Spaces:
Configuration error
Configuration error
File size: 9,767 Bytes
1ba539f |
1 2 3 4 5 6 7 8 9 10 11 12 13 14 15 16 17 18 19 20 21 22 23 24 25 26 27 28 29 30 31 32 33 34 35 36 37 38 39 40 41 42 43 44 45 46 47 48 49 50 51 52 53 54 55 56 57 58 59 60 61 62 63 64 65 66 67 68 69 70 71 72 73 74 75 76 77 78 79 80 81 82 83 84 85 86 87 88 89 90 91 92 93 94 95 96 97 98 99 100 101 102 103 104 105 106 107 108 109 110 111 112 113 114 115 116 117 118 119 120 121 122 123 124 125 126 127 128 129 130 131 132 133 134 135 136 137 138 139 140 141 142 143 144 145 146 147 148 149 150 151 152 153 154 155 156 157 158 159 160 161 162 163 164 165 166 167 168 169 170 171 172 173 174 175 176 177 178 179 180 181 182 183 184 185 186 187 188 189 190 191 192 193 194 195 196 197 198 199 200 201 202 203 |
**News**
* `05/17/2021` To make the comparison on ZJU-MoCap easier, we save quantitative and qualitative results of other methods at [here](https://github.com/zju3dv/neuralbody/blob/master/supplementary_material.md#results-of-other-methods-on-zju-mocap), including Neural Volumes, Multi-view Neural Human Rendering, and Deferred Neural Human Rendering.
* `05/13/2021` To make the following works easier compare with our model, we save our rendering results of ZJU-MoCap at [here](https://zjueducn-my.sharepoint.com/:u:/g/personal/pengsida_zju_edu_cn/Ea3VOUy204VAiVJ-V-OGd9YBxdhbtfpS-U6icD_rDq0mUQ?e=cAcylK) and write a [document](supplementary_material.md) that describes the training and test protocols.
* `05/12/2021` The code supports the test and visualization on unseen human poses.
* `05/12/2021` We update the ZJU-MoCap dataset with better fitted SMPL using [EasyMocap](https://github.com/zju3dv/EasyMocap). We also release a [website](https://zju3dv.github.io/zju_mocap/) for visualization. Please see [here](https://github.com/zju3dv/neuralbody#potential-problems-of-provided-smpl-parameters) for the usage of provided smpl parameters.
# Neural Body: Implicit Neural Representations with Structured Latent Codes for Novel View Synthesis of Dynamic Humans
### [Project Page](https://zju3dv.github.io/neuralbody) | [Video](https://www.youtube.com/watch?v=BPCAMeBCE-8) | [Paper](https://arxiv.org/pdf/2012.15838.pdf) | [Data](https://github.com/zju3dv/neuralbody/blob/master/INSTALL.md#zju-mocap-dataset)
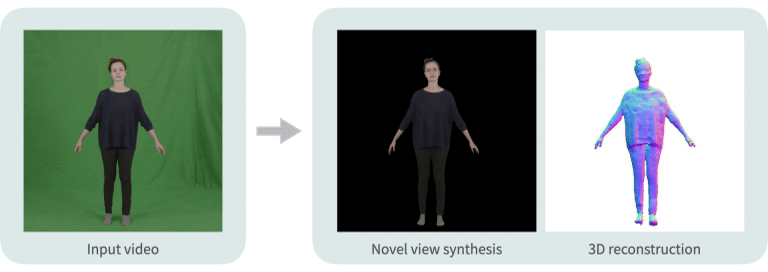
> [Neural Body: Implicit Neural Representations with Structured Latent Codes for Novel View Synthesis of Dynamic Humans](https://arxiv.org/pdf/2012.15838.pdf)
> Sida Peng, Yuanqing Zhang, Yinghao Xu, Qianqian Wang, Qing Shuai, Hujun Bao, Xiaowei Zhou
> CVPR 2021
Any questions or discussions are welcomed!
## Installation
Please see [INSTALL.md](INSTALL.md) for manual installation.
### Installation using docker
Please see [docker/README.md](docker/README.md).
Thanks to [Zhaoyi Wan](https://github.com/wanzysky) for providing the docker implementation.
## Run the code on the custom dataset
Please see [CUSTOM](tools/custom).
## Run the code on People-Snapshot
Please see [INSTALL.md](INSTALL.md) to download the dataset.
We provide the pretrained models at [here](https://zjueducn-my.sharepoint.com/:f:/g/personal/pengsida_zju_edu_cn/Enn43YWDHwBEg-XBqnetFYcBLr3cItZ0qUFU-oKUpDHKXw?e=FObjE9).
### Process People-Snapshot
We already provide some processed data. If you want to process more videos of People-Snapshot, you could use [tools/process_snapshot.py](tools/process_snapshot.py).
You can also visualize smpl parameters of People-Snapshot with [tools/vis_snapshot.py](tools/vis_snapshot.py).
### Visualization on People-Snapshot
Take the visualization on `female-3-casual` as an example. The command lines for visualization are recorded in [visualize.sh](visualize.sh).
1. Download the corresponding pretrained model and put it to `$ROOT/data/trained_model/if_nerf/female3c/latest.pth`.
2. Visualization:
* Visualize novel views of single frame
```
python run.py --type visualize --cfg_file configs/snapshot_exp/snapshot_f3c.yaml exp_name female3c vis_novel_view True num_render_views 144
```
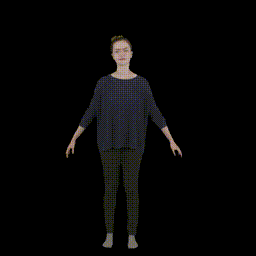
* Visualize views of dynamic humans with fixed camera
```
python run.py --type visualize --cfg_file configs/snapshot_exp/snapshot_f3c.yaml exp_name female3c vis_novel_pose True
```
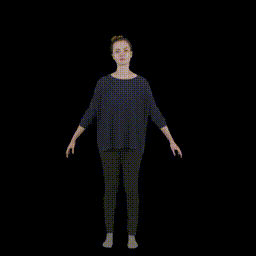
* Visualize mesh
```
# generate meshes
python run.py --type visualize --cfg_file configs/snapshot_exp/snapshot_f3c.yaml exp_name female3c vis_mesh True train.num_workers 0
# visualize a specific mesh
python tools/render_mesh.py --exp_name female3c --dataset people_snapshot --mesh_ind 226
```
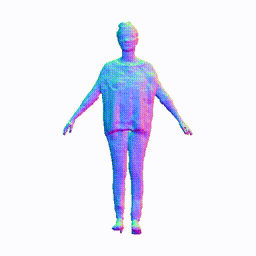
3. The results of visualization are located at `$ROOT/data/render/female3c` and `$ROOT/data/perform/female3c`.
### Training on People-Snapshot
Take the training on `female-3-casual` as an example. The command lines for training are recorded in [train.sh](train.sh).
1. Train:
```
# training
python train_net.py --cfg_file configs/snapshot_exp/snapshot_f3c.yaml exp_name female3c resume False
# distributed training
python -m torch.distributed.launch --nproc_per_node=4 train_net.py --cfg_file configs/snapshot_exp/snapshot_f3c.yaml exp_name female3c resume False gpus "0, 1, 2, 3" distributed True
```
2. Train with white background:
```
# training
python train_net.py --cfg_file configs/snapshot_exp/snapshot_f3c.yaml exp_name female3c resume False white_bkgd True
```
3. Tensorboard:
```
tensorboard --logdir data/record/if_nerf
```
## Run the code on ZJU-MoCap
Please see [INSTALL.md](INSTALL.md) to download the dataset.
We provide the pretrained models at [here](https://zjueducn-my.sharepoint.com/:f:/g/personal/pengsida_zju_edu_cn/Enn43YWDHwBEg-XBqnetFYcBLr3cItZ0qUFU-oKUpDHKXw?e=FObjE9).
### Potential problems of provided smpl parameters
1. The newly fitted parameters locate in `new_params`. Currently, the released pretrained models are trained on previously fitted parameters, which locate in `params`.
2. The smpl parameters of ZJU-MoCap have different definition from the one of MPI's smplx.
* If you want to extract vertices from the provided smpl parameters, please use `zju_smpl/extract_vertices.py`.
* The reason that we use the current definition is described at [here](https://github.com/zju3dv/EasyMocap/blob/master/doc/02_output.md#attention-for-smplsmpl-x-users).
It is okay to train Neural Body with smpl parameters fitted by smplx.
### Test on ZJU-MoCap
The command lines for test are recorded in [test.sh](test.sh).
Take the test on `sequence 313` as an example.
1. Download the corresponding pretrained model and put it to `$ROOT/data/trained_model/if_nerf/xyzc_313/latest.pth`.
2. Test on training human poses:
```
python run.py --type evaluate --cfg_file configs/zju_mocap_exp/latent_xyzc_313.yaml exp_name xyzc_313
```
3. Test on unseen human poses:
```
python run.py --type evaluate --cfg_file configs/zju_mocap_exp/latent_xyzc_313.yaml exp_name xyzc_313 test_novel_pose True
```
### Visualization on ZJU-MoCap
Take the visualization on `sequence 313` as an example. The command lines for visualization are recorded in [visualize.sh](visualize.sh).
1. Download the corresponding pretrained model and put it to `$ROOT/data/trained_model/if_nerf/xyzc_313/latest.pth`.
2. Visualization:
* Visualize novel views of single frame
```
python run.py --type visualize --cfg_file configs/zju_mocap_exp/latent_xyzc_313.yaml exp_name xyzc_313 vis_novel_view True
```
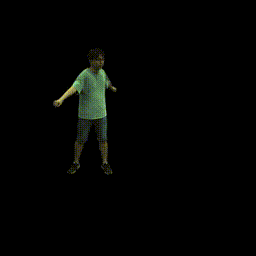
* Visualize novel views of single frame by rotating the SMPL model
```
python run.py --type visualize --cfg_file configs/zju_mocap_exp/latent_xyzc_313.yaml exp_name xyzc_313 vis_novel_view True num_render_views 100
```
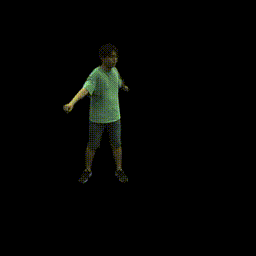
* Visualize views of dynamic humans with fixed camera
```
python run.py --type visualize --cfg_file configs/zju_mocap_exp/latent_xyzc_313.yaml exp_name xyzc_313 vis_novel_pose True num_render_frame 1000 num_render_views 1
```
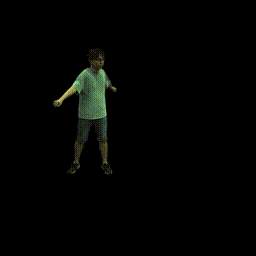
* Visualize views of dynamic humans with rotated camera
```
python run.py --type visualize --cfg_file configs/zju_mocap_exp/latent_xyzc_313.yaml exp_name xyzc_313 vis_novel_pose True num_render_frame 1000
```
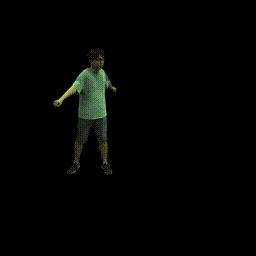
* Visualize mesh
```
# generate meshes
python run.py --type visualize --cfg_file configs/zju_mocap_exp/latent_xyzc_313.yaml exp_name xyzc_313 vis_mesh True train.num_workers 0
# visualize a specific mesh
python tools/render_mesh.py --exp_name xyzc_313 --dataset zju_mocap --mesh_ind 0
```
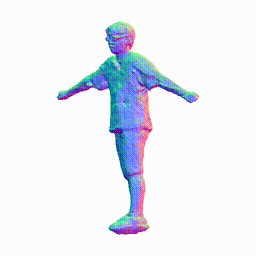
4. The results of visualization are located at `$ROOT/data/render/xyzc_313` and `$ROOT/data/perform/xyzc_313`.
### Training on ZJU-MoCap
Take the training on `sequence 313` as an example. The command lines for training are recorded in [train.sh](train.sh).
1. Train:
```
# training
python train_net.py --cfg_file configs/zju_mocap_exp/latent_xyzc_313.yaml exp_name xyzc_313 resume False
# distributed training
python -m torch.distributed.launch --nproc_per_node=4 train_net.py --cfg_file configs/zju_mocap_exp/latent_xyzc_313.yaml exp_name xyzc_313 resume False gpus "0, 1, 2, 3" distributed True
```
2. Train with white background:
```
# training
python train_net.py --cfg_file configs/zju_mocap_exp/latent_xyzc_313.yaml exp_name xyzc_313 resume False white_bkgd True
```
3. Tensorboard:
```
tensorboard --logdir data/record/if_nerf
```
## Citation
If you find this code useful for your research, please use the following BibTeX entry.
```
@inproceedings{peng2021neural,
title={Neural Body: Implicit Neural Representations with Structured Latent Codes for Novel View Synthesis of Dynamic Humans},
author={Peng, Sida and Zhang, Yuanqing and Xu, Yinghao and Wang, Qianqian and Shuai, Qing and Bao, Hujun and Zhou, Xiaowei},
booktitle={CVPR},
year={2021}
}
```
|