83 84 85 86 87 88 89 90 91 92 93 94 95 96 97 98 99 100 101 102 103 104 105 106 107 108 109 110 111 112 113 114 115 116 117 118 119 120 121 122 123 124 125 126 127 128 129 130 131 132 133 134 135 136 137 138 139 140 141 142 143 144 145 146 147 148 149 150 151 152 153 154 155 156 157 158 159 160 161 162 163 164 165 166 167 168 169 170 171 172 173 174 175 176 177 178 179 180 181 182 183 184 185 186 187 188 189 190 191 192 193 194 195 196 197 198 199 200 201 202 203 204 205 206 207 208 209 210 211 212 213 214 215 216 217 218 219 220 221 222 223 224 225 226 227 228 229 230 231 232 233 234 235 236 237 238 239 240 241 242 243 244 245 246 247 248 249 250 251 252 253 254 255 256 257 258 259 260 261 262 263 264 265 266 267 268 269 270 271 272 273 274 275 276 277 278 279 280 281 282 283 284 285 286 287 288 289 290 291 292 293 294 295 296 297 298 299 300 301 302 303 304 305 306 307 308 309 310 311 312 313 314 315 316 317 318 319 320 321 322 323 324 325 326 327 328 329 330 331 332 333 334 335 336 337 338 339 340 341 342 343 344 345 346 347 348 |
{
"cells": [
{
"cell_type": "markdown",
"metadata": {},
"source": [
"# Comet"
]
},
{
"cell_type": "markdown",
"metadata": {},
"source": [
"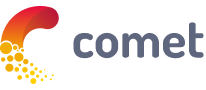"
]
},
{
"cell_type": "markdown",
"metadata": {},
"source": [
"In this guide we will demonstrate how to track your Langchain Experiments, Evaluation Metrics, and LLM Sessions with [Comet](https://www.comet.com/site/?utm_source=langchain&utm_medium=referral&utm_campaign=comet_notebook). \n",
"\n",
"<a target=\"_blank\" href=\"https://colab.research.google.com/github/hwchase17/langchain/blob/master/docs/ecosystem/comet_tracking.ipynb\">\n",
" <img src=\"https://colab.research.google.com/assets/colab-badge.svg\" alt=\"Open In Colab\"/>\n",
"</a>\n",
"\n",
"**Example Project:** [Comet with LangChain](https://www.comet.com/examples/comet-example-langchain/view/b5ZThK6OFdhKWVSP3fDfRtrNF/panels?utm_source=langchain&utm_medium=referral&utm_campaign=comet_notebook)"
]
},
{
"cell_type": "markdown",
"metadata": {},
"source": [
"<img width=\"1280\" alt=\"comet-langchain\" src=\"https://user-images.githubusercontent.com/7529846/230326720-a9711435-9c6f-4edb-a707-94b67271ab25.png\">\n"
]
},
{
"cell_type": "markdown",
"metadata": {},
"source": [
"### Install Comet and Dependencies"
]
},
{
"cell_type": "code",
"execution_count": null,
"metadata": {},
"outputs": [],
"source": [
"%pip install comet_ml langchain openai google-search-results spacy textstat pandas\n",
"\n",
"import sys\n",
"!{sys.executable} -m spacy download en_core_web_sm"
]
},
{
"cell_type": "markdown",
"metadata": {},
"source": [
"### Initialize Comet and Set your Credentials"
]
},
{
"cell_type": "markdown",
"metadata": {},
"source": [
"You can grab your [Comet API Key here](https://www.comet.com/signup?utm_source=langchain&utm_medium=referral&utm_campaign=comet_notebook) or click the link after initializing Comet"
]
},
{
"cell_type": "code",
"execution_count": null,
"metadata": {},
"outputs": [],
"source": [
"import comet_ml\n",
"\n",
"comet_ml.init(project_name=\"comet-example-langchain\")"
]
},
{
"cell_type": "markdown",
"metadata": {},
"source": [
"### Set OpenAI and SerpAPI credentials"
]
},
{
"cell_type": "markdown",
"metadata": {},
"source": [
"You will need an [OpenAI API Key](https://platform.openai.com/account/api-keys) and a [SerpAPI API Key](https://serpapi.com/dashboard) to run the following examples"
]
},
{
"cell_type": "code",
"execution_count": null,
"metadata": {},
"outputs": [],
"source": [
"import os\n",
"\n",
"os.environ[\"OPENAI_API_KEY\"] = \"...\"\n",
"#os.environ[\"OPENAI_ORGANIZATION\"] = \"...\"\n",
"os.environ[\"SERPAPI_API_KEY\"] = \"...\""
]
},
{
"cell_type": "markdown",
"metadata": {},
"source": [
"### Scenario 1: Using just an LLM"
]
},
{
"cell_type": "code",
"execution_count": null,
"metadata": {},
"outputs": [],
"source": [
"from datetime import datetime\n",
"\n",
"from langchain.callbacks import CometCallbackHandler, StdOutCallbackHandler\n",
"from langchain.llms import OpenAI\n",
"\n",
"comet_callback = CometCallbackHandler(\n",
" project_name=\"comet-example-langchain\",\n",
" complexity_metrics=True,\n",
" stream_logs=True,\n",
" tags=[\"llm\"],\n",
" visualizations=[\"dep\"],\n",
")\n",
"callbacks = [StdOutCallbackHandler(), comet_callback]\n",
"llm = OpenAI(temperature=0.9, callbacks=callbacks, verbose=True)\n",
"\n",
"llm_result = llm.generate([\"Tell me a joke\", \"Tell me a poem\", \"Tell me a fact\"] * 3)\n",
"print(\"LLM result\", llm_result)\n",
"comet_callback.flush_tracker(llm, finish=True)"
]
},
{
"cell_type": "markdown",
"metadata": {},
"source": [
"### Scenario 2: Using an LLM in a Chain"
]
},
{
"cell_type": "code",
"execution_count": null,
"metadata": {},
"outputs": [],
"source": [
"from langchain.callbacks import CometCallbackHandler, StdOutCallbackHandler\n",
"from langchain.chains import LLMChain\n",
"from langchain.llms import OpenAI\n",
"from langchain.prompts import PromptTemplate\n",
"\n",
"comet_callback = CometCallbackHandler(\n",
" complexity_metrics=True,\n",
" project_name=\"comet-example-langchain\",\n",
" stream_logs=True,\n",
" tags=[\"synopsis-chain\"],\n",
")\n",
"callbacks = [StdOutCallbackHandler(), comet_callback]\n",
"llm = OpenAI(temperature=0.9, callbacks=callbacks)\n",
"\n",
"template = \"\"\"You are a playwright. Given the title of play, it is your job to write a synopsis for that title.\n",
"Title: {title}\n",
"Playwright: This is a synopsis for the above play:\"\"\"\n",
"prompt_template = PromptTemplate(input_variables=[\"title\"], template=template)\n",
"synopsis_chain = LLMChain(llm=llm, prompt=prompt_template, callbacks=callbacks)\n",
"\n",
"test_prompts = [{\"title\": \"Documentary about Bigfoot in Paris\"}]\n",
"print(synopsis_chain.apply(test_prompts))\n",
"comet_callback.flush_tracker(synopsis_chain, finish=True)"
]
},
{
"cell_type": "markdown",
"metadata": {},
"source": [
"### Scenario 3: Using An Agent with Tools "
]
},
{
"cell_type": "code",
"execution_count": null,
"metadata": {},
"outputs": [],
"source": [
"from langchain.agents import initialize_agent, load_tools\n",
"from langchain.callbacks import CometCallbackHandler, StdOutCallbackHandler\n",
"from langchain.llms import OpenAI\n",
"\n",
"comet_callback = CometCallbackHandler(\n",
" project_name=\"comet-example-langchain\",\n",
" complexity_metrics=True,\n",
" stream_logs=True,\n",
" tags=[\"agent\"],\n",
")\n",
"callbacks = [StdOutCallbackHandler(), comet_callback]\n",
"llm = OpenAI(temperature=0.9, callbacks=callbacks)\n",
"\n",
"tools = load_tools([\"serpapi\", \"llm-math\"], llm=llm, callbacks=callbacks)\n",
"agent = initialize_agent(\n",
" tools,\n",
" llm,\n",
" agent=\"zero-shot-react-description\",\n",
" callbacks=callbacks,\n",
" verbose=True,\n",
")\n",
"agent.run(\n",
" \"Who is Leo DiCaprio's girlfriend? What is her current age raised to the 0.43 power?\"\n",
")\n",
"comet_callback.flush_tracker(agent, finish=True)"
]
},
{
"cell_type": "markdown",
"metadata": {},
"source": [
"### Scenario 4: Using Custom Evaluation Metrics"
]
},
{
"cell_type": "markdown",
"metadata": {},
"source": [
"The `CometCallbackManager` also allows you to define and use Custom Evaluation Metrics to assess generated outputs from your model. Let's take a look at how this works. \n",
"\n",
"\n",
"In the snippet below, we will use the [ROUGE](https://huggingface.co/spaces/evaluate-metric/rouge) metric to evaluate the quality of a generated summary of an input prompt. "
]
},
{
"cell_type": "code",
"execution_count": null,
"metadata": {},
"outputs": [],
"source": [
"%pip install rouge-score"
]
},
{
"cell_type": "code",
"execution_count": null,
"metadata": {},
"outputs": [],
"source": [
"from rouge_score import rouge_scorer\n",
"\n",
"from langchain.callbacks import CometCallbackHandler, StdOutCallbackHandler\n",
"from langchain.chains import LLMChain\n",
"from langchain.llms import OpenAI\n",
"from langchain.prompts import PromptTemplate\n",
"\n",
"\n",
"class Rouge:\n",
" def __init__(self, reference):\n",
" self.reference = reference\n",
" self.scorer = rouge_scorer.RougeScorer([\"rougeLsum\"], use_stemmer=True)\n",
"\n",
" def compute_metric(self, generation, prompt_idx, gen_idx):\n",
" prediction = generation.text\n",
" results = self.scorer.score(target=self.reference, prediction=prediction)\n",
"\n",
" return {\n",
" \"rougeLsum_score\": results[\"rougeLsum\"].fmeasure,\n",
" \"reference\": self.reference,\n",
" }\n",
"\n",
"\n",
"reference = \"\"\"\n",
"The tower is 324 metres (1,063 ft) tall, about the same height as an 81-storey building.\n",
"It was the first structure to reach a height of 300 metres.\n",
"\n",
"It is now taller than the Chrysler Building in New York City by 5.2 metres (17 ft)\n",
"Excluding transmitters, the Eiffel Tower is the second tallest free-standing structure in France .\n",
"\"\"\"\n",
"rouge_score = Rouge(reference=reference)\n",
"\n",
"template = \"\"\"Given the following article, it is your job to write a summary.\n",
"Article:\n",
"{article}\n",
"Summary: This is the summary for the above article:\"\"\"\n",
"prompt_template = PromptTemplate(input_variables=[\"article\"], template=template)\n",
"\n",
"comet_callback = CometCallbackHandler(\n",
" project_name=\"comet-example-langchain\",\n",
" complexity_metrics=False,\n",
" stream_logs=True,\n",
" tags=[\"custom_metrics\"],\n",
" custom_metrics=rouge_score.compute_metric,\n",
")\n",
"callbacks = [StdOutCallbackHandler(), comet_callback]\n",
"llm = OpenAI(temperature=0.9)\n",
"\n",
"synopsis_chain = LLMChain(llm=llm, prompt=prompt_template)\n",
"\n",
"test_prompts = [\n",
" {\n",
" \"article\": \"\"\"\n",
" The tower is 324 metres (1,063 ft) tall, about the same height as\n",
" an 81-storey building, and the tallest structure in Paris. Its base is square,\n",
" measuring 125 metres (410 ft) on each side.\n",
" During its construction, the Eiffel Tower surpassed the\n",
" Washington Monument to become the tallest man-made structure in the world,\n",
" a title it held for 41 years until the Chrysler Building\n",
" in New York City was finished in 1930.\n",
"\n",
" It was the first structure to reach a height of 300 metres.\n",
" Due to the addition of a broadcasting aerial at the top of the tower in 1957,\n",
" it is now taller than the Chrysler Building by 5.2 metres (17 ft).\n",
"\n",
" Excluding transmitters, the Eiffel Tower is the second tallest\n",
" free-standing structure in France after the Millau Viaduct.\n",
" \"\"\"\n",
" }\n",
"]\n",
"print(synopsis_chain.apply(test_prompts, callbacks=callbacks))\n",
"comet_callback.flush_tracker(synopsis_chain, finish=True)"
]
}
],
"metadata": {
"kernelspec": {
"display_name": "Python 3 (ipykernel)",
"language": "python",
"name": "python3"
},
"language_info": {
"codemirror_mode": {
"name": "ipython",
"version": 3
},
"file_extension": ".py",
"mimetype": "text/x-python",
"name": "python",
"nbconvert_exporter": "python",
"pygments_lexer": "ipython3",
"version": "3.9.15"
}
},
"nbformat": 4,
"nbformat_minor": 2
}
|