Spaces:
Running
Running
File size: 10,855 Bytes
8777b6a 599f646 bcb2553 599f646 bcb2553 599f646 bcb2553 599f646 2d0fd49 22294bc bcb2553 22294bc bcb2553 22294bc 2d0fd49 |
1 2 3 4 5 6 7 8 9 10 11 12 13 14 15 16 17 18 19 20 21 22 23 24 25 26 27 28 29 30 31 32 33 34 35 36 37 38 39 40 41 42 43 44 45 46 47 48 49 50 51 52 53 54 55 56 57 58 59 60 61 62 63 64 65 66 67 68 69 70 71 72 73 74 75 76 77 78 79 80 81 82 83 84 85 86 87 88 89 90 91 92 93 94 95 96 97 98 99 100 101 102 103 104 105 106 107 108 109 110 111 112 113 114 115 116 117 118 119 120 121 122 123 124 125 126 127 128 129 130 131 132 133 134 135 136 137 138 139 140 141 142 143 144 145 146 147 148 149 150 151 152 153 154 155 156 157 158 159 160 161 162 163 164 165 166 167 168 169 170 171 172 173 174 175 176 177 178 179 180 181 182 183 184 185 186 187 188 189 190 191 192 193 194 195 196 197 198 199 200 201 202 203 204 205 206 207 208 209 210 211 212 213 214 215 216 217 218 219 220 221 222 223 224 225 226 227 228 229 230 231 232 233 234 235 236 237 238 239 240 241 242 243 244 245 246 247 248 249 250 251 252 253 254 255 256 257 258 259 260 261 262 263 264 265 266 267 268 269 270 271 272 273 274 275 276 277 278 279 |
# InspectorRAGet
InspectorRAGet, an introspection platform for RAG evaluation. InspectorRAGet allows the user to analyze aggregate and instance-level performance of RAG systems, using both human and algorithmic metrics as well as annotator quality.
InspectorRAGet has been developed as a [React](https://react.dev/) web application built with [NextJS 14](https://nextjs.org/) framework and the [Carbon Design System](https://carbondesignsystem.com/).
## 🎥 Demo
[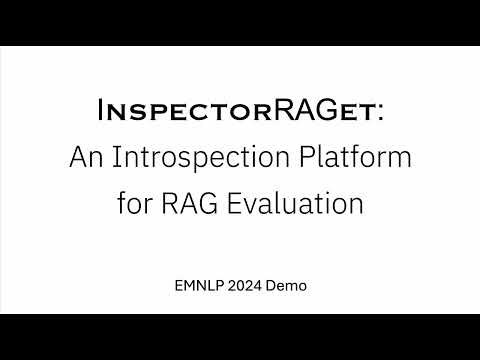](https://www.youtube.com/watch?v=vB7mJnSNx7s)
## 🏗️ Build & Deploy
To install and run InspectorRAGet follow the steps below:
### Installation
We use yarn as a default package manager.
```shell
yarn install
```
⚠️ node version must be `20.12.0` or higher.
### Development server
To start InspectorRAGet in development mode, please run the following command.
```shell
yarn dev
```
### Build
To build a static production bundle, please run the following command.
```shell
yarn dev
```
### Production server
To start InspectorRAGet in production mode, please run the following command.
```shell
yarn start
```
## Usage
Once you have started InspectorRAGet, the next step is import a json file with the evaluation results in the format expected by the platform. You can do this in two ways:
- Use one of our [integration notebooks](#use-inspectorraget-through-integration-notebooks), showing how to use InspectorRAGet in combination with popular evaluation frameworks.
- Manually convert the evaluation results into the expected format by consulting the [documentation of InspectorRAGet's file format](#use-inspectorraget-by-manually-creating-input-file).
## Use InspectorRAGet through integration notebooks
To make it easier to get started, we have created notebooks showcasing how InspectorRAGet can be used in combination with popular evaluation frameworks. Each notebook demonstrates how to use the corresponding framework to run an evaluation experiment and transform its output to the input format expected by InspectorRAGet for analysis. We provide notebooks demonstrating integrations of InspectorRAGet with the following popular frameworks:
| Framework | Description | Integration Notebook |
| --- | --- | --- |
| Language Model Evaluation Harness | Popular evaluation framework used to evaluate language models on different tasks | [LM_Eval_Demonstration.ipynb](notebooks/LM_Eval_Demonstration.ipynb) |
| Ragas | Popular evaluation framework specifically designed for the evaluation of RAG systems through LLM-as-a-judge techniques | [Ragas_Demonstration.ipynb](notebooks/Ragas_Demonstration.ipynb) |
| HuggingFace | Offers libraries and assets (incl. datasets, models, and metric evaluators) that can be used to both create and evaluate RAG systems | [HuggingFace_Demonstration.ipynb](notebooks/HuggingFace_Demonstration.ipynb) |
## Use InspectorRAGet by manually creating input file
If you want to use your own code/framework, not covered by the integration notebooks above, to run the evaluation, you can manually transform the evaluation results to the input format expected by InspectorRAGet, described below. Examples of input files in the expected format can be found in the [data](data) folder.
The experiment results json file expected by InspectorRAGet can be broadly split into six sections along their functional boundaries. The first section captures general details about the experiment in `name`, `description` and `timestamp` fields. The second and third sections describe the
sets of models and metrics used in the experiment via the `models` and `metrics` fields, respectively. The last three sections cover the dataset and the outcome of evaluation experiment in the form of `documents`, `tasks` and `evaluations` fields.
#### 1. Metadata
```json
{
"name": "Sample experiment name",
"description": "Sample example description",
...
```
#### 2. Models
```json
"models": [
{
"model_id": "model_1",
"name": "Model 1",
"owner": "Model 1 owner",
},
{
"model_id": "model_2",
"name": "Model 2",
"owner": "Model 2 owner",
}
],
```
Notes:
1. Each model must have a unique `model_id` and `name`.
#### 3. Metrics
```json
"numerical": [
{
"name": "metric_a",
"display_name": "Metric A",
"description": "Metric A description",
"author": "algorithm | human",
"type": "numerical",
"aggregator": "average",
"range": [0, 1, 0.1]
},
{
"name": "metric_b",
"display_name": "Metric B",
"description": "Metric B description",
"author": "algorithm | human",
"type": "categorical",
"aggregator": "majority | average",
"values": [
{
"value": "value_a",
"display_value": "A",
"numeric_value": 1
},
{
"value": "value_b",
"display_value": "B",
"numeric_value": 0
}
]
},
{
"name": "metric_c",
"display_name": "Metric C",
"description": "Metric C description",
"author": "algorithm | human",
"type": "text"
}
],
```
Notes:
1. Each metric must have a unique name.
2. Metric can be of `numerical`, `categorical`, or `text` type.
3. Numerical type metrics must specify `range` field in `[start, end, bin_size]` format.
4. Categoricl type metrics must specify `values` field where each value must have `value` and `numerical_value` fields.
5. Text type metric are only accesible in instance level view and not used in any experiment level aggregate statistics and visual elements.
#### 4. Documents
```json
"documents": [
{
"document_id": "GUID 1",
"text": "document text 1",
"title": "document title 1"
},
{
"document_id": "GUID 2",
"text": "document text 2",
"title": "document title 2"
},
{
"document_id": "GUID 3",
"text": "document text 3",
"title": "document title 3"
}
],
```
Notes:
1. Each document must have a unique `document_id` field.
2. Each document must have a `text` field.
#### 5. Tasks
```json
"filters": ["category"],
"tasks": [
{
"task_id": "task_1",
"task_type": "rag",
"category": "grounded",
"input": [
{
"speaker": "user",
"text": "Sample user query"
}
],
"contexts": [
{
"document_id": "GUID 1"
}
],
"targets": [
{
"text": "Sample response"
}
]
},
{
"task_id": "task_2",
"task_type": "rag",
"category": "random",
"input": [
{
"speaker": "user",
"text": "Hello"
}
],
"contexts": [
{
"document_id": "GUID 2"
}
],
"targets": [
{
"text": "How can I help you?"
}
]
}
],
```
Notes:
1. Each task must have a unique `task_id`.
2. Task type can be of `question_answering`, `conversation`, or of `rag` type.
3. `input` is an array of utterances. An utterance's speaker could be either `user` or `agent`. Each utterance must have a `text` field.
4. `contexts` field represents a subset of documents from the `documents` field relevant to the `input` and is available to the generative models.
5. `targets` field is an array of expected gold or reference texts.
6. `category` is an optional field that represents the type of task for grouping similar tasks.
7. `filters` is a top-level field (parallel to `tasks`) which specifies an array of fields defined inside `tasks` for filtering tasks during analysis.
#### 6. Evaluations
```json
"evaluations": [
{
"task_id": "task_1 | task_2",
"model_id": "model_1 | model_2",
"model_response": "Model response",
"annotations": {
"metric_a": {
"system": {
"value": 0.233766233766233
}
},
"metric_b": {
"system": {
"value": "value_a | value_b"
}
},
"metric_c": {
"system": {
"value": "text"
}
},
}
}
]
```
Notes:
1. `evaluations` field must contain evaluation for every model defined in `models` section and on every task in `tasks` section. Thus, total number of evaluations is equal to number of models (M) X number of tasks (T) = M X T
2. Each evaluation must be associated with single task and single model.
3. Each evaluation must have model prediction on a task captured in the `model_response` field.
4. `annotations` field captures ratings on the model for a given task and for every metric specified in the `metrics` field.
5. Each metric annotation is a dictionary containing worker ids as keys. In the example above, `system` is a worker id.
6. Annotation from any worker on all metrics must be in the form of a dictionary. At minimum, such dictionary contains `value` key capturing model's rating for the metric by the worker.
## Citation
If you use InspectorRAGet in your research, please cite our paper:
```
@misc{fadnis2024inspectorraget,
title={InspectorRAGet: An Introspection Platform for RAG Evaluation},
author={Kshitij Fadnis and Siva Sankalp Patel and Odellia Boni and Yannis Katsis and Sara Rosenthal and Benjamin Sznajder and Marina Danilevsky},
year={2024},
eprint={2404.17347},
archivePrefix={arXiv},
primaryClass={cs.SE}
}
```
|