Spaces:
Runtime error
Runtime error
File size: 15,666 Bytes
a63d2a4 |
1 2 3 4 5 6 7 8 9 10 11 12 13 14 15 16 17 18 19 20 21 22 23 24 25 26 27 28 29 30 31 32 33 34 35 36 37 38 39 40 41 42 43 44 45 46 47 48 49 50 51 52 53 54 55 56 57 58 59 60 61 62 63 64 65 66 67 68 69 70 71 72 73 74 75 76 77 78 79 80 81 82 83 84 85 86 87 88 89 90 91 92 93 94 95 96 97 98 99 100 101 102 103 104 105 106 107 108 109 110 111 112 113 114 115 116 117 118 119 120 121 122 123 124 125 126 127 128 129 130 131 132 133 134 135 136 137 138 139 140 141 142 143 144 145 146 147 148 149 150 151 152 153 154 155 156 157 158 159 160 161 162 163 164 165 166 167 168 169 170 171 172 173 174 175 176 177 178 179 180 181 182 183 184 185 186 187 188 189 190 191 192 193 194 195 196 197 198 199 200 201 202 203 204 205 206 207 208 209 210 211 212 213 214 215 216 217 218 219 220 221 222 223 224 225 226 227 228 229 230 231 232 233 234 235 236 237 238 239 240 241 242 243 244 245 246 247 248 249 250 251 252 253 254 255 256 257 258 259 |
<!--Copyright 2023 The HuggingFace Team. All rights reserved.
Licensed under the Apache License, Version 2.0 (the "License"); you may not use this file except in compliance with
the License. You may obtain a copy of the License at
http://www.apache.org/licenses/LICENSE-2.0
Unless required by applicable law or agreed to in writing, software distributed under the License is distributed on
an "AS IS" BASIS, WITHOUT WARRANTIES OR CONDITIONS OF ANY KIND, either express or implied. See the License for the
specific language governing permissions and limitations under the License.
-->
# Text-to-Image Generation with Adapter Conditioning
## Overview
[T2I-Adapter: Learning Adapters to Dig out More Controllable Ability for Text-to-Image Diffusion Models](https://arxiv.org/abs/2302.08453) by Chong Mou, Xintao Wang, Liangbin Xie, Jian Zhang, Zhongang Qi, Ying Shan, Xiaohu Qie.
Using the pretrained models we can provide control images (for example, a depth map) to control Stable Diffusion text-to-image generation so that it follows the structure of the depth image and fills in the details.
The abstract of the paper is the following:
*The incredible generative ability of large-scale text-to-image (T2I) models has demonstrated strong power of learning complex structures and meaningful semantics. However, relying solely on text prompts cannot fully take advantage of the knowledge learned by the model, especially when flexible and accurate structure control is needed. In this paper, we aim to ``dig out" the capabilities that T2I models have implicitly learned, and then explicitly use them to control the generation more granularly. Specifically, we propose to learn simple and small T2I-Adapters to align internal knowledge in T2I models with external control signals, while freezing the original large T2I models. In this way, we can train various adapters according to different conditions, and achieve rich control and editing effects. Further, the proposed T2I-Adapters have attractive properties of practical value, such as composability and generalization ability. Extensive experiments demonstrate that our T2I-Adapter has promising generation quality and a wide range of applications.*
This model was contributed by the community contributor [HimariO](https://github.com/HimariO) ❤️ .
## Available Pipelines:
| Pipeline | Tasks | Demo
|---|---|:---:|
| [StableDiffusionAdapterPipeline](https://github.com/huggingface/diffusers/blob/main/src/diffusers/pipelines/stable_diffusion/pipeline_stable_diffusion_adapter.py) | *Text-to-Image Generation with T2I-Adapter Conditioning* | -
| [StableDiffusionXLAdapterPipeline](https://github.com/huggingface/diffusers/blob/main/src/diffusers/pipelines/stable_diffusion/pipeline_stable_diffusion_xl_adapter.py) | *Text-to-Image Generation with T2I-Adapter Conditioning on StableDiffusion-XL* | -
## Usage example with the base model of StableDiffusion-1.4/1.5
In the following we give a simple example of how to use a *T2IAdapter* checkpoint with Diffusers for inference based on StableDiffusion-1.4/1.5.
All adapters use the same pipeline.
1. Images are first converted into the appropriate *control image* format.
2. The *control image* and *prompt* are passed to the [`StableDiffusionAdapterPipeline`].
Let's have a look at a simple example using the [Color Adapter](https://huggingface.co/TencentARC/t2iadapter_color_sd14v1).
```python
from diffusers.utils import load_image
image = load_image("https://huggingface.co/datasets/diffusers/docs-images/resolve/main/t2i-adapter/color_ref.png")
```
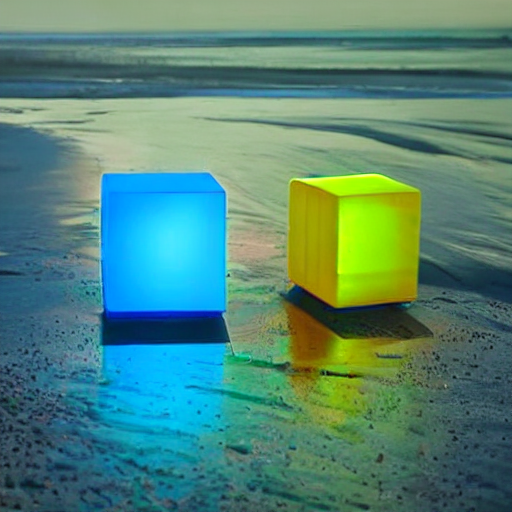
Then we can create our color palette by simply resizing it to 8 by 8 pixels and then scaling it back to original size.
```python
from PIL import Image
color_palette = image.resize((8, 8))
color_palette = color_palette.resize((512, 512), resample=Image.Resampling.NEAREST)
```
Let's take a look at the processed image.
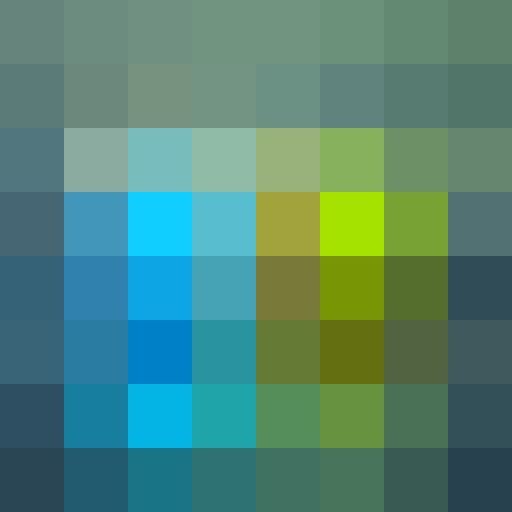
Next, create the adapter pipeline
```py
import torch
from diffusers import StableDiffusionAdapterPipeline, T2IAdapter
adapter = T2IAdapter.from_pretrained("TencentARC/t2iadapter_color_sd14v1", torch_dtype=torch.float16)
pipe = StableDiffusionAdapterPipeline.from_pretrained(
"CompVis/stable-diffusion-v1-4",
adapter=adapter,
torch_dtype=torch.float16,
)
pipe.to("cuda")
```
Finally, pass the prompt and control image to the pipeline
```py
# fix the random seed, so you will get the same result as the example
generator = torch.manual_seed(7)
out_image = pipe(
"At night, glowing cubes in front of the beach",
image=color_palette,
generator=generator,
).images[0]
```
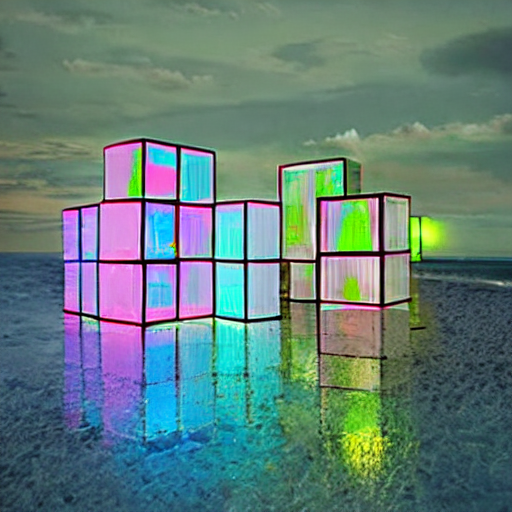
## Usage example with the base model of StableDiffusion-XL
In the following we give a simple example of how to use a *T2IAdapter* checkpoint with Diffusers for inference based on StableDiffusion-XL.
All adapters use the same pipeline.
1. Images are first downloaded into the appropriate *control image* format.
2. The *control image* and *prompt* are passed to the [`StableDiffusionXLAdapterPipeline`].
Let's have a look at a simple example using the [Sketch Adapter](https://huggingface.co/Adapter/t2iadapter/tree/main/sketch_sdxl_1.0).
```python
from diffusers.utils import load_image
sketch_image = load_image("https://huggingface.co/Adapter/t2iadapter/resolve/main/sketch.png").convert("L")
```
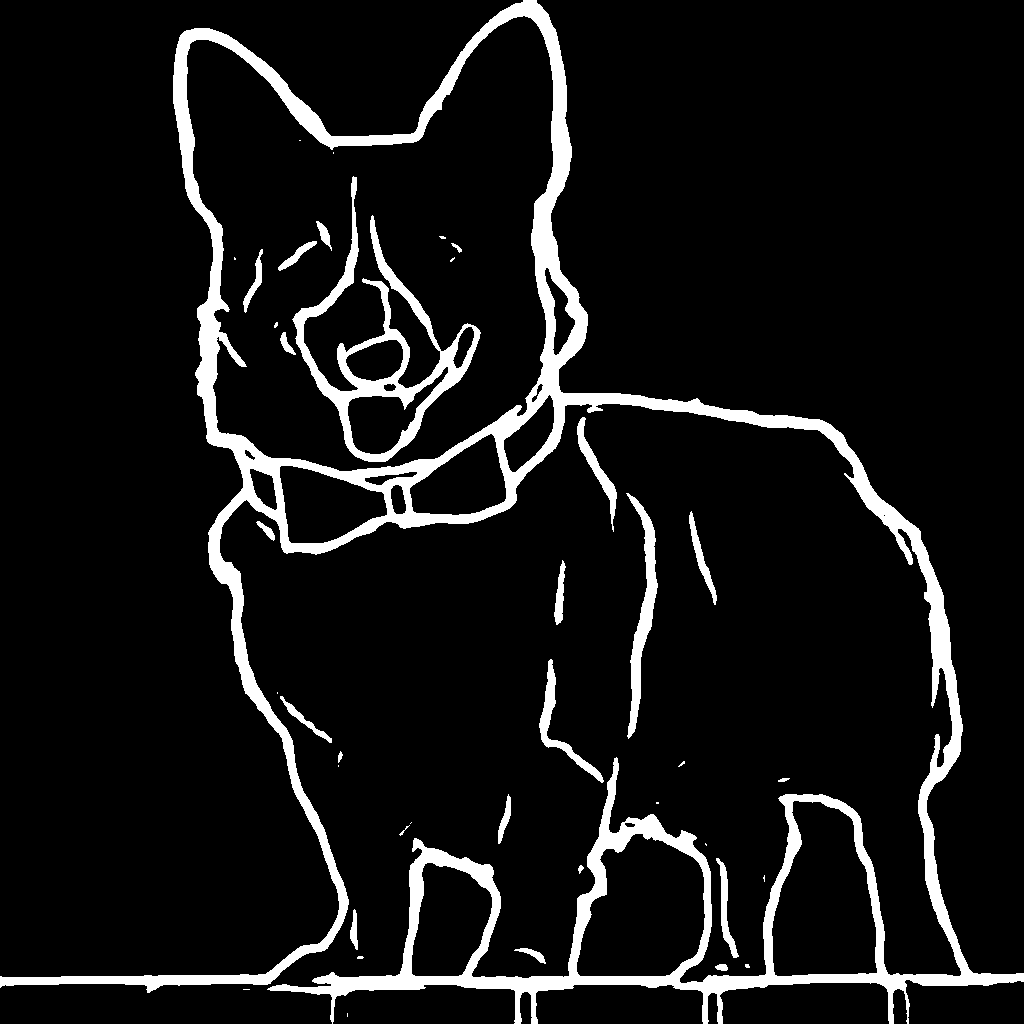
Then, create the adapter pipeline
```py
import torch
from diffusers import (
T2IAdapter,
StableDiffusionXLAdapterPipeline,
DDPMScheduler
)
from diffusers.models.unet_2d_condition import UNet2DConditionModel
model_id = "stabilityai/stable-diffusion-xl-base-1.0"
adapter = T2IAdapter.from_pretrained("Adapter/t2iadapter", subfolder="sketch_sdxl_1.0",torch_dtype=torch.float16, adapter_type="full_adapter_xl")
scheduler = DDPMScheduler.from_pretrained(model_id, subfolder="scheduler")
pipe = StableDiffusionXLAdapterPipeline.from_pretrained(
model_id, adapter=adapter, safety_checker=None, torch_dtype=torch.float16, variant="fp16", scheduler=scheduler
)
pipe.to("cuda")
```
Finally, pass the prompt and control image to the pipeline
```py
# fix the random seed, so you will get the same result as the example
generator = torch.Generator().manual_seed(42)
sketch_image_out = pipe(
prompt="a photo of a dog in real world, high quality",
negative_prompt="extra digit, fewer digits, cropped, worst quality, low quality",
image=sketch_image,
generator=generator,
guidance_scale=7.5
).images[0]
```
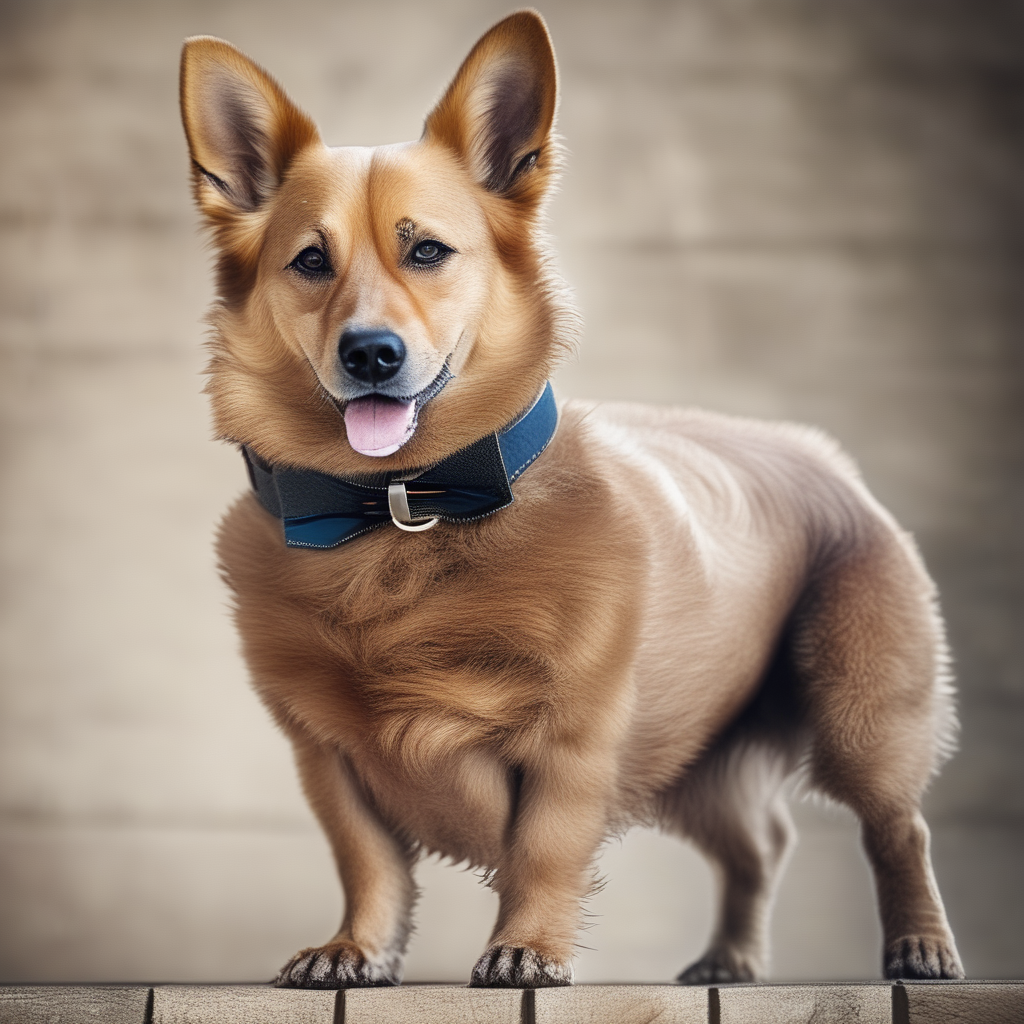
## Available checkpoints
Non-diffusers checkpoints can be found under [TencentARC/T2I-Adapter](https://huggingface.co/TencentARC/T2I-Adapter/tree/main/models).
### T2I-Adapter with Stable Diffusion 1.4
| Model Name | Control Image Overview| Control Image Example | Generated Image Example |
|---|---|---|---|
|[TencentARC/t2iadapter_color_sd14v1](https://huggingface.co/TencentARC/t2iadapter_color_sd14v1)<br/> *Trained with spatial color palette* | A image with 8x8 color palette.|<a href="https://huggingface.co/datasets/diffusers/docs-images/resolve/main/t2i-adapter/color_sample_input.png"><img width="64" style="margin:0;padding:0;" src="https://huggingface.co/datasets/diffusers/docs-images/resolve/main/t2i-adapter/color_sample_input.png"/></a>|<a href="https://huggingface.co/datasets/diffusers/docs-images/resolve/main/t2i-adapter/color_sample_output.png"><img width="64" src="https://huggingface.co/datasets/diffusers/docs-images/resolve/main/t2i-adapter/color_sample_output.png"/></a>|
|[TencentARC/t2iadapter_canny_sd14v1](https://huggingface.co/TencentARC/t2iadapter_canny_sd14v1)<br/> *Trained with canny edge detection* | A monochrome image with white edges on a black background.|<a href="https://huggingface.co/datasets/diffusers/docs-images/resolve/main/t2i-adapter/canny_sample_input.png"><img width="64" style="margin:0;padding:0;" src="https://huggingface.co/datasets/diffusers/docs-images/resolve/main/t2i-adapter/canny_sample_input.png"/></a>|<a href="https://huggingface.co/datasets/diffusers/docs-images/resolve/main/t2i-adapter/canny_sample_output.png"><img width="64" src="https://huggingface.co/datasets/diffusers/docs-images/resolve/main/t2i-adapter/canny_sample_output.png"/></a>|
|[TencentARC/t2iadapter_sketch_sd14v1](https://huggingface.co/TencentARC/t2iadapter_sketch_sd14v1)<br/> *Trained with [PidiNet](https://github.com/zhuoinoulu/pidinet) edge detection* | A hand-drawn monochrome image with white outlines on a black background.|<a href="https://huggingface.co/datasets/diffusers/docs-images/resolve/main/t2i-adapter/sketch_sample_input.png"><img width="64" style="margin:0;padding:0;" src="https://huggingface.co/datasets/diffusers/docs-images/resolve/main/t2i-adapter/sketch_sample_input.png"/></a>|<a href="https://huggingface.co/datasets/diffusers/docs-images/resolve/main/t2i-adapter/sketch_sample_output.png"><img width="64" src="https://huggingface.co/datasets/diffusers/docs-images/resolve/main/t2i-adapter/sketch_sample_output.png"/></a>|
|[TencentARC/t2iadapter_depth_sd14v1](https://huggingface.co/TencentARC/t2iadapter_depth_sd14v1)<br/> *Trained with Midas depth estimation* | A grayscale image with black representing deep areas and white representing shallow areas.|<a href="https://huggingface.co/datasets/diffusers/docs-images/resolve/main/t2i-adapter/depth_sample_input.png"><img width="64" src="https://huggingface.co/datasets/diffusers/docs-images/resolve/main/t2i-adapter/depth_sample_input.png"/></a>|<a href="https://huggingface.co/datasets/diffusers/docs-images/resolve/main/t2i-adapter/depth_sample_output.png"><img width="64" src="https://huggingface.co/datasets/diffusers/docs-images/resolve/main/t2i-adapter/depth_sample_output.png"/></a>|
|[TencentARC/t2iadapter_openpose_sd14v1](https://huggingface.co/TencentARC/t2iadapter_openpose_sd14v1)<br/> *Trained with OpenPose bone image* | A [OpenPose bone](https://github.com/CMU-Perceptual-Computing-Lab/openpose) image.|<a href="https://huggingface.co/datasets/diffusers/docs-images/resolve/main/t2i-adapter/openpose_sample_input.png"><img width="64" src="https://huggingface.co/datasets/diffusers/docs-images/resolve/main/t2i-adapter/openpose_sample_input.png"/></a>|<a href="https://huggingface.co/datasets/diffusers/docs-images/resolve/main/t2i-adapter/openpose_sample_output.png"><img width="64" src="https://huggingface.co/datasets/diffusers/docs-images/resolve/main/t2i-adapter/openpose_sample_output.png"/></a>|
|[TencentARC/t2iadapter_keypose_sd14v1](https://huggingface.co/TencentARC/t2iadapter_keypose_sd14v1)<br/> *Trained with mmpose skeleton image* | A [mmpose skeleton](https://github.com/open-mmlab/mmpose) image.|<a href="https://huggingface.co/datasets/diffusers/docs-images/resolve/main/t2i-adapter/keypose_sample_input.png"><img width="64" src="https://huggingface.co/datasets/diffusers/docs-images/resolve/main/t2i-adapter/keypose_sample_input.png"/></a>|<a href="https://huggingface.co/datasets/diffusers/docs-images/resolve/main/t2i-adapter/keypose_sample_output.png"><img width="64" src="https://huggingface.co/datasets/diffusers/docs-images/resolve/main/t2i-adapter/keypose_sample_output.png"/></a>|
|[TencentARC/t2iadapter_seg_sd14v1](https://huggingface.co/TencentARC/t2iadapter_seg_sd14v1)<br/>*Trained with semantic segmentation* | An [custom](https://github.com/TencentARC/T2I-Adapter/discussions/25) segmentation protocol image.|<a href="https://huggingface.co/datasets/diffusers/docs-images/resolve/main/t2i-adapter/seg_sample_input.png"><img width="64" src="https://huggingface.co/datasets/diffusers/docs-images/resolve/main/t2i-adapter/seg_sample_input.png"/></a>|<a href="https://huggingface.co/datasets/diffusers/docs-images/resolve/main/t2i-adapter/seg_sample_output.png"><img width="64" src="https://huggingface.co/datasets/diffusers/docs-images/resolve/main/t2i-adapter/seg_sample_output.png"/></a> |
|[TencentARC/t2iadapter_canny_sd15v2](https://huggingface.co/TencentARC/t2iadapter_canny_sd15v2)||
|[TencentARC/t2iadapter_depth_sd15v2](https://huggingface.co/TencentARC/t2iadapter_depth_sd15v2)||
|[TencentARC/t2iadapter_sketch_sd15v2](https://huggingface.co/TencentARC/t2iadapter_sketch_sd15v2)||
|[TencentARC/t2iadapter_zoedepth_sd15v1](https://huggingface.co/TencentARC/t2iadapter_zoedepth_sd15v1)||
|[Adapter/t2iadapter, subfolder='sketch_sdxl_1.0'](https://huggingface.co/Adapter/t2iadapter/tree/main/sketch_sdxl_1.0)||
|[Adapter/t2iadapter, subfolder='canny_sdxl_1.0'](https://huggingface.co/Adapter/t2iadapter/tree/main/canny_sdxl_1.0)||
|[Adapter/t2iadapter, subfolder='openpose_sdxl_1.0'](https://huggingface.co/Adapter/t2iadapter/tree/main/openpose_sdxl_1.0)||
## Combining multiple adapters
[`MultiAdapter`] can be used for applying multiple conditionings at once.
Here we use the keypose adapter for the character posture and the depth adapter for creating the scene.
```py
import torch
from PIL import Image
from diffusers.utils import load_image
cond_keypose = load_image(
"https://huggingface.co/datasets/diffusers/docs-images/resolve/main/t2i-adapter/keypose_sample_input.png"
)
cond_depth = load_image(
"https://huggingface.co/datasets/diffusers/docs-images/resolve/main/t2i-adapter/depth_sample_input.png"
)
cond = [[cond_keypose, cond_depth]]
prompt = ["A man walking in an office room with a nice view"]
```
The two control images look as such:
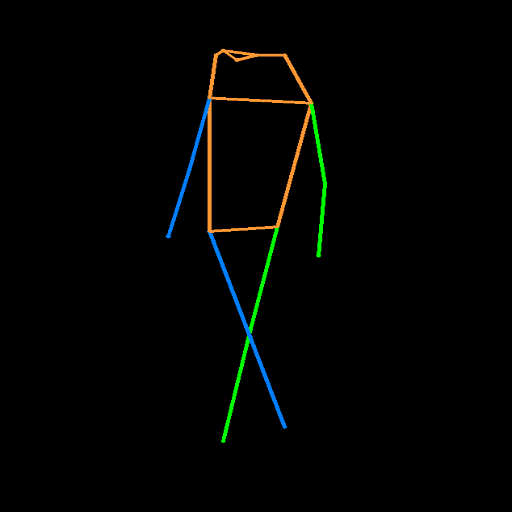
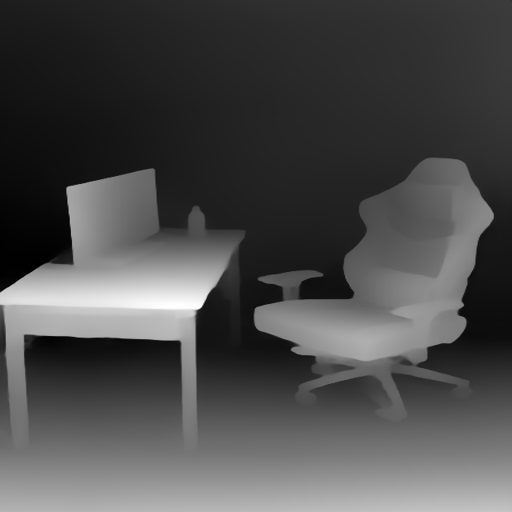
`MultiAdapter` combines keypose and depth adapters.
`adapter_conditioning_scale` balances the relative influence of the different adapters.
```py
from diffusers import StableDiffusionAdapterPipeline, MultiAdapter
adapters = MultiAdapter(
[
T2IAdapter.from_pretrained("TencentARC/t2iadapter_keypose_sd14v1"),
T2IAdapter.from_pretrained("TencentARC/t2iadapter_depth_sd14v1"),
]
)
adapters = adapters.to(torch.float16)
pipe = StableDiffusionAdapterPipeline.from_pretrained(
"CompVis/stable-diffusion-v1-4",
torch_dtype=torch.float16,
adapter=adapters,
)
images = pipe(prompt, cond, adapter_conditioning_scale=[0.8, 0.8])
```
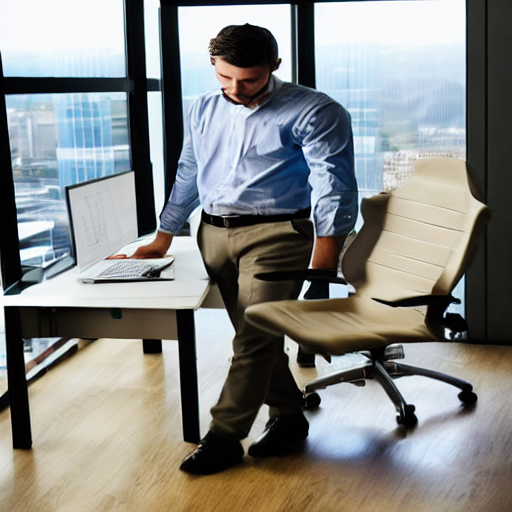
## T2I Adapter vs ControlNet
T2I-Adapter is similar to [ControlNet](https://huggingface.co/docs/diffusers/main/en/api/pipelines/controlnet).
T2i-Adapter uses a smaller auxiliary network which is only run once for the entire diffusion process.
However, T2I-Adapter performs slightly worse than ControlNet.
## StableDiffusionAdapterPipeline
[[autodoc]] StableDiffusionAdapterPipeline
- all
- __call__
- enable_attention_slicing
- disable_attention_slicing
- enable_vae_slicing
- disable_vae_slicing
- enable_xformers_memory_efficient_attention
- disable_xformers_memory_efficient_attention
## StableDiffusionXLAdapterPipeline
[[autodoc]] StableDiffusionXLAdapterPipeline
- all
- __call__
- enable_attention_slicing
- disable_attention_slicing
- enable_vae_slicing
- disable_vae_slicing
- enable_xformers_memory_efficient_attention
- disable_xformers_memory_efficient_attention
|