State-of-the-art Natural Language Processing for TensorFlow 2.0 and PyTorch
-
-
-🤗 Transformers (formerly known as `pytorch-transformers` and `pytorch-pretrained-bert`) provides state-of-the-art general-purpose architectures (BERT, GPT-2, RoBERTa, XLM, DistilBert, XLNet, CTRL...) for Natural Language Understanding (NLU) and Natural Language Generation (NLG) with over 32+ pretrained models in 100+ languages and deep interoperability between TensorFlow 2.0 and PyTorch.
-
-### Features
-
-- As easy to use as pytorch-transformers
-- As powerful and concise as Keras
-- High performance on NLU and NLG tasks
-- Low barrier to entry for educators and practitioners
-
-State-of-the-art NLP for everyone
-- Deep learning researchers
-- Hands-on practitioners
-- AI/ML/NLP teachers and educators
-
-Lower compute costs, smaller carbon footprint
-- Researchers can share trained models instead of always retraining
-- Practitioners can reduce compute time and production costs
-- 10 architectures with over 30 pretrained models, some in more than 100 languages
-
-Choose the right framework for every part of a model's lifetime
-- Train state-of-the-art models in 3 lines of code
-- Deep interoperability between TensorFlow 2.0 and PyTorch models
-- Move a single model between TF2.0/PyTorch frameworks at will
-- Seamlessly pick the right framework for training, evaluation, production
-
-
-| Section | Description |
-|-|-|
-| [Installation](#installation) | How to install the package |
-| [Model architectures](#model-architectures) | Architectures (with pretrained weights) |
-| [Online demo](#online-demo) | Experimenting with this repo’s text generation capabilities |
-| [Quick tour: Usage](#quick-tour) | Tokenizers & models usage: Bert and GPT-2 |
-| [Quick tour: TF 2.0 and PyTorch ](#Quick-tour-TF-20-training-and-PyTorch-interoperability) | Train a TF 2.0 model in 10 lines of code, load it in PyTorch |
-| [Quick tour: pipelines](#quick-tour-of-pipelines) | Using Pipelines: Wrapper around tokenizer and models to use finetuned models |
-| [Quick tour: Fine-tuning/usage scripts](#quick-tour-of-the-fine-tuningusage-scripts) | Using provided scripts: GLUE, SQuAD and Text generation |
-| [Quick tour: Share your models ](#Quick-tour-of-model-sharing) | Upload and share your fine-tuned models with the community |
-| [Migrating from pytorch-transformers to transformers](#Migrating-from-pytorch-transformers-to-transformers) | Migrating your code from pytorch-transformers to transformers |
-| [Migrating from pytorch-pretrained-bert to pytorch-transformers](#Migrating-from-pytorch-pretrained-bert-to-transformers) | Migrating your code from pytorch-pretrained-bert to transformers |
-| [Documentation][(v2.4.0)](https://huggingface.co/transformers/v2.4.0)[(v2.3.0)](https://huggingface.co/transformers/v2.3.0)[(v2.2.0/v2.2.1/v2.2.2)](https://huggingface.co/transformers/v2.2.0) [(v2.1.1)](https://huggingface.co/transformers/v2.1.1) [(v2.0.0)](https://huggingface.co/transformers/v2.0.0) [(v1.2.0)](https://huggingface.co/transformers/v1.2.0) [(v1.1.0)](https://huggingface.co/transformers/v1.1.0) [(v1.0.0)](https://huggingface.co/transformers/v1.0.0) [(master)](https://huggingface.co/transformers) | Full API documentation and more |
-
-## Installation
-
-This repo is tested on Python 3.5+, PyTorch 1.0.0+ and TensorFlow 2.0.0-rc1
-
-You should install 🤗 Transformers in a [virtual environment](https://docs.python.org/3/library/venv.html). If you're unfamiliar with Python virtual environments, check out the [user guide](https://packaging.python.org/guides/installing-using-pip-and-virtual-environments/).
-
-Create a virtual environment with the version of Python you're going to use and activate it.
-
-Now, if you want to use 🤗 Transformers, you can install it with pip. If you'd like to play with the examples, you must install it from source.
-
-### With pip
-
-First you need to install one of, or both, TensorFlow 2.0 and PyTorch.
-Please refer to [TensorFlow installation page](https://www.tensorflow.org/install/pip#tensorflow-2.0-rc-is-available) and/or [PyTorch installation page](https://pytorch.org/get-started/locally/#start-locally) regarding the specific install command for your platform.
-
-When TensorFlow 2.0 and/or PyTorch has been installed, 🤗 Transformers can be installed using pip as follows:
-
-```bash
-pip install transformers
-```
-
-### From source
-
-Here also, you first need to install one of, or both, TensorFlow 2.0 and PyTorch.
-Please refer to [TensorFlow installation page](https://www.tensorflow.org/install/pip#tensorflow-2.0-rc-is-available) and/or [PyTorch installation page](https://pytorch.org/get-started/locally/#start-locally) regarding the specific install command for your platform.
-
-When TensorFlow 2.0 and/or PyTorch has been installed, you can install from source by cloning the repository and running:
-
-```bash
-git clone https://github.com/huggingface/transformers
-cd transformers
-pip install .
-```
-
-When you update the repository, you should upgrade the transformers installation and its dependencies as follows:
-
-```bash
-git pull
-pip install --upgrade .
-```
-
-### Run the examples
-
-Examples are included in the repository but are not shipped with the library.
-
-Therefore, in order to run the latest versions of the examples, you need to install from source, as described above.
-
-Look at the [README](https://github.com/huggingface/transformers/blob/master/examples/README.md) for how to run examples.
-
-### Tests
-
-A series of tests are included for the library and for some example scripts. Library tests can be found in the [tests folder](https://github.com/huggingface/transformers/tree/master/tests) and examples tests in the [examples folder](https://github.com/huggingface/transformers/tree/master/examples).
-
-Depending on which framework is installed (TensorFlow 2.0 and/or PyTorch), the irrelevant tests will be skipped. Ensure that both frameworks are installed if you want to execute all tests.
-
-Here's the easiest way to run tests for the library:
-
-```bash
-pip install -e ".[testing]"
-make test
-```
-
-and for the examples:
-
-```bash
-pip install -e ".[testing]"
-pip install -r examples/requirements.txt
-make test-examples
-```
-
-For details, refer to the [contributing guide](https://github.com/huggingface/transformers/blob/master/CONTRIBUTING.md#tests).
-
-### Do you want to run a Transformer model on a mobile device?
-
-You should check out our [`swift-coreml-transformers`](https://github.com/huggingface/swift-coreml-transformers) repo.
-
-It contains a set of tools to convert PyTorch or TensorFlow 2.0 trained Transformer models (currently contains `GPT-2`, `DistilGPT-2`, `BERT`, and `DistilBERT`) to CoreML models that run on iOS devices.
-
-At some point in the future, you'll be able to seamlessly move from pre-training or fine-tuning models to productizing them in CoreML, or prototype a model or an app in CoreML then research its hyperparameters or architecture from TensorFlow 2.0 and/or PyTorch. Super exciting!
-
-## Model architectures
-
-🤗 Transformers currently provides the following NLU/NLG architectures:
-
-1. **[BERT](https://github.com/google-research/bert)** (from Google) released with the paper [BERT: Pre-training of Deep Bidirectional Transformers for Language Understanding](https://arxiv.org/abs/1810.04805) by Jacob Devlin, Ming-Wei Chang, Kenton Lee and Kristina Toutanova.
-2. **[GPT](https://github.com/openai/finetune-transformer-lm)** (from OpenAI) released with the paper [Improving Language Understanding by Generative Pre-Training](https://blog.openai.com/language-unsupervised/) by Alec Radford, Karthik Narasimhan, Tim Salimans and Ilya Sutskever.
-3. **[GPT-2](https://blog.openai.com/better-language-models/)** (from OpenAI) released with the paper [Language Models are Unsupervised Multitask Learners](https://blog.openai.com/better-language-models/) by Alec Radford*, Jeffrey Wu*, Rewon Child, David Luan, Dario Amodei** and Ilya Sutskever**.
-4. **[Transformer-XL](https://github.com/kimiyoung/transformer-xl)** (from Google/CMU) released with the paper [Transformer-XL: Attentive Language Models Beyond a Fixed-Length Context](https://arxiv.org/abs/1901.02860) by Zihang Dai*, Zhilin Yang*, Yiming Yang, Jaime Carbonell, Quoc V. Le, Ruslan Salakhutdinov.
-5. **[XLNet](https://github.com/zihangdai/xlnet/)** (from Google/CMU) released with the paper [XLNet: Generalized Autoregressive Pretraining for Language Understanding](https://arxiv.org/abs/1906.08237) by Zhilin Yang*, Zihang Dai*, Yiming Yang, Jaime Carbonell, Ruslan Salakhutdinov, Quoc V. Le.
-6. **[XLM](https://github.com/facebookresearch/XLM/)** (from Facebook) released together with the paper [Cross-lingual Language Model Pretraining](https://arxiv.org/abs/1901.07291) by Guillaume Lample and Alexis Conneau.
-7. **[RoBERTa](https://github.com/pytorch/fairseq/tree/master/examples/roberta)** (from Facebook), released together with the paper a [Robustly Optimized BERT Pretraining Approach](https://arxiv.org/abs/1907.11692) by Yinhan Liu, Myle Ott, Naman Goyal, Jingfei Du, Mandar Joshi, Danqi Chen, Omer Levy, Mike Lewis, Luke Zettlemoyer, Veselin Stoyanov.
-8. **[DistilBERT](https://github.com/huggingface/transformers/tree/master/examples/distillation)** (from HuggingFace), released together with the paper [DistilBERT, a distilled version of BERT: smaller, faster, cheaper and lighter](https://arxiv.org/abs/1910.01108) by Victor Sanh, Lysandre Debut and Thomas Wolf. The same method has been applied to compress GPT2 into [DistilGPT2](https://github.com/huggingface/transformers/tree/master/examples/distillation), RoBERTa into [DistilRoBERTa](https://github.com/huggingface/transformers/tree/master/examples/distillation), Multilingual BERT into [DistilmBERT](https://github.com/huggingface/transformers/tree/master/examples/distillation) and a German version of DistilBERT.
-9. **[CTRL](https://github.com/salesforce/ctrl/)** (from Salesforce) released with the paper [CTRL: A Conditional Transformer Language Model for Controllable Generation](https://arxiv.org/abs/1909.05858) by Nitish Shirish Keskar*, Bryan McCann*, Lav R. Varshney, Caiming Xiong and Richard Socher.
-10. **[CamemBERT](https://camembert-model.fr)** (from Inria/Facebook/Sorbonne) released with the paper [CamemBERT: a Tasty French Language Model](https://arxiv.org/abs/1911.03894) by Louis Martin*, Benjamin Muller*, Pedro Javier Ortiz Suárez*, Yoann Dupont, Laurent Romary, Éric Villemonte de la Clergerie, Djamé Seddah and Benoît Sagot.
-11. **[ALBERT](https://github.com/google-research/ALBERT)** (from Google Research and the Toyota Technological Institute at Chicago) released with the paper [ALBERT: A Lite BERT for Self-supervised Learning of Language Representations](https://arxiv.org/abs/1909.11942), by Zhenzhong Lan, Mingda Chen, Sebastian Goodman, Kevin Gimpel, Piyush Sharma, Radu Soricut.
-12. **[T5](https://github.com/google-research/text-to-text-transfer-transformer)** (from Google AI) released with the paper [Exploring the Limits of Transfer Learning with a Unified Text-to-Text Transformer](https://arxiv.org/abs/1910.10683) by Colin Raffel and Noam Shazeer and Adam Roberts and Katherine Lee and Sharan Narang and Michael Matena and Yanqi Zhou and Wei Li and Peter J. Liu.
-13. **[XLM-RoBERTa](https://github.com/pytorch/fairseq/tree/master/examples/xlmr)** (from Facebook AI), released together with the paper [Unsupervised Cross-lingual Representation Learning at Scale](https://arxiv.org/abs/1911.02116) by Alexis Conneau*, Kartikay Khandelwal*, Naman Goyal, Vishrav Chaudhary, Guillaume Wenzek, Francisco Guzmán, Edouard Grave, Myle Ott, Luke Zettlemoyer and Veselin Stoyanov.
-14. **[MMBT](https://github.com/facebookresearch/mmbt/)** (from Facebook), released together with the paper a [Supervised Multimodal Bitransformers for Classifying Images and Text](https://arxiv.org/pdf/1909.02950.pdf) by Douwe Kiela, Suvrat Bhooshan, Hamed Firooz, Davide Testuggine.
-15. **[FlauBERT](https://github.com/getalp/Flaubert)** (from CNRS) released with the paper [FlauBERT: Unsupervised Language Model Pre-training for French](https://arxiv.org/abs/1912.05372) by Hang Le, Loïc Vial, Jibril Frej, Vincent Segonne, Maximin Coavoux, Benjamin Lecouteux, Alexandre Allauzen, Benoît Crabbé, Laurent Besacier, Didier Schwab.
-16. **[Other community models](https://huggingface.co/models)**, contributed by the [community](https://huggingface.co/users).
-17. Want to contribute a new model? We have added a **detailed guide and templates** to guide you in the process of adding a new model. You can find them in the [`templates`](./templates) folder of the repository. Be sure to check the [contributing guidelines](./CONTRIBUTING.md) and contact the maintainers or open an issue to collect feedbacks before starting your PR.
-
-These implementations have been tested on several datasets (see the example scripts) and should match the performances of the original implementations (e.g. ~93 F1 on SQuAD for BERT Whole-Word-Masking, ~88 F1 on RocStories for OpenAI GPT, ~18.3 perplexity on WikiText 103 for Transformer-XL, ~0.916 Peason R coefficient on STS-B for XLNet). You can find more details on the performances in the Examples section of the [documentation](https://huggingface.co/transformers/examples.html).
-
-## Online demo
-
-**[Write With Transformer](https://transformer.huggingface.co)**, built by the Hugging Face team at transformer.huggingface.co, is the official demo of this repo’s text generation capabilities.
-You can use it to experiment with completions generated by `GPT2Model`, `TransfoXLModel`, and `XLNetModel`.
-
-> “🦄 Write with transformer is to writing what calculators are to calculus.”
-
-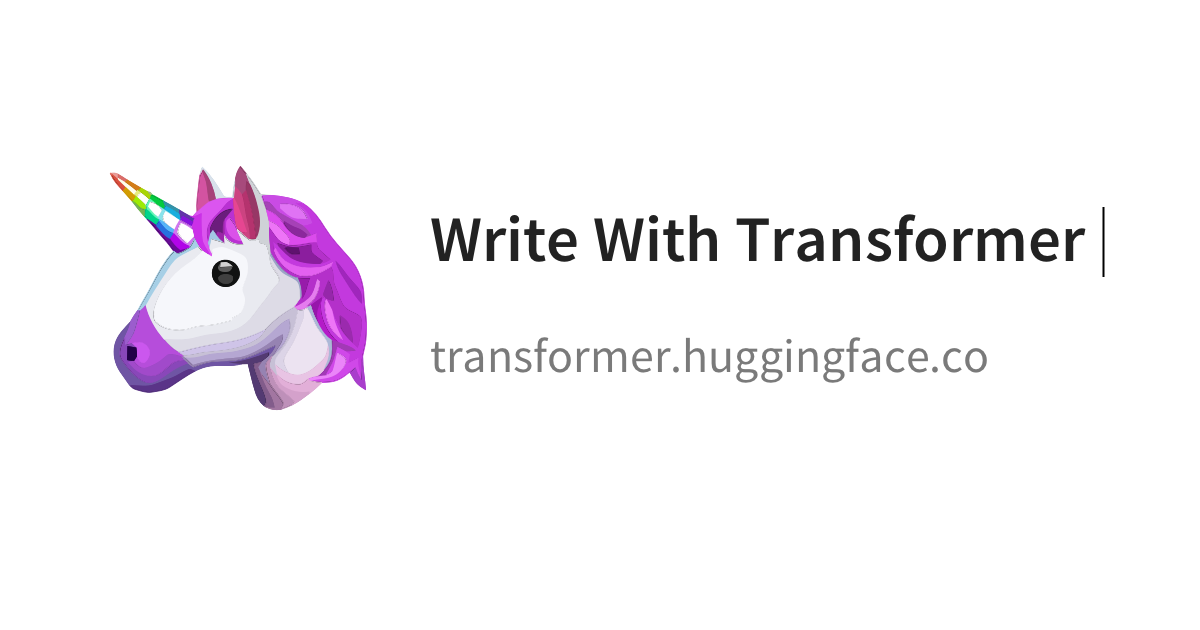
-
-## Quick tour
-
-Let's do a very quick overview of the model architectures in 🤗 Transformers. Detailed examples for each model architecture (Bert, GPT, GPT-2, Transformer-XL, XLNet and XLM) can be found in the [full documentation](https://huggingface.co/transformers/).
-
-```python
-import torch
-from transformers import *
-
-# Transformers has a unified API
-# for 10 transformer architectures and 30 pretrained weights.
-# Model | Tokenizer | Pretrained weights shortcut
-MODELS = [(BertModel, BertTokenizer, 'bert-base-uncased'),
- (OpenAIGPTModel, OpenAIGPTTokenizer, 'openai-gpt'),
- (GPT2Model, GPT2Tokenizer, 'gpt2'),
- (CTRLModel, CTRLTokenizer, 'ctrl'),
- (TransfoXLModel, TransfoXLTokenizer, 'transfo-xl-wt103'),
- (XLNetModel, XLNetTokenizer, 'xlnet-base-cased'),
- (XLMModel, XLMTokenizer, 'xlm-mlm-enfr-1024'),
- (DistilBertModel, DistilBertTokenizer, 'distilbert-base-uncased'),
- (RobertaModel, RobertaTokenizer, 'roberta-base'),
- (XLMRobertaModel, XLMRobertaTokenizer, 'xlm-roberta-base'),
- ]
-
-# To use TensorFlow 2.0 versions of the models, simply prefix the class names with 'TF', e.g. `TFRobertaModel` is the TF 2.0 counterpart of the PyTorch model `RobertaModel`
-
-# Let's encode some text in a sequence of hidden-states using each model:
-for model_class, tokenizer_class, pretrained_weights in MODELS:
- # Load pretrained model/tokenizer
- tokenizer = tokenizer_class.from_pretrained(pretrained_weights)
- model = model_class.from_pretrained(pretrained_weights)
-
- # Encode text
- input_ids = torch.tensor([tokenizer.encode("Here is some text to encode", add_special_tokens=True)]) # Add special tokens takes care of adding [CLS], [SEP], ... tokens in the right way for each model.
- with torch.no_grad():
- last_hidden_states = model(input_ids)[0] # Models outputs are now tuples
-
-# Each architecture is provided with several class for fine-tuning on down-stream tasks, e.g.
-BERT_MODEL_CLASSES = [BertModel, BertForPreTraining, BertForMaskedLM, BertForNextSentencePrediction,
- BertForSequenceClassification, BertForTokenClassification, BertForQuestionAnswering]
-
-# All the classes for an architecture can be initiated from pretrained weights for this architecture
-# Note that additional weights added for fine-tuning are only initialized
-# and need to be trained on the down-stream task
-pretrained_weights = 'bert-base-uncased'
-tokenizer = BertTokenizer.from_pretrained(pretrained_weights)
-for model_class in BERT_MODEL_CLASSES:
- # Load pretrained model/tokenizer
- model = model_class.from_pretrained(pretrained_weights)
-
- # Models can return full list of hidden-states & attentions weights at each layer
- model = model_class.from_pretrained(pretrained_weights,
- output_hidden_states=True,
- output_attentions=True)
- input_ids = torch.tensor([tokenizer.encode("Let's see all hidden-states and attentions on this text")])
- all_hidden_states, all_attentions = model(input_ids)[-2:]
-
- # Models are compatible with Torchscript
- model = model_class.from_pretrained(pretrained_weights, torchscript=True)
- traced_model = torch.jit.trace(model, (input_ids,))
-
- # Simple serialization for models and tokenizers
- model.save_pretrained('./directory/to/save/') # save
- model = model_class.from_pretrained('./directory/to/save/') # re-load
- tokenizer.save_pretrained('./directory/to/save/') # save
- tokenizer = BertTokenizer.from_pretrained('./directory/to/save/') # re-load
-
- # SOTA examples for GLUE, SQUAD, text generation...
-```
-
-## Quick tour TF 2.0 training and PyTorch interoperability
-
-Let's do a quick example of how a TensorFlow 2.0 model can be trained in 12 lines of code with 🤗 Transformers and then loaded in PyTorch for fast inspection/tests.
-
-```python
-import tensorflow as tf
-import tensorflow_datasets
-from transformers import *
-
-# Load dataset, tokenizer, model from pretrained model/vocabulary
-tokenizer = BertTokenizer.from_pretrained('bert-base-cased')
-model = TFBertForSequenceClassification.from_pretrained('bert-base-cased')
-data = tensorflow_datasets.load('glue/mrpc')
-
-# Prepare dataset for GLUE as a tf.data.Dataset instance
-train_dataset = glue_convert_examples_to_features(data['train'], tokenizer, max_length=128, task='mrpc')
-valid_dataset = glue_convert_examples_to_features(data['validation'], tokenizer, max_length=128, task='mrpc')
-train_dataset = train_dataset.shuffle(100).batch(32).repeat(2)
-valid_dataset = valid_dataset.batch(64)
-
-# Prepare training: Compile tf.keras model with optimizer, loss and learning rate schedule
-optimizer = tf.keras.optimizers.Adam(learning_rate=3e-5, epsilon=1e-08, clipnorm=1.0)
-loss = tf.keras.losses.SparseCategoricalCrossentropy(from_logits=True)
-metric = tf.keras.metrics.SparseCategoricalAccuracy('accuracy')
-model.compile(optimizer=optimizer, loss=loss, metrics=[metric])
-
-# Train and evaluate using tf.keras.Model.fit()
-history = model.fit(train_dataset, epochs=2, steps_per_epoch=115,
- validation_data=valid_dataset, validation_steps=7)
-
-# Load the TensorFlow model in PyTorch for inspection
-model.save_pretrained('./save/')
-pytorch_model = BertForSequenceClassification.from_pretrained('./save/', from_tf=True)
-
-# Quickly test a few predictions - MRPC is a paraphrasing task, let's see if our model learned the task
-sentence_0 = "This research was consistent with his findings."
-sentence_1 = "His findings were compatible with this research."
-sentence_2 = "His findings were not compatible with this research."
-inputs_1 = tokenizer.encode_plus(sentence_0, sentence_1, add_special_tokens=True, return_tensors='pt')
-inputs_2 = tokenizer.encode_plus(sentence_0, sentence_2, add_special_tokens=True, return_tensors='pt')
-
-pred_1 = pytorch_model(inputs_1['input_ids'], token_type_ids=inputs_1['token_type_ids'])[0].argmax().item()
-pred_2 = pytorch_model(inputs_2['input_ids'], token_type_ids=inputs_2['token_type_ids'])[0].argmax().item()
-
-print("sentence_1 is", "a paraphrase" if pred_1 else "not a paraphrase", "of sentence_0")
-print("sentence_2 is", "a paraphrase" if pred_2 else "not a paraphrase", "of sentence_0")
-```
-
-## Quick tour of the fine-tuning/usage scripts
-
-**Important**
-Before running the fine-tuning scripts, please read the
-[instructions](#run-the-examples) on how to
-setup your environment to run the examples.
-
-The library comprises several example scripts with SOTA performances for NLU and NLG tasks:
-
-- `run_glue.py`: an example fine-tuning Bert, XLNet and XLM on nine different GLUE tasks (*sequence-level classification*)
-- `run_squad.py`: an example fine-tuning Bert, XLNet and XLM on the question answering dataset SQuAD 2.0 (*token-level classification*)
-- `run_generation.py`: an example using GPT, GPT-2, CTRL, Transformer-XL and XLNet for conditional language generation
-- other model-specific examples (see the documentation).
-
-Here are three quick usage examples for these scripts:
-
-### `run_glue.py`: Fine-tuning on GLUE tasks for sequence classification
-
-The [General Language Understanding Evaluation (GLUE) benchmark](https://gluebenchmark.com/) is a collection of nine sentence- or sentence-pair language understanding tasks for evaluating and analyzing natural language understanding systems.
-
-Before running anyone of these GLUE tasks you should download the
-[GLUE data](https://gluebenchmark.com/tasks) by running
-[this script](https://gist.github.com/W4ngatang/60c2bdb54d156a41194446737ce03e2e)
-and unpack it to some directory `$GLUE_DIR`.
-
-You should also install the additional packages required by the examples:
-
-```shell
-pip install -r ./examples/requirements.txt
-```
-
-```shell
-export GLUE_DIR=/path/to/glue
-export TASK_NAME=MRPC
-
-python ./examples/run_glue.py \
- --model_type bert \
- --model_name_or_path bert-base-uncased \
- --task_name $TASK_NAME \
- --do_train \
- --do_eval \
- --do_lower_case \
- --data_dir $GLUE_DIR/$TASK_NAME \
- --max_seq_length 128 \
- --per_gpu_eval_batch_size=8 \
- --per_gpu_train_batch_size=8 \
- --learning_rate 2e-5 \
- --num_train_epochs 3.0 \
- --output_dir /tmp/$TASK_NAME/
-```
-
-where task name can be one of CoLA, SST-2, MRPC, STS-B, QQP, MNLI, QNLI, RTE, WNLI.
-
-The dev set results will be present within the text file 'eval_results.txt' in the specified output_dir. In case of MNLI, since there are two separate dev sets, matched and mismatched, there will be a separate output folder called '/tmp/MNLI-MM/' in addition to '/tmp/MNLI/'.
-
-#### Fine-tuning XLNet model on the STS-B regression task
-
-This example code fine-tunes XLNet on the STS-B corpus using parallel training on a server with 4 V100 GPUs.
-Parallel training is a simple way to use several GPUs (but is slower and less flexible than distributed training, see below).
-
-```shell
-export GLUE_DIR=/path/to/glue
-
-python ./examples/run_glue.py \
- --model_type xlnet \
- --model_name_or_path xlnet-large-cased \
- --do_train \
- --do_eval \
- --task_name=sts-b \
- --data_dir=${GLUE_DIR}/STS-B \
- --output_dir=./proc_data/sts-b-110 \
- --max_seq_length=128 \
- --per_gpu_eval_batch_size=8 \
- --per_gpu_train_batch_size=8 \
- --gradient_accumulation_steps=1 \
- --max_steps=1200 \
- --model_name=xlnet-large-cased \
- --overwrite_output_dir \
- --overwrite_cache \
- --warmup_steps=120
-```
-
-On this machine we thus have a batch size of 32, please increase `gradient_accumulation_steps` to reach the same batch size if you have a smaller machine. These hyper-parameters should result in a Pearson correlation coefficient of `+0.917` on the development set.
-
-#### Fine-tuning Bert model on the MRPC classification task
-
-This example code fine-tunes the Bert Whole Word Masking model on the Microsoft Research Paraphrase Corpus (MRPC) corpus using distributed training on 8 V100 GPUs to reach a F1 > 92.
-
-```bash
-python -m torch.distributed.launch --nproc_per_node 8 ./examples/run_glue.py \
- --model_type bert \
- --model_name_or_path bert-large-uncased-whole-word-masking \
- --task_name MRPC \
- --do_train \
- --do_eval \
- --do_lower_case \
- --data_dir $GLUE_DIR/MRPC/ \
- --max_seq_length 128 \
- --per_gpu_eval_batch_size=8 \
- --per_gpu_train_batch_size=8 \
- --learning_rate 2e-5 \
- --num_train_epochs 3.0 \
- --output_dir /tmp/mrpc_output/ \
- --overwrite_output_dir \
- --overwrite_cache \
-```
-
-Training with these hyper-parameters gave us the following results:
-
-```bash
- acc = 0.8823529411764706
- acc_and_f1 = 0.901702786377709
- eval_loss = 0.3418912578906332
- f1 = 0.9210526315789473
- global_step = 174
- loss = 0.07231863956341798
-```
-
-### `run_squad.py`: Fine-tuning on SQuAD for question-answering
-
-This example code fine-tunes BERT on the SQuAD dataset using distributed training on 8 V100 GPUs and Bert Whole Word Masking uncased model to reach a F1 > 93 on SQuAD:
-
-```bash
-python -m torch.distributed.launch --nproc_per_node=8 ./examples/run_squad.py \
- --model_type bert \
- --model_name_or_path bert-large-uncased-whole-word-masking \
- --do_train \
- --do_eval \
- --do_lower_case \
- --train_file $SQUAD_DIR/train-v1.1.json \
- --predict_file $SQUAD_DIR/dev-v1.1.json \
- --learning_rate 3e-5 \
- --num_train_epochs 2 \
- --max_seq_length 384 \
- --doc_stride 128 \
- --output_dir ../models/wwm_uncased_finetuned_squad/ \
- --per_gpu_eval_batch_size=3 \
- --per_gpu_train_batch_size=3 \
-```
-
-Training with these hyper-parameters gave us the following results:
-
-```bash
-python $SQUAD_DIR/evaluate-v1.1.py $SQUAD_DIR/dev-v1.1.json ../models/wwm_uncased_finetuned_squad/predictions.json
-{"exact_match": 86.91579943235573, "f1": 93.1532499015869}
-```
-
-This is the model provided as `bert-large-uncased-whole-word-masking-finetuned-squad`.
-
-### `run_generation.py`: Text generation with GPT, GPT-2, CTRL, Transformer-XL and XLNet
-
-A conditional generation script is also included to generate text from a prompt.
-The generation script includes the [tricks](https://github.com/rusiaaman/XLNet-gen#methodology) proposed by Aman Rusia to get high-quality generation with memory models like Transformer-XL and XLNet (include a predefined text to make short inputs longer).
-
-Here is how to run the script with the small version of OpenAI GPT-2 model:
-
-```shell
-python ./examples/run_generation.py \
- --model_type=gpt2 \
- --length=20 \
- --model_name_or_path=gpt2 \
-```
-
-and from the Salesforce CTRL model:
-```shell
-python ./examples/run_generation.py \
- --model_type=ctrl \
- --length=20 \
- --model_name_or_path=ctrl \
- --temperature=0 \
- --repetition_penalty=1.2 \
-```
-
-## Quick tour of model sharing
-
-Starting with `v2.2.2`, you can now upload and share your fine-tuned models with the community, using the CLI that's built-in to the library.
-
-**First, create an account on [https://huggingface.co/join](https://huggingface.co/join)**. Then:
-
-```shell
-transformers-cli login
-# log in using the same credentials as on huggingface.co
-```
-Upload your model:
-```shell
-transformers-cli upload ./path/to/pretrained_model/
-
-# ^^ Upload folder containing weights/tokenizer/config
-# saved via `.save_pretrained()`
-
-transformers-cli upload ./config.json [--filename folder/foobar.json]
-
-# ^^ Upload a single file
-# (you can optionally override its filename, which can be nested inside a folder)
-```
-
-Your model will then be accessible through its identifier, a concatenation of your username and the folder name above:
-```python
-"username/pretrained_model"
-```
-
-Anyone can load it from code:
-```python
-tokenizer = AutoTokenizer.from_pretrained("username/pretrained_model")
-model = AutoModel.from_pretrained("username/pretrained_model")
-```
-
-Finally, list all your files on S3:
-```shell
-transformers-cli s3 ls
-# List all your S3 objects.
-```
-
-You can also delete files:
-
-```shell
-transformers-cli s3 rm …
-```
-
-## Quick tour of pipelines
-
-New in version `v2.3`: `Pipeline` are high-level objects which automatically handle tokenization, running your data through a transformers model
-and outputting the result in a structured object.
-
-You can create `Pipeline` objects for the following down-stream tasks:
-
- - `feature-extraction`: Generates a tensor representation for the input sequence
- - `ner`: Generates named entity mapping for each word in the input sequence.
- - `sentiment-analysis`: Gives the polarity (positive / negative) of the whole input sequence.
- - `text-classification`: Initialize a `TextClassificationPipeline` directly, or see `sentiment-analysis` for an example.
- - `question-answering`: Provided some context and a question refering to the context, it will extract the answer to the question in the context.
- - `fill-mask`: Takes an input sequence containing a masked token (e.g. ``) and return list of most probable filled sequences, with their probabilities.
-
-```python
-from transformers import pipeline
-
-# Allocate a pipeline for sentiment-analysis
-nlp = pipeline('sentiment-analysis')
-nlp('We are very happy to include pipeline into the transformers repository.')
->>> {'label': 'POSITIVE', 'score': 0.99893874}
-
-# Allocate a pipeline for question-answering
-nlp = pipeline('question-answering')
-nlp({
- 'question': 'What is the name of the repository ?',
- 'context': 'Pipeline have been included in the huggingface/transformers repository'
-})
->>> {'score': 0.28756016668193496, 'start': 35, 'end': 59, 'answer': 'huggingface/transformers'}
-```
-
-## Migrating from pytorch-transformers to transformers
-
-Here is a quick summary of what you should take care of when migrating from `pytorch-transformers` to `transformers`.
-
-### Positional order of some models' keywords inputs (`attention_mask`, `token_type_ids`...) changed
-
-To be able to use Torchscript (see #1010, #1204 and #1195) the specific order of some models **keywords inputs** (`attention_mask`, `token_type_ids`...) has been changed.
-
-If you used to call the models with keyword names for keyword arguments, e.g. `model(inputs_ids, attention_mask=attention_mask, token_type_ids=token_type_ids)`, this should not cause any change.
-
-If you used to call the models with positional inputs for keyword arguments, e.g. `model(inputs_ids, attention_mask, token_type_ids)`, you may have to double check the exact order of input arguments.
-
-
-## Migrating from pytorch-pretrained-bert to transformers
-
-Here is a quick summary of what you should take care of when migrating from `pytorch-pretrained-bert` to `transformers`.
-
-### Models always output `tuples`
-
-The main breaking change when migrating from `pytorch-pretrained-bert` to `transformers` is that every model's forward method always outputs a `tuple` with various elements depending on the model and the configuration parameters.
-
-The exact content of the tuples for each model is detailed in the models' docstrings and the [documentation](https://huggingface.co/transformers/).
-
-In pretty much every case, you will be fine by taking the first element of the output as the output you previously used in `pytorch-pretrained-bert`.
-
-Here is a `pytorch-pretrained-bert` to `transformers` conversion example for a `BertForSequenceClassification` classification model:
-
-```python
-# Let's load our model
-model = BertForSequenceClassification.from_pretrained('bert-base-uncased')
-
-# If you used to have this line in pytorch-pretrained-bert:
-loss = model(input_ids, labels=labels)
-
-# Now just use this line in transformers to extract the loss from the output tuple:
-outputs = model(input_ids, labels=labels)
-loss = outputs[0]
-
-# In transformers you can also have access to the logits:
-loss, logits = outputs[:2]
-
-# And even the attention weights if you configure the model to output them (and other outputs too, see the docstrings and documentation)
-model = BertForSequenceClassification.from_pretrained('bert-base-uncased', output_attentions=True)
-outputs = model(input_ids, labels=labels)
-loss, logits, attentions = outputs
-```
-
-### Using hidden states
-
-By enabling the configuration option `output_hidden_states`, it was possible to retrieve the last hidden states of the encoder. In `pytorch-transformers` as well as `transformers` the return value has changed slightly: `all_hidden_states` now also includes the hidden state of the embeddings in addition to those of the encoding layers. This allows users to easily access the embeddings final state.
-
-### Serialization
-
-Breaking change in the `from_pretrained()` method:
-
-1. Models are now set in evaluation mode by default when instantiated with the `from_pretrained()` method. To train them, don't forget to set them back in training mode (`model.train()`) to activate the dropout modules.
-
-2. The additional `*input` and `**kwargs` arguments supplied to the `from_pretrained()` method used to be directly passed to the underlying model's class `__init__()` method. They are now used to update the model configuration attribute instead, which can break derived model classes built based on the previous `BertForSequenceClassification` examples. We are working on a way to mitigate this breaking change in [#866](https://github.com/huggingface/transformers/pull/866) by forwarding the the model's `__init__()` method (i) the provided positional arguments and (ii) the keyword arguments which do not match any configuration class attributes.
-
-Also, while not a breaking change, the serialization methods have been standardized and you probably should switch to the new method `save_pretrained(save_directory)` if you were using any other serialization method before.
-
-Here is an example:
-
-```python
-### Let's load a model and tokenizer
-model = BertForSequenceClassification.from_pretrained('bert-base-uncased')
-tokenizer = BertTokenizer.from_pretrained('bert-base-uncased')
-
-### Do some stuff to our model and tokenizer
-# Ex: add new tokens to the vocabulary and embeddings of our model
-tokenizer.add_tokens(['[SPECIAL_TOKEN_1]', '[SPECIAL_TOKEN_2]'])
-model.resize_token_embeddings(len(tokenizer))
-# Train our model
-train(model)
-
-### Now let's save our model and tokenizer to a directory
-model.save_pretrained('./my_saved_model_directory/')
-tokenizer.save_pretrained('./my_saved_model_directory/')
-
-### Reload the model and the tokenizer
-model = BertForSequenceClassification.from_pretrained('./my_saved_model_directory/')
-tokenizer = BertTokenizer.from_pretrained('./my_saved_model_directory/')
-```
-
-### Optimizers: BertAdam & OpenAIAdam are now AdamW, schedules are standard PyTorch schedules
-
-The two optimizers previously included, `BertAdam` and `OpenAIAdam`, have been replaced by a single `AdamW` optimizer which has a few differences:
-
-- it only implements weights decay correction,
-- schedules are now externals (see below),
-- gradient clipping is now also external (see below).
-
-The new optimizer `AdamW` matches PyTorch `Adam` optimizer API and let you use standard PyTorch or apex methods for the schedule and clipping.
-
-The schedules are now standard [PyTorch learning rate schedulers](https://pytorch.org/docs/stable/optim.html#how-to-adjust-learning-rate) and not part of the optimizer anymore.
-
-Here is a conversion examples from `BertAdam` with a linear warmup and decay schedule to `AdamW` and the same schedule:
-
-```python
-# Parameters:
-lr = 1e-3
-max_grad_norm = 1.0
-num_training_steps = 1000
-num_warmup_steps = 100
-warmup_proportion = float(num_warmup_steps) / float(num_training_steps) # 0.1
-
-### Previously BertAdam optimizer was instantiated like this:
-optimizer = BertAdam(model.parameters(), lr=lr, schedule='warmup_linear', warmup=warmup_proportion, t_total=num_training_steps)
-### and used like this:
-for batch in train_data:
- loss = model(batch)
- loss.backward()
- optimizer.step()
-
-### In Transformers, optimizer and schedules are splitted and instantiated like this:
-optimizer = AdamW(model.parameters(), lr=lr, correct_bias=False) # To reproduce BertAdam specific behavior set correct_bias=False
-scheduler = get_linear_schedule_with_warmup(optimizer, num_warmup_steps=num_warmup_steps, num_training_steps=num_training_steps) # PyTorch scheduler
-### and used like this:
-for batch in train_data:
- model.train()
- loss = model(batch)
- loss.backward()
- torch.nn.utils.clip_grad_norm_(model.parameters(), max_grad_norm) # Gradient clipping is not in AdamW anymore (so you can use amp without issue)
- optimizer.step()
- scheduler.step()
- optimizer.zero_grad()
-```
-
-## Citation
-
-We now have a paper you can cite for the 🤗 Transformers library:
-```
-@article{Wolf2019HuggingFacesTS,
- title={HuggingFace's Transformers: State-of-the-art Natural Language Processing},
- author={Thomas Wolf and Lysandre Debut and Victor Sanh and Julien Chaumond and Clement Delangue and Anthony Moi and Pierric Cistac and Tim Rault and R'emi Louf and Morgan Funtowicz and Jamie Brew},
- journal={ArXiv},
- year={2019},
- volume={abs/1910.03771}
-}
-```
diff --git a/server/transformers/deploy_multi_version_doc.sh b/server/transformers/deploy_multi_version_doc.sh
deleted file mode 100644
index 37c5de114f0cf44a71b8a86ea3fd8eb39ddf1338..0000000000000000000000000000000000000000
--- a/server/transformers/deploy_multi_version_doc.sh
+++ /dev/null
@@ -1,23 +0,0 @@
-cd docs
-
-function deploy_doc(){
- echo "Creating doc at commit $1 and pushing to folder $2"
- git checkout $1
- if [ ! -z "$2" ]
- then
- echo "Pushing version" $2
- make clean && make html && scp -r -oStrictHostKeyChecking=no _build/html $doc:$dir/$2
- else
- echo "Pushing master"
- make clean && make html && scp -r -oStrictHostKeyChecking=no _build/html/* $doc:$dir
- fi
-}
-
-deploy_doc "master"
-deploy_doc "b33a385" v1.0.0
-deploy_doc "fe02e45" v1.1.0
-deploy_doc "89fd345" v1.2.0
-deploy_doc "fc9faa8" v2.0.0
-deploy_doc "3ddce1d" v2.1.1
-deploy_doc "f2f3294" v2.2.0
-deploy_doc "d0f8b9a" v2.3.0
diff --git a/server/transformers/docker/Dockerfile b/server/transformers/docker/Dockerfile
deleted file mode 100644
index fed834ff88e89ee21e0919b068b0ead5b24984c6..0000000000000000000000000000000000000000
--- a/server/transformers/docker/Dockerfile
+++ /dev/null
@@ -1,7 +0,0 @@
-FROM pytorch/pytorch:latest
-
-RUN git clone https://github.com/NVIDIA/apex.git && cd apex && python setup.py install --cuda_ext --cpp_ext
-
-RUN pip install transformers
-
-WORKDIR /workspace
\ No newline at end of file
diff --git a/server/transformers/docs/Makefile b/server/transformers/docs/Makefile
deleted file mode 100644
index 8879933e6cda150267451c9e7d07dd22b7b0d3f1..0000000000000000000000000000000000000000
--- a/server/transformers/docs/Makefile
+++ /dev/null
@@ -1,19 +0,0 @@
-# Minimal makefile for Sphinx documentation
-#
-
-# You can set these variables from the command line.
-SPHINXOPTS =
-SPHINXBUILD = sphinx-build
-SOURCEDIR = source
-BUILDDIR = _build
-
-# Put it first so that "make" without argument is like "make help".
-help:
- @$(SPHINXBUILD) -M help "$(SOURCEDIR)" "$(BUILDDIR)" $(SPHINXOPTS) $(O)
-
-.PHONY: help Makefile
-
-# Catch-all target: route all unknown targets to Sphinx using the new
-# "make mode" option. $(O) is meant as a shortcut for $(SPHINXOPTS).
-%: Makefile
- @$(SPHINXBUILD) -M $@ "$(SOURCEDIR)" "$(BUILDDIR)" $(SPHINXOPTS) $(O)
\ No newline at end of file
diff --git a/server/transformers/docs/README.md b/server/transformers/docs/README.md
deleted file mode 100644
index d1a8b24103ba562cfa630e4926910d3254872a8f..0000000000000000000000000000000000000000
--- a/server/transformers/docs/README.md
+++ /dev/null
@@ -1,67 +0,0 @@
-# Generating the documentation
-
-To generate the documentation, you first have to build it. Several packages are necessary to build the doc,
-you can install them with the following command, at the root of the code repository:
-
-```bash
-pip install -e ".[docs]"
-```
-
-## Packages installed
-
-Here's an overview of all the packages installed. If you ran the previous command installing all packages from
-`requirements.txt`, you do not need to run the following commands.
-
-Building it requires the package `sphinx` that you can
-install using:
-
-```bash
-pip install -U sphinx
-```
-
-You would also need the custom installed [theme](https://github.com/readthedocs/sphinx_rtd_theme) by
-[Read The Docs](https://readthedocs.org/). You can install it using the following command:
-
-```bash
-pip install sphinx_rtd_theme
-```
-
-The third necessary package is the `recommonmark` package to accept Markdown as well as Restructured text:
-
-```bash
-pip install recommonmark
-```
-
-## Building the documentation
-
-Make sure that there is a symlink from the `example` file (in /examples) inside the source folder. Run the following
-command to generate it:
-
-```bash
-ln -s ../../examples/README.md examples.md
-```
-
-Once you have setup `sphinx`, you can build the documentation by running the following command in the `/docs` folder:
-
-```bash
-make html
-```
-
----
-**NOTE**
-
-If you are adding/removing elements from the toc-tree or from any structural item, it is recommended to clean the build
-directory before rebuilding. Run the following command to clean and build:
-
-```bash
-make clean && make html
-```
-
----
-
-It should build the static app that will be available under `/docs/_build/html`
-
-## Adding a new element to the tree (toc-tree)
-
-Accepted files are reStructuredText (.rst) and Markdown (.md). Create a file with its extension and put it
-in the source directory. You can then link it to the toc-tree by putting the filename without the extension.
diff --git a/server/transformers/docs/source/_static/css/Calibre-Light.ttf b/server/transformers/docs/source/_static/css/Calibre-Light.ttf
deleted file mode 100644
index 2e6631909a671e74db99044a7a1dad512df82207..0000000000000000000000000000000000000000
Binary files a/server/transformers/docs/source/_static/css/Calibre-Light.ttf and /dev/null differ
diff --git a/server/transformers/docs/source/_static/css/Calibre-Medium.otf b/server/transformers/docs/source/_static/css/Calibre-Medium.otf
deleted file mode 100644
index f9f11ebe430e3745b7b363078530cd6305f04ebc..0000000000000000000000000000000000000000
Binary files a/server/transformers/docs/source/_static/css/Calibre-Medium.otf and /dev/null differ
diff --git a/server/transformers/docs/source/_static/css/Calibre-Regular.otf b/server/transformers/docs/source/_static/css/Calibre-Regular.otf
deleted file mode 100644
index 3801b704cc8b83ee419b44b160b4d2105f4e52f8..0000000000000000000000000000000000000000
Binary files a/server/transformers/docs/source/_static/css/Calibre-Regular.otf and /dev/null differ
diff --git a/server/transformers/docs/source/_static/css/Calibre-Thin.otf b/server/transformers/docs/source/_static/css/Calibre-Thin.otf
deleted file mode 100644
index 44f93821ee80e78a1a8d9aa92b319d29ea01240c..0000000000000000000000000000000000000000
Binary files a/server/transformers/docs/source/_static/css/Calibre-Thin.otf and /dev/null differ
diff --git a/server/transformers/docs/source/_static/css/code-snippets.css b/server/transformers/docs/source/_static/css/code-snippets.css
deleted file mode 100644
index 43acc6751c5ca59a16889bfffc471eb566f93af5..0000000000000000000000000000000000000000
--- a/server/transformers/docs/source/_static/css/code-snippets.css
+++ /dev/null
@@ -1,12 +0,0 @@
-
-.highlight .c1, .highlight .sd{
- color: #999
-}
-
-.highlight .nn, .highlight .k, .highlight .s1, .highlight .nb, .highlight .bp, .highlight .kc {
- color: #FB8D68;
-}
-
-.highlight .kn, .highlight .nv, .highlight .s2, .highlight .ow {
- color: #6670FF;
-}
\ No newline at end of file
diff --git a/server/transformers/docs/source/_static/css/huggingface.css b/server/transformers/docs/source/_static/css/huggingface.css
deleted file mode 100644
index 3f006a996ba80f53048e01dcad9a28a6f22dc937..0000000000000000000000000000000000000000
--- a/server/transformers/docs/source/_static/css/huggingface.css
+++ /dev/null
@@ -1,196 +0,0 @@
-/* The literal code blocks */
-.rst-content tt.literal, .rst-content tt.literal, .rst-content code.literal {
- color: #6670FF;
-}
-
-/* To keep the logo centered */
-.wy-side-scroll {
- width: auto;
- font-size: 20px;
-}
-
-/* The div that holds the Hugging Face logo */
-.HuggingFaceDiv {
- width: 100%
-}
-
-/* The research field on top of the toc tree */
-.wy-side-nav-search{
- background-color: #6670FF;
-}
-
-/* The toc tree */
-.wy-nav-side{
- background-color: #6670FF;
-}
-
-/* The selected items in the toc tree */
-.wy-menu-vertical li.current{
- background-color: #A6B0FF;
-}
-
-/* When a list item that does belong to the selected block from the toc tree is hovered */
-.wy-menu-vertical li.current a:hover{
- background-color: #B6C0FF;
-}
-
-/* When a list item that does NOT belong to the selected block from the toc tree is hovered. */
-.wy-menu-vertical li a:hover{
- background-color: #A7AFFB;
-}
-
-/* The text items on the toc tree */
-.wy-menu-vertical a {
- color: #FFFFDD;
- font-family: Calibre-Light, sans-serif;
-}
-.wy-menu-vertical header, .wy-menu-vertical p.caption{
- color: white;
- font-family: Calibre-Light, sans-serif;
-}
-
-/* The color inside the selected toc tree block */
-.wy-menu-vertical li.toctree-l2 a, .wy-menu-vertical li.toctree-l3 a, .wy-menu-vertical li.toctree-l4 a {
- color: black;
-}
-
-/* Inside the depth-2 selected toc tree block */
-.wy-menu-vertical li.toctree-l2.current>a {
- background-color: #B6C0FF
-}
-.wy-menu-vertical li.toctree-l2.current li.toctree-l3>a {
- background-color: #C6D0FF
-}
-
-/* Inside the depth-3 selected toc tree block */
-.wy-menu-vertical li.toctree-l3.current li.toctree-l4>a{
- background-color: #D6E0FF
-}
-
-/* Inside code snippets */
-.rst-content dl:not(.docutils) dt{
- font-size: 15px;
-}
-
-/* Links */
-a {
- color: #6670FF;
-}
-
-/* Content bars */
-.rst-content dl:not(.docutils) dt {
- background-color: rgba(251, 141, 104, 0.1);
- border-right: solid 2px #FB8D68;
- border-left: solid 2px #FB8D68;
- color: #FB8D68;
- font-family: Calibre-Light, sans-serif;
- border-top: none;
- font-style: normal !important;
-}
-
-/* Expand button */
-.wy-menu-vertical li.toctree-l2 span.toctree-expand,
-.wy-menu-vertical li.on a span.toctree-expand, .wy-menu-vertical li.current>a span.toctree-expand,
-.wy-menu-vertical li.toctree-l3 span.toctree-expand{
- color: black;
-}
-
-/* Max window size */
-.wy-nav-content{
- max-width: 1200px;
-}
-
-/* Mobile header */
-.wy-nav-top{
- background-color: #6670FF;
-}
-
-
-/* Source spans */
-.rst-content .viewcode-link, .rst-content .viewcode-back{
- color: #6670FF;
- font-size: 110%;
- letter-spacing: 2px;
- text-transform: uppercase;
-}
-
-/* It would be better for table to be visible without horizontal scrolling */
-.wy-table-responsive table td, .wy-table-responsive table th{
- white-space: normal;
-}
-
-.footer {
- margin-top: 20px;
-}
-
-.footer__Social {
- display: flex;
- flex-direction: row;
-}
-
-.footer__CustomImage {
- margin: 2px 5px 0 0;
-}
-
-/* class and method names in doc */
-.rst-content dl:not(.docutils) tt.descname, .rst-content dl:not(.docutils) tt.descclassname, .rst-content dl:not(.docutils) tt.descname, .rst-content dl:not(.docutils) code.descname, .rst-content dl:not(.docutils) tt.descclassname, .rst-content dl:not(.docutils) code.descclassname{
- font-family: Calibre, sans-serif;
- font-size: 20px !important;
-}
-
-/* class name in doc*/
-.rst-content dl:not(.docutils) tt.descname, .rst-content dl:not(.docutils) tt.descname, .rst-content dl:not(.docutils) code.descname{
- margin-right: 10px;
- font-family: Calibre-Medium, sans-serif;
-}
-
-/* Method and class parameters */
-.sig-param{
- line-height: 23px;
-}
-
-/* Class introduction "class" string at beginning */
-.rst-content dl:not(.docutils) .property{
- font-size: 18px;
- color: black;
-}
-
-
-/* FONTS */
-body{
- font-family: Calibre, sans-serif;
- font-size: 16px;
-}
-
-h1 {
- font-family: Calibre-Thin, sans-serif;
- font-size: 70px;
-}
-
-h2, .rst-content .toctree-wrapper p.caption, h3, h4, h5, h6, legend{
- font-family: Calibre-Medium, sans-serif;
-}
-
-@font-face {
- font-family: Calibre-Medium;
- src: url(./Calibre-Medium.otf);
- font-weight:400;
-}
-
-@font-face {
- font-family: Calibre;
- src: url(./Calibre-Regular.otf);
- font-weight:400;
-}
-
-@font-face {
- font-family: Calibre-Light;
- src: url(./Calibre-Light.ttf);
- font-weight:400;
-}
-
-@font-face {
- font-family: Calibre-Thin;
- src: url(./Calibre-Thin.otf);
- font-weight:400;
-}
diff --git a/server/transformers/docs/source/_static/js/custom.js b/server/transformers/docs/source/_static/js/custom.js
deleted file mode 100644
index ec804b3704a1dc8c3eb021ac4fe6412112856722..0000000000000000000000000000000000000000
--- a/server/transformers/docs/source/_static/js/custom.js
+++ /dev/null
@@ -1,79 +0,0 @@
-function addIcon() {
- const huggingFaceLogo = "https://huggingface.co/landing/assets/transformers-docs/huggingface_logo.svg";
- const image = document.createElement("img");
- image.setAttribute("src", huggingFaceLogo);
-
- const div = document.createElement("div");
- div.appendChild(image);
- div.style.textAlign = 'center';
- div.style.paddingTop = '30px';
- div.style.backgroundColor = '#6670FF';
-
- const scrollDiv = document.querySelector(".wy-side-scroll");
- scrollDiv.prepend(div);
-}
-
-function addCustomFooter() {
- const customFooter = document.createElement("div");
- const questionOrIssue = document.createElement("div");
- questionOrIssue.innerHTML = "Stuck? Read our Blog posts or Create an issue";
- customFooter.appendChild(questionOrIssue);
- customFooter.classList.add("footer");
-
- const social = document.createElement("div");
- social.classList.add("footer__Social");
-
- const imageDetails = [
- { link: "https://huggingface.co", imageLink: "https://huggingface.co/landing/assets/transformers-docs/website.svg" },
- { link: "https://twitter.com/huggingface", imageLink: "https://huggingface.co/landing/assets/transformers-docs/twitter.svg" },
- { link: "https://github.com/huggingface", imageLink: "https://huggingface.co/landing/assets/transformers-docs/github.svg" },
- { link: "https://www.linkedin.com/company/huggingface/", imageLink: "https://huggingface.co/landing/assets/transformers-docs/linkedin.svg" }
- ];
-
- imageDetails.forEach(imageLinks => {
- const link = document.createElement("a");
- const image = document.createElement("img");
- image.src = imageLinks.imageLink;
- link.href = imageLinks.link;
- image.style.width = "30px";
- image.classList.add("footer__CustomImage");
- link.appendChild(image);
- social.appendChild(link);
- });
-
- customFooter.appendChild(social);
- document.querySelector("footer").appendChild(customFooter);
-}
-
-function addGithubButton() {
- const div = `
-
- `;
- document.querySelector(".wy-side-nav-search .icon-home").insertAdjacentHTML('afterend', div);
-}
-
-/*!
- * github-buttons v2.2.10
- * (c) 2019 なつき
- * @license BSD-2-Clause
- */
-/**
- * modified to run programmatically
- */
-function parseGithubButtons (){"use strict";var e=window.document,t=e.location,o=window.encodeURIComponent,r=window.decodeURIComponent,n=window.Math,a=window.HTMLElement,i=window.XMLHttpRequest,l="https://unpkg.com/github-buttons@2.2.10/dist/buttons.html",c=i&&i.prototype&&"withCredentials"in i.prototype,d=c&&a&&a.prototype.attachShadow&&!a.prototype.attachShadow.prototype,s=function(e,t,o){e.addEventListener?e.addEventListener(t,o):e.attachEvent("on"+t,o)},u=function(e,t,o){e.removeEventListener?e.removeEventListener(t,o):e.detachEvent("on"+t,o)},h=function(e,t,o){var r=function(n){return u(e,t,r),o(n)};s(e,t,r)},f=function(e,t,o){var r=function(n){if(t.test(e.readyState))return u(e,"readystatechange",r),o(n)};s(e,"readystatechange",r)},p=function(e){return function(t,o,r){var n=e.createElement(t);if(o)for(var a in o){var i=o[a];null!=i&&(null!=n[a]?n[a]=i:n.setAttribute(a,i))}if(r)for(var l=0,c=r.length;l'},eye:{width:16,height:16,path:''},star:{width:14,height:16,path:''},"repo-forked":{width:10,height:16,path:''},"issue-opened":{width:14,height:16,path:''},"cloud-download":{width:16,height:16,path:''}},w={},x=function(e,t,o){var r=p(e.ownerDocument),n=e.appendChild(r("style",{type:"text/css"}));n.styleSheet?n.styleSheet.cssText=m:n.appendChild(e.ownerDocument.createTextNode(m));var a,l,d=r("a",{className:"btn",href:t.href,target:"_blank",innerHTML:(a=t["data-icon"],l=/^large$/i.test(t["data-size"])?16:14,a=(""+a).toLowerCase().replace(/^octicon-/,""),{}.hasOwnProperty.call(v,a)||(a="mark-github"),'"),"aria-label":t["aria-label"]||void 0},[" ",r("span",{},[t["data-text"]||""])]);/\.github\.com$/.test("."+d.hostname)?/^https?:\/\/((gist\.)?github\.com\/[^\/?#]+\/[^\/?#]+\/archive\/|github\.com\/[^\/?#]+\/[^\/?#]+\/releases\/download\/|codeload\.github\.com\/)/.test(d.href)&&(d.target="_top"):(d.href="#",d.target="_self");var u,h,g,x,y=e.appendChild(r("div",{className:"widget"+(/^large$/i.test(t["data-size"])?" lg":"")},[d]));/^(true|1)$/i.test(t["data-show-count"])&&"github.com"===d.hostname&&(u=d.pathname.replace(/^(?!\/)/,"/").match(/^\/([^\/?#]+)(?:\/([^\/?#]+)(?:\/(?:(subscription)|(fork)|(issues)|([^\/?#]+)))?)?(?:[\/?#]|$)/))&&!u[6]?(u[2]?(h="/repos/"+u[1]+"/"+u[2],u[3]?(x="subscribers_count",g="watchers"):u[4]?(x="forks_count",g="network"):u[5]?(x="open_issues_count",g="issues"):(x="stargazers_count",g="stargazers")):(h="/users/"+u[1],g=x="followers"),function(e,t){var o=w[e]||(w[e]=[]);if(!(o.push(t)>1)){var r=b(function(){for(delete w[e];t=o.shift();)t.apply(null,arguments)});if(c){var n=new i;s(n,"abort",r),s(n,"error",r),s(n,"load",function(){var e;try{e=JSON.parse(n.responseText)}catch(e){return void r(e)}r(200!==n.status,e)}),n.open("GET",e),n.send()}else{var a=this||window;a._=function(e){a._=null,r(200!==e.meta.status,e.data)};var l=p(a.document)("script",{async:!0,src:e+(/\?/.test(e)?"&":"?")+"callback=_"}),d=function(){a._&&a._({meta:{}})};s(l,"load",d),s(l,"error",d),l.readyState&&f(l,/de|m/,d),a.document.getElementsByTagName("head")[0].appendChild(l)}}}.call(this,"https://api.github.com"+h,function(e,t){if(!e){var n=t[x];y.appendChild(r("a",{className:"social-count",href:t.html_url+"/"+g,target:"_blank","aria-label":n+" "+x.replace(/_count$/,"").replace("_"," ").slice(0,n<2?-1:void 0)+" on GitHub"},[r("b"),r("i"),r("span",{},[(""+n).replace(/\B(?=(\d{3})+(?!\d))/g,",")])]))}o&&o(y)})):o&&o(y)},y=window.devicePixelRatio||1,C=function(e){return(y>1?n.ceil(n.round(e*y)/y*2)/2:n.ceil(e))||0},F=function(e,t){e.style.width=t[0]+"px",e.style.height=t[1]+"px"},k=function(t,r){if(null!=t&&null!=r)if(t.getAttribute&&(t=function(e){for(var t={href:e.href,title:e.title,"aria-label":e.getAttribute("aria-label")},o=["icon","text","size","show-count"],r=0,n=o.length;r
-
- icon
- Created with Sketch.
-
-
-
-
-
-
-
-
-
-
-
-
-
-
-
-
-
-
-
-
-
-
-
-
-
-
-
-
-
-
-
-
-
-
-
-
-
-
-
-
-
-
-
\ No newline at end of file
diff --git a/server/transformers/docs/source/benchmarks.md b/server/transformers/docs/source/benchmarks.md
deleted file mode 100644
index decbac47b754e895d87b3130f33f1f2195b65036..0000000000000000000000000000000000000000
--- a/server/transformers/docs/source/benchmarks.md
+++ /dev/null
@@ -1,54 +0,0 @@
-# Benchmarks
-
-This section is dedicated to the Benchmarks done by the library, both by maintainers, contributors and users. These
-benchmark will help keep track of the preformance improvements that are brought to our models across versions.
-
-## Benchmarking all models for inference
-
-As of version 2.1 we have benchmarked all models for inference, across many different settings: using PyTorch, with
-and without TorchScript, using TensorFlow, with and without XLA. All of those tests were done across CPUs (except for
-TensorFlow XLA) and GPUs.
-
-The approach is detailed in the [following blogpost](https://medium.com/huggingface/benchmarking-transformers-pytorch-and-tensorflow-e2917fb891c2)
-
-The results are available [here](https://docs.google.com/spreadsheets/d/1sryqufw2D0XlUH4sq3e9Wnxu5EAQkaohzrJbd5HdQ_w/edit?usp=sharing).
-
-## TF2 with mixed precision, XLA, Distribution (@tlkh)
-
-This work was done by [Timothy Liu](https://github.com/tlkh).
-
-There are very positive results to be gained from the various TensorFlow 2.0 features:
-
-- Automatic Mixed Precision (AMP)
-- XLA compiler
-- Distribution strategies (multi-GPU)
-
-The benefits are listed here (tested on CoLA, MRPC, SST-2):
-
-- AMP: Between 1.4x to 1.6x decrease in overall time without change in batch size
-- AMP+XLA: Up to 2.5x decrease in overall time on SST-2 (larger dataset)
-- Distribution: Between 1.4x to 3.4x decrease in overall time on 4xV100
-- Combined: Up to 5.7x decrease in overall training time, or 9.1x training throughput
-
-The model quality (measured by the validation accuracy) fluctuates slightly. Taking an average of 4 training runs
-on a single GPU gives the following results:
-
-- CoLA: AMP results in slighter lower acc (0.820 vs 0.824)
-- MRPC: AMP results in lower acc (0.823 vs 0.835)
-- SST-2: AMP results in slighter lower acc (0.918 vs 0.922)
-
-However, in a distributed setting with 4xV100 (4x batch size), AMP can yield in better results:
-
-CoLA: AMP results in higher acc (0.828 vs 0.812)
-MRPC: AMP results in lower acc (0.817 vs 0.827)
-SST-2: AMP results in slightly lower acc (0.926 vs 0.929)
-
-The benchmark script is available [here](https://github.com/NVAITC/benchmarking/blob/master/tf2/bert_dist.py).
-
-Note: on some tasks (e.g. MRPC), the dataset is too small. The overhead due to the model compilation with XLA as well
-as the distribution strategy setup does not speed things up. The XLA compile time is also the reason why although throughput
-can increase a lot (e.g. 2.7x for single GPU), overall (end-to-end) training speed-up is not as fast (as low as 1.4x)
-
-The benefits as seen on SST-2 (larger dataset) is much clear.
-
-All results can be seen on this [Google Sheet](https://docs.google.com/spreadsheets/d/1538MN224EzjbRL239sqSiUy6YY-rAjHyXhTzz_Zptls/edit#gid=960868445).
diff --git a/server/transformers/docs/source/bertology.rst b/server/transformers/docs/source/bertology.rst
deleted file mode 100644
index c3d1b2f8b83e99510a45623492d0f2cb1a3b2dca..0000000000000000000000000000000000000000
--- a/server/transformers/docs/source/bertology.rst
+++ /dev/null
@@ -1,18 +0,0 @@
-BERTology
----------
-
-There is a growing field of study concerned with investigating the inner working of large-scale transformers like BERT (that some call "BERTology"). Some good examples of this field are:
-
-
-* BERT Rediscovers the Classical NLP Pipeline by Ian Tenney, Dipanjan Das, Ellie Pavlick: https://arxiv.org/abs/1905.05950
-* Are Sixteen Heads Really Better than One? by Paul Michel, Omer Levy, Graham Neubig: https://arxiv.org/abs/1905.10650
-* What Does BERT Look At? An Analysis of BERT's Attention by Kevin Clark, Urvashi Khandelwal, Omer Levy, Christopher D. Manning: https://arxiv.org/abs/1906.04341
-
-In order to help this new field develop, we have included a few additional features in the BERT/GPT/GPT-2 models to help people access the inner representations, mainly adapted from the great work of Paul Michel (https://arxiv.org/abs/1905.10650):
-
-
-* accessing all the hidden-states of BERT/GPT/GPT-2,
-* accessing all the attention weights for each head of BERT/GPT/GPT-2,
-* retrieving heads output values and gradients to be able to compute head importance score and prune head as explained in https://arxiv.org/abs/1905.10650.
-
-To help you understand and use these features, we have added a specific example script: `bertology.py `_ while extract information and prune a model pre-trained on GLUE.
diff --git a/server/transformers/docs/source/conf.py b/server/transformers/docs/source/conf.py
deleted file mode 100644
index 65552cd14b0a88a050b929be2e4f1127a0366175..0000000000000000000000000000000000000000
--- a/server/transformers/docs/source/conf.py
+++ /dev/null
@@ -1,188 +0,0 @@
-# -*- coding: utf-8 -*-
-#
-# Configuration file for the Sphinx documentation builder.
-#
-# This file does only contain a selection of the most common options. For a
-# full list see the documentation:
-# http://www.sphinx-doc.org/en/master/config
-
-# -- Path setup --------------------------------------------------------------
-
-# If extensions (or modules to document with autodoc) are in another directory,
-# add these directories to sys.path here. If the directory is relative to the
-# documentation root, use os.path.abspath to make it absolute, like shown here.
-#
-import os
-import sys
-sys.path.insert(0, os.path.abspath('../../src'))
-
-
-# -- Project information -----------------------------------------------------
-
-project = u'transformers'
-copyright = u'2019, huggingface'
-author = u'huggingface'
-
-# The short X.Y version
-version = u''
-# The full version, including alpha/beta/rc tags
-release = u'2.4.1'
-
-
-# -- General configuration ---------------------------------------------------
-
-# If your documentation needs a minimal Sphinx version, state it here.
-#
-# needs_sphinx = '1.0'
-
-# Add any Sphinx extension module names here, as strings. They can be
-# extensions coming with Sphinx (named 'sphinx.ext.*') or your custom
-# ones.
-extensions = [
- 'sphinx.ext.autodoc',
- 'sphinx.ext.coverage',
- 'sphinx.ext.napoleon',
- 'recommonmark',
- 'sphinx.ext.viewcode',
- 'sphinx_markdown_tables'
-]
-
-# Add any paths that contain templates here, relative to this directory.
-templates_path = ['_templates']
-
-# The suffix(es) of source filenames.
-# You can specify multiple suffix as a list of string:
-#
-source_suffix = ['.rst', '.md']
-# source_suffix = '.rst'
-
-# The master toctree document.
-master_doc = 'index'
-
-# The language for content autogenerated by Sphinx. Refer to documentation
-# for a list of supported languages.
-#
-# This is also used if you do content translation via gettext catalogs.
-# Usually you set "language" from the command line for these cases.
-language = None
-
-# List of patterns, relative to source directory, that match files and
-# directories to ignore when looking for source files.
-# This pattern also affects html_static_path and html_extra_path.
-exclude_patterns = [u'_build', 'Thumbs.db', '.DS_Store']
-
-# The name of the Pygments (syntax highlighting) style to use.
-pygments_style = None
-
-
-# -- Options for HTML output -------------------------------------------------
-
-# The theme to use for HTML and HTML Help pages. See the documentation for
-# a list of builtin themes.
-#
-html_theme = 'sphinx_rtd_theme'
-
-# Theme options are theme-specific and customize the look and feel of a theme
-# further. For a list of options available for each theme, see the
-# documentation.
-#
-html_theme_options = {
- 'analytics_id': 'UA-83738774-2'
-}
-
-# Add any paths that contain custom static files (such as style sheets) here,
-# relative to this directory. They are copied after the builtin static files,
-# so a file named "default.css" will overwrite the builtin "default.css".
-html_static_path = ['_static']
-
-# Custom sidebar templates, must be a dictionary that maps document names
-# to template names.
-#
-# The default sidebars (for documents that don't match any pattern) are
-# defined by theme itself. Builtin themes are using these templates by
-# default: ``['localtoc.html', 'relations.html', 'sourcelink.html',
-# 'searchbox.html']``.
-#
-# html_sidebars = {}
-
-
-# -- Options for HTMLHelp output ---------------------------------------------
-
-# Output file base name for HTML help builder.
-htmlhelp_basename = 'transformersdoc'
-
-
-# -- Options for LaTeX output ------------------------------------------------
-
-latex_elements = {
- # The paper size ('letterpaper' or 'a4paper').
- #
- # 'papersize': 'letterpaper',
-
- # The font size ('10pt', '11pt' or '12pt').
- #
- # 'pointsize': '10pt',
-
- # Additional stuff for the LaTeX preamble.
- #
- # 'preamble': '',
-
- # Latex figure (float) alignment
- #
- # 'figure_align': 'htbp',
-}
-
-# Grouping the document tree into LaTeX files. List of tuples
-# (source start file, target name, title,
-# author, documentclass [howto, manual, or own class]).
-latex_documents = [
- (master_doc, 'transformers.tex', u'transformers Documentation',
- u'huggingface', 'manual'),
-]
-
-
-# -- Options for manual page output ------------------------------------------
-
-# One entry per manual page. List of tuples
-# (source start file, name, description, authors, manual section).
-man_pages = [
- (master_doc, 'transformers', u'transformers Documentation',
- [author], 1)
-]
-
-
-# -- Options for Texinfo output ----------------------------------------------
-
-# Grouping the document tree into Texinfo files. List of tuples
-# (source start file, target name, title, author,
-# dir menu entry, description, category)
-texinfo_documents = [
- (master_doc, 'transformers', u'transformers Documentation',
- author, 'transformers', 'One line description of project.',
- 'Miscellaneous'),
-]
-
-
-# -- Options for Epub output -------------------------------------------------
-
-# Bibliographic Dublin Core info.
-epub_title = project
-
-# The unique identifier of the text. This can be a ISBN number
-# or the project homepage.
-#
-# epub_identifier = ''
-
-# A unique identification for the text.
-#
-# epub_uid = ''
-
-# A list of files that should not be packed into the epub file.
-epub_exclude_files = ['search.html']
-
-def setup(app):
- app.add_stylesheet('css/huggingface.css')
- app.add_stylesheet('css/code-snippets.css')
- app.add_js_file('js/custom.js')
-
-# -- Extension configuration -------------------------------------------------
diff --git a/server/transformers/docs/source/converting_tensorflow_models.rst b/server/transformers/docs/source/converting_tensorflow_models.rst
deleted file mode 100644
index 595f134fb227c20e13e84906e1bf7f4d73231880..0000000000000000000000000000000000000000
--- a/server/transformers/docs/source/converting_tensorflow_models.rst
+++ /dev/null
@@ -1,137 +0,0 @@
-Converting Tensorflow Checkpoints
-================================================
-
-A command-line interface is provided to convert original Bert/GPT/GPT-2/Transformer-XL/XLNet/XLM checkpoints in models than be loaded using the ``from_pretrained`` methods of the library.
-
-.. note::
- Since 2.3.0 the conversion script is now part of the transformers CLI (**transformers-cli**)
- available in any transformers >= 2.3.0 installation.
-
- The documentation below reflects the **transformers-cli convert** command format.
-
-BERT
-^^^^
-
-You can convert any TensorFlow checkpoint for BERT (in particular `the pre-trained models released by Google `_\ ) in a PyTorch save file by using the `convert_tf_checkpoint_to_pytorch.py `_ script.
-
-This CLI takes as input a TensorFlow checkpoint (three files starting with ``bert_model.ckpt``\ ) and the associated configuration file (\ ``bert_config.json``\ ), and creates a PyTorch model for this configuration, loads the weights from the TensorFlow checkpoint in the PyTorch model and saves the resulting model in a standard PyTorch save file that can be imported using ``torch.load()`` (see examples in `run_bert_extract_features.py `_\ , `run_bert_classifier.py `_ and `run_bert_squad.py `_\ ).
-
-You only need to run this conversion script **once** to get a PyTorch model. You can then disregard the TensorFlow checkpoint (the three files starting with ``bert_model.ckpt``\ ) but be sure to keep the configuration file (\ ``bert_config.json``\ ) and the vocabulary file (\ ``vocab.txt``\ ) as these are needed for the PyTorch model too.
-
-To run this specific conversion script you will need to have TensorFlow and PyTorch installed (\ ``pip install tensorflow``\ ). The rest of the repository only requires PyTorch.
-
-Here is an example of the conversion process for a pre-trained ``BERT-Base Uncased`` model:
-
-.. code-block:: shell
-
- export BERT_BASE_DIR=/path/to/bert/uncased_L-12_H-768_A-12
-
-<<<<<<< HEAD
- transformers-cli --model_type bert \
-=======
- transformers-cli convert --model_type bert \
->>>>>>> bfec203d4ed95255619e7e2f28c9040744a16232
- --tf_checkpoint $BERT_BASE_DIR/bert_model.ckpt \
- --config $BERT_BASE_DIR/bert_config.json \
- --pytorch_dump_output $BERT_BASE_DIR/pytorch_model.bin
-
-You can download Google's pre-trained models for the conversion `here `__.
-
-OpenAI GPT
-^^^^^^^^^^
-
-Here is an example of the conversion process for a pre-trained OpenAI GPT model, assuming that your NumPy checkpoint save as the same format than OpenAI pretrained model (see `here `__\ )
-
-.. code-block:: shell
-
- export OPENAI_GPT_CHECKPOINT_FOLDER_PATH=/path/to/openai/pretrained/numpy/weights
-
-<<<<<<< HEAD
- transformers-cli --model_type gpt \
-=======
- transformers-cli convert --model_type gpt \
->>>>>>> bfec203d4ed95255619e7e2f28c9040744a16232
- --tf_checkpoint $OPENAI_GPT_CHECKPOINT_FOLDER_PATH \
- --pytorch_dump_output $PYTORCH_DUMP_OUTPUT \
- [--config OPENAI_GPT_CONFIG] \
- [--finetuning_task_name OPENAI_GPT_FINETUNED_TASK] \
-
-
-OpenAI GPT-2
-^^^^^^^^^^^^
-
-Here is an example of the conversion process for a pre-trained OpenAI GPT-2 model (see `here `__\ )
-
-.. code-block:: shell
-
- export OPENAI_GPT2_CHECKPOINT_PATH=/path/to/gpt2/pretrained/weights
-
-<<<<<<< HEAD
- transformers-cli --model_type gpt2 \
-=======
- transformers-cli convert --model_type gpt2 \
->>>>>>> bfec203d4ed95255619e7e2f28c9040744a16232
- --tf_checkpoint $OPENAI_GPT2_CHECKPOINT_PATH \
- --pytorch_dump_output $PYTORCH_DUMP_OUTPUT \
- [--config OPENAI_GPT2_CONFIG] \
- [--finetuning_task_name OPENAI_GPT2_FINETUNED_TASK]
-
-Transformer-XL
-^^^^^^^^^^^^^^
-
-Here is an example of the conversion process for a pre-trained Transformer-XL model (see `here `__\ )
-
-.. code-block:: shell
-
- export TRANSFO_XL_CHECKPOINT_FOLDER_PATH=/path/to/transfo/xl/checkpoint
-
-<<<<<<< HEAD
- transformers-cli --model_type transfo_xl \
-=======
- transformers-cli convert --model_type transfo_xl \
->>>>>>> bfec203d4ed95255619e7e2f28c9040744a16232
- --tf_checkpoint $TRANSFO_XL_CHECKPOINT_FOLDER_PATH \
- --pytorch_dump_output $PYTORCH_DUMP_OUTPUT \
- [--config TRANSFO_XL_CONFIG] \
- [--finetuning_task_name TRANSFO_XL_FINETUNED_TASK]
-
-
-XLNet
-^^^^^
-
-Here is an example of the conversion process for a pre-trained XLNet model:
-
-.. code-block:: shell
-
- export TRANSFO_XL_CHECKPOINT_PATH=/path/to/xlnet/checkpoint
- export TRANSFO_XL_CONFIG_PATH=/path/to/xlnet/config
-
-<<<<<<< HEAD
- transformers-cli --model_type xlnet \
-=======
- transformers-cli convert --model_type xlnet \
->>>>>>> bfec203d4ed95255619e7e2f28c9040744a16232
- --tf_checkpoint $TRANSFO_XL_CHECKPOINT_PATH \
- --config $TRANSFO_XL_CONFIG_PATH \
- --pytorch_dump_output $PYTORCH_DUMP_OUTPUT \
- [--finetuning_task_name XLNET_FINETUNED_TASK] \
-
-
-XLM
-^^^
-
-Here is an example of the conversion process for a pre-trained XLM model:
-
-.. code-block:: shell
-
- export XLM_CHECKPOINT_PATH=/path/to/xlm/checkpoint
-
-<<<<<<< HEAD
- transformers-cli --model_type xlm \
-=======
- transformers-cli convert --model_type xlm \
->>>>>>> bfec203d4ed95255619e7e2f28c9040744a16232
- --tf_checkpoint $XLM_CHECKPOINT_PATH \
- --pytorch_dump_output $PYTORCH_DUMP_OUTPUT
- [--config XML_CONFIG] \
- [--finetuning_task_name XML_FINETUNED_TASK]
\ No newline at end of file
diff --git a/server/transformers/docs/source/examples.md b/server/transformers/docs/source/examples.md
deleted file mode 120000
index 6fa53604d902346dcd54d7291e2f73a7ef858443..0000000000000000000000000000000000000000
--- a/server/transformers/docs/source/examples.md
+++ /dev/null
@@ -1 +0,0 @@
-../../examples/README.md
\ No newline at end of file
diff --git a/server/transformers/docs/source/glossary.rst b/server/transformers/docs/source/glossary.rst
deleted file mode 100644
index cfd8c50dd6bdb0f752b3edf8ac404518ab3e7f6f..0000000000000000000000000000000000000000
--- a/server/transformers/docs/source/glossary.rst
+++ /dev/null
@@ -1,145 +0,0 @@
-Glossary
-^^^^^^^^^^^^^^^^^^^^^^^^^^^^^^^^^^
-
-Every model is different yet bears similarities with the others. Therefore most models use the same inputs, which are
-detailed here alongside usage examples.
-
-Input IDs
---------------------------
-
-The input ids are often the only required parameters to be passed to the model as input. *They are token indices,
-numerical representations of tokens building the sequences that will be used as input by the model*.
-
-Each tokenizer works differently but the underlying mechanism remains the same. Here's an example using the BERT
-tokenizer, which is a `WordPiece `__ tokenizer:
-
-::
-
- from transformers import BertTokenizer
- tokenizer = BertTokenizer.from_pretrained("bert-base-cased")
-
- sequence = "A Titan RTX has 24GB of VRAM"
-
-The tokenizer takes care of splitting the sequence into tokens available in the tokenizer vocabulary.
-
-::
-
- # Continuation of the previous script
- tokenized_sequence = tokenizer.tokenize(sequence)
- assert tokenized_sequence == ['A', 'Titan', 'R', '##T', '##X', 'has', '24', '##GB', 'of', 'V', '##RA', '##M']
-
-These tokens can then be converted into IDs which are understandable by the model. Several methods are available for
-this, the recommended being `encode` or `encode_plus`, which leverage the Rust implementation of
-`huggingface/tokenizers `__ for peak performance.
-
-::
-
- # Continuation of the previous script
- encoded_sequence = tokenizer.encode(sequence)
- assert encoded_sequence == [101, 138, 18696, 155, 1942, 3190, 1144, 1572, 13745, 1104, 159, 9664, 2107, 102]
-
-The `encode` and `encode_plus` methods automatically add "special tokens" which are special IDs the model uses.
-
-Attention mask
---------------------------
-
-The attention mask is an optional argument used when batching sequences together. This argument indicates to the
-model which tokens should be attended to, and which should not.
-
-For example, consider these two sequences:
-
-::
-
- from transformers import BertTokenizer
- tokenizer = BertTokenizer.from_pretrained("bert-base-cased")
-
- sequence_a = "This is a short sequence."
- sequence_b = "This is a rather long sequence. It is at least longer than the sequence A."
-
- encoded_sequence_a = tokenizer.encode(sequence_a)
- assert len(encoded_sequence_a) == 8
-
- encoded_sequence_b = tokenizer.encode(sequence_b)
- assert len(encoded_sequence_b) == 19
-
-These two sequences have different lengths and therefore can't be put together in a same tensor as-is. The first
-sequence needs to be padded up to the length of the second one, or the second one needs to be truncated down to
-the length of the first one.
-
-In the first case, the list of IDs will be extended by the padding indices:
-
-::
-
- # Continuation of the previous script
- padded_sequence_a = tokenizer.encode(sequence_a, max_length=19, pad_to_max_length=True)
-
- assert padded_sequence_a == [101, 1188, 1110, 170, 1603, 4954, 119, 102, 0, 0, 0, 0, 0, 0, 0, 0, 0, 0, 0]
- assert encoded_sequence_b == [101, 1188, 1110, 170, 1897, 1263, 4954, 119, 1135, 1110, 1120, 1655, 2039, 1190, 1103, 4954, 138, 119, 102]
-
-These can then be converted into a tensor in PyTorch or TensorFlow. The attention mask is a binary tensor indicating
-the position of the padded indices so that the model does not attend to them. For the
-:class:`~transformers.BertTokenizer`, :obj:`1` indicate a value that should be attended to while :obj:`0` indicate
-a padded value.
-
-The method :func:`~transformers.PreTrainedTokenizer.encode_plus` may be used to obtain the attention mask directly:
-
-::
-
- # Continuation of the previous script
- sequence_a_dict = tokenizer.encode_plus(sequence_a, max_length=19, pad_to_max_length=True)
-
- assert sequence_a_dict['input_ids'] == [101, 1188, 1110, 170, 1603, 4954, 119, 102, 0, 0, 0, 0, 0, 0, 0, 0, 0, 0, 0]
- assert sequence_a_dict['attention_mask'] == [1, 1, 1, 1, 1, 1, 1, 1, 0, 0, 0, 0, 0, 0, 0, 0, 0, 0, 0]
-
-
-Token Type IDs
---------------------------
-
-Some models' purpose is to do sequence classification or question answering. These require two different sequences to
-be encoded in the same input IDs. They are usually separated by special tokens, such as the classifier and separator
-tokens. For example, the BERT model builds its two sequence input as such:
-
-::
-
- from transformers import BertTokenizer
- tokenizer = BertTokenizer.from_pretrained("bert-base-cased")
-
- # [CLS] SEQ_A [SEP] SEQ_B [SEP]
-
- sequence_a = "HuggingFace is based in NYC"
- sequence_b = "Where is HuggingFace based?"
-
- encoded_sequence = tokenizer.encode(sequence_a, sequence_b)
- assert tokenizer.decode(encoded_sequence) == "[CLS] HuggingFace is based in NYC [SEP] Where is HuggingFace based? [SEP]"
-
-This is enough for some models to understand where one sequence ends and where another begins. However, other models
-such as BERT have an additional mechanism, which are the segment IDs. The Token Type IDs are a binary mask identifying
-the different sequences in the model.
-
-We can leverage :func:`~transformers.PreTrainedTokenizer.encode_plus` to output the Token Type IDs for us:
-
-::
-
- # Continuation of the previous script
- encoded_dict = tokenizer.encode_plus(sequence_a, sequence_b)
-
- assert encoded_dict['input_ids'] == [101, 20164, 10932, 2271, 7954, 1110, 1359, 1107, 17520, 102, 2777, 1110, 20164, 10932, 2271, 7954, 1359, 136, 102]
- assert encoded_dict['token_type_ids'] == [0, 0, 0, 0, 0, 0, 0, 0, 0, 0, 1, 1, 1, 1, 1, 1, 1, 1, 1]
-
-The first sequence, the "context" used for the question, has all its tokens represented by :obj:`0`, whereas the
-question has all its tokens represented by :obj:`1`. Some models, like :class:`~transformers.XLNetModel` use an
-additional token represented by a :obj:`2`.
-
-
-Position IDs
---------------------------
-
-The position IDs are used by the model to identify which token is at which position. Contrary to RNNs that have the
-position of each token embedded within them, transformers are unaware of the position of each token. The position
-IDs are created for this purpose.
-
-They are an optional parameter. If no position IDs are passed to the model, they are automatically created as absolute
-positional embeddings.
-
-Absolute positional embeddings are selected in the range ``[0, config.max_position_embeddings - 1]``. Some models
-use other types of positional embeddings, such as sinusoidal position embeddings or relative position embeddings.
diff --git a/server/transformers/docs/source/imgs/transformers_logo_name.png b/server/transformers/docs/source/imgs/transformers_logo_name.png
deleted file mode 100644
index 5e4c2dcf575b7f7cf7e64640dee771fc311b7068..0000000000000000000000000000000000000000
Binary files a/server/transformers/docs/source/imgs/transformers_logo_name.png and /dev/null differ
diff --git a/server/transformers/docs/source/imgs/warmup_constant_schedule.png b/server/transformers/docs/source/imgs/warmup_constant_schedule.png
deleted file mode 100644
index e2448e9f2c7999497d3e2d252a5dcb22b0ac7da5..0000000000000000000000000000000000000000
Binary files a/server/transformers/docs/source/imgs/warmup_constant_schedule.png and /dev/null differ
diff --git a/server/transformers/docs/source/imgs/warmup_cosine_hard_restarts_schedule.png b/server/transformers/docs/source/imgs/warmup_cosine_hard_restarts_schedule.png
deleted file mode 100644
index be73605b9c080cdc7cea8b4ff7e29de90db2d9eb..0000000000000000000000000000000000000000
Binary files a/server/transformers/docs/source/imgs/warmup_cosine_hard_restarts_schedule.png and /dev/null differ
diff --git a/server/transformers/docs/source/imgs/warmup_cosine_schedule.png b/server/transformers/docs/source/imgs/warmup_cosine_schedule.png
deleted file mode 100644
index 6d27926ab10e9d2649ce3f28eb9656ea7cd3e9f8..0000000000000000000000000000000000000000
Binary files a/server/transformers/docs/source/imgs/warmup_cosine_schedule.png and /dev/null differ
diff --git a/server/transformers/docs/source/imgs/warmup_cosine_warm_restarts_schedule.png b/server/transformers/docs/source/imgs/warmup_cosine_warm_restarts_schedule.png
deleted file mode 100644
index 71b39bffd3daccf7fc89cad77ef8e03df40bf0ab..0000000000000000000000000000000000000000
Binary files a/server/transformers/docs/source/imgs/warmup_cosine_warm_restarts_schedule.png and /dev/null differ
diff --git a/server/transformers/docs/source/imgs/warmup_linear_schedule.png b/server/transformers/docs/source/imgs/warmup_linear_schedule.png
deleted file mode 100644
index 4e1af31025fafbd9c6b7c74ad6c2948ca2d3ff77..0000000000000000000000000000000000000000
Binary files a/server/transformers/docs/source/imgs/warmup_linear_schedule.png and /dev/null differ
diff --git a/server/transformers/docs/source/index.rst b/server/transformers/docs/source/index.rst
deleted file mode 100644
index f9ff1a0606ce2cf1da2e9f43a8591bfd888fd7f2..0000000000000000000000000000000000000000
--- a/server/transformers/docs/source/index.rst
+++ /dev/null
@@ -1,102 +0,0 @@
-Transformers
-================================================================================================================================================
-
-🤗 Transformers (formerly known as `pytorch-transformers` and `pytorch-pretrained-bert`) provides general-purpose architectures
-(BERT, GPT-2, RoBERTa, XLM, DistilBert, XLNet...) for Natural Language Understanding (NLU) and Natural Language Generation
-(NLG) with over 32+ pretrained models in 100+ languages and deep interoperability between TensorFlow 2.0 and PyTorch.
-
-This is the documentation of our repository `transformers `__.
-
-Features
----------------------------------------------------
-
-- As easy to use as pytorch-transformers
-- As powerful and concise as Keras
-- High performance on NLU and NLG tasks
-- Low barrier to entry for educators and practitioners
-
-State-of-the-art NLP for everyone:
-
-- Deep learning researchers
-- Hands-on practitioners
-- AI/ML/NLP teachers and educators
-
-Lower compute costs, smaller carbon footprint:
-
-- Researchers can share trained models instead of always retraining
-- Practitioners can reduce compute time and production costs
-- 8 architectures with over 30 pretrained models, some in more than 100 languages
-
-Choose the right framework for every part of a model's lifetime:
-
-- Train state-of-the-art models in 3 lines of code
-- Deep interoperability between TensorFlow 2.0 and PyTorch models
-- Move a single model between TF2.0/PyTorch frameworks at will
-- Seamlessly pick the right framework for training, evaluation, production
-
-Contents
----------------------------------
-
-The library currently contains PyTorch and Tensorflow implementations, pre-trained model weights, usage scripts and conversion utilities for the following models:
-
-1. `BERT `_ (from Google) released with the paper `BERT: Pre-training of Deep Bidirectional Transformers for Language Understanding `_ by Jacob Devlin, Ming-Wei Chang, Kenton Lee and Kristina Toutanova.
-2. `GPT `_ (from OpenAI) released with the paper `Improving Language Understanding by Generative Pre-Training `_ by Alec Radford, Karthik Narasimhan, Tim Salimans and Ilya Sutskever.
-3. `GPT-2 `_ (from OpenAI) released with the paper `Language Models are Unsupervised Multitask Learners `_ by Alec Radford*, Jeffrey Wu*, Rewon Child, David Luan, Dario Amodei** and Ilya Sutskever**.
-4. `Transformer-XL `_ (from Google/CMU) released with the paper `Transformer-XL: Attentive Language Models Beyond a Fixed-Length Context `_ by Zihang Dai*, Zhilin Yang*, Yiming Yang, Jaime Carbonell, Quoc V. Le, Ruslan Salakhutdinov.
-5. `XLNet `_ (from Google/CMU) released with the paper `XLNet: Generalized Autoregressive Pretraining for Language Understanding `_ by Zhilin Yang*, Zihang Dai*, Yiming Yang, Jaime Carbonell, Ruslan Salakhutdinov, Quoc V. Le.
-6. `XLM `_ (from Facebook) released together with the paper `Cross-lingual Language Model Pretraining `_ by Guillaume Lample and Alexis Conneau.
-7. `RoBERTa `_ (from Facebook), released together with the paper a `Robustly Optimized BERT Pretraining Approach `_ by Yinhan Liu, Myle Ott, Naman Goyal, Jingfei Du, Mandar Joshi, Danqi Chen, Omer Levy, Mike Lewis, Luke Zettlemoyer, Veselin Stoyanov.
-8. `DistilBERT `_ (from HuggingFace) released together with the paper `DistilBERT, a distilled version of BERT: smaller, faster, cheaper and lighter `_ by Victor Sanh, Lysandre Debut and Thomas Wolf. The same method has been applied to compress GPT2 into `DistilGPT2 `_.
-9. `CTRL `_ (from Salesforce), released together with the paper `CTRL: A Conditional Transformer Language Model for Controllable Generation `_ by Nitish Shirish Keskar*, Bryan McCann*, Lav R. Varshney, Caiming Xiong and Richard Socher.
-10. `CamemBERT `_ (from FAIR, Inria, Sorbonne Université) released together with the paper `CamemBERT: a Tasty French Language Model `_ by Louis Martin, Benjamin Muller, Pedro Javier Ortiz Suarez, Yoann Dupont, Laurent Romary, Eric Villemonte de la Clergerie, Djame Seddah, and Benoît Sagot.
-11. `ALBERT `_ (from Google Research), released together with the paper a `ALBERT: A Lite BERT for Self-supervised Learning of Language Representations `_ by Zhenzhong Lan, Mingda Chen, Sebastian Goodman, Kevin Gimpel, Piyush Sharma, Radu Soricut.
-12. `XLM-RoBERTa `_ (from Facebook AI), released together with the paper `Unsupervised Cross-lingual Representation Learning at Scale `_ by Alexis Conneau*, Kartikay Khandelwal*, Naman Goyal, Vishrav Chaudhary, Guillaume Wenzek, Francisco Guzmán, Edouard Grave, Myle Ott, Luke Zettlemoyer and Veselin Stoyanov.
-13. `FlauBERT `_ (from CNRS) released with the paper `FlauBERT: Unsupervised Language Model Pre-training for French `_ by Hang Le, Loïc Vial, Jibril Frej, Vincent Segonne, Maximin Coavoux, Benjamin Lecouteux, Alexandre Allauzen, Benoît Crabbé, Laurent Besacier, Didier Schwab.
-
-.. toctree::
- :maxdepth: 2
- :caption: Notes
-
- installation
- quickstart
- glossary
- pretrained_models
- model_sharing
- examples
- notebooks
- serialization
- converting_tensorflow_models
- migration
- bertology
- torchscript
- multilingual
- benchmarks
-
-.. toctree::
- :maxdepth: 2
- :caption: Main classes
-
- main_classes/configuration
- main_classes/model
- main_classes/tokenizer
- main_classes/optimizer_schedules
- main_classes/processors
-
-.. toctree::
- :maxdepth: 2
- :caption: Package Reference
-
- model_doc/auto
- model_doc/bert
- model_doc/gpt
- model_doc/transformerxl
- model_doc/gpt2
- model_doc/xlm
- model_doc/xlnet
- model_doc/roberta
- model_doc/distilbert
- model_doc/ctrl
- model_doc/camembert
- model_doc/albert
- model_doc/xlmroberta
- model_doc/flaubert
\ No newline at end of file
diff --git a/server/transformers/docs/source/installation.md b/server/transformers/docs/source/installation.md
deleted file mode 100644
index f4b7781ea9a934a41172605657853fa6bc709cdc..0000000000000000000000000000000000000000
--- a/server/transformers/docs/source/installation.md
+++ /dev/null
@@ -1,51 +0,0 @@
-# Installation
-
-Transformers is tested on Python 3.5+ and PyTorch 1.1.0
-
-## With pip
-
-PyTorch Transformers can be installed using pip as follows:
-
-``` bash
-pip install transformers
-```
-
-## From source
-
-To install from source, clone the repository and install with:
-
-``` bash
-git clone https://github.com/huggingface/transformers.git
-cd transformers
-pip install .
-```
-
-## Tests
-
-An extensive test suite is included to test the library behavior and several examples. Library tests can be found in the [tests folder](https://github.com/huggingface/transformers/tree/master/tests) and examples tests in the [examples folder](https://github.com/huggingface/transformers/tree/master/examples).
-
-Refer to the [contributing guide](https://github.com/huggingface/transformers/blob/master/CONTRIBUTING.md#tests) for details about running tests.
-
-## OpenAI GPT original tokenization workflow
-
-If you want to reproduce the original tokenization process of the `OpenAI GPT` paper, you will need to install `ftfy` and `SpaCy`:
-
-``` bash
-pip install spacy ftfy==4.4.3
-python -m spacy download en
-```
-
-If you don't install `ftfy` and `SpaCy`, the `OpenAI GPT` tokenizer will default to tokenize using BERT's `BasicTokenizer` followed by Byte-Pair Encoding (which should be fine for most usage, don't worry).
-
-## Note on model downloads (Continuous Integration or large-scale deployments)
-
-If you expect to be downloading large volumes of models (more than 1,000) from our hosted bucket (for instance through your CI setup, or a large-scale production deployment), please cache the model files on your end. It will be way faster, and cheaper. Feel free to contact us privately if you need any help.
-
-## Do you want to run a Transformer model on a mobile device?
-
-You should check out our [swift-coreml-transformers](https://github.com/huggingface/swift-coreml-transformers) repo.
-
-It contains a set of tools to convert PyTorch or TensorFlow 2.0 trained Transformer models (currently contains `GPT-2`, `DistilGPT-2`, `BERT`, and `DistilBERT`) to CoreML models that run on iOS devices.
-
-At some point in the future, you'll be able to seamlessly move from pre-training or fine-tuning models in PyTorch to productizing them in CoreML,
-or prototype a model or an app in CoreML then research its hyperparameters or architecture from PyTorch. Super exciting!
diff --git a/server/transformers/docs/source/main_classes/configuration.rst b/server/transformers/docs/source/main_classes/configuration.rst
deleted file mode 100644
index 2131433759c9c16801e31688ac5be37ea4c22d47..0000000000000000000000000000000000000000
--- a/server/transformers/docs/source/main_classes/configuration.rst
+++ /dev/null
@@ -1,10 +0,0 @@
-Configuration
-----------------------------------------------------
-
-The base class ``PretrainedConfig`` implements the common methods for loading/saving a configuration either from a local file or directory, or from a pretrained model configuration provided by the library (downloaded from HuggingFace's AWS S3 repository).
-
-``PretrainedConfig``
-~~~~~~~~~~~~~~~~~~~~~
-
-.. autoclass:: transformers.PretrainedConfig
- :members:
diff --git a/server/transformers/docs/source/main_classes/model.rst b/server/transformers/docs/source/main_classes/model.rst
deleted file mode 100644
index 6e3da45bc2dfa3089e2345b814776ad6790576d1..0000000000000000000000000000000000000000
--- a/server/transformers/docs/source/main_classes/model.rst
+++ /dev/null
@@ -1,21 +0,0 @@
-Models
-----------------------------------------------------
-
-The base class ``PreTrainedModel`` implements the common methods for loading/saving a model either from a local file or directory, or from a pretrained model configuration provided by the library (downloaded from HuggingFace's AWS S3 repository).
-
-``PreTrainedModel`` also implements a few methods which are common among all the models to:
-
-- resize the input token embeddings when new tokens are added to the vocabulary
-- prune the attention heads of the model.
-
-``PreTrainedModel``
-~~~~~~~~~~~~~~~~~~~~~
-
-.. autoclass:: transformers.PreTrainedModel
- :members:
-
-``TFPreTrainedModel``
-~~~~~~~~~~~~~~~~~~~~~
-
-.. autoclass:: transformers.TFPreTrainedModel
- :members:
diff --git a/server/transformers/docs/source/main_classes/optimizer_schedules.rst b/server/transformers/docs/source/main_classes/optimizer_schedules.rst
deleted file mode 100644
index ec4998389b2f37ae89240d56f3a7b325f9e78bd7..0000000000000000000000000000000000000000
--- a/server/transformers/docs/source/main_classes/optimizer_schedules.rst
+++ /dev/null
@@ -1,72 +0,0 @@
-Optimizer
-----------------------------------------------------
-
-The ``.optimization`` module provides:
-
-- an optimizer with weight decay fixed that can be used to fine-tuned models, and
-- several schedules in the form of schedule objects that inherit from ``_LRSchedule``:
-- a gradient accumulation class to accumulate the gradients of multiple batches
-
-``AdamW``
-~~~~~~~~~~~~~~~~
-
-.. autoclass:: transformers.AdamW
- :members:
-
-``AdamWeightDecay``
-~~~~~~~~~~~~~~~~~~~
-
-.. autoclass:: transformers.AdamWeightDecay
- :members:
-
-.. autofunction:: transformers.create_optimizer
-
-Schedules
-----------------------------------------------------
-
-Learning Rate Schedules
-~~~~~~~~~~~~~~~~~~~~~~~~~~~~~~~~~~~~~~~~~~~~~~~~~~~~
-.. autofunction:: transformers.get_constant_schedule
-
-
-.. autofunction:: transformers.get_constant_schedule_with_warmup
-
-.. image:: /imgs/warmup_constant_schedule.png
- :target: /imgs/warmup_constant_schedule.png
- :alt:
-
-
-.. autofunction:: transformers.get_cosine_schedule_with_warmup
-
-.. image:: /imgs/warmup_cosine_schedule.png
- :target: /imgs/warmup_cosine_schedule.png
- :alt:
-
-
-.. autofunction:: transformers.get_cosine_with_hard_restarts_schedule_with_warmup
-
-.. image:: /imgs/warmup_cosine_hard_restarts_schedule.png
- :target: /imgs/warmup_cosine_hard_restarts_schedule.png
- :alt:
-
-
-
-.. autofunction:: transformers.get_linear_schedule_with_warmup
-
-.. image:: /imgs/warmup_linear_schedule.png
- :target: /imgs/warmup_linear_schedule.png
- :alt:
-
-``Warmup``
-~~~~~~~~~~~~~~~~
-
-.. autoclass:: transformers.WarmUp
- :members:
-
-Gradient Strategies
-----------------------------------------------------
-
-``GradientAccumulator``
-~~~~~~~~~~~~~~~~~~~~~~~
-
-.. autoclass:: transformers.GradientAccumulator
diff --git a/server/transformers/docs/source/main_classes/processors.rst b/server/transformers/docs/source/main_classes/processors.rst
deleted file mode 100644
index 46839ce67e6f842e95b83c0086ff82a77a01b60a..0000000000000000000000000000000000000000
--- a/server/transformers/docs/source/main_classes/processors.rst
+++ /dev/null
@@ -1,153 +0,0 @@
-Processors
-----------------------------------------------------
-
-This library includes processors for several traditional tasks. These processors can be used to process a dataset into
-examples that can be fed to a model.
-
-Processors
-~~~~~~~~~~~~~~~~~~~~~
-
-All processors follow the same architecture which is that of the
-:class:`~transformers.data.processors.utils.DataProcessor`. The processor returns a list
-of :class:`~transformers.data.processors.utils.InputExample`. These
-:class:`~transformers.data.processors.utils.InputExample` can be converted to
-:class:`~transformers.data.processors.utils.InputFeatures` in order to be fed to the model.
-
-.. autoclass:: transformers.data.processors.utils.DataProcessor
- :members:
-
-
-.. autoclass:: transformers.data.processors.utils.InputExample
- :members:
-
-
-.. autoclass:: transformers.data.processors.utils.InputFeatures
- :members:
-
-
-GLUE
-~~~~~~~~~~~~~~~~~~~~~
-
-`General Language Understanding Evaluation (GLUE) `__ is a benchmark that evaluates
-the performance of models across a diverse set of existing NLU tasks. It was released together with the paper
-`GLUE: A multi-task benchmark and analysis platform for natural language understanding `__
-
-This library hosts a total of 10 processors for the following tasks: MRPC, MNLI, MNLI (mismatched),
-CoLA, SST2, STSB, QQP, QNLI, RTE and WNLI.
-
-Those processors are:
- - :class:`~transformers.data.processors.utils.MrpcProcessor`
- - :class:`~transformers.data.processors.utils.MnliProcessor`
- - :class:`~transformers.data.processors.utils.MnliMismatchedProcessor`
- - :class:`~transformers.data.processors.utils.Sst2Processor`
- - :class:`~transformers.data.processors.utils.StsbProcessor`
- - :class:`~transformers.data.processors.utils.QqpProcessor`
- - :class:`~transformers.data.processors.utils.QnliProcessor`
- - :class:`~transformers.data.processors.utils.RteProcessor`
- - :class:`~transformers.data.processors.utils.WnliProcessor`
-
-Additionally, the following method can be used to load values from a data file and convert them to a list of
-:class:`~transformers.data.processors.utils.InputExample`.
-
-.. automethod:: transformers.data.processors.glue.glue_convert_examples_to_features
-
-Example usage
-^^^^^^^^^^^^^^^^^^^^^^^^^
-
-An example using these processors is given in the `run_glue.py `__ script.
-
-
-XNLI
-~~~~~~~~~~~~~~~~~~~~~
-
-`The Cross-Lingual NLI Corpus (XNLI) `__ is a benchmark that evaluates
-the quality of cross-lingual text representations.
-XNLI is crowd-sourced dataset based on `MultiNLI `: pairs of text are labeled with textual entailment
-annotations for 15 different languages (including both high-ressource language such as English and low-ressource languages such as Swahili).
-
-It was released together with the paper
-`XNLI: Evaluating Cross-lingual Sentence Representations `__
-
-This library hosts the processor to load the XNLI data:
- - :class:`~transformers.data.processors.utils.XnliProcessor`
-
-Please note that since the gold labels are available on the test set, evaluation is performed on the test set.
-
-An example using these processors is given in the
-`run_xnli.py `__ script.
-
-
-SQuAD
-~~~~~~~~~~~~~~~~~~~~~
-
-`The Stanford Question Answering Dataset (SQuAD) `__ is a benchmark that evaluates
-the performance of models on question answering. Two versions are available, v1.1 and v2.0. The first version (v1.1) was released together with the paper
-`SQuAD: 100,000+ Questions for Machine Comprehension of Text `__. The second version (v2.0) was released alongside
-the paper `Know What You Don't Know: Unanswerable Questions for SQuAD `__.
-
-This library hosts a processor for each of the two versions:
-
-Processors
-^^^^^^^^^^^^^^^^^^^^^^^^^
-
-Those processors are:
- - :class:`~transformers.data.processors.utils.SquadV1Processor`
- - :class:`~transformers.data.processors.utils.SquadV2Processor`
-
-They both inherit from the abstract class :class:`~transformers.data.processors.utils.SquadProcessor`
-
-.. autoclass:: transformers.data.processors.squad.SquadProcessor
- :members:
-
-Additionally, the following method can be used to convert SQuAD examples into :class:`~transformers.data.processors.utils.SquadFeatures`
-that can be used as model inputs.
-
-.. automethod:: transformers.data.processors.squad.squad_convert_examples_to_features
-
-These processors as well as the aforementionned method can be used with files containing the data as well as with the `tensorflow_datasets` package.
-Examples are given below.
-
-
-Example usage
-^^^^^^^^^^^^^^^^^^^^^^^^^
-Here is an example using the processors as well as the conversion method using data files:
-
-Example::
-
- # Loading a V2 processor
- processor = SquadV2Processor()
- examples = processor.get_dev_examples(squad_v2_data_dir)
-
- # Loading a V1 processor
- processor = SquadV1Processor()
- examples = processor.get_dev_examples(squad_v1_data_dir)
-
- features = squad_convert_examples_to_features(
- examples=examples,
- tokenizer=tokenizer,
- max_seq_length=max_seq_length,
- doc_stride=args.doc_stride,
- max_query_length=max_query_length,
- is_training=not evaluate,
- )
-
-Using `tensorflow_datasets` is as easy as using a data file:
-
-Example::
-
- # tensorflow_datasets only handle Squad V1.
- tfds_examples = tfds.load("squad")
- examples = SquadV1Processor().get_examples_from_dataset(tfds_examples, evaluate=evaluate)
-
- features = squad_convert_examples_to_features(
- examples=examples,
- tokenizer=tokenizer,
- max_seq_length=max_seq_length,
- doc_stride=args.doc_stride,
- max_query_length=max_query_length,
- is_training=not evaluate,
- )
-
-
-Another example using these processors is given in the
-`run_squad.py `__ script.
diff --git a/server/transformers/docs/source/main_classes/tokenizer.rst b/server/transformers/docs/source/main_classes/tokenizer.rst
deleted file mode 100644
index c33eb458292716d08ff2a10cccb492107c77a9b0..0000000000000000000000000000000000000000
--- a/server/transformers/docs/source/main_classes/tokenizer.rst
+++ /dev/null
@@ -1,16 +0,0 @@
-Tokenizer
-----------------------------------------------------
-
-The base class ``PreTrainedTokenizer`` implements the common methods for loading/saving a tokenizer either from a local file or directory, or from a pretrained tokenizer provided by the library (downloaded from HuggingFace's AWS S3 repository).
-
-``PreTrainedTokenizer`` is the main entry point into tokenizers as it also implements the main methods for using all the tokenizers:
-
-- tokenizing, converting tokens to ids and back and encoding/decoding,
-- adding new tokens to the vocabulary in a way that is independant of the underlying structure (BPE, SentencePiece...),
-- managing special tokens (adding them, assigning them to roles, making sure they are not split during tokenization)
-
-``PreTrainedTokenizer``
-~~~~~~~~~~~~~~~~~~~~~~~~
-
-.. autoclass:: transformers.PreTrainedTokenizer
- :members:
diff --git a/server/transformers/docs/source/migration.md b/server/transformers/docs/source/migration.md
deleted file mode 100644
index f50d1dff0a8e2a6205c66a6a012d17fb98b19f38..0000000000000000000000000000000000000000
--- a/server/transformers/docs/source/migration.md
+++ /dev/null
@@ -1,109 +0,0 @@
-# Migrating from pytorch-pretrained-bert
-
-
-Here is a quick summary of what you should take care of when migrating from `pytorch-pretrained-bert` to `transformers`
-
-### Models always output `tuples`
-
-The main breaking change when migrating from `pytorch-pretrained-bert` to `transformers` is that the models forward method always outputs a `tuple` with various elements depending on the model and the configuration parameters.
-
-The exact content of the tuples for each model are detailled in the models' docstrings and the [documentation](https://huggingface.co/transformers/).
-
-In pretty much every case, you will be fine by taking the first element of the output as the output you previously used in `pytorch-pretrained-bert`.
-
-Here is a `pytorch-pretrained-bert` to `transformers` conversion example for a `BertForSequenceClassification` classification model:
-
-```python
-# Let's load our model
-model = BertForSequenceClassification.from_pretrained('bert-base-uncased')
-
-# If you used to have this line in pytorch-pretrained-bert:
-loss = model(input_ids, labels=labels)
-
-# Now just use this line in transformers to extract the loss from the output tuple:
-outputs = model(input_ids, labels=labels)
-loss = outputs[0]
-
-# In transformers you can also have access to the logits:
-loss, logits = outputs[:2]
-
-# And even the attention weigths if you configure the model to output them (and other outputs too, see the docstrings and documentation)
-model = BertForSequenceClassification.from_pretrained('bert-base-uncased', output_attentions=True)
-outputs = model(input_ids, labels=labels)
-loss, logits, attentions = outputs
-```
-
-### Serialization
-
-Breaking change in the `from_pretrained()`method:
-
-1. Models are now set in evaluation mode by default when instantiated with the `from_pretrained()` method. To train them don't forget to set them back in training mode (`model.train()`) to activate the dropout modules.
-
-2. The additional `*inputs` and `**kwargs` arguments supplied to the `from_pretrained()` method used to be directly passed to the underlying model's class `__init__()` method. They are now used to update the model configuration attribute first which can break derived model classes build based on the previous `BertForSequenceClassification` examples. More precisely, the positional arguments `*inputs` provided to `from_pretrained()` are directly forwarded the model `__init__()` method while the keyword arguments `**kwargs` (i) which match configuration class attributes are used to update said attributes (ii) which don't match any configuration class attributes are forwarded to the model `__init__()` method.
-
-Also, while not a breaking change, the serialization methods have been standardized and you probably should switch to the new method `save_pretrained(save_directory)` if you were using any other serialization method before.
-
-Here is an example:
-
-```python
-### Let's load a model and tokenizer
-model = BertForSequenceClassification.from_pretrained('bert-base-uncased')
-tokenizer = BertTokenizer.from_pretrained('bert-base-uncased')
-
-### Do some stuff to our model and tokenizer
-# Ex: add new tokens to the vocabulary and embeddings of our model
-tokenizer.add_tokens(['[SPECIAL_TOKEN_1]', '[SPECIAL_TOKEN_2]'])
-model.resize_token_embeddings(len(tokenizer))
-# Train our model
-train(model)
-
-### Now let's save our model and tokenizer to a directory
-model.save_pretrained('./my_saved_model_directory/')
-tokenizer.save_pretrained('./my_saved_model_directory/')
-
-### Reload the model and the tokenizer
-model = BertForSequenceClassification.from_pretrained('./my_saved_model_directory/')
-tokenizer = BertTokenizer.from_pretrained('./my_saved_model_directory/')
-```
-
-### Optimizers: BertAdam & OpenAIAdam are now AdamW, schedules are standard PyTorch schedules
-
-The two optimizers previously included, `BertAdam` and `OpenAIAdam`, have been replaced by a single `AdamW` optimizer which has a few differences:
-
-- it only implements weights decay correction,
-- schedules are now externals (see below),
-- gradient clipping is now also external (see below).
-
-The new optimizer `AdamW` matches PyTorch `Adam` optimizer API and let you use standard PyTorch or apex methods for the schedule and clipping.
-
-The schedules are now standard [PyTorch learning rate schedulers](https://pytorch.org/docs/stable/optim.html#how-to-adjust-learning-rate) and not part of the optimizer anymore.
-
-Here is a conversion examples from `BertAdam` with a linear warmup and decay schedule to `AdamW` and the same schedule:
-
-```python
-# Parameters:
-lr = 1e-3
-max_grad_norm = 1.0
-num_training_steps = 1000
-num_warmup_steps = 100
-warmup_proportion = float(num_warmup_steps) / float(num_training_steps) # 0.1
-
-### Previously BertAdam optimizer was instantiated like this:
-optimizer = BertAdam(model.parameters(), lr=lr, schedule='warmup_linear', warmup=warmup_proportion, num_training_steps=num_training_steps)
-### and used like this:
-for batch in train_data:
- loss = model(batch)
- loss.backward()
- optimizer.step()
-
-### In Transformers, optimizer and schedules are splitted and instantiated like this:
-optimizer = AdamW(model.parameters(), lr=lr, correct_bias=False) # To reproduce BertAdam specific behavior set correct_bias=False
-scheduler = get_linear_schedule_with_warmup(optimizer, num_warmup_steps=num_warmup_steps, num_training_steps=num_training_steps) # PyTorch scheduler
-### and used like this:
-for batch in train_data:
- loss = model(batch)
- loss.backward()
- torch.nn.utils.clip_grad_norm_(model.parameters(), max_grad_norm) # Gradient clipping is not in AdamW anymore (so you can use amp without issue)
- optimizer.step()
- scheduler.step()
-```
diff --git a/server/transformers/docs/source/model_doc/albert.rst b/server/transformers/docs/source/model_doc/albert.rst
deleted file mode 100644
index 06a9b5bfd50b0c7aef601b6693572bef8bc20b82..0000000000000000000000000000000000000000
--- a/server/transformers/docs/source/model_doc/albert.rst
+++ /dev/null
@@ -1,93 +0,0 @@
-ALBERT
-----------------------------------------------------
-
-Overview
-~~~~~~~~~~~~~~~~~~~~~
-
-The ALBERT model was proposed in `ALBERT: A Lite BERT for Self-supervised Learning of Language Representations `_
-by Zhenzhong Lan, Mingda Chen, Sebastian Goodman, Kevin Gimpel, Piyush Sharma, Radu Soricut. It presents
-two parameter-reduction techniques to lower memory consumption and increase the trainig speed of BERT:
-
-- Splitting the embedding matrix into two smaller matrices
-- Using repeating layers split among groups
-
-The abstract from the paper is the following:
-
-*Increasing model size when pretraining natural language representations often results in improved performance on
-downstream tasks. However, at some point further model increases become harder due to GPU/TPU memory limitations,
-longer training times, and unexpected model degradation. To address these problems, we present two parameter-reduction
-techniques to lower memory consumption and increase the training speed of BERT. Comprehensive empirical evidence shows
-that our proposed methods lead to models that scale much better compared to the original BERT. We also use a
-self-supervised loss that focuses on modeling inter-sentence coherence, and show it consistently helps downstream
-tasks with multi-sentence inputs. As a result, our best model establishes new state-of-the-art results on the GLUE,
-RACE, and SQuAD benchmarks while having fewer parameters compared to BERT-large.*
-
-Tips:
-
-- ALBERT is a model with absolute position embeddings so it's usually advised to pad the inputs on
- the right rather than the left.
-- ALBERT uses repeating layers which results in a small memory footprint, however the computational cost remains
- similar to a BERT-like architecture with the same number of hidden layers as it has to iterate through the same
- number of (repeating) layers.
-
-AlbertConfig
-~~~~~~~~~~~~~~~~~~~~~
-
-.. autoclass:: transformers.AlbertConfig
- :members:
-
-
-AlbertTokenizer
-~~~~~~~~~~~~~~~~~~~~~
-
-.. autoclass:: transformers.AlbertTokenizer
- :members:
-
-
-AlbertModel
-~~~~~~~~~~~~~~~~~~~~
-
-.. autoclass:: transformers.AlbertModel
- :members:
-
-
-AlbertForMaskedLM
-~~~~~~~~~~~~~~~~~~~~~~~~~~~~~
-
-.. autoclass:: transformers.AlbertForMaskedLM
- :members:
-
-
-AlbertForSequenceClassification
-~~~~~~~~~~~~~~~~~~~~~~~~~~~~~~~~~~~~~~~~~~~
-
-.. autoclass:: transformers.AlbertForSequenceClassification
- :members:
-
-
-AlbertForQuestionAnswering
-~~~~~~~~~~~~~~~~~~~~~~~~~~~~~~~~~~~~~~~~
-
-.. autoclass:: transformers.AlbertForQuestionAnswering
- :members:
-
-
-TFAlbertModel
-~~~~~~~~~~~~~~~~~~~~
-
-.. autoclass:: transformers.TFAlbertModel
- :members:
-
-
-TFAlbertForMaskedLM
-~~~~~~~~~~~~~~~~~~~~~~~~~~
-
-.. autoclass:: transformers.TFAlbertForMaskedLM
- :members:
-
-
-TFAlbertForSequenceClassification
-~~~~~~~~~~~~~~~~~~~~~~~~~~~~~~~~~~~~~~~~
-
-.. autoclass:: transformers.TFAlbertForSequenceClassification
- :members:
diff --git a/server/transformers/docs/source/model_doc/auto.rst b/server/transformers/docs/source/model_doc/auto.rst
deleted file mode 100644
index 541d03a8e588ecec7fa483c5f48243f49a76d6cc..0000000000000000000000000000000000000000
--- a/server/transformers/docs/source/model_doc/auto.rst
+++ /dev/null
@@ -1,65 +0,0 @@
-AutoModels
------------
-
-In many cases, the architecture you want to use can be guessed from the name or the path of the pretrained model you are supplying to the ``from_pretrained`` method.
-
-AutoClasses are here to do this job for you so that you automatically retrieve the relevant model given the name/path to the pretrained weights/config/vocabulary:
-
-Instantiating one of ``AutoModel``, ``AutoConfig`` and ``AutoTokenizer`` will directly create a class of the relevant architecture (ex: ``model = AutoModel.from_pretrained('bert-base-cased')`` will create a instance of ``BertModel``).
-
-
-``AutoConfig``
-~~~~~~~~~~~~~~~~~~~~~
-
-.. autoclass:: transformers.AutoConfig
- :members:
-
-
-``AutoTokenizer``
-~~~~~~~~~~~~~~~~~~~~~~~~~~~~~
-
-.. autoclass:: transformers.AutoTokenizer
- :members:
-
-
-``AutoModel``
-~~~~~~~~~~~~~~~~~~~~~
-
-.. autoclass:: transformers.AutoModel
- :members:
-
-
-``AutoModelForPreTraining``
-~~~~~~~~~~~~~~~~~~~~~
-
-.. autoclass:: transformers.AutoModelForPreTraining
- :members:
-
-
-``AutoModelWithLMHead``
-~~~~~~~~~~~~~~~~~~~~~
-
-.. autoclass:: transformers.AutoModelWithLMHead
- :members:
-
-
-``AutoModelForSequenceClassification``
-~~~~~~~~~~~~~~~~~~~~~
-
-.. autoclass:: transformers.AutoModelForSequenceClassification
- :members:
-
-
-``AutoModelForQuestionAnswering``
-~~~~~~~~~~~~~~~~~~~~~
-
-.. autoclass:: transformers.AutoModelForQuestionAnswering
- :members:
-
-
-``AutoModelForTokenClassification``
-~~~~~~~~~~~~~~~~~~~~~
-
-.. autoclass:: transformers.AutoModelForTokenClassification
- :members:
-
diff --git a/server/transformers/docs/source/model_doc/bert.rst b/server/transformers/docs/source/model_doc/bert.rst
deleted file mode 100644
index 5e785eed1c9f3fff0063b4d881b86535423093a4..0000000000000000000000000000000000000000
--- a/server/transformers/docs/source/model_doc/bert.rst
+++ /dev/null
@@ -1,162 +0,0 @@
-BERT
-----------------------------------------------------
-
-Overview
-~~~~~~~~~~~~~~~~~~~~~
-
-The BERT model was proposed in `BERT: Pre-training of Deep Bidirectional Transformers for Language Understanding `__
-by Jacob Devlin, Ming-Wei Chang, Kenton Lee and Kristina Toutanova. It's a bidirectional transformer
-pre-trained using a combination of masked language modeling objective and next sentence prediction
-on a large corpus comprising the Toronto Book Corpus and Wikipedia.
-
-The abstract from the paper is the following:
-
-*We introduce a new language representation model called BERT, which stands for Bidirectional Encoder Representations
-from Transformers. Unlike recent language representation models, BERT is designed to pre-train deep bidirectional
-representations from unlabeled text by jointly conditioning on both left and right context in all layers. As a result,
-the pre-trained BERT model can be fine-tuned with just one additional output layer to create state-of-the-art models
-for a wide range of tasks, such as question answering and language inference, without substantial task-specific
-architecture modifications.*
-
-*BERT is conceptually simple and empirically powerful. It obtains new state-of-the-art results on eleven natural
-language processing tasks, including pushing the GLUE score to 80.5% (7.7% point absolute improvement), MultiNLI
-accuracy to 86.7% (4.6% absolute improvement), SQuAD v1.1 question answering Test F1 to 93.2 (1.5 point absolute
-improvement) and SQuAD v2.0 Test F1 to 83.1 (5.1 point absolute improvement).*
-
-Tips:
-
-- BERT is a model with absolute position embeddings so it's usually advised to pad the inputs on
- the right rather than the left.
-- BERT was trained with a masked language modeling (MLM) objective. It is therefore efficient at predicting masked
- tokens and at NLU in general, but is not optimal for text generation. Models trained with a causal language
- modeling (CLM) objective are better in that regard.
-- Alongside MLM, BERT was trained using a next sentence prediction (NSP) objective using the [CLS] token as a sequence
- approximate. The user may use this token (the first token in a sequence built with special tokens) to get a sequence
- prediction rather than a token prediction. However, averaging over the sequence may yield better results than using
- the [CLS] token.
-
-BertConfig
-~~~~~~~~~~~~~~~~~~~~~
-
-.. autoclass:: transformers.BertConfig
- :members:
-
-
-BertTokenizer
-~~~~~~~~~~~~~~~~~~~~~
-
-.. autoclass:: transformers.BertTokenizer
- :members:
-
-
-BertModel
-~~~~~~~~~~~~~~~~~~~~
-
-.. autoclass:: transformers.BertModel
- :members:
-
-
-BertForPreTraining
-~~~~~~~~~~~~~~~~~~~~~~~~~~~~~
-
-.. autoclass:: transformers.BertForPreTraining
- :members:
-
-
-BertForMaskedLM
-~~~~~~~~~~~~~~~~~~~~~~~~~~
-
-.. autoclass:: transformers.BertForMaskedLM
- :members:
-
-
-BertForNextSentencePrediction
-~~~~~~~~~~~~~~~~~~~~~~~~~~~~~~~~~~~~~~~~
-
-.. autoclass:: transformers.BertForNextSentencePrediction
- :members:
-
-
-BertForSequenceClassification
-~~~~~~~~~~~~~~~~~~~~~~~~~~~~~~~~~~~~~~~~
-
-.. autoclass:: transformers.BertForSequenceClassification
- :members:
-
-
-BertForMultipleChoice
-~~~~~~~~~~~~~~~~~~~~~~~~~~~~~~~~
-
-.. autoclass:: transformers.BertForMultipleChoice
- :members:
-
-
-BertForTokenClassification
-~~~~~~~~~~~~~~~~~~~~~~~~~~~~~~~~~~~~~
-
-.. autoclass:: transformers.BertForTokenClassification
- :members:
-
-
-BertForQuestionAnswering
-~~~~~~~~~~~~~~~~~~~~~~~~~~~~~~~~~~~
-
-.. autoclass:: transformers.BertForQuestionAnswering
- :members:
-
-
-TFBertModel
-~~~~~~~~~~~~~~~~~~~~
-
-.. autoclass:: transformers.TFBertModel
- :members:
-
-
-TFBertForPreTraining
-~~~~~~~~~~~~~~~~~~~~~~~~~~~~~
-
-.. autoclass:: transformers.TFBertForPreTraining
- :members:
-
-
-TFBertForMaskedLM
-~~~~~~~~~~~~~~~~~~~~~~~~~~
-
-.. autoclass:: transformers.TFBertForMaskedLM
- :members:
-
-
-TFBertForNextSentencePrediction
-~~~~~~~~~~~~~~~~~~~~~~~~~~~~~~~~~~~~~~~~
-
-.. autoclass:: transformers.TFBertForNextSentencePrediction
- :members:
-
-
-TFBertForSequenceClassification
-~~~~~~~~~~~~~~~~~~~~~~~~~~~~~~~~~~~~~~~~
-
-.. autoclass:: transformers.TFBertForSequenceClassification
- :members:
-
-
-TFBertForMultipleChoice
-~~~~~~~~~~~~~~~~~~~~~~~~~~~~~~~~
-
-.. autoclass:: transformers.TFBertForMultipleChoice
- :members:
-
-
-TFBertForTokenClassification
-~~~~~~~~~~~~~~~~~~~~~~~~~~~~~~~~~~~~~
-
-.. autoclass:: transformers.TFBertForTokenClassification
- :members:
-
-
-TFBertForQuestionAnswering
-~~~~~~~~~~~~~~~~~~~~~~~~~~~~~~~~~~~
-
-.. autoclass:: transformers.TFBertForQuestionAnswering
- :members:
-
diff --git a/server/transformers/docs/source/model_doc/camembert.rst b/server/transformers/docs/source/model_doc/camembert.rst
deleted file mode 100644
index 611d930d6ed8fd16c0b4b6d1d0350683f7778cd1..0000000000000000000000000000000000000000
--- a/server/transformers/docs/source/model_doc/camembert.rst
+++ /dev/null
@@ -1,99 +0,0 @@
-CamemBERT
-----------------------------------------------------
-
-The CamemBERT model was proposed in `CamemBERT: a Tasty French Language Model `__
-by Louis Martin, Benjamin Muller, Pedro Javier Ortiz Suárez, Yoann Dupont, Laurent Romary, Éric Villemonte de la
-Clergerie, Djamé Seddah, and Benoît Sagot. It is based on Facebook's RoBERTa model released in 2019. It is a model
-trained on 138GB of French text.
-
-The abstract from the paper is the following:
-
-*Pretrained language models are now ubiquitous in Natural Language Processing. Despite their success,
-most available models have either been trained on English data or on the concatenation of data in multiple
-languages. This makes practical use of such models --in all languages except English-- very limited. Aiming
-to address this issue for French, we release CamemBERT, a French version of the Bi-directional Encoders for
-Transformers (BERT). We measure the performance of CamemBERT compared to multilingual models in multiple
-downstream tasks, namely part-of-speech tagging, dependency parsing, named-entity recognition, and natural
-language inference. CamemBERT improves the state of the art for most of the tasks considered. We release the
-pretrained model for CamemBERT hoping to foster research and downstream applications for French NLP.*
-
-Tips:
-
-- This implementation is the same as RoBERTa. Refer to the `documentation of RoBERTa <./roberta.html>`__ for usage
- examples as well as the information relative to the inputs and outputs.
-
-CamembertConfig
-~~~~~~~~~~~~~~~~~~~~~~~~~~~~~~~~~~~~~~~~~~
-
-.. autoclass:: transformers.CamembertConfig
- :members:
-
-
-CamembertTokenizer
-~~~~~~~~~~~~~~~~~~~~~~~~~~~~~~~~~~~~~~~~~~
-
-.. autoclass:: transformers.CamembertTokenizer
- :members:
-
-
-CamembertModel
-~~~~~~~~~~~~~~~~~~~~~~~~~~~~~~~~~~~~~~~~~~
-
-.. autoclass:: transformers.CamembertModel
- :members:
-
-
-CamembertForMaskedLM
-~~~~~~~~~~~~~~~~~~~~~~~~~~~~~~~~~~~~~~~~~~
-
-.. autoclass:: transformers.CamembertForMaskedLM
- :members:
-
-
-CamembertForSequenceClassification
-~~~~~~~~~~~~~~~~~~~~~~~~~~~~~~~~~~~~~~~~~~
-
-.. autoclass:: transformers.CamembertForSequenceClassification
- :members:
-
-
-CamembertForMultipleChoice
-~~~~~~~~~~~~~~~~~~~~~~~~~~~~~~~~~~~~~~~~~~
-
-.. autoclass:: transformers.CamembertForMultipleChoice
- :members:
-
-
-CamembertForTokenClassification
-~~~~~~~~~~~~~~~~~~~~~~~~~~~~~~~~~~~~~~~~~~
-
-.. autoclass:: transformers.CamembertForTokenClassification
- :members:
-
-
-TFCamembertModel
-~~~~~~~~~~~~~~~~~~~~~~~~~~~~~~~~~~~~~~~~~~
-
-.. autoclass:: transformers.TFCamembertModel
- :members:
-
-
-TFCamembertForMaskedLM
-~~~~~~~~~~~~~~~~~~~~~~~~~~~~~~~~~~~~~~~~~~
-
-.. autoclass:: transformers.TFCamembertForMaskedLM
- :members:
-
-
-TFCamembertForSequenceClassification
-~~~~~~~~~~~~~~~~~~~~~~~~~~~~~~~~~~~~~~~~~~
-
-.. autoclass:: transformers.TFCamembertForSequenceClassification
- :members:
-
-
-TFCamembertForTokenClassification
-~~~~~~~~~~~~~~~~~~~~~~~~~~~~~~~~~~~~~~~~~~
-
-.. autoclass:: transformers.TFCamembertForTokenClassification
- :members:
diff --git a/server/transformers/docs/source/model_doc/ctrl.rst b/server/transformers/docs/source/model_doc/ctrl.rst
deleted file mode 100644
index a8a04837d75ea068286cb37ab1f3b02ffb4a1ad4..0000000000000000000000000000000000000000
--- a/server/transformers/docs/source/model_doc/ctrl.rst
+++ /dev/null
@@ -1,75 +0,0 @@
-CTRL
-----------------------------------------------------
-
-CTRL model was proposed in `CTRL: A Conditional Transformer Language Model for Controllable Generation `_
-by Nitish Shirish Keskar*, Bryan McCann*, Lav R. Varshney, Caiming Xiong and Richard Socher.
-It's a causal (unidirectional) transformer pre-trained using language modeling on a very large
-corpus of ~140 GB of text data with the first token reserved as a control code (such as Links, Books, Wikipedia etc.).
-
-The abstract from the paper is the following:
-
-*Large-scale language models show promising text generation capabilities, but users cannot easily control particular
-aspects of the generated text. We release CTRL, a 1.63 billion-parameter conditional transformer language model,
-trained to condition on control codes that govern style, content, and task-specific behavior. Control codes were
-derived from structure that naturally co-occurs with raw text, preserving the advantages of unsupervised learning
-while providing more explicit control over text generation. These codes also allow CTRL to predict which parts of
-the training data are most likely given a sequence. This provides a potential method for analyzing large amounts
-of data via model-based source attribution.*
-
-Tips:
-
-- CTRL makes use of control codes to generate text: it requires generations to be started by certain words, sentences
- or links to generate coherent text. Refer to the `original implementation `__
- for more information.
-- CTRL is a model with absolute position embeddings so it's usually advised to pad the inputs on
- the right rather than the left.
-- CTRL was trained with a causal language modeling (CLM) objective and is therefore powerful at predicting the next
- token in a sequence. Leveraging this feature allows CTRL to generate syntactically coherent text as
- it can be observed in the `run_generation.py` example script.
-- The PyTorch models can take the `past` as input, which is the previously computed key/value attention pairs. Using
- this `past` value prevents the model from re-computing pre-computed values in the context of text generation.
- See `reusing the past in generative models <../quickstart.html#using-the-past>`_ for more information on the usage
- of this argument.
-
-
-CTRLConfig
-~~~~~~~~~~~~~~~~~~~~~~~~~~~~~~~~
-
-.. autoclass:: transformers.CTRLConfig
- :members:
-
-
-CTRLTokenizer
-~~~~~~~~~~~~~~~~~~~~~~~~~~~~~~~~
-
-.. autoclass:: transformers.CTRLTokenizer
- :members:
-
-
-CTRLModel
-~~~~~~~~~~~~~~~~~~~~~~~~~~~~~~~
-
-.. autoclass:: transformers.CTRLModel
- :members:
-
-
-CTRLLMHeadModel
-~~~~~~~~~~~~~~~~~~~~~~~~~~~~~~~~~~~~~
-
-.. autoclass:: transformers.CTRLLMHeadModel
- :members:
-
-
-TFCTRLModel
-~~~~~~~~~~~~~~~~~~~~~~~~~~~~~~~
-
-.. autoclass:: transformers.TFCTRLModel
- :members:
-
-
-TFCTRLLMHeadModel
-~~~~~~~~~~~~~~~~~~~~~~~~~~~~~~~~~~~~~
-
-.. autoclass:: transformers.TFCTRLLMHeadModel
- :members:
-
diff --git a/server/transformers/docs/source/model_doc/distilbert.rst b/server/transformers/docs/source/model_doc/distilbert.rst
deleted file mode 100644
index 81d8086c151fd8b864c7dad409da6c970ec39790..0000000000000000000000000000000000000000
--- a/server/transformers/docs/source/model_doc/distilbert.rst
+++ /dev/null
@@ -1,97 +0,0 @@
-DistilBERT
-----------------------------------------------------
-
-The DistilBERT model was proposed in the blog post
-`Smaller, faster, cheaper, lighter: Introducing DistilBERT, a distilled version of BERT `__,
-and the paper `DistilBERT, a distilled version of BERT: smaller, faster, cheaper and lighter `__.
-DistilBERT is a small, fast, cheap and light Transformer model trained by distilling Bert base. It has 40% less
-parameters than `bert-base-uncased`, runs 60% faster while preserving over 95% of Bert's performances as measured on
-the GLUE language understanding benchmark.
-
-The abstract from the paper is the following:
-
-*As Transfer Learning from large-scale pre-trained models becomes more prevalent in Natural Language Processing (NLP),
-operating these large models in on-the-edge and/or under constrained computational training or inference budgets
-remains challenging. In this work, we propose a method to pre-train a smaller general-purpose language representation
-model, called DistilBERT, which can then be fine-tuned with good performances on a wide range of tasks like its larger
-counterparts. While most prior work investigated the use of distillation for building task-specific models, we
-leverage knowledge distillation during the pre-training phase and show that it is possible to reduce the size of a
-BERT model by 40%, while retaining 97% of its language understanding capabilities and being 60% faster. To leverage
-the inductive biases learned by larger models during pre-training, we introduce a triple loss combining language
-modeling, distillation and cosine-distance losses. Our smaller, faster and lighter model is cheaper to pre-train
-and we demonstrate its capabilities for on-device computations in a proof-of-concept experiment and a comparative
-on-device study.*
-
-Tips:
-
-- DistilBert doesn't have `token_type_ids`, you don't need to indicate which token belongs to which segment. Just separate your segments with the separation token `tokenizer.sep_token` (or `[SEP]`)
-- DistilBert doesn't have options to select the input positions (`position_ids` input). This could be added if necessary though, just let's us know if you need this option.
-
-
-DistilBertConfig
-~~~~~~~~~~~~~~~~~~~~~~~~~~~~~~~~
-
-.. autoclass:: transformers.DistilBertConfig
- :members:
-
-
-DistilBertTokenizer
-~~~~~~~~~~~~~~~~~~~~~~~~~~~~~~~~
-
-.. autoclass:: transformers.DistilBertTokenizer
- :members:
-
-
-DistilBertModel
-~~~~~~~~~~~~~~~~~~~~~~~~~~~~~~~
-
-.. autoclass:: transformers.DistilBertModel
- :members:
-
-
-DistilBertForMaskedLM
-~~~~~~~~~~~~~~~~~~~~~~~~~~~~~~~~~~~~~
-
-.. autoclass:: transformers.DistilBertForMaskedLM
- :members:
-
-
-DistilBertForSequenceClassification
-~~~~~~~~~~~~~~~~~~~~~~~~~~~~~~~~~~~~~~~~~~~~~~~~~~~
-
-.. autoclass:: transformers.DistilBertForSequenceClassification
- :members:
-
-
-DistilBertForQuestionAnswering
-~~~~~~~~~~~~~~~~~~~~~~~~~~~~~~~~~~~~~~~~
-
-.. autoclass:: transformers.DistilBertForQuestionAnswering
- :members:
-
-TFDistilBertModel
-~~~~~~~~~~~~~~~~~~~~~~~~~~~~~~~
-
-.. autoclass:: transformers.TFDistilBertModel
- :members:
-
-
-TFDistilBertForMaskedLM
-~~~~~~~~~~~~~~~~~~~~~~~~~~~~~~~~~~~~~
-
-.. autoclass:: transformers.TFDistilBertForMaskedLM
- :members:
-
-
-TFDistilBertForSequenceClassification
-~~~~~~~~~~~~~~~~~~~~~~~~~~~~~~~~~~~~~~~~~~~~~~~~~~~
-
-.. autoclass:: transformers.TFDistilBertForSequenceClassification
- :members:
-
-
-TFDistilBertForQuestionAnswering
-~~~~~~~~~~~~~~~~~~~~~~~~~~~~~~~~~~~~~~~~
-
-.. autoclass:: transformers.TFDistilBertForQuestionAnswering
- :members:
diff --git a/server/transformers/docs/source/model_doc/flaubert.rst b/server/transformers/docs/source/model_doc/flaubert.rst
deleted file mode 100644
index d0211306eed90c781f418327a9ebe5feb359624b..0000000000000000000000000000000000000000
--- a/server/transformers/docs/source/model_doc/flaubert.rst
+++ /dev/null
@@ -1,72 +0,0 @@
-FlauBERT
-----------------------------------------------------
-
-The FlauBERT model was proposed in the paper
-`FlauBERT: Unsupervised Language Model Pre-training for French `__ by Hang Le et al.
-It's a transformer pre-trained using a masked language modeling (MLM) objective (BERT-like).
-
-The abstract from the paper is the following:
-
-*Language models have become a key step to achieve state-of-the art results in many different Natural Language
-Processing (NLP) tasks. Leveraging the huge amount of unlabeled texts nowadays available, they provide an efficient
-way to pre-train continuous word representations that can be fine-tuned for a downstream task, along with their
-contextualization at the sentence level. This has been widely demonstrated for English using contextualized
-representations (Dai and Le, 2015; Peters et al., 2018; Howard and Ruder, 2018; Radford et al., 2018; Devlin et
-al., 2019; Yang et al., 2019b). In this paper, we introduce and share FlauBERT, a model learned on a very large
-and heterogeneous French corpus. Models of different sizes are trained using the new CNRS (French National Centre
-for Scientific Research) Jean Zay supercomputer. We apply our French language models to diverse NLP tasks (text
-classification, paraphrasing, natural language inference, parsing, word sense disambiguation) and show that most
-of the time they outperform other pre-training approaches. Different versions of FlauBERT as well as a unified
-evaluation protocol for the downstream tasks, called FLUE (French Language Understanding Evaluation), are shared
-to the research community for further reproducible experiments in French NLP.*
-
-
-FlaubertConfig
-~~~~~~~~~~~~~~~~~~~~~~~~~~~~~~~~
-
-.. autoclass:: transformers.FlaubertConfig
- :members:
-
-
-FlaubertTokenizer
-~~~~~~~~~~~~~~~~~~~~~~~~~~~~~~~~
-
-.. autoclass:: transformers.FlaubertTokenizer
- :members:
-
-
-FlaubertModel
-~~~~~~~~~~~~~~~~~~~~~~~~~~~~~~~
-
-.. autoclass:: transformers.FlaubertModel
- :members:
-
-
-FlaubertWithLMHeadModel
-~~~~~~~~~~~~~~~~~~~~~~~~~~~~~~~~~~~~~
-
-.. autoclass:: transformers.FlaubertWithLMHeadModel
- :members:
-
-
-FlaubertForSequenceClassification
-~~~~~~~~~~~~~~~~~~~~~~~~~~~~~~~~~~~~~~~~~~~~~~~~~~~
-
-.. autoclass:: transformers.FlaubertForSequenceClassification
- :members:
-
-
-FlaubertForQuestionAnsweringSimple
-~~~~~~~~~~~~~~~~~~~~~~~~~~~~~~~~~~~~~~~~
-
-.. autoclass:: transformers.FlaubertForQuestionAnsweringSimple
- :members:
-
-
-FlaubertForQuestionAnswering
-~~~~~~~~~~~~~~~~~~~~~~~~~~~~~~~~~~~~~~~~
-
-.. autoclass:: transformers.FlaubertForQuestionAnswering
- :members:
-
-
diff --git a/server/transformers/docs/source/model_doc/gpt.rst b/server/transformers/docs/source/model_doc/gpt.rst
deleted file mode 100644
index 9604b39ceae0a435deab58df5ed6648f588c27f5..0000000000000000000000000000000000000000
--- a/server/transformers/docs/source/model_doc/gpt.rst
+++ /dev/null
@@ -1,92 +0,0 @@
-OpenAI GPT
-----------------------------------------------------
-
-Overview
-~~~~~~~~~~~~~~~~~~~~~
-
-OpenAI GPT model was proposed in `Improving Language Understanding by Generative Pre-Training `__
-by Alec Radford, Karthik Narasimhan, Tim Salimans and Ilya Sutskever. It's a causal (unidirectional)
-transformer pre-trained using language modeling on a large corpus will long range dependencies, the Toronto Book Corpus.
-
-The abstract from the paper is the following:
-
-*Natural language understanding comprises a wide range of diverse tasks such
-as textual entailment, question answering, semantic similarity assessment, and
-document classification. Although large unlabeled text corpora are abundant,
-labeled data for learning these specific tasks is scarce, making it challenging for
-discriminatively trained models to perform adequately. We demonstrate that large
-gains on these tasks can be realized by generative pre-training of a language model
-on a diverse corpus of unlabeled text, followed by discriminative fine-tuning on each
-specific task. In contrast to previous approaches, we make use of task-aware input
-transformations during fine-tuning to achieve effective transfer while requiring
-minimal changes to the model architecture. We demonstrate the effectiveness of
-our approach on a wide range of benchmarks for natural language understanding.
-Our general task-agnostic model outperforms discriminatively trained models that
-use architectures specifically crafted for each task, significantly improving upon the
-state of the art in 9 out of the 12 tasks studied.*
-
-Tips:
-
-- GPT is a model with absolute position embeddings so it's usually advised to pad the inputs on
- the right rather than the left.
-- GPT was trained with a causal language modeling (CLM) objective and is therefore powerful at predicting the next
- token in a sequence. Leveraging this feature allows GPT-2 to generate syntactically coherent text as
- it can be observed in the `run_generation.py` example script.
-
-`Write With Transformer `__ is a webapp created and hosted by
-Hugging Face showcasing the generative capabilities of several models. GPT is one of them.
-
-OpenAIGPTConfig
-~~~~~~~~~~~~~~~~~~~~~
-
-.. autoclass:: transformers.OpenAIGPTConfig
- :members:
-
-
-OpenAIGPTTokenizer
-~~~~~~~~~~~~~~~~~~~~~~~~~~
-
-.. autoclass:: transformers.OpenAIGPTTokenizer
- :members:
-
-
-OpenAIGPTModel
-~~~~~~~~~~~~~~~~~~~~~~~~~
-
-.. autoclass:: transformers.OpenAIGPTModel
- :members:
-
-
-OpenAIGPTLMHeadModel
-~~~~~~~~~~~~~~~~~~~~~~~~~~~~~~~~
-
-.. autoclass:: transformers.OpenAIGPTLMHeadModel
- :members:
-
-
-OpenAIGPTDoubleHeadsModel
-~~~~~~~~~~~~~~~~~~~~~~~~~~~~~~~~~~~~~
-
-.. autoclass:: transformers.OpenAIGPTDoubleHeadsModel
- :members:
-
-
-TFOpenAIGPTModel
-~~~~~~~~~~~~~~~~~~~~~~~~~
-
-.. autoclass:: transformers.TFOpenAIGPTModel
- :members:
-
-
-TFOpenAIGPTLMHeadModel
-~~~~~~~~~~~~~~~~~~~~~~~~~~~~~~~~
-
-.. autoclass:: transformers.TFOpenAIGPTLMHeadModel
- :members:
-
-
-TFOpenAIGPTDoubleHeadsModel
-~~~~~~~~~~~~~~~~~~~~~~~~~~~~~~~~~~~~~
-
-.. autoclass:: transformers.TFOpenAIGPTDoubleHeadsModel
- :members:
diff --git a/server/transformers/docs/source/model_doc/gpt2.rst b/server/transformers/docs/source/model_doc/gpt2.rst
deleted file mode 100644
index 54ef3cea08c3d864d23a0bf567789ad899de7081..0000000000000000000000000000000000000000
--- a/server/transformers/docs/source/model_doc/gpt2.rst
+++ /dev/null
@@ -1,91 +0,0 @@
-OpenAI GPT2
-----------------------------------------------------
-
-Overview
-~~~~~~~~~~~~~~~~~~~~~
-
-OpenAI GPT-2 model was proposed in
-`Language Models are Unsupervised Multitask Learners`_
-by Alec Radford*, Jeffrey Wu*, Rewon Child, David Luan, Dario Amodei** and Ilya Sutskever**.
-It's a causal (unidirectional) transformer pre-trained using language modeling on a very large
-corpus of ~40 GB of text data.
-
-The abstract from the paper is the following:
-
-*GPT-2 is a large transformer-based language model with 1.5 billion parameters, trained on a dataset[1]
-of 8 million web pages. GPT-2 is trained with a simple objective: predict the next word, given all of the previous
-words within some text. The diversity of the dataset causes this simple goal to contain naturally occurring
-demonstrations of many tasks across diverse domains. GPT-2 is a direct scale-up of GPT, with more than 10X
-the parameters and trained on more than 10X the amount of data.*
-
-Tips:
-
-- GPT-2 is a model with absolute position embeddings so it's usually advised to pad the inputs on
- the right rather than the left.
-- GPT-2 was trained with a causal language modeling (CLM) objective and is therefore powerful at predicting the next
- token in a sequence. Leveraging this feature allows GPT-2 to generate syntactically coherent text as
- it can be observed in the `run_generation.py` example script.
-- The PyTorch models can take the `past` as input, which is the previously computed key/value attention pairs. Using
- this `past` value prevents the model from re-computing pre-computed values in the context of text generation.
- See `reusing the past in generative models <../quickstart.html#using-the-past>`_ for more information on the usage
- of this argument.
-
-`Write With Transformer `__ is a webapp created and hosted by
-Hugging Face showcasing the generative capabilities of several models. GPT-2 is one of them and is available in five
-different sizes: small, medium, large, xl and a distilled version of the small checkpoint: distilgpt-2.
-
-
-GPT2Config
-~~~~~~~~~~~~~~~~~~~~~
-
-.. autoclass:: transformers.GPT2Config
- :members:
-
-
-GPT2Tokenizer
-~~~~~~~~~~~~~~~~~~~~~
-
-.. autoclass:: transformers.GPT2Tokenizer
- :members:
-
-
-GPT2Model
-~~~~~~~~~~~~~~~~~~~~~
-
-.. autoclass:: transformers.GPT2Model
- :members:
-
-
-GPT2LMHeadModel
-~~~~~~~~~~~~~~~~~~~~~~~~~~~
-
-.. autoclass:: transformers.GPT2LMHeadModel
- :members:
-
-
-GPT2DoubleHeadsModel
-~~~~~~~~~~~~~~~~~~~~~~~~~~~~~~~~
-
-.. autoclass:: transformers.GPT2DoubleHeadsModel
- :members:
-
-
-TFGPT2Model
-~~~~~~~~~~~~~~~~~~~~~
-
-.. autoclass:: transformers.TFGPT2Model
- :members:
-
-
-TFGPT2LMHeadModel
-~~~~~~~~~~~~~~~~~~~~~~~~~~~
-
-.. autoclass:: transformers.TFGPT2LMHeadModel
- :members:
-
-
-TFGPT2DoubleHeadsModel
-~~~~~~~~~~~~~~~~~~~~~~~~~~~~~~~~
-
-.. autoclass:: transformers.TFGPT2DoubleHeadsModel
- :members:
diff --git a/server/transformers/docs/source/model_doc/roberta.rst b/server/transformers/docs/source/model_doc/roberta.rst
deleted file mode 100644
index d3276d55e0bbfa233629d45fe4de8da4eed09331..0000000000000000000000000000000000000000
--- a/server/transformers/docs/source/model_doc/roberta.rst
+++ /dev/null
@@ -1,94 +0,0 @@
-RoBERTa
-----------------------------------------------------
-
-The RoBERTa model was proposed in `RoBERTa: A Robustly Optimized BERT Pretraining Approach `_
-by Yinhan Liu, Myle Ott, Naman Goyal, Jingfei Du, Mandar Joshi, Danqi Chen, Omer Levy, Mike Lewis, Luke Zettlemoyer,
-Veselin Stoyanov. It is based on Google's BERT model released in 2018.
-
-It builds on BERT and modifies key hyperparameters, removing the next-sentence pretraining
-objective and training with much larger mini-batches and learning rates.
-
-The abstract from the paper is the following:
-
-*Language model pretraining has led to significant performance gains but careful comparison between different
-approaches is challenging. Training is computationally expensive, often done on private datasets of different sizes,
-and, as we will show, hyperparameter choices have significant impact on the final results. We present a replication
-study of BERT pretraining (Devlin et al., 2019) that carefully measures the impact of many key hyperparameters and
-training data size. We find that BERT was significantly undertrained, and can match or exceed the performance of
-every model published after it. Our best model achieves state-of-the-art results on GLUE, RACE and SQuAD. These
-results highlight the importance of previously overlooked design choices, and raise questions about the source
-of recently reported improvements. We release our models and code.*
-
-Tips:
-
-- This implementation is the same as :class:`~transformers.BertModel` with a tiny embeddings tweak as well as a
- setup for Roberta pretrained models.
-- `Camembert <./camembert.html>`__ is a wrapper around RoBERTa. Refer to this page for usage examples.
-
-RobertaConfig
-~~~~~~~~~~~~~~~~~~~~~
-
-.. autoclass:: transformers.RobertaConfig
- :members:
-
-
-RobertaTokenizer
-~~~~~~~~~~~~~~~~~~~~~
-
-.. autoclass:: transformers.RobertaTokenizer
- :members:
-
-
-RobertaModel
-~~~~~~~~~~~~~~~~~~~~
-
-.. autoclass:: transformers.RobertaModel
- :members:
-
-
-RobertaForMaskedLM
-~~~~~~~~~~~~~~~~~~~~~~~~~~
-
-.. autoclass:: transformers.RobertaForMaskedLM
- :members:
-
-
-RobertaForSequenceClassification
-~~~~~~~~~~~~~~~~~~~~~~~~~~~~~~~~~~~~~~~~
-
-.. autoclass:: transformers.RobertaForSequenceClassification
- :members:
-
-
-RobertaForTokenClassification
-~~~~~~~~~~~~~~~~~~~~~~~~~~~~~~~~~~~~~~~~
-
-.. autoclass:: transformers.RobertaForTokenClassification
- :members:
-
-TFRobertaModel
-~~~~~~~~~~~~~~~~~~~~
-
-.. autoclass:: transformers.TFRobertaModel
- :members:
-
-
-TFRobertaForMaskedLM
-~~~~~~~~~~~~~~~~~~~~~~~~~~
-
-.. autoclass:: transformers.TFRobertaForMaskedLM
- :members:
-
-
-TFRobertaForSequenceClassification
-~~~~~~~~~~~~~~~~~~~~~~~~~~~~~~~~~~~~~~~~
-
-.. autoclass:: transformers.TFRobertaForSequenceClassification
- :members:
-
-
-TFRobertaForTokenClassification
-~~~~~~~~~~~~~~~~~~~~~~~~~~~~~~~~~~~~~~~~
-
-.. autoclass:: transformers.TFRobertaForTokenClassification
- :members:
diff --git a/server/transformers/docs/source/model_doc/transformerxl.rst b/server/transformers/docs/source/model_doc/transformerxl.rst
deleted file mode 100644
index 5240df3df4aec29fefd7032e39c0bca78a4f379e..0000000000000000000000000000000000000000
--- a/server/transformers/docs/source/model_doc/transformerxl.rst
+++ /dev/null
@@ -1,73 +0,0 @@
-Transformer XL
-----------------------------------------------------
-
-Overview
-~~~~~~~~~~~~~~~~~~~~~
-
-The Transformer-XL model was proposed in
-`Transformer-XL: Attentive Language Models Beyond a Fixed-Length Context `__
-by Zihang Dai*, Zhilin Yang*, Yiming Yang, Jaime Carbonell, Quoc V. Le, Ruslan Salakhutdinov.
-It's a causal (uni-directional) transformer with relative positioning (sinusoïdal) embeddings which can reuse
-previously computed hidden-states to attend to longer context (memory).
-This model also uses adaptive softmax inputs and outputs (tied).
-
-The abstract from the paper is the following:
-
-*Transformers have a potential of learning longer-term dependency, but are limited by a fixed-length context in the
-setting of language modeling. We propose a novel neural architecture Transformer-XL that enables learning dependency
-beyond a fixed length without disrupting temporal coherence. It consists of a segment-level recurrence mechanism and
-a novel positional encoding scheme. Our method not only enables capturing longer-term dependency, but also resolves
-the context fragmentation problem. As a result, Transformer-XL learns dependency that is 80% longer than RNNs and
-450% longer than vanilla Transformers, achieves better performance on both short and long sequences, and is up
-to 1,800+ times faster than vanilla Transformers during evaluation. Notably, we improve the state-of-the-art results
-of bpc/perplexity to 0.99 on enwiki8, 1.08 on text8, 18.3 on WikiText-103, 21.8 on One Billion Word, and 54.5 on
-Penn Treebank (without finetuning). When trained only on WikiText-103, Transformer-XL manages to generate reasonably
-coherent, novel text articles with thousands of tokens.*
-
-Tips:
-
-- Transformer-XL uses relative sinusoidal positional embeddings. Padding can be done on the left or on the right.
- The original implementation trains on SQuAD with padding on the left, therefore the padding defaults are set to left.
-- Transformer-XL is one of the few models that has no sequence length limit.
-
-
-TransfoXLConfig
-~~~~~~~~~~~~~~~~~~~~~
-
-.. autoclass:: transformers.TransfoXLConfig
- :members:
-
-
-TransfoXLTokenizer
-~~~~~~~~~~~~~~~~~~~~~~~~~~
-
-.. autoclass:: transformers.TransfoXLTokenizer
- :members:
-
-
-TransfoXLModel
-~~~~~~~~~~~~~~~~~~~~~~~~~~
-
-.. autoclass:: transformers.TransfoXLModel
- :members:
-
-
-TransfoXLLMHeadModel
-~~~~~~~~~~~~~~~~~~~~~~~~~~~~~~~~
-
-.. autoclass:: transformers.TransfoXLLMHeadModel
- :members:
-
-
-TFTransfoXLModel
-~~~~~~~~~~~~~~~~~~~~~~~~~~
-
-.. autoclass:: transformers.TFTransfoXLModel
- :members:
-
-
-TFTransfoXLLMHeadModel
-~~~~~~~~~~~~~~~~~~~~~~~~~~~~~~~~
-
-.. autoclass:: transformers.TFTransfoXLLMHeadModel
- :members:
diff --git a/server/transformers/docs/source/model_doc/xlm.rst b/server/transformers/docs/source/model_doc/xlm.rst
deleted file mode 100644
index 73466937523efabbc60c821319222c81b817e6df..0000000000000000000000000000000000000000
--- a/server/transformers/docs/source/model_doc/xlm.rst
+++ /dev/null
@@ -1,106 +0,0 @@
-XLM
-----------------------------------------------------
-
-Overview
-~~~~~~~~~~~~~~~~~~~~~
-
-The XLM model was proposed in `Cross-lingual Language Model Pretraining `_
-by Guillaume Lample*, Alexis Conneau*. It's a transformer pre-trained using one of the following objectives:
-
-- a causal language modeling (CLM) objective (next token prediction),
-- a masked language modeling (MLM) objective (Bert-like), or
-- a Translation Language Modeling (TLM) object (extension of Bert's MLM to multiple language inputs)
-
-The abstract from the paper is the following:
-
-*Recent studies have demonstrated the efficiency of generative pretraining for English natural language understanding.
-In this work, we extend this approach to multiple languages and show the effectiveness of cross-lingual pretraining.
-We propose two methods to learn cross-lingual language models (XLMs): one unsupervised that only relies on monolingual
-data, and one supervised that leverages parallel data with a new cross-lingual language model objective. We obtain
-state-of-the-art results on cross-lingual classification, unsupervised and supervised machine translation. On XNLI,
-our approach pushes the state of the art by an absolute gain of 4.9% accuracy. On unsupervised machine translation,
-we obtain 34.3 BLEU on WMT'16 German-English, improving the previous state of the art by more than 9 BLEU. On
-supervised machine translation, we obtain a new state of the art of 38.5 BLEU on WMT'16 Romanian-English, outperforming
-the previous best approach by more than 4 BLEU. Our code and pretrained models will be made publicly available.*
-
-Tips:
-
-- XLM has many different checkpoints, which were trained using different objectives: CLM, MLM or TLM. Make sure to
- select the correct objective for your task (e.g. MLM checkpoints are not suitable for generation).
-- XLM has multilingual checkpoints which leverage a specific `lang` parameter. Check out the
- `multi-lingual <../multilingual.html>`__ page for more information.
-
-
-XLMConfig
-~~~~~~~~~~~~~~~~~~~~~~~~~~~~~~~~~~~~~~~~~~
-
-.. autoclass:: transformers.XLMConfig
- :members:
-
-XLMTokenizer
-~~~~~~~~~~~~~~~~~~~~~~~~~~~~~~~~~~~~~~~~~~
-
-.. autoclass:: transformers.XLMTokenizer
- :members:
-
-XLMModel
-~~~~~~~~~~~~~~~~~~~~~~~~~~~~~~~~~~~~~~~~~~~~~~~
-
-.. autoclass:: transformers.XLMModel
- :members:
-
-
-XLMWithLMHeadModel
-~~~~~~~~~~~~~~~~~~~~~~~~~~~~~~~~~~~~~~~~~~~~~~~
-
-.. autoclass:: transformers.XLMWithLMHeadModel
- :members:
-
-
-XLMForSequenceClassification
-~~~~~~~~~~~~~~~~~~~~~~~~~~~~~~~~~~~~~~~~~~~~~~~~~~~~~
-
-.. autoclass:: transformers.XLMForSequenceClassification
- :members:
-
-
-XLMForQuestionAnsweringSimple
-~~~~~~~~~~~~~~~~~~~~~~~~~~~~~~~~~~~~~~~~~~~~~~~~~~~~~
-
-.. autoclass:: transformers.XLMForQuestionAnsweringSimple
- :members:
-
-
-XLMForQuestionAnswering
-~~~~~~~~~~~~~~~~~~~~~~~~~~~~~~~~~~~~~~~~~~~~~~~~~~~~~
-
-.. autoclass:: transformers.XLMForQuestionAnswering
- :members:
-
-
-TFXLMModel
-~~~~~~~~~~~~~~~~~~~~~~~~~~~~~~~~~~~~~~~~~~~~~~~
-
-.. autoclass:: transformers.TFXLMModel
- :members:
-
-
-TFXLMWithLMHeadModel
-~~~~~~~~~~~~~~~~~~~~~~~~~~~~~~~~~~~~~~~~~~~~~~~
-
-.. autoclass:: transformers.TFXLMWithLMHeadModel
- :members:
-
-
-TFXLMForSequenceClassification
-~~~~~~~~~~~~~~~~~~~~~~~~~~~~~~~~~~~~~~~~~~~~~~~~~~~~~
-
-.. autoclass:: transformers.TFXLMForSequenceClassification
- :members:
-
-
-TFXLMForQuestionAnsweringSimple
-~~~~~~~~~~~~~~~~~~~~~~~~~~~~~~~~~~~~~~~~~~~~~~~~~~~~~
-
-.. autoclass:: transformers.TFXLMForQuestionAnsweringSimple
- :members:
diff --git a/server/transformers/docs/source/model_doc/xlmroberta.rst b/server/transformers/docs/source/model_doc/xlmroberta.rst
deleted file mode 100644
index 8ddb38b1c2159334e4878cdcd0da9c925d7e7aa5..0000000000000000000000000000000000000000
--- a/server/transformers/docs/source/model_doc/xlmroberta.rst
+++ /dev/null
@@ -1,102 +0,0 @@
-XLM-RoBERTa
-------------------------------------------
-
-The XLM-RoBERTa model was proposed in `Unsupervised Cross-lingual Representation Learning at Scale `__
-by Alexis Conneau, Kartikay Khandelwal, Naman Goyal, Vishrav Chaudhary, Guillaume Wenzek, Francisco Guzmán,
-Edouard Grave, Myle Ott, Luke Zettlemoyer and Veselin Stoyanov. It is based on Facebook's RoBERTa model released in 2019.
-It is a large multi-lingual language model, trained on 2.5TB of filtered CommonCrawl data.
-
-The abstract from the paper is the following:
-
-*This paper shows that pretraining multilingual language models at scale leads to significant performance gains for
-a wide range of cross-lingual transfer tasks. We train a Transformer-based masked language model on one hundred
-languages, using more than two terabytes of filtered CommonCrawl data. Our model, dubbed XLM-R, significantly
-outperforms multilingual BERT (mBERT) on a variety of cross-lingual benchmarks, including +13.8% average accuracy
-on XNLI, +12.3% average F1 score on MLQA, and +2.1% average F1 score on NER. XLM-R performs particularly well on
-low-resource languages, improving 11.8% in XNLI accuracy for Swahili and 9.2% for Urdu over the previous XLM model.
-We also present a detailed empirical evaluation of the key factors that are required to achieve these gains,
-including the trade-offs between (1) positive transfer and capacity dilution and (2) the performance of high and
-low resource languages at scale. Finally, we show, for the first time, the possibility of multilingual modeling
-without sacrificing per-language performance; XLM-Ris very competitive with strong monolingual models on the GLUE
-and XNLI benchmarks. We will make XLM-R code, data, and models publicly available.*
-
-Tips:
-
-- This implementation is the same as RoBERTa. Refer to the `documentation of RoBERTa <./roberta.html>`__ for usage
- examples as well as the information relative to the inputs and outputs.
-
-XLMRobertaConfig
-~~~~~~~~~~~~~~~~~~~~~~~~~~~~~~~~~~~~~~~~~~~~~~
-
-.. autoclass:: transformers.XLMRobertaConfig
- :members:
-
-
-XLMRobertaTokenizer
-~~~~~~~~~~~~~~~~~~~~~~~~~~~~~~~~~~~~~~~~~~~~~~
-
-.. autoclass:: transformers.XLMRobertaTokenizer
- :members:
-
-
-XLMRobertaModel
-~~~~~~~~~~~~~~~~~~~~~~~~~~~~~~~~~~~~~~~~~~~~~~
-
-.. autoclass:: transformers.XLMRobertaModel
- :members:
-
-
-XLMRobertaForMaskedLM
-~~~~~~~~~~~~~~~~~~~~~~~~~~~~~~~~~~~~~~~~~~~~~~
-
-.. autoclass:: transformers.XLMRobertaForMaskedLM
- :members:
-
-
-XLMRobertaForSequenceClassification
-~~~~~~~~~~~~~~~~~~~~~~~~~~~~~~~~~~~~~~~~~~~~~~
-
-.. autoclass:: transformers.XLMRobertaForSequenceClassification
- :members:
-
-
-XLMRobertaForMultipleChoice
-~~~~~~~~~~~~~~~~~~~~~~~~~~~~~~~~~~~~~~~~~~~~~~
-
-.. autoclass:: transformers.XLMRobertaForMultipleChoice
- :members:
-
-
-XLMRobertaForTokenClassification
-~~~~~~~~~~~~~~~~~~~~~~~~~~~~~~~~~~~~~~~~~~~~~~
-
-.. autoclass:: transformers.XLMRobertaForTokenClassification
- :members:
-
-
-TFXLMRobertaModel
-~~~~~~~~~~~~~~~~~~~~~~~~~~~~~~~~~~~~~~~~~~~~~~
-
-.. autoclass:: transformers.TFXLMRobertaModel
- :members:
-
-
-TFXLMRobertaForMaskedLM
-~~~~~~~~~~~~~~~~~~~~~~~~~~~~~~~~~~~~~~~~~~~~~~
-
-.. autoclass:: transformers.TFXLMRobertaForMaskedLM
- :members:
-
-
-TFXLMRobertaForSequenceClassification
-~~~~~~~~~~~~~~~~~~~~~~~~~~~~~~~~~~~~~~~~~~~~~~
-
-.. autoclass:: transformers.TFXLMRobertaForSequenceClassification
- :members:
-
-
-TFXLMRobertaForTokenClassification
-~~~~~~~~~~~~~~~~~~~~~~~~~~~~~~~~~~~~~~~~~~~~~~
-
-.. autoclass:: transformers.TFXLMRobertaForTokenClassification
- :members:
diff --git a/server/transformers/docs/source/model_doc/xlnet.rst b/server/transformers/docs/source/model_doc/xlnet.rst
deleted file mode 100644
index 0f8c61098c60bd2195f554dc1d8de8fe164428a7..0000000000000000000000000000000000000000
--- a/server/transformers/docs/source/model_doc/xlnet.rst
+++ /dev/null
@@ -1,124 +0,0 @@
-XLNet
-----------------------------------------------------
-
-Overview
-~~~~~~~~~~~~~~~~~~~~~
-
-The XLNet model was proposed in `XLNet: Generalized Autoregressive Pretraining for Language Understanding `_
-by Zhilin Yang*, Zihang Dai*, Yiming Yang, Jaime Carbonell, Ruslan Salakhutdinov, Quoc V. Le.
-XLnet is an extension of the Transformer-XL model pre-trained using an autoregressive method
-to learn bidirectional contexts by maximizing the expected likelihood over all permutations
-of the input sequence factorization order.
-
-The abstract from the paper is the following:
-
-*With the capability of modeling bidirectional contexts, denoising autoencoding based pretraining like BERT achieves
-better performance than pretraining approaches based on autoregressive language modeling. However, relying on
-corrupting the input with masks, BERT neglects dependency between the masked positions and suffers from a
-pretrain-finetune discrepancy. In light of these pros and cons, we propose XLNet, a generalized autoregressive
-pretraining method that (1) enables learning bidirectional contexts by maximizing the expected likelihood over
-all permutations of the factorization order and (2) overcomes the limitations of BERT thanks to its autoregressive
-formulation. Furthermore, XLNet integrates ideas from Transformer-XL, the state-of-the-art autoregressive model,
-into pretraining. Empirically, under comparable experiment settings, XLNet outperforms BERT on 20 tasks, often by
-a large margin, including question answering, natural language inference, sentiment analysis, and document ranking.*
-
-Tips:
-
-- The specific attention pattern can be controlled at training and test time using the `perm_mask` input.
-- Due to the difficulty of training a fully auto-regressive model over various factorization order,
- XLNet is pretrained using only a sub-set of the output tokens as target which are selected
- with the `target_mapping` input.
-- To use XLNet for sequential decoding (i.e. not in fully bi-directional setting), use the `perm_mask` and
- `target_mapping` inputs to control the attention span and outputs (see examples in `examples/run_generation.py`)
-- XLNet is one of the few models that has no sequence length limit.
-
-
-XLNetConfig
-~~~~~~~~~~~~~~~~~~~~~~~~~~~~~~~~~~~~~~~~~~
-
-.. autoclass:: transformers.XLNetConfig
- :members:
-
-
-XLNetTokenizer
-~~~~~~~~~~~~~~~~~~~~~~~~~~~~~~~~~~~~~~~~~~
-
-.. autoclass:: transformers.XLNetTokenizer
- :members:
-
-
-XLNetModel
-~~~~~~~~~~~~~~~~~~~~~~~~~~~~~~~~~~~~~~~~~~~~~~~
-
-.. autoclass:: transformers.XLNetModel
- :members:
-
-
-XLNetLMHeadModel
-~~~~~~~~~~~~~~~~~~~~~~~~~~~~~~~~~~~~~~~~~~~~~~~
-
-.. autoclass:: transformers.XLNetLMHeadModel
- :members:
-
-
-XLNetForSequenceClassification
-~~~~~~~~~~~~~~~~~~~~~~~~~~~~~~~~~~~~~~~~~~~~~~~~~~~~~
-
-.. autoclass:: transformers.XLNetForSequenceClassification
- :members:
-
-
-XLNetForTokenClassification
-~~~~~~~~~~~~~~~~~~~~~~~~~~~~~~~~~~~~~~~~~~~~~~~~~~~~~
-
-.. autoclass:: transformers.XLNetForTokenClassification
- :members:
-
-
-XLNetForMultipleChoice
-~~~~~~~~~~~~~~~~~~~~~~~~~~~~~~~~~~~~~~~~~~~~~~~~~~~~~
-
-.. autoclass:: transformers.XLNetForMultipleChoice
- :members:
-
-
-XLNetForQuestionAnsweringSimple
-~~~~~~~~~~~~~~~~~~~~~~~~~~~~~~~~~~~~~~~~~~~~~~~~~~~~~
-
-.. autoclass:: transformers.XLNetForQuestionAnsweringSimple
- :members:
-
-
-XLNetForQuestionAnswering
-~~~~~~~~~~~~~~~~~~~~~~~~~~~~~~~~~~~~~~~~~~~~~~~~~~~~~
-
-.. autoclass:: transformers.XLNetForQuestionAnswering
- :members:
-
-
-TFXLNetModel
-~~~~~~~~~~~~~~~~~~~~~~~~~~~~~~~~~~~~~~~~~~~~~~~
-
-.. autoclass:: transformers.TFXLNetModel
- :members:
-
-
-TFXLNetLMHeadModel
-~~~~~~~~~~~~~~~~~~~~~~~~~~~~~~~~~~~~~~~~~~~~~~~
-
-.. autoclass:: transformers.TFXLNetLMHeadModel
- :members:
-
-
-TFXLNetForSequenceClassification
-~~~~~~~~~~~~~~~~~~~~~~~~~~~~~~~~~~~~~~~~~~~~~~~~~~~~~
-
-.. autoclass:: transformers.TFXLNetForSequenceClassification
- :members:
-
-
-TFXLNetForQuestionAnsweringSimple
-~~~~~~~~~~~~~~~~~~~~~~~~~~~~~~~~~~~~~~~~~~~~~~~~~~~~~
-
-.. autoclass:: transformers.TFXLNetForQuestionAnsweringSimple
- :members:
diff --git a/server/transformers/docs/source/model_sharing.md b/server/transformers/docs/source/model_sharing.md
deleted file mode 100644
index 03ea4c3d8060cf64c03771071e4d8cda58bfc1b4..0000000000000000000000000000000000000000
--- a/server/transformers/docs/source/model_sharing.md
+++ /dev/null
@@ -1,45 +0,0 @@
-# Model upload and sharing
-
-Starting with `v2.2.2`, you can now upload and share your fine-tuned models with the community, using the CLI that's built-in to the library.
-
-**First, create an account on [https://huggingface.co/join](https://huggingface.co/join)**. Then:
-
-```shell
-transformers-cli login
-# log in using the same credentials as on huggingface.co
-```
-Upload your model:
-```shell
-transformers-cli upload ./path/to/pretrained_model/
-
-# ^^ Upload folder containing weights/tokenizer/config
-# saved via `.save_pretrained()`
-
-transformers-cli upload ./config.json [--filename folder/foobar.json]
-
-# ^^ Upload a single file
-# (you can optionally override its filename, which can be nested inside a folder)
-```
-
-Your model will then be accessible through its identifier, a concatenation of your username and the folder name above:
-```python
-"username/pretrained_model"
-```
-
-Anyone can load it from code:
-```python
-tokenizer = AutoTokenizer.from_pretrained("username/pretrained_model")
-model = AutoModel.from_pretrained("username/pretrained_model")
-```
-
-Finally, list all your files on S3:
-```shell
-transformers-cli s3 ls
-# List all your S3 objects.
-```
-
-You can also delete files:
-
-```shell
-transformers-cli s3 rm …
-```
\ No newline at end of file
diff --git a/server/transformers/docs/source/multilingual.rst b/server/transformers/docs/source/multilingual.rst
deleted file mode 100644
index f6f72b2434e8480874c4e13f88b6ab156b326ea7..0000000000000000000000000000000000000000
--- a/server/transformers/docs/source/multilingual.rst
+++ /dev/null
@@ -1,103 +0,0 @@
-Multi-lingual models
-================================================
-
-Most of the models available in this library are mono-lingual models (English, Chinese and German). A few
-multi-lingual models are available and have a different mechanisms than mono-lingual models.
-This page details the usage of these models.
-
-The two models that currently support multiple languages are BERT and XLM.
-
-XLM
-^^^^^^^^^^^^^^^^^^^^^^^^^^^^^^^^^^^^^^^^^^^^
-
-XLM has a total of 10 different checkpoints, only one of which is mono-lingual. The 9 remaining model checkpoints can
-be split in two categories: the checkpoints that make use of language embeddings, and those that don't
-
-XLM & Language Embeddings
-------------------------------------------------
-
-This section concerns the following checkpoints:
-
-- ``xlm-mlm-ende-1024`` (Masked language modeling, English-German)
-- ``xlm-mlm-enfr-1024`` (Masked language modeling, English-French)
-- ``xlm-mlm-enro-1024`` (Masked language modeling, English-Romanian)
-- ``xlm-mlm-xnli15-1024`` (Masked language modeling, XNLI languages)
-- ``xlm-mlm-tlm-xnli15-1024`` (Masked language modeling + Translation, XNLI languages)
-- ``xlm-clm-enfr-1024`` (Causal language modeling, English-French)
-- ``xlm-clm-ende-1024`` (Causal language modeling, English-German)
-
-These checkpoints require language embeddings that will specify the language used at inference time. These language
-embeddings are represented as a tensor that is of the same shape as the input ids passed to the model. The values in
-these tensors depend on the language used and are identifiable using the ``lang2id`` and ``id2lang`` attributes
-from the tokenizer.
-
-Here is an example using the ``xlm-clm-enfr-1024`` checkpoint (Causal language modeling, English-French):
-
-
-.. code-block::
-
- import torch
- from transformers import XLMTokenizer, XLMWithLMHeadModel
-
- tokenizer = XLMTokenizer.from_pretrained("xlm-clm-1024-enfr")
-
-
-The different languages this model/tokenizer handles, as well as the ids of these languages are visible using the
-``lang2id`` attribute:
-
-.. code-block::
-
- print(tokenizer.lang2id) # {'en': 0, 'fr': 1}
-
-
-These ids should be used when passing a language parameter during a model pass. Let's define our inputs:
-
-.. code-block::
-
- input_ids = torch.tensor([tokenizer.encode("Wikipedia was used to")]) # batch size of 1
-
-
-We should now define the language embedding by using the previously defined language id. We want to create a tensor
-filled with the appropriate language ids, of the same size as input_ids. For english, the id is 0:
-
-.. code-block::
-
- language_id = tokenizer.lang2id['en'] # 0
- langs = torch.tensor([language_id] * input_ids.shape[1]) # torch.tensor([0, 0, 0, ..., 0])
-
- # We reshape it to be of size (batch_size, sequence_length)
- langs = langs.view(1, -1) # is now of shape [1, sequence_length] (we have a batch size of 1)
-
-
-You can then feed it all as input to your model:
-
-.. code-block::
-
- outputs = model(input_ids, langs=langs)
-
-
-The example `run_generation.py `__
-can generate text using the CLM checkpoints from XLM, using the language embeddings.
-
-XLM without Language Embeddings
-------------------------------------------------
-
-This section concerns the following checkpoints:
-
-- ``xlm-mlm-17-1280`` (Masked language modeling, 17 languages)
-- ``xlm-mlm-100-1280`` (Masked language modeling, 100 languages)
-
-These checkpoints do not require language embeddings at inference time. These models are used to have generic
-sentence representations, differently from previously-mentioned XLM checkpoints.
-
-
-BERT
-^^^^^^^^^^^^^^^^^^^^^^^^^^^^^^^^^^^^^^^^^^^^
-
-BERT has two checkpoints that can be used for multi-lingual tasks:
-
-- ``bert-base-multilingual-uncased`` (Masked language modeling + Next sentence prediction, 102 languages)
-- ``bert-base-multilingual-cased`` (Masked language modeling + Next sentence prediction, 104 languages)
-
-These checkpoints do not require language embeddings at inference time. They should identify the language
-used in the context and infer accordingly.
\ No newline at end of file
diff --git a/server/transformers/docs/source/notebooks.rst b/server/transformers/docs/source/notebooks.rst
deleted file mode 100644
index fe669e8e47f8bf76fa26380e46ea74d951e135be..0000000000000000000000000000000000000000
--- a/server/transformers/docs/source/notebooks.rst
+++ /dev/null
@@ -1,16 +0,0 @@
-Notebooks
-================================================
-
-We include `three Jupyter Notebooks `_ that can be used to check that the predictions of the PyTorch model are identical to the predictions of the original TensorFlow model.
-
-
-*
- The first NoteBook (\ `Comparing-TF-and-PT-models.ipynb `_\ ) extracts the hidden states of a full sequence on each layers of the TensorFlow and the PyTorch models and computes the standard deviation between them. In the given example, we get a standard deviation of 1.5e-7 to 9e-7 on the various hidden state of the models.
-
-*
- The second NoteBook (\ `Comparing-TF-and-PT-models-SQuAD.ipynb `_\ ) compares the loss computed by the TensorFlow and the PyTorch models for identical initialization of the fine-tuning layer of the ``BertForQuestionAnswering`` and computes the standard deviation between them. In the given example, we get a standard deviation of 2.5e-7 between the models.
-
-*
- The third NoteBook (\ `Comparing-TF-and-PT-models-MLM-NSP.ipynb `_\ ) compares the predictions computed by the TensorFlow and the PyTorch models for masked token language modeling using the pre-trained masked language modeling model.
-
-Please follow the instructions given in the notebooks to run and modify them.
diff --git a/server/transformers/docs/source/pretrained_models.rst b/server/transformers/docs/source/pretrained_models.rst
deleted file mode 100644
index e124e414c91a62485712ed08427de24e05bbd861..0000000000000000000000000000000000000000
--- a/server/transformers/docs/source/pretrained_models.rst
+++ /dev/null
@@ -1,272 +0,0 @@
-Pretrained models
-================================================
-
-Here is the full list of the currently provided pretrained models together with a short presentation of each model.
-
-For a list that includes community-uploaded models, refer to `https://huggingface.co/models `__.
-
-+-------------------+------------------------------------------------------------+---------------------------------------------------------------------------------------------------------------------------------------+
-| Architecture | Shortcut name | Details of the model |
-+===================+============================================================+=======================================================================================================================================+
-| BERT | ``bert-base-uncased`` | | 12-layer, 768-hidden, 12-heads, 110M parameters. |
-| | | | Trained on lower-cased English text. |
-| +------------------------------------------------------------+---------------------------------------------------------------------------------------------------------------------------------------+
-| | ``bert-large-uncased`` | | 24-layer, 1024-hidden, 16-heads, 340M parameters. |
-| | | | Trained on lower-cased English text. |
-| +------------------------------------------------------------+---------------------------------------------------------------------------------------------------------------------------------------+
-| | ``bert-base-cased`` | | 12-layer, 768-hidden, 12-heads, 110M parameters. |
-| | | | Trained on cased English text. |
-| +------------------------------------------------------------+---------------------------------------------------------------------------------------------------------------------------------------+
-| | ``bert-large-cased`` | | 24-layer, 1024-hidden, 16-heads, 340M parameters. |
-| | | | Trained on cased English text. |
-| +------------------------------------------------------------+---------------------------------------------------------------------------------------------------------------------------------------+
-| | ``bert-base-multilingual-uncased`` | | (Original, not recommended) 12-layer, 768-hidden, 12-heads, 110M parameters. |
-| | | | Trained on lower-cased text in the top 102 languages with the largest Wikipedias |
-| | | (see `details `__). |
-| +------------------------------------------------------------+---------------------------------------------------------------------------------------------------------------------------------------+
-| | ``bert-base-multilingual-cased`` | | (New, **recommended**) 12-layer, 768-hidden, 12-heads, 110M parameters. |
-| | | | Trained on cased text in the top 104 languages with the largest Wikipedias |
-| | | (see `details `__). |
-| +------------------------------------------------------------+---------------------------------------------------------------------------------------------------------------------------------------+
-| | ``bert-base-chinese`` | | 12-layer, 768-hidden, 12-heads, 110M parameters. |
-| | | | Trained on cased Chinese Simplified and Traditional text. |
-| +------------------------------------------------------------+---------------------------------------------------------------------------------------------------------------------------------------+
-| | ``bert-base-german-cased`` | | 12-layer, 768-hidden, 12-heads, 110M parameters. |
-| | | | Trained on cased German text by Deepset.ai |
-| | | (see `details on deepset.ai website `__). |
-| +------------------------------------------------------------+---------------------------------------------------------------------------------------------------------------------------------------+
-| | ``bert-large-uncased-whole-word-masking`` | | 24-layer, 1024-hidden, 16-heads, 340M parameters. |
-| | | | Trained on lower-cased English text using Whole-Word-Masking |
-| | | (see `details `__). |
-| +------------------------------------------------------------+---------------------------------------------------------------------------------------------------------------------------------------+
-| | ``bert-large-cased-whole-word-masking`` | | 24-layer, 1024-hidden, 16-heads, 340M parameters. |
-| | | | Trained on cased English text using Whole-Word-Masking |
-| | | (see `details `__). |
-| +------------------------------------------------------------+---------------------------------------------------------------------------------------------------------------------------------------+
-| | ``bert-large-uncased-whole-word-masking-finetuned-squad`` | | 24-layer, 1024-hidden, 16-heads, 340M parameters. |
-| | | | The ``bert-large-uncased-whole-word-masking`` model fine-tuned on SQuAD |
-| | | (see details of fine-tuning in the `example section `__). |
-| +------------------------------------------------------------+---------------------------------------------------------------------------------------------------------------------------------------+
-| | ``bert-large-cased-whole-word-masking-finetuned-squad`` | | 24-layer, 1024-hidden, 16-heads, 340M parameters |
-| | | | The ``bert-large-cased-whole-word-masking`` model fine-tuned on SQuAD |
-| | | (see `details of fine-tuning in the example section `__) |
-| +------------------------------------------------------------+---------------------------------------------------------------------------------------------------------------------------------------+
-| | ``bert-base-cased-finetuned-mrpc`` | | 12-layer, 768-hidden, 12-heads, 110M parameters. |
-| | | | The ``bert-base-cased`` model fine-tuned on MRPC |
-| | | (see `details of fine-tuning in the example section `__) |
-| +------------------------------------------------------------+---------------------------------------------------------------------------------------------------------------------------------------+
-| | ``bert-base-german-dbmdz-cased`` | | 12-layer, 768-hidden, 12-heads, 110M parameters. |
-| | | | Trained on cased German text by DBMDZ |
-| | | (see `details on dbmdz repository `__). |
-| +------------------------------------------------------------+---------------------------------------------------------------------------------------------------------------------------------------+
-| | ``bert-base-german-dbmdz-uncased`` | | 12-layer, 768-hidden, 12-heads, 110M parameters. |
-| | | | Trained on uncased German text by DBMDZ |
-| | | (see `details on dbmdz repository `__). |
-| +------------------------------------------------------------+---------------------------------------------------------------------------------------------------------------------------------------+
-| | ``bert-base-japanese`` | | 12-layer, 768-hidden, 12-heads, 110M parameters. |
-| | | | Trained on Japanese text. Text is tokenized with MeCab and WordPiece. |
-| | | | `MeCab `__ is required for tokenization. |
-| | | (see `details on cl-tohoku repository `__). |
-| +------------------------------------------------------------+---------------------------------------------------------------------------------------------------------------------------------------+
-| | ``bert-base-japanese-whole-word-masking`` | | 12-layer, 768-hidden, 12-heads, 110M parameters. |
-| | | | Trained on Japanese text using Whole-Word-Masking. Text is tokenized with MeCab and WordPiece. |
-| | | | `MeCab `__ is required for tokenization. |
-| | | (see `details on cl-tohoku repository `__). |
-| +------------------------------------------------------------+---------------------------------------------------------------------------------------------------------------------------------------+
-| | ``bert-base-japanese-char`` | | 12-layer, 768-hidden, 12-heads, 110M parameters. |
-| | | | Trained on Japanese text. Text is tokenized into characters. |
-| | | (see `details on cl-tohoku repository `__). |
-| +------------------------------------------------------------+---------------------------------------------------------------------------------------------------------------------------------------+
-| | ``bert-base-japanese-char-whole-word-masking`` | | 12-layer, 768-hidden, 12-heads, 110M parameters. |
-| | | | Trained on Japanese text using Whole-Word-Masking. Text is tokenized into characters. |
-| | | (see `details on cl-tohoku repository `__). |
-| +------------------------------------------------------------+---------------------------------------------------------------------------------------------------------------------------------------+
-| | ``bert-base-finnish-cased-v1`` | | 12-layer, 768-hidden, 12-heads, 110M parameters. |
-| | | | Trained on cased Finnish text. |
-| | | (see `details on turkunlp.org `__). |
-| +------------------------------------------------------------+---------------------------------------------------------------------------------------------------------------------------------------+
-| | ``bert-base-finnish-uncased-v1`` | | 12-layer, 768-hidden, 12-heads, 110M parameters. |
-| | | | Trained on uncased Finnish text. |
-| | | (see `details on turkunlp.org `__). |
-| +------------------------------------------------------------+---------------------------------------------------------------------------------------------------------------------------------------+
-| | ``bert-base-dutch-cased`` | | 12-layer, 768-hidden, 12-heads, 110M parameters. |
-| | | | Trained on cased Dutch text. |
-| | | (see `details on wietsedv repository `__). |
-+-------------------+------------------------------------------------------------+---------------------------------------------------------------------------------------------------------------------------------------+
-| GPT | ``openai-gpt`` | | 12-layer, 768-hidden, 12-heads, 110M parameters. |
-| | | | OpenAI GPT English model |
-+-------------------+------------------------------------------------------------+---------------------------------------------------------------------------------------------------------------------------------------+
-| GPT-2 | ``gpt2`` | | 12-layer, 768-hidden, 12-heads, 117M parameters. |
-| | | | OpenAI GPT-2 English model |
-| +------------------------------------------------------------+---------------------------------------------------------------------------------------------------------------------------------------+
-| | ``gpt2-medium`` | | 24-layer, 1024-hidden, 16-heads, 345M parameters. |
-| | | | OpenAI's Medium-sized GPT-2 English model |
-| +------------------------------------------------------------+---------------------------------------------------------------------------------------------------------------------------------------+
-| | ``gpt2-large`` | | 36-layer, 1280-hidden, 20-heads, 774M parameters. |
-| | | | OpenAI's Large-sized GPT-2 English model |
-| +------------------------------------------------------------+---------------------------------------------------------------------------------------------------------------------------------------+
-| | ``gpt2-xl`` | | 48-layer, 1600-hidden, 25-heads, 1558M parameters. |
-| | | | OpenAI's XL-sized GPT-2 English model |
-+-------------------+------------------------------------------------------------+---------------------------------------------------------------------------------------------------------------------------------------+
-| Transformer-XL | ``transfo-xl-wt103`` | | 18-layer, 1024-hidden, 16-heads, 257M parameters. |
-| | | | English model trained on wikitext-103 |
-+-------------------+------------------------------------------------------------+---------------------------------------------------------------------------------------------------------------------------------------+
-| XLNet | ``xlnet-base-cased`` | | 12-layer, 768-hidden, 12-heads, 110M parameters. |
-| | | | XLNet English model |
-| +------------------------------------------------------------+---------------------------------------------------------------------------------------------------------------------------------------+
-| | ``xlnet-large-cased`` | | 24-layer, 1024-hidden, 16-heads, 340M parameters. |
-| | | | XLNet Large English model |
-+-------------------+------------------------------------------------------------+---------------------------------------------------------------------------------------------------------------------------------------+
-| XLM | ``xlm-mlm-en-2048`` | | 12-layer, 2048-hidden, 16-heads |
-| | | | XLM English model |
-| +------------------------------------------------------------+---------------------------------------------------------------------------------------------------------------------------------------+
-| | ``xlm-mlm-ende-1024`` | | 6-layer, 1024-hidden, 8-heads |
-| | | | XLM English-German model trained on the concatenation of English and German wikipedia |
-| +------------------------------------------------------------+---------------------------------------------------------------------------------------------------------------------------------------+
-| | ``xlm-mlm-enfr-1024`` | | 6-layer, 1024-hidden, 8-heads |
-| | | | XLM English-French model trained on the concatenation of English and French wikipedia |
-| +------------------------------------------------------------+---------------------------------------------------------------------------------------------------------------------------------------+
-| | ``xlm-mlm-enro-1024`` | | 6-layer, 1024-hidden, 8-heads |
-| | | | XLM English-Romanian Multi-language model |
-| +------------------------------------------------------------+---------------------------------------------------------------------------------------------------------------------------------------+
-| | ``xlm-mlm-xnli15-1024`` | | 12-layer, 1024-hidden, 8-heads |
-| | | | XLM Model pre-trained with MLM on the `15 XNLI languages `__. |
-| +------------------------------------------------------------+---------------------------------------------------------------------------------------------------------------------------------------+
-| | ``xlm-mlm-tlm-xnli15-1024`` | | 12-layer, 1024-hidden, 8-heads |
-| | | | XLM Model pre-trained with MLM + TLM on the `15 XNLI languages `__. |
-| +------------------------------------------------------------+---------------------------------------------------------------------------------------------------------------------------------------+
-| | ``xlm-clm-enfr-1024`` | | 6-layer, 1024-hidden, 8-heads |
-| | | | XLM English-French model trained with CLM (Causal Language Modeling) on the concatenation of English and French wikipedia |
-| +------------------------------------------------------------+---------------------------------------------------------------------------------------------------------------------------------------+
-| | ``xlm-clm-ende-1024`` | | 6-layer, 1024-hidden, 8-heads |
-| | | | XLM English-German model trained with CLM (Causal Language Modeling) on the concatenation of English and German wikipedia |
-| +------------------------------------------------------------+---------------------------------------------------------------------------------------------------------------------------------------+
-| | ``xlm-mlm-17-1280`` | | 16-layer, 1280-hidden, 16-heads |
-| | | | XLM model trained with MLM (Masked Language Modeling) on 17 languages. |
-| +------------------------------------------------------------+---------------------------------------------------------------------------------------------------------------------------------------+
-| | ``xlm-mlm-100-1280`` | | 16-layer, 1280-hidden, 16-heads |
-| | | | XLM model trained with MLM (Masked Language Modeling) on 100 languages. |
-+-------------------+------------------------------------------------------------+---------------------------------------------------------------------------------------------------------------------------------------+
-| RoBERTa | ``roberta-base`` | | 12-layer, 768-hidden, 12-heads, 125M parameters |
-| | | | RoBERTa using the BERT-base architecture |
-| | | (see `details `__) |
-| +------------------------------------------------------------+---------------------------------------------------------------------------------------------------------------------------------------+
-| | ``roberta-large`` | | 24-layer, 1024-hidden, 16-heads, 355M parameters |
-| | | | RoBERTa using the BERT-large architecture |
-| | | (see `details `__) |
-| +------------------------------------------------------------+---------------------------------------------------------------------------------------------------------------------------------------+
-| | ``roberta-large-mnli`` | | 24-layer, 1024-hidden, 16-heads, 355M parameters |
-| | | | ``roberta-large`` fine-tuned on `MNLI `__. |
-| | | (see `details `__) |
-| +------------------------------------------------------------+---------------------------------------------------------------------------------------------------------------------------------------+
-| | ``distilroberta-base`` | | 6-layer, 768-hidden, 12-heads, 82M parameters |
-| | | | The DistilRoBERTa model distilled from the RoBERTa model `roberta-base` checkpoint. |
-| | | (see `details `__) |
-| +------------------------------------------------------------+---------------------------------------------------------------------------------------------------------------------------------------+
-| | ``roberta-base-openai-detector`` | | 12-layer, 768-hidden, 12-heads, 125M parameters |
-| | | | ``roberta-base`` fine-tuned by OpenAI on the outputs of the 1.5B-parameter GPT-2 model. |
-| | | (see `details `__) |
-| +------------------------------------------------------------+---------------------------------------------------------------------------------------------------------------------------------------+
-| | ``roberta-large-openai-detector`` | | 24-layer, 1024-hidden, 16-heads, 355M parameters |
-| | | | ``roberta-large`` fine-tuned by OpenAI on the outputs of the 1.5B-parameter GPT-2 model. |
-| | | (see `details `__) |
-+-------------------+------------------------------------------------------------+---------------------------------------------------------------------------------------------------------------------------------------+
-| DistilBERT | ``distilbert-base-uncased`` | | 6-layer, 768-hidden, 12-heads, 66M parameters |
-| | | | The DistilBERT model distilled from the BERT model `bert-base-uncased` checkpoint |
-| | | (see `details `__) |
-| +------------------------------------------------------------+---------------------------------------------------------------------------------------------------------------------------------------+
-| | ``distilbert-base-uncased-distilled-squad`` | | 6-layer, 768-hidden, 12-heads, 66M parameters |
-| | | | The DistilBERT model distilled from the BERT model `bert-base-uncased` checkpoint, with an additional linear layer. |
-| | | (see `details `__) |
-| +------------------------------------------------------------+---------------------------------------------------------------------------------------------------------------------------------------+
-| | ``distilgpt2`` | | 6-layer, 768-hidden, 12-heads, 82M parameters |
-| | | | The DistilGPT2 model distilled from the GPT2 model `gpt2` checkpoint. |
-| | | (see `details `__) |
-| +------------------------------------------------------------+---------------------------------------------------------------------------------------------------------------------------------------+
-| | ``distilbert-base-german-cased`` | | 6-layer, 768-hidden, 12-heads, 66M parameters |
-| | | | The German DistilBERT model distilled from the German DBMDZ BERT model `bert-base-german-dbmdz-cased` checkpoint. |
-| | | (see `details `__) |
-| +------------------------------------------------------------+---------------------------------------------------------------------------------------------------------------------------------------+
-| | ``distilbert-base-multilingual-cased`` | | 6-layer, 768-hidden, 12-heads, 134M parameters |
-| | | | The multilingual DistilBERT model distilled from the Multilingual BERT model `bert-base-multilingual-cased` checkpoint. |
-| | | (see `details `__) |
-+-------------------+------------------------------------------------------------+---------------------------------------------------------------------------------------------------------------------------------------+
-| CTRL | ``ctrl`` | | 48-layer, 1280-hidden, 16-heads, 1.6B parameters |
-| | | | Salesforce's Large-sized CTRL English model |
-+-------------------+------------------------------------------------------------+---------------------------------------------------------------------------------------------------------------------------------------+
-| CamemBERT | ``camembert-base`` | | 12-layer, 768-hidden, 12-heads, 110M parameters |
-| | | | CamemBERT using the BERT-base architecture |
-| | | (see `details `__) |
-+-------------------+------------------------------------------------------------+---------------------------------------------------------------------------------------------------------------------------------------+
-| ALBERT | ``albert-base-v1`` | | 12 repeating layers, 128 embedding, 768-hidden, 12-heads, 11M parameters |
-| | | | ALBERT base model |
-| | | (see `details `__) |
-| +------------------------------------------------------------+---------------------------------------------------------------------------------------------------------------------------------------+
-| | ``albert-large-v1`` | | 24 repeating layers, 128 embedding, 1024-hidden, 16-heads, 17M parameters |
-| | | | ALBERT large model |
-| | | (see `details `__) |
-| +------------------------------------------------------------+---------------------------------------------------------------------------------------------------------------------------------------+
-| | ``albert-xlarge-v1`` | | 24 repeating layers, 128 embedding, 2048-hidden, 16-heads, 58M parameters |
-| | | | ALBERT xlarge model |
-| | | (see `details `__) |
-| +------------------------------------------------------------+---------------------------------------------------------------------------------------------------------------------------------------+
-| | ``albert-xxlarge-v1`` | | 12 repeating layer, 128 embedding, 4096-hidden, 64-heads, 223M parameters |
-| | | | ALBERT xxlarge model |
-| | | (see `details `__) |
-| +------------------------------------------------------------+---------------------------------------------------------------------------------------------------------------------------------------+
-| | ``albert-base-v2`` | | 12 repeating layers, 128 embedding, 768-hidden, 12-heads, 11M parameters |
-| | | | ALBERT base model with no dropout, additional training data and longer training |
-| | | (see `details `__) |
-| +------------------------------------------------------------+---------------------------------------------------------------------------------------------------------------------------------------+
-| | ``albert-large-v2`` | | 24 repeating layers, 128 embedding, 1024-hidden, 16-heads, 17M parameters |
-| | | | ALBERT large model with no dropout, additional training data and longer training |
-| | | (see `details `__) |
-| +------------------------------------------------------------+---------------------------------------------------------------------------------------------------------------------------------------+
-| | ``albert-xlarge-v2`` | | 24 repeating layers, 128 embedding, 2048-hidden, 16-heads, 58M parameters |
-| | | | ALBERT xlarge model with no dropout, additional training data and longer training |
-| | | (see `details `__) |
-| +------------------------------------------------------------+---------------------------------------------------------------------------------------------------------------------------------------+
-| | ``albert-xxlarge-v2`` | | 12 repeating layer, 128 embedding, 4096-hidden, 64-heads, 223M parameters |
-| | | | ALBERT xxlarge model with no dropout, additional training data and longer training |
-| | | (see `details `__) |
-+-------------------+------------------------------------------------------------+---------------------------------------------------------------------------------------------------------------------------------------+
-| T5 | ``t5-small`` | | ~60M parameters with 6-layers, 512-hidden-state, 2048 feed-forward hidden-state, 8-heads, |
-| | | | Trained on English text: the Colossal Clean Crawled Corpus (C4) |
-| +------------------------------------------------------------+---------------------------------------------------------------------------------------------------------------------------------------+
-| | ``t5-base`` | | ~220M parameters with 12-layers, 768-hidden-state, 3072 feed-forward hidden-state, 12-heads, |
-| | | | Trained on English text: the Colossal Clean Crawled Corpus (C4) |
-| +------------------------------------------------------------+---------------------------------------------------------------------------------------------------------------------------------------+
-| | ``t5-large`` | | ~770M parameters with 24-layers, 1024-hidden-state, 4096 feed-forward hidden-state, 16-heads, |
-| | | | Trained on English text: the Colossal Clean Crawled Corpus (C4) |
-| +------------------------------------------------------------+---------------------------------------------------------------------------------------------------------------------------------------+
-| | ``t5-3B`` | | ~2.8B parameters with 24-layers, 1024-hidden-state, 16384 feed-forward hidden-state, 32-heads, |
-| | | | Trained on English text: the Colossal Clean Crawled Corpus (C4) |
-| +------------------------------------------------------------+---------------------------------------------------------------------------------------------------------------------------------------+
-| | ``t5-11B`` | | ~11B parameters with 24-layers, 1024-hidden-state, 65536 feed-forward hidden-state, 128-heads, |
-| | | | Trained on English text: the Colossal Clean Crawled Corpus (C4) |
-+-------------------+------------------------------------------------------------+---------------------------------------------------------------------------------------------------------------------------------------+
-| XLM-RoBERTa | ``xlm-roberta-base`` | | ~125M parameters with 12-layers, 768-hidden-state, 3072 feed-forward hidden-state, 8-heads, |
-| | | | Trained on on 2.5 TB of newly created clean CommonCrawl data in 100 languages |
-| +------------------------------------------------------------+---------------------------------------------------------------------------------------------------------------------------------------+
-| | ``xlm-roberta-large`` | | ~355M parameters with 24-layers, 1027-hidden-state, 4096 feed-forward hidden-state, 16-heads, |
-| | | | Trained on 2.5 TB of newly created clean CommonCrawl data in 100 languages |
-+-------------------+------------------------------------------------------------+---------------------------------------------------------------------------------------------------------------------------------------+
-| FlauBERT | ``flaubert-small-cased`` | | 6-layer, 512-hidden, 8-heads, 54M parameters |
-| | | | FlauBERT small architecture |
-| | | (see `details `__) |
-| +------------------------------------------------------------+---------------------------------------------------------------------------------------------------------------------------------------+
-| | ``flaubert-base-uncased`` | | 12-layer, 768-hidden, 12-heads, 137M parameters |
-| | | | FlauBERT base architecture with uncased vocabulary |
-| | | (see `details `__) |
-| +------------------------------------------------------------+---------------------------------------------------------------------------------------------------------------------------------------+
-| | ``flaubert-base-cased`` | | 12-layer, 768-hidden, 12-heads, 138M parameters |
-| | | | FlauBERT base architecture with cased vocabulary |
-| | | (see `details `__) |
-| +------------------------------------------------------------+---------------------------------------------------------------------------------------------------------------------------------------+
-| | ``flaubert-large-cased`` | | 24-layer, 1024-hidden, 16-heads, 373M parameters |
-| | | | FlauBERT large architecture |
-| | | (see `details `__) |
-+-------------------+------------------------------------------------------------+---------------------------------------------------------------------------------------------------------------------------------------+
-
-
-.. `__
diff --git a/server/transformers/docs/source/quickstart.md b/server/transformers/docs/source/quickstart.md
deleted file mode 100644
index 60e2cf3fd84193365abb92432b403386b188f1ac..0000000000000000000000000000000000000000
--- a/server/transformers/docs/source/quickstart.md
+++ /dev/null
@@ -1,315 +0,0 @@
-# Quickstart
-
-## Philosophy
-
-Transformers is an opinionated library built for NLP researchers seeking to use/study/extend large-scale transformers models.
-
-The library was designed with two strong goals in mind:
-
-- be as easy and fast to use as possible:
-
- - we strongly limited the number of user-facing abstractions to learn, in fact there are almost no abstractions, just three standard classes required to use each model: configuration, models and tokenizer,
- - all of these classes can be initialized in a simple and unified way from pretrained instances by using a common `from_pretrained()` instantiation method which will take care of downloading (if needed), caching and loading the related class from a pretrained instance supplied in the library or your own saved instance.
- - as a consequence, this library is NOT a modular toolbox of building blocks for neural nets. If you want to extend/build-upon the library, just use regular Python/PyTorch modules and inherit from the base classes of the library to reuse functionalities like model loading/saving.
-
-- provide state-of-the-art models with performances as close as possible to the original models:
-
- - we provide at least one example for each architecture which reproduces a result provided by the official authors of said architecture,
- - the code is usually as close to the original code base as possible which means some PyTorch code may be not as *pytorchic* as it could be as a result of being converted TensorFlow code.
-
-A few other goals:
-
-- expose the models' internals as consistently as possible:
-
- - we give access, using a single API to the full hidden-states and attention weights,
- - tokenizer and base model's API are standardized to easily switch between models.
-
-- incorporate a subjective selection of promising tools for fine-tuning/investigating these models:
-
- - a simple/consistent way to add new tokens to the vocabulary and embeddings for fine-tuning,
- - simple ways to mask and prune transformer heads.
-
-## Main concepts
-
-The library is build around three type of classes for each models:
-
-- **model classes** which are PyTorch models (`torch.nn.Modules`) of the 8 models architectures currently provided in the library, e.g. `BertModel`
-- **configuration classes** which store all the parameters required to build a model, e.g. `BertConfig`. You don't always need to instantiate these your-self, in particular if you are using a pretrained model without any modification, creating the model will automatically take care of instantiating the configuration (which is part of the model)
-- **tokenizer classes** which store the vocabulary for each model and provide methods for encoding/decoding strings in list of token embeddings indices to be fed to a model, e.g. `BertTokenizer`
-
-All these classes can be instantiated from pretrained instances and saved locally using two methods:
-
-- `from_pretrained()` let you instantiate a model/configuration/tokenizer from a pretrained version either provided by the library itself (currently 27 models are provided as listed [here](https://huggingface.co/transformers/pretrained_models.html)) or stored locally (or on a server) by the user,
-- `save_pretrained()` let you save a model/configuration/tokenizer locally so that it can be reloaded using `from_pretrained()`.
-
-We'll finish this quickstart tour by going through a few simple quick-start examples to see how we can instantiate and use these classes. The rest of the documentation is organized in two parts:
-
-- the **MAIN CLASSES** section details the common functionalities/method/attributes of the three main type of classes (configuration, model, tokenizer) plus some optimization related classes provided as utilities for training,
-- the **PACKAGE REFERENCE** section details all the variants of each class for each model architectures and in particular the input/output that you should expect when calling each of them.
-
-## Quick tour: Usage
-
-Here are two examples showcasing a few `Bert` and `GPT2` classes and pre-trained models.
-
-See full API reference for examples for each model class.
-
-### BERT example
-
-Let's start by preparing a tokenized input (a list of token embeddings indices to be fed to Bert) from a text string using `BertTokenizer`
-
-```python
-import torch
-from transformers import BertTokenizer, BertModel, BertForMaskedLM
-
-# OPTIONAL: if you want to have more information on what's happening under the hood, activate the logger as follows
-import logging
-logging.basicConfig(level=logging.INFO)
-
-# Load pre-trained model tokenizer (vocabulary)
-tokenizer = BertTokenizer.from_pretrained('bert-base-uncased')
-
-# Tokenize input
-text = "[CLS] Who was Jim Henson ? [SEP] Jim Henson was a puppeteer [SEP]"
-tokenized_text = tokenizer.tokenize(text)
-
-# Mask a token that we will try to predict back with `BertForMaskedLM`
-masked_index = 8
-tokenized_text[masked_index] = '[MASK]'
-assert tokenized_text == ['[CLS]', 'who', 'was', 'jim', 'henson', '?', '[SEP]', 'jim', '[MASK]', 'was', 'a', 'puppet', '##eer', '[SEP]']
-
-# Convert token to vocabulary indices
-indexed_tokens = tokenizer.convert_tokens_to_ids(tokenized_text)
-# Define sentence A and B indices associated to 1st and 2nd sentences (see paper)
-segments_ids = [0, 0, 0, 0, 0, 0, 0, 1, 1, 1, 1, 1, 1, 1]
-
-# Convert inputs to PyTorch tensors
-tokens_tensor = torch.tensor([indexed_tokens])
-segments_tensors = torch.tensor([segments_ids])
-```
-
-Let's see how we can use `BertModel` to encode our inputs in hidden-states:
-
-```python
-# Load pre-trained model (weights)
-model = BertModel.from_pretrained('bert-base-uncased')
-
-# Set the model in evaluation mode to deactivate the DropOut modules
-# This is IMPORTANT to have reproducible results during evaluation!
-model.eval()
-
-# If you have a GPU, put everything on cuda
-tokens_tensor = tokens_tensor.to('cuda')
-segments_tensors = segments_tensors.to('cuda')
-model.to('cuda')
-
-# Predict hidden states features for each layer
-with torch.no_grad():
- # See the models docstrings for the detail of the inputs
- outputs = model(tokens_tensor, token_type_ids=segments_tensors)
- # Transformers models always output tuples.
- # See the models docstrings for the detail of all the outputs
- # In our case, the first element is the hidden state of the last layer of the Bert model
- encoded_layers = outputs[0]
-# We have encoded our input sequence in a FloatTensor of shape (batch size, sequence length, model hidden dimension)
-assert tuple(encoded_layers.shape) == (1, len(indexed_tokens), model.config.hidden_size)
-```
-
-And how to use `BertForMaskedLM` to predict a masked token:
-
-```python
-# Load pre-trained model (weights)
-model = BertForMaskedLM.from_pretrained('bert-base-uncased')
-model.eval()
-
-# If you have a GPU, put everything on cuda
-tokens_tensor = tokens_tensor.to('cuda')
-segments_tensors = segments_tensors.to('cuda')
-model.to('cuda')
-
-# Predict all tokens
-with torch.no_grad():
- outputs = model(tokens_tensor, token_type_ids=segments_tensors)
- predictions = outputs[0]
-
-# confirm we were able to predict 'henson'
-predicted_index = torch.argmax(predictions[0, masked_index]).item()
-predicted_token = tokenizer.convert_ids_to_tokens([predicted_index])[0]
-assert predicted_token == 'henson'
-```
-
-### OpenAI GPT-2
-
-Here is a quick-start example using `GPT2Tokenizer` and `GPT2LMHeadModel` class with OpenAI's pre-trained model to predict the next token from a text prompt.
-
-First let's prepare a tokenized input from our text string using `GPT2Tokenizer`
-
-```python
-import torch
-from transformers import GPT2Tokenizer, GPT2LMHeadModel
-
-# OPTIONAL: if you want to have more information on what's happening, activate the logger as follows
-import logging
-logging.basicConfig(level=logging.INFO)
-
-# Load pre-trained model tokenizer (vocabulary)
-tokenizer = GPT2Tokenizer.from_pretrained('gpt2')
-
-# Encode a text inputs
-text = "Who was Jim Henson ? Jim Henson was a"
-indexed_tokens = tokenizer.encode(text)
-
-# Convert indexed tokens in a PyTorch tensor
-tokens_tensor = torch.tensor([indexed_tokens])
-```
-
-Let's see how to use `GPT2LMHeadModel` to generate the next token following our text:
-
-```python
-# Load pre-trained model (weights)
-model = GPT2LMHeadModel.from_pretrained('gpt2')
-
-# Set the model in evaluation mode to deactivate the DropOut modules
-# This is IMPORTANT to have reproducible results during evaluation!
-model.eval()
-
-# If you have a GPU, put everything on cuda
-tokens_tensor = tokens_tensor.to('cuda')
-model.to('cuda')
-
-# Predict all tokens
-with torch.no_grad():
- outputs = model(tokens_tensor)
- predictions = outputs[0]
-
-# get the predicted next sub-word (in our case, the word 'man')
-predicted_index = torch.argmax(predictions[0, -1, :]).item()
-predicted_text = tokenizer.decode(indexed_tokens + [predicted_index])
-assert predicted_text == 'Who was Jim Henson? Jim Henson was a man'
-```
-
-Examples for each model class of each model architecture (Bert, GPT, GPT-2, Transformer-XL, XLNet and XLM) can be found in the [documentation](#documentation).
-
-#### Using the past
-
-GPT-2 as well as some other models (GPT, XLNet, Transfo-XL, CTRL) make use of a `past` or `mems` attribute which can be used to prevent re-computing the key/value pairs when using sequential decoding. It is useful when generating sequences as a big part of the attention mechanism benefits from previous computations.
-
-Here is a fully-working example using the `past` with `GPT2LMHeadModel` and argmax decoding (which should only be used as an example, as argmax decoding introduces a lot of repetition):
-
-```python
-from transformers import GPT2LMHeadModel, GPT2Tokenizer
-import torch
-
-tokenizer = GPT2Tokenizer.from_pretrained("gpt2")
-model = GPT2LMHeadModel.from_pretrained('gpt2')
-
-generated = tokenizer.encode("The Manhattan bridge")
-context = torch.tensor([generated])
-past = None
-
-for i in range(100):
- print(i)
- output, past = model(context, past=past)
- token = torch.argmax(output[0, :])
-
- generated += [token.tolist()]
- context = token.unsqueeze(0)
-
-sequence = tokenizer.decode(generated)
-
-print(sequence)
-```
-
-The model only requires a single token as input as all the previous tokens' key/value pairs are contained in the `past`.
-
-### Model2Model example
-
-Encoder-decoder architectures require two tokenized inputs: one for the encoder and the other one for the decoder. Let's assume that we want to use `Model2Model` for generative question answering, and start by tokenizing the question and answer that will be fed to the model.
-
-```python
-import torch
-from transformers import BertTokenizer, Model2Model
-
-# OPTIONAL: if you want to have more information on what's happening under the hood, activate the logger as follows
-import logging
-logging.basicConfig(level=logging.INFO)
-
-# Load pre-trained model tokenizer (vocabulary)
-tokenizer = BertTokenizer.from_pretrained('bert-base-uncased')
-
-# Encode the input to the encoder (the question)
-question = "Who was Jim Henson?"
-encoded_question = tokenizer.encode(question)
-
-# Encode the input to the decoder (the answer)
-answer = "Jim Henson was a puppeteer"
-encoded_answer = tokenizer.encode(answer)
-
-# Convert inputs to PyTorch tensors
-question_tensor = torch.tensor([encoded_question])
-answer_tensor = torch.tensor([encoded_answer])
-```
-
-Let's see how we can use `Model2Model` to get the value of the loss associated with this (question, answer) pair:
-
-```python
-# In order to compute the loss we need to provide language model
-# labels (the token ids that the model should have produced) to
-# the decoder.
-lm_labels = encoded_answer
-labels_tensor = torch.tensor([lm_labels])
-
-# Load pre-trained model (weights)
-model = Model2Model.from_pretrained('bert-base-uncased')
-
-# Set the model in evaluation mode to deactivate the DropOut modules
-# This is IMPORTANT to have reproducible results during evaluation!
-model.eval()
-
-# If you have a GPU, put everything on cuda
-question_tensor = question_tensor.to('cuda')
-answer_tensor = answer_tensor.to('cuda')
-labels_tensor = labels_tensor.to('cuda')
-model.to('cuda')
-
-# Predict hidden states features for each layer
-with torch.no_grad():
- # See the models docstrings for the detail of the inputs
- outputs = model(question_tensor, answer_tensor, decoder_lm_labels=labels_tensor)
- # Transformers models always output tuples.
- # See the models docstrings for the detail of all the outputs
- # In our case, the first element is the value of the LM loss
- lm_loss = outputs[0]
-```
-
-This loss can be used to fine-tune `Model2Model` on the question answering task. Assuming that we fine-tuned the model, let us now see how to generate an answer:
-
-```python
-# Let's re-use the previous question
-question = "Who was Jim Henson?"
-encoded_question = tokenizer.encode(question)
-question_tensor = torch.tensor([encoded_question])
-
-# This time we try to generate the answer, so we start with an empty sequence
-answer = "[CLS]"
-encoded_answer = tokenizer.encode(answer, add_special_tokens=False)
-answer_tensor = torch.tensor([encoded_answer])
-
-# Load pre-trained model (weights)
-model = Model2Model.from_pretrained('fine-tuned-weights')
-model.eval()
-
-# If you have a GPU, put everything on cuda
-question_tensor = encoded_question.to('cuda')
-answer_tensor = encoded_answer.to('cuda')
-model.to('cuda')
-
-# Predict all tokens
-with torch.no_grad():
- outputs = model(question_tensor, answer_tensor)
- predictions = outputs[0]
-
-# confirm we were able to predict 'jim'
-predicted_index = torch.argmax(predictions[0, -1]).item()
-predicted_token = tokenizer.convert_ids_to_tokens([predicted_index])[0]
-assert predicted_token == 'jim'
-```
diff --git a/server/transformers/docs/source/serialization.rst b/server/transformers/docs/source/serialization.rst
deleted file mode 100644
index d2862dc0b50589a84f3c354c5fc3fdc2638ed010..0000000000000000000000000000000000000000
--- a/server/transformers/docs/source/serialization.rst
+++ /dev/null
@@ -1,190 +0,0 @@
-Loading Google AI or OpenAI pre-trained weights or PyTorch dump
-^^^^^^^^^^^^^^^^^^^^^^^^^^^^^^^^^^^^^^^^^^^^^^^^^^^^^^^^^^^^^^^
-
-``from_pretrained()`` method
-~~~~~~~~~~~~~~~~~~~~~~~~~~~~~~~~~~~~~~~~~~~~~~~~~~~~~~~~~~
-
-To load one of Google AI's, OpenAI's pre-trained models or a PyTorch saved model (an instance of ``BertForPreTraining`` saved with ``torch.save()``\ ), the PyTorch model classes and the tokenizer can be instantiated using the ``from_pretrained()`` method:
-
-.. code-block:: python
-
- model = BERT_CLASS.from_pretrained(PRE_TRAINED_MODEL_NAME_OR_PATH, cache_dir=None, from_tf=False, state_dict=None, *input, **kwargs)
-
-where
-
-
-* ``BERT_CLASS`` is either a tokenizer to load the vocabulary (\ ``BertTokenizer`` or ``OpenAIGPTTokenizer`` classes) or one of the eight BERT or three OpenAI GPT PyTorch model classes (to load the pre-trained weights): ``BertModel``\ , ``BertForMaskedLM``\ , ``BertForNextSentencePrediction``\ , ``BertForPreTraining``\ , ``BertForSequenceClassification``\ , ``BertForTokenClassification``\ , ``BertForMultipleChoice``\ , ``BertForQuestionAnswering``\ , ``OpenAIGPTModel``\ , ``OpenAIGPTLMHeadModel`` or ``OpenAIGPTDoubleHeadsModel``\ , and
-*
- ``PRE_TRAINED_MODEL_NAME_OR_PATH`` is either:
-
-
- *
- the shortcut name of a Google AI's or OpenAI's pre-trained model selected in the list:
-
-
- * ``bert-base-uncased``: 12-layer, 768-hidden, 12-heads, 110M parameters
- * ``bert-large-uncased``: 24-layer, 1024-hidden, 16-heads, 340M parameters
- * ``bert-base-cased``: 12-layer, 768-hidden, 12-heads , 110M parameters
- * ``bert-large-cased``: 24-layer, 1024-hidden, 16-heads, 340M parameters
- * ``bert-base-multilingual-uncased``: (Orig, not recommended) 102 languages, 12-layer, 768-hidden, 12-heads, 110M parameters
- * ``bert-base-multilingual-cased``: **(New, recommended)** 104 languages, 12-layer, 768-hidden, 12-heads, 110M parameters
- * ``bert-base-chinese``: Chinese Simplified and Traditional, 12-layer, 768-hidden, 12-heads, 110M parameters
- * ``bert-base-german-cased``: Trained on German data only, 12-layer, 768-hidden, 12-heads, 110M parameters `Performance Evaluation `__
- * ``bert-large-uncased-whole-word-masking``: 24-layer, 1024-hidden, 16-heads, 340M parameters - Trained with Whole Word Masking (mask all of the the tokens corresponding to a word at once)
- * ``bert-large-cased-whole-word-masking``: 24-layer, 1024-hidden, 16-heads, 340M parameters - Trained with Whole Word Masking (mask all of the the tokens corresponding to a word at once)
- * ``bert-large-uncased-whole-word-masking-finetuned-squad``: The ``bert-large-uncased-whole-word-masking`` model finetuned on SQuAD (using the ``run_bert_squad.py`` examples). Results: *exact_match: 86.91579943235573, f1: 93.1532499015869*
- * ``bert-base-german-dbmdz-cased``: Trained on German data only, 12-layer, 768-hidden, 12-heads, 110M parameters `Performance Evaluation `__
- * ``bert-base-german-dbmdz-uncased``: Trained on (uncased) German data only, 12-layer, 768-hidden, 12-heads, 110M parameters `Performance Evaluation `__
- * ``openai-gpt``: OpenAI GPT English model, 12-layer, 768-hidden, 12-heads, 110M parameters
- * ``gpt2``: OpenAI GPT-2 English model, 12-layer, 768-hidden, 12-heads, 117M parameters
- * ``gpt2-medium``: OpenAI GPT-2 English model, 24-layer, 1024-hidden, 16-heads, 345M parameters
- * ``transfo-xl-wt103``: Transformer-XL English model trained on wikitext-103, 18-layer, 1024-hidden, 16-heads, 257M parameters
-
- *
- a path or url to a pretrained model archive containing:
-
-
- * ``bert_config.json`` or ``openai_gpt_config.json`` a configuration file for the model, and
- * ``pytorch_model.bin`` a PyTorch dump of a pre-trained instance of ``BertForPreTraining``\ , ``OpenAIGPTModel``\ , ``TransfoXLModel``\ , ``GPT2LMHeadModel`` (saved with the usual ``torch.save()``\ )
-
- If ``PRE_TRAINED_MODEL_NAME_OR_PATH`` is a shortcut name, the pre-trained weights will be downloaded from AWS S3 (see the links `here `__\ ) and stored in a cache folder to avoid future download (the cache folder can be found at ``~/.pytorch_pretrained_bert/``\ ).
-
-*
- ``cache_dir`` can be an optional path to a specific directory to download and cache the pre-trained model weights. This option is useful in particular when you are using distributed training: to avoid concurrent access to the same weights you can set for example ``cache_dir='./pretrained_model_{}'.format(args.local_rank)`` (see the section on distributed training for more information).
-
-* ``from_tf``\ : should we load the weights from a locally saved TensorFlow checkpoint
-* ``state_dict``\ : an optional state dictionary (collections.OrderedDict object) to use instead of Google pre-trained models
-* ``*inputs``\ , `**kwargs`: additional input for the specific Bert class (ex: num_labels for BertForSequenceClassification)
-
-``Uncased`` means that the text has been lowercased before WordPiece tokenization, e.g., ``John Smith`` becomes ``john smith``. The Uncased model also strips out any accent markers. ``Cased`` means that the true case and accent markers are preserved. Typically, the Uncased model is better unless you know that case information is important for your task (e.g., Named Entity Recognition or Part-of-Speech tagging). For information about the Multilingual and Chinese model, see the `Multilingual README `__ or the original TensorFlow repository.
-
-When using an ``uncased model``\ , make sure to pass ``--do_lower_case`` to the example training scripts (or pass ``do_lower_case=True`` to FullTokenizer if you're using your own script and loading the tokenizer your-self.).
-
-Examples:
-
-.. code-block:: python
-
- # BERT
- tokenizer = BertTokenizer.from_pretrained('bert-base-uncased', do_lower_case=True, do_basic_tokenize=True)
- model = BertForSequenceClassification.from_pretrained('bert-base-uncased')
-
- # OpenAI GPT
- tokenizer = OpenAIGPTTokenizer.from_pretrained('openai-gpt')
- model = OpenAIGPTModel.from_pretrained('openai-gpt')
-
- # Transformer-XL
- tokenizer = TransfoXLTokenizer.from_pretrained('transfo-xl-wt103')
- model = TransfoXLModel.from_pretrained('transfo-xl-wt103')
-
- # OpenAI GPT-2
- tokenizer = GPT2Tokenizer.from_pretrained('gpt2')
- model = GPT2Model.from_pretrained('gpt2')
-
-Cache directory
-~~~~~~~~~~~~~~~
-
-``pytorch_pretrained_bert`` save the pretrained weights in a cache directory which is located at (in this order of priority):
-
-
-* ``cache_dir`` optional arguments to the ``from_pretrained()`` method (see above),
-* shell environment variable ``PYTORCH_PRETRAINED_BERT_CACHE``\ ,
-* PyTorch cache home + ``/pytorch_pretrained_bert/``
- where PyTorch cache home is defined by (in this order):
-
- * shell environment variable ``ENV_TORCH_HOME``
- * shell environment variable ``ENV_XDG_CACHE_HOME`` + ``/torch/``\ )
- * default: ``~/.cache/torch/``
-
-Usually, if you don't set any specific environment variable, ``pytorch_pretrained_bert`` cache will be at ``~/.cache/torch/pytorch_pretrained_bert/``.
-
-You can alsways safely delete ``pytorch_pretrained_bert`` cache but the pretrained model weights and vocabulary files wil have to be re-downloaded from our S3.
-
-Serialization best-practices
-^^^^^^^^^^^^^^^^^^^^^^^^^^^^^^^^^^
-
-This section explain how you can save and re-load a fine-tuned model (BERT, GPT, GPT-2 and Transformer-XL).
-There are three types of files you need to save to be able to reload a fine-tuned model:
-
-
-* the model itself which should be saved following PyTorch serialization `best practices `__\ ,
-* the configuration file of the model which is saved as a JSON file, and
-* the vocabulary (and the merges for the BPE-based models GPT and GPT-2).
-
-The *default filenames* of these files are as follow:
-
-
-* the model weights file: ``pytorch_model.bin``\ ,
-* the configuration file: ``config.json``\ ,
-* the vocabulary file: ``vocab.txt`` for BERT and Transformer-XL, ``vocab.json`` for GPT/GPT-2 (BPE vocabulary),
-* for GPT/GPT-2 (BPE vocabulary) the additional merges file: ``merges.txt``.
-
-**If you save a model using these *default filenames*\ , you can then re-load the model and tokenizer using the ``from_pretrained()`` method.**
-
-Here is the recommended way of saving the model, configuration and vocabulary to an ``output_dir`` directory and reloading the model and tokenizer afterwards:
-
-.. code-block:: python
-
- from transformers import WEIGHTS_NAME, CONFIG_NAME
-
- output_dir = "./models/"
-
- # Step 1: Save a model, configuration and vocabulary that you have fine-tuned
-
- # If we have a distributed model, save only the encapsulated model
- # (it was wrapped in PyTorch DistributedDataParallel or DataParallel)
- model_to_save = model.module if hasattr(model, 'module') else model
-
- # If we save using the predefined names, we can load using `from_pretrained`
- output_model_file = os.path.join(output_dir, WEIGHTS_NAME)
- output_config_file = os.path.join(output_dir, CONFIG_NAME)
-
- torch.save(model_to_save.state_dict(), output_model_file)
- model_to_save.config.to_json_file(output_config_file)
- tokenizer.save_vocabulary(output_dir)
-
- # Step 2: Re-load the saved model and vocabulary
-
- # Example for a Bert model
- model = BertForQuestionAnswering.from_pretrained(output_dir)
- tokenizer = BertTokenizer.from_pretrained(output_dir, do_lower_case=args.do_lower_case) # Add specific options if needed
- # Example for a GPT model
- model = OpenAIGPTDoubleHeadsModel.from_pretrained(output_dir)
- tokenizer = OpenAIGPTTokenizer.from_pretrained(output_dir)
-
-Here is another way you can save and reload the model if you want to use specific paths for each type of files:
-
-.. code-block:: python
-
- output_model_file = "./models/my_own_model_file.bin"
- output_config_file = "./models/my_own_config_file.bin"
- output_vocab_file = "./models/my_own_vocab_file.bin"
-
- # Step 1: Save a model, configuration and vocabulary that you have fine-tuned
-
- # If we have a distributed model, save only the encapsulated model
- # (it was wrapped in PyTorch DistributedDataParallel or DataParallel)
- model_to_save = model.module if hasattr(model, 'module') else model
-
- torch.save(model_to_save.state_dict(), output_model_file)
- model_to_save.config.to_json_file(output_config_file)
- tokenizer.save_vocabulary(output_vocab_file)
-
- # Step 2: Re-load the saved model and vocabulary
-
- # We didn't save using the predefined WEIGHTS_NAME, CONFIG_NAME names, we cannot load using `from_pretrained`.
- # Here is how to do it in this situation:
-
- # Example for a Bert model
- config = BertConfig.from_json_file(output_config_file)
- model = BertForQuestionAnswering(config)
- state_dict = torch.load(output_model_file)
- model.load_state_dict(state_dict)
- tokenizer = BertTokenizer(output_vocab_file, do_lower_case=args.do_lower_case)
-
- # Example for a GPT model
- config = OpenAIGPTConfig.from_json_file(output_config_file)
- model = OpenAIGPTDoubleHeadsModel(config)
- state_dict = torch.load(output_model_file)
- model.load_state_dict(state_dict)
- tokenizer = OpenAIGPTTokenizer(output_vocab_file)
-
diff --git a/server/transformers/docs/source/torchscript.rst b/server/transformers/docs/source/torchscript.rst
deleted file mode 100644
index fd1eeb53635ff30bac4597d2e0308b9443c6afbe..0000000000000000000000000000000000000000
--- a/server/transformers/docs/source/torchscript.rst
+++ /dev/null
@@ -1,135 +0,0 @@
-TorchScript
-================================================
-
-.. note::
- This is the very beginning of our experiments with TorchScript and we are still exploring its capabilities
- with variable-input-size models. It is a focus of interest to us and we will deepen our analysis in upcoming
- releases, with more code examples, a more flexible implementation, and benchmarks comparing python-based codes
- with compiled TorchScript.
-
-
-According to Pytorch's documentation: "TorchScript is a way to create serializable and optimizable models from PyTorch code".
-Pytorch's two modules `JIT and TRACE `_ allow the developer to export
-their model to be re-used in other programs, such as efficiency-oriented C++ programs.
-
-We have provided an interface that allows the export of `transformers` models to TorchScript so that they can
-be reused in a different environment than a Pytorch-based python program. Here we explain how to use our models so that
-they can be exported, and what to be mindful of when using these models with TorchScript.
-
-Exporting a model needs two things:
-
-* dummy inputs to execute a model forward pass.
-* the model needs to be instantiated with the ``torchscript`` flag.
-
-These necessities imply several things developers should be careful about. These are detailed below.
-
-
-Implications
-^^^^^^^^^^^^^^^^^^^^^^^^^^^^^^^^^^^^^^^^^^^^^^^^^
-
-TorchScript flag and tied weights
-------------------------------------------------
-This flag is necessary because most of the language models in this repository have tied weights between their
-``Embedding`` layer and their ``Decoding`` layer. TorchScript does not allow the export of models that have tied weights,
-it is therefore necessary to untie the weights beforehand.
-
-This implies that models instantiated with the ``torchscript`` flag have their ``Embedding`` layer and ``Decoding`` layer
-separate, which means that they should not be trained down the line. Training would de-synchronize the two layers,
-leading to unexpected results.
-
-This is not the case for models that do not have a Language Model head, as those do not have tied weights. These models
-can be safely exported without the ``torchscript`` flag.
-
-Dummy inputs and standard lengths
-------------------------------------------------
-
-The dummy inputs are used to do a model forward pass. While the inputs' values are propagating through the layers,
-Pytorch keeps track of the different operations executed on each tensor. These recorded operations are then used
-to create the "trace" of the model.
-
-The trace is created relatively to the inputs' dimensions. It is therefore constrained by the dimensions of the dummy
-input, and will not work for any other sequence length or batch size. When trying with a different size, an error such
-as:
-
-``The expanded size of the tensor (3) must match the existing size (7) at non-singleton dimension 2``
-
-will be raised. It is therefore recommended to trace the model with a dummy input size at least as large as the largest
-input that will be fed to the model during inference. Padding can be performed to fill the missing values. As the model
-will have been traced with a large input size however, the dimensions of the different matrix will be large as well,
-resulting in more calculations.
-
-It is recommended to be careful of the total number of operations done on each input and to follow performance closely
-when exporting varying sequence-length models.
-
-Using TorchScript in Python
-^^^^^^^^^^^^^^^^^^^^^^^^^^^^^^^^^^^^^^^^^^^^^^^^^
-
-Below are examples of using the Python to save, load models as well as how to use the trace for inference.
-
-Saving a model
-------------------------------------------------
-
-This snippet shows how to use TorchScript to export a ``BertModel``. Here the ``BertModel`` is instantiated
-according to a ``BertConfig`` class and then saved to disk under the filename ``traced_bert.pt``
-
-.. code-block:: python
-
- from transformers import BertModel, BertTokenizer, BertConfig
- import torch
-
- enc = BertTokenizer.from_pretrained("bert-base-uncased")
-
- # Tokenizing input text
- text = "[CLS] Who was Jim Henson ? [SEP] Jim Henson was a puppeteer [SEP]"
- tokenized_text = enc.tokenize(text)
-
- # Masking one of the input tokens
- masked_index = 8
- tokenized_text[masked_index] = '[MASK]'
- indexed_tokens = enc.convert_tokens_to_ids(tokenized_text)
- segments_ids = [0, 0, 0, 0, 0, 0, 0, 1, 1, 1, 1, 1, 1, 1]
-
- # Creating a dummy input
- tokens_tensor = torch.tensor([indexed_tokens])
- segments_tensors = torch.tensor([segments_ids])
- dummy_input = [tokens_tensor, segments_tensors]
-
- # Initializing the model with the torchscript flag
- # Flag set to True even though it is not necessary as this model does not have an LM Head.
- config = BertConfig(vocab_size_or_config_json_file=32000, hidden_size=768,
- num_hidden_layers=12, num_attention_heads=12, intermediate_size=3072, torchscript=True)
-
- # Instantiating the model
- model = BertModel(config)
-
- # The model needs to be in evaluation mode
- model.eval()
-
- # If you are instantiating the model with `from_pretrained` you can also easily set the TorchScript flag
- model = BertModel.from_pretrained("bert-base-uncased", torchscript=True)
-
- # Creating the trace
- traced_model = torch.jit.trace(model, [tokens_tensor, segments_tensors])
- torch.jit.save(traced_model, "traced_bert.pt")
-
-Loading a model
-------------------------------------------------
-
-This snippet shows how to load the ``BertModel`` that was previously saved to disk under the name ``traced_bert.pt``.
-We are re-using the previously initialised ``dummy_input``.
-
-.. code-block:: python
-
- loaded_model = torch.jit.load("traced_model.pt")
- loaded_model.eval()
-
- all_encoder_layers, pooled_output = loaded_model(dummy_input)
-
-Using a traced model for inference
-------------------------------------------------
-
-Using the traced model for inference is as simple as using its ``__call__`` dunder method:
-
-.. code-block:: python
-
- traced_model(tokens_tensor, segments_tensors)
diff --git a/server/transformers/examples/README.md b/server/transformers/examples/README.md
deleted file mode 100644
index d161d1b832bdd994f08b8564e3ee06fe71524afd..0000000000000000000000000000000000000000
--- a/server/transformers/examples/README.md
+++ /dev/null
@@ -1,801 +0,0 @@
-# Examples
-
-In this section a few examples are put together. All of these examples work for several models, making use of the very
-similar API between the different models.
-
-**Important**
-To run the latest versions of the examples, you have to install from source and install some specific requirements for the examples.
-Execute the following steps in a new virtual environment:
-
-```bash
-git clone https://github.com/huggingface/transformers
-cd transformers
-pip install .
-pip install -r ./examples/requirements.txt
-```
-
-| Section | Description |
-|----------------------------|------------------------------------------------------------------------------------------------------------------------------------------------------------|
-| [TensorFlow 2.0 models on GLUE](#TensorFlow-2.0-Bert-models-on-GLUE) | Examples running BERT TensorFlow 2.0 model on the GLUE tasks.
-| [Language Model fine-tuning](#language-model-fine-tuning) | Fine-tuning the library models for language modeling on a text dataset. Causal language modeling for GPT/GPT-2, masked language modeling for BERT/RoBERTa. |
-| [Language Generation](#language-generation) | Conditional text generation using the auto-regressive models of the library: GPT, GPT-2, Transformer-XL and XLNet. |
-| [GLUE](#glue) | Examples running BERT/XLM/XLNet/RoBERTa on the 9 GLUE tasks. Examples feature distributed training as well as half-precision. |
-| [SQuAD](#squad) | Using BERT/RoBERTa/XLNet/XLM for question answering, examples with distributed training. |
-| [Multiple Choice](#multiple-choice) | Examples running BERT/XLNet/RoBERTa on the SWAG/RACE/ARC tasks.
-| [Named Entity Recognition](#named-entity-recognition) | Using BERT for Named Entity Recognition (NER) on the CoNLL 2003 dataset, examples with distributed training. |
-| [XNLI](#xnli) | Examples running BERT/XLM on the XNLI benchmark. |
-| [Adversarial evaluation of model performances](#adversarial-evaluation-of-model-performances) | Testing a model with adversarial evaluation of natural language
-inference on the Heuristic Analysis for NLI Systems (HANS) dataset (McCoy et al., 2019.) |
-
-## TensorFlow 2.0 Bert models on GLUE
-
-Based on the script [`run_tf_glue.py`](https://github.com/huggingface/transformers/blob/master/examples/run_tf_glue.py).
-
-Fine-tuning the library TensorFlow 2.0 Bert model for sequence classification on the MRPC task of the GLUE benchmark: [General Language Understanding Evaluation](https://gluebenchmark.com/).
-
-This script has an option for mixed precision (Automatic Mixed Precision / AMP) to run models on Tensor Cores (NVIDIA Volta/Turing GPUs) and future hardware and an option for XLA, which uses the XLA compiler to reduce model runtime.
-Options are toggled using `USE_XLA` or `USE_AMP` variables in the script.
-These options and the below benchmark are provided by @tlkh.
-
-Quick benchmarks from the script (no other modifications):
-
-| GPU | Mode | Time (2nd epoch) | Val Acc (3 runs) |
-| --------- | -------- | ----------------------- | ----------------------|
-| Titan V | FP32 | 41s | 0.8438/0.8281/0.8333 |
-| Titan V | AMP | 26s | 0.8281/0.8568/0.8411 |
-| V100 | FP32 | 35s | 0.8646/0.8359/0.8464 |
-| V100 | AMP | 22s | 0.8646/0.8385/0.8411 |
-| 1080 Ti | FP32 | 55s | - |
-
-Mixed precision (AMP) reduces the training time considerably for the same hardware and hyper-parameters (same batch size was used).
-
-## Language model fine-tuning
-
-Based on the script [`run_lm_finetuning.py`](https://github.com/huggingface/transformers/blob/master/examples/run_lm_finetuning.py).
-
-Fine-tuning the library models for language modeling on a text dataset for GPT, GPT-2, BERT and RoBERTa (DistilBERT
-to be added soon). GPT and GPT-2 are fine-tuned using a causal language modeling (CLM) loss while BERT and RoBERTa
-are fine-tuned using a masked language modeling (MLM) loss.
-
-Before running the following example, you should get a file that contains text on which the language model will be
-fine-tuned. A good example of such text is the [WikiText-2 dataset](https://blog.einstein.ai/the-wikitext-long-term-dependency-language-modeling-dataset/).
-
-We will refer to two different files: `$TRAIN_FILE`, which contains text for training, and `$TEST_FILE`, which contains
-text that will be used for evaluation.
-
-### GPT-2/GPT and causal language modeling
-
-The following example fine-tunes GPT-2 on WikiText-2. We're using the raw WikiText-2 (no tokens were replaced before
-the tokenization). The loss here is that of causal language modeling.
-
-```bash
-export TRAIN_FILE=/path/to/dataset/wiki.train.raw
-export TEST_FILE=/path/to/dataset/wiki.test.raw
-
-python run_lm_finetuning.py \
- --output_dir=output \
- --model_type=gpt2 \
- --model_name_or_path=gpt2 \
- --do_train \
- --train_data_file=$TRAIN_FILE \
- --do_eval \
- --eval_data_file=$TEST_FILE
-```
-
-This takes about half an hour to train on a single K80 GPU and about one minute for the evaluation to run. It reaches
-a score of ~20 perplexity once fine-tuned on the dataset.
-
-### RoBERTa/BERT and masked language modeling
-
-The following example fine-tunes RoBERTa on WikiText-2. Here too, we're using the raw WikiText-2. The loss is different
-as BERT/RoBERTa have a bidirectional mechanism; we're therefore using the same loss that was used during their
-pre-training: masked language modeling.
-
-In accordance to the RoBERTa paper, we use dynamic masking rather than static masking. The model may, therefore, converge
-slightly slower (over-fitting takes more epochs).
-
-We use the `--mlm` flag so that the script may change its loss function.
-
-```bash
-export TRAIN_FILE=/path/to/dataset/wiki.train.raw
-export TEST_FILE=/path/to/dataset/wiki.test.raw
-
-python run_lm_finetuning.py \
- --output_dir=output \
- --model_type=roberta \
- --model_name_or_path=roberta-base \
- --do_train \
- --train_data_file=$TRAIN_FILE \
- --do_eval \
- --eval_data_file=$TEST_FILE \
- --mlm
-```
-
-## Language generation
-
-Based on the script [`run_generation.py`](https://github.com/huggingface/transformers/blob/master/examples/run_generation.py).
-
-Conditional text generation using the auto-regressive models of the library: GPT, GPT-2, Transformer-XL, XLNet, CTRL.
-A similar script is used for our official demo [Write With Transfomer](https://transformer.huggingface.co), where you
-can try out the different models available in the library.
-
-Example usage:
-
-```bash
-python run_generation.py \
- --model_type=gpt2 \
- --model_name_or_path=gpt2
-```
-
-## GLUE
-
-Based on the script [`run_glue.py`](https://github.com/huggingface/transformers/blob/master/examples/run_glue.py).
-
-Fine-tuning the library models for sequence classification on the GLUE benchmark: [General Language Understanding
-Evaluation](https://gluebenchmark.com/). This script can fine-tune the following models: BERT, XLM, XLNet and RoBERTa.
-
-GLUE is made up of a total of 9 different tasks. We get the following results on the dev set of the benchmark with an
-uncased BERT base model (the checkpoint `bert-base-uncased`). All experiments ran single V100 GPUs with a total train
-batch sizes between 16 and 64. Some of these tasks have a small dataset and training can lead to high variance in the results
-between different runs. We report the median on 5 runs (with different seeds) for each of the metrics.
-
-| Task | Metric | Result |
-|-------|------------------------------|-------------|
-| CoLA | Matthew's corr | 49.23 |
-| SST-2 | Accuracy | 91.97 |
-| MRPC | F1/Accuracy | 89.47/85.29 |
-| STS-B | Person/Spearman corr. | 83.95/83.70 |
-| QQP | Accuracy/F1 | 88.40/84.31 |
-| MNLI | Matched acc./Mismatched acc. | 80.61/81.08 |
-| QNLI | Accuracy | 87.46 |
-| RTE | Accuracy | 61.73 |
-| WNLI | Accuracy | 45.07 |
-
-Some of these results are significantly different from the ones reported on the test set
-of GLUE benchmark on the website. For QQP and WNLI, please refer to [FAQ #12](https://gluebenchmark.com/faq) on the webite.
-
-Before running anyone of these GLUE tasks you should download the
-[GLUE data](https://gluebenchmark.com/tasks) by running
-[this script](https://gist.github.com/W4ngatang/60c2bdb54d156a41194446737ce03e2e)
-and unpack it to some directory `$GLUE_DIR`.
-
-```bash
-export GLUE_DIR=/path/to/glue
-export TASK_NAME=MRPC
-
-python run_glue.py \
- --model_type bert \
- --model_name_or_path bert-base-cased \
- --task_name $TASK_NAME \
- --do_train \
- --do_eval \
- --do_lower_case \
- --data_dir $GLUE_DIR/$TASK_NAME \
- --max_seq_length 128 \
- --per_gpu_train_batch_size 32 \
- --learning_rate 2e-5 \
- --num_train_epochs 3.0 \
- --output_dir /tmp/$TASK_NAME/
-```
-
-where task name can be one of CoLA, SST-2, MRPC, STS-B, QQP, MNLI, QNLI, RTE, WNLI.
-
-The dev set results will be present within the text file `eval_results.txt` in the specified output_dir.
-In case of MNLI, since there are two separate dev sets (matched and mismatched), there will be a separate
-output folder called `/tmp/MNLI-MM/` in addition to `/tmp/MNLI/`.
-
-The code has not been tested with half-precision training with apex on any GLUE task apart from MRPC, MNLI,
-CoLA, SST-2. The following section provides details on how to run half-precision training with MRPC. With that being
-said, there shouldn’t be any issues in running half-precision training with the remaining GLUE tasks as well,
-since the data processor for each task inherits from the base class DataProcessor.
-
-### MRPC
-
-#### Fine-tuning example
-
-The following examples fine-tune BERT on the Microsoft Research Paraphrase Corpus (MRPC) corpus and runs in less
-than 10 minutes on a single K-80 and in 27 seconds (!) on single tesla V100 16GB with apex installed.
-
-Before running anyone of these GLUE tasks you should download the
-[GLUE data](https://gluebenchmark.com/tasks) by running
-[this script](https://gist.github.com/W4ngatang/60c2bdb54d156a41194446737ce03e2e)
-and unpack it to some directory `$GLUE_DIR`.
-
-```bash
-export GLUE_DIR=/path/to/glue
-
-python run_glue.py \
- --model_type bert \
- --model_name_or_path bert-base-cased \
- --task_name MRPC \
- --do_train \
- --do_eval \
- --do_lower_case \
- --data_dir $GLUE_DIR/MRPC/ \
- --max_seq_length 128 \
- --per_gpu_train_batch_size 32 \
- --learning_rate 2e-5 \
- --num_train_epochs 3.0 \
- --output_dir /tmp/mrpc_output/
-```
-
-Our test ran on a few seeds with [the original implementation hyper-
-parameters](https://github.com/google-research/bert#sentence-and-sentence-pair-classification-tasks) gave evaluation
-results between 84% and 88%.
-
-#### Using Apex and mixed-precision
-
-Using Apex and 16 bit precision, the fine-tuning on MRPC only takes 27 seconds. First install
-[apex](https://github.com/NVIDIA/apex), then run the following example:
-
-```bash
-export GLUE_DIR=/path/to/glue
-
-python run_glue.py \
- --model_type bert \
- --model_name_or_path bert-base-cased \
- --task_name MRPC \
- --do_train \
- --do_eval \
- --do_lower_case \
- --data_dir $GLUE_DIR/MRPC/ \
- --max_seq_length 128 \
- --per_gpu_train_batch_size 32 \
- --learning_rate 2e-5 \
- --num_train_epochs 3.0 \
- --output_dir /tmp/mrpc_output/ \
- --fp16
-```
-
-#### Distributed training
-
-Here is an example using distributed training on 8 V100 GPUs. The model used is the BERT whole-word-masking and it
-reaches F1 > 92 on MRPC.
-
-```bash
-export GLUE_DIR=/path/to/glue
-
-python -m torch.distributed.launch \
- --nproc_per_node 8 run_glue.py \
- --model_type bert \
- --model_name_or_path bert-base-cased \
- --task_name MRPC \
- --do_train \
- --do_eval \
- --do_lower_case \
- --data_dir $GLUE_DIR/MRPC/ \
- --max_seq_length 128 \
- --per_gpu_train_batch_size 8 \
- --learning_rate 2e-5 \
- --num_train_epochs 3.0 \
- --output_dir /tmp/mrpc_output/
-```
-
-Training with these hyper-parameters gave us the following results:
-
-```bash
-acc = 0.8823529411764706
-acc_and_f1 = 0.901702786377709
-eval_loss = 0.3418912578906332
-f1 = 0.9210526315789473
-global_step = 174
-loss = 0.07231863956341798
-```
-
-### MNLI
-
-The following example uses the BERT-large, uncased, whole-word-masking model and fine-tunes it on the MNLI task.
-
-```bash
-export GLUE_DIR=/path/to/glue
-
-python -m torch.distributed.launch \
- --nproc_per_node 8 run_glue.py \
- --model_type bert \
- --model_name_or_path bert-base-cased \
- --task_name mnli \
- --do_train \
- --do_eval \
- --do_lower_case \
- --data_dir $GLUE_DIR/MNLI/ \
- --max_seq_length 128 \
- --per_gpu_train_batch_size 8 \
- --learning_rate 2e-5 \
- --num_train_epochs 3.0 \
- --output_dir output_dir \
-```
-
-The results are the following:
-
-```bash
-***** Eval results *****
- acc = 0.8679706601466992
- eval_loss = 0.4911287787382479
- global_step = 18408
- loss = 0.04755385363816904
-
-***** Eval results *****
- acc = 0.8747965825874695
- eval_loss = 0.45516540421714036
- global_step = 18408
- loss = 0.04755385363816904
-```
-
-## Multiple Choice
-
-Based on the script [`run_multiple_choice.py`]().
-
-#### Fine-tuning on SWAG
-Download [swag](https://github.com/rowanz/swagaf/tree/master/data) data
-
-```bash
-#training on 4 tesla V100(16GB) GPUS
-export SWAG_DIR=/path/to/swag_data_dir
-python ./examples/run_multiple_choice.py \
---model_type roberta \
---task_name swag \
---model_name_or_path roberta-base \
---do_train \
---do_eval \
---do_lower_case \
---data_dir $SWAG_DIR \
---learning_rate 5e-5 \
---num_train_epochs 3 \
---max_seq_length 80 \
---output_dir models_bert/swag_base \
---per_gpu_eval_batch_size=16 \
---per_gpu_train_batch_size=16 \
---gradient_accumulation_steps 2 \
---overwrite_output
-```
-Training with the defined hyper-parameters yields the following results:
-```
-***** Eval results *****
-eval_acc = 0.8338998300509847
-eval_loss = 0.44457291918821606
-```
-
-## SQuAD
-
-Based on the script [`run_squad.py`](https://github.com/huggingface/transformers/blob/master/examples/run_squad.py).
-
-#### Fine-tuning BERT on SQuAD1.0
-
-This example code fine-tunes BERT on the SQuAD1.0 dataset. It runs in 24 min (with BERT-base) or 68 min (with BERT-large)
-on a single tesla V100 16GB. The data for SQuAD can be downloaded with the following links and should be saved in a
-$SQUAD_DIR directory.
-
-* [train-v1.1.json](https://rajpurkar.github.io/SQuAD-explorer/dataset/train-v1.1.json)
-* [dev-v1.1.json](https://rajpurkar.github.io/SQuAD-explorer/dataset/dev-v1.1.json)
-* [evaluate-v1.1.py](https://github.com/allenai/bi-att-flow/blob/master/squad/evaluate-v1.1.py)
-
-And for SQuAD2.0, you need to download:
-
-- [train-v2.0.json](https://rajpurkar.github.io/SQuAD-explorer/dataset/train-v2.0.json)
-- [dev-v2.0.json](https://rajpurkar.github.io/SQuAD-explorer/dataset/dev-v2.0.json)
-- [evaluate-v2.0.py](https://worksheets.codalab.org/rest/bundles/0x6b567e1cf2e041ec80d7098f031c5c9e/contents/blob/)
-
-```bash
-export SQUAD_DIR=/path/to/SQUAD
-
-python run_squad.py \
- --model_type bert \
- --model_name_or_path bert-base-cased \
- --do_train \
- --do_eval \
- --do_lower_case \
- --train_file $SQUAD_DIR/train-v1.1.json \
- --predict_file $SQUAD_DIR/dev-v1.1.json \
- --per_gpu_train_batch_size 12 \
- --learning_rate 3e-5 \
- --num_train_epochs 2.0 \
- --max_seq_length 384 \
- --doc_stride 128 \
- --output_dir /tmp/debug_squad/
-```
-
-Training with the previously defined hyper-parameters yields the following results:
-
-```bash
-f1 = 88.52
-exact_match = 81.22
-```
-
-#### Distributed training
-
-
-Here is an example using distributed training on 8 V100 GPUs and Bert Whole Word Masking uncased model to reach a F1 > 93 on SQuAD1.1:
-
-```bash
-python -m torch.distributed.launch --nproc_per_node=8 ./examples/run_squad.py \
- --model_type bert \
- --model_name_or_path bert-large-uncased-whole-word-masking \
- --do_train \
- --do_eval \
- --do_lower_case \
- --train_file $SQUAD_DIR/train-v1.1.json \
- --predict_file $SQUAD_DIR/dev-v1.1.json \
- --learning_rate 3e-5 \
- --num_train_epochs 2 \
- --max_seq_length 384 \
- --doc_stride 128 \
- --output_dir ./examples/models/wwm_uncased_finetuned_squad/ \
- --per_gpu_eval_batch_size=3 \
- --per_gpu_train_batch_size=3 \
-```
-
-Training with the previously defined hyper-parameters yields the following results:
-
-```bash
-f1 = 93.15
-exact_match = 86.91
-```
-
-This fine-tuned model is available as a checkpoint under the reference
-`bert-large-uncased-whole-word-masking-finetuned-squad`.
-
-#### Fine-tuning XLNet on SQuAD
-
-This example code fine-tunes XLNet on both SQuAD1.0 and SQuAD2.0 dataset. See above to download the data for SQuAD .
-
-##### Command for SQuAD1.0:
-
-```bash
-export SQUAD_DIR=/path/to/SQUAD
-
-python /data/home/hlu/transformers/examples/run_squad.py \
- --model_type xlnet \
- --model_name_or_path xlnet-large-cased \
- --do_train \
- --do_eval \
- --do_lower_case \
- --train_file /data/home/hlu/notebooks/NLP/examples/question_answering/train-v1.1.json \
- --predict_file /data/home/hlu/notebooks/NLP/examples/question_answering/dev-v1.1.json \
- --learning_rate 3e-5 \
- --num_train_epochs 2 \
- --max_seq_length 384 \
- --doc_stride 128 \
- --output_dir ./wwm_cased_finetuned_squad/ \
- --per_gpu_eval_batch_size=4 \
- --per_gpu_train_batch_size=4 \
- --save_steps 5000
-```
-
-##### Command for SQuAD2.0:
-
-```bash
-export SQUAD_DIR=/path/to/SQUAD
-
-python run_squad.py \
- --model_type xlnet \
- --model_name_or_path xlnet-large-cased \
- --do_train \
- --do_eval \
- --version_2_with_negative \
- --train_file $SQUAD_DIR/train-v2.0.json \
- --predict_file $SQUAD_DIR/dev-v2.0.json \
- --learning_rate 3e-5 \
- --num_train_epochs 4 \
- --max_seq_length 384 \
- --doc_stride 128 \
- --output_dir ./wwm_cased_finetuned_squad/ \
- --per_gpu_eval_batch_size=2 \
- --per_gpu_train_batch_size=2 \
- --save_steps 5000
-```
-
-Larger batch size may improve the performance while costing more memory.
-
-##### Results for SQuAD1.0 with the previously defined hyper-parameters:
-
-```python
-{
-"exact": 85.45884578997162,
-"f1": 92.5974600601065,
-"total": 10570,
-"HasAns_exact": 85.45884578997162,
-"HasAns_f1": 92.59746006010651,
-"HasAns_total": 10570
-}
-```
-
-##### Results for SQuAD2.0 with the previously defined hyper-parameters:
-
-```python
-{
-"exact": 80.4177545691906,
-"f1": 84.07154997729623,
-"total": 11873,
-"HasAns_exact": 76.73751686909581,
-"HasAns_f1": 84.05558584352873,
-"HasAns_total": 5928,
-"NoAns_exact": 84.0874684608915,
-"NoAns_f1": 84.0874684608915,
-"NoAns_total": 5945
-}
-```
-
-
-
-## Named Entity Recognition
-
-Based on the scripts [`run_ner.py`](https://github.com/huggingface/transformers/blob/master/examples/run_ner.py) for Pytorch and
-[`run_tf_ner.py`](https://github.com/huggingface/transformers/blob/master/examples/run_tf_ner.py) for Tensorflow 2.
-This example fine-tune Bert Multilingual on GermEval 2014 (German NER).
-Details and results for the fine-tuning provided by @stefan-it.
-
-### Data (Download and pre-processing steps)
-
-Data can be obtained from the [GermEval 2014](https://sites.google.com/site/germeval2014ner/data) shared task page.
-
-Here are the commands for downloading and pre-processing train, dev and test datasets. The original data format has four (tab-separated) columns, in a pre-processing step only the two relevant columns (token and outer span NER annotation) are extracted:
-
-```bash
-curl -L 'https://sites.google.com/site/germeval2014ner/data/NER-de-train.tsv?attredirects=0&d=1' \
-| grep -v "^#" | cut -f 2,3 | tr '\t' ' ' > train.txt.tmp
-curl -L 'https://sites.google.com/site/germeval2014ner/data/NER-de-dev.tsv?attredirects=0&d=1' \
-| grep -v "^#" | cut -f 2,3 | tr '\t' ' ' > dev.txt.tmp
-curl -L 'https://sites.google.com/site/germeval2014ner/data/NER-de-test.tsv?attredirects=0&d=1' \
-| grep -v "^#" | cut -f 2,3 | tr '\t' ' ' > test.txt.tmp
-```
-
-The GermEval 2014 dataset contains some strange "control character" tokens like `'\x96', '\u200e', '\x95', '\xad' or '\x80'`. One problem with these tokens is, that `BertTokenizer` returns an empty token for them, resulting in misaligned `InputExample`s. I wrote a script that a) filters these tokens and b) splits longer sentences into smaller ones (once the max. subtoken length is reached).
-
-```bash
-wget "https://raw.githubusercontent.com/stefan-it/fine-tuned-berts-seq/master/scripts/preprocess.py"
-```
-Let's define some variables that we need for further pre-processing steps and training the model:
-
-```bash
-export MAX_LENGTH=128
-export BERT_MODEL=bert-base-multilingual-cased
-```
-
-Run the pre-processing script on training, dev and test datasets:
-
-```bash
-python3 preprocess.py train.txt.tmp $BERT_MODEL $MAX_LENGTH > train.txt
-python3 preprocess.py dev.txt.tmp $BERT_MODEL $MAX_LENGTH > dev.txt
-python3 preprocess.py test.txt.tmp $BERT_MODEL $MAX_LENGTH > test.txt
-```
-
-The GermEval 2014 dataset has much more labels than CoNLL-2002/2003 datasets, so an own set of labels must be used:
-
-```bash
-cat train.txt dev.txt test.txt | cut -d " " -f 2 | grep -v "^$"| sort | uniq > labels.txt
-```
-
-### Prepare the run
-
-Additional environment variables must be set:
-
-```bash
-export OUTPUT_DIR=germeval-model
-export BATCH_SIZE=32
-export NUM_EPOCHS=3
-export SAVE_STEPS=750
-export SEED=1
-```
-
-### Run the Pytorch version
-
-To start training, just run:
-
-```bash
-python3 run_ner.py --data_dir ./ \
---model_type bert \
---labels ./labels.txt \
---model_name_or_path $BERT_MODEL \
---output_dir $OUTPUT_DIR \
---max_seq_length $MAX_LENGTH \
---num_train_epochs $NUM_EPOCHS \
---per_gpu_train_batch_size $BATCH_SIZE \
---save_steps $SAVE_STEPS \
---seed $SEED \
---do_train \
---do_eval \
---do_predict
-```
-
-If your GPU supports half-precision training, just add the `--fp16` flag. After training, the model will be both evaluated on development and test datasets.
-
-#### Evaluation
-
-Evaluation on development dataset outputs the following for our example:
-
-```bash
-10/04/2019 00:42:06 - INFO - __main__ - ***** Eval results *****
-10/04/2019 00:42:06 - INFO - __main__ - f1 = 0.8623348017621146
-10/04/2019 00:42:06 - INFO - __main__ - loss = 0.07183869666975543
-10/04/2019 00:42:06 - INFO - __main__ - precision = 0.8467916366258111
-10/04/2019 00:42:06 - INFO - __main__ - recall = 0.8784592370979806
-```
-
-On the test dataset the following results could be achieved:
-
-```bash
-10/04/2019 00:42:42 - INFO - __main__ - ***** Eval results *****
-10/04/2019 00:42:42 - INFO - __main__ - f1 = 0.8614389652384803
-10/04/2019 00:42:42 - INFO - __main__ - loss = 0.07064602487454782
-10/04/2019 00:42:42 - INFO - __main__ - precision = 0.8604651162790697
-10/04/2019 00:42:42 - INFO - __main__ - recall = 0.8624150210424085
-```
-
-#### Comparing BERT (large, cased), RoBERTa (large, cased) and DistilBERT (base, uncased)
-
-Here is a small comparison between BERT (large, cased), RoBERTa (large, cased) and DistilBERT (base, uncased) with the same hyperparameters as specified in the [example documentation](https://huggingface.co/transformers/examples.html#named-entity-recognition) (one run):
-
-| Model | F-Score Dev | F-Score Test
-| --------------------------------- | ------- | --------
-| `bert-large-cased` | 95.59 | 91.70
-| `roberta-large` | 95.96 | 91.87
-| `distilbert-base-uncased` | 94.34 | 90.32
-
-### Run the Tensorflow 2 version
-
-To start training, just run:
-
-```bash
-python3 run_tf_ner.py --data_dir ./ \
---model_type bert \
---labels ./labels.txt \
---model_name_or_path $BERT_MODEL \
---output_dir $OUTPUT_DIR \
---max_seq_length $MAX_LENGTH \
---num_train_epochs $NUM_EPOCHS \
---per_device_train_batch_size $BATCH_SIZE \
---save_steps $SAVE_STEPS \
---seed $SEED \
---do_train \
---do_eval \
---do_predict
-```
-
-Such as the Pytorch version, if your GPU supports half-precision training, just add the `--fp16` flag. After training, the model will be both evaluated on development and test datasets.
-
-#### Evaluation
-
-Evaluation on development dataset outputs the following for our example:
-```bash
- precision recall f1-score support
-
- LOCderiv 0.7619 0.6154 0.6809 52
- PERpart 0.8724 0.8997 0.8858 4057
- OTHpart 0.9360 0.9466 0.9413 711
- ORGpart 0.7015 0.6989 0.7002 269
- LOCpart 0.7668 0.8488 0.8057 496
- LOC 0.8745 0.9191 0.8963 235
- ORGderiv 0.7723 0.8571 0.8125 91
- OTHderiv 0.4800 0.6667 0.5581 18
- OTH 0.5789 0.6875 0.6286 16
- PERderiv 0.5385 0.3889 0.4516 18
- PER 0.5000 0.5000 0.5000 2
- ORG 0.0000 0.0000 0.0000 3
-
-micro avg 0.8574 0.8862 0.8715 5968
-macro avg 0.8575 0.8862 0.8713 5968
-```
-
-On the test dataset the following results could be achieved:
-```bash
- precision recall f1-score support
-
- PERpart 0.8847 0.8944 0.8896 9397
- OTHpart 0.9376 0.9353 0.9365 1639
- ORGpart 0.7307 0.7044 0.7173 697
- LOC 0.9133 0.9394 0.9262 561
- LOCpart 0.8058 0.8157 0.8107 1150
- ORG 0.0000 0.0000 0.0000 8
- OTHderiv 0.5882 0.4762 0.5263 42
- PERderiv 0.6571 0.5227 0.5823 44
- OTH 0.4906 0.6667 0.5652 39
- ORGderiv 0.7016 0.7791 0.7383 172
- LOCderiv 0.8256 0.6514 0.7282 109
- PER 0.0000 0.0000 0.0000 11
-
-micro avg 0.8722 0.8774 0.8748 13869
-macro avg 0.8712 0.8774 0.8740 13869
-```
-
-## XNLI
-
-Based on the script [`run_xnli.py`](https://github.com/huggingface/transformers/blob/master/examples/run_xnli.py).
-
-[XNLI](https://www.nyu.edu/projects/bowman/xnli/) is crowd-sourced dataset based on [MultiNLI](http://www.nyu.edu/projects/bowman/multinli/). It is an evaluation benchmark for cross-lingual text representations. Pairs of text are labeled with textual entailment annotations for 15 different languages (including both high-ressource language such as English and low-ressource languages such as Swahili).
-
-#### Fine-tuning on XNLI
-
-This example code fine-tunes mBERT (multi-lingual BERT) on the XNLI dataset. It runs in 106 mins
-on a single tesla V100 16GB. The data for XNLI can be downloaded with the following links and should be both saved (and un-zipped) in a
-`$XNLI_DIR` directory.
-
-* [XNLI 1.0](https://www.nyu.edu/projects/bowman/xnli/XNLI-1.0.zip)
-* [XNLI-MT 1.0](https://www.nyu.edu/projects/bowman/xnli/XNLI-MT-1.0.zip)
-
-```bash
-export XNLI_DIR=/path/to/XNLI
-
-python run_xnli.py \
- --model_type bert \
- --model_name_or_path bert-base-multilingual-cased \
- --language de \
- --train_language en \
- --do_train \
- --do_eval \
- --data_dir $XNLI_DIR \
- --per_gpu_train_batch_size 32 \
- --learning_rate 5e-5 \
- --num_train_epochs 2.0 \
- --max_seq_length 128 \
- --output_dir /tmp/debug_xnli/ \
- --save_steps -1
-```
-
-Training with the previously defined hyper-parameters yields the following results on the **test** set:
-
-```bash
-acc = 0.7093812375249501
-```
-
-## MM-IMDb
-
-Based on the script [`run_mmimdb.py`](https://github.com/huggingface/transformers/blob/master/examples/mm-imdb/run_mmimdb.py).
-
-[MM-IMDb](http://lisi1.unal.edu.co/mmimdb/) is a Multimodal dataset with around 26,000 movies including images, plots and other metadata.
-
-### Training on MM-IMDb
-
-```
-python run_mmimdb.py \
- --data_dir /path/to/mmimdb/dataset/ \
- --model_type bert \
- --model_name_or_path bert-base-uncased \
- --output_dir /path/to/save/dir/ \
- --do_train \
- --do_eval \
- --max_seq_len 512 \
- --gradient_accumulation_steps 20 \
- --num_image_embeds 3 \
- --num_train_epochs 100 \
- --patience 5
-```
-
-## Adversarial evaluation of model performances
-
-Here is an example on evaluating a model using adversarial evaluation of natural language inference with the Heuristic Analysis for NLI Systems (HANS) dataset [McCoy et al., 2019](https://arxiv.org/abs/1902.01007). The example was gracefully provided by [Nafise Sadat Moosavi](https://github.com/ns-moosavi).
-
-The HANS dataset can be downloaded from [this location](https://github.com/tommccoy1/hans).
-
-This is an example of using test_hans.py:
-
-```bash
-export HANS_DIR=path-to-hans
-export MODEL_TYPE=type-of-the-model-e.g.-bert-roberta-xlnet-etc
-export MODEL_PATH=path-to-the-model-directory-that-is-trained-on-NLI-e.g.-by-using-run_glue.py
-
-python examples/test_hans.py \
- --task_name hans \
- --model_type $MODEL_TYPE \
- --do_eval \
- --do_lower_case \
- --data_dir $HANS_DIR \
- --model_name_or_path $MODEL_PATH \
- --max_seq_length 128 \
- -output_dir $MODEL_PATH \
-```
-
-This will create the hans_predictions.txt file in MODEL_PATH, which can then be evaluated using hans/evaluate_heur_output.py from the HANS dataset.
-
-The results of the BERT-base model that is trained on MNLI using batch size 8 and the random seed 42 on the HANS dataset is as follows:
-
-```bash
-Heuristic entailed results:
-lexical_overlap: 0.9702
-subsequence: 0.9942
-constituent: 0.9962
-
-Heuristic non-entailed results:
-lexical_overlap: 0.199
-subsequence: 0.0396
-constituent: 0.118
-```
diff --git a/server/transformers/examples/benchmarks.py b/server/transformers/examples/benchmarks.py
deleted file mode 100644
index 07de19d4b518674bb27dd0b5d2b378bfe934e576..0000000000000000000000000000000000000000
--- a/server/transformers/examples/benchmarks.py
+++ /dev/null
@@ -1,531 +0,0 @@
-# coding=utf-8
-# Copyright 2018 The HuggingFace Inc. team.
-# Copyright (c) 2018, NVIDIA CORPORATION. All rights reserved.
-#
-# Licensed under the Apache License, Version 2.0 (the "License");
-# you may not use this file except in compliance with the License.
-# You may obtain a copy of the License at
-#
-# http://www.apache.org/licenses/LICENSE-2.0
-#
-# Unless required by applicable law or agreed to in writing, software
-# distributed under the License is distributed on an "AS IS" BASIS,
-# WITHOUT WARRANTIES OR CONDITIONS OF ANY KIND, either express or implied.
-# See the License for the specific language governing permissions and
-# limitations under the License.
-""" Benchmarking the library on inference and training """
-
-# If checking the tensors placement
-# tf.debugging.set_log_device_placement(True)
-
-import argparse
-import csv
-import timeit
-from time import time
-from typing import List
-
-from transformers import AutoConfig, AutoTokenizer, is_tf_available, is_torch_available
-
-
-if is_tf_available():
- import tensorflow as tf
- from transformers import TFAutoModel
-
-if is_torch_available():
- import torch
- from transformers import AutoModel
-
-
-input_text = """Bent over their instruments, three hundred Fertilizers were plunged, as
-the Director of Hatcheries and Conditioning entered the room, in the
-
-
-
-scarcely breathing silence, the absent-minded, soliloquizing hum or
-whistle, of absorbed concentration. A troop of newly arrived students,
-very young, pink and callow, followed nervously, rather abjectly, at the
-Director's heels. Each of them carried a notebook, in which, whenever
-the great man spoke, he desperately scribbled. Straight from the
-horse's mouth. It was a rare privilege. The D. H. C. for Central London
-always made a point of personally conducting his new students round
-the various departments.
-
-"Just to give you a general idea," he would explain to them. For of
-course some sort of general idea they must have, if they were to do
-their work intelligently-though as little of one, if they were to be good
-and happy members of society, as possible. For particulars, as every
-one knows, make for virtue and happiness; generalities are intellectu-
-ally necessary evils. Not philosophers but fret-sawyers and stamp col-
-lectors compose the backbone of society.
-
-"To-morrow," he would add, smiling at them with a slightly menacing
-geniality, "you'll be settling down to serious work. You won't have time
-for generalities. Meanwhile ..."
-
-Meanwhile, it was a privilege. Straight from the horse's mouth into the
-notebook. The boys scribbled like mad.
-
-Tall and rather thin but upright, the Director advanced into the room.
-He had a long chin and big rather prominent teeth, just covered, when
-he was not talking, by his full, floridly curved lips. Old, young? Thirty?
-Fifty? Fifty-five? It was hard to say. And anyhow the question didn't
-arise; in this year of stability, A. F. 632, it didn't occur to you to ask it.
-
-"I shall begin at the beginning," said the D.H.C. and the more zealous
-students recorded his intention in their notebooks: Begin at the begin-
-ning. "These," he waved his hand, "are the incubators." And opening
-an insulated door he showed them racks upon racks of numbered test-
-tubes. "The week's supply of ova. Kept," he explained, "at blood heat;
-whereas the male gametes," and here he opened another door, "they
-have to be kept at thirty-five instead of thirty-seven. Full blood heat
-sterilizes." Rams wrapped in theremogene beget no lambs.
-
-Still leaning against the incubators he gave them, while the pencils
-scurried illegibly across the pages, a brief description of the modern
-
-
-
-fertilizing process; spoke first, of course, of its surgical introduc-
-tion-"the operation undergone voluntarily for the good of Society, not
-to mention the fact that it carries a bonus amounting to six months'
-salary"; continued with some account of the technique for preserving
-the excised ovary alive and actively developing; passed on to a consid-
-eration of optimum temperature, salinity, viscosity; referred to the liq-
-uor in which the detached and ripened eggs were kept; and, leading
-his charges to the work tables, actually showed them how this liquor
-was drawn off from the test-tubes; how it was let out drop by drop
-onto the specially warmed slides of the microscopes; how the eggs
-which it contained were inspected for abnormalities, counted and
-transferred to a porous receptacle; how (and he now took them to
-watch the operation) this receptacle was immersed in a warm bouillon
-containing free-swimming spermatozoa-at a minimum concentration
-of one hundred thousand per cubic centimetre, he insisted; and how,
-after ten minutes, the container was lifted out of the liquor and its
-contents re-examined; how, if any of the eggs remained unfertilized, it
-was again immersed, and, if necessary, yet again; how the fertilized
-ova went back to the incubators; where the Alphas and Betas re-
-mained until definitely bottled; while the Gammas, Deltas and Epsilons
-were brought out again, after only thirty-six hours, to undergo Bo-
-kanovsky's Process.
-
-"Bokanovsky's Process," repeated the Director, and the students un-
-derlined the words in their little notebooks.
-
-One egg, one embryo, one adult-normality. But a bokanovskified egg
-will bud, will proliferate, will divide. From eight to ninety-six buds, and
-every bud will grow into a perfectly formed embryo, and every embryo
-into a full-sized adult. Making ninety-six human beings grow where
-only one grew before. Progress.
-
-"Essentially," the D.H.C. concluded, "bokanovskification consists of a
-series of arrests of development. We check the normal growth and,
-paradoxically enough, the egg responds by budding."
-
-Responds by budding. The pencils were busy.
-
-He pointed. On a very slowly moving band a rack-full of test-tubes was
-entering a large metal box, another, rack-full was emerging. Machinery
-faintly purred. It took eight minutes for the tubes to go through, he
-
-
-
-told them. Eight minutes of hard X-rays being about as much as an
-egg can stand. A few died; of the rest, the least susceptible divided
-into two; most put out four buds; some eight; all were returned to the
-incubators, where the buds began to develop; then, after two days,
-were suddenly chilled, chilled and checked. Two, four, eight, the buds
-in their turn budded; and having budded were dosed almost to death
-with alcohol; consequently burgeoned again and having budded-bud
-out of bud out of bud-were thereafter-further arrest being generally
-fatal-left to develop in peace. By which time the original egg was in a
-fair way to becoming anything from eight to ninety-six embryos- a
-prodigious improvement, you will agree, on nature. Identical twins-but
-not in piddling twos and threes as in the old viviparous days, when an
-egg would sometimes accidentally divide; actually by dozens, by
-scores at a time.
-
-"Scores," the Director repeated and flung out his arms, as though he
-were distributing largesse. "Scores."
-
-But one of the students was fool enough to ask where the advantage
-lay.
-
-"My good boy!" The Director wheeled sharply round on him. "Can't you
-see? Can't you see?" He raised a hand; his expression was solemn.
-"Bokanovsky's Process is one of the major instruments of social stabil-
-ity!"
-
-Major instruments of social stability.
-
-Standard men and women; in uniform batches. The whole of a small
-factory staffed with the products of a single bokanovskified egg.
-
-"Ninety-six identical twins working ninety-six identical machines!" The
-voice was almost tremulous with enthusiasm. "You really know where
-you are. For the first time in history." He quoted the planetary motto.
-"Community, Identity, Stability." Grand words. "If we could bo-
-kanovskify indefinitely the whole problem would be solved."
-
-Solved by standard Gammas, unvarying Deltas, uniform Epsilons. Mil-
-lions of identical twins. The principle of mass production at last applied
-to biology.
-
-
-
-"But, alas," the Director shook his head, "we can't bokanovskify indefi-
-nitely."
-
-Ninety-six seemed to be the limit; seventy-two a good average. From
-the same ovary and with gametes of the same male to manufacture as
-many batches of identical twins as possible-that was the best (sadly a
-second best) that they could do. And even that was difficult.
-
-"For in nature it takes thirty years for two hundred eggs to reach ma-
-turity. But our business is to stabilize the population at this moment,
-here and now. Dribbling out twins over a quarter of a century-what
-would be the use of that?"
-
-Obviously, no use at all. But Podsnap's Technique had immensely ac-
-celerated the process of ripening. They could make sure of at least a
-hundred and fifty mature eggs within two years. Fertilize and bo-
-kanovskify-in other words, multiply by seventy-two-and you get an
-average of nearly eleven thousand brothers and sisters in a hundred
-and fifty batches of identical twins, all within two years of the same
-age.
-
-"And in exceptional cases we can make one ovary yield us over fifteen
-thousand adult individuals."
-
-Beckoning to a fair-haired, ruddy young man who happened to be
-passing at the moment. "Mr. Foster," he called. The ruddy young man
-approached. "Can you tell us the record for a single ovary, Mr. Foster?"
-
-"Sixteen thousand and twelve in this Centre," Mr. Foster replied with-
-out hesitation. He spoke very quickly, had a vivacious blue eye, and
-took an evident pleasure in quoting figures. "Sixteen thousand and
-twelve; in one hundred and eighty-nine batches of identicals. But of
-course they've done much better," he rattled on, "in some of the tropi-
-cal Centres. Singapore has often produced over sixteen thousand five
-hundred; and Mombasa has actually touched the seventeen thousand
-mark. But then they have unfair advantages. You should see the way a
-negro ovary responds to pituitary! It's quite astonishing, when you're
-used to working with European material. Still," he added, with a laugh
-(but the light of combat was in his eyes and the lift of his chin was
-challenging), "still, we mean to beat them if we can. I'm working on a
-wonderful Delta-Minus ovary at this moment. Only just eighteen
-
-
-
-months old. Over twelve thousand seven hundred children already, ei-
-ther decanted or in embryo. And still going strong. We'll beat them
-yet."
-
-"That's the spirit I like!" cried the Director, and clapped Mr. Foster on
-the shoulder. "Come along with us, and give these boys the benefit of
-your expert knowledge."
-
-Mr. Foster smiled modestly. "With pleasure." They went.
-In the Bottling Room all was harmonious bustle and ordered activity.
-Flaps of fresh sow's peritoneum ready cut to the proper size came
-shooting up in little lifts from the Organ Store in the sub-basement.
-Whizz and then, click! the lift-hatches hew open; the bottle-liner had
-only to reach out a hand, take the flap, insert, smooth-down, and be-
-fore the lined bottle had had time to travel out of reach along the end-
-less band, whizz, click! another flap of peritoneum had shot up from
-the depths, ready to be slipped into yet another bottle, the next of that
-slow interminable procession on the band.
-
-Next to the Liners stood the Matriculators. The procession advanced;
-one by one the eggs were transferred from their test-tubes to the
-larger containers; deftly the peritoneal lining was slit, the morula
-dropped into place, the saline solution poured in ... and already the
-bottle had passed, and it was the turn of the labellers. Heredity, date
-of fertilization, membership of Bokanovsky Group-details were trans-
-ferred from test-tube to bottle. No longer anonymous, but named,
-identified, the procession marched slowly on; on through an opening in
-the wall, slowly on into the Social Predestination Room.
-"Eighty-eight cubic metres of card-index," said Mr. Foster with relish,
-as they entered."""
-
-
-def create_setup_and_compute(
- model_names: List[str],
- gpu: bool = True,
- tensorflow: bool = False,
- average_over: int = 3,
- torchscript: bool = False,
- xla: bool = False,
- amp: bool = False,
- fp16: bool = False,
- save_to_csv: bool = False,
- csv_filename: str = f"results_{round(time())}.csv",
-):
- if xla:
- tf.config.optimizer.set_jit(True)
- if amp:
- tf.config.optimizer.set_experimental_options({"auto_mixed_precision": True})
-
- if tensorflow:
- dictionary = {model_name: {} for model_name in model_names}
- results = _compute_tensorflow(model_names, dictionary, average_over, amp)
- else:
- device = "cuda" if (gpu and torch.cuda.is_available()) else "cpu"
- dictionary = {model_name: {} for model_name in model_names}
- results = _compute_pytorch(model_names, dictionary, average_over, device, torchscript, fp16)
-
- print("=========== RESULTS ===========")
- for model_name in model_names:
- print("\t" + f"======= MODEL CHECKPOINT: {model_name} =======")
- for batch_size in results[model_name]["bs"]:
- print("\t\t" + f"===== BATCH SIZE: {batch_size} =====")
- for slice_size in results[model_name]["ss"]:
- result = results[model_name]["results"][batch_size][slice_size]
- if isinstance(result, str):
- print(f"\t\t{model_name}/{batch_size}/{slice_size}: " f"{result}")
- else:
- print(f"\t\t{model_name}/{batch_size}/{slice_size}: " f"{(round(1000 * result) / 1000)}" f"s")
-
- if save_to_csv:
- with open(csv_filename, mode="w") as csv_file:
- fieldnames = [
- "model",
- "1x8",
- "1x64",
- "1x128",
- "1x256",
- "1x512",
- "1x1024",
- "2x8",
- "2x64",
- "2x128",
- "2x256",
- "2x512",
- "2x1024",
- "4x8",
- "4x64",
- "4x128",
- "4x256",
- "4x512",
- "4x1024",
- "8x8",
- "8x64",
- "8x128",
- "8x256",
- "8x512",
- "8x1024",
- ]
-
- writer = csv.DictWriter(csv_file, fieldnames=fieldnames)
- writer.writeheader()
-
- for model_name in model_names:
- model_results = {
- f"{bs}x{ss}": results[model_name]["results"][bs][ss]
- for bs in results[model_name]["results"]
- for ss in results[model_name]["results"][bs]
- }
- writer.writerow({"model": model_name, **model_results})
-
-
-def _compute_pytorch(model_names, dictionary, average_over, device, torchscript, fp16):
- for c, model_name in enumerate(model_names):
- print(f"{c + 1} / {len(model_names)}")
- config = AutoConfig.from_pretrained(model_name, torchscript=torchscript)
- model = AutoModel.from_pretrained(model_name, config=config)
- tokenizer = AutoTokenizer.from_pretrained(model_name)
-
- tokenized_sequence = tokenizer.encode(input_text, add_special_tokens=False)
-
- max_input_size = tokenizer.max_model_input_sizes[model_name]
- batch_sizes = [1, 2, 4, 8]
- slice_sizes = [8, 64, 128, 256, 512, 1024]
-
- dictionary[model_name] = {"bs": batch_sizes, "ss": slice_sizes, "results": {}}
- dictionary[model_name]["results"] = {i: {} for i in batch_sizes}
-
- for batch_size in batch_sizes:
- if fp16:
- model.half()
- model.to(device)
- model.eval()
- for slice_size in slice_sizes:
- if max_input_size is not None and slice_size > max_input_size:
- dictionary[model_name]["results"][batch_size][slice_size] = "N/A"
- else:
- sequence = torch.tensor(tokenized_sequence[:slice_size], device=device).repeat(batch_size, 1)
- try:
- if torchscript:
- print("Tracing model with sequence size", sequence.shape)
- inference = torch.jit.trace(model, sequence)
- inference(sequence)
- else:
- inference = model
- inference(sequence)
-
- print("Going through model with sequence of shape", sequence.shape)
- runtimes = timeit.repeat(lambda: inference(sequence), repeat=average_over, number=3)
- average_time = sum(runtimes) / float(len(runtimes)) / 3.0
- dictionary[model_name]["results"][batch_size][slice_size] = average_time
- except RuntimeError as e:
- print("Doesn't fit on GPU.", e)
- torch.cuda.empty_cache()
- dictionary[model_name]["results"][batch_size][slice_size] = "N/A"
- return dictionary
-
-
-def _compute_tensorflow(model_names, dictionary, average_over, amp):
- for c, model_name in enumerate(model_names):
- print(f"{c + 1} / {len(model_names)}")
- config = AutoConfig.from_pretrained(model_name)
- model = TFAutoModel.from_pretrained(model_name, config=config)
- tokenizer = AutoTokenizer.from_pretrained(model_name)
-
- tokenized_sequence = tokenizer.encode(input_text, add_special_tokens=False)
-
- max_input_size = tokenizer.max_model_input_sizes[model_name]
- batch_sizes = [1, 2, 4, 8]
- slice_sizes = [8, 64, 128, 256, 512, 1024]
-
- dictionary[model_name] = {"bs": batch_sizes, "ss": slice_sizes, "results": {}}
- dictionary[model_name]["results"] = {i: {} for i in batch_sizes}
-
- print("Using model", model)
-
- @tf.function
- def inference(inputs):
- return model(inputs)
-
- for batch_size in batch_sizes:
- for slice_size in slice_sizes:
- if max_input_size is not None and slice_size > max_input_size:
- dictionary[model_name]["results"][batch_size][slice_size] = "N/A"
- else:
- sequence = tf.stack(
- [tf.squeeze(tf.constant(tokenized_sequence[:slice_size])[None, :])] * batch_size
- )
-
- try:
- print("Going through model with sequence of shape", sequence.shape)
- # To make sure that the model is traced + that the tensors are on the appropriate device
- inference(sequence)
-
- runtimes = timeit.repeat(lambda: inference(sequence), repeat=average_over, number=3)
- average_time = sum(runtimes) / float(len(runtimes)) / 3.0
- dictionary[model_name]["results"][batch_size][slice_size] = average_time
- except tf.errors.ResourceExhaustedError as e:
- print("Doesn't fit on GPU.", e)
- torch.cuda.empty_cache()
- dictionary[model_name]["results"][batch_size][slice_size] = "N/A"
- return dictionary
-
-
-def main():
- parser = argparse.ArgumentParser()
-
- parser.add_argument(
- "--models",
- required=False,
- type=str,
- default="all",
- help="Model checkpoints to be provided "
- "to the AutoModel classes. Leave "
- "blank to benchmark the base version "
- "of all available model "
- "architectures.",
- )
- parser.add_argument(
- "--torch", required=False, action="store_true", help="Benchmark the Pytorch version of the " "models"
- )
- parser.add_argument(
- "--torch_cuda", required=False, action="store_true", help="Pytorch only: run on available " "cuda devices"
- )
- parser.add_argument(
- "--torchscript",
- required=False,
- action="store_true",
- help="Pytorch only: trace the models " "using torchscript",
- )
- parser.add_argument(
- "--tensorflow",
- required=False,
- action="store_true",
- help="Benchmark the TensorFlow version "
- "of the models. Will run on GPU if "
- "the correct dependencies are "
- "installed",
- )
- parser.add_argument("--xla", required=False, action="store_true", help="TensorFlow only: use XLA acceleration.")
- parser.add_argument(
- "--amp",
- required=False,
- action="store_true",
- help="TensorFlow only: use automatic mixed precision acceleration.",
- )
- parser.add_argument(
- "--fp16", required=False, action="store_true", help="PyTorch only: use FP16 to accelerate inference."
- )
- parser.add_argument(
- "--keras_predict",
- required=False,
- action="store_true",
- help="Whether to use model.predict " "instead of model() to do a " "forward pass.",
- )
- parser.add_argument("--save_to_csv", required=False, action="store_true", help="Save to a CSV file.")
- parser.add_argument(
- "--csv_filename", required=False, default=None, help="CSV filename used if saving results to csv."
- )
- parser.add_argument(
- "--average_over", required=False, default=30, type=int, help="Times an experiment will be run."
- )
-
- args = parser.parse_args()
- if args.models == "all":
- args.models = [
- "gpt2",
- "bert-base-cased",
- "xlnet-base-cased",
- "xlm-mlm-en-2048",
- "transfo-xl-wt103",
- "openai-gpt",
- "distilbert-base-uncased",
- "distilgpt2",
- "roberta-base",
- "ctrl",
- ]
- else:
- args.models = args.models.split()
-
- print("Running with arguments", args)
-
- if args.torch:
- if is_torch_available():
- create_setup_and_compute(
- model_names=args.models,
- tensorflow=False,
- gpu=args.torch_cuda,
- torchscript=args.torchscript,
- fp16=args.fp16,
- save_to_csv=args.save_to_csv,
- csv_filename=args.csv_filename,
- average_over=args.average_over,
- )
- else:
- raise ImportError("Trying to run a PyTorch benchmark but PyTorch was not found in the environment.")
-
- if args.tensorflow:
- if is_tf_available():
- create_setup_and_compute(
- model_names=args.models,
- tensorflow=True,
- xla=args.xla,
- amp=args.amp,
- save_to_csv=args.save_to_csv,
- csv_filename=args.csv_filename,
- average_over=args.average_over,
- )
- else:
- raise ImportError("Trying to run a TensorFlow benchmark but TensorFlow was not found in the environment.")
-
-
-if __name__ == "__main__":
- main()
diff --git a/server/transformers/examples/contrib/README.md b/server/transformers/examples/contrib/README.md
deleted file mode 100644
index f2d0616e629bcc7d7800d1a4b727e725379ac736..0000000000000000000000000000000000000000
--- a/server/transformers/examples/contrib/README.md
+++ /dev/null
@@ -1,5 +0,0 @@
-# Community contributed examples
-
-This folder contains examples which are not actively maintained (mostly contributed by the community).
-
-Using these examples together with a recent version of the library usually requires to make small (sometimes big) adaptations to get the scripts working.
diff --git a/server/transformers/examples/contrib/run_camembert.py b/server/transformers/examples/contrib/run_camembert.py
deleted file mode 100644
index 3da66d419b96885b7d4186619174a548bd0abe20..0000000000000000000000000000000000000000
--- a/server/transformers/examples/contrib/run_camembert.py
+++ /dev/null
@@ -1,43 +0,0 @@
-import torch
-
-from transformers.modeling_camembert import CamembertForMaskedLM
-from transformers.tokenization_camembert import CamembertTokenizer
-
-
-def fill_mask(masked_input, model, tokenizer, topk=5):
- # Adapted from https://github.com/pytorch/fairseq/blob/master/fairseq/models/roberta/hub_interface.py
- assert masked_input.count("") == 1
- input_ids = torch.tensor(tokenizer.encode(masked_input, add_special_tokens=True)).unsqueeze(0) # Batch size 1
- logits = model(input_ids)[0] # The last hidden-state is the first element of the output tuple
- masked_index = (input_ids.squeeze() == tokenizer.mask_token_id).nonzero().item()
- logits = logits[0, masked_index, :]
- prob = logits.softmax(dim=0)
- values, indices = prob.topk(k=topk, dim=0)
- topk_predicted_token_bpe = " ".join(
- [tokenizer.convert_ids_to_tokens(indices[i].item()) for i in range(len(indices))]
- )
- masked_token = tokenizer.mask_token
- topk_filled_outputs = []
- for index, predicted_token_bpe in enumerate(topk_predicted_token_bpe.split(" ")):
- predicted_token = predicted_token_bpe.replace("\u2581", " ")
- if " {0}".format(masked_token) in masked_input:
- topk_filled_outputs.append(
- (
- masked_input.replace(" {0}".format(masked_token), predicted_token),
- values[index].item(),
- predicted_token,
- )
- )
- else:
- topk_filled_outputs.append(
- (masked_input.replace(masked_token, predicted_token), values[index].item(), predicted_token,)
- )
- return topk_filled_outputs
-
-
-tokenizer = CamembertTokenizer.from_pretrained("camembert-base")
-model = CamembertForMaskedLM.from_pretrained("camembert-base")
-model.eval()
-
-masked_input = "Le camembert est :)"
-print(fill_mask(masked_input, model, tokenizer, topk=3))
diff --git a/server/transformers/examples/contrib/run_openai_gpt.py b/server/transformers/examples/contrib/run_openai_gpt.py
deleted file mode 100644
index 136e25821f1c1e4526c7ef6aa6453e6b3d8ff89e..0000000000000000000000000000000000000000
--- a/server/transformers/examples/contrib/run_openai_gpt.py
+++ /dev/null
@@ -1,316 +0,0 @@
-# coding=utf-8
-# Copyright 2018 Google AI, Google Brain and Carnegie Mellon University Authors and the HuggingFace Inc. team.
-# Copyright (c) 2018, NVIDIA CORPORATION. All rights reserved.
-#
-# Licensed under the Apache License, Version 2.0 (the "License");
-# you may not use this file except in compliance with the License.
-# You may obtain a copy of the License at
-#
-# http://www.apache.org/licenses/LICENSE-2.0
-#
-# Unless required by applicable law or agreed to in writing, software
-# distributed under the License is distributed on an "AS IS" BASIS,
-# WITHOUT WARRANTIES OR CONDITIONS OF ANY KIND, either express or implied.
-# See the License for the specific language governing permissions and
-# limitations under the License.
-""" OpenAI GPT model fine-tuning script.
- Adapted from https://github.com/huggingface/pytorch-openai-transformer-lm/blob/master/train.py
- It self adapted from https://github.com/openai/finetune-transformer-lm/blob/master/train.py
-
- This script with default values fine-tunes and evaluate a pretrained OpenAI GPT on the RocStories dataset:
- python run_openai_gpt.py \
- --model_name openai-gpt \
- --do_train \
- --do_eval \
- --train_dataset "$ROC_STORIES_DIR/cloze_test_val__spring2016 - cloze_test_ALL_val.csv" \
- --eval_dataset "$ROC_STORIES_DIR/cloze_test_test__spring2016 - cloze_test_ALL_test.csv" \
- --output_dir ../log \
- --train_batch_size 16 \
-"""
-import argparse
-import csv
-import logging
-import os
-import random
-
-import numpy as np
-import torch
-from torch.utils.data import DataLoader, RandomSampler, SequentialSampler, TensorDataset
-from tqdm import tqdm, trange
-
-from transformers import (
- CONFIG_NAME,
- WEIGHTS_NAME,
- AdamW,
- OpenAIGPTDoubleHeadsModel,
- OpenAIGPTTokenizer,
- get_linear_schedule_with_warmup,
-)
-
-
-logging.basicConfig(
- format="%(asctime)s - %(levelname)s - %(name)s - %(message)s", datefmt="%m/%d/%Y %H:%M:%S", level=logging.INFO
-)
-logger = logging.getLogger(__name__)
-
-
-def accuracy(out, labels):
- outputs = np.argmax(out, axis=1)
- return np.sum(outputs == labels)
-
-
-def load_rocstories_dataset(dataset_path):
- """ Output a list of tuples(story, 1st continuation, 2nd continuation, label) """
- with open(dataset_path, encoding="utf_8") as f:
- f = csv.reader(f)
- output = []
- next(f) # skip the first line
- for line in tqdm(f):
- output.append((" ".join(line[1:5]), line[5], line[6], int(line[-1]) - 1))
- return output
-
-
-def pre_process_datasets(encoded_datasets, input_len, cap_length, start_token, delimiter_token, clf_token):
- """ Pre-process datasets containing lists of tuples(story, 1st continuation, 2nd continuation, label)
-
- To Transformer inputs of shape (n_batch, n_alternative, length) comprising for each batch, continuation:
- input_ids[batch, alternative, :] = [start_token] + story[:cap_length] + [delimiter_token] + cont1[:cap_length] + [clf_token]
- """
- tensor_datasets = []
- for dataset in encoded_datasets:
- n_batch = len(dataset)
- input_ids = np.zeros((n_batch, 2, input_len), dtype=np.int64)
- mc_token_ids = np.zeros((n_batch, 2), dtype=np.int64)
- lm_labels = np.full((n_batch, 2, input_len), fill_value=-100, dtype=np.int64)
- mc_labels = np.zeros((n_batch,), dtype=np.int64)
- for i, (story, cont1, cont2, mc_label), in enumerate(dataset):
- with_cont1 = [start_token] + story[:cap_length] + [delimiter_token] + cont1[:cap_length] + [clf_token]
- with_cont2 = [start_token] + story[:cap_length] + [delimiter_token] + cont2[:cap_length] + [clf_token]
- input_ids[i, 0, : len(with_cont1)] = with_cont1
- input_ids[i, 1, : len(with_cont2)] = with_cont2
- mc_token_ids[i, 0] = len(with_cont1) - 1
- mc_token_ids[i, 1] = len(with_cont2) - 1
- lm_labels[i, 0, : len(with_cont1)] = with_cont1
- lm_labels[i, 1, : len(with_cont2)] = with_cont2
- mc_labels[i] = mc_label
- all_inputs = (input_ids, mc_token_ids, lm_labels, mc_labels)
- tensor_datasets.append(tuple(torch.tensor(t) for t in all_inputs))
- return tensor_datasets
-
-
-def main():
- parser = argparse.ArgumentParser()
- parser.add_argument("--model_name", type=str, default="openai-gpt", help="pretrained model name")
- parser.add_argument("--do_train", action="store_true", help="Whether to run training.")
- parser.add_argument("--do_eval", action="store_true", help="Whether to run eval on the dev set.")
- parser.add_argument(
- "--output_dir",
- default=None,
- type=str,
- required=True,
- help="The output directory where the model predictions and checkpoints will be written.",
- )
- parser.add_argument("--train_dataset", type=str, default="")
- parser.add_argument("--eval_dataset", type=str, default="")
- parser.add_argument("--seed", type=int, default=42)
- parser.add_argument("--num_train_epochs", type=int, default=3)
- parser.add_argument("--train_batch_size", type=int, default=8)
- parser.add_argument("--eval_batch_size", type=int, default=16)
- parser.add_argument("--adam_epsilon", default=1e-8, type=float, help="Epsilon for Adam optimizer.")
- parser.add_argument("--max_grad_norm", type=int, default=1)
- parser.add_argument(
- "--max_steps",
- default=-1,
- type=int,
- help="If > 0: set total number of training \
- steps to perform. Override num_train_epochs.",
- )
- parser.add_argument(
- "--gradient_accumulation_steps",
- type=int,
- default=1,
- help="Number of updates steps to accumulate before\
- performing a backward/update pass.",
- )
- parser.add_argument("--learning_rate", type=float, default=6.25e-5)
- parser.add_argument("--warmup_steps", default=0, type=int, help="Linear warmup over warmup_steps.")
- parser.add_argument("--lr_schedule", type=str, default="warmup_linear")
- parser.add_argument("--weight_decay", type=float, default=0.01)
- parser.add_argument("--lm_coef", type=float, default=0.9)
- parser.add_argument("--n_valid", type=int, default=374)
-
- parser.add_argument("--server_ip", type=str, default="", help="Can be used for distant debugging.")
- parser.add_argument("--server_port", type=str, default="", help="Can be used for distant debugging.")
- args = parser.parse_args()
- print(args)
-
- if args.server_ip and args.server_port:
- # Distant debugging - see https://code.visualstudio.com/docs/python/debugging#_attach-to-a-local-script
- import ptvsd
-
- print("Waiting for debugger attach")
- ptvsd.enable_attach(address=(args.server_ip, args.server_port), redirect_output=True)
- ptvsd.wait_for_attach()
-
- random.seed(args.seed)
- np.random.seed(args.seed)
- torch.manual_seed(args.seed)
- torch.cuda.manual_seed_all(args.seed)
-
- device = torch.device("cuda" if torch.cuda.is_available() else "cpu")
- n_gpu = torch.cuda.device_count()
- logger.info("device: {}, n_gpu {}".format(device, n_gpu))
-
- if not args.do_train and not args.do_eval:
- raise ValueError("At least one of `do_train` or `do_eval` must be True.")
-
- if not os.path.exists(args.output_dir):
- os.makedirs(args.output_dir)
-
- # Load tokenizer and model
- # This loading functions also add new tokens and embeddings called `special tokens`
- # These new embeddings will be fine-tuned on the RocStories dataset
- special_tokens = ["_start_", "_delimiter_", "_classify_"]
- tokenizer = OpenAIGPTTokenizer.from_pretrained(args.model_name)
- tokenizer.add_tokens(special_tokens)
- special_tokens_ids = tokenizer.convert_tokens_to_ids(special_tokens)
- model = OpenAIGPTDoubleHeadsModel.from_pretrained(args.model_name)
- model.resize_token_embeddings(len(tokenizer))
- model.to(device)
-
- # Load and encode the datasets
- def tokenize_and_encode(obj):
- """ Tokenize and encode a nested object """
- if isinstance(obj, str):
- return tokenizer.convert_tokens_to_ids(tokenizer.tokenize(obj))
- elif isinstance(obj, int):
- return obj
- return list(tokenize_and_encode(o) for o in obj)
-
- logger.info("Encoding dataset...")
- train_dataset = load_rocstories_dataset(args.train_dataset)
- eval_dataset = load_rocstories_dataset(args.eval_dataset)
- datasets = (train_dataset, eval_dataset)
- encoded_datasets = tokenize_and_encode(datasets)
-
- # Compute the max input length for the Transformer
- max_length = model.config.n_positions // 2 - 2
- input_length = max(
- len(story[:max_length]) + max(len(cont1[:max_length]), len(cont2[:max_length])) + 3
- for dataset in encoded_datasets
- for story, cont1, cont2, _ in dataset
- )
- input_length = min(input_length, model.config.n_positions) # Max size of input for the pre-trained model
-
- # Prepare inputs tensors and dataloaders
- tensor_datasets = pre_process_datasets(encoded_datasets, input_length, max_length, *special_tokens_ids)
- train_tensor_dataset, eval_tensor_dataset = tensor_datasets[0], tensor_datasets[1]
-
- train_data = TensorDataset(*train_tensor_dataset)
- train_sampler = RandomSampler(train_data)
- train_dataloader = DataLoader(train_data, sampler=train_sampler, batch_size=args.train_batch_size)
-
- eval_data = TensorDataset(*eval_tensor_dataset)
- eval_sampler = SequentialSampler(eval_data)
- eval_dataloader = DataLoader(eval_data, sampler=eval_sampler, batch_size=args.eval_batch_size)
-
- # Prepare optimizer
- if args.do_train:
- if args.max_steps > 0:
- t_total = args.max_steps
- args.num_train_epochs = args.max_steps // (len(train_dataloader) // args.gradient_accumulation_steps) + 1
- else:
- t_total = len(train_dataloader) // args.gradient_accumulation_steps * args.num_train_epochs
-
- param_optimizer = list(model.named_parameters())
- no_decay = ["bias", "LayerNorm.bias", "LayerNorm.weight"]
- optimizer_grouped_parameters = [
- {
- "params": [p for n, p in param_optimizer if not any(nd in n for nd in no_decay)],
- "weight_decay": args.weight_decay,
- },
- {"params": [p for n, p in param_optimizer if any(nd in n for nd in no_decay)], "weight_decay": 0.0},
- ]
- optimizer = AdamW(optimizer_grouped_parameters, lr=args.learning_rate, eps=args.adam_epsilon)
- scheduler = get_linear_schedule_with_warmup(
- optimizer, num_warmup_steps=args.warmup_steps, num_training_steps=t_total
- )
-
- if args.do_train:
- nb_tr_steps, tr_loss, exp_average_loss = 0, 0, None
- model.train()
- for _ in trange(int(args.num_train_epochs), desc="Epoch"):
- tr_loss = 0
- nb_tr_steps = 0
- tqdm_bar = tqdm(train_dataloader, desc="Training")
- for step, batch in enumerate(tqdm_bar):
- batch = tuple(t.to(device) for t in batch)
- input_ids, mc_token_ids, lm_labels, mc_labels = batch
- losses = model(input_ids, mc_token_ids=mc_token_ids, lm_labels=lm_labels, mc_labels=mc_labels)
- loss = args.lm_coef * losses[0] + losses[1]
- loss.backward()
- scheduler.step()
- optimizer.step()
- optimizer.zero_grad()
- tr_loss += loss.item()
- exp_average_loss = (
- loss.item() if exp_average_loss is None else 0.7 * exp_average_loss + 0.3 * loss.item()
- )
- nb_tr_steps += 1
- tqdm_bar.desc = "Training loss: {:.2e} lr: {:.2e}".format(exp_average_loss, scheduler.get_lr()[0])
-
- # Save a trained model
- if args.do_train:
- # Save a trained model, configuration and tokenizer
- model_to_save = model.module if hasattr(model, "module") else model # Only save the model itself
-
- # If we save using the predefined names, we can load using `from_pretrained`
- output_model_file = os.path.join(args.output_dir, WEIGHTS_NAME)
- output_config_file = os.path.join(args.output_dir, CONFIG_NAME)
-
- torch.save(model_to_save.state_dict(), output_model_file)
- model_to_save.config.to_json_file(output_config_file)
- tokenizer.save_vocabulary(args.output_dir)
-
- # Load a trained model and vocabulary that you have fine-tuned
- model = OpenAIGPTDoubleHeadsModel.from_pretrained(args.output_dir)
- tokenizer = OpenAIGPTTokenizer.from_pretrained(args.output_dir)
- model.to(device)
-
- if args.do_eval:
- model.eval()
- eval_loss, eval_accuracy = 0, 0
- nb_eval_steps, nb_eval_examples = 0, 0
- for batch in tqdm(eval_dataloader, desc="Evaluating"):
- batch = tuple(t.to(device) for t in batch)
- input_ids, mc_token_ids, lm_labels, mc_labels = batch
- with torch.no_grad():
- _, mc_loss, _, mc_logits = model(
- input_ids, mc_token_ids=mc_token_ids, lm_labels=lm_labels, mc_labels=mc_labels
- )
-
- mc_logits = mc_logits.detach().cpu().numpy()
- mc_labels = mc_labels.to("cpu").numpy()
- tmp_eval_accuracy = accuracy(mc_logits, mc_labels)
-
- eval_loss += mc_loss.mean().item()
- eval_accuracy += tmp_eval_accuracy
-
- nb_eval_examples += input_ids.size(0)
- nb_eval_steps += 1
-
- eval_loss = eval_loss / nb_eval_steps
- eval_accuracy = eval_accuracy / nb_eval_examples
- train_loss = tr_loss / nb_tr_steps if args.do_train else None
- result = {"eval_loss": eval_loss, "eval_accuracy": eval_accuracy, "train_loss": train_loss}
-
- output_eval_file = os.path.join(args.output_dir, "eval_results.txt")
- with open(output_eval_file, "w") as writer:
- logger.info("***** Eval results *****")
- for key in sorted(result.keys()):
- logger.info(" %s = %s", key, str(result[key]))
- writer.write("%s = %s\n" % (key, str(result[key])))
-
-
-if __name__ == "__main__":
- main()
diff --git a/server/transformers/examples/contrib/run_swag.py b/server/transformers/examples/contrib/run_swag.py
deleted file mode 100644
index 497ddeca9de3e4687017fa0c6526523199693ff5..0000000000000000000000000000000000000000
--- a/server/transformers/examples/contrib/run_swag.py
+++ /dev/null
@@ -1,737 +0,0 @@
-# coding=utf-8
-# Copyright 2018 The Google AI Language Team Authors and The HuggingFace Inc. team.
-# Copyright (c) 2018, NVIDIA CORPORATION. All rights reserved.
-#
-# Licensed under the Apache License, Version 2.0 (the "License");
-# you may not use this file except in compliance with the License.
-# You may obtain a copy of the License at
-#
-# http://www.apache.org/licenses/LICENSE-2.0
-#
-# Unless required by applicable law or agreed to in writing, software
-# distributed under the License is distributed on an "AS IS" BASIS,
-# WITHOUT WARRANTIES OR CONDITIONS OF ANY KIND, either express or implied.
-# See the License for the specific language governing permissions and
-# limitations under the License.
-"""BERT finetuning runner.
- Finetuning the library models for multiple choice on SWAG (Bert).
-"""
-
-
-import argparse
-import csv
-import glob
-import logging
-import os
-import random
-
-import numpy as np
-import torch
-from torch.utils.data import DataLoader, RandomSampler, SequentialSampler, TensorDataset
-from torch.utils.data.distributed import DistributedSampler
-from tqdm import tqdm, trange
-
-from transformers import (
- WEIGHTS_NAME,
- AdamW,
- BertConfig,
- BertForMultipleChoice,
- BertTokenizer,
- get_linear_schedule_with_warmup,
-)
-
-
-try:
- from torch.utils.tensorboard import SummaryWriter
-except ImportError:
- from tensorboardX import SummaryWriter
-
-
-logger = logging.getLogger(__name__)
-
-ALL_MODELS = sum((tuple(conf.pretrained_config_archive_map.keys()) for conf in [BertConfig]), ())
-
-MODEL_CLASSES = {
- "bert": (BertConfig, BertForMultipleChoice, BertTokenizer),
-}
-
-
-class SwagExample(object):
- """A single training/test example for the SWAG dataset."""
-
- def __init__(self, swag_id, context_sentence, start_ending, ending_0, ending_1, ending_2, ending_3, label=None):
- self.swag_id = swag_id
- self.context_sentence = context_sentence
- self.start_ending = start_ending
- self.endings = [
- ending_0,
- ending_1,
- ending_2,
- ending_3,
- ]
- self.label = label
-
- def __str__(self):
- return self.__repr__()
-
- def __repr__(self):
- attributes = [
- "swag_id: {}".format(self.swag_id),
- "context_sentence: {}".format(self.context_sentence),
- "start_ending: {}".format(self.start_ending),
- "ending_0: {}".format(self.endings[0]),
- "ending_1: {}".format(self.endings[1]),
- "ending_2: {}".format(self.endings[2]),
- "ending_3: {}".format(self.endings[3]),
- ]
-
- if self.label is not None:
- attributes.append("label: {}".format(self.label))
-
- return ", ".join(attributes)
-
-
-class InputFeatures(object):
- def __init__(self, example_id, choices_features, label):
- self.example_id = example_id
- self.choices_features = [
- {"input_ids": input_ids, "input_mask": input_mask, "segment_ids": segment_ids}
- for _, input_ids, input_mask, segment_ids in choices_features
- ]
- self.label = label
-
-
-def read_swag_examples(input_file, is_training=True):
- with open(input_file, "r", encoding="utf-8") as f:
- lines = list(csv.reader(f))
-
- if is_training and lines[0][-1] != "label":
- raise ValueError("For training, the input file must contain a label column.")
-
- examples = [
- SwagExample(
- swag_id=line[2],
- context_sentence=line[4],
- start_ending=line[5], # in the swag dataset, the
- # common beginning of each
- # choice is stored in "sent2".
- ending_0=line[7],
- ending_1=line[8],
- ending_2=line[9],
- ending_3=line[10],
- label=int(line[11]) if is_training else None,
- )
- for line in lines[1:] # we skip the line with the column names
- ]
-
- return examples
-
-
-def convert_examples_to_features(examples, tokenizer, max_seq_length, is_training):
- """Loads a data file into a list of `InputBatch`s."""
-
- # Swag is a multiple choice task. To perform this task using Bert,
- # we will use the formatting proposed in "Improving Language
- # Understanding by Generative Pre-Training" and suggested by
- # @jacobdevlin-google in this issue
- # https://github.com/google-research/bert/issues/38.
- #
- # Each choice will correspond to a sample on which we run the
- # inference. For a given Swag example, we will create the 4
- # following inputs:
- # - [CLS] context [SEP] choice_1 [SEP]
- # - [CLS] context [SEP] choice_2 [SEP]
- # - [CLS] context [SEP] choice_3 [SEP]
- # - [CLS] context [SEP] choice_4 [SEP]
- # The model will output a single value for each input. To get the
- # final decision of the model, we will run a softmax over these 4
- # outputs.
- features = []
- for example_index, example in tqdm(enumerate(examples)):
- context_tokens = tokenizer.tokenize(example.context_sentence)
- start_ending_tokens = tokenizer.tokenize(example.start_ending)
-
- choices_features = []
- for ending_index, ending in enumerate(example.endings):
- # We create a copy of the context tokens in order to be
- # able to shrink it according to ending_tokens
- context_tokens_choice = context_tokens[:]
- ending_tokens = start_ending_tokens + tokenizer.tokenize(ending)
- # Modifies `context_tokens_choice` and `ending_tokens` in
- # place so that the total length is less than the
- # specified length. Account for [CLS], [SEP], [SEP] with
- # "- 3"
- _truncate_seq_pair(context_tokens_choice, ending_tokens, max_seq_length - 3)
-
- tokens = ["[CLS]"] + context_tokens_choice + ["[SEP]"] + ending_tokens + ["[SEP]"]
- segment_ids = [0] * (len(context_tokens_choice) + 2) + [1] * (len(ending_tokens) + 1)
-
- input_ids = tokenizer.convert_tokens_to_ids(tokens)
- input_mask = [1] * len(input_ids)
-
- # Zero-pad up to the sequence length.
- padding = [0] * (max_seq_length - len(input_ids))
- input_ids += padding
- input_mask += padding
- segment_ids += padding
-
- assert len(input_ids) == max_seq_length
- assert len(input_mask) == max_seq_length
- assert len(segment_ids) == max_seq_length
-
- choices_features.append((tokens, input_ids, input_mask, segment_ids))
-
- label = example.label
- if example_index < 5:
- logger.info("*** Example ***")
- logger.info("swag_id: {}".format(example.swag_id))
- for choice_idx, (tokens, input_ids, input_mask, segment_ids) in enumerate(choices_features):
- logger.info("choice: {}".format(choice_idx))
- logger.info("tokens: {}".format(" ".join(tokens)))
- logger.info("input_ids: {}".format(" ".join(map(str, input_ids))))
- logger.info("input_mask: {}".format(" ".join(map(str, input_mask))))
- logger.info("segment_ids: {}".format(" ".join(map(str, segment_ids))))
- if is_training:
- logger.info("label: {}".format(label))
-
- features.append(InputFeatures(example_id=example.swag_id, choices_features=choices_features, label=label))
-
- return features
-
-
-def _truncate_seq_pair(tokens_a, tokens_b, max_length):
- """Truncates a sequence pair in place to the maximum length."""
-
- # This is a simple heuristic which will always truncate the longer sequence
- # one token at a time. This makes more sense than truncating an equal percent
- # of tokens from each, since if one sequence is very short then each token
- # that's truncated likely contains more information than a longer sequence.
- while True:
- total_length = len(tokens_a) + len(tokens_b)
- if total_length <= max_length:
- break
- if len(tokens_a) > len(tokens_b):
- tokens_a.pop()
- else:
- tokens_b.pop()
-
-
-def accuracy(out, labels):
- outputs = np.argmax(out, axis=1)
- return np.sum(outputs == labels)
-
-
-def select_field(features, field):
- return [[choice[field] for choice in feature.choices_features] for feature in features]
-
-
-def set_seed(args):
- random.seed(args.seed)
- np.random.seed(args.seed)
- torch.manual_seed(args.seed)
- if args.n_gpu > 0:
- torch.cuda.manual_seed_all(args.seed)
-
-
-def load_and_cache_examples(args, tokenizer, evaluate=False, output_examples=False):
- if args.local_rank not in [-1, 0]:
- torch.distributed.barrier() # Make sure only the first process in distributed training process the dataset, and the others will use the cache
-
- # Load data features from cache or dataset file
- input_file = args.predict_file if evaluate else args.train_file
- cached_features_file = os.path.join(
- os.path.dirname(input_file),
- "cached_{}_{}_{}".format(
- "dev" if evaluate else "train",
- list(filter(None, args.model_name_or_path.split("/"))).pop(),
- str(args.max_seq_length),
- ),
- )
- if os.path.exists(cached_features_file) and not args.overwrite_cache and not output_examples:
- logger.info("Loading features from cached file %s", cached_features_file)
- features = torch.load(cached_features_file)
- else:
- logger.info("Creating features from dataset file at %s", input_file)
- examples = read_swag_examples(input_file)
- features = convert_examples_to_features(examples, tokenizer, args.max_seq_length, not evaluate)
-
- if args.local_rank in [-1, 0]:
- logger.info("Saving features into cached file %s", cached_features_file)
- torch.save(features, cached_features_file)
-
- if args.local_rank == 0:
- torch.distributed.barrier() # Make sure only the first process in distributed training process the dataset, and the others will use the cache
-
- # Convert to Tensors and build dataset
- all_input_ids = torch.tensor(select_field(features, "input_ids"), dtype=torch.long)
- all_input_mask = torch.tensor(select_field(features, "input_mask"), dtype=torch.long)
- all_segment_ids = torch.tensor(select_field(features, "segment_ids"), dtype=torch.long)
- all_label = torch.tensor([f.label for f in features], dtype=torch.long)
-
- if evaluate:
- dataset = TensorDataset(all_input_ids, all_input_mask, all_segment_ids, all_label)
- else:
- dataset = TensorDataset(all_input_ids, all_input_mask, all_segment_ids, all_label)
-
- if output_examples:
- return dataset, examples, features
- return dataset
-
-
-def train(args, train_dataset, model, tokenizer):
- """ Train the model """
- if args.local_rank in [-1, 0]:
- tb_writer = SummaryWriter()
-
- args.train_batch_size = args.per_gpu_train_batch_size * max(1, args.n_gpu)
- train_sampler = RandomSampler(train_dataset) if args.local_rank == -1 else DistributedSampler(train_dataset)
- train_dataloader = DataLoader(train_dataset, sampler=train_sampler, batch_size=args.train_batch_size)
-
- if args.max_steps > 0:
- t_total = args.max_steps
- args.num_train_epochs = args.max_steps // (len(train_dataloader) // args.gradient_accumulation_steps) + 1
- else:
- t_total = len(train_dataloader) // args.gradient_accumulation_steps * args.num_train_epochs
-
- # Prepare optimizer and schedule (linear warmup and decay)
- no_decay = ["bias", "LayerNorm.weight"]
- optimizer_grouped_parameters = [
- {
- "params": [p for n, p in model.named_parameters() if not any(nd in n for nd in no_decay)],
- "weight_decay": args.weight_decay,
- },
- {"params": [p for n, p in model.named_parameters() if any(nd in n for nd in no_decay)], "weight_decay": 0.0},
- ]
- optimizer = AdamW(optimizer_grouped_parameters, lr=args.learning_rate, eps=args.adam_epsilon)
- scheduler = get_linear_schedule_with_warmup(
- optimizer, num_warmup_steps=args.warmup_steps, num_training_steps=t_total
- )
- if args.fp16:
- try:
- from apex import amp
- except ImportError:
- raise ImportError("Please install apex from https://www.github.com/nvidia/apex to use fp16 training.")
- model, optimizer = amp.initialize(model, optimizer, opt_level=args.fp16_opt_level)
-
- # multi-gpu training (should be after apex fp16 initialization)
- if args.n_gpu > 1:
- model = torch.nn.DataParallel(model)
-
- # Distributed training (should be after apex fp16 initialization)
- if args.local_rank != -1:
- model = torch.nn.parallel.DistributedDataParallel(
- model, device_ids=[args.local_rank], output_device=args.local_rank, find_unused_parameters=True
- )
-
- # Train!
- logger.info("***** Running training *****")
- logger.info(" Num examples = %d", len(train_dataset))
- logger.info(" Num Epochs = %d", args.num_train_epochs)
- logger.info(" Instantaneous batch size per GPU = %d", args.per_gpu_train_batch_size)
- logger.info(
- " Total train batch size (w. parallel, distributed & accumulation) = %d",
- args.train_batch_size
- * args.gradient_accumulation_steps
- * (torch.distributed.get_world_size() if args.local_rank != -1 else 1),
- )
- logger.info(" Gradient Accumulation steps = %d", args.gradient_accumulation_steps)
- logger.info(" Total optimization steps = %d", t_total)
-
- global_step = 0
- tr_loss, logging_loss = 0.0, 0.0
- model.zero_grad()
- train_iterator = trange(int(args.num_train_epochs), desc="Epoch", disable=args.local_rank not in [-1, 0])
- set_seed(args) # Added here for reproductibility
- for _ in train_iterator:
- epoch_iterator = tqdm(train_dataloader, desc="Iteration", disable=args.local_rank not in [-1, 0])
- for step, batch in enumerate(epoch_iterator):
- model.train()
- batch = tuple(t.to(args.device) for t in batch)
- inputs = {
- "input_ids": batch[0],
- "attention_mask": batch[1],
- # 'token_type_ids': None if args.model_type == 'xlm' else batch[2],
- "token_type_ids": batch[2],
- "labels": batch[3],
- }
- # if args.model_type in ['xlnet', 'xlm']:
- # inputs.update({'cls_index': batch[5],
- # 'p_mask': batch[6]})
- outputs = model(**inputs)
- loss = outputs[0] # model outputs are always tuple in transformers (see doc)
-
- if args.n_gpu > 1:
- loss = loss.mean() # mean() to average on multi-gpu parallel (not distributed) training
- if args.gradient_accumulation_steps > 1:
- loss = loss / args.gradient_accumulation_steps
-
- if args.fp16:
- with amp.scale_loss(loss, optimizer) as scaled_loss:
- scaled_loss.backward()
- torch.nn.utils.clip_grad_norm_(amp.master_params(optimizer), args.max_grad_norm)
- else:
- loss.backward()
- torch.nn.utils.clip_grad_norm_(model.parameters(), args.max_grad_norm)
-
- tr_loss += loss.item()
- if (step + 1) % args.gradient_accumulation_steps == 0:
- optimizer.step()
- scheduler.step() # Update learning rate schedule
- model.zero_grad()
- global_step += 1
-
- if args.local_rank in [-1, 0] and args.logging_steps > 0 and global_step % args.logging_steps == 0:
- # Log metrics
- if (
- args.local_rank == -1 and args.evaluate_during_training
- ): # Only evaluate when single GPU otherwise metrics may not average well
- results = evaluate(args, model, tokenizer)
- for key, value in results.items():
- tb_writer.add_scalar("eval_{}".format(key), value, global_step)
- tb_writer.add_scalar("lr", scheduler.get_lr()[0], global_step)
- tb_writer.add_scalar("loss", (tr_loss - logging_loss) / args.logging_steps, global_step)
- logging_loss = tr_loss
-
- if args.local_rank in [-1, 0] and args.save_steps > 0 and global_step % args.save_steps == 0:
- # Save model checkpoint
- output_dir = os.path.join(args.output_dir, "checkpoint-{}".format(global_step))
- if not os.path.exists(output_dir):
- os.makedirs(output_dir)
- model_to_save = (
- model.module if hasattr(model, "module") else model
- ) # Take care of distributed/parallel training
- model_to_save.save_pretrained(output_dir)
- tokenizer.save_vocabulary(output_dir)
- torch.save(args, os.path.join(output_dir, "training_args.bin"))
- logger.info("Saving model checkpoint to %s", output_dir)
-
- if args.max_steps > 0 and global_step > args.max_steps:
- epoch_iterator.close()
- break
- if args.max_steps > 0 and global_step > args.max_steps:
- train_iterator.close()
- break
-
- if args.local_rank in [-1, 0]:
- tb_writer.close()
-
- return global_step, tr_loss / global_step
-
-
-def evaluate(args, model, tokenizer, prefix=""):
- dataset, examples, features = load_and_cache_examples(args, tokenizer, evaluate=True, output_examples=True)
-
- if not os.path.exists(args.output_dir) and args.local_rank in [-1, 0]:
- os.makedirs(args.output_dir)
-
- args.eval_batch_size = args.per_gpu_eval_batch_size * max(1, args.n_gpu)
- # Note that DistributedSampler samples randomly
- eval_sampler = SequentialSampler(dataset) if args.local_rank == -1 else DistributedSampler(dataset)
- eval_dataloader = DataLoader(dataset, sampler=eval_sampler, batch_size=args.eval_batch_size)
-
- # Eval!
- logger.info("***** Running evaluation {} *****".format(prefix))
- logger.info(" Num examples = %d", len(dataset))
- logger.info(" Batch size = %d", args.eval_batch_size)
-
- eval_loss, eval_accuracy = 0, 0
- nb_eval_steps, nb_eval_examples = 0, 0
-
- for batch in tqdm(eval_dataloader, desc="Evaluating"):
- model.eval()
- batch = tuple(t.to(args.device) for t in batch)
- with torch.no_grad():
- inputs = {
- "input_ids": batch[0],
- "attention_mask": batch[1],
- # 'token_type_ids': None if args.model_type == 'xlm' else batch[2] # XLM don't use segment_ids
- "token_type_ids": batch[2],
- "labels": batch[3],
- }
-
- # if args.model_type in ['xlnet', 'xlm']:
- # inputs.update({'cls_index': batch[4],
- # 'p_mask': batch[5]})
- outputs = model(**inputs)
- tmp_eval_loss, logits = outputs[:2]
- eval_loss += tmp_eval_loss.mean().item()
-
- logits = logits.detach().cpu().numpy()
- label_ids = inputs["labels"].to("cpu").numpy()
- tmp_eval_accuracy = accuracy(logits, label_ids)
- eval_accuracy += tmp_eval_accuracy
-
- nb_eval_steps += 1
- nb_eval_examples += inputs["input_ids"].size(0)
-
- eval_loss = eval_loss / nb_eval_steps
- eval_accuracy = eval_accuracy / nb_eval_examples
- result = {"eval_loss": eval_loss, "eval_accuracy": eval_accuracy}
-
- output_eval_file = os.path.join(args.output_dir, "eval_results.txt")
- with open(output_eval_file, "w") as writer:
- logger.info("***** Eval results *****")
- for key in sorted(result.keys()):
- logger.info("%s = %s", key, str(result[key]))
- writer.write("%s = %s\n" % (key, str(result[key])))
-
- return result
-
-
-def main():
- parser = argparse.ArgumentParser()
-
- # Required parameters
- parser.add_argument(
- "--train_file", default=None, type=str, required=True, help="SWAG csv for training. E.g., train.csv"
- )
- parser.add_argument(
- "--predict_file",
- default=None,
- type=str,
- required=True,
- help="SWAG csv for predictions. E.g., val.csv or test.csv",
- )
- parser.add_argument(
- "--model_type",
- default=None,
- type=str,
- required=True,
- help="Model type selected in the list: " + ", ".join(MODEL_CLASSES.keys()),
- )
- parser.add_argument(
- "--model_name_or_path",
- default=None,
- type=str,
- required=True,
- help="Path to pre-trained model or shortcut name selected in the list: " + ", ".join(ALL_MODELS),
- )
- parser.add_argument(
- "--output_dir",
- default=None,
- type=str,
- required=True,
- help="The output directory where the model checkpoints and predictions will be written.",
- )
-
- # Other parameters
- parser.add_argument(
- "--config_name", default="", type=str, help="Pretrained config name or path if not the same as model_name"
- )
- parser.add_argument(
- "--tokenizer_name",
- default="",
- type=str,
- help="Pretrained tokenizer name or path if not the same as model_name",
- )
- parser.add_argument(
- "--max_seq_length",
- default=384,
- type=int,
- help="The maximum total input sequence length after tokenization. Sequences "
- "longer than this will be truncated, and sequences shorter than this will be padded.",
- )
- parser.add_argument("--do_train", action="store_true", help="Whether to run training.")
- parser.add_argument("--do_eval", action="store_true", help="Whether to run eval on the dev set.")
- parser.add_argument(
- "--evaluate_during_training", action="store_true", help="Rul evaluation during training at each logging step."
- )
- parser.add_argument(
- "--do_lower_case", action="store_true", help="Set this flag if you are using an uncased model."
- )
-
- parser.add_argument("--per_gpu_train_batch_size", default=8, type=int, help="Batch size per GPU/CPU for training.")
- parser.add_argument(
- "--per_gpu_eval_batch_size", default=8, type=int, help="Batch size per GPU/CPU for evaluation."
- )
- parser.add_argument("--learning_rate", default=5e-5, type=float, help="The initial learning rate for Adam.")
- parser.add_argument(
- "--gradient_accumulation_steps",
- type=int,
- default=1,
- help="Number of updates steps to accumulate before performing a backward/update pass.",
- )
- parser.add_argument("--weight_decay", default=0.0, type=float, help="Weight deay if we apply some.")
- parser.add_argument("--adam_epsilon", default=1e-8, type=float, help="Epsilon for Adam optimizer.")
- parser.add_argument("--max_grad_norm", default=1.0, type=float, help="Max gradient norm.")
- parser.add_argument(
- "--num_train_epochs", default=3.0, type=float, help="Total number of training epochs to perform."
- )
- parser.add_argument(
- "--max_steps",
- default=-1,
- type=int,
- help="If > 0: set total number of training steps to perform. Override num_train_epochs.",
- )
- parser.add_argument("--warmup_steps", default=0, type=int, help="Linear warmup over warmup_steps.")
-
- parser.add_argument("--logging_steps", type=int, default=50, help="Log every X updates steps.")
- parser.add_argument("--save_steps", type=int, default=50, help="Save checkpoint every X updates steps.")
- parser.add_argument(
- "--eval_all_checkpoints",
- action="store_true",
- help="Evaluate all checkpoints starting with the same prefix as model_name ending and ending with step number",
- )
- parser.add_argument("--no_cuda", action="store_true", help="Whether not to use CUDA when available")
- parser.add_argument(
- "--overwrite_output_dir", action="store_true", help="Overwrite the content of the output directory"
- )
- parser.add_argument(
- "--overwrite_cache", action="store_true", help="Overwrite the cached training and evaluation sets"
- )
- parser.add_argument("--seed", type=int, default=42, help="random seed for initialization")
-
- parser.add_argument("--local_rank", type=int, default=-1, help="local_rank for distributed training on gpus")
- parser.add_argument(
- "--fp16",
- action="store_true",
- help="Whether to use 16-bit (mixed) precision (through NVIDIA apex) instead of 32-bit",
- )
- parser.add_argument(
- "--fp16_opt_level",
- type=str,
- default="O1",
- help="For fp16: Apex AMP optimization level selected in ['O0', 'O1', 'O2', and 'O3']."
- "See details at https://nvidia.github.io/apex/amp.html",
- )
- parser.add_argument("--server_ip", type=str, default="", help="Can be used for distant debugging.")
- parser.add_argument("--server_port", type=str, default="", help="Can be used for distant debugging.")
- args = parser.parse_args()
-
- if (
- os.path.exists(args.output_dir)
- and os.listdir(args.output_dir)
- and args.do_train
- and not args.overwrite_output_dir
- ):
- raise ValueError(
- "Output directory ({}) already exists and is not empty. Use --overwrite_output_dir to overcome.".format(
- args.output_dir
- )
- )
-
- # Setup distant debugging if needed
- if args.server_ip and args.server_port:
- # Distant debugging - see https://code.visualstudio.com/docs/python/debugging#_attach-to-a-local-script
- import ptvsd
-
- print("Waiting for debugger attach")
- ptvsd.enable_attach(address=(args.server_ip, args.server_port), redirect_output=True)
- ptvsd.wait_for_attach()
-
- # Setup CUDA, GPU & distributed training
- if args.local_rank == -1 or args.no_cuda:
- device = torch.device("cuda" if torch.cuda.is_available() and not args.no_cuda else "cpu")
- args.n_gpu = torch.cuda.device_count()
- else: # Initializes the distributed backend which will take care of sychronizing nodes/GPUs
- torch.cuda.set_device(args.local_rank)
- device = torch.device("cuda", args.local_rank)
- torch.distributed.init_process_group(backend="nccl")
- args.n_gpu = 1
- args.device = device
-
- # Setup logging
- logging.basicConfig(
- format="%(asctime)s - %(levelname)s - %(name)s - %(message)s",
- datefmt="%m/%d/%Y %H:%M:%S",
- level=logging.INFO if args.local_rank in [-1, 0] else logging.WARN,
- )
- logger.warning(
- "Process rank: %s, device: %s, n_gpu: %s, distributed training: %s, 16-bits training: %s",
- args.local_rank,
- device,
- args.n_gpu,
- bool(args.local_rank != -1),
- args.fp16,
- )
-
- # Set seed
- set_seed(args)
-
- # Load pretrained model and tokenizer
- if args.local_rank not in [-1, 0]:
- torch.distributed.barrier() # Make sure only the first process in distributed training will download model & vocab
-
- args.model_type = args.model_type.lower()
- config_class, model_class, tokenizer_class = MODEL_CLASSES[args.model_type]
- config = config_class.from_pretrained(args.config_name if args.config_name else args.model_name_or_path)
- tokenizer = tokenizer_class.from_pretrained(
- args.tokenizer_name if args.tokenizer_name else args.model_name_or_path, do_lower_case=args.do_lower_case
- )
- model = model_class.from_pretrained(
- args.model_name_or_path, from_tf=bool(".ckpt" in args.model_name_or_path), config=config
- )
-
- if args.local_rank == 0:
- torch.distributed.barrier() # Make sure only the first process in distributed training will download model & vocab
-
- model.to(args.device)
-
- logger.info("Training/evaluation parameters %s", args)
-
- # Training
- if args.do_train:
- train_dataset = load_and_cache_examples(args, tokenizer, evaluate=False, output_examples=False)
- global_step, tr_loss = train(args, train_dataset, model, tokenizer)
- logger.info(" global_step = %s, average loss = %s", global_step, tr_loss)
-
- # Save the trained model and the tokenizer
- if args.local_rank == -1 or torch.distributed.get_rank() == 0:
- # Create output directory if needed
- if not os.path.exists(args.output_dir) and args.local_rank in [-1, 0]:
- os.makedirs(args.output_dir)
-
- logger.info("Saving model checkpoint to %s", args.output_dir)
- # Save a trained model, configuration and tokenizer using `save_pretrained()`.
- # They can then be reloaded using `from_pretrained()`
- model_to_save = (
- model.module if hasattr(model, "module") else model
- ) # Take care of distributed/parallel training
- model_to_save.save_pretrained(args.output_dir)
- tokenizer.save_pretrained(args.output_dir)
-
- # Good practice: save your training arguments together with the trained model
- torch.save(args, os.path.join(args.output_dir, "training_args.bin"))
-
- # Load a trained model and vocabulary that you have fine-tuned
- model = model_class.from_pretrained(args.output_dir)
- tokenizer = tokenizer_class.from_pretrained(args.output_dir)
- model.to(args.device)
-
- # Evaluation - we can ask to evaluate all the checkpoints (sub-directories) in a directory
- results = {}
- if args.do_eval and args.local_rank in [-1, 0]:
- if args.do_train:
- checkpoints = [args.output_dir]
- else:
- # if do_train is False and do_eval is true, load model directly from pretrained.
- checkpoints = [args.model_name_or_path]
-
- if args.eval_all_checkpoints:
- checkpoints = list(
- os.path.dirname(c) for c in sorted(glob.glob(args.output_dir + "/**/" + WEIGHTS_NAME, recursive=True))
- )
- logging.getLogger("transformers.modeling_utils").setLevel(logging.WARN) # Reduce model loading logs
-
- logger.info("Evaluate the following checkpoints: %s", checkpoints)
-
- for checkpoint in checkpoints:
- # Reload the model
- global_step = checkpoint.split("-")[-1] if len(checkpoints) > 1 else ""
- model = model_class.from_pretrained(checkpoint)
- tokenizer = tokenizer_class.from_pretrained(checkpoint)
- model.to(args.device)
-
- # Evaluate
- result = evaluate(args, model, tokenizer, prefix=global_step)
-
- result = dict((k + ("_{}".format(global_step) if global_step else ""), v) for k, v in result.items())
- results.update(result)
-
- logger.info("Results: {}".format(results))
-
- return results
-
-
-if __name__ == "__main__":
- main()
diff --git a/server/transformers/examples/contrib/run_transfo_xl.py b/server/transformers/examples/contrib/run_transfo_xl.py
deleted file mode 100644
index 84e2806a7b2abc8d2b8d082610db060ca1d68c2d..0000000000000000000000000000000000000000
--- a/server/transformers/examples/contrib/run_transfo_xl.py
+++ /dev/null
@@ -1,144 +0,0 @@
-# coding=utf-8
-# Copyright 2018 Google AI, Google Brain and Carnegie Mellon University Authors and the HuggingFace Inc. team.
-# Copyright (c) 2018, NVIDIA CORPORATION. All rights reserved.
-#
-# Licensed under the Apache License, Version 2.0 (the "License");
-# you may not use this file except in compliance with the License.
-# You may obtain a copy of the License at
-#
-# http://www.apache.org/licenses/LICENSE-2.0
-#
-# Unless required by applicable law or agreed to in writing, software
-# distributed under the License is distributed on an "AS IS" BASIS,
-# WITHOUT WARRANTIES OR CONDITIONS OF ANY KIND, either express or implied.
-# See the License for the specific language governing permissions and
-# limitations under the License.
-""" PyTorch Transformer XL model evaluation script.
- Adapted from https://github.com/kimiyoung/transformer-xl.
- In particular https://github.com/kimiyoung/transformer-xl/blob/master/pytorch/eval.py
-
- This script with default values evaluates a pretrained Transformer-XL on WikiText 103
-"""
-
-
-import argparse
-import logging
-import math
-import time
-
-import torch
-
-from transformers import TransfoXLCorpus, TransfoXLLMHeadModel
-
-
-logging.basicConfig(
- format="%(asctime)s - %(levelname)s - %(name)s - %(message)s", datefmt="%m/%d/%Y %H:%M:%S", level=logging.INFO
-)
-logger = logging.getLogger(__name__)
-
-
-def main():
- parser = argparse.ArgumentParser(description="PyTorch Transformer Language Model")
- parser.add_argument("--model_name", type=str, default="transfo-xl-wt103", help="pretrained model name")
- parser.add_argument(
- "--split", type=str, default="test", choices=["all", "valid", "test"], help="which split to evaluate"
- )
- parser.add_argument("--batch_size", type=int, default=10, help="batch size")
- parser.add_argument("--tgt_len", type=int, default=128, help="number of tokens to predict")
- parser.add_argument("--ext_len", type=int, default=0, help="length of the extended context")
- parser.add_argument("--mem_len", type=int, default=1600, help="length of the retained previous heads")
- parser.add_argument("--clamp_len", type=int, default=1000, help="max positional embedding index")
- parser.add_argument("--no_cuda", action="store_true", help="Do not use CUDA even though CUA is available")
- parser.add_argument("--work_dir", type=str, required=True, help="path to the work_dir")
- parser.add_argument("--no_log", action="store_true", help="do not log the eval result")
- parser.add_argument("--same_length", action="store_true", help="set same length attention with masking")
- parser.add_argument("--server_ip", type=str, default="", help="Can be used for distant debugging.")
- parser.add_argument("--server_port", type=str, default="", help="Can be used for distant debugging.")
- args = parser.parse_args()
- assert args.ext_len >= 0, "extended context length must be non-negative"
-
- if args.server_ip and args.server_port:
- # Distant debugging - see https://code.visualstudio.com/docs/python/debugging#_attach-to-a-local-script
- import ptvsd
-
- print("Waiting for debugger attach")
- ptvsd.enable_attach(address=(args.server_ip, args.server_port), redirect_output=True)
- ptvsd.wait_for_attach()
-
- device = torch.device("cuda" if torch.cuda.is_available() and not args.no_cuda else "cpu")
- logger.info("device: {}".format(device))
-
- # Load a pre-processed dataset
- # You can also build the corpus yourself using TransfoXLCorpus methods
- # The pre-processing involve computing word frequencies to prepare the Adaptive input and SoftMax
- # and tokenizing the dataset
- # The pre-processed corpus is a convertion (using the conversion script )
- corpus = TransfoXLCorpus.from_pretrained(args.model_name)
-
- va_iter = corpus.get_iterator("valid", args.batch_size, args.tgt_len, device=device, ext_len=args.ext_len)
- te_iter = corpus.get_iterator("test", args.batch_size, args.tgt_len, device=device, ext_len=args.ext_len)
-
- # Load a pre-trained model
- model = TransfoXLLMHeadModel.from_pretrained(args.model_name)
- model = model.to(device)
-
- logger.info(
- "Evaluating with bsz {} tgt_len {} ext_len {} mem_len {} clamp_len {}".format(
- args.batch_size, args.tgt_len, args.ext_len, args.mem_len, args.clamp_len
- )
- )
-
- model.reset_length(args.tgt_len, args.ext_len, args.mem_len)
- if args.clamp_len > 0:
- model.clamp_len = args.clamp_len
- if args.same_length:
- model.same_length = True
-
- ###############################################################################
- # Evaluation code
- ###############################################################################
- def evaluate(eval_iter):
- # Turn on evaluation mode which disables dropout.
- model.eval()
- total_len, total_loss = 0, 0.0
- start_time = time.time()
- with torch.no_grad():
- mems = None
- for idx, (data, target, seq_len) in enumerate(eval_iter):
- ret = model(data, lm_labels=target, mems=mems)
- loss, _, mems = ret
- loss = loss.mean()
- total_loss += seq_len * loss.item()
- total_len += seq_len
- total_time = time.time() - start_time
- logger.info("Time : {:.2f}s, {:.2f}ms/segment".format(total_time, 1000 * total_time / (idx + 1)))
- return total_loss / total_len
-
- # Run on test data.
- if args.split == "all":
- test_loss = evaluate(te_iter)
- valid_loss = evaluate(va_iter)
- elif args.split == "valid":
- valid_loss = evaluate(va_iter)
- test_loss = None
- elif args.split == "test":
- test_loss = evaluate(te_iter)
- valid_loss = None
-
- def format_log(loss, split):
- log_str = "| {0} loss {1:5.2f} | {0} ppl {2:9.3f} ".format(split, loss, math.exp(loss))
- return log_str
-
- log_str = ""
- if valid_loss is not None:
- log_str += format_log(valid_loss, "valid")
- if test_loss is not None:
- log_str += format_log(test_loss, "test")
-
- logger.info("=" * 100)
- logger.info(log_str)
- logger.info("=" * 100)
-
-
-if __name__ == "__main__":
- main()
diff --git a/server/transformers/examples/distillation/README.md b/server/transformers/examples/distillation/README.md
deleted file mode 100644
index c8fbb01aa43e95b625eaaf92b7d1091d9d6fddaa..0000000000000000000000000000000000000000
--- a/server/transformers/examples/distillation/README.md
+++ /dev/null
@@ -1,186 +0,0 @@
-# Distil*
-
-This folder contains the original code used to train Distil* as well as examples showcasing how to use DistilBERT, DistilRoBERTa and DistilGPT2.
-
-**January 20, 2020 - Bug fixing** We have recently discovered and fixed [a bug](https://github.com/huggingface/transformers/commit/48cbf267c988b56c71a2380f748a3e6092ccaed3) in the evaluation of our `run_*.py` scripts that caused the reported metrics to be over-estimated on average. We have updated all the metrics with the latest runs.
-
-**December 6, 2019 - Update** We release **DistilmBERT**: 92% of `bert-base-multilingual-cased` on XNLI. The model supports 104 different languages listed [here](https://github.com/google-research/bert/blob/master/multilingual.md#list-of-languages).
-
-**November 19, 2019 - Update** We release German **DistilBERT**: 98.8% of `bert-base-german-dbmdz-cased` on NER tasks.
-
-**October 23, 2019 - Update** We release **DistilRoBERTa**: 95% of `RoBERTa-base`'s performance on GLUE, twice as fast as RoBERTa while being 35% smaller.
-
-**October 3, 2019 - Update** We release our [NeurIPS workshop paper](https://arxiv.org/abs/1910.01108) explaining our approach on **DistilBERT**. It includes updated results and further experiments. We applied the same method to GPT2 and release the weights of **DistilGPT2**. DistilGPT2 is two times faster and 33% smaller than GPT2. **The paper superseeds our [previous blogpost](https://medium.com/huggingface/distilbert-8cf3380435b5) with a different distillation loss and better performances. Please use the paper as a reference when comparing/reporting results on DistilBERT.**
-
-**September 19, 2019 - Update:** We fixed bugs in the code and released an upadted version of the weights trained with a modification of the distillation loss. DistilBERT now reaches 99% of `BERT-base`'s performance on GLUE, and 86.9 F1 score on SQuAD v1.1 dev set (compared to 88.5 for `BERT-base`). We will publish a formal write-up of our approach in the near future!
-
-
-## What is Distil*
-
-Distil* is a class of compressed models that started with DistilBERT. DistilBERT stands for Distillated-BERT. DistilBERT is a small, fast, cheap and light Transformer model based on Bert architecture. It has 40% less parameters than `bert-base-uncased`, runs 60% faster while preserving 99% of BERT's performances as measured on the GLUE language understanding benchmark. DistilBERT is trained using knowledge distillation, a technique to compress a large model called the teacher into a smaller model called the student. By distillating Bert, we obtain a smaller Transformer model that bears a lot of similarities with the original BERT model while being lighter, smaller and faster to run. DistilBERT is thus an interesting option to put large-scaled trained Transformer model into production.
-
-We have applied the same method to other Transformer architectures and released the weights:
-- GPT2: on the [WikiText-103](https://blog.einstein.ai/the-wikitext-long-term-dependency-language-modeling-dataset/) benchmark, GPT2 reaches a perplexity on the test set of 16.3 compared to 21.1 for **DistilGPT2** (after fine-tuning on the train set).
-- RoBERTa: **DistilRoBERTa** reaches 95% of `RoBERTa-base`'s performance on GLUE while being twice faster and 35% smaller.
-- German BERT: **German DistilBERT** reaches 99% of `bert-base-german-dbmdz-cased`'s performance on German NER (CoNLL-2003).
-- Multilingual BERT: **DistilmBERT** reaches 92% of Multilingual BERT's performance on XNLI while being twice faster and 25% smaller. The model supports 104 languages listed [here](https://github.com/google-research/bert/blob/master/multilingual.md#list-of-languages).
-
-For more information on DistilBERT, please refer to our [NeurIPS workshop paper](https://arxiv.org/abs/1910.01108).
-
-Here are the results on the dev sets of GLUE:
-
-| Model | Macro-score | CoLA | MNLI | MRPC | QNLI | QQP | RTE | SST-2| STS-B| WNLI |
-| :---: | :---: | :---:| :---:| :---:| :---:| :---:| :---:| :---:| :---:| :---: |
-| BERT-base-uncased | **77.6** | 49.2 | 80.8 | 87.4 | 87.5 | 86.4 | 61.7 | 92.0 | 83.8 | 45.1 |
-| DistilBERT-base-uncased | **76.8** | 43.6 | 79.0 | 87.5 | 85.3 | 84.9 | 59.9 | 90.7 | 81.2 | 56.3 |
-| --- | --- | --- | --- | --- | --- | --- | --- | --- | --- | --- |
-| RoBERTa-base (reported) | **83.2**/**86.4**2 | 63.6 | 87.6 | 90.2 | 92.8 | 91.9 | 78.7 | 94.8 | 91.2 | 57.73 |
-| DistilRoBERTa1 | **79.0**/**82.3**2 | 59.3 | 84.0 | 86.6 | 90.8 | 89.4 | 67.9 | 92.5 | 88.3 | 52.1 |
-
-1 We did not use the MNLI checkpoint for fine-tuning but directy perform transfer learning on the pre-trained DistilRoBERTa.
-
-2 Macro-score computed without WNLI.
-
-3 We compute this score ourselves for completeness.
-
-Here are the results on the *test* sets for 6 of the languages available in XNLI. The results are computed in the zero shot setting (trained on the English portion and evaluated on the target language portion):
-
-| Model | English | Spanish | Chinese | German | Arabic | Urdu |
-| :---: | :---: | :---: | :---: | :---: | :---: | :---:|
-| mBERT base cased (computed) | 82.1 | 74.6 | 69.1 | 72.3 | 66.4 | 58.5 |
-| mBERT base uncased (reported)| 81.4 | 74.3 | 63.8 | 70.5 | 62.1 | 58.3 |
-| DistilmBERT | 78.2 | 69.1 | 64.0 | 66.3 | 59.1 | 54.7 |
-
-## Setup
-
-This part of the library has only be tested with Python3.6+. There are few specific dependencies to install before launching a distillation, you can install them with the command `pip install -r requirements.txt`.
-
-**Important note:** The training scripts have been updated to support PyTorch v1.2.0 (there are breakings changes compared to v1.1.0).
-
-
-## How to use DistilBERT
-
-Transformers includes five pre-trained Distil* models, currently only provided for English and German (we are investigating the possibility to train and release a multilingual version of DistilBERT):
-
-- `distilbert-base-uncased`: DistilBERT English language model pretrained on the same data used to pretrain Bert (concatenation of the Toronto Book Corpus and full English Wikipedia) using distillation with the supervision of the `bert-base-uncased` version of Bert. The model has 6 layers, 768 dimension and 12 heads, totalizing 66M parameters.
-- `distilbert-base-uncased-distilled-squad`: A finetuned version of `distilbert-base-uncased` finetuned using (a second step of) knwoledge distillation on SQuAD 1.0. This model reaches a F1 score of 86.9 on the dev set (for comparison, Bert `bert-base-uncased` version reaches a 88.5 F1 score).
-- `distilbert-base-german-cased`: DistilBERT German language model pretrained on 1/2 of the data used to pretrain Bert using distillation with the supervision of the `bert-base-german-dbmdz-cased` version of German DBMDZ Bert. For NER tasks the model reaches a F1 score of 83.49 on the CoNLL-2003 test set (for comparison, `bert-base-german-dbmdz-cased` reaches a 84.52 F1 score), and a F1 score of 85.23 on the GermEval 2014 test set (`bert-base-german-dbmdz-cased` reaches a 86.89 F1 score).
-- `distilgpt2`: DistilGPT2 English language model pretrained with the supervision of `gpt2` (the smallest version of GPT2) on [OpenWebTextCorpus](https://skylion007.github.io/OpenWebTextCorpus/), a reproduction of OpenAI's WebText dataset. The model has 6 layers, 768 dimension and 12 heads, totalizing 82M parameters (compared to 124M parameters for GPT2). On average, DistilGPT2 is two times faster than GPT2.
-- `distilroberta-base`: DistilRoBERTa English language model pretrained with the supervision of `roberta-base` solely on [OpenWebTextCorpus](https://skylion007.github.io/OpenWebTextCorpus/), a reproduction of OpenAI's WebText dataset (it is ~4 times less training data than the teacher RoBERTa). The model has 6 layers, 768 dimension and 12 heads, totalizing 82M parameters (compared to 125M parameters for RoBERTa-base). On average DistilRoBERTa is twice as fast as Roberta-base.
-- `distilbert-base-multilingual-cased`: DistilmBERT multilingual model pretrained with the supervision of `bert-base-multilingual-cased` on the concatenation of Wikipedia in 104 different languages. The model supports the 104 languages listed [here](https://github.com/google-research/bert/blob/master/multilingual.md#list-of-languages). The model has 6 layers, 768 dimension and 12 heads, totalizing 134M parameters (compared to 177M parameters for mBERT-base). On average DistilmBERT is twice as fast as mBERT-base.
-
-Using DistilBERT is very similar to using BERT. DistilBERT share the same tokenizer as BERT's `bert-base-uncased` even though we provide a link to this tokenizer under the `DistilBertTokenizer` name to have a consistent naming between the library models.
-
-```python
-tokenizer = DistilBertTokenizer.from_pretrained('distilbert-base-uncased')
-model = DistilBertModel.from_pretrained('distilbert-base-uncased')
-
-input_ids = torch.tensor(tokenizer.encode("Hello, my dog is cute")).unsqueeze(0)
-outputs = model(input_ids)
-last_hidden_states = outputs[0] # The last hidden-state is the first element of the output tuple
-```
-
-Similarly, using the other Distil* models simply consists in calling the base classes with a different pretrained checkpoint:
-- DistilGPT2: `model = GPT2Model.from_pretrained('distilgpt2')`
-- DistilRoBERTa: `model = RobertaModel.from_pretrained('distilroberta-base')`
-- DistilmBERT: `model = DistilBertModel.from_pretrained('distilbert-base-multilingual-cased')`
-
-
-## How to train Distil*
-
-In the following, we will explain how you can train DistilBERT.
-
-### A. Preparing the data
-
-The weights we release are trained using a concatenation of Toronto Book Corpus and English Wikipedia (same training data as the English version of BERT).
-
-To avoid processing the data several time, we do it once and for all before the training. From now on, will suppose that you have a text file `dump.txt` which contains one sequence per line (a sequence being composed of one of several coherent sentences).
-
-First, we will binarize the data, i.e. tokenize the data and convert each token in an index in our model's vocabulary.
-
-```bash
-python scripts/binarized_data.py \
- --file_path data/dump.txt \
- --tokenizer_type bert \
- --tokenizer_name bert-base-uncased \
- --dump_file data/binarized_text
-```
-
-Our implementation of masked language modeling loss follows [XLM](https://github.com/facebookresearch/XLM)'s one and smoothes the probability of masking with a factor that put more emphasis on rare words. Thus we count the occurences of each tokens in the data:
-
-```bash
-python scripts/token_counts.py \
- --data_file data/binarized_text.bert-base-uncased.pickle \
- --token_counts_dump data/token_counts.bert-base-uncased.pickle \
- --vocab_size 30522
-```
-
-### B. Training
-
-Training with distillation is really simple once you have pre-processed the data:
-
-```bash
-python train.py \
- --student_type distilbert \
- --student_config training_configs/distilbert-base-uncased.json \
- --teacher_type bert \
- --teacher_name bert-base-uncased \
- --alpha_ce 5.0 --alpha_mlm 2.0 --alpha_cos 1.0 --alpha_clm 0.0 --mlm \
- --freeze_pos_embs \
- --dump_path serialization_dir/my_first_training \
- --data_file data/binarized_text.bert-base-uncased.pickle \
- --token_counts data/token_counts.bert-base-uncased.pickle \
- --force # overwrites the `dump_path` if it already exists.
-```
-
-By default, this will launch a training on a single GPU (even if more are available on the cluster). Other parameters are available in the command line, please look in `train.py` or run `python train.py --help` to list them.
-
-We highly encourage you to use distributed training for training DistilBERT as the training corpus is quite large. Here's an example that runs a distributed training on a single node having 4 GPUs:
-
-```bash
-export NODE_RANK=0
-export N_NODES=1
-
-export N_GPU_NODE=4
-export WORLD_SIZE=4
-export MASTER_PORT=
-export MASTER_ADDR=
-
-pkill -f 'python -u train.py'
-
-python -m torch.distributed.launch \
- --nproc_per_node=$N_GPU_NODE \
- --nnodes=$N_NODES \
- --node_rank $NODE_RANK \
- --master_addr $MASTER_ADDR \
- --master_port $MASTER_PORT \
- train.py \
- --force \
- --n_gpu $WORLD_SIZE \
- --student_type distilbert \
- --student_config training_configs/distilbert-base-uncased.json \
- --teacher_type bert \
- --teacher_name bert-base-uncased \
- --alpha_ce 0.33 --alpha_mlm 0.33 --alpha_cos 0.33 --alpha_clm 0.0 --mlm \
- --freeze_pos_embs \
- --dump_path serialization_dir/my_first_training \
- --data_file data/binarized_text.bert-base-uncased.pickle \
- --token_counts data/token_counts.bert-base-uncased.pickle
-```
-
-**Tips:** Starting distillated training with good initialization of the model weights is crucial to reach decent performance. In our experiments, we initialized our model from a few layers of the teacher (Bert) itself! Please refer to `scripts/extract.py` and `scripts/extract_distilbert.py` to create a valid initialization checkpoint and use `--student_pretrained_weights` argument to use this initialization for the distilled training!
-
-Happy distillation!
-
-## Citation
-
-If you find the ressource useful, you should cite the following paper:
-
-```
-@inproceedings{sanh2019distilbert,
- title={DistilBERT, a distilled version of BERT: smaller, faster, cheaper and lighter},
- author={Sanh, Victor and Debut, Lysandre and Chaumond, Julien and Wolf, Thomas},
- booktitle={NeurIPS EMC^2 Workshop},
- year={2019}
-}
-```
diff --git a/server/transformers/examples/distillation/distiller.py b/server/transformers/examples/distillation/distiller.py
deleted file mode 100644
index 53669623b6f67a0e6c740717ce86409c67b0ad97..0000000000000000000000000000000000000000
--- a/server/transformers/examples/distillation/distiller.py
+++ /dev/null
@@ -1,603 +0,0 @@
-# coding=utf-8
-# Copyright 2019-present, the HuggingFace Inc. team and Facebook, Inc.
-#
-# Licensed under the Apache License, Version 2.0 (the "License");
-# you may not use this file except in compliance with the License.
-# You may obtain a copy of the License at
-#
-# http://www.apache.org/licenses/LICENSE-2.0
-#
-# Unless required by applicable law or agreed to in writing, software
-# distributed under the License is distributed on an "AS IS" BASIS,
-# WITHOUT WARRANTIES OR CONDITIONS OF ANY KIND, either express or implied.
-# See the License for the specific language governing permissions and
-# limitations under the License.
-""" The distiller to distil the student.
- Adapted in part from Facebook, Inc XLM model (https://github.com/facebookresearch/XLM)
-"""
-import math
-import os
-import time
-
-import psutil
-import torch
-import torch.nn as nn
-import torch.nn.functional as F
-from torch.optim import AdamW
-from torch.utils.data import BatchSampler, DataLoader, RandomSampler
-from torch.utils.data.distributed import DistributedSampler
-from tqdm import tqdm
-
-from grouped_batch_sampler import GroupedBatchSampler, create_lengths_groups
-from lm_seqs_dataset import LmSeqsDataset
-from transformers import get_linear_schedule_with_warmup
-from utils import logger
-
-
-try:
- from torch.utils.tensorboard import SummaryWriter
-except ImportError:
- from tensorboardX import SummaryWriter
-
-
-class Distiller:
- def __init__(
- self, params: dict, dataset: LmSeqsDataset, token_probs: torch.tensor, student: nn.Module, teacher: nn.Module
- ):
- logger.info("Initializing Distiller")
- self.params = params
- self.dump_path = params.dump_path
- self.multi_gpu = params.multi_gpu
- self.fp16 = params.fp16
-
- self.student = student
- self.teacher = teacher
-
- self.student_config = student.config
- self.vocab_size = student.config.vocab_size
-
- if params.n_gpu <= 1:
- sampler = RandomSampler(dataset)
- else:
- sampler = DistributedSampler(dataset)
-
- if params.group_by_size:
- groups = create_lengths_groups(lengths=dataset.lengths, k=params.max_model_input_size)
- sampler = GroupedBatchSampler(sampler=sampler, group_ids=groups, batch_size=params.batch_size)
- else:
- sampler = BatchSampler(sampler=sampler, batch_size=params.batch_size, drop_last=False)
-
- self.dataloader = DataLoader(dataset=dataset, batch_sampler=sampler, collate_fn=dataset.batch_sequences)
-
- self.temperature = params.temperature
- assert self.temperature > 0.0
-
- self.alpha_ce = params.alpha_ce
- self.alpha_mlm = params.alpha_mlm
- self.alpha_clm = params.alpha_clm
- self.alpha_mse = params.alpha_mse
- self.alpha_cos = params.alpha_cos
-
- self.mlm = params.mlm
- if self.mlm:
- logger.info(f"Using MLM loss for LM step.")
- self.mlm_mask_prop = params.mlm_mask_prop
- assert 0.0 <= self.mlm_mask_prop <= 1.0
- assert params.word_mask + params.word_keep + params.word_rand == 1.0
- self.pred_probs = torch.FloatTensor([params.word_mask, params.word_keep, params.word_rand])
- self.pred_probs = self.pred_probs.to(f"cuda:{params.local_rank}") if params.n_gpu > 0 else self.pred_probs
- self.token_probs = token_probs.to(f"cuda:{params.local_rank}") if params.n_gpu > 0 else token_probs
- if self.fp16:
- self.pred_probs = self.pred_probs.half()
- self.token_probs = self.token_probs.half()
- else:
- logger.info(f"Using CLM loss for LM step.")
-
- self.epoch = 0
- self.n_iter = 0
- self.n_total_iter = 0
- self.n_sequences_epoch = 0
- self.total_loss_epoch = 0
- self.last_loss = 0
- self.last_loss_ce = 0
- self.last_loss_mlm = 0
- self.last_loss_clm = 0
- if self.alpha_mse > 0.0:
- self.last_loss_mse = 0
- if self.alpha_cos > 0.0:
- self.last_loss_cos = 0
- self.last_log = 0
-
- self.ce_loss_fct = nn.KLDivLoss(reduction="batchmean")
- self.lm_loss_fct = nn.CrossEntropyLoss(ignore_index=-100)
- if self.alpha_mse > 0.0:
- self.mse_loss_fct = nn.MSELoss(reduction="sum")
- if self.alpha_cos > 0.0:
- self.cosine_loss_fct = nn.CosineEmbeddingLoss(reduction="mean")
-
- logger.info("--- Initializing model optimizer")
- assert params.gradient_accumulation_steps >= 1
- self.num_steps_epoch = len(self.dataloader)
- num_train_optimization_steps = (
- int(self.num_steps_epoch / params.gradient_accumulation_steps * params.n_epoch) + 1
- )
-
- no_decay = ["bias", "LayerNorm.weight"]
- optimizer_grouped_parameters = [
- {
- "params": [
- p for n, p in student.named_parameters() if not any(nd in n for nd in no_decay) and p.requires_grad
- ],
- "weight_decay": params.weight_decay,
- },
- {
- "params": [
- p for n, p in student.named_parameters() if any(nd in n for nd in no_decay) and p.requires_grad
- ],
- "weight_decay": 0.0,
- },
- ]
- logger.info(
- "------ Number of trainable parameters (student): %i"
- % sum([p.numel() for p in self.student.parameters() if p.requires_grad])
- )
- logger.info("------ Number of parameters (student): %i" % sum([p.numel() for p in self.student.parameters()]))
- self.optimizer = AdamW(
- optimizer_grouped_parameters, lr=params.learning_rate, eps=params.adam_epsilon, betas=(0.9, 0.98)
- )
-
- warmup_steps = math.ceil(num_train_optimization_steps * params.warmup_prop)
- self.scheduler = get_linear_schedule_with_warmup(
- self.optimizer, num_warmup_steps=warmup_steps, num_training_steps=num_train_optimization_steps
- )
-
- if self.fp16:
- try:
- from apex import amp
- except ImportError:
- raise ImportError("Please install apex from https://www.github.com/nvidia/apex to use fp16 training.")
- logger.info(f"Using fp16 training: {self.params.fp16_opt_level} level")
- self.student, self.optimizer = amp.initialize(
- self.student, self.optimizer, opt_level=self.params.fp16_opt_level
- )
- self.teacher = self.teacher.half()
-
- if self.multi_gpu:
- if self.fp16:
- from apex.parallel import DistributedDataParallel
-
- logger.info("Using apex.parallel.DistributedDataParallel for distributed training.")
- self.student = DistributedDataParallel(self.student)
- else:
- from torch.nn.parallel import DistributedDataParallel
-
- logger.info("Using nn.parallel.DistributedDataParallel for distributed training.")
- self.student = DistributedDataParallel(
- self.student,
- device_ids=[params.local_rank],
- output_device=params.local_rank,
- find_unused_parameters=True,
- )
-
- self.is_master = params.is_master
- if self.is_master:
- logger.info("--- Initializing Tensorboard")
- self.tensorboard = SummaryWriter(log_dir=os.path.join(self.dump_path, "log", "train"))
- self.tensorboard.add_text(tag="config/training", text_string=str(self.params), global_step=0)
- self.tensorboard.add_text(tag="config/student", text_string=str(self.student_config), global_step=0)
-
- def prepare_batch_mlm(self, batch):
- """
- Prepare the batch: from the token_ids and the lenghts, compute the attention mask and the masked label for MLM.
-
- Input:
- ------
- batch: `Tuple`
- token_ids: `torch.tensor(bs, seq_length)` - The token ids for each of the sequence. It is padded.
- lengths: `torch.tensor(bs)` - The lengths of each of the sequences in the batch.
-
- Output:
- -------
- token_ids: `torch.tensor(bs, seq_length)` - The token ids after the modifications for MLM.
- attn_mask: `torch.tensor(bs, seq_length)` - The attention mask for the self-attention.
- mlm_labels: `torch.tensor(bs, seq_length)` - The masked languge modeling labels. There is a -100 where there is nothing to predict.
- """
- token_ids, lengths = batch
- token_ids, lengths = self.round_batch(x=token_ids, lengths=lengths)
- assert token_ids.size(0) == lengths.size(0)
-
- attn_mask = torch.arange(token_ids.size(1), dtype=torch.long, device=lengths.device) < lengths[:, None]
-
- bs, max_seq_len = token_ids.size()
- mlm_labels = token_ids.new(token_ids.size()).copy_(token_ids)
-
- x_prob = self.token_probs[token_ids.flatten()]
- n_tgt = math.ceil(self.mlm_mask_prop * lengths.sum().item())
- tgt_ids = torch.multinomial(x_prob / x_prob.sum(), n_tgt, replacement=False)
- pred_mask = torch.zeros(
- bs * max_seq_len, dtype=torch.bool, device=token_ids.device
- ) # previously `dtype=torch.uint8`, cf pytorch 1.2.0 compatibility
- pred_mask[tgt_ids] = 1
- pred_mask = pred_mask.view(bs, max_seq_len)
-
- pred_mask[token_ids == self.params.special_tok_ids["pad_token"]] = 0
-
- # mask a number of words == 0 [8] (faster with fp16)
- if self.fp16:
- n1 = pred_mask.sum().item()
- if n1 > 8:
- pred_mask = pred_mask.view(-1)
- n2 = max(n1 % 8, 8 * (n1 // 8))
- if n2 != n1:
- pred_mask[torch.nonzero(pred_mask).view(-1)[: n1 - n2]] = 0
- pred_mask = pred_mask.view(bs, max_seq_len)
- assert pred_mask.sum().item() % 8 == 0, pred_mask.sum().item()
-
- _token_ids_real = token_ids[pred_mask]
- _token_ids_rand = _token_ids_real.clone().random_(self.vocab_size)
- _token_ids_mask = _token_ids_real.clone().fill_(self.params.special_tok_ids["mask_token"])
- probs = torch.multinomial(self.pred_probs, len(_token_ids_real), replacement=True)
- _token_ids = (
- _token_ids_mask * (probs == 0).long()
- + _token_ids_real * (probs == 1).long()
- + _token_ids_rand * (probs == 2).long()
- )
- token_ids = token_ids.masked_scatter(pred_mask, _token_ids)
-
- mlm_labels[~pred_mask] = -100 # previously `mlm_labels[1-pred_mask] = -1`, cf pytorch 1.2.0 compatibility
-
- # sanity checks
- assert 0 <= token_ids.min() <= token_ids.max() < self.vocab_size
-
- return token_ids, attn_mask, mlm_labels
-
- def prepare_batch_clm(self, batch):
- """
- Prepare the batch: from the token_ids and the lenghts, compute the attention mask and the labels for CLM.
-
- Input:
- ------
- batch: `Tuple`
- token_ids: `torch.tensor(bs, seq_length)` - The token ids for each of the sequence. It is padded.
- lengths: `torch.tensor(bs)` - The lengths of each of the sequences in the batch.
-
- Output:
- -------
- token_ids: `torch.tensor(bs, seq_length)` - The token ids after the modifications for MLM.
- attn_mask: `torch.tensor(bs, seq_length)` - The attention mask for the self-attention.
- clm_labels: `torch.tensor(bs, seq_length)` - The causal languge modeling labels. There is a -100 where there is nothing to predict.
- """
- token_ids, lengths = batch
- token_ids, lengths = self.round_batch(x=token_ids, lengths=lengths)
- assert token_ids.size(0) == lengths.size(0)
-
- attn_mask = torch.arange(token_ids.size(1), dtype=torch.long, device=lengths.device) < lengths[:, None]
- clm_labels = token_ids.new(token_ids.size()).copy_(token_ids)
- clm_labels[~attn_mask] = -100 # previously `clm_labels[1-attn_mask] = -1`, cf pytorch 1.2.0 compatibility
-
- # sanity checks
- assert 0 <= token_ids.min() <= token_ids.max() < self.vocab_size
-
- return token_ids, attn_mask, clm_labels
-
- def round_batch(self, x: torch.tensor, lengths: torch.tensor):
- """
- For float16 only.
- Sub-sample sentences in a batch, and add padding, so that each dimension is a multiple of 8.
-
- Input:
- ------
- x: `torch.tensor(bs, seq_length)` - The token ids.
- lengths: `torch.tensor(bs, seq_length)` - The lengths of each of the sequence in the batch.
-
- Output:
- -------
- x: `torch.tensor(new_bs, new_seq_length)` - The updated token ids.
- lengths: `torch.tensor(new_bs, new_seq_length)` - The updated lengths.
- """
- if not self.fp16 or len(lengths) < 8:
- return x, lengths
-
- # number of sentences == 0 [8]
- bs1 = len(lengths)
- bs2 = 8 * (bs1 // 8)
- assert bs2 > 0 and bs2 % 8 == 0
- if bs1 != bs2:
- idx = torch.randperm(bs1)[:bs2]
- lengths = lengths[idx]
- slen = lengths.max().item()
- x = x[idx, :slen]
- else:
- idx = None
-
- # sequence length == 0 [8]
- ml1 = x.size(1)
- if ml1 % 8 != 0:
- pad = 8 - (ml1 % 8)
- ml2 = ml1 + pad
- if self.mlm:
- pad_id = self.params.special_tok_ids["pad_token"]
- else:
- pad_id = self.params.special_tok_ids["unk_token"]
- padding_tensor = torch.zeros(bs2, pad, dtype=torch.long, device=x.device).fill_(pad_id)
- x = torch.cat([x, padding_tensor], 1)
- assert x.size() == (bs2, ml2)
-
- assert x.size(0) % 8 == 0
- assert x.size(1) % 8 == 0
- return x, lengths
-
- def train(self):
- """
- The real training loop.
- """
- if self.is_master:
- logger.info("Starting training")
- self.last_log = time.time()
- self.student.train()
- self.teacher.eval()
-
- for _ in range(self.params.n_epoch):
- if self.is_master:
- logger.info(f"--- Starting epoch {self.epoch}/{self.params.n_epoch-1}")
- if self.multi_gpu:
- torch.distributed.barrier()
-
- iter_bar = tqdm(self.dataloader, desc="-Iter", disable=self.params.local_rank not in [-1, 0])
- for batch in iter_bar:
- if self.params.n_gpu > 0:
- batch = tuple(t.to(f"cuda:{self.params.local_rank}") for t in batch)
-
- if self.mlm:
- token_ids, attn_mask, lm_labels = self.prepare_batch_mlm(batch=batch)
- else:
- token_ids, attn_mask, lm_labels = self.prepare_batch_clm(batch=batch)
- self.step(input_ids=token_ids, attention_mask=attn_mask, lm_labels=lm_labels)
-
- iter_bar.update()
- iter_bar.set_postfix(
- {"Last_loss": f"{self.last_loss:.2f}", "Avg_cum_loss": f"{self.total_loss_epoch/self.n_iter:.2f}"}
- )
- iter_bar.close()
-
- if self.is_master:
- logger.info(f"--- Ending epoch {self.epoch}/{self.params.n_epoch-1}")
- self.end_epoch()
-
- if self.is_master:
- logger.info(f"Save very last checkpoint as `pytorch_model.bin`.")
- self.save_checkpoint(checkpoint_name=f"pytorch_model.bin")
- logger.info("Training is finished")
-
- def step(self, input_ids: torch.tensor, attention_mask: torch.tensor, lm_labels: torch.tensor):
- """
- One optimization step: forward of student AND teacher, backward on the loss (for gradient accumulation),
- and possibly a parameter update (depending on the gradient accumulation).
-
- Input:
- ------
- input_ids: `torch.tensor(bs, seq_length)` - The token ids.
- attention_mask: `torch.tensor(bs, seq_length)` - The attention mask for self attention.
- lm_labels: `torch.tensor(bs, seq_length)` - The language modeling labels (mlm labels for MLM and clm labels for CLM).
- """
- if self.mlm:
- s_logits, s_hidden_states = self.student(
- input_ids=input_ids, attention_mask=attention_mask
- ) # (bs, seq_length, voc_size)
- with torch.no_grad():
- t_logits, t_hidden_states = self.teacher(
- input_ids=input_ids, attention_mask=attention_mask
- ) # (bs, seq_length, voc_size)
- else:
- s_logits, _, s_hidden_states = self.student(
- input_ids=input_ids, attention_mask=None
- ) # (bs, seq_length, voc_size)
- with torch.no_grad():
- t_logits, _, t_hidden_states = self.teacher(
- input_ids=input_ids, attention_mask=None
- ) # (bs, seq_length, voc_size)
- assert s_logits.size() == t_logits.size()
-
- # https://github.com/peterliht/knowledge-distillation-pytorch/blob/master/model/net.py#L100
- # https://github.com/peterliht/knowledge-distillation-pytorch/issues/2
- if self.params.restrict_ce_to_mask:
- mask = (lm_labels > -1).unsqueeze(-1).expand_as(s_logits) # (bs, seq_lenth, voc_size)
- else:
- mask = attention_mask.unsqueeze(-1).expand_as(s_logits) # (bs, seq_lenth, voc_size)
- s_logits_slct = torch.masked_select(s_logits, mask) # (bs * seq_length * voc_size) modulo the 1s in mask
- s_logits_slct = s_logits_slct.view(-1, s_logits.size(-1)) # (bs * seq_length, voc_size) modulo the 1s in mask
- t_logits_slct = torch.masked_select(t_logits, mask) # (bs * seq_length * voc_size) modulo the 1s in mask
- t_logits_slct = t_logits_slct.view(-1, s_logits.size(-1)) # (bs * seq_length, voc_size) modulo the 1s in mask
- assert t_logits_slct.size() == s_logits_slct.size()
-
- loss_ce = (
- self.ce_loss_fct(
- F.log_softmax(s_logits_slct / self.temperature, dim=-1),
- F.softmax(t_logits_slct / self.temperature, dim=-1),
- )
- * (self.temperature) ** 2
- )
- loss = self.alpha_ce * loss_ce
-
- if self.alpha_mlm > 0.0:
- loss_mlm = self.lm_loss_fct(s_logits.view(-1, s_logits.size(-1)), lm_labels.view(-1))
- loss += self.alpha_mlm * loss_mlm
- if self.alpha_clm > 0.0:
- shift_logits = s_logits[..., :-1, :].contiguous()
- shift_labels = lm_labels[..., 1:].contiguous()
- loss_clm = self.lm_loss_fct(shift_logits.view(-1, shift_logits.size(-1)), shift_labels.view(-1))
- loss += self.alpha_clm * loss_clm
-
- if self.alpha_mse > 0.0:
- loss_mse = self.mse_loss_fct(s_logits_slct, t_logits_slct) / s_logits_slct.size(
- 0
- ) # Reproducing batchmean reduction
- loss += self.alpha_mse * loss_mse
- if self.alpha_cos > 0.0:
- s_hidden_states = s_hidden_states[-1] # (bs, seq_length, dim)
- t_hidden_states = t_hidden_states[-1] # (bs, seq_length, dim)
- mask = attention_mask.unsqueeze(-1).expand_as(s_hidden_states) # (bs, seq_length, dim)
- assert s_hidden_states.size() == t_hidden_states.size()
- dim = s_hidden_states.size(-1)
-
- s_hidden_states_slct = torch.masked_select(s_hidden_states, mask) # (bs * seq_length * dim)
- s_hidden_states_slct = s_hidden_states_slct.view(-1, dim) # (bs * seq_length, dim)
- t_hidden_states_slct = torch.masked_select(t_hidden_states, mask) # (bs * seq_length * dim)
- t_hidden_states_slct = t_hidden_states_slct.view(-1, dim) # (bs * seq_length, dim)
-
- target = s_hidden_states_slct.new(s_hidden_states_slct.size(0)).fill_(1) # (bs * seq_length,)
- loss_cos = self.cosine_loss_fct(s_hidden_states_slct, t_hidden_states_slct, target)
- loss += self.alpha_cos * loss_cos
-
- self.total_loss_epoch += loss.item()
- self.last_loss = loss.item()
- self.last_loss_ce = loss_ce.item()
- if self.alpha_mlm > 0.0:
- self.last_loss_mlm = loss_mlm.item()
- if self.alpha_clm > 0.0:
- self.last_loss_clm = loss_clm.item()
- if self.alpha_mse > 0.0:
- self.last_loss_mse = loss_mse.item()
- if self.alpha_cos > 0.0:
- self.last_loss_cos = loss_cos.item()
-
- self.optimize(loss)
-
- self.n_sequences_epoch += input_ids.size(0)
-
- def optimize(self, loss):
- """
- Normalization on the loss (gradient accumulation or distributed training), followed by
- backward pass on the loss, possibly followed by a parameter update (depending on the gradient accumulation).
- Also update the metrics for tensorboard.
- """
- # Check for NaN
- if (loss != loss).data.any():
- logger.error("NaN detected")
- exit()
-
- if self.multi_gpu:
- loss = loss.mean()
- if self.params.gradient_accumulation_steps > 1:
- loss = loss / self.params.gradient_accumulation_steps
-
- if self.fp16:
- from apex import amp
-
- with amp.scale_loss(loss, self.optimizer) as scaled_loss:
- scaled_loss.backward()
- else:
- loss.backward()
-
- self.iter()
- if self.n_iter % self.params.gradient_accumulation_steps == 0:
- if self.fp16:
- torch.nn.utils.clip_grad_norm_(amp.master_params(self.optimizer), self.params.max_grad_norm)
- else:
- torch.nn.utils.clip_grad_norm_(self.student.parameters(), self.params.max_grad_norm)
- self.optimizer.step()
- self.optimizer.zero_grad()
- self.scheduler.step()
-
- def iter(self):
- """
- Update global counts, write to tensorboard and save checkpoint.
- """
- self.n_iter += 1
- self.n_total_iter += 1
-
- if self.n_total_iter % self.params.log_interval == 0:
- self.log_tensorboard()
- self.last_log = time.time()
- if self.n_total_iter % self.params.checkpoint_interval == 0:
- self.save_checkpoint()
-
- def log_tensorboard(self):
- """
- Log into tensorboard. Only by the master process.
- """
- if not self.is_master:
- return
-
- for param_name, param in self.student.named_parameters():
- self.tensorboard.add_scalar(
- tag="parameter_mean/" + param_name, scalar_value=param.data.mean(), global_step=self.n_total_iter
- )
- self.tensorboard.add_scalar(
- tag="parameter_std/" + param_name, scalar_value=param.data.std(), global_step=self.n_total_iter
- )
- if param.grad is None:
- continue
- self.tensorboard.add_scalar(
- tag="grad_mean/" + param_name, scalar_value=param.grad.data.mean(), global_step=self.n_total_iter
- )
- self.tensorboard.add_scalar(
- tag="grad_std/" + param_name, scalar_value=param.grad.data.std(), global_step=self.n_total_iter
- )
-
- self.tensorboard.add_scalar(
- tag="losses/cum_avg_loss_epoch",
- scalar_value=self.total_loss_epoch / self.n_iter,
- global_step=self.n_total_iter,
- )
- self.tensorboard.add_scalar(tag="losses/loss", scalar_value=self.last_loss, global_step=self.n_total_iter)
- self.tensorboard.add_scalar(
- tag="losses/loss_ce", scalar_value=self.last_loss_ce, global_step=self.n_total_iter
- )
- if self.alpha_mlm > 0.0:
- self.tensorboard.add_scalar(
- tag="losses/loss_mlm", scalar_value=self.last_loss_mlm, global_step=self.n_total_iter
- )
- if self.alpha_clm > 0.0:
- self.tensorboard.add_scalar(
- tag="losses/loss_clm", scalar_value=self.last_loss_clm, global_step=self.n_total_iter
- )
- if self.alpha_mse > 0.0:
- self.tensorboard.add_scalar(
- tag="losses/loss_mse", scalar_value=self.last_loss_mse, global_step=self.n_total_iter
- )
- if self.alpha_cos > 0.0:
- self.tensorboard.add_scalar(
- tag="losses/loss_cos", scalar_value=self.last_loss_cos, global_step=self.n_total_iter
- )
- self.tensorboard.add_scalar(
- tag="learning_rate/lr", scalar_value=self.scheduler.get_lr()[0], global_step=self.n_total_iter
- )
-
- self.tensorboard.add_scalar(
- tag="global/memory_usage",
- scalar_value=psutil.virtual_memory()._asdict()["used"] / 1_000_000,
- global_step=self.n_total_iter,
- )
- self.tensorboard.add_scalar(
- tag="global/speed", scalar_value=time.time() - self.last_log, global_step=self.n_total_iter
- )
-
- def end_epoch(self):
- """
- Finally arrived at the end of epoch (full pass on dataset).
- Do some tensorboard logging and checkpoint saving.
- """
- logger.info(f"{self.n_sequences_epoch} sequences have been trained during this epoch.")
-
- if self.is_master:
- self.save_checkpoint(checkpoint_name=f"model_epoch_{self.epoch}.pth")
- self.tensorboard.add_scalar(
- tag="epoch/loss", scalar_value=self.total_loss_epoch / self.n_iter, global_step=self.epoch
- )
-
- self.epoch += 1
- self.n_sequences_epoch = 0
- self.n_iter = 0
- self.total_loss_epoch = 0
-
- def save_checkpoint(self, checkpoint_name: str = "checkpoint.pth"):
- """
- Save the current state. Only by the master process.
- """
- if not self.is_master:
- return
- mdl_to_save = self.student.module if hasattr(self.student, "module") else self.student
- mdl_to_save.config.save_pretrained(self.dump_path)
- state_dict = mdl_to_save.state_dict()
- torch.save(state_dict, os.path.join(self.dump_path, checkpoint_name))
diff --git a/server/transformers/examples/distillation/grouped_batch_sampler.py b/server/transformers/examples/distillation/grouped_batch_sampler.py
deleted file mode 100644
index c386c4224d25a9caada95c392269e61699b4b337..0000000000000000000000000000000000000000
--- a/server/transformers/examples/distillation/grouped_batch_sampler.py
+++ /dev/null
@@ -1,108 +0,0 @@
-# coding=utf-8
-# Copyright 2019-present, the HuggingFace Inc. team and Facebook, Inc.
-#
-# Licensed under the Apache License, Version 2.0 (the "License");
-# you may not use this file except in compliance with the License.
-# You may obtain a copy of the License at
-#
-# http://www.apache.org/licenses/LICENSE-2.0
-#
-# Unless required by applicable law or agreed to in writing, software
-# distributed under the License is distributed on an "AS IS" BASIS,
-# WITHOUT WARRANTIES OR CONDITIONS OF ANY KIND, either express or implied.
-# See the License for the specific language governing permissions and
-# limitations under the License.
-""" Adapted from PyTorch Vision (https://github.com/pytorch/vision/blob/master/references/detection/group_by_aspect_ratio.py)
-"""
-import bisect
-import copy
-from collections import defaultdict
-
-import numpy as np
-from torch.utils.data.sampler import BatchSampler, Sampler
-
-from utils import logger
-
-
-def _quantize(x, bins):
- bins = copy.deepcopy(bins)
- bins = sorted(bins)
- quantized = list(map(lambda y: bisect.bisect_right(bins, y), x))
- return quantized
-
-
-def create_lengths_groups(lengths, k=0):
- bins = np.arange(start=3, stop=k, step=4).tolist() if k > 0 else [10]
- groups = _quantize(lengths, bins)
- # count number of elements per group
- counts = np.unique(groups, return_counts=True)[1]
- fbins = [0] + bins + [np.inf]
- logger.info("Using {} as bins for aspect lengths quantization".format(fbins))
- logger.info("Count of instances per bin: {}".format(counts))
- return groups
-
-
-class GroupedBatchSampler(BatchSampler):
- """
- Wraps another sampler to yield a mini-batch of indices.
- It enforces that the batch only contain elements from the same group.
- It also tries to provide mini-batches which follows an ordering which is
- as close as possible to the ordering from the original sampler.
- Arguments:
- sampler (Sampler): Base sampler.
- group_ids (list[int]): If the sampler produces indices in range [0, N),
- `group_ids` must be a list of `N` ints which contains the group id of each sample.
- The group ids must be a continuous set of integers starting from
- 0, i.e. they must be in the range [0, num_groups).
- batch_size (int): Size of mini-batch.
- """
-
- def __init__(self, sampler, group_ids, batch_size):
- if not isinstance(sampler, Sampler):
- raise ValueError(
- "sampler should be an instance of " "torch.utils.data.Sampler, but got sampler={}".format(sampler)
- )
- self.sampler = sampler
- self.group_ids = group_ids
- self.batch_size = batch_size
-
- def __iter__(self):
- buffer_per_group = defaultdict(list)
- samples_per_group = defaultdict(list)
-
- num_batches = 0
- for idx in self.sampler:
- group_id = self.group_ids[idx]
- buffer_per_group[group_id].append(idx)
- samples_per_group[group_id].append(idx)
- if len(buffer_per_group[group_id]) == self.batch_size:
- yield buffer_per_group[group_id] # TODO
- num_batches += 1
- del buffer_per_group[group_id]
- assert len(buffer_per_group[group_id]) < self.batch_size
-
- # now we have run out of elements that satisfy
- # the group criteria, let's return the remaining
- # elements so that the size of the sampler is
- # deterministic
- expected_num_batches = len(self)
- num_remaining = expected_num_batches - num_batches
- if num_remaining > 0:
- # for the remaining batches, group the batches by similar lengths
- batch_idx = []
- for group_id, idxs in sorted(buffer_per_group.items(), key=lambda x: x[0]):
- batch_idx.extend(idxs)
- if len(batch_idx) >= self.batch_size:
- yield batch_idx[: self.batch_size]
- batch_idx = batch_idx[self.batch_size :]
- num_remaining -= 1
- if len(batch_idx) > 0:
- yield batch_idx
- num_remaining -= 1
- assert num_remaining == 0
-
- def __len__(self):
- """
- Return the number of mini-batches rather than the number of samples.
- """
- return (len(self.sampler) + self.batch_size - 1) // self.batch_size
diff --git a/server/transformers/examples/distillation/lm_seqs_dataset.py b/server/transformers/examples/distillation/lm_seqs_dataset.py
deleted file mode 100644
index 8f444f4e0e151f1342016e86ba60199cebc39dec..0000000000000000000000000000000000000000
--- a/server/transformers/examples/distillation/lm_seqs_dataset.py
+++ /dev/null
@@ -1,166 +0,0 @@
-# coding=utf-8
-# Copyright 2019-present, the HuggingFace Inc. team and Facebook, Inc.
-#
-# Licensed under the Apache License, Version 2.0 (the "License");
-# you may not use this file except in compliance with the License.
-# You may obtain a copy of the License at
-#
-# http://www.apache.org/licenses/LICENSE-2.0
-#
-# Unless required by applicable law or agreed to in writing, software
-# distributed under the License is distributed on an "AS IS" BASIS,
-# WITHOUT WARRANTIES OR CONDITIONS OF ANY KIND, either express or implied.
-# See the License for the specific language governing permissions and
-# limitations under the License.
-""" Dataset to distilled models
- adapted in part from Facebook, Inc XLM model (https://github.com/facebookresearch/XLM)
-"""
-import numpy as np
-import torch
-from torch.utils.data import Dataset
-
-from utils import logger
-
-
-class LmSeqsDataset(Dataset):
- """Custom Dataset wrapping language modeling sequences.
-
- Each sample will be retrieved by indexing the list of token_ids and their corresponding lengths.
-
- Input:
- ------
- params: `NameSpace` parameters
- data: `List[np.array[int]]
- """
-
- def __init__(self, params, data):
- self.params = params
-
- self.token_ids = np.array(data)
- self.lengths = np.array([len(t) for t in data])
-
- self.check()
- self.remove_long_sequences()
- self.remove_empty_sequences()
- self.remove_unknown_sequences()
- self.check()
- self.print_statistics()
-
- def __getitem__(self, index):
- return (self.token_ids[index], self.lengths[index])
-
- def __len__(self):
- return len(self.lengths)
-
- def check(self):
- """
- Some sanity checks
- """
- assert len(self.token_ids) == len(self.lengths)
- assert all(self.lengths[i] == len(self.token_ids[i]) for i in range(len(self.lengths)))
-
- def remove_long_sequences(self):
- """
- Sequences that are too long are splitted by chunk of max_model_input_size.
- """
- max_len = self.params.max_model_input_size
- indices = self.lengths > max_len
- logger.info(f"Splitting {sum(indices)} too long sequences.")
-
- def divide_chunks(l, n):
- return [l[i : i + n] for i in range(0, len(l), n)]
-
- new_tok_ids = []
- new_lengths = []
- if self.params.mlm:
- cls_id, sep_id = self.params.special_tok_ids["cls_token"], self.params.special_tok_ids["sep_token"]
- else:
- cls_id, sep_id = self.params.special_tok_ids["bos_token"], self.params.special_tok_ids["eos_token"]
-
- for seq_, len_ in zip(self.token_ids, self.lengths):
- assert (seq_[0] == cls_id) and (seq_[-1] == sep_id), seq_
- if len_ <= max_len:
- new_tok_ids.append(seq_)
- new_lengths.append(len_)
- else:
- sub_seqs = []
- for sub_s in divide_chunks(seq_, max_len - 2):
- if sub_s[0] != cls_id:
- sub_s = np.insert(sub_s, 0, cls_id)
- if sub_s[-1] != sep_id:
- sub_s = np.insert(sub_s, len(sub_s), sep_id)
- assert len(sub_s) <= max_len
- assert (sub_s[0] == cls_id) and (sub_s[-1] == sep_id), sub_s
- sub_seqs.append(sub_s)
-
- new_tok_ids.extend(sub_seqs)
- new_lengths.extend([len(l) for l in sub_seqs])
-
- self.token_ids = np.array(new_tok_ids)
- self.lengths = np.array(new_lengths)
-
- def remove_empty_sequences(self):
- """
- Too short sequences are simply removed. This could be tunedd.
- """
- init_size = len(self)
- indices = self.lengths > 11
- self.token_ids = self.token_ids[indices]
- self.lengths = self.lengths[indices]
- new_size = len(self)
- logger.info(f"Remove {init_size - new_size} too short (<=11 tokens) sequences.")
-
- def remove_unknown_sequences(self):
- """
- Remove sequences with a (too) high level of unknown tokens.
- """
- if "unk_token" not in self.params.special_tok_ids:
- return
- else:
- unk_token_id = self.params.special_tok_ids["unk_token"]
- init_size = len(self)
- unk_occs = np.array([np.count_nonzero(a == unk_token_id) for a in self.token_ids])
- indices = (unk_occs / self.lengths) < 0.5
- self.token_ids = self.token_ids[indices]
- self.lengths = self.lengths[indices]
- new_size = len(self)
- logger.info(f"Remove {init_size - new_size} sequences with a high level of unknown tokens (50%).")
-
- def print_statistics(self):
- """
- Print some statistics on the corpus. Only the master process.
- """
- if not self.params.is_master:
- return
- logger.info(f"{len(self)} sequences")
- # data_len = sum(self.lengths)
- # nb_unique_tokens = len(Counter(list(chain(*self.token_ids))))
- # logger.info(f'{data_len} tokens ({nb_unique_tokens} unique)')
-
- # unk_idx = self.params.special_tok_ids['unk_token']
- # nb_unkown = sum([(t==unk_idx).sum() for t in self.token_ids])
- # logger.info(f'{nb_unkown} unknown tokens (covering {100*nb_unkown/data_len:.2f}% of the data)')
-
- def batch_sequences(self, batch):
- """
- Do the padding and transform into torch.tensor.
- """
- token_ids = [t[0] for t in batch]
- lengths = [t[1] for t in batch]
- assert len(token_ids) == len(lengths)
-
- # Max for paddings
- max_seq_len_ = max(lengths)
-
- # Pad token ids
- if self.params.mlm:
- pad_idx = self.params.special_tok_ids["pad_token"]
- else:
- pad_idx = self.params.special_tok_ids["unk_token"]
- tk_ = [list(t.astype(int)) + [pad_idx] * (max_seq_len_ - len(t)) for t in token_ids]
- assert len(tk_) == len(token_ids)
- assert all(len(t) == max_seq_len_ for t in tk_)
-
- tk_t = torch.tensor(tk_) # (bs, max_seq_len_)
- lg_t = torch.tensor(lengths) # (bs)
- return tk_t, lg_t
diff --git a/server/transformers/examples/distillation/requirements.txt b/server/transformers/examples/distillation/requirements.txt
deleted file mode 100644
index 1f1a1b8a6e1485772d1ed1d46aff415555de0e18..0000000000000000000000000000000000000000
--- a/server/transformers/examples/distillation/requirements.txt
+++ /dev/null
@@ -1,7 +0,0 @@
-transformers
-
-gitpython==3.0.2
-tensorboard>=1.14.0
-tensorboardX==1.8
-psutil==5.6.3
-scipy==1.3.1
diff --git a/server/transformers/examples/distillation/run_squad_w_distillation.py b/server/transformers/examples/distillation/run_squad_w_distillation.py
deleted file mode 100644
index 4900f19ead6915215ac32edaf87935ee6e5e9afc..0000000000000000000000000000000000000000
--- a/server/transformers/examples/distillation/run_squad_w_distillation.py
+++ /dev/null
@@ -1,864 +0,0 @@
-# coding=utf-8
-# Copyright 2018 The Google AI Language Team Authors and The HuggingFace Inc. team.
-# Copyright (c) 2018, NVIDIA CORPORATION. All rights reserved.
-#
-# Licensed under the Apache License, Version 2.0 (the "License");
-# you may not use this file except in compliance with the License.
-# You may obtain a copy of the License at
-#
-# http://www.apache.org/licenses/LICENSE-2.0
-#
-# Unless required by applicable law or agreed to in writing, software
-# distributed under the License is distributed on an "AS IS" BASIS,
-# WITHOUT WARRANTIES OR CONDITIONS OF ANY KIND, either express or implied.
-# See the License for the specific language governing permissions and
-# limitations under the License.
-""" This is the exact same script as `examples/run_squad.py` (as of 2020, January 8th) with an additional and optional step of distillation."""
-
-import argparse
-import glob
-import logging
-import os
-import random
-import timeit
-
-import numpy as np
-import torch
-import torch.nn as nn
-import torch.nn.functional as F
-from torch.utils.data import DataLoader, RandomSampler, SequentialSampler
-from torch.utils.data.distributed import DistributedSampler
-from tqdm import tqdm, trange
-
-from transformers import (
- WEIGHTS_NAME,
- AdamW,
- BertConfig,
- BertForQuestionAnswering,
- BertTokenizer,
- DistilBertConfig,
- DistilBertForQuestionAnswering,
- DistilBertTokenizer,
- XLMConfig,
- XLMForQuestionAnswering,
- XLMTokenizer,
- XLNetConfig,
- XLNetForQuestionAnswering,
- XLNetTokenizer,
- get_linear_schedule_with_warmup,
- squad_convert_examples_to_features,
-)
-from transformers.data.metrics.squad_metrics import (
- compute_predictions_log_probs,
- compute_predictions_logits,
- squad_evaluate,
-)
-from transformers.data.processors.squad import SquadResult, SquadV1Processor, SquadV2Processor
-
-
-try:
- from torch.utils.tensorboard import SummaryWriter
-except ImportError:
- from tensorboardX import SummaryWriter
-
-
-logger = logging.getLogger(__name__)
-
-ALL_MODELS = sum(
- (tuple(conf.pretrained_config_archive_map.keys()) for conf in (BertConfig, XLNetConfig, XLMConfig)), ()
-)
-
-MODEL_CLASSES = {
- "bert": (BertConfig, BertForQuestionAnswering, BertTokenizer),
- "xlnet": (XLNetConfig, XLNetForQuestionAnswering, XLNetTokenizer),
- "xlm": (XLMConfig, XLMForQuestionAnswering, XLMTokenizer),
- "distilbert": (DistilBertConfig, DistilBertForQuestionAnswering, DistilBertTokenizer),
-}
-
-
-def set_seed(args):
- random.seed(args.seed)
- np.random.seed(args.seed)
- torch.manual_seed(args.seed)
- if args.n_gpu > 0:
- torch.cuda.manual_seed_all(args.seed)
-
-
-def to_list(tensor):
- return tensor.detach().cpu().tolist()
-
-
-def train(args, train_dataset, model, tokenizer, teacher=None):
- """ Train the model """
- if args.local_rank in [-1, 0]:
- tb_writer = SummaryWriter()
-
- args.train_batch_size = args.per_gpu_train_batch_size * max(1, args.n_gpu)
- train_sampler = RandomSampler(train_dataset) if args.local_rank == -1 else DistributedSampler(train_dataset)
- train_dataloader = DataLoader(train_dataset, sampler=train_sampler, batch_size=args.train_batch_size)
-
- if args.max_steps > 0:
- t_total = args.max_steps
- args.num_train_epochs = args.max_steps // (len(train_dataloader) // args.gradient_accumulation_steps) + 1
- else:
- t_total = len(train_dataloader) // args.gradient_accumulation_steps * args.num_train_epochs
-
- # Prepare optimizer and schedule (linear warmup and decay)
- no_decay = ["bias", "LayerNorm.weight"]
- optimizer_grouped_parameters = [
- {
- "params": [p for n, p in model.named_parameters() if not any(nd in n for nd in no_decay)],
- "weight_decay": args.weight_decay,
- },
- {"params": [p for n, p in model.named_parameters() if any(nd in n for nd in no_decay)], "weight_decay": 0.0},
- ]
- optimizer = AdamW(optimizer_grouped_parameters, lr=args.learning_rate, eps=args.adam_epsilon)
- scheduler = get_linear_schedule_with_warmup(
- optimizer, num_warmup_steps=args.warmup_steps, num_training_steps=t_total
- )
-
- # Check if saved optimizer or scheduler states exist
- if os.path.isfile(os.path.join(args.model_name_or_path, "optimizer.pt")) and os.path.isfile(
- os.path.join(args.model_name_or_path, "scheduler.pt")
- ):
- # Load in optimizer and scheduler states
- optimizer.load_state_dict(torch.load(os.path.join(args.model_name_or_path, "optimizer.pt")))
- scheduler.load_state_dict(torch.load(os.path.join(args.model_name_or_path, "scheduler.pt")))
-
- if args.fp16:
- try:
- from apex import amp
- except ImportError:
- raise ImportError("Please install apex from https://www.github.com/nvidia/apex to use fp16 training.")
-
- model, optimizer = amp.initialize(model, optimizer, opt_level=args.fp16_opt_level)
-
- # multi-gpu training (should be after apex fp16 initialization)
- if args.n_gpu > 1:
- model = torch.nn.DataParallel(model)
-
- # Distributed training (should be after apex fp16 initialization)
- if args.local_rank != -1:
- model = torch.nn.parallel.DistributedDataParallel(
- model, device_ids=[args.local_rank], output_device=args.local_rank, find_unused_parameters=True
- )
-
- # Train!
- logger.info("***** Running training *****")
- logger.info(" Num examples = %d", len(train_dataset))
- logger.info(" Num Epochs = %d", args.num_train_epochs)
- logger.info(" Instantaneous batch size per GPU = %d", args.per_gpu_train_batch_size)
- logger.info(
- " Total train batch size (w. parallel, distributed & accumulation) = %d",
- args.train_batch_size
- * args.gradient_accumulation_steps
- * (torch.distributed.get_world_size() if args.local_rank != -1 else 1),
- )
- logger.info(" Gradient Accumulation steps = %d", args.gradient_accumulation_steps)
- logger.info(" Total optimization steps = %d", t_total)
-
- global_step = 1
- epochs_trained = 0
- steps_trained_in_current_epoch = 0
- # Check if continuing training from a checkpoint
- if os.path.exists(args.model_name_or_path):
- try:
- # set global_step to gobal_step of last saved checkpoint from model path
- checkpoint_suffix = args.model_name_or_path.split("-")[-1].split("/")[0]
- global_step = int(checkpoint_suffix)
- epochs_trained = global_step // (len(train_dataloader) // args.gradient_accumulation_steps)
- steps_trained_in_current_epoch = global_step % (len(train_dataloader) // args.gradient_accumulation_steps)
-
- logger.info(" Continuing training from checkpoint, will skip to saved global_step")
- logger.info(" Continuing training from epoch %d", epochs_trained)
- logger.info(" Continuing training from global step %d", global_step)
- logger.info(" Will skip the first %d steps in the first epoch", steps_trained_in_current_epoch)
- except ValueError:
- logger.info(" Starting fine-tuning.")
-
- tr_loss, logging_loss = 0.0, 0.0
- model.zero_grad()
- train_iterator = trange(
- epochs_trained, int(args.num_train_epochs), desc="Epoch", disable=args.local_rank not in [-1, 0]
- )
- # Added here for reproductibility
- set_seed(args)
-
- for _ in train_iterator:
- epoch_iterator = tqdm(train_dataloader, desc="Iteration", disable=args.local_rank not in [-1, 0])
- for step, batch in enumerate(epoch_iterator):
-
- # Skip past any already trained steps if resuming training
- if steps_trained_in_current_epoch > 0:
- steps_trained_in_current_epoch -= 1
- continue
-
- model.train()
- if teacher is not None:
- teacher.eval()
- batch = tuple(t.to(args.device) for t in batch)
-
- inputs = {
- "input_ids": batch[0],
- "attention_mask": batch[1],
- "start_positions": batch[3],
- "end_positions": batch[4],
- }
- if args.model_type != "distilbert":
- inputs["token_type_ids"] = None if args.model_type == "xlm" else batch[2]
- if args.model_type in ["xlnet", "xlm"]:
- inputs.update({"cls_index": batch[5], "p_mask": batch[6]})
- if args.version_2_with_negative:
- inputs.update({"is_impossible": batch[7]})
- outputs = model(**inputs)
- loss, start_logits_stu, end_logits_stu = outputs
-
- # Distillation loss
- if teacher is not None:
- if "token_type_ids" not in inputs:
- inputs["token_type_ids"] = None if args.teacher_type == "xlm" else batch[2]
- with torch.no_grad():
- start_logits_tea, end_logits_tea = teacher(
- input_ids=inputs["input_ids"],
- token_type_ids=inputs["token_type_ids"],
- attention_mask=inputs["attention_mask"],
- )
- assert start_logits_tea.size() == start_logits_stu.size()
- assert end_logits_tea.size() == end_logits_stu.size()
-
- loss_fct = nn.KLDivLoss(reduction="batchmean")
- loss_start = loss_fct(
- F.log_softmax(start_logits_stu / args.temperature, dim=-1),
- F.softmax(start_logits_tea / args.temperature, dim=-1),
- ) * (args.temperature ** 2)
- loss_end = loss_fct(
- F.log_softmax(end_logits_stu / args.temperature, dim=-1),
- F.softmax(end_logits_tea / args.temperature, dim=-1),
- ) * (args.temperature ** 2)
- loss_ce = (loss_start + loss_end) / 2.0
-
- loss = args.alpha_ce * loss_ce + args.alpha_squad * loss
-
- if args.n_gpu > 1:
- loss = loss.mean() # mean() to average on multi-gpu parallel (not distributed) training
- if args.gradient_accumulation_steps > 1:
- loss = loss / args.gradient_accumulation_steps
-
- if args.fp16:
- with amp.scale_loss(loss, optimizer) as scaled_loss:
- scaled_loss.backward()
- else:
- loss.backward()
-
- tr_loss += loss.item()
- if (step + 1) % args.gradient_accumulation_steps == 0:
- if args.fp16:
- torch.nn.utils.clip_grad_norm_(amp.master_params(optimizer), args.max_grad_norm)
- else:
- torch.nn.utils.clip_grad_norm_(model.parameters(), args.max_grad_norm)
-
- optimizer.step()
- scheduler.step() # Update learning rate schedule
- model.zero_grad()
- global_step += 1
-
- # Log metrics
- if args.local_rank in [-1, 0] and args.logging_steps > 0 and global_step % args.logging_steps == 0:
- # Only evaluate when single GPU otherwise metrics may not average well
- if args.local_rank == -1 and args.evaluate_during_training:
- results = evaluate(args, model, tokenizer)
- for key, value in results.items():
- tb_writer.add_scalar("eval_{}".format(key), value, global_step)
- tb_writer.add_scalar("lr", scheduler.get_lr()[0], global_step)
- tb_writer.add_scalar("loss", (tr_loss - logging_loss) / args.logging_steps, global_step)
- logging_loss = tr_loss
-
- if args.local_rank in [-1, 0] and args.save_steps > 0 and global_step % args.save_steps == 0:
- # Save model checkpoint
- output_dir = os.path.join(args.output_dir, "checkpoint-{}".format(global_step))
- if not os.path.exists(output_dir):
- os.makedirs(output_dir)
- model_to_save = (
- model.module if hasattr(model, "module") else model
- ) # Take care of distributed/parallel training
- model_to_save.save_pretrained(output_dir)
- tokenizer.save_pretrained(output_dir)
-
- torch.save(args, os.path.join(output_dir, "training_args.bin"))
- logger.info("Saving model checkpoint to %s", output_dir)
-
- torch.save(optimizer.state_dict(), os.path.join(output_dir, "optimizer.pt"))
- torch.save(scheduler.state_dict(), os.path.join(output_dir, "scheduler.pt"))
- logger.info("Saving optimizer and scheduler states to %s", output_dir)
-
- if args.max_steps > 0 and global_step > args.max_steps:
- epoch_iterator.close()
- break
- if args.max_steps > 0 and global_step > args.max_steps:
- train_iterator.close()
- break
-
- if args.local_rank in [-1, 0]:
- tb_writer.close()
-
- return global_step, tr_loss / global_step
-
-
-def evaluate(args, model, tokenizer, prefix=""):
- dataset, examples, features = load_and_cache_examples(args, tokenizer, evaluate=True, output_examples=True)
-
- if not os.path.exists(args.output_dir) and args.local_rank in [-1, 0]:
- os.makedirs(args.output_dir)
-
- args.eval_batch_size = args.per_gpu_eval_batch_size * max(1, args.n_gpu)
-
- # Note that DistributedSampler samples randomly
- eval_sampler = SequentialSampler(dataset)
- eval_dataloader = DataLoader(dataset, sampler=eval_sampler, batch_size=args.eval_batch_size)
-
- # multi-gpu evaluate
- if args.n_gpu > 1 and not isinstance(model, torch.nn.DataParallel):
- model = torch.nn.DataParallel(model)
-
- # Eval!
- logger.info("***** Running evaluation {} *****".format(prefix))
- logger.info(" Num examples = %d", len(dataset))
- logger.info(" Batch size = %d", args.eval_batch_size)
-
- all_results = []
- start_time = timeit.default_timer()
-
- for batch in tqdm(eval_dataloader, desc="Evaluating"):
- model.eval()
- batch = tuple(t.to(args.device) for t in batch)
-
- with torch.no_grad():
- inputs = {"input_ids": batch[0], "attention_mask": batch[1]}
- if args.model_type != "distilbert":
- inputs["token_type_ids"] = None if args.model_type == "xlm" else batch[2] # XLM don't use segment_ids
- example_indices = batch[3]
- if args.model_type in ["xlnet", "xlm"]:
- inputs.update({"cls_index": batch[4], "p_mask": batch[5]})
-
- outputs = model(**inputs)
-
- for i, example_index in enumerate(example_indices):
- eval_feature = features[example_index.item()]
- unique_id = int(eval_feature.unique_id)
-
- output = [to_list(output[i]) for output in outputs]
-
- # Some models (XLNet, XLM) use 5 arguments for their predictions, while the other "simpler"
- # models only use two.
- if len(output) >= 5:
- start_logits = output[0]
- start_top_index = output[1]
- end_logits = output[2]
- end_top_index = output[3]
- cls_logits = output[4]
-
- result = SquadResult(
- unique_id,
- start_logits,
- end_logits,
- start_top_index=start_top_index,
- end_top_index=end_top_index,
- cls_logits=cls_logits,
- )
-
- else:
- start_logits, end_logits = output
- result = SquadResult(unique_id, start_logits, end_logits)
-
- all_results.append(result)
-
- evalTime = timeit.default_timer() - start_time
- logger.info(" Evaluation done in total %f secs (%f sec per example)", evalTime, evalTime / len(dataset))
-
- # Compute predictions
- output_prediction_file = os.path.join(args.output_dir, "predictions_{}.json".format(prefix))
- output_nbest_file = os.path.join(args.output_dir, "nbest_predictions_{}.json".format(prefix))
-
- if args.version_2_with_negative:
- output_null_log_odds_file = os.path.join(args.output_dir, "null_odds_{}.json".format(prefix))
- else:
- output_null_log_odds_file = None
-
- if args.model_type in ["xlnet", "xlm"]:
- # XLNet uses a more complex post-processing procedure
- predictions = compute_predictions_log_probs(
- examples,
- features,
- all_results,
- args.n_best_size,
- args.max_answer_length,
- output_prediction_file,
- output_nbest_file,
- output_null_log_odds_file,
- model.config.start_n_top,
- model.config.end_n_top,
- args.version_2_with_negative,
- tokenizer,
- args.verbose_logging,
- )
- else:
- predictions = compute_predictions_logits(
- examples,
- features,
- all_results,
- args.n_best_size,
- args.max_answer_length,
- args.do_lower_case,
- output_prediction_file,
- output_nbest_file,
- output_null_log_odds_file,
- args.verbose_logging,
- args.version_2_with_negative,
- args.null_score_diff_threshold,
- tokenizer,
- )
-
- # Compute the F1 and exact scores.
- results = squad_evaluate(examples, predictions)
- return results
-
-
-def load_and_cache_examples(args, tokenizer, evaluate=False, output_examples=False):
- if args.local_rank not in [-1, 0] and not evaluate:
- # Make sure only the first process in distributed training process the dataset, and the others will use the cache
- torch.distributed.barrier()
-
- # Load data features from cache or dataset file
- input_file = args.predict_file if evaluate else args.train_file
- cached_features_file = os.path.join(
- os.path.dirname(input_file),
- "cached_distillation_{}_{}_{}".format(
- "dev" if evaluate else "train",
- list(filter(None, args.model_name_or_path.split("/"))).pop(),
- str(args.max_seq_length),
- ),
- )
- if os.path.exists(cached_features_file) and not args.overwrite_cache:
- logger.info("Loading features from cached file %s", cached_features_file)
- features_and_dataset = torch.load(cached_features_file)
-
- try:
- features, dataset, examples = (
- features_and_dataset["features"],
- features_and_dataset["dataset"],
- features_and_dataset["examples"],
- )
- except KeyError:
- raise DeprecationWarning(
- "You seem to be loading features from an older version of this script please delete the "
- "file %s in order for it to be created again" % cached_features_file
- )
- else:
- logger.info("Creating features from dataset file at %s", input_file)
- processor = SquadV2Processor() if args.version_2_with_negative else SquadV1Processor()
- if evaluate:
- examples = processor.get_dev_examples(args.data_dir, filename=args.predict_file)
- else:
- examples = processor.get_train_examples(args.data_dir, filename=args.train_file)
-
- features, dataset = squad_convert_examples_to_features(
- examples=examples,
- tokenizer=tokenizer,
- max_seq_length=args.max_seq_length,
- doc_stride=args.doc_stride,
- max_query_length=args.max_query_length,
- is_training=not evaluate,
- return_dataset="pt",
- threads=args.threads,
- )
-
- if args.local_rank in [-1, 0]:
- logger.info("Saving features into cached file %s", cached_features_file)
- torch.save({"features": features, "dataset": dataset, "examples": examples}, cached_features_file)
-
- if args.local_rank == 0 and not evaluate:
- # Make sure only the first process in distributed training process the dataset, and the others will use the cache
- torch.distributed.barrier()
-
- if output_examples:
- return dataset, examples, features
- return dataset
-
-
-def main():
- parser = argparse.ArgumentParser()
-
- # Required parameters
- parser.add_argument(
- "--model_type",
- default=None,
- type=str,
- required=True,
- help="Model type selected in the list: " + ", ".join(MODEL_CLASSES.keys()),
- )
- parser.add_argument(
- "--model_name_or_path",
- default=None,
- type=str,
- required=True,
- help="Path to pre-trained model or shortcut name selected in the list: " + ", ".join(ALL_MODELS),
- )
- parser.add_argument(
- "--output_dir",
- default=None,
- type=str,
- required=True,
- help="The output directory where the model checkpoints and predictions will be written.",
- )
-
- # Distillation parameters (optional)
- parser.add_argument(
- "--teacher_type",
- default=None,
- type=str,
- help="Teacher type. Teacher tokenizer and student (model) tokenizer must output the same tokenization. Only for distillation.",
- )
- parser.add_argument(
- "--teacher_name_or_path",
- default=None,
- type=str,
- help="Path to the already SQuAD fine-tuned teacher model. Only for distillation.",
- )
- parser.add_argument(
- "--alpha_ce", default=0.5, type=float, help="Distillation loss linear weight. Only for distillation."
- )
- parser.add_argument(
- "--alpha_squad", default=0.5, type=float, help="True SQuAD loss linear weight. Only for distillation."
- )
- parser.add_argument(
- "--temperature", default=2.0, type=float, help="Distillation temperature. Only for distillation."
- )
-
- # Other parameters
- parser.add_argument(
- "--data_dir",
- default=None,
- type=str,
- help="The input data dir. Should contain the .json files for the task."
- + "If no data dir or train/predict files are specified, will run with tensorflow_datasets.",
- )
- parser.add_argument(
- "--train_file",
- default=None,
- type=str,
- help="The input training file. If a data dir is specified, will look for the file there"
- + "If no data dir or train/predict files are specified, will run with tensorflow_datasets.",
- )
- parser.add_argument(
- "--predict_file",
- default=None,
- type=str,
- help="The input evaluation file. If a data dir is specified, will look for the file there"
- + "If no data dir or train/predict files are specified, will run with tensorflow_datasets.",
- )
- parser.add_argument(
- "--config_name", default="", type=str, help="Pretrained config name or path if not the same as model_name"
- )
- parser.add_argument(
- "--tokenizer_name",
- default="",
- type=str,
- help="Pretrained tokenizer name or path if not the same as model_name",
- )
- parser.add_argument(
- "--cache_dir",
- default="",
- type=str,
- help="Where do you want to store the pre-trained models downloaded from s3",
- )
-
- parser.add_argument(
- "--version_2_with_negative",
- action="store_true",
- help="If true, the SQuAD examples contain some that do not have an answer.",
- )
- parser.add_argument(
- "--null_score_diff_threshold",
- type=float,
- default=0.0,
- help="If null_score - best_non_null is greater than the threshold predict null.",
- )
-
- parser.add_argument(
- "--max_seq_length",
- default=384,
- type=int,
- help="The maximum total input sequence length after WordPiece tokenization. Sequences "
- "longer than this will be truncated, and sequences shorter than this will be padded.",
- )
- parser.add_argument(
- "--doc_stride",
- default=128,
- type=int,
- help="When splitting up a long document into chunks, how much stride to take between chunks.",
- )
- parser.add_argument(
- "--max_query_length",
- default=64,
- type=int,
- help="The maximum number of tokens for the question. Questions longer than this will "
- "be truncated to this length.",
- )
- parser.add_argument("--do_train", action="store_true", help="Whether to run training.")
- parser.add_argument("--do_eval", action="store_true", help="Whether to run eval on the dev set.")
- parser.add_argument(
- "--evaluate_during_training", action="store_true", help="Rul evaluation during training at each logging step."
- )
- parser.add_argument(
- "--do_lower_case", action="store_true", help="Set this flag if you are using an uncased model."
- )
-
- parser.add_argument("--per_gpu_train_batch_size", default=8, type=int, help="Batch size per GPU/CPU for training.")
- parser.add_argument(
- "--per_gpu_eval_batch_size", default=8, type=int, help="Batch size per GPU/CPU for evaluation."
- )
- parser.add_argument("--learning_rate", default=5e-5, type=float, help="The initial learning rate for Adam.")
- parser.add_argument(
- "--gradient_accumulation_steps",
- type=int,
- default=1,
- help="Number of updates steps to accumulate before performing a backward/update pass.",
- )
- parser.add_argument("--weight_decay", default=0.0, type=float, help="Weight decay if we apply some.")
- parser.add_argument("--adam_epsilon", default=1e-8, type=float, help="Epsilon for Adam optimizer.")
- parser.add_argument("--max_grad_norm", default=1.0, type=float, help="Max gradient norm.")
- parser.add_argument(
- "--num_train_epochs", default=3.0, type=float, help="Total number of training epochs to perform."
- )
- parser.add_argument(
- "--max_steps",
- default=-1,
- type=int,
- help="If > 0: set total number of training steps to perform. Override num_train_epochs.",
- )
- parser.add_argument("--warmup_steps", default=0, type=int, help="Linear warmup over warmup_steps.")
- parser.add_argument(
- "--n_best_size",
- default=20,
- type=int,
- help="The total number of n-best predictions to generate in the nbest_predictions.json output file.",
- )
- parser.add_argument(
- "--max_answer_length",
- default=30,
- type=int,
- help="The maximum length of an answer that can be generated. This is needed because the start "
- "and end predictions are not conditioned on one another.",
- )
- parser.add_argument(
- "--verbose_logging",
- action="store_true",
- help="If true, all of the warnings related to data processing will be printed. "
- "A number of warnings are expected for a normal SQuAD evaluation.",
- )
-
- parser.add_argument("--logging_steps", type=int, default=50, help="Log every X updates steps.")
- parser.add_argument("--save_steps", type=int, default=50, help="Save checkpoint every X updates steps.")
- parser.add_argument(
- "--eval_all_checkpoints",
- action="store_true",
- help="Evaluate all checkpoints starting with the same prefix as model_name ending and ending with step number",
- )
- parser.add_argument("--no_cuda", action="store_true", help="Whether not to use CUDA when available")
- parser.add_argument(
- "--overwrite_output_dir", action="store_true", help="Overwrite the content of the output directory"
- )
- parser.add_argument(
- "--overwrite_cache", action="store_true", help="Overwrite the cached training and evaluation sets"
- )
- parser.add_argument("--seed", type=int, default=42, help="random seed for initialization")
-
- parser.add_argument("--local_rank", type=int, default=-1, help="local_rank for distributed training on gpus")
- parser.add_argument(
- "--fp16",
- action="store_true",
- help="Whether to use 16-bit (mixed) precision (through NVIDIA apex) instead of 32-bit",
- )
- parser.add_argument(
- "--fp16_opt_level",
- type=str,
- default="O1",
- help="For fp16: Apex AMP optimization level selected in ['O0', 'O1', 'O2', and 'O3']."
- "See details at https://nvidia.github.io/apex/amp.html",
- )
- parser.add_argument("--server_ip", type=str, default="", help="Can be used for distant debugging.")
- parser.add_argument("--server_port", type=str, default="", help="Can be used for distant debugging.")
-
- parser.add_argument("--threads", type=int, default=1, help="multiple threads for converting example to features")
- args = parser.parse_args()
-
- if (
- os.path.exists(args.output_dir)
- and os.listdir(args.output_dir)
- and args.do_train
- and not args.overwrite_output_dir
- ):
- raise ValueError(
- "Output directory ({}) already exists and is not empty. Use --overwrite_output_dir to overcome.".format(
- args.output_dir
- )
- )
-
- # Setup distant debugging if needed
- if args.server_ip and args.server_port:
- # Distant debugging - see https://code.visualstudio.com/docs/python/debugging#_attach-to-a-local-script
- import ptvsd
-
- print("Waiting for debugger attach")
- ptvsd.enable_attach(address=(args.server_ip, args.server_port), redirect_output=True)
- ptvsd.wait_for_attach()
-
- # Setup CUDA, GPU & distributed training
- if args.local_rank == -1 or args.no_cuda:
- device = torch.device("cuda" if torch.cuda.is_available() and not args.no_cuda else "cpu")
- args.n_gpu = torch.cuda.device_count()
- else: # Initializes the distributed backend which will take care of sychronizing nodes/GPUs
- torch.cuda.set_device(args.local_rank)
- device = torch.device("cuda", args.local_rank)
- torch.distributed.init_process_group(backend="nccl")
- args.n_gpu = 1
- args.device = device
-
- # Setup logging
- logging.basicConfig(
- format="%(asctime)s - %(levelname)s - %(name)s - %(message)s",
- datefmt="%m/%d/%Y %H:%M:%S",
- level=logging.INFO if args.local_rank in [-1, 0] else logging.WARN,
- )
- logger.warning(
- "Process rank: %s, device: %s, n_gpu: %s, distributed training: %s, 16-bits training: %s",
- args.local_rank,
- device,
- args.n_gpu,
- bool(args.local_rank != -1),
- args.fp16,
- )
-
- # Set seed
- set_seed(args)
-
- # Load pretrained model and tokenizer
- if args.local_rank not in [-1, 0]:
- # Make sure only the first process in distributed training will download model & vocab
- torch.distributed.barrier()
-
- args.model_type = args.model_type.lower()
- config_class, model_class, tokenizer_class = MODEL_CLASSES[args.model_type]
- config = config_class.from_pretrained(
- args.config_name if args.config_name else args.model_name_or_path,
- cache_dir=args.cache_dir if args.cache_dir else None,
- )
- tokenizer = tokenizer_class.from_pretrained(
- args.tokenizer_name if args.tokenizer_name else args.model_name_or_path,
- do_lower_case=args.do_lower_case,
- cache_dir=args.cache_dir if args.cache_dir else None,
- )
- model = model_class.from_pretrained(
- args.model_name_or_path,
- from_tf=bool(".ckpt" in args.model_name_or_path),
- config=config,
- cache_dir=args.cache_dir if args.cache_dir else None,
- )
-
- if args.teacher_type is not None:
- assert args.teacher_name_or_path is not None
- assert args.alpha_ce > 0.0
- assert args.alpha_ce + args.alpha_squad > 0.0
- assert args.teacher_type != "distilbert", "We constraint teachers not to be of type DistilBERT."
- teacher_config_class, teacher_model_class, _ = MODEL_CLASSES[args.teacher_type]
- teacher_config = teacher_config_class.from_pretrained(
- args.teacher_name_or_path, cache_dir=args.cache_dir if args.cache_dir else None
- )
- teacher = teacher_model_class.from_pretrained(
- args.teacher_name_or_path, config=teacher_config, cache_dir=args.cache_dir if args.cache_dir else None
- )
- teacher.to(args.device)
- else:
- teacher = None
-
- if args.local_rank == 0:
- # Make sure only the first process in distributed training will download model & vocab
- torch.distributed.barrier()
-
- model.to(args.device)
-
- logger.info("Training/evaluation parameters %s", args)
-
- # Before we do anything with models, we want to ensure that we get fp16 execution of torch.einsum if args.fp16 is set.
- # Otherwise it'll default to "promote" mode, and we'll get fp32 operations. Note that running `--fp16_opt_level="O2"` will
- # remove the need for this code, but it is still valid.
- if args.fp16:
- try:
- import apex
-
- apex.amp.register_half_function(torch, "einsum")
- except ImportError:
- raise ImportError("Please install apex from https://www.github.com/nvidia/apex to use fp16 training.")
-
- # Training
- if args.do_train:
- train_dataset = load_and_cache_examples(args, tokenizer, evaluate=False, output_examples=False)
- global_step, tr_loss = train(args, train_dataset, model, tokenizer, teacher=teacher)
- logger.info(" global_step = %s, average loss = %s", global_step, tr_loss)
-
- # Save the trained model and the tokenizer
- if args.do_train and (args.local_rank == -1 or torch.distributed.get_rank() == 0):
- # Create output directory if needed
- if not os.path.exists(args.output_dir) and args.local_rank in [-1, 0]:
- os.makedirs(args.output_dir)
-
- logger.info("Saving model checkpoint to %s", args.output_dir)
- # Save a trained model, configuration and tokenizer using `save_pretrained()`.
- # They can then be reloaded using `from_pretrained()`
- model_to_save = (
- model.module if hasattr(model, "module") else model
- ) # Take care of distributed/parallel training
- model_to_save.save_pretrained(args.output_dir)
- tokenizer.save_pretrained(args.output_dir)
-
- # Good practice: save your training arguments together with the trained model
- torch.save(args, os.path.join(args.output_dir, "training_args.bin"))
-
- # Load a trained model and vocabulary that you have fine-tuned
- model = model_class.from_pretrained(args.output_dir)
- tokenizer = tokenizer_class.from_pretrained(args.output_dir, do_lower_case=args.do_lower_case)
- model.to(args.device)
-
- # Evaluation - we can ask to evaluate all the checkpoints (sub-directories) in a directory
- results = {}
- if args.do_eval and args.local_rank in [-1, 0]:
- if args.do_train:
- logger.info("Loading checkpoints saved during training for evaluation")
- checkpoints = [args.output_dir]
- if args.eval_all_checkpoints:
- checkpoints = list(
- os.path.dirname(c) for c in sorted(glob.glob(args.output_dir + "/**/" + WEIGHTS_NAME, recursive=True))
- )
- logging.getLogger("transformers.modeling_utils").setLevel(logging.WARN) # Reduce model loading logs
-
- logger.info("Evaluate the following checkpoints: %s", checkpoints)
-
- for checkpoint in checkpoints:
- # Reload the model
- global_step = checkpoint.split("-")[-1] if len(checkpoints) > 1 else ""
- model = model_class.from_pretrained(checkpoint)
- model.to(args.device)
-
- # Evaluate
- result = evaluate(args, model, tokenizer, prefix=global_step)
-
- result = dict((k + ("_{}".format(global_step) if global_step else ""), v) for k, v in result.items())
- results.update(result)
-
- logger.info("Results: {}".format(results))
-
- return results
-
-
-if __name__ == "__main__":
- main()
diff --git a/server/transformers/examples/distillation/scripts/binarized_data.py b/server/transformers/examples/distillation/scripts/binarized_data.py
deleted file mode 100644
index 7590cfcbcf97956010fea877402f87d936717690..0000000000000000000000000000000000000000
--- a/server/transformers/examples/distillation/scripts/binarized_data.py
+++ /dev/null
@@ -1,92 +0,0 @@
-# coding=utf-8
-# Copyright 2019-present, the HuggingFace Inc. team.
-#
-# Licensed under the Apache License, Version 2.0 (the "License");
-# you may not use this file except in compliance with the License.
-# You may obtain a copy of the License at
-#
-# http://www.apache.org/licenses/LICENSE-2.0
-#
-# Unless required by applicable law or agreed to in writing, software
-# distributed under the License is distributed on an "AS IS" BASIS,
-# WITHOUT WARRANTIES OR CONDITIONS OF ANY KIND, either express or implied.
-# See the License for the specific language governing permissions and
-# limitations under the License.
-"""
-Preprocessing script before distillation.
-"""
-import argparse
-import logging
-import pickle
-import random
-import time
-
-import numpy as np
-
-from transformers import BertTokenizer, GPT2Tokenizer, RobertaTokenizer
-
-
-logging.basicConfig(
- format="%(asctime)s - %(levelname)s - %(name)s - %(message)s", datefmt="%m/%d/%Y %H:%M:%S", level=logging.INFO
-)
-logger = logging.getLogger(__name__)
-
-
-def main():
- parser = argparse.ArgumentParser(
- description="Preprocess the data to avoid re-doing it several times by (tokenization + token_to_ids)."
- )
- parser.add_argument("--file_path", type=str, default="data/dump.txt", help="The path to the data.")
- parser.add_argument("--tokenizer_type", type=str, default="bert", choices=["bert", "roberta", "gpt2"])
- parser.add_argument("--tokenizer_name", type=str, default="bert-base-uncased", help="The tokenizer to use.")
- parser.add_argument("--dump_file", type=str, default="data/dump", help="The dump file prefix.")
- args = parser.parse_args()
-
- logger.info(f"Loading Tokenizer ({args.tokenizer_name})")
- if args.tokenizer_type == "bert":
- tokenizer = BertTokenizer.from_pretrained(args.tokenizer_name)
- bos = tokenizer.special_tokens_map["cls_token"] # `[CLS]`
- sep = tokenizer.special_tokens_map["sep_token"] # `[SEP]`
- elif args.tokenizer_type == "roberta":
- tokenizer = RobertaTokenizer.from_pretrained(args.tokenizer_name)
- bos = tokenizer.special_tokens_map["cls_token"] # ``
- sep = tokenizer.special_tokens_map["sep_token"] # ``
- elif args.tokenizer_type == "gpt2":
- tokenizer = GPT2Tokenizer.from_pretrained(args.tokenizer_name)
- bos = tokenizer.special_tokens_map["bos_token"] # `<|endoftext|>`
- sep = tokenizer.special_tokens_map["eos_token"] # `<|endoftext|>`
-
- logger.info(f"Loading text from {args.file_path}")
- with open(args.file_path, "r", encoding="utf8") as fp:
- data = fp.readlines()
-
- logger.info(f"Start encoding")
- logger.info(f"{len(data)} examples to process.")
-
- rslt = []
- iter = 0
- interval = 10000
- start = time.time()
- for text in data:
- text = f"{bos} {text.strip()} {sep}"
- token_ids = tokenizer.encode(text, add_special_tokens=False)
- rslt.append(token_ids)
-
- iter += 1
- if iter % interval == 0:
- end = time.time()
- logger.info(f"{iter} examples processed. - {(end-start)/interval:.2f}s/expl")
- start = time.time()
- logger.info("Finished binarization")
- logger.info(f"{len(data)} examples processed.")
-
- dp_file = f"{args.dump_file}.{args.tokenizer_name}.pickle"
- rslt_ = [np.uint16(d) for d in rslt]
- random.shuffle(rslt_)
- logger.info(f"Dump to {dp_file}")
- with open(dp_file, "wb") as handle:
- pickle.dump(rslt_, handle, protocol=pickle.HIGHEST_PROTOCOL)
-
-
-if __name__ == "__main__":
- main()
diff --git a/server/transformers/examples/distillation/scripts/extract.py b/server/transformers/examples/distillation/scripts/extract.py
deleted file mode 100644
index 8d102c0cda8f23cafbfcd05a214791544d8aea99..0000000000000000000000000000000000000000
--- a/server/transformers/examples/distillation/scripts/extract.py
+++ /dev/null
@@ -1,102 +0,0 @@
-# coding=utf-8
-# Copyright 2019-present, the HuggingFace Inc. team.
-#
-# Licensed under the Apache License, Version 2.0 (the "License");
-# you may not use this file except in compliance with the License.
-# You may obtain a copy of the License at
-#
-# http://www.apache.org/licenses/LICENSE-2.0
-#
-# Unless required by applicable law or agreed to in writing, software
-# distributed under the License is distributed on an "AS IS" BASIS,
-# WITHOUT WARRANTIES OR CONDITIONS OF ANY KIND, either express or implied.
-# See the License for the specific language governing permissions and
-# limitations under the License.
-"""
-Preprocessing script before training the distilled model.
-Specific to RoBERTa -> DistilRoBERTa and GPT2 -> DistilGPT2.
-"""
-import argparse
-
-import torch
-
-from transformers import GPT2LMHeadModel, RobertaForMaskedLM
-
-
-if __name__ == "__main__":
- parser = argparse.ArgumentParser(
- description="Extraction some layers of the full RobertaForMaskedLM or GPT2LMHeadModel for Transfer Learned Distillation"
- )
- parser.add_argument("--model_type", default="roberta", choices=["roberta", "gpt2"])
- parser.add_argument("--model_name", default="roberta-large", type=str)
- parser.add_argument("--dump_checkpoint", default="serialization_dir/tf_roberta_048131723.pth", type=str)
- parser.add_argument("--vocab_transform", action="store_true")
- args = parser.parse_args()
-
- if args.model_type == "roberta":
- model = RobertaForMaskedLM.from_pretrained(args.model_name)
- prefix = "roberta"
- elif args.model_type == "gpt2":
- model = GPT2LMHeadModel.from_pretrained(args.model_name)
- prefix = "transformer"
-
- state_dict = model.state_dict()
- compressed_sd = {}
-
- # Embeddings #
- if args.model_type == "gpt2":
- for param_name in ["wte.weight", "wpe.weight"]:
- compressed_sd[f"{prefix}.{param_name}"] = state_dict[f"{prefix}.{param_name}"]
- else:
- for w in ["word_embeddings", "position_embeddings", "token_type_embeddings"]:
- param_name = f"{prefix}.embeddings.{w}.weight"
- compressed_sd[param_name] = state_dict[param_name]
- for w in ["weight", "bias"]:
- param_name = f"{prefix}.embeddings.LayerNorm.{w}"
- compressed_sd[param_name] = state_dict[param_name]
-
- # Transformer Blocks #
- std_idx = 0
- for teacher_idx in [0, 2, 4, 7, 9, 11]:
- if args.model_type == "gpt2":
- for layer in ["ln_1", "attn.c_attn", "attn.c_proj", "ln_2", "mlp.c_fc", "mlp.c_proj"]:
- for w in ["weight", "bias"]:
- compressed_sd[f"{prefix}.h.{std_idx}.{layer}.{w}"] = state_dict[
- f"{prefix}.h.{teacher_idx}.{layer}.{w}"
- ]
- compressed_sd[f"{prefix}.h.{std_idx}.attn.bias"] = state_dict[f"{prefix}.h.{teacher_idx}.attn.bias"]
- else:
- for layer in [
- "attention.self.query",
- "attention.self.key",
- "attention.self.value",
- "attention.output.dense",
- "attention.output.LayerNorm",
- "intermediate.dense",
- "output.dense",
- "output.LayerNorm",
- ]:
- for w in ["weight", "bias"]:
- compressed_sd[f"{prefix}.encoder.layer.{std_idx}.{layer}.{w}"] = state_dict[
- f"{prefix}.encoder.layer.{teacher_idx}.{layer}.{w}"
- ]
- std_idx += 1
-
- # Language Modeling Head ###s
- if args.model_type == "roberta":
- for layer in ["lm_head.decoder.weight", "lm_head.bias"]:
- compressed_sd[f"{layer}"] = state_dict[f"{layer}"]
- if args.vocab_transform:
- for w in ["weight", "bias"]:
- compressed_sd[f"lm_head.dense.{w}"] = state_dict[f"lm_head.dense.{w}"]
- compressed_sd[f"lm_head.layer_norm.{w}"] = state_dict[f"lm_head.layer_norm.{w}"]
- elif args.model_type == "gpt2":
- for w in ["weight", "bias"]:
- compressed_sd[f"{prefix}.ln_f.{w}"] = state_dict[f"{prefix}.ln_f.{w}"]
- compressed_sd[f"lm_head.weight"] = state_dict[f"lm_head.weight"]
-
- print(f"N layers selected for distillation: {std_idx}")
- print(f"Number of params transfered for distillation: {len(compressed_sd.keys())}")
-
- print(f"Save transfered checkpoint to {args.dump_checkpoint}.")
- torch.save(compressed_sd, args.dump_checkpoint)
diff --git a/server/transformers/examples/distillation/scripts/extract_distilbert.py b/server/transformers/examples/distillation/scripts/extract_distilbert.py
deleted file mode 100644
index 972418b56b80bb1e7d2d8f71950bd3654079da31..0000000000000000000000000000000000000000
--- a/server/transformers/examples/distillation/scripts/extract_distilbert.py
+++ /dev/null
@@ -1,92 +0,0 @@
-# coding=utf-8
-# Copyright 2019-present, the HuggingFace Inc. team.
-#
-# Licensed under the Apache License, Version 2.0 (the "License");
-# you may not use this file except in compliance with the License.
-# You may obtain a copy of the License at
-#
-# http://www.apache.org/licenses/LICENSE-2.0
-#
-# Unless required by applicable law or agreed to in writing, software
-# distributed under the License is distributed on an "AS IS" BASIS,
-# WITHOUT WARRANTIES OR CONDITIONS OF ANY KIND, either express or implied.
-# See the License for the specific language governing permissions and
-# limitations under the License.
-"""
-Preprocessing script before training DistilBERT.
-Specific to BERT -> DistilBERT.
-"""
-import argparse
-
-import torch
-
-from transformers import BertForMaskedLM
-
-
-if __name__ == "__main__":
- parser = argparse.ArgumentParser(
- description="Extraction some layers of the full BertForMaskedLM or RObertaForMaskedLM for Transfer Learned Distillation"
- )
- parser.add_argument("--model_type", default="bert", choices=["bert"])
- parser.add_argument("--model_name", default="bert-base-uncased", type=str)
- parser.add_argument("--dump_checkpoint", default="serialization_dir/tf_bert-base-uncased_0247911.pth", type=str)
- parser.add_argument("--vocab_transform", action="store_true")
- args = parser.parse_args()
-
- if args.model_type == "bert":
- model = BertForMaskedLM.from_pretrained(args.model_name)
- prefix = "bert"
- else:
- raise ValueError(f'args.model_type should be "bert".')
-
- state_dict = model.state_dict()
- compressed_sd = {}
-
- for w in ["word_embeddings", "position_embeddings"]:
- compressed_sd[f"distilbert.embeddings.{w}.weight"] = state_dict[f"{prefix}.embeddings.{w}.weight"]
- for w in ["weight", "bias"]:
- compressed_sd[f"distilbert.embeddings.LayerNorm.{w}"] = state_dict[f"{prefix}.embeddings.LayerNorm.{w}"]
-
- std_idx = 0
- for teacher_idx in [0, 2, 4, 7, 9, 11]:
- for w in ["weight", "bias"]:
- compressed_sd[f"distilbert.transformer.layer.{std_idx}.attention.q_lin.{w}"] = state_dict[
- f"{prefix}.encoder.layer.{teacher_idx}.attention.self.query.{w}"
- ]
- compressed_sd[f"distilbert.transformer.layer.{std_idx}.attention.k_lin.{w}"] = state_dict[
- f"{prefix}.encoder.layer.{teacher_idx}.attention.self.key.{w}"
- ]
- compressed_sd[f"distilbert.transformer.layer.{std_idx}.attention.v_lin.{w}"] = state_dict[
- f"{prefix}.encoder.layer.{teacher_idx}.attention.self.value.{w}"
- ]
-
- compressed_sd[f"distilbert.transformer.layer.{std_idx}.attention.out_lin.{w}"] = state_dict[
- f"{prefix}.encoder.layer.{teacher_idx}.attention.output.dense.{w}"
- ]
- compressed_sd[f"distilbert.transformer.layer.{std_idx}.sa_layer_norm.{w}"] = state_dict[
- f"{prefix}.encoder.layer.{teacher_idx}.attention.output.LayerNorm.{w}"
- ]
-
- compressed_sd[f"distilbert.transformer.layer.{std_idx}.ffn.lin1.{w}"] = state_dict[
- f"{prefix}.encoder.layer.{teacher_idx}.intermediate.dense.{w}"
- ]
- compressed_sd[f"distilbert.transformer.layer.{std_idx}.ffn.lin2.{w}"] = state_dict[
- f"{prefix}.encoder.layer.{teacher_idx}.output.dense.{w}"
- ]
- compressed_sd[f"distilbert.transformer.layer.{std_idx}.output_layer_norm.{w}"] = state_dict[
- f"{prefix}.encoder.layer.{teacher_idx}.output.LayerNorm.{w}"
- ]
- std_idx += 1
-
- compressed_sd[f"vocab_projector.weight"] = state_dict[f"cls.predictions.decoder.weight"]
- compressed_sd[f"vocab_projector.bias"] = state_dict[f"cls.predictions.bias"]
- if args.vocab_transform:
- for w in ["weight", "bias"]:
- compressed_sd[f"vocab_transform.{w}"] = state_dict[f"cls.predictions.transform.dense.{w}"]
- compressed_sd[f"vocab_layer_norm.{w}"] = state_dict[f"cls.predictions.transform.LayerNorm.{w}"]
-
- print(f"N layers selected for distillation: {std_idx}")
- print(f"Number of params transfered for distillation: {len(compressed_sd.keys())}")
-
- print(f"Save transfered checkpoint to {args.dump_checkpoint}.")
- torch.save(compressed_sd, args.dump_checkpoint)
diff --git a/server/transformers/examples/distillation/scripts/token_counts.py b/server/transformers/examples/distillation/scripts/token_counts.py
deleted file mode 100644
index 0238bf66f865be5d32bff6783a8cb048563adc2b..0000000000000000000000000000000000000000
--- a/server/transformers/examples/distillation/scripts/token_counts.py
+++ /dev/null
@@ -1,56 +0,0 @@
-# coding=utf-8
-# Copyright 2019-present, the HuggingFace Inc. team.
-#
-# Licensed under the Apache License, Version 2.0 (the "License");
-# you may not use this file except in compliance with the License.
-# You may obtain a copy of the License at
-#
-# http://www.apache.org/licenses/LICENSE-2.0
-#
-# Unless required by applicable law or agreed to in writing, software
-# distributed under the License is distributed on an "AS IS" BASIS,
-# WITHOUT WARRANTIES OR CONDITIONS OF ANY KIND, either express or implied.
-# See the License for the specific language governing permissions and
-# limitations under the License.
-"""
-Preprocessing script before training the distilled model.
-"""
-import argparse
-import logging
-import pickle
-from collections import Counter
-
-
-logging.basicConfig(
- format="%(asctime)s - %(levelname)s - %(name)s - %(message)s", datefmt="%m/%d/%Y %H:%M:%S", level=logging.INFO
-)
-logger = logging.getLogger(__name__)
-
-if __name__ == "__main__":
- parser = argparse.ArgumentParser(
- description="Token Counts for smoothing the masking probabilities in MLM (cf XLM/word2vec)"
- )
- parser.add_argument(
- "--data_file", type=str, default="data/dump.bert-base-uncased.pickle", help="The binarized dataset."
- )
- parser.add_argument(
- "--token_counts_dump", type=str, default="data/token_counts.bert-base-uncased.pickle", help="The dump file."
- )
- parser.add_argument("--vocab_size", default=30522, type=int)
- args = parser.parse_args()
-
- logger.info(f"Loading data from {args.data_file}")
- with open(args.data_file, "rb") as fp:
- data = pickle.load(fp)
-
- logger.info("Counting occurences for MLM.")
- counter = Counter()
- for tk_ids in data:
- counter.update(tk_ids)
- counts = [0] * args.vocab_size
- for k, v in counter.items():
- counts[k] = v
-
- logger.info(f"Dump to {args.token_counts_dump}")
- with open(args.token_counts_dump, "wb") as handle:
- pickle.dump(counts, handle, protocol=pickle.HIGHEST_PROTOCOL)
diff --git a/server/transformers/examples/distillation/train.py b/server/transformers/examples/distillation/train.py
deleted file mode 100644
index 670d03ea16edf345e5f2a60b16988a8d3fffde6c..0000000000000000000000000000000000000000
--- a/server/transformers/examples/distillation/train.py
+++ /dev/null
@@ -1,322 +0,0 @@
-# coding=utf-8
-# Copyright 2019-present, the HuggingFace Inc. team.
-#
-# Licensed under the Apache License, Version 2.0 (the "License");
-# you may not use this file except in compliance with the License.
-# You may obtain a copy of the License at
-#
-# http://www.apache.org/licenses/LICENSE-2.0
-#
-# Unless required by applicable law or agreed to in writing, software
-# distributed under the License is distributed on an "AS IS" BASIS,
-# WITHOUT WARRANTIES OR CONDITIONS OF ANY KIND, either express or implied.
-# See the License for the specific language governing permissions and
-# limitations under the License.
-"""
-Training the distilled model.
-Supported architectures include: BERT -> DistilBERT, RoBERTa -> DistilRoBERTa, GPT2 -> DistilGPT2.
-"""
-import argparse
-import json
-import os
-import pickle
-import shutil
-
-import numpy as np
-import torch
-
-from distiller import Distiller
-from lm_seqs_dataset import LmSeqsDataset
-from transformers import (
- BertConfig,
- BertForMaskedLM,
- BertTokenizer,
- DistilBertConfig,
- DistilBertForMaskedLM,
- DistilBertTokenizer,
- GPT2Config,
- GPT2LMHeadModel,
- GPT2Tokenizer,
- RobertaConfig,
- RobertaForMaskedLM,
- RobertaTokenizer,
-)
-from utils import git_log, init_gpu_params, logger, set_seed
-
-
-MODEL_CLASSES = {
- "distilbert": (DistilBertConfig, DistilBertForMaskedLM, DistilBertTokenizer),
- "roberta": (RobertaConfig, RobertaForMaskedLM, RobertaTokenizer),
- "bert": (BertConfig, BertForMaskedLM, BertTokenizer),
- "gpt2": (GPT2Config, GPT2LMHeadModel, GPT2Tokenizer),
-}
-
-
-def sanity_checks(args):
- """
- A bunch of args sanity checks to perform even starting...
- """
- assert (args.mlm and args.alpha_mlm > 0.0) or (not args.mlm and args.alpha_mlm == 0.0)
- assert (args.alpha_mlm > 0.0 and args.alpha_clm == 0.0) or (args.alpha_mlm == 0.0 and args.alpha_clm > 0.0)
- if args.mlm:
- assert os.path.isfile(args.token_counts)
- assert (args.student_type in ["roberta", "distilbert"]) and (args.teacher_type in ["roberta", "bert"])
- else:
- assert (args.student_type in ["gpt2"]) and (args.teacher_type in ["gpt2"])
-
- assert args.teacher_type == args.student_type or (
- args.student_type == "distilbert" and args.teacher_type == "bert"
- )
- assert os.path.isfile(args.student_config)
- if args.student_pretrained_weights is not None:
- assert os.path.isfile(args.student_pretrained_weights)
-
- if args.freeze_token_type_embds:
- assert args.student_type in ["roberta"]
-
- assert args.alpha_ce >= 0.0
- assert args.alpha_mlm >= 0.0
- assert args.alpha_clm >= 0.0
- assert args.alpha_mse >= 0.0
- assert args.alpha_cos >= 0.0
- assert args.alpha_ce + args.alpha_mlm + args.alpha_clm + args.alpha_mse + args.alpha_cos > 0.0
-
-
-def freeze_pos_embeddings(student, args):
- if args.student_type == "roberta":
- student.roberta.embeddings.position_embeddings.weight.requires_grad = False
- elif args.student_type == "gpt2":
- student.transformer.wpe.weight.requires_grad = False
-
-
-def freeze_token_type_embeddings(student, args):
- if args.student_type == "roberta":
- student.roberta.embeddings.token_type_embeddings.weight.requires_grad = False
-
-
-def main():
- parser = argparse.ArgumentParser(description="Training")
- parser.add_argument("--force", action="store_true", help="Overwrite dump_path if it already exists.")
-
- parser.add_argument(
- "--dump_path", type=str, required=True, help="The output directory (log, checkpoints, parameters, etc.)"
- )
- parser.add_argument(
- "--data_file",
- type=str,
- required=True,
- help="The binarized file (tokenized + tokens_to_ids) and grouped by sequence.",
- )
-
- parser.add_argument(
- "--student_type",
- type=str,
- choices=["distilbert", "roberta", "gpt2"],
- required=True,
- help="The student type (DistilBERT, RoBERTa).",
- )
- parser.add_argument("--student_config", type=str, required=True, help="Path to the student configuration.")
- parser.add_argument(
- "--student_pretrained_weights", default=None, type=str, help="Load student initialization checkpoint."
- )
-
- parser.add_argument(
- "--teacher_type", choices=["bert", "roberta", "gpt2"], required=True, help="Teacher type (BERT, RoBERTa)."
- )
- parser.add_argument("--teacher_name", type=str, required=True, help="The teacher model.")
-
- parser.add_argument("--temperature", default=2.0, type=float, help="Temperature for the softmax temperature.")
- parser.add_argument(
- "--alpha_ce", default=0.5, type=float, help="Linear weight for the distillation loss. Must be >=0."
- )
- parser.add_argument(
- "--alpha_mlm",
- default=0.0,
- type=float,
- help="Linear weight for the MLM loss. Must be >=0. Should be used in coonjunction with `mlm` flag.",
- )
- parser.add_argument("--alpha_clm", default=0.5, type=float, help="Linear weight for the CLM loss. Must be >=0.")
- parser.add_argument("--alpha_mse", default=0.0, type=float, help="Linear weight of the MSE loss. Must be >=0.")
- parser.add_argument(
- "--alpha_cos", default=0.0, type=float, help="Linear weight of the cosine embedding loss. Must be >=0."
- )
-
- parser.add_argument(
- "--mlm", action="store_true", help="The LM step: MLM or CLM. If `mlm` is True, the MLM is used over CLM."
- )
- parser.add_argument(
- "--mlm_mask_prop",
- default=0.15,
- type=float,
- help="Proportion of tokens for which we need to make a prediction.",
- )
- parser.add_argument("--word_mask", default=0.8, type=float, help="Proportion of tokens to mask out.")
- parser.add_argument("--word_keep", default=0.1, type=float, help="Proportion of tokens to keep.")
- parser.add_argument("--word_rand", default=0.1, type=float, help="Proportion of tokens to randomly replace.")
- parser.add_argument(
- "--mlm_smoothing",
- default=0.7,
- type=float,
- help="Smoothing parameter to emphasize more rare tokens (see XLM, similar to word2vec).",
- )
- parser.add_argument("--token_counts", type=str, help="The token counts in the data_file for MLM.")
-
- parser.add_argument(
- "--restrict_ce_to_mask",
- action="store_true",
- help="If true, compute the distilation loss only the [MLM] prediction distribution.",
- )
- parser.add_argument(
- "--freeze_pos_embs",
- action="store_true",
- help="Freeze positional embeddings during distillation. For student_type in ['roberta', 'gpt2'] only.",
- )
- parser.add_argument(
- "--freeze_token_type_embds",
- action="store_true",
- help="Freeze token type embeddings during distillation if existent. For student_type in ['roberta'] only.",
- )
-
- parser.add_argument("--n_epoch", type=int, default=3, help="Number of pass on the whole dataset.")
- parser.add_argument("--batch_size", type=int, default=5, help="Batch size (for each process).")
- parser.add_argument(
- "--group_by_size",
- action="store_false",
- help="If true, group sequences that have similar length into the same batch. Default is true.",
- )
-
- parser.add_argument(
- "--gradient_accumulation_steps",
- type=int,
- default=50,
- help="Gradient accumulation for larger training batches.",
- )
- parser.add_argument("--warmup_prop", default=0.05, type=float, help="Linear warmup proportion.")
- parser.add_argument("--weight_decay", default=0.0, type=float, help="Weight deay if we apply some.")
- parser.add_argument("--learning_rate", default=5e-4, type=float, help="The initial learning rate for Adam.")
- parser.add_argument("--adam_epsilon", default=1e-6, type=float, help="Epsilon for Adam optimizer.")
- parser.add_argument("--max_grad_norm", default=5.0, type=float, help="Max gradient norm.")
- parser.add_argument("--initializer_range", default=0.02, type=float, help="Random initialization range.")
-
- parser.add_argument(
- "--fp16",
- action="store_true",
- help="Whether to use 16-bit (mixed) precision (through NVIDIA apex) instead of 32-bit",
- )
- parser.add_argument(
- "--fp16_opt_level",
- type=str,
- default="O1",
- help="For fp16: Apex AMP optimization level selected in ['O0', 'O1', 'O2', and 'O3']."
- "See details at https://nvidia.github.io/apex/amp.html",
- )
- parser.add_argument("--n_gpu", type=int, default=1, help="Number of GPUs in the node.")
- parser.add_argument("--local_rank", type=int, default=-1, help="Distributed training - Local rank")
- parser.add_argument("--seed", type=int, default=56, help="Random seed")
-
- parser.add_argument("--log_interval", type=int, default=500, help="Tensorboard logging interval.")
- parser.add_argument("--checkpoint_interval", type=int, default=4000, help="Checkpoint interval.")
- args = parser.parse_args()
- sanity_checks(args)
-
- # ARGS #
- init_gpu_params(args)
- set_seed(args)
- if args.is_master:
- if os.path.exists(args.dump_path):
- if not args.force:
- raise ValueError(
- f"Serialization dir {args.dump_path} already exists, but you have not precised wheter to overwrite it"
- "Use `--force` if you want to overwrite it"
- )
- else:
- shutil.rmtree(args.dump_path)
-
- if not os.path.exists(args.dump_path):
- os.makedirs(args.dump_path)
- logger.info(f"Experiment will be dumped and logged in {args.dump_path}")
-
- # SAVE PARAMS #
- logger.info(f"Param: {args}")
- with open(os.path.join(args.dump_path, "parameters.json"), "w") as f:
- json.dump(vars(args), f, indent=4)
- git_log(args.dump_path)
-
- student_config_class, student_model_class, _ = MODEL_CLASSES[args.student_type]
- teacher_config_class, teacher_model_class, teacher_tokenizer_class = MODEL_CLASSES[args.teacher_type]
-
- # TOKENIZER #
- tokenizer = teacher_tokenizer_class.from_pretrained(args.teacher_name)
- special_tok_ids = {}
- for tok_name, tok_symbol in tokenizer.special_tokens_map.items():
- idx = tokenizer.all_special_tokens.index(tok_symbol)
- special_tok_ids[tok_name] = tokenizer.all_special_ids[idx]
- logger.info(f"Special tokens {special_tok_ids}")
- args.special_tok_ids = special_tok_ids
- args.max_model_input_size = tokenizer.max_model_input_sizes[args.teacher_name]
-
- # DATA LOADER #
- logger.info(f"Loading data from {args.data_file}")
- with open(args.data_file, "rb") as fp:
- data = pickle.load(fp)
-
- if args.mlm:
- logger.info(f"Loading token counts from {args.token_counts} (already pre-computed)")
- with open(args.token_counts, "rb") as fp:
- counts = pickle.load(fp)
-
- token_probs = np.maximum(counts, 1) ** -args.mlm_smoothing
- for idx in special_tok_ids.values():
- token_probs[idx] = 0.0 # do not predict special tokens
- token_probs = torch.from_numpy(token_probs)
- else:
- token_probs = None
-
- train_lm_seq_dataset = LmSeqsDataset(params=args, data=data)
- logger.info(f"Data loader created.")
-
- # STUDENT #
- logger.info(f"Loading student config from {args.student_config}")
- stu_architecture_config = student_config_class.from_pretrained(args.student_config)
- stu_architecture_config.output_hidden_states = True
-
- if args.student_pretrained_weights is not None:
- logger.info(f"Loading pretrained weights from {args.student_pretrained_weights}")
- student = student_model_class.from_pretrained(args.student_pretrained_weights, config=stu_architecture_config)
- else:
- student = student_model_class(stu_architecture_config)
-
- if args.n_gpu > 0:
- student.to(f"cuda:{args.local_rank}")
- logger.info(f"Student loaded.")
-
- # TEACHER #
- teacher = teacher_model_class.from_pretrained(args.teacher_name, output_hidden_states=True)
- if args.n_gpu > 0:
- teacher.to(f"cuda:{args.local_rank}")
- logger.info(f"Teacher loaded from {args.teacher_name}.")
-
- # FREEZING #
- if args.freeze_pos_embs:
- freeze_pos_embeddings(student, args)
- if args.freeze_token_type_embds:
- freeze_token_type_embeddings(student, args)
-
- # SANITY CHECKS #
- assert student.config.vocab_size == teacher.config.vocab_size
- assert student.config.hidden_size == teacher.config.hidden_size
- assert student.config.max_position_embeddings == teacher.config.max_position_embeddings
- if args.mlm:
- assert token_probs.size(0) == stu_architecture_config.vocab_size
-
- # DISTILLER #
- torch.cuda.empty_cache()
- distiller = Distiller(
- params=args, dataset=train_lm_seq_dataset, token_probs=token_probs, student=student, teacher=teacher
- )
- distiller.train()
- logger.info("Let's go get some drinks.")
-
-
-if __name__ == "__main__":
- main()
diff --git a/server/transformers/examples/distillation/training_configs/distilbert-base-multilingual-cased.json b/server/transformers/examples/distillation/training_configs/distilbert-base-multilingual-cased.json
deleted file mode 100644
index f76e7febcba536f7ee6137e70ffca0acae649bea..0000000000000000000000000000000000000000
--- a/server/transformers/examples/distillation/training_configs/distilbert-base-multilingual-cased.json
+++ /dev/null
@@ -1,15 +0,0 @@
-{
- "activation": "gelu",
- "attention_dropout": 0.1,
- "dim": 768,
- "dropout": 0.1,
- "hidden_dim": 3072,
- "initializer_range": 0.02,
- "max_position_embeddings": 512,
- "n_heads": 12,
- "n_layers": 6,
- "sinusoidal_pos_embds": true,
- "tie_weights_": true,
- "vocab_size": 119547
- }
-
\ No newline at end of file
diff --git a/server/transformers/examples/distillation/training_configs/distilbert-base-uncased.json b/server/transformers/examples/distillation/training_configs/distilbert-base-uncased.json
deleted file mode 100644
index 15d1e7fe00e63100b602a0d7db0cdbf16f7e6ff0..0000000000000000000000000000000000000000
--- a/server/transformers/examples/distillation/training_configs/distilbert-base-uncased.json
+++ /dev/null
@@ -1,15 +0,0 @@
-{
- "activation": "gelu",
- "attention_dropout": 0.1,
- "dim": 768,
- "dropout": 0.1,
- "hidden_dim": 3072,
- "initializer_range": 0.02,
- "max_position_embeddings": 512,
- "n_heads": 12,
- "n_layers": 6,
- "sinusoidal_pos_embds": true,
- "tie_weights_": true,
- "vocab_size": 30522
- }
-
\ No newline at end of file
diff --git a/server/transformers/examples/distillation/training_configs/distilgpt2.json b/server/transformers/examples/distillation/training_configs/distilgpt2.json
deleted file mode 100644
index 8616e8e60fd522461462444f81f7259fe904f104..0000000000000000000000000000000000000000
--- a/server/transformers/examples/distillation/training_configs/distilgpt2.json
+++ /dev/null
@@ -1,10 +0,0 @@
-{
- "initializer_range": 0.02,
- "layer_norm_epsilon": 0.00001,
- "n_ctx": 1024,
- "n_embd": 768,
- "n_head": 12,
- "n_layer": 6,
- "n_positions": 1024,
- "vocab_size": 50257
-}
\ No newline at end of file
diff --git a/server/transformers/examples/distillation/training_configs/distilroberta-base.json b/server/transformers/examples/distillation/training_configs/distilroberta-base.json
deleted file mode 100644
index 2d90ef6380a0e4d54dbab8b1a151f7162665c0da..0000000000000000000000000000000000000000
--- a/server/transformers/examples/distillation/training_configs/distilroberta-base.json
+++ /dev/null
@@ -1,14 +0,0 @@
-{
- "vocab_size": 50265,
- "hidden_size": 768,
- "num_hidden_layers": 6,
- "num_attention_heads": 12,
- "intermediate_size": 3072,
- "hidden_act": "gelu",
- "hidden_dropout_prob": 0.1,
- "attention_probs_dropout_prob": 0.1,
- "max_position_embeddings": 514,
- "type_vocab_size": 1,
- "initializer_range": 0.02,
- "layer_norm_eps": 0.00001
-}
\ No newline at end of file
diff --git a/server/transformers/examples/distillation/utils.py b/server/transformers/examples/distillation/utils.py
deleted file mode 100644
index 211e7c61dacf1c252104cb9f67759ca5e29cf23c..0000000000000000000000000000000000000000
--- a/server/transformers/examples/distillation/utils.py
+++ /dev/null
@@ -1,132 +0,0 @@
-# coding=utf-8
-# Copyright 2019-present, the HuggingFace Inc. team and Facebook, Inc.
-#
-# Licensed under the Apache License, Version 2.0 (the "License");
-# you may not use this file except in compliance with the License.
-# You may obtain a copy of the License at
-#
-# http://www.apache.org/licenses/LICENSE-2.0
-#
-# Unless required by applicable law or agreed to in writing, software
-# distributed under the License is distributed on an "AS IS" BASIS,
-# WITHOUT WARRANTIES OR CONDITIONS OF ANY KIND, either express or implied.
-# See the License for the specific language governing permissions and
-# limitations under the License.
-""" Utils to train DistilBERT
- adapted in part from Facebook, Inc XLM model (https://github.com/facebookresearch/XLM)
-"""
-import json
-import logging
-import os
-import socket
-
-import git
-import numpy as np
-import torch
-
-
-logging.basicConfig(
- format="%(asctime)s - %(levelname)s - %(name)s - PID: %(process)d - %(message)s",
- datefmt="%m/%d/%Y %H:%M:%S",
- level=logging.INFO,
-)
-logger = logging.getLogger(__name__)
-
-
-def git_log(folder_path: str):
- """
- Log commit info.
- """
- repo = git.Repo(search_parent_directories=True)
- repo_infos = {
- "repo_id": str(repo),
- "repo_sha": str(repo.head.object.hexsha),
- "repo_branch": str(repo.active_branch),
- }
-
- with open(os.path.join(folder_path, "git_log.json"), "w") as f:
- json.dump(repo_infos, f, indent=4)
-
-
-def init_gpu_params(params):
- """
- Handle single and multi-GPU / multi-node.
- """
- if params.n_gpu <= 0:
- params.local_rank = 0
- params.master_port = -1
- params.is_master = True
- params.multi_gpu = False
- return
-
- assert torch.cuda.is_available()
-
- logger.info("Initializing GPUs")
- if params.n_gpu > 1:
- assert params.local_rank != -1
-
- params.world_size = int(os.environ["WORLD_SIZE"])
- params.n_gpu_per_node = int(os.environ["N_GPU_NODE"])
- params.global_rank = int(os.environ["RANK"])
-
- # number of nodes / node ID
- params.n_nodes = params.world_size // params.n_gpu_per_node
- params.node_id = params.global_rank // params.n_gpu_per_node
- params.multi_gpu = True
-
- assert params.n_nodes == int(os.environ["N_NODES"])
- assert params.node_id == int(os.environ["NODE_RANK"])
-
- # local job (single GPU)
- else:
- assert params.local_rank == -1
-
- params.n_nodes = 1
- params.node_id = 0
- params.local_rank = 0
- params.global_rank = 0
- params.world_size = 1
- params.n_gpu_per_node = 1
- params.multi_gpu = False
-
- # sanity checks
- assert params.n_nodes >= 1
- assert 0 <= params.node_id < params.n_nodes
- assert 0 <= params.local_rank <= params.global_rank < params.world_size
- assert params.world_size == params.n_nodes * params.n_gpu_per_node
-
- # define whether this is the master process / if we are in multi-node distributed mode
- params.is_master = params.node_id == 0 and params.local_rank == 0
- params.multi_node = params.n_nodes > 1
-
- # summary
- PREFIX = f"--- Global rank: {params.global_rank} - "
- logger.info(PREFIX + "Number of nodes: %i" % params.n_nodes)
- logger.info(PREFIX + "Node ID : %i" % params.node_id)
- logger.info(PREFIX + "Local rank : %i" % params.local_rank)
- logger.info(PREFIX + "World size : %i" % params.world_size)
- logger.info(PREFIX + "GPUs per node : %i" % params.n_gpu_per_node)
- logger.info(PREFIX + "Master : %s" % str(params.is_master))
- logger.info(PREFIX + "Multi-node : %s" % str(params.multi_node))
- logger.info(PREFIX + "Multi-GPU : %s" % str(params.multi_gpu))
- logger.info(PREFIX + "Hostname : %s" % socket.gethostname())
-
- # set GPU device
- torch.cuda.set_device(params.local_rank)
-
- # initialize multi-GPU
- if params.multi_gpu:
- logger.info("Initializing PyTorch distributed")
- torch.distributed.init_process_group(
- init_method="env://", backend="nccl",
- )
-
-
-def set_seed(args):
- """
- Set the random seed.
- """
- np.random.seed(args.seed)
- torch.manual_seed(args.seed)
- if args.n_gpu > 0:
- torch.cuda.manual_seed_all(args.seed)
diff --git a/server/transformers/examples/hans/hans_processors.py b/server/transformers/examples/hans/hans_processors.py
deleted file mode 100644
index ff75a0acd18c5da6d5da08ea20d603753bc0ff80..0000000000000000000000000000000000000000
--- a/server/transformers/examples/hans/hans_processors.py
+++ /dev/null
@@ -1,221 +0,0 @@
-# coding=utf-8
-# Copyright 2018 The Google AI Language Team Authors and The HuggingFace Inc. team.
-# Copyright (c) 2018, NVIDIA CORPORATION. All rights reserved.
-#
-# Licensed under the Apache License, Version 2.0 (the "License");
-# you may not use this file except in compliance with the License.
-# You may obtain a copy of the License at
-#
-# http://www.apache.org/licenses/LICENSE-2.0
-#
-# Unless required by applicable law or agreed to in writing, software
-# distributed under the License is distributed on an "AS IS" BASIS,
-# WITHOUT WARRANTIES OR CONDITIONS OF ANY KIND, either express or implied.
-# See the License for the specific language governing permissions and
-# limitations under the License.
-""" GLUE processors and helpers """
-
-import logging
-import os
-
-from transformers.file_utils import is_tf_available
-from utils_hans import DataProcessor, InputExample, InputFeatures
-
-
-if is_tf_available():
- import tensorflow as tf
-
-logger = logging.getLogger(__name__)
-
-
-def hans_convert_examples_to_features(
- examples,
- tokenizer,
- max_length=512,
- task=None,
- label_list=None,
- output_mode=None,
- pad_on_left=False,
- pad_token=0,
- pad_token_segment_id=0,
- mask_padding_with_zero=True,
-):
- """
- Loads a data file into a list of ``InputFeatures``
-
- Args:
- examples: List of ``InputExamples`` or ``tf.data.Dataset`` containing the examples.
- tokenizer: Instance of a tokenizer that will tokenize the examples
- max_length: Maximum example length
- task: HANS
- label_list: List of labels. Can be obtained from the processor using the ``processor.get_labels()`` method
- output_mode: String indicating the output mode. Either ``regression`` or ``classification``
- pad_on_left: If set to ``True``, the examples will be padded on the left rather than on the right (default)
- pad_token: Padding token
- pad_token_segment_id: The segment ID for the padding token (It is usually 0, but can vary such as for XLNet where it is 4)
- mask_padding_with_zero: If set to ``True``, the attention mask will be filled by ``1`` for actual values
- and by ``0`` for padded values. If set to ``False``, inverts it (``1`` for padded values, ``0`` for
- actual values)
-
- Returns:
- If the ``examples`` input is a ``tf.data.Dataset``, will return a ``tf.data.Dataset``
- containing the task-specific features. If the input is a list of ``InputExamples``, will return
- a list of task-specific ``InputFeatures`` which can be fed to the model.
-
- """
- is_tf_dataset = False
- if is_tf_available() and isinstance(examples, tf.data.Dataset):
- is_tf_dataset = True
-
- if task is not None:
- processor = glue_processors[task]()
- if label_list is None:
- label_list = processor.get_labels()
- logger.info("Using label list %s for task %s" % (label_list, task))
- if output_mode is None:
- output_mode = glue_output_modes[task]
- logger.info("Using output mode %s for task %s" % (output_mode, task))
-
- label_map = {label: i for i, label in enumerate(label_list)}
-
- features = []
- for (ex_index, example) in enumerate(examples):
- if ex_index % 10000 == 0:
- logger.info("Writing example %d" % (ex_index))
- if is_tf_dataset:
- example = processor.get_example_from_tensor_dict(example)
- example = processor.tfds_map(example)
-
- inputs = tokenizer.encode_plus(example.text_a, example.text_b, add_special_tokens=True, max_length=max_length,)
- input_ids, token_type_ids = inputs["input_ids"], inputs["token_type_ids"]
-
- # The mask has 1 for real tokens and 0 for padding tokens. Only real
- # tokens are attended to.
- attention_mask = [1 if mask_padding_with_zero else 0] * len(input_ids)
-
- # Zero-pad up to the sequence length.
- padding_length = max_length - len(input_ids)
- if pad_on_left:
- input_ids = ([pad_token] * padding_length) + input_ids
- attention_mask = ([0 if mask_padding_with_zero else 1] * padding_length) + attention_mask
- token_type_ids = ([pad_token_segment_id] * padding_length) + token_type_ids
- else:
- input_ids = input_ids + ([pad_token] * padding_length)
- attention_mask = attention_mask + ([0 if mask_padding_with_zero else 1] * padding_length)
- token_type_ids = token_type_ids + ([pad_token_segment_id] * padding_length)
-
- assert len(input_ids) == max_length, "Error with input length {} vs {}".format(len(input_ids), max_length)
- assert len(attention_mask) == max_length, "Error with input length {} vs {}".format(
- len(attention_mask), max_length
- )
- assert len(token_type_ids) == max_length, "Error with input length {} vs {}".format(
- len(token_type_ids), max_length
- )
-
- if output_mode == "classification":
- label = label_map[example.label] if example.label in label_map else 0
- elif output_mode == "regression":
- label = float(example.label)
- else:
- raise KeyError(output_mode)
- pairID = str(example.pairID)
-
- if ex_index < 10:
- logger.info("*** Example ***")
- logger.info("text_a: %s" % (example.text_a))
- logger.info("text_b: %s" % (example.text_b))
- logger.info("guid: %s" % (example.guid))
- logger.info("input_ids: %s" % " ".join([str(x) for x in input_ids]))
- logger.info("attention_mask: %s" % " ".join([str(x) for x in attention_mask]))
- logger.info("token_type_ids: %s" % " ".join([str(x) for x in token_type_ids]))
- logger.info("label: %s (id = %d)" % (example.label, label))
-
- features.append(
- InputFeatures(
- input_ids=input_ids,
- attention_mask=attention_mask,
- token_type_ids=token_type_ids,
- label=label,
- pairID=pairID,
- )
- )
-
- if is_tf_available() and is_tf_dataset:
-
- def gen():
- for ex in features:
- yield (
- {
- "input_ids": ex.input_ids,
- "attention_mask": ex.attention_mask,
- "token_type_ids": ex.token_type_ids,
- },
- ex.label,
- )
-
- return tf.data.Dataset.from_generator(
- gen,
- ({"input_ids": tf.int32, "attention_mask": tf.int32, "token_type_ids": tf.int32}, tf.int64),
- (
- {
- "input_ids": tf.TensorShape([None]),
- "attention_mask": tf.TensorShape([None]),
- "token_type_ids": tf.TensorShape([None]),
- },
- tf.TensorShape([]),
- ),
- )
-
- return features
-
-
-class HansProcessor(DataProcessor):
- """Processor for the HANS data set."""
-
- def get_example_from_tensor_dict(self, tensor_dict):
- """See base class."""
- return InputExample(
- tensor_dict["idx"].numpy(),
- tensor_dict["premise"].numpy().decode("utf-8"),
- tensor_dict["hypothesis"].numpy().decode("utf-8"),
- str(tensor_dict["label"].numpy()),
- )
-
- def get_train_examples(self, data_dir):
- """See base class."""
- return self._create_examples(self._read_tsv(os.path.join(data_dir, "heuristics_train_set.txt")), "train")
-
- def get_dev_examples(self, data_dir):
- """See base class."""
- return self._create_examples(self._read_tsv(os.path.join(data_dir, "heuristics_evaluation_set.txt")), "dev")
-
- def get_labels(self):
- """See base class."""
- return ["contradiction", "entailment", "neutral"]
-
- def _create_examples(self, lines, set_type):
- """Creates examples for the training and dev sets."""
- examples = []
- for (i, line) in enumerate(lines):
- if i == 0:
- continue
- guid = "%s-%s" % (set_type, line[0])
- text_a = line[5]
- text_b = line[6]
- pairID = line[7][2:] if line[7].startswith("ex") else line[7]
- label = line[-1]
- examples.append(InputExample(guid=guid, text_a=text_a, text_b=text_b, label=label, pairID=pairID))
- return examples
-
-
-glue_tasks_num_labels = {
- "hans": 3,
-}
-
-glue_processors = {
- "hans": HansProcessor,
-}
-
-glue_output_modes = {
- "hans": "classification",
-}
diff --git a/server/transformers/examples/hans/test_hans.py b/server/transformers/examples/hans/test_hans.py
deleted file mode 100644
index 40c2a1bd3a1e015213bec1e0418ca9ac5d42ba3d..0000000000000000000000000000000000000000
--- a/server/transformers/examples/hans/test_hans.py
+++ /dev/null
@@ -1,643 +0,0 @@
-# coding=utf-8
-# Copyright 2018 The Google AI Language Team Authors and The HuggingFace Inc. team.
-# Copyright (c) 2018, NVIDIA CORPORATION. All rights reserved.
-#
-# Licensed under the Apache License, Version 2.0 (the "License");
-# you may not use this file except in compliance with the License.
-# You may obtain a copy of the License at
-#
-# http://www.apache.org/licenses/LICENSE-2.0
-#
-# Unless required by applicable law or agreed to in writing, software
-# distributed under the License is distributed on an "AS IS" BASIS,
-# WITHOUT WARRANTIES OR CONDITIONS OF ANY KIND, either express or implied.
-# See the License for the specific language governing permissions and
-# limitations under the License.
-""" Finetuning the library models for sequence classification on GLUE (Bert, XLM, XLNet, RoBERTa)."""
-
-from __future__ import absolute_import, division, print_function
-
-import argparse
-import glob
-import logging
-import os
-import random
-
-import numpy as np
-import torch
-from torch.utils.data import DataLoader, RandomSampler, SequentialSampler, TensorDataset
-from torch.utils.data.distributed import DistributedSampler
-from tqdm import tqdm, trange
-
-from hans_processors import glue_output_modes as output_modes
-from hans_processors import glue_processors as processors
-from hans_processors import hans_convert_examples_to_features as convert_examples_to_features
-from transformers import (
- WEIGHTS_NAME,
- AdamW,
- AlbertConfig,
- AlbertForSequenceClassification,
- AlbertTokenizer,
- BertConfig,
- BertForSequenceClassification,
- BertTokenizer,
- DistilBertConfig,
- DistilBertForSequenceClassification,
- DistilBertTokenizer,
- RobertaConfig,
- RobertaForSequenceClassification,
- RobertaTokenizer,
- XLMConfig,
- XLMForSequenceClassification,
- XLMTokenizer,
- XLNetConfig,
- XLNetForSequenceClassification,
- XLNetTokenizer,
- get_linear_schedule_with_warmup,
-)
-
-
-try:
- from torch.utils.tensorboard import SummaryWriter
-except ImportError:
- from tensorboardX import SummaryWriter
-
-
-logger = logging.getLogger(__name__)
-
-ALL_MODELS = sum(
- (
- tuple(conf.pretrained_config_archive_map.keys())
- for conf in (BertConfig, XLNetConfig, XLMConfig, RobertaConfig, DistilBertConfig)
- ),
- (),
-)
-
-MODEL_CLASSES = {
- "bert": (BertConfig, BertForSequenceClassification, BertTokenizer),
- "xlnet": (XLNetConfig, XLNetForSequenceClassification, XLNetTokenizer),
- "xlm": (XLMConfig, XLMForSequenceClassification, XLMTokenizer),
- "roberta": (RobertaConfig, RobertaForSequenceClassification, RobertaTokenizer),
- "distilbert": (DistilBertConfig, DistilBertForSequenceClassification, DistilBertTokenizer),
- "albert": (AlbertConfig, AlbertForSequenceClassification, AlbertTokenizer),
-}
-
-
-def set_seed(args):
- random.seed(args.seed)
- np.random.seed(args.seed)
- torch.manual_seed(args.seed)
- if args.n_gpu > 0:
- torch.cuda.manual_seed_all(args.seed)
-
-
-def train(args, train_dataset, model, tokenizer):
- """ Train the model """
- if args.local_rank in [-1, 0]:
- tb_writer = SummaryWriter()
-
- args.train_batch_size = args.per_gpu_train_batch_size * max(1, args.n_gpu)
- train_sampler = RandomSampler(train_dataset) if args.local_rank == -1 else DistributedSampler(train_dataset)
- train_dataloader = DataLoader(train_dataset, sampler=train_sampler, batch_size=args.train_batch_size)
-
- if args.max_steps > 0:
- t_total = args.max_steps
- args.num_train_epochs = args.max_steps // (len(train_dataloader) // args.gradient_accumulation_steps) + 1
- else:
- t_total = len(train_dataloader) // args.gradient_accumulation_steps * args.num_train_epochs
-
- # Prepare optimizer and schedule (linear warmup and decay)
- no_decay = ["bias", "LayerNorm.weight"]
- optimizer_grouped_parameters = [
- {
- "params": [p for n, p in model.named_parameters() if not any(nd in n for nd in no_decay)],
- "weight_decay": args.weight_decay,
- },
- {"params": [p for n, p in model.named_parameters() if any(nd in n for nd in no_decay)], "weight_decay": 0.0},
- ]
-
- optimizer = AdamW(optimizer_grouped_parameters, lr=args.learning_rate, eps=args.adam_epsilon)
- scheduler = get_linear_schedule_with_warmup(
- optimizer, num_warmup_steps=args.warmup_steps, num_training_steps=t_total
- )
- if args.fp16:
- try:
- from apex import amp
- except ImportError:
- raise ImportError("Please install apex from https://www.github.com/nvidia/apex to use fp16 training.")
- model, optimizer = amp.initialize(model, optimizer, opt_level=args.fp16_opt_level)
-
- # multi-gpu training (should be after apex fp16 initialization)
- if args.n_gpu > 1:
- model = torch.nn.DataParallel(model)
-
- # Distributed training (should be after apex fp16 initialization)
- if args.local_rank != -1:
- model = torch.nn.parallel.DistributedDataParallel(
- model, device_ids=[args.local_rank], output_device=args.local_rank, find_unused_parameters=True
- )
-
- # Train!
- logger.info("***** Running training *****")
- logger.info(" Num examples = %d", len(train_dataset))
- logger.info(" Num Epochs = %d", args.num_train_epochs)
- logger.info(" Instantaneous batch size per GPU = %d", args.per_gpu_train_batch_size)
- logger.info(
- " Total train batch size (w. parallel, distributed & accumulation) = %d",
- args.train_batch_size
- * args.gradient_accumulation_steps
- * (torch.distributed.get_world_size() if args.local_rank != -1 else 1),
- )
- logger.info(" Gradient Accumulation steps = %d", args.gradient_accumulation_steps)
- logger.info(" Total optimization steps = %d", t_total)
-
- global_step = 0
- tr_loss, logging_loss = 0.0, 0.0
- model.zero_grad()
- train_iterator = trange(int(args.num_train_epochs), desc="Epoch", disable=args.local_rank not in [-1, 0])
- set_seed(args) # Added here for reproductibility (even between python 2 and 3)
- for _ in train_iterator:
- epoch_iterator = tqdm(train_dataloader, desc="Iteration", disable=args.local_rank not in [-1, 0])
- for step, batch in enumerate(epoch_iterator):
- model.train()
- batch = tuple(t.to(args.device) for t in batch)
- inputs = {"input_ids": batch[0], "attention_mask": batch[1], "labels": batch[3]}
- if args.model_type != "distilbert":
- inputs["token_type_ids"] = (
- batch[2] if args.model_type in ["bert", "xlnet"] else None
- ) # XLM, DistilBERT and RoBERTa don't use segment_ids
- outputs = model(**inputs)
- loss = outputs[0] # model outputs are always tuple in transformers (see doc)
-
- if args.n_gpu > 1:
- loss = loss.mean() # mean() to average on multi-gpu parallel training
- if args.gradient_accumulation_steps > 1:
- loss = loss / args.gradient_accumulation_steps
-
- if args.fp16:
- with amp.scale_loss(loss, optimizer) as scaled_loss:
- scaled_loss.backward()
- else:
- loss.backward()
-
- tr_loss += loss.item()
- if (step + 1) % args.gradient_accumulation_steps == 0:
- if args.fp16:
- torch.nn.utils.clip_grad_norm_(amp.master_params(optimizer), args.max_grad_norm)
- else:
- torch.nn.utils.clip_grad_norm_(model.parameters(), args.max_grad_norm)
-
- optimizer.step()
- scheduler.step() # Update learning rate schedule
- model.zero_grad()
- global_step += 1
-
- if args.local_rank in [-1, 0] and args.logging_steps > 0 and global_step % args.logging_steps == 0:
- logs = {}
- if (
- args.local_rank == -1 and args.evaluate_during_training
- ): # Only evaluate when single GPU otherwise metrics may not average well
- results = evaluate(args, model, tokenizer)
- for key, value in results.items():
- eval_key = "eval_{}".format(key)
- logs[eval_key] = value
-
- loss_scalar = (tr_loss - logging_loss) / args.logging_steps
- learning_rate_scalar = scheduler.get_lr()[0]
- logs["learning_rate"] = learning_rate_scalar
- logs["loss"] = loss_scalar
- logging_loss = tr_loss
-
- for key, value in logs.items():
- tb_writer.add_scalar(key, value, global_step)
- # print(json.dumps({**logs, **{'step': global_step}}))
-
- if args.local_rank in [-1, 0] and args.save_steps > 0 and global_step % args.save_steps == 0:
- # Save model checkpoint
- output_dir = os.path.join(args.output_dir, "checkpoint-{}".format(global_step))
- if not os.path.exists(output_dir):
- os.makedirs(output_dir)
- model_to_save = (
- model.module if hasattr(model, "module") else model
- ) # Take care of distributed/parallel training
- model_to_save.save_pretrained(output_dir)
- torch.save(args, os.path.join(output_dir, "training_args.bin"))
- logger.info("Saving model checkpoint to %s", output_dir)
-
- if args.max_steps > 0 and global_step > args.max_steps:
- epoch_iterator.close()
- break
- if args.max_steps > 0 and global_step > args.max_steps:
- train_iterator.close()
- break
-
- if args.local_rank in [-1, 0]:
- tb_writer.close()
-
- return global_step, tr_loss / global_step
-
-
-def evaluate(args, model, tokenizer, prefix=""):
- # Loop to handle MNLI double evaluation (matched, mis-matched)
- eval_task_names = ("mnli", "mnli-mm") if args.task_name == "mnli" else (args.task_name,)
- eval_outputs_dirs = (args.output_dir, args.output_dir + "-MM") if args.task_name == "mnli" else (args.output_dir,)
-
- results = {}
- for eval_task, eval_output_dir in zip(eval_task_names, eval_outputs_dirs):
- eval_dataset, label_list = load_and_cache_examples(args, eval_task, tokenizer, evaluate=True)
-
- if not os.path.exists(eval_output_dir) and args.local_rank in [-1, 0]:
- os.makedirs(eval_output_dir)
-
- args.eval_batch_size = args.per_gpu_eval_batch_size * max(1, args.n_gpu)
- # Note that DistributedSampler samples randomly
- eval_sampler = SequentialSampler(eval_dataset)
- eval_dataloader = DataLoader(eval_dataset, sampler=eval_sampler, batch_size=args.eval_batch_size)
-
- # multi-gpu eval
- if args.n_gpu > 1:
- model = torch.nn.DataParallel(model)
-
- # Eval!
- logger.info("***** Running evaluation {} *****".format(prefix))
- logger.info(" Num examples = %d", len(eval_dataset))
- logger.info(" Batch size = %d", args.eval_batch_size)
- eval_loss = 0.0
- nb_eval_steps = 0
- preds = None
- out_label_ids = None
- for batch in tqdm(eval_dataloader, desc="Evaluating"):
- model.eval()
- batch = tuple(t.to(args.device) for t in batch)
-
- with torch.no_grad():
- inputs = {"input_ids": batch[0], "attention_mask": batch[1], "labels": batch[3]}
- if args.model_type != "distilbert":
- inputs["token_type_ids"] = (
- batch[2] if args.model_type in ["bert", "xlnet"] else None
- ) # XLM, DistilBERT and RoBERTa don't use segment_ids
- outputs = model(**inputs)
- tmp_eval_loss, logits = outputs[:2]
-
- eval_loss += tmp_eval_loss.mean().item()
- nb_eval_steps += 1
- if preds is None:
- preds = logits.detach().cpu().numpy()
- out_label_ids = inputs["labels"].detach().cpu().numpy()
- pair_ids = batch[4].detach().cpu().numpy()
- else:
- preds = np.append(preds, logits.detach().cpu().numpy(), axis=0)
- out_label_ids = np.append(out_label_ids, inputs["labels"].detach().cpu().numpy(), axis=0)
- pair_ids = np.append(pair_ids, batch[4].detach().cpu().numpy(), axis=0)
-
- eval_loss = eval_loss / nb_eval_steps
- if args.output_mode == "classification":
- preds = np.argmax(preds, axis=1)
- elif args.output_mode == "regression":
- preds = np.squeeze(preds)
-
- output_eval_file = os.path.join(eval_output_dir, "hans_predictions.txt")
- with open(output_eval_file, "w") as writer:
- writer.write("pairID,gld_label\n")
- for pid, pred in zip(pair_ids, preds):
- writer.write("ex" + str(pid) + "," + label_list[int(pred)] + "\n")
-
- return results
-
-
-def load_and_cache_examples(args, task, tokenizer, evaluate=False):
- if args.local_rank not in [-1, 0] and not evaluate:
- torch.distributed.barrier() # Make sure only the first process in distributed training process the dataset, and the others will use the cache
-
- processor = processors[task]()
- output_mode = output_modes[task]
- # Load data features from cache or dataset file
- cached_features_file = os.path.join(
- args.data_dir,
- "cached_{}_{}_{}_{}".format(
- "dev" if evaluate else "train",
- list(filter(None, args.model_name_or_path.split("/"))).pop(),
- str(args.max_seq_length),
- str(task),
- ),
- )
-
- label_list = processor.get_labels()
-
- if os.path.exists(cached_features_file) and not args.overwrite_cache:
- logger.info("Loading features from cached file %s", cached_features_file)
- features = torch.load(cached_features_file)
- else:
- logger.info("Creating features from dataset file at %s", args.data_dir)
- if task in ["mnli", "mnli-mm"] and args.model_type in ["roberta"]:
- # HACK(label indices are swapped in RoBERTa pretrained model)
- label_list[1], label_list[2] = label_list[2], label_list[1]
- examples = (
- processor.get_dev_examples(args.data_dir) if evaluate else processor.get_train_examples(args.data_dir)
- )
- features = convert_examples_to_features(
- examples,
- tokenizer,
- label_list=label_list,
- max_length=args.max_seq_length,
- output_mode=output_mode,
- pad_on_left=bool(args.model_type in ["xlnet"]), # pad on the left for xlnet
- pad_token=tokenizer.convert_tokens_to_ids([tokenizer.pad_token])[0],
- pad_token_segment_id=4 if args.model_type in ["xlnet"] else 0,
- )
- if args.local_rank in [-1, 0]:
- logger.info("Saving features into cached file %s", cached_features_file)
- torch.save(features, cached_features_file)
-
- if args.local_rank == 0 and not evaluate:
- torch.distributed.barrier() # Make sure only the first process in distributed training process the dataset, and the others will use the cache
-
- # Convert to Tensors and build dataset
- all_input_ids = torch.tensor([f.input_ids for f in features], dtype=torch.long)
- all_attention_mask = torch.tensor([f.attention_mask for f in features], dtype=torch.long)
- all_token_type_ids = torch.tensor([f.token_type_ids for f in features], dtype=torch.long)
- if output_mode == "classification":
- all_labels = torch.tensor([f.label for f in features], dtype=torch.long)
- elif output_mode == "regression":
- all_labels = torch.tensor([f.label for f in features], dtype=torch.float)
- all_pair_ids = torch.tensor([int(f.pairID) for f in features], dtype=torch.long)
-
- dataset = TensorDataset(all_input_ids, all_attention_mask, all_token_type_ids, all_labels, all_pair_ids)
- return dataset, label_list
-
-
-def main():
- parser = argparse.ArgumentParser()
-
- # Required parameters
- parser.add_argument(
- "--data_dir",
- default=None,
- type=str,
- required=True,
- help="The input data dir. Should contain the .tsv files (or other data files) for the task.",
- )
- parser.add_argument(
- "--model_type",
- default=None,
- type=str,
- required=True,
- help="Model type selected in the list: " + ", ".join(MODEL_CLASSES.keys()),
- )
- parser.add_argument(
- "--model_name_or_path",
- default=None,
- type=str,
- required=True,
- help="Path to pre-trained model or shortcut name selected in the list: " + ", ".join(ALL_MODELS),
- )
- parser.add_argument(
- "--task_name",
- default=None,
- type=str,
- required=True,
- help="The name of the task to train selected in the list: " + ", ".join(processors.keys()),
- )
- parser.add_argument(
- "--output_dir",
- default=None,
- type=str,
- required=True,
- help="The output directory where the model predictions and checkpoints will be written.",
- )
-
- # Other parameters
- parser.add_argument(
- "--config_name", default="", type=str, help="Pretrained config name or path if not the same as model_name"
- )
- parser.add_argument(
- "--tokenizer_name",
- default="",
- type=str,
- help="Pretrained tokenizer name or path if not the same as model_name",
- )
- parser.add_argument(
- "--cache_dir",
- default="",
- type=str,
- help="Where do you want to store the pre-trained models downloaded from s3",
- )
- parser.add_argument(
- "--max_seq_length",
- default=128,
- type=int,
- help="The maximum total input sequence length after tokenization. Sequences longer "
- "than this will be truncated, sequences shorter will be padded.",
- )
- parser.add_argument("--do_train", action="store_true", help="Whether to run training.")
- parser.add_argument("--do_eval", action="store_true", help="Whether to run eval on the dev set.")
- parser.add_argument(
- "--evaluate_during_training", action="store_true", help="Rul evaluation during training at each logging step."
- )
- parser.add_argument(
- "--do_lower_case", action="store_true", help="Set this flag if you are using an uncased model."
- )
-
- parser.add_argument("--per_gpu_train_batch_size", default=8, type=int, help="Batch size per GPU/CPU for training.")
- parser.add_argument(
- "--per_gpu_eval_batch_size", default=8, type=int, help="Batch size per GPU/CPU for evaluation."
- )
- parser.add_argument(
- "--gradient_accumulation_steps",
- type=int,
- default=1,
- help="Number of updates steps to accumulate before performing a backward/update pass.",
- )
- parser.add_argument("--learning_rate", default=5e-5, type=float, help="The initial learning rate for Adam.")
- parser.add_argument("--weight_decay", default=0.0, type=float, help="Weight decay if we apply some.")
- parser.add_argument("--adam_epsilon", default=1e-8, type=float, help="Epsilon for Adam optimizer.")
- parser.add_argument("--max_grad_norm", default=1.0, type=float, help="Max gradient norm.")
- parser.add_argument(
- "--num_train_epochs", default=3.0, type=float, help="Total number of training epochs to perform."
- )
- parser.add_argument(
- "--max_steps",
- default=-1,
- type=int,
- help="If > 0: set total number of training steps to perform. Override num_train_epochs.",
- )
- parser.add_argument("--warmup_steps", default=0, type=int, help="Linear warmup over warmup_steps.")
-
- parser.add_argument("--logging_steps", type=int, default=50, help="Log every X updates steps.")
- parser.add_argument("--save_steps", type=int, default=50, help="Save checkpoint every X updates steps.")
- parser.add_argument(
- "--eval_all_checkpoints",
- action="store_true",
- help="Evaluate all checkpoints starting with the same prefix as model_name ending and ending with step number",
- )
- parser.add_argument("--no_cuda", action="store_true", help="Avoid using CUDA when available")
- parser.add_argument(
- "--overwrite_output_dir", action="store_true", help="Overwrite the content of the output directory"
- )
- parser.add_argument(
- "--overwrite_cache", action="store_true", help="Overwrite the cached training and evaluation sets"
- )
- parser.add_argument("--seed", type=int, default=42, help="random seed for initialization")
-
- parser.add_argument(
- "--fp16",
- action="store_true",
- help="Whether to use 16-bit (mixed) precision (through NVIDIA apex) instead of 32-bit",
- )
- parser.add_argument(
- "--fp16_opt_level",
- type=str,
- default="O1",
- help="For fp16: Apex AMP optimization level selected in ['O0', 'O1', 'O2', and 'O3']."
- "See details at https://nvidia.github.io/apex/amp.html",
- )
- parser.add_argument("--local_rank", type=int, default=-1, help="For distributed training: local_rank")
- parser.add_argument("--server_ip", type=str, default="", help="For distant debugging.")
- parser.add_argument("--server_port", type=str, default="", help="For distant debugging.")
- args = parser.parse_args()
-
- if (
- os.path.exists(args.output_dir)
- and os.listdir(args.output_dir)
- and args.do_train
- and not args.overwrite_output_dir
- ):
- raise ValueError(
- "Output directory ({}) already exists and is not empty. Use --overwrite_output_dir to overcome.".format(
- args.output_dir
- )
- )
-
- # Setup distant debugging if needed
- if args.server_ip and args.server_port:
- # Distant debugging - see https://code.visualstudio.com/docs/python/debugging#_attach-to-a-local-script
- import ptvsd
-
- print("Waiting for debugger attach")
- ptvsd.enable_attach(address=(args.server_ip, args.server_port), redirect_output=True)
- ptvsd.wait_for_attach()
-
- # Setup CUDA, GPU & distributed training
- if args.local_rank == -1 or args.no_cuda:
- device = torch.device("cuda" if torch.cuda.is_available() and not args.no_cuda else "cpu")
- args.n_gpu = torch.cuda.device_count()
- else: # Initializes the distributed backend which will take care of sychronizing nodes/GPUs
- torch.cuda.set_device(args.local_rank)
- device = torch.device("cuda", args.local_rank)
- torch.distributed.init_process_group(backend="nccl")
- args.n_gpu = 1
- args.device = device
-
- # Setup logging
- logging.basicConfig(
- format="%(asctime)s - %(levelname)s - %(name)s - %(message)s",
- datefmt="%m/%d/%Y %H:%M:%S",
- level=logging.INFO if args.local_rank in [-1, 0] else logging.WARN,
- )
- logger.warning(
- "Process rank: %s, device: %s, n_gpu: %s, distributed training: %s, 16-bits training: %s",
- args.local_rank,
- device,
- args.n_gpu,
- bool(args.local_rank != -1),
- args.fp16,
- )
-
- # Set seed
- set_seed(args)
-
- # Prepare GLUE task
- args.task_name = args.task_name.lower()
- if args.task_name not in processors:
- raise ValueError("Task not found: %s" % (args.task_name))
- processor = processors[args.task_name]()
- args.output_mode = output_modes[args.task_name]
- label_list = processor.get_labels()
- num_labels = len(label_list)
-
- # Load pretrained model and tokenizer
- if args.local_rank not in [-1, 0]:
- torch.distributed.barrier() # Make sure only the first process in distributed training will download model & vocab
-
- args.model_type = args.model_type.lower()
- config_class, model_class, tokenizer_class = MODEL_CLASSES[args.model_type]
- config = config_class.from_pretrained(
- args.config_name if args.config_name else args.model_name_or_path,
- num_labels=num_labels,
- finetuning_task=args.task_name,
- cache_dir=args.cache_dir if args.cache_dir else None,
- )
- tokenizer = tokenizer_class.from_pretrained(
- args.tokenizer_name if args.tokenizer_name else args.model_name_or_path,
- do_lower_case=args.do_lower_case,
- cache_dir=args.cache_dir if args.cache_dir else None,
- )
- model = model_class.from_pretrained(
- args.model_name_or_path,
- from_tf=bool(".ckpt" in args.model_name_or_path),
- config=config,
- cache_dir=args.cache_dir if args.cache_dir else None,
- )
-
- if args.local_rank == 0:
- torch.distributed.barrier() # Make sure only the first process in distributed training will download model & vocab
-
- model.to(args.device)
-
- logger.info("Training/evaluation parameters %s", args)
-
- # Training
- if args.do_train:
- train_dataset, _ = load_and_cache_examples(args, args.task_name, tokenizer, evaluate=False)
- global_step, tr_loss = train(args, train_dataset, model, tokenizer)
- logger.info(" global_step = %s, average loss = %s", global_step, tr_loss)
-
- # Saving best-practices: if you use defaults names for the model, you can reload it using from_pretrained()
- if args.do_train and (args.local_rank == -1 or torch.distributed.get_rank() == 0):
- # Create output directory if needed
- if not os.path.exists(args.output_dir) and args.local_rank in [-1, 0]:
- os.makedirs(args.output_dir)
-
- logger.info("Saving model checkpoint to %s", args.output_dir)
- # Save a trained model, configuration and tokenizer using `save_pretrained()`.
- # They can then be reloaded using `from_pretrained()`
- model_to_save = (
- model.module if hasattr(model, "module") else model
- ) # Take care of distributed/parallel training
- model_to_save.save_pretrained(args.output_dir)
- tokenizer.save_pretrained(args.output_dir)
-
- # Good practice: save your training arguments together with the trained model
- torch.save(args, os.path.join(args.output_dir, "training_args.bin"))
-
- # Load a trained model and vocabulary that you have fine-tuned
- model = model_class.from_pretrained(args.output_dir)
- tokenizer = tokenizer_class.from_pretrained(args.output_dir)
- model.to(args.device)
-
- # Evaluation
- results = {}
- if args.do_eval and args.local_rank in [-1, 0]:
- tokenizer = tokenizer_class.from_pretrained(args.output_dir, do_lower_case=args.do_lower_case)
- checkpoints = [args.output_dir]
- if args.eval_all_checkpoints:
- checkpoints = list(
- os.path.dirname(c) for c in sorted(glob.glob(args.output_dir + "/**/" + WEIGHTS_NAME, recursive=True))
- )
- logging.getLogger("transformers.modeling_utils").setLevel(logging.WARN) # Reduce logging
- logger.info("Evaluate the following checkpoints: %s", checkpoints)
- for checkpoint in checkpoints:
- global_step = checkpoint.split("-")[-1] if len(checkpoints) > 1 else ""
- prefix = checkpoint.split("/")[-1] if checkpoint.find("checkpoint") != -1 else ""
-
- model = model_class.from_pretrained(checkpoint)
- model.to(args.device)
- result = evaluate(args, model, tokenizer, prefix=prefix)
- result = dict((k + "_{}".format(global_step), v) for k, v in result.items())
- results.update(result)
-
- return results
-
-
-if __name__ == "__main__":
- main()
diff --git a/server/transformers/examples/hans/utils_hans.py b/server/transformers/examples/hans/utils_hans.py
deleted file mode 100644
index 8d0b42165caff48c66e85799c235a2d94647366e..0000000000000000000000000000000000000000
--- a/server/transformers/examples/hans/utils_hans.py
+++ /dev/null
@@ -1,121 +0,0 @@
-# coding=utf-8
-# Copyright 2018 The Google AI Language Team Authors and The HuggingFace Inc. team.
-# Copyright (c) 2018, NVIDIA CORPORATION. All rights reserved.
-#
-# Licensed under the Apache License, Version 2.0 (the "License");
-# you may not use this file except in compliance with the License.
-# You may obtain a copy of the License at
-#
-# http://www.apache.org/licenses/LICENSE-2.0
-#
-# Unless required by applicable law or agreed to in writing, software
-# distributed under the License is distributed on an "AS IS" BASIS,
-# WITHOUT WARRANTIES OR CONDITIONS OF ANY KIND, either express or implied.
-# See the License for the specific language governing permissions and
-# limitations under the License.
-
-import copy
-import csv
-import json
-
-
-class InputExample(object):
- """
- A single training/test example for simple sequence classification.
-
- Args:
- guid: Unique id for the example.
- text_a: string. The untokenized text of the first sequence. For single
- sequence tasks, only this sequence must be specified.
- text_b: (Optional) string. The untokenized text of the second sequence.
- Only must be specified for sequence pair tasks.
- label: (Optional) string. The label of the example. This should be
- specified for train and dev examples, but not for test examples.
- """
-
- def __init__(self, guid, text_a, text_b=None, label=None, pairID=None):
- self.guid = guid
- self.text_a = text_a
- self.text_b = text_b
- self.label = label
- self.pairID = pairID
-
- def __repr__(self):
- return str(self.to_json_string())
-
- def to_dict(self):
- """Serializes this instance to a Python dictionary."""
- output = copy.deepcopy(self.__dict__)
- return output
-
- def to_json_string(self):
- """Serializes this instance to a JSON string."""
- return json.dumps(self.to_dict(), indent=2, sort_keys=True) + "\n"
-
-
-class InputFeatures(object):
- """
- A single set of features of data.
-
- Args:
- input_ids: Indices of input sequence tokens in the vocabulary.
- attention_mask: Mask to avoid performing attention on padding token indices.
- Mask values selected in ``[0, 1]``:
- Usually ``1`` for tokens that are NOT MASKED, ``0`` for MASKED (padded) tokens.
- token_type_ids: Segment token indices to indicate first and second portions of the inputs.
- label: Label corresponding to the input
- """
-
- def __init__(self, input_ids, attention_mask, token_type_ids, label, pairID=None):
- self.input_ids = input_ids
- self.attention_mask = attention_mask
- self.token_type_ids = token_type_ids
- self.label = label
- self.pairID = pairID
-
- def __repr__(self):
- return str(self.to_json_string())
-
- def to_dict(self):
- """Serializes this instance to a Python dictionary."""
- output = copy.deepcopy(self.__dict__)
- return output
-
- def to_json_string(self):
- """Serializes this instance to a JSON string."""
- return json.dumps(self.to_dict(), indent=2, sort_keys=True) + "\n"
-
-
-class DataProcessor(object):
- """Base class for data converters for sequence classification data sets."""
-
- def get_example_from_tensor_dict(self, tensor_dict):
- """Gets an example from a dict with tensorflow tensors
-
- Args:
- tensor_dict: Keys and values should match the corresponding Glue
- tensorflow_dataset examples.
- """
- raise NotImplementedError()
-
- def get_train_examples(self, data_dir):
- """Gets a collection of `InputExample`s for the train set."""
- raise NotImplementedError()
-
- def get_dev_examples(self, data_dir):
- """Gets a collection of `InputExample`s for the dev set."""
- raise NotImplementedError()
-
- def get_labels(self):
- """Gets the list of labels for this data set."""
- raise NotImplementedError()
-
- @classmethod
- def _read_tsv(cls, input_file, quotechar=None):
- """Reads a tab separated value file."""
- with open(input_file, "r", encoding="utf-8-sig") as f:
- reader = csv.reader(f, delimiter="\t", quotechar=quotechar)
- lines = []
- for line in reader:
- lines.append(line)
- return lines
diff --git a/server/transformers/examples/mm-imdb/run_mmimdb.py b/server/transformers/examples/mm-imdb/run_mmimdb.py
deleted file mode 100644
index c7e9f7b47e0226cff61d0a01de7d4a2365021f70..0000000000000000000000000000000000000000
--- a/server/transformers/examples/mm-imdb/run_mmimdb.py
+++ /dev/null
@@ -1,614 +0,0 @@
-# coding=utf-8
-# Copyright (c) Facebook, Inc. and its affiliates.
-# Copyright (c) HuggingFace Inc. team.
-#
-# Licensed under the Apache License, Version 2.0 (the "License");
-# you may not use this file except in compliance with the License.
-# You may obtain a copy of the License at
-#
-# http://www.apache.org/licenses/LICENSE-2.0
-#
-# Unless required by applicable law or agreed to in writing, software
-# distributed under the License is distributed on an "AS IS" BASIS,
-# WITHOUT WARRANTIES OR CONDITIONS OF ANY KIND, either express or implied.
-# See the License for the specific language governing permissions and
-# limitations under the License.
-""" Finetuning the library models for multimodal multiclass prediction on MM-IMDB dataset."""
-
-
-import argparse
-import glob
-import json
-import logging
-import os
-import random
-
-import numpy as np
-import torch
-import torch.nn as nn
-from sklearn.metrics import f1_score
-from torch.utils.data import DataLoader, RandomSampler, SequentialSampler
-from torch.utils.data.distributed import DistributedSampler
-from tqdm import tqdm, trange
-
-from transformers import (
- WEIGHTS_NAME,
- AdamW,
- AlbertConfig,
- AlbertModel,
- AlbertTokenizer,
- BertConfig,
- BertModel,
- BertTokenizer,
- DistilBertConfig,
- DistilBertModel,
- DistilBertTokenizer,
- MMBTConfig,
- MMBTForClassification,
- RobertaConfig,
- RobertaModel,
- RobertaTokenizer,
- XLMConfig,
- XLMModel,
- XLMTokenizer,
- XLNetConfig,
- XLNetModel,
- XLNetTokenizer,
- get_linear_schedule_with_warmup,
-)
-from utils_mmimdb import ImageEncoder, JsonlDataset, collate_fn, get_image_transforms, get_mmimdb_labels
-
-
-try:
- from torch.utils.tensorboard import SummaryWriter
-except ImportError:
- from tensorboardX import SummaryWriter
-
-
-logger = logging.getLogger(__name__)
-
-ALL_MODELS = sum(
- (
- tuple(conf.pretrained_config_archive_map.keys())
- for conf in (BertConfig, XLNetConfig, XLMConfig, RobertaConfig, DistilBertConfig)
- ),
- (),
-)
-
-MODEL_CLASSES = {
- "bert": (BertConfig, BertModel, BertTokenizer),
- "xlnet": (XLNetConfig, XLNetModel, XLNetTokenizer),
- "xlm": (XLMConfig, XLMModel, XLMTokenizer),
- "roberta": (RobertaConfig, RobertaModel, RobertaTokenizer),
- "distilbert": (DistilBertConfig, DistilBertModel, DistilBertTokenizer),
- "albert": (AlbertConfig, AlbertModel, AlbertTokenizer),
-}
-
-
-def set_seed(args):
- random.seed(args.seed)
- np.random.seed(args.seed)
- torch.manual_seed(args.seed)
- if args.n_gpu > 0:
- torch.cuda.manual_seed_all(args.seed)
-
-
-def train(args, train_dataset, model, tokenizer, criterion):
- """ Train the model """
- if args.local_rank in [-1, 0]:
- tb_writer = SummaryWriter()
-
- args.train_batch_size = args.per_gpu_train_batch_size * max(1, args.n_gpu)
- train_sampler = RandomSampler(train_dataset) if args.local_rank == -1 else DistributedSampler(train_dataset)
- train_dataloader = DataLoader(
- train_dataset,
- sampler=train_sampler,
- batch_size=args.train_batch_size,
- collate_fn=collate_fn,
- num_workers=args.num_workers,
- )
-
- if args.max_steps > 0:
- t_total = args.max_steps
- args.num_train_epochs = args.max_steps // (len(train_dataloader) // args.gradient_accumulation_steps) + 1
- else:
- t_total = len(train_dataloader) // args.gradient_accumulation_steps * args.num_train_epochs
-
- # Prepare optimizer and schedule (linear warmup and decay)
- no_decay = ["bias", "LayerNorm.weight"]
- optimizer_grouped_parameters = [
- {
- "params": [p for n, p in model.named_parameters() if not any(nd in n for nd in no_decay)],
- "weight_decay": args.weight_decay,
- },
- {"params": [p for n, p in model.named_parameters() if any(nd in n for nd in no_decay)], "weight_decay": 0.0},
- ]
-
- optimizer = AdamW(optimizer_grouped_parameters, lr=args.learning_rate, eps=args.adam_epsilon)
- scheduler = get_linear_schedule_with_warmup(
- optimizer, num_warmup_steps=args.warmup_steps, num_training_steps=t_total
- )
- if args.fp16:
- try:
- from apex import amp
- except ImportError:
- raise ImportError("Please install apex from https://www.github.com/nvidia/apex to use fp16 training.")
- model, optimizer = amp.initialize(model, optimizer, opt_level=args.fp16_opt_level)
-
- # multi-gpu training (should be after apex fp16 initialization)
- if args.n_gpu > 1:
- model = torch.nn.DataParallel(model)
-
- # Distributed training (should be after apex fp16 initialization)
- if args.local_rank != -1:
- model = torch.nn.parallel.DistributedDataParallel(
- model, device_ids=[args.local_rank], output_device=args.local_rank, find_unused_parameters=True
- )
-
- # Train!
- logger.info("***** Running training *****")
- logger.info(" Num examples = %d", len(train_dataset))
- logger.info(" Num Epochs = %d", args.num_train_epochs)
- logger.info(" Instantaneous batch size per GPU = %d", args.per_gpu_train_batch_size)
- logger.info(
- " Total train batch size (w. parallel, distributed & accumulation) = %d",
- args.train_batch_size
- * args.gradient_accumulation_steps
- * (torch.distributed.get_world_size() if args.local_rank != -1 else 1),
- )
- logger.info(" Gradient Accumulation steps = %d", args.gradient_accumulation_steps)
- logger.info(" Total optimization steps = %d", t_total)
-
- global_step = 0
- tr_loss, logging_loss = 0.0, 0.0
- best_f1, n_no_improve = 0, 0
- model.zero_grad()
- train_iterator = trange(int(args.num_train_epochs), desc="Epoch", disable=args.local_rank not in [-1, 0])
- set_seed(args) # Added here for reproductibility
- for _ in train_iterator:
- epoch_iterator = tqdm(train_dataloader, desc="Iteration", disable=args.local_rank not in [-1, 0])
- for step, batch in enumerate(epoch_iterator):
- model.train()
- batch = tuple(t.to(args.device) for t in batch)
- labels = batch[5]
- inputs = {
- "input_ids": batch[0],
- "input_modal": batch[2],
- "attention_mask": batch[1],
- "modal_start_tokens": batch[3],
- "modal_end_tokens": batch[4],
- }
- outputs = model(**inputs)
- logits = outputs[0] # model outputs are always tuple in transformers (see doc)
- loss = criterion(logits, labels)
-
- if args.n_gpu > 1:
- loss = loss.mean() # mean() to average on multi-gpu parallel training
- if args.gradient_accumulation_steps > 1:
- loss = loss / args.gradient_accumulation_steps
-
- if args.fp16:
- with amp.scale_loss(loss, optimizer) as scaled_loss:
- scaled_loss.backward()
- else:
- loss.backward()
-
- tr_loss += loss.item()
- if (step + 1) % args.gradient_accumulation_steps == 0:
- if args.fp16:
- torch.nn.utils.clip_grad_norm_(amp.master_params(optimizer), args.max_grad_norm)
- else:
- torch.nn.utils.clip_grad_norm_(model.parameters(), args.max_grad_norm)
-
- optimizer.step()
- scheduler.step() # Update learning rate schedule
- model.zero_grad()
- global_step += 1
-
- if args.local_rank in [-1, 0] and args.logging_steps > 0 and global_step % args.logging_steps == 0:
- logs = {}
- if (
- args.local_rank == -1 and args.evaluate_during_training
- ): # Only evaluate when single GPU otherwise metrics may not average well
- results = evaluate(args, model, tokenizer, criterion)
- for key, value in results.items():
- eval_key = "eval_{}".format(key)
- logs[eval_key] = value
-
- loss_scalar = (tr_loss - logging_loss) / args.logging_steps
- learning_rate_scalar = scheduler.get_lr()[0]
- logs["learning_rate"] = learning_rate_scalar
- logs["loss"] = loss_scalar
- logging_loss = tr_loss
-
- for key, value in logs.items():
- tb_writer.add_scalar(key, value, global_step)
- print(json.dumps({**logs, **{"step": global_step}}))
-
- if args.local_rank in [-1, 0] and args.save_steps > 0 and global_step % args.save_steps == 0:
- # Save model checkpoint
- output_dir = os.path.join(args.output_dir, "checkpoint-{}".format(global_step))
- if not os.path.exists(output_dir):
- os.makedirs(output_dir)
- model_to_save = (
- model.module if hasattr(model, "module") else model
- ) # Take care of distributed/parallel training
- torch.save(model_to_save.state_dict(), os.path.join(output_dir, WEIGHTS_NAME))
- torch.save(args, os.path.join(output_dir, "training_args.bin"))
- logger.info("Saving model checkpoint to %s", output_dir)
-
- if args.max_steps > 0 and global_step > args.max_steps:
- epoch_iterator.close()
- break
- if args.max_steps > 0 and global_step > args.max_steps:
- train_iterator.close()
- break
-
- if args.local_rank == -1:
- results = evaluate(args, model, tokenizer, criterion)
- if results["micro_f1"] > best_f1:
- best_f1 = results["micro_f1"]
- n_no_improve = 0
- else:
- n_no_improve += 1
-
- if n_no_improve > args.patience:
- train_iterator.close()
- break
-
- if args.local_rank in [-1, 0]:
- tb_writer.close()
-
- return global_step, tr_loss / global_step
-
-
-def evaluate(args, model, tokenizer, criterion, prefix=""):
- # Loop to handle MNLI double evaluation (matched, mis-matched)
- eval_output_dir = args.output_dir
- eval_dataset = load_examples(args, tokenizer, evaluate=True)
-
- if not os.path.exists(eval_output_dir) and args.local_rank in [-1, 0]:
- os.makedirs(eval_output_dir)
-
- args.eval_batch_size = args.per_gpu_eval_batch_size * max(1, args.n_gpu)
- # Note that DistributedSampler samples randomly
- eval_sampler = SequentialSampler(eval_dataset)
- eval_dataloader = DataLoader(
- eval_dataset, sampler=eval_sampler, batch_size=args.eval_batch_size, collate_fn=collate_fn
- )
-
- # multi-gpu eval
- if args.n_gpu > 1:
- model = torch.nn.DataParallel(model)
-
- # Eval!
- logger.info("***** Running evaluation {} *****".format(prefix))
- logger.info(" Num examples = %d", len(eval_dataset))
- logger.info(" Batch size = %d", args.eval_batch_size)
- eval_loss = 0.0
- nb_eval_steps = 0
- preds = None
- out_label_ids = None
- for batch in tqdm(eval_dataloader, desc="Evaluating"):
- model.eval()
- batch = tuple(t.to(args.device) for t in batch)
-
- with torch.no_grad():
- batch = tuple(t.to(args.device) for t in batch)
- labels = batch[5]
- inputs = {
- "input_ids": batch[0],
- "input_modal": batch[2],
- "attention_mask": batch[1],
- "modal_start_tokens": batch[3],
- "modal_end_tokens": batch[4],
- }
- outputs = model(**inputs)
- logits = outputs[0] # model outputs are always tuple in transformers (see doc)
- tmp_eval_loss = criterion(logits, labels)
- eval_loss += tmp_eval_loss.mean().item()
- nb_eval_steps += 1
- if preds is None:
- preds = torch.sigmoid(logits).detach().cpu().numpy() > 0.5
- out_label_ids = labels.detach().cpu().numpy()
- else:
- preds = np.append(preds, torch.sigmoid(logits).detach().cpu().numpy() > 0.5, axis=0)
- out_label_ids = np.append(out_label_ids, labels.detach().cpu().numpy(), axis=0)
-
- eval_loss = eval_loss / nb_eval_steps
- result = {
- "loss": eval_loss,
- "macro_f1": f1_score(out_label_ids, preds, average="macro"),
- "micro_f1": f1_score(out_label_ids, preds, average="micro"),
- }
-
- output_eval_file = os.path.join(eval_output_dir, prefix, "eval_results.txt")
- with open(output_eval_file, "w") as writer:
- logger.info("***** Eval results {} *****".format(prefix))
- for key in sorted(result.keys()):
- logger.info(" %s = %s", key, str(result[key]))
- writer.write("%s = %s\n" % (key, str(result[key])))
-
- return result
-
-
-def load_examples(args, tokenizer, evaluate=False):
- path = os.path.join(args.data_dir, "dev.jsonl" if evaluate else "train.jsonl")
- transforms = get_image_transforms()
- labels = get_mmimdb_labels()
- dataset = JsonlDataset(path, tokenizer, transforms, labels, args.max_seq_length - args.num_image_embeds - 2)
- return dataset
-
-
-def main():
- parser = argparse.ArgumentParser()
-
- # Required parameters
- parser.add_argument(
- "--data_dir",
- default=None,
- type=str,
- required=True,
- help="The input data dir. Should contain the .jsonl files for MMIMDB.",
- )
- parser.add_argument(
- "--model_type",
- default=None,
- type=str,
- required=True,
- help="Model type selected in the list: " + ", ".join(MODEL_CLASSES.keys()),
- )
- parser.add_argument(
- "--model_name_or_path",
- default=None,
- type=str,
- required=True,
- help="Path to pre-trained model or shortcut name selected in the list: " + ", ".join(ALL_MODELS),
- )
- parser.add_argument(
- "--output_dir",
- default=None,
- type=str,
- required=True,
- help="The output directory where the model predictions and checkpoints will be written.",
- )
-
- # Other parameters
- parser.add_argument(
- "--config_name", default="", type=str, help="Pretrained config name or path if not the same as model_name"
- )
- parser.add_argument(
- "--tokenizer_name",
- default="",
- type=str,
- help="Pretrained tokenizer name or path if not the same as model_name",
- )
- parser.add_argument(
- "--cache_dir",
- default="",
- type=str,
- help="Where do you want to store the pre-trained models downloaded from s3",
- )
- parser.add_argument(
- "--max_seq_length",
- default=128,
- type=int,
- help="The maximum total input sequence length after tokenization. Sequences longer "
- "than this will be truncated, sequences shorter will be padded.",
- )
- parser.add_argument(
- "--num_image_embeds", default=1, type=int, help="Number of Image Embeddings from the Image Encoder"
- )
- parser.add_argument("--do_train", action="store_true", help="Whether to run training.")
- parser.add_argument("--do_eval", action="store_true", help="Whether to run eval on the dev set.")
- parser.add_argument(
- "--evaluate_during_training", action="store_true", help="Rul evaluation during training at each logging step."
- )
- parser.add_argument(
- "--do_lower_case", action="store_true", help="Set this flag if you are using an uncased model."
- )
-
- parser.add_argument("--per_gpu_train_batch_size", default=8, type=int, help="Batch size per GPU/CPU for training.")
- parser.add_argument(
- "--per_gpu_eval_batch_size", default=8, type=int, help="Batch size per GPU/CPU for evaluation."
- )
- parser.add_argument(
- "--gradient_accumulation_steps",
- type=int,
- default=1,
- help="Number of updates steps to accumulate before performing a backward/update pass.",
- )
- parser.add_argument("--learning_rate", default=5e-5, type=float, help="The initial learning rate for Adam.")
- parser.add_argument("--weight_decay", default=0.0, type=float, help="Weight deay if we apply some.")
- parser.add_argument("--adam_epsilon", default=1e-8, type=float, help="Epsilon for Adam optimizer.")
- parser.add_argument("--max_grad_norm", default=1.0, type=float, help="Max gradient norm.")
- parser.add_argument(
- "--num_train_epochs", default=3.0, type=float, help="Total number of training epochs to perform."
- )
- parser.add_argument("--patience", default=5, type=int, help="Patience for Early Stopping.")
- parser.add_argument(
- "--max_steps",
- default=-1,
- type=int,
- help="If > 0: set total number of training steps to perform. Override num_train_epochs.",
- )
- parser.add_argument("--warmup_steps", default=0, type=int, help="Linear warmup over warmup_steps.")
-
- parser.add_argument("--logging_steps", type=int, default=50, help="Log every X updates steps.")
- parser.add_argument("--save_steps", type=int, default=50, help="Save checkpoint every X updates steps.")
- parser.add_argument(
- "--eval_all_checkpoints",
- action="store_true",
- help="Evaluate all checkpoints starting with the same prefix as model_name ending and ending with step number",
- )
- parser.add_argument("--no_cuda", action="store_true", help="Avoid using CUDA when available")
- parser.add_argument("--num_workers", type=int, default=8, help="number of worker threads for dataloading")
- parser.add_argument(
- "--overwrite_output_dir", action="store_true", help="Overwrite the content of the output directory"
- )
- parser.add_argument(
- "--overwrite_cache", action="store_true", help="Overwrite the cached training and evaluation sets"
- )
- parser.add_argument("--seed", type=int, default=42, help="random seed for initialization")
-
- parser.add_argument(
- "--fp16",
- action="store_true",
- help="Whether to use 16-bit (mixed) precision (through NVIDIA apex) instead of 32-bit",
- )
- parser.add_argument(
- "--fp16_opt_level",
- type=str,
- default="O1",
- help="For fp16: Apex AMP optimization level selected in ['O0', 'O1', 'O2', and 'O3']."
- "See details at https://nvidia.github.io/apex/amp.html",
- )
- parser.add_argument("--local_rank", type=int, default=-1, help="For distributed training: local_rank")
- parser.add_argument("--server_ip", type=str, default="", help="For distant debugging.")
- parser.add_argument("--server_port", type=str, default="", help="For distant debugging.")
- args = parser.parse_args()
-
- if (
- os.path.exists(args.output_dir)
- and os.listdir(args.output_dir)
- and args.do_train
- and not args.overwrite_output_dir
- ):
- raise ValueError(
- "Output directory ({}) already exists and is not empty. Use --overwrite_output_dir to overcome.".format(
- args.output_dir
- )
- )
-
- # Setup distant debugging if needed
- if args.server_ip and args.server_port:
- # Distant debugging - see https://code.visualstudio.com/docs/python/debugging#_attach-to-a-local-script
- import ptvsd
-
- print("Waiting for debugger attach")
- ptvsd.enable_attach(address=(args.server_ip, args.server_port), redirect_output=True)
- ptvsd.wait_for_attach()
-
- # Setup CUDA, GPU & distributed training
- if args.local_rank == -1 or args.no_cuda:
- device = torch.device("cuda" if torch.cuda.is_available() and not args.no_cuda else "cpu")
- args.n_gpu = torch.cuda.device_count()
- else: # Initializes the distributed backend which will take care of sychronizing nodes/GPUs
- torch.cuda.set_device(args.local_rank)
- device = torch.device("cuda", args.local_rank)
- torch.distributed.init_process_group(backend="nccl")
- args.n_gpu = 1
-
- args.device = device
-
- # Setup logging
- logging.basicConfig(
- format="%(asctime)s - %(levelname)s - %(name)s - %(message)s",
- datefmt="%m/%d/%Y %H:%M:%S",
- level=logging.INFO if args.local_rank in [-1, 0] else logging.WARN,
- )
- logger.warning(
- "Process rank: %s, device: %s, n_gpu: %s, distributed training: %s, 16-bits training: %s",
- args.local_rank,
- device,
- args.n_gpu,
- bool(args.local_rank != -1),
- args.fp16,
- )
-
- # Set seed
- set_seed(args)
-
- # Load pretrained model and tokenizer
- if args.local_rank not in [-1, 0]:
- torch.distributed.barrier() # Make sure only the first process in distributed training will download model & vocab
-
- # Setup model
- labels = get_mmimdb_labels()
- num_labels = len(labels)
- args.model_type = args.model_type.lower()
- config_class, model_class, tokenizer_class = MODEL_CLASSES[args.model_type]
- transformer_config = config_class.from_pretrained(
- args.config_name if args.config_name else args.model_name_or_path
- )
- tokenizer = tokenizer_class.from_pretrained(
- args.tokenizer_name if args.tokenizer_name else args.model_name_or_path,
- do_lower_case=args.do_lower_case,
- cache_dir=args.cache_dir if args.cache_dir else None,
- )
- transformer = model_class.from_pretrained(
- args.model_name_or_path, config=transformer_config, cache_dir=args.cache_dir if args.cache_dir else None
- )
- img_encoder = ImageEncoder(args)
- config = MMBTConfig(transformer_config, num_labels=num_labels)
- model = MMBTForClassification(config, transformer, img_encoder)
-
- if args.local_rank == 0:
- torch.distributed.barrier() # Make sure only the first process in distributed training will download model & vocab
-
- model.to(args.device)
-
- logger.info("Training/evaluation parameters %s", args)
-
- # Training
- if args.do_train:
- train_dataset = load_examples(args, tokenizer, evaluate=False)
- label_frequences = train_dataset.get_label_frequencies()
- label_frequences = [label_frequences[l] for l in labels]
- label_weights = (
- torch.tensor(label_frequences, device=args.device, dtype=torch.float) / len(train_dataset)
- ) ** -1
- criterion = nn.BCEWithLogitsLoss(pos_weight=label_weights)
- global_step, tr_loss = train(args, train_dataset, model, tokenizer, criterion)
- logger.info(" global_step = %s, average loss = %s", global_step, tr_loss)
-
- # Saving best-practices: if you use defaults names for the model, you can reload it using from_pretrained()
- if args.do_train and (args.local_rank == -1 or torch.distributed.get_rank() == 0):
- # Create output directory if needed
- if not os.path.exists(args.output_dir) and args.local_rank in [-1, 0]:
- os.makedirs(args.output_dir)
-
- logger.info("Saving model checkpoint to %s", args.output_dir)
- # Save a trained model, configuration and tokenizer using `save_pretrained()`.
- # They can then be reloaded using `from_pretrained()`
- model_to_save = (
- model.module if hasattr(model, "module") else model
- ) # Take care of distributed/parallel training
- torch.save(model_to_save.state_dict(), os.path.join(args.output_dir, WEIGHTS_NAME))
- tokenizer.save_pretrained(args.output_dir)
-
- # Good practice: save your training arguments together with the trained model
- torch.save(args, os.path.join(args.output_dir, "training_args.bin"))
-
- # Load a trained model and vocabulary that you have fine-tuned
- model = MMBTForClassification(config, transformer, img_encoder)
- model.load_state_dict(torch.load(os.path.join(args.output_dir, WEIGHTS_NAME)))
- tokenizer = tokenizer_class.from_pretrained(args.output_dir)
- model.to(args.device)
-
- # Evaluation
- results = {}
- if args.do_eval and args.local_rank in [-1, 0]:
- tokenizer = tokenizer_class.from_pretrained(args.output_dir, do_lower_case=args.do_lower_case)
- checkpoints = [args.output_dir]
- if args.eval_all_checkpoints:
- checkpoints = list(
- os.path.dirname(c) for c in sorted(glob.glob(args.output_dir + "/**/" + WEIGHTS_NAME, recursive=True))
- )
- logging.getLogger("transformers.modeling_utils").setLevel(logging.WARN) # Reduce logging
- logger.info("Evaluate the following checkpoints: %s", checkpoints)
- for checkpoint in checkpoints:
- global_step = checkpoint.split("-")[-1] if len(checkpoints) > 1 else ""
- prefix = checkpoint.split("/")[-1] if checkpoint.find("checkpoint") != -1 else ""
- model = MMBTForClassification(config, transformer, img_encoder)
- model.load_state_dict(torch.load(checkpoint))
- model.to(args.device)
- result = evaluate(args, model, tokenizer, criterion, prefix=prefix)
- result = dict((k + "_{}".format(global_step), v) for k, v in result.items())
- results.update(result)
-
- return results
-
-
-if __name__ == "__main__":
- main()
diff --git a/server/transformers/examples/mm-imdb/utils_mmimdb.py b/server/transformers/examples/mm-imdb/utils_mmimdb.py
deleted file mode 100644
index 5df0a886eca0ec0f98e8f1224e8772485df8650f..0000000000000000000000000000000000000000
--- a/server/transformers/examples/mm-imdb/utils_mmimdb.py
+++ /dev/null
@@ -1,143 +0,0 @@
-# coding=utf-8
-# Copyright (c) Facebook, Inc. and its affiliates.
-# Copyright (c) HuggingFace Inc. team.
-#
-# Licensed under the Apache License, Version 2.0 (the "License");
-# you may not use this file except in compliance with the License.
-# You may obtain a copy of the License at
-#
-# http://www.apache.org/licenses/LICENSE-2.0
-#
-# Unless required by applicable law or agreed to in writing, software
-# distributed under the License is distributed on an "AS IS" BASIS,
-# WITHOUT WARRANTIES OR CONDITIONS OF ANY KIND, either express or implied.
-# See the License for the specific language governing permissions and
-# limitations under the License.
-
-import json
-import os
-from collections import Counter
-
-import torch
-import torch.nn as nn
-import torchvision
-import torchvision.transforms as transforms
-from PIL import Image
-from torch.utils.data import Dataset
-
-
-POOLING_BREAKDOWN = {1: (1, 1), 2: (2, 1), 3: (3, 1), 4: (2, 2), 5: (5, 1), 6: (3, 2), 7: (7, 1), 8: (4, 2), 9: (3, 3)}
-
-
-class ImageEncoder(nn.Module):
- def __init__(self, args):
- super().__init__()
- model = torchvision.models.resnet152(pretrained=True)
- modules = list(model.children())[:-2]
- self.model = nn.Sequential(*modules)
- self.pool = nn.AdaptiveAvgPool2d(POOLING_BREAKDOWN[args.num_image_embeds])
-
- def forward(self, x):
- # Bx3x224x224 -> Bx2048x7x7 -> Bx2048xN -> BxNx2048
- out = self.pool(self.model(x))
- out = torch.flatten(out, start_dim=2)
- out = out.transpose(1, 2).contiguous()
- return out # BxNx2048
-
-
-class JsonlDataset(Dataset):
- def __init__(self, data_path, tokenizer, transforms, labels, max_seq_length):
- self.data = [json.loads(l) for l in open(data_path)]
- self.data_dir = os.path.dirname(data_path)
- self.tokenizer = tokenizer
- self.labels = labels
- self.n_classes = len(labels)
- self.max_seq_length = max_seq_length
-
- self.transforms = transforms
-
- def __len__(self):
- return len(self.data)
-
- def __getitem__(self, index):
- sentence = torch.LongTensor(self.tokenizer.encode(self.data[index]["text"], add_special_tokens=True))
- start_token, sentence, end_token = sentence[0], sentence[1:-1], sentence[-1]
- sentence = sentence[: self.max_seq_length]
-
- label = torch.zeros(self.n_classes)
- label[[self.labels.index(tgt) for tgt in self.data[index]["label"]]] = 1
-
- image = Image.open(os.path.join(self.data_dir, self.data[index]["img"])).convert("RGB")
- image = self.transforms(image)
-
- return {
- "image_start_token": start_token,
- "image_end_token": end_token,
- "sentence": sentence,
- "image": image,
- "label": label,
- }
-
- def get_label_frequencies(self):
- label_freqs = Counter()
- for row in self.data:
- label_freqs.update(row["label"])
- return label_freqs
-
-
-def collate_fn(batch):
- lens = [len(row["sentence"]) for row in batch]
- bsz, max_seq_len = len(batch), max(lens)
-
- mask_tensor = torch.zeros(bsz, max_seq_len, dtype=torch.long)
- text_tensor = torch.zeros(bsz, max_seq_len, dtype=torch.long)
-
- for i_batch, (input_row, length) in enumerate(zip(batch, lens)):
- text_tensor[i_batch, :length] = input_row["sentence"]
- mask_tensor[i_batch, :length] = 1
-
- img_tensor = torch.stack([row["image"] for row in batch])
- tgt_tensor = torch.stack([row["label"] for row in batch])
- img_start_token = torch.stack([row["image_start_token"] for row in batch])
- img_end_token = torch.stack([row["image_end_token"] for row in batch])
-
- return text_tensor, mask_tensor, img_tensor, img_start_token, img_end_token, tgt_tensor
-
-
-def get_mmimdb_labels():
- return [
- "Crime",
- "Drama",
- "Thriller",
- "Action",
- "Comedy",
- "Romance",
- "Documentary",
- "Short",
- "Mystery",
- "History",
- "Family",
- "Adventure",
- "Fantasy",
- "Sci-Fi",
- "Western",
- "Horror",
- "Sport",
- "War",
- "Music",
- "Musical",
- "Animation",
- "Biography",
- "Film-Noir",
- ]
-
-
-def get_image_transforms():
- return transforms.Compose(
- [
- transforms.Resize(256),
- transforms.CenterCrop(224),
- transforms.ToTensor(),
- transforms.Normalize(mean=[0.46777044, 0.44531429, 0.40661017], std=[0.12221994, 0.12145835, 0.14380469],),
- ]
- )
diff --git a/server/transformers/examples/pplm/README.md b/server/transformers/examples/pplm/README.md
deleted file mode 100644
index ed105f95cf42a3f7b19624b1c478d9caba56c6ab..0000000000000000000000000000000000000000
--- a/server/transformers/examples/pplm/README.md
+++ /dev/null
@@ -1,54 +0,0 @@
-# Plug and Play Language Models: a Simple Approach to Controlled Text Generation
-
-Authors: [Sumanth Dathathri](https://dathath.github.io/), [Andrea Madotto](https://andreamad8.github.io/), Janice Lan, Jane Hung, Eric Frank, [Piero Molino](https://w4nderlu.st/), [Jason Yosinski](http://yosinski.com/), and [Rosanne Liu](http://www.rosanneliu.com/)
-
-This folder contains the original code used to run the Plug and Play Language Model (PPLM).
-
-Paper link: https://arxiv.org/abs/1912.02164
-
-Blog link: https://eng.uber.com/pplm
-
-Please check out the repo under uber-research for more information: https://github.com/uber-research/PPLM
-
-
-## Setup
-
-```bash
-git clone https://github.com/huggingface/transformers && cd transformers
-pip install .
-pip install nltk torchtext # additional requirements.
-cd examples/pplm
-```
-
-## PPLM-BoW
-
-### Example command for bag-of-words control
-
-```bash
-python run_pplm.py -B military --cond_text "The potato" --length 50 --gamma 1.5 --num_iterations 3 --num_samples 10 --stepsize 0.03 --window_length 5 --kl_scale 0.01 --gm_scale 0.99 --colorama --sample
-```
-
-### Tuning hyperparameters for bag-of-words control
-
-1. Increase `--stepsize` to intensify topic control, and decrease its value to soften the control. `--stepsize 0` recovers the original uncontrolled GPT-2 model.
-
-2. If the language being generated is repetitive (For e.g. "science science experiment experiment"), there are several options to consider:
- a) Reduce the `--stepsize`
- b) Increase `--kl_scale` (the KL-loss coefficient) or decrease `--gm_scale` (the gm-scaling term)
- c) Add `--grad-length xx` where xx is an (integer <= length, e.g. `--grad-length 30`).
-
-
-## PPLM-Discrim
-
-### Example command for discriminator based sentiment control
-
-```bash
-python run_pplm.py -D sentiment --class_label 2 --cond_text "My dog died" --length 50 --gamma 1.0 --num_iterations 10 --num_samples 10 --stepsize 0.04 --kl_scale 0.01 --gm_scale 0.95 --sample
-```
-
-### Tuning hyperparameters for discriminator control
-
-1. Increase `--stepsize` to intensify topic control, and decrease its value to soften the control. `--stepsize 0` recovers the original uncontrolled GPT-2 model.
-
-2. Use `--class_label 3` for negative, and `--class_label 2` for positive
-
diff --git a/server/transformers/examples/pplm/imgs/headfigure.png b/server/transformers/examples/pplm/imgs/headfigure.png
deleted file mode 100644
index f4c11ad54d10b300e2051ef6ba2d209447bc92e4..0000000000000000000000000000000000000000
Binary files a/server/transformers/examples/pplm/imgs/headfigure.png and /dev/null differ
diff --git a/server/transformers/examples/pplm/imgs/wooly.png b/server/transformers/examples/pplm/imgs/wooly.png
deleted file mode 100644
index 190d3afd49f1795245772a5d8b81a50b821d17b4..0000000000000000000000000000000000000000
Binary files a/server/transformers/examples/pplm/imgs/wooly.png and /dev/null differ
diff --git a/server/transformers/examples/pplm/pplm_classification_head.py b/server/transformers/examples/pplm/pplm_classification_head.py
deleted file mode 100644
index e85ba608b225c5489aa26481fa04c0f626dabfce..0000000000000000000000000000000000000000
--- a/server/transformers/examples/pplm/pplm_classification_head.py
+++ /dev/null
@@ -1,19 +0,0 @@
-import torch
-
-
-class ClassificationHead(torch.nn.Module):
- """Classification Head for transformer encoders"""
-
- def __init__(self, class_size, embed_size):
- super().__init__()
- self.class_size = class_size
- self.embed_size = embed_size
- # self.mlp1 = torch.nn.Linear(embed_size, embed_size)
- # self.mlp2 = (torch.nn.Linear(embed_size, class_size))
- self.mlp = torch.nn.Linear(embed_size, class_size)
-
- def forward(self, hidden_state):
- # hidden_state = F.relu(self.mlp1(hidden_state))
- # hidden_state = self.mlp2(hidden_state)
- logits = self.mlp(hidden_state)
- return logits
diff --git a/server/transformers/examples/pplm/run_pplm.py b/server/transformers/examples/pplm/run_pplm.py
deleted file mode 100644
index b334a0098cc913393c51ed06a16e8209422c4b81..0000000000000000000000000000000000000000
--- a/server/transformers/examples/pplm/run_pplm.py
+++ /dev/null
@@ -1,794 +0,0 @@
-#! /usr/bin/env python3
-# coding=utf-8
-
-# Copyright (c) 2019 Uber Technologies, Inc.
-#
-# Licensed under the Apache License, Version 2.0 (the "License");
-# you may not use this file except in compliance with the License.
-# You may obtain a copy of the License at
-#
-# http://www.apache.org/licenses/LICENSE-2.0
-#
-# Unless required by applicable law or agreed to in writing, software
-# distributed under the License is distributed on an "AS IS" BASIS,
-# WITHOUT WARRANTIES OR CONDITIONS OF ANY KIND, either express or implied.
-# See the License for the specific language governing permissions and
-# limitations under the License.
-
-"""
-Example command with bag of words:
-python examples/run_pplm.py -B space --cond_text "The president" --length 100 --gamma 1.5 --num_iterations 3 --num_samples 10 --stepsize 0.01 --window_length 5 --kl_scale 0.01 --gm_scale 0.95
-
-Example command with discriminator:
-python examples/run_pplm.py -D sentiment --class_label 3 --cond_text "The lake" --length 10 --gamma 1.0 --num_iterations 30 --num_samples 10 --stepsize 0.01 --kl_scale 0.01 --gm_scale 0.95
-"""
-
-import argparse
-import json
-from operator import add
-from typing import List, Optional, Tuple, Union
-
-import numpy as np
-import torch
-import torch.nn.functional as F
-from torch.autograd import Variable
-from tqdm import trange
-
-from pplm_classification_head import ClassificationHead
-from transformers import GPT2Tokenizer
-from transformers.file_utils import cached_path
-from transformers.modeling_gpt2 import GPT2LMHeadModel
-
-
-PPLM_BOW = 1
-PPLM_DISCRIM = 2
-PPLM_BOW_DISCRIM = 3
-SMALL_CONST = 1e-15
-BIG_CONST = 1e10
-
-BAG_OF_WORDS_ARCHIVE_MAP = {
- "legal": "https://s3.amazonaws.com/models.huggingface.co/bert/pplm/bow/legal.txt",
- "military": "https://s3.amazonaws.com/models.huggingface.co/bert/pplm/bow/military.txt",
- "politics": "https://s3.amazonaws.com/models.huggingface.co/bert/pplm/bow/politics.txt",
- "religion": "https://s3.amazonaws.com/models.huggingface.co/bert/pplm/bow/religion.txt",
- "science": "https://s3.amazonaws.com/models.huggingface.co/bert/pplm/bow/science.txt",
- "space": "https://s3.amazonaws.com/models.huggingface.co/bert/pplm/bow/space.txt",
- "technology": "https://s3.amazonaws.com/models.huggingface.co/bert/pplm/bow/technology.txt",
-}
-
-DISCRIMINATOR_MODELS_PARAMS = {
- "clickbait": {
- "url": "https://s3.amazonaws.com/models.huggingface.co/bert/pplm/discriminators/clickbait_classifier_head.pt",
- "class_size": 2,
- "embed_size": 1024,
- "class_vocab": {"non_clickbait": 0, "clickbait": 1},
- "default_class": 1,
- "pretrained_model": "gpt2-medium",
- },
- "sentiment": {
- "url": "https://s3.amazonaws.com/models.huggingface.co/bert/pplm/discriminators/SST_classifier_head.pt",
- "class_size": 5,
- "embed_size": 1024,
- "class_vocab": {"very_positive": 2, "very_negative": 3},
- "default_class": 3,
- "pretrained_model": "gpt2-medium",
- },
-}
-
-
-def to_var(x, requires_grad=False, volatile=False, device="cuda"):
- if torch.cuda.is_available() and device == "cuda":
- x = x.cuda()
- elif device != "cuda":
- x = x.to(device)
- return Variable(x, requires_grad=requires_grad, volatile=volatile)
-
-
-def top_k_filter(logits, k, probs=False):
- """
- Masks everything but the k top entries as -infinity (1e10).
- Used to mask logits such that e^-infinity -> 0 won't contribute to the
- sum of the denominator.
- """
- if k == 0:
- return logits
- else:
- values = torch.topk(logits, k)[0]
- batch_mins = values[:, -1].view(-1, 1).expand_as(logits)
- if probs:
- return torch.where(logits < batch_mins, torch.ones_like(logits) * 0.0, logits)
- return torch.where(logits < batch_mins, torch.ones_like(logits) * -BIG_CONST, logits)
-
-
-def perturb_past(
- past,
- model,
- last,
- unpert_past=None,
- unpert_logits=None,
- accumulated_hidden=None,
- grad_norms=None,
- stepsize=0.01,
- one_hot_bows_vectors=None,
- classifier=None,
- class_label=None,
- loss_type=0,
- num_iterations=3,
- horizon_length=1,
- window_length=0,
- decay=False,
- gamma=1.5,
- kl_scale=0.01,
- device="cuda",
-):
- # Generate inital perturbed past
- grad_accumulator = [(np.zeros(p.shape).astype("float32")) for p in past]
-
- if accumulated_hidden is None:
- accumulated_hidden = 0
-
- if decay:
- decay_mask = torch.arange(0.0, 1.0 + SMALL_CONST, 1.0 / (window_length))[1:]
- else:
- decay_mask = 1.0
-
- # TODO fix this comment (SUMANTH)
- # Generate a mask is gradient perturbated is based on a past window
- _, _, _, curr_length, _ = past[0].shape
-
- if curr_length > window_length and window_length > 0:
- ones_key_val_shape = tuple(past[0].shape[:-2]) + tuple([window_length]) + tuple(past[0].shape[-1:])
-
- zeros_key_val_shape = (
- tuple(past[0].shape[:-2]) + tuple([curr_length - window_length]) + tuple(past[0].shape[-1:])
- )
-
- ones_mask = torch.ones(ones_key_val_shape)
- ones_mask = decay_mask * ones_mask.permute(0, 1, 2, 4, 3)
- ones_mask = ones_mask.permute(0, 1, 2, 4, 3)
-
- window_mask = torch.cat((ones_mask, torch.zeros(zeros_key_val_shape)), dim=-2).to(device)
- else:
- window_mask = torch.ones_like(past[0]).to(device)
-
- # accumulate perturbations for num_iterations
- loss_per_iter = []
- new_accumulated_hidden = None
- for i in range(num_iterations):
- print("Iteration ", i + 1)
- curr_perturbation = [
- to_var(torch.from_numpy(p_), requires_grad=True, device=device) for p_ in grad_accumulator
- ]
-
- # Compute hidden using perturbed past
- perturbed_past = list(map(add, past, curr_perturbation))
- _, _, _, curr_length, _ = curr_perturbation[0].shape
- all_logits, _, all_hidden = model(last, past=perturbed_past)
- hidden = all_hidden[-1]
- new_accumulated_hidden = accumulated_hidden + torch.sum(hidden, dim=1).detach()
- # TODO: Check the layer-norm consistency of this with trained discriminator (Sumanth)
- logits = all_logits[:, -1, :]
- probs = F.softmax(logits, dim=-1)
-
- loss = 0.0
- loss_list = []
- if loss_type == PPLM_BOW or loss_type == PPLM_BOW_DISCRIM:
- for one_hot_bow in one_hot_bows_vectors:
- bow_logits = torch.mm(probs, torch.t(one_hot_bow))
- bow_loss = -torch.log(torch.sum(bow_logits))
- loss += bow_loss
- loss_list.append(bow_loss)
- print(" pplm_bow_loss:", loss.data.cpu().numpy())
-
- if loss_type == 2 or loss_type == 3:
- ce_loss = torch.nn.CrossEntropyLoss()
- # TODO why we need to do this assignment and not just using unpert_past? (Sumanth)
- curr_unpert_past = unpert_past
- curr_probs = torch.unsqueeze(probs, dim=1)
- wte = model.resize_token_embeddings()
- for _ in range(horizon_length):
- inputs_embeds = torch.matmul(curr_probs, wte.weight.data)
- _, curr_unpert_past, curr_all_hidden = model(past=curr_unpert_past, inputs_embeds=inputs_embeds)
- curr_hidden = curr_all_hidden[-1]
- new_accumulated_hidden = new_accumulated_hidden + torch.sum(curr_hidden, dim=1)
-
- prediction = classifier(new_accumulated_hidden / (curr_length + 1 + horizon_length))
-
- label = torch.tensor(prediction.shape[0] * [class_label], device=device, dtype=torch.long)
- discrim_loss = ce_loss(prediction, label)
- print(" pplm_discrim_loss:", discrim_loss.data.cpu().numpy())
- loss += discrim_loss
- loss_list.append(discrim_loss)
-
- kl_loss = 0.0
- if kl_scale > 0.0:
- unpert_probs = F.softmax(unpert_logits[:, -1, :], dim=-1)
- unpert_probs = unpert_probs + SMALL_CONST * (unpert_probs <= SMALL_CONST).float().to(device).detach()
- correction = SMALL_CONST * (probs <= SMALL_CONST).float().to(device).detach()
- corrected_probs = probs + correction.detach()
- kl_loss = kl_scale * ((corrected_probs * (corrected_probs / unpert_probs).log()).sum())
- print(" kl_loss", kl_loss.data.cpu().numpy())
- loss += kl_loss
-
- loss_per_iter.append(loss.data.cpu().numpy())
- print(" pplm_loss", (loss - kl_loss).data.cpu().numpy())
-
- # compute gradients
- loss.backward()
-
- # calculate gradient norms
- if grad_norms is not None and loss_type == PPLM_BOW:
- grad_norms = [
- torch.max(grad_norms[index], torch.norm(p_.grad * window_mask))
- for index, p_ in enumerate(curr_perturbation)
- ]
- else:
- grad_norms = [
- (torch.norm(p_.grad * window_mask) + SMALL_CONST) for index, p_ in enumerate(curr_perturbation)
- ]
-
- # normalize gradients
- grad = [
- -stepsize * (p_.grad * window_mask / grad_norms[index] ** gamma).data.cpu().numpy()
- for index, p_ in enumerate(curr_perturbation)
- ]
-
- # accumulate gradient
- grad_accumulator = list(map(add, grad, grad_accumulator))
-
- # reset gradients, just to make sure
- for p_ in curr_perturbation:
- p_.grad.data.zero_()
-
- # removing past from the graph
- new_past = []
- for p_ in past:
- new_past.append(p_.detach())
- past = new_past
-
- # apply the accumulated perturbations to the past
- grad_accumulator = [to_var(torch.from_numpy(p_), requires_grad=True, device=device) for p_ in grad_accumulator]
- pert_past = list(map(add, past, grad_accumulator))
-
- return pert_past, new_accumulated_hidden, grad_norms, loss_per_iter
-
-
-def get_classifier(
- name: Optional[str], class_label: Union[str, int], device: str
-) -> Tuple[Optional[ClassificationHead], Optional[int]]:
- if name is None:
- return None, None
-
- params = DISCRIMINATOR_MODELS_PARAMS[name]
- classifier = ClassificationHead(class_size=params["class_size"], embed_size=params["embed_size"]).to(device)
- if "url" in params:
- resolved_archive_file = cached_path(params["url"])
- elif "path" in params:
- resolved_archive_file = params["path"]
- else:
- raise ValueError("Either url or path have to be specified " "in the discriminator model parameters")
- classifier.load_state_dict(torch.load(resolved_archive_file, map_location=device))
- classifier.eval()
-
- if isinstance(class_label, str):
- if class_label in params["class_vocab"]:
- label_id = params["class_vocab"][class_label]
- else:
- label_id = params["default_class"]
- print("class_label {} not in class_vocab".format(class_label))
- print("available values are: {}".format(params["class_vocab"]))
- print("using default class {}".format(label_id))
-
- elif isinstance(class_label, int):
- if class_label in set(params["class_vocab"].values()):
- label_id = class_label
- else:
- label_id = params["default_class"]
- print("class_label {} not in class_vocab".format(class_label))
- print("available values are: {}".format(params["class_vocab"]))
- print("using default class {}".format(label_id))
-
- else:
- label_id = params["default_class"]
-
- return classifier, label_id
-
-
-def get_bag_of_words_indices(bag_of_words_ids_or_paths: List[str], tokenizer) -> List[List[List[int]]]:
- bow_indices = []
- for id_or_path in bag_of_words_ids_or_paths:
- if id_or_path in BAG_OF_WORDS_ARCHIVE_MAP:
- filepath = cached_path(BAG_OF_WORDS_ARCHIVE_MAP[id_or_path])
- else:
- filepath = id_or_path
- with open(filepath, "r") as f:
- words = f.read().strip().split("\n")
- bow_indices.append([tokenizer.encode(word.strip(), add_prefix_space=True) for word in words])
- return bow_indices
-
-
-def build_bows_one_hot_vectors(bow_indices, tokenizer, device="cuda"):
- if bow_indices is None:
- return None
-
- one_hot_bows_vectors = []
- for single_bow in bow_indices:
- single_bow = list(filter(lambda x: len(x) <= 1, single_bow))
- single_bow = torch.tensor(single_bow).to(device)
- num_words = single_bow.shape[0]
- one_hot_bow = torch.zeros(num_words, tokenizer.vocab_size).to(device)
- one_hot_bow.scatter_(1, single_bow, 1)
- one_hot_bows_vectors.append(one_hot_bow)
- return one_hot_bows_vectors
-
-
-def full_text_generation(
- model,
- tokenizer,
- context=None,
- num_samples=1,
- device="cuda",
- bag_of_words=None,
- discrim=None,
- class_label=None,
- length=100,
- stepsize=0.02,
- temperature=1.0,
- top_k=10,
- sample=False,
- num_iterations=3,
- grad_length=10000,
- horizon_length=1,
- window_length=0,
- decay=False,
- gamma=1.5,
- gm_scale=0.9,
- kl_scale=0.01,
- repetition_penalty=1.0,
- **kwargs
-):
- classifier, class_id = get_classifier(discrim, class_label, device)
-
- bow_indices = []
- if bag_of_words:
- bow_indices = get_bag_of_words_indices(bag_of_words.split(";"), tokenizer)
-
- if bag_of_words and classifier:
- print("Both PPLM-BoW and PPLM-Discrim are on. This is not optimized.")
- loss_type = PPLM_BOW_DISCRIM
-
- elif bag_of_words:
- loss_type = PPLM_BOW
- print("Using PPLM-BoW")
-
- elif classifier is not None:
- loss_type = PPLM_DISCRIM
- print("Using PPLM-Discrim")
-
- else:
- raise Exception("Specify either a bag of words or a discriminator")
-
- unpert_gen_tok_text, _, _ = generate_text_pplm(
- model=model,
- tokenizer=tokenizer,
- context=context,
- device=device,
- length=length,
- sample=sample,
- perturb=False,
- repetition_penalty=repetition_penalty,
- )
- if device == "cuda":
- torch.cuda.empty_cache()
-
- pert_gen_tok_texts = []
- discrim_losses = []
- losses_in_time = []
-
- for i in range(num_samples):
- pert_gen_tok_text, discrim_loss, loss_in_time = generate_text_pplm(
- model=model,
- tokenizer=tokenizer,
- context=context,
- device=device,
- perturb=True,
- bow_indices=bow_indices,
- classifier=classifier,
- class_label=class_id,
- loss_type=loss_type,
- length=length,
- stepsize=stepsize,
- temperature=temperature,
- top_k=top_k,
- sample=sample,
- num_iterations=num_iterations,
- grad_length=grad_length,
- horizon_length=horizon_length,
- window_length=window_length,
- decay=decay,
- gamma=gamma,
- gm_scale=gm_scale,
- kl_scale=kl_scale,
- repetition_penalty=repetition_penalty,
- )
- pert_gen_tok_texts.append(pert_gen_tok_text)
- if classifier is not None:
- discrim_losses.append(discrim_loss.data.cpu().numpy())
- losses_in_time.append(loss_in_time)
-
- if device == "cuda":
- torch.cuda.empty_cache()
-
- return unpert_gen_tok_text, pert_gen_tok_texts, discrim_losses, losses_in_time
-
-
-def generate_text_pplm(
- model,
- tokenizer,
- context=None,
- past=None,
- device="cuda",
- perturb=True,
- bow_indices=None,
- classifier=None,
- class_label=None,
- loss_type=0,
- length=100,
- stepsize=0.02,
- temperature=1.0,
- top_k=10,
- sample=False,
- num_iterations=3,
- grad_length=10000,
- horizon_length=1,
- window_length=0,
- decay=False,
- gamma=1.5,
- gm_scale=0.9,
- kl_scale=0.01,
- repetition_penalty=1.0,
-):
- output_so_far = None
- if context:
- context_t = torch.tensor(context, device=device, dtype=torch.long)
- while len(context_t.shape) < 2:
- context_t = context_t.unsqueeze(0)
- output_so_far = context_t
-
- # collect one hot vectors for bags of words
- one_hot_bows_vectors = build_bows_one_hot_vectors(bow_indices, tokenizer, device)
-
- grad_norms = None
- last = None
- unpert_discrim_loss = 0
- loss_in_time = []
- for i in trange(length, ascii=True):
-
- # Get past/probs for current output, except for last word
- # Note that GPT takes 2 inputs: past + current_token
-
- # run model forward to obtain unperturbed
- if past is None and output_so_far is not None:
- last = output_so_far[:, -1:]
- if output_so_far.shape[1] > 1:
- _, past, _ = model(output_so_far[:, :-1])
-
- unpert_logits, unpert_past, unpert_all_hidden = model(output_so_far)
- unpert_last_hidden = unpert_all_hidden[-1]
-
- # check if we are abowe grad max length
- if i >= grad_length:
- current_stepsize = stepsize * 0
- else:
- current_stepsize = stepsize
-
- # modify the past if necessary
- if not perturb or num_iterations == 0:
- pert_past = past
-
- else:
- accumulated_hidden = unpert_last_hidden[:, :-1, :]
- accumulated_hidden = torch.sum(accumulated_hidden, dim=1)
-
- if past is not None:
- pert_past, _, grad_norms, loss_this_iter = perturb_past(
- past,
- model,
- last,
- unpert_past=unpert_past,
- unpert_logits=unpert_logits,
- accumulated_hidden=accumulated_hidden,
- grad_norms=grad_norms,
- stepsize=current_stepsize,
- one_hot_bows_vectors=one_hot_bows_vectors,
- classifier=classifier,
- class_label=class_label,
- loss_type=loss_type,
- num_iterations=num_iterations,
- horizon_length=horizon_length,
- window_length=window_length,
- decay=decay,
- gamma=gamma,
- kl_scale=kl_scale,
- device=device,
- )
- loss_in_time.append(loss_this_iter)
- else:
- pert_past = past
-
- pert_logits, past, pert_all_hidden = model(last, past=pert_past)
- pert_logits = pert_logits[:, -1, :] / temperature # + SMALL_CONST
-
- for token_idx in set(output_so_far[0].tolist()):
- if pert_logits[0, token_idx] < 0:
- pert_logits[0, token_idx] *= repetition_penalty
- else:
- pert_logits[0, token_idx] /= repetition_penalty
-
- pert_probs = F.softmax(pert_logits, dim=-1)
-
- if classifier is not None:
- ce_loss = torch.nn.CrossEntropyLoss()
- prediction = classifier(torch.mean(unpert_last_hidden, dim=1))
- label = torch.tensor([class_label], device=device, dtype=torch.long)
- unpert_discrim_loss = ce_loss(prediction, label)
- print("unperturbed discrim loss", unpert_discrim_loss.data.cpu().numpy())
- else:
- unpert_discrim_loss = 0
-
- # Fuse the modified model and original model
- if perturb:
-
- unpert_probs = F.softmax(unpert_logits[:, -1, :], dim=-1)
-
- pert_probs = (pert_probs ** gm_scale) * (unpert_probs ** (1 - gm_scale)) # + SMALL_CONST
- pert_probs = top_k_filter(pert_probs, k=top_k, probs=True) # + SMALL_CONST
-
- # rescale
- if torch.sum(pert_probs) <= 1:
- pert_probs = pert_probs / torch.sum(pert_probs)
-
- else:
- pert_logits = top_k_filter(pert_logits, k=top_k) # + SMALL_CONST
- pert_probs = F.softmax(pert_logits, dim=-1)
-
- # sample or greedy
- if sample:
- last = torch.multinomial(pert_probs, num_samples=1)
-
- else:
- _, last = torch.topk(pert_probs, k=1, dim=-1)
-
- # update context/output_so_far appending the new token
- output_so_far = last if output_so_far is None else torch.cat((output_so_far, last), dim=1)
-
- print(tokenizer.decode(output_so_far.tolist()[0]))
-
- return output_so_far, unpert_discrim_loss, loss_in_time
-
-
-def set_generic_model_params(discrim_weights, discrim_meta):
- if discrim_weights is None:
- raise ValueError("When using a generic discriminator, " "discrim_weights need to be specified")
- if discrim_meta is None:
- raise ValueError("When using a generic discriminator, " "discrim_meta need to be specified")
-
- with open(discrim_meta, "r") as discrim_meta_file:
- meta = json.load(discrim_meta_file)
- meta["path"] = discrim_weights
- DISCRIMINATOR_MODELS_PARAMS["generic"] = meta
-
-
-def run_pplm_example(
- pretrained_model="gpt2-medium",
- cond_text="",
- uncond=False,
- num_samples=1,
- bag_of_words=None,
- discrim=None,
- discrim_weights=None,
- discrim_meta=None,
- class_label=-1,
- length=100,
- stepsize=0.02,
- temperature=1.0,
- top_k=10,
- sample=False,
- num_iterations=3,
- grad_length=10000,
- horizon_length=1,
- window_length=0,
- decay=False,
- gamma=1.5,
- gm_scale=0.9,
- kl_scale=0.01,
- seed=0,
- no_cuda=False,
- colorama=False,
- repetition_penalty=1.0,
-):
- # set Random seed
- torch.manual_seed(seed)
- np.random.seed(seed)
-
- # set the device
- device = "cuda" if torch.cuda.is_available() and not no_cuda else "cpu"
-
- if discrim == "generic":
- set_generic_model_params(discrim_weights, discrim_meta)
-
- if discrim is not None:
- pretrained_model = DISCRIMINATOR_MODELS_PARAMS[discrim]["pretrained_model"]
- print("discrim = {}, pretrained_model set " "to discriminator's = {}".format(discrim, pretrained_model))
-
- # load pretrained model
- model = GPT2LMHeadModel.from_pretrained(pretrained_model, output_hidden_states=True)
- model.to(device)
- model.eval()
-
- # load tokenizer
- tokenizer = GPT2Tokenizer.from_pretrained(pretrained_model)
-
- # Freeze GPT-2 weights
- for param in model.parameters():
- param.requires_grad = False
-
- # figure out conditioning text
- if uncond:
- tokenized_cond_text = tokenizer.encode([tokenizer.bos_token])
- else:
- raw_text = cond_text
- while not raw_text:
- print("Did you forget to add `--cond_text`? ")
- raw_text = input("Model prompt >>> ")
- tokenized_cond_text = tokenizer.encode(tokenizer.bos_token + raw_text)
-
- print("= Prefix of sentence =")
- print(tokenizer.decode(tokenized_cond_text))
- print()
-
- # generate unperturbed and perturbed texts
-
- # full_text_generation returns:
- # unpert_gen_tok_text, pert_gen_tok_texts, discrim_losses, losses_in_time
- unpert_gen_tok_text, pert_gen_tok_texts, _, _ = full_text_generation(
- model=model,
- tokenizer=tokenizer,
- context=tokenized_cond_text,
- device=device,
- num_samples=num_samples,
- bag_of_words=bag_of_words,
- discrim=discrim,
- class_label=class_label,
- length=length,
- stepsize=stepsize,
- temperature=temperature,
- top_k=top_k,
- sample=sample,
- num_iterations=num_iterations,
- grad_length=grad_length,
- horizon_length=horizon_length,
- window_length=window_length,
- decay=decay,
- gamma=gamma,
- gm_scale=gm_scale,
- kl_scale=kl_scale,
- repetition_penalty=repetition_penalty,
- )
-
- # untokenize unperturbed text
- unpert_gen_text = tokenizer.decode(unpert_gen_tok_text.tolist()[0])
-
- print("=" * 80)
- print("= Unperturbed generated text =")
- print(unpert_gen_text)
- print()
-
- generated_texts = []
-
- bow_word_ids = set()
- if bag_of_words and colorama:
- bow_indices = get_bag_of_words_indices(bag_of_words.split(";"), tokenizer)
- for single_bow_list in bow_indices:
- # filtering all words in the list composed of more than 1 token
- filtered = list(filter(lambda x: len(x) <= 1, single_bow_list))
- # w[0] because we are sure w has only 1 item because previous fitler
- bow_word_ids.update(w[0] for w in filtered)
-
- # iterate through the perturbed texts
- for i, pert_gen_tok_text in enumerate(pert_gen_tok_texts):
- try:
- # untokenize unperturbed text
- if colorama:
- import colorama
-
- pert_gen_text = ""
- for word_id in pert_gen_tok_text.tolist()[0]:
- if word_id in bow_word_ids:
- pert_gen_text += "{}{}{}".format(
- colorama.Fore.RED, tokenizer.decode([word_id]), colorama.Style.RESET_ALL
- )
- else:
- pert_gen_text += tokenizer.decode([word_id])
- else:
- pert_gen_text = tokenizer.decode(pert_gen_tok_text.tolist()[0])
-
- print("= Perturbed generated text {} =".format(i + 1))
- print(pert_gen_text)
- print()
- except Exception as exc:
- print("Ignoring error while generating perturbed text:", exc)
-
- # keep the prefix, perturbed seq, original seq for each index
- generated_texts.append((tokenized_cond_text, pert_gen_tok_text, unpert_gen_tok_text))
-
- return
-
-
-if __name__ == "__main__":
- parser = argparse.ArgumentParser()
- parser.add_argument(
- "--pretrained_model",
- "-M",
- type=str,
- default="gpt2-medium",
- help="pretrained model name or path to local checkpoint",
- )
- parser.add_argument("--cond_text", type=str, default="The lake", help="Prefix texts to condition on")
- parser.add_argument("--uncond", action="store_true", help="Generate from end-of-text as prefix")
- parser.add_argument(
- "--num_samples", type=int, default=1, help="Number of samples to generate from the modified latents",
- )
- parser.add_argument(
- "--bag_of_words",
- "-B",
- type=str,
- default=None,
- help="Bags of words used for PPLM-BoW. "
- "Either a BOW id (see list in code) or a filepath. "
- "Multiple BoWs separated by ;",
- )
- parser.add_argument(
- "--discrim",
- "-D",
- type=str,
- default=None,
- choices=("clickbait", "sentiment", "toxicity", "generic"),
- help="Discriminator to use",
- )
- parser.add_argument("--discrim_weights", type=str, default=None, help="Weights for the generic discriminator")
- parser.add_argument(
- "--discrim_meta", type=str, default=None, help="Meta information for the generic discriminator"
- )
- parser.add_argument(
- "--class_label", type=int, default=-1, help="Class label used for the discriminator",
- )
- parser.add_argument("--length", type=int, default=100)
- parser.add_argument("--stepsize", type=float, default=0.02)
- parser.add_argument("--temperature", type=float, default=1.0)
- parser.add_argument("--top_k", type=int, default=10)
- parser.add_argument("--sample", action="store_true", help="Generate from end-of-text as prefix")
- parser.add_argument("--num_iterations", type=int, default=3)
- parser.add_argument("--grad_length", type=int, default=10000)
- parser.add_argument(
- "--window_length",
- type=int,
- default=0,
- help="Length of past which is being optimized; " "0 corresponds to infinite window length",
- )
- parser.add_argument(
- "--horizon_length", type=int, default=1, help="Length of future to optimize over",
- )
- parser.add_argument("--decay", action="store_true", help="whether to decay or not")
- parser.add_argument("--gamma", type=float, default=1.5)
- parser.add_argument("--gm_scale", type=float, default=0.9)
- parser.add_argument("--kl_scale", type=float, default=0.01)
- parser.add_argument("--seed", type=int, default=0)
- parser.add_argument("--no_cuda", action="store_true", help="no cuda")
- parser.add_argument("--colorama", action="store_true", help="colors keywords")
- parser.add_argument(
- "--repetition_penalty", type=float, default=1.0, help="Penalize repetition. More than 1.0 -> less repetition",
- )
-
- args = parser.parse_args()
- run_pplm_example(**vars(args))
diff --git a/server/transformers/examples/pplm/run_pplm_discrim_train.py b/server/transformers/examples/pplm/run_pplm_discrim_train.py
deleted file mode 100644
index ce6f583dc6d8bfe3c0d4612ce76adbeaaf7572e4..0000000000000000000000000000000000000000
--- a/server/transformers/examples/pplm/run_pplm_discrim_train.py
+++ /dev/null
@@ -1,517 +0,0 @@
-#! /usr/bin/env python3
-# coding=utf-8
-
-# Copyright (c) 2019 Uber Technologies, Inc.
-#
-# Licensed under the Apache License, Version 2.0 (the "License");
-# you may not use this file except in compliance with the License.
-# You may obtain a copy of the License at
-#
-# http://www.apache.org/licenses/LICENSE-2.0
-#
-# Unless required by applicable law or agreed to in writing, software
-# distributed under the License is distributed on an "AS IS" BASIS,
-# WITHOUT WARRANTIES OR CONDITIONS OF ANY KIND, either express or implied.
-# See the License for the specific language governing permissions and
-# limitations under the License.
-
-import argparse
-import csv
-import json
-import math
-import time
-
-import numpy as np
-import torch
-import torch.nn.functional as F
-import torch.optim as optim
-import torch.utils.data as data
-from nltk.tokenize.treebank import TreebankWordDetokenizer
-from torchtext import data as torchtext_data
-from torchtext import datasets
-from tqdm import tqdm, trange
-
-from pplm_classification_head import ClassificationHead
-from transformers import GPT2LMHeadModel, GPT2Tokenizer
-
-
-torch.manual_seed(0)
-np.random.seed(0)
-EPSILON = 1e-10
-example_sentence = "This is incredible! I love it, this is the best chicken I have ever had."
-max_length_seq = 100
-
-
-class Discriminator(torch.nn.Module):
- """Transformer encoder followed by a Classification Head"""
-
- def __init__(self, class_size, pretrained_model="gpt2-medium", cached_mode=False, device="cpu"):
- super().__init__()
- self.tokenizer = GPT2Tokenizer.from_pretrained(pretrained_model)
- self.encoder = GPT2LMHeadModel.from_pretrained(pretrained_model)
- self.embed_size = self.encoder.transformer.config.hidden_size
- self.classifier_head = ClassificationHead(class_size=class_size, embed_size=self.embed_size)
- self.cached_mode = cached_mode
- self.device = device
-
- def get_classifier(self):
- return self.classifier_head
-
- def train_custom(self):
- for param in self.encoder.parameters():
- param.requires_grad = False
- self.classifier_head.train()
-
- def avg_representation(self, x):
- mask = x.ne(0).unsqueeze(2).repeat(1, 1, self.embed_size).float().to(self.device).detach()
- hidden, _ = self.encoder.transformer(x)
- masked_hidden = hidden * mask
- avg_hidden = torch.sum(masked_hidden, dim=1) / (torch.sum(mask, dim=1).detach() + EPSILON)
- return avg_hidden
-
- def forward(self, x):
- if self.cached_mode:
- avg_hidden = x.to(self.device)
- else:
- avg_hidden = self.avg_representation(x.to(self.device))
-
- logits = self.classifier_head(avg_hidden)
- probs = F.log_softmax(logits, dim=-1)
-
- return probs
-
-
-class Dataset(data.Dataset):
- def __init__(self, X, y):
- """Reads source and target sequences from txt files."""
- self.X = X
- self.y = y
-
- def __len__(self):
- return len(self.X)
-
- def __getitem__(self, index):
- """Returns one data pair (source and target)."""
- data = {}
- data["X"] = self.X[index]
- data["y"] = self.y[index]
- return data
-
-
-def collate_fn(data):
- def pad_sequences(sequences):
- lengths = [len(seq) for seq in sequences]
-
- padded_sequences = torch.zeros(len(sequences), max(lengths)).long() # padding value = 0
-
- for i, seq in enumerate(sequences):
- end = lengths[i]
- padded_sequences[i, :end] = seq[:end]
-
- return padded_sequences, lengths
-
- item_info = {}
- for key in data[0].keys():
- item_info[key] = [d[key] for d in data]
-
- x_batch, _ = pad_sequences(item_info["X"])
- y_batch = torch.tensor(item_info["y"], dtype=torch.long)
-
- return x_batch, y_batch
-
-
-def cached_collate_fn(data):
- item_info = {}
- for key in data[0].keys():
- item_info[key] = [d[key] for d in data]
-
- x_batch = torch.cat(item_info["X"], 0)
- y_batch = torch.tensor(item_info["y"], dtype=torch.long)
-
- return x_batch, y_batch
-
-
-def train_epoch(data_loader, discriminator, optimizer, epoch=0, log_interval=10, device="cpu"):
- samples_so_far = 0
- discriminator.train_custom()
- for batch_idx, (input_t, target_t) in enumerate(data_loader):
- input_t, target_t = input_t.to(device), target_t.to(device)
-
- optimizer.zero_grad()
-
- output_t = discriminator(input_t)
- loss = F.nll_loss(output_t, target_t)
- loss.backward(retain_graph=True)
- optimizer.step()
-
- samples_so_far += len(input_t)
-
- if batch_idx % log_interval == 0:
- print(
- "Train Epoch: {} [{}/{} ({:.0f}%)]\tLoss: {:.6f}".format(
- epoch + 1,
- samples_so_far,
- len(data_loader.dataset),
- 100 * samples_so_far / len(data_loader.dataset),
- loss.item(),
- )
- )
-
-
-def evaluate_performance(data_loader, discriminator, device="cpu"):
- discriminator.eval()
- test_loss = 0
- correct = 0
- with torch.no_grad():
- for input_t, target_t in data_loader:
- input_t, target_t = input_t.to(device), target_t.to(device)
- output_t = discriminator(input_t)
- # sum up batch loss
- test_loss += F.nll_loss(output_t, target_t, reduction="sum").item()
- # get the index of the max log-probability
- pred_t = output_t.argmax(dim=1, keepdim=True)
- correct += pred_t.eq(target_t.view_as(pred_t)).sum().item()
-
- test_loss /= len(data_loader.dataset)
-
- print(
- "Performance on test set: "
- "Average loss: {:.4f}, Accuracy: {}/{} ({:.0f}%)".format(
- test_loss, correct, len(data_loader.dataset), 100.0 * correct / len(data_loader.dataset)
- )
- )
-
-
-def predict(input_sentence, model, classes, cached=False, device="cpu"):
- input_t = model.tokenizer.encode(input_sentence)
- input_t = torch.tensor([input_t], dtype=torch.long, device=device)
- if cached:
- input_t = model.avg_representation(input_t)
-
- log_probs = model(input_t).data.cpu().numpy().flatten().tolist()
- print("Input sentence:", input_sentence)
- print(
- "Predictions:",
- ", ".join("{}: {:.4f}".format(c, math.exp(log_prob)) for c, log_prob in zip(classes, log_probs)),
- )
-
-
-def get_cached_data_loader(dataset, batch_size, discriminator, shuffle=False, device="cpu"):
- data_loader = torch.utils.data.DataLoader(dataset=dataset, batch_size=batch_size, collate_fn=collate_fn)
-
- xs = []
- ys = []
- for batch_idx, (x, y) in enumerate(tqdm(data_loader, ascii=True)):
- with torch.no_grad():
- x = x.to(device)
- avg_rep = discriminator.avg_representation(x).cpu().detach()
- avg_rep_list = torch.unbind(avg_rep.unsqueeze(1))
- xs += avg_rep_list
- ys += y.cpu().numpy().tolist()
-
- data_loader = torch.utils.data.DataLoader(
- dataset=Dataset(xs, ys), batch_size=batch_size, shuffle=shuffle, collate_fn=cached_collate_fn
- )
-
- return data_loader
-
-
-def train_discriminator(
- dataset,
- dataset_fp=None,
- pretrained_model="gpt2-medium",
- epochs=10,
- batch_size=64,
- log_interval=10,
- save_model=False,
- cached=False,
- no_cuda=False,
-):
- device = "cuda" if torch.cuda.is_available() and not no_cuda else "cpu"
-
- print("Preprocessing {} dataset...".format(dataset))
- start = time.time()
-
- if dataset == "SST":
- idx2class = ["positive", "negative", "very positive", "very negative", "neutral"]
- class2idx = {c: i for i, c in enumerate(idx2class)}
-
- discriminator = Discriminator(
- class_size=len(idx2class), pretrained_model=pretrained_model, cached_mode=cached, device=device
- ).to(device)
-
- text = torchtext_data.Field()
- label = torchtext_data.Field(sequential=False)
- train_data, val_data, test_data = datasets.SST.splits(text, label, fine_grained=True, train_subtrees=True,)
-
- x = []
- y = []
- for i in trange(len(train_data), ascii=True):
- seq = TreebankWordDetokenizer().detokenize(vars(train_data[i])["text"])
- seq = discriminator.tokenizer.encode(seq)
- seq = torch.tensor([50256] + seq, device=device, dtype=torch.long)
- x.append(seq)
- y.append(class2idx[vars(train_data[i])["label"]])
- train_dataset = Dataset(x, y)
-
- test_x = []
- test_y = []
- for i in trange(len(test_data), ascii=True):
- seq = TreebankWordDetokenizer().detokenize(vars(test_data[i])["text"])
- seq = discriminator.tokenizer.encode(seq)
- seq = torch.tensor([50256] + seq, device=device, dtype=torch.long)
- test_x.append(seq)
- test_y.append(class2idx[vars(test_data[i])["label"]])
- test_dataset = Dataset(test_x, test_y)
-
- discriminator_meta = {
- "class_size": len(idx2class),
- "embed_size": discriminator.embed_size,
- "pretrained_model": pretrained_model,
- "class_vocab": class2idx,
- "default_class": 2,
- }
-
- elif dataset == "clickbait":
- idx2class = ["non_clickbait", "clickbait"]
- class2idx = {c: i for i, c in enumerate(idx2class)}
-
- discriminator = Discriminator(
- class_size=len(idx2class), pretrained_model=pretrained_model, cached_mode=cached, device=device
- ).to(device)
-
- with open("datasets/clickbait/clickbait_train_prefix.txt") as f:
- data = []
- for i, line in enumerate(f):
- try:
- data.append(eval(line))
- except Exception:
- print("Error evaluating line {}: {}".format(i, line))
- continue
- x = []
- y = []
- with open("datasets/clickbait/clickbait_train_prefix.txt") as f:
- for i, line in enumerate(tqdm(f, ascii=True)):
- try:
- d = eval(line)
- seq = discriminator.tokenizer.encode(d["text"])
-
- if len(seq) < max_length_seq:
- seq = torch.tensor([50256] + seq, device=device, dtype=torch.long)
- else:
- print("Line {} is longer than maximum length {}".format(i, max_length_seq))
- continue
- x.append(seq)
- y.append(d["label"])
- except Exception:
- print("Error evaluating / tokenizing" " line {}, skipping it".format(i))
- pass
-
- full_dataset = Dataset(x, y)
- train_size = int(0.9 * len(full_dataset))
- test_size = len(full_dataset) - train_size
- train_dataset, test_dataset = torch.utils.data.random_split(full_dataset, [train_size, test_size])
-
- discriminator_meta = {
- "class_size": len(idx2class),
- "embed_size": discriminator.embed_size,
- "pretrained_model": pretrained_model,
- "class_vocab": class2idx,
- "default_class": 1,
- }
-
- elif dataset == "toxic":
- idx2class = ["non_toxic", "toxic"]
- class2idx = {c: i for i, c in enumerate(idx2class)}
-
- discriminator = Discriminator(
- class_size=len(idx2class), pretrained_model=pretrained_model, cached_mode=cached, device=device
- ).to(device)
-
- x = []
- y = []
- with open("datasets/toxic/toxic_train.txt") as f:
- for i, line in enumerate(tqdm(f, ascii=True)):
- try:
- d = eval(line)
- seq = discriminator.tokenizer.encode(d["text"])
-
- if len(seq) < max_length_seq:
- seq = torch.tensor([50256] + seq, device=device, dtype=torch.long)
- else:
- print("Line {} is longer than maximum length {}".format(i, max_length_seq))
- continue
- x.append(seq)
- y.append(int(np.sum(d["label"]) > 0))
- except Exception:
- print("Error evaluating / tokenizing" " line {}, skipping it".format(i))
- pass
-
- full_dataset = Dataset(x, y)
- train_size = int(0.9 * len(full_dataset))
- test_size = len(full_dataset) - train_size
- train_dataset, test_dataset = torch.utils.data.random_split(full_dataset, [train_size, test_size])
-
- discriminator_meta = {
- "class_size": len(idx2class),
- "embed_size": discriminator.embed_size,
- "pretrained_model": pretrained_model,
- "class_vocab": class2idx,
- "default_class": 0,
- }
-
- else: # if dataset == "generic":
- # This assumes the input dataset is a TSV with the following structure:
- # class \t text
-
- if dataset_fp is None:
- raise ValueError("When generic dataset is selected, " "dataset_fp needs to be specified aswell.")
-
- classes = set()
- with open(dataset_fp) as f:
- csv_reader = csv.reader(f, delimiter="\t")
- for row in tqdm(csv_reader, ascii=True):
- if row:
- classes.add(row[0])
-
- idx2class = sorted(classes)
- class2idx = {c: i for i, c in enumerate(idx2class)}
-
- discriminator = Discriminator(
- class_size=len(idx2class), pretrained_model=pretrained_model, cached_mode=cached, device=device
- ).to(device)
-
- x = []
- y = []
- with open(dataset_fp) as f:
- csv_reader = csv.reader(f, delimiter="\t")
- for i, row in enumerate(tqdm(csv_reader, ascii=True)):
- if row:
- label = row[0]
- text = row[1]
-
- try:
- seq = discriminator.tokenizer.encode(text)
- if len(seq) < max_length_seq:
- seq = torch.tensor([50256] + seq, device=device, dtype=torch.long)
-
- else:
- print("Line {} is longer than maximum length {}".format(i, max_length_seq))
- continue
-
- x.append(seq)
- y.append(class2idx[label])
-
- except Exception:
- print("Error tokenizing line {}, skipping it".format(i))
- pass
-
- full_dataset = Dataset(x, y)
- train_size = int(0.9 * len(full_dataset))
- test_size = len(full_dataset) - train_size
- train_dataset, test_dataset = torch.utils.data.random_split(full_dataset, [train_size, test_size])
-
- discriminator_meta = {
- "class_size": len(idx2class),
- "embed_size": discriminator.embed_size,
- "pretrained_model": pretrained_model,
- "class_vocab": class2idx,
- "default_class": 0,
- }
-
- end = time.time()
- print("Preprocessed {} data points".format(len(train_dataset) + len(test_dataset)))
- print("Data preprocessing took: {:.3f}s".format(end - start))
-
- if cached:
- print("Building representation cache...")
-
- start = time.time()
-
- train_loader = get_cached_data_loader(train_dataset, batch_size, discriminator, shuffle=True, device=device)
-
- test_loader = get_cached_data_loader(test_dataset, batch_size, discriminator, device=device)
-
- end = time.time()
- print("Building representation cache took: {:.3f}s".format(end - start))
-
- else:
- train_loader = torch.utils.data.DataLoader(
- dataset=train_dataset, batch_size=batch_size, shuffle=True, collate_fn=collate_fn
- )
- test_loader = torch.utils.data.DataLoader(dataset=test_dataset, batch_size=batch_size, collate_fn=collate_fn)
-
- if save_model:
- with open("{}_classifier_head_meta.json".format(dataset), "w") as meta_file:
- json.dump(discriminator_meta, meta_file)
-
- optimizer = optim.Adam(discriminator.parameters(), lr=0.0001)
-
- for epoch in range(epochs):
- start = time.time()
- print("\nEpoch", epoch + 1)
-
- train_epoch(
- discriminator=discriminator,
- data_loader=train_loader,
- optimizer=optimizer,
- epoch=epoch,
- log_interval=log_interval,
- device=device,
- )
- evaluate_performance(data_loader=test_loader, discriminator=discriminator, device=device)
-
- end = time.time()
- print("Epoch took: {:.3f}s".format(end - start))
-
- print("\nExample prediction")
- predict(example_sentence, discriminator, idx2class, cached=cached, device=device)
-
- if save_model:
- # torch.save(discriminator.state_dict(),
- # "{}_discriminator_{}.pt".format(
- # args.dataset, epoch + 1
- # ))
- torch.save(
- discriminator.get_classifier().state_dict(),
- "{}_classifier_head_epoch_{}.pt".format(dataset, epoch + 1),
- )
-
-
-if __name__ == "__main__":
- parser = argparse.ArgumentParser(description="Train a discriminator on top of GPT-2 representations")
- parser.add_argument(
- "--dataset",
- type=str,
- default="SST",
- choices=("SST", "clickbait", "toxic", "generic"),
- help="dataset to train the discriminator on."
- "In case of generic, the dataset is expected"
- "to be a TSBV file with structure: class \\t text",
- )
- parser.add_argument(
- "--dataset_fp",
- type=str,
- default="",
- help="File path of the dataset to use. " "Needed only in case of generic datadset",
- )
- parser.add_argument(
- "--pretrained_model", type=str, default="gpt2-medium", help="Pretrained model to use as encoder"
- )
- parser.add_argument("--epochs", type=int, default=10, metavar="N", help="Number of training epochs")
- parser.add_argument(
- "--batch_size", type=int, default=64, metavar="N", help="input batch size for training (default: 64)"
- )
- parser.add_argument(
- "--log_interval",
- type=int,
- default=10,
- metavar="N",
- help="how many batches to wait before logging training status",
- )
- parser.add_argument("--save_model", action="store_true", help="whether to save the model")
- parser.add_argument("--cached", action="store_true", help="whether to cache the input representations")
- parser.add_argument("--no_cuda", action="store_true", help="use to turn off cuda")
- args = parser.parse_args()
-
- train_discriminator(**(vars(args)))
diff --git a/server/transformers/examples/requirements.txt b/server/transformers/examples/requirements.txt
deleted file mode 100644
index 36229755e81885681fd14a80eff8325cbc6053f5..0000000000000000000000000000000000000000
--- a/server/transformers/examples/requirements.txt
+++ /dev/null
@@ -1,4 +0,0 @@
-tensorboardX
-tensorboard
-scikit-learn
-seqeval
diff --git a/server/transformers/examples/run_bertology.py b/server/transformers/examples/run_bertology.py
deleted file mode 100644
index acac56128a05f6a8c05149234e474dc35ef348df..0000000000000000000000000000000000000000
--- a/server/transformers/examples/run_bertology.py
+++ /dev/null
@@ -1,426 +0,0 @@
-#!/usr/bin/env python3
-# Copyright 2018 CMU and The HuggingFace Inc. team.
-#
-# Licensed under the Apache License, Version 2.0 (the "License");
-# you may not use this file except in compliance with the License.
-# You may obtain a copy of the License at
-#
-# http://www.apache.org/licenses/LICENSE-2.0
-#
-# Unless required by applicable law or agreed to in writing, software
-# distributed under the License is distributed on an "AS IS" BASIS,
-# WITHOUT WARRANTIES OR CONDITIONS OF ANY KIND, either express or implied.
-# See the License for the specific language governing permissions and
-# limitations under the License.
-""" Bertology: this script shows how you can explore the internals of the models in the library to:
- - compute the entropy of the head attentions
- - compute the importance of each head
- - prune (remove) the low importance head.
- Some parts of this script are adapted from the code of Michel et al. (http://arxiv.org/abs/1905.10650)
- which is available at https://github.com/pmichel31415/are-16-heads-really-better-than-1
-"""
-import argparse
-import logging
-import os
-from datetime import datetime
-
-import numpy as np
-import torch
-from torch.utils.data import DataLoader, SequentialSampler, Subset
-from torch.utils.data.distributed import DistributedSampler
-from tqdm import tqdm
-
-from run_glue import ALL_MODELS, MODEL_CLASSES, load_and_cache_examples, set_seed
-from transformers import glue_compute_metrics as compute_metrics
-from transformers import glue_output_modes as output_modes
-from transformers import glue_processors as processors
-
-
-logger = logging.getLogger(__name__)
-
-
-def entropy(p):
- """ Compute the entropy of a probability distribution """
- plogp = p * torch.log(p)
- plogp[p == 0] = 0
- return -plogp.sum(dim=-1)
-
-
-def print_2d_tensor(tensor):
- """ Print a 2D tensor """
- logger.info("lv, h >\t" + "\t".join(f"{x + 1}" for x in range(len(tensor))))
- for row in range(len(tensor)):
- if tensor.dtype != torch.long:
- logger.info(f"layer {row + 1}:\t" + "\t".join(f"{x:.5f}" for x in tensor[row].cpu().data))
- else:
- logger.info(f"layer {row + 1}:\t" + "\t".join(f"{x:d}" for x in tensor[row].cpu().data))
-
-
-def compute_heads_importance(
- args, model, eval_dataloader, compute_entropy=True, compute_importance=True, head_mask=None
-):
- """ This method shows how to compute:
- - head attention entropy
- - head importance scores according to http://arxiv.org/abs/1905.10650
- """
- # Prepare our tensors
- n_layers, n_heads = model.bert.config.num_hidden_layers, model.bert.config.num_attention_heads
- head_importance = torch.zeros(n_layers, n_heads).to(args.device)
- attn_entropy = torch.zeros(n_layers, n_heads).to(args.device)
-
- if head_mask is None:
- head_mask = torch.ones(n_layers, n_heads).to(args.device)
- head_mask.requires_grad_(requires_grad=True)
- preds = None
- labels = None
- tot_tokens = 0.0
-
- for step, batch in enumerate(tqdm(eval_dataloader, desc="Iteration", disable=args.local_rank not in [-1, 0])):
- batch = tuple(t.to(args.device) for t in batch)
- input_ids, input_mask, segment_ids, label_ids = batch
-
- # Do a forward pass (not with torch.no_grad() since we need gradients for importance score - see below)
- outputs = model(
- input_ids, token_type_ids=segment_ids, attention_mask=input_mask, labels=label_ids, head_mask=head_mask
- )
- loss, logits, all_attentions = (
- outputs[0],
- outputs[1],
- outputs[-1],
- ) # Loss and logits are the first, attention the last
- loss.backward() # Backpropagate to populate the gradients in the head mask
-
- if compute_entropy:
- for layer, attn in enumerate(all_attentions):
- masked_entropy = entropy(attn.detach()) * input_mask.float().unsqueeze(1)
- attn_entropy[layer] += masked_entropy.sum(-1).sum(0).detach()
-
- if compute_importance:
- head_importance += head_mask.grad.abs().detach()
-
- # Also store our logits/labels if we want to compute metrics afterwards
- if preds is None:
- preds = logits.detach().cpu().numpy()
- labels = label_ids.detach().cpu().numpy()
- else:
- preds = np.append(preds, logits.detach().cpu().numpy(), axis=0)
- labels = np.append(labels, label_ids.detach().cpu().numpy(), axis=0)
-
- tot_tokens += input_mask.float().detach().sum().data
-
- # Normalize
- attn_entropy /= tot_tokens
- head_importance /= tot_tokens
- # Layerwise importance normalization
- if not args.dont_normalize_importance_by_layer:
- exponent = 2
- norm_by_layer = torch.pow(torch.pow(head_importance, exponent).sum(-1), 1 / exponent)
- head_importance /= norm_by_layer.unsqueeze(-1) + 1e-20
-
- if not args.dont_normalize_global_importance:
- head_importance = (head_importance - head_importance.min()) / (head_importance.max() - head_importance.min())
-
- # Print/save matrices
- np.save(os.path.join(args.output_dir, "attn_entropy.npy"), attn_entropy.detach().cpu().numpy())
- np.save(os.path.join(args.output_dir, "head_importance.npy"), head_importance.detach().cpu().numpy())
-
- logger.info("Attention entropies")
- print_2d_tensor(attn_entropy)
- logger.info("Head importance scores")
- print_2d_tensor(head_importance)
- logger.info("Head ranked by importance scores")
- head_ranks = torch.zeros(head_importance.numel(), dtype=torch.long, device=args.device)
- head_ranks[head_importance.view(-1).sort(descending=True)[1]] = torch.arange(
- head_importance.numel(), device=args.device
- )
- head_ranks = head_ranks.view_as(head_importance)
- print_2d_tensor(head_ranks)
-
- return attn_entropy, head_importance, preds, labels
-
-
-def mask_heads(args, model, eval_dataloader):
- """ This method shows how to mask head (set some heads to zero), to test the effect on the network,
- based on the head importance scores, as described in Michel et al. (http://arxiv.org/abs/1905.10650)
- """
- _, head_importance, preds, labels = compute_heads_importance(args, model, eval_dataloader, compute_entropy=False)
- preds = np.argmax(preds, axis=1) if args.output_mode == "classification" else np.squeeze(preds)
- original_score = compute_metrics(args.task_name, preds, labels)[args.metric_name]
- logger.info("Pruning: original score: %f, threshold: %f", original_score, original_score * args.masking_threshold)
-
- new_head_mask = torch.ones_like(head_importance)
- num_to_mask = max(1, int(new_head_mask.numel() * args.masking_amount))
-
- current_score = original_score
- while current_score >= original_score * args.masking_threshold:
- head_mask = new_head_mask.clone() # save current head mask
- # heads from least important to most - keep only not-masked heads
- head_importance[head_mask == 0.0] = float("Inf")
- current_heads_to_mask = head_importance.view(-1).sort()[1]
-
- if len(current_heads_to_mask) <= num_to_mask:
- break
-
- # mask heads
- current_heads_to_mask = current_heads_to_mask[:num_to_mask]
- logger.info("Heads to mask: %s", str(current_heads_to_mask.tolist()))
- new_head_mask = new_head_mask.view(-1)
- new_head_mask[current_heads_to_mask] = 0.0
- new_head_mask = new_head_mask.view_as(head_mask)
- print_2d_tensor(new_head_mask)
-
- # Compute metric and head importance again
- _, head_importance, preds, labels = compute_heads_importance(
- args, model, eval_dataloader, compute_entropy=False, head_mask=new_head_mask
- )
- preds = np.argmax(preds, axis=1) if args.output_mode == "classification" else np.squeeze(preds)
- current_score = compute_metrics(args.task_name, preds, labels)[args.metric_name]
- logger.info(
- "Masking: current score: %f, remaning heads %d (%.1f percents)",
- current_score,
- new_head_mask.sum(),
- new_head_mask.sum() / new_head_mask.numel() * 100,
- )
-
- logger.info("Final head mask")
- print_2d_tensor(head_mask)
- np.save(os.path.join(args.output_dir, "head_mask.npy"), head_mask.detach().cpu().numpy())
-
- return head_mask
-
-
-def prune_heads(args, model, eval_dataloader, head_mask):
- """ This method shows how to prune head (remove heads weights) based on
- the head importance scores as described in Michel et al. (http://arxiv.org/abs/1905.10650)
- """
- # Try pruning and test time speedup
- # Pruning is like masking but we actually remove the masked weights
- before_time = datetime.now()
- _, _, preds, labels = compute_heads_importance(
- args, model, eval_dataloader, compute_entropy=False, compute_importance=False, head_mask=head_mask
- )
- preds = np.argmax(preds, axis=1) if args.output_mode == "classification" else np.squeeze(preds)
- score_masking = compute_metrics(args.task_name, preds, labels)[args.metric_name]
- original_time = datetime.now() - before_time
-
- original_num_params = sum(p.numel() for p in model.parameters())
- heads_to_prune = dict((layer, (1 - head_mask[layer].long()).nonzero().tolist()) for layer in range(len(head_mask)))
- assert sum(len(h) for h in heads_to_prune.values()) == (1 - head_mask.long()).sum().item()
- model.prune_heads(heads_to_prune)
- pruned_num_params = sum(p.numel() for p in model.parameters())
-
- before_time = datetime.now()
- _, _, preds, labels = compute_heads_importance(
- args, model, eval_dataloader, compute_entropy=False, compute_importance=False, head_mask=None
- )
- preds = np.argmax(preds, axis=1) if args.output_mode == "classification" else np.squeeze(preds)
- score_pruning = compute_metrics(args.task_name, preds, labels)[args.metric_name]
- new_time = datetime.now() - before_time
-
- logger.info(
- "Pruning: original num of params: %.2e, after pruning %.2e (%.1f percents)",
- original_num_params,
- pruned_num_params,
- pruned_num_params / original_num_params * 100,
- )
- logger.info("Pruning: score with masking: %f score with pruning: %f", score_masking, score_pruning)
- logger.info("Pruning: speed ratio (new timing / original timing): %f percents", original_time / new_time * 100)
-
-
-def main():
- parser = argparse.ArgumentParser()
- # Required parameters
- parser.add_argument(
- "--data_dir",
- default=None,
- type=str,
- required=True,
- help="The input data dir. Should contain the .tsv files (or other data files) for the task.",
- )
- parser.add_argument(
- "--model_name_or_path",
- default=None,
- type=str,
- required=True,
- help="Path to pre-trained model or shortcut name selected in the list: " + ", ".join(ALL_MODELS),
- )
- parser.add_argument(
- "--task_name",
- default=None,
- type=str,
- required=True,
- help="The name of the task to train selected in the list: " + ", ".join(processors.keys()),
- )
- parser.add_argument(
- "--output_dir",
- default=None,
- type=str,
- required=True,
- help="The output directory where the model predictions and checkpoints will be written.",
- )
-
- # Other parameters
- parser.add_argument(
- "--config_name",
- default="",
- type=str,
- help="Pretrained config name or path if not the same as model_name_or_path",
- )
- parser.add_argument(
- "--tokenizer_name",
- default="",
- type=str,
- help="Pretrained tokenizer name or path if not the same as model_name_or_path",
- )
- parser.add_argument(
- "--cache_dir",
- default="",
- type=str,
- help="Where do you want to store the pre-trained models downloaded from s3",
- )
- parser.add_argument(
- "--data_subset", type=int, default=-1, help="If > 0: limit the data to a subset of data_subset instances."
- )
- parser.add_argument(
- "--overwrite_output_dir", action="store_true", help="Whether to overwrite data in output directory"
- )
- parser.add_argument(
- "--overwrite_cache", action="store_true", help="Overwrite the cached training and evaluation sets"
- )
-
- parser.add_argument(
- "--dont_normalize_importance_by_layer", action="store_true", help="Don't normalize importance score by layers"
- )
- parser.add_argument(
- "--dont_normalize_global_importance",
- action="store_true",
- help="Don't normalize all importance scores between 0 and 1",
- )
-
- parser.add_argument(
- "--try_masking", action="store_true", help="Whether to try to mask head until a threshold of accuracy."
- )
- parser.add_argument(
- "--masking_threshold",
- default=0.9,
- type=float,
- help="masking threshold in term of metrics (stop masking when metric < threshold * original metric value).",
- )
- parser.add_argument(
- "--masking_amount", default=0.1, type=float, help="Amount to heads to masking at each masking step."
- )
- parser.add_argument("--metric_name", default="acc", type=str, help="Metric to use for head masking.")
-
- parser.add_argument(
- "--max_seq_length",
- default=128,
- type=int,
- help="The maximum total input sequence length after WordPiece tokenization. \n"
- "Sequences longer than this will be truncated, sequences shorter padded.",
- )
- parser.add_argument("--batch_size", default=1, type=int, help="Batch size.")
-
- parser.add_argument("--seed", type=int, default=42)
- parser.add_argument("--local_rank", type=int, default=-1, help="local_rank for distributed training on gpus")
- parser.add_argument("--no_cuda", action="store_true", help="Whether not to use CUDA when available")
- parser.add_argument("--server_ip", type=str, default="", help="Can be used for distant debugging.")
- parser.add_argument("--server_port", type=str, default="", help="Can be used for distant debugging.")
- args = parser.parse_args()
-
- if args.server_ip and args.server_port:
- # Distant debugging - see https://code.visualstudio.com/docs/python/debugging#_attach-to-a-local-script
- import ptvsd
-
- print("Waiting for debugger attach")
- ptvsd.enable_attach(address=(args.server_ip, args.server_port), redirect_output=True)
- ptvsd.wait_for_attach()
-
- # Setup devices and distributed training
- if args.local_rank == -1 or args.no_cuda:
- args.device = torch.device("cuda" if torch.cuda.is_available() and not args.no_cuda else "cpu")
- args.n_gpu = torch.cuda.device_count()
- else:
- torch.cuda.set_device(args.local_rank)
- args.device = torch.device("cuda", args.local_rank)
- args.n_gpu = 1
- torch.distributed.init_process_group(backend="nccl") # Initializes the distributed backend
-
- # Setup logging
- logging.basicConfig(level=logging.INFO if args.local_rank in [-1, 0] else logging.WARN)
- logger.info("device: {} n_gpu: {}, distributed: {}".format(args.device, args.n_gpu, bool(args.local_rank != -1)))
-
- # Set seeds
- set_seed(args)
-
- # Prepare GLUE task
- args.task_name = args.task_name.lower()
- if args.task_name not in processors:
- raise ValueError("Task not found: %s" % (args.task_name))
- processor = processors[args.task_name]()
- args.output_mode = output_modes[args.task_name]
- label_list = processor.get_labels()
- num_labels = len(label_list)
-
- # Load pretrained model and tokenizer
- if args.local_rank not in [-1, 0]:
- torch.distributed.barrier() # Make sure only the first process in distributed training will download model & vocab
-
- args.model_type = ""
- for key in MODEL_CLASSES:
- if key in args.model_name_or_path.lower():
- args.model_type = key # take the first match in model types
- break
- config_class, model_class, tokenizer_class = MODEL_CLASSES[args.model_type]
- config = config_class.from_pretrained(
- args.config_name if args.config_name else args.model_name_or_path,
- num_labels=num_labels,
- finetuning_task=args.task_name,
- output_attentions=True,
- cache_dir=args.cache_dir if args.cache_dir else None,
- )
- tokenizer = tokenizer_class.from_pretrained(
- args.tokenizer_name if args.tokenizer_name else args.model_name_or_path,
- cache_dir=args.cache_dir if args.cache_dir else None,
- )
- model = model_class.from_pretrained(
- args.model_name_or_path,
- from_tf=bool(".ckpt" in args.model_name_or_path),
- config=config,
- cache_dir=args.cache_dir if args.cache_dir else None,
- )
-
- if args.local_rank == 0:
- torch.distributed.barrier() # Make sure only the first process in distributed training will download model & vocab
-
- # Distributed and parallel training
- model.to(args.device)
- if args.local_rank != -1:
- model = torch.nn.parallel.DistributedDataParallel(
- model, device_ids=[args.local_rank], output_device=args.local_rank, find_unused_parameters=True
- )
- elif args.n_gpu > 1:
- model = torch.nn.DataParallel(model)
-
- # Print/save training arguments
- torch.save(args, os.path.join(args.output_dir, "run_args.bin"))
- logger.info("Training/evaluation parameters %s", args)
-
- # Prepare dataset for the GLUE task
- eval_data = load_and_cache_examples(args, args.task_name, tokenizer, evaluate=True)
- if args.data_subset > 0:
- eval_data = Subset(eval_data, list(range(min(args.data_subset, len(eval_data)))))
- eval_sampler = SequentialSampler(eval_data) if args.local_rank == -1 else DistributedSampler(eval_data)
- eval_dataloader = DataLoader(eval_data, sampler=eval_sampler, batch_size=args.batch_size)
-
- # Compute head entropy and importance score
- compute_heads_importance(args, model, eval_dataloader)
-
- # Try head masking (set heads to zero until the score goes under a threshole)
- # and head pruning (remove masked heads and see the effect on the network)
- if args.try_masking and args.masking_threshold > 0.0 and args.masking_threshold < 1.0:
- head_mask = mask_heads(args, model, eval_dataloader)
- prune_heads(args, model, eval_dataloader, head_mask)
-
-
-if __name__ == "__main__":
- main()
diff --git a/server/transformers/examples/run_generation.py b/server/transformers/examples/run_generation.py
deleted file mode 100644
index d074c9e2642c753bec2766f9317fd511c6a4e3a4..0000000000000000000000000000000000000000
--- a/server/transformers/examples/run_generation.py
+++ /dev/null
@@ -1,238 +0,0 @@
-#!/usr/bin/env python3
-# coding=utf-8
-# Copyright 2018 Google AI, Google Brain and Carnegie Mellon University Authors and the HuggingFace Inc. team.
-# Copyright (c) 2018, NVIDIA CORPORATION. All rights reserved.
-#
-# Licensed under the Apache License, Version 2.0 (the "License");
-# you may not use this file except in compliance with the License.
-# You may obtain a copy of the License at
-#
-# http://www.apache.org/licenses/LICENSE-2.0
-#
-# Unless required by applicable law or agreed to in writing, software
-# distributed under the License is distributed on an "AS IS" BASIS,
-# WITHOUT WARRANTIES OR CONDITIONS OF ANY KIND, either express or implied.
-# See the License for the specific language governing permissions and
-# limitations under the License.
-""" Conditional text generation with the auto-regressive models of the library (GPT/GPT-2/CTRL/Transformer-XL/XLNet)
-"""
-
-
-import argparse
-import logging
-
-import numpy as np
-import torch
-
-from transformers import (
- CTRLLMHeadModel,
- CTRLTokenizer,
- GPT2LMHeadModel,
- GPT2Tokenizer,
- OpenAIGPTLMHeadModel,
- OpenAIGPTTokenizer,
- TransfoXLLMHeadModel,
- TransfoXLTokenizer,
- XLMTokenizer,
- XLMWithLMHeadModel,
- XLNetLMHeadModel,
- XLNetTokenizer,
-)
-
-
-logging.basicConfig(
- format="%(asctime)s - %(levelname)s - %(name)s - %(message)s", datefmt="%m/%d/%Y %H:%M:%S", level=logging.INFO,
-)
-logger = logging.getLogger(__name__)
-
-MAX_LENGTH = int(10000) # Hardcoded max length to avoid infinite loop
-
-MODEL_CLASSES = {
- "gpt2": (GPT2LMHeadModel, GPT2Tokenizer),
- "ctrl": (CTRLLMHeadModel, CTRLTokenizer),
- "openai-gpt": (OpenAIGPTLMHeadModel, OpenAIGPTTokenizer),
- "xlnet": (XLNetLMHeadModel, XLNetTokenizer),
- "transfo-xl": (TransfoXLLMHeadModel, TransfoXLTokenizer),
- "xlm": (XLMWithLMHeadModel, XLMTokenizer),
-}
-
-# Padding text to help Transformer-XL and XLNet with short prompts as proposed by Aman Rusia
-# in https://github.com/rusiaaman/XLNet-gen#methodology
-# and https://medium.com/@amanrusia/xlnet-speaks-comparison-to-gpt-2-ea1a4e9ba39e
-PADDING_TEXT = """ In 1991, the remains of Russian Tsar Nicholas II and his family
-(except for Alexei and Maria) are discovered.
-The voice of Nicholas's young son, Tsarevich Alexei Nikolaevich, narrates the
-remainder of the story. 1883 Western Siberia,
-a young Grigori Rasputin is asked by his father and a group of men to perform magic.
-Rasputin has a vision and denounces one of the men as a horse thief. Although his
-father initially slaps him for making such an accusation, Rasputin watches as the
-man is chased outside and beaten. Twenty years later, Rasputin sees a vision of
-the Virgin Mary, prompting him to become a priest. Rasputin quickly becomes famous,
-with people, even a bishop, begging for his blessing. """
-
-
-def set_seed(args):
- np.random.seed(args.seed)
- torch.manual_seed(args.seed)
- if args.n_gpu > 0:
- torch.cuda.manual_seed_all(args.seed)
-
-
-#
-# Functions to prepare models' input
-#
-
-
-def prepare_ctrl_input(args, _, tokenizer, prompt_text):
- if args.temperature > 0.7:
- logger.info("CTRL typically works better with lower temperatures (and lower top_k).")
-
- encoded_prompt = tokenizer.encode(prompt_text, add_special_tokens=False)
- if not any(encoded_prompt[0] == x for x in tokenizer.control_codes.values()):
- logger.info("WARNING! You are not starting your generation from a control code so you won't get good results")
- return prompt_text
-
-
-def prepare_xlm_input(args, model, tokenizer, prompt_text):
- # kwargs = {"language": None, "mask_token_id": None}
-
- # Set the language
- use_lang_emb = hasattr(model.config, "use_lang_emb") and model.config.use_lang_emb
- if hasattr(model.config, "lang2id") and use_lang_emb:
- available_languages = model.config.lang2id.keys()
- if args.xlm_language in available_languages:
- language = args.xlm_language
- else:
- language = None
- while language not in available_languages:
- language = input("Using XLM. Select language in " + str(list(available_languages)) + " >>> ")
- # kwargs["language"] = tokenizer.lang2id[language]
-
- # TODO fix mask_token_id setup when configurations will be synchronized between models and tokenizers
- # XLM masked-language modeling (MLM) models need masked token
- # is_xlm_mlm = "mlm" in args.model_name_or_path
- # if is_xlm_mlm:
- # kwargs["mask_token_id"] = tokenizer.mask_token_id
-
- return prompt_text
-
-
-def prepare_xlnet_input(args, _, tokenizer, prompt_text):
- prompt_text = (args.padding_text if args.padding_text else PADDING_TEXT) + prompt_text
- return prompt_text, {}
-
-
-def prepare_transfoxl_input(args, _, tokenizer, prompt_text):
- prompt_text = (args.padding_text if args.padding_text else PADDING_TEXT) + prompt_text
- return prompt_text, {}
-
-
-PREPROCESSING_FUNCTIONS = {
- "ctrl": prepare_ctrl_input,
- "xlm": prepare_xlm_input,
- "xlnet": prepare_xlnet_input,
- "transfo-xl": prepare_transfoxl_input,
-}
-
-
-def adjust_length_to_model(length, max_sequence_length):
- if length < 0 and max_sequence_length > 0:
- length = max_sequence_length
- elif 0 < max_sequence_length < length:
- length = max_sequence_length # No generation bigger than model size
- elif length < 0:
- length = MAX_LENGTH # avoid infinite loop
- return length
-
-
-def main():
- parser = argparse.ArgumentParser()
- parser.add_argument(
- "--model_type",
- default=None,
- type=str,
- required=True,
- help="Model type selected in the list: " + ", ".join(MODEL_CLASSES.keys()),
- )
- parser.add_argument(
- "--model_name_or_path",
- default=None,
- type=str,
- required=True,
- help="Path to pre-trained model or shortcut name selected in the list: " + ", ".join(MODEL_CLASSES.keys()),
- )
-
- parser.add_argument("--prompt", type=str, default="")
- parser.add_argument("--length", type=int, default=20)
- parser.add_argument("--stop_token", type=str, default=None, help="Token at which text generation is stopped")
-
- parser.add_argument(
- "--temperature",
- type=float,
- default=1.0,
- help="temperature of 1.0 has no effect, lower tend toward greedy sampling",
- )
- parser.add_argument(
- "--repetition_penalty", type=float, default=1.0, help="primarily useful for CTRL model; in that case, use 1.2"
- )
- parser.add_argument("--k", type=int, default=0)
- parser.add_argument("--p", type=float, default=0.9)
-
- parser.add_argument("--padding_text", type=str, default="", help="Padding text for Transfo-XL and XLNet.")
- parser.add_argument("--xlm_language", type=str, default="", help="Optional language when used with the XLM model.")
-
- parser.add_argument("--seed", type=int, default=42, help="random seed for initialization")
- parser.add_argument("--no_cuda", action="store_true", help="Avoid using CUDA when available")
- args = parser.parse_args()
-
- args.device = torch.device("cuda" if torch.cuda.is_available() and not args.no_cuda else "cpu")
- args.n_gpu = torch.cuda.device_count()
-
- set_seed(args)
-
- # Initialize the model and tokenizer
- try:
- args.model_type = args.model_type.lower()
- model_class, tokenizer_class = MODEL_CLASSES[args.model_type]
- except KeyError:
- raise KeyError("the model {} you specified is not supported. You are welcome to add it and open a PR :)")
-
- tokenizer = tokenizer_class.from_pretrained(args.model_name_or_path)
- model = model_class.from_pretrained(args.model_name_or_path)
- model.to(args.device)
-
- args.length = adjust_length_to_model(args.length, max_sequence_length=model.config.max_position_embeddings)
- logger.info(args)
-
- prompt_text = args.prompt if args.prompt else input("Model prompt >>> ")
-
- # Different models need different input formatting and/or extra arguments
- requires_preprocessing = args.model_type in PREPROCESSING_FUNCTIONS.keys()
- if requires_preprocessing:
- prepare_input = PREPROCESSING_FUNCTIONS.get(args.model_type)
- prompt_text = prepare_input(args, model, tokenizer, prompt_text)
- encoded_prompt = tokenizer.encode(prompt_text, add_special_tokens=False, return_tensors="pt")
- encoded_prompt = encoded_prompt.to(args.device)
-
- output_sequences = model.generate(
- input_ids=encoded_prompt,
- max_length=args.length,
- temperature=args.temperature,
- top_k=args.k,
- top_p=args.p,
- repetition_penalty=args.repetition_penalty,
- do_sample=True,
- )
-
- # Batch size == 1. to add more examples please use num_return_sequences > 1
- generated_sequence = output_sequences[0].tolist()
- text = tokenizer.decode(generated_sequence, clean_up_tokenization_spaces=True)
- text = text[: text.find(args.stop_token) if args.stop_token else None]
-
- print(text)
-
- return text
-
-
-if __name__ == "__main__":
- main()
diff --git a/server/transformers/examples/run_glue.py b/server/transformers/examples/run_glue.py
deleted file mode 100644
index dc8f66434bb8377050ea02396c0bcbe8e96fb1ff..0000000000000000000000000000000000000000
--- a/server/transformers/examples/run_glue.py
+++ /dev/null
@@ -1,698 +0,0 @@
-# coding=utf-8
-# Copyright 2018 The Google AI Language Team Authors and The HuggingFace Inc. team.
-# Copyright (c) 2018, NVIDIA CORPORATION. All rights reserved.
-#
-# Licensed under the Apache License, Version 2.0 (the "License");
-# you may not use this file except in compliance with the License.
-# You may obtain a copy of the License at
-#
-# http://www.apache.org/licenses/LICENSE-2.0
-#
-# Unless required by applicable law or agreed to in writing, software
-# distributed under the License is distributed on an "AS IS" BASIS,
-# WITHOUT WARRANTIES OR CONDITIONS OF ANY KIND, either express or implied.
-# See the License for the specific language governing permissions and
-# limitations under the License.
-""" Finetuning the library models for sequence classification on GLUE (Bert, XLM, XLNet, RoBERTa, Albert, XLM-RoBERTa)."""
-
-
-import argparse
-import glob
-import json
-import logging
-import os
-import random
-
-import numpy as np
-import torch
-from torch.utils.data import DataLoader, RandomSampler, SequentialSampler, TensorDataset
-from torch.utils.data.distributed import DistributedSampler
-from tqdm import tqdm, trange
-
-from transformers import (
- WEIGHTS_NAME,
- AdamW,
- AlbertConfig,
- AlbertForSequenceClassification,
- AlbertTokenizer,
- BertConfig,
- BertForSequenceClassification,
- BertTokenizer,
- DistilBertConfig,
- DistilBertForSequenceClassification,
- DistilBertTokenizer,
- FlaubertConfig,
- FlaubertForSequenceClassification,
- FlaubertTokenizer,
- RobertaConfig,
- RobertaForSequenceClassification,
- RobertaTokenizer,
- XLMConfig,
- XLMForSequenceClassification,
- XLMRobertaConfig,
- XLMRobertaForSequenceClassification,
- XLMRobertaTokenizer,
- XLMTokenizer,
- XLNetConfig,
- XLNetForSequenceClassification,
- XLNetTokenizer,
- get_linear_schedule_with_warmup,
-)
-from transformers import glue_compute_metrics as compute_metrics
-from transformers import glue_convert_examples_to_features as convert_examples_to_features
-from transformers import glue_output_modes as output_modes
-from transformers import glue_processors as processors
-
-
-try:
- from torch.utils.tensorboard import SummaryWriter
-except ImportError:
- from tensorboardX import SummaryWriter
-
-
-logger = logging.getLogger(__name__)
-
-ALL_MODELS = sum(
- (
- tuple(conf.pretrained_config_archive_map.keys())
- for conf in (
- BertConfig,
- XLNetConfig,
- XLMConfig,
- RobertaConfig,
- DistilBertConfig,
- AlbertConfig,
- XLMRobertaConfig,
- FlaubertConfig,
- )
- ),
- (),
-)
-
-MODEL_CLASSES = {
- "bert": (BertConfig, BertForSequenceClassification, BertTokenizer),
- "xlnet": (XLNetConfig, XLNetForSequenceClassification, XLNetTokenizer),
- "xlm": (XLMConfig, XLMForSequenceClassification, XLMTokenizer),
- "roberta": (RobertaConfig, RobertaForSequenceClassification, RobertaTokenizer),
- "distilbert": (DistilBertConfig, DistilBertForSequenceClassification, DistilBertTokenizer),
- "albert": (AlbertConfig, AlbertForSequenceClassification, AlbertTokenizer),
- "xlmroberta": (XLMRobertaConfig, XLMRobertaForSequenceClassification, XLMRobertaTokenizer),
- "flaubert": (FlaubertConfig, FlaubertForSequenceClassification, FlaubertTokenizer),
-}
-
-
-def set_seed(args):
- random.seed(args.seed)
- np.random.seed(args.seed)
- torch.manual_seed(args.seed)
- if args.n_gpu > 0:
- torch.cuda.manual_seed_all(args.seed)
-
-
-def train(args, train_dataset, model, tokenizer):
- """ Train the model """
- if args.local_rank in [-1, 0]:
- tb_writer = SummaryWriter()
-
- args.train_batch_size = args.per_gpu_train_batch_size * max(1, args.n_gpu)
- train_sampler = RandomSampler(train_dataset) if args.local_rank == -1 else DistributedSampler(train_dataset)
- train_dataloader = DataLoader(train_dataset, sampler=train_sampler, batch_size=args.train_batch_size)
-
- if args.max_steps > 0:
- t_total = args.max_steps
- args.num_train_epochs = args.max_steps // (len(train_dataloader) // args.gradient_accumulation_steps) + 1
- else:
- t_total = len(train_dataloader) // args.gradient_accumulation_steps * args.num_train_epochs
-
- # Prepare optimizer and schedule (linear warmup and decay)
- no_decay = ["bias", "LayerNorm.weight"]
- optimizer_grouped_parameters = [
- {
- "params": [p for n, p in model.named_parameters() if not any(nd in n for nd in no_decay)],
- "weight_decay": args.weight_decay,
- },
- {"params": [p for n, p in model.named_parameters() if any(nd in n for nd in no_decay)], "weight_decay": 0.0},
- ]
-
- optimizer = AdamW(optimizer_grouped_parameters, lr=args.learning_rate, eps=args.adam_epsilon)
- scheduler = get_linear_schedule_with_warmup(
- optimizer, num_warmup_steps=args.warmup_steps, num_training_steps=t_total
- )
-
- # Check if saved optimizer or scheduler states exist
- if os.path.isfile(os.path.join(args.model_name_or_path, "optimizer.pt")) and os.path.isfile(
- os.path.join(args.model_name_or_path, "scheduler.pt")
- ):
- # Load in optimizer and scheduler states
- optimizer.load_state_dict(torch.load(os.path.join(args.model_name_or_path, "optimizer.pt")))
- scheduler.load_state_dict(torch.load(os.path.join(args.model_name_or_path, "scheduler.pt")))
-
- if args.fp16:
- try:
- from apex import amp
- except ImportError:
- raise ImportError("Please install apex from https://www.github.com/nvidia/apex to use fp16 training.")
- model, optimizer = amp.initialize(model, optimizer, opt_level=args.fp16_opt_level)
-
- # multi-gpu training (should be after apex fp16 initialization)
- if args.n_gpu > 1:
- model = torch.nn.DataParallel(model)
-
- # Distributed training (should be after apex fp16 initialization)
- if args.local_rank != -1:
- model = torch.nn.parallel.DistributedDataParallel(
- model, device_ids=[args.local_rank], output_device=args.local_rank, find_unused_parameters=True,
- )
-
- # Train!
- logger.info("***** Running training *****")
- logger.info(" Num examples = %d", len(train_dataset))
- logger.info(" Num Epochs = %d", args.num_train_epochs)
- logger.info(" Instantaneous batch size per GPU = %d", args.per_gpu_train_batch_size)
- logger.info(
- " Total train batch size (w. parallel, distributed & accumulation) = %d",
- args.train_batch_size
- * args.gradient_accumulation_steps
- * (torch.distributed.get_world_size() if args.local_rank != -1 else 1),
- )
- logger.info(" Gradient Accumulation steps = %d", args.gradient_accumulation_steps)
- logger.info(" Total optimization steps = %d", t_total)
-
- global_step = 0
- epochs_trained = 0
- steps_trained_in_current_epoch = 0
- # Check if continuing training from a checkpoint
- if os.path.exists(args.model_name_or_path):
- # set global_step to gobal_step of last saved checkpoint from model path
- global_step = int(args.model_name_or_path.split("-")[-1].split("/")[0])
- epochs_trained = global_step // (len(train_dataloader) // args.gradient_accumulation_steps)
- steps_trained_in_current_epoch = global_step % (len(train_dataloader) // args.gradient_accumulation_steps)
-
- logger.info(" Continuing training from checkpoint, will skip to saved global_step")
- logger.info(" Continuing training from epoch %d", epochs_trained)
- logger.info(" Continuing training from global step %d", global_step)
- logger.info(" Will skip the first %d steps in the first epoch", steps_trained_in_current_epoch)
-
- tr_loss, logging_loss = 0.0, 0.0
- model.zero_grad()
- train_iterator = trange(
- epochs_trained, int(args.num_train_epochs), desc="Epoch", disable=args.local_rank not in [-1, 0],
- )
- set_seed(args) # Added here for reproductibility
- for _ in train_iterator:
- epoch_iterator = tqdm(train_dataloader, desc="Iteration", disable=args.local_rank not in [-1, 0])
- for step, batch in enumerate(epoch_iterator):
-
- # Skip past any already trained steps if resuming training
- if steps_trained_in_current_epoch > 0:
- steps_trained_in_current_epoch -= 1
- continue
-
- model.train()
- batch = tuple(t.to(args.device) for t in batch)
- inputs = {"input_ids": batch[0], "attention_mask": batch[1], "labels": batch[3]}
- if args.model_type != "distilbert":
- inputs["token_type_ids"] = (
- batch[2] if args.model_type in ["bert", "xlnet", "albert"] else None
- ) # XLM, DistilBERT, RoBERTa, and XLM-RoBERTa don't use segment_ids
- outputs = model(**inputs)
- loss = outputs[0] # model outputs are always tuple in transformers (see doc)
-
- if args.n_gpu > 1:
- loss = loss.mean() # mean() to average on multi-gpu parallel training
- if args.gradient_accumulation_steps > 1:
- loss = loss / args.gradient_accumulation_steps
-
- if args.fp16:
- with amp.scale_loss(loss, optimizer) as scaled_loss:
- scaled_loss.backward()
- else:
- loss.backward()
-
- tr_loss += loss.item()
- if (step + 1) % args.gradient_accumulation_steps == 0:
- if args.fp16:
- torch.nn.utils.clip_grad_norm_(amp.master_params(optimizer), args.max_grad_norm)
- else:
- torch.nn.utils.clip_grad_norm_(model.parameters(), args.max_grad_norm)
-
- optimizer.step()
- scheduler.step() # Update learning rate schedule
- model.zero_grad()
- global_step += 1
-
- if args.local_rank in [-1, 0] and args.logging_steps > 0 and global_step % args.logging_steps == 0:
- logs = {}
- if (
- args.local_rank == -1 and args.evaluate_during_training
- ): # Only evaluate when single GPU otherwise metrics may not average well
- results = evaluate(args, model, tokenizer)
- for key, value in results.items():
- eval_key = "eval_{}".format(key)
- logs[eval_key] = value
-
- loss_scalar = (tr_loss - logging_loss) / args.logging_steps
- learning_rate_scalar = scheduler.get_lr()[0]
- logs["learning_rate"] = learning_rate_scalar
- logs["loss"] = loss_scalar
- logging_loss = tr_loss
-
- for key, value in logs.items():
- tb_writer.add_scalar(key, value, global_step)
- print(json.dumps({**logs, **{"step": global_step}}))
-
- if args.local_rank in [-1, 0] and args.save_steps > 0 and global_step % args.save_steps == 0:
- # Save model checkpoint
- output_dir = os.path.join(args.output_dir, "checkpoint-{}".format(global_step))
- if not os.path.exists(output_dir):
- os.makedirs(output_dir)
- model_to_save = (
- model.module if hasattr(model, "module") else model
- ) # Take care of distributed/parallel training
- model_to_save.save_pretrained(output_dir)
- tokenizer.save_pretrained(output_dir)
-
- torch.save(args, os.path.join(output_dir, "training_args.bin"))
- logger.info("Saving model checkpoint to %s", output_dir)
-
- torch.save(optimizer.state_dict(), os.path.join(output_dir, "optimizer.pt"))
- torch.save(scheduler.state_dict(), os.path.join(output_dir, "scheduler.pt"))
- logger.info("Saving optimizer and scheduler states to %s", output_dir)
-
- if args.max_steps > 0 and global_step > args.max_steps:
- epoch_iterator.close()
- break
- if args.max_steps > 0 and global_step > args.max_steps:
- train_iterator.close()
- break
-
- if args.local_rank in [-1, 0]:
- tb_writer.close()
-
- return global_step, tr_loss / global_step
-
-
-def evaluate(args, model, tokenizer, prefix=""):
- # Loop to handle MNLI double evaluation (matched, mis-matched)
- eval_task_names = ("mnli", "mnli-mm") if args.task_name == "mnli" else (args.task_name,)
- eval_outputs_dirs = (args.output_dir, args.output_dir + "-MM") if args.task_name == "mnli" else (args.output_dir,)
-
- results = {}
- for eval_task, eval_output_dir in zip(eval_task_names, eval_outputs_dirs):
- eval_dataset = load_and_cache_examples(args, eval_task, tokenizer, evaluate=True)
-
- if not os.path.exists(eval_output_dir) and args.local_rank in [-1, 0]:
- os.makedirs(eval_output_dir)
-
- args.eval_batch_size = args.per_gpu_eval_batch_size * max(1, args.n_gpu)
- # Note that DistributedSampler samples randomly
- eval_sampler = SequentialSampler(eval_dataset)
- eval_dataloader = DataLoader(eval_dataset, sampler=eval_sampler, batch_size=args.eval_batch_size)
-
- # multi-gpu eval
- if args.n_gpu > 1:
- model = torch.nn.DataParallel(model)
-
- # Eval!
- logger.info("***** Running evaluation {} *****".format(prefix))
- logger.info(" Num examples = %d", len(eval_dataset))
- logger.info(" Batch size = %d", args.eval_batch_size)
- eval_loss = 0.0
- nb_eval_steps = 0
- preds = None
- out_label_ids = None
- for batch in tqdm(eval_dataloader, desc="Evaluating"):
- model.eval()
- batch = tuple(t.to(args.device) for t in batch)
-
- with torch.no_grad():
- inputs = {"input_ids": batch[0], "attention_mask": batch[1], "labels": batch[3]}
- if args.model_type != "distilbert":
- inputs["token_type_ids"] = (
- batch[2] if args.model_type in ["bert", "xlnet", "albert"] else None
- ) # XLM, DistilBERT, RoBERTa, and XLM-RoBERTa don't use segment_ids
- outputs = model(**inputs)
- tmp_eval_loss, logits = outputs[:2]
-
- eval_loss += tmp_eval_loss.mean().item()
- nb_eval_steps += 1
- if preds is None:
- preds = logits.detach().cpu().numpy()
- out_label_ids = inputs["labels"].detach().cpu().numpy()
- else:
- preds = np.append(preds, logits.detach().cpu().numpy(), axis=0)
- out_label_ids = np.append(out_label_ids, inputs["labels"].detach().cpu().numpy(), axis=0)
-
- eval_loss = eval_loss / nb_eval_steps
- if args.output_mode == "classification":
- preds = np.argmax(preds, axis=1)
- elif args.output_mode == "regression":
- preds = np.squeeze(preds)
- result = compute_metrics(eval_task, preds, out_label_ids)
- results.update(result)
-
- output_eval_file = os.path.join(eval_output_dir, prefix, "eval_results.txt")
- with open(output_eval_file, "w") as writer:
- logger.info("***** Eval results {} *****".format(prefix))
- for key in sorted(result.keys()):
- logger.info(" %s = %s", key, str(result[key]))
- writer.write("%s = %s\n" % (key, str(result[key])))
-
- return results
-
-
-def load_and_cache_examples(args, task, tokenizer, evaluate=False):
- if args.local_rank not in [-1, 0] and not evaluate:
- torch.distributed.barrier() # Make sure only the first process in distributed training process the dataset, and the others will use the cache
-
- processor = processors[task]()
- output_mode = output_modes[task]
- # Load data features from cache or dataset file
- cached_features_file = os.path.join(
- args.data_dir,
- "cached_{}_{}_{}_{}".format(
- "dev" if evaluate else "train",
- list(filter(None, args.model_name_or_path.split("/"))).pop(),
- str(args.max_seq_length),
- str(task),
- ),
- )
- if os.path.exists(cached_features_file) and not args.overwrite_cache:
- logger.info("Loading features from cached file %s", cached_features_file)
- features = torch.load(cached_features_file)
- else:
- logger.info("Creating features from dataset file at %s", args.data_dir)
- label_list = processor.get_labels()
- if task in ["mnli", "mnli-mm"] and args.model_type in ["roberta", "xlmroberta"]:
- # HACK(label indices are swapped in RoBERTa pretrained model)
- label_list[1], label_list[2] = label_list[2], label_list[1]
- examples = (
- processor.get_dev_examples(args.data_dir) if evaluate else processor.get_train_examples(args.data_dir)
- )
- features = convert_examples_to_features(
- examples,
- tokenizer,
- label_list=label_list,
- max_length=args.max_seq_length,
- output_mode=output_mode,
- pad_on_left=bool(args.model_type in ["xlnet"]), # pad on the left for xlnet
- pad_token=tokenizer.convert_tokens_to_ids([tokenizer.pad_token])[0],
- pad_token_segment_id=4 if args.model_type in ["xlnet"] else 0,
- )
- if args.local_rank in [-1, 0]:
- logger.info("Saving features into cached file %s", cached_features_file)
- torch.save(features, cached_features_file)
-
- if args.local_rank == 0 and not evaluate:
- torch.distributed.barrier() # Make sure only the first process in distributed training process the dataset, and the others will use the cache
-
- # Convert to Tensors and build dataset
- all_input_ids = torch.tensor([f.input_ids for f in features], dtype=torch.long)
- all_attention_mask = torch.tensor([f.attention_mask for f in features], dtype=torch.long)
- all_token_type_ids = torch.tensor([f.token_type_ids for f in features], dtype=torch.long)
- if output_mode == "classification":
- all_labels = torch.tensor([f.label for f in features], dtype=torch.long)
- elif output_mode == "regression":
- all_labels = torch.tensor([f.label for f in features], dtype=torch.float)
-
- dataset = TensorDataset(all_input_ids, all_attention_mask, all_token_type_ids, all_labels)
- return dataset
-
-
-def main():
- parser = argparse.ArgumentParser()
-
- # Required parameters
- parser.add_argument(
- "--data_dir",
- default=None,
- type=str,
- required=True,
- help="The input data dir. Should contain the .tsv files (or other data files) for the task.",
- )
- parser.add_argument(
- "--model_type",
- default=None,
- type=str,
- required=True,
- help="Model type selected in the list: " + ", ".join(MODEL_CLASSES.keys()),
- )
- parser.add_argument(
- "--model_name_or_path",
- default=None,
- type=str,
- required=True,
- help="Path to pre-trained model or shortcut name selected in the list: " + ", ".join(ALL_MODELS),
- )
- parser.add_argument(
- "--task_name",
- default=None,
- type=str,
- required=True,
- help="The name of the task to train selected in the list: " + ", ".join(processors.keys()),
- )
- parser.add_argument(
- "--output_dir",
- default=None,
- type=str,
- required=True,
- help="The output directory where the model predictions and checkpoints will be written.",
- )
-
- # Other parameters
- parser.add_argument(
- "--config_name", default="", type=str, help="Pretrained config name or path if not the same as model_name",
- )
- parser.add_argument(
- "--tokenizer_name",
- default="",
- type=str,
- help="Pretrained tokenizer name or path if not the same as model_name",
- )
- parser.add_argument(
- "--cache_dir",
- default="",
- type=str,
- help="Where do you want to store the pre-trained models downloaded from s3",
- )
- parser.add_argument(
- "--max_seq_length",
- default=128,
- type=int,
- help="The maximum total input sequence length after tokenization. Sequences longer "
- "than this will be truncated, sequences shorter will be padded.",
- )
- parser.add_argument("--do_train", action="store_true", help="Whether to run training.")
- parser.add_argument("--do_eval", action="store_true", help="Whether to run eval on the dev set.")
- parser.add_argument(
- "--evaluate_during_training", action="store_true", help="Run evaluation during training at each logging step.",
- )
- parser.add_argument(
- "--do_lower_case", action="store_true", help="Set this flag if you are using an uncased model.",
- )
-
- parser.add_argument(
- "--per_gpu_train_batch_size", default=8, type=int, help="Batch size per GPU/CPU for training.",
- )
- parser.add_argument(
- "--per_gpu_eval_batch_size", default=8, type=int, help="Batch size per GPU/CPU for evaluation.",
- )
- parser.add_argument(
- "--gradient_accumulation_steps",
- type=int,
- default=1,
- help="Number of updates steps to accumulate before performing a backward/update pass.",
- )
- parser.add_argument("--learning_rate", default=5e-5, type=float, help="The initial learning rate for Adam.")
- parser.add_argument("--weight_decay", default=0.0, type=float, help="Weight decay if we apply some.")
- parser.add_argument("--adam_epsilon", default=1e-8, type=float, help="Epsilon for Adam optimizer.")
- parser.add_argument("--max_grad_norm", default=1.0, type=float, help="Max gradient norm.")
- parser.add_argument(
- "--num_train_epochs", default=3.0, type=float, help="Total number of training epochs to perform.",
- )
- parser.add_argument(
- "--max_steps",
- default=-1,
- type=int,
- help="If > 0: set total number of training steps to perform. Override num_train_epochs.",
- )
- parser.add_argument("--warmup_steps", default=0, type=int, help="Linear warmup over warmup_steps.")
-
- parser.add_argument("--logging_steps", type=int, default=500, help="Log every X updates steps.")
- parser.add_argument("--save_steps", type=int, default=500, help="Save checkpoint every X updates steps.")
- parser.add_argument(
- "--eval_all_checkpoints",
- action="store_true",
- help="Evaluate all checkpoints starting with the same prefix as model_name ending and ending with step number",
- )
- parser.add_argument("--no_cuda", action="store_true", help="Avoid using CUDA when available")
- parser.add_argument(
- "--overwrite_output_dir", action="store_true", help="Overwrite the content of the output directory",
- )
- parser.add_argument(
- "--overwrite_cache", action="store_true", help="Overwrite the cached training and evaluation sets",
- )
- parser.add_argument("--seed", type=int, default=42, help="random seed for initialization")
-
- parser.add_argument(
- "--fp16",
- action="store_true",
- help="Whether to use 16-bit (mixed) precision (through NVIDIA apex) instead of 32-bit",
- )
- parser.add_argument(
- "--fp16_opt_level",
- type=str,
- default="O1",
- help="For fp16: Apex AMP optimization level selected in ['O0', 'O1', 'O2', and 'O3']."
- "See details at https://nvidia.github.io/apex/amp.html",
- )
- parser.add_argument("--local_rank", type=int, default=-1, help="For distributed training: local_rank")
- parser.add_argument("--server_ip", type=str, default="", help="For distant debugging.")
- parser.add_argument("--server_port", type=str, default="", help="For distant debugging.")
- args = parser.parse_args()
-
- if (
- os.path.exists(args.output_dir)
- and os.listdir(args.output_dir)
- and args.do_train
- and not args.overwrite_output_dir
- ):
- raise ValueError(
- "Output directory ({}) already exists and is not empty. Use --overwrite_output_dir to overcome.".format(
- args.output_dir
- )
- )
-
- # Setup distant debugging if needed
- if args.server_ip and args.server_port:
- # Distant debugging - see https://code.visualstudio.com/docs/python/debugging#_attach-to-a-local-script
- import ptvsd
-
- print("Waiting for debugger attach")
- ptvsd.enable_attach(address=(args.server_ip, args.server_port), redirect_output=True)
- ptvsd.wait_for_attach()
-
- # Setup CUDA, GPU & distributed training
- if args.local_rank == -1 or args.no_cuda:
- device = torch.device("cuda" if torch.cuda.is_available() and not args.no_cuda else "cpu")
- args.n_gpu = torch.cuda.device_count()
- else: # Initializes the distributed backend which will take care of sychronizing nodes/GPUs
- torch.cuda.set_device(args.local_rank)
- device = torch.device("cuda", args.local_rank)
- torch.distributed.init_process_group(backend="nccl")
- args.n_gpu = 1
- args.device = device
-
- # Setup logging
- logging.basicConfig(
- format="%(asctime)s - %(levelname)s - %(name)s - %(message)s",
- datefmt="%m/%d/%Y %H:%M:%S",
- level=logging.INFO if args.local_rank in [-1, 0] else logging.WARN,
- )
- logger.warning(
- "Process rank: %s, device: %s, n_gpu: %s, distributed training: %s, 16-bits training: %s",
- args.local_rank,
- device,
- args.n_gpu,
- bool(args.local_rank != -1),
- args.fp16,
- )
-
- # Set seed
- set_seed(args)
-
- # Prepare GLUE task
- args.task_name = args.task_name.lower()
- if args.task_name not in processors:
- raise ValueError("Task not found: %s" % (args.task_name))
- processor = processors[args.task_name]()
- args.output_mode = output_modes[args.task_name]
- label_list = processor.get_labels()
- num_labels = len(label_list)
-
- # Load pretrained model and tokenizer
- if args.local_rank not in [-1, 0]:
- torch.distributed.barrier() # Make sure only the first process in distributed training will download model & vocab
-
- args.model_type = args.model_type.lower()
- config_class, model_class, tokenizer_class = MODEL_CLASSES[args.model_type]
- config = config_class.from_pretrained(
- args.config_name if args.config_name else args.model_name_or_path,
- num_labels=num_labels,
- finetuning_task=args.task_name,
- cache_dir=args.cache_dir if args.cache_dir else None,
- )
- tokenizer = tokenizer_class.from_pretrained(
- args.tokenizer_name if args.tokenizer_name else args.model_name_or_path,
- do_lower_case=args.do_lower_case,
- cache_dir=args.cache_dir if args.cache_dir else None,
- )
- model = model_class.from_pretrained(
- args.model_name_or_path,
- from_tf=bool(".ckpt" in args.model_name_or_path),
- config=config,
- cache_dir=args.cache_dir if args.cache_dir else None,
- )
-
- if args.local_rank == 0:
- torch.distributed.barrier() # Make sure only the first process in distributed training will download model & vocab
-
- model.to(args.device)
-
- logger.info("Training/evaluation parameters %s", args)
-
- # Training
- if args.do_train:
- train_dataset = load_and_cache_examples(args, args.task_name, tokenizer, evaluate=False)
- global_step, tr_loss = train(args, train_dataset, model, tokenizer)
- logger.info(" global_step = %s, average loss = %s", global_step, tr_loss)
-
- # Saving best-practices: if you use defaults names for the model, you can reload it using from_pretrained()
- if args.do_train and (args.local_rank == -1 or torch.distributed.get_rank() == 0):
- # Create output directory if needed
- if not os.path.exists(args.output_dir) and args.local_rank in [-1, 0]:
- os.makedirs(args.output_dir)
-
- logger.info("Saving model checkpoint to %s", args.output_dir)
- # Save a trained model, configuration and tokenizer using `save_pretrained()`.
- # They can then be reloaded using `from_pretrained()`
- model_to_save = (
- model.module if hasattr(model, "module") else model
- ) # Take care of distributed/parallel training
- model_to_save.save_pretrained(args.output_dir)
- tokenizer.save_pretrained(args.output_dir)
-
- # Good practice: save your training arguments together with the trained model
- torch.save(args, os.path.join(args.output_dir, "training_args.bin"))
-
- # Load a trained model and vocabulary that you have fine-tuned
- model = model_class.from_pretrained(args.output_dir)
- tokenizer = tokenizer_class.from_pretrained(args.output_dir)
- model.to(args.device)
-
- # Evaluation
- results = {}
- if args.do_eval and args.local_rank in [-1, 0]:
- tokenizer = tokenizer_class.from_pretrained(args.output_dir, do_lower_case=args.do_lower_case)
- checkpoints = [args.output_dir]
- if args.eval_all_checkpoints:
- checkpoints = list(
- os.path.dirname(c) for c in sorted(glob.glob(args.output_dir + "/**/" + WEIGHTS_NAME, recursive=True))
- )
- logging.getLogger("transformers.modeling_utils").setLevel(logging.WARN) # Reduce logging
- logger.info("Evaluate the following checkpoints: %s", checkpoints)
- for checkpoint in checkpoints:
- global_step = checkpoint.split("-")[-1] if len(checkpoints) > 1 else ""
- prefix = checkpoint.split("/")[-1] if checkpoint.find("checkpoint") != -1 else ""
-
- model = model_class.from_pretrained(checkpoint)
- model.to(args.device)
- result = evaluate(args, model, tokenizer, prefix=prefix)
- result = dict((k + "_{}".format(global_step), v) for k, v in result.items())
- results.update(result)
-
- return results
-
-
-if __name__ == "__main__":
- main()
diff --git a/server/transformers/examples/run_lm_finetuning.py b/server/transformers/examples/run_lm_finetuning.py
deleted file mode 100644
index 663881649d815772a0e4ff02367992fba3883425..0000000000000000000000000000000000000000
--- a/server/transformers/examples/run_lm_finetuning.py
+++ /dev/null
@@ -1,790 +0,0 @@
-# coding=utf-8
-# Copyright 2018 The Google AI Language Team Authors and The HuggingFace Inc. team.
-# Copyright (c) 2018, NVIDIA CORPORATION. All rights reserved.
-#
-# Licensed under the Apache License, Version 2.0 (the "License");
-# you may not use this file except in compliance with the License.
-# You may obtain a copy of the License at
-#
-# http://www.apache.org/licenses/LICENSE-2.0
-#
-# Unless required by applicable law or agreed to in writing, software
-# distributed under the License is distributed on an "AS IS" BASIS,
-# WITHOUT WARRANTIES OR CONDITIONS OF ANY KIND, either express or implied.
-# See the License for the specific language governing permissions and
-# limitations under the License.
-"""
-Fine-tuning the library models for language modeling on a text file (GPT, GPT-2, BERT, RoBERTa).
-GPT and GPT-2 are fine-tuned using a causal language modeling (CLM) loss while BERT and RoBERTa are fine-tuned
-using a masked language modeling (MLM) loss.
-"""
-
-
-import argparse
-import glob
-import logging
-import os
-import pickle
-import random
-import re
-import shutil
-from typing import Dict, List, Tuple
-
-import numpy as np
-import torch
-from torch.nn.utils.rnn import pad_sequence
-from torch.utils.data import DataLoader, Dataset, RandomSampler, SequentialSampler
-from torch.utils.data.distributed import DistributedSampler
-from tqdm import tqdm, trange
-
-from transformers import (
- WEIGHTS_NAME,
- AdamW,
- BertConfig,
- BertForMaskedLM,
- BertTokenizer,
- CamembertConfig,
- CamembertForMaskedLM,
- CamembertTokenizer,
- DistilBertConfig,
- DistilBertForMaskedLM,
- DistilBertTokenizer,
- GPT2Config,
- GPT2LMHeadModel,
- GPT2Tokenizer,
- OpenAIGPTConfig,
- OpenAIGPTLMHeadModel,
- OpenAIGPTTokenizer,
- PreTrainedModel,
- PreTrainedTokenizer,
- RobertaConfig,
- RobertaForMaskedLM,
- RobertaTokenizer,
- get_linear_schedule_with_warmup,
-)
-
-
-try:
- from torch.utils.tensorboard import SummaryWriter
-except ImportError:
- from tensorboardX import SummaryWriter
-
-
-logger = logging.getLogger(__name__)
-
-
-MODEL_CLASSES = {
- "gpt2": (GPT2Config, GPT2LMHeadModel, GPT2Tokenizer),
- "openai-gpt": (OpenAIGPTConfig, OpenAIGPTLMHeadModel, OpenAIGPTTokenizer),
- "bert": (BertConfig, BertForMaskedLM, BertTokenizer),
- "roberta": (RobertaConfig, RobertaForMaskedLM, RobertaTokenizer),
- "distilbert": (DistilBertConfig, DistilBertForMaskedLM, DistilBertTokenizer),
- "camembert": (CamembertConfig, CamembertForMaskedLM, CamembertTokenizer),
-}
-
-
-class TextDataset(Dataset):
- def __init__(self, tokenizer: PreTrainedTokenizer, args, file_path: str, block_size=512):
- assert os.path.isfile(file_path)
- directory, filename = os.path.split(file_path)
- cached_features_file = os.path.join(
- directory, args.model_type + "_cached_lm_" + str(block_size) + "_" + filename
- )
-
- if os.path.exists(cached_features_file) and not args.overwrite_cache:
- logger.info("Loading features from cached file %s", cached_features_file)
- with open(cached_features_file, "rb") as handle:
- self.examples = pickle.load(handle)
- else:
- logger.info("Creating features from dataset file at %s", directory)
-
- self.examples = []
- with open(file_path, encoding="utf-8") as f:
- text = f.read()
-
- tokenized_text = tokenizer.convert_tokens_to_ids(tokenizer.tokenize(text))
-
- for i in range(0, len(tokenized_text) - block_size + 1, block_size): # Truncate in block of block_size
- self.examples.append(tokenizer.build_inputs_with_special_tokens(tokenized_text[i : i + block_size]))
- # Note that we are loosing the last truncated example here for the sake of simplicity (no padding)
- # If your dataset is small, first you should loook for a bigger one :-) and second you
- # can change this behavior by adding (model specific) padding.
-
- logger.info("Saving features into cached file %s", cached_features_file)
- with open(cached_features_file, "wb") as handle:
- pickle.dump(self.examples, handle, protocol=pickle.HIGHEST_PROTOCOL)
-
- def __len__(self):
- return len(self.examples)
-
- def __getitem__(self, item):
- return torch.tensor(self.examples[item])
-
-
-class LineByLineTextDataset(Dataset):
- def __init__(self, tokenizer: PreTrainedTokenizer, args, file_path: str, block_size=512):
- assert os.path.isfile(file_path)
- # Here, we do not cache the features, operating under the assumption
- # that we will soon use fast multithreaded tokenizers from the
- # `tokenizers` repo everywhere =)
- logger.info("Creating features from dataset file at %s", file_path)
-
- with open(file_path, encoding="utf-8") as f:
- lines = [line for line in f.read().splitlines() if len(line) > 0]
-
- self.examples = tokenizer.batch_encode_plus(lines, max_length=block_size)["input_ids"]
-
- def __len__(self):
- return len(self.examples)
-
- def __getitem__(self, i):
- return torch.tensor(self.examples[i])
-
-
-def load_and_cache_examples(args, tokenizer, evaluate=False):
- file_path = args.eval_data_file if evaluate else args.train_data_file
- if args.line_by_line:
- return LineByLineTextDataset(tokenizer, args, file_path=file_path, block_size=args.block_size)
- else:
- return TextDataset(tokenizer, args, file_path=file_path, block_size=args.block_size)
-
-
-def set_seed(args):
- random.seed(args.seed)
- np.random.seed(args.seed)
- torch.manual_seed(args.seed)
- if args.n_gpu > 0:
- torch.cuda.manual_seed_all(args.seed)
-
-
-def _sorted_checkpoints(args, checkpoint_prefix="checkpoint", use_mtime=False) -> List[str]:
- ordering_and_checkpoint_path = []
-
- glob_checkpoints = glob.glob(os.path.join(args.output_dir, "{}-*".format(checkpoint_prefix)))
-
- for path in glob_checkpoints:
- if use_mtime:
- ordering_and_checkpoint_path.append((os.path.getmtime(path), path))
- else:
- regex_match = re.match(".*{}-([0-9]+)".format(checkpoint_prefix), path)
- if regex_match and regex_match.groups():
- ordering_and_checkpoint_path.append((int(regex_match.groups()[0]), path))
-
- checkpoints_sorted = sorted(ordering_and_checkpoint_path)
- checkpoints_sorted = [checkpoint[1] for checkpoint in checkpoints_sorted]
- return checkpoints_sorted
-
-
-def _rotate_checkpoints(args, checkpoint_prefix="checkpoint", use_mtime=False) -> None:
- if not args.save_total_limit:
- return
- if args.save_total_limit <= 0:
- return
-
- # Check if we should delete older checkpoint(s)
- checkpoints_sorted = _sorted_checkpoints(args, checkpoint_prefix, use_mtime)
- if len(checkpoints_sorted) <= args.save_total_limit:
- return
-
- number_of_checkpoints_to_delete = max(0, len(checkpoints_sorted) - args.save_total_limit)
- checkpoints_to_be_deleted = checkpoints_sorted[:number_of_checkpoints_to_delete]
- for checkpoint in checkpoints_to_be_deleted:
- logger.info("Deleting older checkpoint [{}] due to args.save_total_limit".format(checkpoint))
- shutil.rmtree(checkpoint)
-
-
-def mask_tokens(inputs: torch.Tensor, tokenizer: PreTrainedTokenizer, args) -> Tuple[torch.Tensor, torch.Tensor]:
- """ Prepare masked tokens inputs/labels for masked language modeling: 80% MASK, 10% random, 10% original. """
- labels = inputs.clone()
- # We sample a few tokens in each sequence for masked-LM training (with probability args.mlm_probability defaults to 0.15 in Bert/RoBERTa)
- probability_matrix = torch.full(labels.shape, args.mlm_probability)
- special_tokens_mask = [
- tokenizer.get_special_tokens_mask(val, already_has_special_tokens=True) for val in labels.tolist()
- ]
- probability_matrix.masked_fill_(torch.tensor(special_tokens_mask, dtype=torch.bool), value=0.0)
- if tokenizer._pad_token is not None:
- padding_mask = labels.eq(tokenizer.pad_token_id)
- probability_matrix.masked_fill_(padding_mask, value=0.0)
- masked_indices = torch.bernoulli(probability_matrix).bool()
- labels[~masked_indices] = -100 # We only compute loss on masked tokens
-
- # 80% of the time, we replace masked input tokens with tokenizer.mask_token ([MASK])
- indices_replaced = torch.bernoulli(torch.full(labels.shape, 0.8)).bool() & masked_indices
- inputs[indices_replaced] = tokenizer.convert_tokens_to_ids(tokenizer.mask_token)
-
- # 10% of the time, we replace masked input tokens with random word
- indices_random = torch.bernoulli(torch.full(labels.shape, 0.5)).bool() & masked_indices & ~indices_replaced
- random_words = torch.randint(len(tokenizer), labels.shape, dtype=torch.long)
- inputs[indices_random] = random_words[indices_random]
-
- # The rest of the time (10% of the time) we keep the masked input tokens unchanged
- return inputs, labels
-
-
-def train(args, train_dataset, model: PreTrainedModel, tokenizer: PreTrainedTokenizer) -> Tuple[int, float]:
- """ Train the model """
- if args.local_rank in [-1, 0]:
- tb_writer = SummaryWriter()
-
- args.train_batch_size = args.per_gpu_train_batch_size * max(1, args.n_gpu)
-
- def collate(examples: List[torch.Tensor]):
- if tokenizer._pad_token is None:
- return pad_sequence(examples, batch_first=True)
- return pad_sequence(examples, batch_first=True, padding_value=tokenizer.pad_token_id)
-
- train_sampler = RandomSampler(train_dataset) if args.local_rank == -1 else DistributedSampler(train_dataset)
- train_dataloader = DataLoader(
- train_dataset, sampler=train_sampler, batch_size=args.train_batch_size, collate_fn=collate
- )
-
- if args.max_steps > 0:
- t_total = args.max_steps
- args.num_train_epochs = args.max_steps // (len(train_dataloader) // args.gradient_accumulation_steps) + 1
- else:
- t_total = len(train_dataloader) // args.gradient_accumulation_steps * args.num_train_epochs
-
- # Prepare optimizer and schedule (linear warmup and decay)
- no_decay = ["bias", "LayerNorm.weight"]
- optimizer_grouped_parameters = [
- {
- "params": [p for n, p in model.named_parameters() if not any(nd in n for nd in no_decay)],
- "weight_decay": args.weight_decay,
- },
- {"params": [p for n, p in model.named_parameters() if any(nd in n for nd in no_decay)], "weight_decay": 0.0},
- ]
- optimizer = AdamW(optimizer_grouped_parameters, lr=args.learning_rate, eps=args.adam_epsilon)
- scheduler = get_linear_schedule_with_warmup(
- optimizer, num_warmup_steps=args.warmup_steps, num_training_steps=t_total
- )
-
- # Check if saved optimizer or scheduler states exist
- if (
- args.model_name_or_path
- and os.path.isfile(os.path.join(args.model_name_or_path, "optimizer.pt"))
- and os.path.isfile(os.path.join(args.model_name_or_path, "scheduler.pt"))
- ):
- # Load in optimizer and scheduler states
- optimizer.load_state_dict(torch.load(os.path.join(args.model_name_or_path, "optimizer.pt")))
- scheduler.load_state_dict(torch.load(os.path.join(args.model_name_or_path, "scheduler.pt")))
-
- if args.fp16:
- try:
- from apex import amp
- except ImportError:
- raise ImportError("Please install apex from https://www.github.com/nvidia/apex to use fp16 training.")
- model, optimizer = amp.initialize(model, optimizer, opt_level=args.fp16_opt_level)
-
- # multi-gpu training (should be after apex fp16 initialization)
- if args.n_gpu > 1:
- model = torch.nn.DataParallel(model)
-
- # Distributed training (should be after apex fp16 initialization)
- if args.local_rank != -1:
- model = torch.nn.parallel.DistributedDataParallel(
- model, device_ids=[args.local_rank], output_device=args.local_rank, find_unused_parameters=True
- )
-
- # Train!
- logger.info("***** Running training *****")
- logger.info(" Num examples = %d", len(train_dataset))
- logger.info(" Num Epochs = %d", args.num_train_epochs)
- logger.info(" Instantaneous batch size per GPU = %d", args.per_gpu_train_batch_size)
- logger.info(
- " Total train batch size (w. parallel, distributed & accumulation) = %d",
- args.train_batch_size
- * args.gradient_accumulation_steps
- * (torch.distributed.get_world_size() if args.local_rank != -1 else 1),
- )
- logger.info(" Gradient Accumulation steps = %d", args.gradient_accumulation_steps)
- logger.info(" Total optimization steps = %d", t_total)
-
- global_step = 0
- epochs_trained = 0
- steps_trained_in_current_epoch = 0
- # Check if continuing training from a checkpoint
- if args.model_name_or_path and os.path.exists(args.model_name_or_path):
- try:
- # set global_step to gobal_step of last saved checkpoint from model path
- checkpoint_suffix = args.model_name_or_path.split("-")[-1].split("/")[0]
- global_step = int(checkpoint_suffix)
- epochs_trained = global_step // (len(train_dataloader) // args.gradient_accumulation_steps)
- steps_trained_in_current_epoch = global_step % (len(train_dataloader) // args.gradient_accumulation_steps)
-
- logger.info(" Continuing training from checkpoint, will skip to saved global_step")
- logger.info(" Continuing training from epoch %d", epochs_trained)
- logger.info(" Continuing training from global step %d", global_step)
- logger.info(" Will skip the first %d steps in the first epoch", steps_trained_in_current_epoch)
- except ValueError:
- logger.info(" Starting fine-tuning.")
-
- tr_loss, logging_loss = 0.0, 0.0
-
- model_to_resize = model.module if hasattr(model, "module") else model # Take care of distributed/parallel training
- model_to_resize.resize_token_embeddings(len(tokenizer))
-
- model.zero_grad()
- train_iterator = trange(
- epochs_trained, int(args.num_train_epochs), desc="Epoch", disable=args.local_rank not in [-1, 0]
- )
- set_seed(args) # Added here for reproducibility
- for _ in train_iterator:
- epoch_iterator = tqdm(train_dataloader, desc="Iteration", disable=args.local_rank not in [-1, 0])
- for step, batch in enumerate(epoch_iterator):
-
- # Skip past any already trained steps if resuming training
- if steps_trained_in_current_epoch > 0:
- steps_trained_in_current_epoch -= 1
- continue
-
- inputs, labels = mask_tokens(batch, tokenizer, args) if args.mlm else (batch, batch)
- inputs = inputs.to(args.device)
- labels = labels.to(args.device)
- model.train()
- outputs = model(inputs, masked_lm_labels=labels) if args.mlm else model(inputs, labels=labels)
- loss = outputs[0] # model outputs are always tuple in transformers (see doc)
-
- if args.n_gpu > 1:
- loss = loss.mean() # mean() to average on multi-gpu parallel training
- if args.gradient_accumulation_steps > 1:
- loss = loss / args.gradient_accumulation_steps
-
- if args.fp16:
- with amp.scale_loss(loss, optimizer) as scaled_loss:
- scaled_loss.backward()
- else:
- loss.backward()
-
- tr_loss += loss.item()
- if (step + 1) % args.gradient_accumulation_steps == 0:
- if args.fp16:
- torch.nn.utils.clip_grad_norm_(amp.master_params(optimizer), args.max_grad_norm)
- else:
- torch.nn.utils.clip_grad_norm_(model.parameters(), args.max_grad_norm)
- optimizer.step()
- scheduler.step() # Update learning rate schedule
- model.zero_grad()
- global_step += 1
-
- if args.local_rank in [-1, 0] and args.logging_steps > 0 and global_step % args.logging_steps == 0:
- # Log metrics
- if (
- args.local_rank == -1 and args.evaluate_during_training
- ): # Only evaluate when single GPU otherwise metrics may not average well
- results = evaluate(args, model, tokenizer)
- for key, value in results.items():
- tb_writer.add_scalar("eval_{}".format(key), value, global_step)
- tb_writer.add_scalar("lr", scheduler.get_lr()[0], global_step)
- tb_writer.add_scalar("loss", (tr_loss - logging_loss) / args.logging_steps, global_step)
- logging_loss = tr_loss
-
- if args.local_rank in [-1, 0] and args.save_steps > 0 and global_step % args.save_steps == 0:
- checkpoint_prefix = "checkpoint"
- # Save model checkpoint
- output_dir = os.path.join(args.output_dir, "{}-{}".format(checkpoint_prefix, global_step))
- os.makedirs(output_dir, exist_ok=True)
- model_to_save = (
- model.module if hasattr(model, "module") else model
- ) # Take care of distributed/parallel training
- model_to_save.save_pretrained(output_dir)
- tokenizer.save_pretrained(output_dir)
-
- torch.save(args, os.path.join(output_dir, "training_args.bin"))
- logger.info("Saving model checkpoint to %s", output_dir)
-
- _rotate_checkpoints(args, checkpoint_prefix)
-
- torch.save(optimizer.state_dict(), os.path.join(output_dir, "optimizer.pt"))
- torch.save(scheduler.state_dict(), os.path.join(output_dir, "scheduler.pt"))
- logger.info("Saving optimizer and scheduler states to %s", output_dir)
-
- if args.max_steps > 0 and global_step > args.max_steps:
- epoch_iterator.close()
- break
- if args.max_steps > 0 and global_step > args.max_steps:
- train_iterator.close()
- break
-
- if args.local_rank in [-1, 0]:
- tb_writer.close()
-
- return global_step, tr_loss / global_step
-
-
-def evaluate(args, model: PreTrainedModel, tokenizer: PreTrainedTokenizer, prefix="") -> Dict:
- # Loop to handle MNLI double evaluation (matched, mis-matched)
- eval_output_dir = args.output_dir
-
- eval_dataset = load_and_cache_examples(args, tokenizer, evaluate=True)
-
- if args.local_rank in [-1, 0]:
- os.makedirs(eval_output_dir, exist_ok=True)
-
- args.eval_batch_size = args.per_gpu_eval_batch_size * max(1, args.n_gpu)
- # Note that DistributedSampler samples randomly
-
- def collate(examples: List[torch.Tensor]):
- if tokenizer._pad_token is None:
- return pad_sequence(examples, batch_first=True)
- return pad_sequence(examples, batch_first=True, padding_value=tokenizer.pad_token_id)
-
- eval_sampler = SequentialSampler(eval_dataset)
- eval_dataloader = DataLoader(
- eval_dataset, sampler=eval_sampler, batch_size=args.eval_batch_size, collate_fn=collate
- )
-
- # multi-gpu evaluate
- if args.n_gpu > 1:
- model = torch.nn.DataParallel(model)
-
- # Eval!
- logger.info("***** Running evaluation {} *****".format(prefix))
- logger.info(" Num examples = %d", len(eval_dataset))
- logger.info(" Batch size = %d", args.eval_batch_size)
- eval_loss = 0.0
- nb_eval_steps = 0
- model.eval()
-
- for batch in tqdm(eval_dataloader, desc="Evaluating"):
- inputs, labels = mask_tokens(batch, tokenizer, args) if args.mlm else (batch, batch)
- inputs = inputs.to(args.device)
- labels = labels.to(args.device)
-
- with torch.no_grad():
- outputs = model(inputs, masked_lm_labels=labels) if args.mlm else model(inputs, labels=labels)
- lm_loss = outputs[0]
- eval_loss += lm_loss.mean().item()
- nb_eval_steps += 1
-
- eval_loss = eval_loss / nb_eval_steps
- perplexity = torch.exp(torch.tensor(eval_loss))
-
- result = {"perplexity": perplexity}
-
- output_eval_file = os.path.join(eval_output_dir, prefix, "eval_results.txt")
- with open(output_eval_file, "w") as writer:
- logger.info("***** Eval results {} *****".format(prefix))
- for key in sorted(result.keys()):
- logger.info(" %s = %s", key, str(result[key]))
- writer.write("%s = %s\n" % (key, str(result[key])))
-
- return result
-
-
-def main():
- parser = argparse.ArgumentParser()
-
- # Required parameters
- parser.add_argument(
- "--train_data_file", default=None, type=str, required=True, help="The input training data file (a text file)."
- )
- parser.add_argument(
- "--output_dir",
- type=str,
- required=True,
- help="The output directory where the model predictions and checkpoints will be written.",
- )
- parser.add_argument(
- "--model_type", type=str, required=True, help="The model architecture to be trained or fine-tuned.",
- )
-
- # Other parameters
- parser.add_argument(
- "--eval_data_file",
- default=None,
- type=str,
- help="An optional input evaluation data file to evaluate the perplexity on (a text file).",
- )
- parser.add_argument(
- "--line_by_line",
- action="store_true",
- help="Whether distinct lines of text in the dataset are to be handled as distinct sequences.",
- )
- parser.add_argument(
- "--should_continue", action="store_true", help="Whether to continue from latest checkpoint in output_dir"
- )
- parser.add_argument(
- "--model_name_or_path",
- default=None,
- type=str,
- help="The model checkpoint for weights initialization. Leave None if you want to train a model from scratch.",
- )
-
- parser.add_argument(
- "--mlm", action="store_true", help="Train with masked-language modeling loss instead of language modeling."
- )
- parser.add_argument(
- "--mlm_probability", type=float, default=0.15, help="Ratio of tokens to mask for masked language modeling loss"
- )
-
- parser.add_argument(
- "--config_name",
- default=None,
- type=str,
- help="Optional pretrained config name or path if not the same as model_name_or_path. If both are None, initialize a new config.",
- )
- parser.add_argument(
- "--tokenizer_name",
- default=None,
- type=str,
- help="Optional pretrained tokenizer name or path if not the same as model_name_or_path. If both are None, initialize a new tokenizer.",
- )
- parser.add_argument(
- "--cache_dir",
- default=None,
- type=str,
- help="Optional directory to store the pre-trained models downloaded from s3 (instead of the default one)",
- )
- parser.add_argument(
- "--block_size",
- default=-1,
- type=int,
- help="Optional input sequence length after tokenization."
- "The training dataset will be truncated in block of this size for training."
- "Default to the model max input length for single sentence inputs (take into account special tokens).",
- )
- parser.add_argument("--do_train", action="store_true", help="Whether to run training.")
- parser.add_argument("--do_eval", action="store_true", help="Whether to run eval on the dev set.")
- parser.add_argument(
- "--evaluate_during_training", action="store_true", help="Run evaluation during training at each logging step."
- )
-
- parser.add_argument("--per_gpu_train_batch_size", default=4, type=int, help="Batch size per GPU/CPU for training.")
- parser.add_argument(
- "--per_gpu_eval_batch_size", default=4, type=int, help="Batch size per GPU/CPU for evaluation."
- )
- parser.add_argument(
- "--gradient_accumulation_steps",
- type=int,
- default=1,
- help="Number of updates steps to accumulate before performing a backward/update pass.",
- )
- parser.add_argument("--learning_rate", default=5e-5, type=float, help="The initial learning rate for Adam.")
- parser.add_argument("--weight_decay", default=0.0, type=float, help="Weight decay if we apply some.")
- parser.add_argument("--adam_epsilon", default=1e-8, type=float, help="Epsilon for Adam optimizer.")
- parser.add_argument("--max_grad_norm", default=1.0, type=float, help="Max gradient norm.")
- parser.add_argument(
- "--num_train_epochs", default=1.0, type=float, help="Total number of training epochs to perform."
- )
- parser.add_argument(
- "--max_steps",
- default=-1,
- type=int,
- help="If > 0: set total number of training steps to perform. Override num_train_epochs.",
- )
- parser.add_argument("--warmup_steps", default=0, type=int, help="Linear warmup over warmup_steps.")
-
- parser.add_argument("--logging_steps", type=int, default=500, help="Log every X updates steps.")
- parser.add_argument("--save_steps", type=int, default=500, help="Save checkpoint every X updates steps.")
- parser.add_argument(
- "--save_total_limit",
- type=int,
- default=None,
- help="Limit the total amount of checkpoints, delete the older checkpoints in the output_dir, does not delete by default",
- )
- parser.add_argument(
- "--eval_all_checkpoints",
- action="store_true",
- help="Evaluate all checkpoints starting with the same prefix as model_name_or_path ending and ending with step number",
- )
- parser.add_argument("--no_cuda", action="store_true", help="Avoid using CUDA when available")
- parser.add_argument(
- "--overwrite_output_dir", action="store_true", help="Overwrite the content of the output directory"
- )
- parser.add_argument(
- "--overwrite_cache", action="store_true", help="Overwrite the cached training and evaluation sets"
- )
- parser.add_argument("--seed", type=int, default=42, help="random seed for initialization")
-
- parser.add_argument(
- "--fp16",
- action="store_true",
- help="Whether to use 16-bit (mixed) precision (through NVIDIA apex) instead of 32-bit",
- )
- parser.add_argument(
- "--fp16_opt_level",
- type=str,
- default="O1",
- help="For fp16: Apex AMP optimization level selected in ['O0', 'O1', 'O2', and 'O3']."
- "See details at https://nvidia.github.io/apex/amp.html",
- )
- parser.add_argument("--local_rank", type=int, default=-1, help="For distributed training: local_rank")
- parser.add_argument("--server_ip", type=str, default="", help="For distant debugging.")
- parser.add_argument("--server_port", type=str, default="", help="For distant debugging.")
- args = parser.parse_args()
-
- if args.model_type in ["bert", "roberta", "distilbert", "camembert"] and not args.mlm:
- raise ValueError(
- "BERT and RoBERTa-like models do not have LM heads but masked LM heads. They must be run using the --mlm "
- "flag (masked language modeling)."
- )
- if args.eval_data_file is None and args.do_eval:
- raise ValueError(
- "Cannot do evaluation without an evaluation data file. Either supply a file to --eval_data_file "
- "or remove the --do_eval argument."
- )
- if args.should_continue:
- sorted_checkpoints = _sorted_checkpoints(args)
- if len(sorted_checkpoints) == 0:
- raise ValueError("Used --should_continue but no checkpoint was found in --output_dir.")
- else:
- args.model_name_or_path = sorted_checkpoints[-1]
-
- if (
- os.path.exists(args.output_dir)
- and os.listdir(args.output_dir)
- and args.do_train
- and not args.overwrite_output_dir
- ):
- raise ValueError(
- "Output directory ({}) already exists and is not empty. Use --overwrite_output_dir to overcome.".format(
- args.output_dir
- )
- )
-
- # Setup distant debugging if needed
- if args.server_ip and args.server_port:
- # Distant debugging - see https://code.visualstudio.com/docs/python/debugging#_attach-to-a-local-script
- import ptvsd
-
- print("Waiting for debugger attach")
- ptvsd.enable_attach(address=(args.server_ip, args.server_port), redirect_output=True)
- ptvsd.wait_for_attach()
-
- # Setup CUDA, GPU & distributed training
- if args.local_rank == -1 or args.no_cuda:
- device = torch.device("cuda" if torch.cuda.is_available() and not args.no_cuda else "cpu")
- args.n_gpu = torch.cuda.device_count()
- else: # Initializes the distributed backend which will take care of sychronizing nodes/GPUs
- torch.cuda.set_device(args.local_rank)
- device = torch.device("cuda", args.local_rank)
- torch.distributed.init_process_group(backend="nccl")
- args.n_gpu = 1
- args.device = device
-
- # Setup logging
- logging.basicConfig(
- format="%(asctime)s - %(levelname)s - %(name)s - %(message)s",
- datefmt="%m/%d/%Y %H:%M:%S",
- level=logging.INFO if args.local_rank in [-1, 0] else logging.WARN,
- )
- logger.warning(
- "Process rank: %s, device: %s, n_gpu: %s, distributed training: %s, 16-bits training: %s",
- args.local_rank,
- device,
- args.n_gpu,
- bool(args.local_rank != -1),
- args.fp16,
- )
-
- # Set seed
- set_seed(args)
-
- # Load pretrained model and tokenizer
- if args.local_rank not in [-1, 0]:
- torch.distributed.barrier() # Barrier to make sure only the first process in distributed training download model & vocab
-
- config_class, model_class, tokenizer_class = MODEL_CLASSES[args.model_type]
-
- if args.config_name:
- config = config_class.from_pretrained(args.config_name, cache_dir=args.cache_dir)
- elif args.model_name_or_path:
- config = config_class.from_pretrained(args.model_name_or_path, cache_dir=args.cache_dir)
- else:
- config = config_class()
-
- if args.tokenizer_name:
- tokenizer = tokenizer_class.from_pretrained(args.tokenizer_name, cache_dir=args.cache_dir)
- elif args.model_name_or_path:
- tokenizer = tokenizer_class.from_pretrained(args.model_name_or_path, cache_dir=args.cache_dir)
- else:
- raise ValueError(
- "You are instantiating a new {} tokenizer. This is not supported, but you can do it from another script, save it,"
- "and load it from here, using --tokenizer_name".format(tokenizer_class.__name__)
- )
-
- if args.block_size <= 0:
- args.block_size = tokenizer.max_len_single_sentence
- # Our input block size will be the max possible for the model
- else:
- args.block_size = min(args.block_size, tokenizer.max_len_single_sentence)
-
- if args.model_name_or_path:
- model = model_class.from_pretrained(
- args.model_name_or_path,
- from_tf=bool(".ckpt" in args.model_name_or_path),
- config=config,
- cache_dir=args.cache_dir,
- )
- else:
- logger.info("Training new model from scratch")
- model = model_class(config=config)
-
- model.to(args.device)
-
- if args.local_rank == 0:
- torch.distributed.barrier() # End of barrier to make sure only the first process in distributed training download model & vocab
-
- logger.info("Training/evaluation parameters %s", args)
-
- # Training
- if args.do_train:
- if args.local_rank not in [-1, 0]:
- torch.distributed.barrier() # Barrier to make sure only the first process in distributed training process the dataset, and the others will use the cache
-
- train_dataset = load_and_cache_examples(args, tokenizer, evaluate=False)
-
- if args.local_rank == 0:
- torch.distributed.barrier()
-
- global_step, tr_loss = train(args, train_dataset, model, tokenizer)
- logger.info(" global_step = %s, average loss = %s", global_step, tr_loss)
-
- # Saving best-practices: if you use save_pretrained for the model and tokenizer, you can reload them using from_pretrained()
- if args.do_train and (args.local_rank == -1 or torch.distributed.get_rank() == 0):
- # Create output directory if needed
- if args.local_rank in [-1, 0]:
- os.makedirs(args.output_dir, exist_ok=True)
-
- logger.info("Saving model checkpoint to %s", args.output_dir)
- # Save a trained model, configuration and tokenizer using `save_pretrained()`.
- # They can then be reloaded using `from_pretrained()`
- model_to_save = (
- model.module if hasattr(model, "module") else model
- ) # Take care of distributed/parallel training
- model_to_save.save_pretrained(args.output_dir)
- tokenizer.save_pretrained(args.output_dir)
-
- # Good practice: save your training arguments together with the trained model
- torch.save(args, os.path.join(args.output_dir, "training_args.bin"))
-
- # Load a trained model and vocabulary that you have fine-tuned
- model = model_class.from_pretrained(args.output_dir)
- tokenizer = tokenizer_class.from_pretrained(args.output_dir)
- model.to(args.device)
-
- # Evaluation
- results = {}
- if args.do_eval and args.local_rank in [-1, 0]:
- checkpoints = [args.output_dir]
- if args.eval_all_checkpoints:
- checkpoints = list(
- os.path.dirname(c) for c in sorted(glob.glob(args.output_dir + "/**/" + WEIGHTS_NAME, recursive=True))
- )
- logging.getLogger("transformers.modeling_utils").setLevel(logging.WARN) # Reduce logging
- logger.info("Evaluate the following checkpoints: %s", checkpoints)
- for checkpoint in checkpoints:
- global_step = checkpoint.split("-")[-1] if len(checkpoints) > 1 else ""
- prefix = checkpoint.split("/")[-1] if checkpoint.find("checkpoint") != -1 else ""
-
- model = model_class.from_pretrained(checkpoint)
- model.to(args.device)
- result = evaluate(args, model, tokenizer, prefix=prefix)
- result = dict((k + "_{}".format(global_step), v) for k, v in result.items())
- results.update(result)
-
- return results
-
-
-if __name__ == "__main__":
- main()
diff --git a/server/transformers/examples/run_multiple_choice.py b/server/transformers/examples/run_multiple_choice.py
deleted file mode 100644
index 72337c110fcb9fed295af13d4bd26906a9a55100..0000000000000000000000000000000000000000
--- a/server/transformers/examples/run_multiple_choice.py
+++ /dev/null
@@ -1,678 +0,0 @@
-# coding=utf-8
-# Copyright 2018 The Google AI Language Team Authors and The HuggingFace Inc. team.
-# Copyright (c) 2018, NVIDIA CORPORATION. All rights reserved.
-#
-# Licensed under the Apache License, Version 2.0 (the "License");
-# you may not use this file except in compliance with the License.
-# You may obtain a copy of the License at
-#
-# http://www.apache.org/licenses/LICENSE-2.0
-#
-# Unless required by applicable law or agreed to in writing, software
-# distributed under the License is distributed on an "AS IS" BASIS,
-# WITHOUT WARRANTIES OR CONDITIONS OF ANY KIND, either express or implied.
-# See the License for the specific language governing permissions and
-# limitations under the License.
-""" Finetuning the library models for multiple choice (Bert, Roberta, XLNet)."""
-
-
-import argparse
-import glob
-import logging
-import os
-import random
-
-import numpy as np
-import torch
-from torch.utils.data import DataLoader, RandomSampler, SequentialSampler, TensorDataset
-from torch.utils.data.distributed import DistributedSampler
-from tqdm import tqdm, trange
-
-from transformers import (
- WEIGHTS_NAME,
- AdamW,
- BertConfig,
- BertForMultipleChoice,
- BertTokenizer,
- RobertaConfig,
- RobertaForMultipleChoice,
- RobertaTokenizer,
- XLNetConfig,
- XLNetForMultipleChoice,
- XLNetTokenizer,
- get_linear_schedule_with_warmup,
-)
-from utils_multiple_choice import convert_examples_to_features, processors
-
-
-try:
- from torch.utils.tensorboard import SummaryWriter
-except ImportError:
- from tensorboardX import SummaryWriter
-
-
-logger = logging.getLogger(__name__)
-
-ALL_MODELS = sum(
- (tuple(conf.pretrained_config_archive_map.keys()) for conf in (BertConfig, XLNetConfig, RobertaConfig)), ()
-)
-
-MODEL_CLASSES = {
- "bert": (BertConfig, BertForMultipleChoice, BertTokenizer),
- "xlnet": (XLNetConfig, XLNetForMultipleChoice, XLNetTokenizer),
- "roberta": (RobertaConfig, RobertaForMultipleChoice, RobertaTokenizer),
-}
-
-
-def select_field(features, field):
- return [[choice[field] for choice in feature.choices_features] for feature in features]
-
-
-def simple_accuracy(preds, labels):
- return (preds == labels).mean()
-
-
-def set_seed(args):
- random.seed(args.seed)
- np.random.seed(args.seed)
- torch.manual_seed(args.seed)
- if args.n_gpu > 0:
- torch.cuda.manual_seed_all(args.seed)
-
-
-def train(args, train_dataset, model, tokenizer):
- """ Train the model """
- if args.local_rank in [-1, 0]:
- tb_writer = SummaryWriter()
-
- args.train_batch_size = args.per_gpu_train_batch_size * max(1, args.n_gpu)
- train_sampler = RandomSampler(train_dataset) if args.local_rank == -1 else DistributedSampler(train_dataset)
- train_dataloader = DataLoader(train_dataset, sampler=train_sampler, batch_size=args.train_batch_size)
-
- if args.max_steps > 0:
- t_total = args.max_steps
- args.num_train_epochs = args.max_steps // (len(train_dataloader) // args.gradient_accumulation_steps) + 1
- else:
- t_total = len(train_dataloader) // args.gradient_accumulation_steps * args.num_train_epochs
-
- # Prepare optimizer and schedule (linear warmup and decay)
- no_decay = ["bias", "LayerNorm.weight"]
- optimizer_grouped_parameters = [
- {
- "params": [p for n, p in model.named_parameters() if not any(nd in n for nd in no_decay)],
- "weight_decay": args.weight_decay,
- },
- {"params": [p for n, p in model.named_parameters() if any(nd in n for nd in no_decay)], "weight_decay": 0.0},
- ]
- optimizer = AdamW(optimizer_grouped_parameters, lr=args.learning_rate, eps=args.adam_epsilon)
- scheduler = get_linear_schedule_with_warmup(
- optimizer, num_warmup_steps=args.warmup_steps, num_training_steps=t_total
- )
- if args.fp16:
- try:
- from apex import amp
- except ImportError:
- raise ImportError("Please install apex from https://www.github.com/nvidia/apex to use fp16 training.")
- model, optimizer = amp.initialize(model, optimizer, opt_level=args.fp16_opt_level)
-
- # multi-gpu training (should be after apex fp16 initialization)
- if args.n_gpu > 1:
- model = torch.nn.DataParallel(model)
-
- # Distributed training (should be after apex fp16 initialization)
- if args.local_rank != -1:
- model = torch.nn.parallel.DistributedDataParallel(
- model, device_ids=[args.local_rank], output_device=args.local_rank, find_unused_parameters=True
- )
-
- # Train!
- logger.info("***** Running training *****")
- logger.info(" Num examples = %d", len(train_dataset))
- logger.info(" Num Epochs = %d", args.num_train_epochs)
- logger.info(" Instantaneous batch size per GPU = %d", args.per_gpu_train_batch_size)
- logger.info(
- " Total train batch size (w. parallel, distributed & accumulation) = %d",
- args.train_batch_size
- * args.gradient_accumulation_steps
- * (torch.distributed.get_world_size() if args.local_rank != -1 else 1),
- )
- logger.info(" Gradient Accumulation steps = %d", args.gradient_accumulation_steps)
- logger.info(" Total optimization steps = %d", t_total)
-
- global_step = 0
- tr_loss, logging_loss = 0.0, 0.0
- best_dev_acc = 0.0
- best_steps = 0
- model.zero_grad()
- train_iterator = trange(int(args.num_train_epochs), desc="Epoch", disable=args.local_rank not in [-1, 0])
- set_seed(args) # Added here for reproductibility
- for _ in train_iterator:
- epoch_iterator = tqdm(train_dataloader, desc="Iteration", disable=args.local_rank not in [-1, 0])
- for step, batch in enumerate(epoch_iterator):
- model.train()
- batch = tuple(t.to(args.device) for t in batch)
- inputs = {
- "input_ids": batch[0],
- "attention_mask": batch[1],
- "token_type_ids": batch[2]
- if args.model_type in ["bert", "xlnet"]
- else None, # XLM don't use segment_ids
- "labels": batch[3],
- }
- outputs = model(**inputs)
- loss = outputs[0] # model outputs are always tuple in transformers (see doc)
-
- if args.n_gpu > 1:
- loss = loss.mean() # mean() to average on multi-gpu parallel training
- if args.gradient_accumulation_steps > 1:
- loss = loss / args.gradient_accumulation_steps
-
- if args.fp16:
- with amp.scale_loss(loss, optimizer) as scaled_loss:
- scaled_loss.backward()
- torch.nn.utils.clip_grad_norm_(amp.master_params(optimizer), args.max_grad_norm)
- else:
- loss.backward()
- torch.nn.utils.clip_grad_norm_(model.parameters(), args.max_grad_norm)
-
- tr_loss += loss.item()
- if (step + 1) % args.gradient_accumulation_steps == 0:
-
- optimizer.step()
- scheduler.step() # Update learning rate schedule
- model.zero_grad()
- global_step += 1
-
- if args.local_rank in [-1, 0] and args.logging_steps > 0 and global_step % args.logging_steps == 0:
- # Log metrics
- if (
- args.local_rank == -1 and args.evaluate_during_training
- ): # Only evaluate when single GPU otherwise metrics may not average well
- results = evaluate(args, model, tokenizer)
- for key, value in results.items():
- tb_writer.add_scalar("eval_{}".format(key), value, global_step)
- if results["eval_acc"] > best_dev_acc:
- best_dev_acc = results["eval_acc"]
- best_steps = global_step
- if args.do_test:
- results_test = evaluate(args, model, tokenizer, test=True)
- for key, value in results_test.items():
- tb_writer.add_scalar("test_{}".format(key), value, global_step)
- logger.info(
- "test acc: %s, loss: %s, global steps: %s",
- str(results_test["eval_acc"]),
- str(results_test["eval_loss"]),
- str(global_step),
- )
- tb_writer.add_scalar("lr", scheduler.get_lr()[0], global_step)
- tb_writer.add_scalar("loss", (tr_loss - logging_loss) / args.logging_steps, global_step)
- logger.info(
- "Average loss: %s at global step: %s",
- str((tr_loss - logging_loss) / args.logging_steps),
- str(global_step),
- )
- logging_loss = tr_loss
-
- if args.local_rank in [-1, 0] and args.save_steps > 0 and global_step % args.save_steps == 0:
- # Save model checkpoint
- output_dir = os.path.join(args.output_dir, "checkpoint-{}".format(global_step))
- if not os.path.exists(output_dir):
- os.makedirs(output_dir)
- model_to_save = (
- model.module if hasattr(model, "module") else model
- ) # Take care of distributed/parallel training
- model_to_save.save_pretrained(output_dir)
- tokenizer.save_vocabulary(output_dir)
- torch.save(args, os.path.join(output_dir, "training_args.bin"))
- logger.info("Saving model checkpoint to %s", output_dir)
-
- if args.max_steps > 0 and global_step > args.max_steps:
- epoch_iterator.close()
- break
- if args.max_steps > 0 and global_step > args.max_steps:
- train_iterator.close()
- break
-
- if args.local_rank in [-1, 0]:
- tb_writer.close()
-
- return global_step, tr_loss / global_step, best_steps
-
-
-def evaluate(args, model, tokenizer, prefix="", test=False):
- eval_task_names = (args.task_name,)
- eval_outputs_dirs = (args.output_dir,)
-
- results = {}
- for eval_task, eval_output_dir in zip(eval_task_names, eval_outputs_dirs):
- eval_dataset = load_and_cache_examples(args, eval_task, tokenizer, evaluate=not test, test=test)
-
- if not os.path.exists(eval_output_dir) and args.local_rank in [-1, 0]:
- os.makedirs(eval_output_dir)
-
- args.eval_batch_size = args.per_gpu_eval_batch_size * max(1, args.n_gpu)
- # Note that DistributedSampler samples randomly
- eval_sampler = SequentialSampler(eval_dataset)
- eval_dataloader = DataLoader(eval_dataset, sampler=eval_sampler, batch_size=args.eval_batch_size)
-
- # multi-gpu evaluate
- if args.n_gpu > 1:
- model = torch.nn.DataParallel(model)
-
- # Eval!
- logger.info("***** Running evaluation {} *****".format(prefix))
- logger.info(" Num examples = %d", len(eval_dataset))
- logger.info(" Batch size = %d", args.eval_batch_size)
- eval_loss = 0.0
- nb_eval_steps = 0
- preds = None
- out_label_ids = None
- for batch in tqdm(eval_dataloader, desc="Evaluating"):
- model.eval()
- batch = tuple(t.to(args.device) for t in batch)
-
- with torch.no_grad():
- inputs = {
- "input_ids": batch[0],
- "attention_mask": batch[1],
- "token_type_ids": batch[2]
- if args.model_type in ["bert", "xlnet"]
- else None, # XLM don't use segment_ids
- "labels": batch[3],
- }
- outputs = model(**inputs)
- tmp_eval_loss, logits = outputs[:2]
-
- eval_loss += tmp_eval_loss.mean().item()
- nb_eval_steps += 1
- if preds is None:
- preds = logits.detach().cpu().numpy()
- out_label_ids = inputs["labels"].detach().cpu().numpy()
- else:
- preds = np.append(preds, logits.detach().cpu().numpy(), axis=0)
- out_label_ids = np.append(out_label_ids, inputs["labels"].detach().cpu().numpy(), axis=0)
-
- eval_loss = eval_loss / nb_eval_steps
- preds = np.argmax(preds, axis=1)
- acc = simple_accuracy(preds, out_label_ids)
- result = {"eval_acc": acc, "eval_loss": eval_loss}
- results.update(result)
-
- output_eval_file = os.path.join(eval_output_dir, "is_test_" + str(test).lower() + "_eval_results.txt")
-
- with open(output_eval_file, "w") as writer:
- logger.info("***** Eval results {} *****".format(str(prefix) + " is test:" + str(test)))
- writer.write("model =%s\n" % str(args.model_name_or_path))
- writer.write(
- "total batch size=%d\n"
- % (
- args.per_gpu_train_batch_size
- * args.gradient_accumulation_steps
- * (torch.distributed.get_world_size() if args.local_rank != -1 else 1)
- )
- )
- writer.write("train num epochs=%d\n" % args.num_train_epochs)
- writer.write("fp16 =%s\n" % args.fp16)
- writer.write("max seq length =%d\n" % args.max_seq_length)
- for key in sorted(result.keys()):
- logger.info(" %s = %s", key, str(result[key]))
- writer.write("%s = %s\n" % (key, str(result[key])))
- return results
-
-
-def load_and_cache_examples(args, task, tokenizer, evaluate=False, test=False):
- if args.local_rank not in [-1, 0]:
- torch.distributed.barrier() # Make sure only the first process in distributed training process the dataset, and the others will use the cache
-
- processor = processors[task]()
- # Load data features from cache or dataset file
- if evaluate:
- cached_mode = "dev"
- elif test:
- cached_mode = "test"
- else:
- cached_mode = "train"
- assert not (evaluate and test)
- cached_features_file = os.path.join(
- args.data_dir,
- "cached_{}_{}_{}_{}".format(
- cached_mode,
- list(filter(None, args.model_name_or_path.split("/"))).pop(),
- str(args.max_seq_length),
- str(task),
- ),
- )
- if os.path.exists(cached_features_file) and not args.overwrite_cache:
- logger.info("Loading features from cached file %s", cached_features_file)
- features = torch.load(cached_features_file)
- else:
- logger.info("Creating features from dataset file at %s", args.data_dir)
- label_list = processor.get_labels()
- if evaluate:
- examples = processor.get_dev_examples(args.data_dir)
- elif test:
- examples = processor.get_test_examples(args.data_dir)
- else:
- examples = processor.get_train_examples(args.data_dir)
- logger.info("Training number: %s", str(len(examples)))
- features = convert_examples_to_features(
- examples,
- label_list,
- args.max_seq_length,
- tokenizer,
- pad_on_left=bool(args.model_type in ["xlnet"]), # pad on the left for xlnet
- pad_token_segment_id=4 if args.model_type in ["xlnet"] else 0,
- )
- if args.local_rank in [-1, 0]:
- logger.info("Saving features into cached file %s", cached_features_file)
- torch.save(features, cached_features_file)
-
- if args.local_rank == 0:
- torch.distributed.barrier() # Make sure only the first process in distributed training process the dataset, and the others will use the cache
-
- # Convert to Tensors and build dataset
- all_input_ids = torch.tensor(select_field(features, "input_ids"), dtype=torch.long)
- all_input_mask = torch.tensor(select_field(features, "input_mask"), dtype=torch.long)
- all_segment_ids = torch.tensor(select_field(features, "segment_ids"), dtype=torch.long)
- all_label_ids = torch.tensor([f.label for f in features], dtype=torch.long)
-
- dataset = TensorDataset(all_input_ids, all_input_mask, all_segment_ids, all_label_ids)
- return dataset
-
-
-def main():
- parser = argparse.ArgumentParser()
-
- # Required parameters
- parser.add_argument(
- "--data_dir",
- default=None,
- type=str,
- required=True,
- help="The input data dir. Should contain the .tsv files (or other data files) for the task.",
- )
- parser.add_argument(
- "--model_type",
- default=None,
- type=str,
- required=True,
- help="Model type selected in the list: " + ", ".join(MODEL_CLASSES.keys()),
- )
- parser.add_argument(
- "--model_name_or_path",
- default=None,
- type=str,
- required=True,
- help="Path to pre-trained model or shortcut name selected in the list: " + ", ".join(ALL_MODELS),
- )
- parser.add_argument(
- "--task_name",
- default=None,
- type=str,
- required=True,
- help="The name of the task to train selected in the list: " + ", ".join(processors.keys()),
- )
- parser.add_argument(
- "--output_dir",
- default=None,
- type=str,
- required=True,
- help="The output directory where the model predictions and checkpoints will be written.",
- )
-
- # Other parameters
- parser.add_argument(
- "--config_name", default="", type=str, help="Pretrained config name or path if not the same as model_name"
- )
- parser.add_argument(
- "--tokenizer_name",
- default="",
- type=str,
- help="Pretrained tokenizer name or path if not the same as model_name",
- )
- parser.add_argument(
- "--cache_dir",
- default="",
- type=str,
- help="Where do you want to store the pre-trained models downloaded from s3",
- )
- parser.add_argument(
- "--max_seq_length",
- default=128,
- type=int,
- help="The maximum total input sequence length after tokenization. Sequences longer "
- "than this will be truncated, sequences shorter will be padded.",
- )
- parser.add_argument("--do_train", action="store_true", help="Whether to run training.")
- parser.add_argument("--do_eval", action="store_true", help="Whether to run eval on the dev set.")
- parser.add_argument("--do_test", action="store_true", help="Whether to run test on the test set")
- parser.add_argument(
- "--evaluate_during_training", action="store_true", help="Run evaluation during training at each logging step."
- )
- parser.add_argument(
- "--do_lower_case", action="store_true", help="Set this flag if you are using an uncased model."
- )
-
- parser.add_argument("--per_gpu_train_batch_size", default=8, type=int, help="Batch size per GPU/CPU for training.")
- parser.add_argument(
- "--per_gpu_eval_batch_size", default=8, type=int, help="Batch size per GPU/CPU for evaluation."
- )
- parser.add_argument(
- "--gradient_accumulation_steps",
- type=int,
- default=1,
- help="Number of updates steps to accumulate before performing a backward/update pass.",
- )
- parser.add_argument("--learning_rate", default=5e-5, type=float, help="The initial learning rate for Adam.")
- parser.add_argument("--weight_decay", default=0.0, type=float, help="Weight deay if we apply some.")
- parser.add_argument("--adam_epsilon", default=1e-8, type=float, help="Epsilon for Adam optimizer.")
- parser.add_argument("--max_grad_norm", default=1.0, type=float, help="Max gradient norm.")
- parser.add_argument(
- "--num_train_epochs", default=3.0, type=float, help="Total number of training epochs to perform."
- )
- parser.add_argument(
- "--max_steps",
- default=-1,
- type=int,
- help="If > 0: set total number of training steps to perform. Override num_train_epochs.",
- )
- parser.add_argument("--warmup_steps", default=0, type=int, help="Linear warmup over warmup_steps.")
-
- parser.add_argument("--logging_steps", type=int, default=500, help="Log every X updates steps.")
- parser.add_argument("--save_steps", type=int, default=500, help="Save checkpoint every X updates steps.")
- parser.add_argument(
- "--eval_all_checkpoints",
- action="store_true",
- help="Evaluate all checkpoints starting with the same prefix as model_name ending and ending with step number",
- )
- parser.add_argument("--no_cuda", action="store_true", help="Avoid using CUDA when available")
- parser.add_argument(
- "--overwrite_output_dir", action="store_true", help="Overwrite the content of the output directory"
- )
- parser.add_argument(
- "--overwrite_cache", action="store_true", help="Overwrite the cached training and evaluation sets"
- )
- parser.add_argument("--seed", type=int, default=42, help="random seed for initialization")
-
- parser.add_argument(
- "--fp16",
- action="store_true",
- help="Whether to use 16-bit (mixed) precision (through NVIDIA apex) instead of 32-bit",
- )
- parser.add_argument(
- "--fp16_opt_level",
- type=str,
- default="O1",
- help="For fp16: Apex AMP optimization level selected in ['O0', 'O1', 'O2', and 'O3']."
- "See details at https://nvidia.github.io/apex/amp.html",
- )
- parser.add_argument("--local_rank", type=int, default=-1, help="For distributed training: local_rank")
- parser.add_argument("--server_ip", type=str, default="", help="For distant debugging.")
- parser.add_argument("--server_port", type=str, default="", help="For distant debugging.")
- args = parser.parse_args()
-
- if (
- os.path.exists(args.output_dir)
- and os.listdir(args.output_dir)
- and args.do_train
- and not args.overwrite_output_dir
- ):
- raise ValueError(
- "Output directory ({}) already exists and is not empty. Use --overwrite_output_dir to overcome.".format(
- args.output_dir
- )
- )
-
- # Setup distant debugging if needed
- if args.server_ip and args.server_port:
- # Distant debugging - see https://code.visualstudio.com/docs/python/debugging#_attach-to-a-local-script
- import ptvsd
-
- print("Waiting for debugger attach")
- ptvsd.enable_attach(address=(args.server_ip, args.server_port), redirect_output=True)
- ptvsd.wait_for_attach()
-
- # Setup CUDA, GPU & distributed training
- if args.local_rank == -1 or args.no_cuda:
- device = torch.device("cuda" if torch.cuda.is_available() and not args.no_cuda else "cpu")
- args.n_gpu = torch.cuda.device_count()
- else: # Initializes the distributed backend which will take care of sychronizing nodes/GPUs
- torch.cuda.set_device(args.local_rank)
- device = torch.device("cuda", args.local_rank)
- torch.distributed.init_process_group(backend="nccl")
- args.n_gpu = 1
- args.device = device
-
- # Setup logging
- logging.basicConfig(
- format="%(asctime)s - %(levelname)s - %(name)s - %(message)s",
- datefmt="%m/%d/%Y %H:%M:%S",
- level=logging.INFO if args.local_rank in [-1, 0] else logging.WARN,
- )
- logger.warning(
- "Process rank: %s, device: %s, n_gpu: %s, distributed training: %s, 16-bits training: %s",
- args.local_rank,
- device,
- args.n_gpu,
- bool(args.local_rank != -1),
- args.fp16,
- )
-
- # Set seed
- set_seed(args)
-
- # Prepare GLUE task
- args.task_name = args.task_name.lower()
- if args.task_name not in processors:
- raise ValueError("Task not found: %s" % (args.task_name))
- processor = processors[args.task_name]()
- label_list = processor.get_labels()
- num_labels = len(label_list)
-
- # Load pretrained model and tokenizer
- if args.local_rank not in [-1, 0]:
- torch.distributed.barrier() # Make sure only the first process in distributed training will download model & vocab
-
- args.model_type = args.model_type.lower()
- config_class, model_class, tokenizer_class = MODEL_CLASSES[args.model_type]
- config = config_class.from_pretrained(
- args.config_name if args.config_name else args.model_name_or_path,
- num_labels=num_labels,
- finetuning_task=args.task_name,
- cache_dir=args.cache_dir if args.cache_dir else None,
- )
- tokenizer = tokenizer_class.from_pretrained(
- args.tokenizer_name if args.tokenizer_name else args.model_name_or_path,
- do_lower_case=args.do_lower_case,
- cache_dir=args.cache_dir if args.cache_dir else None,
- )
- model = model_class.from_pretrained(
- args.model_name_or_path,
- from_tf=bool(".ckpt" in args.model_name_or_path),
- config=config,
- cache_dir=args.cache_dir if args.cache_dir else None,
- )
-
- if args.local_rank == 0:
- torch.distributed.barrier() # Make sure only the first process in distributed training will download model & vocab
-
- model.to(args.device)
-
- logger.info("Training/evaluation parameters %s", args)
- best_steps = 0
-
- # Training
- if args.do_train:
- train_dataset = load_and_cache_examples(args, args.task_name, tokenizer, evaluate=False)
- global_step, tr_loss, best_steps = train(args, train_dataset, model, tokenizer)
- logger.info(" global_step = %s, average loss = %s", global_step, tr_loss)
-
- # Saving best-practices: if you use defaults names for the model, you can reload it using from_pretrained()
- if args.do_train and (args.local_rank == -1 or torch.distributed.get_rank() == 0):
- # Create output directory if needed
- if not os.path.exists(args.output_dir) and args.local_rank in [-1, 0]:
- os.makedirs(args.output_dir)
-
- logger.info("Saving model checkpoint to %s", args.output_dir)
- # Save a trained model, configuration and tokenizer using `save_pretrained()`.
- # They can then be reloaded using `from_pretrained()`
- model_to_save = (
- model.module if hasattr(model, "module") else model
- ) # Take care of distributed/parallel training
- model_to_save.save_pretrained(args.output_dir)
- tokenizer.save_pretrained(args.output_dir)
-
- # Good practice: save your training arguments together with the trained model
- torch.save(args, os.path.join(args.output_dir, "training_args.bin"))
-
- # Load a trained model and vocabulary that you have fine-tuned
- model = model_class.from_pretrained(args.output_dir)
- tokenizer = tokenizer_class.from_pretrained(args.output_dir)
- model.to(args.device)
-
- # Evaluation
- results = {}
- if args.do_eval and args.local_rank in [-1, 0]:
- if not args.do_train:
- args.output_dir = args.model_name_or_path
- checkpoints = [args.output_dir]
- if args.eval_all_checkpoints:
- checkpoints = list(
- os.path.dirname(c) for c in sorted(glob.glob(args.output_dir + "/**/" + WEIGHTS_NAME, recursive=True))
- )
- logging.getLogger("transformers.modeling_utils").setLevel(logging.WARN) # Reduce logging
- logger.info("Evaluate the following checkpoints: %s", checkpoints)
- for checkpoint in checkpoints:
- global_step = checkpoint.split("-")[-1] if len(checkpoints) > 1 else ""
- prefix = checkpoint.split("/")[-1] if checkpoint.find("checkpoint") != -1 else ""
-
- model = model_class.from_pretrained(checkpoint)
- model.to(args.device)
- result = evaluate(args, model, tokenizer, prefix=prefix)
- result = dict((k + "_{}".format(global_step), v) for k, v in result.items())
- results.update(result)
-
- if args.do_test and args.local_rank in [-1, 0]:
- if not args.do_train:
- args.output_dir = args.model_name_or_path
- checkpoints = [args.output_dir]
- # if args.eval_all_checkpoints: # can not use this to do test!!
- # checkpoints = list(os.path.dirname(c) for c in sorted(glob.glob(args.output_dir + '/**/' + WEIGHTS_NAME, recursive=True)))
- # logging.getLogger("transformers.modeling_utils").setLevel(logging.WARN) # Reduce logging
- logger.info("Evaluate the following checkpoints: %s", checkpoints)
- for checkpoint in checkpoints:
- global_step = checkpoint.split("-")[-1] if len(checkpoints) > 1 else ""
- prefix = checkpoint.split("/")[-1] if checkpoint.find("checkpoint") != -1 else ""
-
- model = model_class.from_pretrained(checkpoint)
- model.to(args.device)
- result = evaluate(args, model, tokenizer, prefix=prefix, test=True)
- result = dict((k + "_{}".format(global_step), v) for k, v in result.items())
- results.update(result)
- if best_steps:
- logger.info("best steps of eval acc is the following checkpoints: %s", best_steps)
- return results
-
-
-if __name__ == "__main__":
- main()
diff --git a/server/transformers/examples/run_ner.py b/server/transformers/examples/run_ner.py
deleted file mode 100644
index a2937985ecbef23b6daf020ff9e68898584e4298..0000000000000000000000000000000000000000
--- a/server/transformers/examples/run_ner.py
+++ /dev/null
@@ -1,685 +0,0 @@
-# coding=utf-8
-# Copyright 2018 The Google AI Language Team Authors and The HuggingFace Inc. team.
-# Copyright (c) 2018, NVIDIA CORPORATION. All rights reserved.
-#
-# Licensed under the Apache License, Version 2.0 (the "License");
-# you may not use this file except in compliance with the License.
-# You may obtain a copy of the License at
-#
-# http://www.apache.org/licenses/LICENSE-2.0
-#
-# Unless required by applicable law or agreed to in writing, software
-# distributed under the License is distributed on an "AS IS" BASIS,
-# WITHOUT WARRANTIES OR CONDITIONS OF ANY KIND, either express or implied.
-# See the License for the specific language governing permissions and
-# limitations under the License.
-""" Fine-tuning the library models for named entity recognition on CoNLL-2003 (Bert or Roberta). """
-
-
-import argparse
-import glob
-import logging
-import os
-import random
-
-import numpy as np
-import torch
-from seqeval.metrics import f1_score, precision_score, recall_score
-from torch.nn import CrossEntropyLoss
-from torch.utils.data import DataLoader, RandomSampler, SequentialSampler, TensorDataset
-from torch.utils.data.distributed import DistributedSampler
-from tqdm import tqdm, trange
-
-from transformers import (
- WEIGHTS_NAME,
- AdamW,
- BertConfig,
- BertForTokenClassification,
- BertTokenizer,
- CamembertConfig,
- CamembertForTokenClassification,
- CamembertTokenizer,
- DistilBertConfig,
- DistilBertForTokenClassification,
- DistilBertTokenizer,
- RobertaConfig,
- RobertaForTokenClassification,
- RobertaTokenizer,
- XLMRobertaConfig,
- XLMRobertaForTokenClassification,
- XLMRobertaTokenizer,
- get_linear_schedule_with_warmup,
-)
-from utils_ner import convert_examples_to_features, get_labels, read_examples_from_file
-
-
-try:
- from torch.utils.tensorboard import SummaryWriter
-except ImportError:
- from tensorboardX import SummaryWriter
-
-
-logger = logging.getLogger(__name__)
-
-ALL_MODELS = sum(
- (
- tuple(conf.pretrained_config_archive_map.keys())
- for conf in (BertConfig, RobertaConfig, DistilBertConfig, CamembertConfig, XLMRobertaConfig)
- ),
- (),
-)
-
-MODEL_CLASSES = {
- "bert": (BertConfig, BertForTokenClassification, BertTokenizer),
- "roberta": (RobertaConfig, RobertaForTokenClassification, RobertaTokenizer),
- "distilbert": (DistilBertConfig, DistilBertForTokenClassification, DistilBertTokenizer),
- "camembert": (CamembertConfig, CamembertForTokenClassification, CamembertTokenizer),
- "xlmroberta": (XLMRobertaConfig, XLMRobertaForTokenClassification, XLMRobertaTokenizer),
-}
-
-
-def set_seed(args):
- random.seed(args.seed)
- np.random.seed(args.seed)
- torch.manual_seed(args.seed)
- if args.n_gpu > 0:
- torch.cuda.manual_seed_all(args.seed)
-
-
-def train(args, train_dataset, model, tokenizer, labels, pad_token_label_id):
- """ Train the model """
- if args.local_rank in [-1, 0]:
- tb_writer = SummaryWriter()
-
- args.train_batch_size = args.per_gpu_train_batch_size * max(1, args.n_gpu)
- train_sampler = RandomSampler(train_dataset) if args.local_rank == -1 else DistributedSampler(train_dataset)
- train_dataloader = DataLoader(train_dataset, sampler=train_sampler, batch_size=args.train_batch_size)
-
- if args.max_steps > 0:
- t_total = args.max_steps
- args.num_train_epochs = args.max_steps // (len(train_dataloader) // args.gradient_accumulation_steps) + 1
- else:
- t_total = len(train_dataloader) // args.gradient_accumulation_steps * args.num_train_epochs
-
- # Prepare optimizer and schedule (linear warmup and decay)
- no_decay = ["bias", "LayerNorm.weight"]
- optimizer_grouped_parameters = [
- {
- "params": [p for n, p in model.named_parameters() if not any(nd in n for nd in no_decay)],
- "weight_decay": args.weight_decay,
- },
- {"params": [p for n, p in model.named_parameters() if any(nd in n for nd in no_decay)], "weight_decay": 0.0},
- ]
- optimizer = AdamW(optimizer_grouped_parameters, lr=args.learning_rate, eps=args.adam_epsilon)
- scheduler = get_linear_schedule_with_warmup(
- optimizer, num_warmup_steps=args.warmup_steps, num_training_steps=t_total
- )
-
- # Check if saved optimizer or scheduler states exist
- if os.path.isfile(os.path.join(args.model_name_or_path, "optimizer.pt")) and os.path.isfile(
- os.path.join(args.model_name_or_path, "scheduler.pt")
- ):
- # Load in optimizer and scheduler states
- optimizer.load_state_dict(torch.load(os.path.join(args.model_name_or_path, "optimizer.pt")))
- scheduler.load_state_dict(torch.load(os.path.join(args.model_name_or_path, "scheduler.pt")))
-
- if args.fp16:
- try:
- from apex import amp
- except ImportError:
- raise ImportError("Please install apex from https://www.github.com/nvidia/apex to use fp16 training.")
- model, optimizer = amp.initialize(model, optimizer, opt_level=args.fp16_opt_level)
-
- # multi-gpu training (should be after apex fp16 initialization)
- if args.n_gpu > 1:
- model = torch.nn.DataParallel(model)
-
- # Distributed training (should be after apex fp16 initialization)
- if args.local_rank != -1:
- model = torch.nn.parallel.DistributedDataParallel(
- model, device_ids=[args.local_rank], output_device=args.local_rank, find_unused_parameters=True
- )
-
- # Train!
- logger.info("***** Running training *****")
- logger.info(" Num examples = %d", len(train_dataset))
- logger.info(" Num Epochs = %d", args.num_train_epochs)
- logger.info(" Instantaneous batch size per GPU = %d", args.per_gpu_train_batch_size)
- logger.info(
- " Total train batch size (w. parallel, distributed & accumulation) = %d",
- args.train_batch_size
- * args.gradient_accumulation_steps
- * (torch.distributed.get_world_size() if args.local_rank != -1 else 1),
- )
- logger.info(" Gradient Accumulation steps = %d", args.gradient_accumulation_steps)
- logger.info(" Total optimization steps = %d", t_total)
-
- global_step = 0
- epochs_trained = 0
- steps_trained_in_current_epoch = 0
- # Check if continuing training from a checkpoint
- if os.path.exists(args.model_name_or_path):
- # set global_step to gobal_step of last saved checkpoint from model path
- global_step = int(args.model_name_or_path.split("-")[-1].split("/")[0])
- epochs_trained = global_step // (len(train_dataloader) // args.gradient_accumulation_steps)
- steps_trained_in_current_epoch = global_step % (len(train_dataloader) // args.gradient_accumulation_steps)
-
- logger.info(" Continuing training from checkpoint, will skip to saved global_step")
- logger.info(" Continuing training from epoch %d", epochs_trained)
- logger.info(" Continuing training from global step %d", global_step)
- logger.info(" Will skip the first %d steps in the first epoch", steps_trained_in_current_epoch)
-
- tr_loss, logging_loss = 0.0, 0.0
- model.zero_grad()
- train_iterator = trange(
- epochs_trained, int(args.num_train_epochs), desc="Epoch", disable=args.local_rank not in [-1, 0]
- )
- set_seed(args) # Added here for reproductibility
- for _ in train_iterator:
- epoch_iterator = tqdm(train_dataloader, desc="Iteration", disable=args.local_rank not in [-1, 0])
- for step, batch in enumerate(epoch_iterator):
-
- # Skip past any already trained steps if resuming training
- if steps_trained_in_current_epoch > 0:
- steps_trained_in_current_epoch -= 1
- continue
-
- model.train()
- batch = tuple(t.to(args.device) for t in batch)
- inputs = {"input_ids": batch[0], "attention_mask": batch[1], "labels": batch[3]}
- if args.model_type != "distilbert":
- inputs["token_type_ids"] = (
- batch[2] if args.model_type in ["bert", "xlnet"] else None
- ) # XLM and RoBERTa don"t use segment_ids
-
- outputs = model(**inputs)
- loss = outputs[0] # model outputs are always tuple in pytorch-transformers (see doc)
-
- if args.n_gpu > 1:
- loss = loss.mean() # mean() to average on multi-gpu parallel training
- if args.gradient_accumulation_steps > 1:
- loss = loss / args.gradient_accumulation_steps
-
- if args.fp16:
- with amp.scale_loss(loss, optimizer) as scaled_loss:
- scaled_loss.backward()
- else:
- loss.backward()
-
- tr_loss += loss.item()
- if (step + 1) % args.gradient_accumulation_steps == 0:
- if args.fp16:
- torch.nn.utils.clip_grad_norm_(amp.master_params(optimizer), args.max_grad_norm)
- else:
- torch.nn.utils.clip_grad_norm_(model.parameters(), args.max_grad_norm)
-
- scheduler.step() # Update learning rate schedule
- optimizer.step()
- model.zero_grad()
- global_step += 1
-
- if args.local_rank in [-1, 0] and args.logging_steps > 0 and global_step % args.logging_steps == 0:
- # Log metrics
- if (
- args.local_rank == -1 and args.evaluate_during_training
- ): # Only evaluate when single GPU otherwise metrics may not average well
- results, _ = evaluate(args, model, tokenizer, labels, pad_token_label_id, mode="dev")
- for key, value in results.items():
- tb_writer.add_scalar("eval_{}".format(key), value, global_step)
- tb_writer.add_scalar("lr", scheduler.get_lr()[0], global_step)
- tb_writer.add_scalar("loss", (tr_loss - logging_loss) / args.logging_steps, global_step)
- logging_loss = tr_loss
-
- if args.local_rank in [-1, 0] and args.save_steps > 0 and global_step % args.save_steps == 0:
- # Save model checkpoint
- output_dir = os.path.join(args.output_dir, "checkpoint-{}".format(global_step))
- if not os.path.exists(output_dir):
- os.makedirs(output_dir)
- model_to_save = (
- model.module if hasattr(model, "module") else model
- ) # Take care of distributed/parallel training
- model_to_save.save_pretrained(output_dir)
- tokenizer.save_pretrained(output_dir)
-
- torch.save(args, os.path.join(output_dir, "training_args.bin"))
- logger.info("Saving model checkpoint to %s", output_dir)
-
- torch.save(optimizer.state_dict(), os.path.join(output_dir, "optimizer.pt"))
- torch.save(scheduler.state_dict(), os.path.join(output_dir, "scheduler.pt"))
- logger.info("Saving optimizer and scheduler states to %s", output_dir)
-
- if args.max_steps > 0 and global_step > args.max_steps:
- epoch_iterator.close()
- break
- if args.max_steps > 0 and global_step > args.max_steps:
- train_iterator.close()
- break
-
- if args.local_rank in [-1, 0]:
- tb_writer.close()
-
- return global_step, tr_loss / global_step
-
-
-def evaluate(args, model, tokenizer, labels, pad_token_label_id, mode, prefix=""):
- eval_dataset = load_and_cache_examples(args, tokenizer, labels, pad_token_label_id, mode=mode)
-
- args.eval_batch_size = args.per_gpu_eval_batch_size * max(1, args.n_gpu)
- # Note that DistributedSampler samples randomly
- eval_sampler = SequentialSampler(eval_dataset) if args.local_rank == -1 else DistributedSampler(eval_dataset)
- eval_dataloader = DataLoader(eval_dataset, sampler=eval_sampler, batch_size=args.eval_batch_size)
-
- # multi-gpu evaluate
- if args.n_gpu > 1:
- model = torch.nn.DataParallel(model)
-
- # Eval!
- logger.info("***** Running evaluation %s *****", prefix)
- logger.info(" Num examples = %d", len(eval_dataset))
- logger.info(" Batch size = %d", args.eval_batch_size)
- eval_loss = 0.0
- nb_eval_steps = 0
- preds = None
- out_label_ids = None
- model.eval()
- for batch in tqdm(eval_dataloader, desc="Evaluating"):
- batch = tuple(t.to(args.device) for t in batch)
-
- with torch.no_grad():
- inputs = {"input_ids": batch[0], "attention_mask": batch[1], "labels": batch[3]}
- if args.model_type != "distilbert":
- inputs["token_type_ids"] = (
- batch[2] if args.model_type in ["bert", "xlnet"] else None
- ) # XLM and RoBERTa don"t use segment_ids
- outputs = model(**inputs)
- tmp_eval_loss, logits = outputs[:2]
-
- if args.n_gpu > 1:
- tmp_eval_loss = tmp_eval_loss.mean() # mean() to average on multi-gpu parallel evaluating
-
- eval_loss += tmp_eval_loss.item()
- nb_eval_steps += 1
- if preds is None:
- preds = logits.detach().cpu().numpy()
- out_label_ids = inputs["labels"].detach().cpu().numpy()
- else:
- preds = np.append(preds, logits.detach().cpu().numpy(), axis=0)
- out_label_ids = np.append(out_label_ids, inputs["labels"].detach().cpu().numpy(), axis=0)
-
- eval_loss = eval_loss / nb_eval_steps
- preds = np.argmax(preds, axis=2)
-
- label_map = {i: label for i, label in enumerate(labels)}
-
- out_label_list = [[] for _ in range(out_label_ids.shape[0])]
- preds_list = [[] for _ in range(out_label_ids.shape[0])]
-
- for i in range(out_label_ids.shape[0]):
- for j in range(out_label_ids.shape[1]):
- if out_label_ids[i, j] != pad_token_label_id:
- out_label_list[i].append(label_map[out_label_ids[i][j]])
- preds_list[i].append(label_map[preds[i][j]])
-
- results = {
- "loss": eval_loss,
- "precision": precision_score(out_label_list, preds_list),
- "recall": recall_score(out_label_list, preds_list),
- "f1": f1_score(out_label_list, preds_list),
- }
-
- logger.info("***** Eval results %s *****", prefix)
- for key in sorted(results.keys()):
- logger.info(" %s = %s", key, str(results[key]))
-
- return results, preds_list
-
-
-def load_and_cache_examples(args, tokenizer, labels, pad_token_label_id, mode):
- if args.local_rank not in [-1, 0] and not evaluate:
- torch.distributed.barrier() # Make sure only the first process in distributed training process the dataset, and the others will use the cache
-
- # Load data features from cache or dataset file
- cached_features_file = os.path.join(
- args.data_dir,
- "cached_{}_{}_{}".format(
- mode, list(filter(None, args.model_name_or_path.split("/"))).pop(), str(args.max_seq_length)
- ),
- )
- if os.path.exists(cached_features_file) and not args.overwrite_cache:
- logger.info("Loading features from cached file %s", cached_features_file)
- features = torch.load(cached_features_file)
- else:
- logger.info("Creating features from dataset file at %s", args.data_dir)
- examples = read_examples_from_file(args.data_dir, mode)
- features = convert_examples_to_features(
- examples,
- labels,
- args.max_seq_length,
- tokenizer,
- cls_token_at_end=bool(args.model_type in ["xlnet"]),
- # xlnet has a cls token at the end
- cls_token=tokenizer.cls_token,
- cls_token_segment_id=2 if args.model_type in ["xlnet"] else 0,
- sep_token=tokenizer.sep_token,
- sep_token_extra=bool(args.model_type in ["roberta"]),
- # roberta uses an extra separator b/w pairs of sentences, cf. github.com/pytorch/fairseq/commit/1684e166e3da03f5b600dbb7855cb98ddfcd0805
- pad_on_left=bool(args.model_type in ["xlnet"]),
- # pad on the left for xlnet
- pad_token=tokenizer.convert_tokens_to_ids([tokenizer.pad_token])[0],
- pad_token_segment_id=4 if args.model_type in ["xlnet"] else 0,
- pad_token_label_id=pad_token_label_id,
- )
- if args.local_rank in [-1, 0]:
- logger.info("Saving features into cached file %s", cached_features_file)
- torch.save(features, cached_features_file)
-
- if args.local_rank == 0 and not evaluate:
- torch.distributed.barrier() # Make sure only the first process in distributed training process the dataset, and the others will use the cache
-
- # Convert to Tensors and build dataset
- all_input_ids = torch.tensor([f.input_ids for f in features], dtype=torch.long)
- all_input_mask = torch.tensor([f.input_mask for f in features], dtype=torch.long)
- all_segment_ids = torch.tensor([f.segment_ids for f in features], dtype=torch.long)
- all_label_ids = torch.tensor([f.label_ids for f in features], dtype=torch.long)
-
- dataset = TensorDataset(all_input_ids, all_input_mask, all_segment_ids, all_label_ids)
- return dataset
-
-
-def main():
- parser = argparse.ArgumentParser()
-
- # Required parameters
- parser.add_argument(
- "--data_dir",
- default=None,
- type=str,
- required=True,
- help="The input data dir. Should contain the training files for the CoNLL-2003 NER task.",
- )
- parser.add_argument(
- "--model_type",
- default=None,
- type=str,
- required=True,
- help="Model type selected in the list: " + ", ".join(MODEL_CLASSES.keys()),
- )
- parser.add_argument(
- "--model_name_or_path",
- default=None,
- type=str,
- required=True,
- help="Path to pre-trained model or shortcut name selected in the list: " + ", ".join(ALL_MODELS),
- )
- parser.add_argument(
- "--output_dir",
- default=None,
- type=str,
- required=True,
- help="The output directory where the model predictions and checkpoints will be written.",
- )
-
- # Other parameters
- parser.add_argument(
- "--labels",
- default="",
- type=str,
- help="Path to a file containing all labels. If not specified, CoNLL-2003 labels are used.",
- )
- parser.add_argument(
- "--config_name", default="", type=str, help="Pretrained config name or path if not the same as model_name"
- )
- parser.add_argument(
- "--tokenizer_name",
- default="",
- type=str,
- help="Pretrained tokenizer name or path if not the same as model_name",
- )
- parser.add_argument(
- "--cache_dir",
- default="",
- type=str,
- help="Where do you want to store the pre-trained models downloaded from s3",
- )
- parser.add_argument(
- "--max_seq_length",
- default=128,
- type=int,
- help="The maximum total input sequence length after tokenization. Sequences longer "
- "than this will be truncated, sequences shorter will be padded.",
- )
- parser.add_argument("--do_train", action="store_true", help="Whether to run training.")
- parser.add_argument("--do_eval", action="store_true", help="Whether to run eval on the dev set.")
- parser.add_argument("--do_predict", action="store_true", help="Whether to run predictions on the test set.")
- parser.add_argument(
- "--evaluate_during_training",
- action="store_true",
- help="Whether to run evaluation during training at each logging step.",
- )
- parser.add_argument(
- "--do_lower_case", action="store_true", help="Set this flag if you are using an uncased model."
- )
-
- parser.add_argument("--per_gpu_train_batch_size", default=8, type=int, help="Batch size per GPU/CPU for training.")
- parser.add_argument(
- "--per_gpu_eval_batch_size", default=8, type=int, help="Batch size per GPU/CPU for evaluation."
- )
- parser.add_argument(
- "--gradient_accumulation_steps",
- type=int,
- default=1,
- help="Number of updates steps to accumulate before performing a backward/update pass.",
- )
- parser.add_argument("--learning_rate", default=5e-5, type=float, help="The initial learning rate for Adam.")
- parser.add_argument("--weight_decay", default=0.0, type=float, help="Weight decay if we apply some.")
- parser.add_argument("--adam_epsilon", default=1e-8, type=float, help="Epsilon for Adam optimizer.")
- parser.add_argument("--max_grad_norm", default=1.0, type=float, help="Max gradient norm.")
- parser.add_argument(
- "--num_train_epochs", default=3.0, type=float, help="Total number of training epochs to perform."
- )
- parser.add_argument(
- "--max_steps",
- default=-1,
- type=int,
- help="If > 0: set total number of training steps to perform. Override num_train_epochs.",
- )
- parser.add_argument("--warmup_steps", default=0, type=int, help="Linear warmup over warmup_steps.")
-
- parser.add_argument("--logging_steps", type=int, default=500, help="Log every X updates steps.")
- parser.add_argument("--save_steps", type=int, default=500, help="Save checkpoint every X updates steps.")
- parser.add_argument(
- "--eval_all_checkpoints",
- action="store_true",
- help="Evaluate all checkpoints starting with the same prefix as model_name ending and ending with step number",
- )
- parser.add_argument("--no_cuda", action="store_true", help="Avoid using CUDA when available")
- parser.add_argument(
- "--overwrite_output_dir", action="store_true", help="Overwrite the content of the output directory"
- )
- parser.add_argument(
- "--overwrite_cache", action="store_true", help="Overwrite the cached training and evaluation sets"
- )
- parser.add_argument("--seed", type=int, default=42, help="random seed for initialization")
-
- parser.add_argument(
- "--fp16",
- action="store_true",
- help="Whether to use 16-bit (mixed) precision (through NVIDIA apex) instead of 32-bit",
- )
- parser.add_argument(
- "--fp16_opt_level",
- type=str,
- default="O1",
- help="For fp16: Apex AMP optimization level selected in ['O0', 'O1', 'O2', and 'O3']."
- "See details at https://nvidia.github.io/apex/amp.html",
- )
- parser.add_argument("--local_rank", type=int, default=-1, help="For distributed training: local_rank")
- parser.add_argument("--server_ip", type=str, default="", help="For distant debugging.")
- parser.add_argument("--server_port", type=str, default="", help="For distant debugging.")
- args = parser.parse_args()
-
- if (
- os.path.exists(args.output_dir)
- and os.listdir(args.output_dir)
- and args.do_train
- and not args.overwrite_output_dir
- ):
- raise ValueError(
- "Output directory ({}) already exists and is not empty. Use --overwrite_output_dir to overcome.".format(
- args.output_dir
- )
- )
-
- # Setup distant debugging if needed
- if args.server_ip and args.server_port:
- # Distant debugging - see https://code.visualstudio.com/docs/python/debugging#_attach-to-a-local-script
- import ptvsd
-
- print("Waiting for debugger attach")
- ptvsd.enable_attach(address=(args.server_ip, args.server_port), redirect_output=True)
- ptvsd.wait_for_attach()
-
- # Setup CUDA, GPU & distributed training
- if args.local_rank == -1 or args.no_cuda:
- device = torch.device("cuda" if torch.cuda.is_available() and not args.no_cuda else "cpu")
- args.n_gpu = torch.cuda.device_count()
- else: # Initializes the distributed backend which will take care of sychronizing nodes/GPUs
- torch.cuda.set_device(args.local_rank)
- device = torch.device("cuda", args.local_rank)
- torch.distributed.init_process_group(backend="nccl")
- args.n_gpu = 1
- args.device = device
-
- # Setup logging
- logging.basicConfig(
- format="%(asctime)s - %(levelname)s - %(name)s - %(message)s",
- datefmt="%m/%d/%Y %H:%M:%S",
- level=logging.INFO if args.local_rank in [-1, 0] else logging.WARN,
- )
- logger.warning(
- "Process rank: %s, device: %s, n_gpu: %s, distributed training: %s, 16-bits training: %s",
- args.local_rank,
- device,
- args.n_gpu,
- bool(args.local_rank != -1),
- args.fp16,
- )
-
- # Set seed
- set_seed(args)
-
- # Prepare CONLL-2003 task
- labels = get_labels(args.labels)
- num_labels = len(labels)
- # Use cross entropy ignore index as padding label id so that only real label ids contribute to the loss later
- pad_token_label_id = CrossEntropyLoss().ignore_index
-
- # Load pretrained model and tokenizer
- if args.local_rank not in [-1, 0]:
- torch.distributed.barrier() # Make sure only the first process in distributed training will download model & vocab
-
- args.model_type = args.model_type.lower()
- config_class, model_class, tokenizer_class = MODEL_CLASSES[args.model_type]
- config = config_class.from_pretrained(
- args.config_name if args.config_name else args.model_name_or_path,
- num_labels=num_labels,
- cache_dir=args.cache_dir if args.cache_dir else None,
- )
- tokenizer = tokenizer_class.from_pretrained(
- args.tokenizer_name if args.tokenizer_name else args.model_name_or_path,
- do_lower_case=args.do_lower_case,
- cache_dir=args.cache_dir if args.cache_dir else None,
- )
- model = model_class.from_pretrained(
- args.model_name_or_path,
- from_tf=bool(".ckpt" in args.model_name_or_path),
- config=config,
- cache_dir=args.cache_dir if args.cache_dir else None,
- )
-
- if args.local_rank == 0:
- torch.distributed.barrier() # Make sure only the first process in distributed training will download model & vocab
-
- model.to(args.device)
-
- logger.info("Training/evaluation parameters %s", args)
-
- # Training
- if args.do_train:
- train_dataset = load_and_cache_examples(args, tokenizer, labels, pad_token_label_id, mode="train")
- global_step, tr_loss = train(args, train_dataset, model, tokenizer, labels, pad_token_label_id)
- logger.info(" global_step = %s, average loss = %s", global_step, tr_loss)
-
- # Saving best-practices: if you use defaults names for the model, you can reload it using from_pretrained()
- if args.do_train and (args.local_rank == -1 or torch.distributed.get_rank() == 0):
- # Create output directory if needed
- if not os.path.exists(args.output_dir) and args.local_rank in [-1, 0]:
- os.makedirs(args.output_dir)
-
- logger.info("Saving model checkpoint to %s", args.output_dir)
- # Save a trained model, configuration and tokenizer using `save_pretrained()`.
- # They can then be reloaded using `from_pretrained()`
- model_to_save = (
- model.module if hasattr(model, "module") else model
- ) # Take care of distributed/parallel training
- model_to_save.save_pretrained(args.output_dir)
- tokenizer.save_pretrained(args.output_dir)
-
- # Good practice: save your training arguments together with the trained model
- torch.save(args, os.path.join(args.output_dir, "training_args.bin"))
-
- # Evaluation
- results = {}
- if args.do_eval and args.local_rank in [-1, 0]:
- tokenizer = tokenizer_class.from_pretrained(args.output_dir, do_lower_case=args.do_lower_case)
- checkpoints = [args.output_dir]
- if args.eval_all_checkpoints:
- checkpoints = list(
- os.path.dirname(c) for c in sorted(glob.glob(args.output_dir + "/**/" + WEIGHTS_NAME, recursive=True))
- )
- logging.getLogger("pytorch_transformers.modeling_utils").setLevel(logging.WARN) # Reduce logging
- logger.info("Evaluate the following checkpoints: %s", checkpoints)
- for checkpoint in checkpoints:
- global_step = checkpoint.split("-")[-1] if len(checkpoints) > 1 else ""
- model = model_class.from_pretrained(checkpoint)
- model.to(args.device)
- result, _ = evaluate(args, model, tokenizer, labels, pad_token_label_id, mode="dev", prefix=global_step)
- if global_step:
- result = {"{}_{}".format(global_step, k): v for k, v in result.items()}
- results.update(result)
- output_eval_file = os.path.join(args.output_dir, "eval_results.txt")
- with open(output_eval_file, "w") as writer:
- for key in sorted(results.keys()):
- writer.write("{} = {}\n".format(key, str(results[key])))
-
- if args.do_predict and args.local_rank in [-1, 0]:
- tokenizer = tokenizer_class.from_pretrained(args.output_dir, do_lower_case=args.do_lower_case)
- model = model_class.from_pretrained(args.output_dir)
- model.to(args.device)
- result, predictions = evaluate(args, model, tokenizer, labels, pad_token_label_id, mode="test")
- # Save results
- output_test_results_file = os.path.join(args.output_dir, "test_results.txt")
- with open(output_test_results_file, "w") as writer:
- for key in sorted(result.keys()):
- writer.write("{} = {}\n".format(key, str(result[key])))
- # Save predictions
- output_test_predictions_file = os.path.join(args.output_dir, "test_predictions.txt")
- with open(output_test_predictions_file, "w") as writer:
- with open(os.path.join(args.data_dir, "test.txt"), "r") as f:
- example_id = 0
- for line in f:
- if line.startswith("-DOCSTART-") or line == "" or line == "\n":
- writer.write(line)
- if not predictions[example_id]:
- example_id += 1
- elif predictions[example_id]:
- output_line = line.split()[0] + " " + predictions[example_id].pop(0) + "\n"
- writer.write(output_line)
- else:
- logger.warning("Maximum sequence length exceeded: No prediction for '%s'.", line.split()[0])
-
- return results
-
-
-if __name__ == "__main__":
- main()
diff --git a/server/transformers/examples/run_squad.py b/server/transformers/examples/run_squad.py
deleted file mode 100644
index 86d00bd7701bba2f499a6b2123f71147dcb02461..0000000000000000000000000000000000000000
--- a/server/transformers/examples/run_squad.py
+++ /dev/null
@@ -1,837 +0,0 @@
-# coding=utf-8
-# Copyright 2018 The Google AI Language Team Authors and The HuggingFace Inc. team.
-# Copyright (c) 2018, NVIDIA CORPORATION. All rights reserved.
-#
-# Licensed under the Apache License, Version 2.0 (the "License");
-# you may not use this file except in compliance with the License.
-# You may obtain a copy of the License at
-#
-# http://www.apache.org/licenses/LICENSE-2.0
-#
-# Unless required by applicable law or agreed to in writing, software
-# distributed under the License is distributed on an "AS IS" BASIS,
-# WITHOUT WARRANTIES OR CONDITIONS OF ANY KIND, either express or implied.
-# See the License for the specific language governing permissions and
-# limitations under the License.
-""" Finetuning the library models for question-answering on SQuAD (DistilBERT, Bert, XLM, XLNet)."""
-
-
-import argparse
-import glob
-import logging
-import os
-import random
-import timeit
-
-import numpy as np
-import torch
-from torch.utils.data import DataLoader, RandomSampler, SequentialSampler
-from torch.utils.data.distributed import DistributedSampler
-from tqdm import tqdm, trange
-
-from transformers import (
- WEIGHTS_NAME,
- AdamW,
- AlbertConfig,
- AlbertForQuestionAnswering,
- AlbertTokenizer,
- BertConfig,
- BertForQuestionAnswering,
- BertTokenizer,
- DistilBertConfig,
- DistilBertForQuestionAnswering,
- DistilBertTokenizer,
- RobertaConfig,
- RobertaForQuestionAnswering,
- RobertaTokenizer,
- XLMConfig,
- XLMForQuestionAnswering,
- XLMTokenizer,
- XLNetConfig,
- XLNetForQuestionAnswering,
- XLNetTokenizer,
- get_linear_schedule_with_warmup,
- squad_convert_examples_to_features,
-)
-from transformers.data.metrics.squad_metrics import (
- compute_predictions_log_probs,
- compute_predictions_logits,
- squad_evaluate,
-)
-from transformers.data.processors.squad import SquadResult, SquadV1Processor, SquadV2Processor
-
-
-try:
- from torch.utils.tensorboard import SummaryWriter
-except ImportError:
- from tensorboardX import SummaryWriter
-
-
-logger = logging.getLogger(__name__)
-
-ALL_MODELS = sum(
- (tuple(conf.pretrained_config_archive_map.keys()) for conf in (BertConfig, RobertaConfig, XLNetConfig, XLMConfig)),
- (),
-)
-
-MODEL_CLASSES = {
- "bert": (BertConfig, BertForQuestionAnswering, BertTokenizer),
- "roberta": (RobertaConfig, RobertaForQuestionAnswering, RobertaTokenizer),
- "xlnet": (XLNetConfig, XLNetForQuestionAnswering, XLNetTokenizer),
- "xlm": (XLMConfig, XLMForQuestionAnswering, XLMTokenizer),
- "distilbert": (DistilBertConfig, DistilBertForQuestionAnswering, DistilBertTokenizer),
- "albert": (AlbertConfig, AlbertForQuestionAnswering, AlbertTokenizer),
-}
-
-
-def set_seed(args):
- random.seed(args.seed)
- np.random.seed(args.seed)
- torch.manual_seed(args.seed)
- if args.n_gpu > 0:
- torch.cuda.manual_seed_all(args.seed)
-
-
-def to_list(tensor):
- return tensor.detach().cpu().tolist()
-
-
-def train(args, train_dataset, model, tokenizer):
- """ Train the model """
- if args.local_rank in [-1, 0]:
- tb_writer = SummaryWriter()
-
- args.train_batch_size = args.per_gpu_train_batch_size * max(1, args.n_gpu)
- train_sampler = RandomSampler(train_dataset) if args.local_rank == -1 else DistributedSampler(train_dataset)
- train_dataloader = DataLoader(train_dataset, sampler=train_sampler, batch_size=args.train_batch_size)
-
- if args.max_steps > 0:
- t_total = args.max_steps
- args.num_train_epochs = args.max_steps // (len(train_dataloader) // args.gradient_accumulation_steps) + 1
- else:
- t_total = len(train_dataloader) // args.gradient_accumulation_steps * args.num_train_epochs
-
- # Prepare optimizer and schedule (linear warmup and decay)
- no_decay = ["bias", "LayerNorm.weight"]
- optimizer_grouped_parameters = [
- {
- "params": [p for n, p in model.named_parameters() if not any(nd in n for nd in no_decay)],
- "weight_decay": args.weight_decay,
- },
- {"params": [p for n, p in model.named_parameters() if any(nd in n for nd in no_decay)], "weight_decay": 0.0},
- ]
- optimizer = AdamW(optimizer_grouped_parameters, lr=args.learning_rate, eps=args.adam_epsilon)
- scheduler = get_linear_schedule_with_warmup(
- optimizer, num_warmup_steps=args.warmup_steps, num_training_steps=t_total
- )
-
- # Check if saved optimizer or scheduler states exist
- if os.path.isfile(os.path.join(args.model_name_or_path, "optimizer.pt")) and os.path.isfile(
- os.path.join(args.model_name_or_path, "scheduler.pt")
- ):
- # Load in optimizer and scheduler states
- optimizer.load_state_dict(torch.load(os.path.join(args.model_name_or_path, "optimizer.pt")))
- scheduler.load_state_dict(torch.load(os.path.join(args.model_name_or_path, "scheduler.pt")))
-
- if args.fp16:
- try:
- from apex import amp
- except ImportError:
- raise ImportError("Please install apex from https://www.github.com/nvidia/apex to use fp16 training.")
-
- model, optimizer = amp.initialize(model, optimizer, opt_level=args.fp16_opt_level)
-
- # multi-gpu training (should be after apex fp16 initialization)
- if args.n_gpu > 1:
- model = torch.nn.DataParallel(model)
-
- # Distributed training (should be after apex fp16 initialization)
- if args.local_rank != -1:
- model = torch.nn.parallel.DistributedDataParallel(
- model, device_ids=[args.local_rank], output_device=args.local_rank, find_unused_parameters=True
- )
-
- # Train!
- logger.info("***** Running training *****")
- logger.info(" Num examples = %d", len(train_dataset))
- logger.info(" Num Epochs = %d", args.num_train_epochs)
- logger.info(" Instantaneous batch size per GPU = %d", args.per_gpu_train_batch_size)
- logger.info(
- " Total train batch size (w. parallel, distributed & accumulation) = %d",
- args.train_batch_size
- * args.gradient_accumulation_steps
- * (torch.distributed.get_world_size() if args.local_rank != -1 else 1),
- )
- logger.info(" Gradient Accumulation steps = %d", args.gradient_accumulation_steps)
- logger.info(" Total optimization steps = %d", t_total)
-
- global_step = 1
- epochs_trained = 0
- steps_trained_in_current_epoch = 0
- # Check if continuing training from a checkpoint
- if os.path.exists(args.model_name_or_path):
- try:
- # set global_step to gobal_step of last saved checkpoint from model path
- checkpoint_suffix = args.model_name_or_path.split("-")[-1].split("/")[0]
- global_step = int(checkpoint_suffix)
- epochs_trained = global_step // (len(train_dataloader) // args.gradient_accumulation_steps)
- steps_trained_in_current_epoch = global_step % (len(train_dataloader) // args.gradient_accumulation_steps)
-
- logger.info(" Continuing training from checkpoint, will skip to saved global_step")
- logger.info(" Continuing training from epoch %d", epochs_trained)
- logger.info(" Continuing training from global step %d", global_step)
- logger.info(" Will skip the first %d steps in the first epoch", steps_trained_in_current_epoch)
- except ValueError:
- logger.info(" Starting fine-tuning.")
-
- tr_loss, logging_loss = 0.0, 0.0
- model.zero_grad()
- train_iterator = trange(
- epochs_trained, int(args.num_train_epochs), desc="Epoch", disable=args.local_rank not in [-1, 0]
- )
- # Added here for reproductibility
- set_seed(args)
-
- for _ in train_iterator:
- epoch_iterator = tqdm(train_dataloader, desc="Iteration", disable=args.local_rank not in [-1, 0])
- for step, batch in enumerate(epoch_iterator):
-
- # Skip past any already trained steps if resuming training
- if steps_trained_in_current_epoch > 0:
- steps_trained_in_current_epoch -= 1
- continue
-
- model.train()
- batch = tuple(t.to(args.device) for t in batch)
-
- inputs = {
- "input_ids": batch[0],
- "attention_mask": batch[1],
- "token_type_ids": batch[2],
- "start_positions": batch[3],
- "end_positions": batch[4],
- }
-
- if args.model_type in ["xlm", "roberta", "distilbert"]:
- del inputs["token_type_ids"]
-
- if args.model_type in ["xlnet", "xlm"]:
- inputs.update({"cls_index": batch[5], "p_mask": batch[6]})
- if args.version_2_with_negative:
- inputs.update({"is_impossible": batch[7]})
- outputs = model(**inputs)
- # model outputs are always tuple in transformers (see doc)
- loss = outputs[0]
-
- if args.n_gpu > 1:
- loss = loss.mean() # mean() to average on multi-gpu parallel (not distributed) training
- if args.gradient_accumulation_steps > 1:
- loss = loss / args.gradient_accumulation_steps
-
- if args.fp16:
- with amp.scale_loss(loss, optimizer) as scaled_loss:
- scaled_loss.backward()
- else:
- loss.backward()
-
- tr_loss += loss.item()
- if (step + 1) % args.gradient_accumulation_steps == 0:
- if args.fp16:
- torch.nn.utils.clip_grad_norm_(amp.master_params(optimizer), args.max_grad_norm)
- else:
- torch.nn.utils.clip_grad_norm_(model.parameters(), args.max_grad_norm)
-
- optimizer.step()
- scheduler.step() # Update learning rate schedule
- model.zero_grad()
- global_step += 1
-
- # Log metrics
- if args.local_rank in [-1, 0] and args.logging_steps > 0 and global_step % args.logging_steps == 0:
- # Only evaluate when single GPU otherwise metrics may not average well
- if args.local_rank == -1 and args.evaluate_during_training:
- results = evaluate(args, model, tokenizer)
- for key, value in results.items():
- tb_writer.add_scalar("eval_{}".format(key), value, global_step)
- tb_writer.add_scalar("lr", scheduler.get_lr()[0], global_step)
- tb_writer.add_scalar("loss", (tr_loss - logging_loss) / args.logging_steps, global_step)
- logging_loss = tr_loss
-
- # Save model checkpoint
- if args.local_rank in [-1, 0] and args.save_steps > 0 and global_step % args.save_steps == 0:
- output_dir = os.path.join(args.output_dir, "checkpoint-{}".format(global_step))
- if not os.path.exists(output_dir):
- os.makedirs(output_dir)
- # Take care of distributed/parallel training
- model_to_save = model.module if hasattr(model, "module") else model
- model_to_save.save_pretrained(output_dir)
- tokenizer.save_pretrained(output_dir)
-
- torch.save(args, os.path.join(output_dir, "training_args.bin"))
- logger.info("Saving model checkpoint to %s", output_dir)
-
- torch.save(optimizer.state_dict(), os.path.join(output_dir, "optimizer.pt"))
- torch.save(scheduler.state_dict(), os.path.join(output_dir, "scheduler.pt"))
- logger.info("Saving optimizer and scheduler states to %s", output_dir)
-
- if args.max_steps > 0 and global_step > args.max_steps:
- epoch_iterator.close()
- break
- if args.max_steps > 0 and global_step > args.max_steps:
- train_iterator.close()
- break
-
- if args.local_rank in [-1, 0]:
- tb_writer.close()
-
- return global_step, tr_loss / global_step
-
-
-def evaluate(args, model, tokenizer, prefix=""):
- dataset, examples, features = load_and_cache_examples(args, tokenizer, evaluate=True, output_examples=True)
-
- if not os.path.exists(args.output_dir) and args.local_rank in [-1, 0]:
- os.makedirs(args.output_dir)
-
- args.eval_batch_size = args.per_gpu_eval_batch_size * max(1, args.n_gpu)
-
- # Note that DistributedSampler samples randomly
- eval_sampler = SequentialSampler(dataset)
- eval_dataloader = DataLoader(dataset, sampler=eval_sampler, batch_size=args.eval_batch_size)
-
- # multi-gpu evaluate
- if args.n_gpu > 1 and not isinstance(model, torch.nn.DataParallel):
- model = torch.nn.DataParallel(model)
-
- # Eval!
- logger.info("***** Running evaluation {} *****".format(prefix))
- logger.info(" Num examples = %d", len(dataset))
- logger.info(" Batch size = %d", args.eval_batch_size)
-
- all_results = []
- start_time = timeit.default_timer()
-
- for batch in tqdm(eval_dataloader, desc="Evaluating"):
- model.eval()
- batch = tuple(t.to(args.device) for t in batch)
-
- with torch.no_grad():
- inputs = {
- "input_ids": batch[0],
- "attention_mask": batch[1],
- "token_type_ids": batch[2],
- }
-
- if args.model_type in ["xlm", "roberta", "distilbert"]:
- del inputs["token_type_ids"]
-
- example_indices = batch[3]
-
- # XLNet and XLM use more arguments for their predictions
- if args.model_type in ["xlnet", "xlm"]:
- inputs.update({"cls_index": batch[4], "p_mask": batch[5]})
-
- outputs = model(**inputs)
-
- for i, example_index in enumerate(example_indices):
- eval_feature = features[example_index.item()]
- unique_id = int(eval_feature.unique_id)
-
- output = [to_list(output[i]) for output in outputs]
-
- # Some models (XLNet, XLM) use 5 arguments for their predictions, while the other "simpler"
- # models only use two.
- if len(output) >= 5:
- start_logits = output[0]
- start_top_index = output[1]
- end_logits = output[2]
- end_top_index = output[3]
- cls_logits = output[4]
-
- result = SquadResult(
- unique_id,
- start_logits,
- end_logits,
- start_top_index=start_top_index,
- end_top_index=end_top_index,
- cls_logits=cls_logits,
- )
-
- else:
- start_logits, end_logits = output
- result = SquadResult(unique_id, start_logits, end_logits)
-
- all_results.append(result)
-
- evalTime = timeit.default_timer() - start_time
- logger.info(" Evaluation done in total %f secs (%f sec per example)", evalTime, evalTime / len(dataset))
-
- # Compute predictions
- output_prediction_file = os.path.join(args.output_dir, "predictions_{}.json".format(prefix))
- output_nbest_file = os.path.join(args.output_dir, "nbest_predictions_{}.json".format(prefix))
-
- if args.version_2_with_negative:
- output_null_log_odds_file = os.path.join(args.output_dir, "null_odds_{}.json".format(prefix))
- else:
- output_null_log_odds_file = None
-
- # XLNet and XLM use a more complex post-processing procedure
- if args.model_type in ["xlnet", "xlm"]:
- start_n_top = model.config.start_n_top if hasattr(model, "config") else model.module.config.start_n_top
- end_n_top = model.config.end_n_top if hasattr(model, "config") else model.module.config.end_n_top
-
- predictions = compute_predictions_log_probs(
- examples,
- features,
- all_results,
- args.n_best_size,
- args.max_answer_length,
- output_prediction_file,
- output_nbest_file,
- output_null_log_odds_file,
- start_n_top,
- end_n_top,
- args.version_2_with_negative,
- tokenizer,
- args.verbose_logging,
- )
- else:
- predictions = compute_predictions_logits(
- examples,
- features,
- all_results,
- args.n_best_size,
- args.max_answer_length,
- args.do_lower_case,
- output_prediction_file,
- output_nbest_file,
- output_null_log_odds_file,
- args.verbose_logging,
- args.version_2_with_negative,
- args.null_score_diff_threshold,
- tokenizer,
- )
-
- # Compute the F1 and exact scores.
- results = squad_evaluate(examples, predictions)
- return results
-
-
-def load_and_cache_examples(args, tokenizer, evaluate=False, output_examples=False):
- if args.local_rank not in [-1, 0] and not evaluate:
- # Make sure only the first process in distributed training process the dataset, and the others will use the cache
- torch.distributed.barrier()
-
- # Load data features from cache or dataset file
- input_dir = args.data_dir if args.data_dir else "."
- cached_features_file = os.path.join(
- input_dir,
- "cached_{}_{}_{}".format(
- "dev" if evaluate else "train",
- list(filter(None, args.model_name_or_path.split("/"))).pop(),
- str(args.max_seq_length),
- ),
- )
-
- # Init features and dataset from cache if it exists
- if os.path.exists(cached_features_file) and not args.overwrite_cache:
- logger.info("Loading features from cached file %s", cached_features_file)
- features_and_dataset = torch.load(cached_features_file)
- features, dataset, examples = (
- features_and_dataset["features"],
- features_and_dataset["dataset"],
- features_and_dataset["examples"],
- )
- else:
- logger.info("Creating features from dataset file at %s", input_dir)
-
- if not args.data_dir and ((evaluate and not args.predict_file) or (not evaluate and not args.train_file)):
- try:
- import tensorflow_datasets as tfds
- except ImportError:
- raise ImportError("If not data_dir is specified, tensorflow_datasets needs to be installed.")
-
- if args.version_2_with_negative:
- logger.warn("tensorflow_datasets does not handle version 2 of SQuAD.")
-
- tfds_examples = tfds.load("squad")
- examples = SquadV1Processor().get_examples_from_dataset(tfds_examples, evaluate=evaluate)
- else:
- processor = SquadV2Processor() if args.version_2_with_negative else SquadV1Processor()
- if evaluate:
- examples = processor.get_dev_examples(args.data_dir, filename=args.predict_file)
- else:
- examples = processor.get_train_examples(args.data_dir, filename=args.train_file)
-
- features, dataset = squad_convert_examples_to_features(
- examples=examples,
- tokenizer=tokenizer,
- max_seq_length=args.max_seq_length,
- doc_stride=args.doc_stride,
- max_query_length=args.max_query_length,
- is_training=not evaluate,
- return_dataset="pt",
- threads=args.threads,
- )
-
- if args.local_rank in [-1, 0]:
- logger.info("Saving features into cached file %s", cached_features_file)
- torch.save({"features": features, "dataset": dataset, "examples": examples}, cached_features_file)
-
- if args.local_rank == 0 and not evaluate:
- # Make sure only the first process in distributed training process the dataset, and the others will use the cache
- torch.distributed.barrier()
-
- if output_examples:
- return dataset, examples, features
- return dataset
-
-
-def main():
- parser = argparse.ArgumentParser()
-
- # Required parameters
- parser.add_argument(
- "--model_type",
- default=None,
- type=str,
- required=True,
- help="Model type selected in the list: " + ", ".join(MODEL_CLASSES.keys()),
- )
- parser.add_argument(
- "--model_name_or_path",
- default=None,
- type=str,
- required=True,
- help="Path to pre-trained model or shortcut name selected in the list: " + ", ".join(ALL_MODELS),
- )
- parser.add_argument(
- "--output_dir",
- default=None,
- type=str,
- required=True,
- help="The output directory where the model checkpoints and predictions will be written.",
- )
-
- # Other parameters
- parser.add_argument(
- "--data_dir",
- default=None,
- type=str,
- help="The input data dir. Should contain the .json files for the task."
- + "If no data dir or train/predict files are specified, will run with tensorflow_datasets.",
- )
- parser.add_argument(
- "--train_file",
- default=None,
- type=str,
- help="The input training file. If a data dir is specified, will look for the file there"
- + "If no data dir or train/predict files are specified, will run with tensorflow_datasets.",
- )
- parser.add_argument(
- "--predict_file",
- default=None,
- type=str,
- help="The input evaluation file. If a data dir is specified, will look for the file there"
- + "If no data dir or train/predict files are specified, will run with tensorflow_datasets.",
- )
- parser.add_argument(
- "--config_name", default="", type=str, help="Pretrained config name or path if not the same as model_name"
- )
- parser.add_argument(
- "--tokenizer_name",
- default="",
- type=str,
- help="Pretrained tokenizer name or path if not the same as model_name",
- )
- parser.add_argument(
- "--cache_dir",
- default="",
- type=str,
- help="Where do you want to store the pre-trained models downloaded from s3",
- )
-
- parser.add_argument(
- "--version_2_with_negative",
- action="store_true",
- help="If true, the SQuAD examples contain some that do not have an answer.",
- )
- parser.add_argument(
- "--null_score_diff_threshold",
- type=float,
- default=0.0,
- help="If null_score - best_non_null is greater than the threshold predict null.",
- )
-
- parser.add_argument(
- "--max_seq_length",
- default=384,
- type=int,
- help="The maximum total input sequence length after WordPiece tokenization. Sequences "
- "longer than this will be truncated, and sequences shorter than this will be padded.",
- )
- parser.add_argument(
- "--doc_stride",
- default=128,
- type=int,
- help="When splitting up a long document into chunks, how much stride to take between chunks.",
- )
- parser.add_argument(
- "--max_query_length",
- default=64,
- type=int,
- help="The maximum number of tokens for the question. Questions longer than this will "
- "be truncated to this length.",
- )
- parser.add_argument("--do_train", action="store_true", help="Whether to run training.")
- parser.add_argument("--do_eval", action="store_true", help="Whether to run eval on the dev set.")
- parser.add_argument(
- "--evaluate_during_training", action="store_true", help="Run evaluation during training at each logging step."
- )
- parser.add_argument(
- "--do_lower_case", action="store_true", help="Set this flag if you are using an uncased model."
- )
-
- parser.add_argument("--per_gpu_train_batch_size", default=8, type=int, help="Batch size per GPU/CPU for training.")
- parser.add_argument(
- "--per_gpu_eval_batch_size", default=8, type=int, help="Batch size per GPU/CPU for evaluation."
- )
- parser.add_argument("--learning_rate", default=5e-5, type=float, help="The initial learning rate for Adam.")
- parser.add_argument(
- "--gradient_accumulation_steps",
- type=int,
- default=1,
- help="Number of updates steps to accumulate before performing a backward/update pass.",
- )
- parser.add_argument("--weight_decay", default=0.0, type=float, help="Weight decay if we apply some.")
- parser.add_argument("--adam_epsilon", default=1e-8, type=float, help="Epsilon for Adam optimizer.")
- parser.add_argument("--max_grad_norm", default=1.0, type=float, help="Max gradient norm.")
- parser.add_argument(
- "--num_train_epochs", default=3.0, type=float, help="Total number of training epochs to perform."
- )
- parser.add_argument(
- "--max_steps",
- default=-1,
- type=int,
- help="If > 0: set total number of training steps to perform. Override num_train_epochs.",
- )
- parser.add_argument("--warmup_steps", default=0, type=int, help="Linear warmup over warmup_steps.")
- parser.add_argument(
- "--n_best_size",
- default=20,
- type=int,
- help="The total number of n-best predictions to generate in the nbest_predictions.json output file.",
- )
- parser.add_argument(
- "--max_answer_length",
- default=30,
- type=int,
- help="The maximum length of an answer that can be generated. This is needed because the start "
- "and end predictions are not conditioned on one another.",
- )
- parser.add_argument(
- "--verbose_logging",
- action="store_true",
- help="If true, all of the warnings related to data processing will be printed. "
- "A number of warnings are expected for a normal SQuAD evaluation.",
- )
-
- parser.add_argument("--logging_steps", type=int, default=500, help="Log every X updates steps.")
- parser.add_argument("--save_steps", type=int, default=500, help="Save checkpoint every X updates steps.")
- parser.add_argument(
- "--eval_all_checkpoints",
- action="store_true",
- help="Evaluate all checkpoints starting with the same prefix as model_name ending and ending with step number",
- )
- parser.add_argument("--no_cuda", action="store_true", help="Whether not to use CUDA when available")
- parser.add_argument(
- "--overwrite_output_dir", action="store_true", help="Overwrite the content of the output directory"
- )
- parser.add_argument(
- "--overwrite_cache", action="store_true", help="Overwrite the cached training and evaluation sets"
- )
- parser.add_argument("--seed", type=int, default=42, help="random seed for initialization")
-
- parser.add_argument("--local_rank", type=int, default=-1, help="local_rank for distributed training on gpus")
- parser.add_argument(
- "--fp16",
- action="store_true",
- help="Whether to use 16-bit (mixed) precision (through NVIDIA apex) instead of 32-bit",
- )
- parser.add_argument(
- "--fp16_opt_level",
- type=str,
- default="O1",
- help="For fp16: Apex AMP optimization level selected in ['O0', 'O1', 'O2', and 'O3']."
- "See details at https://nvidia.github.io/apex/amp.html",
- )
- parser.add_argument("--server_ip", type=str, default="", help="Can be used for distant debugging.")
- parser.add_argument("--server_port", type=str, default="", help="Can be used for distant debugging.")
-
- parser.add_argument("--threads", type=int, default=1, help="multiple threads for converting example to features")
- args = parser.parse_args()
-
- if args.doc_stride >= args.max_seq_length - args.max_query_length:
- logger.warning(
- "WARNING - You've set a doc stride which may be superior to the document length in some "
- "examples. This could result in errors when building features from the examples. Please reduce the doc "
- "stride or increase the maximum length to ensure the features are correctly built."
- )
-
- if (
- os.path.exists(args.output_dir)
- and os.listdir(args.output_dir)
- and args.do_train
- and not args.overwrite_output_dir
- ):
- raise ValueError(
- "Output directory ({}) already exists and is not empty. Use --overwrite_output_dir to overcome.".format(
- args.output_dir
- )
- )
-
- # Setup distant debugging if needed
- if args.server_ip and args.server_port:
- # Distant debugging - see https://code.visualstudio.com/docs/python/debugging#_attach-to-a-local-script
- import ptvsd
-
- print("Waiting for debugger attach")
- ptvsd.enable_attach(address=(args.server_ip, args.server_port), redirect_output=True)
- ptvsd.wait_for_attach()
-
- # Setup CUDA, GPU & distributed training
- if args.local_rank == -1 or args.no_cuda:
- device = torch.device("cuda" if torch.cuda.is_available() and not args.no_cuda else "cpu")
- args.n_gpu = torch.cuda.device_count()
- else: # Initializes the distributed backend which will take care of sychronizing nodes/GPUs
- torch.cuda.set_device(args.local_rank)
- device = torch.device("cuda", args.local_rank)
- torch.distributed.init_process_group(backend="nccl")
- args.n_gpu = 1
- args.device = device
-
- # Setup logging
- logging.basicConfig(
- format="%(asctime)s - %(levelname)s - %(name)s - %(message)s",
- datefmt="%m/%d/%Y %H:%M:%S",
- level=logging.INFO if args.local_rank in [-1, 0] else logging.WARN,
- )
- logger.warning(
- "Process rank: %s, device: %s, n_gpu: %s, distributed training: %s, 16-bits training: %s",
- args.local_rank,
- device,
- args.n_gpu,
- bool(args.local_rank != -1),
- args.fp16,
- )
-
- # Set seed
- set_seed(args)
-
- # Load pretrained model and tokenizer
- if args.local_rank not in [-1, 0]:
- # Make sure only the first process in distributed training will download model & vocab
- torch.distributed.barrier()
-
- args.model_type = args.model_type.lower()
- config_class, model_class, tokenizer_class = MODEL_CLASSES[args.model_type]
- config = config_class.from_pretrained(
- args.config_name if args.config_name else args.model_name_or_path,
- cache_dir=args.cache_dir if args.cache_dir else None,
- )
- tokenizer = tokenizer_class.from_pretrained(
- args.tokenizer_name if args.tokenizer_name else args.model_name_or_path,
- do_lower_case=args.do_lower_case,
- cache_dir=args.cache_dir if args.cache_dir else None,
- )
- model = model_class.from_pretrained(
- args.model_name_or_path,
- from_tf=bool(".ckpt" in args.model_name_or_path),
- config=config,
- cache_dir=args.cache_dir if args.cache_dir else None,
- )
-
- if args.local_rank == 0:
- # Make sure only the first process in distributed training will download model & vocab
- torch.distributed.barrier()
-
- model.to(args.device)
-
- logger.info("Training/evaluation parameters %s", args)
-
- # Before we do anything with models, we want to ensure that we get fp16 execution of torch.einsum if args.fp16 is set.
- # Otherwise it'll default to "promote" mode, and we'll get fp32 operations. Note that running `--fp16_opt_level="O2"` will
- # remove the need for this code, but it is still valid.
- if args.fp16:
- try:
- import apex
-
- apex.amp.register_half_function(torch, "einsum")
- except ImportError:
- raise ImportError("Please install apex from https://www.github.com/nvidia/apex to use fp16 training.")
-
- # Training
- if args.do_train:
- train_dataset = load_and_cache_examples(args, tokenizer, evaluate=False, output_examples=False)
- global_step, tr_loss = train(args, train_dataset, model, tokenizer)
- logger.info(" global_step = %s, average loss = %s", global_step, tr_loss)
-
- # Save the trained model and the tokenizer
- if args.do_train and (args.local_rank == -1 or torch.distributed.get_rank() == 0):
- # Create output directory if needed
- if not os.path.exists(args.output_dir) and args.local_rank in [-1, 0]:
- os.makedirs(args.output_dir)
-
- logger.info("Saving model checkpoint to %s", args.output_dir)
- # Save a trained model, configuration and tokenizer using `save_pretrained()`.
- # They can then be reloaded using `from_pretrained()`
- # Take care of distributed/parallel training
- model_to_save = model.module if hasattr(model, "module") else model
- model_to_save.save_pretrained(args.output_dir)
- tokenizer.save_pretrained(args.output_dir)
-
- # Good practice: save your training arguments together with the trained model
- torch.save(args, os.path.join(args.output_dir, "training_args.bin"))
-
- # Load a trained model and vocabulary that you have fine-tuned
- model = model_class.from_pretrained(args.output_dir) # , force_download=True)
- tokenizer = tokenizer_class.from_pretrained(args.output_dir, do_lower_case=args.do_lower_case)
- model.to(args.device)
-
- # Evaluation - we can ask to evaluate all the checkpoints (sub-directories) in a directory
- results = {}
- if args.do_eval and args.local_rank in [-1, 0]:
- if args.do_train:
- logger.info("Loading checkpoints saved during training for evaluation")
- checkpoints = [args.output_dir]
- if args.eval_all_checkpoints:
- checkpoints = list(
- os.path.dirname(c)
- for c in sorted(glob.glob(args.output_dir + "/**/" + WEIGHTS_NAME, recursive=True))
- )
- logging.getLogger("transformers.modeling_utils").setLevel(logging.WARN) # Reduce model loading logs
- else:
- logger.info("Loading checkpoint %s for evaluation", args.model_name_or_path)
- checkpoints = [args.model_name_or_path]
-
- logger.info("Evaluate the following checkpoints: %s", checkpoints)
-
- for checkpoint in checkpoints:
- # Reload the model
- global_step = checkpoint.split("-")[-1] if len(checkpoints) > 1 else ""
- model = model_class.from_pretrained(checkpoint) # , force_download=True)
- model.to(args.device)
-
- # Evaluate
- result = evaluate(args, model, tokenizer, prefix=global_step)
-
- result = dict((k + ("_{}".format(global_step) if global_step else ""), v) for k, v in result.items())
- results.update(result)
-
- logger.info("Results: {}".format(results))
-
- return results
-
-
-if __name__ == "__main__":
- main()
diff --git a/server/transformers/examples/run_tf_glue.py b/server/transformers/examples/run_tf_glue.py
deleted file mode 100644
index dae11d22b365be2a417179b689110a44714c7d54..0000000000000000000000000000000000000000
--- a/server/transformers/examples/run_tf_glue.py
+++ /dev/null
@@ -1,105 +0,0 @@
-import os
-
-import tensorflow as tf
-import tensorflow_datasets
-
-from transformers import (
- BertConfig,
- BertForSequenceClassification,
- BertTokenizer,
- TFBertForSequenceClassification,
- glue_convert_examples_to_features,
- glue_processors,
-)
-
-
-# script parameters
-BATCH_SIZE = 32
-EVAL_BATCH_SIZE = BATCH_SIZE * 2
-USE_XLA = False
-USE_AMP = False
-EPOCHS = 3
-
-TASK = "mrpc"
-
-if TASK == "sst-2":
- TFDS_TASK = "sst2"
-elif TASK == "sts-b":
- TFDS_TASK = "stsb"
-else:
- TFDS_TASK = TASK
-
-num_labels = len(glue_processors[TASK]().get_labels())
-print(num_labels)
-
-tf.config.optimizer.set_jit(USE_XLA)
-tf.config.optimizer.set_experimental_options({"auto_mixed_precision": USE_AMP})
-
-# Load tokenizer and model from pretrained model/vocabulary. Specify the number of labels to classify (2+: classification, 1: regression)
-config = BertConfig.from_pretrained("bert-base-cased", num_labels=num_labels)
-tokenizer = BertTokenizer.from_pretrained("bert-base-cased")
-model = TFBertForSequenceClassification.from_pretrained("bert-base-cased", config=config)
-
-# Load dataset via TensorFlow Datasets
-data, info = tensorflow_datasets.load(f"glue/{TFDS_TASK}", with_info=True)
-train_examples = info.splits["train"].num_examples
-
-# MNLI expects either validation_matched or validation_mismatched
-valid_examples = info.splits["validation"].num_examples
-
-# Prepare dataset for GLUE as a tf.data.Dataset instance
-train_dataset = glue_convert_examples_to_features(data["train"], tokenizer, 128, TASK)
-
-# MNLI expects either validation_matched or validation_mismatched
-valid_dataset = glue_convert_examples_to_features(data["validation"], tokenizer, 128, TASK)
-train_dataset = train_dataset.shuffle(128).batch(BATCH_SIZE).repeat(-1)
-valid_dataset = valid_dataset.batch(EVAL_BATCH_SIZE)
-
-# Prepare training: Compile tf.keras model with optimizer, loss and learning rate schedule
-opt = tf.keras.optimizers.Adam(learning_rate=3e-5, epsilon=1e-08)
-if USE_AMP:
- # loss scaling is currently required when using mixed precision
- opt = tf.keras.mixed_precision.experimental.LossScaleOptimizer(opt, "dynamic")
-
-
-if num_labels == 1:
- loss = tf.keras.losses.MeanSquaredError()
-else:
- loss = tf.keras.losses.SparseCategoricalCrossentropy(from_logits=True)
-
-metric = tf.keras.metrics.SparseCategoricalAccuracy("accuracy")
-model.compile(optimizer=opt, loss=loss, metrics=[metric])
-
-# Train and evaluate using tf.keras.Model.fit()
-train_steps = train_examples // BATCH_SIZE
-valid_steps = valid_examples // EVAL_BATCH_SIZE
-
-history = model.fit(
- train_dataset,
- epochs=EPOCHS,
- steps_per_epoch=train_steps,
- validation_data=valid_dataset,
- validation_steps=valid_steps,
-)
-
-# Save TF2 model
-os.makedirs("./save/", exist_ok=True)
-model.save_pretrained("./save/")
-
-if TASK == "mrpc":
- # Load the TensorFlow model in PyTorch for inspection
- # This is to demo the interoperability between the two frameworks, you don't have to
- # do this in real life (you can run the inference on the TF model).
- pytorch_model = BertForSequenceClassification.from_pretrained("./save/", from_tf=True)
-
- # Quickly test a few predictions - MRPC is a paraphrasing task, let's see if our model learned the task
- sentence_0 = "This research was consistent with his findings."
- sentence_1 = "His findings were compatible with this research."
- sentence_2 = "His findings were not compatible with this research."
- inputs_1 = tokenizer.encode_plus(sentence_0, sentence_1, add_special_tokens=True, return_tensors="pt")
- inputs_2 = tokenizer.encode_plus(sentence_0, sentence_2, add_special_tokens=True, return_tensors="pt")
-
- pred_1 = pytorch_model(**inputs_1)[0].argmax().item()
- pred_2 = pytorch_model(**inputs_2)[0].argmax().item()
- print("sentence_1 is", "a paraphrase" if pred_1 else "not a paraphrase", "of sentence_0")
- print("sentence_2 is", "a paraphrase" if pred_2 else "not a paraphrase", "of sentence_0")
diff --git a/server/transformers/examples/run_tf_ner.py b/server/transformers/examples/run_tf_ner.py
deleted file mode 100644
index ef970d839016a49c8af076bacf386904ded9221e..0000000000000000000000000000000000000000
--- a/server/transformers/examples/run_tf_ner.py
+++ /dev/null
@@ -1,655 +0,0 @@
-# coding=utf-8
-import collections
-import datetime
-import glob
-import math
-import os
-import re
-
-import numpy as np
-import tensorflow as tf
-from absl import app, flags, logging
-from seqeval import metrics
-
-from transformers import (
- TF2_WEIGHTS_NAME,
- BertConfig,
- BertTokenizer,
- DistilBertConfig,
- DistilBertTokenizer,
- GradientAccumulator,
- RobertaConfig,
- RobertaTokenizer,
- TFBertForTokenClassification,
- TFDistilBertForTokenClassification,
- TFRobertaForTokenClassification,
- create_optimizer,
-)
-from utils_ner import convert_examples_to_features, get_labels, read_examples_from_file
-
-
-try:
- from fastprogress import master_bar, progress_bar
-except ImportError:
- from fastprogress.fastprogress import master_bar, progress_bar
-
-
-ALL_MODELS = sum(
- (tuple(conf.pretrained_config_archive_map.keys()) for conf in (BertConfig, RobertaConfig, DistilBertConfig)), ()
-)
-
-MODEL_CLASSES = {
- "bert": (BertConfig, TFBertForTokenClassification, BertTokenizer),
- "roberta": (RobertaConfig, TFRobertaForTokenClassification, RobertaTokenizer),
- "distilbert": (DistilBertConfig, TFDistilBertForTokenClassification, DistilBertTokenizer),
-}
-
-
-flags.DEFINE_string(
- "data_dir", None, "The input data dir. Should contain the .conll files (or other data files) " "for the task."
-)
-
-flags.DEFINE_string("model_type", None, "Model type selected in the list: " + ", ".join(MODEL_CLASSES.keys()))
-
-flags.DEFINE_string(
- "model_name_or_path",
- None,
- "Path to pre-trained model or shortcut name selected in the list: " + ", ".join(ALL_MODELS),
-)
-
-flags.DEFINE_string("output_dir", None, "The output directory where the model checkpoints will be written.")
-
-flags.DEFINE_string(
- "labels", "", "Path to a file containing all labels. If not specified, CoNLL-2003 labels are used."
-)
-
-flags.DEFINE_string("config_name", "", "Pretrained config name or path if not the same as model_name")
-
-flags.DEFINE_string("tokenizer_name", "", "Pretrained tokenizer name or path if not the same as model_name")
-
-flags.DEFINE_string("cache_dir", "", "Where do you want to store the pre-trained models downloaded from s3")
-
-flags.DEFINE_integer(
- "max_seq_length",
- 128,
- "The maximum total input sentence length after tokenization. "
- "Sequences longer than this will be truncated, sequences shorter "
- "will be padded.",
-)
-
-flags.DEFINE_string(
- "tpu",
- None,
- "The Cloud TPU to use for training. This should be either the name "
- "used when creating the Cloud TPU, or a grpc://ip.address.of.tpu:8470 "
- "url.",
-)
-
-flags.DEFINE_integer("num_tpu_cores", 8, "Total number of TPU cores to use.")
-
-flags.DEFINE_boolean("do_train", False, "Whether to run training.")
-
-flags.DEFINE_boolean("do_eval", False, "Whether to run eval on the dev set.")
-
-flags.DEFINE_boolean("do_predict", False, "Whether to run predictions on the test set.")
-
-flags.DEFINE_boolean(
- "evaluate_during_training", False, "Whether to run evaluation during training at each logging step."
-)
-
-flags.DEFINE_boolean("do_lower_case", False, "Set this flag if you are using an uncased model.")
-
-flags.DEFINE_integer("per_device_train_batch_size", 8, "Batch size per GPU/CPU/TPU for training.")
-
-flags.DEFINE_integer("per_device_eval_batch_size", 8, "Batch size per GPU/CPU/TPU for evaluation.")
-
-flags.DEFINE_integer(
- "gradient_accumulation_steps", 1, "Number of updates steps to accumulate before performing a backward/update pass."
-)
-
-flags.DEFINE_float("learning_rate", 5e-5, "The initial learning rate for Adam.")
-
-flags.DEFINE_float("weight_decay", 0.0, "Weight decay if we apply some.")
-
-flags.DEFINE_float("adam_epsilon", 1e-8, "Epsilon for Adam optimizer.")
-
-flags.DEFINE_float("max_grad_norm", 1.0, "Max gradient norm.")
-
-flags.DEFINE_integer("num_train_epochs", 3, "Total number of training epochs to perform.")
-
-flags.DEFINE_integer(
- "max_steps", -1, "If > 0: set total number of training steps to perform. Override num_train_epochs."
-)
-
-flags.DEFINE_integer("warmup_steps", 0, "Linear warmup over warmup_steps.")
-
-flags.DEFINE_integer("logging_steps", 50, "Log every X updates steps.")
-
-flags.DEFINE_integer("save_steps", 50, "Save checkpoint every X updates steps.")
-
-flags.DEFINE_boolean(
- "eval_all_checkpoints",
- False,
- "Evaluate all checkpoints starting with the same prefix as model_name ending and ending with step number",
-)
-
-flags.DEFINE_boolean("no_cuda", False, "Avoid using CUDA when available")
-
-flags.DEFINE_boolean("overwrite_output_dir", False, "Overwrite the content of the output directory")
-
-flags.DEFINE_boolean("overwrite_cache", False, "Overwrite the cached training and evaluation sets")
-
-flags.DEFINE_integer("seed", 42, "random seed for initialization")
-
-flags.DEFINE_boolean("fp16", False, "Whether to use 16-bit (mixed) precision instead of 32-bit")
-
-flags.DEFINE_string(
- "gpus",
- "0",
- "Comma separated list of gpus devices. If only one, switch to single "
- "gpu strategy, if None takes all the gpus available.",
-)
-
-
-def train(
- args, strategy, train_dataset, tokenizer, model, num_train_examples, labels, train_batch_size, pad_token_label_id
-):
- if args["max_steps"] > 0:
- num_train_steps = args["max_steps"] * args["gradient_accumulation_steps"]
- args["num_train_epochs"] = 1
- else:
- num_train_steps = (
- math.ceil(num_train_examples / train_batch_size)
- // args["gradient_accumulation_steps"]
- * args["num_train_epochs"]
- )
-
- writer = tf.summary.create_file_writer("/tmp/mylogs")
-
- with strategy.scope():
- loss_fct = tf.keras.losses.SparseCategoricalCrossentropy(reduction=tf.keras.losses.Reduction.NONE)
- optimizer = create_optimizer(args["learning_rate"], num_train_steps, args["warmup_steps"])
-
- if args["fp16"]:
- optimizer = tf.keras.mixed_precision.experimental.LossScaleOptimizer(optimizer, "dynamic")
-
- loss_metric = tf.keras.metrics.Mean(name="loss", dtype=tf.float32)
- gradient_accumulator = GradientAccumulator()
-
- logging.info("***** Running training *****")
- logging.info(" Num examples = %d", num_train_examples)
- logging.info(" Num Epochs = %d", args["num_train_epochs"])
- logging.info(" Instantaneous batch size per device = %d", args["per_device_train_batch_size"])
- logging.info(
- " Total train batch size (w. parallel, distributed & accumulation) = %d",
- train_batch_size * args["gradient_accumulation_steps"],
- )
- logging.info(" Gradient Accumulation steps = %d", args["gradient_accumulation_steps"])
- logging.info(" Total training steps = %d", num_train_steps)
-
- model.summary()
-
- @tf.function
- def apply_gradients():
- grads_and_vars = []
-
- for gradient, variable in zip(gradient_accumulator.gradients, model.trainable_variables):
- if gradient is not None:
- scaled_gradient = gradient / (args["n_device"] * args["gradient_accumulation_steps"])
- grads_and_vars.append((scaled_gradient, variable))
- else:
- grads_and_vars.append((gradient, variable))
-
- optimizer.apply_gradients(grads_and_vars, args["max_grad_norm"])
- gradient_accumulator.reset()
-
- @tf.function
- def train_step(train_features, train_labels):
- def step_fn(train_features, train_labels):
- inputs = {"attention_mask": train_features["input_mask"], "training": True}
-
- if args["model_type"] != "distilbert":
- inputs["token_type_ids"] = (
- train_features["segment_ids"] if args["model_type"] in ["bert", "xlnet"] else None
- )
-
- with tf.GradientTape() as tape:
- logits = model(train_features["input_ids"], **inputs)[0]
- logits = tf.reshape(logits, (-1, len(labels) + 1))
- active_loss = tf.reshape(train_features["input_mask"], (-1,))
- active_logits = tf.boolean_mask(logits, active_loss)
- train_labels = tf.reshape(train_labels, (-1,))
- active_labels = tf.boolean_mask(train_labels, active_loss)
- cross_entropy = loss_fct(active_labels, active_logits)
- loss = tf.reduce_sum(cross_entropy) * (1.0 / train_batch_size)
- grads = tape.gradient(loss, model.trainable_variables)
-
- gradient_accumulator(grads)
-
- return cross_entropy
-
- per_example_losses = strategy.experimental_run_v2(step_fn, args=(train_features, train_labels))
- mean_loss = strategy.reduce(tf.distribute.ReduceOp.MEAN, per_example_losses, axis=0)
-
- return mean_loss
-
- current_time = datetime.datetime.now()
- train_iterator = master_bar(range(args["num_train_epochs"]))
- global_step = 0
- logging_loss = 0.0
-
- for epoch in train_iterator:
- epoch_iterator = progress_bar(
- train_dataset, total=num_train_steps, parent=train_iterator, display=args["n_device"] > 1
- )
- step = 1
-
- with strategy.scope():
- for train_features, train_labels in epoch_iterator:
- loss = train_step(train_features, train_labels)
-
- if step % args["gradient_accumulation_steps"] == 0:
- strategy.experimental_run_v2(apply_gradients)
-
- loss_metric(loss)
-
- global_step += 1
-
- if args["logging_steps"] > 0 and global_step % args["logging_steps"] == 0:
- # Log metrics
- if (
- args["n_device"] == 1 and args["evaluate_during_training"]
- ): # Only evaluate when single GPU otherwise metrics may not average well
- y_true, y_pred, eval_loss = evaluate(
- args, strategy, model, tokenizer, labels, pad_token_label_id, mode="dev"
- )
- report = metrics.classification_report(y_true, y_pred, digits=4)
-
- logging.info("Eval at step " + str(global_step) + "\n" + report)
- logging.info("eval_loss: " + str(eval_loss))
-
- precision = metrics.precision_score(y_true, y_pred)
- recall = metrics.recall_score(y_true, y_pred)
- f1 = metrics.f1_score(y_true, y_pred)
-
- with writer.as_default():
- tf.summary.scalar("eval_loss", eval_loss, global_step)
- tf.summary.scalar("precision", precision, global_step)
- tf.summary.scalar("recall", recall, global_step)
- tf.summary.scalar("f1", f1, global_step)
-
- lr = optimizer.learning_rate
- learning_rate = lr(step)
-
- with writer.as_default():
- tf.summary.scalar("lr", learning_rate, global_step)
- tf.summary.scalar(
- "loss", (loss_metric.result() - logging_loss) / args["logging_steps"], global_step
- )
-
- logging_loss = loss_metric.result()
-
- with writer.as_default():
- tf.summary.scalar("loss", loss_metric.result(), step=step)
-
- if args["save_steps"] > 0 and global_step % args["save_steps"] == 0:
- # Save model checkpoint
- output_dir = os.path.join(args["output_dir"], "checkpoint-{}".format(global_step))
-
- if not os.path.exists(output_dir):
- os.makedirs(output_dir)
-
- model.save_pretrained(output_dir)
- logging.info("Saving model checkpoint to %s", output_dir)
-
- train_iterator.child.comment = f"loss : {loss_metric.result()}"
- step += 1
-
- train_iterator.write(f"loss epoch {epoch + 1}: {loss_metric.result()}")
-
- loss_metric.reset_states()
-
- logging.info(" Training took time = {}".format(datetime.datetime.now() - current_time))
-
-
-def evaluate(args, strategy, model, tokenizer, labels, pad_token_label_id, mode):
- eval_batch_size = args["per_device_eval_batch_size"] * args["n_device"]
- eval_dataset, size = load_and_cache_examples(
- args, tokenizer, labels, pad_token_label_id, eval_batch_size, mode=mode
- )
- eval_dataset = strategy.experimental_distribute_dataset(eval_dataset)
- preds = None
- num_eval_steps = math.ceil(size / eval_batch_size)
- master = master_bar(range(1))
- eval_iterator = progress_bar(eval_dataset, total=num_eval_steps, parent=master, display=args["n_device"] > 1)
- loss_fct = tf.keras.losses.SparseCategoricalCrossentropy(reduction=tf.keras.losses.Reduction.NONE)
- loss = 0.0
-
- logging.info("***** Running evaluation *****")
- logging.info(" Num examples = %d", size)
- logging.info(" Batch size = %d", eval_batch_size)
-
- for eval_features, eval_labels in eval_iterator:
- inputs = {"attention_mask": eval_features["input_mask"], "training": False}
-
- if args["model_type"] != "distilbert":
- inputs["token_type_ids"] = (
- eval_features["segment_ids"] if args["model_type"] in ["bert", "xlnet"] else None
- )
-
- with strategy.scope():
- logits = model(eval_features["input_ids"], **inputs)[0]
- tmp_logits = tf.reshape(logits, (-1, len(labels) + 1))
- active_loss = tf.reshape(eval_features["input_mask"], (-1,))
- active_logits = tf.boolean_mask(tmp_logits, active_loss)
- tmp_eval_labels = tf.reshape(eval_labels, (-1,))
- active_labels = tf.boolean_mask(tmp_eval_labels, active_loss)
- cross_entropy = loss_fct(active_labels, active_logits)
- loss += tf.reduce_sum(cross_entropy) * (1.0 / eval_batch_size)
-
- if preds is None:
- preds = logits.numpy()
- label_ids = eval_labels.numpy()
- else:
- preds = np.append(preds, logits.numpy(), axis=0)
- label_ids = np.append(label_ids, eval_labels.numpy(), axis=0)
-
- preds = np.argmax(preds, axis=2)
- y_pred = [[] for _ in range(label_ids.shape[0])]
- y_true = [[] for _ in range(label_ids.shape[0])]
- loss = loss / num_eval_steps
-
- for i in range(label_ids.shape[0]):
- for j in range(label_ids.shape[1]):
- if label_ids[i, j] != pad_token_label_id:
- y_pred[i].append(labels[preds[i, j] - 1])
- y_true[i].append(labels[label_ids[i, j] - 1])
-
- return y_true, y_pred, loss.numpy()
-
-
-def load_cache(cached_file, max_seq_length):
- name_to_features = {
- "input_ids": tf.io.FixedLenFeature([max_seq_length], tf.int64),
- "input_mask": tf.io.FixedLenFeature([max_seq_length], tf.int64),
- "segment_ids": tf.io.FixedLenFeature([max_seq_length], tf.int64),
- "label_ids": tf.io.FixedLenFeature([max_seq_length], tf.int64),
- }
-
- def _decode_record(record):
- example = tf.io.parse_single_example(record, name_to_features)
- features = {}
- features["input_ids"] = example["input_ids"]
- features["input_mask"] = example["input_mask"]
- features["segment_ids"] = example["segment_ids"]
-
- return features, example["label_ids"]
-
- d = tf.data.TFRecordDataset(cached_file)
- d = d.map(_decode_record, num_parallel_calls=4)
- count = d.reduce(0, lambda x, _: x + 1)
-
- return d, count.numpy()
-
-
-def save_cache(features, cached_features_file):
- writer = tf.io.TFRecordWriter(cached_features_file)
-
- for (ex_index, feature) in enumerate(features):
- if ex_index % 5000 == 0:
- logging.info("Writing example %d of %d" % (ex_index, len(features)))
-
- def create_int_feature(values):
- f = tf.train.Feature(int64_list=tf.train.Int64List(value=list(values)))
- return f
-
- record_feature = collections.OrderedDict()
- record_feature["input_ids"] = create_int_feature(feature.input_ids)
- record_feature["input_mask"] = create_int_feature(feature.input_mask)
- record_feature["segment_ids"] = create_int_feature(feature.segment_ids)
- record_feature["label_ids"] = create_int_feature(feature.label_ids)
-
- tf_example = tf.train.Example(features=tf.train.Features(feature=record_feature))
-
- writer.write(tf_example.SerializeToString())
-
- writer.close()
-
-
-def load_and_cache_examples(args, tokenizer, labels, pad_token_label_id, batch_size, mode):
- drop_remainder = True if args["tpu"] or mode == "train" else False
-
- # Load data features from cache or dataset file
- cached_features_file = os.path.join(
- args["data_dir"],
- "cached_{}_{}_{}.tf_record".format(
- mode, list(filter(None, args["model_name_or_path"].split("/"))).pop(), str(args["max_seq_length"])
- ),
- )
- if os.path.exists(cached_features_file) and not args["overwrite_cache"]:
- logging.info("Loading features from cached file %s", cached_features_file)
- dataset, size = load_cache(cached_features_file, args["max_seq_length"])
- else:
- logging.info("Creating features from dataset file at %s", args["data_dir"])
- examples = read_examples_from_file(args["data_dir"], mode)
- features = convert_examples_to_features(
- examples,
- labels,
- args["max_seq_length"],
- tokenizer,
- cls_token_at_end=bool(args["model_type"] in ["xlnet"]),
- # xlnet has a cls token at the end
- cls_token=tokenizer.cls_token,
- cls_token_segment_id=2 if args["model_type"] in ["xlnet"] else 0,
- sep_token=tokenizer.sep_token,
- sep_token_extra=bool(args["model_type"] in ["roberta"]),
- # roberta uses an extra separator b/w pairs of sentences, cf. github.com/pytorch/fairseq/commit/1684e166e3da03f5b600dbb7855cb98ddfcd0805
- pad_on_left=bool(args["model_type"] in ["xlnet"]),
- # pad on the left for xlnet
- pad_token=tokenizer.convert_tokens_to_ids([tokenizer.pad_token])[0],
- pad_token_segment_id=4 if args["model_type"] in ["xlnet"] else 0,
- pad_token_label_id=pad_token_label_id,
- )
- logging.info("Saving features into cached file %s", cached_features_file)
- save_cache(features, cached_features_file)
- dataset, size = load_cache(cached_features_file, args["max_seq_length"])
-
- if mode == "train":
- dataset = dataset.repeat()
- dataset = dataset.shuffle(buffer_size=8192, seed=args["seed"])
-
- dataset = dataset.batch(batch_size, drop_remainder)
- dataset = dataset.prefetch(buffer_size=batch_size)
-
- return dataset, size
-
-
-def main(_):
- logging.set_verbosity(logging.INFO)
- args = flags.FLAGS.flag_values_dict()
-
- if (
- os.path.exists(args["output_dir"])
- and os.listdir(args["output_dir"])
- and args["do_train"]
- and not args["overwrite_output_dir"]
- ):
- raise ValueError(
- "Output directory ({}) already exists and is not empty. Use --overwrite_output_dir to overcome.".format(
- args["output_dir"]
- )
- )
-
- if args["fp16"]:
- tf.config.optimizer.set_experimental_options({"auto_mixed_precision": True})
-
- if args["tpu"]:
- resolver = tf.distribute.cluster_resolver.TPUClusterResolver(tpu=args["tpu"])
- tf.config.experimental_connect_to_cluster(resolver)
- tf.tpu.experimental.initialize_tpu_system(resolver)
- strategy = tf.distribute.experimental.TPUStrategy(resolver)
- args["n_device"] = args["num_tpu_cores"]
- elif len(args["gpus"].split(",")) > 1:
- args["n_device"] = len([f"/gpu:{gpu}" for gpu in args["gpus"].split(",")])
- strategy = tf.distribute.MirroredStrategy(devices=[f"/gpu:{gpu}" for gpu in args["gpus"].split(",")])
- elif args["no_cuda"]:
- args["n_device"] = 1
- strategy = tf.distribute.OneDeviceStrategy(device="/cpu:0")
- else:
- args["n_device"] = len(args["gpus"].split(","))
- strategy = tf.distribute.OneDeviceStrategy(device="/gpu:" + args["gpus"].split(",")[0])
-
- logging.warning(
- "n_device: %s, distributed training: %s, 16-bits training: %s",
- args["n_device"],
- bool(args["n_device"] > 1),
- args["fp16"],
- )
-
- labels = get_labels(args["labels"])
- num_labels = len(labels) + 1
- pad_token_label_id = 0
- config_class, model_class, tokenizer_class = MODEL_CLASSES[args["model_type"]]
- config = config_class.from_pretrained(
- args["config_name"] if args["config_name"] else args["model_name_or_path"],
- num_labels=num_labels,
- cache_dir=args["cache_dir"] if args["cache_dir"] else None,
- )
-
- logging.info("Training/evaluation parameters %s", args)
-
- # Training
- if args["do_train"]:
- tokenizer = tokenizer_class.from_pretrained(
- args["tokenizer_name"] if args["tokenizer_name"] else args["model_name_or_path"],
- do_lower_case=args["do_lower_case"],
- cache_dir=args["cache_dir"] if args["cache_dir"] else None,
- )
-
- with strategy.scope():
- model = model_class.from_pretrained(
- args["model_name_or_path"],
- from_pt=bool(".bin" in args["model_name_or_path"]),
- config=config,
- cache_dir=args["cache_dir"] if args["cache_dir"] else None,
- )
- model.layers[-1].activation = tf.keras.activations.softmax
-
- train_batch_size = args["per_device_train_batch_size"] * args["n_device"]
- train_dataset, num_train_examples = load_and_cache_examples(
- args, tokenizer, labels, pad_token_label_id, train_batch_size, mode="train"
- )
- train_dataset = strategy.experimental_distribute_dataset(train_dataset)
- train(
- args,
- strategy,
- train_dataset,
- tokenizer,
- model,
- num_train_examples,
- labels,
- train_batch_size,
- pad_token_label_id,
- )
-
- if not os.path.exists(args["output_dir"]):
- os.makedirs(args["output_dir"])
-
- logging.info("Saving model to %s", args["output_dir"])
-
- model.save_pretrained(args["output_dir"])
- tokenizer.save_pretrained(args["output_dir"])
-
- # Evaluation
- if args["do_eval"]:
- tokenizer = tokenizer_class.from_pretrained(args["output_dir"], do_lower_case=args["do_lower_case"])
- checkpoints = []
- results = []
-
- if args["eval_all_checkpoints"]:
- checkpoints = list(
- os.path.dirname(c)
- for c in sorted(
- glob.glob(args["output_dir"] + "/**/" + TF2_WEIGHTS_NAME, recursive=True),
- key=lambda f: int("".join(filter(str.isdigit, f)) or -1),
- )
- )
-
- logging.info("Evaluate the following checkpoints: %s", checkpoints)
-
- if len(checkpoints) == 0:
- checkpoints.append(args["output_dir"])
-
- for checkpoint in checkpoints:
- global_step = checkpoint.split("-")[-1] if re.match(".*checkpoint-[0-9]", checkpoint) else "final"
-
- with strategy.scope():
- model = model_class.from_pretrained(checkpoint)
-
- y_true, y_pred, eval_loss = evaluate(
- args, strategy, model, tokenizer, labels, pad_token_label_id, mode="dev"
- )
- report = metrics.classification_report(y_true, y_pred, digits=4)
-
- if global_step:
- results.append({global_step + "_report": report, global_step + "_loss": eval_loss})
-
- output_eval_file = os.path.join(args["output_dir"], "eval_results.txt")
-
- with tf.io.gfile.GFile(output_eval_file, "w") as writer:
- for res in results:
- for key, val in res.items():
- if "loss" in key:
- logging.info(key + " = " + str(val))
- writer.write(key + " = " + str(val))
- writer.write("\n")
- else:
- logging.info(key)
- logging.info("\n" + report)
- writer.write(key + "\n")
- writer.write(report)
- writer.write("\n")
-
- if args["do_predict"]:
- tokenizer = tokenizer_class.from_pretrained(args["output_dir"], do_lower_case=args["do_lower_case"])
- model = model_class.from_pretrained(args["output_dir"])
- eval_batch_size = args["per_device_eval_batch_size"] * args["n_device"]
- predict_dataset, _ = load_and_cache_examples(
- args, tokenizer, labels, pad_token_label_id, eval_batch_size, mode="test"
- )
- y_true, y_pred, pred_loss = evaluate(args, strategy, model, tokenizer, labels, pad_token_label_id, mode="test")
- output_test_results_file = os.path.join(args["output_dir"], "test_results.txt")
- output_test_predictions_file = os.path.join(args["output_dir"], "test_predictions.txt")
- report = metrics.classification_report(y_true, y_pred, digits=4)
-
- with tf.io.gfile.GFile(output_test_results_file, "w") as writer:
- report = metrics.classification_report(y_true, y_pred, digits=4)
-
- logging.info("\n" + report)
-
- writer.write(report)
- writer.write("\n\nloss = " + str(pred_loss))
-
- with tf.io.gfile.GFile(output_test_predictions_file, "w") as writer:
- with tf.io.gfile.GFile(os.path.join(args["data_dir"], "test.txt"), "r") as f:
- example_id = 0
-
- for line in f:
- if line.startswith("-DOCSTART-") or line == "" or line == "\n":
- writer.write(line)
-
- if not y_pred[example_id]:
- example_id += 1
- elif y_pred[example_id]:
- output_line = line.split()[0] + " " + y_pred[example_id].pop(0) + "\n"
- writer.write(output_line)
- else:
- logging.warning("Maximum sequence length exceeded: No prediction for '%s'.", line.split()[0])
-
-
-if __name__ == "__main__":
- flags.mark_flag_as_required("data_dir")
- flags.mark_flag_as_required("output_dir")
- flags.mark_flag_as_required("model_name_or_path")
- flags.mark_flag_as_required("model_type")
- app.run(main)
diff --git a/server/transformers/examples/run_xnli.py b/server/transformers/examples/run_xnli.py
deleted file mode 100644
index e995d27f1bd945e9c40915e9bdbe94970b6b62c4..0000000000000000000000000000000000000000
--- a/server/transformers/examples/run_xnli.py
+++ /dev/null
@@ -1,653 +0,0 @@
-# coding=utf-8
-# Copyright 2018 The Google AI Language Team Authors and The HuggingFace Inc. team.
-# Copyright (c) 2018, NVIDIA CORPORATION. All rights reserved.
-#
-# Licensed under the Apache License, Version 2.0 (the "License");
-# you may not use this file except in compliance with the License.
-# You may obtain a copy of the License at
-#
-# http://www.apache.org/licenses/LICENSE-2.0
-#
-# Unless required by applicable law or agreed to in writing, software
-# distributed under the License is distributed on an "AS IS" BASIS,
-# WITHOUT WARRANTIES OR CONDITIONS OF ANY KIND, either express or implied.
-# See the License for the specific language governing permissions and
-# limitations under the License.
-""" Finetuning multi-lingual models on XNLI (Bert, DistilBERT, XLM).
- Adapted from `examples/run_glue.py`"""
-
-
-import argparse
-import glob
-import logging
-import os
-import random
-
-import numpy as np
-import torch
-from torch.utils.data import DataLoader, RandomSampler, SequentialSampler, TensorDataset
-from torch.utils.data.distributed import DistributedSampler
-from tqdm import tqdm, trange
-
-from transformers import (
- WEIGHTS_NAME,
- AdamW,
- BertConfig,
- BertForSequenceClassification,
- BertTokenizer,
- DistilBertConfig,
- DistilBertForSequenceClassification,
- DistilBertTokenizer,
- XLMConfig,
- XLMForSequenceClassification,
- XLMTokenizer,
- get_linear_schedule_with_warmup,
-)
-from transformers import glue_convert_examples_to_features as convert_examples_to_features
-from transformers import xnli_compute_metrics as compute_metrics
-from transformers import xnli_output_modes as output_modes
-from transformers import xnli_processors as processors
-
-
-try:
- from torch.utils.tensorboard import SummaryWriter
-except ImportError:
- from tensorboardX import SummaryWriter
-
-
-logger = logging.getLogger(__name__)
-
-ALL_MODELS = sum(
- (tuple(conf.pretrained_config_archive_map.keys()) for conf in (BertConfig, DistilBertConfig, XLMConfig)), ()
-)
-
-MODEL_CLASSES = {
- "bert": (BertConfig, BertForSequenceClassification, BertTokenizer),
- "xlm": (XLMConfig, XLMForSequenceClassification, XLMTokenizer),
- "distilbert": (DistilBertConfig, DistilBertForSequenceClassification, DistilBertTokenizer),
-}
-
-
-def set_seed(args):
- random.seed(args.seed)
- np.random.seed(args.seed)
- torch.manual_seed(args.seed)
- if args.n_gpu > 0:
- torch.cuda.manual_seed_all(args.seed)
-
-
-def train(args, train_dataset, model, tokenizer):
- """ Train the model """
- if args.local_rank in [-1, 0]:
- tb_writer = SummaryWriter()
-
- args.train_batch_size = args.per_gpu_train_batch_size * max(1, args.n_gpu)
- train_sampler = RandomSampler(train_dataset) if args.local_rank == -1 else DistributedSampler(train_dataset)
- train_dataloader = DataLoader(train_dataset, sampler=train_sampler, batch_size=args.train_batch_size)
-
- if args.max_steps > 0:
- t_total = args.max_steps
- args.num_train_epochs = args.max_steps // (len(train_dataloader) // args.gradient_accumulation_steps) + 1
- else:
- t_total = len(train_dataloader) // args.gradient_accumulation_steps * args.num_train_epochs
-
- # Prepare optimizer and schedule (linear warmup and decay)
- no_decay = ["bias", "LayerNorm.weight"]
- optimizer_grouped_parameters = [
- {
- "params": [p for n, p in model.named_parameters() if not any(nd in n for nd in no_decay)],
- "weight_decay": args.weight_decay,
- },
- {"params": [p for n, p in model.named_parameters() if any(nd in n for nd in no_decay)], "weight_decay": 0.0},
- ]
- optimizer = AdamW(optimizer_grouped_parameters, lr=args.learning_rate, eps=args.adam_epsilon)
- scheduler = get_linear_schedule_with_warmup(
- optimizer, num_warmup_steps=args.warmup_steps, num_training_steps=t_total
- )
-
- # Check if saved optimizer or scheduler states exist
- if os.path.isfile(os.path.join(args.model_name_or_path, "optimizer.pt")) and os.path.isfile(
- os.path.join(args.model_name_or_path, "scheduler.pt")
- ):
- # Load in optimizer and scheduler states
- optimizer.load_state_dict(torch.load(os.path.join(args.model_name_or_path, "optimizer.pt")))
- scheduler.load_state_dict(torch.load(os.path.join(args.model_name_or_path, "scheduler.pt")))
-
- if args.fp16:
- try:
- from apex import amp
- except ImportError:
- raise ImportError("Please install apex from https://www.github.com/nvidia/apex to use fp16 training.")
- model, optimizer = amp.initialize(model, optimizer, opt_level=args.fp16_opt_level)
-
- # multi-gpu training (should be after apex fp16 initialization)
- if args.n_gpu > 1:
- model = torch.nn.DataParallel(model)
-
- # Distributed training (should be after apex fp16 initialization)
- if args.local_rank != -1:
- model = torch.nn.parallel.DistributedDataParallel(
- model, device_ids=[args.local_rank], output_device=args.local_rank, find_unused_parameters=True
- )
-
- # Train!
- logger.info("***** Running training *****")
- logger.info(" Num examples = %d", len(train_dataset))
- logger.info(" Num Epochs = %d", args.num_train_epochs)
- logger.info(" Instantaneous batch size per GPU = %d", args.per_gpu_train_batch_size)
- logger.info(
- " Total train batch size (w. parallel, distributed & accumulation) = %d",
- args.train_batch_size
- * args.gradient_accumulation_steps
- * (torch.distributed.get_world_size() if args.local_rank != -1 else 1),
- )
- logger.info(" Gradient Accumulation steps = %d", args.gradient_accumulation_steps)
- logger.info(" Total optimization steps = %d", t_total)
-
- global_step = 0
- epochs_trained = 0
- steps_trained_in_current_epoch = 0
- # Check if continuing training from a checkpoint
- if os.path.exists(args.model_name_or_path):
- # set global_step to gobal_step of last saved checkpoint from model path
- global_step = int(args.model_name_or_path.split("-")[-1].split("/")[0])
- epochs_trained = global_step // (len(train_dataloader) // args.gradient_accumulation_steps)
- steps_trained_in_current_epoch = global_step % (len(train_dataloader) // args.gradient_accumulation_steps)
-
- logger.info(" Continuing training from checkpoint, will skip to saved global_step")
- logger.info(" Continuing training from epoch %d", epochs_trained)
- logger.info(" Continuing training from global step %d", global_step)
- logger.info(" Will skip the first %d steps in the first epoch", steps_trained_in_current_epoch)
-
- tr_loss, logging_loss = 0.0, 0.0
- model.zero_grad()
- train_iterator = trange(
- epochs_trained, int(args.num_train_epochs), desc="Epoch", disable=args.local_rank not in [-1, 0]
- )
- set_seed(args) # Added here for reproductibility
- for _ in train_iterator:
- epoch_iterator = tqdm(train_dataloader, desc="Iteration", disable=args.local_rank not in [-1, 0])
- for step, batch in enumerate(epoch_iterator):
- # Skip past any already trained steps if resuming training
- if steps_trained_in_current_epoch > 0:
- steps_trained_in_current_epoch -= 1
- continue
-
- model.train()
- batch = tuple(t.to(args.device) for t in batch)
- inputs = {"input_ids": batch[0], "attention_mask": batch[1], "labels": batch[3]}
- if args.model_type != "distilbert":
- inputs["token_type_ids"] = (
- batch[2] if args.model_type in ["bert"] else None
- ) # XLM and DistilBERT don't use segment_ids
- outputs = model(**inputs)
- loss = outputs[0] # model outputs are always tuple in transformers (see doc)
-
- if args.n_gpu > 1:
- loss = loss.mean() # mean() to average on multi-gpu parallel training
- if args.gradient_accumulation_steps > 1:
- loss = loss / args.gradient_accumulation_steps
-
- if args.fp16:
- with amp.scale_loss(loss, optimizer) as scaled_loss:
- scaled_loss.backward()
- else:
- loss.backward()
-
- tr_loss += loss.item()
- if (step + 1) % args.gradient_accumulation_steps == 0:
- if args.fp16:
- torch.nn.utils.clip_grad_norm_(amp.master_params(optimizer), args.max_grad_norm)
- else:
- torch.nn.utils.clip_grad_norm_(model.parameters(), args.max_grad_norm)
-
- optimizer.step()
- scheduler.step() # Update learning rate schedule
- model.zero_grad()
- global_step += 1
-
- if args.local_rank in [-1, 0] and args.logging_steps > 0 and global_step % args.logging_steps == 0:
- # Log metrics
- if (
- args.local_rank == -1 and args.evaluate_during_training
- ): # Only evaluate when single GPU otherwise metrics may not average well
- results = evaluate(args, model, tokenizer)
- for key, value in results.items():
- tb_writer.add_scalar("eval_{}".format(key), value, global_step)
- tb_writer.add_scalar("lr", scheduler.get_lr()[0], global_step)
- tb_writer.add_scalar("loss", (tr_loss - logging_loss) / args.logging_steps, global_step)
- logging_loss = tr_loss
-
- if args.local_rank in [-1, 0] and args.save_steps > 0 and global_step % args.save_steps == 0:
- # Save model checkpoint
- output_dir = os.path.join(args.output_dir, "checkpoint-{}".format(global_step))
- if not os.path.exists(output_dir):
- os.makedirs(output_dir)
- model_to_save = (
- model.module if hasattr(model, "module") else model
- ) # Take care of distributed/parallel training
- model_to_save.save_pretrained(output_dir)
- tokenizer.save_pretrained(output_dir)
-
- torch.save(args, os.path.join(output_dir, "training_args.bin"))
- logger.info("Saving model checkpoint to %s", output_dir)
-
- torch.save(optimizer.state_dict(), os.path.join(output_dir, "optimizer.pt"))
- torch.save(scheduler.state_dict(), os.path.join(output_dir, "scheduler.pt"))
- logger.info("Saving optimizer and scheduler states to %s", output_dir)
-
- if args.max_steps > 0 and global_step > args.max_steps:
- epoch_iterator.close()
- break
- if args.max_steps > 0 and global_step > args.max_steps:
- train_iterator.close()
- break
-
- if args.local_rank in [-1, 0]:
- tb_writer.close()
-
- return global_step, tr_loss / global_step
-
-
-def evaluate(args, model, tokenizer, prefix=""):
- eval_task_names = (args.task_name,)
- eval_outputs_dirs = (args.output_dir,)
-
- results = {}
- for eval_task, eval_output_dir in zip(eval_task_names, eval_outputs_dirs):
- eval_dataset = load_and_cache_examples(args, eval_task, tokenizer, evaluate=True)
-
- if not os.path.exists(eval_output_dir) and args.local_rank in [-1, 0]:
- os.makedirs(eval_output_dir)
-
- args.eval_batch_size = args.per_gpu_eval_batch_size * max(1, args.n_gpu)
- # Note that DistributedSampler samples randomly
- eval_sampler = SequentialSampler(eval_dataset)
- eval_dataloader = DataLoader(eval_dataset, sampler=eval_sampler, batch_size=args.eval_batch_size)
-
- # multi-gpu eval
- if args.n_gpu > 1:
- model = torch.nn.DataParallel(model)
-
- # Eval!
- logger.info("***** Running evaluation {} *****".format(prefix))
- logger.info(" Num examples = %d", len(eval_dataset))
- logger.info(" Batch size = %d", args.eval_batch_size)
- eval_loss = 0.0
- nb_eval_steps = 0
- preds = None
- out_label_ids = None
- for batch in tqdm(eval_dataloader, desc="Evaluating"):
- model.eval()
- batch = tuple(t.to(args.device) for t in batch)
-
- with torch.no_grad():
- inputs = {"input_ids": batch[0], "attention_mask": batch[1], "labels": batch[3]}
- if args.model_type != "distilbert":
- inputs["token_type_ids"] = (
- batch[2] if args.model_type in ["bert"] else None
- ) # XLM and DistilBERT don't use segment_ids
- outputs = model(**inputs)
- tmp_eval_loss, logits = outputs[:2]
-
- eval_loss += tmp_eval_loss.mean().item()
- nb_eval_steps += 1
- if preds is None:
- preds = logits.detach().cpu().numpy()
- out_label_ids = inputs["labels"].detach().cpu().numpy()
- else:
- preds = np.append(preds, logits.detach().cpu().numpy(), axis=0)
- out_label_ids = np.append(out_label_ids, inputs["labels"].detach().cpu().numpy(), axis=0)
-
- eval_loss = eval_loss / nb_eval_steps
- if args.output_mode == "classification":
- preds = np.argmax(preds, axis=1)
- else:
- raise ValueError("No other `output_mode` for XNLI.")
- result = compute_metrics(eval_task, preds, out_label_ids)
- results.update(result)
-
- output_eval_file = os.path.join(eval_output_dir, prefix, "eval_results.txt")
- with open(output_eval_file, "w") as writer:
- logger.info("***** Eval results {} *****".format(prefix))
- for key in sorted(result.keys()):
- logger.info(" %s = %s", key, str(result[key]))
- writer.write("%s = %s\n" % (key, str(result[key])))
-
- return results
-
-
-def load_and_cache_examples(args, task, tokenizer, evaluate=False):
- if args.local_rank not in [-1, 0] and not evaluate:
- torch.distributed.barrier() # Make sure only the first process in distributed training process the dataset, and the others will use the cache
-
- processor = processors[task](language=args.language, train_language=args.train_language)
- output_mode = output_modes[task]
- # Load data features from cache or dataset file
- cached_features_file = os.path.join(
- args.data_dir,
- "cached_{}_{}_{}_{}_{}".format(
- "test" if evaluate else "train",
- list(filter(None, args.model_name_or_path.split("/"))).pop(),
- str(args.max_seq_length),
- str(task),
- str(args.train_language if (not evaluate and args.train_language is not None) else args.language),
- ),
- )
- if os.path.exists(cached_features_file) and not args.overwrite_cache:
- logger.info("Loading features from cached file %s", cached_features_file)
- features = torch.load(cached_features_file)
- else:
- logger.info("Creating features from dataset file at %s", args.data_dir)
- label_list = processor.get_labels()
- examples = (
- processor.get_test_examples(args.data_dir) if evaluate else processor.get_train_examples(args.data_dir)
- )
- features = convert_examples_to_features(
- examples,
- tokenizer,
- label_list=label_list,
- max_length=args.max_seq_length,
- output_mode=output_mode,
- pad_on_left=False,
- pad_token=tokenizer.convert_tokens_to_ids([tokenizer.pad_token])[0],
- pad_token_segment_id=0,
- )
- if args.local_rank in [-1, 0]:
- logger.info("Saving features into cached file %s", cached_features_file)
- torch.save(features, cached_features_file)
-
- if args.local_rank == 0 and not evaluate:
- torch.distributed.barrier() # Make sure only the first process in distributed training process the dataset, and the others will use the cache
-
- # Convert to Tensors and build dataset
- all_input_ids = torch.tensor([f.input_ids for f in features], dtype=torch.long)
- all_attention_mask = torch.tensor([f.attention_mask for f in features], dtype=torch.long)
- all_token_type_ids = torch.tensor([f.token_type_ids for f in features], dtype=torch.long)
- if output_mode == "classification":
- all_labels = torch.tensor([f.label for f in features], dtype=torch.long)
- else:
- raise ValueError("No other `output_mode` for XNLI.")
-
- dataset = TensorDataset(all_input_ids, all_attention_mask, all_token_type_ids, all_labels)
- return dataset
-
-
-def main():
- parser = argparse.ArgumentParser()
-
- # Required parameters
- parser.add_argument(
- "--data_dir",
- default=None,
- type=str,
- required=True,
- help="The input data dir. Should contain the .tsv files (or other data files) for the task.",
- )
- parser.add_argument(
- "--model_type",
- default=None,
- type=str,
- required=True,
- help="Model type selected in the list: " + ", ".join(MODEL_CLASSES.keys()),
- )
- parser.add_argument(
- "--model_name_or_path",
- default=None,
- type=str,
- required=True,
- help="Path to pre-trained model or shortcut name selected in the list: " + ", ".join(ALL_MODELS),
- )
- parser.add_argument(
- "--language",
- default=None,
- type=str,
- required=True,
- help="Evaluation language. Also train language if `train_language` is set to None.",
- )
- parser.add_argument(
- "--train_language", default=None, type=str, help="Train language if is different of the evaluation language."
- )
- parser.add_argument(
- "--output_dir",
- default=None,
- type=str,
- required=True,
- help="The output directory where the model predictions and checkpoints will be written.",
- )
-
- # Other parameters
- parser.add_argument(
- "--config_name", default="", type=str, help="Pretrained config name or path if not the same as model_name"
- )
- parser.add_argument(
- "--tokenizer_name",
- default="",
- type=str,
- help="Pretrained tokenizer name or path if not the same as model_name",
- )
- parser.add_argument(
- "--cache_dir",
- default="",
- type=str,
- help="Where do you want to store the pre-trained models downloaded from s3",
- )
- parser.add_argument(
- "--max_seq_length",
- default=128,
- type=int,
- help="The maximum total input sequence length after tokenization. Sequences longer "
- "than this will be truncated, sequences shorter will be padded.",
- )
- parser.add_argument("--do_train", action="store_true", help="Whether to run training.")
- parser.add_argument("--do_eval", action="store_true", help="Whether to run eval on the test set.")
- parser.add_argument(
- "--evaluate_during_training", action="store_true", help="Rul evaluation during training at each logging step."
- )
- parser.add_argument(
- "--do_lower_case", action="store_true", help="Set this flag if you are using an uncased model."
- )
-
- parser.add_argument("--per_gpu_train_batch_size", default=8, type=int, help="Batch size per GPU/CPU for training.")
- parser.add_argument(
- "--per_gpu_eval_batch_size", default=8, type=int, help="Batch size per GPU/CPU for evaluation."
- )
- parser.add_argument(
- "--gradient_accumulation_steps",
- type=int,
- default=1,
- help="Number of updates steps to accumulate before performing a backward/update pass.",
- )
- parser.add_argument("--learning_rate", default=5e-5, type=float, help="The initial learning rate for Adam.")
- parser.add_argument("--weight_decay", default=0.0, type=float, help="Weight deay if we apply some.")
- parser.add_argument("--adam_epsilon", default=1e-8, type=float, help="Epsilon for Adam optimizer.")
- parser.add_argument("--max_grad_norm", default=1.0, type=float, help="Max gradient norm.")
- parser.add_argument(
- "--num_train_epochs", default=3.0, type=float, help="Total number of training epochs to perform."
- )
- parser.add_argument(
- "--max_steps",
- default=-1,
- type=int,
- help="If > 0: set total number of training steps to perform. Override num_train_epochs.",
- )
- parser.add_argument("--warmup_steps", default=0, type=int, help="Linear warmup over warmup_steps.")
-
- parser.add_argument("--logging_steps", type=int, default=500, help="Log every X updates steps.")
- parser.add_argument("--save_steps", type=int, default=500, help="Save checkpoint every X updates steps.")
- parser.add_argument(
- "--eval_all_checkpoints",
- action="store_true",
- help="Evaluate all checkpoints starting with the same prefix as model_name ending and ending with step number",
- )
- parser.add_argument("--no_cuda", action="store_true", help="Avoid using CUDA when available")
- parser.add_argument(
- "--overwrite_output_dir", action="store_true", help="Overwrite the content of the output directory"
- )
- parser.add_argument(
- "--overwrite_cache", action="store_true", help="Overwrite the cached training and evaluation sets"
- )
- parser.add_argument("--seed", type=int, default=42, help="random seed for initialization")
-
- parser.add_argument(
- "--fp16",
- action="store_true",
- help="Whether to use 16-bit (mixed) precision (through NVIDIA apex) instead of 32-bit",
- )
- parser.add_argument(
- "--fp16_opt_level",
- type=str,
- default="O1",
- help="For fp16: Apex AMP optimization level selected in ['O0', 'O1', 'O2', and 'O3']."
- "See details at https://nvidia.github.io/apex/amp.html",
- )
- parser.add_argument("--local_rank", type=int, default=-1, help="For distributed training: local_rank")
- parser.add_argument("--server_ip", type=str, default="", help="For distant debugging.")
- parser.add_argument("--server_port", type=str, default="", help="For distant debugging.")
- args = parser.parse_args()
-
- if (
- os.path.exists(args.output_dir)
- and os.listdir(args.output_dir)
- and args.do_train
- and not args.overwrite_output_dir
- ):
- raise ValueError(
- "Output directory ({}) already exists and is not empty. Use --overwrite_output_dir to overcome.".format(
- args.output_dir
- )
- )
-
- # Setup distant debugging if needed
- if args.server_ip and args.server_port:
- # Distant debugging - see https://code.visualstudio.com/docs/python/debugging#_attach-to-a-local-script
- import ptvsd
-
- print("Waiting for debugger attach")
- ptvsd.enable_attach(address=(args.server_ip, args.server_port), redirect_output=True)
- ptvsd.wait_for_attach()
-
- # Setup CUDA, GPU & distributed training
- if args.local_rank == -1 or args.no_cuda:
- device = torch.device("cuda" if torch.cuda.is_available() and not args.no_cuda else "cpu")
- args.n_gpu = torch.cuda.device_count()
- else: # Initializes the distributed backend which will take care of sychronizing nodes/GPUs
- torch.cuda.set_device(args.local_rank)
- device = torch.device("cuda", args.local_rank)
- torch.distributed.init_process_group(backend="nccl")
- args.n_gpu = 1
- args.device = device
-
- # Setup logging
- logging.basicConfig(
- format="%(asctime)s - %(levelname)s - %(name)s - %(message)s",
- datefmt="%m/%d/%Y %H:%M:%S",
- level=logging.INFO if args.local_rank in [-1, 0] else logging.WARN,
- )
- logger.warning(
- "Process rank: %s, device: %s, n_gpu: %s, distributed training: %s, 16-bits training: %s",
- args.local_rank,
- device,
- args.n_gpu,
- bool(args.local_rank != -1),
- args.fp16,
- )
-
- # Set seed
- set_seed(args)
-
- # Prepare XNLI task
- args.task_name = "xnli"
- if args.task_name not in processors:
- raise ValueError("Task not found: %s" % (args.task_name))
- processor = processors[args.task_name](language=args.language, train_language=args.train_language)
- args.output_mode = output_modes[args.task_name]
- label_list = processor.get_labels()
- num_labels = len(label_list)
-
- # Load pretrained model and tokenizer
- if args.local_rank not in [-1, 0]:
- torch.distributed.barrier() # Make sure only the first process in distributed training will download model & vocab
-
- args.model_type = args.model_type.lower()
- config_class, model_class, tokenizer_class = MODEL_CLASSES[args.model_type]
- config = config_class.from_pretrained(
- args.config_name if args.config_name else args.model_name_or_path,
- num_labels=num_labels,
- finetuning_task=args.task_name,
- cache_dir=args.cache_dir if args.cache_dir else None,
- )
- tokenizer = tokenizer_class.from_pretrained(
- args.tokenizer_name if args.tokenizer_name else args.model_name_or_path,
- do_lower_case=args.do_lower_case,
- cache_dir=args.cache_dir if args.cache_dir else None,
- )
- model = model_class.from_pretrained(
- args.model_name_or_path,
- from_tf=bool(".ckpt" in args.model_name_or_path),
- config=config,
- cache_dir=args.cache_dir if args.cache_dir else None,
- )
-
- if args.local_rank == 0:
- torch.distributed.barrier() # Make sure only the first process in distributed training will download model & vocab
-
- model.to(args.device)
-
- logger.info("Training/evaluation parameters %s", args)
-
- # Training
- if args.do_train:
- train_dataset = load_and_cache_examples(args, args.task_name, tokenizer, evaluate=False)
- global_step, tr_loss = train(args, train_dataset, model, tokenizer)
- logger.info(" global_step = %s, average loss = %s", global_step, tr_loss)
-
- # Saving best-practices: if you use defaults names for the model, you can reload it using from_pretrained()
- if args.do_train and (args.local_rank == -1 or torch.distributed.get_rank() == 0):
- # Create output directory if needed
- if not os.path.exists(args.output_dir) and args.local_rank in [-1, 0]:
- os.makedirs(args.output_dir)
-
- logger.info("Saving model checkpoint to %s", args.output_dir)
- # Save a trained model, configuration and tokenizer using `save_pretrained()`.
- # They can then be reloaded using `from_pretrained()`
- model_to_save = (
- model.module if hasattr(model, "module") else model
- ) # Take care of distributed/parallel training
- model_to_save.save_pretrained(args.output_dir)
- tokenizer.save_pretrained(args.output_dir)
-
- # Good practice: save your training arguments together with the trained model
- torch.save(args, os.path.join(args.output_dir, "training_args.bin"))
-
- # Load a trained model and vocabulary that you have fine-tuned
- model = model_class.from_pretrained(args.output_dir)
- tokenizer = tokenizer_class.from_pretrained(args.output_dir)
- model.to(args.device)
-
- # Evaluation
- results = {}
- if args.do_eval and args.local_rank in [-1, 0]:
- tokenizer = tokenizer_class.from_pretrained(args.output_dir, do_lower_case=args.do_lower_case)
- checkpoints = [args.output_dir]
- if args.eval_all_checkpoints:
- checkpoints = list(
- os.path.dirname(c) for c in sorted(glob.glob(args.output_dir + "/**/" + WEIGHTS_NAME, recursive=True))
- )
- logging.getLogger("transformers.modeling_utils").setLevel(logging.WARN) # Reduce logging
- logger.info("Evaluate the following checkpoints: %s", checkpoints)
- for checkpoint in checkpoints:
- global_step = checkpoint.split("-")[-1] if len(checkpoints) > 1 else ""
- prefix = checkpoint.split("/")[-1] if checkpoint.find("checkpoint") != -1 else ""
-
- model = model_class.from_pretrained(checkpoint)
- model.to(args.device)
- result = evaluate(args, model, tokenizer, prefix=prefix)
- result = dict((k + "_{}".format(global_step), v) for k, v in result.items())
- results.update(result)
-
- return results
-
-
-if __name__ == "__main__":
- main()
diff --git a/server/transformers/examples/summarization/README.md b/server/transformers/examples/summarization/README.md
deleted file mode 100644
index 250c4bcfe8471a85690be3393b1f4e00124a4442..0000000000000000000000000000000000000000
--- a/server/transformers/examples/summarization/README.md
+++ /dev/null
@@ -1,61 +0,0 @@
-# Text Summarization with Pretrained Encoders
-
-This folder contains part of the code necessary to reproduce the results on abstractive summarization from the article [Text Summarization with Pretrained Encoders](https://arxiv.org/pdf/1908.08345.pdf) by [Yang Liu](https://nlp-yang.github.io/) and [Mirella Lapata](https://homepages.inf.ed.ac.uk/mlap/). It can also be used to summarize any document.
-
-The original code can be found on the Yang Liu's [github repository](https://github.com/nlpyang/PreSumm).
-
-The model is loaded with the pre-trained weights for the abstractive summarization model trained on the CNN/Daily Mail dataset with an extractive and then abstractive tasks.
-
-## Setup
-
-```
-git clone https://github.com/huggingface/transformers && cd transformers
-pip install .
-pip install nltk py-rouge
-cd examples/summarization
-```
-
-## Reproduce the authors' results on ROUGE
-
-To be able to reproduce the authors' results on the CNN/Daily Mail dataset you first need to download both CNN and Daily Mail datasets [from Kyunghyun Cho's website](https://cs.nyu.edu/~kcho/DMQA/) (the links next to "Stories") in the same folder. Then uncompress the archives by running:
-
-```bash
-tar -xvf cnn_stories.tgz && tar -xvf dailymail_stories.tgz
-```
-
-And move all the stories to the same folder. We will refer as `$DATA_PATH` the path to where you uncompressed both archive. Then run the following in the same folder as `run_summarization.py`:
-
-```bash
-python run_summarization.py \
- --documents_dir $DATA_PATH \
- --summaries_output_dir $SUMMARIES_PATH \ # optional
- --no_cuda false \
- --batch_size 4 \
- --min_length 50 \
- --max_length 200 \
- --beam_size 5 \
- --alpha 0.95 \
- --block_trigram true \
- --compute_rouge true
-```
-
-The scripts executes on GPU if one is available and if `no_cuda` is not set to `true`. Inference on multiple GPUs is not suported yet. The ROUGE scores will be displayed in the console at the end of evaluation and written in a `rouge_scores.txt` file. The script takes 30 hours to compute with a single Tesla V100 GPU and a batch size of 10 (300,000 texts to summarize).
-
-## Summarize any text
-
-Put the documents that you would like to summarize in a folder (the path to which is referred to as `$DATA_PATH` below) and run the following in the same folder as `run_summarization.py`:
-
-```bash
-python run_summarization.py \
- --documents_dir $DATA_PATH \
- --summaries_output_dir $SUMMARIES_PATH \ # optional
- --no_cuda false \
- --batch_size 4 \
- --min_length 50 \
- --max_length 200 \
- --beam_size 5 \
- --alpha 0.95 \
- --block_trigram true \
-```
-
-You may want to play around with `min_length`, `max_length` and `alpha` to suit your use case. If you want to compute ROUGE on another dataset you will need to tweak the stories/summaries import in `utils_summarization.py` and tell it where to fetch the reference summaries.
diff --git a/server/transformers/examples/summarization/configuration_bertabs.py b/server/transformers/examples/summarization/configuration_bertabs.py
deleted file mode 100644
index c976180b2fc4d76e29f557e38bcf0708dc4ccbc0..0000000000000000000000000000000000000000
--- a/server/transformers/examples/summarization/configuration_bertabs.py
+++ /dev/null
@@ -1,98 +0,0 @@
-# coding=utf-8
-# Copyright 2019 The HuggingFace Inc. team.
-# Copyright (c) 2018, NVIDIA CORPORATION. All rights reserved.
-#
-# Licensed under the Apache License, Version 2.0 (the "License");
-# you may not use this file except in compliance with the License.
-# You may obtain a copy of the License at
-#
-# http://www.apache.org/licenses/LICENSE-2.0
-#
-# Unless required by applicable law or agreed to in writing, software
-# distributed under the License is distributed on an "AS IS" BASIS,
-# WITHOUT WARRANTIES OR CONDITIONS OF ANY KIND, either express or implied.
-# See the License for the specific language governing permissions and
-# limitations under the License.
-""" BertAbs configuration """
-import logging
-
-from transformers import PretrainedConfig
-
-
-logger = logging.getLogger(__name__)
-
-
-BERTABS_FINETUNED_CONFIG_MAP = {
- "bertabs-finetuned-cnndm": "https://s3.amazonaws.com/models.huggingface.co/bert/remi/bertabs-finetuned-cnndm-extractive-abstractive-summarization-config.json",
-}
-
-
-class BertAbsConfig(PretrainedConfig):
- r""" Class to store the configuration of the BertAbs model.
-
- Arguments:
- vocab_size: int
- Number of tokens in the vocabulary.
- max_pos: int
- The maximum sequence length that this model will be used with.
- enc_layer: int
- The numner of hidden layers in the Transformer encoder.
- enc_hidden_size: int
- The size of the encoder's layers.
- enc_heads: int
- The number of attention heads for each attention layer in the encoder.
- enc_ff_size: int
- The size of the encoder's feed-forward layers.
- enc_dropout: int
- The dropout probabilitiy for all fully connected layers in the
- embeddings, layers, pooler and also the attention probabilities in
- the encoder.
- dec_layer: int
- The numner of hidden layers in the decoder.
- dec_hidden_size: int
- The size of the decoder's layers.
- dec_heads: int
- The number of attention heads for each attention layer in the decoder.
- dec_ff_size: int
- The size of the decoder's feed-forward layers.
- dec_dropout: int
- The dropout probabilitiy for all fully connected layers in the
- embeddings, layers, pooler and also the attention probabilities in
- the decoder.
- """
-
- pretrained_config_archive_map = BERTABS_FINETUNED_CONFIG_MAP
- model_type = "bertabs"
-
- def __init__(
- self,
- vocab_size=30522,
- max_pos=512,
- enc_layers=6,
- enc_hidden_size=512,
- enc_heads=8,
- enc_ff_size=512,
- enc_dropout=0.2,
- dec_layers=6,
- dec_hidden_size=768,
- dec_heads=8,
- dec_ff_size=2048,
- dec_dropout=0.2,
- **kwargs,
- ):
- super().__init__(**kwargs)
-
- self.vocab_size = vocab_size
- self.max_pos = max_pos
-
- self.enc_layers = enc_layers
- self.enc_hidden_size = enc_hidden_size
- self.enc_heads = enc_heads
- self.enc_ff_size = enc_ff_size
- self.enc_dropout = enc_dropout
-
- self.dec_layers = dec_layers
- self.dec_hidden_size = dec_hidden_size
- self.dec_heads = dec_heads
- self.dec_ff_size = dec_ff_size
- self.dec_dropout = dec_dropout
diff --git a/server/transformers/examples/summarization/convert_bertabs_original_pytorch_checkpoint.py b/server/transformers/examples/summarization/convert_bertabs_original_pytorch_checkpoint.py
deleted file mode 100644
index a1cbd64dd8e9923d11d525e08cab8cd79ef50461..0000000000000000000000000000000000000000
--- a/server/transformers/examples/summarization/convert_bertabs_original_pytorch_checkpoint.py
+++ /dev/null
@@ -1,176 +0,0 @@
-# coding=utf-8
-# Copyright 2018 The HuggingFace Inc. team.
-#
-# Licensed under the Apache License, Version 2.0 (the "License");
-# you may not use this file except in compliance with the License.
-# You may obtain a copy of the License at
-#
-# http://www.apache.org/licenses/LICENSE-2.0
-#
-# Unless required by applicable law or agreed to in writing, software
-# distributed under the License is distributed on an "AS IS" BASIS,
-# WITHOUT WARRANTIES OR CONDITIONS OF ANY KIND, either express or implied.
-# See the License for the specific language governing permissions and
-# limitations under the License.
-""" Convert BertExtAbs's checkpoints.
-
-The script looks like it is doing something trivial but it is not. The "weights"
-proposed by the authors are actually the entire model pickled. We need to load
-the model within the original codebase to be able to only save its `state_dict`.
-"""
-
-import argparse
-import logging
-from collections import namedtuple
-
-import torch
-
-from model_bertabs import BertAbsSummarizer
-from models.model_builder import AbsSummarizer # The authors' implementation
-from transformers import BertTokenizer
-
-
-logging.basicConfig(level=logging.INFO)
-logger = logging.getLogger(__name__)
-
-
-SAMPLE_TEXT = "Hello world! cécé herlolip"
-
-
-BertAbsConfig = namedtuple(
- "BertAbsConfig",
- [
- "temp_dir",
- "large",
- "use_bert_emb",
- "finetune_bert",
- "encoder",
- "share_emb",
- "max_pos",
- "enc_layers",
- "enc_hidden_size",
- "enc_heads",
- "enc_ff_size",
- "enc_dropout",
- "dec_layers",
- "dec_hidden_size",
- "dec_heads",
- "dec_ff_size",
- "dec_dropout",
- ],
-)
-
-
-def convert_bertabs_checkpoints(path_to_checkpoints, dump_path):
- """ Copy/paste and tweak the pre-trained weights provided by the creators
- of BertAbs for the internal architecture.
- """
-
- # Instantiate the authors' model with the pre-trained weights
- config = BertAbsConfig(
- temp_dir=".",
- finetune_bert=False,
- large=False,
- share_emb=True,
- use_bert_emb=False,
- encoder="bert",
- max_pos=512,
- enc_layers=6,
- enc_hidden_size=512,
- enc_heads=8,
- enc_ff_size=512,
- enc_dropout=0.2,
- dec_layers=6,
- dec_hidden_size=768,
- dec_heads=8,
- dec_ff_size=2048,
- dec_dropout=0.2,
- )
- checkpoints = torch.load(path_to_checkpoints, lambda storage, loc: storage)
- original = AbsSummarizer(config, torch.device("cpu"), checkpoints)
- original.eval()
-
- new_model = BertAbsSummarizer(config, torch.device("cpu"))
- new_model.eval()
-
- # -------------------
- # Convert the weights
- # -------------------
-
- logging.info("convert the model")
- new_model.bert.load_state_dict(original.bert.state_dict())
- new_model.decoder.load_state_dict(original.decoder.state_dict())
- new_model.generator.load_state_dict(original.generator.state_dict())
-
- # ----------------------------------
- # Make sure the outpus are identical
- # ----------------------------------
-
- logging.info("Make sure that the models' outputs are identical")
- tokenizer = BertTokenizer.from_pretrained("bert-base-uncased")
-
- # prepare the model inputs
- encoder_input_ids = tokenizer.encode("This is sample éàalj'-.")
- encoder_input_ids.extend([tokenizer.pad_token_id] * (512 - len(encoder_input_ids)))
- encoder_input_ids = torch.tensor(encoder_input_ids).unsqueeze(0)
- decoder_input_ids = tokenizer.encode("This is sample 3 éàalj'-.")
- decoder_input_ids.extend([tokenizer.pad_token_id] * (512 - len(decoder_input_ids)))
- decoder_input_ids = torch.tensor(decoder_input_ids).unsqueeze(0)
-
- # failsafe to make sure the weights reset does not affect the
- # loaded weights.
- assert torch.max(torch.abs(original.generator[0].weight - new_model.generator[0].weight)) == 0
-
- # forward pass
- src = encoder_input_ids
- tgt = decoder_input_ids
- segs = token_type_ids = None
- clss = None
- mask_src = encoder_attention_mask = None
- mask_tgt = decoder_attention_mask = None
- mask_cls = None
-
- # The original model does not apply the geneator layer immediatly but rather in
- # the beam search (where it combines softmax + linear layer). Since we already
- # apply the softmax in our generation process we only apply the linear layer here.
- # We make sure that the outputs of the full stack are identical
- output_original_model = original(src, tgt, segs, clss, mask_src, mask_tgt, mask_cls)[0]
- output_original_generator = original.generator(output_original_model)
-
- output_converted_model = new_model(
- encoder_input_ids, decoder_input_ids, token_type_ids, encoder_attention_mask, decoder_attention_mask
- )[0]
- output_converted_generator = new_model.generator(output_converted_model)
-
- maximum_absolute_difference = torch.max(torch.abs(output_converted_model - output_original_model)).item()
- print("Maximum absolute difference beween weights: {:.2f}".format(maximum_absolute_difference))
- maximum_absolute_difference = torch.max(torch.abs(output_converted_generator - output_original_generator)).item()
- print("Maximum absolute difference beween weights: {:.2f}".format(maximum_absolute_difference))
-
- are_identical = torch.allclose(output_converted_model, output_original_model, atol=1e-3)
- if are_identical:
- logging.info("all weights are equal up to 1e-3")
- else:
- raise ValueError("the weights are different. The new model is likely different from the original one.")
-
- # The model has been saved with torch.save(model) and this is bound to the exact
- # directory structure. We save the state_dict instead.
- logging.info("saving the model's state dictionary")
- torch.save(
- new_model.state_dict(), "bertabs-finetuned-cnndm-extractive-abstractive-summarization-pytorch_model.bin"
- )
-
-
-if __name__ == "__main__":
- parser = argparse.ArgumentParser()
- parser.add_argument(
- "--bertabs_checkpoint_path", default=None, type=str, required=True, help="Path the official PyTorch dump.",
- )
- parser.add_argument(
- "--pytorch_dump_folder_path", default=None, type=str, required=True, help="Path to the output PyTorch model.",
- )
- args = parser.parse_args()
-
- convert_bertabs_checkpoints(
- args.bertabs_checkpoint_path, args.pytorch_dump_folder_path,
- )
diff --git a/server/transformers/examples/summarization/modeling_bertabs.py b/server/transformers/examples/summarization/modeling_bertabs.py
deleted file mode 100644
index bad412baac1dd38d3bf5742a629ee83a9b6c7b0b..0000000000000000000000000000000000000000
--- a/server/transformers/examples/summarization/modeling_bertabs.py
+++ /dev/null
@@ -1,1027 +0,0 @@
-# MIT License
-
-# Copyright (c) 2019 Yang Liu and the HuggingFace team
-
-# Permission is hereby granted, free of charge, to any person obtaining a copy
-# of this software and associated documentation files (the "Software"), to deal
-# in the Software without restriction, including without limitation the rights
-# to use, copy, modify, merge, publish, distribute, sublicense, and/or sell
-# copies of the Software, and to permit persons to whom the Software is
-# furnished to do so, subject to the following conditions:
-
-# The above copyright notice and this permission notice shall be included in all
-# copies or substantial portions of the Software.
-
-# THE SOFTWARE IS PROVIDED "AS IS", WITHOUT WARRANTY OF ANY KIND, EXPRESS OR
-# IMPLIED, INCLUDING BUT NOT LIMITED TO THE WARRANTIES OF MERCHANTABILITY,
-# FITNESS FOR A PARTICULAR PURPOSE AND NONINFRINGEMENT. IN NO EVENT SHALL THE
-# AUTHORS OR COPYRIGHT HOLDERS BE LIABLE FOR ANY CLAIM, DAMAGES OR OTHER
-# LIABILITY, WHETHER IN AN ACTION OF CONTRACT, TORT OR OTHERWISE, ARISING FROM,
-# OUT OF OR IN CONNECTION WITH THE SOFTWARE OR THE USE OR OTHER DEALINGS IN THE
-# SOFTWARE.
-import copy
-import math
-
-import numpy as np
-import torch
-from torch import nn
-from torch.nn.init import xavier_uniform_
-
-from configuration_bertabs import BertAbsConfig
-from transformers import BertConfig, BertModel, PreTrainedModel
-
-
-MAX_SIZE = 5000
-
-BERTABS_FINETUNED_MODEL_MAP = {
- "bertabs-finetuned-cnndm": "https://s3.amazonaws.com/models.huggingface.co/bert/remi/bertabs-finetuned-cnndm-extractive-abstractive-summarization-pytorch_model.bin",
-}
-
-
-class BertAbsPreTrainedModel(PreTrainedModel):
- config_class = BertAbsConfig
- pretrained_model_archive_map = BERTABS_FINETUNED_MODEL_MAP
- load_tf_weights = False
- base_model_prefix = "bert"
-
-
-class BertAbs(BertAbsPreTrainedModel):
- def __init__(self, args, checkpoint=None, bert_extractive_checkpoint=None):
- super().__init__(args)
- self.args = args
- self.bert = Bert()
-
- # If pre-trained weights are passed for Bert, load these.
- load_bert_pretrained_extractive = True if bert_extractive_checkpoint else False
- if load_bert_pretrained_extractive:
- self.bert.model.load_state_dict(
- dict([(n[11:], p) for n, p in bert_extractive_checkpoint.items() if n.startswith("bert.model")]),
- strict=True,
- )
-
- self.vocab_size = self.bert.model.config.vocab_size
-
- if args.max_pos > 512:
- my_pos_embeddings = nn.Embedding(args.max_pos, self.bert.model.config.hidden_size)
- my_pos_embeddings.weight.data[:512] = self.bert.model.embeddings.position_embeddings.weight.data
- my_pos_embeddings.weight.data[512:] = self.bert.model.embeddings.position_embeddings.weight.data[-1][
- None, :
- ].repeat(args.max_pos - 512, 1)
- self.bert.model.embeddings.position_embeddings = my_pos_embeddings
- tgt_embeddings = nn.Embedding(self.vocab_size, self.bert.model.config.hidden_size, padding_idx=0)
-
- tgt_embeddings.weight = copy.deepcopy(self.bert.model.embeddings.word_embeddings.weight)
-
- self.decoder = TransformerDecoder(
- self.args.dec_layers,
- self.args.dec_hidden_size,
- heads=self.args.dec_heads,
- d_ff=self.args.dec_ff_size,
- dropout=self.args.dec_dropout,
- embeddings=tgt_embeddings,
- vocab_size=self.vocab_size,
- )
-
- gen_func = nn.LogSoftmax(dim=-1)
- self.generator = nn.Sequential(nn.Linear(args.dec_hidden_size, args.vocab_size), gen_func)
- self.generator[0].weight = self.decoder.embeddings.weight
-
- load_from_checkpoints = False if checkpoint is None else True
- if load_from_checkpoints:
- self.load_state_dict(checkpoint)
-
- def init_weights(self):
- for module in self.decoder.modules():
- if isinstance(module, (nn.Linear, nn.Embedding)):
- module.weight.data.normal_(mean=0.0, std=0.02)
- elif isinstance(module, nn.LayerNorm):
- module.bias.data.zero_()
- module.weight.data.fill_(1.0)
- if isinstance(module, nn.Linear) and module.bias is not None:
- module.bias.data.zero_()
- for p in self.generator.parameters():
- if p.dim() > 1:
- xavier_uniform_(p)
- else:
- p.data.zero_()
-
- def forward(
- self, encoder_input_ids, decoder_input_ids, token_type_ids, encoder_attention_mask, decoder_attention_mask,
- ):
- encoder_output = self.bert(
- input_ids=encoder_input_ids, token_type_ids=token_type_ids, attention_mask=encoder_attention_mask,
- )
- encoder_hidden_states = encoder_output[0]
- dec_state = self.decoder.init_decoder_state(encoder_input_ids, encoder_hidden_states)
- decoder_outputs, _ = self.decoder(decoder_input_ids[:, :-1], encoder_hidden_states, dec_state)
- return decoder_outputs
-
-
-class Bert(nn.Module):
- """ This class is not really necessary and should probably disappear.
- """
-
- def __init__(self):
- super().__init__()
- config = BertConfig.from_pretrained("bert-base-uncased")
- self.model = BertModel(config)
-
- def forward(self, input_ids, attention_mask=None, token_type_ids=None, **kwargs):
- self.eval()
- with torch.no_grad():
- encoder_outputs, _ = self.model(
- input_ids, token_type_ids=token_type_ids, attention_mask=attention_mask, **kwargs
- )
- return encoder_outputs
-
-
-class TransformerDecoder(nn.Module):
- """
- The Transformer decoder from "Attention is All You Need".
-
- Args:
- num_layers (int): number of encoder layers.
- d_model (int): size of the model
- heads (int): number of heads
- d_ff (int): size of the inner FF layer
- dropout (float): dropout parameters
- embeddings (:obj:`onmt.modules.Embeddings`):
- embeddings to use, should have positional encodings
- attn_type (str): if using a seperate copy attention
- """
-
- def __init__(self, num_layers, d_model, heads, d_ff, dropout, embeddings, vocab_size):
- super().__init__()
-
- # Basic attributes.
- self.decoder_type = "transformer"
- self.num_layers = num_layers
- self.embeddings = embeddings
- self.pos_emb = PositionalEncoding(dropout, self.embeddings.embedding_dim)
-
- # Build TransformerDecoder.
- self.transformer_layers = nn.ModuleList(
- [TransformerDecoderLayer(d_model, heads, d_ff, dropout) for _ in range(num_layers)]
- )
-
- self.layer_norm = nn.LayerNorm(d_model, eps=1e-6)
-
- # forward(input_ids, attention_mask, encoder_hidden_states, encoder_attention_mask)
- # def forward(self, input_ids, state, attention_mask=None, memory_lengths=None,
- # step=None, cache=None, encoder_attention_mask=None, encoder_hidden_states=None, memory_masks=None):
- def forward(
- self,
- input_ids,
- encoder_hidden_states=None,
- state=None,
- attention_mask=None,
- memory_lengths=None,
- step=None,
- cache=None,
- encoder_attention_mask=None,
- ):
- """
- See :obj:`onmt.modules.RNNDecoderBase.forward()`
- memory_bank = encoder_hidden_states
- """
- # Name conversion
- tgt = input_ids
- memory_bank = encoder_hidden_states
- memory_mask = encoder_attention_mask
-
- # src_words = state.src
- src_words = state.src
- src_batch, src_len = src_words.size()
-
- padding_idx = self.embeddings.padding_idx
-
- # Decoder padding mask
- tgt_words = tgt
- tgt_batch, tgt_len = tgt_words.size()
- tgt_pad_mask = tgt_words.data.eq(padding_idx).unsqueeze(1).expand(tgt_batch, tgt_len, tgt_len)
-
- # Encoder padding mask
- if memory_mask is not None:
- src_len = memory_mask.size(-1)
- src_pad_mask = memory_mask.expand(src_batch, tgt_len, src_len)
- else:
- src_pad_mask = src_words.data.eq(padding_idx).unsqueeze(1).expand(src_batch, tgt_len, src_len)
-
- # Pass through the embeddings
- emb = self.embeddings(input_ids)
- output = self.pos_emb(emb, step)
- assert emb.dim() == 3 # len x batch x embedding_dim
-
- if state.cache is None:
- saved_inputs = []
-
- for i in range(self.num_layers):
- prev_layer_input = None
- if state.cache is None:
- if state.previous_input is not None:
- prev_layer_input = state.previous_layer_inputs[i]
-
- output, all_input = self.transformer_layers[i](
- output,
- memory_bank,
- src_pad_mask,
- tgt_pad_mask,
- previous_input=prev_layer_input,
- layer_cache=state.cache["layer_{}".format(i)] if state.cache is not None else None,
- step=step,
- )
- if state.cache is None:
- saved_inputs.append(all_input)
-
- if state.cache is None:
- saved_inputs = torch.stack(saved_inputs)
-
- output = self.layer_norm(output)
-
- if state.cache is None:
- state = state.update_state(tgt, saved_inputs)
-
- # Decoders in transformers return a tuple. Beam search will fail
- # if we don't follow this convention.
- return output, state # , state
-
- def init_decoder_state(self, src, memory_bank, with_cache=False):
- """ Init decoder state """
- state = TransformerDecoderState(src)
- if with_cache:
- state._init_cache(memory_bank, self.num_layers)
- return state
-
-
-class PositionalEncoding(nn.Module):
- def __init__(self, dropout, dim, max_len=5000):
- pe = torch.zeros(max_len, dim)
- position = torch.arange(0, max_len).unsqueeze(1)
- div_term = torch.exp((torch.arange(0, dim, 2, dtype=torch.float) * -(math.log(10000.0) / dim)))
- pe[:, 0::2] = torch.sin(position.float() * div_term)
- pe[:, 1::2] = torch.cos(position.float() * div_term)
- pe = pe.unsqueeze(0)
- super().__init__()
- self.register_buffer("pe", pe)
- self.dropout = nn.Dropout(p=dropout)
- self.dim = dim
-
- def forward(self, emb, step=None):
- emb = emb * math.sqrt(self.dim)
- if step:
- emb = emb + self.pe[:, step][:, None, :]
-
- else:
- emb = emb + self.pe[:, : emb.size(1)]
- emb = self.dropout(emb)
- return emb
-
- def get_emb(self, emb):
- return self.pe[:, : emb.size(1)]
-
-
-class TransformerDecoderLayer(nn.Module):
- """
- Args:
- d_model (int): the dimension of keys/values/queries in
- MultiHeadedAttention, also the input size of
- the first-layer of the PositionwiseFeedForward.
- heads (int): the number of heads for MultiHeadedAttention.
- d_ff (int): the second-layer of the PositionwiseFeedForward.
- dropout (float): dropout probability(0-1.0).
- self_attn_type (string): type of self-attention scaled-dot, average
- """
-
- def __init__(self, d_model, heads, d_ff, dropout):
- super().__init__()
-
- self.self_attn = MultiHeadedAttention(heads, d_model, dropout=dropout)
-
- self.context_attn = MultiHeadedAttention(heads, d_model, dropout=dropout)
- self.feed_forward = PositionwiseFeedForward(d_model, d_ff, dropout)
- self.layer_norm_1 = nn.LayerNorm(d_model, eps=1e-6)
- self.layer_norm_2 = nn.LayerNorm(d_model, eps=1e-6)
- self.drop = nn.Dropout(dropout)
- mask = self._get_attn_subsequent_mask(MAX_SIZE)
- # Register self.mask as a buffer in TransformerDecoderLayer, so
- # it gets TransformerDecoderLayer's cuda behavior automatically.
- self.register_buffer("mask", mask)
-
- def forward(
- self, inputs, memory_bank, src_pad_mask, tgt_pad_mask, previous_input=None, layer_cache=None, step=None,
- ):
- """
- Args:
- inputs (`FloatTensor`): `[batch_size x 1 x model_dim]`
- memory_bank (`FloatTensor`): `[batch_size x src_len x model_dim]`
- src_pad_mask (`LongTensor`): `[batch_size x 1 x src_len]`
- tgt_pad_mask (`LongTensor`): `[batch_size x 1 x 1]`
-
- Returns:
- (`FloatTensor`, `FloatTensor`, `FloatTensor`):
-
- * output `[batch_size x 1 x model_dim]`
- * attn `[batch_size x 1 x src_len]`
- * all_input `[batch_size x current_step x model_dim]`
-
- """
- dec_mask = torch.gt(tgt_pad_mask + self.mask[:, : tgt_pad_mask.size(1), : tgt_pad_mask.size(1)], 0)
- input_norm = self.layer_norm_1(inputs)
- all_input = input_norm
- if previous_input is not None:
- all_input = torch.cat((previous_input, input_norm), dim=1)
- dec_mask = None
-
- query = self.self_attn(all_input, all_input, input_norm, mask=dec_mask, layer_cache=layer_cache, type="self",)
-
- query = self.drop(query) + inputs
-
- query_norm = self.layer_norm_2(query)
- mid = self.context_attn(
- memory_bank, memory_bank, query_norm, mask=src_pad_mask, layer_cache=layer_cache, type="context",
- )
- output = self.feed_forward(self.drop(mid) + query)
-
- return output, all_input
- # return output
-
- def _get_attn_subsequent_mask(self, size):
- """
- Get an attention mask to avoid using the subsequent info.
-
- Args:
- size: int
-
- Returns:
- (`LongTensor`):
-
- * subsequent_mask `[1 x size x size]`
- """
- attn_shape = (1, size, size)
- subsequent_mask = np.triu(np.ones(attn_shape), k=1).astype("uint8")
- subsequent_mask = torch.from_numpy(subsequent_mask)
- return subsequent_mask
-
-
-class MultiHeadedAttention(nn.Module):
- """
- Multi-Head Attention module from
- "Attention is All You Need"
- :cite:`DBLP:journals/corr/VaswaniSPUJGKP17`.
-
- Similar to standard `dot` attention but uses
- multiple attention distributions simulataneously
- to select relevant items.
-
- .. mermaid::
-
- graph BT
- A[key]
- B[value]
- C[query]
- O[output]
- subgraph Attn
- D[Attn 1]
- E[Attn 2]
- F[Attn N]
- end
- A --> D
- C --> D
- A --> E
- C --> E
- A --> F
- C --> F
- D --> O
- E --> O
- F --> O
- B --> O
-
- Also includes several additional tricks.
-
- Args:
- head_count (int): number of parallel heads
- model_dim (int): the dimension of keys/values/queries,
- must be divisible by head_count
- dropout (float): dropout parameter
- """
-
- def __init__(self, head_count, model_dim, dropout=0.1, use_final_linear=True):
- assert model_dim % head_count == 0
- self.dim_per_head = model_dim // head_count
- self.model_dim = model_dim
-
- super().__init__()
- self.head_count = head_count
-
- self.linear_keys = nn.Linear(model_dim, head_count * self.dim_per_head)
- self.linear_values = nn.Linear(model_dim, head_count * self.dim_per_head)
- self.linear_query = nn.Linear(model_dim, head_count * self.dim_per_head)
- self.softmax = nn.Softmax(dim=-1)
- self.dropout = nn.Dropout(dropout)
- self.use_final_linear = use_final_linear
- if self.use_final_linear:
- self.final_linear = nn.Linear(model_dim, model_dim)
-
- def forward(
- self, key, value, query, mask=None, layer_cache=None, type=None, predefined_graph_1=None,
- ):
- """
- Compute the context vector and the attention vectors.
-
- Args:
- key (`FloatTensor`): set of `key_len`
- key vectors `[batch, key_len, dim]`
- value (`FloatTensor`): set of `key_len`
- value vectors `[batch, key_len, dim]`
- query (`FloatTensor`): set of `query_len`
- query vectors `[batch, query_len, dim]`
- mask: binary mask indicating which keys have
- non-zero attention `[batch, query_len, key_len]`
- Returns:
- (`FloatTensor`, `FloatTensor`) :
-
- * output context vectors `[batch, query_len, dim]`
- * one of the attention vectors `[batch, query_len, key_len]`
- """
- batch_size = key.size(0)
- dim_per_head = self.dim_per_head
- head_count = self.head_count
-
- def shape(x):
- """ projection """
- return x.view(batch_size, -1, head_count, dim_per_head).transpose(1, 2)
-
- def unshape(x):
- """ compute context """
- return x.transpose(1, 2).contiguous().view(batch_size, -1, head_count * dim_per_head)
-
- # 1) Project key, value, and query.
- if layer_cache is not None:
- if type == "self":
- query, key, value = (
- self.linear_query(query),
- self.linear_keys(query),
- self.linear_values(query),
- )
-
- key = shape(key)
- value = shape(value)
-
- if layer_cache is not None:
- device = key.device
- if layer_cache["self_keys"] is not None:
- key = torch.cat((layer_cache["self_keys"].to(device), key), dim=2)
- if layer_cache["self_values"] is not None:
- value = torch.cat((layer_cache["self_values"].to(device), value), dim=2)
- layer_cache["self_keys"] = key
- layer_cache["self_values"] = value
- elif type == "context":
- query = self.linear_query(query)
- if layer_cache is not None:
- if layer_cache["memory_keys"] is None:
- key, value = self.linear_keys(key), self.linear_values(value)
- key = shape(key)
- value = shape(value)
- else:
- key, value = (
- layer_cache["memory_keys"],
- layer_cache["memory_values"],
- )
- layer_cache["memory_keys"] = key
- layer_cache["memory_values"] = value
- else:
- key, value = self.linear_keys(key), self.linear_values(value)
- key = shape(key)
- value = shape(value)
- else:
- key = self.linear_keys(key)
- value = self.linear_values(value)
- query = self.linear_query(query)
- key = shape(key)
- value = shape(value)
-
- query = shape(query)
-
- # 2) Calculate and scale scores.
- query = query / math.sqrt(dim_per_head)
- scores = torch.matmul(query, key.transpose(2, 3))
-
- if mask is not None:
- mask = mask.unsqueeze(1).expand_as(scores)
- scores = scores.masked_fill(mask, -1e18)
-
- # 3) Apply attention dropout and compute context vectors.
-
- attn = self.softmax(scores)
-
- if predefined_graph_1 is not None:
- attn_masked = attn[:, -1] * predefined_graph_1
- attn_masked = attn_masked / (torch.sum(attn_masked, 2).unsqueeze(2) + 1e-9)
-
- attn = torch.cat([attn[:, :-1], attn_masked.unsqueeze(1)], 1)
-
- drop_attn = self.dropout(attn)
- if self.use_final_linear:
- context = unshape(torch.matmul(drop_attn, value))
- output = self.final_linear(context)
- return output
- else:
- context = torch.matmul(drop_attn, value)
- return context
-
-
-class DecoderState(object):
- """Interface for grouping together the current state of a recurrent
- decoder. In the simplest case just represents the hidden state of
- the model. But can also be used for implementing various forms of
- input_feeding and non-recurrent models.
-
- Modules need to implement this to utilize beam search decoding.
- """
-
- def detach(self):
- """ Need to document this """
- self.hidden = tuple([_.detach() for _ in self.hidden])
- self.input_feed = self.input_feed.detach()
-
- def beam_update(self, idx, positions, beam_size):
- """ Need to document this """
- for e in self._all:
- sizes = e.size()
- br = sizes[1]
- if len(sizes) == 3:
- sent_states = e.view(sizes[0], beam_size, br // beam_size, sizes[2])[:, :, idx]
- else:
- sent_states = e.view(sizes[0], beam_size, br // beam_size, sizes[2], sizes[3])[:, :, idx]
-
- sent_states.data.copy_(sent_states.data.index_select(1, positions))
-
- def map_batch_fn(self, fn):
- raise NotImplementedError()
-
-
-class TransformerDecoderState(DecoderState):
- """ Transformer Decoder state base class """
-
- def __init__(self, src):
- """
- Args:
- src (FloatTensor): a sequence of source words tensors
- with optional feature tensors, of size (len x batch).
- """
- self.src = src
- self.previous_input = None
- self.previous_layer_inputs = None
- self.cache = None
-
- @property
- def _all(self):
- """
- Contains attributes that need to be updated in self.beam_update().
- """
- if self.previous_input is not None and self.previous_layer_inputs is not None:
- return (self.previous_input, self.previous_layer_inputs, self.src)
- else:
- return (self.src,)
-
- def detach(self):
- if self.previous_input is not None:
- self.previous_input = self.previous_input.detach()
- if self.previous_layer_inputs is not None:
- self.previous_layer_inputs = self.previous_layer_inputs.detach()
- self.src = self.src.detach()
-
- def update_state(self, new_input, previous_layer_inputs):
- state = TransformerDecoderState(self.src)
- state.previous_input = new_input
- state.previous_layer_inputs = previous_layer_inputs
- return state
-
- def _init_cache(self, memory_bank, num_layers):
- self.cache = {}
-
- for l in range(num_layers):
- layer_cache = {"memory_keys": None, "memory_values": None}
- layer_cache["self_keys"] = None
- layer_cache["self_values"] = None
- self.cache["layer_{}".format(l)] = layer_cache
-
- def repeat_beam_size_times(self, beam_size):
- """ Repeat beam_size times along batch dimension. """
- self.src = self.src.data.repeat(1, beam_size, 1)
-
- def map_batch_fn(self, fn):
- def _recursive_map(struct, batch_dim=0):
- for k, v in struct.items():
- if v is not None:
- if isinstance(v, dict):
- _recursive_map(v)
- else:
- struct[k] = fn(v, batch_dim)
-
- self.src = fn(self.src, 0)
- if self.cache is not None:
- _recursive_map(self.cache)
-
-
-def gelu(x):
- return 0.5 * x * (1 + torch.tanh(math.sqrt(2 / math.pi) * (x + 0.044715 * torch.pow(x, 3))))
-
-
-class PositionwiseFeedForward(nn.Module):
- """ A two-layer Feed-Forward-Network with residual layer norm.
-
- Args:
- d_model (int): the size of input for the first-layer of the FFN.
- d_ff (int): the hidden layer size of the second-layer
- of the FNN.
- dropout (float): dropout probability in :math:`[0, 1)`.
- """
-
- def __init__(self, d_model, d_ff, dropout=0.1):
- super().__init__()
- self.w_1 = nn.Linear(d_model, d_ff)
- self.w_2 = nn.Linear(d_ff, d_model)
- self.layer_norm = nn.LayerNorm(d_model, eps=1e-6)
- self.actv = gelu
- self.dropout_1 = nn.Dropout(dropout)
- self.dropout_2 = nn.Dropout(dropout)
-
- def forward(self, x):
- inter = self.dropout_1(self.actv(self.w_1(self.layer_norm(x))))
- output = self.dropout_2(self.w_2(inter))
- return output + x
-
-
-#
-# TRANSLATOR
-# The following code is used to generate summaries using the
-# pre-trained weights and beam search.
-#
-
-
-def build_predictor(args, tokenizer, symbols, model, logger=None):
- # we should be able to refactor the global scorer a lot
- scorer = GNMTGlobalScorer(args.alpha, length_penalty="wu")
- translator = Translator(args, model, tokenizer, symbols, global_scorer=scorer, logger=logger)
- return translator
-
-
-class GNMTGlobalScorer(object):
- """
- NMT re-ranking score from
- "Google's Neural Machine Translation System" :cite:`wu2016google`
-
- Args:
- alpha (float): length parameter
- beta (float): coverage parameter
- """
-
- def __init__(self, alpha, length_penalty):
- self.alpha = alpha
- penalty_builder = PenaltyBuilder(length_penalty)
- self.length_penalty = penalty_builder.length_penalty()
-
- def score(self, beam, logprobs):
- """
- Rescores a prediction based on penalty functions
- """
- normalized_probs = self.length_penalty(beam, logprobs, self.alpha)
- return normalized_probs
-
-
-class PenaltyBuilder(object):
- """
- Returns the Length and Coverage Penalty function for Beam Search.
-
- Args:
- length_pen (str): option name of length pen
- cov_pen (str): option name of cov pen
- """
-
- def __init__(self, length_pen):
- self.length_pen = length_pen
-
- def length_penalty(self):
- if self.length_pen == "wu":
- return self.length_wu
- elif self.length_pen == "avg":
- return self.length_average
- else:
- return self.length_none
-
- """
- Below are all the different penalty terms implemented so far
- """
-
- def length_wu(self, beam, logprobs, alpha=0.0):
- """
- NMT length re-ranking score from
- "Google's Neural Machine Translation System" :cite:`wu2016google`.
- """
-
- modifier = ((5 + len(beam.next_ys)) ** alpha) / ((5 + 1) ** alpha)
- return logprobs / modifier
-
- def length_average(self, beam, logprobs, alpha=0.0):
- """
- Returns the average probability of tokens in a sequence.
- """
- return logprobs / len(beam.next_ys)
-
- def length_none(self, beam, logprobs, alpha=0.0, beta=0.0):
- """
- Returns unmodified scores.
- """
- return logprobs
-
-
-class Translator(object):
- """
- Uses a model to translate a batch of sentences.
-
- Args:
- model (:obj:`onmt.modules.NMTModel`):
- NMT model to use for translation
- fields (dict of Fields): data fields
- beam_size (int): size of beam to use
- n_best (int): number of translations produced
- max_length (int): maximum length output to produce
- global_scores (:obj:`GlobalScorer`):
- object to rescore final translations
- copy_attn (bool): use copy attention during translation
- beam_trace (bool): trace beam search for debugging
- logger(logging.Logger): logger.
- """
-
- def __init__(self, args, model, vocab, symbols, global_scorer=None, logger=None):
- self.logger = logger
-
- self.args = args
- self.model = model
- self.generator = self.model.generator
- self.vocab = vocab
- self.symbols = symbols
- self.start_token = symbols["BOS"]
- self.end_token = symbols["EOS"]
-
- self.global_scorer = global_scorer
- self.beam_size = args.beam_size
- self.min_length = args.min_length
- self.max_length = args.max_length
-
- def translate(self, batch, step, attn_debug=False):
- """ Generates summaries from one batch of data.
- """
- self.model.eval()
- with torch.no_grad():
- batch_data = self.translate_batch(batch)
- translations = self.from_batch(batch_data)
- return translations
-
- def translate_batch(self, batch, fast=False):
- """
- Translate a batch of sentences.
-
- Mostly a wrapper around :obj:`Beam`.
-
- Args:
- batch (:obj:`Batch`): a batch from a dataset object
- data (:obj:`Dataset`): the dataset object
- fast (bool): enables fast beam search (may not support all features)
-
- Todo:
- Shouldn't need the original dataset.
- """
- with torch.no_grad():
- return self._fast_translate_batch(batch, self.max_length, min_length=self.min_length)
-
- # Where the beam search lives
- # I have no idea why it is being called from the method above
- def _fast_translate_batch(self, batch, max_length, min_length=0):
- """ Beam Search using the encoder inputs contained in `batch`.
- """
-
- # The batch object is funny
- # Instead of just looking at the size of the arguments we encapsulate
- # a size argument.
- # Where is it defined?
- beam_size = self.beam_size
- batch_size = batch.batch_size
- src = batch.src
- segs = batch.segs
- mask_src = batch.mask_src
-
- src_features = self.model.bert(src, segs, mask_src)
- dec_states = self.model.decoder.init_decoder_state(src, src_features, with_cache=True)
- device = src_features.device
-
- # Tile states and memory beam_size times.
- dec_states.map_batch_fn(lambda state, dim: tile(state, beam_size, dim=dim))
- src_features = tile(src_features, beam_size, dim=0)
- batch_offset = torch.arange(batch_size, dtype=torch.long, device=device)
- beam_offset = torch.arange(0, batch_size * beam_size, step=beam_size, dtype=torch.long, device=device)
- alive_seq = torch.full([batch_size * beam_size, 1], self.start_token, dtype=torch.long, device=device)
-
- # Give full probability to the first beam on the first step.
- topk_log_probs = torch.tensor([0.0] + [float("-inf")] * (beam_size - 1), device=device).repeat(batch_size)
-
- # Structure that holds finished hypotheses.
- hypotheses = [[] for _ in range(batch_size)] # noqa: F812
-
- results = {}
- results["predictions"] = [[] for _ in range(batch_size)] # noqa: F812
- results["scores"] = [[] for _ in range(batch_size)] # noqa: F812
- results["gold_score"] = [0] * batch_size
- results["batch"] = batch
-
- for step in range(max_length):
- decoder_input = alive_seq[:, -1].view(1, -1)
-
- # Decoder forward.
- decoder_input = decoder_input.transpose(0, 1)
-
- dec_out, dec_states = self.model.decoder(decoder_input, src_features, dec_states, step=step)
-
- # Generator forward.
- log_probs = self.generator.forward(dec_out.transpose(0, 1).squeeze(0))
- vocab_size = log_probs.size(-1)
-
- if step < min_length:
- log_probs[:, self.end_token] = -1e20
-
- # Multiply probs by the beam probability.
- log_probs += topk_log_probs.view(-1).unsqueeze(1)
-
- alpha = self.global_scorer.alpha
- length_penalty = ((5.0 + (step + 1)) / 6.0) ** alpha
-
- # Flatten probs into a list of possibilities.
- curr_scores = log_probs / length_penalty
-
- if self.args.block_trigram:
- cur_len = alive_seq.size(1)
- if cur_len > 3:
- for i in range(alive_seq.size(0)):
- fail = False
- words = [int(w) for w in alive_seq[i]]
- words = [self.vocab.ids_to_tokens[w] for w in words]
- words = " ".join(words).replace(" ##", "").split()
- if len(words) <= 3:
- continue
- trigrams = [(words[i - 1], words[i], words[i + 1]) for i in range(1, len(words) - 1)]
- trigram = tuple(trigrams[-1])
- if trigram in trigrams[:-1]:
- fail = True
- if fail:
- curr_scores[i] = -10e20
-
- curr_scores = curr_scores.reshape(-1, beam_size * vocab_size)
- topk_scores, topk_ids = curr_scores.topk(beam_size, dim=-1)
-
- # Recover log probs.
- topk_log_probs = topk_scores * length_penalty
-
- # Resolve beam origin and true word ids.
- topk_beam_index = topk_ids.div(vocab_size)
- topk_ids = topk_ids.fmod(vocab_size)
-
- # Map beam_index to batch_index in the flat representation.
- batch_index = topk_beam_index + beam_offset[: topk_beam_index.size(0)].unsqueeze(1)
- select_indices = batch_index.view(-1)
-
- # Append last prediction.
- alive_seq = torch.cat([alive_seq.index_select(0, select_indices), topk_ids.view(-1, 1)], -1)
-
- is_finished = topk_ids.eq(self.end_token)
- if step + 1 == max_length:
- is_finished.fill_(1)
- # End condition is top beam is finished.
- end_condition = is_finished[:, 0].eq(1)
- # Save finished hypotheses.
- if is_finished.any():
- predictions = alive_seq.view(-1, beam_size, alive_seq.size(-1))
- for i in range(is_finished.size(0)):
- b = batch_offset[i]
- if end_condition[i]:
- is_finished[i].fill_(1)
- finished_hyp = is_finished[i].nonzero().view(-1)
- # Store finished hypotheses for this batch.
- for j in finished_hyp:
- hypotheses[b].append((topk_scores[i, j], predictions[i, j, 1:]))
- # If the batch reached the end, save the n_best hypotheses.
- if end_condition[i]:
- best_hyp = sorted(hypotheses[b], key=lambda x: x[0], reverse=True)
- score, pred = best_hyp[0]
-
- results["scores"][b].append(score)
- results["predictions"][b].append(pred)
- non_finished = end_condition.eq(0).nonzero().view(-1)
- # If all sentences are translated, no need to go further.
- if len(non_finished) == 0:
- break
- # Remove finished batches for the next step.
- topk_log_probs = topk_log_probs.index_select(0, non_finished)
- batch_index = batch_index.index_select(0, non_finished)
- batch_offset = batch_offset.index_select(0, non_finished)
- alive_seq = predictions.index_select(0, non_finished).view(-1, alive_seq.size(-1))
- # Reorder states.
- select_indices = batch_index.view(-1)
- src_features = src_features.index_select(0, select_indices)
- dec_states.map_batch_fn(lambda state, dim: state.index_select(dim, select_indices))
-
- return results
-
- def from_batch(self, translation_batch):
- batch = translation_batch["batch"]
- assert len(translation_batch["gold_score"]) == len(translation_batch["predictions"])
- batch_size = batch.batch_size
-
- preds, _, _, tgt_str, src = (
- translation_batch["predictions"],
- translation_batch["scores"],
- translation_batch["gold_score"],
- batch.tgt_str,
- batch.src,
- )
-
- translations = []
- for b in range(batch_size):
- pred_sents = self.vocab.convert_ids_to_tokens([int(n) for n in preds[b][0]])
- pred_sents = " ".join(pred_sents).replace(" ##", "")
- gold_sent = " ".join(tgt_str[b].split())
- raw_src = [self.vocab.ids_to_tokens[int(t)] for t in src[b]][:500]
- raw_src = " ".join(raw_src)
- translation = (pred_sents, gold_sent, raw_src)
- translations.append(translation)
-
- return translations
-
-
-def tile(x, count, dim=0):
- """
- Tiles x on dimension dim count times.
- """
- perm = list(range(len(x.size())))
- if dim != 0:
- perm[0], perm[dim] = perm[dim], perm[0]
- x = x.permute(perm).contiguous()
- out_size = list(x.size())
- out_size[0] *= count
- batch = x.size(0)
- x = x.view(batch, -1).transpose(0, 1).repeat(count, 1).transpose(0, 1).contiguous().view(*out_size)
- if dim != 0:
- x = x.permute(perm).contiguous()
- return x
-
-
-#
-# Optimizer for training. We keep this here in case we want to add
-# a finetuning script.
-#
-
-
-class BertSumOptimizer(object):
- """ Specific optimizer for BertSum.
-
- As described in [1], the authors fine-tune BertSum for abstractive
- summarization using two Adam Optimizers with different warm-up steps and
- learning rate. They also use a custom learning rate scheduler.
-
- [1] Liu, Yang, and Mirella Lapata. "Text summarization with pretrained encoders."
- arXiv preprint arXiv:1908.08345 (2019).
- """
-
- def __init__(self, model, lr, warmup_steps, beta_1=0.99, beta_2=0.999, eps=1e-8):
- self.encoder = model.encoder
- self.decoder = model.decoder
- self.lr = lr
- self.warmup_steps = warmup_steps
-
- self.optimizers = {
- "encoder": torch.optim.Adam(
- model.encoder.parameters(), lr=lr["encoder"], betas=(beta_1, beta_2), eps=eps,
- ),
- "decoder": torch.optim.Adam(
- model.decoder.parameters(), lr=lr["decoder"], betas=(beta_1, beta_2), eps=eps,
- ),
- }
-
- self._step = 0
- self.current_learning_rates = {}
-
- def _update_rate(self, stack):
- return self.lr[stack] * min(self._step ** (-0.5), self._step * self.warmup_steps[stack] ** (-1.5))
-
- def zero_grad(self):
- self.optimizer_decoder.zero_grad()
- self.optimizer_encoder.zero_grad()
-
- def step(self):
- self._step += 1
- for stack, optimizer in self.optimizers.items():
- new_rate = self._update_rate(stack)
- for param_group in optimizer.param_groups:
- param_group["lr"] = new_rate
- optimizer.step()
- self.current_learning_rates[stack] = new_rate
diff --git a/server/transformers/examples/summarization/requirements.txt b/server/transformers/examples/summarization/requirements.txt
deleted file mode 100644
index f984af489cfc4f7210524cd4efce58766404e04c..0000000000000000000000000000000000000000
--- a/server/transformers/examples/summarization/requirements.txt
+++ /dev/null
@@ -1,5 +0,0 @@
-transformers
-
-# For ROUGE
-nltk
-py-rouge
diff --git a/server/transformers/examples/summarization/run_summarization.py b/server/transformers/examples/summarization/run_summarization.py
deleted file mode 100644
index 4afa97b5a963a909d9f1465dbd5f96e1f23c7987..0000000000000000000000000000000000000000
--- a/server/transformers/examples/summarization/run_summarization.py
+++ /dev/null
@@ -1,323 +0,0 @@
-#! /usr/bin/python3
-import argparse
-import logging
-import os
-import sys
-from collections import namedtuple
-
-import torch
-from torch.utils.data import DataLoader, SequentialSampler
-from tqdm import tqdm
-
-from modeling_bertabs import BertAbs, build_predictor
-from transformers import BertTokenizer
-from utils_summarization import (
- SummarizationDataset,
- build_mask,
- compute_token_type_ids,
- encode_for_summarization,
- fit_to_block_size,
-)
-
-
-logger = logging.getLogger(__name__)
-logging.basicConfig(stream=sys.stdout, level=logging.INFO)
-
-
-Batch = namedtuple("Batch", ["document_names", "batch_size", "src", "segs", "mask_src", "tgt_str"])
-
-
-def evaluate(args):
- tokenizer = BertTokenizer.from_pretrained("bert-base-uncased", do_lower_case=True)
- model = BertAbs.from_pretrained("bertabs-finetuned-cnndm")
- model.to(args.device)
- model.eval()
-
- symbols = {
- "BOS": tokenizer.vocab["[unused0]"],
- "EOS": tokenizer.vocab["[unused1]"],
- "PAD": tokenizer.vocab["[PAD]"],
- }
-
- if args.compute_rouge:
- reference_summaries = []
- generated_summaries = []
-
- import rouge
- import nltk
-
- nltk.download("punkt")
- rouge_evaluator = rouge.Rouge(
- metrics=["rouge-n", "rouge-l"],
- max_n=2,
- limit_length=True,
- length_limit=args.beam_size,
- length_limit_type="words",
- apply_avg=True,
- apply_best=False,
- alpha=0.5, # Default F1_score
- weight_factor=1.2,
- stemming=True,
- )
-
- # these (unused) arguments are defined to keep the compatibility
- # with the legacy code and will be deleted in a next iteration.
- args.result_path = ""
- args.temp_dir = ""
-
- data_iterator = build_data_iterator(args, tokenizer)
- predictor = build_predictor(args, tokenizer, symbols, model)
-
- logger.info("***** Running evaluation *****")
- logger.info(" Number examples = %d", len(data_iterator.dataset))
- logger.info(" Batch size = %d", args.batch_size)
- logger.info("")
- logger.info("***** Beam Search parameters *****")
- logger.info(" Beam size = %d", args.beam_size)
- logger.info(" Minimum length = %d", args.min_length)
- logger.info(" Maximum length = %d", args.max_length)
- logger.info(" Alpha (length penalty) = %.2f", args.alpha)
- logger.info(" Trigrams %s be blocked", ("will" if args.block_trigram else "will NOT"))
-
- for batch in tqdm(data_iterator):
- batch_data = predictor.translate_batch(batch)
- translations = predictor.from_batch(batch_data)
- summaries = [format_summary(t) for t in translations]
- save_summaries(summaries, args.summaries_output_dir, batch.document_names)
-
- if args.compute_rouge:
- reference_summaries += batch.tgt_str
- generated_summaries += summaries
-
- if args.compute_rouge:
- scores = rouge_evaluator.get_scores(generated_summaries, reference_summaries)
- str_scores = format_rouge_scores(scores)
- save_rouge_scores(str_scores)
- print(str_scores)
-
-
-def save_summaries(summaries, path, original_document_name):
- """ Write the summaries in fies that are prefixed by the original
- files' name with the `_summary` appended.
-
- Attributes:
- original_document_names: List[string]
- Name of the document that was summarized.
- path: string
- Path were the summaries will be written
- summaries: List[string]
- The summaries that we produced.
- """
- for summary, document_name in zip(summaries, original_document_name):
- # Prepare the summary file's name
- if "." in document_name:
- bare_document_name = ".".join(document_name.split(".")[:-1])
- extension = document_name.split(".")[-1]
- name = bare_document_name + "_summary." + extension
- else:
- name = document_name + "_summary"
-
- file_path = os.path.join(path, name)
- with open(file_path, "w") as output:
- output.write(summary)
-
-
-def format_summary(translation):
- """ Transforms the output of the `from_batch` function
- into nicely formatted summaries.
- """
- raw_summary, _, _ = translation
- summary = (
- raw_summary.replace("[unused0]", "")
- .replace("[unused3]", "")
- .replace("[PAD]", "")
- .replace("[unused1]", "")
- .replace(r" +", " ")
- .replace(" [unused2] ", ". ")
- .replace("[unused2]", "")
- .strip()
- )
-
- return summary
-
-
-def format_rouge_scores(scores):
- return """\n
-****** ROUGE SCORES ******
-
-** ROUGE 1
-F1 >> {:.3f}
-Precision >> {:.3f}
-Recall >> {:.3f}
-
-** ROUGE 2
-F1 >> {:.3f}
-Precision >> {:.3f}
-Recall >> {:.3f}
-
-** ROUGE L
-F1 >> {:.3f}
-Precision >> {:.3f}
-Recall >> {:.3f}""".format(
- scores["rouge-1"]["f"],
- scores["rouge-1"]["p"],
- scores["rouge-1"]["r"],
- scores["rouge-2"]["f"],
- scores["rouge-2"]["p"],
- scores["rouge-2"]["r"],
- scores["rouge-l"]["f"],
- scores["rouge-l"]["p"],
- scores["rouge-l"]["r"],
- )
-
-
-def save_rouge_scores(str_scores):
- with open("rouge_scores.txt", "w") as output:
- output.write(str_scores)
-
-
-#
-# LOAD the dataset
-#
-
-
-def build_data_iterator(args, tokenizer):
- dataset = load_and_cache_examples(args, tokenizer)
- sampler = SequentialSampler(dataset)
-
- def collate_fn(data):
- return collate(data, tokenizer, block_size=512, device=args.device)
-
- iterator = DataLoader(dataset, sampler=sampler, batch_size=args.batch_size, collate_fn=collate_fn,)
-
- return iterator
-
-
-def load_and_cache_examples(args, tokenizer):
- dataset = SummarizationDataset(args.documents_dir)
- return dataset
-
-
-def collate(data, tokenizer, block_size, device):
- """ Collate formats the data passed to the data loader.
-
- In particular we tokenize the data batch after batch to avoid keeping them
- all in memory. We output the data as a namedtuple to fit the original BertAbs's
- API.
- """
- data = [x for x in data if not len(x[1]) == 0] # remove empty_files
- names = [name for name, _, _ in data]
- summaries = [" ".join(summary_list) for _, _, summary_list in data]
-
- encoded_text = [encode_for_summarization(story, summary, tokenizer) for _, story, summary in data]
- encoded_stories = torch.tensor(
- [fit_to_block_size(story, block_size, tokenizer.pad_token_id) for story, _ in encoded_text]
- )
- encoder_token_type_ids = compute_token_type_ids(encoded_stories, tokenizer.cls_token_id)
- encoder_mask = build_mask(encoded_stories, tokenizer.pad_token_id)
-
- batch = Batch(
- document_names=names,
- batch_size=len(encoded_stories),
- src=encoded_stories.to(device),
- segs=encoder_token_type_ids.to(device),
- mask_src=encoder_mask.to(device),
- tgt_str=summaries,
- )
-
- return batch
-
-
-def decode_summary(summary_tokens, tokenizer):
- """ Decode the summary and return it in a format
- suitable for evaluation.
- """
- summary_tokens = summary_tokens.to("cpu").numpy()
- summary = tokenizer.decode(summary_tokens)
- sentences = summary.split(".")
- sentences = [s + "." for s in sentences]
- return sentences
-
-
-def main():
- """ The main function defines the interface with the users.
- """
- parser = argparse.ArgumentParser()
- parser.add_argument(
- "--documents_dir",
- default=None,
- type=str,
- required=True,
- help="The folder where the documents to summarize are located.",
- )
- parser.add_argument(
- "--summaries_output_dir",
- default=None,
- type=str,
- required=False,
- help="The folder in wich the summaries should be written. Defaults to the folder where the documents are",
- )
- parser.add_argument(
- "--compute_rouge",
- default=False,
- type=bool,
- required=False,
- help="Compute the ROUGE metrics during evaluation. Only available for the CNN/DailyMail dataset.",
- )
- # EVALUATION options
- parser.add_argument(
- "--no_cuda", default=False, type=bool, help="Whether to force the execution on CPU.",
- )
- parser.add_argument(
- "--batch_size", default=4, type=int, help="Batch size per GPU/CPU for training.",
- )
- # BEAM SEARCH arguments
- parser.add_argument(
- "--min_length", default=50, type=int, help="Minimum number of tokens for the summaries.",
- )
- parser.add_argument(
- "--max_length", default=200, type=int, help="Maixmum number of tokens for the summaries.",
- )
- parser.add_argument(
- "--beam_size", default=5, type=int, help="The number of beams to start with for each example.",
- )
- parser.add_argument(
- "--alpha", default=0.95, type=float, help="The value of alpha for the length penalty in the beam search.",
- )
- parser.add_argument(
- "--block_trigram",
- default=True,
- type=bool,
- help="Whether to block the existence of repeating trigrams in the text generated by beam search.",
- )
- args = parser.parse_args()
-
- # Select device (distibuted not available)
- args.device = torch.device("cuda" if torch.cuda.is_available() and not args.no_cuda else "cpu")
-
- # Check the existence of directories
- if not args.summaries_output_dir:
- args.summaries_output_dir = args.documents_dir
-
- if not documents_dir_is_valid(args.documents_dir):
- raise FileNotFoundError(
- "We could not find the directory you specified for the documents to summarize, or it was empty. Please specify a valid path."
- )
- os.makedirs(args.summaries_output_dir, exist_ok=True)
-
- evaluate(args)
-
-
-def documents_dir_is_valid(path):
- if not os.path.exists(path):
- return False
-
- file_list = os.listdir(path)
- if len(file_list) == 0:
- return False
-
- return True
-
-
-if __name__ == "__main__":
- main()
diff --git a/server/transformers/examples/summarization/test_utils_summarization.py b/server/transformers/examples/summarization/test_utils_summarization.py
deleted file mode 100644
index d562ad04b7be01be4dbc54d71fcbf019ed6929e1..0000000000000000000000000000000000000000
--- a/server/transformers/examples/summarization/test_utils_summarization.py
+++ /dev/null
@@ -1,100 +0,0 @@
-# coding=utf-8
-# Copyright 2019 HuggingFace Inc.
-#
-# Licensed under the Apache License, Version 2.0 (the "License");
-# you may not use this file except in compliance with the License.
-# You may obtain a copy of the License at
-#
-# http://www.apache.org/licenses/LICENSE-2.0
-#
-# Unless required by applicable law or agreed to in writing, software
-# distributed under the License is distributed on an "AS IS" BASIS,
-# WITHOUT WARRANTIES OR CONDITIONS OF ANY KIND, either express or implied.
-# See the License for the specific language governing permissions and
-# limitations under the License.
-import unittest
-
-import numpy as np
-import torch
-
-from utils_summarization import build_mask, compute_token_type_ids, fit_to_block_size, process_story
-
-
-class SummarizationDataProcessingTest(unittest.TestCase):
- def setUp(self):
- self.block_size = 10
-
- def test_fit_to_block_sequence_too_small(self):
- """ Pad the sequence with 0 if the sequence is smaller than the block size."""
- sequence = [1, 2, 3, 4]
- expected_output = [1, 2, 3, 4, 0, 0, 0, 0, 0, 0]
- self.assertEqual(fit_to_block_size(sequence, self.block_size, 0), expected_output)
-
- def test_fit_to_block_sequence_fit_exactly(self):
- """ Do nothing if the sequence is the right size. """
- sequence = [1, 2, 3, 4, 5, 6, 7, 8, 9, 10]
- expected_output = [1, 2, 3, 4, 5, 6, 7, 8, 9, 10]
- self.assertEqual(fit_to_block_size(sequence, self.block_size, 0), expected_output)
-
- def test_fit_to_block_sequence_too_big(self):
- """ Truncate the sequence if it is too long. """
- sequence = [1, 2, 3, 4, 5, 6, 7, 8, 9, 10, 11, 12, 13]
- expected_output = [1, 2, 3, 4, 5, 6, 7, 8, 9, 10]
- self.assertEqual(fit_to_block_size(sequence, self.block_size, 0), expected_output)
-
- def test_process_story_no_highlights(self):
- """ Processing a story with no highlights returns an empty list for the summary.
- """
- raw_story = """It was the year of Our Lord one thousand seven hundred and
- seventy-five.\n\nSpiritual revelations were conceded to England at that
- favoured period, as at this."""
- _, summary_lines = process_story(raw_story)
- self.assertEqual(summary_lines, [])
-
- def test_process_empty_story(self):
- """ An empty story returns an empty collection of lines.
- """
- raw_story = ""
- story_lines, summary_lines = process_story(raw_story)
- self.assertEqual(story_lines, [])
- self.assertEqual(summary_lines, [])
-
- def test_process_story_with_missing_period(self):
- raw_story = (
- "It was the year of Our Lord one thousand seven hundred and "
- "seventy-five\n\nSpiritual revelations were conceded to England "
- "at that favoured period, as at this.\n@highlight\n\nIt was the best of times"
- )
- story_lines, summary_lines = process_story(raw_story)
-
- expected_story_lines = [
- "It was the year of Our Lord one thousand seven hundred and seventy-five.",
- "Spiritual revelations were conceded to England at that favoured period, as at this.",
- ]
- self.assertEqual(expected_story_lines, story_lines)
-
- expected_summary_lines = ["It was the best of times."]
- self.assertEqual(expected_summary_lines, summary_lines)
-
- def test_build_mask_no_padding(self):
- sequence = torch.tensor([1, 2, 3, 4])
- expected = torch.tensor([1, 1, 1, 1])
- np.testing.assert_array_equal(build_mask(sequence, 0).numpy(), expected.numpy())
-
- def test_build_mask(self):
- sequence = torch.tensor([1, 2, 3, 4, 23, 23, 23])
- expected = torch.tensor([1, 1, 1, 1, 0, 0, 0])
- np.testing.assert_array_equal(build_mask(sequence, 23).numpy(), expected.numpy())
-
- def test_build_mask_with_padding_equal_to_one(self):
- sequence = torch.tensor([8, 2, 3, 4, 1, 1, 1])
- expected = torch.tensor([1, 1, 1, 1, 0, 0, 0])
- np.testing.assert_array_equal(build_mask(sequence, 1).numpy(), expected.numpy())
-
- def test_compute_token_type_ids(self):
- separator = 101
- batch = torch.tensor([[1, 2, 3, 4, 5, 6], [1, 2, 3, 101, 5, 6], [1, 101, 3, 4, 101, 6]])
- expected = torch.tensor([[1, 1, 1, 1, 1, 1], [1, 1, 1, 0, 0, 0], [1, 0, 0, 0, 1, 1]])
-
- result = compute_token_type_ids(batch, separator)
- np.testing.assert_array_equal(result, expected)
diff --git a/server/transformers/examples/summarization/utils_summarization.py b/server/transformers/examples/summarization/utils_summarization.py
deleted file mode 100644
index 529eeb3efa05a323d3177ea60e0055a2f29dfbbb..0000000000000000000000000000000000000000
--- a/server/transformers/examples/summarization/utils_summarization.py
+++ /dev/null
@@ -1,167 +0,0 @@
-import os
-from collections import deque
-
-import torch
-from torch.utils.data import Dataset
-
-
-# ------------
-# Data loading
-# ------------
-
-
-class SummarizationDataset(Dataset):
- """ Abstracts the dataset used to train seq2seq models.
-
- The class will process the documents that are located in the specified
- folder. The preprocessing will work on any document that is reasonably
- formatted. On the CNN/DailyMail dataset it will extract both the story
- and the summary.
-
- CNN/Daily News:
-
- The CNN/Daily News raw datasets are downloaded from [1]. The stories are
- stored in different files; the summary appears at the end of the story as
- sentences that are prefixed by the special `@highlight` line. To process
- the data, untar both datasets in the same folder, and pass the path to this
- folder as the "data_dir argument. The formatting code was inspired by [2].
-
- [1] https://cs.nyu.edu/~kcho/
- [2] https://github.com/abisee/cnn-dailymail/
- """
-
- def __init__(self, path="", prefix="train"):
- """ We initialize the class by listing all the documents to summarize.
- Files are not read in memory due to the size of some datasets (like CNN/DailyMail).
- """
- assert os.path.isdir(path)
-
- self.documents = []
- story_filenames_list = os.listdir(path)
- for story_filename in story_filenames_list:
- if "summary" in story_filename:
- continue
- path_to_story = os.path.join(path, story_filename)
- if not os.path.isfile(path_to_story):
- continue
- self.documents.append(path_to_story)
-
- def __len__(self):
- """ Returns the number of documents. """
- return len(self.documents)
-
- def __getitem__(self, idx):
- document_path = self.documents[idx]
- document_name = document_path.split("/")[-1]
- with open(document_path, encoding="utf-8") as source:
- raw_story = source.read()
- story_lines, summary_lines = process_story(raw_story)
- return document_name, story_lines, summary_lines
-
-
-def process_story(raw_story):
- """ Extract the story and summary from a story file.
-
- Attributes:
- raw_story (str): content of the story file as an utf-8 encoded string.
-
- Raises:
- IndexError: If the stoy is empty or contains no highlights.
- """
- nonempty_lines = list(filter(lambda x: len(x) != 0, [line.strip() for line in raw_story.split("\n")]))
-
- # for some unknown reason some lines miss a period, add it
- nonempty_lines = [_add_missing_period(line) for line in nonempty_lines]
-
- # gather article lines
- story_lines = []
- lines = deque(nonempty_lines)
- while True:
- try:
- element = lines.popleft()
- if element.startswith("@highlight"):
- break
- story_lines.append(element)
- except IndexError:
- # if "@highlight" is absent from the file we pop
- # all elements until there is None, raising an exception.
- return story_lines, []
-
- # gather summary lines
- summary_lines = list(filter(lambda t: not t.startswith("@highlight"), lines))
-
- return story_lines, summary_lines
-
-
-def _add_missing_period(line):
- END_TOKENS = [".", "!", "?", "...", "'", "`", '"', "\u2019", "\u2019", ")"]
- if line.startswith("@highlight"):
- return line
- if line[-1] in END_TOKENS:
- return line
- return line + "."
-
-
-# --------------------------
-# Encoding and preprocessing
-# --------------------------
-
-
-def fit_to_block_size(sequence, block_size, pad_token_id):
- """ Adapt the source and target sequences' lengths to the block size.
- If the sequence is shorter we append padding token to the right of the sequence.
- """
- if len(sequence) > block_size:
- return sequence[:block_size]
- else:
- sequence.extend([pad_token_id] * (block_size - len(sequence)))
- return sequence
-
-
-def build_mask(sequence, pad_token_id):
- """ Builds the mask. The attention mechanism will only attend to positions
- with value 1. """
- mask = torch.ones_like(sequence)
- idx_pad_tokens = sequence == pad_token_id
- mask[idx_pad_tokens] = 0
- return mask
-
-
-def encode_for_summarization(story_lines, summary_lines, tokenizer):
- """ Encode the story and summary lines, and join them
- as specified in [1] by using `[SEP] [CLS]` tokens to separate
- sentences.
- """
- story_lines_token_ids = [tokenizer.encode(line) for line in story_lines]
- story_token_ids = [token for sentence in story_lines_token_ids for token in sentence]
- summary_lines_token_ids = [tokenizer.encode(line) for line in summary_lines]
- summary_token_ids = [token for sentence in summary_lines_token_ids for token in sentence]
-
- return story_token_ids, summary_token_ids
-
-
-def compute_token_type_ids(batch, separator_token_id):
- """ Segment embeddings as described in [1]
-
- The values {0,1} were found in the repository [2].
-
- Attributes:
- batch: torch.Tensor, size [batch_size, block_size]
- Batch of input.
- separator_token_id: int
- The value of the token that separates the segments.
-
- [1] Liu, Yang, and Mirella Lapata. "Text summarization with pretrained encoders."
- arXiv preprint arXiv:1908.08345 (2019).
- [2] https://github.com/nlpyang/PreSumm (/src/prepro/data_builder.py, commit fac1217)
- """
- batch_embeddings = []
- for sequence in batch:
- sentence_num = -1
- embeddings = []
- for s in sequence:
- if s == separator_token_id:
- sentence_num += 1
- embeddings.append(sentence_num % 2)
- batch_embeddings.append(embeddings)
- return torch.tensor(batch_embeddings)
diff --git a/server/transformers/examples/test_examples.py b/server/transformers/examples/test_examples.py
deleted file mode 100644
index a31c243dd84a0c5eebb7e6e9f39a77a342fb2ccc..0000000000000000000000000000000000000000
--- a/server/transformers/examples/test_examples.py
+++ /dev/null
@@ -1,100 +0,0 @@
-# coding=utf-8
-# Copyright 2018 HuggingFace Inc..
-#
-# Licensed under the Apache License, Version 2.0 (the "License");
-# you may not use this file except in compliance with the License.
-# You may obtain a copy of the License at
-#
-# http://www.apache.org/licenses/LICENSE-2.0
-#
-# Unless required by applicable law or agreed to in writing, software
-# distributed under the License is distributed on an "AS IS" BASIS,
-# WITHOUT WARRANTIES OR CONDITIONS OF ANY KIND, either express or implied.
-# See the License for the specific language governing permissions and
-# limitations under the License.
-
-
-import argparse
-import logging
-import sys
-import unittest
-from unittest.mock import patch
-
-import run_generation
-import run_glue
-import run_squad
-
-
-logging.basicConfig(level=logging.DEBUG)
-
-logger = logging.getLogger()
-
-
-def get_setup_file():
- parser = argparse.ArgumentParser()
- parser.add_argument("-f")
- args = parser.parse_args()
- return args.f
-
-
-class ExamplesTests(unittest.TestCase):
- def test_run_glue(self):
- stream_handler = logging.StreamHandler(sys.stdout)
- logger.addHandler(stream_handler)
-
- testargs = [
- "run_glue.py",
- "--data_dir=./examples/tests_samples/MRPC/",
- "--task_name=mrpc",
- "--do_train",
- "--do_eval",
- "--output_dir=./examples/tests_samples/temp_dir",
- "--per_gpu_train_batch_size=2",
- "--per_gpu_eval_batch_size=1",
- "--learning_rate=1e-4",
- "--max_steps=10",
- "--warmup_steps=2",
- "--overwrite_output_dir",
- "--seed=42",
- ]
- model_type, model_name = ("--model_type=bert", "--model_name_or_path=bert-base-uncased")
- with patch.object(sys, "argv", testargs + [model_type, model_name]):
- result = run_glue.main()
- for value in result.values():
- self.assertGreaterEqual(value, 0.75)
-
- def test_run_squad(self):
- stream_handler = logging.StreamHandler(sys.stdout)
- logger.addHandler(stream_handler)
-
- testargs = [
- "run_squad.py",
- "--data_dir=./examples/tests_samples/SQUAD",
- "--model_name=bert-base-uncased",
- "--output_dir=./examples/tests_samples/temp_dir",
- "--max_steps=10",
- "--warmup_steps=2",
- "--do_train",
- "--do_eval",
- "--version_2_with_negative",
- "--learning_rate=2e-4",
- "--per_gpu_train_batch_size=2",
- "--per_gpu_eval_batch_size=1",
- "--overwrite_output_dir",
- "--seed=42",
- ]
- model_type, model_name = ("--model_type=bert", "--model_name_or_path=bert-base-uncased")
- with patch.object(sys, "argv", testargs + [model_type, model_name]):
- result = run_squad.main()
- self.assertGreaterEqual(result["f1"], 30)
- self.assertGreaterEqual(result["exact"], 30)
-
- def test_generation(self):
- stream_handler = logging.StreamHandler(sys.stdout)
- logger.addHandler(stream_handler)
-
- testargs = ["run_generation.py", "--prompt=Hello", "--length=10", "--seed=42"]
- model_type, model_name = ("--model_type=openai-gpt", "--model_name_or_path=openai-gpt")
- with patch.object(sys, "argv", testargs + [model_type, model_name]):
- result = run_generation.main()
- self.assertGreaterEqual(len(result), 10)
diff --git a/server/transformers/examples/tests_samples/.gitignore b/server/transformers/examples/tests_samples/.gitignore
deleted file mode 100644
index c8ce21fe2411c3dc3022e26ccf4e11cc6b58a01d..0000000000000000000000000000000000000000
--- a/server/transformers/examples/tests_samples/.gitignore
+++ /dev/null
@@ -1,6 +0,0 @@
-*.*
-cache*
-temp*
-!*.tsv
-!*.json
-!.gitignore
\ No newline at end of file
diff --git a/server/transformers/examples/tests_samples/MRPC/dev.tsv b/server/transformers/examples/tests_samples/MRPC/dev.tsv
deleted file mode 100644
index 5b814856c63f44ef8c082726ae19285a4faec26c..0000000000000000000000000000000000000000
--- a/server/transformers/examples/tests_samples/MRPC/dev.tsv
+++ /dev/null
@@ -1,7 +0,0 @@
-Quality #1 ID #2 ID #1 String #2 String
-1 1355540 1355592 He said the foodservice pie business doesn 't fit the company 's long-term growth strategy . " The foodservice pie business does not fit our long-term growth strategy .
-0 2029631 2029565 Magnarelli said Racicot hated the Iraqi regime and looked forward to using his long years of training in the war . His wife said he was " 100 percent behind George Bush " and looked forward to using his years of training in the war .
-0 487993 487952 The dollar was at 116.92 yen against the yen , flat on the session , and at 1.2891 against the Swiss franc , also flat . The dollar was at 116.78 yen JPY = , virtually flat on the session , and at 1.2871 against the Swiss franc CHF = , down 0.1 percent .
-1 1989515 1989458 The AFL-CIO is waiting until October to decide if it will endorse a candidate . The AFL-CIO announced Wednesday that it will decide in October whether to endorse a candidate before the primaries .
-0 1783137 1782659 No dates have been set for the civil or the criminal trial . No dates have been set for the criminal or civil cases , but Shanley has pleaded not guilty .
-1 3039165 3039036 Wal-Mart said it would check all of its million-plus domestic workers to ensure they were legally employed . It has also said it would review all of its domestic employees more than 1 million to ensure they have legal status .
diff --git a/server/transformers/examples/tests_samples/MRPC/train.tsv b/server/transformers/examples/tests_samples/MRPC/train.tsv
deleted file mode 100644
index 5b814856c63f44ef8c082726ae19285a4faec26c..0000000000000000000000000000000000000000
--- a/server/transformers/examples/tests_samples/MRPC/train.tsv
+++ /dev/null
@@ -1,7 +0,0 @@
-Quality #1 ID #2 ID #1 String #2 String
-1 1355540 1355592 He said the foodservice pie business doesn 't fit the company 's long-term growth strategy . " The foodservice pie business does not fit our long-term growth strategy .
-0 2029631 2029565 Magnarelli said Racicot hated the Iraqi regime and looked forward to using his long years of training in the war . His wife said he was " 100 percent behind George Bush " and looked forward to using his years of training in the war .
-0 487993 487952 The dollar was at 116.92 yen against the yen , flat on the session , and at 1.2891 against the Swiss franc , also flat . The dollar was at 116.78 yen JPY = , virtually flat on the session , and at 1.2871 against the Swiss franc CHF = , down 0.1 percent .
-1 1989515 1989458 The AFL-CIO is waiting until October to decide if it will endorse a candidate . The AFL-CIO announced Wednesday that it will decide in October whether to endorse a candidate before the primaries .
-0 1783137 1782659 No dates have been set for the civil or the criminal trial . No dates have been set for the criminal or civil cases , but Shanley has pleaded not guilty .
-1 3039165 3039036 Wal-Mart said it would check all of its million-plus domestic workers to ensure they were legally employed . It has also said it would review all of its domestic employees more than 1 million to ensure they have legal status .
diff --git a/server/transformers/examples/tests_samples/SQUAD/dev-v2.0.json b/server/transformers/examples/tests_samples/SQUAD/dev-v2.0.json
deleted file mode 100644
index 834d9ee6602b300ea45c67212800b0bbf6d1129e..0000000000000000000000000000000000000000
--- a/server/transformers/examples/tests_samples/SQUAD/dev-v2.0.json
+++ /dev/null
@@ -1,140 +0,0 @@
-{
- "version": "v2.0",
- "data": [{
- "title": "Normans",
- "paragraphs": [{
- "qas": [{
- "question": "In what country is Normandy located?",
- "id": "56ddde6b9a695914005b9628",
- "answers": [{
- "text": "France",
- "answer_start": 159
- }],
- "is_impossible": false
- }, {
- "question": "When were the Normans in Normandy?",
- "id": "56ddde6b9a695914005b9629",
- "answers": [{
- "text": "10th and 11th centuries",
- "answer_start": 94
- }],
- "is_impossible": false
- }, {
- "question": "From which countries did the Norse originate?",
- "id": "56ddde6b9a695914005b962a",
- "answers": [{
- "text": "Denmark, Iceland and Norway",
- "answer_start": 256
- }],
- "is_impossible": false
- }, {
- "plausible_answers": [{
- "text": "Rollo",
- "answer_start": 308
- }],
- "question": "Who did King Charles III swear fealty to?",
- "id": "5ad39d53604f3c001a3fe8d3",
- "answers": [],
- "is_impossible": true
- }, {
- "plausible_answers": [{
- "text": "10th century",
- "answer_start": 671
- }],
- "question": "When did the Frankish identity emerge?",
- "id": "5ad39d53604f3c001a3fe8d4",
- "answers": [],
- "is_impossible": true
- }],
- "context": "The Normans (Norman: Nourmands; French: Normands; Latin: Normanni) were the people who in the 10th and 11th centuries gave their name to Normandy, a region in France. They were descended from Norse (\"Norman\" comes from \"Norseman\") raiders and pirates from Denmark, Iceland and Norway who, under their leader Rollo, agreed to swear fealty to King Charles III of West Francia. Through generations of assimilation and mixing with the native Frankish and Roman-Gaulish populations, their descendants would gradually merge with the Carolingian-based cultures of West Francia. The distinct cultural and ethnic identity of the Normans emerged initially in the first half of the 10th century, and it continued to evolve over the succeeding centuries."
- }, {
- "qas": [{
- "question": "Who was the duke in the battle of Hastings?",
- "id": "56dddf4066d3e219004dad5f",
- "answers": [{
- "text": "William the Conqueror",
- "answer_start": 1022
- }],
- "is_impossible": false
- }, {
- "plausible_answers": [{
- "text": "Antioch",
- "answer_start": 1295
- }],
- "question": "What principality did William the conquerer found?",
- "id": "5ad3a266604f3c001a3fea2b",
- "answers": [],
- "is_impossible": true
- }],
- "context": "The Norman dynasty had a major political, cultural and military impact on medieval Europe and even the Near East. The Normans were famed for their martial spirit and eventually for their Christian piety, becoming exponents of the Catholic orthodoxy into which they assimilated. They adopted the Gallo-Romance language of the Frankish land they settled, their dialect becoming known as Norman, Normaund or Norman French, an important literary language. The Duchy of Normandy, which they formed by treaty with the French crown, was a great fief of medieval France, and under Richard I of Normandy was forged into a cohesive and formidable principality in feudal tenure. The Normans are noted both for their culture, such as their unique Romanesque architecture and musical traditions, and for their significant military accomplishments and innovations. Norman adventurers founded the Kingdom of Sicily under Roger II after conquering southern Italy on the Saracens and Byzantines, and an expedition on behalf of their duke, William the Conqueror, led to the Norman conquest of England at the Battle of Hastings in 1066. Norman cultural and military influence spread from these new European centres to the Crusader states of the Near East, where their prince Bohemond I founded the Principality of Antioch in the Levant, to Scotland and Wales in Great Britain, to Ireland, and to the coasts of north Africa and the Canary Islands."
- }]
- }, {
- "title": "Computational_complexity_theory",
- "paragraphs": [{
- "qas": [{
- "question": "What branch of theoretical computer science deals with broadly classifying computational problems by difficulty and class of relationship?",
- "id": "56e16182e3433e1400422e28",
- "answers": [{
- "text": "Computational complexity theory",
- "answer_start": 0
- }],
- "is_impossible": false
- }, {
- "plausible_answers": [{
- "text": "algorithm",
- "answer_start": 472
- }],
- "question": "What is a manual application of mathematical steps?",
- "id": "5ad5316b5b96ef001a10ab76",
- "answers": [],
- "is_impossible": true
- }],
- "context": "Computational complexity theory is a branch of the theory of computation in theoretical computer science that focuses on classifying computational problems according to their inherent difficulty, and relating those classes to each other. A computational problem is understood to be a task that is in principle amenable to being solved by a computer, which is equivalent to stating that the problem may be solved by mechanical application of mathematical steps, such as an algorithm."
- }, {
- "qas": [{
- "question": "What measure of a computational problem broadly defines the inherent difficulty of the solution?",
- "id": "56e16839cd28a01900c67887",
- "answers": [{
- "text": "if its solution requires significant resources",
- "answer_start": 46
- }],
- "is_impossible": false
- }, {
- "question": "What method is used to intuitively assess or quantify the amount of resources required to solve a computational problem?",
- "id": "56e16839cd28a01900c67888",
- "answers": [{
- "text": "mathematical models of computation",
- "answer_start": 176
- }],
- "is_impossible": false
- }, {
- "question": "What are two basic primary resources used to guage complexity?",
- "id": "56e16839cd28a01900c67889",
- "answers": [{
- "text": "time and storage",
- "answer_start": 305
- }],
- "is_impossible": false
- }, {
- "plausible_answers": [{
- "text": "the number of gates in a circuit",
- "answer_start": 436
- }],
- "question": "What unit is measured to determine circuit simplicity?",
- "id": "5ad532575b96ef001a10ab7f",
- "answers": [],
- "is_impossible": true
- }, {
- "plausible_answers": [{
- "text": "the number of processors",
- "answer_start": 502
- }],
- "question": "What number is used in perpendicular computing?",
- "id": "5ad532575b96ef001a10ab80",
- "answers": [],
- "is_impossible": true
- }],
- "context": "A problem is regarded as inherently difficult if its solution requires significant resources, whatever the algorithm used. The theory formalizes this intuition, by introducing mathematical models of computation to study these problems and quantifying the amount of resources needed to solve them, such as time and storage. Other complexity measures are also used, such as the amount of communication (used in communication complexity), the number of gates in a circuit (used in circuit complexity) and the number of processors (used in parallel computing). One of the roles of computational complexity theory is to determine the practical limits on what computers can and cannot do."
- }]
- }]
-}
\ No newline at end of file
diff --git a/server/transformers/examples/tests_samples/SQUAD/train-v2.0.json b/server/transformers/examples/tests_samples/SQUAD/train-v2.0.json
deleted file mode 100644
index 834d9ee6602b300ea45c67212800b0bbf6d1129e..0000000000000000000000000000000000000000
--- a/server/transformers/examples/tests_samples/SQUAD/train-v2.0.json
+++ /dev/null
@@ -1,140 +0,0 @@
-{
- "version": "v2.0",
- "data": [{
- "title": "Normans",
- "paragraphs": [{
- "qas": [{
- "question": "In what country is Normandy located?",
- "id": "56ddde6b9a695914005b9628",
- "answers": [{
- "text": "France",
- "answer_start": 159
- }],
- "is_impossible": false
- }, {
- "question": "When were the Normans in Normandy?",
- "id": "56ddde6b9a695914005b9629",
- "answers": [{
- "text": "10th and 11th centuries",
- "answer_start": 94
- }],
- "is_impossible": false
- }, {
- "question": "From which countries did the Norse originate?",
- "id": "56ddde6b9a695914005b962a",
- "answers": [{
- "text": "Denmark, Iceland and Norway",
- "answer_start": 256
- }],
- "is_impossible": false
- }, {
- "plausible_answers": [{
- "text": "Rollo",
- "answer_start": 308
- }],
- "question": "Who did King Charles III swear fealty to?",
- "id": "5ad39d53604f3c001a3fe8d3",
- "answers": [],
- "is_impossible": true
- }, {
- "plausible_answers": [{
- "text": "10th century",
- "answer_start": 671
- }],
- "question": "When did the Frankish identity emerge?",
- "id": "5ad39d53604f3c001a3fe8d4",
- "answers": [],
- "is_impossible": true
- }],
- "context": "The Normans (Norman: Nourmands; French: Normands; Latin: Normanni) were the people who in the 10th and 11th centuries gave their name to Normandy, a region in France. They were descended from Norse (\"Norman\" comes from \"Norseman\") raiders and pirates from Denmark, Iceland and Norway who, under their leader Rollo, agreed to swear fealty to King Charles III of West Francia. Through generations of assimilation and mixing with the native Frankish and Roman-Gaulish populations, their descendants would gradually merge with the Carolingian-based cultures of West Francia. The distinct cultural and ethnic identity of the Normans emerged initially in the first half of the 10th century, and it continued to evolve over the succeeding centuries."
- }, {
- "qas": [{
- "question": "Who was the duke in the battle of Hastings?",
- "id": "56dddf4066d3e219004dad5f",
- "answers": [{
- "text": "William the Conqueror",
- "answer_start": 1022
- }],
- "is_impossible": false
- }, {
- "plausible_answers": [{
- "text": "Antioch",
- "answer_start": 1295
- }],
- "question": "What principality did William the conquerer found?",
- "id": "5ad3a266604f3c001a3fea2b",
- "answers": [],
- "is_impossible": true
- }],
- "context": "The Norman dynasty had a major political, cultural and military impact on medieval Europe and even the Near East. The Normans were famed for their martial spirit and eventually for their Christian piety, becoming exponents of the Catholic orthodoxy into which they assimilated. They adopted the Gallo-Romance language of the Frankish land they settled, their dialect becoming known as Norman, Normaund or Norman French, an important literary language. The Duchy of Normandy, which they formed by treaty with the French crown, was a great fief of medieval France, and under Richard I of Normandy was forged into a cohesive and formidable principality in feudal tenure. The Normans are noted both for their culture, such as their unique Romanesque architecture and musical traditions, and for their significant military accomplishments and innovations. Norman adventurers founded the Kingdom of Sicily under Roger II after conquering southern Italy on the Saracens and Byzantines, and an expedition on behalf of their duke, William the Conqueror, led to the Norman conquest of England at the Battle of Hastings in 1066. Norman cultural and military influence spread from these new European centres to the Crusader states of the Near East, where their prince Bohemond I founded the Principality of Antioch in the Levant, to Scotland and Wales in Great Britain, to Ireland, and to the coasts of north Africa and the Canary Islands."
- }]
- }, {
- "title": "Computational_complexity_theory",
- "paragraphs": [{
- "qas": [{
- "question": "What branch of theoretical computer science deals with broadly classifying computational problems by difficulty and class of relationship?",
- "id": "56e16182e3433e1400422e28",
- "answers": [{
- "text": "Computational complexity theory",
- "answer_start": 0
- }],
- "is_impossible": false
- }, {
- "plausible_answers": [{
- "text": "algorithm",
- "answer_start": 472
- }],
- "question": "What is a manual application of mathematical steps?",
- "id": "5ad5316b5b96ef001a10ab76",
- "answers": [],
- "is_impossible": true
- }],
- "context": "Computational complexity theory is a branch of the theory of computation in theoretical computer science that focuses on classifying computational problems according to their inherent difficulty, and relating those classes to each other. A computational problem is understood to be a task that is in principle amenable to being solved by a computer, which is equivalent to stating that the problem may be solved by mechanical application of mathematical steps, such as an algorithm."
- }, {
- "qas": [{
- "question": "What measure of a computational problem broadly defines the inherent difficulty of the solution?",
- "id": "56e16839cd28a01900c67887",
- "answers": [{
- "text": "if its solution requires significant resources",
- "answer_start": 46
- }],
- "is_impossible": false
- }, {
- "question": "What method is used to intuitively assess or quantify the amount of resources required to solve a computational problem?",
- "id": "56e16839cd28a01900c67888",
- "answers": [{
- "text": "mathematical models of computation",
- "answer_start": 176
- }],
- "is_impossible": false
- }, {
- "question": "What are two basic primary resources used to guage complexity?",
- "id": "56e16839cd28a01900c67889",
- "answers": [{
- "text": "time and storage",
- "answer_start": 305
- }],
- "is_impossible": false
- }, {
- "plausible_answers": [{
- "text": "the number of gates in a circuit",
- "answer_start": 436
- }],
- "question": "What unit is measured to determine circuit simplicity?",
- "id": "5ad532575b96ef001a10ab7f",
- "answers": [],
- "is_impossible": true
- }, {
- "plausible_answers": [{
- "text": "the number of processors",
- "answer_start": 502
- }],
- "question": "What number is used in perpendicular computing?",
- "id": "5ad532575b96ef001a10ab80",
- "answers": [],
- "is_impossible": true
- }],
- "context": "A problem is regarded as inherently difficult if its solution requires significant resources, whatever the algorithm used. The theory formalizes this intuition, by introducing mathematical models of computation to study these problems and quantifying the amount of resources needed to solve them, such as time and storage. Other complexity measures are also used, such as the amount of communication (used in communication complexity), the number of gates in a circuit (used in circuit complexity) and the number of processors (used in parallel computing). One of the roles of computational complexity theory is to determine the practical limits on what computers can and cannot do."
- }]
- }]
-}
\ No newline at end of file
diff --git a/server/transformers/examples/utils_multiple_choice.py b/server/transformers/examples/utils_multiple_choice.py
deleted file mode 100644
index 8e19c51414168f91ac6fd5358b6b30048377ed99..0000000000000000000000000000000000000000
--- a/server/transformers/examples/utils_multiple_choice.py
+++ /dev/null
@@ -1,373 +0,0 @@
-# coding=utf-8
-# Copyright 2018 The Google AI Language Team Authors and The HuggingFace Inc. team.
-# Copyright (c) 2018, NVIDIA CORPORATION. All rights reserved.
-#
-# Licensed under the Apache License, Version 2.0 (the "License");
-# you may not use this file except in compliance with the License.
-# You may obtain a copy of the License at
-#
-# http://www.apache.org/licenses/LICENSE-2.0
-#
-# Unless required by applicable law or agreed to in writing, software
-# distributed under the License is distributed on an "AS IS" BASIS,
-# WITHOUT WARRANTIES OR CONDITIONS OF ANY KIND, either express or implied.
-# See the License for the specific language governing permissions and
-# limitations under the License.
-""" Multiple choice fine-tuning: utilities to work with multiple choice tasks of reading comprehension """
-
-
-import csv
-import glob
-import json
-import logging
-import os
-from typing import List
-
-import tqdm
-
-from transformers import PreTrainedTokenizer
-
-
-logger = logging.getLogger(__name__)
-
-
-class InputExample(object):
- """A single training/test example for multiple choice"""
-
- def __init__(self, example_id, question, contexts, endings, label=None):
- """Constructs a InputExample.
-
- Args:
- example_id: Unique id for the example.
- contexts: list of str. The untokenized text of the first sequence (context of corresponding question).
- question: string. The untokenized text of the second sequence (question).
- endings: list of str. multiple choice's options. Its length must be equal to contexts' length.
- label: (Optional) string. The label of the example. This should be
- specified for train and dev examples, but not for test examples.
- """
- self.example_id = example_id
- self.question = question
- self.contexts = contexts
- self.endings = endings
- self.label = label
-
-
-class InputFeatures(object):
- def __init__(self, example_id, choices_features, label):
- self.example_id = example_id
- self.choices_features = [
- {"input_ids": input_ids, "input_mask": input_mask, "segment_ids": segment_ids}
- for input_ids, input_mask, segment_ids in choices_features
- ]
- self.label = label
-
-
-class DataProcessor(object):
- """Base class for data converters for multiple choice data sets."""
-
- def get_train_examples(self, data_dir):
- """Gets a collection of `InputExample`s for the train set."""
- raise NotImplementedError()
-
- def get_dev_examples(self, data_dir):
- """Gets a collection of `InputExample`s for the dev set."""
- raise NotImplementedError()
-
- def get_test_examples(self, data_dir):
- """Gets a collection of `InputExample`s for the test set."""
- raise NotImplementedError()
-
- def get_labels(self):
- """Gets the list of labels for this data set."""
- raise NotImplementedError()
-
-
-class RaceProcessor(DataProcessor):
- """Processor for the RACE data set."""
-
- def get_train_examples(self, data_dir):
- """See base class."""
- logger.info("LOOKING AT {} train".format(data_dir))
- high = os.path.join(data_dir, "train/high")
- middle = os.path.join(data_dir, "train/middle")
- high = self._read_txt(high)
- middle = self._read_txt(middle)
- return self._create_examples(high + middle, "train")
-
- def get_dev_examples(self, data_dir):
- """See base class."""
- logger.info("LOOKING AT {} dev".format(data_dir))
- high = os.path.join(data_dir, "dev/high")
- middle = os.path.join(data_dir, "dev/middle")
- high = self._read_txt(high)
- middle = self._read_txt(middle)
- return self._create_examples(high + middle, "dev")
-
- def get_test_examples(self, data_dir):
- """See base class."""
- logger.info("LOOKING AT {} test".format(data_dir))
- high = os.path.join(data_dir, "test/high")
- middle = os.path.join(data_dir, "test/middle")
- high = self._read_txt(high)
- middle = self._read_txt(middle)
- return self._create_examples(high + middle, "test")
-
- def get_labels(self):
- """See base class."""
- return ["0", "1", "2", "3"]
-
- def _read_txt(self, input_dir):
- lines = []
- files = glob.glob(input_dir + "/*txt")
- for file in tqdm.tqdm(files, desc="read files"):
- with open(file, "r", encoding="utf-8") as fin:
- data_raw = json.load(fin)
- data_raw["race_id"] = file
- lines.append(data_raw)
- return lines
-
- def _create_examples(self, lines, set_type):
- """Creates examples for the training and dev sets."""
- examples = []
- for (_, data_raw) in enumerate(lines):
- race_id = "%s-%s" % (set_type, data_raw["race_id"])
- article = data_raw["article"]
- for i in range(len(data_raw["answers"])):
- truth = str(ord(data_raw["answers"][i]) - ord("A"))
- question = data_raw["questions"][i]
- options = data_raw["options"][i]
-
- examples.append(
- InputExample(
- example_id=race_id,
- question=question,
- contexts=[article, article, article, article], # this is not efficient but convenient
- endings=[options[0], options[1], options[2], options[3]],
- label=truth,
- )
- )
- return examples
-
-
-class SwagProcessor(DataProcessor):
- """Processor for the SWAG data set."""
-
- def get_train_examples(self, data_dir):
- """See base class."""
- logger.info("LOOKING AT {} train".format(data_dir))
- return self._create_examples(self._read_csv(os.path.join(data_dir, "train.csv")), "train")
-
- def get_dev_examples(self, data_dir):
- """See base class."""
- logger.info("LOOKING AT {} dev".format(data_dir))
- return self._create_examples(self._read_csv(os.path.join(data_dir, "val.csv")), "dev")
-
- def get_test_examples(self, data_dir):
- """See base class."""
- logger.info("LOOKING AT {} dev".format(data_dir))
- raise ValueError(
- "For swag testing, the input file does not contain a label column. It can not be tested in current code"
- "setting!"
- )
- return self._create_examples(self._read_csv(os.path.join(data_dir, "test.csv")), "test")
-
- def get_labels(self):
- """See base class."""
- return ["0", "1", "2", "3"]
-
- def _read_csv(self, input_file):
- with open(input_file, "r", encoding="utf-8") as f:
- return list(csv.reader(f))
-
- def _create_examples(self, lines: List[List[str]], type: str):
- """Creates examples for the training and dev sets."""
- if type == "train" and lines[0][-1] != "label":
- raise ValueError("For training, the input file must contain a label column.")
-
- examples = [
- InputExample(
- example_id=line[2],
- question=line[5], # in the swag dataset, the
- # common beginning of each
- # choice is stored in "sent2".
- contexts=[line[4], line[4], line[4], line[4]],
- endings=[line[7], line[8], line[9], line[10]],
- label=line[11],
- )
- for line in lines[1:] # we skip the line with the column names
- ]
-
- return examples
-
-
-class ArcProcessor(DataProcessor):
- """Processor for the ARC data set (request from allennlp)."""
-
- def get_train_examples(self, data_dir):
- """See base class."""
- logger.info("LOOKING AT {} train".format(data_dir))
- return self._create_examples(self._read_json(os.path.join(data_dir, "train.jsonl")), "train")
-
- def get_dev_examples(self, data_dir):
- """See base class."""
- logger.info("LOOKING AT {} dev".format(data_dir))
- return self._create_examples(self._read_json(os.path.join(data_dir, "dev.jsonl")), "dev")
-
- def get_test_examples(self, data_dir):
- logger.info("LOOKING AT {} test".format(data_dir))
- return self._create_examples(self._read_json(os.path.join(data_dir, "test.jsonl")), "test")
-
- def get_labels(self):
- """See base class."""
- return ["0", "1", "2", "3"]
-
- def _read_json(self, input_file):
- with open(input_file, "r", encoding="utf-8") as fin:
- lines = fin.readlines()
- return lines
-
- def _create_examples(self, lines, type):
- """Creates examples for the training and dev sets."""
-
- # There are two types of labels. They should be normalized
- def normalize(truth):
- if truth in "ABCD":
- return ord(truth) - ord("A")
- elif truth in "1234":
- return int(truth) - 1
- else:
- logger.info("truth ERROR! %s", str(truth))
- return None
-
- examples = []
- three_choice = 0
- four_choice = 0
- five_choice = 0
- other_choices = 0
- # we deleted example which has more than or less than four choices
- for line in tqdm.tqdm(lines, desc="read arc data"):
- data_raw = json.loads(line.strip("\n"))
- if len(data_raw["question"]["choices"]) == 3:
- three_choice += 1
- continue
- elif len(data_raw["question"]["choices"]) == 5:
- five_choice += 1
- continue
- elif len(data_raw["question"]["choices"]) != 4:
- other_choices += 1
- continue
- four_choice += 1
- truth = str(normalize(data_raw["answerKey"]))
- assert truth != "None"
- question_choices = data_raw["question"]
- question = question_choices["stem"]
- id = data_raw["id"]
- options = question_choices["choices"]
- if len(options) == 4:
- examples.append(
- InputExample(
- example_id=id,
- question=question,
- contexts=[
- options[0]["para"].replace("_", ""),
- options[1]["para"].replace("_", ""),
- options[2]["para"].replace("_", ""),
- options[3]["para"].replace("_", ""),
- ],
- endings=[options[0]["text"], options[1]["text"], options[2]["text"], options[3]["text"]],
- label=truth,
- )
- )
-
- if type == "train":
- assert len(examples) > 1
- assert examples[0].label is not None
- logger.info("len examples: %s}", str(len(examples)))
- logger.info("Three choices: %s", str(three_choice))
- logger.info("Five choices: %s", str(five_choice))
- logger.info("Other choices: %s", str(other_choices))
- logger.info("four choices: %s", str(four_choice))
-
- return examples
-
-
-def convert_examples_to_features(
- examples: List[InputExample],
- label_list: List[str],
- max_length: int,
- tokenizer: PreTrainedTokenizer,
- pad_token_segment_id=0,
- pad_on_left=False,
- pad_token=0,
- mask_padding_with_zero=True,
-) -> List[InputFeatures]:
- """
- Loads a data file into a list of `InputFeatures`
- """
-
- label_map = {label: i for i, label in enumerate(label_list)}
-
- features = []
- for (ex_index, example) in tqdm.tqdm(enumerate(examples), desc="convert examples to features"):
- if ex_index % 10000 == 0:
- logger.info("Writing example %d of %d" % (ex_index, len(examples)))
- choices_features = []
- for ending_idx, (context, ending) in enumerate(zip(example.contexts, example.endings)):
- text_a = context
- if example.question.find("_") != -1:
- # this is for cloze question
- text_b = example.question.replace("_", ending)
- else:
- text_b = example.question + " " + ending
-
- inputs = tokenizer.encode_plus(text_a, text_b, add_special_tokens=True, max_length=max_length,)
- if "num_truncated_tokens" in inputs and inputs["num_truncated_tokens"] > 0:
- logger.info(
- "Attention! you are cropping tokens (swag task is ok). "
- "If you are training ARC and RACE and you are poping question + options,"
- "you need to try to use a bigger max seq length!"
- )
-
- input_ids, token_type_ids = inputs["input_ids"], inputs["token_type_ids"]
-
- # The mask has 1 for real tokens and 0 for padding tokens. Only real
- # tokens are attended to.
- attention_mask = [1 if mask_padding_with_zero else 0] * len(input_ids)
-
- # Zero-pad up to the sequence length.
- padding_length = max_length - len(input_ids)
- if pad_on_left:
- input_ids = ([pad_token] * padding_length) + input_ids
- attention_mask = ([0 if mask_padding_with_zero else 1] * padding_length) + attention_mask
- token_type_ids = ([pad_token_segment_id] * padding_length) + token_type_ids
- else:
- input_ids = input_ids + ([pad_token] * padding_length)
- attention_mask = attention_mask + ([0 if mask_padding_with_zero else 1] * padding_length)
- token_type_ids = token_type_ids + ([pad_token_segment_id] * padding_length)
-
- assert len(input_ids) == max_length
- assert len(attention_mask) == max_length
- assert len(token_type_ids) == max_length
- choices_features.append((input_ids, attention_mask, token_type_ids))
-
- label = label_map[example.label]
-
- if ex_index < 2:
- logger.info("*** Example ***")
- logger.info("race_id: {}".format(example.example_id))
- for choice_idx, (input_ids, attention_mask, token_type_ids) in enumerate(choices_features):
- logger.info("choice: {}".format(choice_idx))
- logger.info("input_ids: {}".format(" ".join(map(str, input_ids))))
- logger.info("attention_mask: {}".format(" ".join(map(str, attention_mask))))
- logger.info("token_type_ids: {}".format(" ".join(map(str, token_type_ids))))
- logger.info("label: {}".format(label))
-
- features.append(InputFeatures(example_id=example.example_id, choices_features=choices_features, label=label,))
-
- return features
-
-
-processors = {"race": RaceProcessor, "swag": SwagProcessor, "arc": ArcProcessor}
-
-
-MULTIPLE_CHOICE_TASKS_NUM_LABELS = {"race", 4, "swag", 4, "arc", 4}
diff --git a/server/transformers/examples/utils_ner.py b/server/transformers/examples/utils_ner.py
deleted file mode 100644
index 510749c2f59c3e734dd5d07b3b2ad00cf4789849..0000000000000000000000000000000000000000
--- a/server/transformers/examples/utils_ner.py
+++ /dev/null
@@ -1,207 +0,0 @@
-# coding=utf-8
-# Copyright 2018 The Google AI Language Team Authors and The HuggingFace Inc. team.
-# Copyright (c) 2018, NVIDIA CORPORATION. All rights reserved.
-#
-# Licensed under the Apache License, Version 2.0 (the "License");
-# you may not use this file except in compliance with the License.
-# You may obtain a copy of the License at
-#
-# http://www.apache.org/licenses/LICENSE-2.0
-#
-# Unless required by applicable law or agreed to in writing, software
-# distributed under the License is distributed on an "AS IS" BASIS,
-# WITHOUT WARRANTIES OR CONDITIONS OF ANY KIND, either express or implied.
-# See the License for the specific language governing permissions and
-# limitations under the License.
-""" Named entity recognition fine-tuning: utilities to work with CoNLL-2003 task. """
-
-
-import logging
-import os
-
-
-logger = logging.getLogger(__name__)
-
-
-class InputExample(object):
- """A single training/test example for token classification."""
-
- def __init__(self, guid, words, labels):
- """Constructs a InputExample.
-
- Args:
- guid: Unique id for the example.
- words: list. The words of the sequence.
- labels: (Optional) list. The labels for each word of the sequence. This should be
- specified for train and dev examples, but not for test examples.
- """
- self.guid = guid
- self.words = words
- self.labels = labels
-
-
-class InputFeatures(object):
- """A single set of features of data."""
-
- def __init__(self, input_ids, input_mask, segment_ids, label_ids):
- self.input_ids = input_ids
- self.input_mask = input_mask
- self.segment_ids = segment_ids
- self.label_ids = label_ids
-
-
-def read_examples_from_file(data_dir, mode):
- file_path = os.path.join(data_dir, "{}.txt".format(mode))
- guid_index = 1
- examples = []
- with open(file_path, encoding="utf-8") as f:
- words = []
- labels = []
- for line in f:
- if line.startswith("-DOCSTART-") or line == "" or line == "\n":
- if words:
- examples.append(InputExample(guid="{}-{}".format(mode, guid_index), words=words, labels=labels))
- guid_index += 1
- words = []
- labels = []
- else:
- splits = line.split(" ")
- words.append(splits[0])
- if len(splits) > 1:
- labels.append(splits[-1].replace("\n", ""))
- else:
- # Examples could have no label for mode = "test"
- labels.append("O")
- if words:
- examples.append(InputExample(guid="{}-{}".format(mode, guid_index), words=words, labels=labels))
- return examples
-
-
-def convert_examples_to_features(
- examples,
- label_list,
- max_seq_length,
- tokenizer,
- cls_token_at_end=False,
- cls_token="[CLS]",
- cls_token_segment_id=1,
- sep_token="[SEP]",
- sep_token_extra=False,
- pad_on_left=False,
- pad_token=0,
- pad_token_segment_id=0,
- pad_token_label_id=-100,
- sequence_a_segment_id=0,
- mask_padding_with_zero=True,
-):
- """ Loads a data file into a list of `InputBatch`s
- `cls_token_at_end` define the location of the CLS token:
- - False (Default, BERT/XLM pattern): [CLS] + A + [SEP] + B + [SEP]
- - True (XLNet/GPT pattern): A + [SEP] + B + [SEP] + [CLS]
- `cls_token_segment_id` define the segment id associated to the CLS token (0 for BERT, 2 for XLNet)
- """
-
- label_map = {label: i for i, label in enumerate(label_list)}
-
- features = []
- for (ex_index, example) in enumerate(examples):
- if ex_index % 10000 == 0:
- logger.info("Writing example %d of %d", ex_index, len(examples))
-
- tokens = []
- label_ids = []
- for word, label in zip(example.words, example.labels):
- word_tokens = tokenizer.tokenize(word)
- tokens.extend(word_tokens)
- # Use the real label id for the first token of the word, and padding ids for the remaining tokens
- label_ids.extend([label_map[label]] + [pad_token_label_id] * (len(word_tokens) - 1))
-
- # Account for [CLS] and [SEP] with "- 2" and with "- 3" for RoBERTa.
- special_tokens_count = 3 if sep_token_extra else 2
- if len(tokens) > max_seq_length - special_tokens_count:
- tokens = tokens[: (max_seq_length - special_tokens_count)]
- label_ids = label_ids[: (max_seq_length - special_tokens_count)]
-
- # The convention in BERT is:
- # (a) For sequence pairs:
- # tokens: [CLS] is this jack ##son ##ville ? [SEP] no it is not . [SEP]
- # type_ids: 0 0 0 0 0 0 0 0 1 1 1 1 1 1
- # (b) For single sequences:
- # tokens: [CLS] the dog is hairy . [SEP]
- # type_ids: 0 0 0 0 0 0 0
- #
- # Where "type_ids" are used to indicate whether this is the first
- # sequence or the second sequence. The embedding vectors for `type=0` and
- # `type=1` were learned during pre-training and are added to the wordpiece
- # embedding vector (and position vector). This is not *strictly* necessary
- # since the [SEP] token unambiguously separates the sequences, but it makes
- # it easier for the model to learn the concept of sequences.
- #
- # For classification tasks, the first vector (corresponding to [CLS]) is
- # used as as the "sentence vector". Note that this only makes sense because
- # the entire model is fine-tuned.
- tokens += [sep_token]
- label_ids += [pad_token_label_id]
- if sep_token_extra:
- # roberta uses an extra separator b/w pairs of sentences
- tokens += [sep_token]
- label_ids += [pad_token_label_id]
- segment_ids = [sequence_a_segment_id] * len(tokens)
-
- if cls_token_at_end:
- tokens += [cls_token]
- label_ids += [pad_token_label_id]
- segment_ids += [cls_token_segment_id]
- else:
- tokens = [cls_token] + tokens
- label_ids = [pad_token_label_id] + label_ids
- segment_ids = [cls_token_segment_id] + segment_ids
-
- input_ids = tokenizer.convert_tokens_to_ids(tokens)
-
- # The mask has 1 for real tokens and 0 for padding tokens. Only real
- # tokens are attended to.
- input_mask = [1 if mask_padding_with_zero else 0] * len(input_ids)
-
- # Zero-pad up to the sequence length.
- padding_length = max_seq_length - len(input_ids)
- if pad_on_left:
- input_ids = ([pad_token] * padding_length) + input_ids
- input_mask = ([0 if mask_padding_with_zero else 1] * padding_length) + input_mask
- segment_ids = ([pad_token_segment_id] * padding_length) + segment_ids
- label_ids = ([pad_token_label_id] * padding_length) + label_ids
- else:
- input_ids += [pad_token] * padding_length
- input_mask += [0 if mask_padding_with_zero else 1] * padding_length
- segment_ids += [pad_token_segment_id] * padding_length
- label_ids += [pad_token_label_id] * padding_length
-
- assert len(input_ids) == max_seq_length
- assert len(input_mask) == max_seq_length
- assert len(segment_ids) == max_seq_length
- assert len(label_ids) == max_seq_length
-
- if ex_index < 5:
- logger.info("*** Example ***")
- logger.info("guid: %s", example.guid)
- logger.info("tokens: %s", " ".join([str(x) for x in tokens]))
- logger.info("input_ids: %s", " ".join([str(x) for x in input_ids]))
- logger.info("input_mask: %s", " ".join([str(x) for x in input_mask]))
- logger.info("segment_ids: %s", " ".join([str(x) for x in segment_ids]))
- logger.info("label_ids: %s", " ".join([str(x) for x in label_ids]))
-
- features.append(
- InputFeatures(input_ids=input_ids, input_mask=input_mask, segment_ids=segment_ids, label_ids=label_ids)
- )
- return features
-
-
-def get_labels(path):
- if path:
- with open(path, "r") as f:
- labels = f.read().splitlines()
- if "O" not in labels:
- labels = ["O"] + labels
- return labels
- else:
- return ["O", "B-MISC", "I-MISC", "B-PER", "I-PER", "B-ORG", "I-ORG", "B-LOC", "I-LOC"]
diff --git a/server/transformers/hubconf.py b/server/transformers/hubconf.py
deleted file mode 100644
index 4e5c1b4b01d3f4b93a58f3f3a66b297b516c1205..0000000000000000000000000000000000000000
--- a/server/transformers/hubconf.py
+++ /dev/null
@@ -1,120 +0,0 @@
-from transformers import (
- AutoConfig,
- AutoModel,
- AutoModelForQuestionAnswering,
- AutoModelForSequenceClassification,
- AutoModelWithLMHead,
- AutoTokenizer,
-)
-from transformers.file_utils import add_start_docstrings
-
-
-dependencies = ["torch", "tqdm", "boto3", "requests", "regex", "sentencepiece", "sacremoses"]
-
-
-@add_start_docstrings(AutoConfig.__doc__)
-def config(*args, **kwargs):
- r"""
- # Using torch.hub !
- import torch
-
- config = torch.hub.load('huggingface/transformers', 'config', 'bert-base-uncased') # Download configuration from S3 and cache.
- config = torch.hub.load('huggingface/transformers', 'config', './test/bert_saved_model/') # E.g. config (or model) was saved using `save_pretrained('./test/saved_model/')`
- config = torch.hub.load('huggingface/transformers', 'config', './test/bert_saved_model/my_configuration.json')
- config = torch.hub.load('huggingface/transformers', 'config', 'bert-base-uncased', output_attention=True, foo=False)
- assert config.output_attention == True
- config, unused_kwargs = torch.hub.load('huggingface/transformers', 'config', 'bert-base-uncased', output_attention=True, foo=False, return_unused_kwargs=True)
- assert config.output_attention == True
- assert unused_kwargs == {'foo': False}
-
- """
-
- return AutoConfig.from_pretrained(*args, **kwargs)
-
-
-@add_start_docstrings(AutoTokenizer.__doc__)
-def tokenizer(*args, **kwargs):
- r"""
- # Using torch.hub !
- import torch
-
- tokenizer = torch.hub.load('huggingface/transformers', 'tokenizer', 'bert-base-uncased') # Download vocabulary from S3 and cache.
- tokenizer = torch.hub.load('huggingface/transformers', 'tokenizer', './test/bert_saved_model/') # E.g. tokenizer was saved using `save_pretrained('./test/saved_model/')`
-
- """
-
- return AutoTokenizer.from_pretrained(*args, **kwargs)
-
-
-@add_start_docstrings(AutoModel.__doc__)
-def model(*args, **kwargs):
- r"""
- # Using torch.hub !
- import torch
-
- model = torch.hub.load('huggingface/transformers', 'model', 'bert-base-uncased') # Download model and configuration from S3 and cache.
- model = torch.hub.load('huggingface/transformers', 'model', './test/bert_model/') # E.g. model was saved using `save_pretrained('./test/saved_model/')`
- model = torch.hub.load('huggingface/transformers', 'model', 'bert-base-uncased', output_attention=True) # Update configuration during loading
- assert model.config.output_attention == True
- # Loading from a TF checkpoint file instead of a PyTorch model (slower)
- config = AutoConfig.from_json_file('./tf_model/bert_tf_model_config.json')
- model = torch.hub.load('huggingface/transformers', 'model', './tf_model/bert_tf_checkpoint.ckpt.index', from_tf=True, config=config)
-
- """
-
- return AutoModel.from_pretrained(*args, **kwargs)
-
-
-@add_start_docstrings(AutoModelWithLMHead.__doc__)
-def modelWithLMHead(*args, **kwargs):
- r"""
- # Using torch.hub !
- import torch
-
- model = torch.hub.load('huggingface/transformers', 'modelWithLMHead', 'bert-base-uncased') # Download model and configuration from S3 and cache.
- model = torch.hub.load('huggingface/transformers', 'modelWithLMHead', './test/bert_model/') # E.g. model was saved using `save_pretrained('./test/saved_model/')`
- model = torch.hub.load('huggingface/transformers', 'modelWithLMHead', 'bert-base-uncased', output_attention=True) # Update configuration during loading
- assert model.config.output_attention == True
- # Loading from a TF checkpoint file instead of a PyTorch model (slower)
- config = AutoConfig.from_json_file('./tf_model/bert_tf_model_config.json')
- model = torch.hub.load('huggingface/transformers', 'modelWithLMHead', './tf_model/bert_tf_checkpoint.ckpt.index', from_tf=True, config=config)
-
- """
- return AutoModelWithLMHead.from_pretrained(*args, **kwargs)
-
-
-@add_start_docstrings(AutoModelForSequenceClassification.__doc__)
-def modelForSequenceClassification(*args, **kwargs):
- r"""
- # Using torch.hub !
- import torch
-
- model = torch.hub.load('huggingface/transformers', 'modelForSequenceClassification', 'bert-base-uncased') # Download model and configuration from S3 and cache.
- model = torch.hub.load('huggingface/transformers', 'modelForSequenceClassification', './test/bert_model/') # E.g. model was saved using `save_pretrained('./test/saved_model/')`
- model = torch.hub.load('huggingface/transformers', 'modelForSequenceClassification', 'bert-base-uncased', output_attention=True) # Update configuration during loading
- assert model.config.output_attention == True
- # Loading from a TF checkpoint file instead of a PyTorch model (slower)
- config = AutoConfig.from_json_file('./tf_model/bert_tf_model_config.json')
- model = torch.hub.load('huggingface/transformers', 'modelForSequenceClassification', './tf_model/bert_tf_checkpoint.ckpt.index', from_tf=True, config=config)
-
- """
-
- return AutoModelForSequenceClassification.from_pretrained(*args, **kwargs)
-
-
-@add_start_docstrings(AutoModelForQuestionAnswering.__doc__)
-def modelForQuestionAnswering(*args, **kwargs):
- r"""
- # Using torch.hub !
- import torch
-
- model = torch.hub.load('huggingface/transformers', 'modelForQuestionAnswering', 'bert-base-uncased') # Download model and configuration from S3 and cache.
- model = torch.hub.load('huggingface/transformers', 'modelForQuestionAnswering', './test/bert_model/') # E.g. model was saved using `save_pretrained('./test/saved_model/')`
- model = torch.hub.load('huggingface/transformers', 'modelForQuestionAnswering', 'bert-base-uncased', output_attention=True) # Update configuration during loading
- assert model.config.output_attention == True
- # Loading from a TF checkpoint file instead of a PyTorch model (slower)
- config = AutoConfig.from_json_file('./tf_model/bert_tf_model_config.json')
- model = torch.hub.load('huggingface/transformers', 'modelForQuestionAnswering', './tf_model/bert_tf_checkpoint.ckpt.index', from_tf=True, config=config)
-
- """
- return AutoModelForQuestionAnswering.from_pretrained(*args, **kwargs)
diff --git a/server/transformers/model_cards/KB/albert-base-swedish-cased-alpha/README.md b/server/transformers/model_cards/KB/albert-base-swedish-cased-alpha/README.md
deleted file mode 100644
index aa6ae466a44ad83dc94f902c8f75c33f32eab03d..0000000000000000000000000000000000000000
--- a/server/transformers/model_cards/KB/albert-base-swedish-cased-alpha/README.md
+++ /dev/null
@@ -1,117 +0,0 @@
-# Swedish BERT Models
-
-The National Library of Sweden / KBLab releases three pretrained language models based on BERT and ALBERT. The models are trained on aproximately 15-20GB of text (200M sentences, 3000M tokens) from various sources (books, news, government publications, swedish wikipedia and internet forums) aiming to provide a representative BERT model for Swedish text. A more complete description will be published later on.
-
-The following three models are currently available:
-
-- **bert-base-swedish-cased** (*v1*) - A BERT trained with the same hyperparameters as first published by Google.
-- **bert-base-swedish-cased-ner** (*experimental*) - a BERT fine-tuned for NER using SUC 3.0.
-- **albert-base-swedish-cased-alpha** (*alpha*) - A first attempt at an ALBERT for Swedish.
-
-All models are cased and trained with whole word masking.
-
-## Files
-
-| **name** | **files** |
-|---------------------------------|-----------|
-| bert-base-swedish-cased | [config](https://s3.amazonaws.com/models.huggingface.co/bert/KB/bert-base-swedish-cased/config.json), [vocab](https://s3.amazonaws.com/models.huggingface.co/bert/KB/bert-base-swedish-cased/vocab.txt), [pytorch_model.bin](https://s3.amazonaws.com/models.huggingface.co/bert/KB/bert-base-swedish-cased/pytorch_model.bin) |
-| bert-base-swedish-cased-ner | [config](https://s3.amazonaws.com/models.huggingface.co/bert/KB/bert-base-swedish-cased-ner/config.json), [vocab](https://s3.amazonaws.com/models.huggingface.co/bert/KB/bert-base-swedish-cased-ner/vocab.txt) [pytorch_model.bin](https://s3.amazonaws.com/models.huggingface.co/bert/KB/bert-base-swedish-cased-ner/pytorch_model.bin) |
-| albert-base-swedish-cased-alpha | [config](https://s3.amazonaws.com/models.huggingface.co/bert/KB/albert-base-swedish-cased-alpha/config.json), [sentencepiece model](https://s3.amazonaws.com/models.huggingface.co/bert/KB/albert-base-swedish-cased-alpha/spiece.model), [pytorch_model.bin](https://s3.amazonaws.com/models.huggingface.co/bert/KB/albert-base-swedish-cased-alpha/pytorch_model.bin) |
-
-TensorFlow model weights will be released soon.
-
-## Usage requirements / installation instructions
-
-The examples below require Huggingface Transformers 2.4.1 and Pytorch 1.3.1 or greater. For Transformers<2.4.0 the tokenizer must be instantiated manually and the `do_lower_case` flag parameter set to `False` and `keep_accents` to `True` (for ALBERT).
-
-To create an environment where the examples can be run, run the following in an terminal on your OS of choice.
-
-```
-# git clone https://github.com/Kungbib/swedish-bert-models
-# cd swedish-bert-models
-# python3 -m venv venv
-# source venv/bin/activate
-# pip install --upgrade pip
-# pip install -r requirements.txt
-```
-
-### BERT Base Swedish
-
-A standard BERT base for Swedish trained on a variety of sources. Vocabulary size is ~50k. Using Huggingface Transformers the model can be loaded in Python as follows:
-
-```python
-from transformers import AutoModel,AutoTokenizer
-
-tok = AutoTokenizer.from_pretrained('KB/bert-base-swedish-cased')
-model = AutoModel.from_pretrained('KB/bert-base-swedish-cased')
-```
-
-
-### BERT base fine-tuned for Swedish NER
-
-This model is fine-tuned on the SUC 3.0 dataset. Using the Huggingface pipeline the model can be easily instantiated. For Transformer<2.4.1 it seems the tokenizer must be loaded separately to disable lower-casing of input strings:
-
-```python
-from transformers import pipeline
-
-nlp = pipeline('ner', model='KB/bert-base-swedish-cased-ner', tokenizer='KB/bert-base-swedish-cased-ner')
-
-nlp('Idag släpper KB tre språkmodeller.')
-```
-
-Running the Python code above should produce in something like the result below. Entity types used are `TME` for time, `PRS` for personal names, `LOC` for locations, `EVN` for events and `ORG` for organisations. These labels are subject to change.
-
-```python
-[ { 'word': 'Idag', 'score': 0.9998126029968262, 'entity': 'TME' },
- { 'word': 'KB', 'score': 0.9814832210540771, 'entity': 'ORG' } ]
-```
-
-The BERT tokenizer often splits words into multiple tokens, with the subparts starting with `##`, for example the string `Engelbert kör Volvo till Herrängens fotbollsklubb` gets tokenized as `Engel ##bert kör Volvo till Herr ##ängens fotbolls ##klubb`. To glue parts back together one can use something like this:
-
-```python
-text = 'Engelbert tar Volvon till Tele2 Arena för att titta på Djurgården IF ' +\
- 'som spelar fotboll i VM klockan två på kvällen.'
-
-l = []
-for token in nlp(text):
- if token['word'].startswith('##'):
- l[-1]['word'] += token['word'][2:]
- else:
- l += [ token ]
-
-print(l)
-```
-
-Which should result in the following (though less cleanly formated):
-
-```python
-[ { 'word': 'Engelbert', 'score': 0.99..., 'entity': 'PRS'},
- { 'word': 'Volvon', 'score': 0.99..., 'entity': 'OBJ'},
- { 'word': 'Tele2', 'score': 0.99..., 'entity': 'LOC'},
- { 'word': 'Arena', 'score': 0.99..., 'entity': 'LOC'},
- { 'word': 'Djurgården', 'score': 0.99..., 'entity': 'ORG'},
- { 'word': 'IF', 'score': 0.99..., 'entity': 'ORG'},
- { 'word': 'VM', 'score': 0.99..., 'entity': 'EVN'},
- { 'word': 'klockan', 'score': 0.99..., 'entity': 'TME'},
- { 'word': 'två', 'score': 0.99..., 'entity': 'TME'},
- { 'word': 'på', 'score': 0.99..., 'entity': 'TME'},
- { 'word': 'kvällen', 'score': 0.54..., 'entity': 'TME'} ]
-```
-
-### ALBERT base
-
-The easisest way to do this is, again, using Huggingface Transformers:
-
-```python
-from transformers import AutoModel,AutoTokenizer
-
-tok = AutoTokenizer.from_pretrained('KB/albert-base-swedish-cased-alpha'),
-model = AutoModel.from_pretrained('KB/albert-base-swedish-cased-alpha')
-```
-
-## Acknowledgements ❤️
-
-- Resources from Stockholms University, Umeå University and Swedish Language Bank at Gothenburg University were used when fine-tuning BERT for NER.
-- Model pretraining was made partly in-house at the KBLab and partly (for material without active copyright) with the support of Cloud TPUs from Google's TensorFlow Research Cloud (TFRC).
-- Models are hosted on S3 by Huggingface 🤗
-
diff --git a/server/transformers/model_cards/KB/bert-base-swedish-cased-ner/README.md b/server/transformers/model_cards/KB/bert-base-swedish-cased-ner/README.md
deleted file mode 100644
index aa6ae466a44ad83dc94f902c8f75c33f32eab03d..0000000000000000000000000000000000000000
--- a/server/transformers/model_cards/KB/bert-base-swedish-cased-ner/README.md
+++ /dev/null
@@ -1,117 +0,0 @@
-# Swedish BERT Models
-
-The National Library of Sweden / KBLab releases three pretrained language models based on BERT and ALBERT. The models are trained on aproximately 15-20GB of text (200M sentences, 3000M tokens) from various sources (books, news, government publications, swedish wikipedia and internet forums) aiming to provide a representative BERT model for Swedish text. A more complete description will be published later on.
-
-The following three models are currently available:
-
-- **bert-base-swedish-cased** (*v1*) - A BERT trained with the same hyperparameters as first published by Google.
-- **bert-base-swedish-cased-ner** (*experimental*) - a BERT fine-tuned for NER using SUC 3.0.
-- **albert-base-swedish-cased-alpha** (*alpha*) - A first attempt at an ALBERT for Swedish.
-
-All models are cased and trained with whole word masking.
-
-## Files
-
-| **name** | **files** |
-|---------------------------------|-----------|
-| bert-base-swedish-cased | [config](https://s3.amazonaws.com/models.huggingface.co/bert/KB/bert-base-swedish-cased/config.json), [vocab](https://s3.amazonaws.com/models.huggingface.co/bert/KB/bert-base-swedish-cased/vocab.txt), [pytorch_model.bin](https://s3.amazonaws.com/models.huggingface.co/bert/KB/bert-base-swedish-cased/pytorch_model.bin) |
-| bert-base-swedish-cased-ner | [config](https://s3.amazonaws.com/models.huggingface.co/bert/KB/bert-base-swedish-cased-ner/config.json), [vocab](https://s3.amazonaws.com/models.huggingface.co/bert/KB/bert-base-swedish-cased-ner/vocab.txt) [pytorch_model.bin](https://s3.amazonaws.com/models.huggingface.co/bert/KB/bert-base-swedish-cased-ner/pytorch_model.bin) |
-| albert-base-swedish-cased-alpha | [config](https://s3.amazonaws.com/models.huggingface.co/bert/KB/albert-base-swedish-cased-alpha/config.json), [sentencepiece model](https://s3.amazonaws.com/models.huggingface.co/bert/KB/albert-base-swedish-cased-alpha/spiece.model), [pytorch_model.bin](https://s3.amazonaws.com/models.huggingface.co/bert/KB/albert-base-swedish-cased-alpha/pytorch_model.bin) |
-
-TensorFlow model weights will be released soon.
-
-## Usage requirements / installation instructions
-
-The examples below require Huggingface Transformers 2.4.1 and Pytorch 1.3.1 or greater. For Transformers<2.4.0 the tokenizer must be instantiated manually and the `do_lower_case` flag parameter set to `False` and `keep_accents` to `True` (for ALBERT).
-
-To create an environment where the examples can be run, run the following in an terminal on your OS of choice.
-
-```
-# git clone https://github.com/Kungbib/swedish-bert-models
-# cd swedish-bert-models
-# python3 -m venv venv
-# source venv/bin/activate
-# pip install --upgrade pip
-# pip install -r requirements.txt
-```
-
-### BERT Base Swedish
-
-A standard BERT base for Swedish trained on a variety of sources. Vocabulary size is ~50k. Using Huggingface Transformers the model can be loaded in Python as follows:
-
-```python
-from transformers import AutoModel,AutoTokenizer
-
-tok = AutoTokenizer.from_pretrained('KB/bert-base-swedish-cased')
-model = AutoModel.from_pretrained('KB/bert-base-swedish-cased')
-```
-
-
-### BERT base fine-tuned for Swedish NER
-
-This model is fine-tuned on the SUC 3.0 dataset. Using the Huggingface pipeline the model can be easily instantiated. For Transformer<2.4.1 it seems the tokenizer must be loaded separately to disable lower-casing of input strings:
-
-```python
-from transformers import pipeline
-
-nlp = pipeline('ner', model='KB/bert-base-swedish-cased-ner', tokenizer='KB/bert-base-swedish-cased-ner')
-
-nlp('Idag släpper KB tre språkmodeller.')
-```
-
-Running the Python code above should produce in something like the result below. Entity types used are `TME` for time, `PRS` for personal names, `LOC` for locations, `EVN` for events and `ORG` for organisations. These labels are subject to change.
-
-```python
-[ { 'word': 'Idag', 'score': 0.9998126029968262, 'entity': 'TME' },
- { 'word': 'KB', 'score': 0.9814832210540771, 'entity': 'ORG' } ]
-```
-
-The BERT tokenizer often splits words into multiple tokens, with the subparts starting with `##`, for example the string `Engelbert kör Volvo till Herrängens fotbollsklubb` gets tokenized as `Engel ##bert kör Volvo till Herr ##ängens fotbolls ##klubb`. To glue parts back together one can use something like this:
-
-```python
-text = 'Engelbert tar Volvon till Tele2 Arena för att titta på Djurgården IF ' +\
- 'som spelar fotboll i VM klockan två på kvällen.'
-
-l = []
-for token in nlp(text):
- if token['word'].startswith('##'):
- l[-1]['word'] += token['word'][2:]
- else:
- l += [ token ]
-
-print(l)
-```
-
-Which should result in the following (though less cleanly formated):
-
-```python
-[ { 'word': 'Engelbert', 'score': 0.99..., 'entity': 'PRS'},
- { 'word': 'Volvon', 'score': 0.99..., 'entity': 'OBJ'},
- { 'word': 'Tele2', 'score': 0.99..., 'entity': 'LOC'},
- { 'word': 'Arena', 'score': 0.99..., 'entity': 'LOC'},
- { 'word': 'Djurgården', 'score': 0.99..., 'entity': 'ORG'},
- { 'word': 'IF', 'score': 0.99..., 'entity': 'ORG'},
- { 'word': 'VM', 'score': 0.99..., 'entity': 'EVN'},
- { 'word': 'klockan', 'score': 0.99..., 'entity': 'TME'},
- { 'word': 'två', 'score': 0.99..., 'entity': 'TME'},
- { 'word': 'på', 'score': 0.99..., 'entity': 'TME'},
- { 'word': 'kvällen', 'score': 0.54..., 'entity': 'TME'} ]
-```
-
-### ALBERT base
-
-The easisest way to do this is, again, using Huggingface Transformers:
-
-```python
-from transformers import AutoModel,AutoTokenizer
-
-tok = AutoTokenizer.from_pretrained('KB/albert-base-swedish-cased-alpha'),
-model = AutoModel.from_pretrained('KB/albert-base-swedish-cased-alpha')
-```
-
-## Acknowledgements ❤️
-
-- Resources from Stockholms University, Umeå University and Swedish Language Bank at Gothenburg University were used when fine-tuning BERT for NER.
-- Model pretraining was made partly in-house at the KBLab and partly (for material without active copyright) with the support of Cloud TPUs from Google's TensorFlow Research Cloud (TFRC).
-- Models are hosted on S3 by Huggingface 🤗
-
diff --git a/server/transformers/model_cards/KB/bert-base-swedish-cased/README.md b/server/transformers/model_cards/KB/bert-base-swedish-cased/README.md
deleted file mode 100644
index aa6ae466a44ad83dc94f902c8f75c33f32eab03d..0000000000000000000000000000000000000000
--- a/server/transformers/model_cards/KB/bert-base-swedish-cased/README.md
+++ /dev/null
@@ -1,117 +0,0 @@
-# Swedish BERT Models
-
-The National Library of Sweden / KBLab releases three pretrained language models based on BERT and ALBERT. The models are trained on aproximately 15-20GB of text (200M sentences, 3000M tokens) from various sources (books, news, government publications, swedish wikipedia and internet forums) aiming to provide a representative BERT model for Swedish text. A more complete description will be published later on.
-
-The following three models are currently available:
-
-- **bert-base-swedish-cased** (*v1*) - A BERT trained with the same hyperparameters as first published by Google.
-- **bert-base-swedish-cased-ner** (*experimental*) - a BERT fine-tuned for NER using SUC 3.0.
-- **albert-base-swedish-cased-alpha** (*alpha*) - A first attempt at an ALBERT for Swedish.
-
-All models are cased and trained with whole word masking.
-
-## Files
-
-| **name** | **files** |
-|---------------------------------|-----------|
-| bert-base-swedish-cased | [config](https://s3.amazonaws.com/models.huggingface.co/bert/KB/bert-base-swedish-cased/config.json), [vocab](https://s3.amazonaws.com/models.huggingface.co/bert/KB/bert-base-swedish-cased/vocab.txt), [pytorch_model.bin](https://s3.amazonaws.com/models.huggingface.co/bert/KB/bert-base-swedish-cased/pytorch_model.bin) |
-| bert-base-swedish-cased-ner | [config](https://s3.amazonaws.com/models.huggingface.co/bert/KB/bert-base-swedish-cased-ner/config.json), [vocab](https://s3.amazonaws.com/models.huggingface.co/bert/KB/bert-base-swedish-cased-ner/vocab.txt) [pytorch_model.bin](https://s3.amazonaws.com/models.huggingface.co/bert/KB/bert-base-swedish-cased-ner/pytorch_model.bin) |
-| albert-base-swedish-cased-alpha | [config](https://s3.amazonaws.com/models.huggingface.co/bert/KB/albert-base-swedish-cased-alpha/config.json), [sentencepiece model](https://s3.amazonaws.com/models.huggingface.co/bert/KB/albert-base-swedish-cased-alpha/spiece.model), [pytorch_model.bin](https://s3.amazonaws.com/models.huggingface.co/bert/KB/albert-base-swedish-cased-alpha/pytorch_model.bin) |
-
-TensorFlow model weights will be released soon.
-
-## Usage requirements / installation instructions
-
-The examples below require Huggingface Transformers 2.4.1 and Pytorch 1.3.1 or greater. For Transformers<2.4.0 the tokenizer must be instantiated manually and the `do_lower_case` flag parameter set to `False` and `keep_accents` to `True` (for ALBERT).
-
-To create an environment where the examples can be run, run the following in an terminal on your OS of choice.
-
-```
-# git clone https://github.com/Kungbib/swedish-bert-models
-# cd swedish-bert-models
-# python3 -m venv venv
-# source venv/bin/activate
-# pip install --upgrade pip
-# pip install -r requirements.txt
-```
-
-### BERT Base Swedish
-
-A standard BERT base for Swedish trained on a variety of sources. Vocabulary size is ~50k. Using Huggingface Transformers the model can be loaded in Python as follows:
-
-```python
-from transformers import AutoModel,AutoTokenizer
-
-tok = AutoTokenizer.from_pretrained('KB/bert-base-swedish-cased')
-model = AutoModel.from_pretrained('KB/bert-base-swedish-cased')
-```
-
-
-### BERT base fine-tuned for Swedish NER
-
-This model is fine-tuned on the SUC 3.0 dataset. Using the Huggingface pipeline the model can be easily instantiated. For Transformer<2.4.1 it seems the tokenizer must be loaded separately to disable lower-casing of input strings:
-
-```python
-from transformers import pipeline
-
-nlp = pipeline('ner', model='KB/bert-base-swedish-cased-ner', tokenizer='KB/bert-base-swedish-cased-ner')
-
-nlp('Idag släpper KB tre språkmodeller.')
-```
-
-Running the Python code above should produce in something like the result below. Entity types used are `TME` for time, `PRS` for personal names, `LOC` for locations, `EVN` for events and `ORG` for organisations. These labels are subject to change.
-
-```python
-[ { 'word': 'Idag', 'score': 0.9998126029968262, 'entity': 'TME' },
- { 'word': 'KB', 'score': 0.9814832210540771, 'entity': 'ORG' } ]
-```
-
-The BERT tokenizer often splits words into multiple tokens, with the subparts starting with `##`, for example the string `Engelbert kör Volvo till Herrängens fotbollsklubb` gets tokenized as `Engel ##bert kör Volvo till Herr ##ängens fotbolls ##klubb`. To glue parts back together one can use something like this:
-
-```python
-text = 'Engelbert tar Volvon till Tele2 Arena för att titta på Djurgården IF ' +\
- 'som spelar fotboll i VM klockan två på kvällen.'
-
-l = []
-for token in nlp(text):
- if token['word'].startswith('##'):
- l[-1]['word'] += token['word'][2:]
- else:
- l += [ token ]
-
-print(l)
-```
-
-Which should result in the following (though less cleanly formated):
-
-```python
-[ { 'word': 'Engelbert', 'score': 0.99..., 'entity': 'PRS'},
- { 'word': 'Volvon', 'score': 0.99..., 'entity': 'OBJ'},
- { 'word': 'Tele2', 'score': 0.99..., 'entity': 'LOC'},
- { 'word': 'Arena', 'score': 0.99..., 'entity': 'LOC'},
- { 'word': 'Djurgården', 'score': 0.99..., 'entity': 'ORG'},
- { 'word': 'IF', 'score': 0.99..., 'entity': 'ORG'},
- { 'word': 'VM', 'score': 0.99..., 'entity': 'EVN'},
- { 'word': 'klockan', 'score': 0.99..., 'entity': 'TME'},
- { 'word': 'två', 'score': 0.99..., 'entity': 'TME'},
- { 'word': 'på', 'score': 0.99..., 'entity': 'TME'},
- { 'word': 'kvällen', 'score': 0.54..., 'entity': 'TME'} ]
-```
-
-### ALBERT base
-
-The easisest way to do this is, again, using Huggingface Transformers:
-
-```python
-from transformers import AutoModel,AutoTokenizer
-
-tok = AutoTokenizer.from_pretrained('KB/albert-base-swedish-cased-alpha'),
-model = AutoModel.from_pretrained('KB/albert-base-swedish-cased-alpha')
-```
-
-## Acknowledgements ❤️
-
-- Resources from Stockholms University, Umeå University and Swedish Language Bank at Gothenburg University were used when fine-tuning BERT for NER.
-- Model pretraining was made partly in-house at the KBLab and partly (for material without active copyright) with the support of Cloud TPUs from Google's TensorFlow Research Cloud (TFRC).
-- Models are hosted on S3 by Huggingface 🤗
-
diff --git a/server/transformers/model_cards/Musixmatch/umberto-commoncrawl-cased-v1/README.md b/server/transformers/model_cards/Musixmatch/umberto-commoncrawl-cased-v1/README.md
deleted file mode 100644
index aacd4d9e3cae5df88d4afe929d728cd38885c1c1..0000000000000000000000000000000000000000
--- a/server/transformers/model_cards/Musixmatch/umberto-commoncrawl-cased-v1/README.md
+++ /dev/null
@@ -1,114 +0,0 @@
-# UmBERTo Commoncrawl Cased
-
-[UmBERTo](https://github.com/musixmatchresearch/umberto) is a Roberta-based Language Model trained on large Italian Corpora and uses two innovative approaches: SentencePiece and Whole Word Masking. Now available at [github.com/huggingface/transformers](https://huggingface.co/Musixmatch/umberto-commoncrawl-cased-v1)
-
-
-
- Marco Lodola, Monument to Umberto Eco, Alessandria 2019
-
-
-## Dataset
-UmBERTo-Commoncrawl-Cased utilizes the Italian subcorpus of [OSCAR](https://traces1.inria.fr/oscar/) as training set of the language model. We used deduplicated version of the Italian corpus that consists in 70 GB of plain text data, 210M sentences with 11B words where the sentences have been filtered and shuffled at line level in order to be used for NLP research.
-
-## Pre-trained model
-
-| Model | WWM | Cased | Tokenizer | Vocab Size | Train Steps | Download |
-| ------ | ------ | ------ | ------ | ------ |------ | ------ |
-| `umberto-commoncrawl-cased-v1` | YES | YES | SPM | 32K | 125k | [Link](http://bit.ly/35zO7GH) |
-
-This model was trained with [SentencePiece](https://github.com/google/sentencepiece) and Whole Word Masking.
-
-## Downstream Tasks
-These results refers to umberto-commoncrawl-cased model. All details are at [Umberto](https://github.com/musixmatchresearch/umberto) Official Page.
-
-#### Named Entity Recognition (NER)
-
-| Dataset | F1 | Precision | Recall | Accuracy |
-| ------ | ------ | ------ | ------ | ------ |
-| **ICAB-EvalITA07** | **87.565** | 86.596 | 88.556 | 98.690 |
-| **WikiNER-ITA** | **92.531** | 92.509 | 92.553 | 99.136 |
-
-#### Part of Speech (POS)
-
-| Dataset | F1 | Precision | Recall | Accuracy |
-| ------ | ------ | ------ | ------ | ------ |
-| **UD_Italian-ISDT** | 98.870 | 98.861 | 98.879 | **98.977** |
-| **UD_Italian-ParTUT** | 98.786 | 98.812 | 98.760 | **98.903** |
-
-
-
-## Usage
-
-##### Load UmBERTo with AutoModel, Autotokenizer:
-
-```python
-
-import torch
-from transformers import AutoTokenizer, AutoModel
-
-tokenizer = AutoTokenizer.from_pretrained("Musixmatch/umberto-commoncrawl-cased-v1")
-umberto = AutoModel.from_pretrained("Musixmatch/umberto-commoncrawl-cased-v1")
-
-encoded_input = tokenizer.encode("Umberto Eco è stato un grande scrittore")
-input_ids = torch.tensor(encoded_input).unsqueeze(0) # Batch size 1
-outputs = umberto(input_ids)
-last_hidden_states = outputs[0] # The last hidden-state is the first element of the output
-```
-
-##### Predict masked token:
-
-```python
-from transformers import pipeline
-
-fill_mask = pipeline(
- "fill-mask",
- model="Musixmatch/umberto-commoncrawl-cased-v1",
- tokenizer="Musixmatch/umberto-commoncrawl-cased-v1"
-)
-
-result = fill_mask("Umberto Eco è un grande scrittore")
-# {'sequence': ' Umberto Eco è considerato un grande scrittore', 'score': 0.18599839508533478, 'token': 5032}
-# {'sequence': ' Umberto Eco è stato un grande scrittore', 'score': 0.17816807329654694, 'token': 471}
-# {'sequence': ' Umberto Eco è sicuramente un grande scrittore', 'score': 0.16565583646297455, 'token': 2654}
-# {'sequence': ' Umberto Eco è indubbiamente un grande scrittore', 'score': 0.0932890921831131, 'token': 17908}
-# {'sequence': ' Umberto Eco è certamente un grande scrittore', 'score': 0.054701317101716995, 'token': 5269}
-```
-
-
-## Citation
-All of the original datasets are publicly available or were released with the owners' grant. The datasets are all released under a CC0 or CCBY license.
-
-* UD Italian-ISDT Dataset [Github](https://github.com/UniversalDependencies/UD_Italian-ISDT)
-* UD Italian-ParTUT Dataset [Github](https://github.com/UniversalDependencies/UD_Italian-ParTUT)
-* I-CAB (Italian Content Annotation Bank), EvalITA [Page](http://www.evalita.it/)
-* WIKINER [Page](https://figshare.com/articles/Learning_multilingual_named_entity_recognition_from_Wikipedia/5462500) , [Paper](https://www.sciencedirect.com/science/article/pii/S0004370212000276?via%3Dihub)
-
-```
-@inproceedings {magnini2006annotazione,
- title = {Annotazione di contenuti concettuali in un corpus italiano: I - CAB},
- author = {Magnini,Bernardo and Cappelli,Amedeo and Pianta,Emanuele and Speranza,Manuela and Bartalesi Lenzi,V and Sprugnoli,Rachele and Romano,Lorenza and Girardi,Christian and Negri,Matteo},
- booktitle = {Proc.of SILFI 2006},
- year = {2006}
-}
-@inproceedings {magnini2006cab,
- title = {I - CAB: the Italian Content Annotation Bank.},
- author = {Magnini,Bernardo and Pianta,Emanuele and Girardi,Christian and Negri,Matteo and Romano,Lorenza and Speranza,Manuela and Lenzi,Valentina Bartalesi and Sprugnoli,Rachele},
- booktitle = {LREC},
- pages = {963--968},
- year = {2006},
- organization = {Citeseer}
-}
-```
-
-## Authors
-
-**Loreto Parisi**: `loreto at musixmatch dot com`, [loretoparisi](https://github.com/loretoparisi)
-**Simone Francia**: `simone.francia at musixmatch dot com`, [simonefrancia](https://github.com/simonefrancia)
-**Paolo Magnani**: `paul.magnani95 at gmail dot com`, [paulthemagno](https://github.com/paulthemagno)
-
-## About Musixmatch AI
-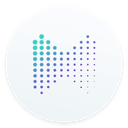
-We do Machine Learning and Artificial Intelligence @[musixmatch](https://twitter.com/Musixmatch)
-Follow us on [Twitter](https://twitter.com/musixmatchai) [Github](https://github.com/musixmatchresearch)
-
-
diff --git a/server/transformers/model_cards/Musixmatch/umberto-wikipedia-uncased-v1/README.md b/server/transformers/model_cards/Musixmatch/umberto-wikipedia-uncased-v1/README.md
deleted file mode 100644
index fd94e5e13daaa931940fe1a15c7d3e5b96d40f8a..0000000000000000000000000000000000000000
--- a/server/transformers/model_cards/Musixmatch/umberto-wikipedia-uncased-v1/README.md
+++ /dev/null
@@ -1,113 +0,0 @@
-# UmBERTo Wikipedia Uncased
-
-[UmBERTo](https://github.com/musixmatchresearch/umberto) is a Roberta-based Language Model trained on large Italian Corpora and uses two innovative approaches: SentencePiece and Whole Word Masking. Now available at [github.com/huggingface/transformers](https://huggingface.co/Musixmatch/umberto-commoncrawl-cased-v1)
-
-
-
- Marco Lodola, Monument to Umberto Eco, Alessandria 2019
-
-
-## Dataset
-UmBERTo-Wikipedia-Uncased Training is trained on a relative small corpus (~7GB) extracted from [Wikipedia-ITA](https://linguatools.org/tools/corpora/wikipedia-monolingual-corpora/).
-
-## Pre-trained model
-
-| Model | WWM | Cased | Tokenizer | Vocab Size | Train Steps | Download |
-| ------ | ------ | ------ | ------ | ------ |------ | ------ |
-| `umberto-wikipedia-uncased-v1` | YES | YES | SPM | 32K | 100k | [Link](http://bit.ly/35wbSj6) |
-
-This model was trained with [SentencePiece](https://github.com/google/sentencepiece) and Whole Word Masking.
-
-## Downstream Tasks
-These results refers to umberto-wikipedia-uncased model. All details are at [Umberto](https://github.com/musixmatchresearch/umberto) Official Page.
-
-#### Named Entity Recognition (NER)
-
-| Dataset | F1 | Precision | Recall | Accuracy |
-| ------ | ------ | ------ | ------ | ----- |
-| **ICAB-EvalITA07** | **86.240** | 85.939 | 86.544 | 98.534 |
-| **WikiNER-ITA** | **90.483** | 90.328 | 90.638 | 98.661 |
-
-#### Part of Speech (POS)
-
-| Dataset | F1 | Precision | Recall | Accuracy |
-| ------ | ------ | ------ | ------ | ------ |
-| **UD_Italian-ISDT** | 98.563 | 98.508 | 98.618 | **98.717** |
-| **UD_Italian-ParTUT** | 97.810 | 97.835 | 97.784 | **98.060** |
-
-
-
-## Usage
-
-##### Load UmBERTo Wikipedia Uncased with AutoModel, Autotokenizer:
-
-```python
-
-import torch
-from transformers import AutoTokenizer, AutoModel
-
-tokenizer = AutoTokenizer.from_pretrained("Musixmatch/umberto-wikipedia-uncased-v1")
-umberto = AutoModel.from_pretrained("Musixmatch/umberto-wikipedia-uncased-v1")
-
-encoded_input = tokenizer.encode("Umberto Eco è stato un grande scrittore")
-input_ids = torch.tensor(encoded_input).unsqueeze(0) # Batch size 1
-outputs = umberto(input_ids)
-last_hidden_states = outputs[0] # The last hidden-state is the first element of the output
-```
-
-##### Predict masked token:
-
-```python
-from transformers import pipeline
-
-fill_mask = pipeline(
- "fill-mask",
- model="Musixmatch/umberto-wikipedia-uncased-v1",
- tokenizer="Musixmatch/umberto-wikipedia-uncased-v1"
-)
-
-result = fill_mask("Umberto Eco è un grande scrittore")
-# {'sequence': ' umberto eco è stato un grande scrittore', 'score': 0.5784581303596497, 'token': 361}
-# {'sequence': ' umberto eco è anche un grande scrittore', 'score': 0.33813193440437317, 'token': 269}
-# {'sequence': ' umberto eco è considerato un grande scrittore', 'score': 0.027196012437343597, 'token': 3236}
-# {'sequence': ' umberto eco è diventato un grande scrittore', 'score': 0.013716378249228, 'token': 5742}
-# {'sequence': ' umberto eco è inoltre un grande scrittore', 'score': 0.010662357322871685, 'token': 1030}
-```
-
-
-## Citation
-All of the original datasets are publicly available or were released with the owners' grant. The datasets are all released under a CC0 or CCBY license.
-
-* UD Italian-ISDT Dataset [Github](https://github.com/UniversalDependencies/UD_Italian-ISDT)
-* UD Italian-ParTUT Dataset [Github](https://github.com/UniversalDependencies/UD_Italian-ParTUT)
-* I-CAB (Italian Content Annotation Bank), EvalITA [Page](http://www.evalita.it/)
-* WIKINER [Page](https://figshare.com/articles/Learning_multilingual_named_entity_recognition_from_Wikipedia/5462500) , [Paper](https://www.sciencedirect.com/science/article/pii/S0004370212000276?via%3Dihub)
-
-```
-@inproceedings {magnini2006annotazione,
- title = {Annotazione di contenuti concettuali in un corpus italiano: I - CAB},
- author = {Magnini,Bernardo and Cappelli,Amedeo and Pianta,Emanuele and Speranza,Manuela and Bartalesi Lenzi,V and Sprugnoli,Rachele and Romano,Lorenza and Girardi,Christian and Negri,Matteo},
- booktitle = {Proc.of SILFI 2006},
- year = {2006}
-}
-@inproceedings {magnini2006cab,
- title = {I - CAB: the Italian Content Annotation Bank.},
- author = {Magnini,Bernardo and Pianta,Emanuele and Girardi,Christian and Negri,Matteo and Romano,Lorenza and Speranza,Manuela and Lenzi,Valentina Bartalesi and Sprugnoli,Rachele},
- booktitle = {LREC},
- pages = {963--968},
- year = {2006},
- organization = {Citeseer}
-}
-```
-
-## Authors
-
-**Loreto Parisi**: `loreto at musixmatch dot com`, [loretoparisi](https://github.com/loretoparisi)
-**Simone Francia**: `simone.francia at musixmatch dot com`, [simonefrancia](https://github.com/simonefrancia)
-**Paolo Magnani**: `paul.magnani95 at gmail dot com`, [paulthemagno](https://github.com/paulthemagno)
-
-## About Musixmatch AI
-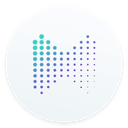
-We do Machine Learning and Artificial Intelligence @[musixmatch](https://twitter.com/Musixmatch)
-Follow us on [Twitter](https://twitter.com/musixmatchai) [Github](https://github.com/musixmatchresearch)
-
diff --git a/server/transformers/model_cards/dbmdz/bert-base-german-cased/README.md b/server/transformers/model_cards/dbmdz/bert-base-german-cased/README.md
deleted file mode 100644
index fccd05054577d6633cf6c6ed2193e875b0a0a560..0000000000000000000000000000000000000000
--- a/server/transformers/model_cards/dbmdz/bert-base-german-cased/README.md
+++ /dev/null
@@ -1,66 +0,0 @@
-# 🤗 + 📚 dbmdz German BERT models
-
-In this repository the MDZ Digital Library team (dbmdz) at the Bavarian State
-Library open sources another German BERT models 🎉
-
-# German BERT
-
-## Stats
-
-In addition to the recently released [German BERT](https://deepset.ai/german-bert)
-model by [deepset](https://deepset.ai/) we provide another German-language model.
-
-The source data for the model consists of a recent Wikipedia dump, EU Bookshop corpus,
-Open Subtitles, CommonCrawl, ParaCrawl and News Crawl. This results in a dataset with
-a size of 16GB and 2,350,234,427 tokens.
-
-For sentence splitting, we use [spacy](https://spacy.io/). Our preprocessing steps
-(sentence piece model for vocab generation) follow those used for training
-[SciBERT](https://github.com/allenai/scibert). The model is trained with an initial
-sequence length of 512 subwords and was performed for 1.5M steps.
-
-This release includes both cased and uncased models.
-
-## Model weights
-
-Currently only PyTorch-[Transformers](https://github.com/huggingface/transformers)
-compatible weights are available. If you need access to TensorFlow checkpoints,
-please raise an issue!
-
-| Model | Downloads
-| -------------------------------- | ---------------------------------------------------------------------------------------------------------------
-| `bert-base-german-dbmdz-cased` | [`config.json`](https://s3.amazonaws.com/models.huggingface.co/bert/bert-base-german-dbmdz-cased-config.json) • [`pytorch_model.bin`](https://s3.amazonaws.com/models.huggingface.co/bert/bert-base-german-dbmdz-cased-pytorch_model.bin) • [`vocab.txt`](https://s3.amazonaws.com/models.huggingface.co/bert/bert-base-german-dbmdz-cased-vocab.txt)
-| `bert-base-german-dbmdz-uncased` | [`config.json`](https://s3.amazonaws.com/models.huggingface.co/bert/bert-base-german-dbmdz-uncased-config.json) • [`pytorch_model.bin`](https://s3.amazonaws.com/models.huggingface.co/bert/bert-base-german-dbmdz-uncased-pytorch_model.bin) • [`vocab.txt`](https://s3.amazonaws.com/models.huggingface.co/bert/bert-base-german-dbmdz-uncased-vocab.txt)
-
-## Usage
-
-With Transformers >= 2.3 our German BERT models can be loaded like:
-
-```python
-from transformers import AutoModel, AutoTokenizer
-
-tokenizer = AutoTokenizer.from_pretrained("dbmdz/bert-base-german-cased")
-model = AutoModel.from_pretrained("dbmdz/bert-base-german-cased")
-```
-
-## Results
-
-For results on downstream tasks like NER or PoS tagging, please refer to
-[this repository](https://github.com/stefan-it/fine-tuned-berts-seq).
-
-# Huggingface model hub
-
-All models are available on the [Huggingface model hub](https://huggingface.co/dbmdz).
-
-# Contact (Bugs, Feedback, Contribution and more)
-
-For questions about our BERT models just open an issue
-[here](https://github.com/dbmdz/berts/issues/new) 🤗
-
-# Acknowledgments
-
-Research supported with Cloud TPUs from Google's TensorFlow Research Cloud (TFRC).
-Thanks for providing access to the TFRC ❤️
-
-Thanks to the generous support from the [Hugging Face](https://huggingface.co/) team,
-it is possible to download both cased and uncased models from their S3 storage 🤗
diff --git a/server/transformers/model_cards/dbmdz/bert-base-german-uncased/README.md b/server/transformers/model_cards/dbmdz/bert-base-german-uncased/README.md
deleted file mode 100644
index fccd05054577d6633cf6c6ed2193e875b0a0a560..0000000000000000000000000000000000000000
--- a/server/transformers/model_cards/dbmdz/bert-base-german-uncased/README.md
+++ /dev/null
@@ -1,66 +0,0 @@
-# 🤗 + 📚 dbmdz German BERT models
-
-In this repository the MDZ Digital Library team (dbmdz) at the Bavarian State
-Library open sources another German BERT models 🎉
-
-# German BERT
-
-## Stats
-
-In addition to the recently released [German BERT](https://deepset.ai/german-bert)
-model by [deepset](https://deepset.ai/) we provide another German-language model.
-
-The source data for the model consists of a recent Wikipedia dump, EU Bookshop corpus,
-Open Subtitles, CommonCrawl, ParaCrawl and News Crawl. This results in a dataset with
-a size of 16GB and 2,350,234,427 tokens.
-
-For sentence splitting, we use [spacy](https://spacy.io/). Our preprocessing steps
-(sentence piece model for vocab generation) follow those used for training
-[SciBERT](https://github.com/allenai/scibert). The model is trained with an initial
-sequence length of 512 subwords and was performed for 1.5M steps.
-
-This release includes both cased and uncased models.
-
-## Model weights
-
-Currently only PyTorch-[Transformers](https://github.com/huggingface/transformers)
-compatible weights are available. If you need access to TensorFlow checkpoints,
-please raise an issue!
-
-| Model | Downloads
-| -------------------------------- | ---------------------------------------------------------------------------------------------------------------
-| `bert-base-german-dbmdz-cased` | [`config.json`](https://s3.amazonaws.com/models.huggingface.co/bert/bert-base-german-dbmdz-cased-config.json) • [`pytorch_model.bin`](https://s3.amazonaws.com/models.huggingface.co/bert/bert-base-german-dbmdz-cased-pytorch_model.bin) • [`vocab.txt`](https://s3.amazonaws.com/models.huggingface.co/bert/bert-base-german-dbmdz-cased-vocab.txt)
-| `bert-base-german-dbmdz-uncased` | [`config.json`](https://s3.amazonaws.com/models.huggingface.co/bert/bert-base-german-dbmdz-uncased-config.json) • [`pytorch_model.bin`](https://s3.amazonaws.com/models.huggingface.co/bert/bert-base-german-dbmdz-uncased-pytorch_model.bin) • [`vocab.txt`](https://s3.amazonaws.com/models.huggingface.co/bert/bert-base-german-dbmdz-uncased-vocab.txt)
-
-## Usage
-
-With Transformers >= 2.3 our German BERT models can be loaded like:
-
-```python
-from transformers import AutoModel, AutoTokenizer
-
-tokenizer = AutoTokenizer.from_pretrained("dbmdz/bert-base-german-cased")
-model = AutoModel.from_pretrained("dbmdz/bert-base-german-cased")
-```
-
-## Results
-
-For results on downstream tasks like NER or PoS tagging, please refer to
-[this repository](https://github.com/stefan-it/fine-tuned-berts-seq).
-
-# Huggingface model hub
-
-All models are available on the [Huggingface model hub](https://huggingface.co/dbmdz).
-
-# Contact (Bugs, Feedback, Contribution and more)
-
-For questions about our BERT models just open an issue
-[here](https://github.com/dbmdz/berts/issues/new) 🤗
-
-# Acknowledgments
-
-Research supported with Cloud TPUs from Google's TensorFlow Research Cloud (TFRC).
-Thanks for providing access to the TFRC ❤️
-
-Thanks to the generous support from the [Hugging Face](https://huggingface.co/) team,
-it is possible to download both cased and uncased models from their S3 storage 🤗
diff --git a/server/transformers/model_cards/dbmdz/bert-base-italian-cased/README.md b/server/transformers/model_cards/dbmdz/bert-base-italian-cased/README.md
deleted file mode 100644
index 549c1133af281477b2b62101b39862cf010e8d2f..0000000000000000000000000000000000000000
--- a/server/transformers/model_cards/dbmdz/bert-base-italian-cased/README.md
+++ /dev/null
@@ -1,73 +0,0 @@
-# 🤗 + 📚 dbmdz BERT models
-
-In this repository the MDZ Digital Library team (dbmdz) at the Bavarian State
-Library open sources Italian BERT models 🎉
-
-# Italian BERT
-
-The source data for the Italian BERT model consists of a recent Wikipedia dump and
-various texts from the [OPUS corpora](http://opus.nlpl.eu/) collection. The final
-training corpus has a size of 13GB and 2,050,057,573 tokens.
-
-For sentence splitting, we use NLTK (faster compared to spacy).
-Our cased and uncased models are training with an initial sequence length of 512
-subwords for ~2-3M steps.
-
-For the XXL Italian models, we use the same training data from OPUS and extend
-it with data from the Italian part of the [OSCAR corpus](https://traces1.inria.fr/oscar/).
-Thus, the final training corpus has a size of 81GB and 13,138,379,147 tokens.
-
-## Model weights
-
-Currently only PyTorch-[Transformers](https://github.com/huggingface/transformers)
-compatible weights are available. If you need access to TensorFlow checkpoints,
-please raise an issue!
-
-| Model | Downloads
-| --------------------------------------- | ---------------------------------------------------------------------------------------------------------------
-| `dbmdz/bert-base-italian-cased` | [`config.json`](https://cdn.huggingface.co/dbmdz/bert-base-italian-cased/config.json) • [`pytorch_model.bin`](https://cdn.huggingface.co/dbmdz/bert-base-italian-cased/pytorch_model.bin) • [`vocab.txt`](https://cdn.huggingface.co/dbmdz/bert-base-italian-cased/vocab.txt)
-| `dbmdz/bert-base-italian-uncased` | [`config.json`](https://cdn.huggingface.co/dbmdz/bert-base-italian-uncased/config.json) • [`pytorch_model.bin`](https://cdn.huggingface.co/dbmdz/bert-base-italian-uncased/pytorch_model.bin) • [`vocab.txt`](https://cdn.huggingface.co/dbmdz/bert-base-italian-uncased/vocab.txt)
-| `dbmdz/bert-base-italian-xxl-cased` | [`config.json`](https://cdn.huggingface.co/dbmdz/bert-base-italian-xxl-cased/config.json) • [`pytorch_model.bin`](https://cdn.huggingface.co/dbmdz/bert-base-italian-xxl-cased/pytorch_model.bin) • [`vocab.txt`](https://cdn.huggingface.co/dbmdz/bert-base-italian-xxl-cased/vocab.txt)
-| `dbmdz/bert-base-italian-xxl-uncased` | [`config.json`](https://cdn.huggingface.co/dbmdz/bert-base-italian-xxl-uncased/config.json) • [`pytorch_model.bin`](https://cdn.huggingface.co/dbmdz/bert-base-italian-xxl-uncased/pytorch_model.bin) • [`vocab.txt`](https://cdn.huggingface.co/dbmdz/bert-base-italian-xxl-uncased/vocab.txt)
-
-## Results
-
-For results on downstream tasks like NER or PoS tagging, please refer to
-[this repository](https://github.com/stefan-it/fine-tuned-berts-seq).
-
-## Usage
-
-With Transformers >= 2.3 our Italian BERT models can be loaded like:
-
-```python
-from transformers import AutoModel, AutoTokenizer
-
-tokenizer = AutoTokenizer.from_pretrained("dbmdz/bert-base-italian-cased")
-model = AutoModel.from_pretrained("dbmdz/bert-base-italian-cased")
-```
-
-To load the (recommended) Italian XXL BERT models, just use:
-
-```python
-from transformers import AutoModel, AutoTokenizer
-
-tokenizer = AutoTokenizer.from_pretrained("dbmdz/bert-base-italian-xxl-cased")
-model = AutoModel.from_pretrained("dbmdz/bert-base-italian-xxl-cased")
-```
-
-# Huggingface model hub
-
-All models are available on the [Huggingface model hub](https://huggingface.co/dbmdz).
-
-# Contact (Bugs, Feedback, Contribution and more)
-
-For questions about our BERT models just open an issue
-[here](https://github.com/dbmdz/berts/issues/new) 🤗
-
-# Acknowledgments
-
-Research supported with Cloud TPUs from Google's TensorFlow Research Cloud (TFRC).
-Thanks for providing access to the TFRC ❤️
-
-Thanks to the generous support from the [Hugging Face](https://huggingface.co/) team,
-it is possible to download both cased and uncased models from their S3 storage 🤗
diff --git a/server/transformers/model_cards/dbmdz/bert-base-italian-uncased/README.md b/server/transformers/model_cards/dbmdz/bert-base-italian-uncased/README.md
deleted file mode 100644
index 549c1133af281477b2b62101b39862cf010e8d2f..0000000000000000000000000000000000000000
--- a/server/transformers/model_cards/dbmdz/bert-base-italian-uncased/README.md
+++ /dev/null
@@ -1,73 +0,0 @@
-# 🤗 + 📚 dbmdz BERT models
-
-In this repository the MDZ Digital Library team (dbmdz) at the Bavarian State
-Library open sources Italian BERT models 🎉
-
-# Italian BERT
-
-The source data for the Italian BERT model consists of a recent Wikipedia dump and
-various texts from the [OPUS corpora](http://opus.nlpl.eu/) collection. The final
-training corpus has a size of 13GB and 2,050,057,573 tokens.
-
-For sentence splitting, we use NLTK (faster compared to spacy).
-Our cased and uncased models are training with an initial sequence length of 512
-subwords for ~2-3M steps.
-
-For the XXL Italian models, we use the same training data from OPUS and extend
-it with data from the Italian part of the [OSCAR corpus](https://traces1.inria.fr/oscar/).
-Thus, the final training corpus has a size of 81GB and 13,138,379,147 tokens.
-
-## Model weights
-
-Currently only PyTorch-[Transformers](https://github.com/huggingface/transformers)
-compatible weights are available. If you need access to TensorFlow checkpoints,
-please raise an issue!
-
-| Model | Downloads
-| --------------------------------------- | ---------------------------------------------------------------------------------------------------------------
-| `dbmdz/bert-base-italian-cased` | [`config.json`](https://cdn.huggingface.co/dbmdz/bert-base-italian-cased/config.json) • [`pytorch_model.bin`](https://cdn.huggingface.co/dbmdz/bert-base-italian-cased/pytorch_model.bin) • [`vocab.txt`](https://cdn.huggingface.co/dbmdz/bert-base-italian-cased/vocab.txt)
-| `dbmdz/bert-base-italian-uncased` | [`config.json`](https://cdn.huggingface.co/dbmdz/bert-base-italian-uncased/config.json) • [`pytorch_model.bin`](https://cdn.huggingface.co/dbmdz/bert-base-italian-uncased/pytorch_model.bin) • [`vocab.txt`](https://cdn.huggingface.co/dbmdz/bert-base-italian-uncased/vocab.txt)
-| `dbmdz/bert-base-italian-xxl-cased` | [`config.json`](https://cdn.huggingface.co/dbmdz/bert-base-italian-xxl-cased/config.json) • [`pytorch_model.bin`](https://cdn.huggingface.co/dbmdz/bert-base-italian-xxl-cased/pytorch_model.bin) • [`vocab.txt`](https://cdn.huggingface.co/dbmdz/bert-base-italian-xxl-cased/vocab.txt)
-| `dbmdz/bert-base-italian-xxl-uncased` | [`config.json`](https://cdn.huggingface.co/dbmdz/bert-base-italian-xxl-uncased/config.json) • [`pytorch_model.bin`](https://cdn.huggingface.co/dbmdz/bert-base-italian-xxl-uncased/pytorch_model.bin) • [`vocab.txt`](https://cdn.huggingface.co/dbmdz/bert-base-italian-xxl-uncased/vocab.txt)
-
-## Results
-
-For results on downstream tasks like NER or PoS tagging, please refer to
-[this repository](https://github.com/stefan-it/fine-tuned-berts-seq).
-
-## Usage
-
-With Transformers >= 2.3 our Italian BERT models can be loaded like:
-
-```python
-from transformers import AutoModel, AutoTokenizer
-
-tokenizer = AutoTokenizer.from_pretrained("dbmdz/bert-base-italian-cased")
-model = AutoModel.from_pretrained("dbmdz/bert-base-italian-cased")
-```
-
-To load the (recommended) Italian XXL BERT models, just use:
-
-```python
-from transformers import AutoModel, AutoTokenizer
-
-tokenizer = AutoTokenizer.from_pretrained("dbmdz/bert-base-italian-xxl-cased")
-model = AutoModel.from_pretrained("dbmdz/bert-base-italian-xxl-cased")
-```
-
-# Huggingface model hub
-
-All models are available on the [Huggingface model hub](https://huggingface.co/dbmdz).
-
-# Contact (Bugs, Feedback, Contribution and more)
-
-For questions about our BERT models just open an issue
-[here](https://github.com/dbmdz/berts/issues/new) 🤗
-
-# Acknowledgments
-
-Research supported with Cloud TPUs from Google's TensorFlow Research Cloud (TFRC).
-Thanks for providing access to the TFRC ❤️
-
-Thanks to the generous support from the [Hugging Face](https://huggingface.co/) team,
-it is possible to download both cased and uncased models from their S3 storage 🤗
diff --git a/server/transformers/model_cards/dbmdz/bert-base-italian-xxl-cased/README.md b/server/transformers/model_cards/dbmdz/bert-base-italian-xxl-cased/README.md
deleted file mode 100644
index 549c1133af281477b2b62101b39862cf010e8d2f..0000000000000000000000000000000000000000
--- a/server/transformers/model_cards/dbmdz/bert-base-italian-xxl-cased/README.md
+++ /dev/null
@@ -1,73 +0,0 @@
-# 🤗 + 📚 dbmdz BERT models
-
-In this repository the MDZ Digital Library team (dbmdz) at the Bavarian State
-Library open sources Italian BERT models 🎉
-
-# Italian BERT
-
-The source data for the Italian BERT model consists of a recent Wikipedia dump and
-various texts from the [OPUS corpora](http://opus.nlpl.eu/) collection. The final
-training corpus has a size of 13GB and 2,050,057,573 tokens.
-
-For sentence splitting, we use NLTK (faster compared to spacy).
-Our cased and uncased models are training with an initial sequence length of 512
-subwords for ~2-3M steps.
-
-For the XXL Italian models, we use the same training data from OPUS and extend
-it with data from the Italian part of the [OSCAR corpus](https://traces1.inria.fr/oscar/).
-Thus, the final training corpus has a size of 81GB and 13,138,379,147 tokens.
-
-## Model weights
-
-Currently only PyTorch-[Transformers](https://github.com/huggingface/transformers)
-compatible weights are available. If you need access to TensorFlow checkpoints,
-please raise an issue!
-
-| Model | Downloads
-| --------------------------------------- | ---------------------------------------------------------------------------------------------------------------
-| `dbmdz/bert-base-italian-cased` | [`config.json`](https://cdn.huggingface.co/dbmdz/bert-base-italian-cased/config.json) • [`pytorch_model.bin`](https://cdn.huggingface.co/dbmdz/bert-base-italian-cased/pytorch_model.bin) • [`vocab.txt`](https://cdn.huggingface.co/dbmdz/bert-base-italian-cased/vocab.txt)
-| `dbmdz/bert-base-italian-uncased` | [`config.json`](https://cdn.huggingface.co/dbmdz/bert-base-italian-uncased/config.json) • [`pytorch_model.bin`](https://cdn.huggingface.co/dbmdz/bert-base-italian-uncased/pytorch_model.bin) • [`vocab.txt`](https://cdn.huggingface.co/dbmdz/bert-base-italian-uncased/vocab.txt)
-| `dbmdz/bert-base-italian-xxl-cased` | [`config.json`](https://cdn.huggingface.co/dbmdz/bert-base-italian-xxl-cased/config.json) • [`pytorch_model.bin`](https://cdn.huggingface.co/dbmdz/bert-base-italian-xxl-cased/pytorch_model.bin) • [`vocab.txt`](https://cdn.huggingface.co/dbmdz/bert-base-italian-xxl-cased/vocab.txt)
-| `dbmdz/bert-base-italian-xxl-uncased` | [`config.json`](https://cdn.huggingface.co/dbmdz/bert-base-italian-xxl-uncased/config.json) • [`pytorch_model.bin`](https://cdn.huggingface.co/dbmdz/bert-base-italian-xxl-uncased/pytorch_model.bin) • [`vocab.txt`](https://cdn.huggingface.co/dbmdz/bert-base-italian-xxl-uncased/vocab.txt)
-
-## Results
-
-For results on downstream tasks like NER or PoS tagging, please refer to
-[this repository](https://github.com/stefan-it/fine-tuned-berts-seq).
-
-## Usage
-
-With Transformers >= 2.3 our Italian BERT models can be loaded like:
-
-```python
-from transformers import AutoModel, AutoTokenizer
-
-tokenizer = AutoTokenizer.from_pretrained("dbmdz/bert-base-italian-cased")
-model = AutoModel.from_pretrained("dbmdz/bert-base-italian-cased")
-```
-
-To load the (recommended) Italian XXL BERT models, just use:
-
-```python
-from transformers import AutoModel, AutoTokenizer
-
-tokenizer = AutoTokenizer.from_pretrained("dbmdz/bert-base-italian-xxl-cased")
-model = AutoModel.from_pretrained("dbmdz/bert-base-italian-xxl-cased")
-```
-
-# Huggingface model hub
-
-All models are available on the [Huggingface model hub](https://huggingface.co/dbmdz).
-
-# Contact (Bugs, Feedback, Contribution and more)
-
-For questions about our BERT models just open an issue
-[here](https://github.com/dbmdz/berts/issues/new) 🤗
-
-# Acknowledgments
-
-Research supported with Cloud TPUs from Google's TensorFlow Research Cloud (TFRC).
-Thanks for providing access to the TFRC ❤️
-
-Thanks to the generous support from the [Hugging Face](https://huggingface.co/) team,
-it is possible to download both cased and uncased models from their S3 storage 🤗
diff --git a/server/transformers/model_cards/dbmdz/bert-base-italian-xxl-uncased/README.md b/server/transformers/model_cards/dbmdz/bert-base-italian-xxl-uncased/README.md
deleted file mode 100644
index 549c1133af281477b2b62101b39862cf010e8d2f..0000000000000000000000000000000000000000
--- a/server/transformers/model_cards/dbmdz/bert-base-italian-xxl-uncased/README.md
+++ /dev/null
@@ -1,73 +0,0 @@
-# 🤗 + 📚 dbmdz BERT models
-
-In this repository the MDZ Digital Library team (dbmdz) at the Bavarian State
-Library open sources Italian BERT models 🎉
-
-# Italian BERT
-
-The source data for the Italian BERT model consists of a recent Wikipedia dump and
-various texts from the [OPUS corpora](http://opus.nlpl.eu/) collection. The final
-training corpus has a size of 13GB and 2,050,057,573 tokens.
-
-For sentence splitting, we use NLTK (faster compared to spacy).
-Our cased and uncased models are training with an initial sequence length of 512
-subwords for ~2-3M steps.
-
-For the XXL Italian models, we use the same training data from OPUS and extend
-it with data from the Italian part of the [OSCAR corpus](https://traces1.inria.fr/oscar/).
-Thus, the final training corpus has a size of 81GB and 13,138,379,147 tokens.
-
-## Model weights
-
-Currently only PyTorch-[Transformers](https://github.com/huggingface/transformers)
-compatible weights are available. If you need access to TensorFlow checkpoints,
-please raise an issue!
-
-| Model | Downloads
-| --------------------------------------- | ---------------------------------------------------------------------------------------------------------------
-| `dbmdz/bert-base-italian-cased` | [`config.json`](https://cdn.huggingface.co/dbmdz/bert-base-italian-cased/config.json) • [`pytorch_model.bin`](https://cdn.huggingface.co/dbmdz/bert-base-italian-cased/pytorch_model.bin) • [`vocab.txt`](https://cdn.huggingface.co/dbmdz/bert-base-italian-cased/vocab.txt)
-| `dbmdz/bert-base-italian-uncased` | [`config.json`](https://cdn.huggingface.co/dbmdz/bert-base-italian-uncased/config.json) • [`pytorch_model.bin`](https://cdn.huggingface.co/dbmdz/bert-base-italian-uncased/pytorch_model.bin) • [`vocab.txt`](https://cdn.huggingface.co/dbmdz/bert-base-italian-uncased/vocab.txt)
-| `dbmdz/bert-base-italian-xxl-cased` | [`config.json`](https://cdn.huggingface.co/dbmdz/bert-base-italian-xxl-cased/config.json) • [`pytorch_model.bin`](https://cdn.huggingface.co/dbmdz/bert-base-italian-xxl-cased/pytorch_model.bin) • [`vocab.txt`](https://cdn.huggingface.co/dbmdz/bert-base-italian-xxl-cased/vocab.txt)
-| `dbmdz/bert-base-italian-xxl-uncased` | [`config.json`](https://cdn.huggingface.co/dbmdz/bert-base-italian-xxl-uncased/config.json) • [`pytorch_model.bin`](https://cdn.huggingface.co/dbmdz/bert-base-italian-xxl-uncased/pytorch_model.bin) • [`vocab.txt`](https://cdn.huggingface.co/dbmdz/bert-base-italian-xxl-uncased/vocab.txt)
-
-## Results
-
-For results on downstream tasks like NER or PoS tagging, please refer to
-[this repository](https://github.com/stefan-it/fine-tuned-berts-seq).
-
-## Usage
-
-With Transformers >= 2.3 our Italian BERT models can be loaded like:
-
-```python
-from transformers import AutoModel, AutoTokenizer
-
-tokenizer = AutoTokenizer.from_pretrained("dbmdz/bert-base-italian-cased")
-model = AutoModel.from_pretrained("dbmdz/bert-base-italian-cased")
-```
-
-To load the (recommended) Italian XXL BERT models, just use:
-
-```python
-from transformers import AutoModel, AutoTokenizer
-
-tokenizer = AutoTokenizer.from_pretrained("dbmdz/bert-base-italian-xxl-cased")
-model = AutoModel.from_pretrained("dbmdz/bert-base-italian-xxl-cased")
-```
-
-# Huggingface model hub
-
-All models are available on the [Huggingface model hub](https://huggingface.co/dbmdz).
-
-# Contact (Bugs, Feedback, Contribution and more)
-
-For questions about our BERT models just open an issue
-[here](https://github.com/dbmdz/berts/issues/new) 🤗
-
-# Acknowledgments
-
-Research supported with Cloud TPUs from Google's TensorFlow Research Cloud (TFRC).
-Thanks for providing access to the TFRC ❤️
-
-Thanks to the generous support from the [Hugging Face](https://huggingface.co/) team,
-it is possible to download both cased and uncased models from their S3 storage 🤗
diff --git a/server/transformers/model_cards/henryk/bert-base-multilingual-cased-finetuned-dutch-squad2/README.md b/server/transformers/model_cards/henryk/bert-base-multilingual-cased-finetuned-dutch-squad2/README.md
deleted file mode 100644
index 3d366061a4cb3ff9ade87e83382813a7b5de0855..0000000000000000000000000000000000000000
--- a/server/transformers/model_cards/henryk/bert-base-multilingual-cased-finetuned-dutch-squad2/README.md
+++ /dev/null
@@ -1,46 +0,0 @@
-# Multilingual + Dutch SQuAD2.0
-
-This model is the multilingual model provided by the Google research team with a fine-tuned dutch Q&A downstream task.
-
-## Details of the language model(bert-base-multilingual-cased)
-
-Language model ([**bert-base-multilingual-cased**](https://github.com/google-research/bert/blob/master/multilingual.md)):
-12-layer, 768-hidden, 12-heads, 110M parameters.
-Trained on cased text in the top 104 languages with the largest Wikipedias.
-
-## Details of the downstream task - Dataset
-Using the `mtranslate` Python module, [**SQuAD2.0**](https://rajpurkar.github.io/SQuAD-explorer/) was machine-translated. In order to find the start tokens the direct translations of the answers were searched in the corresponding paragraphs. Since the answer could not always be found in the text, due to the different translations depending on the context (missing context in the pure answer), a loss of question-answer examples occurred. This is a potential problem where errors can occur in the data set (but in the end it was a quick and dirty solution that worked well enough for my task).
-
-| Dataset | # Q&A |
-| ---------------------- | ----- |
-| SQuAD2.0 Train | 130 K |
-| Dutch SQuAD2.0 Train | 99 K |
-| SQuAD2.0 Dev | 12 K |
-| Dutch SQuAD2.0 Dev | 10 K |
-
-## Model training
-
-The model was trained on a Tesla V100 GPU with the following command:
-
-```python
-export SQUAD_DIR=path/to/nl_squad
-
-python run_squad.py \
- --model_type bert \
- --model_name_or_path bert-base-multilingual-cased \
- --version_2_with_negative \
- --do_train \
- --do_eval \
- --train_file $SQUAD_DIR/train_nl-v2.0.json \
- --predict_file $SQUAD_DIR/dev_nl-v2.0.json \
- --per_gpu_train_batch_size 12 \
- --learning_rate 3e-5 \
- --num_train_epochs 2.0 \
- --max_seq_length 384 \
- --doc_stride 128 \
- --output_dir /tmp/output_dir/
-```
-
-**Results**:
-
-{'exact': **67.38**, 'f1': **71.36**}
\ No newline at end of file
diff --git a/server/transformers/model_cards/jplu/tf-camembert-base/README.md b/server/transformers/model_cards/jplu/tf-camembert-base/README.md
deleted file mode 100644
index be8e1380e83936540e872f6f061f28380422f423..0000000000000000000000000000000000000000
--- a/server/transformers/model_cards/jplu/tf-camembert-base/README.md
+++ /dev/null
@@ -1,31 +0,0 @@
-# Tensorflow CamemBERT
-
-In this repository you will find different versions of the CamemBERT model for Tensorflow.
-
-## CamemBERT
-
-[CamemBERT](https://camembert-model.fr/) is a state-of-the-art language model for French based on the RoBERTa architecture pretrained on the French subcorpus of the newly available multilingual corpus OSCAR.
-
-## Model Weights
-
-| Model | Downloads
-| -------------------------------- | ---------------------------------------------------------------------------------------------------------------
-| `jplu/tf-camembert-base` | [`config.json`](https://s3.amazonaws.com/models.huggingface.co/bert/jplu/tf-camembert-base/config.json) • [`tf_model.h5`](https://s3.amazonaws.com/models.huggingface.co/bert/jplu/tf-camembert-base/tf_model.h5)
-
-## Usage
-
-With Transformers >= 2.4 the Tensorflow models of CamemBERT can be loaded like:
-
-```python
-from transformers import TFCamembertModel
-
-model = TFCamembertModel.from_pretrained("jplu/tf-camembert-base")
-```
-
-## Huggingface model hub
-
-All models are available on the [Huggingface model hub](https://huggingface.co/jplu).
-
-## Acknowledgments
-
-Thanks to all the Huggingface team for the support and their amazing library!
diff --git a/server/transformers/model_cards/jplu/tf-xlm-roberta-base/README.md b/server/transformers/model_cards/jplu/tf-xlm-roberta-base/README.md
deleted file mode 100644
index 39569c71c9f83c5258ccc2c6a52de803decfbc38..0000000000000000000000000000000000000000
--- a/server/transformers/model_cards/jplu/tf-xlm-roberta-base/README.md
+++ /dev/null
@@ -1,36 +0,0 @@
-# Tensorflow XLM-RoBERTa
-
-In this repository you will find different versions of the XLM-RoBERTa model for Tensorflow.
-
-## XLM-RoBERTa
-
-[XLM-RoBERTa](https://ai.facebook.com/blog/-xlm-r-state-of-the-art-cross-lingual-understanding-through-self-supervision/) is a scaled cross lingual sentence encoder. It is trained on 2.5T of data across 100 languages data filtered from Common Crawl. XLM-R achieves state-of-the-arts results on multiple cross lingual benchmarks.
-
-## Model Weights
-
-| Model | Downloads
-| -------------------------------- | ---------------------------------------------------------------------------------------------------------------
-| `jplu/tf-xlm-roberta-base` | [`config.json`](https://s3.amazonaws.com/models.huggingface.co/bert/jplu/tf-xlm-roberta-base/config.json) • [`tf_model.h5`](https://s3.amazonaws.com/models.huggingface.co/bert/jplu/tf-xlm-roberta-base/tf_model.h5)
-| `jplu/tf-xlm-roberta-large` | [`config.json`](https://s3.amazonaws.com/models.huggingface.co/bert/jplu/tf-xlm-roberta-large/config.json) • [`tf_model.h5`](https://s3.amazonaws.com/models.huggingface.co/bert/jplu/tf-xlm-roberta-large/tf_model.h5)
-
-## Usage
-
-With Transformers >= 2.4 the Tensorflow models of XLM-RoBERTa can be loaded like:
-
-```python
-from transformers import TFXLMRobertaModel
-
-model = TFXLMRobertaModel.from_pretrained("jplu/tf-xlm-roberta-base")
-```
-Or
-```
-model = TFXLMRobertaModel.from_pretrained("jplu/tf-xlm-roberta-large")
-```
-
-## Huggingface model hub
-
-All models are available on the [Huggingface model hub](https://huggingface.co/jplu).
-
-## Acknowledgments
-
-Thanks to all the Huggingface team for the support and their amazing library!
diff --git a/server/transformers/model_cards/jplu/tf-xlm-roberta-large/README.md b/server/transformers/model_cards/jplu/tf-xlm-roberta-large/README.md
deleted file mode 100644
index 39569c71c9f83c5258ccc2c6a52de803decfbc38..0000000000000000000000000000000000000000
--- a/server/transformers/model_cards/jplu/tf-xlm-roberta-large/README.md
+++ /dev/null
@@ -1,36 +0,0 @@
-# Tensorflow XLM-RoBERTa
-
-In this repository you will find different versions of the XLM-RoBERTa model for Tensorflow.
-
-## XLM-RoBERTa
-
-[XLM-RoBERTa](https://ai.facebook.com/blog/-xlm-r-state-of-the-art-cross-lingual-understanding-through-self-supervision/) is a scaled cross lingual sentence encoder. It is trained on 2.5T of data across 100 languages data filtered from Common Crawl. XLM-R achieves state-of-the-arts results on multiple cross lingual benchmarks.
-
-## Model Weights
-
-| Model | Downloads
-| -------------------------------- | ---------------------------------------------------------------------------------------------------------------
-| `jplu/tf-xlm-roberta-base` | [`config.json`](https://s3.amazonaws.com/models.huggingface.co/bert/jplu/tf-xlm-roberta-base/config.json) • [`tf_model.h5`](https://s3.amazonaws.com/models.huggingface.co/bert/jplu/tf-xlm-roberta-base/tf_model.h5)
-| `jplu/tf-xlm-roberta-large` | [`config.json`](https://s3.amazonaws.com/models.huggingface.co/bert/jplu/tf-xlm-roberta-large/config.json) • [`tf_model.h5`](https://s3.amazonaws.com/models.huggingface.co/bert/jplu/tf-xlm-roberta-large/tf_model.h5)
-
-## Usage
-
-With Transformers >= 2.4 the Tensorflow models of XLM-RoBERTa can be loaded like:
-
-```python
-from transformers import TFXLMRobertaModel
-
-model = TFXLMRobertaModel.from_pretrained("jplu/tf-xlm-roberta-base")
-```
-Or
-```
-model = TFXLMRobertaModel.from_pretrained("jplu/tf-xlm-roberta-large")
-```
-
-## Huggingface model hub
-
-All models are available on the [Huggingface model hub](https://huggingface.co/jplu).
-
-## Acknowledgments
-
-Thanks to all the Huggingface team for the support and their amazing library!
diff --git a/server/transformers/model_cards/julien-c/bert-xsmall-dummy/README.md b/server/transformers/model_cards/julien-c/bert-xsmall-dummy/README.md
deleted file mode 100644
index 36eef6232722f15d84f08d414020550d1af36f9a..0000000000000000000000000000000000000000
--- a/server/transformers/model_cards/julien-c/bert-xsmall-dummy/README.md
+++ /dev/null
@@ -1,25 +0,0 @@
-## How to build a dummy model
-
-
-```python
-from transformers.configuration_bert import BertConfig
-from transformers.modeling_bert import BertForMaskedLM
-from transformers.modeling_tf_bert import TFBertForMaskedLM
-from transformers.tokenization_bert import BertTokenizer
-
-
-SMALL_MODEL_IDENTIFIER = "julien-c/bert-xsmall-dummy"
-DIRNAME = "./bert-xsmall-dummy"
-
-config = BertConfig(10, 20, 1, 1, 40)
-
-model = BertForMaskedLM(config)
-model.save_pretrained(DIRNAME)
-
-tf_model = TFBertForMaskedLM.from_pretrained(DIRNAME, from_pt=True)
-tf_model.save_pretrained(DIRNAME)
-
-# Slightly different for tokenizer.
-# tokenizer = BertTokenizer.from_pretrained(DIRNAME)
-# tokenizer.save_pretrained()
-```
diff --git a/server/transformers/model_cards/julien-c/dummy-unknown/README.md b/server/transformers/model_cards/julien-c/dummy-unknown/README.md
deleted file mode 100644
index 9cdc3d24375813a747b340b31ece2a24a9124f39..0000000000000000000000000000000000000000
--- a/server/transformers/model_cards/julien-c/dummy-unknown/README.md
+++ /dev/null
@@ -1,52 +0,0 @@
-
-```python
-import json
-import os
-from transformers.configuration_roberta import RobertaConfig
-from transformers import RobertaForMaskedLM, TFRobertaForMaskedLM
-
-DIRNAME = "./dummy-unknown"
-
-
-config = RobertaConfig(10, 20, 1, 1, 40)
-
-model = RobertaForMaskedLM(config)
-model.save_pretrained(DIRNAME)
-
-tf_model = TFRobertaForMaskedLM.from_pretrained(DIRNAME, from_pt=True)
-tf_model.save_pretrained(DIRNAME)
-
-# Tokenizer:
-
-vocab = [
- "l",
- "o",
- "w",
- "e",
- "r",
- "s",
- "t",
- "i",
- "d",
- "n",
- "\u0120",
- "\u0120l",
- "\u0120n",
- "\u0120lo",
- "\u0120low",
- "er",
- "\u0120lowest",
- "\u0120newer",
- "\u0120wider",
- "",
-]
-vocab_tokens = dict(zip(vocab, range(len(vocab))))
-merges = ["#version: 0.2", "\u0120 l", "\u0120l o", "\u0120lo w", "e r", ""]
-
-vocab_file = os.path.join(DIRNAME, "vocab.json")
-merges_file = os.path.join(DIRNAME, "merges.txt")
-with open(vocab_file, "w", encoding="utf-8") as fp:
- fp.write(json.dumps(vocab_tokens) + "\n")
-with open(merges_file, "w", encoding="utf-8") as fp:
- fp.write("\n".join(merges))
-```
diff --git a/server/transformers/notebooks/Comparing-PT-and-TF-models.ipynb b/server/transformers/notebooks/Comparing-PT-and-TF-models.ipynb
deleted file mode 100644
index 321c2ebe30e21531e894a8057e6c520736eb3b19..0000000000000000000000000000000000000000
--- a/server/transformers/notebooks/Comparing-PT-and-TF-models.ipynb
+++ /dev/null
@@ -1,1630 +0,0 @@
-{
- "cells": [
- {
- "cell_type": "markdown",
- "metadata": {},
- "source": [
- "# Pytorch to Tensorflow Conversion Test Notebook\n",
- "\n",
- "To run this notebook follow these steps, modifying the **Config** section as necessary:\n",
- "\n",
- "1. Point `pt_model_dir` to your local directory containing the pytorch Bert model to be converted.\n",
- "2. Point `tf_bert_dir` to your clone of Google's Bert implementation which can be found here: https://github.com/google-research/bert.\n",
- "\n",
- "Note: \n",
- "1. This feature currently only supports the base BERT models (uncased/cased).\n",
- "2. Tensorflow model will be dumped in `tf_model_dir`."
- ]
- },
- {
- "cell_type": "markdown",
- "metadata": {},
- "source": [
- "## Config"
- ]
- },
- {
- "cell_type": "code",
- "execution_count": 1,
- "metadata": {},
- "outputs": [],
- "source": [
- "import os\n",
- "import sys\n",
- "\n",
- "model_cls = 'BertModel'\n",
- "model_typ = 'bert-base-uncased'\n",
- "token_cls = 'BertTokenizer'\n",
- "max_seq = 12\n",
- "CLS = \"[CLS]\"\n",
- "SEP = \"[SEP]\"\n",
- "MASK = \"[MASK]\"\n",
- "CLS_IDX = 0\n",
- "layer_idxs = tuple(range(12))\n",
- "input_text = \"jim henson was a puppeteer\"\n",
- "\n",
- "pt_model_dir = \"/home/ubuntu/.pytorch-pretrained-BERT-cache/{}\".format(model_typ)\n",
- "tf_bert_dir = \"/home/ubuntu/bert\"\n",
- "\n",
- "pt_vocab_file = os.path.join(pt_model_dir, \"vocab.txt\")\n",
- "pt_init_ckpt = os.path.join(pt_model_dir, model_typ.replace(\"-\", \"_\") + \".bin\")\n",
- "tf_model_dir = os.path.join(pt_model_dir, 'tf')\n",
- "tf_vocab_file = os.path.join(tf_model_dir, \"vocab.txt\")\n",
- "tf_init_ckpt = os.path.join(tf_model_dir, model_typ.replace(\"-\", \"_\") + \".ckpt\")\n",
- "tf_config_file = os.path.join(tf_model_dir, \"bert_config.json\")\n",
- "\n",
- "if not os.path.isdir(tf_model_dir): \n",
- " os.makedirs(tf_model_dir, exist_ok=True)"
- ]
- },
- {
- "cell_type": "markdown",
- "metadata": {},
- "source": [
- "### Tokenization"
- ]
- },
- {
- "cell_type": "code",
- "execution_count": 2,
- "metadata": {},
- "outputs": [],
- "source": [
- "def tokenize(text, tokenizer):\n",
- " text = text.strip().lower()\n",
- " tok_ids = tokenizer.tokenize(text)\n",
- " if len(tok_ids) > max_seq - 2:\n",
- " tok_ids = tok_ids[:max_seq - 2]\n",
- " tok_ids.insert(CLS_IDX, CLS)\n",
- " tok_ids.append(SEP)\n",
- " input_ids = tokenizer.convert_tokens_to_ids(tok_ids)\n",
- " mask_ids = [1] * len(input_ids)\n",
- " seg_ids = [0] * len(input_ids)\n",
- " padding = [0] * (max_seq - len(input_ids))\n",
- " input_ids += padding\n",
- " mask_ids += padding\n",
- " seg_ids += padding\n",
- " return input_ids, mask_ids, seg_ids"
- ]
- },
- {
- "cell_type": "markdown",
- "metadata": {},
- "source": [
- "## Pytorch execution"
- ]
- },
- {
- "cell_type": "code",
- "execution_count": 3,
- "metadata": {},
- "outputs": [
- {
- "name": "stderr",
- "output_type": "stream",
- "text": [
- "100%|██████████| 231508/231508 [00:00<00:00, 41092464.26B/s]\n",
- "100%|██████████| 407873900/407873900 [00:07<00:00, 58092479.52B/s]\n"
- ]
- },
- {
- "name": "stdout",
- "output_type": "stream",
- "text": [
- "Pytorch embedding shape: (1, 768)\n"
- ]
- }
- ],
- "source": [
- "import numpy as np\n",
- "import torch\n",
- "from pytorch_pretrained_bert import (BertConfig,\n",
- " BertModel, \n",
- " BertTokenizer, \n",
- " BertForSequenceClassification)\n",
- "\n",
- "# Save Vocab\n",
- "pt_tokenizer = BertTokenizer.from_pretrained(\n",
- " pretrained_model_name_or_path=model_typ, \n",
- " cache_dir=pt_model_dir)\n",
- "pt_tokenizer.save_vocabulary(pt_model_dir)\n",
- "pt_tokenizer.save_vocabulary(tf_model_dir)\n",
- "\n",
- "# Save Model\n",
- "pt_model = BertModel.from_pretrained(\n",
- " pretrained_model_name_or_path=model_typ, \n",
- " cache_dir=pt_model_dir).to('cpu')\n",
- "pt_model.eval()\n",
- "pt_model.config.hidden_dropout_prob = 0.0\n",
- "pt_model.config.attention_probs_dropout_prob = 0.0\n",
- "pt_model.config.to_json_file(tf_config_file)\n",
- "torch.save(pt_model.state_dict(), pt_init_ckpt)\n",
- "\n",
- "# Inputs\n",
- "input_ids_pt, mask_ids_pt, seg_ids_pt = tokenize(input_text, pt_tokenizer)\n",
- "\n",
- "# PT Embedding\n",
- "tok_tensor = torch.tensor(input_ids_pt).to('cpu').unsqueeze(0)\n",
- "seg_tensor = torch.tensor(seg_ids_pt).to('cpu').unsqueeze(0)\n",
- "msk_tensor = torch.tensor(mask_ids_pt).to('cpu').unsqueeze(0)\n",
- "attn_blks, nsp_logits = pt_model(tok_tensor, seg_tensor, msk_tensor)\n",
- "pt_embedding = nsp_logits.detach().numpy() \n",
- "print(\"Pytorch embedding shape: {}\".format(pt_embedding.shape))"
- ]
- },
- {
- "cell_type": "markdown",
- "metadata": {},
- "source": [
- "## Pytorch → Tensorflow conversion"
- ]
- },
- {
- "cell_type": "code",
- "execution_count": 4,
- "metadata": {},
- "outputs": [
- {
- "name": "stdout",
- "output_type": "stream",
- "text": [
- "WARNING:tensorflow:From /home/ubuntu/anaconda3/envs/nlp/lib/python3.6/site-packages/tensorflow/python/framework/op_def_library.py:263: colocate_with (from tensorflow.python.framework.ops) is deprecated and will be removed in a future version.\n",
- "Instructions for updating:\n",
- "Colocations handled automatically by placer.\n",
- "bert/embeddings/word_embeddings initialized\n",
- "bert/embeddings/position_embeddings initialized\n",
- "bert/embeddings/token_type_embeddings initialized\n",
- "bert/embeddings/LayerNorm/gamma initialized\n",
- "bert/embeddings/LayerNorm/beta initialized\n",
- "bert/encoder/layer_0/attention/self/query/kernel initialized\n",
- "bert/encoder/layer_0/attention/self/query/bias initialized\n",
- "bert/encoder/layer_0/attention/self/key/kernel initialized\n",
- "bert/encoder/layer_0/attention/self/key/bias initialized\n",
- "bert/encoder/layer_0/attention/self/value/kernel initialized\n",
- "bert/encoder/layer_0/attention/self/value/bias initialized\n",
- "bert/encoder/layer_0/attention/output/dense/kernel initialized\n",
- "bert/encoder/layer_0/attention/output/dense/bias initialized\n",
- "bert/encoder/layer_0/attention/output/LayerNorm/gamma initialized\n",
- "bert/encoder/layer_0/attention/output/LayerNorm/beta initialized\n",
- "bert/encoder/layer_0/intermediate/dense/kernel initialized\n",
- "bert/encoder/layer_0/intermediate/dense/bias initialized\n",
- "bert/encoder/layer_0/output/dense/kernel initialized\n",
- "bert/encoder/layer_0/output/dense/bias initialized\n",
- "bert/encoder/layer_0/output/LayerNorm/gamma initialized\n",
- "bert/encoder/layer_0/output/LayerNorm/beta initialized\n",
- "bert/encoder/layer_1/attention/self/query/kernel initialized\n",
- "bert/encoder/layer_1/attention/self/query/bias initialized\n",
- "bert/encoder/layer_1/attention/self/key/kernel initialized\n",
- "bert/encoder/layer_1/attention/self/key/bias initialized\n",
- "bert/encoder/layer_1/attention/self/value/kernel initialized\n",
- "bert/encoder/layer_1/attention/self/value/bias initialized\n",
- "bert/encoder/layer_1/attention/output/dense/kernel initialized\n",
- "bert/encoder/layer_1/attention/output/dense/bias initialized\n",
- "bert/encoder/layer_1/attention/output/LayerNorm/gamma initialized\n",
- "bert/encoder/layer_1/attention/output/LayerNorm/beta initialized\n",
- "bert/encoder/layer_1/intermediate/dense/kernel initialized\n",
- "bert/encoder/layer_1/intermediate/dense/bias initialized\n",
- "bert/encoder/layer_1/output/dense/kernel initialized\n",
- "bert/encoder/layer_1/output/dense/bias initialized\n",
- "bert/encoder/layer_1/output/LayerNorm/gamma initialized\n",
- "bert/encoder/layer_1/output/LayerNorm/beta initialized\n",
- "bert/encoder/layer_2/attention/self/query/kernel initialized\n",
- "bert/encoder/layer_2/attention/self/query/bias initialized\n",
- "bert/encoder/layer_2/attention/self/key/kernel initialized\n",
- "bert/encoder/layer_2/attention/self/key/bias initialized\n",
- "bert/encoder/layer_2/attention/self/value/kernel initialized\n",
- "bert/encoder/layer_2/attention/self/value/bias initialized\n",
- "bert/encoder/layer_2/attention/output/dense/kernel initialized\n",
- "bert/encoder/layer_2/attention/output/dense/bias initialized\n",
- "bert/encoder/layer_2/attention/output/LayerNorm/gamma initialized\n",
- "bert/encoder/layer_2/attention/output/LayerNorm/beta initialized\n",
- "bert/encoder/layer_2/intermediate/dense/kernel initialized\n",
- "bert/encoder/layer_2/intermediate/dense/bias initialized\n",
- "bert/encoder/layer_2/output/dense/kernel initialized\n",
- "bert/encoder/layer_2/output/dense/bias initialized\n",
- "bert/encoder/layer_2/output/LayerNorm/gamma initialized\n",
- "bert/encoder/layer_2/output/LayerNorm/beta initialized\n",
- "bert/encoder/layer_3/attention/self/query/kernel initialized\n",
- "bert/encoder/layer_3/attention/self/query/bias initialized\n",
- "bert/encoder/layer_3/attention/self/key/kernel initialized\n",
- "bert/encoder/layer_3/attention/self/key/bias initialized\n",
- "bert/encoder/layer_3/attention/self/value/kernel initialized\n",
- "bert/encoder/layer_3/attention/self/value/bias initialized\n",
- "bert/encoder/layer_3/attention/output/dense/kernel initialized\n",
- "bert/encoder/layer_3/attention/output/dense/bias initialized\n",
- "bert/encoder/layer_3/attention/output/LayerNorm/gamma initialized\n",
- "bert/encoder/layer_3/attention/output/LayerNorm/beta initialized\n",
- "bert/encoder/layer_3/intermediate/dense/kernel initialized\n",
- "bert/encoder/layer_3/intermediate/dense/bias initialized\n",
- "bert/encoder/layer_3/output/dense/kernel initialized\n",
- "bert/encoder/layer_3/output/dense/bias initialized\n",
- "bert/encoder/layer_3/output/LayerNorm/gamma initialized\n",
- "bert/encoder/layer_3/output/LayerNorm/beta initialized\n",
- "bert/encoder/layer_4/attention/self/query/kernel initialized\n",
- "bert/encoder/layer_4/attention/self/query/bias initialized\n",
- "bert/encoder/layer_4/attention/self/key/kernel initialized\n",
- "bert/encoder/layer_4/attention/self/key/bias initialized\n",
- "bert/encoder/layer_4/attention/self/value/kernel initialized\n",
- "bert/encoder/layer_4/attention/self/value/bias initialized\n",
- "bert/encoder/layer_4/attention/output/dense/kernel initialized\n",
- "bert/encoder/layer_4/attention/output/dense/bias initialized\n",
- "bert/encoder/layer_4/attention/output/LayerNorm/gamma initialized\n",
- "bert/encoder/layer_4/attention/output/LayerNorm/beta initialized\n",
- "bert/encoder/layer_4/intermediate/dense/kernel initialized\n",
- "bert/encoder/layer_4/intermediate/dense/bias initialized\n",
- "bert/encoder/layer_4/output/dense/kernel initialized\n",
- "bert/encoder/layer_4/output/dense/bias initialized\n",
- "bert/encoder/layer_4/output/LayerNorm/gamma initialized\n",
- "bert/encoder/layer_4/output/LayerNorm/beta initialized\n",
- "bert/encoder/layer_5/attention/self/query/kernel initialized\n",
- "bert/encoder/layer_5/attention/self/query/bias initialized\n",
- "bert/encoder/layer_5/attention/self/key/kernel initialized\n",
- "bert/encoder/layer_5/attention/self/key/bias initialized\n",
- "bert/encoder/layer_5/attention/self/value/kernel initialized\n",
- "bert/encoder/layer_5/attention/self/value/bias initialized\n",
- "bert/encoder/layer_5/attention/output/dense/kernel initialized\n",
- "bert/encoder/layer_5/attention/output/dense/bias initialized\n",
- "bert/encoder/layer_5/attention/output/LayerNorm/gamma initialized\n",
- "bert/encoder/layer_5/attention/output/LayerNorm/beta initialized\n",
- "bert/encoder/layer_5/intermediate/dense/kernel initialized\n",
- "bert/encoder/layer_5/intermediate/dense/bias initialized\n",
- "bert/encoder/layer_5/output/dense/kernel initialized\n",
- "bert/encoder/layer_5/output/dense/bias initialized\n",
- "bert/encoder/layer_5/output/LayerNorm/gamma initialized\n",
- "bert/encoder/layer_5/output/LayerNorm/beta initialized\n",
- "bert/encoder/layer_6/attention/self/query/kernel initialized\n",
- "bert/encoder/layer_6/attention/self/query/bias initialized\n",
- "bert/encoder/layer_6/attention/self/key/kernel initialized\n",
- "bert/encoder/layer_6/attention/self/key/bias initialized\n",
- "bert/encoder/layer_6/attention/self/value/kernel initialized\n",
- "bert/encoder/layer_6/attention/self/value/bias initialized\n",
- "bert/encoder/layer_6/attention/output/dense/kernel initialized\n",
- "bert/encoder/layer_6/attention/output/dense/bias initialized\n",
- "bert/encoder/layer_6/attention/output/LayerNorm/gamma initialized\n",
- "bert/encoder/layer_6/attention/output/LayerNorm/beta initialized\n",
- "bert/encoder/layer_6/intermediate/dense/kernel initialized\n",
- "bert/encoder/layer_6/intermediate/dense/bias initialized\n",
- "bert/encoder/layer_6/output/dense/kernel initialized\n",
- "bert/encoder/layer_6/output/dense/bias initialized\n",
- "bert/encoder/layer_6/output/LayerNorm/gamma initialized\n",
- "bert/encoder/layer_6/output/LayerNorm/beta initialized\n",
- "bert/encoder/layer_7/attention/self/query/kernel initialized\n",
- "bert/encoder/layer_7/attention/self/query/bias initialized\n",
- "bert/encoder/layer_7/attention/self/key/kernel initialized\n",
- "bert/encoder/layer_7/attention/self/key/bias initialized\n",
- "bert/encoder/layer_7/attention/self/value/kernel initialized\n",
- "bert/encoder/layer_7/attention/self/value/bias initialized\n",
- "bert/encoder/layer_7/attention/output/dense/kernel initialized\n",
- "bert/encoder/layer_7/attention/output/dense/bias initialized\n",
- "bert/encoder/layer_7/attention/output/LayerNorm/gamma initialized\n",
- "bert/encoder/layer_7/attention/output/LayerNorm/beta initialized\n",
- "bert/encoder/layer_7/intermediate/dense/kernel initialized\n",
- "bert/encoder/layer_7/intermediate/dense/bias initialized\n",
- "bert/encoder/layer_7/output/dense/kernel initialized\n",
- "bert/encoder/layer_7/output/dense/bias initialized\n",
- "bert/encoder/layer_7/output/LayerNorm/gamma initialized\n",
- "bert/encoder/layer_7/output/LayerNorm/beta initialized\n",
- "bert/encoder/layer_8/attention/self/query/kernel initialized\n",
- "bert/encoder/layer_8/attention/self/query/bias initialized\n",
- "bert/encoder/layer_8/attention/self/key/kernel initialized\n",
- "bert/encoder/layer_8/attention/self/key/bias initialized\n",
- "bert/encoder/layer_8/attention/self/value/kernel initialized\n",
- "bert/encoder/layer_8/attention/self/value/bias initialized\n",
- "bert/encoder/layer_8/attention/output/dense/kernel initialized\n",
- "bert/encoder/layer_8/attention/output/dense/bias initialized\n",
- "bert/encoder/layer_8/attention/output/LayerNorm/gamma initialized\n",
- "bert/encoder/layer_8/attention/output/LayerNorm/beta initialized\n",
- "bert/encoder/layer_8/intermediate/dense/kernel initialized\n",
- "bert/encoder/layer_8/intermediate/dense/bias initialized\n",
- "bert/encoder/layer_8/output/dense/kernel initialized\n",
- "bert/encoder/layer_8/output/dense/bias initialized\n",
- "bert/encoder/layer_8/output/LayerNorm/gamma initialized\n",
- "bert/encoder/layer_8/output/LayerNorm/beta initialized\n",
- "bert/encoder/layer_9/attention/self/query/kernel initialized\n",
- "bert/encoder/layer_9/attention/self/query/bias initialized\n",
- "bert/encoder/layer_9/attention/self/key/kernel initialized\n",
- "bert/encoder/layer_9/attention/self/key/bias initialized\n",
- "bert/encoder/layer_9/attention/self/value/kernel initialized\n",
- "bert/encoder/layer_9/attention/self/value/bias initialized\n",
- "bert/encoder/layer_9/attention/output/dense/kernel initialized\n",
- "bert/encoder/layer_9/attention/output/dense/bias initialized\n",
- "bert/encoder/layer_9/attention/output/LayerNorm/gamma initialized\n",
- "bert/encoder/layer_9/attention/output/LayerNorm/beta initialized\n",
- "bert/encoder/layer_9/intermediate/dense/kernel initialized\n",
- "bert/encoder/layer_9/intermediate/dense/bias initialized\n",
- "bert/encoder/layer_9/output/dense/kernel initialized\n",
- "bert/encoder/layer_9/output/dense/bias initialized\n",
- "bert/encoder/layer_9/output/LayerNorm/gamma initialized\n",
- "bert/encoder/layer_9/output/LayerNorm/beta initialized\n",
- "bert/encoder/layer_10/attention/self/query/kernel initialized\n",
- "bert/encoder/layer_10/attention/self/query/bias initialized\n",
- "bert/encoder/layer_10/attention/self/key/kernel initialized\n",
- "bert/encoder/layer_10/attention/self/key/bias initialized\n",
- "bert/encoder/layer_10/attention/self/value/kernel initialized\n",
- "bert/encoder/layer_10/attention/self/value/bias initialized\n",
- "bert/encoder/layer_10/attention/output/dense/kernel initialized\n",
- "bert/encoder/layer_10/attention/output/dense/bias initialized\n",
- "bert/encoder/layer_10/attention/output/LayerNorm/gamma initialized\n",
- "bert/encoder/layer_10/attention/output/LayerNorm/beta initialized\n",
- "bert/encoder/layer_10/intermediate/dense/kernel initialized\n",
- "bert/encoder/layer_10/intermediate/dense/bias initialized\n",
- "bert/encoder/layer_10/output/dense/kernel initialized\n",
- "bert/encoder/layer_10/output/dense/bias initialized\n",
- "bert/encoder/layer_10/output/LayerNorm/gamma initialized\n",
- "bert/encoder/layer_10/output/LayerNorm/beta initialized\n",
- "bert/encoder/layer_11/attention/self/query/kernel initialized\n",
- "bert/encoder/layer_11/attention/self/query/bias initialized\n",
- "bert/encoder/layer_11/attention/self/key/kernel initialized\n",
- "bert/encoder/layer_11/attention/self/key/bias initialized\n",
- "bert/encoder/layer_11/attention/self/value/kernel initialized\n",
- "bert/encoder/layer_11/attention/self/value/bias initialized\n",
- "bert/encoder/layer_11/attention/output/dense/kernel initialized\n",
- "bert/encoder/layer_11/attention/output/dense/bias initialized\n",
- "bert/encoder/layer_11/attention/output/LayerNorm/gamma initialized\n",
- "bert/encoder/layer_11/attention/output/LayerNorm/beta initialized\n",
- "bert/encoder/layer_11/intermediate/dense/kernel initialized\n",
- "bert/encoder/layer_11/intermediate/dense/bias initialized\n",
- "bert/encoder/layer_11/output/dense/kernel initialized\n",
- "bert/encoder/layer_11/output/dense/bias initialized\n",
- "bert/encoder/layer_11/output/LayerNorm/gamma initialized\n",
- "bert/encoder/layer_11/output/LayerNorm/beta initialized\n",
- "bert/pooler/dense/kernel initialized\n",
- "bert/pooler/dense/bias initialized\n"
- ]
- }
- ],
- "source": [
- "from pytorch_pretrained_bert.convert_pytorch_checkpoint_to_tf import main\n",
- "\n",
- "main([\n",
- " '--model_name', model_typ, \n",
- " '--pytorch_model_path', pt_init_ckpt,\n",
- " '--tf_cache_dir', tf_model_dir,\n",
- " '--cache_dir', pt_model_dir\n",
- "])"
- ]
- },
- {
- "cell_type": "markdown",
- "metadata": {},
- "source": [
- "## Tensorflow execution"
- ]
- },
- {
- "cell_type": "code",
- "execution_count": 5,
- "metadata": {},
- "outputs": [
- {
- "name": "stdout",
- "output_type": "stream",
- "text": [
- "\n",
- "WARNING: The TensorFlow contrib module will not be included in TensorFlow 2.0.\n",
- "For more information, please see:\n",
- " * https://github.com/tensorflow/community/blob/master/rfcs/20180907-contrib-sunset.md\n",
- " * https://github.com/tensorflow/addons\n",
- "If you depend on functionality not listed there, please file an issue.\n",
- "\n",
- "WARNING:tensorflow:From /home/ubuntu/bert/modeling.py:671: dense (from tensorflow.python.layers.core) is deprecated and will be removed in a future version.\n",
- "Instructions for updating:\n",
- "Use keras.layers.dense instead.\n",
- "WARNING:tensorflow:From /home/ubuntu/anaconda3/envs/nlp/lib/python3.6/site-packages/tensorflow/python/training/saver.py:1266: checkpoint_exists (from tensorflow.python.training.checkpoint_management) is deprecated and will be removed in a future version.\n",
- "Instructions for updating:\n",
- "Use standard file APIs to check for files with this prefix.\n",
- "INFO:tensorflow:Restoring parameters from /home/ubuntu/.pytorch-pretrained-BERT-cache/bert-base-uncased/tf/bert_base_uncased.ckpt\n",
- "Tensorflow embedding shape: (1, 768)\n"
- ]
- }
- ],
- "source": [
- "import tensorflow as tf\n",
- "sys.path.insert(0, tf_bert_dir)\n",
- "import modeling\n",
- "import tokenization\n",
- "\n",
- "tf.reset_default_graph()\n",
- "\n",
- "# Process text\n",
- "tf_tokenizer = tokenization.FullTokenizer(vocab_file=tf_vocab_file)\n",
- "\n",
- "# Graph inputs\n",
- "input_ids_tf, mask_ids_tf, seg_ids_tf = tokenize(input_text, tf_tokenizer)\n",
- "config = modeling.BertConfig.from_json_file(\n",
- " os.path.join(tf_model_dir, 'bert_config.json'))\n",
- "input_tensor = tf.placeholder(\n",
- " dtype=tf.int32,\n",
- " shape=[1, None],\n",
- " name='input_ids')\n",
- "mask_tensor = tf.placeholder(\n",
- " dtype=tf.int32,\n",
- " shape=[1, None],\n",
- " name='mask_ids')\n",
- "seg_tensor = tf.placeholder(\n",
- " dtype=tf.int32,\n",
- " shape=[1, None],\n",
- " name='seg_ids')\n",
- "tf_model = modeling.BertModel(\n",
- " config=config,\n",
- " is_training=False,\n",
- " input_ids=input_tensor,\n",
- " input_mask=mask_tensor,\n",
- " token_type_ids=seg_tensor,\n",
- " use_one_hot_embeddings=False)\n",
- "output_layer = tf_model.get_pooled_output()\n",
- "\n",
- "# Load tf model\n",
- "session = tf.Session(config=tf.ConfigProto(allow_soft_placement=True))\n",
- "vars_to_load = [v for v in tf.global_variables()]\n",
- "session.run(tf.variables_initializer(var_list=vars_to_load))\n",
- "saver = tf.train.Saver(vars_to_load)\n",
- "saver.restore(session, save_path=tf_init_ckpt)\n",
- "\n",
- "# TF Embedding\n",
- "fetches = output_layer\n",
- "feed_dict = {\n",
- " input_tensor: [input_ids_tf],\n",
- " mask_tensor: [mask_ids_tf],\n",
- " seg_tensor: [seg_ids_tf]\n",
- "}\n",
- "tf_embedding = session.run(fetches=fetches, feed_dict=feed_dict)\n",
- "print(\"Tensorflow embedding shape: {}\".format(tf_embedding.shape))"
- ]
- },
- {
- "cell_type": "markdown",
- "metadata": {},
- "source": [
- "## Compare Tokenization"
- ]
- },
- {
- "cell_type": "code",
- "execution_count": 6,
- "metadata": {},
- "outputs": [
- {
- "name": "stdout",
- "output_type": "stream",
- "text": [
- "TOKEN_IDS_PT: [101, 3958, 27227, 2001, 1037, 13997, 11510, 102, 0, 0, 0, 0]\n",
- "TOKEN_IDS_TF: [101, 3958, 27227, 2001, 1037, 13997, 11510, 102, 0, 0, 0, 0]\n",
- "SEG_IDS_PT: [0, 0, 0, 0, 0, 0, 0, 0, 0, 0, 0, 0]\n",
- "SEG_IDS_TF: [0, 0, 0, 0, 0, 0, 0, 0, 0, 0, 0, 0]\n",
- "MASK_IDS_PT: [1, 1, 1, 1, 1, 1, 1, 1, 0, 0, 0, 0]\n",
- "MASK_IDS_TF: [1, 1, 1, 1, 1, 1, 1, 1, 0, 0, 0, 0]\n"
- ]
- }
- ],
- "source": [
- "print(\"TOKEN_IDS_PT: {}\".format(input_ids_pt))\n",
- "print(\"TOKEN_IDS_TF: {}\".format(input_ids_tf))\n",
- "print(\"SEG_IDS_PT: {}\".format(seg_ids_pt))\n",
- "print(\"SEG_IDS_TF: {}\".format(seg_ids_tf))\n",
- "print(\"MASK_IDS_PT: {}\".format(mask_ids_pt))\n",
- "print(\"MASK_IDS_TF: {}\".format(mask_ids_tf))"
- ]
- },
- {
- "cell_type": "markdown",
- "metadata": {},
- "source": [
- "## Compare Model Weights"
- ]
- },
- {
- "cell_type": "code",
- "execution_count": 7,
- "metadata": {},
- "outputs": [
- {
- "name": "stdout",
- "output_type": "stream",
- "text": [
- "bert/embeddings/word_embeddings\n",
- "|sum(pt_wts - tf_wts)| = 0.0\n",
- "PT: shape: (30522, 768) values: [-0.01018257 -0.06154883 -0.02649689 -0.0420608 0.00116716]\n",
- "TF: shape: (30522, 768) values: [-0.01018257 -0.06154883 -0.02649689 -0.0420608 0.00116716]\n",
- "\n",
- "bert/embeddings/token_type_embeddings\n",
- "|sum(pt_wts - tf_wts)| = 0.0\n",
- "PT: shape: (2, 768) values: [0.00043164 0.01098826 0.00370439 0.00150542 0.00057812]\n",
- "TF: shape: (2, 768) values: [0.00043164 0.01098826 0.00370439 0.00150542 0.00057812]\n",
- "\n",
- "bert/embeddings/position_embeddings\n",
- "|sum(pt_wts - tf_wts)| = 0.0\n",
- "PT: shape: (512, 768) values: [ 0.01750538 -0.02563101 -0.03664156 -0.02528613 0.00797095]\n",
- "TF: shape: (512, 768) values: [ 0.01750538 -0.02563101 -0.03664156 -0.02528613 0.00797095]\n",
- "\n",
- "bert/embeddings/LayerNorm/beta\n",
- "|sum(pt_wts - tf_wts)| = 0.0\n",
- "PT: shape: (768,) values: [-0.02591471 -0.0195513 0.02423946 0.08904593 -0.06281059]\n",
- "TF: shape: (768,) values: [-0.02591471 -0.0195513 0.02423946 0.08904593 -0.06281059]\n",
- "\n",
- "bert/embeddings/LayerNorm/gamma\n",
- "|sum(pt_wts - tf_wts)| = 0.0\n",
- "PT: shape: (768,) values: [0.9260566 0.8851115 0.85807985 0.8616906 0.8937205 ]\n",
- "TF: shape: (768,) values: [0.9260566 0.8851115 0.85807985 0.8616906 0.8937205 ]\n",
- "\n",
- "bert/encoder/layer_0/attention/self/query/kernel\n",
- "|sum(pt_wts - tf_wts)| = 0.0\n",
- "PT: shape: (768, 768) values: [-0.01640572 -0.03257025 0.01046295 -0.04442816 -0.02256124]\n",
- "TF: shape: (768, 768) values: [-0.01640572 -0.03257025 0.01046295 -0.04442816 -0.02256124]\n",
- "\n",
- "bert/encoder/layer_0/attention/self/query/bias\n",
- "|sum(pt_wts - tf_wts)| = 0.0\n",
- "PT: shape: (768,) values: [ 0.58488506 -0.3312432 -0.43010172 0.37446147 -0.29811692]\n",
- "TF: shape: (768,) values: [ 0.58488506 -0.3312432 -0.43010172 0.37446147 -0.29811692]\n",
- "\n",
- "bert/encoder/layer_0/attention/self/key/kernel\n",
- "|sum(pt_wts - tf_wts)| = 0.0\n",
- "PT: shape: (768, 768) values: [ 0.00807745 0.02652155 -0.01866494 0.01797846 0.00450485]\n",
- "TF: shape: (768, 768) values: [ 0.00807745 0.02652155 -0.01866494 0.01797846 0.00450485]\n",
- "\n",
- "bert/encoder/layer_0/attention/self/key/bias\n",
- "|sum(pt_wts - tf_wts)| = 0.0\n",
- "PT: shape: (768,) values: [ 0.00104306 0.00035106 -0.0024626 -0.00010567 -0.00119283]\n",
- "TF: shape: (768,) values: [ 0.00104306 0.00035106 -0.0024626 -0.00010567 -0.00119283]\n",
- "\n",
- "bert/encoder/layer_0/attention/self/value/kernel\n",
- "|sum(pt_wts - tf_wts)| = 0.0\n",
- "PT: shape: (768, 768) values: [ 0.01144261 -0.02663044 0.01911472 -0.02206182 -0.00287949]\n",
- "TF: shape: (768, 768) values: [ 0.01144261 -0.02663044 0.01911472 -0.02206182 -0.00287949]\n",
- "\n",
- "bert/encoder/layer_0/attention/self/value/bias\n",
- "|sum(pt_wts - tf_wts)| = 0.0\n",
- "PT: shape: (768,) values: [-0.01184616 -0.01596605 -0.00251847 0.01736802 0.00449983]\n",
- "TF: shape: (768,) values: [-0.01184616 -0.01596605 -0.00251847 0.01736802 0.00449983]\n",
- "\n",
- "bert/encoder/layer_0/attention/output/dense/kernel\n",
- "|sum(pt_wts - tf_wts)| = 0.0\n",
- "PT: shape: (768, 768) values: [ 0.00581949 0.03170148 -0.06135742 -0.01706108 -0.00759045]\n",
- "TF: shape: (768, 768) values: [ 0.00581949 0.03170148 -0.06135742 -0.01706108 -0.00759045]\n",
- "\n",
- "bert/encoder/layer_0/attention/output/dense/bias\n",
- "|sum(pt_wts - tf_wts)| = 0.0\n",
- "PT: shape: (768,) values: [ 0.00511063 -0.0166625 0.02812938 -0.01166061 0.01942627]\n",
- "TF: shape: (768,) values: [ 0.00511063 -0.0166625 0.02812938 -0.01166061 0.01942627]\n",
- "\n",
- "bert/encoder/layer_0/attention/output/LayerNorm/beta\n",
- "|sum(pt_wts - tf_wts)| = 0.0\n",
- "PT: shape: (768,) values: [ 0.25779155 -0.03077853 -0.2772697 -0.38847703 0.36841765]\n",
- "TF: shape: (768,) values: [ 0.25779155 -0.03077853 -0.2772697 -0.38847703 0.36841765]\n",
- "\n",
- "bert/encoder/layer_0/attention/output/LayerNorm/gamma\n",
- "|sum(pt_wts - tf_wts)| = 0.0\n",
- "PT: shape: (768,) values: [0.9803408 0.959969 0.96368986 0.9603653 0.9801324 ]\n",
- "TF: shape: (768,) values: [0.9803408 0.959969 0.96368986 0.9603653 0.9801324 ]\n",
- "\n",
- "bert/encoder/layer_0/intermediate/dense/kernel\n",
- "|sum(pt_wts - tf_wts)| = 0.0\n",
- "PT: shape: (768, 3072) values: [-0.01010427 -0.060398 -0.01468864 0.00311493 0.02862451]\n",
- "TF: shape: (768, 3072) values: [-0.01010427 -0.060398 -0.01468864 0.00311493 0.02862451]\n",
- "\n",
- "bert/encoder/layer_0/intermediate/dense/bias\n",
- "|sum(pt_wts - tf_wts)| = 0.0\n",
- "PT: shape: (3072,) values: [-0.11498757 -0.09629171 -0.12399033 -0.129036 -0.06369043]\n",
- "TF: shape: (3072,) values: [-0.11498757 -0.09629171 -0.12399033 -0.129036 -0.06369043]\n",
- "\n",
- "bert/encoder/layer_0/output/dense/kernel\n",
- "|sum(pt_wts - tf_wts)| = 0.0\n",
- "PT: shape: (3072, 768) values: [-0.03710171 0.0648794 0.00758566 -0.05224452 -0.04348791]\n",
- "TF: shape: (3072, 768) values: [-0.03710171 0.0648794 0.00758566 -0.05224452 -0.04348791]\n",
- "\n",
- "bert/encoder/layer_0/output/dense/bias\n",
- "|sum(pt_wts - tf_wts)| = 0.0\n",
- "PT: shape: (768,) values: [-0.04801027 0.19766568 0.02154854 0.02880666 0.0444298 ]\n",
- "TF: shape: (768,) values: [-0.04801027 0.19766568 0.02154854 0.02880666 0.0444298 ]\n",
- "\n",
- "bert/encoder/layer_0/output/LayerNorm/beta\n",
- "|sum(pt_wts - tf_wts)| = 0.0\n",
- "PT: shape: (768,) values: [-0.10142924 -0.00499344 0.04274083 0.09324206 -0.10700516]\n",
- "TF: shape: (768,) values: [-0.10142924 -0.00499344 0.04274083 0.09324206 -0.10700516]\n",
- "\n",
- "bert/encoder/layer_0/output/LayerNorm/gamma\n",
- "|sum(pt_wts - tf_wts)| = 0.0\n",
- "PT: shape: (768,) values: [0.7835125 0.8072406 0.7670588 0.73706394 0.76303864]\n",
- "TF: shape: (768,) values: [0.7835125 0.8072406 0.7670588 0.73706394 0.76303864]\n",
- "\n",
- "bert/encoder/layer_1/attention/self/query/kernel\n",
- "|sum(pt_wts - tf_wts)| = 0.0\n",
- "PT: shape: (768, 768) values: [ 0.03132744 -0.01340016 -0.07761582 0.0655639 -0.00337808]\n",
- "TF: shape: (768, 768) values: [ 0.03132744 -0.01340016 -0.07761582 0.0655639 -0.00337808]\n",
- "\n",
- "bert/encoder/layer_1/attention/self/query/bias\n",
- "|sum(pt_wts - tf_wts)| = 0.0\n",
- "PT: shape: (768,) values: [-0.27827993 0.17387655 -0.2497937 -0.8809636 0.41262135]\n",
- "TF: shape: (768,) values: [-0.27827993 0.17387655 -0.2497937 -0.8809636 0.41262135]\n",
- "\n",
- "bert/encoder/layer_1/attention/self/key/kernel\n",
- "|sum(pt_wts - tf_wts)| = 0.0\n",
- "PT: shape: (768, 768) values: [-0.03353037 0.04007257 0.05320328 -0.02166729 -0.03581231]\n",
- "TF: shape: (768, 768) values: [-0.03353037 0.04007257 0.05320328 -0.02166729 -0.03581231]\n",
- "\n",
- "bert/encoder/layer_1/attention/self/key/bias\n",
- "|sum(pt_wts - tf_wts)| = 0.0\n",
- "PT: shape: (768,) values: [-0.00504407 0.00136887 -0.00394336 0.00646125 -0.00148919]\n",
- "TF: shape: (768,) values: [-0.00504407 0.00136887 -0.00394336 0.00646125 -0.00148919]\n",
- "\n",
- "bert/encoder/layer_1/attention/self/value/kernel\n",
- "|sum(pt_wts - tf_wts)| = 0.0\n",
- "PT: shape: (768, 768) values: [-0.00464159 0.06674305 -0.00970626 -0.0276653 -0.01597566]\n",
- "TF: shape: (768, 768) values: [-0.00464159 0.06674305 -0.00970626 -0.0276653 -0.01597566]\n",
- "\n",
- "bert/encoder/layer_1/attention/self/value/bias\n",
- "|sum(pt_wts - tf_wts)| = 0.0\n",
- "PT: shape: (768,) values: [ 0.00381288 0.02650839 -0.0059689 -0.00508269 -0.01293722]\n",
- "TF: shape: (768,) values: [ 0.00381288 0.02650839 -0.0059689 -0.00508269 -0.01293722]\n",
- "\n",
- "bert/encoder/layer_1/attention/output/dense/kernel\n",
- "|sum(pt_wts - tf_wts)| = 0.0\n",
- "PT: shape: (768, 768) values: [-0.01390745 -0.01100563 0.01303005 -0.01969771 0.0125082 ]\n",
- "TF: shape: (768, 768) values: [-0.01390745 -0.01100563 0.01303005 -0.01969771 0.0125082 ]\n",
- "\n",
- "bert/encoder/layer_1/attention/output/dense/bias\n",
- "|sum(pt_wts - tf_wts)| = 0.0\n",
- "PT: shape: (768,) values: [0.02946591 0.05715097 0.01293636 0.01920356 0.00805334]\n",
- "TF: shape: (768,) values: [0.02946591 0.05715097 0.01293636 0.01920356 0.00805334]\n",
- "\n",
- "bert/encoder/layer_1/attention/output/LayerNorm/beta\n",
- "|sum(pt_wts - tf_wts)| = 0.0\n",
- "PT: shape: (768,) values: [ 0.08583715 0.14199966 -0.0856637 -0.18797271 0.21056814]\n",
- "TF: shape: (768,) values: [ 0.08583715 0.14199966 -0.0856637 -0.18797271 0.21056814]\n",
- "\n",
- "bert/encoder/layer_1/attention/output/LayerNorm/gamma\n",
- "|sum(pt_wts - tf_wts)| = 0.0\n",
- "PT: shape: (768,) values: [0.896962 0.87148863 0.8531161 0.8690647 0.9488987 ]\n",
- "TF: shape: (768,) values: [0.896962 0.87148863 0.8531161 0.8690647 0.9488987 ]\n",
- "\n",
- "bert/encoder/layer_1/intermediate/dense/kernel\n",
- "|sum(pt_wts - tf_wts)| = 0.0\n",
- "PT: shape: (768, 3072) values: [ 0.01841293 -0.02650284 -0.09708428 -0.01734244 -0.05529237]\n",
- "TF: shape: (768, 3072) values: [ 0.01841293 -0.02650284 -0.09708428 -0.01734244 -0.05529237]\n",
- "\n",
- "bert/encoder/layer_1/intermediate/dense/bias\n",
- "|sum(pt_wts - tf_wts)| = 0.0\n",
- "PT: shape: (3072,) values: [-0.15203774 -0.10449131 -0.08440229 -0.09323178 -0.08511415]\n",
- "TF: shape: (3072,) values: [-0.15203774 -0.10449131 -0.08440229 -0.09323178 -0.08511415]\n",
- "\n",
- "bert/encoder/layer_1/output/dense/kernel\n",
- "|sum(pt_wts - tf_wts)| = 0.0\n",
- "PT: shape: (3072, 768) values: [-0.02372648 0.03326349 0.08291997 -0.01519038 0.01868557]\n",
- "TF: shape: (3072, 768) values: [-0.02372648 0.03326349 0.08291997 -0.01519038 0.01868557]\n",
- "\n",
- "bert/encoder/layer_1/output/dense/bias\n",
- "|sum(pt_wts - tf_wts)| = 0.0\n",
- "PT: shape: (768,) values: [-0.02514724 0.09868994 -0.027811 0.03749462 0.01086514]\n",
- "TF: shape: (768,) values: [-0.02514724 0.09868994 -0.027811 0.03749462 0.01086514]\n",
- "\n",
- "bert/encoder/layer_1/output/LayerNorm/beta\n",
- "|sum(pt_wts - tf_wts)| = 0.0\n",
- "PT: shape: (768,) values: [-0.07662535 -0.10506564 0.03191236 0.07633785 -0.11187791]\n",
- "TF: shape: (768,) values: [-0.07662535 -0.10506564 0.03191236 0.07633785 -0.11187791]\n",
- "\n",
- "bert/encoder/layer_1/output/LayerNorm/gamma\n",
- "|sum(pt_wts - tf_wts)| = 0.0\n",
- "PT: shape: (768,) values: [0.9017883 0.8868776 0.8862677 0.85865664 0.87496454]\n",
- "TF: shape: (768,) values: [0.9017883 0.8868776 0.8862677 0.85865664 0.87496454]\n",
- "\n",
- "bert/encoder/layer_2/attention/self/query/kernel\n",
- "|sum(pt_wts - tf_wts)| = 0.0\n",
- "PT: shape: (768, 768) values: [ 0.08433672 0.09580533 0.07543895 -0.01126779 -0.01354045]\n",
- "TF: shape: (768, 768) values: [ 0.08433672 0.09580533 0.07543895 -0.01126779 -0.01354045]\n",
- "\n",
- "bert/encoder/layer_2/attention/self/query/bias\n",
- "|sum(pt_wts - tf_wts)| = 0.0\n",
- "PT: shape: (768,) values: [ 0.0371241 0.03406003 0.27713948 -0.21613775 -0.05275448]\n",
- "TF: shape: (768,) values: [ 0.0371241 0.03406003 0.27713948 -0.21613775 -0.05275448]\n",
- "\n",
- "bert/encoder/layer_2/attention/self/key/kernel\n",
- "|sum(pt_wts - tf_wts)| = 0.0\n",
- "PT: shape: (768, 768) values: [ 0.04794507 0.02517631 -0.01319554 -0.02094732 0.09073472]\n",
- "TF: shape: (768, 768) values: [ 0.04794507 0.02517631 -0.01319554 -0.02094732 0.09073472]\n",
- "\n",
- "bert/encoder/layer_2/attention/self/key/bias\n",
- "|sum(pt_wts - tf_wts)| = 0.0\n",
- "PT: shape: (768,) values: [-0.00037404 -0.00125881 -0.00114734 -0.00157741 0.00037122]\n",
- "TF: shape: (768,) values: [-0.00037404 -0.00125881 -0.00114734 -0.00157741 0.00037122]\n",
- "\n",
- "bert/encoder/layer_2/attention/self/value/kernel\n",
- "|sum(pt_wts - tf_wts)| = 0.0\n",
- "PT: shape: (768, 768) values: [-0.01119406 -0.01488636 -0.02960914 0.04746444 0.00428481]\n",
- "TF: shape: (768, 768) values: [-0.01119406 -0.01488636 -0.02960914 0.04746444 0.00428481]\n",
- "\n",
- "bert/encoder/layer_2/attention/self/value/bias\n",
- "|sum(pt_wts - tf_wts)| = 0.0\n",
- "PT: shape: (768,) values: [-0.02728729 0.04979054 0.08326469 0.04150949 0.600959 ]\n",
- "TF: shape: (768,) values: [-0.02728729 0.04979054 0.08326469 0.04150949 0.600959 ]\n",
- "\n",
- "bert/encoder/layer_2/attention/output/dense/kernel\n",
- "|sum(pt_wts - tf_wts)| = 0.0\n",
- "PT: shape: (768, 768) values: [ 0.00517425 0.01197957 0.0393172 -0.0063884 -0.02673388]\n",
- "TF: shape: (768, 768) values: [ 0.00517425 0.01197957 0.0393172 -0.0063884 -0.02673388]\n",
- "\n",
- "bert/encoder/layer_2/attention/output/dense/bias\n",
- "|sum(pt_wts - tf_wts)| = 0.0\n",
- "PT: shape: (768,) values: [ 0.01754025 0.1226335 -0.05733554 0.06844623 0.00879776]\n",
- "TF: shape: (768,) values: [ 0.01754025 0.1226335 -0.05733554 0.06844623 0.00879776]\n",
- "\n",
- "bert/encoder/layer_2/attention/output/LayerNorm/beta\n",
- "|sum(pt_wts - tf_wts)| = 0.0\n",
- "PT: shape: (768,) values: [ 0.1490809 0.12386955 -0.19382021 -0.26515856 0.32723007]\n",
- "TF: shape: (768,) values: [ 0.1490809 0.12386955 -0.19382021 -0.26515856 0.32723007]\n",
- "\n",
- "bert/encoder/layer_2/attention/output/LayerNorm/gamma\n",
- "|sum(pt_wts - tf_wts)| = 0.0\n",
- "PT: shape: (768,) values: [0.8983343 0.88877076 0.86283594 0.8584952 0.9587886 ]\n",
- "TF: shape: (768,) values: [0.8983343 0.88877076 0.86283594 0.8584952 0.9587886 ]\n",
- "\n",
- "bert/encoder/layer_2/intermediate/dense/kernel\n",
- "|sum(pt_wts - tf_wts)| = 0.0\n",
- "PT: shape: (768, 3072) values: [-0.01619919 0.00662888 0.01492284 -0.01280748 0.01318596]\n",
- "TF: shape: (768, 3072) values: [-0.01619919 0.00662888 0.01492284 -0.01280748 0.01318596]\n",
- "\n",
- "bert/encoder/layer_2/intermediate/dense/bias\n",
- "|sum(pt_wts - tf_wts)| = 0.0\n",
- "PT: shape: (3072,) values: [-0.08474881 -0.12850781 -0.11550345 -0.09513011 -0.02519853]\n",
- "TF: shape: (3072,) values: [-0.08474881 -0.12850781 -0.11550345 -0.09513011 -0.02519853]\n",
- "\n",
- "bert/encoder/layer_2/output/dense/kernel\n",
- "|sum(pt_wts - tf_wts)| = 0.0\n",
- "PT: shape: (3072, 768) values: [-0.07225161 -0.0129784 0.00618811 -0.01593373 -0.02160194]\n",
- "TF: shape: (3072, 768) values: [-0.07225161 -0.0129784 0.00618811 -0.01593373 -0.02160194]\n",
- "\n",
- "bert/encoder/layer_2/output/dense/bias\n",
- "|sum(pt_wts - tf_wts)| = 0.0\n",
- "PT: shape: (768,) values: [-0.06319264 0.06169628 -0.03041368 0.00924282 0.06277442]\n",
- "TF: shape: (768,) values: [-0.06319264 0.06169628 -0.03041368 0.00924282 0.06277442]\n",
- "\n",
- "bert/encoder/layer_2/output/LayerNorm/beta\n",
- "|sum(pt_wts - tf_wts)| = 0.0\n",
- "PT: shape: (768,) values: [-0.1139038 -0.11665309 0.07883061 0.07796711 -0.14219187]\n",
- "TF: shape: (768,) values: [-0.1139038 -0.11665309 0.07883061 0.07796711 -0.14219187]\n",
- "\n",
- "bert/encoder/layer_2/output/LayerNorm/gamma\n",
- "|sum(pt_wts - tf_wts)| = 0.0\n",
- "PT: shape: (768,) values: [0.8813261 0.85744697 0.8511922 0.85261875 0.8329574 ]\n",
- "TF: shape: (768,) values: [0.8813261 0.85744697 0.8511922 0.85261875 0.8329574 ]\n",
- "\n",
- "bert/encoder/layer_3/attention/self/query/kernel\n",
- "|sum(pt_wts - tf_wts)| = 0.0\n",
- "PT: shape: (768, 768) values: [ 0.05855456 -0.00111438 -0.00828963 0.04117409 -0.07591715]\n",
- "TF: shape: (768, 768) values: [ 0.05855456 -0.00111438 -0.00828963 0.04117409 -0.07591715]\n",
- "\n",
- "bert/encoder/layer_3/attention/self/query/bias\n",
- "|sum(pt_wts - tf_wts)| = 0.0\n",
- "PT: shape: (768,) values: [ 0.09740101 -0.19290674 0.04332267 0.17937997 -0.08023558]\n",
- "TF: shape: (768,) values: [ 0.09740101 -0.19290674 0.04332267 0.17937997 -0.08023558]\n",
- "\n",
- "bert/encoder/layer_3/attention/self/key/kernel\n",
- "|sum(pt_wts - tf_wts)| = 0.0\n",
- "PT: shape: (768, 768) values: [ 0.02562077 0.02507281 -0.03361562 0.05613289 -0.05435724]\n",
- "TF: shape: (768, 768) values: [ 0.02562077 0.02507281 -0.03361562 0.05613289 -0.05435724]\n",
- "\n",
- "bert/encoder/layer_3/attention/self/key/bias\n",
- "|sum(pt_wts - tf_wts)| = 0.0\n",
- "PT: shape: (768,) values: [ 0.00188639 -0.00379197 -0.01020415 0.00969649 -0.00094182]\n",
- "TF: shape: (768,) values: [ 0.00188639 -0.00379197 -0.01020415 0.00969649 -0.00094182]\n",
- "\n",
- "bert/encoder/layer_3/attention/self/value/kernel\n",
- "|sum(pt_wts - tf_wts)| = 0.0\n",
- "PT: shape: (768, 768) values: [-0.00539032 0.00959642 0.01325458 0.00490616 0.0129908 ]\n",
- "TF: shape: (768, 768) values: [-0.00539032 0.00959642 0.01325458 0.00490616 0.0129908 ]\n",
- "\n",
- "bert/encoder/layer_3/attention/self/value/bias\n",
- "|sum(pt_wts - tf_wts)| = 0.0\n",
- "PT: shape: (768,) values: [0.04573824 0.05405985 0.00681163 0.00655945 0.01141771]\n",
- "TF: shape: (768,) values: [0.04573824 0.05405985 0.00681163 0.00655945 0.01141771]\n",
- "\n",
- "bert/encoder/layer_3/attention/output/dense/kernel\n",
- "|sum(pt_wts - tf_wts)| = 0.0\n",
- "PT: shape: (768, 768) values: [ 0.01850341 0.03148198 0.02705758 -0.0004669 0.01367511]\n",
- "TF: shape: (768, 768) values: [ 0.01850341 0.03148198 0.02705758 -0.0004669 0.01367511]\n",
- "\n",
- "bert/encoder/layer_3/attention/output/dense/bias\n",
- "|sum(pt_wts - tf_wts)| = 0.0\n",
- "PT: shape: (768,) values: [ 0.01981483 0.03566506 -0.05016088 0.02958186 0.04989756]\n",
- "TF: shape: (768,) values: [ 0.01981483 0.03566506 -0.05016088 0.02958186 0.04989756]\n",
- "\n",
- "bert/encoder/layer_3/attention/output/LayerNorm/beta\n",
- "|sum(pt_wts - tf_wts)| = 0.0\n",
- "PT: shape: (768,) values: [ 0.09815404 0.00063774 -0.01257733 -0.26485074 0.22568701]\n",
- "TF: shape: (768,) values: [ 0.09815404 0.00063774 -0.01257733 -0.26485074 0.22568701]\n",
- "\n",
- "bert/encoder/layer_3/attention/output/LayerNorm/gamma\n",
- "|sum(pt_wts - tf_wts)| = 0.0\n",
- "PT: shape: (768,) values: [0.91457725 0.88453823 0.8340887 0.84203583 0.95247847]\n",
- "TF: shape: (768,) values: [0.91457725 0.88453823 0.8340887 0.84203583 0.95247847]\n",
- "\n",
- "bert/encoder/layer_3/intermediate/dense/kernel\n",
- "|sum(pt_wts - tf_wts)| = 0.0\n",
- "PT: shape: (768, 3072) values: [-0.02733567 0.03307878 -0.01331292 -0.00032527 0.03252084]\n",
- "TF: shape: (768, 3072) values: [-0.02733567 0.03307878 -0.01331292 -0.00032527 0.03252084]\n",
- "\n",
- "bert/encoder/layer_3/intermediate/dense/bias\n",
- "|sum(pt_wts - tf_wts)| = 0.0\n",
- "PT: shape: (3072,) values: [-0.11436842 -0.15038085 -0.07842971 0.01335877 -0.09492484]\n",
- "TF: shape: (3072,) values: [-0.11436842 -0.15038085 -0.07842971 0.01335877 -0.09492484]\n",
- "\n",
- "bert/encoder/layer_3/output/dense/kernel\n",
- "|sum(pt_wts - tf_wts)| = 0.0\n",
- "PT: shape: (3072, 768) values: [-0.01751153 0.01631314 -0.02660011 0.03569947 -0.01394763]\n",
- "TF: shape: (3072, 768) values: [-0.01751153 0.01631314 -0.02660011 0.03569947 -0.01394763]\n",
- "\n",
- "bert/encoder/layer_3/output/dense/bias\n",
- "|sum(pt_wts - tf_wts)| = 0.0\n",
- "PT: shape: (768,) values: [-0.03873252 0.08414765 -0.0399323 0.01997361 0.12924597]\n",
- "TF: shape: (768,) values: [-0.03873252 0.08414765 -0.0399323 0.01997361 0.12924597]\n",
- "\n",
- "bert/encoder/layer_3/output/LayerNorm/beta\n",
- "|sum(pt_wts - tf_wts)| = 0.0\n",
- "PT: shape: (768,) values: [-0.08049371 -0.06923949 -0.03357155 0.05231095 -0.09717073]\n",
- "TF: shape: (768,) values: [-0.08049371 -0.06923949 -0.03357155 0.05231095 -0.09717073]\n",
- "\n",
- "bert/encoder/layer_3/output/LayerNorm/gamma\n",
- "|sum(pt_wts - tf_wts)| = 0.0\n",
- "PT: shape: (768,) values: [0.827748 0.83012533 0.82399255 0.81772 0.80794513]\n",
- "TF: shape: (768,) values: [0.827748 0.83012533 0.82399255 0.81772 0.80794513]\n",
- "\n",
- "bert/encoder/layer_4/attention/self/query/kernel\n",
- "|sum(pt_wts - tf_wts)| = 0.0\n",
- "PT: shape: (768, 768) values: [ 0.08296382 0.02076941 0.06525186 -0.02659729 0.03491377]\n",
- "TF: shape: (768, 768) values: [ 0.08296382 0.02076941 0.06525186 -0.02659729 0.03491377]\n",
- "\n",
- "bert/encoder/layer_4/attention/self/query/bias\n",
- "|sum(pt_wts - tf_wts)| = 0.0\n",
- "PT: shape: (768,) values: [ 0.07045844 -0.13412629 -0.0514146 0.00061329 0.1248519 ]\n",
- "TF: shape: (768,) values: [ 0.07045844 -0.13412629 -0.0514146 0.00061329 0.1248519 ]\n",
- "\n",
- "bert/encoder/layer_4/attention/self/key/kernel\n",
- "|sum(pt_wts - tf_wts)| = 0.0\n",
- "PT: shape: (768, 768) values: [ 0.06941643 0.08133814 -0.0453992 0.0668715 -0.06014847]\n",
- "TF: shape: (768, 768) values: [ 0.06941643 0.08133814 -0.0453992 0.0668715 -0.06014847]\n",
- "\n",
- "bert/encoder/layer_4/attention/self/key/bias\n",
- "|sum(pt_wts - tf_wts)| = 0.0\n",
- "PT: shape: (768,) values: [-0.00588725 -0.00235185 0.00281131 0.00173088 -0.00546653]\n",
- "TF: shape: (768,) values: [-0.00588725 -0.00235185 0.00281131 0.00173088 -0.00546653]\n",
- "\n",
- "bert/encoder/layer_4/attention/self/value/kernel\n",
- "|sum(pt_wts - tf_wts)| = 0.0\n",
- "PT: shape: (768, 768) values: [ 0.06889665 0.06645385 0.01232084 0.0132611 -0.01595679]\n",
- "TF: shape: (768, 768) values: [ 0.06889665 0.06645385 0.01232084 0.0132611 -0.01595679]\n",
- "\n",
- "bert/encoder/layer_4/attention/self/value/bias\n",
- "|sum(pt_wts - tf_wts)| = 0.0\n",
- "PT: shape: (768,) values: [-0.01126871 -0.02704018 0.0301532 0.02332082 -0.04233487]\n",
- "TF: shape: (768,) values: [-0.01126871 -0.02704018 0.0301532 0.02332082 -0.04233487]\n",
- "\n",
- "bert/encoder/layer_4/attention/output/dense/kernel\n",
- "|sum(pt_wts - tf_wts)| = 0.0\n",
- "PT: shape: (768, 768) values: [ 0.02285513 -0.04172142 -0.0146292 0.04862929 -0.0442014 ]\n",
- "TF: shape: (768, 768) values: [ 0.02285513 -0.04172142 -0.0146292 0.04862929 -0.0442014 ]\n",
- "\n",
- "bert/encoder/layer_4/attention/output/dense/bias\n",
- "|sum(pt_wts - tf_wts)| = 0.0\n",
- "PT: shape: (768,) values: [ 0.03054528 0.00479777 -0.02729505 -0.0325212 -0.00525727]\n",
- "TF: shape: (768,) values: [ 0.03054528 0.00479777 -0.02729505 -0.0325212 -0.00525727]\n",
- "\n",
- "bert/encoder/layer_4/attention/output/LayerNorm/beta\n",
- "|sum(pt_wts - tf_wts)| = 0.0\n",
- "PT: shape: (768,) values: [ 0.00903359 0.0052285 -0.02841488 -0.22355485 0.28281343]\n",
- "TF: shape: (768,) values: [ 0.00903359 0.0052285 -0.02841488 -0.22355485 0.28281343]\n",
- "\n",
- "bert/encoder/layer_4/attention/output/LayerNorm/gamma\n",
- "|sum(pt_wts - tf_wts)| = 0.0\n",
- "PT: shape: (768,) values: [0.8849676 0.86927813 0.8114595 0.80269504 0.94864094]\n",
- "TF: shape: (768,) values: [0.8849676 0.86927813 0.8114595 0.80269504 0.94864094]\n",
- "\n",
- "bert/encoder/layer_4/intermediate/dense/kernel\n",
- "|sum(pt_wts - tf_wts)| = 0.0\n",
- "PT: shape: (768, 3072) values: [-0.00639783 0.06198016 -0.03184223 0.00485356 -0.02453273]\n",
- "TF: shape: (768, 3072) values: [-0.00639783 0.06198016 -0.03184223 0.00485356 -0.02453273]\n",
- "\n",
- "bert/encoder/layer_4/intermediate/dense/bias\n",
- "|sum(pt_wts - tf_wts)| = 0.0\n",
- "PT: shape: (3072,) values: [-0.08770327 -0.11779705 -0.11764182 -0.00192611 -0.1335473 ]\n",
- "TF: shape: (3072,) values: [-0.08770327 -0.11779705 -0.11764182 -0.00192611 -0.1335473 ]\n",
- "\n",
- "bert/encoder/layer_4/output/dense/kernel\n",
- "|sum(pt_wts - tf_wts)| = 0.0\n",
- "PT: shape: (3072, 768) values: [-0.05421264 0.0221118 -0.02674172 0.03672203 -0.02399626]\n",
- "TF: shape: (3072, 768) values: [-0.05421264 0.0221118 -0.02674172 0.03672203 -0.02399626]\n",
- "\n",
- "bert/encoder/layer_4/output/dense/bias\n",
- "|sum(pt_wts - tf_wts)| = 0.0\n",
- "PT: shape: (768,) values: [-0.05068972 0.04838871 0.01156022 0.05381602 0.08857913]\n",
- "TF: shape: (768,) values: [-0.05068972 0.04838871 0.01156022 0.05381602 0.08857913]\n",
- "\n",
- "bert/encoder/layer_4/output/LayerNorm/beta\n",
- "|sum(pt_wts - tf_wts)| = 0.0\n",
- "PT: shape: (768,) values: [-0.04338909 -0.0781464 -0.01518662 0.04936362 -0.12378412]\n",
- "TF: shape: (768,) values: [-0.04338909 -0.0781464 -0.01518662 0.04936362 -0.12378412]\n",
- "\n",
- "bert/encoder/layer_4/output/LayerNorm/gamma\n",
- "|sum(pt_wts - tf_wts)| = 0.0\n",
- "PT: shape: (768,) values: [0.8734387 0.8576282 0.8339444 0.8450325 0.8105372]\n",
- "TF: shape: (768,) values: [0.8734387 0.8576282 0.8339444 0.8450325 0.8105372]\n",
- "\n",
- "bert/encoder/layer_5/attention/self/query/kernel\n",
- "|sum(pt_wts - tf_wts)| = 0.0\n",
- "PT: shape: (768, 768) values: [-0.00858843 -0.03920127 0.02552994 -0.02786552 0.02436485]\n",
- "TF: shape: (768, 768) values: [-0.00858843 -0.03920127 0.02552994 -0.02786552 0.02436485]\n",
- "\n",
- "bert/encoder/layer_5/attention/self/query/bias\n",
- "|sum(pt_wts - tf_wts)| = 0.0\n",
- "PT: shape: (768,) values: [-0.00859117 -0.01642405 -0.04391079 0.01085692 0.02925887]\n",
- "TF: shape: (768,) values: [-0.00859117 -0.01642405 -0.04391079 0.01085692 0.02925887]\n",
- "\n",
- "bert/encoder/layer_5/attention/self/key/kernel\n",
- "|sum(pt_wts - tf_wts)| = 0.0\n",
- "PT: shape: (768, 768) values: [ 0.00352847 0.02330176 -0.00369894 -0.03904612 0.00294574]\n",
- "TF: shape: (768, 768) values: [ 0.00352847 0.02330176 -0.00369894 -0.03904612 0.00294574]\n",
- "\n",
- "bert/encoder/layer_5/attention/self/key/bias\n",
- "|sum(pt_wts - tf_wts)| = 0.0\n",
- "PT: shape: (768,) values: [-0.01087186 -0.01176561 0.00016575 -0.01163023 0.00946616]\n",
- "TF: shape: (768,) values: [-0.01087186 -0.01176561 0.00016575 -0.01163023 0.00946616]\n",
- "\n",
- "bert/encoder/layer_5/attention/self/value/kernel\n",
- "|sum(pt_wts - tf_wts)| = 0.0\n",
- "PT: shape: (768, 768) values: [ 0.06134222 0.04238288 0.02796064 -0.01284983 0.03683741]\n",
- "TF: shape: (768, 768) values: [ 0.06134222 0.04238288 0.02796064 -0.01284983 0.03683741]\n",
- "\n",
- "bert/encoder/layer_5/attention/self/value/bias\n",
- "|sum(pt_wts - tf_wts)| = 0.0\n",
- "PT: shape: (768,) values: [ 0.05061118 -0.02954445 -0.0034053 -0.00025261 0.0437019 ]\n",
- "TF: shape: (768,) values: [ 0.05061118 -0.02954445 -0.0034053 -0.00025261 0.0437019 ]\n",
- "\n",
- "bert/encoder/layer_5/attention/output/dense/kernel\n",
- "|sum(pt_wts - tf_wts)| = 0.0\n",
- "PT: shape: (768, 768) values: [-0.00739815 0.0533964 -0.03736389 -0.04999201 0.01693069]\n",
- "TF: shape: (768, 768) values: [-0.00739815 0.0533964 -0.03736389 -0.04999201 0.01693069]\n",
- "\n",
- "bert/encoder/layer_5/attention/output/dense/bias\n",
- "|sum(pt_wts - tf_wts)| = 0.0\n",
- "PT: shape: (768,) values: [-0.0021682 0.01711399 -0.04201518 0.01605333 0.00552063]\n",
- "TF: shape: (768,) values: [-0.0021682 0.01711399 -0.04201518 0.01605333 0.00552063]\n",
- "\n",
- "bert/encoder/layer_5/attention/output/LayerNorm/beta\n",
- "|sum(pt_wts - tf_wts)| = 0.0\n",
- "PT: shape: (768,) values: [-0.06841327 -0.0146848 0.09792476 -0.23284538 0.2785602 ]\n",
- "TF: shape: (768,) values: [-0.06841327 -0.0146848 0.09792476 -0.23284538 0.2785602 ]\n",
- "\n",
- "bert/encoder/layer_5/attention/output/LayerNorm/gamma\n",
- "|sum(pt_wts - tf_wts)| = 0.0\n",
- "PT: shape: (768,) values: [0.8908311 0.87884724 0.81637293 0.8047641 0.96539867]\n",
- "TF: shape: (768,) values: [0.8908311 0.87884724 0.81637293 0.8047641 0.96539867]\n",
- "\n",
- "bert/encoder/layer_5/intermediate/dense/kernel\n",
- "|sum(pt_wts - tf_wts)| = 0.0\n",
- "PT: shape: (768, 3072) values: [-0.03246041 0.07251058 -0.08201726 0.00772481 0.02532209]\n",
- "TF: shape: (768, 3072) values: [-0.03246041 0.07251058 -0.08201726 0.00772481 0.02532209]\n",
- "\n",
- "bert/encoder/layer_5/intermediate/dense/bias\n",
- "|sum(pt_wts - tf_wts)| = 0.0\n",
- "PT: shape: (3072,) values: [-0.09689714 -0.27696273 -0.13047501 -0.10892326 -0.1057625 ]\n",
- "TF: shape: (3072,) values: [-0.09689714 -0.27696273 -0.13047501 -0.10892326 -0.1057625 ]\n",
- "\n",
- "bert/encoder/layer_5/output/dense/kernel\n",
- "|sum(pt_wts - tf_wts)| = 0.0\n",
- "PT: shape: (3072, 768) values: [ 0.0642072 -0.01738782 -0.05095377 0.00523853 0.04425264]\n",
- "TF: shape: (3072, 768) values: [ 0.0642072 -0.01738782 -0.05095377 0.00523853 0.04425264]\n",
- "\n",
- "bert/encoder/layer_5/output/dense/bias\n",
- "|sum(pt_wts - tf_wts)| = 0.0\n",
- "PT: shape: (768,) values: [-0.0007217 0.06006297 0.0016595 0.03848181 0.06703516]\n",
- "TF: shape: (768,) values: [-0.0007217 0.06006297 0.0016595 0.03848181 0.06703516]\n",
- "\n",
- "bert/encoder/layer_5/output/LayerNorm/beta\n",
- "|sum(pt_wts - tf_wts)| = 0.0\n",
- "PT: shape: (768,) values: [-0.00278729 -0.05594506 -0.0631047 0.06023621 -0.18672828]\n",
- "TF: shape: (768,) values: [-0.00278729 -0.05594506 -0.0631047 0.06023621 -0.18672828]\n",
- "\n",
- "bert/encoder/layer_5/output/LayerNorm/gamma\n",
- "|sum(pt_wts - tf_wts)| = 0.0\n",
- "PT: shape: (768,) values: [0.8621183 0.8515807 0.82654256 0.81729776 0.7985204 ]\n",
- "TF: shape: (768,) values: [0.8621183 0.8515807 0.82654256 0.81729776 0.7985204 ]\n",
- "\n",
- "bert/encoder/layer_6/attention/self/query/kernel\n",
- "|sum(pt_wts - tf_wts)| = 0.0\n",
- "PT: shape: (768, 768) values: [-0.02527807 -0.01429243 0.01467054 0.08624706 -0.00188593]\n",
- "TF: shape: (768, 768) values: [-0.02527807 -0.01429243 0.01467054 0.08624706 -0.00188593]\n",
- "\n",
- "bert/encoder/layer_6/attention/self/query/bias\n",
- "|sum(pt_wts - tf_wts)| = 0.0\n",
- "PT: shape: (768,) values: [-0.17319514 0.27564248 0.16801168 -0.10946485 0.1643271 ]\n",
- "TF: shape: (768,) values: [-0.17319514 0.27564248 0.16801168 -0.10946485 0.1643271 ]\n",
- "\n",
- "bert/encoder/layer_6/attention/self/key/kernel\n",
- "|sum(pt_wts - tf_wts)| = 0.0\n",
- "PT: shape: (768, 768) values: [ 0.05886372 0.00706217 0.0398422 0.00882155 -0.04571463]\n",
- "TF: shape: (768, 768) values: [ 0.05886372 0.00706217 0.0398422 0.00882155 -0.04571463]\n",
- "\n",
- "bert/encoder/layer_6/attention/self/key/bias\n",
- "|sum(pt_wts - tf_wts)| = 0.0\n",
- "PT: shape: (768,) values: [-0.00424696 -0.0001192 0.0046079 -0.00315606 0.00434314]\n",
- "TF: shape: (768,) values: [-0.00424696 -0.0001192 0.0046079 -0.00315606 0.00434314]\n",
- "\n",
- "bert/encoder/layer_6/attention/self/value/kernel\n",
- "|sum(pt_wts - tf_wts)| = 0.0\n",
- "PT: shape: (768, 768) values: [-0.01720381 0.01170722 0.02346902 -0.02284313 -0.03173028]\n",
- "TF: shape: (768, 768) values: [-0.01720381 0.01170722 0.02346902 -0.02284313 -0.03173028]\n",
- "\n",
- "bert/encoder/layer_6/attention/self/value/bias\n",
- "|sum(pt_wts - tf_wts)| = 0.0\n",
- "PT: shape: (768,) values: [-0.03492057 0.01813157 -0.00182878 -0.01420629 -0.00508944]\n",
- "TF: shape: (768,) values: [-0.03492057 0.01813157 -0.00182878 -0.01420629 -0.00508944]\n",
- "\n",
- "bert/encoder/layer_6/attention/output/dense/kernel\n",
- "|sum(pt_wts - tf_wts)| = 0.0\n",
- "PT: shape: (768, 768) values: [ 0.0323688 -0.00689882 0.07379091 0.01121114 -0.02059202]\n",
- "TF: shape: (768, 768) values: [ 0.0323688 -0.00689882 0.07379091 0.01121114 -0.02059202]\n",
- "\n",
- "bert/encoder/layer_6/attention/output/dense/bias\n",
- "|sum(pt_wts - tf_wts)| = 0.0\n",
- "PT: shape: (768,) values: [-0.00648672 -0.05935453 -0.05673229 -0.01152384 -0.02766573]\n",
- "TF: shape: (768,) values: [-0.00648672 -0.05935453 -0.05673229 -0.01152384 -0.02766573]\n",
- "\n",
- "bert/encoder/layer_6/attention/output/LayerNorm/beta\n",
- "|sum(pt_wts - tf_wts)| = 0.0\n",
- "PT: shape: (768,) values: [-0.06793639 0.03157783 0.15647687 -0.15025291 0.14727171]\n",
- "TF: shape: (768,) values: [-0.06793639 0.03157783 0.15647687 -0.15025291 0.14727171]\n",
- "\n",
- "bert/encoder/layer_6/attention/output/LayerNorm/gamma\n",
- "|sum(pt_wts - tf_wts)| = 0.0\n",
- "PT: shape: (768,) values: [0.8882361 0.8704905 0.80289173 0.77365315 0.92333615]\n",
- "TF: shape: (768,) values: [0.8882361 0.8704905 0.80289173 0.77365315 0.92333615]\n",
- "\n",
- "bert/encoder/layer_6/intermediate/dense/kernel\n",
- "|sum(pt_wts - tf_wts)| = 0.0\n",
- "PT: shape: (768, 3072) values: [ 0.04492201 0.05160861 0.09041415 -0.00742628 0.048133 ]\n",
- "TF: shape: (768, 3072) values: [ 0.04492201 0.05160861 0.09041415 -0.00742628 0.048133 ]\n",
- "\n",
- "bert/encoder/layer_6/intermediate/dense/bias\n",
- "|sum(pt_wts - tf_wts)| = 0.0\n",
- "PT: shape: (3072,) values: [-0.09301704 -0.158612 -0.10633879 -0.09706812 -0.17319229]\n",
- "TF: shape: (3072,) values: [-0.09301704 -0.158612 -0.10633879 -0.09706812 -0.17319229]\n",
- "\n",
- "bert/encoder/layer_6/output/dense/kernel\n",
- "|sum(pt_wts - tf_wts)| = 0.0\n",
- "PT: shape: (3072, 768) values: [-0.00085372 -0.00974195 0.00684915 0.00038686 0.06610142]\n",
- "TF: shape: (3072, 768) values: [-0.00085372 -0.00974195 0.00684915 0.00038686 0.06610142]\n",
- "\n",
- "bert/encoder/layer_6/output/dense/bias\n",
- "|sum(pt_wts - tf_wts)| = 0.0\n",
- "PT: shape: (768,) values: [-0.03254414 0.05681704 0.03720434 0.01936359 0.09134153]\n",
- "TF: shape: (768,) values: [-0.03254414 0.05681704 0.03720434 0.01936359 0.09134153]\n",
- "\n",
- "bert/encoder/layer_6/output/LayerNorm/beta\n",
- "|sum(pt_wts - tf_wts)| = 0.0\n",
- "PT: shape: (768,) values: [-0.0117129 -0.03209404 -0.08646043 0.03760341 -0.13841423]\n",
- "TF: shape: (768,) values: [-0.0117129 -0.03209404 -0.08646043 0.03760341 -0.13841423]\n",
- "\n",
- "bert/encoder/layer_6/output/LayerNorm/gamma\n",
- "|sum(pt_wts - tf_wts)| = 0.0\n",
- "PT: shape: (768,) values: [0.8674175 0.8657014 0.8151861 0.82301307 0.8305737 ]\n",
- "TF: shape: (768,) values: [0.8674175 0.8657014 0.8151861 0.82301307 0.8305737 ]\n",
- "\n",
- "bert/encoder/layer_7/attention/self/query/kernel\n",
- "|sum(pt_wts - tf_wts)| = 0.0\n",
- "PT: shape: (768, 768) values: [-0.00075523 -0.01501983 0.04090893 0.01884826 0.04670674]\n",
- "TF: shape: (768, 768) values: [-0.00075523 -0.01501983 0.04090893 0.01884826 0.04670674]\n",
- "\n",
- "bert/encoder/layer_7/attention/self/query/bias\n",
- "|sum(pt_wts - tf_wts)| = 0.0\n",
- "PT: shape: (768,) values: [ 0.0010344 -0.00423982 0.3117479 0.04494623 -0.01260845]\n",
- "TF: shape: (768,) values: [ 0.0010344 -0.00423982 0.3117479 0.04494623 -0.01260845]\n",
- "\n",
- "bert/encoder/layer_7/attention/self/key/kernel\n",
- "|sum(pt_wts - tf_wts)| = 0.0\n",
- "PT: shape: (768, 768) values: [ 0.02781927 -0.00906972 0.02121989 0.0298591 0.05854786]\n",
- "TF: shape: (768, 768) values: [ 0.02781927 -0.00906972 0.02121989 0.0298591 0.05854786]\n",
- "\n",
- "bert/encoder/layer_7/attention/self/key/bias\n",
- "|sum(pt_wts - tf_wts)| = 0.0\n",
- "PT: shape: (768,) values: [-0.00074918 0.00731079 0.00089338 0.00345652 0.00043817]\n",
- "TF: shape: (768,) values: [-0.00074918 0.00731079 0.00089338 0.00345652 0.00043817]\n",
- "\n",
- "bert/encoder/layer_7/attention/self/value/kernel\n",
- "|sum(pt_wts - tf_wts)| = 0.0\n",
- "PT: shape: (768, 768) values: [-0.01080035 -0.03468366 0.03167168 0.01583073 0.0327719 ]\n",
- "TF: shape: (768, 768) values: [-0.01080035 -0.03468366 0.03167168 0.01583073 0.0327719 ]\n",
- "\n",
- "bert/encoder/layer_7/attention/self/value/bias\n",
- "|sum(pt_wts - tf_wts)| = 0.0\n",
- "PT: shape: (768,) values: [-0.02824226 0.01605172 0.00067929 -0.04553111 0.0076044 ]\n",
- "TF: shape: (768,) values: [-0.02824226 0.01605172 0.00067929 -0.04553111 0.0076044 ]\n",
- "\n",
- "bert/encoder/layer_7/attention/output/dense/kernel\n",
- "|sum(pt_wts - tf_wts)| = 0.0\n",
- "PT: shape: (768, 768) values: [-0.05496112 0.01006968 0.02206531 -0.01873116 0.02149118]\n",
- "TF: shape: (768, 768) values: [-0.05496112 0.01006968 0.02206531 -0.01873116 0.02149118]\n",
- "\n",
- "bert/encoder/layer_7/attention/output/dense/bias\n",
- "|sum(pt_wts - tf_wts)| = 0.0\n",
- "PT: shape: (768,) values: [ 0.00349772 -0.05831751 -0.0594084 -0.0342187 0.02965918]\n",
- "TF: shape: (768,) values: [ 0.00349772 -0.05831751 -0.0594084 -0.0342187 0.02965918]\n",
- "\n",
- "bert/encoder/layer_7/attention/output/LayerNorm/beta\n",
- "|sum(pt_wts - tf_wts)| = 0.0\n",
- "PT: shape: (768,) values: [-0.02826844 0.04427591 0.05678326 -0.0475907 0.16136196]\n",
- "TF: shape: (768,) values: [-0.02826844 0.04427591 0.05678326 -0.0475907 0.16136196]\n",
- "\n",
- "bert/encoder/layer_7/attention/output/LayerNorm/gamma\n",
- "|sum(pt_wts - tf_wts)| = 0.0\n",
- "PT: shape: (768,) values: [0.8742141 0.870608 0.79147685 0.7595279 0.9223656 ]\n",
- "TF: shape: (768,) values: [0.8742141 0.870608 0.79147685 0.7595279 0.9223656 ]\n",
- "\n",
- "bert/encoder/layer_7/intermediate/dense/kernel\n",
- "|sum(pt_wts - tf_wts)| = 0.0\n",
- "PT: shape: (768, 3072) values: [ 0.03598932 -0.12225644 0.03019998 0.05691092 0.03717208]\n",
- "TF: shape: (768, 3072) values: [ 0.03598932 -0.12225644 0.03019998 0.05691092 0.03717208]\n",
- "\n",
- "bert/encoder/layer_7/intermediate/dense/bias\n",
- "|sum(pt_wts - tf_wts)| = 0.0\n",
- "PT: shape: (3072,) values: [-0.12465011 -0.08639494 -0.06206005 -0.08012587 -0.08773767]\n",
- "TF: shape: (3072,) values: [-0.12465011 -0.08639494 -0.06206005 -0.08012587 -0.08773767]\n",
- "\n",
- "bert/encoder/layer_7/output/dense/kernel\n",
- "|sum(pt_wts - tf_wts)| = 0.0\n",
- "PT: shape: (3072, 768) values: [-0.02190432 -0.02279165 0.03279508 0.01011065 -0.07793335]\n",
- "TF: shape: (3072, 768) values: [-0.02190432 -0.02279165 0.03279508 0.01011065 -0.07793335]\n",
- "\n",
- "bert/encoder/layer_7/output/dense/bias\n",
- "|sum(pt_wts - tf_wts)| = 0.0\n",
- "PT: shape: (768,) values: [-0.04282642 0.03700675 0.06142357 -0.04787201 0.02958163]\n",
- "TF: shape: (768,) values: [-0.04282642 0.03700675 0.06142357 -0.04787201 0.02958163]\n",
- "\n",
- "bert/encoder/layer_7/output/LayerNorm/beta\n",
- "|sum(pt_wts - tf_wts)| = 0.0\n",
- "PT: shape: (768,) values: [-0.03142036 -0.04358427 -0.05132087 -0.01788123 -0.16399944]\n",
- "TF: shape: (768,) values: [-0.03142036 -0.04358427 -0.05132087 -0.01788123 -0.16399944]\n",
- "\n",
- "bert/encoder/layer_7/output/LayerNorm/gamma\n",
- "|sum(pt_wts - tf_wts)| = 0.0\n",
- "PT: shape: (768,) values: [0.83858097 0.8179645 0.80693793 0.81225365 0.7844832 ]\n",
- "TF: shape: (768,) values: [0.83858097 0.8179645 0.80693793 0.81225365 0.7844832 ]\n",
- "\n",
- "bert/encoder/layer_8/attention/self/query/kernel\n",
- "|sum(pt_wts - tf_wts)| = 0.0\n",
- "PT: shape: (768, 768) values: [0.0448719 0.02289526 0.03083764 0.03048073 0.02436891]\n",
- "TF: shape: (768, 768) values: [0.0448719 0.02289526 0.03083764 0.03048073 0.02436891]\n",
- "\n",
- "bert/encoder/layer_8/attention/self/query/bias\n",
- "|sum(pt_wts - tf_wts)| = 0.0\n",
- "PT: shape: (768,) values: [-0.25132924 -0.23753347 0.02581017 0.00901509 0.18424493]\n",
- "TF: shape: (768,) values: [-0.25132924 -0.23753347 0.02581017 0.00901509 0.18424493]\n",
- "\n",
- "bert/encoder/layer_8/attention/self/key/kernel\n",
- "|sum(pt_wts - tf_wts)| = 0.0\n",
- "PT: shape: (768, 768) values: [-0.01999719 0.00711403 0.03949134 -0.0102224 0.03152475]\n",
- "TF: shape: (768, 768) values: [-0.01999719 0.00711403 0.03949134 -0.0102224 0.03152475]\n",
- "\n",
- "bert/encoder/layer_8/attention/self/key/bias\n",
- "|sum(pt_wts - tf_wts)| = 0.0\n",
- "PT: shape: (768,) values: [ 5.5668897e-05 3.4638541e-03 -1.7605867e-03 -6.1321147e-03\n",
- " -4.4074579e-04]\n",
- "TF: shape: (768,) values: [ 5.5668897e-05 3.4638541e-03 -1.7605867e-03 -6.1321147e-03\n",
- " -4.4074579e-04]\n",
- "\n",
- "bert/encoder/layer_8/attention/self/value/kernel\n",
- "|sum(pt_wts - tf_wts)| = 0.0\n",
- "PT: shape: (768, 768) values: [-0.00736056 -0.01795213 0.00104576 -0.00034653 0.03190543]\n",
- "TF: shape: (768, 768) values: [-0.00736056 -0.01795213 0.00104576 -0.00034653 0.03190543]\n",
- "\n",
- "bert/encoder/layer_8/attention/self/value/bias\n",
- "|sum(pt_wts - tf_wts)| = 0.0\n",
- "PT: shape: (768,) values: [ 0.02892835 0.00642501 -0.03608712 0.00264269 -0.0245198 ]\n",
- "TF: shape: (768,) values: [ 0.02892835 0.00642501 -0.03608712 0.00264269 -0.0245198 ]\n",
- "\n",
- "bert/encoder/layer_8/attention/output/dense/kernel\n",
- "|sum(pt_wts - tf_wts)| = 0.0\n",
- "PT: shape: (768, 768) values: [ 0.03971623 0.05307067 -0.01298818 0.00946693 -0.00121235]\n",
- "TF: shape: (768, 768) values: [ 0.03971623 0.05307067 -0.01298818 0.00946693 -0.00121235]\n",
- "\n",
- "bert/encoder/layer_8/attention/output/dense/bias\n",
- "|sum(pt_wts - tf_wts)| = 0.0\n",
- "PT: shape: (768,) values: [ 0.01468131 -0.05406622 -0.06289103 0.004484 0.0240819 ]\n",
- "TF: shape: (768,) values: [ 0.01468131 -0.05406622 -0.06289103 0.004484 0.0240819 ]\n",
- "\n",
- "bert/encoder/layer_8/attention/output/LayerNorm/beta\n",
- "|sum(pt_wts - tf_wts)| = 0.0\n",
- "PT: shape: (768,) values: [-0.06004262 0.0457275 0.08688109 -0.14416659 -0.05500487]\n",
- "TF: shape: (768,) values: [-0.06004262 0.0457275 0.08688109 -0.14416659 -0.05500487]\n",
- "\n",
- "bert/encoder/layer_8/attention/output/LayerNorm/gamma\n",
- "|sum(pt_wts - tf_wts)| = 0.0\n",
- "PT: shape: (768,) values: [0.8907534 0.89116573 0.811639 0.7810443 0.9045574 ]\n",
- "TF: shape: (768,) values: [0.8907534 0.89116573 0.811639 0.7810443 0.9045574 ]\n",
- "\n",
- "bert/encoder/layer_8/intermediate/dense/kernel\n",
- "|sum(pt_wts - tf_wts)| = 0.0\n",
- "PT: shape: (768, 3072) values: [-0.01962814 -0.01482586 -0.02292624 0.03397145 0.02457482]\n",
- "TF: shape: (768, 3072) values: [-0.01962814 -0.01482586 -0.02292624 0.03397145 0.02457482]\n",
- "\n",
- "bert/encoder/layer_8/intermediate/dense/bias\n",
- "|sum(pt_wts - tf_wts)| = 0.0\n",
- "PT: shape: (3072,) values: [-0.08129632 -0.1691108 -0.10681771 -0.10392351 -0.13120006]\n",
- "TF: shape: (3072,) values: [-0.08129632 -0.1691108 -0.10681771 -0.10392351 -0.13120006]\n",
- "\n",
- "bert/encoder/layer_8/output/dense/kernel\n",
- "|sum(pt_wts - tf_wts)| = 0.0\n",
- "PT: shape: (3072, 768) values: [-0.04683433 -0.02690669 0.02979059 0.02223369 -0.00130287]\n",
- "TF: shape: (3072, 768) values: [-0.04683433 -0.02690669 0.02979059 0.02223369 -0.00130287]\n",
- "\n",
- "bert/encoder/layer_8/output/dense/bias\n",
- "|sum(pt_wts - tf_wts)| = 0.0\n",
- "PT: shape: (768,) values: [-0.09155537 -0.04465394 0.05649116 -0.09628641 0.11875238]\n",
- "TF: shape: (768,) values: [-0.09155537 -0.04465394 0.05649116 -0.09628641 0.11875238]\n",
- "\n",
- "bert/encoder/layer_8/output/LayerNorm/beta\n",
- "|sum(pt_wts - tf_wts)| = 0.0\n",
- "PT: shape: (768,) values: [-0.06043394 -0.06657387 -0.05341128 -0.00374733 -0.10855272]\n",
- "TF: shape: (768,) values: [-0.06043394 -0.06657387 -0.05341128 -0.00374733 -0.10855272]\n",
- "\n",
- "bert/encoder/layer_8/output/LayerNorm/gamma\n",
- "|sum(pt_wts - tf_wts)| = 0.0\n",
- "PT: shape: (768,) values: [0.84467345 0.84421015 0.82582206 0.84553087 0.8207573 ]\n",
- "TF: shape: (768,) values: [0.84467345 0.84421015 0.82582206 0.84553087 0.8207573 ]\n",
- "\n",
- "bert/encoder/layer_9/attention/self/query/kernel\n",
- "|sum(pt_wts - tf_wts)| = 0.0\n",
- "PT: shape: (768, 768) values: [ 0.08004542 -0.0143706 -0.04219061 -0.05175152 -0.01147588]\n",
- "TF: shape: (768, 768) values: [ 0.08004542 -0.0143706 -0.04219061 -0.05175152 -0.01147588]\n",
- "\n",
- "bert/encoder/layer_9/attention/self/query/bias\n",
- "|sum(pt_wts - tf_wts)| = 0.0\n",
- "PT: shape: (768,) values: [-0.14508031 0.40926442 -0.3281781 -0.02869792 -0.26104516]\n",
- "TF: shape: (768,) values: [-0.14508031 0.40926442 -0.3281781 -0.02869792 -0.26104516]\n",
- "\n",
- "bert/encoder/layer_9/attention/self/key/kernel\n",
- "|sum(pt_wts - tf_wts)| = 0.0\n",
- "PT: shape: (768, 768) values: [-0.01337681 0.00615428 -0.0455939 0.03379053 -0.01992556]\n",
- "TF: shape: (768, 768) values: [-0.01337681 0.00615428 -0.0455939 0.03379053 -0.01992556]\n",
- "\n",
- "bert/encoder/layer_9/attention/self/key/bias\n",
- "|sum(pt_wts - tf_wts)| = 0.0\n",
- "PT: shape: (768,) values: [-0.0051302 0.0083288 0.00377641 0.00928865 -0.00418182]\n",
- "TF: shape: (768,) values: [-0.0051302 0.0083288 0.00377641 0.00928865 -0.00418182]\n",
- "\n",
- "bert/encoder/layer_9/attention/self/value/kernel\n",
- "|sum(pt_wts - tf_wts)| = 0.0\n",
- "PT: shape: (768, 768) values: [-0.02485976 -0.0301923 0.00984638 -0.02495162 0.01074037]\n",
- "TF: shape: (768, 768) values: [-0.02485976 -0.0301923 0.00984638 -0.02495162 0.01074037]\n",
- "\n",
- "bert/encoder/layer_9/attention/self/value/bias\n",
- "|sum(pt_wts - tf_wts)| = 0.0\n",
- "PT: shape: (768,) values: [-0.04229928 -0.02636711 0.0060447 0.00222829 0.04979481]\n",
- "TF: shape: (768,) values: [-0.04229928 -0.02636711 0.0060447 0.00222829 0.04979481]\n",
- "\n",
- "bert/encoder/layer_9/attention/output/dense/kernel\n",
- "|sum(pt_wts - tf_wts)| = 0.0\n",
- "PT: shape: (768, 768) values: [-0.01258144 0.00871274 0.00482882 -0.00675888 -0.04390825]\n",
- "TF: shape: (768, 768) values: [-0.01258144 0.00871274 0.00482882 -0.00675888 -0.04390825]\n",
- "\n",
- "bert/encoder/layer_9/attention/output/dense/bias\n",
- "|sum(pt_wts - tf_wts)| = 0.0\n",
- "PT: shape: (768,) values: [ 0.02457753 0.05051134 -0.06890804 -0.00962795 0.00864793]\n",
- "TF: shape: (768,) values: [ 0.02457753 0.05051134 -0.06890804 -0.00962795 0.00864793]\n",
- "\n",
- "bert/encoder/layer_9/attention/output/LayerNorm/beta\n",
- "|sum(pt_wts - tf_wts)| = 0.0\n",
- "PT: shape: (768,) values: [-0.08963391 -0.06362236 0.0676669 -0.09895685 0.08318913]\n",
- "TF: shape: (768,) values: [-0.08963391 -0.06362236 0.0676669 -0.09895685 0.08318913]\n",
- "\n",
- "bert/encoder/layer_9/attention/output/LayerNorm/gamma\n",
- "|sum(pt_wts - tf_wts)| = 0.0\n",
- "PT: shape: (768,) values: [0.85100883 0.82569736 0.7927931 0.7660444 0.8912934 ]\n",
- "TF: shape: (768,) values: [0.85100883 0.82569736 0.7927931 0.7660444 0.8912934 ]\n",
- "\n",
- "bert/encoder/layer_9/intermediate/dense/kernel\n",
- "|sum(pt_wts - tf_wts)| = 0.0\n",
- "PT: shape: (768, 3072) values: [ 0.06290598 0.0203122 -0.05384256 0.05442941 0.00484769]\n",
- "TF: shape: (768, 3072) values: [ 0.06290598 0.0203122 -0.05384256 0.05442941 0.00484769]\n",
- "\n",
- "bert/encoder/layer_9/intermediate/dense/bias\n",
- "|sum(pt_wts - tf_wts)| = 0.0\n",
- "PT: shape: (3072,) values: [-0.10818483 -0.00169527 -0.08962701 -0.10280421 -0.14310956]\n",
- "TF: shape: (3072,) values: [-0.10818483 -0.00169527 -0.08962701 -0.10280421 -0.14310956]\n",
- "\n",
- "bert/encoder/layer_9/output/dense/kernel\n",
- "|sum(pt_wts - tf_wts)| = 0.0\n",
- "PT: shape: (3072, 768) values: [ 0.05487705 0.01644666 0.00436198 -0.00490768 -0.03238423]\n",
- "TF: shape: (3072, 768) values: [ 0.05487705 0.01644666 0.00436198 -0.00490768 -0.03238423]\n",
- "\n",
- "bert/encoder/layer_9/output/dense/bias\n",
- "|sum(pt_wts - tf_wts)| = 0.0\n",
- "PT: shape: (768,) values: [-0.08755219 -0.01910074 -0.02988298 -0.08150438 0.09897955]\n",
- "TF: shape: (768,) values: [-0.08755219 -0.01910074 -0.02988298 -0.08150438 0.09897955]\n",
- "\n",
- "bert/encoder/layer_9/output/LayerNorm/beta\n",
- "|sum(pt_wts - tf_wts)| = 0.0\n",
- "PT: shape: (768,) values: [-0.04136161 -0.02113917 -0.07581077 -0.00809791 -0.09790538]\n",
- "TF: shape: (768,) values: [-0.04136161 -0.02113917 -0.07581077 -0.00809791 -0.09790538]\n",
- "\n",
- "bert/encoder/layer_9/output/LayerNorm/gamma\n",
- "|sum(pt_wts - tf_wts)| = 0.0\n",
- "PT: shape: (768,) values: [0.8250572 0.83477134 0.7794141 0.81264955 0.7827918 ]\n",
- "TF: shape: (768,) values: [0.8250572 0.83477134 0.7794141 0.81264955 0.7827918 ]\n",
- "\n",
- "bert/encoder/layer_10/attention/self/query/kernel\n",
- "|sum(pt_wts - tf_wts)| = 0.0\n",
- "PT: shape: (768, 768) values: [ 0.00071212 -0.00853064 0.01776993 0.03189976 0.02183623]\n",
- "TF: shape: (768, 768) values: [ 0.00071212 -0.00853064 0.01776993 0.03189976 0.02183623]\n",
- "\n",
- "bert/encoder/layer_10/attention/self/query/bias\n",
- "|sum(pt_wts - tf_wts)| = 0.0\n",
- "PT: shape: (768,) values: [-0.03667567 -0.01449654 -0.03822913 0.00118343 -0.05489838]\n",
- "TF: shape: (768,) values: [-0.03667567 -0.01449654 -0.03822913 0.00118343 -0.05489838]\n",
- "\n",
- "bert/encoder/layer_10/attention/self/key/kernel\n",
- "|sum(pt_wts - tf_wts)| = 0.0\n",
- "PT: shape: (768, 768) values: [-0.0494106 0.05531096 -0.02459413 -0.06019118 -0.02829785]\n",
- "TF: shape: (768, 768) values: [-0.0494106 0.05531096 -0.02459413 -0.06019118 -0.02829785]\n",
- "\n",
- "bert/encoder/layer_10/attention/self/key/bias\n",
- "|sum(pt_wts - tf_wts)| = 0.0\n",
- "PT: shape: (768,) values: [-0.00692997 0.00855893 0.00670777 -0.0052475 -0.00017074]\n",
- "TF: shape: (768,) values: [-0.00692997 0.00855893 0.00670777 -0.0052475 -0.00017074]\n",
- "\n",
- "bert/encoder/layer_10/attention/self/value/kernel\n",
- "|sum(pt_wts - tf_wts)| = 0.0\n",
- "PT: shape: (768, 768) values: [ 0.01911842 0.04858809 -0.02608485 0.00794924 -0.02246636]\n",
- "TF: shape: (768, 768) values: [ 0.01911842 0.04858809 -0.02608485 0.00794924 -0.02246636]\n",
- "\n",
- "bert/encoder/layer_10/attention/self/value/bias\n",
- "|sum(pt_wts - tf_wts)| = 0.0\n",
- "PT: shape: (768,) values: [-0.0133503 -0.01224133 -0.0051834 -0.00232528 0.00148614]\n",
- "TF: shape: (768,) values: [-0.0133503 -0.01224133 -0.0051834 -0.00232528 0.00148614]\n",
- "\n",
- "bert/encoder/layer_10/attention/output/dense/kernel\n",
- "|sum(pt_wts - tf_wts)| = 0.0\n",
- "PT: shape: (768, 768) values: [-0.05904732 0.02616 0.00794104 -0.02889086 -0.03692576]\n",
- "TF: shape: (768, 768) values: [-0.05904732 0.02616 0.00794104 -0.02889086 -0.03692576]\n",
- "\n",
- "bert/encoder/layer_10/attention/output/dense/bias\n",
- "|sum(pt_wts - tf_wts)| = 0.0\n",
- "PT: shape: (768,) values: [0.02089205 0.01458059 0.05217785 0.0324267 0.00907548]\n",
- "TF: shape: (768,) values: [0.02089205 0.01458059 0.05217785 0.0324267 0.00907548]\n",
- "\n",
- "bert/encoder/layer_10/attention/output/LayerNorm/beta\n",
- "|sum(pt_wts - tf_wts)| = 0.0\n",
- "PT: shape: (768,) values: [-0.10986238 -0.04332284 0.02603893 -0.06236923 0.14469369]\n",
- "TF: shape: (768,) values: [-0.10986238 -0.04332284 0.02603893 -0.06236923 0.14469369]\n",
- "\n",
- "bert/encoder/layer_10/attention/output/LayerNorm/gamma\n",
- "|sum(pt_wts - tf_wts)| = 0.0\n",
- "PT: shape: (768,) values: [0.8515822 0.81392974 0.836747 0.78040504 0.88091415]\n",
- "TF: shape: (768,) values: [0.8515822 0.81392974 0.836747 0.78040504 0.88091415]\n",
- "\n",
- "bert/encoder/layer_10/intermediate/dense/kernel\n",
- "|sum(pt_wts - tf_wts)| = 0.0\n",
- "PT: shape: (768, 3072) values: [-0.07061081 0.06997397 0.01433633 0.04150929 0.02865192]\n",
- "TF: shape: (768, 3072) values: [-0.07061081 0.06997397 0.01433633 0.04150929 0.02865192]\n",
- "\n",
- "bert/encoder/layer_10/intermediate/dense/bias\n",
- "|sum(pt_wts - tf_wts)| = 0.0\n",
- "PT: shape: (3072,) values: [-0.13879126 -0.06401426 -0.1408043 -0.15043251 -0.10193057]\n",
- "TF: shape: (3072,) values: [-0.13879126 -0.06401426 -0.1408043 -0.15043251 -0.10193057]\n",
- "\n",
- "bert/encoder/layer_10/output/dense/kernel\n",
- "|sum(pt_wts - tf_wts)| = 0.0\n",
- "PT: shape: (3072, 768) values: [ 0.02918765 0.02609882 -0.02259856 0.01636725 -0.00038442]\n",
- "TF: shape: (3072, 768) values: [ 0.02918765 0.02609882 -0.02259856 0.01636725 -0.00038442]\n",
- "\n",
- "bert/encoder/layer_10/output/dense/bias\n",
- "|sum(pt_wts - tf_wts)| = 0.0\n",
- "PT: shape: (768,) values: [-0.01799502 0.10970547 -0.02384165 -0.03350981 0.10491351]\n",
- "TF: shape: (768,) values: [-0.01799502 0.10970547 -0.02384165 -0.03350981 0.10491351]\n",
- "\n",
- "bert/encoder/layer_10/output/LayerNorm/beta\n",
- "|sum(pt_wts - tf_wts)| = 0.0\n",
- "PT: shape: (768,) values: [ 0.00999107 -0.0217309 -0.0854177 -0.01109101 -0.07902174]\n",
- "TF: shape: (768,) values: [ 0.00999107 -0.0217309 -0.0854177 -0.01109101 -0.07902174]\n",
- "\n",
- "bert/encoder/layer_10/output/LayerNorm/gamma\n",
- "|sum(pt_wts - tf_wts)| = 0.0\n",
- "PT: shape: (768,) values: [0.8272796 0.8597452 0.79116803 0.81267637 0.8273501 ]\n",
- "TF: shape: (768,) values: [0.8272796 0.8597452 0.79116803 0.81267637 0.8273501 ]\n",
- "\n",
- "bert/encoder/layer_11/attention/self/query/kernel\n",
- "|sum(pt_wts - tf_wts)| = 0.0\n",
- "PT: shape: (768, 768) values: [-0.04141425 -0.06491017 -0.03202523 0.06226195 0.02193764]\n",
- "TF: shape: (768, 768) values: [-0.04141425 -0.06491017 -0.03202523 0.06226195 0.02193764]\n",
- "\n",
- "bert/encoder/layer_11/attention/self/query/bias\n",
- "|sum(pt_wts - tf_wts)| = 0.0\n",
- "PT: shape: (768,) values: [ 0.0501296 0.11886728 0.2186807 0.08720991 -0.20476632]\n",
- "TF: shape: (768,) values: [ 0.0501296 0.11886728 0.2186807 0.08720991 -0.20476632]\n",
- "\n",
- "bert/encoder/layer_11/attention/self/key/kernel\n",
- "|sum(pt_wts - tf_wts)| = 0.0\n",
- "PT: shape: (768, 768) values: [ 0.02634268 -0.01357682 -0.06076496 0.04210597 0.01783857]\n",
- "TF: shape: (768, 768) values: [ 0.02634268 -0.01357682 -0.06076496 0.04210597 0.01783857]\n",
- "\n",
- "bert/encoder/layer_11/attention/self/key/bias\n",
- "|sum(pt_wts - tf_wts)| = 0.0\n",
- "PT: shape: (768,) values: [-0.0007798 -0.00065806 -0.00010521 0.00119144 -0.00180091]\n",
- "TF: shape: (768,) values: [-0.0007798 -0.00065806 -0.00010521 0.00119144 -0.00180091]\n",
- "\n",
- "bert/encoder/layer_11/attention/self/value/kernel\n",
- "|sum(pt_wts - tf_wts)| = 0.0\n",
- "PT: shape: (768, 768) values: [ 0.03520973 -0.00678078 -0.02883583 -0.01011515 0.04519828]\n",
- "TF: shape: (768, 768) values: [ 0.03520973 -0.00678078 -0.02883583 -0.01011515 0.04519828]\n",
- "\n",
- "bert/encoder/layer_11/attention/self/value/bias\n",
- "|sum(pt_wts - tf_wts)| = 0.0\n",
- "PT: shape: (768,) values: [ 0.01502306 -0.00530942 0.00023572 0.00205218 -0.00578036]\n",
- "TF: shape: (768,) values: [ 0.01502306 -0.00530942 0.00023572 0.00205218 -0.00578036]\n",
- "\n",
- "bert/encoder/layer_11/attention/output/dense/kernel\n",
- "|sum(pt_wts - tf_wts)| = 0.0\n",
- "PT: shape: (768, 768) values: [ 0.02361419 0.03112707 -0.00063031 0.04209773 -0.02434015]\n",
- "TF: shape: (768, 768) values: [ 0.02361419 0.03112707 -0.00063031 0.04209773 -0.02434015]\n",
- "\n",
- "bert/encoder/layer_11/attention/output/dense/bias\n",
- "|sum(pt_wts - tf_wts)| = 0.0\n",
- "PT: shape: (768,) values: [ 0.02566087 0.0028438 -0.00475678 0.02149458 -0.01755187]\n",
- "TF: shape: (768,) values: [ 0.02566087 0.0028438 -0.00475678 0.02149458 -0.01755187]\n",
- "\n",
- "bert/encoder/layer_11/attention/output/LayerNorm/beta\n",
- "|sum(pt_wts - tf_wts)| = 0.0\n",
- "PT: shape: (768,) values: [-0.03134411 0.01207957 -0.04636396 -0.03013046 0.07944281]\n",
- "TF: shape: (768,) values: [-0.03134411 0.01207957 -0.04636396 -0.03013046 0.07944281]\n",
- "\n",
- "bert/encoder/layer_11/attention/output/LayerNorm/gamma\n",
- "|sum(pt_wts - tf_wts)| = 0.0\n",
- "PT: shape: (768,) values: [0.85203767 0.8020145 0.8554237 0.8150477 0.8441815 ]\n",
- "TF: shape: (768,) values: [0.85203767 0.8020145 0.8554237 0.8150477 0.8441815 ]\n",
- "\n",
- "bert/encoder/layer_11/intermediate/dense/kernel\n",
- "|sum(pt_wts - tf_wts)| = 0.0\n",
- "PT: shape: (768, 3072) values: [ 0.05871898 -0.01124212 0.00206979 -0.04366514 -0.00716808]\n",
- "TF: shape: (768, 3072) values: [ 0.05871898 -0.01124212 0.00206979 -0.04366514 -0.00716808]\n",
- "\n",
- "bert/encoder/layer_11/intermediate/dense/bias\n",
- "|sum(pt_wts - tf_wts)| = 0.0\n",
- "PT: shape: (3072,) values: [-0.09762521 -0.06175711 -0.05153917 -0.08580919 -0.09734315]\n",
- "TF: shape: (3072,) values: [-0.09762521 -0.06175711 -0.05153917 -0.08580919 -0.09734315]\n",
- "\n",
- "bert/encoder/layer_11/output/dense/kernel\n",
- "|sum(pt_wts - tf_wts)| = 0.0\n",
- "PT: shape: (3072, 768) values: [-0.022382 0.01073206 -0.01357213 0.02484621 0.01403091]\n",
- "TF: shape: (3072, 768) values: [-0.022382 0.01073206 -0.01357213 0.02484621 0.01403091]\n",
- "\n",
- "bert/encoder/layer_11/output/dense/bias\n",
- "|sum(pt_wts - tf_wts)| = 0.0\n",
- "PT: shape: (768,) values: [-0.06574099 0.04207807 0.01201084 0.00229322 0.05551811]\n",
- "TF: shape: (768,) values: [-0.06574099 0.04207807 0.01201084 0.00229322 0.05551811]\n",
- "\n",
- "bert/encoder/layer_11/output/LayerNorm/beta\n",
- "|sum(pt_wts - tf_wts)| = 0.0\n",
- "PT: shape: (768,) values: [-0.00634605 -0.01989403 0.04628465 0.01585056 -0.04256899]\n",
- "TF: shape: (768,) values: [-0.00634605 -0.01989403 0.04628465 0.01585056 -0.04256899]\n",
- "\n",
- "bert/encoder/layer_11/output/LayerNorm/gamma\n",
- "|sum(pt_wts - tf_wts)| = 0.0\n",
- "PT: shape: (768,) values: [0.6384234 0.6300364 0.66570055 0.6126921 0.63756436]\n",
- "TF: shape: (768,) values: [0.6384234 0.6300364 0.66570055 0.6126921 0.63756436]\n",
- "\n",
- "bert/pooler/dense/kernel\n",
- "|sum(pt_wts - tf_wts)| = 0.0\n",
- "PT: shape: (768, 768) values: [-0.00127425 0.00199868 -0.03863145 -0.00139355 0.00691627]\n",
- "TF: shape: (768, 768) values: [-0.00127425 0.00199868 -0.03863145 -0.00139355 0.00691627]\n",
- "\n",
- "bert/pooler/dense/bias\n",
- "|sum(pt_wts - tf_wts)| = 0.0\n",
- "PT: shape: (768,) values: [-0.03597581 -0.00389536 0.05181352 0.02224747 -0.00493723]\n",
- "TF: shape: (768,) values: [-0.03597581 -0.00389536 0.05181352 0.02224747 -0.00493723]\n",
- "\n"
- ]
- }
- ],
- "source": [
- "tensors_to_transopse = (\n",
- " \"dense.weight\",\n",
- " \"attention.self.query\",\n",
- " \"attention.self.key\",\n",
- " \"attention.self.value\"\n",
- ")\n",
- "var_map = (\n",
- " ('layer.', 'layer_'),\n",
- " ('word_embeddings.weight', 'word_embeddings'),\n",
- " ('position_embeddings.weight', 'position_embeddings'),\n",
- " ('token_type_embeddings.weight', 'token_type_embeddings'),\n",
- " ('.', '/'),\n",
- " ('LayerNorm/weight', 'LayerNorm/gamma'),\n",
- " ('LayerNorm/bias', 'LayerNorm/beta'),\n",
- " ('weight', 'kernel')\n",
- ")\n",
- "\n",
- "def to_tf_var_name(name:str):\n",
- " for patt, repl in iter(var_map):\n",
- " name = name.replace(patt, repl)\n",
- " return 'bert/{}'.format(name)\n",
- "\n",
- "tf_vars = {v.name: session.run(fetches=v) for v in tf.global_variables()}\n",
- "pt_vars = {}\n",
- "for v, T in pt_model.state_dict().items():\n",
- " T = T.detach().numpy()\n",
- " if any([x in v for x in tensors_to_transopse]):\n",
- " T = T.T\n",
- " pt_vars.update({to_tf_var_name(v): T})\n",
- "\n",
- "for var_name in tf_vars:\n",
- " \n",
- " pt = pt_vars[var_name.strip(\":0\")]\n",
- " tf = tf_vars[var_name]\n",
- "\n",
- " print(var_name.strip(\":0\"))\n",
- " \n",
- " # Assert equivalence\n",
- " print(\"|sum(pt_wts - tf_wts)| = {}\".format(\n",
- " np.abs(np.sum(pt - tf, keepdims=False))\n",
- " ))\n",
- " assert not np.sum(pt - tf, keepdims=False)\n",
- " \n",
- " if len(pt.shape) == 2:\n",
- " print(\"PT: shape: {0} values: {1}\".format(pt.shape, pt[0, :5]))\n",
- " print(\"TF: shape: {0} values: {1}\".format(tf.shape, tf[0, :5]))\n",
- " else:\n",
- " print(\"PT: shape: {0} values: {1}\".format(pt.shape, pt[:5]))\n",
- " print(\"TF: shape: {0} values: {1}\".format(tf.shape, tf[:5]))\n",
- " print()"
- ]
- },
- {
- "cell_type": "markdown",
- "metadata": {},
- "source": [
- "## Compare Layer-12 Projections"
- ]
- },
- {
- "cell_type": "code",
- "execution_count": 8,
- "metadata": {},
- "outputs": [
- {
- "name": "stdout",
- "output_type": "stream",
- "text": [
- "MSE: 2.7155439966009e-05\n",
- "PT-values: [-0.876663 -0.41088238 -0.12200808 0.44941 0.19445966]\n",
- "TF-values: [-0.8742865 -0.40621698 -0.10585472 0.444904 0.1825743 ]\n"
- ]
- }
- ],
- "source": [
- "# Mean Squared Error (MSE) between last projection of each model\n",
- "MSE = np.mean((pt_embedding - tf_embedding) ** 2, keepdims=False)\n",
- "print(\"MSE: {}\".format(MSE))\n",
- "print(\"PT-values: {}\".format(pt_embedding[0, :5]))\n",
- "print(\"TF-values: {}\".format(tf_embedding[0, :5]))"
- ]
- }
- ],
- "metadata": {
- "kernelspec": {
- "display_name": "nlp",
- "language": "python",
- "name": "nlp"
- },
- "language_info": {
- "codemirror_mode": {
- "name": "ipython",
- "version": 3
- },
- "file_extension": ".py",
- "mimetype": "text/x-python",
- "name": "python",
- "nbconvert_exporter": "python",
- "pygments_lexer": "ipython3",
- "version": "3.6.8"
- }
- },
- "nbformat": 4,
- "nbformat_minor": 2
-}
diff --git a/server/transformers/notebooks/Comparing-TF-and-PT-models-MLM-NSP.ipynb b/server/transformers/notebooks/Comparing-TF-and-PT-models-MLM-NSP.ipynb
deleted file mode 100644
index 809f6ea6e0f3267e50d01ee6aedee5d6316f2665..0000000000000000000000000000000000000000
--- a/server/transformers/notebooks/Comparing-TF-and-PT-models-MLM-NSP.ipynb
+++ /dev/null
@@ -1,4815 +0,0 @@
-{
- "cells": [
- {
- "cell_type": "markdown",
- "metadata": {},
- "source": [
- "# Comparing TensorFlow (original) and PyTorch models\n",
- "\n",
- "You can use this small notebook to check the conversion of the model's weights from the TensorFlow model to the PyTorch model. In the following, we compare the weights of the last layer on a simple example (in `input.txt`) but both models returns all the hidden layers so you can check every stage of the model.\n",
- "\n",
- "To run this notebook, follow these instructions:\n",
- "- make sure that your Python environment has both TensorFlow and PyTorch installed,\n",
- "- download the original TensorFlow implementation,\n",
- "- download a pre-trained TensorFlow model as indicaded in the TensorFlow implementation readme,\n",
- "- run the script `convert_tf_checkpoint_to_pytorch.py` as indicated in the `README` to convert the pre-trained TensorFlow model to PyTorch.\n",
- "\n",
- "If needed change the relative paths indicated in this notebook (at the beggining of Sections 1 and 2) to point to the relevent models and code."
- ]
- },
- {
- "cell_type": "code",
- "execution_count": 1,
- "metadata": {
- "ExecuteTime": {
- "end_time": "2018-11-16T10:02:26.999106Z",
- "start_time": "2018-11-16T10:02:26.985709Z"
- }
- },
- "outputs": [],
- "source": [
- "import os\n",
- "os.chdir('../')"
- ]
- },
- {
- "cell_type": "markdown",
- "metadata": {},
- "source": [
- "## 1/ TensorFlow code"
- ]
- },
- {
- "cell_type": "code",
- "execution_count": 2,
- "metadata": {
- "ExecuteTime": {
- "end_time": "2018-11-16T10:02:27.664528Z",
- "start_time": "2018-11-16T10:02:27.651019Z"
- }
- },
- "outputs": [],
- "source": [
- "original_tf_inplem_dir = \"./tensorflow_code/\"\n",
- "model_dir = \"../google_models/uncased_L-12_H-768_A-12/\"\n",
- "\n",
- "vocab_file = model_dir + \"vocab.txt\"\n",
- "bert_config_file = model_dir + \"bert_config.json\"\n",
- "init_checkpoint = model_dir + \"bert_model.ckpt\"\n",
- "\n",
- "input_file = \"./samples/input.txt\"\n",
- "max_seq_length = 128\n",
- "max_predictions_per_seq = 20\n",
- "\n",
- "masked_lm_positions = [6]"
- ]
- },
- {
- "cell_type": "code",
- "execution_count": 3,
- "metadata": {
- "ExecuteTime": {
- "end_time": "2018-11-16T10:02:30.202182Z",
- "start_time": "2018-11-16T10:02:28.112570Z"
- }
- },
- "outputs": [],
- "source": [
- "import importlib.util\n",
- "import sys\n",
- "import tensorflow as tf\n",
- "import pytorch_transformers as ppb\n",
- "\n",
- "def del_all_flags(FLAGS):\n",
- " flags_dict = FLAGS._flags() \n",
- " keys_list = [keys for keys in flags_dict] \n",
- " for keys in keys_list:\n",
- " FLAGS.__delattr__(keys)\n",
- "\n",
- "del_all_flags(tf.flags.FLAGS)\n",
- "import tensorflow_code.extract_features as ef\n",
- "del_all_flags(tf.flags.FLAGS)\n",
- "import tensorflow_code.modeling as tfm\n",
- "del_all_flags(tf.flags.FLAGS)\n",
- "import tensorflow_code.tokenization as tft\n",
- "del_all_flags(tf.flags.FLAGS)\n",
- "import tensorflow_code.run_pretraining as rp\n",
- "del_all_flags(tf.flags.FLAGS)\n",
- "import tensorflow_code.create_pretraining_data as cpp"
- ]
- },
- {
- "cell_type": "code",
- "execution_count": 4,
- "metadata": {
- "ExecuteTime": {
- "end_time": "2018-11-16T10:02:30.238027Z",
- "start_time": "2018-11-16T10:02:30.204943Z"
- },
- "code_folding": [
- 15
- ]
- },
- "outputs": [],
- "source": [
- "import re\n",
- "class InputExample(object):\n",
- " \"\"\"A single instance example.\"\"\"\n",
- "\n",
- " def __init__(self, tokens, segment_ids, masked_lm_positions,\n",
- " masked_lm_labels, is_random_next):\n",
- " self.tokens = tokens\n",
- " self.segment_ids = segment_ids\n",
- " self.masked_lm_positions = masked_lm_positions\n",
- " self.masked_lm_labels = masked_lm_labels\n",
- " self.is_random_next = is_random_next\n",
- " def __repr__(self):\n",
- " return '\\n'.join(k + \":\" + str(v) for k, v in self.__dict__.items())\n",
- "\n",
- "\n",
- "def read_examples(input_file, tokenizer, masked_lm_positions):\n",
- " \"\"\"Read a list of `InputExample`s from an input file.\"\"\"\n",
- " examples = []\n",
- " unique_id = 0\n",
- " with tf.gfile.GFile(input_file, \"r\") as reader:\n",
- " while True:\n",
- " line = reader.readline()\n",
- " if not line:\n",
- " break\n",
- " line = line.strip()\n",
- " text_a = None\n",
- " text_b = None\n",
- " m = re.match(r\"^(.*) \\|\\|\\| (.*)$\", line)\n",
- " if m is None:\n",
- " text_a = line\n",
- " else:\n",
- " text_a = m.group(1)\n",
- " text_b = m.group(2)\n",
- " tokens_a = tokenizer.tokenize(text_a)\n",
- " tokens_b = None\n",
- " if text_b:\n",
- " tokens_b = tokenizer.tokenize(text_b)\n",
- " tokens = tokens_a + tokens_b\n",
- " masked_lm_labels = []\n",
- " for m_pos in masked_lm_positions:\n",
- " masked_lm_labels.append(tokens[m_pos])\n",
- " tokens[m_pos] = '[MASK]'\n",
- " examples.append(\n",
- " InputExample(\n",
- " tokens = tokens,\n",
- " segment_ids = [0] * len(tokens_a) + [1] * len(tokens_b),\n",
- " masked_lm_positions = masked_lm_positions,\n",
- " masked_lm_labels = masked_lm_labels,\n",
- " is_random_next = False))\n",
- " unique_id += 1\n",
- " return examples"
- ]
- },
- {
- "cell_type": "code",
- "execution_count": 5,
- "metadata": {
- "ExecuteTime": {
- "end_time": "2018-11-16T10:02:30.304018Z",
- "start_time": "2018-11-16T10:02:30.240189Z"
- }
- },
- "outputs": [
- {
- "name": "stdout",
- "output_type": "stream",
- "text": [
- "tokens:['who', 'was', 'jim', 'henson', '?', 'jim', '[MASK]', 'was', 'a', 'puppet', '##eer']\n",
- "segment_ids:[0, 0, 0, 0, 0, 1, 1, 1, 1, 1, 1]\n",
- "masked_lm_positions:[6]\n",
- "masked_lm_labels:['henson']\n",
- "is_random_next:False\n"
- ]
- }
- ],
- "source": [
- "bert_config = tfm.BertConfig.from_json_file(bert_config_file)\n",
- "tokenizer = ppb.BertTokenizer(\n",
- " vocab_file=vocab_file, do_lower_case=True)\n",
- "examples = read_examples(input_file, tokenizer, masked_lm_positions=masked_lm_positions)\n",
- "\n",
- "print(examples[0])"
- ]
- },
- {
- "cell_type": "code",
- "execution_count": 6,
- "metadata": {
- "ExecuteTime": {
- "end_time": "2018-11-16T10:02:33.324167Z",
- "start_time": "2018-11-16T10:02:33.291909Z"
- },
- "code_folding": [
- 16
- ]
- },
- "outputs": [],
- "source": [
- "class InputFeatures(object):\n",
- " \"\"\"A single set of features of data.\"\"\"\n",
- "\n",
- " def __init__(self, input_ids, input_mask, segment_ids, masked_lm_positions,\n",
- " masked_lm_ids, masked_lm_weights, next_sentence_label):\n",
- " self.input_ids = input_ids\n",
- " self.input_mask = input_mask\n",
- " self.segment_ids = segment_ids\n",
- " self.masked_lm_positions = masked_lm_positions\n",
- " self.masked_lm_ids = masked_lm_ids\n",
- " self.masked_lm_weights = masked_lm_weights\n",
- " self.next_sentence_labels = next_sentence_label\n",
- "\n",
- " def __repr__(self):\n",
- " return '\\n'.join(k + \":\" + str(v) for k, v in self.__dict__.items())\n",
- "\n",
- "def pretraining_convert_examples_to_features(instances, tokenizer, max_seq_length,\n",
- " max_predictions_per_seq):\n",
- " \"\"\"Create TF example files from `TrainingInstance`s.\"\"\"\n",
- " features = []\n",
- " for (inst_index, instance) in enumerate(instances):\n",
- " input_ids = tokenizer.convert_tokens_to_ids(instance.tokens)\n",
- " input_mask = [1] * len(input_ids)\n",
- " segment_ids = list(instance.segment_ids)\n",
- " assert len(input_ids) <= max_seq_length\n",
- "\n",
- " while len(input_ids) < max_seq_length:\n",
- " input_ids.append(0)\n",
- " input_mask.append(0)\n",
- " segment_ids.append(0)\n",
- "\n",
- " assert len(input_ids) == max_seq_length\n",
- " assert len(input_mask) == max_seq_length\n",
- " assert len(segment_ids) == max_seq_length\n",
- "\n",
- " masked_lm_positions = list(instance.masked_lm_positions)\n",
- " masked_lm_ids = tokenizer.convert_tokens_to_ids(instance.masked_lm_labels)\n",
- " masked_lm_weights = [1.0] * len(masked_lm_ids)\n",
- "\n",
- " while len(masked_lm_positions) < max_predictions_per_seq:\n",
- " masked_lm_positions.append(0)\n",
- " masked_lm_ids.append(0)\n",
- " masked_lm_weights.append(0.0)\n",
- "\n",
- " next_sentence_label = 1 if instance.is_random_next else 0\n",
- "\n",
- " features.append(\n",
- " InputFeatures(input_ids, input_mask, segment_ids,\n",
- " masked_lm_positions, masked_lm_ids,\n",
- " masked_lm_weights, next_sentence_label))\n",
- "\n",
- " if inst_index < 5:\n",
- " tf.logging.info(\"*** Example ***\")\n",
- " tf.logging.info(\"tokens: %s\" % \" \".join(\n",
- " [str(x) for x in instance.tokens]))\n",
- " tf.logging.info(\"features: %s\" % str(features[-1]))\n",
- " return features"
- ]
- },
- {
- "cell_type": "code",
- "execution_count": 7,
- "metadata": {
- "ExecuteTime": {
- "end_time": "2018-11-16T10:02:34.185367Z",
- "start_time": "2018-11-16T10:02:34.155046Z"
- }
- },
- "outputs": [
- {
- "name": "stdout",
- "output_type": "stream",
- "text": [
- "INFO:tensorflow:*** Example ***\n"
- ]
- },
- {
- "name": "stderr",
- "output_type": "stream",
- "text": [
- "11/16/2018 11:02:34 - INFO - tensorflow - *** Example ***\n"
- ]
- },
- {
- "name": "stdout",
- "output_type": "stream",
- "text": [
- "INFO:tensorflow:tokens: who was jim henson ? jim [MASK] was a puppet ##eer\n"
- ]
- },
- {
- "name": "stderr",
- "output_type": "stream",
- "text": [
- "11/16/2018 11:02:34 - INFO - tensorflow - tokens: who was jim henson ? jim [MASK] was a puppet ##eer\n"
- ]
- },
- {
- "name": "stdout",
- "output_type": "stream",
- "text": [
- "INFO:tensorflow:features: input_ids:[2040, 2001, 3958, 27227, 1029, 3958, 103, 2001, 1037, 13997, 11510, 0, 0, 0, 0, 0, 0, 0, 0, 0, 0, 0, 0, 0, 0, 0, 0, 0, 0, 0, 0, 0, 0, 0, 0, 0, 0, 0, 0, 0, 0, 0, 0, 0, 0, 0, 0, 0, 0, 0, 0, 0, 0, 0, 0, 0, 0, 0, 0, 0, 0, 0, 0, 0, 0, 0, 0, 0, 0, 0, 0, 0, 0, 0, 0, 0, 0, 0, 0, 0, 0, 0, 0, 0, 0, 0, 0, 0, 0, 0, 0, 0, 0, 0, 0, 0, 0, 0, 0, 0, 0, 0, 0, 0, 0, 0, 0, 0, 0, 0, 0, 0, 0, 0, 0, 0, 0, 0, 0, 0, 0, 0, 0, 0, 0, 0, 0, 0]\n",
- "input_mask:[1, 1, 1, 1, 1, 1, 1, 1, 1, 1, 1, 0, 0, 0, 0, 0, 0, 0, 0, 0, 0, 0, 0, 0, 0, 0, 0, 0, 0, 0, 0, 0, 0, 0, 0, 0, 0, 0, 0, 0, 0, 0, 0, 0, 0, 0, 0, 0, 0, 0, 0, 0, 0, 0, 0, 0, 0, 0, 0, 0, 0, 0, 0, 0, 0, 0, 0, 0, 0, 0, 0, 0, 0, 0, 0, 0, 0, 0, 0, 0, 0, 0, 0, 0, 0, 0, 0, 0, 0, 0, 0, 0, 0, 0, 0, 0, 0, 0, 0, 0, 0, 0, 0, 0, 0, 0, 0, 0, 0, 0, 0, 0, 0, 0, 0, 0, 0, 0, 0, 0, 0, 0, 0, 0, 0, 0, 0, 0]\n",
- "segment_ids:[0, 0, 0, 0, 0, 1, 1, 1, 1, 1, 1, 0, 0, 0, 0, 0, 0, 0, 0, 0, 0, 0, 0, 0, 0, 0, 0, 0, 0, 0, 0, 0, 0, 0, 0, 0, 0, 0, 0, 0, 0, 0, 0, 0, 0, 0, 0, 0, 0, 0, 0, 0, 0, 0, 0, 0, 0, 0, 0, 0, 0, 0, 0, 0, 0, 0, 0, 0, 0, 0, 0, 0, 0, 0, 0, 0, 0, 0, 0, 0, 0, 0, 0, 0, 0, 0, 0, 0, 0, 0, 0, 0, 0, 0, 0, 0, 0, 0, 0, 0, 0, 0, 0, 0, 0, 0, 0, 0, 0, 0, 0, 0, 0, 0, 0, 0, 0, 0, 0, 0, 0, 0, 0, 0, 0, 0, 0, 0]\n",
- "masked_lm_positions:[6, 0, 0, 0, 0, 0, 0, 0, 0, 0, 0, 0, 0, 0, 0, 0, 0, 0, 0, 0]\n",
- "masked_lm_ids:[27227, 0, 0, 0, 0, 0, 0, 0, 0, 0, 0, 0, 0, 0, 0, 0, 0, 0, 0, 0]\n",
- "masked_lm_weights:[1.0, 0.0, 0.0, 0.0, 0.0, 0.0, 0.0, 0.0, 0.0, 0.0, 0.0, 0.0, 0.0, 0.0, 0.0, 0.0, 0.0, 0.0, 0.0, 0.0]\n",
- "next_sentence_labels:0\n"
- ]
- },
- {
- "name": "stderr",
- "output_type": "stream",
- "text": [
- "11/16/2018 11:02:34 - INFO - tensorflow - features: input_ids:[2040, 2001, 3958, 27227, 1029, 3958, 103, 2001, 1037, 13997, 11510, 0, 0, 0, 0, 0, 0, 0, 0, 0, 0, 0, 0, 0, 0, 0, 0, 0, 0, 0, 0, 0, 0, 0, 0, 0, 0, 0, 0, 0, 0, 0, 0, 0, 0, 0, 0, 0, 0, 0, 0, 0, 0, 0, 0, 0, 0, 0, 0, 0, 0, 0, 0, 0, 0, 0, 0, 0, 0, 0, 0, 0, 0, 0, 0, 0, 0, 0, 0, 0, 0, 0, 0, 0, 0, 0, 0, 0, 0, 0, 0, 0, 0, 0, 0, 0, 0, 0, 0, 0, 0, 0, 0, 0, 0, 0, 0, 0, 0, 0, 0, 0, 0, 0, 0, 0, 0, 0, 0, 0, 0, 0, 0, 0, 0, 0, 0, 0]\n",
- "input_mask:[1, 1, 1, 1, 1, 1, 1, 1, 1, 1, 1, 0, 0, 0, 0, 0, 0, 0, 0, 0, 0, 0, 0, 0, 0, 0, 0, 0, 0, 0, 0, 0, 0, 0, 0, 0, 0, 0, 0, 0, 0, 0, 0, 0, 0, 0, 0, 0, 0, 0, 0, 0, 0, 0, 0, 0, 0, 0, 0, 0, 0, 0, 0, 0, 0, 0, 0, 0, 0, 0, 0, 0, 0, 0, 0, 0, 0, 0, 0, 0, 0, 0, 0, 0, 0, 0, 0, 0, 0, 0, 0, 0, 0, 0, 0, 0, 0, 0, 0, 0, 0, 0, 0, 0, 0, 0, 0, 0, 0, 0, 0, 0, 0, 0, 0, 0, 0, 0, 0, 0, 0, 0, 0, 0, 0, 0, 0, 0]\n",
- "segment_ids:[0, 0, 0, 0, 0, 1, 1, 1, 1, 1, 1, 0, 0, 0, 0, 0, 0, 0, 0, 0, 0, 0, 0, 0, 0, 0, 0, 0, 0, 0, 0, 0, 0, 0, 0, 0, 0, 0, 0, 0, 0, 0, 0, 0, 0, 0, 0, 0, 0, 0, 0, 0, 0, 0, 0, 0, 0, 0, 0, 0, 0, 0, 0, 0, 0, 0, 0, 0, 0, 0, 0, 0, 0, 0, 0, 0, 0, 0, 0, 0, 0, 0, 0, 0, 0, 0, 0, 0, 0, 0, 0, 0, 0, 0, 0, 0, 0, 0, 0, 0, 0, 0, 0, 0, 0, 0, 0, 0, 0, 0, 0, 0, 0, 0, 0, 0, 0, 0, 0, 0, 0, 0, 0, 0, 0, 0, 0, 0]\n",
- "masked_lm_positions:[6, 0, 0, 0, 0, 0, 0, 0, 0, 0, 0, 0, 0, 0, 0, 0, 0, 0, 0, 0]\n",
- "masked_lm_ids:[27227, 0, 0, 0, 0, 0, 0, 0, 0, 0, 0, 0, 0, 0, 0, 0, 0, 0, 0, 0]\n",
- "masked_lm_weights:[1.0, 0.0, 0.0, 0.0, 0.0, 0.0, 0.0, 0.0, 0.0, 0.0, 0.0, 0.0, 0.0, 0.0, 0.0, 0.0, 0.0, 0.0, 0.0, 0.0]\n",
- "next_sentence_labels:0\n"
- ]
- }
- ],
- "source": [
- "features = pretraining_convert_examples_to_features(\n",
- " instances=examples, max_seq_length=max_seq_length, \n",
- " max_predictions_per_seq=max_predictions_per_seq, tokenizer=tokenizer)"
- ]
- },
- {
- "cell_type": "code",
- "execution_count": 8,
- "metadata": {
- "ExecuteTime": {
- "end_time": "2018-11-16T10:02:34.912005Z",
- "start_time": "2018-11-16T10:02:34.882111Z"
- }
- },
- "outputs": [],
- "source": [
- "def input_fn_builder(features, seq_length, max_predictions_per_seq, tokenizer):\n",
- " \"\"\"Creates an `input_fn` closure to be passed to TPUEstimator.\"\"\"\n",
- "\n",
- " all_input_ids = []\n",
- " all_input_mask = []\n",
- " all_segment_ids = []\n",
- " all_masked_lm_positions = []\n",
- " all_masked_lm_ids = []\n",
- " all_masked_lm_weights = []\n",
- " all_next_sentence_labels = []\n",
- "\n",
- " for feature in features:\n",
- " all_input_ids.append(feature.input_ids)\n",
- " all_input_mask.append(feature.input_mask)\n",
- " all_segment_ids.append(feature.segment_ids)\n",
- " all_masked_lm_positions.append(feature.masked_lm_positions)\n",
- " all_masked_lm_ids.append(feature.masked_lm_ids)\n",
- " all_masked_lm_weights.append(feature.masked_lm_weights)\n",
- " all_next_sentence_labels.append(feature.next_sentence_labels)\n",
- "\n",
- " def input_fn(params):\n",
- " \"\"\"The actual input function.\"\"\"\n",
- " batch_size = params[\"batch_size\"]\n",
- "\n",
- " num_examples = len(features)\n",
- "\n",
- " # This is for demo purposes and does NOT scale to large data sets. We do\n",
- " # not use Dataset.from_generator() because that uses tf.py_func which is\n",
- " # not TPU compatible. The right way to load data is with TFRecordReader.\n",
- " d = tf.data.Dataset.from_tensor_slices({\n",
- " \"input_ids\":\n",
- " tf.constant(\n",
- " all_input_ids, shape=[num_examples, seq_length],\n",
- " dtype=tf.int32),\n",
- " \"input_mask\":\n",
- " tf.constant(\n",
- " all_input_mask,\n",
- " shape=[num_examples, seq_length],\n",
- " dtype=tf.int32),\n",
- " \"segment_ids\":\n",
- " tf.constant(\n",
- " all_segment_ids,\n",
- " shape=[num_examples, seq_length],\n",
- " dtype=tf.int32),\n",
- " \"masked_lm_positions\":\n",
- " tf.constant(\n",
- " all_masked_lm_positions,\n",
- " shape=[num_examples, max_predictions_per_seq],\n",
- " dtype=tf.int32),\n",
- " \"masked_lm_ids\":\n",
- " tf.constant(\n",
- " all_masked_lm_ids,\n",
- " shape=[num_examples, max_predictions_per_seq],\n",
- " dtype=tf.int32),\n",
- " \"masked_lm_weights\":\n",
- " tf.constant(\n",
- " all_masked_lm_weights,\n",
- " shape=[num_examples, max_predictions_per_seq],\n",
- " dtype=tf.float32),\n",
- " \"next_sentence_labels\":\n",
- " tf.constant(\n",
- " all_next_sentence_labels,\n",
- " shape=[num_examples, 1],\n",
- " dtype=tf.int32),\n",
- " })\n",
- "\n",
- " d = d.batch(batch_size=batch_size, drop_remainder=False)\n",
- " return d\n",
- "\n",
- " return input_fn\n"
- ]
- },
- {
- "cell_type": "code",
- "execution_count": 9,
- "metadata": {
- "ExecuteTime": {
- "end_time": "2018-11-16T10:02:35.671603Z",
- "start_time": "2018-11-16T10:02:35.626167Z"
- },
- "code_folding": [
- 64,
- 77
- ]
- },
- "outputs": [],
- "source": [
- "def model_fn_builder(bert_config, init_checkpoint, learning_rate,\n",
- " num_train_steps, num_warmup_steps, use_tpu,\n",
- " use_one_hot_embeddings):\n",
- " \"\"\"Returns `model_fn` closure for TPUEstimator.\"\"\"\n",
- "\n",
- " def model_fn(features, labels, mode, params): # pylint: disable=unused-argument\n",
- " \"\"\"The `model_fn` for TPUEstimator.\"\"\"\n",
- "\n",
- " tf.logging.info(\"*** Features ***\")\n",
- " for name in sorted(features.keys()):\n",
- " tf.logging.info(\" name = %s, shape = %s\" % (name, features[name].shape))\n",
- "\n",
- " input_ids = features[\"input_ids\"]\n",
- " input_mask = features[\"input_mask\"]\n",
- " segment_ids = features[\"segment_ids\"]\n",
- " masked_lm_positions = features[\"masked_lm_positions\"]\n",
- " masked_lm_ids = features[\"masked_lm_ids\"]\n",
- " masked_lm_weights = features[\"masked_lm_weights\"]\n",
- " next_sentence_labels = features[\"next_sentence_labels\"]\n",
- "\n",
- " is_training = (mode == tf.estimator.ModeKeys.TRAIN)\n",
- "\n",
- " model = tfm.BertModel(\n",
- " config=bert_config,\n",
- " is_training=is_training,\n",
- " input_ids=input_ids,\n",
- " input_mask=input_mask,\n",
- " token_type_ids=segment_ids,\n",
- " use_one_hot_embeddings=use_one_hot_embeddings)\n",
- "\n",
- " (masked_lm_loss,\n",
- " masked_lm_example_loss, masked_lm_log_probs) = rp.get_masked_lm_output(\n",
- " bert_config, model.get_sequence_output(), model.get_embedding_table(),\n",
- " masked_lm_positions, masked_lm_ids, masked_lm_weights)\n",
- "\n",
- " (next_sentence_loss, next_sentence_example_loss,\n",
- " next_sentence_log_probs) = rp.get_next_sentence_output(\n",
- " bert_config, model.get_pooled_output(), next_sentence_labels)\n",
- "\n",
- " total_loss = masked_lm_loss + next_sentence_loss\n",
- "\n",
- " tvars = tf.trainable_variables()\n",
- "\n",
- " initialized_variable_names = {}\n",
- " scaffold_fn = None\n",
- " if init_checkpoint:\n",
- " (assignment_map,\n",
- " initialized_variable_names) = tfm.get_assigment_map_from_checkpoint(\n",
- " tvars, init_checkpoint)\n",
- " if use_tpu:\n",
- "\n",
- " def tpu_scaffold():\n",
- " tf.train.init_from_checkpoint(init_checkpoint, assignment_map)\n",
- " return tf.train.Scaffold()\n",
- "\n",
- " scaffold_fn = tpu_scaffold\n",
- " else:\n",
- " tf.train.init_from_checkpoint(init_checkpoint, assignment_map)\n",
- "\n",
- " tf.logging.info(\"**** Trainable Variables ****\")\n",
- " for var in tvars:\n",
- " init_string = \"\"\n",
- " if var.name in initialized_variable_names:\n",
- " init_string = \", *INIT_FROM_CKPT*\"\n",
- " tf.logging.info(\" name = %s, shape = %s%s\", var.name, var.shape,\n",
- " init_string)\n",
- "\n",
- " output_spec = None\n",
- " if mode == tf.estimator.ModeKeys.TRAIN:\n",
- " masked_lm_positions = features[\"masked_lm_positions\"]\n",
- " masked_lm_ids = features[\"masked_lm_ids\"]\n",
- " masked_lm_weights = features[\"masked_lm_weights\"]\n",
- " next_sentence_labels = features[\"next_sentence_labels\"]\n",
- " train_op = optimization.create_optimizer(\n",
- " total_loss, learning_rate, num_train_steps, num_warmup_steps, use_tpu)\n",
- "\n",
- " output_spec = tf.contrib.tpu.TPUEstimatorSpec(\n",
- " mode=mode,\n",
- " loss=total_loss,\n",
- " train_op=train_op,\n",
- " scaffold_fn=scaffold_fn)\n",
- " elif mode == tf.estimator.ModeKeys.EVAL:\n",
- " masked_lm_positions = features[\"masked_lm_positions\"]\n",
- " masked_lm_ids = features[\"masked_lm_ids\"]\n",
- " masked_lm_weights = features[\"masked_lm_weights\"]\n",
- " next_sentence_labels = features[\"next_sentence_labels\"]\n",
- "\n",
- " def metric_fn(masked_lm_example_loss, masked_lm_log_probs, masked_lm_ids,\n",
- " masked_lm_weights, next_sentence_example_loss,\n",
- " next_sentence_log_probs, next_sentence_labels):\n",
- " \"\"\"Computes the loss and accuracy of the model.\"\"\"\n",
- " masked_lm_log_probs = tf.reshape(masked_lm_log_probs,\n",
- " [-1, masked_lm_log_probs.shape[-1]])\n",
- " masked_lm_predictions = tf.argmax(\n",
- " masked_lm_log_probs, axis=-1, output_type=tf.int32)\n",
- " masked_lm_example_loss = tf.reshape(masked_lm_example_loss, [-1])\n",
- " masked_lm_ids = tf.reshape(masked_lm_ids, [-1])\n",
- " masked_lm_weights = tf.reshape(masked_lm_weights, [-1])\n",
- " masked_lm_accuracy = tf.metrics.accuracy(\n",
- " labels=masked_lm_ids,\n",
- " predictions=masked_lm_predictions,\n",
- " weights=masked_lm_weights)\n",
- " masked_lm_mean_loss = tf.metrics.mean(\n",
- " values=masked_lm_example_loss, weights=masked_lm_weights)\n",
- "\n",
- " next_sentence_log_probs = tf.reshape(\n",
- " next_sentence_log_probs, [-1, next_sentence_log_probs.shape[-1]])\n",
- " next_sentence_predictions = tf.argmax(\n",
- " next_sentence_log_probs, axis=-1, output_type=tf.int32)\n",
- " next_sentence_labels = tf.reshape(next_sentence_labels, [-1])\n",
- " next_sentence_accuracy = tf.metrics.accuracy(\n",
- " labels=next_sentence_labels, predictions=next_sentence_predictions)\n",
- " next_sentence_mean_loss = tf.metrics.mean(\n",
- " values=next_sentence_example_loss)\n",
- "\n",
- " return {\n",
- " \"masked_lm_accuracy\": masked_lm_accuracy,\n",
- " \"masked_lm_loss\": masked_lm_mean_loss,\n",
- " \"next_sentence_accuracy\": next_sentence_accuracy,\n",
- " \"next_sentence_loss\": next_sentence_mean_loss,\n",
- " }\n",
- "\n",
- " eval_metrics = (metric_fn, [\n",
- " masked_lm_example_loss, masked_lm_log_probs, masked_lm_ids,\n",
- " masked_lm_weights, next_sentence_example_loss,\n",
- " next_sentence_log_probs, next_sentence_labels\n",
- " ])\n",
- " output_spec = tf.contrib.tpu.TPUEstimatorSpec(\n",
- " mode=mode,\n",
- " loss=total_loss,\n",
- " eval_metrics=eval_metrics,\n",
- " scaffold_fn=scaffold_fn)\n",
- " elif mode == tf.estimator.ModeKeys.PREDICT:\n",
- " masked_lm_log_probs = tf.reshape(masked_lm_log_probs,\n",
- " [-1, masked_lm_log_probs.shape[-1]])\n",
- " masked_lm_predictions = tf.argmax(\n",
- " masked_lm_log_probs, axis=-1, output_type=tf.int32)\n",
- "\n",
- " next_sentence_log_probs = tf.reshape(\n",
- " next_sentence_log_probs, [-1, next_sentence_log_probs.shape[-1]])\n",
- " next_sentence_predictions = tf.argmax(\n",
- " next_sentence_log_probs, axis=-1, output_type=tf.int32)\n",
- "\n",
- " masked_lm_predictions = tf.reshape(masked_lm_predictions,\n",
- " [1, masked_lm_positions.shape[-1]])\n",
- " next_sentence_predictions = tf.reshape(next_sentence_predictions,\n",
- " [1, 1])\n",
- "\n",
- " predictions = {\n",
- " \"masked_lm_predictions\": masked_lm_predictions,\n",
- " \"next_sentence_predictions\": next_sentence_predictions\n",
- " }\n",
- "\n",
- " output_spec = tf.contrib.tpu.TPUEstimatorSpec(\n",
- " mode=mode, predictions=predictions, scaffold_fn=scaffold_fn)\n",
- " return output_spec\n",
- " else:\n",
- " raise ValueError(\"Only TRAIN, EVAL and PREDICT modes are supported: %s\" % (mode))\n",
- "\n",
- " return output_spec\n",
- "\n",
- " return model_fn"
- ]
- },
- {
- "cell_type": "code",
- "execution_count": 10,
- "metadata": {
- "ExecuteTime": {
- "end_time": "2018-11-16T10:02:40.328700Z",
- "start_time": "2018-11-16T10:02:36.289676Z"
- }
- },
- "outputs": [
- {
- "name": "stdout",
- "output_type": "stream",
- "text": [
- "WARNING:tensorflow:Estimator's model_fn (.model_fn at 0x12a864ae8>) includes params argument, but params are not passed to Estimator.\n"
- ]
- },
- {
- "name": "stderr",
- "output_type": "stream",
- "text": [
- "11/16/2018 11:02:40 - WARNING - tensorflow - Estimator's model_fn (.model_fn at 0x12a864ae8>) includes params argument, but params are not passed to Estimator.\n"
- ]
- },
- {
- "name": "stdout",
- "output_type": "stream",
- "text": [
- "WARNING:tensorflow:Using temporary folder as model directory: /var/folders/yx/cw8n_njx3js5jksyw_qlp8p00000gn/T/tmp4x8r3x3d\n"
- ]
- },
- {
- "name": "stderr",
- "output_type": "stream",
- "text": [
- "11/16/2018 11:02:40 - WARNING - tensorflow - Using temporary folder as model directory: /var/folders/yx/cw8n_njx3js5jksyw_qlp8p00000gn/T/tmp4x8r3x3d\n"
- ]
- },
- {
- "name": "stdout",
- "output_type": "stream",
- "text": [
- "INFO:tensorflow:Using config: {'_model_dir': '/var/folders/yx/cw8n_njx3js5jksyw_qlp8p00000gn/T/tmp4x8r3x3d', '_tf_random_seed': None, '_save_summary_steps': 100, '_save_checkpoints_steps': None, '_save_checkpoints_secs': 600, '_session_config': allow_soft_placement: true\n",
- "graph_options {\n",
- " rewrite_options {\n",
- " meta_optimizer_iterations: ONE\n",
- " }\n",
- "}\n",
- ", '_keep_checkpoint_max': 5, '_keep_checkpoint_every_n_hours': 10000, '_log_step_count_steps': None, '_train_distribute': None, '_device_fn': None, '_protocol': None, '_eval_distribute': None, '_experimental_distribute': None, '_service': None, '_cluster_spec': , '_task_type': 'worker', '_task_id': 0, '_global_id_in_cluster': 0, '_master': '', '_evaluation_master': '', '_is_chief': True, '_num_ps_replicas': 0, '_num_worker_replicas': 1, '_tpu_config': TPUConfig(iterations_per_loop=2, num_shards=1, num_cores_per_replica=None, per_host_input_for_training=3, tpu_job_name=None, initial_infeed_sleep_secs=None, input_partition_dims=None), '_cluster': None}\n"
- ]
- },
- {
- "name": "stderr",
- "output_type": "stream",
- "text": [
- "11/16/2018 11:02:40 - INFO - tensorflow - Using config: {'_model_dir': '/var/folders/yx/cw8n_njx3js5jksyw_qlp8p00000gn/T/tmp4x8r3x3d', '_tf_random_seed': None, '_save_summary_steps': 100, '_save_checkpoints_steps': None, '_save_checkpoints_secs': 600, '_session_config': allow_soft_placement: true\n",
- "graph_options {\n",
- " rewrite_options {\n",
- " meta_optimizer_iterations: ONE\n",
- " }\n",
- "}\n",
- ", '_keep_checkpoint_max': 5, '_keep_checkpoint_every_n_hours': 10000, '_log_step_count_steps': None, '_train_distribute': None, '_device_fn': None, '_protocol': None, '_eval_distribute': None, '_experimental_distribute': None, '_service': None, '_cluster_spec': , '_task_type': 'worker', '_task_id': 0, '_global_id_in_cluster': 0, '_master': '', '_evaluation_master': '', '_is_chief': True, '_num_ps_replicas': 0, '_num_worker_replicas': 1, '_tpu_config': TPUConfig(iterations_per_loop=2, num_shards=1, num_cores_per_replica=None, per_host_input_for_training=3, tpu_job_name=None, initial_infeed_sleep_secs=None, input_partition_dims=None), '_cluster': None}\n"
- ]
- },
- {
- "name": "stdout",
- "output_type": "stream",
- "text": [
- "WARNING:tensorflow:Setting TPUConfig.num_shards==1 is an unsupported behavior. Please fix as soon as possible (leaving num_shards as None.\n"
- ]
- },
- {
- "name": "stderr",
- "output_type": "stream",
- "text": [
- "11/16/2018 11:02:40 - WARNING - tensorflow - Setting TPUConfig.num_shards==1 is an unsupported behavior. Please fix as soon as possible (leaving num_shards as None.\n"
- ]
- },
- {
- "name": "stdout",
- "output_type": "stream",
- "text": [
- "INFO:tensorflow:_TPUContext: eval_on_tpu True\n"
- ]
- },
- {
- "name": "stderr",
- "output_type": "stream",
- "text": [
- "11/16/2018 11:02:40 - INFO - tensorflow - _TPUContext: eval_on_tpu True\n"
- ]
- },
- {
- "name": "stdout",
- "output_type": "stream",
- "text": [
- "WARNING:tensorflow:eval_on_tpu ignored because use_tpu is False.\n"
- ]
- },
- {
- "name": "stderr",
- "output_type": "stream",
- "text": [
- "11/16/2018 11:02:40 - WARNING - tensorflow - eval_on_tpu ignored because use_tpu is False.\n"
- ]
- }
- ],
- "source": [
- "is_per_host = tf.contrib.tpu.InputPipelineConfig.PER_HOST_V2\n",
- "run_config = tf.contrib.tpu.RunConfig(\n",
- " master=None,\n",
- " tpu_config=tf.contrib.tpu.TPUConfig(\n",
- " num_shards=1,\n",
- " per_host_input_for_training=is_per_host))\n",
- "\n",
- "model_fn = model_fn_builder(\n",
- " bert_config=bert_config,\n",
- " init_checkpoint=init_checkpoint,\n",
- " learning_rate=0,\n",
- " num_train_steps=1,\n",
- " num_warmup_steps=1,\n",
- " use_tpu=False,\n",
- " use_one_hot_embeddings=False)\n",
- "\n",
- "# If TPU is not available, this will fall back to normal Estimator on CPU\n",
- "# or GPU.\n",
- "estimator = tf.contrib.tpu.TPUEstimator(\n",
- " use_tpu=False,\n",
- " model_fn=model_fn,\n",
- " config=run_config,\n",
- " predict_batch_size=1)\n",
- "\n",
- "input_fn = input_fn_builder(\n",
- " features=features, seq_length=max_seq_length, max_predictions_per_seq=max_predictions_per_seq,\n",
- "tokenizer=tokenizer)"
- ]
- },
- {
- "cell_type": "code",
- "execution_count": 11,
- "metadata": {
- "ExecuteTime": {
- "end_time": "2018-11-16T10:02:46.596956Z",
- "start_time": "2018-11-16T10:02:40.331008Z"
- }
- },
- "outputs": [
- {
- "name": "stdout",
- "output_type": "stream",
- "text": [
- "INFO:tensorflow:Could not find trained model in model_dir: /var/folders/yx/cw8n_njx3js5jksyw_qlp8p00000gn/T/tmp4x8r3x3d, running initialization to predict.\n"
- ]
- },
- {
- "name": "stderr",
- "output_type": "stream",
- "text": [
- "11/16/2018 11:02:40 - INFO - tensorflow - Could not find trained model in model_dir: /var/folders/yx/cw8n_njx3js5jksyw_qlp8p00000gn/T/tmp4x8r3x3d, running initialization to predict.\n"
- ]
- },
- {
- "name": "stdout",
- "output_type": "stream",
- "text": [
- "INFO:tensorflow:Calling model_fn.\n"
- ]
- },
- {
- "name": "stderr",
- "output_type": "stream",
- "text": [
- "11/16/2018 11:02:40 - INFO - tensorflow - Calling model_fn.\n"
- ]
- },
- {
- "name": "stdout",
- "output_type": "stream",
- "text": [
- "INFO:tensorflow:Running infer on CPU\n"
- ]
- },
- {
- "name": "stderr",
- "output_type": "stream",
- "text": [
- "11/16/2018 11:02:40 - INFO - tensorflow - Running infer on CPU\n"
- ]
- },
- {
- "name": "stdout",
- "output_type": "stream",
- "text": [
- "INFO:tensorflow:*** Features ***\n"
- ]
- },
- {
- "name": "stderr",
- "output_type": "stream",
- "text": [
- "11/16/2018 11:02:40 - INFO - tensorflow - *** Features ***\n"
- ]
- },
- {
- "name": "stdout",
- "output_type": "stream",
- "text": [
- "INFO:tensorflow: name = input_ids, shape = (?, 128)\n"
- ]
- },
- {
- "name": "stderr",
- "output_type": "stream",
- "text": [
- "11/16/2018 11:02:40 - INFO - tensorflow - name = input_ids, shape = (?, 128)\n"
- ]
- },
- {
- "name": "stdout",
- "output_type": "stream",
- "text": [
- "INFO:tensorflow: name = input_mask, shape = (?, 128)\n"
- ]
- },
- {
- "name": "stderr",
- "output_type": "stream",
- "text": [
- "11/16/2018 11:02:40 - INFO - tensorflow - name = input_mask, shape = (?, 128)\n"
- ]
- },
- {
- "name": "stdout",
- "output_type": "stream",
- "text": [
- "INFO:tensorflow: name = masked_lm_ids, shape = (?, 20)\n"
- ]
- },
- {
- "name": "stderr",
- "output_type": "stream",
- "text": [
- "11/16/2018 11:02:40 - INFO - tensorflow - name = masked_lm_ids, shape = (?, 20)\n"
- ]
- },
- {
- "name": "stdout",
- "output_type": "stream",
- "text": [
- "INFO:tensorflow: name = masked_lm_positions, shape = (?, 20)\n"
- ]
- },
- {
- "name": "stderr",
- "output_type": "stream",
- "text": [
- "11/16/2018 11:02:40 - INFO - tensorflow - name = masked_lm_positions, shape = (?, 20)\n"
- ]
- },
- {
- "name": "stdout",
- "output_type": "stream",
- "text": [
- "INFO:tensorflow: name = masked_lm_weights, shape = (?, 20)\n"
- ]
- },
- {
- "name": "stderr",
- "output_type": "stream",
- "text": [
- "11/16/2018 11:02:40 - INFO - tensorflow - name = masked_lm_weights, shape = (?, 20)\n"
- ]
- },
- {
- "name": "stdout",
- "output_type": "stream",
- "text": [
- "INFO:tensorflow: name = next_sentence_labels, shape = (?, 1)\n"
- ]
- },
- {
- "name": "stderr",
- "output_type": "stream",
- "text": [
- "11/16/2018 11:02:40 - INFO - tensorflow - name = next_sentence_labels, shape = (?, 1)\n"
- ]
- },
- {
- "name": "stdout",
- "output_type": "stream",
- "text": [
- "INFO:tensorflow: name = segment_ids, shape = (?, 128)\n"
- ]
- },
- {
- "name": "stderr",
- "output_type": "stream",
- "text": [
- "11/16/2018 11:02:40 - INFO - tensorflow - name = segment_ids, shape = (?, 128)\n"
- ]
- },
- {
- "name": "stdout",
- "output_type": "stream",
- "text": [
- "INFO:tensorflow:**** Trainable Variables ****\n"
- ]
- },
- {
- "name": "stderr",
- "output_type": "stream",
- "text": [
- "11/16/2018 11:02:43 - INFO - tensorflow - **** Trainable Variables ****\n"
- ]
- },
- {
- "name": "stdout",
- "output_type": "stream",
- "text": [
- "INFO:tensorflow: name = bert/embeddings/word_embeddings:0, shape = (30522, 768), *INIT_FROM_CKPT*\n"
- ]
- },
- {
- "name": "stderr",
- "output_type": "stream",
- "text": [
- "11/16/2018 11:02:43 - INFO - tensorflow - name = bert/embeddings/word_embeddings:0, shape = (30522, 768), *INIT_FROM_CKPT*\n"
- ]
- },
- {
- "name": "stdout",
- "output_type": "stream",
- "text": [
- "INFO:tensorflow: name = bert/embeddings/token_type_embeddings:0, shape = (2, 768), *INIT_FROM_CKPT*\n"
- ]
- },
- {
- "name": "stderr",
- "output_type": "stream",
- "text": [
- "11/16/2018 11:02:43 - INFO - tensorflow - name = bert/embeddings/token_type_embeddings:0, shape = (2, 768), *INIT_FROM_CKPT*\n"
- ]
- },
- {
- "name": "stdout",
- "output_type": "stream",
- "text": [
- "INFO:tensorflow: name = bert/embeddings/position_embeddings:0, shape = (512, 768), *INIT_FROM_CKPT*\n"
- ]
- },
- {
- "name": "stderr",
- "output_type": "stream",
- "text": [
- "11/16/2018 11:02:43 - INFO - tensorflow - name = bert/embeddings/position_embeddings:0, shape = (512, 768), *INIT_FROM_CKPT*\n"
- ]
- },
- {
- "name": "stdout",
- "output_type": "stream",
- "text": [
- "INFO:tensorflow: name = bert/embeddings/LayerNorm/beta:0, shape = (768,), *INIT_FROM_CKPT*\n"
- ]
- },
- {
- "name": "stderr",
- "output_type": "stream",
- "text": [
- "11/16/2018 11:02:43 - INFO - tensorflow - name = bert/embeddings/LayerNorm/beta:0, shape = (768,), *INIT_FROM_CKPT*\n"
- ]
- },
- {
- "name": "stdout",
- "output_type": "stream",
- "text": [
- "INFO:tensorflow: name = bert/embeddings/LayerNorm/gamma:0, shape = (768,), *INIT_FROM_CKPT*\n"
- ]
- },
- {
- "name": "stderr",
- "output_type": "stream",
- "text": [
- "11/16/2018 11:02:43 - INFO - tensorflow - name = bert/embeddings/LayerNorm/gamma:0, shape = (768,), *INIT_FROM_CKPT*\n"
- ]
- },
- {
- "name": "stdout",
- "output_type": "stream",
- "text": [
- "INFO:tensorflow: name = bert/encoder/layer_0/attention/self/query/kernel:0, shape = (768, 768), *INIT_FROM_CKPT*\n"
- ]
- },
- {
- "name": "stderr",
- "output_type": "stream",
- "text": [
- "11/16/2018 11:02:43 - INFO - tensorflow - name = bert/encoder/layer_0/attention/self/query/kernel:0, shape = (768, 768), *INIT_FROM_CKPT*\n"
- ]
- },
- {
- "name": "stdout",
- "output_type": "stream",
- "text": [
- "INFO:tensorflow: name = bert/encoder/layer_0/attention/self/query/bias:0, shape = (768,), *INIT_FROM_CKPT*\n"
- ]
- },
- {
- "name": "stderr",
- "output_type": "stream",
- "text": [
- "11/16/2018 11:02:43 - INFO - tensorflow - name = bert/encoder/layer_0/attention/self/query/bias:0, shape = (768,), *INIT_FROM_CKPT*\n"
- ]
- },
- {
- "name": "stdout",
- "output_type": "stream",
- "text": [
- "INFO:tensorflow: name = bert/encoder/layer_0/attention/self/key/kernel:0, shape = (768, 768), *INIT_FROM_CKPT*\n"
- ]
- },
- {
- "name": "stderr",
- "output_type": "stream",
- "text": [
- "11/16/2018 11:02:43 - INFO - tensorflow - name = bert/encoder/layer_0/attention/self/key/kernel:0, shape = (768, 768), *INIT_FROM_CKPT*\n"
- ]
- },
- {
- "name": "stdout",
- "output_type": "stream",
- "text": [
- "INFO:tensorflow: name = bert/encoder/layer_0/attention/self/key/bias:0, shape = (768,), *INIT_FROM_CKPT*\n"
- ]
- },
- {
- "name": "stderr",
- "output_type": "stream",
- "text": [
- "11/16/2018 11:02:43 - INFO - tensorflow - name = bert/encoder/layer_0/attention/self/key/bias:0, shape = (768,), *INIT_FROM_CKPT*\n"
- ]
- },
- {
- "name": "stdout",
- "output_type": "stream",
- "text": [
- "INFO:tensorflow: name = bert/encoder/layer_0/attention/self/value/kernel:0, shape = (768, 768), *INIT_FROM_CKPT*\n"
- ]
- },
- {
- "name": "stderr",
- "output_type": "stream",
- "text": [
- "11/16/2018 11:02:43 - INFO - tensorflow - name = bert/encoder/layer_0/attention/self/value/kernel:0, shape = (768, 768), *INIT_FROM_CKPT*\n"
- ]
- },
- {
- "name": "stdout",
- "output_type": "stream",
- "text": [
- "INFO:tensorflow: name = bert/encoder/layer_0/attention/self/value/bias:0, shape = (768,), *INIT_FROM_CKPT*\n"
- ]
- },
- {
- "name": "stderr",
- "output_type": "stream",
- "text": [
- "11/16/2018 11:02:43 - INFO - tensorflow - name = bert/encoder/layer_0/attention/self/value/bias:0, shape = (768,), *INIT_FROM_CKPT*\n"
- ]
- },
- {
- "name": "stdout",
- "output_type": "stream",
- "text": [
- "INFO:tensorflow: name = bert/encoder/layer_0/attention/output/dense/kernel:0, shape = (768, 768), *INIT_FROM_CKPT*\n"
- ]
- },
- {
- "name": "stderr",
- "output_type": "stream",
- "text": [
- "11/16/2018 11:02:43 - INFO - tensorflow - name = bert/encoder/layer_0/attention/output/dense/kernel:0, shape = (768, 768), *INIT_FROM_CKPT*\n"
- ]
- },
- {
- "name": "stdout",
- "output_type": "stream",
- "text": [
- "INFO:tensorflow: name = bert/encoder/layer_0/attention/output/dense/bias:0, shape = (768,), *INIT_FROM_CKPT*\n"
- ]
- },
- {
- "name": "stderr",
- "output_type": "stream",
- "text": [
- "11/16/2018 11:02:43 - INFO - tensorflow - name = bert/encoder/layer_0/attention/output/dense/bias:0, shape = (768,), *INIT_FROM_CKPT*\n"
- ]
- },
- {
- "name": "stdout",
- "output_type": "stream",
- "text": [
- "INFO:tensorflow: name = bert/encoder/layer_0/attention/output/LayerNorm/beta:0, shape = (768,), *INIT_FROM_CKPT*\n"
- ]
- },
- {
- "name": "stderr",
- "output_type": "stream",
- "text": [
- "11/16/2018 11:02:43 - INFO - tensorflow - name = bert/encoder/layer_0/attention/output/LayerNorm/beta:0, shape = (768,), *INIT_FROM_CKPT*\n"
- ]
- },
- {
- "name": "stdout",
- "output_type": "stream",
- "text": [
- "INFO:tensorflow: name = bert/encoder/layer_0/attention/output/LayerNorm/gamma:0, shape = (768,), *INIT_FROM_CKPT*\n"
- ]
- },
- {
- "name": "stderr",
- "output_type": "stream",
- "text": [
- "11/16/2018 11:02:43 - INFO - tensorflow - name = bert/encoder/layer_0/attention/output/LayerNorm/gamma:0, shape = (768,), *INIT_FROM_CKPT*\n"
- ]
- },
- {
- "name": "stdout",
- "output_type": "stream",
- "text": [
- "INFO:tensorflow: name = bert/encoder/layer_0/intermediate/dense/kernel:0, shape = (768, 3072), *INIT_FROM_CKPT*\n"
- ]
- },
- {
- "name": "stderr",
- "output_type": "stream",
- "text": [
- "11/16/2018 11:02:43 - INFO - tensorflow - name = bert/encoder/layer_0/intermediate/dense/kernel:0, shape = (768, 3072), *INIT_FROM_CKPT*\n"
- ]
- },
- {
- "name": "stdout",
- "output_type": "stream",
- "text": [
- "INFO:tensorflow: name = bert/encoder/layer_0/intermediate/dense/bias:0, shape = (3072,), *INIT_FROM_CKPT*\n"
- ]
- },
- {
- "name": "stderr",
- "output_type": "stream",
- "text": [
- "11/16/2018 11:02:43 - INFO - tensorflow - name = bert/encoder/layer_0/intermediate/dense/bias:0, shape = (3072,), *INIT_FROM_CKPT*\n"
- ]
- },
- {
- "name": "stdout",
- "output_type": "stream",
- "text": [
- "INFO:tensorflow: name = bert/encoder/layer_0/output/dense/kernel:0, shape = (3072, 768), *INIT_FROM_CKPT*\n"
- ]
- },
- {
- "name": "stderr",
- "output_type": "stream",
- "text": [
- "11/16/2018 11:02:43 - INFO - tensorflow - name = bert/encoder/layer_0/output/dense/kernel:0, shape = (3072, 768), *INIT_FROM_CKPT*\n"
- ]
- },
- {
- "name": "stdout",
- "output_type": "stream",
- "text": [
- "INFO:tensorflow: name = bert/encoder/layer_0/output/dense/bias:0, shape = (768,), *INIT_FROM_CKPT*\n"
- ]
- },
- {
- "name": "stderr",
- "output_type": "stream",
- "text": [
- "11/16/2018 11:02:43 - INFO - tensorflow - name = bert/encoder/layer_0/output/dense/bias:0, shape = (768,), *INIT_FROM_CKPT*\n"
- ]
- },
- {
- "name": "stdout",
- "output_type": "stream",
- "text": [
- "INFO:tensorflow: name = bert/encoder/layer_0/output/LayerNorm/beta:0, shape = (768,), *INIT_FROM_CKPT*\n"
- ]
- },
- {
- "name": "stderr",
- "output_type": "stream",
- "text": [
- "11/16/2018 11:02:43 - INFO - tensorflow - name = bert/encoder/layer_0/output/LayerNorm/beta:0, shape = (768,), *INIT_FROM_CKPT*\n"
- ]
- },
- {
- "name": "stdout",
- "output_type": "stream",
- "text": [
- "INFO:tensorflow: name = bert/encoder/layer_0/output/LayerNorm/gamma:0, shape = (768,), *INIT_FROM_CKPT*\n"
- ]
- },
- {
- "name": "stderr",
- "output_type": "stream",
- "text": [
- "11/16/2018 11:02:43 - INFO - tensorflow - name = bert/encoder/layer_0/output/LayerNorm/gamma:0, shape = (768,), *INIT_FROM_CKPT*\n"
- ]
- },
- {
- "name": "stdout",
- "output_type": "stream",
- "text": [
- "INFO:tensorflow: name = bert/encoder/layer_1/attention/self/query/kernel:0, shape = (768, 768), *INIT_FROM_CKPT*\n"
- ]
- },
- {
- "name": "stderr",
- "output_type": "stream",
- "text": [
- "11/16/2018 11:02:43 - INFO - tensorflow - name = bert/encoder/layer_1/attention/self/query/kernel:0, shape = (768, 768), *INIT_FROM_CKPT*\n"
- ]
- },
- {
- "name": "stdout",
- "output_type": "stream",
- "text": [
- "INFO:tensorflow: name = bert/encoder/layer_1/attention/self/query/bias:0, shape = (768,), *INIT_FROM_CKPT*\n"
- ]
- },
- {
- "name": "stderr",
- "output_type": "stream",
- "text": [
- "11/16/2018 11:02:43 - INFO - tensorflow - name = bert/encoder/layer_1/attention/self/query/bias:0, shape = (768,), *INIT_FROM_CKPT*\n"
- ]
- },
- {
- "name": "stdout",
- "output_type": "stream",
- "text": [
- "INFO:tensorflow: name = bert/encoder/layer_1/attention/self/key/kernel:0, shape = (768, 768), *INIT_FROM_CKPT*\n"
- ]
- },
- {
- "name": "stderr",
- "output_type": "stream",
- "text": [
- "11/16/2018 11:02:43 - INFO - tensorflow - name = bert/encoder/layer_1/attention/self/key/kernel:0, shape = (768, 768), *INIT_FROM_CKPT*\n"
- ]
- },
- {
- "name": "stdout",
- "output_type": "stream",
- "text": [
- "INFO:tensorflow: name = bert/encoder/layer_1/attention/self/key/bias:0, shape = (768,), *INIT_FROM_CKPT*\n"
- ]
- },
- {
- "name": "stderr",
- "output_type": "stream",
- "text": [
- "11/16/2018 11:02:43 - INFO - tensorflow - name = bert/encoder/layer_1/attention/self/key/bias:0, shape = (768,), *INIT_FROM_CKPT*\n"
- ]
- },
- {
- "name": "stdout",
- "output_type": "stream",
- "text": [
- "INFO:tensorflow: name = bert/encoder/layer_1/attention/self/value/kernel:0, shape = (768, 768), *INIT_FROM_CKPT*\n"
- ]
- },
- {
- "name": "stderr",
- "output_type": "stream",
- "text": [
- "11/16/2018 11:02:43 - INFO - tensorflow - name = bert/encoder/layer_1/attention/self/value/kernel:0, shape = (768, 768), *INIT_FROM_CKPT*\n"
- ]
- },
- {
- "name": "stdout",
- "output_type": "stream",
- "text": [
- "INFO:tensorflow: name = bert/encoder/layer_1/attention/self/value/bias:0, shape = (768,), *INIT_FROM_CKPT*\n"
- ]
- },
- {
- "name": "stderr",
- "output_type": "stream",
- "text": [
- "11/16/2018 11:02:43 - INFO - tensorflow - name = bert/encoder/layer_1/attention/self/value/bias:0, shape = (768,), *INIT_FROM_CKPT*\n"
- ]
- },
- {
- "name": "stdout",
- "output_type": "stream",
- "text": [
- "INFO:tensorflow: name = bert/encoder/layer_1/attention/output/dense/kernel:0, shape = (768, 768), *INIT_FROM_CKPT*\n"
- ]
- },
- {
- "name": "stderr",
- "output_type": "stream",
- "text": [
- "11/16/2018 11:02:43 - INFO - tensorflow - name = bert/encoder/layer_1/attention/output/dense/kernel:0, shape = (768, 768), *INIT_FROM_CKPT*\n"
- ]
- },
- {
- "name": "stdout",
- "output_type": "stream",
- "text": [
- "INFO:tensorflow: name = bert/encoder/layer_1/attention/output/dense/bias:0, shape = (768,), *INIT_FROM_CKPT*\n"
- ]
- },
- {
- "name": "stderr",
- "output_type": "stream",
- "text": [
- "11/16/2018 11:02:43 - INFO - tensorflow - name = bert/encoder/layer_1/attention/output/dense/bias:0, shape = (768,), *INIT_FROM_CKPT*\n"
- ]
- },
- {
- "name": "stdout",
- "output_type": "stream",
- "text": [
- "INFO:tensorflow: name = bert/encoder/layer_1/attention/output/LayerNorm/beta:0, shape = (768,), *INIT_FROM_CKPT*\n"
- ]
- },
- {
- "name": "stderr",
- "output_type": "stream",
- "text": [
- "11/16/2018 11:02:43 - INFO - tensorflow - name = bert/encoder/layer_1/attention/output/LayerNorm/beta:0, shape = (768,), *INIT_FROM_CKPT*\n"
- ]
- },
- {
- "name": "stdout",
- "output_type": "stream",
- "text": [
- "INFO:tensorflow: name = bert/encoder/layer_1/attention/output/LayerNorm/gamma:0, shape = (768,), *INIT_FROM_CKPT*\n"
- ]
- },
- {
- "name": "stderr",
- "output_type": "stream",
- "text": [
- "11/16/2018 11:02:43 - INFO - tensorflow - name = bert/encoder/layer_1/attention/output/LayerNorm/gamma:0, shape = (768,), *INIT_FROM_CKPT*\n"
- ]
- },
- {
- "name": "stdout",
- "output_type": "stream",
- "text": [
- "INFO:tensorflow: name = bert/encoder/layer_1/intermediate/dense/kernel:0, shape = (768, 3072), *INIT_FROM_CKPT*\n"
- ]
- },
- {
- "name": "stderr",
- "output_type": "stream",
- "text": [
- "11/16/2018 11:02:43 - INFO - tensorflow - name = bert/encoder/layer_1/intermediate/dense/kernel:0, shape = (768, 3072), *INIT_FROM_CKPT*\n"
- ]
- },
- {
- "name": "stdout",
- "output_type": "stream",
- "text": [
- "INFO:tensorflow: name = bert/encoder/layer_1/intermediate/dense/bias:0, shape = (3072,), *INIT_FROM_CKPT*\n"
- ]
- },
- {
- "name": "stderr",
- "output_type": "stream",
- "text": [
- "11/16/2018 11:02:43 - INFO - tensorflow - name = bert/encoder/layer_1/intermediate/dense/bias:0, shape = (3072,), *INIT_FROM_CKPT*\n"
- ]
- },
- {
- "name": "stdout",
- "output_type": "stream",
- "text": [
- "INFO:tensorflow: name = bert/encoder/layer_1/output/dense/kernel:0, shape = (3072, 768), *INIT_FROM_CKPT*\n"
- ]
- },
- {
- "name": "stderr",
- "output_type": "stream",
- "text": [
- "11/16/2018 11:02:43 - INFO - tensorflow - name = bert/encoder/layer_1/output/dense/kernel:0, shape = (3072, 768), *INIT_FROM_CKPT*\n"
- ]
- },
- {
- "name": "stdout",
- "output_type": "stream",
- "text": [
- "INFO:tensorflow: name = bert/encoder/layer_1/output/dense/bias:0, shape = (768,), *INIT_FROM_CKPT*\n"
- ]
- },
- {
- "name": "stderr",
- "output_type": "stream",
- "text": [
- "11/16/2018 11:02:43 - INFO - tensorflow - name = bert/encoder/layer_1/output/dense/bias:0, shape = (768,), *INIT_FROM_CKPT*\n"
- ]
- },
- {
- "name": "stdout",
- "output_type": "stream",
- "text": [
- "INFO:tensorflow: name = bert/encoder/layer_1/output/LayerNorm/beta:0, shape = (768,), *INIT_FROM_CKPT*\n"
- ]
- },
- {
- "name": "stderr",
- "output_type": "stream",
- "text": [
- "11/16/2018 11:02:43 - INFO - tensorflow - name = bert/encoder/layer_1/output/LayerNorm/beta:0, shape = (768,), *INIT_FROM_CKPT*\n"
- ]
- },
- {
- "name": "stdout",
- "output_type": "stream",
- "text": [
- "INFO:tensorflow: name = bert/encoder/layer_1/output/LayerNorm/gamma:0, shape = (768,), *INIT_FROM_CKPT*\n"
- ]
- },
- {
- "name": "stderr",
- "output_type": "stream",
- "text": [
- "11/16/2018 11:02:43 - INFO - tensorflow - name = bert/encoder/layer_1/output/LayerNorm/gamma:0, shape = (768,), *INIT_FROM_CKPT*\n"
- ]
- },
- {
- "name": "stdout",
- "output_type": "stream",
- "text": [
- "INFO:tensorflow: name = bert/encoder/layer_2/attention/self/query/kernel:0, shape = (768, 768), *INIT_FROM_CKPT*\n"
- ]
- },
- {
- "name": "stderr",
- "output_type": "stream",
- "text": [
- "11/16/2018 11:02:43 - INFO - tensorflow - name = bert/encoder/layer_2/attention/self/query/kernel:0, shape = (768, 768), *INIT_FROM_CKPT*\n"
- ]
- },
- {
- "name": "stdout",
- "output_type": "stream",
- "text": [
- "INFO:tensorflow: name = bert/encoder/layer_2/attention/self/query/bias:0, shape = (768,), *INIT_FROM_CKPT*\n"
- ]
- },
- {
- "name": "stderr",
- "output_type": "stream",
- "text": [
- "11/16/2018 11:02:43 - INFO - tensorflow - name = bert/encoder/layer_2/attention/self/query/bias:0, shape = (768,), *INIT_FROM_CKPT*\n"
- ]
- },
- {
- "name": "stdout",
- "output_type": "stream",
- "text": [
- "INFO:tensorflow: name = bert/encoder/layer_2/attention/self/key/kernel:0, shape = (768, 768), *INIT_FROM_CKPT*\n"
- ]
- },
- {
- "name": "stderr",
- "output_type": "stream",
- "text": [
- "11/16/2018 11:02:43 - INFO - tensorflow - name = bert/encoder/layer_2/attention/self/key/kernel:0, shape = (768, 768), *INIT_FROM_CKPT*\n"
- ]
- },
- {
- "name": "stdout",
- "output_type": "stream",
- "text": [
- "INFO:tensorflow: name = bert/encoder/layer_2/attention/self/key/bias:0, shape = (768,), *INIT_FROM_CKPT*\n"
- ]
- },
- {
- "name": "stderr",
- "output_type": "stream",
- "text": [
- "11/16/2018 11:02:43 - INFO - tensorflow - name = bert/encoder/layer_2/attention/self/key/bias:0, shape = (768,), *INIT_FROM_CKPT*\n"
- ]
- },
- {
- "name": "stdout",
- "output_type": "stream",
- "text": [
- "INFO:tensorflow: name = bert/encoder/layer_2/attention/self/value/kernel:0, shape = (768, 768), *INIT_FROM_CKPT*\n"
- ]
- },
- {
- "name": "stderr",
- "output_type": "stream",
- "text": [
- "11/16/2018 11:02:43 - INFO - tensorflow - name = bert/encoder/layer_2/attention/self/value/kernel:0, shape = (768, 768), *INIT_FROM_CKPT*\n"
- ]
- },
- {
- "name": "stdout",
- "output_type": "stream",
- "text": [
- "INFO:tensorflow: name = bert/encoder/layer_2/attention/self/value/bias:0, shape = (768,), *INIT_FROM_CKPT*\n"
- ]
- },
- {
- "name": "stderr",
- "output_type": "stream",
- "text": [
- "11/16/2018 11:02:43 - INFO - tensorflow - name = bert/encoder/layer_2/attention/self/value/bias:0, shape = (768,), *INIT_FROM_CKPT*\n"
- ]
- },
- {
- "name": "stdout",
- "output_type": "stream",
- "text": [
- "INFO:tensorflow: name = bert/encoder/layer_2/attention/output/dense/kernel:0, shape = (768, 768), *INIT_FROM_CKPT*\n"
- ]
- },
- {
- "name": "stderr",
- "output_type": "stream",
- "text": [
- "11/16/2018 11:02:43 - INFO - tensorflow - name = bert/encoder/layer_2/attention/output/dense/kernel:0, shape = (768, 768), *INIT_FROM_CKPT*\n"
- ]
- },
- {
- "name": "stdout",
- "output_type": "stream",
- "text": [
- "INFO:tensorflow: name = bert/encoder/layer_2/attention/output/dense/bias:0, shape = (768,), *INIT_FROM_CKPT*\n"
- ]
- },
- {
- "name": "stderr",
- "output_type": "stream",
- "text": [
- "11/16/2018 11:02:43 - INFO - tensorflow - name = bert/encoder/layer_2/attention/output/dense/bias:0, shape = (768,), *INIT_FROM_CKPT*\n"
- ]
- },
- {
- "name": "stdout",
- "output_type": "stream",
- "text": [
- "INFO:tensorflow: name = bert/encoder/layer_2/attention/output/LayerNorm/beta:0, shape = (768,), *INIT_FROM_CKPT*\n"
- ]
- },
- {
- "name": "stderr",
- "output_type": "stream",
- "text": [
- "11/16/2018 11:02:43 - INFO - tensorflow - name = bert/encoder/layer_2/attention/output/LayerNorm/beta:0, shape = (768,), *INIT_FROM_CKPT*\n"
- ]
- },
- {
- "name": "stdout",
- "output_type": "stream",
- "text": [
- "INFO:tensorflow: name = bert/encoder/layer_2/attention/output/LayerNorm/gamma:0, shape = (768,), *INIT_FROM_CKPT*\n"
- ]
- },
- {
- "name": "stderr",
- "output_type": "stream",
- "text": [
- "11/16/2018 11:02:43 - INFO - tensorflow - name = bert/encoder/layer_2/attention/output/LayerNorm/gamma:0, shape = (768,), *INIT_FROM_CKPT*\n"
- ]
- },
- {
- "name": "stdout",
- "output_type": "stream",
- "text": [
- "INFO:tensorflow: name = bert/encoder/layer_2/intermediate/dense/kernel:0, shape = (768, 3072), *INIT_FROM_CKPT*\n"
- ]
- },
- {
- "name": "stderr",
- "output_type": "stream",
- "text": [
- "11/16/2018 11:02:43 - INFO - tensorflow - name = bert/encoder/layer_2/intermediate/dense/kernel:0, shape = (768, 3072), *INIT_FROM_CKPT*\n"
- ]
- },
- {
- "name": "stdout",
- "output_type": "stream",
- "text": [
- "INFO:tensorflow: name = bert/encoder/layer_2/intermediate/dense/bias:0, shape = (3072,), *INIT_FROM_CKPT*\n"
- ]
- },
- {
- "name": "stderr",
- "output_type": "stream",
- "text": [
- "11/16/2018 11:02:43 - INFO - tensorflow - name = bert/encoder/layer_2/intermediate/dense/bias:0, shape = (3072,), *INIT_FROM_CKPT*\n"
- ]
- },
- {
- "name": "stdout",
- "output_type": "stream",
- "text": [
- "INFO:tensorflow: name = bert/encoder/layer_2/output/dense/kernel:0, shape = (3072, 768), *INIT_FROM_CKPT*\n"
- ]
- },
- {
- "name": "stderr",
- "output_type": "stream",
- "text": [
- "11/16/2018 11:02:43 - INFO - tensorflow - name = bert/encoder/layer_2/output/dense/kernel:0, shape = (3072, 768), *INIT_FROM_CKPT*\n"
- ]
- },
- {
- "name": "stdout",
- "output_type": "stream",
- "text": [
- "INFO:tensorflow: name = bert/encoder/layer_2/output/dense/bias:0, shape = (768,), *INIT_FROM_CKPT*\n"
- ]
- },
- {
- "name": "stderr",
- "output_type": "stream",
- "text": [
- "11/16/2018 11:02:43 - INFO - tensorflow - name = bert/encoder/layer_2/output/dense/bias:0, shape = (768,), *INIT_FROM_CKPT*\n"
- ]
- },
- {
- "name": "stdout",
- "output_type": "stream",
- "text": [
- "INFO:tensorflow: name = bert/encoder/layer_2/output/LayerNorm/beta:0, shape = (768,), *INIT_FROM_CKPT*\n"
- ]
- },
- {
- "name": "stderr",
- "output_type": "stream",
- "text": [
- "11/16/2018 11:02:43 - INFO - tensorflow - name = bert/encoder/layer_2/output/LayerNorm/beta:0, shape = (768,), *INIT_FROM_CKPT*\n"
- ]
- },
- {
- "name": "stdout",
- "output_type": "stream",
- "text": [
- "INFO:tensorflow: name = bert/encoder/layer_2/output/LayerNorm/gamma:0, shape = (768,), *INIT_FROM_CKPT*\n"
- ]
- },
- {
- "name": "stderr",
- "output_type": "stream",
- "text": [
- "11/16/2018 11:02:43 - INFO - tensorflow - name = bert/encoder/layer_2/output/LayerNorm/gamma:0, shape = (768,), *INIT_FROM_CKPT*\n"
- ]
- },
- {
- "name": "stdout",
- "output_type": "stream",
- "text": [
- "INFO:tensorflow: name = bert/encoder/layer_3/attention/self/query/kernel:0, shape = (768, 768), *INIT_FROM_CKPT*\n"
- ]
- },
- {
- "name": "stderr",
- "output_type": "stream",
- "text": [
- "11/16/2018 11:02:43 - INFO - tensorflow - name = bert/encoder/layer_3/attention/self/query/kernel:0, shape = (768, 768), *INIT_FROM_CKPT*\n"
- ]
- },
- {
- "name": "stdout",
- "output_type": "stream",
- "text": [
- "INFO:tensorflow: name = bert/encoder/layer_3/attention/self/query/bias:0, shape = (768,), *INIT_FROM_CKPT*\n"
- ]
- },
- {
- "name": "stderr",
- "output_type": "stream",
- "text": [
- "11/16/2018 11:02:43 - INFO - tensorflow - name = bert/encoder/layer_3/attention/self/query/bias:0, shape = (768,), *INIT_FROM_CKPT*\n"
- ]
- },
- {
- "name": "stdout",
- "output_type": "stream",
- "text": [
- "INFO:tensorflow: name = bert/encoder/layer_3/attention/self/key/kernel:0, shape = (768, 768), *INIT_FROM_CKPT*\n"
- ]
- },
- {
- "name": "stderr",
- "output_type": "stream",
- "text": [
- "11/16/2018 11:02:43 - INFO - tensorflow - name = bert/encoder/layer_3/attention/self/key/kernel:0, shape = (768, 768), *INIT_FROM_CKPT*\n"
- ]
- },
- {
- "name": "stdout",
- "output_type": "stream",
- "text": [
- "INFO:tensorflow: name = bert/encoder/layer_3/attention/self/key/bias:0, shape = (768,), *INIT_FROM_CKPT*\n"
- ]
- },
- {
- "name": "stderr",
- "output_type": "stream",
- "text": [
- "11/16/2018 11:02:43 - INFO - tensorflow - name = bert/encoder/layer_3/attention/self/key/bias:0, shape = (768,), *INIT_FROM_CKPT*\n"
- ]
- },
- {
- "name": "stdout",
- "output_type": "stream",
- "text": [
- "INFO:tensorflow: name = bert/encoder/layer_3/attention/self/value/kernel:0, shape = (768, 768), *INIT_FROM_CKPT*\n"
- ]
- },
- {
- "name": "stderr",
- "output_type": "stream",
- "text": [
- "11/16/2018 11:02:43 - INFO - tensorflow - name = bert/encoder/layer_3/attention/self/value/kernel:0, shape = (768, 768), *INIT_FROM_CKPT*\n"
- ]
- },
- {
- "name": "stdout",
- "output_type": "stream",
- "text": [
- "INFO:tensorflow: name = bert/encoder/layer_3/attention/self/value/bias:0, shape = (768,), *INIT_FROM_CKPT*\n"
- ]
- },
- {
- "name": "stderr",
- "output_type": "stream",
- "text": [
- "11/16/2018 11:02:43 - INFO - tensorflow - name = bert/encoder/layer_3/attention/self/value/bias:0, shape = (768,), *INIT_FROM_CKPT*\n"
- ]
- },
- {
- "name": "stdout",
- "output_type": "stream",
- "text": [
- "INFO:tensorflow: name = bert/encoder/layer_3/attention/output/dense/kernel:0, shape = (768, 768), *INIT_FROM_CKPT*\n"
- ]
- },
- {
- "name": "stderr",
- "output_type": "stream",
- "text": [
- "11/16/2018 11:02:43 - INFO - tensorflow - name = bert/encoder/layer_3/attention/output/dense/kernel:0, shape = (768, 768), *INIT_FROM_CKPT*\n"
- ]
- },
- {
- "name": "stdout",
- "output_type": "stream",
- "text": [
- "INFO:tensorflow: name = bert/encoder/layer_3/attention/output/dense/bias:0, shape = (768,), *INIT_FROM_CKPT*\n"
- ]
- },
- {
- "name": "stderr",
- "output_type": "stream",
- "text": [
- "11/16/2018 11:02:43 - INFO - tensorflow - name = bert/encoder/layer_3/attention/output/dense/bias:0, shape = (768,), *INIT_FROM_CKPT*\n"
- ]
- },
- {
- "name": "stdout",
- "output_type": "stream",
- "text": [
- "INFO:tensorflow: name = bert/encoder/layer_3/attention/output/LayerNorm/beta:0, shape = (768,), *INIT_FROM_CKPT*\n"
- ]
- },
- {
- "name": "stderr",
- "output_type": "stream",
- "text": [
- "11/16/2018 11:02:43 - INFO - tensorflow - name = bert/encoder/layer_3/attention/output/LayerNorm/beta:0, shape = (768,), *INIT_FROM_CKPT*\n"
- ]
- },
- {
- "name": "stdout",
- "output_type": "stream",
- "text": [
- "INFO:tensorflow: name = bert/encoder/layer_3/attention/output/LayerNorm/gamma:0, shape = (768,), *INIT_FROM_CKPT*\n"
- ]
- },
- {
- "name": "stderr",
- "output_type": "stream",
- "text": [
- "11/16/2018 11:02:43 - INFO - tensorflow - name = bert/encoder/layer_3/attention/output/LayerNorm/gamma:0, shape = (768,), *INIT_FROM_CKPT*\n"
- ]
- },
- {
- "name": "stdout",
- "output_type": "stream",
- "text": [
- "INFO:tensorflow: name = bert/encoder/layer_3/intermediate/dense/kernel:0, shape = (768, 3072), *INIT_FROM_CKPT*\n"
- ]
- },
- {
- "name": "stderr",
- "output_type": "stream",
- "text": [
- "11/16/2018 11:02:43 - INFO - tensorflow - name = bert/encoder/layer_3/intermediate/dense/kernel:0, shape = (768, 3072), *INIT_FROM_CKPT*\n"
- ]
- },
- {
- "name": "stdout",
- "output_type": "stream",
- "text": [
- "INFO:tensorflow: name = bert/encoder/layer_3/intermediate/dense/bias:0, shape = (3072,), *INIT_FROM_CKPT*\n"
- ]
- },
- {
- "name": "stderr",
- "output_type": "stream",
- "text": [
- "11/16/2018 11:02:43 - INFO - tensorflow - name = bert/encoder/layer_3/intermediate/dense/bias:0, shape = (3072,), *INIT_FROM_CKPT*\n"
- ]
- },
- {
- "name": "stdout",
- "output_type": "stream",
- "text": [
- "INFO:tensorflow: name = bert/encoder/layer_3/output/dense/kernel:0, shape = (3072, 768), *INIT_FROM_CKPT*\n"
- ]
- },
- {
- "name": "stderr",
- "output_type": "stream",
- "text": [
- "11/16/2018 11:02:43 - INFO - tensorflow - name = bert/encoder/layer_3/output/dense/kernel:0, shape = (3072, 768), *INIT_FROM_CKPT*\n"
- ]
- },
- {
- "name": "stdout",
- "output_type": "stream",
- "text": [
- "INFO:tensorflow: name = bert/encoder/layer_3/output/dense/bias:0, shape = (768,), *INIT_FROM_CKPT*\n"
- ]
- },
- {
- "name": "stderr",
- "output_type": "stream",
- "text": [
- "11/16/2018 11:02:43 - INFO - tensorflow - name = bert/encoder/layer_3/output/dense/bias:0, shape = (768,), *INIT_FROM_CKPT*\n"
- ]
- },
- {
- "name": "stdout",
- "output_type": "stream",
- "text": [
- "INFO:tensorflow: name = bert/encoder/layer_3/output/LayerNorm/beta:0, shape = (768,), *INIT_FROM_CKPT*\n"
- ]
- },
- {
- "name": "stderr",
- "output_type": "stream",
- "text": [
- "11/16/2018 11:02:43 - INFO - tensorflow - name = bert/encoder/layer_3/output/LayerNorm/beta:0, shape = (768,), *INIT_FROM_CKPT*\n"
- ]
- },
- {
- "name": "stdout",
- "output_type": "stream",
- "text": [
- "INFO:tensorflow: name = bert/encoder/layer_3/output/LayerNorm/gamma:0, shape = (768,), *INIT_FROM_CKPT*\n"
- ]
- },
- {
- "name": "stderr",
- "output_type": "stream",
- "text": [
- "11/16/2018 11:02:43 - INFO - tensorflow - name = bert/encoder/layer_3/output/LayerNorm/gamma:0, shape = (768,), *INIT_FROM_CKPT*\n"
- ]
- },
- {
- "name": "stdout",
- "output_type": "stream",
- "text": [
- "INFO:tensorflow: name = bert/encoder/layer_4/attention/self/query/kernel:0, shape = (768, 768), *INIT_FROM_CKPT*\n"
- ]
- },
- {
- "name": "stderr",
- "output_type": "stream",
- "text": [
- "11/16/2018 11:02:43 - INFO - tensorflow - name = bert/encoder/layer_4/attention/self/query/kernel:0, shape = (768, 768), *INIT_FROM_CKPT*\n"
- ]
- },
- {
- "name": "stdout",
- "output_type": "stream",
- "text": [
- "INFO:tensorflow: name = bert/encoder/layer_4/attention/self/query/bias:0, shape = (768,), *INIT_FROM_CKPT*\n"
- ]
- },
- {
- "name": "stderr",
- "output_type": "stream",
- "text": [
- "11/16/2018 11:02:43 - INFO - tensorflow - name = bert/encoder/layer_4/attention/self/query/bias:0, shape = (768,), *INIT_FROM_CKPT*\n"
- ]
- },
- {
- "name": "stdout",
- "output_type": "stream",
- "text": [
- "INFO:tensorflow: name = bert/encoder/layer_4/attention/self/key/kernel:0, shape = (768, 768), *INIT_FROM_CKPT*\n"
- ]
- },
- {
- "name": "stderr",
- "output_type": "stream",
- "text": [
- "11/16/2018 11:02:43 - INFO - tensorflow - name = bert/encoder/layer_4/attention/self/key/kernel:0, shape = (768, 768), *INIT_FROM_CKPT*\n"
- ]
- },
- {
- "name": "stdout",
- "output_type": "stream",
- "text": [
- "INFO:tensorflow: name = bert/encoder/layer_4/attention/self/key/bias:0, shape = (768,), *INIT_FROM_CKPT*\n"
- ]
- },
- {
- "name": "stderr",
- "output_type": "stream",
- "text": [
- "11/16/2018 11:02:43 - INFO - tensorflow - name = bert/encoder/layer_4/attention/self/key/bias:0, shape = (768,), *INIT_FROM_CKPT*\n"
- ]
- },
- {
- "name": "stdout",
- "output_type": "stream",
- "text": [
- "INFO:tensorflow: name = bert/encoder/layer_4/attention/self/value/kernel:0, shape = (768, 768), *INIT_FROM_CKPT*\n"
- ]
- },
- {
- "name": "stderr",
- "output_type": "stream",
- "text": [
- "11/16/2018 11:02:43 - INFO - tensorflow - name = bert/encoder/layer_4/attention/self/value/kernel:0, shape = (768, 768), *INIT_FROM_CKPT*\n"
- ]
- },
- {
- "name": "stdout",
- "output_type": "stream",
- "text": [
- "INFO:tensorflow: name = bert/encoder/layer_4/attention/self/value/bias:0, shape = (768,), *INIT_FROM_CKPT*\n"
- ]
- },
- {
- "name": "stderr",
- "output_type": "stream",
- "text": [
- "11/16/2018 11:02:43 - INFO - tensorflow - name = bert/encoder/layer_4/attention/self/value/bias:0, shape = (768,), *INIT_FROM_CKPT*\n"
- ]
- },
- {
- "name": "stdout",
- "output_type": "stream",
- "text": [
- "INFO:tensorflow: name = bert/encoder/layer_4/attention/output/dense/kernel:0, shape = (768, 768), *INIT_FROM_CKPT*\n"
- ]
- },
- {
- "name": "stderr",
- "output_type": "stream",
- "text": [
- "11/16/2018 11:02:43 - INFO - tensorflow - name = bert/encoder/layer_4/attention/output/dense/kernel:0, shape = (768, 768), *INIT_FROM_CKPT*\n"
- ]
- },
- {
- "name": "stdout",
- "output_type": "stream",
- "text": [
- "INFO:tensorflow: name = bert/encoder/layer_4/attention/output/dense/bias:0, shape = (768,), *INIT_FROM_CKPT*\n"
- ]
- },
- {
- "name": "stderr",
- "output_type": "stream",
- "text": [
- "11/16/2018 11:02:43 - INFO - tensorflow - name = bert/encoder/layer_4/attention/output/dense/bias:0, shape = (768,), *INIT_FROM_CKPT*\n"
- ]
- },
- {
- "name": "stdout",
- "output_type": "stream",
- "text": [
- "INFO:tensorflow: name = bert/encoder/layer_4/attention/output/LayerNorm/beta:0, shape = (768,), *INIT_FROM_CKPT*\n"
- ]
- },
- {
- "name": "stderr",
- "output_type": "stream",
- "text": [
- "11/16/2018 11:02:43 - INFO - tensorflow - name = bert/encoder/layer_4/attention/output/LayerNorm/beta:0, shape = (768,), *INIT_FROM_CKPT*\n"
- ]
- },
- {
- "name": "stdout",
- "output_type": "stream",
- "text": [
- "INFO:tensorflow: name = bert/encoder/layer_4/attention/output/LayerNorm/gamma:0, shape = (768,), *INIT_FROM_CKPT*\n"
- ]
- },
- {
- "name": "stderr",
- "output_type": "stream",
- "text": [
- "11/16/2018 11:02:43 - INFO - tensorflow - name = bert/encoder/layer_4/attention/output/LayerNorm/gamma:0, shape = (768,), *INIT_FROM_CKPT*\n"
- ]
- },
- {
- "name": "stdout",
- "output_type": "stream",
- "text": [
- "INFO:tensorflow: name = bert/encoder/layer_4/intermediate/dense/kernel:0, shape = (768, 3072), *INIT_FROM_CKPT*\n"
- ]
- },
- {
- "name": "stderr",
- "output_type": "stream",
- "text": [
- "11/16/2018 11:02:43 - INFO - tensorflow - name = bert/encoder/layer_4/intermediate/dense/kernel:0, shape = (768, 3072), *INIT_FROM_CKPT*\n"
- ]
- },
- {
- "name": "stdout",
- "output_type": "stream",
- "text": [
- "INFO:tensorflow: name = bert/encoder/layer_4/intermediate/dense/bias:0, shape = (3072,), *INIT_FROM_CKPT*\n"
- ]
- },
- {
- "name": "stderr",
- "output_type": "stream",
- "text": [
- "11/16/2018 11:02:43 - INFO - tensorflow - name = bert/encoder/layer_4/intermediate/dense/bias:0, shape = (3072,), *INIT_FROM_CKPT*\n"
- ]
- },
- {
- "name": "stdout",
- "output_type": "stream",
- "text": [
- "INFO:tensorflow: name = bert/encoder/layer_4/output/dense/kernel:0, shape = (3072, 768), *INIT_FROM_CKPT*\n"
- ]
- },
- {
- "name": "stderr",
- "output_type": "stream",
- "text": [
- "11/16/2018 11:02:43 - INFO - tensorflow - name = bert/encoder/layer_4/output/dense/kernel:0, shape = (3072, 768), *INIT_FROM_CKPT*\n"
- ]
- },
- {
- "name": "stdout",
- "output_type": "stream",
- "text": [
- "INFO:tensorflow: name = bert/encoder/layer_4/output/dense/bias:0, shape = (768,), *INIT_FROM_CKPT*\n"
- ]
- },
- {
- "name": "stderr",
- "output_type": "stream",
- "text": [
- "11/16/2018 11:02:43 - INFO - tensorflow - name = bert/encoder/layer_4/output/dense/bias:0, shape = (768,), *INIT_FROM_CKPT*\n"
- ]
- },
- {
- "name": "stdout",
- "output_type": "stream",
- "text": [
- "INFO:tensorflow: name = bert/encoder/layer_4/output/LayerNorm/beta:0, shape = (768,), *INIT_FROM_CKPT*\n"
- ]
- },
- {
- "name": "stderr",
- "output_type": "stream",
- "text": [
- "11/16/2018 11:02:43 - INFO - tensorflow - name = bert/encoder/layer_4/output/LayerNorm/beta:0, shape = (768,), *INIT_FROM_CKPT*\n"
- ]
- },
- {
- "name": "stdout",
- "output_type": "stream",
- "text": [
- "INFO:tensorflow: name = bert/encoder/layer_4/output/LayerNorm/gamma:0, shape = (768,), *INIT_FROM_CKPT*\n"
- ]
- },
- {
- "name": "stderr",
- "output_type": "stream",
- "text": [
- "11/16/2018 11:02:43 - INFO - tensorflow - name = bert/encoder/layer_4/output/LayerNorm/gamma:0, shape = (768,), *INIT_FROM_CKPT*\n"
- ]
- },
- {
- "name": "stdout",
- "output_type": "stream",
- "text": [
- "INFO:tensorflow: name = bert/encoder/layer_5/attention/self/query/kernel:0, shape = (768, 768), *INIT_FROM_CKPT*\n"
- ]
- },
- {
- "name": "stderr",
- "output_type": "stream",
- "text": [
- "11/16/2018 11:02:43 - INFO - tensorflow - name = bert/encoder/layer_5/attention/self/query/kernel:0, shape = (768, 768), *INIT_FROM_CKPT*\n"
- ]
- },
- {
- "name": "stdout",
- "output_type": "stream",
- "text": [
- "INFO:tensorflow: name = bert/encoder/layer_5/attention/self/query/bias:0, shape = (768,), *INIT_FROM_CKPT*\n"
- ]
- },
- {
- "name": "stderr",
- "output_type": "stream",
- "text": [
- "11/16/2018 11:02:43 - INFO - tensorflow - name = bert/encoder/layer_5/attention/self/query/bias:0, shape = (768,), *INIT_FROM_CKPT*\n"
- ]
- },
- {
- "name": "stdout",
- "output_type": "stream",
- "text": [
- "INFO:tensorflow: name = bert/encoder/layer_5/attention/self/key/kernel:0, shape = (768, 768), *INIT_FROM_CKPT*\n"
- ]
- },
- {
- "name": "stderr",
- "output_type": "stream",
- "text": [
- "11/16/2018 11:02:43 - INFO - tensorflow - name = bert/encoder/layer_5/attention/self/key/kernel:0, shape = (768, 768), *INIT_FROM_CKPT*\n"
- ]
- },
- {
- "name": "stdout",
- "output_type": "stream",
- "text": [
- "INFO:tensorflow: name = bert/encoder/layer_5/attention/self/key/bias:0, shape = (768,), *INIT_FROM_CKPT*\n"
- ]
- },
- {
- "name": "stderr",
- "output_type": "stream",
- "text": [
- "11/16/2018 11:02:43 - INFO - tensorflow - name = bert/encoder/layer_5/attention/self/key/bias:0, shape = (768,), *INIT_FROM_CKPT*\n"
- ]
- },
- {
- "name": "stdout",
- "output_type": "stream",
- "text": [
- "INFO:tensorflow: name = bert/encoder/layer_5/attention/self/value/kernel:0, shape = (768, 768), *INIT_FROM_CKPT*\n"
- ]
- },
- {
- "name": "stderr",
- "output_type": "stream",
- "text": [
- "11/16/2018 11:02:43 - INFO - tensorflow - name = bert/encoder/layer_5/attention/self/value/kernel:0, shape = (768, 768), *INIT_FROM_CKPT*\n"
- ]
- },
- {
- "name": "stdout",
- "output_type": "stream",
- "text": [
- "INFO:tensorflow: name = bert/encoder/layer_5/attention/self/value/bias:0, shape = (768,), *INIT_FROM_CKPT*\n"
- ]
- },
- {
- "name": "stderr",
- "output_type": "stream",
- "text": [
- "11/16/2018 11:02:43 - INFO - tensorflow - name = bert/encoder/layer_5/attention/self/value/bias:0, shape = (768,), *INIT_FROM_CKPT*\n"
- ]
- },
- {
- "name": "stdout",
- "output_type": "stream",
- "text": [
- "INFO:tensorflow: name = bert/encoder/layer_5/attention/output/dense/kernel:0, shape = (768, 768), *INIT_FROM_CKPT*\n"
- ]
- },
- {
- "name": "stderr",
- "output_type": "stream",
- "text": [
- "11/16/2018 11:02:43 - INFO - tensorflow - name = bert/encoder/layer_5/attention/output/dense/kernel:0, shape = (768, 768), *INIT_FROM_CKPT*\n"
- ]
- },
- {
- "name": "stdout",
- "output_type": "stream",
- "text": [
- "INFO:tensorflow: name = bert/encoder/layer_5/attention/output/dense/bias:0, shape = (768,), *INIT_FROM_CKPT*\n"
- ]
- },
- {
- "name": "stderr",
- "output_type": "stream",
- "text": [
- "11/16/2018 11:02:43 - INFO - tensorflow - name = bert/encoder/layer_5/attention/output/dense/bias:0, shape = (768,), *INIT_FROM_CKPT*\n"
- ]
- },
- {
- "name": "stdout",
- "output_type": "stream",
- "text": [
- "INFO:tensorflow: name = bert/encoder/layer_5/attention/output/LayerNorm/beta:0, shape = (768,), *INIT_FROM_CKPT*\n"
- ]
- },
- {
- "name": "stderr",
- "output_type": "stream",
- "text": [
- "11/16/2018 11:02:43 - INFO - tensorflow - name = bert/encoder/layer_5/attention/output/LayerNorm/beta:0, shape = (768,), *INIT_FROM_CKPT*\n"
- ]
- },
- {
- "name": "stdout",
- "output_type": "stream",
- "text": [
- "INFO:tensorflow: name = bert/encoder/layer_5/attention/output/LayerNorm/gamma:0, shape = (768,), *INIT_FROM_CKPT*\n"
- ]
- },
- {
- "name": "stderr",
- "output_type": "stream",
- "text": [
- "11/16/2018 11:02:43 - INFO - tensorflow - name = bert/encoder/layer_5/attention/output/LayerNorm/gamma:0, shape = (768,), *INIT_FROM_CKPT*\n"
- ]
- },
- {
- "name": "stdout",
- "output_type": "stream",
- "text": [
- "INFO:tensorflow: name = bert/encoder/layer_5/intermediate/dense/kernel:0, shape = (768, 3072), *INIT_FROM_CKPT*\n"
- ]
- },
- {
- "name": "stderr",
- "output_type": "stream",
- "text": [
- "11/16/2018 11:02:43 - INFO - tensorflow - name = bert/encoder/layer_5/intermediate/dense/kernel:0, shape = (768, 3072), *INIT_FROM_CKPT*\n"
- ]
- },
- {
- "name": "stdout",
- "output_type": "stream",
- "text": [
- "INFO:tensorflow: name = bert/encoder/layer_5/intermediate/dense/bias:0, shape = (3072,), *INIT_FROM_CKPT*\n"
- ]
- },
- {
- "name": "stderr",
- "output_type": "stream",
- "text": [
- "11/16/2018 11:02:43 - INFO - tensorflow - name = bert/encoder/layer_5/intermediate/dense/bias:0, shape = (3072,), *INIT_FROM_CKPT*\n"
- ]
- },
- {
- "name": "stdout",
- "output_type": "stream",
- "text": [
- "INFO:tensorflow: name = bert/encoder/layer_5/output/dense/kernel:0, shape = (3072, 768), *INIT_FROM_CKPT*\n"
- ]
- },
- {
- "name": "stderr",
- "output_type": "stream",
- "text": [
- "11/16/2018 11:02:43 - INFO - tensorflow - name = bert/encoder/layer_5/output/dense/kernel:0, shape = (3072, 768), *INIT_FROM_CKPT*\n"
- ]
- },
- {
- "name": "stdout",
- "output_type": "stream",
- "text": [
- "INFO:tensorflow: name = bert/encoder/layer_5/output/dense/bias:0, shape = (768,), *INIT_FROM_CKPT*\n"
- ]
- },
- {
- "name": "stderr",
- "output_type": "stream",
- "text": [
- "11/16/2018 11:02:43 - INFO - tensorflow - name = bert/encoder/layer_5/output/dense/bias:0, shape = (768,), *INIT_FROM_CKPT*\n"
- ]
- },
- {
- "name": "stdout",
- "output_type": "stream",
- "text": [
- "INFO:tensorflow: name = bert/encoder/layer_5/output/LayerNorm/beta:0, shape = (768,), *INIT_FROM_CKPT*\n"
- ]
- },
- {
- "name": "stderr",
- "output_type": "stream",
- "text": [
- "11/16/2018 11:02:43 - INFO - tensorflow - name = bert/encoder/layer_5/output/LayerNorm/beta:0, shape = (768,), *INIT_FROM_CKPT*\n"
- ]
- },
- {
- "name": "stdout",
- "output_type": "stream",
- "text": [
- "INFO:tensorflow: name = bert/encoder/layer_5/output/LayerNorm/gamma:0, shape = (768,), *INIT_FROM_CKPT*\n"
- ]
- },
- {
- "name": "stderr",
- "output_type": "stream",
- "text": [
- "11/16/2018 11:02:43 - INFO - tensorflow - name = bert/encoder/layer_5/output/LayerNorm/gamma:0, shape = (768,), *INIT_FROM_CKPT*\n"
- ]
- },
- {
- "name": "stdout",
- "output_type": "stream",
- "text": [
- "INFO:tensorflow: name = bert/encoder/layer_6/attention/self/query/kernel:0, shape = (768, 768), *INIT_FROM_CKPT*\n"
- ]
- },
- {
- "name": "stderr",
- "output_type": "stream",
- "text": [
- "11/16/2018 11:02:43 - INFO - tensorflow - name = bert/encoder/layer_6/attention/self/query/kernel:0, shape = (768, 768), *INIT_FROM_CKPT*\n"
- ]
- },
- {
- "name": "stdout",
- "output_type": "stream",
- "text": [
- "INFO:tensorflow: name = bert/encoder/layer_6/attention/self/query/bias:0, shape = (768,), *INIT_FROM_CKPT*\n"
- ]
- },
- {
- "name": "stderr",
- "output_type": "stream",
- "text": [
- "11/16/2018 11:02:43 - INFO - tensorflow - name = bert/encoder/layer_6/attention/self/query/bias:0, shape = (768,), *INIT_FROM_CKPT*\n"
- ]
- },
- {
- "name": "stdout",
- "output_type": "stream",
- "text": [
- "INFO:tensorflow: name = bert/encoder/layer_6/attention/self/key/kernel:0, shape = (768, 768), *INIT_FROM_CKPT*\n"
- ]
- },
- {
- "name": "stderr",
- "output_type": "stream",
- "text": [
- "11/16/2018 11:02:43 - INFO - tensorflow - name = bert/encoder/layer_6/attention/self/key/kernel:0, shape = (768, 768), *INIT_FROM_CKPT*\n"
- ]
- },
- {
- "name": "stdout",
- "output_type": "stream",
- "text": [
- "INFO:tensorflow: name = bert/encoder/layer_6/attention/self/key/bias:0, shape = (768,), *INIT_FROM_CKPT*\n"
- ]
- },
- {
- "name": "stderr",
- "output_type": "stream",
- "text": [
- "11/16/2018 11:02:43 - INFO - tensorflow - name = bert/encoder/layer_6/attention/self/key/bias:0, shape = (768,), *INIT_FROM_CKPT*\n"
- ]
- },
- {
- "name": "stdout",
- "output_type": "stream",
- "text": [
- "INFO:tensorflow: name = bert/encoder/layer_6/attention/self/value/kernel:0, shape = (768, 768), *INIT_FROM_CKPT*\n"
- ]
- },
- {
- "name": "stderr",
- "output_type": "stream",
- "text": [
- "11/16/2018 11:02:43 - INFO - tensorflow - name = bert/encoder/layer_6/attention/self/value/kernel:0, shape = (768, 768), *INIT_FROM_CKPT*\n"
- ]
- },
- {
- "name": "stdout",
- "output_type": "stream",
- "text": [
- "INFO:tensorflow: name = bert/encoder/layer_6/attention/self/value/bias:0, shape = (768,), *INIT_FROM_CKPT*\n"
- ]
- },
- {
- "name": "stderr",
- "output_type": "stream",
- "text": [
- "11/16/2018 11:02:43 - INFO - tensorflow - name = bert/encoder/layer_6/attention/self/value/bias:0, shape = (768,), *INIT_FROM_CKPT*\n"
- ]
- },
- {
- "name": "stdout",
- "output_type": "stream",
- "text": [
- "INFO:tensorflow: name = bert/encoder/layer_6/attention/output/dense/kernel:0, shape = (768, 768), *INIT_FROM_CKPT*\n"
- ]
- },
- {
- "name": "stderr",
- "output_type": "stream",
- "text": [
- "11/16/2018 11:02:43 - INFO - tensorflow - name = bert/encoder/layer_6/attention/output/dense/kernel:0, shape = (768, 768), *INIT_FROM_CKPT*\n"
- ]
- },
- {
- "name": "stdout",
- "output_type": "stream",
- "text": [
- "INFO:tensorflow: name = bert/encoder/layer_6/attention/output/dense/bias:0, shape = (768,), *INIT_FROM_CKPT*\n"
- ]
- },
- {
- "name": "stderr",
- "output_type": "stream",
- "text": [
- "11/16/2018 11:02:43 - INFO - tensorflow - name = bert/encoder/layer_6/attention/output/dense/bias:0, shape = (768,), *INIT_FROM_CKPT*\n"
- ]
- },
- {
- "name": "stdout",
- "output_type": "stream",
- "text": [
- "INFO:tensorflow: name = bert/encoder/layer_6/attention/output/LayerNorm/beta:0, shape = (768,), *INIT_FROM_CKPT*\n"
- ]
- },
- {
- "name": "stderr",
- "output_type": "stream",
- "text": [
- "11/16/2018 11:02:43 - INFO - tensorflow - name = bert/encoder/layer_6/attention/output/LayerNorm/beta:0, shape = (768,), *INIT_FROM_CKPT*\n"
- ]
- },
- {
- "name": "stdout",
- "output_type": "stream",
- "text": [
- "INFO:tensorflow: name = bert/encoder/layer_6/attention/output/LayerNorm/gamma:0, shape = (768,), *INIT_FROM_CKPT*\n"
- ]
- },
- {
- "name": "stderr",
- "output_type": "stream",
- "text": [
- "11/16/2018 11:02:43 - INFO - tensorflow - name = bert/encoder/layer_6/attention/output/LayerNorm/gamma:0, shape = (768,), *INIT_FROM_CKPT*\n"
- ]
- },
- {
- "name": "stdout",
- "output_type": "stream",
- "text": [
- "INFO:tensorflow: name = bert/encoder/layer_6/intermediate/dense/kernel:0, shape = (768, 3072), *INIT_FROM_CKPT*\n"
- ]
- },
- {
- "name": "stderr",
- "output_type": "stream",
- "text": [
- "11/16/2018 11:02:43 - INFO - tensorflow - name = bert/encoder/layer_6/intermediate/dense/kernel:0, shape = (768, 3072), *INIT_FROM_CKPT*\n"
- ]
- },
- {
- "name": "stdout",
- "output_type": "stream",
- "text": [
- "INFO:tensorflow: name = bert/encoder/layer_6/intermediate/dense/bias:0, shape = (3072,), *INIT_FROM_CKPT*\n"
- ]
- },
- {
- "name": "stderr",
- "output_type": "stream",
- "text": [
- "11/16/2018 11:02:43 - INFO - tensorflow - name = bert/encoder/layer_6/intermediate/dense/bias:0, shape = (3072,), *INIT_FROM_CKPT*\n"
- ]
- },
- {
- "name": "stdout",
- "output_type": "stream",
- "text": [
- "INFO:tensorflow: name = bert/encoder/layer_6/output/dense/kernel:0, shape = (3072, 768), *INIT_FROM_CKPT*\n"
- ]
- },
- {
- "name": "stderr",
- "output_type": "stream",
- "text": [
- "11/16/2018 11:02:43 - INFO - tensorflow - name = bert/encoder/layer_6/output/dense/kernel:0, shape = (3072, 768), *INIT_FROM_CKPT*\n"
- ]
- },
- {
- "name": "stdout",
- "output_type": "stream",
- "text": [
- "INFO:tensorflow: name = bert/encoder/layer_6/output/dense/bias:0, shape = (768,), *INIT_FROM_CKPT*\n"
- ]
- },
- {
- "name": "stderr",
- "output_type": "stream",
- "text": [
- "11/16/2018 11:02:43 - INFO - tensorflow - name = bert/encoder/layer_6/output/dense/bias:0, shape = (768,), *INIT_FROM_CKPT*\n"
- ]
- },
- {
- "name": "stdout",
- "output_type": "stream",
- "text": [
- "INFO:tensorflow: name = bert/encoder/layer_6/output/LayerNorm/beta:0, shape = (768,), *INIT_FROM_CKPT*\n"
- ]
- },
- {
- "name": "stderr",
- "output_type": "stream",
- "text": [
- "11/16/2018 11:02:43 - INFO - tensorflow - name = bert/encoder/layer_6/output/LayerNorm/beta:0, shape = (768,), *INIT_FROM_CKPT*\n"
- ]
- },
- {
- "name": "stdout",
- "output_type": "stream",
- "text": [
- "INFO:tensorflow: name = bert/encoder/layer_6/output/LayerNorm/gamma:0, shape = (768,), *INIT_FROM_CKPT*\n"
- ]
- },
- {
- "name": "stderr",
- "output_type": "stream",
- "text": [
- "11/16/2018 11:02:43 - INFO - tensorflow - name = bert/encoder/layer_6/output/LayerNorm/gamma:0, shape = (768,), *INIT_FROM_CKPT*\n"
- ]
- },
- {
- "name": "stdout",
- "output_type": "stream",
- "text": [
- "INFO:tensorflow: name = bert/encoder/layer_7/attention/self/query/kernel:0, shape = (768, 768), *INIT_FROM_CKPT*\n"
- ]
- },
- {
- "name": "stderr",
- "output_type": "stream",
- "text": [
- "11/16/2018 11:02:43 - INFO - tensorflow - name = bert/encoder/layer_7/attention/self/query/kernel:0, shape = (768, 768), *INIT_FROM_CKPT*\n"
- ]
- },
- {
- "name": "stdout",
- "output_type": "stream",
- "text": [
- "INFO:tensorflow: name = bert/encoder/layer_7/attention/self/query/bias:0, shape = (768,), *INIT_FROM_CKPT*\n"
- ]
- },
- {
- "name": "stderr",
- "output_type": "stream",
- "text": [
- "11/16/2018 11:02:43 - INFO - tensorflow - name = bert/encoder/layer_7/attention/self/query/bias:0, shape = (768,), *INIT_FROM_CKPT*\n"
- ]
- },
- {
- "name": "stdout",
- "output_type": "stream",
- "text": [
- "INFO:tensorflow: name = bert/encoder/layer_7/attention/self/key/kernel:0, shape = (768, 768), *INIT_FROM_CKPT*\n"
- ]
- },
- {
- "name": "stderr",
- "output_type": "stream",
- "text": [
- "11/16/2018 11:02:43 - INFO - tensorflow - name = bert/encoder/layer_7/attention/self/key/kernel:0, shape = (768, 768), *INIT_FROM_CKPT*\n"
- ]
- },
- {
- "name": "stdout",
- "output_type": "stream",
- "text": [
- "INFO:tensorflow: name = bert/encoder/layer_7/attention/self/key/bias:0, shape = (768,), *INIT_FROM_CKPT*\n"
- ]
- },
- {
- "name": "stderr",
- "output_type": "stream",
- "text": [
- "11/16/2018 11:02:43 - INFO - tensorflow - name = bert/encoder/layer_7/attention/self/key/bias:0, shape = (768,), *INIT_FROM_CKPT*\n"
- ]
- },
- {
- "name": "stdout",
- "output_type": "stream",
- "text": [
- "INFO:tensorflow: name = bert/encoder/layer_7/attention/self/value/kernel:0, shape = (768, 768), *INIT_FROM_CKPT*\n"
- ]
- },
- {
- "name": "stderr",
- "output_type": "stream",
- "text": [
- "11/16/2018 11:02:43 - INFO - tensorflow - name = bert/encoder/layer_7/attention/self/value/kernel:0, shape = (768, 768), *INIT_FROM_CKPT*\n"
- ]
- },
- {
- "name": "stdout",
- "output_type": "stream",
- "text": [
- "INFO:tensorflow: name = bert/encoder/layer_7/attention/self/value/bias:0, shape = (768,), *INIT_FROM_CKPT*\n"
- ]
- },
- {
- "name": "stderr",
- "output_type": "stream",
- "text": [
- "11/16/2018 11:02:43 - INFO - tensorflow - name = bert/encoder/layer_7/attention/self/value/bias:0, shape = (768,), *INIT_FROM_CKPT*\n"
- ]
- },
- {
- "name": "stdout",
- "output_type": "stream",
- "text": [
- "INFO:tensorflow: name = bert/encoder/layer_7/attention/output/dense/kernel:0, shape = (768, 768), *INIT_FROM_CKPT*\n"
- ]
- },
- {
- "name": "stderr",
- "output_type": "stream",
- "text": [
- "11/16/2018 11:02:43 - INFO - tensorflow - name = bert/encoder/layer_7/attention/output/dense/kernel:0, shape = (768, 768), *INIT_FROM_CKPT*\n"
- ]
- },
- {
- "name": "stdout",
- "output_type": "stream",
- "text": [
- "INFO:tensorflow: name = bert/encoder/layer_7/attention/output/dense/bias:0, shape = (768,), *INIT_FROM_CKPT*\n"
- ]
- },
- {
- "name": "stderr",
- "output_type": "stream",
- "text": [
- "11/16/2018 11:02:43 - INFO - tensorflow - name = bert/encoder/layer_7/attention/output/dense/bias:0, shape = (768,), *INIT_FROM_CKPT*\n"
- ]
- },
- {
- "name": "stdout",
- "output_type": "stream",
- "text": [
- "INFO:tensorflow: name = bert/encoder/layer_7/attention/output/LayerNorm/beta:0, shape = (768,), *INIT_FROM_CKPT*\n"
- ]
- },
- {
- "name": "stderr",
- "output_type": "stream",
- "text": [
- "11/16/2018 11:02:43 - INFO - tensorflow - name = bert/encoder/layer_7/attention/output/LayerNorm/beta:0, shape = (768,), *INIT_FROM_CKPT*\n"
- ]
- },
- {
- "name": "stdout",
- "output_type": "stream",
- "text": [
- "INFO:tensorflow: name = bert/encoder/layer_7/attention/output/LayerNorm/gamma:0, shape = (768,), *INIT_FROM_CKPT*\n"
- ]
- },
- {
- "name": "stderr",
- "output_type": "stream",
- "text": [
- "11/16/2018 11:02:43 - INFO - tensorflow - name = bert/encoder/layer_7/attention/output/LayerNorm/gamma:0, shape = (768,), *INIT_FROM_CKPT*\n"
- ]
- },
- {
- "name": "stdout",
- "output_type": "stream",
- "text": [
- "INFO:tensorflow: name = bert/encoder/layer_7/intermediate/dense/kernel:0, shape = (768, 3072), *INIT_FROM_CKPT*\n"
- ]
- },
- {
- "name": "stderr",
- "output_type": "stream",
- "text": [
- "11/16/2018 11:02:43 - INFO - tensorflow - name = bert/encoder/layer_7/intermediate/dense/kernel:0, shape = (768, 3072), *INIT_FROM_CKPT*\n"
- ]
- },
- {
- "name": "stdout",
- "output_type": "stream",
- "text": [
- "INFO:tensorflow: name = bert/encoder/layer_7/intermediate/dense/bias:0, shape = (3072,), *INIT_FROM_CKPT*\n"
- ]
- },
- {
- "name": "stderr",
- "output_type": "stream",
- "text": [
- "11/16/2018 11:02:43 - INFO - tensorflow - name = bert/encoder/layer_7/intermediate/dense/bias:0, shape = (3072,), *INIT_FROM_CKPT*\n"
- ]
- },
- {
- "name": "stdout",
- "output_type": "stream",
- "text": [
- "INFO:tensorflow: name = bert/encoder/layer_7/output/dense/kernel:0, shape = (3072, 768), *INIT_FROM_CKPT*\n"
- ]
- },
- {
- "name": "stderr",
- "output_type": "stream",
- "text": [
- "11/16/2018 11:02:43 - INFO - tensorflow - name = bert/encoder/layer_7/output/dense/kernel:0, shape = (3072, 768), *INIT_FROM_CKPT*\n"
- ]
- },
- {
- "name": "stdout",
- "output_type": "stream",
- "text": [
- "INFO:tensorflow: name = bert/encoder/layer_7/output/dense/bias:0, shape = (768,), *INIT_FROM_CKPT*\n"
- ]
- },
- {
- "name": "stderr",
- "output_type": "stream",
- "text": [
- "11/16/2018 11:02:43 - INFO - tensorflow - name = bert/encoder/layer_7/output/dense/bias:0, shape = (768,), *INIT_FROM_CKPT*\n"
- ]
- },
- {
- "name": "stdout",
- "output_type": "stream",
- "text": [
- "INFO:tensorflow: name = bert/encoder/layer_7/output/LayerNorm/beta:0, shape = (768,), *INIT_FROM_CKPT*\n"
- ]
- },
- {
- "name": "stderr",
- "output_type": "stream",
- "text": [
- "11/16/2018 11:02:43 - INFO - tensorflow - name = bert/encoder/layer_7/output/LayerNorm/beta:0, shape = (768,), *INIT_FROM_CKPT*\n"
- ]
- },
- {
- "name": "stdout",
- "output_type": "stream",
- "text": [
- "INFO:tensorflow: name = bert/encoder/layer_7/output/LayerNorm/gamma:0, shape = (768,), *INIT_FROM_CKPT*\n"
- ]
- },
- {
- "name": "stderr",
- "output_type": "stream",
- "text": [
- "11/16/2018 11:02:43 - INFO - tensorflow - name = bert/encoder/layer_7/output/LayerNorm/gamma:0, shape = (768,), *INIT_FROM_CKPT*\n"
- ]
- },
- {
- "name": "stdout",
- "output_type": "stream",
- "text": [
- "INFO:tensorflow: name = bert/encoder/layer_8/attention/self/query/kernel:0, shape = (768, 768), *INIT_FROM_CKPT*\n"
- ]
- },
- {
- "name": "stderr",
- "output_type": "stream",
- "text": [
- "11/16/2018 11:02:43 - INFO - tensorflow - name = bert/encoder/layer_8/attention/self/query/kernel:0, shape = (768, 768), *INIT_FROM_CKPT*\n"
- ]
- },
- {
- "name": "stdout",
- "output_type": "stream",
- "text": [
- "INFO:tensorflow: name = bert/encoder/layer_8/attention/self/query/bias:0, shape = (768,), *INIT_FROM_CKPT*\n"
- ]
- },
- {
- "name": "stderr",
- "output_type": "stream",
- "text": [
- "11/16/2018 11:02:43 - INFO - tensorflow - name = bert/encoder/layer_8/attention/self/query/bias:0, shape = (768,), *INIT_FROM_CKPT*\n"
- ]
- },
- {
- "name": "stdout",
- "output_type": "stream",
- "text": [
- "INFO:tensorflow: name = bert/encoder/layer_8/attention/self/key/kernel:0, shape = (768, 768), *INIT_FROM_CKPT*\n"
- ]
- },
- {
- "name": "stderr",
- "output_type": "stream",
- "text": [
- "11/16/2018 11:02:43 - INFO - tensorflow - name = bert/encoder/layer_8/attention/self/key/kernel:0, shape = (768, 768), *INIT_FROM_CKPT*\n"
- ]
- },
- {
- "name": "stdout",
- "output_type": "stream",
- "text": [
- "INFO:tensorflow: name = bert/encoder/layer_8/attention/self/key/bias:0, shape = (768,), *INIT_FROM_CKPT*\n"
- ]
- },
- {
- "name": "stderr",
- "output_type": "stream",
- "text": [
- "11/16/2018 11:02:43 - INFO - tensorflow - name = bert/encoder/layer_8/attention/self/key/bias:0, shape = (768,), *INIT_FROM_CKPT*\n"
- ]
- },
- {
- "name": "stdout",
- "output_type": "stream",
- "text": [
- "INFO:tensorflow: name = bert/encoder/layer_8/attention/self/value/kernel:0, shape = (768, 768), *INIT_FROM_CKPT*\n"
- ]
- },
- {
- "name": "stderr",
- "output_type": "stream",
- "text": [
- "11/16/2018 11:02:43 - INFO - tensorflow - name = bert/encoder/layer_8/attention/self/value/kernel:0, shape = (768, 768), *INIT_FROM_CKPT*\n"
- ]
- },
- {
- "name": "stdout",
- "output_type": "stream",
- "text": [
- "INFO:tensorflow: name = bert/encoder/layer_8/attention/self/value/bias:0, shape = (768,), *INIT_FROM_CKPT*\n"
- ]
- },
- {
- "name": "stderr",
- "output_type": "stream",
- "text": [
- "11/16/2018 11:02:43 - INFO - tensorflow - name = bert/encoder/layer_8/attention/self/value/bias:0, shape = (768,), *INIT_FROM_CKPT*\n"
- ]
- },
- {
- "name": "stdout",
- "output_type": "stream",
- "text": [
- "INFO:tensorflow: name = bert/encoder/layer_8/attention/output/dense/kernel:0, shape = (768, 768), *INIT_FROM_CKPT*\n"
- ]
- },
- {
- "name": "stderr",
- "output_type": "stream",
- "text": [
- "11/16/2018 11:02:43 - INFO - tensorflow - name = bert/encoder/layer_8/attention/output/dense/kernel:0, shape = (768, 768), *INIT_FROM_CKPT*\n"
- ]
- },
- {
- "name": "stdout",
- "output_type": "stream",
- "text": [
- "INFO:tensorflow: name = bert/encoder/layer_8/attention/output/dense/bias:0, shape = (768,), *INIT_FROM_CKPT*\n"
- ]
- },
- {
- "name": "stderr",
- "output_type": "stream",
- "text": [
- "11/16/2018 11:02:43 - INFO - tensorflow - name = bert/encoder/layer_8/attention/output/dense/bias:0, shape = (768,), *INIT_FROM_CKPT*\n"
- ]
- },
- {
- "name": "stdout",
- "output_type": "stream",
- "text": [
- "INFO:tensorflow: name = bert/encoder/layer_8/attention/output/LayerNorm/beta:0, shape = (768,), *INIT_FROM_CKPT*\n"
- ]
- },
- {
- "name": "stderr",
- "output_type": "stream",
- "text": [
- "11/16/2018 11:02:43 - INFO - tensorflow - name = bert/encoder/layer_8/attention/output/LayerNorm/beta:0, shape = (768,), *INIT_FROM_CKPT*\n"
- ]
- },
- {
- "name": "stdout",
- "output_type": "stream",
- "text": [
- "INFO:tensorflow: name = bert/encoder/layer_8/attention/output/LayerNorm/gamma:0, shape = (768,), *INIT_FROM_CKPT*\n"
- ]
- },
- {
- "name": "stderr",
- "output_type": "stream",
- "text": [
- "11/16/2018 11:02:43 - INFO - tensorflow - name = bert/encoder/layer_8/attention/output/LayerNorm/gamma:0, shape = (768,), *INIT_FROM_CKPT*\n"
- ]
- },
- {
- "name": "stdout",
- "output_type": "stream",
- "text": [
- "INFO:tensorflow: name = bert/encoder/layer_8/intermediate/dense/kernel:0, shape = (768, 3072), *INIT_FROM_CKPT*\n"
- ]
- },
- {
- "name": "stderr",
- "output_type": "stream",
- "text": [
- "11/16/2018 11:02:43 - INFO - tensorflow - name = bert/encoder/layer_8/intermediate/dense/kernel:0, shape = (768, 3072), *INIT_FROM_CKPT*\n"
- ]
- },
- {
- "name": "stdout",
- "output_type": "stream",
- "text": [
- "INFO:tensorflow: name = bert/encoder/layer_8/intermediate/dense/bias:0, shape = (3072,), *INIT_FROM_CKPT*\n"
- ]
- },
- {
- "name": "stderr",
- "output_type": "stream",
- "text": [
- "11/16/2018 11:02:43 - INFO - tensorflow - name = bert/encoder/layer_8/intermediate/dense/bias:0, shape = (3072,), *INIT_FROM_CKPT*\n"
- ]
- },
- {
- "name": "stdout",
- "output_type": "stream",
- "text": [
- "INFO:tensorflow: name = bert/encoder/layer_8/output/dense/kernel:0, shape = (3072, 768), *INIT_FROM_CKPT*\n"
- ]
- },
- {
- "name": "stderr",
- "output_type": "stream",
- "text": [
- "11/16/2018 11:02:43 - INFO - tensorflow - name = bert/encoder/layer_8/output/dense/kernel:0, shape = (3072, 768), *INIT_FROM_CKPT*\n"
- ]
- },
- {
- "name": "stdout",
- "output_type": "stream",
- "text": [
- "INFO:tensorflow: name = bert/encoder/layer_8/output/dense/bias:0, shape = (768,), *INIT_FROM_CKPT*\n"
- ]
- },
- {
- "name": "stderr",
- "output_type": "stream",
- "text": [
- "11/16/2018 11:02:43 - INFO - tensorflow - name = bert/encoder/layer_8/output/dense/bias:0, shape = (768,), *INIT_FROM_CKPT*\n"
- ]
- },
- {
- "name": "stdout",
- "output_type": "stream",
- "text": [
- "INFO:tensorflow: name = bert/encoder/layer_8/output/LayerNorm/beta:0, shape = (768,), *INIT_FROM_CKPT*\n"
- ]
- },
- {
- "name": "stderr",
- "output_type": "stream",
- "text": [
- "11/16/2018 11:02:43 - INFO - tensorflow - name = bert/encoder/layer_8/output/LayerNorm/beta:0, shape = (768,), *INIT_FROM_CKPT*\n"
- ]
- },
- {
- "name": "stdout",
- "output_type": "stream",
- "text": [
- "INFO:tensorflow: name = bert/encoder/layer_8/output/LayerNorm/gamma:0, shape = (768,), *INIT_FROM_CKPT*\n"
- ]
- },
- {
- "name": "stderr",
- "output_type": "stream",
- "text": [
- "11/16/2018 11:02:43 - INFO - tensorflow - name = bert/encoder/layer_8/output/LayerNorm/gamma:0, shape = (768,), *INIT_FROM_CKPT*\n"
- ]
- },
- {
- "name": "stdout",
- "output_type": "stream",
- "text": [
- "INFO:tensorflow: name = bert/encoder/layer_9/attention/self/query/kernel:0, shape = (768, 768), *INIT_FROM_CKPT*\n"
- ]
- },
- {
- "name": "stderr",
- "output_type": "stream",
- "text": [
- "11/16/2018 11:02:43 - INFO - tensorflow - name = bert/encoder/layer_9/attention/self/query/kernel:0, shape = (768, 768), *INIT_FROM_CKPT*\n"
- ]
- },
- {
- "name": "stdout",
- "output_type": "stream",
- "text": [
- "INFO:tensorflow: name = bert/encoder/layer_9/attention/self/query/bias:0, shape = (768,), *INIT_FROM_CKPT*\n"
- ]
- },
- {
- "name": "stderr",
- "output_type": "stream",
- "text": [
- "11/16/2018 11:02:43 - INFO - tensorflow - name = bert/encoder/layer_9/attention/self/query/bias:0, shape = (768,), *INIT_FROM_CKPT*\n"
- ]
- },
- {
- "name": "stdout",
- "output_type": "stream",
- "text": [
- "INFO:tensorflow: name = bert/encoder/layer_9/attention/self/key/kernel:0, shape = (768, 768), *INIT_FROM_CKPT*\n"
- ]
- },
- {
- "name": "stderr",
- "output_type": "stream",
- "text": [
- "11/16/2018 11:02:43 - INFO - tensorflow - name = bert/encoder/layer_9/attention/self/key/kernel:0, shape = (768, 768), *INIT_FROM_CKPT*\n"
- ]
- },
- {
- "name": "stdout",
- "output_type": "stream",
- "text": [
- "INFO:tensorflow: name = bert/encoder/layer_9/attention/self/key/bias:0, shape = (768,), *INIT_FROM_CKPT*\n"
- ]
- },
- {
- "name": "stderr",
- "output_type": "stream",
- "text": [
- "11/16/2018 11:02:43 - INFO - tensorflow - name = bert/encoder/layer_9/attention/self/key/bias:0, shape = (768,), *INIT_FROM_CKPT*\n"
- ]
- },
- {
- "name": "stdout",
- "output_type": "stream",
- "text": [
- "INFO:tensorflow: name = bert/encoder/layer_9/attention/self/value/kernel:0, shape = (768, 768), *INIT_FROM_CKPT*\n"
- ]
- },
- {
- "name": "stderr",
- "output_type": "stream",
- "text": [
- "11/16/2018 11:02:43 - INFO - tensorflow - name = bert/encoder/layer_9/attention/self/value/kernel:0, shape = (768, 768), *INIT_FROM_CKPT*\n"
- ]
- },
- {
- "name": "stdout",
- "output_type": "stream",
- "text": [
- "INFO:tensorflow: name = bert/encoder/layer_9/attention/self/value/bias:0, shape = (768,), *INIT_FROM_CKPT*\n"
- ]
- },
- {
- "name": "stderr",
- "output_type": "stream",
- "text": [
- "11/16/2018 11:02:43 - INFO - tensorflow - name = bert/encoder/layer_9/attention/self/value/bias:0, shape = (768,), *INIT_FROM_CKPT*\n"
- ]
- },
- {
- "name": "stdout",
- "output_type": "stream",
- "text": [
- "INFO:tensorflow: name = bert/encoder/layer_9/attention/output/dense/kernel:0, shape = (768, 768), *INIT_FROM_CKPT*\n"
- ]
- },
- {
- "name": "stderr",
- "output_type": "stream",
- "text": [
- "11/16/2018 11:02:43 - INFO - tensorflow - name = bert/encoder/layer_9/attention/output/dense/kernel:0, shape = (768, 768), *INIT_FROM_CKPT*\n"
- ]
- },
- {
- "name": "stdout",
- "output_type": "stream",
- "text": [
- "INFO:tensorflow: name = bert/encoder/layer_9/attention/output/dense/bias:0, shape = (768,), *INIT_FROM_CKPT*\n"
- ]
- },
- {
- "name": "stderr",
- "output_type": "stream",
- "text": [
- "11/16/2018 11:02:43 - INFO - tensorflow - name = bert/encoder/layer_9/attention/output/dense/bias:0, shape = (768,), *INIT_FROM_CKPT*\n"
- ]
- },
- {
- "name": "stdout",
- "output_type": "stream",
- "text": [
- "INFO:tensorflow: name = bert/encoder/layer_9/attention/output/LayerNorm/beta:0, shape = (768,), *INIT_FROM_CKPT*\n"
- ]
- },
- {
- "name": "stderr",
- "output_type": "stream",
- "text": [
- "11/16/2018 11:02:43 - INFO - tensorflow - name = bert/encoder/layer_9/attention/output/LayerNorm/beta:0, shape = (768,), *INIT_FROM_CKPT*\n"
- ]
- },
- {
- "name": "stdout",
- "output_type": "stream",
- "text": [
- "INFO:tensorflow: name = bert/encoder/layer_9/attention/output/LayerNorm/gamma:0, shape = (768,), *INIT_FROM_CKPT*\n"
- ]
- },
- {
- "name": "stderr",
- "output_type": "stream",
- "text": [
- "11/16/2018 11:02:43 - INFO - tensorflow - name = bert/encoder/layer_9/attention/output/LayerNorm/gamma:0, shape = (768,), *INIT_FROM_CKPT*\n"
- ]
- },
- {
- "name": "stdout",
- "output_type": "stream",
- "text": [
- "INFO:tensorflow: name = bert/encoder/layer_9/intermediate/dense/kernel:0, shape = (768, 3072), *INIT_FROM_CKPT*\n"
- ]
- },
- {
- "name": "stderr",
- "output_type": "stream",
- "text": [
- "11/16/2018 11:02:43 - INFO - tensorflow - name = bert/encoder/layer_9/intermediate/dense/kernel:0, shape = (768, 3072), *INIT_FROM_CKPT*\n"
- ]
- },
- {
- "name": "stdout",
- "output_type": "stream",
- "text": [
- "INFO:tensorflow: name = bert/encoder/layer_9/intermediate/dense/bias:0, shape = (3072,), *INIT_FROM_CKPT*\n"
- ]
- },
- {
- "name": "stderr",
- "output_type": "stream",
- "text": [
- "11/16/2018 11:02:43 - INFO - tensorflow - name = bert/encoder/layer_9/intermediate/dense/bias:0, shape = (3072,), *INIT_FROM_CKPT*\n"
- ]
- },
- {
- "name": "stdout",
- "output_type": "stream",
- "text": [
- "INFO:tensorflow: name = bert/encoder/layer_9/output/dense/kernel:0, shape = (3072, 768), *INIT_FROM_CKPT*\n"
- ]
- },
- {
- "name": "stderr",
- "output_type": "stream",
- "text": [
- "11/16/2018 11:02:43 - INFO - tensorflow - name = bert/encoder/layer_9/output/dense/kernel:0, shape = (3072, 768), *INIT_FROM_CKPT*\n"
- ]
- },
- {
- "name": "stdout",
- "output_type": "stream",
- "text": [
- "INFO:tensorflow: name = bert/encoder/layer_9/output/dense/bias:0, shape = (768,), *INIT_FROM_CKPT*\n"
- ]
- },
- {
- "name": "stderr",
- "output_type": "stream",
- "text": [
- "11/16/2018 11:02:43 - INFO - tensorflow - name = bert/encoder/layer_9/output/dense/bias:0, shape = (768,), *INIT_FROM_CKPT*\n"
- ]
- },
- {
- "name": "stdout",
- "output_type": "stream",
- "text": [
- "INFO:tensorflow: name = bert/encoder/layer_9/output/LayerNorm/beta:0, shape = (768,), *INIT_FROM_CKPT*\n"
- ]
- },
- {
- "name": "stderr",
- "output_type": "stream",
- "text": [
- "11/16/2018 11:02:43 - INFO - tensorflow - name = bert/encoder/layer_9/output/LayerNorm/beta:0, shape = (768,), *INIT_FROM_CKPT*\n"
- ]
- },
- {
- "name": "stdout",
- "output_type": "stream",
- "text": [
- "INFO:tensorflow: name = bert/encoder/layer_9/output/LayerNorm/gamma:0, shape = (768,), *INIT_FROM_CKPT*\n"
- ]
- },
- {
- "name": "stderr",
- "output_type": "stream",
- "text": [
- "11/16/2018 11:02:43 - INFO - tensorflow - name = bert/encoder/layer_9/output/LayerNorm/gamma:0, shape = (768,), *INIT_FROM_CKPT*\n"
- ]
- },
- {
- "name": "stdout",
- "output_type": "stream",
- "text": [
- "INFO:tensorflow: name = bert/encoder/layer_10/attention/self/query/kernel:0, shape = (768, 768), *INIT_FROM_CKPT*\n"
- ]
- },
- {
- "name": "stderr",
- "output_type": "stream",
- "text": [
- "11/16/2018 11:02:43 - INFO - tensorflow - name = bert/encoder/layer_10/attention/self/query/kernel:0, shape = (768, 768), *INIT_FROM_CKPT*\n"
- ]
- },
- {
- "name": "stdout",
- "output_type": "stream",
- "text": [
- "INFO:tensorflow: name = bert/encoder/layer_10/attention/self/query/bias:0, shape = (768,), *INIT_FROM_CKPT*\n"
- ]
- },
- {
- "name": "stderr",
- "output_type": "stream",
- "text": [
- "11/16/2018 11:02:43 - INFO - tensorflow - name = bert/encoder/layer_10/attention/self/query/bias:0, shape = (768,), *INIT_FROM_CKPT*\n"
- ]
- },
- {
- "name": "stdout",
- "output_type": "stream",
- "text": [
- "INFO:tensorflow: name = bert/encoder/layer_10/attention/self/key/kernel:0, shape = (768, 768), *INIT_FROM_CKPT*\n"
- ]
- },
- {
- "name": "stderr",
- "output_type": "stream",
- "text": [
- "11/16/2018 11:02:43 - INFO - tensorflow - name = bert/encoder/layer_10/attention/self/key/kernel:0, shape = (768, 768), *INIT_FROM_CKPT*\n"
- ]
- },
- {
- "name": "stdout",
- "output_type": "stream",
- "text": [
- "INFO:tensorflow: name = bert/encoder/layer_10/attention/self/key/bias:0, shape = (768,), *INIT_FROM_CKPT*\n"
- ]
- },
- {
- "name": "stderr",
- "output_type": "stream",
- "text": [
- "11/16/2018 11:02:43 - INFO - tensorflow - name = bert/encoder/layer_10/attention/self/key/bias:0, shape = (768,), *INIT_FROM_CKPT*\n"
- ]
- },
- {
- "name": "stdout",
- "output_type": "stream",
- "text": [
- "INFO:tensorflow: name = bert/encoder/layer_10/attention/self/value/kernel:0, shape = (768, 768), *INIT_FROM_CKPT*\n"
- ]
- },
- {
- "name": "stderr",
- "output_type": "stream",
- "text": [
- "11/16/2018 11:02:43 - INFO - tensorflow - name = bert/encoder/layer_10/attention/self/value/kernel:0, shape = (768, 768), *INIT_FROM_CKPT*\n"
- ]
- },
- {
- "name": "stdout",
- "output_type": "stream",
- "text": [
- "INFO:tensorflow: name = bert/encoder/layer_10/attention/self/value/bias:0, shape = (768,), *INIT_FROM_CKPT*\n"
- ]
- },
- {
- "name": "stderr",
- "output_type": "stream",
- "text": [
- "11/16/2018 11:02:43 - INFO - tensorflow - name = bert/encoder/layer_10/attention/self/value/bias:0, shape = (768,), *INIT_FROM_CKPT*\n"
- ]
- },
- {
- "name": "stdout",
- "output_type": "stream",
- "text": [
- "INFO:tensorflow: name = bert/encoder/layer_10/attention/output/dense/kernel:0, shape = (768, 768), *INIT_FROM_CKPT*\n"
- ]
- },
- {
- "name": "stderr",
- "output_type": "stream",
- "text": [
- "11/16/2018 11:02:43 - INFO - tensorflow - name = bert/encoder/layer_10/attention/output/dense/kernel:0, shape = (768, 768), *INIT_FROM_CKPT*\n"
- ]
- },
- {
- "name": "stdout",
- "output_type": "stream",
- "text": [
- "INFO:tensorflow: name = bert/encoder/layer_10/attention/output/dense/bias:0, shape = (768,), *INIT_FROM_CKPT*\n"
- ]
- },
- {
- "name": "stderr",
- "output_type": "stream",
- "text": [
- "11/16/2018 11:02:43 - INFO - tensorflow - name = bert/encoder/layer_10/attention/output/dense/bias:0, shape = (768,), *INIT_FROM_CKPT*\n"
- ]
- },
- {
- "name": "stdout",
- "output_type": "stream",
- "text": [
- "INFO:tensorflow: name = bert/encoder/layer_10/attention/output/LayerNorm/beta:0, shape = (768,), *INIT_FROM_CKPT*\n"
- ]
- },
- {
- "name": "stderr",
- "output_type": "stream",
- "text": [
- "11/16/2018 11:02:43 - INFO - tensorflow - name = bert/encoder/layer_10/attention/output/LayerNorm/beta:0, shape = (768,), *INIT_FROM_CKPT*\n"
- ]
- },
- {
- "name": "stdout",
- "output_type": "stream",
- "text": [
- "INFO:tensorflow: name = bert/encoder/layer_10/attention/output/LayerNorm/gamma:0, shape = (768,), *INIT_FROM_CKPT*\n"
- ]
- },
- {
- "name": "stderr",
- "output_type": "stream",
- "text": [
- "11/16/2018 11:02:43 - INFO - tensorflow - name = bert/encoder/layer_10/attention/output/LayerNorm/gamma:0, shape = (768,), *INIT_FROM_CKPT*\n"
- ]
- },
- {
- "name": "stdout",
- "output_type": "stream",
- "text": [
- "INFO:tensorflow: name = bert/encoder/layer_10/intermediate/dense/kernel:0, shape = (768, 3072), *INIT_FROM_CKPT*\n"
- ]
- },
- {
- "name": "stderr",
- "output_type": "stream",
- "text": [
- "11/16/2018 11:02:43 - INFO - tensorflow - name = bert/encoder/layer_10/intermediate/dense/kernel:0, shape = (768, 3072), *INIT_FROM_CKPT*\n"
- ]
- },
- {
- "name": "stdout",
- "output_type": "stream",
- "text": [
- "INFO:tensorflow: name = bert/encoder/layer_10/intermediate/dense/bias:0, shape = (3072,), *INIT_FROM_CKPT*\n"
- ]
- },
- {
- "name": "stderr",
- "output_type": "stream",
- "text": [
- "11/16/2018 11:02:43 - INFO - tensorflow - name = bert/encoder/layer_10/intermediate/dense/bias:0, shape = (3072,), *INIT_FROM_CKPT*\n"
- ]
- },
- {
- "name": "stdout",
- "output_type": "stream",
- "text": [
- "INFO:tensorflow: name = bert/encoder/layer_10/output/dense/kernel:0, shape = (3072, 768), *INIT_FROM_CKPT*\n"
- ]
- },
- {
- "name": "stderr",
- "output_type": "stream",
- "text": [
- "11/16/2018 11:02:43 - INFO - tensorflow - name = bert/encoder/layer_10/output/dense/kernel:0, shape = (3072, 768), *INIT_FROM_CKPT*\n"
- ]
- },
- {
- "name": "stdout",
- "output_type": "stream",
- "text": [
- "INFO:tensorflow: name = bert/encoder/layer_10/output/dense/bias:0, shape = (768,), *INIT_FROM_CKPT*\n"
- ]
- },
- {
- "name": "stderr",
- "output_type": "stream",
- "text": [
- "11/16/2018 11:02:43 - INFO - tensorflow - name = bert/encoder/layer_10/output/dense/bias:0, shape = (768,), *INIT_FROM_CKPT*\n"
- ]
- },
- {
- "name": "stdout",
- "output_type": "stream",
- "text": [
- "INFO:tensorflow: name = bert/encoder/layer_10/output/LayerNorm/beta:0, shape = (768,), *INIT_FROM_CKPT*\n"
- ]
- },
- {
- "name": "stderr",
- "output_type": "stream",
- "text": [
- "11/16/2018 11:02:43 - INFO - tensorflow - name = bert/encoder/layer_10/output/LayerNorm/beta:0, shape = (768,), *INIT_FROM_CKPT*\n"
- ]
- },
- {
- "name": "stdout",
- "output_type": "stream",
- "text": [
- "INFO:tensorflow: name = bert/encoder/layer_10/output/LayerNorm/gamma:0, shape = (768,), *INIT_FROM_CKPT*\n"
- ]
- },
- {
- "name": "stderr",
- "output_type": "stream",
- "text": [
- "11/16/2018 11:02:43 - INFO - tensorflow - name = bert/encoder/layer_10/output/LayerNorm/gamma:0, shape = (768,), *INIT_FROM_CKPT*\n"
- ]
- },
- {
- "name": "stdout",
- "output_type": "stream",
- "text": [
- "INFO:tensorflow: name = bert/encoder/layer_11/attention/self/query/kernel:0, shape = (768, 768), *INIT_FROM_CKPT*\n"
- ]
- },
- {
- "name": "stderr",
- "output_type": "stream",
- "text": [
- "11/16/2018 11:02:43 - INFO - tensorflow - name = bert/encoder/layer_11/attention/self/query/kernel:0, shape = (768, 768), *INIT_FROM_CKPT*\n"
- ]
- },
- {
- "name": "stdout",
- "output_type": "stream",
- "text": [
- "INFO:tensorflow: name = bert/encoder/layer_11/attention/self/query/bias:0, shape = (768,), *INIT_FROM_CKPT*\n"
- ]
- },
- {
- "name": "stderr",
- "output_type": "stream",
- "text": [
- "11/16/2018 11:02:43 - INFO - tensorflow - name = bert/encoder/layer_11/attention/self/query/bias:0, shape = (768,), *INIT_FROM_CKPT*\n"
- ]
- },
- {
- "name": "stdout",
- "output_type": "stream",
- "text": [
- "INFO:tensorflow: name = bert/encoder/layer_11/attention/self/key/kernel:0, shape = (768, 768), *INIT_FROM_CKPT*\n"
- ]
- },
- {
- "name": "stderr",
- "output_type": "stream",
- "text": [
- "11/16/2018 11:02:43 - INFO - tensorflow - name = bert/encoder/layer_11/attention/self/key/kernel:0, shape = (768, 768), *INIT_FROM_CKPT*\n"
- ]
- },
- {
- "name": "stdout",
- "output_type": "stream",
- "text": [
- "INFO:tensorflow: name = bert/encoder/layer_11/attention/self/key/bias:0, shape = (768,), *INIT_FROM_CKPT*\n"
- ]
- },
- {
- "name": "stderr",
- "output_type": "stream",
- "text": [
- "11/16/2018 11:02:43 - INFO - tensorflow - name = bert/encoder/layer_11/attention/self/key/bias:0, shape = (768,), *INIT_FROM_CKPT*\n"
- ]
- },
- {
- "name": "stdout",
- "output_type": "stream",
- "text": [
- "INFO:tensorflow: name = bert/encoder/layer_11/attention/self/value/kernel:0, shape = (768, 768), *INIT_FROM_CKPT*\n"
- ]
- },
- {
- "name": "stderr",
- "output_type": "stream",
- "text": [
- "11/16/2018 11:02:43 - INFO - tensorflow - name = bert/encoder/layer_11/attention/self/value/kernel:0, shape = (768, 768), *INIT_FROM_CKPT*\n"
- ]
- },
- {
- "name": "stdout",
- "output_type": "stream",
- "text": [
- "INFO:tensorflow: name = bert/encoder/layer_11/attention/self/value/bias:0, shape = (768,), *INIT_FROM_CKPT*\n"
- ]
- },
- {
- "name": "stderr",
- "output_type": "stream",
- "text": [
- "11/16/2018 11:02:43 - INFO - tensorflow - name = bert/encoder/layer_11/attention/self/value/bias:0, shape = (768,), *INIT_FROM_CKPT*\n"
- ]
- },
- {
- "name": "stdout",
- "output_type": "stream",
- "text": [
- "INFO:tensorflow: name = bert/encoder/layer_11/attention/output/dense/kernel:0, shape = (768, 768), *INIT_FROM_CKPT*\n"
- ]
- },
- {
- "name": "stderr",
- "output_type": "stream",
- "text": [
- "11/16/2018 11:02:43 - INFO - tensorflow - name = bert/encoder/layer_11/attention/output/dense/kernel:0, shape = (768, 768), *INIT_FROM_CKPT*\n"
- ]
- },
- {
- "name": "stdout",
- "output_type": "stream",
- "text": [
- "INFO:tensorflow: name = bert/encoder/layer_11/attention/output/dense/bias:0, shape = (768,), *INIT_FROM_CKPT*\n"
- ]
- },
- {
- "name": "stderr",
- "output_type": "stream",
- "text": [
- "11/16/2018 11:02:43 - INFO - tensorflow - name = bert/encoder/layer_11/attention/output/dense/bias:0, shape = (768,), *INIT_FROM_CKPT*\n"
- ]
- },
- {
- "name": "stdout",
- "output_type": "stream",
- "text": [
- "INFO:tensorflow: name = bert/encoder/layer_11/attention/output/LayerNorm/beta:0, shape = (768,), *INIT_FROM_CKPT*\n"
- ]
- },
- {
- "name": "stderr",
- "output_type": "stream",
- "text": [
- "11/16/2018 11:02:43 - INFO - tensorflow - name = bert/encoder/layer_11/attention/output/LayerNorm/beta:0, shape = (768,), *INIT_FROM_CKPT*\n"
- ]
- },
- {
- "name": "stdout",
- "output_type": "stream",
- "text": [
- "INFO:tensorflow: name = bert/encoder/layer_11/attention/output/LayerNorm/gamma:0, shape = (768,), *INIT_FROM_CKPT*\n"
- ]
- },
- {
- "name": "stderr",
- "output_type": "stream",
- "text": [
- "11/16/2018 11:02:43 - INFO - tensorflow - name = bert/encoder/layer_11/attention/output/LayerNorm/gamma:0, shape = (768,), *INIT_FROM_CKPT*\n"
- ]
- },
- {
- "name": "stdout",
- "output_type": "stream",
- "text": [
- "INFO:tensorflow: name = bert/encoder/layer_11/intermediate/dense/kernel:0, shape = (768, 3072), *INIT_FROM_CKPT*\n"
- ]
- },
- {
- "name": "stderr",
- "output_type": "stream",
- "text": [
- "11/16/2018 11:02:43 - INFO - tensorflow - name = bert/encoder/layer_11/intermediate/dense/kernel:0, shape = (768, 3072), *INIT_FROM_CKPT*\n"
- ]
- },
- {
- "name": "stdout",
- "output_type": "stream",
- "text": [
- "INFO:tensorflow: name = bert/encoder/layer_11/intermediate/dense/bias:0, shape = (3072,), *INIT_FROM_CKPT*\n"
- ]
- },
- {
- "name": "stderr",
- "output_type": "stream",
- "text": [
- "11/16/2018 11:02:43 - INFO - tensorflow - name = bert/encoder/layer_11/intermediate/dense/bias:0, shape = (3072,), *INIT_FROM_CKPT*\n"
- ]
- },
- {
- "name": "stdout",
- "output_type": "stream",
- "text": [
- "INFO:tensorflow: name = bert/encoder/layer_11/output/dense/kernel:0, shape = (3072, 768), *INIT_FROM_CKPT*\n"
- ]
- },
- {
- "name": "stderr",
- "output_type": "stream",
- "text": [
- "11/16/2018 11:02:43 - INFO - tensorflow - name = bert/encoder/layer_11/output/dense/kernel:0, shape = (3072, 768), *INIT_FROM_CKPT*\n"
- ]
- },
- {
- "name": "stdout",
- "output_type": "stream",
- "text": [
- "INFO:tensorflow: name = bert/encoder/layer_11/output/dense/bias:0, shape = (768,), *INIT_FROM_CKPT*\n"
- ]
- },
- {
- "name": "stderr",
- "output_type": "stream",
- "text": [
- "11/16/2018 11:02:43 - INFO - tensorflow - name = bert/encoder/layer_11/output/dense/bias:0, shape = (768,), *INIT_FROM_CKPT*\n"
- ]
- },
- {
- "name": "stdout",
- "output_type": "stream",
- "text": [
- "INFO:tensorflow: name = bert/encoder/layer_11/output/LayerNorm/beta:0, shape = (768,), *INIT_FROM_CKPT*\n"
- ]
- },
- {
- "name": "stderr",
- "output_type": "stream",
- "text": [
- "11/16/2018 11:02:43 - INFO - tensorflow - name = bert/encoder/layer_11/output/LayerNorm/beta:0, shape = (768,), *INIT_FROM_CKPT*\n"
- ]
- },
- {
- "name": "stdout",
- "output_type": "stream",
- "text": [
- "INFO:tensorflow: name = bert/encoder/layer_11/output/LayerNorm/gamma:0, shape = (768,), *INIT_FROM_CKPT*\n"
- ]
- },
- {
- "name": "stderr",
- "output_type": "stream",
- "text": [
- "11/16/2018 11:02:43 - INFO - tensorflow - name = bert/encoder/layer_11/output/LayerNorm/gamma:0, shape = (768,), *INIT_FROM_CKPT*\n"
- ]
- },
- {
- "name": "stdout",
- "output_type": "stream",
- "text": [
- "INFO:tensorflow: name = bert/pooler/dense/kernel:0, shape = (768, 768), *INIT_FROM_CKPT*\n"
- ]
- },
- {
- "name": "stderr",
- "output_type": "stream",
- "text": [
- "11/16/2018 11:02:43 - INFO - tensorflow - name = bert/pooler/dense/kernel:0, shape = (768, 768), *INIT_FROM_CKPT*\n"
- ]
- },
- {
- "name": "stdout",
- "output_type": "stream",
- "text": [
- "INFO:tensorflow: name = bert/pooler/dense/bias:0, shape = (768,), *INIT_FROM_CKPT*\n"
- ]
- },
- {
- "name": "stderr",
- "output_type": "stream",
- "text": [
- "11/16/2018 11:02:43 - INFO - tensorflow - name = bert/pooler/dense/bias:0, shape = (768,), *INIT_FROM_CKPT*\n"
- ]
- },
- {
- "name": "stdout",
- "output_type": "stream",
- "text": [
- "INFO:tensorflow: name = cls/predictions/transform/dense/kernel:0, shape = (768, 768), *INIT_FROM_CKPT*\n"
- ]
- },
- {
- "name": "stderr",
- "output_type": "stream",
- "text": [
- "11/16/2018 11:02:43 - INFO - tensorflow - name = cls/predictions/transform/dense/kernel:0, shape = (768, 768), *INIT_FROM_CKPT*\n"
- ]
- },
- {
- "name": "stdout",
- "output_type": "stream",
- "text": [
- "INFO:tensorflow: name = cls/predictions/transform/dense/bias:0, shape = (768,), *INIT_FROM_CKPT*\n"
- ]
- },
- {
- "name": "stderr",
- "output_type": "stream",
- "text": [
- "11/16/2018 11:02:43 - INFO - tensorflow - name = cls/predictions/transform/dense/bias:0, shape = (768,), *INIT_FROM_CKPT*\n"
- ]
- },
- {
- "name": "stdout",
- "output_type": "stream",
- "text": [
- "INFO:tensorflow: name = cls/predictions/transform/LayerNorm/beta:0, shape = (768,), *INIT_FROM_CKPT*\n"
- ]
- },
- {
- "name": "stderr",
- "output_type": "stream",
- "text": [
- "11/16/2018 11:02:43 - INFO - tensorflow - name = cls/predictions/transform/LayerNorm/beta:0, shape = (768,), *INIT_FROM_CKPT*\n"
- ]
- },
- {
- "name": "stdout",
- "output_type": "stream",
- "text": [
- "INFO:tensorflow: name = cls/predictions/transform/LayerNorm/gamma:0, shape = (768,), *INIT_FROM_CKPT*\n"
- ]
- },
- {
- "name": "stderr",
- "output_type": "stream",
- "text": [
- "11/16/2018 11:02:43 - INFO - tensorflow - name = cls/predictions/transform/LayerNorm/gamma:0, shape = (768,), *INIT_FROM_CKPT*\n"
- ]
- },
- {
- "name": "stdout",
- "output_type": "stream",
- "text": [
- "INFO:tensorflow: name = cls/predictions/output_bias:0, shape = (30522,), *INIT_FROM_CKPT*\n"
- ]
- },
- {
- "name": "stderr",
- "output_type": "stream",
- "text": [
- "11/16/2018 11:02:43 - INFO - tensorflow - name = cls/predictions/output_bias:0, shape = (30522,), *INIT_FROM_CKPT*\n"
- ]
- },
- {
- "name": "stdout",
- "output_type": "stream",
- "text": [
- "INFO:tensorflow: name = cls/seq_relationship/output_weights:0, shape = (2, 768), *INIT_FROM_CKPT*\n"
- ]
- },
- {
- "name": "stderr",
- "output_type": "stream",
- "text": [
- "11/16/2018 11:02:43 - INFO - tensorflow - name = cls/seq_relationship/output_weights:0, shape = (2, 768), *INIT_FROM_CKPT*\n"
- ]
- },
- {
- "name": "stdout",
- "output_type": "stream",
- "text": [
- "INFO:tensorflow: name = cls/seq_relationship/output_bias:0, shape = (2,), *INIT_FROM_CKPT*\n"
- ]
- },
- {
- "name": "stderr",
- "output_type": "stream",
- "text": [
- "11/16/2018 11:02:43 - INFO - tensorflow - name = cls/seq_relationship/output_bias:0, shape = (2,), *INIT_FROM_CKPT*\n"
- ]
- },
- {
- "name": "stdout",
- "output_type": "stream",
- "text": [
- "INFO:tensorflow:Done calling model_fn.\n"
- ]
- },
- {
- "name": "stderr",
- "output_type": "stream",
- "text": [
- "11/16/2018 11:02:43 - INFO - tensorflow - Done calling model_fn.\n"
- ]
- },
- {
- "name": "stdout",
- "output_type": "stream",
- "text": [
- "INFO:tensorflow:Graph was finalized.\n"
- ]
- },
- {
- "name": "stderr",
- "output_type": "stream",
- "text": [
- "11/16/2018 11:02:44 - INFO - tensorflow - Graph was finalized.\n"
- ]
- },
- {
- "name": "stdout",
- "output_type": "stream",
- "text": [
- "INFO:tensorflow:Running local_init_op.\n"
- ]
- },
- {
- "name": "stderr",
- "output_type": "stream",
- "text": [
- "11/16/2018 11:02:45 - INFO - tensorflow - Running local_init_op.\n"
- ]
- },
- {
- "name": "stdout",
- "output_type": "stream",
- "text": [
- "INFO:tensorflow:Done running local_init_op.\n"
- ]
- },
- {
- "name": "stderr",
- "output_type": "stream",
- "text": [
- "11/16/2018 11:02:45 - INFO - tensorflow - Done running local_init_op.\n"
- ]
- },
- {
- "name": "stdout",
- "output_type": "stream",
- "text": [
- "INFO:tensorflow:prediction_loop marked as finished\n"
- ]
- },
- {
- "name": "stderr",
- "output_type": "stream",
- "text": [
- "11/16/2018 11:02:46 - INFO - tensorflow - prediction_loop marked as finished\n"
- ]
- },
- {
- "name": "stdout",
- "output_type": "stream",
- "text": [
- "INFO:tensorflow:prediction_loop marked as finished\n"
- ]
- },
- {
- "name": "stderr",
- "output_type": "stream",
- "text": [
- "11/16/2018 11:02:46 - INFO - tensorflow - prediction_loop marked as finished\n"
- ]
- }
- ],
- "source": [
- "tensorflow_all_out = []\n",
- "for result in estimator.predict(input_fn, yield_single_examples=True):\n",
- " tensorflow_all_out.append(result)"
- ]
- },
- {
- "cell_type": "code",
- "execution_count": 12,
- "metadata": {
- "ExecuteTime": {
- "end_time": "2018-11-16T10:02:46.634304Z",
- "start_time": "2018-11-16T10:02:46.598800Z"
- }
- },
- "outputs": [
- {
- "name": "stdout",
- "output_type": "stream",
- "text": [
- "1\n",
- "2\n",
- "dict_keys(['masked_lm_predictions', 'next_sentence_predictions'])\n",
- "masked_lm_predictions [27227 1010 1010 1010 1010 1010 1010 1010 1010 1010 1010 1010\n",
- " 1010 1010 1010 1010 1010 1010 1010 1010]\n",
- "predicted token ['henson', ',', ',', ',', ',', ',', ',', ',', ',', ',', ',', ',', ',', ',', ',', ',', ',', ',', ',', ',']\n"
- ]
- }
- ],
- "source": [
- "print(len(tensorflow_all_out))\n",
- "print(len(tensorflow_all_out[0]))\n",
- "print(tensorflow_all_out[0].keys())\n",
- "print(\"masked_lm_predictions\", tensorflow_all_out[0]['masked_lm_predictions'])\n",
- "print(\"predicted token\", tokenizer.convert_ids_to_tokens(tensorflow_all_out[0]['masked_lm_predictions']))"
- ]
- },
- {
- "cell_type": "code",
- "execution_count": 13,
- "metadata": {
- "ExecuteTime": {
- "end_time": "2018-11-16T10:02:46.671229Z",
- "start_time": "2018-11-16T10:02:46.637102Z"
- }
- },
- "outputs": [
- {
- "name": "stdout",
- "output_type": "stream",
- "text": [
- "tensorflow_output: ['henson']\n"
- ]
- }
- ],
- "source": [
- "tensorflow_outputs = tokenizer.convert_ids_to_tokens(tensorflow_all_out[0]['masked_lm_predictions'])[:len(masked_lm_positions)]\n",
- "print(\"tensorflow_output:\", tensorflow_outputs)"
- ]
- },
- {
- "cell_type": "markdown",
- "metadata": {},
- "source": [
- "## 2/ PyTorch code"
- ]
- },
- {
- "cell_type": "code",
- "execution_count": 14,
- "metadata": {
- "ExecuteTime": {
- "end_time": "2018-11-16T10:03:03.556557Z",
- "start_time": "2018-11-16T10:03:03.519654Z"
- }
- },
- "outputs": [],
- "source": [
- "from examples import extract_features\n",
- "from examples.extract_features import *"
- ]
- },
- {
- "cell_type": "code",
- "execution_count": 15,
- "metadata": {
- "ExecuteTime": {
- "end_time": "2018-11-16T10:03:03.952710Z",
- "start_time": "2018-11-16T10:03:03.921917Z"
- }
- },
- "outputs": [],
- "source": [
- "init_checkpoint_pt = \"../google_models/uncased_L-12_H-768_A-12/pytorch_model.bin\""
- ]
- },
- {
- "cell_type": "code",
- "execution_count": 16,
- "metadata": {
- "ExecuteTime": {
- "end_time": "2018-11-16T10:03:12.307673Z",
- "start_time": "2018-11-16T10:03:04.439317Z"
- },
- "scrolled": true
- },
- "outputs": [
- {
- "name": "stderr",
- "output_type": "stream",
- "text": [
- "11/16/2018 11:03:05 - INFO - pytorch_transformers.modeling_bert - loading archive file https://s3.amazonaws.com/models.huggingface.co/bert/bert-base-uncased.tar.gz from cache at /Users/thomaswolf/.pytorch_transformers/9c41111e2de84547a463fd39217199738d1e3deb72d4fec4399e6e241983c6f0.ae3cef932725ca7a30cdcb93fc6e09150a55e2a130ec7af63975a16c153ae2ba\n",
- "11/16/2018 11:03:05 - INFO - pytorch_transformers.modeling_bert - extracting archive file /Users/thomaswolf/.pytorch_transformers/9c41111e2de84547a463fd39217199738d1e3deb72d4fec4399e6e241983c6f0.ae3cef932725ca7a30cdcb93fc6e09150a55e2a130ec7af63975a16c153ae2ba to temp dir /var/folders/yx/cw8n_njx3js5jksyw_qlp8p00000gn/T/tmpaqgsm566\n",
- "11/16/2018 11:03:08 - INFO - pytorch_transformers.modeling_bert - Model config {\n",
- " \"attention_probs_dropout_prob\": 0.1,\n",
- " \"hidden_act\": \"gelu\",\n",
- " \"hidden_dropout_prob\": 0.1,\n",
- " \"hidden_size\": 768,\n",
- " \"initializer_range\": 0.02,\n",
- " \"intermediate_size\": 3072,\n",
- " \"max_position_embeddings\": 512,\n",
- " \"num_attention_heads\": 12,\n",
- " \"num_hidden_layers\": 12,\n",
- " \"type_vocab_size\": 2,\n",
- " \"vocab_size\": 30522\n",
- "}\n",
- "\n"
- ]
- },
- {
- "data": {
- "text/plain": [
- "BertForPreTraining(\n",
- " (bert): BertModel(\n",
- " (embeddings): BertEmbeddings(\n",
- " (word_embeddings): Embedding(30522, 768)\n",
- " (position_embeddings): Embedding(512, 768)\n",
- " (token_type_embeddings): Embedding(2, 768)\n",
- " (LayerNorm): BertLayerNorm()\n",
- " (dropout): Dropout(p=0.1)\n",
- " )\n",
- " (encoder): BertEncoder(\n",
- " (layer): ModuleList(\n",
- " (0): BertLayer(\n",
- " (attention): BertAttention(\n",
- " (self): BertSelfAttention(\n",
- " (query): Linear(in_features=768, out_features=768, bias=True)\n",
- " (key): Linear(in_features=768, out_features=768, bias=True)\n",
- " (value): Linear(in_features=768, out_features=768, bias=True)\n",
- " (dropout): Dropout(p=0.1)\n",
- " )\n",
- " (output): BertSelfOutput(\n",
- " (dense): Linear(in_features=768, out_features=768, bias=True)\n",
- " (LayerNorm): BertLayerNorm()\n",
- " (dropout): Dropout(p=0.1)\n",
- " )\n",
- " )\n",
- " (intermediate): BertIntermediate(\n",
- " (dense): Linear(in_features=768, out_features=3072, bias=True)\n",
- " )\n",
- " (output): BertOutput(\n",
- " (dense): Linear(in_features=3072, out_features=768, bias=True)\n",
- " (LayerNorm): BertLayerNorm()\n",
- " (dropout): Dropout(p=0.1)\n",
- " )\n",
- " )\n",
- " (1): BertLayer(\n",
- " (attention): BertAttention(\n",
- " (self): BertSelfAttention(\n",
- " (query): Linear(in_features=768, out_features=768, bias=True)\n",
- " (key): Linear(in_features=768, out_features=768, bias=True)\n",
- " (value): Linear(in_features=768, out_features=768, bias=True)\n",
- " (dropout): Dropout(p=0.1)\n",
- " )\n",
- " (output): BertSelfOutput(\n",
- " (dense): Linear(in_features=768, out_features=768, bias=True)\n",
- " (LayerNorm): BertLayerNorm()\n",
- " (dropout): Dropout(p=0.1)\n",
- " )\n",
- " )\n",
- " (intermediate): BertIntermediate(\n",
- " (dense): Linear(in_features=768, out_features=3072, bias=True)\n",
- " )\n",
- " (output): BertOutput(\n",
- " (dense): Linear(in_features=3072, out_features=768, bias=True)\n",
- " (LayerNorm): BertLayerNorm()\n",
- " (dropout): Dropout(p=0.1)\n",
- " )\n",
- " )\n",
- " (2): BertLayer(\n",
- " (attention): BertAttention(\n",
- " (self): BertSelfAttention(\n",
- " (query): Linear(in_features=768, out_features=768, bias=True)\n",
- " (key): Linear(in_features=768, out_features=768, bias=True)\n",
- " (value): Linear(in_features=768, out_features=768, bias=True)\n",
- " (dropout): Dropout(p=0.1)\n",
- " )\n",
- " (output): BertSelfOutput(\n",
- " (dense): Linear(in_features=768, out_features=768, bias=True)\n",
- " (LayerNorm): BertLayerNorm()\n",
- " (dropout): Dropout(p=0.1)\n",
- " )\n",
- " )\n",
- " (intermediate): BertIntermediate(\n",
- " (dense): Linear(in_features=768, out_features=3072, bias=True)\n",
- " )\n",
- " (output): BertOutput(\n",
- " (dense): Linear(in_features=3072, out_features=768, bias=True)\n",
- " (LayerNorm): BertLayerNorm()\n",
- " (dropout): Dropout(p=0.1)\n",
- " )\n",
- " )\n",
- " (3): BertLayer(\n",
- " (attention): BertAttention(\n",
- " (self): BertSelfAttention(\n",
- " (query): Linear(in_features=768, out_features=768, bias=True)\n",
- " (key): Linear(in_features=768, out_features=768, bias=True)\n",
- " (value): Linear(in_features=768, out_features=768, bias=True)\n",
- " (dropout): Dropout(p=0.1)\n",
- " )\n",
- " (output): BertSelfOutput(\n",
- " (dense): Linear(in_features=768, out_features=768, bias=True)\n",
- " (LayerNorm): BertLayerNorm()\n",
- " (dropout): Dropout(p=0.1)\n",
- " )\n",
- " )\n",
- " (intermediate): BertIntermediate(\n",
- " (dense): Linear(in_features=768, out_features=3072, bias=True)\n",
- " )\n",
- " (output): BertOutput(\n",
- " (dense): Linear(in_features=3072, out_features=768, bias=True)\n",
- " (LayerNorm): BertLayerNorm()\n",
- " (dropout): Dropout(p=0.1)\n",
- " )\n",
- " )\n",
- " (4): BertLayer(\n",
- " (attention): BertAttention(\n",
- " (self): BertSelfAttention(\n",
- " (query): Linear(in_features=768, out_features=768, bias=True)\n",
- " (key): Linear(in_features=768, out_features=768, bias=True)\n",
- " (value): Linear(in_features=768, out_features=768, bias=True)\n",
- " (dropout): Dropout(p=0.1)\n",
- " )\n",
- " (output): BertSelfOutput(\n",
- " (dense): Linear(in_features=768, out_features=768, bias=True)\n",
- " (LayerNorm): BertLayerNorm()\n",
- " (dropout): Dropout(p=0.1)\n",
- " )\n",
- " )\n",
- " (intermediate): BertIntermediate(\n",
- " (dense): Linear(in_features=768, out_features=3072, bias=True)\n",
- " )\n",
- " (output): BertOutput(\n",
- " (dense): Linear(in_features=3072, out_features=768, bias=True)\n",
- " (LayerNorm): BertLayerNorm()\n",
- " (dropout): Dropout(p=0.1)\n",
- " )\n",
- " )\n",
- " (5): BertLayer(\n",
- " (attention): BertAttention(\n",
- " (self): BertSelfAttention(\n",
- " (query): Linear(in_features=768, out_features=768, bias=True)\n",
- " (key): Linear(in_features=768, out_features=768, bias=True)\n",
- " (value): Linear(in_features=768, out_features=768, bias=True)\n",
- " (dropout): Dropout(p=0.1)\n",
- " )\n",
- " (output): BertSelfOutput(\n",
- " (dense): Linear(in_features=768, out_features=768, bias=True)\n",
- " (LayerNorm): BertLayerNorm()\n",
- " (dropout): Dropout(p=0.1)\n",
- " )\n",
- " )\n",
- " (intermediate): BertIntermediate(\n",
- " (dense): Linear(in_features=768, out_features=3072, bias=True)\n",
- " )\n",
- " (output): BertOutput(\n",
- " (dense): Linear(in_features=3072, out_features=768, bias=True)\n",
- " (LayerNorm): BertLayerNorm()\n",
- " (dropout): Dropout(p=0.1)\n",
- " )\n",
- " )\n",
- " (6): BertLayer(\n",
- " (attention): BertAttention(\n",
- " (self): BertSelfAttention(\n",
- " (query): Linear(in_features=768, out_features=768, bias=True)\n",
- " (key): Linear(in_features=768, out_features=768, bias=True)\n",
- " (value): Linear(in_features=768, out_features=768, bias=True)\n",
- " (dropout): Dropout(p=0.1)\n",
- " )\n",
- " (output): BertSelfOutput(\n",
- " (dense): Linear(in_features=768, out_features=768, bias=True)\n",
- " (LayerNorm): BertLayerNorm()\n",
- " (dropout): Dropout(p=0.1)\n",
- " )\n",
- " )\n",
- " (intermediate): BertIntermediate(\n",
- " (dense): Linear(in_features=768, out_features=3072, bias=True)\n",
- " )\n",
- " (output): BertOutput(\n",
- " (dense): Linear(in_features=3072, out_features=768, bias=True)\n",
- " (LayerNorm): BertLayerNorm()\n",
- " (dropout): Dropout(p=0.1)\n",
- " )\n",
- " )\n",
- " (7): BertLayer(\n",
- " (attention): BertAttention(\n",
- " (self): BertSelfAttention(\n",
- " (query): Linear(in_features=768, out_features=768, bias=True)\n",
- " (key): Linear(in_features=768, out_features=768, bias=True)\n",
- " (value): Linear(in_features=768, out_features=768, bias=True)\n",
- " (dropout): Dropout(p=0.1)\n",
- " )\n",
- " (output): BertSelfOutput(\n",
- " (dense): Linear(in_features=768, out_features=768, bias=True)\n",
- " (LayerNorm): BertLayerNorm()\n",
- " (dropout): Dropout(p=0.1)\n",
- " )\n",
- " )\n",
- " (intermediate): BertIntermediate(\n",
- " (dense): Linear(in_features=768, out_features=3072, bias=True)\n",
- " )\n",
- " (output): BertOutput(\n",
- " (dense): Linear(in_features=3072, out_features=768, bias=True)\n",
- " (LayerNorm): BertLayerNorm()\n",
- " (dropout): Dropout(p=0.1)\n",
- " )\n",
- " )\n",
- " (8): BertLayer(\n",
- " (attention): BertAttention(\n",
- " (self): BertSelfAttention(\n",
- " (query): Linear(in_features=768, out_features=768, bias=True)\n",
- " (key): Linear(in_features=768, out_features=768, bias=True)\n",
- " (value): Linear(in_features=768, out_features=768, bias=True)\n",
- " (dropout): Dropout(p=0.1)\n",
- " )\n",
- " (output): BertSelfOutput(\n",
- " (dense): Linear(in_features=768, out_features=768, bias=True)\n",
- " (LayerNorm): BertLayerNorm()\n",
- " (dropout): Dropout(p=0.1)\n",
- " )\n",
- " )\n",
- " (intermediate): BertIntermediate(\n",
- " (dense): Linear(in_features=768, out_features=3072, bias=True)\n",
- " )\n",
- " (output): BertOutput(\n",
- " (dense): Linear(in_features=3072, out_features=768, bias=True)\n",
- " (LayerNorm): BertLayerNorm()\n",
- " (dropout): Dropout(p=0.1)\n",
- " )\n",
- " )\n",
- " (9): BertLayer(\n",
- " (attention): BertAttention(\n",
- " (self): BertSelfAttention(\n",
- " (query): Linear(in_features=768, out_features=768, bias=True)\n",
- " (key): Linear(in_features=768, out_features=768, bias=True)\n",
- " (value): Linear(in_features=768, out_features=768, bias=True)\n",
- " (dropout): Dropout(p=0.1)\n",
- " )\n",
- " (output): BertSelfOutput(\n",
- " (dense): Linear(in_features=768, out_features=768, bias=True)\n",
- " (LayerNorm): BertLayerNorm()\n",
- " (dropout): Dropout(p=0.1)\n",
- " )\n",
- " )\n",
- " (intermediate): BertIntermediate(\n",
- " (dense): Linear(in_features=768, out_features=3072, bias=True)\n",
- " )\n",
- " (output): BertOutput(\n",
- " (dense): Linear(in_features=3072, out_features=768, bias=True)\n",
- " (LayerNorm): BertLayerNorm()\n",
- " (dropout): Dropout(p=0.1)\n",
- " )\n",
- " )\n",
- " (10): BertLayer(\n",
- " (attention): BertAttention(\n",
- " (self): BertSelfAttention(\n",
- " (query): Linear(in_features=768, out_features=768, bias=True)\n",
- " (key): Linear(in_features=768, out_features=768, bias=True)\n",
- " (value): Linear(in_features=768, out_features=768, bias=True)\n",
- " (dropout): Dropout(p=0.1)\n",
- " )\n",
- " (output): BertSelfOutput(\n",
- " (dense): Linear(in_features=768, out_features=768, bias=True)\n",
- " (LayerNorm): BertLayerNorm()\n",
- " (dropout): Dropout(p=0.1)\n",
- " )\n",
- " )\n",
- " (intermediate): BertIntermediate(\n",
- " (dense): Linear(in_features=768, out_features=3072, bias=True)\n",
- " )\n",
- " (output): BertOutput(\n",
- " (dense): Linear(in_features=3072, out_features=768, bias=True)\n",
- " (LayerNorm): BertLayerNorm()\n",
- " (dropout): Dropout(p=0.1)\n",
- " )\n",
- " )\n",
- " (11): BertLayer(\n",
- " (attention): BertAttention(\n",
- " (self): BertSelfAttention(\n",
- " (query): Linear(in_features=768, out_features=768, bias=True)\n",
- " (key): Linear(in_features=768, out_features=768, bias=True)\n",
- " (value): Linear(in_features=768, out_features=768, bias=True)\n",
- " (dropout): Dropout(p=0.1)\n",
- " )\n",
- " (output): BertSelfOutput(\n",
- " (dense): Linear(in_features=768, out_features=768, bias=True)\n",
- " (LayerNorm): BertLayerNorm()\n",
- " (dropout): Dropout(p=0.1)\n",
- " )\n",
- " )\n",
- " (intermediate): BertIntermediate(\n",
- " (dense): Linear(in_features=768, out_features=3072, bias=True)\n",
- " )\n",
- " (output): BertOutput(\n",
- " (dense): Linear(in_features=3072, out_features=768, bias=True)\n",
- " (LayerNorm): BertLayerNorm()\n",
- " (dropout): Dropout(p=0.1)\n",
- " )\n",
- " )\n",
- " )\n",
- " )\n",
- " (pooler): BertPooler(\n",
- " (dense): Linear(in_features=768, out_features=768, bias=True)\n",
- " (activation): Tanh()\n",
- " )\n",
- " )\n",
- " (cls): BertPreTrainingHeads(\n",
- " (predictions): BertLMPredictionHead(\n",
- " (transform): BertPredictionHeadTransform(\n",
- " (dense): Linear(in_features=768, out_features=768, bias=True)\n",
- " (LayerNorm): BertLayerNorm()\n",
- " )\n",
- " (decoder): Linear(in_features=768, out_features=30522, bias=False)\n",
- " )\n",
- " (seq_relationship): Linear(in_features=768, out_features=2, bias=True)\n",
- " )\n",
- ")"
- ]
- },
- "execution_count": 16,
- "metadata": {},
- "output_type": "execute_result"
- }
- ],
- "source": [
- "device = torch.device(\"cpu\")\n",
- "model = ppb.BertForPreTraining.from_pretrained('bert-base-uncased')\n",
- "model.to(device)"
- ]
- },
- {
- "cell_type": "code",
- "execution_count": 17,
- "metadata": {
- "ExecuteTime": {
- "end_time": "2018-11-16T10:03:12.351625Z",
- "start_time": "2018-11-16T10:03:12.310736Z"
- },
- "code_folding": []
- },
- "outputs": [
- {
- "data": {
- "text/plain": [
- "BertForPreTraining(\n",
- " (bert): BertModel(\n",
- " (embeddings): BertEmbeddings(\n",
- " (word_embeddings): Embedding(30522, 768)\n",
- " (position_embeddings): Embedding(512, 768)\n",
- " (token_type_embeddings): Embedding(2, 768)\n",
- " (LayerNorm): BertLayerNorm()\n",
- " (dropout): Dropout(p=0.1)\n",
- " )\n",
- " (encoder): BertEncoder(\n",
- " (layer): ModuleList(\n",
- " (0): BertLayer(\n",
- " (attention): BertAttention(\n",
- " (self): BertSelfAttention(\n",
- " (query): Linear(in_features=768, out_features=768, bias=True)\n",
- " (key): Linear(in_features=768, out_features=768, bias=True)\n",
- " (value): Linear(in_features=768, out_features=768, bias=True)\n",
- " (dropout): Dropout(p=0.1)\n",
- " )\n",
- " (output): BertSelfOutput(\n",
- " (dense): Linear(in_features=768, out_features=768, bias=True)\n",
- " (LayerNorm): BertLayerNorm()\n",
- " (dropout): Dropout(p=0.1)\n",
- " )\n",
- " )\n",
- " (intermediate): BertIntermediate(\n",
- " (dense): Linear(in_features=768, out_features=3072, bias=True)\n",
- " )\n",
- " (output): BertOutput(\n",
- " (dense): Linear(in_features=3072, out_features=768, bias=True)\n",
- " (LayerNorm): BertLayerNorm()\n",
- " (dropout): Dropout(p=0.1)\n",
- " )\n",
- " )\n",
- " (1): BertLayer(\n",
- " (attention): BertAttention(\n",
- " (self): BertSelfAttention(\n",
- " (query): Linear(in_features=768, out_features=768, bias=True)\n",
- " (key): Linear(in_features=768, out_features=768, bias=True)\n",
- " (value): Linear(in_features=768, out_features=768, bias=True)\n",
- " (dropout): Dropout(p=0.1)\n",
- " )\n",
- " (output): BertSelfOutput(\n",
- " (dense): Linear(in_features=768, out_features=768, bias=True)\n",
- " (LayerNorm): BertLayerNorm()\n",
- " (dropout): Dropout(p=0.1)\n",
- " )\n",
- " )\n",
- " (intermediate): BertIntermediate(\n",
- " (dense): Linear(in_features=768, out_features=3072, bias=True)\n",
- " )\n",
- " (output): BertOutput(\n",
- " (dense): Linear(in_features=3072, out_features=768, bias=True)\n",
- " (LayerNorm): BertLayerNorm()\n",
- " (dropout): Dropout(p=0.1)\n",
- " )\n",
- " )\n",
- " (2): BertLayer(\n",
- " (attention): BertAttention(\n",
- " (self): BertSelfAttention(\n",
- " (query): Linear(in_features=768, out_features=768, bias=True)\n",
- " (key): Linear(in_features=768, out_features=768, bias=True)\n",
- " (value): Linear(in_features=768, out_features=768, bias=True)\n",
- " (dropout): Dropout(p=0.1)\n",
- " )\n",
- " (output): BertSelfOutput(\n",
- " (dense): Linear(in_features=768, out_features=768, bias=True)\n",
- " (LayerNorm): BertLayerNorm()\n",
- " (dropout): Dropout(p=0.1)\n",
- " )\n",
- " )\n",
- " (intermediate): BertIntermediate(\n",
- " (dense): Linear(in_features=768, out_features=3072, bias=True)\n",
- " )\n",
- " (output): BertOutput(\n",
- " (dense): Linear(in_features=3072, out_features=768, bias=True)\n",
- " (LayerNorm): BertLayerNorm()\n",
- " (dropout): Dropout(p=0.1)\n",
- " )\n",
- " )\n",
- " (3): BertLayer(\n",
- " (attention): BertAttention(\n",
- " (self): BertSelfAttention(\n",
- " (query): Linear(in_features=768, out_features=768, bias=True)\n",
- " (key): Linear(in_features=768, out_features=768, bias=True)\n",
- " (value): Linear(in_features=768, out_features=768, bias=True)\n",
- " (dropout): Dropout(p=0.1)\n",
- " )\n",
- " (output): BertSelfOutput(\n",
- " (dense): Linear(in_features=768, out_features=768, bias=True)\n",
- " (LayerNorm): BertLayerNorm()\n",
- " (dropout): Dropout(p=0.1)\n",
- " )\n",
- " )\n",
- " (intermediate): BertIntermediate(\n",
- " (dense): Linear(in_features=768, out_features=3072, bias=True)\n",
- " )\n",
- " (output): BertOutput(\n",
- " (dense): Linear(in_features=3072, out_features=768, bias=True)\n",
- " (LayerNorm): BertLayerNorm()\n",
- " (dropout): Dropout(p=0.1)\n",
- " )\n",
- " )\n",
- " (4): BertLayer(\n",
- " (attention): BertAttention(\n",
- " (self): BertSelfAttention(\n",
- " (query): Linear(in_features=768, out_features=768, bias=True)\n",
- " (key): Linear(in_features=768, out_features=768, bias=True)\n",
- " (value): Linear(in_features=768, out_features=768, bias=True)\n",
- " (dropout): Dropout(p=0.1)\n",
- " )\n",
- " (output): BertSelfOutput(\n",
- " (dense): Linear(in_features=768, out_features=768, bias=True)\n",
- " (LayerNorm): BertLayerNorm()\n",
- " (dropout): Dropout(p=0.1)\n",
- " )\n",
- " )\n",
- " (intermediate): BertIntermediate(\n",
- " (dense): Linear(in_features=768, out_features=3072, bias=True)\n",
- " )\n",
- " (output): BertOutput(\n",
- " (dense): Linear(in_features=3072, out_features=768, bias=True)\n",
- " (LayerNorm): BertLayerNorm()\n",
- " (dropout): Dropout(p=0.1)\n",
- " )\n",
- " )\n",
- " (5): BertLayer(\n",
- " (attention): BertAttention(\n",
- " (self): BertSelfAttention(\n",
- " (query): Linear(in_features=768, out_features=768, bias=True)\n",
- " (key): Linear(in_features=768, out_features=768, bias=True)\n",
- " (value): Linear(in_features=768, out_features=768, bias=True)\n",
- " (dropout): Dropout(p=0.1)\n",
- " )\n",
- " (output): BertSelfOutput(\n",
- " (dense): Linear(in_features=768, out_features=768, bias=True)\n",
- " (LayerNorm): BertLayerNorm()\n",
- " (dropout): Dropout(p=0.1)\n",
- " )\n",
- " )\n",
- " (intermediate): BertIntermediate(\n",
- " (dense): Linear(in_features=768, out_features=3072, bias=True)\n",
- " )\n",
- " (output): BertOutput(\n",
- " (dense): Linear(in_features=3072, out_features=768, bias=True)\n",
- " (LayerNorm): BertLayerNorm()\n",
- " (dropout): Dropout(p=0.1)\n",
- " )\n",
- " )\n",
- " (6): BertLayer(\n",
- " (attention): BertAttention(\n",
- " (self): BertSelfAttention(\n",
- " (query): Linear(in_features=768, out_features=768, bias=True)\n",
- " (key): Linear(in_features=768, out_features=768, bias=True)\n",
- " (value): Linear(in_features=768, out_features=768, bias=True)\n",
- " (dropout): Dropout(p=0.1)\n",
- " )\n",
- " (output): BertSelfOutput(\n",
- " (dense): Linear(in_features=768, out_features=768, bias=True)\n",
- " (LayerNorm): BertLayerNorm()\n",
- " (dropout): Dropout(p=0.1)\n",
- " )\n",
- " )\n",
- " (intermediate): BertIntermediate(\n",
- " (dense): Linear(in_features=768, out_features=3072, bias=True)\n",
- " )\n",
- " (output): BertOutput(\n",
- " (dense): Linear(in_features=3072, out_features=768, bias=True)\n",
- " (LayerNorm): BertLayerNorm()\n",
- " (dropout): Dropout(p=0.1)\n",
- " )\n",
- " )\n",
- " (7): BertLayer(\n",
- " (attention): BertAttention(\n",
- " (self): BertSelfAttention(\n",
- " (query): Linear(in_features=768, out_features=768, bias=True)\n",
- " (key): Linear(in_features=768, out_features=768, bias=True)\n",
- " (value): Linear(in_features=768, out_features=768, bias=True)\n",
- " (dropout): Dropout(p=0.1)\n",
- " )\n",
- " (output): BertSelfOutput(\n",
- " (dense): Linear(in_features=768, out_features=768, bias=True)\n",
- " (LayerNorm): BertLayerNorm()\n",
- " (dropout): Dropout(p=0.1)\n",
- " )\n",
- " )\n",
- " (intermediate): BertIntermediate(\n",
- " (dense): Linear(in_features=768, out_features=3072, bias=True)\n",
- " )\n",
- " (output): BertOutput(\n",
- " (dense): Linear(in_features=3072, out_features=768, bias=True)\n",
- " (LayerNorm): BertLayerNorm()\n",
- " (dropout): Dropout(p=0.1)\n",
- " )\n",
- " )\n",
- " (8): BertLayer(\n",
- " (attention): BertAttention(\n",
- " (self): BertSelfAttention(\n",
- " (query): Linear(in_features=768, out_features=768, bias=True)\n",
- " (key): Linear(in_features=768, out_features=768, bias=True)\n",
- " (value): Linear(in_features=768, out_features=768, bias=True)\n",
- " (dropout): Dropout(p=0.1)\n",
- " )\n",
- " (output): BertSelfOutput(\n",
- " (dense): Linear(in_features=768, out_features=768, bias=True)\n",
- " (LayerNorm): BertLayerNorm()\n",
- " (dropout): Dropout(p=0.1)\n",
- " )\n",
- " )\n",
- " (intermediate): BertIntermediate(\n",
- " (dense): Linear(in_features=768, out_features=3072, bias=True)\n",
- " )\n",
- " (output): BertOutput(\n",
- " (dense): Linear(in_features=3072, out_features=768, bias=True)\n",
- " (LayerNorm): BertLayerNorm()\n",
- " (dropout): Dropout(p=0.1)\n",
- " )\n",
- " )\n",
- " (9): BertLayer(\n",
- " (attention): BertAttention(\n",
- " (self): BertSelfAttention(\n",
- " (query): Linear(in_features=768, out_features=768, bias=True)\n",
- " (key): Linear(in_features=768, out_features=768, bias=True)\n",
- " (value): Linear(in_features=768, out_features=768, bias=True)\n",
- " (dropout): Dropout(p=0.1)\n",
- " )\n",
- " (output): BertSelfOutput(\n",
- " (dense): Linear(in_features=768, out_features=768, bias=True)\n",
- " (LayerNorm): BertLayerNorm()\n",
- " (dropout): Dropout(p=0.1)\n",
- " )\n",
- " )\n",
- " (intermediate): BertIntermediate(\n",
- " (dense): Linear(in_features=768, out_features=3072, bias=True)\n",
- " )\n",
- " (output): BertOutput(\n",
- " (dense): Linear(in_features=3072, out_features=768, bias=True)\n",
- " (LayerNorm): BertLayerNorm()\n",
- " (dropout): Dropout(p=0.1)\n",
- " )\n",
- " )\n",
- " (10): BertLayer(\n",
- " (attention): BertAttention(\n",
- " (self): BertSelfAttention(\n",
- " (query): Linear(in_features=768, out_features=768, bias=True)\n",
- " (key): Linear(in_features=768, out_features=768, bias=True)\n",
- " (value): Linear(in_features=768, out_features=768, bias=True)\n",
- " (dropout): Dropout(p=0.1)\n",
- " )\n",
- " (output): BertSelfOutput(\n",
- " (dense): Linear(in_features=768, out_features=768, bias=True)\n",
- " (LayerNorm): BertLayerNorm()\n",
- " (dropout): Dropout(p=0.1)\n",
- " )\n",
- " )\n",
- " (intermediate): BertIntermediate(\n",
- " (dense): Linear(in_features=768, out_features=3072, bias=True)\n",
- " )\n",
- " (output): BertOutput(\n",
- " (dense): Linear(in_features=3072, out_features=768, bias=True)\n",
- " (LayerNorm): BertLayerNorm()\n",
- " (dropout): Dropout(p=0.1)\n",
- " )\n",
- " )\n",
- " (11): BertLayer(\n",
- " (attention): BertAttention(\n",
- " (self): BertSelfAttention(\n",
- " (query): Linear(in_features=768, out_features=768, bias=True)\n",
- " (key): Linear(in_features=768, out_features=768, bias=True)\n",
- " (value): Linear(in_features=768, out_features=768, bias=True)\n",
- " (dropout): Dropout(p=0.1)\n",
- " )\n",
- " (output): BertSelfOutput(\n",
- " (dense): Linear(in_features=768, out_features=768, bias=True)\n",
- " (LayerNorm): BertLayerNorm()\n",
- " (dropout): Dropout(p=0.1)\n",
- " )\n",
- " )\n",
- " (intermediate): BertIntermediate(\n",
- " (dense): Linear(in_features=768, out_features=3072, bias=True)\n",
- " )\n",
- " (output): BertOutput(\n",
- " (dense): Linear(in_features=3072, out_features=768, bias=True)\n",
- " (LayerNorm): BertLayerNorm()\n",
- " (dropout): Dropout(p=0.1)\n",
- " )\n",
- " )\n",
- " )\n",
- " )\n",
- " (pooler): BertPooler(\n",
- " (dense): Linear(in_features=768, out_features=768, bias=True)\n",
- " (activation): Tanh()\n",
- " )\n",
- " )\n",
- " (cls): BertPreTrainingHeads(\n",
- " (predictions): BertLMPredictionHead(\n",
- " (transform): BertPredictionHeadTransform(\n",
- " (dense): Linear(in_features=768, out_features=768, bias=True)\n",
- " (LayerNorm): BertLayerNorm()\n",
- " )\n",
- " (decoder): Linear(in_features=768, out_features=30522, bias=False)\n",
- " )\n",
- " (seq_relationship): Linear(in_features=768, out_features=2, bias=True)\n",
- " )\n",
- ")"
- ]
- },
- "execution_count": 17,
- "metadata": {},
- "output_type": "execute_result"
- }
- ],
- "source": [
- "all_input_ids = torch.tensor([f.input_ids for f in features], dtype=torch.long)\n",
- "all_input_mask = torch.tensor([f.input_mask for f in features], dtype=torch.long)\n",
- "all_segment_ids = torch.tensor([f.segment_ids for f in features], dtype=torch.long)\n",
- "all_masked_lm_positions = torch.tensor([f.masked_lm_positions for f in features], dtype=torch.long)\n",
- "\n",
- "eval_data = TensorDataset(all_input_ids, all_input_mask, all_segment_ids, all_masked_lm_positions)\n",
- "eval_sampler = SequentialSampler(eval_data)\n",
- "eval_dataloader = DataLoader(eval_data, sampler=eval_sampler, batch_size=1)\n",
- "\n",
- "model.eval()"
- ]
- },
- {
- "cell_type": "code",
- "execution_count": 18,
- "metadata": {
- "ExecuteTime": {
- "end_time": "2018-11-16T10:03:12.792741Z",
- "start_time": "2018-11-16T10:03:12.354253Z"
- }
- },
- "outputs": [
- {
- "name": "stdout",
- "output_type": "stream",
- "text": [
- "tensor([[ 2040, 2001, 3958, 27227, 1029, 3958, 103, 2001, 1037, 13997,\n",
- " 11510, 0, 0, 0, 0, 0, 0, 0, 0, 0,\n",
- " 0, 0, 0, 0, 0, 0, 0, 0, 0, 0,\n",
- " 0, 0, 0, 0, 0, 0, 0, 0, 0, 0,\n",
- " 0, 0, 0, 0, 0, 0, 0, 0, 0, 0,\n",
- " 0, 0, 0, 0, 0, 0, 0, 0, 0, 0,\n",
- " 0, 0, 0, 0, 0, 0, 0, 0, 0, 0,\n",
- " 0, 0, 0, 0, 0, 0, 0, 0, 0, 0,\n",
- " 0, 0, 0, 0, 0, 0, 0, 0, 0, 0,\n",
- " 0, 0, 0, 0, 0, 0, 0, 0, 0, 0,\n",
- " 0, 0, 0, 0, 0, 0, 0, 0, 0, 0,\n",
- " 0, 0, 0, 0, 0, 0, 0, 0, 0, 0,\n",
- " 0, 0, 0, 0, 0, 0, 0, 0]])\n",
- "tensor([[1, 1, 1, 1, 1, 1, 1, 1, 1, 1, 1, 0, 0, 0, 0, 0, 0, 0, 0, 0, 0, 0, 0, 0,\n",
- " 0, 0, 0, 0, 0, 0, 0, 0, 0, 0, 0, 0, 0, 0, 0, 0, 0, 0, 0, 0, 0, 0, 0, 0,\n",
- " 0, 0, 0, 0, 0, 0, 0, 0, 0, 0, 0, 0, 0, 0, 0, 0, 0, 0, 0, 0, 0, 0, 0, 0,\n",
- " 0, 0, 0, 0, 0, 0, 0, 0, 0, 0, 0, 0, 0, 0, 0, 0, 0, 0, 0, 0, 0, 0, 0, 0,\n",
- " 0, 0, 0, 0, 0, 0, 0, 0, 0, 0, 0, 0, 0, 0, 0, 0, 0, 0, 0, 0, 0, 0, 0, 0,\n",
- " 0, 0, 0, 0, 0, 0, 0, 0]])\n",
- "tensor([[0, 0, 0, 0, 0, 1, 1, 1, 1, 1, 1, 0, 0, 0, 0, 0, 0, 0, 0, 0, 0, 0, 0, 0,\n",
- " 0, 0, 0, 0, 0, 0, 0, 0, 0, 0, 0, 0, 0, 0, 0, 0, 0, 0, 0, 0, 0, 0, 0, 0,\n",
- " 0, 0, 0, 0, 0, 0, 0, 0, 0, 0, 0, 0, 0, 0, 0, 0, 0, 0, 0, 0, 0, 0, 0, 0,\n",
- " 0, 0, 0, 0, 0, 0, 0, 0, 0, 0, 0, 0, 0, 0, 0, 0, 0, 0, 0, 0, 0, 0, 0, 0,\n",
- " 0, 0, 0, 0, 0, 0, 0, 0, 0, 0, 0, 0, 0, 0, 0, 0, 0, 0, 0, 0, 0, 0, 0, 0,\n",
- " 0, 0, 0, 0, 0, 0, 0, 0]])\n",
- "(1, 20, 30522)\n",
- "[27227, 1010, 1010, 1010, 1010, 1010, 1010, 1010, 1010, 1010, 1010, 1010, 1010, 1010, 1010, 1010, 1010, 1010, 1010, 1010]\n"
- ]
- }
- ],
- "source": [
- "import numpy as np\n",
- "pytorch_all_out = []\n",
- "for input_ids, input_mask, segment_ids, tensor_masked_lm_positions in eval_dataloader:\n",
- " print(input_ids)\n",
- " print(input_mask)\n",
- " print(segment_ids)\n",
- " input_ids = input_ids.to(device)\n",
- " input_mask = input_mask.to(device)\n",
- " segment_ids = segment_ids.to(device)\n",
- "\n",
- " prediction_scores, _ = model(input_ids, token_type_ids=segment_ids, attention_mask=input_mask)\n",
- " prediction_scores = prediction_scores[0, tensor_masked_lm_positions].detach().cpu().numpy()\n",
- " print(prediction_scores.shape)\n",
- " masked_lm_predictions = np.argmax(prediction_scores, axis=-1).squeeze().tolist()\n",
- " print(masked_lm_predictions)\n",
- " pytorch_all_out.append(masked_lm_predictions)"
- ]
- },
- {
- "cell_type": "code",
- "execution_count": 19,
- "metadata": {
- "ExecuteTime": {
- "end_time": "2018-11-16T10:03:12.828439Z",
- "start_time": "2018-11-16T10:03:12.795420Z"
- }
- },
- "outputs": [
- {
- "name": "stdout",
- "output_type": "stream",
- "text": [
- "pytorch_output: ['henson']\n",
- "tensorflow_output: ['henson']\n"
- ]
- }
- ],
- "source": [
- "pytorch_outputs = tokenizer.convert_ids_to_tokens(pytorch_all_out[0])[:len(masked_lm_positions)]\n",
- "print(\"pytorch_output:\", pytorch_outputs)\n",
- "print(\"tensorflow_output:\", tensorflow_outputs)"
- ]
- },
- {
- "cell_type": "code",
- "execution_count": null,
- "metadata": {},
- "outputs": [],
- "source": []
- }
- ],
- "metadata": {
- "hide_input": false,
- "kernelspec": {
- "display_name": "Python [default]",
- "language": "python",
- "name": "python3"
- },
- "language_info": {
- "codemirror_mode": {
- "name": "ipython",
- "version": 3
- },
- "file_extension": ".py",
- "mimetype": "text/x-python",
- "name": "python",
- "nbconvert_exporter": "python",
- "pygments_lexer": "ipython3",
- "version": "3.6.7"
- },
- "toc": {
- "colors": {
- "hover_highlight": "#DAA520",
- "running_highlight": "#FF0000",
- "selected_highlight": "#FFD700"
- },
- "moveMenuLeft": true,
- "nav_menu": {
- "height": "48px",
- "width": "252px"
- },
- "navigate_menu": true,
- "number_sections": true,
- "sideBar": true,
- "threshold": 4,
- "toc_cell": false,
- "toc_section_display": "block",
- "toc_window_display": false
- }
- },
- "nbformat": 4,
- "nbformat_minor": 2
-}
diff --git a/server/transformers/notebooks/Comparing-TF-and-PT-models-SQuAD.ipynb b/server/transformers/notebooks/Comparing-TF-and-PT-models-SQuAD.ipynb
deleted file mode 100644
index a75e052643f59bd80617f0682101267d1a0e134b..0000000000000000000000000000000000000000
--- a/server/transformers/notebooks/Comparing-TF-and-PT-models-SQuAD.ipynb
+++ /dev/null
@@ -1,1644 +0,0 @@
-{
- "cells": [
- {
- "cell_type": "markdown",
- "metadata": {},
- "source": [
- "# Comparing TensorFlow (original) and PyTorch model on the SQuAD task\n",
- "\n",
- "You can use this small notebook to check the loss computation from the TensorFlow model to the PyTorch model. In the following, we compare the total loss computed by the models starting from identical initializations (position prediction linear layers with weights at 1 and bias at 0).\n",
- "\n",
- "To run this notebook, follow these instructions:\n",
- "- make sure that your Python environment has both TensorFlow and PyTorch installed,\n",
- "- download the original TensorFlow implementation,\n",
- "- download a pre-trained TensorFlow model as indicaded in the TensorFlow implementation readme,\n",
- "- run the script `convert_tf_checkpoint_to_pytorch.py` as indicated in the `README` to convert the pre-trained TensorFlow model to PyTorch.\n",
- "\n",
- "If needed change the relative paths indicated in this notebook (at the beggining of Sections 1 and 2) to point to the relevent models and code."
- ]
- },
- {
- "cell_type": "code",
- "execution_count": 1,
- "metadata": {
- "ExecuteTime": {
- "end_time": "2018-11-06T10:11:33.636911Z",
- "start_time": "2018-11-06T10:11:33.623091Z"
- }
- },
- "outputs": [],
- "source": [
- "import os\n",
- "os.chdir('../')"
- ]
- },
- {
- "cell_type": "markdown",
- "metadata": {},
- "source": [
- "## 1/ TensorFlow code"
- ]
- },
- {
- "cell_type": "code",
- "execution_count": 2,
- "metadata": {
- "ExecuteTime": {
- "end_time": "2018-11-06T10:11:33.651792Z",
- "start_time": "2018-11-06T10:11:33.638984Z"
- }
- },
- "outputs": [],
- "source": [
- "original_tf_inplem_dir = \"./tensorflow_code/\"\n",
- "model_dir = \"../google_models/uncased_L-12_H-768_A-12/\"\n",
- "\n",
- "vocab_file = model_dir + \"vocab.txt\"\n",
- "bert_config_file = model_dir + \"bert_config.json\"\n",
- "init_checkpoint = model_dir + \"bert_model.ckpt\"\n",
- "\n",
- "input_file = \"../data/squad_data/train-v1.1.json\"\n",
- "max_seq_length = 384\n",
- "outside_pos = max_seq_length + 10\n",
- "doc_stride = 128\n",
- "max_query_length = 64\n",
- "max_answer_length = 30\n",
- "output_dir = \"/tmp/squad_base/\"\n",
- "learning_rate = 3e-5"
- ]
- },
- {
- "cell_type": "code",
- "execution_count": 3,
- "metadata": {
- "ExecuteTime": {
- "end_time": "2018-11-06T10:11:35.165788Z",
- "start_time": "2018-11-06T10:11:33.653401Z"
- }
- },
- "outputs": [],
- "source": [
- "import importlib.util\n",
- "import sys\n",
- "\n",
- "spec = importlib.util.spec_from_file_location('*', original_tf_inplem_dir + '/modeling.py')\n",
- "module = importlib.util.module_from_spec(spec)\n",
- "spec.loader.exec_module(module)\n",
- "sys.modules['modeling_tensorflow'] = module\n",
- "\n",
- "spec = importlib.util.spec_from_file_location('*', original_tf_inplem_dir + '/run_bert_squad.py')\n",
- "module = importlib.util.module_from_spec(spec)\n",
- "spec.loader.exec_module(module)\n",
- "sys.modules['run_squad_tensorflow'] = module\n",
- "import modeling_tensorflow\n",
- "from run_squad_tensorflow import *"
- ]
- },
- {
- "cell_type": "code",
- "execution_count": 4,
- "metadata": {
- "ExecuteTime": {
- "end_time": "2018-11-06T10:11:37.494391Z",
- "start_time": "2018-11-06T10:11:35.168615Z"
- }
- },
- "outputs": [
- {
- "name": "stdout",
- "output_type": "stream",
- "text": [
- "INFO:tensorflow:*** Example ***\n",
- "INFO:tensorflow:unique_id: 1000000000\n",
- "INFO:tensorflow:example_index: 0\n",
- "INFO:tensorflow:doc_span_index: 0\n",
- "INFO:tensorflow:tokens: [CLS] to whom did the virgin mary allegedly appear in 1858 in lou ##rdes france ? [SEP] architectural ##ly , the school has a catholic character . atop the main building ' s gold dome is a golden statue of the virgin mary . immediately in front of the main building and facing it , is a copper statue of christ with arms up ##rai ##sed with the legend \" ve ##ni ##te ad me om ##nes \" . next to the main building is the basilica of the sacred heart . immediately behind the basilica is the gr ##otto , a marian place of prayer and reflection . it is a replica of the gr ##otto at lou ##rdes , france where the virgin mary reputed ##ly appeared to saint bern ##ade ##tte so ##ub ##iro ##us in 1858 . at the end of the main drive ( and in a direct line that connects through 3 statues and the gold dome ) , is a simple , modern stone statue of mary . [SEP]\n",
- "INFO:tensorflow:token_to_orig_map: 17:0 18:0 19:0 20:1 21:2 22:3 23:4 24:5 25:6 26:6 27:7 28:8 29:9 30:10 31:10 32:10 33:11 34:12 35:13 36:14 37:15 38:16 39:17 40:18 41:19 42:20 43:20 44:21 45:22 46:23 47:24 48:25 49:26 50:27 51:28 52:29 53:30 54:30 55:31 56:32 57:33 58:34 59:35 60:36 61:37 62:38 63:39 64:39 65:39 66:40 67:41 68:42 69:43 70:43 71:43 72:43 73:44 74:45 75:46 76:46 77:46 78:46 79:47 80:48 81:49 82:50 83:51 84:52 85:53 86:54 87:55 88:56 89:57 90:58 91:58 92:59 93:60 94:61 95:62 96:63 97:64 98:65 99:65 100:65 101:66 102:67 103:68 104:69 105:70 106:71 107:72 108:72 109:73 110:74 111:75 112:76 113:77 114:78 115:79 116:79 117:80 118:81 119:81 120:81 121:82 122:83 123:84 124:85 125:86 126:87 127:87 128:88 129:89 130:90 131:91 132:91 133:91 134:92 135:92 136:92 137:92 138:93 139:94 140:94 141:95 142:96 143:97 144:98 145:99 146:100 147:101 148:102 149:102 150:103 151:104 152:105 153:106 154:107 155:108 156:109 157:110 158:111 159:112 160:113 161:114 162:115 163:115 164:115 165:116 166:117 167:118 168:118 169:119 170:120 171:121 172:122 173:123 174:123\n",
- "INFO:tensorflow:token_is_max_context: 17:True 18:True 19:True 20:True 21:True 22:True 23:True 24:True 25:True 26:True 27:True 28:True 29:True 30:True 31:True 32:True 33:True 34:True 35:True 36:True 37:True 38:True 39:True 40:True 41:True 42:True 43:True 44:True 45:True 46:True 47:True 48:True 49:True 50:True 51:True 52:True 53:True 54:True 55:True 56:True 57:True 58:True 59:True 60:True 61:True 62:True 63:True 64:True 65:True 66:True 67:True 68:True 69:True 70:True 71:True 72:True 73:True 74:True 75:True 76:True 77:True 78:True 79:True 80:True 81:True 82:True 83:True 84:True 85:True 86:True 87:True 88:True 89:True 90:True 91:True 92:True 93:True 94:True 95:True 96:True 97:True 98:True 99:True 100:True 101:True 102:True 103:True 104:True 105:True 106:True 107:True 108:True 109:True 110:True 111:True 112:True 113:True 114:True 115:True 116:True 117:True 118:True 119:True 120:True 121:True 122:True 123:True 124:True 125:True 126:True 127:True 128:True 129:True 130:True 131:True 132:True 133:True 134:True 135:True 136:True 137:True 138:True 139:True 140:True 141:True 142:True 143:True 144:True 145:True 146:True 147:True 148:True 149:True 150:True 151:True 152:True 153:True 154:True 155:True 156:True 157:True 158:True 159:True 160:True 161:True 162:True 163:True 164:True 165:True 166:True 167:True 168:True 169:True 170:True 171:True 172:True 173:True 174:True\n",
- "INFO:tensorflow:input_ids: 101 2000 3183 2106 1996 6261 2984 9382 3711 1999 8517 1999 10223 26371 2605 1029 102 6549 2135 1010 1996 2082 2038 1037 3234 2839 1012 10234 1996 2364 2311 1005 1055 2751 8514 2003 1037 3585 6231 1997 1996 6261 2984 1012 3202 1999 2392 1997 1996 2364 2311 1998 5307 2009 1010 2003 1037 6967 6231 1997 4828 2007 2608 2039 14995 6924 2007 1996 5722 1000 2310 3490 2618 4748 2033 18168 5267 1000 1012 2279 2000 1996 2364 2311 2003 1996 13546 1997 1996 6730 2540 1012 3202 2369 1996 13546 2003 1996 24665 23052 1010 1037 14042 2173 1997 7083 1998 9185 1012 2009 2003 1037 15059 1997 1996 24665 23052 2012 10223 26371 1010 2605 2073 1996 6261 2984 22353 2135 2596 2000 3002 16595 9648 4674 2061 12083 9711 2271 1999 8517 1012 2012 1996 2203 1997 1996 2364 3298 1006 1998 1999 1037 3622 2240 2008 8539 2083 1017 11342 1998 1996 2751 8514 1007 1010 2003 1037 3722 1010 2715 2962 6231 1997 2984 1012 102 0 0 0 0 0 0 0 0 0 0 0 0 0 0 0 0 0 0 0 0 0 0 0 0 0 0 0 0 0 0 0 0 0 0 0 0 0 0 0 0 0 0 0 0 0 0 0 0 0 0 0 0 0 0 0 0 0 0 0 0 0 0 0 0 0 0 0 0 0 0 0 0 0 0 0 0 0 0 0 0 0 0 0 0 0 0 0 0 0 0 0 0 0 0 0 0 0 0 0 0 0 0 0 0 0 0 0 0 0 0 0 0 0 0 0 0 0 0 0 0 0 0 0 0 0 0 0 0 0 0 0 0 0 0 0 0 0 0 0 0 0 0 0 0 0 0 0 0 0 0 0 0 0 0 0 0 0 0 0 0 0 0 0 0 0 0 0 0 0 0 0 0 0 0 0 0 0 0 0 0 0 0 0 0 0 0 0 0 0 0 0 0 0 0 0 0 0 0 0 0 0 0 0 0 0 0 0 0\n",
- "INFO:tensorflow:input_mask: 1 1 1 1 1 1 1 1 1 1 1 1 1 1 1 1 1 1 1 1 1 1 1 1 1 1 1 1 1 1 1 1 1 1 1 1 1 1 1 1 1 1 1 1 1 1 1 1 1 1 1 1 1 1 1 1 1 1 1 1 1 1 1 1 1 1 1 1 1 1 1 1 1 1 1 1 1 1 1 1 1 1 1 1 1 1 1 1 1 1 1 1 1 1 1 1 1 1 1 1 1 1 1 1 1 1 1 1 1 1 1 1 1 1 1 1 1 1 1 1 1 1 1 1 1 1 1 1 1 1 1 1 1 1 1 1 1 1 1 1 1 1 1 1 1 1 1 1 1 1 1 1 1 1 1 1 1 1 1 1 1 1 1 1 1 1 1 1 1 1 1 1 1 1 1 1 0 0 0 0 0 0 0 0 0 0 0 0 0 0 0 0 0 0 0 0 0 0 0 0 0 0 0 0 0 0 0 0 0 0 0 0 0 0 0 0 0 0 0 0 0 0 0 0 0 0 0 0 0 0 0 0 0 0 0 0 0 0 0 0 0 0 0 0 0 0 0 0 0 0 0 0 0 0 0 0 0 0 0 0 0 0 0 0 0 0 0 0 0 0 0 0 0 0 0 0 0 0 0 0 0 0 0 0 0 0 0 0 0 0 0 0 0 0 0 0 0 0 0 0 0 0 0 0 0 0 0 0 0 0 0 0 0 0 0 0 0 0 0 0 0 0 0 0 0 0 0 0 0 0 0 0 0 0 0 0 0 0 0 0 0 0 0 0 0 0 0 0 0 0 0 0 0 0 0 0 0 0 0 0 0 0 0 0 0 0 0 0 0 0 0 0 0 0 0 0 0 0 0 0 0 0 0 0\n",
- "INFO:tensorflow:segment_ids: 0 0 0 0 0 0 0 0 0 0 0 0 0 0 0 0 0 1 1 1 1 1 1 1 1 1 1 1 1 1 1 1 1 1 1 1 1 1 1 1 1 1 1 1 1 1 1 1 1 1 1 1 1 1 1 1 1 1 1 1 1 1 1 1 1 1 1 1 1 1 1 1 1 1 1 1 1 1 1 1 1 1 1 1 1 1 1 1 1 1 1 1 1 1 1 1 1 1 1 1 1 1 1 1 1 1 1 1 1 1 1 1 1 1 1 1 1 1 1 1 1 1 1 1 1 1 1 1 1 1 1 1 1 1 1 1 1 1 1 1 1 1 1 1 1 1 1 1 1 1 1 1 1 1 1 1 1 1 1 1 1 1 1 1 1 1 1 1 1 1 1 1 1 1 1 1 0 0 0 0 0 0 0 0 0 0 0 0 0 0 0 0 0 0 0 0 0 0 0 0 0 0 0 0 0 0 0 0 0 0 0 0 0 0 0 0 0 0 0 0 0 0 0 0 0 0 0 0 0 0 0 0 0 0 0 0 0 0 0 0 0 0 0 0 0 0 0 0 0 0 0 0 0 0 0 0 0 0 0 0 0 0 0 0 0 0 0 0 0 0 0 0 0 0 0 0 0 0 0 0 0 0 0 0 0 0 0 0 0 0 0 0 0 0 0 0 0 0 0 0 0 0 0 0 0 0 0 0 0 0 0 0 0 0 0 0 0 0 0 0 0 0 0 0 0 0 0 0 0 0 0 0 0 0 0 0 0 0 0 0 0 0 0 0 0 0 0 0 0 0 0 0 0 0 0 0 0 0 0 0 0 0 0 0 0 0 0 0 0 0 0 0 0 0 0 0 0 0 0 0 0 0 0 0\n",
- "INFO:tensorflow:start_position: 130\n",
- "INFO:tensorflow:end_position: 137\n",
- "INFO:tensorflow:answer: saint bern ##ade ##tte so ##ub ##iro ##us\n",
- "INFO:tensorflow:*** Example ***\n",
- "INFO:tensorflow:unique_id: 1000000001\n",
- "INFO:tensorflow:example_index: 1\n",
- "INFO:tensorflow:doc_span_index: 0\n",
- "INFO:tensorflow:tokens: [CLS] what is in front of the notre dame main building ? [SEP] architectural ##ly , the school has a catholic character . atop the main building ' s gold dome is a golden statue of the virgin mary . immediately in front of the main building and facing it , is a copper statue of christ with arms up ##rai ##sed with the legend \" ve ##ni ##te ad me om ##nes \" . next to the main building is the basilica of the sacred heart . immediately behind the basilica is the gr ##otto , a marian place of prayer and reflection . it is a replica of the gr ##otto at lou ##rdes , france where the virgin mary reputed ##ly appeared to saint bern ##ade ##tte so ##ub ##iro ##us in 1858 . at the end of the main drive ( and in a direct line that connects through 3 statues and the gold dome ) , is a simple , modern stone statue of mary . [SEP]\n",
- "INFO:tensorflow:token_to_orig_map: 13:0 14:0 15:0 16:1 17:2 18:3 19:4 20:5 21:6 22:6 23:7 24:8 25:9 26:10 27:10 28:10 29:11 30:12 31:13 32:14 33:15 34:16 35:17 36:18 37:19 38:20 39:20 40:21 41:22 42:23 43:24 44:25 45:26 46:27 47:28 48:29 49:30 50:30 51:31 52:32 53:33 54:34 55:35 56:36 57:37 58:38 59:39 60:39 61:39 62:40 63:41 64:42 65:43 66:43 67:43 68:43 69:44 70:45 71:46 72:46 73:46 74:46 75:47 76:48 77:49 78:50 79:51 80:52 81:53 82:54 83:55 84:56 85:57 86:58 87:58 88:59 89:60 90:61 91:62 92:63 93:64 94:65 95:65 96:65 97:66 98:67 99:68 100:69 101:70 102:71 103:72 104:72 105:73 106:74 107:75 108:76 109:77 110:78 111:79 112:79 113:80 114:81 115:81 116:81 117:82 118:83 119:84 120:85 121:86 122:87 123:87 124:88 125:89 126:90 127:91 128:91 129:91 130:92 131:92 132:92 133:92 134:93 135:94 136:94 137:95 138:96 139:97 140:98 141:99 142:100 143:101 144:102 145:102 146:103 147:104 148:105 149:106 150:107 151:108 152:109 153:110 154:111 155:112 156:113 157:114 158:115 159:115 160:115 161:116 162:117 163:118 164:118 165:119 166:120 167:121 168:122 169:123 170:123\n"
- ]
- },
- {
- "name": "stdout",
- "output_type": "stream",
- "text": [
- "INFO:tensorflow:token_is_max_context: 13:True 14:True 15:True 16:True 17:True 18:True 19:True 20:True 21:True 22:True 23:True 24:True 25:True 26:True 27:True 28:True 29:True 30:True 31:True 32:True 33:True 34:True 35:True 36:True 37:True 38:True 39:True 40:True 41:True 42:True 43:True 44:True 45:True 46:True 47:True 48:True 49:True 50:True 51:True 52:True 53:True 54:True 55:True 56:True 57:True 58:True 59:True 60:True 61:True 62:True 63:True 64:True 65:True 66:True 67:True 68:True 69:True 70:True 71:True 72:True 73:True 74:True 75:True 76:True 77:True 78:True 79:True 80:True 81:True 82:True 83:True 84:True 85:True 86:True 87:True 88:True 89:True 90:True 91:True 92:True 93:True 94:True 95:True 96:True 97:True 98:True 99:True 100:True 101:True 102:True 103:True 104:True 105:True 106:True 107:True 108:True 109:True 110:True 111:True 112:True 113:True 114:True 115:True 116:True 117:True 118:True 119:True 120:True 121:True 122:True 123:True 124:True 125:True 126:True 127:True 128:True 129:True 130:True 131:True 132:True 133:True 134:True 135:True 136:True 137:True 138:True 139:True 140:True 141:True 142:True 143:True 144:True 145:True 146:True 147:True 148:True 149:True 150:True 151:True 152:True 153:True 154:True 155:True 156:True 157:True 158:True 159:True 160:True 161:True 162:True 163:True 164:True 165:True 166:True 167:True 168:True 169:True 170:True\n",
- "INFO:tensorflow:input_ids: 101 2054 2003 1999 2392 1997 1996 10289 8214 2364 2311 1029 102 6549 2135 1010 1996 2082 2038 1037 3234 2839 1012 10234 1996 2364 2311 1005 1055 2751 8514 2003 1037 3585 6231 1997 1996 6261 2984 1012 3202 1999 2392 1997 1996 2364 2311 1998 5307 2009 1010 2003 1037 6967 6231 1997 4828 2007 2608 2039 14995 6924 2007 1996 5722 1000 2310 3490 2618 4748 2033 18168 5267 1000 1012 2279 2000 1996 2364 2311 2003 1996 13546 1997 1996 6730 2540 1012 3202 2369 1996 13546 2003 1996 24665 23052 1010 1037 14042 2173 1997 7083 1998 9185 1012 2009 2003 1037 15059 1997 1996 24665 23052 2012 10223 26371 1010 2605 2073 1996 6261 2984 22353 2135 2596 2000 3002 16595 9648 4674 2061 12083 9711 2271 1999 8517 1012 2012 1996 2203 1997 1996 2364 3298 1006 1998 1999 1037 3622 2240 2008 8539 2083 1017 11342 1998 1996 2751 8514 1007 1010 2003 1037 3722 1010 2715 2962 6231 1997 2984 1012 102 0 0 0 0 0 0 0 0 0 0 0 0 0 0 0 0 0 0 0 0 0 0 0 0 0 0 0 0 0 0 0 0 0 0 0 0 0 0 0 0 0 0 0 0 0 0 0 0 0 0 0 0 0 0 0 0 0 0 0 0 0 0 0 0 0 0 0 0 0 0 0 0 0 0 0 0 0 0 0 0 0 0 0 0 0 0 0 0 0 0 0 0 0 0 0 0 0 0 0 0 0 0 0 0 0 0 0 0 0 0 0 0 0 0 0 0 0 0 0 0 0 0 0 0 0 0 0 0 0 0 0 0 0 0 0 0 0 0 0 0 0 0 0 0 0 0 0 0 0 0 0 0 0 0 0 0 0 0 0 0 0 0 0 0 0 0 0 0 0 0 0 0 0 0 0 0 0 0 0 0 0 0 0 0 0 0 0 0 0 0 0 0 0 0 0 0 0 0 0 0 0 0 0 0 0 0 0 0 0 0 0 0\n",
- "INFO:tensorflow:input_mask: 1 1 1 1 1 1 1 1 1 1 1 1 1 1 1 1 1 1 1 1 1 1 1 1 1 1 1 1 1 1 1 1 1 1 1 1 1 1 1 1 1 1 1 1 1 1 1 1 1 1 1 1 1 1 1 1 1 1 1 1 1 1 1 1 1 1 1 1 1 1 1 1 1 1 1 1 1 1 1 1 1 1 1 1 1 1 1 1 1 1 1 1 1 1 1 1 1 1 1 1 1 1 1 1 1 1 1 1 1 1 1 1 1 1 1 1 1 1 1 1 1 1 1 1 1 1 1 1 1 1 1 1 1 1 1 1 1 1 1 1 1 1 1 1 1 1 1 1 1 1 1 1 1 1 1 1 1 1 1 1 1 1 1 1 1 1 1 1 1 1 1 1 0 0 0 0 0 0 0 0 0 0 0 0 0 0 0 0 0 0 0 0 0 0 0 0 0 0 0 0 0 0 0 0 0 0 0 0 0 0 0 0 0 0 0 0 0 0 0 0 0 0 0 0 0 0 0 0 0 0 0 0 0 0 0 0 0 0 0 0 0 0 0 0 0 0 0 0 0 0 0 0 0 0 0 0 0 0 0 0 0 0 0 0 0 0 0 0 0 0 0 0 0 0 0 0 0 0 0 0 0 0 0 0 0 0 0 0 0 0 0 0 0 0 0 0 0 0 0 0 0 0 0 0 0 0 0 0 0 0 0 0 0 0 0 0 0 0 0 0 0 0 0 0 0 0 0 0 0 0 0 0 0 0 0 0 0 0 0 0 0 0 0 0 0 0 0 0 0 0 0 0 0 0 0 0 0 0 0 0 0 0 0 0 0 0 0 0 0 0 0 0 0 0 0 0 0 0 0 0 0 0 0 0\n",
- "INFO:tensorflow:segment_ids: 0 0 0 0 0 0 0 0 0 0 0 0 0 1 1 1 1 1 1 1 1 1 1 1 1 1 1 1 1 1 1 1 1 1 1 1 1 1 1 1 1 1 1 1 1 1 1 1 1 1 1 1 1 1 1 1 1 1 1 1 1 1 1 1 1 1 1 1 1 1 1 1 1 1 1 1 1 1 1 1 1 1 1 1 1 1 1 1 1 1 1 1 1 1 1 1 1 1 1 1 1 1 1 1 1 1 1 1 1 1 1 1 1 1 1 1 1 1 1 1 1 1 1 1 1 1 1 1 1 1 1 1 1 1 1 1 1 1 1 1 1 1 1 1 1 1 1 1 1 1 1 1 1 1 1 1 1 1 1 1 1 1 1 1 1 1 1 1 1 1 1 1 0 0 0 0 0 0 0 0 0 0 0 0 0 0 0 0 0 0 0 0 0 0 0 0 0 0 0 0 0 0 0 0 0 0 0 0 0 0 0 0 0 0 0 0 0 0 0 0 0 0 0 0 0 0 0 0 0 0 0 0 0 0 0 0 0 0 0 0 0 0 0 0 0 0 0 0 0 0 0 0 0 0 0 0 0 0 0 0 0 0 0 0 0 0 0 0 0 0 0 0 0 0 0 0 0 0 0 0 0 0 0 0 0 0 0 0 0 0 0 0 0 0 0 0 0 0 0 0 0 0 0 0 0 0 0 0 0 0 0 0 0 0 0 0 0 0 0 0 0 0 0 0 0 0 0 0 0 0 0 0 0 0 0 0 0 0 0 0 0 0 0 0 0 0 0 0 0 0 0 0 0 0 0 0 0 0 0 0 0 0 0 0 0 0 0 0 0 0 0 0 0 0 0 0 0 0 0 0 0 0 0 0\n",
- "INFO:tensorflow:start_position: 52\n",
- "INFO:tensorflow:end_position: 56\n",
- "INFO:tensorflow:answer: a copper statue of christ\n",
- "INFO:tensorflow:*** Example ***\n",
- "INFO:tensorflow:unique_id: 1000000002\n",
- "INFO:tensorflow:example_index: 2\n",
- "INFO:tensorflow:doc_span_index: 0\n",
- "INFO:tensorflow:tokens: [CLS] the basilica of the sacred heart at notre dame is beside to which structure ? [SEP] architectural ##ly , the school has a catholic character . atop the main building ' s gold dome is a golden statue of the virgin mary . immediately in front of the main building and facing it , is a copper statue of christ with arms up ##rai ##sed with the legend \" ve ##ni ##te ad me om ##nes \" . next to the main building is the basilica of the sacred heart . immediately behind the basilica is the gr ##otto , a marian place of prayer and reflection . it is a replica of the gr ##otto at lou ##rdes , france where the virgin mary reputed ##ly appeared to saint bern ##ade ##tte so ##ub ##iro ##us in 1858 . at the end of the main drive ( and in a direct line that connects through 3 statues and the gold dome ) , is a simple , modern stone statue of mary . [SEP]\n",
- "INFO:tensorflow:token_to_orig_map: 17:0 18:0 19:0 20:1 21:2 22:3 23:4 24:5 25:6 26:6 27:7 28:8 29:9 30:10 31:10 32:10 33:11 34:12 35:13 36:14 37:15 38:16 39:17 40:18 41:19 42:20 43:20 44:21 45:22 46:23 47:24 48:25 49:26 50:27 51:28 52:29 53:30 54:30 55:31 56:32 57:33 58:34 59:35 60:36 61:37 62:38 63:39 64:39 65:39 66:40 67:41 68:42 69:43 70:43 71:43 72:43 73:44 74:45 75:46 76:46 77:46 78:46 79:47 80:48 81:49 82:50 83:51 84:52 85:53 86:54 87:55 88:56 89:57 90:58 91:58 92:59 93:60 94:61 95:62 96:63 97:64 98:65 99:65 100:65 101:66 102:67 103:68 104:69 105:70 106:71 107:72 108:72 109:73 110:74 111:75 112:76 113:77 114:78 115:79 116:79 117:80 118:81 119:81 120:81 121:82 122:83 123:84 124:85 125:86 126:87 127:87 128:88 129:89 130:90 131:91 132:91 133:91 134:92 135:92 136:92 137:92 138:93 139:94 140:94 141:95 142:96 143:97 144:98 145:99 146:100 147:101 148:102 149:102 150:103 151:104 152:105 153:106 154:107 155:108 156:109 157:110 158:111 159:112 160:113 161:114 162:115 163:115 164:115 165:116 166:117 167:118 168:118 169:119 170:120 171:121 172:122 173:123 174:123\n",
- "INFO:tensorflow:token_is_max_context: 17:True 18:True 19:True 20:True 21:True 22:True 23:True 24:True 25:True 26:True 27:True 28:True 29:True 30:True 31:True 32:True 33:True 34:True 35:True 36:True 37:True 38:True 39:True 40:True 41:True 42:True 43:True 44:True 45:True 46:True 47:True 48:True 49:True 50:True 51:True 52:True 53:True 54:True 55:True 56:True 57:True 58:True 59:True 60:True 61:True 62:True 63:True 64:True 65:True 66:True 67:True 68:True 69:True 70:True 71:True 72:True 73:True 74:True 75:True 76:True 77:True 78:True 79:True 80:True 81:True 82:True 83:True 84:True 85:True 86:True 87:True 88:True 89:True 90:True 91:True 92:True 93:True 94:True 95:True 96:True 97:True 98:True 99:True 100:True 101:True 102:True 103:True 104:True 105:True 106:True 107:True 108:True 109:True 110:True 111:True 112:True 113:True 114:True 115:True 116:True 117:True 118:True 119:True 120:True 121:True 122:True 123:True 124:True 125:True 126:True 127:True 128:True 129:True 130:True 131:True 132:True 133:True 134:True 135:True 136:True 137:True 138:True 139:True 140:True 141:True 142:True 143:True 144:True 145:True 146:True 147:True 148:True 149:True 150:True 151:True 152:True 153:True 154:True 155:True 156:True 157:True 158:True 159:True 160:True 161:True 162:True 163:True 164:True 165:True 166:True 167:True 168:True 169:True 170:True 171:True 172:True 173:True 174:True\n",
- "INFO:tensorflow:input_ids: 101 1996 13546 1997 1996 6730 2540 2012 10289 8214 2003 3875 2000 2029 3252 1029 102 6549 2135 1010 1996 2082 2038 1037 3234 2839 1012 10234 1996 2364 2311 1005 1055 2751 8514 2003 1037 3585 6231 1997 1996 6261 2984 1012 3202 1999 2392 1997 1996 2364 2311 1998 5307 2009 1010 2003 1037 6967 6231 1997 4828 2007 2608 2039 14995 6924 2007 1996 5722 1000 2310 3490 2618 4748 2033 18168 5267 1000 1012 2279 2000 1996 2364 2311 2003 1996 13546 1997 1996 6730 2540 1012 3202 2369 1996 13546 2003 1996 24665 23052 1010 1037 14042 2173 1997 7083 1998 9185 1012 2009 2003 1037 15059 1997 1996 24665 23052 2012 10223 26371 1010 2605 2073 1996 6261 2984 22353 2135 2596 2000 3002 16595 9648 4674 2061 12083 9711 2271 1999 8517 1012 2012 1996 2203 1997 1996 2364 3298 1006 1998 1999 1037 3622 2240 2008 8539 2083 1017 11342 1998 1996 2751 8514 1007 1010 2003 1037 3722 1010 2715 2962 6231 1997 2984 1012 102 0 0 0 0 0 0 0 0 0 0 0 0 0 0 0 0 0 0 0 0 0 0 0 0 0 0 0 0 0 0 0 0 0 0 0 0 0 0 0 0 0 0 0 0 0 0 0 0 0 0 0 0 0 0 0 0 0 0 0 0 0 0 0 0 0 0 0 0 0 0 0 0 0 0 0 0 0 0 0 0 0 0 0 0 0 0 0 0 0 0 0 0 0 0 0 0 0 0 0 0 0 0 0 0 0 0 0 0 0 0 0 0 0 0 0 0 0 0 0 0 0 0 0 0 0 0 0 0 0 0 0 0 0 0 0 0 0 0 0 0 0 0 0 0 0 0 0 0 0 0 0 0 0 0 0 0 0 0 0 0 0 0 0 0 0 0 0 0 0 0 0 0 0 0 0 0 0 0 0 0 0 0 0 0 0 0 0 0 0 0 0 0 0 0 0 0 0 0 0 0 0 0 0 0 0 0 0 0\n"
- ]
- },
- {
- "name": "stdout",
- "output_type": "stream",
- "text": [
- "INFO:tensorflow:input_mask: 1 1 1 1 1 1 1 1 1 1 1 1 1 1 1 1 1 1 1 1 1 1 1 1 1 1 1 1 1 1 1 1 1 1 1 1 1 1 1 1 1 1 1 1 1 1 1 1 1 1 1 1 1 1 1 1 1 1 1 1 1 1 1 1 1 1 1 1 1 1 1 1 1 1 1 1 1 1 1 1 1 1 1 1 1 1 1 1 1 1 1 1 1 1 1 1 1 1 1 1 1 1 1 1 1 1 1 1 1 1 1 1 1 1 1 1 1 1 1 1 1 1 1 1 1 1 1 1 1 1 1 1 1 1 1 1 1 1 1 1 1 1 1 1 1 1 1 1 1 1 1 1 1 1 1 1 1 1 1 1 1 1 1 1 1 1 1 1 1 1 1 1 1 1 1 1 0 0 0 0 0 0 0 0 0 0 0 0 0 0 0 0 0 0 0 0 0 0 0 0 0 0 0 0 0 0 0 0 0 0 0 0 0 0 0 0 0 0 0 0 0 0 0 0 0 0 0 0 0 0 0 0 0 0 0 0 0 0 0 0 0 0 0 0 0 0 0 0 0 0 0 0 0 0 0 0 0 0 0 0 0 0 0 0 0 0 0 0 0 0 0 0 0 0 0 0 0 0 0 0 0 0 0 0 0 0 0 0 0 0 0 0 0 0 0 0 0 0 0 0 0 0 0 0 0 0 0 0 0 0 0 0 0 0 0 0 0 0 0 0 0 0 0 0 0 0 0 0 0 0 0 0 0 0 0 0 0 0 0 0 0 0 0 0 0 0 0 0 0 0 0 0 0 0 0 0 0 0 0 0 0 0 0 0 0 0 0 0 0 0 0 0 0 0 0 0 0 0 0 0 0 0 0 0\n",
- "INFO:tensorflow:segment_ids: 0 0 0 0 0 0 0 0 0 0 0 0 0 0 0 0 0 1 1 1 1 1 1 1 1 1 1 1 1 1 1 1 1 1 1 1 1 1 1 1 1 1 1 1 1 1 1 1 1 1 1 1 1 1 1 1 1 1 1 1 1 1 1 1 1 1 1 1 1 1 1 1 1 1 1 1 1 1 1 1 1 1 1 1 1 1 1 1 1 1 1 1 1 1 1 1 1 1 1 1 1 1 1 1 1 1 1 1 1 1 1 1 1 1 1 1 1 1 1 1 1 1 1 1 1 1 1 1 1 1 1 1 1 1 1 1 1 1 1 1 1 1 1 1 1 1 1 1 1 1 1 1 1 1 1 1 1 1 1 1 1 1 1 1 1 1 1 1 1 1 1 1 1 1 1 1 0 0 0 0 0 0 0 0 0 0 0 0 0 0 0 0 0 0 0 0 0 0 0 0 0 0 0 0 0 0 0 0 0 0 0 0 0 0 0 0 0 0 0 0 0 0 0 0 0 0 0 0 0 0 0 0 0 0 0 0 0 0 0 0 0 0 0 0 0 0 0 0 0 0 0 0 0 0 0 0 0 0 0 0 0 0 0 0 0 0 0 0 0 0 0 0 0 0 0 0 0 0 0 0 0 0 0 0 0 0 0 0 0 0 0 0 0 0 0 0 0 0 0 0 0 0 0 0 0 0 0 0 0 0 0 0 0 0 0 0 0 0 0 0 0 0 0 0 0 0 0 0 0 0 0 0 0 0 0 0 0 0 0 0 0 0 0 0 0 0 0 0 0 0 0 0 0 0 0 0 0 0 0 0 0 0 0 0 0 0 0 0 0 0 0 0 0 0 0 0 0 0 0 0 0 0 0 0\n",
- "INFO:tensorflow:start_position: 81\n",
- "INFO:tensorflow:end_position: 83\n",
- "INFO:tensorflow:answer: the main building\n",
- "INFO:tensorflow:*** Example ***\n",
- "INFO:tensorflow:unique_id: 1000000003\n",
- "INFO:tensorflow:example_index: 3\n",
- "INFO:tensorflow:doc_span_index: 0\n",
- "INFO:tensorflow:tokens: [CLS] what is the gr ##otto at notre dame ? [SEP] architectural ##ly , the school has a catholic character . atop the main building ' s gold dome is a golden statue of the virgin mary . immediately in front of the main building and facing it , is a copper statue of christ with arms up ##rai ##sed with the legend \" ve ##ni ##te ad me om ##nes \" . next to the main building is the basilica of the sacred heart . immediately behind the basilica is the gr ##otto , a marian place of prayer and reflection . it is a replica of the gr ##otto at lou ##rdes , france where the virgin mary reputed ##ly appeared to saint bern ##ade ##tte so ##ub ##iro ##us in 1858 . at the end of the main drive ( and in a direct line that connects through 3 statues and the gold dome ) , is a simple , modern stone statue of mary . [SEP]\n",
- "INFO:tensorflow:token_to_orig_map: 11:0 12:0 13:0 14:1 15:2 16:3 17:4 18:5 19:6 20:6 21:7 22:8 23:9 24:10 25:10 26:10 27:11 28:12 29:13 30:14 31:15 32:16 33:17 34:18 35:19 36:20 37:20 38:21 39:22 40:23 41:24 42:25 43:26 44:27 45:28 46:29 47:30 48:30 49:31 50:32 51:33 52:34 53:35 54:36 55:37 56:38 57:39 58:39 59:39 60:40 61:41 62:42 63:43 64:43 65:43 66:43 67:44 68:45 69:46 70:46 71:46 72:46 73:47 74:48 75:49 76:50 77:51 78:52 79:53 80:54 81:55 82:56 83:57 84:58 85:58 86:59 87:60 88:61 89:62 90:63 91:64 92:65 93:65 94:65 95:66 96:67 97:68 98:69 99:70 100:71 101:72 102:72 103:73 104:74 105:75 106:76 107:77 108:78 109:79 110:79 111:80 112:81 113:81 114:81 115:82 116:83 117:84 118:85 119:86 120:87 121:87 122:88 123:89 124:90 125:91 126:91 127:91 128:92 129:92 130:92 131:92 132:93 133:94 134:94 135:95 136:96 137:97 138:98 139:99 140:100 141:101 142:102 143:102 144:103 145:104 146:105 147:106 148:107 149:108 150:109 151:110 152:111 153:112 154:113 155:114 156:115 157:115 158:115 159:116 160:117 161:118 162:118 163:119 164:120 165:121 166:122 167:123 168:123\n",
- "INFO:tensorflow:token_is_max_context: 11:True 12:True 13:True 14:True 15:True 16:True 17:True 18:True 19:True 20:True 21:True 22:True 23:True 24:True 25:True 26:True 27:True 28:True 29:True 30:True 31:True 32:True 33:True 34:True 35:True 36:True 37:True 38:True 39:True 40:True 41:True 42:True 43:True 44:True 45:True 46:True 47:True 48:True 49:True 50:True 51:True 52:True 53:True 54:True 55:True 56:True 57:True 58:True 59:True 60:True 61:True 62:True 63:True 64:True 65:True 66:True 67:True 68:True 69:True 70:True 71:True 72:True 73:True 74:True 75:True 76:True 77:True 78:True 79:True 80:True 81:True 82:True 83:True 84:True 85:True 86:True 87:True 88:True 89:True 90:True 91:True 92:True 93:True 94:True 95:True 96:True 97:True 98:True 99:True 100:True 101:True 102:True 103:True 104:True 105:True 106:True 107:True 108:True 109:True 110:True 111:True 112:True 113:True 114:True 115:True 116:True 117:True 118:True 119:True 120:True 121:True 122:True 123:True 124:True 125:True 126:True 127:True 128:True 129:True 130:True 131:True 132:True 133:True 134:True 135:True 136:True 137:True 138:True 139:True 140:True 141:True 142:True 143:True 144:True 145:True 146:True 147:True 148:True 149:True 150:True 151:True 152:True 153:True 154:True 155:True 156:True 157:True 158:True 159:True 160:True 161:True 162:True 163:True 164:True 165:True 166:True 167:True 168:True\n",
- "INFO:tensorflow:input_ids: 101 2054 2003 1996 24665 23052 2012 10289 8214 1029 102 6549 2135 1010 1996 2082 2038 1037 3234 2839 1012 10234 1996 2364 2311 1005 1055 2751 8514 2003 1037 3585 6231 1997 1996 6261 2984 1012 3202 1999 2392 1997 1996 2364 2311 1998 5307 2009 1010 2003 1037 6967 6231 1997 4828 2007 2608 2039 14995 6924 2007 1996 5722 1000 2310 3490 2618 4748 2033 18168 5267 1000 1012 2279 2000 1996 2364 2311 2003 1996 13546 1997 1996 6730 2540 1012 3202 2369 1996 13546 2003 1996 24665 23052 1010 1037 14042 2173 1997 7083 1998 9185 1012 2009 2003 1037 15059 1997 1996 24665 23052 2012 10223 26371 1010 2605 2073 1996 6261 2984 22353 2135 2596 2000 3002 16595 9648 4674 2061 12083 9711 2271 1999 8517 1012 2012 1996 2203 1997 1996 2364 3298 1006 1998 1999 1037 3622 2240 2008 8539 2083 1017 11342 1998 1996 2751 8514 1007 1010 2003 1037 3722 1010 2715 2962 6231 1997 2984 1012 102 0 0 0 0 0 0 0 0 0 0 0 0 0 0 0 0 0 0 0 0 0 0 0 0 0 0 0 0 0 0 0 0 0 0 0 0 0 0 0 0 0 0 0 0 0 0 0 0 0 0 0 0 0 0 0 0 0 0 0 0 0 0 0 0 0 0 0 0 0 0 0 0 0 0 0 0 0 0 0 0 0 0 0 0 0 0 0 0 0 0 0 0 0 0 0 0 0 0 0 0 0 0 0 0 0 0 0 0 0 0 0 0 0 0 0 0 0 0 0 0 0 0 0 0 0 0 0 0 0 0 0 0 0 0 0 0 0 0 0 0 0 0 0 0 0 0 0 0 0 0 0 0 0 0 0 0 0 0 0 0 0 0 0 0 0 0 0 0 0 0 0 0 0 0 0 0 0 0 0 0 0 0 0 0 0 0 0 0 0 0 0 0 0 0 0 0 0 0 0 0 0 0 0 0 0 0 0 0 0 0 0 0 0 0\n",
- "INFO:tensorflow:input_mask: 1 1 1 1 1 1 1 1 1 1 1 1 1 1 1 1 1 1 1 1 1 1 1 1 1 1 1 1 1 1 1 1 1 1 1 1 1 1 1 1 1 1 1 1 1 1 1 1 1 1 1 1 1 1 1 1 1 1 1 1 1 1 1 1 1 1 1 1 1 1 1 1 1 1 1 1 1 1 1 1 1 1 1 1 1 1 1 1 1 1 1 1 1 1 1 1 1 1 1 1 1 1 1 1 1 1 1 1 1 1 1 1 1 1 1 1 1 1 1 1 1 1 1 1 1 1 1 1 1 1 1 1 1 1 1 1 1 1 1 1 1 1 1 1 1 1 1 1 1 1 1 1 1 1 1 1 1 1 1 1 1 1 1 1 1 1 1 1 1 1 0 0 0 0 0 0 0 0 0 0 0 0 0 0 0 0 0 0 0 0 0 0 0 0 0 0 0 0 0 0 0 0 0 0 0 0 0 0 0 0 0 0 0 0 0 0 0 0 0 0 0 0 0 0 0 0 0 0 0 0 0 0 0 0 0 0 0 0 0 0 0 0 0 0 0 0 0 0 0 0 0 0 0 0 0 0 0 0 0 0 0 0 0 0 0 0 0 0 0 0 0 0 0 0 0 0 0 0 0 0 0 0 0 0 0 0 0 0 0 0 0 0 0 0 0 0 0 0 0 0 0 0 0 0 0 0 0 0 0 0 0 0 0 0 0 0 0 0 0 0 0 0 0 0 0 0 0 0 0 0 0 0 0 0 0 0 0 0 0 0 0 0 0 0 0 0 0 0 0 0 0 0 0 0 0 0 0 0 0 0 0 0 0 0 0 0 0 0 0 0 0 0 0 0 0 0 0 0 0 0 0 0 0 0\n",
- "INFO:tensorflow:segment_ids: 0 0 0 0 0 0 0 0 0 0 0 1 1 1 1 1 1 1 1 1 1 1 1 1 1 1 1 1 1 1 1 1 1 1 1 1 1 1 1 1 1 1 1 1 1 1 1 1 1 1 1 1 1 1 1 1 1 1 1 1 1 1 1 1 1 1 1 1 1 1 1 1 1 1 1 1 1 1 1 1 1 1 1 1 1 1 1 1 1 1 1 1 1 1 1 1 1 1 1 1 1 1 1 1 1 1 1 1 1 1 1 1 1 1 1 1 1 1 1 1 1 1 1 1 1 1 1 1 1 1 1 1 1 1 1 1 1 1 1 1 1 1 1 1 1 1 1 1 1 1 1 1 1 1 1 1 1 1 1 1 1 1 1 1 1 1 1 1 1 1 0 0 0 0 0 0 0 0 0 0 0 0 0 0 0 0 0 0 0 0 0 0 0 0 0 0 0 0 0 0 0 0 0 0 0 0 0 0 0 0 0 0 0 0 0 0 0 0 0 0 0 0 0 0 0 0 0 0 0 0 0 0 0 0 0 0 0 0 0 0 0 0 0 0 0 0 0 0 0 0 0 0 0 0 0 0 0 0 0 0 0 0 0 0 0 0 0 0 0 0 0 0 0 0 0 0 0 0 0 0 0 0 0 0 0 0 0 0 0 0 0 0 0 0 0 0 0 0 0 0 0 0 0 0 0 0 0 0 0 0 0 0 0 0 0 0 0 0 0 0 0 0 0 0 0 0 0 0 0 0 0 0 0 0 0 0 0 0 0 0 0 0 0 0 0 0 0 0 0 0 0 0 0 0 0 0 0 0 0 0 0 0 0 0 0 0 0 0 0 0 0 0 0 0 0 0 0 0 0 0 0 0 0 0\n",
- "INFO:tensorflow:start_position: 95\n",
- "INFO:tensorflow:end_position: 101\n",
- "INFO:tensorflow:answer: a marian place of prayer and reflection\n",
- "INFO:tensorflow:*** Example ***\n"
- ]
- },
- {
- "name": "stdout",
- "output_type": "stream",
- "text": [
- "INFO:tensorflow:unique_id: 1000000004\n",
- "INFO:tensorflow:example_index: 4\n",
- "INFO:tensorflow:doc_span_index: 0\n",
- "INFO:tensorflow:tokens: [CLS] what sits on top of the main building at notre dame ? [SEP] architectural ##ly , the school has a catholic character . atop the main building ' s gold dome is a golden statue of the virgin mary . immediately in front of the main building and facing it , is a copper statue of christ with arms up ##rai ##sed with the legend \" ve ##ni ##te ad me om ##nes \" . next to the main building is the basilica of the sacred heart . immediately behind the basilica is the gr ##otto , a marian place of prayer and reflection . it is a replica of the gr ##otto at lou ##rdes , france where the virgin mary reputed ##ly appeared to saint bern ##ade ##tte so ##ub ##iro ##us in 1858 . at the end of the main drive ( and in a direct line that connects through 3 statues and the gold dome ) , is a simple , modern stone statue of mary . [SEP]\n",
- "INFO:tensorflow:token_to_orig_map: 14:0 15:0 16:0 17:1 18:2 19:3 20:4 21:5 22:6 23:6 24:7 25:8 26:9 27:10 28:10 29:10 30:11 31:12 32:13 33:14 34:15 35:16 36:17 37:18 38:19 39:20 40:20 41:21 42:22 43:23 44:24 45:25 46:26 47:27 48:28 49:29 50:30 51:30 52:31 53:32 54:33 55:34 56:35 57:36 58:37 59:38 60:39 61:39 62:39 63:40 64:41 65:42 66:43 67:43 68:43 69:43 70:44 71:45 72:46 73:46 74:46 75:46 76:47 77:48 78:49 79:50 80:51 81:52 82:53 83:54 84:55 85:56 86:57 87:58 88:58 89:59 90:60 91:61 92:62 93:63 94:64 95:65 96:65 97:65 98:66 99:67 100:68 101:69 102:70 103:71 104:72 105:72 106:73 107:74 108:75 109:76 110:77 111:78 112:79 113:79 114:80 115:81 116:81 117:81 118:82 119:83 120:84 121:85 122:86 123:87 124:87 125:88 126:89 127:90 128:91 129:91 130:91 131:92 132:92 133:92 134:92 135:93 136:94 137:94 138:95 139:96 140:97 141:98 142:99 143:100 144:101 145:102 146:102 147:103 148:104 149:105 150:106 151:107 152:108 153:109 154:110 155:111 156:112 157:113 158:114 159:115 160:115 161:115 162:116 163:117 164:118 165:118 166:119 167:120 168:121 169:122 170:123 171:123\n",
- "INFO:tensorflow:token_is_max_context: 14:True 15:True 16:True 17:True 18:True 19:True 20:True 21:True 22:True 23:True 24:True 25:True 26:True 27:True 28:True 29:True 30:True 31:True 32:True 33:True 34:True 35:True 36:True 37:True 38:True 39:True 40:True 41:True 42:True 43:True 44:True 45:True 46:True 47:True 48:True 49:True 50:True 51:True 52:True 53:True 54:True 55:True 56:True 57:True 58:True 59:True 60:True 61:True 62:True 63:True 64:True 65:True 66:True 67:True 68:True 69:True 70:True 71:True 72:True 73:True 74:True 75:True 76:True 77:True 78:True 79:True 80:True 81:True 82:True 83:True 84:True 85:True 86:True 87:True 88:True 89:True 90:True 91:True 92:True 93:True 94:True 95:True 96:True 97:True 98:True 99:True 100:True 101:True 102:True 103:True 104:True 105:True 106:True 107:True 108:True 109:True 110:True 111:True 112:True 113:True 114:True 115:True 116:True 117:True 118:True 119:True 120:True 121:True 122:True 123:True 124:True 125:True 126:True 127:True 128:True 129:True 130:True 131:True 132:True 133:True 134:True 135:True 136:True 137:True 138:True 139:True 140:True 141:True 142:True 143:True 144:True 145:True 146:True 147:True 148:True 149:True 150:True 151:True 152:True 153:True 154:True 155:True 156:True 157:True 158:True 159:True 160:True 161:True 162:True 163:True 164:True 165:True 166:True 167:True 168:True 169:True 170:True 171:True\n",
- "INFO:tensorflow:input_ids: 101 2054 7719 2006 2327 1997 1996 2364 2311 2012 10289 8214 1029 102 6549 2135 1010 1996 2082 2038 1037 3234 2839 1012 10234 1996 2364 2311 1005 1055 2751 8514 2003 1037 3585 6231 1997 1996 6261 2984 1012 3202 1999 2392 1997 1996 2364 2311 1998 5307 2009 1010 2003 1037 6967 6231 1997 4828 2007 2608 2039 14995 6924 2007 1996 5722 1000 2310 3490 2618 4748 2033 18168 5267 1000 1012 2279 2000 1996 2364 2311 2003 1996 13546 1997 1996 6730 2540 1012 3202 2369 1996 13546 2003 1996 24665 23052 1010 1037 14042 2173 1997 7083 1998 9185 1012 2009 2003 1037 15059 1997 1996 24665 23052 2012 10223 26371 1010 2605 2073 1996 6261 2984 22353 2135 2596 2000 3002 16595 9648 4674 2061 12083 9711 2271 1999 8517 1012 2012 1996 2203 1997 1996 2364 3298 1006 1998 1999 1037 3622 2240 2008 8539 2083 1017 11342 1998 1996 2751 8514 1007 1010 2003 1037 3722 1010 2715 2962 6231 1997 2984 1012 102 0 0 0 0 0 0 0 0 0 0 0 0 0 0 0 0 0 0 0 0 0 0 0 0 0 0 0 0 0 0 0 0 0 0 0 0 0 0 0 0 0 0 0 0 0 0 0 0 0 0 0 0 0 0 0 0 0 0 0 0 0 0 0 0 0 0 0 0 0 0 0 0 0 0 0 0 0 0 0 0 0 0 0 0 0 0 0 0 0 0 0 0 0 0 0 0 0 0 0 0 0 0 0 0 0 0 0 0 0 0 0 0 0 0 0 0 0 0 0 0 0 0 0 0 0 0 0 0 0 0 0 0 0 0 0 0 0 0 0 0 0 0 0 0 0 0 0 0 0 0 0 0 0 0 0 0 0 0 0 0 0 0 0 0 0 0 0 0 0 0 0 0 0 0 0 0 0 0 0 0 0 0 0 0 0 0 0 0 0 0 0 0 0 0 0 0 0 0 0 0 0 0 0 0 0 0 0 0 0 0 0\n",
- "INFO:tensorflow:input_mask: 1 1 1 1 1 1 1 1 1 1 1 1 1 1 1 1 1 1 1 1 1 1 1 1 1 1 1 1 1 1 1 1 1 1 1 1 1 1 1 1 1 1 1 1 1 1 1 1 1 1 1 1 1 1 1 1 1 1 1 1 1 1 1 1 1 1 1 1 1 1 1 1 1 1 1 1 1 1 1 1 1 1 1 1 1 1 1 1 1 1 1 1 1 1 1 1 1 1 1 1 1 1 1 1 1 1 1 1 1 1 1 1 1 1 1 1 1 1 1 1 1 1 1 1 1 1 1 1 1 1 1 1 1 1 1 1 1 1 1 1 1 1 1 1 1 1 1 1 1 1 1 1 1 1 1 1 1 1 1 1 1 1 1 1 1 1 1 1 1 1 1 1 1 0 0 0 0 0 0 0 0 0 0 0 0 0 0 0 0 0 0 0 0 0 0 0 0 0 0 0 0 0 0 0 0 0 0 0 0 0 0 0 0 0 0 0 0 0 0 0 0 0 0 0 0 0 0 0 0 0 0 0 0 0 0 0 0 0 0 0 0 0 0 0 0 0 0 0 0 0 0 0 0 0 0 0 0 0 0 0 0 0 0 0 0 0 0 0 0 0 0 0 0 0 0 0 0 0 0 0 0 0 0 0 0 0 0 0 0 0 0 0 0 0 0 0 0 0 0 0 0 0 0 0 0 0 0 0 0 0 0 0 0 0 0 0 0 0 0 0 0 0 0 0 0 0 0 0 0 0 0 0 0 0 0 0 0 0 0 0 0 0 0 0 0 0 0 0 0 0 0 0 0 0 0 0 0 0 0 0 0 0 0 0 0 0 0 0 0 0 0 0 0 0 0 0 0 0 0 0 0 0 0 0\n",
- "INFO:tensorflow:segment_ids: 0 0 0 0 0 0 0 0 0 0 0 0 0 0 1 1 1 1 1 1 1 1 1 1 1 1 1 1 1 1 1 1 1 1 1 1 1 1 1 1 1 1 1 1 1 1 1 1 1 1 1 1 1 1 1 1 1 1 1 1 1 1 1 1 1 1 1 1 1 1 1 1 1 1 1 1 1 1 1 1 1 1 1 1 1 1 1 1 1 1 1 1 1 1 1 1 1 1 1 1 1 1 1 1 1 1 1 1 1 1 1 1 1 1 1 1 1 1 1 1 1 1 1 1 1 1 1 1 1 1 1 1 1 1 1 1 1 1 1 1 1 1 1 1 1 1 1 1 1 1 1 1 1 1 1 1 1 1 1 1 1 1 1 1 1 1 1 1 1 1 1 1 1 0 0 0 0 0 0 0 0 0 0 0 0 0 0 0 0 0 0 0 0 0 0 0 0 0 0 0 0 0 0 0 0 0 0 0 0 0 0 0 0 0 0 0 0 0 0 0 0 0 0 0 0 0 0 0 0 0 0 0 0 0 0 0 0 0 0 0 0 0 0 0 0 0 0 0 0 0 0 0 0 0 0 0 0 0 0 0 0 0 0 0 0 0 0 0 0 0 0 0 0 0 0 0 0 0 0 0 0 0 0 0 0 0 0 0 0 0 0 0 0 0 0 0 0 0 0 0 0 0 0 0 0 0 0 0 0 0 0 0 0 0 0 0 0 0 0 0 0 0 0 0 0 0 0 0 0 0 0 0 0 0 0 0 0 0 0 0 0 0 0 0 0 0 0 0 0 0 0 0 0 0 0 0 0 0 0 0 0 0 0 0 0 0 0 0 0 0 0 0 0 0 0 0 0 0 0 0 0 0 0 0\n",
- "INFO:tensorflow:start_position: 33\n",
- "INFO:tensorflow:end_position: 39\n",
- "INFO:tensorflow:answer: a golden statue of the virgin mary\n",
- "INFO:tensorflow:*** Example ***\n",
- "INFO:tensorflow:unique_id: 1000000005\n",
- "INFO:tensorflow:example_index: 5\n",
- "INFO:tensorflow:doc_span_index: 0\n",
- "INFO:tensorflow:tokens: [CLS] when did the scholastic magazine of notre dame begin publishing ? [SEP] as at most other universities , notre dame ' s students run a number of news media outlets . the nine student - run outlets include three newspapers , both a radio and television station , and several magazines and journals . begun as a one - page journal in september 1876 , the scholastic magazine is issued twice monthly and claims to be the oldest continuous collegiate publication in the united states . the other magazine , the jug ##gler , is released twice a year and focuses on student literature and artwork . the dome yearbook is published annually . the newspapers have varying publication interests , with the observer published daily and mainly reporting university and other news , and staffed by students from both notre dame and saint mary ' s college . unlike scholastic and the dome , the observer is an independent publication and does not have a faculty advisor or any editorial oversight from the university . in 1987 , when some students believed that the observer began to show a conservative bias , a liberal newspaper , common sense was published . likewise , in 2003 , when other students believed that the paper showed a liberal bias , the conservative paper irish rover went into production . neither paper is published as often as the observer ; however , all three are distributed to all students . finally , in spring 2008 an undergraduate journal for political science research , beyond politics , made its debut . [SEP]\n",
- "INFO:tensorflow:token_to_orig_map: 13:0 14:1 15:2 16:3 17:4 18:4 19:5 20:6 21:6 22:6 23:7 24:8 25:9 26:10 27:11 28:12 29:13 30:14 31:14 32:15 33:16 34:17 35:17 36:17 37:18 38:19 39:20 40:21 41:21 42:22 43:23 44:24 45:25 46:26 47:27 48:27 49:28 50:29 51:30 52:31 53:32 54:32 55:33 56:34 57:35 58:36 59:36 60:36 61:37 62:38 63:39 64:40 65:40 66:41 67:42 68:43 69:44 70:45 71:46 72:47 73:48 74:49 75:50 76:51 77:52 78:53 79:54 80:55 81:56 82:57 83:58 84:59 85:60 86:60 87:61 88:62 89:63 90:63 91:64 92:65 93:65 94:65 95:66 96:67 97:68 98:69 99:70 100:71 101:72 102:73 103:74 104:75 105:76 106:77 107:77 108:78 109:79 110:80 111:81 112:82 113:83 114:83 115:84 116:85 117:86 118:87 119:88 120:89 121:89 122:90 123:91 124:92 125:93 126:94 127:95 128:96 129:97 130:98 131:99 132:100 133:101 134:101 135:102 136:103 137:104 138:105 139:106 140:107 141:108 142:109 143:110 144:111 145:112 146:112 147:112 148:113 149:113 150:114 151:115 152:116 153:117 154:118 155:118 156:119 157:120 158:121 159:122 160:123 161:124 162:125 163:126 164:127 165:128 166:129 167:130 168:131 169:132 170:133 171:134 172:135 173:136 174:137 175:138 176:138 177:139 178:140 179:140 180:141 181:142 182:143 183:144 184:145 185:146 186:147 187:148 188:149 189:150 190:151 191:152 192:153 193:153 194:154 195:155 196:156 197:156 198:157 199:158 200:159 201:160 202:160 203:161 204:161 205:162 206:163 207:163 208:164 209:165 210:166 211:167 212:168 213:169 214:170 215:171 216:172 217:173 218:174 219:174 220:175 221:176 222:177 223:178 224:179 225:180 226:181 227:182 228:182 229:183 230:184 231:185 232:186 233:187 234:188 235:189 236:190 237:191 238:191 239:192 240:192 241:193 242:194 243:195 244:196 245:197 246:198 247:199 248:199 249:200 250:200 251:201 252:202 253:203 254:204 255:205 256:206 257:207 258:208 259:209 260:210 261:210 262:211 263:212 264:212 265:213 266:214 267:215 268:215\n"
- ]
- },
- {
- "name": "stdout",
- "output_type": "stream",
- "text": [
- "INFO:tensorflow:token_is_max_context: 13:True 14:True 15:True 16:True 17:True 18:True 19:True 20:True 21:True 22:True 23:True 24:True 25:True 26:True 27:True 28:True 29:True 30:True 31:True 32:True 33:True 34:True 35:True 36:True 37:True 38:True 39:True 40:True 41:True 42:True 43:True 44:True 45:True 46:True 47:True 48:True 49:True 50:True 51:True 52:True 53:True 54:True 55:True 56:True 57:True 58:True 59:True 60:True 61:True 62:True 63:True 64:True 65:True 66:True 67:True 68:True 69:True 70:True 71:True 72:True 73:True 74:True 75:True 76:True 77:True 78:True 79:True 80:True 81:True 82:True 83:True 84:True 85:True 86:True 87:True 88:True 89:True 90:True 91:True 92:True 93:True 94:True 95:True 96:True 97:True 98:True 99:True 100:True 101:True 102:True 103:True 104:True 105:True 106:True 107:True 108:True 109:True 110:True 111:True 112:True 113:True 114:True 115:True 116:True 117:True 118:True 119:True 120:True 121:True 122:True 123:True 124:True 125:True 126:True 127:True 128:True 129:True 130:True 131:True 132:True 133:True 134:True 135:True 136:True 137:True 138:True 139:True 140:True 141:True 142:True 143:True 144:True 145:True 146:True 147:True 148:True 149:True 150:True 151:True 152:True 153:True 154:True 155:True 156:True 157:True 158:True 159:True 160:True 161:True 162:True 163:True 164:True 165:True 166:True 167:True 168:True 169:True 170:True 171:True 172:True 173:True 174:True 175:True 176:True 177:True 178:True 179:True 180:True 181:True 182:True 183:True 184:True 185:True 186:True 187:True 188:True 189:True 190:True 191:True 192:True 193:True 194:True 195:True 196:True 197:True 198:True 199:True 200:True 201:True 202:True 203:True 204:True 205:True 206:True 207:True 208:True 209:True 210:True 211:True 212:True 213:True 214:True 215:True 216:True 217:True 218:True 219:True 220:True 221:True 222:True 223:True 224:True 225:True 226:True 227:True 228:True 229:True 230:True 231:True 232:True 233:True 234:True 235:True 236:True 237:True 238:True 239:True 240:True 241:True 242:True 243:True 244:True 245:True 246:True 247:True 248:True 249:True 250:True 251:True 252:True 253:True 254:True 255:True 256:True 257:True 258:True 259:True 260:True 261:True 262:True 263:True 264:True 265:True 266:True 267:True 268:True\n",
- "INFO:tensorflow:input_ids: 101 2043 2106 1996 24105 2932 1997 10289 8214 4088 4640 1029 102 2004 2012 2087 2060 5534 1010 10289 8214 1005 1055 2493 2448 1037 2193 1997 2739 2865 11730 1012 1996 3157 3076 1011 2448 11730 2421 2093 6399 1010 2119 1037 2557 1998 2547 2276 1010 1998 2195 7298 1998 9263 1012 5625 2004 1037 2028 1011 3931 3485 1999 2244 7326 1010 1996 24105 2932 2003 3843 3807 7058 1998 4447 2000 2022 1996 4587 7142 9234 4772 1999 1996 2142 2163 1012 1996 2060 2932 1010 1996 26536 17420 1010 2003 2207 3807 1037 2095 1998 7679 2006 3076 3906 1998 8266 1012 1996 8514 24803 2003 2405 6604 1012 1996 6399 2031 9671 4772 5426 1010 2007 1996 9718 2405 3679 1998 3701 7316 2118 1998 2060 2739 1010 1998 21121 2011 2493 2013 2119 10289 8214 1998 3002 2984 1005 1055 2267 1012 4406 24105 1998 1996 8514 1010 1996 9718 2003 2019 2981 4772 1998 2515 2025 2031 1037 4513 8619 2030 2151 8368 15709 2013 1996 2118 1012 1999 3055 1010 2043 2070 2493 3373 2008 1996 9718 2211 2000 2265 1037 4603 13827 1010 1037 4314 3780 1010 2691 3168 2001 2405 1012 10655 1010 1999 2494 1010 2043 2060 2493 3373 2008 1996 3259 3662 1037 4314 13827 1010 1996 4603 3259 3493 13631 2253 2046 2537 1012 4445 3259 2003 2405 2004 2411 2004 1996 9718 1025 2174 1010 2035 2093 2024 5500 2000 2035 2493 1012 2633 1010 1999 3500 2263 2019 8324 3485 2005 2576 2671 2470 1010 3458 4331 1010 2081 2049 2834 1012 102 0 0 0 0 0 0 0 0 0 0 0 0 0 0 0 0 0 0 0 0 0 0 0 0 0 0 0 0 0 0 0 0 0 0 0 0 0 0 0 0 0 0 0 0 0 0 0 0 0 0 0 0 0 0 0 0 0 0 0 0 0 0 0 0 0 0 0 0 0 0 0 0 0 0 0 0 0 0 0 0 0 0 0 0 0 0 0 0 0 0 0 0 0 0 0 0 0 0 0 0 0 0 0 0 0 0 0 0 0 0 0 0 0 0\n",
- "INFO:tensorflow:input_mask: 1 1 1 1 1 1 1 1 1 1 1 1 1 1 1 1 1 1 1 1 1 1 1 1 1 1 1 1 1 1 1 1 1 1 1 1 1 1 1 1 1 1 1 1 1 1 1 1 1 1 1 1 1 1 1 1 1 1 1 1 1 1 1 1 1 1 1 1 1 1 1 1 1 1 1 1 1 1 1 1 1 1 1 1 1 1 1 1 1 1 1 1 1 1 1 1 1 1 1 1 1 1 1 1 1 1 1 1 1 1 1 1 1 1 1 1 1 1 1 1 1 1 1 1 1 1 1 1 1 1 1 1 1 1 1 1 1 1 1 1 1 1 1 1 1 1 1 1 1 1 1 1 1 1 1 1 1 1 1 1 1 1 1 1 1 1 1 1 1 1 1 1 1 1 1 1 1 1 1 1 1 1 1 1 1 1 1 1 1 1 1 1 1 1 1 1 1 1 1 1 1 1 1 1 1 1 1 1 1 1 1 1 1 1 1 1 1 1 1 1 1 1 1 1 1 1 1 1 1 1 1 1 1 1 1 1 1 1 1 1 1 1 1 1 1 1 1 1 1 1 1 1 1 1 1 1 1 1 1 1 1 1 1 1 1 1 1 1 1 1 0 0 0 0 0 0 0 0 0 0 0 0 0 0 0 0 0 0 0 0 0 0 0 0 0 0 0 0 0 0 0 0 0 0 0 0 0 0 0 0 0 0 0 0 0 0 0 0 0 0 0 0 0 0 0 0 0 0 0 0 0 0 0 0 0 0 0 0 0 0 0 0 0 0 0 0 0 0 0 0 0 0 0 0 0 0 0 0 0 0 0 0 0 0 0 0 0 0 0 0 0 0 0 0 0 0 0 0 0 0 0 0 0 0\n",
- "INFO:tensorflow:segment_ids: 0 0 0 0 0 0 0 0 0 0 0 0 0 1 1 1 1 1 1 1 1 1 1 1 1 1 1 1 1 1 1 1 1 1 1 1 1 1 1 1 1 1 1 1 1 1 1 1 1 1 1 1 1 1 1 1 1 1 1 1 1 1 1 1 1 1 1 1 1 1 1 1 1 1 1 1 1 1 1 1 1 1 1 1 1 1 1 1 1 1 1 1 1 1 1 1 1 1 1 1 1 1 1 1 1 1 1 1 1 1 1 1 1 1 1 1 1 1 1 1 1 1 1 1 1 1 1 1 1 1 1 1 1 1 1 1 1 1 1 1 1 1 1 1 1 1 1 1 1 1 1 1 1 1 1 1 1 1 1 1 1 1 1 1 1 1 1 1 1 1 1 1 1 1 1 1 1 1 1 1 1 1 1 1 1 1 1 1 1 1 1 1 1 1 1 1 1 1 1 1 1 1 1 1 1 1 1 1 1 1 1 1 1 1 1 1 1 1 1 1 1 1 1 1 1 1 1 1 1 1 1 1 1 1 1 1 1 1 1 1 1 1 1 1 1 1 1 1 1 1 1 1 1 1 1 1 1 1 1 1 1 1 1 1 1 1 1 1 1 1 0 0 0 0 0 0 0 0 0 0 0 0 0 0 0 0 0 0 0 0 0 0 0 0 0 0 0 0 0 0 0 0 0 0 0 0 0 0 0 0 0 0 0 0 0 0 0 0 0 0 0 0 0 0 0 0 0 0 0 0 0 0 0 0 0 0 0 0 0 0 0 0 0 0 0 0 0 0 0 0 0 0 0 0 0 0 0 0 0 0 0 0 0 0 0 0 0 0 0 0 0 0 0 0 0 0 0 0 0 0 0 0 0 0\n",
- "INFO:tensorflow:start_position: 63\n",
- "INFO:tensorflow:end_position: 64\n",
- "INFO:tensorflow:answer: september 1876\n",
- "INFO:tensorflow:*** Example ***\n",
- "INFO:tensorflow:unique_id: 1000000006\n",
- "INFO:tensorflow:example_index: 6\n",
- "INFO:tensorflow:doc_span_index: 0\n",
- "INFO:tensorflow:tokens: [CLS] how often is notre dame ' s the jug ##gler published ? [SEP] as at most other universities , notre dame ' s students run a number of news media outlets . the nine student - run outlets include three newspapers , both a radio and television station , and several magazines and journals . begun as a one - page journal in september 1876 , the scholastic magazine is issued twice monthly and claims to be the oldest continuous collegiate publication in the united states . the other magazine , the jug ##gler , is released twice a year and focuses on student literature and artwork . the dome yearbook is published annually . the newspapers have varying publication interests , with the observer published daily and mainly reporting university and other news , and staffed by students from both notre dame and saint mary ' s college . unlike scholastic and the dome , the observer is an independent publication and does not have a faculty advisor or any editorial oversight from the university . in 1987 , when some students believed that the observer began to show a conservative bias , a liberal newspaper , common sense was published . likewise , in 2003 , when other students believed that the paper showed a liberal bias , the conservative paper irish rover went into production . neither paper is published as often as the observer ; however , all three are distributed to all students . finally , in spring 2008 an undergraduate journal for political science research , beyond politics , made its debut . [SEP]\n",
- "INFO:tensorflow:token_to_orig_map: 14:0 15:1 16:2 17:3 18:4 19:4 20:5 21:6 22:6 23:6 24:7 25:8 26:9 27:10 28:11 29:12 30:13 31:14 32:14 33:15 34:16 35:17 36:17 37:17 38:18 39:19 40:20 41:21 42:21 43:22 44:23 45:24 46:25 47:26 48:27 49:27 50:28 51:29 52:30 53:31 54:32 55:32 56:33 57:34 58:35 59:36 60:36 61:36 62:37 63:38 64:39 65:40 66:40 67:41 68:42 69:43 70:44 71:45 72:46 73:47 74:48 75:49 76:50 77:51 78:52 79:53 80:54 81:55 82:56 83:57 84:58 85:59 86:60 87:60 88:61 89:62 90:63 91:63 92:64 93:65 94:65 95:65 96:66 97:67 98:68 99:69 100:70 101:71 102:72 103:73 104:74 105:75 106:76 107:77 108:77 109:78 110:79 111:80 112:81 113:82 114:83 115:83 116:84 117:85 118:86 119:87 120:88 121:89 122:89 123:90 124:91 125:92 126:93 127:94 128:95 129:96 130:97 131:98 132:99 133:100 134:101 135:101 136:102 137:103 138:104 139:105 140:106 141:107 142:108 143:109 144:110 145:111 146:112 147:112 148:112 149:113 150:113 151:114 152:115 153:116 154:117 155:118 156:118 157:119 158:120 159:121 160:122 161:123 162:124 163:125 164:126 165:127 166:128 167:129 168:130 169:131 170:132 171:133 172:134 173:135 174:136 175:137 176:138 177:138 178:139 179:140 180:140 181:141 182:142 183:143 184:144 185:145 186:146 187:147 188:148 189:149 190:150 191:151 192:152 193:153 194:153 195:154 196:155 197:156 198:156 199:157 200:158 201:159 202:160 203:160 204:161 205:161 206:162 207:163 208:163 209:164 210:165 211:166 212:167 213:168 214:169 215:170 216:171 217:172 218:173 219:174 220:174 221:175 222:176 223:177 224:178 225:179 226:180 227:181 228:182 229:182 230:183 231:184 232:185 233:186 234:187 235:188 236:189 237:190 238:191 239:191 240:192 241:192 242:193 243:194 244:195 245:196 246:197 247:198 248:199 249:199 250:200 251:200 252:201 253:202 254:203 255:204 256:205 257:206 258:207 259:208 260:209 261:210 262:210 263:211 264:212 265:212 266:213 267:214 268:215 269:215\n"
- ]
- },
- {
- "name": "stdout",
- "output_type": "stream",
- "text": [
- "INFO:tensorflow:token_is_max_context: 14:True 15:True 16:True 17:True 18:True 19:True 20:True 21:True 22:True 23:True 24:True 25:True 26:True 27:True 28:True 29:True 30:True 31:True 32:True 33:True 34:True 35:True 36:True 37:True 38:True 39:True 40:True 41:True 42:True 43:True 44:True 45:True 46:True 47:True 48:True 49:True 50:True 51:True 52:True 53:True 54:True 55:True 56:True 57:True 58:True 59:True 60:True 61:True 62:True 63:True 64:True 65:True 66:True 67:True 68:True 69:True 70:True 71:True 72:True 73:True 74:True 75:True 76:True 77:True 78:True 79:True 80:True 81:True 82:True 83:True 84:True 85:True 86:True 87:True 88:True 89:True 90:True 91:True 92:True 93:True 94:True 95:True 96:True 97:True 98:True 99:True 100:True 101:True 102:True 103:True 104:True 105:True 106:True 107:True 108:True 109:True 110:True 111:True 112:True 113:True 114:True 115:True 116:True 117:True 118:True 119:True 120:True 121:True 122:True 123:True 124:True 125:True 126:True 127:True 128:True 129:True 130:True 131:True 132:True 133:True 134:True 135:True 136:True 137:True 138:True 139:True 140:True 141:True 142:True 143:True 144:True 145:True 146:True 147:True 148:True 149:True 150:True 151:True 152:True 153:True 154:True 155:True 156:True 157:True 158:True 159:True 160:True 161:True 162:True 163:True 164:True 165:True 166:True 167:True 168:True 169:True 170:True 171:True 172:True 173:True 174:True 175:True 176:True 177:True 178:True 179:True 180:True 181:True 182:True 183:True 184:True 185:True 186:True 187:True 188:True 189:True 190:True 191:True 192:True 193:True 194:True 195:True 196:True 197:True 198:True 199:True 200:True 201:True 202:True 203:True 204:True 205:True 206:True 207:True 208:True 209:True 210:True 211:True 212:True 213:True 214:True 215:True 216:True 217:True 218:True 219:True 220:True 221:True 222:True 223:True 224:True 225:True 226:True 227:True 228:True 229:True 230:True 231:True 232:True 233:True 234:True 235:True 236:True 237:True 238:True 239:True 240:True 241:True 242:True 243:True 244:True 245:True 246:True 247:True 248:True 249:True 250:True 251:True 252:True 253:True 254:True 255:True 256:True 257:True 258:True 259:True 260:True 261:True 262:True 263:True 264:True 265:True 266:True 267:True 268:True 269:True\n",
- "INFO:tensorflow:input_ids: 101 2129 2411 2003 10289 8214 1005 1055 1996 26536 17420 2405 1029 102 2004 2012 2087 2060 5534 1010 10289 8214 1005 1055 2493 2448 1037 2193 1997 2739 2865 11730 1012 1996 3157 3076 1011 2448 11730 2421 2093 6399 1010 2119 1037 2557 1998 2547 2276 1010 1998 2195 7298 1998 9263 1012 5625 2004 1037 2028 1011 3931 3485 1999 2244 7326 1010 1996 24105 2932 2003 3843 3807 7058 1998 4447 2000 2022 1996 4587 7142 9234 4772 1999 1996 2142 2163 1012 1996 2060 2932 1010 1996 26536 17420 1010 2003 2207 3807 1037 2095 1998 7679 2006 3076 3906 1998 8266 1012 1996 8514 24803 2003 2405 6604 1012 1996 6399 2031 9671 4772 5426 1010 2007 1996 9718 2405 3679 1998 3701 7316 2118 1998 2060 2739 1010 1998 21121 2011 2493 2013 2119 10289 8214 1998 3002 2984 1005 1055 2267 1012 4406 24105 1998 1996 8514 1010 1996 9718 2003 2019 2981 4772 1998 2515 2025 2031 1037 4513 8619 2030 2151 8368 15709 2013 1996 2118 1012 1999 3055 1010 2043 2070 2493 3373 2008 1996 9718 2211 2000 2265 1037 4603 13827 1010 1037 4314 3780 1010 2691 3168 2001 2405 1012 10655 1010 1999 2494 1010 2043 2060 2493 3373 2008 1996 3259 3662 1037 4314 13827 1010 1996 4603 3259 3493 13631 2253 2046 2537 1012 4445 3259 2003 2405 2004 2411 2004 1996 9718 1025 2174 1010 2035 2093 2024 5500 2000 2035 2493 1012 2633 1010 1999 3500 2263 2019 8324 3485 2005 2576 2671 2470 1010 3458 4331 1010 2081 2049 2834 1012 102 0 0 0 0 0 0 0 0 0 0 0 0 0 0 0 0 0 0 0 0 0 0 0 0 0 0 0 0 0 0 0 0 0 0 0 0 0 0 0 0 0 0 0 0 0 0 0 0 0 0 0 0 0 0 0 0 0 0 0 0 0 0 0 0 0 0 0 0 0 0 0 0 0 0 0 0 0 0 0 0 0 0 0 0 0 0 0 0 0 0 0 0 0 0 0 0 0 0 0 0 0 0 0 0 0 0 0 0 0 0 0 0 0\n",
- "INFO:tensorflow:input_mask: 1 1 1 1 1 1 1 1 1 1 1 1 1 1 1 1 1 1 1 1 1 1 1 1 1 1 1 1 1 1 1 1 1 1 1 1 1 1 1 1 1 1 1 1 1 1 1 1 1 1 1 1 1 1 1 1 1 1 1 1 1 1 1 1 1 1 1 1 1 1 1 1 1 1 1 1 1 1 1 1 1 1 1 1 1 1 1 1 1 1 1 1 1 1 1 1 1 1 1 1 1 1 1 1 1 1 1 1 1 1 1 1 1 1 1 1 1 1 1 1 1 1 1 1 1 1 1 1 1 1 1 1 1 1 1 1 1 1 1 1 1 1 1 1 1 1 1 1 1 1 1 1 1 1 1 1 1 1 1 1 1 1 1 1 1 1 1 1 1 1 1 1 1 1 1 1 1 1 1 1 1 1 1 1 1 1 1 1 1 1 1 1 1 1 1 1 1 1 1 1 1 1 1 1 1 1 1 1 1 1 1 1 1 1 1 1 1 1 1 1 1 1 1 1 1 1 1 1 1 1 1 1 1 1 1 1 1 1 1 1 1 1 1 1 1 1 1 1 1 1 1 1 1 1 1 1 1 1 1 1 1 1 1 1 1 1 1 1 1 1 1 0 0 0 0 0 0 0 0 0 0 0 0 0 0 0 0 0 0 0 0 0 0 0 0 0 0 0 0 0 0 0 0 0 0 0 0 0 0 0 0 0 0 0 0 0 0 0 0 0 0 0 0 0 0 0 0 0 0 0 0 0 0 0 0 0 0 0 0 0 0 0 0 0 0 0 0 0 0 0 0 0 0 0 0 0 0 0 0 0 0 0 0 0 0 0 0 0 0 0 0 0 0 0 0 0 0 0 0 0 0 0 0 0\n",
- "INFO:tensorflow:segment_ids: 0 0 0 0 0 0 0 0 0 0 0 0 0 0 1 1 1 1 1 1 1 1 1 1 1 1 1 1 1 1 1 1 1 1 1 1 1 1 1 1 1 1 1 1 1 1 1 1 1 1 1 1 1 1 1 1 1 1 1 1 1 1 1 1 1 1 1 1 1 1 1 1 1 1 1 1 1 1 1 1 1 1 1 1 1 1 1 1 1 1 1 1 1 1 1 1 1 1 1 1 1 1 1 1 1 1 1 1 1 1 1 1 1 1 1 1 1 1 1 1 1 1 1 1 1 1 1 1 1 1 1 1 1 1 1 1 1 1 1 1 1 1 1 1 1 1 1 1 1 1 1 1 1 1 1 1 1 1 1 1 1 1 1 1 1 1 1 1 1 1 1 1 1 1 1 1 1 1 1 1 1 1 1 1 1 1 1 1 1 1 1 1 1 1 1 1 1 1 1 1 1 1 1 1 1 1 1 1 1 1 1 1 1 1 1 1 1 1 1 1 1 1 1 1 1 1 1 1 1 1 1 1 1 1 1 1 1 1 1 1 1 1 1 1 1 1 1 1 1 1 1 1 1 1 1 1 1 1 1 1 1 1 1 1 1 1 1 1 1 1 1 0 0 0 0 0 0 0 0 0 0 0 0 0 0 0 0 0 0 0 0 0 0 0 0 0 0 0 0 0 0 0 0 0 0 0 0 0 0 0 0 0 0 0 0 0 0 0 0 0 0 0 0 0 0 0 0 0 0 0 0 0 0 0 0 0 0 0 0 0 0 0 0 0 0 0 0 0 0 0 0 0 0 0 0 0 0 0 0 0 0 0 0 0 0 0 0 0 0 0 0 0 0 0 0 0 0 0 0 0 0 0 0 0\n",
- "INFO:tensorflow:start_position: 98\n",
- "INFO:tensorflow:end_position: 98\n",
- "INFO:tensorflow:answer: twice\n",
- "INFO:tensorflow:*** Example ***\n",
- "INFO:tensorflow:unique_id: 1000000007\n",
- "INFO:tensorflow:example_index: 7\n",
- "INFO:tensorflow:doc_span_index: 0\n",
- "INFO:tensorflow:tokens: [CLS] what is the daily student paper at notre dame called ? [SEP] as at most other universities , notre dame ' s students run a number of news media outlets . the nine student - run outlets include three newspapers , both a radio and television station , and several magazines and journals . begun as a one - page journal in september 1876 , the scholastic magazine is issued twice monthly and claims to be the oldest continuous collegiate publication in the united states . the other magazine , the jug ##gler , is released twice a year and focuses on student literature and artwork . the dome yearbook is published annually . the newspapers have varying publication interests , with the observer published daily and mainly reporting university and other news , and staffed by students from both notre dame and saint mary ' s college . unlike scholastic and the dome , the observer is an independent publication and does not have a faculty advisor or any editorial oversight from the university . in 1987 , when some students believed that the observer began to show a conservative bias , a liberal newspaper , common sense was published . likewise , in 2003 , when other students believed that the paper showed a liberal bias , the conservative paper irish rover went into production . neither paper is published as often as the observer ; however , all three are distributed to all students . finally , in spring 2008 an undergraduate journal for political science research , beyond politics , made its debut . [SEP]\n",
- "INFO:tensorflow:token_to_orig_map: 13:0 14:1 15:2 16:3 17:4 18:4 19:5 20:6 21:6 22:6 23:7 24:8 25:9 26:10 27:11 28:12 29:13 30:14 31:14 32:15 33:16 34:17 35:17 36:17 37:18 38:19 39:20 40:21 41:21 42:22 43:23 44:24 45:25 46:26 47:27 48:27 49:28 50:29 51:30 52:31 53:32 54:32 55:33 56:34 57:35 58:36 59:36 60:36 61:37 62:38 63:39 64:40 65:40 66:41 67:42 68:43 69:44 70:45 71:46 72:47 73:48 74:49 75:50 76:51 77:52 78:53 79:54 80:55 81:56 82:57 83:58 84:59 85:60 86:60 87:61 88:62 89:63 90:63 91:64 92:65 93:65 94:65 95:66 96:67 97:68 98:69 99:70 100:71 101:72 102:73 103:74 104:75 105:76 106:77 107:77 108:78 109:79 110:80 111:81 112:82 113:83 114:83 115:84 116:85 117:86 118:87 119:88 120:89 121:89 122:90 123:91 124:92 125:93 126:94 127:95 128:96 129:97 130:98 131:99 132:100 133:101 134:101 135:102 136:103 137:104 138:105 139:106 140:107 141:108 142:109 143:110 144:111 145:112 146:112 147:112 148:113 149:113 150:114 151:115 152:116 153:117 154:118 155:118 156:119 157:120 158:121 159:122 160:123 161:124 162:125 163:126 164:127 165:128 166:129 167:130 168:131 169:132 170:133 171:134 172:135 173:136 174:137 175:138 176:138 177:139 178:140 179:140 180:141 181:142 182:143 183:144 184:145 185:146 186:147 187:148 188:149 189:150 190:151 191:152 192:153 193:153 194:154 195:155 196:156 197:156 198:157 199:158 200:159 201:160 202:160 203:161 204:161 205:162 206:163 207:163 208:164 209:165 210:166 211:167 212:168 213:169 214:170 215:171 216:172 217:173 218:174 219:174 220:175 221:176 222:177 223:178 224:179 225:180 226:181 227:182 228:182 229:183 230:184 231:185 232:186 233:187 234:188 235:189 236:190 237:191 238:191 239:192 240:192 241:193 242:194 243:195 244:196 245:197 246:198 247:199 248:199 249:200 250:200 251:201 252:202 253:203 254:204 255:205 256:206 257:207 258:208 259:209 260:210 261:210 262:211 263:212 264:212 265:213 266:214 267:215 268:215\n"
- ]
- },
- {
- "name": "stdout",
- "output_type": "stream",
- "text": [
- "INFO:tensorflow:token_is_max_context: 13:True 14:True 15:True 16:True 17:True 18:True 19:True 20:True 21:True 22:True 23:True 24:True 25:True 26:True 27:True 28:True 29:True 30:True 31:True 32:True 33:True 34:True 35:True 36:True 37:True 38:True 39:True 40:True 41:True 42:True 43:True 44:True 45:True 46:True 47:True 48:True 49:True 50:True 51:True 52:True 53:True 54:True 55:True 56:True 57:True 58:True 59:True 60:True 61:True 62:True 63:True 64:True 65:True 66:True 67:True 68:True 69:True 70:True 71:True 72:True 73:True 74:True 75:True 76:True 77:True 78:True 79:True 80:True 81:True 82:True 83:True 84:True 85:True 86:True 87:True 88:True 89:True 90:True 91:True 92:True 93:True 94:True 95:True 96:True 97:True 98:True 99:True 100:True 101:True 102:True 103:True 104:True 105:True 106:True 107:True 108:True 109:True 110:True 111:True 112:True 113:True 114:True 115:True 116:True 117:True 118:True 119:True 120:True 121:True 122:True 123:True 124:True 125:True 126:True 127:True 128:True 129:True 130:True 131:True 132:True 133:True 134:True 135:True 136:True 137:True 138:True 139:True 140:True 141:True 142:True 143:True 144:True 145:True 146:True 147:True 148:True 149:True 150:True 151:True 152:True 153:True 154:True 155:True 156:True 157:True 158:True 159:True 160:True 161:True 162:True 163:True 164:True 165:True 166:True 167:True 168:True 169:True 170:True 171:True 172:True 173:True 174:True 175:True 176:True 177:True 178:True 179:True 180:True 181:True 182:True 183:True 184:True 185:True 186:True 187:True 188:True 189:True 190:True 191:True 192:True 193:True 194:True 195:True 196:True 197:True 198:True 199:True 200:True 201:True 202:True 203:True 204:True 205:True 206:True 207:True 208:True 209:True 210:True 211:True 212:True 213:True 214:True 215:True 216:True 217:True 218:True 219:True 220:True 221:True 222:True 223:True 224:True 225:True 226:True 227:True 228:True 229:True 230:True 231:True 232:True 233:True 234:True 235:True 236:True 237:True 238:True 239:True 240:True 241:True 242:True 243:True 244:True 245:True 246:True 247:True 248:True 249:True 250:True 251:True 252:True 253:True 254:True 255:True 256:True 257:True 258:True 259:True 260:True 261:True 262:True 263:True 264:True 265:True 266:True 267:True 268:True\n",
- "INFO:tensorflow:input_ids: 101 2054 2003 1996 3679 3076 3259 2012 10289 8214 2170 1029 102 2004 2012 2087 2060 5534 1010 10289 8214 1005 1055 2493 2448 1037 2193 1997 2739 2865 11730 1012 1996 3157 3076 1011 2448 11730 2421 2093 6399 1010 2119 1037 2557 1998 2547 2276 1010 1998 2195 7298 1998 9263 1012 5625 2004 1037 2028 1011 3931 3485 1999 2244 7326 1010 1996 24105 2932 2003 3843 3807 7058 1998 4447 2000 2022 1996 4587 7142 9234 4772 1999 1996 2142 2163 1012 1996 2060 2932 1010 1996 26536 17420 1010 2003 2207 3807 1037 2095 1998 7679 2006 3076 3906 1998 8266 1012 1996 8514 24803 2003 2405 6604 1012 1996 6399 2031 9671 4772 5426 1010 2007 1996 9718 2405 3679 1998 3701 7316 2118 1998 2060 2739 1010 1998 21121 2011 2493 2013 2119 10289 8214 1998 3002 2984 1005 1055 2267 1012 4406 24105 1998 1996 8514 1010 1996 9718 2003 2019 2981 4772 1998 2515 2025 2031 1037 4513 8619 2030 2151 8368 15709 2013 1996 2118 1012 1999 3055 1010 2043 2070 2493 3373 2008 1996 9718 2211 2000 2265 1037 4603 13827 1010 1037 4314 3780 1010 2691 3168 2001 2405 1012 10655 1010 1999 2494 1010 2043 2060 2493 3373 2008 1996 3259 3662 1037 4314 13827 1010 1996 4603 3259 3493 13631 2253 2046 2537 1012 4445 3259 2003 2405 2004 2411 2004 1996 9718 1025 2174 1010 2035 2093 2024 5500 2000 2035 2493 1012 2633 1010 1999 3500 2263 2019 8324 3485 2005 2576 2671 2470 1010 3458 4331 1010 2081 2049 2834 1012 102 0 0 0 0 0 0 0 0 0 0 0 0 0 0 0 0 0 0 0 0 0 0 0 0 0 0 0 0 0 0 0 0 0 0 0 0 0 0 0 0 0 0 0 0 0 0 0 0 0 0 0 0 0 0 0 0 0 0 0 0 0 0 0 0 0 0 0 0 0 0 0 0 0 0 0 0 0 0 0 0 0 0 0 0 0 0 0 0 0 0 0 0 0 0 0 0 0 0 0 0 0 0 0 0 0 0 0 0 0 0 0 0 0 0\n",
- "INFO:tensorflow:input_mask: 1 1 1 1 1 1 1 1 1 1 1 1 1 1 1 1 1 1 1 1 1 1 1 1 1 1 1 1 1 1 1 1 1 1 1 1 1 1 1 1 1 1 1 1 1 1 1 1 1 1 1 1 1 1 1 1 1 1 1 1 1 1 1 1 1 1 1 1 1 1 1 1 1 1 1 1 1 1 1 1 1 1 1 1 1 1 1 1 1 1 1 1 1 1 1 1 1 1 1 1 1 1 1 1 1 1 1 1 1 1 1 1 1 1 1 1 1 1 1 1 1 1 1 1 1 1 1 1 1 1 1 1 1 1 1 1 1 1 1 1 1 1 1 1 1 1 1 1 1 1 1 1 1 1 1 1 1 1 1 1 1 1 1 1 1 1 1 1 1 1 1 1 1 1 1 1 1 1 1 1 1 1 1 1 1 1 1 1 1 1 1 1 1 1 1 1 1 1 1 1 1 1 1 1 1 1 1 1 1 1 1 1 1 1 1 1 1 1 1 1 1 1 1 1 1 1 1 1 1 1 1 1 1 1 1 1 1 1 1 1 1 1 1 1 1 1 1 1 1 1 1 1 1 1 1 1 1 1 1 1 1 1 1 1 1 1 1 1 1 1 0 0 0 0 0 0 0 0 0 0 0 0 0 0 0 0 0 0 0 0 0 0 0 0 0 0 0 0 0 0 0 0 0 0 0 0 0 0 0 0 0 0 0 0 0 0 0 0 0 0 0 0 0 0 0 0 0 0 0 0 0 0 0 0 0 0 0 0 0 0 0 0 0 0 0 0 0 0 0 0 0 0 0 0 0 0 0 0 0 0 0 0 0 0 0 0 0 0 0 0 0 0 0 0 0 0 0 0 0 0 0 0 0 0\n",
- "INFO:tensorflow:segment_ids: 0 0 0 0 0 0 0 0 0 0 0 0 0 1 1 1 1 1 1 1 1 1 1 1 1 1 1 1 1 1 1 1 1 1 1 1 1 1 1 1 1 1 1 1 1 1 1 1 1 1 1 1 1 1 1 1 1 1 1 1 1 1 1 1 1 1 1 1 1 1 1 1 1 1 1 1 1 1 1 1 1 1 1 1 1 1 1 1 1 1 1 1 1 1 1 1 1 1 1 1 1 1 1 1 1 1 1 1 1 1 1 1 1 1 1 1 1 1 1 1 1 1 1 1 1 1 1 1 1 1 1 1 1 1 1 1 1 1 1 1 1 1 1 1 1 1 1 1 1 1 1 1 1 1 1 1 1 1 1 1 1 1 1 1 1 1 1 1 1 1 1 1 1 1 1 1 1 1 1 1 1 1 1 1 1 1 1 1 1 1 1 1 1 1 1 1 1 1 1 1 1 1 1 1 1 1 1 1 1 1 1 1 1 1 1 1 1 1 1 1 1 1 1 1 1 1 1 1 1 1 1 1 1 1 1 1 1 1 1 1 1 1 1 1 1 1 1 1 1 1 1 1 1 1 1 1 1 1 1 1 1 1 1 1 1 1 1 1 1 1 0 0 0 0 0 0 0 0 0 0 0 0 0 0 0 0 0 0 0 0 0 0 0 0 0 0 0 0 0 0 0 0 0 0 0 0 0 0 0 0 0 0 0 0 0 0 0 0 0 0 0 0 0 0 0 0 0 0 0 0 0 0 0 0 0 0 0 0 0 0 0 0 0 0 0 0 0 0 0 0 0 0 0 0 0 0 0 0 0 0 0 0 0 0 0 0 0 0 0 0 0 0 0 0 0 0 0 0 0 0 0 0 0 0\n",
- "INFO:tensorflow:start_position: 123\n",
- "INFO:tensorflow:end_position: 124\n",
- "INFO:tensorflow:answer: the observer\n",
- "INFO:tensorflow:*** Example ***\n",
- "INFO:tensorflow:unique_id: 1000000008\n",
- "INFO:tensorflow:example_index: 8\n",
- "INFO:tensorflow:doc_span_index: 0\n",
- "INFO:tensorflow:tokens: [CLS] how many student news papers are found at notre dame ? [SEP] as at most other universities , notre dame ' s students run a number of news media outlets . the nine student - run outlets include three newspapers , both a radio and television station , and several magazines and journals . begun as a one - page journal in september 1876 , the scholastic magazine is issued twice monthly and claims to be the oldest continuous collegiate publication in the united states . the other magazine , the jug ##gler , is released twice a year and focuses on student literature and artwork . the dome yearbook is published annually . the newspapers have varying publication interests , with the observer published daily and mainly reporting university and other news , and staffed by students from both notre dame and saint mary ' s college . unlike scholastic and the dome , the observer is an independent publication and does not have a faculty advisor or any editorial oversight from the university . in 1987 , when some students believed that the observer began to show a conservative bias , a liberal newspaper , common sense was published . likewise , in 2003 , when other students believed that the paper showed a liberal bias , the conservative paper irish rover went into production . neither paper is published as often as the observer ; however , all three are distributed to all students . finally , in spring 2008 an undergraduate journal for political science research , beyond politics , made its debut . [SEP]\n",
- "INFO:tensorflow:token_to_orig_map: 13:0 14:1 15:2 16:3 17:4 18:4 19:5 20:6 21:6 22:6 23:7 24:8 25:9 26:10 27:11 28:12 29:13 30:14 31:14 32:15 33:16 34:17 35:17 36:17 37:18 38:19 39:20 40:21 41:21 42:22 43:23 44:24 45:25 46:26 47:27 48:27 49:28 50:29 51:30 52:31 53:32 54:32 55:33 56:34 57:35 58:36 59:36 60:36 61:37 62:38 63:39 64:40 65:40 66:41 67:42 68:43 69:44 70:45 71:46 72:47 73:48 74:49 75:50 76:51 77:52 78:53 79:54 80:55 81:56 82:57 83:58 84:59 85:60 86:60 87:61 88:62 89:63 90:63 91:64 92:65 93:65 94:65 95:66 96:67 97:68 98:69 99:70 100:71 101:72 102:73 103:74 104:75 105:76 106:77 107:77 108:78 109:79 110:80 111:81 112:82 113:83 114:83 115:84 116:85 117:86 118:87 119:88 120:89 121:89 122:90 123:91 124:92 125:93 126:94 127:95 128:96 129:97 130:98 131:99 132:100 133:101 134:101 135:102 136:103 137:104 138:105 139:106 140:107 141:108 142:109 143:110 144:111 145:112 146:112 147:112 148:113 149:113 150:114 151:115 152:116 153:117 154:118 155:118 156:119 157:120 158:121 159:122 160:123 161:124 162:125 163:126 164:127 165:128 166:129 167:130 168:131 169:132 170:133 171:134 172:135 173:136 174:137 175:138 176:138 177:139 178:140 179:140 180:141 181:142 182:143 183:144 184:145 185:146 186:147 187:148 188:149 189:150 190:151 191:152 192:153 193:153 194:154 195:155 196:156 197:156 198:157 199:158 200:159 201:160 202:160 203:161 204:161 205:162 206:163 207:163 208:164 209:165 210:166 211:167 212:168 213:169 214:170 215:171 216:172 217:173 218:174 219:174 220:175 221:176 222:177 223:178 224:179 225:180 226:181 227:182 228:182 229:183 230:184 231:185 232:186 233:187 234:188 235:189 236:190 237:191 238:191 239:192 240:192 241:193 242:194 243:195 244:196 245:197 246:198 247:199 248:199 249:200 250:200 251:201 252:202 253:203 254:204 255:205 256:206 257:207 258:208 259:209 260:210 261:210 262:211 263:212 264:212 265:213 266:214 267:215 268:215\n"
- ]
- },
- {
- "name": "stdout",
- "output_type": "stream",
- "text": [
- "INFO:tensorflow:token_is_max_context: 13:True 14:True 15:True 16:True 17:True 18:True 19:True 20:True 21:True 22:True 23:True 24:True 25:True 26:True 27:True 28:True 29:True 30:True 31:True 32:True 33:True 34:True 35:True 36:True 37:True 38:True 39:True 40:True 41:True 42:True 43:True 44:True 45:True 46:True 47:True 48:True 49:True 50:True 51:True 52:True 53:True 54:True 55:True 56:True 57:True 58:True 59:True 60:True 61:True 62:True 63:True 64:True 65:True 66:True 67:True 68:True 69:True 70:True 71:True 72:True 73:True 74:True 75:True 76:True 77:True 78:True 79:True 80:True 81:True 82:True 83:True 84:True 85:True 86:True 87:True 88:True 89:True 90:True 91:True 92:True 93:True 94:True 95:True 96:True 97:True 98:True 99:True 100:True 101:True 102:True 103:True 104:True 105:True 106:True 107:True 108:True 109:True 110:True 111:True 112:True 113:True 114:True 115:True 116:True 117:True 118:True 119:True 120:True 121:True 122:True 123:True 124:True 125:True 126:True 127:True 128:True 129:True 130:True 131:True 132:True 133:True 134:True 135:True 136:True 137:True 138:True 139:True 140:True 141:True 142:True 143:True 144:True 145:True 146:True 147:True 148:True 149:True 150:True 151:True 152:True 153:True 154:True 155:True 156:True 157:True 158:True 159:True 160:True 161:True 162:True 163:True 164:True 165:True 166:True 167:True 168:True 169:True 170:True 171:True 172:True 173:True 174:True 175:True 176:True 177:True 178:True 179:True 180:True 181:True 182:True 183:True 184:True 185:True 186:True 187:True 188:True 189:True 190:True 191:True 192:True 193:True 194:True 195:True 196:True 197:True 198:True 199:True 200:True 201:True 202:True 203:True 204:True 205:True 206:True 207:True 208:True 209:True 210:True 211:True 212:True 213:True 214:True 215:True 216:True 217:True 218:True 219:True 220:True 221:True 222:True 223:True 224:True 225:True 226:True 227:True 228:True 229:True 230:True 231:True 232:True 233:True 234:True 235:True 236:True 237:True 238:True 239:True 240:True 241:True 242:True 243:True 244:True 245:True 246:True 247:True 248:True 249:True 250:True 251:True 252:True 253:True 254:True 255:True 256:True 257:True 258:True 259:True 260:True 261:True 262:True 263:True 264:True 265:True 266:True 267:True 268:True\n",
- "INFO:tensorflow:input_ids: 101 2129 2116 3076 2739 4981 2024 2179 2012 10289 8214 1029 102 2004 2012 2087 2060 5534 1010 10289 8214 1005 1055 2493 2448 1037 2193 1997 2739 2865 11730 1012 1996 3157 3076 1011 2448 11730 2421 2093 6399 1010 2119 1037 2557 1998 2547 2276 1010 1998 2195 7298 1998 9263 1012 5625 2004 1037 2028 1011 3931 3485 1999 2244 7326 1010 1996 24105 2932 2003 3843 3807 7058 1998 4447 2000 2022 1996 4587 7142 9234 4772 1999 1996 2142 2163 1012 1996 2060 2932 1010 1996 26536 17420 1010 2003 2207 3807 1037 2095 1998 7679 2006 3076 3906 1998 8266 1012 1996 8514 24803 2003 2405 6604 1012 1996 6399 2031 9671 4772 5426 1010 2007 1996 9718 2405 3679 1998 3701 7316 2118 1998 2060 2739 1010 1998 21121 2011 2493 2013 2119 10289 8214 1998 3002 2984 1005 1055 2267 1012 4406 24105 1998 1996 8514 1010 1996 9718 2003 2019 2981 4772 1998 2515 2025 2031 1037 4513 8619 2030 2151 8368 15709 2013 1996 2118 1012 1999 3055 1010 2043 2070 2493 3373 2008 1996 9718 2211 2000 2265 1037 4603 13827 1010 1037 4314 3780 1010 2691 3168 2001 2405 1012 10655 1010 1999 2494 1010 2043 2060 2493 3373 2008 1996 3259 3662 1037 4314 13827 1010 1996 4603 3259 3493 13631 2253 2046 2537 1012 4445 3259 2003 2405 2004 2411 2004 1996 9718 1025 2174 1010 2035 2093 2024 5500 2000 2035 2493 1012 2633 1010 1999 3500 2263 2019 8324 3485 2005 2576 2671 2470 1010 3458 4331 1010 2081 2049 2834 1012 102 0 0 0 0 0 0 0 0 0 0 0 0 0 0 0 0 0 0 0 0 0 0 0 0 0 0 0 0 0 0 0 0 0 0 0 0 0 0 0 0 0 0 0 0 0 0 0 0 0 0 0 0 0 0 0 0 0 0 0 0 0 0 0 0 0 0 0 0 0 0 0 0 0 0 0 0 0 0 0 0 0 0 0 0 0 0 0 0 0 0 0 0 0 0 0 0 0 0 0 0 0 0 0 0 0 0 0 0 0 0 0 0 0 0\n",
- "INFO:tensorflow:input_mask: 1 1 1 1 1 1 1 1 1 1 1 1 1 1 1 1 1 1 1 1 1 1 1 1 1 1 1 1 1 1 1 1 1 1 1 1 1 1 1 1 1 1 1 1 1 1 1 1 1 1 1 1 1 1 1 1 1 1 1 1 1 1 1 1 1 1 1 1 1 1 1 1 1 1 1 1 1 1 1 1 1 1 1 1 1 1 1 1 1 1 1 1 1 1 1 1 1 1 1 1 1 1 1 1 1 1 1 1 1 1 1 1 1 1 1 1 1 1 1 1 1 1 1 1 1 1 1 1 1 1 1 1 1 1 1 1 1 1 1 1 1 1 1 1 1 1 1 1 1 1 1 1 1 1 1 1 1 1 1 1 1 1 1 1 1 1 1 1 1 1 1 1 1 1 1 1 1 1 1 1 1 1 1 1 1 1 1 1 1 1 1 1 1 1 1 1 1 1 1 1 1 1 1 1 1 1 1 1 1 1 1 1 1 1 1 1 1 1 1 1 1 1 1 1 1 1 1 1 1 1 1 1 1 1 1 1 1 1 1 1 1 1 1 1 1 1 1 1 1 1 1 1 1 1 1 1 1 1 1 1 1 1 1 1 1 1 1 1 1 1 0 0 0 0 0 0 0 0 0 0 0 0 0 0 0 0 0 0 0 0 0 0 0 0 0 0 0 0 0 0 0 0 0 0 0 0 0 0 0 0 0 0 0 0 0 0 0 0 0 0 0 0 0 0 0 0 0 0 0 0 0 0 0 0 0 0 0 0 0 0 0 0 0 0 0 0 0 0 0 0 0 0 0 0 0 0 0 0 0 0 0 0 0 0 0 0 0 0 0 0 0 0 0 0 0 0 0 0 0 0 0 0 0 0\n",
- "INFO:tensorflow:segment_ids: 0 0 0 0 0 0 0 0 0 0 0 0 0 1 1 1 1 1 1 1 1 1 1 1 1 1 1 1 1 1 1 1 1 1 1 1 1 1 1 1 1 1 1 1 1 1 1 1 1 1 1 1 1 1 1 1 1 1 1 1 1 1 1 1 1 1 1 1 1 1 1 1 1 1 1 1 1 1 1 1 1 1 1 1 1 1 1 1 1 1 1 1 1 1 1 1 1 1 1 1 1 1 1 1 1 1 1 1 1 1 1 1 1 1 1 1 1 1 1 1 1 1 1 1 1 1 1 1 1 1 1 1 1 1 1 1 1 1 1 1 1 1 1 1 1 1 1 1 1 1 1 1 1 1 1 1 1 1 1 1 1 1 1 1 1 1 1 1 1 1 1 1 1 1 1 1 1 1 1 1 1 1 1 1 1 1 1 1 1 1 1 1 1 1 1 1 1 1 1 1 1 1 1 1 1 1 1 1 1 1 1 1 1 1 1 1 1 1 1 1 1 1 1 1 1 1 1 1 1 1 1 1 1 1 1 1 1 1 1 1 1 1 1 1 1 1 1 1 1 1 1 1 1 1 1 1 1 1 1 1 1 1 1 1 1 1 1 1 1 1 0 0 0 0 0 0 0 0 0 0 0 0 0 0 0 0 0 0 0 0 0 0 0 0 0 0 0 0 0 0 0 0 0 0 0 0 0 0 0 0 0 0 0 0 0 0 0 0 0 0 0 0 0 0 0 0 0 0 0 0 0 0 0 0 0 0 0 0 0 0 0 0 0 0 0 0 0 0 0 0 0 0 0 0 0 0 0 0 0 0 0 0 0 0 0 0 0 0 0 0 0 0 0 0 0 0 0 0 0 0 0 0 0 0\n",
- "INFO:tensorflow:start_position: 39\n",
- "INFO:tensorflow:end_position: 39\n",
- "INFO:tensorflow:answer: three\n",
- "INFO:tensorflow:*** Example ***\n",
- "INFO:tensorflow:unique_id: 1000000009\n",
- "INFO:tensorflow:example_index: 9\n",
- "INFO:tensorflow:doc_span_index: 0\n",
- "INFO:tensorflow:tokens: [CLS] in what year did the student paper common sense begin publication at notre dame ? [SEP] as at most other universities , notre dame ' s students run a number of news media outlets . the nine student - run outlets include three newspapers , both a radio and television station , and several magazines and journals . begun as a one - page journal in september 1876 , the scholastic magazine is issued twice monthly and claims to be the oldest continuous collegiate publication in the united states . the other magazine , the jug ##gler , is released twice a year and focuses on student literature and artwork . the dome yearbook is published annually . the newspapers have varying publication interests , with the observer published daily and mainly reporting university and other news , and staffed by students from both notre dame and saint mary ' s college . unlike scholastic and the dome , the observer is an independent publication and does not have a faculty advisor or any editorial oversight from the university . in 1987 , when some students believed that the observer began to show a conservative bias , a liberal newspaper , common sense was published . likewise , in 2003 , when other students believed that the paper showed a liberal bias , the conservative paper irish rover went into production . neither paper is published as often as the observer ; however , all three are distributed to all students . finally , in spring 2008 an undergraduate journal for political science research , beyond politics , made its debut . [SEP]\n",
- "INFO:tensorflow:token_to_orig_map: 17:0 18:1 19:2 20:3 21:4 22:4 23:5 24:6 25:6 26:6 27:7 28:8 29:9 30:10 31:11 32:12 33:13 34:14 35:14 36:15 37:16 38:17 39:17 40:17 41:18 42:19 43:20 44:21 45:21 46:22 47:23 48:24 49:25 50:26 51:27 52:27 53:28 54:29 55:30 56:31 57:32 58:32 59:33 60:34 61:35 62:36 63:36 64:36 65:37 66:38 67:39 68:40 69:40 70:41 71:42 72:43 73:44 74:45 75:46 76:47 77:48 78:49 79:50 80:51 81:52 82:53 83:54 84:55 85:56 86:57 87:58 88:59 89:60 90:60 91:61 92:62 93:63 94:63 95:64 96:65 97:65 98:65 99:66 100:67 101:68 102:69 103:70 104:71 105:72 106:73 107:74 108:75 109:76 110:77 111:77 112:78 113:79 114:80 115:81 116:82 117:83 118:83 119:84 120:85 121:86 122:87 123:88 124:89 125:89 126:90 127:91 128:92 129:93 130:94 131:95 132:96 133:97 134:98 135:99 136:100 137:101 138:101 139:102 140:103 141:104 142:105 143:106 144:107 145:108 146:109 147:110 148:111 149:112 150:112 151:112 152:113 153:113 154:114 155:115 156:116 157:117 158:118 159:118 160:119 161:120 162:121 163:122 164:123 165:124 166:125 167:126 168:127 169:128 170:129 171:130 172:131 173:132 174:133 175:134 176:135 177:136 178:137 179:138 180:138 181:139 182:140 183:140 184:141 185:142 186:143 187:144 188:145 189:146 190:147 191:148 192:149 193:150 194:151 195:152 196:153 197:153 198:154 199:155 200:156 201:156 202:157 203:158 204:159 205:160 206:160 207:161 208:161 209:162 210:163 211:163 212:164 213:165 214:166 215:167 216:168 217:169 218:170 219:171 220:172 221:173 222:174 223:174 224:175 225:176 226:177 227:178 228:179 229:180 230:181 231:182 232:182 233:183 234:184 235:185 236:186 237:187 238:188 239:189 240:190 241:191 242:191 243:192 244:192 245:193 246:194 247:195 248:196 249:197 250:198 251:199 252:199 253:200 254:200 255:201 256:202 257:203 258:204 259:205 260:206 261:207 262:208 263:209 264:210 265:210 266:211 267:212 268:212 269:213 270:214 271:215 272:215\n"
- ]
- },
- {
- "name": "stdout",
- "output_type": "stream",
- "text": [
- "INFO:tensorflow:token_is_max_context: 17:True 18:True 19:True 20:True 21:True 22:True 23:True 24:True 25:True 26:True 27:True 28:True 29:True 30:True 31:True 32:True 33:True 34:True 35:True 36:True 37:True 38:True 39:True 40:True 41:True 42:True 43:True 44:True 45:True 46:True 47:True 48:True 49:True 50:True 51:True 52:True 53:True 54:True 55:True 56:True 57:True 58:True 59:True 60:True 61:True 62:True 63:True 64:True 65:True 66:True 67:True 68:True 69:True 70:True 71:True 72:True 73:True 74:True 75:True 76:True 77:True 78:True 79:True 80:True 81:True 82:True 83:True 84:True 85:True 86:True 87:True 88:True 89:True 90:True 91:True 92:True 93:True 94:True 95:True 96:True 97:True 98:True 99:True 100:True 101:True 102:True 103:True 104:True 105:True 106:True 107:True 108:True 109:True 110:True 111:True 112:True 113:True 114:True 115:True 116:True 117:True 118:True 119:True 120:True 121:True 122:True 123:True 124:True 125:True 126:True 127:True 128:True 129:True 130:True 131:True 132:True 133:True 134:True 135:True 136:True 137:True 138:True 139:True 140:True 141:True 142:True 143:True 144:True 145:True 146:True 147:True 148:True 149:True 150:True 151:True 152:True 153:True 154:True 155:True 156:True 157:True 158:True 159:True 160:True 161:True 162:True 163:True 164:True 165:True 166:True 167:True 168:True 169:True 170:True 171:True 172:True 173:True 174:True 175:True 176:True 177:True 178:True 179:True 180:True 181:True 182:True 183:True 184:True 185:True 186:True 187:True 188:True 189:True 190:True 191:True 192:True 193:True 194:True 195:True 196:True 197:True 198:True 199:True 200:True 201:True 202:True 203:True 204:True 205:True 206:True 207:True 208:True 209:True 210:True 211:True 212:True 213:True 214:True 215:True 216:True 217:True 218:True 219:True 220:True 221:True 222:True 223:True 224:True 225:True 226:True 227:True 228:True 229:True 230:True 231:True 232:True 233:True 234:True 235:True 236:True 237:True 238:True 239:True 240:True 241:True 242:True 243:True 244:True 245:True 246:True 247:True 248:True 249:True 250:True 251:True 252:True 253:True 254:True 255:True 256:True 257:True 258:True 259:True 260:True 261:True 262:True 263:True 264:True 265:True 266:True 267:True 268:True 269:True 270:True 271:True 272:True\n",
- "INFO:tensorflow:input_ids: 101 1999 2054 2095 2106 1996 3076 3259 2691 3168 4088 4772 2012 10289 8214 1029 102 2004 2012 2087 2060 5534 1010 10289 8214 1005 1055 2493 2448 1037 2193 1997 2739 2865 11730 1012 1996 3157 3076 1011 2448 11730 2421 2093 6399 1010 2119 1037 2557 1998 2547 2276 1010 1998 2195 7298 1998 9263 1012 5625 2004 1037 2028 1011 3931 3485 1999 2244 7326 1010 1996 24105 2932 2003 3843 3807 7058 1998 4447 2000 2022 1996 4587 7142 9234 4772 1999 1996 2142 2163 1012 1996 2060 2932 1010 1996 26536 17420 1010 2003 2207 3807 1037 2095 1998 7679 2006 3076 3906 1998 8266 1012 1996 8514 24803 2003 2405 6604 1012 1996 6399 2031 9671 4772 5426 1010 2007 1996 9718 2405 3679 1998 3701 7316 2118 1998 2060 2739 1010 1998 21121 2011 2493 2013 2119 10289 8214 1998 3002 2984 1005 1055 2267 1012 4406 24105 1998 1996 8514 1010 1996 9718 2003 2019 2981 4772 1998 2515 2025 2031 1037 4513 8619 2030 2151 8368 15709 2013 1996 2118 1012 1999 3055 1010 2043 2070 2493 3373 2008 1996 9718 2211 2000 2265 1037 4603 13827 1010 1037 4314 3780 1010 2691 3168 2001 2405 1012 10655 1010 1999 2494 1010 2043 2060 2493 3373 2008 1996 3259 3662 1037 4314 13827 1010 1996 4603 3259 3493 13631 2253 2046 2537 1012 4445 3259 2003 2405 2004 2411 2004 1996 9718 1025 2174 1010 2035 2093 2024 5500 2000 2035 2493 1012 2633 1010 1999 3500 2263 2019 8324 3485 2005 2576 2671 2470 1010 3458 4331 1010 2081 2049 2834 1012 102 0 0 0 0 0 0 0 0 0 0 0 0 0 0 0 0 0 0 0 0 0 0 0 0 0 0 0 0 0 0 0 0 0 0 0 0 0 0 0 0 0 0 0 0 0 0 0 0 0 0 0 0 0 0 0 0 0 0 0 0 0 0 0 0 0 0 0 0 0 0 0 0 0 0 0 0 0 0 0 0 0 0 0 0 0 0 0 0 0 0 0 0 0 0 0 0 0 0 0 0 0 0 0 0 0 0 0 0 0 0\n",
- "INFO:tensorflow:input_mask: 1 1 1 1 1 1 1 1 1 1 1 1 1 1 1 1 1 1 1 1 1 1 1 1 1 1 1 1 1 1 1 1 1 1 1 1 1 1 1 1 1 1 1 1 1 1 1 1 1 1 1 1 1 1 1 1 1 1 1 1 1 1 1 1 1 1 1 1 1 1 1 1 1 1 1 1 1 1 1 1 1 1 1 1 1 1 1 1 1 1 1 1 1 1 1 1 1 1 1 1 1 1 1 1 1 1 1 1 1 1 1 1 1 1 1 1 1 1 1 1 1 1 1 1 1 1 1 1 1 1 1 1 1 1 1 1 1 1 1 1 1 1 1 1 1 1 1 1 1 1 1 1 1 1 1 1 1 1 1 1 1 1 1 1 1 1 1 1 1 1 1 1 1 1 1 1 1 1 1 1 1 1 1 1 1 1 1 1 1 1 1 1 1 1 1 1 1 1 1 1 1 1 1 1 1 1 1 1 1 1 1 1 1 1 1 1 1 1 1 1 1 1 1 1 1 1 1 1 1 1 1 1 1 1 1 1 1 1 1 1 1 1 1 1 1 1 1 1 1 1 1 1 1 1 1 1 1 1 1 1 1 1 1 1 1 1 1 1 1 1 1 1 1 1 0 0 0 0 0 0 0 0 0 0 0 0 0 0 0 0 0 0 0 0 0 0 0 0 0 0 0 0 0 0 0 0 0 0 0 0 0 0 0 0 0 0 0 0 0 0 0 0 0 0 0 0 0 0 0 0 0 0 0 0 0 0 0 0 0 0 0 0 0 0 0 0 0 0 0 0 0 0 0 0 0 0 0 0 0 0 0 0 0 0 0 0 0 0 0 0 0 0 0 0 0 0 0 0 0 0 0 0 0 0\n",
- "INFO:tensorflow:segment_ids: 0 0 0 0 0 0 0 0 0 0 0 0 0 0 0 0 0 1 1 1 1 1 1 1 1 1 1 1 1 1 1 1 1 1 1 1 1 1 1 1 1 1 1 1 1 1 1 1 1 1 1 1 1 1 1 1 1 1 1 1 1 1 1 1 1 1 1 1 1 1 1 1 1 1 1 1 1 1 1 1 1 1 1 1 1 1 1 1 1 1 1 1 1 1 1 1 1 1 1 1 1 1 1 1 1 1 1 1 1 1 1 1 1 1 1 1 1 1 1 1 1 1 1 1 1 1 1 1 1 1 1 1 1 1 1 1 1 1 1 1 1 1 1 1 1 1 1 1 1 1 1 1 1 1 1 1 1 1 1 1 1 1 1 1 1 1 1 1 1 1 1 1 1 1 1 1 1 1 1 1 1 1 1 1 1 1 1 1 1 1 1 1 1 1 1 1 1 1 1 1 1 1 1 1 1 1 1 1 1 1 1 1 1 1 1 1 1 1 1 1 1 1 1 1 1 1 1 1 1 1 1 1 1 1 1 1 1 1 1 1 1 1 1 1 1 1 1 1 1 1 1 1 1 1 1 1 1 1 1 1 1 1 1 1 1 1 1 1 1 1 1 1 1 1 0 0 0 0 0 0 0 0 0 0 0 0 0 0 0 0 0 0 0 0 0 0 0 0 0 0 0 0 0 0 0 0 0 0 0 0 0 0 0 0 0 0 0 0 0 0 0 0 0 0 0 0 0 0 0 0 0 0 0 0 0 0 0 0 0 0 0 0 0 0 0 0 0 0 0 0 0 0 0 0 0 0 0 0 0 0 0 0 0 0 0 0 0 0 0 0 0 0 0 0 0 0 0 0 0 0 0 0 0 0\n",
- "INFO:tensorflow:start_position: 182\n",
- "INFO:tensorflow:end_position: 182\n",
- "INFO:tensorflow:answer: 1987\n",
- "INFO:tensorflow:*** Example ***\n",
- "INFO:tensorflow:unique_id: 1000000010\n",
- "INFO:tensorflow:example_index: 10\n",
- "INFO:tensorflow:doc_span_index: 0\n",
- "INFO:tensorflow:tokens: [CLS] where is the headquarters of the congregation of the holy cross ? [SEP] the university is the major seat of the congregation of holy cross ( albeit not its official headquarters , which are in rome ) . its main seminary , more ##au seminary , is located on the campus across st . joseph lake from the main building . old college , the oldest building on campus and located near the shore of st . mary lake , houses undergraduate seminar ##ians . retired priests and brothers reside in fatima house ( a former retreat center ) , holy cross house , as well as col ##umb ##a hall near the gr ##otto . the university through the more ##au seminary has ties to theologian frederick bu ##ech ##ner . while not catholic , bu ##ech ##ner has praised writers from notre dame and more ##au seminary created a bu ##ech ##ner prize for preaching . [SEP]\n",
- "INFO:tensorflow:token_to_orig_map: 14:0 15:1 16:2 17:3 18:4 19:5 20:6 21:7 22:8 23:9 24:10 25:11 26:12 27:12 28:13 29:14 30:15 31:16 32:16 33:17 34:18 35:19 36:20 37:20 38:20 39:21 40:22 41:23 42:23 43:24 44:24 45:25 46:25 47:26 48:27 49:28 50:29 51:30 52:31 53:32 54:32 55:33 56:34 57:35 58:36 59:37 60:38 61:38 62:39 63:40 64:40 65:41 66:42 67:43 68:44 69:45 70:46 71:47 72:48 73:49 74:50 75:51 76:52 77:52 78:53 79:54 80:54 81:55 82:56 83:57 84:57 85:57 86:58 87:59 88:60 89:61 90:62 91:63 92:64 93:65 94:66 95:66 96:67 97:68 98:69 99:69 100:69 101:70 102:71 103:72 104:72 105:73 106:74 107:75 108:76 109:76 110:76 111:77 112:78 113:79 114:80 115:80 116:80 117:81 118:82 119:83 120:84 121:85 122:85 123:86 124:87 125:88 126:89 127:90 128:91 129:92 130:92 131:92 132:92 133:93 134:94 135:95 136:95 137:96 138:96 139:96 140:97 141:98 142:99 143:100 144:101 145:102 146:103 147:104 148:104 149:105 150:106 151:107 152:108 153:108 154:108 155:109 156:110 157:111 158:111\n",
- "INFO:tensorflow:token_is_max_context: 14:True 15:True 16:True 17:True 18:True 19:True 20:True 21:True 22:True 23:True 24:True 25:True 26:True 27:True 28:True 29:True 30:True 31:True 32:True 33:True 34:True 35:True 36:True 37:True 38:True 39:True 40:True 41:True 42:True 43:True 44:True 45:True 46:True 47:True 48:True 49:True 50:True 51:True 52:True 53:True 54:True 55:True 56:True 57:True 58:True 59:True 60:True 61:True 62:True 63:True 64:True 65:True 66:True 67:True 68:True 69:True 70:True 71:True 72:True 73:True 74:True 75:True 76:True 77:True 78:True 79:True 80:True 81:True 82:True 83:True 84:True 85:True 86:True 87:True 88:True 89:True 90:True 91:True 92:True 93:True 94:True 95:True 96:True 97:True 98:True 99:True 100:True 101:True 102:True 103:True 104:True 105:True 106:True 107:True 108:True 109:True 110:True 111:True 112:True 113:True 114:True 115:True 116:True 117:True 118:True 119:True 120:True 121:True 122:True 123:True 124:True 125:True 126:True 127:True 128:True 129:True 130:True 131:True 132:True 133:True 134:True 135:True 136:True 137:True 138:True 139:True 140:True 141:True 142:True 143:True 144:True 145:True 146:True 147:True 148:True 149:True 150:True 151:True 152:True 153:True 154:True 155:True 156:True 157:True 158:True\n"
- ]
- },
- {
- "name": "stdout",
- "output_type": "stream",
- "text": [
- "INFO:tensorflow:input_ids: 101 2073 2003 1996 4075 1997 1996 7769 1997 1996 4151 2892 1029 102 1996 2118 2003 1996 2350 2835 1997 1996 7769 1997 4151 2892 1006 12167 2025 2049 2880 4075 1010 2029 2024 1999 4199 1007 1012 2049 2364 8705 1010 2062 4887 8705 1010 2003 2284 2006 1996 3721 2408 2358 1012 3312 2697 2013 1996 2364 2311 1012 2214 2267 1010 1996 4587 2311 2006 3721 1998 2284 2379 1996 5370 1997 2358 1012 2984 2697 1010 3506 8324 18014 7066 1012 3394 8656 1998 3428 13960 1999 27596 2160 1006 1037 2280 7822 2415 1007 1010 4151 2892 2160 1010 2004 2092 2004 8902 25438 2050 2534 2379 1996 24665 23052 1012 1996 2118 2083 1996 2062 4887 8705 2038 7208 2000 17200 5406 20934 15937 3678 1012 2096 2025 3234 1010 20934 15937 3678 2038 5868 4898 2013 10289 8214 1998 2062 4887 8705 2580 1037 20934 15937 3678 3396 2005 17979 1012 102 0 0 0 0 0 0 0 0 0 0 0 0 0 0 0 0 0 0 0 0 0 0 0 0 0 0 0 0 0 0 0 0 0 0 0 0 0 0 0 0 0 0 0 0 0 0 0 0 0 0 0 0 0 0 0 0 0 0 0 0 0 0 0 0 0 0 0 0 0 0 0 0 0 0 0 0 0 0 0 0 0 0 0 0 0 0 0 0 0 0 0 0 0 0 0 0 0 0 0 0 0 0 0 0 0 0 0 0 0 0 0 0 0 0 0 0 0 0 0 0 0 0 0 0 0 0 0 0 0 0 0 0 0 0 0 0 0 0 0 0 0 0 0 0 0 0 0 0 0 0 0 0 0 0 0 0 0 0 0 0 0 0 0 0 0 0 0 0 0 0 0 0 0 0 0 0 0 0 0 0 0 0 0 0 0 0 0 0 0 0 0 0 0 0 0 0 0 0 0 0 0 0 0 0 0 0 0 0 0 0 0 0 0 0 0 0 0 0 0 0 0 0 0 0\n",
- "INFO:tensorflow:input_mask: 1 1 1 1 1 1 1 1 1 1 1 1 1 1 1 1 1 1 1 1 1 1 1 1 1 1 1 1 1 1 1 1 1 1 1 1 1 1 1 1 1 1 1 1 1 1 1 1 1 1 1 1 1 1 1 1 1 1 1 1 1 1 1 1 1 1 1 1 1 1 1 1 1 1 1 1 1 1 1 1 1 1 1 1 1 1 1 1 1 1 1 1 1 1 1 1 1 1 1 1 1 1 1 1 1 1 1 1 1 1 1 1 1 1 1 1 1 1 1 1 1 1 1 1 1 1 1 1 1 1 1 1 1 1 1 1 1 1 1 1 1 1 1 1 1 1 1 1 1 1 1 1 1 1 1 1 1 1 1 1 0 0 0 0 0 0 0 0 0 0 0 0 0 0 0 0 0 0 0 0 0 0 0 0 0 0 0 0 0 0 0 0 0 0 0 0 0 0 0 0 0 0 0 0 0 0 0 0 0 0 0 0 0 0 0 0 0 0 0 0 0 0 0 0 0 0 0 0 0 0 0 0 0 0 0 0 0 0 0 0 0 0 0 0 0 0 0 0 0 0 0 0 0 0 0 0 0 0 0 0 0 0 0 0 0 0 0 0 0 0 0 0 0 0 0 0 0 0 0 0 0 0 0 0 0 0 0 0 0 0 0 0 0 0 0 0 0 0 0 0 0 0 0 0 0 0 0 0 0 0 0 0 0 0 0 0 0 0 0 0 0 0 0 0 0 0 0 0 0 0 0 0 0 0 0 0 0 0 0 0 0 0 0 0 0 0 0 0 0 0 0 0 0 0 0 0 0 0 0 0 0 0 0 0 0 0 0 0 0 0 0 0 0 0 0 0 0 0 0 0 0 0 0 0\n",
- "INFO:tensorflow:segment_ids: 0 0 0 0 0 0 0 0 0 0 0 0 0 0 1 1 1 1 1 1 1 1 1 1 1 1 1 1 1 1 1 1 1 1 1 1 1 1 1 1 1 1 1 1 1 1 1 1 1 1 1 1 1 1 1 1 1 1 1 1 1 1 1 1 1 1 1 1 1 1 1 1 1 1 1 1 1 1 1 1 1 1 1 1 1 1 1 1 1 1 1 1 1 1 1 1 1 1 1 1 1 1 1 1 1 1 1 1 1 1 1 1 1 1 1 1 1 1 1 1 1 1 1 1 1 1 1 1 1 1 1 1 1 1 1 1 1 1 1 1 1 1 1 1 1 1 1 1 1 1 1 1 1 1 1 1 1 1 1 1 0 0 0 0 0 0 0 0 0 0 0 0 0 0 0 0 0 0 0 0 0 0 0 0 0 0 0 0 0 0 0 0 0 0 0 0 0 0 0 0 0 0 0 0 0 0 0 0 0 0 0 0 0 0 0 0 0 0 0 0 0 0 0 0 0 0 0 0 0 0 0 0 0 0 0 0 0 0 0 0 0 0 0 0 0 0 0 0 0 0 0 0 0 0 0 0 0 0 0 0 0 0 0 0 0 0 0 0 0 0 0 0 0 0 0 0 0 0 0 0 0 0 0 0 0 0 0 0 0 0 0 0 0 0 0 0 0 0 0 0 0 0 0 0 0 0 0 0 0 0 0 0 0 0 0 0 0 0 0 0 0 0 0 0 0 0 0 0 0 0 0 0 0 0 0 0 0 0 0 0 0 0 0 0 0 0 0 0 0 0 0 0 0 0 0 0 0 0 0 0 0 0 0 0 0 0 0 0 0 0 0 0 0 0 0 0 0 0 0 0 0 0 0 0\n",
- "INFO:tensorflow:start_position: 36\n",
- "INFO:tensorflow:end_position: 36\n",
- "INFO:tensorflow:answer: rome\n",
- "INFO:tensorflow:*** Example ***\n",
- "INFO:tensorflow:unique_id: 1000000011\n",
- "INFO:tensorflow:example_index: 11\n",
- "INFO:tensorflow:doc_span_index: 0\n",
- "INFO:tensorflow:tokens: [CLS] what is the primary seminary of the congregation of the holy cross ? [SEP] the university is the major seat of the congregation of holy cross ( albeit not its official headquarters , which are in rome ) . its main seminary , more ##au seminary , is located on the campus across st . joseph lake from the main building . old college , the oldest building on campus and located near the shore of st . mary lake , houses undergraduate seminar ##ians . retired priests and brothers reside in fatima house ( a former retreat center ) , holy cross house , as well as col ##umb ##a hall near the gr ##otto . the university through the more ##au seminary has ties to theologian frederick bu ##ech ##ner . while not catholic , bu ##ech ##ner has praised writers from notre dame and more ##au seminary created a bu ##ech ##ner prize for preaching . [SEP]\n",
- "INFO:tensorflow:token_to_orig_map: 15:0 16:1 17:2 18:3 19:4 20:5 21:6 22:7 23:8 24:9 25:10 26:11 27:12 28:12 29:13 30:14 31:15 32:16 33:16 34:17 35:18 36:19 37:20 38:20 39:20 40:21 41:22 42:23 43:23 44:24 45:24 46:25 47:25 48:26 49:27 50:28 51:29 52:30 53:31 54:32 55:32 56:33 57:34 58:35 59:36 60:37 61:38 62:38 63:39 64:40 65:40 66:41 67:42 68:43 69:44 70:45 71:46 72:47 73:48 74:49 75:50 76:51 77:52 78:52 79:53 80:54 81:54 82:55 83:56 84:57 85:57 86:57 87:58 88:59 89:60 90:61 91:62 92:63 93:64 94:65 95:66 96:66 97:67 98:68 99:69 100:69 101:69 102:70 103:71 104:72 105:72 106:73 107:74 108:75 109:76 110:76 111:76 112:77 113:78 114:79 115:80 116:80 117:80 118:81 119:82 120:83 121:84 122:85 123:85 124:86 125:87 126:88 127:89 128:90 129:91 130:92 131:92 132:92 133:92 134:93 135:94 136:95 137:95 138:96 139:96 140:96 141:97 142:98 143:99 144:100 145:101 146:102 147:103 148:104 149:104 150:105 151:106 152:107 153:108 154:108 155:108 156:109 157:110 158:111 159:111\n",
- "INFO:tensorflow:token_is_max_context: 15:True 16:True 17:True 18:True 19:True 20:True 21:True 22:True 23:True 24:True 25:True 26:True 27:True 28:True 29:True 30:True 31:True 32:True 33:True 34:True 35:True 36:True 37:True 38:True 39:True 40:True 41:True 42:True 43:True 44:True 45:True 46:True 47:True 48:True 49:True 50:True 51:True 52:True 53:True 54:True 55:True 56:True 57:True 58:True 59:True 60:True 61:True 62:True 63:True 64:True 65:True 66:True 67:True 68:True 69:True 70:True 71:True 72:True 73:True 74:True 75:True 76:True 77:True 78:True 79:True 80:True 81:True 82:True 83:True 84:True 85:True 86:True 87:True 88:True 89:True 90:True 91:True 92:True 93:True 94:True 95:True 96:True 97:True 98:True 99:True 100:True 101:True 102:True 103:True 104:True 105:True 106:True 107:True 108:True 109:True 110:True 111:True 112:True 113:True 114:True 115:True 116:True 117:True 118:True 119:True 120:True 121:True 122:True 123:True 124:True 125:True 126:True 127:True 128:True 129:True 130:True 131:True 132:True 133:True 134:True 135:True 136:True 137:True 138:True 139:True 140:True 141:True 142:True 143:True 144:True 145:True 146:True 147:True 148:True 149:True 150:True 151:True 152:True 153:True 154:True 155:True 156:True 157:True 158:True 159:True\n",
- "INFO:tensorflow:input_ids: 101 2054 2003 1996 3078 8705 1997 1996 7769 1997 1996 4151 2892 1029 102 1996 2118 2003 1996 2350 2835 1997 1996 7769 1997 4151 2892 1006 12167 2025 2049 2880 4075 1010 2029 2024 1999 4199 1007 1012 2049 2364 8705 1010 2062 4887 8705 1010 2003 2284 2006 1996 3721 2408 2358 1012 3312 2697 2013 1996 2364 2311 1012 2214 2267 1010 1996 4587 2311 2006 3721 1998 2284 2379 1996 5370 1997 2358 1012 2984 2697 1010 3506 8324 18014 7066 1012 3394 8656 1998 3428 13960 1999 27596 2160 1006 1037 2280 7822 2415 1007 1010 4151 2892 2160 1010 2004 2092 2004 8902 25438 2050 2534 2379 1996 24665 23052 1012 1996 2118 2083 1996 2062 4887 8705 2038 7208 2000 17200 5406 20934 15937 3678 1012 2096 2025 3234 1010 20934 15937 3678 2038 5868 4898 2013 10289 8214 1998 2062 4887 8705 2580 1037 20934 15937 3678 3396 2005 17979 1012 102 0 0 0 0 0 0 0 0 0 0 0 0 0 0 0 0 0 0 0 0 0 0 0 0 0 0 0 0 0 0 0 0 0 0 0 0 0 0 0 0 0 0 0 0 0 0 0 0 0 0 0 0 0 0 0 0 0 0 0 0 0 0 0 0 0 0 0 0 0 0 0 0 0 0 0 0 0 0 0 0 0 0 0 0 0 0 0 0 0 0 0 0 0 0 0 0 0 0 0 0 0 0 0 0 0 0 0 0 0 0 0 0 0 0 0 0 0 0 0 0 0 0 0 0 0 0 0 0 0 0 0 0 0 0 0 0 0 0 0 0 0 0 0 0 0 0 0 0 0 0 0 0 0 0 0 0 0 0 0 0 0 0 0 0 0 0 0 0 0 0 0 0 0 0 0 0 0 0 0 0 0 0 0 0 0 0 0 0 0 0 0 0 0 0 0 0 0 0 0 0 0 0 0 0 0 0 0 0 0 0 0 0 0 0 0 0 0 0 0 0 0 0 0\n",
- "INFO:tensorflow:input_mask: 1 1 1 1 1 1 1 1 1 1 1 1 1 1 1 1 1 1 1 1 1 1 1 1 1 1 1 1 1 1 1 1 1 1 1 1 1 1 1 1 1 1 1 1 1 1 1 1 1 1 1 1 1 1 1 1 1 1 1 1 1 1 1 1 1 1 1 1 1 1 1 1 1 1 1 1 1 1 1 1 1 1 1 1 1 1 1 1 1 1 1 1 1 1 1 1 1 1 1 1 1 1 1 1 1 1 1 1 1 1 1 1 1 1 1 1 1 1 1 1 1 1 1 1 1 1 1 1 1 1 1 1 1 1 1 1 1 1 1 1 1 1 1 1 1 1 1 1 1 1 1 1 1 1 1 1 1 1 1 1 1 0 0 0 0 0 0 0 0 0 0 0 0 0 0 0 0 0 0 0 0 0 0 0 0 0 0 0 0 0 0 0 0 0 0 0 0 0 0 0 0 0 0 0 0 0 0 0 0 0 0 0 0 0 0 0 0 0 0 0 0 0 0 0 0 0 0 0 0 0 0 0 0 0 0 0 0 0 0 0 0 0 0 0 0 0 0 0 0 0 0 0 0 0 0 0 0 0 0 0 0 0 0 0 0 0 0 0 0 0 0 0 0 0 0 0 0 0 0 0 0 0 0 0 0 0 0 0 0 0 0 0 0 0 0 0 0 0 0 0 0 0 0 0 0 0 0 0 0 0 0 0 0 0 0 0 0 0 0 0 0 0 0 0 0 0 0 0 0 0 0 0 0 0 0 0 0 0 0 0 0 0 0 0 0 0 0 0 0 0 0 0 0 0 0 0 0 0 0 0 0 0 0 0 0 0 0 0 0 0 0 0 0 0 0 0 0 0 0 0 0 0 0 0\n"
- ]
- },
- {
- "name": "stdout",
- "output_type": "stream",
- "text": [
- "INFO:tensorflow:segment_ids: 0 0 0 0 0 0 0 0 0 0 0 0 0 0 0 1 1 1 1 1 1 1 1 1 1 1 1 1 1 1 1 1 1 1 1 1 1 1 1 1 1 1 1 1 1 1 1 1 1 1 1 1 1 1 1 1 1 1 1 1 1 1 1 1 1 1 1 1 1 1 1 1 1 1 1 1 1 1 1 1 1 1 1 1 1 1 1 1 1 1 1 1 1 1 1 1 1 1 1 1 1 1 1 1 1 1 1 1 1 1 1 1 1 1 1 1 1 1 1 1 1 1 1 1 1 1 1 1 1 1 1 1 1 1 1 1 1 1 1 1 1 1 1 1 1 1 1 1 1 1 1 1 1 1 1 1 1 1 1 1 1 0 0 0 0 0 0 0 0 0 0 0 0 0 0 0 0 0 0 0 0 0 0 0 0 0 0 0 0 0 0 0 0 0 0 0 0 0 0 0 0 0 0 0 0 0 0 0 0 0 0 0 0 0 0 0 0 0 0 0 0 0 0 0 0 0 0 0 0 0 0 0 0 0 0 0 0 0 0 0 0 0 0 0 0 0 0 0 0 0 0 0 0 0 0 0 0 0 0 0 0 0 0 0 0 0 0 0 0 0 0 0 0 0 0 0 0 0 0 0 0 0 0 0 0 0 0 0 0 0 0 0 0 0 0 0 0 0 0 0 0 0 0 0 0 0 0 0 0 0 0 0 0 0 0 0 0 0 0 0 0 0 0 0 0 0 0 0 0 0 0 0 0 0 0 0 0 0 0 0 0 0 0 0 0 0 0 0 0 0 0 0 0 0 0 0 0 0 0 0 0 0 0 0 0 0 0 0 0 0 0 0 0 0 0 0 0 0 0 0 0 0 0 0\n",
- "INFO:tensorflow:start_position: 44\n",
- "INFO:tensorflow:end_position: 46\n",
- "INFO:tensorflow:answer: more ##au seminary\n",
- "INFO:tensorflow:*** Example ***\n",
- "INFO:tensorflow:unique_id: 1000000012\n",
- "INFO:tensorflow:example_index: 12\n",
- "INFO:tensorflow:doc_span_index: 0\n",
- "INFO:tensorflow:tokens: [CLS] what is the oldest structure at notre dame ? [SEP] the university is the major seat of the congregation of holy cross ( albeit not its official headquarters , which are in rome ) . its main seminary , more ##au seminary , is located on the campus across st . joseph lake from the main building . old college , the oldest building on campus and located near the shore of st . mary lake , houses undergraduate seminar ##ians . retired priests and brothers reside in fatima house ( a former retreat center ) , holy cross house , as well as col ##umb ##a hall near the gr ##otto . the university through the more ##au seminary has ties to theologian frederick bu ##ech ##ner . while not catholic , bu ##ech ##ner has praised writers from notre dame and more ##au seminary created a bu ##ech ##ner prize for preaching . [SEP]\n",
- "INFO:tensorflow:token_to_orig_map: 11:0 12:1 13:2 14:3 15:4 16:5 17:6 18:7 19:8 20:9 21:10 22:11 23:12 24:12 25:13 26:14 27:15 28:16 29:16 30:17 31:18 32:19 33:20 34:20 35:20 36:21 37:22 38:23 39:23 40:24 41:24 42:25 43:25 44:26 45:27 46:28 47:29 48:30 49:31 50:32 51:32 52:33 53:34 54:35 55:36 56:37 57:38 58:38 59:39 60:40 61:40 62:41 63:42 64:43 65:44 66:45 67:46 68:47 69:48 70:49 71:50 72:51 73:52 74:52 75:53 76:54 77:54 78:55 79:56 80:57 81:57 82:57 83:58 84:59 85:60 86:61 87:62 88:63 89:64 90:65 91:66 92:66 93:67 94:68 95:69 96:69 97:69 98:70 99:71 100:72 101:72 102:73 103:74 104:75 105:76 106:76 107:76 108:77 109:78 110:79 111:80 112:80 113:80 114:81 115:82 116:83 117:84 118:85 119:85 120:86 121:87 122:88 123:89 124:90 125:91 126:92 127:92 128:92 129:92 130:93 131:94 132:95 133:95 134:96 135:96 136:96 137:97 138:98 139:99 140:100 141:101 142:102 143:103 144:104 145:104 146:105 147:106 148:107 149:108 150:108 151:108 152:109 153:110 154:111 155:111\n",
- "INFO:tensorflow:token_is_max_context: 11:True 12:True 13:True 14:True 15:True 16:True 17:True 18:True 19:True 20:True 21:True 22:True 23:True 24:True 25:True 26:True 27:True 28:True 29:True 30:True 31:True 32:True 33:True 34:True 35:True 36:True 37:True 38:True 39:True 40:True 41:True 42:True 43:True 44:True 45:True 46:True 47:True 48:True 49:True 50:True 51:True 52:True 53:True 54:True 55:True 56:True 57:True 58:True 59:True 60:True 61:True 62:True 63:True 64:True 65:True 66:True 67:True 68:True 69:True 70:True 71:True 72:True 73:True 74:True 75:True 76:True 77:True 78:True 79:True 80:True 81:True 82:True 83:True 84:True 85:True 86:True 87:True 88:True 89:True 90:True 91:True 92:True 93:True 94:True 95:True 96:True 97:True 98:True 99:True 100:True 101:True 102:True 103:True 104:True 105:True 106:True 107:True 108:True 109:True 110:True 111:True 112:True 113:True 114:True 115:True 116:True 117:True 118:True 119:True 120:True 121:True 122:True 123:True 124:True 125:True 126:True 127:True 128:True 129:True 130:True 131:True 132:True 133:True 134:True 135:True 136:True 137:True 138:True 139:True 140:True 141:True 142:True 143:True 144:True 145:True 146:True 147:True 148:True 149:True 150:True 151:True 152:True 153:True 154:True 155:True\n",
- "INFO:tensorflow:input_ids: 101 2054 2003 1996 4587 3252 2012 10289 8214 1029 102 1996 2118 2003 1996 2350 2835 1997 1996 7769 1997 4151 2892 1006 12167 2025 2049 2880 4075 1010 2029 2024 1999 4199 1007 1012 2049 2364 8705 1010 2062 4887 8705 1010 2003 2284 2006 1996 3721 2408 2358 1012 3312 2697 2013 1996 2364 2311 1012 2214 2267 1010 1996 4587 2311 2006 3721 1998 2284 2379 1996 5370 1997 2358 1012 2984 2697 1010 3506 8324 18014 7066 1012 3394 8656 1998 3428 13960 1999 27596 2160 1006 1037 2280 7822 2415 1007 1010 4151 2892 2160 1010 2004 2092 2004 8902 25438 2050 2534 2379 1996 24665 23052 1012 1996 2118 2083 1996 2062 4887 8705 2038 7208 2000 17200 5406 20934 15937 3678 1012 2096 2025 3234 1010 20934 15937 3678 2038 5868 4898 2013 10289 8214 1998 2062 4887 8705 2580 1037 20934 15937 3678 3396 2005 17979 1012 102 0 0 0 0 0 0 0 0 0 0 0 0 0 0 0 0 0 0 0 0 0 0 0 0 0 0 0 0 0 0 0 0 0 0 0 0 0 0 0 0 0 0 0 0 0 0 0 0 0 0 0 0 0 0 0 0 0 0 0 0 0 0 0 0 0 0 0 0 0 0 0 0 0 0 0 0 0 0 0 0 0 0 0 0 0 0 0 0 0 0 0 0 0 0 0 0 0 0 0 0 0 0 0 0 0 0 0 0 0 0 0 0 0 0 0 0 0 0 0 0 0 0 0 0 0 0 0 0 0 0 0 0 0 0 0 0 0 0 0 0 0 0 0 0 0 0 0 0 0 0 0 0 0 0 0 0 0 0 0 0 0 0 0 0 0 0 0 0 0 0 0 0 0 0 0 0 0 0 0 0 0 0 0 0 0 0 0 0 0 0 0 0 0 0 0 0 0 0 0 0 0 0 0 0 0 0 0 0 0 0 0 0 0 0 0 0 0 0 0 0 0 0 0 0 0 0 0\n",
- "INFO:tensorflow:input_mask: 1 1 1 1 1 1 1 1 1 1 1 1 1 1 1 1 1 1 1 1 1 1 1 1 1 1 1 1 1 1 1 1 1 1 1 1 1 1 1 1 1 1 1 1 1 1 1 1 1 1 1 1 1 1 1 1 1 1 1 1 1 1 1 1 1 1 1 1 1 1 1 1 1 1 1 1 1 1 1 1 1 1 1 1 1 1 1 1 1 1 1 1 1 1 1 1 1 1 1 1 1 1 1 1 1 1 1 1 1 1 1 1 1 1 1 1 1 1 1 1 1 1 1 1 1 1 1 1 1 1 1 1 1 1 1 1 1 1 1 1 1 1 1 1 1 1 1 1 1 1 1 1 1 1 1 1 1 0 0 0 0 0 0 0 0 0 0 0 0 0 0 0 0 0 0 0 0 0 0 0 0 0 0 0 0 0 0 0 0 0 0 0 0 0 0 0 0 0 0 0 0 0 0 0 0 0 0 0 0 0 0 0 0 0 0 0 0 0 0 0 0 0 0 0 0 0 0 0 0 0 0 0 0 0 0 0 0 0 0 0 0 0 0 0 0 0 0 0 0 0 0 0 0 0 0 0 0 0 0 0 0 0 0 0 0 0 0 0 0 0 0 0 0 0 0 0 0 0 0 0 0 0 0 0 0 0 0 0 0 0 0 0 0 0 0 0 0 0 0 0 0 0 0 0 0 0 0 0 0 0 0 0 0 0 0 0 0 0 0 0 0 0 0 0 0 0 0 0 0 0 0 0 0 0 0 0 0 0 0 0 0 0 0 0 0 0 0 0 0 0 0 0 0 0 0 0 0 0 0 0 0 0 0 0 0 0 0 0 0 0 0 0 0 0 0 0 0 0 0 0 0 0 0 0\n",
- "INFO:tensorflow:segment_ids: 0 0 0 0 0 0 0 0 0 0 0 1 1 1 1 1 1 1 1 1 1 1 1 1 1 1 1 1 1 1 1 1 1 1 1 1 1 1 1 1 1 1 1 1 1 1 1 1 1 1 1 1 1 1 1 1 1 1 1 1 1 1 1 1 1 1 1 1 1 1 1 1 1 1 1 1 1 1 1 1 1 1 1 1 1 1 1 1 1 1 1 1 1 1 1 1 1 1 1 1 1 1 1 1 1 1 1 1 1 1 1 1 1 1 1 1 1 1 1 1 1 1 1 1 1 1 1 1 1 1 1 1 1 1 1 1 1 1 1 1 1 1 1 1 1 1 1 1 1 1 1 1 1 1 1 1 1 0 0 0 0 0 0 0 0 0 0 0 0 0 0 0 0 0 0 0 0 0 0 0 0 0 0 0 0 0 0 0 0 0 0 0 0 0 0 0 0 0 0 0 0 0 0 0 0 0 0 0 0 0 0 0 0 0 0 0 0 0 0 0 0 0 0 0 0 0 0 0 0 0 0 0 0 0 0 0 0 0 0 0 0 0 0 0 0 0 0 0 0 0 0 0 0 0 0 0 0 0 0 0 0 0 0 0 0 0 0 0 0 0 0 0 0 0 0 0 0 0 0 0 0 0 0 0 0 0 0 0 0 0 0 0 0 0 0 0 0 0 0 0 0 0 0 0 0 0 0 0 0 0 0 0 0 0 0 0 0 0 0 0 0 0 0 0 0 0 0 0 0 0 0 0 0 0 0 0 0 0 0 0 0 0 0 0 0 0 0 0 0 0 0 0 0 0 0 0 0 0 0 0 0 0 0 0 0 0 0 0 0 0 0 0 0 0 0 0 0 0 0 0 0 0 0 0\n",
- "INFO:tensorflow:start_position: 59\n",
- "INFO:tensorflow:end_position: 60\n",
- "INFO:tensorflow:answer: old college\n",
- "INFO:tensorflow:*** Example ***\n",
- "INFO:tensorflow:unique_id: 1000000013\n",
- "INFO:tensorflow:example_index: 13\n",
- "INFO:tensorflow:doc_span_index: 0\n",
- "INFO:tensorflow:tokens: [CLS] what individuals live at fatima house at notre dame ? [SEP] the university is the major seat of the congregation of holy cross ( albeit not its official headquarters , which are in rome ) . its main seminary , more ##au seminary , is located on the campus across st . joseph lake from the main building . old college , the oldest building on campus and located near the shore of st . mary lake , houses undergraduate seminar ##ians . retired priests and brothers reside in fatima house ( a former retreat center ) , holy cross house , as well as col ##umb ##a hall near the gr ##otto . the university through the more ##au seminary has ties to theologian frederick bu ##ech ##ner . while not catholic , bu ##ech ##ner has praised writers from notre dame and more ##au seminary created a bu ##ech ##ner prize for preaching . [SEP]\n",
- "INFO:tensorflow:token_to_orig_map: 12:0 13:1 14:2 15:3 16:4 17:5 18:6 19:7 20:8 21:9 22:10 23:11 24:12 25:12 26:13 27:14 28:15 29:16 30:16 31:17 32:18 33:19 34:20 35:20 36:20 37:21 38:22 39:23 40:23 41:24 42:24 43:25 44:25 45:26 46:27 47:28 48:29 49:30 50:31 51:32 52:32 53:33 54:34 55:35 56:36 57:37 58:38 59:38 60:39 61:40 62:40 63:41 64:42 65:43 66:44 67:45 68:46 69:47 70:48 71:49 72:50 73:51 74:52 75:52 76:53 77:54 78:54 79:55 80:56 81:57 82:57 83:57 84:58 85:59 86:60 87:61 88:62 89:63 90:64 91:65 92:66 93:66 94:67 95:68 96:69 97:69 98:69 99:70 100:71 101:72 102:72 103:73 104:74 105:75 106:76 107:76 108:76 109:77 110:78 111:79 112:80 113:80 114:80 115:81 116:82 117:83 118:84 119:85 120:85 121:86 122:87 123:88 124:89 125:90 126:91 127:92 128:92 129:92 130:92 131:93 132:94 133:95 134:95 135:96 136:96 137:96 138:97 139:98 140:99 141:100 142:101 143:102 144:103 145:104 146:104 147:105 148:106 149:107 150:108 151:108 152:108 153:109 154:110 155:111 156:111\n"
- ]
- },
- {
- "name": "stdout",
- "output_type": "stream",
- "text": [
- "INFO:tensorflow:token_is_max_context: 12:True 13:True 14:True 15:True 16:True 17:True 18:True 19:True 20:True 21:True 22:True 23:True 24:True 25:True 26:True 27:True 28:True 29:True 30:True 31:True 32:True 33:True 34:True 35:True 36:True 37:True 38:True 39:True 40:True 41:True 42:True 43:True 44:True 45:True 46:True 47:True 48:True 49:True 50:True 51:True 52:True 53:True 54:True 55:True 56:True 57:True 58:True 59:True 60:True 61:True 62:True 63:True 64:True 65:True 66:True 67:True 68:True 69:True 70:True 71:True 72:True 73:True 74:True 75:True 76:True 77:True 78:True 79:True 80:True 81:True 82:True 83:True 84:True 85:True 86:True 87:True 88:True 89:True 90:True 91:True 92:True 93:True 94:True 95:True 96:True 97:True 98:True 99:True 100:True 101:True 102:True 103:True 104:True 105:True 106:True 107:True 108:True 109:True 110:True 111:True 112:True 113:True 114:True 115:True 116:True 117:True 118:True 119:True 120:True 121:True 122:True 123:True 124:True 125:True 126:True 127:True 128:True 129:True 130:True 131:True 132:True 133:True 134:True 135:True 136:True 137:True 138:True 139:True 140:True 141:True 142:True 143:True 144:True 145:True 146:True 147:True 148:True 149:True 150:True 151:True 152:True 153:True 154:True 155:True 156:True\n",
- "INFO:tensorflow:input_ids: 101 2054 3633 2444 2012 27596 2160 2012 10289 8214 1029 102 1996 2118 2003 1996 2350 2835 1997 1996 7769 1997 4151 2892 1006 12167 2025 2049 2880 4075 1010 2029 2024 1999 4199 1007 1012 2049 2364 8705 1010 2062 4887 8705 1010 2003 2284 2006 1996 3721 2408 2358 1012 3312 2697 2013 1996 2364 2311 1012 2214 2267 1010 1996 4587 2311 2006 3721 1998 2284 2379 1996 5370 1997 2358 1012 2984 2697 1010 3506 8324 18014 7066 1012 3394 8656 1998 3428 13960 1999 27596 2160 1006 1037 2280 7822 2415 1007 1010 4151 2892 2160 1010 2004 2092 2004 8902 25438 2050 2534 2379 1996 24665 23052 1012 1996 2118 2083 1996 2062 4887 8705 2038 7208 2000 17200 5406 20934 15937 3678 1012 2096 2025 3234 1010 20934 15937 3678 2038 5868 4898 2013 10289 8214 1998 2062 4887 8705 2580 1037 20934 15937 3678 3396 2005 17979 1012 102 0 0 0 0 0 0 0 0 0 0 0 0 0 0 0 0 0 0 0 0 0 0 0 0 0 0 0 0 0 0 0 0 0 0 0 0 0 0 0 0 0 0 0 0 0 0 0 0 0 0 0 0 0 0 0 0 0 0 0 0 0 0 0 0 0 0 0 0 0 0 0 0 0 0 0 0 0 0 0 0 0 0 0 0 0 0 0 0 0 0 0 0 0 0 0 0 0 0 0 0 0 0 0 0 0 0 0 0 0 0 0 0 0 0 0 0 0 0 0 0 0 0 0 0 0 0 0 0 0 0 0 0 0 0 0 0 0 0 0 0 0 0 0 0 0 0 0 0 0 0 0 0 0 0 0 0 0 0 0 0 0 0 0 0 0 0 0 0 0 0 0 0 0 0 0 0 0 0 0 0 0 0 0 0 0 0 0 0 0 0 0 0 0 0 0 0 0 0 0 0 0 0 0 0 0 0 0 0 0 0 0 0 0 0 0 0 0 0 0 0 0 0 0 0 0 0\n",
- "INFO:tensorflow:input_mask: 1 1 1 1 1 1 1 1 1 1 1 1 1 1 1 1 1 1 1 1 1 1 1 1 1 1 1 1 1 1 1 1 1 1 1 1 1 1 1 1 1 1 1 1 1 1 1 1 1 1 1 1 1 1 1 1 1 1 1 1 1 1 1 1 1 1 1 1 1 1 1 1 1 1 1 1 1 1 1 1 1 1 1 1 1 1 1 1 1 1 1 1 1 1 1 1 1 1 1 1 1 1 1 1 1 1 1 1 1 1 1 1 1 1 1 1 1 1 1 1 1 1 1 1 1 1 1 1 1 1 1 1 1 1 1 1 1 1 1 1 1 1 1 1 1 1 1 1 1 1 1 1 1 1 1 1 1 1 0 0 0 0 0 0 0 0 0 0 0 0 0 0 0 0 0 0 0 0 0 0 0 0 0 0 0 0 0 0 0 0 0 0 0 0 0 0 0 0 0 0 0 0 0 0 0 0 0 0 0 0 0 0 0 0 0 0 0 0 0 0 0 0 0 0 0 0 0 0 0 0 0 0 0 0 0 0 0 0 0 0 0 0 0 0 0 0 0 0 0 0 0 0 0 0 0 0 0 0 0 0 0 0 0 0 0 0 0 0 0 0 0 0 0 0 0 0 0 0 0 0 0 0 0 0 0 0 0 0 0 0 0 0 0 0 0 0 0 0 0 0 0 0 0 0 0 0 0 0 0 0 0 0 0 0 0 0 0 0 0 0 0 0 0 0 0 0 0 0 0 0 0 0 0 0 0 0 0 0 0 0 0 0 0 0 0 0 0 0 0 0 0 0 0 0 0 0 0 0 0 0 0 0 0 0 0 0 0 0 0 0 0 0 0 0 0 0 0 0 0 0 0 0 0 0\n",
- "INFO:tensorflow:segment_ids: 0 0 0 0 0 0 0 0 0 0 0 0 1 1 1 1 1 1 1 1 1 1 1 1 1 1 1 1 1 1 1 1 1 1 1 1 1 1 1 1 1 1 1 1 1 1 1 1 1 1 1 1 1 1 1 1 1 1 1 1 1 1 1 1 1 1 1 1 1 1 1 1 1 1 1 1 1 1 1 1 1 1 1 1 1 1 1 1 1 1 1 1 1 1 1 1 1 1 1 1 1 1 1 1 1 1 1 1 1 1 1 1 1 1 1 1 1 1 1 1 1 1 1 1 1 1 1 1 1 1 1 1 1 1 1 1 1 1 1 1 1 1 1 1 1 1 1 1 1 1 1 1 1 1 1 1 1 1 0 0 0 0 0 0 0 0 0 0 0 0 0 0 0 0 0 0 0 0 0 0 0 0 0 0 0 0 0 0 0 0 0 0 0 0 0 0 0 0 0 0 0 0 0 0 0 0 0 0 0 0 0 0 0 0 0 0 0 0 0 0 0 0 0 0 0 0 0 0 0 0 0 0 0 0 0 0 0 0 0 0 0 0 0 0 0 0 0 0 0 0 0 0 0 0 0 0 0 0 0 0 0 0 0 0 0 0 0 0 0 0 0 0 0 0 0 0 0 0 0 0 0 0 0 0 0 0 0 0 0 0 0 0 0 0 0 0 0 0 0 0 0 0 0 0 0 0 0 0 0 0 0 0 0 0 0 0 0 0 0 0 0 0 0 0 0 0 0 0 0 0 0 0 0 0 0 0 0 0 0 0 0 0 0 0 0 0 0 0 0 0 0 0 0 0 0 0 0 0 0 0 0 0 0 0 0 0 0 0 0 0 0 0 0 0 0 0 0 0 0 0 0 0 0 0\n",
- "INFO:tensorflow:start_position: 84\n",
- "INFO:tensorflow:end_position: 87\n",
- "INFO:tensorflow:answer: retired priests and brothers\n",
- "INFO:tensorflow:*** Example ***\n",
- "INFO:tensorflow:unique_id: 1000000014\n",
- "INFO:tensorflow:example_index: 14\n",
- "INFO:tensorflow:doc_span_index: 0\n",
- "INFO:tensorflow:tokens: [CLS] which prize did frederick bu ##ech ##ner create ? [SEP] the university is the major seat of the congregation of holy cross ( albeit not its official headquarters , which are in rome ) . its main seminary , more ##au seminary , is located on the campus across st . joseph lake from the main building . old college , the oldest building on campus and located near the shore of st . mary lake , houses undergraduate seminar ##ians . retired priests and brothers reside in fatima house ( a former retreat center ) , holy cross house , as well as col ##umb ##a hall near the gr ##otto . the university through the more ##au seminary has ties to theologian frederick bu ##ech ##ner . while not catholic , bu ##ech ##ner has praised writers from notre dame and more ##au seminary created a bu ##ech ##ner prize for preaching . [SEP]\n",
- "INFO:tensorflow:token_to_orig_map: 11:0 12:1 13:2 14:3 15:4 16:5 17:6 18:7 19:8 20:9 21:10 22:11 23:12 24:12 25:13 26:14 27:15 28:16 29:16 30:17 31:18 32:19 33:20 34:20 35:20 36:21 37:22 38:23 39:23 40:24 41:24 42:25 43:25 44:26 45:27 46:28 47:29 48:30 49:31 50:32 51:32 52:33 53:34 54:35 55:36 56:37 57:38 58:38 59:39 60:40 61:40 62:41 63:42 64:43 65:44 66:45 67:46 68:47 69:48 70:49 71:50 72:51 73:52 74:52 75:53 76:54 77:54 78:55 79:56 80:57 81:57 82:57 83:58 84:59 85:60 86:61 87:62 88:63 89:64 90:65 91:66 92:66 93:67 94:68 95:69 96:69 97:69 98:70 99:71 100:72 101:72 102:73 103:74 104:75 105:76 106:76 107:76 108:77 109:78 110:79 111:80 112:80 113:80 114:81 115:82 116:83 117:84 118:85 119:85 120:86 121:87 122:88 123:89 124:90 125:91 126:92 127:92 128:92 129:92 130:93 131:94 132:95 133:95 134:96 135:96 136:96 137:97 138:98 139:99 140:100 141:101 142:102 143:103 144:104 145:104 146:105 147:106 148:107 149:108 150:108 151:108 152:109 153:110 154:111 155:111\n",
- "INFO:tensorflow:token_is_max_context: 11:True 12:True 13:True 14:True 15:True 16:True 17:True 18:True 19:True 20:True 21:True 22:True 23:True 24:True 25:True 26:True 27:True 28:True 29:True 30:True 31:True 32:True 33:True 34:True 35:True 36:True 37:True 38:True 39:True 40:True 41:True 42:True 43:True 44:True 45:True 46:True 47:True 48:True 49:True 50:True 51:True 52:True 53:True 54:True 55:True 56:True 57:True 58:True 59:True 60:True 61:True 62:True 63:True 64:True 65:True 66:True 67:True 68:True 69:True 70:True 71:True 72:True 73:True 74:True 75:True 76:True 77:True 78:True 79:True 80:True 81:True 82:True 83:True 84:True 85:True 86:True 87:True 88:True 89:True 90:True 91:True 92:True 93:True 94:True 95:True 96:True 97:True 98:True 99:True 100:True 101:True 102:True 103:True 104:True 105:True 106:True 107:True 108:True 109:True 110:True 111:True 112:True 113:True 114:True 115:True 116:True 117:True 118:True 119:True 120:True 121:True 122:True 123:True 124:True 125:True 126:True 127:True 128:True 129:True 130:True 131:True 132:True 133:True 134:True 135:True 136:True 137:True 138:True 139:True 140:True 141:True 142:True 143:True 144:True 145:True 146:True 147:True 148:True 149:True 150:True 151:True 152:True 153:True 154:True 155:True\n",
- "INFO:tensorflow:input_ids: 101 2029 3396 2106 5406 20934 15937 3678 3443 1029 102 1996 2118 2003 1996 2350 2835 1997 1996 7769 1997 4151 2892 1006 12167 2025 2049 2880 4075 1010 2029 2024 1999 4199 1007 1012 2049 2364 8705 1010 2062 4887 8705 1010 2003 2284 2006 1996 3721 2408 2358 1012 3312 2697 2013 1996 2364 2311 1012 2214 2267 1010 1996 4587 2311 2006 3721 1998 2284 2379 1996 5370 1997 2358 1012 2984 2697 1010 3506 8324 18014 7066 1012 3394 8656 1998 3428 13960 1999 27596 2160 1006 1037 2280 7822 2415 1007 1010 4151 2892 2160 1010 2004 2092 2004 8902 25438 2050 2534 2379 1996 24665 23052 1012 1996 2118 2083 1996 2062 4887 8705 2038 7208 2000 17200 5406 20934 15937 3678 1012 2096 2025 3234 1010 20934 15937 3678 2038 5868 4898 2013 10289 8214 1998 2062 4887 8705 2580 1037 20934 15937 3678 3396 2005 17979 1012 102 0 0 0 0 0 0 0 0 0 0 0 0 0 0 0 0 0 0 0 0 0 0 0 0 0 0 0 0 0 0 0 0 0 0 0 0 0 0 0 0 0 0 0 0 0 0 0 0 0 0 0 0 0 0 0 0 0 0 0 0 0 0 0 0 0 0 0 0 0 0 0 0 0 0 0 0 0 0 0 0 0 0 0 0 0 0 0 0 0 0 0 0 0 0 0 0 0 0 0 0 0 0 0 0 0 0 0 0 0 0 0 0 0 0 0 0 0 0 0 0 0 0 0 0 0 0 0 0 0 0 0 0 0 0 0 0 0 0 0 0 0 0 0 0 0 0 0 0 0 0 0 0 0 0 0 0 0 0 0 0 0 0 0 0 0 0 0 0 0 0 0 0 0 0 0 0 0 0 0 0 0 0 0 0 0 0 0 0 0 0 0 0 0 0 0 0 0 0 0 0 0 0 0 0 0 0 0 0 0 0 0 0 0 0 0 0 0 0 0 0 0 0 0 0 0 0 0\n"
- ]
- },
- {
- "name": "stdout",
- "output_type": "stream",
- "text": [
- "INFO:tensorflow:input_mask: 1 1 1 1 1 1 1 1 1 1 1 1 1 1 1 1 1 1 1 1 1 1 1 1 1 1 1 1 1 1 1 1 1 1 1 1 1 1 1 1 1 1 1 1 1 1 1 1 1 1 1 1 1 1 1 1 1 1 1 1 1 1 1 1 1 1 1 1 1 1 1 1 1 1 1 1 1 1 1 1 1 1 1 1 1 1 1 1 1 1 1 1 1 1 1 1 1 1 1 1 1 1 1 1 1 1 1 1 1 1 1 1 1 1 1 1 1 1 1 1 1 1 1 1 1 1 1 1 1 1 1 1 1 1 1 1 1 1 1 1 1 1 1 1 1 1 1 1 1 1 1 1 1 1 1 1 1 0 0 0 0 0 0 0 0 0 0 0 0 0 0 0 0 0 0 0 0 0 0 0 0 0 0 0 0 0 0 0 0 0 0 0 0 0 0 0 0 0 0 0 0 0 0 0 0 0 0 0 0 0 0 0 0 0 0 0 0 0 0 0 0 0 0 0 0 0 0 0 0 0 0 0 0 0 0 0 0 0 0 0 0 0 0 0 0 0 0 0 0 0 0 0 0 0 0 0 0 0 0 0 0 0 0 0 0 0 0 0 0 0 0 0 0 0 0 0 0 0 0 0 0 0 0 0 0 0 0 0 0 0 0 0 0 0 0 0 0 0 0 0 0 0 0 0 0 0 0 0 0 0 0 0 0 0 0 0 0 0 0 0 0 0 0 0 0 0 0 0 0 0 0 0 0 0 0 0 0 0 0 0 0 0 0 0 0 0 0 0 0 0 0 0 0 0 0 0 0 0 0 0 0 0 0 0 0 0 0 0 0 0 0 0 0 0 0 0 0 0 0 0 0 0 0 0\n",
- "INFO:tensorflow:segment_ids: 0 0 0 0 0 0 0 0 0 0 0 1 1 1 1 1 1 1 1 1 1 1 1 1 1 1 1 1 1 1 1 1 1 1 1 1 1 1 1 1 1 1 1 1 1 1 1 1 1 1 1 1 1 1 1 1 1 1 1 1 1 1 1 1 1 1 1 1 1 1 1 1 1 1 1 1 1 1 1 1 1 1 1 1 1 1 1 1 1 1 1 1 1 1 1 1 1 1 1 1 1 1 1 1 1 1 1 1 1 1 1 1 1 1 1 1 1 1 1 1 1 1 1 1 1 1 1 1 1 1 1 1 1 1 1 1 1 1 1 1 1 1 1 1 1 1 1 1 1 1 1 1 1 1 1 1 1 0 0 0 0 0 0 0 0 0 0 0 0 0 0 0 0 0 0 0 0 0 0 0 0 0 0 0 0 0 0 0 0 0 0 0 0 0 0 0 0 0 0 0 0 0 0 0 0 0 0 0 0 0 0 0 0 0 0 0 0 0 0 0 0 0 0 0 0 0 0 0 0 0 0 0 0 0 0 0 0 0 0 0 0 0 0 0 0 0 0 0 0 0 0 0 0 0 0 0 0 0 0 0 0 0 0 0 0 0 0 0 0 0 0 0 0 0 0 0 0 0 0 0 0 0 0 0 0 0 0 0 0 0 0 0 0 0 0 0 0 0 0 0 0 0 0 0 0 0 0 0 0 0 0 0 0 0 0 0 0 0 0 0 0 0 0 0 0 0 0 0 0 0 0 0 0 0 0 0 0 0 0 0 0 0 0 0 0 0 0 0 0 0 0 0 0 0 0 0 0 0 0 0 0 0 0 0 0 0 0 0 0 0 0 0 0 0 0 0 0 0 0 0 0 0 0 0\n",
- "INFO:tensorflow:start_position: 149\n",
- "INFO:tensorflow:end_position: 154\n",
- "INFO:tensorflow:answer: bu ##ech ##ner prize for preaching\n",
- "INFO:tensorflow:*** Example ***\n",
- "INFO:tensorflow:unique_id: 1000000015\n",
- "INFO:tensorflow:example_index: 15\n",
- "INFO:tensorflow:doc_span_index: 0\n",
- "INFO:tensorflow:tokens: [CLS] how many bs level degrees are offered in the college of engineering at notre dame ? [SEP] the college of engineering was established in 1920 , however , early courses in civil and mechanical engineering were a part of the college of science since the 1870s . today the college , housed in the fitzpatrick , cu ##shing , and st ##ins ##on - re ##mic ##k halls of engineering , includes five departments of study – aerospace and mechanical engineering , chemical and bio ##mo ##le ##cular engineering , civil engineering and geological sciences , computer science and engineering , and electrical engineering – with eight b . s . degrees offered . additionally , the college offers five - year dual degree programs with the colleges of arts and letters and of business awarding additional b . a . and master of business administration ( mba ) degrees , respectively . [SEP]\n",
- "INFO:tensorflow:token_to_orig_map: 18:0 19:1 20:2 21:3 22:4 23:5 24:6 25:7 26:7 27:8 28:8 29:9 30:10 31:11 32:12 33:13 34:14 35:15 36:16 37:17 38:18 39:19 40:20 41:21 42:22 43:23 44:24 45:25 46:26 47:26 48:27 49:28 50:29 51:29 52:30 53:31 54:32 55:33 56:33 57:34 58:34 59:34 60:35 61:36 62:36 63:36 64:36 65:36 66:36 67:36 68:37 69:38 70:39 71:39 72:40 73:41 74:42 75:43 76:44 77:45 78:46 79:47 80:48 81:49 82:49 83:50 84:51 85:52 86:52 87:52 88:52 89:53 90:53 91:54 92:55 93:56 94:57 95:58 96:58 97:59 98:60 99:61 100:62 101:62 102:63 103:64 104:65 105:66 106:67 107:68 108:69 109:69 110:69 111:69 112:70 113:71 114:71 115:72 116:72 117:73 118:74 119:75 120:76 121:76 122:76 123:77 124:78 125:79 126:80 127:81 128:82 129:83 130:84 131:85 132:86 133:87 134:88 135:89 136:90 137:91 138:92 139:92 140:92 141:92 142:93 143:94 144:95 145:96 146:97 147:98 148:98 149:98 150:99 151:99 152:100 153:100\n",
- "INFO:tensorflow:token_is_max_context: 18:True 19:True 20:True 21:True 22:True 23:True 24:True 25:True 26:True 27:True 28:True 29:True 30:True 31:True 32:True 33:True 34:True 35:True 36:True 37:True 38:True 39:True 40:True 41:True 42:True 43:True 44:True 45:True 46:True 47:True 48:True 49:True 50:True 51:True 52:True 53:True 54:True 55:True 56:True 57:True 58:True 59:True 60:True 61:True 62:True 63:True 64:True 65:True 66:True 67:True 68:True 69:True 70:True 71:True 72:True 73:True 74:True 75:True 76:True 77:True 78:True 79:True 80:True 81:True 82:True 83:True 84:True 85:True 86:True 87:True 88:True 89:True 90:True 91:True 92:True 93:True 94:True 95:True 96:True 97:True 98:True 99:True 100:True 101:True 102:True 103:True 104:True 105:True 106:True 107:True 108:True 109:True 110:True 111:True 112:True 113:True 114:True 115:True 116:True 117:True 118:True 119:True 120:True 121:True 122:True 123:True 124:True 125:True 126:True 127:True 128:True 129:True 130:True 131:True 132:True 133:True 134:True 135:True 136:True 137:True 138:True 139:True 140:True 141:True 142:True 143:True 144:True 145:True 146:True 147:True 148:True 149:True 150:True 151:True 152:True 153:True\n",
- "INFO:tensorflow:input_ids: 101 2129 2116 18667 2504 5445 2024 3253 1999 1996 2267 1997 3330 2012 10289 8214 1029 102 1996 2267 1997 3330 2001 2511 1999 4444 1010 2174 1010 2220 5352 1999 2942 1998 6228 3330 2020 1037 2112 1997 1996 2267 1997 2671 2144 1996 14896 1012 2651 1996 2267 1010 7431 1999 1996 26249 1010 12731 12227 1010 1998 2358 7076 2239 1011 2128 7712 2243 9873 1997 3330 1010 2950 2274 7640 1997 2817 1516 13395 1998 6228 3330 1010 5072 1998 16012 5302 2571 15431 3330 1010 2942 3330 1998 9843 4163 1010 3274 2671 1998 3330 1010 1998 5992 3330 1516 2007 2809 1038 1012 1055 1012 5445 3253 1012 5678 1010 1996 2267 4107 2274 1011 2095 7037 3014 3454 2007 1996 6667 1997 2840 1998 4144 1998 1997 2449 21467 3176 1038 1012 1037 1012 1998 3040 1997 2449 3447 1006 15038 1007 5445 1010 4414 1012 102 0 0 0 0 0 0 0 0 0 0 0 0 0 0 0 0 0 0 0 0 0 0 0 0 0 0 0 0 0 0 0 0 0 0 0 0 0 0 0 0 0 0 0 0 0 0 0 0 0 0 0 0 0 0 0 0 0 0 0 0 0 0 0 0 0 0 0 0 0 0 0 0 0 0 0 0 0 0 0 0 0 0 0 0 0 0 0 0 0 0 0 0 0 0 0 0 0 0 0 0 0 0 0 0 0 0 0 0 0 0 0 0 0 0 0 0 0 0 0 0 0 0 0 0 0 0 0 0 0 0 0 0 0 0 0 0 0 0 0 0 0 0 0 0 0 0 0 0 0 0 0 0 0 0 0 0 0 0 0 0 0 0 0 0 0 0 0 0 0 0 0 0 0 0 0 0 0 0 0 0 0 0 0 0 0 0 0 0 0 0 0 0 0 0 0 0 0 0 0 0 0 0 0 0 0 0 0 0 0 0 0 0 0 0 0 0 0 0 0 0 0 0 0 0 0 0 0 0 0\n",
- "INFO:tensorflow:input_mask: 1 1 1 1 1 1 1 1 1 1 1 1 1 1 1 1 1 1 1 1 1 1 1 1 1 1 1 1 1 1 1 1 1 1 1 1 1 1 1 1 1 1 1 1 1 1 1 1 1 1 1 1 1 1 1 1 1 1 1 1 1 1 1 1 1 1 1 1 1 1 1 1 1 1 1 1 1 1 1 1 1 1 1 1 1 1 1 1 1 1 1 1 1 1 1 1 1 1 1 1 1 1 1 1 1 1 1 1 1 1 1 1 1 1 1 1 1 1 1 1 1 1 1 1 1 1 1 1 1 1 1 1 1 1 1 1 1 1 1 1 1 1 1 1 1 1 1 1 1 1 1 1 1 1 1 0 0 0 0 0 0 0 0 0 0 0 0 0 0 0 0 0 0 0 0 0 0 0 0 0 0 0 0 0 0 0 0 0 0 0 0 0 0 0 0 0 0 0 0 0 0 0 0 0 0 0 0 0 0 0 0 0 0 0 0 0 0 0 0 0 0 0 0 0 0 0 0 0 0 0 0 0 0 0 0 0 0 0 0 0 0 0 0 0 0 0 0 0 0 0 0 0 0 0 0 0 0 0 0 0 0 0 0 0 0 0 0 0 0 0 0 0 0 0 0 0 0 0 0 0 0 0 0 0 0 0 0 0 0 0 0 0 0 0 0 0 0 0 0 0 0 0 0 0 0 0 0 0 0 0 0 0 0 0 0 0 0 0 0 0 0 0 0 0 0 0 0 0 0 0 0 0 0 0 0 0 0 0 0 0 0 0 0 0 0 0 0 0 0 0 0 0 0 0 0 0 0 0 0 0 0 0 0 0 0 0 0 0 0 0 0 0 0 0 0 0 0 0 0 0 0 0 0 0\n",
- "INFO:tensorflow:segment_ids: 0 0 0 0 0 0 0 0 0 0 0 0 0 0 0 0 0 0 1 1 1 1 1 1 1 1 1 1 1 1 1 1 1 1 1 1 1 1 1 1 1 1 1 1 1 1 1 1 1 1 1 1 1 1 1 1 1 1 1 1 1 1 1 1 1 1 1 1 1 1 1 1 1 1 1 1 1 1 1 1 1 1 1 1 1 1 1 1 1 1 1 1 1 1 1 1 1 1 1 1 1 1 1 1 1 1 1 1 1 1 1 1 1 1 1 1 1 1 1 1 1 1 1 1 1 1 1 1 1 1 1 1 1 1 1 1 1 1 1 1 1 1 1 1 1 1 1 1 1 1 1 1 1 1 1 0 0 0 0 0 0 0 0 0 0 0 0 0 0 0 0 0 0 0 0 0 0 0 0 0 0 0 0 0 0 0 0 0 0 0 0 0 0 0 0 0 0 0 0 0 0 0 0 0 0 0 0 0 0 0 0 0 0 0 0 0 0 0 0 0 0 0 0 0 0 0 0 0 0 0 0 0 0 0 0 0 0 0 0 0 0 0 0 0 0 0 0 0 0 0 0 0 0 0 0 0 0 0 0 0 0 0 0 0 0 0 0 0 0 0 0 0 0 0 0 0 0 0 0 0 0 0 0 0 0 0 0 0 0 0 0 0 0 0 0 0 0 0 0 0 0 0 0 0 0 0 0 0 0 0 0 0 0 0 0 0 0 0 0 0 0 0 0 0 0 0 0 0 0 0 0 0 0 0 0 0 0 0 0 0 0 0 0 0 0 0 0 0 0 0 0 0 0 0 0 0 0 0 0 0 0 0 0 0 0 0 0 0 0 0 0 0 0 0 0 0 0 0 0 0 0 0 0 0\n",
- "INFO:tensorflow:start_position: 107\n",
- "INFO:tensorflow:end_position: 107\n",
- "INFO:tensorflow:answer: eight\n",
- "INFO:tensorflow:*** Example ***\n",
- "INFO:tensorflow:unique_id: 1000000016\n",
- "INFO:tensorflow:example_index: 16\n",
- "INFO:tensorflow:doc_span_index: 0\n",
- "INFO:tensorflow:tokens: [CLS] in what year was the college of engineering at notre dame formed ? [SEP] the college of engineering was established in 1920 , however , early courses in civil and mechanical engineering were a part of the college of science since the 1870s . today the college , housed in the fitzpatrick , cu ##shing , and st ##ins ##on - re ##mic ##k halls of engineering , includes five departments of study – aerospace and mechanical engineering , chemical and bio ##mo ##le ##cular engineering , civil engineering and geological sciences , computer science and engineering , and electrical engineering – with eight b . s . degrees offered . additionally , the college offers five - year dual degree programs with the colleges of arts and letters and of business awarding additional b . a . and master of business administration ( mba ) degrees , respectively . [SEP]\n"
- ]
- },
- {
- "name": "stdout",
- "output_type": "stream",
- "text": [
- "INFO:tensorflow:token_to_orig_map: 15:0 16:1 17:2 18:3 19:4 20:5 21:6 22:7 23:7 24:8 25:8 26:9 27:10 28:11 29:12 30:13 31:14 32:15 33:16 34:17 35:18 36:19 37:20 38:21 39:22 40:23 41:24 42:25 43:26 44:26 45:27 46:28 47:29 48:29 49:30 50:31 51:32 52:33 53:33 54:34 55:34 56:34 57:35 58:36 59:36 60:36 61:36 62:36 63:36 64:36 65:37 66:38 67:39 68:39 69:40 70:41 71:42 72:43 73:44 74:45 75:46 76:47 77:48 78:49 79:49 80:50 81:51 82:52 83:52 84:52 85:52 86:53 87:53 88:54 89:55 90:56 91:57 92:58 93:58 94:59 95:60 96:61 97:62 98:62 99:63 100:64 101:65 102:66 103:67 104:68 105:69 106:69 107:69 108:69 109:70 110:71 111:71 112:72 113:72 114:73 115:74 116:75 117:76 118:76 119:76 120:77 121:78 122:79 123:80 124:81 125:82 126:83 127:84 128:85 129:86 130:87 131:88 132:89 133:90 134:91 135:92 136:92 137:92 138:92 139:93 140:94 141:95 142:96 143:97 144:98 145:98 146:98 147:99 148:99 149:100 150:100\n",
- "INFO:tensorflow:token_is_max_context: 15:True 16:True 17:True 18:True 19:True 20:True 21:True 22:True 23:True 24:True 25:True 26:True 27:True 28:True 29:True 30:True 31:True 32:True 33:True 34:True 35:True 36:True 37:True 38:True 39:True 40:True 41:True 42:True 43:True 44:True 45:True 46:True 47:True 48:True 49:True 50:True 51:True 52:True 53:True 54:True 55:True 56:True 57:True 58:True 59:True 60:True 61:True 62:True 63:True 64:True 65:True 66:True 67:True 68:True 69:True 70:True 71:True 72:True 73:True 74:True 75:True 76:True 77:True 78:True 79:True 80:True 81:True 82:True 83:True 84:True 85:True 86:True 87:True 88:True 89:True 90:True 91:True 92:True 93:True 94:True 95:True 96:True 97:True 98:True 99:True 100:True 101:True 102:True 103:True 104:True 105:True 106:True 107:True 108:True 109:True 110:True 111:True 112:True 113:True 114:True 115:True 116:True 117:True 118:True 119:True 120:True 121:True 122:True 123:True 124:True 125:True 126:True 127:True 128:True 129:True 130:True 131:True 132:True 133:True 134:True 135:True 136:True 137:True 138:True 139:True 140:True 141:True 142:True 143:True 144:True 145:True 146:True 147:True 148:True 149:True 150:True\n",
- "INFO:tensorflow:input_ids: 101 1999 2054 2095 2001 1996 2267 1997 3330 2012 10289 8214 2719 1029 102 1996 2267 1997 3330 2001 2511 1999 4444 1010 2174 1010 2220 5352 1999 2942 1998 6228 3330 2020 1037 2112 1997 1996 2267 1997 2671 2144 1996 14896 1012 2651 1996 2267 1010 7431 1999 1996 26249 1010 12731 12227 1010 1998 2358 7076 2239 1011 2128 7712 2243 9873 1997 3330 1010 2950 2274 7640 1997 2817 1516 13395 1998 6228 3330 1010 5072 1998 16012 5302 2571 15431 3330 1010 2942 3330 1998 9843 4163 1010 3274 2671 1998 3330 1010 1998 5992 3330 1516 2007 2809 1038 1012 1055 1012 5445 3253 1012 5678 1010 1996 2267 4107 2274 1011 2095 7037 3014 3454 2007 1996 6667 1997 2840 1998 4144 1998 1997 2449 21467 3176 1038 1012 1037 1012 1998 3040 1997 2449 3447 1006 15038 1007 5445 1010 4414 1012 102 0 0 0 0 0 0 0 0 0 0 0 0 0 0 0 0 0 0 0 0 0 0 0 0 0 0 0 0 0 0 0 0 0 0 0 0 0 0 0 0 0 0 0 0 0 0 0 0 0 0 0 0 0 0 0 0 0 0 0 0 0 0 0 0 0 0 0 0 0 0 0 0 0 0 0 0 0 0 0 0 0 0 0 0 0 0 0 0 0 0 0 0 0 0 0 0 0 0 0 0 0 0 0 0 0 0 0 0 0 0 0 0 0 0 0 0 0 0 0 0 0 0 0 0 0 0 0 0 0 0 0 0 0 0 0 0 0 0 0 0 0 0 0 0 0 0 0 0 0 0 0 0 0 0 0 0 0 0 0 0 0 0 0 0 0 0 0 0 0 0 0 0 0 0 0 0 0 0 0 0 0 0 0 0 0 0 0 0 0 0 0 0 0 0 0 0 0 0 0 0 0 0 0 0 0 0 0 0 0 0 0 0 0 0 0 0 0 0 0 0 0 0 0 0 0 0 0 0 0 0 0 0\n",
- "INFO:tensorflow:input_mask: 1 1 1 1 1 1 1 1 1 1 1 1 1 1 1 1 1 1 1 1 1 1 1 1 1 1 1 1 1 1 1 1 1 1 1 1 1 1 1 1 1 1 1 1 1 1 1 1 1 1 1 1 1 1 1 1 1 1 1 1 1 1 1 1 1 1 1 1 1 1 1 1 1 1 1 1 1 1 1 1 1 1 1 1 1 1 1 1 1 1 1 1 1 1 1 1 1 1 1 1 1 1 1 1 1 1 1 1 1 1 1 1 1 1 1 1 1 1 1 1 1 1 1 1 1 1 1 1 1 1 1 1 1 1 1 1 1 1 1 1 1 1 1 1 1 1 1 1 1 1 1 1 0 0 0 0 0 0 0 0 0 0 0 0 0 0 0 0 0 0 0 0 0 0 0 0 0 0 0 0 0 0 0 0 0 0 0 0 0 0 0 0 0 0 0 0 0 0 0 0 0 0 0 0 0 0 0 0 0 0 0 0 0 0 0 0 0 0 0 0 0 0 0 0 0 0 0 0 0 0 0 0 0 0 0 0 0 0 0 0 0 0 0 0 0 0 0 0 0 0 0 0 0 0 0 0 0 0 0 0 0 0 0 0 0 0 0 0 0 0 0 0 0 0 0 0 0 0 0 0 0 0 0 0 0 0 0 0 0 0 0 0 0 0 0 0 0 0 0 0 0 0 0 0 0 0 0 0 0 0 0 0 0 0 0 0 0 0 0 0 0 0 0 0 0 0 0 0 0 0 0 0 0 0 0 0 0 0 0 0 0 0 0 0 0 0 0 0 0 0 0 0 0 0 0 0 0 0 0 0 0 0 0 0 0 0 0 0 0 0 0 0 0 0 0 0 0 0 0 0 0 0 0 0\n",
- "INFO:tensorflow:segment_ids: 0 0 0 0 0 0 0 0 0 0 0 0 0 0 0 1 1 1 1 1 1 1 1 1 1 1 1 1 1 1 1 1 1 1 1 1 1 1 1 1 1 1 1 1 1 1 1 1 1 1 1 1 1 1 1 1 1 1 1 1 1 1 1 1 1 1 1 1 1 1 1 1 1 1 1 1 1 1 1 1 1 1 1 1 1 1 1 1 1 1 1 1 1 1 1 1 1 1 1 1 1 1 1 1 1 1 1 1 1 1 1 1 1 1 1 1 1 1 1 1 1 1 1 1 1 1 1 1 1 1 1 1 1 1 1 1 1 1 1 1 1 1 1 1 1 1 1 1 1 1 1 1 0 0 0 0 0 0 0 0 0 0 0 0 0 0 0 0 0 0 0 0 0 0 0 0 0 0 0 0 0 0 0 0 0 0 0 0 0 0 0 0 0 0 0 0 0 0 0 0 0 0 0 0 0 0 0 0 0 0 0 0 0 0 0 0 0 0 0 0 0 0 0 0 0 0 0 0 0 0 0 0 0 0 0 0 0 0 0 0 0 0 0 0 0 0 0 0 0 0 0 0 0 0 0 0 0 0 0 0 0 0 0 0 0 0 0 0 0 0 0 0 0 0 0 0 0 0 0 0 0 0 0 0 0 0 0 0 0 0 0 0 0 0 0 0 0 0 0 0 0 0 0 0 0 0 0 0 0 0 0 0 0 0 0 0 0 0 0 0 0 0 0 0 0 0 0 0 0 0 0 0 0 0 0 0 0 0 0 0 0 0 0 0 0 0 0 0 0 0 0 0 0 0 0 0 0 0 0 0 0 0 0 0 0 0 0 0 0 0 0 0 0 0 0 0 0 0 0 0 0 0 0 0\n",
- "INFO:tensorflow:start_position: 22\n",
- "INFO:tensorflow:end_position: 22\n",
- "INFO:tensorflow:answer: 1920\n",
- "INFO:tensorflow:*** Example ***\n",
- "INFO:tensorflow:unique_id: 1000000017\n",
- "INFO:tensorflow:example_index: 17\n",
- "INFO:tensorflow:doc_span_index: 0\n",
- "INFO:tensorflow:tokens: [CLS] before the creation of the college of engineering similar studies were carried out at which notre dame college ? [SEP] the college of engineering was established in 1920 , however , early courses in civil and mechanical engineering were a part of the college of science since the 1870s . today the college , housed in the fitzpatrick , cu ##shing , and st ##ins ##on - re ##mic ##k halls of engineering , includes five departments of study – aerospace and mechanical engineering , chemical and bio ##mo ##le ##cular engineering , civil engineering and geological sciences , computer science and engineering , and electrical engineering – with eight b . s . degrees offered . additionally , the college offers five - year dual degree programs with the colleges of arts and letters and of business awarding additional b . a . and master of business administration ( mba ) degrees , respectively . [SEP]\n",
- "INFO:tensorflow:token_to_orig_map: 21:0 22:1 23:2 24:3 25:4 26:5 27:6 28:7 29:7 30:8 31:8 32:9 33:10 34:11 35:12 36:13 37:14 38:15 39:16 40:17 41:18 42:19 43:20 44:21 45:22 46:23 47:24 48:25 49:26 50:26 51:27 52:28 53:29 54:29 55:30 56:31 57:32 58:33 59:33 60:34 61:34 62:34 63:35 64:36 65:36 66:36 67:36 68:36 69:36 70:36 71:37 72:38 73:39 74:39 75:40 76:41 77:42 78:43 79:44 80:45 81:46 82:47 83:48 84:49 85:49 86:50 87:51 88:52 89:52 90:52 91:52 92:53 93:53 94:54 95:55 96:56 97:57 98:58 99:58 100:59 101:60 102:61 103:62 104:62 105:63 106:64 107:65 108:66 109:67 110:68 111:69 112:69 113:69 114:69 115:70 116:71 117:71 118:72 119:72 120:73 121:74 122:75 123:76 124:76 125:76 126:77 127:78 128:79 129:80 130:81 131:82 132:83 133:84 134:85 135:86 136:87 137:88 138:89 139:90 140:91 141:92 142:92 143:92 144:92 145:93 146:94 147:95 148:96 149:97 150:98 151:98 152:98 153:99 154:99 155:100 156:100\n",
- "INFO:tensorflow:token_is_max_context: 21:True 22:True 23:True 24:True 25:True 26:True 27:True 28:True 29:True 30:True 31:True 32:True 33:True 34:True 35:True 36:True 37:True 38:True 39:True 40:True 41:True 42:True 43:True 44:True 45:True 46:True 47:True 48:True 49:True 50:True 51:True 52:True 53:True 54:True 55:True 56:True 57:True 58:True 59:True 60:True 61:True 62:True 63:True 64:True 65:True 66:True 67:True 68:True 69:True 70:True 71:True 72:True 73:True 74:True 75:True 76:True 77:True 78:True 79:True 80:True 81:True 82:True 83:True 84:True 85:True 86:True 87:True 88:True 89:True 90:True 91:True 92:True 93:True 94:True 95:True 96:True 97:True 98:True 99:True 100:True 101:True 102:True 103:True 104:True 105:True 106:True 107:True 108:True 109:True 110:True 111:True 112:True 113:True 114:True 115:True 116:True 117:True 118:True 119:True 120:True 121:True 122:True 123:True 124:True 125:True 126:True 127:True 128:True 129:True 130:True 131:True 132:True 133:True 134:True 135:True 136:True 137:True 138:True 139:True 140:True 141:True 142:True 143:True 144:True 145:True 146:True 147:True 148:True 149:True 150:True 151:True 152:True 153:True 154:True 155:True 156:True\n",
- "INFO:tensorflow:input_ids: 101 2077 1996 4325 1997 1996 2267 1997 3330 2714 2913 2020 3344 2041 2012 2029 10289 8214 2267 1029 102 1996 2267 1997 3330 2001 2511 1999 4444 1010 2174 1010 2220 5352 1999 2942 1998 6228 3330 2020 1037 2112 1997 1996 2267 1997 2671 2144 1996 14896 1012 2651 1996 2267 1010 7431 1999 1996 26249 1010 12731 12227 1010 1998 2358 7076 2239 1011 2128 7712 2243 9873 1997 3330 1010 2950 2274 7640 1997 2817 1516 13395 1998 6228 3330 1010 5072 1998 16012 5302 2571 15431 3330 1010 2942 3330 1998 9843 4163 1010 3274 2671 1998 3330 1010 1998 5992 3330 1516 2007 2809 1038 1012 1055 1012 5445 3253 1012 5678 1010 1996 2267 4107 2274 1011 2095 7037 3014 3454 2007 1996 6667 1997 2840 1998 4144 1998 1997 2449 21467 3176 1038 1012 1037 1012 1998 3040 1997 2449 3447 1006 15038 1007 5445 1010 4414 1012 102 0 0 0 0 0 0 0 0 0 0 0 0 0 0 0 0 0 0 0 0 0 0 0 0 0 0 0 0 0 0 0 0 0 0 0 0 0 0 0 0 0 0 0 0 0 0 0 0 0 0 0 0 0 0 0 0 0 0 0 0 0 0 0 0 0 0 0 0 0 0 0 0 0 0 0 0 0 0 0 0 0 0 0 0 0 0 0 0 0 0 0 0 0 0 0 0 0 0 0 0 0 0 0 0 0 0 0 0 0 0 0 0 0 0 0 0 0 0 0 0 0 0 0 0 0 0 0 0 0 0 0 0 0 0 0 0 0 0 0 0 0 0 0 0 0 0 0 0 0 0 0 0 0 0 0 0 0 0 0 0 0 0 0 0 0 0 0 0 0 0 0 0 0 0 0 0 0 0 0 0 0 0 0 0 0 0 0 0 0 0 0 0 0 0 0 0 0 0 0 0 0 0 0 0 0 0 0 0 0 0 0 0 0 0 0 0 0 0 0 0 0 0 0 0 0 0\n"
- ]
- },
- {
- "name": "stdout",
- "output_type": "stream",
- "text": [
- "INFO:tensorflow:input_mask: 1 1 1 1 1 1 1 1 1 1 1 1 1 1 1 1 1 1 1 1 1 1 1 1 1 1 1 1 1 1 1 1 1 1 1 1 1 1 1 1 1 1 1 1 1 1 1 1 1 1 1 1 1 1 1 1 1 1 1 1 1 1 1 1 1 1 1 1 1 1 1 1 1 1 1 1 1 1 1 1 1 1 1 1 1 1 1 1 1 1 1 1 1 1 1 1 1 1 1 1 1 1 1 1 1 1 1 1 1 1 1 1 1 1 1 1 1 1 1 1 1 1 1 1 1 1 1 1 1 1 1 1 1 1 1 1 1 1 1 1 1 1 1 1 1 1 1 1 1 1 1 1 1 1 1 1 1 1 0 0 0 0 0 0 0 0 0 0 0 0 0 0 0 0 0 0 0 0 0 0 0 0 0 0 0 0 0 0 0 0 0 0 0 0 0 0 0 0 0 0 0 0 0 0 0 0 0 0 0 0 0 0 0 0 0 0 0 0 0 0 0 0 0 0 0 0 0 0 0 0 0 0 0 0 0 0 0 0 0 0 0 0 0 0 0 0 0 0 0 0 0 0 0 0 0 0 0 0 0 0 0 0 0 0 0 0 0 0 0 0 0 0 0 0 0 0 0 0 0 0 0 0 0 0 0 0 0 0 0 0 0 0 0 0 0 0 0 0 0 0 0 0 0 0 0 0 0 0 0 0 0 0 0 0 0 0 0 0 0 0 0 0 0 0 0 0 0 0 0 0 0 0 0 0 0 0 0 0 0 0 0 0 0 0 0 0 0 0 0 0 0 0 0 0 0 0 0 0 0 0 0 0 0 0 0 0 0 0 0 0 0 0 0 0 0 0 0 0 0 0 0 0 0 0\n",
- "INFO:tensorflow:segment_ids: 0 0 0 0 0 0 0 0 0 0 0 0 0 0 0 0 0 0 0 0 0 1 1 1 1 1 1 1 1 1 1 1 1 1 1 1 1 1 1 1 1 1 1 1 1 1 1 1 1 1 1 1 1 1 1 1 1 1 1 1 1 1 1 1 1 1 1 1 1 1 1 1 1 1 1 1 1 1 1 1 1 1 1 1 1 1 1 1 1 1 1 1 1 1 1 1 1 1 1 1 1 1 1 1 1 1 1 1 1 1 1 1 1 1 1 1 1 1 1 1 1 1 1 1 1 1 1 1 1 1 1 1 1 1 1 1 1 1 1 1 1 1 1 1 1 1 1 1 1 1 1 1 1 1 1 1 1 1 0 0 0 0 0 0 0 0 0 0 0 0 0 0 0 0 0 0 0 0 0 0 0 0 0 0 0 0 0 0 0 0 0 0 0 0 0 0 0 0 0 0 0 0 0 0 0 0 0 0 0 0 0 0 0 0 0 0 0 0 0 0 0 0 0 0 0 0 0 0 0 0 0 0 0 0 0 0 0 0 0 0 0 0 0 0 0 0 0 0 0 0 0 0 0 0 0 0 0 0 0 0 0 0 0 0 0 0 0 0 0 0 0 0 0 0 0 0 0 0 0 0 0 0 0 0 0 0 0 0 0 0 0 0 0 0 0 0 0 0 0 0 0 0 0 0 0 0 0 0 0 0 0 0 0 0 0 0 0 0 0 0 0 0 0 0 0 0 0 0 0 0 0 0 0 0 0 0 0 0 0 0 0 0 0 0 0 0 0 0 0 0 0 0 0 0 0 0 0 0 0 0 0 0 0 0 0 0 0 0 0 0 0 0 0 0 0 0 0 0 0 0 0 0 0 0\n",
- "INFO:tensorflow:start_position: 43\n",
- "INFO:tensorflow:end_position: 46\n",
- "INFO:tensorflow:answer: the college of science\n",
- "INFO:tensorflow:*** Example ***\n",
- "INFO:tensorflow:unique_id: 1000000018\n",
- "INFO:tensorflow:example_index: 18\n",
- "INFO:tensorflow:doc_span_index: 0\n",
- "INFO:tensorflow:tokens: [CLS] how many departments are within the st ##ins ##on - re ##mic ##k hall of engineering ? [SEP] the college of engineering was established in 1920 , however , early courses in civil and mechanical engineering were a part of the college of science since the 1870s . today the college , housed in the fitzpatrick , cu ##shing , and st ##ins ##on - re ##mic ##k halls of engineering , includes five departments of study – aerospace and mechanical engineering , chemical and bio ##mo ##le ##cular engineering , civil engineering and geological sciences , computer science and engineering , and electrical engineering – with eight b . s . degrees offered . additionally , the college offers five - year dual degree programs with the colleges of arts and letters and of business awarding additional b . a . and master of business administration ( mba ) degrees , respectively . [SEP]\n",
- "INFO:tensorflow:token_to_orig_map: 19:0 20:1 21:2 22:3 23:4 24:5 25:6 26:7 27:7 28:8 29:8 30:9 31:10 32:11 33:12 34:13 35:14 36:15 37:16 38:17 39:18 40:19 41:20 42:21 43:22 44:23 45:24 46:25 47:26 48:26 49:27 50:28 51:29 52:29 53:30 54:31 55:32 56:33 57:33 58:34 59:34 60:34 61:35 62:36 63:36 64:36 65:36 66:36 67:36 68:36 69:37 70:38 71:39 72:39 73:40 74:41 75:42 76:43 77:44 78:45 79:46 80:47 81:48 82:49 83:49 84:50 85:51 86:52 87:52 88:52 89:52 90:53 91:53 92:54 93:55 94:56 95:57 96:58 97:58 98:59 99:60 100:61 101:62 102:62 103:63 104:64 105:65 106:66 107:67 108:68 109:69 110:69 111:69 112:69 113:70 114:71 115:71 116:72 117:72 118:73 119:74 120:75 121:76 122:76 123:76 124:77 125:78 126:79 127:80 128:81 129:82 130:83 131:84 132:85 133:86 134:87 135:88 136:89 137:90 138:91 139:92 140:92 141:92 142:92 143:93 144:94 145:95 146:96 147:97 148:98 149:98 150:98 151:99 152:99 153:100 154:100\n",
- "INFO:tensorflow:token_is_max_context: 19:True 20:True 21:True 22:True 23:True 24:True 25:True 26:True 27:True 28:True 29:True 30:True 31:True 32:True 33:True 34:True 35:True 36:True 37:True 38:True 39:True 40:True 41:True 42:True 43:True 44:True 45:True 46:True 47:True 48:True 49:True 50:True 51:True 52:True 53:True 54:True 55:True 56:True 57:True 58:True 59:True 60:True 61:True 62:True 63:True 64:True 65:True 66:True 67:True 68:True 69:True 70:True 71:True 72:True 73:True 74:True 75:True 76:True 77:True 78:True 79:True 80:True 81:True 82:True 83:True 84:True 85:True 86:True 87:True 88:True 89:True 90:True 91:True 92:True 93:True 94:True 95:True 96:True 97:True 98:True 99:True 100:True 101:True 102:True 103:True 104:True 105:True 106:True 107:True 108:True 109:True 110:True 111:True 112:True 113:True 114:True 115:True 116:True 117:True 118:True 119:True 120:True 121:True 122:True 123:True 124:True 125:True 126:True 127:True 128:True 129:True 130:True 131:True 132:True 133:True 134:True 135:True 136:True 137:True 138:True 139:True 140:True 141:True 142:True 143:True 144:True 145:True 146:True 147:True 148:True 149:True 150:True 151:True 152:True 153:True 154:True\n",
- "INFO:tensorflow:input_ids: 101 2129 2116 7640 2024 2306 1996 2358 7076 2239 1011 2128 7712 2243 2534 1997 3330 1029 102 1996 2267 1997 3330 2001 2511 1999 4444 1010 2174 1010 2220 5352 1999 2942 1998 6228 3330 2020 1037 2112 1997 1996 2267 1997 2671 2144 1996 14896 1012 2651 1996 2267 1010 7431 1999 1996 26249 1010 12731 12227 1010 1998 2358 7076 2239 1011 2128 7712 2243 9873 1997 3330 1010 2950 2274 7640 1997 2817 1516 13395 1998 6228 3330 1010 5072 1998 16012 5302 2571 15431 3330 1010 2942 3330 1998 9843 4163 1010 3274 2671 1998 3330 1010 1998 5992 3330 1516 2007 2809 1038 1012 1055 1012 5445 3253 1012 5678 1010 1996 2267 4107 2274 1011 2095 7037 3014 3454 2007 1996 6667 1997 2840 1998 4144 1998 1997 2449 21467 3176 1038 1012 1037 1012 1998 3040 1997 2449 3447 1006 15038 1007 5445 1010 4414 1012 102 0 0 0 0 0 0 0 0 0 0 0 0 0 0 0 0 0 0 0 0 0 0 0 0 0 0 0 0 0 0 0 0 0 0 0 0 0 0 0 0 0 0 0 0 0 0 0 0 0 0 0 0 0 0 0 0 0 0 0 0 0 0 0 0 0 0 0 0 0 0 0 0 0 0 0 0 0 0 0 0 0 0 0 0 0 0 0 0 0 0 0 0 0 0 0 0 0 0 0 0 0 0 0 0 0 0 0 0 0 0 0 0 0 0 0 0 0 0 0 0 0 0 0 0 0 0 0 0 0 0 0 0 0 0 0 0 0 0 0 0 0 0 0 0 0 0 0 0 0 0 0 0 0 0 0 0 0 0 0 0 0 0 0 0 0 0 0 0 0 0 0 0 0 0 0 0 0 0 0 0 0 0 0 0 0 0 0 0 0 0 0 0 0 0 0 0 0 0 0 0 0 0 0 0 0 0 0 0 0 0 0 0 0 0 0 0 0 0 0 0 0 0 0 0 0 0 0 0\n",
- "INFO:tensorflow:input_mask: 1 1 1 1 1 1 1 1 1 1 1 1 1 1 1 1 1 1 1 1 1 1 1 1 1 1 1 1 1 1 1 1 1 1 1 1 1 1 1 1 1 1 1 1 1 1 1 1 1 1 1 1 1 1 1 1 1 1 1 1 1 1 1 1 1 1 1 1 1 1 1 1 1 1 1 1 1 1 1 1 1 1 1 1 1 1 1 1 1 1 1 1 1 1 1 1 1 1 1 1 1 1 1 1 1 1 1 1 1 1 1 1 1 1 1 1 1 1 1 1 1 1 1 1 1 1 1 1 1 1 1 1 1 1 1 1 1 1 1 1 1 1 1 1 1 1 1 1 1 1 1 1 1 1 1 1 0 0 0 0 0 0 0 0 0 0 0 0 0 0 0 0 0 0 0 0 0 0 0 0 0 0 0 0 0 0 0 0 0 0 0 0 0 0 0 0 0 0 0 0 0 0 0 0 0 0 0 0 0 0 0 0 0 0 0 0 0 0 0 0 0 0 0 0 0 0 0 0 0 0 0 0 0 0 0 0 0 0 0 0 0 0 0 0 0 0 0 0 0 0 0 0 0 0 0 0 0 0 0 0 0 0 0 0 0 0 0 0 0 0 0 0 0 0 0 0 0 0 0 0 0 0 0 0 0 0 0 0 0 0 0 0 0 0 0 0 0 0 0 0 0 0 0 0 0 0 0 0 0 0 0 0 0 0 0 0 0 0 0 0 0 0 0 0 0 0 0 0 0 0 0 0 0 0 0 0 0 0 0 0 0 0 0 0 0 0 0 0 0 0 0 0 0 0 0 0 0 0 0 0 0 0 0 0 0 0 0 0 0 0 0 0 0 0 0 0 0 0 0 0 0 0 0 0\n",
- "INFO:tensorflow:segment_ids: 0 0 0 0 0 0 0 0 0 0 0 0 0 0 0 0 0 0 0 1 1 1 1 1 1 1 1 1 1 1 1 1 1 1 1 1 1 1 1 1 1 1 1 1 1 1 1 1 1 1 1 1 1 1 1 1 1 1 1 1 1 1 1 1 1 1 1 1 1 1 1 1 1 1 1 1 1 1 1 1 1 1 1 1 1 1 1 1 1 1 1 1 1 1 1 1 1 1 1 1 1 1 1 1 1 1 1 1 1 1 1 1 1 1 1 1 1 1 1 1 1 1 1 1 1 1 1 1 1 1 1 1 1 1 1 1 1 1 1 1 1 1 1 1 1 1 1 1 1 1 1 1 1 1 1 1 0 0 0 0 0 0 0 0 0 0 0 0 0 0 0 0 0 0 0 0 0 0 0 0 0 0 0 0 0 0 0 0 0 0 0 0 0 0 0 0 0 0 0 0 0 0 0 0 0 0 0 0 0 0 0 0 0 0 0 0 0 0 0 0 0 0 0 0 0 0 0 0 0 0 0 0 0 0 0 0 0 0 0 0 0 0 0 0 0 0 0 0 0 0 0 0 0 0 0 0 0 0 0 0 0 0 0 0 0 0 0 0 0 0 0 0 0 0 0 0 0 0 0 0 0 0 0 0 0 0 0 0 0 0 0 0 0 0 0 0 0 0 0 0 0 0 0 0 0 0 0 0 0 0 0 0 0 0 0 0 0 0 0 0 0 0 0 0 0 0 0 0 0 0 0 0 0 0 0 0 0 0 0 0 0 0 0 0 0 0 0 0 0 0 0 0 0 0 0 0 0 0 0 0 0 0 0 0 0 0 0 0 0 0 0 0 0 0 0 0 0 0 0 0 0 0 0 0\n",
- "INFO:tensorflow:start_position: 74\n",
- "INFO:tensorflow:end_position: 74\n",
- "INFO:tensorflow:answer: five\n",
- "INFO:tensorflow:*** Example ***\n",
- "INFO:tensorflow:unique_id: 1000000019\n",
- "INFO:tensorflow:example_index: 19\n",
- "INFO:tensorflow:doc_span_index: 0\n",
- "INFO:tensorflow:tokens: [CLS] the college of science began to offer civil engineering courses beginning at what time at notre dame ? [SEP] the college of engineering was established in 1920 , however , early courses in civil and mechanical engineering were a part of the college of science since the 1870s . today the college , housed in the fitzpatrick , cu ##shing , and st ##ins ##on - re ##mic ##k halls of engineering , includes five departments of study – aerospace and mechanical engineering , chemical and bio ##mo ##le ##cular engineering , civil engineering and geological sciences , computer science and engineering , and electrical engineering – with eight b . s . degrees offered . additionally , the college offers five - year dual degree programs with the colleges of arts and letters and of business awarding additional b . a . and master of business administration ( mba ) degrees , respectively . [SEP]\n"
- ]
- },
- {
- "name": "stdout",
- "output_type": "stream",
- "text": [
- "INFO:tensorflow:token_to_orig_map: 20:0 21:1 22:2 23:3 24:4 25:5 26:6 27:7 28:7 29:8 30:8 31:9 32:10 33:11 34:12 35:13 36:14 37:15 38:16 39:17 40:18 41:19 42:20 43:21 44:22 45:23 46:24 47:25 48:26 49:26 50:27 51:28 52:29 53:29 54:30 55:31 56:32 57:33 58:33 59:34 60:34 61:34 62:35 63:36 64:36 65:36 66:36 67:36 68:36 69:36 70:37 71:38 72:39 73:39 74:40 75:41 76:42 77:43 78:44 79:45 80:46 81:47 82:48 83:49 84:49 85:50 86:51 87:52 88:52 89:52 90:52 91:53 92:53 93:54 94:55 95:56 96:57 97:58 98:58 99:59 100:60 101:61 102:62 103:62 104:63 105:64 106:65 107:66 108:67 109:68 110:69 111:69 112:69 113:69 114:70 115:71 116:71 117:72 118:72 119:73 120:74 121:75 122:76 123:76 124:76 125:77 126:78 127:79 128:80 129:81 130:82 131:83 132:84 133:85 134:86 135:87 136:88 137:89 138:90 139:91 140:92 141:92 142:92 143:92 144:93 145:94 146:95 147:96 148:97 149:98 150:98 151:98 152:99 153:99 154:100 155:100\n",
- "INFO:tensorflow:token_is_max_context: 20:True 21:True 22:True 23:True 24:True 25:True 26:True 27:True 28:True 29:True 30:True 31:True 32:True 33:True 34:True 35:True 36:True 37:True 38:True 39:True 40:True 41:True 42:True 43:True 44:True 45:True 46:True 47:True 48:True 49:True 50:True 51:True 52:True 53:True 54:True 55:True 56:True 57:True 58:True 59:True 60:True 61:True 62:True 63:True 64:True 65:True 66:True 67:True 68:True 69:True 70:True 71:True 72:True 73:True 74:True 75:True 76:True 77:True 78:True 79:True 80:True 81:True 82:True 83:True 84:True 85:True 86:True 87:True 88:True 89:True 90:True 91:True 92:True 93:True 94:True 95:True 96:True 97:True 98:True 99:True 100:True 101:True 102:True 103:True 104:True 105:True 106:True 107:True 108:True 109:True 110:True 111:True 112:True 113:True 114:True 115:True 116:True 117:True 118:True 119:True 120:True 121:True 122:True 123:True 124:True 125:True 126:True 127:True 128:True 129:True 130:True 131:True 132:True 133:True 134:True 135:True 136:True 137:True 138:True 139:True 140:True 141:True 142:True 143:True 144:True 145:True 146:True 147:True 148:True 149:True 150:True 151:True 152:True 153:True 154:True 155:True\n",
- "INFO:tensorflow:input_ids: 101 1996 2267 1997 2671 2211 2000 3749 2942 3330 5352 2927 2012 2054 2051 2012 10289 8214 1029 102 1996 2267 1997 3330 2001 2511 1999 4444 1010 2174 1010 2220 5352 1999 2942 1998 6228 3330 2020 1037 2112 1997 1996 2267 1997 2671 2144 1996 14896 1012 2651 1996 2267 1010 7431 1999 1996 26249 1010 12731 12227 1010 1998 2358 7076 2239 1011 2128 7712 2243 9873 1997 3330 1010 2950 2274 7640 1997 2817 1516 13395 1998 6228 3330 1010 5072 1998 16012 5302 2571 15431 3330 1010 2942 3330 1998 9843 4163 1010 3274 2671 1998 3330 1010 1998 5992 3330 1516 2007 2809 1038 1012 1055 1012 5445 3253 1012 5678 1010 1996 2267 4107 2274 1011 2095 7037 3014 3454 2007 1996 6667 1997 2840 1998 4144 1998 1997 2449 21467 3176 1038 1012 1037 1012 1998 3040 1997 2449 3447 1006 15038 1007 5445 1010 4414 1012 102 0 0 0 0 0 0 0 0 0 0 0 0 0 0 0 0 0 0 0 0 0 0 0 0 0 0 0 0 0 0 0 0 0 0 0 0 0 0 0 0 0 0 0 0 0 0 0 0 0 0 0 0 0 0 0 0 0 0 0 0 0 0 0 0 0 0 0 0 0 0 0 0 0 0 0 0 0 0 0 0 0 0 0 0 0 0 0 0 0 0 0 0 0 0 0 0 0 0 0 0 0 0 0 0 0 0 0 0 0 0 0 0 0 0 0 0 0 0 0 0 0 0 0 0 0 0 0 0 0 0 0 0 0 0 0 0 0 0 0 0 0 0 0 0 0 0 0 0 0 0 0 0 0 0 0 0 0 0 0 0 0 0 0 0 0 0 0 0 0 0 0 0 0 0 0 0 0 0 0 0 0 0 0 0 0 0 0 0 0 0 0 0 0 0 0 0 0 0 0 0 0 0 0 0 0 0 0 0 0 0 0 0 0 0 0 0 0 0 0 0 0 0 0 0 0 0 0\n",
- "INFO:tensorflow:input_mask: 1 1 1 1 1 1 1 1 1 1 1 1 1 1 1 1 1 1 1 1 1 1 1 1 1 1 1 1 1 1 1 1 1 1 1 1 1 1 1 1 1 1 1 1 1 1 1 1 1 1 1 1 1 1 1 1 1 1 1 1 1 1 1 1 1 1 1 1 1 1 1 1 1 1 1 1 1 1 1 1 1 1 1 1 1 1 1 1 1 1 1 1 1 1 1 1 1 1 1 1 1 1 1 1 1 1 1 1 1 1 1 1 1 1 1 1 1 1 1 1 1 1 1 1 1 1 1 1 1 1 1 1 1 1 1 1 1 1 1 1 1 1 1 1 1 1 1 1 1 1 1 1 1 1 1 1 1 0 0 0 0 0 0 0 0 0 0 0 0 0 0 0 0 0 0 0 0 0 0 0 0 0 0 0 0 0 0 0 0 0 0 0 0 0 0 0 0 0 0 0 0 0 0 0 0 0 0 0 0 0 0 0 0 0 0 0 0 0 0 0 0 0 0 0 0 0 0 0 0 0 0 0 0 0 0 0 0 0 0 0 0 0 0 0 0 0 0 0 0 0 0 0 0 0 0 0 0 0 0 0 0 0 0 0 0 0 0 0 0 0 0 0 0 0 0 0 0 0 0 0 0 0 0 0 0 0 0 0 0 0 0 0 0 0 0 0 0 0 0 0 0 0 0 0 0 0 0 0 0 0 0 0 0 0 0 0 0 0 0 0 0 0 0 0 0 0 0 0 0 0 0 0 0 0 0 0 0 0 0 0 0 0 0 0 0 0 0 0 0 0 0 0 0 0 0 0 0 0 0 0 0 0 0 0 0 0 0 0 0 0 0 0 0 0 0 0 0 0 0 0 0 0 0 0\n",
- "INFO:tensorflow:segment_ids: 0 0 0 0 0 0 0 0 0 0 0 0 0 0 0 0 0 0 0 0 1 1 1 1 1 1 1 1 1 1 1 1 1 1 1 1 1 1 1 1 1 1 1 1 1 1 1 1 1 1 1 1 1 1 1 1 1 1 1 1 1 1 1 1 1 1 1 1 1 1 1 1 1 1 1 1 1 1 1 1 1 1 1 1 1 1 1 1 1 1 1 1 1 1 1 1 1 1 1 1 1 1 1 1 1 1 1 1 1 1 1 1 1 1 1 1 1 1 1 1 1 1 1 1 1 1 1 1 1 1 1 1 1 1 1 1 1 1 1 1 1 1 1 1 1 1 1 1 1 1 1 1 1 1 1 1 1 0 0 0 0 0 0 0 0 0 0 0 0 0 0 0 0 0 0 0 0 0 0 0 0 0 0 0 0 0 0 0 0 0 0 0 0 0 0 0 0 0 0 0 0 0 0 0 0 0 0 0 0 0 0 0 0 0 0 0 0 0 0 0 0 0 0 0 0 0 0 0 0 0 0 0 0 0 0 0 0 0 0 0 0 0 0 0 0 0 0 0 0 0 0 0 0 0 0 0 0 0 0 0 0 0 0 0 0 0 0 0 0 0 0 0 0 0 0 0 0 0 0 0 0 0 0 0 0 0 0 0 0 0 0 0 0 0 0 0 0 0 0 0 0 0 0 0 0 0 0 0 0 0 0 0 0 0 0 0 0 0 0 0 0 0 0 0 0 0 0 0 0 0 0 0 0 0 0 0 0 0 0 0 0 0 0 0 0 0 0 0 0 0 0 0 0 0 0 0 0 0 0 0 0 0 0 0 0 0 0 0 0 0 0 0 0 0 0 0 0 0 0 0 0 0 0 0\n",
- "INFO:tensorflow:start_position: 47\n",
- "INFO:tensorflow:end_position: 48\n",
- "INFO:tensorflow:answer: the 1870s\n"
- ]
- }
- ],
- "source": [
- "bert_config = modeling_tensorflow.BertConfig.from_json_file(bert_config_file)\n",
- "tokenizer = tokenization.BertTokenizer(\n",
- " vocab_file=vocab_file, do_lower_case=True)\n",
- "\n",
- "eval_examples = read_squad_examples(\n",
- " input_file=input_file, is_training=True, max_num=16)\n",
- "\n",
- "eval_features = convert_examples_to_features(\n",
- " examples=eval_examples,\n",
- " tokenizer=tokenizer,\n",
- " max_seq_length=max_seq_length,\n",
- " doc_stride=doc_stride,\n",
- " max_query_length=max_query_length,\n",
- " is_training=True)\n",
- "\n",
- "# You can use that to test the behavior of the models when target are outside of the model input sequence\n",
- "# for feature in eval_features:\n",
- "# feature.start_position = outside_pos\n",
- "# feature.end_position = outside_pos"
- ]
- },
- {
- "cell_type": "code",
- "execution_count": 5,
- "metadata": {
- "ExecuteTime": {
- "end_time": "2018-11-06T10:11:37.525632Z",
- "start_time": "2018-11-06T10:11:37.498695Z"
- }
- },
- "outputs": [],
- "source": [
- "eval_unique_id_to_feature = {}\n",
- "for eval_feature in eval_features:\n",
- " eval_unique_id_to_feature[eval_feature.unique_id] = eval_feature"
- ]
- },
- {
- "cell_type": "code",
- "execution_count": 6,
- "metadata": {
- "ExecuteTime": {
- "end_time": "2018-11-06T10:11:37.558325Z",
- "start_time": "2018-11-06T10:11:37.527972Z"
- }
- },
- "outputs": [],
- "source": [
- "def input_fn_builder(features, seq_length, drop_remainder):\n",
- " \"\"\"Creates an `input_fn` closure to be passed to TPUEstimator.\"\"\"\n",
- "\n",
- " all_unique_ids = []\n",
- " all_input_ids = []\n",
- " all_input_mask = []\n",
- " all_segment_ids = []\n",
- " all_start_positions = []\n",
- " all_end_positions = []\n",
- "\n",
- " for feature in features:\n",
- " all_unique_ids.append(feature.unique_id)\n",
- " all_input_ids.append(feature.input_ids)\n",
- " all_input_mask.append(feature.input_mask)\n",
- " all_segment_ids.append(feature.segment_ids)\n",
- " all_start_positions.append(feature.start_position)\n",
- " all_end_positions.append(feature.end_position)\n",
- "\n",
- " def input_fn(params):\n",
- " \"\"\"The actual input function.\"\"\"\n",
- " batch_size = params[\"batch_size\"]\n",
- "\n",
- " num_examples = len(features)\n",
- "\n",
- " # This is for demo purposes and does NOT scale to large data sets. We do\n",
- " # not use Dataset.from_generator() because that uses tf.py_func which is\n",
- " # not TPU compatible. The right way to load data is with TFRecordReader.\n",
- " feature_map = {\n",
- " \"unique_ids\":\n",
- " tf.constant(all_unique_ids, shape=[num_examples], dtype=tf.int32),\n",
- " \"input_ids\":\n",
- " tf.constant(\n",
- " all_input_ids, shape=[num_examples, seq_length],\n",
- " dtype=tf.int32),\n",
- " \"input_mask\":\n",
- " tf.constant(\n",
- " all_input_mask,\n",
- " shape=[num_examples, seq_length],\n",
- " dtype=tf.int32),\n",
- " \"segment_ids\":\n",
- " tf.constant(\n",
- " all_segment_ids,\n",
- " shape=[num_examples, seq_length],\n",
- " dtype=tf.int32),\n",
- " \"start_positions\":\n",
- " tf.constant(\n",
- " all_start_positions,\n",
- " shape=[num_examples],\n",
- " dtype=tf.int32),\n",
- " \"end_positions\":\n",
- " tf.constant(\n",
- " all_end_positions,\n",
- " shape=[num_examples],\n",
- " dtype=tf.int32),\n",
- " }\n",
- "\n",
- " d = tf.data.Dataset.from_tensor_slices(feature_map)\n",
- " d = d.repeat()\n",
- " d = d.batch(batch_size=batch_size, drop_remainder=drop_remainder)\n",
- " return d\n",
- "\n",
- " return input_fn"
- ]
- },
- {
- "cell_type": "code",
- "execution_count": 7,
- "metadata": {
- "ExecuteTime": {
- "end_time": "2018-11-06T10:11:37.601666Z",
- "start_time": "2018-11-06T10:11:37.560082Z"
- }
- },
- "outputs": [],
- "source": [
- "def model_fn_builder(bert_config, init_checkpoint, learning_rate,\n",
- " num_train_steps, num_warmup_steps, use_tpu,\n",
- " use_one_hot_embeddings):\n",
- " \"\"\"Returns `model_fn` closure for TPUEstimator.\"\"\"\n",
- "\n",
- " def model_fn(features, labels, mode, params): # pylint: disable=unused-argument\n",
- " \"\"\"The `model_fn` for TPUEstimator.\"\"\"\n",
- "\n",
- " tf.logging.info(\"*** Features ***\")\n",
- " for name in sorted(features.keys()):\n",
- " tf.logging.info(\" name = %s, shape = %s\" % (name, features[name].shape))\n",
- "\n",
- " unique_ids = features[\"unique_ids\"]\n",
- " input_ids = features[\"input_ids\"]\n",
- " input_mask = features[\"input_mask\"]\n",
- " segment_ids = features[\"segment_ids\"]\n",
- "\n",
- " is_training = (mode == tf.estimator.ModeKeys.TRAIN)\n",
- "\n",
- " (start_logits, end_logits) = create_model(\n",
- " bert_config=bert_config,\n",
- " is_training=is_training,\n",
- " input_ids=input_ids,\n",
- " input_mask=input_mask,\n",
- " segment_ids=segment_ids,\n",
- " use_one_hot_embeddings=use_one_hot_embeddings)\n",
- "\n",
- " tvars = tf.trainable_variables()\n",
- "\n",
- " initialized_variable_names = {}\n",
- " scaffold_fn = None\n",
- " if init_checkpoint:\n",
- " (assignment_map,\n",
- " initialized_variable_names) = modeling_tensorflow.get_assigment_map_from_checkpoint(\n",
- " tvars, init_checkpoint)\n",
- " if use_tpu:\n",
- "\n",
- " def tpu_scaffold():\n",
- " tf.train.init_from_checkpoint(init_checkpoint, assignment_map)\n",
- " return tf.train.Scaffold()\n",
- "\n",
- " scaffold_fn = tpu_scaffold\n",
- " else:\n",
- " tf.train.init_from_checkpoint(init_checkpoint, assignment_map)\n",
- "\n",
- " tf.logging.info(\"**** Trainable Variables ****\")\n",
- " for var in tvars:\n",
- " init_string = \"\"\n",
- " if var.name in initialized_variable_names:\n",
- " init_string = \", *INIT_FROM_CKPT*\"\n",
- " tf.logging.info(\" name = %s, shape = %s%s\", var.name, var.shape,\n",
- " init_string)\n",
- "\n",
- " output_spec = None\n",
- " if mode == tf.estimator.ModeKeys.TRAIN:\n",
- " seq_length = modeling_tensorflow.get_shape_list(input_ids)[1]\n",
- "\n",
- " def compute_loss(logits, positions):\n",
- " one_hot_positions = tf.one_hot(\n",
- " positions, depth=seq_length, dtype=tf.float32)\n",
- " log_probs = tf.nn.log_softmax(logits, axis=-1)\n",
- " loss = -tf.reduce_mean(\n",
- " tf.reduce_sum(one_hot_positions * log_probs, axis=-1))\n",
- " return loss\n",
- "\n",
- " start_positions = features[\"start_positions\"]\n",
- " end_positions = features[\"end_positions\"]\n",
- "\n",
- " start_loss = compute_loss(start_logits, start_positions)\n",
- " end_loss = compute_loss(end_logits, end_positions)\n",
- "\n",
- " total_loss = (start_loss + end_loss) / 2.0\n",
- "\n",
- " train_op = optimization.create_optimizer(\n",
- " total_loss, learning_rate, num_train_steps, num_warmup_steps, use_tpu)\n",
- "\n",
- " output_spec = tf.contrib.tpu.TPUEstimatorSpec(\n",
- " mode=mode,\n",
- " loss=total_loss,\n",
- " train_op=train_op,\n",
- " scaffold_fn=scaffold_fn)\n",
- " elif mode == tf.estimator.ModeKeys.PREDICT:\n",
- " batch_size = modeling_tensorflow.get_shape_list(start_logits)[0]\n",
- " seq_length = modeling_tensorflow.get_shape_list(input_ids)[1]\n",
- "\n",
- " def compute_loss(logits, positions):\n",
- " one_hot_positions = tf.one_hot(\n",
- " positions, depth=seq_length, dtype=tf.float32)\n",
- " log_probs = tf.nn.log_softmax(logits, axis=-1)\n",
- " loss = -tf.reduce_mean(\n",
- " tf.reduce_sum(one_hot_positions * log_probs, axis=-1))\n",
- " return loss\n",
- "\n",
- " start_positions = features[\"start_positions\"]\n",
- " end_positions = features[\"end_positions\"]\n",
- "\n",
- " start_loss = compute_loss(start_logits, start_positions)\n",
- " end_loss = compute_loss(end_logits, end_positions)\n",
- "\n",
- " total_loss = (start_loss + end_loss) / 2.0\n",
- "\n",
- " predictions = {\n",
- " \"unique_ids\": unique_ids,\n",
- " \"start_logits\": start_logits,\n",
- " \"end_logits\": end_logits,\n",
- " \"total_loss\": tf.reshape(total_loss, [batch_size, 1]),\n",
- " \"start_loss\": tf.reshape(start_loss, [batch_size, 1]),\n",
- " \"end_loss\": tf.reshape(end_loss, [batch_size, 1]),\n",
- " }\n",
- " output_spec = tf.contrib.tpu.TPUEstimatorSpec(\n",
- " mode=mode, predictions=predictions, scaffold_fn=scaffold_fn)\n",
- " else:\n",
- " raise ValueError(\n",
- " \"Only TRAIN and PREDICT modes are supported: %s\" % (mode))\n",
- "\n",
- " return output_spec\n",
- "\n",
- " return model_fn"
- ]
- },
- {
- "cell_type": "code",
- "execution_count": 8,
- "metadata": {
- "ExecuteTime": {
- "end_time": "2018-11-06T10:11:41.104542Z",
- "start_time": "2018-11-06T10:11:37.603474Z"
- }
- },
- "outputs": [
- {
- "name": "stdout",
- "output_type": "stream",
- "text": [
- "WARNING:tensorflow:Estimator's model_fn (.model_fn at 0x120df3f28>) includes params argument, but params are not passed to Estimator.\n",
- "INFO:tensorflow:Using config: {'_model_dir': '/tmp/squad_base/', '_tf_random_seed': None, '_save_summary_steps': 100, '_save_checkpoints_steps': 1000, '_save_checkpoints_secs': None, '_session_config': allow_soft_placement: true\n",
- "graph_options {\n",
- " rewrite_options {\n",
- " meta_optimizer_iterations: ONE\n",
- " }\n",
- "}\n",
- ", '_keep_checkpoint_max': 5, '_keep_checkpoint_every_n_hours': 10000, '_log_step_count_steps': None, '_train_distribute': None, '_device_fn': None, '_protocol': None, '_eval_distribute': None, '_experimental_distribute': None, '_service': None, '_cluster_spec': , '_task_type': 'worker', '_task_id': 0, '_global_id_in_cluster': 0, '_master': '', '_evaluation_master': '', '_is_chief': True, '_num_ps_replicas': 0, '_num_worker_replicas': 1, '_tpu_config': TPUConfig(iterations_per_loop=1000, num_shards=8, num_cores_per_replica=None, per_host_input_for_training=3, tpu_job_name=None, initial_infeed_sleep_secs=None, input_partition_dims=None), '_cluster': None}\n",
- "INFO:tensorflow:_TPUContext: eval_on_tpu True\n",
- "WARNING:tensorflow:eval_on_tpu ignored because use_tpu is False.\n"
- ]
- }
- ],
- "source": [
- "is_per_host = tf.contrib.tpu.InputPipelineConfig.PER_HOST_V2\n",
- "run_config = tf.contrib.tpu.RunConfig(\n",
- " cluster=None,\n",
- " master=None,\n",
- " model_dir=output_dir,\n",
- " save_checkpoints_steps=1000,\n",
- " tpu_config=tf.contrib.tpu.TPUConfig(\n",
- " iterations_per_loop=1000,\n",
- " num_shards=8,\n",
- " per_host_input_for_training=is_per_host))\n",
- "\n",
- "model_fn = model_fn_builder(\n",
- " bert_config=bert_config,\n",
- " init_checkpoint=init_checkpoint,\n",
- " learning_rate=learning_rate,\n",
- " num_train_steps=None,\n",
- " num_warmup_steps=None,\n",
- " use_tpu=False,\n",
- " use_one_hot_embeddings=False)\n",
- "\n",
- "estimator = tf.contrib.tpu.TPUEstimator(\n",
- " use_tpu=False,\n",
- " model_fn=model_fn,\n",
- " config=run_config,\n",
- " train_batch_size=12,\n",
- " predict_batch_size=1)\n",
- "\n",
- "predict_input_fn = input_fn_builder(\n",
- " features=eval_features,\n",
- " seq_length=max_seq_length,\n",
- " drop_remainder=True)"
- ]
- },
- {
- "cell_type": "code",
- "execution_count": 9,
- "metadata": {
- "ExecuteTime": {
- "end_time": "2018-11-06T10:11:47.857601Z",
- "start_time": "2018-11-06T10:11:41.106219Z"
- }
- },
- "outputs": [
- {
- "name": "stdout",
- "output_type": "stream",
- "text": [
- "INFO:tensorflow:Could not find trained model in model_dir: /tmp/squad_base/, running initialization to predict.\n",
- "INFO:tensorflow:Calling model_fn.\n",
- "INFO:tensorflow:Running infer on CPU\n",
- "INFO:tensorflow:*** Features ***\n",
- "INFO:tensorflow: name = end_positions, shape = (1,)\n",
- "INFO:tensorflow: name = input_ids, shape = (1, 384)\n",
- "INFO:tensorflow: name = input_mask, shape = (1, 384)\n",
- "INFO:tensorflow: name = segment_ids, shape = (1, 384)\n",
- "INFO:tensorflow: name = start_positions, shape = (1,)\n",
- "INFO:tensorflow: name = unique_ids, shape = (1,)\n",
- "INFO:tensorflow:**** Trainable Variables ****\n",
- "INFO:tensorflow: name = bert/embeddings/word_embeddings:0, shape = (30522, 768), *INIT_FROM_CKPT*\n",
- "INFO:tensorflow: name = bert/embeddings/token_type_embeddings:0, shape = (2, 768), *INIT_FROM_CKPT*\n",
- "INFO:tensorflow: name = bert/embeddings/position_embeddings:0, shape = (512, 768), *INIT_FROM_CKPT*\n",
- "INFO:tensorflow: name = bert/embeddings/LayerNorm/beta:0, shape = (768,), *INIT_FROM_CKPT*\n",
- "INFO:tensorflow: name = bert/embeddings/LayerNorm/gamma:0, shape = (768,), *INIT_FROM_CKPT*\n",
- "INFO:tensorflow: name = bert/encoder/layer_0/attention/self/query/kernel:0, shape = (768, 768), *INIT_FROM_CKPT*\n",
- "INFO:tensorflow: name = bert/encoder/layer_0/attention/self/query/bias:0, shape = (768,), *INIT_FROM_CKPT*\n",
- "INFO:tensorflow: name = bert/encoder/layer_0/attention/self/key/kernel:0, shape = (768, 768), *INIT_FROM_CKPT*\n",
- "INFO:tensorflow: name = bert/encoder/layer_0/attention/self/key/bias:0, shape = (768,), *INIT_FROM_CKPT*\n",
- "INFO:tensorflow: name = bert/encoder/layer_0/attention/self/value/kernel:0, shape = (768, 768), *INIT_FROM_CKPT*\n",
- "INFO:tensorflow: name = bert/encoder/layer_0/attention/self/value/bias:0, shape = (768,), *INIT_FROM_CKPT*\n",
- "INFO:tensorflow: name = bert/encoder/layer_0/attention/output/dense/kernel:0, shape = (768, 768), *INIT_FROM_CKPT*\n",
- "INFO:tensorflow: name = bert/encoder/layer_0/attention/output/dense/bias:0, shape = (768,), *INIT_FROM_CKPT*\n",
- "INFO:tensorflow: name = bert/encoder/layer_0/attention/output/LayerNorm/beta:0, shape = (768,), *INIT_FROM_CKPT*\n",
- "INFO:tensorflow: name = bert/encoder/layer_0/attention/output/LayerNorm/gamma:0, shape = (768,), *INIT_FROM_CKPT*\n",
- "INFO:tensorflow: name = bert/encoder/layer_0/intermediate/dense/kernel:0, shape = (768, 3072), *INIT_FROM_CKPT*\n",
- "INFO:tensorflow: name = bert/encoder/layer_0/intermediate/dense/bias:0, shape = (3072,), *INIT_FROM_CKPT*\n",
- "INFO:tensorflow: name = bert/encoder/layer_0/output/dense/kernel:0, shape = (3072, 768), *INIT_FROM_CKPT*\n",
- "INFO:tensorflow: name = bert/encoder/layer_0/output/dense/bias:0, shape = (768,), *INIT_FROM_CKPT*\n",
- "INFO:tensorflow: name = bert/encoder/layer_0/output/LayerNorm/beta:0, shape = (768,), *INIT_FROM_CKPT*\n",
- "INFO:tensorflow: name = bert/encoder/layer_0/output/LayerNorm/gamma:0, shape = (768,), *INIT_FROM_CKPT*\n",
- "INFO:tensorflow: name = bert/encoder/layer_1/attention/self/query/kernel:0, shape = (768, 768), *INIT_FROM_CKPT*\n",
- "INFO:tensorflow: name = bert/encoder/layer_1/attention/self/query/bias:0, shape = (768,), *INIT_FROM_CKPT*\n",
- "INFO:tensorflow: name = bert/encoder/layer_1/attention/self/key/kernel:0, shape = (768, 768), *INIT_FROM_CKPT*\n",
- "INFO:tensorflow: name = bert/encoder/layer_1/attention/self/key/bias:0, shape = (768,), *INIT_FROM_CKPT*\n",
- "INFO:tensorflow: name = bert/encoder/layer_1/attention/self/value/kernel:0, shape = (768, 768), *INIT_FROM_CKPT*\n",
- "INFO:tensorflow: name = bert/encoder/layer_1/attention/self/value/bias:0, shape = (768,), *INIT_FROM_CKPT*\n",
- "INFO:tensorflow: name = bert/encoder/layer_1/attention/output/dense/kernel:0, shape = (768, 768), *INIT_FROM_CKPT*\n",
- "INFO:tensorflow: name = bert/encoder/layer_1/attention/output/dense/bias:0, shape = (768,), *INIT_FROM_CKPT*\n",
- "INFO:tensorflow: name = bert/encoder/layer_1/attention/output/LayerNorm/beta:0, shape = (768,), *INIT_FROM_CKPT*\n",
- "INFO:tensorflow: name = bert/encoder/layer_1/attention/output/LayerNorm/gamma:0, shape = (768,), *INIT_FROM_CKPT*\n",
- "INFO:tensorflow: name = bert/encoder/layer_1/intermediate/dense/kernel:0, shape = (768, 3072), *INIT_FROM_CKPT*\n",
- "INFO:tensorflow: name = bert/encoder/layer_1/intermediate/dense/bias:0, shape = (3072,), *INIT_FROM_CKPT*\n",
- "INFO:tensorflow: name = bert/encoder/layer_1/output/dense/kernel:0, shape = (3072, 768), *INIT_FROM_CKPT*\n",
- "INFO:tensorflow: name = bert/encoder/layer_1/output/dense/bias:0, shape = (768,), *INIT_FROM_CKPT*\n",
- "INFO:tensorflow: name = bert/encoder/layer_1/output/LayerNorm/beta:0, shape = (768,), *INIT_FROM_CKPT*\n",
- "INFO:tensorflow: name = bert/encoder/layer_1/output/LayerNorm/gamma:0, shape = (768,), *INIT_FROM_CKPT*\n",
- "INFO:tensorflow: name = bert/encoder/layer_2/attention/self/query/kernel:0, shape = (768, 768), *INIT_FROM_CKPT*\n",
- "INFO:tensorflow: name = bert/encoder/layer_2/attention/self/query/bias:0, shape = (768,), *INIT_FROM_CKPT*\n",
- "INFO:tensorflow: name = bert/encoder/layer_2/attention/self/key/kernel:0, shape = (768, 768), *INIT_FROM_CKPT*\n",
- "INFO:tensorflow: name = bert/encoder/layer_2/attention/self/key/bias:0, shape = (768,), *INIT_FROM_CKPT*\n",
- "INFO:tensorflow: name = bert/encoder/layer_2/attention/self/value/kernel:0, shape = (768, 768), *INIT_FROM_CKPT*\n",
- "INFO:tensorflow: name = bert/encoder/layer_2/attention/self/value/bias:0, shape = (768,), *INIT_FROM_CKPT*\n",
- "INFO:tensorflow: name = bert/encoder/layer_2/attention/output/dense/kernel:0, shape = (768, 768), *INIT_FROM_CKPT*\n",
- "INFO:tensorflow: name = bert/encoder/layer_2/attention/output/dense/bias:0, shape = (768,), *INIT_FROM_CKPT*\n",
- "INFO:tensorflow: name = bert/encoder/layer_2/attention/output/LayerNorm/beta:0, shape = (768,), *INIT_FROM_CKPT*\n",
- "INFO:tensorflow: name = bert/encoder/layer_2/attention/output/LayerNorm/gamma:0, shape = (768,), *INIT_FROM_CKPT*\n",
- "INFO:tensorflow: name = bert/encoder/layer_2/intermediate/dense/kernel:0, shape = (768, 3072), *INIT_FROM_CKPT*\n",
- "INFO:tensorflow: name = bert/encoder/layer_2/intermediate/dense/bias:0, shape = (3072,), *INIT_FROM_CKPT*\n",
- "INFO:tensorflow: name = bert/encoder/layer_2/output/dense/kernel:0, shape = (3072, 768), *INIT_FROM_CKPT*\n",
- "INFO:tensorflow: name = bert/encoder/layer_2/output/dense/bias:0, shape = (768,), *INIT_FROM_CKPT*\n",
- "INFO:tensorflow: name = bert/encoder/layer_2/output/LayerNorm/beta:0, shape = (768,), *INIT_FROM_CKPT*\n",
- "INFO:tensorflow: name = bert/encoder/layer_2/output/LayerNorm/gamma:0, shape = (768,), *INIT_FROM_CKPT*\n",
- "INFO:tensorflow: name = bert/encoder/layer_3/attention/self/query/kernel:0, shape = (768, 768), *INIT_FROM_CKPT*\n",
- "INFO:tensorflow: name = bert/encoder/layer_3/attention/self/query/bias:0, shape = (768,), *INIT_FROM_CKPT*\n",
- "INFO:tensorflow: name = bert/encoder/layer_3/attention/self/key/kernel:0, shape = (768, 768), *INIT_FROM_CKPT*\n",
- "INFO:tensorflow: name = bert/encoder/layer_3/attention/self/key/bias:0, shape = (768,), *INIT_FROM_CKPT*\n",
- "INFO:tensorflow: name = bert/encoder/layer_3/attention/self/value/kernel:0, shape = (768, 768), *INIT_FROM_CKPT*\n",
- "INFO:tensorflow: name = bert/encoder/layer_3/attention/self/value/bias:0, shape = (768,), *INIT_FROM_CKPT*\n",
- "INFO:tensorflow: name = bert/encoder/layer_3/attention/output/dense/kernel:0, shape = (768, 768), *INIT_FROM_CKPT*\n",
- "INFO:tensorflow: name = bert/encoder/layer_3/attention/output/dense/bias:0, shape = (768,), *INIT_FROM_CKPT*\n",
- "INFO:tensorflow: name = bert/encoder/layer_3/attention/output/LayerNorm/beta:0, shape = (768,), *INIT_FROM_CKPT*\n",
- "INFO:tensorflow: name = bert/encoder/layer_3/attention/output/LayerNorm/gamma:0, shape = (768,), *INIT_FROM_CKPT*\n",
- "INFO:tensorflow: name = bert/encoder/layer_3/intermediate/dense/kernel:0, shape = (768, 3072), *INIT_FROM_CKPT*\n",
- "INFO:tensorflow: name = bert/encoder/layer_3/intermediate/dense/bias:0, shape = (3072,), *INIT_FROM_CKPT*\n",
- "INFO:tensorflow: name = bert/encoder/layer_3/output/dense/kernel:0, shape = (3072, 768), *INIT_FROM_CKPT*\n",
- "INFO:tensorflow: name = bert/encoder/layer_3/output/dense/bias:0, shape = (768,), *INIT_FROM_CKPT*\n",
- "INFO:tensorflow: name = bert/encoder/layer_3/output/LayerNorm/beta:0, shape = (768,), *INIT_FROM_CKPT*\n",
- "INFO:tensorflow: name = bert/encoder/layer_3/output/LayerNorm/gamma:0, shape = (768,), *INIT_FROM_CKPT*\n",
- "INFO:tensorflow: name = bert/encoder/layer_4/attention/self/query/kernel:0, shape = (768, 768), *INIT_FROM_CKPT*\n"
- ]
- },
- {
- "name": "stdout",
- "output_type": "stream",
- "text": [
- "INFO:tensorflow: name = bert/encoder/layer_4/attention/self/query/bias:0, shape = (768,), *INIT_FROM_CKPT*\n",
- "INFO:tensorflow: name = bert/encoder/layer_4/attention/self/key/kernel:0, shape = (768, 768), *INIT_FROM_CKPT*\n",
- "INFO:tensorflow: name = bert/encoder/layer_4/attention/self/key/bias:0, shape = (768,), *INIT_FROM_CKPT*\n",
- "INFO:tensorflow: name = bert/encoder/layer_4/attention/self/value/kernel:0, shape = (768, 768), *INIT_FROM_CKPT*\n",
- "INFO:tensorflow: name = bert/encoder/layer_4/attention/self/value/bias:0, shape = (768,), *INIT_FROM_CKPT*\n",
- "INFO:tensorflow: name = bert/encoder/layer_4/attention/output/dense/kernel:0, shape = (768, 768), *INIT_FROM_CKPT*\n",
- "INFO:tensorflow: name = bert/encoder/layer_4/attention/output/dense/bias:0, shape = (768,), *INIT_FROM_CKPT*\n",
- "INFO:tensorflow: name = bert/encoder/layer_4/attention/output/LayerNorm/beta:0, shape = (768,), *INIT_FROM_CKPT*\n",
- "INFO:tensorflow: name = bert/encoder/layer_4/attention/output/LayerNorm/gamma:0, shape = (768,), *INIT_FROM_CKPT*\n",
- "INFO:tensorflow: name = bert/encoder/layer_4/intermediate/dense/kernel:0, shape = (768, 3072), *INIT_FROM_CKPT*\n",
- "INFO:tensorflow: name = bert/encoder/layer_4/intermediate/dense/bias:0, shape = (3072,), *INIT_FROM_CKPT*\n",
- "INFO:tensorflow: name = bert/encoder/layer_4/output/dense/kernel:0, shape = (3072, 768), *INIT_FROM_CKPT*\n",
- "INFO:tensorflow: name = bert/encoder/layer_4/output/dense/bias:0, shape = (768,), *INIT_FROM_CKPT*\n",
- "INFO:tensorflow: name = bert/encoder/layer_4/output/LayerNorm/beta:0, shape = (768,), *INIT_FROM_CKPT*\n",
- "INFO:tensorflow: name = bert/encoder/layer_4/output/LayerNorm/gamma:0, shape = (768,), *INIT_FROM_CKPT*\n",
- "INFO:tensorflow: name = bert/encoder/layer_5/attention/self/query/kernel:0, shape = (768, 768), *INIT_FROM_CKPT*\n",
- "INFO:tensorflow: name = bert/encoder/layer_5/attention/self/query/bias:0, shape = (768,), *INIT_FROM_CKPT*\n",
- "INFO:tensorflow: name = bert/encoder/layer_5/attention/self/key/kernel:0, shape = (768, 768), *INIT_FROM_CKPT*\n",
- "INFO:tensorflow: name = bert/encoder/layer_5/attention/self/key/bias:0, shape = (768,), *INIT_FROM_CKPT*\n",
- "INFO:tensorflow: name = bert/encoder/layer_5/attention/self/value/kernel:0, shape = (768, 768), *INIT_FROM_CKPT*\n",
- "INFO:tensorflow: name = bert/encoder/layer_5/attention/self/value/bias:0, shape = (768,), *INIT_FROM_CKPT*\n",
- "INFO:tensorflow: name = bert/encoder/layer_5/attention/output/dense/kernel:0, shape = (768, 768), *INIT_FROM_CKPT*\n",
- "INFO:tensorflow: name = bert/encoder/layer_5/attention/output/dense/bias:0, shape = (768,), *INIT_FROM_CKPT*\n",
- "INFO:tensorflow: name = bert/encoder/layer_5/attention/output/LayerNorm/beta:0, shape = (768,), *INIT_FROM_CKPT*\n",
- "INFO:tensorflow: name = bert/encoder/layer_5/attention/output/LayerNorm/gamma:0, shape = (768,), *INIT_FROM_CKPT*\n",
- "INFO:tensorflow: name = bert/encoder/layer_5/intermediate/dense/kernel:0, shape = (768, 3072), *INIT_FROM_CKPT*\n",
- "INFO:tensorflow: name = bert/encoder/layer_5/intermediate/dense/bias:0, shape = (3072,), *INIT_FROM_CKPT*\n",
- "INFO:tensorflow: name = bert/encoder/layer_5/output/dense/kernel:0, shape = (3072, 768), *INIT_FROM_CKPT*\n",
- "INFO:tensorflow: name = bert/encoder/layer_5/output/dense/bias:0, shape = (768,), *INIT_FROM_CKPT*\n",
- "INFO:tensorflow: name = bert/encoder/layer_5/output/LayerNorm/beta:0, shape = (768,), *INIT_FROM_CKPT*\n",
- "INFO:tensorflow: name = bert/encoder/layer_5/output/LayerNorm/gamma:0, shape = (768,), *INIT_FROM_CKPT*\n",
- "INFO:tensorflow: name = bert/encoder/layer_6/attention/self/query/kernel:0, shape = (768, 768), *INIT_FROM_CKPT*\n",
- "INFO:tensorflow: name = bert/encoder/layer_6/attention/self/query/bias:0, shape = (768,), *INIT_FROM_CKPT*\n",
- "INFO:tensorflow: name = bert/encoder/layer_6/attention/self/key/kernel:0, shape = (768, 768), *INIT_FROM_CKPT*\n",
- "INFO:tensorflow: name = bert/encoder/layer_6/attention/self/key/bias:0, shape = (768,), *INIT_FROM_CKPT*\n",
- "INFO:tensorflow: name = bert/encoder/layer_6/attention/self/value/kernel:0, shape = (768, 768), *INIT_FROM_CKPT*\n",
- "INFO:tensorflow: name = bert/encoder/layer_6/attention/self/value/bias:0, shape = (768,), *INIT_FROM_CKPT*\n",
- "INFO:tensorflow: name = bert/encoder/layer_6/attention/output/dense/kernel:0, shape = (768, 768), *INIT_FROM_CKPT*\n",
- "INFO:tensorflow: name = bert/encoder/layer_6/attention/output/dense/bias:0, shape = (768,), *INIT_FROM_CKPT*\n",
- "INFO:tensorflow: name = bert/encoder/layer_6/attention/output/LayerNorm/beta:0, shape = (768,), *INIT_FROM_CKPT*\n",
- "INFO:tensorflow: name = bert/encoder/layer_6/attention/output/LayerNorm/gamma:0, shape = (768,), *INIT_FROM_CKPT*\n",
- "INFO:tensorflow: name = bert/encoder/layer_6/intermediate/dense/kernel:0, shape = (768, 3072), *INIT_FROM_CKPT*\n",
- "INFO:tensorflow: name = bert/encoder/layer_6/intermediate/dense/bias:0, shape = (3072,), *INIT_FROM_CKPT*\n",
- "INFO:tensorflow: name = bert/encoder/layer_6/output/dense/kernel:0, shape = (3072, 768), *INIT_FROM_CKPT*\n",
- "INFO:tensorflow: name = bert/encoder/layer_6/output/dense/bias:0, shape = (768,), *INIT_FROM_CKPT*\n",
- "INFO:tensorflow: name = bert/encoder/layer_6/output/LayerNorm/beta:0, shape = (768,), *INIT_FROM_CKPT*\n",
- "INFO:tensorflow: name = bert/encoder/layer_6/output/LayerNorm/gamma:0, shape = (768,), *INIT_FROM_CKPT*\n",
- "INFO:tensorflow: name = bert/encoder/layer_7/attention/self/query/kernel:0, shape = (768, 768), *INIT_FROM_CKPT*\n",
- "INFO:tensorflow: name = bert/encoder/layer_7/attention/self/query/bias:0, shape = (768,), *INIT_FROM_CKPT*\n",
- "INFO:tensorflow: name = bert/encoder/layer_7/attention/self/key/kernel:0, shape = (768, 768), *INIT_FROM_CKPT*\n",
- "INFO:tensorflow: name = bert/encoder/layer_7/attention/self/key/bias:0, shape = (768,), *INIT_FROM_CKPT*\n",
- "INFO:tensorflow: name = bert/encoder/layer_7/attention/self/value/kernel:0, shape = (768, 768), *INIT_FROM_CKPT*\n",
- "INFO:tensorflow: name = bert/encoder/layer_7/attention/self/value/bias:0, shape = (768,), *INIT_FROM_CKPT*\n",
- "INFO:tensorflow: name = bert/encoder/layer_7/attention/output/dense/kernel:0, shape = (768, 768), *INIT_FROM_CKPT*\n",
- "INFO:tensorflow: name = bert/encoder/layer_7/attention/output/dense/bias:0, shape = (768,), *INIT_FROM_CKPT*\n",
- "INFO:tensorflow: name = bert/encoder/layer_7/attention/output/LayerNorm/beta:0, shape = (768,), *INIT_FROM_CKPT*\n",
- "INFO:tensorflow: name = bert/encoder/layer_7/attention/output/LayerNorm/gamma:0, shape = (768,), *INIT_FROM_CKPT*\n",
- "INFO:tensorflow: name = bert/encoder/layer_7/intermediate/dense/kernel:0, shape = (768, 3072), *INIT_FROM_CKPT*\n",
- "INFO:tensorflow: name = bert/encoder/layer_7/intermediate/dense/bias:0, shape = (3072,), *INIT_FROM_CKPT*\n",
- "INFO:tensorflow: name = bert/encoder/layer_7/output/dense/kernel:0, shape = (3072, 768), *INIT_FROM_CKPT*\n",
- "INFO:tensorflow: name = bert/encoder/layer_7/output/dense/bias:0, shape = (768,), *INIT_FROM_CKPT*\n",
- "INFO:tensorflow: name = bert/encoder/layer_7/output/LayerNorm/beta:0, shape = (768,), *INIT_FROM_CKPT*\n",
- "INFO:tensorflow: name = bert/encoder/layer_7/output/LayerNorm/gamma:0, shape = (768,), *INIT_FROM_CKPT*\n",
- "INFO:tensorflow: name = bert/encoder/layer_8/attention/self/query/kernel:0, shape = (768, 768), *INIT_FROM_CKPT*\n",
- "INFO:tensorflow: name = bert/encoder/layer_8/attention/self/query/bias:0, shape = (768,), *INIT_FROM_CKPT*\n",
- "INFO:tensorflow: name = bert/encoder/layer_8/attention/self/key/kernel:0, shape = (768, 768), *INIT_FROM_CKPT*\n",
- "INFO:tensorflow: name = bert/encoder/layer_8/attention/self/key/bias:0, shape = (768,), *INIT_FROM_CKPT*\n",
- "INFO:tensorflow: name = bert/encoder/layer_8/attention/self/value/kernel:0, shape = (768, 768), *INIT_FROM_CKPT*\n",
- "INFO:tensorflow: name = bert/encoder/layer_8/attention/self/value/bias:0, shape = (768,), *INIT_FROM_CKPT*\n",
- "INFO:tensorflow: name = bert/encoder/layer_8/attention/output/dense/kernel:0, shape = (768, 768), *INIT_FROM_CKPT*\n",
- "INFO:tensorflow: name = bert/encoder/layer_8/attention/output/dense/bias:0, shape = (768,), *INIT_FROM_CKPT*\n",
- "INFO:tensorflow: name = bert/encoder/layer_8/attention/output/LayerNorm/beta:0, shape = (768,), *INIT_FROM_CKPT*\n",
- "INFO:tensorflow: name = bert/encoder/layer_8/attention/output/LayerNorm/gamma:0, shape = (768,), *INIT_FROM_CKPT*\n",
- "INFO:tensorflow: name = bert/encoder/layer_8/intermediate/dense/kernel:0, shape = (768, 3072), *INIT_FROM_CKPT*\n",
- "INFO:tensorflow: name = bert/encoder/layer_8/intermediate/dense/bias:0, shape = (3072,), *INIT_FROM_CKPT*\n"
- ]
- },
- {
- "name": "stdout",
- "output_type": "stream",
- "text": [
- "INFO:tensorflow: name = bert/encoder/layer_8/output/dense/kernel:0, shape = (3072, 768), *INIT_FROM_CKPT*\n",
- "INFO:tensorflow: name = bert/encoder/layer_8/output/dense/bias:0, shape = (768,), *INIT_FROM_CKPT*\n",
- "INFO:tensorflow: name = bert/encoder/layer_8/output/LayerNorm/beta:0, shape = (768,), *INIT_FROM_CKPT*\n",
- "INFO:tensorflow: name = bert/encoder/layer_8/output/LayerNorm/gamma:0, shape = (768,), *INIT_FROM_CKPT*\n",
- "INFO:tensorflow: name = bert/encoder/layer_9/attention/self/query/kernel:0, shape = (768, 768), *INIT_FROM_CKPT*\n",
- "INFO:tensorflow: name = bert/encoder/layer_9/attention/self/query/bias:0, shape = (768,), *INIT_FROM_CKPT*\n",
- "INFO:tensorflow: name = bert/encoder/layer_9/attention/self/key/kernel:0, shape = (768, 768), *INIT_FROM_CKPT*\n",
- "INFO:tensorflow: name = bert/encoder/layer_9/attention/self/key/bias:0, shape = (768,), *INIT_FROM_CKPT*\n",
- "INFO:tensorflow: name = bert/encoder/layer_9/attention/self/value/kernel:0, shape = (768, 768), *INIT_FROM_CKPT*\n",
- "INFO:tensorflow: name = bert/encoder/layer_9/attention/self/value/bias:0, shape = (768,), *INIT_FROM_CKPT*\n",
- "INFO:tensorflow: name = bert/encoder/layer_9/attention/output/dense/kernel:0, shape = (768, 768), *INIT_FROM_CKPT*\n",
- "INFO:tensorflow: name = bert/encoder/layer_9/attention/output/dense/bias:0, shape = (768,), *INIT_FROM_CKPT*\n",
- "INFO:tensorflow: name = bert/encoder/layer_9/attention/output/LayerNorm/beta:0, shape = (768,), *INIT_FROM_CKPT*\n",
- "INFO:tensorflow: name = bert/encoder/layer_9/attention/output/LayerNorm/gamma:0, shape = (768,), *INIT_FROM_CKPT*\n",
- "INFO:tensorflow: name = bert/encoder/layer_9/intermediate/dense/kernel:0, shape = (768, 3072), *INIT_FROM_CKPT*\n",
- "INFO:tensorflow: name = bert/encoder/layer_9/intermediate/dense/bias:0, shape = (3072,), *INIT_FROM_CKPT*\n",
- "INFO:tensorflow: name = bert/encoder/layer_9/output/dense/kernel:0, shape = (3072, 768), *INIT_FROM_CKPT*\n",
- "INFO:tensorflow: name = bert/encoder/layer_9/output/dense/bias:0, shape = (768,), *INIT_FROM_CKPT*\n",
- "INFO:tensorflow: name = bert/encoder/layer_9/output/LayerNorm/beta:0, shape = (768,), *INIT_FROM_CKPT*\n",
- "INFO:tensorflow: name = bert/encoder/layer_9/output/LayerNorm/gamma:0, shape = (768,), *INIT_FROM_CKPT*\n",
- "INFO:tensorflow: name = bert/encoder/layer_10/attention/self/query/kernel:0, shape = (768, 768), *INIT_FROM_CKPT*\n",
- "INFO:tensorflow: name = bert/encoder/layer_10/attention/self/query/bias:0, shape = (768,), *INIT_FROM_CKPT*\n",
- "INFO:tensorflow: name = bert/encoder/layer_10/attention/self/key/kernel:0, shape = (768, 768), *INIT_FROM_CKPT*\n",
- "INFO:tensorflow: name = bert/encoder/layer_10/attention/self/key/bias:0, shape = (768,), *INIT_FROM_CKPT*\n",
- "INFO:tensorflow: name = bert/encoder/layer_10/attention/self/value/kernel:0, shape = (768, 768), *INIT_FROM_CKPT*\n",
- "INFO:tensorflow: name = bert/encoder/layer_10/attention/self/value/bias:0, shape = (768,), *INIT_FROM_CKPT*\n",
- "INFO:tensorflow: name = bert/encoder/layer_10/attention/output/dense/kernel:0, shape = (768, 768), *INIT_FROM_CKPT*\n",
- "INFO:tensorflow: name = bert/encoder/layer_10/attention/output/dense/bias:0, shape = (768,), *INIT_FROM_CKPT*\n",
- "INFO:tensorflow: name = bert/encoder/layer_10/attention/output/LayerNorm/beta:0, shape = (768,), *INIT_FROM_CKPT*\n",
- "INFO:tensorflow: name = bert/encoder/layer_10/attention/output/LayerNorm/gamma:0, shape = (768,), *INIT_FROM_CKPT*\n",
- "INFO:tensorflow: name = bert/encoder/layer_10/intermediate/dense/kernel:0, shape = (768, 3072), *INIT_FROM_CKPT*\n",
- "INFO:tensorflow: name = bert/encoder/layer_10/intermediate/dense/bias:0, shape = (3072,), *INIT_FROM_CKPT*\n",
- "INFO:tensorflow: name = bert/encoder/layer_10/output/dense/kernel:0, shape = (3072, 768), *INIT_FROM_CKPT*\n",
- "INFO:tensorflow: name = bert/encoder/layer_10/output/dense/bias:0, shape = (768,), *INIT_FROM_CKPT*\n",
- "INFO:tensorflow: name = bert/encoder/layer_10/output/LayerNorm/beta:0, shape = (768,), *INIT_FROM_CKPT*\n",
- "INFO:tensorflow: name = bert/encoder/layer_10/output/LayerNorm/gamma:0, shape = (768,), *INIT_FROM_CKPT*\n",
- "INFO:tensorflow: name = bert/encoder/layer_11/attention/self/query/kernel:0, shape = (768, 768), *INIT_FROM_CKPT*\n",
- "INFO:tensorflow: name = bert/encoder/layer_11/attention/self/query/bias:0, shape = (768,), *INIT_FROM_CKPT*\n",
- "INFO:tensorflow: name = bert/encoder/layer_11/attention/self/key/kernel:0, shape = (768, 768), *INIT_FROM_CKPT*\n",
- "INFO:tensorflow: name = bert/encoder/layer_11/attention/self/key/bias:0, shape = (768,), *INIT_FROM_CKPT*\n",
- "INFO:tensorflow: name = bert/encoder/layer_11/attention/self/value/kernel:0, shape = (768, 768), *INIT_FROM_CKPT*\n",
- "INFO:tensorflow: name = bert/encoder/layer_11/attention/self/value/bias:0, shape = (768,), *INIT_FROM_CKPT*\n",
- "INFO:tensorflow: name = bert/encoder/layer_11/attention/output/dense/kernel:0, shape = (768, 768), *INIT_FROM_CKPT*\n",
- "INFO:tensorflow: name = bert/encoder/layer_11/attention/output/dense/bias:0, shape = (768,), *INIT_FROM_CKPT*\n",
- "INFO:tensorflow: name = bert/encoder/layer_11/attention/output/LayerNorm/beta:0, shape = (768,), *INIT_FROM_CKPT*\n",
- "INFO:tensorflow: name = bert/encoder/layer_11/attention/output/LayerNorm/gamma:0, shape = (768,), *INIT_FROM_CKPT*\n",
- "INFO:tensorflow: name = bert/encoder/layer_11/intermediate/dense/kernel:0, shape = (768, 3072), *INIT_FROM_CKPT*\n",
- "INFO:tensorflow: name = bert/encoder/layer_11/intermediate/dense/bias:0, shape = (3072,), *INIT_FROM_CKPT*\n",
- "INFO:tensorflow: name = bert/encoder/layer_11/output/dense/kernel:0, shape = (3072, 768), *INIT_FROM_CKPT*\n",
- "INFO:tensorflow: name = bert/encoder/layer_11/output/dense/bias:0, shape = (768,), *INIT_FROM_CKPT*\n",
- "INFO:tensorflow: name = bert/encoder/layer_11/output/LayerNorm/beta:0, shape = (768,), *INIT_FROM_CKPT*\n",
- "INFO:tensorflow: name = bert/encoder/layer_11/output/LayerNorm/gamma:0, shape = (768,), *INIT_FROM_CKPT*\n",
- "INFO:tensorflow: name = bert/pooler/dense/kernel:0, shape = (768, 768), *INIT_FROM_CKPT*\n",
- "INFO:tensorflow: name = bert/pooler/dense/bias:0, shape = (768,), *INIT_FROM_CKPT*\n",
- "INFO:tensorflow: name = cls/squad/output_weights:0, shape = (2, 768)\n",
- "INFO:tensorflow: name = cls/squad/output_bias:0, shape = (2,)\n",
- "INFO:tensorflow:Done calling model_fn.\n",
- "INFO:tensorflow:Graph was finalized.\n",
- "INFO:tensorflow:Running local_init_op.\n",
- "INFO:tensorflow:Done running local_init_op.\n",
- "INFO:tensorflow:prediction_loop marked as finished\n"
- ]
- }
- ],
- "source": [
- "tensorflow_all_out = []\n",
- "tensorflow_all_results = []\n",
- "for result in estimator.predict(predict_input_fn, yield_single_examples=True):\n",
- " unique_id = int(result[\"unique_ids\"])\n",
- " eval_feature = eval_unique_id_to_feature[unique_id]\n",
- " start_logits = result[\"start_logits\"]\n",
- " end_logits = result[\"end_logits\"]\n",
- " total_loss = result[\"total_loss\"]\n",
- " start_loss = result[\"start_loss\"]\n",
- " end_loss = result[\"end_loss\"]\n",
- "\n",
- " output_json = collections.OrderedDict()\n",
- " output_json[\"linex_index\"] = unique_id\n",
- " output_json[\"tokens\"] = [token for (i, token) in enumerate(eval_feature.tokens)]\n",
- " output_json[\"start_logits\"] = [round(float(x), 6) for x in start_logits.flat]\n",
- " output_json[\"end_logits\"] = [round(float(x), 6) for x in end_logits.flat]\n",
- " output_json[\"total_loss\"] = [round(float(x), 6) for x in total_loss.flat]\n",
- " output_json[\"start_loss\"] = [round(float(x), 6) for x in start_loss.flat]\n",
- " output_json[\"end_loss\"] = [round(float(x), 6) for x in end_loss.flat]\n",
- " tensorflow_all_out.append(output_json)\n",
- " tensorflow_all_results.append(RawResult(\n",
- " unique_id=unique_id,\n",
- " start_logits=start_logits,\n",
- " end_logits=end_logits))\n",
- " break"
- ]
- },
- {
- "cell_type": "code",
- "execution_count": 10,
- "metadata": {
- "ExecuteTime": {
- "end_time": "2018-11-06T10:11:47.912836Z",
- "start_time": "2018-11-06T10:11:47.859679Z"
- },
- "code_folding": []
- },
- "outputs": [],
- "source": [
- "def _get_best_indexes(logits, n_best_size):\n",
- " \"\"\"Get the n-best logits from a list.\"\"\"\n",
- " index_and_score = sorted(enumerate(logits), key=lambda x: x[1], reverse=True)\n",
- "\n",
- " best_indexes = []\n",
- " for i in range(len(index_and_score)):\n",
- " if i >= n_best_size:\n",
- " break\n",
- " best_indexes.append(index_and_score[i][0])\n",
- " return best_indexes\n",
- "\n",
- "def _compute_softmax(scores):\n",
- " \"\"\"Compute softmax probability over raw logits.\"\"\"\n",
- " if not scores:\n",
- " return []\n",
- "\n",
- " max_score = None\n",
- " for score in scores:\n",
- " if max_score is None or score > max_score:\n",
- " max_score = score\n",
- "\n",
- " exp_scores = []\n",
- " total_sum = 0.0\n",
- " for score in scores:\n",
- " x = math.exp(score - max_score)\n",
- " exp_scores.append(x)\n",
- " total_sum += x\n",
- "\n",
- " probs = []\n",
- " for score in exp_scores:\n",
- " probs.append(score / total_sum)\n",
- " return probs\n",
- "\n",
- "\n",
- "def compute_predictions(all_examples, all_features, all_results, n_best_size,\n",
- " max_answer_length, do_lower_case):\n",
- " \"\"\"Compute final predictions.\"\"\"\n",
- " example_index_to_features = collections.defaultdict(list)\n",
- " for feature in all_features:\n",
- " example_index_to_features[feature.example_index].append(feature)\n",
- "\n",
- " unique_id_to_result = {}\n",
- " for result in all_results:\n",
- " unique_id_to_result[result.unique_id] = result\n",
- "\n",
- " _PrelimPrediction = collections.namedtuple( # pylint: disable=invalid-name\n",
- " \"PrelimPrediction\",\n",
- " [\"feature_index\", \"start_index\", \"end_index\", \"start_logit\", \"end_logit\"])\n",
- "\n",
- " all_predictions = collections.OrderedDict()\n",
- " all_nbest_json = collections.OrderedDict()\n",
- " for (example_index, example) in enumerate(all_examples):\n",
- " features = example_index_to_features[example_index]\n",
- "\n",
- " prelim_predictions = []\n",
- " for (feature_index, feature) in enumerate(features):\n",
- " result = unique_id_to_result[feature.unique_id]\n",
- "\n",
- " start_indexes = _get_best_indexes(result.start_logits, n_best_size)\n",
- " end_indexes = _get_best_indexes(result.end_logits, n_best_size)\n",
- " for start_index in start_indexes:\n",
- " for end_index in end_indexes:\n",
- " # We could hypothetically create invalid predictions, e.g., predict\n",
- " # that the start of the span is in the question. We throw out all\n",
- " # invalid predictions.\n",
- " if start_index >= len(feature.tokens):\n",
- " continue\n",
- " if end_index >= len(feature.tokens):\n",
- " continue\n",
- " if start_index not in feature.token_to_orig_map:\n",
- " continue\n",
- " if end_index not in feature.token_to_orig_map:\n",
- " continue\n",
- " if not feature.token_is_max_context.get(start_index, False):\n",
- " continue\n",
- " if end_index < start_index:\n",
- " continue\n",
- " length = end_index - start_index + 1\n",
- " if length > max_answer_length:\n",
- " continue\n",
- " prelim_predictions.append(\n",
- " _PrelimPrediction(\n",
- " feature_index=feature_index,\n",
- " start_index=start_index,\n",
- " end_index=end_index,\n",
- " start_logit=result.start_logits[start_index],\n",
- " end_logit=result.end_logits[end_index]))\n",
- "\n",
- " prelim_predictions = sorted(\n",
- " prelim_predictions,\n",
- " key=lambda x: (x.start_logit + x.end_logit),\n",
- " reverse=True)\n",
- "\n",
- " _NbestPrediction = collections.namedtuple( # pylint: disable=invalid-name\n",
- " \"NbestPrediction\", [\"text\", \"start_logit\", \"end_logit\"])\n",
- "\n",
- " seen_predictions = {}\n",
- " nbest = []\n",
- " for pred in prelim_predictions:\n",
- " if len(nbest) >= n_best_size:\n",
- " break\n",
- " feature = features[pred.feature_index]\n",
- "\n",
- " tok_tokens = feature.tokens[pred.start_index:(pred.end_index + 1)]\n",
- " orig_doc_start = feature.token_to_orig_map[pred.start_index]\n",
- " orig_doc_end = feature.token_to_orig_map[pred.end_index]\n",
- " orig_tokens = example.doc_tokens[orig_doc_start:(orig_doc_end + 1)]\n",
- " tok_text = \" \".join(tok_tokens)\n",
- "\n",
- " # De-tokenize WordPieces that have been split off.\n",
- " tok_text = tok_text.replace(\" ##\", \"\")\n",
- " tok_text = tok_text.replace(\"##\", \"\")\n",
- "\n",
- " # Clean whitespace\n",
- " tok_text = tok_text.strip()\n",
- " tok_text = \" \".join(tok_text.split())\n",
- " orig_text = \" \".join(orig_tokens)\n",
- "\n",
- " final_text = get_final_text(tok_text, orig_text, do_lower_case)\n",
- " if final_text in seen_predictions:\n",
- " continue\n",
- "\n",
- " seen_predictions[final_text] = True\n",
- " nbest.append(\n",
- " _NbestPrediction(\n",
- " text=final_text,\n",
- " start_logit=pred.start_logit,\n",
- " end_logit=pred.end_logit))\n",
- "\n",
- " # In very rare edge cases we could have no valid predictions. So we\n",
- " # just create a nonce prediction in this case to avoid failure.\n",
- " if not nbest:\n",
- " nbest.append(\n",
- " _NbestPrediction(text=\"empty\", start_logit=0.0, end_logit=0.0))\n",
- "\n",
- " assert len(nbest) >= 1\n",
- "\n",
- " total_scores = []\n",
- " for entry in nbest:\n",
- " total_scores.append(entry.start_logit + entry.end_logit)\n",
- "\n",
- " probs = _compute_softmax(total_scores)\n",
- "\n",
- " nbest_json = []\n",
- " for (i, entry) in enumerate(nbest):\n",
- " output = collections.OrderedDict()\n",
- " output[\"text\"] = entry.text\n",
- " output[\"probability\"] = probs[i]\n",
- " output[\"start_logit\"] = entry.start_logit\n",
- " output[\"end_logit\"] = entry.end_logit\n",
- " nbest_json.append(output)\n",
- "\n",
- " assert len(nbest_json) >= 1\n",
- "\n",
- " all_predictions[example.qas_id] = nbest_json[0][\"text\"]\n",
- " all_nbest_json[example.qas_id] = nbest_json\n",
- "\n",
- " return all_predictions, all_nbest_json"
- ]
- },
- {
- "cell_type": "code",
- "execution_count": 11,
- "metadata": {
- "ExecuteTime": {
- "end_time": "2018-11-06T10:11:47.953205Z",
- "start_time": "2018-11-06T10:11:47.914751Z"
- }
- },
- "outputs": [],
- "source": [
- "all_predictions, all_nbest_json = compute_predictions(eval_examples[:1], eval_features[:1], tensorflow_all_results, 20, max_answer_length, True)"
- ]
- },
- {
- "cell_type": "code",
- "execution_count": 12,
- "metadata": {
- "ExecuteTime": {
- "end_time": "2018-11-06T10:11:47.994647Z",
- "start_time": "2018-11-06T10:11:47.955015Z"
- }
- },
- "outputs": [
- {
- "data": {
- "text/plain": [
- "OrderedDict([('5733be284776f41900661182',\n",
- " [OrderedDict([('text', 'empty'),\n",
- " ('probability', 1.0),\n",
- " ('start_logit', 0.0),\n",
- " ('end_logit', 0.0)])])])"
- ]
- },
- "execution_count": 12,
- "metadata": {},
- "output_type": "execute_result"
- }
- ],
- "source": [
- "all_nbest_json"
- ]
- },
- {
- "cell_type": "code",
- "execution_count": 13,
- "metadata": {
- "ExecuteTime": {
- "end_time": "2018-11-06T10:11:48.028473Z",
- "start_time": "2018-11-06T10:11:47.996311Z"
- }
- },
- "outputs": [
- {
- "name": "stdout",
- "output_type": "stream",
- "text": [
- "1\n",
- "7\n",
- "odict_keys(['linex_index', 'tokens', 'start_logits', 'end_logits', 'total_loss', 'start_loss', 'end_loss'])\n",
- "number of tokens 176\n",
- "number of start_logits 384\n",
- "shape of end_logits 384\n"
- ]
- }
- ],
- "source": [
- "print(len(tensorflow_all_out))\n",
- "print(len(tensorflow_all_out[0]))\n",
- "print(tensorflow_all_out[0].keys())\n",
- "print(\"number of tokens\", len(tensorflow_all_out[0]['tokens']))\n",
- "print(\"number of start_logits\", len(tensorflow_all_out[0]['start_logits']))\n",
- "print(\"shape of end_logits\", len(tensorflow_all_out[0]['end_logits']))"
- ]
- },
- {
- "cell_type": "code",
- "execution_count": 14,
- "metadata": {
- "ExecuteTime": {
- "end_time": "2018-11-06T10:11:48.060658Z",
- "start_time": "2018-11-06T10:11:48.030289Z"
- }
- },
- "outputs": [],
- "source": [
- "tensorflow_outputs = [tensorflow_all_out[0]['start_logits'], tensorflow_all_out[0]['end_logits'],\n",
- " tensorflow_all_out[0]['total_loss'], tensorflow_all_out[0]['start_loss'],\n",
- " tensorflow_all_out[0]['end_loss']]"
- ]
- },
- {
- "cell_type": "markdown",
- "metadata": {},
- "source": [
- "## 2/ PyTorch code"
- ]
- },
- {
- "cell_type": "code",
- "execution_count": 15,
- "metadata": {
- "ExecuteTime": {
- "end_time": "2018-11-06T10:11:48.478814Z",
- "start_time": "2018-11-06T10:11:48.062585Z"
- }
- },
- "outputs": [],
- "source": [
- "import modeling\n",
- "from run_squad import *"
- ]
- },
- {
- "cell_type": "code",
- "execution_count": 16,
- "metadata": {
- "ExecuteTime": {
- "end_time": "2018-11-06T10:11:48.512607Z",
- "start_time": "2018-11-06T10:11:48.480729Z"
- }
- },
- "outputs": [],
- "source": [
- "init_checkpoint_pt = \"../google_models/uncased_L-12_H-768_A-12/pytorch_model.bin\""
- ]
- },
- {
- "cell_type": "code",
- "execution_count": 17,
- "metadata": {
- "ExecuteTime": {
- "end_time": "2018-11-06T10:11:51.023405Z",
- "start_time": "2018-11-06T10:11:48.514306Z"
- },
- "scrolled": true
- },
- "outputs": [
- {
- "data": {
- "text/plain": [
- "tensor([0., 0.])"
- ]
- },
- "execution_count": 17,
- "metadata": {},
- "output_type": "execute_result"
- }
- ],
- "source": [
- "device = torch.device(\"cpu\")\n",
- "model = modeling.BertForQuestionAnswering(bert_config)\n",
- "model.bert.load_state_dict(torch.load(init_checkpoint_pt, map_location='cpu'))\n",
- "model.to(device)\n",
- "model.qa_outputs.weight.data.fill_(1.0)\n",
- "model.qa_outputs.bias.data.zero_()"
- ]
- },
- {
- "cell_type": "code",
- "execution_count": 18,
- "metadata": {
- "ExecuteTime": {
- "end_time": "2018-11-06T10:11:51.079364Z",
- "start_time": "2018-11-06T10:11:51.028228Z"
- },
- "code_folding": []
- },
- "outputs": [],
- "source": [
- "all_input_ids = torch.tensor([f.input_ids for f in eval_features], dtype=torch.long)\n",
- "all_input_mask = torch.tensor([f.input_mask for f in eval_features], dtype=torch.long)\n",
- "all_segment_ids = torch.tensor([f.segment_ids for f in eval_features], dtype=torch.long)\n",
- "all_example_index = torch.arange(all_input_ids.size(0), dtype=torch.long)\n",
- "all_start_positions = torch.tensor([[f.start_position] for f in eval_features], dtype=torch.long)\n",
- "all_end_positions = torch.tensor([[f.end_position] for f in eval_features], dtype=torch.long)\n",
- "\n",
- "eval_data = TensorDataset(all_input_ids, all_input_mask, all_segment_ids,\n",
- " all_start_positions, all_end_positions, all_example_index)\n",
- "eval_sampler = SequentialSampler(eval_data)\n",
- "eval_dataloader = DataLoader(eval_data, sampler=eval_sampler, batch_size=1)\n",
- "\n",
- "model.eval()\n",
- "None"
- ]
- },
- {
- "cell_type": "code",
- "execution_count": 19,
- "metadata": {
- "ExecuteTime": {
- "end_time": "2018-11-06T10:11:51.114686Z",
- "start_time": "2018-11-06T10:11:51.081474Z"
- }
- },
- "outputs": [
- {
- "name": "stdout",
- "output_type": "stream",
- "text": [
- "[torch.Size([1, 384]), torch.Size([1, 384]), torch.Size([1, 384]), torch.Size([1, 1]), torch.Size([1, 1]), torch.Size([1])]\n"
- ]
- },
- {
- "data": {
- "text/plain": [
- "torch.Size([1, 1])"
- ]
- },
- "execution_count": 19,
- "metadata": {},
- "output_type": "execute_result"
- }
- ],
- "source": [
- "batch = iter(eval_dataloader).next()\n",
- "input_ids, input_mask, segment_ids, start_positions, end_positions, example_index = batch\n",
- "print([t.shape for t in batch])\n",
- "start_positions.size()"
- ]
- },
- {
- "cell_type": "code",
- "execution_count": 20,
- "metadata": {
- "ExecuteTime": {
- "end_time": "2018-11-06T10:11:52.298367Z",
- "start_time": "2018-11-06T10:11:51.116219Z"
- }
- },
- "outputs": [
- {
- "name": "stderr",
- "output_type": "stream",
- "text": [
- "Evaluating: 0%| | 0/270 [00:00, ?it/s]\n"
- ]
- }
- ],
- "source": [
- "pytorch_all_out = []\n",
- "for batch in tqdm(eval_dataloader, desc=\"Evaluating\"):\n",
- " input_ids, input_mask, segment_ids, start_positions, end_positions, example_index = batch\n",
- " input_ids = input_ids.to(device)\n",
- " input_mask = input_mask.to(device)\n",
- " segment_ids = segment_ids.to(device)\n",
- " start_positions = start_positions.to(device)\n",
- " end_positions = end_positions.to(device)\n",
- "\n",
- " total_loss, (start_logits, end_logits) = model(input_ids, segment_ids, input_mask, start_positions, end_positions)\n",
- " \n",
- " eval_feature = eval_features[example_index.item()]\n",
- "\n",
- " output_json = collections.OrderedDict()\n",
- " output_json[\"linex_index\"] = unique_id\n",
- " output_json[\"tokens\"] = [token for (i, token) in enumerate(eval_feature.tokens)]\n",
- " output_json[\"total_loss\"] = total_loss.detach().cpu().numpy()\n",
- " output_json[\"start_logits\"] = start_logits.detach().cpu().numpy()\n",
- " output_json[\"end_logits\"] = end_logits.detach().cpu().numpy()\n",
- " pytorch_all_out.append(output_json)\n",
- " break"
- ]
- },
- {
- "cell_type": "code",
- "execution_count": 21,
- "metadata": {
- "ExecuteTime": {
- "end_time": "2018-11-06T10:11:52.339553Z",
- "start_time": "2018-11-06T10:11:52.300335Z"
- }
- },
- "outputs": [
- {
- "name": "stdout",
- "output_type": "stream",
- "text": [
- "1\n",
- "5\n",
- "odict_keys(['linex_index', 'tokens', 'total_loss', 'start_logits', 'end_logits'])\n",
- "number of tokens 176\n",
- "number of start_logits 1\n",
- "number of end_logits 1\n"
- ]
- }
- ],
- "source": [
- "print(len(pytorch_all_out))\n",
- "print(len(pytorch_all_out[0]))\n",
- "print(pytorch_all_out[0].keys())\n",
- "print(\"number of tokens\", len(pytorch_all_out[0]['tokens']))\n",
- "print(\"number of start_logits\", len(pytorch_all_out[0]['start_logits']))\n",
- "print(\"number of end_logits\", len(pytorch_all_out[0]['end_logits']))"
- ]
- },
- {
- "cell_type": "code",
- "execution_count": 22,
- "metadata": {
- "ExecuteTime": {
- "end_time": "2018-11-06T10:11:52.372827Z",
- "start_time": "2018-11-06T10:11:52.341393Z"
- }
- },
- "outputs": [],
- "source": [
- "pytorch_outputs = [pytorch_all_out[0]['start_logits'], pytorch_all_out[0]['end_logits'], pytorch_all_out[0]['total_loss']]"
- ]
- },
- {
- "cell_type": "markdown",
- "metadata": {},
- "source": [
- "## 3/ Comparing the standard deviation of start_logits, end_logits and loss of both models"
- ]
- },
- {
- "cell_type": "code",
- "execution_count": 23,
- "metadata": {
- "ExecuteTime": {
- "end_time": "2018-11-06T10:11:52.402814Z",
- "start_time": "2018-11-06T10:11:52.374329Z"
- }
- },
- "outputs": [],
- "source": [
- "import numpy as np"
- ]
- },
- {
- "cell_type": "code",
- "execution_count": 24,
- "metadata": {
- "ExecuteTime": {
- "end_time": "2018-11-06T10:11:52.434743Z",
- "start_time": "2018-11-06T10:11:52.404345Z"
- }
- },
- "outputs": [
- {
- "name": "stdout",
- "output_type": "stream",
- "text": [
- "shape tensorflow layer, shape pytorch layer, standard deviation\n",
- "((384,), (1, 384), 5.244962470555037e-06)\n",
- "((384,), (1, 384), 5.244962470555037e-06)\n",
- "((1,), (), 4.560241698925438e-06)\n"
- ]
- }
- ],
- "source": [
- "print('shape tensorflow layer, shape pytorch layer, standard deviation')\n",
- "print('\\n'.join(list(str((np.array(tensorflow_outputs[i]).shape,\n",
- " np.array(pytorch_outputs[i]).shape, \n",
- " np.sqrt(np.mean((np.array(tensorflow_outputs[i]) - np.array(pytorch_outputs[i]))**2.0)))) for i in range(3))))"
- ]
- },
- {
- "cell_type": "code",
- "execution_count": 27,
- "metadata": {
- "ExecuteTime": {
- "end_time": "2018-11-06T10:12:54.200059Z",
- "start_time": "2018-11-06T10:12:54.167355Z"
- }
- },
- "outputs": [
- {
- "name": "stdout",
- "output_type": "stream",
- "text": [
- "Total loss of the TF model 9.06024 - Total loss of the PT model 9.0602445602417\n"
- ]
- }
- ],
- "source": [
- "print(\"Total loss of the TF model {} - Total loss of the PT model {}\".format(tensorflow_outputs[2][0], pytorch_outputs[2]))"
- ]
- },
- {
- "cell_type": "code",
- "execution_count": null,
- "metadata": {},
- "outputs": [],
- "source": []
- }
- ],
- "metadata": {
- "hide_input": false,
- "kernelspec": {
- "display_name": "Python [default]",
- "language": "python",
- "name": "python3"
- },
- "language_info": {
- "codemirror_mode": {
- "name": "ipython",
- "version": 3
- },
- "file_extension": ".py",
- "mimetype": "text/x-python",
- "name": "python",
- "nbconvert_exporter": "python",
- "pygments_lexer": "ipython3",
- "version": "3.6.7"
- },
- "toc": {
- "colors": {
- "hover_highlight": "#DAA520",
- "running_highlight": "#FF0000",
- "selected_highlight": "#FFD700"
- },
- "moveMenuLeft": true,
- "nav_menu": {
- "height": "48px",
- "width": "252px"
- },
- "navigate_menu": true,
- "number_sections": true,
- "sideBar": true,
- "threshold": 4,
- "toc_cell": false,
- "toc_section_display": "block",
- "toc_window_display": false
- }
- },
- "nbformat": 4,
- "nbformat_minor": 2
-}
diff --git a/server/transformers/notebooks/Comparing-TF-and-PT-models.ipynb b/server/transformers/notebooks/Comparing-TF-and-PT-models.ipynb
deleted file mode 100644
index b7382e4652bc5c1b80c4664811b1f45375483512..0000000000000000000000000000000000000000
--- a/server/transformers/notebooks/Comparing-TF-and-PT-models.ipynb
+++ /dev/null
@@ -1,1318 +0,0 @@
-{
- "cells": [
- {
- "cell_type": "markdown",
- "metadata": {},
- "source": [
- "# Comparing TensorFlow (original) and PyTorch models\n",
- "\n",
- "You can use this small notebook to check the conversion of the model's weights from the TensorFlow model to the PyTorch model. In the following, we compare the weights of the last layer on a simple example (in `input.txt`) but both models returns all the hidden layers so you can check every stage of the model.\n",
- "\n",
- "To run this notebook, follow these instructions:\n",
- "- make sure that your Python environment has both TensorFlow and PyTorch installed,\n",
- "- download the original TensorFlow implementation,\n",
- "- download a pre-trained TensorFlow model as indicaded in the TensorFlow implementation readme,\n",
- "- run the script `convert_tf_checkpoint_to_pytorch.py` as indicated in the `README` to convert the pre-trained TensorFlow model to PyTorch.\n",
- "\n",
- "If needed change the relative paths indicated in this notebook (at the beggining of Sections 1 and 2) to point to the relevent models and code."
- ]
- },
- {
- "cell_type": "code",
- "execution_count": 1,
- "metadata": {
- "ExecuteTime": {
- "end_time": "2018-11-15T14:56:48.412622Z",
- "start_time": "2018-11-15T14:56:48.400110Z"
- }
- },
- "outputs": [],
- "source": [
- "import os\n",
- "os.chdir('../')"
- ]
- },
- {
- "cell_type": "markdown",
- "metadata": {},
- "source": [
- "## 1/ TensorFlow code"
- ]
- },
- {
- "cell_type": "code",
- "execution_count": 2,
- "metadata": {
- "ExecuteTime": {
- "end_time": "2018-11-15T14:56:49.483829Z",
- "start_time": "2018-11-15T14:56:49.471296Z"
- }
- },
- "outputs": [],
- "source": [
- "original_tf_inplem_dir = \"./tensorflow_code/\"\n",
- "model_dir = \"../google_models/uncased_L-12_H-768_A-12/\"\n",
- "\n",
- "vocab_file = model_dir + \"vocab.txt\"\n",
- "bert_config_file = model_dir + \"bert_config.json\"\n",
- "init_checkpoint = model_dir + \"bert_model.ckpt\"\n",
- "\n",
- "input_file = \"./samples/input.txt\"\n",
- "max_seq_length = 128"
- ]
- },
- {
- "cell_type": "code",
- "execution_count": 6,
- "metadata": {
- "ExecuteTime": {
- "end_time": "2018-11-15T14:57:51.597932Z",
- "start_time": "2018-11-15T14:57:51.549466Z"
- }
- },
- "outputs": [
- {
- "ename": "DuplicateFlagError",
- "evalue": "The flag 'input_file' is defined twice. First from *, Second from *. Description from first occurrence: (no help available)",
- "output_type": "error",
- "traceback": [
- "\u001b[0;31m---------------------------------------------------------------------------\u001b[0m",
- "\u001b[0;31mDuplicateFlagError\u001b[0m Traceback (most recent call last)",
- "\u001b[0;32m\u001b[0m in \u001b[0;36m\u001b[0;34m\u001b[0m\n\u001b[1;32m 4\u001b[0m \u001b[0mspec\u001b[0m \u001b[0;34m=\u001b[0m \u001b[0mimportlib\u001b[0m\u001b[0;34m.\u001b[0m\u001b[0mutil\u001b[0m\u001b[0;34m.\u001b[0m\u001b[0mspec_from_file_location\u001b[0m\u001b[0;34m(\u001b[0m\u001b[0;34m'*'\u001b[0m\u001b[0;34m,\u001b[0m \u001b[0moriginal_tf_inplem_dir\u001b[0m \u001b[0;34m+\u001b[0m \u001b[0;34m'/extract_features_tensorflow.py'\u001b[0m\u001b[0;34m)\u001b[0m\u001b[0;34m\u001b[0m\u001b[0;34m\u001b[0m\u001b[0m\n\u001b[1;32m 5\u001b[0m \u001b[0mmodule\u001b[0m \u001b[0;34m=\u001b[0m \u001b[0mimportlib\u001b[0m\u001b[0;34m.\u001b[0m\u001b[0mutil\u001b[0m\u001b[0;34m.\u001b[0m\u001b[0mmodule_from_spec\u001b[0m\u001b[0;34m(\u001b[0m\u001b[0mspec\u001b[0m\u001b[0;34m)\u001b[0m\u001b[0;34m\u001b[0m\u001b[0;34m\u001b[0m\u001b[0m\n\u001b[0;32m----> 6\u001b[0;31m \u001b[0mspec\u001b[0m\u001b[0;34m.\u001b[0m\u001b[0mloader\u001b[0m\u001b[0;34m.\u001b[0m\u001b[0mexec_module\u001b[0m\u001b[0;34m(\u001b[0m\u001b[0mmodule\u001b[0m\u001b[0;34m)\u001b[0m\u001b[0;34m\u001b[0m\u001b[0;34m\u001b[0m\u001b[0m\n\u001b[0m\u001b[1;32m 7\u001b[0m \u001b[0msys\u001b[0m\u001b[0;34m.\u001b[0m\u001b[0mmodules\u001b[0m\u001b[0;34m[\u001b[0m\u001b[0;34m'extract_features_tensorflow'\u001b[0m\u001b[0;34m]\u001b[0m \u001b[0;34m=\u001b[0m \u001b[0mmodule\u001b[0m\u001b[0;34m\u001b[0m\u001b[0;34m\u001b[0m\u001b[0m\n\u001b[1;32m 8\u001b[0m \u001b[0;34m\u001b[0m\u001b[0m\n",
- "\u001b[0;32m~/miniconda3/envs/bert/lib/python3.6/importlib/_bootstrap_external.py\u001b[0m in \u001b[0;36mexec_module\u001b[0;34m(self, module)\u001b[0m\n",
- "\u001b[0;32m~/miniconda3/envs/bert/lib/python3.6/importlib/_bootstrap.py\u001b[0m in \u001b[0;36m_call_with_frames_removed\u001b[0;34m(f, *args, **kwds)\u001b[0m\n",
- "\u001b[0;32m~/Documents/Thomas/Code/HF/BERT/pytorch-pretrained-BERT/tensorflow_code/extract_features_tensorflow.py\u001b[0m in \u001b[0;36m\u001b[0;34m\u001b[0m\n\u001b[1;32m 32\u001b[0m \u001b[0mFLAGS\u001b[0m \u001b[0;34m=\u001b[0m \u001b[0mflags\u001b[0m\u001b[0;34m.\u001b[0m\u001b[0mFLAGS\u001b[0m\u001b[0;34m\u001b[0m\u001b[0;34m\u001b[0m\u001b[0m\n\u001b[1;32m 33\u001b[0m \u001b[0;34m\u001b[0m\u001b[0m\n\u001b[0;32m---> 34\u001b[0;31m \u001b[0mflags\u001b[0m\u001b[0;34m.\u001b[0m\u001b[0mDEFINE_string\u001b[0m\u001b[0;34m(\u001b[0m\u001b[0;34m\"input_file\"\u001b[0m\u001b[0;34m,\u001b[0m \u001b[0;32mNone\u001b[0m\u001b[0;34m,\u001b[0m \u001b[0;34m\"\"\u001b[0m\u001b[0;34m)\u001b[0m\u001b[0;34m\u001b[0m\u001b[0;34m\u001b[0m\u001b[0m\n\u001b[0m\u001b[1;32m 35\u001b[0m \u001b[0;34m\u001b[0m\u001b[0m\n\u001b[1;32m 36\u001b[0m \u001b[0mflags\u001b[0m\u001b[0;34m.\u001b[0m\u001b[0mDEFINE_string\u001b[0m\u001b[0;34m(\u001b[0m\u001b[0;34m\"output_file\"\u001b[0m\u001b[0;34m,\u001b[0m \u001b[0;32mNone\u001b[0m\u001b[0;34m,\u001b[0m \u001b[0;34m\"\"\u001b[0m\u001b[0;34m)\u001b[0m\u001b[0;34m\u001b[0m\u001b[0;34m\u001b[0m\u001b[0m\n",
- "\u001b[0;32m~/miniconda3/envs/bert/lib/python3.6/site-packages/tensorflow/python/platform/flags.py\u001b[0m in \u001b[0;36mwrapper\u001b[0;34m(*args, **kwargs)\u001b[0m\n\u001b[1;32m 56\u001b[0m \u001b[0;34m'Use of the keyword argument names (flag_name, default_value, '\u001b[0m\u001b[0;34m\u001b[0m\u001b[0;34m\u001b[0m\u001b[0m\n\u001b[1;32m 57\u001b[0m 'docstring) is deprecated, please use (name, default, help) instead.')\n\u001b[0;32m---> 58\u001b[0;31m \u001b[0;32mreturn\u001b[0m \u001b[0moriginal_function\u001b[0m\u001b[0;34m(\u001b[0m\u001b[0;34m*\u001b[0m\u001b[0margs\u001b[0m\u001b[0;34m,\u001b[0m \u001b[0;34m**\u001b[0m\u001b[0mkwargs\u001b[0m\u001b[0;34m)\u001b[0m\u001b[0;34m\u001b[0m\u001b[0;34m\u001b[0m\u001b[0m\n\u001b[0m\u001b[1;32m 59\u001b[0m \u001b[0;34m\u001b[0m\u001b[0m\n\u001b[1;32m 60\u001b[0m \u001b[0;32mreturn\u001b[0m \u001b[0mtf_decorator\u001b[0m\u001b[0;34m.\u001b[0m\u001b[0mmake_decorator\u001b[0m\u001b[0;34m(\u001b[0m\u001b[0moriginal_function\u001b[0m\u001b[0;34m,\u001b[0m \u001b[0mwrapper\u001b[0m\u001b[0;34m)\u001b[0m\u001b[0;34m\u001b[0m\u001b[0;34m\u001b[0m\u001b[0m\n",
- "\u001b[0;32m~/miniconda3/envs/bert/lib/python3.6/site-packages/absl/flags/_defines.py\u001b[0m in \u001b[0;36mDEFINE_string\u001b[0;34m(name, default, help, flag_values, **args)\u001b[0m\n\u001b[1;32m 239\u001b[0m \u001b[0mparser\u001b[0m \u001b[0;34m=\u001b[0m \u001b[0m_argument_parser\u001b[0m\u001b[0;34m.\u001b[0m\u001b[0mArgumentParser\u001b[0m\u001b[0;34m(\u001b[0m\u001b[0;34m)\u001b[0m\u001b[0;34m\u001b[0m\u001b[0;34m\u001b[0m\u001b[0m\n\u001b[1;32m 240\u001b[0m \u001b[0mserializer\u001b[0m \u001b[0;34m=\u001b[0m \u001b[0m_argument_parser\u001b[0m\u001b[0;34m.\u001b[0m\u001b[0mArgumentSerializer\u001b[0m\u001b[0;34m(\u001b[0m\u001b[0;34m)\u001b[0m\u001b[0;34m\u001b[0m\u001b[0;34m\u001b[0m\u001b[0m\n\u001b[0;32m--> 241\u001b[0;31m \u001b[0mDEFINE\u001b[0m\u001b[0;34m(\u001b[0m\u001b[0mparser\u001b[0m\u001b[0;34m,\u001b[0m \u001b[0mname\u001b[0m\u001b[0;34m,\u001b[0m \u001b[0mdefault\u001b[0m\u001b[0;34m,\u001b[0m \u001b[0mhelp\u001b[0m\u001b[0;34m,\u001b[0m \u001b[0mflag_values\u001b[0m\u001b[0;34m,\u001b[0m \u001b[0mserializer\u001b[0m\u001b[0;34m,\u001b[0m \u001b[0;34m**\u001b[0m\u001b[0margs\u001b[0m\u001b[0;34m)\u001b[0m\u001b[0;34m\u001b[0m\u001b[0;34m\u001b[0m\u001b[0m\n\u001b[0m\u001b[1;32m 242\u001b[0m \u001b[0;34m\u001b[0m\u001b[0m\n\u001b[1;32m 243\u001b[0m \u001b[0;34m\u001b[0m\u001b[0m\n",
- "\u001b[0;32m~/miniconda3/envs/bert/lib/python3.6/site-packages/absl/flags/_defines.py\u001b[0m in \u001b[0;36mDEFINE\u001b[0;34m(parser, name, default, help, flag_values, serializer, module_name, **args)\u001b[0m\n\u001b[1;32m 80\u001b[0m \"\"\"\n\u001b[1;32m 81\u001b[0m DEFINE_flag(_flag.Flag(parser, serializer, name, default, help, **args),\n\u001b[0;32m---> 82\u001b[0;31m flag_values, module_name)\n\u001b[0m\u001b[1;32m 83\u001b[0m \u001b[0;34m\u001b[0m\u001b[0m\n\u001b[1;32m 84\u001b[0m \u001b[0;34m\u001b[0m\u001b[0m\n",
- "\u001b[0;32m~/miniconda3/envs/bert/lib/python3.6/site-packages/absl/flags/_defines.py\u001b[0m in \u001b[0;36mDEFINE_flag\u001b[0;34m(flag, flag_values, module_name)\u001b[0m\n\u001b[1;32m 102\u001b[0m \u001b[0;31m# Copying the reference to flag_values prevents pychecker warnings.\u001b[0m\u001b[0;34m\u001b[0m\u001b[0;34m\u001b[0m\u001b[0;34m\u001b[0m\u001b[0m\n\u001b[1;32m 103\u001b[0m \u001b[0mfv\u001b[0m \u001b[0;34m=\u001b[0m \u001b[0mflag_values\u001b[0m\u001b[0;34m\u001b[0m\u001b[0;34m\u001b[0m\u001b[0m\n\u001b[0;32m--> 104\u001b[0;31m \u001b[0mfv\u001b[0m\u001b[0;34m[\u001b[0m\u001b[0mflag\u001b[0m\u001b[0;34m.\u001b[0m\u001b[0mname\u001b[0m\u001b[0;34m]\u001b[0m \u001b[0;34m=\u001b[0m \u001b[0mflag\u001b[0m\u001b[0;34m\u001b[0m\u001b[0;34m\u001b[0m\u001b[0m\n\u001b[0m\u001b[1;32m 105\u001b[0m \u001b[0;31m# Tell flag_values who's defining the flag.\u001b[0m\u001b[0;34m\u001b[0m\u001b[0;34m\u001b[0m\u001b[0;34m\u001b[0m\u001b[0m\n\u001b[1;32m 106\u001b[0m \u001b[0;32mif\u001b[0m \u001b[0mmodule_name\u001b[0m\u001b[0;34m:\u001b[0m\u001b[0;34m\u001b[0m\u001b[0;34m\u001b[0m\u001b[0m\n",
- "\u001b[0;32m~/miniconda3/envs/bert/lib/python3.6/site-packages/absl/flags/_flagvalues.py\u001b[0m in \u001b[0;36m__setitem__\u001b[0;34m(self, name, flag)\u001b[0m\n\u001b[1;32m 427\u001b[0m \u001b[0;31m# module is simply being imported a subsequent time.\u001b[0m\u001b[0;34m\u001b[0m\u001b[0;34m\u001b[0m\u001b[0;34m\u001b[0m\u001b[0m\n\u001b[1;32m 428\u001b[0m \u001b[0;32mreturn\u001b[0m\u001b[0;34m\u001b[0m\u001b[0;34m\u001b[0m\u001b[0m\n\u001b[0;32m--> 429\u001b[0;31m \u001b[0;32mraise\u001b[0m \u001b[0m_exceptions\u001b[0m\u001b[0;34m.\u001b[0m\u001b[0mDuplicateFlagError\u001b[0m\u001b[0;34m.\u001b[0m\u001b[0mfrom_flag\u001b[0m\u001b[0;34m(\u001b[0m\u001b[0mname\u001b[0m\u001b[0;34m,\u001b[0m \u001b[0mself\u001b[0m\u001b[0;34m)\u001b[0m\u001b[0;34m\u001b[0m\u001b[0;34m\u001b[0m\u001b[0m\n\u001b[0m\u001b[1;32m 430\u001b[0m \u001b[0mshort_name\u001b[0m \u001b[0;34m=\u001b[0m \u001b[0mflag\u001b[0m\u001b[0;34m.\u001b[0m\u001b[0mshort_name\u001b[0m\u001b[0;34m\u001b[0m\u001b[0;34m\u001b[0m\u001b[0m\n\u001b[1;32m 431\u001b[0m \u001b[0;31m# If a new flag overrides an old one, we need to cleanup the old flag's\u001b[0m\u001b[0;34m\u001b[0m\u001b[0;34m\u001b[0m\u001b[0;34m\u001b[0m\u001b[0m\n",
- "\u001b[0;31mDuplicateFlagError\u001b[0m: The flag 'input_file' is defined twice. First from *, Second from *. Description from first occurrence: (no help available)"
- ]
- }
- ],
- "source": [
- "import importlib.util\n",
- "import sys\n",
- "\n",
- "spec = importlib.util.spec_from_file_location('*', original_tf_inplem_dir + '/extract_features_tensorflow.py')\n",
- "module = importlib.util.module_from_spec(spec)\n",
- "spec.loader.exec_module(module)\n",
- "sys.modules['extract_features_tensorflow'] = module\n",
- "\n",
- "from extract_features_tensorflow import *"
- ]
- },
- {
- "cell_type": "code",
- "execution_count": 8,
- "metadata": {
- "ExecuteTime": {
- "end_time": "2018-11-15T14:58:05.650987Z",
- "start_time": "2018-11-15T14:58:05.541620Z"
- }
- },
- "outputs": [
- {
- "name": "stdout",
- "output_type": "stream",
- "text": [
- "INFO:tensorflow:*** Example ***\n",
- "INFO:tensorflow:unique_id: 0\n",
- "INFO:tensorflow:tokens: [CLS] who was jim henson ? [SEP] jim henson was a puppet ##eer [SEP]\n",
- "INFO:tensorflow:input_ids: 101 2040 2001 3958 27227 1029 102 3958 27227 2001 1037 13997 11510 102 0 0 0 0 0 0 0 0 0 0 0 0 0 0 0 0 0 0 0 0 0 0 0 0 0 0 0 0 0 0 0 0 0 0 0 0 0 0 0 0 0 0 0 0 0 0 0 0 0 0 0 0 0 0 0 0 0 0 0 0 0 0 0 0 0 0 0 0 0 0 0 0 0 0 0 0 0 0 0 0 0 0 0 0 0 0 0 0 0 0 0 0 0 0 0 0 0 0 0 0 0 0 0 0 0 0 0 0 0 0 0 0 0 0\n",
- "INFO:tensorflow:input_mask: 1 1 1 1 1 1 1 1 1 1 1 1 1 1 0 0 0 0 0 0 0 0 0 0 0 0 0 0 0 0 0 0 0 0 0 0 0 0 0 0 0 0 0 0 0 0 0 0 0 0 0 0 0 0 0 0 0 0 0 0 0 0 0 0 0 0 0 0 0 0 0 0 0 0 0 0 0 0 0 0 0 0 0 0 0 0 0 0 0 0 0 0 0 0 0 0 0 0 0 0 0 0 0 0 0 0 0 0 0 0 0 0 0 0 0 0 0 0 0 0 0 0 0 0 0 0 0 0\n",
- "INFO:tensorflow:input_type_ids: 0 0 0 0 0 0 0 1 1 1 1 1 1 1 0 0 0 0 0 0 0 0 0 0 0 0 0 0 0 0 0 0 0 0 0 0 0 0 0 0 0 0 0 0 0 0 0 0 0 0 0 0 0 0 0 0 0 0 0 0 0 0 0 0 0 0 0 0 0 0 0 0 0 0 0 0 0 0 0 0 0 0 0 0 0 0 0 0 0 0 0 0 0 0 0 0 0 0 0 0 0 0 0 0 0 0 0 0 0 0 0 0 0 0 0 0 0 0 0 0 0 0 0 0 0 0 0 0\n"
- ]
- }
- ],
- "source": [
- "layer_indexes = list(range(12))\n",
- "bert_config = modeling.BertConfig.from_json_file(bert_config_file)\n",
- "tokenizer = tokenization.FullTokenizer(\n",
- " vocab_file=vocab_file, do_lower_case=True)\n",
- "examples = read_examples(input_file)\n",
- "\n",
- "features = convert_examples_to_features(\n",
- " examples=examples, seq_length=max_seq_length, tokenizer=tokenizer)\n",
- "unique_id_to_feature = {}\n",
- "for feature in features:\n",
- " unique_id_to_feature[feature.unique_id] = feature"
- ]
- },
- {
- "cell_type": "code",
- "execution_count": 9,
- "metadata": {
- "ExecuteTime": {
- "end_time": "2018-11-15T14:58:11.562443Z",
- "start_time": "2018-11-15T14:58:08.036485Z"
- }
- },
- "outputs": [
- {
- "name": "stdout",
- "output_type": "stream",
- "text": [
- "WARNING:tensorflow:Estimator's model_fn (.model_fn at 0x11ea7f1e0>) includes params argument, but params are not passed to Estimator.\n",
- "WARNING:tensorflow:Using temporary folder as model directory: /var/folders/yx/cw8n_njx3js5jksyw_qlp8p00000gn/T/tmphs4_nsq9\n",
- "INFO:tensorflow:Using config: {'_model_dir': '/var/folders/yx/cw8n_njx3js5jksyw_qlp8p00000gn/T/tmphs4_nsq9', '_tf_random_seed': None, '_save_summary_steps': 100, '_save_checkpoints_steps': None, '_save_checkpoints_secs': 600, '_session_config': allow_soft_placement: true\n",
- "graph_options {\n",
- " rewrite_options {\n",
- " meta_optimizer_iterations: ONE\n",
- " }\n",
- "}\n",
- ", '_keep_checkpoint_max': 5, '_keep_checkpoint_every_n_hours': 10000, '_log_step_count_steps': None, '_train_distribute': None, '_device_fn': None, '_protocol': None, '_eval_distribute': None, '_experimental_distribute': None, '_service': None, '_cluster_spec': , '_task_type': 'worker', '_task_id': 0, '_global_id_in_cluster': 0, '_master': '', '_evaluation_master': '', '_is_chief': True, '_num_ps_replicas': 0, '_num_worker_replicas': 1, '_tpu_config': TPUConfig(iterations_per_loop=2, num_shards=1, num_cores_per_replica=None, per_host_input_for_training=3, tpu_job_name=None, initial_infeed_sleep_secs=None, input_partition_dims=None), '_cluster': None}\n",
- "WARNING:tensorflow:Setting TPUConfig.num_shards==1 is an unsupported behavior. Please fix as soon as possible (leaving num_shards as None.\n",
- "INFO:tensorflow:_TPUContext: eval_on_tpu True\n",
- "WARNING:tensorflow:eval_on_tpu ignored because use_tpu is False.\n"
- ]
- }
- ],
- "source": [
- "is_per_host = tf.contrib.tpu.InputPipelineConfig.PER_HOST_V2\n",
- "run_config = tf.contrib.tpu.RunConfig(\n",
- " master=None,\n",
- " tpu_config=tf.contrib.tpu.TPUConfig(\n",
- " num_shards=1,\n",
- " per_host_input_for_training=is_per_host))\n",
- "\n",
- "model_fn = model_fn_builder(\n",
- " bert_config=bert_config,\n",
- " init_checkpoint=init_checkpoint,\n",
- " layer_indexes=layer_indexes,\n",
- " use_tpu=False,\n",
- " use_one_hot_embeddings=False)\n",
- "\n",
- "# If TPU is not available, this will fall back to normal Estimator on CPU\n",
- "# or GPU.\n",
- "estimator = tf.contrib.tpu.TPUEstimator(\n",
- " use_tpu=False,\n",
- " model_fn=model_fn,\n",
- " config=run_config,\n",
- " predict_batch_size=1)\n",
- "\n",
- "input_fn = input_fn_builder(\n",
- " features=features, seq_length=max_seq_length)"
- ]
- },
- {
- "cell_type": "code",
- "execution_count": 10,
- "metadata": {
- "ExecuteTime": {
- "end_time": "2018-11-15T14:58:21.736543Z",
- "start_time": "2018-11-15T14:58:16.723829Z"
- }
- },
- "outputs": [
- {
- "name": "stdout",
- "output_type": "stream",
- "text": [
- "INFO:tensorflow:Could not find trained model in model_dir: /var/folders/yx/cw8n_njx3js5jksyw_qlp8p00000gn/T/tmphs4_nsq9, running initialization to predict.\n",
- "INFO:tensorflow:Calling model_fn.\n",
- "INFO:tensorflow:Running infer on CPU\n",
- "INFO:tensorflow:Done calling model_fn.\n",
- "INFO:tensorflow:Graph was finalized.\n",
- "INFO:tensorflow:Running local_init_op.\n",
- "INFO:tensorflow:Done running local_init_op.\n",
- "extracting layer 0\n",
- "extracting layer 1\n",
- "extracting layer 2\n",
- "extracting layer 3\n",
- "extracting layer 4\n",
- "extracting layer 5\n",
- "extracting layer 6\n",
- "extracting layer 7\n",
- "extracting layer 8\n",
- "extracting layer 9\n",
- "extracting layer 10\n",
- "extracting layer 11\n",
- "INFO:tensorflow:prediction_loop marked as finished\n",
- "INFO:tensorflow:prediction_loop marked as finished\n"
- ]
- }
- ],
- "source": [
- "tensorflow_all_out = []\n",
- "for result in estimator.predict(input_fn, yield_single_examples=True):\n",
- " unique_id = int(result[\"unique_id\"])\n",
- " feature = unique_id_to_feature[unique_id]\n",
- " output_json = collections.OrderedDict()\n",
- " output_json[\"linex_index\"] = unique_id\n",
- " tensorflow_all_out_features = []\n",
- " # for (i, token) in enumerate(feature.tokens):\n",
- " all_layers = []\n",
- " for (j, layer_index) in enumerate(layer_indexes):\n",
- " print(\"extracting layer {}\".format(j))\n",
- " layer_output = result[\"layer_output_%d\" % j]\n",
- " layers = collections.OrderedDict()\n",
- " layers[\"index\"] = layer_index\n",
- " layers[\"values\"] = layer_output\n",
- " all_layers.append(layers)\n",
- " tensorflow_out_features = collections.OrderedDict()\n",
- " tensorflow_out_features[\"layers\"] = all_layers\n",
- " tensorflow_all_out_features.append(tensorflow_out_features)\n",
- "\n",
- " output_json[\"features\"] = tensorflow_all_out_features\n",
- " tensorflow_all_out.append(output_json)"
- ]
- },
- {
- "cell_type": "code",
- "execution_count": 11,
- "metadata": {
- "ExecuteTime": {
- "end_time": "2018-11-15T14:58:23.970714Z",
- "start_time": "2018-11-15T14:58:23.931930Z"
- }
- },
- "outputs": [
- {
- "name": "stdout",
- "output_type": "stream",
- "text": [
- "1\n",
- "2\n",
- "odict_keys(['linex_index', 'features'])\n",
- "number of tokens 1\n",
- "number of layers 12\n"
- ]
- },
- {
- "data": {
- "text/plain": [
- "(128, 768)"
- ]
- },
- "execution_count": 11,
- "metadata": {},
- "output_type": "execute_result"
- }
- ],
- "source": [
- "print(len(tensorflow_all_out))\n",
- "print(len(tensorflow_all_out[0]))\n",
- "print(tensorflow_all_out[0].keys())\n",
- "print(\"number of tokens\", len(tensorflow_all_out[0]['features']))\n",
- "print(\"number of layers\", len(tensorflow_all_out[0]['features'][0]['layers']))\n",
- "tensorflow_all_out[0]['features'][0]['layers'][0]['values'].shape"
- ]
- },
- {
- "cell_type": "code",
- "execution_count": 12,
- "metadata": {
- "ExecuteTime": {
- "end_time": "2018-11-15T14:58:25.547012Z",
- "start_time": "2018-11-15T14:58:25.516076Z"
- }
- },
- "outputs": [],
- "source": [
- "tensorflow_outputs = list(tensorflow_all_out[0]['features'][0]['layers'][t]['values'] for t in layer_indexes)"
- ]
- },
- {
- "cell_type": "markdown",
- "metadata": {},
- "source": [
- "## 2/ PyTorch code"
- ]
- },
- {
- "cell_type": "code",
- "execution_count": null,
- "metadata": {},
- "outputs": [],
- "source": [
- "os.chdir('./examples')"
- ]
- },
- {
- "cell_type": "code",
- "execution_count": 17,
- "metadata": {
- "ExecuteTime": {
- "end_time": "2018-11-15T15:03:49.528679Z",
- "start_time": "2018-11-15T15:03:49.497697Z"
- }
- },
- "outputs": [],
- "source": [
- "import extract_features\n",
- "import pytorch_transformers as ppb\n",
- "from extract_features import *"
- ]
- },
- {
- "cell_type": "code",
- "execution_count": 25,
- "metadata": {
- "ExecuteTime": {
- "end_time": "2018-11-15T15:21:18.001177Z",
- "start_time": "2018-11-15T15:21:17.970369Z"
- }
- },
- "outputs": [],
- "source": [
- "init_checkpoint_pt = \"../../google_models/uncased_L-12_H-768_A-12/\""
- ]
- },
- {
- "cell_type": "code",
- "execution_count": 26,
- "metadata": {
- "ExecuteTime": {
- "end_time": "2018-11-15T15:21:20.893669Z",
- "start_time": "2018-11-15T15:21:18.786623Z"
- },
- "scrolled": true
- },
- "outputs": [
- {
- "name": "stderr",
- "output_type": "stream",
- "text": [
- "11/15/2018 16:21:18 - INFO - pytorch_transformers.modeling_bert - loading archive file ../../google_models/uncased_L-12_H-768_A-12/\n",
- "11/15/2018 16:21:18 - INFO - pytorch_transformers.modeling_bert - Model config {\n",
- " \"attention_probs_dropout_prob\": 0.1,\n",
- " \"hidden_act\": \"gelu\",\n",
- " \"hidden_dropout_prob\": 0.1,\n",
- " \"hidden_size\": 768,\n",
- " \"initializer_range\": 0.02,\n",
- " \"intermediate_size\": 3072,\n",
- " \"max_position_embeddings\": 512,\n",
- " \"num_attention_heads\": 12,\n",
- " \"num_hidden_layers\": 12,\n",
- " \"type_vocab_size\": 2,\n",
- " \"vocab_size\": 30522\n",
- "}\n",
- "\n"
- ]
- },
- {
- "data": {
- "text/plain": [
- "BertModel(\n",
- " (embeddings): BertEmbeddings(\n",
- " (word_embeddings): Embedding(30522, 768)\n",
- " (position_embeddings): Embedding(512, 768)\n",
- " (token_type_embeddings): Embedding(2, 768)\n",
- " (LayerNorm): BertLayerNorm()\n",
- " (dropout): Dropout(p=0.1)\n",
- " )\n",
- " (encoder): BertEncoder(\n",
- " (layer): ModuleList(\n",
- " (0): BertLayer(\n",
- " (attention): BertAttention(\n",
- " (self): BertSelfAttention(\n",
- " (query): Linear(in_features=768, out_features=768, bias=True)\n",
- " (key): Linear(in_features=768, out_features=768, bias=True)\n",
- " (value): Linear(in_features=768, out_features=768, bias=True)\n",
- " (dropout): Dropout(p=0.1)\n",
- " )\n",
- " (output): BertSelfOutput(\n",
- " (dense): Linear(in_features=768, out_features=768, bias=True)\n",
- " (LayerNorm): BertLayerNorm()\n",
- " (dropout): Dropout(p=0.1)\n",
- " )\n",
- " )\n",
- " (intermediate): BertIntermediate(\n",
- " (dense): Linear(in_features=768, out_features=3072, bias=True)\n",
- " )\n",
- " (output): BertOutput(\n",
- " (dense): Linear(in_features=3072, out_features=768, bias=True)\n",
- " (LayerNorm): BertLayerNorm()\n",
- " (dropout): Dropout(p=0.1)\n",
- " )\n",
- " )\n",
- " (1): BertLayer(\n",
- " (attention): BertAttention(\n",
- " (self): BertSelfAttention(\n",
- " (query): Linear(in_features=768, out_features=768, bias=True)\n",
- " (key): Linear(in_features=768, out_features=768, bias=True)\n",
- " (value): Linear(in_features=768, out_features=768, bias=True)\n",
- " (dropout): Dropout(p=0.1)\n",
- " )\n",
- " (output): BertSelfOutput(\n",
- " (dense): Linear(in_features=768, out_features=768, bias=True)\n",
- " (LayerNorm): BertLayerNorm()\n",
- " (dropout): Dropout(p=0.1)\n",
- " )\n",
- " )\n",
- " (intermediate): BertIntermediate(\n",
- " (dense): Linear(in_features=768, out_features=3072, bias=True)\n",
- " )\n",
- " (output): BertOutput(\n",
- " (dense): Linear(in_features=3072, out_features=768, bias=True)\n",
- " (LayerNorm): BertLayerNorm()\n",
- " (dropout): Dropout(p=0.1)\n",
- " )\n",
- " )\n",
- " (2): BertLayer(\n",
- " (attention): BertAttention(\n",
- " (self): BertSelfAttention(\n",
- " (query): Linear(in_features=768, out_features=768, bias=True)\n",
- " (key): Linear(in_features=768, out_features=768, bias=True)\n",
- " (value): Linear(in_features=768, out_features=768, bias=True)\n",
- " (dropout): Dropout(p=0.1)\n",
- " )\n",
- " (output): BertSelfOutput(\n",
- " (dense): Linear(in_features=768, out_features=768, bias=True)\n",
- " (LayerNorm): BertLayerNorm()\n",
- " (dropout): Dropout(p=0.1)\n",
- " )\n",
- " )\n",
- " (intermediate): BertIntermediate(\n",
- " (dense): Linear(in_features=768, out_features=3072, bias=True)\n",
- " )\n",
- " (output): BertOutput(\n",
- " (dense): Linear(in_features=3072, out_features=768, bias=True)\n",
- " (LayerNorm): BertLayerNorm()\n",
- " (dropout): Dropout(p=0.1)\n",
- " )\n",
- " )\n",
- " (3): BertLayer(\n",
- " (attention): BertAttention(\n",
- " (self): BertSelfAttention(\n",
- " (query): Linear(in_features=768, out_features=768, bias=True)\n",
- " (key): Linear(in_features=768, out_features=768, bias=True)\n",
- " (value): Linear(in_features=768, out_features=768, bias=True)\n",
- " (dropout): Dropout(p=0.1)\n",
- " )\n",
- " (output): BertSelfOutput(\n",
- " (dense): Linear(in_features=768, out_features=768, bias=True)\n",
- " (LayerNorm): BertLayerNorm()\n",
- " (dropout): Dropout(p=0.1)\n",
- " )\n",
- " )\n",
- " (intermediate): BertIntermediate(\n",
- " (dense): Linear(in_features=768, out_features=3072, bias=True)\n",
- " )\n",
- " (output): BertOutput(\n",
- " (dense): Linear(in_features=3072, out_features=768, bias=True)\n",
- " (LayerNorm): BertLayerNorm()\n",
- " (dropout): Dropout(p=0.1)\n",
- " )\n",
- " )\n",
- " (4): BertLayer(\n",
- " (attention): BertAttention(\n",
- " (self): BertSelfAttention(\n",
- " (query): Linear(in_features=768, out_features=768, bias=True)\n",
- " (key): Linear(in_features=768, out_features=768, bias=True)\n",
- " (value): Linear(in_features=768, out_features=768, bias=True)\n",
- " (dropout): Dropout(p=0.1)\n",
- " )\n",
- " (output): BertSelfOutput(\n",
- " (dense): Linear(in_features=768, out_features=768, bias=True)\n",
- " (LayerNorm): BertLayerNorm()\n",
- " (dropout): Dropout(p=0.1)\n",
- " )\n",
- " )\n",
- " (intermediate): BertIntermediate(\n",
- " (dense): Linear(in_features=768, out_features=3072, bias=True)\n",
- " )\n",
- " (output): BertOutput(\n",
- " (dense): Linear(in_features=3072, out_features=768, bias=True)\n",
- " (LayerNorm): BertLayerNorm()\n",
- " (dropout): Dropout(p=0.1)\n",
- " )\n",
- " )\n",
- " (5): BertLayer(\n",
- " (attention): BertAttention(\n",
- " (self): BertSelfAttention(\n",
- " (query): Linear(in_features=768, out_features=768, bias=True)\n",
- " (key): Linear(in_features=768, out_features=768, bias=True)\n",
- " (value): Linear(in_features=768, out_features=768, bias=True)\n",
- " (dropout): Dropout(p=0.1)\n",
- " )\n",
- " (output): BertSelfOutput(\n",
- " (dense): Linear(in_features=768, out_features=768, bias=True)\n",
- " (LayerNorm): BertLayerNorm()\n",
- " (dropout): Dropout(p=0.1)\n",
- " )\n",
- " )\n",
- " (intermediate): BertIntermediate(\n",
- " (dense): Linear(in_features=768, out_features=3072, bias=True)\n",
- " )\n",
- " (output): BertOutput(\n",
- " (dense): Linear(in_features=3072, out_features=768, bias=True)\n",
- " (LayerNorm): BertLayerNorm()\n",
- " (dropout): Dropout(p=0.1)\n",
- " )\n",
- " )\n",
- " (6): BertLayer(\n",
- " (attention): BertAttention(\n",
- " (self): BertSelfAttention(\n",
- " (query): Linear(in_features=768, out_features=768, bias=True)\n",
- " (key): Linear(in_features=768, out_features=768, bias=True)\n",
- " (value): Linear(in_features=768, out_features=768, bias=True)\n",
- " (dropout): Dropout(p=0.1)\n",
- " )\n",
- " (output): BertSelfOutput(\n",
- " (dense): Linear(in_features=768, out_features=768, bias=True)\n",
- " (LayerNorm): BertLayerNorm()\n",
- " (dropout): Dropout(p=0.1)\n",
- " )\n",
- " )\n",
- " (intermediate): BertIntermediate(\n",
- " (dense): Linear(in_features=768, out_features=3072, bias=True)\n",
- " )\n",
- " (output): BertOutput(\n",
- " (dense): Linear(in_features=3072, out_features=768, bias=True)\n",
- " (LayerNorm): BertLayerNorm()\n",
- " (dropout): Dropout(p=0.1)\n",
- " )\n",
- " )\n",
- " (7): BertLayer(\n",
- " (attention): BertAttention(\n",
- " (self): BertSelfAttention(\n",
- " (query): Linear(in_features=768, out_features=768, bias=True)\n",
- " (key): Linear(in_features=768, out_features=768, bias=True)\n",
- " (value): Linear(in_features=768, out_features=768, bias=True)\n",
- " (dropout): Dropout(p=0.1)\n",
- " )\n",
- " (output): BertSelfOutput(\n",
- " (dense): Linear(in_features=768, out_features=768, bias=True)\n",
- " (LayerNorm): BertLayerNorm()\n",
- " (dropout): Dropout(p=0.1)\n",
- " )\n",
- " )\n",
- " (intermediate): BertIntermediate(\n",
- " (dense): Linear(in_features=768, out_features=3072, bias=True)\n",
- " )\n",
- " (output): BertOutput(\n",
- " (dense): Linear(in_features=3072, out_features=768, bias=True)\n",
- " (LayerNorm): BertLayerNorm()\n",
- " (dropout): Dropout(p=0.1)\n",
- " )\n",
- " )\n",
- " (8): BertLayer(\n",
- " (attention): BertAttention(\n",
- " (self): BertSelfAttention(\n",
- " (query): Linear(in_features=768, out_features=768, bias=True)\n",
- " (key): Linear(in_features=768, out_features=768, bias=True)\n",
- " (value): Linear(in_features=768, out_features=768, bias=True)\n",
- " (dropout): Dropout(p=0.1)\n",
- " )\n",
- " (output): BertSelfOutput(\n",
- " (dense): Linear(in_features=768, out_features=768, bias=True)\n",
- " (LayerNorm): BertLayerNorm()\n",
- " (dropout): Dropout(p=0.1)\n",
- " )\n",
- " )\n",
- " (intermediate): BertIntermediate(\n",
- " (dense): Linear(in_features=768, out_features=3072, bias=True)\n",
- " )\n",
- " (output): BertOutput(\n",
- " (dense): Linear(in_features=3072, out_features=768, bias=True)\n",
- " (LayerNorm): BertLayerNorm()\n",
- " (dropout): Dropout(p=0.1)\n",
- " )\n",
- " )\n",
- " (9): BertLayer(\n",
- " (attention): BertAttention(\n",
- " (self): BertSelfAttention(\n",
- " (query): Linear(in_features=768, out_features=768, bias=True)\n",
- " (key): Linear(in_features=768, out_features=768, bias=True)\n",
- " (value): Linear(in_features=768, out_features=768, bias=True)\n",
- " (dropout): Dropout(p=0.1)\n",
- " )\n",
- " (output): BertSelfOutput(\n",
- " (dense): Linear(in_features=768, out_features=768, bias=True)\n",
- " (LayerNorm): BertLayerNorm()\n",
- " (dropout): Dropout(p=0.1)\n",
- " )\n",
- " )\n",
- " (intermediate): BertIntermediate(\n",
- " (dense): Linear(in_features=768, out_features=3072, bias=True)\n",
- " )\n",
- " (output): BertOutput(\n",
- " (dense): Linear(in_features=3072, out_features=768, bias=True)\n",
- " (LayerNorm): BertLayerNorm()\n",
- " (dropout): Dropout(p=0.1)\n",
- " )\n",
- " )\n",
- " (10): BertLayer(\n",
- " (attention): BertAttention(\n",
- " (self): BertSelfAttention(\n",
- " (query): Linear(in_features=768, out_features=768, bias=True)\n",
- " (key): Linear(in_features=768, out_features=768, bias=True)\n",
- " (value): Linear(in_features=768, out_features=768, bias=True)\n",
- " (dropout): Dropout(p=0.1)\n",
- " )\n",
- " (output): BertSelfOutput(\n",
- " (dense): Linear(in_features=768, out_features=768, bias=True)\n",
- " (LayerNorm): BertLayerNorm()\n",
- " (dropout): Dropout(p=0.1)\n",
- " )\n",
- " )\n",
- " (intermediate): BertIntermediate(\n",
- " (dense): Linear(in_features=768, out_features=3072, bias=True)\n",
- " )\n",
- " (output): BertOutput(\n",
- " (dense): Linear(in_features=3072, out_features=768, bias=True)\n",
- " (LayerNorm): BertLayerNorm()\n",
- " (dropout): Dropout(p=0.1)\n",
- " )\n",
- " )\n",
- " (11): BertLayer(\n",
- " (attention): BertAttention(\n",
- " (self): BertSelfAttention(\n",
- " (query): Linear(in_features=768, out_features=768, bias=True)\n",
- " (key): Linear(in_features=768, out_features=768, bias=True)\n",
- " (value): Linear(in_features=768, out_features=768, bias=True)\n",
- " (dropout): Dropout(p=0.1)\n",
- " )\n",
- " (output): BertSelfOutput(\n",
- " (dense): Linear(in_features=768, out_features=768, bias=True)\n",
- " (LayerNorm): BertLayerNorm()\n",
- " (dropout): Dropout(p=0.1)\n",
- " )\n",
- " )\n",
- " (intermediate): BertIntermediate(\n",
- " (dense): Linear(in_features=768, out_features=3072, bias=True)\n",
- " )\n",
- " (output): BertOutput(\n",
- " (dense): Linear(in_features=3072, out_features=768, bias=True)\n",
- " (LayerNorm): BertLayerNorm()\n",
- " (dropout): Dropout(p=0.1)\n",
- " )\n",
- " )\n",
- " )\n",
- " )\n",
- " (pooler): BertPooler(\n",
- " (dense): Linear(in_features=768, out_features=768, bias=True)\n",
- " (activation): Tanh()\n",
- " )\n",
- ")"
- ]
- },
- "execution_count": 26,
- "metadata": {},
- "output_type": "execute_result"
- }
- ],
- "source": [
- "device = torch.device(\"cpu\")\n",
- "model = ppb.BertModel.from_pretrained(init_checkpoint_pt)\n",
- "model.to(device)"
- ]
- },
- {
- "cell_type": "code",
- "execution_count": 27,
- "metadata": {
- "ExecuteTime": {
- "end_time": "2018-11-15T15:21:26.963427Z",
- "start_time": "2018-11-15T15:21:26.922494Z"
- },
- "code_folding": []
- },
- "outputs": [
- {
- "data": {
- "text/plain": [
- "BertModel(\n",
- " (embeddings): BertEmbeddings(\n",
- " (word_embeddings): Embedding(30522, 768)\n",
- " (position_embeddings): Embedding(512, 768)\n",
- " (token_type_embeddings): Embedding(2, 768)\n",
- " (LayerNorm): BertLayerNorm()\n",
- " (dropout): Dropout(p=0.1)\n",
- " )\n",
- " (encoder): BertEncoder(\n",
- " (layer): ModuleList(\n",
- " (0): BertLayer(\n",
- " (attention): BertAttention(\n",
- " (self): BertSelfAttention(\n",
- " (query): Linear(in_features=768, out_features=768, bias=True)\n",
- " (key): Linear(in_features=768, out_features=768, bias=True)\n",
- " (value): Linear(in_features=768, out_features=768, bias=True)\n",
- " (dropout): Dropout(p=0.1)\n",
- " )\n",
- " (output): BertSelfOutput(\n",
- " (dense): Linear(in_features=768, out_features=768, bias=True)\n",
- " (LayerNorm): BertLayerNorm()\n",
- " (dropout): Dropout(p=0.1)\n",
- " )\n",
- " )\n",
- " (intermediate): BertIntermediate(\n",
- " (dense): Linear(in_features=768, out_features=3072, bias=True)\n",
- " )\n",
- " (output): BertOutput(\n",
- " (dense): Linear(in_features=3072, out_features=768, bias=True)\n",
- " (LayerNorm): BertLayerNorm()\n",
- " (dropout): Dropout(p=0.1)\n",
- " )\n",
- " )\n",
- " (1): BertLayer(\n",
- " (attention): BertAttention(\n",
- " (self): BertSelfAttention(\n",
- " (query): Linear(in_features=768, out_features=768, bias=True)\n",
- " (key): Linear(in_features=768, out_features=768, bias=True)\n",
- " (value): Linear(in_features=768, out_features=768, bias=True)\n",
- " (dropout): Dropout(p=0.1)\n",
- " )\n",
- " (output): BertSelfOutput(\n",
- " (dense): Linear(in_features=768, out_features=768, bias=True)\n",
- " (LayerNorm): BertLayerNorm()\n",
- " (dropout): Dropout(p=0.1)\n",
- " )\n",
- " )\n",
- " (intermediate): BertIntermediate(\n",
- " (dense): Linear(in_features=768, out_features=3072, bias=True)\n",
- " )\n",
- " (output): BertOutput(\n",
- " (dense): Linear(in_features=3072, out_features=768, bias=True)\n",
- " (LayerNorm): BertLayerNorm()\n",
- " (dropout): Dropout(p=0.1)\n",
- " )\n",
- " )\n",
- " (2): BertLayer(\n",
- " (attention): BertAttention(\n",
- " (self): BertSelfAttention(\n",
- " (query): Linear(in_features=768, out_features=768, bias=True)\n",
- " (key): Linear(in_features=768, out_features=768, bias=True)\n",
- " (value): Linear(in_features=768, out_features=768, bias=True)\n",
- " (dropout): Dropout(p=0.1)\n",
- " )\n",
- " (output): BertSelfOutput(\n",
- " (dense): Linear(in_features=768, out_features=768, bias=True)\n",
- " (LayerNorm): BertLayerNorm()\n",
- " (dropout): Dropout(p=0.1)\n",
- " )\n",
- " )\n",
- " (intermediate): BertIntermediate(\n",
- " (dense): Linear(in_features=768, out_features=3072, bias=True)\n",
- " )\n",
- " (output): BertOutput(\n",
- " (dense): Linear(in_features=3072, out_features=768, bias=True)\n",
- " (LayerNorm): BertLayerNorm()\n",
- " (dropout): Dropout(p=0.1)\n",
- " )\n",
- " )\n",
- " (3): BertLayer(\n",
- " (attention): BertAttention(\n",
- " (self): BertSelfAttention(\n",
- " (query): Linear(in_features=768, out_features=768, bias=True)\n",
- " (key): Linear(in_features=768, out_features=768, bias=True)\n",
- " (value): Linear(in_features=768, out_features=768, bias=True)\n",
- " (dropout): Dropout(p=0.1)\n",
- " )\n",
- " (output): BertSelfOutput(\n",
- " (dense): Linear(in_features=768, out_features=768, bias=True)\n",
- " (LayerNorm): BertLayerNorm()\n",
- " (dropout): Dropout(p=0.1)\n",
- " )\n",
- " )\n",
- " (intermediate): BertIntermediate(\n",
- " (dense): Linear(in_features=768, out_features=3072, bias=True)\n",
- " )\n",
- " (output): BertOutput(\n",
- " (dense): Linear(in_features=3072, out_features=768, bias=True)\n",
- " (LayerNorm): BertLayerNorm()\n",
- " (dropout): Dropout(p=0.1)\n",
- " )\n",
- " )\n",
- " (4): BertLayer(\n",
- " (attention): BertAttention(\n",
- " (self): BertSelfAttention(\n",
- " (query): Linear(in_features=768, out_features=768, bias=True)\n",
- " (key): Linear(in_features=768, out_features=768, bias=True)\n",
- " (value): Linear(in_features=768, out_features=768, bias=True)\n",
- " (dropout): Dropout(p=0.1)\n",
- " )\n",
- " (output): BertSelfOutput(\n",
- " (dense): Linear(in_features=768, out_features=768, bias=True)\n",
- " (LayerNorm): BertLayerNorm()\n",
- " (dropout): Dropout(p=0.1)\n",
- " )\n",
- " )\n",
- " (intermediate): BertIntermediate(\n",
- " (dense): Linear(in_features=768, out_features=3072, bias=True)\n",
- " )\n",
- " (output): BertOutput(\n",
- " (dense): Linear(in_features=3072, out_features=768, bias=True)\n",
- " (LayerNorm): BertLayerNorm()\n",
- " (dropout): Dropout(p=0.1)\n",
- " )\n",
- " )\n",
- " (5): BertLayer(\n",
- " (attention): BertAttention(\n",
- " (self): BertSelfAttention(\n",
- " (query): Linear(in_features=768, out_features=768, bias=True)\n",
- " (key): Linear(in_features=768, out_features=768, bias=True)\n",
- " (value): Linear(in_features=768, out_features=768, bias=True)\n",
- " (dropout): Dropout(p=0.1)\n",
- " )\n",
- " (output): BertSelfOutput(\n",
- " (dense): Linear(in_features=768, out_features=768, bias=True)\n",
- " (LayerNorm): BertLayerNorm()\n",
- " (dropout): Dropout(p=0.1)\n",
- " )\n",
- " )\n",
- " (intermediate): BertIntermediate(\n",
- " (dense): Linear(in_features=768, out_features=3072, bias=True)\n",
- " )\n",
- " (output): BertOutput(\n",
- " (dense): Linear(in_features=3072, out_features=768, bias=True)\n",
- " (LayerNorm): BertLayerNorm()\n",
- " (dropout): Dropout(p=0.1)\n",
- " )\n",
- " )\n",
- " (6): BertLayer(\n",
- " (attention): BertAttention(\n",
- " (self): BertSelfAttention(\n",
- " (query): Linear(in_features=768, out_features=768, bias=True)\n",
- " (key): Linear(in_features=768, out_features=768, bias=True)\n",
- " (value): Linear(in_features=768, out_features=768, bias=True)\n",
- " (dropout): Dropout(p=0.1)\n",
- " )\n",
- " (output): BertSelfOutput(\n",
- " (dense): Linear(in_features=768, out_features=768, bias=True)\n",
- " (LayerNorm): BertLayerNorm()\n",
- " (dropout): Dropout(p=0.1)\n",
- " )\n",
- " )\n",
- " (intermediate): BertIntermediate(\n",
- " (dense): Linear(in_features=768, out_features=3072, bias=True)\n",
- " )\n",
- " (output): BertOutput(\n",
- " (dense): Linear(in_features=3072, out_features=768, bias=True)\n",
- " (LayerNorm): BertLayerNorm()\n",
- " (dropout): Dropout(p=0.1)\n",
- " )\n",
- " )\n",
- " (7): BertLayer(\n",
- " (attention): BertAttention(\n",
- " (self): BertSelfAttention(\n",
- " (query): Linear(in_features=768, out_features=768, bias=True)\n",
- " (key): Linear(in_features=768, out_features=768, bias=True)\n",
- " (value): Linear(in_features=768, out_features=768, bias=True)\n",
- " (dropout): Dropout(p=0.1)\n",
- " )\n",
- " (output): BertSelfOutput(\n",
- " (dense): Linear(in_features=768, out_features=768, bias=True)\n",
- " (LayerNorm): BertLayerNorm()\n",
- " (dropout): Dropout(p=0.1)\n",
- " )\n",
- " )\n",
- " (intermediate): BertIntermediate(\n",
- " (dense): Linear(in_features=768, out_features=3072, bias=True)\n",
- " )\n",
- " (output): BertOutput(\n",
- " (dense): Linear(in_features=3072, out_features=768, bias=True)\n",
- " (LayerNorm): BertLayerNorm()\n",
- " (dropout): Dropout(p=0.1)\n",
- " )\n",
- " )\n",
- " (8): BertLayer(\n",
- " (attention): BertAttention(\n",
- " (self): BertSelfAttention(\n",
- " (query): Linear(in_features=768, out_features=768, bias=True)\n",
- " (key): Linear(in_features=768, out_features=768, bias=True)\n",
- " (value): Linear(in_features=768, out_features=768, bias=True)\n",
- " (dropout): Dropout(p=0.1)\n",
- " )\n",
- " (output): BertSelfOutput(\n",
- " (dense): Linear(in_features=768, out_features=768, bias=True)\n",
- " (LayerNorm): BertLayerNorm()\n",
- " (dropout): Dropout(p=0.1)\n",
- " )\n",
- " )\n",
- " (intermediate): BertIntermediate(\n",
- " (dense): Linear(in_features=768, out_features=3072, bias=True)\n",
- " )\n",
- " (output): BertOutput(\n",
- " (dense): Linear(in_features=3072, out_features=768, bias=True)\n",
- " (LayerNorm): BertLayerNorm()\n",
- " (dropout): Dropout(p=0.1)\n",
- " )\n",
- " )\n",
- " (9): BertLayer(\n",
- " (attention): BertAttention(\n",
- " (self): BertSelfAttention(\n",
- " (query): Linear(in_features=768, out_features=768, bias=True)\n",
- " (key): Linear(in_features=768, out_features=768, bias=True)\n",
- " (value): Linear(in_features=768, out_features=768, bias=True)\n",
- " (dropout): Dropout(p=0.1)\n",
- " )\n",
- " (output): BertSelfOutput(\n",
- " (dense): Linear(in_features=768, out_features=768, bias=True)\n",
- " (LayerNorm): BertLayerNorm()\n",
- " (dropout): Dropout(p=0.1)\n",
- " )\n",
- " )\n",
- " (intermediate): BertIntermediate(\n",
- " (dense): Linear(in_features=768, out_features=3072, bias=True)\n",
- " )\n",
- " (output): BertOutput(\n",
- " (dense): Linear(in_features=3072, out_features=768, bias=True)\n",
- " (LayerNorm): BertLayerNorm()\n",
- " (dropout): Dropout(p=0.1)\n",
- " )\n",
- " )\n",
- " (10): BertLayer(\n",
- " (attention): BertAttention(\n",
- " (self): BertSelfAttention(\n",
- " (query): Linear(in_features=768, out_features=768, bias=True)\n",
- " (key): Linear(in_features=768, out_features=768, bias=True)\n",
- " (value): Linear(in_features=768, out_features=768, bias=True)\n",
- " (dropout): Dropout(p=0.1)\n",
- " )\n",
- " (output): BertSelfOutput(\n",
- " (dense): Linear(in_features=768, out_features=768, bias=True)\n",
- " (LayerNorm): BertLayerNorm()\n",
- " (dropout): Dropout(p=0.1)\n",
- " )\n",
- " )\n",
- " (intermediate): BertIntermediate(\n",
- " (dense): Linear(in_features=768, out_features=3072, bias=True)\n",
- " )\n",
- " (output): BertOutput(\n",
- " (dense): Linear(in_features=3072, out_features=768, bias=True)\n",
- " (LayerNorm): BertLayerNorm()\n",
- " (dropout): Dropout(p=0.1)\n",
- " )\n",
- " )\n",
- " (11): BertLayer(\n",
- " (attention): BertAttention(\n",
- " (self): BertSelfAttention(\n",
- " (query): Linear(in_features=768, out_features=768, bias=True)\n",
- " (key): Linear(in_features=768, out_features=768, bias=True)\n",
- " (value): Linear(in_features=768, out_features=768, bias=True)\n",
- " (dropout): Dropout(p=0.1)\n",
- " )\n",
- " (output): BertSelfOutput(\n",
- " (dense): Linear(in_features=768, out_features=768, bias=True)\n",
- " (LayerNorm): BertLayerNorm()\n",
- " (dropout): Dropout(p=0.1)\n",
- " )\n",
- " )\n",
- " (intermediate): BertIntermediate(\n",
- " (dense): Linear(in_features=768, out_features=3072, bias=True)\n",
- " )\n",
- " (output): BertOutput(\n",
- " (dense): Linear(in_features=3072, out_features=768, bias=True)\n",
- " (LayerNorm): BertLayerNorm()\n",
- " (dropout): Dropout(p=0.1)\n",
- " )\n",
- " )\n",
- " )\n",
- " )\n",
- " (pooler): BertPooler(\n",
- " (dense): Linear(in_features=768, out_features=768, bias=True)\n",
- " (activation): Tanh()\n",
- " )\n",
- ")"
- ]
- },
- "execution_count": 27,
- "metadata": {},
- "output_type": "execute_result"
- }
- ],
- "source": [
- "all_input_ids = torch.tensor([f.input_ids for f in features], dtype=torch.long)\n",
- "all_input_mask = torch.tensor([f.input_mask for f in features], dtype=torch.long)\n",
- "all_input_type_ids = torch.tensor([f.input_type_ids for f in features], dtype=torch.long)\n",
- "all_example_index = torch.arange(all_input_ids.size(0), dtype=torch.long)\n",
- "\n",
- "eval_data = TensorDataset(all_input_ids, all_input_mask, all_input_type_ids, all_example_index)\n",
- "eval_sampler = SequentialSampler(eval_data)\n",
- "eval_dataloader = DataLoader(eval_data, sampler=eval_sampler, batch_size=1)\n",
- "\n",
- "model.eval()"
- ]
- },
- {
- "cell_type": "code",
- "execution_count": 28,
- "metadata": {
- "ExecuteTime": {
- "end_time": "2018-11-15T15:21:30.718724Z",
- "start_time": "2018-11-15T15:21:30.329205Z"
- }
- },
- "outputs": [
- {
- "name": "stdout",
- "output_type": "stream",
- "text": [
- "tensor([[ 101, 2040, 2001, 3958, 27227, 1029, 102, 3958, 27227, 2001,\n",
- " 1037, 13997, 11510, 102, 0, 0, 0, 0, 0, 0,\n",
- " 0, 0, 0, 0, 0, 0, 0, 0, 0, 0,\n",
- " 0, 0, 0, 0, 0, 0, 0, 0, 0, 0,\n",
- " 0, 0, 0, 0, 0, 0, 0, 0, 0, 0,\n",
- " 0, 0, 0, 0, 0, 0, 0, 0, 0, 0,\n",
- " 0, 0, 0, 0, 0, 0, 0, 0, 0, 0,\n",
- " 0, 0, 0, 0, 0, 0, 0, 0, 0, 0,\n",
- " 0, 0, 0, 0, 0, 0, 0, 0, 0, 0,\n",
- " 0, 0, 0, 0, 0, 0, 0, 0, 0, 0,\n",
- " 0, 0, 0, 0, 0, 0, 0, 0, 0, 0,\n",
- " 0, 0, 0, 0, 0, 0, 0, 0, 0, 0,\n",
- " 0, 0, 0, 0, 0, 0, 0, 0]])\n",
- "tensor([[1, 1, 1, 1, 1, 1, 1, 1, 1, 1, 1, 1, 1, 1, 0, 0, 0, 0, 0, 0, 0, 0, 0, 0,\n",
- " 0, 0, 0, 0, 0, 0, 0, 0, 0, 0, 0, 0, 0, 0, 0, 0, 0, 0, 0, 0, 0, 0, 0, 0,\n",
- " 0, 0, 0, 0, 0, 0, 0, 0, 0, 0, 0, 0, 0, 0, 0, 0, 0, 0, 0, 0, 0, 0, 0, 0,\n",
- " 0, 0, 0, 0, 0, 0, 0, 0, 0, 0, 0, 0, 0, 0, 0, 0, 0, 0, 0, 0, 0, 0, 0, 0,\n",
- " 0, 0, 0, 0, 0, 0, 0, 0, 0, 0, 0, 0, 0, 0, 0, 0, 0, 0, 0, 0, 0, 0, 0, 0,\n",
- " 0, 0, 0, 0, 0, 0, 0, 0]])\n",
- "tensor([0])\n",
- "layer 0 0\n",
- "layer 1 1\n",
- "layer 2 2\n",
- "layer 3 3\n",
- "layer 4 4\n",
- "layer 5 5\n",
- "layer 6 6\n",
- "layer 7 7\n",
- "layer 8 8\n",
- "layer 9 9\n",
- "layer 10 10\n",
- "layer 11 11\n"
- ]
- }
- ],
- "source": [
- "layer_indexes = list(range(12))\n",
- "\n",
- "pytorch_all_out = []\n",
- "for input_ids, input_mask, input_type_ids, example_indices in eval_dataloader:\n",
- " print(input_ids)\n",
- " print(input_mask)\n",
- " print(example_indices)\n",
- " input_ids = input_ids.to(device)\n",
- " input_mask = input_mask.to(device)\n",
- "\n",
- " all_encoder_layers, _ = model(input_ids, token_type_ids=input_type_ids, attention_mask=input_mask)\n",
- "\n",
- " for b, example_index in enumerate(example_indices):\n",
- " feature = features[example_index.item()]\n",
- " unique_id = int(feature.unique_id)\n",
- " # feature = unique_id_to_feature[unique_id]\n",
- " output_json = collections.OrderedDict()\n",
- " output_json[\"linex_index\"] = unique_id\n",
- " all_out_features = []\n",
- " # for (i, token) in enumerate(feature.tokens):\n",
- " all_layers = []\n",
- " for (j, layer_index) in enumerate(layer_indexes):\n",
- " print(\"layer\", j, layer_index)\n",
- " layer_output = all_encoder_layers[int(layer_index)].detach().cpu().numpy()\n",
- " layer_output = layer_output[b]\n",
- " layers = collections.OrderedDict()\n",
- " layers[\"index\"] = layer_index\n",
- " layer_output = layer_output\n",
- " layers[\"values\"] = layer_output if not isinstance(layer_output, (int, float)) else [layer_output]\n",
- " all_layers.append(layers)\n",
- "\n",
- " out_features = collections.OrderedDict()\n",
- " out_features[\"layers\"] = all_layers\n",
- " all_out_features.append(out_features)\n",
- " output_json[\"features\"] = all_out_features\n",
- " pytorch_all_out.append(output_json)"
- ]
- },
- {
- "cell_type": "code",
- "execution_count": 29,
- "metadata": {
- "ExecuteTime": {
- "end_time": "2018-11-15T15:21:35.703615Z",
- "start_time": "2018-11-15T15:21:35.666150Z"
- }
- },
- "outputs": [
- {
- "name": "stdout",
- "output_type": "stream",
- "text": [
- "1\n",
- "2\n",
- "odict_keys(['linex_index', 'features'])\n",
- "number of tokens 1\n",
- "number of layers 12\n",
- "hidden_size 128\n"
- ]
- },
- {
- "data": {
- "text/plain": [
- "(128, 768)"
- ]
- },
- "execution_count": 29,
- "metadata": {},
- "output_type": "execute_result"
- }
- ],
- "source": [
- "print(len(pytorch_all_out))\n",
- "print(len(pytorch_all_out[0]))\n",
- "print(pytorch_all_out[0].keys())\n",
- "print(\"number of tokens\", len(pytorch_all_out))\n",
- "print(\"number of layers\", len(pytorch_all_out[0]['features'][0]['layers']))\n",
- "print(\"hidden_size\", len(pytorch_all_out[0]['features'][0]['layers'][0]['values']))\n",
- "pytorch_all_out[0]['features'][0]['layers'][0]['values'].shape"
- ]
- },
- {
- "cell_type": "code",
- "execution_count": 30,
- "metadata": {
- "ExecuteTime": {
- "end_time": "2018-11-15T15:21:36.999073Z",
- "start_time": "2018-11-15T15:21:36.966762Z"
- }
- },
- "outputs": [
- {
- "name": "stdout",
- "output_type": "stream",
- "text": [
- "(128, 768)\n",
- "(128, 768)\n"
- ]
- }
- ],
- "source": [
- "pytorch_outputs = list(pytorch_all_out[0]['features'][0]['layers'][t]['values'] for t in layer_indexes)\n",
- "print(pytorch_outputs[0].shape)\n",
- "print(pytorch_outputs[1].shape)"
- ]
- },
- {
- "cell_type": "code",
- "execution_count": 31,
- "metadata": {
- "ExecuteTime": {
- "end_time": "2018-11-15T15:21:37.936522Z",
- "start_time": "2018-11-15T15:21:37.905269Z"
- }
- },
- "outputs": [
- {
- "name": "stdout",
- "output_type": "stream",
- "text": [
- "(128, 768)\n",
- "(128, 768)\n"
- ]
- }
- ],
- "source": [
- "print(tensorflow_outputs[0].shape)\n",
- "print(tensorflow_outputs[1].shape)"
- ]
- },
- {
- "cell_type": "markdown",
- "metadata": {},
- "source": [
- "## 3/ Comparing the standard deviation on the last layer of both models"
- ]
- },
- {
- "cell_type": "code",
- "execution_count": 32,
- "metadata": {
- "ExecuteTime": {
- "end_time": "2018-11-15T15:21:39.437137Z",
- "start_time": "2018-11-15T15:21:39.406150Z"
- }
- },
- "outputs": [],
- "source": [
- "import numpy as np"
- ]
- },
- {
- "cell_type": "code",
- "execution_count": 33,
- "metadata": {
- "ExecuteTime": {
- "end_time": "2018-11-15T15:21:40.181870Z",
- "start_time": "2018-11-15T15:21:40.137023Z"
- }
- },
- "outputs": [
- {
- "name": "stdout",
- "output_type": "stream",
- "text": [
- "shape tensorflow layer, shape pytorch layer, standard deviation\n",
- "((128, 768), (128, 768), 1.5258875e-07)\n",
- "((128, 768), (128, 768), 2.342731e-07)\n",
- "((128, 768), (128, 768), 2.801949e-07)\n",
- "((128, 768), (128, 768), 3.5904986e-07)\n",
- "((128, 768), (128, 768), 4.2842768e-07)\n",
- "((128, 768), (128, 768), 5.127951e-07)\n",
- "((128, 768), (128, 768), 6.14668e-07)\n",
- "((128, 768), (128, 768), 7.063922e-07)\n",
- "((128, 768), (128, 768), 7.906173e-07)\n",
- "((128, 768), (128, 768), 8.475192e-07)\n",
- "((128, 768), (128, 768), 8.975489e-07)\n",
- "((128, 768), (128, 768), 4.1671223e-07)\n"
- ]
- }
- ],
- "source": [
- "print('shape tensorflow layer, shape pytorch layer, standard deviation')\n",
- "print('\\n'.join(list(str((np.array(tensorflow_outputs[i]).shape,\n",
- " np.array(pytorch_outputs[i]).shape, \n",
- " np.sqrt(np.mean((np.array(tensorflow_outputs[i]) - np.array(pytorch_outputs[i]))**2.0)))) for i in range(12))))"
- ]
- },
- {
- "cell_type": "code",
- "execution_count": null,
- "metadata": {},
- "outputs": [],
- "source": []
- }
- ],
- "metadata": {
- "hide_input": false,
- "kernelspec": {
- "display_name": "Python [default]",
- "language": "python",
- "name": "python3"
- },
- "language_info": {
- "codemirror_mode": {
- "name": "ipython",
- "version": 3
- },
- "file_extension": ".py",
- "mimetype": "text/x-python",
- "name": "python",
- "nbconvert_exporter": "python",
- "pygments_lexer": "ipython3",
- "version": "3.6.7"
- },
- "toc": {
- "colors": {
- "hover_highlight": "#DAA520",
- "running_highlight": "#FF0000",
- "selected_highlight": "#FFD700"
- },
- "moveMenuLeft": true,
- "nav_menu": {
- "height": "48px",
- "width": "252px"
- },
- "navigate_menu": true,
- "number_sections": true,
- "sideBar": true,
- "threshold": 4,
- "toc_cell": false,
- "toc_section_display": "block",
- "toc_window_display": false
- }
- },
- "nbformat": 4,
- "nbformat_minor": 2
-}
diff --git a/server/transformers/notebooks/Test Models.ipynb b/server/transformers/notebooks/Test Models.ipynb
deleted file mode 100644
index 18ec939217d2178010e65a1378b7563db2fd59a5..0000000000000000000000000000000000000000
--- a/server/transformers/notebooks/Test Models.ipynb
+++ /dev/null
@@ -1,526 +0,0 @@
-{
- "cells": [
- {
- "cell_type": "code",
- "execution_count": 2,
- "metadata": {},
- "outputs": [],
- "source": [
- "%reload_ext autoreload\n",
- "%autoreload 2"
- ]
- },
- {
- "cell_type": "code",
- "execution_count": 3,
- "metadata": {},
- "outputs": [],
- "source": [
- "import transformers\n",
- "import torch"
- ]
- },
- {
- "cell_type": "code",
- "execution_count": 67,
- "metadata": {},
- "outputs": [],
- "source": [
- "from transformers import AutoModel, AutoTokenizer, BertModel, DistilBertModel, RobertaModel, GPT2Model"
- ]
- },
- {
- "cell_type": "code",
- "execution_count": 113,
- "metadata": {},
- "outputs": [],
- "source": [
- "mname = 'bert-base-uncased'\n",
- "sentence = 'The count went forward with his original plan'\n",
- "t_class = BertModel\n",
- "\n",
- "def test_model(t_class, mname, sentence):\n",
- " m = t_class.from_pretrained(mname, output_hidden_states=True, output_past=False, output_attentions=True, output_additional_info=True)\n",
- " t = AutoTokenizer.from_pretrained(mname)\n",
- " input_ids = t.encode(sentence)\n",
- " outputs = m(torch.tensor(input_ids).unsqueeze(0))\n",
- " return outputs\n"
- ]
- },
- {
- "cell_type": "code",
- "execution_count": 58,
- "metadata": {},
- "outputs": [],
- "source": [
- "mname = 'bert-base-uncased'\n",
- "sentence = 'The count went forward with his original plan'\n",
- "t_class = BertModel\n",
- "out = test_model(t_class, mname, sentence)"
- ]
- },
- {
- "cell_type": "code",
- "execution_count": 59,
- "metadata": {},
- "outputs": [],
- "source": [
- "mname = 'distilbert-base-uncased'\n",
- "sentence = 'The count went forward with his original plan'\n",
- "t_class = DistilBertModel\n",
- "out = test_model(t_class, mname, sentence)"
- ]
- },
- {
- "cell_type": "code",
- "execution_count": 65,
- "metadata": {},
- "outputs": [],
- "source": [
- "mname = 'roberta-base'\n",
- "sentence = 'The count went forward with his original plan'\n",
- "t_class = RobertaModel\n",
- "out = test_model(t_class, mname, sentence)"
- ]
- },
- {
- "cell_type": "code",
- "execution_count": 122,
- "metadata": {},
- "outputs": [
- {
- "name": "stdout",
- "output_type": "stream",
- "text": [
- "CONTEXTS: torch.Size([1, 16, 12, 64])\n",
- "CONTEXTS: torch.Size([1, 16, 12, 64])\n",
- "CONTEXTS: torch.Size([1, 16, 12, 64])\n",
- "CONTEXTS: torch.Size([1, 16, 12, 64])\n",
- "CONTEXTS: torch.Size([1, 16, 12, 64])\n",
- "CONTEXTS: torch.Size([1, 16, 12, 64])\n",
- "CONTEXTS: torch.Size([1, 16, 12, 64])\n",
- "CONTEXTS: torch.Size([1, 16, 12, 64])\n",
- "CONTEXTS: torch.Size([1, 16, 12, 64])\n",
- "CONTEXTS: torch.Size([1, 16, 12, 64])\n",
- "CONTEXTS: torch.Size([1, 16, 12, 64])\n",
- "CONTEXTS: torch.Size([1, 16, 12, 64])\n"
- ]
- }
- ],
- "source": [
- "mname = 'gpt2'\n",
- "sentence = 'The count went forward with his original plan to take over the mighty world of Disney'\n",
- "t_class = GPT2Model\n",
- "out = test_model(t_class, mname, sentence)"
- ]
- },
- {
- "cell_type": "code",
- "execution_count": 123,
- "metadata": {},
- "outputs": [
- {
- "data": {
- "text/plain": [
- "4"
- ]
- },
- "execution_count": 123,
- "metadata": {},
- "output_type": "execute_result"
- }
- ],
- "source": [
- "len(out)"
- ]
- },
- {
- "cell_type": "code",
- "execution_count": 124,
- "metadata": {},
- "outputs": [
- {
- "data": {
- "text/plain": [
- "torch.Size([1, 16, 12, 64])"
- ]
- },
- "execution_count": 124,
- "metadata": {},
- "output_type": "execute_result"
- }
- ],
- "source": [
- "out[-1][0].shape"
- ]
- },
- {
- "cell_type": "code",
- "execution_count": 120,
- "metadata": {},
- "outputs": [
- {
- "data": {
- "text/plain": [
- "torch.Size([1, 16, 768])"
- ]
- },
- "execution_count": 120,
- "metadata": {},
- "output_type": "execute_result"
- }
- ],
- "source": [
- "out[1][0].shape"
- ]
- },
- {
- "cell_type": "code",
- "execution_count": 107,
- "metadata": {},
- "outputs": [
- {
- "data": {
- "text/plain": [
- "12"
- ]
- },
- "execution_count": 107,
- "metadata": {},
- "output_type": "execute_result"
- }
- ],
- "source": [
- "m = t_class.from_pretrained(mname)\n",
- "\n",
- "m.config.n_head"
- ]
- },
- {
- "cell_type": "code",
- "execution_count": 109,
- "metadata": {},
- "outputs": [
- {
- "data": {
- "text/plain": [
- "GPT2Config {\n",
- " \"attn_pdrop\": 0.1,\n",
- " \"bos_token_id\": 0,\n",
- " \"do_sample\": false,\n",
- " \"embd_pdrop\": 0.1,\n",
- " \"eos_token_ids\": 0,\n",
- " \"finetuning_task\": null,\n",
- " \"id2label\": {\n",
- " \"0\": \"LABEL_0\",\n",
- " \"1\": \"LABEL_1\"\n",
- " },\n",
- " \"initializer_range\": 0.02,\n",
- " \"is_decoder\": false,\n",
- " \"label2id\": {\n",
- " \"LABEL_0\": 0,\n",
- " \"LABEL_1\": 1\n",
- " },\n",
- " \"layer_norm_epsilon\": 1e-05,\n",
- " \"length_penalty\": 1.0,\n",
- " \"max_length\": 20,\n",
- " \"model_type\": \"gpt2\",\n",
- " \"n_ctx\": 1024,\n",
- " \"n_embd\": 768,\n",
- " \"n_head\": 12,\n",
- " \"n_layer\": 12,\n",
- " \"n_positions\": 1024,\n",
- " \"num_beams\": 1,\n",
- " \"num_labels\": 2,\n",
- " \"num_return_sequences\": 1,\n",
- " \"output_additional_info\": false,\n",
- " \"output_attentions\": false,\n",
- " \"output_hidden_states\": false,\n",
- " \"output_past\": true,\n",
- " \"pad_token_id\": 0,\n",
- " \"pruned_heads\": {},\n",
- " \"repetition_penalty\": 1.0,\n",
- " \"resid_pdrop\": 0.1,\n",
- " \"summary_activation\": null,\n",
- " \"summary_first_dropout\": 0.1,\n",
- " \"summary_proj_to_labels\": true,\n",
- " \"summary_type\": \"cls_index\",\n",
- " \"summary_use_proj\": true,\n",
- " \"temperature\": 1.0,\n",
- " \"top_k\": 50,\n",
- " \"top_p\": 1.0,\n",
- " \"torchscript\": false,\n",
- " \"use_bfloat16\": false,\n",
- " \"vocab_size\": 50257\n",
- "}"
- ]
- },
- "execution_count": 109,
- "metadata": {},
- "output_type": "execute_result"
- }
- ],
- "source": [
- "m.config"
- ]
- },
- {
- "cell_type": "code",
- "execution_count": 85,
- "metadata": {},
- "outputs": [
- {
- "data": {
- "text/plain": [
- "torch.Size([1, 12, 8, 8])"
- ]
- },
- "execution_count": 85,
- "metadata": {},
- "output_type": "execute_result"
- }
- ],
- "source": [
- "out[-1][1].shape"
- ]
- },
- {
- "cell_type": "code",
- "execution_count": 77,
- "metadata": {},
- "outputs": [
- {
- "data": {
- "text/plain": [
- "torch.Size([2, 1, 12, 8, 64])"
- ]
- },
- "execution_count": 77,
- "metadata": {},
- "output_type": "execute_result"
- }
- ],
- "source": [
- "out[1][0].shape"
- ]
- },
- {
- "cell_type": "markdown",
- "metadata": {},
- "source": [
- "# Tokenizing smiles"
- ]
- },
- {
- "cell_type": "code",
- "execution_count": 5,
- "metadata": {},
- "outputs": [
- {
- "name": "stdout",
- "output_type": "stream",
- "text": [
- "['C', 'O', '.', 'C', 'O', 'C', '(', '=', 'O', ')', 'C', '(', 'C', ')', '(', 'C', ')', 'c', '1', 'c', 'c', 'c', '(', 'C', '(', '=', 'O', ')', 'C', 'C', 'C', 'N', '2', 'C', 'C', 'C', '(', 'C', '(', 'O', ')', '(', 'c', '3', 'c', 'c', 'c', 'c', 'c', '3', ')', 'c', '3', 'c', 'c', 'c', 'c', 'c', '3', ')', 'C', 'C', '2', ')', 'c', 'c', '1', '.', 'Cl', '.', 'O', '[Na]', '>>', 'C', 'C', '(', 'C', ')', '(', 'C', '(', '=', 'O', ')', 'O', ')', 'c', '1', 'c', 'c', 'c', '(', 'C', '(', '=', 'O', ')', 'C', 'C', 'C', 'N', '2', 'C', 'C', 'C', '(', 'C', '(', 'O', ')', '(', 'c', '3', 'c', 'c', 'c', 'c', 'c', '3', ')', 'c', '3', 'c', 'c', 'c', 'c', 'c', '3', ')', 'C', 'C', '2', ')', 'c', 'c', '1']\n"
- ]
- }
- ],
- "source": [
- "import re\n",
- "import regex\n",
- "\n",
- "def tokenize_smiles(smiles: str) -> str:\n",
- " \"\"\"\n",
- " Tokenize a SMILES molecule or reaction\n",
- " \"\"\"\n",
- " pattern = r\"(\\[[^\\]]+]|Br?|Cl?|N|O|S|P|F|I|b|c|n|o|s|p|\\(|\\)|\\.|=|#|-|\\+|\\\\|\\/|:|~|@|\\?|>>?|\\*|\\$|\\%[0-9]{2}|\\%\\([0-9]{3}\\)|[0-9])\"\n",
- " regex = re.compile(pattern)\n",
- " tokens = [token for token in regex.findall(smiles)]\n",
- " if smiles != ''.join(tokens):\n",
- " raise \n",
- "# return ' '.join(tokens)\n",
- " return tokens\n",
- "\n",
- "\n",
- "rxn = 'CO.COC(=O)C(C)(C)c1ccc(C(=O)CCCN2CCC(C(O)(c3ccccc3)c3ccccc3)CC2)cc1.Cl.O[Na]>>CC(C)(C(=O)O)c1ccc(C(=O)CCCN2CCC(C(O)(c3ccccc3)c3ccccc3)CC2)cc1'\n",
- "tokenized_rxn = tokenize_smiles(rxn)\n",
- "print(tokenized_rxn)"
- ]
- },
- {
- "cell_type": "code",
- "execution_count": 8,
- "metadata": {},
- "outputs": [
- {
- "data": {
- "text/plain": [
- "['C',\n",
- " 'O',\n",
- " '.',\n",
- " 'C',\n",
- " 'O',\n",
- " 'C',\n",
- " '(',\n",
- " '=',\n",
- " 'O',\n",
- " ')',\n",
- " 'C',\n",
- " '(',\n",
- " 'C',\n",
- " ')',\n",
- " '(',\n",
- " 'C',\n",
- " ')',\n",
- " 'c',\n",
- " '1',\n",
- " 'c',\n",
- " 'c',\n",
- " 'c',\n",
- " '(',\n",
- " 'C',\n",
- " '(',\n",
- " '=',\n",
- " 'O',\n",
- " ')',\n",
- " 'C',\n",
- " 'C',\n",
- " 'C',\n",
- " 'N',\n",
- " '2',\n",
- " 'C',\n",
- " 'C',\n",
- " 'C',\n",
- " '(',\n",
- " 'C',\n",
- " '(',\n",
- " 'O',\n",
- " ')',\n",
- " '(',\n",
- " 'c',\n",
- " '3',\n",
- " 'c',\n",
- " 'c',\n",
- " 'c',\n",
- " 'c',\n",
- " 'c',\n",
- " '3',\n",
- " ')',\n",
- " 'c',\n",
- " '3',\n",
- " 'c',\n",
- " 'c',\n",
- " 'c',\n",
- " 'c',\n",
- " 'c',\n",
- " '3',\n",
- " ')',\n",
- " 'C',\n",
- " 'C',\n",
- " '2',\n",
- " ')',\n",
- " 'c',\n",
- " 'c',\n",
- " '1',\n",
- " '.',\n",
- " 'Cl',\n",
- " '.',\n",
- " 'O',\n",
- " '[Na]',\n",
- " '>>',\n",
- " 'C',\n",
- " 'C',\n",
- " '(',\n",
- " 'C',\n",
- " ')',\n",
- " '(',\n",
- " 'C',\n",
- " '(',\n",
- " '=',\n",
- " 'O',\n",
- " ')',\n",
- " 'O',\n",
- " ')',\n",
- " 'c',\n",
- " '1',\n",
- " 'c',\n",
- " 'c',\n",
- " 'c',\n",
- " '(',\n",
- " 'C',\n",
- " '(',\n",
- " '=',\n",
- " 'O',\n",
- " ')',\n",
- " 'C',\n",
- " 'C',\n",
- " 'C',\n",
- " 'N',\n",
- " '2',\n",
- " 'C',\n",
- " 'C',\n",
- " 'C',\n",
- " '(',\n",
- " 'C',\n",
- " '(',\n",
- " 'O',\n",
- " ')',\n",
- " '(',\n",
- " 'c',\n",
- " '3',\n",
- " 'c',\n",
- " 'c',\n",
- " 'c',\n",
- " 'c',\n",
- " 'c',\n",
- " '3',\n",
- " ')',\n",
- " 'c',\n",
- " '3',\n",
- " 'c',\n",
- " 'c',\n",
- " 'c',\n",
- " 'c',\n",
- " 'c',\n",
- " '3',\n",
- " ')',\n",
- " 'C',\n",
- " 'C',\n",
- " '2',\n",
- " ')',\n",
- " 'c',\n",
- " 'c',\n",
- " '1']"
- ]
- },
- "execution_count": 8,
- "metadata": {},
- "output_type": "execute_result"
- }
- ],
- "source": [
- "tokenized_rxn"
- ]
- }
- ],
- "metadata": {
- "kernelspec": {
- "display_name": "Python [conda env:tformers] *",
- "language": "python",
- "name": "conda-env-tformers-py"
- },
- "language_info": {
- "codemirror_mode": {
- "name": "ipython",
- "version": 3
- },
- "file_extension": ".py",
- "mimetype": "text/x-python",
- "name": "python",
- "nbconvert_exporter": "python",
- "pygments_lexer": "ipython3",
- "version": "3.7.6"
- }
- },
- "nbformat": 4,
- "nbformat_minor": 4
-}
diff --git a/server/transformers/setup.cfg b/server/transformers/setup.cfg
deleted file mode 100644
index e69f8d5551226f7a92bff57d2614af0533abd35b..0000000000000000000000000000000000000000
--- a/server/transformers/setup.cfg
+++ /dev/null
@@ -1,34 +0,0 @@
-[isort]
-ensure_newline_before_comments = True
-force_grid_wrap = 0
-include_trailing_comma = True
-known_first_party = transformers
-known_third_party =
- absl
- fairseq
- fastprogress
- git
- h5py
- MeCab
- nltk
- numpy
- packaging
- PIL
- psutil
- seqeval
- sklearn
- tensorboardX
- tensorflow
- tensorflow_datasets
- torch
- torchtext
- torchvision
-
-line_length = 119
-lines_after_imports = 2
-multi_line_output = 3
-use_parentheses = True
-
-[flake8]
-ignore = E203, E501, W503
-max-line-length = 119
diff --git a/server/transformers/setup.py b/server/transformers/setup.py
deleted file mode 100644
index b36d51e719bc6c0428dce7237c797db45e195ce1..0000000000000000000000000000000000000000
--- a/server/transformers/setup.py
+++ /dev/null
@@ -1,123 +0,0 @@
-"""
-Simple check list from AllenNLP repo: https://github.com/allenai/allennlp/blob/master/setup.py
-
-To create the package for pypi.
-
-1. Change the version in __init__.py, setup.py as well as docs/source/conf.py.
-
-2. Commit these changes with the message: "Release: VERSION"
-
-3. Add a tag in git to mark the release: "git tag VERSION -m'Adds tag VERSION for pypi' "
- Push the tag to git: git push --tags origin master
-
-4. Build both the sources and the wheel. Do not change anything in setup.py between
- creating the wheel and the source distribution (obviously).
-
- For the wheel, run: "python setup.py bdist_wheel" in the top level directory.
- (this will build a wheel for the python version you use to build it).
-
- For the sources, run: "python setup.py sdist"
- You should now have a /dist directory with both .whl and .tar.gz source versions.
-
-5. Check that everything looks correct by uploading the package to the pypi test server:
-
- twine upload dist/* -r pypitest
- (pypi suggest using twine as other methods upload files via plaintext.)
- You may have to specify the repository url, use the following command then:
- twine upload dist/* -r pypitest --repository-url=https://test.pypi.org/legacy/
-
- Check that you can install it in a virtualenv by running:
- pip install -i https://testpypi.python.org/pypi transformers
-
-6. Upload the final version to actual pypi:
- twine upload dist/* -r pypi
-
-7. Copy the release notes from RELEASE.md to the tag in github once everything is looking hunky-dory.
-
-"""
-
-import shutil
-from pathlib import Path
-
-from setuptools import find_packages, setup
-
-
-# Remove stale transformers.egg-info directory to avoid https://github.com/pypa/pip/issues/5466
-stale_egg_info = Path(__file__).parent / "transformers.egg-info"
-if stale_egg_info.exists():
- print(
- (
- "Warning: {} exists.\n\n"
- "If you recently updated transformers to 3.0 or later, this is expected,\n"
- "but it may prevent transformers from installing in editable mode.\n\n"
- "This directory is automatically generated by Python's packaging tools.\n"
- "I will remove it now.\n\n"
- "See https://github.com/pypa/pip/issues/5466 for details.\n"
- ).format(stale_egg_info)
- )
- shutil.rmtree(stale_egg_info)
-
-
-extras = {}
-
-extras["mecab"] = ["mecab-python3"]
-extras["sklearn"] = ["scikit-learn"]
-extras["tf"] = ["tensorflow"]
-extras["torch"] = ["torch"]
-
-extras["serving"] = ["pydantic", "uvicorn", "fastapi", "starlette"]
-extras["all"] = extras["serving"] + ["tensorflow", "torch"]
-
-extras["testing"] = ["pytest", "pytest-xdist"]
-extras["quality"] = ["black", "isort", "flake8"]
-extras["docs"] = ["recommonmark", "sphinx", "sphinx-markdown-tables", "sphinx-rtd-theme"]
-extras["dev"] = extras["testing"] + extras["quality"] + ["mecab-python3", "scikit-learn", "tensorflow", "torch"]
-
-setup(
- name="transformers",
- version="2.4.1",
- author="Thomas Wolf, Lysandre Debut, Victor Sanh, Julien Chaumond, Google AI Language Team Authors, Open AI team Authors, Facebook AI Authors, Carnegie Mellon University Authors",
- author_email="thomas@huggingface.co",
- description="State-of-the-art Natural Language Processing for TensorFlow 2.0 and PyTorch",
- long_description=open("README.md", "r", encoding="utf-8").read(),
- long_description_content_type="text/markdown",
- keywords="NLP deep learning transformer pytorch tensorflow BERT GPT GPT-2 google openai CMU",
- license="Apache",
- url="https://github.com/huggingface/transformers",
- package_dir={"": "src"},
- packages=find_packages("src"),
- install_requires=[
- "numpy",
- "tokenizers == 0.0.11",
- # accessing files from S3 directly
- "boto3",
- # filesystem locks e.g. to prevent parallel downloads
- "filelock",
- # for downloading models over HTTPS
- "requests",
- # progress bars in model download and training scripts
- "tqdm >= 4.27",
- # for OpenAI GPT
- "regex != 2019.12.17",
- # for XLNet
- "sentencepiece",
- # for XLM
- "sacremoses",
- ],
- extras_require=extras,
- scripts=["transformers-cli"],
- python_requires=">=3.5.0",
- classifiers=[
- "Development Status :: 5 - Production/Stable",
- "Intended Audience :: Developers",
- "Intended Audience :: Education",
- "Intended Audience :: Science/Research",
- "License :: OSI Approved :: Apache Software License",
- "Operating System :: OS Independent",
- "Programming Language :: Python :: 3",
- "Programming Language :: Python :: 3.5",
- "Programming Language :: Python :: 3.6",
- "Programming Language :: Python :: 3.7",
- "Topic :: Scientific/Engineering :: Artificial Intelligence",
- ],
-)
diff --git a/server/transformers/src/transformers/__init__.py b/server/transformers/src/transformers/__init__.py
deleted file mode 100755
index 3cbbf815d65167b8b35f41b220a102dc8f3f84dd..0000000000000000000000000000000000000000
--- a/server/transformers/src/transformers/__init__.py
+++ /dev/null
@@ -1,429 +0,0 @@
-# flake8: noqa
-# There's no way to ignore "F401 '...' imported but unused" warnings in this
-# module, but to preserve other warnings. So, don't check this module at all.
-
-__version__ = "2.4.1"
-
-# Work around to update TensorFlow's absl.logging threshold which alters the
-# default Python logging output behavior when present.
-# see: https://github.com/abseil/abseil-py/issues/99
-# and: https://github.com/tensorflow/tensorflow/issues/26691#issuecomment-500369493
-try:
- import absl.logging
-except ImportError:
- pass
-else:
- absl.logging.set_verbosity("info")
- absl.logging.set_stderrthreshold("info")
- absl.logging._warn_preinit_stderr = False
-
-import logging
-
-from .configuration_albert import ALBERT_PRETRAINED_CONFIG_ARCHIVE_MAP, AlbertConfig
-from .configuration_auto import ALL_PRETRAINED_CONFIG_ARCHIVE_MAP, AutoConfig
-from .configuration_bert import BERT_PRETRAINED_CONFIG_ARCHIVE_MAP, BertConfig
-from .configuration_camembert import CAMEMBERT_PRETRAINED_CONFIG_ARCHIVE_MAP, CamembertConfig
-from .configuration_ctrl import CTRL_PRETRAINED_CONFIG_ARCHIVE_MAP, CTRLConfig
-from .configuration_distilbert import DISTILBERT_PRETRAINED_CONFIG_ARCHIVE_MAP, DistilBertConfig
-from .configuration_flaubert import FLAUBERT_PRETRAINED_CONFIG_ARCHIVE_MAP, FlaubertConfig
-from .configuration_gpt2 import GPT2_PRETRAINED_CONFIG_ARCHIVE_MAP, GPT2Config
-from .configuration_mmbt import MMBTConfig
-from .configuration_openai import OPENAI_GPT_PRETRAINED_CONFIG_ARCHIVE_MAP, OpenAIGPTConfig
-from .configuration_roberta import ROBERTA_PRETRAINED_CONFIG_ARCHIVE_MAP, RobertaConfig
-from .configuration_t5 import T5_PRETRAINED_CONFIG_ARCHIVE_MAP, T5Config
-from .configuration_transfo_xl import TRANSFO_XL_PRETRAINED_CONFIG_ARCHIVE_MAP, TransfoXLConfig
-
-# Configurations
-from .configuration_utils import PretrainedConfig
-from .configuration_xlm import XLM_PRETRAINED_CONFIG_ARCHIVE_MAP, XLMConfig
-from .configuration_xlm_roberta import XLM_ROBERTA_PRETRAINED_CONFIG_ARCHIVE_MAP, XLMRobertaConfig
-from .configuration_xlnet import XLNET_PRETRAINED_CONFIG_ARCHIVE_MAP, XLNetConfig
-from .data import (
- DataProcessor,
- InputExample,
- InputFeatures,
- SingleSentenceClassificationProcessor,
- SquadExample,
- SquadFeatures,
- SquadV1Processor,
- SquadV2Processor,
- glue_convert_examples_to_features,
- glue_output_modes,
- glue_processors,
- glue_tasks_num_labels,
- is_sklearn_available,
- squad_convert_examples_to_features,
- xnli_output_modes,
- xnli_processors,
- xnli_tasks_num_labels,
-)
-
-# Files and general utilities
-from .file_utils import (
- CONFIG_NAME,
- MODEL_CARD_NAME,
- PYTORCH_PRETRAINED_BERT_CACHE,
- PYTORCH_TRANSFORMERS_CACHE,
- TF2_WEIGHTS_NAME,
- TF_WEIGHTS_NAME,
- TRANSFORMERS_CACHE,
- WEIGHTS_NAME,
- add_end_docstrings,
- add_start_docstrings,
- cached_path,
- is_tf_available,
- is_torch_available,
-)
-
-# Model Cards
-from .modelcard import ModelCard
-
-# TF 2.0 <=> PyTorch conversion utilities
-from .modeling_tf_pytorch_utils import (
- convert_tf_weight_name_to_pt_weight_name,
- load_pytorch_checkpoint_in_tf2_model,
- load_pytorch_model_in_tf2_model,
- load_pytorch_weights_in_tf2_model,
- load_tf2_checkpoint_in_pytorch_model,
- load_tf2_model_in_pytorch_model,
- load_tf2_weights_in_pytorch_model,
-)
-
-# Pipelines
-from .pipelines import (
- CsvPipelineDataFormat,
- FeatureExtractionPipeline,
- FillMaskPipeline,
- JsonPipelineDataFormat,
- NerPipeline,
- PipedPipelineDataFormat,
- Pipeline,
- PipelineDataFormat,
- QuestionAnsweringPipeline,
- TextClassificationPipeline,
- pipeline,
-)
-from .tokenization_albert import AlbertTokenizer
-from .tokenization_auto import AutoTokenizer
-from .tokenization_bert import BasicTokenizer, BertTokenizer, BertTokenizerFast, WordpieceTokenizer
-from .tokenization_bert_japanese import BertJapaneseTokenizer, CharacterTokenizer, MecabTokenizer
-from .tokenization_camembert import CamembertTokenizer
-from .tokenization_ctrl import CTRLTokenizer
-from .tokenization_distilbert import DistilBertTokenizer
-from .tokenization_flaubert import FlaubertTokenizer
-from .tokenization_gpt2 import GPT2Tokenizer, GPT2TokenizerFast
-from .tokenization_openai import OpenAIGPTTokenizer
-from .tokenization_roberta import RobertaTokenizer
-from .tokenization_t5 import T5Tokenizer
-from .tokenization_transfo_xl import TransfoXLCorpus, TransfoXLTokenizer
-
-# Tokenizers
-from .tokenization_utils import PreTrainedTokenizer
-from .tokenization_xlm import XLMTokenizer
-from .tokenization_xlm_roberta import XLMRobertaTokenizer
-from .tokenization_xlnet import SPIECE_UNDERLINE, XLNetTokenizer
-
-
-logger = logging.getLogger(__name__) # pylint: disable=invalid-name
-
-
-if is_sklearn_available():
- from .data import glue_compute_metrics, xnli_compute_metrics
-
-
-# Modeling
-if is_torch_available():
- from .modeling_utils import PreTrainedModel, prune_layer, Conv1D
- from .modeling_auto import (
- AutoModel,
- AutoModelForPreTraining,
- AutoModelForSequenceClassification,
- AutoModelForQuestionAnswering,
- AutoModelWithLMHead,
- AutoModelForTokenClassification,
- ALL_PRETRAINED_MODEL_ARCHIVE_MAP,
- )
-
- from .modeling_bert import (
- BertPreTrainedModel,
- BertModel,
- BertForPreTraining,
- BertForMaskedLM,
- BertForNextSentencePrediction,
- BertForSequenceClassification,
- BertForMultipleChoice,
- BertForTokenClassification,
- BertForQuestionAnswering,
- load_tf_weights_in_bert,
- BERT_PRETRAINED_MODEL_ARCHIVE_MAP,
- )
- from .modeling_openai import (
- OpenAIGPTPreTrainedModel,
- OpenAIGPTModel,
- OpenAIGPTLMHeadModel,
- OpenAIGPTDoubleHeadsModel,
- load_tf_weights_in_openai_gpt,
- OPENAI_GPT_PRETRAINED_MODEL_ARCHIVE_MAP,
- )
- from .modeling_transfo_xl import (
- TransfoXLPreTrainedModel,
- TransfoXLModel,
- TransfoXLLMHeadModel,
- AdaptiveEmbedding,
- load_tf_weights_in_transfo_xl,
- TRANSFO_XL_PRETRAINED_MODEL_ARCHIVE_MAP,
- )
- from .modeling_gpt2 import (
- GPT2PreTrainedModel,
- GPT2Model,
- GPT2LMHeadModel,
- GPT2DoubleHeadsModel,
- load_tf_weights_in_gpt2,
- GPT2_PRETRAINED_MODEL_ARCHIVE_MAP,
- )
- from .modeling_ctrl import CTRLPreTrainedModel, CTRLModel, CTRLLMHeadModel, CTRL_PRETRAINED_MODEL_ARCHIVE_MAP
- from .modeling_xlnet import (
- XLNetPreTrainedModel,
- XLNetModel,
- XLNetLMHeadModel,
- XLNetForSequenceClassification,
- XLNetForTokenClassification,
- XLNetForMultipleChoice,
- XLNetForQuestionAnsweringSimple,
- XLNetForQuestionAnswering,
- load_tf_weights_in_xlnet,
- XLNET_PRETRAINED_MODEL_ARCHIVE_MAP,
- )
- from .modeling_xlm import (
- XLMPreTrainedModel,
- XLMModel,
- XLMWithLMHeadModel,
- XLMForSequenceClassification,
- XLMForQuestionAnswering,
- XLMForQuestionAnsweringSimple,
- XLM_PRETRAINED_MODEL_ARCHIVE_MAP,
- )
- from .modeling_roberta import (
- RobertaForMaskedLM,
- RobertaModel,
- RobertaForSequenceClassification,
- RobertaForMultipleChoice,
- RobertaForTokenClassification,
- RobertaForQuestionAnswering,
- ROBERTA_PRETRAINED_MODEL_ARCHIVE_MAP,
- )
- from .modeling_camembert import (
- CamembertForMaskedLM,
- CamembertModel,
- CamembertForSequenceClassification,
- CamembertForTokenClassification,
- CAMEMBERT_PRETRAINED_MODEL_ARCHIVE_MAP,
- )
- from .modeling_distilbert import (
- DistilBertPreTrainedModel,
- DistilBertForMaskedLM,
- DistilBertModel,
- DistilBertForSequenceClassification,
- DistilBertForQuestionAnswering,
- DistilBertForTokenClassification,
- DISTILBERT_PRETRAINED_MODEL_ARCHIVE_MAP,
- )
- from .modeling_camembert import (
- CamembertForMaskedLM,
- CamembertModel,
- CamembertForSequenceClassification,
- CamembertForMultipleChoice,
- CamembertForTokenClassification,
- CAMEMBERT_PRETRAINED_MODEL_ARCHIVE_MAP,
- )
- from .modeling_encoder_decoder import PreTrainedEncoderDecoder, Model2Model
- from .modeling_t5 import (
- T5PreTrainedModel,
- T5Model,
- T5WithLMHeadModel,
- load_tf_weights_in_t5,
- T5_PRETRAINED_MODEL_ARCHIVE_MAP,
- )
- from .modeling_albert import (
- AlbertPreTrainedModel,
- AlbertModel,
- AlbertForMaskedLM,
- AlbertForSequenceClassification,
- AlbertForQuestionAnswering,
- load_tf_weights_in_albert,
- ALBERT_PRETRAINED_MODEL_ARCHIVE_MAP,
- )
- from .modeling_xlm_roberta import (
- XLMRobertaForMaskedLM,
- XLMRobertaModel,
- XLMRobertaForMultipleChoice,
- XLMRobertaForSequenceClassification,
- XLMRobertaForTokenClassification,
- XLM_ROBERTA_PRETRAINED_MODEL_ARCHIVE_MAP,
- )
- from .modeling_mmbt import ModalEmbeddings, MMBTModel, MMBTForClassification
-
- from .modeling_flaubert import (
- FlaubertModel,
- FlaubertWithLMHeadModel,
- FlaubertForSequenceClassification,
- FlaubertForQuestionAnswering,
- FlaubertForQuestionAnsweringSimple,
- FLAUBERT_PRETRAINED_MODEL_ARCHIVE_MAP,
- )
-
- # Optimization
- from .optimization import (
- AdamW,
- get_constant_schedule,
- get_constant_schedule_with_warmup,
- get_cosine_schedule_with_warmup,
- get_cosine_with_hard_restarts_schedule_with_warmup,
- get_linear_schedule_with_warmup,
- )
-
-
-# TensorFlow
-if is_tf_available():
- from .modeling_tf_utils import TFPreTrainedModel, TFSharedEmbeddings, TFSequenceSummary, shape_list
- from .modeling_tf_auto import (
- TFAutoModel,
- TFAutoModelForPreTraining,
- TFAutoModelForSequenceClassification,
- TFAutoModelForQuestionAnswering,
- TFAutoModelWithLMHead,
- TFAutoModelForTokenClassification,
- TF_ALL_PRETRAINED_MODEL_ARCHIVE_MAP,
- )
-
- from .modeling_tf_bert import (
- TFBertPreTrainedModel,
- TFBertMainLayer,
- TFBertEmbeddings,
- TFBertModel,
- TFBertForPreTraining,
- TFBertForMaskedLM,
- TFBertForNextSentencePrediction,
- TFBertForSequenceClassification,
- TFBertForMultipleChoice,
- TFBertForTokenClassification,
- TFBertForQuestionAnswering,
- TF_BERT_PRETRAINED_MODEL_ARCHIVE_MAP,
- )
-
- from .modeling_tf_gpt2 import (
- TFGPT2PreTrainedModel,
- TFGPT2MainLayer,
- TFGPT2Model,
- TFGPT2LMHeadModel,
- TFGPT2DoubleHeadsModel,
- TF_GPT2_PRETRAINED_MODEL_ARCHIVE_MAP,
- )
-
- from .modeling_tf_openai import (
- TFOpenAIGPTPreTrainedModel,
- TFOpenAIGPTMainLayer,
- TFOpenAIGPTModel,
- TFOpenAIGPTLMHeadModel,
- TFOpenAIGPTDoubleHeadsModel,
- TF_OPENAI_GPT_PRETRAINED_MODEL_ARCHIVE_MAP,
- )
-
- from .modeling_tf_transfo_xl import (
- TFTransfoXLPreTrainedModel,
- TFTransfoXLMainLayer,
- TFTransfoXLModel,
- TFTransfoXLLMHeadModel,
- TF_TRANSFO_XL_PRETRAINED_MODEL_ARCHIVE_MAP,
- )
-
- from .modeling_tf_xlnet import (
- TFXLNetPreTrainedModel,
- TFXLNetMainLayer,
- TFXLNetModel,
- TFXLNetLMHeadModel,
- TFXLNetForSequenceClassification,
- TFXLNetForTokenClassification,
- TFXLNetForQuestionAnsweringSimple,
- TF_XLNET_PRETRAINED_MODEL_ARCHIVE_MAP,
- )
-
- from .modeling_tf_xlm import (
- TFXLMPreTrainedModel,
- TFXLMMainLayer,
- TFXLMModel,
- TFXLMWithLMHeadModel,
- TFXLMForSequenceClassification,
- TFXLMForQuestionAnsweringSimple,
- TF_XLM_PRETRAINED_MODEL_ARCHIVE_MAP,
- )
-
- from .modeling_tf_xlm_roberta import (
- TFXLMRobertaForMaskedLM,
- TFXLMRobertaModel,
- TFXLMRobertaForSequenceClassification,
- TFXLMRobertaForTokenClassification,
- TF_XLM_ROBERTA_PRETRAINED_MODEL_ARCHIVE_MAP,
- )
-
- from .modeling_tf_roberta import (
- TFRobertaPreTrainedModel,
- TFRobertaMainLayer,
- TFRobertaModel,
- TFRobertaForMaskedLM,
- TFRobertaForSequenceClassification,
- TFRobertaForTokenClassification,
- TF_ROBERTA_PRETRAINED_MODEL_ARCHIVE_MAP,
- )
-
- from .modeling_tf_camembert import (
- TFCamembertModel,
- TFCamembertForMaskedLM,
- TFCamembertForSequenceClassification,
- TFCamembertForTokenClassification,
- TF_CAMEMBERT_PRETRAINED_MODEL_ARCHIVE_MAP,
- )
-
- from .modeling_tf_distilbert import (
- TFDistilBertPreTrainedModel,
- TFDistilBertMainLayer,
- TFDistilBertModel,
- TFDistilBertForMaskedLM,
- TFDistilBertForSequenceClassification,
- TFDistilBertForTokenClassification,
- TFDistilBertForQuestionAnswering,
- TF_DISTILBERT_PRETRAINED_MODEL_ARCHIVE_MAP,
- )
-
- from .modeling_tf_ctrl import (
- TFCTRLPreTrainedModel,
- TFCTRLModel,
- TFCTRLLMHeadModel,
- TF_CTRL_PRETRAINED_MODEL_ARCHIVE_MAP,
- )
-
- from .modeling_tf_albert import (
- TFAlbertPreTrainedModel,
- TFAlbertModel,
- TFAlbertForMaskedLM,
- TFAlbertForSequenceClassification,
- TF_ALBERT_PRETRAINED_MODEL_ARCHIVE_MAP,
- )
-
- from .modeling_tf_t5 import (
- TFT5PreTrainedModel,
- TFT5Model,
- TFT5WithLMHeadModel,
- TF_T5_PRETRAINED_MODEL_ARCHIVE_MAP,
- )
-
- # Optimization
- from .optimization_tf import WarmUp, create_optimizer, AdamWeightDecay, GradientAccumulator
-
-
-if not is_tf_available() and not is_torch_available():
- logger.warning(
- "Neither PyTorch nor TensorFlow >= 2.0 have been found."
- "Models won't be available and only tokenizers, configuration"
- "and file/data utilities can be used."
- )
diff --git a/server/transformers/src/transformers/commands/__init__.py b/server/transformers/src/transformers/commands/__init__.py
deleted file mode 100644
index 13171f42853e27083c89bc7d2a648a2ba3287c20..0000000000000000000000000000000000000000
--- a/server/transformers/src/transformers/commands/__init__.py
+++ /dev/null
@@ -1,13 +0,0 @@
-from abc import ABC, abstractmethod
-from argparse import ArgumentParser
-
-
-class BaseTransformersCLICommand(ABC):
- @staticmethod
- @abstractmethod
- def register_subcommand(parser: ArgumentParser):
- raise NotImplementedError()
-
- @abstractmethod
- def run(self):
- raise NotImplementedError()
diff --git a/server/transformers/src/transformers/commands/convert.py b/server/transformers/src/transformers/commands/convert.py
deleted file mode 100644
index a31ef53b624dec01e849e58a05f1e7591acdb1ab..0000000000000000000000000000000000000000
--- a/server/transformers/src/transformers/commands/convert.py
+++ /dev/null
@@ -1,144 +0,0 @@
-from argparse import ArgumentParser, Namespace
-from logging import getLogger
-
-from transformers.commands import BaseTransformersCLICommand
-
-
-def convert_command_factory(args: Namespace):
- """
- Factory function used to convert a model TF 1.0 checkpoint in a PyTorch checkpoint.
- :return: ServeCommand
- """
- return ConvertCommand(
- args.model_type, args.tf_checkpoint, args.pytorch_dump_output, args.config, args.finetuning_task_name
- )
-
-
-class ConvertCommand(BaseTransformersCLICommand):
- @staticmethod
- def register_subcommand(parser: ArgumentParser):
- """
- Register this command to argparse so it's available for the transformer-cli
- :param parser: Root parser to register command-specific arguments
- :return:
- """
- train_parser = parser.add_parser(
- "convert",
- help="CLI tool to run convert model from original "
- "author checkpoints to Transformers PyTorch checkpoints.",
- )
- train_parser.add_argument("--model_type", type=str, required=True, help="Model's type.")
- train_parser.add_argument(
- "--tf_checkpoint", type=str, required=True, help="TensorFlow checkpoint path or folder."
- )
- train_parser.add_argument(
- "--pytorch_dump_output", type=str, required=True, help="Path to the PyTorch savd model output."
- )
- train_parser.add_argument("--config", type=str, default="", help="Configuration file path or folder.")
- train_parser.add_argument(
- "--finetuning_task_name",
- type=str,
- default=None,
- help="Optional fine-tuning task name if the TF model was a finetuned model.",
- )
- train_parser.set_defaults(func=convert_command_factory)
-
- def __init__(
- self,
- model_type: str,
- tf_checkpoint: str,
- pytorch_dump_output: str,
- config: str,
- finetuning_task_name: str,
- *args
- ):
- self._logger = getLogger("transformers-cli/converting")
-
- self._logger.info("Loading model {}".format(model_type))
- self._model_type = model_type
- self._tf_checkpoint = tf_checkpoint
- self._pytorch_dump_output = pytorch_dump_output
- self._config = config
- self._finetuning_task_name = finetuning_task_name
-
- def run(self):
- if self._model_type == "bert":
- try:
- from transformers.convert_bert_original_tf_checkpoint_to_pytorch import (
- convert_tf_checkpoint_to_pytorch,
- )
- except ImportError:
- msg = (
- "transformers can only be used from the commandline to convert TensorFlow models in PyTorch, "
- "In that case, it requires TensorFlow to be installed. Please see "
- "https://www.tensorflow.org/install/ for installation instructions."
- )
- raise ImportError(msg)
-
- convert_tf_checkpoint_to_pytorch(self._tf_checkpoint, self._config, self._pytorch_dump_output)
- elif self._model_type == "gpt":
- from transformers.convert_openai_original_tf_checkpoint_to_pytorch import (
- convert_openai_checkpoint_to_pytorch,
- )
-
- convert_openai_checkpoint_to_pytorch(self._tf_checkpoint, self._config, self._pytorch_dump_output)
- elif self._model_type == "transfo_xl":
- try:
- from transformers.convert_transfo_xl_original_tf_checkpoint_to_pytorch import (
- convert_transfo_xl_checkpoint_to_pytorch,
- )
- except ImportError:
- msg = (
- "transformers can only be used from the commandline to convert TensorFlow models in PyTorch, "
- "In that case, it requires TensorFlow to be installed. Please see "
- "https://www.tensorflow.org/install/ for installation instructions."
- )
- raise ImportError(msg)
-
- if "ckpt" in self._tf_checkpoint.lower():
- TF_CHECKPOINT = self._tf_checkpoint
- TF_DATASET_FILE = ""
- else:
- TF_DATASET_FILE = self._tf_checkpoint
- TF_CHECKPOINT = ""
- convert_transfo_xl_checkpoint_to_pytorch(
- TF_CHECKPOINT, self._config, self._pytorch_dump_output, TF_DATASET_FILE
- )
- elif self._model_type == "gpt2":
- try:
- from transformers.convert_gpt2_original_tf_checkpoint_to_pytorch import (
- convert_gpt2_checkpoint_to_pytorch,
- )
- except ImportError:
- msg = (
- "transformers can only be used from the commandline to convert TensorFlow models in PyTorch, "
- "In that case, it requires TensorFlow to be installed. Please see "
- "https://www.tensorflow.org/install/ for installation instructions."
- )
- raise ImportError(msg)
-
- convert_gpt2_checkpoint_to_pytorch(self._tf_checkpoint, self._config, self._pytorch_dump_output)
- elif self._model_type == "xlnet":
- try:
- from transformers.convert_xlnet_original_tf_checkpoint_to_pytorch import (
- convert_xlnet_checkpoint_to_pytorch,
- )
- except ImportError:
- msg = (
- "transformers can only be used from the commandline to convert TensorFlow models in PyTorch, "
- "In that case, it requires TensorFlow to be installed. Please see "
- "https://www.tensorflow.org/install/ for installation instructions."
- )
- raise ImportError(msg)
-
- convert_xlnet_checkpoint_to_pytorch(
- self._tf_checkpoint, self._config, self._pytorch_dump_output, self._finetuning_task_name
- )
- elif self._model_type == "xlm":
- from transformers.convert_xlm_original_pytorch_checkpoint_to_pytorch import (
- convert_xlm_checkpoint_to_pytorch,
- )
-
- convert_xlm_checkpoint_to_pytorch(self._tf_checkpoint, self._pytorch_dump_output)
- else:
- raise ValueError("--model_type should be selected in the list [bert, gpt, gpt2, transfo_xl, xlnet, xlm]")
diff --git a/server/transformers/src/transformers/commands/download.py b/server/transformers/src/transformers/commands/download.py
deleted file mode 100644
index acfb3eeb927f6d2d30e8fb49d00183fc53de8770..0000000000000000000000000000000000000000
--- a/server/transformers/src/transformers/commands/download.py
+++ /dev/null
@@ -1,32 +0,0 @@
-from argparse import ArgumentParser
-
-from transformers.commands import BaseTransformersCLICommand
-
-
-def download_command_factory(args):
- return DownloadCommand(args.model, args.cache_dir, args.force)
-
-
-class DownloadCommand(BaseTransformersCLICommand):
- @staticmethod
- def register_subcommand(parser: ArgumentParser):
- download_parser = parser.add_parser("download")
- download_parser.add_argument(
- "--cache-dir", type=str, default=None, help="Path to location to store the models"
- )
- download_parser.add_argument(
- "--force", action="store_true", help="Force the model to be download even if already in cache-dir"
- )
- download_parser.add_argument("model", type=str, help="Name of the model to download")
- download_parser.set_defaults(func=download_command_factory)
-
- def __init__(self, model: str, cache: str, force: bool):
- self._model = model
- self._cache = cache
- self._force = force
-
- def run(self):
- from transformers import AutoModel, AutoTokenizer
-
- AutoModel.from_pretrained(self._model, cache_dir=self._cache, force_download=self._force)
- AutoTokenizer.from_pretrained(self._model, cache_dir=self._cache, force_download=self._force)
diff --git a/server/transformers/src/transformers/commands/env.py b/server/transformers/src/transformers/commands/env.py
deleted file mode 100644
index efc8fbb683c61bea4896023caabe9cba2c2ea583..0000000000000000000000000000000000000000
--- a/server/transformers/src/transformers/commands/env.py
+++ /dev/null
@@ -1,58 +0,0 @@
-import platform
-from argparse import ArgumentParser
-
-from transformers import __version__ as version
-from transformers import is_tf_available, is_torch_available
-from transformers.commands import BaseTransformersCLICommand
-
-
-def info_command_factory(_):
- return EnvironmentCommand()
-
-
-class EnvironmentCommand(BaseTransformersCLICommand):
- @staticmethod
- def register_subcommand(parser: ArgumentParser):
- download_parser = parser.add_parser("env")
- download_parser.set_defaults(func=info_command_factory)
-
- def run(self):
- pt_version = "not installed"
- pt_cuda_available = "NA"
- if is_torch_available():
- import torch
-
- pt_version = torch.__version__
- pt_cuda_available = torch.cuda.is_available()
-
- tf_version = "not installed"
- tf_cuda_available = "NA"
- if is_tf_available():
- import tensorflow as tf
-
- tf_version = tf.__version__
- try:
- # deprecated in v2.1
- tf_cuda_available = tf.test.is_gpu_available()
- except AttributeError:
- # returns list of devices, convert to bool
- tf_cuda_available = bool(tf.config.list_physical_devices("GPU"))
-
- info = {
- "`transformers` version": version,
- "Platform": platform.platform(),
- "Python version": platform.python_version(),
- "PyTorch version (GPU?)": "{} ({})".format(pt_version, pt_cuda_available),
- "Tensorflow version (GPU?)": "{} ({})".format(tf_version, tf_cuda_available),
- "Using GPU in script?": "",
- "Using distributed or parallel set-up in script?": "",
- }
-
- print("\nCopy-and-paste the text below in your GitHub issue and FILL OUT the two last points.\n")
- print(self.format_dict(info))
-
- return info
-
- @staticmethod
- def format_dict(d):
- return "\n".join(["- {}: {}".format(prop, val) for prop, val in d.items()]) + "\n"
diff --git a/server/transformers/src/transformers/commands/run.py b/server/transformers/src/transformers/commands/run.py
deleted file mode 100644
index fdc88c55e4a847a160bf9549d8d44d5ea0b6c570..0000000000000000000000000000000000000000
--- a/server/transformers/src/transformers/commands/run.py
+++ /dev/null
@@ -1,96 +0,0 @@
-import logging
-from argparse import ArgumentParser
-
-from transformers.commands import BaseTransformersCLICommand
-from transformers.pipelines import SUPPORTED_TASKS, Pipeline, PipelineDataFormat, pipeline
-
-
-logger = logging.getLogger(__name__) # pylint: disable=invalid-name
-
-
-def try_infer_format_from_ext(path: str):
- if not path:
- return "pipe"
-
- for ext in PipelineDataFormat.SUPPORTED_FORMATS:
- if path.endswith(ext):
- return ext
-
- raise Exception(
- "Unable to determine file format from file extension {}. "
- "Please provide the format through --format {}".format(path, PipelineDataFormat.SUPPORTED_FORMATS)
- )
-
-
-def run_command_factory(args):
- nlp = pipeline(
- task=args.task,
- model=args.model if args.model else None,
- config=args.config,
- tokenizer=args.tokenizer,
- device=args.device,
- )
- format = try_infer_format_from_ext(args.input) if args.format == "infer" else args.format
- reader = PipelineDataFormat.from_str(
- format=format,
- output_path=args.output,
- input_path=args.input,
- column=args.column if args.column else nlp.default_input_names,
- overwrite=args.overwrite,
- )
- return RunCommand(nlp, reader)
-
-
-class RunCommand(BaseTransformersCLICommand):
- def __init__(self, nlp: Pipeline, reader: PipelineDataFormat):
- self._nlp = nlp
- self._reader = reader
-
- @staticmethod
- def register_subcommand(parser: ArgumentParser):
- run_parser = parser.add_parser("run", help="Run a pipeline through the CLI")
- run_parser.add_argument("--task", choices=SUPPORTED_TASKS.keys(), help="Task to run")
- run_parser.add_argument("--input", type=str, help="Path to the file to use for inference")
- run_parser.add_argument("--output", type=str, help="Path to the file that will be used post to write results.")
- run_parser.add_argument("--model", type=str, help="Name or path to the model to instantiate.")
- run_parser.add_argument("--config", type=str, help="Name or path to the model's config to instantiate.")
- run_parser.add_argument(
- "--tokenizer", type=str, help="Name of the tokenizer to use. (default: same as the model name)"
- )
- run_parser.add_argument(
- "--column",
- type=str,
- help="Name of the column to use as input. (For multi columns input as QA use column1,columns2)",
- )
- run_parser.add_argument(
- "--format",
- type=str,
- default="infer",
- choices=PipelineDataFormat.SUPPORTED_FORMATS,
- help="Input format to read from",
- )
- run_parser.add_argument(
- "--device",
- type=int,
- default=-1,
- help="Indicate the device to run onto, -1 indicates CPU, >= 0 indicates GPU (default: -1)",
- )
- run_parser.add_argument("--overwrite", action="store_true", help="Allow overwriting the output file.")
- run_parser.set_defaults(func=run_command_factory)
-
- def run(self):
- nlp, outputs = self._nlp, []
-
- for entry in self._reader:
- output = nlp(**entry) if self._reader.is_multi_columns else nlp(entry)
- if isinstance(output, dict):
- outputs.append(output)
- else:
- outputs += output
-
- # Saving data
- if self._nlp.binary_output:
- binary_path = self._reader.save_binary(outputs)
- logger.warning("Current pipeline requires output to be in binary format, saving at {}".format(binary_path))
- else:
- self._reader.save(outputs)
diff --git a/server/transformers/src/transformers/commands/serving.py b/server/transformers/src/transformers/commands/serving.py
deleted file mode 100644
index f45d0b0987d5ec68f6001351539405912e16337a..0000000000000000000000000000000000000000
--- a/server/transformers/src/transformers/commands/serving.py
+++ /dev/null
@@ -1,214 +0,0 @@
-import logging
-from argparse import ArgumentParser, Namespace
-from typing import Any, List, Optional
-
-from transformers import Pipeline
-from transformers.commands import BaseTransformersCLICommand
-from transformers.pipelines import SUPPORTED_TASKS, pipeline
-
-
-try:
- from uvicorn import run
- from fastapi import FastAPI, HTTPException, Body
- from fastapi.routing import APIRoute
- from pydantic import BaseModel
- from starlette.responses import JSONResponse
-
- _serve_dependencies_installed = True
-except (ImportError, AttributeError):
- BaseModel = object
-
- def Body(*x, **y):
- pass
-
- _serve_dependencies_installed = False
-
-
-logger = logging.getLogger("transformers-cli/serving")
-
-
-def serve_command_factory(args: Namespace):
- """
- Factory function used to instantiate serving server from provided command line arguments.
- :return: ServeCommand
- """
- nlp = pipeline(
- task=args.task,
- model=args.model if args.model else None,
- config=args.config,
- tokenizer=args.tokenizer,
- device=args.device,
- )
- return ServeCommand(nlp, args.host, args.port, args.workers)
-
-
-class ServeModelInfoResult(BaseModel):
- """
- Expose model information
- """
-
- infos: dict
-
-
-class ServeTokenizeResult(BaseModel):
- """
- Tokenize result model
- """
-
- tokens: List[str]
- tokens_ids: Optional[List[int]]
-
-
-class ServeDeTokenizeResult(BaseModel):
- """
- DeTokenize result model
- """
-
- text: str
-
-
-class ServeForwardResult(BaseModel):
- """
- Forward result model
- """
-
- output: Any
-
-
-class ServeCommand(BaseTransformersCLICommand):
- @staticmethod
- def register_subcommand(parser: ArgumentParser):
- """
- Register this command to argparse so it's available for the transformer-cli
- :param parser: Root parser to register command-specific arguments
- :return:
- """
- serve_parser = parser.add_parser(
- "serve", help="CLI tool to run inference requests through REST and GraphQL endpoints."
- )
- serve_parser.add_argument(
- "--task", type=str, choices=SUPPORTED_TASKS.keys(), help="The task to run the pipeline on"
- )
- serve_parser.add_argument("--host", type=str, default="localhost", help="Interface the server will listen on.")
- serve_parser.add_argument("--port", type=int, default=8888, help="Port the serving will listen to.")
- serve_parser.add_argument("--workers", type=int, default=1, help="Number of http workers")
- serve_parser.add_argument("--model", type=str, help="Model's name or path to stored model.")
- serve_parser.add_argument("--config", type=str, help="Model's config name or path to stored model.")
- serve_parser.add_argument("--tokenizer", type=str, help="Tokenizer name to use.")
- serve_parser.add_argument(
- "--device",
- type=int,
- default=-1,
- help="Indicate the device to run onto, -1 indicates CPU, >= 0 indicates GPU (default: -1)",
- )
- serve_parser.set_defaults(func=serve_command_factory)
-
- def __init__(self, pipeline: Pipeline, host: str, port: int, workers: int):
-
- self._pipeline = pipeline
-
- self.host = host
- self.port = port
- self.workers = workers
-
- if not _serve_dependencies_installed:
- raise RuntimeError(
- "Using serve command requires FastAPI and unicorn. "
- 'Please install transformers with [serving]: pip install "transformers[serving]".'
- "Or install FastAPI and unicorn separately."
- )
- else:
- logger.info("Serving model over {}:{}".format(host, port))
- self._app = FastAPI(
- routes=[
- APIRoute(
- "/",
- self.model_info,
- response_model=ServeModelInfoResult,
- response_class=JSONResponse,
- methods=["GET"],
- ),
- APIRoute(
- "/tokenize",
- self.tokenize,
- response_model=ServeTokenizeResult,
- response_class=JSONResponse,
- methods=["POST"],
- ),
- APIRoute(
- "/detokenize",
- self.detokenize,
- response_model=ServeDeTokenizeResult,
- response_class=JSONResponse,
- methods=["POST"],
- ),
- APIRoute(
- "/forward",
- self.forward,
- response_model=ServeForwardResult,
- response_class=JSONResponse,
- methods=["POST"],
- ),
- ],
- timeout=600,
- )
-
- def run(self):
- run(self._app, host=self.host, port=self.port, workers=self.workers)
-
- def model_info(self):
- return ServeModelInfoResult(infos=vars(self._pipeline.model.config))
-
- def tokenize(self, text_input: str = Body(None, embed=True), return_ids: bool = Body(False, embed=True)):
- """
- Tokenize the provided input and eventually returns corresponding tokens id:
- - **text_input**: String to tokenize
- - **return_ids**: Boolean flags indicating if the tokens have to be converted to their integer mapping.
- """
- try:
- tokens_txt = self._pipeline.tokenizer.tokenize(text_input)
-
- if return_ids:
- tokens_ids = self._pipeline.tokenizer.convert_tokens_to_ids(tokens_txt)
- return ServeTokenizeResult(tokens=tokens_txt, tokens_ids=tokens_ids)
- else:
- return ServeTokenizeResult(tokens=tokens_txt)
-
- except Exception as e:
- raise HTTPException(status_code=500, detail={"model": "", "error": str(e)})
-
- def detokenize(
- self,
- tokens_ids: List[int] = Body(None, embed=True),
- skip_special_tokens: bool = Body(False, embed=True),
- cleanup_tokenization_spaces: bool = Body(True, embed=True),
- ):
- """
- Detokenize the provided tokens ids to readable text:
- - **tokens_ids**: List of tokens ids
- - **skip_special_tokens**: Flag indicating to not try to decode special tokens
- - **cleanup_tokenization_spaces**: Flag indicating to remove all leading/trailing spaces and intermediate ones.
- """
- try:
- decoded_str = self._pipeline.tokenizer.decode(tokens_ids, skip_special_tokens, cleanup_tokenization_spaces)
- return ServeDeTokenizeResult(model="", text=decoded_str)
- except Exception as e:
- raise HTTPException(status_code=500, detail={"model": "", "error": str(e)})
-
- async def forward(self, inputs=Body(None, embed=True)):
- """
- **inputs**:
- **attention_mask**:
- **tokens_type_ids**:
- """
-
- # Check we don't have empty string
- if len(inputs) == 0:
- return ServeForwardResult(output=[], attention=[])
-
- try:
- # Forward through the model
- output = self._pipeline(inputs)
- return ServeForwardResult(output=output)
- except Exception as e:
- raise HTTPException(500, {"error": str(e)})
diff --git a/server/transformers/src/transformers/commands/train.py b/server/transformers/src/transformers/commands/train.py
deleted file mode 100644
index afa035c9401d57221c02a4dd87069488c9435184..0000000000000000000000000000000000000000
--- a/server/transformers/src/transformers/commands/train.py
+++ /dev/null
@@ -1,144 +0,0 @@
-import os
-from argparse import ArgumentParser, Namespace
-from logging import getLogger
-
-from transformers import SingleSentenceClassificationProcessor as Processor
-from transformers import TextClassificationPipeline, is_tf_available, is_torch_available
-from transformers.commands import BaseTransformersCLICommand
-
-
-if not is_tf_available() and not is_torch_available():
- raise RuntimeError("At least one of PyTorch or TensorFlow 2.0+ should be installed to use CLI training")
-
-# TF training parameters
-USE_XLA = False
-USE_AMP = False
-
-
-def train_command_factory(args: Namespace):
- """
- Factory function used to instantiate serving server from provided command line arguments.
- :return: ServeCommand
- """
- return TrainCommand(args)
-
-
-class TrainCommand(BaseTransformersCLICommand):
- @staticmethod
- def register_subcommand(parser: ArgumentParser):
- """
- Register this command to argparse so it's available for the transformer-cli
- :param parser: Root parser to register command-specific arguments
- :return:
- """
- train_parser = parser.add_parser("train", help="CLI tool to train a model on a task.")
-
- train_parser.add_argument(
- "--train_data",
- type=str,
- required=True,
- help="path to train (and optionally evaluation) dataset as a csv with "
- "tab separated labels and sentences.",
- )
- train_parser.add_argument(
- "--column_label", type=int, default=0, help="Column of the dataset csv file with example labels."
- )
- train_parser.add_argument(
- "--column_text", type=int, default=1, help="Column of the dataset csv file with example texts."
- )
- train_parser.add_argument(
- "--column_id", type=int, default=2, help="Column of the dataset csv file with example ids."
- )
- train_parser.add_argument(
- "--skip_first_row", action="store_true", help="Skip the first row of the csv file (headers)."
- )
-
- train_parser.add_argument("--validation_data", type=str, default="", help="path to validation dataset.")
- train_parser.add_argument(
- "--validation_split",
- type=float,
- default=0.1,
- help="if validation dataset is not provided, fraction of train dataset " "to use as validation dataset.",
- )
-
- train_parser.add_argument("--output", type=str, default="./", help="path to saved the trained model.")
-
- train_parser.add_argument(
- "--task", type=str, default="text_classification", help="Task to train the model on."
- )
- train_parser.add_argument(
- "--model", type=str, default="bert-base-uncased", help="Model's name or path to stored model."
- )
- train_parser.add_argument("--train_batch_size", type=int, default=32, help="Batch size for training.")
- train_parser.add_argument("--valid_batch_size", type=int, default=64, help="Batch size for validation.")
- train_parser.add_argument("--learning_rate", type=float, default=3e-5, help="Learning rate.")
- train_parser.add_argument("--adam_epsilon", type=float, default=1e-08, help="Epsilon for Adam optimizer.")
- train_parser.set_defaults(func=train_command_factory)
-
- def __init__(self, args: Namespace):
- self.logger = getLogger("transformers-cli/training")
-
- self.framework = "tf" if is_tf_available() else "torch"
-
- os.makedirs(args.output, exist_ok=True)
- assert os.path.isdir(args.output)
- self.output = args.output
-
- self.column_label = args.column_label
- self.column_text = args.column_text
- self.column_id = args.column_id
-
- self.logger.info("Loading {} pipeline for {}".format(args.task, args.model))
- if args.task == "text_classification":
- self.pipeline = TextClassificationPipeline.from_pretrained(args.model)
- elif args.task == "token_classification":
- raise NotImplementedError
- elif args.task == "question_answering":
- raise NotImplementedError
-
- self.logger.info("Loading dataset from {}".format(args.train_data))
- self.train_dataset = Processor.create_from_csv(
- args.train_data,
- column_label=args.column_label,
- column_text=args.column_text,
- column_id=args.column_id,
- skip_first_row=args.skip_first_row,
- )
- self.valid_dataset = None
- if args.validation_data:
- self.logger.info("Loading validation dataset from {}".format(args.validation_data))
- self.valid_dataset = Processor.create_from_csv(
- args.validation_data,
- column_label=args.column_label,
- column_text=args.column_text,
- column_id=args.column_id,
- skip_first_row=args.skip_first_row,
- )
-
- self.validation_split = args.validation_split
- self.train_batch_size = args.train_batch_size
- self.valid_batch_size = args.valid_batch_size
- self.learning_rate = args.learning_rate
- self.adam_epsilon = args.adam_epsilon
-
- def run(self):
- if self.framework == "tf":
- return self.run_tf()
- return self.run_torch()
-
- def run_torch(self):
- raise NotImplementedError
-
- def run_tf(self):
- self.pipeline.fit(
- self.train_dataset,
- validation_data=self.valid_dataset,
- validation_split=self.validation_split,
- learning_rate=self.learning_rate,
- adam_epsilon=self.adam_epsilon,
- train_batch_size=self.train_batch_size,
- valid_batch_size=self.valid_batch_size,
- )
-
- # Save trained pipeline
- self.pipeline.save_pretrained(self.output)
diff --git a/server/transformers/src/transformers/commands/user.py b/server/transformers/src/transformers/commands/user.py
deleted file mode 100644
index 47c7860114b34b3996dfc1f11fc19b384d3bf8c9..0000000000000000000000000000000000000000
--- a/server/transformers/src/transformers/commands/user.py
+++ /dev/null
@@ -1,209 +0,0 @@
-import os
-import sys
-from argparse import ArgumentParser
-from getpass import getpass
-from typing import List, Union
-
-from requests.exceptions import HTTPError
-
-from transformers.commands import BaseTransformersCLICommand
-from transformers.hf_api import HfApi, HfFolder
-
-
-UPLOAD_MAX_FILES = 15
-
-
-class UserCommands(BaseTransformersCLICommand):
- @staticmethod
- def register_subcommand(parser: ArgumentParser):
- login_parser = parser.add_parser("login", help="Log in using the same credentials as on huggingface.co")
- login_parser.set_defaults(func=lambda args: LoginCommand(args))
- whoami_parser = parser.add_parser("whoami", help="Find out which huggingface.co account you are logged in as.")
- whoami_parser.set_defaults(func=lambda args: WhoamiCommand(args))
- logout_parser = parser.add_parser("logout", help="Log out")
- logout_parser.set_defaults(func=lambda args: LogoutCommand(args))
- # s3
- s3_parser = parser.add_parser("s3", help="{ls, rm} Commands to interact with the files you upload on S3.")
- s3_subparsers = s3_parser.add_subparsers(help="s3 related commands")
- ls_parser = s3_subparsers.add_parser("ls")
- ls_parser.set_defaults(func=lambda args: ListObjsCommand(args))
- rm_parser = s3_subparsers.add_parser("rm")
- rm_parser.add_argument("filename", type=str, help="individual object filename to delete from S3.")
- rm_parser.set_defaults(func=lambda args: DeleteObjCommand(args))
- # upload
- upload_parser = parser.add_parser("upload")
- upload_parser.add_argument("path", type=str, help="Local path of the folder or individual file to upload.")
- upload_parser.add_argument(
- "--filename", type=str, default=None, help="Optional: override individual object filename on S3."
- )
- upload_parser.set_defaults(func=lambda args: UploadCommand(args))
-
-
-class ANSI:
- """
- Helper for en.wikipedia.org/wiki/ANSI_escape_code
- """
-
- _bold = "\u001b[1m"
- _reset = "\u001b[0m"
-
- @classmethod
- def bold(cls, s):
- return "{}{}{}".format(cls._bold, s, cls._reset)
-
-
-class BaseUserCommand:
- def __init__(self, args):
- self.args = args
- self._api = HfApi()
-
-
-class LoginCommand(BaseUserCommand):
- def run(self):
- print(
- """
- _| _| _| _| _|_|_| _|_|_| _|_|_| _| _| _|_|_| _|_|_|_| _|_| _|_|_| _|_|_|_|
- _| _| _| _| _| _| _| _|_| _| _| _| _| _| _| _|
- _|_|_|_| _| _| _| _|_| _| _|_| _| _| _| _| _| _|_| _|_|_| _|_|_|_| _| _|_|_|
- _| _| _| _| _| _| _| _| _| _| _|_| _| _| _| _| _| _| _|
- _| _| _|_| _|_|_| _|_|_| _|_|_| _| _| _|_|_| _| _| _| _|_|_| _|_|_|_|
-
- """
- )
- username = input("Username: ")
- password = getpass()
- try:
- token = self._api.login(username, password)
- except HTTPError as e:
- # probably invalid credentials, display error message.
- print(e)
- exit(1)
- HfFolder.save_token(token)
- print("Login successful")
- print("Your token:", token, "\n")
- print("Your token has been saved to", HfFolder.path_token)
-
-
-class WhoamiCommand(BaseUserCommand):
- def run(self):
- token = HfFolder.get_token()
- if token is None:
- print("Not logged in")
- exit()
- try:
- user = self._api.whoami(token)
- print(user)
- except HTTPError as e:
- print(e)
-
-
-class LogoutCommand(BaseUserCommand):
- def run(self):
- token = HfFolder.get_token()
- if token is None:
- print("Not logged in")
- exit()
- HfFolder.delete_token()
- self._api.logout(token)
- print("Successfully logged out.")
-
-
-class ListObjsCommand(BaseUserCommand):
- def tabulate(self, rows: List[List[Union[str, int]]], headers: List[str]) -> str:
- """
- Inspired by:
- stackoverflow.com/a/8356620/593036
- stackoverflow.com/questions/9535954/printing-lists-as-tabular-data
- """
- col_widths = [max(len(str(x)) for x in col) for col in zip(*rows, headers)]
- row_format = ("{{:{}}} " * len(headers)).format(*col_widths)
- lines = []
- lines.append(row_format.format(*headers))
- lines.append(row_format.format(*["-" * w for w in col_widths]))
- for row in rows:
- lines.append(row_format.format(*row))
- return "\n".join(lines)
-
- def run(self):
- token = HfFolder.get_token()
- if token is None:
- print("Not logged in")
- exit(1)
- try:
- objs = self._api.list_objs(token)
- except HTTPError as e:
- print(e)
- exit(1)
- if len(objs) == 0:
- print("No shared file yet")
- exit()
- rows = [[obj.filename, obj.LastModified, obj.ETag, obj.Size] for obj in objs]
- print(self.tabulate(rows, headers=["Filename", "LastModified", "ETag", "Size"]))
-
-
-class DeleteObjCommand(BaseUserCommand):
- def run(self):
- token = HfFolder.get_token()
- if token is None:
- print("Not logged in")
- exit(1)
- try:
- self._api.delete_obj(token, filename=self.args.filename)
- except HTTPError as e:
- print(e)
- exit(1)
- print("Done")
-
-
-class UploadCommand(BaseUserCommand):
- def walk_dir(self, rel_path):
- """
- Recursively list all files in a folder.
- """
- entries: List[os.DirEntry] = list(os.scandir(rel_path))
- files = [(os.path.join(os.getcwd(), f.path), f.path) for f in entries if f.is_file()] # (filepath, filename)
- for f in entries:
- if f.is_dir():
- files += self.walk_dir(f.path)
- return files
-
- def run(self):
- token = HfFolder.get_token()
- if token is None:
- print("Not logged in")
- exit(1)
- local_path = os.path.abspath(self.args.path)
- if os.path.isdir(local_path):
- if self.args.filename is not None:
- raise ValueError("Cannot specify a filename override when uploading a folder.")
- rel_path = os.path.basename(local_path)
- files = self.walk_dir(rel_path)
- elif os.path.isfile(local_path):
- filename = self.args.filename if self.args.filename is not None else os.path.basename(local_path)
- files = [(local_path, filename)]
- else:
- raise ValueError("Not a valid file or directory: {}".format(local_path))
-
- if sys.platform == "win32":
- files = [(filepath, filename.replace(os.sep, "/")) for filepath, filename in files]
-
- if len(files) > UPLOAD_MAX_FILES:
- print(
- "About to upload {} files to S3. This is probably wrong. Please filter files before uploading.".format(
- ANSI.bold(len(files))
- )
- )
- exit(1)
-
- for filepath, filename in files:
- print("About to upload file {} to S3 under filename {}".format(ANSI.bold(filepath), ANSI.bold(filename)))
-
- choice = input("Proceed? [Y/n] ").lower()
- if not (choice == "" or choice == "y" or choice == "yes"):
- print("Abort")
- exit()
- print(ANSI.bold("Uploading... This might take a while if files are large"))
- for filepath, filename in files:
- access_url = self._api.presign_and_upload(token=token, filename=filename, filepath=filepath)
- print("Your file now lives at:")
- print(access_url)
diff --git a/server/transformers/src/transformers/configuration_albert.py b/server/transformers/src/transformers/configuration_albert.py
deleted file mode 100644
index 3419753cb1ff1065978b5eead21ce10e64706a1d..0000000000000000000000000000000000000000
--- a/server/transformers/src/transformers/configuration_albert.py
+++ /dev/null
@@ -1,146 +0,0 @@
-# coding=utf-8
-# Copyright 2018 The Google AI Language Team Authors and The HuggingFace Inc. team.
-# Copyright (c) 2018, NVIDIA CORPORATION. All rights reserved.
-#
-# Licensed under the Apache License, Version 2.0 (the "License");
-# you may not use this file except in compliance with the License.
-# You may obtain a copy of the License at
-#
-# http://www.apache.org/licenses/LICENSE-2.0
-#
-# Unless required by applicable law or agreed to in writing, software
-# distributed under the License is distributed on an "AS IS" BASIS,
-# WITHOUT WARRANTIES OR CONDITIONS OF ANY KIND, either express or implied.
-# See the License for the specific language governing permissions and
-# limitations under the License.
-""" ALBERT model configuration """
-
-from .configuration_utils import PretrainedConfig
-
-
-ALBERT_PRETRAINED_CONFIG_ARCHIVE_MAP = {
- "albert-base-v1": "https://s3.amazonaws.com/models.huggingface.co/bert/albert-base-config.json",
- "albert-large-v1": "https://s3.amazonaws.com/models.huggingface.co/bert/albert-large-config.json",
- "albert-xlarge-v1": "https://s3.amazonaws.com/models.huggingface.co/bert/albert-xlarge-config.json",
- "albert-xxlarge-v1": "https://s3.amazonaws.com/models.huggingface.co/bert/albert-xxlarge-config.json",
- "albert-base-v2": "https://s3.amazonaws.com/models.huggingface.co/bert/albert-base-v2-config.json",
- "albert-large-v2": "https://s3.amazonaws.com/models.huggingface.co/bert/albert-large-v2-config.json",
- "albert-xlarge-v2": "https://s3.amazonaws.com/models.huggingface.co/bert/albert-xlarge-v2-config.json",
- "albert-xxlarge-v2": "https://s3.amazonaws.com/models.huggingface.co/bert/albert-xxlarge-v2-config.json",
-}
-
-
-class AlbertConfig(PretrainedConfig):
- r"""
- This is the configuration class to store the configuration of an :class:`~transformers.AlbertModel`.
- It is used to instantiate an ALBERT model according to the specified arguments, defining the model
- architecture. Instantiating a configuration with the defaults will yield a similar configuration to that of
- the ALBERT `xxlarge `__ architecture.
-
- Configuration objects inherit from :class:`~transformers.PretrainedConfig` and can be used
- to control the model outputs. Read the documentation from :class:`~transformers.PretrainedConfig`
- for more information.
-
-
- Args:
- vocab_size (:obj:`int`, optional, defaults to 30000):
- Vocabulary size of the ALBERT model. Defines the different tokens that
- can be represented by the `inputs_ids` passed to the forward method of :class:`~transformers.AlbertModel`.
- embedding_size (:obj:`int`, optional, defaults to 128):
- Dimensionality of vocabulary embeddings.
- hidden_size (:obj:`int`, optional, defaults to 4096):
- Dimensionality of the encoder layers and the pooler layer.
- num_hidden_layers (:obj:`int`, optional, defaults to 12):
- Number of hidden layers in the Transformer encoder.
- num_hidden_groups (:obj:`int`, optional, defaults to 1):
- Number of groups for the hidden layers, parameters in the same group are shared.
- num_attention_heads (:obj:`int`, optional, defaults to 64):
- Number of attention heads for each attention layer in the Transformer encoder.
- intermediate_size (:obj:`int`, optional, defaults to 16384):
- The dimensionality of the "intermediate" (i.e., feed-forward) layer in the Transformer encoder.
- inner_group_num (:obj:`int`, optional, defaults to 1):
- The number of inner repetition of attention and ffn.
- hidden_act (:obj:`str` or :obj:`function`, optional, defaults to "gelu_new"):
- The non-linear activation function (function or string) in the encoder and pooler.
- If string, "gelu", "relu", "swish" and "gelu_new" are supported.
- hidden_dropout_prob (:obj:`float`, optional, defaults to 0):
- The dropout probability for all fully connected layers in the embeddings, encoder, and pooler.
- attention_probs_dropout_prob (:obj:`float`, optional, defaults to 0):
- The dropout ratio for the attention probabilities.
- max_position_embeddings (:obj:`int`, optional, defaults to 512):
- The maximum sequence length that this model might ever be used with. Typically set this to something
- large (e.g., 512 or 1024 or 2048).
- type_vocab_size (:obj:`int`, optional, defaults to 2):
- The vocabulary size of the `token_type_ids` passed into :class:`~transformers.AlbertModel`.
- initializer_range (:obj:`float`, optional, defaults to 0.02):
- The standard deviation of the truncated_normal_initializer for initializing all weight matrices.
- layer_norm_eps (:obj:`float`, optional, defaults to 1e-12):
- The epsilon used by the layer normalization layers.
- classifier_dropout_prob (:obj:`float`, optional, defaults to 0.1):
- The dropout ratio for attached classifiers.
-
- Example::
-
- from transformers import AlbertConfig, AlbertModel
- # Initializing an ALBERT-xxlarge style configuration
- albert_xxlarge_configuration = AlbertConfig()
-
- # Initializing an ALBERT-base style configuration
- albert_base_configuration = AlbertConfig(
- hidden_size=768,
- num_attention_heads=12,
- intermediate_size=3072,
- )
-
- # Initializing a model from the ALBERT-base style configuration
- model = AlbertModel(albert_xxlarge_configuration)
-
- # Accessing the model configuration
- configuration = model.config
-
- Attributes:
- pretrained_config_archive_map (Dict[str, str]):
- A dictionary containing all the available pre-trained checkpoints.
- """
-
- pretrained_config_archive_map = ALBERT_PRETRAINED_CONFIG_ARCHIVE_MAP
- model_type = "albert"
-
- def __init__(
- self,
- vocab_size=30000,
- embedding_size=128,
- hidden_size=4096,
- num_hidden_layers=12,
- num_hidden_groups=1,
- num_attention_heads=64,
- intermediate_size=16384,
- inner_group_num=1,
- hidden_act="gelu_new",
- hidden_dropout_prob=0,
- attention_probs_dropout_prob=0,
- max_position_embeddings=512,
- type_vocab_size=2,
- initializer_range=0.02,
- layer_norm_eps=1e-12,
- classifier_dropout_prob=0.1,
- **kwargs
- ):
- super().__init__(**kwargs)
-
- self.vocab_size = vocab_size
- self.embedding_size = embedding_size
- self.hidden_size = hidden_size
- self.num_hidden_layers = num_hidden_layers
- self.num_hidden_groups = num_hidden_groups
- self.num_attention_heads = num_attention_heads
- self.inner_group_num = inner_group_num
- self.hidden_act = hidden_act
- self.intermediate_size = intermediate_size
- self.hidden_dropout_prob = hidden_dropout_prob
- self.attention_probs_dropout_prob = attention_probs_dropout_prob
- self.max_position_embeddings = max_position_embeddings
- self.type_vocab_size = type_vocab_size
- self.initializer_range = initializer_range
- self.layer_norm_eps = layer_norm_eps
- self.classifier_dropout_prob = classifier_dropout_prob
diff --git a/server/transformers/src/transformers/configuration_auto.py b/server/transformers/src/transformers/configuration_auto.py
deleted file mode 100644
index 4fd23fee26019594b9636ea5ed8ba7804d3ace95..0000000000000000000000000000000000000000
--- a/server/transformers/src/transformers/configuration_auto.py
+++ /dev/null
@@ -1,196 +0,0 @@
-# coding=utf-8
-# Copyright 2018 The HuggingFace Inc. team.
-#
-# Licensed under the Apache License, Version 2.0 (the "License");
-# you may not use this file except in compliance with the License.
-# You may obtain a copy of the License at
-#
-# http://www.apache.org/licenses/LICENSE-2.0
-#
-# Unless required by applicable law or agreed to in writing, software
-# distributed under the License is distributed on an "AS IS" BASIS,
-# WITHOUT WARRANTIES OR CONDITIONS OF ANY KIND, either express or implied.
-# See the License for the specific language governing permissions and
-# limitations under the License.
-""" Auto Config class. """
-
-
-import logging
-from collections import OrderedDict
-
-from .configuration_albert import ALBERT_PRETRAINED_CONFIG_ARCHIVE_MAP, AlbertConfig
-from .configuration_bert import BERT_PRETRAINED_CONFIG_ARCHIVE_MAP, BertConfig
-from .configuration_camembert import CAMEMBERT_PRETRAINED_CONFIG_ARCHIVE_MAP, CamembertConfig
-from .configuration_ctrl import CTRL_PRETRAINED_CONFIG_ARCHIVE_MAP, CTRLConfig
-from .configuration_distilbert import DISTILBERT_PRETRAINED_CONFIG_ARCHIVE_MAP, DistilBertConfig
-from .configuration_flaubert import FLAUBERT_PRETRAINED_CONFIG_ARCHIVE_MAP, FlaubertConfig
-from .configuration_gpt2 import GPT2_PRETRAINED_CONFIG_ARCHIVE_MAP, GPT2Config
-from .configuration_openai import OPENAI_GPT_PRETRAINED_CONFIG_ARCHIVE_MAP, OpenAIGPTConfig
-from .configuration_roberta import ROBERTA_PRETRAINED_CONFIG_ARCHIVE_MAP, RobertaConfig
-from .configuration_t5 import T5_PRETRAINED_CONFIG_ARCHIVE_MAP, T5Config
-from .configuration_transfo_xl import TRANSFO_XL_PRETRAINED_CONFIG_ARCHIVE_MAP, TransfoXLConfig
-from .configuration_utils import PretrainedConfig
-from .configuration_xlm import XLM_PRETRAINED_CONFIG_ARCHIVE_MAP, XLMConfig
-from .configuration_xlm_roberta import XLM_ROBERTA_PRETRAINED_CONFIG_ARCHIVE_MAP, XLMRobertaConfig
-from .configuration_xlnet import XLNET_PRETRAINED_CONFIG_ARCHIVE_MAP, XLNetConfig
-
-
-logger = logging.getLogger(__name__)
-
-
-ALL_PRETRAINED_CONFIG_ARCHIVE_MAP = dict(
- (key, value)
- for pretrained_map in [
- BERT_PRETRAINED_CONFIG_ARCHIVE_MAP,
- OPENAI_GPT_PRETRAINED_CONFIG_ARCHIVE_MAP,
- TRANSFO_XL_PRETRAINED_CONFIG_ARCHIVE_MAP,
- GPT2_PRETRAINED_CONFIG_ARCHIVE_MAP,
- CTRL_PRETRAINED_CONFIG_ARCHIVE_MAP,
- XLNET_PRETRAINED_CONFIG_ARCHIVE_MAP,
- XLM_PRETRAINED_CONFIG_ARCHIVE_MAP,
- ROBERTA_PRETRAINED_CONFIG_ARCHIVE_MAP,
- DISTILBERT_PRETRAINED_CONFIG_ARCHIVE_MAP,
- ALBERT_PRETRAINED_CONFIG_ARCHIVE_MAP,
- CAMEMBERT_PRETRAINED_CONFIG_ARCHIVE_MAP,
- T5_PRETRAINED_CONFIG_ARCHIVE_MAP,
- XLM_ROBERTA_PRETRAINED_CONFIG_ARCHIVE_MAP,
- FLAUBERT_PRETRAINED_CONFIG_ARCHIVE_MAP,
- ]
- for key, value, in pretrained_map.items()
-)
-
-
-CONFIG_MAPPING = OrderedDict(
- [
- ("t5", T5Config,),
- ("distilbert", DistilBertConfig,),
- ("albert", AlbertConfig,),
- ("camembert", CamembertConfig,),
- ("xlm-roberta", XLMRobertaConfig,),
- ("roberta", RobertaConfig,),
- ("flaubert", FlaubertConfig,),
- ("bert", BertConfig,),
- ("openai-gpt", OpenAIGPTConfig,),
- ("gpt2", GPT2Config,),
- ("transfo-xl", TransfoXLConfig,),
- ("xlnet", XLNetConfig,),
- ("xlm", XLMConfig,),
- ("ctrl", CTRLConfig,),
- ]
-)
-
-
-class AutoConfig:
- r"""
- :class:`~transformers.AutoConfig` is a generic configuration class
- that will be instantiated as one of the configuration classes of the library
- when created with the :func:`~transformers.AutoConfig.from_pretrained` class method.
-
- The :func:`~transformers.AutoConfig.from_pretrained` method takes care of returning the correct model class instance
- based on the `model_type` property of the config object, or when it's missing,
- falling back to using pattern matching on the `pretrained_model_name_or_path` string.
- """
-
- def __init__(self):
- raise EnvironmentError(
- "AutoConfig is designed to be instantiated "
- "using the `AutoConfig.from_pretrained(pretrained_model_name_or_path)` method."
- )
-
- @classmethod
- def for_model(cls, model_type, *args, **kwargs):
- for pattern, config_class in CONFIG_MAPPING.items():
- if pattern in model_type:
- return config_class(*args, **kwargs)
- raise ValueError(
- "Unrecognized model identifier in {}. Should contain one of {}".format(
- model_type, ", ".join(CONFIG_MAPPING.keys())
- )
- )
-
- @classmethod
- def from_pretrained(cls, pretrained_model_name_or_path, **kwargs):
- r""" Instantiates one of the configuration classes of the library
- from a pre-trained model configuration.
-
- The configuration class to instantiate is selected
- based on the `model_type` property of the config object, or when it's missing,
- falling back to using pattern matching on the `pretrained_model_name_or_path` string.
- - contains `t5`: :class:`~transformers.T5Config` (T5 model)
- - contains `distilbert`: :class:`~transformers.DistilBertConfig` (DistilBERT model)
- - contains `albert`: :class:`~transformers.AlbertConfig` (ALBERT model)
- - contains `camembert`: :class:`~transformers.CamembertConfig` (CamemBERT model)
- - contains `xlm-roberta`: :class:`~transformers.XLMRobertaConfig` (XLM-RoBERTa model)
- - contains `roberta`: :class:`~transformers.RobertaConfig` (RoBERTa model)
- - contains `bert`: :class:`~transformers.BertConfig` (Bert model)
- - contains `openai-gpt`: :class:`~transformers.OpenAIGPTConfig` (OpenAI GPT model)
- - contains `gpt2`: :class:`~transformers.GPT2Config` (OpenAI GPT-2 model)
- - contains `transfo-xl`: :class:`~transformers.TransfoXLConfig` (Transformer-XL model)
- - contains `xlnet`: :class:`~transformers.XLNetConfig` (XLNet model)
- - contains `xlm`: :class:`~transformers.XLMConfig` (XLM model)
- - contains `ctrl` : :class:`~transformers.CTRLConfig` (CTRL model)
- - contains `flaubert` : :class:`~transformers.FlaubertConfig` (Flaubert model)
-
-
- Args:
- pretrained_model_name_or_path (:obj:`string`):
- Is either: \
- - a string with the `shortcut name` of a pre-trained model configuration to load from cache or download, e.g.: ``bert-base-uncased``.
- - a string with the `identifier name` of a pre-trained model configuration that was user-uploaded to our S3, e.g.: ``dbmdz/bert-base-german-cased``.
- - a path to a `directory` containing a configuration file saved using the :func:`~transformers.PretrainedConfig.save_pretrained` method, e.g.: ``./my_model_directory/``.
- - a path or url to a saved configuration JSON `file`, e.g.: ``./my_model_directory/configuration.json``.
-
- cache_dir (:obj:`string`, optional, defaults to `None`):
- Path to a directory in which a downloaded pre-trained model
- configuration should be cached if the standard cache should not be used.
-
- force_download (:obj:`boolean`, optional, defaults to `False`):
- Force to (re-)download the model weights and configuration files and override the cached versions if they exist.
-
- resume_download (:obj:`boolean`, optional, defaults to `False`):
- Do not delete incompletely received file. Attempt to resume the download if such a file exists.
-
- proxies (:obj:`Dict[str, str]`, optional, defaults to `None`):
- A dictionary of proxy servers to use by protocol or endpoint, e.g.: :obj:`{'http': 'foo.bar:3128', 'http://hostname': 'foo.bar:4012'}`.
- The proxies are used on each request. See `the requests documentation `__ for usage.
-
- return_unused_kwargs (:obj:`boolean`, optional, defaults to `False`):
- - If False, then this function returns just the final configuration object.
- - If True, then this functions returns a tuple `(config, unused_kwargs)` where `unused_kwargs` is a dictionary consisting of the key/value pairs whose keys are not configuration attributes: ie the part of kwargs which has not been used to update `config` and is otherwise ignored.
-
- kwargs (:obj:`Dict[str, any]`, optional, defaults to `{}`): key/value pairs with which to update the configuration object after loading.
- - The values in kwargs of any keys which are configuration attributes will be used to override the loaded values.
- - Behavior concerning key/value pairs whose keys are *not* configuration attributes is controlled by the `return_unused_kwargs` keyword parameter.
-
-
- Examples::
-
- config = AutoConfig.from_pretrained('bert-base-uncased') # Download configuration from S3 and cache.
- config = AutoConfig.from_pretrained('./test/bert_saved_model/') # E.g. config (or model) was saved using `save_pretrained('./test/saved_model/')`
- config = AutoConfig.from_pretrained('./test/bert_saved_model/my_configuration.json')
- config = AutoConfig.from_pretrained('bert-base-uncased', output_attention=True, foo=False)
- assert config.output_attention == True
- config, unused_kwargs = AutoConfig.from_pretrained('bert-base-uncased', output_attention=True,
- foo=False, return_unused_kwargs=True)
- assert config.output_attention == True
- assert unused_kwargs == {'foo': False}
-
- """
- config_dict, _ = PretrainedConfig.get_config_dict(
- pretrained_model_name_or_path, pretrained_config_archive_map=ALL_PRETRAINED_CONFIG_ARCHIVE_MAP, **kwargs
- )
-
- if "model_type" in config_dict:
- config_class = CONFIG_MAPPING[config_dict["model_type"]]
- return config_class.from_dict(config_dict, **kwargs)
- else:
- # Fallback: use pattern matching on the string.
- for pattern, config_class in CONFIG_MAPPING.items():
- if pattern in pretrained_model_name_or_path:
- return config_class.from_dict(config_dict, **kwargs)
-
- raise ValueError(
- "Unrecognized model in {}. "
- "Should have a `model_type` key in its config.json, or contain one of the following strings "
- "in its name: {}".format(pretrained_model_name_or_path, ", ".join(CONFIG_MAPPING.keys()))
- )
diff --git a/server/transformers/src/transformers/configuration_bert.py b/server/transformers/src/transformers/configuration_bert.py
deleted file mode 100644
index d668d04cb8ee19207f9ec7c6695365503a477b87..0000000000000000000000000000000000000000
--- a/server/transformers/src/transformers/configuration_bert.py
+++ /dev/null
@@ -1,142 +0,0 @@
-# coding=utf-8
-# Copyright 2018 The Google AI Language Team Authors and The HuggingFace Inc. team.
-# Copyright (c) 2018, NVIDIA CORPORATION. All rights reserved.
-#
-# Licensed under the Apache License, Version 2.0 (the "License");
-# you may not use this file except in compliance with the License.
-# You may obtain a copy of the License at
-#
-# http://www.apache.org/licenses/LICENSE-2.0
-#
-# Unless required by applicable law or agreed to in writing, software
-# distributed under the License is distributed on an "AS IS" BASIS,
-# WITHOUT WARRANTIES OR CONDITIONS OF ANY KIND, either express or implied.
-# See the License for the specific language governing permissions and
-# limitations under the License.
-""" BERT model configuration """
-
-
-import logging
-
-from .configuration_utils import PretrainedConfig
-
-
-logger = logging.getLogger(__name__)
-
-BERT_PRETRAINED_CONFIG_ARCHIVE_MAP = {
- "bert-base-uncased": "https://s3.amazonaws.com/models.huggingface.co/bert/bert-base-uncased-config.json",
- "bert-large-uncased": "https://s3.amazonaws.com/models.huggingface.co/bert/bert-large-uncased-config.json",
- "bert-base-cased": "https://s3.amazonaws.com/models.huggingface.co/bert/bert-base-cased-config.json",
- "bert-large-cased": "https://s3.amazonaws.com/models.huggingface.co/bert/bert-large-cased-config.json",
- "bert-base-multilingual-uncased": "https://s3.amazonaws.com/models.huggingface.co/bert/bert-base-multilingual-uncased-config.json",
- "bert-base-multilingual-cased": "https://s3.amazonaws.com/models.huggingface.co/bert/bert-base-multilingual-cased-config.json",
- "bert-base-chinese": "https://s3.amazonaws.com/models.huggingface.co/bert/bert-base-chinese-config.json",
- "bert-base-german-cased": "https://s3.amazonaws.com/models.huggingface.co/bert/bert-base-german-cased-config.json",
- "bert-large-uncased-whole-word-masking": "https://s3.amazonaws.com/models.huggingface.co/bert/bert-large-uncased-whole-word-masking-config.json",
- "bert-large-cased-whole-word-masking": "https://s3.amazonaws.com/models.huggingface.co/bert/bert-large-cased-whole-word-masking-config.json",
- "bert-large-uncased-whole-word-masking-finetuned-squad": "https://s3.amazonaws.com/models.huggingface.co/bert/bert-large-uncased-whole-word-masking-finetuned-squad-config.json",
- "bert-large-cased-whole-word-masking-finetuned-squad": "https://s3.amazonaws.com/models.huggingface.co/bert/bert-large-cased-whole-word-masking-finetuned-squad-config.json",
- "bert-base-cased-finetuned-mrpc": "https://s3.amazonaws.com/models.huggingface.co/bert/bert-base-cased-finetuned-mrpc-config.json",
- "bert-base-german-dbmdz-cased": "https://s3.amazonaws.com/models.huggingface.co/bert/bert-base-german-dbmdz-cased-config.json",
- "bert-base-german-dbmdz-uncased": "https://s3.amazonaws.com/models.huggingface.co/bert/bert-base-german-dbmdz-uncased-config.json",
- "bert-base-japanese": "https://s3.amazonaws.com/models.huggingface.co/bert/cl-tohoku/bert-base-japanese-config.json",
- "bert-base-japanese-whole-word-masking": "https://s3.amazonaws.com/models.huggingface.co/bert/cl-tohoku/bert-base-japanese-whole-word-masking-config.json",
- "bert-base-japanese-char": "https://s3.amazonaws.com/models.huggingface.co/bert/cl-tohoku/bert-base-japanese-char-config.json",
- "bert-base-japanese-char-whole-word-masking": "https://s3.amazonaws.com/models.huggingface.co/bert/cl-tohoku/bert-base-japanese-char-whole-word-masking-config.json",
- "bert-base-finnish-cased-v1": "https://s3.amazonaws.com/models.huggingface.co/bert/TurkuNLP/bert-base-finnish-cased-v1/config.json",
- "bert-base-finnish-uncased-v1": "https://s3.amazonaws.com/models.huggingface.co/bert/TurkuNLP/bert-base-finnish-uncased-v1/config.json",
- "bert-base-dutch-cased": "https://s3.amazonaws.com/models.huggingface.co/bert/wietsedv/bert-base-dutch-cased/config.json",
-}
-
-
-class BertConfig(PretrainedConfig):
- r"""
- This is the configuration class to store the configuration of a :class:`~transformers.BertModel`.
- It is used to instantiate an BERT model according to the specified arguments, defining the model
- architecture. Instantiating a configuration with the defaults will yield a similar configuration to that of
- the BERT `bert-base-uncased `__ architecture.
-
- Configuration objects inherit from :class:`~transformers.PretrainedConfig` and can be used
- to control the model outputs. Read the documentation from :class:`~transformers.PretrainedConfig`
- for more information.
-
-
- Args:
- vocab_size (:obj:`int`, optional, defaults to 30522):
- Vocabulary size of the BERT model. Defines the different tokens that
- can be represented by the `inputs_ids` passed to the forward method of :class:`~transformers.BertModel`.
- hidden_size (:obj:`int`, optional, defaults to 768):
- Dimensionality of the encoder layers and the pooler layer.
- num_hidden_layers (:obj:`int`, optional, defaults to 12):
- Number of hidden layers in the Transformer encoder.
- num_attention_heads (:obj:`int`, optional, defaults to 12):
- Number of attention heads for each attention layer in the Transformer encoder.
- intermediate_size (:obj:`int`, optional, defaults to 3072):
- Dimensionality of the "intermediate" (i.e., feed-forward) layer in the Transformer encoder.
- hidden_act (:obj:`str` or :obj:`function`, optional, defaults to "gelu"):
- The non-linear activation function (function or string) in the encoder and pooler.
- If string, "gelu", "relu", "swish" and "gelu_new" are supported.
- hidden_dropout_prob (:obj:`float`, optional, defaults to 0.1):
- The dropout probabilitiy for all fully connected layers in the embeddings, encoder, and pooler.
- attention_probs_dropout_prob (:obj:`float`, optional, defaults to 0.1):
- The dropout ratio for the attention probabilities.
- max_position_embeddings (:obj:`int`, optional, defaults to 512):
- The maximum sequence length that this model might ever be used with.
- Typically set this to something large just in case (e.g., 512 or 1024 or 2048).
- type_vocab_size (:obj:`int`, optional, defaults to 2):
- The vocabulary size of the `token_type_ids` passed into :class:`~transformers.BertModel`.
- initializer_range (:obj:`float`, optional, defaults to 0.02):
- The standard deviation of the truncated_normal_initializer for initializing all weight matrices.
- layer_norm_eps (:obj:`float`, optional, defaults to 1e-12):
- The epsilon used by the layer normalization layers.
-
- Example::
-
- from transformers import BertModel, BertConfig
-
- # Initializing a BERT bert-base-uncased style configuration
- configuration = BertConfig()
-
- # Initializing a model from the bert-base-uncased style configuration
- model = BertModel(configuration)
-
- # Accessing the model configuration
- configuration = model.config
-
- Attributes:
- pretrained_config_archive_map (Dict[str, str]):
- A dictionary containing all the available pre-trained checkpoints.
- """
- pretrained_config_archive_map = BERT_PRETRAINED_CONFIG_ARCHIVE_MAP
- model_type = "bert"
-
- def __init__(
- self,
- vocab_size=30522,
- hidden_size=768,
- num_hidden_layers=12,
- num_attention_heads=12,
- intermediate_size=3072,
- hidden_act="gelu",
- hidden_dropout_prob=0.1,
- attention_probs_dropout_prob=0.1,
- max_position_embeddings=512,
- type_vocab_size=2,
- initializer_range=0.02,
- layer_norm_eps=1e-12,
- **kwargs
- ):
- super().__init__(**kwargs)
-
- self.vocab_size = vocab_size
- self.hidden_size = hidden_size
- self.num_hidden_layers = num_hidden_layers
- self.num_attention_heads = num_attention_heads
- self.hidden_act = hidden_act
- self.intermediate_size = intermediate_size
- self.hidden_dropout_prob = hidden_dropout_prob
- self.attention_probs_dropout_prob = attention_probs_dropout_prob
- self.max_position_embeddings = max_position_embeddings
- self.type_vocab_size = type_vocab_size
- self.initializer_range = initializer_range
- self.layer_norm_eps = layer_norm_eps
diff --git a/server/transformers/src/transformers/configuration_camembert.py b/server/transformers/src/transformers/configuration_camembert.py
deleted file mode 100644
index f930fe2ece43706ece61d5f135088c3e7e89e7bb..0000000000000000000000000000000000000000
--- a/server/transformers/src/transformers/configuration_camembert.py
+++ /dev/null
@@ -1,40 +0,0 @@
-# coding=utf-8
-# Copyright 2018 The Google AI Language Team Authors and The HuggingFace Inc. team.
-# Copyright (c) 2018, NVIDIA CORPORATION. All rights reserved.
-#
-# Licensed under the Apache License, Version 2.0 (the "License");
-# you may not use this file except in compliance with the License.
-# You may obtain a copy of the License at
-#
-# http://www.apache.org/licenses/LICENSE-2.0
-#
-# Unless required by applicable law or agreed to in writing, software
-# distributed under the License is distributed on an "AS IS" BASIS,
-# WITHOUT WARRANTIES OR CONDITIONS OF ANY KIND, either express or implied.
-# See the License for the specific language governing permissions and
-# limitations under the License.
-""" CamemBERT configuration """
-
-
-import logging
-
-from .configuration_roberta import RobertaConfig
-
-
-logger = logging.getLogger(__name__)
-
-CAMEMBERT_PRETRAINED_CONFIG_ARCHIVE_MAP = {
- "camembert-base": "https://s3.amazonaws.com/models.huggingface.co/bert/camembert-base-config.json",
- "umberto-commoncrawl-cased-v1": "https://s3.amazonaws.com/models.huggingface.co/bert/Musixmatch/umberto-commoncrawl-cased-v1/config.json",
- "umberto-wikipedia-uncased-v1": "https://s3.amazonaws.com/models.huggingface.co/bert/Musixmatch/umberto-wikipedia-uncased-v1/config.json",
-}
-
-
-class CamembertConfig(RobertaConfig):
- """
- This class overrides :class:`~transformers.RobertaConfig`. Please check the
- superclass for the appropriate documentation alongside usage examples.
- """
-
- pretrained_config_archive_map = CAMEMBERT_PRETRAINED_CONFIG_ARCHIVE_MAP
- model_type = "camembert"
diff --git a/server/transformers/src/transformers/configuration_ctrl.py b/server/transformers/src/transformers/configuration_ctrl.py
deleted file mode 100644
index 4daba2a97ab1578b0bdfbcf674e4cf3ebe28cb3d..0000000000000000000000000000000000000000
--- a/server/transformers/src/transformers/configuration_ctrl.py
+++ /dev/null
@@ -1,143 +0,0 @@
-# coding=utf-8
-# Copyright 2018 Salesforce and HuggingFace Inc. team.
-# Copyright (c) 2018, NVIDIA CORPORATION. All rights reserved.
-# Licensed under the Apache License, Version 2.0 (the "License");
-# you may not use this file except in compliance with the License.
-# You may obtain a copy of the License at
-#
-# http://www.apache.org/licenses/LICENSE-2.0
-#
-# Unless required by applicable law or agreed to in writing, software
-# distributed under the License is distributed on an "AS IS" BASIS,
-# WITHOUT WARRANTIES OR CONDITIONS OF ANY KIND, either express or implied.
-# See the License for the specific language governing permissions and
-# limitations under the License.
-""" Salesforce CTRL configuration """
-
-
-import logging
-
-from .configuration_utils import PretrainedConfig
-
-
-logger = logging.getLogger(__name__)
-
-CTRL_PRETRAINED_CONFIG_ARCHIVE_MAP = {"ctrl": "https://storage.googleapis.com/sf-ctrl/pytorch/ctrl-config.json"}
-
-
-class CTRLConfig(PretrainedConfig):
- """
- This is the configuration class to store the configuration of an :class:`~transformers.CTRLModel`.
- It is used to instantiate an CTRL model according to the specified arguments, defining the model
- architecture. Instantiating a configuration with the defaults will yield a similar configuration to that of
- the `ctrl `__ architecture from SalesForce.
-
- Configuration objects inherit from :class:`~transformers.PretrainedConfig` and can be used
- to control the model outputs. Read the documentation from :class:`~transformers.PretrainedConfig`
- for more information.
-
- Args:
- vocab_size (:obj:`int`, optional, defaults to 246534):
- Vocabulary size of the CTRL model. Defines the different tokens that
- can be represented by the `inputs_ids` passed to the forward method of :class:`~transformers.CTRLModel`.
- n_positions (:obj:`int`, optional, defaults to 256):
- The maximum sequence length that this model might ever be used with.
- Typically set this to something large just in case (e.g., 512 or 1024 or 2048).
- n_ctx (:obj:`int`, optional, defaults to 256):
- Dimensionality of the causal mask (usually same as n_positions).
- n_embd (:obj:`int`, optional, defaults to 1280):
- Dimensionality of the embeddings and hidden states.
- dff (:obj:`int`, optional, defaults to 8192):
- Dimensionality of the inner dimension of the FFN.
- n_layer (:obj:`int`, optional, defaults to 48):
- Number of hidden layers in the Transformer encoder.
- n_head (:obj:`int`, optional, defaults to 16):
- Number of attention heads for each attention layer in the Transformer encoder.
- resid_pdrop (:obj:`float`, optional, defaults to 0.1):
- The dropout probability for all fully connected layers in the embeddings, encoder, and pooler.
- embd_pdrop (:obj:`int`, optional, defaults to 0.1):
- The dropout ratio for the embeddings.
- attn_pdrop (:obj:`float`, optional, defaults to 0.1):
- The dropout ratio for the attention.
- layer_norm_epsilon (:obj:`float`, optional, defaults to 1e-6):
- The epsilon to use in the layer normalization layers
- initializer_range (:obj:`float`, optional, defaults to 0.02):
- The standard deviation of the truncated_normal_initializer for initializing all weight matrices.
-
- Example::
-
- from transformers import CTRLModel, CTRLConfig
-
- # Initializing a CTRL configuration
- configuration = CTRLConfig()
-
- # Initializing a model from the configuration
- model = CTRLModel(configuration)
-
- # Accessing the model configuration
- configuration = model.config
-
- Attributes:
- pretrained_config_archive_map (Dict[str, str]):
- A dictionary containing all the available pre-trained checkpoints.
- """
-
- pretrained_config_archive_map = CTRL_PRETRAINED_CONFIG_ARCHIVE_MAP
- model_type = "ctrl"
-
- def __init__(
- self,
- vocab_size=246534,
- n_positions=256,
- n_ctx=256,
- n_embd=1280,
- dff=8192,
- n_layer=48,
- n_head=16,
- resid_pdrop=0.1,
- embd_pdrop=0.1,
- attn_pdrop=0.1,
- layer_norm_epsilon=1e-6,
- initializer_range=0.02,
- summary_type="cls_index",
- summary_use_proj=True,
- summary_activation=None,
- summary_proj_to_labels=True,
- summary_first_dropout=0.1,
- **kwargs
- ):
- super().__init__(**kwargs)
- self.vocab_size = vocab_size
- self.n_ctx = n_ctx
- self.n_positions = n_positions
- self.n_embd = n_embd
- self.n_layer = n_layer
- self.n_head = n_head
- self.dff = dff
- self.resid_pdrop = resid_pdrop
- self.embd_pdrop = embd_pdrop
- self.attn_pdrop = attn_pdrop
- self.layer_norm_epsilon = layer_norm_epsilon
- self.initializer_range = initializer_range
-
- self.summary_type = summary_type
- self.summary_use_proj = summary_use_proj
- self.summary_activation = summary_activation
- self.summary_first_dropout = summary_first_dropout
- self.summary_proj_to_labels = summary_proj_to_labels
-
- @property
- def max_position_embeddings(self):
- return self.n_positions
-
- @property
- def hidden_size(self):
- return self.n_embd
-
- @property
- def num_attention_heads(self):
- return self.n_head
-
- @property
- def num_hidden_layers(self):
- return self.n_layer
diff --git a/server/transformers/src/transformers/configuration_distilbert.py b/server/transformers/src/transformers/configuration_distilbert.py
deleted file mode 100644
index b3386e0ab81c6115d641f8b50f7fca70a1bfe212..0000000000000000000000000000000000000000
--- a/server/transformers/src/transformers/configuration_distilbert.py
+++ /dev/null
@@ -1,141 +0,0 @@
-# coding=utf-8
-# Copyright 2019-present, the HuggingFace Inc. team, The Google AI Language Team and Facebook, Inc.
-#
-# Licensed under the Apache License, Version 2.0 (the "License");
-# you may not use this file except in compliance with the License.
-# You may obtain a copy of the License at
-#
-# http://www.apache.org/licenses/LICENSE-2.0
-#
-# Unless required by applicable law or agreed to in writing, software
-# distributed under the License is distributed on an "AS IS" BASIS,
-# WITHOUT WARRANTIES OR CONDITIONS OF ANY KIND, either express or implied.
-# See the License for the specific language governing permissions and
-# limitations under the License.
-""" DistilBERT model configuration """
-
-
-import logging
-
-from .configuration_utils import PretrainedConfig
-
-
-logger = logging.getLogger(__name__)
-
-DISTILBERT_PRETRAINED_CONFIG_ARCHIVE_MAP = {
- "distilbert-base-uncased": "https://s3.amazonaws.com/models.huggingface.co/bert/distilbert-base-uncased-config.json",
- "distilbert-base-uncased-distilled-squad": "https://s3.amazonaws.com/models.huggingface.co/bert/distilbert-base-uncased-distilled-squad-config.json",
- "distilbert-base-german-cased": "https://s3.amazonaws.com/models.huggingface.co/bert/distilbert-base-german-cased-config.json",
- "distilbert-base-multilingual-cased": "https://s3.amazonaws.com/models.huggingface.co/bert/distilbert-base-multilingual-cased-config.json",
- "distilbert-base-uncased-finetuned-sst-2-english": "https://s3.amazonaws.com/models.huggingface.co/bert/distilbert-base-uncased-finetuned-sst-2-english-config.json",
-}
-
-
-class DistilBertConfig(PretrainedConfig):
- r"""
- This is the configuration class to store the configuration of a :class:`~transformers.DistilBertModel`.
- It is used to instantiate a DistilBERT model according to the specified arguments, defining the model
- architecture. Instantiating a configuration with the defaults will yield a similar configuration to that of
- the DistilBERT `distilbert-base-uncased `__ architecture.
-
- Configuration objects inherit from :class:`~transformers.PretrainedConfig` and can be used
- to control the model outputs. Read the documentation from :class:`~transformers.PretrainedConfig`
- for more information.
-
-
- Args:
- vocab_size (:obj:`int`, optional, defaults to 30522):
- Vocabulary size of the DistilBERT model. Defines the different tokens that
- can be represented by the `inputs_ids` passed to the forward method of :class:`~transformers.BertModel`.
- max_position_embeddings (:obj:`int`, optional, defaults to 512):
- The maximum sequence length that this model might ever be used with.
- Typically set this to something large just in case (e.g., 512 or 1024 or 2048).
- sinusoidal_pos_embds (:obj:`boolean`, optional, defaults to :obj:`False`):
- Whether to use sinusoidal positional embeddings.
- n_layers (:obj:`int`, optional, defaults to 6):
- Number of hidden layers in the Transformer encoder.
- n_heads (:obj:`int`, optional, defaults to 12):
- Number of attention heads for each attention layer in the Transformer encoder.
- dim (:obj:`int`, optional, defaults to 768):
- Dimensionality of the encoder layers and the pooler layer.
- intermediate_size (:obj:`int`, optional, defaults to 3072):
- The size of the "intermediate" (i.e., feed-forward) layer in the Transformer encoder.
- dropout (:obj:`float`, optional, defaults to 0.1):
- The dropout probabilitiy for all fully connected layers in the embeddings, encoder, and pooler.
- attention_dropout (:obj:`float`, optional, defaults to 0.1):
- The dropout ratio for the attention probabilities.
- activation (:obj:`str` or :obj:`function`, optional, defaults to "gelu"):
- The non-linear activation function (function or string) in the encoder and pooler.
- If string, "gelu", "relu", "swish" and "gelu_new" are supported.
- initializer_range (:obj:`float`, optional, defaults to 0.02):
- The standard deviation of the truncated_normal_initializer for initializing all weight matrices.
- qa_dropout (:obj:`float`, optional, defaults to 0.1):
- The dropout probabilities used in the question answering model
- :class:`~tranformers.DistilBertForQuestionAnswering`.
- seq_classif_dropout (:obj:`float`, optional, defaults to 0.2):
- The dropout probabilities used in the sequence classification model
- :class:`~tranformers.DistilBertForSequenceClassification`.
-
- Example::
-
- from transformers import DistilBertModel, DistilBertConfig
-
- # Initializing a DistilBERT configuration
- configuration = DistilBertConfig()
-
- # Initializing a model from the configuration
- model = DistilBertModel(configuration)
-
- # Accessing the model configuration
- configuration = model.config
-
- Attributes:
- pretrained_config_archive_map (Dict[str, str]):
- A dictionary containing all the available pre-trained checkpoints.
- """
- pretrained_config_archive_map = DISTILBERT_PRETRAINED_CONFIG_ARCHIVE_MAP
- model_type = "distilbert"
-
- def __init__(
- self,
- vocab_size=30522,
- max_position_embeddings=512,
- sinusoidal_pos_embds=False,
- n_layers=6,
- n_heads=12,
- dim=768,
- hidden_dim=4 * 768,
- dropout=0.1,
- attention_dropout=0.1,
- activation="gelu",
- initializer_range=0.02,
- qa_dropout=0.1,
- seq_classif_dropout=0.2,
- **kwargs
- ):
- super().__init__(**kwargs)
- self.vocab_size = vocab_size
- self.max_position_embeddings = max_position_embeddings
- self.sinusoidal_pos_embds = sinusoidal_pos_embds
- self.n_layers = n_layers
- self.n_heads = n_heads
- self.dim = dim
- self.hidden_dim = hidden_dim
- self.dropout = dropout
- self.attention_dropout = attention_dropout
- self.activation = activation
- self.initializer_range = initializer_range
- self.qa_dropout = qa_dropout
- self.seq_classif_dropout = seq_classif_dropout
-
- @property
- def hidden_size(self):
- return self.dim
-
- @property
- def num_attention_heads(self):
- return self.n_heads
-
- @property
- def num_hidden_layers(self):
- return self.n_layers
diff --git a/server/transformers/src/transformers/configuration_flaubert.py b/server/transformers/src/transformers/configuration_flaubert.py
deleted file mode 100644
index 511033081996d6d794ff86ecde0e1ca106a9e283..0000000000000000000000000000000000000000
--- a/server/transformers/src/transformers/configuration_flaubert.py
+++ /dev/null
@@ -1,152 +0,0 @@
-# coding=utf-8
-# Copyright 2019-present CNRS, Facebook Inc. and the HuggingFace Inc. team.
-#
-# Licensed under the Apache License, Version 2.0 (the "License");
-# you may not use this file except in compliance with the License.
-# You may obtain a copy of the License at
-#
-# http://www.apache.org/licenses/LICENSE-2.0
-#
-# Unless required by applicable law or agreed to in writing, software
-# distributed under the License is distributed on an "AS IS" BASIS,
-# WITHOUT WARRANTIES OR CONDITIONS OF ANY KIND, either express or implied.
-# See the License for the specific language governing permissions and
-# limitations under the License.
-""" Flaubert configuration, based on XLM. """
-
-
-import logging
-
-from .configuration_xlm import XLMConfig
-
-
-logger = logging.getLogger(__name__)
-
-FLAUBERT_PRETRAINED_CONFIG_ARCHIVE_MAP = {
- "flaubert-small-cased": "https://s3.amazonaws.com/models.huggingface.co/bert/flaubert/flaubert_small_cased/config.json",
- "flaubert-base-uncased": "https://s3.amazonaws.com/models.huggingface.co/bert/flaubert/flaubert_base_uncased/config.json",
- "flaubert-base-cased": "https://s3.amazonaws.com/models.huggingface.co/bert/flaubert/flaubert_base_cased/config.json",
- "flaubert-large-cased": "https://s3.amazonaws.com/models.huggingface.co/bert/flaubert/flaubert_large_cased/config.json",
-}
-
-
-class FlaubertConfig(XLMConfig):
- """
- Configuration class to store the configuration of a `FlaubertModel`.
- This is the configuration class to store the configuration of a :class:`~transformers.XLMModel`.
- It is used to instantiate an XLM model according to the specified arguments, defining the model
- architecture. Instantiating a configuration with the defaults will yield a similar configuration to that of
- the `xlm-mlm-en-2048 `__ architecture.
-
- Configuration objects inherit from :class:`~transformers.PretrainedConfig` and can be used
- to control the model outputs. Read the documentation from :class:`~transformers.PretrainedConfig`
- for more information.
-
- Args:
- pre_norm (:obj:`bool`, `optional`, defaults to :obj:`False`):
- Whether to apply the layer normalization before or after the feed forward layer following the
- attention in each layer (Vaswani et al., Tensor2Tensor for Neural Machine Translation. 2018)
- layerdrop (:obj:`float`, `optional`, defaults to 0.0):
- Probability to drop layers during training (Fan et al., Reducing Transformer Depth on Demand
- with Structured Dropout. ICLR 2020)
- vocab_size (:obj:`int`, optional, defaults to 30145):
- Vocabulary size of the Flaubert model. Defines the different tokens that
- can be represented by the `inputs_ids` passed to the forward method of :class:`~transformers.FlaubertModel`.
- emb_dim (:obj:`int`, optional, defaults to 2048):
- Dimensionality of the encoder layers and the pooler layer.
- n_layer (:obj:`int`, optional, defaults to 12):
- Number of hidden layers in the Transformer encoder.
- n_head (:obj:`int`, optional, defaults to 16):
- Number of attention heads for each attention layer in the Transformer encoder.
- dropout (:obj:`float`, optional, defaults to 0.1):
- The dropout probability for all fully connected
- layers in the embeddings, encoder, and pooler.
- attention_dropout (:obj:`float`, optional, defaults to 0.1):
- The dropout probability for the attention mechanism
- gelu_activation (:obj:`boolean`, optional, defaults to :obj:`True`):
- The non-linear activation function (function or string) in the
- encoder and pooler. If set to `True`, "gelu" will be used instead of "relu".
- sinusoidal_embeddings (:obj:`boolean`, optional, defaults to :obj:`False`):
- Whether to use sinusoidal positional embeddings instead of absolute positional embeddings.
- causal (:obj:`boolean`, optional, defaults to :obj:`False`):
- Set this to `True` for the model to behave in a causal manner.
- Causal models use a triangular attention mask in order to only attend to the left-side context instead
- if a bidirectional context.
- asm (:obj:`boolean`, optional, defaults to :obj:`False`):
- Whether to use an adaptive log softmax projection layer instead of a linear layer for the prediction
- layer.
- n_langs (:obj:`int`, optional, defaults to 1):
- The number of languages the model handles. Set to 1 for monolingual models.
- use_lang_emb (:obj:`boolean`, optional, defaults to :obj:`True`)
- Whether to use language embeddings. Some models use additional language embeddings, see
- `the multilingual models page `__
- for information on how to use them.
- max_position_embeddings (:obj:`int`, optional, defaults to 512):
- The maximum sequence length that this model might
- ever be used with. Typically set this to something large just in case
- (e.g., 512 or 1024 or 2048).
- embed_init_std (:obj:`float`, optional, defaults to 2048^-0.5):
- The standard deviation of the truncated_normal_initializer for
- initializing the embedding matrices.
- init_std (:obj:`int`, optional, defaults to 50257):
- The standard deviation of the truncated_normal_initializer for
- initializing all weight matrices except the embedding matrices.
- layer_norm_eps (:obj:`float`, optional, defaults to 1e-12):
- The epsilon used by the layer normalization layers.
- bos_index (:obj:`int`, optional, defaults to 0):
- The index of the beginning of sentence token in the vocabulary.
- eos_index (:obj:`int`, optional, defaults to 1):
- The index of the end of sentence token in the vocabulary.
- pad_index (:obj:`int`, optional, defaults to 2):
- The index of the padding token in the vocabulary.
- unk_index (:obj:`int`, optional, defaults to 3):
- The index of the unknown token in the vocabulary.
- mask_index (:obj:`int`, optional, defaults to 5):
- The index of the masking token in the vocabulary.
- is_encoder(:obj:`boolean`, optional, defaults to :obj:`True`):
- Whether the initialized model should be a transformer encoder or decoder as seen in Vaswani et al.
- summary_type (:obj:`string`, optional, defaults to "first"):
- Argument used when doing sequence summary. Used in for the multiple choice head in
- :class:`~transformers.XLMForSequenceClassification`.
- Is one of the following options:
- - 'last' => take the last token hidden state (like XLNet)
- - 'first' => take the first token hidden state (like Bert)
- - 'mean' => take the mean of all tokens hidden states
- - 'cls_index' => supply a Tensor of classification token position (GPT/GPT-2)
- - 'attn' => Not implemented now, use multi-head attention
- summary_use_proj (:obj:`boolean`, optional, defaults to :obj:`True`):
- Argument used when doing sequence summary. Used in for the multiple choice head in
- :class:`~transformers.XLMForSequenceClassification`.
- Add a projection after the vector extraction
- summary_activation (:obj:`string` or :obj:`None`, optional, defaults to :obj:`None`):
- Argument used when doing sequence summary. Used in for the multiple choice head in
- :class:`~transformers.XLMForSequenceClassification`.
- 'tanh' => add a tanh activation to the output, Other => no activation.
- summary_proj_to_labels (:obj:`boolean`, optional, defaults to :obj:`True`):
- Argument used when doing sequence summary. Used in for the multiple choice head in
- :class:`~transformers.XLMForSequenceClassification`.
- If True, the projection outputs to config.num_labels classes (otherwise to hidden_size). Default: False.
- summary_first_dropout (:obj:`float`, optional, defaults to 0.1):
- Argument used when doing sequence summary. Used in for the multiple choice head in
- :class:`~transformers.XLMForSequenceClassification`.
- Add a dropout before the projection and activation
- start_n_top (:obj:`int`, optional, defaults to 5):
- Used in the SQuAD evaluation script for XLM and XLNet.
- end_n_top (:obj:`int`, optional, defaults to 5):
- Used in the SQuAD evaluation script for XLM and XLNet.
- mask_token_id (:obj:`int`, optional, defaults to 0):
- Model agnostic parameter to identify masked tokens when generating text in an MLM context.
- lang_id (:obj:`int`, optional, defaults to 1):
- The ID of the language used by the model. This parameter is used when generating
- text in a given language.
- """
-
- pretrained_config_archive_map = FLAUBERT_PRETRAINED_CONFIG_ARCHIVE_MAP
- model_type = "flaubert"
-
- def __init__(self, layerdrop=0.0, pre_norm=False, **kwargs):
- """Constructs FlaubertConfig.
- """
- super().__init__(**kwargs)
- self.layerdrop = layerdrop
- self.pre_norm = pre_norm
diff --git a/server/transformers/src/transformers/configuration_gpt2.py b/server/transformers/src/transformers/configuration_gpt2.py
deleted file mode 100644
index 7fff0b6c4918f08b4817b3aa0fb16a0723db2de0..0000000000000000000000000000000000000000
--- a/server/transformers/src/transformers/configuration_gpt2.py
+++ /dev/null
@@ -1,172 +0,0 @@
-# coding=utf-8
-# Copyright 2018 The OpenAI Team Authors and HuggingFace Inc. team.
-# Copyright (c) 2018, NVIDIA CORPORATION. All rights reserved.
-#
-# Licensed under the Apache License, Version 2.0 (the "License");
-# you may not use this file except in compliance with the License.
-# You may obtain a copy of the License at
-#
-# http://www.apache.org/licenses/LICENSE-2.0
-#
-# Unless required by applicable law or agreed to in writing, software
-# distributed under the License is distributed on an "AS IS" BASIS,
-# WITHOUT WARRANTIES OR CONDITIONS OF ANY KIND, either express or implied.
-# See the License for the specific language governing permissions and
-# limitations under the License.
-""" OpenAI GPT-2 configuration """
-
-
-import logging
-
-from .configuration_utils import PretrainedConfig
-
-
-logger = logging.getLogger(__name__)
-
-GPT2_PRETRAINED_CONFIG_ARCHIVE_MAP = {
- "gpt2": "https://s3.amazonaws.com/models.huggingface.co/bert/gpt2-config.json",
- "gpt2-medium": "https://s3.amazonaws.com/models.huggingface.co/bert/gpt2-medium-config.json",
- "gpt2-large": "https://s3.amazonaws.com/models.huggingface.co/bert/gpt2-large-config.json",
- "gpt2-xl": "https://s3.amazonaws.com/models.huggingface.co/bert/gpt2-xl-config.json",
- "distilgpt2": "https://s3.amazonaws.com/models.huggingface.co/bert/distilgpt2-config.json",
-}
-
-
-class GPT2Config(PretrainedConfig):
- """
- This is the configuration class to store the configuration of a :class:`~transformers.GPT2Model`.
- It is used to instantiate an GPT-2 model according to the specified arguments, defining the model
- architecture. Instantiating a configuration with the defaults will yield a similar configuration to that of
- the GPT-2 `small `__ architecture.
-
- Configuration objects inherit from :class:`~transformers.PretrainedConfig` and can be used
- to control the model outputs. Read the documentation from :class:`~transformers.PretrainedConfig`
- for more information.
-
-
- Args:
- vocab_size (:obj:`int`, optional, defaults to 50257):
- Vocabulary size of the GPT-2 model. Defines the different tokens that
- can be represented by the `inputs_ids` passed to the forward method of :class:`~transformers.GPT2Model`.
- n_positions (:obj:`int`, optional, defaults to 1024):
- The maximum sequence length that this model might ever be used with.
- Typically set this to something large just in case (e.g., 512 or 1024 or 2048).
- n_ctx (:obj:`int`, optional, defaults to 1024):
- Dimensionality of the causal mask (usually same as n_positions).
- n_embd (:obj:`int`, optional, defaults to 768):
- Dimensionality of the embeddings and hidden states.
- n_layer (:obj:`int`, optional, defaults to 12):
- Number of hidden layers in the Transformer encoder.
- n_head (:obj:`int`, optional, defaults to 12):
- Number of attention heads for each attention layer in the Transformer encoder.
- resid_pdrop (:obj:`float`, optional, defaults to 0.1):
- The dropout probability for all fully connected layers in the embeddings, encoder, and pooler.
- embd_pdrop (:obj:`int`, optional, defaults to 0.1):
- The dropout ratio for the embeddings.
- attn_pdrop (:obj:`float`, optional, defaults to 0.1):
- The dropout ratio for the attention.
- layer_norm_epsilon (:obj:`float`, optional, defaults to 1e-5):
- The epsilon to use in the layer normalization layers
- initializer_range (:obj:`float`, optional, defaults to 16):
- The standard deviation of the truncated_normal_initializer for initializing all weight matrices.
- summary_type (:obj:`string`, optional, defaults to "cls_index"):
- Argument used when doing sequence summary. Used in for the multiple choice head in
- :class:`~transformers.GPT2DoubleHeadsModel`.
- Is one of the following options:
- - 'last' => take the last token hidden state (like XLNet)
- - 'first' => take the first token hidden state (like Bert)
- - 'mean' => take the mean of all tokens hidden states
- - 'cls_index' => supply a Tensor of classification token position (GPT/GPT-2)
- - 'attn' => Not implemented now, use multi-head attention
- summary_use_proj (:obj:`boolean`, optional, defaults to :obj:`True`):
- Argument used when doing sequence summary. Used in for the multiple choice head in
- :class:`~transformers.GPT2DoubleHeadsModel`.
- Add a projection after the vector extraction
- summary_activation (:obj:`string` or :obj:`None`, optional, defaults to :obj:`None`):
- Argument used when doing sequence summary. Used in for the multiple choice head in
- :class:`~transformers.GPT2DoubleHeadsModel`.
- 'tanh' => add a tanh activation to the output, Other => no activation.
- summary_proj_to_labels (:obj:`boolean`, optional, defaults to :obj:`True`):
- Argument used when doing sequence summary. Used in for the multiple choice head in
- :class:`~transformers.GPT2DoubleHeadsModel`.
- If True, the projection outputs to config.num_labels classes (otherwise to hidden_size). Default: False.
- summary_first_dropout (:obj:`float`, optional, defaults to 0.1):
- Argument used when doing sequence summary. Used in for the multiple choice head in
- :class:`~transformers.GPT2DoubleHeadsModel`.
- Add a dropout before the projection and activation
-
- Example::
-
- from transformers import GPT2Model, GPT2Config
-
- # Initializing a GPT2 configuration
- configuration = GPT2Config()
-
- # Initializing a model from the configuration
- model = GPT2Model(configuration)
-
- # Accessing the model configuration
- configuration = model.config
-
- Attributes:
- pretrained_config_archive_map (Dict[str, str]):
- A dictionary containing all the available pre-trained checkpoints.
- """
-
- pretrained_config_archive_map = GPT2_PRETRAINED_CONFIG_ARCHIVE_MAP
- model_type = "gpt2"
-
- def __init__(
- self,
- vocab_size=50257,
- n_positions=1024,
- n_ctx=1024,
- n_embd=768,
- n_layer=12,
- n_head=12,
- resid_pdrop=0.1,
- embd_pdrop=0.1,
- attn_pdrop=0.1,
- layer_norm_epsilon=1e-5,
- initializer_range=0.02,
- summary_type="cls_index",
- summary_use_proj=True,
- summary_activation=None,
- summary_proj_to_labels=True,
- summary_first_dropout=0.1,
- **kwargs
- ):
- super().__init__(**kwargs)
-
- self.vocab_size = vocab_size
- self.n_ctx = n_ctx
- self.n_positions = n_positions
- self.n_embd = n_embd
- self.n_layer = n_layer
- self.n_head = n_head
- self.resid_pdrop = resid_pdrop
- self.embd_pdrop = embd_pdrop
- self.attn_pdrop = attn_pdrop
- self.layer_norm_epsilon = layer_norm_epsilon
- self.initializer_range = initializer_range
- self.summary_type = summary_type
- self.summary_use_proj = summary_use_proj
- self.summary_activation = summary_activation
- self.summary_first_dropout = summary_first_dropout
- self.summary_proj_to_labels = summary_proj_to_labels
-
- @property
- def max_position_embeddings(self):
- return self.n_positions
-
- @property
- def hidden_size(self):
- return self.n_embd
-
- @property
- def num_attention_heads(self):
- return self.n_head
-
- @property
- def num_hidden_layers(self):
- return self.n_layer
diff --git a/server/transformers/src/transformers/configuration_mmbt.py b/server/transformers/src/transformers/configuration_mmbt.py
deleted file mode 100644
index 56a35e237c07400fe714940d9c85b0700893fbd1..0000000000000000000000000000000000000000
--- a/server/transformers/src/transformers/configuration_mmbt.py
+++ /dev/null
@@ -1,42 +0,0 @@
-# coding=utf-8
-# Copyright (c) Facebook, Inc. and its affiliates.
-# Copyright (c) HuggingFace Inc. team.
-#
-# Licensed under the Apache License, Version 2.0 (the "License");
-# you may not use this file except in compliance with the License.
-# You may obtain a copy of the License at
-#
-# http://www.apache.org/licenses/LICENSE-2.0
-#
-# Unless required by applicable law or agreed to in writing, software
-# distributed under the License is distributed on an "AS IS" BASIS,
-# WITHOUT WARRANTIES OR CONDITIONS OF ANY KIND, either express or implied.
-# See the License for the specific language governing permissions and
-# limitations under the License.
-""" MMBT configuration """
-
-
-import logging
-
-
-logger = logging.getLogger(__name__)
-
-
-class MMBTConfig(object):
- """Configuration class to store the configuration of a `MMBT Model`.
-
- Args:
- config (:obj:`~transformers.PreTrainedConfig`):
- Config of the underlying Transformer models. Its values are
- copied over to use a single config.
- num_labels (:obj:`int` or :obj:`None`, optional, defaults to `None`):
- Size of final Linear layer for classification.
- modal_hidden_size (:obj:`int`, optional, defautls to 2048):
- Embedding dimension of the non-text modality encoder.
- """
-
- def __init__(self, config, num_labels=None, modal_hidden_size=2048):
- self.__dict__ = config.__dict__
- self.modal_hidden_size = modal_hidden_size
- if num_labels:
- self.num_labels = num_labels
diff --git a/server/transformers/src/transformers/configuration_openai.py b/server/transformers/src/transformers/configuration_openai.py
deleted file mode 100644
index d4a965bde14eadacc9665521fe300373f9ccf688..0000000000000000000000000000000000000000
--- a/server/transformers/src/transformers/configuration_openai.py
+++ /dev/null
@@ -1,176 +0,0 @@
-# coding=utf-8
-# Copyright 2018 The OpenAI Team Authors and HuggingFace Inc. team.
-# Copyright (c) 2018, NVIDIA CORPORATION. All rights reserved.
-#
-# Licensed under the Apache License, Version 2.0 (the "License");
-# you may not use this file except in compliance with the License.
-# You may obtain a copy of the License at
-#
-# http://www.apache.org/licenses/LICENSE-2.0
-#
-# Unless required by applicable law or agreed to in writing, software
-# distributed under the License is distributed on an "AS IS" BASIS,
-# WITHOUT WARRANTIES OR CONDITIONS OF ANY KIND, either express or implied.
-# See the License for the specific language governing permissions and
-# limitations under the License.
-""" OpenAI GPT configuration """
-
-
-import logging
-
-from .configuration_utils import PretrainedConfig
-
-
-logger = logging.getLogger(__name__)
-
-OPENAI_GPT_PRETRAINED_CONFIG_ARCHIVE_MAP = {
- "openai-gpt": "https://s3.amazonaws.com/models.huggingface.co/bert/openai-gpt-config.json"
-}
-
-
-class OpenAIGPTConfig(PretrainedConfig):
- """
- This is the configuration class to store the configuration of an :class:`~transformers.OpenAIGPTModel`.
- It is used to instantiate an GPT model according to the specified arguments, defining the model
- architecture. Instantiating a configuration with the defaults will yield a similar configuration to that of
- the `GPT `__ architecture from OpenAI.
-
- Configuration objects inherit from :class:`~transformers.PretrainedConfig` and can be used
- to control the model outputs. Read the documentation from :class:`~transformers.PretrainedConfig`
- for more information.
-
- Args:
- vocab_size (:obj:`int`, optional, defaults to 40478):
- Vocabulary size of the GPT model. Defines the different tokens that
- can be represented by the `inputs_ids` passed to the forward method of :class:`~transformers.CTRLModel`.
- n_positions (:obj:`int`, optional, defaults to 512):
- The maximum sequence length that this model might ever be used with.
- Typically set this to something large just in case (e.g., 512 or 1024 or 2048).
- n_ctx (:obj:`int`, optional, defaults to 512):
- Dimensionality of the causal mask (usually same as n_positions).
- n_embd (:obj:`int`, optional, defaults to 768):
- Dimensionality of the embeddings and hidden states.
- n_layer (:obj:`int`, optional, defaults to 12):
- Number of hidden layers in the Transformer encoder.
- n_head (:obj:`int`, optional, defaults to 12):
- Number of attention heads for each attention layer in the Transformer encoder.
- afn (:obj:`str` or :obj:`function`, optional, defaults to "gelu"):
- The non-linear activation function (function or string) in the encoder and pooler.
- If string, "gelu", "relu", "swish" and "gelu_new" are supported.
- resid_pdrop (:obj:`float`, optional, defaults to 0.1):
- The dropout probability for all fully connected layers in the embeddings, encoder, and pooler.
- embd_pdrop (:obj:`int`, optional, defaults to 0.1):
- The dropout ratio for the embeddings.
- attn_pdrop (:obj:`float`, optional, defaults to 0.1):
- The dropout ratio for the attention.
- layer_norm_epsilon (:obj:`float`, optional, defaults to 1e-5):
- The epsilon to use in the layer normalization layers
- initializer_range (:obj:`float`, optional, defaults to 0.02):
- The standard deviation of the truncated_normal_initializer for initializing all weight matrices.
- predict_special_tokens (:obj:`boolean`, optional, defaults to :obj:`True`):
- Whether special tokens should be predicted when the model is has a language modeling head.
- summary_type (:obj:`string`, optional, defaults to "cls_index"):
- Argument used when doing sequence summary. Used in for the multiple choice head in
- :class:`~transformers.OpenAIGPTDoubleHeadsModel`.
- Is one of the following options:
- - 'last' => take the last token hidden state (like XLNet)
- - 'first' => take the first token hidden state (like Bert)
- - 'mean' => take the mean of all tokens hidden states
- - 'cls_index' => supply a Tensor of classification token position (GPT/GPT-2)
- - 'attn' => Not implemented now, use multi-head attention
- summary_use_proj (:obj:`boolean`, optional, defaults to :obj:`True`):
- Argument used when doing sequence summary. Used in for the multiple choice head in
- :class:`~transformers.OpenAIGPTDoubleHeadsModel`.
- Add a projection after the vector extraction
- summary_activation (:obj:`string` or :obj:`None`, optional, defaults to :obj:`None`):
- Argument used when doing sequence summary. Used in for the multiple choice head in
- :class:`~transformers.OpenAIGPTDoubleHeadsModel`.
- 'tanh' => add a tanh activation to the output, Other => no activation.
- summary_proj_to_labels (:obj:`boolean`, optional, defaults to :obj:`True`):
- Argument used when doing sequence summary. Used in for the multiple choice head in
- :class:`~transformers.OpenAIGPTDoubleHeadsModel`.
- If True, the projection outputs to config.num_labels classes (otherwise to hidden_size). Default: False.
- summary_first_dropout (:obj:`float`, optional, defaults to 0.1):
- Argument used when doing sequence summary. Used in for the multiple choice head in
- :class:`~transformers.OpenAIGPTDoubleHeadsModel`.
- Add a dropout before the projection and activation
-
- Example::
-
- from transformers import OpenAIGPTConfig, OpenAIGPTModel
-
- # Initializing a GPT configuration
- configuration = OpenAIGPTConfig()
-
- # Initializing a model from the configuration
- model = OpenAIGPTModel(configuration)
-
- # Accessing the model configuration
- configuration = model.config
-
- Attributes:
- pretrained_config_archive_map (Dict[str, str]):
- A dictionary containing all the available pre-trained checkpoints.
- """
-
- pretrained_config_archive_map = OPENAI_GPT_PRETRAINED_CONFIG_ARCHIVE_MAP
- model_type = "openai-gpt"
-
- def __init__(
- self,
- vocab_size=40478,
- n_positions=512,
- n_ctx=512,
- n_embd=768,
- n_layer=12,
- n_head=12,
- afn="gelu",
- resid_pdrop=0.1,
- embd_pdrop=0.1,
- attn_pdrop=0.1,
- layer_norm_epsilon=1e-5,
- initializer_range=0.02,
- predict_special_tokens=True,
- summary_type="cls_index",
- summary_use_proj=True,
- summary_activation=None,
- summary_proj_to_labels=True,
- summary_first_dropout=0.1,
- **kwargs
- ):
- super().__init__(**kwargs)
-
- self.vocab_size = vocab_size
- self.n_ctx = n_ctx
- self.n_positions = n_positions
- self.n_embd = n_embd
- self.n_layer = n_layer
- self.n_head = n_head
- self.afn = afn
- self.resid_pdrop = resid_pdrop
- self.embd_pdrop = embd_pdrop
- self.attn_pdrop = attn_pdrop
- self.layer_norm_epsilon = layer_norm_epsilon
- self.initializer_range = initializer_range
- self.predict_special_tokens = predict_special_tokens
- self.summary_type = summary_type
- self.summary_use_proj = summary_use_proj
- self.summary_activation = summary_activation
- self.summary_first_dropout = summary_first_dropout
- self.summary_proj_to_labels = summary_proj_to_labels
-
- @property
- def max_position_embeddings(self):
- return self.n_positions
-
- @property
- def hidden_size(self):
- return self.n_embd
-
- @property
- def num_attention_heads(self):
- return self.n_head
-
- @property
- def num_hidden_layers(self):
- return self.n_layer
diff --git a/server/transformers/src/transformers/configuration_roberta.py b/server/transformers/src/transformers/configuration_roberta.py
deleted file mode 100644
index 655fe03b71424a64c009f5c4a289ec23ca5ed354..0000000000000000000000000000000000000000
--- a/server/transformers/src/transformers/configuration_roberta.py
+++ /dev/null
@@ -1,68 +0,0 @@
-# coding=utf-8
-# Copyright 2018 The Google AI Language Team Authors and The HuggingFace Inc. team.
-# Copyright (c) 2018, NVIDIA CORPORATION. All rights reserved.
-#
-# Licensed under the Apache License, Version 2.0 (the "License");
-# you may not use this file except in compliance with the License.
-# You may obtain a copy of the License at
-#
-# http://www.apache.org/licenses/LICENSE-2.0
-#
-# Unless required by applicable law or agreed to in writing, software
-# distributed under the License is distributed on an "AS IS" BASIS,
-# WITHOUT WARRANTIES OR CONDITIONS OF ANY KIND, either express or implied.
-# See the License for the specific language governing permissions and
-# limitations under the License.
-""" RoBERTa configuration """
-
-
-import logging
-
-from .configuration_bert import BertConfig
-
-
-logger = logging.getLogger(__name__)
-
-ROBERTA_PRETRAINED_CONFIG_ARCHIVE_MAP = {
- "roberta-base": "https://s3.amazonaws.com/models.huggingface.co/bert/roberta-base-config.json",
- "roberta-large": "https://s3.amazonaws.com/models.huggingface.co/bert/roberta-large-config.json",
- "roberta-large-mnli": "https://s3.amazonaws.com/models.huggingface.co/bert/roberta-large-mnli-config.json",
- "distilroberta-base": "https://s3.amazonaws.com/models.huggingface.co/bert/distilroberta-base-config.json",
- "roberta-base-openai-detector": "https://s3.amazonaws.com/models.huggingface.co/bert/roberta-base-openai-detector-config.json",
- "roberta-large-openai-detector": "https://s3.amazonaws.com/models.huggingface.co/bert/roberta-large-openai-detector-config.json",
-}
-
-
-class RobertaConfig(BertConfig):
- r"""
- This is the configuration class to store the configuration of an :class:`~transformers.RobertaModel`.
- It is used to instantiate an RoBERTa model according to the specified arguments, defining the model
- architecture. Instantiating a configuration with the defaults will yield a similar configuration to that of
- the BERT `bert-base-uncased `__ architecture.
-
- Configuration objects inherit from :class:`~transformers.PretrainedConfig` and can be used
- to control the model outputs. Read the documentation from :class:`~transformers.PretrainedConfig`
- for more information.
-
- The :class:`~transformers.RobertaConfig` class directly inherits :class:`~transformers.BertConfig`.
- It reuses the same defaults. Please check the parent class for more information.
-
- Example::
-
- from transformers import RobertaConfig, RobertaModel
-
- # Initializing a RoBERTa configuration
- configuration = RobertaConfig()
-
- # Initializing a model from the configuration
- model = RobertaModel(configuration)
-
- # Accessing the model configuration
- configuration = model.config
-
- Attributes:
- pretrained_config_archive_map (Dict[str, str]):
- A dictionary containing all the available pre-trained checkpoints.
- """
- pretrained_config_archive_map = ROBERTA_PRETRAINED_CONFIG_ARCHIVE_MAP
- model_type = "roberta"
diff --git a/server/transformers/src/transformers/configuration_t5.py b/server/transformers/src/transformers/configuration_t5.py
deleted file mode 100644
index 39dd7b4e249bf70428d6209d03ad6696f23faf89..0000000000000000000000000000000000000000
--- a/server/transformers/src/transformers/configuration_t5.py
+++ /dev/null
@@ -1,107 +0,0 @@
-# coding=utf-8
-# Copyright 2010, The T5 Authors and HuggingFace Inc.
-#
-# Licensed under the Apache License, Version 2.0 (the "License");
-# you may not use this file except in compliance with the License.
-# You may obtain a copy of the License at
-#
-# http://www.apache.org/licenses/LICENSE-2.0
-#
-# Unless required by applicable law or agreed to in writing, software
-# distributed under the License is distributed on an "AS IS" BASIS,
-# WITHOUT WARRANTIES OR CONDITIONS OF ANY KIND, either express or implied.
-# See the License for the specific language governing permissions and
-# limitations under the License.
-""" T5 model configuration """
-
-
-import logging
-
-from .configuration_utils import PretrainedConfig
-
-
-logger = logging.getLogger(__name__)
-
-T5_PRETRAINED_CONFIG_ARCHIVE_MAP = {
- "t5-small": "https://s3.amazonaws.com/models.huggingface.co/bert/t5-small-config.json",
- "t5-base": "https://s3.amazonaws.com/models.huggingface.co/bert/t5-base-config.json",
- "t5-large": "https://s3.amazonaws.com/models.huggingface.co/bert/t5-large-config.json",
- "t5-3b": "https://s3.amazonaws.com/models.huggingface.co/bert/t5-3b-config.json",
- "t5-11b": "https://s3.amazonaws.com/models.huggingface.co/bert/t5-11b-config.json",
-}
-
-
-class T5Config(PretrainedConfig):
- r"""
- :class:`~transformers.T5Config` is the configuration class to store the configuration of a
- `T5Model`.
-
-
- Arguments:
- vocab_size_or_config_json_file: Vocabulary size of `inputs_ids` in `T5Model`.
- hidden_size: Size of the encoder layers and the pooler layer.
- num_hidden_layers: Number of hidden layers in the Transformer encoder.
- num_attention_heads: Number of attention heads for each attention layer in
- the Transformer encoder.
- intermediate_size: The size of the "intermediate" (i.e., feed-forward)
- layer in the Transformer encoder.
- hidden_act: The non-linear activation function (function or string) in the
- encoder and pooler. If string, "gelu", "relu", "swish" and "gelu_new" are supported.
- hidden_dropout_prob: The dropout probabilitiy for all fully connected
- layers in the embeddings, encoder, and pooler.
- attention_probs_dropout_prob: The dropout ratio for the attention
- probabilities.
- max_position_embeddings: The maximum sequence length that this model might
- ever be used with. Typically set this to something large just in case
- (e.g., 512 or 1024 or 2048).
- type_vocab_size: The vocabulary size of the `token_type_ids` passed into
- `T5Model`.
- initializer_factor: A factor for initializing all weight matrices (should be kept to 1.0, used for initialization testing).
- layer_norm_eps: The epsilon used by LayerNorm.
- """
- pretrained_config_archive_map = T5_PRETRAINED_CONFIG_ARCHIVE_MAP
- model_type = "t5"
-
- def __init__(
- self,
- vocab_size=32128,
- n_positions=512,
- d_model=512,
- d_kv=64,
- d_ff=2048,
- num_layers=6,
- num_heads=8,
- relative_attention_num_buckets=32,
- dropout_rate=0.1,
- layer_norm_epsilon=1e-6,
- initializer_factor=1.0,
- **kwargs
- ):
- super().__init__(**kwargs)
- self.vocab_size = vocab_size
- self.n_positions = n_positions
- self.d_model = d_model
- self.d_kv = d_kv
- self.d_ff = d_ff
- self.num_layers = num_layers
- self.num_heads = num_heads
- self.relative_attention_num_buckets = relative_attention_num_buckets
- self.dropout_rate = dropout_rate
- self.layer_norm_epsilon = layer_norm_epsilon
- self.initializer_factor = initializer_factor
-
- @property
- def max_position_embeddings(self):
- return self.n_positions
-
- @property
- def hidden_size(self):
- return self.d_model
-
- @property
- def num_attention_heads(self):
- return self.num_heads
-
- @property
- def num_hidden_layers(self):
- return self.num_layers
diff --git a/server/transformers/src/transformers/configuration_transfo_xl.py b/server/transformers/src/transformers/configuration_transfo_xl.py
deleted file mode 100644
index ebcc4af4f74de5e0efd13a886b38f79e47b6fbd6..0000000000000000000000000000000000000000
--- a/server/transformers/src/transformers/configuration_transfo_xl.py
+++ /dev/null
@@ -1,211 +0,0 @@
-# coding=utf-8
-# Copyright 2018 Google AI, Google Brain and Carnegie Mellon University Authors and the HuggingFace Inc. team.
-# Copyright (c) 2018, NVIDIA CORPORATION. All rights reserved.
-#
-# Licensed under the Apache License, Version 2.0 (the "License");
-# you may not use this file except in compliance with the License.
-# You may obtain a copy of the License at
-#
-# http://www.apache.org/licenses/LICENSE-2.0
-#
-# Unless required by applicable law or agreed to in writing, software
-# distributed under the License is distributed on an "AS IS" BASIS,
-# WITHOUT WARRANTIES OR CONDITIONS OF ANY KIND, either express or implied.
-# See the License for the specific language governing permissions and
-# limitations under the License.
-""" Transformer XL configuration """
-
-
-import logging
-
-from .configuration_utils import PretrainedConfig
-
-
-logger = logging.getLogger(__name__)
-
-TRANSFO_XL_PRETRAINED_CONFIG_ARCHIVE_MAP = {
- "transfo-xl-wt103": "https://s3.amazonaws.com/models.huggingface.co/bert/transfo-xl-wt103-config.json",
-}
-
-
-class TransfoXLConfig(PretrainedConfig):
- """
- This is the configuration class to store the configuration of an :class:`~transformers.TransfoXLModel`.
- It is used to instantiate a Transformer XL model according to the specified arguments, defining the model
- architecture. Instantiating a configuration with the defaults will yield a similar configuration to that of
- the `Transformer XL `__ architecture.
-
- Configuration objects inherit from :class:`~transformers.PretrainedConfig` and can be used
- to control the model outputs. Read the documentation from :class:`~transformers.PretrainedConfig`
- for more information.
-
- Args:
- vocab_size (:obj:`int`, optional, defaults to 267735):
- Vocabulary size of the Transformer XL model. Defines the different tokens that
- can be represented by the `inputs_ids` passed to the forward method of :class:`~transformers.TransfoXLModel`.
- cutoffs (:obj:`List[int]`, optional, defaults to :obj:`[20000, 40000, 200000]`):
- Cutoffs for the adaptive softmax
- d_model (:obj:`int`, optional, defaults to 1024):
- Dimensionality of the model's hidden states.
- d_embed (:obj:`int`, optional, defaults to 1024):
- Dimensionality of the embeddings
- n_head (:obj:`int`, optional, defaults to 16):
- Number of attention heads for each attention layer in the Transformer encoder.
- d_head (:obj:`int`, optional, defaults to 64):
- Dimensionality of the model's heads.
- d_inner (:obj:`int`, optional, defaults to 4096):
- Inner dimension in FF
- div_val (:obj:`int`, optional, defaults to 4):
- Divident value for adapative input and softmax
- pre_lnorm (:obj:`boolean`, optional, defaults to :obj:`False`):
- Apply LayerNorm to the input instead of the output
- n_layer (:obj:`int`, optional, defaults to 18):
- Number of hidden layers in the Transformer encoder.
- tgt_len (:obj:`int`, optional, defaults to 128):
- Number of tokens to predict
- ext_len (:obj:`int`, optional, defaults to 0):
- Length of the extended context
- mem_len (:obj:`int`, optional, defaults to 1600):
- Length of the retained previous heads
- clamp_len (:obj:`int`, optional, defaults to 1000):
- use the same pos embeddings after clamp_len
- same_length (:obj:`boolean`, optional, defaults to :obj:`True`):
- Use the same attn length for all tokens
- proj_share_all_but_first (:obj:`boolean`, optional, defaults to :obj:`True`):
- True to share all but first projs, False not to share.
- attn_type (:obj:`int`, optional, defaults to 0):
- Attention type. 0 for Transformer-XL, 1 for Shaw et al, 2 for Vaswani et al, 3 for Al Rfou et al.
- sample_softmax (:obj:`int`, optional, defaults to -1):
- number of samples in sampled softmax
- adaptive (:obj:`boolean`, optional, defaults to :obj:`True`):
- use adaptive softmax
- tie_weight (:obj:`boolean`, optional, defaults to :obj:`True`):
- tie the word embedding and softmax weights
- dropout (:obj:`float`, optional, defaults to 0.1):
- The dropout probabilitiy for all fully connected layers in the embeddings, encoder, and pooler.
- dropatt (:obj:`float`, optional, defaults to 0):
- The dropout ratio for the attention probabilities.
- untie_r (:obj:`boolean`, optional, defaults to :obj:`True`):
- Untie relative position biases
- init (:obj:`string`, optional, defaults to `normal`):
- Parameter initializer to use
- init_range (:obj:`float`, optional, defaults to 0.01):
- Parameters initialized by U(-init_range, init_range).
- proj_init_std (:obj:`float`, optional, defaults to 0.01):
- Parameters initialized by N(0, init_std)
- init_std (:obj:`float`, optional, defaults to 0.02):
- Parameters initialized by N(0, init_std)
- layer_norm_epsilon (:obj:`float`, optional, defaults to 1e-5):
- The epsilon to use in the layer normalization layers
-
- Example::
-
- from transformers import TransfoXLConfig, TransfoXLModel
-
- # Initializing a Transformer XL configuration
- configuration = TransfoXLConfig()
-
- # Initializing a model from the configuration
- model = TransfoXLModel(configuration)
-
- # Accessing the model configuration
- configuration = model.config
-
- Attributes:
- pretrained_config_archive_map (Dict[str, str]):
- A dictionary containing all the available pre-trained checkpoints.
- """
-
- pretrained_config_archive_map = TRANSFO_XL_PRETRAINED_CONFIG_ARCHIVE_MAP
- model_type = "transfo-xl"
-
- def __init__(
- self,
- vocab_size=267735,
- cutoffs=[20000, 40000, 200000],
- d_model=1024,
- d_embed=1024,
- n_head=16,
- d_head=64,
- d_inner=4096,
- div_val=4,
- pre_lnorm=False,
- n_layer=18,
- tgt_len=128,
- ext_len=0,
- mem_len=1600,
- clamp_len=1000,
- same_length=True,
- proj_share_all_but_first=True,
- attn_type=0,
- sample_softmax=-1,
- adaptive=True,
- tie_weight=True,
- dropout=0.1,
- dropatt=0.0,
- untie_r=True,
- init="normal",
- init_range=0.01,
- proj_init_std=0.01,
- init_std=0.02,
- layer_norm_epsilon=1e-5,
- **kwargs
- ):
- super().__init__(**kwargs)
-
- self.vocab_size = vocab_size
- self.cutoffs = []
- self.cutoffs.extend(cutoffs)
- self.tie_weight = tie_weight
- if proj_share_all_but_first:
- self.tie_projs = [False] + [True] * len(self.cutoffs)
- else:
- self.tie_projs = [False] + [False] * len(self.cutoffs)
- self.d_model = d_model
- self.d_embed = d_embed
- self.d_head = d_head
- self.d_inner = d_inner
- self.div_val = div_val
- self.pre_lnorm = pre_lnorm
- self.n_layer = n_layer
- self.n_head = n_head
- self.tgt_len = tgt_len
- self.ext_len = ext_len
- self.mem_len = mem_len
- self.same_length = same_length
- self.attn_type = attn_type
- self.clamp_len = clamp_len
- self.sample_softmax = sample_softmax
- self.adaptive = adaptive
- self.dropout = dropout
- self.dropatt = dropatt
- self.untie_r = untie_r
- self.init = init
- self.init_range = init_range
- self.proj_init_std = proj_init_std
- self.init_std = init_std
- self.layer_norm_epsilon = layer_norm_epsilon
-
- @property
- def max_position_embeddings(self):
- return self.tgt_len + self.ext_len + self.mem_len
-
- @property
- def n_token(self): # Backward compatibility
- return self.vocab_size
-
- @n_token.setter
- def n_token(self, value): # Backward compatibility
- self.vocab_size = value
-
- @property
- def hidden_size(self):
- return self.d_model
-
- @property
- def num_attention_heads(self):
- return self.n_head
-
- @property
- def num_hidden_layers(self):
- return self.n_layer
diff --git a/server/transformers/src/transformers/configuration_utils.py b/server/transformers/src/transformers/configuration_utils.py
deleted file mode 100644
index 97b68ce16d5fd1f9de98b6f40a7686fa34d52e08..0000000000000000000000000000000000000000
--- a/server/transformers/src/transformers/configuration_utils.py
+++ /dev/null
@@ -1,355 +0,0 @@
-# coding=utf-8
-# Copyright 2018 The Google AI Language Team Authors and The HuggingFace Inc. team.
-# Copyright (c) 2018, NVIDIA CORPORATION. All rights reserved.
-#
-# Licensed under the Apache License, Version 2.0 (the "License");
-# you may not use this file except in compliance with the License.
-# You may obtain a copy of the License at
-#
-# http://www.apache.org/licenses/LICENSE-2.0
-#
-# Unless required by applicable law or agreed to in writing, software
-# distributed under the License is distributed on an "AS IS" BASIS,
-# WITHOUT WARRANTIES OR CONDITIONS OF ANY KIND, either express or implied.
-# See the License for the specific language governing permissions and
-# limitations under the License.
-""" Configuration base class and utilities."""
-
-
-import copy
-import json
-import logging
-import os
-from typing import Dict, Optional, Tuple
-
-from .file_utils import CONFIG_NAME, cached_path, hf_bucket_url, is_remote_url
-
-
-logger = logging.getLogger(__name__)
-
-
-class PretrainedConfig(object):
- r""" Base class for all configuration classes.
- Handles a few parameters common to all models' configurations as well as methods for loading/downloading/saving configurations.
-
- Note:
- A configuration file can be loaded and saved to disk. Loading the configuration file and using this file to initialize a model does **not** load the model weights.
- It only affects the model's configuration.
-
- Class attributes (overridden by derived classes):
- - ``pretrained_config_archive_map``: a python ``dict`` with `shortcut names` (string) as keys and `url` (string) of associated pretrained model configurations as values.
- - ``model_type``: a string that identifies the model type, that we serialize into the JSON file, and that we use to recreate the correct object in :class:`~transformers.AutoConfig`.
-
- Args:
- finetuning_task (:obj:`string` or :obj:`None`, `optional`, defaults to :obj:`None`):
- Name of the task used to fine-tune the model. This can be used when converting from an original (TensorFlow or PyTorch) checkpoint.
- num_labels (:obj:`int`, `optional`, defaults to `2`):
- Number of classes to use when the model is a classification model (sequences/tokens)
- output_attentions (:obj:`bool`, `optional`, defaults to :obj:`False`):
- Should the model returns attentions weights.
- output_hidden_states (:obj:`string`, `optional`, defaults to :obj:`False`):
- Should the model returns all hidden-states.
- torchscript (:obj:`bool`, `optional`, defaults to :obj:`False`):
- Is the model used with Torchscript (for PyTorch models).
- """
- pretrained_config_archive_map = {} # type: Dict[str, str]
- model_type = "" # type: str
-
- def __init__(self, **kwargs):
- # Attributes with defaults
- self.output_attentions = kwargs.pop("output_attentions", False)
- self.output_hidden_states = kwargs.pop("output_hidden_states", False)
- self.output_additional_info = kwargs.pop("output_additional_info", False)
- self.output_past = kwargs.pop("output_past", True) # Not used by all models
- self.torchscript = kwargs.pop("torchscript", False) # Only used by PyTorch models
- self.use_bfloat16 = kwargs.pop("use_bfloat16", False)
- self.pruned_heads = kwargs.pop("pruned_heads", {})
-
- # Is decoder is used in encoder-decoder models to differentiate encoder from decoder
- self.is_decoder = kwargs.pop("is_decoder", False)
-
- # Parameters for sequence generation
- self.max_length = kwargs.pop("max_length", 20)
- self.do_sample = kwargs.pop("do_sample", False)
- self.num_beams = kwargs.pop("num_beams", 1)
- self.temperature = kwargs.pop("temperature", 1.0)
- self.top_k = kwargs.pop("top_k", 50)
- self.top_p = kwargs.pop("top_p", 1.0)
- self.repetition_penalty = kwargs.pop("repetition_penalty", 1.0)
- self.bos_token_id = kwargs.pop("bos_token_id", 0)
- self.pad_token_id = kwargs.pop("pad_token_id", 0)
- self.eos_token_ids = kwargs.pop("eos_token_ids", 0)
- self.length_penalty = kwargs.pop("length_penalty", 1.0)
- self.num_return_sequences = kwargs.pop("num_return_sequences", 1)
-
- # Fine-tuning task arguments
- self.architectures = kwargs.pop("architectures", None)
- self.finetuning_task = kwargs.pop("finetuning_task", None)
- self.num_labels = kwargs.pop("num_labels", 2)
- self.id2label = kwargs.pop("id2label", {i: "LABEL_{}".format(i) for i in range(self.num_labels)})
- self.id2label = dict((int(key), value) for key, value in self.id2label.items())
- self.label2id = kwargs.pop("label2id", dict(zip(self.id2label.values(), self.id2label.keys())))
- self.label2id = dict((key, int(value)) for key, value in self.label2id.items())
-
- # Additional attributes without default values
- for key, value in kwargs.items():
- try:
- setattr(self, key, value)
- except AttributeError as err:
- logger.error("Can't set {} with value {} for {}".format(key, value, self))
- raise err
-
- def save_pretrained(self, save_directory):
- """
- Save a configuration object to the directory `save_directory`, so that it
- can be re-loaded using the :func:`~transformers.PretrainedConfig.from_pretrained` class method.
-
- Args:
- save_directory (:obj:`string`):
- Directory where the configuration JSON file will be saved.
- """
- assert os.path.isdir(
- save_directory
- ), "Saving path should be a directory where the model and configuration can be saved"
-
- # If we save using the predefined names, we can load using `from_pretrained`
- output_config_file = os.path.join(save_directory, CONFIG_NAME)
-
- self.to_json_file(output_config_file)
- logger.info("Configuration saved in {}".format(output_config_file))
-
- @classmethod
- def from_pretrained(cls, pretrained_model_name_or_path, **kwargs) -> "PretrainedConfig":
- r"""
-
- Instantiate a :class:`~transformers.PretrainedConfig` (or a derived class) from a pre-trained model configuration.
-
- Args:
- pretrained_model_name_or_path (:obj:`string`):
- either:
- - a string with the `shortcut name` of a pre-trained model configuration to load from cache or
- download, e.g.: ``bert-base-uncased``.
- - a string with the `identifier name` of a pre-trained model configuration that was user-uploaded to
- our S3, e.g.: ``dbmdz/bert-base-german-cased``.
- - a path to a `directory` containing a configuration file saved using the
- :func:`~transformers.PretrainedConfig.save_pretrained` method, e.g.: ``./my_model_directory/``.
- - a path or url to a saved configuration JSON `file`, e.g.:
- ``./my_model_directory/configuration.json``.
- cache_dir (:obj:`string`, `optional`):
- Path to a directory in which a downloaded pre-trained model
- configuration should be cached if the standard cache should not be used.
- kwargs (:obj:`Dict[str, any]`, `optional`):
- The values in kwargs of any keys which are configuration attributes will be used to override the loaded
- values. Behavior concerning key/value pairs whose keys are *not* configuration attributes is
- controlled by the `return_unused_kwargs` keyword parameter.
- force_download (:obj:`bool`, `optional`, defaults to :obj:`False`):
- Force to (re-)download the model weights and configuration files and override the cached versions if they exist.
- resume_download (:obj:`bool`, `optional`, defaults to :obj:`False`):
- Do not delete incompletely recieved file. Attempt to resume the download if such a file exists.
- proxies (:obj:`Dict`, `optional`):
- A dictionary of proxy servers to use by protocol or endpoint, e.g.:
- :obj:`{'http': 'foo.bar:3128', 'http://hostname': 'foo.bar:4012'}.`
- The proxies are used on each request.
- return_unused_kwargs: (`optional`) bool:
- If False, then this function returns just the final configuration object.
- If True, then this functions returns a :obj:`Tuple(config, unused_kwargs)` where `unused_kwargs` is a
- dictionary consisting of the key/value pairs whose keys are not configuration attributes: ie the part
- of kwargs which has not been used to update `config` and is otherwise ignored.
-
- Returns:
- :class:`PretrainedConfig`: An instance of a configuration object
-
- Examples::
-
- # We can't instantiate directly the base class `PretrainedConfig` so let's show the examples on a
- # derived class: BertConfig
- config = BertConfig.from_pretrained('bert-base-uncased') # Download configuration from S3 and cache.
- config = BertConfig.from_pretrained('./test/saved_model/') # E.g. config (or model) was saved using `save_pretrained('./test/saved_model/')`
- config = BertConfig.from_pretrained('./test/saved_model/my_configuration.json')
- config = BertConfig.from_pretrained('bert-base-uncased', output_attention=True, foo=False)
- assert config.output_attention == True
- config, unused_kwargs = BertConfig.from_pretrained('bert-base-uncased', output_attention=True,
- foo=False, return_unused_kwargs=True)
- assert config.output_attention == True
- assert unused_kwargs == {'foo': False}
-
- """
- config_dict, kwargs = cls.get_config_dict(pretrained_model_name_or_path, **kwargs)
- return cls.from_dict(config_dict, **kwargs)
-
- @classmethod
- def get_config_dict(
- cls, pretrained_model_name_or_path: str, pretrained_config_archive_map: Optional[Dict] = None, **kwargs
- ) -> Tuple[Dict, Dict]:
- """
- From a `pretrained_model_name_or_path`, resolve to a dictionary of parameters, to be used
- for instantiating a Config using `from_dict`.
-
- Parameters:
- pretrained_model_name_or_path (:obj:`string`):
- The identifier of the pre-trained checkpoint from which we want the dictionary of parameters.
- pretrained_config_archive_map: (:obj:`Dict[str, str]`, `optional`) Dict:
- A map of `shortcut names` to `url`. By default, will use the current class attribute.
-
- Returns:
- :obj:`Tuple[Dict, Dict]`: The dictionary that will be used to instantiate the configuration object.
-
- """
- cache_dir = kwargs.pop("cache_dir", None)
- force_download = kwargs.pop("force_download", False)
- resume_download = kwargs.pop("resume_download", False)
- proxies = kwargs.pop("proxies", None)
-
- if pretrained_config_archive_map is None:
- pretrained_config_archive_map = cls.pretrained_config_archive_map
-
- if pretrained_model_name_or_path in pretrained_config_archive_map:
- config_file = pretrained_config_archive_map[pretrained_model_name_or_path]
- elif os.path.isdir(pretrained_model_name_or_path):
- config_file = os.path.join(pretrained_model_name_or_path, CONFIG_NAME)
- elif os.path.isfile(pretrained_model_name_or_path) or is_remote_url(pretrained_model_name_or_path):
- config_file = pretrained_model_name_or_path
- else:
- config_file = hf_bucket_url(pretrained_model_name_or_path, postfix=CONFIG_NAME)
-
- try:
- # Load from URL or cache if already cached
- resolved_config_file = cached_path(
- config_file,
- cache_dir=cache_dir,
- force_download=force_download,
- proxies=proxies,
- resume_download=resume_download,
- )
- # Load config dict
- if resolved_config_file is None:
- raise EnvironmentError
- config_dict = cls._dict_from_json_file(resolved_config_file)
-
- except EnvironmentError:
- if pretrained_model_name_or_path in pretrained_config_archive_map:
- msg = "Couldn't reach server at '{}' to download pretrained model configuration file.".format(
- config_file
- )
- else:
- msg = (
- "Model name '{}' was not found in model name list. "
- "We assumed '{}' was a path, a model identifier, or url to a configuration file named {} or "
- "a directory containing such a file but couldn't find any such file at this path or url.".format(
- pretrained_model_name_or_path, config_file, CONFIG_NAME,
- )
- )
- raise EnvironmentError(msg)
-
- except json.JSONDecodeError:
- msg = (
- "Couldn't reach server at '{}' to download configuration file or "
- "configuration file is not a valid JSON file. "
- "Please check network or file content here: {}.".format(config_file, resolved_config_file)
- )
- raise EnvironmentError(msg)
-
- if resolved_config_file == config_file:
- logger.info("loading configuration file {}".format(config_file))
- else:
- logger.info("loading configuration file {} from cache at {}".format(config_file, resolved_config_file))
-
- return config_dict, kwargs
-
- @classmethod
- def from_dict(cls, config_dict: Dict, **kwargs) -> "PretrainedConfig":
- """
- Constructs a `Config` from a Python dictionary of parameters.
-
- Args:
- config_dict (:obj:`Dict[str, any]`):
- Dictionary that will be used to instantiate the configuration object. Such a dictionary can be retrieved
- from a pre-trained checkpoint by leveraging the :func:`~transformers.PretrainedConfig.get_config_dict`
- method.
- kwargs (:obj:`Dict[str, any]`):
- Additional parameters from which to initialize the configuration object.
-
- Returns:
- :class:`PretrainedConfig`: An instance of a configuration object
- """
- return_unused_kwargs = kwargs.pop("return_unused_kwargs", False)
-
- config = cls(**config_dict)
-
- if hasattr(config, "pruned_heads"):
- config.pruned_heads = dict((int(key), value) for key, value in config.pruned_heads.items())
-
- # Update config with kwargs if needed
- to_remove = []
- for key, value in kwargs.items():
- if hasattr(config, key):
- setattr(config, key, value)
- to_remove.append(key)
- for key in to_remove:
- kwargs.pop(key, None)
-
- logger.info("Model config %s", str(config))
- if return_unused_kwargs:
- return config, kwargs
- else:
- return config
-
- @classmethod
- def from_json_file(cls, json_file: str) -> "PretrainedConfig":
- """
- Constructs a `Config` from the path to a json file of parameters.
-
- Args:
- json_file (:obj:`string`):
- Path to the JSON file containing the parameters.
-
- Returns:
- :class:`PretrainedConfig`: An instance of a configuration object
-
- """
- config_dict = cls._dict_from_json_file(json_file)
- return cls(**config_dict)
-
- @classmethod
- def _dict_from_json_file(cls, json_file: str):
- with open(json_file, "r", encoding="utf-8") as reader:
- text = reader.read()
- return json.loads(text)
-
- def __eq__(self, other):
- return self.__dict__ == other.__dict__
-
- def __repr__(self):
- return "{} {}".format(self.__class__.__name__, self.to_json_string())
-
- def to_dict(self):
- """
- Serializes this instance to a Python dictionary.
-
- Returns:
- :obj:`Dict[str, any]`: Dictionary of all the attributes that make up this configuration instance,
- """
- output = copy.deepcopy(self.__dict__)
- if hasattr(self.__class__, "model_type"):
- output["model_type"] = self.__class__.model_type
- return output
-
- def to_json_string(self):
- """
- Serializes this instance to a JSON string.
-
- Returns:
- :obj:`string`: String containing all the attributes that make up this configuration instance in JSON format.
- """
- return json.dumps(self.to_dict(), indent=2, sort_keys=True) + "\n"
-
- def to_json_file(self, json_file_path):
- """
- Save this instance to a json file.
-
- Args:
- json_file_path (:obj:`string`):
- Path to the JSON file in which this configuration instance's parameters will be saved.
- """
- with open(json_file_path, "w", encoding="utf-8") as writer:
- writer.write(self.to_json_string())
diff --git a/server/transformers/src/transformers/configuration_xlm.py b/server/transformers/src/transformers/configuration_xlm.py
deleted file mode 100644
index c4d61808d6ece169c071b944068a899231b9b28f..0000000000000000000000000000000000000000
--- a/server/transformers/src/transformers/configuration_xlm.py
+++ /dev/null
@@ -1,254 +0,0 @@
-# coding=utf-8
-# Copyright 2019-present, Facebook, Inc and the HuggingFace Inc. team.
-#
-# Licensed under the Apache License, Version 2.0 (the "License");
-# you may not use this file except in compliance with the License.
-# You may obtain a copy of the License at
-#
-# http://www.apache.org/licenses/LICENSE-2.0
-#
-# Unless required by applicable law or agreed to in writing, software
-# distributed under the License is distributed on an "AS IS" BASIS,
-# WITHOUT WARRANTIES OR CONDITIONS OF ANY KIND, either express or implied.
-# See the License for the specific language governing permissions and
-# limitations under the License.
-""" XLM configuration """
-
-
-import logging
-
-from .configuration_utils import PretrainedConfig
-
-
-logger = logging.getLogger(__name__)
-
-XLM_PRETRAINED_CONFIG_ARCHIVE_MAP = {
- "xlm-mlm-en-2048": "https://s3.amazonaws.com/models.huggingface.co/bert/xlm-mlm-en-2048-config.json",
- "xlm-mlm-ende-1024": "https://s3.amazonaws.com/models.huggingface.co/bert/xlm-mlm-ende-1024-config.json",
- "xlm-mlm-enfr-1024": "https://s3.amazonaws.com/models.huggingface.co/bert/xlm-mlm-enfr-1024-config.json",
- "xlm-mlm-enro-1024": "https://s3.amazonaws.com/models.huggingface.co/bert/xlm-mlm-enro-1024-config.json",
- "xlm-mlm-tlm-xnli15-1024": "https://s3.amazonaws.com/models.huggingface.co/bert/xlm-mlm-tlm-xnli15-1024-config.json",
- "xlm-mlm-xnli15-1024": "https://s3.amazonaws.com/models.huggingface.co/bert/xlm-mlm-xnli15-1024-config.json",
- "xlm-clm-enfr-1024": "https://s3.amazonaws.com/models.huggingface.co/bert/xlm-clm-enfr-1024-config.json",
- "xlm-clm-ende-1024": "https://s3.amazonaws.com/models.huggingface.co/bert/xlm-clm-ende-1024-config.json",
- "xlm-mlm-17-1280": "https://s3.amazonaws.com/models.huggingface.co/bert/xlm-mlm-17-1280-config.json",
- "xlm-mlm-100-1280": "https://s3.amazonaws.com/models.huggingface.co/bert/xlm-mlm-100-1280-config.json",
-}
-
-
-class XLMConfig(PretrainedConfig):
- """
- This is the configuration class to store the configuration of a :class:`~transformers.XLMModel`.
- It is used to instantiate an XLM model according to the specified arguments, defining the model
- architecture. Instantiating a configuration with the defaults will yield a similar configuration to that of
- the `xlm-mlm-en-2048 `__ architecture.
-
- Configuration objects inherit from :class:`~transformers.PretrainedConfig` and can be used
- to control the model outputs. Read the documentation from :class:`~transformers.PretrainedConfig`
- for more information.
-
- Args:
- vocab_size (:obj:`int`, optional, defaults to 30145):
- Vocabulary size of the XLM model. Defines the different tokens that
- can be represented by the `inputs_ids` passed to the forward method of :class:`~transformers.XLMModel`.
- emb_dim (:obj:`int`, optional, defaults to 2048):
- Dimensionality of the encoder layers and the pooler layer.
- n_layer (:obj:`int`, optional, defaults to 12):
- Number of hidden layers in the Transformer encoder.
- n_head (:obj:`int`, optional, defaults to 16):
- Number of attention heads for each attention layer in the Transformer encoder.
- dropout (:obj:`float`, optional, defaults to 0.1):
- The dropout probability for all fully connected
- layers in the embeddings, encoder, and pooler.
- attention_dropout (:obj:`float`, optional, defaults to 0.1):
- The dropout probability for the attention mechanism
- gelu_activation (:obj:`boolean`, optional, defaults to :obj:`True`):
- The non-linear activation function (function or string) in the
- encoder and pooler. If set to `True`, "gelu" will be used instead of "relu".
- sinusoidal_embeddings (:obj:`boolean`, optional, defaults to :obj:`False`):
- Whether to use sinusoidal positional embeddings instead of absolute positional embeddings.
- causal (:obj:`boolean`, optional, defaults to :obj:`False`):
- Set this to `True` for the model to behave in a causal manner.
- Causal models use a triangular attention mask in order to only attend to the left-side context instead
- if a bidirectional context.
- asm (:obj:`boolean`, optional, defaults to :obj:`False`):
- Whether to use an adaptive log softmax projection layer instead of a linear layer for the prediction
- layer.
- n_langs (:obj:`int`, optional, defaults to 1):
- The number of languages the model handles. Set to 1 for monolingual models.
- use_lang_emb (:obj:`boolean`, optional, defaults to :obj:`True`)
- Whether to use language embeddings. Some models use additional language embeddings, see
- `the multilingual models page `__
- for information on how to use them.
- max_position_embeddings (:obj:`int`, optional, defaults to 512):
- The maximum sequence length that this model might
- ever be used with. Typically set this to something large just in case
- (e.g., 512 or 1024 or 2048).
- embed_init_std (:obj:`float`, optional, defaults to 2048^-0.5):
- The standard deviation of the truncated_normal_initializer for
- initializing the embedding matrices.
- init_std (:obj:`int`, optional, defaults to 50257):
- The standard deviation of the truncated_normal_initializer for
- initializing all weight matrices except the embedding matrices.
- layer_norm_eps (:obj:`float`, optional, defaults to 1e-12):
- The epsilon used by the layer normalization layers.
- bos_index (:obj:`int`, optional, defaults to 0):
- The index of the beginning of sentence token in the vocabulary.
- eos_index (:obj:`int`, optional, defaults to 1):
- The index of the end of sentence token in the vocabulary.
- pad_index (:obj:`int`, optional, defaults to 2):
- The index of the padding token in the vocabulary.
- unk_index (:obj:`int`, optional, defaults to 3):
- The index of the unknown token in the vocabulary.
- mask_index (:obj:`int`, optional, defaults to 5):
- The index of the masking token in the vocabulary.
- is_encoder(:obj:`boolean`, optional, defaults to :obj:`True`):
- Whether the initialized model should be a transformer encoder or decoder as seen in Vaswani et al.
- summary_type (:obj:`string`, optional, defaults to "first"):
- Argument used when doing sequence summary. Used in for the multiple choice head in
- :class:`~transformers.XLMForSequenceClassification`.
- Is one of the following options:
- - 'last' => take the last token hidden state (like XLNet)
- - 'first' => take the first token hidden state (like Bert)
- - 'mean' => take the mean of all tokens hidden states
- - 'cls_index' => supply a Tensor of classification token position (GPT/GPT-2)
- - 'attn' => Not implemented now, use multi-head attention
- summary_use_proj (:obj:`boolean`, optional, defaults to :obj:`True`):
- Argument used when doing sequence summary. Used in for the multiple choice head in
- :class:`~transformers.XLMForSequenceClassification`.
- Add a projection after the vector extraction
- summary_activation (:obj:`string` or :obj:`None`, optional, defaults to :obj:`None`):
- Argument used when doing sequence summary. Used in for the multiple choice head in
- :class:`~transformers.XLMForSequenceClassification`.
- 'tanh' => add a tanh activation to the output, Other => no activation.
- summary_proj_to_labels (:obj:`boolean`, optional, defaults to :obj:`True`):
- Argument used when doing sequence summary. Used in for the multiple choice head in
- :class:`~transformers.XLMForSequenceClassification`.
- If True, the projection outputs to config.num_labels classes (otherwise to hidden_size). Default: False.
- summary_first_dropout (:obj:`float`, optional, defaults to 0.1):
- Argument used when doing sequence summary. Used in for the multiple choice head in
- :class:`~transformers.XLMForSequenceClassification`.
- Add a dropout before the projection and activation
- start_n_top (:obj:`int`, optional, defaults to 5):
- Used in the SQuAD evaluation script for XLM and XLNet.
- end_n_top (:obj:`int`, optional, defaults to 5):
- Used in the SQuAD evaluation script for XLM and XLNet.
- mask_token_id (:obj:`int`, optional, defaults to 0):
- Model agnostic parameter to identify masked tokens when generating text in an MLM context.
- lang_id (:obj:`int`, optional, defaults to 1):
- The ID of the language used by the model. This parameter is used when generating
- text in a given language.
-
- Example::
-
- from transformers import XLMConfig, XLMModel
-
- # Initializing a XLM configuration
- configuration = XLMConfig()
-
- # Initializing a model from the configuration
- model = XLMModel(configuration)
-
- # Accessing the model configuration
- configuration = model.config
-
- Attributes:
- pretrained_config_archive_map (Dict[str, str]):
- A dictionary containing all the available pre-trained checkpoints.
- """
-
- pretrained_config_archive_map = XLM_PRETRAINED_CONFIG_ARCHIVE_MAP
- model_type = "xlm"
-
- def __init__(
- self,
- vocab_size=30145,
- emb_dim=2048,
- n_layers=12,
- n_heads=16,
- dropout=0.1,
- attention_dropout=0.1,
- gelu_activation=True,
- sinusoidal_embeddings=False,
- causal=False,
- asm=False,
- n_langs=1,
- use_lang_emb=True,
- max_position_embeddings=512,
- embed_init_std=2048 ** -0.5,
- layer_norm_eps=1e-12,
- init_std=0.02,
- bos_index=0,
- eos_index=1,
- pad_index=2,
- unk_index=3,
- mask_index=5,
- is_encoder=True,
- summary_type="first",
- summary_use_proj=True,
- summary_activation=None,
- summary_proj_to_labels=True,
- summary_first_dropout=0.1,
- start_n_top=5,
- end_n_top=5,
- mask_token_id=0,
- lang_id=0,
- **kwargs
- ):
- """Constructs XLMConfig.
- """
- super().__init__(**kwargs)
- self.vocab_size = vocab_size
- self.emb_dim = emb_dim
- self.n_layers = n_layers
- self.n_heads = n_heads
- self.dropout = dropout
- self.attention_dropout = attention_dropout
- self.gelu_activation = gelu_activation
- self.sinusoidal_embeddings = sinusoidal_embeddings
- self.causal = causal
- self.asm = asm
- self.n_langs = n_langs
- self.use_lang_emb = use_lang_emb
- self.layer_norm_eps = layer_norm_eps
- self.bos_index = bos_index
- self.eos_index = eos_index
- self.pad_index = pad_index
- self.unk_index = unk_index
- self.mask_index = mask_index
- self.is_encoder = is_encoder
- self.max_position_embeddings = max_position_embeddings
- self.embed_init_std = embed_init_std
- self.init_std = init_std
- self.summary_type = summary_type
- self.summary_use_proj = summary_use_proj
- self.summary_activation = summary_activation
- self.summary_proj_to_labels = summary_proj_to_labels
- self.summary_first_dropout = summary_first_dropout
- self.start_n_top = start_n_top
- self.end_n_top = end_n_top
- self.mask_token_id = mask_token_id
- self.lang_id = lang_id
-
- if "n_words" in kwargs:
- self.n_words = kwargs["n_words"]
-
- @property
- def n_words(self): # For backward compatibility
- return self.vocab_size
-
- @n_words.setter
- def n_words(self, value): # For backward compatibility
- self.vocab_size = value
-
- @property
- def hidden_size(self):
- return self.emb_dim
-
- @property
- def num_attention_heads(self):
- return self.n_heads
-
- @property
- def num_hidden_layers(self):
- return self.n_layers
diff --git a/server/transformers/src/transformers/configuration_xlm_roberta.py b/server/transformers/src/transformers/configuration_xlm_roberta.py
deleted file mode 100644
index 330bc0d41f125399dd95bcf25a13ca1c75f272b0..0000000000000000000000000000000000000000
--- a/server/transformers/src/transformers/configuration_xlm_roberta.py
+++ /dev/null
@@ -1,43 +0,0 @@
-# coding=utf-8
-# Copyright 2018 The Google AI Language Team Authors and The HuggingFace Inc. team.
-# Copyright (c) 2018, NVIDIA CORPORATION. All rights reserved.
-#
-# Licensed under the Apache License, Version 2.0 (the "License");
-# you may not use this file except in compliance with the License.
-# You may obtain a copy of the License at
-#
-# http://www.apache.org/licenses/LICENSE-2.0
-#
-# Unless required by applicable law or agreed to in writing, software
-# distributed under the License is distributed on an "AS IS" BASIS,
-# WITHOUT WARRANTIES OR CONDITIONS OF ANY KIND, either express or implied.
-# See the License for the specific language governing permissions and
-# limitations under the License.
-""" XLM-RoBERTa configuration """
-
-
-import logging
-
-from .configuration_roberta import RobertaConfig
-
-
-logger = logging.getLogger(__name__)
-
-XLM_ROBERTA_PRETRAINED_CONFIG_ARCHIVE_MAP = {
- "xlm-roberta-base": "https://s3.amazonaws.com/models.huggingface.co/bert/xlm-roberta-base-config.json",
- "xlm-roberta-large": "https://s3.amazonaws.com/models.huggingface.co/bert/xlm-roberta-large-config.json",
- "xlm-roberta-large-finetuned-conll02-dutch": "https://s3.amazonaws.com/models.huggingface.co/bert/xlm-roberta-large-finetuned-conll02-dutch-config.json",
- "xlm-roberta-large-finetuned-conll02-spanish": "https://s3.amazonaws.com/models.huggingface.co/bert/xlm-roberta-large-finetuned-conll02-spanish-config.json",
- "xlm-roberta-large-finetuned-conll03-english": "https://s3.amazonaws.com/models.huggingface.co/bert/xlm-roberta-large-finetuned-conll03-english-config.json",
- "xlm-roberta-large-finetuned-conll03-german": "https://s3.amazonaws.com/models.huggingface.co/bert/xlm-roberta-large-finetuned-conll03-german-config.json",
-}
-
-
-class XLMRobertaConfig(RobertaConfig):
- """
- This class overrides :class:`~transformers.RobertaConfig`. Please check the
- superclass for the appropriate documentation alongside usage examples.
- """
-
- pretrained_config_archive_map = XLM_ROBERTA_PRETRAINED_CONFIG_ARCHIVE_MAP
- model_type = "xlm-roberta"
diff --git a/server/transformers/src/transformers/configuration_xlnet.py b/server/transformers/src/transformers/configuration_xlnet.py
deleted file mode 100644
index 42f6a00c5fd77a4d8528f9762169af3a2cb1ad26..0000000000000000000000000000000000000000
--- a/server/transformers/src/transformers/configuration_xlnet.py
+++ /dev/null
@@ -1,213 +0,0 @@
-# coding=utf-8
-# Copyright 2018 Google AI, Google Brain and Carnegie Mellon University Authors and the HuggingFace Inc. team.
-# Copyright (c) 2018, NVIDIA CORPORATION. All rights reserved.
-#
-# Licensed under the Apache License, Version 2.0 (the "License");
-# you may not use this file except in compliance with the License.
-# You may obtain a copy of the License at
-#
-# http://www.apache.org/licenses/LICENSE-2.0
-#
-# Unless required by applicable law or agreed to in writing, software
-# distributed under the License is distributed on an "AS IS" BASIS,
-# WITHOUT WARRANTIES OR CONDITIONS OF ANY KIND, either express or implied.
-# See the License for the specific language governing permissions and
-# limitations under the License.
-""" XLNet configuration """
-
-
-import logging
-
-from .configuration_utils import PretrainedConfig
-
-
-logger = logging.getLogger(__name__)
-
-XLNET_PRETRAINED_CONFIG_ARCHIVE_MAP = {
- "xlnet-base-cased": "https://s3.amazonaws.com/models.huggingface.co/bert/xlnet-base-cased-config.json",
- "xlnet-large-cased": "https://s3.amazonaws.com/models.huggingface.co/bert/xlnet-large-cased-config.json",
-}
-
-
-class XLNetConfig(PretrainedConfig):
- """
- This is the configuration class to store the configuration of a :class:`~transformers.XLNetModel`.
- It is used to instantiate an XLNet model according to the specified arguments, defining the model
- architecture. Instantiating a configuration with the defaults will yield a similar configuration to that of
- the `xlnet-large-cased `__ architecture.
-
- Configuration objects inherit from :class:`~transformers.PretrainedConfig` and can be used
- to control the model outputs. Read the documentation from :class:`~transformers.PretrainedConfig`
- for more information.
-
- Args:
- vocab_size (:obj:`int`, optional, defaults to 32000):
- Vocabulary size of the XLNet model. Defines the different tokens that
- can be represented by the `inputs_ids` passed to the forward method of :class:`~transformers.XLNetModel`.
- d_model (:obj:`int`, optional, defaults to 1024):
- Dimensionality of the encoder layers and the pooler layer.
- n_layer (:obj:`int`, optional, defaults to 24):
- Number of hidden layers in the Transformer encoder.
- n_head (:obj:`int`, optional, defaults to 16):
- Number of attention heads for each attention layer in the Transformer encoder.
- d_inner (:obj:`int`, optional, defaults to 4096):
- Dimensionality of the "intermediate" (i.e., feed-forward) layer in the Transformer encoder.
- ff_activation (:obj:`string`, optional, defaults to "gelu"):
- The non-linear activation function (function or string) in the
- encoder and pooler. If string, "gelu", "relu" and "swish" are supported.
- untie_r (:obj:`boolean`, optional, defaults to :obj:`True`):
- Untie relative position biases
- attn_type (:obj:`string`, optional, defaults to "bi"):
- The attention type used by the model. Set 'bi' for XLNet, 'uni' for Transformer-XL.
- initializer_range (:obj:`float`, optional, defaults to 0.02):
- The standard deviation of the truncated_normal_initializer for initializing all weight matrices.
- layer_norm_eps (:obj:`float`, optional, defaults to 1e-12):
- The epsilon used by the layer normalization layers.
- dropout (:obj:`float`, optional, defaults to 0.1):
- The dropout probability for all fully connected layers in the embeddings, encoder, and pooler.
- mem_len (:obj:`int` or :obj:`None`, optional, defaults to :obj:`None`):
- The number of tokens to cache. The key/value pairs that have already been pre-computed
- in a previous forward pass won't be re-computed. See the
- `quickstart `__
- for more information.
- reuse_len (:obj:`int` or :obj:`None`, optional, defaults to :obj:`None`):
- The number of tokens in the current batch to be cached and reused in the future.
- bi_data (:obj:`boolean`, optional, defaults to :obj:`False`):
- Whether to use bidirectional input pipeline. Usually set to `True` during
- pretraining and `False` during finetuning.
- clamp_len (:obj:`int`, optional, defaults to -1):
- Clamp all relative distances larger than clamp_len.
- Setting this attribute to -1 means no clamping.
- same_length (:obj:`boolean`, optional, defaults to :obj:`False`):
- Whether to use the same attention length for each token.
- summary_type (:obj:`string`, optional, defaults to "last"):
- Argument used when doing sequence summary. Used in for the multiple choice head in
- :class:transformers.XLNetForSequenceClassification` and :class:`~transformers.XLNetForMultipleChoice`.
- Is one of the following options:
- - 'last' => take the last token hidden state (like XLNet)
- - 'first' => take the first token hidden state (like Bert)
- - 'mean' => take the mean of all tokens hidden states
- - 'cls_index' => supply a Tensor of classification token position (GPT/GPT-2)
- - 'attn' => Not implemented now, use multi-head attention
- summary_use_proj (:obj:`boolean`, optional, defaults to :obj:`True`):
- Argument used when doing sequence summary. Used in for the multiple choice head in
- :class:`~transformers.XLNetForSequenceClassification` and :class:`~transformers.XLNetForMultipleChoice`.
- Add a projection after the vector extraction
- summary_activation (:obj:`string` or :obj:`None`, optional, defaults to :obj:`None`):
- Argument used when doing sequence summary. Used in for the multiple choice head in
- :class:`~transformers.XLNetForSequenceClassification` and :class:`~transformers.XLNetForMultipleChoice`.
- 'tanh' => add a tanh activation to the output, Other => no activation.
- summary_proj_to_labels (:obj:`boolean`, optional, defaults to :obj:`True`):
- Argument used when doing sequence summary. Used in for the multiple choice head in
- :class:`~transformers.XLNetForSequenceClassification` and :class:`~transformers.XLNetForMultipleChoice`.
- If True, the projection outputs to config.num_labels classes (otherwise to hidden_size). Default: False.
- summary_last_dropout (:obj:`float`, optional, defaults to 0.1):
- Argument used when doing sequence summary. Used in for the multiple choice head in
- :class:`~transformers.XLNetForSequenceClassification` and :class:`~transformers.XLNetForMultipleChoice`.
- Add a dropout after the projection and activation
- start_n_top (:obj:`int`, optional, defaults to 5):
- Used in the SQuAD evaluation script for XLM and XLNet.
- end_n_top (:obj:`int`, optional, defaults to 5):
- Used in the SQuAD evaluation script for XLM and XLNet.
-
- Example::
-
- from transformers import XLNetConfig, XLNetModel
-
- # Initializing a XLNet configuration
- configuration = XLNetConfig()
-
- # Initializing a model from the configuration
- model = XLNetModel(configuration)
-
- # Accessing the model configuration
- configuration = model.config
-
- Attributes:
- pretrained_config_archive_map (Dict[str, str]):
- A dictionary containing all the available pre-trained checkpoints.
- """
-
- pretrained_config_archive_map = XLNET_PRETRAINED_CONFIG_ARCHIVE_MAP
- model_type = "xlnet"
-
- def __init__(
- self,
- vocab_size=32000,
- d_model=1024,
- n_layer=24,
- n_head=16,
- d_inner=4096,
- ff_activation="gelu",
- untie_r=True,
- attn_type="bi",
- initializer_range=0.02,
- layer_norm_eps=1e-12,
- dropout=0.1,
- mem_len=None,
- reuse_len=None,
- bi_data=False,
- clamp_len=-1,
- same_length=False,
- summary_type="last",
- summary_use_proj=True,
- summary_activation="tanh",
- summary_last_dropout=0.1,
- start_n_top=5,
- end_n_top=5,
- **kwargs
- ):
- """Constructs XLNetConfig.
- """
- super().__init__(**kwargs)
- self.vocab_size = vocab_size
- self.d_model = d_model
- self.n_layer = n_layer
- self.n_head = n_head
- assert d_model % n_head == 0
- self.d_head = d_model // n_head
- self.ff_activation = ff_activation
- self.d_inner = d_inner
- self.untie_r = untie_r
- self.attn_type = attn_type
-
- self.initializer_range = initializer_range
- self.layer_norm_eps = layer_norm_eps
-
- self.dropout = dropout
- self.mem_len = mem_len
- self.reuse_len = reuse_len
- self.bi_data = bi_data
- self.clamp_len = clamp_len
- self.same_length = same_length
-
- self.summary_type = summary_type
- self.summary_use_proj = summary_use_proj
- self.summary_activation = summary_activation
- self.summary_last_dropout = summary_last_dropout
- self.start_n_top = start_n_top
- self.end_n_top = end_n_top
-
- @property
- def max_position_embeddings(self):
- return -1
-
- @property
- def n_token(self): # Backward compatibility
- return self.vocab_size
-
- @n_token.setter
- def n_token(self, value): # Backward compatibility
- self.vocab_size = value
-
- @property
- def hidden_size(self):
- return self.d_model
-
- @property
- def num_attention_heads(self):
- return self.n_head
-
- @property
- def num_hidden_layers(self):
- return self.n_layer
diff --git a/server/transformers/src/transformers/convert_albert_original_tf_checkpoint_to_pytorch.py b/server/transformers/src/transformers/convert_albert_original_tf_checkpoint_to_pytorch.py
deleted file mode 100644
index 88658d5a9fd77771b675c0e7c825845c03c0312f..0000000000000000000000000000000000000000
--- a/server/transformers/src/transformers/convert_albert_original_tf_checkpoint_to_pytorch.py
+++ /dev/null
@@ -1,61 +0,0 @@
-# coding=utf-8
-# Copyright 2018 The HuggingFace Inc. team.
-#
-# Licensed under the Apache License, Version 2.0 (the "License");
-# you may not use this file except in compliance with the License.
-# You may obtain a copy of the License at
-#
-# http://www.apache.org/licenses/LICENSE-2.0
-#
-# Unless required by applicable law or agreed to in writing, software
-# distributed under the License is distributed on an "AS IS" BASIS,
-# WITHOUT WARRANTIES OR CONDITIONS OF ANY KIND, either express or implied.
-# See the License for the specific language governing permissions and
-# limitations under the License.
-"""Convert ALBERT checkpoint."""
-
-
-import argparse
-import logging
-
-import torch
-
-from transformers import AlbertConfig, AlbertForMaskedLM, load_tf_weights_in_albert
-
-
-logging.basicConfig(level=logging.INFO)
-
-
-def convert_tf_checkpoint_to_pytorch(tf_checkpoint_path, albert_config_file, pytorch_dump_path):
- # Initialise PyTorch model
- config = AlbertConfig.from_json_file(albert_config_file)
- print("Building PyTorch model from configuration: {}".format(str(config)))
- model = AlbertForMaskedLM(config)
-
- # Load weights from tf checkpoint
- load_tf_weights_in_albert(model, config, tf_checkpoint_path)
-
- # Save pytorch-model
- print("Save PyTorch model to {}".format(pytorch_dump_path))
- torch.save(model.state_dict(), pytorch_dump_path)
-
-
-if __name__ == "__main__":
- parser = argparse.ArgumentParser()
- # Required parameters
- parser.add_argument(
- "--tf_checkpoint_path", default=None, type=str, required=True, help="Path to the TensorFlow checkpoint path."
- )
- parser.add_argument(
- "--albert_config_file",
- default=None,
- type=str,
- required=True,
- help="The config json file corresponding to the pre-trained ALBERT model. \n"
- "This specifies the model architecture.",
- )
- parser.add_argument(
- "--pytorch_dump_path", default=None, type=str, required=True, help="Path to the output PyTorch model."
- )
- args = parser.parse_args()
- convert_tf_checkpoint_to_pytorch(args.tf_checkpoint_path, args.albert_config_file, args.pytorch_dump_path)
diff --git a/server/transformers/src/transformers/convert_bert_original_tf_checkpoint_to_pytorch.py b/server/transformers/src/transformers/convert_bert_original_tf_checkpoint_to_pytorch.py
deleted file mode 100755
index 806ace556a80feba96cd2e1a2fbb97d4ae6d5f5e..0000000000000000000000000000000000000000
--- a/server/transformers/src/transformers/convert_bert_original_tf_checkpoint_to_pytorch.py
+++ /dev/null
@@ -1,61 +0,0 @@
-# coding=utf-8
-# Copyright 2018 The HuggingFace Inc. team.
-#
-# Licensed under the Apache License, Version 2.0 (the "License");
-# you may not use this file except in compliance with the License.
-# You may obtain a copy of the License at
-#
-# http://www.apache.org/licenses/LICENSE-2.0
-#
-# Unless required by applicable law or agreed to in writing, software
-# distributed under the License is distributed on an "AS IS" BASIS,
-# WITHOUT WARRANTIES OR CONDITIONS OF ANY KIND, either express or implied.
-# See the License for the specific language governing permissions and
-# limitations under the License.
-"""Convert BERT checkpoint."""
-
-
-import argparse
-import logging
-
-import torch
-
-from transformers import BertConfig, BertForPreTraining, load_tf_weights_in_bert
-
-
-logging.basicConfig(level=logging.INFO)
-
-
-def convert_tf_checkpoint_to_pytorch(tf_checkpoint_path, bert_config_file, pytorch_dump_path):
- # Initialise PyTorch model
- config = BertConfig.from_json_file(bert_config_file)
- print("Building PyTorch model from configuration: {}".format(str(config)))
- model = BertForPreTraining(config)
-
- # Load weights from tf checkpoint
- load_tf_weights_in_bert(model, config, tf_checkpoint_path)
-
- # Save pytorch-model
- print("Save PyTorch model to {}".format(pytorch_dump_path))
- torch.save(model.state_dict(), pytorch_dump_path)
-
-
-if __name__ == "__main__":
- parser = argparse.ArgumentParser()
- # Required parameters
- parser.add_argument(
- "--tf_checkpoint_path", default=None, type=str, required=True, help="Path to the TensorFlow checkpoint path."
- )
- parser.add_argument(
- "--bert_config_file",
- default=None,
- type=str,
- required=True,
- help="The config json file corresponding to the pre-trained BERT model. \n"
- "This specifies the model architecture.",
- )
- parser.add_argument(
- "--pytorch_dump_path", default=None, type=str, required=True, help="Path to the output PyTorch model."
- )
- args = parser.parse_args()
- convert_tf_checkpoint_to_pytorch(args.tf_checkpoint_path, args.bert_config_file, args.pytorch_dump_path)
diff --git a/server/transformers/src/transformers/convert_bert_pytorch_checkpoint_to_original_tf.py b/server/transformers/src/transformers/convert_bert_pytorch_checkpoint_to_original_tf.py
deleted file mode 100644
index c451521a461b67ae26a830dbe17b45fbd141a463..0000000000000000000000000000000000000000
--- a/server/transformers/src/transformers/convert_bert_pytorch_checkpoint_to_original_tf.py
+++ /dev/null
@@ -1,112 +0,0 @@
-# coding=utf-8
-# Copyright 2018 The HuggingFace Inc. team.
-#
-# Licensed under the Apache License, Version 2.0 (the "License");
-# you may not use this file except in compliance with the License.
-# You may obtain a copy of the License at
-#
-# http://www.apache.org/licenses/LICENSE-2.0
-#
-# Unless required by applicable law or agreed to in writing, software
-# distributed under the License is distributed on an "AS IS" BASIS,
-# WITHOUT WARRANTIES OR CONDITIONS OF ANY KIND, either express or implied.
-# See the License for the specific language governing permissions and
-# limitations under the License.
-
-"""Convert Huggingface Pytorch checkpoint to Tensorflow checkpoint."""
-
-import argparse
-import os
-
-import numpy as np
-import tensorflow as tf
-import torch
-
-from transformers import BertModel
-
-
-def convert_pytorch_checkpoint_to_tf(model: BertModel, ckpt_dir: str, model_name: str):
-
- """
- :param model:BertModel Pytorch model instance to be converted
- :param ckpt_dir: Tensorflow model directory
- :param model_name: model name
- :return:
-
- Currently supported HF models:
- Y BertModel
- N BertForMaskedLM
- N BertForPreTraining
- N BertForMultipleChoice
- N BertForNextSentencePrediction
- N BertForSequenceClassification
- N BertForQuestionAnswering
- """
-
- tensors_to_transpose = ("dense.weight", "attention.self.query", "attention.self.key", "attention.self.value")
-
- var_map = (
- ("layer.", "layer_"),
- ("word_embeddings.weight", "word_embeddings"),
- ("position_embeddings.weight", "position_embeddings"),
- ("token_type_embeddings.weight", "token_type_embeddings"),
- (".", "/"),
- ("LayerNorm/weight", "LayerNorm/gamma"),
- ("LayerNorm/bias", "LayerNorm/beta"),
- ("weight", "kernel"),
- )
-
- if not os.path.isdir(ckpt_dir):
- os.makedirs(ckpt_dir)
-
- state_dict = model.state_dict()
-
- def to_tf_var_name(name: str):
- for patt, repl in iter(var_map):
- name = name.replace(patt, repl)
- return "bert/{}".format(name)
-
- def create_tf_var(tensor: np.ndarray, name: str, session: tf.Session):
- tf_dtype = tf.dtypes.as_dtype(tensor.dtype)
- tf_var = tf.get_variable(dtype=tf_dtype, shape=tensor.shape, name=name, initializer=tf.zeros_initializer())
- session.run(tf.variables_initializer([tf_var]))
- session.run(tf_var)
- return tf_var
-
- tf.reset_default_graph()
- with tf.Session() as session:
- for var_name in state_dict:
- tf_name = to_tf_var_name(var_name)
- torch_tensor = state_dict[var_name].numpy()
- if any([x in var_name for x in tensors_to_transpose]):
- torch_tensor = torch_tensor.T
- tf_var = create_tf_var(tensor=torch_tensor, name=tf_name, session=session)
- tf.keras.backend.set_value(tf_var, torch_tensor)
- tf_weight = session.run(tf_var)
- print("Successfully created {}: {}".format(tf_name, np.allclose(tf_weight, torch_tensor)))
-
- saver = tf.train.Saver(tf.trainable_variables())
- saver.save(session, os.path.join(ckpt_dir, model_name.replace("-", "_") + ".ckpt"))
-
-
-def main(raw_args=None):
- parser = argparse.ArgumentParser()
- parser.add_argument("--model_name", type=str, required=True, help="model name e.g. bert-base-uncased")
- parser.add_argument(
- "--cache_dir", type=str, default=None, required=False, help="Directory containing pytorch model"
- )
- parser.add_argument("--pytorch_model_path", type=str, required=True, help="/path/to/.bin")
- parser.add_argument("--tf_cache_dir", type=str, required=True, help="Directory in which to save tensorflow model")
- args = parser.parse_args(raw_args)
-
- model = BertModel.from_pretrained(
- pretrained_model_name_or_path=args.model_name,
- state_dict=torch.load(args.pytorch_model_path),
- cache_dir=args.cache_dir,
- )
-
- convert_pytorch_checkpoint_to_tf(model=model, ckpt_dir=args.tf_cache_dir, model_name=args.model_name)
-
-
-if __name__ == "__main__":
- main()
diff --git a/server/transformers/src/transformers/convert_gpt2_original_tf_checkpoint_to_pytorch.py b/server/transformers/src/transformers/convert_gpt2_original_tf_checkpoint_to_pytorch.py
deleted file mode 100755
index d86b6b0c8861d6f0d7d60be6256fa7342a3affea..0000000000000000000000000000000000000000
--- a/server/transformers/src/transformers/convert_gpt2_original_tf_checkpoint_to_pytorch.py
+++ /dev/null
@@ -1,67 +0,0 @@
-# coding=utf-8
-# Copyright 2018 The HuggingFace Inc. team.
-#
-# Licensed under the Apache License, Version 2.0 (the "License");
-# you may not use this file except in compliance with the License.
-# You may obtain a copy of the License at
-#
-# http://www.apache.org/licenses/LICENSE-2.0
-#
-# Unless required by applicable law or agreed to in writing, software
-# distributed under the License is distributed on an "AS IS" BASIS,
-# WITHOUT WARRANTIES OR CONDITIONS OF ANY KIND, either express or implied.
-# See the License for the specific language governing permissions and
-# limitations under the License.
-"""Convert OpenAI GPT checkpoint."""
-
-
-import argparse
-import logging
-
-import torch
-
-from transformers import CONFIG_NAME, WEIGHTS_NAME, GPT2Config, GPT2Model, load_tf_weights_in_gpt2
-
-
-logging.basicConfig(level=logging.INFO)
-
-
-def convert_gpt2_checkpoint_to_pytorch(gpt2_checkpoint_path, gpt2_config_file, pytorch_dump_folder_path):
- # Construct model
- if gpt2_config_file == "":
- config = GPT2Config()
- else:
- config = GPT2Config.from_json_file(gpt2_config_file)
- model = GPT2Model(config)
-
- # Load weights from numpy
- load_tf_weights_in_gpt2(model, config, gpt2_checkpoint_path)
-
- # Save pytorch-model
- pytorch_weights_dump_path = pytorch_dump_folder_path + "/" + WEIGHTS_NAME
- pytorch_config_dump_path = pytorch_dump_folder_path + "/" + CONFIG_NAME
- print("Save PyTorch model to {}".format(pytorch_weights_dump_path))
- torch.save(model.state_dict(), pytorch_weights_dump_path)
- print("Save configuration file to {}".format(pytorch_config_dump_path))
- with open(pytorch_config_dump_path, "w", encoding="utf-8") as f:
- f.write(config.to_json_string())
-
-
-if __name__ == "__main__":
- parser = argparse.ArgumentParser()
- # Required parameters
- parser.add_argument(
- "--gpt2_checkpoint_path", default=None, type=str, required=True, help="Path to the TensorFlow checkpoint path."
- )
- parser.add_argument(
- "--pytorch_dump_folder_path", default=None, type=str, required=True, help="Path to the output PyTorch model."
- )
- parser.add_argument(
- "--gpt2_config_file",
- default="",
- type=str,
- help="An optional config json file corresponding to the pre-trained OpenAI model. \n"
- "This specifies the model architecture.",
- )
- args = parser.parse_args()
- convert_gpt2_checkpoint_to_pytorch(args.gpt2_checkpoint_path, args.gpt2_config_file, args.pytorch_dump_folder_path)
diff --git a/server/transformers/src/transformers/convert_openai_original_tf_checkpoint_to_pytorch.py b/server/transformers/src/transformers/convert_openai_original_tf_checkpoint_to_pytorch.py
deleted file mode 100755
index a1e1b80272005ee42dc74fc6696a8f867510dd20..0000000000000000000000000000000000000000
--- a/server/transformers/src/transformers/convert_openai_original_tf_checkpoint_to_pytorch.py
+++ /dev/null
@@ -1,73 +0,0 @@
-# coding=utf-8
-# Copyright 2018 The HuggingFace Inc. team.
-#
-# Licensed under the Apache License, Version 2.0 (the "License");
-# you may not use this file except in compliance with the License.
-# You may obtain a copy of the License at
-#
-# http://www.apache.org/licenses/LICENSE-2.0
-#
-# Unless required by applicable law or agreed to in writing, software
-# distributed under the License is distributed on an "AS IS" BASIS,
-# WITHOUT WARRANTIES OR CONDITIONS OF ANY KIND, either express or implied.
-# See the License for the specific language governing permissions and
-# limitations under the License.
-"""Convert OpenAI GPT checkpoint."""
-
-
-import argparse
-import logging
-
-import torch
-
-from transformers import CONFIG_NAME, WEIGHTS_NAME, OpenAIGPTConfig, OpenAIGPTModel, load_tf_weights_in_openai_gpt
-
-
-logging.basicConfig(level=logging.INFO)
-
-
-def convert_openai_checkpoint_to_pytorch(openai_checkpoint_folder_path, openai_config_file, pytorch_dump_folder_path):
- # Construct model
- if openai_config_file == "":
- config = OpenAIGPTConfig()
- else:
- config = OpenAIGPTConfig.from_json_file(openai_config_file)
- model = OpenAIGPTModel(config)
-
- # Load weights from numpy
- load_tf_weights_in_openai_gpt(model, config, openai_checkpoint_folder_path)
-
- # Save pytorch-model
- pytorch_weights_dump_path = pytorch_dump_folder_path + "/" + WEIGHTS_NAME
- pytorch_config_dump_path = pytorch_dump_folder_path + "/" + CONFIG_NAME
- print("Save PyTorch model to {}".format(pytorch_weights_dump_path))
- torch.save(model.state_dict(), pytorch_weights_dump_path)
- print("Save configuration file to {}".format(pytorch_config_dump_path))
- with open(pytorch_config_dump_path, "w", encoding="utf-8") as f:
- f.write(config.to_json_string())
-
-
-if __name__ == "__main__":
- parser = argparse.ArgumentParser()
- # Required parameters
- parser.add_argument(
- "--openai_checkpoint_folder_path",
- default=None,
- type=str,
- required=True,
- help="Path to the TensorFlow checkpoint path.",
- )
- parser.add_argument(
- "--pytorch_dump_folder_path", default=None, type=str, required=True, help="Path to the output PyTorch model."
- )
- parser.add_argument(
- "--openai_config_file",
- default="",
- type=str,
- help="An optional config json file corresponding to the pre-trained OpenAI model. \n"
- "This specifies the model architecture.",
- )
- args = parser.parse_args()
- convert_openai_checkpoint_to_pytorch(
- args.openai_checkpoint_folder_path, args.openai_config_file, args.pytorch_dump_folder_path
- )
diff --git a/server/transformers/src/transformers/convert_pytorch_checkpoint_to_tf2.py b/server/transformers/src/transformers/convert_pytorch_checkpoint_to_tf2.py
deleted file mode 100644
index a8032f2662e7071b0593117ab9adb0654908504d..0000000000000000000000000000000000000000
--- a/server/transformers/src/transformers/convert_pytorch_checkpoint_to_tf2.py
+++ /dev/null
@@ -1,500 +0,0 @@
-# coding=utf-8
-# Copyright 2018 The HuggingFace Inc. team.
-#
-# Licensed under the Apache License, Version 2.0 (the "License");
-# you may not use this file except in compliance with the License.
-# You may obtain a copy of the License at
-#
-# http://www.apache.org/licenses/LICENSE-2.0
-#
-# Unless required by applicable law or agreed to in writing, software
-# distributed under the License is distributed on an "AS IS" BASIS,
-# WITHOUT WARRANTIES OR CONDITIONS OF ANY KIND, either express or implied.
-# See the License for the specific language governing permissions and
-# limitations under the License.
-""" Convert pytorch checkpoints to TensorFlow """
-
-
-import argparse
-import logging
-import os
-
-from transformers import (
- ALBERT_PRETRAINED_CONFIG_ARCHIVE_MAP,
- BERT_PRETRAINED_CONFIG_ARCHIVE_MAP,
- CAMEMBERT_PRETRAINED_CONFIG_ARCHIVE_MAP,
- CTRL_PRETRAINED_CONFIG_ARCHIVE_MAP,
- DISTILBERT_PRETRAINED_CONFIG_ARCHIVE_MAP,
- GPT2_PRETRAINED_CONFIG_ARCHIVE_MAP,
- OPENAI_GPT_PRETRAINED_CONFIG_ARCHIVE_MAP,
- ROBERTA_PRETRAINED_CONFIG_ARCHIVE_MAP,
- T5_PRETRAINED_CONFIG_ARCHIVE_MAP,
- TRANSFO_XL_PRETRAINED_CONFIG_ARCHIVE_MAP,
- XLM_PRETRAINED_CONFIG_ARCHIVE_MAP,
- XLM_ROBERTA_PRETRAINED_CONFIG_ARCHIVE_MAP,
- XLNET_PRETRAINED_CONFIG_ARCHIVE_MAP,
- AlbertConfig,
- BertConfig,
- CamembertConfig,
- CTRLConfig,
- DistilBertConfig,
- GPT2Config,
- OpenAIGPTConfig,
- RobertaConfig,
- T5Config,
- TFAlbertForMaskedLM,
- TFBertForPreTraining,
- TFBertForQuestionAnswering,
- TFBertForSequenceClassification,
- TFCamembertForMaskedLM,
- TFCTRLLMHeadModel,
- TFDistilBertForMaskedLM,
- TFDistilBertForQuestionAnswering,
- TFGPT2LMHeadModel,
- TFOpenAIGPTLMHeadModel,
- TFRobertaForMaskedLM,
- TFRobertaForSequenceClassification,
- TFT5WithLMHeadModel,
- TFTransfoXLLMHeadModel,
- TFXLMRobertaForMaskedLM,
- TFXLMWithLMHeadModel,
- TFXLNetLMHeadModel,
- TransfoXLConfig,
- XLMConfig,
- XLMRobertaConfig,
- XLNetConfig,
- cached_path,
- is_torch_available,
- load_pytorch_checkpoint_in_tf2_model,
-)
-
-
-if is_torch_available():
- import torch
- import numpy as np
- from transformers import (
- BertForPreTraining,
- BertForQuestionAnswering,
- BertForSequenceClassification,
- BERT_PRETRAINED_MODEL_ARCHIVE_MAP,
- GPT2LMHeadModel,
- GPT2_PRETRAINED_MODEL_ARCHIVE_MAP,
- XLNetLMHeadModel,
- XLNET_PRETRAINED_MODEL_ARCHIVE_MAP,
- XLMWithLMHeadModel,
- XLM_PRETRAINED_MODEL_ARCHIVE_MAP,
- XLM_ROBERTA_PRETRAINED_MODEL_ARCHIVE_MAP,
- XLMRobertaForMaskedLM,
- TransfoXLLMHeadModel,
- TRANSFO_XL_PRETRAINED_MODEL_ARCHIVE_MAP,
- OpenAIGPTLMHeadModel,
- OPENAI_GPT_PRETRAINED_MODEL_ARCHIVE_MAP,
- RobertaForMaskedLM,
- RobertaForSequenceClassification,
- ROBERTA_PRETRAINED_MODEL_ARCHIVE_MAP,
- CamembertForMaskedLM,
- CamembertForSequenceClassification,
- CAMEMBERT_PRETRAINED_MODEL_ARCHIVE_MAP,
- DistilBertForMaskedLM,
- DistilBertForQuestionAnswering,
- DistilBertForSequenceClassification,
- DISTILBERT_PRETRAINED_MODEL_ARCHIVE_MAP,
- CTRLLMHeadModel,
- CTRL_PRETRAINED_MODEL_ARCHIVE_MAP,
- AlbertForMaskedLM,
- ALBERT_PRETRAINED_MODEL_ARCHIVE_MAP,
- T5WithLMHeadModel,
- T5_PRETRAINED_MODEL_ARCHIVE_MAP,
- )
-else:
- (
- BertForPreTraining,
- BertForQuestionAnswering,
- BertForSequenceClassification,
- BERT_PRETRAINED_MODEL_ARCHIVE_MAP,
- GPT2LMHeadModel,
- GPT2_PRETRAINED_MODEL_ARCHIVE_MAP,
- XLNetLMHeadModel,
- XLNET_PRETRAINED_MODEL_ARCHIVE_MAP,
- XLMWithLMHeadModel,
- XLM_PRETRAINED_MODEL_ARCHIVE_MAP,
- XLM_ROBERTA_PRETRAINED_MODEL_ARCHIVE_MAP,
- XLMRobertaForMaskedLM,
- TransfoXLLMHeadModel,
- TRANSFO_XL_PRETRAINED_MODEL_ARCHIVE_MAP,
- OpenAIGPTLMHeadModel,
- OPENAI_GPT_PRETRAINED_MODEL_ARCHIVE_MAP,
- RobertaForMaskedLM,
- RobertaForSequenceClassification,
- ROBERTA_PRETRAINED_MODEL_ARCHIVE_MAP,
- CamembertForMaskedLM,
- CamembertForSequenceClassification,
- CAMEMBERT_PRETRAINED_MODEL_ARCHIVE_MAP,
- DistilBertForMaskedLM,
- DistilBertForSequenceClassification,
- DistilBertForQuestionAnswering,
- DISTILBERT_PRETRAINED_MODEL_ARCHIVE_MAP,
- CTRLLMHeadModel,
- CTRL_PRETRAINED_MODEL_ARCHIVE_MAP,
- AlbertForMaskedLM,
- ALBERT_PRETRAINED_MODEL_ARCHIVE_MAP,
- T5WithLMHeadModel,
- T5_PRETRAINED_MODEL_ARCHIVE_MAP,
- ) = (
- None,
- None,
- None,
- None,
- None,
- None,
- None,
- None,
- None,
- None,
- None,
- None,
- None,
- None,
- None,
- None,
- None,
- None,
- None,
- None,
- None,
- None,
- None,
- None,
- None,
- None,
- None,
- None,
- None,
- None,
- None,
- None,
- )
-
-
-logging.basicConfig(level=logging.INFO)
-
-MODEL_CLASSES = {
- "bert": (
- BertConfig,
- TFBertForPreTraining,
- BertForPreTraining,
- BERT_PRETRAINED_MODEL_ARCHIVE_MAP,
- BERT_PRETRAINED_CONFIG_ARCHIVE_MAP,
- ),
- "bert-large-uncased-whole-word-masking-finetuned-squad": (
- BertConfig,
- TFBertForQuestionAnswering,
- BertForQuestionAnswering,
- BERT_PRETRAINED_MODEL_ARCHIVE_MAP,
- BERT_PRETRAINED_CONFIG_ARCHIVE_MAP,
- ),
- "bert-large-cased-whole-word-masking-finetuned-squad": (
- BertConfig,
- TFBertForQuestionAnswering,
- BertForQuestionAnswering,
- BERT_PRETRAINED_MODEL_ARCHIVE_MAP,
- BERT_PRETRAINED_CONFIG_ARCHIVE_MAP,
- ),
- "bert-base-cased-finetuned-mrpc": (
- BertConfig,
- TFBertForSequenceClassification,
- BertForSequenceClassification,
- BERT_PRETRAINED_MODEL_ARCHIVE_MAP,
- BERT_PRETRAINED_CONFIG_ARCHIVE_MAP,
- ),
- "gpt2": (
- GPT2Config,
- TFGPT2LMHeadModel,
- GPT2LMHeadModel,
- GPT2_PRETRAINED_MODEL_ARCHIVE_MAP,
- GPT2_PRETRAINED_CONFIG_ARCHIVE_MAP,
- ),
- "xlnet": (
- XLNetConfig,
- TFXLNetLMHeadModel,
- XLNetLMHeadModel,
- XLNET_PRETRAINED_MODEL_ARCHIVE_MAP,
- XLNET_PRETRAINED_CONFIG_ARCHIVE_MAP,
- ),
- "xlm": (
- XLMConfig,
- TFXLMWithLMHeadModel,
- XLMWithLMHeadModel,
- XLM_PRETRAINED_MODEL_ARCHIVE_MAP,
- XLM_PRETRAINED_CONFIG_ARCHIVE_MAP,
- ),
- "xlm-roberta": (
- XLMRobertaConfig,
- TFXLMRobertaForMaskedLM,
- XLMRobertaForMaskedLM,
- XLM_ROBERTA_PRETRAINED_MODEL_ARCHIVE_MAP,
- XLM_ROBERTA_PRETRAINED_CONFIG_ARCHIVE_MAP,
- ),
- "transfo-xl": (
- TransfoXLConfig,
- TFTransfoXLLMHeadModel,
- TransfoXLLMHeadModel,
- TRANSFO_XL_PRETRAINED_MODEL_ARCHIVE_MAP,
- TRANSFO_XL_PRETRAINED_CONFIG_ARCHIVE_MAP,
- ),
- "openai-gpt": (
- OpenAIGPTConfig,
- TFOpenAIGPTLMHeadModel,
- OpenAIGPTLMHeadModel,
- OPENAI_GPT_PRETRAINED_MODEL_ARCHIVE_MAP,
- OPENAI_GPT_PRETRAINED_CONFIG_ARCHIVE_MAP,
- ),
- "roberta": (
- RobertaConfig,
- TFRobertaForMaskedLM,
- RobertaForMaskedLM,
- ROBERTA_PRETRAINED_MODEL_ARCHIVE_MAP,
- ROBERTA_PRETRAINED_CONFIG_ARCHIVE_MAP,
- ),
- "roberta-large-mnli": (
- RobertaConfig,
- TFRobertaForSequenceClassification,
- RobertaForSequenceClassification,
- ROBERTA_PRETRAINED_MODEL_ARCHIVE_MAP,
- ROBERTA_PRETRAINED_CONFIG_ARCHIVE_MAP,
- ),
- "camembert": (
- CamembertConfig,
- TFCamembertForMaskedLM,
- CamembertForMaskedLM,
- CAMEMBERT_PRETRAINED_MODEL_ARCHIVE_MAP,
- CAMEMBERT_PRETRAINED_CONFIG_ARCHIVE_MAP,
- ),
- "distilbert": (
- DistilBertConfig,
- TFDistilBertForMaskedLM,
- DistilBertForMaskedLM,
- DISTILBERT_PRETRAINED_MODEL_ARCHIVE_MAP,
- DISTILBERT_PRETRAINED_CONFIG_ARCHIVE_MAP,
- ),
- "distilbert-base-uncased-distilled-squad": (
- DistilBertConfig,
- TFDistilBertForQuestionAnswering,
- DistilBertForQuestionAnswering,
- DISTILBERT_PRETRAINED_MODEL_ARCHIVE_MAP,
- DISTILBERT_PRETRAINED_CONFIG_ARCHIVE_MAP,
- ),
- "ctrl": (
- CTRLConfig,
- TFCTRLLMHeadModel,
- CTRLLMHeadModel,
- CTRL_PRETRAINED_MODEL_ARCHIVE_MAP,
- CTRL_PRETRAINED_CONFIG_ARCHIVE_MAP,
- ),
- "albert": (
- AlbertConfig,
- TFAlbertForMaskedLM,
- AlbertForMaskedLM,
- ALBERT_PRETRAINED_MODEL_ARCHIVE_MAP,
- ALBERT_PRETRAINED_CONFIG_ARCHIVE_MAP,
- ),
- "t5": (
- T5Config,
- TFT5WithLMHeadModel,
- T5WithLMHeadModel,
- T5_PRETRAINED_MODEL_ARCHIVE_MAP,
- T5_PRETRAINED_CONFIG_ARCHIVE_MAP,
- ),
-}
-
-
-def convert_pt_checkpoint_to_tf(
- model_type, pytorch_checkpoint_path, config_file, tf_dump_path, compare_with_pt_model=False, use_cached_models=True
-):
- if model_type not in MODEL_CLASSES:
- raise ValueError("Unrecognized model type, should be one of {}.".format(list(MODEL_CLASSES.keys())))
-
- config_class, model_class, pt_model_class, aws_model_maps, aws_config_map = MODEL_CLASSES[model_type]
-
- # Initialise TF model
- if config_file in aws_config_map:
- config_file = cached_path(aws_config_map[config_file], force_download=not use_cached_models)
- config = config_class.from_json_file(config_file)
- config.output_hidden_states = True
- config.output_attentions = True
- print("Building TensorFlow model from configuration: {}".format(str(config)))
- tf_model = model_class(config)
-
- # Load weights from tf checkpoint
- if pytorch_checkpoint_path in aws_model_maps:
- pytorch_checkpoint_path = cached_path(
- aws_model_maps[pytorch_checkpoint_path], force_download=not use_cached_models
- )
- # Load PyTorch checkpoint in tf2 model:
- tf_model = load_pytorch_checkpoint_in_tf2_model(tf_model, pytorch_checkpoint_path)
-
- if compare_with_pt_model:
- tfo = tf_model(tf_model.dummy_inputs, training=False) # build the network
-
- state_dict = torch.load(pytorch_checkpoint_path, map_location="cpu")
- pt_model = pt_model_class.from_pretrained(
- pretrained_model_name_or_path=None, config=config, state_dict=state_dict
- )
-
- with torch.no_grad():
- pto = pt_model(**pt_model.dummy_inputs)
-
- np_pt = pto[0].numpy()
- np_tf = tfo[0].numpy()
- diff = np.amax(np.abs(np_pt - np_tf))
- print("Max absolute difference between models outputs {}".format(diff))
- assert diff <= 2e-2, "Error, model absolute difference is >2e-2: {}".format(diff)
-
- # Save pytorch-model
- print("Save TensorFlow model to {}".format(tf_dump_path))
- tf_model.save_weights(tf_dump_path, save_format="h5")
-
-
-def convert_all_pt_checkpoints_to_tf(
- args_model_type,
- tf_dump_path,
- model_shortcut_names_or_path=None,
- config_shortcut_names_or_path=None,
- compare_with_pt_model=False,
- use_cached_models=False,
- remove_cached_files=False,
- only_convert_finetuned_models=False,
-):
- assert os.path.isdir(args.tf_dump_path), "--tf_dump_path should be a directory"
-
- if args_model_type is None:
- model_types = list(MODEL_CLASSES.keys())
- else:
- model_types = [args_model_type]
-
- for j, model_type in enumerate(model_types, start=1):
- print("=" * 100)
- print(" Converting model type {}/{}: {}".format(j, len(model_types), model_type))
- print("=" * 100)
- if model_type not in MODEL_CLASSES:
- raise ValueError(
- "Unrecognized model type {}, should be one of {}.".format(model_type, list(MODEL_CLASSES.keys()))
- )
-
- config_class, model_class, pt_model_class, aws_model_maps, aws_config_map = MODEL_CLASSES[model_type]
-
- if model_shortcut_names_or_path is None:
- model_shortcut_names_or_path = list(aws_model_maps.keys())
- if config_shortcut_names_or_path is None:
- config_shortcut_names_or_path = model_shortcut_names_or_path
-
- for i, (model_shortcut_name, config_shortcut_name) in enumerate(
- zip(model_shortcut_names_or_path, config_shortcut_names_or_path), start=1
- ):
- print("-" * 100)
- if "-squad" in model_shortcut_name or "-mrpc" in model_shortcut_name or "-mnli" in model_shortcut_name:
- if not only_convert_finetuned_models:
- print(" Skipping finetuned checkpoint {}".format(model_shortcut_name))
- continue
- model_type = model_shortcut_name
- elif only_convert_finetuned_models:
- print(" Skipping not finetuned checkpoint {}".format(model_shortcut_name))
- continue
- print(
- " Converting checkpoint {}/{}: {} - model_type {}".format(
- i, len(aws_config_map), model_shortcut_name, model_type
- )
- )
- print("-" * 100)
-
- if config_shortcut_name in aws_config_map:
- config_file = cached_path(aws_config_map[config_shortcut_name], force_download=not use_cached_models)
- else:
- config_file = cached_path(config_shortcut_name, force_download=not use_cached_models)
-
- if model_shortcut_name in aws_model_maps:
- model_file = cached_path(aws_model_maps[model_shortcut_name], force_download=not use_cached_models)
- else:
- model_file = cached_path(model_shortcut_name, force_download=not use_cached_models)
-
- if os.path.isfile(model_shortcut_name):
- model_shortcut_name = "converted_model"
-
- convert_pt_checkpoint_to_tf(
- model_type=model_type,
- pytorch_checkpoint_path=model_file,
- config_file=config_file,
- tf_dump_path=os.path.join(tf_dump_path, model_shortcut_name + "-tf_model.h5"),
- compare_with_pt_model=compare_with_pt_model,
- )
- if remove_cached_files:
- os.remove(config_file)
- os.remove(model_file)
-
-
-if __name__ == "__main__":
- parser = argparse.ArgumentParser()
- # Required parameters
- parser.add_argument(
- "--tf_dump_path", default=None, type=str, required=True, help="Path to the output Tensorflow dump file."
- )
- parser.add_argument(
- "--model_type",
- default=None,
- type=str,
- help="Model type selected in the list of {}. If not given, will download and convert all the models from AWS.".format(
- list(MODEL_CLASSES.keys())
- ),
- )
- parser.add_argument(
- "--pytorch_checkpoint_path",
- default=None,
- type=str,
- help="Path to the PyTorch checkpoint path or shortcut name to download from AWS. "
- "If not given, will download and convert all the checkpoints from AWS.",
- )
- parser.add_argument(
- "--config_file",
- default=None,
- type=str,
- help="The config json file corresponding to the pre-trained model. \n"
- "This specifies the model architecture. If not given and "
- "--pytorch_checkpoint_path is not given or is a shortcut name"
- "use the configuration associated to the shortcut name on the AWS",
- )
- parser.add_argument(
- "--compare_with_pt_model", action="store_true", help="Compare Tensorflow and PyTorch model predictions."
- )
- parser.add_argument(
- "--use_cached_models",
- action="store_true",
- help="Use cached models if possible instead of updating to latest checkpoint versions.",
- )
- parser.add_argument(
- "--remove_cached_files",
- action="store_true",
- help="Remove pytorch models after conversion (save memory when converting in batches).",
- )
- parser.add_argument("--only_convert_finetuned_models", action="store_true", help="Only convert finetuned models.")
- args = parser.parse_args()
-
- # if args.pytorch_checkpoint_path is not None:
- # convert_pt_checkpoint_to_tf(args.model_type.lower(),
- # args.pytorch_checkpoint_path,
- # args.config_file if args.config_file is not None else args.pytorch_checkpoint_path,
- # args.tf_dump_path,
- # compare_with_pt_model=args.compare_with_pt_model,
- # use_cached_models=args.use_cached_models)
- # else:
- convert_all_pt_checkpoints_to_tf(
- args.model_type.lower() if args.model_type is not None else None,
- args.tf_dump_path,
- model_shortcut_names_or_path=[args.pytorch_checkpoint_path]
- if args.pytorch_checkpoint_path is not None
- else None,
- config_shortcut_names_or_path=[args.config_file] if args.config_file is not None else None,
- compare_with_pt_model=args.compare_with_pt_model,
- use_cached_models=args.use_cached_models,
- remove_cached_files=args.remove_cached_files,
- only_convert_finetuned_models=args.only_convert_finetuned_models,
- )
diff --git a/server/transformers/src/transformers/convert_roberta_original_pytorch_checkpoint_to_pytorch.py b/server/transformers/src/transformers/convert_roberta_original_pytorch_checkpoint_to_pytorch.py
deleted file mode 100644
index df4c3414360851a5e1fca1dab0543a5712a34522..0000000000000000000000000000000000000000
--- a/server/transformers/src/transformers/convert_roberta_original_pytorch_checkpoint_to_pytorch.py
+++ /dev/null
@@ -1,177 +0,0 @@
-# coding=utf-8
-# Copyright 2018 The HuggingFace Inc. team.
-#
-# Licensed under the Apache License, Version 2.0 (the "License");
-# you may not use this file except in compliance with the License.
-# You may obtain a copy of the License at
-#
-# http://www.apache.org/licenses/LICENSE-2.0
-#
-# Unless required by applicable law or agreed to in writing, software
-# distributed under the License is distributed on an "AS IS" BASIS,
-# WITHOUT WARRANTIES OR CONDITIONS OF ANY KIND, either express or implied.
-# See the License for the specific language governing permissions and
-# limitations under the License.
-"""Convert RoBERTa checkpoint."""
-
-
-import argparse
-import logging
-import pathlib
-
-import fairseq
-import torch
-from fairseq.models.roberta import RobertaModel as FairseqRobertaModel
-from fairseq.modules import TransformerSentenceEncoderLayer
-from packaging import version
-
-from transformers.modeling_bert import (
- BertConfig,
- BertIntermediate,
- BertLayer,
- BertOutput,
- BertSelfAttention,
- BertSelfOutput,
-)
-from transformers.modeling_roberta import RobertaForMaskedLM, RobertaForSequenceClassification
-
-
-if version.parse(fairseq.__version__) < version.parse("0.9.0"):
- raise Exception("requires fairseq >= 0.9.0")
-
-
-logging.basicConfig(level=logging.INFO)
-logger = logging.getLogger(__name__)
-
-SAMPLE_TEXT = "Hello world! cécé herlolip"
-
-
-def convert_roberta_checkpoint_to_pytorch(roberta_checkpoint_path, pytorch_dump_folder_path, classification_head):
- """
- Copy/paste/tweak roberta's weights to our BERT structure.
- """
- roberta = FairseqRobertaModel.from_pretrained(roberta_checkpoint_path)
- roberta.eval() # disable dropout
- roberta_sent_encoder = roberta.model.decoder.sentence_encoder
- config = BertConfig(
- vocab_size=roberta_sent_encoder.embed_tokens.num_embeddings,
- hidden_size=roberta.args.encoder_embed_dim,
- num_hidden_layers=roberta.args.encoder_layers,
- num_attention_heads=roberta.args.encoder_attention_heads,
- intermediate_size=roberta.args.encoder_ffn_embed_dim,
- max_position_embeddings=514,
- type_vocab_size=1,
- layer_norm_eps=1e-5, # PyTorch default used in fairseq
- )
- if classification_head:
- config.num_labels = roberta.args.num_classes
- print("Our BERT config:", config)
-
- model = RobertaForSequenceClassification(config) if classification_head else RobertaForMaskedLM(config)
- model.eval()
-
- # Now let's copy all the weights.
- # Embeddings
- model.roberta.embeddings.word_embeddings.weight = roberta_sent_encoder.embed_tokens.weight
- model.roberta.embeddings.position_embeddings.weight = roberta_sent_encoder.embed_positions.weight
- model.roberta.embeddings.token_type_embeddings.weight.data = torch.zeros_like(
- model.roberta.embeddings.token_type_embeddings.weight
- ) # just zero them out b/c RoBERTa doesn't use them.
- model.roberta.embeddings.LayerNorm.weight = roberta_sent_encoder.emb_layer_norm.weight
- model.roberta.embeddings.LayerNorm.bias = roberta_sent_encoder.emb_layer_norm.bias
-
- for i in range(config.num_hidden_layers):
- # Encoder: start of layer
- layer: BertLayer = model.roberta.encoder.layer[i]
- roberta_layer: TransformerSentenceEncoderLayer = roberta_sent_encoder.layers[i]
-
- # self attention
- self_attn: BertSelfAttention = layer.attention.self
- assert (
- roberta_layer.self_attn.k_proj.weight.data.shape
- == roberta_layer.self_attn.q_proj.weight.data.shape
- == roberta_layer.self_attn.v_proj.weight.data.shape
- == torch.Size((config.hidden_size, config.hidden_size))
- )
-
- self_attn.query.weight.data = roberta_layer.self_attn.q_proj.weight
- self_attn.query.bias.data = roberta_layer.self_attn.q_proj.bias
- self_attn.key.weight.data = roberta_layer.self_attn.k_proj.weight
- self_attn.key.bias.data = roberta_layer.self_attn.k_proj.bias
- self_attn.value.weight.data = roberta_layer.self_attn.v_proj.weight
- self_attn.value.bias.data = roberta_layer.self_attn.v_proj.bias
-
- # self-attention output
- self_output: BertSelfOutput = layer.attention.output
- assert self_output.dense.weight.shape == roberta_layer.self_attn.out_proj.weight.shape
- self_output.dense.weight = roberta_layer.self_attn.out_proj.weight
- self_output.dense.bias = roberta_layer.self_attn.out_proj.bias
- self_output.LayerNorm.weight = roberta_layer.self_attn_layer_norm.weight
- self_output.LayerNorm.bias = roberta_layer.self_attn_layer_norm.bias
-
- # intermediate
- intermediate: BertIntermediate = layer.intermediate
- assert intermediate.dense.weight.shape == roberta_layer.fc1.weight.shape
- intermediate.dense.weight = roberta_layer.fc1.weight
- intermediate.dense.bias = roberta_layer.fc1.bias
-
- # output
- bert_output: BertOutput = layer.output
- assert bert_output.dense.weight.shape == roberta_layer.fc2.weight.shape
- bert_output.dense.weight = roberta_layer.fc2.weight
- bert_output.dense.bias = roberta_layer.fc2.bias
- bert_output.LayerNorm.weight = roberta_layer.final_layer_norm.weight
- bert_output.LayerNorm.bias = roberta_layer.final_layer_norm.bias
- # end of layer
-
- if classification_head:
- model.classifier.dense.weight = roberta.model.classification_heads["mnli"].dense.weight
- model.classifier.dense.bias = roberta.model.classification_heads["mnli"].dense.bias
- model.classifier.out_proj.weight = roberta.model.classification_heads["mnli"].out_proj.weight
- model.classifier.out_proj.bias = roberta.model.classification_heads["mnli"].out_proj.bias
- else:
- # LM Head
- model.lm_head.dense.weight = roberta.model.decoder.lm_head.dense.weight
- model.lm_head.dense.bias = roberta.model.decoder.lm_head.dense.bias
- model.lm_head.layer_norm.weight = roberta.model.decoder.lm_head.layer_norm.weight
- model.lm_head.layer_norm.bias = roberta.model.decoder.lm_head.layer_norm.bias
- model.lm_head.decoder.weight = roberta.model.decoder.lm_head.weight
- model.lm_head.bias = roberta.model.decoder.lm_head.bias
-
- # Let's check that we get the same results.
- input_ids: torch.Tensor = roberta.encode(SAMPLE_TEXT).unsqueeze(0) # batch of size 1
-
- our_output = model(input_ids)[0]
- if classification_head:
- their_output = roberta.model.classification_heads["mnli"](roberta.extract_features(input_ids))
- else:
- their_output = roberta.model(input_ids)[0]
- print(our_output.shape, their_output.shape)
- max_absolute_diff = torch.max(torch.abs(our_output - their_output)).item()
- print(f"max_absolute_diff = {max_absolute_diff}") # ~ 1e-7
- success = torch.allclose(our_output, their_output, atol=1e-3)
- print("Do both models output the same tensors?", "🔥" if success else "💩")
- if not success:
- raise Exception("Something went wRoNg")
-
- pathlib.Path(pytorch_dump_folder_path).mkdir(parents=True, exist_ok=True)
- print(f"Saving model to {pytorch_dump_folder_path}")
- model.save_pretrained(pytorch_dump_folder_path)
-
-
-if __name__ == "__main__":
- parser = argparse.ArgumentParser()
- # Required parameters
- parser.add_argument(
- "--roberta_checkpoint_path", default=None, type=str, required=True, help="Path the official PyTorch dump."
- )
- parser.add_argument(
- "--pytorch_dump_folder_path", default=None, type=str, required=True, help="Path to the output PyTorch model."
- )
- parser.add_argument(
- "--classification_head", action="store_true", help="Whether to convert a final classification head."
- )
- args = parser.parse_args()
- convert_roberta_checkpoint_to_pytorch(
- args.roberta_checkpoint_path, args.pytorch_dump_folder_path, args.classification_head
- )
diff --git a/server/transformers/src/transformers/convert_t5_original_tf_checkpoint_to_pytorch.py b/server/transformers/src/transformers/convert_t5_original_tf_checkpoint_to_pytorch.py
deleted file mode 100755
index e497a5a64163c80c6a9f1eb94ab62452e26dc108..0000000000000000000000000000000000000000
--- a/server/transformers/src/transformers/convert_t5_original_tf_checkpoint_to_pytorch.py
+++ /dev/null
@@ -1,61 +0,0 @@
-# coding=utf-8
-# Copyright 2018 The T5 authors and HuggingFace Inc. team.
-#
-# Licensed under the Apache License, Version 2.0 (the "License");
-# you may not use this file except in compliance with the License.
-# You may obtain a copy of the License at
-#
-# http://www.apache.org/licenses/LICENSE-2.0
-#
-# Unless required by applicable law or agreed to in writing, software
-# distributed under the License is distributed on an "AS IS" BASIS,
-# WITHOUT WARRANTIES OR CONDITIONS OF ANY KIND, either express or implied.
-# See the License for the specific language governing permissions and
-# limitations under the License.
-"""Convert T5 checkpoint."""
-
-
-import argparse
-import logging
-
-import torch
-
-from transformers import T5Config, T5Model, load_tf_weights_in_t5
-
-
-logging.basicConfig(level=logging.INFO)
-
-
-def convert_tf_checkpoint_to_pytorch(tf_checkpoint_path, config_file, pytorch_dump_path):
- # Initialise PyTorch model
- config = T5Config.from_json_file(config_file)
- print("Building PyTorch model from configuration: {}".format(str(config)))
- model = T5Model(config)
-
- # Load weights from tf checkpoint
- load_tf_weights_in_t5(model, config, tf_checkpoint_path)
-
- # Save pytorch-model
- print("Save PyTorch model to {}".format(pytorch_dump_path))
- torch.save(model.state_dict(), pytorch_dump_path)
-
-
-if __name__ == "__main__":
- parser = argparse.ArgumentParser()
- # Required parameters
- parser.add_argument(
- "--tf_checkpoint_path", default=None, type=str, required=True, help="Path to the TensorFlow checkpoint path."
- )
- parser.add_argument(
- "--config_file",
- default=None,
- type=str,
- required=True,
- help="The config json file corresponding to the pre-trained T5 model. \n"
- "This specifies the model architecture.",
- )
- parser.add_argument(
- "--pytorch_dump_path", default=None, type=str, required=True, help="Path to the output PyTorch model."
- )
- args = parser.parse_args()
- convert_tf_checkpoint_to_pytorch(args.tf_checkpoint_path, args.config_file, args.pytorch_dump_path)
diff --git a/server/transformers/src/transformers/convert_transfo_xl_original_tf_checkpoint_to_pytorch.py b/server/transformers/src/transformers/convert_transfo_xl_original_tf_checkpoint_to_pytorch.py
deleted file mode 100755
index 3a9048ba8e831446330fad4cde255d566d4f9e7c..0000000000000000000000000000000000000000
--- a/server/transformers/src/transformers/convert_transfo_xl_original_tf_checkpoint_to_pytorch.py
+++ /dev/null
@@ -1,125 +0,0 @@
-# coding=utf-8
-# Copyright 2018 The HuggingFace Inc. team.
-#
-# Licensed under the Apache License, Version 2.0 (the "License");
-# you may not use this file except in compliance with the License.
-# You may obtain a copy of the License at
-#
-# http://www.apache.org/licenses/LICENSE-2.0
-#
-# Unless required by applicable law or agreed to in writing, software
-# distributed under the License is distributed on an "AS IS" BASIS,
-# WITHOUT WARRANTIES OR CONDITIONS OF ANY KIND, either express or implied.
-# See the License for the specific language governing permissions and
-# limitations under the License.
-"""Convert Transformer XL checkpoint and datasets."""
-
-
-import argparse
-import logging
-import os
-import pickle
-import sys
-
-import torch
-
-import transformers.tokenization_transfo_xl as data_utils
-from transformers import (
- CONFIG_NAME,
- WEIGHTS_NAME,
- TransfoXLConfig,
- TransfoXLLMHeadModel,
- load_tf_weights_in_transfo_xl,
-)
-from transformers.tokenization_transfo_xl import CORPUS_NAME, VOCAB_FILES_NAMES
-
-
-logging.basicConfig(level=logging.INFO)
-
-# We do this to be able to load python 2 datasets pickles
-# See e.g. https://stackoverflow.com/questions/2121874/python-pickling-after-changing-a-modules-directory/2121918#2121918
-data_utils.Vocab = data_utils.TransfoXLTokenizer
-data_utils.Corpus = data_utils.TransfoXLCorpus
-sys.modules["data_utils"] = data_utils
-sys.modules["vocabulary"] = data_utils
-
-
-def convert_transfo_xl_checkpoint_to_pytorch(
- tf_checkpoint_path, transfo_xl_config_file, pytorch_dump_folder_path, transfo_xl_dataset_file
-):
- if transfo_xl_dataset_file:
- # Convert a pre-processed corpus (see original TensorFlow repo)
- with open(transfo_xl_dataset_file, "rb") as fp:
- corpus = pickle.load(fp, encoding="latin1")
- # Save vocabulary and dataset cache as Dictionaries (should be better than pickles for the long-term)
- pytorch_vocab_dump_path = pytorch_dump_folder_path + "/" + VOCAB_FILES_NAMES["pretrained_vocab_file"]
- print("Save vocabulary to {}".format(pytorch_vocab_dump_path))
- corpus_vocab_dict = corpus.vocab.__dict__
- torch.save(corpus_vocab_dict, pytorch_vocab_dump_path)
-
- corpus_dict_no_vocab = corpus.__dict__
- corpus_dict_no_vocab.pop("vocab", None)
- pytorch_dataset_dump_path = pytorch_dump_folder_path + "/" + CORPUS_NAME
- print("Save dataset to {}".format(pytorch_dataset_dump_path))
- torch.save(corpus_dict_no_vocab, pytorch_dataset_dump_path)
-
- if tf_checkpoint_path:
- # Convert a pre-trained TensorFlow model
- config_path = os.path.abspath(transfo_xl_config_file)
- tf_path = os.path.abspath(tf_checkpoint_path)
-
- print("Converting Transformer XL checkpoint from {} with config at {}".format(tf_path, config_path))
- # Initialise PyTorch model
- if transfo_xl_config_file == "":
- config = TransfoXLConfig()
- else:
- config = TransfoXLConfig.from_json_file(transfo_xl_config_file)
- print("Building PyTorch model from configuration: {}".format(str(config)))
- model = TransfoXLLMHeadModel(config)
-
- model = load_tf_weights_in_transfo_xl(model, config, tf_path)
- # Save pytorch-model
- pytorch_weights_dump_path = os.path.join(pytorch_dump_folder_path, WEIGHTS_NAME)
- pytorch_config_dump_path = os.path.join(pytorch_dump_folder_path, CONFIG_NAME)
- print("Save PyTorch model to {}".format(os.path.abspath(pytorch_weights_dump_path)))
- torch.save(model.state_dict(), pytorch_weights_dump_path)
- print("Save configuration file to {}".format(os.path.abspath(pytorch_config_dump_path)))
- with open(pytorch_config_dump_path, "w", encoding="utf-8") as f:
- f.write(config.to_json_string())
-
-
-if __name__ == "__main__":
- parser = argparse.ArgumentParser()
- parser.add_argument(
- "--pytorch_dump_folder_path",
- default=None,
- type=str,
- required=True,
- help="Path to the folder to store the PyTorch model or dataset/vocab.",
- )
- parser.add_argument(
- "--tf_checkpoint_path",
- default="",
- type=str,
- help="An optional path to a TensorFlow checkpoint path to be converted.",
- )
- parser.add_argument(
- "--transfo_xl_config_file",
- default="",
- type=str,
- help="An optional config json file corresponding to the pre-trained BERT model. \n"
- "This specifies the model architecture.",
- )
- parser.add_argument(
- "--transfo_xl_dataset_file",
- default="",
- type=str,
- help="An optional dataset file to be converted in a vocabulary.",
- )
- args = parser.parse_args()
- convert_transfo_xl_checkpoint_to_pytorch(
- args.tf_checkpoint_path,
- args.transfo_xl_config_file,
- args.pytorch_dump_folder_path,
- args.transfo_xl_dataset_file,
- )
diff --git a/server/transformers/src/transformers/convert_xlm_original_pytorch_checkpoint_to_pytorch.py b/server/transformers/src/transformers/convert_xlm_original_pytorch_checkpoint_to_pytorch.py
deleted file mode 100755
index 7d66dc5b3132c0a635d50f14693bd815da1bd180..0000000000000000000000000000000000000000
--- a/server/transformers/src/transformers/convert_xlm_original_pytorch_checkpoint_to_pytorch.py
+++ /dev/null
@@ -1,79 +0,0 @@
-# coding=utf-8
-# Copyright 2018 The HuggingFace Inc. team.
-#
-# Licensed under the Apache License, Version 2.0 (the "License");
-# you may not use this file except in compliance with the License.
-# You may obtain a copy of the License at
-#
-# http://www.apache.org/licenses/LICENSE-2.0
-#
-# Unless required by applicable law or agreed to in writing, software
-# distributed under the License is distributed on an "AS IS" BASIS,
-# WITHOUT WARRANTIES OR CONDITIONS OF ANY KIND, either express or implied.
-# See the License for the specific language governing permissions and
-# limitations under the License.
-"""Convert OpenAI GPT checkpoint."""
-
-
-import argparse
-import json
-import logging
-
-import numpy
-import torch
-
-from transformers import CONFIG_NAME, WEIGHTS_NAME
-from transformers.tokenization_xlm import VOCAB_FILES_NAMES
-
-
-logging.basicConfig(level=logging.INFO)
-
-
-def convert_xlm_checkpoint_to_pytorch(xlm_checkpoint_path, pytorch_dump_folder_path):
- # Load checkpoint
- chkpt = torch.load(xlm_checkpoint_path, map_location="cpu")
-
- state_dict = chkpt["model"]
-
- # We have the base model one level deeper than the original XLM repository
- two_levels_state_dict = {}
- for k, v in state_dict.items():
- if "pred_layer" in k:
- two_levels_state_dict[k] = v
- else:
- two_levels_state_dict["transformer." + k] = v
-
- config = chkpt["params"]
- config = dict((n, v) for n, v in config.items() if not isinstance(v, (torch.FloatTensor, numpy.ndarray)))
-
- vocab = chkpt["dico_word2id"]
- vocab = dict((s + "" if s.find("@@") == -1 and i > 13 else s.replace("@@", ""), i) for s, i in vocab.items())
-
- # Save pytorch-model
- pytorch_weights_dump_path = pytorch_dump_folder_path + "/" + WEIGHTS_NAME
- pytorch_config_dump_path = pytorch_dump_folder_path + "/" + CONFIG_NAME
- pytorch_vocab_dump_path = pytorch_dump_folder_path + "/" + VOCAB_FILES_NAMES["vocab_file"]
-
- print("Save PyTorch model to {}".format(pytorch_weights_dump_path))
- torch.save(two_levels_state_dict, pytorch_weights_dump_path)
-
- print("Save configuration file to {}".format(pytorch_config_dump_path))
- with open(pytorch_config_dump_path, "w", encoding="utf-8") as f:
- f.write(json.dumps(config, indent=2) + "\n")
-
- print("Save vocab file to {}".format(pytorch_config_dump_path))
- with open(pytorch_vocab_dump_path, "w", encoding="utf-8") as f:
- f.write(json.dumps(vocab, indent=2) + "\n")
-
-
-if __name__ == "__main__":
- parser = argparse.ArgumentParser()
- # Required parameters
- parser.add_argument(
- "--xlm_checkpoint_path", default=None, type=str, required=True, help="Path the official PyTorch dump."
- )
- parser.add_argument(
- "--pytorch_dump_folder_path", default=None, type=str, required=True, help="Path to the output PyTorch model."
- )
- args = parser.parse_args()
- convert_xlm_checkpoint_to_pytorch(args.xlm_checkpoint_path, args.pytorch_dump_folder_path)
diff --git a/server/transformers/src/transformers/convert_xlnet_original_tf_checkpoint_to_pytorch.py b/server/transformers/src/transformers/convert_xlnet_original_tf_checkpoint_to_pytorch.py
deleted file mode 100755
index 51eed0e1214aa0bce2d1adffabb0b599d0dfa0fa..0000000000000000000000000000000000000000
--- a/server/transformers/src/transformers/convert_xlnet_original_tf_checkpoint_to_pytorch.py
+++ /dev/null
@@ -1,114 +0,0 @@
-# coding=utf-8
-# Copyright 2018 The HuggingFace Inc. team.
-#
-# Licensed under the Apache License, Version 2.0 (the "License");
-# you may not use this file except in compliance with the License.
-# You may obtain a copy of the License at
-#
-# http://www.apache.org/licenses/LICENSE-2.0
-#
-# Unless required by applicable law or agreed to in writing, software
-# distributed under the License is distributed on an "AS IS" BASIS,
-# WITHOUT WARRANTIES OR CONDITIONS OF ANY KIND, either express or implied.
-# See the License for the specific language governing permissions and
-# limitations under the License.
-"""Convert BERT checkpoint."""
-
-
-import argparse
-import logging
-import os
-
-import torch
-
-from transformers import (
- CONFIG_NAME,
- WEIGHTS_NAME,
- XLNetConfig,
- XLNetForQuestionAnswering,
- XLNetForSequenceClassification,
- XLNetLMHeadModel,
- load_tf_weights_in_xlnet,
-)
-
-
-GLUE_TASKS_NUM_LABELS = {
- "cola": 2,
- "mnli": 3,
- "mrpc": 2,
- "sst-2": 2,
- "sts-b": 1,
- "qqp": 2,
- "qnli": 2,
- "rte": 2,
- "wnli": 2,
-}
-
-
-logging.basicConfig(level=logging.INFO)
-
-
-def convert_xlnet_checkpoint_to_pytorch(
- tf_checkpoint_path, bert_config_file, pytorch_dump_folder_path, finetuning_task=None
-):
- # Initialise PyTorch model
- config = XLNetConfig.from_json_file(bert_config_file)
-
- finetuning_task = finetuning_task.lower() if finetuning_task is not None else ""
- if finetuning_task in GLUE_TASKS_NUM_LABELS:
- print("Building PyTorch XLNetForSequenceClassification model from configuration: {}".format(str(config)))
- config.finetuning_task = finetuning_task
- config.num_labels = GLUE_TASKS_NUM_LABELS[finetuning_task]
- model = XLNetForSequenceClassification(config)
- elif "squad" in finetuning_task:
- config.finetuning_task = finetuning_task
- model = XLNetForQuestionAnswering(config)
- else:
- model = XLNetLMHeadModel(config)
-
- # Load weights from tf checkpoint
- load_tf_weights_in_xlnet(model, config, tf_checkpoint_path)
-
- # Save pytorch-model
- pytorch_weights_dump_path = os.path.join(pytorch_dump_folder_path, WEIGHTS_NAME)
- pytorch_config_dump_path = os.path.join(pytorch_dump_folder_path, CONFIG_NAME)
- print("Save PyTorch model to {}".format(os.path.abspath(pytorch_weights_dump_path)))
- torch.save(model.state_dict(), pytorch_weights_dump_path)
- print("Save configuration file to {}".format(os.path.abspath(pytorch_config_dump_path)))
- with open(pytorch_config_dump_path, "w", encoding="utf-8") as f:
- f.write(config.to_json_string())
-
-
-if __name__ == "__main__":
- parser = argparse.ArgumentParser()
- # Required parameters
- parser.add_argument(
- "--tf_checkpoint_path", default=None, type=str, required=True, help="Path to the TensorFlow checkpoint path."
- )
- parser.add_argument(
- "--xlnet_config_file",
- default=None,
- type=str,
- required=True,
- help="The config json file corresponding to the pre-trained XLNet model. \n"
- "This specifies the model architecture.",
- )
- parser.add_argument(
- "--pytorch_dump_folder_path",
- default=None,
- type=str,
- required=True,
- help="Path to the folder to store the PyTorch model or dataset/vocab.",
- )
- parser.add_argument(
- "--finetuning_task",
- default=None,
- type=str,
- help="Name of a task on which the XLNet TensorFloaw model was fine-tuned",
- )
- args = parser.parse_args()
- print(args)
-
- convert_xlnet_checkpoint_to_pytorch(
- args.tf_checkpoint_path, args.xlnet_config_file, args.pytorch_dump_folder_path, args.finetuning_task
- )
diff --git a/server/transformers/src/transformers/data/__init__.py b/server/transformers/src/transformers/data/__init__.py
deleted file mode 100644
index 8d5f6b85b0292359a77a08b2b7f8d8d334f4202b..0000000000000000000000000000000000000000
--- a/server/transformers/src/transformers/data/__init__.py
+++ /dev/null
@@ -1,27 +0,0 @@
-# flake8: noqa
-# There's no way to ignore "F401 '...' imported but unused" warnings in this
-# module, but to preserve other warnings. So, don't check this module at all.
-
-from .metrics import is_sklearn_available
-from .processors import (
- DataProcessor,
- InputExample,
- InputFeatures,
- SingleSentenceClassificationProcessor,
- SquadExample,
- SquadFeatures,
- SquadV1Processor,
- SquadV2Processor,
- glue_convert_examples_to_features,
- glue_output_modes,
- glue_processors,
- glue_tasks_num_labels,
- squad_convert_examples_to_features,
- xnli_output_modes,
- xnli_processors,
- xnli_tasks_num_labels,
-)
-
-
-if is_sklearn_available():
- from .metrics import glue_compute_metrics, xnli_compute_metrics
diff --git a/server/transformers/src/transformers/data/metrics/__init__.py b/server/transformers/src/transformers/data/metrics/__init__.py
deleted file mode 100644
index 6c29c2313dd4bde827b724e1b0b24b2e300047da..0000000000000000000000000000000000000000
--- a/server/transformers/src/transformers/data/metrics/__init__.py
+++ /dev/null
@@ -1,85 +0,0 @@
-# coding=utf-8
-# Copyright 2018 The Google AI Language Team Authors and The HuggingFace Inc. team.
-# Copyright (c) 2018, NVIDIA CORPORATION. All rights reserved.
-#
-# Licensed under the Apache License, Version 2.0 (the "License");
-# you may not use this file except in compliance with the License.
-# You may obtain a copy of the License at
-#
-# http://www.apache.org/licenses/LICENSE-2.0
-#
-# Unless required by applicable law or agreed to in writing, software
-# distributed under the License is distributed on an "AS IS" BASIS,
-# WITHOUT WARRANTIES OR CONDITIONS OF ANY KIND, either express or implied.
-# See the License for the specific language governing permissions and
-# limitations under the License.
-
-try:
- from scipy.stats import pearsonr, spearmanr
- from sklearn.metrics import matthews_corrcoef, f1_score
-
- _has_sklearn = True
-except (AttributeError, ImportError):
- _has_sklearn = False
-
-
-def is_sklearn_available():
- return _has_sklearn
-
-
-if _has_sklearn:
-
- def simple_accuracy(preds, labels):
- return (preds == labels).mean()
-
- def acc_and_f1(preds, labels):
- acc = simple_accuracy(preds, labels)
- f1 = f1_score(y_true=labels, y_pred=preds)
- return {
- "acc": acc,
- "f1": f1,
- "acc_and_f1": (acc + f1) / 2,
- }
-
- def pearson_and_spearman(preds, labels):
- pearson_corr = pearsonr(preds, labels)[0]
- spearman_corr = spearmanr(preds, labels)[0]
- return {
- "pearson": pearson_corr,
- "spearmanr": spearman_corr,
- "corr": (pearson_corr + spearman_corr) / 2,
- }
-
- def glue_compute_metrics(task_name, preds, labels):
- assert len(preds) == len(labels)
- if task_name == "cola":
- return {"mcc": matthews_corrcoef(labels, preds)}
- elif task_name == "sst-2":
- return {"acc": simple_accuracy(preds, labels)}
- elif task_name == "mrpc":
- return acc_and_f1(preds, labels)
- elif task_name == "sts-b":
- return pearson_and_spearman(preds, labels)
- elif task_name == "qqp":
- return acc_and_f1(preds, labels)
- elif task_name == "mnli":
- return {"acc": simple_accuracy(preds, labels)}
- elif task_name == "mnli-mm":
- return {"acc": simple_accuracy(preds, labels)}
- elif task_name == "qnli":
- return {"acc": simple_accuracy(preds, labels)}
- elif task_name == "rte":
- return {"acc": simple_accuracy(preds, labels)}
- elif task_name == "wnli":
- return {"acc": simple_accuracy(preds, labels)}
- elif task_name == "hans":
- return {"acc": simple_accuracy(preds, labels)}
- else:
- raise KeyError(task_name)
-
- def xnli_compute_metrics(task_name, preds, labels):
- assert len(preds) == len(labels)
- if task_name == "xnli":
- return {"acc": simple_accuracy(preds, labels)}
- else:
- raise KeyError(task_name)
diff --git a/server/transformers/src/transformers/data/metrics/squad_metrics.py b/server/transformers/src/transformers/data/metrics/squad_metrics.py
deleted file mode 100644
index 54fdeb7c7ea1a4d69d7b380aba0f781153fb2ec7..0000000000000000000000000000000000000000
--- a/server/transformers/src/transformers/data/metrics/squad_metrics.py
+++ /dev/null
@@ -1,757 +0,0 @@
-""" Very heavily inspired by the official evaluation script for SQuAD version 2.0 which was
-modified by XLNet authors to update `find_best_threshold` scripts for SQuAD V2.0
-
-In addition to basic functionality, we also compute additional statistics and
-plot precision-recall curves if an additional na_prob.json file is provided.
-This file is expected to map question ID's to the model's predicted probability
-that a question is unanswerable.
-"""
-
-
-import collections
-import json
-import logging
-import math
-import re
-import string
-
-from transformers.tokenization_bert import BasicTokenizer
-
-
-logger = logging.getLogger(__name__)
-
-
-def normalize_answer(s):
- """Lower text and remove punctuation, articles and extra whitespace."""
-
- def remove_articles(text):
- regex = re.compile(r"\b(a|an|the)\b", re.UNICODE)
- return re.sub(regex, " ", text)
-
- def white_space_fix(text):
- return " ".join(text.split())
-
- def remove_punc(text):
- exclude = set(string.punctuation)
- return "".join(ch for ch in text if ch not in exclude)
-
- def lower(text):
- return text.lower()
-
- return white_space_fix(remove_articles(remove_punc(lower(s))))
-
-
-def get_tokens(s):
- if not s:
- return []
- return normalize_answer(s).split()
-
-
-def compute_exact(a_gold, a_pred):
- return int(normalize_answer(a_gold) == normalize_answer(a_pred))
-
-
-def compute_f1(a_gold, a_pred):
- gold_toks = get_tokens(a_gold)
- pred_toks = get_tokens(a_pred)
- common = collections.Counter(gold_toks) & collections.Counter(pred_toks)
- num_same = sum(common.values())
- if len(gold_toks) == 0 or len(pred_toks) == 0:
- # If either is no-answer, then F1 is 1 if they agree, 0 otherwise
- return int(gold_toks == pred_toks)
- if num_same == 0:
- return 0
- precision = 1.0 * num_same / len(pred_toks)
- recall = 1.0 * num_same / len(gold_toks)
- f1 = (2 * precision * recall) / (precision + recall)
- return f1
-
-
-def get_raw_scores(examples, preds):
- """
- Computes the exact and f1 scores from the examples and the model predictions
- """
- exact_scores = {}
- f1_scores = {}
-
- for example in examples:
- qas_id = example.qas_id
- gold_answers = [answer["text"] for answer in example.answers if normalize_answer(answer["text"])]
-
- if not gold_answers:
- # For unanswerable questions, only correct answer is empty string
- gold_answers = [""]
-
- if qas_id not in preds:
- print("Missing prediction for %s" % qas_id)
- continue
-
- prediction = preds[qas_id]
- exact_scores[qas_id] = max(compute_exact(a, prediction) for a in gold_answers)
- f1_scores[qas_id] = max(compute_f1(a, prediction) for a in gold_answers)
-
- return exact_scores, f1_scores
-
-
-def apply_no_ans_threshold(scores, na_probs, qid_to_has_ans, na_prob_thresh):
- new_scores = {}
- for qid, s in scores.items():
- pred_na = na_probs[qid] > na_prob_thresh
- if pred_na:
- new_scores[qid] = float(not qid_to_has_ans[qid])
- else:
- new_scores[qid] = s
- return new_scores
-
-
-def make_eval_dict(exact_scores, f1_scores, qid_list=None):
- if not qid_list:
- total = len(exact_scores)
- return collections.OrderedDict(
- [
- ("exact", 100.0 * sum(exact_scores.values()) / total),
- ("f1", 100.0 * sum(f1_scores.values()) / total),
- ("total", total),
- ]
- )
- else:
- total = len(qid_list)
- return collections.OrderedDict(
- [
- ("exact", 100.0 * sum(exact_scores[k] for k in qid_list) / total),
- ("f1", 100.0 * sum(f1_scores[k] for k in qid_list) / total),
- ("total", total),
- ]
- )
-
-
-def merge_eval(main_eval, new_eval, prefix):
- for k in new_eval:
- main_eval["%s_%s" % (prefix, k)] = new_eval[k]
-
-
-def find_best_thresh_v2(preds, scores, na_probs, qid_to_has_ans):
- num_no_ans = sum(1 for k in qid_to_has_ans if not qid_to_has_ans[k])
- cur_score = num_no_ans
- best_score = cur_score
- best_thresh = 0.0
- qid_list = sorted(na_probs, key=lambda k: na_probs[k])
- for i, qid in enumerate(qid_list):
- if qid not in scores:
- continue
- if qid_to_has_ans[qid]:
- diff = scores[qid]
- else:
- if preds[qid]:
- diff = -1
- else:
- diff = 0
- cur_score += diff
- if cur_score > best_score:
- best_score = cur_score
- best_thresh = na_probs[qid]
-
- has_ans_score, has_ans_cnt = 0, 0
- for qid in qid_list:
- if not qid_to_has_ans[qid]:
- continue
- has_ans_cnt += 1
-
- if qid not in scores:
- continue
- has_ans_score += scores[qid]
-
- return 100.0 * best_score / len(scores), best_thresh, 1.0 * has_ans_score / has_ans_cnt
-
-
-def find_all_best_thresh_v2(main_eval, preds, exact_raw, f1_raw, na_probs, qid_to_has_ans):
- best_exact, exact_thresh, has_ans_exact = find_best_thresh_v2(preds, exact_raw, na_probs, qid_to_has_ans)
- best_f1, f1_thresh, has_ans_f1 = find_best_thresh_v2(preds, f1_raw, na_probs, qid_to_has_ans)
- main_eval["best_exact"] = best_exact
- main_eval["best_exact_thresh"] = exact_thresh
- main_eval["best_f1"] = best_f1
- main_eval["best_f1_thresh"] = f1_thresh
- main_eval["has_ans_exact"] = has_ans_exact
- main_eval["has_ans_f1"] = has_ans_f1
-
-
-def find_best_thresh(preds, scores, na_probs, qid_to_has_ans):
- num_no_ans = sum(1 for k in qid_to_has_ans if not qid_to_has_ans[k])
- cur_score = num_no_ans
- best_score = cur_score
- best_thresh = 0.0
- qid_list = sorted(na_probs, key=lambda k: na_probs[k])
- for _, qid in enumerate(qid_list):
- if qid not in scores:
- continue
- if qid_to_has_ans[qid]:
- diff = scores[qid]
- else:
- if preds[qid]:
- diff = -1
- else:
- diff = 0
- cur_score += diff
- if cur_score > best_score:
- best_score = cur_score
- best_thresh = na_probs[qid]
- return 100.0 * best_score / len(scores), best_thresh
-
-
-def find_all_best_thresh(main_eval, preds, exact_raw, f1_raw, na_probs, qid_to_has_ans):
- best_exact, exact_thresh = find_best_thresh(preds, exact_raw, na_probs, qid_to_has_ans)
- best_f1, f1_thresh = find_best_thresh(preds, f1_raw, na_probs, qid_to_has_ans)
-
- main_eval["best_exact"] = best_exact
- main_eval["best_exact_thresh"] = exact_thresh
- main_eval["best_f1"] = best_f1
- main_eval["best_f1_thresh"] = f1_thresh
-
-
-def squad_evaluate(examples, preds, no_answer_probs=None, no_answer_probability_threshold=1.0):
- qas_id_to_has_answer = {example.qas_id: bool(example.answers) for example in examples}
- has_answer_qids = [qas_id for qas_id, has_answer in qas_id_to_has_answer.items() if has_answer]
- no_answer_qids = [qas_id for qas_id, has_answer in qas_id_to_has_answer.items() if not has_answer]
-
- if no_answer_probs is None:
- no_answer_probs = {k: 0.0 for k in preds}
-
- exact, f1 = get_raw_scores(examples, preds)
-
- exact_threshold = apply_no_ans_threshold(
- exact, no_answer_probs, qas_id_to_has_answer, no_answer_probability_threshold
- )
- f1_threshold = apply_no_ans_threshold(f1, no_answer_probs, qas_id_to_has_answer, no_answer_probability_threshold)
-
- evaluation = make_eval_dict(exact_threshold, f1_threshold)
-
- if has_answer_qids:
- has_ans_eval = make_eval_dict(exact_threshold, f1_threshold, qid_list=has_answer_qids)
- merge_eval(evaluation, has_ans_eval, "HasAns")
-
- if no_answer_qids:
- no_ans_eval = make_eval_dict(exact_threshold, f1_threshold, qid_list=no_answer_qids)
- merge_eval(evaluation, no_ans_eval, "NoAns")
-
- if no_answer_probs:
- find_all_best_thresh(evaluation, preds, exact, f1, no_answer_probs, qas_id_to_has_answer)
-
- return evaluation
-
-
-def get_final_text(pred_text, orig_text, do_lower_case, verbose_logging=False):
- """Project the tokenized prediction back to the original text."""
-
- # When we created the data, we kept track of the alignment between original
- # (whitespace tokenized) tokens and our WordPiece tokenized tokens. So
- # now `orig_text` contains the span of our original text corresponding to the
- # span that we predicted.
- #
- # However, `orig_text` may contain extra characters that we don't want in
- # our prediction.
- #
- # For example, let's say:
- # pred_text = steve smith
- # orig_text = Steve Smith's
- #
- # We don't want to return `orig_text` because it contains the extra "'s".
- #
- # We don't want to return `pred_text` because it's already been normalized
- # (the SQuAD eval script also does punctuation stripping/lower casing but
- # our tokenizer does additional normalization like stripping accent
- # characters).
- #
- # What we really want to return is "Steve Smith".
- #
- # Therefore, we have to apply a semi-complicated alignment heuristic between
- # `pred_text` and `orig_text` to get a character-to-character alignment. This
- # can fail in certain cases in which case we just return `orig_text`.
-
- def _strip_spaces(text):
- ns_chars = []
- ns_to_s_map = collections.OrderedDict()
- for (i, c) in enumerate(text):
- if c == " ":
- continue
- ns_to_s_map[len(ns_chars)] = i
- ns_chars.append(c)
- ns_text = "".join(ns_chars)
- return (ns_text, ns_to_s_map)
-
- # We first tokenize `orig_text`, strip whitespace from the result
- # and `pred_text`, and check if they are the same length. If they are
- # NOT the same length, the heuristic has failed. If they are the same
- # length, we assume the characters are one-to-one aligned.
- tokenizer = BasicTokenizer(do_lower_case=do_lower_case)
-
- tok_text = " ".join(tokenizer.tokenize(orig_text))
-
- start_position = tok_text.find(pred_text)
- if start_position == -1:
- if verbose_logging:
- logger.info("Unable to find text: '%s' in '%s'" % (pred_text, orig_text))
- return orig_text
- end_position = start_position + len(pred_text) - 1
-
- (orig_ns_text, orig_ns_to_s_map) = _strip_spaces(orig_text)
- (tok_ns_text, tok_ns_to_s_map) = _strip_spaces(tok_text)
-
- if len(orig_ns_text) != len(tok_ns_text):
- if verbose_logging:
- logger.info("Length not equal after stripping spaces: '%s' vs '%s'", orig_ns_text, tok_ns_text)
- return orig_text
-
- # We then project the characters in `pred_text` back to `orig_text` using
- # the character-to-character alignment.
- tok_s_to_ns_map = {}
- for (i, tok_index) in tok_ns_to_s_map.items():
- tok_s_to_ns_map[tok_index] = i
-
- orig_start_position = None
- if start_position in tok_s_to_ns_map:
- ns_start_position = tok_s_to_ns_map[start_position]
- if ns_start_position in orig_ns_to_s_map:
- orig_start_position = orig_ns_to_s_map[ns_start_position]
-
- if orig_start_position is None:
- if verbose_logging:
- logger.info("Couldn't map start position")
- return orig_text
-
- orig_end_position = None
- if end_position in tok_s_to_ns_map:
- ns_end_position = tok_s_to_ns_map[end_position]
- if ns_end_position in orig_ns_to_s_map:
- orig_end_position = orig_ns_to_s_map[ns_end_position]
-
- if orig_end_position is None:
- if verbose_logging:
- logger.info("Couldn't map end position")
- return orig_text
-
- output_text = orig_text[orig_start_position : (orig_end_position + 1)]
- return output_text
-
-
-def _get_best_indexes(logits, n_best_size):
- """Get the n-best logits from a list."""
- index_and_score = sorted(enumerate(logits), key=lambda x: x[1], reverse=True)
-
- best_indexes = []
- for i in range(len(index_and_score)):
- if i >= n_best_size:
- break
- best_indexes.append(index_and_score[i][0])
- return best_indexes
-
-
-def _compute_softmax(scores):
- """Compute softmax probability over raw logits."""
- if not scores:
- return []
-
- max_score = None
- for score in scores:
- if max_score is None or score > max_score:
- max_score = score
-
- exp_scores = []
- total_sum = 0.0
- for score in scores:
- x = math.exp(score - max_score)
- exp_scores.append(x)
- total_sum += x
-
- probs = []
- for score in exp_scores:
- probs.append(score / total_sum)
- return probs
-
-
-def compute_predictions_logits(
- all_examples,
- all_features,
- all_results,
- n_best_size,
- max_answer_length,
- do_lower_case,
- output_prediction_file,
- output_nbest_file,
- output_null_log_odds_file,
- verbose_logging,
- version_2_with_negative,
- null_score_diff_threshold,
- tokenizer,
-):
- """Write final predictions to the json file and log-odds of null if needed."""
- logger.info("Writing predictions to: %s" % (output_prediction_file))
- logger.info("Writing nbest to: %s" % (output_nbest_file))
-
- example_index_to_features = collections.defaultdict(list)
- for feature in all_features:
- example_index_to_features[feature.example_index].append(feature)
-
- unique_id_to_result = {}
- for result in all_results:
- unique_id_to_result[result.unique_id] = result
-
- _PrelimPrediction = collections.namedtuple( # pylint: disable=invalid-name
- "PrelimPrediction", ["feature_index", "start_index", "end_index", "start_logit", "end_logit"]
- )
-
- all_predictions = collections.OrderedDict()
- all_nbest_json = collections.OrderedDict()
- scores_diff_json = collections.OrderedDict()
-
- for (example_index, example) in enumerate(all_examples):
- features = example_index_to_features[example_index]
-
- prelim_predictions = []
- # keep track of the minimum score of null start+end of position 0
- score_null = 1000000 # large and positive
- min_null_feature_index = 0 # the paragraph slice with min null score
- null_start_logit = 0 # the start logit at the slice with min null score
- null_end_logit = 0 # the end logit at the slice with min null score
- for (feature_index, feature) in enumerate(features):
- result = unique_id_to_result[feature.unique_id]
- start_indexes = _get_best_indexes(result.start_logits, n_best_size)
- end_indexes = _get_best_indexes(result.end_logits, n_best_size)
- # if we could have irrelevant answers, get the min score of irrelevant
- if version_2_with_negative:
- feature_null_score = result.start_logits[0] + result.end_logits[0]
- if feature_null_score < score_null:
- score_null = feature_null_score
- min_null_feature_index = feature_index
- null_start_logit = result.start_logits[0]
- null_end_logit = result.end_logits[0]
- for start_index in start_indexes:
- for end_index in end_indexes:
- # We could hypothetically create invalid predictions, e.g., predict
- # that the start of the span is in the question. We throw out all
- # invalid predictions.
- if start_index >= len(feature.tokens):
- continue
- if end_index >= len(feature.tokens):
- continue
- if start_index not in feature.token_to_orig_map:
- continue
- if end_index not in feature.token_to_orig_map:
- continue
- if not feature.token_is_max_context.get(start_index, False):
- continue
- if end_index < start_index:
- continue
- length = end_index - start_index + 1
- if length > max_answer_length:
- continue
- prelim_predictions.append(
- _PrelimPrediction(
- feature_index=feature_index,
- start_index=start_index,
- end_index=end_index,
- start_logit=result.start_logits[start_index],
- end_logit=result.end_logits[end_index],
- )
- )
- if version_2_with_negative:
- prelim_predictions.append(
- _PrelimPrediction(
- feature_index=min_null_feature_index,
- start_index=0,
- end_index=0,
- start_logit=null_start_logit,
- end_logit=null_end_logit,
- )
- )
- prelim_predictions = sorted(prelim_predictions, key=lambda x: (x.start_logit + x.end_logit), reverse=True)
-
- _NbestPrediction = collections.namedtuple( # pylint: disable=invalid-name
- "NbestPrediction", ["text", "start_logit", "end_logit"]
- )
-
- seen_predictions = {}
- nbest = []
- for pred in prelim_predictions:
- if len(nbest) >= n_best_size:
- break
- feature = features[pred.feature_index]
- if pred.start_index > 0: # this is a non-null prediction
- tok_tokens = feature.tokens[pred.start_index : (pred.end_index + 1)]
- orig_doc_start = feature.token_to_orig_map[pred.start_index]
- orig_doc_end = feature.token_to_orig_map[pred.end_index]
- orig_tokens = example.doc_tokens[orig_doc_start : (orig_doc_end + 1)]
-
- tok_text = tokenizer.convert_tokens_to_string(tok_tokens)
-
- # tok_text = " ".join(tok_tokens)
- #
- # # De-tokenize WordPieces that have been split off.
- # tok_text = tok_text.replace(" ##", "")
- # tok_text = tok_text.replace("##", "")
-
- # Clean whitespace
- tok_text = tok_text.strip()
- tok_text = " ".join(tok_text.split())
- orig_text = " ".join(orig_tokens)
-
- final_text = get_final_text(tok_text, orig_text, do_lower_case, verbose_logging)
- if final_text in seen_predictions:
- continue
-
- seen_predictions[final_text] = True
- else:
- final_text = ""
- seen_predictions[final_text] = True
-
- nbest.append(_NbestPrediction(text=final_text, start_logit=pred.start_logit, end_logit=pred.end_logit))
- # if we didn't include the empty option in the n-best, include it
- if version_2_with_negative:
- if "" not in seen_predictions:
- nbest.append(_NbestPrediction(text="", start_logit=null_start_logit, end_logit=null_end_logit))
-
- # In very rare edge cases we could only have single null prediction.
- # So we just create a nonce prediction in this case to avoid failure.
- if len(nbest) == 1:
- nbest.insert(0, _NbestPrediction(text="empty", start_logit=0.0, end_logit=0.0))
-
- # In very rare edge cases we could have no valid predictions. So we
- # just create a nonce prediction in this case to avoid failure.
- if not nbest:
- nbest.append(_NbestPrediction(text="empty", start_logit=0.0, end_logit=0.0))
-
- assert len(nbest) >= 1
-
- total_scores = []
- best_non_null_entry = None
- for entry in nbest:
- total_scores.append(entry.start_logit + entry.end_logit)
- if not best_non_null_entry:
- if entry.text:
- best_non_null_entry = entry
-
- probs = _compute_softmax(total_scores)
-
- nbest_json = []
- for (i, entry) in enumerate(nbest):
- output = collections.OrderedDict()
- output["text"] = entry.text
- output["probability"] = probs[i]
- output["start_logit"] = entry.start_logit
- output["end_logit"] = entry.end_logit
- nbest_json.append(output)
-
- assert len(nbest_json) >= 1
-
- if not version_2_with_negative:
- all_predictions[example.qas_id] = nbest_json[0]["text"]
- else:
- # predict "" iff the null score - the score of best non-null > threshold
- score_diff = score_null - best_non_null_entry.start_logit - (best_non_null_entry.end_logit)
- scores_diff_json[example.qas_id] = score_diff
- if score_diff > null_score_diff_threshold:
- all_predictions[example.qas_id] = ""
- else:
- all_predictions[example.qas_id] = best_non_null_entry.text
- all_nbest_json[example.qas_id] = nbest_json
-
- with open(output_prediction_file, "w") as writer:
- writer.write(json.dumps(all_predictions, indent=4) + "\n")
-
- with open(output_nbest_file, "w") as writer:
- writer.write(json.dumps(all_nbest_json, indent=4) + "\n")
-
- if version_2_with_negative:
- with open(output_null_log_odds_file, "w") as writer:
- writer.write(json.dumps(scores_diff_json, indent=4) + "\n")
-
- return all_predictions
-
-
-def compute_predictions_log_probs(
- all_examples,
- all_features,
- all_results,
- n_best_size,
- max_answer_length,
- output_prediction_file,
- output_nbest_file,
- output_null_log_odds_file,
- start_n_top,
- end_n_top,
- version_2_with_negative,
- tokenizer,
- verbose_logging,
-):
- """ XLNet write prediction logic (more complex than Bert's).
- Write final predictions to the json file and log-odds of null if needed.
-
- Requires utils_squad_evaluate.py
- """
- _PrelimPrediction = collections.namedtuple( # pylint: disable=invalid-name
- "PrelimPrediction", ["feature_index", "start_index", "end_index", "start_log_prob", "end_log_prob"]
- )
-
- _NbestPrediction = collections.namedtuple( # pylint: disable=invalid-name
- "NbestPrediction", ["text", "start_log_prob", "end_log_prob"]
- )
-
- logger.info("Writing predictions to: %s", output_prediction_file)
- # logger.info("Writing nbest to: %s" % (output_nbest_file))
-
- example_index_to_features = collections.defaultdict(list)
- for feature in all_features:
- example_index_to_features[feature.example_index].append(feature)
-
- unique_id_to_result = {}
- for result in all_results:
- unique_id_to_result[result.unique_id] = result
-
- all_predictions = collections.OrderedDict()
- all_nbest_json = collections.OrderedDict()
- scores_diff_json = collections.OrderedDict()
-
- for (example_index, example) in enumerate(all_examples):
- features = example_index_to_features[example_index]
-
- prelim_predictions = []
- # keep track of the minimum score of null start+end of position 0
- score_null = 1000000 # large and positive
-
- for (feature_index, feature) in enumerate(features):
- result = unique_id_to_result[feature.unique_id]
-
- cur_null_score = result.cls_logits
-
- # if we could have irrelevant answers, get the min score of irrelevant
- score_null = min(score_null, cur_null_score)
-
- for i in range(start_n_top):
- for j in range(end_n_top):
- start_log_prob = result.start_logits[i]
- start_index = result.start_top_index[i]
-
- j_index = i * end_n_top + j
-
- end_log_prob = result.end_logits[j_index]
- end_index = result.end_top_index[j_index]
-
- # We could hypothetically create invalid predictions, e.g., predict
- # that the start of the span is in the question. We throw out all
- # invalid predictions.
- if start_index >= feature.paragraph_len - 1:
- continue
- if end_index >= feature.paragraph_len - 1:
- continue
-
- if not feature.token_is_max_context.get(start_index, False):
- continue
- if end_index < start_index:
- continue
- length = end_index - start_index + 1
- if length > max_answer_length:
- continue
-
- prelim_predictions.append(
- _PrelimPrediction(
- feature_index=feature_index,
- start_index=start_index,
- end_index=end_index,
- start_log_prob=start_log_prob,
- end_log_prob=end_log_prob,
- )
- )
-
- prelim_predictions = sorted(
- prelim_predictions, key=lambda x: (x.start_log_prob + x.end_log_prob), reverse=True
- )
-
- seen_predictions = {}
- nbest = []
- for pred in prelim_predictions:
- if len(nbest) >= n_best_size:
- break
- feature = features[pred.feature_index]
-
- # XLNet un-tokenizer
- # Let's keep it simple for now and see if we need all this later.
- #
- # tok_start_to_orig_index = feature.tok_start_to_orig_index
- # tok_end_to_orig_index = feature.tok_end_to_orig_index
- # start_orig_pos = tok_start_to_orig_index[pred.start_index]
- # end_orig_pos = tok_end_to_orig_index[pred.end_index]
- # paragraph_text = example.paragraph_text
- # final_text = paragraph_text[start_orig_pos: end_orig_pos + 1].strip()
-
- # Previously used Bert untokenizer
- tok_tokens = feature.tokens[pred.start_index : (pred.end_index + 1)]
- orig_doc_start = feature.token_to_orig_map[pred.start_index]
- orig_doc_end = feature.token_to_orig_map[pred.end_index]
- orig_tokens = example.doc_tokens[orig_doc_start : (orig_doc_end + 1)]
- tok_text = tokenizer.convert_tokens_to_string(tok_tokens)
-
- # Clean whitespace
- tok_text = tok_text.strip()
- tok_text = " ".join(tok_text.split())
- orig_text = " ".join(orig_tokens)
-
- if hasattr(tokenizer, "do_lower_case"):
- do_lower_case = tokenizer.do_lower_case
- else:
- do_lower_case = tokenizer.do_lowercase_and_remove_accent
-
- final_text = get_final_text(tok_text, orig_text, do_lower_case, verbose_logging)
-
- if final_text in seen_predictions:
- continue
-
- seen_predictions[final_text] = True
-
- nbest.append(
- _NbestPrediction(text=final_text, start_log_prob=pred.start_log_prob, end_log_prob=pred.end_log_prob)
- )
-
- # In very rare edge cases we could have no valid predictions. So we
- # just create a nonce prediction in this case to avoid failure.
- if not nbest:
- nbest.append(_NbestPrediction(text="", start_log_prob=-1e6, end_log_prob=-1e6))
-
- total_scores = []
- best_non_null_entry = None
- for entry in nbest:
- total_scores.append(entry.start_log_prob + entry.end_log_prob)
- if not best_non_null_entry:
- best_non_null_entry = entry
-
- probs = _compute_softmax(total_scores)
-
- nbest_json = []
- for (i, entry) in enumerate(nbest):
- output = collections.OrderedDict()
- output["text"] = entry.text
- output["probability"] = probs[i]
- output["start_log_prob"] = entry.start_log_prob
- output["end_log_prob"] = entry.end_log_prob
- nbest_json.append(output)
-
- assert len(nbest_json) >= 1
- assert best_non_null_entry is not None
-
- score_diff = score_null
- scores_diff_json[example.qas_id] = score_diff
- # note(zhiliny): always predict best_non_null_entry
- # and the evaluation script will search for the best threshold
- all_predictions[example.qas_id] = best_non_null_entry.text
-
- all_nbest_json[example.qas_id] = nbest_json
-
- with open(output_prediction_file, "w") as writer:
- writer.write(json.dumps(all_predictions, indent=4) + "\n")
-
- with open(output_nbest_file, "w") as writer:
- writer.write(json.dumps(all_nbest_json, indent=4) + "\n")
-
- if version_2_with_negative:
- with open(output_null_log_odds_file, "w") as writer:
- writer.write(json.dumps(scores_diff_json, indent=4) + "\n")
-
- return all_predictions
diff --git a/server/transformers/src/transformers/data/processors/__init__.py b/server/transformers/src/transformers/data/processors/__init__.py
deleted file mode 100644
index 4cb37faf2511f8ee48d7efb83ff38fca92cae892..0000000000000000000000000000000000000000
--- a/server/transformers/src/transformers/data/processors/__init__.py
+++ /dev/null
@@ -1,8 +0,0 @@
-# flake8: noqa
-# There's no way to ignore "F401 '...' imported but unused" warnings in this
-# module, but to preserve other warnings. So, don't check this module at all.
-
-from .glue import glue_convert_examples_to_features, glue_output_modes, glue_processors, glue_tasks_num_labels
-from .squad import SquadExample, SquadFeatures, SquadV1Processor, SquadV2Processor, squad_convert_examples_to_features
-from .utils import DataProcessor, InputExample, InputFeatures, SingleSentenceClassificationProcessor
-from .xnli import xnli_output_modes, xnli_processors, xnli_tasks_num_labels
diff --git a/server/transformers/src/transformers/data/processors/glue.py b/server/transformers/src/transformers/data/processors/glue.py
deleted file mode 100644
index 87885577fabb564556626dbaee549ad2bb0be4fb..0000000000000000000000000000000000000000
--- a/server/transformers/src/transformers/data/processors/glue.py
+++ /dev/null
@@ -1,555 +0,0 @@
-# coding=utf-8
-# Copyright 2018 The Google AI Language Team Authors and The HuggingFace Inc. team.
-# Copyright (c) 2018, NVIDIA CORPORATION. All rights reserved.
-#
-# Licensed under the Apache License, Version 2.0 (the "License");
-# you may not use this file except in compliance with the License.
-# You may obtain a copy of the License at
-#
-# http://www.apache.org/licenses/LICENSE-2.0
-#
-# Unless required by applicable law or agreed to in writing, software
-# distributed under the License is distributed on an "AS IS" BASIS,
-# WITHOUT WARRANTIES OR CONDITIONS OF ANY KIND, either express or implied.
-# See the License for the specific language governing permissions and
-# limitations under the License.
-""" GLUE processors and helpers """
-
-import logging
-import os
-
-from ...file_utils import is_tf_available
-from .utils import DataProcessor, InputExample, InputFeatures
-
-
-if is_tf_available():
- import tensorflow as tf
-
-logger = logging.getLogger(__name__)
-
-
-def glue_convert_examples_to_features(
- examples,
- tokenizer,
- max_length=512,
- task=None,
- label_list=None,
- output_mode=None,
- pad_on_left=False,
- pad_token=0,
- pad_token_segment_id=0,
- mask_padding_with_zero=True,
-):
- """
- Loads a data file into a list of ``InputFeatures``
-
- Args:
- examples: List of ``InputExamples`` or ``tf.data.Dataset`` containing the examples.
- tokenizer: Instance of a tokenizer that will tokenize the examples
- max_length: Maximum example length
- task: GLUE task
- label_list: List of labels. Can be obtained from the processor using the ``processor.get_labels()`` method
- output_mode: String indicating the output mode. Either ``regression`` or ``classification``
- pad_on_left: If set to ``True``, the examples will be padded on the left rather than on the right (default)
- pad_token: Padding token
- pad_token_segment_id: The segment ID for the padding token (It is usually 0, but can vary such as for XLNet where it is 4)
- mask_padding_with_zero: If set to ``True``, the attention mask will be filled by ``1`` for actual values
- and by ``0`` for padded values. If set to ``False``, inverts it (``1`` for padded values, ``0`` for
- actual values)
-
- Returns:
- If the ``examples`` input is a ``tf.data.Dataset``, will return a ``tf.data.Dataset``
- containing the task-specific features. If the input is a list of ``InputExamples``, will return
- a list of task-specific ``InputFeatures`` which can be fed to the model.
-
- """
- is_tf_dataset = False
- if is_tf_available() and isinstance(examples, tf.data.Dataset):
- is_tf_dataset = True
-
- if task is not None:
- processor = glue_processors[task]()
- if label_list is None:
- label_list = processor.get_labels()
- logger.info("Using label list %s for task %s" % (label_list, task))
- if output_mode is None:
- output_mode = glue_output_modes[task]
- logger.info("Using output mode %s for task %s" % (output_mode, task))
-
- label_map = {label: i for i, label in enumerate(label_list)}
-
- features = []
- for (ex_index, example) in enumerate(examples):
- len_examples = 0
- if is_tf_dataset:
- example = processor.get_example_from_tensor_dict(example)
- example = processor.tfds_map(example)
- len_examples = tf.data.experimental.cardinality(examples)
- else:
- len_examples = len(examples)
- if ex_index % 10000 == 0:
- logger.info("Writing example %d/%d" % (ex_index, len_examples))
-
- inputs = tokenizer.encode_plus(example.text_a, example.text_b, add_special_tokens=True, max_length=max_length,)
- input_ids, token_type_ids = inputs["input_ids"], inputs["token_type_ids"]
-
- # The mask has 1 for real tokens and 0 for padding tokens. Only real
- # tokens are attended to.
- attention_mask = [1 if mask_padding_with_zero else 0] * len(input_ids)
-
- # Zero-pad up to the sequence length.
- padding_length = max_length - len(input_ids)
- if pad_on_left:
- input_ids = ([pad_token] * padding_length) + input_ids
- attention_mask = ([0 if mask_padding_with_zero else 1] * padding_length) + attention_mask
- token_type_ids = ([pad_token_segment_id] * padding_length) + token_type_ids
- else:
- input_ids = input_ids + ([pad_token] * padding_length)
- attention_mask = attention_mask + ([0 if mask_padding_with_zero else 1] * padding_length)
- token_type_ids = token_type_ids + ([pad_token_segment_id] * padding_length)
-
- assert len(input_ids) == max_length, "Error with input length {} vs {}".format(len(input_ids), max_length)
- assert len(attention_mask) == max_length, "Error with input length {} vs {}".format(
- len(attention_mask), max_length
- )
- assert len(token_type_ids) == max_length, "Error with input length {} vs {}".format(
- len(token_type_ids), max_length
- )
-
- if output_mode == "classification":
- label = label_map[example.label]
- elif output_mode == "regression":
- label = float(example.label)
- else:
- raise KeyError(output_mode)
-
- if ex_index < 5:
- logger.info("*** Example ***")
- logger.info("guid: %s" % (example.guid))
- logger.info("input_ids: %s" % " ".join([str(x) for x in input_ids]))
- logger.info("attention_mask: %s" % " ".join([str(x) for x in attention_mask]))
- logger.info("token_type_ids: %s" % " ".join([str(x) for x in token_type_ids]))
- logger.info("label: %s (id = %d)" % (example.label, label))
-
- features.append(
- InputFeatures(
- input_ids=input_ids, attention_mask=attention_mask, token_type_ids=token_type_ids, label=label
- )
- )
-
- if is_tf_available() and is_tf_dataset:
-
- def gen():
- for ex in features:
- yield (
- {
- "input_ids": ex.input_ids,
- "attention_mask": ex.attention_mask,
- "token_type_ids": ex.token_type_ids,
- },
- ex.label,
- )
-
- return tf.data.Dataset.from_generator(
- gen,
- ({"input_ids": tf.int32, "attention_mask": tf.int32, "token_type_ids": tf.int32}, tf.int64),
- (
- {
- "input_ids": tf.TensorShape([None]),
- "attention_mask": tf.TensorShape([None]),
- "token_type_ids": tf.TensorShape([None]),
- },
- tf.TensorShape([]),
- ),
- )
-
- return features
-
-
-class MrpcProcessor(DataProcessor):
- """Processor for the MRPC data set (GLUE version)."""
-
- def get_example_from_tensor_dict(self, tensor_dict):
- """See base class."""
- return InputExample(
- tensor_dict["idx"].numpy(),
- tensor_dict["sentence1"].numpy().decode("utf-8"),
- tensor_dict["sentence2"].numpy().decode("utf-8"),
- str(tensor_dict["label"].numpy()),
- )
-
- def get_train_examples(self, data_dir):
- """See base class."""
- logger.info("LOOKING AT {}".format(os.path.join(data_dir, "train.tsv")))
- return self._create_examples(self._read_tsv(os.path.join(data_dir, "train.tsv")), "train")
-
- def get_dev_examples(self, data_dir):
- """See base class."""
- return self._create_examples(self._read_tsv(os.path.join(data_dir, "dev.tsv")), "dev")
-
- def get_labels(self):
- """See base class."""
- return ["0", "1"]
-
- def _create_examples(self, lines, set_type):
- """Creates examples for the training and dev sets."""
- examples = []
- for (i, line) in enumerate(lines):
- if i == 0:
- continue
- guid = "%s-%s" % (set_type, i)
- text_a = line[3]
- text_b = line[4]
- label = line[0]
- examples.append(InputExample(guid=guid, text_a=text_a, text_b=text_b, label=label))
- return examples
-
-
-class MnliProcessor(DataProcessor):
- """Processor for the MultiNLI data set (GLUE version)."""
-
- def get_example_from_tensor_dict(self, tensor_dict):
- """See base class."""
- return InputExample(
- tensor_dict["idx"].numpy(),
- tensor_dict["premise"].numpy().decode("utf-8"),
- tensor_dict["hypothesis"].numpy().decode("utf-8"),
- str(tensor_dict["label"].numpy()),
- )
-
- def get_train_examples(self, data_dir):
- """See base class."""
- return self._create_examples(self._read_tsv(os.path.join(data_dir, "train.tsv")), "train")
-
- def get_dev_examples(self, data_dir):
- """See base class."""
- return self._create_examples(self._read_tsv(os.path.join(data_dir, "dev_matched.tsv")), "dev_matched")
-
- def get_labels(self):
- """See base class."""
- return ["contradiction", "entailment", "neutral"]
-
- def _create_examples(self, lines, set_type):
- """Creates examples for the training and dev sets."""
- examples = []
- for (i, line) in enumerate(lines):
- if i == 0:
- continue
- guid = "%s-%s" % (set_type, line[0])
- text_a = line[8]
- text_b = line[9]
- label = line[-1]
- examples.append(InputExample(guid=guid, text_a=text_a, text_b=text_b, label=label))
- return examples
-
-
-class MnliMismatchedProcessor(MnliProcessor):
- """Processor for the MultiNLI Mismatched data set (GLUE version)."""
-
- def get_dev_examples(self, data_dir):
- """See base class."""
- return self._create_examples(self._read_tsv(os.path.join(data_dir, "dev_mismatched.tsv")), "dev_matched")
-
-
-class ColaProcessor(DataProcessor):
- """Processor for the CoLA data set (GLUE version)."""
-
- def get_example_from_tensor_dict(self, tensor_dict):
- """See base class."""
- return InputExample(
- tensor_dict["idx"].numpy(),
- tensor_dict["sentence"].numpy().decode("utf-8"),
- None,
- str(tensor_dict["label"].numpy()),
- )
-
- def get_train_examples(self, data_dir):
- """See base class."""
- return self._create_examples(self._read_tsv(os.path.join(data_dir, "train.tsv")), "train")
-
- def get_dev_examples(self, data_dir):
- """See base class."""
- return self._create_examples(self._read_tsv(os.path.join(data_dir, "dev.tsv")), "dev")
-
- def get_labels(self):
- """See base class."""
- return ["0", "1"]
-
- def _create_examples(self, lines, set_type):
- """Creates examples for the training and dev sets."""
- examples = []
- for (i, line) in enumerate(lines):
- guid = "%s-%s" % (set_type, i)
- text_a = line[3]
- label = line[1]
- examples.append(InputExample(guid=guid, text_a=text_a, text_b=None, label=label))
- return examples
-
-
-class Sst2Processor(DataProcessor):
- """Processor for the SST-2 data set (GLUE version)."""
-
- def get_example_from_tensor_dict(self, tensor_dict):
- """See base class."""
- return InputExample(
- tensor_dict["idx"].numpy(),
- tensor_dict["sentence"].numpy().decode("utf-8"),
- None,
- str(tensor_dict["label"].numpy()),
- )
-
- def get_train_examples(self, data_dir):
- """See base class."""
- return self._create_examples(self._read_tsv(os.path.join(data_dir, "train.tsv")), "train")
-
- def get_dev_examples(self, data_dir):
- """See base class."""
- return self._create_examples(self._read_tsv(os.path.join(data_dir, "dev.tsv")), "dev")
-
- def get_labels(self):
- """See base class."""
- return ["0", "1"]
-
- def _create_examples(self, lines, set_type):
- """Creates examples for the training and dev sets."""
- examples = []
- for (i, line) in enumerate(lines):
- if i == 0:
- continue
- guid = "%s-%s" % (set_type, i)
- text_a = line[0]
- label = line[1]
- examples.append(InputExample(guid=guid, text_a=text_a, text_b=None, label=label))
- return examples
-
-
-class StsbProcessor(DataProcessor):
- """Processor for the STS-B data set (GLUE version)."""
-
- def get_example_from_tensor_dict(self, tensor_dict):
- """See base class."""
- return InputExample(
- tensor_dict["idx"].numpy(),
- tensor_dict["sentence1"].numpy().decode("utf-8"),
- tensor_dict["sentence2"].numpy().decode("utf-8"),
- str(tensor_dict["label"].numpy()),
- )
-
- def get_train_examples(self, data_dir):
- """See base class."""
- return self._create_examples(self._read_tsv(os.path.join(data_dir, "train.tsv")), "train")
-
- def get_dev_examples(self, data_dir):
- """See base class."""
- return self._create_examples(self._read_tsv(os.path.join(data_dir, "dev.tsv")), "dev")
-
- def get_labels(self):
- """See base class."""
- return [None]
-
- def _create_examples(self, lines, set_type):
- """Creates examples for the training and dev sets."""
- examples = []
- for (i, line) in enumerate(lines):
- if i == 0:
- continue
- guid = "%s-%s" % (set_type, line[0])
- text_a = line[7]
- text_b = line[8]
- label = line[-1]
- examples.append(InputExample(guid=guid, text_a=text_a, text_b=text_b, label=label))
- return examples
-
-
-class QqpProcessor(DataProcessor):
- """Processor for the QQP data set (GLUE version)."""
-
- def get_example_from_tensor_dict(self, tensor_dict):
- """See base class."""
- return InputExample(
- tensor_dict["idx"].numpy(),
- tensor_dict["question1"].numpy().decode("utf-8"),
- tensor_dict["question2"].numpy().decode("utf-8"),
- str(tensor_dict["label"].numpy()),
- )
-
- def get_train_examples(self, data_dir):
- """See base class."""
- return self._create_examples(self._read_tsv(os.path.join(data_dir, "train.tsv")), "train")
-
- def get_dev_examples(self, data_dir):
- """See base class."""
- return self._create_examples(self._read_tsv(os.path.join(data_dir, "dev.tsv")), "dev")
-
- def get_labels(self):
- """See base class."""
- return ["0", "1"]
-
- def _create_examples(self, lines, set_type):
- """Creates examples for the training and dev sets."""
- examples = []
- for (i, line) in enumerate(lines):
- if i == 0:
- continue
- guid = "%s-%s" % (set_type, line[0])
- try:
- text_a = line[3]
- text_b = line[4]
- label = line[5]
- except IndexError:
- continue
- examples.append(InputExample(guid=guid, text_a=text_a, text_b=text_b, label=label))
- return examples
-
-
-class QnliProcessor(DataProcessor):
- """Processor for the QNLI data set (GLUE version)."""
-
- def get_example_from_tensor_dict(self, tensor_dict):
- """See base class."""
- return InputExample(
- tensor_dict["idx"].numpy(),
- tensor_dict["question"].numpy().decode("utf-8"),
- tensor_dict["sentence"].numpy().decode("utf-8"),
- str(tensor_dict["label"].numpy()),
- )
-
- def get_train_examples(self, data_dir):
- """See base class."""
- return self._create_examples(self._read_tsv(os.path.join(data_dir, "train.tsv")), "train")
-
- def get_dev_examples(self, data_dir):
- """See base class."""
- return self._create_examples(self._read_tsv(os.path.join(data_dir, "dev.tsv")), "dev_matched")
-
- def get_labels(self):
- """See base class."""
- return ["entailment", "not_entailment"]
-
- def _create_examples(self, lines, set_type):
- """Creates examples for the training and dev sets."""
- examples = []
- for (i, line) in enumerate(lines):
- if i == 0:
- continue
- guid = "%s-%s" % (set_type, line[0])
- text_a = line[1]
- text_b = line[2]
- label = line[-1]
- examples.append(InputExample(guid=guid, text_a=text_a, text_b=text_b, label=label))
- return examples
-
-
-class RteProcessor(DataProcessor):
- """Processor for the RTE data set (GLUE version)."""
-
- def get_example_from_tensor_dict(self, tensor_dict):
- """See base class."""
- return InputExample(
- tensor_dict["idx"].numpy(),
- tensor_dict["sentence1"].numpy().decode("utf-8"),
- tensor_dict["sentence2"].numpy().decode("utf-8"),
- str(tensor_dict["label"].numpy()),
- )
-
- def get_train_examples(self, data_dir):
- """See base class."""
- return self._create_examples(self._read_tsv(os.path.join(data_dir, "train.tsv")), "train")
-
- def get_dev_examples(self, data_dir):
- """See base class."""
- return self._create_examples(self._read_tsv(os.path.join(data_dir, "dev.tsv")), "dev")
-
- def get_labels(self):
- """See base class."""
- return ["entailment", "not_entailment"]
-
- def _create_examples(self, lines, set_type):
- """Creates examples for the training and dev sets."""
- examples = []
- for (i, line) in enumerate(lines):
- if i == 0:
- continue
- guid = "%s-%s" % (set_type, line[0])
- text_a = line[1]
- text_b = line[2]
- label = line[-1]
- examples.append(InputExample(guid=guid, text_a=text_a, text_b=text_b, label=label))
- return examples
-
-
-class WnliProcessor(DataProcessor):
- """Processor for the WNLI data set (GLUE version)."""
-
- def get_example_from_tensor_dict(self, tensor_dict):
- """See base class."""
- return InputExample(
- tensor_dict["idx"].numpy(),
- tensor_dict["sentence1"].numpy().decode("utf-8"),
- tensor_dict["sentence2"].numpy().decode("utf-8"),
- str(tensor_dict["label"].numpy()),
- )
-
- def get_train_examples(self, data_dir):
- """See base class."""
- return self._create_examples(self._read_tsv(os.path.join(data_dir, "train.tsv")), "train")
-
- def get_dev_examples(self, data_dir):
- """See base class."""
- return self._create_examples(self._read_tsv(os.path.join(data_dir, "dev.tsv")), "dev")
-
- def get_labels(self):
- """See base class."""
- return ["0", "1"]
-
- def _create_examples(self, lines, set_type):
- """Creates examples for the training and dev sets."""
- examples = []
- for (i, line) in enumerate(lines):
- if i == 0:
- continue
- guid = "%s-%s" % (set_type, line[0])
- text_a = line[1]
- text_b = line[2]
- label = line[-1]
- examples.append(InputExample(guid=guid, text_a=text_a, text_b=text_b, label=label))
- return examples
-
-
-glue_tasks_num_labels = {
- "cola": 2,
- "mnli": 3,
- "mrpc": 2,
- "sst-2": 2,
- "sts-b": 1,
- "qqp": 2,
- "qnli": 2,
- "rte": 2,
- "wnli": 2,
-}
-
-glue_processors = {
- "cola": ColaProcessor,
- "mnli": MnliProcessor,
- "mnli-mm": MnliMismatchedProcessor,
- "mrpc": MrpcProcessor,
- "sst-2": Sst2Processor,
- "sts-b": StsbProcessor,
- "qqp": QqpProcessor,
- "qnli": QnliProcessor,
- "rte": RteProcessor,
- "wnli": WnliProcessor,
-}
-
-glue_output_modes = {
- "cola": "classification",
- "mnli": "classification",
- "mnli-mm": "classification",
- "mrpc": "classification",
- "sst-2": "classification",
- "sts-b": "regression",
- "qqp": "classification",
- "qnli": "classification",
- "rte": "classification",
- "wnli": "classification",
-}
diff --git a/server/transformers/src/transformers/data/processors/squad.py b/server/transformers/src/transformers/data/processors/squad.py
deleted file mode 100644
index f2e63e939497399c8d942bdb7012c88cb5d39927..0000000000000000000000000000000000000000
--- a/server/transformers/src/transformers/data/processors/squad.py
+++ /dev/null
@@ -1,710 +0,0 @@
-import json
-import logging
-import os
-from functools import partial
-from multiprocessing import Pool, cpu_count
-
-import numpy as np
-from tqdm import tqdm
-
-from ...file_utils import is_tf_available, is_torch_available
-from ...tokenization_bert import whitespace_tokenize
-from .utils import DataProcessor
-
-
-if is_torch_available():
- import torch
- from torch.utils.data import TensorDataset
-
-if is_tf_available():
- import tensorflow as tf
-
-logger = logging.getLogger(__name__)
-
-
-def _improve_answer_span(doc_tokens, input_start, input_end, tokenizer, orig_answer_text):
- """Returns tokenized answer spans that better match the annotated answer."""
- tok_answer_text = " ".join(tokenizer.tokenize(orig_answer_text))
-
- for new_start in range(input_start, input_end + 1):
- for new_end in range(input_end, new_start - 1, -1):
- text_span = " ".join(doc_tokens[new_start : (new_end + 1)])
- if text_span == tok_answer_text:
- return (new_start, new_end)
-
- return (input_start, input_end)
-
-
-def _check_is_max_context(doc_spans, cur_span_index, position):
- """Check if this is the 'max context' doc span for the token."""
- best_score = None
- best_span_index = None
- for (span_index, doc_span) in enumerate(doc_spans):
- end = doc_span.start + doc_span.length - 1
- if position < doc_span.start:
- continue
- if position > end:
- continue
- num_left_context = position - doc_span.start
- num_right_context = end - position
- score = min(num_left_context, num_right_context) + 0.01 * doc_span.length
- if best_score is None or score > best_score:
- best_score = score
- best_span_index = span_index
-
- return cur_span_index == best_span_index
-
-
-def _new_check_is_max_context(doc_spans, cur_span_index, position):
- """Check if this is the 'max context' doc span for the token."""
- # if len(doc_spans) == 1:
- # return True
- best_score = None
- best_span_index = None
- for (span_index, doc_span) in enumerate(doc_spans):
- end = doc_span["start"] + doc_span["length"] - 1
- if position < doc_span["start"]:
- continue
- if position > end:
- continue
- num_left_context = position - doc_span["start"]
- num_right_context = end - position
- score = min(num_left_context, num_right_context) + 0.01 * doc_span["length"]
- if best_score is None or score > best_score:
- best_score = score
- best_span_index = span_index
-
- return cur_span_index == best_span_index
-
-
-def _is_whitespace(c):
- if c == " " or c == "\t" or c == "\r" or c == "\n" or ord(c) == 0x202F:
- return True
- return False
-
-
-def squad_convert_example_to_features(example, max_seq_length, doc_stride, max_query_length, is_training):
- features = []
- if is_training and not example.is_impossible:
- # Get start and end position
- start_position = example.start_position
- end_position = example.end_position
-
- # If the answer cannot be found in the text, then skip this example.
- actual_text = " ".join(example.doc_tokens[start_position : (end_position + 1)])
- cleaned_answer_text = " ".join(whitespace_tokenize(example.answer_text))
- if actual_text.find(cleaned_answer_text) == -1:
- logger.warning("Could not find answer: '%s' vs. '%s'", actual_text, cleaned_answer_text)
- return []
-
- tok_to_orig_index = []
- orig_to_tok_index = []
- all_doc_tokens = []
- for (i, token) in enumerate(example.doc_tokens):
- orig_to_tok_index.append(len(all_doc_tokens))
- sub_tokens = tokenizer.tokenize(token)
- for sub_token in sub_tokens:
- tok_to_orig_index.append(i)
- all_doc_tokens.append(sub_token)
-
- if is_training and not example.is_impossible:
- tok_start_position = orig_to_tok_index[example.start_position]
- if example.end_position < len(example.doc_tokens) - 1:
- tok_end_position = orig_to_tok_index[example.end_position + 1] - 1
- else:
- tok_end_position = len(all_doc_tokens) - 1
-
- (tok_start_position, tok_end_position) = _improve_answer_span(
- all_doc_tokens, tok_start_position, tok_end_position, tokenizer, example.answer_text
- )
-
- spans = []
-
- truncated_query = tokenizer.encode(example.question_text, add_special_tokens=False, max_length=max_query_length)
- sequence_added_tokens = (
- tokenizer.max_len - tokenizer.max_len_single_sentence + 1
- if "roberta" in str(type(tokenizer))
- else tokenizer.max_len - tokenizer.max_len_single_sentence
- )
- sequence_pair_added_tokens = tokenizer.max_len - tokenizer.max_len_sentences_pair
-
- span_doc_tokens = all_doc_tokens
- while len(spans) * doc_stride < len(all_doc_tokens):
-
- encoded_dict = tokenizer.encode_plus(
- truncated_query if tokenizer.padding_side == "right" else span_doc_tokens,
- span_doc_tokens if tokenizer.padding_side == "right" else truncated_query,
- max_length=max_seq_length,
- return_overflowing_tokens=True,
- pad_to_max_length=True,
- stride=max_seq_length - doc_stride - len(truncated_query) - sequence_pair_added_tokens,
- truncation_strategy="only_second" if tokenizer.padding_side == "right" else "only_first",
- )
-
- paragraph_len = min(
- len(all_doc_tokens) - len(spans) * doc_stride,
- max_seq_length - len(truncated_query) - sequence_pair_added_tokens,
- )
-
- if tokenizer.pad_token_id in encoded_dict["input_ids"]:
- non_padded_ids = encoded_dict["input_ids"][: encoded_dict["input_ids"].index(tokenizer.pad_token_id)]
- else:
- non_padded_ids = encoded_dict["input_ids"]
-
- tokens = tokenizer.convert_ids_to_tokens(non_padded_ids)
-
- token_to_orig_map = {}
- for i in range(paragraph_len):
- index = len(truncated_query) + sequence_added_tokens + i if tokenizer.padding_side == "right" else i
- token_to_orig_map[index] = tok_to_orig_index[len(spans) * doc_stride + i]
-
- encoded_dict["paragraph_len"] = paragraph_len
- encoded_dict["tokens"] = tokens
- encoded_dict["token_to_orig_map"] = token_to_orig_map
- encoded_dict["truncated_query_with_special_tokens_length"] = len(truncated_query) + sequence_added_tokens
- encoded_dict["token_is_max_context"] = {}
- encoded_dict["start"] = len(spans) * doc_stride
- encoded_dict["length"] = paragraph_len
-
- spans.append(encoded_dict)
-
- if "overflowing_tokens" not in encoded_dict:
- break
- span_doc_tokens = encoded_dict["overflowing_tokens"]
-
- for doc_span_index in range(len(spans)):
- for j in range(spans[doc_span_index]["paragraph_len"]):
- is_max_context = _new_check_is_max_context(spans, doc_span_index, doc_span_index * doc_stride + j)
- index = (
- j
- if tokenizer.padding_side == "left"
- else spans[doc_span_index]["truncated_query_with_special_tokens_length"] + j
- )
- spans[doc_span_index]["token_is_max_context"][index] = is_max_context
-
- for span in spans:
- # Identify the position of the CLS token
- cls_index = span["input_ids"].index(tokenizer.cls_token_id)
-
- # p_mask: mask with 1 for token than cannot be in the answer (0 for token which can be in an answer)
- # Original TF implem also keep the classification token (set to 0) (not sure why...)
- p_mask = np.array(span["token_type_ids"])
-
- p_mask = np.minimum(p_mask, 1)
-
- if tokenizer.padding_side == "right":
- # Limit positive values to one
- p_mask = 1 - p_mask
-
- p_mask[np.where(np.array(span["input_ids"]) == tokenizer.sep_token_id)[0]] = 1
-
- # Set the CLS index to '0'
- p_mask[cls_index] = 0
-
- span_is_impossible = example.is_impossible
- start_position = 0
- end_position = 0
- if is_training and not span_is_impossible:
- # For training, if our document chunk does not contain an annotation
- # we throw it out, since there is nothing to predict.
- doc_start = span["start"]
- doc_end = span["start"] + span["length"] - 1
- out_of_span = False
-
- if not (tok_start_position >= doc_start and tok_end_position <= doc_end):
- out_of_span = True
-
- if out_of_span:
- start_position = cls_index
- end_position = cls_index
- span_is_impossible = True
- else:
- if tokenizer.padding_side == "left":
- doc_offset = 0
- else:
- doc_offset = len(truncated_query) + sequence_added_tokens
-
- start_position = tok_start_position - doc_start + doc_offset
- end_position = tok_end_position - doc_start + doc_offset
-
- features.append(
- SquadFeatures(
- span["input_ids"],
- span["attention_mask"],
- span["token_type_ids"],
- cls_index,
- p_mask.tolist(),
- example_index=0, # Can not set unique_id and example_index here. They will be set after multiple processing.
- unique_id=0,
- paragraph_len=span["paragraph_len"],
- token_is_max_context=span["token_is_max_context"],
- tokens=span["tokens"],
- token_to_orig_map=span["token_to_orig_map"],
- start_position=start_position,
- end_position=end_position,
- is_impossible=span_is_impossible,
- )
- )
- return features
-
-
-def squad_convert_example_to_features_init(tokenizer_for_convert):
- global tokenizer
- tokenizer = tokenizer_for_convert
-
-
-def squad_convert_examples_to_features(
- examples, tokenizer, max_seq_length, doc_stride, max_query_length, is_training, return_dataset=False, threads=1
-):
- """
- Converts a list of examples into a list of features that can be directly given as input to a model.
- It is model-dependant and takes advantage of many of the tokenizer's features to create the model's inputs.
-
- Args:
- examples: list of :class:`~transformers.data.processors.squad.SquadExample`
- tokenizer: an instance of a child of :class:`~transformers.PreTrainedTokenizer`
- max_seq_length: The maximum sequence length of the inputs.
- doc_stride: The stride used when the context is too large and is split across several features.
- max_query_length: The maximum length of the query.
- is_training: whether to create features for model evaluation or model training.
- return_dataset: Default False. Either 'pt' or 'tf'.
- if 'pt': returns a torch.data.TensorDataset,
- if 'tf': returns a tf.data.Dataset
- threads: multiple processing threadsa-smi
-
-
- Returns:
- list of :class:`~transformers.data.processors.squad.SquadFeatures`
-
- Example::
-
- processor = SquadV2Processor()
- examples = processor.get_dev_examples(data_dir)
-
- features = squad_convert_examples_to_features(
- examples=examples,
- tokenizer=tokenizer,
- max_seq_length=args.max_seq_length,
- doc_stride=args.doc_stride,
- max_query_length=args.max_query_length,
- is_training=not evaluate,
- )
- """
-
- # Defining helper methods
- features = []
- threads = min(threads, cpu_count())
- with Pool(threads, initializer=squad_convert_example_to_features_init, initargs=(tokenizer,)) as p:
- annotate_ = partial(
- squad_convert_example_to_features,
- max_seq_length=max_seq_length,
- doc_stride=doc_stride,
- max_query_length=max_query_length,
- is_training=is_training,
- )
- features = list(
- tqdm(
- p.imap(annotate_, examples, chunksize=32),
- total=len(examples),
- desc="convert squad examples to features",
- )
- )
- new_features = []
- unique_id = 1000000000
- example_index = 0
- for example_features in tqdm(features, total=len(features), desc="add example index and unique id"):
- if not example_features:
- continue
- for example_feature in example_features:
- example_feature.example_index = example_index
- example_feature.unique_id = unique_id
- new_features.append(example_feature)
- unique_id += 1
- example_index += 1
- features = new_features
- del new_features
- if return_dataset == "pt":
- if not is_torch_available():
- raise RuntimeError("PyTorch must be installed to return a PyTorch dataset.")
-
- # Convert to Tensors and build dataset
- all_input_ids = torch.tensor([f.input_ids for f in features], dtype=torch.long)
- all_attention_masks = torch.tensor([f.attention_mask for f in features], dtype=torch.long)
- all_token_type_ids = torch.tensor([f.token_type_ids for f in features], dtype=torch.long)
- all_cls_index = torch.tensor([f.cls_index for f in features], dtype=torch.long)
- all_p_mask = torch.tensor([f.p_mask for f in features], dtype=torch.float)
- all_is_impossible = torch.tensor([f.is_impossible for f in features], dtype=torch.float)
-
- if not is_training:
- all_example_index = torch.arange(all_input_ids.size(0), dtype=torch.long)
- dataset = TensorDataset(
- all_input_ids, all_attention_masks, all_token_type_ids, all_example_index, all_cls_index, all_p_mask
- )
- else:
- all_start_positions = torch.tensor([f.start_position for f in features], dtype=torch.long)
- all_end_positions = torch.tensor([f.end_position for f in features], dtype=torch.long)
- dataset = TensorDataset(
- all_input_ids,
- all_attention_masks,
- all_token_type_ids,
- all_start_positions,
- all_end_positions,
- all_cls_index,
- all_p_mask,
- all_is_impossible,
- )
-
- return features, dataset
- elif return_dataset == "tf":
- if not is_tf_available():
- raise RuntimeError("TensorFlow must be installed to return a TensorFlow dataset.")
-
- def gen():
- for ex in features:
- yield (
- {
- "input_ids": ex.input_ids,
- "attention_mask": ex.attention_mask,
- "token_type_ids": ex.token_type_ids,
- },
- {
- "start_position": ex.start_position,
- "end_position": ex.end_position,
- "cls_index": ex.cls_index,
- "p_mask": ex.p_mask,
- "is_impossible": ex.is_impossible,
- },
- )
-
- return tf.data.Dataset.from_generator(
- gen,
- (
- {"input_ids": tf.int32, "attention_mask": tf.int32, "token_type_ids": tf.int32},
- {
- "start_position": tf.int64,
- "end_position": tf.int64,
- "cls_index": tf.int64,
- "p_mask": tf.int32,
- "is_impossible": tf.int32,
- },
- ),
- (
- {
- "input_ids": tf.TensorShape([None]),
- "attention_mask": tf.TensorShape([None]),
- "token_type_ids": tf.TensorShape([None]),
- },
- {
- "start_position": tf.TensorShape([]),
- "end_position": tf.TensorShape([]),
- "cls_index": tf.TensorShape([]),
- "p_mask": tf.TensorShape([None]),
- "is_impossible": tf.TensorShape([]),
- },
- ),
- )
-
- return features
-
-
-class SquadProcessor(DataProcessor):
- """
- Processor for the SQuAD data set.
- Overriden by SquadV1Processor and SquadV2Processor, used by the version 1.1 and version 2.0 of SQuAD, respectively.
- """
-
- train_file = None
- dev_file = None
-
- def _get_example_from_tensor_dict(self, tensor_dict, evaluate=False):
- if not evaluate:
- answer = tensor_dict["answers"]["text"][0].numpy().decode("utf-8")
- answer_start = tensor_dict["answers"]["answer_start"][0].numpy()
- answers = []
- else:
- answers = [
- {"answer_start": start.numpy(), "text": text.numpy().decode("utf-8")}
- for start, text in zip(tensor_dict["answers"]["answer_start"], tensor_dict["answers"]["text"])
- ]
-
- answer = None
- answer_start = None
-
- return SquadExample(
- qas_id=tensor_dict["id"].numpy().decode("utf-8"),
- question_text=tensor_dict["question"].numpy().decode("utf-8"),
- context_text=tensor_dict["context"].numpy().decode("utf-8"),
- answer_text=answer,
- start_position_character=answer_start,
- title=tensor_dict["title"].numpy().decode("utf-8"),
- answers=answers,
- )
-
- def get_examples_from_dataset(self, dataset, evaluate=False):
- """
- Creates a list of :class:`~transformers.data.processors.squad.SquadExample` using a TFDS dataset.
-
- Args:
- dataset: The tfds dataset loaded from `tensorflow_datasets.load("squad")`
- evaluate: boolean specifying if in evaluation mode or in training mode
-
- Returns:
- List of SquadExample
-
- Examples::
-
- import tensorflow_datasets as tfds
- dataset = tfds.load("squad")
-
- training_examples = get_examples_from_dataset(dataset, evaluate=False)
- evaluation_examples = get_examples_from_dataset(dataset, evaluate=True)
- """
-
- if evaluate:
- dataset = dataset["validation"]
- else:
- dataset = dataset["train"]
-
- examples = []
- for tensor_dict in tqdm(dataset):
- examples.append(self._get_example_from_tensor_dict(tensor_dict, evaluate=evaluate))
-
- return examples
-
- def get_train_examples(self, data_dir, filename=None):
- """
- Returns the training examples from the data directory.
-
- Args:
- data_dir: Directory containing the data files used for training and evaluating.
- filename: None by default, specify this if the training file has a different name than the original one
- which is `train-v1.1.json` and `train-v2.0.json` for squad versions 1.1 and 2.0 respectively.
-
- """
- if data_dir is None:
- data_dir = ""
-
- if self.train_file is None:
- raise ValueError("SquadProcessor should be instantiated via SquadV1Processor or SquadV2Processor")
-
- with open(
- os.path.join(data_dir, self.train_file if filename is None else filename), "r", encoding="utf-8"
- ) as reader:
- input_data = json.load(reader)["data"]
- return self._create_examples(input_data, "train")
-
- def get_dev_examples(self, data_dir, filename=None):
- """
- Returns the evaluation example from the data directory.
-
- Args:
- data_dir: Directory containing the data files used for training and evaluating.
- filename: None by default, specify this if the evaluation file has a different name than the original one
- which is `train-v1.1.json` and `train-v2.0.json` for squad versions 1.1 and 2.0 respectively.
- """
- if data_dir is None:
- data_dir = ""
-
- if self.dev_file is None:
- raise ValueError("SquadProcessor should be instantiated via SquadV1Processor or SquadV2Processor")
-
- with open(
- os.path.join(data_dir, self.dev_file if filename is None else filename), "r", encoding="utf-8"
- ) as reader:
- input_data = json.load(reader)["data"]
- return self._create_examples(input_data, "dev")
-
- def _create_examples(self, input_data, set_type):
- is_training = set_type == "train"
- examples = []
- for entry in tqdm(input_data):
- title = entry["title"]
- for paragraph in entry["paragraphs"]:
- context_text = paragraph["context"]
- for qa in paragraph["qas"]:
- qas_id = qa["id"]
- question_text = qa["question"]
- start_position_character = None
- answer_text = None
- answers = []
-
- if "is_impossible" in qa:
- is_impossible = qa["is_impossible"]
- else:
- is_impossible = False
-
- if not is_impossible:
- if is_training:
- answer = qa["answers"][0]
- answer_text = answer["text"]
- start_position_character = answer["answer_start"]
- else:
- answers = qa["answers"]
-
- example = SquadExample(
- qas_id=qas_id,
- question_text=question_text,
- context_text=context_text,
- answer_text=answer_text,
- start_position_character=start_position_character,
- title=title,
- is_impossible=is_impossible,
- answers=answers,
- )
-
- examples.append(example)
- return examples
-
-
-class SquadV1Processor(SquadProcessor):
- train_file = "train-v1.1.json"
- dev_file = "dev-v1.1.json"
-
-
-class SquadV2Processor(SquadProcessor):
- train_file = "train-v2.0.json"
- dev_file = "dev-v2.0.json"
-
-
-class SquadExample(object):
- """
- A single training/test example for the Squad dataset, as loaded from disk.
-
- Args:
- qas_id: The example's unique identifier
- question_text: The question string
- context_text: The context string
- answer_text: The answer string
- start_position_character: The character position of the start of the answer
- title: The title of the example
- answers: None by default, this is used during evaluation. Holds answers as well as their start positions.
- is_impossible: False by default, set to True if the example has no possible answer.
- """
-
- def __init__(
- self,
- qas_id,
- question_text,
- context_text,
- answer_text,
- start_position_character,
- title,
- answers=[],
- is_impossible=False,
- ):
- self.qas_id = qas_id
- self.question_text = question_text
- self.context_text = context_text
- self.answer_text = answer_text
- self.title = title
- self.is_impossible = is_impossible
- self.answers = answers
-
- self.start_position, self.end_position = 0, 0
-
- doc_tokens = []
- char_to_word_offset = []
- prev_is_whitespace = True
-
- # Split on whitespace so that different tokens may be attributed to their original position.
- for c in self.context_text:
- if _is_whitespace(c):
- prev_is_whitespace = True
- else:
- if prev_is_whitespace:
- doc_tokens.append(c)
- else:
- doc_tokens[-1] += c
- prev_is_whitespace = False
- char_to_word_offset.append(len(doc_tokens) - 1)
-
- self.doc_tokens = doc_tokens
- self.char_to_word_offset = char_to_word_offset
-
- # Start end end positions only has a value during evaluation.
- if start_position_character is not None and not is_impossible:
- self.start_position = char_to_word_offset[start_position_character]
- self.end_position = char_to_word_offset[
- min(start_position_character + len(answer_text) - 1, len(char_to_word_offset) - 1)
- ]
-
-
-class SquadFeatures(object):
- """
- Single squad example features to be fed to a model.
- Those features are model-specific and can be crafted from :class:`~transformers.data.processors.squad.SquadExample`
- using the :method:`~transformers.data.processors.squad.squad_convert_examples_to_features` method.
-
- Args:
- input_ids: Indices of input sequence tokens in the vocabulary.
- attention_mask: Mask to avoid performing attention on padding token indices.
- token_type_ids: Segment token indices to indicate first and second portions of the inputs.
- cls_index: the index of the CLS token.
- p_mask: Mask identifying tokens that can be answers vs. tokens that cannot.
- Mask with 1 for tokens than cannot be in the answer and 0 for token that can be in an answer
- example_index: the index of the example
- unique_id: The unique Feature identifier
- paragraph_len: The length of the context
- token_is_max_context: List of booleans identifying which tokens have their maximum context in this feature object.
- If a token does not have their maximum context in this feature object, it means that another feature object
- has more information related to that token and should be prioritized over this feature for that token.
- tokens: list of tokens corresponding to the input ids
- token_to_orig_map: mapping between the tokens and the original text, needed in order to identify the answer.
- start_position: start of the answer token index
- end_position: end of the answer token index
- """
-
- def __init__(
- self,
- input_ids,
- attention_mask,
- token_type_ids,
- cls_index,
- p_mask,
- example_index,
- unique_id,
- paragraph_len,
- token_is_max_context,
- tokens,
- token_to_orig_map,
- start_position,
- end_position,
- is_impossible,
- ):
- self.input_ids = input_ids
- self.attention_mask = attention_mask
- self.token_type_ids = token_type_ids
- self.cls_index = cls_index
- self.p_mask = p_mask
-
- self.example_index = example_index
- self.unique_id = unique_id
- self.paragraph_len = paragraph_len
- self.token_is_max_context = token_is_max_context
- self.tokens = tokens
- self.token_to_orig_map = token_to_orig_map
-
- self.start_position = start_position
- self.end_position = end_position
- self.is_impossible = is_impossible
-
-
-class SquadResult(object):
- """
- Constructs a SquadResult which can be used to evaluate a model's output on the SQuAD dataset.
-
- Args:
- unique_id: The unique identifier corresponding to that example.
- start_logits: The logits corresponding to the start of the answer
- end_logits: The logits corresponding to the end of the answer
- """
-
- def __init__(self, unique_id, start_logits, end_logits, start_top_index=None, end_top_index=None, cls_logits=None):
- self.start_logits = start_logits
- self.end_logits = end_logits
- self.unique_id = unique_id
-
- if start_top_index:
- self.start_top_index = start_top_index
- self.end_top_index = end_top_index
- self.cls_logits = cls_logits
diff --git a/server/transformers/src/transformers/data/processors/utils.py b/server/transformers/src/transformers/data/processors/utils.py
deleted file mode 100644
index 4cc931cdf9ccded2abfec08d9d5044c4acafb7ac..0000000000000000000000000000000000000000
--- a/server/transformers/src/transformers/data/processors/utils.py
+++ /dev/null
@@ -1,353 +0,0 @@
-# coding=utf-8
-# Copyright 2018 The Google AI Language Team Authors and The HuggingFace Inc. team.
-# Copyright (c) 2018, NVIDIA CORPORATION. All rights reserved.
-#
-# Licensed under the Apache License, Version 2.0 (the "License");
-# you may not use this file except in compliance with the License.
-# You may obtain a copy of the License at
-#
-# http://www.apache.org/licenses/LICENSE-2.0
-#
-# Unless required by applicable law or agreed to in writing, software
-# distributed under the License is distributed on an "AS IS" BASIS,
-# WITHOUT WARRANTIES OR CONDITIONS OF ANY KIND, either express or implied.
-# See the License for the specific language governing permissions and
-# limitations under the License.
-
-import copy
-import csv
-import json
-import logging
-
-from ...file_utils import is_tf_available, is_torch_available
-
-
-logger = logging.getLogger(__name__)
-
-
-class InputExample(object):
- """
- A single training/test example for simple sequence classification.
-
- Args:
- guid: Unique id for the example.
- text_a: string. The untokenized text of the first sequence. For single
- sequence tasks, only this sequence must be specified.
- text_b: (Optional) string. The untokenized text of the second sequence.
- Only must be specified for sequence pair tasks.
- label: (Optional) string. The label of the example. This should be
- specified for train and dev examples, but not for test examples.
- """
-
- def __init__(self, guid, text_a, text_b=None, label=None):
- self.guid = guid
- self.text_a = text_a
- self.text_b = text_b
- self.label = label
-
- def __repr__(self):
- return str(self.to_json_string())
-
- def to_dict(self):
- """Serializes this instance to a Python dictionary."""
- output = copy.deepcopy(self.__dict__)
- return output
-
- def to_json_string(self):
- """Serializes this instance to a JSON string."""
- return json.dumps(self.to_dict(), indent=2, sort_keys=True) + "\n"
-
-
-class InputFeatures(object):
- """
- A single set of features of data.
-
- Args:
- input_ids: Indices of input sequence tokens in the vocabulary.
- attention_mask: Mask to avoid performing attention on padding token indices.
- Mask values selected in ``[0, 1]``:
- Usually ``1`` for tokens that are NOT MASKED, ``0`` for MASKED (padded) tokens.
- token_type_ids: Segment token indices to indicate first and second portions of the inputs.
- label: Label corresponding to the input
- """
-
- def __init__(self, input_ids, attention_mask=None, token_type_ids=None, label=None):
- self.input_ids = input_ids
- self.attention_mask = attention_mask
- self.token_type_ids = token_type_ids
- self.label = label
-
- def __repr__(self):
- return str(self.to_json_string())
-
- def to_dict(self):
- """Serializes this instance to a Python dictionary."""
- output = copy.deepcopy(self.__dict__)
- return output
-
- def to_json_string(self):
- """Serializes this instance to a JSON string."""
- return json.dumps(self.to_dict(), indent=2, sort_keys=True) + "\n"
-
-
-class DataProcessor(object):
- """Base class for data converters for sequence classification data sets."""
-
- def get_example_from_tensor_dict(self, tensor_dict):
- """Gets an example from a dict with tensorflow tensors
- Args:
- tensor_dict: Keys and values should match the corresponding Glue
- tensorflow_dataset examples.
- """
- raise NotImplementedError()
-
- def get_train_examples(self, data_dir):
- """Gets a collection of `InputExample`s for the train set."""
- raise NotImplementedError()
-
- def get_dev_examples(self, data_dir):
- """Gets a collection of `InputExample`s for the dev set."""
- raise NotImplementedError()
-
- def get_labels(self):
- """Gets the list of labels for this data set."""
- raise NotImplementedError()
-
- def tfds_map(self, example):
- """Some tensorflow_datasets datasets are not formatted the same way the GLUE datasets are.
- This method converts examples to the correct format."""
- if len(self.get_labels()) > 1:
- example.label = self.get_labels()[int(example.label)]
- return example
-
- @classmethod
- def _read_tsv(cls, input_file, quotechar=None):
- """Reads a tab separated value file."""
- with open(input_file, "r", encoding="utf-8-sig") as f:
- return list(csv.reader(f, delimiter="\t", quotechar=quotechar))
-
-
-class SingleSentenceClassificationProcessor(DataProcessor):
- """ Generic processor for a single sentence classification data set."""
-
- def __init__(self, labels=None, examples=None, mode="classification", verbose=False):
- self.labels = [] if labels is None else labels
- self.examples = [] if examples is None else examples
- self.mode = mode
- self.verbose = verbose
-
- def __len__(self):
- return len(self.examples)
-
- def __getitem__(self, idx):
- if isinstance(idx, slice):
- return SingleSentenceClassificationProcessor(labels=self.labels, examples=self.examples[idx])
- return self.examples[idx]
-
- @classmethod
- def create_from_csv(
- cls, file_name, split_name="", column_label=0, column_text=1, column_id=None, skip_first_row=False, **kwargs
- ):
- processor = cls(**kwargs)
- processor.add_examples_from_csv(
- file_name,
- split_name=split_name,
- column_label=column_label,
- column_text=column_text,
- column_id=column_id,
- skip_first_row=skip_first_row,
- overwrite_labels=True,
- overwrite_examples=True,
- )
- return processor
-
- @classmethod
- def create_from_examples(cls, texts_or_text_and_labels, labels=None, **kwargs):
- processor = cls(**kwargs)
- processor.add_examples(texts_or_text_and_labels, labels=labels)
- return processor
-
- def add_examples_from_csv(
- self,
- file_name,
- split_name="",
- column_label=0,
- column_text=1,
- column_id=None,
- skip_first_row=False,
- overwrite_labels=False,
- overwrite_examples=False,
- ):
- lines = self._read_tsv(file_name)
- if skip_first_row:
- lines = lines[1:]
- texts = []
- labels = []
- ids = []
- for (i, line) in enumerate(lines):
- texts.append(line[column_text])
- labels.append(line[column_label])
- if column_id is not None:
- ids.append(line[column_id])
- else:
- guid = "%s-%s" % (split_name, i) if split_name else "%s" % i
- ids.append(guid)
-
- return self.add_examples(
- texts, labels, ids, overwrite_labels=overwrite_labels, overwrite_examples=overwrite_examples
- )
-
- def add_examples(
- self, texts_or_text_and_labels, labels=None, ids=None, overwrite_labels=False, overwrite_examples=False
- ):
- assert labels is None or len(texts_or_text_and_labels) == len(labels)
- assert ids is None or len(texts_or_text_and_labels) == len(ids)
- if ids is None:
- ids = [None] * len(texts_or_text_and_labels)
- if labels is None:
- labels = [None] * len(texts_or_text_and_labels)
- examples = []
- added_labels = set()
- for (text_or_text_and_label, label, guid) in zip(texts_or_text_and_labels, labels, ids):
- if isinstance(text_or_text_and_label, (tuple, list)) and label is None:
- text, label = text_or_text_and_label
- else:
- text = text_or_text_and_label
- added_labels.add(label)
- examples.append(InputExample(guid=guid, text_a=text, text_b=None, label=label))
-
- # Update examples
- if overwrite_examples:
- self.examples = examples
- else:
- self.examples.extend(examples)
-
- # Update labels
- if overwrite_labels:
- self.labels = list(added_labels)
- else:
- self.labels = list(set(self.labels).union(added_labels))
-
- return self.examples
-
- def get_features(
- self,
- tokenizer,
- max_length=None,
- pad_on_left=False,
- pad_token=0,
- mask_padding_with_zero=True,
- return_tensors=None,
- ):
- """
- Convert examples in a list of ``InputFeatures``
-
- Args:
- tokenizer: Instance of a tokenizer that will tokenize the examples
- max_length: Maximum example length
- task: GLUE task
- label_list: List of labels. Can be obtained from the processor using the ``processor.get_labels()`` method
- output_mode: String indicating the output mode. Either ``regression`` or ``classification``
- pad_on_left: If set to ``True``, the examples will be padded on the left rather than on the right (default)
- pad_token: Padding token
- mask_padding_with_zero: If set to ``True``, the attention mask will be filled by ``1`` for actual values
- and by ``0`` for padded values. If set to ``False``, inverts it (``1`` for padded values, ``0`` for
- actual values)
-
- Returns:
- If the ``examples`` input is a ``tf.data.Dataset``, will return a ``tf.data.Dataset``
- containing the task-specific features. If the input is a list of ``InputExamples``, will return
- a list of task-specific ``InputFeatures`` which can be fed to the model.
-
- """
- if max_length is None:
- max_length = tokenizer.max_len
-
- label_map = {label: i for i, label in enumerate(self.labels)}
-
- all_input_ids = []
- for (ex_index, example) in enumerate(self.examples):
- if ex_index % 10000 == 0:
- logger.info("Tokenizing example %d", ex_index)
-
- input_ids = tokenizer.encode(
- example.text_a, add_special_tokens=True, max_length=min(max_length, tokenizer.max_len),
- )
- all_input_ids.append(input_ids)
-
- batch_length = max(len(input_ids) for input_ids in all_input_ids)
-
- features = []
- for (ex_index, (input_ids, example)) in enumerate(zip(all_input_ids, self.examples)):
- if ex_index % 10000 == 0:
- logger.info("Writing example %d/%d" % (ex_index, len(self.examples)))
- # The mask has 1 for real tokens and 0 for padding tokens. Only real
- # tokens are attended to.
- attention_mask = [1 if mask_padding_with_zero else 0] * len(input_ids)
-
- # Zero-pad up to the sequence length.
- padding_length = batch_length - len(input_ids)
- if pad_on_left:
- input_ids = ([pad_token] * padding_length) + input_ids
- attention_mask = ([0 if mask_padding_with_zero else 1] * padding_length) + attention_mask
- else:
- input_ids = input_ids + ([pad_token] * padding_length)
- attention_mask = attention_mask + ([0 if mask_padding_with_zero else 1] * padding_length)
-
- assert len(input_ids) == batch_length, "Error with input length {} vs {}".format(
- len(input_ids), batch_length
- )
- assert len(attention_mask) == batch_length, "Error with input length {} vs {}".format(
- len(attention_mask), batch_length
- )
-
- if self.mode == "classification":
- label = label_map[example.label]
- elif self.mode == "regression":
- label = float(example.label)
- else:
- raise ValueError(self.mode)
-
- if ex_index < 5 and self.verbose:
- logger.info("*** Example ***")
- logger.info("guid: %s" % (example.guid))
- logger.info("input_ids: %s" % " ".join([str(x) for x in input_ids]))
- logger.info("attention_mask: %s" % " ".join([str(x) for x in attention_mask]))
- logger.info("label: %s (id = %d)" % (example.label, label))
-
- features.append(InputFeatures(input_ids=input_ids, attention_mask=attention_mask, label=label))
-
- if return_tensors is None:
- return features
- elif return_tensors == "tf":
- if not is_tf_available():
- raise RuntimeError("return_tensors set to 'tf' but TensorFlow 2.0 can't be imported")
- import tensorflow as tf
-
- def gen():
- for ex in features:
- yield ({"input_ids": ex.input_ids, "attention_mask": ex.attention_mask}, ex.label)
-
- dataset = tf.data.Dataset.from_generator(
- gen,
- ({"input_ids": tf.int32, "attention_mask": tf.int32}, tf.int64),
- ({"input_ids": tf.TensorShape([None]), "attention_mask": tf.TensorShape([None])}, tf.TensorShape([])),
- )
- return dataset
- elif return_tensors == "pt":
- if not is_torch_available():
- raise RuntimeError("return_tensors set to 'pt' but PyTorch can't be imported")
- import torch
- from torch.utils.data import TensorDataset
-
- all_input_ids = torch.tensor([f.input_ids for f in features], dtype=torch.long)
- all_attention_mask = torch.tensor([f.attention_mask for f in features], dtype=torch.long)
- if self.mode == "classification":
- all_labels = torch.tensor([f.label for f in features], dtype=torch.long)
- elif self.mode == "regression":
- all_labels = torch.tensor([f.label for f in features], dtype=torch.float)
-
- dataset = TensorDataset(all_input_ids, all_attention_mask, all_labels)
- return dataset
- else:
- raise ValueError("return_tensors should be one of 'tf' or 'pt'")
diff --git a/server/transformers/src/transformers/data/processors/xnli.py b/server/transformers/src/transformers/data/processors/xnli.py
deleted file mode 100644
index 6a744c6280145efb3b305c775db9931dcc8f3e25..0000000000000000000000000000000000000000
--- a/server/transformers/src/transformers/data/processors/xnli.py
+++ /dev/null
@@ -1,85 +0,0 @@
-# coding=utf-8
-# Copyright 2018 The Google AI Language Team Authors and The HuggingFace Inc. team.
-# Copyright (c) 2018, NVIDIA CORPORATION. All rights reserved.
-#
-# Licensed under the Apache License, Version 2.0 (the "License");
-# you may not use this file except in compliance with the License.
-# You may obtain a copy of the License at
-#
-# http://www.apache.org/licenses/LICENSE-2.0
-#
-# Unless required by applicable law or agreed to in writing, software
-# distributed under the License is distributed on an "AS IS" BASIS,
-# WITHOUT WARRANTIES OR CONDITIONS OF ANY KIND, either express or implied.
-# See the License for the specific language governing permissions and
-# limitations under the License.
-""" XNLI utils (dataset loading and evaluation) """
-
-
-import logging
-import os
-
-from .utils import DataProcessor, InputExample
-
-
-logger = logging.getLogger(__name__)
-
-
-class XnliProcessor(DataProcessor):
- """Processor for the XNLI dataset.
- Adapted from https://github.com/google-research/bert/blob/f39e881b169b9d53bea03d2d341b31707a6c052b/run_classifier.py#L207"""
-
- def __init__(self, language, train_language=None):
- self.language = language
- self.train_language = train_language
-
- def get_train_examples(self, data_dir):
- """See base class."""
- lg = self.language if self.train_language is None else self.train_language
- lines = self._read_tsv(os.path.join(data_dir, "XNLI-MT-1.0/multinli/multinli.train.{}.tsv".format(lg)))
- examples = []
- for (i, line) in enumerate(lines):
- if i == 0:
- continue
- guid = "%s-%s" % ("train", i)
- text_a = line[0]
- text_b = line[1]
- label = "contradiction" if line[2] == "contradictory" else line[2]
- assert isinstance(text_a, str) and isinstance(text_b, str) and isinstance(label, str)
- examples.append(InputExample(guid=guid, text_a=text_a, text_b=text_b, label=label))
- return examples
-
- def get_test_examples(self, data_dir):
- """See base class."""
- lines = self._read_tsv(os.path.join(data_dir, "XNLI-1.0/xnli.test.tsv"))
- examples = []
- for (i, line) in enumerate(lines):
- if i == 0:
- continue
- language = line[0]
- if language != self.language:
- continue
- guid = "%s-%s" % ("test", i)
- text_a = line[6]
- text_b = line[7]
- label = line[1]
- assert isinstance(text_a, str) and isinstance(text_b, str) and isinstance(label, str)
- examples.append(InputExample(guid=guid, text_a=text_a, text_b=text_b, label=label))
- return examples
-
- def get_labels(self):
- """See base class."""
- return ["contradiction", "entailment", "neutral"]
-
-
-xnli_processors = {
- "xnli": XnliProcessor,
-}
-
-xnli_output_modes = {
- "xnli": "classification",
-}
-
-xnli_tasks_num_labels = {
- "xnli": 3,
-}
diff --git a/server/transformers/src/transformers/file_utils.py b/server/transformers/src/transformers/file_utils.py
deleted file mode 100644
index 8aafa95f432aaad668bb8acddd063ed8d2b265b3..0000000000000000000000000000000000000000
--- a/server/transformers/src/transformers/file_utils.py
+++ /dev/null
@@ -1,432 +0,0 @@
-"""
-Utilities for working with the local dataset cache.
-This file is adapted from the AllenNLP library at https://github.com/allenai/allennlp
-Copyright by the AllenNLP authors.
-"""
-
-import fnmatch
-import json
-import logging
-import os
-import sys
-import tempfile
-from contextlib import contextmanager
-from functools import partial, wraps
-from hashlib import sha256
-from typing import Optional
-from urllib.parse import urlparse
-
-import boto3
-import requests
-from botocore.config import Config
-from botocore.exceptions import ClientError
-from filelock import FileLock
-from tqdm.auto import tqdm
-
-from . import __version__
-
-
-logger = logging.getLogger(__name__) # pylint: disable=invalid-name
-
-try:
- USE_TF = os.environ.get("USE_TF", "AUTO").upper()
- USE_TORCH = os.environ.get("USE_TORCH", "AUTO").upper()
- if USE_TORCH in ("1", "ON", "YES", "AUTO") and USE_TF not in ("1", "ON", "YES"):
- import torch
-
- _torch_available = True # pylint: disable=invalid-name
- logger.info("PyTorch version {} available.".format(torch.__version__))
- else:
- logger.info("Disabling PyTorch because USE_TF is set")
- _torch_available = False
-except ImportError:
- _torch_available = False # pylint: disable=invalid-name
-
-try:
- USE_TF = os.environ.get("USE_TF", "AUTO").upper()
- USE_TORCH = os.environ.get("USE_TORCH", "AUTO").upper()
-
- if USE_TF in ("1", "ON", "YES", "AUTO") and USE_TORCH not in ("1", "ON", "YES"):
- import tensorflow as tf
-
- assert hasattr(tf, "__version__") and int(tf.__version__[0]) >= 2
- _tf_available = True # pylint: disable=invalid-name
- logger.info("TensorFlow version {} available.".format(tf.__version__))
- else:
- logger.info("Disabling Tensorflow because USE_TORCH is set")
- _tf_available = False
-except (ImportError, AssertionError):
- _tf_available = False # pylint: disable=invalid-name
-
-try:
- from torch.hub import _get_torch_home
-
- torch_cache_home = _get_torch_home()
-except ImportError:
- torch_cache_home = os.path.expanduser(
- os.getenv("TORCH_HOME", os.path.join(os.getenv("XDG_CACHE_HOME", "~/.cache"), "torch"))
- )
-default_cache_path = os.path.join(torch_cache_home, "transformers")
-
-try:
- from pathlib import Path
-
- PYTORCH_PRETRAINED_BERT_CACHE = Path(
- os.getenv("PYTORCH_TRANSFORMERS_CACHE", os.getenv("PYTORCH_PRETRAINED_BERT_CACHE", default_cache_path))
- )
-except (AttributeError, ImportError):
- PYTORCH_PRETRAINED_BERT_CACHE = os.getenv(
- "PYTORCH_TRANSFORMERS_CACHE", os.getenv("PYTORCH_PRETRAINED_BERT_CACHE", default_cache_path)
- )
-
-PYTORCH_TRANSFORMERS_CACHE = PYTORCH_PRETRAINED_BERT_CACHE # Kept for backward compatibility
-TRANSFORMERS_CACHE = PYTORCH_PRETRAINED_BERT_CACHE # Kept for backward compatibility
-
-WEIGHTS_NAME = "pytorch_model.bin"
-TF2_WEIGHTS_NAME = "tf_model.h5"
-TF_WEIGHTS_NAME = "model.ckpt"
-CONFIG_NAME = "config.json"
-MODEL_CARD_NAME = "modelcard.json"
-
-
-MULTIPLE_CHOICE_DUMMY_INPUTS = [[[0], [1]], [[0], [1]]]
-DUMMY_INPUTS = [[7, 6, 0, 0, 1], [1, 2, 3, 0, 0], [0, 0, 0, 4, 5]]
-DUMMY_MASK = [[1, 1, 1, 1, 1], [1, 1, 1, 0, 0], [0, 0, 0, 1, 1]]
-
-S3_BUCKET_PREFIX = "https://s3.amazonaws.com/models.huggingface.co/bert"
-CLOUDFRONT_DISTRIB_PREFIX = "https://d2ws9o8vfrpkyk.cloudfront.net"
-
-
-def is_torch_available():
- return _torch_available
-
-
-def is_tf_available():
- return _tf_available
-
-
-def add_start_docstrings(*docstr):
- def docstring_decorator(fn):
- fn.__doc__ = "".join(docstr) + (fn.__doc__ if fn.__doc__ is not None else "")
- return fn
-
- return docstring_decorator
-
-
-def add_start_docstrings_to_callable(*docstr):
- def docstring_decorator(fn):
- class_name = ":class:`~transformers.{}`".format(fn.__qualname__.split(".")[0])
- intro = " The {} forward method, overrides the :func:`__call__` special method.".format(class_name)
- note = r"""
-
- .. note::
- Although the recipe for forward pass needs to be defined within
- this function, one should call the :class:`Module` instance afterwards
- instead of this since the former takes care of running the
- pre and post processing steps while the latter silently ignores them.
- """
- fn.__doc__ = intro + note + "".join(docstr) + (fn.__doc__ if fn.__doc__ is not None else "")
- return fn
-
- return docstring_decorator
-
-
-def add_end_docstrings(*docstr):
- def docstring_decorator(fn):
- fn.__doc__ = fn.__doc__ + "".join(docstr)
- return fn
-
- return docstring_decorator
-
-
-def is_remote_url(url_or_filename):
- parsed = urlparse(url_or_filename)
- return parsed.scheme in ("http", "https", "s3")
-
-
-def hf_bucket_url(identifier, postfix=None, cdn=False) -> str:
- endpoint = CLOUDFRONT_DISTRIB_PREFIX if cdn else S3_BUCKET_PREFIX
- if postfix is None:
- return "/".join((endpoint, identifier))
- else:
- return "/".join((endpoint, identifier, postfix))
-
-
-def url_to_filename(url, etag=None):
- """
- Convert `url` into a hashed filename in a repeatable way.
- If `etag` is specified, append its hash to the url's, delimited
- by a period.
- If the url ends with .h5 (Keras HDF5 weights) adds '.h5' to the name
- so that TF 2.0 can identify it as a HDF5 file
- (see https://github.com/tensorflow/tensorflow/blob/00fad90125b18b80fe054de1055770cfb8fe4ba3/tensorflow/python/keras/engine/network.py#L1380)
- """
- url_bytes = url.encode("utf-8")
- url_hash = sha256(url_bytes)
- filename = url_hash.hexdigest()
-
- if etag:
- etag_bytes = etag.encode("utf-8")
- etag_hash = sha256(etag_bytes)
- filename += "." + etag_hash.hexdigest()
-
- if url.endswith(".h5"):
- filename += ".h5"
-
- return filename
-
-
-def filename_to_url(filename, cache_dir=None):
- """
- Return the url and etag (which may be ``None``) stored for `filename`.
- Raise ``EnvironmentError`` if `filename` or its stored metadata do not exist.
- """
- if cache_dir is None:
- cache_dir = TRANSFORMERS_CACHE
- if isinstance(cache_dir, Path):
- cache_dir = str(cache_dir)
-
- cache_path = os.path.join(cache_dir, filename)
- if not os.path.exists(cache_path):
- raise EnvironmentError("file {} not found".format(cache_path))
-
- meta_path = cache_path + ".json"
- if not os.path.exists(meta_path):
- raise EnvironmentError("file {} not found".format(meta_path))
-
- with open(meta_path, encoding="utf-8") as meta_file:
- metadata = json.load(meta_file)
- url = metadata["url"]
- etag = metadata["etag"]
-
- return url, etag
-
-
-def cached_path(
- url_or_filename, cache_dir=None, force_download=False, proxies=None, resume_download=False, user_agent=None
-) -> Optional[str]:
- """
- Given something that might be a URL (or might be a local path),
- determine which. If it's a URL, download the file and cache it, and
- return the path to the cached file. If it's already a local path,
- make sure the file exists and then return the path.
- Args:
- cache_dir: specify a cache directory to save the file to (overwrite the default cache dir).
- force_download: if True, re-dowload the file even if it's already cached in the cache dir.
- resume_download: if True, resume the download if incompletly recieved file is found.
- user_agent: Optional string or dict that will be appended to the user-agent on remote requests.
-
- Return:
- None in case of non-recoverable file (non-existent or inaccessible url + no cache on disk).
- Local path (string) otherwise
- """
- if cache_dir is None:
- cache_dir = TRANSFORMERS_CACHE
- if isinstance(url_or_filename, Path):
- url_or_filename = str(url_or_filename)
- if isinstance(cache_dir, Path):
- cache_dir = str(cache_dir)
-
- if is_remote_url(url_or_filename):
- # URL, so get it from the cache (downloading if necessary)
- return get_from_cache(
- url_or_filename,
- cache_dir=cache_dir,
- force_download=force_download,
- proxies=proxies,
- resume_download=resume_download,
- user_agent=user_agent,
- )
- elif os.path.exists(url_or_filename):
- # File, and it exists.
- return url_or_filename
- elif urlparse(url_or_filename).scheme == "":
- # File, but it doesn't exist.
- raise EnvironmentError("file {} not found".format(url_or_filename))
- else:
- # Something unknown
- raise ValueError("unable to parse {} as a URL or as a local path".format(url_or_filename))
-
-
-def split_s3_path(url):
- """Split a full s3 path into the bucket name and path."""
- parsed = urlparse(url)
- if not parsed.netloc or not parsed.path:
- raise ValueError("bad s3 path {}".format(url))
- bucket_name = parsed.netloc
- s3_path = parsed.path
- # Remove '/' at beginning of path.
- if s3_path.startswith("/"):
- s3_path = s3_path[1:]
- return bucket_name, s3_path
-
-
-def s3_request(func):
- """
- Wrapper function for s3 requests in order to create more helpful error
- messages.
- """
-
- @wraps(func)
- def wrapper(url, *args, **kwargs):
- try:
- return func(url, *args, **kwargs)
- except ClientError as exc:
- if int(exc.response["Error"]["Code"]) == 404:
- raise EnvironmentError("file {} not found".format(url))
- else:
- raise
-
- return wrapper
-
-
-@s3_request
-def s3_etag(url, proxies=None):
- """Check ETag on S3 object."""
- s3_resource = boto3.resource("s3", config=Config(proxies=proxies))
- bucket_name, s3_path = split_s3_path(url)
- s3_object = s3_resource.Object(bucket_name, s3_path)
- return s3_object.e_tag
-
-
-@s3_request
-def s3_get(url, temp_file, proxies=None):
- """Pull a file directly from S3."""
- s3_resource = boto3.resource("s3", config=Config(proxies=proxies))
- bucket_name, s3_path = split_s3_path(url)
- s3_resource.Bucket(bucket_name).download_fileobj(s3_path, temp_file)
-
-
-def http_get(url, temp_file, proxies=None, resume_size=0, user_agent=None):
- ua = "transformers/{}; python/{}".format(__version__, sys.version.split()[0])
- if is_torch_available():
- ua += "; torch/{}".format(torch.__version__)
- if is_tf_available():
- ua += "; tensorflow/{}".format(tf.__version__)
- if isinstance(user_agent, dict):
- ua += "; " + "; ".join("{}/{}".format(k, v) for k, v in user_agent.items())
- elif isinstance(user_agent, str):
- ua += "; " + user_agent
- headers = {"user-agent": ua}
- if resume_size > 0:
- headers["Range"] = "bytes=%d-" % (resume_size,)
- response = requests.get(url, stream=True, proxies=proxies, headers=headers)
- if response.status_code == 416: # Range not satisfiable
- return
- content_length = response.headers.get("Content-Length")
- total = resume_size + int(content_length) if content_length is not None else None
- progress = tqdm(
- unit="B",
- unit_scale=True,
- total=total,
- initial=resume_size,
- desc="Downloading",
- disable=bool(logger.getEffectiveLevel() == logging.NOTSET),
- )
- for chunk in response.iter_content(chunk_size=1024):
- if chunk: # filter out keep-alive new chunks
- progress.update(len(chunk))
- temp_file.write(chunk)
- progress.close()
-
-
-def get_from_cache(
- url, cache_dir=None, force_download=False, proxies=None, etag_timeout=10, resume_download=False, user_agent=None
-) -> Optional[str]:
- """
- Given a URL, look for the corresponding file in the local cache.
- If it's not there, download it. Then return the path to the cached file.
-
- Return:
- None in case of non-recoverable file (non-existent or inaccessible url + no cache on disk).
- Local path (string) otherwise
- """
- if cache_dir is None:
- cache_dir = TRANSFORMERS_CACHE
- if isinstance(cache_dir, Path):
- cache_dir = str(cache_dir)
-
- os.makedirs(cache_dir, exist_ok=True)
-
- # Get eTag to add to filename, if it exists.
- if url.startswith("s3://"):
- etag = s3_etag(url, proxies=proxies)
- else:
- try:
- response = requests.head(url, allow_redirects=True, proxies=proxies, timeout=etag_timeout)
- if response.status_code != 200:
- etag = None
- else:
- etag = response.headers.get("ETag")
- except (EnvironmentError, requests.exceptions.Timeout):
- etag = None
-
- filename = url_to_filename(url, etag)
-
- # get cache path to put the file
- cache_path = os.path.join(cache_dir, filename)
-
- # etag is None = we don't have a connection, or url doesn't exist, or is otherwise inaccessible.
- # try to get the last downloaded one
- if etag is None:
- if os.path.exists(cache_path):
- return cache_path
- else:
- matching_files = [
- file
- for file in fnmatch.filter(os.listdir(cache_dir), filename + ".*")
- if not file.endswith(".json") and not file.endswith(".lock")
- ]
- if len(matching_files) > 0:
- return os.path.join(cache_dir, matching_files[-1])
- else:
- return None
-
- # From now on, etag is not None.
- if os.path.exists(cache_path) and not force_download:
- return cache_path
-
- # Prevent parallel downloads of the same file with a lock.
- lock_path = cache_path + ".lock"
- with FileLock(lock_path):
-
- if resume_download:
- incomplete_path = cache_path + ".incomplete"
-
- @contextmanager
- def _resumable_file_manager():
- with open(incomplete_path, "a+b") as f:
- yield f
-
- temp_file_manager = _resumable_file_manager
- if os.path.exists(incomplete_path):
- resume_size = os.stat(incomplete_path).st_size
- else:
- resume_size = 0
- else:
- temp_file_manager = partial(tempfile.NamedTemporaryFile, dir=cache_dir, delete=False)
- resume_size = 0
-
- # Download to temporary file, then copy to cache dir once finished.
- # Otherwise you get corrupt cache entries if the download gets interrupted.
- with temp_file_manager() as temp_file:
- logger.info("%s not found in cache or force_download set to True, downloading to %s", url, temp_file.name)
-
- # GET file object
- if url.startswith("s3://"):
- if resume_download:
- logger.warn('Warning: resumable downloads are not implemented for "s3://" urls')
- s3_get(url, temp_file, proxies=proxies)
- else:
- http_get(url, temp_file, proxies=proxies, resume_size=resume_size, user_agent=user_agent)
-
- logger.info("storing %s in cache at %s", url, cache_path)
- os.rename(temp_file.name, cache_path)
-
- logger.info("creating metadata file for %s", cache_path)
- meta = {"url": url, "etag": etag}
- meta_path = cache_path + ".json"
- with open(meta_path, "w") as meta_file:
- json.dump(meta, meta_file)
-
- return cache_path
diff --git a/server/transformers/src/transformers/hf_api.py b/server/transformers/src/transformers/hf_api.py
deleted file mode 100644
index c8da5615e5db698f0d36b8627c7972d91ab3af63..0000000000000000000000000000000000000000
--- a/server/transformers/src/transformers/hf_api.py
+++ /dev/null
@@ -1,189 +0,0 @@
-# coding=utf-8
-# Copyright 2019-present, the HuggingFace Inc. team.
-#
-# Licensed under the Apache License, Version 2.0 (the "License");
-# you may not use this file except in compliance with the License.
-# You may obtain a copy of the License at
-#
-# http://www.apache.org/licenses/LICENSE-2.0
-#
-# Unless required by applicable law or agreed to in writing, software
-# distributed under the License is distributed on an "AS IS" BASIS,
-# WITHOUT WARRANTIES OR CONDITIONS OF ANY KIND, either express or implied.
-# See the License for the specific language governing permissions and
-# limitations under the License.
-
-
-import io
-import os
-from os.path import expanduser
-from typing import List
-
-import requests
-from tqdm import tqdm
-
-
-ENDPOINT = "https://huggingface.co"
-
-
-class S3Obj:
- def __init__(self, filename: str, LastModified: str, ETag: str, Size: int, **kwargs):
- self.filename = filename
- self.LastModified = LastModified
- self.ETag = ETag
- self.Size = Size
-
-
-class PresignedUrl:
- def __init__(self, write: str, access: str, type: str, **kwargs):
- self.write = write
- self.access = access
- self.type = type # mime-type to send to S3.
-
-
-class HfApi:
- def __init__(self, endpoint=None):
- self.endpoint = endpoint if endpoint is not None else ENDPOINT
-
- def login(self, username: str, password: str) -> str:
- """
- Call HF API to sign in a user and get a token if credentials are valid.
-
- Outputs:
- token if credentials are valid
-
- Throws:
- requests.exceptions.HTTPError if credentials are invalid
- """
- path = "{}/api/login".format(self.endpoint)
- r = requests.post(path, json={"username": username, "password": password})
- r.raise_for_status()
- d = r.json()
- return d["token"]
-
- def whoami(self, token: str) -> str:
- """
- Call HF API to know "whoami"
- """
- path = "{}/api/whoami".format(self.endpoint)
- r = requests.get(path, headers={"authorization": "Bearer {}".format(token)})
- r.raise_for_status()
- d = r.json()
- return d["user"]
-
- def logout(self, token: str) -> None:
- """
- Call HF API to log out.
- """
- path = "{}/api/logout".format(self.endpoint)
- r = requests.post(path, headers={"authorization": "Bearer {}".format(token)})
- r.raise_for_status()
-
- def presign(self, token: str, filename: str) -> PresignedUrl:
- """
- Call HF API to get a presigned url to upload `filename` to S3.
- """
- path = "{}/api/presign".format(self.endpoint)
- r = requests.post(path, headers={"authorization": "Bearer {}".format(token)}, json={"filename": filename})
- r.raise_for_status()
- d = r.json()
- return PresignedUrl(**d)
-
- def presign_and_upload(self, token: str, filename: str, filepath: str) -> str:
- """
- Get a presigned url, then upload file to S3.
-
- Outputs:
- url: Read-only url for the stored file on S3.
- """
- urls = self.presign(token, filename=filename)
- # streaming upload:
- # https://2.python-requests.org/en/master/user/advanced/#streaming-uploads
- #
- # Even though we presign with the correct content-type,
- # the client still has to specify it when uploading the file.
- with open(filepath, "rb") as f:
- pf = TqdmProgressFileReader(f)
- data = f if pf.total_size > 0 else ""
-
- r = requests.put(urls.write, data=data, headers={"content-type": urls.type})
- r.raise_for_status()
- pf.close()
- return urls.access
-
- def list_objs(self, token: str) -> List[S3Obj]:
- """
- Call HF API to list all stored files for user.
- """
- path = "{}/api/listObjs".format(self.endpoint)
- r = requests.get(path, headers={"authorization": "Bearer {}".format(token)})
- r.raise_for_status()
- d = r.json()
- return [S3Obj(**x) for x in d]
-
- def delete_obj(self, token: str, filename: str):
- """
- Call HF API to delete a file stored by user
- """
- path = "{}/api/deleteObj".format(self.endpoint)
- r = requests.delete(path, headers={"authorization": "Bearer {}".format(token)}, json={"filename": filename})
- r.raise_for_status()
-
-
-class TqdmProgressFileReader:
- """
- Wrap an io.BufferedReader `f` (such as the output of `open(…, "rb")`)
- and override `f.read()` so as to display a tqdm progress bar.
-
- see github.com/huggingface/transformers/pull/2078#discussion_r354739608
- for implementation details.
- """
-
- def __init__(self, f: io.BufferedReader):
- self.f = f
- self.total_size = os.fstat(f.fileno()).st_size
- self.pbar = tqdm(total=self.total_size, leave=False)
- self.read = f.read
- f.read = self._read
-
- def _read(self, n=-1):
- self.pbar.update(n)
- return self.read(n)
-
- def close(self):
- self.pbar.close()
-
-
-class HfFolder:
- path_token = expanduser("~/.huggingface/token")
-
- @classmethod
- def save_token(cls, token):
- """
- Save token, creating folder as needed.
- """
- os.makedirs(os.path.dirname(cls.path_token), exist_ok=True)
- with open(cls.path_token, "w+") as f:
- f.write(token)
-
- @classmethod
- def get_token(cls):
- """
- Get token or None if not existent.
- """
- try:
- with open(cls.path_token, "r") as f:
- return f.read()
- except FileNotFoundError:
- pass
-
- @classmethod
- def delete_token(cls):
- """
- Delete token.
- Do not fail if token does not exist.
- """
- try:
- os.remove(cls.path_token)
- except FileNotFoundError:
- pass
diff --git a/server/transformers/src/transformers/modelcard.py b/server/transformers/src/transformers/modelcard.py
deleted file mode 100644
index 7661a3615485c1c15b642688bf7b5064236a2cf7..0000000000000000000000000000000000000000
--- a/server/transformers/src/transformers/modelcard.py
+++ /dev/null
@@ -1,242 +0,0 @@
-# coding=utf-8
-# Copyright 2018 The HuggingFace Inc. team.
-#
-# Licensed under the Apache License, Version 2.0 (the "License");
-# you may not use this file except in compliance with the License.
-# You may obtain a copy of the License at
-#
-# http://www.apache.org/licenses/LICENSE-2.0
-#
-# Unless required by applicable law or agreed to in writing, software
-# distributed under the License is distributed on an "AS IS" BASIS,
-# WITHOUT WARRANTIES OR CONDITIONS OF ANY KIND, either express or implied.
-# See the License for the specific language governing permissions and
-# limitations under the License.
-""" Configuration base class and utilities."""
-
-
-import copy
-import json
-import logging
-import os
-
-from .configuration_auto import ALL_PRETRAINED_CONFIG_ARCHIVE_MAP
-from .file_utils import (
- CONFIG_NAME,
- MODEL_CARD_NAME,
- TF2_WEIGHTS_NAME,
- WEIGHTS_NAME,
- cached_path,
- hf_bucket_url,
- is_remote_url,
-)
-
-
-logger = logging.getLogger(__name__)
-
-
-class ModelCard(object):
- r""" Model Card class.
- Store model card as well as methods for loading/downloading/saving model cards.
-
- Please read the following paper for details and explanation on the sections:
- "Model Cards for Model Reporting"
- by Margaret Mitchell, Simone Wu,
- Andrew Zaldivar, Parker Barnes, Lucy Vasserman, Ben Hutchinson, Elena Spitzer,
- Inioluwa Deborah Raji and Timnit Gebru for the proposal behind model cards.
- Link: https://arxiv.org/abs/1810.03993
-
- Note:
- A model card can be loaded and saved to disk.
-
- Parameters:
- """
-
- def __init__(self, **kwargs):
- # Recomended attributes from https://arxiv.org/abs/1810.03993 (see papers)
- self.model_details = kwargs.pop("model_details", {})
- self.intended_use = kwargs.pop("intended_use", {})
- self.factors = kwargs.pop("factors", {})
- self.metrics = kwargs.pop("metrics", {})
- self.evaluation_data = kwargs.pop("evaluation_data", {})
- self.training_data = kwargs.pop("training_data", {})
- self.quantitative_analyses = kwargs.pop("quantitative_analyses", {})
- self.ethical_considerations = kwargs.pop("ethical_considerations", {})
- self.caveats_and_recommendations = kwargs.pop("caveats_and_recommendations", {})
-
- # Open additional attributes
- for key, value in kwargs.items():
- try:
- setattr(self, key, value)
- except AttributeError as err:
- logger.error("Can't set {} with value {} for {}".format(key, value, self))
- raise err
-
- def save_pretrained(self, save_directory_or_file):
- """ Save a model card object to the directory or file `save_directory_or_file`.
- """
- if os.path.isdir(save_directory_or_file):
- # If we save using the predefined names, we can load using `from_pretrained`
- output_model_card_file = os.path.join(save_directory_or_file, MODEL_CARD_NAME)
- else:
- output_model_card_file = save_directory_or_file
-
- self.to_json_file(output_model_card_file)
- logger.info("Model card saved in {}".format(output_model_card_file))
-
- @classmethod
- def from_pretrained(cls, pretrained_model_name_or_path, **kwargs):
- r""" Instantiate a :class:`~transformers.ModelCard` from a pre-trained model model card.
-
- Parameters:
- pretrained_model_name_or_path: either:
-
- - a string with the `shortcut name` of a pre-trained model card to load from cache or download, e.g.: ``bert-base-uncased``.
- - a string with the `identifier name` of a pre-trained model card that was user-uploaded to our S3, e.g.: ``dbmdz/bert-base-german-cased``.
- - a path to a `directory` containing a mode card file saved using the :func:`~transformers.ModelCard.save_pretrained` method, e.g.: ``./my_model_directory/``.
- - a path or url to a saved model card JSON `file`, e.g.: ``./my_model_directory/modelcard.json``.
-
- cache_dir: (`optional`) string:
- Path to a directory in which a downloaded pre-trained model
- card should be cached if the standard cache should not be used.
-
- kwargs: (`optional`) dict: key/value pairs with which to update the ModelCard object after loading.
-
- - The values in kwargs of any keys which are model card attributes will be used to override the loaded values.
- - Behavior concerning key/value pairs whose keys are *not* model card attributes is controlled by the `return_unused_kwargs` keyword parameter.
-
- proxies: (`optional`) dict, default None:
- A dictionary of proxy servers to use by protocol or endpoint, e.g.: {'http': 'foo.bar:3128', 'http://hostname': 'foo.bar:4012'}.
- The proxies are used on each request.
-
- find_from_standard_name: (`optional`) boolean, default True:
- If the pretrained_model_name_or_path ends with our standard model or config filenames, replace them with our standard modelcard filename.
- Can be used to directly feed a model/config url and access the colocated modelcard.
-
- return_unused_kwargs: (`optional`) bool:
-
- - If False, then this function returns just the final model card object.
- - If True, then this functions returns a tuple `(model card, unused_kwargs)` where `unused_kwargs` is a dictionary consisting of the key/value pairs whose keys are not model card attributes: ie the part of kwargs which has not been used to update `ModelCard` and is otherwise ignored.
-
- Examples::
-
- modelcard = ModelCard.from_pretrained('bert-base-uncased') # Download model card from S3 and cache.
- modelcard = ModelCard.from_pretrained('./test/saved_model/') # E.g. model card was saved using `save_pretrained('./test/saved_model/')`
- modelcard = ModelCard.from_pretrained('./test/saved_model/modelcard.json')
- modelcard = ModelCard.from_pretrained('bert-base-uncased', output_attention=True, foo=False)
-
- """
- cache_dir = kwargs.pop("cache_dir", None)
- proxies = kwargs.pop("proxies", None)
- find_from_standard_name = kwargs.pop("find_from_standard_name", True)
- return_unused_kwargs = kwargs.pop("return_unused_kwargs", False)
-
- if pretrained_model_name_or_path in ALL_PRETRAINED_CONFIG_ARCHIVE_MAP:
- # For simplicity we use the same pretrained url than the configuration files
- # but with a different suffix (modelcard.json). This suffix is replaced below.
- model_card_file = ALL_PRETRAINED_CONFIG_ARCHIVE_MAP[pretrained_model_name_or_path]
- elif os.path.isdir(pretrained_model_name_or_path):
- model_card_file = os.path.join(pretrained_model_name_or_path, MODEL_CARD_NAME)
- elif os.path.isfile(pretrained_model_name_or_path) or is_remote_url(pretrained_model_name_or_path):
- model_card_file = pretrained_model_name_or_path
- else:
- model_card_file = hf_bucket_url(pretrained_model_name_or_path, postfix=MODEL_CARD_NAME)
-
- if find_from_standard_name or pretrained_model_name_or_path in ALL_PRETRAINED_CONFIG_ARCHIVE_MAP:
- model_card_file = model_card_file.replace(CONFIG_NAME, MODEL_CARD_NAME)
- model_card_file = model_card_file.replace(WEIGHTS_NAME, MODEL_CARD_NAME)
- model_card_file = model_card_file.replace(TF2_WEIGHTS_NAME, MODEL_CARD_NAME)
-
- try:
- # Load from URL or cache if already cached
- resolved_model_card_file = cached_path(
- model_card_file, cache_dir=cache_dir, force_download=True, proxies=proxies, resume_download=False
- )
- if resolved_model_card_file is None:
- raise EnvironmentError
- if resolved_model_card_file == model_card_file:
- logger.info("loading model card file {}".format(model_card_file))
- else:
- logger.info(
- "loading model card file {} from cache at {}".format(model_card_file, resolved_model_card_file)
- )
- # Load model card
- modelcard = cls.from_json_file(resolved_model_card_file)
-
- except EnvironmentError:
- if pretrained_model_name_or_path in ALL_PRETRAINED_CONFIG_ARCHIVE_MAP:
- logger.warning("Couldn't reach server at '{}' to download model card file.".format(model_card_file))
- else:
- logger.warning(
- "Model name '{}' was not found in model name list ({}). "
- "We assumed '{}' was a path or url to a model card file named {} or "
- "a directory containing such a file but couldn't find any such file at this path or url.".format(
- pretrained_model_name_or_path,
- ", ".join(ALL_PRETRAINED_CONFIG_ARCHIVE_MAP.keys()),
- model_card_file,
- MODEL_CARD_NAME,
- )
- )
- logger.warning("Creating an empty model card.")
-
- # We fall back on creating an empty model card
- modelcard = cls()
-
- except json.JSONDecodeError:
- logger.warning(
- "Couldn't reach server at '{}' to download model card file or "
- "model card file is not a valid JSON file. "
- "Please check network or file content here: {}.".format(model_card_file, resolved_model_card_file)
- )
- logger.warning("Creating an empty model card.")
-
- # We fall back on creating an empty model card
- modelcard = cls()
-
- # Update model card with kwargs if needed
- to_remove = []
- for key, value in kwargs.items():
- if hasattr(modelcard, key):
- setattr(modelcard, key, value)
- to_remove.append(key)
- for key in to_remove:
- kwargs.pop(key, None)
-
- logger.info("Model card: %s", str(modelcard))
- if return_unused_kwargs:
- return modelcard, kwargs
- else:
- return modelcard
-
- @classmethod
- def from_dict(cls, json_object):
- """Constructs a `ModelCard` from a Python dictionary of parameters."""
- return cls(**json_object)
-
- @classmethod
- def from_json_file(cls, json_file):
- """Constructs a `ModelCard` from a json file of parameters."""
- with open(json_file, "r", encoding="utf-8") as reader:
- text = reader.read()
- dict_obj = json.loads(text)
- return cls(**dict_obj)
-
- def __eq__(self, other):
- return self.__dict__ == other.__dict__
-
- def __repr__(self):
- return str(self.to_json_string())
-
- def to_dict(self):
- """Serializes this instance to a Python dictionary."""
- output = copy.deepcopy(self.__dict__)
- return output
-
- def to_json_string(self):
- """Serializes this instance to a JSON string."""
- return json.dumps(self.to_dict(), indent=2, sort_keys=True) + "\n"
-
- def to_json_file(self, json_file_path):
- """ Save this instance to a json file."""
- with open(json_file_path, "w", encoding="utf-8") as writer:
- writer.write(self.to_json_string())
diff --git a/server/transformers/src/transformers/modeling_albert.py b/server/transformers/src/transformers/modeling_albert.py
deleted file mode 100644
index d2a5d4878e5e4496b29c4d13dc156dc8b1126bd7..0000000000000000000000000000000000000000
--- a/server/transformers/src/transformers/modeling_albert.py
+++ /dev/null
@@ -1,892 +0,0 @@
-# coding=utf-8
-# Copyright 2018 Google AI, Google Brain and the HuggingFace Inc. team.
-#
-# Licensed under the Apache License, Version 2.0 (the "License");
-# you may not use this file except in compliance with the License.
-# You may obtain a copy of the License at
-#
-# http://www.apache.org/licenses/LICENSE-2.0
-#
-# Unless required by applicable law or agreed to in writing, software
-# distributed under the License is distributed on an "AS IS" BASIS,
-# WITHOUT WARRANTIES OR CONDITIONS OF ANY KIND, either express or implied.
-# See the License for the specific language governing permissions and
-# limitations under the License.
-"""PyTorch ALBERT model. """
-
-import logging
-import math
-import os
-
-import torch
-import torch.nn as nn
-from torch.nn import CrossEntropyLoss, MSELoss
-
-from transformers.configuration_albert import AlbertConfig
-from transformers.modeling_bert import ACT2FN, BertEmbeddings, BertSelfAttention, prune_linear_layer
-from transformers.modeling_utils import PreTrainedModel
-
-from .file_utils import add_start_docstrings, add_start_docstrings_to_callable
-
-
-logger = logging.getLogger(__name__)
-
-
-ALBERT_PRETRAINED_MODEL_ARCHIVE_MAP = {
- "albert-base-v1": "https://s3.amazonaws.com/models.huggingface.co/bert/albert-base-pytorch_model.bin",
- "albert-large-v1": "https://s3.amazonaws.com/models.huggingface.co/bert/albert-large-pytorch_model.bin",
- "albert-xlarge-v1": "https://s3.amazonaws.com/models.huggingface.co/bert/albert-xlarge-pytorch_model.bin",
- "albert-xxlarge-v1": "https://s3.amazonaws.com/models.huggingface.co/bert/albert-xxlarge-pytorch_model.bin",
- "albert-base-v2": "https://s3.amazonaws.com/models.huggingface.co/bert/albert-base-v2-pytorch_model.bin",
- "albert-large-v2": "https://s3.amazonaws.com/models.huggingface.co/bert/albert-large-v2-pytorch_model.bin",
- "albert-xlarge-v2": "https://s3.amazonaws.com/models.huggingface.co/bert/albert-xlarge-v2-pytorch_model.bin",
- "albert-xxlarge-v2": "https://s3.amazonaws.com/models.huggingface.co/bert/albert-xxlarge-v2-pytorch_model.bin",
-}
-
-
-def load_tf_weights_in_albert(model, config, tf_checkpoint_path):
- """ Load tf checkpoints in a pytorch model."""
- try:
- import re
- import numpy as np
- import tensorflow as tf
- except ImportError:
- logger.error(
- "Loading a TensorFlow model in PyTorch, requires TensorFlow to be installed. Please see "
- "https://www.tensorflow.org/install/ for installation instructions."
- )
- raise
- tf_path = os.path.abspath(tf_checkpoint_path)
- logger.info("Converting TensorFlow checkpoint from {}".format(tf_path))
- # Load weights from TF model
- init_vars = tf.train.list_variables(tf_path)
- names = []
- arrays = []
- for name, shape in init_vars:
- logger.info("Loading TF weight {} with shape {}".format(name, shape))
- array = tf.train.load_variable(tf_path, name)
- names.append(name)
- arrays.append(array)
-
- for name, array in zip(names, arrays):
- print(name)
-
- for name, array in zip(names, arrays):
- original_name = name
-
- # If saved from the TF HUB module
- name = name.replace("module/", "")
-
- # Renaming and simplifying
- name = name.replace("ffn_1", "ffn")
- name = name.replace("bert/", "albert/")
- name = name.replace("attention_1", "attention")
- name = name.replace("transform/", "")
- name = name.replace("LayerNorm_1", "full_layer_layer_norm")
- name = name.replace("LayerNorm", "attention/LayerNorm")
- name = name.replace("transformer/", "")
-
- # The feed forward layer had an 'intermediate' step which has been abstracted away
- name = name.replace("intermediate/dense/", "")
- name = name.replace("ffn/intermediate/output/dense/", "ffn_output/")
-
- # ALBERT attention was split between self and output which have been abstracted away
- name = name.replace("/output/", "/")
- name = name.replace("/self/", "/")
-
- # The pooler is a linear layer
- name = name.replace("pooler/dense", "pooler")
-
- # The classifier was simplified to predictions from cls/predictions
- name = name.replace("cls/predictions", "predictions")
- name = name.replace("predictions/attention", "predictions")
-
- # Naming was changed to be more explicit
- name = name.replace("embeddings/attention", "embeddings")
- name = name.replace("inner_group_", "albert_layers/")
- name = name.replace("group_", "albert_layer_groups/")
-
- # Classifier
- if len(name.split("/")) == 1 and ("output_bias" in name or "output_weights" in name):
- name = "classifier/" + name
-
- # No ALBERT model currently handles the next sentence prediction task
- if "seq_relationship" in name:
- continue
-
- name = name.split("/")
-
- # Ignore the gradients applied by the LAMB/ADAM optimizers.
- if "adam_m" in name or "adam_v" in name or "global_step" in name:
- logger.info("Skipping {}".format("/".join(name)))
- continue
-
- pointer = model
- for m_name in name:
- if re.fullmatch(r"[A-Za-z]+_\d+", m_name):
- scope_names = re.split(r"_(\d+)", m_name)
- else:
- scope_names = [m_name]
-
- if scope_names[0] == "kernel" or scope_names[0] == "gamma":
- pointer = getattr(pointer, "weight")
- elif scope_names[0] == "output_bias" or scope_names[0] == "beta":
- pointer = getattr(pointer, "bias")
- elif scope_names[0] == "output_weights":
- pointer = getattr(pointer, "weight")
- elif scope_names[0] == "squad":
- pointer = getattr(pointer, "classifier")
- else:
- try:
- pointer = getattr(pointer, scope_names[0])
- except AttributeError:
- logger.info("Skipping {}".format("/".join(name)))
- continue
- if len(scope_names) >= 2:
- num = int(scope_names[1])
- pointer = pointer[num]
-
- if m_name[-11:] == "_embeddings":
- pointer = getattr(pointer, "weight")
- elif m_name == "kernel":
- array = np.transpose(array)
- try:
- assert pointer.shape == array.shape
- except AssertionError as e:
- e.args += (pointer.shape, array.shape)
- raise
- print("Initialize PyTorch weight {} from {}".format(name, original_name))
- pointer.data = torch.from_numpy(array)
-
- return model
-
-
-class AlbertEmbeddings(BertEmbeddings):
- """
- Construct the embeddings from word, position and token_type embeddings.
- """
-
- def __init__(self, config):
- super().__init__(config)
-
- self.word_embeddings = nn.Embedding(config.vocab_size, config.embedding_size, padding_idx=0)
- self.position_embeddings = nn.Embedding(config.max_position_embeddings, config.embedding_size)
- self.token_type_embeddings = nn.Embedding(config.type_vocab_size, config.embedding_size)
- self.LayerNorm = torch.nn.LayerNorm(config.embedding_size, eps=config.layer_norm_eps)
-
-
-class AlbertAttention(BertSelfAttention):
- def __init__(self, config):
- super().__init__(config)
-
- self.output_attentions = config.output_attentions
- self.num_attention_heads = config.num_attention_heads
- self.hidden_size = config.hidden_size
- self.attention_head_size = config.hidden_size // config.num_attention_heads
- self.dropout = nn.Dropout(config.attention_probs_dropout_prob)
- self.dense = nn.Linear(config.hidden_size, config.hidden_size)
- self.LayerNorm = nn.LayerNorm(config.hidden_size, eps=config.layer_norm_eps)
- self.pruned_heads = set()
-
- def prune_heads(self, heads):
- if len(heads) == 0:
- return
- mask = torch.ones(self.num_attention_heads, self.attention_head_size)
- heads = set(heads) - self.pruned_heads # Convert to set and emove already pruned heads
- for head in heads:
- # Compute how many pruned heads are before the head and move the index accordingly
- head = head - sum(1 if h < head else 0 for h in self.pruned_heads)
- mask[head] = 0
- mask = mask.view(-1).contiguous().eq(1)
- index = torch.arange(len(mask))[mask].long()
-
- # Prune linear layers
- self.query = prune_linear_layer(self.query, index)
- self.key = prune_linear_layer(self.key, index)
- self.value = prune_linear_layer(self.value, index)
- self.dense = prune_linear_layer(self.dense, index, dim=1)
-
- # Update hyper params and store pruned heads
- self.num_attention_heads = self.num_attention_heads - len(heads)
- self.all_head_size = self.attention_head_size * self.num_attention_heads
- self.pruned_heads = self.pruned_heads.union(heads)
-
- def forward(self, input_ids, attention_mask=None, head_mask=None):
- mixed_query_layer = self.query(input_ids)
- mixed_key_layer = self.key(input_ids)
- mixed_value_layer = self.value(input_ids)
-
- query_layer = self.transpose_for_scores(mixed_query_layer)
- key_layer = self.transpose_for_scores(mixed_key_layer)
- value_layer = self.transpose_for_scores(mixed_value_layer)
-
- # Take the dot product between "query" and "key" to get the raw attention scores.
- attention_scores = torch.matmul(query_layer, key_layer.transpose(-1, -2))
- attention_scores = attention_scores / math.sqrt(self.attention_head_size)
- if attention_mask is not None:
- # Apply the attention mask is (precomputed for all layers in BertModel forward() function)
- attention_scores = attention_scores + attention_mask
-
- # Normalize the attention scores to probabilities.
- attention_probs = nn.Softmax(dim=-1)(attention_scores)
-
- # This is actually dropping out entire tokens to attend to, which might
- # seem a bit unusual, but is taken from the original Transformer paper.
- attention_probs = self.dropout(attention_probs)
-
- # Mask heads if we want to
- if head_mask is not None:
- attention_probs = attention_probs * head_mask
-
- context_layer = torch.matmul(attention_probs, value_layer)
-
- context_layer = context_layer.permute(0, 2, 1, 3).contiguous()
-
- # Should find a better way to do this
- w = (
- self.dense.weight.t()
- .view(self.num_attention_heads, self.attention_head_size, self.hidden_size)
- .to(context_layer.dtype)
- )
- b = self.dense.bias.to(context_layer.dtype)
-
- projected_context_layer = torch.einsum("bfnd,ndh->bfh", context_layer, w) + b
- projected_context_layer_dropout = self.dropout(projected_context_layer)
- layernormed_context_layer = self.LayerNorm(input_ids + projected_context_layer_dropout)
- return (layernormed_context_layer, attention_probs) if self.output_attentions else (layernormed_context_layer,)
-
-
-class AlbertLayer(nn.Module):
- def __init__(self, config):
- super().__init__()
-
- self.config = config
- self.full_layer_layer_norm = nn.LayerNorm(config.hidden_size, eps=config.layer_norm_eps)
- self.attention = AlbertAttention(config)
- self.ffn = nn.Linear(config.hidden_size, config.intermediate_size)
- self.ffn_output = nn.Linear(config.intermediate_size, config.hidden_size)
- self.activation = ACT2FN[config.hidden_act]
-
- def forward(self, hidden_states, attention_mask=None, head_mask=None):
- attention_output = self.attention(hidden_states, attention_mask, head_mask)
- ffn_output = self.ffn(attention_output[0])
- ffn_output = self.activation(ffn_output)
- ffn_output = self.ffn_output(ffn_output)
- hidden_states = self.full_layer_layer_norm(ffn_output + attention_output[0])
-
- return (hidden_states,) + attention_output[1:] # add attentions if we output them
-
-
-class AlbertLayerGroup(nn.Module):
- def __init__(self, config):
- super().__init__()
-
- self.output_attentions = config.output_attentions
- self.output_hidden_states = config.output_hidden_states
- self.albert_layers = nn.ModuleList([AlbertLayer(config) for _ in range(config.inner_group_num)])
-
- def forward(self, hidden_states, attention_mask=None, head_mask=None):
- layer_hidden_states = ()
- layer_attentions = ()
-
- for layer_index, albert_layer in enumerate(self.albert_layers):
- layer_output = albert_layer(hidden_states, attention_mask, head_mask[layer_index])
- hidden_states = layer_output[0]
-
- if self.output_attentions:
- layer_attentions = layer_attentions + (layer_output[1],)
-
- if self.output_hidden_states:
- layer_hidden_states = layer_hidden_states + (hidden_states,)
-
- outputs = (hidden_states,)
- if self.output_hidden_states:
- outputs = outputs + (layer_hidden_states,)
- if self.output_attentions:
- outputs = outputs + (layer_attentions,)
- return outputs # last-layer hidden state, (layer hidden states), (layer attentions)
-
-
-class AlbertTransformer(nn.Module):
- def __init__(self, config):
- super().__init__()
-
- self.config = config
- self.output_attentions = config.output_attentions
- self.output_hidden_states = config.output_hidden_states
- self.embedding_hidden_mapping_in = nn.Linear(config.embedding_size, config.hidden_size)
- self.albert_layer_groups = nn.ModuleList([AlbertLayerGroup(config) for _ in range(config.num_hidden_groups)])
-
- def forward(self, hidden_states, attention_mask=None, head_mask=None):
- hidden_states = self.embedding_hidden_mapping_in(hidden_states)
-
- all_attentions = ()
-
- if self.output_hidden_states:
- all_hidden_states = (hidden_states,)
-
- for i in range(self.config.num_hidden_layers):
- # Number of layers in a hidden group
- layers_per_group = int(self.config.num_hidden_layers / self.config.num_hidden_groups)
-
- # Index of the hidden group
- group_idx = int(i / (self.config.num_hidden_layers / self.config.num_hidden_groups))
-
- layer_group_output = self.albert_layer_groups[group_idx](
- hidden_states,
- attention_mask,
- head_mask[group_idx * layers_per_group : (group_idx + 1) * layers_per_group],
- )
- hidden_states = layer_group_output[0]
-
- if self.output_attentions:
- all_attentions = all_attentions + layer_group_output[-1]
-
- if self.output_hidden_states:
- all_hidden_states = all_hidden_states + (hidden_states,)
-
- outputs = (hidden_states,)
- if self.output_hidden_states:
- outputs = outputs + (all_hidden_states,)
- if self.output_attentions:
- outputs = outputs + (all_attentions,)
- return outputs # last-layer hidden state, (all hidden states), (all attentions)
-
-
-class AlbertPreTrainedModel(PreTrainedModel):
- """ An abstract class to handle weights initialization and
- a simple interface for downloading and loading pretrained models.
- """
-
- config_class = AlbertConfig
- pretrained_model_archive_map = ALBERT_PRETRAINED_MODEL_ARCHIVE_MAP
- base_model_prefix = "albert"
-
- def _init_weights(self, module):
- """ Initialize the weights.
- """
- if isinstance(module, (nn.Linear, nn.Embedding)):
- # Slightly different from the TF version which uses truncated_normal for initialization
- # cf https://github.com/pytorch/pytorch/pull/5617
- module.weight.data.normal_(mean=0.0, std=self.config.initializer_range)
- if isinstance(module, (nn.Linear)) and module.bias is not None:
- module.bias.data.zero_()
- elif isinstance(module, nn.LayerNorm):
- module.bias.data.zero_()
- module.weight.data.fill_(1.0)
-
-
-ALBERT_START_DOCSTRING = r"""
-
- This model is a PyTorch `torch.nn.Module `_ sub-class.
- Use it as a regular PyTorch Module and refer to the PyTorch documentation for all matter related to general
- usage and behavior.
-
- Args:
- config (:class:`~transformers.AlbertConfig`): Model configuration class with all the parameters of the model.
- Initializing with a config file does not load the weights associated with the model, only the configuration.
- Check out the :meth:`~transformers.PreTrainedModel.from_pretrained` method to load the model weights.
-"""
-
-ALBERT_INPUTS_DOCSTRING = r"""
- Args:
- input_ids (:obj:`torch.LongTensor` of shape :obj:`(batch_size, sequence_length)`):
- Indices of input sequence tokens in the vocabulary.
-
- Indices can be obtained using :class:`transformers.AlbertTokenizer`.
- See :func:`transformers.PreTrainedTokenizer.encode` and
- :func:`transformers.PreTrainedTokenizer.encode_plus` for details.
-
- `What are input IDs? <../glossary.html#input-ids>`__
- attention_mask (:obj:`torch.FloatTensor` of shape :obj:`(batch_size, sequence_length)`, `optional`, defaults to :obj:`None`):
- Mask to avoid performing attention on padding token indices.
- Mask values selected in ``[0, 1]``:
- ``1`` for tokens that are NOT MASKED, ``0`` for MASKED tokens.
-
- `What are attention masks? <../glossary.html#attention-mask>`__
- token_type_ids (:obj:`torch.LongTensor` of shape :obj:`(batch_size, sequence_length)`, `optional`, defaults to :obj:`None`):
- Segment token indices to indicate first and second portions of the inputs.
- Indices are selected in ``[0, 1]``: ``0`` corresponds to a `sentence A` token, ``1``
- corresponds to a `sentence B` token
-
- `What are token type IDs? <../glossary.html#token-type-ids>`_
- position_ids (:obj:`torch.LongTensor` of shape :obj:`(batch_size, sequence_length)`, `optional`, defaults to :obj:`None`):
- Indices of positions of each input sequence tokens in the position embeddings.
- Selected in the range ``[0, config.max_position_embeddings - 1]``.
-
- `What are position IDs? <../glossary.html#position-ids>`_
- head_mask (:obj:`torch.FloatTensor` of shape :obj:`(num_heads,)` or :obj:`(num_layers, num_heads)`, `optional`, defaults to :obj:`None`):
- Mask to nullify selected heads of the self-attention modules.
- Mask values selected in ``[0, 1]``:
- :obj:`1` indicates the head is **not masked**, :obj:`0` indicates the head is **masked**.
- input_embeds (:obj:`torch.FloatTensor` of shape :obj:`(batch_size, sequence_length, hidden_size)`, `optional`, defaults to :obj:`None`):
- Optionally, instead of passing :obj:`input_ids` you can choose to directly pass an embedded representation.
- This is useful if you want more control over how to convert `input_ids` indices into associated vectors
- than the model's internal embedding lookup matrix.
-"""
-
-
-@add_start_docstrings(
- "The bare ALBERT Model transformer outputting raw hidden-states without any specific head on top.",
- ALBERT_START_DOCSTRING,
-)
-class AlbertModel(AlbertPreTrainedModel):
-
- config_class = AlbertConfig
- pretrained_model_archive_map = ALBERT_PRETRAINED_MODEL_ARCHIVE_MAP
- load_tf_weights = load_tf_weights_in_albert
- base_model_prefix = "albert"
-
- def __init__(self, config):
- super().__init__(config)
-
- self.config = config
- self.embeddings = AlbertEmbeddings(config)
- self.encoder = AlbertTransformer(config)
- self.pooler = nn.Linear(config.hidden_size, config.hidden_size)
- self.pooler_activation = nn.Tanh()
-
- self.init_weights()
-
- def get_input_embeddings(self):
- return self.embeddings.word_embeddings
-
- def set_input_embeddings(self, value):
- self.embeddings.word_embeddings = value
-
- def _resize_token_embeddings(self, new_num_tokens):
- old_embeddings = self.embeddings.word_embeddings
- new_embeddings = self._get_resized_embeddings(old_embeddings, new_num_tokens)
- self.embeddings.word_embeddings = new_embeddings
- return self.embeddings.word_embeddings
-
- def _prune_heads(self, heads_to_prune):
- """ Prunes heads of the model.
- heads_to_prune: dict of {layer_num: list of heads to prune in this layer}
- ALBERT has a different architecture in that its layers are shared across groups, which then has inner groups.
- If an ALBERT model has 12 hidden layers and 2 hidden groups, with two inner groups, there
- is a total of 4 different layers.
-
- These layers are flattened: the indices [0,1] correspond to the two inner groups of the first hidden layer,
- while [2,3] correspond to the two inner groups of the second hidden layer.
-
- Any layer with in index other than [0,1,2,3] will result in an error.
- See base class PreTrainedModel for more information about head pruning
- """
- for layer, heads in heads_to_prune.items():
- group_idx = int(layer / self.config.inner_group_num)
- inner_group_idx = int(layer - group_idx * self.config.inner_group_num)
- self.encoder.albert_layer_groups[group_idx].albert_layers[inner_group_idx].attention.prune_heads(heads)
-
- @add_start_docstrings_to_callable(ALBERT_INPUTS_DOCSTRING)
- def forward(
- self,
- input_ids=None,
- attention_mask=None,
- token_type_ids=None,
- position_ids=None,
- head_mask=None,
- inputs_embeds=None,
- ):
- r"""
- Return:
- :obj:`tuple(torch.FloatTensor)` comprising various elements depending on the configuration (:class:`~transformers.AlbertConfig`) and inputs:
- last_hidden_state (:obj:`torch.FloatTensor` of shape :obj:`(batch_size, sequence_length, hidden_size)`):
- Sequence of hidden-states at the output of the last layer of the model.
- pooler_output (:obj:`torch.FloatTensor`: of shape :obj:`(batch_size, hidden_size)`):
- Last layer hidden-state of the first token of the sequence (classification token)
- further processed by a Linear layer and a Tanh activation function. The Linear
- layer weights are trained from the next sentence prediction (classification)
- objective during pre-training.
-
- This output is usually *not* a good summary
- of the semantic content of the input, you're often better with averaging or pooling
- the sequence of hidden-states for the whole input sequence.
- hidden_states (:obj:`tuple(torch.FloatTensor)`, `optional`, returned when ``config.output_hidden_states=True``):
- Tuple of :obj:`torch.FloatTensor` (one for the output of the embeddings + one for the output of each layer)
- of shape :obj:`(batch_size, sequence_length, hidden_size)`.
-
- Hidden-states of the model at the output of each layer plus the initial embedding outputs.
- attentions (:obj:`tuple(torch.FloatTensor)`, `optional`, returned when ``config.output_attentions=True``):
- Tuple of :obj:`torch.FloatTensor` (one for each layer) of shape
- :obj:`(batch_size, num_heads, sequence_length, sequence_length)`.
-
- Attentions weights after the attention softmax, used to compute the weighted average in the self-attention
- heads.
-
- Example::
-
- from transformers import AlbertModel, AlbertTokenizer
- import torch
-
- tokenizer = AlbertTokenizer.from_pretrained('albert-base-v2')
- model = AlbertModel.from_pretrained('albert-base-v2')
- input_ids = torch.tensor(tokenizer.encode("Hello, my dog is cute", add_special_tokens=True)).unsqueeze(0) # Batch size 1
- outputs = model(input_ids)
- last_hidden_states = outputs[0] # The last hidden-state is the first element of the output tuple
-
- """
-
- if input_ids is not None and inputs_embeds is not None:
- raise ValueError("You cannot specify both input_ids and inputs_embeds at the same time")
- elif input_ids is not None:
- input_shape = input_ids.size()
- elif inputs_embeds is not None:
- input_shape = inputs_embeds.size()[:-1]
- else:
- raise ValueError("You have to specify either input_ids or inputs_embeds")
-
- device = input_ids.device if input_ids is not None else inputs_embeds.device
-
- if attention_mask is None:
- attention_mask = torch.ones(input_shape, device=device)
- if token_type_ids is None:
- token_type_ids = torch.zeros(input_shape, dtype=torch.long, device=device)
-
- extended_attention_mask = attention_mask.unsqueeze(1).unsqueeze(2)
- extended_attention_mask = extended_attention_mask.to(dtype=next(self.parameters()).dtype) # fp16 compatibility
- extended_attention_mask = (1.0 - extended_attention_mask) * -10000.0
- if head_mask is not None:
- if head_mask.dim() == 1:
- head_mask = head_mask.unsqueeze(0).unsqueeze(0).unsqueeze(-1).unsqueeze(-1)
- head_mask = head_mask.expand(self.config.num_hidden_layers, -1, -1, -1, -1)
- elif head_mask.dim() == 2:
- head_mask = (
- head_mask.unsqueeze(1).unsqueeze(-1).unsqueeze(-1)
- ) # We can specify head_mask for each layer
- head_mask = head_mask.to(
- dtype=next(self.parameters()).dtype
- ) # switch to fload if need + fp16 compatibility
- else:
- head_mask = [None] * self.config.num_hidden_layers
-
- embedding_output = self.embeddings(
- input_ids, position_ids=position_ids, token_type_ids=token_type_ids, inputs_embeds=inputs_embeds
- )
- encoder_outputs = self.encoder(embedding_output, extended_attention_mask, head_mask=head_mask)
-
- sequence_output = encoder_outputs[0]
-
- pooled_output = self.pooler_activation(self.pooler(sequence_output[:, 0]))
-
- outputs = (sequence_output, pooled_output) + encoder_outputs[
- 1:
- ] # add hidden_states and attentions if they are here
- return outputs
-
-
-class AlbertMLMHead(nn.Module):
- def __init__(self, config):
- super().__init__()
-
- self.LayerNorm = nn.LayerNorm(config.embedding_size)
- self.bias = nn.Parameter(torch.zeros(config.vocab_size))
- self.dense = nn.Linear(config.hidden_size, config.embedding_size)
- self.decoder = nn.Linear(config.embedding_size, config.vocab_size)
- self.activation = ACT2FN[config.hidden_act]
-
- # Need a link between the two variables so that the bias is correctly resized with `resize_token_embeddings`
- self.decoder.bias = self.bias
-
- def forward(self, hidden_states):
- hidden_states = self.dense(hidden_states)
- hidden_states = self.activation(hidden_states)
- hidden_states = self.LayerNorm(hidden_states)
- hidden_states = self.decoder(hidden_states)
-
- prediction_scores = hidden_states + self.bias
-
- return prediction_scores
-
-
-@add_start_docstrings(
- "Albert Model with a `language modeling` head on top.", ALBERT_START_DOCSTRING,
-)
-class AlbertForMaskedLM(AlbertPreTrainedModel):
- def __init__(self, config):
- super().__init__(config)
-
- self.albert = AlbertModel(config)
- self.predictions = AlbertMLMHead(config)
-
- self.init_weights()
- self.tie_weights()
-
- def tie_weights(self):
- self._tie_or_clone_weights(self.predictions.decoder, self.albert.embeddings.word_embeddings)
-
- def get_output_embeddings(self):
- return self.predictions.decoder
-
- @add_start_docstrings_to_callable(ALBERT_INPUTS_DOCSTRING)
- def forward(
- self,
- input_ids=None,
- attention_mask=None,
- token_type_ids=None,
- position_ids=None,
- head_mask=None,
- inputs_embeds=None,
- masked_lm_labels=None,
- ):
- r"""
- masked_lm_labels (:obj:`torch.LongTensor` of shape :obj:`(batch_size, sequence_length)`, `optional`, defaults to :obj:`None`):
- Labels for computing the masked language modeling loss.
- Indices should be in ``[-100, 0, ..., config.vocab_size]`` (see ``input_ids`` docstring)
- Tokens with indices set to ``-100`` are ignored (masked), the loss is only computed for the tokens with
- labels in ``[0, ..., config.vocab_size]``
-
- Returns:
- :obj:`tuple(torch.FloatTensor)` comprising various elements depending on the configuration (:class:`~transformers.AlbertConfig`) and inputs:
- loss (`optional`, returned when ``masked_lm_labels`` is provided) ``torch.FloatTensor`` of shape ``(1,)``:
- Masked language modeling loss.
- prediction_scores (:obj:`torch.FloatTensor` of shape :obj:`(batch_size, sequence_length, config.vocab_size)`)
- Prediction scores of the language modeling head (scores for each vocabulary token before SoftMax).
- hidden_states (:obj:`tuple(torch.FloatTensor)`, `optional`, returned when ``config.output_hidden_states=True``):
- Tuple of :obj:`torch.FloatTensor` (one for the output of the embeddings + one for the output of each layer)
- of shape :obj:`(batch_size, sequence_length, hidden_size)`.
-
- Hidden-states of the model at the output of each layer plus the initial embedding outputs.
- attentions (:obj:`tuple(torch.FloatTensor)`, `optional`, returned when ``config.output_attentions=True``):
- Tuple of :obj:`torch.FloatTensor` (one for each layer) of shape
- :obj:`(batch_size, num_heads, sequence_length, sequence_length)`.
-
- Attentions weights after the attention softmax, used to compute the weighted average in the self-attention
- heads.
-
- Example::
-
- from transformers import AlbertTokenizer, AlbertForMaskedLM
- import torch
-
- tokenizer = AlbertTokenizer.from_pretrained('albert-base-v2')
- model = AlbertForMaskedLM.from_pretrained('albert-base-v2')
- input_ids = torch.tensor(tokenizer.encode("Hello, my dog is cute", add_special_tokens=True)).unsqueeze(0) # Batch size 1
- outputs = model(input_ids, masked_lm_labels=input_ids)
- loss, prediction_scores = outputs[:2]
-
- """
- outputs = self.albert(
- input_ids=input_ids,
- attention_mask=attention_mask,
- token_type_ids=token_type_ids,
- position_ids=position_ids,
- head_mask=head_mask,
- inputs_embeds=inputs_embeds,
- )
- sequence_outputs = outputs[0]
-
- prediction_scores = self.predictions(sequence_outputs)
-
- outputs = (prediction_scores,) + outputs[2:] # Add hidden states and attention if they are here
- if masked_lm_labels is not None:
- loss_fct = CrossEntropyLoss()
- masked_lm_loss = loss_fct(prediction_scores.view(-1, self.config.vocab_size), masked_lm_labels.view(-1))
- outputs = (masked_lm_loss,) + outputs
-
- return outputs
-
-
-@add_start_docstrings(
- """Albert Model transformer with a sequence classification/regression head on top (a linear layer on top of
- the pooled output) e.g. for GLUE tasks. """,
- ALBERT_START_DOCSTRING,
-)
-class AlbertForSequenceClassification(AlbertPreTrainedModel):
- def __init__(self, config):
- super().__init__(config)
- self.num_labels = config.num_labels
-
- self.albert = AlbertModel(config)
- self.dropout = nn.Dropout(config.classifier_dropout_prob)
- self.classifier = nn.Linear(config.hidden_size, self.config.num_labels)
-
- self.init_weights()
-
- @add_start_docstrings_to_callable(ALBERT_INPUTS_DOCSTRING)
- def forward(
- self,
- input_ids=None,
- attention_mask=None,
- token_type_ids=None,
- position_ids=None,
- head_mask=None,
- inputs_embeds=None,
- labels=None,
- ):
- r"""
- labels (:obj:`torch.LongTensor` of shape :obj:`(batch_size,)`, `optional`, defaults to :obj:`None`):
- Labels for computing the sequence classification/regression loss.
- Indices should be in ``[0, ..., config.num_labels - 1]``.
- If ``config.num_labels == 1`` a regression loss is computed (Mean-Square loss),
- If ``config.num_labels > 1`` a classification loss is computed (Cross-Entropy).
-
- Returns:
- :obj:`tuple(torch.FloatTensor)` comprising various elements depending on the configuration (:class:`~transformers.AlbertConfig`) and inputs:
- loss: (`optional`, returned when ``labels`` is provided) ``torch.FloatTensor`` of shape ``(1,)``:
- Classification (or regression if config.num_labels==1) loss.
- logits ``torch.FloatTensor`` of shape ``(batch_size, config.num_labels)``
- Classification (or regression if config.num_labels==1) scores (before SoftMax).
- hidden_states (:obj:`tuple(torch.FloatTensor)`, `optional`, returned when ``config.output_hidden_states=True``):
- Tuple of :obj:`torch.FloatTensor` (one for the output of the embeddings + one for the output of each layer)
- of shape :obj:`(batch_size, sequence_length, hidden_size)`.
-
- Hidden-states of the model at the output of each layer plus the initial embedding outputs.
- attentions (:obj:`tuple(torch.FloatTensor)`, `optional`, returned when ``config.output_attentions=True``):
- Tuple of :obj:`torch.FloatTensor` (one for each layer) of shape
- :obj:`(batch_size, num_heads, sequence_length, sequence_length)`.
-
- Attentions weights after the attention softmax, used to compute the weighted average in the self-attention
- heads.
-
- Examples::
-
- from transformers import AlbertTokenizer, AlbertForSequenceClassification
- import torch
-
- tokenizer = AlbertTokenizer.from_pretrained('albert-base-v2')
- model = AlbertForSequenceClassification.from_pretrained('albert-base-v2')
- input_ids = torch.tensor(tokenizer.encode("Hello, my dog is cute")).unsqueeze(0) # Batch size 1
- labels = torch.tensor([1]).unsqueeze(0) # Batch size 1
- outputs = model(input_ids, labels=labels)
- loss, logits = outputs[:2]
-
- """
-
- outputs = self.albert(
- input_ids=input_ids,
- attention_mask=attention_mask,
- token_type_ids=token_type_ids,
- position_ids=position_ids,
- head_mask=head_mask,
- inputs_embeds=inputs_embeds,
- )
-
- pooled_output = outputs[1]
-
- pooled_output = self.dropout(pooled_output)
- logits = self.classifier(pooled_output)
-
- outputs = (logits,) + outputs[2:] # add hidden states and attention if they are here
-
- if labels is not None:
- if self.num_labels == 1:
- # We are doing regression
- loss_fct = MSELoss()
- loss = loss_fct(logits.view(-1), labels.view(-1))
- else:
- loss_fct = CrossEntropyLoss()
- loss = loss_fct(logits.view(-1, self.num_labels), labels.view(-1))
- outputs = (loss,) + outputs
-
- return outputs # (loss), logits, (hidden_states), (attentions)
-
-
-@add_start_docstrings(
- """Albert Model with a span classification head on top for extractive question-answering tasks like SQuAD (a linear layers on top of
- the hidden-states output to compute `span start logits` and `span end logits`). """,
- ALBERT_START_DOCSTRING,
-)
-class AlbertForQuestionAnswering(AlbertPreTrainedModel):
- def __init__(self, config):
- super().__init__(config)
- self.num_labels = config.num_labels
-
- self.albert = AlbertModel(config)
- self.qa_outputs = nn.Linear(config.hidden_size, config.num_labels)
-
- self.init_weights()
-
- @add_start_docstrings_to_callable(ALBERT_INPUTS_DOCSTRING)
- def forward(
- self,
- input_ids=None,
- attention_mask=None,
- token_type_ids=None,
- position_ids=None,
- head_mask=None,
- inputs_embeds=None,
- start_positions=None,
- end_positions=None,
- ):
- r"""
- start_positions (:obj:`torch.LongTensor` of shape :obj:`(batch_size,)`, `optional`, defaults to :obj:`None`):
- Labels for position (index) of the start of the labelled span for computing the token classification loss.
- Positions are clamped to the length of the sequence (`sequence_length`).
- Position outside of the sequence are not taken into account for computing the loss.
- end_positions (:obj:`torch.LongTensor` of shape :obj:`(batch_size,)`, `optional`, defaults to :obj:`None`):
- Labels for position (index) of the end of the labelled span for computing the token classification loss.
- Positions are clamped to the length of the sequence (`sequence_length`).
- Position outside of the sequence are not taken into account for computing the loss.
-
- Returns:
- :obj:`tuple(torch.FloatTensor)` comprising various elements depending on the configuration (:class:`~transformers.AlbertConfig`) and inputs:
- loss: (`optional`, returned when ``labels`` is provided) ``torch.FloatTensor`` of shape ``(1,)``:
- Total span extraction loss is the sum of a Cross-Entropy for the start and end positions.
- start_scores ``torch.FloatTensor`` of shape ``(batch_size, sequence_length,)``
- Span-start scores (before SoftMax).
- end_scores: ``torch.FloatTensor`` of shape ``(batch_size, sequence_length,)``
- Span-end scores (before SoftMax).
- hidden_states (:obj:`tuple(torch.FloatTensor)`, `optional`, returned when ``config.output_hidden_states=True``):
- Tuple of :obj:`torch.FloatTensor` (one for the output of the embeddings + one for the output of each layer)
- of shape :obj:`(batch_size, sequence_length, hidden_size)`.
-
- Hidden-states of the model at the output of each layer plus the initial embedding outputs.
- attentions (:obj:`tuple(torch.FloatTensor)`, `optional`, returned when ``config.output_attentions=True``):
- Tuple of :obj:`torch.FloatTensor` (one for each layer) of shape
- :obj:`(batch_size, num_heads, sequence_length, sequence_length)`.
-
- Attentions weights after the attention softmax, used to compute the weighted average in the self-attention
- heads.
-
- Examples::
-
- # The checkpoint albert-base-v2 is not fine-tuned for question answering. Please see the
- # examples/run_squad.py example to see how to fine-tune a model to a question answering task.
-
- from transformers import AlbertTokenizer, AlbertForQuestionAnswering
- import torch
-
- tokenizer = AlbertTokenizer.from_pretrained('albert-base-v2')
- model = AlbertForQuestionAnswering.from_pretrained('albert-base-v2')
- question, text = "Who was Jim Henson?", "Jim Henson was a nice puppet"
- input_dict = tokenizer.encode_plus(question, text, return_tensors='pt')
- start_scores, end_scores = model(**input_dict)
-
- """
-
- outputs = self.albert(
- input_ids=input_ids,
- attention_mask=attention_mask,
- token_type_ids=token_type_ids,
- position_ids=position_ids,
- head_mask=head_mask,
- inputs_embeds=inputs_embeds,
- )
-
- sequence_output = outputs[0]
-
- logits = self.qa_outputs(sequence_output)
- start_logits, end_logits = logits.split(1, dim=-1)
- start_logits = start_logits.squeeze(-1)
- end_logits = end_logits.squeeze(-1)
-
- outputs = (start_logits, end_logits,) + outputs[2:]
- if start_positions is not None and end_positions is not None:
- # If we are on multi-GPU, split add a dimension
- if len(start_positions.size()) > 1:
- start_positions = start_positions.squeeze(-1)
- if len(end_positions.size()) > 1:
- end_positions = end_positions.squeeze(-1)
- # sometimes the start/end positions are outside our model inputs, we ignore these terms
- ignored_index = start_logits.size(1)
- start_positions.clamp_(0, ignored_index)
- end_positions.clamp_(0, ignored_index)
-
- loss_fct = CrossEntropyLoss(ignore_index=ignored_index)
- start_loss = loss_fct(start_logits, start_positions)
- end_loss = loss_fct(end_logits, end_positions)
- total_loss = (start_loss + end_loss) / 2
- outputs = (total_loss,) + outputs
-
- return outputs # (loss), start_logits, end_logits, (hidden_states), (attentions)
diff --git a/server/transformers/src/transformers/modeling_auto.py b/server/transformers/src/transformers/modeling_auto.py
deleted file mode 100644
index fbc8bc03ad38c225754c3444253b1424ebec9e32..0000000000000000000000000000000000000000
--- a/server/transformers/src/transformers/modeling_auto.py
+++ /dev/null
@@ -1,1128 +0,0 @@
-# coding=utf-8
-# Copyright 2018 The HuggingFace Inc. team.
-#
-# Licensed under the Apache License, Version 2.0 (the "License");
-# you may not use this file except in compliance with the License.
-# You may obtain a copy of the License at
-#
-# http://www.apache.org/licenses/LICENSE-2.0
-#
-# Unless required by applicable law or agreed to in writing, software
-# distributed under the License is distributed on an "AS IS" BASIS,
-# WITHOUT WARRANTIES OR CONDITIONS OF ANY KIND, either express or implied.
-# See the License for the specific language governing permissions and
-# limitations under the License.
-""" Auto Model class. """
-
-
-import logging
-from collections import OrderedDict
-
-from .configuration_auto import (
- AlbertConfig,
- AutoConfig,
- BertConfig,
- CamembertConfig,
- CTRLConfig,
- DistilBertConfig,
- FlaubertConfig,
- GPT2Config,
- OpenAIGPTConfig,
- RobertaConfig,
- T5Config,
- TransfoXLConfig,
- XLMConfig,
- XLMRobertaConfig,
- XLNetConfig,
-)
-from .configuration_utils import PretrainedConfig
-from .modeling_albert import (
- ALBERT_PRETRAINED_MODEL_ARCHIVE_MAP,
- AlbertForMaskedLM,
- AlbertForQuestionAnswering,
- AlbertForSequenceClassification,
- AlbertModel,
-)
-from .modeling_bert import (
- BERT_PRETRAINED_MODEL_ARCHIVE_MAP,
- BertForMaskedLM,
- BertForPreTraining,
- BertForQuestionAnswering,
- BertForSequenceClassification,
- BertForTokenClassification,
- BertModel,
-)
-from .modeling_camembert import (
- CAMEMBERT_PRETRAINED_MODEL_ARCHIVE_MAP,
- CamembertForMaskedLM,
- CamembertForSequenceClassification,
- CamembertForTokenClassification,
- CamembertModel,
-)
-from .modeling_ctrl import CTRL_PRETRAINED_MODEL_ARCHIVE_MAP, CTRLLMHeadModel, CTRLModel
-from .modeling_distilbert import (
- DISTILBERT_PRETRAINED_MODEL_ARCHIVE_MAP,
- DistilBertForMaskedLM,
- DistilBertForQuestionAnswering,
- DistilBertForSequenceClassification,
- DistilBertForTokenClassification,
- DistilBertModel,
-)
-from .modeling_flaubert import (
- FLAUBERT_PRETRAINED_MODEL_ARCHIVE_MAP,
- FlaubertForQuestionAnswering,
- FlaubertForSequenceClassification,
- FlaubertModel,
- FlaubertWithLMHeadModel,
-)
-from .modeling_gpt2 import GPT2_PRETRAINED_MODEL_ARCHIVE_MAP, GPT2LMHeadModel, GPT2Model
-from .modeling_openai import OPENAI_GPT_PRETRAINED_MODEL_ARCHIVE_MAP, OpenAIGPTLMHeadModel, OpenAIGPTModel
-from .modeling_roberta import (
- ROBERTA_PRETRAINED_MODEL_ARCHIVE_MAP,
- RobertaForMaskedLM,
- RobertaForQuestionAnswering,
- RobertaForSequenceClassification,
- RobertaForTokenClassification,
- RobertaModel,
-)
-from .modeling_t5 import T5_PRETRAINED_MODEL_ARCHIVE_MAP, T5Model, T5WithLMHeadModel
-from .modeling_transfo_xl import TRANSFO_XL_PRETRAINED_MODEL_ARCHIVE_MAP, TransfoXLLMHeadModel, TransfoXLModel
-from .modeling_xlm import (
- XLM_PRETRAINED_MODEL_ARCHIVE_MAP,
- XLMForQuestionAnswering,
- XLMForSequenceClassification,
- XLMModel,
- XLMWithLMHeadModel,
-)
-from .modeling_xlm_roberta import (
- XLM_ROBERTA_PRETRAINED_MODEL_ARCHIVE_MAP,
- XLMRobertaForMaskedLM,
- XLMRobertaForSequenceClassification,
- XLMRobertaForTokenClassification,
- XLMRobertaModel,
-)
-from .modeling_xlnet import (
- XLNET_PRETRAINED_MODEL_ARCHIVE_MAP,
- XLNetForQuestionAnswering,
- XLNetForSequenceClassification,
- XLNetForTokenClassification,
- XLNetLMHeadModel,
- XLNetModel,
-)
-
-
-logger = logging.getLogger(__name__)
-
-
-ALL_PRETRAINED_MODEL_ARCHIVE_MAP = dict(
- (key, value)
- for pretrained_map in [
- BERT_PRETRAINED_MODEL_ARCHIVE_MAP,
- OPENAI_GPT_PRETRAINED_MODEL_ARCHIVE_MAP,
- TRANSFO_XL_PRETRAINED_MODEL_ARCHIVE_MAP,
- GPT2_PRETRAINED_MODEL_ARCHIVE_MAP,
- CTRL_PRETRAINED_MODEL_ARCHIVE_MAP,
- XLNET_PRETRAINED_MODEL_ARCHIVE_MAP,
- XLM_PRETRAINED_MODEL_ARCHIVE_MAP,
- ROBERTA_PRETRAINED_MODEL_ARCHIVE_MAP,
- DISTILBERT_PRETRAINED_MODEL_ARCHIVE_MAP,
- ALBERT_PRETRAINED_MODEL_ARCHIVE_MAP,
- CAMEMBERT_PRETRAINED_MODEL_ARCHIVE_MAP,
- T5_PRETRAINED_MODEL_ARCHIVE_MAP,
- FLAUBERT_PRETRAINED_MODEL_ARCHIVE_MAP,
- XLM_ROBERTA_PRETRAINED_MODEL_ARCHIVE_MAP,
- ]
- for key, value, in pretrained_map.items()
-)
-
-MODEL_MAPPING = OrderedDict(
- [
- (T5Config, T5Model),
- (DistilBertConfig, DistilBertModel),
- (AlbertConfig, AlbertModel),
- (CamembertConfig, CamembertModel),
- (XLMRobertaConfig, XLMRobertaModel),
- (RobertaConfig, RobertaModel),
- (BertConfig, BertModel),
- (OpenAIGPTConfig, OpenAIGPTModel),
- (GPT2Config, GPT2Model),
- (TransfoXLConfig, TransfoXLModel),
- (XLNetConfig, XLNetModel),
- (FlaubertConfig, FlaubertModel),
- (XLMConfig, XLMModel),
- (CTRLConfig, CTRLModel),
- ]
-)
-
-MODEL_FOR_PRETRAINING_MAPPING = OrderedDict(
- [
- (T5Config, T5WithLMHeadModel),
- (DistilBertConfig, DistilBertForMaskedLM),
- (AlbertConfig, AlbertForMaskedLM),
- (CamembertConfig, CamembertForMaskedLM),
- (XLMRobertaConfig, XLMRobertaForMaskedLM),
- (RobertaConfig, RobertaForMaskedLM),
- (BertConfig, BertForPreTraining),
- (OpenAIGPTConfig, OpenAIGPTLMHeadModel),
- (GPT2Config, GPT2LMHeadModel),
- (TransfoXLConfig, TransfoXLLMHeadModel),
- (XLNetConfig, XLNetLMHeadModel),
- (FlaubertConfig, FlaubertWithLMHeadModel),
- (XLMConfig, XLMWithLMHeadModel),
- (CTRLConfig, CTRLLMHeadModel),
- ]
-)
-
-MODEL_WITH_LM_HEAD_MAPPING = OrderedDict(
- [
- (T5Config, T5WithLMHeadModel),
- (DistilBertConfig, DistilBertForMaskedLM),
- (AlbertConfig, AlbertForMaskedLM),
- (CamembertConfig, CamembertForMaskedLM),
- (XLMRobertaConfig, XLMRobertaForMaskedLM),
- (RobertaConfig, RobertaForMaskedLM),
- (BertConfig, BertForMaskedLM),
- (OpenAIGPTConfig, OpenAIGPTLMHeadModel),
- (GPT2Config, GPT2LMHeadModel),
- (TransfoXLConfig, TransfoXLLMHeadModel),
- (XLNetConfig, XLNetLMHeadModel),
- (FlaubertConfig, FlaubertWithLMHeadModel),
- (XLMConfig, XLMWithLMHeadModel),
- (CTRLConfig, CTRLLMHeadModel),
- ]
-)
-
-MODEL_FOR_SEQUENCE_CLASSIFICATION_MAPPING = OrderedDict(
- [
- (DistilBertConfig, DistilBertForSequenceClassification),
- (AlbertConfig, AlbertForSequenceClassification),
- (CamembertConfig, CamembertForSequenceClassification),
- (XLMRobertaConfig, XLMRobertaForSequenceClassification),
- (RobertaConfig, RobertaForSequenceClassification),
- (BertConfig, BertForSequenceClassification),
- (XLNetConfig, XLNetForSequenceClassification),
- (FlaubertConfig, FlaubertForSequenceClassification),
- (XLMConfig, XLMForSequenceClassification),
- ]
-)
-
-MODEL_FOR_QUESTION_ANSWERING_MAPPING = OrderedDict(
- [
- (DistilBertConfig, DistilBertForQuestionAnswering),
- (AlbertConfig, AlbertForQuestionAnswering),
- (RobertaConfig, RobertaForQuestionAnswering),
- (BertConfig, BertForQuestionAnswering),
- (XLNetConfig, XLNetForQuestionAnswering),
- (FlaubertConfig, FlaubertForQuestionAnswering),
- (XLMConfig, XLMForQuestionAnswering),
- ]
-)
-
-MODEL_FOR_TOKEN_CLASSIFICATION_MAPPING = OrderedDict(
- [
- (DistilBertConfig, DistilBertForTokenClassification),
- (CamembertConfig, CamembertForTokenClassification),
- (XLMRobertaConfig, XLMRobertaForTokenClassification),
- (RobertaConfig, RobertaForTokenClassification),
- (BertConfig, BertForTokenClassification),
- (XLNetConfig, XLNetForTokenClassification),
- ]
-)
-
-
-class AutoModel(object):
- r"""
- :class:`~transformers.AutoModel` is a generic model class
- that will be instantiated as one of the base model classes of the library
- when created with the `AutoModel.from_pretrained(pretrained_model_name_or_path)`
- or the `AutoModel.from_config(config)` class methods.
-
- This class cannot be instantiated using `__init__()` (throws an error).
- """
-
- def __init__(self):
- raise EnvironmentError(
- "AutoModel is designed to be instantiated "
- "using the `AutoModel.from_pretrained(pretrained_model_name_or_path)` or "
- "`AutoModel.from_config(config)` methods."
- )
-
- @classmethod
- def from_config(cls, config):
- r""" Instantiates one of the base model classes of the library
- from a configuration.
-
- Args:
- config (:class:`~transformers.PretrainedConfig`):
- The model class to instantiate is selected based on the configuration class:
-
- - isInstance of `distilbert` configuration class: :class:`~transformers.DistilBertModel` (DistilBERT model)
- - isInstance of `roberta` configuration class: :class:`~transformers.RobertaModel` (RoBERTa model)
- - isInstance of `bert` configuration class: :class:`~transformers.BertModel` (Bert model)
- - isInstance of `openai-gpt` configuration class: :class:`~transformers.OpenAIGPTModel` (OpenAI GPT model)
- - isInstance of `gpt2` configuration class: :class:`~transformers.GPT2Model` (OpenAI GPT-2 model)
- - isInstance of `ctrl` configuration class: :class:`~transformers.CTRLModel` (Salesforce CTRL model)
- - isInstance of `transfo-xl` configuration class: :class:`~transformers.TransfoXLModel` (Transformer-XL model)
- - isInstance of `xlnet` configuration class: :class:`~transformers.XLNetModel` (XLNet model)
- - isInstance of `xlm` configuration class: :class:`~transformers.XLMModel` (XLM model)
- - isInstance of `flaubert` configuration class: :class:`~transformers.FlaubertModel` (XLM model)
-
- Examples::
-
- config = BertConfig.from_pretrained('bert-base-uncased') # Download configuration from S3 and cache.
- model = AutoModel.from_config(config) # E.g. model was saved using `save_pretrained('./test/saved_model/')`
- """
- for config_class, model_class in MODEL_MAPPING.items():
- if isinstance(config, config_class):
- return model_class(config)
- raise ValueError(
- "Unrecognized configuration class {} for this kind of AutoModel: {}.\n"
- "Model type should be one of {}.".format(
- config.__class__, cls.__name__, ", ".join(c.__name__ for c in MODEL_MAPPING.keys())
- )
- )
-
- @classmethod
- def from_pretrained(cls, pretrained_model_name_or_path, *model_args, **kwargs):
- r""" Instantiates one of the base model classes of the library
- from a pre-trained model configuration.
-
- The `from_pretrained()` method takes care of returning the correct model class instance
- based on the `model_type` property of the config object, or when it's missing,
- falling back to using pattern matching on the `pretrained_model_name_or_path` string.
-
- The base model class to instantiate is selected as the first pattern matching
- in the `pretrained_model_name_or_path` string (in the following order):
- - contains `t5`: :class:`~transformers.T5Model` (T5 model)
- - contains `distilbert`: :class:`~transformers.DistilBertModel` (DistilBERT model)
- - contains `albert`: :class:`~transformers.AlbertModel` (ALBERT model)
- - contains `camembert`: :class:`~transformers.CamembertModel` (CamemBERT model)
- - contains `xlm-roberta`: :class:`~transformers.XLMRobertaModel` (XLM-RoBERTa model)
- - contains `roberta`: :class:`~transformers.RobertaModel` (RoBERTa model)
- - contains `bert`: :class:`~transformers.BertModel` (Bert model)
- - contains `openai-gpt`: :class:`~transformers.OpenAIGPTModel` (OpenAI GPT model)
- - contains `gpt2`: :class:`~transformers.GPT2Model` (OpenAI GPT-2 model)
- - contains `transfo-xl`: :class:`~transformers.TransfoXLModel` (Transformer-XL model)
- - contains `xlnet`: :class:`~transformers.XLNetModel` (XLNet model)
- - contains `xlm`: :class:`~transformers.XLMModel` (XLM model)
- - contains `ctrl`: :class:`~transformers.CTRLModel` (Salesforce CTRL model)
- - contains `flaubert`: :class:`~transformers.Flaubert` (Flaubert model)
-
- The model is set in evaluation mode by default using `model.eval()` (Dropout modules are deactivated)
- To train the model, you should first set it back in training mode with `model.train()`
-
- Args:
- pretrained_model_name_or_path: either:
-
- - a string with the `shortcut name` of a pre-trained model to load from cache or download, e.g.: ``bert-base-uncased``.
- - a string with the `identifier name` of a pre-trained model that was user-uploaded to our S3, e.g.: ``dbmdz/bert-base-german-cased``.
- - a path to a `directory` containing model weights saved using :func:`~transformers.PreTrainedModel.save_pretrained`, e.g.: ``./my_model_directory/``.
- - a path or url to a `tensorflow index checkpoint file` (e.g. `./tf_model/model.ckpt.index`). In this case, ``from_tf`` should be set to True and a configuration object should be provided as ``config`` argument. This loading path is slower than converting the TensorFlow checkpoint in a PyTorch model using the provided conversion scripts and loading the PyTorch model afterwards.
-
- model_args: (`optional`) Sequence of positional arguments:
- All remaning positional arguments will be passed to the underlying model's ``__init__`` method
-
- config: (`optional`) instance of a class derived from :class:`~transformers.PretrainedConfig`:
- Configuration for the model to use instead of an automatically loaded configuation. Configuration can be automatically loaded when:
-
- - the model is a model provided by the library (loaded with the ``shortcut-name`` string of a pretrained model), or
- - the model was saved using :func:`~transformers.PreTrainedModel.save_pretrained` and is reloaded by suppling the save directory.
- - the model is loaded by suppling a local directory as ``pretrained_model_name_or_path`` and a configuration JSON file named `config.json` is found in the directory.
-
- state_dict: (`optional`) dict:
- an optional state dictionnary for the model to use instead of a state dictionary loaded from saved weights file.
- This option can be used if you want to create a model from a pretrained configuration but load your own weights.
- In this case though, you should check if using :func:`~transformers.PreTrainedModel.save_pretrained` and :func:`~transformers.PreTrainedModel.from_pretrained` is not a simpler option.
-
- cache_dir: (`optional`) string:
- Path to a directory in which a downloaded pre-trained model
- configuration should be cached if the standard cache should not be used.
-
- force_download: (`optional`) boolean, default False:
- Force to (re-)download the model weights and configuration files and override the cached versions if they exists.
-
- resume_download: (`optional`) boolean, default False:
- Do not delete incompletely recieved file. Attempt to resume the download if such a file exists.
-
- proxies: (`optional`) dict, default None:
- A dictionary of proxy servers to use by protocol or endpoint, e.g.: {'http': 'foo.bar:3128', 'http://hostname': 'foo.bar:4012'}.
- The proxies are used on each request.
-
- output_loading_info: (`optional`) boolean:
- Set to ``True`` to also return a dictionnary containing missing keys, unexpected keys and error messages.
-
- kwargs: (`optional`) Remaining dictionary of keyword arguments:
- Can be used to update the configuration object (after it being loaded) and initiate the model. (e.g. ``output_attention=True``). Behave differently depending on whether a `config` is provided or automatically loaded:
-
- - If a configuration is provided with ``config``, ``**kwargs`` will be directly passed to the underlying model's ``__init__`` method (we assume all relevant updates to the configuration have already been done)
- - If a configuration is not provided, ``kwargs`` will be first passed to the configuration class initialization function (:func:`~transformers.PretrainedConfig.from_pretrained`). Each key of ``kwargs`` that corresponds to a configuration attribute will be used to override said attribute with the supplied ``kwargs`` value. Remaining keys that do not correspond to any configuration attribute will be passed to the underlying model's ``__init__`` function.
-
- Examples::
-
- model = AutoModel.from_pretrained('bert-base-uncased') # Download model and configuration from S3 and cache.
- model = AutoModel.from_pretrained('./test/bert_model/') # E.g. model was saved using `save_pretrained('./test/saved_model/')`
- model = AutoModel.from_pretrained('bert-base-uncased', output_attention=True) # Update configuration during loading
- assert model.config.output_attention == True
- # Loading from a TF checkpoint file instead of a PyTorch model (slower)
- config = AutoConfig.from_json_file('./tf_model/bert_tf_model_config.json')
- model = AutoModel.from_pretrained('./tf_model/bert_tf_checkpoint.ckpt.index', from_tf=True, config=config)
-
- """
- config = kwargs.pop("config", None)
- if not isinstance(config, PretrainedConfig):
- config = AutoConfig.from_pretrained(pretrained_model_name_or_path, **kwargs)
-
- for config_class, model_class in MODEL_MAPPING.items():
- if isinstance(config, config_class):
- return model_class.from_pretrained(pretrained_model_name_or_path, *model_args, config=config, **kwargs)
- raise ValueError(
- "Unrecognized configuration class {} for this kind of AutoModel: {}.\n"
- "Model type should be one of {}.".format(
- config.__class__, cls.__name__, ", ".join(c.__name__ for c in MODEL_MAPPING.keys())
- )
- )
-
-
-class AutoModelForPreTraining(object):
- r"""
- :class:`~transformers.AutoModelForPreTraining` is a generic model class
- that will be instantiated as one of the model classes of the library -with the architecture used for pretraining this model– when created with the `AutoModelForPreTraining.from_pretrained(pretrained_model_name_or_path)`
- class method.
-
- This class cannot be instantiated using `__init__()` (throws an error).
- """
-
- def __init__(self):
- raise EnvironmentError(
- "AutoModelForPreTraining is designed to be instantiated "
- "using the `AutoModelForPreTraining.from_pretrained(pretrained_model_name_or_path)` or "
- "`AutoModelForPreTraining.from_config(config)` methods."
- )
-
- @classmethod
- def from_config(cls, config):
- r""" Instantiates one of the base model classes of the library
- from a configuration.
-
- Args:
- config (:class:`~transformers.PretrainedConfig`):
- The model class to instantiate is selected based on the configuration class:
-
- - isInstance of `distilbert` configuration class: :class:`~transformers.DistilBertModelForMaskedLM` (DistilBERT model)
- - isInstance of `roberta` configuration class: :class:`~transformers.RobertaModelForMaskedLM` (RoBERTa model)
- - isInstance of `bert` configuration class: :class:`~transformers.BertForPreTraining` (Bert model)
- - isInstance of `openai-gpt` configuration class: :class:`~transformers.OpenAIGPTLMHeadModel` (OpenAI GPT model)
- - isInstance of `gpt2` configuration class: :class:`~transformers.GPT2ModelLMHeadModel` (OpenAI GPT-2 model)
- - isInstance of `ctrl` configuration class: :class:`~transformers.CTRLModelLMHeadModel` (Salesforce CTRL model)
- - isInstance of `transfo-xl` configuration class: :class:`~transformers.TransfoXLLMHeadModel` (Transformer-XL model)
- - isInstance of `xlnet` configuration class: :class:`~transformers.XLNetLMHeadModel` (XLNet model)
- - isInstance of `xlm` configuration class: :class:`~transformers.XLMWithLMHeadModel` (XLM model)
- - isInstance of `flaubert` configuration class: :class:`~transformers.FlaubertWithLMHeadModel` (Flaubert model)
-
- Examples::
-
- config = BertConfig.from_pretrained('bert-base-uncased') # Download configuration from S3 and cache.
- model = AutoModelForPreTraining.from_config(config) # E.g. model was saved using `save_pretrained('./test/saved_model/')`
- """
- for config_class, model_class in MODEL_FOR_PRETRAINING_MAPPING.items():
- if isinstance(config, config_class):
- return model_class(config)
- raise ValueError(
- "Unrecognized configuration class {} for this kind of AutoModel: {}.\n"
- "Model type should be one of {}.".format(
- config.__class__, cls.__name__, ", ".join(c.__name__ for c in MODEL_FOR_PRETRAINING_MAPPING.keys())
- )
- )
-
- @classmethod
- def from_pretrained(cls, pretrained_model_name_or_path, *model_args, **kwargs):
- r""" Instantiates one of the model classes of the library -with the architecture used for pretraining this model– from a pre-trained model configuration.
-
- The `from_pretrained()` method takes care of returning the correct model class instance
- based on the `model_type` property of the config object, or when it's missing,
- falling back to using pattern matching on the `pretrained_model_name_or_path` string.
-
- The model class to instantiate is selected as the first pattern matching
- in the `pretrained_model_name_or_path` string (in the following order):
- - contains `t5`: :class:`~transformers.T5ModelWithLMHead` (T5 model)
- - contains `distilbert`: :class:`~transformers.DistilBertForMaskedLM` (DistilBERT model)
- - contains `albert`: :class:`~transformers.AlbertForMaskedLM` (ALBERT model)
- - contains `camembert`: :class:`~transformers.CamembertForMaskedLM` (CamemBERT model)
- - contains `xlm-roberta`: :class:`~transformers.XLMRobertaForMaskedLM` (XLM-RoBERTa model)
- - contains `roberta`: :class:`~transformers.RobertaForMaskedLM` (RoBERTa model)
- - contains `bert`: :class:`~transformers.BertForPreTraining` (Bert model)
- - contains `openai-gpt`: :class:`~transformers.OpenAIGPTLMHeadModel` (OpenAI GPT model)
- - contains `gpt2`: :class:`~transformers.GPT2LMHeadModel` (OpenAI GPT-2 model)
- - contains `transfo-xl`: :class:`~transformers.TransfoXLLMHeadModel` (Transformer-XL model)
- - contains `xlnet`: :class:`~transformers.XLNetLMHeadModel` (XLNet model)
- - contains `xlm`: :class:`~transformers.XLMWithLMHeadModel` (XLM model)
- - contains `ctrl`: :class:`~transformers.CTRLLMHeadModel` (Salesforce CTRL model)
- - contains `flaubert`: :class:`~transformers.FlaubertWithLMHeadModel` (Flaubert model)
-
- The model is set in evaluation mode by default using `model.eval()` (Dropout modules are deactivated)
- To train the model, you should first set it back in training mode with `model.train()`
-
- Args:
- pretrained_model_name_or_path:
- Either:
-
- - a string with the `shortcut name` of a pre-trained model to load from cache or download, e.g.: ``bert-base-uncased``.
- - a string with the `identifier name` of a pre-trained model that was user-uploaded to our S3, e.g.: ``dbmdz/bert-base-german-cased``.
- - a path to a `directory` containing model weights saved using :func:`~transformers.PreTrainedModel.save_pretrained`, e.g.: ``./my_model_directory/``.
- - a path or url to a `tensorflow index checkpoint file` (e.g. `./tf_model/model.ckpt.index`). In this case, ``from_tf`` should be set to True and a configuration object should be provided as ``config`` argument. This loading path is slower than converting the TensorFlow checkpoint in a PyTorch model using the provided conversion scripts and loading the PyTorch model afterwards.
- model_args: (`optional`) Sequence of positional arguments:
- All remaning positional arguments will be passed to the underlying model's ``__init__`` method
- config: (`optional`) instance of a class derived from :class:`~transformers.PretrainedConfig`:
- Configuration for the model to use instead of an automatically loaded configuation. Configuration can be automatically loaded when:
-
- - the model is a model provided by the library (loaded with the ``shortcut-name`` string of a pretrained model), or
- - the model was saved using :func:`~transformers.PreTrainedModel.save_pretrained` and is reloaded by suppling the save directory.
- - the model is loaded by suppling a local directory as ``pretrained_model_name_or_path`` and a configuration JSON file named `config.json` is found in the directory.
-
- state_dict: (`optional`) dict:
- an optional state dictionnary for the model to use instead of a state dictionary loaded from saved weights file.
- This option can be used if you want to create a model from a pretrained configuration but load your own weights.
- In this case though, you should check if using :func:`~transformers.PreTrainedModel.save_pretrained` and :func:`~transformers.PreTrainedModel.from_pretrained` is not a simpler option.
- cache_dir: (`optional`) string:
- Path to a directory in which a downloaded pre-trained model
- configuration should be cached if the standard cache should not be used.
- force_download: (`optional`) boolean, default False:
- Force to (re-)download the model weights and configuration files and override the cached versions if they exists.
- resume_download: (`optional`) boolean, default False:
- Do not delete incompletely received file. Attempt to resume the download if such a file exists.
- proxies: (`optional`) dict, default None:
- A dictionary of proxy servers to use by protocol or endpoint, e.g.: {'http': 'foo.bar:3128', 'http://hostname': 'foo.bar:4012'}.
- The proxies are used on each request.
- output_loading_info: (`optional`) boolean:
- Set to ``True`` to also return a dictionnary containing missing keys, unexpected keys and error messages.
- kwargs: (`optional`) Remaining dictionary of keyword arguments:
- Can be used to update the configuration object (after it being loaded) and initiate the model.
- (e.g. ``output_attention=True``). Behave differently depending on whether a `config` is provided or
- automatically loaded:
-
- - If a configuration is provided with ``config``, ``**kwargs`` will be directly passed to the
- underlying model's ``__init__`` method (we assume all relevant updates to the configuration have
- already been done)
- - If a configuration is not provided, ``kwargs`` will be first passed to the configuration class
- initialization function (:func:`~transformers.PretrainedConfig.from_pretrained`). Each key of
- ``kwargs`` that corresponds to a configuration attribute will be used to override said attribute
- with the supplied ``kwargs`` value. Remaining keys that do not correspond to any configuration
- attribute will be passed to the underlying model's ``__init__`` function.
-
- Examples::
-
- model = AutoModelForPreTraining.from_pretrained('bert-base-uncased') # Download model and configuration from S3 and cache.
- model = AutoModelForPreTraining.from_pretrained('./test/bert_model/') # E.g. model was saved using `save_pretrained('./test/saved_model/')`
- model = AutoModelForPreTraining.from_pretrained('bert-base-uncased', output_attention=True) # Update configuration during loading
- assert model.config.output_attention == True
- # Loading from a TF checkpoint file instead of a PyTorch model (slower)
- config = AutoConfig.from_json_file('./tf_model/bert_tf_model_config.json')
- model = AutoModelForPreTraining.from_pretrained('./tf_model/bert_tf_checkpoint.ckpt.index', from_tf=True, config=config)
-
- """
- config = kwargs.pop("config", None)
- if not isinstance(config, PretrainedConfig):
- config = AutoConfig.from_pretrained(pretrained_model_name_or_path, **kwargs)
-
- for config_class, model_class in MODEL_FOR_PRETRAINING_MAPPING.items():
- if isinstance(config, config_class):
- return model_class.from_pretrained(pretrained_model_name_or_path, *model_args, config=config, **kwargs)
- raise ValueError(
- "Unrecognized configuration class {} for this kind of AutoModel: {}.\n"
- "Model type should be one of {}.".format(
- config.__class__, cls.__name__, ", ".join(c.__name__ for c in MODEL_FOR_PRETRAINING_MAPPING.keys())
- )
- )
-
-
-class AutoModelWithLMHead(object):
- r"""
- :class:`~transformers.AutoModelWithLMHead` is a generic model class
- that will be instantiated as one of the language modeling model classes of the library
- when created with the `AutoModelWithLMHead.from_pretrained(pretrained_model_name_or_path)`
- class method.
-
- This class cannot be instantiated using `__init__()` (throws an error).
- """
-
- def __init__(self):
- raise EnvironmentError(
- "AutoModelWithLMHead is designed to be instantiated "
- "using the `AutoModelWithLMHead.from_pretrained(pretrained_model_name_or_path)` or "
- "`AutoModelWithLMHead.from_config(config)` methods."
- )
-
- @classmethod
- def from_config(cls, config):
- r""" Instantiates one of the base model classes of the library
- from a configuration.
-
- Args:
- config (:class:`~transformers.PretrainedConfig`):
- The model class to instantiate is selected based on the configuration class:
-
- - isInstance of `distilbert` configuration class: :class:`~transformers.DistilBertModelForMaskedLM` (DistilBERT model)
- - isInstance of `roberta` configuration class: :class:`~transformers.RobertaModelForMaskedLM` (RoBERTa model)
- - isInstance of `bert` configuration class: :class:`~transformers.BertModelForMaskedLM` (Bert model)
- - isInstance of `openai-gpt` configuration class: :class:`~transformers.OpenAIGPTLMHeadModel` (OpenAI GPT model)
- - isInstance of `gpt2` configuration class: :class:`~transformers.GPT2ModelLMHeadModel` (OpenAI GPT-2 model)
- - isInstance of `ctrl` configuration class: :class:`~transformers.CTRLModelLMHeadModel` (Salesforce CTRL model)
- - isInstance of `transfo-xl` configuration class: :class:`~transformers.TransfoXLLMHeadModel` (Transformer-XL model)
- - isInstance of `xlnet` configuration class: :class:`~transformers.XLNetLMHeadModel` (XLNet model)
- - isInstance of `xlm` configuration class: :class:`~transformers.XLMWithLMHeadModel` (XLM model)
- - isInstance of `flaubert` configuration class: :class:`~transformers.FlaubertWithLMHeadModel` (Flaubert model)
-
- Examples::
-
- config = BertConfig.from_pretrained('bert-base-uncased') # Download configuration from S3 and cache.
- model = AutoModelWithLMHead.from_config(config) # E.g. model was saved using `save_pretrained('./test/saved_model/')`
- """
- for config_class, model_class in MODEL_WITH_LM_HEAD_MAPPING.items():
- if isinstance(config, config_class):
- return model_class(config)
- raise ValueError(
- "Unrecognized configuration class {} for this kind of AutoModel: {}.\n"
- "Model type should be one of {}.".format(
- config.__class__, cls.__name__, ", ".join(c.__name__ for c in MODEL_WITH_LM_HEAD_MAPPING.keys())
- )
- )
-
- @classmethod
- def from_pretrained(cls, pretrained_model_name_or_path, *model_args, **kwargs):
- r""" Instantiates one of the language modeling model classes of the library
- from a pre-trained model configuration.
-
- The `from_pretrained()` method takes care of returning the correct model class instance
- based on the `model_type` property of the config object, or when it's missing,
- falling back to using pattern matching on the `pretrained_model_name_or_path` string.
-
- The model class to instantiate is selected as the first pattern matching
- in the `pretrained_model_name_or_path` string (in the following order):
- - contains `t5`: :class:`~transformers.T5ModelWithLMHead` (T5 model)
- - contains `distilbert`: :class:`~transformers.DistilBertForMaskedLM` (DistilBERT model)
- - contains `albert`: :class:`~transformers.AlbertForMaskedLM` (ALBERT model)
- - contains `camembert`: :class:`~transformers.CamembertForMaskedLM` (CamemBERT model)
- - contains `xlm-roberta`: :class:`~transformers.XLMRobertaForMaskedLM` (XLM-RoBERTa model)
- - contains `roberta`: :class:`~transformers.RobertaForMaskedLM` (RoBERTa model)
- - contains `bert`: :class:`~transformers.BertForMaskedLM` (Bert model)
- - contains `openai-gpt`: :class:`~transformers.OpenAIGPTLMHeadModel` (OpenAI GPT model)
- - contains `gpt2`: :class:`~transformers.GPT2LMHeadModel` (OpenAI GPT-2 model)
- - contains `transfo-xl`: :class:`~transformers.TransfoXLLMHeadModel` (Transformer-XL model)
- - contains `xlnet`: :class:`~transformers.XLNetLMHeadModel` (XLNet model)
- - contains `xlm`: :class:`~transformers.XLMWithLMHeadModel` (XLM model)
- - contains `ctrl`: :class:`~transformers.CTRLLMHeadModel` (Salesforce CTRL model)
- - contains `flaubert`: :class:`~transformers.FlaubertWithLMHeadModel` (Flaubert model)
-
- The model is set in evaluation mode by default using `model.eval()` (Dropout modules are deactivated)
- To train the model, you should first set it back in training mode with `model.train()`
-
- Args:
- pretrained_model_name_or_path:
- Either:
-
- - a string with the `shortcut name` of a pre-trained model to load from cache or download, e.g.: ``bert-base-uncased``.
- - a string with the `identifier name` of a pre-trained model that was user-uploaded to our S3, e.g.: ``dbmdz/bert-base-german-cased``.
- - a path to a `directory` containing model weights saved using :func:`~transformers.PreTrainedModel.save_pretrained`, e.g.: ``./my_model_directory/``.
- - a path or url to a `tensorflow index checkpoint file` (e.g. `./tf_model/model.ckpt.index`). In this case, ``from_tf`` should be set to True and a configuration object should be provided as ``config`` argument. This loading path is slower than converting the TensorFlow checkpoint in a PyTorch model using the provided conversion scripts and loading the PyTorch model afterwards.
- model_args: (`optional`) Sequence of positional arguments:
- All remaning positional arguments will be passed to the underlying model's ``__init__`` method
- config: (`optional`) instance of a class derived from :class:`~transformers.PretrainedConfig`:
- Configuration for the model to use instead of an automatically loaded configuation. Configuration can be automatically loaded when:
-
- - the model is a model provided by the library (loaded with the ``shortcut-name`` string of a pretrained model), or
- - the model was saved using :func:`~transformers.PreTrainedModel.save_pretrained` and is reloaded by suppling the save directory.
- - the model is loaded by suppling a local directory as ``pretrained_model_name_or_path`` and a configuration JSON file named `config.json` is found in the directory.
-
- state_dict: (`optional`) dict:
- an optional state dictionnary for the model to use instead of a state dictionary loaded from saved weights file.
- This option can be used if you want to create a model from a pretrained configuration but load your own weights.
- In this case though, you should check if using :func:`~transformers.PreTrainedModel.save_pretrained` and :func:`~transformers.PreTrainedModel.from_pretrained` is not a simpler option.
- cache_dir: (`optional`) string:
- Path to a directory in which a downloaded pre-trained model
- configuration should be cached if the standard cache should not be used.
- force_download: (`optional`) boolean, default False:
- Force to (re-)download the model weights and configuration files and override the cached versions if they exists.
- resume_download: (`optional`) boolean, default False:
- Do not delete incompletely received file. Attempt to resume the download if such a file exists.
- proxies: (`optional`) dict, default None:
- A dictionary of proxy servers to use by protocol or endpoint, e.g.: {'http': 'foo.bar:3128', 'http://hostname': 'foo.bar:4012'}.
- The proxies are used on each request.
- output_loading_info: (`optional`) boolean:
- Set to ``True`` to also return a dictionnary containing missing keys, unexpected keys and error messages.
- kwargs: (`optional`) Remaining dictionary of keyword arguments:
- Can be used to update the configuration object (after it being loaded) and initiate the model.
- (e.g. ``output_attention=True``). Behave differently depending on whether a `config` is provided or
- automatically loaded:
-
- - If a configuration is provided with ``config``, ``**kwargs`` will be directly passed to the
- underlying model's ``__init__`` method (we assume all relevant updates to the configuration have
- already been done)
- - If a configuration is not provided, ``kwargs`` will be first passed to the configuration class
- initialization function (:func:`~transformers.PretrainedConfig.from_pretrained`). Each key of
- ``kwargs`` that corresponds to a configuration attribute will be used to override said attribute
- with the supplied ``kwargs`` value. Remaining keys that do not correspond to any configuration
- attribute will be passed to the underlying model's ``__init__`` function.
-
- Examples::
-
- model = AutoModelWithLMHead.from_pretrained('bert-base-uncased') # Download model and configuration from S3 and cache.
- model = AutoModelWithLMHead.from_pretrained('./test/bert_model/') # E.g. model was saved using `save_pretrained('./test/saved_model/')`
- model = AutoModelWithLMHead.from_pretrained('bert-base-uncased', output_attention=True) # Update configuration during loading
- assert model.config.output_attention == True
- # Loading from a TF checkpoint file instead of a PyTorch model (slower)
- config = AutoConfig.from_json_file('./tf_model/bert_tf_model_config.json')
- model = AutoModelWithLMHead.from_pretrained('./tf_model/bert_tf_checkpoint.ckpt.index', from_tf=True, config=config)
-
- """
- config = kwargs.pop("config", None)
- if not isinstance(config, PretrainedConfig):
- config = AutoConfig.from_pretrained(pretrained_model_name_or_path, **kwargs)
-
- for config_class, model_class in MODEL_WITH_LM_HEAD_MAPPING.items():
- if isinstance(config, config_class):
- return model_class.from_pretrained(pretrained_model_name_or_path, *model_args, config=config, **kwargs)
- raise ValueError(
- "Unrecognized configuration class {} for this kind of AutoModel: {}.\n"
- "Model type should be one of {}.".format(
- config.__class__, cls.__name__, ", ".join(c.__name__ for c in MODEL_WITH_LM_HEAD_MAPPING.keys())
- )
- )
-
-
-class AutoModelForSequenceClassification(object):
- r"""
- :class:`~transformers.AutoModelForSequenceClassification` is a generic model class
- that will be instantiated as one of the sequence classification model classes of the library
- when created with the `AutoModelForSequenceClassification.from_pretrained(pretrained_model_name_or_path)`
- class method.
-
- This class cannot be instantiated using `__init__()` (throws an error).
- """
-
- def __init__(self):
- raise EnvironmentError(
- "AutoModelForSequenceClassification is designed to be instantiated "
- "using the `AutoModelForSequenceClassification.from_pretrained(pretrained_model_name_or_path)` or "
- "`AutoModelForSequenceClassification.from_config(config)` methods."
- )
-
- @classmethod
- def from_config(cls, config):
- r""" Instantiates one of the base model classes of the library
- from a configuration.
-
- Args:
- config (:class:`~transformers.PretrainedConfig`):
- The model class to instantiate is selected based on the configuration class:
-
- - isInstance of `distilbert` configuration class: :class:`~transformers.DistilBertModelForSequenceClassification` (DistilBERT model)
- - isInstance of `albert` configuration class: :class:`~transformers.AlbertModelForSequenceClassification` (ALBERT model)
- - isInstance of `camembert` configuration class: :class:`~transformers.CamembertModelForSequenceClassification` (CamemBERT model)
- - isInstance of `xlm roberta` configuration class: :class:`~transformers.XLMRobertaModelForSequenceClassification` (XLM-RoBERTa model)
- - isInstance of `roberta` configuration class: :class:`~transformers.RobertaModelForSequenceClassification` (RoBERTa model)
- - isInstance of `bert` configuration class: :class:`~transformers.BertModelForSequenceClassification` (Bert model)
- - isInstance of `xlnet` configuration class: :class:`~transformers.XLNetModelForSequenceClassification` (XLNet model)
- - isInstance of `xlm` configuration class: :class:`~transformers.XLMModelForSequenceClassification` (XLM model)
- - isInstance of `flaubert` configuration class: :class:`~transformers.FlaubertForSequenceClassification` (Flaubert model)
-
-
- Examples::
-
- config = BertConfig.from_pretrained('bert-base-uncased') # Download configuration from S3 and cache.
- model = AutoModelForSequenceClassification.from_config(config) # E.g. model was saved using `save_pretrained('./test/saved_model/')`
- """
- for config_class, model_class in MODEL_FOR_SEQUENCE_CLASSIFICATION_MAPPING.items():
- if isinstance(config, config_class):
- return model_class(config)
- raise ValueError(
- "Unrecognized configuration class {} for this kind of AutoModel: {}.\n"
- "Model type should be one of {}.".format(
- config.__class__,
- cls.__name__,
- ", ".join(c.__name__ for c in MODEL_FOR_SEQUENCE_CLASSIFICATION_MAPPING.keys()),
- )
- )
-
- @classmethod
- def from_pretrained(cls, pretrained_model_name_or_path, *model_args, **kwargs):
- r""" Instantiates one of the sequence classification model classes of the library
- from a pre-trained model configuration.
-
- The `from_pretrained()` method takes care of returning the correct model class instance
- based on the `model_type` property of the config object, or when it's missing,
- falling back to using pattern matching on the `pretrained_model_name_or_path` string.
-
- The model class to instantiate is selected as the first pattern matching
- in the `pretrained_model_name_or_path` string (in the following order):
- - contains `distilbert`: :class:`~transformers.DistilBertForSequenceClassification` (DistilBERT model)
- - contains `albert`: :class:`~transformers.AlbertForSequenceClassification` (ALBERT model)
- - contains `camembert`: :class:`~transformers.CamembertForSequenceClassification` (CamemBERT model)
- - contains `xlm-roberta`: :class:`~transformers.XLMRobertaForSequenceClassification` (XLM-RoBERTa model)
- - contains `roberta`: :class:`~transformers.RobertaForSequenceClassification` (RoBERTa model)
- - contains `bert`: :class:`~transformers.BertForSequenceClassification` (Bert model)
- - contains `xlnet`: :class:`~transformers.XLNetForSequenceClassification` (XLNet model)
- - contains `flaubert`: :class:`~transformers.FlaubertForSequenceClassification` (Flaubert model)
-
- The model is set in evaluation mode by default using `model.eval()` (Dropout modules are deactivated)
- To train the model, you should first set it back in training mode with `model.train()`
-
- Args:
- pretrained_model_name_or_path: either:
-
- - a string with the `shortcut name` of a pre-trained model to load from cache or download, e.g.: ``bert-base-uncased``.
- - a string with the `identifier name` of a pre-trained model that was user-uploaded to our S3, e.g.: ``dbmdz/bert-base-german-cased``.
- - a path to a `directory` containing model weights saved using :func:`~transformers.PreTrainedModel.save_pretrained`, e.g.: ``./my_model_directory/``.
- - a path or url to a `tensorflow index checkpoint file` (e.g. `./tf_model/model.ckpt.index`). In this case, ``from_tf`` should be set to True and a configuration object should be provided as ``config`` argument. This loading path is slower than converting the TensorFlow checkpoint in a PyTorch model using the provided conversion scripts and loading the PyTorch model afterwards.
-
- model_args: (`optional`) Sequence of positional arguments:
- All remaining positional arguments will be passed to the underlying model's ``__init__`` method
-
- config: (`optional`) instance of a class derived from :class:`~transformers.PretrainedConfig`:
- Configuration for the model to use instead of an automatically loaded configuation. Configuration can be automatically loaded when:
-
- - the model is a model provided by the library (loaded with the ``shortcut-name`` string of a pretrained model), or
- - the model was saved using :func:`~transformers.PreTrainedModel.save_pretrained` and is reloaded by suppling the save directory.
- - the model is loaded by suppling a local directory as ``pretrained_model_name_or_path`` and a configuration JSON file named `config.json` is found in the directory.
-
- state_dict: (`optional`) dict:
- an optional state dictionnary for the model to use instead of a state dictionary loaded from saved weights file.
- This option can be used if you want to create a model from a pretrained configuration but load your own weights.
- In this case though, you should check if using :func:`~transformers.PreTrainedModel.save_pretrained` and :func:`~transformers.PreTrainedModel.from_pretrained` is not a simpler option.
-
- cache_dir: (`optional`) string:
- Path to a directory in which a downloaded pre-trained model
- configuration should be cached if the standard cache should not be used.
-
- force_download: (`optional`) boolean, default False:
- Force to (re-)download the model weights and configuration files and override the cached versions if they exists.
-
- resume_download: (`optional`) boolean, default False:
- Do not delete incompletely recieved file. Attempt to resume the download if such a file exists.
-
- proxies: (`optional`) dict, default None:
- A dictionary of proxy servers to use by protocol or endpoint, e.g.: {'http': 'foo.bar:3128', 'http://hostname': 'foo.bar:4012'}.
- The proxies are used on each request.
-
- output_loading_info: (`optional`) boolean:
- Set to ``True`` to also return a dictionnary containing missing keys, unexpected keys and error messages.
-
- kwargs: (`optional`) Remaining dictionary of keyword arguments:
- Can be used to update the configuration object (after it being loaded) and initiate the model. (e.g. ``output_attention=True``). Behave differently depending on whether a `config` is provided or automatically loaded:
-
- - If a configuration is provided with ``config``, ``**kwargs`` will be directly passed to the underlying model's ``__init__`` method (we assume all relevant updates to the configuration have already been done)
- - If a configuration is not provided, ``kwargs`` will be first passed to the configuration class initialization function (:func:`~transformers.PretrainedConfig.from_pretrained`). Each key of ``kwargs`` that corresponds to a configuration attribute will be used to override said attribute with the supplied ``kwargs`` value. Remaining keys that do not correspond to any configuration attribute will be passed to the underlying model's ``__init__`` function.
-
- Examples::
-
- model = AutoModelForSequenceClassification.from_pretrained('bert-base-uncased') # Download model and configuration from S3 and cache.
- model = AutoModelForSequenceClassification.from_pretrained('./test/bert_model/') # E.g. model was saved using `save_pretrained('./test/saved_model/')`
- model = AutoModelForSequenceClassification.from_pretrained('bert-base-uncased', output_attention=True) # Update configuration during loading
- assert model.config.output_attention == True
- # Loading from a TF checkpoint file instead of a PyTorch model (slower)
- config = AutoConfig.from_json_file('./tf_model/bert_tf_model_config.json')
- model = AutoModelForSequenceClassification.from_pretrained('./tf_model/bert_tf_checkpoint.ckpt.index', from_tf=True, config=config)
-
- """
- config = kwargs.pop("config", None)
- if not isinstance(config, PretrainedConfig):
- config = AutoConfig.from_pretrained(pretrained_model_name_or_path, **kwargs)
-
- for config_class, model_class in MODEL_FOR_SEQUENCE_CLASSIFICATION_MAPPING.items():
- if isinstance(config, config_class):
- return model_class.from_pretrained(pretrained_model_name_or_path, *model_args, config=config, **kwargs)
- raise ValueError(
- "Unrecognized configuration class {} for this kind of AutoModel: {}.\n"
- "Model type should be one of {}.".format(
- config.__class__,
- cls.__name__,
- ", ".join(c.__name__ for c in MODEL_FOR_SEQUENCE_CLASSIFICATION_MAPPING.keys()),
- )
- )
-
-
-class AutoModelForQuestionAnswering(object):
- r"""
- :class:`~transformers.AutoModelForQuestionAnswering` is a generic model class
- that will be instantiated as one of the question answering model classes of the library
- when created with the `AutoModelForQuestionAnswering.from_pretrained(pretrained_model_name_or_path)`
- class method.
-
- This class cannot be instantiated using `__init__()` (throws an error).
- """
-
- def __init__(self):
- raise EnvironmentError(
- "AutoModelForQuestionAnswering is designed to be instantiated "
- "using the `AutoModelForQuestionAnswering.from_pretrained(pretrained_model_name_or_path)` or "
- "`AutoModelForQuestionAnswering.from_config(config)` methods."
- )
-
- @classmethod
- def from_config(cls, config):
- r""" Instantiates one of the base model classes of the library
- from a configuration.
-
- Args:
- config (:class:`~transformers.PretrainedConfig`):
- The model class to instantiate is selected based on the configuration class:
-
- - isInstance of `distilbert` configuration class: :class:`~transformers.DistilBertModelForQuestionAnswering` (DistilBERT model)
- - isInstance of `albert` configuration class: :class:`~transformers.AlbertModelForQuestionAnswering` (ALBERT model)
- - isInstance of `bert` configuration class: :class:`~transformers.BertModelForQuestionAnswering` (Bert model)
- - isInstance of `xlnet` configuration class: :class:`~transformers.XLNetModelForQuestionAnswering` (XLNet model)
- - isInstance of `xlm` configuration class: :class:`~transformers.XLMModelForQuestionAnswering` (XLM model)
- - isInstance of `flaubert` configuration class: :class:`~transformers.FlaubertForQuestionAnswering` (XLM model)
-
- Examples::
-
- config = BertConfig.from_pretrained('bert-base-uncased') # Download configuration from S3 and cache.
- model = AutoModelForSequenceClassification.from_config(config) # E.g. model was saved using `save_pretrained('./test/saved_model/')`
- """
- for config_class, model_class in MODEL_FOR_QUESTION_ANSWERING_MAPPING.items():
- if isinstance(config, config_class):
- return model_class(config)
-
- raise ValueError(
- "Unrecognized configuration class {} for this kind of AutoModel: {}.\n"
- "Model type should be one of {}.".format(
- config.__class__,
- cls.__name__,
- ", ".join(c.__name__ for c in MODEL_FOR_QUESTION_ANSWERING_MAPPING.keys()),
- )
- )
-
- @classmethod
- def from_pretrained(cls, pretrained_model_name_or_path, *model_args, **kwargs):
- r""" Instantiates one of the question answering model classes of the library
- from a pre-trained model configuration.
-
- The `from_pretrained()` method takes care of returning the correct model class instance
- based on the `model_type` property of the config object, or when it's missing,
- falling back to using pattern matching on the `pretrained_model_name_or_path` string.
-
- The model class to instantiate is selected as the first pattern matching
- in the `pretrained_model_name_or_path` string (in the following order):
- - contains `distilbert`: :class:`~transformers.DistilBertForQuestionAnswering` (DistilBERT model)
- - contains `albert`: :class:`~transformers.AlbertForQuestionAnswering` (ALBERT model)
- - contains `bert`: :class:`~transformers.BertForQuestionAnswering` (Bert model)
- - contains `xlnet`: :class:`~transformers.XLNetForQuestionAnswering` (XLNet model)
- - contains `xlm`: :class:`~transformers.XLMForQuestionAnswering` (XLM model)
- - contains `flaubert`: :class:`~transformers.FlaubertForQuestionAnswering` (XLM model)
-
- The model is set in evaluation mode by default using `model.eval()` (Dropout modules are deactivated)
- To train the model, you should first set it back in training mode with `model.train()`
-
- Args:
- pretrained_model_name_or_path: either:
-
- - a string with the `shortcut name` of a pre-trained model to load from cache or download, e.g.: ``bert-base-uncased``.
- - a string with the `identifier name` of a pre-trained model that was user-uploaded to our S3, e.g.: ``dbmdz/bert-base-german-cased``.
- - a path to a `directory` containing model weights saved using :func:`~transformers.PreTrainedModel.save_pretrained`, e.g.: ``./my_model_directory/``.
- - a path or url to a `tensorflow index checkpoint file` (e.g. `./tf_model/model.ckpt.index`). In this case, ``from_tf`` should be set to True and a configuration object should be provided as ``config`` argument. This loading path is slower than converting the TensorFlow checkpoint in a PyTorch model using the provided conversion scripts and loading the PyTorch model afterwards.
-
- model_args: (`optional`) Sequence of positional arguments:
- All remaning positional arguments will be passed to the underlying model's ``__init__`` method
-
- config: (`optional`) instance of a class derived from :class:`~transformers.PretrainedConfig`:
- Configuration for the model to use instead of an automatically loaded configuation. Configuration can be automatically loaded when:
-
- - the model is a model provided by the library (loaded with the ``shortcut-name`` string of a pretrained model), or
- - the model was saved using :func:`~transformers.PreTrainedModel.save_pretrained` and is reloaded by suppling the save directory.
- - the model is loaded by suppling a local directory as ``pretrained_model_name_or_path`` and a configuration JSON file named `config.json` is found in the directory.
-
- state_dict: (`optional`) dict:
- an optional state dictionnary for the model to use instead of a state dictionary loaded from saved weights file.
- This option can be used if you want to create a model from a pretrained configuration but load your own weights.
- In this case though, you should check if using :func:`~transformers.PreTrainedModel.save_pretrained` and :func:`~transformers.PreTrainedModel.from_pretrained` is not a simpler option.
-
- cache_dir: (`optional`) string:
- Path to a directory in which a downloaded pre-trained model
- configuration should be cached if the standard cache should not be used.
-
- force_download: (`optional`) boolean, default False:
- Force to (re-)download the model weights and configuration files and override the cached versions if they exists.
-
- proxies: (`optional`) dict, default None:
- A dictionary of proxy servers to use by protocol or endpoint, e.g.: {'http': 'foo.bar:3128', 'http://hostname': 'foo.bar:4012'}.
- The proxies are used on each request.
-
- output_loading_info: (`optional`) boolean:
- Set to ``True`` to also return a dictionnary containing missing keys, unexpected keys and error messages.
-
- kwargs: (`optional`) Remaining dictionary of keyword arguments:
- Can be used to update the configuration object (after it being loaded) and initiate the model. (e.g. ``output_attention=True``). Behave differently depending on whether a `config` is provided or automatically loaded:
-
- - If a configuration is provided with ``config``, ``**kwargs`` will be directly passed to the underlying model's ``__init__`` method (we assume all relevant updates to the configuration have already been done)
- - If a configuration is not provided, ``kwargs`` will be first passed to the configuration class initialization function (:func:`~transformers.PretrainedConfig.from_pretrained`). Each key of ``kwargs`` that corresponds to a configuration attribute will be used to override said attribute with the supplied ``kwargs`` value. Remaining keys that do not correspond to any configuration attribute will be passed to the underlying model's ``__init__`` function.
-
- Examples::
-
- model = AutoModelForQuestionAnswering.from_pretrained('bert-base-uncased') # Download model and configuration from S3 and cache.
- model = AutoModelForQuestionAnswering.from_pretrained('./test/bert_model/') # E.g. model was saved using `save_pretrained('./test/saved_model/')`
- model = AutoModelForQuestionAnswering.from_pretrained('bert-base-uncased', output_attention=True) # Update configuration during loading
- assert model.config.output_attention == True
- # Loading from a TF checkpoint file instead of a PyTorch model (slower)
- config = AutoConfig.from_json_file('./tf_model/bert_tf_model_config.json')
- model = AutoModelForQuestionAnswering.from_pretrained('./tf_model/bert_tf_checkpoint.ckpt.index', from_tf=True, config=config)
-
- """
- config = kwargs.pop("config", None)
- if not isinstance(config, PretrainedConfig):
- config = AutoConfig.from_pretrained(pretrained_model_name_or_path, **kwargs)
-
- for config_class, model_class in MODEL_FOR_QUESTION_ANSWERING_MAPPING.items():
- if isinstance(config, config_class):
- return model_class.from_pretrained(pretrained_model_name_or_path, *model_args, config=config, **kwargs)
-
- raise ValueError(
- "Unrecognized configuration class {} for this kind of AutoModel: {}.\n"
- "Model type should be one of {}.".format(
- config.__class__,
- cls.__name__,
- ", ".join(c.__name__ for c in MODEL_FOR_QUESTION_ANSWERING_MAPPING.keys()),
- )
- )
-
-
-class AutoModelForTokenClassification:
- r"""
- :class:`~transformers.AutoModelForTokenClassification` is a generic model class
- that will be instantiated as one of the token classification model classes of the library
- when created with the `AutoModelForTokenClassification.from_pretrained(pretrained_model_name_or_path)`
- class method.
-
- This class cannot be instantiated using `__init__()` (throws an error).
- """
-
- def __init__(self):
- raise EnvironmentError(
- "AutoModelForTokenClassification is designed to be instantiated "
- "using the `AutoModelForTokenClassification.from_pretrained(pretrained_model_name_or_path)` or "
- "`AutoModelForTokenClassification.from_config(config)` methods."
- )
-
- @classmethod
- def from_config(cls, config):
- r""" Instantiates one of the base model classes of the library
- from a configuration.
-
- Args:
- config (:class:`~transformers.PretrainedConfig`):
- The model class to instantiate is selected based on the configuration class:
-
- - isInstance of `distilbert` configuration class: :class:`~transformers.DistilBertModelForTokenClassification` (DistilBERT model)
- - isInstance of `xlm roberta` configuration class: :class:`~transformers.XLMRobertaModelForTokenClassification` (XLMRoberta model)
- - isInstance of `bert` configuration class: :class:`~transformers.BertModelForTokenClassification` (Bert model)
- - isInstance of `xlnet` configuration class: :class:`~transformers.XLNetModelForTokenClassification` (XLNet model)
- - isInstance of `camembert` configuration class: :class:`~transformers.CamembertModelForTokenClassification` (Camembert model)
- - isInstance of `roberta` configuration class: :class:`~transformers.RobertaModelForTokenClassification` (Roberta model)
-
- Examples::
-
- config = BertConfig.from_pretrained('bert-base-uncased') # Download configuration from S3 and cache.
- model = AutoModelForTokenClassification.from_config(config) # E.g. model was saved using `save_pretrained('./test/saved_model/')`
- """
- for config_class, model_class in MODEL_FOR_TOKEN_CLASSIFICATION_MAPPING.items():
- if isinstance(config, config_class):
- return model_class(config)
-
- raise ValueError(
- "Unrecognized configuration class {} for this kind of AutoModel: {}.\n"
- "Model type should be one of {}.".format(
- config.__class__,
- cls.__name__,
- ", ".join(c.__name__ for c in MODEL_FOR_TOKEN_CLASSIFICATION_MAPPING.keys()),
- )
- )
-
- @classmethod
- def from_pretrained(cls, pretrained_model_name_or_path, *model_args, **kwargs):
- r""" Instantiates one of the question answering model classes of the library
- from a pre-trained model configuration.
-
- The `from_pretrained()` method takes care of returning the correct model class instance
- based on the `model_type` property of the config object, or when it's missing,
- falling back to using pattern matching on the `pretrained_model_name_or_path` string.
-
- The model class to instantiate is selected as the first pattern matching
- in the `pretrained_model_name_or_path` string (in the following order):
- - contains `distilbert`: :class:`~transformers.DistilBertForTokenClassification` (DistilBERT model)
- - contains `xlm-roberta`: :class:`~transformers.XLMRobertaForTokenClassification` (XLM-RoBERTa?Para model)
- - contains `camembert`: :class:`~transformers.CamembertForTokenClassification` (Camembert model)
- - contains `bert`: :class:`~transformers.BertForTokenClassification` (Bert model)
- - contains `xlnet`: :class:`~transformers.XLNetForTokenClassification` (XLNet model)
- - contains `roberta`: :class:`~transformers.RobertaForTokenClassification` (Roberta model)
-
- The model is set in evaluation mode by default using `model.eval()` (Dropout modules are deactivated)
- To train the model, you should first set it back in training mode with `model.train()`
-
- Args:
- pretrained_model_name_or_path:
- Either:
-
- - a string with the `shortcut name` of a pre-trained model to load from cache or download, e.g.: ``bert-base-uncased``.
- - a path to a `directory` containing model weights saved using :func:`~transformers.PreTrainedModel.save_pretrained`, e.g.: ``./my_model_directory/``.
- - a path or url to a `tensorflow index checkpoint file` (e.g. `./tf_model/model.ckpt.index`). In this case, ``from_tf`` should be set to True and a configuration object should be provided as ``config`` argument. This loading path is slower than converting the TensorFlow checkpoint in a PyTorch model using the provided conversion scripts and loading the PyTorch model afterwards.
-
- model_args: (`optional`) Sequence of positional arguments:
- All remaning positional arguments will be passed to the underlying model's ``__init__`` method
-
- config: (`optional`) instance of a class derived from :class:`~transformers.PretrainedConfig`:
- Configuration for the model to use instead of an automatically loaded configuation. Configuration can be automatically loaded when:
-
- - the model is a model provided by the library (loaded with the ``shortcut-name`` string of a pretrained model), or
- - the model was saved using :func:`~transformers.PreTrainedModel.save_pretrained` and is reloaded by suppling the save directory.
- - the model is loaded by suppling a local directory as ``pretrained_model_name_or_path`` and a configuration JSON file named `config.json` is found in the directory.
-
- state_dict: (`optional`) dict:
- an optional state dictionnary for the model to use instead of a state dictionary loaded from saved weights file.
- This option can be used if you want to create a model from a pretrained configuration but load your own weights.
- In this case though, you should check if using :func:`~transformers.PreTrainedModel.save_pretrained` and :func:`~transformers.PreTrainedModel.from_pretrained` is not a simpler option.
-
- cache_dir: (`optional`) string:
- Path to a directory in which a downloaded pre-trained model
- configuration should be cached if the standard cache should not be used.
-
- force_download: (`optional`) boolean, default False:
- Force to (re-)download the model weights and configuration files and override the cached versions if they exists.
-
- proxies: (`optional`) dict, default None:
- A dictionary of proxy servers to use by protocol or endpoint, e.g.: {'http': 'foo.bar:3128', 'http://hostname': 'foo.bar:4012'}.
- The proxies are used on each request.
-
- output_loading_info: (`optional`) boolean:
- Set to ``True`` to also return a dictionnary containing missing keys, unexpected keys and error messages.
-
- kwargs: (`optional`) Remaining dictionary of keyword arguments:
- Can be used to update the configuration object (after it being loaded) and initiate the model. (e.g. ``output_attention=True``). Behave differently depending on whether a `config` is provided or automatically loaded:
-
- - If a configuration is provided with ``config``, ``**kwargs`` will be directly passed to the underlying model's ``__init__`` method (we assume all relevant updates to the configuration have already been done)
- - If a configuration is not provided, ``kwargs`` will be first passed to the configuration class initialization function (:func:`~transformers.PretrainedConfig.from_pretrained`). Each key of ``kwargs`` that corresponds to a configuration attribute will be used to override said attribute with the supplied ``kwargs`` value. Remaining keys that do not correspond to any configuration attribute will be passed to the underlying model's ``__init__`` function.
-
- Examples::
-
- model = AutoModelForTokenClassification.from_pretrained('bert-base-uncased') # Download model and configuration from S3 and cache.
- model = AutoModelForTokenClassification.from_pretrained('./test/bert_model/') # E.g. model was saved using `save_pretrained('./test/saved_model/')`
- model = AutoModelForTokenClassification.from_pretrained('bert-base-uncased', output_attention=True) # Update configuration during loading
- assert model.config.output_attention == True
- # Loading from a TF checkpoint file instead of a PyTorch model (slower)
- config = AutoConfig.from_json_file('./tf_model/bert_tf_model_config.json')
- model = AutoModelForTokenClassification.from_pretrained('./tf_model/bert_tf_checkpoint.ckpt.index', from_tf=True, config=config)
-
- """
- config = kwargs.pop("config", None)
- if not isinstance(config, PretrainedConfig):
- config = AutoConfig.from_pretrained(pretrained_model_name_or_path, **kwargs)
-
- for config_class, model_class in MODEL_FOR_TOKEN_CLASSIFICATION_MAPPING.items():
- if isinstance(config, config_class):
- return model_class.from_pretrained(pretrained_model_name_or_path, *model_args, config=config, **kwargs)
-
- raise ValueError(
- "Unrecognized configuration class {} for this kind of AutoModel: {}.\n"
- "Model type should be one of {}.".format(
- config.__class__,
- cls.__name__,
- ", ".join(c.__name__ for c in MODEL_FOR_TOKEN_CLASSIFICATION_MAPPING.keys()),
- )
- )
diff --git a/server/transformers/src/transformers/modeling_bert.py b/server/transformers/src/transformers/modeling_bert.py
deleted file mode 100644
index caa056b64cb9869634c973cf525cdd4c6c7f88c9..0000000000000000000000000000000000000000
--- a/server/transformers/src/transformers/modeling_bert.py
+++ /dev/null
@@ -1,1535 +0,0 @@
-# coding=utf-8
-# Copyright 2018 The Google AI Language Team Authors and The HuggingFace Inc. team.
-# Copyright (c) 2018, NVIDIA CORPORATION. All rights reserved.
-#
-# Licensed under the Apache License, Version 2.0 (the "License");
-# you may not use this file except in compliance with the License.
-# You may obtain a copy of the License at
-#
-# http://www.apache.org/licenses/LICENSE-2.0
-#
-# Unless required by applicable law or agreed to in writing, software
-# distributed under the License is distributed on an "AS IS" BASIS,
-# WITHOUT WARRANTIES OR CONDITIONS OF ANY KIND, either express or implied.
-# See the License for the specific language governing permissions and
-# limitations under the License.
-
-"""PyTorch BERT model. """
-
-
-import logging
-import math
-import os
-
-import torch
-from torch import nn
-from torch.nn import CrossEntropyLoss, MSELoss
-
-from .configuration_bert import BertConfig
-from .modeling_utils import PreTrainedModel, prune_linear_layer, transpose_iterable
-from .file_utils import add_start_docstrings, add_start_docstrings_to_callable
-
-logger = logging.getLogger(__name__)
-
-BERT_PRETRAINED_MODEL_ARCHIVE_MAP = {
- "bert-base-uncased": "https://s3.amazonaws.com/models.huggingface.co/bert/bert-base-uncased-pytorch_model.bin",
- "bert-large-uncased": "https://s3.amazonaws.com/models.huggingface.co/bert/bert-large-uncased-pytorch_model.bin",
- "bert-base-cased": "https://s3.amazonaws.com/models.huggingface.co/bert/bert-base-cased-pytorch_model.bin",
- "bert-large-cased": "https://s3.amazonaws.com/models.huggingface.co/bert/bert-large-cased-pytorch_model.bin",
- "bert-base-multilingual-uncased": "https://s3.amazonaws.com/models.huggingface.co/bert/bert-base-multilingual-uncased-pytorch_model.bin",
- "bert-base-multilingual-cased": "https://s3.amazonaws.com/models.huggingface.co/bert/bert-base-multilingual-cased-pytorch_model.bin",
- "bert-base-chinese": "https://s3.amazonaws.com/models.huggingface.co/bert/bert-base-chinese-pytorch_model.bin",
- "bert-base-german-cased": "https://s3.amazonaws.com/models.huggingface.co/bert/bert-base-german-cased-pytorch_model.bin",
- "bert-large-uncased-whole-word-masking": "https://s3.amazonaws.com/models.huggingface.co/bert/bert-large-uncased-whole-word-masking-pytorch_model.bin",
- "bert-large-cased-whole-word-masking": "https://s3.amazonaws.com/models.huggingface.co/bert/bert-large-cased-whole-word-masking-pytorch_model.bin",
- "bert-large-uncased-whole-word-masking-finetuned-squad": "https://s3.amazonaws.com/models.huggingface.co/bert/bert-large-uncased-whole-word-masking-finetuned-squad-pytorch_model.bin",
- "bert-large-cased-whole-word-masking-finetuned-squad": "https://s3.amazonaws.com/models.huggingface.co/bert/bert-large-cased-whole-word-masking-finetuned-squad-pytorch_model.bin",
- "bert-base-cased-finetuned-mrpc": "https://s3.amazonaws.com/models.huggingface.co/bert/bert-base-cased-finetuned-mrpc-pytorch_model.bin",
- "bert-base-german-dbmdz-cased": "https://s3.amazonaws.com/models.huggingface.co/bert/bert-base-german-dbmdz-cased-pytorch_model.bin",
- "bert-base-german-dbmdz-uncased": "https://s3.amazonaws.com/models.huggingface.co/bert/bert-base-german-dbmdz-uncased-pytorch_model.bin",
- "bert-base-japanese": "https://s3.amazonaws.com/models.huggingface.co/bert/cl-tohoku/bert-base-japanese-pytorch_model.bin",
- "bert-base-japanese-whole-word-masking": "https://s3.amazonaws.com/models.huggingface.co/bert/cl-tohoku/bert-base-japanese-whole-word-masking-pytorch_model.bin",
- "bert-base-japanese-char": "https://s3.amazonaws.com/models.huggingface.co/bert/cl-tohoku/bert-base-japanese-char-pytorch_model.bin",
- "bert-base-japanese-char-whole-word-masking": "https://s3.amazonaws.com/models.huggingface.co/bert/cl-tohoku/bert-base-japanese-char-whole-word-masking-pytorch_model.bin",
- "bert-base-finnish-cased-v1": "https://s3.amazonaws.com/models.huggingface.co/bert/TurkuNLP/bert-base-finnish-cased-v1/pytorch_model.bin",
- "bert-base-finnish-uncased-v1": "https://s3.amazonaws.com/models.huggingface.co/bert/TurkuNLP/bert-base-finnish-uncased-v1/pytorch_model.bin",
- "bert-base-dutch-cased": "https://s3.amazonaws.com/models.huggingface.co/bert/wietsedv/bert-base-dutch-cased/pytorch_model.bin",
-}
-
-
-def load_tf_weights_in_bert(model, config, tf_checkpoint_path):
- """ Load tf checkpoints in a pytorch model.
- """
- try:
- import re
- import numpy as np
- import tensorflow as tf
- except ImportError:
- logger.error(
- "Loading a TensorFlow model in PyTorch, requires TensorFlow to be installed. Please see "
- "https://www.tensorflow.org/install/ for installation instructions."
- )
- raise
- tf_path = os.path.abspath(tf_checkpoint_path)
- logger.info("Converting TensorFlow checkpoint from {}".format(tf_path))
- # Load weights from TF model
- init_vars = tf.train.list_variables(tf_path)
- names = []
- arrays = []
- for name, shape in init_vars:
- logger.info("Loading TF weight {} with shape {}".format(name, shape))
- array = tf.train.load_variable(tf_path, name)
- names.append(name)
- arrays.append(array)
-
- for name, array in zip(names, arrays):
- name = name.split("/")
- # adam_v and adam_m are variables used in AdamWeightDecayOptimizer to calculated m and v
- # which are not required for using pretrained model
- if any(n in ["adam_v", "adam_m", "global_step"] for n in name):
- logger.info("Skipping {}".format("/".join(name)))
- continue
- pointer = model
- for m_name in name:
- if re.fullmatch(r"[A-Za-z]+_\d+", m_name):
- scope_names = re.split(r"_(\d+)", m_name)
- else:
- scope_names = [m_name]
- if scope_names[0] == "kernel" or scope_names[0] == "gamma":
- pointer = getattr(pointer, "weight")
- elif scope_names[0] == "output_bias" or scope_names[0] == "beta":
- pointer = getattr(pointer, "bias")
- elif scope_names[0] == "output_weights":
- pointer = getattr(pointer, "weight")
- elif scope_names[0] == "squad":
- pointer = getattr(pointer, "classifier")
- else:
- try:
- pointer = getattr(pointer, scope_names[0])
- except AttributeError:
- logger.info("Skipping {}".format("/".join(name)))
- continue
- if len(scope_names) >= 2:
- num = int(scope_names[1])
- pointer = pointer[num]
- if m_name[-11:] == "_embeddings":
- pointer = getattr(pointer, "weight")
- elif m_name == "kernel":
- array = np.transpose(array)
- try:
- assert pointer.shape == array.shape
- except AssertionError as e:
- e.args += (pointer.shape, array.shape)
- raise
- logger.info("Initialize PyTorch weight {}".format(name))
- pointer.data = torch.from_numpy(array)
- return model
-
-
-def gelu(x):
- """ Original Implementation of the gelu activation function in Google Bert repo when initially created.
- For information: OpenAI GPT's gelu is slightly different (and gives slightly different results):
- 0.5 * x * (1 + torch.tanh(math.sqrt(2 / math.pi) * (x + 0.044715 * torch.pow(x, 3))))
- Also see https://arxiv.org/abs/1606.08415
- """
- return x * 0.5 * (1.0 + torch.erf(x / math.sqrt(2.0)))
-
-
-def gelu_new(x):
- """ Implementation of the gelu activation function currently in Google Bert repo (identical to OpenAI GPT).
- Also see https://arxiv.org/abs/1606.08415
- """
- return 0.5 * x * (1 + torch.tanh(math.sqrt(2 / math.pi) * (x + 0.044715 * torch.pow(x, 3))))
-
-
-def swish(x):
- return x * torch.sigmoid(x)
-
-
-def mish(x):
- return x * torch.tanh(nn.functional.softplus(x))
-
-
-ACT2FN = {"gelu": gelu, "relu": torch.nn.functional.relu, "swish": swish, "gelu_new": gelu_new, "mish": mish}
-
-
-BertLayerNorm = torch.nn.LayerNorm
-
-
-class BertEmbeddings(nn.Module):
- """Construct the embeddings from word, position and token_type embeddings.
- """
-
- def __init__(self, config):
- super().__init__()
- self.word_embeddings = nn.Embedding(config.vocab_size, config.hidden_size, padding_idx=0)
- self.position_embeddings = nn.Embedding(config.max_position_embeddings, config.hidden_size)
- self.token_type_embeddings = nn.Embedding(config.type_vocab_size, config.hidden_size)
-
- # self.LayerNorm is not snake-cased to stick with TensorFlow model variable name and be able to load
- # any TensorFlow checkpoint file
- self.LayerNorm = BertLayerNorm(config.hidden_size, eps=config.layer_norm_eps)
- self.dropout = nn.Dropout(config.hidden_dropout_prob)
-
- def forward(self, input_ids=None, token_type_ids=None, position_ids=None, inputs_embeds=None):
- if input_ids is not None:
- input_shape = input_ids.size()
- else:
- input_shape = inputs_embeds.size()[:-1]
-
- seq_length = input_shape[1]
- device = input_ids.device if input_ids is not None else inputs_embeds.device
- if position_ids is None:
- position_ids = torch.arange(seq_length, dtype=torch.long, device=device)
- position_ids = position_ids.unsqueeze(0).expand(input_shape)
- if token_type_ids is None:
- token_type_ids = torch.zeros(input_shape, dtype=torch.long, device=device)
-
- if inputs_embeds is None:
- inputs_embeds = self.word_embeddings(input_ids)
- position_embeddings = self.position_embeddings(position_ids)
- token_type_embeddings = self.token_type_embeddings(token_type_ids)
-
- embeddings = inputs_embeds + position_embeddings + token_type_embeddings
- embeddings = self.LayerNorm(embeddings)
- embeddings = self.dropout(embeddings)
- return embeddings
-
-
-class BertSelfAttention(nn.Module):
- def __init__(self, config):
- super().__init__()
- if config.hidden_size % config.num_attention_heads != 0:
- raise ValueError(
- "The hidden size (%d) is not a multiple of the number of attention "
- "heads (%d)" % (config.hidden_size, config.num_attention_heads)
- )
- self.output_attentions = config.output_attentions
- self.output_additional_info = config.output_additional_info
-
- self.num_attention_heads = config.num_attention_heads
- self.attention_head_size = int(config.hidden_size / config.num_attention_heads)
- self.all_head_size = self.num_attention_heads * self.attention_head_size
-
- self.query = nn.Linear(config.hidden_size, self.all_head_size)
- self.key = nn.Linear(config.hidden_size, self.all_head_size)
- self.value = nn.Linear(config.hidden_size, self.all_head_size)
-
- self.dropout = nn.Dropout(config.attention_probs_dropout_prob)
-
- def transpose_for_scores(self, x):
- new_x_shape = x.size()[:-1] + (self.num_attention_heads, self.attention_head_size)
- x = x.view(*new_x_shape)
- return x.permute(0, 2, 1, 3)
-
- def forward(
- self,
- hidden_states,
- attention_mask=None,
- head_mask=None,
- encoder_hidden_states=None,
- encoder_attention_mask=None,
- ):
- mixed_query_layer = self.query(hidden_states)
-
- # If this is instantiated as a cross-attention module, the keys
- # and values come from an encoder; the attention mask needs to be
- # such that the encoder's padding tokens are not attended to.
- if encoder_hidden_states is not None:
- mixed_key_layer = self.key(encoder_hidden_states)
- mixed_value_layer = self.value(encoder_hidden_states)
- attention_mask = encoder_attention_mask
- else:
- mixed_key_layer = self.key(hidden_states)
- mixed_value_layer = self.value(hidden_states)
-
- query_layer = self.transpose_for_scores(mixed_query_layer)
- key_layer = self.transpose_for_scores(mixed_key_layer)
- value_layer = self.transpose_for_scores(mixed_value_layer)
-
- # Take the dot product between "query" and "key" to get the raw attention scores.
- attention_scores = torch.matmul(query_layer, key_layer.transpose(-1, -2))
- attention_scores = attention_scores / math.sqrt(self.attention_head_size)
- if attention_mask is not None:
- # Apply the attention mask is (precomputed for all layers in BertModel forward() function)
- attention_scores = attention_scores + attention_mask
-
- # Normalize the attention scores to probabilities.
- attention_probs = nn.Softmax(dim=-1)(attention_scores)
-
- # This is actually dropping out entire tokens to attend to, which might
- # seem a bit unusual, but is taken from the original Transformer paper.
- attention_probs = self.dropout(attention_probs)
-
- # Mask heads if we want to
- if head_mask is not None:
- attention_probs = attention_probs * head_mask
-
- context_layer = torch.matmul(attention_probs, value_layer)
-
- context_layer = context_layer.permute(0, 2, 1, 3).contiguous()
- new_context_layer_shape = context_layer.size()[:-2] + (self.all_head_size,)
- new_context_layer = context_layer.view(*new_context_layer_shape)
-
- outputs = (new_context_layer,)
- if self.output_attentions:
- outputs += (attention_probs,)
- if self.output_additional_info: # Only support additional info if attentions are desired
- outputs += (context_layer,)
-
- return outputs
-
-
-class BertSelfOutput(nn.Module):
- def __init__(self, config):
- super().__init__()
- self.dense = nn.Linear(config.hidden_size, config.hidden_size)
- self.LayerNorm = BertLayerNorm(config.hidden_size, eps=config.layer_norm_eps)
- self.dropout = nn.Dropout(config.hidden_dropout_prob)
-
- def forward(self, hidden_states, input_tensor):
- hidden_states = self.dense(hidden_states)
- hidden_states = self.dropout(hidden_states)
- hidden_states = self.LayerNorm(hidden_states + input_tensor)
- return hidden_states
-
-
-class BertAttention(nn.Module):
- def __init__(self, config):
- super().__init__()
- self.self = BertSelfAttention(config)
- self.output = BertSelfOutput(config)
- self.pruned_heads = set()
-
- def prune_heads(self, heads):
- if len(heads) == 0:
- return
- mask = torch.ones(self.self.num_attention_heads, self.self.attention_head_size)
- heads = set(heads) - self.pruned_heads # Convert to set and remove already pruned heads
- for head in heads:
- # Compute how many pruned heads are before the head and move the index accordingly
- head = head - sum(1 if h < head else 0 for h in self.pruned_heads)
- mask[head] = 0
- mask = mask.view(-1).contiguous().eq(1)
- index = torch.arange(len(mask))[mask].long()
-
- # Prune linear layers
- self.self.query = prune_linear_layer(self.self.query, index)
- self.self.key = prune_linear_layer(self.self.key, index)
- self.self.value = prune_linear_layer(self.self.value, index)
- self.output.dense = prune_linear_layer(self.output.dense, index, dim=1)
-
- # Update hyper params and store pruned heads
- self.self.num_attention_heads = self.self.num_attention_heads - len(heads)
- self.self.all_head_size = self.self.attention_head_size * self.self.num_attention_heads
- self.pruned_heads = self.pruned_heads.union(heads)
-
- def forward(
- self,
- hidden_states,
- attention_mask=None,
- head_mask=None,
- encoder_hidden_states=None,
- encoder_attention_mask=None,
- ):
- self_outputs = self.self(
- hidden_states, attention_mask, head_mask, encoder_hidden_states, encoder_attention_mask
- )
- attention_output = self.output(self_outputs[0], hidden_states)
- outputs = (attention_output,) + self_outputs[1:] # add attentions if we output them
- return outputs
-
-
-class BertIntermediate(nn.Module):
- def __init__(self, config):
- super().__init__()
- self.dense = nn.Linear(config.hidden_size, config.intermediate_size)
- if isinstance(config.hidden_act, str):
- self.intermediate_act_fn = ACT2FN[config.hidden_act]
- else:
- self.intermediate_act_fn = config.hidden_act
-
- def forward(self, hidden_states):
- hidden_states = self.dense(hidden_states)
- hidden_states = self.intermediate_act_fn(hidden_states)
- return hidden_states
-
-
-class BertOutput(nn.Module):
- def __init__(self, config):
- super().__init__()
- self.dense = nn.Linear(config.intermediate_size, config.hidden_size)
- self.LayerNorm = BertLayerNorm(config.hidden_size, eps=config.layer_norm_eps)
- self.dropout = nn.Dropout(config.hidden_dropout_prob)
-
- def forward(self, hidden_states, input_tensor):
- hidden_states = self.dense(hidden_states)
- hidden_states = self.dropout(hidden_states)
- hidden_states = self.LayerNorm(hidden_states + input_tensor)
- return hidden_states
-
-
-class BertLayer(nn.Module):
- def __init__(self, config):
- super().__init__()
- self.attention = BertAttention(config)
- self.is_decoder = config.is_decoder
- if self.is_decoder:
- self.crossattention = BertAttention(config)
- self.intermediate = BertIntermediate(config)
- self.output = BertOutput(config)
-
- def forward(
- self,
- hidden_states,
- attention_mask=None,
- head_mask=None,
- encoder_hidden_states=None,
- encoder_attention_mask=None,
- ):
- self_attention_outputs = self.attention(hidden_states, attention_mask, head_mask)
- attention_output = self_attention_outputs[0]
- outputs = self_attention_outputs[1:] # add self attentions if we output attention weights
-
- if self.is_decoder and encoder_hidden_states is not None:
- cross_attention_outputs = self.crossattention(
- attention_output, attention_mask, head_mask, encoder_hidden_states, encoder_attention_mask
- )
- attention_output = cross_attention_outputs[0]
- outputs = outputs + cross_attention_outputs[1:] # add cross attentions if we output attention weights
-
- intermediate_output = self.intermediate(attention_output)
- layer_output = self.output(intermediate_output, attention_output)
- outputs = (layer_output,) + outputs
- return outputs
-
-
-class BertEncoder(nn.Module):
- def __init__(self, config):
- super().__init__()
- self.output_attentions = config.output_attentions
- self.output_hidden_states = config.output_hidden_states
- self.output_additional_info = config.output_additional_info
- self.layer = nn.ModuleList([BertLayer(config) for _ in range(config.num_hidden_layers)])
-
- def forward(
- self,
- hidden_states,
- attention_mask=None,
- head_mask=None,
- encoder_hidden_states=None,
- encoder_attention_mask=None,
- ):
- all_hidden_states = ()
- all_attentions = ()
- all_additional_info = ()
- for i, layer_module in enumerate(self.layer):
- if self.output_hidden_states:
- all_hidden_states = all_hidden_states + (hidden_states,)
-
- layer_outputs = layer_module(
- hidden_states, attention_mask, head_mask[i], encoder_hidden_states, encoder_attention_mask
- )
- hidden_states = layer_outputs[0]
-
- if self.output_attentions:
- all_attentions = all_attentions + (layer_outputs[1],)
- if self.output_additional_info:
- all_additional_info = all_additional_info + (layer_outputs[2],)
-
- # Add last layer
- if self.output_hidden_states:
- all_hidden_states = all_hidden_states + (hidden_states,)
-
- outputs = (hidden_states,)
- if self.output_hidden_states:
- outputs = outputs + (all_hidden_states,)
- if self.output_attentions:
- outputs = outputs + (all_attentions,)
- if self.output_additional_info:
- outputs = outputs + (all_additional_info,)
-
- return outputs # last-layer hidden state, (all hidden states), (all attentions)
-
-
-class BertPooler(nn.Module):
- def __init__(self, config):
- super().__init__()
- self.dense = nn.Linear(config.hidden_size, config.hidden_size)
- self.activation = nn.Tanh()
-
- def forward(self, hidden_states):
- # We "pool" the model by simply taking the hidden state corresponding
- # to the first token.
- first_token_tensor = hidden_states[:, 0]
- pooled_output = self.dense(first_token_tensor)
- pooled_output = self.activation(pooled_output)
- return pooled_output
-
-
-class BertPredictionHeadTransform(nn.Module):
- def __init__(self, config):
- super().__init__()
- self.dense = nn.Linear(config.hidden_size, config.hidden_size)
- if isinstance(config.hidden_act, str):
- self.transform_act_fn = ACT2FN[config.hidden_act]
- else:
- self.transform_act_fn = config.hidden_act
- self.LayerNorm = BertLayerNorm(config.hidden_size, eps=config.layer_norm_eps)
-
- def forward(self, hidden_states):
- hidden_states = self.dense(hidden_states)
- hidden_states = self.transform_act_fn(hidden_states)
- hidden_states = self.LayerNorm(hidden_states)
- return hidden_states
-
-
-class BertLMPredictionHead(nn.Module):
- def __init__(self, config):
- super().__init__()
- self.transform = BertPredictionHeadTransform(config)
-
- # The output weights are the same as the input embeddings, but there is
- # an output-only bias for each token.
- self.decoder = nn.Linear(config.hidden_size, config.vocab_size, bias=False)
-
- self.bias = nn.Parameter(torch.zeros(config.vocab_size))
-
- # Need a link between the two variables so that the bias is correctly resized with `resize_token_embeddings`
- self.decoder.bias = self.bias
-
- def forward(self, hidden_states):
- hidden_states = self.transform(hidden_states)
- hidden_states = self.decoder(hidden_states) + self.bias
- return hidden_states
-
-
-class BertOnlyMLMHead(nn.Module):
- def __init__(self, config):
- super().__init__()
- self.predictions = BertLMPredictionHead(config)
-
- def forward(self, sequence_output):
- prediction_scores = self.predictions(sequence_output)
- return prediction_scores
-
-
-class BertOnlyNSPHead(nn.Module):
- def __init__(self, config):
- super().__init__()
- self.seq_relationship = nn.Linear(config.hidden_size, 2)
-
- def forward(self, pooled_output):
- seq_relationship_score = self.seq_relationship(pooled_output)
- return seq_relationship_score
-
-
-class BertPreTrainingHeads(nn.Module):
- def __init__(self, config):
- super().__init__()
- self.predictions = BertLMPredictionHead(config)
- self.seq_relationship = nn.Linear(config.hidden_size, 2)
-
- def forward(self, sequence_output, pooled_output):
- prediction_scores = self.predictions(sequence_output)
- seq_relationship_score = self.seq_relationship(pooled_output)
- return prediction_scores, seq_relationship_score
-
-
-class BertPreTrainedModel(PreTrainedModel):
- """ An abstract class to handle weights initialization and
- a simple interface for downloading and loading pretrained models.
- """
-
- config_class = BertConfig
- pretrained_model_archive_map = BERT_PRETRAINED_MODEL_ARCHIVE_MAP
- load_tf_weights = load_tf_weights_in_bert
- base_model_prefix = "bert"
-
- def _init_weights(self, module):
- """ Initialize the weights """
- if isinstance(module, (nn.Linear, nn.Embedding)):
- # Slightly different from the TF version which uses truncated_normal for initialization
- # cf https://github.com/pytorch/pytorch/pull/5617
- module.weight.data.normal_(mean=0.0, std=self.config.initializer_range)
- elif isinstance(module, BertLayerNorm):
- module.bias.data.zero_()
- module.weight.data.fill_(1.0)
- if isinstance(module, nn.Linear) and module.bias is not None:
- module.bias.data.zero_()
-
-
-BERT_START_DOCSTRING = r"""
- This model is a PyTorch `torch.nn.Module `_ sub-class.
- Use it as a regular PyTorch Module and refer to the PyTorch documentation for all matter related to general
- usage and behavior.
-
- Parameters:
- config (:class:`~transformers.BertConfig`): Model configuration class with all the parameters of the model.
- Initializing with a config file does not load the weights associated with the model, only the configuration.
- Check out the :meth:`~transformers.PreTrainedModel.from_pretrained` method to load the model weights.
-"""
-
-BERT_INPUTS_DOCSTRING = r"""
- Args:
- input_ids (:obj:`torch.LongTensor` of shape :obj:`(batch_size, sequence_length)`):
- Indices of input sequence tokens in the vocabulary.
-
- Indices can be obtained using :class:`transformers.BertTokenizer`.
- See :func:`transformers.PreTrainedTokenizer.encode` and
- :func:`transformers.PreTrainedTokenizer.encode_plus` for details.
-
- `What are input IDs? <../glossary.html#input-ids>`__
- attention_mask (:obj:`torch.FloatTensor` of shape :obj:`(batch_size, sequence_length)`, `optional`, defaults to :obj:`None`):
- Mask to avoid performing attention on padding token indices.
- Mask values selected in ``[0, 1]``:
- ``1`` for tokens that are NOT MASKED, ``0`` for MASKED tokens.
-
- `What are attention masks? <../glossary.html#attention-mask>`__
- token_type_ids (:obj:`torch.LongTensor` of shape :obj:`(batch_size, sequence_length)`, `optional`, defaults to :obj:`None`):
- Segment token indices to indicate first and second portions of the inputs.
- Indices are selected in ``[0, 1]``: ``0`` corresponds to a `sentence A` token, ``1``
- corresponds to a `sentence B` token
-
- `What are token type IDs? <../glossary.html#token-type-ids>`_
- position_ids (:obj:`torch.LongTensor` of shape :obj:`(batch_size, sequence_length)`, `optional`, defaults to :obj:`None`):
- Indices of positions of each input sequence tokens in the position embeddings.
- Selected in the range ``[0, config.max_position_embeddings - 1]``.
-
- `What are position IDs? <../glossary.html#position-ids>`_
- head_mask (:obj:`torch.FloatTensor` of shape :obj:`(num_heads,)` or :obj:`(num_layers, num_heads)`, `optional`, defaults to :obj:`None`):
- Mask to nullify selected heads of the self-attention modules.
- Mask values selected in ``[0, 1]``:
- :obj:`1` indicates the head is **not masked**, :obj:`0` indicates the head is **masked**.
- inputs_embeds (:obj:`torch.FloatTensor` of shape :obj:`(batch_size, sequence_length, hidden_size)`, `optional`, defaults to :obj:`None`):
- Optionally, instead of passing :obj:`input_ids` you can choose to directly pass an embedded representation.
- This is useful if you want more control over how to convert `input_ids` indices into associated vectors
- than the model's internal embedding lookup matrix.
- encoder_hidden_states (:obj:`torch.FloatTensor` of shape :obj:`(batch_size, sequence_length, hidden_size)`, `optional`, defaults to :obj:`None`):
- Sequence of hidden-states at the output of the last layer of the encoder. Used in the cross-attention
- if the model is configured as a decoder.
- encoder_attention_mask (:obj:`torch.FloatTensor` of shape :obj:`(batch_size, sequence_length)`, `optional`, defaults to :obj:`None`):
- Mask to avoid performing attention on the padding token indices of the encoder input. This mask
- is used in the cross-attention if the model is configured as a decoder.
- Mask values selected in ``[0, 1]``:
- ``1`` for tokens that are NOT MASKED, ``0`` for MASKED tokens.
-"""
-
-
-@add_start_docstrings(
- "The bare Bert Model transformer outputting raw hidden-states without any specific head on top.",
- BERT_START_DOCSTRING,
-)
-class BertModel(BertPreTrainedModel):
- """
-
- The model can behave as an encoder (with only self-attention) as well
- as a decoder, in which case a layer of cross-attention is added between
- the self-attention layers, following the architecture described in `Attention is all you need`_ by Ashish Vaswani,
- Noam Shazeer, Niki Parmar, Jakob Uszkoreit, Llion Jones, Aidan N. Gomez, Lukasz Kaiser and Illia Polosukhin.
-
- To behave as an decoder the model needs to be initialized with the
- :obj:`is_decoder` argument of the configuration set to :obj:`True`; an
- :obj:`encoder_hidden_states` is expected as an input to the forward pass.
-
- .. _`Attention is all you need`:
- https://arxiv.org/abs/1706.03762
-
- """
-
- def __init__(self, config):
- super().__init__(config)
- self.config = config
-
- self.embeddings = BertEmbeddings(config)
- self.encoder = BertEncoder(config)
- self.pooler = BertPooler(config)
-
- self.init_weights()
-
- def get_input_embeddings(self):
- return self.embeddings.word_embeddings
-
- def set_input_embeddings(self, value):
- self.embeddings.word_embeddings = value
-
- def _prune_heads(self, heads_to_prune):
- """ Prunes heads of the model.
- heads_to_prune: dict of {layer_num: list of heads to prune in this layer}
- See base class PreTrainedModel
- """
- for layer, heads in heads_to_prune.items():
- self.encoder.layer[layer].attention.prune_heads(heads)
-
- @add_start_docstrings_to_callable(BERT_INPUTS_DOCSTRING)
- def forward(
- self,
- input_ids=None,
- attention_mask=None,
- token_type_ids=None,
- position_ids=None,
- head_mask=None,
- inputs_embeds=None,
- encoder_hidden_states=None,
- encoder_attention_mask=None,
- ):
- r"""
- Return:
- :obj:`tuple(torch.FloatTensor)` comprising various elements depending on the configuration (:class:`~transformers.BertConfig`) and inputs:
- last_hidden_state (:obj:`torch.FloatTensor` of shape :obj:`(batch_size, sequence_length, hidden_size)`):
- Sequence of hidden-states at the output of the last layer of the model.
- pooler_output (:obj:`torch.FloatTensor`: of shape :obj:`(batch_size, hidden_size)`):
- Last layer hidden-state of the first token of the sequence (classification token)
- further processed by a Linear layer and a Tanh activation function. The Linear
- layer weights are trained from the next sentence prediction (classification)
- objective during pre-training.
-
- This output is usually *not* a good summary
- of the semantic content of the input, you're often better with averaging or pooling
- the sequence of hidden-states for the whole input sequence.
- hidden_states (:obj:`tuple(torch.FloatTensor)`, `optional`, returned when ``config.output_hidden_states=True``):
- Tuple of :obj:`torch.FloatTensor` (one for the output of the embeddings + one for the output of each layer)
- of shape :obj:`(batch_size, sequence_length, hidden_size)`.
-
- Hidden-states of the model at the output of each layer plus the initial embedding outputs.
- attentions (:obj:`tuple(torch.FloatTensor)`, `optional`, returned when ``config.output_attentions=True``):
- Tuple of :obj:`torch.FloatTensor` (one for each layer) of shape
- :obj:`(batch_size, num_heads, sequence_length, sequence_length)`.
-
- Attentions weights after the attention softmax, used to compute the weighted average in the self-attention
- heads.
-
- Examples::
-
- from transformers import BertModel, BertTokenizer
- import torch
-
- tokenizer = BertTokenizer.from_pretrained('bert-base-uncased')
- model = BertModel.from_pretrained('bert-base-uncased')
-
- input_ids = torch.tensor(tokenizer.encode("Hello, my dog is cute", add_special_tokens=True)).unsqueeze(0) # Batch size 1
- outputs = model(input_ids)
-
- last_hidden_states = outputs[0] # The last hidden-state is the first element of the output tuple
-
- """
-
- if input_ids is not None and inputs_embeds is not None:
- raise ValueError("You cannot specify both input_ids and inputs_embeds at the same time")
- elif input_ids is not None:
- input_shape = input_ids.size()
- elif inputs_embeds is not None:
- input_shape = inputs_embeds.size()[:-1]
- else:
- raise ValueError("You have to specify either input_ids or inputs_embeds")
-
- device = input_ids.device if input_ids is not None else inputs_embeds.device
-
- if attention_mask is None:
- attention_mask = torch.ones(input_shape, device=device)
- if token_type_ids is None:
- token_type_ids = torch.zeros(input_shape, dtype=torch.long, device=device)
-
- # We can provide a self-attention mask of dimensions [batch_size, from_seq_length, to_seq_length]
- # ourselves in which case we just need to make it broadcastable to all heads.
- if attention_mask.dim() == 3:
- extended_attention_mask = attention_mask[:, None, :, :]
- elif attention_mask.dim() == 2:
- # Provided a padding mask of dimensions [batch_size, seq_length]
- # - if the model is a decoder, apply a causal mask in addition to the padding mask
- # - if the model is an encoder, make the mask broadcastable to [batch_size, num_heads, seq_length, seq_length]
- if self.config.is_decoder:
- batch_size, seq_length = input_shape
- seq_ids = torch.arange(seq_length, device=device)
- causal_mask = seq_ids[None, None, :].repeat(batch_size, seq_length, 1) <= seq_ids[None, :, None]
- causal_mask = causal_mask.to(
- torch.long
- ) # not converting to long will cause errors with pytorch version < 1.3
- extended_attention_mask = causal_mask[:, None, :, :] * attention_mask[:, None, None, :]
- else:
- extended_attention_mask = attention_mask[:, None, None, :]
- else:
- raise ValueError(
- "Wrong shape for input_ids (shape {}) or attention_mask (shape {})".format(
- input_shape, attention_mask.shape
- )
- )
-
- # Since attention_mask is 1.0 for positions we want to attend and 0.0 for
- # masked positions, this operation will create a tensor which is 0.0 for
- # positions we want to attend and -10000.0 for masked positions.
- # Since we are adding it to the raw scores before the softmax, this is
- # effectively the same as removing these entirely.
- extended_attention_mask = extended_attention_mask.to(dtype=next(self.parameters()).dtype) # fp16 compatibility
- extended_attention_mask = (1.0 - extended_attention_mask) * -10000.0
-
- # If a 2D ou 3D attention mask is provided for the cross-attention
- # we need to make broadcastabe to [batch_size, num_heads, seq_length, seq_length]
- if self.config.is_decoder and encoder_hidden_states is not None:
- encoder_batch_size, encoder_sequence_length, _ = encoder_hidden_states.size()
- encoder_hidden_shape = (encoder_batch_size, encoder_sequence_length)
- if encoder_attention_mask is None:
- encoder_attention_mask = torch.ones(encoder_hidden_shape, device=device)
-
- if encoder_attention_mask.dim() == 3:
- encoder_extended_attention_mask = encoder_attention_mask[:, None, :, :]
- elif encoder_attention_mask.dim() == 2:
- encoder_extended_attention_mask = encoder_attention_mask[:, None, None, :]
- else:
- raise ValueError(
- "Wrong shape for encoder_hidden_shape (shape {}) or encoder_attention_mask (shape {})".format(
- encoder_hidden_shape, encoder_attention_mask.shape
- )
- )
-
- encoder_extended_attention_mask = encoder_extended_attention_mask.to(
- dtype=next(self.parameters()).dtype
- ) # fp16 compatibility
- encoder_extended_attention_mask = (1.0 - encoder_extended_attention_mask) * -10000.0
- else:
- encoder_extended_attention_mask = None
-
- # Prepare head mask if needed
- # 1.0 in head_mask indicate we keep the head
- # attention_probs has shape bsz x n_heads x N x N
- # input head_mask has shape [num_heads] or [num_hidden_layers x num_heads]
- # and head_mask is converted to shape [num_hidden_layers x batch x num_heads x seq_length x seq_length]
- if head_mask is not None:
- if head_mask.dim() == 1:
- head_mask = head_mask.unsqueeze(0).unsqueeze(0).unsqueeze(-1).unsqueeze(-1)
- head_mask = head_mask.expand(self.config.num_hidden_layers, -1, -1, -1, -1)
- elif head_mask.dim() == 2:
- head_mask = (
- head_mask.unsqueeze(1).unsqueeze(-1).unsqueeze(-1)
- ) # We can specify head_mask for each layer
- head_mask = head_mask.to(
- dtype=next(self.parameters()).dtype
- ) # switch to fload if need + fp16 compatibility
- else:
- head_mask = [None] * self.config.num_hidden_layers
-
- embedding_output = self.embeddings(
- input_ids=input_ids, position_ids=position_ids, token_type_ids=token_type_ids, inputs_embeds=inputs_embeds
- )
- encoder_outputs = self.encoder(
- embedding_output,
- attention_mask=extended_attention_mask,
- head_mask=head_mask,
- encoder_hidden_states=encoder_hidden_states,
- encoder_attention_mask=encoder_extended_attention_mask,
- )
- sequence_output = encoder_outputs[0]
- pooled_output = self.pooler(sequence_output)
-
- outputs = (sequence_output, pooled_output,) + encoder_outputs[
- 1:
- ] # add hidden_states and attentions if they are here
- return outputs # sequence_output, pooled_output, (hidden_states), (attentions)
-
-
-@add_start_docstrings(
- """Bert Model with two heads on top as done during the pre-training: a `masked language modeling` head and
- a `next sentence prediction (classification)` head. """,
- BERT_START_DOCSTRING,
-)
-class BertForPreTraining(BertPreTrainedModel):
- def __init__(self, config):
- super().__init__(config)
-
- self.bert = BertModel(config)
- self.cls = BertPreTrainingHeads(config)
-
- self.init_weights()
-
- def get_output_embeddings(self):
- return self.cls.predictions.decoder
-
- @add_start_docstrings_to_callable(BERT_INPUTS_DOCSTRING)
- def forward(
- self,
- input_ids=None,
- attention_mask=None,
- token_type_ids=None,
- position_ids=None,
- head_mask=None,
- inputs_embeds=None,
- masked_lm_labels=None,
- next_sentence_label=None,
- ):
- r"""
- masked_lm_labels (``torch.LongTensor`` of shape ``(batch_size, sequence_length)``, `optional`, defaults to :obj:`None`):
- Labels for computing the masked language modeling loss.
- Indices should be in ``[-100, 0, ..., config.vocab_size]`` (see ``input_ids`` docstring)
- Tokens with indices set to ``-100`` are ignored (masked), the loss is only computed for the tokens with labels
- in ``[0, ..., config.vocab_size]``
- next_sentence_label (``torch.LongTensor`` of shape ``(batch_size,)``, `optional`, defaults to :obj:`None`):
- Labels for computing the next sequence prediction (classification) loss. Input should be a sequence pair (see :obj:`input_ids` docstring)
- Indices should be in ``[0, 1]``.
- ``0`` indicates sequence B is a continuation of sequence A,
- ``1`` indicates sequence B is a random sequence.
-
- Returns:
- :obj:`tuple(torch.FloatTensor)` comprising various elements depending on the configuration (:class:`~transformers.BertConfig`) and inputs:
- loss (`optional`, returned when ``masked_lm_labels`` is provided) ``torch.FloatTensor`` of shape ``(1,)``:
- Total loss as the sum of the masked language modeling loss and the next sequence prediction (classification) loss.
- prediction_scores (:obj:`torch.FloatTensor` of shape :obj:`(batch_size, sequence_length, config.vocab_size)`)
- Prediction scores of the language modeling head (scores for each vocabulary token before SoftMax).
- seq_relationship_scores (:obj:`torch.FloatTensor` of shape :obj:`(batch_size, sequence_length, 2)`):
- Prediction scores of the next sequence prediction (classification) head (scores of True/False
- continuation before SoftMax).
- hidden_states (:obj:`tuple(torch.FloatTensor)`, `optional`, returned when :obj:`config.output_hidden_states=True`):
- Tuple of :obj:`torch.FloatTensor` (one for the output of the embeddings + one for the output of each layer)
- of shape :obj:`(batch_size, sequence_length, hidden_size)`.
-
- Hidden-states of the model at the output of each layer plus the initial embedding outputs.
- attentions (:obj:`tuple(torch.FloatTensor)`, `optional`, returned when ``config.output_attentions=True``):
- Tuple of :obj:`torch.FloatTensor` (one for each layer) of shape
- :obj:`(batch_size, num_heads, sequence_length, sequence_length)`.
-
- Attentions weights after the attention softmax, used to compute the weighted average in the self-attention
- heads.
-
-
- Examples::
-
- from transformers import BertTokenizer, BertForPreTraining
- import torch
-
- tokenizer = BertTokenizer.from_pretrained('bert-base-uncased')
- model = BertForPreTraining.from_pretrained('bert-base-uncased')
-
- input_ids = torch.tensor(tokenizer.encode("Hello, my dog is cute", add_special_tokens=True)).unsqueeze(0) # Batch size 1
- outputs = model(input_ids)
-
- prediction_scores, seq_relationship_scores = outputs[:2]
-
- """
-
- outputs = self.bert(
- input_ids,
- attention_mask=attention_mask,
- token_type_ids=token_type_ids,
- position_ids=position_ids,
- head_mask=head_mask,
- inputs_embeds=inputs_embeds,
- )
-
- sequence_output, pooled_output = outputs[:2]
- prediction_scores, seq_relationship_score = self.cls(sequence_output, pooled_output)
-
- outputs = (prediction_scores, seq_relationship_score,) + outputs[
- 2:
- ] # add hidden states and attention if they are here
-
- if masked_lm_labels is not None and next_sentence_label is not None:
- loss_fct = CrossEntropyLoss()
- masked_lm_loss = loss_fct(prediction_scores.view(-1, self.config.vocab_size), masked_lm_labels.view(-1))
- next_sentence_loss = loss_fct(seq_relationship_score.view(-1, 2), next_sentence_label.view(-1))
- total_loss = masked_lm_loss + next_sentence_loss
- outputs = (total_loss,) + outputs
-
- return outputs # (loss), prediction_scores, seq_relationship_score, (hidden_states), (attentions)
-
-
-@add_start_docstrings("""Bert Model with a `language modeling` head on top. """, BERT_START_DOCSTRING)
-class BertForMaskedLM(BertPreTrainedModel):
- def __init__(self, config):
- super().__init__(config)
-
- self.bert = BertModel(config)
- self.cls = BertOnlyMLMHead(config)
-
- self.init_weights()
-
- def get_output_embeddings(self):
- return self.cls.predictions.decoder
-
- @add_start_docstrings_to_callable(BERT_INPUTS_DOCSTRING)
- def forward(
- self,
- input_ids=None,
- attention_mask=None,
- token_type_ids=None,
- position_ids=None,
- head_mask=None,
- inputs_embeds=None,
- masked_lm_labels=None,
- encoder_hidden_states=None,
- encoder_attention_mask=None,
- lm_labels=None,
- ):
- r"""
- masked_lm_labels (:obj:`torch.LongTensor` of shape :obj:`(batch_size, sequence_length)`, `optional`, defaults to :obj:`None`):
- Labels for computing the masked language modeling loss.
- Indices should be in ``[-100, 0, ..., config.vocab_size]`` (see ``input_ids`` docstring)
- Tokens with indices set to ``-100`` are ignored (masked), the loss is only computed for the tokens with labels
- in ``[0, ..., config.vocab_size]``
- lm_labels (:obj:`torch.LongTensor` of shape :obj:`(batch_size, sequence_length)`, `optional`, defaults to :obj:`None`):
- Labels for computing the left-to-right language modeling loss (next word prediction).
- Indices should be in ``[-100, 0, ..., config.vocab_size]`` (see ``input_ids`` docstring)
- Tokens with indices set to ``-100`` are ignored (masked), the loss is only computed for the tokens with labels
- in ``[0, ..., config.vocab_size]``
-
- Returns:
- :obj:`tuple(torch.FloatTensor)` comprising various elements depending on the configuration (:class:`~transformers.BertConfig`) and inputs:
- masked_lm_loss (`optional`, returned when ``masked_lm_labels`` is provided) ``torch.FloatTensor`` of shape ``(1,)``:
- Masked language modeling loss.
- ltr_lm_loss (:obj:`torch.FloatTensor` of shape :obj:`(1,)`, `optional`, returned when :obj:`lm_labels` is provided):
- Next token prediction loss.
- prediction_scores (:obj:`torch.FloatTensor` of shape :obj:`(batch_size, sequence_length, config.vocab_size)`)
- Prediction scores of the language modeling head (scores for each vocabulary token before SoftMax).
- hidden_states (:obj:`tuple(torch.FloatTensor)`, `optional`, returned when ``config.output_hidden_states=True``):
- Tuple of :obj:`torch.FloatTensor` (one for the output of the embeddings + one for the output of each layer)
- of shape :obj:`(batch_size, sequence_length, hidden_size)`.
-
- Hidden-states of the model at the output of each layer plus the initial embedding outputs.
- attentions (:obj:`tuple(torch.FloatTensor)`, `optional`, returned when ``config.output_attentions=True``):
- Tuple of :obj:`torch.FloatTensor` (one for each layer) of shape
- :obj:`(batch_size, num_heads, sequence_length, sequence_length)`.
-
- Attentions weights after the attention softmax, used to compute the weighted average in the self-attention
- heads.
-
- Examples::
-
- from transformers import BertTokenizer, BertForMaskedLM
- import torch
-
- tokenizer = BertTokenizer.from_pretrained('bert-base-uncased')
- model = BertForMaskedLM.from_pretrained('bert-base-uncased')
-
- input_ids = torch.tensor(tokenizer.encode("Hello, my dog is cute", add_special_tokens=True)).unsqueeze(0) # Batch size 1
- outputs = model(input_ids, masked_lm_labels=input_ids)
-
- loss, prediction_scores = outputs[:2]
-
- """
-
- outputs = self.bert(
- input_ids,
- attention_mask=attention_mask,
- token_type_ids=token_type_ids,
- position_ids=position_ids,
- head_mask=head_mask,
- inputs_embeds=inputs_embeds,
- encoder_hidden_states=encoder_hidden_states,
- encoder_attention_mask=encoder_attention_mask,
- )
-
- sequence_output = outputs[0]
- prediction_scores = self.cls(sequence_output)
-
- outputs = (prediction_scores,) + outputs[2:] # Add hidden states and attention if they are here
-
- # Although this may seem awkward, BertForMaskedLM supports two scenarios:
- # 1. If a tensor that contains the indices of masked labels is provided,
- # the cross-entropy is the MLM cross-entropy that measures the likelihood
- # of predictions for masked words.
- # 2. If `lm_labels` is provided we are in a causal scenario where we
- # try to predict the next token for each input in the decoder.
- if masked_lm_labels is not None:
- loss_fct = CrossEntropyLoss() # -100 index = padding token
- masked_lm_loss = loss_fct(prediction_scores.view(-1, self.config.vocab_size), masked_lm_labels.view(-1))
- outputs = (masked_lm_loss,) + outputs
-
- if lm_labels is not None:
- # we are doing next-token prediction; shift prediction scores and input ids by one
- prediction_scores = prediction_scores[:, :-1, :].contiguous()
- lm_labels = lm_labels[:, 1:].contiguous()
- loss_fct = CrossEntropyLoss()
- ltr_lm_loss = loss_fct(prediction_scores.view(-1, self.config.vocab_size), lm_labels.view(-1))
- outputs = (ltr_lm_loss,) + outputs
-
- return outputs # (masked_lm_loss), (ltr_lm_loss), prediction_scores, (hidden_states), (attentions)
-
-
-@add_start_docstrings(
- """Bert Model with a `next sentence prediction (classification)` head on top. """, BERT_START_DOCSTRING,
-)
-class BertForNextSentencePrediction(BertPreTrainedModel):
- def __init__(self, config):
- super().__init__(config)
-
- self.bert = BertModel(config)
- self.cls = BertOnlyNSPHead(config)
-
- self.init_weights()
-
- @add_start_docstrings_to_callable(BERT_INPUTS_DOCSTRING)
- def forward(
- self,
- input_ids=None,
- attention_mask=None,
- token_type_ids=None,
- position_ids=None,
- head_mask=None,
- inputs_embeds=None,
- next_sentence_label=None,
- ):
- r"""
- next_sentence_label (:obj:`torch.LongTensor` of shape :obj:`(batch_size,)`, `optional`, defaults to :obj:`None`):
- Labels for computing the next sequence prediction (classification) loss. Input should be a sequence pair (see ``input_ids`` docstring)
- Indices should be in ``[0, 1]``.
- ``0`` indicates sequence B is a continuation of sequence A,
- ``1`` indicates sequence B is a random sequence.
-
- Returns:
- :obj:`tuple(torch.FloatTensor)` comprising various elements depending on the configuration (:class:`~transformers.BertConfig`) and inputs:
- loss (:obj:`torch.FloatTensor` of shape :obj:`(1,)`, `optional`, returned when :obj:`next_sentence_label` is provided):
- Next sequence prediction (classification) loss.
- seq_relationship_scores (:obj:`torch.FloatTensor` of shape :obj:`(batch_size, sequence_length, 2)`):
- Prediction scores of the next sequence prediction (classification) head (scores of True/False continuation before SoftMax).
- hidden_states (:obj:`tuple(torch.FloatTensor)`, `optional`, returned when ``config.output_hidden_states=True``):
- Tuple of :obj:`torch.FloatTensor` (one for the output of the embeddings + one for the output of each layer)
- of shape :obj:`(batch_size, sequence_length, hidden_size)`.
-
- Hidden-states of the model at the output of each layer plus the initial embedding outputs.
- attentions (:obj:`tuple(torch.FloatTensor)`, `optional`, returned when ``config.output_attentions=True``):
- Tuple of :obj:`torch.FloatTensor` (one for each layer) of shape
- :obj:`(batch_size, num_heads, sequence_length, sequence_length)`.
-
- Attentions weights after the attention softmax, used to compute the weighted average in the self-attention
- heads.
-
- Examples::
-
- from transformers import BertTokenizer, BertForNextSentencePrediction
- import torch
-
- tokenizer = BertTokenizer.from_pretrained('bert-base-uncased')
- model = BertForNextSentencePrediction.from_pretrained('bert-base-uncased')
-
- input_ids = torch.tensor(tokenizer.encode("Hello, my dog is cute", add_special_tokens=True)).unsqueeze(0) # Batch size 1
- outputs = model(input_ids)
-
- seq_relationship_scores = outputs[0]
-
- """
-
- outputs = self.bert(
- input_ids,
- attention_mask=attention_mask,
- token_type_ids=token_type_ids,
- position_ids=position_ids,
- head_mask=head_mask,
- inputs_embeds=inputs_embeds,
- )
-
- pooled_output = outputs[1]
-
- seq_relationship_score = self.cls(pooled_output)
-
- outputs = (seq_relationship_score,) + outputs[2:] # add hidden states and attention if they are here
- if next_sentence_label is not None:
- loss_fct = CrossEntropyLoss()
- next_sentence_loss = loss_fct(seq_relationship_score.view(-1, 2), next_sentence_label.view(-1))
- outputs = (next_sentence_loss,) + outputs
-
- return outputs # (next_sentence_loss), seq_relationship_score, (hidden_states), (attentions)
-
-
-@add_start_docstrings(
- """Bert Model transformer with a sequence classification/regression head on top (a linear layer on top of
- the pooled output) e.g. for GLUE tasks. """,
- BERT_START_DOCSTRING,
-)
-class BertForSequenceClassification(BertPreTrainedModel):
- def __init__(self, config):
- super().__init__(config)
- self.num_labels = config.num_labels
-
- self.bert = BertModel(config)
- self.dropout = nn.Dropout(config.hidden_dropout_prob)
- self.classifier = nn.Linear(config.hidden_size, self.config.num_labels)
-
- self.init_weights()
-
- @add_start_docstrings_to_callable(BERT_INPUTS_DOCSTRING)
- def forward(
- self,
- input_ids=None,
- attention_mask=None,
- token_type_ids=None,
- position_ids=None,
- head_mask=None,
- inputs_embeds=None,
- labels=None,
- ):
- r"""
- labels (:obj:`torch.LongTensor` of shape :obj:`(batch_size,)`, `optional`, defaults to :obj:`None`):
- Labels for computing the sequence classification/regression loss.
- Indices should be in :obj:`[0, ..., config.num_labels - 1]`.
- If :obj:`config.num_labels == 1` a regression loss is computed (Mean-Square loss),
- If :obj:`config.num_labels > 1` a classification loss is computed (Cross-Entropy).
-
- Returns:
- :obj:`tuple(torch.FloatTensor)` comprising various elements depending on the configuration (:class:`~transformers.BertConfig`) and inputs:
- loss (:obj:`torch.FloatTensor` of shape :obj:`(1,)`, `optional`, returned when :obj:`label` is provided):
- Classification (or regression if config.num_labels==1) loss.
- logits (:obj:`torch.FloatTensor` of shape :obj:`(batch_size, config.num_labels)`):
- Classification (or regression if config.num_labels==1) scores (before SoftMax).
- hidden_states (:obj:`tuple(torch.FloatTensor)`, `optional`, returned when ``config.output_hidden_states=True``):
- Tuple of :obj:`torch.FloatTensor` (one for the output of the embeddings + one for the output of each layer)
- of shape :obj:`(batch_size, sequence_length, hidden_size)`.
-
- Hidden-states of the model at the output of each layer plus the initial embedding outputs.
- attentions (:obj:`tuple(torch.FloatTensor)`, `optional`, returned when ``config.output_attentions=True``):
- Tuple of :obj:`torch.FloatTensor` (one for each layer) of shape
- :obj:`(batch_size, num_heads, sequence_length, sequence_length)`.
-
- Attentions weights after the attention softmax, used to compute the weighted average in the self-attention
- heads.
-
- Examples::
-
- from transformers import BertTokenizer, BertForSequenceClassification
- import torch
-
- tokenizer = BertTokenizer.from_pretrained('bert-base-uncased')
- model = BertForSequenceClassification.from_pretrained('bert-base-uncased')
-
- input_ids = torch.tensor(tokenizer.encode("Hello, my dog is cute", add_special_tokens=True)).unsqueeze(0) # Batch size 1
- labels = torch.tensor([1]).unsqueeze(0) # Batch size 1
- outputs = model(input_ids, labels=labels)
-
- loss, logits = outputs[:2]
-
- """
-
- outputs = self.bert(
- input_ids,
- attention_mask=attention_mask,
- token_type_ids=token_type_ids,
- position_ids=position_ids,
- head_mask=head_mask,
- inputs_embeds=inputs_embeds,
- )
-
- pooled_output = outputs[1]
-
- pooled_output = self.dropout(pooled_output)
- logits = self.classifier(pooled_output)
-
- outputs = (logits,) + outputs[2:] # add hidden states and attention if they are here
-
- if labels is not None:
- if self.num_labels == 1:
- # We are doing regression
- loss_fct = MSELoss()
- loss = loss_fct(logits.view(-1), labels.view(-1))
- else:
- loss_fct = CrossEntropyLoss()
- loss = loss_fct(logits.view(-1, self.num_labels), labels.view(-1))
- outputs = (loss,) + outputs
-
- return outputs # (loss), logits, (hidden_states), (attentions)
-
-
-@add_start_docstrings(
- """Bert Model with a multiple choice classification head on top (a linear layer on top of
- the pooled output and a softmax) e.g. for RocStories/SWAG tasks. """,
- BERT_START_DOCSTRING,
-)
-class BertForMultipleChoice(BertPreTrainedModel):
- def __init__(self, config):
- super().__init__(config)
-
- self.bert = BertModel(config)
- self.dropout = nn.Dropout(config.hidden_dropout_prob)
- self.classifier = nn.Linear(config.hidden_size, 1)
-
- self.init_weights()
-
- @add_start_docstrings_to_callable(BERT_INPUTS_DOCSTRING)
- def forward(
- self,
- input_ids=None,
- attention_mask=None,
- token_type_ids=None,
- position_ids=None,
- head_mask=None,
- inputs_embeds=None,
- labels=None,
- ):
- r"""
- labels (:obj:`torch.LongTensor` of shape :obj:`(batch_size,)`, `optional`, defaults to :obj:`None`):
- Labels for computing the multiple choice classification loss.
- Indices should be in ``[0, ..., num_choices]`` where `num_choices` is the size of the second dimension
- of the input tensors. (see `input_ids` above)
-
- Returns:
- :obj:`tuple(torch.FloatTensor)` comprising various elements depending on the configuration (:class:`~transformers.BertConfig`) and inputs:
- loss (:obj:`torch.FloatTensor`` of shape ``(1,)`, `optional`, returned when :obj:`labels` is provided):
- Classification loss.
- classification_scores (:obj:`torch.FloatTensor` of shape :obj:`(batch_size, num_choices)`):
- `num_choices` is the second dimension of the input tensors. (see `input_ids` above).
-
- Classification scores (before SoftMax).
- hidden_states (:obj:`tuple(torch.FloatTensor)`, `optional`, returned when ``config.output_hidden_states=True``):
- Tuple of :obj:`torch.FloatTensor` (one for the output of the embeddings + one for the output of each layer)
- of shape :obj:`(batch_size, sequence_length, hidden_size)`.
-
- Hidden-states of the model at the output of each layer plus the initial embedding outputs.
- attentions (:obj:`tuple(torch.FloatTensor)`, `optional`, returned when ``config.output_attentions=True``):
- Tuple of :obj:`torch.FloatTensor` (one for each layer) of shape
- :obj:`(batch_size, num_heads, sequence_length, sequence_length)`.
-
- Attentions weights after the attention softmax, used to compute the weighted average in the self-attention
- heads.
-
- Examples::
-
- from transformers import BertTokenizer, BertForMultipleChoice
- import torch
-
- tokenizer = BertTokenizer.from_pretrained('bert-base-uncased')
- model = BertForMultipleChoice.from_pretrained('bert-base-uncased')
- choices = ["Hello, my dog is cute", "Hello, my cat is amazing"]
-
- input_ids = torch.tensor([tokenizer.encode(s, add_special_tokens=True) for s in choices]).unsqueeze(0) # Batch size 1, 2 choices
- labels = torch.tensor(1).unsqueeze(0) # Batch size 1
- outputs = model(input_ids, labels=labels)
-
- loss, classification_scores = outputs[:2]
-
- """
- num_choices = input_ids.shape[1]
-
- input_ids = input_ids.view(-1, input_ids.size(-1))
- attention_mask = attention_mask.view(-1, attention_mask.size(-1)) if attention_mask is not None else None
- token_type_ids = token_type_ids.view(-1, token_type_ids.size(-1)) if token_type_ids is not None else None
- position_ids = position_ids.view(-1, position_ids.size(-1)) if position_ids is not None else None
-
- outputs = self.bert(
- input_ids,
- attention_mask=attention_mask,
- token_type_ids=token_type_ids,
- position_ids=position_ids,
- head_mask=head_mask,
- inputs_embeds=inputs_embeds,
- )
-
- pooled_output = outputs[1]
-
- pooled_output = self.dropout(pooled_output)
- logits = self.classifier(pooled_output)
- reshaped_logits = logits.view(-1, num_choices)
-
- outputs = (reshaped_logits,) + outputs[2:] # add hidden states and attention if they are here
-
- if labels is not None:
- loss_fct = CrossEntropyLoss()
- loss = loss_fct(reshaped_logits, labels)
- outputs = (loss,) + outputs
-
- return outputs # (loss), reshaped_logits, (hidden_states), (attentions)
-
-
-@add_start_docstrings(
- """Bert Model with a token classification head on top (a linear layer on top of
- the hidden-states output) e.g. for Named-Entity-Recognition (NER) tasks. """,
- BERT_START_DOCSTRING,
-)
-class BertForTokenClassification(BertPreTrainedModel):
- def __init__(self, config):
- super().__init__(config)
- self.num_labels = config.num_labels
-
- self.bert = BertModel(config)
- self.dropout = nn.Dropout(config.hidden_dropout_prob)
- self.classifier = nn.Linear(config.hidden_size, config.num_labels)
-
- self.init_weights()
-
- @add_start_docstrings_to_callable(BERT_INPUTS_DOCSTRING)
- def forward(
- self,
- input_ids=None,
- attention_mask=None,
- token_type_ids=None,
- position_ids=None,
- head_mask=None,
- inputs_embeds=None,
- labels=None,
- ):
- r"""
- labels (:obj:`torch.LongTensor` of shape :obj:`(batch_size, sequence_length)`, `optional`, defaults to :obj:`None`):
- Labels for computing the token classification loss.
- Indices should be in ``[0, ..., config.num_labels - 1]``.
-
- Returns:
- :obj:`tuple(torch.FloatTensor)` comprising various elements depending on the configuration (:class:`~transformers.BertConfig`) and inputs:
- loss (:obj:`torch.FloatTensor` of shape :obj:`(1,)`, `optional`, returned when ``labels`` is provided) :
- Classification loss.
- scores (:obj:`torch.FloatTensor` of shape :obj:`(batch_size, sequence_length, config.num_labels)`)
- Classification scores (before SoftMax).
- hidden_states (:obj:`tuple(torch.FloatTensor)`, `optional`, returned when ``config.output_hidden_states=True``):
- Tuple of :obj:`torch.FloatTensor` (one for the output of the embeddings + one for the output of each layer)
- of shape :obj:`(batch_size, sequence_length, hidden_size)`.
-
- Hidden-states of the model at the output of each layer plus the initial embedding outputs.
- attentions (:obj:`tuple(torch.FloatTensor)`, `optional`, returned when ``config.output_attentions=True``):
- Tuple of :obj:`torch.FloatTensor` (one for each layer) of shape
- :obj:`(batch_size, num_heads, sequence_length, sequence_length)`.
-
- Attentions weights after the attention softmax, used to compute the weighted average in the self-attention
- heads.
-
- Examples::
-
- from transformers import BertTokenizer, BertForTokenClassification
- import torch
-
- tokenizer = BertTokenizer.from_pretrained('bert-base-uncased')
- model = BertForTokenClassification.from_pretrained('bert-base-uncased')
-
- input_ids = torch.tensor(tokenizer.encode("Hello, my dog is cute", add_special_tokens=True)).unsqueeze(0) # Batch size 1
- labels = torch.tensor([1] * input_ids.size(1)).unsqueeze(0) # Batch size 1
- outputs = model(input_ids, labels=labels)
-
- loss, scores = outputs[:2]
-
- """
-
- outputs = self.bert(
- input_ids,
- attention_mask=attention_mask,
- token_type_ids=token_type_ids,
- position_ids=position_ids,
- head_mask=head_mask,
- inputs_embeds=inputs_embeds,
- )
-
- sequence_output = outputs[0]
-
- sequence_output = self.dropout(sequence_output)
- logits = self.classifier(sequence_output)
-
- outputs = (logits,) + outputs[2:] # add hidden states and attention if they are here
- if labels is not None:
- loss_fct = CrossEntropyLoss()
- # Only keep active parts of the loss
- if attention_mask is not None:
- active_loss = attention_mask.view(-1) == 1
- active_logits = logits.view(-1, self.num_labels)[active_loss]
- active_labels = labels.view(-1)[active_loss]
- loss = loss_fct(active_logits, active_labels)
- else:
- loss = loss_fct(logits.view(-1, self.num_labels), labels.view(-1))
- outputs = (loss,) + outputs
-
- return outputs # (loss), scores, (hidden_states), (attentions)
-
-
-@add_start_docstrings(
- """Bert Model with a span classification head on top for extractive question-answering tasks like SQuAD (a linear
- layers on top of the hidden-states output to compute `span start logits` and `span end logits`). """,
- BERT_START_DOCSTRING,
-)
-class BertForQuestionAnswering(BertPreTrainedModel):
- def __init__(self, config):
- super(BertForQuestionAnswering, self).__init__(config)
- self.num_labels = config.num_labels
-
- self.bert = BertModel(config)
- self.qa_outputs = nn.Linear(config.hidden_size, config.num_labels)
-
- self.init_weights()
-
- @add_start_docstrings_to_callable(BERT_INPUTS_DOCSTRING)
- def forward(
- self,
- input_ids=None,
- attention_mask=None,
- token_type_ids=None,
- position_ids=None,
- head_mask=None,
- inputs_embeds=None,
- start_positions=None,
- end_positions=None,
- ):
- r"""
- start_positions (:obj:`torch.LongTensor` of shape :obj:`(batch_size,)`, `optional`, defaults to :obj:`None`):
- Labels for position (index) of the start of the labelled span for computing the token classification loss.
- Positions are clamped to the length of the sequence (`sequence_length`).
- Position outside of the sequence are not taken into account for computing the loss.
- end_positions (:obj:`torch.LongTensor` of shape :obj:`(batch_size,)`, `optional`, defaults to :obj:`None`):
- Labels for position (index) of the end of the labelled span for computing the token classification loss.
- Positions are clamped to the length of the sequence (`sequence_length`).
- Position outside of the sequence are not taken into account for computing the loss.
-
- Returns:
- :obj:`tuple(torch.FloatTensor)` comprising various elements depending on the configuration (:class:`~transformers.BertConfig`) and inputs:
- loss (:obj:`torch.FloatTensor` of shape :obj:`(1,)`, `optional`, returned when :obj:`labels` is provided):
- Total span extraction loss is the sum of a Cross-Entropy for the start and end positions.
- start_scores (:obj:`torch.FloatTensor` of shape :obj:`(batch_size, sequence_length,)`):
- Span-start scores (before SoftMax).
- end_scores (:obj:`torch.FloatTensor` of shape :obj:`(batch_size, sequence_length,)`):
- Span-end scores (before SoftMax).
- hidden_states (:obj:`tuple(torch.FloatTensor)`, `optional`, returned when ``config.output_hidden_states=True``):
- Tuple of :obj:`torch.FloatTensor` (one for the output of the embeddings + one for the output of each layer)
- of shape :obj:`(batch_size, sequence_length, hidden_size)`.
-
- Hidden-states of the model at the output of each layer plus the initial embedding outputs.
- attentions (:obj:`tuple(torch.FloatTensor)`, `optional`, returned when ``config.output_attentions=True``):
- Tuple of :obj:`torch.FloatTensor` (one for each layer) of shape
- :obj:`(batch_size, num_heads, sequence_length, sequence_length)`.
-
- Attentions weights after the attention softmax, used to compute the weighted average in the self-attention
- heads.
-
- Examples::
-
- from transformers import BertTokenizer, BertForQuestionAnswering
- import torch
-
- tokenizer = BertTokenizer.from_pretrained('bert-base-uncased')
- model = BertForQuestionAnswering.from_pretrained('bert-large-uncased-whole-word-masking-finetuned-squad')
-
- question, text = "Who was Jim Henson?", "Jim Henson was a nice puppet"
- input_ids = tokenizer.encode(question, text)
- token_type_ids = [0 if i <= input_ids.index(102) else 1 for i in range(len(input_ids))]
- start_scores, end_scores = model(torch.tensor([input_ids]), token_type_ids=torch.tensor([token_type_ids]))
-
- all_tokens = tokenizer.convert_ids_to_tokens(input_ids)
- answer = ' '.join(all_tokens[torch.argmax(start_scores) : torch.argmax(end_scores)+1])
-
- assert answer == "a nice puppet"
-
- """
-
- outputs = self.bert(
- input_ids,
- attention_mask=attention_mask,
- token_type_ids=token_type_ids,
- position_ids=position_ids,
- head_mask=head_mask,
- inputs_embeds=inputs_embeds,
- )
-
- sequence_output = outputs[0]
-
- logits = self.qa_outputs(sequence_output)
- start_logits, end_logits = logits.split(1, dim=-1)
- start_logits = start_logits.squeeze(-1)
- end_logits = end_logits.squeeze(-1)
-
- outputs = (start_logits, end_logits,) + outputs[2:]
- if start_positions is not None and end_positions is not None:
- # If we are on multi-GPU, split add a dimension
- if len(start_positions.size()) > 1:
- start_positions = start_positions.squeeze(-1)
- if len(end_positions.size()) > 1:
- end_positions = end_positions.squeeze(-1)
- # sometimes the start/end positions are outside our model inputs, we ignore these terms
- ignored_index = start_logits.size(1)
- start_positions.clamp_(0, ignored_index)
- end_positions.clamp_(0, ignored_index)
-
- loss_fct = CrossEntropyLoss(ignore_index=ignored_index)
- start_loss = loss_fct(start_logits, start_positions)
- end_loss = loss_fct(end_logits, end_positions)
- total_loss = (start_loss + end_loss) / 2
- outputs = (total_loss,) + outputs
-
- return outputs # (loss), start_logits, end_logits, (hidden_states), (attentions)
diff --git a/server/transformers/src/transformers/modeling_camembert.py b/server/transformers/src/transformers/modeling_camembert.py
deleted file mode 100644
index 12877dff16fa22b32e6efa8f0870cc4abed93d54..0000000000000000000000000000000000000000
--- a/server/transformers/src/transformers/modeling_camembert.py
+++ /dev/null
@@ -1,123 +0,0 @@
-# coding=utf-8
-# Copyright 2019 Inria, Facebook AI Research and the HuggingFace Inc. team.
-# Copyright (c) 2018, NVIDIA CORPORATION. All rights reserved.
-#
-# Licensed under the Apache License, Version 2.0 (the "License");
-# you may not use this file except in compliance with the License.
-# You may obtain a copy of the License at
-#
-# http://www.apache.org/licenses/LICENSE-2.0
-#
-# Unless required by applicable law or agreed to in writing, software
-# distributed under the License is distributed on an "AS IS" BASIS,
-# WITHOUT WARRANTIES OR CONDITIONS OF ANY KIND, either express or implied.
-# See the License for the specific language governing permissions and
-# limitations under the License.
-"""PyTorch CamemBERT model. """
-
-
-import logging
-
-from .configuration_camembert import CamembertConfig
-from .file_utils import add_start_docstrings
-from .modeling_roberta import (
- RobertaForMaskedLM,
- RobertaForMultipleChoice,
- RobertaForSequenceClassification,
- RobertaForTokenClassification,
- RobertaModel,
-)
-
-
-logger = logging.getLogger(__name__)
-
-CAMEMBERT_PRETRAINED_MODEL_ARCHIVE_MAP = {
- "camembert-base": "https://s3.amazonaws.com/models.huggingface.co/bert/camembert-base-pytorch_model.bin",
- "umberto-commoncrawl-cased-v1": "https://s3.amazonaws.com/models.huggingface.co/bert/Musixmatch/umberto-commoncrawl-cased-v1/pytorch_model.bin",
- "umberto-wikipedia-uncased-v1": "https://s3.amazonaws.com/models.huggingface.co/bert/Musixmatch/umberto-wikipedia-uncased-v1/pytorch_model.bin",
-}
-
-
-CAMEMBERT_START_DOCSTRING = r"""
-
- This model is a PyTorch `torch.nn.Module `_ sub-class.
- Use it as a regular PyTorch Module and refer to the PyTorch documentation for all matter related to general
- usage and behavior.
-
- Parameters:
- config (:class:`~transformers.CamembertConfig`): Model configuration class with all the parameters of the
- model. Initializing with a config file does not load the weights associated with the model, only the configuration.
- Check out the :meth:`~transformers.PreTrainedModel.from_pretrained` method to load the model weights.
-"""
-
-
-@add_start_docstrings(
- "The bare CamemBERT Model transformer outputting raw hidden-states without any specific head on top.",
- CAMEMBERT_START_DOCSTRING,
-)
-class CamembertModel(RobertaModel):
- """
- This class overrides :class:`~transformers.RobertaModel`. Please check the
- superclass for the appropriate documentation alongside usage examples.
- """
-
- config_class = CamembertConfig
- pretrained_model_archive_map = CAMEMBERT_PRETRAINED_MODEL_ARCHIVE_MAP
-
-
-@add_start_docstrings(
- """CamemBERT Model with a `language modeling` head on top. """, CAMEMBERT_START_DOCSTRING,
-)
-class CamembertForMaskedLM(RobertaForMaskedLM):
- """
- This class overrides :class:`~transformers.RobertaForMaskedLM`. Please check the
- superclass for the appropriate documentation alongside usage examples.
- """
-
- config_class = CamembertConfig
- pretrained_model_archive_map = CAMEMBERT_PRETRAINED_MODEL_ARCHIVE_MAP
-
-
-@add_start_docstrings(
- """CamemBERT Model transformer with a sequence classification/regression head on top (a linear layer
- on top of the pooled output) e.g. for GLUE tasks. """,
- CAMEMBERT_START_DOCSTRING,
-)
-class CamembertForSequenceClassification(RobertaForSequenceClassification):
- """
- This class overrides :class:`~transformers.RobertaForSequenceClassification`. Please check the
- superclass for the appropriate documentation alongside usage examples.
- """
-
- config_class = CamembertConfig
- pretrained_model_archive_map = CAMEMBERT_PRETRAINED_MODEL_ARCHIVE_MAP
-
-
-@add_start_docstrings(
- """CamemBERT Model with a multiple choice classification head on top (a linear layer on top of
- the pooled output and a softmax) e.g. for RocStories/SWAG tasks. """,
- CAMEMBERT_START_DOCSTRING,
-)
-class CamembertForMultipleChoice(RobertaForMultipleChoice):
- """
- This class overrides :class:`~transformers.RobertaForMultipleChoice`. Please check the
- superclass for the appropriate documentation alongside usage examples.
- """
-
- config_class = CamembertConfig
- pretrained_model_archive_map = CAMEMBERT_PRETRAINED_MODEL_ARCHIVE_MAP
-
-
-@add_start_docstrings(
- """CamemBERT Model with a token classification head on top (a linear layer on top of
- the hidden-states output) e.g. for Named-Entity-Recognition (NER) tasks. """,
- CAMEMBERT_START_DOCSTRING,
-)
-class CamembertForTokenClassification(RobertaForTokenClassification):
- """
- This class overrides :class:`~transformers.RobertaForTokenClassification`. Please check the
- superclass for the appropriate documentation alongside usage examples.
- """
-
- config_class = CamembertConfig
- pretrained_model_archive_map = CAMEMBERT_PRETRAINED_MODEL_ARCHIVE_MAP
diff --git a/server/transformers/src/transformers/modeling_ctrl.py b/server/transformers/src/transformers/modeling_ctrl.py
deleted file mode 100644
index 40e076a4982ef388986b1f04aea8954f97624295..0000000000000000000000000000000000000000
--- a/server/transformers/src/transformers/modeling_ctrl.py
+++ /dev/null
@@ -1,546 +0,0 @@
-# coding=utf-8
-# Copyright 2018 Salesforce and HuggingFace Inc. team.
-# Copyright (c) 2018, NVIDIA CORPORATION. All rights reserved.
-#
-# Licensed under the Apache License, Version 2.0 (the "License");
-# you may not use this file except in compliance with the License.
-# You may obtain a copy of the License at
-#
-# http://www.apache.org/licenses/LICENSE-2.0
-#
-# Unless required by applicable law or agreed to in writing, software
-# distributed under the License is distributed on an "AS IS" BASIS,
-# WITHOUT WARRANTIES OR CONDITIONS OF ANY KIND, either express or implied.
-# See the License for the specific language governing permissions and
-# limitations under the License.
-""" PyTorch CTRL model."""
-
-
-import logging
-
-import numpy as np
-import torch
-import torch.nn as nn
-from torch.nn import CrossEntropyLoss
-
-from .configuration_ctrl import CTRLConfig
-from .file_utils import add_start_docstrings, add_start_docstrings_to_callable
-from .modeling_utils import Conv1D, PreTrainedModel
-
-
-logger = logging.getLogger(__name__)
-
-CTRL_PRETRAINED_MODEL_ARCHIVE_MAP = {"ctrl": "https://storage.googleapis.com/sf-ctrl/pytorch/seqlen256_v1.bin"}
-
-
-def angle_defn(pos, i, d_model_size):
- angle_rates = 1 / torch.pow(10000, (2 * (i // 2)) / d_model_size)
- return pos * angle_rates
-
-
-def positional_encoding(position, d_model_size, dtype):
- # create the sinusoidal pattern for the positional encoding
- angle_rads = angle_defn(
- torch.arange(position, dtype=dtype).unsqueeze(1),
- torch.arange(d_model_size, dtype=dtype).unsqueeze(0),
- d_model_size,
- )
-
- sines = torch.sin(angle_rads[:, 0::2])
- cosines = torch.cos(angle_rads[:, 1::2])
-
- pos_encoding = torch.cat([sines, cosines], dim=-1)
- return pos_encoding
-
-
-def scaled_dot_product_attention(q, k, v, mask, attention_mask=None, head_mask=None):
- # calculate attention
- matmul_qk = torch.matmul(q, k.permute(0, 1, 3, 2))
-
- dk = k.shape[-1]
- scaled_attention_logits = matmul_qk / np.sqrt(dk)
-
- if mask is not None:
- nd, ns = scaled_attention_logits.size(-2), scaled_attention_logits.size(-1)
- scaled_attention_logits += mask[ns - nd : ns, :ns] * -1e4
-
- if attention_mask is not None:
- # Apply the attention mask
- scaled_attention_logits = scaled_attention_logits + attention_mask
-
- attention_weights = torch.softmax(scaled_attention_logits, dim=-1)
-
- # Mask heads if we want to
- if head_mask is not None:
- attention_weights = attention_weights * head_mask
-
- output = torch.matmul(attention_weights, v)
-
- return output, attention_weights
-
-
-class MultiHeadAttention(torch.nn.Module):
- def __init__(self, d_model_size, num_heads, output_attentions=False):
- super().__init__()
- self.output_attentions = output_attentions
- self.num_heads = num_heads
- self.d_model_size = d_model_size
-
- self.depth = int(d_model_size / self.num_heads)
-
- self.Wq = torch.nn.Linear(d_model_size, d_model_size)
- self.Wk = torch.nn.Linear(d_model_size, d_model_size)
- self.Wv = torch.nn.Linear(d_model_size, d_model_size)
-
- self.dense = torch.nn.Linear(d_model_size, d_model_size)
-
- def split_into_heads(self, x, batch_size):
- x = x.reshape(batch_size, -1, self.num_heads, self.depth)
- return x.permute([0, 2, 1, 3])
-
- def forward(self, v, k, q, mask, layer_past=None, attention_mask=None, head_mask=None):
- batch_size = q.shape[0]
-
- q = self.Wq(q)
- k = self.Wk(k)
- v = self.Wv(v)
-
- q = self.split_into_heads(q, batch_size)
- k = self.split_into_heads(k, batch_size)
- v = self.split_into_heads(v, batch_size)
- if layer_past is not None:
- past_key, past_value = layer_past[0], layer_past[1]
- k = torch.cat((past_key, k), dim=-2)
- v = torch.cat((past_value, v), dim=-2)
- present = torch.stack((k, v))
-
- output = scaled_dot_product_attention(q, k, v, mask, attention_mask, head_mask)
- scaled_attention = output[0].permute([0, 2, 1, 3])
- attn = output[1]
- original_size_attention = scaled_attention.reshape(batch_size, -1, self.d_model_size)
- output = self.dense(original_size_attention)
-
- outputs = (output, present)
- if self.output_attentions:
- outputs = outputs + (attn,)
- return outputs
-
-
-def point_wise_feed_forward_network(d_model_size, dff):
- return torch.nn.Sequential(torch.nn.Linear(d_model_size, dff), torch.nn.ReLU(), torch.nn.Linear(dff, d_model_size))
-
-
-class EncoderLayer(torch.nn.Module):
- def __init__(self, d_model_size, num_heads, dff, rate=0.1, output_attentions=False):
- super().__init__()
-
- self.multi_head_attention = MultiHeadAttention(d_model_size, num_heads, output_attentions)
- self.ffn = point_wise_feed_forward_network(d_model_size, dff)
-
- self.layernorm1 = torch.nn.LayerNorm(d_model_size, eps=1e-6)
- self.layernorm2 = torch.nn.LayerNorm(d_model_size, eps=1e-6)
-
- self.dropout1 = torch.nn.Dropout(rate)
- self.dropout2 = torch.nn.Dropout(rate)
-
- def forward(self, x, mask, layer_past=None, attention_mask=None, head_mask=None):
- normed = self.layernorm1(x)
- attn_outputs = self.multi_head_attention(
- normed, normed, normed, mask, layer_past=layer_past, attention_mask=attention_mask, head_mask=head_mask
- )
- attn_output = attn_outputs[0]
- attn_output = self.dropout1(attn_output)
- out1 = x + attn_output
-
- out2 = self.layernorm2(out1)
- ffn_output = self.ffn(out2)
- ffn_output = self.dropout2(ffn_output)
- out2 = out1 + ffn_output
-
- outputs = (out2,) + attn_outputs[1:]
- return outputs
-
-
-class CTRLPreTrainedModel(PreTrainedModel):
- """ An abstract class to handle weights initialization and
- a simple interface for downloading and loading pretrained models.
- """
-
- config_class = CTRLConfig
- pretrained_model_archive_map = CTRL_PRETRAINED_MODEL_ARCHIVE_MAP
- base_model_prefix = "transformer"
-
- def _init_weights(self, module):
- """ Initialize the weights.
- """
- if isinstance(module, (nn.Linear, nn.Embedding, Conv1D)):
- # Slightly different from the TF version which uses truncated_normal for initialization
- # cf https://github.com/pytorch/pytorch/pull/5617
- module.weight.data.normal_(mean=0.0, std=self.config.initializer_range)
- if isinstance(module, (nn.Linear, Conv1D)) and module.bias is not None:
- module.bias.data.zero_()
- elif isinstance(module, nn.LayerNorm):
- module.bias.data.zero_()
- module.weight.data.fill_(1.0)
-
-
-CTRL_START_DOCSTRING = r"""
- This model is a PyTorch `torch.nn.Module `_ sub-class.
- Use it as a regular PyTorch Module and refer to the PyTorch documentation for all matter related to general
- usage and behavior.
-
- Parameters:
- config (:class:`~transformers.CTRLConfig`): Model configuration class with all the parameters of the model.
- Initializing with a config file does not load the weights associated with the model, only the configuration.
- Check out the :meth:`~transformers.PreTrainedModel.from_pretrained` method to load the model weights.
-"""
-
-CTRL_INPUTS_DOCSTRING = r"""
- Args:
- input_ids (:obj:`torch.LongTensor` of shape :obj:`(batch_size, sequence_length)`):
- Indices of input sequence tokens in the vocabulary.
-
- Indices can be obtained using :class:`transformers.CTRLTokenizer`.
- See :func:`transformers.PreTrainedTokenizer.encode` and
- :func:`transformers.PreTrainedTokenizer.encode_plus` for details.
-
- `What are input IDs? <../glossary.html#input-ids>`__
- past (:obj:`List[torch.FloatTensor]` of length :obj:`config.n_layers`):
- Contains pre-computed hidden-states (key and values in the attention blocks) as computed by the model
- (see `past` output below). Can be used to speed up sequential decoding. The token ids which have their past given to this model
- should not be passed as input ids as they have already been computed.
- attention_mask (:obj:`torch.FloatTensor` of shape :obj:`(batch_size, sequence_length)`, `optional`, defaults to :obj:`None`):
- Mask to avoid performing attention on padding token indices.
- Mask values selected in ``[0, 1]``:
- ``1`` for tokens that are NOT MASKED, ``0`` for MASKED tokens.
-
- `What are attention masks? <../glossary.html#attention-mask>`__
- token_type_ids (:obj:`torch.LongTensor` of shape :obj:`(batch_size, sequence_length)`, `optional`, defaults to :obj:`None`):
- Segment token indices to indicate first and second portions of the inputs.
- Indices are selected in ``[0, 1]``: ``0`` corresponds to a `sentence A` token, ``1``
- corresponds to a `sentence B` token
-
- `What are token type IDs? <../glossary.html#token-type-ids>`_
- position_ids (:obj:`torch.LongTensor` of shape :obj:`(batch_size, sequence_length)`, `optional`, defaults to :obj:`None`):
- Indices of positions of each input sequence tokens in the position embeddings.
- Selected in the range ``[0, config.max_position_embeddings - 1]``.
-
- `What are position IDs? <../glossary.html#position-ids>`_
- head_mask (:obj:`torch.FloatTensor` of shape :obj:`(num_heads,)` or :obj:`(num_layers, num_heads)`, `optional`, defaults to :obj:`None`):
- Mask to nullify selected heads of the self-attention modules.
- Mask values selected in ``[0, 1]``:
- :obj:`1` indicates the head is **not masked**, :obj:`0` indicates the head is **masked**.
- input_embeds (:obj:`torch.FloatTensor` of shape :obj:`(batch_size, sequence_length, hidden_size)`, `optional`, defaults to :obj:`None`):
- Optionally, instead of passing :obj:`input_ids` you can choose to directly pass an embedded representation.
- This is useful if you want more control over how to convert `input_ids` indices into associated vectors
- than the model's internal embedding lookup matrix.
-"""
-
-
-@add_start_docstrings(
- "The bare CTRL Model transformer outputting raw hidden-states without any specific head on top.",
- CTRL_START_DOCSTRING,
-)
-class CTRLModel(CTRLPreTrainedModel):
- def __init__(self, config):
- super().__init__(config)
- self.output_hidden_states = config.output_hidden_states
- self.output_attentions = config.output_attentions
- self.output_past = config.output_past
-
- self.d_model_size = config.n_embd
- self.num_layers = config.n_layer
-
- self.pos_encoding = positional_encoding(config.n_positions, self.d_model_size, torch.float)
-
- self.w = nn.Embedding(config.vocab_size, config.n_embd)
-
- self.dropout = nn.Dropout(config.embd_pdrop)
- self.h = nn.ModuleList(
- [
- EncoderLayer(config.n_embd, config.n_head, config.dff, config.resid_pdrop, config.output_attentions)
- for _ in range(config.n_layer)
- ]
- )
- self.layernorm = nn.LayerNorm(config.n_embd, eps=config.layer_norm_epsilon)
-
- self.init_weights()
-
- def get_input_embeddings(self):
- return self.w
-
- def set_input_embeddings(self, new_embeddings):
- self.w = new_embeddings
-
- def _prune_heads(self, heads_to_prune):
- """ Prunes heads of the model.
- heads_to_prune: dict of {layer_num: list of heads to prune in this layer}
- """
- for layer, heads in heads_to_prune.items():
- self.h[layer].attn.prune_heads(heads)
-
- @add_start_docstrings_to_callable(CTRL_INPUTS_DOCSTRING)
- def forward(
- self,
- input_ids=None,
- past=None,
- attention_mask=None,
- token_type_ids=None,
- position_ids=None,
- head_mask=None,
- inputs_embeds=None,
- ):
- r"""
- Return:
- :obj:`tuple(torch.FloatTensor)` comprising various elements depending on the configuration (:class:`~transformers.CTRLConfig`) and inputs:
- last_hidden_state (:obj:`torch.FloatTensor` of shape :obj:`(batch_size, sequence_length, hidden_size)`):
- Sequence of hidden-states at the last layer of the model.
- past (:obj:`List[torch.FloatTensor]` of length :obj:`config.n_layers` with each tensor of shape :obj:`(2, batch_size, num_heads, sequence_length, embed_size_per_head)`):
- Contains pre-computed hidden-states (key and values in the attention blocks).
- Can be used (see `past` input) to speed up sequential decoding. The token ids which have their past given to this model
- should not be passed as input ids as they have already been computed.
- hidden_states (:obj:`tuple(torch.FloatTensor)`, `optional`, returned when ``config.output_hidden_states=True``):
- Tuple of :obj:`torch.FloatTensor` (one for the output of the embeddings + one for the output of each layer)
- of shape :obj:`(batch_size, sequence_length, hidden_size)`.
-
- Hidden-states of the model at the output of each layer plus the initial embedding outputs.
- attentions (:obj:`tuple(torch.FloatTensor)`, `optional`, returned when ``config.output_attentions=True``):
- Tuple of :obj:`torch.FloatTensor` (one for each layer) of shape
- :obj:`(batch_size, num_heads, sequence_length, sequence_length)`.
-
- Attentions weights after the attention softmax, used to compute the weighted average in the self-attention
- heads.
-
- Examples::
-
- from transformers import CTRLTokenizer, CTRLModel
- import torch
-
- tokenizer = CTRLTokenizer.from_pretrained('ctrl')
- model = CTRLModel.from_pretrained('ctrl')
-
- input_ids = torch.tensor(tokenizer.encode("Links Hello, my dog is cute", add_special_tokens=True)).unsqueeze(0) # Batch size 1
- outputs = model(input_ids)
-
- last_hidden_states = outputs[0] # The last hidden-state is the first element of the output tuple
-
- """
- if input_ids is not None and inputs_embeds is not None:
- raise ValueError("You cannot specify both input_ids and inputs_embeds at the same time")
- elif input_ids is not None:
- input_shape = input_ids.size()
- input_ids = input_ids.view(-1, input_shape[-1])
- elif inputs_embeds is not None:
- input_shape = inputs_embeds.size()[:-1]
- else:
- raise ValueError("You have to specify either input_ids or inputs_embeds")
-
- if past is None:
- past_length = 0
- past = [None] * len(self.h)
- else:
- past_length = past[0][0].size(-2)
- if position_ids is None:
- device = input_ids.device if input_ids is not None else inputs_embeds.device
- position_ids = torch.arange(past_length, input_shape[-1] + past_length, dtype=torch.long, device=device)
- position_ids = position_ids.unsqueeze(0).view(-1, input_shape[-1])
-
- # Attention mask.
- if attention_mask is not None:
- attention_mask = attention_mask.view(-1, input_shape[-1])
- # We create a 3D attention mask from a 2D tensor mask.
- # Sizes are [batch_size, 1, 1, to_seq_length]
- # So we can broadcast to [batch_size, num_heads, from_seq_length, to_seq_length]
- # this attention mask is more simple than the triangular masking of causal attention
- # used in OpenAI GPT, we just need to prepare the broadcast dimension here.
- attention_mask = attention_mask.unsqueeze(1).unsqueeze(2)
-
- # Since attention_mask is 1.0 for positions we want to attend and 0.0 for
- # masked positions, this operation will create a tensor which is 0.0 for
- # positions we want to attend and -10000.0 for masked positions.
- # Since we are adding it to the raw scores before the softmax, this is
- # effectively the same as removing these entirely.
- attention_mask = attention_mask.to(dtype=next(self.parameters()).dtype) # fp16 compatibility
- attention_mask = (1.0 - attention_mask) * -10000.0
-
- # Prepare head mask if needed
- # 1.0 in head_mask indicate we keep the head
- # attention_probs has shape bsz x n_heads x N x N
- # head_mask has shape n_layer x batch x n_heads x N x N
- if head_mask is not None:
- if head_mask.dim() == 1:
- head_mask = head_mask.unsqueeze(0).unsqueeze(0).unsqueeze(-1).unsqueeze(-1)
- head_mask = head_mask.expand(self.config.n_layer, -1, -1, -1, -1)
- elif head_mask.dim() == 2:
- head_mask = (
- head_mask.unsqueeze(1).unsqueeze(-1).unsqueeze(-1)
- ) # We can specify head_mask for each layer
- head_mask = head_mask.to(
- dtype=next(self.parameters()).dtype
- ) # switch to fload if need + fp16 compatibility
- else:
- head_mask = [None] * self.config.n_layer
-
- if token_type_ids is not None:
- token_type_ids = token_type_ids.view(-1, input_shape[-1])
- token_type_embeds = self.w(token_type_ids)
- token_type_embeds *= np.sqrt(self.d_model_size)
- else:
- token_type_embeds = 0
- position_ids = position_ids.view(-1, input_shape[-1])
-
- if inputs_embeds is None:
- inputs_embeds = self.w(input_ids)
- # inputs_embeds = embedded.unsqueeze(0) if len(input_ids.shape)<2 else embedded
- seq_len = input_shape[-1]
- mask = torch.triu(torch.ones(seq_len + past_length, seq_len + past_length), 1).to(inputs_embeds.device)
-
- inputs_embeds *= np.sqrt(self.d_model_size)
-
- pos_embeds = self.pos_encoding[position_ids, :].to(inputs_embeds.device)
-
- hidden_states = inputs_embeds + pos_embeds + token_type_embeds
-
- hidden_states = self.dropout(hidden_states)
-
- output_shape = input_shape + (inputs_embeds.size(-1),)
- presents = ()
- all_hidden_states = ()
- all_attentions = []
- for i, (h, layer_past) in enumerate(zip(self.h, past)):
- if self.output_hidden_states:
- all_hidden_states = all_hidden_states + (hidden_states.view(*output_shape),)
- outputs = h(
- hidden_states, mask, layer_past=layer_past, attention_mask=attention_mask, head_mask=head_mask[i]
- )
- hidden_states, present = outputs[:2]
- if self.output_past:
- presents = presents + (present,)
-
- if self.output_attentions:
- all_attentions.append(outputs[2])
-
- hidden_states = self.layernorm(hidden_states)
- hidden_states = hidden_states.view(*output_shape)
- if self.output_hidden_states:
- all_hidden_states = all_hidden_states + (hidden_states,)
-
- outputs = (hidden_states,)
- if self.output_past:
- outputs = outputs + (presents,)
- if self.output_hidden_states:
- outputs = outputs + (all_hidden_states,)
- if self.output_attentions:
- # let the number of heads free (-1) so we can extract attention even after head pruning
- attention_output_shape = input_shape[:-1] + (-1,) + all_attentions[0].shape[-2:]
- all_attentions = tuple(t.view(*attention_output_shape) for t in all_attentions)
- outputs = outputs + (all_attentions,)
- return outputs
-
-
-@add_start_docstrings(
- """The CTRL Model transformer with a language modeling head on top
- (linear layer with weights tied to the input embeddings). """,
- CTRL_START_DOCSTRING,
-)
-class CTRLLMHeadModel(CTRLPreTrainedModel):
- def __init__(self, config):
- super().__init__(config)
- self.transformer = CTRLModel(config)
- self.lm_head = nn.Linear(config.n_embd, config.vocab_size, bias=True)
-
- self.init_weights()
-
- def get_output_embeddings(self):
- return self.lm_head
-
- def prepare_inputs_for_generation(self, input_ids, **kwargs):
- # only last token for inputs_ids if past is defined in kwargs
- if "past" in kwargs and kwargs["past"]:
- input_ids = input_ids[:, -1].unsqueeze(-1)
-
- inputs = {"input_ids": input_ids}
- inputs.update(kwargs)
- return inputs
-
- @add_start_docstrings_to_callable(CTRL_INPUTS_DOCSTRING)
- def forward(
- self,
- input_ids=None,
- past=None,
- attention_mask=None,
- token_type_ids=None,
- position_ids=None,
- head_mask=None,
- inputs_embeds=None,
- labels=None,
- ):
- r"""
- labels (:obj:`torch.LongTensor` of shape :obj:`(batch_size, sequence_length)`, `optional`, defaults to :obj:`None`):
- Labels for language modeling.
- Note that the labels **are shifted** inside the model, i.e. you can set ``lm_labels = input_ids``
- Indices are selected in ``[-100, 0, ..., config.vocab_size]``
- All labels set to ``-100`` are ignored (masked), the loss is only
- computed for labels in ``[0, ..., config.vocab_size]``
-
- Return:
- :obj:`tuple(torch.FloatTensor)` comprising various elements depending on the configuration (:class:`~transformers.CTRLConfig`) and inputs:
- loss (:obj:`torch.FloatTensor` of shape `(1,)`, `optional`, returned when ``labels`` is provided)
- Language modeling loss.
- prediction_scores (:obj:`torch.FloatTensor` of shape :obj:`(batch_size, sequence_length, config.vocab_size)`):
- Prediction scores of the language modeling head (scores for each vocabulary token before SoftMax).
- past (:obj:`List[torch.FloatTensor]` of length :obj:`config.n_layers` with each tensor of shape :obj:`(2, batch_size, num_heads, sequence_length, embed_size_per_head)`):
- Contains pre-computed hidden-states (key and values in the attention blocks).
- Can be used (see `past` input) to speed up sequential decoding. The token ids which have their past given to this model
- should not be passed as input ids as they have already been computed.
- hidden_states (:obj:`tuple(torch.FloatTensor)`, `optional`, returned when ``config.output_hidden_states=True``):
- Tuple of :obj:`torch.FloatTensor` (one for the output of the embeddings + one for the output of each layer)
- of shape :obj:`(batch_size, sequence_length, hidden_size)`.
-
- Hidden-states of the model at the output of each layer plus the initial embedding outputs.
- attentions (:obj:`tuple(torch.FloatTensor)`, `optional`, returned when ``config.output_attentions=True``):
- Tuple of :obj:`torch.FloatTensor` (one for each layer) of shape
- :obj:`(batch_size, num_heads, sequence_length, sequence_length)`.
-
- Attentions weights after the attention softmax, used to compute the weighted average in the self-attention
- heads.
-
- Examples::
-
- import torch
- from transformers import CTRLTokenizer, CTRLLMHeadModel
-
- tokenizer = CTRLTokenizer.from_pretrained('ctrl')
- model = CTRLLMHeadModel.from_pretrained('ctrl')
-
- input_ids = torch.tensor(tokenizer.encode("Links Hello, my dog is cute", add_special_tokens=True)).unsqueeze(0) # Batch size 1
- outputs = model(input_ids, labels=input_ids)
- loss, logits = outputs[:2]
-
- """
- transformer_outputs = self.transformer(
- input_ids,
- past=past,
- attention_mask=attention_mask,
- token_type_ids=token_type_ids,
- position_ids=position_ids,
- head_mask=head_mask,
- inputs_embeds=inputs_embeds,
- )
-
- hidden_states = transformer_outputs[0]
-
- lm_logits = self.lm_head(hidden_states)
-
- outputs = (lm_logits,) + transformer_outputs[1:]
-
- if labels is not None:
- # Shift so that tokens < n predict n
- shift_logits = lm_logits[..., :-1, :].contiguous()
- shift_labels = labels[..., 1:].contiguous()
- # Flatten the tokens
- loss_fct = CrossEntropyLoss()
- loss = loss_fct(shift_logits.view(-1, shift_logits.size(-1)), shift_labels.view(-1))
- outputs = (loss,) + outputs
-
- return outputs # (loss), lm_logits, presents, (all hidden_states), (attentions)
diff --git a/server/transformers/src/transformers/modeling_distilbert.py b/server/transformers/src/transformers/modeling_distilbert.py
deleted file mode 100644
index be876f362f339f0a9b5ec4ff795d8196d8e53b9e..0000000000000000000000000000000000000000
--- a/server/transformers/src/transformers/modeling_distilbert.py
+++ /dev/null
@@ -1,841 +0,0 @@
-# coding=utf-8
-# Copyright 2019-present, the HuggingFace Inc. team, The Google AI Language Team and Facebook, Inc.
-#
-# Licensed under the Apache License, Version 2.0 (the "License");
-# you may not use this file except in compliance with the License.
-# You may obtain a copy of the License at
-#
-# http://www.apache.org/licenses/LICENSE-2.0
-#
-# Unless required by applicable law or agreed to in writing, software
-# distributed under the License is distributed on an "AS IS" BASIS,
-# WITHOUT WARRANTIES OR CONDITIONS OF ANY KIND, either express or implied.
-# See the License for the specific language governing permissions and
-# limitations under the License.
-""" PyTorch DistilBERT model
- adapted in part from Facebook, Inc XLM model (https://github.com/facebookresearch/XLM)
- and in part from HuggingFace PyTorch version of Google AI Bert model (https://github.com/google-research/bert)
-"""
-
-
-import copy
-import logging
-import math
-
-import numpy as np
-import torch
-import torch.nn as nn
-from torch.nn import CrossEntropyLoss
-
-from .configuration_distilbert import DistilBertConfig
-from .file_utils import add_start_docstrings, add_start_docstrings_to_callable
-from .modeling_utils import PreTrainedModel, prune_linear_layer, transpose_iterable
-
-
-logger = logging.getLogger(__name__)
-
-
-DISTILBERT_PRETRAINED_MODEL_ARCHIVE_MAP = {
- "distilbert-base-uncased": "https://s3.amazonaws.com/models.huggingface.co/bert/distilbert-base-uncased-pytorch_model.bin",
- "distilbert-base-uncased-distilled-squad": "https://s3.amazonaws.com/models.huggingface.co/bert/distilbert-base-uncased-distilled-squad-pytorch_model.bin",
- "distilbert-base-german-cased": "https://s3.amazonaws.com/models.huggingface.co/bert/distilbert-base-german-cased-pytorch_model.bin",
- "distilbert-base-multilingual-cased": "https://s3.amazonaws.com/models.huggingface.co/bert/distilbert-base-multilingual-cased-pytorch_model.bin",
- "distilbert-base-uncased-finetuned-sst-2-english": "https://s3.amazonaws.com/models.huggingface.co/bert/distilbert-base-uncased-finetuned-sst-2-english-pytorch_model.bin",
-}
-
-
-# UTILS AND BUILDING BLOCKS OF THE ARCHITECTURE #
-def gelu(x):
- return 0.5 * x * (1.0 + torch.erf(x / math.sqrt(2.0)))
-
-
-def create_sinusoidal_embeddings(n_pos, dim, out):
- position_enc = np.array([[pos / np.power(10000, 2 * (j // 2) / dim) for j in range(dim)] for pos in range(n_pos)])
- out[:, 0::2] = torch.FloatTensor(np.sin(position_enc[:, 0::2]))
- out[:, 1::2] = torch.FloatTensor(np.cos(position_enc[:, 1::2]))
- out.detach_()
- out.requires_grad = False
-
-
-class Embeddings(nn.Module):
- def __init__(self, config):
- super().__init__()
- self.word_embeddings = nn.Embedding(config.vocab_size, config.dim, padding_idx=0)
- self.position_embeddings = nn.Embedding(config.max_position_embeddings, config.dim)
- if config.sinusoidal_pos_embds:
- create_sinusoidal_embeddings(
- n_pos=config.max_position_embeddings, dim=config.dim, out=self.position_embeddings.weight
- )
-
- self.LayerNorm = nn.LayerNorm(config.dim, eps=1e-12)
- self.dropout = nn.Dropout(config.dropout)
-
- def forward(self, input_ids):
- """
- Parameters
- ----------
- input_ids: torch.tensor(bs, max_seq_length)
- The token ids to embed.
-
- Outputs
- -------
- embeddings: torch.tensor(bs, max_seq_length, dim)
- The embedded tokens (plus position embeddings, no token_type embeddings)
- """
- seq_length = input_ids.size(1)
- position_ids = torch.arange(seq_length, dtype=torch.long, device=input_ids.device) # (max_seq_length)
- position_ids = position_ids.unsqueeze(0).expand_as(input_ids) # (bs, max_seq_length)
-
- word_embeddings = self.word_embeddings(input_ids) # (bs, max_seq_length, dim)
- position_embeddings = self.position_embeddings(position_ids) # (bs, max_seq_length, dim)
-
- embeddings = word_embeddings + position_embeddings # (bs, max_seq_length, dim)
- embeddings = self.LayerNorm(embeddings) # (bs, max_seq_length, dim)
- embeddings = self.dropout(embeddings) # (bs, max_seq_length, dim)
- return embeddings
-
-
-class MultiHeadSelfAttention(nn.Module):
- def __init__(self, config):
- super().__init__()
-
- self.n_heads = config.n_heads
- self.dim = config.dim
- self.dropout = nn.Dropout(p=config.attention_dropout)
- self.output_attentions = config.output_attentions
- self.output_additional_info = config.output_additional_info
-
- assert self.dim % self.n_heads == 0
-
- self.q_lin = nn.Linear(in_features=config.dim, out_features=config.dim)
- self.k_lin = nn.Linear(in_features=config.dim, out_features=config.dim)
- self.v_lin = nn.Linear(in_features=config.dim, out_features=config.dim)
- self.out_lin = nn.Linear(in_features=config.dim, out_features=config.dim)
-
- self.pruned_heads = set()
-
- def prune_heads(self, heads):
- attention_head_size = self.dim // self.n_heads
- if len(heads) == 0:
- return
- mask = torch.ones(self.n_heads, attention_head_size)
- heads = set(heads) - self.pruned_heads
- for head in heads:
- head -= sum(1 if h < head else 0 for h in self.pruned_heads)
- mask[head] = 0
- mask = mask.view(-1).contiguous().eq(1)
- index = torch.arange(len(mask))[mask].long()
- # Prune linear layers
- self.q_lin = prune_linear_layer(self.q_lin, index)
- self.k_lin = prune_linear_layer(self.k_lin, index)
- self.v_lin = prune_linear_layer(self.v_lin, index)
- self.out_lin = prune_linear_layer(self.out_lin, index, dim=1)
- # Update hyper params
- self.n_heads = self.n_heads - len(heads)
- self.dim = attention_head_size * self.n_heads
- self.pruned_heads = self.pruned_heads.union(heads)
-
- def forward(self, query, key, value, mask, head_mask=None):
- """
- Parameters
- ----------
- query: torch.tensor(bs, seq_length, dim)
- key: torch.tensor(bs, seq_length, dim)
- value: torch.tensor(bs, seq_length, dim)
- mask: torch.tensor(bs, seq_length)
-
- Outputs
- -------
- weights: torch.tensor(bs, n_heads, seq_length, seq_length)
- Attention weights
- context: torch.tensor(bs, seq_length, dim)
- Contextualized layer. Optional: only if `output_attentions=True`
- """
- bs, q_length, dim = query.size()
- k_length = key.size(1)
- # assert dim == self.dim, 'Dimensions do not match: %s input vs %s configured' % (dim, self.dim)
- # assert key.size() == value.size()
-
- dim_per_head = self.dim // self.n_heads
-
- mask_reshp = (bs, 1, 1, k_length)
-
- def shape(x):
- """ separate heads """
- return x.view(bs, -1, self.n_heads, dim_per_head).transpose(1, 2)
-
- def unshape(x):
- """ group heads """
- return x.transpose(1, 2).contiguous().view(bs, -1, self.n_heads * dim_per_head)
-
- q = shape(self.q_lin(query)) # (bs, n_heads, q_length, dim_per_head)
- k = shape(self.k_lin(key)) # (bs, n_heads, k_length, dim_per_head)
- v = shape(self.v_lin(value)) # (bs, n_heads, k_length, dim_per_head)
-
- q = q / math.sqrt(dim_per_head) # (bs, n_heads, q_length, dim_per_head)
- scores = torch.matmul(q, k.transpose(2, 3)) # (bs, n_heads, q_length, k_length)
- mask = (mask == 0).view(mask_reshp).expand_as(scores) # (bs, n_heads, q_length, k_length)
- scores.masked_fill_(mask, -float("inf")) # (bs, n_heads, q_length, k_length)
-
- weights = nn.Softmax(dim=-1)(scores) # (bs, n_heads, q_length, k_length)
- weights = self.dropout(weights) # (bs, n_heads, q_length, k_length)
-
- # Mask heads if we want to
- if head_mask is not None:
- weights = weights * head_mask
-
- context = torch.matmul(weights, v) # (bs, n_heads, q_length, dim_per_head)
- new_context = unshape(context) # (bs, q_length, dim)
- new_context = self.out_lin(new_context) # (bs, q_length, dim)
-
- output = (new_context,)
-
- if self.output_attentions:
- output += (weights,)
-
- if self.output_additional_info:
- output += (context,)
-
- return output
-
-
-class FFN(nn.Module):
- def __init__(self, config):
- super().__init__()
- self.dropout = nn.Dropout(p=config.dropout)
- self.lin1 = nn.Linear(in_features=config.dim, out_features=config.hidden_dim)
- self.lin2 = nn.Linear(in_features=config.hidden_dim, out_features=config.dim)
- assert config.activation in ["relu", "gelu"], "activation ({}) must be in ['relu', 'gelu']".format(
- config.activation
- )
- self.activation = gelu if config.activation == "gelu" else nn.ReLU()
-
- def forward(self, input):
- x = self.lin1(input)
- x = self.activation(x)
- x = self.lin2(x)
- x = self.dropout(x)
- return x
-
-
-class TransformerBlock(nn.Module):
- def __init__(self, config):
- super().__init__()
-
- self.n_heads = config.n_heads
- self.dim = config.dim
- self.hidden_dim = config.hidden_dim
- self.dropout = nn.Dropout(p=config.dropout)
- self.activation = config.activation
- self.output_attentions = config.output_attentions
- self.output_additional_info = config.output_additional_info
-
- assert config.dim % config.n_heads == 0
-
- self.attention = MultiHeadSelfAttention(config)
- self.sa_layer_norm = nn.LayerNorm(normalized_shape=config.dim, eps=1e-12)
-
- self.ffn = FFN(config)
- self.output_layer_norm = nn.LayerNorm(normalized_shape=config.dim, eps=1e-12)
-
- def forward(self, x, attn_mask=None, head_mask=None):
- """
- Parameters
- ----------
- x: torch.tensor(bs, seq_length, dim)
- attn_mask: torch.tensor(bs, seq_length)
-
- Outputs
- -------
- sa_weights: torch.tensor(bs, n_heads, seq_length, seq_length)
- The attention weights
- ffn_output: torch.tensor(bs, seq_length, dim)
- The output of the transformer block contextualization.
- """
- # Self-Attention
- sa_raw_output = self.attention(query=x, key=x, value=x, mask=attn_mask, head_mask=head_mask)
- assert type(sa_raw_output) == tuple, "Expected output to be a tuple"
- sa_output = sa_raw_output[0]
- if self.output_attentions:
- sa_weights = sa_raw_output[1]# (bs, seq_length, dim), (bs, n_heads, seq_length, seq_length)
- if self.output_additional_info:
- sa_additional_info = sa_raw_output[2]
-
- sa_output = self.sa_layer_norm(sa_output + x) # (bs, seq_length, dim)
-
- # Feed Forward Network
- ffn_output = self.ffn(sa_output) # (bs, seq_length, dim)
- ffn_output = self.output_layer_norm(ffn_output + sa_output) # (bs, seq_length, dim)
-
- output = (ffn_output,)
- output = output + sa_raw_output[1:]
- return output
-
-
-class Transformer(nn.Module):
- def __init__(self, config):
- super().__init__()
- self.n_layers = config.n_layers
- self.output_attentions = config.output_attentions
- self.output_hidden_states = config.output_hidden_states
- self.output_additional_info = config.output_additional_info
-
- layer = TransformerBlock(config)
- self.layer = nn.ModuleList([copy.deepcopy(layer) for _ in range(config.n_layers)])
-
- def forward(self, x, attn_mask=None, head_mask=None):
- """
- Parameters
- ----------
- x: torch.tensor(bs, seq_length, dim)
- Input sequence embedded.
- attn_mask: torch.tensor(bs, seq_length)
- Attention mask on the sequence.
-
- Outputs
- -------
- hidden_state: torch.tensor(bs, seq_length, dim)
- Sequence of hiddens states in the last (top) layer
- all_hidden_states: Tuple[torch.tensor(bs, seq_length, dim)]
- Tuple of length n_layers with the hidden states from each layer.
- Optional: only if output_hidden_states=True
- all_attentions: Tuple[torch.tensor(bs, n_heads, seq_length, seq_length)]
- Tuple of length n_layers with the attention weights from each layer
- Optional: only if output_attentions=True
- """
- all_hidden_states = ()
- all_attentions = ()
- all_additional_info = ()
-
- hidden_state = x
- for i, layer_module in enumerate(self.layer):
- if self.output_hidden_states:
- all_hidden_states = all_hidden_states + (hidden_state,)
-
- layer_outputs = layer_module(x=hidden_state, attn_mask=attn_mask, head_mask=head_mask[i])
- hidden_state = layer_outputs[0]
-
- if self.output_attentions:
- all_attentions = all_attentions + (layer_outputs[1],)
- if self.output_additional_info:
- all_additional_info = all_additional_info + (layer_outputs[2],)
-
- outputs = (hidden_state,)
- if self.output_hidden_states:
- # Add last layer
- all_hidden_states = all_hidden_states + (hidden_state,)
- outputs = outputs + (all_hidden_states,)
-
- if self.output_attentions:
- outputs = outputs + (all_attentions,)
- if self.output_additional_info:
- outputs = outputs + (all_additional_info,)
- return outputs # last-layer hidden state, (all hidden states), (all attentions)
-
-
-# INTERFACE FOR ENCODER AND TASK SPECIFIC MODEL #
-class DistilBertPreTrainedModel(PreTrainedModel):
- """ An abstract class to handle weights initialization and
- a simple interface for downloading and loading pretrained models.
- """
-
- config_class = DistilBertConfig
- pretrained_model_archive_map = DISTILBERT_PRETRAINED_MODEL_ARCHIVE_MAP
- load_tf_weights = None
- base_model_prefix = "distilbert"
-
- def _init_weights(self, module):
- """ Initialize the weights.
- """
- if isinstance(module, nn.Embedding):
- if module.weight.requires_grad:
- module.weight.data.normal_(mean=0.0, std=self.config.initializer_range)
- if isinstance(module, nn.Linear):
- module.weight.data.normal_(mean=0.0, std=self.config.initializer_range)
- elif isinstance(module, nn.LayerNorm):
- module.bias.data.zero_()
- module.weight.data.fill_(1.0)
- if isinstance(module, nn.Linear) and module.bias is not None:
- module.bias.data.zero_()
-
-
-DISTILBERT_START_DOCSTRING = r"""
-
- This model is a PyTorch `torch.nn.Module `_ sub-class.
- Use it as a regular PyTorch Module and refer to the PyTorch documentation for all matter related to general
- usage and behavior.
-
- Parameters:
- config (:class:`~transformers.DistilBertConfig`): Model configuration class with all the parameters of the model.
- Initializing with a config file does not load the weights associated with the model, only the configuration.
- Check out the :meth:`~transformers.PreTrainedModel.from_pretrained` method to load the model weights.
-"""
-
-DISTILBERT_INPUTS_DOCSTRING = r"""
- Args:
- input_ids (:obj:`torch.LongTensor` of shape :obj:`(batch_size, sequence_length)`):
- Indices of input sequence tokens in the vocabulary.
-
- Indices can be obtained using :class:`transformers.DistilBertTokenizer`.
- See :func:`transformers.PreTrainedTokenizer.encode` and
- :func:`transformers.PreTrainedTokenizer.encode_plus` for details.
-
- `What are input IDs? <../glossary.html#input-ids>`__
- attention_mask (:obj:`torch.FloatTensor` of shape :obj:`(batch_size, sequence_length)`, `optional`, defaults to :obj:`None`):
- Mask to avoid performing attention on padding token indices.
- Mask values selected in ``[0, 1]``:
- ``1`` for tokens that are NOT MASKED, ``0`` for MASKED tokens.
-
- `What are attention masks? <../glossary.html#attention-mask>`__
- head_mask (:obj:`torch.FloatTensor` of shape :obj:`(num_heads,)` or :obj:`(num_layers, num_heads)`, `optional`, defaults to :obj:`None`):
- Mask to nullify selected heads of the self-attention modules.
- Mask values selected in ``[0, 1]``:
- :obj:`1` indicates the head is **not masked**, :obj:`0` indicates the head is **masked**.
- inputs_embeds (:obj:`torch.FloatTensor` of shape :obj:`(batch_size, sequence_length, hidden_size)`, `optional`, defaults to :obj:`None`):
- Optionally, instead of passing :obj:`input_ids` you can choose to directly pass an embedded representation.
- This is useful if you want more control over how to convert `input_ids` indices into associated vectors
- than the model's internal embedding lookup matrix.
-"""
-
-
-@add_start_docstrings(
- "The bare DistilBERT encoder/transformer outputting raw hidden-states without any specific head on top.",
- DISTILBERT_START_DOCSTRING,
-)
-class DistilBertModel(DistilBertPreTrainedModel):
- def __init__(self, config):
- super().__init__(config)
-
- self.embeddings = Embeddings(config) # Embeddings
- self.transformer = Transformer(config) # Encoder
-
- self.init_weights()
-
- def get_input_embeddings(self):
- return self.embeddings.word_embeddings
-
- def set_input_embeddings(self, new_embeddings):
- self.embeddings.word_embeddings = new_embeddings
-
- def _prune_heads(self, heads_to_prune):
- """ Prunes heads of the model.
- heads_to_prune: dict of {layer_num: list of heads to prune in this layer}
- See base class PreTrainedModel
- """
- for layer, heads in heads_to_prune.items():
- self.transformer.layer[layer].attention.prune_heads(heads)
-
- @add_start_docstrings_to_callable(DISTILBERT_INPUTS_DOCSTRING)
- def forward(self, input_ids=None, attention_mask=None, head_mask=None, inputs_embeds=None):
- r"""
- Return:
- :obj:`tuple(torch.FloatTensor)` comprising various elements depending on the configuration (:class:`~transformers.DistilBertConfig`) and inputs:
- last_hidden_state (:obj:`torch.FloatTensor` of shape :obj:`(batch_size, sequence_length, hidden_size)`):
- Sequence of hidden-states at the output of the last layer of the model.
- hidden_states (:obj:`tuple(torch.FloatTensor)`, `optional`, returned when ``config.output_hidden_states=True``):
- Tuple of :obj:`torch.FloatTensor` (one for the output of the embeddings + one for the output of each layer)
- of shape :obj:`(batch_size, sequence_length, hidden_size)`.
-
- Hidden-states of the model at the output of each layer plus the initial embedding outputs.
- attentions (:obj:`tuple(torch.FloatTensor)`, `optional`, returned when ``config.output_attentions=True``):
- Tuple of :obj:`torch.FloatTensor` (one for each layer) of shape
- :obj:`(batch_size, num_heads, sequence_length, sequence_length)`.
-
- Attentions weights after the attention softmax, used to compute the weighted average in the self-attention
- heads.
-
- Examples::
-
- from transformers import DistilBertTokenizer, DistilBertModel
- import torch
-
- tokenizer = DistilBertTokenizer.from_pretrained('distilbert-base-uncased')
- model = DistilBertModel.from_pretrained('distilbert-base-uncased')
-
- input_ids = torch.tensor(tokenizer.encode("Hello, my dog is cute", add_special_tokens=True)).unsqueeze(0) # Batch size 1
- outputs = model(input_ids)
-
- last_hidden_states = outputs[0] # The last hidden-state is the first element of the output tuple
-
- """
- if input_ids is not None and inputs_embeds is not None:
- raise ValueError("You cannot specify both input_ids and inputs_embeds at the same time")
- elif input_ids is not None:
- input_shape = input_ids.size()
- elif inputs_embeds is not None:
- input_shape = inputs_embeds.size()[:-1]
- else:
- raise ValueError("You have to specify either input_ids or inputs_embeds")
-
- device = input_ids.device if input_ids is not None else inputs_embeds.device
-
- if attention_mask is None:
- attention_mask = torch.ones(input_shape, device=device) # (bs, seq_length)
-
- # Prepare head mask if needed
- # 1.0 in head_mask indicate we keep the head
- # attention_probs has shape bsz x n_heads x N x N
- # input head_mask has shape [num_heads] or [num_hidden_layers x num_heads]
- # and head_mask is converted to shape [num_hidden_layers x batch x num_heads x seq_length x seq_length]
- if head_mask is not None:
- if head_mask.dim() == 1:
- head_mask = head_mask.unsqueeze(0).unsqueeze(0).unsqueeze(-1).unsqueeze(-1)
- head_mask = head_mask.expand(self.config.num_hidden_layers, -1, -1, -1, -1)
- elif head_mask.dim() == 2:
- head_mask = (
- head_mask.unsqueeze(1).unsqueeze(-1).unsqueeze(-1)
- ) # We can specify head_mask for each layer
- head_mask = head_mask.to(
- dtype=next(self.parameters()).dtype
- ) # switch to fload if need + fp16 compatibility
- else:
- head_mask = [None] * self.config.num_hidden_layers
-
- if inputs_embeds is None:
- inputs_embeds = self.embeddings(input_ids) # (bs, seq_length, dim)
- tfmr_output = self.transformer(x=inputs_embeds, attn_mask=attention_mask, head_mask=head_mask)
- hidden_state = tfmr_output[0]
- output = (hidden_state,) + tfmr_output[1:]
-
- return output # last-layer hidden-state, (all hidden_states), (all attentions), (all additional info)
-
-
-@add_start_docstrings(
- """DistilBert Model with a `masked language modeling` head on top. """, DISTILBERT_START_DOCSTRING,
-)
-class DistilBertForMaskedLM(DistilBertPreTrainedModel):
- def __init__(self, config):
- super().__init__(config)
- self.output_attentions = config.output_attentions
- self.output_hidden_states = config.output_hidden_states
-
- self.distilbert = DistilBertModel(config)
- self.vocab_transform = nn.Linear(config.dim, config.dim)
- self.vocab_layer_norm = nn.LayerNorm(config.dim, eps=1e-12)
- self.vocab_projector = nn.Linear(config.dim, config.vocab_size)
-
- self.init_weights()
-
- self.mlm_loss_fct = nn.CrossEntropyLoss()
-
- def get_output_embeddings(self):
- return self.vocab_projector
-
- @add_start_docstrings_to_callable(DISTILBERT_INPUTS_DOCSTRING)
- def forward(self, input_ids=None, attention_mask=None, head_mask=None, inputs_embeds=None, masked_lm_labels=None):
- r"""
- masked_lm_labels (:obj:`torch.LongTensor` of shape :obj:`(batch_size, sequence_length)`, `optional`, defaults to :obj:`None`):
- Labels for computing the masked language modeling loss.
- Indices should be in ``[-100, 0, ..., config.vocab_size]`` (see ``input_ids`` docstring)
- Tokens with indices set to ``-100`` are ignored (masked), the loss is only computed for the tokens with labels
- in ``[0, ..., config.vocab_size]``
-
- Returns:
- :obj:`tuple(torch.FloatTensor)` comprising various elements depending on the configuration (:class:`~transformers.DistilBertConfig`) and inputs:
- loss (`optional`, returned when ``masked_lm_labels`` is provided) ``torch.FloatTensor`` of shape ``(1,)``:
- Masked language modeling loss.
- prediction_scores (:obj:`torch.FloatTensor` of shape :obj:`(batch_size, sequence_length, config.vocab_size)`)
- Prediction scores of the language modeling head (scores for each vocabulary token before SoftMax).
- hidden_states (:obj:`tuple(torch.FloatTensor)`, `optional`, returned when ``config.output_hidden_states=True``):
- Tuple of :obj:`torch.FloatTensor` (one for the output of the embeddings + one for the output of each layer)
- of shape :obj:`(batch_size, sequence_length, hidden_size)`.
-
- Hidden-states of the model at the output of each layer plus the initial embedding outputs.
- attentions (:obj:`tuple(torch.FloatTensor)`, `optional`, returned when ``config.output_attentions=True``):
- Tuple of :obj:`torch.FloatTensor` (one for each layer) of shape
- :obj:`(batch_size, num_heads, sequence_length, sequence_length)`.
-
- Attentions weights after the attention softmax, used to compute the weighted average in the self-attention
- heads.
-
- Examples::
-
- from transformers import DistilBertTokenizer, DistilBertForMaskedLM
- import torch
-
- tokenizer = DistilBertTokenizer.from_pretrained('distilbert-base-uncased')
- model = DistilBertForMaskedLM.from_pretrained('distilbert-base-uncased')
- input_ids = torch.tensor(tokenizer.encode("Hello, my dog is cute", add_special_tokens=True)).unsqueeze(0) # Batch size 1
- outputs = model(input_ids, masked_lm_labels=input_ids)
- loss, prediction_scores = outputs[:2]
-
- """
- dlbrt_output = self.distilbert(
- input_ids=input_ids, attention_mask=attention_mask, head_mask=head_mask, inputs_embeds=inputs_embeds
- )
- hidden_states = dlbrt_output[0] # (bs, seq_length, dim)
- prediction_logits = self.vocab_transform(hidden_states) # (bs, seq_length, dim)
- prediction_logits = gelu(prediction_logits) # (bs, seq_length, dim)
- prediction_logits = self.vocab_layer_norm(prediction_logits) # (bs, seq_length, dim)
- prediction_logits = self.vocab_projector(prediction_logits) # (bs, seq_length, vocab_size)
-
- outputs = (prediction_logits,) + dlbrt_output[1:]
- if masked_lm_labels is not None:
- mlm_loss = self.mlm_loss_fct(
- prediction_logits.view(-1, prediction_logits.size(-1)), masked_lm_labels.view(-1)
- )
- outputs = (mlm_loss,) + outputs
-
- return outputs # (mlm_loss), prediction_logits, (all hidden_states), (all attentions)
-
-
-@add_start_docstrings(
- """DistilBert Model transformer with a sequence classification/regression head on top (a linear layer on top of
- the pooled output) e.g. for GLUE tasks. """,
- DISTILBERT_START_DOCSTRING,
-)
-class DistilBertForSequenceClassification(DistilBertPreTrainedModel):
- def __init__(self, config):
- super().__init__(config)
- self.num_labels = config.num_labels
-
- self.distilbert = DistilBertModel(config)
- self.pre_classifier = nn.Linear(config.dim, config.dim)
- self.classifier = nn.Linear(config.dim, config.num_labels)
- self.dropout = nn.Dropout(config.seq_classif_dropout)
-
- self.init_weights()
-
- @add_start_docstrings_to_callable(DISTILBERT_INPUTS_DOCSTRING)
- def forward(self, input_ids=None, attention_mask=None, head_mask=None, inputs_embeds=None, labels=None):
- r"""
- labels (:obj:`torch.LongTensor` of shape :obj:`(batch_size,)`, `optional`, defaults to :obj:`None`):
- Labels for computing the sequence classification/regression loss.
- Indices should be in :obj:`[0, ..., config.num_labels - 1]`.
- If :obj:`config.num_labels == 1` a regression loss is computed (Mean-Square loss),
- If :obj:`config.num_labels > 1` a classification loss is computed (Cross-Entropy).
-
- Returns:
- :obj:`tuple(torch.FloatTensor)` comprising various elements depending on the configuration (:class:`~transformers.DistilBertConfig`) and inputs:
- loss (:obj:`torch.FloatTensor` of shape :obj:`(1,)`, `optional`, returned when :obj:`label` is provided):
- Classification (or regression if config.num_labels==1) loss.
- logits (:obj:`torch.FloatTensor` of shape :obj:`(batch_size, config.num_labels)`):
- Classification (or regression if config.num_labels==1) scores (before SoftMax).
- hidden_states (:obj:`tuple(torch.FloatTensor)`, `optional`, returned when ``config.output_hidden_states=True``):
- Tuple of :obj:`torch.FloatTensor` (one for the output of the embeddings + one for the output of each layer)
- of shape :obj:`(batch_size, sequence_length, hidden_size)`.
-
- Hidden-states of the model at the output of each layer plus the initial embedding outputs.
- attentions (:obj:`tuple(torch.FloatTensor)`, `optional`, returned when ``config.output_attentions=True``):
- Tuple of :obj:`torch.FloatTensor` (one for each layer) of shape
- :obj:`(batch_size, num_heads, sequence_length, sequence_length)`.
-
- Attentions weights after the attention softmax, used to compute the weighted average in the self-attention
- heads.
-
- Examples::
-
- from transformers import DistilBertTokenizer, DistilBertForSequenceClassification
- import torch
-
- tokenizer = DistilBertTokenizer.from_pretrained('distilbert-base-uncased')
- model = DistilBertForSequenceClassification.from_pretrained('distilbert-base-uncased')
- input_ids = torch.tensor(tokenizer.encode("Hello, my dog is cute", add_special_tokens=True)).unsqueeze(0) # Batch size 1
- labels = torch.tensor([1]).unsqueeze(0) # Batch size 1
- outputs = model(input_ids, labels=labels)
- loss, logits = outputs[:2]
-
- """
- distilbert_output = self.distilbert(
- input_ids=input_ids, attention_mask=attention_mask, head_mask=head_mask, inputs_embeds=inputs_embeds
- )
- hidden_state = distilbert_output[0] # (bs, seq_len, dim)
- pooled_output = hidden_state[:, 0] # (bs, dim)
- pooled_output = self.pre_classifier(pooled_output) # (bs, dim)
- pooled_output = nn.ReLU()(pooled_output) # (bs, dim)
- pooled_output = self.dropout(pooled_output) # (bs, dim)
- logits = self.classifier(pooled_output) # (bs, dim)
-
- outputs = (logits,) + distilbert_output[1:]
- if labels is not None:
- if self.num_labels == 1:
- loss_fct = nn.MSELoss()
- loss = loss_fct(logits.view(-1), labels.view(-1))
- else:
- loss_fct = nn.CrossEntropyLoss()
- loss = loss_fct(logits.view(-1, self.num_labels), labels.view(-1))
- outputs = (loss,) + outputs
-
- return outputs # (loss), logits, (hidden_states), (attentions)
-
-
-@add_start_docstrings(
- """DistilBert Model with a span classification head on top for extractive question-answering tasks like SQuAD (a linear layers on top of
- the hidden-states output to compute `span start logits` and `span end logits`). """,
- DISTILBERT_START_DOCSTRING,
-)
-class DistilBertForQuestionAnswering(DistilBertPreTrainedModel):
- def __init__(self, config):
- super().__init__(config)
-
- self.distilbert = DistilBertModel(config)
- self.qa_outputs = nn.Linear(config.dim, config.num_labels)
- assert config.num_labels == 2
- self.dropout = nn.Dropout(config.qa_dropout)
-
- self.init_weights()
-
- @add_start_docstrings_to_callable(DISTILBERT_INPUTS_DOCSTRING)
- def forward(
- self,
- input_ids=None,
- attention_mask=None,
- head_mask=None,
- inputs_embeds=None,
- start_positions=None,
- end_positions=None,
- ):
- r"""
- start_positions (:obj:`torch.LongTensor` of shape :obj:`(batch_size,)`, `optional`, defaults to :obj:`None`):
- Labels for position (index) of the start of the labelled span for computing the token classification loss.
- Positions are clamped to the length of the sequence (`sequence_length`).
- Position outside of the sequence are not taken into account for computing the loss.
- end_positions (:obj:`torch.LongTensor` of shape :obj:`(batch_size,)`, `optional`, defaults to :obj:`None`):
- Labels for position (index) of the end of the labelled span for computing the token classification loss.
- Positions are clamped to the length of the sequence (`sequence_length`).
- Position outside of the sequence are not taken into account for computing the loss.
-
- Returns:
- :obj:`tuple(torch.FloatTensor)` comprising various elements depending on the configuration (:class:`~transformers.DistilBertConfig`) and inputs:
- loss (:obj:`torch.FloatTensor` of shape :obj:`(1,)`, `optional`, returned when :obj:`labels` is provided):
- Total span extraction loss is the sum of a Cross-Entropy for the start and end positions.
- start_scores (:obj:`torch.FloatTensor` of shape :obj:`(batch_size, sequence_length,)`):
- Span-start scores (before SoftMax).
- end_scores (:obj:`torch.FloatTensor` of shape :obj:`(batch_size, sequence_length,)`):
- Span-end scores (before SoftMax).
- hidden_states (:obj:`tuple(torch.FloatTensor)`, `optional`, returned when ``config.output_hidden_states=True``):
- Tuple of :obj:`torch.FloatTensor` (one for the output of the embeddings + one for the output of each layer)
- of shape :obj:`(batch_size, sequence_length, hidden_size)`.
-
- Hidden-states of the model at the output of each layer plus the initial embedding outputs.
- attentions (:obj:`tuple(torch.FloatTensor)`, `optional`, returned when ``config.output_attentions=True``):
- Tuple of :obj:`torch.FloatTensor` (one for each layer) of shape
- :obj:`(batch_size, num_heads, sequence_length, sequence_length)`.
-
- Attentions weights after the attention softmax, used to compute the weighted average in the self-attention
- heads.
-
- Examples::
-
- from transformers import DistilBertTokenizer, DistilBertForQuestionAnswering
- import torch
-
- tokenizer = DistilBertTokenizer.from_pretrained('distilbert-base-uncased')
- model = DistilBertForQuestionAnswering.from_pretrained('distilbert-base-uncased')
- input_ids = torch.tensor(tokenizer.encode("Hello, my dog is cute", add_special_tokens=True)).unsqueeze(0) # Batch size 1
- start_positions = torch.tensor([1])
- end_positions = torch.tensor([3])
- outputs = model(input_ids, start_positions=start_positions, end_positions=end_positions)
- loss, start_scores, end_scores = outputs[:3]
-
- """
- distilbert_output = self.distilbert(
- input_ids=input_ids, attention_mask=attention_mask, head_mask=head_mask, inputs_embeds=inputs_embeds
- )
- hidden_states = distilbert_output[0] # (bs, max_query_len, dim)
-
- hidden_states = self.dropout(hidden_states) # (bs, max_query_len, dim)
- logits = self.qa_outputs(hidden_states) # (bs, max_query_len, 2)
- start_logits, end_logits = logits.split(1, dim=-1)
- start_logits = start_logits.squeeze(-1) # (bs, max_query_len)
- end_logits = end_logits.squeeze(-1) # (bs, max_query_len)
-
- outputs = (start_logits, end_logits,) + distilbert_output[1:]
- if start_positions is not None and end_positions is not None:
- # If we are on multi-GPU, split add a dimension
- if len(start_positions.size()) > 1:
- start_positions = start_positions.squeeze(-1)
- if len(end_positions.size()) > 1:
- end_positions = end_positions.squeeze(-1)
- # sometimes the start/end positions are outside our model inputs, we ignore these terms
- ignored_index = start_logits.size(1)
- start_positions.clamp_(0, ignored_index)
- end_positions.clamp_(0, ignored_index)
-
- loss_fct = nn.CrossEntropyLoss(ignore_index=ignored_index)
- start_loss = loss_fct(start_logits, start_positions)
- end_loss = loss_fct(end_logits, end_positions)
- total_loss = (start_loss + end_loss) / 2
- outputs = (total_loss,) + outputs
-
- return outputs # (loss), start_logits, end_logits, (hidden_states), (attentions)
-
-
-@add_start_docstrings(
- """DistilBert Model with a token classification head on top (a linear layer on top of
- the hidden-states output) e.g. for Named-Entity-Recognition (NER) tasks. """,
- DISTILBERT_START_DOCSTRING,
-)
-class DistilBertForTokenClassification(DistilBertPreTrainedModel):
- def __init__(self, config):
- super().__init__(config)
- self.num_labels = config.num_labels
-
- self.distilbert = DistilBertModel(config)
- self.dropout = nn.Dropout(config.dropout)
- self.classifier = nn.Linear(config.hidden_size, config.num_labels)
-
- self.init_weights()
-
- @add_start_docstrings_to_callable(DISTILBERT_INPUTS_DOCSTRING)
- def forward(self, input_ids=None, attention_mask=None, head_mask=None, inputs_embeds=None, labels=None):
- r"""
- labels (:obj:`torch.LongTensor` of shape :obj:`(batch_size, sequence_length)`, `optional`, defaults to :obj:`None`):
- Labels for computing the token classification loss.
- Indices should be in ``[0, ..., config.num_labels - 1]``.
-
- Returns:
- :obj:`tuple(torch.FloatTensor)` comprising various elements depending on the configuration (:class:`~transformers.DistilBertConfig`) and inputs:
- loss (:obj:`torch.FloatTensor` of shape :obj:`(1,)`, `optional`, returned when ``labels`` is provided) :
- Classification loss.
- scores (:obj:`torch.FloatTensor` of shape :obj:`(batch_size, sequence_length, config.num_labels)`)
- Classification scores (before SoftMax).
- hidden_states (:obj:`tuple(torch.FloatTensor)`, `optional`, returned when ``config.output_hidden_states=True``):
- Tuple of :obj:`torch.FloatTensor` (one for the output of the embeddings + one for the output of each layer)
- of shape :obj:`(batch_size, sequence_length, hidden_size)`.
-
- Hidden-states of the model at the output of each layer plus the initial embedding outputs.
- attentions (:obj:`tuple(torch.FloatTensor)`, `optional`, returned when ``config.output_attentions=True``):
- Tuple of :obj:`torch.FloatTensor` (one for each layer) of shape
- :obj:`(batch_size, num_heads, sequence_length, sequence_length)`.
-
- Attentions weights after the attention softmax, used to compute the weighted average in the self-attention
- heads.
-
- Examples::
-
- from transformers import DistilBertTokenizer, DistilBertForTokenClassification
- import torch
-
- tokenizer = DistilBertTokenizer.from_pretrained('distilbert-base-uncased')
- model = DistilBertForTokenClassification.from_pretrained('distilbert-base-uncased')
- input_ids = torch.tensor(tokenizer.encode("Hello, my dog is cute")).unsqueeze(0) # Batch size 1
- labels = torch.tensor([1] * input_ids.size(1)).unsqueeze(0) # Batch size 1
- outputs = model(input_ids, labels=labels)
- loss, scores = outputs[:2]
-
- """
-
- outputs = self.distilbert(
- input_ids, attention_mask=attention_mask, head_mask=head_mask, inputs_embeds=inputs_embeds
- )
-
- sequence_output = outputs[0]
-
- sequence_output = self.dropout(sequence_output)
- logits = self.classifier(sequence_output)
-
- outputs = (logits,) + outputs[2:] # add hidden states and attention if they are here
- if labels is not None:
- loss_fct = CrossEntropyLoss()
- # Only keep active parts of the loss
- if attention_mask is not None:
- active_loss = attention_mask.view(-1) == 1
- active_logits = logits.view(-1, self.num_labels)[active_loss]
- active_labels = labels.view(-1)[active_loss]
- loss = loss_fct(active_logits, active_labels)
- else:
- loss = loss_fct(logits.view(-1, self.num_labels), labels.view(-1))
- outputs = (loss,) + outputs
-
- return outputs # (loss), scores, (hidden_states), (attentions)
diff --git a/server/transformers/src/transformers/modeling_encoder_decoder.py b/server/transformers/src/transformers/modeling_encoder_decoder.py
deleted file mode 100644
index 0951baff7d4c3b207013850437b815cfddba7c9e..0000000000000000000000000000000000000000
--- a/server/transformers/src/transformers/modeling_encoder_decoder.py
+++ /dev/null
@@ -1,350 +0,0 @@
-# coding=utf-8
-# Copyright 2018 The HuggingFace Inc. team.
-#
-# Licensed under the Apache License, Version 2.0 (the "License");
-# you may not use this file except in compliance with the License.
-# You may obtain a copy of the License at
-#
-# http://www.apache.org/licenses/LICENSE-2.0
-#
-# Unless required by applicable law or agreed to in writing, software
-# distributed under the License is distributed on an "AS IS" BASIS,
-# WITHOUT WARRANTIES OR CONDITIONS OF ANY KIND, either express or implied.
-# See the License for the specific language governing permissions and
-# limitations under the License.
-""" Classes to support Encoder-Decoder architectures """
-
-
-import logging
-import os
-
-import torch
-from torch import nn
-
-from .modeling_auto import AutoModel, AutoModelWithLMHead
-
-
-logger = logging.getLogger(__name__)
-
-
-class PreTrainedEncoderDecoder(nn.Module):
- r"""
- :class:`~transformers.PreTrainedEncoderDecoder` is a generic model class that will be
- instantiated as a transformer architecture with one of the base model
- classes of the library as encoder and (optionally) another one as
- decoder when created with the `AutoModel.from_pretrained(pretrained_model_name_or_path)`
- class method.
- """
-
- def __init__(self, encoder, decoder):
- super().__init__()
- self.encoder = encoder
- self.decoder = decoder
-
- @classmethod
- def from_pretrained(
- cls,
- encoder_pretrained_model_name_or_path=None,
- decoder_pretrained_model_name_or_path=None,
- *model_args,
- **kwargs
- ):
- r""" Instantiates an encoder and a decoder from one or two base classes of the library from pre-trained model checkpoints.
-
-
- The model is set in evaluation mode by default using `model.eval()` (Dropout modules are deactivated)
- To train the model, you need to first set it back in training mode with `model.train()`
-
- Params:
- encoder_pretrained_model_name_or_path: information necessary to initiate the encoder. Either:
-
- - a string with the `shortcut name` of a pre-trained model to load from cache or download, e.g.: ``bert-base-uncased``.
- - a string with the `identifier name` of a pre-trained model that was user-uploaded to our S3, e.g.: ``dbmdz/bert-base-german-cased``.
- - a path to a `directory` containing model weights saved using :func:`~transformers.PreTrainedModel.save_pretrained`, e.g.: ``./my_model_directory/encoder``.
- - a path or url to a `tensorflow index checkpoint file` (e.g. `./tf_model/model.ckpt.index`). In this case, ``from_tf`` should be set to True and a configuration object should be provided as ``config`` argument. This loading path is slower than converting the TensorFlow checkpoint in a PyTorch model using the provided conversion scripts and loading the PyTorch model afterwards.
-
- decoder_pretrained_model_name_or_path: information necessary to initiate the decoder. Either:
-
- - a string with the `shortcut name` of a pre-trained model to load from cache or download, e.g.: ``bert-base-uncased``.
- - a string with the `identifier name` of a pre-trained model that was user-uploaded to our S3, e.g.: ``dbmdz/bert-base-german-cased``.
- - a path to a `directory` containing model weights saved using :func:`~transformers.PreTrainedModel.save_pretrained`, e.g.: ``./my_model_directory/decoder``.
- - a path or url to a `tensorflow index checkpoint file` (e.g. `./tf_model/model.ckpt.index`). In this case, ``from_tf`` should be set to True and a configuration object should be provided as ``config`` argument. This loading path is slower than converting the TensorFlow checkpoint in a PyTorch model using the provided conversion scripts and loading the PyTorch model afterwards.
-
- model_args: (`optional`) Sequence of positional arguments:
- All remaning positional arguments will be passed to the underlying model's ``__init__`` method
-
- config: (`optional`) instance of a class derived from :class:`~transformers.PretrainedConfig`:
- Configuration for the model to use instead of an automatically loaded configuation. Configuration can be automatically loaded when:
-
- - the model is a model provided by the library (loaded with the ``shortcut-name`` string of a pretrained model), or
- - the model was saved using :func:`~transformers.PreTrainedModel.save_pretrained` and is reloaded by suppling the save directory.
- - the model is loaded by suppling a local directory as ``pretrained_model_name_or_path`` and a configuration JSON file named `config.json` is found in the directory.
-
- state_dict: (`optional`) dict:
- an optional state dictionnary for the model to use instead of a state dictionary loaded from saved weights file.
- This option can be used if you want to create a model from a pretrained configuration but load your own weights.
- In this case though, you should check if using :func:`~transformers.PreTrainedModel.save_pretrained` and :func:`~transformers.PreTrainedModel.from_pretrained` is not a simpler option.
-
- cache_dir: (`optional`) string:
- Path to a directory in which a downloaded pre-trained model
- configuration should be cached if the standard cache should not be used.
-
- force_download: (`optional`) boolean, default False:
- Force to (re-)download the model weights and configuration files and override the cached versions if they exists.
-
- proxies: (`optional`) dict, default None:
- A dictionary of proxy servers to use by protocol or endpoint, e.g.: {'http': 'foo.bar:3128', 'http://hostname': 'foo.bar:4012'}.
- The proxies are used on each request.
-
- output_loading_info: (`optional`) boolean:
- Set to ``True`` to also return a dictionnary containing missing keys, unexpected keys and error messages.
-
- kwargs: (`optional`) Remaining dictionary of keyword arguments.
- Can be used to update the configuration object (after it being loaded) and initiate the model. (e.g. ``output_attention=True``). Behave differently depending on whether a `config` is provided or automatically loaded:
-
- - If a configuration is provided with ``config``, ``**kwargs`` will be directly passed to the underlying model's ``__init__`` method (we assume all relevant updates to the configuration have already been done)
- - If a configuration is not provided, ``kwargs`` will be first passed to the configuration class initialization function (:func:`~transformers.PretrainedConfig.from_pretrained`). Each key of ``kwargs`` that corresponds to a configuration attribute will be used to override said attribute with the supplied ``kwargs`` value. Remaining keys that do not correspond to any configuration attribute will be passed to the underlying model's ``__init__`` function.
-
- You can specify kwargs sepcific for the encoder and decoder by prefixing the key with `encoder_` and `decoder_` respectively. (e.g. ``decoder_output_attention=True``). The remaining kwargs will be passed to both encoders and decoders.
-
- Examples::
-
- # For example purposes. Not runnable.
- model = PreTrainedEncoderDecoder.from_pretained('bert-base-uncased', 'bert-base-uncased') # initialize Bert2Bert
- """
-
- # keyword arguments come in 3 flavors: encoder-specific (prefixed by
- # `encoder_`), decoder-specific (prefixed by `decoder_`) and those
- # that apply to the model as a whole.
- # We let the specific kwargs override the common ones in case of conflict.
- kwargs_common = {
- argument: value
- for argument, value in kwargs.items()
- if not argument.startswith("encoder_") and not argument.startswith("decoder_")
- }
- kwargs_decoder = kwargs_common.copy()
- kwargs_encoder = kwargs_common.copy()
- kwargs_encoder.update(
- {
- argument[len("encoder_") :]: value
- for argument, value in kwargs.items()
- if argument.startswith("encoder_")
- }
- )
- kwargs_decoder.update(
- {
- argument[len("decoder_") :]: value
- for argument, value in kwargs.items()
- if argument.startswith("decoder_")
- }
- )
-
- # Load and initialize the encoder and decoder
- # The distinction between encoder and decoder at the model level is made
- # by the value of the flag `is_decoder` that we need to set correctly.
- encoder = kwargs_encoder.pop("model", None)
- if encoder is None:
- encoder = AutoModel.from_pretrained(encoder_pretrained_model_name_or_path, *model_args, **kwargs_encoder)
- encoder.config.is_decoder = False
-
- decoder = kwargs_decoder.pop("model", None)
- if decoder is None:
- decoder = AutoModelWithLMHead.from_pretrained(decoder_pretrained_model_name_or_path, **kwargs_decoder)
- decoder.config.is_decoder = True
-
- model = cls(encoder, decoder)
-
- return model
-
- def save_pretrained(self, save_directory):
- """ Save a Seq2Seq model and its configuration file in a format such
- that it can be loaded using `:func:`~transformers.PreTrainedEncoderDecoder.from_pretrained`
-
- We save the encoder' and decoder's parameters in two separate directories.
- """
-
- # If the root output directory does not exist, create it
- if not os.path.exists(save_directory):
- os.mkdir(save_directory)
-
- # Check whether the output directory is empty or not
- sub_directories = [
- directory
- for directory in os.listdir(save_directory)
- if os.path.isdir(os.path.join(save_directory, directory))
- ]
-
- if len(sub_directories) > 0:
- if "encoder" in sub_directories and "decoder" in sub_directories:
- print(
- "WARNING: there is an older version of encoder-decoder saved in"
- + " the output directory. The default behaviour is to overwrite them."
- )
-
- # Empty the output directory
- for directory_to_remove in sub_directories:
- # Remove all files into the subdirectory
- files_to_remove = os.listdir(os.path.join(save_directory, directory_to_remove))
- for file_to_remove in files_to_remove:
- os.remove(os.path.join(save_directory, directory_to_remove, file_to_remove))
- # Remove the subdirectory itself
- os.rmdir(os.path.join(save_directory, directory_to_remove))
-
- assert len(os.listdir(save_directory)) == 0 # sanity check
-
- # Create the "encoder" directory inside the output directory and save the encoder into it
- if not os.path.exists(os.path.join(save_directory, "encoder")):
- os.mkdir(os.path.join(save_directory, "encoder"))
- self.encoder.save_pretrained(os.path.join(save_directory, "encoder"))
-
- # Create the "encoder" directory inside the output directory and save the decoder into it
- if not os.path.exists(os.path.join(save_directory, "decoder")):
- os.mkdir(os.path.join(save_directory, "decoder"))
- self.decoder.save_pretrained(os.path.join(save_directory, "decoder"))
-
- def forward(self, encoder_input_ids, decoder_input_ids, **kwargs):
- """ The forward pass on a seq2eq depends what we are performing:
-
- - During training we perform one forward pass through both the encoder
- and decoder;
- - During prediction, we perform one forward pass through the encoder,
- and then perform several forward passes with the encoder's hidden
- state through the decoder to decode a full sequence.
-
- Therefore, we skip the forward pass on the encoder if an argument named
- `encoder_hidden_state` is passed to this function.
-
- Params:
- encoder_input_ids: ``torch.LongTensor`` of shape ``(batch_size, sequence_length)``
- Indices of encoder input sequence tokens in the vocabulary.
- decoder_input_ids: ``torch.LongTensor`` of shape ``(batch_size, sequence_length)``
- Indices of decoder input sequence tokens in the vocabulary.
- kwargs: (`optional`) Remaining dictionary of keyword arguments.
- """
- kwargs_encoder, kwargs_decoder = self.prepare_model_kwargs(**kwargs)
-
- # Encode if needed (training, first prediction pass)
- encoder_hidden_states = kwargs_encoder.pop("hidden_states", None)
- if encoder_hidden_states is None:
- encoder_outputs = self.encoder(encoder_input_ids, **kwargs_encoder)
- encoder_hidden_states = encoder_outputs[0]
- else:
- encoder_outputs = ()
-
- kwargs_decoder["encoder_hidden_states"] = encoder_hidden_states
- decoder_outputs = self.decoder(decoder_input_ids, encoder_hidden_states, **kwargs_decoder)
-
- return decoder_outputs + encoder_outputs
-
- @staticmethod
- def prepare_model_kwargs(**kwargs):
- """ Prepare the encoder and decoder's keyword arguments.
-
- Keyword arguments come in 3 flavors:
- - encoder-specific (prefixed by `encoder_`)
- - decoder-specific (prefixed by `decoder_`)
- - those that apply to the model as whole.
-
- We let the specific kwargs override the common ones in case of
- conflict.
- """
- kwargs_common = {
- argument: value
- for argument, value in kwargs.items()
- if not argument.startswith("encoder_") and not argument.startswith("decoder_")
- }
- decoder_kwargs = kwargs_common.copy()
- encoder_kwargs = kwargs_common.copy()
- encoder_kwargs.update(
- {
- argument[len("encoder_") :]: value
- for argument, value in kwargs.items()
- if argument.startswith("encoder_")
- }
- )
- decoder_kwargs.update(
- {
- argument[len("decoder_") :]: value
- for argument, value in kwargs.items()
- if argument.startswith("decoder_")
- }
- )
- decoder_kwargs["encoder_attention_mask"] = encoder_kwargs.get("attention_mask", None)
- return encoder_kwargs, decoder_kwargs
-
-
-class Model2Model(PreTrainedEncoderDecoder):
- r"""
- :class:`~transformers.Model2Model` instantiates a Seq2Seq2 model
- where both of the encoder and decoder are of the same family. If the
- name of or that path to a pretrained model is specified the encoder and
- the decoder will be initialized with the pretrained weight (the
- cross-attention will be intialized randomly if its weights are not
- present).
-
- It is possible to override this behavior and initialize, say, the decoder randomly
- by creating it beforehand as follows
-
- config = BertConfig.from_pretrained()
- decoder = BertForMaskedLM(config)
- model = Model2Model.from_pretrained('bert-base-uncased', decoder_model=decoder)
- """
-
- def __init__(self, *args, **kwargs):
- super().__init__(*args, **kwargs)
- self.tie_weights()
-
- def tie_weights(self):
- """ Tying the encoder and decoders' embeddings together.
-
- We need for each to get down to the embedding weights. However the
- different model classes are inconsistent to that respect:
- - BertModel: embeddings.word_embeddings
- - RoBERTa: embeddings.word_embeddings
- - XLMModel: embeddings
- - GPT2: wte
- - BertForMaskedLM: bert.embeddings.word_embeddings
- - RobertaForMaskedLM: roberta.embeddings.word_embeddings
-
- argument of the XEmbedding layer for each model, but it is "blocked"
- by a model-specific keyword (bert, )...
- """
- # self._tie_or_clone_weights(self.encoder, self.decoder)
- pass
-
- @classmethod
- def from_pretrained(cls, pretrained_model_name_or_path, *args, **kwargs):
-
- if (
- "bert" not in pretrained_model_name_or_path
- or "roberta" in pretrained_model_name_or_path
- or "distilbert" in pretrained_model_name_or_path
- ):
- raise ValueError("Only the Bert model is currently supported.")
-
- model = super().from_pretrained(
- encoder_pretrained_model_name_or_path=pretrained_model_name_or_path,
- decoder_pretrained_model_name_or_path=pretrained_model_name_or_path,
- *args,
- **kwargs,
- )
-
- return model
-
-
-class Model2LSTM(PreTrainedEncoderDecoder):
- @classmethod
- def from_pretrained(cls, *args, **kwargs):
- if kwargs.get("decoder_model", None) is None:
- # We will create a randomly initilized LSTM model as decoder
- if "decoder_config" not in kwargs:
- raise ValueError(
- "To load an LSTM in Encoder-Decoder model, please supply either: "
- " - a torch.nn.LSTM model as `decoder_model` parameter (`decoder_model=lstm_model`), or"
- " - a dictionary of configuration parameters that will be used to initialize a"
- " torch.nn.LSTM model as `decoder_config` keyword argument. "
- " E.g. `decoder_config={'input_size': 768, 'hidden_size': 768, 'num_layers': 2}`"
- )
- kwargs["decoder_model"] = torch.nn.LSTM(kwargs.pop("decoder_config"))
- model = super().from_pretrained(*args, **kwargs)
- return model
diff --git a/server/transformers/src/transformers/modeling_flaubert.py b/server/transformers/src/transformers/modeling_flaubert.py
deleted file mode 100644
index 6ec64ba8cc32990b63eceff4dd551fe261a83d63..0000000000000000000000000000000000000000
--- a/server/transformers/src/transformers/modeling_flaubert.py
+++ /dev/null
@@ -1,385 +0,0 @@
-# coding=utf-8
-# Copyright 2019-present CNRS, Facebook Inc. and the HuggingFace Inc. team.
-#
-# Licensed under the Apache License, Version 2.0 (the "License");
-# you may not use this file except in compliance with the License.
-# You may obtain a copy of the License at
-#
-# http://www.apache.org/licenses/LICENSE-2.0
-#
-# Unless required by applicable law or agreed to in writing, software
-# distributed under the License is distributed on an "AS IS" BASIS,
-# WITHOUT WARRANTIES OR CONDITIONS OF ANY KIND, either express or implied.
-# See the License for the specific language governing permissions and
-# limitations under the License.
-""" PyTorch Flaubert model, based on XLM. """
-
-
-import logging
-import random
-
-import torch
-from torch.nn import functional as F
-
-from .configuration_flaubert import FlaubertConfig
-from .file_utils import add_start_docstrings, add_start_docstrings_to_callable
-from .modeling_xlm import (
- XLMForQuestionAnswering,
- XLMForQuestionAnsweringSimple,
- XLMForSequenceClassification,
- XLMModel,
- XLMWithLMHeadModel,
- get_masks,
-)
-
-
-logger = logging.getLogger(__name__)
-
-FLAUBERT_PRETRAINED_MODEL_ARCHIVE_MAP = {
- "flaubert-small-cased": "https://s3.amazonaws.com/models.huggingface.co/bert/flaubert/flaubert_small_cased/pytorch_model.bin",
- "flaubert-base-uncased": "https://s3.amazonaws.com/models.huggingface.co/bert/flaubert/flaubert_base_uncased/pytorch_model.bin",
- "flaubert-base-cased": "https://s3.amazonaws.com/models.huggingface.co/bert/flaubert/flaubert_base_cased/pytorch_model.bin",
- "flaubert-large-cased": "https://s3.amazonaws.com/models.huggingface.co/bert/flaubert/flaubert_large_cased/pytorch_model.bin",
-}
-
-
-FLAUBERT_START_DOCSTRING = r"""
-
- This model is a PyTorch `torch.nn.Module `_ sub-class.
- Use it as a regular PyTorch Module and refer to the PyTorch documentation for all matter related to general
- usage and behavior.
-
- Parameters:
- config (:class:`~transformers.FlaubertConfig`): Model configuration class with all the parameters of the model.
- Initializing with a config file does not load the weights associated with the model, only the configuration.
- Check out the :meth:`~transformers.PreTrainedModel.from_pretrained` method to load the model weights.
-"""
-
-FLAUBERT_INPUTS_DOCSTRING = r"""
- Args:
- input_ids (:obj:`torch.LongTensor` of shape :obj:`(batch_size, sequence_length)`):
- Indices of input sequence tokens in the vocabulary.
-
- Indices can be obtained using :class:`transformers.BertTokenizer`.
- See :func:`transformers.PreTrainedTokenizer.encode` and
- :func:`transformers.PreTrainedTokenizer.encode_plus` for details.
-
- `What are input IDs? <../glossary.html#input-ids>`__
- attention_mask (:obj:`torch.FloatTensor` of shape :obj:`(batch_size, sequence_length)`, `optional`, defaults to :obj:`None`):
- Mask to avoid performing attention on padding token indices.
- Mask values selected in ``[0, 1]``:
- ``1`` for tokens that are NOT MASKED, ``0`` for MASKED tokens.
-
- `What are attention masks? <../glossary.html#attention-mask>`__
- token_type_ids (:obj:`torch.LongTensor` of shape :obj:`(batch_size, sequence_length)`, `optional`, defaults to :obj:`None`):
- Segment token indices to indicate first and second portions of the inputs.
- Indices are selected in ``[0, 1]``: ``0`` corresponds to a `sentence A` token, ``1``
- corresponds to a `sentence B` token
-
- `What are token type IDs? <../glossary.html#token-type-ids>`_
- position_ids (:obj:`torch.LongTensor` of shape :obj:`(batch_size, sequence_length)`, `optional`, defaults to :obj:`None`):
- Indices of positions of each input sequence tokens in the position embeddings.
- Selected in the range ``[0, config.max_position_embeddings - 1]``.
-
- `What are position IDs? <../glossary.html#position-ids>`_
- lengths (:obj:`torch.LongTensor` of shape :obj:`(batch_size,)`, `optional`, defaults to :obj:`None`):
- Length of each sentence that can be used to avoid performing attention on padding token indices.
- You can also use `attention_mask` for the same result (see above), kept here for compatbility.
- Indices selected in ``[0, ..., input_ids.size(-1)]``:
- cache (:obj:`Dict[str, torch.FloatTensor]`, `optional`, defaults to :obj:`None`):
- dictionary with ``torch.FloatTensor`` that contains pre-computed
- hidden-states (key and values in the attention blocks) as computed by the model
- (see `cache` output below). Can be used to speed up sequential decoding.
- The dictionary object will be modified in-place during the forward pass to add newly computed hidden-states.
- head_mask (:obj:`torch.FloatTensor` of shape :obj:`(num_heads,)` or :obj:`(num_layers, num_heads)`, `optional`, defaults to :obj:`None`):
- Mask to nullify selected heads of the self-attention modules.
- Mask values selected in ``[0, 1]``:
- :obj:`1` indicates the head is **not masked**, :obj:`0` indicates the head is **masked**.
- input_embeds (:obj:`torch.FloatTensor` of shape :obj:`(batch_size, sequence_length, hidden_size)`, `optional`, defaults to :obj:`None`):
- Optionally, instead of passing :obj:`input_ids` you can choose to directly pass an embedded representation.
- This is useful if you want more control over how to convert `input_ids` indices into associated vectors
- than the model's internal embedding lookup matrix.
-"""
-
-
-@add_start_docstrings(
- "The bare Flaubert Model transformer outputting raw hidden-states without any specific head on top.",
- FLAUBERT_START_DOCSTRING,
-)
-class FlaubertModel(XLMModel):
-
- config_class = FlaubertConfig
- pretrained_model_archive_map = FLAUBERT_PRETRAINED_MODEL_ARCHIVE_MAP
-
- def __init__(self, config): # , dico, is_encoder, with_output):
- super(FlaubertModel, self).__init__(config)
- self.layerdrop = getattr(config, "layerdrop", 0.0)
- self.pre_norm = getattr(config, "pre_norm", False)
-
- @add_start_docstrings_to_callable(FLAUBERT_INPUTS_DOCSTRING)
- def forward(
- self,
- input_ids=None,
- attention_mask=None,
- langs=None,
- token_type_ids=None,
- position_ids=None,
- lengths=None,
- cache=None,
- head_mask=None,
- inputs_embeds=None,
- ):
- r"""
- Return:
- :obj:`tuple(torch.FloatTensor)` comprising various elements depending on the configuration (:class:`~transformers.XLMConfig`) and inputs:
- last_hidden_state (:obj:`torch.FloatTensor` of shape :obj:`(batch_size, sequence_length, hidden_size)`):
- Sequence of hidden-states at the output of the last layer of the model.
- hidden_states (:obj:`tuple(torch.FloatTensor)`, `optional`, returned when ``config.output_hidden_states=True``):
- Tuple of :obj:`torch.FloatTensor` (one for the output of the embeddings + one for the output of each layer)
- of shape :obj:`(batch_size, sequence_length, hidden_size)`.
-
- Hidden-states of the model at the output of each layer plus the initial embedding outputs.
- attentions (:obj:`tuple(torch.FloatTensor)`, `optional`, returned when ``config.output_attentions=True``):
- Tuple of :obj:`torch.FloatTensor` (one for each layer) of shape
- :obj:`(batch_size, num_heads, sequence_length, sequence_length)`.
-
- Attentions weights after the attention softmax, used to compute the weighted average in the self-attention
- heads.
-
- Examples::
-
- tokenizer = FlaubertTokenizer.from_pretrained('flaubert-base-cased')
- model = FlaubertModel.from_pretrained('flaubert-base-cased')
- input_ids = torch.tensor(tokenizer.encode("Le chat manges une pomme.", add_special_tokens=True)).unsqueeze(0) # Batch size 1
- outputs = model(input_ids)
- last_hidden_states = outputs[0] # The last hidden-state is the first element of the output tuple
-
- """
- # removed: src_enc=None, src_len=None
- if input_ids is not None:
- bs, slen = input_ids.size()
- else:
- bs, slen = inputs_embeds.size()[:-1]
-
- if lengths is None:
- if input_ids is not None:
- lengths = (input_ids != self.pad_index).sum(dim=1).long()
- else:
- lengths = torch.LongTensor([slen] * bs)
- # mask = input_ids != self.pad_index
-
- # check inputs
- assert lengths.size(0) == bs
- assert lengths.max().item() <= slen
- # input_ids = input_ids.transpose(0, 1) # batch size as dimension 0
- # assert (src_enc is None) == (src_len is None)
- # if src_enc is not None:
- # assert self.is_decoder
- # assert src_enc.size(0) == bs
-
- # generate masks
- mask, attn_mask = get_masks(slen, lengths, self.causal, padding_mask=attention_mask)
- # if self.is_decoder and src_enc is not None:
- # src_mask = torch.arange(src_len.max(), dtype=torch.long, device=lengths.device) < src_len[:, None]
-
- device = input_ids.device if input_ids is not None else inputs_embeds.device
-
- # position_ids
- if position_ids is None:
- position_ids = torch.arange(slen, dtype=torch.long, device=device)
- position_ids = position_ids.unsqueeze(0).expand((bs, slen))
- else:
- assert position_ids.size() == (bs, slen) # (slen, bs)
- # position_ids = position_ids.transpose(0, 1)
-
- # langs
- if langs is not None:
- assert langs.size() == (bs, slen) # (slen, bs)
- # langs = langs.transpose(0, 1)
-
- # Prepare head mask if needed
- # 1.0 in head_mask indicate we keep the head
- # attention_probs has shape bsz x n_heads x N x N
- # input head_mask has shape [num_heads] or [num_hidden_layers x num_heads]
- # and head_mask is converted to shape [num_hidden_layers x batch x num_heads x qlen x klen]
- if head_mask is not None:
- if head_mask.dim() == 1:
- head_mask = head_mask.unsqueeze(0).unsqueeze(0).unsqueeze(-1).unsqueeze(-1)
- head_mask = head_mask.expand(self.n_layers, -1, -1, -1, -1)
- elif head_mask.dim() == 2:
- head_mask = (
- head_mask.unsqueeze(1).unsqueeze(-1).unsqueeze(-1)
- ) # We can specify head_mask for each layer
- head_mask = head_mask.to(
- dtype=next(self.parameters()).dtype
- ) # switch to fload if need + fp16 compatibility
- else:
- head_mask = [None] * self.n_layers
-
- # do not recompute cached elements
- if cache is not None and input_ids is not None:
- _slen = slen - cache["slen"]
- input_ids = input_ids[:, -_slen:]
- position_ids = position_ids[:, -_slen:]
- if langs is not None:
- langs = langs[:, -_slen:]
- mask = mask[:, -_slen:]
- attn_mask = attn_mask[:, -_slen:]
-
- # embeddings
- if inputs_embeds is None:
- inputs_embeds = self.embeddings(input_ids)
-
- tensor = inputs_embeds + self.position_embeddings(position_ids).expand_as(inputs_embeds)
- if langs is not None and self.use_lang_emb:
- tensor = tensor + self.lang_embeddings(langs)
- if token_type_ids is not None:
- tensor = tensor + self.embeddings(token_type_ids)
- tensor = self.layer_norm_emb(tensor)
- tensor = F.dropout(tensor, p=self.dropout, training=self.training)
- tensor *= mask.unsqueeze(-1).to(tensor.dtype)
-
- # transformer layers
- hidden_states = ()
- attentions = ()
- for i in range(self.n_layers):
- # LayerDrop
- dropout_probability = random.uniform(0, 1)
- if self.training and (dropout_probability < self.layerdrop):
- continue
-
- if self.output_hidden_states:
- hidden_states = hidden_states + (tensor,)
-
- # self attention
- if not self.pre_norm:
- attn_outputs = self.attentions[i](tensor, attn_mask, cache=cache, head_mask=head_mask[i])
- attn = attn_outputs[0]
- if self.output_attentions:
- attentions = attentions + (attn_outputs[1],)
- attn = F.dropout(attn, p=self.dropout, training=self.training)
- tensor = tensor + attn
- tensor = self.layer_norm1[i](tensor)
- else:
- tensor_normalized = self.layer_norm1[i](tensor)
- attn_outputs = self.attentions[i](tensor_normalized, attn_mask, cache=cache, head_mask=head_mask[i])
- attn = attn_outputs[0]
- if self.output_attentions:
- attentions = attentions + (attn_outputs[1],)
- attn = F.dropout(attn, p=self.dropout, training=self.training)
- tensor = tensor + attn
-
- # encoder attention (for decoder only)
- # if self.is_decoder and src_enc is not None:
- # attn = self.encoder_attn[i](tensor, src_mask, kv=src_enc, cache=cache)
- # attn = F.dropout(attn, p=self.dropout, training=self.training)
- # tensor = tensor + attn
- # tensor = self.layer_norm15[i](tensor)
-
- # FFN
- if not self.pre_norm:
- tensor = tensor + self.ffns[i](tensor)
- tensor = self.layer_norm2[i](tensor)
- else:
- tensor_normalized = self.layer_norm2[i](tensor)
- tensor = tensor + self.ffns[i](tensor_normalized)
-
- tensor *= mask.unsqueeze(-1).to(tensor.dtype)
-
- # Add last hidden state
- if self.output_hidden_states:
- hidden_states = hidden_states + (tensor,)
-
- # update cache length
- if cache is not None:
- cache["slen"] += tensor.size(1)
-
- # move back sequence length to dimension 0
- # tensor = tensor.transpose(0, 1)
-
- outputs = (tensor,)
- if self.output_hidden_states:
- outputs = outputs + (hidden_states,)
- if self.output_attentions:
- outputs = outputs + (attentions,)
- return outputs # outputs, (hidden_states), (attentions)
-
-
-@add_start_docstrings(
- """The Flaubert Model transformer with a language modeling head on top
- (linear layer with weights tied to the input embeddings). """,
- FLAUBERT_START_DOCSTRING,
-)
-class FlaubertWithLMHeadModel(XLMWithLMHeadModel):
- """
- This class overrides :class:`~transformers.XLMWithLMHeadModel`. Please check the
- superclass for the appropriate documentation alongside usage examples.
- """
-
- config_class = FlaubertConfig
- pretrained_model_archive_map = FLAUBERT_PRETRAINED_MODEL_ARCHIVE_MAP
-
- def __init__(self, config):
- super(FlaubertWithLMHeadModel, self).__init__(config)
- self.transformer = FlaubertModel(config)
- self.init_weights()
-
-
-@add_start_docstrings(
- """Flaubert Model with a sequence classification/regression head on top (a linear layer on top of
- the pooled output) e.g. for GLUE tasks. """,
- FLAUBERT_START_DOCSTRING,
-)
-class FlaubertForSequenceClassification(XLMForSequenceClassification):
- """
- This class overrides :class:`~transformers.XLMForSequenceClassification`. Please check the
- superclass for the appropriate documentation alongside usage examples.
- """
-
- config_class = FlaubertConfig
- pretrained_model_archive_map = FLAUBERT_PRETRAINED_MODEL_ARCHIVE_MAP
-
- def __init__(self, config):
- super(FlaubertForSequenceClassification, self).__init__(config)
- self.transformer = FlaubertModel(config)
- self.init_weights()
-
-
-@add_start_docstrings(
- """Flaubert Model with a span classification head on top for extractive question-answering tasks like SQuAD (a linear layers on top of
- the hidden-states output to compute `span start logits` and `span end logits`). """,
- FLAUBERT_START_DOCSTRING,
-)
-class FlaubertForQuestionAnsweringSimple(XLMForQuestionAnsweringSimple):
- """
- This class overrides :class:`~transformers.XLMForQuestionAnsweringSimple`. Please check the
- superclass for the appropriate documentation alongside usage examples.
- """
-
- config_class = FlaubertConfig
- pretrained_model_archive_map = FLAUBERT_PRETRAINED_MODEL_ARCHIVE_MAP
-
- def __init__(self, config):
- super(FlaubertForQuestionAnsweringSimple, self).__init__(config)
- self.transformer = FlaubertModel(config)
- self.init_weights()
-
-
-@add_start_docstrings(
- """Flaubert Model with a beam-search span classification head on top for extractive question-answering tasks like SQuAD (a linear layers on top of
- the hidden-states output to compute `span start logits` and `span end logits`). """,
- FLAUBERT_START_DOCSTRING,
-)
-class FlaubertForQuestionAnswering(XLMForQuestionAnswering):
- """
- This class overrides :class:`~transformers.XLMForQuestionAnswering`. Please check the
- superclass for the appropriate documentation alongside usage examples.
- """
-
- config_class = FlaubertConfig
- pretrained_model_archive_map = FLAUBERT_PRETRAINED_MODEL_ARCHIVE_MAP
-
- def __init__(self, config):
- super(FlaubertForQuestionAnswering, self).__init__(config)
- self.transformer = FlaubertModel(config)
- self.init_weights()
diff --git a/server/transformers/src/transformers/modeling_gpt2.py b/server/transformers/src/transformers/modeling_gpt2.py
deleted file mode 100644
index 77027acd53b63a9c688a9ecdc00e54f5c3d737b5..0000000000000000000000000000000000000000
--- a/server/transformers/src/transformers/modeling_gpt2.py
+++ /dev/null
@@ -1,757 +0,0 @@
-# coding=utf-8
-# Copyright 2018 The OpenAI Team Authors and HuggingFace Inc. team.
-# Copyright (c) 2018, NVIDIA CORPORATION. All rights reserved.
-#
-# Licensed under the Apache License, Version 2.0 (the "License");
-# you may not use this file except in compliance with the License.
-# You may obtain a copy of the License at
-#
-# http://www.apache.org/licenses/LICENSE-2.0
-#
-# Unless required by applicable law or agreed to in writing, software
-# distributed under the License is distributed on an "AS IS" BASIS,
-# WITHOUT WARRANTIES OR CONDITIONS OF ANY KIND, either express or implied.
-# See the License for the specific language governing permissions and
-# limitations under the License.
-"""PyTorch OpenAI GPT-2 model."""
-
-
-import logging
-import math
-import os
-
-import torch
-import torch.nn as nn
-from torch.nn import CrossEntropyLoss
-
-from .configuration_gpt2 import GPT2Config
-from .file_utils import add_start_docstrings, add_start_docstrings_to_callable
-from .modeling_utils import Conv1D, PreTrainedModel, SequenceSummary, prune_conv1d_layer, transpose_iterable
-
-
-logger = logging.getLogger(__name__)
-
-GPT2_PRETRAINED_MODEL_ARCHIVE_MAP = {
- "gpt2": "https://s3.amazonaws.com/models.huggingface.co/bert/gpt2-pytorch_model.bin",
- "gpt2-medium": "https://s3.amazonaws.com/models.huggingface.co/bert/gpt2-medium-pytorch_model.bin",
- "gpt2-large": "https://s3.amazonaws.com/models.huggingface.co/bert/gpt2-large-pytorch_model.bin",
- "gpt2-xl": "https://s3.amazonaws.com/models.huggingface.co/bert/gpt2-xl-pytorch_model.bin",
- "distilgpt2": "https://s3.amazonaws.com/models.huggingface.co/bert/distilgpt2-pytorch_model.bin",
-}
-
-
-def load_tf_weights_in_gpt2(model, config, gpt2_checkpoint_path):
- """ Load tf checkpoints in a pytorch model
- """
- try:
- import re
- import tensorflow as tf
- except ImportError:
- logger.error(
- "Loading a TensorFlow model in PyTorch, requires TensorFlow to be installed. Please see "
- "https://www.tensorflow.org/install/ for installation instructions."
- )
- raise
- tf_path = os.path.abspath(gpt2_checkpoint_path)
- logger.info("Converting TensorFlow checkpoint from {}".format(tf_path))
- # Load weights from TF model
- init_vars = tf.train.list_variables(tf_path)
- names = []
- arrays = []
- for name, shape in init_vars:
- logger.info("Loading TF weight {} with shape {}".format(name, shape))
- array = tf.train.load_variable(tf_path, name)
- names.append(name)
- arrays.append(array.squeeze())
-
- for name, array in zip(names, arrays):
- name = name[6:] # skip "model/"
- name = name.split("/")
- pointer = model
- for m_name in name:
- if re.fullmatch(r"[A-Za-z]+\d+", m_name):
- scope_names = re.split(r"(\d+)", m_name)
- else:
- scope_names = [m_name]
- if scope_names[0] == "w" or scope_names[0] == "g":
- pointer = getattr(pointer, "weight")
- elif scope_names[0] == "b":
- pointer = getattr(pointer, "bias")
- elif scope_names[0] == "wpe" or scope_names[0] == "wte":
- pointer = getattr(pointer, scope_names[0])
- pointer = getattr(pointer, "weight")
- else:
- pointer = getattr(pointer, scope_names[0])
- if len(scope_names) >= 2:
- num = int(scope_names[1])
- pointer = pointer[num]
- try:
- assert pointer.shape == array.shape
- except AssertionError as e:
- e.args += (pointer.shape, array.shape)
- raise
- logger.info("Initialize PyTorch weight {}".format(name))
- pointer.data = torch.from_numpy(array)
- return model
-
-
-def gelu(x):
- return 0.5 * x * (1 + torch.tanh(math.sqrt(2 / math.pi) * (x + 0.044715 * torch.pow(x, 3))))
-
-
-class Attention(nn.Module):
- def __init__(self, nx, n_ctx, config, scale=False):
- super().__init__()
- self.output_attentions = config.output_attentions
- self.output_additional_info = config.output_additional_info
-
- n_state = nx # in Attention: n_state=768 (nx=n_embd)
- # [switch nx => n_state from Block to Attention to keep identical to TF implem]
- assert n_state % config.n_head == 0
- self.register_buffer("bias", torch.tril(torch.ones(n_ctx, n_ctx)).view(1, 1, n_ctx, n_ctx))
- self.n_head = config.n_head
- self.split_size = n_state
- self.scale = scale
-
- self.c_attn = Conv1D(n_state * 3, nx)
- self.c_proj = Conv1D(n_state, nx)
- self.attn_dropout = nn.Dropout(config.attn_pdrop)
- self.resid_dropout = nn.Dropout(config.resid_pdrop)
- self.pruned_heads = set()
-
- def prune_heads(self, heads):
- if len(heads) == 0:
- return
- mask = torch.ones(self.n_head, self.split_size // self.n_head)
- heads = set(heads) - self.pruned_heads # Convert to set and emove already pruned heads
- for head in heads:
- # Compute how many pruned heads are before the head and move the index accordingly
- head = head - sum(1 if h < head else 0 for h in self.pruned_heads)
- mask[head] = 0
- mask = mask.view(-1).contiguous().eq(1)
- index = torch.arange(len(mask))[mask].long()
- index_attn = torch.cat([index, index + self.split_size, index + (2 * self.split_size)])
-
- # Prune conv1d layers
- self.c_attn = prune_conv1d_layer(self.c_attn, index_attn, dim=1)
- self.c_proj = prune_conv1d_layer(self.c_proj, index, dim=0)
-
- # Update hyper params
- self.split_size = (self.split_size // self.n_head) * (self.n_head - len(heads))
- self.n_head = self.n_head - len(heads)
- self.pruned_heads = self.pruned_heads.union(heads)
-
- def _attn(self, q, k, v, attention_mask=None, head_mask=None):
- w = torch.matmul(q, k)
- if self.scale:
- w = w / math.sqrt(v.size(-1))
- nd, ns = w.size(-2), w.size(-1)
- b = self.bias[:, :, ns - nd : ns, :ns]
- w = w * b - 1e4 * (1 - b)
-
- if attention_mask is not None:
- # Apply the attention mask
- w = w + attention_mask
-
- w = nn.Softmax(dim=-1)(w)
- w = self.attn_dropout(w)
-
- # Mask heads if we want to
- if head_mask is not None:
- w = w * head_mask
-
- contexts = torch.matmul(w, v)
- outputs = [contexts]
- if self.output_attentions:
- outputs.append(w)
-
- if self.output_additional_info:
- contexts = contexts.permute(0, 2, 1, 3).contiguous()
- print("CONTEXTS: ", contexts.shape)
- outputs.append(contexts)
-
- return outputs
-
- def merge_heads(self, x):
- x = x.permute(0, 2, 1, 3).contiguous()
- new_x_shape = x.size()[:-2] + (x.size(-2) * x.size(-1),)
- return x.view(*new_x_shape) # in Tensorflow implem: fct merge_states
-
- def split_heads(self, x, k=False):
- new_x_shape = x.size()[:-1] + (self.n_head, x.size(-1) // self.n_head)
- x = x.view(*new_x_shape) # in Tensorflow implem: fct split_states
- if k:
- return x.permute(0, 2, 3, 1) # (batch, head, head_features, seq_length)
- else:
- return x.permute(0, 2, 1, 3) # (batch, head, seq_length, head_features)
-
- def forward(self, x, layer_past=None, attention_mask=None, head_mask=None):
- x = self.c_attn(x)
- query, key, value = x.split(self.split_size, dim=2)
- query = self.split_heads(query)
- key = self.split_heads(key, k=True)
- value = self.split_heads(value)
- if layer_past is not None:
- past_key, past_value = layer_past[0].transpose(-2, -1), layer_past[1] # transpose back cf below
- key = torch.cat((past_key, key), dim=-1)
- value = torch.cat((past_value, value), dim=-2)
- present = torch.stack((key.transpose(-2, -1), value)) # transpose to have same shapes for stacking
-
- attn_outputs = self._attn(query, key, value, attention_mask, head_mask)
- a = attn_outputs[0]
-
- a = self.merge_heads(a)
- a = self.c_proj(a)
- a = self.resid_dropout(a)
-
- outputs = [a, present] + attn_outputs[1:]
- return outputs # a, present, (attentions), (contexts)
-
-
-class MLP(nn.Module):
- def __init__(self, n_state, config): # in MLP: n_state=3072 (4 * n_embd)
- super().__init__()
- nx = config.n_embd
- self.c_fc = Conv1D(n_state, nx)
- self.c_proj = Conv1D(nx, n_state)
- self.act = gelu
- self.dropout = nn.Dropout(config.resid_pdrop)
-
- def forward(self, x):
- h = self.act(self.c_fc(x))
- h2 = self.c_proj(h)
- return self.dropout(h2)
-
-
-class Block(nn.Module):
- def __init__(self, n_ctx, config, scale=False):
- super().__init__()
- nx = config.n_embd
- self.ln_1 = nn.LayerNorm(nx, eps=config.layer_norm_epsilon)
- self.attn = Attention(nx, n_ctx, config, scale)
- self.ln_2 = nn.LayerNorm(nx, eps=config.layer_norm_epsilon)
- self.mlp = MLP(4 * nx, config)
-
- def forward(self, x, layer_past=None, attention_mask=None, head_mask=None):
- output_attn = self.attn(
- self.ln_1(x), layer_past=layer_past, attention_mask=attention_mask, head_mask=head_mask
- )
- a = output_attn[0] # output_attn: a, present, (attentions)
-
- x = x + a
- m = self.mlp(self.ln_2(x))
- x = x + m
-
- outputs = [x] + output_attn[1:]
- return outputs # x, present, (attentions), (?contexts)
-
-
-class GPT2PreTrainedModel(PreTrainedModel):
- """ An abstract class to handle weights initialization and
- a simple interface for downloading and loading pretrained models.
- """
-
- config_class = GPT2Config
- pretrained_model_archive_map = GPT2_PRETRAINED_MODEL_ARCHIVE_MAP
- load_tf_weights = load_tf_weights_in_gpt2
- base_model_prefix = "transformer"
-
- def __init__(self, *inputs, **kwargs):
- super().__init__(*inputs, **kwargs)
-
- def _init_weights(self, module):
- """ Initialize the weights.
- """
- if isinstance(module, (nn.Linear, nn.Embedding, Conv1D)):
- # Slightly different from the TF version which uses truncated_normal for initialization
- # cf https://github.com/pytorch/pytorch/pull/5617
- module.weight.data.normal_(mean=0.0, std=self.config.initializer_range)
- if isinstance(module, (nn.Linear, Conv1D)) and module.bias is not None:
- module.bias.data.zero_()
- elif isinstance(module, nn.LayerNorm):
- module.bias.data.zero_()
- module.weight.data.fill_(1.0)
-
-
-GPT2_START_DOCSTRING = r"""
-
- This model is a PyTorch `torch.nn.Module `_ sub-class.
- Use it as a regular PyTorch Module and refer to the PyTorch documentation for all matter related to general
- usage and behavior.
-
- Parameters:
- config (:class:`~transformers.GPT2Config`): Model configuration class with all the parameters of the model.
- Initializing with a config file does not load the weights associated with the model, only the configuration.
- Check out the :meth:`~transformers.PreTrainedModel.from_pretrained` method to load the model weights.
-"""
-
-GPT2_INPUTS_DOCSTRING = r"""
- Args:
- input_ids (:obj:`torch.LongTensor` of shape :obj:`(batch_size, sequence_length)`):
- Indices of input sequence tokens in the vocabulary.
-
- Indices can be obtained using :class:`transformers.GPT2Tokenizer`.
- See :func:`transformers.PreTrainedTokenizer.encode` and
- :func:`transformers.PreTrainedTokenizer.encode_plus` for details.
-
- `What are input IDs? <../glossary.html#input-ids>`__
- past (:obj:`List[torch.FloatTensor]` of length :obj:`config.n_layers`):
- Contains pre-computed hidden-states (key and values in the attention blocks) as computed by the model
- (see `past` output below). Can be used to speed up sequential decoding. The token ids which have their past given to this model
- should not be passed as input ids as they have already been computed.
- attention_mask (:obj:`torch.FloatTensor` of shape :obj:`(batch_size, sequence_length)`, `optional`, defaults to :obj:`None`):
- Mask to avoid performing attention on padding token indices.
- Mask values selected in ``[0, 1]``:
- ``1`` for tokens that are NOT MASKED, ``0`` for MASKED tokens.
-
- `What are attention masks? <../glossary.html#attention-mask>`__
- token_type_ids (:obj:`torch.LongTensor` of shape :obj:`(batch_size, sequence_length)`, `optional`, defaults to :obj:`None`):
- Segment token indices to indicate first and second portions of the inputs.
- Indices are selected in ``[0, 1]``: ``0`` corresponds to a `sentence A` token, ``1``
- corresponds to a `sentence B` token
-
- `What are token type IDs? <../glossary.html#token-type-ids>`_
- position_ids (:obj:`torch.LongTensor` of shape :obj:`(batch_size, sequence_length)`, `optional`, defaults to :obj:`None`):
- Indices of positions of each input sequence tokens in the position embeddings.
- Selected in the range ``[0, config.max_position_embeddings - 1]``.
-
- `What are position IDs? <../glossary.html#position-ids>`_
- head_mask (:obj:`torch.FloatTensor` of shape :obj:`(num_heads,)` or :obj:`(num_layers, num_heads)`, `optional`, defaults to :obj:`None`):
- Mask to nullify selected heads of the self-attention modules.
- Mask values selected in ``[0, 1]``:
- :obj:`1` indicates the head is **not masked**, :obj:`0` indicates the head is **masked**.
- input_embeds (:obj:`torch.FloatTensor` of shape :obj:`(batch_size, sequence_length, hidden_size)`, `optional`, defaults to :obj:`None`):
- Optionally, instead of passing :obj:`input_ids` you can choose to directly pass an embedded representation.
- This is useful if you want more control over how to convert `input_ids` indices into associated vectors
- than the model's internal embedding lookup matrix.
-"""
-
-
-@add_start_docstrings(
- "The bare GPT2 Model transformer outputting raw hidden-states without any specific head on top.",
- GPT2_START_DOCSTRING,
-)
-class GPT2Model(GPT2PreTrainedModel):
- def __init__(self, config):
- super().__init__(config)
- self.output_hidden_states = config.output_hidden_states
- self.output_attentions = config.output_attentions
- self.output_additional_info = config.output_additional_info
- self.output_past = config.output_past
-
- self.wte = nn.Embedding(config.vocab_size, config.n_embd)
- self.wpe = nn.Embedding(config.n_positions, config.n_embd)
- self.drop = nn.Dropout(config.embd_pdrop)
- self.h = nn.ModuleList([Block(config.n_ctx, config, scale=True) for _ in range(config.n_layer)])
- self.ln_f = nn.LayerNorm(config.n_embd, eps=config.layer_norm_epsilon)
-
- self.init_weights()
-
- def get_input_embeddings(self):
- return self.wte
-
- def set_input_embeddings(self, new_embeddings):
- self.wte = new_embeddings
-
- def _prune_heads(self, heads_to_prune):
- """ Prunes heads of the model.
- heads_to_prune: dict of {layer_num: list of heads to prune in this layer}
- """
- for layer, heads in heads_to_prune.items():
- self.h[layer].attn.prune_heads(heads)
-
- @add_start_docstrings_to_callable(GPT2_INPUTS_DOCSTRING)
- def forward(
- self,
- input_ids=None,
- past=None,
- attention_mask=None,
- token_type_ids=None,
- position_ids=None,
- head_mask=None,
- inputs_embeds=None,
- ):
- r"""
- Return:
- :obj:`tuple(torch.FloatTensor)` comprising various elements depending on the configuration (:class:`~transformers.GPT2Config`) and inputs:
- last_hidden_state (:obj:`torch.FloatTensor` of shape :obj:`(batch_size, sequence_length, hidden_size)`):
- Sequence of hidden-states at the last layer of the model.
- past (:obj:`List[torch.FloatTensor]` of length :obj:`config.n_layers` with each tensor of shape :obj:`(2, batch_size, num_heads, sequence_length, embed_size_per_head)`):
- Contains pre-computed hidden-states (key and values in the attention blocks).
- Can be used (see `past` input) to speed up sequential decoding. The token ids which have their past given to this model
- should not be passed as input ids as they have already been computed.
- hidden_states (:obj:`tuple(torch.FloatTensor)`, `optional`, returned when ``config.output_hidden_states=True``):
- Tuple of :obj:`torch.FloatTensor` (one for the output of the embeddings + one for the output of each layer)
- of shape :obj:`(batch_size, sequence_length, hidden_size)`.
-
- Hidden-states of the model at the output of each layer plus the initial embedding outputs.
- attentions (:obj:`tuple(torch.FloatTensor)`, `optional`, returned when ``config.output_attentions=True``):
- Tuple of :obj:`torch.FloatTensor` (one for each layer) of shape
- :obj:`(batch_size, num_heads, sequence_length, sequence_length)`.
-
- Attentions weights after the attention softmax, used to compute the weighted average in the self-attention
- heads.
-
- Examples::
-
- from transformers import GPT2Tokenizer, GPT2Model
- import torch
-
- tokenizer = GPT2Tokenizer.from_pretrained('gpt2')
- model = GPT2Model.from_pretrained('gpt2')
- input_ids = torch.tensor(tokenizer.encode("Hello, my dog is cute", add_special_tokens=True)).unsqueeze(0) # Batch size 1
- outputs = model(input_ids)
- last_hidden_states = outputs[0] # The last hidden-state is the first element of the output tuple
-
- """
- if input_ids is not None and inputs_embeds is not None:
- raise ValueError("You cannot specify both input_ids and inputs_embeds at the same time")
- elif input_ids is not None:
- input_shape = input_ids.size()
- input_ids = input_ids.view(-1, input_shape[-1])
- elif inputs_embeds is not None:
- input_shape = inputs_embeds.size()[:-1]
- else:
- raise ValueError("You have to specify either input_ids or inputs_embeds")
-
- if token_type_ids is not None:
- token_type_ids = token_type_ids.view(-1, input_shape[-1])
- if position_ids is not None:
- position_ids = position_ids.view(-1, input_shape[-1])
-
- if past is None:
- past_length = 0
- past = [None] * len(self.h)
- else:
- past_length = past[0][0].size(-2)
- if position_ids is None:
- device = input_ids.device if input_ids is not None else inputs_embeds.device
- position_ids = torch.arange(past_length, input_shape[-1] + past_length, dtype=torch.long, device=device)
- position_ids = position_ids.unsqueeze(0).view(-1, input_shape[-1])
-
- # Attention mask.
- if attention_mask is not None:
- attention_mask = attention_mask.view(-1, input_shape[-1])
- # We create a 3D attention mask from a 2D tensor mask.
- # Sizes are [batch_size, 1, 1, to_seq_length]
- # So we can broadcast to [batch_size, num_heads, from_seq_length, to_seq_length]
- # this attention mask is more simple than the triangular masking of causal attention
- # used in OpenAI GPT, we just need to prepare the broadcast dimension here.
- attention_mask = attention_mask.unsqueeze(1).unsqueeze(2)
-
- # Since attention_mask is 1.0 for positions we want to attend and 0.0 for
- # masked positions, this operation will create a tensor which is 0.0 for
- # positions we want to attend and -10000.0 for masked positions.
- # Since we are adding it to the raw scores before the softmax, this is
- # effectively the same as removing these entirely.
- attention_mask = attention_mask.to(dtype=next(self.parameters()).dtype) # fp16 compatibility
- attention_mask = (1.0 - attention_mask) * -10000.0
-
- # Prepare head mask if needed
- # 1.0 in head_mask indicate we keep the head
- # attention_probs has shape bsz x n_heads x N x N
- # head_mask has shape n_layer x batch x n_heads x N x N
- if head_mask is not None:
- if head_mask.dim() == 1:
- head_mask = head_mask.unsqueeze(0).unsqueeze(0).unsqueeze(-1).unsqueeze(-1)
- head_mask = head_mask.expand(self.config.n_layer, -1, -1, -1, -1)
- elif head_mask.dim() == 2:
- head_mask = (
- head_mask.unsqueeze(1).unsqueeze(-1).unsqueeze(-1)
- ) # We can specify head_mask for each layer
- head_mask = head_mask.to(
- dtype=next(self.parameters()).dtype
- ) # switch to fload if need + fp16 compatibility
- else:
- head_mask = [None] * self.config.n_layer
-
- if inputs_embeds is None:
- inputs_embeds = self.wte(input_ids)
- position_embeds = self.wpe(position_ids)
- if token_type_ids is not None:
- token_type_embeds = self.wte(token_type_ids)
- else:
- token_type_embeds = 0
- hidden_states = inputs_embeds + position_embeds + token_type_embeds
- hidden_states = self.drop(hidden_states)
-
- output_shape = input_shape + (hidden_states.size(-1),)
-
- presents = ()
- all_attentions = []
- all_hidden_states = ()
- all_additional_info = ()
- for i, (block, layer_past) in enumerate(zip(self.h, past)):
- if self.output_hidden_states:
- all_hidden_states = all_hidden_states + (hidden_states.view(*output_shape),)
-
- outputs = block(
- hidden_states, layer_past=layer_past, attention_mask=attention_mask, head_mask=head_mask[i]
- )
-
- hidden_states, present = outputs[:2]
- if self.output_past:
- presents = presents + (present,)
-
- if self.output_attentions:
- all_attentions.append(outputs[2])
- if self.output_additional_info:
- all_additional_info = all_additional_info + (outputs[3],)
-
- hidden_states = self.ln_f(hidden_states)
-
- hidden_states = hidden_states.view(*output_shape)
- # Add last hidden state
- if self.output_hidden_states:
- all_hidden_states = all_hidden_states + (hidden_states,)
-
- outputs = (hidden_states,)
- if self.output_past:
- outputs = outputs + (presents,)
- if self.output_hidden_states:
- outputs = outputs + (all_hidden_states,)
- if self.output_attentions:
- # let the number of heads free (-1) so we can extract attention even after head pruning
- attention_output_shape = input_shape[:-1] + (-1,) + all_attentions[0].shape[-2:]
- all_attentions = tuple(t.view(*attention_output_shape) for t in all_attentions)
- outputs = outputs + (all_attentions,)
- if self.output_additional_info:
- outputs = outputs + (all_additional_info,)
-
- return outputs # last hidden state, (presents), (all hidden_states), (attentions), (contexts)
-
-
-@add_start_docstrings(
- """The GPT2 Model transformer with a language modeling head on top
- (linear layer with weights tied to the input embeddings). """,
- GPT2_START_DOCSTRING,
-)
-class GPT2LMHeadModel(GPT2PreTrainedModel):
- def __init__(self, config):
- super().__init__(config)
- self.transformer = GPT2Model(config)
- self.lm_head = nn.Linear(config.n_embd, config.vocab_size, bias=False)
-
- self.init_weights()
-
- def get_output_embeddings(self):
- return self.lm_head
-
- def prepare_inputs_for_generation(self, input_ids, **kwargs):
- # only last token for inputs_ids if past is defined in kwargs
- if "past" in kwargs and kwargs["past"]:
- input_ids = input_ids[:, -1].unsqueeze(-1)
-
- inputs = {"input_ids": input_ids}
- inputs.update(kwargs)
- return inputs
-
- @add_start_docstrings_to_callable(GPT2_INPUTS_DOCSTRING)
- def forward(
- self,
- input_ids=None,
- past=None,
- attention_mask=None,
- token_type_ids=None,
- position_ids=None,
- head_mask=None,
- inputs_embeds=None,
- labels=None,
- ):
- r"""
- labels (:obj:`torch.LongTensor` of shape :obj:`(batch_size, sequence_length)`, `optional`, defaults to :obj:`None`):
- Labels for language modeling.
- Note that the labels **are shifted** inside the model, i.e. you can set ``lm_labels = input_ids``
- Indices are selected in ``[-100, 0, ..., config.vocab_size]``
- All labels set to ``-100`` are ignored (masked), the loss is only
- computed for labels in ``[0, ..., config.vocab_size]``
-
- Return:
- :obj:`tuple(torch.FloatTensor)` comprising various elements depending on the configuration (:class:`~transformers.GPT2Config`) and inputs:
- loss (:obj:`torch.FloatTensor` of shape `(1,)`, `optional`, returned when ``labels`` is provided)
- Language modeling loss.
- prediction_scores (:obj:`torch.FloatTensor` of shape :obj:`(batch_size, sequence_length, config.vocab_size)`):
- Prediction scores of the language modeling head (scores for each vocabulary token before SoftMax).
- past (:obj:`List[torch.FloatTensor]` of length :obj:`config.n_layers` with each tensor of shape :obj:`(2, batch_size, num_heads, sequence_length, embed_size_per_head)`):
- Contains pre-computed hidden-states (key and values in the attention blocks).
- Can be used (see `past` input) to speed up sequential decoding. The token ids which have their past given to this model
- should not be passed as input ids as they have already been computed.
- hidden_states (:obj:`tuple(torch.FloatTensor)`, `optional`, returned when ``config.output_hidden_states=True``):
- Tuple of :obj:`torch.FloatTensor` (one for the output of the embeddings + one for the output of each layer)
- of shape :obj:`(batch_size, sequence_length, hidden_size)`.
-
- Hidden-states of the model at the output of each layer plus the initial embedding outputs.
- attentions (:obj:`tuple(torch.FloatTensor)`, `optional`, returned when ``config.output_attentions=True``):
- Tuple of :obj:`torch.FloatTensor` (one for each layer) of shape
- :obj:`(batch_size, num_heads, sequence_length, sequence_length)`.
-
- Attentions weights after the attention softmax, used to compute the weighted average in the self-attention
- heads.
-
- Examples::
-
- import torch
- from transformers import GPT2Tokenizer, GPT2LMHeadModel
-
- tokenizer = GPT2Tokenizer.from_pretrained('gpt2')
- model = GPT2LMHeadModel.from_pretrained('gpt2')
-
- input_ids = torch.tensor(tokenizer.encode("Hello, my dog is cute", add_special_tokens=True)).unsqueeze(0) # Batch size 1
- outputs = model(input_ids, labels=input_ids)
- loss, logits = outputs[:2]
-
- """
- transformer_outputs = self.transformer(
- input_ids,
- past=past,
- attention_mask=attention_mask,
- token_type_ids=token_type_ids,
- position_ids=position_ids,
- head_mask=head_mask,
- inputs_embeds=inputs_embeds,
- )
- hidden_states = transformer_outputs[0]
-
- lm_logits = self.lm_head(hidden_states)
-
- outputs = (lm_logits,) + transformer_outputs[1:]
- if labels is not None:
- # Shift so that tokens < n predict n
- shift_logits = lm_logits[..., :-1, :].contiguous()
- shift_labels = labels[..., 1:].contiguous()
- # Flatten the tokens
- loss_fct = CrossEntropyLoss()
- loss = loss_fct(shift_logits.view(-1, shift_logits.size(-1)), shift_labels.view(-1))
- outputs = (loss,) + outputs
-
- return outputs # (loss), lm_logits, presents, (all hidden_states), (attentions)
-
-
-@add_start_docstrings(
- """The GPT2 Model transformer with a language modeling and a multiple-choice classification
- head on top e.g. for RocStories/SWAG tasks. The two heads are two linear layers.
- The language modeling head has its weights tied to the input embeddings,
- the classification head takes as input the input of a specified classification token index in the input sequence).
-""",
- GPT2_START_DOCSTRING,
-)
-class GPT2DoubleHeadsModel(GPT2PreTrainedModel):
- def __init__(self, config):
- super().__init__(config)
- config.num_labels = 1
- self.transformer = GPT2Model(config)
- self.lm_head = nn.Linear(config.n_embd, config.vocab_size, bias=False)
- self.multiple_choice_head = SequenceSummary(config)
-
- self.init_weights()
-
- def get_output_embeddings(self):
- return self.lm_head
-
- @add_start_docstrings_to_callable(GPT2_INPUTS_DOCSTRING)
- def forward(
- self,
- input_ids=None,
- past=None,
- attention_mask=None,
- token_type_ids=None,
- position_ids=None,
- head_mask=None,
- inputs_embeds=None,
- mc_token_ids=None,
- lm_labels=None,
- mc_labels=None,
- ):
- r"""
- mc_token_ids (:obj:`torch.LongTensor` of shape :obj:`(batch_size, num_choices)`, `optional`, default to index of the last token of the input)
- Index of the classification token in each input sequence.
- Selected in the range ``[0, input_ids.size(-1) - 1[``.
- lm_labels (:obj:`torch.LongTensor` of shape :obj:`(batch_size, sequence_length)`, `optional`, defaults to :obj:`None`)
- Labels for language modeling.
- Note that the labels **are shifted** inside the model, i.e. you can set ``lm_labels = input_ids``
- Indices are selected in ``[-1, 0, ..., config.vocab_size]``
- All labels set to ``-100`` are ignored (masked), the loss is only
- computed for labels in ``[0, ..., config.vocab_size]``
- mc_labels (:obj:`torch.LongTensor` of shape :obj:`(batch_size)`, `optional`, defaults to :obj:`None`)
- Labels for computing the multiple choice classification loss.
- Indices should be in ``[0, ..., num_choices]`` where `num_choices` is the size of the second dimension
- of the input tensors. (see `input_ids` above)
-
- Return:
- :obj:`tuple(torch.FloatTensor)` comprising various elements depending on the configuration (:class:`~transformers.GPT2Config`) and inputs:
- lm_loss (:obj:`torch.FloatTensor` of shape :obj:`(1,)`, `optional`, returned when ``lm_labels`` is provided):
- Language modeling loss.
- mc_loss (:obj:`torch.FloatTensor` of shape :obj:`(1,)`, `optional`, returned when :obj:`multiple_choice_labels` is provided):
- Multiple choice classification loss.
- lm_prediction_scores (:obj:`torch.FloatTensor` of shape :obj:`(batch_size, num_choices, sequence_length, config.vocab_size)`):
- Prediction scores of the language modeling head (scores for each vocabulary token before SoftMax).
- mc_prediction_scores (:obj:`torch.FloatTensor` of shape :obj:`(batch_size, num_choices)`):
- Prediction scores of the multiple choice classification head (scores for each choice before SoftMax).
- past (:obj:`List[torch.FloatTensor]` of length :obj:`config.n_layers` with each tensor of shape :obj:`(2, batch_size, num_heads, sequence_length, embed_size_per_head)`):
- Contains pre-computed hidden-states (key and values in the attention blocks).
- Can be used (see `past` input) to speed up sequential decoding. The token ids which have their past given to this model
- should not be passed as input ids as they have already been computed.
- hidden_states (:obj:`tuple(torch.FloatTensor)`, `optional`, returned when ``config.output_hidden_states=True``):
- Tuple of :obj:`torch.FloatTensor` (one for the output of the embeddings + one for the output of each layer)
- of shape :obj:`(batch_size, sequence_length, hidden_size)`.
-
- Hidden-states of the model at the output of each layer plus the initial embedding outputs.
- attentions (:obj:`tuple(torch.FloatTensor)`, `optional`, returned when ``config.output_attentions=True``):
- Tuple of :obj:`torch.FloatTensor` (one for each layer) of shape
- :obj:`(batch_size, num_heads, sequence_length, sequence_length)`.
-
- Attentions weights after the attention softmax, used to compute the weighted average in the self-attention
- heads.
-
- Examples::
-
- import torch
- from transformers import GPT2Tokenizer, GPT2DoubleHeadsModel
-
- tokenizer = GPT2Tokenizer.from_pretrained('gpt2')
- model = GPT2DoubleHeadsModel.from_pretrained('gpt2')
-
- # Add a [CLS] to the vocabulary (we should train it also!)
- tokenizer.add_special_tokens({'cls_token': '[CLS]'})
- model.resize_token_embeddings(len(tokenizer)) # Update the model embeddings with the new vocabulary size
- print(tokenizer.cls_token_id, len(tokenizer)) # The newly token the last token of the vocabulary
-
- choices = ["Hello, my dog is cute [CLS]", "Hello, my cat is cute [CLS]"]
- encoded_choices = [tokenizer.encode(s) for s in choices]
- cls_token_location = [tokens.index(tokenizer.cls_token_id) for tokens in encoded_choices]
-
- input_ids = torch.tensor(encoded_choices).unsqueeze(0) # Batch size: 1, number of choices: 2
- mc_token_ids = torch.tensor([cls_token_location]) # Batch size: 1
-
- outputs = model(input_ids, mc_token_ids=mc_token_ids)
- lm_prediction_scores, mc_prediction_scores = outputs[:2]
-
- """
- transformer_outputs = self.transformer(
- input_ids,
- past=past,
- attention_mask=attention_mask,
- token_type_ids=token_type_ids,
- position_ids=position_ids,
- head_mask=head_mask,
- inputs_embeds=inputs_embeds,
- )
-
- hidden_states = transformer_outputs[0]
-
- lm_logits = self.lm_head(hidden_states)
- mc_logits = self.multiple_choice_head(hidden_states, mc_token_ids).squeeze(-1)
-
- outputs = (lm_logits, mc_logits) + transformer_outputs[1:]
- if mc_labels is not None:
- loss_fct = CrossEntropyLoss()
- loss = loss_fct(mc_logits.view(-1, mc_logits.size(-1)), mc_labels.view(-1))
- outputs = (loss,) + outputs
- if lm_labels is not None:
- shift_logits = lm_logits[..., :-1, :].contiguous()
- shift_labels = lm_labels[..., 1:].contiguous()
- loss_fct = CrossEntropyLoss()
- loss = loss_fct(shift_logits.view(-1, shift_logits.size(-1)), shift_labels.view(-1))
- outputs = (loss,) + outputs
-
- return outputs # (lm loss), (mc loss), lm logits, mc logits, presents, (all hidden_states), (attentions)
diff --git a/server/transformers/src/transformers/modeling_mmbt.py b/server/transformers/src/transformers/modeling_mmbt.py
deleted file mode 100644
index a3aae3896585a454473cd34735e0606c155ce075..0000000000000000000000000000000000000000
--- a/server/transformers/src/transformers/modeling_mmbt.py
+++ /dev/null
@@ -1,419 +0,0 @@
-# coding=utf-8
-# Copyright (c) Facebook, Inc. and its affiliates.
-# Copyright (c) HuggingFace Inc. team.
-#
-# Licensed under the Apache License, Version 2.0 (the "License");
-# you may not use this file except in compliance with the License.
-# You may obtain a copy of the License at
-#
-# http://www.apache.org/licenses/LICENSE-2.0
-#
-# Unless required by applicable law or agreed to in writing, software
-# distributed under the License is distributed on an "AS IS" BASIS,
-# WITHOUT WARRANTIES OR CONDITIONS OF ANY KIND, either express or implied.
-# See the License for the specific language governing permissions and
-# limitations under the License.
-"""PyTorch MMBT model. """
-
-
-import logging
-
-import torch
-import torch.nn as nn
-from torch.nn import CrossEntropyLoss, MSELoss
-
-from .file_utils import add_start_docstrings
-
-
-logger = logging.getLogger(__name__)
-
-
-class ModalEmbeddings(nn.Module):
- """Generic Modal Embeddings which takes in an encoder, and a transformer embedding.
- """
-
- def __init__(self, config, encoder, embeddings):
- super().__init__()
- self.config = config
- self.encoder = encoder
- self.proj_embeddings = nn.Linear(config.modal_hidden_size, config.hidden_size)
- self.position_embeddings = embeddings.position_embeddings
- self.token_type_embeddings = embeddings.token_type_embeddings
- self.word_embeddings = embeddings.word_embeddings
- self.LayerNorm = embeddings.LayerNorm
- self.dropout = nn.Dropout(p=config.hidden_dropout_prob)
-
- def forward(self, input_modal, start_token=None, end_token=None, position_ids=None, token_type_ids=None):
- token_embeddings = self.proj_embeddings(self.encoder(input_modal))
- seq_length = token_embeddings.size(1)
-
- if start_token is not None:
- start_token_embeds = self.word_embeddings(start_token)
- seq_length += 1
- token_embeddings = torch.cat([start_token_embeds.unsqueeze(1), token_embeddings], dim=1)
-
- if end_token is not None:
- end_token_embeds = self.word_embeddings(end_token)
- seq_length += 1
- token_embeddings = torch.cat([token_embeddings, end_token_embeds.unsqueeze(1)], dim=1)
-
- if position_ids is None:
- position_ids = torch.arange(seq_length, dtype=torch.long, device=input_modal.device)
- position_ids = position_ids.unsqueeze(0).expand(input_modal.size(0), seq_length)
-
- if token_type_ids is None:
- token_type_ids = torch.zeros(
- (input_modal.size(0), seq_length), dtype=torch.long, device=input_modal.device
- )
-
- position_embeddings = self.position_embeddings(position_ids)
- token_type_embeddings = self.token_type_embeddings(token_type_ids)
- embeddings = token_embeddings + position_embeddings + token_type_embeddings
- embeddings = self.LayerNorm(embeddings)
- embeddings = self.dropout(embeddings)
- return embeddings
-
-
-MMBT_START_DOCSTRING = r""" MMBT model was proposed in
- `Supervised Multimodal Bitransformers for Classifying Images and Text`_
- by Douwe Kiela, Suvrat Bhooshan, Hamed Firooz, Davide Testuggine.
- It's a supervised multimodal bitransformer model that fuses information from text and other image encoders,
- and obtain state-of-the-art performance on various multimodal classification benchmark tasks.
-
- This model is a PyTorch `torch.nn.Module`_ sub-class. Use it as a regular PyTorch Module and
- refer to the PyTorch documentation for all matter related to general usage and behavior.
-
- .. _`Supervised Multimodal Bitransformers for Classifying Images and Text`:
- https://github.com/facebookresearch/mmbt
-
- .. _`torch.nn.Module`:
- https://pytorch.org/docs/stable/nn.html#module
-
- Parameters:
- config (:class:`~transformers.MMBTConfig`): Model configuration class with all the parameters of the model.
- Initializing with a config file does not load the weights associated with the model, only the configuration.
- transformer (:class: `~nn.Module`): A text transformer that is used by MMBT.
- It should have embeddings, encoder, and pooler attributes.
- encoder (:class: `~nn.Module`): Encoder for the second modality.
- It should take in a batch of modal inputs and return k, n dimension embeddings.
-"""
-
-MMBT_INPUTS_DOCSTRING = r""" Inputs:
- **input_modal**: ``torch.FloatTensor`` of shape ``(batch_size, ***)``:
- The other modality data. It will be the shape that the encoder for that type expects.
- e.g. With an Image Encoder, the shape would be (batch_size, channels, height, width)
- **input_ids**: ``torch.LongTensor`` of shape ``(batch_size, sequence_length)``:
- Indices of input sequence tokens in the vocabulary.
- It does not expect [CLS] token to be added as it's appended to the end of other modality embeddings.
- See :func:`transformers.PreTrainedTokenizer.encode` and
- :func:`transformers.PreTrainedTokenizer.convert_tokens_to_ids` for details.
- **modal_start_tokens**: (`optional`) ``torch.LongTensor`` of shape ``(batch_size,)``:
- Optional start token to be added to Other Modality Embedding. [CLS] Most commonly used for Classification tasks.
- **modal_end_tokens**: (`optional`) ``torch.LongTensor`` of shape ``(batch_size,)``:
- Optional end token to be added to Other Modality Embedding. [SEP] Most commonly used.
- **attention_mask**: (`optional`) ``torch.FloatTensor`` of shape ``(batch_size, sequence_length)``:
- Mask to avoid performing attention on padding token indices.
- Mask values selected in ``[0, 1]``:
- ``1`` for tokens that are NOT MASKED, ``0`` for MASKED tokens.
- **token_type_ids**: (`optional`) ``torch.LongTensor`` of shape ``(batch_size, sequence_length)``:
- Segment token indices to indicate different portions of the inputs.
- **modal_token_type_ids**: (`optional`) ``torch.LongTensor`` of shape ``(batch_size, modal_sequence_length)``:
- Segment token indices to indicate different portions of the non-text modality.
- The embeddings from these tokens will be summed with the respective token embeddings for the non-text modality.
- **position_ids**: (`optional`) ``torch.LongTensor`` of shape ``(batch_size, sequence_length)``:
- Indices of positions of each input sequence tokens in the position embeddings.
- **modal_position_ids**: (`optional`) ``torch.LongTensor`` of shape ``(batch_size, modal_sequence_length)``:
- Indices of positions of each input sequence tokens in the position embeddings for the non-text modality.
- **head_mask**: (`optional`) ``torch.FloatTensor`` of shape ``(num_heads,)`` or ``(num_layers, num_heads)``:
- Mask to nullify selected heads of the self-attention modules.
- Mask values selected in ``[0, 1]``:
- ``1`` indicates the head is **not masked**, ``0`` indicates the head is **masked**.
- **inputs_embeds**: (`optional`) ``torch.FloatTensor`` of shape ``(batch_size, sequence_length, embedding_dim)``:
- Optionally, instead of passing ``input_ids`` you can choose to directly pass an embedded representation.
- This is useful if you want more control over how to convert `input_ids` indices into associated vectors
- than the model's internal embedding lookup matrix.
- **encoder_hidden_states**: (`optional`) ``torch.FloatTensor`` of shape ``(batch_size, sequence_length, hidden_size)``:
- Sequence of hidden-states at the output of the last layer of the encoder. Used in the cross-attention if the model
- is configured as a decoder.
- **encoder_attention_mask**: (`optional`) ``torch.FloatTensor`` of shape ``(batch_size, sequence_length)``:
- Mask to avoid performing attention on the padding token indices of the encoder input. This mask
- is used in the cross-attention if the model is configured as a decoder.
- Mask values selected in ``[0, 1]``:
- ``1`` for tokens that are NOT MASKED, ``0`` for MASKED tokens.
-"""
-
-
-@add_start_docstrings(
- "The bare MMBT Model outputting raw hidden-states without any specific head on top.",
- MMBT_START_DOCSTRING,
- MMBT_INPUTS_DOCSTRING,
-)
-class MMBTModel(nn.Module):
- r"""
- Outputs: `Tuple` comprising various elements depending on the configuration (config) and inputs:
- **last_hidden_state**: ``torch.FloatTensor`` of shape ``(batch_size, sequence_length, hidden_size)``
- Sequence of hidden-states at the output of the last layer of the model.
- **pooler_output**: ``torch.FloatTensor`` of shape ``(batch_size, hidden_size)``
- Last layer hidden-state of the first token of the sequence (classification token)
- further processed by a Linear layer and a Tanh activation function. The Linear
- layer weights are trained from the next sentence prediction (classification)
- objective during Bert pretraining. This output is usually *not* a good summary
- of the semantic content of the input, you're often better with averaging or pooling
- the sequence of hidden-states for the whole input sequence.
- **hidden_states**: (`optional`, returned when ``config.output_hidden_states=True``)
- list of ``torch.FloatTensor`` (one for the output of each layer + the output of the embeddings)
- of shape ``(batch_size, sequence_length, hidden_size)``:
- Hidden-states of the model at the output of each layer plus the initial embedding outputs.
- **attentions**: (`optional`, returned when ``config.output_attentions=True``)
- list of ``torch.FloatTensor`` (one for each layer) of shape ``(batch_size, num_heads, sequence_length, sequence_length)``:
- Attentions weights after the attention softmax, used to compute the weighted average in the self-attention heads.
-
- Examples::
-
- # For example purposes. Not runnable.
- transformer = BertModel.from_pretrained('bert-base-uncased')
- encoder = ImageEncoder(args)
- mmbt = MMBTModel(config, transformer, encoder)
- """
-
- def __init__(self, config, transformer, encoder):
- super().__init__()
- self.config = config
- self.transformer = transformer
- self.modal_encoder = ModalEmbeddings(config, encoder, transformer.embeddings)
-
- def forward(
- self,
- input_modal,
- input_ids=None,
- modal_start_tokens=None,
- modal_end_tokens=None,
- attention_mask=None,
- token_type_ids=None,
- modal_token_type_ids=None,
- position_ids=None,
- modal_position_ids=None,
- head_mask=None,
- inputs_embeds=None,
- encoder_hidden_states=None,
- encoder_attention_mask=None,
- ):
-
- if input_ids is not None and inputs_embeds is not None:
- raise ValueError("You cannot specify both input_ids and inputs_embeds at the same time")
- elif input_ids is not None:
- input_txt_shape = input_ids.size()
- elif inputs_embeds is not None:
- input_txt_shape = inputs_embeds.size()[:-1]
- else:
- raise ValueError("You have to specify either input_ids or inputs_embeds")
-
- device = input_ids.device if input_ids is not None else inputs_embeds.device
-
- modal_embeddings = self.modal_encoder(
- input_modal,
- start_token=modal_start_tokens,
- end_token=modal_end_tokens,
- position_ids=modal_position_ids,
- token_type_ids=modal_token_type_ids,
- )
-
- input_modal_shape = modal_embeddings.size()[:-1]
-
- if token_type_ids is None:
- token_type_ids = torch.ones(input_txt_shape, dtype=torch.long, device=device)
-
- txt_embeddings = self.transformer.embeddings(
- input_ids=input_ids, position_ids=position_ids, token_type_ids=token_type_ids, inputs_embeds=inputs_embeds
- )
-
- embedding_output = torch.cat([modal_embeddings, txt_embeddings], 1)
-
- input_shape = embedding_output.size()[:-1]
-
- if attention_mask is None:
- attention_mask = torch.ones(input_shape, device=device)
- else:
- attention_mask = torch.cat(
- [torch.ones(input_modal_shape, device=device, dtype=torch.long), attention_mask], dim=1
- )
-
- if encoder_attention_mask is None:
- encoder_attention_mask = torch.ones(input_shape, device=device)
- else:
- encoder_attention_mask = torch.cat(
- [torch.ones(input_modal_shape, device=device), encoder_attention_mask], dim=1
- )
-
- # We can provide a self-attention mask of dimensions [batch_size, from_seq_length, to_seq_length]
- # ourselves in which case we just need to make it broadcastable to all heads.
- if attention_mask.dim() == 3:
- extended_attention_mask = attention_mask[:, None, :, :]
-
- # Provided a padding mask of dimensions [batch_size, seq_length]
- # - if the model is a decoder, apply a causal mask in addition to the padding mask
- # - if the model is an encoder, make the mask broadcastable to [batch_size, num_heads, seq_length, seq_length]
- if attention_mask.dim() == 2:
- if self.config.is_decoder:
- batch_size, seq_length = input_shape
- seq_ids = torch.arange(seq_length, device=device)
- causal_mask = seq_ids[None, None, :].repeat(batch_size, seq_length, 1) <= seq_ids[None, :, None]
- extended_attention_mask = causal_mask[:, None, :, :] * attention_mask[:, None, None, :]
- else:
- extended_attention_mask = attention_mask[:, None, None, :]
-
- # Since attention_mask is 1.0 for positions we want to attend and 0.0 for
- # masked positions, this operation will create a tensor which is 0.0 for
- # positions we want to attend and -10000.0 for masked positions.
- # Since we are adding it to the raw scores before the softmax, this is
- # effectively the same as removing these entirely.
- extended_attention_mask = extended_attention_mask.to(dtype=next(self.parameters()).dtype) # fp16 compatibility
- extended_attention_mask = (1.0 - extended_attention_mask) * -10000.0
-
- # If a 2D ou 3D attention mask is provided for the cross-attention
- # we need to make broadcastabe to [batch_size, num_heads, seq_length, seq_length]
- if encoder_attention_mask.dim() == 3:
- encoder_extended_attention_mask = encoder_attention_mask[:, None, :, :]
- if encoder_attention_mask.dim() == 2:
- encoder_extended_attention_mask = encoder_attention_mask[:, None, None, :]
-
- encoder_extended_attention_mask = encoder_extended_attention_mask.to(
- dtype=next(self.parameters()).dtype
- ) # fp16 compatibility
- encoder_extended_attention_mask = (1.0 - encoder_extended_attention_mask) * -10000.0
-
- # Prepare head mask if needed
- # 1.0 in head_mask indicate we keep the head
- # attention_probs has shape bsz x n_heads x N x N
- # input head_mask has shape [num_heads] or [num_hidden_layers x num_heads]
- # and head_mask is converted to shape [num_hidden_layers x batch x num_heads x seq_length x seq_length]
- if head_mask is not None:
- if head_mask.dim() == 1:
- head_mask = head_mask.unsqueeze(0).unsqueeze(0).unsqueeze(-1).unsqueeze(-1)
- head_mask = head_mask.expand(self.config.num_hidden_layers, -1, -1, -1, -1)
- elif head_mask.dim() == 2:
- head_mask = (
- head_mask.unsqueeze(1).unsqueeze(-1).unsqueeze(-1)
- ) # We can specify head_mask for each layer
- head_mask = head_mask.to(
- dtype=next(self.parameters()).dtype
- ) # switch to fload if need + fp16 compatibility
- else:
- head_mask = [None] * self.config.num_hidden_layers
-
- encoder_outputs = self.transformer.encoder(
- embedding_output,
- attention_mask=extended_attention_mask,
- head_mask=head_mask,
- encoder_hidden_states=encoder_hidden_states,
- encoder_attention_mask=encoder_extended_attention_mask,
- )
-
- sequence_output = encoder_outputs[0]
- pooled_output = self.transformer.pooler(sequence_output)
-
- outputs = (sequence_output, pooled_output,) + encoder_outputs[
- 1:
- ] # add hidden_states and attentions if they are here
- return outputs # sequence_output, pooled_output, (hidden_states), (attentions)
-
- def get_input_embeddings(self):
- return self.embeddings.word_embeddings
-
- def set_input_embeddings(self, value):
- self.embeddings.word_embeddings = value
-
-
-@add_start_docstrings(
- """MMBT Model with a sequence classification/regression head on top (a linear layer on top of
- the pooled output)""",
- MMBT_START_DOCSTRING,
- MMBT_INPUTS_DOCSTRING,
-)
-class MMBTForClassification(nn.Module):
- r"""
- **labels**: (`optional`) ``torch.LongTensor`` of shape ``(batch_size,)``:
- Labels for computing the sequence classification/regression loss.
- Indices should be in ``[0, ..., config.num_labels - 1]``.
- If ``config.num_labels == 1`` a regression loss is computed (Mean-Square loss),
- If ``config.num_labels > 1`` a classification loss is computed (Cross-Entropy).
-
- Outputs: `Tuple` comprising various elements depending on the configuration (config) and inputs:
- **loss**: (`optional`, returned when ``labels`` is provided) ``torch.FloatTensor`` of shape ``(1,)``:
- Classification (or regression if config.num_labels==1) loss.
- **logits**: ``torch.FloatTensor`` of shape ``(batch_size, config.num_labels)``
- Classification (or regression if config.num_labels==1) scores (before SoftMax).
- **hidden_states**: (`optional`, returned when ``config.output_hidden_states=True``)
- list of ``torch.FloatTensor`` (one for the output of each layer + the output of the embeddings)
- of shape ``(batch_size, sequence_length, hidden_size)``:
- Hidden-states of the model at the output of each layer plus the initial embedding outputs.
- **attentions**: (`optional`, returned when ``config.output_attentions=True``)
- list of ``torch.FloatTensor`` (one for each layer) of shape ``(batch_size, num_heads, sequence_length, sequence_length)``:
- Attentions weights after the attention softmax, used to compute the weighted average in the self-attention heads.
-
- Examples::
-
- # For example purposes. Not runnable.
- transformer = BertModel.from_pretrained('bert-base-uncased')
- encoder = ImageEncoder(args)
- model = MMBTForClassification(config, transformer, encoder)
- outputs = model(input_modal, input_ids, labels=labels)
- loss, logits = outputs[:2]
- """
-
- def __init__(self, config, transformer, encoder):
- super().__init__()
- self.num_labels = config.num_labels
-
- self.mmbt = MMBTModel(config, transformer, encoder)
- self.dropout = nn.Dropout(config.hidden_dropout_prob)
- self.classifier = nn.Linear(config.hidden_size, config.num_labels)
-
- def forward(
- self,
- input_modal,
- input_ids=None,
- modal_start_tokens=None,
- modal_end_tokens=None,
- attention_mask=None,
- token_type_ids=None,
- modal_token_type_ids=None,
- position_ids=None,
- modal_position_ids=None,
- head_mask=None,
- inputs_embeds=None,
- labels=None,
- ):
-
- outputs = self.mmbt(
- input_modal=input_modal,
- input_ids=input_ids,
- modal_start_tokens=modal_start_tokens,
- modal_end_tokens=modal_end_tokens,
- attention_mask=attention_mask,
- token_type_ids=token_type_ids,
- modal_token_type_ids=modal_token_type_ids,
- position_ids=position_ids,
- modal_position_ids=modal_position_ids,
- head_mask=head_mask,
- inputs_embeds=inputs_embeds,
- )
-
- pooled_output = outputs[1]
-
- pooled_output = self.dropout(pooled_output)
- logits = self.classifier(pooled_output)
-
- outputs = (logits,) + outputs[2:] # add hidden states and attention if they are here
-
- if labels is not None:
- if self.num_labels == 1:
- # We are doing regression
- loss_fct = MSELoss()
- loss = loss_fct(logits.view(-1), labels.view(-1))
- else:
- loss_fct = CrossEntropyLoss()
- loss = loss_fct(logits.view(-1, self.num_labels), labels.view(-1))
- outputs = (loss,) + outputs
-
- return outputs # (loss), logits, (hidden_states), (attentions)
diff --git a/server/transformers/src/transformers/modeling_openai.py b/server/transformers/src/transformers/modeling_openai.py
deleted file mode 100644
index 70abd5a1dc5fd060066fd34f02dbfbe20e343434..0000000000000000000000000000000000000000
--- a/server/transformers/src/transformers/modeling_openai.py
+++ /dev/null
@@ -1,700 +0,0 @@
-# coding=utf-8
-# Copyright 2018 The OpenAI Team Authors and HuggingFace Inc. team.
-# Copyright (c) 2018, NVIDIA CORPORATION. All rights reserved.
-#
-# Licensed under the Apache License, Version 2.0 (the "License");
-# you may not use this file except in compliance with the License.
-# You may obtain a copy of the License at
-#
-# http://www.apache.org/licenses/LICENSE-2.0
-#
-# Unless required by applicable law or agreed to in writing, software
-# distributed under the License is distributed on an "AS IS" BASIS,
-# WITHOUT WARRANTIES OR CONDITIONS OF ANY KIND, either express or implied.
-# See the License for the specific language governing permissions and
-# limitations under the License.
-"""PyTorch OpenAI GPT model."""
-
-
-import json
-import logging
-import math
-import os
-
-import torch
-import torch.nn as nn
-from torch.nn import CrossEntropyLoss
-
-from .configuration_openai import OpenAIGPTConfig
-from .file_utils import add_start_docstrings, add_start_docstrings_to_callable
-from .modeling_utils import Conv1D, PreTrainedModel, SequenceSummary, prune_conv1d_layer
-
-
-logger = logging.getLogger(__name__)
-
-OPENAI_GPT_PRETRAINED_MODEL_ARCHIVE_MAP = {
- "openai-gpt": "https://s3.amazonaws.com/models.huggingface.co/bert/openai-gpt-pytorch_model.bin"
-}
-
-
-def load_tf_weights_in_openai_gpt(model, config, openai_checkpoint_folder_path):
- """ Load tf pre-trained weights in a pytorch model (from NumPy arrays here)
- """
- import re
- import numpy as np
-
- if ".ckpt" in openai_checkpoint_folder_path:
- openai_checkpoint_folder_path = os.path.dirname(openai_checkpoint_folder_path)
-
- logger.info("Loading weights from {}".format(openai_checkpoint_folder_path))
-
- with open(openai_checkpoint_folder_path + "/parameters_names.json", "r", encoding="utf-8") as names_handle:
- names = json.load(names_handle)
- with open(openai_checkpoint_folder_path + "/params_shapes.json", "r", encoding="utf-8") as shapes_handle:
- shapes = json.load(shapes_handle)
- offsets = np.cumsum([np.prod(shape) for shape in shapes])
- init_params = [np.load(openai_checkpoint_folder_path + "/params_{}.npy".format(n)) for n in range(10)]
- init_params = np.split(np.concatenate(init_params, 0), offsets)[:-1]
- init_params = [param.reshape(shape) for param, shape in zip(init_params, shapes)]
-
- # This was used when we had a single embedding matrix for positions and tokens
- # init_params[0] = np.concatenate([init_params[1], init_params[0]], 0)
- # del init_params[1]
- init_params = [arr.squeeze() for arr in init_params]
-
- try:
- assert model.tokens_embed.weight.shape == init_params[1].shape
- assert model.positions_embed.weight.shape == init_params[0].shape
- except AssertionError as e:
- e.args += (model.tokens_embed.weight.shape, init_params[1].shape)
- e.args += (model.positions_embed.weight.shape, init_params[0].shape)
- raise
-
- model.tokens_embed.weight.data = torch.from_numpy(init_params[1])
- model.positions_embed.weight.data = torch.from_numpy(init_params[0])
- names.pop(0)
- # Pop position and token embedding arrays
- init_params.pop(0)
- init_params.pop(0)
-
- for name, array in zip(names, init_params): # names[1:n_transfer], init_params[1:n_transfer]):
- name = name[6:] # skip "model/"
- assert name[-2:] == ":0"
- name = name[:-2]
- name = name.split("/")
- pointer = model
- for m_name in name:
- if re.fullmatch(r"[A-Za-z]+\d+", m_name):
- scope_names = re.split(r"(\d+)", m_name)
- else:
- scope_names = [m_name]
- if scope_names[0] == "g":
- pointer = getattr(pointer, "weight")
- elif scope_names[0] == "b":
- pointer = getattr(pointer, "bias")
- elif scope_names[0] == "w":
- pointer = getattr(pointer, "weight")
- else:
- pointer = getattr(pointer, scope_names[0])
- if len(scope_names) >= 2:
- num = int(scope_names[1])
- pointer = pointer[num]
- try:
- assert pointer.shape == array.shape
- except AssertionError as e:
- e.args += (pointer.shape, array.shape)
- raise
- try:
- assert pointer.shape == array.shape
- except AssertionError as e:
- e.args += (pointer.shape, array.shape)
- raise
- logger.info("Initialize PyTorch weight {}".format(name))
- pointer.data = torch.from_numpy(array)
- return model
-
-
-def gelu(x):
- return 0.5 * x * (1 + torch.tanh(math.sqrt(2 / math.pi) * (x + 0.044715 * torch.pow(x, 3))))
-
-
-def swish(x):
- return x * torch.sigmoid(x)
-
-
-ACT_FNS = {"relu": nn.ReLU, "swish": swish, "gelu": gelu}
-
-
-class Attention(nn.Module):
- def __init__(self, nx, n_ctx, config, scale=False):
- super().__init__()
- n_state = nx # in Attention: n_state=768 (nx=n_embd)
- # [switch nx => n_state from Block to Attention to keep identical to TF implem]
- assert n_state % config.n_head == 0
- self.register_buffer("bias", torch.tril(torch.ones(n_ctx, n_ctx)).view(1, 1, n_ctx, n_ctx))
- self.n_head = config.n_head
- self.split_size = n_state
- self.scale = scale
-
- self.output_attentions = config.output_attentions
-
- self.c_attn = Conv1D(n_state * 3, nx)
- self.c_proj = Conv1D(n_state, nx)
- self.attn_dropout = nn.Dropout(config.attn_pdrop)
- self.resid_dropout = nn.Dropout(config.resid_pdrop)
- self.pruned_heads = set()
-
- def prune_heads(self, heads):
- if len(heads) == 0:
- return
- mask = torch.ones(self.n_head, self.split_size // self.n_head)
- heads = set(heads) - self.pruned_heads
- for head in heads:
- head -= sum(1 if h < head else 0 for h in self.pruned_heads)
- mask[head] = 0
- mask = mask.view(-1).contiguous().eq(1)
- index = torch.arange(len(mask))[mask].long()
- index_attn = torch.cat([index, index + self.split_size, index + (2 * self.split_size)])
- # Prune conv1d layers
- self.c_attn = prune_conv1d_layer(self.c_attn, index_attn, dim=1)
- self.c_proj = prune_conv1d_layer(self.c_proj, index, dim=0)
- # Update hyper params
- self.split_size = (self.split_size // self.n_head) * (self.n_head - len(heads))
- self.n_head = self.n_head - len(heads)
- self.pruned_heads = self.pruned_heads.union(heads)
-
- def _attn(self, q, k, v, attention_mask=None, head_mask=None):
- w = torch.matmul(q, k)
- if self.scale:
- w = w / math.sqrt(v.size(-1))
- # w = w * self.bias + -1e9 * (1 - self.bias) # TF implem method: mask_attn_weights
- # XD: self.b may be larger than w, so we need to crop it
- b = self.bias[:, :, : w.size(-2), : w.size(-1)]
- w = w * b + -1e4 * (1 - b)
-
- if attention_mask is not None:
- # Apply the attention mask
- w = w + attention_mask
-
- w = nn.Softmax(dim=-1)(w)
- w = self.attn_dropout(w)
-
- # Mask heads if we want to
- if head_mask is not None:
- w = w * head_mask
-
- outputs = [torch.matmul(w, v)]
- if self.output_attentions:
- outputs.append(w)
- return outputs
-
- def merge_heads(self, x):
- x = x.permute(0, 2, 1, 3).contiguous()
- new_x_shape = x.size()[:-2] + (x.size(-2) * x.size(-1),)
- return x.view(*new_x_shape) # in Tensorflow implem: fct merge_states
-
- def split_heads(self, x, k=False):
- new_x_shape = x.size()[:-1] + (self.n_head, x.size(-1) // self.n_head)
- x = x.view(*new_x_shape) # in Tensorflow implem: fct split_states
- if k:
- return x.permute(0, 2, 3, 1)
- else:
- return x.permute(0, 2, 1, 3)
-
- def forward(self, x, attention_mask=None, head_mask=None):
- x = self.c_attn(x)
- query, key, value = x.split(self.split_size, dim=2)
- query = self.split_heads(query)
- key = self.split_heads(key, k=True)
- value = self.split_heads(value)
-
- attn_outputs = self._attn(query, key, value, attention_mask, head_mask)
- a = attn_outputs[0]
-
- a = self.merge_heads(a)
- a = self.c_proj(a)
- a = self.resid_dropout(a)
-
- outputs = [a] + attn_outputs[1:]
- return outputs # a, (attentions)
-
-
-class MLP(nn.Module):
- def __init__(self, n_state, config): # in MLP: n_state=3072 (4 * n_embd)
- super().__init__()
- nx = config.n_embd
- self.c_fc = Conv1D(n_state, nx)
- self.c_proj = Conv1D(nx, n_state)
- self.act = ACT_FNS[config.afn]
- self.dropout = nn.Dropout(config.resid_pdrop)
-
- def forward(self, x):
- h = self.act(self.c_fc(x))
- h2 = self.c_proj(h)
- return self.dropout(h2)
-
-
-class Block(nn.Module):
- def __init__(self, n_ctx, config, scale=False):
- super().__init__()
- nx = config.n_embd
- self.attn = Attention(nx, n_ctx, config, scale)
- self.ln_1 = nn.LayerNorm(nx, eps=config.layer_norm_epsilon)
- self.mlp = MLP(4 * nx, config)
- self.ln_2 = nn.LayerNorm(nx, eps=config.layer_norm_epsilon)
-
- def forward(self, x, attention_mask=None, head_mask=None):
- attn_outputs = self.attn(x, attention_mask=attention_mask, head_mask=head_mask)
- a = attn_outputs[0]
-
- n = self.ln_1(x + a)
- m = self.mlp(n)
- h = self.ln_2(n + m)
-
- outputs = [h] + attn_outputs[1:]
- return outputs
-
-
-class OpenAIGPTPreTrainedModel(PreTrainedModel):
- """ An abstract class to handle weights initialization and
- a simple interface for downloading and loading pretrained models.
- """
-
- config_class = OpenAIGPTConfig
- pretrained_model_archive_map = OPENAI_GPT_PRETRAINED_MODEL_ARCHIVE_MAP
- load_tf_weights = load_tf_weights_in_openai_gpt
- base_model_prefix = "transformer"
-
- def _init_weights(self, module):
- """ Initialize the weights.
- """
- if isinstance(module, (nn.Linear, nn.Embedding, Conv1D)):
- # Slightly different from the TF version which uses truncated_normal for initialization
- # cf https://github.com/pytorch/pytorch/pull/5617
- module.weight.data.normal_(mean=0.0, std=self.config.initializer_range)
- if isinstance(module, (nn.Linear, Conv1D)) and module.bias is not None:
- module.bias.data.zero_()
- elif isinstance(module, nn.LayerNorm):
- module.bias.data.zero_()
- module.weight.data.fill_(1.0)
-
-
-OPENAI_GPT_START_DOCSTRING = r"""
-
- This model is a PyTorch `torch.nn.Module `_ sub-class.
- Use it as a regular PyTorch Module and refer to the PyTorch documentation for all matter related to general
- usage and behavior.
-
- Parameters:
- config (:class:`~transformers.OpenAIGPTConfig`): Model configuration class with all the parameters of the model.
- Initializing with a config file does not load the weights associated with the model, only the configuration.
- Check out the :meth:`~transformers.PreTrainedModel.from_pretrained` method to load the model weights.
-"""
-
-OPENAI_GPT_INPUTS_DOCSTRING = r"""
- Args:
- input_ids (:obj:`torch.LongTensor` of shape :obj:`(batch_size, sequence_length)`):
- Indices of input sequence tokens in the vocabulary.
-
- Indices can be obtained using :class:`transformers.OpenAIGPTTokenizer`.
- See :func:`transformers.PreTrainedTokenizer.encode` and
- :func:`transformers.PreTrainedTokenizer.encode_plus` for details.
-
- `What are input IDs? <../glossary.html#input-ids>`__
- attention_mask (:obj:`torch.FloatTensor` of shape :obj:`(batch_size, sequence_length)`, `optional`, defaults to :obj:`None`):
- Mask to avoid performing attention on padding token indices.
- Mask values selected in ``[0, 1]``:
- ``1`` for tokens that are NOT MASKED, ``0`` for MASKED tokens.
-
- `What are attention masks? <../glossary.html#attention-mask>`__
- token_type_ids (:obj:`torch.LongTensor` of shape :obj:`(batch_size, sequence_length)`, `optional`, defaults to :obj:`None`):
- Segment token indices to indicate first and second portions of the inputs.
- Indices are selected in ``[0, 1]``: ``0`` corresponds to a `sentence A` token, ``1``
- corresponds to a `sentence B` token
-
- `What are token type IDs? <../glossary.html#token-type-ids>`_
- position_ids (:obj:`torch.LongTensor` of shape :obj:`(batch_size, sequence_length)`, `optional`, defaults to :obj:`None`):
- Indices of positions of each input sequence tokens in the position embeddings.
- Selected in the range ``[0, config.max_position_embeddings - 1]``.
-
- `What are position IDs? <../glossary.html#position-ids>`_
- head_mask (:obj:`torch.FloatTensor` of shape :obj:`(num_heads,)` or :obj:`(num_layers, num_heads)`, `optional`, defaults to :obj:`None`):
- Mask to nullify selected heads of the self-attention modules.
- Mask values selected in ``[0, 1]``:
- :obj:`1` indicates the head is **not masked**, :obj:`0` indicates the head is **masked**.
- input_embeds (:obj:`torch.FloatTensor` of shape :obj:`(batch_size, sequence_length, hidden_size)`, `optional`, defaults to :obj:`None`):
- Optionally, instead of passing :obj:`input_ids` you can choose to directly pass an embedded representation.
- This is useful if you want more control over how to convert `input_ids` indices into associated vectors
- than the model's internal embedding lookup matrix.
-"""
-
-
-@add_start_docstrings(
- "The bare OpenAI GPT transformer model outputting raw hidden-states without any specific head on top.",
- OPENAI_GPT_START_DOCSTRING,
-)
-class OpenAIGPTModel(OpenAIGPTPreTrainedModel):
- def __init__(self, config):
- super().__init__(config)
- self.output_attentions = config.output_attentions
- self.output_hidden_states = config.output_hidden_states
-
- self.tokens_embed = nn.Embedding(config.vocab_size, config.n_embd)
- self.positions_embed = nn.Embedding(config.n_positions, config.n_embd)
- self.drop = nn.Dropout(config.embd_pdrop)
- self.h = nn.ModuleList([Block(config.n_ctx, config, scale=True) for _ in range(config.n_layer)])
-
- self.init_weights()
-
- def get_input_embeddings(self):
- return self.tokens_embed
-
- def set_input_embeddings(self, new_embeddings):
- self.tokens_embed = new_embeddings
-
- def _prune_heads(self, heads_to_prune):
- """ Prunes heads of the model.
- heads_to_prune: dict of {layer_num: list of heads to prune in this layer}
- """
- for layer, heads in heads_to_prune.items():
- self.h[layer].attn.prune_heads(heads)
-
- @add_start_docstrings_to_callable(OPENAI_GPT_INPUTS_DOCSTRING)
- def forward(
- self,
- input_ids=None,
- attention_mask=None,
- token_type_ids=None,
- position_ids=None,
- head_mask=None,
- inputs_embeds=None,
- ):
- r"""
- Return:
- :obj:`tuple(torch.FloatTensor)` comprising various elements depending on the configuration (:class:`~transformers.OpenAIGPTConfig`) and inputs:
- last_hidden_state (:obj:`torch.FloatTensor` of shape :obj:`(batch_size, sequence_length, hidden_size)`):
- Sequence of hidden-states at the last layer of the model.
- hidden_states (:obj:`tuple(torch.FloatTensor)`, `optional`, returned when ``config.output_hidden_states=True``):
- Tuple of :obj:`torch.FloatTensor` (one for the output of the embeddings + one for the output of each layer)
- of shape :obj:`(batch_size, sequence_length, hidden_size)`.
-
- Hidden-states of the model at the output of each layer plus the initial embedding outputs.
- attentions (:obj:`tuple(torch.FloatTensor)`, `optional`, returned when ``config.output_attentions=True``):
- Tuple of :obj:`torch.FloatTensor` (one for each layer) of shape
- :obj:`(batch_size, num_heads, sequence_length, sequence_length)`.
-
- Attentions weights after the attention softmax, used to compute the weighted average in the self-attention
- heads.
-
- Examples::
-
- from transformers import OpenAIGPTTokenizer, OpenAIGPTModel
- import torch
-
- tokenizer = OpenAIGPTTokenizer.from_pretrained('openai-gpt')
- model = OpenAIGPTModel.from_pretrained('openai-gpt')
- input_ids = torch.tensor(tokenizer.encode("Hello, my dog is cute", add_special_tokens=True)).unsqueeze(0) # Batch size 1
- outputs = model(input_ids)
- last_hidden_states = outputs[0] # The last hidden-state is the first element of the output tuple
-
- """
- if input_ids is not None and inputs_embeds is not None:
- raise ValueError("You cannot specify both input_ids and inputs_embeds at the same time")
- elif input_ids is not None:
- input_shape = input_ids.size()
- input_ids = input_ids.view(-1, input_shape[-1])
- elif inputs_embeds is not None:
- input_shape = inputs_embeds.size()[:-1]
- else:
- raise ValueError("You have to specify either input_ids or inputs_embeds")
-
- if position_ids is None:
- # Code is different from when we had a single embedding matrice from position and token embeddings
- device = input_ids.device if input_ids is not None else inputs_embeds.device
- position_ids = torch.arange(input_shape[-1], dtype=torch.long, device=device)
- position_ids = position_ids.unsqueeze(0).view(-1, input_shape[-1])
-
- # Attention mask.
- if attention_mask is not None:
- # We create a 3D attention mask from a 2D tensor mask.
- # Sizes are [batch_size, 1, 1, to_seq_length]
- # So we can broadcast to [batch_size, num_heads, from_seq_length, to_seq_length]
- # this attention mask is more simple than the triangular masking of causal attention
- # used in OpenAI GPT, we just need to prepare the broadcast dimension here.
- attention_mask = attention_mask.unsqueeze(1).unsqueeze(2)
-
- # Since attention_mask is 1.0 for positions we want to attend and 0.0 for
- # masked positions, this operation will create a tensor which is 0.0 for
- # positions we want to attend and -10000.0 for masked positions.
- # Since we are adding it to the raw scores before the softmax, this is
- # effectively the same as removing these entirely.
- attention_mask = attention_mask.to(dtype=next(self.parameters()).dtype) # fp16 compatibility
- attention_mask = (1.0 - attention_mask) * -10000.0
-
- # Prepare head mask if needed
- # 1.0 in head_mask indicate we keep the head
- # attention_probs has shape bsz x n_heads x N x N
- # head_mask has shape n_layer x batch x n_heads x N x N
- if head_mask is not None:
- if head_mask.dim() == 1:
- head_mask = head_mask.unsqueeze(0).unsqueeze(0).unsqueeze(-1).unsqueeze(-1)
- head_mask = head_mask.expand(self.config.n_layer, -1, -1, -1, -1)
- elif head_mask.dim() == 2:
- head_mask = (
- head_mask.unsqueeze(1).unsqueeze(-1).unsqueeze(-1)
- ) # We can specify head_mask for each layer
- head_mask = head_mask.to(
- dtype=next(self.parameters()).dtype
- ) # switch to fload if need + fp16 compatibility
- else:
- head_mask = [None] * self.config.n_layer
-
- if inputs_embeds is None:
- inputs_embeds = self.tokens_embed(input_ids)
- position_embeds = self.positions_embed(position_ids)
- if token_type_ids is not None:
- token_type_ids = token_type_ids.view(-1, token_type_ids.size(-1))
- token_type_embeds = self.tokens_embed(token_type_ids)
- else:
- token_type_embeds = 0
- hidden_states = inputs_embeds + position_embeds + token_type_embeds
- hidden_states = self.drop(hidden_states)
-
- output_shape = input_shape + (hidden_states.size(-1),)
-
- all_attentions = ()
- all_hidden_states = ()
- for i, block in enumerate(self.h):
- if self.output_hidden_states:
- all_hidden_states = all_hidden_states + (hidden_states.view(*output_shape),)
-
- outputs = block(hidden_states, attention_mask, head_mask[i])
- hidden_states = outputs[0]
- if self.output_attentions:
- all_attentions = all_attentions + (outputs[1],)
-
- # Add last layer
- if self.output_hidden_states:
- all_hidden_states = all_hidden_states + (hidden_states.view(*output_shape),)
-
- outputs = (hidden_states.view(*output_shape),)
- if self.output_hidden_states:
- outputs = outputs + (all_hidden_states,)
- if self.output_attentions:
- outputs = outputs + (all_attentions,)
- return outputs # last hidden state, (all hidden states), (all attentions)
-
-
-@add_start_docstrings(
- """OpenAI GPT Model transformer with a language modeling head on top
- (linear layer with weights tied to the input embeddings). """,
- OPENAI_GPT_START_DOCSTRING,
-)
-class OpenAIGPTLMHeadModel(OpenAIGPTPreTrainedModel):
- def __init__(self, config):
- super().__init__(config)
- self.transformer = OpenAIGPTModel(config)
- self.lm_head = nn.Linear(config.n_embd, config.vocab_size, bias=False)
-
- self.init_weights()
-
- def get_output_embeddings(self):
- return self.lm_head
-
- @add_start_docstrings_to_callable(OPENAI_GPT_INPUTS_DOCSTRING)
- def forward(
- self,
- input_ids=None,
- attention_mask=None,
- token_type_ids=None,
- position_ids=None,
- head_mask=None,
- inputs_embeds=None,
- labels=None,
- ):
- r"""
- labels (:obj:`torch.LongTensor` of shape :obj:`(batch_size, sequence_length)`, `optional`, defaults to :obj:`None`):
- Labels for language modeling.
- Note that the labels **are shifted** inside the model, i.e. you can set ``lm_labels = input_ids``
- Indices are selected in ``[-100, 0, ..., config.vocab_size]``
- All labels set to ``-100`` are ignored (masked), the loss is only
- computed for labels in ``[0, ..., config.vocab_size]``
-
- Return:
- :obj:`tuple(torch.FloatTensor)` comprising various elements depending on the configuration (:class:`~transformers.OpenAIGPTConfig`) and inputs:
- loss (:obj:`torch.FloatTensor` of shape `(1,)`, `optional`, returned when ``labels`` is provided)
- Language modeling loss.
- prediction_scores (:obj:`torch.FloatTensor` of shape :obj:`(batch_size, sequence_length, config.vocab_size)`):
- Prediction scores of the language modeling head (scores for each vocabulary token before SoftMax).
- past (:obj:`List[torch.FloatTensor]` of length :obj:`config.n_layers` with each tensor of shape :obj:`(2, batch_size, num_heads, sequence_length, embed_size_per_head)`):
- Contains pre-computed hidden-states (key and values in the attention blocks).
- Can be used (see `past` input) to speed up sequential decoding. The token ids which have their past given to this model
- should not be passed as input ids as they have already been computed.
- hidden_states (:obj:`tuple(torch.FloatTensor)`, `optional`, returned when ``config.output_hidden_states=True``):
- Tuple of :obj:`torch.FloatTensor` (one for the output of the embeddings + one for the output of each layer)
- of shape :obj:`(batch_size, sequence_length, hidden_size)`.
-
- Hidden-states of the model at the output of each layer plus the initial embedding outputs.
- attentions (:obj:`tuple(torch.FloatTensor)`, `optional`, returned when ``config.output_attentions=True``):
- Tuple of :obj:`torch.FloatTensor` (one for each layer) of shape
- :obj:`(batch_size, num_heads, sequence_length, sequence_length)`.
-
- Attentions weights after the attention softmax, used to compute the weighted average in the self-attention
- heads.
-
- Examples::
-
- from transformers import OpenAIGPTTokenizer, OpenAIGPTLMHeadModel
- import torch
-
- tokenizer = OpenAIGPTTokenizer.from_pretrained('openai-gpt')
- model = OpenAIGPTLMHeadModel.from_pretrained('openai-gpt')
- input_ids = torch.tensor(tokenizer.encode("Hello, my dog is cute", add_special_tokens=True)).unsqueeze(0) # Batch size 1
- outputs = model(input_ids, labels=input_ids)
- loss, logits = outputs[:2]
-
- """
- transformer_outputs = self.transformer(
- input_ids,
- attention_mask=attention_mask,
- token_type_ids=token_type_ids,
- position_ids=position_ids,
- head_mask=head_mask,
- inputs_embeds=inputs_embeds,
- )
- hidden_states = transformer_outputs[0]
- lm_logits = self.lm_head(hidden_states)
-
- outputs = (lm_logits,) + transformer_outputs[1:]
- if labels is not None:
- # Shift so that tokens < n predict n
- shift_logits = lm_logits[..., :-1, :].contiguous()
- shift_labels = labels[..., 1:].contiguous()
- # Flatten the tokens
- loss_fct = CrossEntropyLoss()
- loss = loss_fct(shift_logits.view(-1, shift_logits.size(-1)), shift_labels.view(-1))
- outputs = (loss,) + outputs
-
- return outputs # (loss), lm_logits, (all hidden states), (all attentions)
-
-
-@add_start_docstrings(
- """OpenAI GPT Model transformer with a language modeling and a multiple-choice classification
- head on top e.g. for RocStories/SWAG tasks. The two heads are two linear layers.
- The language modeling head has its weights tied to the input embeddings,
- the classification head takes as input the input of a specified classification token index in the input sequence).
-""",
- OPENAI_GPT_START_DOCSTRING,
-)
-class OpenAIGPTDoubleHeadsModel(OpenAIGPTPreTrainedModel):
- def __init__(self, config):
- super().__init__(config)
-
- config.num_labels = 1
- self.transformer = OpenAIGPTModel(config)
- self.lm_head = nn.Linear(config.n_embd, config.vocab_size, bias=False)
- self.multiple_choice_head = SequenceSummary(config)
-
- self.init_weights()
-
- def get_output_embeddings(self):
- return self.lm_head
-
- @add_start_docstrings_to_callable(OPENAI_GPT_INPUTS_DOCSTRING)
- def forward(
- self,
- input_ids=None,
- attention_mask=None,
- token_type_ids=None,
- position_ids=None,
- head_mask=None,
- inputs_embeds=None,
- mc_token_ids=None,
- lm_labels=None,
- mc_labels=None,
- ):
- r"""
- mc_token_ids (:obj:`torch.LongTensor` of shape :obj:`(batch_size, num_choices)`, `optional`, default to index of the last token of the input)
- Index of the classification token in each input sequence.
- Selected in the range ``[0, input_ids.size(-1) - 1[``.
- lm_labels (:obj:`torch.LongTensor` of shape :obj:`(batch_size, sequence_length)`, `optional`, defaults to :obj:`None`)
- Labels for language modeling.
- Note that the labels **are shifted** inside the model, i.e. you can set ``lm_labels = input_ids``
- Indices are selected in ``[-1, 0, ..., config.vocab_size]``
- All labels set to ``-100`` are ignored (masked), the loss is only
- computed for labels in ``[0, ..., config.vocab_size]``
- mc_labels (:obj:`torch.LongTensor` of shape :obj:`(batch_size)`, `optional`, defaults to :obj:`None`)
- Labels for computing the multiple choice classification loss.
- Indices should be in ``[0, ..., num_choices]`` where `num_choices` is the size of the second dimension
- of the input tensors. (see `input_ids` above)
-
- Return:
- :obj:`tuple(torch.FloatTensor)` comprising various elements depending on the configuration (:class:`~transformers.OpenAIGPTConfig`) and inputs:
- lm_loss (:obj:`torch.FloatTensor` of shape :obj:`(1,)`, `optional`, returned when ``lm_labels`` is provided):
- Language modeling loss.
- mc_loss (:obj:`torch.FloatTensor` of shape :obj:`(1,)`, `optional`, returned when :obj:`multiple_choice_labels` is provided):
- Multiple choice classification loss.
- lm_prediction_scores (:obj:`torch.FloatTensor` of shape :obj:`(batch_size, num_choices, sequence_length, config.vocab_size)`):
- Prediction scores of the language modeling head (scores for each vocabulary token before SoftMax).
- mc_prediction_scores (:obj:`torch.FloatTensor` of shape :obj:`(batch_size, num_choices)`):
- Prediction scores of the multiple choice classification head (scores for each choice before SoftMax).
- past (:obj:`List[torch.FloatTensor]` of length :obj:`config.n_layers` with each tensor of shape :obj:`(2, batch_size, num_heads, sequence_length, embed_size_per_head)`):
- Contains pre-computed hidden-states (key and values in the attention blocks).
- Can be used (see `past` input) to speed up sequential decoding. The token ids which have their past given to this model
- should not be passed as input ids as they have already been computed.
- hidden_states (:obj:`tuple(torch.FloatTensor)`, `optional`, returned when ``config.output_hidden_states=True``):
- Tuple of :obj:`torch.FloatTensor` (one for the output of the embeddings + one for the output of each layer)
- of shape :obj:`(batch_size, sequence_length, hidden_size)`.
-
- Hidden-states of the model at the output of each layer plus the initial embedding outputs.
- attentions (:obj:`tuple(torch.FloatTensor)`, `optional`, returned when ``config.output_attentions=True``):
- Tuple of :obj:`torch.FloatTensor` (one for each layer) of shape
- :obj:`(batch_size, num_heads, sequence_length, sequence_length)`.
-
- Attentions weights after the attention softmax, used to compute the weighted average in the self-attention
- heads.
-
- Examples::
-
- from transformers import OpenAIGPTTokenizer, OpenAIGPTDoubleHeadsModel
- import torch
-
- tokenizer = OpenAIGPTTokenizer.from_pretrained('openai-gpt')
- model = OpenAIGPTDoubleHeadsModel.from_pretrained('openai-gpt')
- tokenizer.add_special_tokens({'cls_token': '[CLS]'}) # Add a [CLS] to the vocabulary (we should train it also!)
- model.resize_token_embeddings(len(tokenizer))
-
- choices = ["Hello, my dog is cute [CLS]", "Hello, my cat is cute [CLS]"]
- input_ids = torch.tensor([tokenizer.encode(s) for s in choices]).unsqueeze(0) # Batch size 1, 2 choices
- mc_token_ids = torch.tensor([input_ids.size(-1)-1, input_ids.size(-1)-1]).unsqueeze(0) # Batch size 1
-
- outputs = model(input_ids, mc_token_ids=mc_token_ids)
- lm_prediction_scores, mc_prediction_scores = outputs[:2]
-
- """
- transformer_outputs = self.transformer(
- input_ids,
- attention_mask=attention_mask,
- token_type_ids=token_type_ids,
- position_ids=position_ids,
- head_mask=head_mask,
- inputs_embeds=inputs_embeds,
- )
- hidden_states = transformer_outputs[0]
-
- lm_logits = self.lm_head(hidden_states)
- mc_logits = self.multiple_choice_head(hidden_states, mc_token_ids).squeeze(-1)
-
- outputs = (lm_logits, mc_logits) + transformer_outputs[1:]
- if mc_labels is not None:
- loss_fct = CrossEntropyLoss()
- loss = loss_fct(mc_logits.view(-1, mc_logits.size(-1)), mc_labels.view(-1))
- outputs = (loss,) + outputs
- if lm_labels is not None:
- shift_logits = lm_logits[..., :-1, :].contiguous()
- shift_labels = lm_labels[..., 1:].contiguous()
- loss_fct = CrossEntropyLoss()
- loss = loss_fct(shift_logits.view(-1, shift_logits.size(-1)), shift_labels.view(-1))
- outputs = (loss,) + outputs
-
- return outputs # (lm loss), (mc loss), lm logits, mc logits, (all hidden_states), (attentions)
diff --git a/server/transformers/src/transformers/modeling_roberta.py b/server/transformers/src/transformers/modeling_roberta.py
deleted file mode 100644
index 50de77b85c1428e770798dbc71b944882f4d55bd..0000000000000000000000000000000000000000
--- a/server/transformers/src/transformers/modeling_roberta.py
+++ /dev/null
@@ -1,705 +0,0 @@
-# coding=utf-8
-# Copyright 2018 The Google AI Language Team Authors and The HuggingFace Inc. team.
-# Copyright (c) 2018, NVIDIA CORPORATION. All rights reserved.
-#
-# Licensed under the Apache License, Version 2.0 (the "License");
-# you may not use this file except in compliance with the License.
-# You may obtain a copy of the License at
-#
-# http://www.apache.org/licenses/LICENSE-2.0
-#
-# Unless required by applicable law or agreed to in writing, software
-# distributed under the License is distributed on an "AS IS" BASIS,
-# WITHOUT WARRANTIES OR CONDITIONS OF ANY KIND, either express or implied.
-# See the License for the specific language governing permissions and
-# limitations under the License.
-"""PyTorch RoBERTa model. """
-
-
-import logging
-
-import torch
-import torch.nn as nn
-from torch.nn import CrossEntropyLoss, MSELoss
-
-from .configuration_roberta import RobertaConfig
-from .file_utils import add_start_docstrings, add_start_docstrings_to_callable
-from .modeling_bert import BertEmbeddings, BertLayerNorm, BertModel, BertPreTrainedModel, gelu
-
-
-logger = logging.getLogger(__name__)
-
-ROBERTA_PRETRAINED_MODEL_ARCHIVE_MAP = {
- "roberta-base": "https://s3.amazonaws.com/models.huggingface.co/bert/roberta-base-pytorch_model.bin",
- "roberta-large": "https://s3.amazonaws.com/models.huggingface.co/bert/roberta-large-pytorch_model.bin",
- "roberta-large-mnli": "https://s3.amazonaws.com/models.huggingface.co/bert/roberta-large-mnli-pytorch_model.bin",
- "distilroberta-base": "https://s3.amazonaws.com/models.huggingface.co/bert/distilroberta-base-pytorch_model.bin",
- "roberta-base-openai-detector": "https://s3.amazonaws.com/models.huggingface.co/bert/roberta-base-openai-detector-pytorch_model.bin",
- "roberta-large-openai-detector": "https://s3.amazonaws.com/models.huggingface.co/bert/roberta-large-openai-detector-pytorch_model.bin",
-}
-
-
-class RobertaEmbeddings(BertEmbeddings):
- """
- Same as BertEmbeddings with a tiny tweak for positional embeddings indexing.
- """
-
- def __init__(self, config):
- super().__init__(config)
- self.padding_idx = 1
- self.word_embeddings = nn.Embedding(config.vocab_size, config.hidden_size, padding_idx=self.padding_idx)
- self.position_embeddings = nn.Embedding(
- config.max_position_embeddings, config.hidden_size, padding_idx=self.padding_idx
- )
-
- def forward(self, input_ids=None, token_type_ids=None, position_ids=None, inputs_embeds=None):
- if position_ids is None:
- if input_ids is not None:
- # Create the position ids from the input token ids. Any padded tokens remain padded.
- position_ids = self.create_position_ids_from_input_ids(input_ids).to(input_ids.device)
- else:
- position_ids = self.create_position_ids_from_inputs_embeds(inputs_embeds)
-
- return super().forward(
- input_ids, token_type_ids=token_type_ids, position_ids=position_ids, inputs_embeds=inputs_embeds
- )
-
- def create_position_ids_from_input_ids(self, x):
- """ Replace non-padding symbols with their position numbers. Position numbers begin at
- padding_idx+1. Padding symbols are ignored. This is modified from fairseq's
- `utils.make_positions`.
-
- :param torch.Tensor x:
- :return torch.Tensor:
- """
- mask = x.ne(self.padding_idx).long()
- incremental_indicies = torch.cumsum(mask, dim=1) * mask
- return incremental_indicies + self.padding_idx
-
- def create_position_ids_from_inputs_embeds(self, inputs_embeds):
- """ We are provided embeddings directly. We cannot infer which are padded so just generate
- sequential position ids.
-
- :param torch.Tensor inputs_embeds:
- :return torch.Tensor:
- """
- input_shape = inputs_embeds.size()[:-1]
- sequence_length = input_shape[1]
-
- position_ids = torch.arange(
- self.padding_idx + 1, sequence_length + self.padding_idx + 1, dtype=torch.long, device=inputs_embeds.device
- )
- return position_ids.unsqueeze(0).expand(input_shape)
-
-
-ROBERTA_START_DOCSTRING = r"""
-
- This model is a PyTorch `torch.nn.Module `_ sub-class.
- Use it as a regular PyTorch Module and refer to the PyTorch documentation for all matter related to general
- usage and behavior.
-
- Parameters:
- config (:class:`~transformers.RobertaConfig`): Model configuration class with all the parameters of the
- model. Initializing with a config file does not load the weights associated with the model, only the configuration.
- Check out the :meth:`~transformers.PreTrainedModel.from_pretrained` method to load the model weights.
-"""
-
-ROBERTA_INPUTS_DOCSTRING = r"""
- Args:
- input_ids (:obj:`torch.LongTensor` of shape :obj:`(batch_size, sequence_length)`):
- Indices of input sequence tokens in the vocabulary.
-
- Indices can be obtained using :class:`transformers.RobertaTokenizer`.
- See :func:`transformers.PreTrainedTokenizer.encode` and
- :func:`transformers.PreTrainedTokenizer.encode_plus` for details.
-
- `What are input IDs? <../glossary.html#input-ids>`__
- attention_mask (:obj:`torch.FloatTensor` of shape :obj:`(batch_size, sequence_length)`, `optional`, defaults to :obj:`None`):
- Mask to avoid performing attention on padding token indices.
- Mask values selected in ``[0, 1]``:
- ``1`` for tokens that are NOT MASKED, ``0`` for MASKED tokens.
-
- `What are attention masks? <../glossary.html#attention-mask>`__
- token_type_ids (:obj:`torch.LongTensor` of shape :obj:`(batch_size, sequence_length)`, `optional`, defaults to :obj:`None`):
- Segment token indices to indicate first and second portions of the inputs.
- Indices are selected in ``[0, 1]``: ``0`` corresponds to a `sentence A` token, ``1``
- corresponds to a `sentence B` token
-
- `What are token type IDs? <../glossary.html#token-type-ids>`_
- position_ids (:obj:`torch.LongTensor` of shape :obj:`(batch_size, sequence_length)`, `optional`, defaults to :obj:`None`):
- Indices of positions of each input sequence tokens in the position embeddings.
- Selected in the range ``[0, config.max_position_embeddings - 1]``.
-
- `What are position IDs? <../glossary.html#position-ids>`_
- head_mask (:obj:`torch.FloatTensor` of shape :obj:`(num_heads,)` or :obj:`(num_layers, num_heads)`, `optional`, defaults to :obj:`None`):
- Mask to nullify selected heads of the self-attention modules.
- Mask values selected in ``[0, 1]``:
- :obj:`1` indicates the head is **not masked**, :obj:`0` indicates the head is **masked**.
- inputs_embeds (:obj:`torch.FloatTensor` of shape :obj:`(batch_size, sequence_length, hidden_size)`, `optional`, defaults to :obj:`None`):
- Optionally, instead of passing :obj:`input_ids` you can choose to directly pass an embedded representation.
- This is useful if you want more control over how to convert `input_ids` indices into associated vectors
- than the model's internal embedding lookup matrix.
-"""
-
-
-@add_start_docstrings(
- "The bare RoBERTa Model transformer outputting raw hidden-states without any specific head on top.",
- ROBERTA_START_DOCSTRING,
-)
-class RobertaModel(BertModel):
- """
- This class overrides :class:`~transformers.BertModel`. Please check the
- superclass for the appropriate documentation alongside usage examples.
- """
-
- config_class = RobertaConfig
- pretrained_model_archive_map = ROBERTA_PRETRAINED_MODEL_ARCHIVE_MAP
- base_model_prefix = "roberta"
-
- def __init__(self, config):
- super().__init__(config)
-
- self.embeddings = RobertaEmbeddings(config)
- self.init_weights()
-
- def get_input_embeddings(self):
- return self.embeddings.word_embeddings
-
- def set_input_embeddings(self, value):
- self.embeddings.word_embeddings = value
-
-
-@add_start_docstrings("""RoBERTa Model with a `language modeling` head on top. """, ROBERTA_START_DOCSTRING)
-class RobertaForMaskedLM(BertPreTrainedModel):
- config_class = RobertaConfig
- pretrained_model_archive_map = ROBERTA_PRETRAINED_MODEL_ARCHIVE_MAP
- base_model_prefix = "roberta"
-
- def __init__(self, config):
- super().__init__(config)
-
- self.roberta = RobertaModel(config)
- self.lm_head = RobertaLMHead(config)
-
- self.init_weights()
-
- def get_output_embeddings(self):
- return self.lm_head.decoder
-
- @add_start_docstrings_to_callable(ROBERTA_INPUTS_DOCSTRING)
- def forward(
- self,
- input_ids=None,
- attention_mask=None,
- token_type_ids=None,
- position_ids=None,
- head_mask=None,
- inputs_embeds=None,
- masked_lm_labels=None,
- ):
- r"""
- masked_lm_labels (:obj:`torch.LongTensor` of shape :obj:`(batch_size, sequence_length)`, `optional`, defaults to :obj:`None`):
- Labels for computing the masked language modeling loss.
- Indices should be in ``[-100, 0, ..., config.vocab_size]`` (see ``input_ids`` docstring)
- Tokens with indices set to ``-100`` are ignored (masked), the loss is only computed for the tokens with labels
- in ``[0, ..., config.vocab_size]``
-
- Returns:
- :obj:`tuple(torch.FloatTensor)` comprising various elements depending on the configuration (:class:`~transformers.RobertaConfig`) and inputs:
- masked_lm_loss (`optional`, returned when ``masked_lm_labels`` is provided) ``torch.FloatTensor`` of shape ``(1,)``:
- Masked language modeling loss.
- prediction_scores (:obj:`torch.FloatTensor` of shape :obj:`(batch_size, sequence_length, config.vocab_size)`)
- Prediction scores of the language modeling head (scores for each vocabulary token before SoftMax).
- hidden_states (:obj:`tuple(torch.FloatTensor)`, `optional`, returned when ``config.output_hidden_states=True``):
- Tuple of :obj:`torch.FloatTensor` (one for the output of the embeddings + one for the output of each layer)
- of shape :obj:`(batch_size, sequence_length, hidden_size)`.
-
- Hidden-states of the model at the output of each layer plus the initial embedding outputs.
- attentions (:obj:`tuple(torch.FloatTensor)`, `optional`, returned when ``config.output_attentions=True``):
- Tuple of :obj:`torch.FloatTensor` (one for each layer) of shape
- :obj:`(batch_size, num_heads, sequence_length, sequence_length)`.
-
- Attentions weights after the attention softmax, used to compute the weighted average in the self-attention
- heads.
-
- Examples::
-
- from transformers import RobertaTokenizer, RobertaForMaskedLM
- import torch
-
- tokenizer = RobertaTokenizer.from_pretrained('roberta-base')
- model = RobertaForMaskedLM.from_pretrained('roberta-base')
- input_ids = torch.tensor(tokenizer.encode("Hello, my dog is cute", add_special_tokens=True)).unsqueeze(0) # Batch size 1
- outputs = model(input_ids, masked_lm_labels=input_ids)
- loss, prediction_scores = outputs[:2]
-
- """
- outputs = self.roberta(
- input_ids,
- attention_mask=attention_mask,
- token_type_ids=token_type_ids,
- position_ids=position_ids,
- head_mask=head_mask,
- inputs_embeds=inputs_embeds,
- )
- sequence_output = outputs[0]
- prediction_scores = self.lm_head(sequence_output)
-
- outputs = (prediction_scores,) + outputs[2:] # Add hidden states and attention if they are here
-
- if masked_lm_labels is not None:
- loss_fct = CrossEntropyLoss()
- masked_lm_loss = loss_fct(prediction_scores.view(-1, self.config.vocab_size), masked_lm_labels.view(-1))
- outputs = (masked_lm_loss,) + outputs
-
- return outputs # (masked_lm_loss), prediction_scores, (hidden_states), (attentions)
-
-
-class RobertaLMHead(nn.Module):
- """Roberta Head for masked language modeling."""
-
- def __init__(self, config):
- super().__init__()
- self.dense = nn.Linear(config.hidden_size, config.hidden_size)
- self.layer_norm = BertLayerNorm(config.hidden_size, eps=config.layer_norm_eps)
-
- self.decoder = nn.Linear(config.hidden_size, config.vocab_size, bias=False)
- self.bias = nn.Parameter(torch.zeros(config.vocab_size))
-
- # Need a link between the two variables so that the bias is correctly resized with `resize_token_embeddings`
- self.decoder.bias = self.bias
-
- def forward(self, features, **kwargs):
- x = self.dense(features)
- x = gelu(x)
- x = self.layer_norm(x)
-
- # project back to size of vocabulary with bias
- x = self.decoder(x) + self.bias
-
- return x
-
-
-@add_start_docstrings(
- """RoBERTa Model transformer with a sequence classification/regression head on top (a linear layer
- on top of the pooled output) e.g. for GLUE tasks. """,
- ROBERTA_START_DOCSTRING,
-)
-class RobertaForSequenceClassification(BertPreTrainedModel):
- config_class = RobertaConfig
- pretrained_model_archive_map = ROBERTA_PRETRAINED_MODEL_ARCHIVE_MAP
- base_model_prefix = "roberta"
-
- def __init__(self, config):
- super().__init__(config)
- self.num_labels = config.num_labels
-
- self.roberta = RobertaModel(config)
- self.classifier = RobertaClassificationHead(config)
-
- @add_start_docstrings_to_callable(ROBERTA_INPUTS_DOCSTRING)
- def forward(
- self,
- input_ids=None,
- attention_mask=None,
- token_type_ids=None,
- position_ids=None,
- head_mask=None,
- inputs_embeds=None,
- labels=None,
- ):
- r"""
- labels (:obj:`torch.LongTensor` of shape :obj:`(batch_size,)`, `optional`, defaults to :obj:`None`):
- Labels for computing the sequence classification/regression loss.
- Indices should be in :obj:`[0, ..., config.num_labels - 1]`.
- If :obj:`config.num_labels == 1` a regression loss is computed (Mean-Square loss),
- If :obj:`config.num_labels > 1` a classification loss is computed (Cross-Entropy).
-
- Returns:
- :obj:`tuple(torch.FloatTensor)` comprising various elements depending on the configuration (:class:`~transformers.RobertaConfig`) and inputs:
- loss (:obj:`torch.FloatTensor` of shape :obj:`(1,)`, `optional`, returned when :obj:`label` is provided):
- Classification (or regression if config.num_labels==1) loss.
- logits (:obj:`torch.FloatTensor` of shape :obj:`(batch_size, config.num_labels)`):
- Classification (or regression if config.num_labels==1) scores (before SoftMax).
- hidden_states (:obj:`tuple(torch.FloatTensor)`, `optional`, returned when ``config.output_hidden_states=True``):
- Tuple of :obj:`torch.FloatTensor` (one for the output of the embeddings + one for the output of each layer)
- of shape :obj:`(batch_size, sequence_length, hidden_size)`.
-
- Hidden-states of the model at the output of each layer plus the initial embedding outputs.
- attentions (:obj:`tuple(torch.FloatTensor)`, `optional`, returned when ``config.output_attentions=True``):
- Tuple of :obj:`torch.FloatTensor` (one for each layer) of shape
- :obj:`(batch_size, num_heads, sequence_length, sequence_length)`.
-
- Attentions weights after the attention softmax, used to compute the weighted average in the self-attention
- heads.
-
- Examples::
-
- from transformers import RobertaTokenizer, RobertaForSequenceClassification
- import torch
-
- tokenizer = RobertaTokenizer.from_pretrained('roberta-base')
- model = RobertaForSequenceClassification.from_pretrained('roberta-base')
- input_ids = torch.tensor(tokenizer.encode("Hello, my dog is cute", add_special_tokens=True)).unsqueeze(0) # Batch size 1
- labels = torch.tensor([1]).unsqueeze(0) # Batch size 1
- outputs = model(input_ids, labels=labels)
- loss, logits = outputs[:2]
-
- """
- outputs = self.roberta(
- input_ids,
- attention_mask=attention_mask,
- token_type_ids=token_type_ids,
- position_ids=position_ids,
- head_mask=head_mask,
- inputs_embeds=inputs_embeds,
- )
- sequence_output = outputs[0]
- logits = self.classifier(sequence_output)
-
- outputs = (logits,) + outputs[2:]
- if labels is not None:
- if self.num_labels == 1:
- # We are doing regression
- loss_fct = MSELoss()
- loss = loss_fct(logits.view(-1), labels.view(-1))
- else:
- loss_fct = CrossEntropyLoss()
- loss = loss_fct(logits.view(-1, self.num_labels), labels.view(-1))
- outputs = (loss,) + outputs
-
- return outputs # (loss), logits, (hidden_states), (attentions)
-
-
-@add_start_docstrings(
- """Roberta Model with a multiple choice classification head on top (a linear layer on top of
- the pooled output and a softmax) e.g. for RocStories/SWAG tasks. """,
- ROBERTA_START_DOCSTRING,
-)
-class RobertaForMultipleChoice(BertPreTrainedModel):
- config_class = RobertaConfig
- pretrained_model_archive_map = ROBERTA_PRETRAINED_MODEL_ARCHIVE_MAP
- base_model_prefix = "roberta"
-
- def __init__(self, config):
- super().__init__(config)
-
- self.roberta = RobertaModel(config)
- self.dropout = nn.Dropout(config.hidden_dropout_prob)
- self.classifier = nn.Linear(config.hidden_size, 1)
-
- self.init_weights()
-
- @add_start_docstrings_to_callable(ROBERTA_INPUTS_DOCSTRING)
- def forward(
- self,
- input_ids=None,
- token_type_ids=None,
- attention_mask=None,
- labels=None,
- position_ids=None,
- head_mask=None,
- inputs_embeds=None,
- ):
- r"""
- labels (:obj:`torch.LongTensor` of shape :obj:`(batch_size,)`, `optional`, defaults to :obj:`None`):
- Labels for computing the multiple choice classification loss.
- Indices should be in ``[0, ..., num_choices]`` where `num_choices` is the size of the second dimension
- of the input tensors. (see `input_ids` above)
-
- Returns:
- :obj:`tuple(torch.FloatTensor)` comprising various elements depending on the configuration (:class:`~transformers.RobertaConfig`) and inputs:
- loss (:obj:`torch.FloatTensor`` of shape ``(1,)`, `optional`, returned when :obj:`labels` is provided):
- Classification loss.
- classification_scores (:obj:`torch.FloatTensor` of shape :obj:`(batch_size, num_choices)`):
- `num_choices` is the second dimension of the input tensors. (see `input_ids` above).
-
- Classification scores (before SoftMax).
- hidden_states (:obj:`tuple(torch.FloatTensor)`, `optional`, returned when ``config.output_hidden_states=True``):
- Tuple of :obj:`torch.FloatTensor` (one for the output of the embeddings + one for the output of each layer)
- of shape :obj:`(batch_size, sequence_length, hidden_size)`.
-
- Hidden-states of the model at the output of each layer plus the initial embedding outputs.
- attentions (:obj:`tuple(torch.FloatTensor)`, `optional`, returned when ``config.output_attentions=True``):
- Tuple of :obj:`torch.FloatTensor` (one for each layer) of shape
- :obj:`(batch_size, num_heads, sequence_length, sequence_length)`.
-
- Attentions weights after the attention softmax, used to compute the weighted average in the self-attention
- heads.
-
- Examples::
-
- from transformers import RobertaTokenizer, RobertaForMultipleChoice
- import torch
-
- tokenizer = RobertaTokenizer.from_pretrained('roberta-base')
- model = RobertaForMultipleChoice.from_pretrained('roberta-base')
- choices = ["Hello, my dog is cute", "Hello, my cat is amazing"]
- input_ids = torch.tensor([tokenizer.encode(s, add_special_tokens=True) for s in choices]).unsqueeze(0) # Batch size 1, 2 choices
- labels = torch.tensor(1).unsqueeze(0) # Batch size 1
- outputs = model(input_ids, labels=labels)
- loss, classification_scores = outputs[:2]
-
- """
- num_choices = input_ids.shape[1]
-
- flat_input_ids = input_ids.view(-1, input_ids.size(-1))
- flat_position_ids = position_ids.view(-1, position_ids.size(-1)) if position_ids is not None else None
- flat_token_type_ids = token_type_ids.view(-1, token_type_ids.size(-1)) if token_type_ids is not None else None
- flat_attention_mask = attention_mask.view(-1, attention_mask.size(-1)) if attention_mask is not None else None
- outputs = self.roberta(
- flat_input_ids,
- position_ids=flat_position_ids,
- token_type_ids=flat_token_type_ids,
- attention_mask=flat_attention_mask,
- head_mask=head_mask,
- )
- pooled_output = outputs[1]
-
- pooled_output = self.dropout(pooled_output)
- logits = self.classifier(pooled_output)
- reshaped_logits = logits.view(-1, num_choices)
-
- outputs = (reshaped_logits,) + outputs[2:] # add hidden states and attention if they are here
-
- if labels is not None:
- loss_fct = CrossEntropyLoss()
- loss = loss_fct(reshaped_logits, labels)
- outputs = (loss,) + outputs
-
- return outputs # (loss), reshaped_logits, (hidden_states), (attentions)
-
-
-@add_start_docstrings(
- """Roberta Model with a token classification head on top (a linear layer on top of
- the hidden-states output) e.g. for Named-Entity-Recognition (NER) tasks. """,
- ROBERTA_START_DOCSTRING,
-)
-class RobertaForTokenClassification(BertPreTrainedModel):
- config_class = RobertaConfig
- pretrained_model_archive_map = ROBERTA_PRETRAINED_MODEL_ARCHIVE_MAP
- base_model_prefix = "roberta"
-
- def __init__(self, config):
- super().__init__(config)
- self.num_labels = config.num_labels
-
- self.roberta = RobertaModel(config)
- self.dropout = nn.Dropout(config.hidden_dropout_prob)
- self.classifier = nn.Linear(config.hidden_size, config.num_labels)
-
- self.init_weights()
-
- @add_start_docstrings_to_callable(ROBERTA_INPUTS_DOCSTRING)
- def forward(
- self,
- input_ids=None,
- attention_mask=None,
- token_type_ids=None,
- position_ids=None,
- head_mask=None,
- inputs_embeds=None,
- labels=None,
- ):
- r"""
- labels (:obj:`torch.LongTensor` of shape :obj:`(batch_size, sequence_length)`, `optional`, defaults to :obj:`None`):
- Labels for computing the token classification loss.
- Indices should be in ``[0, ..., config.num_labels - 1]``.
-
- Returns:
- :obj:`tuple(torch.FloatTensor)` comprising various elements depending on the configuration (:class:`~transformers.RobertaConfig`) and inputs:
- loss (:obj:`torch.FloatTensor` of shape :obj:`(1,)`, `optional`, returned when ``labels`` is provided) :
- Classification loss.
- scores (:obj:`torch.FloatTensor` of shape :obj:`(batch_size, sequence_length, config.num_labels)`)
- Classification scores (before SoftMax).
- hidden_states (:obj:`tuple(torch.FloatTensor)`, `optional`, returned when ``config.output_hidden_states=True``):
- Tuple of :obj:`torch.FloatTensor` (one for the output of the embeddings + one for the output of each layer)
- of shape :obj:`(batch_size, sequence_length, hidden_size)`.
-
- Hidden-states of the model at the output of each layer plus the initial embedding outputs.
- attentions (:obj:`tuple(torch.FloatTensor)`, `optional`, returned when ``config.output_attentions=True``):
- Tuple of :obj:`torch.FloatTensor` (one for each layer) of shape
- :obj:`(batch_size, num_heads, sequence_length, sequence_length)`.
-
- Attentions weights after the attention softmax, used to compute the weighted average in the self-attention
- heads.
-
- Examples::
-
- from transformers import RobertaTokenizer, RobertaForTokenClassification
- import torch
-
- tokenizer = RobertaTokenizer.from_pretrained('roberta-base')
- model = RobertaForTokenClassification.from_pretrained('roberta-base')
- input_ids = torch.tensor(tokenizer.encode("Hello, my dog is cute", add_special_tokens=True)).unsqueeze(0) # Batch size 1
- labels = torch.tensor([1] * input_ids.size(1)).unsqueeze(0) # Batch size 1
- outputs = model(input_ids, labels=labels)
- loss, scores = outputs[:2]
-
- """
-
- outputs = self.roberta(
- input_ids,
- attention_mask=attention_mask,
- token_type_ids=token_type_ids,
- position_ids=position_ids,
- head_mask=head_mask,
- inputs_embeds=inputs_embeds,
- )
-
- sequence_output = outputs[0]
-
- sequence_output = self.dropout(sequence_output)
- logits = self.classifier(sequence_output)
-
- outputs = (logits,) + outputs[2:] # add hidden states and attention if they are here
- if labels is not None:
- loss_fct = CrossEntropyLoss()
- # Only keep active parts of the loss
- if attention_mask is not None:
- active_loss = attention_mask.view(-1) == 1
- active_logits = logits.view(-1, self.num_labels)[active_loss]
- active_labels = labels.view(-1)[active_loss]
- loss = loss_fct(active_logits, active_labels)
- else:
- loss = loss_fct(logits.view(-1, self.num_labels), labels.view(-1))
- outputs = (loss,) + outputs
-
- return outputs # (loss), scores, (hidden_states), (attentions)
-
-
-class RobertaClassificationHead(nn.Module):
- """Head for sentence-level classification tasks."""
-
- def __init__(self, config):
- super().__init__()
- self.dense = nn.Linear(config.hidden_size, config.hidden_size)
- self.dropout = nn.Dropout(config.hidden_dropout_prob)
- self.out_proj = nn.Linear(config.hidden_size, config.num_labels)
-
- def forward(self, features, **kwargs):
- x = features[:, 0, :] # take token (equiv. to [CLS])
- x = self.dropout(x)
- x = self.dense(x)
- x = torch.tanh(x)
- x = self.dropout(x)
- x = self.out_proj(x)
- return x
-
-
-@add_start_docstrings(
- """Roberta Model with a span classification head on top for extractive question-answering tasks like SQuAD (a linear layers on top of
- the hidden-states output to compute `span start logits` and `span end logits`). """,
- ROBERTA_START_DOCSTRING,
-)
-class RobertaForQuestionAnswering(BertPreTrainedModel):
- config_class = RobertaConfig
- pretrained_model_archive_map = ROBERTA_PRETRAINED_MODEL_ARCHIVE_MAP
- base_model_prefix = "roberta"
-
- def __init__(self, config):
- super().__init__(config)
- self.num_labels = config.num_labels
-
- self.roberta = RobertaModel(config)
- self.qa_outputs = nn.Linear(config.hidden_size, config.num_labels)
-
- self.init_weights()
-
- @add_start_docstrings_to_callable(ROBERTA_INPUTS_DOCSTRING)
- def forward(
- self,
- input_ids,
- attention_mask=None,
- token_type_ids=None,
- position_ids=None,
- head_mask=None,
- inputs_embeds=None,
- start_positions=None,
- end_positions=None,
- ):
- r"""
- start_positions (:obj:`torch.LongTensor` of shape :obj:`(batch_size,)`, `optional`, defaults to :obj:`None`):
- Labels for position (index) of the start of the labelled span for computing the token classification loss.
- Positions are clamped to the length of the sequence (`sequence_length`).
- Position outside of the sequence are not taken into account for computing the loss.
- end_positions (:obj:`torch.LongTensor` of shape :obj:`(batch_size,)`, `optional`, defaults to :obj:`None`):
- Labels for position (index) of the end of the labelled span for computing the token classification loss.
- Positions are clamped to the length of the sequence (`sequence_length`).
- Position outside of the sequence are not taken into account for computing the loss.
-
- Returns:
- :obj:`tuple(torch.FloatTensor)` comprising various elements depending on the configuration (:class:`~transformers.RobertaConfig`) and inputs:
- loss (:obj:`torch.FloatTensor` of shape :obj:`(1,)`, `optional`, returned when :obj:`labels` is provided):
- Total span extraction loss is the sum of a Cross-Entropy for the start and end positions.
- start_scores (:obj:`torch.FloatTensor` of shape :obj:`(batch_size, sequence_length,)`):
- Span-start scores (before SoftMax).
- end_scores (:obj:`torch.FloatTensor` of shape :obj:`(batch_size, sequence_length,)`):
- Span-end scores (before SoftMax).
- hidden_states (:obj:`tuple(torch.FloatTensor)`, `optional`, returned when ``config.output_hidden_states=True``):
- Tuple of :obj:`torch.FloatTensor` (one for the output of the embeddings + one for the output of each layer)
- of shape :obj:`(batch_size, sequence_length, hidden_size)`.
-
- Hidden-states of the model at the output of each layer plus the initial embedding outputs.
- attentions (:obj:`tuple(torch.FloatTensor)`, `optional`, returned when ``config.output_attentions=True``):
- Tuple of :obj:`torch.FloatTensor` (one for each layer) of shape
- :obj:`(batch_size, num_heads, sequence_length, sequence_length)`.
-
- Attentions weights after the attention softmax, used to compute the weighted average in the self-attention
- heads.
-
- Examples::
-
- # The checkpoint roberta-large is not fine-tuned for question answering. Please see the
- # examples/run_squad.py example to see how to fine-tune a model to a question answering task.
-
- from transformers import RobertaTokenizer, RobertaForQuestionAnswering
- import torch
-
- tokenizer = RobertaTokenizer.from_pretrained('roberta-base')
- model = RobertaForQuestionAnswering.from_pretrained('roberta-base')
-
- question, text = "Who was Jim Henson?", "Jim Henson was a nice puppet"
- input_ids = tokenizer.encode(question, text)
- start_scores, end_scores = model(torch.tensor([input_ids]))
-
- all_tokens = tokenizer.convert_ids_to_tokens(input_ids)
- answer = ' '.join(all_tokens[torch.argmax(start_scores) : torch.argmax(end_scores)+1])
-
- """
-
- outputs = self.roberta(
- input_ids,
- attention_mask=attention_mask,
- token_type_ids=token_type_ids,
- position_ids=position_ids,
- head_mask=head_mask,
- inputs_embeds=inputs_embeds,
- )
-
- sequence_output = outputs[0]
-
- logits = self.qa_outputs(sequence_output)
- start_logits, end_logits = logits.split(1, dim=-1)
- start_logits = start_logits.squeeze(-1)
- end_logits = end_logits.squeeze(-1)
-
- outputs = (start_logits, end_logits,) + outputs[2:]
- if start_positions is not None and end_positions is not None:
- # If we are on multi-GPU, split add a dimension
- if len(start_positions.size()) > 1:
- start_positions = start_positions.squeeze(-1)
- if len(end_positions.size()) > 1:
- end_positions = end_positions.squeeze(-1)
- # sometimes the start/end positions are outside our model inputs, we ignore these terms
- ignored_index = start_logits.size(1)
- start_positions.clamp_(0, ignored_index)
- end_positions.clamp_(0, ignored_index)
-
- loss_fct = CrossEntropyLoss(ignore_index=ignored_index)
- start_loss = loss_fct(start_logits, start_positions)
- end_loss = loss_fct(end_logits, end_positions)
- total_loss = (start_loss + end_loss) / 2
- outputs = (total_loss,) + outputs
-
- return outputs # (loss), start_logits, end_logits, (hidden_states), (attentions)
diff --git a/server/transformers/src/transformers/modeling_t5.py b/server/transformers/src/transformers/modeling_t5.py
deleted file mode 100644
index 405ebe56674ee80d6414b218d5f9d4e16907ce97..0000000000000000000000000000000000000000
--- a/server/transformers/src/transformers/modeling_t5.py
+++ /dev/null
@@ -1,915 +0,0 @@
-# coding=utf-8
-# Copyright 2018 Mesh TensorFlow authors, T5 Authors and HuggingFace Inc. team.
-#
-# Licensed under the Apache License, Version 2.0 (the "License");
-# you may not use this file except in compliance with the License.
-# You may obtain a copy of the License at
-#
-# http://www.apache.org/licenses/LICENSE-2.0
-#
-# Unless required by applicable law or agreed to in writing, software
-# distributed under the License is distributed on an "AS IS" BASIS,
-# WITHOUT WARRANTIES OR CONDITIONS OF ANY KIND, either express or implied.
-# See the License for the specific language governing permissions and
-# limitations under the License.
-""" PyTorch T5 model. """
-
-
-import copy
-import itertools
-import logging
-import math
-import os
-
-import torch
-import torch.nn.functional as F
-from torch import nn
-from torch.nn import CrossEntropyLoss
-
-from .configuration_t5 import T5Config
-from .file_utils import DUMMY_INPUTS, DUMMY_MASK, add_start_docstrings
-from .modeling_utils import PreTrainedModel, prune_linear_layer
-
-
-logger = logging.getLogger(__name__)
-
-####################################################
-# This dict contrains shortcut names and associated url
-# for the pretrained weights provided with the models
-####################################################
-T5_PRETRAINED_MODEL_ARCHIVE_MAP = {
- "t5-small": "https://s3.amazonaws.com/models.huggingface.co/bert/t5-small-pytorch_model.bin",
- "t5-base": "https://s3.amazonaws.com/models.huggingface.co/bert/t5-base-pytorch_model.bin",
- "t5-large": "https://s3.amazonaws.com/models.huggingface.co/bert/t5-large-pytorch_model.bin",
- "t5-3b": "https://s3.amazonaws.com/models.huggingface.co/bert/t5-3b-pytorch_model.bin",
- "t5-11b": "https://s3.amazonaws.com/models.huggingface.co/bert/t5-11b-pytorch_model.bin",
-}
-
-
-####################################################
-# This is a conversion method from TF 1.0 to PyTorch
-# More details: https://medium.com/huggingface/from-tensorflow-to-pytorch-265f40ef2a28
-####################################################
-def load_tf_weights_in_t5(model, config, tf_checkpoint_path):
- """ Load tf checkpoints in a pytorch model.
- """
- try:
- import re
- import numpy as np
- import tensorflow as tf
- except ImportError:
- logger.error(
- "Loading a TensorFlow model in PyTorch, requires TensorFlow to be installed. Please see "
- "https://www.tensorflow.org/install/ for installation instructions."
- )
- raise
- tf_path = os.path.abspath(tf_checkpoint_path)
- logger.info("Converting TensorFlow checkpoint from {}".format(tf_path))
- # Load weights from TF model
- init_vars = tf.train.list_variables(tf_path)
- names = []
- tf_weights = {}
- for name, shape in init_vars:
- logger.info("Loading TF weight {} with shape {}".format(name, shape))
- array = tf.train.load_variable(tf_path, name)
- names.append(name)
- tf_weights[name] = array
-
- for txt_name in names:
- name = txt_name.split("/")
- # adam_v and adam_m are variables used in AdamWeightDecayOptimizer to calculated m and v
- # which are not required for using pretrained model
- if any(n in ["adam_v", "adam_m", "global_step"] for n in name):
- logger.info("Skipping {}".format("/".join(name)))
- tf_weights.pop(txt_name, None)
- continue
- if "_slot_" in name[-1]:
- logger.info("Skipping {}".format("/".join(name)))
- tf_weights.pop(txt_name, None)
- continue
- pointer = model
- array = tf_weights[txt_name]
- for m_name in name:
- if re.fullmatch(r"[A-Za-z]+_\d+", m_name):
- scope_names = re.split(r"_(\d+)", m_name)
- else:
- scope_names = [m_name]
- if scope_names[0] in ["kernel", "scale", "embedding"]:
- pointer = getattr(pointer, "weight")
- # elif scope_names[0] == 'scale':
- # pointer = getattr(pointer, 'weight')
- # elif scope_names[0] == 'output_bias' or scope_names[0] == 'beta':
- # pointer = getattr(pointer, 'bias')
- # elif scope_names[0] == 'squad':
- # pointer = getattr(pointer, 'classifier')
- else:
- try:
- pointer = getattr(pointer, scope_names[0])
- except AttributeError:
- logger.info("Skipping {}".format("/".join(name)))
- continue
- if len(scope_names) >= 2:
- num = int(scope_names[1])
- pointer = pointer[num]
- if scope_names[0] not in ["kernel", "scale", "embedding"]:
- pointer = getattr(pointer, "weight")
- if scope_names[0] != "embedding":
- logger.info("Transposing numpy weight of shape {} for {}".format(array.shape, name))
- array = np.transpose(array)
- try:
- assert pointer.shape == array.shape
- except AssertionError as e:
- e.args += (pointer.shape, array.shape)
- raise
- logger.info("Initialize PyTorch weight {}".format(name))
- pointer.data = torch.from_numpy(array.astype(np.float32))
- tf_weights.pop(txt_name, None)
-
- logger.info("Weights not copied to PyTorch model: {}".format(", ".join(tf_weights.keys())))
- # logger.info("Weights not copied to PyTorch model: {}".format(', '.join(tf_weights.keys())))
- return model
-
-
-####################################################
-# PyTorch Models are constructed by sub-classing
-# - torch.nn.Module for the layers and
-# - PreTrainedModel for the models (it-self a sub-class of torch.nn.Module)
-####################################################
-
-
-class T5LayerNorm(nn.Module):
- def __init__(self, hidden_size, eps=1e-6):
- """ Construct a layernorm module in the T5 style
- No bias and no substraction of mean.
- """
- super().__init__()
- self.weight = nn.Parameter(torch.ones(hidden_size))
- self.variance_epsilon = eps
-
- def forward(self, x):
- variance = x.pow(2).mean(-1, keepdim=True)
- x = x / torch.sqrt(variance + self.variance_epsilon)
- return self.weight * x
-
-
-class T5DenseReluDense(nn.Module):
- def __init__(self, config):
- super().__init__()
- self.wi = nn.Linear(config.d_model, config.d_ff, bias=False)
- self.wo = nn.Linear(config.d_ff, config.d_model, bias=False)
- self.dropout = nn.Dropout(config.dropout_rate)
-
- def forward(self, hidden_states):
- h = self.wi(hidden_states)
- h = F.relu(h)
- h = self.dropout(h)
- h = self.wo(h)
- return h
-
-
-class T5LayerFF(nn.Module):
- def __init__(self, config):
- super().__init__()
- self.DenseReluDense = T5DenseReluDense(config)
- self.layer_norm = T5LayerNorm(config.d_model, eps=config.layer_norm_epsilon)
- self.dropout = nn.Dropout(config.dropout_rate)
-
- def forward(self, hidden_states):
- norm_x = self.layer_norm(hidden_states)
- y = self.DenseReluDense(norm_x)
- layer_output = hidden_states + self.dropout(y)
- return layer_output
-
-
-class T5Attention(nn.Module):
- NEW_ID = itertools.count()
-
- def __init__(self, config, has_relative_attention_bias=False):
- super().__init__()
- self.layer_id = next(T5Attention.NEW_ID)
- self.is_decoder = config.is_decoder
- self.has_relative_attention_bias = has_relative_attention_bias
-
- self.output_attentions = config.output_attentions
- self.relative_attention_num_buckets = config.relative_attention_num_buckets
- self.d_model = config.d_model
- self.d_kv = config.d_kv
- self.n_heads = config.num_heads
- self.dropout = config.dropout_rate
- self.inner_dim = self.n_heads * self.d_kv
-
- # Mesh TensorFlow initialization to avoid scaling before softmax
- self.q = nn.Linear(self.d_model, self.inner_dim, bias=False)
- self.k = nn.Linear(self.d_model, self.inner_dim, bias=False)
- self.v = nn.Linear(self.d_model, self.inner_dim, bias=False)
- self.o = nn.Linear(self.inner_dim, self.d_model, bias=False)
-
- if self.has_relative_attention_bias:
- self.relative_attention_bias = nn.Embedding(self.relative_attention_num_buckets, self.n_heads)
- self.pruned_heads = set()
-
- def prune_heads(self, heads):
- if len(heads) == 0:
- return
- mask = torch.ones(self.n_heads, self.d_kv)
- heads = set(heads) - self.pruned_heads
- for head in heads:
- head -= sum(1 if h < head else 0 for h in self.pruned_heads)
- mask[head] = 0
- mask = mask.view(-1).contiguous().eq(1)
- index = torch.arange(len(mask))[mask].long()
- # Prune linear layers
- self.q = prune_linear_layer(self.q, index)
- self.k = prune_linear_layer(self.k, index)
- self.v = prune_linear_layer(self.v, index)
- self.o = prune_linear_layer(self.o, index, dim=1)
- # Update hyper params
- self.n_heads = self.n_heads - len(heads)
- self.inner_dim = self.d_kv * self.n_heads
- self.pruned_heads = self.pruned_heads.union(heads)
-
- @staticmethod
- def _relative_position_bucket(relative_position, bidirectional=True, num_buckets=32, max_distance=128):
- """
- Adapted from Mesh Tensorflow:
- https://github.com/tensorflow/mesh/blob/0cb87fe07da627bf0b7e60475d59f95ed6b5be3d/mesh_tensorflow/transformer/transformer_layers.py#L593
-
- Translate relative position to a bucket number for relative attention.
- The relative position is defined as memory_position - query_position, i.e.
- the distance in tokens from the attending position to the attended-to
- position. If bidirectional=False, then positive relative positions are
- invalid.
- We use smaller buckets for small absolute relative_position and larger buckets
- for larger absolute relative_positions. All relative positions >=max_distance
- map to the same bucket. All relative positions <=-max_distance map to the
- same bucket. This should allow for more graceful generalization to longer
- sequences than the model has been trained on.
- Args:
- relative_position: an int32 Tensor
- bidirectional: a boolean - whether the attention is bidirectional
- num_buckets: an integer
- max_distance: an integer
- Returns:
- a Tensor with the same shape as relative_position, containing int32
- values in the range [0, num_buckets)
- """
- ret = 0
- n = -relative_position
- if bidirectional:
- num_buckets //= 2
- ret += (n < 0).to(torch.long) * num_buckets # mtf.to_int32(mtf.less(n, 0)) * num_buckets
- n = torch.abs(n)
- else:
- n = torch.max(n, torch.zeros_like(n))
- # now n is in the range [0, inf)
-
- # half of the buckets are for exact increments in positions
- max_exact = num_buckets // 2
- is_small = n < max_exact
-
- # The other half of the buckets are for logarithmically bigger bins in positions up to max_distance
- val_if_large = max_exact + (
- torch.log(n.float() / max_exact) / math.log(max_distance / max_exact) * (num_buckets - max_exact)
- ).to(torch.long)
- val_if_large = torch.min(val_if_large, torch.full_like(val_if_large, num_buckets - 1))
-
- ret += torch.where(is_small, n, val_if_large)
- return ret
-
- def compute_bias(self, qlen, klen):
- """ Compute binned relative position bias """
- context_position = torch.arange(qlen, dtype=torch.long)[:, None]
- memory_position = torch.arange(klen, dtype=torch.long)[None, :]
- relative_position = memory_position - context_position # shape (qlen, klen)
- rp_bucket = self._relative_position_bucket(
- relative_position, # shape (qlen, klen)
- bidirectional=not self.is_decoder,
- num_buckets=self.relative_attention_num_buckets,
- )
- rp_bucket = rp_bucket.to(self.relative_attention_bias.weight.device)
- values = self.relative_attention_bias(rp_bucket) # shape (qlen, klen, num_heads)
- values = values.permute([2, 0, 1]).unsqueeze(0) # shape (1, num_heads, qlen, klen)
- return values
-
- def forward(self, input, mask=None, kv=None, position_bias=None, cache=None, head_mask=None):
- """
- Self-attention (if kv is None) or attention over source sentence (provided by kv).
- """
- # Input is (bs, qlen, dim)
- # Mask is (bs, klen) (non-causal) or (bs, klen, klen)
- bs, qlen, dim = input.size()
- if kv is None:
- klen = qlen if cache is None else cache["slen"] + qlen
- else:
- klen = kv.size(1)
-
- def shape(x):
- """ projection """
- return x.view(bs, -1, self.n_heads, self.d_kv).transpose(1, 2)
-
- def unshape(x):
- """ compute context """
- return x.transpose(1, 2).contiguous().view(bs, -1, self.inner_dim)
-
- q = shape(self.q(input)) # (bs, n_heads, qlen, dim_per_head)
- if kv is None:
- k = shape(self.k(input)) # (bs, n_heads, qlen, dim_per_head)
- v = shape(self.v(input)) # (bs, n_heads, qlen, dim_per_head)
- elif cache is None or self.layer_id not in cache:
- k = v = kv
- k = shape(self.k(k)) # (bs, n_heads, qlen, dim_per_head)
- v = shape(self.v(v)) # (bs, n_heads, qlen, dim_per_head)
-
- if cache is not None:
- if self.layer_id in cache:
- if kv is None:
- k_, v_ = cache[self.layer_id]
- k = torch.cat([k_, k], dim=2) # (bs, n_heads, klen, dim_per_head)
- v = torch.cat([v_, v], dim=2) # (bs, n_heads, klen, dim_per_head)
- else:
- k, v = cache[self.layer_id]
- cache[self.layer_id] = (k, v)
-
- # q = q / math.sqrt(dim_per_head) # No scaling in T5
- scores = torch.einsum("bnqd,bnkd->bnqk", q, k) # (bs, n_heads, qlen, klen)
-
- if position_bias is None:
- if not self.has_relative_attention_bias:
- raise ValueError("No position_bias provided and no weights to compute position_bias")
- position_bias = self.compute_bias(qlen, klen)
- if mask is not None:
- position_bias = position_bias + mask # (bs, n_heads, qlen, klen)
-
- scores += position_bias
- weights = F.softmax(scores.float(), dim=-1).type_as(scores) # (bs, n_heads, qlen, klen)
- weights = F.dropout(weights, p=self.dropout, training=self.training) # (bs, n_heads, qlen, klen)
-
- # Mask heads if we want to
- if head_mask is not None:
- weights = weights * head_mask
-
- context = torch.matmul(weights, v) # (bs, n_heads, qlen, dim_per_head)
- context = unshape(context) # (bs, qlen, dim)
-
- context = self.o(context)
-
- outputs = (context,)
- if self.output_attentions:
- outputs = outputs + (weights,)
- if self.has_relative_attention_bias:
- outputs = outputs + (position_bias,)
- return outputs
-
-
-class T5LayerSelfAttention(nn.Module):
- def __init__(self, config, has_relative_attention_bias=False):
- super().__init__()
- self.SelfAttention = T5Attention(config, has_relative_attention_bias=has_relative_attention_bias)
- self.layer_norm = T5LayerNorm(config.d_model, eps=config.layer_norm_epsilon)
- self.dropout = nn.Dropout(config.dropout_rate)
-
- def forward(self, hidden_states, attention_mask=None, position_bias=None, head_mask=None):
- norm_x = self.layer_norm(hidden_states)
- attention_output = self.SelfAttention(
- norm_x, mask=attention_mask, position_bias=position_bias, head_mask=head_mask
- )
- y = attention_output[0]
- layer_output = hidden_states + self.dropout(y)
- outputs = (layer_output,) + attention_output[1:] # add attentions if we output them
- return outputs
-
-
-class T5LayerCrossAttention(nn.Module):
- def __init__(self, config, has_relative_attention_bias=False):
- super().__init__()
- self.EncDecAttention = T5Attention(config, has_relative_attention_bias=has_relative_attention_bias)
- self.layer_norm = T5LayerNorm(config.d_model, eps=config.layer_norm_epsilon)
- self.dropout = nn.Dropout(config.dropout_rate)
-
- def forward(self, hidden_states, kv, attention_mask=None, position_bias=None, head_mask=None):
- norm_x = self.layer_norm(hidden_states)
- attention_output = self.EncDecAttention(
- norm_x, mask=attention_mask, kv=kv, position_bias=position_bias, head_mask=head_mask
- )
- y = attention_output[0]
- layer_output = hidden_states + self.dropout(y)
- outputs = (layer_output,) + attention_output[1:] # add attentions if we output them
- return outputs
-
-
-class T5Block(nn.Module):
- def __init__(self, config, has_relative_attention_bias=False):
- super().__init__()
- self.is_decoder = config.is_decoder
- self.layer = nn.ModuleList()
- self.layer.append(T5LayerSelfAttention(config, has_relative_attention_bias=has_relative_attention_bias))
- if self.is_decoder:
- self.layer.append(T5LayerCrossAttention(config, has_relative_attention_bias=has_relative_attention_bias))
- self.layer.append(T5LayerFF(config))
- else:
- self.layer.append(T5LayerFF(config))
-
- def forward(
- self,
- hidden_states,
- attention_mask=None,
- position_bias=None,
- encoder_hidden_states=None,
- encoder_attention_mask=None,
- encoder_decoder_position_bias=None,
- head_mask=None,
- ):
- self_attention_outputs = self.layer[0](
- hidden_states, attention_mask=attention_mask, position_bias=position_bias, head_mask=head_mask
- )
- hidden_states = self_attention_outputs[0]
- outputs = self_attention_outputs[1:] # Keep self-attention outputs and relative position weights
-
- if not self.is_decoder:
- hidden_states = self.layer[1](hidden_states)
- else:
- cross_attention_outputs = self.layer[1](
- hidden_states,
- kv=encoder_hidden_states,
- attention_mask=encoder_attention_mask,
- position_bias=encoder_decoder_position_bias,
- head_mask=head_mask,
- )
- hidden_states = cross_attention_outputs[0]
- outputs = (
- outputs + cross_attention_outputs[1:]
- ) # Keep cross-attention outputs and relative position weights
- hidden_states = self.layer[2](hidden_states)
-
- outputs = (hidden_states,) + outputs # add attentions if we output them
- return outputs # hidden-states, (self-attention weights), (self-attention position bias), (cross-attention weights), (cross-attention position bias)
-
-
-class T5PreTrainedModel(PreTrainedModel):
- """ An abstract class to handle weights initialization and
- a simple interface for downloading and loading pretrained models.
- """
-
- config_class = T5Config
- pretrained_model_archive_map = T5_PRETRAINED_MODEL_ARCHIVE_MAP
- load_tf_weights = load_tf_weights_in_t5
- base_model_prefix = "transformer"
-
- @property
- def dummy_inputs(self):
- input_ids = torch.tensor(DUMMY_INPUTS)
- input_mask = torch.tensor(DUMMY_MASK)
- dummy_inputs = {
- "decoder_input_ids": input_ids,
- "encoder_input_ids": input_ids,
- "decoder_attention_mask": input_mask,
- }
- return dummy_inputs
-
- def _init_weights(self, module):
- """ Initialize the weights """
- factor = self.config.initializer_factor # Used for testing weights initialization
- if isinstance(module, T5LayerNorm):
- module.weight.data.fill_(factor * 1.0)
- elif isinstance(module, (T5Model, T5WithLMHeadModel)):
- # Mesh TensorFlow embeddings initialization
- # See https://github.com/tensorflow/mesh/blob/fa19d69eafc9a482aff0b59ddd96b025c0cb207d/mesh_tensorflow/layers.py#L1624
- module.shared.weight.data.normal_(mean=0.0, std=factor * 1.0)
- elif isinstance(module, T5DenseReluDense):
- # Mesh TensorFlow FF initialization
- # See https://github.com/tensorflow/mesh/blob/master/mesh_tensorflow/transformer/transformer_layers.py#L56
- # and https://github.com/tensorflow/mesh/blob/fa19d69eafc9a482aff0b59ddd96b025c0cb207d/mesh_tensorflow/layers.py#L89
- module.wi.weight.data.normal_(mean=0.0, std=factor * ((self.config.d_model) ** -0.5))
- if hasattr(module.wi, "bias") and module.wi.bias is not None:
- module.wi.bias.data.zero_()
- module.wo.weight.data.normal_(mean=0.0, std=factor * ((self.config.d_ff) ** -0.5))
- if hasattr(module.wo, "bias") and module.wo.bias is not None:
- module.wo.bias.data.zero_()
- elif isinstance(module, T5Attention):
- # Mesh TensorFlow attention initialization to avoid scaling before softmax
- # See https://github.com/tensorflow/mesh/blob/fa19d69eafc9a482aff0b59ddd96b025c0cb207d/mesh_tensorflow/transformer/attention.py#L136
- d_model = self.config.d_model
- d_kv = self.config.d_kv
- n_heads = self.config.num_heads
- module.q.weight.data.normal_(mean=0.0, std=factor * ((d_model * d_kv) ** -0.5))
- module.k.weight.data.normal_(mean=0.0, std=factor * (d_model ** -0.5))
- module.v.weight.data.normal_(mean=0.0, std=factor * (d_model ** -0.5))
- module.o.weight.data.normal_(mean=0.0, std=factor * ((n_heads * d_kv) ** -0.5))
- if module.has_relative_attention_bias:
- module.relative_attention_bias.weight.data.normal_(mean=0.0, std=factor * ((d_model) ** -0.5))
-
-
-class T5Stack(T5PreTrainedModel):
- def __init__(self, config):
- super().__init__(config)
- self.output_attentions = config.output_attentions
- self.output_hidden_states = config.output_hidden_states
- self.is_decoder = config.is_decoder
-
- self.block = nn.ModuleList(
- [T5Block(config, has_relative_attention_bias=bool(i == 0)) for i in range(config.num_layers)]
- )
- self.final_layer_norm = T5LayerNorm(config.d_model, eps=config.layer_norm_epsilon)
- self.dropout = nn.Dropout(config.dropout_rate)
-
- self.init_weights()
-
- def forward(
- self,
- hidden_states,
- attention_mask=None,
- encoder_hidden_states=None,
- encoder_attention_mask=None,
- head_mask=None,
- ):
-
- batch_size, seq_length = hidden_states.shape[0], hidden_states.shape[1]
- if attention_mask is None:
- attention_mask = torch.ones(batch_size, seq_length).to(hidden_states.device)
- if self.is_decoder and encoder_attention_mask is None:
- encoder_seq_length = encoder_hidden_states.shape[1]
- encoder_attention_mask = torch.ones(batch_size, encoder_seq_length).to(hidden_states.device)
-
- # We can provide a self-attention mask of dimensions [batch_size, from_seq_length, to_seq_length]
- # ourselves in which case we just need to make it broadcastable to all heads.
- if attention_mask.dim() == 3:
- extended_attention_mask = attention_mask[:, None, :, :]
- elif attention_mask.dim() == 2:
- # Provided a padding mask of dimensions [batch_size, seq_length]
- # - if the model is a decoder, apply a causal mask in addition to the padding mask
- # - if the model is an encoder, make the mask broadcastable to [batch_size, num_heads, seq_length, seq_length]
- if self.config.is_decoder:
- seq_ids = torch.arange(seq_length, device=hidden_states.device)
- causal_mask = seq_ids[None, None, :].repeat(batch_size, seq_length, 1) <= seq_ids[None, :, None]
- causal_mask = causal_mask.to(attention_mask)
- extended_attention_mask = causal_mask[:, None, :, :] * attention_mask[:, None, None, :]
- else:
- extended_attention_mask = attention_mask[:, None, None, :]
-
- # Since attention_mask is 1.0 for positions we want to attend and 0.0 for
- # masked positions, this operation will create a tensor which is 0.0 for
- # positions we want to attend and -1e9 for masked positions.
- # Since we are adding it to the raw scores before the softmax, this is
- # effectively the same as removing these entirely.
-
- # T5 has a mask that can compare sequence ids, we can simulate this here with this transposition
- # Cf. https://github.com/tensorflow/mesh/blob/8d2465e9bc93129b913b5ccc6a59aa97abd96ec6/mesh_tensorflow/transformer/transformer_layers.py#L270
- # extended_attention_mask = (extended_attention_mask == extended_attention_mask.transpose(-1, -2))
-
- extended_attention_mask = extended_attention_mask.to(dtype=next(self.parameters()).dtype) # fp16 compatibility
- extended_attention_mask = (1.0 - extended_attention_mask) * -1e9
-
- if self.is_decoder:
- # If a 2D ou 3D attention mask is provided for the cross-attention
- # we need to make broadcastabe to [batch_size, num_heads, seq_length, seq_length]
- if encoder_attention_mask.dim() == 3:
- encoder_extended_attention_mask = encoder_attention_mask[:, None, :, :]
- if encoder_attention_mask.dim() == 2:
- encoder_extended_attention_mask = encoder_attention_mask[:, None, None, :]
-
- # T5 has a mask that can compare sequence ids, we can simulate this here with this transposition
- # Cf. https://github.com/tensorflow/mesh/blob/8d2465e9bc93129b913b5ccc6a59aa97abd96ec6/mesh_tensorflow/transformer/transformer_layers.py#L270
- # encoder_extended_attention_mask = (encoder_extended_attention_mask == encoder_extended_attention_mask.transpose(-1, -2))
-
- encoder_extended_attention_mask = encoder_extended_attention_mask.to(
- dtype=next(self.parameters()).dtype
- ) # fp16 compatibility
- encoder_extended_attention_mask = (1.0 - encoder_extended_attention_mask) * -1e9
- else:
- encoder_extended_attention_mask = None
-
- # Prepare head mask if needed
- # 1.0 in head_mask indicate we keep the head
- # attention_probs has shape bsz x n_heads x N x N
- # input head_mask has shape [num_heads] or [num_hidden_layers x num_heads]
- # and head_mask is converted to shape [num_hidden_layers x batch x num_heads x seq_length x seq_length]
- if head_mask is not None:
- if head_mask.dim() == 1:
- head_mask = head_mask.unsqueeze(0).unsqueeze(0).unsqueeze(-1).unsqueeze(-1)
- head_mask = head_mask.expand(self.config.num_layers, -1, -1, -1, -1)
- elif head_mask.dim() == 2:
- head_mask = (
- head_mask.unsqueeze(1).unsqueeze(-1).unsqueeze(-1)
- ) # We can specify head_mask for each layer
- head_mask = head_mask.to(
- dtype=next(self.parameters()).dtype
- ) # switch to fload if need + fp16 compatibility
- else:
- head_mask = [None] * self.config.num_layers
-
- all_hidden_states = ()
- all_attentions = ()
- position_bias = None
- encoder_decoder_position_bias = None
-
- hidden_states = self.dropout(hidden_states)
- for i, layer_module in enumerate(self.block):
- if self.output_hidden_states:
- all_hidden_states = all_hidden_states + (hidden_states,)
-
- layer_outputs = layer_module(
- hidden_states,
- attention_mask=extended_attention_mask,
- position_bias=position_bias,
- encoder_hidden_states=encoder_hidden_states,
- encoder_attention_mask=encoder_extended_attention_mask,
- encoder_decoder_position_bias=encoder_decoder_position_bias,
- head_mask=head_mask[i],
- )
- # layer_outputs is a tuple with:
- # hidden-states, (self-attention weights), (self-attention position bias), (cross-attention weights), (cross-attention position bias)
- hidden_states = layer_outputs[0]
- if i == 0:
- # We share the position biases between the layers - the first layer store them
- # layer_outputs = hidden-states, (self-attention weights), (self-attention position bias), (cross-attention weights), (cross-attention position bias)
- position_bias = layer_outputs[2 if self.output_attentions else 1]
- if self.is_decoder:
- encoder_decoder_position_bias = layer_outputs[4 if self.output_attentions else 2]
-
- if self.output_attentions:
- all_attentions = all_attentions + (layer_outputs[1],) # We keep only self-attention weights for now
-
- hidden_states = self.final_layer_norm(hidden_states)
- hidden_states = self.dropout(hidden_states)
-
- # Add last layer
- if self.output_hidden_states:
- all_hidden_states = all_hidden_states + (hidden_states,)
-
- outputs = (hidden_states,)
- if self.output_hidden_states:
- outputs = outputs + (all_hidden_states,)
- if self.output_attentions:
- outputs = outputs + (all_attentions,)
- return outputs # last-layer hidden state, (all hidden states), (all attentions)
-
-
-T5_START_DOCSTRING = r""" The T5 model was proposed in
- `Exploring the Limits of Transfer Learning with a Unified Text-to-Text Transformer`_
- by Colin Raffel, Noam Shazeer, Adam Roberts, Katherine Lee, Sharan Narang, Michael Matena, Yanqi Zhou, Wei Li, Peter J. Liu.
- It's an encoder decoder transformer pre-trained in a text-to-text denoising generative setting.
-
- This model is a PyTorch `torch.nn.Module`_ sub-class. Use it as a regular PyTorch Module and
- refer to the PyTorch documentation for all matter related to general usage and behavior.
-
- .. _`Exploring the Limits of Transfer Learning with a Unified Text-to-Text Transformer`:
- https://arxiv.org/abs/1910.10683
-
- .. _`torch.nn.Module`:
- https://pytorch.org/docs/stable/nn.html#module
-
- Parameters:
- config (:class:`~transformers.T5Config`): Model configuration class with all the parameters of the model.
- Initializing with a config file does not load the weights associated with the model, only the configuration.
- Check out the :meth:`~transformers.PreTrainedModel.from_pretrained` method to load the model weights.
-"""
-
-T5_INPUTS_DOCSTRING = r"""
- Inputs:
- **input_ids**: ``torch.LongTensor`` of shape ``(batch_size, sequence_length)``:
- Indices of input sequence tokens in the vocabulary.
- To match pre-training, T5 input sequence should be formatted with [CLS] and [SEP] tokens as follows:
-
- (a) For sequence pairs:
-
- ``tokens: [CLS] is this jack ##son ##ville ? [SEP] no it is not . [SEP]``
-
- (b) For single sequences:
-
- ``tokens: [CLS] the dog is hairy . [SEP]``
-
- T5 is a model with relative position embeddings so you should be able to pad the inputs on
- the right or the left.
-
- Indices can be obtained using :class:`transformers.T5Tokenizer`.
- See :func:`transformers.PreTrainedTokenizer.encode` and
- :func:`transformers.PreTrainedTokenizer.convert_tokens_to_ids` for details.
- **attention_mask**: (`optional`) ``torch.FloatTensor`` of shape ``(batch_size, sequence_length)``:
- Mask to avoid performing attention on padding token indices.
- Mask values selected in ``[0, 1]``:
- ``1`` for tokens that are NOT MASKED, ``0`` for MASKED tokens.
- **head_mask**: (`optional`) ``torch.FloatTensor`` of shape ``(num_heads,)`` or ``(num_layers, num_heads)``:
- Mask to nullify selected heads of the self-attention modules.
- Mask values selected in ``[0, 1]``:
- ``1`` indicates the head is **not masked**, ``0`` indicates the head is **masked**.
-"""
-
-
-@add_start_docstrings(
- "The bare T5 Model transformer outputting raw hidden-states" "without any specific head on top.",
- T5_START_DOCSTRING,
- T5_INPUTS_DOCSTRING,
-)
-class T5Model(T5PreTrainedModel):
- r"""
- Outputs: `Tuple` comprising various elements depending on the configuration (config) and inputs:
- **last_hidden_state**: ``torch.FloatTensor`` of shape ``(batch_size, sequence_length, hidden_size)``
- Sequence of hidden-states at the output of the last layer of the model.
- **hidden_states**: (`optional`, returned when ``config.output_hidden_states=True``)
- list of ``torch.FloatTensor`` (one for the output of each layer + the output of the embeddings)
- of shape ``(batch_size, sequence_length, hidden_size)``:
- Hidden-states of the model at the output of each layer plus the initial embedding outputs.
- **attentions**: (`optional`, returned when ``config.output_attentions=True``)
- list of ``torch.FloatTensor`` (one for each layer) of shape ``(batch_size, num_heads, sequence_length, sequence_length)``:
- Attentions weights after the attention softmax, used to compute the weighted average in the self-attention heads.
-
- Examples::
-
- tokenizer = T5Tokenizer.from_pretrained('t5-small')
- model = T5Model.from_pretrained('t5-small')
- input_ids = torch.tensor(tokenizer.encode("Hello, my dog is cute")).unsqueeze(0) # Batch size 1
- outputs = model(input_ids=input_ids)
- last_hidden_states = outputs[0] # The last hidden-state is the first element of the output tuple
-
- """
-
- def __init__(self, config):
- super().__init__(config)
- self.shared = nn.Embedding(config.vocab_size, config.d_model)
-
- encoder_config = copy.deepcopy(config)
- self.encoder = T5Stack(encoder_config)
-
- decoder_config = copy.deepcopy(config)
- decoder_config.is_decoder = True
- self.decoder = T5Stack(decoder_config)
-
- self.init_weights()
-
- def get_input_embeddings(self):
- return self.shared
-
- def set_input_embeddings(self, new_embeddings):
- self.shared = new_embeddings
-
- def _prune_heads(self, heads_to_prune):
- """ Prunes heads of the model.
- heads_to_prune: dict of {layer_num: list of heads to prune in this layer}
- See base class PreTrainedModel
- """
- for layer, heads in heads_to_prune.items():
- self.encoder.layer[layer].attention.prune_heads(heads)
-
- def forward(self, **kwargs):
- # keyword arguments come in 3 flavors: encoder-specific (prefixed by
- # `encoder_`), decoder-specific (prefixed by `decoder_`) and those
- # that apply to the model as whole.
- # We let the specific kwargs override the common ones in case of conflict.
- kwargs_common = dict(
- (k, v) for k, v in kwargs.items() if not k.startswith("encoder_") and not k.startswith("decoder_")
- )
- kwargs_encoder = kwargs_common.copy()
- kwargs_decoder = kwargs_common.copy()
- kwargs_encoder.update(dict((k[len("encoder_") :], v) for k, v in kwargs.items() if k.startswith("encoder_")))
- kwargs_decoder.update(dict((k[len("decoder_") :], v) for k, v in kwargs.items() if k.startswith("decoder_")))
-
- # Encode if needed (training, first prediction pass)
- encoder_hidden_states = kwargs_encoder.pop("hidden_states", None)
- encoder_attention_mask = kwargs_encoder.get("attention_mask", None)
- if encoder_hidden_states is None:
- # Convert encoder inputs in embeddings if needed
- hidden_states = kwargs_encoder.pop("inputs_embeds", None)
- if hidden_states is None:
- encoder_inputs_ids = kwargs_encoder.pop("input_ids")
- hidden_states = self.shared(encoder_inputs_ids) # Convert inputs in embeddings
-
- if encoder_attention_mask is not None:
- # Apply masking
- encoder_attention_mask = (encoder_attention_mask != 0).to(hidden_states)
- hidden_states = hidden_states * encoder_attention_mask.unsqueeze(-1)
-
- encoder_outputs = self.encoder(hidden_states, **kwargs_encoder)
- encoder_hidden_states = encoder_outputs[0]
- else:
- encoder_outputs = ()
-
- # Decode
- # Convert decoder inputs in embeddings if needed
- hidden_states = kwargs_decoder.pop("inputs_embeds", None)
- if hidden_states is None:
- decoder_inputs_ids = kwargs_decoder.pop("input_ids")
- hidden_states = self.shared(decoder_inputs_ids)
-
- kwargs_decoder["encoder_hidden_states"] = encoder_hidden_states
- kwargs_decoder["encoder_attention_mask"] = encoder_attention_mask
- decoder_outputs = self.decoder(hidden_states, **kwargs_decoder)
-
- return decoder_outputs + encoder_outputs
-
-
-@add_start_docstrings("""T5 Model with a `language modeling` head on top. """, T5_START_DOCSTRING, T5_INPUTS_DOCSTRING)
-class T5WithLMHeadModel(T5PreTrainedModel):
- r"""
- **lm_labels**: (`optional`) ``torch.LongTensor`` of shape ``(batch_size, sequence_length)``:
- Labels for computing the masked language modeling loss.
- Indices should either be in ``[0, ..., config.vocab_size]`` or -100 (see ``input_ids`` docstring).
- Tokens with indices set to ``-100`` are ignored (masked), the loss is only computed for the tokens with labels
- in ``[0, ..., config.vocab_size]``.
-
- Outputs: `Tuple` comprising various elements depending on the configuration (config) and inputs:
- **loss**: (`optional`, returned when ``lm_labels`` is provided) ``torch.FloatTensor`` of shape ``(1,)``:
- Masked language modeling loss.
- **prediction_scores**: ``torch.FloatTensor`` of shape ``(batch_size, sequence_length, config.vocab_size)``
- Prediction scores of the language modeling head (scores for each vocabulary token before SoftMax).
- **hidden_states**: (`optional`, returned when ``config.output_hidden_states=True``)
- list of ``torch.FloatTensor`` (one for the output of each layer + the output of the embeddings)
- of shape ``(batch_size, sequence_length, hidden_size)``:
- Hidden-states of the model at the output of each layer plus the initial embedding outputs.
- **attentions**: (`optional`, returned when ``config.output_attentions=True``)
- list of ``torch.FloatTensor`` (one for each layer) of shape ``(batch_size, num_heads, sequence_length, sequence_length)``:
- Attentions weights after the attention softmax, used to compute the weighted average in the self-attention heads.
-
- Examples::
-
- tokenizer = T5Tokenizer.from_pretrained('t5-small')
- model = T5WithLMHeadModel.from_pretrained('t5-small')
- input_ids = torch.tensor(tokenizer.encode("Hello, my dog is cute")).unsqueeze(0) # Batch size 1
- outputs = model(input_ids=input_ids, lm_labels=input_ids)
- loss, prediction_scores = outputs[:2]
-
- """
-
- def __init__(self, config):
- super().__init__(config)
- self.model_dim = config.d_model
-
- self.shared = nn.Embedding(config.vocab_size, config.d_model)
-
- encoder_config = copy.deepcopy(config)
- self.encoder = T5Stack(encoder_config)
-
- decoder_config = copy.deepcopy(config)
- decoder_config.is_decoder = True
- self.decoder = T5Stack(decoder_config)
-
- self.lm_head = nn.Linear(config.d_model, config.vocab_size, bias=False)
-
- self.init_weights()
-
- def get_input_embeddings(self):
- return self.shared
-
- def set_input_embeddings(self, new_embeddings):
- self.shared = new_embeddings
-
- def get_output_embeddings(self):
- return self.lm_head
-
- def forward(self, **kwargs):
- # keyword arguments come in 3 flavors: encoder-specific (prefixed by
- # `encoder_`), decoder-specific (prefixed by `decoder_`) and those
- # that apply to the model as whole.
- # We let the specific kwargs override the common ones in case of conflict.
-
- lm_labels = kwargs.pop("decoder_lm_labels", None)
-
- kwargs_common = dict(
- (k, v) for k, v in kwargs.items() if not k.startswith("encoder_") and not k.startswith("decoder_")
- )
- kwargs_encoder = kwargs_common.copy()
- kwargs_decoder = kwargs_common.copy()
- kwargs_encoder.update(dict((k[len("encoder_") :], v) for k, v in kwargs.items() if k.startswith("encoder_")))
- kwargs_decoder.update(dict((k[len("decoder_") :], v) for k, v in kwargs.items() if k.startswith("decoder_")))
-
- # Encode if needed (training, first prediction pass)
- encoder_hidden_states = kwargs_encoder.pop("hidden_states", None)
- if encoder_hidden_states is None:
- # Convert encoder inputs in embeddings if needed
- hidden_states = kwargs_encoder.pop("inputs_embeds", None)
- if hidden_states is None:
- encoder_inputs_ids = kwargs_encoder.pop("input_ids")
- hidden_states = self.shared(encoder_inputs_ids) # Convert inputs in embeddings
-
- encoder_outputs = self.encoder(hidden_states, **kwargs_encoder)
- encoder_hidden_states = encoder_outputs[0]
- else:
- encoder_outputs = ()
-
- # Decode
- # Convert decoder inputs in embeddings if needed
- hidden_states = kwargs_decoder.pop("inputs_embeds", None)
- if hidden_states is None:
- decoder_inputs_ids = kwargs_decoder.pop("input_ids")
- hidden_states = self.shared(decoder_inputs_ids)
-
- kwargs_decoder["encoder_hidden_states"] = encoder_hidden_states
- kwargs_decoder["encoder_attention_mask"] = kwargs_encoder.get("attention_mask", None)
- decoder_outputs = self.decoder(hidden_states, **kwargs_decoder)
-
- sequence_output = decoder_outputs[0]
- # Rescale output before projecting on vocab
- # See https://github.com/tensorflow/mesh/blob/fa19d69eafc9a482aff0b59ddd96b025c0cb207d/mesh_tensorflow/transformer/transformer.py#L586
- sequence_output = sequence_output * (self.model_dim ** -0.5)
- lm_logits = self.lm_head(sequence_output)
-
- decoder_outputs = (lm_logits,) + decoder_outputs[1:] # Add hidden states and attention if they are here
- if lm_labels is not None:
- shift_logits = lm_logits[..., :-1, :].contiguous()
- shift_labels = lm_labels[..., 1:].contiguous()
- loss_fct = CrossEntropyLoss(ignore_index=-100)
- loss = loss_fct(shift_logits.view(-1, shift_logits.size(-1)), shift_labels.view(-1))
- decoder_outputs = (
- loss,
- ) + decoder_outputs # TODO(thom): Add z_loss https://github.com/tensorflow/mesh/blob/fa19d69eafc9a482aff0b59ddd96b025c0cb207d/mesh_tensorflow/layers.py#L666
-
- return decoder_outputs + encoder_outputs
diff --git a/server/transformers/src/transformers/modeling_tf_albert.py b/server/transformers/src/transformers/modeling_tf_albert.py
deleted file mode 100644
index 2a1d3f1c4d8ae1844c8de05dbac242ba6d85f042..0000000000000000000000000000000000000000
--- a/server/transformers/src/transformers/modeling_tf_albert.py
+++ /dev/null
@@ -1,814 +0,0 @@
-# coding=utf-8
-# Copyright 2018 The OpenAI Team Authors and HuggingFace Inc. team.
-# Copyright (c) 2018, NVIDIA CORPORATION. All rights reserved.
-#
-# Licensed under the Apache License, Version 2.0 (the "License");
-# you may not use this file except in compliance with the License.
-# You may obtain a copy of the License at
-#
-# http://www.apache.org/licenses/LICENSE-2.0
-#
-# Unless required by applicable law or agreed to in writing, software
-# distributed under the License is distributed on an "AS IS" BASIS,
-# WITHOUT WARRANTIES OR CONDITIONS OF ANY KIND, either express or implied.
-# See the License for the specific language governing permissions and
-# limitations under the License.
-""" TF 2.0 ALBERT model. """
-
-
-import logging
-
-import tensorflow as tf
-
-from .configuration_albert import AlbertConfig
-from .file_utils import add_start_docstrings, add_start_docstrings_to_callable
-from .modeling_tf_bert import ACT2FN, TFBertSelfAttention
-from .modeling_tf_utils import TFPreTrainedModel, get_initializer, shape_list
-
-
-logger = logging.getLogger(__name__)
-
-TF_ALBERT_PRETRAINED_MODEL_ARCHIVE_MAP = {
- "albert-base-v1": "https://s3.amazonaws.com/models.huggingface.co/bert/albert-base-v1-tf_model.h5",
- "albert-large-v1": "https://s3.amazonaws.com/models.huggingface.co/bert/albert-large-v1-tf_model.h5",
- "albert-xlarge-v1": "https://s3.amazonaws.com/models.huggingface.co/bert/albert-xlarge-v1-tf_model.h5",
- "albert-xxlarge-v1": "https://s3.amazonaws.com/models.huggingface.co/bert/albert-xxlarge-v1-tf_model.h5",
- "albert-base-v2": "https://s3.amazonaws.com/models.huggingface.co/bert/albert-base-v2-tf_model.h5",
- "albert-large-v2": "https://s3.amazonaws.com/models.huggingface.co/bert/albert-large-v2-tf_model.h5",
- "albert-xlarge-v2": "https://s3.amazonaws.com/models.huggingface.co/bert/albert-xlarge-v2-tf_model.h5",
- "albert-xxlarge-v2": "https://s3.amazonaws.com/models.huggingface.co/bert/albert-xxlarge-v2-tf_model.h5",
-}
-
-
-class TFAlbertEmbeddings(tf.keras.layers.Layer):
- """Construct the embeddings from word, position and token_type embeddings.
- """
-
- def __init__(self, config, **kwargs):
- super().__init__(**kwargs)
-
- self.config = config
- self.position_embeddings = tf.keras.layers.Embedding(
- config.max_position_embeddings,
- config.embedding_size,
- embeddings_initializer=get_initializer(self.config.initializer_range),
- name="position_embeddings",
- )
- self.token_type_embeddings = tf.keras.layers.Embedding(
- config.type_vocab_size,
- config.embedding_size,
- embeddings_initializer=get_initializer(self.config.initializer_range),
- name="token_type_embeddings",
- )
-
- # self.LayerNorm is not snake-cased to stick with TensorFlow model variable name and be able to load
- # any TensorFlow checkpoint file
- self.LayerNorm = tf.keras.layers.LayerNormalization(epsilon=config.layer_norm_eps, name="LayerNorm")
- self.dropout = tf.keras.layers.Dropout(config.hidden_dropout_prob)
-
- def build(self, input_shape):
- """Build shared word embedding layer """
- with tf.name_scope("word_embeddings"):
- # Create and initialize weights. The random normal initializer was chosen
- # arbitrarily, and works well.
- self.word_embeddings = self.add_weight(
- "weight",
- shape=[self.config.vocab_size, self.config.embedding_size],
- initializer=get_initializer(self.config.initializer_range),
- )
- super().build(input_shape)
-
- def call(self, inputs, mode="embedding", training=False):
- """Get token embeddings of inputs.
- Args:
- inputs: list of three int64 tensors with shape [batch_size, length]: (input_ids, position_ids, token_type_ids)
- mode: string, a valid value is one of "embedding" and "linear".
- Returns:
- outputs: (1) If mode == "embedding", output embedding tensor, float32 with
- shape [batch_size, length, embedding_size]; (2) mode == "linear", output
- linear tensor, float32 with shape [batch_size, length, vocab_size].
- Raises:
- ValueError: if mode is not valid.
-
- Shared weights logic adapted from
- https://github.com/tensorflow/models/blob/a009f4fb9d2fc4949e32192a944688925ef78659/official/transformer/v2/embedding_layer.py#L24
- """
- if mode == "embedding":
- return self._embedding(inputs, training=training)
- elif mode == "linear":
- return self._linear(inputs)
- else:
- raise ValueError("mode {} is not valid.".format(mode))
-
- def _embedding(self, inputs, training=False):
- """Applies embedding based on inputs tensor."""
- input_ids, position_ids, token_type_ids, inputs_embeds = inputs
-
- if input_ids is not None:
- input_shape = shape_list(input_ids)
- else:
- input_shape = shape_list(inputs_embeds)[:-1]
-
- seq_length = input_shape[1]
- if position_ids is None:
- position_ids = tf.range(seq_length, dtype=tf.int32)[tf.newaxis, :]
- if token_type_ids is None:
- token_type_ids = tf.fill(input_shape, 0)
-
- if inputs_embeds is None:
- inputs_embeds = tf.gather(self.word_embeddings, input_ids)
- position_embeddings = self.position_embeddings(position_ids)
- token_type_embeddings = self.token_type_embeddings(token_type_ids)
-
- embeddings = inputs_embeds + position_embeddings + token_type_embeddings
- embeddings = self.LayerNorm(embeddings)
- embeddings = self.dropout(embeddings, training=training)
- return embeddings
-
- def _linear(self, inputs):
- """Computes logits by running inputs through a linear layer.
- Args:
- inputs: A float32 tensor with shape [batch_size, length, embedding_size]
- Returns:
- float32 tensor with shape [batch_size, length, vocab_size].
- """
- batch_size = shape_list(inputs)[0]
- length = shape_list(inputs)[1]
- x = tf.reshape(inputs, [-1, self.config.embedding_size])
- logits = tf.matmul(x, self.word_embeddings, transpose_b=True)
- return tf.reshape(logits, [batch_size, length, self.config.vocab_size])
-
-
-class TFAlbertSelfAttention(tf.keras.layers.Layer):
- def __init__(self, config, **kwargs):
- super().__init__(**kwargs)
- if config.hidden_size % config.num_attention_heads != 0:
- raise ValueError(
- "The hidden size (%d) is not a multiple of the number of attention "
- "heads (%d)" % (config.hidden_size, config.num_attention_heads)
- )
- self.output_attentions = config.output_attentions
-
- self.num_attention_heads = config.num_attention_heads
- assert config.hidden_size % config.num_attention_heads == 0
- self.attention_head_size = int(config.hidden_size / config.num_attention_heads)
- self.all_head_size = self.num_attention_heads * self.attention_head_size
-
- self.query = tf.keras.layers.Dense(
- self.all_head_size, kernel_initializer=get_initializer(config.initializer_range), name="query"
- )
- self.key = tf.keras.layers.Dense(
- self.all_head_size, kernel_initializer=get_initializer(config.initializer_range), name="key"
- )
- self.value = tf.keras.layers.Dense(
- self.all_head_size, kernel_initializer=get_initializer(config.initializer_range), name="value"
- )
-
- self.dropout = tf.keras.layers.Dropout(config.attention_probs_dropout_prob)
-
- def transpose_for_scores(self, x, batch_size):
- x = tf.reshape(x, (batch_size, -1, self.num_attention_heads, self.attention_head_size))
- return tf.transpose(x, perm=[0, 2, 1, 3])
-
- def call(self, inputs, training=False):
- hidden_states, attention_mask, head_mask = inputs
-
- batch_size = shape_list(hidden_states)[0]
- mixed_query_layer = self.query(hidden_states)
- mixed_key_layer = self.key(hidden_states)
- mixed_value_layer = self.value(hidden_states)
-
- query_layer = self.transpose_for_scores(mixed_query_layer, batch_size)
- key_layer = self.transpose_for_scores(mixed_key_layer, batch_size)
- value_layer = self.transpose_for_scores(mixed_value_layer, batch_size)
-
- # Take the dot product between "query" and "key" to get the raw attention scores.
- # (batch size, num_heads, seq_len_q, seq_len_k)
- attention_scores = tf.matmul(query_layer, key_layer, transpose_b=True)
- # scale attention_scores
- dk = tf.cast(shape_list(key_layer)[-1], tf.float32)
- attention_scores = attention_scores / tf.math.sqrt(dk)
-
- if attention_mask is not None:
- # Apply the attention mask is (precomputed for all layers in TFAlbertModel call() function)
- attention_scores = attention_scores + attention_mask
-
- # Normalize the attention scores to probabilities.
- attention_probs = tf.nn.softmax(attention_scores, axis=-1)
-
- # This is actually dropping out entire tokens to attend to, which might
- # seem a bit unusual, but is taken from the original Transformer paper.
- attention_probs = self.dropout(attention_probs, training=training)
-
- # Mask heads if we want to
- if head_mask is not None:
- attention_probs = attention_probs * head_mask
-
- context_layer = tf.matmul(attention_probs, value_layer)
-
- context_layer = tf.transpose(context_layer, perm=[0, 2, 1, 3])
- context_layer = tf.reshape(
- context_layer, (batch_size, -1, self.all_head_size)
- ) # (batch_size, seq_len_q, all_head_size)
-
- outputs = (context_layer, attention_probs) if self.output_attentions else (context_layer,)
- return outputs
-
-
-class TFAlbertSelfOutput(tf.keras.layers.Layer):
- def __init__(self, config, **kwargs):
- super().__init__(**kwargs)
- self.dense = tf.keras.layers.Dense(
- config.hidden_size, kernel_initializer=get_initializer(config.initializer_range), name="dense"
- )
- self.LayerNorm = tf.keras.layers.LayerNormalization(epsilon=config.layer_norm_eps, name="LayerNorm")
- self.dropout = tf.keras.layers.Dropout(config.hidden_dropout_prob)
-
- def call(self, inputs, training=False):
- hidden_states, input_tensor = inputs
-
- hidden_states = self.dense(hidden_states)
- hidden_states = self.dropout(hidden_states, training=training)
- hidden_states = self.LayerNorm(hidden_states + input_tensor)
- return hidden_states
-
-
-class TFAlbertAttention(TFBertSelfAttention):
- def __init__(self, config, **kwargs):
- super().__init__(config, **kwargs)
-
- self.hidden_size = config.hidden_size
- self.dense = tf.keras.layers.Dense(
- config.hidden_size, kernel_initializer=get_initializer(config.initializer_range), name="dense"
- )
- self.LayerNorm = tf.keras.layers.LayerNormalization(epsilon=config.layer_norm_eps, name="LayerNorm")
- self.pruned_heads = set()
-
- def prune_heads(self, heads):
- raise NotImplementedError
-
- def call(self, inputs, training=False):
- input_tensor, attention_mask, head_mask = inputs
-
- batch_size = shape_list(input_tensor)[0]
- mixed_query_layer = self.query(input_tensor)
- mixed_key_layer = self.key(input_tensor)
- mixed_value_layer = self.value(input_tensor)
-
- query_layer = self.transpose_for_scores(mixed_query_layer, batch_size)
- key_layer = self.transpose_for_scores(mixed_key_layer, batch_size)
- value_layer = self.transpose_for_scores(mixed_value_layer, batch_size)
-
- # Take the dot product between "query" and "key" to get the raw attention scores.
- # (batch size, num_heads, seq_len_q, seq_len_k)
- attention_scores = tf.matmul(query_layer, key_layer, transpose_b=True)
- # scale attention_scores
- dk = tf.cast(shape_list(key_layer)[-1], tf.float32)
- attention_scores = attention_scores / tf.math.sqrt(dk)
-
- if attention_mask is not None:
- # Apply the attention mask is (precomputed for all layers in TFBertModel call() function)
- attention_scores = attention_scores + attention_mask
-
- # Normalize the attention scores to probabilities.
- attention_probs = tf.nn.softmax(attention_scores, axis=-1)
-
- # This is actually dropping out entire tokens to attend to, which might
- # seem a bit unusual, but is taken from the original Transformer paper.
- attention_probs = self.dropout(attention_probs, training=training)
-
- # Mask heads if we want to
- if head_mask is not None:
- attention_probs = attention_probs * head_mask
-
- context_layer = tf.matmul(attention_probs, value_layer)
-
- context_layer = tf.transpose(context_layer, perm=[0, 2, 1, 3])
- context_layer = tf.reshape(
- context_layer, (batch_size, -1, self.all_head_size)
- ) # (batch_size, seq_len_q, all_head_size)
-
- self_outputs = (context_layer, attention_probs) if self.output_attentions else (context_layer,)
-
- hidden_states = self_outputs[0]
-
- hidden_states = self.dense(hidden_states)
- hidden_states = self.dropout(hidden_states, training=training)
- attention_output = self.LayerNorm(hidden_states + input_tensor)
-
- # add attentions if we output them
- outputs = (attention_output,) + self_outputs[1:]
- return outputs
-
-
-class TFAlbertLayer(tf.keras.layers.Layer):
- def __init__(self, config, **kwargs):
- super().__init__(**kwargs)
- self.attention = TFAlbertAttention(config, name="attention")
-
- self.ffn = tf.keras.layers.Dense(
- config.intermediate_size, kernel_initializer=get_initializer(config.initializer_range), name="ffn"
- )
-
- if isinstance(config.hidden_act, str):
- self.activation = ACT2FN[config.hidden_act]
- else:
- self.activation = config.hidden_act
-
- self.ffn_output = tf.keras.layers.Dense(
- config.hidden_size, kernel_initializer=get_initializer(config.initializer_range), name="ffn_output"
- )
- self.full_layer_layer_norm = tf.keras.layers.LayerNormalization(
- epsilon=config.layer_norm_eps, name="full_layer_layer_norm"
- )
- self.dropout = tf.keras.layers.Dropout(config.hidden_dropout_prob)
-
- def call(self, inputs, training=False):
- hidden_states, attention_mask, head_mask = inputs
-
- attention_outputs = self.attention([hidden_states, attention_mask, head_mask], training=training)
- ffn_output = self.ffn(attention_outputs[0])
- ffn_output = self.activation(ffn_output)
- ffn_output = self.ffn_output(ffn_output)
-
- hidden_states = self.dropout(hidden_states, training=training)
- hidden_states = self.full_layer_layer_norm(ffn_output + attention_outputs[0])
-
- # add attentions if we output them
- outputs = (hidden_states,) + attention_outputs[1:]
- return outputs
-
-
-class TFAlbertLayerGroup(tf.keras.layers.Layer):
- def __init__(self, config, **kwargs):
- super().__init__(**kwargs)
-
- self.output_attentions = config.output_attentions
- self.output_hidden_states = config.output_hidden_states
- self.albert_layers = [
- TFAlbertLayer(config, name="albert_layers_._{}".format(i)) for i in range(config.inner_group_num)
- ]
-
- def call(self, inputs, training=False):
- hidden_states, attention_mask, head_mask = inputs
-
- layer_hidden_states = ()
- layer_attentions = ()
-
- for layer_index, albert_layer in enumerate(self.albert_layers):
- layer_output = albert_layer([hidden_states, attention_mask, head_mask[layer_index]], training=training)
- hidden_states = layer_output[0]
-
- if self.output_attentions:
- layer_attentions = layer_attentions + (layer_output[1],)
-
- if self.output_hidden_states:
- layer_hidden_states = layer_hidden_states + (hidden_states,)
-
- outputs = (hidden_states,)
- if self.output_hidden_states:
- outputs = outputs + (layer_hidden_states,)
- if self.output_attentions:
- outputs = outputs + (layer_attentions,)
- # last-layer hidden state, (layer hidden states), (layer attentions)
- return outputs
-
-
-class TFAlbertTransformer(tf.keras.layers.Layer):
- def __init__(self, config, **kwargs):
- super().__init__(**kwargs)
-
- self.config = config
- self.output_attentions = config.output_attentions
- self.output_hidden_states = config.output_hidden_states
- self.embedding_hidden_mapping_in = tf.keras.layers.Dense(
- config.hidden_size,
- kernel_initializer=get_initializer(config.initializer_range),
- name="embedding_hidden_mapping_in",
- )
- self.albert_layer_groups = [
- TFAlbertLayerGroup(config, name="albert_layer_groups_._{}".format(i))
- for i in range(config.num_hidden_groups)
- ]
-
- def call(self, inputs, training=False):
- hidden_states, attention_mask, head_mask = inputs
-
- hidden_states = self.embedding_hidden_mapping_in(hidden_states)
- all_attentions = ()
-
- if self.output_hidden_states:
- all_hidden_states = (hidden_states,)
-
- for i in range(self.config.num_hidden_layers):
- # Number of layers in a hidden group
- layers_per_group = int(self.config.num_hidden_layers / self.config.num_hidden_groups)
-
- # Index of the hidden group
- group_idx = int(i / (self.config.num_hidden_layers / self.config.num_hidden_groups))
-
- layer_group_output = self.albert_layer_groups[group_idx](
- [
- hidden_states,
- attention_mask,
- head_mask[group_idx * layers_per_group : (group_idx + 1) * layers_per_group],
- ],
- training=training,
- )
- hidden_states = layer_group_output[0]
-
- if self.output_attentions:
- all_attentions = all_attentions + layer_group_output[-1]
-
- if self.output_hidden_states:
- all_hidden_states = all_hidden_states + (hidden_states,)
-
- outputs = (hidden_states,)
- if self.output_hidden_states:
- outputs = outputs + (all_hidden_states,)
- if self.output_attentions:
- outputs = outputs + (all_attentions,)
-
- # last-layer hidden state, (all hidden states), (all attentions)
- return outputs
-
-
-class TFAlbertPreTrainedModel(TFPreTrainedModel):
- """ An abstract class to handle weights initialization and
- a simple interface for downloading and loading pretrained models.
- """
-
- config_class = AlbertConfig
- pretrained_model_archive_map = TF_ALBERT_PRETRAINED_MODEL_ARCHIVE_MAP
- base_model_prefix = "albert"
-
-
-class TFAlbertMLMHead(tf.keras.layers.Layer):
- def __init__(self, config, input_embeddings, **kwargs):
- super().__init__(**kwargs)
- self.vocab_size = config.vocab_size
-
- self.dense = tf.keras.layers.Dense(
- config.embedding_size, kernel_initializer=get_initializer(config.initializer_range), name="dense"
- )
- if isinstance(config.hidden_act, str):
- self.activation = ACT2FN[config.hidden_act]
- else:
- self.activation = config.hidden_act
-
- self.LayerNorm = tf.keras.layers.LayerNormalization(epsilon=config.layer_norm_eps, name="LayerNorm")
-
- # The output weights are the same as the input embeddings, but there is
- # an output-only bias for each token.
- self.decoder = input_embeddings
-
- def build(self, input_shape):
- self.bias = self.add_weight(shape=(self.vocab_size,), initializer="zeros", trainable=True, name="bias")
- self.decoder_bias = self.add_weight(
- shape=(self.vocab_size,), initializer="zeros", trainable=True, name="decoder/bias"
- )
- super().build(input_shape)
-
- def call(self, hidden_states):
- hidden_states = self.dense(hidden_states)
- hidden_states = self.activation(hidden_states)
- hidden_states = self.LayerNorm(hidden_states)
- hidden_states = self.decoder(hidden_states, mode="linear") + self.decoder_bias
- hidden_states = hidden_states + self.bias
- return hidden_states
-
-
-ALBERT_START_DOCSTRING = r"""
- This model is a `tf.keras.Model `__ sub-class.
- Use it as a regular TF 2.0 Keras Model and
- refer to the TF 2.0 documentation for all matter related to general usage and behavior.
-
- .. _`ALBERT: A Lite BERT for Self-supervised Learning of Language Representations`:
- https://arxiv.org/abs/1909.11942
-
- .. _`tf.keras.Model`:
- https://www.tensorflow.org/versions/r2.0/api_docs/python/tf/keras/Model
-
- .. note::
-
- TF 2.0 models accepts two formats as inputs:
-
- - having all inputs as keyword arguments (like PyTorch models), or
- - having all inputs as a list, tuple or dict in the first positional arguments.
-
- This second option is useful when using :obj:`tf.keras.Model.fit()` method which currently requires having
- all the tensors in the first argument of the model call function: :obj:`model(inputs)`.
-
- If you choose this second option, there are three possibilities you can use to gather all the input Tensors
- in the first positional argument :
-
- - a single Tensor with input_ids only and nothing else: :obj:`model(inputs_ids)`
- - a list of varying length with one or several input Tensors IN THE ORDER given in the docstring:
- :obj:`model([input_ids, attention_mask])` or :obj:`model([input_ids, attention_mask, token_type_ids])`
- - a dictionary with one or several input Tensors associated to the input names given in the docstring:
- :obj:`model({'input_ids': input_ids, 'token_type_ids': token_type_ids})`
-
- Args:
- config (:class:`~transformers.AlbertConfig`): Model configuration class with all the parameters of the model.
- Initializing with a config file does not load the weights associated with the model, only the configuration.
- Check out the :meth:`~transformers.PreTrainedModel.from_pretrained` method to load the model weights.
-"""
-
-ALBERT_INPUTS_DOCSTRING = r"""
- Args:
- input_ids (:obj:`Numpy array` or :obj:`tf.Tensor` of shape :obj:`(batch_size, sequence_length)`):
- Indices of input sequence tokens in the vocabulary.
-
- Indices can be obtained using :class:`transformers.AlbertTokenizer`.
- See :func:`transformers.PreTrainedTokenizer.encode` and
- :func:`transformers.PreTrainedTokenizer.encode_plus` for details.
-
- `What are input IDs? <../glossary.html#input-ids>`__
- attention_mask (:obj:`Numpy array` or :obj:`tf.Tensor` of shape :obj:`(batch_size, sequence_length)`, `optional, defaults to :obj:`None`):
- Mask to avoid performing attention on padding token indices.
- Mask values selected in ``[0, 1]``:
- ``1`` for tokens that are NOT MASKED, ``0`` for MASKED tokens.
-
- `What are attention masks? <../glossary.html#attention-mask>`__
- token_type_ids (:obj:`Numpy array` or :obj:`tf.Tensor` of shape :obj:`(batch_size, sequence_length)`, `optional`, defaults to :obj:`None`):
- Segment token indices to indicate first and second portions of the inputs.
- Indices are selected in ``[0, 1]``: ``0`` corresponds to a `sentence A` token, ``1``
- corresponds to a `sentence B` token
-
- `What are token type IDs? <../glossary.html#token-type-ids>`_
- position_ids (:obj:`Numpy array` or :obj:`tf.Tensor` of shape :obj:`(batch_size, sequence_length)`, `optional`, defaults to :obj:`None`):
- Indices of positions of each input sequence tokens in the position embeddings.
- Selected in the range ``[0, config.max_position_embeddings - 1]``.
-
- `What are position IDs? <../glossary.html#position-ids>`_
- head_mask (:obj:`Numpy array` or :obj:`tf.Tensor` of shape :obj:`(num_heads,)` or :obj:`(num_layers, num_heads)`, `optional`, defaults to :obj:`None`):
- Mask to nullify selected heads of the self-attention modules.
- Mask values selected in ``[0, 1]``:
- ``1`` indicates the head is **not masked**, ``0`` indicates the head is **masked**.
- input_embeds (:obj:`tf.Tensor` of shape :obj:`(batch_size, sequence_length, hidden_size)`, `optional`, defaults to :obj:`None`):
- Optionally, instead of passing :obj:`input_ids` you can choose to directly pass an embedded representation.
- This is useful if you want more control over how to convert `input_ids` indices into associated vectors
- than the model's internal embedding lookup matrix.
- training (:obj:`boolean`, `optional`, defaults to :obj:`False`):
- Whether to activate dropout modules (if set to :obj:`True`) during training or to de-activate them
- (if set to :obj:`False`) for evaluation.
-"""
-
-
-@add_start_docstrings(
- "The bare Albert Model transformer outputing raw hidden-states without any specific head on top.",
- ALBERT_START_DOCSTRING,
-)
-class TFAlbertModel(TFAlbertPreTrainedModel):
- def __init__(self, config, **kwargs):
- super().__init__(config, **kwargs)
- self.num_hidden_layers = config.num_hidden_layers
-
- self.embeddings = TFAlbertEmbeddings(config, name="embeddings")
- self.encoder = TFAlbertTransformer(config, name="encoder")
- self.pooler = tf.keras.layers.Dense(
- config.hidden_size,
- kernel_initializer=get_initializer(config.initializer_range),
- activation="tanh",
- name="pooler",
- )
-
- def get_input_embeddings(self):
- return self.embeddings
-
- def _resize_token_embeddings(self, new_num_tokens):
- raise NotImplementedError
-
- def _prune_heads(self, heads_to_prune):
- """ Prunes heads of the model.
- heads_to_prune: dict of {layer_num: list of heads to prune in this layer}
- See base class PreTrainedModel
- """
- raise NotImplementedError
-
- @add_start_docstrings_to_callable(ALBERT_INPUTS_DOCSTRING)
- def call(
- self,
- inputs,
- attention_mask=None,
- token_type_ids=None,
- position_ids=None,
- head_mask=None,
- inputs_embeds=None,
- training=False,
- ):
- r"""
- Returns:
- :obj:`tuple(tf.Tensor)` comprising various elements depending on the configuration (:class:`~transformers.AlbertConfig`) and inputs:
- last_hidden_state (:obj:`tf.Tensor` of shape :obj:`(batch_size, sequence_length, hidden_size)`):
- Sequence of hidden-states at the output of the last layer of the model.
- pooler_output (:obj:`tf.Tensor` of shape :obj:`(batch_size, hidden_size)`):
- Last layer hidden-state of the first token of the sequence (classification token)
- further processed by a Linear layer and a Tanh activation function. The Linear
- layer weights are trained from the next sentence prediction (classification)
- objective during Albert pretraining. This output is usually *not* a good summary
- of the semantic content of the input, you're often better with averaging or pooling
- the sequence of hidden-states for the whole input sequence.
- hidden_states (:obj:`tuple(tf.Tensor)`, `optional`, returned when :obj:`config.output_hidden_states=True`):
- tuple of :obj:`tf.Tensor` (one for the output of the embeddings + one for the output of each layer)
- of shape :obj:`(batch_size, sequence_length, hidden_size)`.
-
- Hidden-states of the model at the output of each layer plus the initial embedding outputs.
- attentions (:obj:`tuple(tf.Tensor)`, `optional`, returned when ``config.output_attentions=True``):
- tuple of :obj:`tf.Tensor` (one for each layer) of shape
- :obj:`(batch_size, num_heads, sequence_length, sequence_length)`:
-
- Attentions weights after the attention softmax, used to compute the weighted average in the self-attention heads.
-
- Examples::
-
- import tensorflow as tf
- from transformers import AlbertTokenizer, TFAlbertModel
-
- tokenizer = AlbertTokenizer.from_pretrained('albert-base-v2')
- model = TFAlbertModel.from_pretrained('albert-base-v2')
- input_ids = tf.constant(tokenizer.encode("Hello, my dog is cute"))[None, :] # Batch size 1
- outputs = model(input_ids)
- last_hidden_states = outputs[0] # The last hidden-state is the first element of the output tuple
-
- """
- if isinstance(inputs, (tuple, list)):
- input_ids = inputs[0]
- attention_mask = inputs[1] if len(inputs) > 1 else attention_mask
- token_type_ids = inputs[2] if len(inputs) > 2 else token_type_ids
- position_ids = inputs[3] if len(inputs) > 3 else position_ids
- head_mask = inputs[4] if len(inputs) > 4 else head_mask
- inputs_embeds = inputs[5] if len(inputs) > 5 else inputs_embeds
- assert len(inputs) <= 6, "Too many inputs."
- elif isinstance(inputs, dict):
- input_ids = inputs.get("input_ids")
- attention_mask = inputs.get("attention_mask", attention_mask)
- token_type_ids = inputs.get("token_type_ids", token_type_ids)
- position_ids = inputs.get("position_ids", position_ids)
- head_mask = inputs.get("head_mask", head_mask)
- inputs_embeds = inputs.get("inputs_embeds", inputs_embeds)
- assert len(inputs) <= 6, "Too many inputs."
- else:
- input_ids = inputs
-
- if input_ids is not None and inputs_embeds is not None:
- raise ValueError("You cannot specify both input_ids and inputs_embeds at the same time")
- elif input_ids is not None:
- input_shape = shape_list(input_ids)
- elif inputs_embeds is not None:
- input_shape = shape_list(inputs_embeds)[:-1]
- else:
- raise ValueError("You have to specify either input_ids or inputs_embeds")
-
- if attention_mask is None:
- attention_mask = tf.fill(input_shape, 1)
- if token_type_ids is None:
- token_type_ids = tf.fill(input_shape, 0)
-
- # We create a 3D attention mask from a 2D tensor mask.
- # Sizes are [batch_size, 1, 1, to_seq_length]
- # So we can broadcast to [batch_size, num_heads, from_seq_length, to_seq_length]
- # this attention mask is more simple than the triangular masking of causal attention
- # used in OpenAI GPT, we just need to prepare the broadcast dimension here.
- extended_attention_mask = attention_mask[:, tf.newaxis, tf.newaxis, :]
-
- # Since attention_mask is 1.0 for positions we want to attend and 0.0 for
- # masked positions, this operation will create a tensor which is 0.0 for
- # positions we want to attend and -10000.0 for masked positions.
- # Since we are adding it to the raw scores before the softmax, this is
- # effectively the same as removing these entirely.
-
- extended_attention_mask = tf.cast(extended_attention_mask, tf.float32)
- extended_attention_mask = (1.0 - extended_attention_mask) * -10000.0
-
- # Prepare head mask if needed
- # 1.0 in head_mask indicate we keep the head
- # attention_probs has shape bsz x n_heads x N x N
- # input head_mask has shape [num_heads] or [num_hidden_layers x num_heads]
- # and head_mask is converted to shape [num_hidden_layers x batch x num_heads x seq_length x seq_length]
- if head_mask is not None:
- raise NotImplementedError
- else:
- head_mask = [None] * self.num_hidden_layers
- # head_mask = tf.constant([0] * self.num_hidden_layers)
-
- embedding_output = self.embeddings([input_ids, position_ids, token_type_ids, inputs_embeds], training=training)
- encoder_outputs = self.encoder([embedding_output, extended_attention_mask, head_mask], training=training)
-
- sequence_output = encoder_outputs[0]
- pooled_output = self.pooler(sequence_output[:, 0])
-
- # add hidden_states and attentions if they are here
- outputs = (sequence_output, pooled_output,) + encoder_outputs[1:]
- # sequence_output, pooled_output, (hidden_states), (attentions)
- return outputs
-
-
-@add_start_docstrings("""Albert Model with a `language modeling` head on top. """, ALBERT_START_DOCSTRING)
-class TFAlbertForMaskedLM(TFAlbertPreTrainedModel):
- def __init__(self, config, *inputs, **kwargs):
- super(TFAlbertForMaskedLM, self).__init__(config, *inputs, **kwargs)
-
- self.albert = TFAlbertModel(config, name="albert")
- self.predictions = TFAlbertMLMHead(config, self.albert.embeddings, name="predictions")
-
- def get_output_embeddings(self):
- return self.albert.embeddings
-
- @add_start_docstrings_to_callable(ALBERT_INPUTS_DOCSTRING)
- def call(self, inputs, **kwargs):
- r"""
- Returns:
- :obj:`tuple(tf.Tensor)` comprising various elements depending on the configuration (:class:`~transformers.AlbertConfig`) and inputs:
- prediction_scores (:obj:`Numpy array` or :obj:`tf.Tensor` of shape :obj:`(batch_size, sequence_length, config.vocab_size)`
- Prediction scores of the language modeling head (scores for each vocabulary token before SoftMax).
- hidden_states (:obj:`tuple(tf.Tensor)`, `optional`, returned when :obj:`config.output_hidden_states=True`):
- tuple of :obj:`tf.Tensor` (one for the output of the embeddings + one for the output of each layer)
- of shape :obj:`(batch_size, sequence_length, hidden_size)`.
-
- Hidden-states of the model at the output of each layer plus the initial embedding outputs.
- attentions (:obj:`tuple(tf.Tensor)`, `optional`, returned when ``config.output_attentions=True``):
- tuple of :obj:`tf.Tensor` (one for each layer) of shape
- :obj:`(batch_size, num_heads, sequence_length, sequence_length)`:
-
- Attentions weights after the attention softmax, used to compute the weighted average in the self-attention heads.
-
- Examples::
-
- import tensorflow as tf
- from transformers import AlbertTokenizer, TFAlbertForMaskedLM
-
- tokenizer = AlbertTokenizer.from_pretrained('albert-base-v2')
- model = TFAlbertForMaskedLM.from_pretrained('albert-base-v2')
- input_ids = tf.constant(tokenizer.encode("Hello, my dog is cute"))[None, :] # Batch size 1
- outputs = model(input_ids)
- prediction_scores = outputs[0]
-
- """
- outputs = self.albert(inputs, **kwargs)
-
- sequence_output = outputs[0]
- prediction_scores = self.predictions(sequence_output, training=kwargs.get("training", False))
-
- # Add hidden states and attention if they are here
- outputs = (prediction_scores,) + outputs[2:]
-
- return outputs # prediction_scores, (hidden_states), (attentions)
-
-
-@add_start_docstrings(
- """Albert Model transformer with a sequence classification/regression head on top (a linear layer on top of
- the pooled output) e.g. for GLUE tasks. """,
- ALBERT_START_DOCSTRING,
-)
-class TFAlbertForSequenceClassification(TFAlbertPreTrainedModel):
- def __init__(self, config, *inputs, **kwargs):
- super(TFAlbertForSequenceClassification, self).__init__(config, *inputs, **kwargs)
- self.num_labels = config.num_labels
-
- self.albert = TFAlbertModel(config, name="albert")
- self.dropout = tf.keras.layers.Dropout(config.hidden_dropout_prob)
- self.classifier = tf.keras.layers.Dense(
- config.num_labels, kernel_initializer=get_initializer(config.initializer_range), name="classifier"
- )
-
- @add_start_docstrings_to_callable(ALBERT_INPUTS_DOCSTRING)
- def call(self, inputs, **kwargs):
- r"""
- Returns:
- :obj:`tuple(tf.Tensor)` comprising various elements depending on the configuration (:class:`~transformers.AlbertConfig`) and inputs:
- logits (:obj:`Numpy array` or :obj:`tf.Tensor` of shape :obj:`(batch_size, config.num_labels)`)
- Classification (or regression if config.num_labels==1) scores (before SoftMax).
- hidden_states (:obj:`tuple(tf.Tensor)`, `optional`, returned when :obj:`config.output_hidden_states=True`):
- tuple of :obj:`tf.Tensor` (one for the output of the embeddings + one for the output of each layer)
- of shape :obj:`(batch_size, sequence_length, hidden_size)`.
-
- Hidden-states of the model at the output of each layer plus the initial embedding outputs.
- attentions (:obj:`tuple(tf.Tensor)`, `optional`, returned when ``config.output_attentions=True``):
- tuple of :obj:`tf.Tensor` (one for each layer) of shape
- :obj:`(batch_size, num_heads, sequence_length, sequence_length)`:
-
- Attentions weights after the attention softmax, used to compute the weighted average in the self-attention heads.
-
- Examples::
-
- import tensorflow as tf
- from transformers import AlbertTokenizer, TFAlbertForSequenceClassification
-
- tokenizer = AlbertTokenizer.from_pretrained('albert-base-v2')
- model = TFAlbertForSequenceClassification.from_pretrained('albert-base-v2')
- input_ids = tf.constant(tokenizer.encode("Hello, my dog is cute"))[None, :] # Batch size 1
- outputs = model(input_ids)
- logits = outputs[0]
-
- """
- outputs = self.albert(inputs, **kwargs)
-
- pooled_output = outputs[1]
-
- pooled_output = self.dropout(pooled_output, training=kwargs.get("training", False))
- logits = self.classifier(pooled_output)
-
- outputs = (logits,) + outputs[2:] # add hidden states and attention if they are here
-
- return outputs # logits, (hidden_states), (attentions)
diff --git a/server/transformers/src/transformers/modeling_tf_auto.py b/server/transformers/src/transformers/modeling_tf_auto.py
deleted file mode 100644
index dd661006d09b1638488657aa0fd9d5cc801dad07..0000000000000000000000000000000000000000
--- a/server/transformers/src/transformers/modeling_tf_auto.py
+++ /dev/null
@@ -1,1092 +0,0 @@
-# coding=utf-8
-# Copyright 2018 The HuggingFace Inc. team.
-#
-# Licensed under the Apache License, Version 2.0 (the "License");
-# you may not use this file except in compliance with the License.
-# You may obtain a copy of the License at
-#
-# http://www.apache.org/licenses/LICENSE-2.0
-#
-# Unless required by applicable law or agreed to in writing, software
-# distributed under the License is distributed on an "AS IS" BASIS,
-# WITHOUT WARRANTIES OR CONDITIONS OF ANY KIND, either express or implied.
-# See the License for the specific language governing permissions and
-# limitations under the License.
-""" Auto Model class. """
-
-
-import logging
-from collections import OrderedDict
-
-from .configuration_auto import (
- AlbertConfig,
- AutoConfig,
- BertConfig,
- CTRLConfig,
- DistilBertConfig,
- GPT2Config,
- OpenAIGPTConfig,
- RobertaConfig,
- T5Config,
- TransfoXLConfig,
- XLMConfig,
- XLNetConfig,
-)
-from .configuration_utils import PretrainedConfig
-from .modeling_tf_albert import (
- TF_ALBERT_PRETRAINED_MODEL_ARCHIVE_MAP,
- TFAlbertForMaskedLM,
- TFAlbertForSequenceClassification,
- TFAlbertModel,
-)
-from .modeling_tf_bert import (
- TF_BERT_PRETRAINED_MODEL_ARCHIVE_MAP,
- TFBertForMaskedLM,
- TFBertForPreTraining,
- TFBertForQuestionAnswering,
- TFBertForSequenceClassification,
- TFBertForTokenClassification,
- TFBertModel,
-)
-from .modeling_tf_ctrl import TF_CTRL_PRETRAINED_MODEL_ARCHIVE_MAP, TFCTRLLMHeadModel, TFCTRLModel
-from .modeling_tf_distilbert import (
- TF_DISTILBERT_PRETRAINED_MODEL_ARCHIVE_MAP,
- TFDistilBertForMaskedLM,
- TFDistilBertForQuestionAnswering,
- TFDistilBertForSequenceClassification,
- TFDistilBertForTokenClassification,
- TFDistilBertModel,
-)
-from .modeling_tf_gpt2 import TF_GPT2_PRETRAINED_MODEL_ARCHIVE_MAP, TFGPT2LMHeadModel, TFGPT2Model
-from .modeling_tf_openai import TF_OPENAI_GPT_PRETRAINED_MODEL_ARCHIVE_MAP, TFOpenAIGPTLMHeadModel, TFOpenAIGPTModel
-from .modeling_tf_roberta import (
- TF_ROBERTA_PRETRAINED_MODEL_ARCHIVE_MAP,
- TFRobertaForMaskedLM,
- TFRobertaForSequenceClassification,
- TFRobertaForTokenClassification,
- TFRobertaModel,
-)
-from .modeling_tf_t5 import TF_T5_PRETRAINED_MODEL_ARCHIVE_MAP, TFT5Model, TFT5WithLMHeadModel
-from .modeling_tf_transfo_xl import (
- TF_TRANSFO_XL_PRETRAINED_MODEL_ARCHIVE_MAP,
- TFTransfoXLLMHeadModel,
- TFTransfoXLModel,
-)
-from .modeling_tf_xlm import (
- TF_XLM_PRETRAINED_MODEL_ARCHIVE_MAP,
- TFXLMForQuestionAnsweringSimple,
- TFXLMForSequenceClassification,
- TFXLMModel,
- TFXLMWithLMHeadModel,
-)
-from .modeling_tf_xlnet import (
- TF_XLNET_PRETRAINED_MODEL_ARCHIVE_MAP,
- TFXLNetForQuestionAnsweringSimple,
- TFXLNetForSequenceClassification,
- TFXLNetForTokenClassification,
- TFXLNetLMHeadModel,
- TFXLNetModel,
-)
-
-
-logger = logging.getLogger(__name__)
-
-
-TF_ALL_PRETRAINED_MODEL_ARCHIVE_MAP = dict(
- (key, value)
- for pretrained_map in [
- TF_BERT_PRETRAINED_MODEL_ARCHIVE_MAP,
- TF_OPENAI_GPT_PRETRAINED_MODEL_ARCHIVE_MAP,
- TF_TRANSFO_XL_PRETRAINED_MODEL_ARCHIVE_MAP,
- TF_GPT2_PRETRAINED_MODEL_ARCHIVE_MAP,
- TF_CTRL_PRETRAINED_MODEL_ARCHIVE_MAP,
- TF_XLNET_PRETRAINED_MODEL_ARCHIVE_MAP,
- TF_XLM_PRETRAINED_MODEL_ARCHIVE_MAP,
- TF_ROBERTA_PRETRAINED_MODEL_ARCHIVE_MAP,
- TF_DISTILBERT_PRETRAINED_MODEL_ARCHIVE_MAP,
- TF_ALBERT_PRETRAINED_MODEL_ARCHIVE_MAP,
- TF_T5_PRETRAINED_MODEL_ARCHIVE_MAP,
- ]
- for key, value, in pretrained_map.items()
-)
-
-TF_MODEL_MAPPING = OrderedDict(
- [
- (T5Config, TFT5Model),
- (DistilBertConfig, TFDistilBertModel),
- (AlbertConfig, TFAlbertModel),
- (RobertaConfig, TFRobertaModel),
- (BertConfig, TFBertModel),
- (OpenAIGPTConfig, TFOpenAIGPTModel),
- (GPT2Config, TFGPT2Model),
- (TransfoXLConfig, TFTransfoXLModel),
- (XLNetConfig, TFXLNetModel),
- (XLMConfig, TFXLMModel),
- (CTRLConfig, TFCTRLModel),
- ]
-)
-
-TF_MODEL_FOR_PRETRAINING_MAPPING = OrderedDict(
- [
- (T5Config, TFT5WithLMHeadModel),
- (DistilBertConfig, TFDistilBertForMaskedLM),
- (AlbertConfig, TFAlbertForMaskedLM),
- (RobertaConfig, TFRobertaForMaskedLM),
- (BertConfig, TFBertForPreTraining),
- (OpenAIGPTConfig, TFOpenAIGPTLMHeadModel),
- (GPT2Config, TFGPT2LMHeadModel),
- (TransfoXLConfig, TFTransfoXLLMHeadModel),
- (XLNetConfig, TFXLNetLMHeadModel),
- (XLMConfig, TFXLMWithLMHeadModel),
- (CTRLConfig, TFCTRLLMHeadModel),
- ]
-)
-
-TF_MODEL_WITH_LM_HEAD_MAPPING = OrderedDict(
- [
- (T5Config, TFT5WithLMHeadModel),
- (DistilBertConfig, TFDistilBertForMaskedLM),
- (AlbertConfig, TFAlbertForMaskedLM),
- (RobertaConfig, TFRobertaForMaskedLM),
- (BertConfig, TFBertForMaskedLM),
- (OpenAIGPTConfig, TFOpenAIGPTLMHeadModel),
- (GPT2Config, TFGPT2LMHeadModel),
- (TransfoXLConfig, TFTransfoXLLMHeadModel),
- (XLNetConfig, TFXLNetLMHeadModel),
- (XLMConfig, TFXLMWithLMHeadModel),
- (CTRLConfig, TFCTRLLMHeadModel),
- ]
-)
-
-TF_MODEL_FOR_SEQUENCE_CLASSIFICATION_MAPPING = OrderedDict(
- [
- (DistilBertConfig, TFDistilBertForSequenceClassification),
- (AlbertConfig, TFAlbertForSequenceClassification),
- (RobertaConfig, TFRobertaForSequenceClassification),
- (BertConfig, TFBertForSequenceClassification),
- (XLNetConfig, TFXLNetForSequenceClassification),
- (XLMConfig, TFXLMForSequenceClassification),
- ]
-)
-
-TF_MODEL_FOR_QUESTION_ANSWERING_MAPPING = OrderedDict(
- [
- (DistilBertConfig, TFDistilBertForQuestionAnswering),
- (BertConfig, TFBertForQuestionAnswering),
- (XLNetConfig, TFXLNetForQuestionAnsweringSimple),
- (XLMConfig, TFXLMForQuestionAnsweringSimple),
- ]
-)
-
-TF_MODEL_FOR_TOKEN_CLASSIFICATION_MAPPING = OrderedDict(
- [
- (DistilBertConfig, TFDistilBertForTokenClassification),
- (RobertaConfig, TFRobertaForTokenClassification),
- (BertConfig, TFBertForTokenClassification),
- (XLNetConfig, TFXLNetForTokenClassification),
- ]
-)
-
-
-class TFAutoModel(object):
- r"""
- :class:`~transformers.TFAutoModel` is a generic model class
- that will be instantiated as one of the base model classes of the library
- when created with the `TFAutoModel.from_pretrained(pretrained_model_name_or_path)`
- class method.
-
- The `from_pretrained()` method takes care of returning the correct model class instance
- based on the `model_type` property of the config object, or when it's missing,
- falling back to using pattern matching on the `pretrained_model_name_or_path` string.
-
- The base model class to instantiate is selected as the first pattern matching
- in the `pretrained_model_name_or_path` string (in the following order):
- - contains `t5`: TFT5Model (T5 model)
- - contains `distilbert`: TFDistilBertModel (DistilBERT model)
- - contains `roberta`: TFRobertaModel (RoBERTa model)
- - contains `bert`: TFBertModel (Bert model)
- - contains `openai-gpt`: TFOpenAIGPTModel (OpenAI GPT model)
- - contains `gpt2`: TFGPT2Model (OpenAI GPT-2 model)
- - contains `transfo-xl`: TFTransfoXLModel (Transformer-XL model)
- - contains `xlnet`: TFXLNetModel (XLNet model)
- - contains `xlm`: TFXLMModel (XLM model)
- - contains `ctrl`: TFCTRLModel (CTRL model)
-
- This class cannot be instantiated using `__init__()` (throws an error).
- """
-
- def __init__(self):
- raise EnvironmentError(
- "TFAutoModel is designed to be instantiated "
- "using the `TFAutoModel.from_pretrained(pretrained_model_name_or_path)` or "
- "`TFAutoModel.from_config(config)` methods."
- )
-
- @classmethod
- def from_config(cls, config):
- r""" Instantiates one of the base model classes of the library
- from a configuration.
-
- config: (`optional`) instance of a class derived from :class:`~transformers.PretrainedConfig`:
- The model class to instantiate is selected based on the configuration class:
- - isInstance of `distilbert` configuration class: TFDistilBertModel (DistilBERT model)
- - isInstance of `roberta` configuration class: TFRobertaModel (RoBERTa model)
- - isInstance of `bert` configuration class: TFBertModel (Bert model)
- - isInstance of `openai-gpt` configuration class: TFOpenAIGPTModel (OpenAI GPT model)
- - isInstance of `gpt2` configuration class: TFGPT2Model (OpenAI GPT-2 model)
- - isInstance of `ctrl` configuration class: TFCTRLModel (Salesforce CTRL model)
- - isInstance of `transfo-xl` configuration class: TFTransfoXLModel (Transformer-XL model)
- - isInstance of `xlnet` configuration class: TFXLNetModel (XLNet model)
- - isInstance of `xlm` configuration class: TFXLMModel (XLM model)
-
- Examples::
-
- config = BertConfig.from_pretrained('bert-base-uncased') # Download configuration from S3 and cache.
- model = TFAutoModel.from_config(config) # E.g. model was saved using `save_pretrained('./test/saved_model/')`
- """
- for config_class, model_class in TF_MODEL_MAPPING.items():
- if isinstance(config, config_class):
- return model_class(config)
- raise ValueError(
- "Unrecognized configuration class {} for this kind of TFAutoModel: {}.\n"
- "Model type should be one of {}.".format(
- config.__class__, cls.__name__, ", ".join(c.__name__ for c in TF_MODEL_MAPPING.keys())
- )
- )
-
- @classmethod
- def from_pretrained(cls, pretrained_model_name_or_path, *model_args, **kwargs):
- r""" Instantiates one of the base model classes of the library
- from a pre-trained model configuration.
-
- The model class to instantiate is selected as the first pattern matching
- in the `pretrained_model_name_or_path` string (in the following order):
- - contains `t5`: TFT5Model (T5 model)
- - contains `distilbert`: TFDistilBertModel (DistilBERT model)
- - contains `roberta`: TFRobertaModel (RoBERTa model)
- - contains `bert`: TFTFBertModel (Bert model)
- - contains `openai-gpt`: TFOpenAIGPTModel (OpenAI GPT model)
- - contains `gpt2`: TFGPT2Model (OpenAI GPT-2 model)
- - contains `transfo-xl`: TFTransfoXLModel (Transformer-XL model)
- - contains `xlnet`: TFXLNetModel (XLNet model)
- - contains `ctrl`: TFCTRLModel (CTRL model)
-
- Params:
- pretrained_model_name_or_path: either:
-
- - a string with the `shortcut name` of a pre-trained model to load from cache or download, e.g.: ``bert-base-uncased``.
- - a string with the `identifier name` of a pre-trained model that was user-uploaded to our S3, e.g.: ``dbmdz/bert-base-german-cased``.
- - a path to a `directory` containing model weights saved using :func:`~transformers.PreTrainedModel.save_pretrained`, e.g.: ``./my_model_directory/``.
- - a path or url to a `PyTorch, TF 1.X or TF 2.0 checkpoint file` (e.g. `./tf_model/model.ckpt.index`). In the case of a PyTorch checkpoint, ``from_pt`` should be set to True and a configuration object should be provided as ``config`` argument.
-
- from_pt: (`Optional`) Boolean
- Set to True if the Checkpoint is a PyTorch checkpoint.
-
- model_args: (`optional`) Sequence of positional arguments:
- All remaning positional arguments will be passed to the underlying model's ``__init__`` method
-
- config: (`optional`) instance of a class derived from :class:`~transformers.PretrainedConfig`:
- Configuration for the model to use instead of an automatically loaded configuation. Configuration can be automatically loaded when:
-
- - the model is a model provided by the library (loaded with the ``shortcut-name`` string of a pretrained model), or
- - the model was saved using :func:`~transformers.PreTrainedModel.save_pretrained` and is reloaded by suppling the save directory.
- - the model is loaded by suppling a local directory as ``pretrained_model_name_or_path`` and a configuration JSON file named `config.json` is found in the directory.
-
- state_dict: (`optional`) dict:
- an optional state dictionnary for the model to use instead of a state dictionary loaded from saved weights file.
- This option can be used if you want to create a model from a pretrained configuration but load your own weights.
- In this case though, you should check if using :func:`~transformers.PreTrainedModel.save_pretrained` and :func:`~transformers.PreTrainedModel.from_pretrained` is not a simpler option.
-
- cache_dir: (`optional`) string:
- Path to a directory in which a downloaded pre-trained model
- configuration should be cached if the standard cache should not be used.
-
- force_download: (`optional`) boolean, default False:
- Force to (re-)download the model weights and configuration files and override the cached versions if they exists.
-
- resume_download: (`optional`) boolean, default False:
- Do not delete incompletely recieved file. Attempt to resume the download if such a file exists.
-
- proxies: (`optional`) dict, default None:
- A dictionary of proxy servers to use by protocol or endpoint, e.g.: {'http': 'foo.bar:3128', 'http://hostname': 'foo.bar:4012'}.
- The proxies are used on each request.
-
- output_loading_info: (`optional`) boolean:
- Set to ``True`` to also return a dictionnary containing missing keys, unexpected keys and error messages.
-
- kwargs: (`optional`) Remaining dictionary of keyword arguments:
- Can be used to update the configuration object (after it being loaded) and initiate the model. (e.g. ``output_attention=True``). Behave differently depending on whether a `config` is provided or automatically loaded:
-
- - If a configuration is provided with ``config``, ``**kwargs`` will be directly passed to the underlying model's ``__init__`` method (we assume all relevant updates to the configuration have already been done)
- - If a configuration is not provided, ``kwargs`` will be first passed to the configuration class initialization function (:func:`~transformers.PretrainedConfig.from_pretrained`). Each key of ``kwargs`` that corresponds to a configuration attribute will be used to override said attribute with the supplied ``kwargs`` value. Remaining keys that do not correspond to any configuration attribute will be passed to the underlying model's ``__init__`` function.
-
- Examples::
-
- model = TFAutoModel.from_pretrained('bert-base-uncased') # Download model and configuration from S3 and cache.
- model = TFAutoModel.from_pretrained('./test/bert_model/') # E.g. model was saved using `save_pretrained('./test/saved_model/')`
- model = TFAutoModel.from_pretrained('bert-base-uncased', output_attention=True) # Update configuration during loading
- assert model.config.output_attention == True
- # Loading from a TF checkpoint file instead of a PyTorch model (slower)
- config = AutoConfig.from_json_file('./tf_model/bert_tf_model_config.json')
- model = TFAutoModel.from_pretrained('./pt_model/bert_pytorch_model.bin', from_pt=True, config=config)
-
- """
- config = kwargs.pop("config", None)
- if not isinstance(config, PretrainedConfig):
- config = AutoConfig.from_pretrained(pretrained_model_name_or_path, **kwargs)
-
- for config_class, model_class in TF_MODEL_MAPPING.items():
- if isinstance(config, config_class):
- return model_class.from_pretrained(pretrained_model_name_or_path, *model_args, config=config, **kwargs)
- raise ValueError(
- "Unrecognized configuration class {} for this kind of TFAutoModel: {}.\n"
- "Model type should be one of {}.".format(
- config.__class__, cls.__name__, ", ".join(c.__name__ for c in TF_MODEL_MAPPING.keys())
- )
- )
-
-
-class TFAutoModelForPreTraining(object):
- r"""
- :class:`~transformers.TFAutoModelForPreTraining` is a generic model class
- that will be instantiated as one of the model classes of the library -with the architecture used for pretraining this model– when created with the `TFAutoModelForPreTraining.from_pretrained(pretrained_model_name_or_path)`
- class method.
-
- This class cannot be instantiated using `__init__()` (throws an error).
- """
-
- def __init__(self):
- raise EnvironmentError(
- "TFAutoModelForPreTraining is designed to be instantiated "
- "using the `TFAutoModelForPreTraining.from_pretrained(pretrained_model_name_or_path)` or "
- "`TFAutoModelForPreTraining.from_config(config)` methods."
- )
-
- @classmethod
- def from_config(cls, config):
- r""" Instantiates one of the base model classes of the library
- from a configuration.
-
- Args:
- config (:class:`~transformers.PretrainedConfig`):
- The model class to instantiate is selected based on the configuration class:
-
- - isInstance of `distilbert` configuration class: :class:`~transformers.TFDistilBertModelForMaskedLM` (DistilBERT model)
- - isInstance of `roberta` configuration class: :class:`~transformers.TFRobertaModelForMaskedLM` (RoBERTa model)
- - isInstance of `bert` configuration class: :class:`~transformers.TFBertForPreTraining` (Bert model)
- - isInstance of `openai-gpt` configuration class: :class:`~transformers.TFOpenAIGPTLMHeadModel` (OpenAI GPT model)
- - isInstance of `gpt2` configuration class: :class:`~transformers.TFGPT2ModelLMHeadModel` (OpenAI GPT-2 model)
- - isInstance of `ctrl` configuration class: :class:`~transformers.TFCTRLModelLMHeadModel` (Salesforce CTRL model)
- - isInstance of `transfo-xl` configuration class: :class:`~transformers.TFTransfoXLLMHeadModel` (Transformer-XL model)
- - isInstance of `xlnet` configuration class: :class:`~transformers.TFXLNetLMHeadModel` (XLNet model)
- - isInstance of `xlm` configuration class: :class:`~transformers.TFXLMWithLMHeadModel` (XLM model)
-
- Examples::
-
- config = BertConfig.from_pretrained('bert-base-uncased') # Download configuration from S3 and cache.
- model = TFAutoModelForPreTraining.from_config(config) # E.g. model was saved using `save_pretrained('./test/saved_model/')`
- """
- for config_class, model_class in TF_MODEL_FOR_PRETRAINING_MAPPING.items():
- if isinstance(config, config_class):
- return model_class(config)
- raise ValueError(
- "Unrecognized configuration class {} for this kind of AutoModel: {}.\n"
- "Model type should be one of {}.".format(
- config.__class__, cls.__name__, ", ".join(c.__name__ for c in TF_MODEL_FOR_PRETRAINING_MAPPING.keys())
- )
- )
-
- @classmethod
- def from_pretrained(cls, pretrained_model_name_or_path, *model_args, **kwargs):
- r""" Instantiates one of the model classes of the library -with the architecture used for pretraining this model– from a pre-trained model configuration.
-
- The `from_pretrained()` method takes care of returning the correct model class instance
- based on the `model_type` property of the config object, or when it's missing,
- falling back to using pattern matching on the `pretrained_model_name_or_path` string.
-
- The model class to instantiate is selected as the first pattern matching
- in the `pretrained_model_name_or_path` string (in the following order):
- - contains `t5`: :class:`~transformers.TFT5ModelWithLMHead` (T5 model)
- - contains `distilbert`: :class:`~transformers.TFDistilBertForMaskedLM` (DistilBERT model)
- - contains `albert`: :class:`~transformers.TFAlbertForMaskedLM` (ALBERT model)
- - contains `roberta`: :class:`~transformers.TFRobertaForMaskedLM` (RoBERTa model)
- - contains `bert`: :class:`~transformers.TFBertForPreTraining` (Bert model)
- - contains `openai-gpt`: :class:`~transformers.TFOpenAIGPTLMHeadModel` (OpenAI GPT model)
- - contains `gpt2`: :class:`~transformers.TFGPT2LMHeadModel` (OpenAI GPT-2 model)
- - contains `transfo-xl`: :class:`~transformers.TFTransfoXLLMHeadModel` (Transformer-XL model)
- - contains `xlnet`: :class:`~transformers.TFXLNetLMHeadModel` (XLNet model)
- - contains `xlm`: :class:`~transformers.TFXLMWithLMHeadModel` (XLM model)
- - contains `ctrl`: :class:`~transformers.TFCTRLLMHeadModel` (Salesforce CTRL model)
-
- The model is set in evaluation mode by default using `model.eval()` (Dropout modules are deactivated)
- To train the model, you should first set it back in training mode with `model.train()`
-
- Args:
- pretrained_model_name_or_path:
- Either:
-
- - a string with the `shortcut name` of a pre-trained model to load from cache or download, e.g.: ``bert-base-uncased``.
- - a string with the `identifier name` of a pre-trained model that was user-uploaded to our S3, e.g.: ``dbmdz/bert-base-german-cased``.
- - a path to a `directory` containing model weights saved using :func:`~transformers.PreTrainedModel.save_pretrained`, e.g.: ``./my_model_directory/``.
- - a path or url to a `tensorflow index checkpoint file` (e.g. `./tf_model/model.ckpt.index`). In this case, ``from_tf`` should be set to True and a configuration object should be provided as ``config`` argument. This loading path is slower than converting the TensorFlow checkpoint in a PyTorch model using the provided conversion scripts and loading the PyTorch model afterwards.
- model_args: (`optional`) Sequence of positional arguments:
- All remaning positional arguments will be passed to the underlying model's ``__init__`` method
- config: (`optional`) instance of a class derived from :class:`~transformers.PretrainedConfig`:
- Configuration for the model to use instead of an automatically loaded configuation. Configuration can be automatically loaded when:
-
- - the model is a model provided by the library (loaded with the ``shortcut-name`` string of a pretrained model), or
- - the model was saved using :func:`~transformers.PreTrainedModel.save_pretrained` and is reloaded by suppling the save directory.
- - the model is loaded by suppling a local directory as ``pretrained_model_name_or_path`` and a configuration JSON file named `config.json` is found in the directory.
-
- state_dict: (`optional`) dict:
- an optional state dictionnary for the model to use instead of a state dictionary loaded from saved weights file.
- This option can be used if you want to create a model from a pretrained configuration but load your own weights.
- In this case though, you should check if using :func:`~transformers.PreTrainedModel.save_pretrained` and :func:`~transformers.PreTrainedModel.from_pretrained` is not a simpler option.
- cache_dir: (`optional`) string:
- Path to a directory in which a downloaded pre-trained model
- configuration should be cached if the standard cache should not be used.
- force_download: (`optional`) boolean, default False:
- Force to (re-)download the model weights and configuration files and override the cached versions if they exists.
- resume_download: (`optional`) boolean, default False:
- Do not delete incompletely received file. Attempt to resume the download if such a file exists.
- proxies: (`optional`) dict, default None:
- A dictionary of proxy servers to use by protocol or endpoint, e.g.: {'http': 'foo.bar:3128', 'http://hostname': 'foo.bar:4012'}.
- The proxies are used on each request.
- output_loading_info: (`optional`) boolean:
- Set to ``True`` to also return a dictionnary containing missing keys, unexpected keys and error messages.
- kwargs: (`optional`) Remaining dictionary of keyword arguments:
- Can be used to update the configuration object (after it being loaded) and initiate the model.
- (e.g. ``output_attention=True``). Behave differently depending on whether a `config` is provided or
- automatically loaded:
-
- - If a configuration is provided with ``config``, ``**kwargs`` will be directly passed to the
- underlying model's ``__init__`` method (we assume all relevant updates to the configuration have
- already been done)
- - If a configuration is not provided, ``kwargs`` will be first passed to the configuration class
- initialization function (:func:`~transformers.PretrainedConfig.from_pretrained`). Each key of
- ``kwargs`` that corresponds to a configuration attribute will be used to override said attribute
- with the supplied ``kwargs`` value. Remaining keys that do not correspond to any configuration
- attribute will be passed to the underlying model's ``__init__`` function.
-
- Examples::
-
- model = TFAutoModelForPreTraining.from_pretrained('bert-base-uncased') # Download model and configuration from S3 and cache.
- model = TFAutoModelForPreTraining.from_pretrained('./test/bert_model/') # E.g. model was saved using `save_pretrained('./test/saved_model/')`
- model = TFAutoModelForPreTraining.from_pretrained('bert-base-uncased', output_attention=True) # Update configuration during loading
- assert model.config.output_attention == True
- # Loading from a TF checkpoint file instead of a PyTorch model (slower)
- config = AutoConfig.from_json_file('./tf_model/bert_tf_model_config.json')
- model = TFAutoModelForPreTraining.from_pretrained('./tf_model/bert_tf_checkpoint.ckpt.index', from_tf=True, config=config)
-
- """
- config = kwargs.pop("config", None)
- if not isinstance(config, PretrainedConfig):
- config = AutoConfig.from_pretrained(pretrained_model_name_or_path, **kwargs)
-
- for config_class, model_class in TF_MODEL_FOR_PRETRAINING_MAPPING.items():
- if isinstance(config, config_class):
- return model_class.from_pretrained(pretrained_model_name_or_path, *model_args, config=config, **kwargs)
- raise ValueError(
- "Unrecognized configuration class {} for this kind of AutoModel: {}.\n"
- "Model type should be one of {}.".format(
- config.__class__, cls.__name__, ", ".join(c.__name__ for c in TF_MODEL_FOR_PRETRAINING_MAPPING.keys())
- )
- )
-
-
-class TFAutoModelWithLMHead(object):
- r"""
- :class:`~transformers.TFAutoModelWithLMHead` is a generic model class
- that will be instantiated as one of the language modeling model classes of the library
- when created with the `TFAutoModelWithLMHead.from_pretrained(pretrained_model_name_or_path)`
- class method.
-
- The `from_pretrained()` method takes care of returning the correct model class instance
- based on the `model_type` property of the config object, or when it's missing,
- falling back to using pattern matching on the `pretrained_model_name_or_path` string.
-
- The model class to instantiate is selected as the first pattern matching
- in the `pretrained_model_name_or_path` string (in the following order):
- - contains `t5`: TFT5WithLMHeadModel (T5 model)
- - contains `distilbert`: TFDistilBertForMaskedLM (DistilBERT model)
- - contains `roberta`: TFRobertaForMaskedLM (RoBERTa model)
- - contains `bert`: TFBertForMaskedLM (Bert model)
- - contains `openai-gpt`: TFOpenAIGPTLMHeadModel (OpenAI GPT model)
- - contains `gpt2`: TFGPT2LMHeadModel (OpenAI GPT-2 model)
- - contains `transfo-xl`: TFTransfoXLLMHeadModel (Transformer-XL model)
- - contains `xlnet`: TFXLNetLMHeadModel (XLNet model)
- - contains `xlm`: TFXLMWithLMHeadModel (XLM model)
- - contains `ctrl`: TFCTRLLMHeadModel (CTRL model)
-
- This class cannot be instantiated using `__init__()` (throws an error).
- """
-
- def __init__(self):
- raise EnvironmentError(
- "TFAutoModelWithLMHead is designed to be instantiated "
- "using the `TFAutoModelWithLMHead.from_pretrained(pretrained_model_name_or_path)` or "
- "`TFAutoModelWithLMHead.from_config(config)` methods."
- )
-
- @classmethod
- def from_config(cls, config):
- r""" Instantiates one of the base model classes of the library
- from a configuration.
-
- config: (`optional`) instance of a class derived from :class:`~transformers.PretrainedConfig`:
- The model class to instantiate is selected based on the configuration class:
- - isInstance of `distilbert` configuration class: DistilBertModel (DistilBERT model)
- - isInstance of `roberta` configuration class: RobertaModel (RoBERTa model)
- - isInstance of `bert` configuration class: BertModel (Bert model)
- - isInstance of `openai-gpt` configuration class: OpenAIGPTModel (OpenAI GPT model)
- - isInstance of `gpt2` configuration class: GPT2Model (OpenAI GPT-2 model)
- - isInstance of `ctrl` configuration class: CTRLModel (Salesforce CTRL model)
- - isInstance of `transfo-xl` configuration class: TransfoXLModel (Transformer-XL model)
- - isInstance of `xlnet` configuration class: XLNetModel (XLNet model)
- - isInstance of `xlm` configuration class: XLMModel (XLM model)
-
- Examples::
-
- config = BertConfig.from_pretrained('bert-base-uncased') # Download configuration from S3 and cache.
- model = TFAutoModelWithLMHead.from_config(config) # E.g. model was saved using `save_pretrained('./test/saved_model/')`
- """
- for config_class, model_class in TF_MODEL_WITH_LM_HEAD_MAPPING.items():
- if isinstance(config, config_class):
- return model_class(config)
- raise ValueError(
- "Unrecognized configuration class {} for this kind of TFAutoModel: {}.\n"
- "Model type should be one of {}.".format(
- config.__class__, cls.__name__, ", ".join(c.__name__ for c in TF_MODEL_WITH_LM_HEAD_MAPPING.keys())
- )
- )
-
- @classmethod
- def from_pretrained(cls, pretrained_model_name_or_path, *model_args, **kwargs):
- r""" Instantiates one of the language modeling model classes of the library
- from a pre-trained model configuration.
-
- The `from_pretrained()` method takes care of returning the correct model class instance
- based on the `model_type` property of the config object, or when it's missing,
- falling back to using pattern matching on the `pretrained_model_name_or_path` string.
-
- The model class to instantiate is selected as the first pattern matching
- in the `pretrained_model_name_or_path` string (in the following order):
- - contains `t5`: TFT5WithLMHeadModel (T5 model)
- - contains `distilbert`: TFDistilBertForMaskedLM (DistilBERT model)
- - contains `roberta`: TFRobertaForMaskedLM (RoBERTa model)
- - contains `bert`: TFBertForMaskedLM (Bert model)
- - contains `openai-gpt`: TFOpenAIGPTLMHeadModel (OpenAI GPT model)
- - contains `gpt2`: TFGPT2LMHeadModel (OpenAI GPT-2 model)
- - contains `transfo-xl`: TFTransfoXLLMHeadModel (Transformer-XL model)
- - contains `xlnet`: TFXLNetLMHeadModel (XLNet model)
- - contains `xlm`: TFXLMWithLMHeadModel (XLM model)
- - contains `ctrl`: TFCTRLLMHeadModel (CTRL model)
-
- Params:
- pretrained_model_name_or_path: either:
-
- - a string with the `shortcut name` of a pre-trained model to load from cache or download, e.g.: ``bert-base-uncased``.
- - a string with the `identifier name` of a pre-trained model that was user-uploaded to our S3, e.g.: ``dbmdz/bert-base-german-cased``.
- - a path to a `directory` containing model weights saved using :func:`~transformers.PreTrainedModel.save_pretrained`, e.g.: ``./my_model_directory/``.
- - a path or url to a `PyTorch, TF 1.X or TF 2.0 checkpoint file` (e.g. `./tf_model/model.ckpt.index`). In the case of a PyTorch checkpoint, ``from_pt`` should be set to True and a configuration object should be provided as ``config`` argument.
-
- from_pt: (`Optional`) Boolean
- Set to True if the Checkpoint is a PyTorch checkpoint.
-
- model_args: (`optional`) Sequence of positional arguments:
- All remaning positional arguments will be passed to the underlying model's ``__init__`` method
-
- config: (`optional`) instance of a class derived from :class:`~transformers.PretrainedConfig`:
- Configuration for the model to use instead of an automatically loaded configuation. Configuration can be automatically loaded when:
-
- - the model is a model provided by the library (loaded with the ``shortcut-name`` string of a pretrained model), or
- - the model was saved using :func:`~transformers.PreTrainedModel.save_pretrained` and is reloaded by suppling the save directory.
- - the model is loaded by suppling a local directory as ``pretrained_model_name_or_path`` and a configuration JSON file named `config.json` is found in the directory.
-
- state_dict: (`optional`) dict:
- an optional state dictionnary for the model to use instead of a state dictionary loaded from saved weights file.
- This option can be used if you want to create a model from a pretrained configuration but load your own weights.
- In this case though, you should check if using :func:`~transformers.PreTrainedModel.save_pretrained` and :func:`~transformers.PreTrainedModel.from_pretrained` is not a simpler option.
-
- cache_dir: (`optional`) string:
- Path to a directory in which a downloaded pre-trained model
- configuration should be cached if the standard cache should not be used.
-
- force_download: (`optional`) boolean, default False:
- Force to (re-)download the model weights and configuration files and override the cached versions if they exists.
-
- resume_download: (`optional`) boolean, default False:
- Do not delete incompletely recieved file. Attempt to resume the download if such a file exists.
-
- proxies: (`optional`) dict, default None:
- A dictionary of proxy servers to use by protocol or endpoint, e.g.: {'http': 'foo.bar:3128', 'http://hostname': 'foo.bar:4012'}.
- The proxies are used on each request.
-
- output_loading_info: (`optional`) boolean:
- Set to ``True`` to also return a dictionnary containing missing keys, unexpected keys and error messages.
-
- kwargs: (`optional`) Remaining dictionary of keyword arguments:
- Can be used to update the configuration object (after it being loaded) and initiate the model. (e.g. ``output_attention=True``). Behave differently depending on whether a `config` is provided or automatically loaded:
-
- - If a configuration is provided with ``config``, ``**kwargs`` will be directly passed to the underlying model's ``__init__`` method (we assume all relevant updates to the configuration have already been done)
- - If a configuration is not provided, ``kwargs`` will be first passed to the configuration class initialization function (:func:`~transformers.PretrainedConfig.from_pretrained`). Each key of ``kwargs`` that corresponds to a configuration attribute will be used to override said attribute with the supplied ``kwargs`` value. Remaining keys that do not correspond to any configuration attribute will be passed to the underlying model's ``__init__`` function.
-
- Examples::
-
- model = TFAutoModelWithLMHead.from_pretrained('bert-base-uncased') # Download model and configuration from S3 and cache.
- model = TFAutoModelWithLMHead.from_pretrained('./test/bert_model/') # E.g. model was saved using `save_pretrained('./test/saved_model/')`
- model = TFAutoModelWithLMHead.from_pretrained('bert-base-uncased', output_attention=True) # Update configuration during loading
- assert model.config.output_attention == True
- # Loading from a TF checkpoint file instead of a PyTorch model (slower)
- config = AutoConfig.from_json_file('./tf_model/bert_tf_model_config.json')
- model = TFAutoModelWithLMHead.from_pretrained('./pt_model/bert_pytorch_model.bin', from_pt=True, config=config)
-
- """
- config = kwargs.pop("config", None)
- if not isinstance(config, PretrainedConfig):
- config = AutoConfig.from_pretrained(pretrained_model_name_or_path, **kwargs)
-
- for config_class, model_class in TF_MODEL_WITH_LM_HEAD_MAPPING.items():
- if isinstance(config, config_class):
- return model_class.from_pretrained(pretrained_model_name_or_path, *model_args, config=config, **kwargs)
- raise ValueError(
- "Unrecognized configuration class {} for this kind of TFAutoModel: {}.\n"
- "Model type should be one of {}.".format(
- config.__class__, cls.__name__, ", ".join(c.__name__ for c in TF_MODEL_WITH_LM_HEAD_MAPPING.keys())
- )
- )
-
-
-class TFAutoModelForSequenceClassification(object):
- r"""
- :class:`~transformers.TFAutoModelForSequenceClassification` is a generic model class
- that will be instantiated as one of the sequence classification model classes of the library
- when created with the `TFAutoModelForSequenceClassification.from_pretrained(pretrained_model_name_or_path)`
- class method.
-
- The `from_pretrained()` method takes care of returning the correct model class instance
- based on the `model_type` property of the config object, or when it's missing,
- falling back to using pattern matching on the `pretrained_model_name_or_path` string.
-
- The model class to instantiate is selected as the first pattern matching
- in the `pretrained_model_name_or_path` string (in the following order):
- - contains `distilbert`: TFDistilBertForSequenceClassification (DistilBERT model)
- - contains `roberta`: TFRobertaForSequenceClassification (RoBERTa model)
- - contains `bert`: TFBertForSequenceClassification (Bert model)
- - contains `xlnet`: TFXLNetForSequenceClassification (XLNet model)
- - contains `xlm`: TFXLMForSequenceClassification (XLM model)
-
- This class cannot be instantiated using `__init__()` (throws an error).
- """
-
- def __init__(self):
- raise EnvironmentError(
- "TFAutoModelForSequenceClassification is designed to be instantiated "
- "using the `TFAutoModelForSequenceClassification.from_pretrained(pretrained_model_name_or_path)` or "
- "`TFAutoModelForSequenceClassification.from_config(config)` methods."
- )
-
- @classmethod
- def from_config(cls, config):
- r""" Instantiates one of the base model classes of the library
- from a configuration.
-
- config: (`optional`) instance of a class derived from :class:`~transformers.PretrainedConfig`:
- The model class to instantiate is selected based on the configuration class:
- - isInstance of `distilbert` configuration class: DistilBertModel (DistilBERT model)
- - isInstance of `roberta` configuration class: RobertaModel (RoBERTa model)
- - isInstance of `bert` configuration class: BertModel (Bert model)
- - isInstance of `xlnet` configuration class: XLNetModel (XLNet model)
- - isInstance of `xlm` configuration class: XLMModel (XLM model)
-
- Examples::
-
- config = BertConfig.from_pretrained('bert-base-uncased') # Download configuration from S3 and cache.
- model = AutoModelForSequenceClassification.from_config(config) # E.g. model was saved using `save_pretrained('./test/saved_model/')`
- """
- for config_class, model_class in TF_MODEL_FOR_SEQUENCE_CLASSIFICATION_MAPPING.items():
- if isinstance(config, config_class):
- return model_class(config)
- raise ValueError(
- "Unrecognized configuration class {} for this kind of TFAutoModel: {}.\n"
- "Model type should be one of {}.".format(
- config.__class__,
- cls.__name__,
- ", ".join(c.__name__ for c in TF_MODEL_FOR_SEQUENCE_CLASSIFICATION_MAPPING.keys()),
- )
- )
-
- @classmethod
- def from_pretrained(cls, pretrained_model_name_or_path, *model_args, **kwargs):
- r""" Instantiates one of the sequence classification model classes of the library
- from a pre-trained model configuration.
-
- The `from_pretrained()` method takes care of returning the correct model class instance
- based on the `model_type` property of the config object, or when it's missing,
- falling back to using pattern matching on the `pretrained_model_name_or_path` string.
-
- The model class to instantiate is selected as the first pattern matching
- in the `pretrained_model_name_or_path` string (in the following order):
- - contains `distilbert`: TFDistilBertForSequenceClassification (DistilBERT model)
- - contains `roberta`: TFRobertaForSequenceClassification (RoBERTa model)
- - contains `bert`: TFBertForSequenceClassification (Bert model)
- - contains `xlnet`: TFXLNetForSequenceClassification (XLNet model)
- - contains `xlm`: TFXLMForSequenceClassification (XLM model)
-
- The model is set in evaluation mode by default using `model.eval()` (Dropout modules are deactivated)
- To train the model, you should first set it back in training mode with `model.train()`
-
- Params:
- pretrained_model_name_or_path: either:
-
- - a string with the `shortcut name` of a pre-trained model to load from cache or download, e.g.: ``bert-base-uncased``.
- - a string with the `identifier name` of a pre-trained model that was user-uploaded to our S3, e.g.: ``dbmdz/bert-base-german-cased``.
- - a path to a `directory` containing model weights saved using :func:`~transformers.PreTrainedModel.save_pretrained`, e.g.: ``./my_model_directory/``.
- - a path or url to a `PyTorch, TF 1.X or TF 2.0 checkpoint file` (e.g. `./tf_model/model.ckpt.index`). In the case of a PyTorch checkpoint, ``from_pt`` should be set to True and a configuration object should be provided as ``config`` argument.
-
- from_pt: (`Optional`) Boolean
- Set to True if the Checkpoint is a PyTorch checkpoint.
-
- model_args: (`optional`) Sequence of positional arguments:
- All remaning positional arguments will be passed to the underlying model's ``__init__`` method
-
- config: (`optional`) instance of a class derived from :class:`~transformers.PretrainedConfig`:
- Configuration for the model to use instead of an automatically loaded configuation. Configuration can be automatically loaded when:
-
- - the model is a model provided by the library (loaded with the ``shortcut-name`` string of a pretrained model), or
- - the model was saved using :func:`~transformers.PreTrainedModel.save_pretrained` and is reloaded by suppling the save directory.
- - the model is loaded by suppling a local directory as ``pretrained_model_name_or_path`` and a configuration JSON file named `config.json` is found in the directory.
-
- state_dict: (`optional`) dict:
- an optional state dictionnary for the model to use instead of a state dictionary loaded from saved weights file.
- This option can be used if you want to create a model from a pretrained configuration but load your own weights.
- In this case though, you should check if using :func:`~transformers.PreTrainedModel.save_pretrained` and :func:`~transformers.PreTrainedModel.from_pretrained` is not a simpler option.
-
- cache_dir: (`optional`) string:
- Path to a directory in which a downloaded pre-trained model
- configuration should be cached if the standard cache should not be used.
-
- force_download: (`optional`) boolean, default False:
- Force to (re-)download the model weights and configuration files and override the cached versions if they exists.
-
- resume_download: (`optional`) boolean, default False:
- Do not delete incompletely recieved file. Attempt to resume the download if such a file exists.
-
- proxies: (`optional`) dict, default None:
- A dictionary of proxy servers to use by protocol or endpoint, e.g.: {'http': 'foo.bar:3128', 'http://hostname': 'foo.bar:4012'}.
- The proxies are used on each request.
-
- output_loading_info: (`optional`) boolean:
- Set to ``True`` to also return a dictionnary containing missing keys, unexpected keys and error messages.
-
- kwargs: (`optional`) Remaining dictionary of keyword arguments:
- Can be used to update the configuration object (after it being loaded) and initiate the model. (e.g. ``output_attention=True``). Behave differently depending on whether a `config` is provided or automatically loaded:
-
- - If a configuration is provided with ``config``, ``**kwargs`` will be directly passed to the underlying model's ``__init__`` method (we assume all relevant updates to the configuration have already been done)
- - If a configuration is not provided, ``kwargs`` will be first passed to the configuration class initialization function (:func:`~transformers.PretrainedConfig.from_pretrained`). Each key of ``kwargs`` that corresponds to a configuration attribute will be used to override said attribute with the supplied ``kwargs`` value. Remaining keys that do not correspond to any configuration attribute will be passed to the underlying model's ``__init__`` function.
-
- Examples::
-
- model = TFAutoModelForSequenceClassification.from_pretrained('bert-base-uncased') # Download model and configuration from S3 and cache.
- model = TFAutoModelForSequenceClassification.from_pretrained('./test/bert_model/') # E.g. model was saved using `save_pretrained('./test/saved_model/')`
- model = TFAutoModelForSequenceClassification.from_pretrained('bert-base-uncased', output_attention=True) # Update configuration during loading
- assert model.config.output_attention == True
- # Loading from a TF checkpoint file instead of a PyTorch model (slower)
- config = AutoConfig.from_json_file('./tf_model/bert_tf_model_config.json')
- model = TFAutoModelForSequenceClassification.from_pretrained('./pt_model/bert_pytorch_model.bin', from_pt=True, config=config)
-
- """
- config = kwargs.pop("config", None)
- if not isinstance(config, PretrainedConfig):
- config = AutoConfig.from_pretrained(pretrained_model_name_or_path, **kwargs)
-
- for config_class, model_class in TF_MODEL_FOR_SEQUENCE_CLASSIFICATION_MAPPING.items():
- if isinstance(config, config_class):
- return model_class.from_pretrained(pretrained_model_name_or_path, *model_args, config=config, **kwargs)
- raise ValueError(
- "Unrecognized configuration class {} for this kind of TFAutoModel: {}.\n"
- "Model type should be one of {}.".format(
- config.__class__,
- cls.__name__,
- ", ".join(c.__name__ for c in TF_MODEL_FOR_SEQUENCE_CLASSIFICATION_MAPPING.keys()),
- )
- )
-
-
-class TFAutoModelForQuestionAnswering(object):
- r"""
- :class:`~transformers.TFAutoModelForQuestionAnswering` is a generic model class
- that will be instantiated as one of the question answering model classes of the library
- when created with the `TFAutoModelForQuestionAnswering.from_pretrained(pretrained_model_name_or_path)`
- class method.
-
- The `from_pretrained()` method takes care of returning the correct model class instance
- based on the `model_type` property of the config object, or when it's missing,
- falling back to using pattern matching on the `pretrained_model_name_or_path` string.
-
- The model class to instantiate is selected as the first pattern matching
- in the `pretrained_model_name_or_path` string (in the following order):
- - contains `distilbert`: TFDistilBertForQuestionAnswering (DistilBERT model)
- - contains `bert`: TFBertForQuestionAnswering (Bert model)
- - contains `xlnet`: TFXLNetForQuestionAnswering (XLNet model)
- - contains `xlm`: TFXLMForQuestionAnswering (XLM model)
-
- This class cannot be instantiated using `__init__()` (throws an error).
- """
-
- def __init__(self):
- raise EnvironmentError(
- "TFAutoModelForQuestionAnswering is designed to be instantiated "
- "using the `TFAutoModelForQuestionAnswering.from_pretrained(pretrained_model_name_or_path)` or "
- "`TFAutoModelForQuestionAnswering.from_config(config)` methods."
- )
-
- @classmethod
- def from_config(cls, config):
- r""" Instantiates one of the base model classes of the library
- from a configuration.
-
- config: (`optional`) instance of a class derived from :class:`~transformers.PretrainedConfig`:
- The model class to instantiate is selected based on the configuration class:
- - isInstance of `distilbert` configuration class: DistilBertModel (DistilBERT model)
- - isInstance of `bert` configuration class: BertModel (Bert model)
- - isInstance of `xlnet` configuration class: XLNetModel (XLNet model)
- - isInstance of `xlm` configuration class: XLMModel (XLM model)
-
- Examples::
-
- config = BertConfig.from_pretrained('bert-base-uncased') # Download configuration from S3 and cache.
- model = AutoModelForSequenceClassification.from_config(config) # E.g. model was saved using `save_pretrained('./test/saved_model/')`
- """
- for config_class, model_class in TF_MODEL_FOR_QUESTION_ANSWERING_MAPPING.items():
- if isinstance(config, config_class):
- return model_class(config)
- raise ValueError(
- "Unrecognized configuration class {} for this kind of TFAutoModel: {}.\n"
- "Model type should be one of {}.".format(
- config.__class__,
- cls.__name__,
- ", ".join(c.__name__ for c in TF_MODEL_FOR_QUESTION_ANSWERING_MAPPING.keys()),
- )
- )
-
- @classmethod
- def from_pretrained(cls, pretrained_model_name_or_path, *model_args, **kwargs):
- r""" Instantiates one of the question answering model classes of the library
- from a pre-trained model configuration.
-
- The `from_pretrained()` method takes care of returning the correct model class instance
- based on the `model_type` property of the config object, or when it's missing,
- falling back to using pattern matching on the `pretrained_model_name_or_path` string.
-
- The model class to instantiate is selected as the first pattern matching
- in the `pretrained_model_name_or_path` string (in the following order):
- - contains `distilbert`: TFDistilBertForQuestionAnswering (DistilBERT model)
- - contains `bert`: TFBertForQuestionAnswering (Bert model)
- - contains `xlnet`: TFXLNetForQuestionAnswering (XLNet model)
- - contains `xlm`: TFXLMForQuestionAnswering (XLM model)
-
- The model is set in evaluation mode by default using `model.eval()` (Dropout modules are deactivated)
- To train the model, you should first set it back in training mode with `model.train()`
-
- Params:
- pretrained_model_name_or_path: either:
-
- - a string with the `shortcut name` of a pre-trained model to load from cache or download, e.g.: ``bert-base-uncased``.
- - a string with the `identifier name` of a pre-trained model that was user-uploaded to our S3, e.g.: ``dbmdz/bert-base-german-cased``.
- - a path to a `directory` containing model weights saved using :func:`~transformers.PreTrainedModel.save_pretrained`, e.g.: ``./my_model_directory/``.
- - a path or url to a `PyTorch, TF 1.X or TF 2.0 checkpoint file` (e.g. `./tf_model/model.ckpt.index`). In the case of a PyTorch checkpoint, ``from_pt`` should be set to True and a configuration object should be provided as ``config`` argument.
-
- from_pt: (`Optional`) Boolean
- Set to True if the Checkpoint is a PyTorch checkpoint.
-
- model_args: (`optional`) Sequence of positional arguments:
- All remaning positional arguments will be passed to the underlying model's ``__init__`` method
-
- config: (`optional`) instance of a class derived from :class:`~transformers.PretrainedConfig`:
- Configuration for the model to use instead of an automatically loaded configuation. Configuration can be automatically loaded when:
-
- - the model is a model provided by the library (loaded with the ``shortcut-name`` string of a pretrained model), or
- - the model was saved using :func:`~transformers.PreTrainedModel.save_pretrained` and is reloaded by suppling the save directory.
- - the model is loaded by suppling a local directory as ``pretrained_model_name_or_path`` and a configuration JSON file named `config.json` is found in the directory.
-
- state_dict: (`optional`) dict:
- an optional state dictionnary for the model to use instead of a state dictionary loaded from saved weights file.
- This option can be used if you want to create a model from a pretrained configuration but load your own weights.
- In this case though, you should check if using :func:`~transformers.PreTrainedModel.save_pretrained` and :func:`~transformers.PreTrainedModel.from_pretrained` is not a simpler option.
-
- cache_dir: (`optional`) string:
- Path to a directory in which a downloaded pre-trained model
- configuration should be cached if the standard cache should not be used.
-
- force_download: (`optional`) boolean, default False:
- Force to (re-)download the model weights and configuration files and override the cached versions if they exists.
-
- resume_download: (`optional`) boolean, default False:
- Do not delete incompletely recieved file. Attempt to resume the download if such a file exists.
-
- proxies: (`optional`) dict, default None:
- A dictionary of proxy servers to use by protocol or endpoint, e.g.: {'http': 'foo.bar:3128', 'http://hostname': 'foo.bar:4012'}.
- The proxies are used on each request.
-
- output_loading_info: (`optional`) boolean:
- Set to ``True`` to also return a dictionnary containing missing keys, unexpected keys and error messages.
-
- kwargs: (`optional`) Remaining dictionary of keyword arguments:
- Can be used to update the configuration object (after it being loaded) and initiate the model. (e.g. ``output_attention=True``). Behave differently depending on whether a `config` is provided or automatically loaded:
-
- - If a configuration is provided with ``config``, ``**kwargs`` will be directly passed to the underlying model's ``__init__`` method (we assume all relevant updates to the configuration have already been done)
- - If a configuration is not provided, ``kwargs`` will be first passed to the configuration class initialization function (:func:`~transformers.PretrainedConfig.from_pretrained`). Each key of ``kwargs`` that corresponds to a configuration attribute will be used to override said attribute with the supplied ``kwargs`` value. Remaining keys that do not correspond to any configuration attribute will be passed to the underlying model's ``__init__`` function.
-
- Examples::
-
- model = TFAutoModelForQuestionAnswering.from_pretrained('bert-base-uncased') # Download model and configuration from S3 and cache.
- model = TFAutoModelForQuestionAnswering.from_pretrained('./test/bert_model/') # E.g. model was saved using `save_pretrained('./test/saved_model/')`
- model = TFAutoModelForQuestionAnswering.from_pretrained('bert-base-uncased', output_attention=True) # Update configuration during loading
- assert model.config.output_attention == True
- # Loading from a TF checkpoint file instead of a PyTorch model (slower)
- config = AutoConfig.from_json_file('./tf_model/bert_tf_model_config.json')
- model = TFAutoModelForQuestionAnswering.from_pretrained('./pt_model/bert_pytorch_model.bin', from_pt=True, config=config)
-
- """
- config = kwargs.pop("config", None)
- if not isinstance(config, PretrainedConfig):
- config = AutoConfig.from_pretrained(pretrained_model_name_or_path, **kwargs)
-
- for config_class, model_class in TF_MODEL_FOR_QUESTION_ANSWERING_MAPPING.items():
- if isinstance(config, config_class):
- return model_class.from_pretrained(pretrained_model_name_or_path, *model_args, config=config, **kwargs)
- raise ValueError(
- "Unrecognized configuration class {} for this kind of TFAutoModel: {}.\n"
- "Model type should be one of {}.".format(
- config.__class__,
- cls.__name__,
- ", ".join(c.__name__ for c in TF_MODEL_FOR_QUESTION_ANSWERING_MAPPING.keys()),
- )
- )
-
-
-class TFAutoModelForTokenClassification:
- def __init__(self):
- raise EnvironmentError(
- "TFAutoModelForTokenClassification is designed to be instantiated "
- "using the `TFAutoModelForTokenClassification.from_pretrained(pretrained_model_name_or_path)` or "
- "`AutoModelForTokenClassification.from_config(config)` methods."
- )
-
- @classmethod
- def from_config(cls, config):
- r""" Instantiates one of the base model classes of the library
- from a configuration.
-
- config: (`optional`) instance of a class derived from :class:`~transformers.PretrainedConfig`:
- The model class to instantiate is selected based on the configuration class:
- - isInstance of `bert` configuration class: BertModel (Bert model)
- - isInstance of `xlnet` configuration class: XLNetModel (XLNet model)
- - isInstance of `distilbert` configuration class: DistilBertModel (DistilBert model)
- - isInstance of `roberta` configuration class: RobteraModel (Roberta model)
-
- Examples::
-
- config = BertConfig.from_pretrained('bert-base-uncased') # Download configuration from S3 and cache.
- model = TFAutoModelForTokenClassification.from_config(config) # E.g. model was saved using `save_pretrained('./test/saved_model/')`
- """
- for config_class, model_class in TF_MODEL_FOR_TOKEN_CLASSIFICATION_MAPPING.items():
- if isinstance(config, config_class):
- return model_class(config)
- raise ValueError(
- "Unrecognized configuration class {} for this kind of TFAutoModel: {}.\n"
- "Model type should be one of {}.".format(
- config.__class__,
- cls.__name__,
- ", ".join(c.__name__ for c in TF_MODEL_FOR_TOKEN_CLASSIFICATION_MAPPING.keys()),
- )
- )
-
- @classmethod
- def from_pretrained(cls, pretrained_model_name_or_path, *model_args, **kwargs):
- r""" Instantiates one of the question answering model classes of the library
- from a pre-trained model configuration.
-
- The `from_pretrained()` method takes care of returning the correct model class instance
- based on the `model_type` property of the config object, or when it's missing,
- falling back to using pattern matching on the `pretrained_model_name_or_path` string.
-
- The model class to instantiate is selected as the first pattern matching
- in the `pretrained_model_name_or_path` string (in the following order):
- - contains `bert`: BertForTokenClassification (Bert model)
- - contains `xlnet`: XLNetForTokenClassification (XLNet model)
- - contains `distilbert`: DistilBertForTokenClassification (DistilBert model)
- - contains `roberta`: RobertaForTokenClassification (Roberta model)
-
- The model is set in evaluation mode by default using `model.eval()` (Dropout modules are deactivated)
- To train the model, you should first set it back in training mode with `model.train()`
-
- Params:
- pretrained_model_name_or_path: either:
-
- - a string with the `shortcut name` of a pre-trained model to load from cache or download, e.g.: ``bert-base-uncased``.
- - a path to a `directory` containing model weights saved using :func:`~transformers.PreTrainedModel.save_pretrained`, e.g.: ``./my_model_directory/``.
- - a path or url to a `tensorflow index checkpoint file` (e.g. `./tf_model/model.ckpt.index`). In this case, ``from_tf`` should be set to True and a configuration object should be provided as ``config`` argument. This loading path is slower than converting the TensorFlow checkpoint in a PyTorch model using the provided conversion scripts and loading the PyTorch model afterwards.
-
- model_args: (`optional`) Sequence of positional arguments:
- All remaning positional arguments will be passed to the underlying model's ``__init__`` method
-
- config: (`optional`) instance of a class derived from :class:`~transformers.PretrainedConfig`:
- Configuration for the model to use instead of an automatically loaded configuation. Configuration can be automatically loaded when:
-
- - the model is a model provided by the library (loaded with the ``shortcut-name`` string of a pretrained model), or
- - the model was saved using :func:`~transformers.PreTrainedModel.save_pretrained` and is reloaded by suppling the save directory.
- - the model is loaded by suppling a local directory as ``pretrained_model_name_or_path`` and a configuration JSON file named `config.json` is found in the directory.
-
- state_dict: (`optional`) dict:
- an optional state dictionnary for the model to use instead of a state dictionary loaded from saved weights file.
- This option can be used if you want to create a model from a pretrained configuration but load your own weights.
- In this case though, you should check if using :func:`~transformers.PreTrainedModel.save_pretrained` and :func:`~transformers.PreTrainedModel.from_pretrained` is not a simpler option.
-
- cache_dir: (`optional`) string:
- Path to a directory in which a downloaded pre-trained model
- configuration should be cached if the standard cache should not be used.
-
- force_download: (`optional`) boolean, default False:
- Force to (re-)download the model weights and configuration files and override the cached versions if they exists.
-
- proxies: (`optional`) dict, default None:
- A dictionary of proxy servers to use by protocol or endpoint, e.g.: {'http': 'foo.bar:3128', 'http://hostname': 'foo.bar:4012'}.
- The proxies are used on each request.
-
- output_loading_info: (`optional`) boolean:
- Set to ``True`` to also return a dictionnary containing missing keys, unexpected keys and error messages.
-
- kwargs: (`optional`) Remaining dictionary of keyword arguments:
- Can be used to update the configuration object (after it being loaded) and initiate the model. (e.g. ``output_attention=True``). Behave differently depending on whether a `config` is provided or automatically loaded:
-
- - If a configuration is provided with ``config``, ``**kwargs`` will be directly passed to the underlying model's ``__init__`` method (we assume all relevant updates to the configuration have already been done)
- - If a configuration is not provided, ``kwargs`` will be first passed to the configuration class initialization function (:func:`~transformers.PretrainedConfig.from_pretrained`). Each key of ``kwargs`` that corresponds to a configuration attribute will be used to override said attribute with the supplied ``kwargs`` value. Remaining keys that do not correspond to any configuration attribute will be passed to the underlying model's ``__init__`` function.
-
- Examples::
-
- model = TFAutoModelForTokenClassification.from_pretrained('bert-base-uncased') # Download model and configuration from S3 and cache.
- model = TFAutoModelForTokenClassification.from_pretrained('./test/bert_model/') # E.g. model was saved using `save_pretrained('./test/saved_model/')`
- model = TFAutoModelForTokenClassification.from_pretrained('bert-base-uncased', output_attention=True) # Update configuration during loading
- assert model.config.output_attention == True
- # Loading from a TF checkpoint file instead of a PyTorch model (slower)
- config = AutoConfig.from_json_file('./tf_model/bert_tf_model_config.json')
- model = TFAutoModelForTokenClassification.from_pretrained('./tf_model/bert_tf_checkpoint.ckpt.index', from_tf=True, config=config)
-
- """
- config = kwargs.pop("config", None)
- if not isinstance(config, PretrainedConfig):
- config = AutoConfig.from_pretrained(pretrained_model_name_or_path, **kwargs)
-
- for config_class, model_class in TF_MODEL_FOR_TOKEN_CLASSIFICATION_MAPPING.items():
- if isinstance(config, config_class):
- return model_class.from_pretrained(pretrained_model_name_or_path, *model_args, config=config, **kwargs)
- raise ValueError(
- "Unrecognized configuration class {} for this kind of TFAutoModel: {}.\n"
- "Model type should be one of {}.".format(
- config.__class__,
- cls.__name__,
- ", ".join(c.__name__ for c in TF_MODEL_FOR_TOKEN_CLASSIFICATION_MAPPING.keys()),
- )
- )
diff --git a/server/transformers/src/transformers/modeling_tf_bert.py b/server/transformers/src/transformers/modeling_tf_bert.py
deleted file mode 100644
index 01bc1c2be73afbf5cf44cd94c75db33c957d69f5..0000000000000000000000000000000000000000
--- a/server/transformers/src/transformers/modeling_tf_bert.py
+++ /dev/null
@@ -1,1163 +0,0 @@
-# coding=utf-8
-# Copyright 2018 The Google AI Language Team Authors and The HuggingFace Inc. team.
-# Copyright (c) 2018, NVIDIA CORPORATION. All rights reserved.
-#
-# Licensed under the Apache License, Version 2.0 (the "License");
-# you may not use this file except in compliance with the License.
-# You may obtain a copy of the License at
-#
-# http://www.apache.org/licenses/LICENSE-2.0
-#
-# Unless required by applicable law or agreed to in writing, software
-# distributed under the License is distributed on an "AS IS" BASIS,
-# WITHOUT WARRANTIES OR CONDITIONS OF ANY KIND, either express or implied.
-# See the License for the specific language governing permissions and
-# limitations under the License.
-""" TF 2.0 BERT model. """
-
-
-import logging
-
-import numpy as np
-import tensorflow as tf
-
-from .configuration_bert import BertConfig
-from .file_utils import MULTIPLE_CHOICE_DUMMY_INPUTS, add_start_docstrings, add_start_docstrings_to_callable
-from .modeling_tf_utils import TFPreTrainedModel, get_initializer, shape_list
-
-
-logger = logging.getLogger(__name__)
-
-
-TF_BERT_PRETRAINED_MODEL_ARCHIVE_MAP = {
- "bert-base-uncased": "https://s3.amazonaws.com/models.huggingface.co/bert/bert-base-uncased-tf_model.h5",
- "bert-large-uncased": "https://s3.amazonaws.com/models.huggingface.co/bert/bert-large-uncased-tf_model.h5",
- "bert-base-cased": "https://s3.amazonaws.com/models.huggingface.co/bert/bert-base-cased-tf_model.h5",
- "bert-large-cased": "https://s3.amazonaws.com/models.huggingface.co/bert/bert-large-cased-tf_model.h5",
- "bert-base-multilingual-uncased": "https://s3.amazonaws.com/models.huggingface.co/bert/bert-base-multilingual-uncased-tf_model.h5",
- "bert-base-multilingual-cased": "https://s3.amazonaws.com/models.huggingface.co/bert/bert-base-multilingual-cased-tf_model.h5",
- "bert-base-chinese": "https://s3.amazonaws.com/models.huggingface.co/bert/bert-base-chinese-tf_model.h5",
- "bert-base-german-cased": "https://s3.amazonaws.com/models.huggingface.co/bert/bert-base-german-cased-tf_model.h5",
- "bert-large-uncased-whole-word-masking": "https://s3.amazonaws.com/models.huggingface.co/bert/bert-large-uncased-whole-word-masking-tf_model.h5",
- "bert-large-cased-whole-word-masking": "https://s3.amazonaws.com/models.huggingface.co/bert/bert-large-cased-whole-word-masking-tf_model.h5",
- "bert-large-uncased-whole-word-masking-finetuned-squad": "https://s3.amazonaws.com/models.huggingface.co/bert/bert-large-uncased-whole-word-masking-finetuned-squad-tf_model.h5",
- "bert-large-cased-whole-word-masking-finetuned-squad": "https://s3.amazonaws.com/models.huggingface.co/bert/bert-large-cased-whole-word-masking-finetuned-squad-tf_model.h5",
- "bert-base-cased-finetuned-mrpc": "https://s3.amazonaws.com/models.huggingface.co/bert/bert-base-cased-finetuned-mrpc-tf_model.h5",
- "bert-base-japanese": "https://s3.amazonaws.com/models.huggingface.co/bert/cl-tohoku/bert-base-japanese-tf_model.h5",
- "bert-base-japanese-whole-word-masking": "https://s3.amazonaws.com/models.huggingface.co/bert/cl-tohoku/bert-base-japanese-whole-word-masking-tf_model.h5",
- "bert-base-japanese-char": "https://s3.amazonaws.com/models.huggingface.co/bert/cl-tohoku/bert-base-japanese-char-tf_model.h5",
- "bert-base-japanese-char-whole-word-masking": "https://s3.amazonaws.com/models.huggingface.co/bert/cl-tohoku/bert-base-japanese-char-whole-word-masking-tf_model.h5",
- "bert-base-finnish-cased-v1": "https://s3.amazonaws.com/models.huggingface.co/bert/TurkuNLP/bert-base-finnish-cased-v1/tf_model.h5",
- "bert-base-finnish-uncased-v1": "https://s3.amazonaws.com/models.huggingface.co/bert/TurkuNLP/bert-base-finnish-uncased-v1/tf_model.h5",
- "bert-base-dutch-cased": "https://s3.amazonaws.com/models.huggingface.co/bert/wietsedv/bert-base-dutch-cased/tf_model.h5",
-}
-
-
-def gelu(x):
- """ Gaussian Error Linear Unit.
- Original Implementation of the gelu activation function in Google Bert repo when initially created.
- For information: OpenAI GPT's gelu is slightly different (and gives slightly different results):
- 0.5 * x * (1 + torch.tanh(math.sqrt(2 / math.pi) * (x + 0.044715 * torch.pow(x, 3))))
- Also see https://arxiv.org/abs/1606.08415
- """
- cdf = 0.5 * (1.0 + tf.math.erf(x / tf.math.sqrt(2.0)))
- return x * cdf
-
-
-def gelu_new(x):
- """Gaussian Error Linear Unit.
- This is a smoother version of the RELU.
- Original paper: https://arxiv.org/abs/1606.08415
- Args:
- x: float Tensor to perform activation.
- Returns:
- `x` with the GELU activation applied.
- """
- cdf = 0.5 * (1.0 + tf.tanh((np.sqrt(2 / np.pi) * (x + 0.044715 * tf.pow(x, 3)))))
- return x * cdf
-
-
-def swish(x):
- return x * tf.sigmoid(x)
-
-
-ACT2FN = {
- "gelu": tf.keras.layers.Activation(gelu),
- "relu": tf.keras.activations.relu,
- "swish": tf.keras.layers.Activation(swish),
- "gelu_new": tf.keras.layers.Activation(gelu_new),
-}
-
-
-class TFBertEmbeddings(tf.keras.layers.Layer):
- """Construct the embeddings from word, position and token_type embeddings.
- """
-
- def __init__(self, config, **kwargs):
- super().__init__(**kwargs)
- self.vocab_size = config.vocab_size
- self.hidden_size = config.hidden_size
- self.initializer_range = config.initializer_range
-
- self.position_embeddings = tf.keras.layers.Embedding(
- config.max_position_embeddings,
- config.hidden_size,
- embeddings_initializer=get_initializer(self.initializer_range),
- name="position_embeddings",
- )
- self.token_type_embeddings = tf.keras.layers.Embedding(
- config.type_vocab_size,
- config.hidden_size,
- embeddings_initializer=get_initializer(self.initializer_range),
- name="token_type_embeddings",
- )
-
- # self.LayerNorm is not snake-cased to stick with TensorFlow model variable name and be able to load
- # any TensorFlow checkpoint file
- self.LayerNorm = tf.keras.layers.LayerNormalization(epsilon=config.layer_norm_eps, name="LayerNorm")
- self.dropout = tf.keras.layers.Dropout(config.hidden_dropout_prob)
-
- def build(self, input_shape):
- """Build shared word embedding layer """
- with tf.name_scope("word_embeddings"):
- # Create and initialize weights. The random normal initializer was chosen
- # arbitrarily, and works well.
- self.word_embeddings = self.add_weight(
- "weight",
- shape=[self.vocab_size, self.hidden_size],
- initializer=get_initializer(self.initializer_range),
- )
- super().build(input_shape)
-
- def call(self, inputs, mode="embedding", training=False):
- """Get token embeddings of inputs.
- Args:
- inputs: list of three int64 tensors with shape [batch_size, length]: (input_ids, position_ids, token_type_ids)
- mode: string, a valid value is one of "embedding" and "linear".
- Returns:
- outputs: (1) If mode == "embedding", output embedding tensor, float32 with
- shape [batch_size, length, embedding_size]; (2) mode == "linear", output
- linear tensor, float32 with shape [batch_size, length, vocab_size].
- Raises:
- ValueError: if mode is not valid.
-
- Shared weights logic adapted from
- https://github.com/tensorflow/models/blob/a009f4fb9d2fc4949e32192a944688925ef78659/official/transformer/v2/embedding_layer.py#L24
- """
- if mode == "embedding":
- return self._embedding(inputs, training=training)
- elif mode == "linear":
- return self._linear(inputs)
- else:
- raise ValueError("mode {} is not valid.".format(mode))
-
- def _embedding(self, inputs, training=False):
- """Applies embedding based on inputs tensor."""
- input_ids, position_ids, token_type_ids, inputs_embeds = inputs
-
- if input_ids is not None:
- input_shape = shape_list(input_ids)
- else:
- input_shape = shape_list(inputs_embeds)[:-1]
-
- seq_length = input_shape[1]
- if position_ids is None:
- position_ids = tf.range(seq_length, dtype=tf.int32)[tf.newaxis, :]
- if token_type_ids is None:
- token_type_ids = tf.fill(input_shape, 0)
-
- if inputs_embeds is None:
- inputs_embeds = tf.gather(self.word_embeddings, input_ids)
- position_embeddings = self.position_embeddings(position_ids)
- token_type_embeddings = self.token_type_embeddings(token_type_ids)
-
- embeddings = inputs_embeds + position_embeddings + token_type_embeddings
- embeddings = self.LayerNorm(embeddings)
- embeddings = self.dropout(embeddings, training=training)
- return embeddings
-
- def _linear(self, inputs):
- """Computes logits by running inputs through a linear layer.
- Args:
- inputs: A float32 tensor with shape [batch_size, length, hidden_size]
- Returns:
- float32 tensor with shape [batch_size, length, vocab_size].
- """
- batch_size = shape_list(inputs)[0]
- length = shape_list(inputs)[1]
-
- x = tf.reshape(inputs, [-1, self.hidden_size])
- logits = tf.matmul(x, self.word_embeddings, transpose_b=True)
-
- return tf.reshape(logits, [batch_size, length, self.vocab_size])
-
-
-class TFBertSelfAttention(tf.keras.layers.Layer):
- def __init__(self, config, **kwargs):
- super().__init__(**kwargs)
- if config.hidden_size % config.num_attention_heads != 0:
- raise ValueError(
- "The hidden size (%d) is not a multiple of the number of attention "
- "heads (%d)" % (config.hidden_size, config.num_attention_heads)
- )
- self.output_attentions = config.output_attentions
-
- self.num_attention_heads = config.num_attention_heads
- assert config.hidden_size % config.num_attention_heads == 0
- self.attention_head_size = int(config.hidden_size / config.num_attention_heads)
- self.all_head_size = self.num_attention_heads * self.attention_head_size
-
- self.query = tf.keras.layers.Dense(
- self.all_head_size, kernel_initializer=get_initializer(config.initializer_range), name="query"
- )
- self.key = tf.keras.layers.Dense(
- self.all_head_size, kernel_initializer=get_initializer(config.initializer_range), name="key"
- )
- self.value = tf.keras.layers.Dense(
- self.all_head_size, kernel_initializer=get_initializer(config.initializer_range), name="value"
- )
-
- self.dropout = tf.keras.layers.Dropout(config.attention_probs_dropout_prob)
-
- def transpose_for_scores(self, x, batch_size):
- x = tf.reshape(x, (batch_size, -1, self.num_attention_heads, self.attention_head_size))
- return tf.transpose(x, perm=[0, 2, 1, 3])
-
- def call(self, inputs, training=False):
- hidden_states, attention_mask, head_mask = inputs
-
- batch_size = shape_list(hidden_states)[0]
- mixed_query_layer = self.query(hidden_states)
- mixed_key_layer = self.key(hidden_states)
- mixed_value_layer = self.value(hidden_states)
-
- query_layer = self.transpose_for_scores(mixed_query_layer, batch_size)
- key_layer = self.transpose_for_scores(mixed_key_layer, batch_size)
- value_layer = self.transpose_for_scores(mixed_value_layer, batch_size)
-
- # Take the dot product between "query" and "key" to get the raw attention scores.
- attention_scores = tf.matmul(
- query_layer, key_layer, transpose_b=True
- ) # (batch size, num_heads, seq_len_q, seq_len_k)
- dk = tf.cast(shape_list(key_layer)[-1], tf.float32) # scale attention_scores
- attention_scores = attention_scores / tf.math.sqrt(dk)
-
- if attention_mask is not None:
- # Apply the attention mask is (precomputed for all layers in TFBertModel call() function)
- attention_scores = attention_scores + attention_mask
-
- # Normalize the attention scores to probabilities.
- attention_probs = tf.nn.softmax(attention_scores, axis=-1)
-
- # This is actually dropping out entire tokens to attend to, which might
- # seem a bit unusual, but is taken from the original Transformer paper.
- attention_probs = self.dropout(attention_probs, training=training)
-
- # Mask heads if we want to
- if head_mask is not None:
- attention_probs = attention_probs * head_mask
-
- context_layer = tf.matmul(attention_probs, value_layer)
-
- context_layer = tf.transpose(context_layer, perm=[0, 2, 1, 3])
- context_layer = tf.reshape(
- context_layer, (batch_size, -1, self.all_head_size)
- ) # (batch_size, seq_len_q, all_head_size)
-
- outputs = (context_layer, attention_probs) if self.output_attentions else (context_layer,)
- return outputs
-
-
-class TFBertSelfOutput(tf.keras.layers.Layer):
- def __init__(self, config, **kwargs):
- super().__init__(**kwargs)
- self.dense = tf.keras.layers.Dense(
- config.hidden_size, kernel_initializer=get_initializer(config.initializer_range), name="dense"
- )
- self.LayerNorm = tf.keras.layers.LayerNormalization(epsilon=config.layer_norm_eps, name="LayerNorm")
- self.dropout = tf.keras.layers.Dropout(config.hidden_dropout_prob)
-
- def call(self, inputs, training=False):
- hidden_states, input_tensor = inputs
-
- hidden_states = self.dense(hidden_states)
- hidden_states = self.dropout(hidden_states, training=training)
- hidden_states = self.LayerNorm(hidden_states + input_tensor)
- return hidden_states
-
-
-class TFBertAttention(tf.keras.layers.Layer):
- def __init__(self, config, **kwargs):
- super().__init__(**kwargs)
- self.self_attention = TFBertSelfAttention(config, name="self")
- self.dense_output = TFBertSelfOutput(config, name="output")
-
- def prune_heads(self, heads):
- raise NotImplementedError
-
- def call(self, inputs, training=False):
- input_tensor, attention_mask, head_mask = inputs
-
- self_outputs = self.self_attention([input_tensor, attention_mask, head_mask], training=training)
- attention_output = self.dense_output([self_outputs[0], input_tensor], training=training)
- outputs = (attention_output,) + self_outputs[1:] # add attentions if we output them
- return outputs
-
-
-class TFBertIntermediate(tf.keras.layers.Layer):
- def __init__(self, config, **kwargs):
- super().__init__(**kwargs)
- self.dense = tf.keras.layers.Dense(
- config.intermediate_size, kernel_initializer=get_initializer(config.initializer_range), name="dense"
- )
- if isinstance(config.hidden_act, str):
- self.intermediate_act_fn = ACT2FN[config.hidden_act]
- else:
- self.intermediate_act_fn = config.hidden_act
-
- def call(self, hidden_states):
- hidden_states = self.dense(hidden_states)
- hidden_states = self.intermediate_act_fn(hidden_states)
- return hidden_states
-
-
-class TFBertOutput(tf.keras.layers.Layer):
- def __init__(self, config, **kwargs):
- super().__init__(**kwargs)
- self.dense = tf.keras.layers.Dense(
- config.hidden_size, kernel_initializer=get_initializer(config.initializer_range), name="dense"
- )
- self.LayerNorm = tf.keras.layers.LayerNormalization(epsilon=config.layer_norm_eps, name="LayerNorm")
- self.dropout = tf.keras.layers.Dropout(config.hidden_dropout_prob)
-
- def call(self, inputs, training=False):
- hidden_states, input_tensor = inputs
-
- hidden_states = self.dense(hidden_states)
- hidden_states = self.dropout(hidden_states, training=training)
- hidden_states = self.LayerNorm(hidden_states + input_tensor)
- return hidden_states
-
-
-class TFBertLayer(tf.keras.layers.Layer):
- def __init__(self, config, **kwargs):
- super().__init__(**kwargs)
- self.attention = TFBertAttention(config, name="attention")
- self.intermediate = TFBertIntermediate(config, name="intermediate")
- self.bert_output = TFBertOutput(config, name="output")
-
- def call(self, inputs, training=False):
- hidden_states, attention_mask, head_mask = inputs
-
- attention_outputs = self.attention([hidden_states, attention_mask, head_mask], training=training)
- attention_output = attention_outputs[0]
- intermediate_output = self.intermediate(attention_output)
- layer_output = self.bert_output([intermediate_output, attention_output], training=training)
- outputs = (layer_output,) + attention_outputs[1:] # add attentions if we output them
- return outputs
-
-
-class TFBertEncoder(tf.keras.layers.Layer):
- def __init__(self, config, **kwargs):
- super().__init__(**kwargs)
- self.output_attentions = config.output_attentions
- self.output_hidden_states = config.output_hidden_states
- self.layer = [TFBertLayer(config, name="layer_._{}".format(i)) for i in range(config.num_hidden_layers)]
-
- def call(self, inputs, training=False):
- hidden_states, attention_mask, head_mask = inputs
-
- all_hidden_states = ()
- all_attentions = ()
- for i, layer_module in enumerate(self.layer):
- if self.output_hidden_states:
- all_hidden_states = all_hidden_states + (hidden_states,)
-
- layer_outputs = layer_module([hidden_states, attention_mask, head_mask[i]], training=training)
- hidden_states = layer_outputs[0]
-
- if self.output_attentions:
- all_attentions = all_attentions + (layer_outputs[1],)
-
- # Add last layer
- if self.output_hidden_states:
- all_hidden_states = all_hidden_states + (hidden_states,)
-
- outputs = (hidden_states,)
- if self.output_hidden_states:
- outputs = outputs + (all_hidden_states,)
- if self.output_attentions:
- outputs = outputs + (all_attentions,)
- return outputs # outputs, (hidden states), (attentions)
-
-
-class TFBertPooler(tf.keras.layers.Layer):
- def __init__(self, config, **kwargs):
- super().__init__(**kwargs)
- self.dense = tf.keras.layers.Dense(
- config.hidden_size,
- kernel_initializer=get_initializer(config.initializer_range),
- activation="tanh",
- name="dense",
- )
-
- def call(self, hidden_states):
- # We "pool" the model by simply taking the hidden state corresponding
- # to the first token.
- first_token_tensor = hidden_states[:, 0]
- pooled_output = self.dense(first_token_tensor)
- return pooled_output
-
-
-class TFBertPredictionHeadTransform(tf.keras.layers.Layer):
- def __init__(self, config, **kwargs):
- super().__init__(**kwargs)
- self.dense = tf.keras.layers.Dense(
- config.hidden_size, kernel_initializer=get_initializer(config.initializer_range), name="dense"
- )
- if isinstance(config.hidden_act, str):
- self.transform_act_fn = ACT2FN[config.hidden_act]
- else:
- self.transform_act_fn = config.hidden_act
- self.LayerNorm = tf.keras.layers.LayerNormalization(epsilon=config.layer_norm_eps, name="LayerNorm")
-
- def call(self, hidden_states):
- hidden_states = self.dense(hidden_states)
- hidden_states = self.transform_act_fn(hidden_states)
- hidden_states = self.LayerNorm(hidden_states)
- return hidden_states
-
-
-class TFBertLMPredictionHead(tf.keras.layers.Layer):
- def __init__(self, config, input_embeddings, **kwargs):
- super().__init__(**kwargs)
- self.vocab_size = config.vocab_size
- self.transform = TFBertPredictionHeadTransform(config, name="transform")
-
- # The output weights are the same as the input embeddings, but there is
- # an output-only bias for each token.
- self.input_embeddings = input_embeddings
-
- def build(self, input_shape):
- self.bias = self.add_weight(shape=(self.vocab_size,), initializer="zeros", trainable=True, name="bias")
- super().build(input_shape)
-
- def call(self, hidden_states):
- hidden_states = self.transform(hidden_states)
- hidden_states = self.input_embeddings(hidden_states, mode="linear")
- hidden_states = hidden_states + self.bias
- return hidden_states
-
-
-class TFBertMLMHead(tf.keras.layers.Layer):
- def __init__(self, config, input_embeddings, **kwargs):
- super().__init__(**kwargs)
- self.predictions = TFBertLMPredictionHead(config, input_embeddings, name="predictions")
-
- def call(self, sequence_output):
- prediction_scores = self.predictions(sequence_output)
- return prediction_scores
-
-
-class TFBertNSPHead(tf.keras.layers.Layer):
- def __init__(self, config, **kwargs):
- super().__init__(**kwargs)
- self.seq_relationship = tf.keras.layers.Dense(
- 2, kernel_initializer=get_initializer(config.initializer_range), name="seq_relationship"
- )
-
- def call(self, pooled_output):
- seq_relationship_score = self.seq_relationship(pooled_output)
- return seq_relationship_score
-
-
-class TFBertMainLayer(tf.keras.layers.Layer):
- def __init__(self, config, **kwargs):
- super().__init__(**kwargs)
- self.num_hidden_layers = config.num_hidden_layers
-
- self.embeddings = TFBertEmbeddings(config, name="embeddings")
- self.encoder = TFBertEncoder(config, name="encoder")
- self.pooler = TFBertPooler(config, name="pooler")
-
- def get_input_embeddings(self):
- return self.embeddings
-
- def _resize_token_embeddings(self, new_num_tokens):
- raise NotImplementedError
-
- def _prune_heads(self, heads_to_prune):
- """ Prunes heads of the model.
- heads_to_prune: dict of {layer_num: list of heads to prune in this layer}
- See base class PreTrainedModel
- """
- raise NotImplementedError
-
- def call(
- self,
- inputs,
- attention_mask=None,
- token_type_ids=None,
- position_ids=None,
- head_mask=None,
- inputs_embeds=None,
- training=False,
- ):
- if isinstance(inputs, (tuple, list)):
- input_ids = inputs[0]
- attention_mask = inputs[1] if len(inputs) > 1 else attention_mask
- token_type_ids = inputs[2] if len(inputs) > 2 else token_type_ids
- position_ids = inputs[3] if len(inputs) > 3 else position_ids
- head_mask = inputs[4] if len(inputs) > 4 else head_mask
- inputs_embeds = inputs[5] if len(inputs) > 5 else inputs_embeds
- assert len(inputs) <= 6, "Too many inputs."
- elif isinstance(inputs, dict):
- input_ids = inputs.get("input_ids")
- attention_mask = inputs.get("attention_mask", attention_mask)
- token_type_ids = inputs.get("token_type_ids", token_type_ids)
- position_ids = inputs.get("position_ids", position_ids)
- head_mask = inputs.get("head_mask", head_mask)
- inputs_embeds = inputs.get("inputs_embeds", inputs_embeds)
- assert len(inputs) <= 6, "Too many inputs."
- else:
- input_ids = inputs
-
- if input_ids is not None and inputs_embeds is not None:
- raise ValueError("You cannot specify both input_ids and inputs_embeds at the same time")
- elif input_ids is not None:
- input_shape = shape_list(input_ids)
- elif inputs_embeds is not None:
- input_shape = shape_list(inputs_embeds)[:-1]
- else:
- raise ValueError("You have to specify either input_ids or inputs_embeds")
-
- if attention_mask is None:
- attention_mask = tf.fill(input_shape, 1)
- if token_type_ids is None:
- token_type_ids = tf.fill(input_shape, 0)
-
- # We create a 3D attention mask from a 2D tensor mask.
- # Sizes are [batch_size, 1, 1, to_seq_length]
- # So we can broadcast to [batch_size, num_heads, from_seq_length, to_seq_length]
- # this attention mask is more simple than the triangular masking of causal attention
- # used in OpenAI GPT, we just need to prepare the broadcast dimension here.
- extended_attention_mask = attention_mask[:, tf.newaxis, tf.newaxis, :]
-
- # Since attention_mask is 1.0 for positions we want to attend and 0.0 for
- # masked positions, this operation will create a tensor which is 0.0 for
- # positions we want to attend and -10000.0 for masked positions.
- # Since we are adding it to the raw scores before the softmax, this is
- # effectively the same as removing these entirely.
-
- extended_attention_mask = tf.cast(extended_attention_mask, tf.float32)
- extended_attention_mask = (1.0 - extended_attention_mask) * -10000.0
-
- # Prepare head mask if needed
- # 1.0 in head_mask indicate we keep the head
- # attention_probs has shape bsz x n_heads x N x N
- # input head_mask has shape [num_heads] or [num_hidden_layers x num_heads]
- # and head_mask is converted to shape [num_hidden_layers x batch x num_heads x seq_length x seq_length]
- if head_mask is not None:
- raise NotImplementedError
- else:
- head_mask = [None] * self.num_hidden_layers
- # head_mask = tf.constant([0] * self.num_hidden_layers)
-
- embedding_output = self.embeddings([input_ids, position_ids, token_type_ids, inputs_embeds], training=training)
- encoder_outputs = self.encoder([embedding_output, extended_attention_mask, head_mask], training=training)
-
- sequence_output = encoder_outputs[0]
- pooled_output = self.pooler(sequence_output)
-
- outputs = (sequence_output, pooled_output,) + encoder_outputs[
- 1:
- ] # add hidden_states and attentions if they are here
- return outputs # sequence_output, pooled_output, (hidden_states), (attentions)
-
-
-class TFBertPreTrainedModel(TFPreTrainedModel):
- """ An abstract class to handle weights initialization and
- a simple interface for downloading and loading pretrained models.
- """
-
- config_class = BertConfig
- pretrained_model_archive_map = TF_BERT_PRETRAINED_MODEL_ARCHIVE_MAP
- base_model_prefix = "bert"
-
-
-BERT_START_DOCSTRING = r"""
- This model is a `tf.keras.Model `__ sub-class.
- Use it as a regular TF 2.0 Keras Model and
- refer to the TF 2.0 documentation for all matter related to general usage and behavior.
-
- .. note::
-
- TF 2.0 models accepts two formats as inputs:
-
- - having all inputs as keyword arguments (like PyTorch models), or
- - having all inputs as a list, tuple or dict in the first positional arguments.
-
- This second option is useful when using :obj:`tf.keras.Model.fit()` method which currently requires having
- all the tensors in the first argument of the model call function: :obj:`model(inputs)`.
-
- If you choose this second option, there are three possibilities you can use to gather all the input Tensors
- in the first positional argument :
-
- - a single Tensor with input_ids only and nothing else: :obj:`model(inputs_ids)`
- - a list of varying length with one or several input Tensors IN THE ORDER given in the docstring:
- :obj:`model([input_ids, attention_mask])` or :obj:`model([input_ids, attention_mask, token_type_ids])`
- - a dictionary with one or several input Tensors associated to the input names given in the docstring:
- :obj:`model({'input_ids': input_ids, 'token_type_ids': token_type_ids})`
-
- Parameters:
- config (:class:`~transformers.BertConfig`): Model configuration class with all the parameters of the model.
- Initializing with a config file does not load the weights associated with the model, only the configuration.
- Check out the :meth:`~transformers.PreTrainedModel.from_pretrained` method to load the model weights.
-"""
-
-BERT_INPUTS_DOCSTRING = r"""
- Args:
- input_ids (:obj:`Numpy array` or :obj:`tf.Tensor` of shape :obj:`(batch_size, sequence_length)`):
- Indices of input sequence tokens in the vocabulary.
-
- Indices can be obtained using :class:`transformers.BertTokenizer`.
- See :func:`transformers.PreTrainedTokenizer.encode` and
- :func:`transformers.PreTrainedTokenizer.encode_plus` for details.
-
- `What are input IDs? <../glossary.html#input-ids>`__
- attention_mask (:obj:`Numpy array` or :obj:`tf.Tensor` of shape :obj:`(batch_size, sequence_length)`, `optional`, defaults to :obj:`None`):
- Mask to avoid performing attention on padding token indices.
- Mask values selected in ``[0, 1]``:
- ``1`` for tokens that are NOT MASKED, ``0`` for MASKED tokens.
-
- `What are attention masks? <../glossary.html#attention-mask>`__
- token_type_ids (:obj:`Numpy array` or :obj:`tf.Tensor` of shape :obj:`(batch_size, sequence_length)`, `optional`, defaults to :obj:`None`):
- Segment token indices to indicate first and second portions of the inputs.
- Indices are selected in ``[0, 1]``: ``0`` corresponds to a `sentence A` token, ``1``
- corresponds to a `sentence B` token
-
- `What are token type IDs? <../glossary.html#token-type-ids>`__
- position_ids (:obj:`Numpy array` or :obj:`tf.Tensor` of shape :obj:`(batch_size, sequence_length)`, `optional`, defaults to :obj:`None`):
- Indices of positions of each input sequence tokens in the position embeddings.
- Selected in the range ``[0, config.max_position_embeddings - 1]``.
-
- `What are position IDs? <../glossary.html#position-ids>`__
- head_mask (:obj:`Numpy array` or :obj:`tf.Tensor` of shape :obj:`(num_heads,)` or :obj:`(num_layers, num_heads)`, `optional`, defaults to :obj:`None`):
- Mask to nullify selected heads of the self-attention modules.
- Mask values selected in ``[0, 1]``:
- :obj:`1` indicates the head is **not masked**, :obj:`0` indicates the head is **masked**.
- inputs_embeds (:obj:`Numpy array` or :obj:`tf.Tensor` of shape :obj:`(batch_size, sequence_length, embedding_dim)`, `optional`, defaults to :obj:`None`):
- Optionally, instead of passing :obj:`input_ids` you can choose to directly pass an embedded representation.
- This is useful if you want more control over how to convert `input_ids` indices into associated vectors
- than the model's internal embedding lookup matrix.
- training (:obj:`boolean`, `optional`, defaults to :obj:`False`):
- Whether to activate dropout modules (if set to :obj:`True`) during training or to de-activate them
- (if set to :obj:`False`) for evaluation.
-"""
-
-
-@add_start_docstrings(
- "The bare Bert Model transformer outputing raw hidden-states without any specific head on top.",
- BERT_START_DOCSTRING,
-)
-class TFBertModel(TFBertPreTrainedModel):
- def __init__(self, config, *inputs, **kwargs):
- super().__init__(config, *inputs, **kwargs)
- self.bert = TFBertMainLayer(config, name="bert")
-
- @add_start_docstrings_to_callable(BERT_INPUTS_DOCSTRING)
- def call(self, inputs, **kwargs):
- r"""
- Returns:
- :obj:`tuple(torch.FloatTensor)` comprising various elements depending on the configuration (:class:`~transformers.BertConfig`) and inputs:
- last_hidden_state (:obj:`tf.Tensor` of shape :obj:`(batch_size, sequence_length, hidden_size)`):
- Sequence of hidden-states at the output of the last layer of the model.
- pooler_output (:obj:`tf.Tensor` of shape :obj:`(batch_size, hidden_size)`):
- Last layer hidden-state of the first token of the sequence (classification token)
- further processed by a Linear layer and a Tanh activation function. The Linear
- layer weights are trained from the next sentence prediction (classification)
- objective during Bert pretraining. This output is usually *not* a good summary
- of the semantic content of the input, you're often better with averaging or pooling
- the sequence of hidden-states for the whole input sequence.
- hidden_states (:obj:`tuple(tf.Tensor)`, `optional`, returned when :obj:`config.output_hidden_states=True`):
- tuple of :obj:`tf.Tensor` (one for the output of the embeddings + one for the output of each layer)
- of shape :obj:`(batch_size, sequence_length, hidden_size)`.
-
- Hidden-states of the model at the output of each layer plus the initial embedding outputs.
- attentions (:obj:`tuple(tf.Tensor)`, `optional`, returned when ``config.output_attentions=True``):
- tuple of :obj:`tf.Tensor` (one for each layer) of shape
- :obj:`(batch_size, num_heads, sequence_length, sequence_length)`:
-
- Attentions weights after the attention softmax, used to compute the weighted average in the self-attention heads.
-
- Examples::
-
- import tensorflow as tf
- from transformers import BertTokenizer, TFBertModel
-
- tokenizer = BertTokenizer.from_pretrained('bert-base-uncased')
- model = TFBertModel.from_pretrained('bert-base-uncased')
- input_ids = tf.constant(tokenizer.encode("Hello, my dog is cute", add_special_tokens=True))[None, :] # Batch size 1
- outputs = model(input_ids)
- last_hidden_states = outputs[0] # The last hidden-state is the first element of the output tuple
- """
- outputs = self.bert(inputs, **kwargs)
- return outputs
-
-
-@add_start_docstrings(
- """Bert Model with two heads on top as done during the pre-training:
- a `masked language modeling` head and a `next sentence prediction (classification)` head. """,
- BERT_START_DOCSTRING,
-)
-class TFBertForPreTraining(TFBertPreTrainedModel):
- def __init__(self, config, *inputs, **kwargs):
- super().__init__(config, *inputs, **kwargs)
-
- self.bert = TFBertMainLayer(config, name="bert")
- self.nsp = TFBertNSPHead(config, name="nsp___cls")
- self.mlm = TFBertMLMHead(config, self.bert.embeddings, name="mlm___cls")
-
- def get_output_embeddings(self):
- return self.bert.embeddings
-
- @add_start_docstrings_to_callable(BERT_INPUTS_DOCSTRING)
- def call(self, inputs, **kwargs):
- r"""
- Return:
- :obj:`tuple(torch.FloatTensor)` comprising various elements depending on the configuration (:class:`~transformers.BertConfig`) and inputs:
- prediction_scores (:obj:`tf.Tensor` of shape :obj:`(batch_size, sequence_length, config.vocab_size)`):
- Prediction scores of the language modeling head (scores for each vocabulary token before SoftMax).
- seq_relationship_scores (:obj:`tf.Tensor` of shape :obj:`(batch_size, sequence_length, 2)`):
- Prediction scores of the next sequence prediction (classification) head (scores of True/False continuation before SoftMax).
- hidden_states (:obj:`tuple(tf.Tensor)`, `optional`, returned when :obj:`config.output_hidden_states=True`):
- tuple of :obj:`tf.Tensor` (one for the output of the embeddings + one for the output of each layer)
- of shape :obj:`(batch_size, sequence_length, hidden_size)`.
-
- Hidden-states of the model at the output of each layer plus the initial embedding outputs.
- attentions (:obj:`tuple(tf.Tensor)`, `optional`, returned when ``config.output_attentions=True``):
- tuple of :obj:`tf.Tensor` (one for each layer) of shape
- :obj:`(batch_size, num_heads, sequence_length, sequence_length)`:
-
- Attentions weights after the attention softmax, used to compute the weighted average in the self-attention heads.
-
- Examples::
-
- import tensorflow as tf
- from transformers import BertTokenizer, TFBertForPreTraining
-
- tokenizer = BertTokenizer.from_pretrained('bert-base-uncased')
- model = TFBertForPreTraining.from_pretrained('bert-base-uncased')
- input_ids = tf.constant(tokenizer.encode("Hello, my dog is cute", add_special_tokens=True))[None, :] # Batch size 1
- outputs = model(input_ids)
- prediction_scores, seq_relationship_scores = outputs[:2]
-
- """
- outputs = self.bert(inputs, **kwargs)
-
- sequence_output, pooled_output = outputs[:2]
- prediction_scores = self.mlm(sequence_output, training=kwargs.get("training", False))
- seq_relationship_score = self.nsp(pooled_output)
-
- outputs = (prediction_scores, seq_relationship_score,) + outputs[
- 2:
- ] # add hidden states and attention if they are here
-
- return outputs # prediction_scores, seq_relationship_score, (hidden_states), (attentions)
-
-
-@add_start_docstrings("""Bert Model with a `language modeling` head on top. """, BERT_START_DOCSTRING)
-class TFBertForMaskedLM(TFBertPreTrainedModel):
- def __init__(self, config, *inputs, **kwargs):
- super().__init__(config, *inputs, **kwargs)
-
- self.bert = TFBertMainLayer(config, name="bert")
- self.mlm = TFBertMLMHead(config, self.bert.embeddings, name="mlm___cls")
-
- def get_output_embeddings(self):
- return self.bert.embeddings
-
- @add_start_docstrings_to_callable(BERT_INPUTS_DOCSTRING)
- def call(self, inputs, **kwargs):
- r"""
- Return:
- :obj:`tuple(torch.FloatTensor)` comprising various elements depending on the configuration (:class:`~transformers.BertConfig`) and inputs:
- prediction_scores (:obj:`Numpy array` or :obj:`tf.Tensor` of shape :obj:`(batch_size, sequence_length, config.vocab_size)`):
- Prediction scores of the language modeling head (scores for each vocabulary token before SoftMax).
- hidden_states (:obj:`tuple(tf.Tensor)`, `optional`, returned when :obj:`config.output_hidden_states=True`):
- tuple of :obj:`tf.Tensor` (one for the output of the embeddings + one for the output of each layer)
- of shape :obj:`(batch_size, sequence_length, hidden_size)`.
-
- Hidden-states of the model at the output of each layer plus the initial embedding outputs.
- attentions (:obj:`tuple(tf.Tensor)`, `optional`, returned when ``config.output_attentions=True``):
- tuple of :obj:`tf.Tensor` (one for each layer) of shape
- :obj:`(batch_size, num_heads, sequence_length, sequence_length)`:
-
- Attentions weights after the attention softmax, used to compute the weighted average in the self-attention heads.
-
- Examples::
-
- import tensorflow as tf
- from transformers import BertTokenizer, TFBertForMaskedLM
-
- tokenizer = BertTokenizer.from_pretrained('bert-base-uncased')
- model = TFBertForMaskedLM.from_pretrained('bert-base-uncased')
- input_ids = tf.constant(tokenizer.encode("Hello, my dog is cute", add_special_tokens=True))[None, :] # Batch size 1
- outputs = model(input_ids)
- prediction_scores = outputs[0]
-
- """
- outputs = self.bert(inputs, **kwargs)
-
- sequence_output = outputs[0]
- prediction_scores = self.mlm(sequence_output, training=kwargs.get("training", False))
-
- outputs = (prediction_scores,) + outputs[2:] # Add hidden states and attention if they are here
-
- return outputs # prediction_scores, (hidden_states), (attentions)
-
-
-@add_start_docstrings(
- """Bert Model with a `next sentence prediction (classification)` head on top. """, BERT_START_DOCSTRING,
-)
-class TFBertForNextSentencePrediction(TFBertPreTrainedModel):
- def __init__(self, config, *inputs, **kwargs):
- super().__init__(config, *inputs, **kwargs)
-
- self.bert = TFBertMainLayer(config, name="bert")
- self.nsp = TFBertNSPHead(config, name="nsp___cls")
-
- @add_start_docstrings_to_callable(BERT_INPUTS_DOCSTRING)
- def call(self, inputs, **kwargs):
- r"""
- Return:
- :obj:`tuple(torch.FloatTensor)` comprising various elements depending on the configuration (:class:`~transformers.BertConfig`) and inputs:
- seq_relationship_scores (:obj:`Numpy array` or :obj:`tf.Tensor` of shape :obj:`(batch_size, sequence_length, 2)`)
- Prediction scores of the next sequence prediction (classification) head (scores of True/False continuation before SoftMax).
- hidden_states (:obj:`tuple(tf.Tensor)`, `optional`, returned when :obj:`config.output_hidden_states=True`):
- tuple of :obj:`tf.Tensor` (one for the output of the embeddings + one for the output of each layer)
- of shape :obj:`(batch_size, sequence_length, hidden_size)`.
-
- Hidden-states of the model at the output of each layer plus the initial embedding outputs.
- attentions (:obj:`tuple(tf.Tensor)`, `optional`, returned when ``config.output_attentions=True``):
- tuple of :obj:`tf.Tensor` (one for each layer) of shape
- :obj:`(batch_size, num_heads, sequence_length, sequence_length)`:
-
- Attentions weights after the attention softmax, used to compute the weighted average in the self-attention heads.
-
- Examples::
-
- import tensorflow as tf
- from transformers import BertTokenizer, TFBertForNextSentencePrediction
-
- tokenizer = BertTokenizer.from_pretrained('bert-base-uncased')
- model = TFBertForNextSentencePrediction.from_pretrained('bert-base-uncased')
- input_ids = tf.constant(tokenizer.encode("Hello, my dog is cute", add_special_tokens=True))[None, :] # Batch size 1
- outputs = model(input_ids)
- seq_relationship_scores = outputs[0]
-
- """
- outputs = self.bert(inputs, **kwargs)
-
- pooled_output = outputs[1]
- seq_relationship_score = self.nsp(pooled_output)
-
- outputs = (seq_relationship_score,) + outputs[2:] # add hidden states and attention if they are here
-
- return outputs # seq_relationship_score, (hidden_states), (attentions)
-
-
-@add_start_docstrings(
- """Bert Model transformer with a sequence classification/regression head on top (a linear layer on top of
- the pooled output) e.g. for GLUE tasks. """,
- BERT_START_DOCSTRING,
-)
-class TFBertForSequenceClassification(TFBertPreTrainedModel):
- def __init__(self, config, *inputs, **kwargs):
- super().__init__(config, *inputs, **kwargs)
- self.num_labels = config.num_labels
-
- self.bert = TFBertMainLayer(config, name="bert")
- self.dropout = tf.keras.layers.Dropout(config.hidden_dropout_prob)
- self.classifier = tf.keras.layers.Dense(
- config.num_labels, kernel_initializer=get_initializer(config.initializer_range), name="classifier"
- )
-
- @add_start_docstrings_to_callable(BERT_INPUTS_DOCSTRING)
- def call(self, inputs, **kwargs):
- r"""
- Return:
- :obj:`tuple(torch.FloatTensor)` comprising various elements depending on the configuration (:class:`~transformers.BertConfig`) and inputs:
- logits (:obj:`Numpy array` or :obj:`tf.Tensor` of shape :obj:`(batch_size, config.num_labels)`):
- Classification (or regression if config.num_labels==1) scores (before SoftMax).
- hidden_states (:obj:`tuple(tf.Tensor)`, `optional`, returned when :obj:`config.output_hidden_states=True`):
- tuple of :obj:`tf.Tensor` (one for the output of the embeddings + one for the output of each layer)
- of shape :obj:`(batch_size, sequence_length, hidden_size)`.
-
- Hidden-states of the model at the output of each layer plus the initial embedding outputs.
- attentions (:obj:`tuple(tf.Tensor)`, `optional`, returned when ``config.output_attentions=True``):
- tuple of :obj:`tf.Tensor` (one for each layer) of shape
- :obj:`(batch_size, num_heads, sequence_length, sequence_length)`:
-
- Attentions weights after the attention softmax, used to compute the weighted average in the self-attention heads.
-
- Examples::
-
- import tensorflow as tf
- from transformers import BertTokenizer, TFBertForSequenceClassification
-
- tokenizer = BertTokenizer.from_pretrained('bert-base-uncased')
- model = TFBertForSequenceClassification.from_pretrained('bert-base-uncased')
- input_ids = tf.constant(tokenizer.encode("Hello, my dog is cute", add_special_tokens=True))[None, :] # Batch size 1
- outputs = model(input_ids)
- logits = outputs[0]
-
- """
- outputs = self.bert(inputs, **kwargs)
-
- pooled_output = outputs[1]
-
- pooled_output = self.dropout(pooled_output, training=kwargs.get("training", False))
- logits = self.classifier(pooled_output)
-
- outputs = (logits,) + outputs[2:] # add hidden states and attention if they are here
-
- return outputs # logits, (hidden_states), (attentions)
-
-
-@add_start_docstrings(
- """Bert Model with a multiple choice classification head on top (a linear layer on top of
- the pooled output and a softmax) e.g. for RocStories/SWAG tasks. """,
- BERT_START_DOCSTRING,
-)
-class TFBertForMultipleChoice(TFBertPreTrainedModel):
- def __init__(self, config, *inputs, **kwargs):
- super().__init__(config, *inputs, **kwargs)
-
- self.bert = TFBertMainLayer(config, name="bert")
- self.dropout = tf.keras.layers.Dropout(config.hidden_dropout_prob)
- self.classifier = tf.keras.layers.Dense(
- 1, kernel_initializer=get_initializer(config.initializer_range), name="classifier"
- )
-
- @property
- def dummy_inputs(self):
- """ Dummy inputs to build the network.
-
- Returns:
- tf.Tensor with dummy inputs
- """
- return {"input_ids": tf.constant(MULTIPLE_CHOICE_DUMMY_INPUTS)}
-
- @add_start_docstrings_to_callable(BERT_INPUTS_DOCSTRING)
- def call(
- self,
- inputs,
- attention_mask=None,
- token_type_ids=None,
- position_ids=None,
- head_mask=None,
- inputs_embeds=None,
- training=False,
- ):
- r"""
- Return:
- :obj:`tuple(torch.FloatTensor)` comprising various elements depending on the configuration (:class:`~transformers.BertConfig`) and inputs:
- classification_scores (:obj:`Numpy array` or :obj:`tf.Tensor` of shape :obj:`(batch_size, num_choices)`:
- `num_choices` is the size of the second dimension of the input tensors. (see `input_ids` above).
-
- Classification scores (before SoftMax).
- hidden_states (:obj:`tuple(tf.Tensor)`, `optional`, returned when :obj:`config.output_hidden_states=True`):
- tuple of :obj:`tf.Tensor` (one for the output of the embeddings + one for the output of each layer)
- of shape :obj:`(batch_size, sequence_length, hidden_size)`.
-
- Hidden-states of the model at the output of each layer plus the initial embedding outputs.
- attentions (:obj:`tuple(tf.Tensor)`, `optional`, returned when ``config.output_attentions=True``):
- tuple of :obj:`tf.Tensor` (one for each layer) of shape
- :obj:`(batch_size, num_heads, sequence_length, sequence_length)`:
-
- Attentions weights after the attention softmax, used to compute the weighted average in the self-attention heads.
-
- Examples::
-
- import tensorflow as tf
- from transformers import BertTokenizer, TFBertForMultipleChoice
-
- tokenizer = BertTokenizer.from_pretrained('bert-base-uncased')
- model = TFBertForMultipleChoice.from_pretrained('bert-base-uncased')
- choices = ["Hello, my dog is cute", "Hello, my cat is amazing"]
- input_ids = tf.constant([tokenizer.encode(s) for s in choices])[None, :] # Batch size 1, 2 choices
- outputs = model(input_ids)
- classification_scores = outputs[0]
-
- """
- if isinstance(inputs, (tuple, list)):
- input_ids = inputs[0]
- attention_mask = inputs[1] if len(inputs) > 1 else attention_mask
- token_type_ids = inputs[2] if len(inputs) > 2 else token_type_ids
- position_ids = inputs[3] if len(inputs) > 3 else position_ids
- head_mask = inputs[4] if len(inputs) > 4 else head_mask
- inputs_embeds = inputs[5] if len(inputs) > 5 else inputs_embeds
- assert len(inputs) <= 6, "Too many inputs."
- elif isinstance(inputs, dict):
- input_ids = inputs.get("input_ids")
- attention_mask = inputs.get("attention_mask", attention_mask)
- token_type_ids = inputs.get("token_type_ids", token_type_ids)
- position_ids = inputs.get("position_ids", position_ids)
- head_mask = inputs.get("head_mask", head_mask)
- inputs_embeds = inputs.get("inputs_embeds", inputs_embeds)
- assert len(inputs) <= 6, "Too many inputs."
- else:
- input_ids = inputs
-
- if input_ids is not None:
- num_choices = shape_list(input_ids)[1]
- seq_length = shape_list(input_ids)[2]
- else:
- num_choices = shape_list(inputs_embeds)[1]
- seq_length = shape_list(inputs_embeds)[2]
-
- flat_input_ids = tf.reshape(input_ids, (-1, seq_length)) if input_ids is not None else None
- flat_attention_mask = tf.reshape(attention_mask, (-1, seq_length)) if attention_mask is not None else None
- flat_token_type_ids = tf.reshape(token_type_ids, (-1, seq_length)) if token_type_ids is not None else None
- flat_position_ids = tf.reshape(position_ids, (-1, seq_length)) if position_ids is not None else None
-
- flat_inputs = [
- flat_input_ids,
- flat_attention_mask,
- flat_token_type_ids,
- flat_position_ids,
- head_mask,
- inputs_embeds,
- ]
-
- outputs = self.bert(flat_inputs, training=training)
-
- pooled_output = outputs[1]
-
- pooled_output = self.dropout(pooled_output, training=training)
- logits = self.classifier(pooled_output)
- reshaped_logits = tf.reshape(logits, (-1, num_choices))
-
- outputs = (reshaped_logits,) + outputs[2:] # add hidden states and attention if they are here
-
- return outputs # reshaped_logits, (hidden_states), (attentions)
-
-
-@add_start_docstrings(
- """Bert Model with a token classification head on top (a linear layer on top of
- the hidden-states output) e.g. for Named-Entity-Recognition (NER) tasks. """,
- BERT_START_DOCSTRING,
-)
-class TFBertForTokenClassification(TFBertPreTrainedModel):
- def __init__(self, config, *inputs, **kwargs):
- super().__init__(config, *inputs, **kwargs)
- self.num_labels = config.num_labels
-
- self.bert = TFBertMainLayer(config, name="bert")
- self.dropout = tf.keras.layers.Dropout(config.hidden_dropout_prob)
- self.classifier = tf.keras.layers.Dense(
- config.num_labels, kernel_initializer=get_initializer(config.initializer_range), name="classifier"
- )
-
- @add_start_docstrings_to_callable(BERT_INPUTS_DOCSTRING)
- def call(self, inputs, **kwargs):
- r"""
- Return:
- :obj:`tuple(torch.FloatTensor)` comprising various elements depending on the configuration (:class:`~transformers.BertConfig`) and inputs:
- scores (:obj:`Numpy array` or :obj:`tf.Tensor` of shape :obj:`(batch_size, sequence_length, config.num_labels)`):
- Classification scores (before SoftMax).
- hidden_states (:obj:`tuple(tf.Tensor)`, `optional`, returned when :obj:`config.output_hidden_states=True`):
- tuple of :obj:`tf.Tensor` (one for the output of the embeddings + one for the output of each layer)
- of shape :obj:`(batch_size, sequence_length, hidden_size)`.
-
- Hidden-states of the model at the output of each layer plus the initial embedding outputs.
- attentions (:obj:`tuple(tf.Tensor)`, `optional`, returned when ``config.output_attentions=True``):
- tuple of :obj:`tf.Tensor` (one for each layer) of shape
- :obj:`(batch_size, num_heads, sequence_length, sequence_length)`:
-
- Attentions weights after the attention softmax, used to compute the weighted average in the self-attention heads.
-
- Examples::
-
- import tensorflow as tf
- from transformers import BertTokenizer, TFBertForTokenClassification
-
- tokenizer = BertTokenizer.from_pretrained('bert-base-uncased')
- model = TFBertForTokenClassification.from_pretrained('bert-base-uncased')
- input_ids = tf.constant(tokenizer.encode("Hello, my dog is cute", add_special_tokens=True))[None, :] # Batch size 1
- outputs = model(input_ids)
- scores = outputs[0]
-
- """
- outputs = self.bert(inputs, **kwargs)
-
- sequence_output = outputs[0]
-
- sequence_output = self.dropout(sequence_output, training=kwargs.get("training", False))
- logits = self.classifier(sequence_output)
-
- outputs = (logits,) + outputs[2:] # add hidden states and attention if they are here
-
- return outputs # scores, (hidden_states), (attentions)
-
-
-@add_start_docstrings(
- """Bert Model with a span classification head on top for extractive question-answering tasks like SQuAD (a linear layers on top of
- the hidden-states output to compute `span start logits` and `span end logits`). """,
- BERT_START_DOCSTRING,
-)
-class TFBertForQuestionAnswering(TFBertPreTrainedModel):
- def __init__(self, config, *inputs, **kwargs):
- super().__init__(config, *inputs, **kwargs)
- self.num_labels = config.num_labels
-
- self.bert = TFBertMainLayer(config, name="bert")
- self.qa_outputs = tf.keras.layers.Dense(
- config.num_labels, kernel_initializer=get_initializer(config.initializer_range), name="qa_outputs"
- )
-
- @add_start_docstrings_to_callable(BERT_INPUTS_DOCSTRING)
- def call(self, inputs, **kwargs):
- r"""
- Return:
- :obj:`tuple(torch.FloatTensor)` comprising various elements depending on the configuration (:class:`~transformers.BertConfig`) and inputs:
- start_scores (:obj:`Numpy array` or :obj:`tf.Tensor` of shape :obj:`(batch_size, sequence_length,)`):
- Span-start scores (before SoftMax).
- end_scores (:obj:`Numpy array` or :obj:`tf.Tensor` of shape :obj:`(batch_size, sequence_length,)`):
- Span-end scores (before SoftMax).
- hidden_states (:obj:`tuple(tf.Tensor)`, `optional`, returned when :obj:`config.output_hidden_states=True`):
- tuple of :obj:`tf.Tensor` (one for the output of the embeddings + one for the output of each layer)
- of shape :obj:`(batch_size, sequence_length, hidden_size)`.
-
- Hidden-states of the model at the output of each layer plus the initial embedding outputs.
- attentions (:obj:`tuple(tf.Tensor)`, `optional`, returned when ``config.output_attentions=True``):
- tuple of :obj:`tf.Tensor` (one for each layer) of shape
- :obj:`(batch_size, num_heads, sequence_length, sequence_length)`:
-
- Attentions weights after the attention softmax, used to compute the weighted average in the self-attention heads.
-
- Examples::
-
- import tensorflow as tf
- from transformers import BertTokenizer, TFBertForQuestionAnswering
-
- tokenizer = BertTokenizer.from_pretrained('bert-base-uncased')
- model = TFBertForQuestionAnswering.from_pretrained('bert-base-uncased')
- input_ids = tf.constant(tokenizer.encode("Hello, my dog is cute", add_special_tokens=True))[None, :] # Batch size 1
- outputs = model(input_ids)
- start_scores, end_scores = outputs[:2]
-
- """
- outputs = self.bert(inputs, **kwargs)
-
- sequence_output = outputs[0]
-
- logits = self.qa_outputs(sequence_output)
- start_logits, end_logits = tf.split(logits, 2, axis=-1)
- start_logits = tf.squeeze(start_logits, axis=-1)
- end_logits = tf.squeeze(end_logits, axis=-1)
-
- outputs = (start_logits, end_logits,) + outputs[2:]
-
- return outputs # start_logits, end_logits, (hidden_states), (attentions)
diff --git a/server/transformers/src/transformers/modeling_tf_camembert.py b/server/transformers/src/transformers/modeling_tf_camembert.py
deleted file mode 100644
index d6317cacfb5fc0fb2f05d99f33c7e2871fda2a2c..0000000000000000000000000000000000000000
--- a/server/transformers/src/transformers/modeling_tf_camembert.py
+++ /dev/null
@@ -1,118 +0,0 @@
-# coding=utf-8
-# Copyright 2018 The Google AI Language Team Authors and The HuggingFace Inc. team.
-# Copyright (c) 2018, NVIDIA CORPORATION. All rights reserved.
-#
-# Licensed under the Apache License, Version 2.0 (the "License");
-# you may not use this file except in compliance with the License.
-# You may obtain a copy of the License at
-#
-# http://www.apache.org/licenses/LICENSE-2.0
-#
-# Unless required by applicable law or agreed to in writing, software
-# distributed under the License is distributed on an "AS IS" BASIS,
-# WITHOUT WARRANTIES OR CONDITIONS OF ANY KIND, either express or implied.
-# See the License for the specific language governing permissions and
-# limitations under the License.
-""" TF 2.0 RoBERTa model. """
-
-
-import logging
-
-from .configuration_camembert import CamembertConfig
-from .file_utils import add_start_docstrings
-from .modeling_tf_roberta import (
- TFRobertaForMaskedLM,
- TFRobertaForSequenceClassification,
- TFRobertaForTokenClassification,
- TFRobertaModel,
-)
-
-
-logger = logging.getLogger(__name__)
-
-TF_CAMEMBERT_PRETRAINED_MODEL_ARCHIVE_MAP = {}
-
-
-CAMEMBERT_START_DOCSTRING = r"""
-
- .. note::
-
- TF 2.0 models accepts two formats as inputs:
-
- - having all inputs as keyword arguments (like PyTorch models), or
- - having all inputs as a list, tuple or dict in the first positional arguments.
-
- This second option is useful when using :obj:`tf.keras.Model.fit()` method which currently requires having
- all the tensors in the first argument of the model call function: :obj:`model(inputs)`.
-
- If you choose this second option, there are three possibilities you can use to gather all the input Tensors
- in the first positional argument :
-
- - a single Tensor with input_ids only and nothing else: :obj:`model(inputs_ids)`
- - a list of varying length with one or several input Tensors IN THE ORDER given in the docstring:
- :obj:`model([input_ids, attention_mask])` or :obj:`model([input_ids, attention_mask, token_type_ids])`
- - a dictionary with one or several input Tensors associated to the input names given in the docstring:
- :obj:`model({'input_ids': input_ids, 'token_type_ids': token_type_ids})`
-
- Parameters:
- config (:class:`~transformers.CamembertConfig`): Model configuration class with all the parameters of the
- model. Initializing with a config file does not load the weights associated with the model, only the configuration.
- Check out the :meth:`~transformers.PreTrainedModel.from_pretrained` method to load the model weights.
-"""
-
-
-@add_start_docstrings(
- "The bare CamemBERT Model transformer outputting raw hidden-states without any specific head on top.",
- CAMEMBERT_START_DOCSTRING,
-)
-class TFCamembertModel(TFRobertaModel):
- """
- This class overrides :class:`~transformers.TFRobertaModel`. Please check the
- superclass for the appropriate documentation alongside usage examples.
- """
-
- config_class = CamembertConfig
- pretrained_model_archive_map = TF_CAMEMBERT_PRETRAINED_MODEL_ARCHIVE_MAP
-
-
-@add_start_docstrings(
- """CamemBERT Model with a `language modeling` head on top. """, CAMEMBERT_START_DOCSTRING,
-)
-class TFCamembertForMaskedLM(TFRobertaForMaskedLM):
- """
- This class overrides :class:`~transformers.TFRobertaForMaskedLM`. Please check the
- superclass for the appropriate documentation alongside usage examples.
- """
-
- config_class = CamembertConfig
- pretrained_model_archive_map = TF_CAMEMBERT_PRETRAINED_MODEL_ARCHIVE_MAP
-
-
-@add_start_docstrings(
- """CamemBERT Model transformer with a sequence classification/regression head on top (a linear layer
- on top of the pooled output) e.g. for GLUE tasks. """,
- CAMEMBERT_START_DOCSTRING,
-)
-class TFCamembertForSequenceClassification(TFRobertaForSequenceClassification):
- """
- This class overrides :class:`~transformers.TFRobertaForSequenceClassification`. Please check the
- superclass for the appropriate documentation alongside usage examples.
- """
-
- config_class = CamembertConfig
- pretrained_model_archive_map = TF_CAMEMBERT_PRETRAINED_MODEL_ARCHIVE_MAP
-
-
-@add_start_docstrings(
- """CamemBERT Model with a token classification head on top (a linear layer on top of
- the hidden-states output) e.g. for Named-Entity-Recognition (NER) tasks. """,
- CAMEMBERT_START_DOCSTRING,
-)
-class TFCamembertForTokenClassification(TFRobertaForTokenClassification):
- """
- This class overrides :class:`~transformers.TFRobertaForTokenClassification`. Please check the
- superclass for the appropriate documentation alongside usage examples.
- """
-
- config_class = CamembertConfig
- pretrained_model_archive_map = TF_CAMEMBERT_PRETRAINED_MODEL_ARCHIVE_MAP
diff --git a/server/transformers/src/transformers/modeling_tf_ctrl.py b/server/transformers/src/transformers/modeling_tf_ctrl.py
deleted file mode 100644
index 78e0c1113a8b9a796513711efa0ab682a4cd97e6..0000000000000000000000000000000000000000
--- a/server/transformers/src/transformers/modeling_tf_ctrl.py
+++ /dev/null
@@ -1,551 +0,0 @@
-# coding=utf-8
-# Copyright 2018 Salesforce and HuggingFace Inc. team.
-# Copyright (c) 2018, NVIDIA CORPORATION. All rights reserved.
-#
-# Licensed under the Apache License, Version 2.0 (the "License");
-# you may not use this file except in compliance with the License.
-# You may obtain a copy of the License at
-#
-# http://www.apache.org/licenses/LICENSE-2.0
-#
-# Unless required by applicable law or agreed to in writing, software
-# distributed under the License is distributed on an "AS IS" BASIS,
-# WITHOUT WARRANTIES OR CONDITIONS OF ANY KIND, either express or implied.
-# See the License for the specific language governing permissions and
-# limitations under the License.
-""" TF 2.0 CTRL model."""
-
-
-import logging
-
-import numpy as np
-import tensorflow as tf
-
-from .configuration_ctrl import CTRLConfig
-from .file_utils import add_start_docstrings, add_start_docstrings_to_callable
-from .modeling_tf_utils import TFPreTrainedModel, TFSharedEmbeddings, shape_list
-
-
-logger = logging.getLogger(__name__)
-
-TF_CTRL_PRETRAINED_MODEL_ARCHIVE_MAP = {"ctrl": "https://s3.amazonaws.com/models.huggingface.co/bert/ctrl-tf_model.h5"}
-
-
-def angle_defn(pos, i, d_model_size):
- angle_rates = 1 / np.power(10000, (2 * (i // 2)) / np.float32(d_model_size))
- return pos * angle_rates
-
-
-def positional_encoding(position, d_model_size):
- # create the sinusoidal pattern for the positional encoding
- angle_rads = angle_defn(np.arange(position)[:, np.newaxis], np.arange(d_model_size)[np.newaxis, :], d_model_size)
-
- sines = np.sin(angle_rads[:, 0::2])
- cosines = np.cos(angle_rads[:, 1::2])
-
- # pos_encoding = tf.cast(np.concatenate([sines, cosines], axis=-1)[np.newaxis, ...], dtype=tf.float32)
- pos_encoding = tf.cast(np.concatenate([sines, cosines], axis=-1), dtype=tf.float32)
- return pos_encoding
-
-
-def scaled_dot_product_attention(q, k, v, mask, attention_mask=None, head_mask=None):
- # calculate attention
- matmul_qk = tf.matmul(q, k, transpose_b=True)
-
- dk = tf.cast(shape_list(k)[-1], tf.float32)
- scaled_attention_logits = matmul_qk / tf.math.sqrt(dk)
-
- if mask is not None:
- scaled_attention_logits += mask * -1e4
-
- if attention_mask is not None:
- # Apply the attention mask
- scaled_attention_logits = scaled_attention_logits + attention_mask
-
- attention_weights = tf.nn.softmax(scaled_attention_logits, axis=-1)
-
- # Mask heads if we want to
- if head_mask is not None:
- attention_weights = attention_weights * head_mask
-
- output = tf.matmul(attention_weights, v)
-
- return output, attention_weights
-
-
-class TFMultiHeadAttention(tf.keras.layers.Layer):
- def __init__(self, d_model_size, num_heads, output_attentions=False, **kwargs):
- super().__init__(**kwargs)
- self.output_attentions = output_attentions
- self.num_heads = num_heads
- self.d_model_size = d_model_size
-
- self.depth = int(d_model_size / self.num_heads)
-
- self.Wq = tf.keras.layers.Dense(d_model_size, name="Wq")
- self.Wk = tf.keras.layers.Dense(d_model_size, name="Wk")
- self.Wv = tf.keras.layers.Dense(d_model_size, name="Wv")
-
- self.dense = tf.keras.layers.Dense(d_model_size, name="dense")
-
- def split_into_heads(self, x, batch_size):
- x = tf.reshape(x, (batch_size, -1, self.num_heads, self.depth))
- return tf.transpose(x, perm=[0, 2, 1, 3])
-
- def call(self, inputs, training=False):
- v, k, q, mask, layer_past, attention_mask, head_mask = inputs
- batch_size = shape_list(q)[0]
-
- q = self.Wq(q)
- k = self.Wk(k)
- v = self.Wv(v)
-
- q = self.split_into_heads(q, batch_size)
- k = self.split_into_heads(k, batch_size)
- v = self.split_into_heads(v, batch_size)
- if layer_past is not None:
- past_key, past_value = tf.unstack(layer_past, axis=1)
- k = tf.concat((past_key, k), dim=-2)
- v = tf.concat((past_value, v), dim=-2)
- present = tf.stack((k, v), axis=1)
-
- output = scaled_dot_product_attention(q, k, v, mask, attention_mask, head_mask)
- scaled_attention = tf.transpose(output[0], perm=[0, 2, 1, 3])
- attn = output[1]
- original_size_attention = tf.reshape(scaled_attention, (batch_size, -1, self.d_model_size))
- output = self.dense(original_size_attention)
-
- outputs = (output, present)
- if self.output_attentions:
- outputs = outputs + (attn,)
- return outputs
-
-
-def point_wise_feed_forward_network(d_model_size, dff, name=""):
- return tf.keras.Sequential(
- [tf.keras.layers.Dense(dff, activation="relu", name="0"), tf.keras.layers.Dense(d_model_size, name="2")],
- name="ffn",
- )
-
-
-class TFEncoderLayer(tf.keras.layers.Layer):
- def __init__(
- self, d_model_size, num_heads, dff, rate=0.1, layer_norm_epsilon=1e-6, output_attentions=False, **kwargs
- ):
- super().__init__(**kwargs)
-
- self.multi_head_attention = TFMultiHeadAttention(
- d_model_size, num_heads, output_attentions, name="multi_head_attention"
- )
- self.ffn = point_wise_feed_forward_network(d_model_size, dff, name="ffn")
-
- self.layernorm1 = tf.keras.layers.LayerNormalization(epsilon=layer_norm_epsilon, name="layernorm1")
- self.layernorm2 = tf.keras.layers.LayerNormalization(epsilon=layer_norm_epsilon, name="layernorm2")
-
- self.dropout1 = tf.keras.layers.Dropout(rate)
- self.dropout2 = tf.keras.layers.Dropout(rate)
-
- def call(self, inputs, training=False):
- x, mask, layer_past, attention_mask, head_mask = inputs
- normed = self.layernorm1(x)
- attn_outputs = self.multi_head_attention(
- [normed, normed, normed, mask, layer_past, attention_mask, head_mask], training=training
- )
- attn_output = attn_outputs[0]
- attn_output = self.dropout1(attn_output, training=training)
- out1 = x + attn_output
-
- out2 = self.layernorm2(out1)
- ffn_output = self.ffn(out2)
- ffn_output = self.dropout2(ffn_output, training=training)
- out2 = out1 + ffn_output
-
- outputs = (out2,) + attn_outputs[1:]
- return outputs
-
-
-class TFCTRLMainLayer(tf.keras.layers.Layer):
- def __init__(self, config, **kwargs):
- super().__init__(**kwargs)
- self.output_hidden_states = config.output_hidden_states
- self.output_attentions = config.output_attentions
- self.output_past = config.output_past
-
- self.d_model_size = config.n_embd
- self.num_layers = config.n_layer
-
- self.pos_encoding = positional_encoding(config.n_positions, self.d_model_size)
-
- self.w = TFSharedEmbeddings(
- config.vocab_size, config.n_embd, initializer_range=config.initializer_range, name="w"
- )
-
- self.dropout = tf.keras.layers.Dropout(config.embd_pdrop)
- self.h = [
- TFEncoderLayer(
- config.n_embd,
- config.n_head,
- config.dff,
- config.resid_pdrop,
- config.layer_norm_epsilon,
- config.output_attentions,
- name="h_._{}".format(i),
- )
- for i in range(config.n_layer)
- ]
- self.layernorm = tf.keras.layers.LayerNormalization(epsilon=config.layer_norm_epsilon, name="layernorm")
-
- def get_input_embeddings(self):
- return self.w
-
- def _resize_token_embeddings(self, new_num_tokens):
- raise NotImplementedError
-
- def _prune_heads(self, heads_to_prune):
- """ Prunes heads of the model.
- heads_to_prune: dict of {layer_num: list of heads to prune in this layer}
- """
- raise NotImplementedError
-
- def call(
- self,
- inputs,
- past=None,
- attention_mask=None,
- token_type_ids=None,
- position_ids=None,
- head_mask=None,
- inputs_embeds=None,
- training=False,
- ):
- if isinstance(inputs, (tuple, list)):
- input_ids = inputs[0]
- past = inputs[1] if len(inputs) > 1 else past
- attention_mask = inputs[2] if len(inputs) > 2 else attention_mask
- token_type_ids = inputs[3] if len(inputs) > 3 else token_type_ids
- position_ids = inputs[4] if len(inputs) > 4 else position_ids
- head_mask = inputs[5] if len(inputs) > 5 else head_mask
- inputs_embeds = inputs[6] if len(inputs) > 6 else inputs_embeds
- assert len(inputs) <= 7, "Too many inputs."
- elif isinstance(inputs, dict):
- input_ids = inputs.get("input_ids")
- past = inputs.get("past", past)
- attention_mask = inputs.get("attention_mask", attention_mask)
- token_type_ids = inputs.get("token_type_ids", token_type_ids)
- position_ids = inputs.get("position_ids", position_ids)
- head_mask = inputs.get("head_mask", head_mask)
- inputs_embeds = inputs.get("inputs_embeds", inputs_embeds)
- assert len(inputs) <= 7, "Too many inputs."
- else:
- input_ids = inputs
-
- if input_ids is not None and inputs_embeds is not None:
- raise ValueError("You cannot specify both input_ids and inputs_embeds at the same time")
- elif input_ids is not None:
- input_shape = shape_list(input_ids)
- input_ids = tf.reshape(input_ids, [-1, input_shape[-1]])
- elif inputs_embeds is not None:
- input_shape = shape_list(inputs_embeds)[:-1]
- else:
- raise ValueError("You have to specify either input_ids or inputs_embeds")
-
- if past is None:
- past_length = 0
- past = [None] * len(self.h)
- else:
- past_length = shape_list(past[0][0])[-2]
- if position_ids is None:
- position_ids = tf.range(past_length, input_shape[-1] + past_length, dtype=tf.int32)[tf.newaxis, :]
- position_ids = tf.tile(position_ids, [input_shape[0], 1])
-
- # Attention mask.
- if attention_mask is not None:
- # We create a 3D attention mask from a 2D tensor mask.
- # Sizes are [batch_size, 1, 1, to_seq_length]
- # So we can broadcast to [batch_size, num_heads, from_seq_length, to_seq_length]
- # this attention mask is more simple than the triangular masking of causal attention
- # used in OpenAI GPT, we just need to prepare the broadcast dimension here.
- attention_mask = attention_mask[:, tf.newaxis, tf.newaxis, :]
-
- # Since attention_mask is 1.0 for positions we want to attend and 0.0 for
- # masked positions, this operation will create a tensor which is 0.0 for
- # positions we want to attend and -10000.0 for masked positions.
- # Since we are adding it to the raw scores before the softmax, this is
- # effectively the same as removing these entirely.
-
- attention_mask = tf.cast(attention_mask, tf.float32)
- attention_mask = (1.0 - attention_mask) * -10000.0
- else:
- attention_mask = None
-
- # Prepare head mask if needed
- # 1.0 in head_mask indicate we keep the head
- # attention_probs has shape bsz x n_heads x N x N
- # head_mask has shape n_layer x batch x n_heads x N x N
- if head_mask is not None:
- raise NotImplementedError
- else:
- head_mask = [None] * self.num_layers
-
- if token_type_ids is not None:
- token_type_ids = tf.reshape(token_type_ids, [-1, shape_list(token_type_ids)[-1]])
- token_type_embeds = self.w(token_type_ids, mode="embedding")
- token_type_embeds *= tf.math.sqrt(tf.cast(self.d_model_size, tf.float32))
- else:
- token_type_embeds = 0
- position_ids = tf.reshape(position_ids, [-1, shape_list(position_ids)[-1]])
-
- if inputs_embeds is None:
- inputs_embeds = self.w(input_ids, mode="embedding")
- seq_len = input_shape[-1]
- mask = 1 - tf.linalg.band_part(tf.ones((seq_len, seq_len)), -1, 0)
-
- inputs_embeds *= tf.math.sqrt(tf.cast(self.d_model_size, tf.float32))
-
- pos_embeds = tf.gather(self.pos_encoding, position_ids)
-
- hidden_states = inputs_embeds + pos_embeds + token_type_embeds
-
- hidden_states = self.dropout(hidden_states, training=training)
-
- output_shape = input_shape + [shape_list(hidden_states)[-1]]
- presents = ()
- all_hidden_states = ()
- all_attentions = []
- for i, (h, layer_past) in enumerate(zip(self.h, past)):
- if self.output_hidden_states:
- all_hidden_states = all_hidden_states + (tf.reshape(hidden_states, output_shape),)
- outputs = h([hidden_states, mask, layer_past, attention_mask, head_mask[i]], training=training)
- hidden_states, present = outputs[:2]
-
- if self.output_past:
- presents = presents + (present,)
-
- if self.output_attentions:
- all_attentions.append(outputs[2])
-
- hidden_states = self.layernorm(hidden_states)
- hidden_states = tf.reshape(hidden_states, output_shape)
- if self.output_hidden_states:
- all_hidden_states = all_hidden_states + (hidden_states,)
-
- outputs = (hidden_states,)
- if self.output_past:
- outputs = outputs + (presents,)
- if self.output_hidden_states:
- outputs = outputs + (all_hidden_states,)
- if self.output_attentions:
- # let the number of heads free (-1) so we can extract attention even after head pruning
- attention_output_shape = input_shape[:-1] + [-1] + shape_list(all_attentions[0])[-2:]
- all_attentions = tuple(tf.reshape(t, attention_output_shape) for t in all_attentions)
- outputs = outputs + (all_attentions,)
- return outputs
-
-
-class TFCTRLPreTrainedModel(TFPreTrainedModel):
- """ An abstract class to handle weights initialization and
- a simple interface for downloading and loading pretrained models.
- """
-
- config_class = CTRLConfig
- pretrained_model_archive_map = TF_CTRL_PRETRAINED_MODEL_ARCHIVE_MAP
- base_model_prefix = "transformer"
-
-
-CTRL_START_DOCSTRING = r"""
-
- .. note::
- TF 2.0 models accepts two formats as inputs:
-
- - having all inputs as keyword arguments (like PyTorch models), or
- - having all inputs as a list, tuple or dict in the first positional arguments.
-
- This second option is useful when using :obj:`tf.keras.Model.fit()` method which currently requires having
- all the tensors in the first argument of the model call function: :obj:`model(inputs)`.
-
- If you choose this second option, there are three possibilities you can use to gather all the input Tensors
- in the first positional argument :
-
- - a single Tensor with input_ids only and nothing else: :obj:`model(inputs_ids)`
- - a list of varying length with one or several input Tensors IN THE ORDER given in the docstring:
- :obj:`model([input_ids, attention_mask])` or :obj:`model([input_ids, attention_mask, token_type_ids])`
- - a dictionary with one or several input Tensors associated to the input names given in the docstring:
- :obj:`model({'input_ids': input_ids, 'token_type_ids': token_type_ids})`
-
- Parameters:
- config (:class:`~transformers.CTRLConfig`): Model configuration class with all the parameters of the model.
- Initializing with a config file does not load the weights associated with the model, only the configuration.
- Check out the :meth:`~transformers.PreTrainedModel.from_pretrained` method to load the model weights.
-"""
-
-CTRL_INPUTS_DOCSTRING = r"""
- Args:
- input_ids (:obj:`Numpy array` or :obj:`tf.Tensor` of shape :obj:`(batch_size, sequence_length)`):
- Indices of input sequence tokens in the vocabulary.
-
- Indices can be obtained using :class:`transformers.CTRLTokenizer`.
- See :func:`transformers.PreTrainedTokenizer.encode` and
- :func:`transformers.PreTrainedTokenizer.encode_plus` for details.
-
- `What are input IDs? <../glossary.html#input-ids>`__
- past (:obj:`List[tf.Tensor]` of length :obj:`config.n_layers`):
- Contains pre-computed hidden-states (key and values in the attention blocks) as computed by the model
- (see `past` output below). Can be used to speed up sequential decoding. The token ids which have their past given to this model
- should not be passed as input ids as they have already been computed.
- attention_mask (:obj:`tf.Tensor` or :obj:`Numpy array` of shape :obj:`(batch_size, sequence_length)`, `optional`, defaults to :obj:`None`):
- Mask to avoid performing attention on padding token indices.
- Mask values selected in ``[0, 1]``:
- ``1`` for tokens that are NOT MASKED, ``0`` for MASKED tokens.
-
- `What are attention masks? <../glossary.html#attention-mask>`__
- token_type_ids (:obj:`tf.Tensor` or :obj:`Numpy array` of shape :obj:`(batch_size, sequence_length)`, `optional`, defaults to :obj:`None`):
- Segment token indices to indicate first and second portions of the inputs.
- Indices are selected in ``[0, 1]``: ``0`` corresponds to a `sentence A` token, ``1``
- corresponds to a `sentence B` token
-
- `What are token type IDs? <../glossary.html#token-type-ids>`_
- position_ids (:obj:`tf.Tensor` or :obj:`Numpy array` of shape :obj:`(batch_size, sequence_length)`, `optional`, defaults to :obj:`None`):
- Indices of positions of each input sequence tokens in the position embeddings.
- Selected in the range ``[0, config.max_position_embeddings - 1]``.
-
- `What are position IDs? <../glossary.html#position-ids>`_
- head_mask (:obj:`tf.Tensor` or :obj:`Numpy array` of shape :obj:`(num_heads,)` or :obj:`(num_layers, num_heads)`, `optional`, defaults to :obj:`None`):
- Mask to nullify selected heads of the self-attention modules.
- Mask values selected in ``[0, 1]``:
- :obj:`1` indicates the head is **not masked**, :obj:`0` indicates the head is **masked**.
- input_embeds (:obj:`tf.Tensor` or :obj:`Numpy array` of shape :obj:`(batch_size, sequence_length, hidden_size)`, `optional`, defaults to :obj:`None`):
- Optionally, instead of passing :obj:`input_ids` you can choose to directly pass an embedded representation.
- This is useful if you want more control over how to convert `input_ids` indices into associated vectors
- than the model's internal embedding lookup matrix.
- training (:obj:`boolean`, `optional`, defaults to :obj:`False`):
- Whether to activate dropout modules (if set to :obj:`True`) during training or to de-activate them
- (if set to :obj:`False`) for evaluation.
-"""
-
-
-@add_start_docstrings(
- "The bare CTRL Model transformer outputting raw hidden-states without any specific head on top.",
- CTRL_START_DOCSTRING,
-)
-class TFCTRLModel(TFCTRLPreTrainedModel):
- def __init__(self, config, *inputs, **kwargs):
- super().__init__(config, *inputs, **kwargs)
- self.transformer = TFCTRLMainLayer(config, name="transformer")
-
- @add_start_docstrings_to_callable(CTRL_INPUTS_DOCSTRING)
- def call(self, inputs, **kwargs):
- r"""
- Return:
- :obj:`tuple(tf.Tensor)` comprising various elements depending on the configuration (:class:`~transformers.CTRLConfig`) and inputs:
- last_hidden_state (:obj:`tf.Tensor` of shape :obj:`(batch_size, sequence_length, hidden_size)`):
- Sequence of hidden-states at the last layer of the model.
- past (:obj:`List[tf.Tensor]` of length :obj:`config.n_layers` with each tensor of shape :obj:`(2, batch_size, num_heads, sequence_length, embed_size_per_head)`):
- Contains pre-computed hidden-states (key and values in the attention blocks).
- Can be used (see `past` input) to speed up sequential decoding. The token ids which have their past given to this model
- should not be passed as input ids as they have already been computed.
- hidden_states (:obj:`tuple(tf.Tensor)` `optional`, returned when ``config.output_hidden_states=True``):
- Tuple of :obj:`tf.Tensor` (one for the output of the embeddings + one for the output of each layer)
- of shape :obj:`(batch_size, sequence_length, hidden_size)`.
-
- Hidden-states of the model at the output of each layer plus the initial embedding outputs.
- attentions (:obj:`tuple(tf.Tensor)`, `optional`, returned when ``config.output_attentions=True``):
- Tuple of :obj:`tf.Tensor` (one for each layer) of shape
- :obj:`(batch_size, num_heads, sequence_length, sequence_length)`.
-
- Attentions weights after the attention softmax, used to compute the weighted average in the self-attention
- heads.
-
- Examples::
-
- import tensorflow as tf
- from transformers import CTRLTokenizer, TFCTRLModel
-
- tokenizer = CTRLTokenizer.from_pretrained('ctrl')
- model = TFCTRLModel.from_pretrained('ctrl')
- input_ids = tf.constant(tokenizer.encode("Hello, my dog is cute", add_special_tokens=True))[None, :] # Batch size 1
- outputs = model(input_ids)
- last_hidden_states = outputs[0] # The last hidden-state is the first element of the output tuple
-
- """
- outputs = self.transformer(inputs, **kwargs)
- return outputs
-
-
-class TFCTRLLMHead(tf.keras.layers.Layer):
- def __init__(self, config, input_embeddings, **kwargs):
- super().__init__(**kwargs)
- self.vocab_size = config.vocab_size
-
- # The output weights are the same as the input embeddings, but there is
- # an output-only bias for each token.
- self.input_embeddings = input_embeddings
-
- def build(self, input_shape):
- self.bias = self.add_weight(shape=(self.vocab_size,), initializer="zeros", trainable=True, name="bias")
- super().build(input_shape)
-
- def call(self, hidden_states):
- hidden_states = self.input_embeddings(hidden_states, mode="linear")
- hidden_states = hidden_states + self.bias
- return hidden_states
-
-
-@add_start_docstrings(
- """The CTRL Model transformer with a language modeling head on top
- (linear layer with weights tied to the input embeddings). """,
- CTRL_START_DOCSTRING,
-)
-class TFCTRLLMHeadModel(TFCTRLPreTrainedModel):
- def __init__(self, config, *inputs, **kwargs):
- super().__init__(config, *inputs, **kwargs)
- self.transformer = TFCTRLMainLayer(config, name="transformer")
-
- self.lm_head = TFCTRLLMHead(config, self.transformer.w, name="lm_head")
-
- def get_output_embeddings(self):
- return self.lm_head.input_embeddings
-
- @add_start_docstrings_to_callable(CTRL_INPUTS_DOCSTRING)
- def call(self, inputs, **kwargs):
- r"""
- Return:
- :obj:`tuple(tf.Tensor)` comprising various elements depending on the configuration (:class:`~transformers.CTRLConfig`) and inputs:
- prediction_scores (:obj:`tf.Tensor` of shape :obj:`(batch_size, sequence_length, config.vocab_size)`):
- Prediction scores of the language modeling head (scores for each vocabulary token before SoftMax).
- past (:obj:`List[tf.Tensor]` of length :obj:`config.n_layers` with each tensor of shape :obj:`(2, batch_size, num_heads, sequence_length, embed_size_per_head)`):
- Contains pre-computed hidden-states (key and values in the attention blocks).
- Can be used (see `past` input) to speed up sequential decoding. The token ids which have their past given to this model
- should not be passed as input ids as they have already been computed.
- hidden_states (:obj:`tuple(tf.Tensor)`, `optional`, returned when ``config.output_hidden_states=True``):
- Tuple of :obj:`tf.Tensor` (one for the output of the embeddings + one for the output of each layer)
- of shape :obj:`(batch_size, sequence_length, hidden_size)`.
-
- Hidden-states of the model at the output of each layer plus the initial embedding outputs.
- attentions (:obj:`tuple(tf.Tensor)`, `optional`, returned when ``config.output_attentions=True``):
- Tuple of :obj:`tf.Tensor` (one for each layer) of shape
- :obj:`(batch_size, num_heads, sequence_length, sequence_length)`.
-
- Attentions weights after the attention softmax, used to compute the weighted average in the self-attention
- heads.
-
- Examples::
-
- import tensorflow as tf
- from transformers import CTRLTokenizer, TFCTRLLMHeadModel
-
- tokenizer = CTRLTokenizer.from_pretrained('ctrl')
- model = TFCTRLLMHeadModel.from_pretrained('ctrl')
-
- input_ids = tf.constant([tokenizer.encode("Links Hello, my dog is cute", add_special_tokens=True)])
- outputs = model(input_ids)
- loss, logits = outputs[:2]
-
- """
- transformer_outputs = self.transformer(inputs, **kwargs)
- hidden_states = transformer_outputs[0]
-
- lm_logits = self.lm_head(hidden_states)
-
- outputs = (lm_logits,) + transformer_outputs[1:]
-
- return outputs # lm_logits, presents, (all hidden_states), (attentions)
diff --git a/server/transformers/src/transformers/modeling_tf_distilbert.py b/server/transformers/src/transformers/modeling_tf_distilbert.py
deleted file mode 100644
index 1dc8301730e8141e47d3883d8f843625b676cdd5..0000000000000000000000000000000000000000
--- a/server/transformers/src/transformers/modeling_tf_distilbert.py
+++ /dev/null
@@ -1,838 +0,0 @@
-# coding=utf-8
-# Copyright 2019-present, the HuggingFace Inc. team, The Google AI Language Team and Facebook, Inc.
-#
-# Licensed under the Apache License, Version 2.0 (the "License");
-# you may not use this file except in compliance with the License.
-# You may obtain a copy of the License at
-#
-# http://www.apache.org/licenses/LICENSE-2.0
-#
-# Unless required by applicable law or agreed to in writing, software
-# distributed under the License is distributed on an "AS IS" BASIS,
-# WITHOUT WARRANTIES OR CONDITIONS OF ANY KIND, either express or implied.
-# See the License for the specific language governing permissions and
-# limitations under the License.
-""" TF 2.0 DistilBERT model
-"""
-
-
-import logging
-import math
-
-import numpy as np
-import tensorflow as tf
-
-from .configuration_distilbert import DistilBertConfig
-from .file_utils import add_start_docstrings, add_start_docstrings_to_callable
-from .modeling_tf_utils import TFPreTrainedModel, TFSharedEmbeddings, get_initializer, shape_list
-
-
-logger = logging.getLogger(__name__)
-
-
-TF_DISTILBERT_PRETRAINED_MODEL_ARCHIVE_MAP = {
- "distilbert-base-uncased": "https://s3.amazonaws.com/models.huggingface.co/bert/distilbert-base-uncased-tf_model.h5",
- "distilbert-base-uncased-distilled-squad": "https://s3.amazonaws.com/models.huggingface.co/bert/distilbert-base-uncased-distilled-squad-tf_model.h5",
- "distilbert-base-multilingual-cased": "https://s3.amazonaws.com/models.huggingface.co/bert/distilbert-base-multilingual-cased-tf_model.h5",
- "distilbert-base-uncased-finetuned-sst-2-english": "https://s3.amazonaws.com/models.huggingface.co/bert/distilbert-base-uncased-finetuned-sst-2-english-tf_model.h5",
-}
-
-
-# UTILS AND BUILDING BLOCKS OF THE ARCHITECTURE #
-def gelu(x):
- """ Gaussian Error Linear Unit.
- Original Implementation of the gelu activation function in Google Bert repo when initially created.
- For information: OpenAI GPT's gelu is slightly different (and gives slightly different results):
- 0.5 * x * (1 + torch.tanh(math.sqrt(2 / math.pi) * (x + 0.044715 * torch.pow(x, 3))))
- Also see https://arxiv.org/abs/1606.08415
- """
- cdf = 0.5 * (1.0 + tf.math.erf(x / tf.math.sqrt(2.0)))
- return x * cdf
-
-
-def gelu_new(x):
- """Gaussian Error Linear Unit.
- This is a smoother version of the RELU.
- Original paper: https://arxiv.org/abs/1606.08415
- Args:
- x: float Tensor to perform activation.
- Returns:
- `x` with the GELU activation applied.
- """
- cdf = 0.5 * (1.0 + tf.tanh((np.sqrt(2 / np.pi) * (x + 0.044715 * tf.pow(x, 3)))))
- return x * cdf
-
-
-class TFEmbeddings(tf.keras.layers.Layer):
- def __init__(self, config, **kwargs):
- super().__init__(**kwargs)
- self.vocab_size = config.vocab_size
- self.dim = config.dim
- self.initializer_range = config.initializer_range
- self.word_embeddings = TFSharedEmbeddings(
- config.vocab_size, config.dim, initializer_range=config.initializer_range, name="word_embeddings"
- ) # padding_idx=0)
- self.position_embeddings = tf.keras.layers.Embedding(
- config.max_position_embeddings,
- config.dim,
- embeddings_initializer=get_initializer(config.initializer_range),
- name="position_embeddings",
- )
- if config.sinusoidal_pos_embds:
- raise NotImplementedError
-
- self.LayerNorm = tf.keras.layers.LayerNormalization(epsilon=1e-12, name="LayerNorm")
- self.dropout = tf.keras.layers.Dropout(config.dropout)
-
- def build(self, input_shape):
- """Build shared word embedding layer """
- with tf.name_scope("word_embeddings"):
- # Create and initialize weights. The random normal initializer was chosen
- # arbitrarily, and works well.
- self.word_embeddings = self.add_weight(
- "weight", shape=[self.vocab_size, self.dim], initializer=get_initializer(self.initializer_range)
- )
- super().build(input_shape)
-
- def call(self, inputs, inputs_embeds=None, mode="embedding", training=False):
- """Get token embeddings of inputs.
- Args:
- inputs: list of three int64 tensors with shape [batch_size, length]: (input_ids, position_ids, token_type_ids)
- mode: string, a valid value is one of "embedding" and "linear".
- Returns:
- outputs: (1) If mode == "embedding", output embedding tensor, float32 with
- shape [batch_size, length, embedding_size]; (2) mode == "linear", output
- linear tensor, float32 with shape [batch_size, length, vocab_size].
- Raises:
- ValueError: if mode is not valid.
-
- Shared weights logic adapted from
- https://github.com/tensorflow/models/blob/a009f4fb9d2fc4949e32192a944688925ef78659/official/transformer/v2/embedding_layer.py#L24
- """
- if mode == "embedding":
- return self._embedding(inputs, inputs_embeds=inputs_embeds, training=training)
- elif mode == "linear":
- return self._linear(inputs)
- else:
- raise ValueError("mode {} is not valid.".format(mode))
-
- def _embedding(self, inputs, inputs_embeds=None, training=False):
- """
- Parameters
- ----------
- input_ids: tf.Tensor(bs, max_seq_length)
- The token ids to embed.
-
- Outputs
- -------
- embeddings: tf.Tensor(bs, max_seq_length, dim)
- The embedded tokens (plus position embeddings, no token_type embeddings)
- """
- if not isinstance(inputs, (tuple, list)):
- input_ids = inputs
- position_ids = None
- else:
- input_ids, position_ids = inputs
-
- if input_ids is not None:
- seq_length = shape_list(input_ids)[1]
- else:
- seq_length = shape_list(inputs_embeds)[1]
-
- if position_ids is None:
- position_ids = tf.range(seq_length, dtype=tf.int32)[tf.newaxis, :]
-
- if inputs_embeds is None:
- inputs_embeds = tf.gather(self.word_embeddings, input_ids)
- position_embeddings = self.position_embeddings(position_ids) # (bs, max_seq_length, dim)
-
- embeddings = inputs_embeds + position_embeddings # (bs, max_seq_length, dim)
- embeddings = self.LayerNorm(embeddings) # (bs, max_seq_length, dim)
- embeddings = self.dropout(embeddings, training=training) # (bs, max_seq_length, dim)
- return embeddings
-
- def _linear(self, inputs):
- """Computes logits by running inputs through a linear layer.
- Args:
- inputs: A float32 tensor with shape [batch_size, length, hidden_size]
- Returns:
- float32 tensor with shape [batch_size, length, vocab_size].
- """
- batch_size = shape_list(inputs)[0]
- length = shape_list(inputs)[1]
-
- x = tf.reshape(inputs, [-1, self.dim])
- logits = tf.matmul(x, self.word_embeddings, transpose_b=True)
-
- return tf.reshape(logits, [batch_size, length, self.vocab_size])
-
-
-class TFMultiHeadSelfAttention(tf.keras.layers.Layer):
- def __init__(self, config, **kwargs):
- super().__init__(**kwargs)
-
- self.n_heads = config.n_heads
- self.dim = config.dim
- self.dropout = tf.keras.layers.Dropout(config.attention_dropout)
- self.output_attentions = config.output_attentions
-
- assert self.dim % self.n_heads == 0
-
- self.q_lin = tf.keras.layers.Dense(
- config.dim, kernel_initializer=get_initializer(config.initializer_range), name="q_lin"
- )
- self.k_lin = tf.keras.layers.Dense(
- config.dim, kernel_initializer=get_initializer(config.initializer_range), name="k_lin"
- )
- self.v_lin = tf.keras.layers.Dense(
- config.dim, kernel_initializer=get_initializer(config.initializer_range), name="v_lin"
- )
- self.out_lin = tf.keras.layers.Dense(
- config.dim, kernel_initializer=get_initializer(config.initializer_range), name="out_lin"
- )
-
- self.pruned_heads = set()
-
- def prune_heads(self, heads):
- raise NotImplementedError
-
- def call(self, inputs, training=False):
- """
- Parameters
- ----------
- query: tf.Tensor(bs, seq_length, dim)
- key: tf.Tensor(bs, seq_length, dim)
- value: tf.Tensor(bs, seq_length, dim)
- mask: tf.Tensor(bs, seq_length)
-
- Outputs
- -------
- weights: tf.Tensor(bs, n_heads, seq_length, seq_length)
- Attention weights
- context: tf.Tensor(bs, seq_length, dim)
- Contextualized layer. Optional: only if `output_attentions=True`
- """
- query, key, value, mask, head_mask = inputs
- bs, q_length, dim = shape_list(query)
- k_length = shape_list(key)[1]
- # assert dim == self.dim, 'Dimensions do not match: %s input vs %s configured' % (dim, self.dim)
- # assert key.size() == value.size()
-
- dim_per_head = self.dim // self.n_heads
-
- mask_reshape = [bs, 1, 1, k_length]
-
- def shape(x):
- """ separate heads """
- return tf.transpose(tf.reshape(x, (bs, -1, self.n_heads, dim_per_head)), perm=(0, 2, 1, 3))
-
- def unshape(x):
- """ group heads """
- return tf.reshape(tf.transpose(x, perm=(0, 2, 1, 3)), (bs, -1, self.n_heads * dim_per_head))
-
- q = shape(self.q_lin(query)) # (bs, n_heads, q_length, dim_per_head)
- k = shape(self.k_lin(key)) # (bs, n_heads, k_length, dim_per_head)
- v = shape(self.v_lin(value)) # (bs, n_heads, k_length, dim_per_head)
-
- q = q / math.sqrt(dim_per_head) # (bs, n_heads, q_length, dim_per_head)
- scores = tf.matmul(q, k, transpose_b=True) # (bs, n_heads, q_length, k_length)
- mask = tf.reshape(mask, mask_reshape) # (bs, n_heads, qlen, klen)
- # scores.masked_fill_(mask, -float('inf')) # (bs, n_heads, q_length, k_length)
- scores = scores - 1e30 * (1.0 - mask)
-
- weights = tf.nn.softmax(scores, axis=-1) # (bs, n_heads, qlen, klen)
- weights = self.dropout(weights, training=training) # (bs, n_heads, qlen, klen)
-
- # Mask heads if we want to
- if head_mask is not None:
- weights = weights * head_mask
-
- context = tf.matmul(weights, v) # (bs, n_heads, qlen, dim_per_head)
- context = unshape(context) # (bs, q_length, dim)
- context = self.out_lin(context) # (bs, q_length, dim)
-
- if self.output_attentions:
- return (context, weights)
- else:
- return (context,)
-
-
-class TFFFN(tf.keras.layers.Layer):
- def __init__(self, config, **kwargs):
- super().__init__(**kwargs)
- self.dropout = tf.keras.layers.Dropout(config.dropout)
- self.lin1 = tf.keras.layers.Dense(
- config.hidden_dim, kernel_initializer=get_initializer(config.initializer_range), name="lin1"
- )
- self.lin2 = tf.keras.layers.Dense(
- config.dim, kernel_initializer=get_initializer(config.initializer_range), name="lin2"
- )
- assert config.activation in ["relu", "gelu"], "activation ({}) must be in ['relu', 'gelu']".format(
- config.activation
- )
- self.activation = (
- tf.keras.layers.Activation(gelu) if config.activation == "gelu" else tf.keras.activations.relu
- )
-
- def call(self, input, training=False):
- x = self.lin1(input)
- x = self.activation(x)
- x = self.lin2(x)
- x = self.dropout(x, training=training)
- return x
-
-
-class TFTransformerBlock(tf.keras.layers.Layer):
- def __init__(self, config, **kwargs):
- super().__init__(**kwargs)
-
- self.n_heads = config.n_heads
- self.dim = config.dim
- self.hidden_dim = config.hidden_dim
- self.dropout = tf.keras.layers.Dropout(config.dropout)
- self.activation = config.activation
- self.output_attentions = config.output_attentions
-
- assert config.dim % config.n_heads == 0
-
- self.attention = TFMultiHeadSelfAttention(config, name="attention")
- self.sa_layer_norm = tf.keras.layers.LayerNormalization(epsilon=1e-12, name="sa_layer_norm")
-
- self.ffn = TFFFN(config, name="ffn")
- self.output_layer_norm = tf.keras.layers.LayerNormalization(epsilon=1e-12, name="output_layer_norm")
-
- def call(self, inputs, training=False): # removed: src_enc=None, src_len=None
- """
- Parameters
- ----------
- x: tf.Tensor(bs, seq_length, dim)
- attn_mask: tf.Tensor(bs, seq_length)
-
- Outputs
- -------
- sa_weights: tf.Tensor(bs, n_heads, seq_length, seq_length)
- The attention weights
- ffn_output: tf.Tensor(bs, seq_length, dim)
- The output of the transformer block contextualization.
- """
- x, attn_mask, head_mask = inputs
-
- # Self-Attention
- sa_output = self.attention([x, x, x, attn_mask, head_mask], training=training)
- if self.output_attentions:
- sa_output, sa_weights = sa_output # (bs, seq_length, dim), (bs, n_heads, seq_length, seq_length)
- else: # To handle these `output_attention` or `output_hidden_states` cases returning tuples
- # assert type(sa_output) == tuple
- sa_output = sa_output[0]
- sa_output = self.sa_layer_norm(sa_output + x) # (bs, seq_length, dim)
-
- # Feed Forward Network
- ffn_output = self.ffn(sa_output, training=training) # (bs, seq_length, dim)
- ffn_output = self.output_layer_norm(ffn_output + sa_output) # (bs, seq_length, dim)
-
- output = (ffn_output,)
- if self.output_attentions:
- output = (sa_weights,) + output
- return output
-
-
-class TFTransformer(tf.keras.layers.Layer):
- def __init__(self, config, **kwargs):
- super().__init__(**kwargs)
- self.n_layers = config.n_layers
- self.output_attentions = config.output_attentions
- self.output_hidden_states = config.output_hidden_states
-
- self.layer = [TFTransformerBlock(config, name="layer_._{}".format(i)) for i in range(config.n_layers)]
-
- def call(self, inputs, training=False):
- """
- Parameters
- ----------
- x: tf.Tensor(bs, seq_length, dim)
- Input sequence embedded.
- attn_mask: tf.Tensor(bs, seq_length)
- Attention mask on the sequence.
-
- Outputs
- -------
- hidden_state: tf.Tensor(bs, seq_length, dim)
- Sequence of hiddens states in the last (top) layer
- all_hidden_states: Tuple[tf.Tensor(bs, seq_length, dim)]
- Tuple of length n_layers with the hidden states from each layer.
- Optional: only if output_hidden_states=True
- all_attentions: Tuple[tf.Tensor(bs, n_heads, seq_length, seq_length)]
- Tuple of length n_layers with the attention weights from each layer
- Optional: only if output_attentions=True
- """
- x, attn_mask, head_mask = inputs
-
- all_hidden_states = ()
- all_attentions = ()
-
- hidden_state = x
- for i, layer_module in enumerate(self.layer):
- if self.output_hidden_states:
- all_hidden_states = all_hidden_states + (hidden_state,)
-
- layer_outputs = layer_module([hidden_state, attn_mask, head_mask[i]], training=training)
- hidden_state = layer_outputs[-1]
-
- if self.output_attentions:
- assert len(layer_outputs) == 2
- attentions = layer_outputs[0]
- all_attentions = all_attentions + (attentions,)
- else:
- assert len(layer_outputs) == 1
-
- # Add last layer
- if self.output_hidden_states:
- all_hidden_states = all_hidden_states + (hidden_state,)
-
- outputs = (hidden_state,)
- if self.output_hidden_states:
- outputs = outputs + (all_hidden_states,)
- if self.output_attentions:
- outputs = outputs + (all_attentions,)
- return outputs # last-layer hidden state, (all hidden states), (all attentions)
-
-
-class TFDistilBertMainLayer(tf.keras.layers.Layer):
- def __init__(self, config, **kwargs):
- super().__init__(**kwargs)
- self.num_hidden_layers = config.num_hidden_layers
-
- self.embeddings = TFEmbeddings(config, name="embeddings") # Embeddings
- self.transformer = TFTransformer(config, name="transformer") # Encoder
-
- def get_input_embeddings(self):
- return self.embeddings
-
- def _resize_token_embeddings(self, new_num_tokens):
- raise NotImplementedError
-
- def _prune_heads(self, heads_to_prune):
- raise NotImplementedError
-
- def call(self, inputs, attention_mask=None, head_mask=None, inputs_embeds=None, training=False):
- if isinstance(inputs, (tuple, list)):
- input_ids = inputs[0]
- attention_mask = inputs[1] if len(inputs) > 1 else attention_mask
- head_mask = inputs[2] if len(inputs) > 2 else head_mask
- inputs_embeds = inputs[3] if len(inputs) > 3 else inputs_embeds
- assert len(inputs) <= 4, "Too many inputs."
- elif isinstance(inputs, dict):
- input_ids = inputs.get("input_ids")
- attention_mask = inputs.get("attention_mask", attention_mask)
- head_mask = inputs.get("head_mask", head_mask)
- inputs_embeds = inputs.get("inputs_embeds", inputs_embeds)
- assert len(inputs) <= 4, "Too many inputs."
- else:
- input_ids = inputs
-
- if input_ids is not None and inputs_embeds is not None:
- raise ValueError("You cannot specify both input_ids and inputs_embeds at the same time")
- elif input_ids is not None:
- input_shape = shape_list(input_ids)
- elif inputs_embeds is not None:
- input_shape = shape_list(inputs_embeds)[:-1]
- else:
- raise ValueError("You have to specify either input_ids or inputs_embeds")
-
- if attention_mask is None:
- attention_mask = tf.ones(input_shape) # (bs, seq_length)
- attention_mask = tf.cast(attention_mask, dtype=tf.float32)
-
- # Prepare head mask if needed
- # 1.0 in head_mask indicate we keep the head
- # attention_probs has shape bsz x n_heads x N x N
- # input head_mask has shape [num_heads] or [num_hidden_layers x num_heads]
- # and head_mask is converted to shape [num_hidden_layers x batch x num_heads x seq_length x seq_length]
- if head_mask is not None:
- raise NotImplementedError
- else:
- head_mask = [None] * self.num_hidden_layers
-
- embedding_output = self.embeddings(input_ids, inputs_embeds=inputs_embeds) # (bs, seq_length, dim)
- tfmr_output = self.transformer([embedding_output, attention_mask, head_mask], training=training)
-
- return tfmr_output # last-layer hidden-state, (all hidden_states), (all attentions)
-
-
-# INTERFACE FOR ENCODER AND TASK SPECIFIC MODEL #
-class TFDistilBertPreTrainedModel(TFPreTrainedModel):
- """ An abstract class to handle weights initialization and
- a simple interface for downloading and loading pretrained models.
- """
-
- config_class = DistilBertConfig
- pretrained_model_archive_map = TF_DISTILBERT_PRETRAINED_MODEL_ARCHIVE_MAP
- base_model_prefix = "distilbert"
-
-
-DISTILBERT_START_DOCSTRING = r"""
- This model is a `tf.keras.Model `__ sub-class.
- Use it as a regular TF 2.0 Keras Model and
- refer to the TF 2.0 documentation for all matter related to general usage and behavior.
-
- .. note::
-
- TF 2.0 models accepts two formats as inputs:
-
- - having all inputs as keyword arguments (like PyTorch models), or
- - having all inputs as a list, tuple or dict in the first positional arguments.
-
- This second option is useful when using :obj:`tf.keras.Model.fit()` method which currently requires having
- all the tensors in the first argument of the model call function: :obj:`model(inputs)`.
-
- If you choose this second option, there are three possibilities you can use to gather all the input Tensors
- in the first positional argument :
-
- - a single Tensor with input_ids only and nothing else: :obj:`model(inputs_ids)`
- - a list of varying length with one or several input Tensors IN THE ORDER given in the docstring:
- :obj:`model([input_ids, attention_mask])` or :obj:`model([input_ids, attention_mask, token_type_ids])`
- - a dictionary with one or several input Tensors associated to the input names given in the docstring:
- :obj:`model({'input_ids': input_ids, 'token_type_ids': token_type_ids})`
-
- Parameters:
- config (:class:`~transformers.DistilBertConfig`): Model configuration class with all the parameters of the model.
- Initializing with a config file does not load the weights associated with the model, only the configuration.
- Check out the :meth:`~transformers.PreTrainedModel.from_pretrained` method to load the model weights.
-"""
-
-DISTILBERT_INPUTS_DOCSTRING = r"""
- Args:
- input_ids (:obj:`Numpy array` or :obj:`tf.Tensor` of shape :obj:`(batch_size, sequence_length)`):
- Indices of input sequence tokens in the vocabulary.
-
- Indices can be obtained using :class:`transformers.BertTokenizer`.
- See :func:`transformers.PreTrainedTokenizer.encode` and
- :func:`transformers.PreTrainedTokenizer.encode_plus` for details.
-
- `What are input IDs? <../glossary.html#input-ids>`__
- attention_mask (:obj:`Numpy array` or :obj:`tf.Tensor` of shape :obj:`(batch_size, sequence_length)`, `optional`, defaults to :obj:`None`):
- Mask to avoid performing attention on padding token indices.
- Mask values selected in ``[0, 1]``:
- ``1`` for tokens that are NOT MASKED, ``0`` for MASKED tokens.
-
- `What are attention masks? <../glossary.html#attention-mask>`__
- head_mask (:obj:`Numpy array` or :obj:`tf.Tensor` of shape :obj:`(num_heads,)` or :obj:`(num_layers, num_heads)`, `optional`, defaults to :obj:`None`):
- Mask to nullify selected heads of the self-attention modules.
- Mask values selected in ``[0, 1]``:
- :obj:`1` indicates the head is **not masked**, :obj:`0` indicates the head is **masked**.
- inputs_embeds (:obj:`Numpy array` or :obj:`tf.Tensor` of shape :obj:`(batch_size, sequence_length, embedding_dim)`, `optional`, defaults to :obj:`None`):
- Optionally, instead of passing :obj:`input_ids` you can choose to directly pass an embedded representation.
- This is useful if you want more control over how to convert `input_ids` indices into associated vectors
- than the model's internal embedding lookup matrix.
- training (:obj:`boolean`, `optional`, defaults to :obj:`False`):
- Whether to activate dropout modules (if set to :obj:`True`) during training or to de-activate them
- (if set to :obj:`False`) for evaluation.
-
-"""
-
-
-@add_start_docstrings(
- "The bare DistilBERT encoder/transformer outputing raw hidden-states without any specific head on top.",
- DISTILBERT_START_DOCSTRING,
-)
-class TFDistilBertModel(TFDistilBertPreTrainedModel):
- def __init__(self, config, *inputs, **kwargs):
- super().__init__(config, *inputs, **kwargs)
- self.distilbert = TFDistilBertMainLayer(config, name="distilbert") # Embeddings
-
- @add_start_docstrings_to_callable(DISTILBERT_INPUTS_DOCSTRING)
- def call(self, inputs, **kwargs):
- r"""
- Returns:
- :obj:`tuple(torch.FloatTensor)` comprising various elements depending on the configuration (:class:`~transformers,DistilBertConfig`) and inputs:
- last_hidden_state (:obj:`tf.Tensor` of shape :obj:`(batch_size, sequence_length, hidden_size)`):
- Sequence of hidden-states at the output of the last layer of the model.
- hidden_states (:obj:`tuple(tf.Tensor)`, `optional`, returned when :obj:`config.output_hidden_states=True`):
- tuple of :obj:`tf.Tensor` (one for the output of the embeddings + one for the output of each layer)
- of shape :obj:`(batch_size, sequence_length, hidden_size)`.
-
- Hidden-states of the model at the output of each layer plus the initial embedding outputs.
- attentions (:obj:`tuple(tf.Tensor)`, `optional`, returned when ``config.output_attentions=True``):
- tuple of :obj:`tf.Tensor` (one for each layer) of shape
- :obj:`(batch_size, num_heads, sequence_length, sequence_length)`:
-
- Attentions weights after the attention softmax, used to compute the weighted average in the self-attention heads.
-
- Examples::
-
- import tensorflow as tf
- from transformers import DistilBertTokenizer, TFDistilBertModel
-
- tokenizer = DistilBertTokenizer.from_pretrained('distilbert-base-uncased')
- model = TFDistilBertModel.from_pretrained('distilbert-base-uncased')
- input_ids = tf.constant(tokenizer.encode("Hello, my dog is cute"))[None, :] # Batch size 1
- outputs = model(input_ids)
- last_hidden_states = outputs[0] # The last hidden-state is the first element of the output tuple
- """
- outputs = self.distilbert(inputs, **kwargs)
- return outputs
-
-
-class TFDistilBertLMHead(tf.keras.layers.Layer):
- def __init__(self, config, input_embeddings, **kwargs):
- super().__init__(**kwargs)
- self.vocab_size = config.vocab_size
-
- # The output weights are the same as the input embeddings, but there is
- # an output-only bias for each token.
- self.input_embeddings = input_embeddings
-
- def build(self, input_shape):
- self.bias = self.add_weight(shape=(self.vocab_size,), initializer="zeros", trainable=True, name="bias")
- super().build(input_shape)
-
- def call(self, hidden_states):
- hidden_states = self.input_embeddings(hidden_states, mode="linear")
- hidden_states = hidden_states + self.bias
- return hidden_states
-
-
-@add_start_docstrings(
- """DistilBert Model with a `masked language modeling` head on top. """, DISTILBERT_START_DOCSTRING,
-)
-class TFDistilBertForMaskedLM(TFDistilBertPreTrainedModel):
- def __init__(self, config, *inputs, **kwargs):
- super().__init__(config, *inputs, **kwargs)
- self.output_attentions = config.output_attentions
- self.output_hidden_states = config.output_hidden_states
- self.vocab_size = config.vocab_size
-
- self.distilbert = TFDistilBertMainLayer(config, name="distilbert")
- self.vocab_transform = tf.keras.layers.Dense(
- config.dim, kernel_initializer=get_initializer(config.initializer_range), name="vocab_transform"
- )
- self.act = tf.keras.layers.Activation(gelu)
- self.vocab_layer_norm = tf.keras.layers.LayerNormalization(epsilon=1e-12, name="vocab_layer_norm")
- self.vocab_projector = TFDistilBertLMHead(config, self.distilbert.embeddings, name="vocab_projector")
-
- def get_output_embeddings(self):
- return self.vocab_projector.input_embeddings
-
- @add_start_docstrings_to_callable(DISTILBERT_INPUTS_DOCSTRING)
- def call(self, inputs, **kwargs):
- r"""
-
- Returns:
- :obj:`tuple(torch.FloatTensor)` comprising various elements depending on the configuration (:class:`~transformers,DistilBertConfig`) and inputs:
- prediction_scores (:obj:`Numpy array` or :obj:`tf.Tensor` of shape :obj:`(batch_size, sequence_length, config.vocab_size)`):
- Prediction scores of the language modeling head (scores for each vocabulary token before SoftMax).
- hidden_states (:obj:`tuple(tf.Tensor)`, `optional`, returned when :obj:`config.output_hidden_states=True`):
- tuple of :obj:`tf.Tensor` (one for the output of the embeddings + one for the output of each layer)
- of shape :obj:`(batch_size, sequence_length, hidden_size)`.
-
- Hidden-states of the model at the output of each layer plus the initial embedding outputs.
- attentions (:obj:`tuple(tf.Tensor)`, `optional`, returned when ``config.output_attentions=True``):
- tuple of :obj:`tf.Tensor` (one for each layer) of shape
- :obj:`(batch_size, num_heads, sequence_length, sequence_length)`:
-
- Attentions weights after the attention softmax, used to compute the weighted average in the self-attention heads.
-
- Examples::
-
- import tensorflow as tf
- from transformers import DistilBertTokenizer, TFDistilBertForMaskedLM
-
- tokenizer = DistilBertTokenizer.from_pretrained('distilbert-base-uncased')
- model = TFDistilBertForMaskedLM.from_pretrained('distilbert-base-uncased')
- input_ids = tf.constant(tokenizer.encode("Hello, my dog is cute"))[None, :] # Batch size 1
- outputs = model(input_ids)
- prediction_scores = outputs[0]
-
- """
- distilbert_output = self.distilbert(inputs, **kwargs)
-
- hidden_states = distilbert_output[0] # (bs, seq_length, dim)
- prediction_logits = self.vocab_transform(hidden_states) # (bs, seq_length, dim)
- prediction_logits = self.act(prediction_logits) # (bs, seq_length, dim)
- prediction_logits = self.vocab_layer_norm(prediction_logits) # (bs, seq_length, dim)
- prediction_logits = self.vocab_projector(prediction_logits)
-
- outputs = (prediction_logits,) + distilbert_output[1:]
- return outputs # logits, (hidden_states), (attentions)
-
-
-@add_start_docstrings(
- """DistilBert Model transformer with a sequence classification/regression head on top (a linear layer on top of
- the pooled output) e.g. for GLUE tasks. """,
- DISTILBERT_START_DOCSTRING,
-)
-class TFDistilBertForSequenceClassification(TFDistilBertPreTrainedModel):
- def __init__(self, config, *inputs, **kwargs):
- super().__init__(config, *inputs, **kwargs)
- self.num_labels = config.num_labels
-
- self.distilbert = TFDistilBertMainLayer(config, name="distilbert")
- self.pre_classifier = tf.keras.layers.Dense(
- config.dim,
- kernel_initializer=get_initializer(config.initializer_range),
- activation="relu",
- name="pre_classifier",
- )
- self.classifier = tf.keras.layers.Dense(
- config.num_labels, kernel_initializer=get_initializer(config.initializer_range), name="classifier"
- )
- self.dropout = tf.keras.layers.Dropout(config.seq_classif_dropout)
-
- @add_start_docstrings_to_callable(DISTILBERT_INPUTS_DOCSTRING)
- def call(self, inputs, **kwargs):
- r"""
- Returns:
- :obj:`tuple(torch.FloatTensor)` comprising various elements depending on the configuration (:class:`~transformers,DistilBertConfig`) and inputs:
- logits (:obj:`Numpy array` or :obj:`tf.Tensor` of shape :obj:`(batch_size, config.num_labels)`):
- Classification (or regression if config.num_labels==1) scores (before SoftMax).
- hidden_states (:obj:`tuple(tf.Tensor)`, `optional`, returned when :obj:`config.output_hidden_states=True`):
- tuple of :obj:`tf.Tensor` (one for the output of the embeddings + one for the output of each layer)
- of shape :obj:`(batch_size, sequence_length, hidden_size)`.
-
- Hidden-states of the model at the output of each layer plus the initial embedding outputs.
- attentions (:obj:`tuple(tf.Tensor)`, `optional`, returned when ``config.output_attentions=True``):
- tuple of :obj:`tf.Tensor` (one for each layer) of shape
- :obj:`(batch_size, num_heads, sequence_length, sequence_length)`:
-
- Attentions weights after the attention softmax, used to compute the weighted average in the self-attention heads.
-
- Examples::
-
- import tensorflow as tf
- from transformers import DistilBertTokenizer, TFDistilBertForSequenceClassification
-
- tokenizer = DistilBertTokenizer.from_pretrained('distilbert-base-uncased')
- model = TFDistilBertForSequenceClassification.from_pretrained('distilbert-base-uncased')
- input_ids = tf.constant(tokenizer.encode("Hello, my dog is cute"))[None, :] # Batch size 1
- outputs = model(input_ids)
- logits = outputs[0]
-
- """
- distilbert_output = self.distilbert(inputs, **kwargs)
-
- hidden_state = distilbert_output[0] # (bs, seq_len, dim)
- pooled_output = hidden_state[:, 0] # (bs, dim)
- pooled_output = self.pre_classifier(pooled_output) # (bs, dim)
- pooled_output = self.dropout(pooled_output, training=kwargs.get("training", False)) # (bs, dim)
- logits = self.classifier(pooled_output) # (bs, dim)
-
- outputs = (logits,) + distilbert_output[1:]
- return outputs # logits, (hidden_states), (attentions)
-
-
-@add_start_docstrings(
- """DistilBert Model with a token classification head on top (a linear layer on top of
- the hidden-states output) e.g. for Named-Entity-Recognition (NER) tasks. """,
- DISTILBERT_START_DOCSTRING,
-)
-class TFDistilBertForTokenClassification(TFDistilBertPreTrainedModel):
- def __init__(self, config, *inputs, **kwargs):
- super().__init__(config, *inputs, **kwargs)
- self.num_labels = config.num_labels
-
- self.distilbert = TFDistilBertMainLayer(config, name="distilbert")
- self.dropout = tf.keras.layers.Dropout(config.dropout)
- self.classifier = tf.keras.layers.Dense(
- config.num_labels, kernel_initializer=get_initializer(config.initializer_range), name="classifier"
- )
-
- @add_start_docstrings_to_callable(DISTILBERT_INPUTS_DOCSTRING)
- def call(self, inputs, **kwargs):
- r"""
- Returns:
- :obj:`tuple(torch.FloatTensor)` comprising various elements depending on the configuration (:class:`~transformers,DistilBertConfig`) and inputs:
- scores (:obj:`Numpy array` or :obj:`tf.Tensor` of shape :obj:`(batch_size, sequence_length, config.num_labels)`):
- Classification scores (before SoftMax).
- hidden_states (:obj:`tuple(tf.Tensor)`, `optional`, returned when :obj:`config.output_hidden_states=True`):
- tuple of :obj:`tf.Tensor` (one for the output of the embeddings + one for the output of each layer)
- of shape :obj:`(batch_size, sequence_length, hidden_size)`.
-
- Hidden-states of the model at the output of each layer plus the initial embedding outputs.
- attentions (:obj:`tuple(tf.Tensor)`, `optional`, returned when ``config.output_attentions=True``):
- tuple of :obj:`tf.Tensor` (one for each layer) of shape
- :obj:`(batch_size, num_heads, sequence_length, sequence_length)`:
-
- Attentions weights after the attention softmax, used to compute the weighted average in the self-attention heads.
-
- Examples::
-
- import tensorflow as tf
- from transformers import DistilBertTokenizer, TFDistilBertForTokenClassification
-
- tokenizer = DistilBertTokenizer.from_pretrained('distilbert-base-uncased')
- model = TFDistilBertForTokenClassification.from_pretrained('distilbert-base-uncased')
- input_ids = tf.constant(tokenizer.encode("Hello, my dog is cute"))[None, :] # Batch size 1
- outputs = model(input_ids)
- scores = outputs[0]
- """
- outputs = self.distilbert(inputs, **kwargs)
-
- sequence_output = outputs[0]
-
- sequence_output = self.dropout(sequence_output, training=kwargs.get("training", False))
- logits = self.classifier(sequence_output)
-
- outputs = (logits,) + outputs[2:] # add hidden states and attention if they are here
-
- return outputs # scores, (hidden_states), (attentions)
-
-
-@add_start_docstrings(
- """DistilBert Model with a span classification head on top for extractive question-answering tasks like SQuAD (a linear layers on top of
- the hidden-states output to compute `span start logits` and `span end logits`). """,
- DISTILBERT_START_DOCSTRING,
-)
-class TFDistilBertForQuestionAnswering(TFDistilBertPreTrainedModel):
- def __init__(self, config, *inputs, **kwargs):
- super().__init__(config, *inputs, **kwargs)
-
- self.distilbert = TFDistilBertMainLayer(config, name="distilbert")
- self.qa_outputs = tf.keras.layers.Dense(
- config.num_labels, kernel_initializer=get_initializer(config.initializer_range), name="qa_outputs"
- )
- assert config.num_labels == 2
- self.dropout = tf.keras.layers.Dropout(config.qa_dropout)
-
- @add_start_docstrings_to_callable(DISTILBERT_INPUTS_DOCSTRING)
- def call(self, inputs, **kwargs):
- r"""
- Return:
- :obj:`tuple(torch.FloatTensor)` comprising various elements depending on the configuration (:class:`~transformers,DistilBertConfig`) and inputs:
- start_scores (:obj:`Numpy array` or :obj:`tf.Tensor` of shape :obj:`(batch_size, sequence_length,)`):
- Span-start scores (before SoftMax).
- end_scores (:obj:`Numpy array` or :obj:`tf.Tensor` of shape :obj:`(batch_size, sequence_length,)`):
- Span-end scores (before SoftMax).
- hidden_states (:obj:`tuple(tf.Tensor)`, `optional`, returned when :obj:`config.output_hidden_states=True`):
- tuple of :obj:`tf.Tensor` (one for the output of the embeddings + one for the output of each layer)
- of shape :obj:`(batch_size, sequence_length, hidden_size)`.
-
- Hidden-states of the model at the output of each layer plus the initial embedding outputs.
- attentions (:obj:`tuple(tf.Tensor)`, `optional`, returned when ``config.output_attentions=True``):
- tuple of :obj:`tf.Tensor` (one for each layer) of shape
- :obj:`(batch_size, num_heads, sequence_length, sequence_length)`:
-
- Attentions weights after the attention softmax, used to compute the weighted average in the self-attention heads.
-
- Examples::
-
- import tensorflow as tf
- from transformers import DistilBertTokenizer, TFDistilBertForQuestionAnswering
-
- tokenizer = DistilBertTokenizer.from_pretrained('distilbert-base-uncased')
- model = TFDistilBertForQuestionAnswering.from_pretrained('distilbert-base-uncased')
- input_ids = tf.constant(tokenizer.encode("Hello, my dog is cute"))[None, :] # Batch size 1
- outputs = model(input_ids)
- start_scores, end_scores = outputs[:2]
-
- """
- distilbert_output = self.distilbert(inputs, **kwargs)
-
- hidden_states = distilbert_output[0] # (bs, max_query_len, dim)
- hidden_states = self.dropout(hidden_states, training=kwargs.get("training", False)) # (bs, max_query_len, dim)
- logits = self.qa_outputs(hidden_states) # (bs, max_query_len, 2)
- start_logits, end_logits = tf.split(logits, 2, axis=-1)
- start_logits = tf.squeeze(start_logits, axis=-1)
- end_logits = tf.squeeze(end_logits, axis=-1)
-
- outputs = (start_logits, end_logits,) + distilbert_output[1:]
- return outputs # start_logits, end_logits, (hidden_states), (attentions)
diff --git a/server/transformers/src/transformers/modeling_tf_gpt2.py b/server/transformers/src/transformers/modeling_tf_gpt2.py
deleted file mode 100644
index 11566609533684885c9c78c6738930ebc55ebfd7..0000000000000000000000000000000000000000
--- a/server/transformers/src/transformers/modeling_tf_gpt2.py
+++ /dev/null
@@ -1,694 +0,0 @@
-# coding=utf-8
-# Copyright 2018 The OpenAI Team Authors and HuggingFace Inc. team.
-# Copyright (c) 2018, NVIDIA CORPORATION. All rights reserved.
-#
-# Licensed under the Apache License, Version 2.0 (the "License");
-# you may not use this file except in compliance with the License.
-# You may obtain a copy of the License at
-#
-# http://www.apache.org/licenses/LICENSE-2.0
-#
-# Unless required by applicable law or agreed to in writing, software
-# distributed under the License is distributed on an "AS IS" BASIS,
-# WITHOUT WARRANTIES OR CONDITIONS OF ANY KIND, either express or implied.
-# See the License for the specific language governing permissions and
-# limitations under the License.
-""" TF 2.0 OpenAI GPT-2 model. """
-
-
-import logging
-
-import numpy as np
-import tensorflow as tf
-
-from .configuration_gpt2 import GPT2Config
-from .file_utils import add_start_docstrings, add_start_docstrings_to_callable
-from .modeling_tf_utils import (
- TFConv1D,
- TFPreTrainedModel,
- TFSequenceSummary,
- TFSharedEmbeddings,
- get_initializer,
- shape_list,
-)
-
-
-logger = logging.getLogger(__name__)
-
-TF_GPT2_PRETRAINED_MODEL_ARCHIVE_MAP = {
- "gpt2": "https://s3.amazonaws.com/models.huggingface.co/bert/gpt2-tf_model.h5",
- "gpt2-medium": "https://s3.amazonaws.com/models.huggingface.co/bert/gpt2-medium-tf_model.h5",
- "gpt2-large": "https://s3.amazonaws.com/models.huggingface.co/bert/gpt2-large-tf_model.h5",
- "distilgpt2": "https://s3.amazonaws.com/models.huggingface.co/bert/distilgpt2-tf_model.h5",
-}
-
-
-def gelu(x):
- """Gaussian Error Linear Unit.
- This is a smoother version of the RELU.
- Original paper: https://arxiv.org/abs/1606.08415
- Args:
- x: float Tensor to perform activation.
- Returns:
- `x` with the GELU activation applied.
- """
- cdf = 0.5 * (1.0 + tf.tanh((np.sqrt(2 / np.pi) * (x + 0.044715 * tf.pow(x, 3)))))
- return x * cdf
-
-
-class TFAttention(tf.keras.layers.Layer):
- def __init__(self, nx, n_ctx, config, scale=False, **kwargs):
- super().__init__(**kwargs)
- self.output_attentions = config.output_attentions
-
- n_state = nx # in Attention: n_state=768 (nx=n_embd)
- # [switch nx => n_state from Block to Attention to keep identical to TF implem]
- assert n_state % config.n_head == 0
- self.n_ctx = n_ctx
- self.n_head = config.n_head
- self.split_size = n_state
- self.scale = scale
-
- self.c_attn = TFConv1D(n_state * 3, nx, initializer_range=config.initializer_range, name="c_attn")
- self.c_proj = TFConv1D(n_state, nx, initializer_range=config.initializer_range, name="c_proj")
- self.attn_dropout = tf.keras.layers.Dropout(config.attn_pdrop)
- self.resid_dropout = tf.keras.layers.Dropout(config.resid_pdrop)
- self.pruned_heads = set()
-
- def prune_heads(self, heads):
- pass
-
- @staticmethod
- def causal_attention_mask(nd, ns, dtype):
- """1's in the lower triangle, counting from the lower right corner.
- Same as tf.matrix_band_part(tf.ones([nd, ns]), -1, ns-nd), but doesn't produce garbage on TPUs.
- """
- i = tf.range(nd)[:, None]
- j = tf.range(ns)
- m = i >= j - ns + nd
- return tf.cast(m, dtype)
-
- def _attn(self, inputs, training=False):
- q, k, v, attention_mask, head_mask = inputs
- # q, k, v have shape [batch, heads, sequence, features]
- w = tf.matmul(q, k, transpose_b=True)
- if self.scale:
- dk = tf.cast(shape_list(k)[-1], tf.float32) # scale attention_scores
- w = w / tf.math.sqrt(dk)
-
- # w has shape [batch, heads, dst_sequence, src_sequence], where information flows from src to dst.
- _, _, nd, ns = shape_list(w)
- b = self.causal_attention_mask(nd, ns, dtype=w.dtype)
- b = tf.reshape(b, [1, 1, nd, ns])
- w = w * b - 1e4 * (1 - b)
-
- if attention_mask is not None:
- # Apply the attention mask
- w = w + attention_mask
-
- w = tf.nn.softmax(w, axis=-1)
- w = self.attn_dropout(w, training=training)
-
- # Mask heads if we want to
- if head_mask is not None:
- w = w * head_mask
-
- outputs = [tf.matmul(w, v)]
- if self.output_attentions:
- outputs.append(w)
- return outputs
-
- def merge_heads(self, x):
- x = tf.transpose(x, [0, 2, 1, 3])
- x_shape = shape_list(x)
- new_x_shape = x_shape[:-2] + [x_shape[-2] * x_shape[-1]]
- return tf.reshape(x, new_x_shape)
-
- def split_heads(self, x):
- x_shape = shape_list(x)
- new_x_shape = x_shape[:-1] + [self.n_head, x_shape[-1] // self.n_head]
- x = tf.reshape(x, new_x_shape)
- return tf.transpose(x, (0, 2, 1, 3)) # (batch, head, seq_length, head_features)
-
- def call(self, inputs, training=False):
- x, layer_past, attention_mask, head_mask = inputs
-
- x = self.c_attn(x)
- query, key, value = tf.split(x, 3, axis=2)
- query = self.split_heads(query)
- key = self.split_heads(key)
- value = self.split_heads(value)
- if layer_past is not None:
- past_key, past_value = tf.unstack(layer_past, axis=1)
- key = tf.concat([past_key, key], axis=-2)
- value = tf.concat([past_value, value], axis=-2)
- present = tf.stack([key, value], axis=1)
-
- attn_outputs = self._attn([query, key, value, attention_mask, head_mask], training=training)
- a = attn_outputs[0]
-
- a = self.merge_heads(a)
- a = self.c_proj(a)
- a = self.resid_dropout(a, training=training)
-
- outputs = [a, present] + attn_outputs[1:]
- return outputs # a, present, (attentions)
-
-
-class TFMLP(tf.keras.layers.Layer):
- def __init__(self, n_state, config, **kwargs):
- super().__init__(**kwargs)
- nx = config.n_embd
- self.c_fc = TFConv1D(n_state, nx, initializer_range=config.initializer_range, name="c_fc")
- self.c_proj = TFConv1D(nx, n_state, initializer_range=config.initializer_range, name="c_proj")
- self.act = gelu
- self.dropout = tf.keras.layers.Dropout(config.resid_pdrop)
-
- def call(self, x, training=False):
- h = self.act(self.c_fc(x))
- h2 = self.c_proj(h)
- h2 = self.dropout(h2, training=training)
- return h2
-
-
-class TFBlock(tf.keras.layers.Layer):
- def __init__(self, n_ctx, config, scale=False, **kwargs):
- super().__init__(**kwargs)
- nx = config.n_embd
- self.ln_1 = tf.keras.layers.LayerNormalization(epsilon=config.layer_norm_epsilon, name="ln_1")
- self.attn = TFAttention(nx, n_ctx, config, scale, name="attn")
- self.ln_2 = tf.keras.layers.LayerNormalization(epsilon=config.layer_norm_epsilon, name="ln_2")
- self.mlp = TFMLP(4 * nx, config, name="mlp")
-
- def call(self, inputs, training=False):
- x, layer_past, attention_mask, head_mask = inputs
-
- a = self.ln_1(x)
- output_attn = self.attn([a, layer_past, attention_mask, head_mask], training=training)
- a = output_attn[0] # output_attn: a, present, (attentions)
- x = x + a
-
- m = self.ln_2(x)
- m = self.mlp(m, training=training)
- x = x + m
-
- outputs = [x] + output_attn[1:]
- return outputs # x, present, (attentions)
-
-
-class TFGPT2MainLayer(tf.keras.layers.Layer):
- def __init__(self, config, *inputs, **kwargs):
- super().__init__(config, *inputs, **kwargs)
- self.output_hidden_states = config.output_hidden_states
- self.output_attentions = config.output_attentions
- self.num_hidden_layers = config.n_layer
- self.vocab_size = config.vocab_size
- self.n_embd = config.n_embd
-
- self.wte = TFSharedEmbeddings(
- config.vocab_size, config.hidden_size, initializer_range=config.initializer_range, name="wte"
- )
- self.wpe = tf.keras.layers.Embedding(
- config.n_positions,
- config.n_embd,
- embeddings_initializer=get_initializer(config.initializer_range),
- name="wpe",
- )
- self.drop = tf.keras.layers.Dropout(config.embd_pdrop)
- self.h = [TFBlock(config.n_ctx, config, scale=True, name="h_._{}".format(i)) for i in range(config.n_layer)]
- self.ln_f = tf.keras.layers.LayerNormalization(epsilon=config.layer_norm_epsilon, name="ln_f")
-
- def get_input_embeddings(self):
- return self.wte
-
- def _resize_token_embeddings(self, new_num_tokens):
- raise NotImplementedError
-
- def _prune_heads(self, heads_to_prune):
- """ Prunes heads of the model.
- heads_to_prune: dict of {layer_num: list of heads to prune in this layer}
- """
- raise NotImplementedError
-
- def call(
- self,
- inputs,
- past=None,
- attention_mask=None,
- token_type_ids=None,
- position_ids=None,
- head_mask=None,
- inputs_embeds=None,
- training=False,
- ):
- if isinstance(inputs, (tuple, list)):
- input_ids = inputs[0]
- past = inputs[1] if len(inputs) > 1 else past
- attention_mask = inputs[2] if len(inputs) > 2 else attention_mask
- token_type_ids = inputs[3] if len(inputs) > 3 else token_type_ids
- position_ids = inputs[4] if len(inputs) > 4 else position_ids
- head_mask = inputs[5] if len(inputs) > 5 else head_mask
- inputs_embeds = inputs[6] if len(inputs) > 6 else inputs_embeds
- assert len(inputs) <= 7, "Too many inputs."
- elif isinstance(inputs, dict):
- input_ids = inputs.get("input_ids")
- past = inputs.get("past", past)
- attention_mask = inputs.get("attention_mask", attention_mask)
- token_type_ids = inputs.get("token_type_ids", token_type_ids)
- position_ids = inputs.get("position_ids", position_ids)
- head_mask = inputs.get("head_mask", head_mask)
- inputs_embeds = inputs.get("inputs_embeds", inputs_embeds)
- assert len(inputs) <= 7, "Too many inputs."
- else:
- input_ids = inputs
-
- if input_ids is not None and inputs_embeds is not None:
- raise ValueError("You cannot specify both input_ids and inputs_embeds at the same time")
- elif input_ids is not None:
- input_shape = shape_list(input_ids)
- input_ids = tf.reshape(input_ids, [-1, input_shape[-1]])
- elif inputs_embeds is not None:
- input_shape = shape_list(inputs_embeds)[:-1]
- else:
- raise ValueError("You have to specify either input_ids or inputs_embeds")
-
- if past is None:
- past_length = 0
- past = [None] * len(self.h)
- else:
- past_length = shape_list(past[0][0])[-2]
- if position_ids is None:
- position_ids = tf.range(past_length, input_shape[-1] + past_length, dtype=tf.int32)[tf.newaxis, :]
-
- if attention_mask is not None:
- # We create a 3D attention mask from a 2D tensor mask.
- # Sizes are [batch_size, 1, 1, to_seq_length]
- # So we can broadcast to [batch_size, num_heads, from_seq_length, to_seq_length]
- # this attention mask is more simple than the triangular masking of causal attention
- # used in OpenAI GPT, we just need to prepare the broadcast dimension here.
- attention_mask = attention_mask[:, tf.newaxis, tf.newaxis, :]
-
- # Since attention_mask is 1.0 for positions we want to attend and 0.0 for
- # masked positions, this operation will create a tensor which is 0.0 for
- # positions we want to attend and -10000.0 for masked positions.
- # Since we are adding it to the raw scores before the softmax, this is
- # effectively the same as removing these entirely.
-
- attention_mask = tf.cast(attention_mask, tf.float32)
- attention_mask = (1.0 - attention_mask) * -10000.0
- else:
- attention_mask = None
-
- # Prepare head mask if needed
- # 1.0 in head_mask indicate we keep the head
- # attention_probs has shape bsz x n_heads x N x N
- # input head_mask has shape [num_heads] or [num_hidden_layers x num_heads]
- # and head_mask is converted to shape [num_hidden_layers x batch x num_heads x seq_length x seq_length]
- if head_mask is not None:
- raise NotImplementedError
- else:
- head_mask = [None] * self.num_hidden_layers
- # head_mask = tf.constant([0] * self.num_hidden_layers)
-
- position_ids = tf.reshape(position_ids, [-1, shape_list(position_ids)[-1]])
-
- if inputs_embeds is None:
- inputs_embeds = self.wte(input_ids, mode="embedding")
- position_embeds = self.wpe(position_ids)
- if token_type_ids is not None:
- token_type_ids = tf.reshape(token_type_ids, [-1, shape_list(token_type_ids)[-1]])
- token_type_embeds = self.wte(token_type_ids, mode="embedding")
- else:
- token_type_embeds = 0
- hidden_states = inputs_embeds + position_embeds + token_type_embeds
- hidden_states = self.drop(hidden_states, training=training)
-
- output_shape = input_shape + [shape_list(hidden_states)[-1]]
-
- presents = ()
- all_attentions = []
- all_hidden_states = ()
- for i, (block, layer_past) in enumerate(zip(self.h, past)):
- if self.output_hidden_states:
- all_hidden_states = all_hidden_states + (tf.reshape(hidden_states, output_shape),)
-
- outputs = block([hidden_states, layer_past, attention_mask, head_mask[i]], training=training)
-
- hidden_states, present = outputs[:2]
- presents = presents + (present,)
-
- if self.output_attentions:
- all_attentions.append(outputs[2])
-
- hidden_states = self.ln_f(hidden_states)
-
- hidden_states = tf.reshape(hidden_states, output_shape)
- # Add last hidden state
- if self.output_hidden_states:
- all_hidden_states = all_hidden_states + (hidden_states,)
-
- outputs = (hidden_states, presents)
- if self.output_hidden_states:
- outputs = outputs + (all_hidden_states,)
- if self.output_attentions:
- # let the number of heads free (-1) so we can extract attention even after head pruning
- attention_output_shape = input_shape[:-1] + [-1] + shape_list(all_attentions[0])[-2:]
- all_attentions = tuple(tf.reshape(t, attention_output_shape) for t in all_attentions)
- outputs = outputs + (all_attentions,)
- return outputs # last hidden state, presents, (all hidden_states), (attentions)
-
-
-class TFGPT2PreTrainedModel(TFPreTrainedModel):
- """ An abstract class to handle weights initialization and
- a simple interface for downloading and loading pretrained models.
- """
-
- config_class = GPT2Config
- pretrained_model_archive_map = TF_GPT2_PRETRAINED_MODEL_ARCHIVE_MAP
- base_model_prefix = "transformer"
-
-
-GPT2_START_DOCSTRING = r"""
-
- .. note::
- TF 2.0 models accepts two formats as inputs:
-
- - having all inputs as keyword arguments (like PyTorch models), or
- - having all inputs as a list, tuple or dict in the first positional arguments.
-
- This second option is useful when using :obj:`tf.keras.Model.fit()` method which currently requires having
- all the tensors in the first argument of the model call function: :obj:`model(inputs)`.
-
- If you choose this second option, there are three possibilities you can use to gather all the input Tensors
- in the first positional argument :
-
- - a single Tensor with input_ids only and nothing else: :obj:`model(inputs_ids)`
- - a list of varying length with one or several input Tensors IN THE ORDER given in the docstring:
- :obj:`model([input_ids, attention_mask])` or :obj:`model([input_ids, attention_mask, token_type_ids])`
- - a dictionary with one or several input Tensors associated to the input names given in the docstring:
- :obj:`model({'input_ids': input_ids, 'token_type_ids': token_type_ids})`
-
- Parameters:
- config (:class:`~transformers.GPT2Config`): Model configuration class with all the parameters of the model.
- Initializing with a config file does not load the weights associated with the model, only the configuration.
- Check out the :meth:`~transformers.PreTrainedModel.from_pretrained` method to load the model weights.
-"""
-
-GPT2_INPUTS_DOCSTRING = r"""
- Args:
- input_ids (:obj:`Numpy array` or :obj:`tf.Tensor` of shape :obj:`(batch_size, sequence_length)`):
- Indices of input sequence tokens in the vocabulary.
-
- Indices can be obtained using :class:`transformers.GPT2Tokenizer`.
- See :func:`transformers.PreTrainedTokenizer.encode` and
- :func:`transformers.PreTrainedTokenizer.encode_plus` for details.
-
- `What are input IDs? <../glossary.html#input-ids>`__
- past (:obj:`List[tf.Tensor]` of length :obj:`config.n_layers`):
- Contains pre-computed hidden-states (key and values in the attention blocks) as computed by the model
- (see `past` output below). Can be used to speed up sequential decoding. The token ids which have their past given to this model
- should not be passed as input ids as they have already been computed.
- attention_mask (:obj:`tf.Tensor` or :obj:`Numpy array` of shape :obj:`(batch_size, sequence_length)`, `optional`, defaults to :obj:`None`):
- Mask to avoid performing attention on padding token indices.
- Mask values selected in ``[0, 1]``:
- ``1`` for tokens that are NOT MASKED, ``0`` for MASKED tokens.
-
- `What are attention masks? <../glossary.html#attention-mask>`__
- token_type_ids (:obj:`tf.Tensor` or :obj:`Numpy array` of shape :obj:`(batch_size, sequence_length)`, `optional`, defaults to :obj:`None`):
- Segment token indices to indicate first and second portions of the inputs.
- Indices are selected in ``[0, 1]``: ``0`` corresponds to a `sentence A` token, ``1``
- corresponds to a `sentence B` token
-
- `What are token type IDs? <../glossary.html#token-type-ids>`_
- position_ids (:obj:`tf.Tensor` or :obj:`Numpy array` of shape :obj:`(batch_size, sequence_length)`, `optional`, defaults to :obj:`None`):
- Indices of positions of each input sequence tokens in the position embeddings.
- Selected in the range ``[0, config.max_position_embeddings - 1]``.
-
- `What are position IDs? <../glossary.html#position-ids>`_
- head_mask (:obj:`tf.Tensor` or :obj:`Numpy array` of shape :obj:`(num_heads,)` or :obj:`(num_layers, num_heads)`, `optional`, defaults to :obj:`None`):
- Mask to nullify selected heads of the self-attention modules.
- Mask values selected in ``[0, 1]``:
- :obj:`1` indicates the head is **not masked**, :obj:`0` indicates the head is **masked**.
- input_embeds (:obj:`tf.Tensor` or :obj:`Numpy array` of shape :obj:`(batch_size, sequence_length, hidden_size)`, `optional`, defaults to :obj:`None`):
- Optionally, instead of passing :obj:`input_ids` you can choose to directly pass an embedded representation.
- This is useful if you want more control over how to convert `input_ids` indices into associated vectors
- than the model's internal embedding lookup matrix.
- training (:obj:`boolean`, `optional`, defaults to :obj:`False`):
- Whether to activate dropout modules (if set to :obj:`True`) during training or to de-activate them
- (if set to :obj:`False`) for evaluation.
-"""
-
-
-@add_start_docstrings(
- "The bare GPT2 Model transformer outputing raw hidden-states without any specific head on top.",
- GPT2_START_DOCSTRING,
-)
-class TFGPT2Model(TFGPT2PreTrainedModel):
- def __init__(self, config, *inputs, **kwargs):
- super().__init__(config, *inputs, **kwargs)
- self.transformer = TFGPT2MainLayer(config, name="transformer")
-
- @add_start_docstrings_to_callable(GPT2_INPUTS_DOCSTRING)
- def call(self, inputs, **kwargs):
- r"""
- Return:
- :obj:`tuple(tf.Tensor)` comprising various elements depending on the configuration (:class:`~transformers.GPT2Config`) and inputs:
- last_hidden_state (:obj:`tf.Tensor` of shape :obj:`(batch_size, sequence_length, hidden_size)`):
- Sequence of hidden-states at the last layer of the model.
- past (:obj:`List[tf.Tensor]` of length :obj:`config.n_layers` with each tensor of shape :obj:`(2, batch_size, num_heads, sequence_length, embed_size_per_head)`):
- Contains pre-computed hidden-states (key and values in the attention blocks).
- Can be used (see `past` input) to speed up sequential decoding. The token ids which have their past given to this model
- should not be passed as input ids as they have already been computed.
- hidden_states (:obj:`tuple(tf.Tensor)` `optional`, returned when ``config.output_hidden_states=True``):
- Tuple of :obj:`tf.Tensor` (one for the output of the embeddings + one for the output of each layer)
- of shape :obj:`(batch_size, sequence_length, hidden_size)`.
-
- Hidden-states of the model at the output of each layer plus the initial embedding outputs.
- attentions (:obj:`tuple(tf.Tensor)`, `optional`, returned when ``config.output_attentions=True``):
- Tuple of :obj:`tf.Tensor` (one for each layer) of shape
- :obj:`(batch_size, num_heads, sequence_length, sequence_length)`.
-
- Attentions weights after the attention softmax, used to compute the weighted average in the self-attention
- heads.
-
- Examples::
-
- import tensorflow as tf
- from transformers import GPT2Tokenizer, TFGPT2Model
-
- tokenizer = GPT2Tokenizer.from_pretrained('gpt2')
- model = TFGPT2Model.from_pretrained('gpt2')
- input_ids = tf.constant(tokenizer.encode("Hello, my dog is cute", add_special_tokens=True))[None, :] # Batch size 1
- outputs = model(input_ids)
- last_hidden_states = outputs[0] # The last hidden-state is the first element of the output tuple
-
- """
- outputs = self.transformer(inputs, **kwargs)
- return outputs
-
-
-@add_start_docstrings(
- """The GPT2 Model transformer with a language modeling head on top
- (linear layer with weights tied to the input embeddings). """,
- GPT2_START_DOCSTRING,
-)
-class TFGPT2LMHeadModel(TFGPT2PreTrainedModel):
- def __init__(self, config, *inputs, **kwargs):
- super().__init__(config, *inputs, **kwargs)
- self.transformer = TFGPT2MainLayer(config, name="transformer")
-
- def get_output_embeddings(self):
- return self.transformer.wte
-
- @add_start_docstrings_to_callable(GPT2_INPUTS_DOCSTRING)
- def call(self, inputs, **kwargs):
- r"""
- Return:
- :obj:`tuple(tf.Tensor)` comprising various elements depending on the configuration (:class:`~transformers.GPT2Config`) and inputs:
- prediction_scores (:obj:`tf.Tensor` of shape :obj:`(batch_size, sequence_length, config.vocab_size)`):
- Prediction scores of the language modeling head (scores for each vocabulary token before SoftMax).
- past (:obj:`List[tf.Tensor]` of length :obj:`config.n_layers` with each tensor of shape :obj:`(2, batch_size, num_heads, sequence_length, embed_size_per_head)`):
- Contains pre-computed hidden-states (key and values in the attention blocks).
- Can be used (see `past` input) to speed up sequential decoding. The token ids which have their past given to this model
- should not be passed as input ids as they have already been computed.
- hidden_states (:obj:`tuple(tf.Tensor)`, `optional`, returned when ``config.output_hidden_states=True``):
- Tuple of :obj:`tf.Tensor` (one for the output of the embeddings + one for the output of each layer)
- of shape :obj:`(batch_size, sequence_length, hidden_size)`.
-
- Hidden-states of the model at the output of each layer plus the initial embedding outputs.
- attentions (:obj:`tuple(tf.Tensor)`, `optional`, returned when ``config.output_attentions=True``):
- Tuple of :obj:`tf.Tensor` (one for each layer) of shape
- :obj:`(batch_size, num_heads, sequence_length, sequence_length)`.
-
- Attentions weights after the attention softmax, used to compute the weighted average in the self-attention
- heads.
-
- Examples::
-
- import tensorflow as tf
- from transformers import GPT2Tokenizer, TFGPT2LMHeadModel
-
- tokenizer = GPT2Tokenizer.from_pretrained('gpt2')
- model = TFGPT2LMHeadModel.from_pretrained('gpt2')
-
- input_ids = tf.constant(tokenizer.encode("Hello, my dog is cute", add_special_tokens=True))[None, :] # Batch size 1
- outputs = model(input_ids)
- logits = outputs[0]
-
- """
- transformer_outputs = self.transformer(inputs, **kwargs)
- hidden_states = transformer_outputs[0]
-
- lm_logits = self.transformer.wte(hidden_states, mode="linear")
-
- outputs = (lm_logits,) + transformer_outputs[1:]
-
- return outputs # lm_logits, presents, (all hidden_states), (attentions)
-
-
-@add_start_docstrings(
- """The GPT2 Model transformer with a language modeling and a multiple-choice classification
- head on top e.g. for RocStories/SWAG tasks. The two heads are two linear layers.
- The language modeling head has its weights tied to the input embeddings,
- the classification head takes as input the input of a specified classification token index in the input sequence).
-""",
- GPT2_START_DOCSTRING,
-)
-class TFGPT2DoubleHeadsModel(TFGPT2PreTrainedModel):
- def __init__(self, config, *inputs, **kwargs):
- super().__init__(config, *inputs, **kwargs)
- config.num_labels = 1
- self.transformer = TFGPT2MainLayer(config, name="transformer")
- self.multiple_choice_head = TFSequenceSummary(
- config, initializer_range=config.initializer_range, name="multiple_choice_head"
- )
-
- def get_output_embeddings(self):
- return self.transformer.wte
-
- @add_start_docstrings_to_callable(GPT2_INPUTS_DOCSTRING)
- def call(
- self,
- inputs,
- past=None,
- attention_mask=None,
- token_type_ids=None,
- position_ids=None,
- head_mask=None,
- inputs_embeds=None,
- mc_token_ids=None,
- training=False,
- ):
- r"""
- mc_token_ids (:obj:`tf.Tensor` or :obj:`Numpy array` of shape :obj:`(batch_size, num_choices)`, `optional`, default to index of the last token of the input)
- Index of the classification token in each input sequence.
- Selected in the range ``[0, input_ids.size(-1) - 1[``.
-
- Return:
- :obj:`tuple(tf.Tensor)` comprising various elements depending on the configuration (:class:`~transformers.GPT2Config`) and inputs:
- lm_prediction_scores (:obj:`tf.Tensor` of shape :obj:`(batch_size, num_choices, sequence_length, config.vocab_size)`):
- Prediction scores of the language modeling head (scores for each vocabulary token before SoftMax).
- mc_prediction_scores (:obj:`tf.Tensor` of shape :obj:`(batch_size, num_choices)`):
- Prediction scores of the multiple choice classification head (scores for each choice before SoftMax).
- past (:obj:`List[tf.Tensor]` of length :obj:`config.n_layers` with each tensor of shape :obj:`(2, batch_size, num_heads, sequence_length, embed_size_per_head)`):
- Contains pre-computed hidden-states (key and values in the attention blocks).
- Can be used (see `past` input) to speed up sequential decoding. The token ids which have their past given to this model
- should not be passed as input ids as they have already been computed.
- hidden_states (:obj:`tuple(tf.Tensor)`, `optional`, returned when ``config.output_hidden_states=True``):
- Tuple of :obj:`tf.Tensor` (one for the output of the embeddings + one for the output of each layer)
- of shape :obj:`(batch_size, sequence_length, hidden_size)`.
-
- Hidden-states of the model at the output of each layer plus the initial embedding outputs.
- attentions (:obj:`tuple(tf.Tensor)`, `optional`, returned when ``config.output_attentions=True``):
- Tuple of :obj:`tf.Tensor` (one for each layer) of shape
- :obj:`(batch_size, num_heads, sequence_length, sequence_length)`.
-
- Attentions weights after the attention softmax, used to compute the weighted average in the self-attention
- heads.
-
-
- Examples::
-
- # For example purposes. Not runnable.
- import tensorflow as tf
- from transformers import GPT2Tokenizer, TFGPT2DoubleHeadsModel
-
- tokenizer = GPT2Tokenizer.from_pretrained('gpt2')
- model = TFGPT2DoubleHeadsModel.from_pretrained('gpt2')
-
- # Add a [CLS] to the vocabulary (we should train it also!)
- # This option is currently not implemented in TF 2.0
- raise NotImplementedError
- tokenizer.add_special_tokens({'cls_token': '[CLS]'})
- model.resize_token_embeddings(len(tokenizer)) # Update the model embeddings with the new vocabulary size
- print(tokenizer.cls_token_id, len(tokenizer)) # The newly token the last token of the vocabulary
-
- choices = ["Hello, my dog is cute [CLS]", "Hello, my cat is cute [CLS]"]
- encoded_choices = [tokenizer.encode(s) for s in choices]
- cls_token_location = [tokens.index(tokenizer.cls_token_id) for tokens in encoded_choices]
-
- input_ids = tf.constant(encoded_choices)[None, :] # Batch size: 1, number of choices: 2
- mc_token_ids = tf.constant([cls_token_location]) # Batch size: 1
-
- outputs = model(input_ids, mc_token_ids=mc_token_ids)
- lm_prediction_scores, mc_prediction_scores = outputs[:2]
-
- """
- if isinstance(inputs, (tuple, list)):
- input_ids = inputs[0]
- past = inputs[1] if len(inputs) > 1 else past
- attention_mask = inputs[2] if len(inputs) > 2 else attention_mask
- token_type_ids = inputs[3] if len(inputs) > 3 else token_type_ids
- position_ids = inputs[4] if len(inputs) > 4 else position_ids
- head_mask = inputs[5] if len(inputs) > 5 else head_mask
- inputs_embeds = inputs[6] if len(inputs) > 6 else inputs_embeds
- mc_token_ids = inputs[7] if len(inputs) > 7 else mc_token_ids
- assert len(inputs) <= 8, "Too many inputs."
- elif isinstance(inputs, dict):
- input_ids = inputs.get("input_ids")
- past = inputs.get("past", past)
- attention_mask = inputs.get("attention_mask", attention_mask)
- token_type_ids = inputs.get("token_type_ids", token_type_ids)
- position_ids = inputs.get("position_ids", position_ids)
- head_mask = inputs.get("head_mask", head_mask)
- inputs_embeds = inputs.get("inputs_embeds", inputs_embeds)
- mc_token_ids = inputs.get("mc_token_ids", mc_token_ids)
- assert len(inputs) <= 8, "Too many inputs."
- else:
- input_ids = inputs
-
- if input_ids is not None:
- input_shapes = shape_list(input_ids)
- else:
- input_shapes = shape_list(inputs_embeds)[:-1]
-
- seq_length = input_shapes[-1]
-
- flat_input_ids = tf.reshape(input_ids, (-1, seq_length)) if input_ids is not None else None
- flat_attention_mask = tf.reshape(attention_mask, (-1, seq_length)) if attention_mask is not None else None
- flat_token_type_ids = tf.reshape(token_type_ids, (-1, seq_length)) if token_type_ids is not None else None
- flat_position_ids = tf.reshape(position_ids, (-1, seq_length)) if position_ids is not None else None
-
- flat_inputs = [
- flat_input_ids,
- past,
- flat_attention_mask,
- flat_token_type_ids,
- flat_position_ids,
- head_mask,
- inputs_embeds,
- ]
-
- transformer_outputs = self.transformer(flat_inputs, training=training)
- hidden_states = transformer_outputs[0]
-
- hidden_states = tf.reshape(hidden_states, input_shapes + shape_list(hidden_states)[-1:])
-
- lm_logits = self.transformer.wte(hidden_states, mode="linear")
- mc_logits = self.multiple_choice_head([hidden_states, mc_token_ids], training=training)
-
- mc_logits = tf.squeeze(mc_logits, axis=-1)
-
- outputs = (lm_logits, mc_logits) + transformer_outputs[1:]
-
- return outputs # lm logits, mc logits, presents, (all hidden_states), (attentions)
diff --git a/server/transformers/src/transformers/modeling_tf_openai.py b/server/transformers/src/transformers/modeling_tf_openai.py
deleted file mode 100644
index f04104db8352dfbd4f189572554b5a6c1cfa6b50..0000000000000000000000000000000000000000
--- a/server/transformers/src/transformers/modeling_tf_openai.py
+++ /dev/null
@@ -1,661 +0,0 @@
-# coding=utf-8
-# Copyright 2018 The OpenAI Team Authors and HuggingFace Inc. team.
-# Copyright (c) 2018, NVIDIA CORPORATION. All rights reserved.
-#
-# Licensed under the Apache License, Version 2.0 (the "License");
-# you may not use this file except in compliance with the License.
-# You may obtain a copy of the License at
-#
-# http://www.apache.org/licenses/LICENSE-2.0
-#
-# Unless required by applicable law or agreed to in writing, software
-# distributed under the License is distributed on an "AS IS" BASIS,
-# WITHOUT WARRANTIES OR CONDITIONS OF ANY KIND, either express or implied.
-# See the License for the specific language governing permissions and
-# limitations under the License.
-""" TF 2.0 OpenAI GPT model."""
-
-
-import logging
-
-import numpy as np
-import tensorflow as tf
-
-from .configuration_openai import OpenAIGPTConfig
-from .file_utils import add_start_docstrings, add_start_docstrings_to_callable
-from .modeling_tf_utils import (
- TFConv1D,
- TFPreTrainedModel,
- TFSequenceSummary,
- TFSharedEmbeddings,
- get_initializer,
- shape_list,
-)
-
-
-logger = logging.getLogger(__name__)
-
-TF_OPENAI_GPT_PRETRAINED_MODEL_ARCHIVE_MAP = {
- "openai-gpt": "https://s3.amazonaws.com/models.huggingface.co/bert/openai-gpt-tf_model.h5"
-}
-
-
-def gelu(x):
- """Gaussian Error Linear Unit.
- This is a smoother version of the RELU.
- Original paper: https://arxiv.org/abs/1606.08415
- Args:
- x: float Tensor to perform activation.
- Returns:
- `x` with the GELU activation applied.
- """
- cdf = 0.5 * (1.0 + tf.tanh((np.sqrt(2 / np.pi) * (x + 0.044715 * tf.pow(x, 3)))))
- return x * cdf
-
-
-def swish(x):
- return x * tf.math.sigmoid(x)
-
-
-ACT_FNS = {
- "gelu": tf.keras.layers.Activation(gelu),
- "relu": tf.keras.activations.relu,
- "swish": tf.keras.layers.Activation(swish),
-}
-
-
-class TFAttention(tf.keras.layers.Layer):
- def __init__(self, nx, n_ctx, config, scale=False, **kwargs):
- super().__init__(**kwargs)
- self.output_attentions = config.output_attentions
-
- n_state = nx # in Attention: n_state=768 (nx=n_embd)
- # [switch nx => n_state from Block to Attention to keep identical to TF implem]
- assert n_state % config.n_head == 0
- self.n_ctx = n_ctx
- self.n_head = config.n_head
- self.split_size = n_state
- self.scale = scale
-
- self.c_attn = TFConv1D(n_state * 3, nx, initializer_range=config.initializer_range, name="c_attn")
- self.c_proj = TFConv1D(n_state, nx, initializer_range=config.initializer_range, name="c_proj")
- self.attn_dropout = tf.keras.layers.Dropout(config.attn_pdrop)
- self.resid_dropout = tf.keras.layers.Dropout(config.resid_pdrop)
- self.pruned_heads = set()
-
- def prune_heads(self, heads):
- pass
-
- @staticmethod
- def causal_attention_mask(nd, ns, dtype):
- """1's in the lower triangle, counting from the lower right corner.
- Same as tf.matrix_band_part(tf.ones([nd, ns]), -1, ns-nd), but doesn't produce garbage on TPUs.
- """
- i = tf.range(nd)[:, None]
- j = tf.range(ns)
- m = i >= j - ns + nd
- return tf.cast(m, dtype)
-
- def _attn(self, inputs, training=False):
- q, k, v, attention_mask, head_mask = inputs
- # q, k, v have shape [batch, heads, sequence, features]
- w = tf.matmul(q, k, transpose_b=True)
- if self.scale:
- dk = tf.cast(shape_list(k)[-1], tf.float32) # scale attention_scores
- w = w / tf.math.sqrt(dk)
-
- # w has shape [batch, heads, dst_sequence, src_sequence], where information flows from src to dst.
- _, _, nd, ns = shape_list(w)
- b = self.causal_attention_mask(nd, ns, dtype=w.dtype)
- b = tf.reshape(b, [1, 1, nd, ns])
- w = w * b - 1e4 * (1 - b)
-
- if attention_mask is not None:
- # Apply the attention mask
- w = w + attention_mask
-
- w = tf.nn.softmax(w, axis=-1)
- w = self.attn_dropout(w, training=training)
-
- # Mask heads if we want to
- if head_mask is not None:
- w = w * head_mask
-
- outputs = [tf.matmul(w, v)]
- if self.output_attentions:
- outputs.append(w)
- return outputs
-
- def merge_heads(self, x):
- x = tf.transpose(x, [0, 2, 1, 3])
- x_shape = shape_list(x)
- new_x_shape = x_shape[:-2] + [x_shape[-2] * x_shape[-1]]
- return tf.reshape(x, new_x_shape)
-
- def split_heads(self, x):
- x_shape = shape_list(x)
- new_x_shape = x_shape[:-1] + [self.n_head, x_shape[-1] // self.n_head]
- x = tf.reshape(x, new_x_shape)
- return tf.transpose(x, (0, 2, 1, 3)) # (batch, head, seq_length, head_features)
-
- def call(self, inputs, training=False):
- x, attention_mask, head_mask = inputs
-
- x = self.c_attn(x)
- query, key, value = tf.split(x, 3, axis=2)
- query = self.split_heads(query)
- key = self.split_heads(key)
- value = self.split_heads(value)
-
- attn_outputs = self._attn([query, key, value, attention_mask, head_mask], training=training)
- a = attn_outputs[0]
-
- a = self.merge_heads(a)
- a = self.c_proj(a)
- a = self.resid_dropout(a, training=training)
-
- outputs = [a] + attn_outputs[1:]
- return outputs # a, (attentions)
-
-
-class TFMLP(tf.keras.layers.Layer):
- def __init__(self, n_state, config, **kwargs):
- super().__init__(**kwargs)
- nx = config.n_embd
- self.c_fc = TFConv1D(n_state, nx, initializer_range=config.initializer_range, name="c_fc")
- self.c_proj = TFConv1D(nx, n_state, initializer_range=config.initializer_range, name="c_proj")
- self.act = gelu
- self.dropout = tf.keras.layers.Dropout(config.resid_pdrop)
-
- def call(self, x, training=False):
- h = self.act(self.c_fc(x))
- h2 = self.c_proj(h)
- h2 = self.dropout(h2, training=training)
- return h2
-
-
-class TFBlock(tf.keras.layers.Layer):
- def __init__(self, n_ctx, config, scale=False, **kwargs):
- super().__init__(**kwargs)
- nx = config.n_embd
- self.attn = TFAttention(nx, n_ctx, config, scale, name="attn")
- self.ln_1 = tf.keras.layers.LayerNormalization(epsilon=config.layer_norm_epsilon, name="ln_1")
- self.mlp = TFMLP(4 * nx, config, name="mlp")
- self.ln_2 = tf.keras.layers.LayerNormalization(epsilon=config.layer_norm_epsilon, name="ln_2")
-
- def call(self, inputs, training=False):
- x, attention_mask, head_mask = inputs
-
- output_attn = self.attn([x, attention_mask, head_mask], training=training)
- a = output_attn[0] # output_attn: a, (attentions)
-
- n = self.ln_1(x + a)
- m = self.mlp(n, training=training)
- h = self.ln_2(n + m)
-
- outputs = [h] + output_attn[1:]
- return outputs # x, (attentions)
-
-
-class TFOpenAIGPTMainLayer(tf.keras.layers.Layer):
- def __init__(self, config, *inputs, **kwargs):
- super().__init__(config, *inputs, **kwargs)
- self.output_hidden_states = config.output_hidden_states
- self.output_attentions = config.output_attentions
- self.num_hidden_layers = config.n_layer
- self.vocab_size = config.vocab_size
- self.n_embd = config.n_embd
-
- self.tokens_embed = TFSharedEmbeddings(
- config.vocab_size, config.n_embd, initializer_range=config.initializer_range, name="tokens_embed"
- )
- self.positions_embed = tf.keras.layers.Embedding(
- config.n_positions,
- config.n_embd,
- embeddings_initializer=get_initializer(config.initializer_range),
- name="positions_embed",
- )
- self.drop = tf.keras.layers.Dropout(config.embd_pdrop)
- self.h = [TFBlock(config.n_ctx, config, scale=True, name="h_._{}".format(i)) for i in range(config.n_layer)]
-
- def get_input_embeddings(self):
- return self.tokens_embed
-
- def _resize_token_embeddings(self, new_num_tokens):
- raise NotImplementedError
-
- def _prune_heads(self, heads_to_prune):
- """ Prunes heads of the model.
- heads_to_prune: dict of {layer_num: list of heads to prune in this layer}
- """
- raise NotImplementedError
-
- def call(
- self,
- inputs,
- attention_mask=None,
- token_type_ids=None,
- position_ids=None,
- head_mask=None,
- inputs_embeds=None,
- training=False,
- ):
- if isinstance(inputs, (tuple, list)):
- input_ids = inputs[0]
- attention_mask = inputs[1] if len(inputs) > 1 else attention_mask
- token_type_ids = inputs[2] if len(inputs) > 2 else token_type_ids
- position_ids = inputs[3] if len(inputs) > 3 else position_ids
- head_mask = inputs[4] if len(inputs) > 4 else head_mask
- inputs_embeds = inputs[5] if len(inputs) > 5 else inputs_embeds
- assert len(inputs) <= 6, "Too many inputs."
- elif isinstance(inputs, dict):
- input_ids = inputs.get("input_ids")
- attention_mask = inputs.get("attention_mask", attention_mask)
- token_type_ids = inputs.get("token_type_ids", token_type_ids)
- position_ids = inputs.get("position_ids", position_ids)
- head_mask = inputs.get("head_mask", head_mask)
- inputs_embeds = inputs.get("inputs_embeds", inputs_embeds)
- assert len(inputs) <= 6, "Too many inputs."
- else:
- input_ids = inputs
-
- if input_ids is not None and inputs_embeds is not None:
- raise ValueError("You cannot specify both input_ids and inputs_embeds at the same time")
- elif input_ids is not None:
- input_shape = shape_list(input_ids)
- input_ids = tf.reshape(input_ids, [-1, input_shape[-1]])
- elif inputs_embeds is not None:
- input_shape = shape_list(inputs_embeds)[:-1]
- else:
- raise ValueError("You have to specify either input_ids or inputs_embeds")
-
- if position_ids is None:
- position_ids = tf.range(input_shape[-1], dtype=tf.int32)[tf.newaxis, :]
-
- if attention_mask is not None:
- # We create a 3D attention mask from a 2D tensor mask.
- # Sizes are [batch_size, 1, 1, to_seq_length]
- # So we can broadcast to [batch_size, num_heads, from_seq_length, to_seq_length]
- # this attention mask is more simple than the triangular masking of causal attention
- # used in OpenAI GPT, we just need to prepare the broadcast dimension here.
- attention_mask = attention_mask[:, tf.newaxis, tf.newaxis, :]
-
- # Since attention_mask is 1.0 for positions we want to attend and 0.0 for
- # masked positions, this operation will create a tensor which is 0.0 for
- # positions we want to attend and -10000.0 for masked positions.
- # Since we are adding it to the raw scores before the softmax, this is
- # effectively the same as removing these entirely.
-
- attention_mask = tf.cast(attention_mask, tf.float32)
- attention_mask = (1.0 - attention_mask) * -10000.0
- else:
- attention_mask = None
-
- # Prepare head mask if needed
- # 1.0 in head_mask indicate we keep the head
- # attention_probs has shape bsz x n_heads x N x N
- # input head_mask has shape [num_heads] or [num_hidden_layers x num_heads]
- # and head_mask is converted to shape [num_hidden_layers x batch x num_heads x seq_length x seq_length]
- if head_mask is not None:
- raise NotImplementedError
- else:
- head_mask = [None] * self.num_hidden_layers
- # head_mask = tf.constant([0] * self.num_hidden_layers)
-
- position_ids = tf.reshape(position_ids, [-1, shape_list(position_ids)[-1]])
-
- if inputs_embeds is None:
- inputs_embeds = self.tokens_embed(input_ids, mode="embedding")
- position_embeds = self.positions_embed(position_ids)
- if token_type_ids is not None:
- token_type_ids = tf.reshape(token_type_ids, [-1, shape_list(token_type_ids)[-1]])
- token_type_embeds = self.tokens_embed(token_type_ids, mode="embedding")
- else:
- token_type_embeds = 0
- hidden_states = inputs_embeds + position_embeds + token_type_embeds
- hidden_states = self.drop(hidden_states, training=training)
-
- output_shape = input_shape + [shape_list(hidden_states)[-1]]
-
- all_attentions = []
- all_hidden_states = ()
- for i, block in enumerate(self.h):
- if self.output_hidden_states:
- all_hidden_states = all_hidden_states + (tf.reshape(hidden_states, output_shape),)
-
- outputs = block([hidden_states, attention_mask, head_mask[i]], training=training)
- hidden_states = outputs[0]
- if self.output_attentions:
- all_attentions.append(outputs[1])
-
- hidden_states = tf.reshape(hidden_states, output_shape)
- # Add last hidden state
- if self.output_hidden_states:
- all_hidden_states = all_hidden_states + (hidden_states,)
-
- outputs = (hidden_states,)
- if self.output_hidden_states:
- outputs = outputs + (all_hidden_states,)
- if self.output_attentions:
- # let the number of heads free (-1) so we can extract attention even after head pruning
- attention_output_shape = input_shape[:-1] + [-1] + shape_list(all_attentions[0])[-2:]
- all_attentions = tuple(tf.reshape(t, attention_output_shape) for t in all_attentions)
- outputs = outputs + (all_attentions,)
- return outputs # last hidden state, (all hidden_states), (attentions)
-
-
-class TFOpenAIGPTPreTrainedModel(TFPreTrainedModel):
- """ An abstract class to handle weights initialization and
- a simple interface for downloading and loading pretrained models.
- """
-
- config_class = OpenAIGPTConfig
- pretrained_model_archive_map = TF_OPENAI_GPT_PRETRAINED_MODEL_ARCHIVE_MAP
- base_model_prefix = "transformer"
-
-
-OPENAI_GPT_START_DOCSTRING = r"""
-
- .. note::
- TF 2.0 models accepts two formats as inputs:
-
- - having all inputs as keyword arguments (like PyTorch models), or
- - having all inputs as a list, tuple or dict in the first positional arguments.
-
- This second option is useful when using :obj:`tf.keras.Model.fit()` method which currently requires having
- all the tensors in the first argument of the model call function: :obj:`model(inputs)`.
-
- If you choose this second option, there are three possibilities you can use to gather all the input Tensors
- in the first positional argument :
-
- - a single Tensor with input_ids only and nothing else: :obj:`model(inputs_ids)`
- - a list of varying length with one or several input Tensors IN THE ORDER given in the docstring:
- :obj:`model([input_ids, attention_mask])` or :obj:`model([input_ids, attention_mask, token_type_ids])`
- - a dictionary with one or several input Tensors associated to the input names given in the docstring:
- :obj:`model({'input_ids': input_ids, 'token_type_ids': token_type_ids})`
-
-
- Parameters:
- config (:class:`~transformers.OpenAIGPTConfig`): Model configuration class with all the parameters of the model.
- Initializing with a config file does not load the weights associated with the model, only the configuration.
- Check out the :meth:`~transformers.PreTrainedModel.from_pretrained` method to load the model weights.
-"""
-
-OPENAI_GPT_INPUTS_DOCSTRING = r"""
- Args:
- input_ids (:obj:`Numpy array` or :obj:`tf.Tensor` of shape :obj:`(batch_size, sequence_length)`):
- Indices of input sequence tokens in the vocabulary.
-
- Indices can be obtained using :class:`transformers.GPT2Tokenizer`.
- See :func:`transformers.PreTrainedTokenizer.encode` and
- :func:`transformers.PreTrainedTokenizer.encode_plus` for details.
-
- `What are input IDs? <../glossary.html#input-ids>`__
- attention_mask (:obj:`tf.Tensor` or :obj:`Numpy array` of shape :obj:`(batch_size, sequence_length)`, `optional`, defaults to :obj:`None`):
- Mask to avoid performing attention on padding token indices.
- Mask values selected in ``[0, 1]``:
- ``1`` for tokens that are NOT MASKED, ``0`` for MASKED tokens.
-
- `What are attention masks? <../glossary.html#attention-mask>`__
- token_type_ids (:obj:`tf.Tensor` or :obj:`Numpy array` of shape :obj:`(batch_size, sequence_length)`, `optional`, defaults to :obj:`None`):
- Segment token indices to indicate first and second portions of the inputs.
- Indices are selected in ``[0, 1]``: ``0`` corresponds to a `sentence A` token, ``1``
- corresponds to a `sentence B` token
-
- `What are token type IDs? <../glossary.html#token-type-ids>`_
- position_ids (:obj:`tf.Tensor` or :obj:`Numpy array` of shape :obj:`(batch_size, sequence_length)`, `optional`, defaults to :obj:`None`):
- Indices of positions of each input sequence tokens in the position embeddings.
- Selected in the range ``[0, config.max_position_embeddings - 1]``.
-
- `What are position IDs? <../glossary.html#position-ids>`_
- head_mask (:obj:`tf.Tensor` or :obj:`Numpy array` of shape :obj:`(num_heads,)` or :obj:`(num_layers, num_heads)`, `optional`, defaults to :obj:`None`):
- Mask to nullify selected heads of the self-attention modules.
- Mask values selected in ``[0, 1]``:
- :obj:`1` indicates the head is **not masked**, :obj:`0` indicates the head is **masked**.
- input_embeds (:obj:`tf.Tensor` or :obj:`Numpy array` of shape :obj:`(batch_size, sequence_length, hidden_size)`, `optional`, defaults to :obj:`None`):
- Optionally, instead of passing :obj:`input_ids` you can choose to directly pass an embedded representation.
- This is useful if you want more control over how to convert `input_ids` indices into associated vectors
- than the model's internal embedding lookup matrix.
- training (:obj:`boolean`, `optional`, defaults to :obj:`False`):
- Whether to activate dropout modules (if set to :obj:`True`) during training or to de-activate them
- (if set to :obj:`False`) for evaluation.
-"""
-
-
-@add_start_docstrings(
- "The bare OpenAI GPT transformer model outputing raw hidden-states without any specific head on top.",
- OPENAI_GPT_START_DOCSTRING,
-)
-class TFOpenAIGPTModel(TFOpenAIGPTPreTrainedModel):
- def __init__(self, config, *inputs, **kwargs):
- super().__init__(config, *inputs, **kwargs)
- self.transformer = TFOpenAIGPTMainLayer(config, name="transformer")
-
- @add_start_docstrings_to_callable(OPENAI_GPT_INPUTS_DOCSTRING)
- def call(self, inputs, **kwargs):
- r"""
- Return:
- :obj:`tuple(tf.Tensor)` comprising various elements depending on the configuration (:class:`~transformers.OpenAIGPTConfig`) and inputs:
- last_hidden_state (:obj:`tf.Tensor` of shape :obj:`(batch_size, sequence_length, hidden_size)`):
- Sequence of hidden-states at the last layer of the model.
- hidden_states (:obj:`tuple(tf.Tensor)` `optional`, returned when ``config.output_hidden_states=True``):
- Tuple of :obj:`tf.Tensor` (one for the output of the embeddings + one for the output of each layer)
- of shape :obj:`(batch_size, sequence_length, hidden_size)`.
-
- Hidden-states of the model at the output of each layer plus the initial embedding outputs.
- attentions (:obj:`tuple(tf.Tensor)`, `optional`, returned when ``config.output_attentions=True``):
- Tuple of :obj:`tf.Tensor` (one for each layer) of shape
- :obj:`(batch_size, num_heads, sequence_length, sequence_length)`.
-
- Attentions weights after the attention softmax, used to compute the weighted average in the self-attention
- heads.
-
- Examples::
-
- import tensorflow as tf
- from transformers import OpenAIGPTTokenizer, TFOpenAIGPTModel
-
- tokenizer = OpenAIGPTTokenizer.from_pretrained('openai-gpt')
- model = TFOpenAIGPTModel.from_pretrained('openai-gpt')
- input_ids = tf.constant(tokenizer.encode("Hello, my dog is cute", add_special_tokens=True))[None, :] # Batch size 1
- outputs = model(input_ids)
- last_hidden_states = outputs[0] # The last hidden-state is the first element of the output tuple
-
- """
- outputs = self.transformer(inputs, **kwargs)
- return outputs
-
-
-@add_start_docstrings(
- """OpenAI GPT Model transformer with a language modeling head on top
- (linear layer with weights tied to the input embeddings). """,
- OPENAI_GPT_START_DOCSTRING,
-)
-class TFOpenAIGPTLMHeadModel(TFOpenAIGPTPreTrainedModel):
- def __init__(self, config, *inputs, **kwargs):
- super().__init__(config, *inputs, **kwargs)
- self.transformer = TFOpenAIGPTMainLayer(config, name="transformer")
-
- def get_output_embeddings(self):
- return self.transformer.tokens_embed
-
- @add_start_docstrings_to_callable(OPENAI_GPT_INPUTS_DOCSTRING)
- def call(self, inputs, **kwargs):
- r"""
- Return:
- :obj:`tuple(tf.Tensor)` comprising various elements depending on the configuration (:class:`~transformers.OpenAIGPTConfig`) and inputs:
- prediction_scores (:obj:`tf.Tensor` of shape :obj:`(batch_size, sequence_length, config.vocab_size)`):
- Prediction scores of the language modeling head (scores for each vocabulary token before SoftMax).
- hidden_states (:obj:`tuple(tf.Tensor)`, `optional`, returned when ``config.output_hidden_states=True``):
- Tuple of :obj:`tf.Tensor` (one for the output of the embeddings + one for the output of each layer)
- of shape :obj:`(batch_size, sequence_length, hidden_size)`.
-
- Hidden-states of the model at the output of each layer plus the initial embedding outputs.
- attentions (:obj:`tuple(tf.Tensor)`, `optional`, returned when ``config.output_attentions=True``):
- Tuple of :obj:`tf.Tensor` (one for each layer) of shape
- :obj:`(batch_size, num_heads, sequence_length, sequence_length)`.
-
- Attentions weights after the attention softmax, used to compute the weighted average in the self-attention
- heads.
-
- Examples::
-
- import tensorflow as tf
- from transformers import OpenAIGPTTokenizer, TFOpenAIGPTLMHeadModel
-
- tokenizer = OpenAIGPTTokenizer.from_pretrained('openai-gpt')
- model = TFOpenAIGPTLMHeadModel.from_pretrained('openai-gpt')
- input_ids = tf.constant(tokenizer.encode("Hello, my dog is cute", add_special_tokens=True))[None, :] # Batch size 1
- outputs = model(input_ids)
- logits = outputs[0]
-
- """
- transformer_outputs = self.transformer(inputs, **kwargs)
- hidden_states = transformer_outputs[0]
-
- lm_logits = self.transformer.tokens_embed(hidden_states, mode="linear")
-
- outputs = (lm_logits,) + transformer_outputs[1:]
-
- return outputs # lm_logits, (all hidden_states), (attentions)
-
-
-@add_start_docstrings(
- """OpenAI GPT Model transformer with a language modeling and a multiple-choice classification
- head on top e.g. for RocStories/SWAG tasks. The two heads are two linear layers.
- The language modeling head has its weights tied to the input embeddings,
- the classification head takes as input the input of a specified classification token index in the input sequence).
-""",
- OPENAI_GPT_START_DOCSTRING,
-)
-class TFOpenAIGPTDoubleHeadsModel(TFOpenAIGPTPreTrainedModel):
- def __init__(self, config, *inputs, **kwargs):
- super().__init__(config, *inputs, **kwargs)
- config.num_labels = 1
- self.transformer = TFOpenAIGPTMainLayer(config, name="transformer")
- self.multiple_choice_head = TFSequenceSummary(
- config, initializer_range=config.initializer_range, name="multiple_choice_head"
- )
-
- def get_output_embeddings(self):
- return self.transformer.tokens_embed
-
- @add_start_docstrings_to_callable(OPENAI_GPT_INPUTS_DOCSTRING)
- def call(
- self,
- inputs,
- attention_mask=None,
- token_type_ids=None,
- position_ids=None,
- head_mask=None,
- inputs_embeds=None,
- mc_token_ids=None,
- training=False,
- ):
- r"""
- mc_token_ids (:obj:`tf.Tensor` or :obj:`Numpy array` of shape :obj:`(batch_size, num_choices)`, `optional`, default to index of the last token of the input)
- Index of the classification token in each input sequence.
- Selected in the range ``[0, input_ids.size(-1) - 1[``.
-
- Return:
- :obj:`tuple(tf.Tensor)` comprising various elements depending on the configuration (:class:`~transformers.OpenAIGPTConfig`) and inputs:
- lm_prediction_scores (:obj:`tf.Tensor` of shape :obj:`(batch_size, num_choices, sequence_length, config.vocab_size)`):
- Prediction scores of the language modeling head (scores for each vocabulary token before SoftMax).
- mc_prediction_scores (:obj:`tf.Tensor` of shape :obj:`(batch_size, num_choices)`):
- Prediction scores of the multiple choice classification head (scores for each choice before SoftMax).
- past (:obj:`List[tf.Tensor]` of length :obj:`config.n_layers` with each tensor of shape :obj:`(2, batch_size, num_heads, sequence_length, embed_size_per_head)`):
- Contains pre-computed hidden-states (key and values in the attention blocks).
- Can be used (see `past` input) to speed up sequential decoding. The token ids which have their past given to this model
- should not be passed as input ids as they have already been computed.
- hidden_states (:obj:`tuple(tf.Tensor)`, `optional`, returned when ``config.output_hidden_states=True``):
- Tuple of :obj:`tf.Tensor` (one for the output of the embeddings + one for the output of each layer)
- of shape :obj:`(batch_size, sequence_length, hidden_size)`.
-
- Hidden-states of the model at the output of each layer plus the initial embedding outputs.
- attentions (:obj:`tuple(tf.Tensor)`, `optional`, returned when ``config.output_attentions=True``):
- Tuple of :obj:`tf.Tensor` (one for each layer) of shape
- :obj:`(batch_size, num_heads, sequence_length, sequence_length)`.
-
- Attentions weights after the attention softmax, used to compute the weighted average in the self-attention
- heads.
-
-
- Examples::
-
- # For example purposes. Not runnable.
- import tensorflow as tf
- from transformers import OpenAIGPTTokenizer, TFOpenAIGPTDoubleHeadsModel
-
- tokenizer = OpenAIGPTTokenizer.from_pretrained('openai-gpt')
- model = TFOpenAIGPTDoubleHeadsModel.from_pretrained('openai-gpt')
-
- # Add a [CLS] to the vocabulary (we should train it also!)
- # This option is currently not implemented in TF 2.0
- raise NotImplementedError
- tokenizer.add_special_tokens({'cls_token': '[CLS]'})
- model.resize_token_embeddings(len(tokenizer)) # Update the model embeddings with the new vocabulary size
- print(tokenizer.cls_token_id, len(tokenizer)) # The newly token the last token of the vocabulary
-
- choices = ["Hello, my dog is cute [CLS]", "Hello, my cat is cute [CLS]"]
- input_ids = tf.constant([tokenizer.encode(s) for s in choices])[None, :] # Batch size 1, 2 choices
- mc_token_ids = tf.constant([input_ids.size(-1), input_ids.size(-1)])[None, :] # Batch size 1
- outputs = model(input_ids, mc_token_ids=mc_token_ids)
- lm_prediction_scores, mc_prediction_scores = outputs[:2]
-
- """
-
- if isinstance(inputs, (tuple, list)):
- input_ids = inputs[0]
- attention_mask = inputs[1] if len(inputs) > 1 else attention_mask
- token_type_ids = inputs[2] if len(inputs) > 2 else token_type_ids
- position_ids = inputs[3] if len(inputs) > 3 else position_ids
- head_mask = inputs[4] if len(inputs) > 4 else head_mask
- inputs_embeds = inputs[5] if len(inputs) > 5 else inputs_embeds
- mc_token_ids = inputs[6] if len(inputs) > 6 else mc_token_ids
- assert len(inputs) <= 7, "Too many inputs."
- elif isinstance(inputs, dict):
- input_ids = inputs.get("input_ids")
- attention_mask = inputs.get("attention_mask", attention_mask)
- token_type_ids = inputs.get("token_type_ids", token_type_ids)
- position_ids = inputs.get("position_ids", position_ids)
- head_mask = inputs.get("head_mask", head_mask)
- inputs_embeds = inputs.get("inputs_embeds", inputs_embeds)
- mc_token_ids = inputs.get("mc_token_ids", mc_token_ids)
- assert len(inputs) <= 7, "Too many inputs."
- else:
- input_ids = inputs
-
- if input_ids is not None:
- input_shapes = shape_list(input_ids)
- else:
- input_shapes = shape_list(inputs_embeds)[:-1]
-
- seq_length = input_shapes[-1]
-
- flat_input_ids = tf.reshape(input_ids, (-1, seq_length)) if input_ids is not None else None
- flat_attention_mask = tf.reshape(attention_mask, (-1, seq_length)) if attention_mask is not None else None
- flat_token_type_ids = tf.reshape(token_type_ids, (-1, seq_length)) if token_type_ids is not None else None
- flat_position_ids = tf.reshape(position_ids, (-1, seq_length)) if position_ids is not None else None
-
- flat_inputs = [
- flat_input_ids,
- flat_attention_mask,
- flat_token_type_ids,
- flat_position_ids,
- head_mask,
- inputs_embeds,
- ]
-
- transformer_outputs = self.transformer(flat_inputs, training=training)
- hidden_states = transformer_outputs[0]
-
- hidden_states = tf.reshape(hidden_states, input_shapes + shape_list(hidden_states)[-1:])
-
- lm_logits = self.transformer.tokens_embed(hidden_states, mode="linear")
- mc_logits = self.multiple_choice_head([hidden_states, mc_token_ids], training=training)
-
- mc_logits = tf.squeeze(mc_logits, axis=-1)
-
- outputs = (lm_logits, mc_logits) + transformer_outputs[1:]
-
- return outputs # lm logits, mc logits, (all hidden_states), (attentions)
diff --git a/server/transformers/src/transformers/modeling_tf_pytorch_utils.py b/server/transformers/src/transformers/modeling_tf_pytorch_utils.py
deleted file mode 100644
index 81290326c9beb0af3fd98f2bdd52b65974d13cd3..0000000000000000000000000000000000000000
--- a/server/transformers/src/transformers/modeling_tf_pytorch_utils.py
+++ /dev/null
@@ -1,329 +0,0 @@
-# coding=utf-8
-# Copyright 2018 The Google AI Language Team Authors and The HuggingFace Inc. team.
-# Copyright (c) 2018, NVIDIA CORPORATION. All rights reserved.
-#
-# Licensed under the Apache License, Version 2.0 (the "License");
-# you may not use this file except in compliance with the License.
-# You may obtain a copy of the License at
-#
-# http://www.apache.org/licenses/LICENSE-2.0
-#
-# Unless required by applicable law or agreed to in writing, software
-# distributed under the License is distributed on an "AS IS" BASIS,
-# WITHOUT WARRANTIES OR CONDITIONS OF ANY KIND, either express or implied.
-# See the License for the specific language governing permissions and
-# limitations under the License.
-""" PyTorch - TF 2.0 general utilities."""
-
-
-import logging
-import os
-import re
-
-import numpy
-
-
-logger = logging.getLogger(__name__)
-
-
-def convert_tf_weight_name_to_pt_weight_name(tf_name, start_prefix_to_remove=""):
- """ Convert a TF 2.0 model variable name in a pytorch model weight name.
-
- Conventions for TF2.0 scopes -> PyTorch attribute names conversions:
- - '$1___$2' is replaced by $2 (can be used to duplicate or remove layers in TF2.0 vs PyTorch)
- - '_._' is replaced by a new level separation (can be used to convert TF2.0 lists in PyTorch nn.ModulesList)
-
- return tuple with:
- - pytorch model weight name
- - transpose: boolean indicating weither TF2.0 and PyTorch weights matrices are transposed with regards to each other
- """
- tf_name = tf_name.replace(":0", "") # device ids
- tf_name = re.sub(
- r"/[^/]*___([^/]*)/", r"/\1/", tf_name
- ) # '$1___$2' is replaced by $2 (can be used to duplicate or remove layers in TF2.0 vs PyTorch)
- tf_name = tf_name.replace(
- "_._", "/"
- ) # '_._' is replaced by a level separation (can be used to convert TF2.0 lists in PyTorch nn.ModulesList)
- tf_name = re.sub(r"//+", "/", tf_name) # Remove empty levels at the end
- tf_name = tf_name.split("/") # Convert from TF2.0 '/' separators to PyTorch '.' separators
- tf_name = tf_name[1:] # Remove level zero
-
- # When should we transpose the weights
- transpose = bool(tf_name[-1] == "kernel" or "emb_projs" in tf_name or "out_projs" in tf_name)
-
- # Convert standard TF2.0 names in PyTorch names
- if tf_name[-1] == "kernel" or tf_name[-1] == "embeddings" or tf_name[-1] == "gamma":
- tf_name[-1] = "weight"
- if tf_name[-1] == "beta":
- tf_name[-1] = "bias"
-
- # Remove prefix if needed
- tf_name = ".".join(tf_name)
- if start_prefix_to_remove:
- tf_name = tf_name.replace(start_prefix_to_remove, "", 1)
-
- return tf_name, transpose
-
-
-#####################
-# PyTorch => TF 2.0 #
-#####################
-
-
-def load_pytorch_checkpoint_in_tf2_model(tf_model, pytorch_checkpoint_path, tf_inputs=None, allow_missing_keys=False):
- """ Load pytorch checkpoints in a TF 2.0 model
- """
- try:
- import tensorflow as tf # noqa: F401
- import torch # noqa: F401
- except ImportError:
- logger.error(
- "Loading a PyTorch model in TensorFlow, requires both PyTorch and TensorFlow to be installed. Please see "
- "https://pytorch.org/ and https://www.tensorflow.org/install/ for installation instructions."
- )
- raise
-
- pt_path = os.path.abspath(pytorch_checkpoint_path)
- logger.info("Loading PyTorch weights from {}".format(pt_path))
-
- pt_state_dict = torch.load(pt_path, map_location="cpu")
- logger.info("PyTorch checkpoint contains {:,} parameters".format(sum(t.numel() for t in pt_state_dict.values())))
-
- return load_pytorch_weights_in_tf2_model(
- tf_model, pt_state_dict, tf_inputs=tf_inputs, allow_missing_keys=allow_missing_keys
- )
-
-
-def load_pytorch_model_in_tf2_model(tf_model, pt_model, tf_inputs=None, allow_missing_keys=False):
- """ Load pytorch checkpoints in a TF 2.0 model
- """
- pt_state_dict = pt_model.state_dict()
-
- return load_pytorch_weights_in_tf2_model(
- tf_model, pt_state_dict, tf_inputs=tf_inputs, allow_missing_keys=allow_missing_keys
- )
-
-
-def load_pytorch_weights_in_tf2_model(tf_model, pt_state_dict, tf_inputs=None, allow_missing_keys=False):
- """ Load pytorch state_dict in a TF 2.0 model.
- """
- try:
- import torch # noqa: F401
- import tensorflow as tf # noqa: F401
- from tensorflow.python.keras import backend as K
- except ImportError:
- logger.error(
- "Loading a PyTorch model in TensorFlow, requires both PyTorch and TensorFlow to be installed. Please see "
- "https://pytorch.org/ and https://www.tensorflow.org/install/ for installation instructions."
- )
- raise
-
- if tf_inputs is None:
- tf_inputs = tf_model.dummy_inputs
-
- if tf_inputs is not None:
- tf_model(tf_inputs, training=False) # Make sure model is built
-
- # Adapt state dict - TODO remove this and update the AWS weights files instead
- # Convert old format to new format if needed from a PyTorch state_dict
- old_keys = []
- new_keys = []
- for key in pt_state_dict.keys():
- new_key = None
- if "gamma" in key:
- new_key = key.replace("gamma", "weight")
- if "beta" in key:
- new_key = key.replace("beta", "bias")
- if new_key:
- old_keys.append(key)
- new_keys.append(new_key)
- for old_key, new_key in zip(old_keys, new_keys):
- pt_state_dict[new_key] = pt_state_dict.pop(old_key)
-
- # Make sure we are able to load PyTorch base models as well as derived models (with heads)
- # TF models always have a prefix, some of PyTorch models (base ones) don't
- start_prefix_to_remove = ""
- if not any(s.startswith(tf_model.base_model_prefix) for s in pt_state_dict.keys()):
- start_prefix_to_remove = tf_model.base_model_prefix + "."
-
- symbolic_weights = tf_model.trainable_weights + tf_model.non_trainable_weights
- tf_loaded_numel = 0
- weight_value_tuples = []
- all_pytorch_weights = set(list(pt_state_dict.keys()))
- for symbolic_weight in symbolic_weights:
- sw_name = symbolic_weight.name
- name, transpose = convert_tf_weight_name_to_pt_weight_name(
- sw_name, start_prefix_to_remove=start_prefix_to_remove
- )
-
- # Find associated numpy array in pytorch model state dict
- if name not in pt_state_dict:
- if allow_missing_keys:
- continue
- raise AttributeError("{} not found in PyTorch model".format(name))
-
- array = pt_state_dict[name].numpy()
-
- if transpose:
- array = numpy.transpose(array)
-
- if len(symbolic_weight.shape) < len(array.shape):
- array = numpy.squeeze(array)
- elif len(symbolic_weight.shape) > len(array.shape):
- array = numpy.expand_dims(array, axis=0)
-
- try:
- assert list(symbolic_weight.shape) == list(array.shape)
- except AssertionError as e:
- e.args += (symbolic_weight.shape, array.shape)
- raise e
-
- tf_loaded_numel += array.size
- # logger.warning("Initialize TF weight {}".format(symbolic_weight.name))
-
- weight_value_tuples.append((symbolic_weight, array))
- all_pytorch_weights.discard(name)
-
- K.batch_set_value(weight_value_tuples)
-
- if tf_inputs is not None:
- tf_model(tf_inputs, training=False) # Make sure restore ops are run
-
- logger.info("Loaded {:,} parameters in the TF 2.0 model.".format(tf_loaded_numel))
-
- logger.info("Weights or buffers not loaded from PyTorch model: {}".format(all_pytorch_weights))
-
- return tf_model
-
-
-#####################
-# TF 2.0 => PyTorch #
-#####################
-
-
-def load_tf2_checkpoint_in_pytorch_model(pt_model, tf_checkpoint_path, tf_inputs=None, allow_missing_keys=False):
- """ Load TF 2.0 HDF5 checkpoint in a PyTorch model
- We use HDF5 to easily do transfer learning
- (see https://github.com/tensorflow/tensorflow/blob/ee16fcac960ae660e0e4496658a366e2f745e1f0/tensorflow/python/keras/engine/network.py#L1352-L1357).
- """
- try:
- import tensorflow as tf # noqa: F401
- import torch # noqa: F401
- except ImportError:
- logger.error(
- "Loading a TensorFlow model in PyTorch, requires both PyTorch and TensorFlow to be installed. Please see "
- "https://pytorch.org/ and https://www.tensorflow.org/install/ for installation instructions."
- )
- raise
-
- import transformers
-
- logger.info("Loading TensorFlow weights from {}".format(tf_checkpoint_path))
-
- # Instantiate and load the associated TF 2.0 model
- tf_model_class_name = "TF" + pt_model.__class__.__name__ # Add "TF" at the beggining
- tf_model_class = getattr(transformers, tf_model_class_name)
- tf_model = tf_model_class(pt_model.config)
-
- if tf_inputs is None:
- tf_inputs = tf_model.dummy_inputs
-
- if tf_inputs is not None:
- tf_model(tf_inputs, training=False) # Make sure model is built
-
- tf_model.load_weights(tf_checkpoint_path, by_name=True)
-
- return load_tf2_model_in_pytorch_model(pt_model, tf_model, allow_missing_keys=allow_missing_keys)
-
-
-def load_tf2_model_in_pytorch_model(pt_model, tf_model, allow_missing_keys=False):
- """ Load TF 2.0 model in a pytorch model
- """
- weights = tf_model.weights
-
- return load_tf2_weights_in_pytorch_model(pt_model, weights, allow_missing_keys=allow_missing_keys)
-
-
-def load_tf2_weights_in_pytorch_model(pt_model, tf_weights, allow_missing_keys=False):
- """ Load TF2.0 symbolic weights in a PyTorch model
- """
- try:
- import tensorflow as tf # noqa: F401
- import torch # noqa: F401
- except ImportError:
- logger.error(
- "Loading a TensorFlow model in PyTorch, requires both PyTorch and TensorFlow to be installed. Please see "
- "https://pytorch.org/ and https://www.tensorflow.org/install/ for installation instructions."
- )
- raise
-
- new_pt_params_dict = {}
- current_pt_params_dict = dict(pt_model.named_parameters())
-
- # Make sure we are able to load PyTorch base models as well as derived models (with heads)
- # TF models always have a prefix, some of PyTorch models (base ones) don't
- start_prefix_to_remove = ""
- if not any(s.startswith(pt_model.base_model_prefix) for s in current_pt_params_dict.keys()):
- start_prefix_to_remove = pt_model.base_model_prefix + "."
-
- # Build a map from potential PyTorch weight names to TF 2.0 Variables
- tf_weights_map = {}
- for tf_weight in tf_weights:
- pt_name, transpose = convert_tf_weight_name_to_pt_weight_name(
- tf_weight.name, start_prefix_to_remove=start_prefix_to_remove
- )
- tf_weights_map[pt_name] = (tf_weight.numpy(), transpose)
-
- all_tf_weights = set(list(tf_weights_map.keys()))
- loaded_pt_weights_data_ptr = {}
- missing_keys_pt = []
- for pt_weight_name, pt_weight in current_pt_params_dict.items():
- # Handle PyTorch shared weight ()not duplicated in TF 2.0
- if pt_weight.data_ptr() in loaded_pt_weights_data_ptr:
- new_pt_params_dict[pt_weight_name] = loaded_pt_weights_data_ptr[pt_weight.data_ptr()]
- continue
-
- # Find associated numpy array in pytorch model state dict
- if pt_weight_name not in tf_weights_map:
- if allow_missing_keys:
- missing_keys_pt.append(pt_weight_name)
- continue
- raise AttributeError("{} not found in TF 2.0 model".format(pt_weight_name))
-
- array, transpose = tf_weights_map[pt_weight_name]
-
- if transpose:
- array = numpy.transpose(array)
-
- if len(pt_weight.shape) < len(array.shape):
- array = numpy.squeeze(array)
- elif len(pt_weight.shape) > len(array.shape):
- array = numpy.expand_dims(array, axis=0)
-
- try:
- assert list(pt_weight.shape) == list(array.shape)
- except AssertionError as e:
- e.args += (pt_weight.shape, array.shape)
- raise e
-
- # logger.warning("Initialize PyTorch weight {}".format(pt_weight_name))
-
- new_pt_params_dict[pt_weight_name] = torch.from_numpy(array)
- loaded_pt_weights_data_ptr[pt_weight.data_ptr()] = torch.from_numpy(array)
- all_tf_weights.discard(pt_weight_name)
-
- missing_keys, unexpected_keys = pt_model.load_state_dict(new_pt_params_dict, strict=False)
- missing_keys += missing_keys_pt
-
- if len(missing_keys) > 0:
- logger.info(
- "Weights of {} not initialized from TF 2.0 model: {}".format(pt_model.__class__.__name__, missing_keys)
- )
- if len(unexpected_keys) > 0:
- logger.info(
- "Weights from TF 2.0 model not used in {}: {}".format(pt_model.__class__.__name__, unexpected_keys)
- )
-
- logger.info("Weights or buffers not loaded from TF 2.0 model: {}".format(all_tf_weights))
-
- return pt_model
diff --git a/server/transformers/src/transformers/modeling_tf_roberta.py b/server/transformers/src/transformers/modeling_tf_roberta.py
deleted file mode 100644
index 31fb43f1cc6a5479d845f4fa2d242a124a70ccf2..0000000000000000000000000000000000000000
--- a/server/transformers/src/transformers/modeling_tf_roberta.py
+++ /dev/null
@@ -1,444 +0,0 @@
-# coding=utf-8
-# Copyright 2018 The Google AI Language Team Authors and The HuggingFace Inc. team.
-# Copyright (c) 2018, NVIDIA CORPORATION. All rights reserved.
-#
-# Licensed under the Apache License, Version 2.0 (the "License");
-# you may not use this file except in compliance with the License.
-# You may obtain a copy of the License at
-#
-# http://www.apache.org/licenses/LICENSE-2.0
-#
-# Unless required by applicable law or agreed to in writing, software
-# distributed under the License is distributed on an "AS IS" BASIS,
-# WITHOUT WARRANTIES OR CONDITIONS OF ANY KIND, either express or implied.
-# See the License for the specific language governing permissions and
-# limitations under the License.
-""" TF 2.0 RoBERTa model. """
-
-
-import logging
-
-import tensorflow as tf
-
-from .configuration_roberta import RobertaConfig
-from .file_utils import add_start_docstrings, add_start_docstrings_to_callable
-from .modeling_tf_bert import TFBertEmbeddings, TFBertMainLayer, gelu
-from .modeling_tf_utils import TFPreTrainedModel, get_initializer, shape_list
-
-
-logger = logging.getLogger(__name__)
-
-TF_ROBERTA_PRETRAINED_MODEL_ARCHIVE_MAP = {
- "roberta-base": "https://s3.amazonaws.com/models.huggingface.co/bert/roberta-base-tf_model.h5",
- "roberta-large": "https://s3.amazonaws.com/models.huggingface.co/bert/roberta-large-tf_model.h5",
- "roberta-large-mnli": "https://s3.amazonaws.com/models.huggingface.co/bert/roberta-large-mnli-tf_model.h5",
- "distilroberta-base": "https://s3.amazonaws.com/models.huggingface.co/bert/distilroberta-base-tf_model.h5",
-}
-
-
-class TFRobertaEmbeddings(TFBertEmbeddings):
- """
- Same as BertEmbeddings with a tiny tweak for positional embeddings indexing.
- """
-
- def __init__(self, config, **kwargs):
- super().__init__(config, **kwargs)
- self.padding_idx = 1
-
- def create_position_ids_from_input_ids(self, x):
- """ Replace non-padding symbols with their position numbers. Position numbers begin at
- padding_idx+1. Padding symbols are ignored. This is modified from fairseq's
- `utils.make_positions`.
- :param torch.Tensor x:
- :return torch.Tensor:
- """
- mask = tf.cast(tf.math.not_equal(x, self.padding_idx), dtype=tf.int32)
- incremental_indicies = tf.math.cumsum(mask, axis=1) * mask
- return incremental_indicies + self.padding_idx
-
- def create_position_ids_from_inputs_embeds(self, inputs_embeds):
- """ We are provided embeddings directly. We cannot infer which are padded so just generate
- sequential position ids.
- :param torch.Tensor inputs_embeds:
- :return torch.Tensor:
- """
- seq_length = shape_list(inputs_embeds)[1]
-
- position_ids = tf.range(self.padding_idx + 1, seq_length + self.padding_idx + 1, dtype=tf.int32)[tf.newaxis, :]
- return position_ids
-
- def _embedding(self, inputs, training=False):
- """Applies embedding based on inputs tensor."""
- input_ids, position_ids, token_type_ids, inputs_embeds = inputs
-
- if position_ids is None:
- if input_ids is not None:
- # Create the position ids from the input token ids. Any padded tokens remain padded.
- position_ids = self.create_position_ids_from_input_ids(input_ids)
- else:
- position_ids = self.create_position_ids_from_inputs_embeds(inputs_embeds)
-
- return super()._embedding([input_ids, position_ids, token_type_ids, inputs_embeds], training=training)
-
-
-class TFRobertaMainLayer(TFBertMainLayer):
- """
- Same as TFBertMainLayer but uses TFRobertaEmbeddings.
- """
-
- def __init__(self, config, **kwargs):
- super().__init__(config, **kwargs)
- self.embeddings = TFRobertaEmbeddings(config, name="embeddings")
-
- def get_input_embeddings(self):
- return self.embeddings
-
-
-class TFRobertaPreTrainedModel(TFPreTrainedModel):
- """ An abstract class to handle weights initialization and
- a simple interface for downloading and loading pretrained models.
- """
-
- config_class = RobertaConfig
- pretrained_model_archive_map = TF_ROBERTA_PRETRAINED_MODEL_ARCHIVE_MAP
- base_model_prefix = "roberta"
-
-
-ROBERTA_START_DOCSTRING = r"""
- This model is a `tf.keras.Model `__ sub-class.
- Use it as a regular TF 2.0 Keras Model and
- refer to the TF 2.0 documentation for all matter related to general usage and behavior.
-
- .. note::
-
- TF 2.0 models accepts two formats as inputs:
-
- - having all inputs as keyword arguments (like PyTorch models), or
- - having all inputs as a list, tuple or dict in the first positional arguments.
-
- This second option is useful when using :obj:`tf.keras.Model.fit()` method which currently requires having
- all the tensors in the first argument of the model call function: :obj:`model(inputs)`.
-
- If you choose this second option, there are three possibilities you can use to gather all the input Tensors
- in the first positional argument :
-
- - a single Tensor with input_ids only and nothing else: :obj:`model(inputs_ids)`
- - a list of varying length with one or several input Tensors IN THE ORDER given in the docstring:
- :obj:`model([input_ids, attention_mask])` or :obj:`model([input_ids, attention_mask, token_type_ids])`
- - a dictionary with one or several input Tensors associated to the input names given in the docstring:
- :obj:`model({'input_ids': input_ids, 'token_type_ids': token_type_ids})`
-
- Parameters:
- config (:class:`~transformers.RobertaConfig`): Model configuration class with all the parameters of the
- model. Initializing with a config file does not load the weights associated with the model, only the configuration.
- Check out the :meth:`~transformers.PreTrainedModel.from_pretrained` method to load the model weights.
-"""
-
-ROBERTA_INPUTS_DOCSTRING = r"""
- Args:
- input_ids (:obj:`Numpy array` or :obj:`tf.Tensor` of shape :obj:`(batch_size, sequence_length)`):
- Indices of input sequence tokens in the vocabulary.
-
- Indices can be obtained using :class:`transformers.RobertaTokenizer`.
- See :func:`transformers.PreTrainedTokenizer.encode` and
- :func:`transformers.PreTrainedTokenizer.encode_plus` for details.
-
- `What are input IDs? <../glossary.html#input-ids>`__
- attention_mask (:obj:`Numpy array` or :obj:`tf.Tensor` of shape :obj:`(batch_size, sequence_length)`, `optional`, defaults to :obj:`None`):
- Mask to avoid performing attention on padding token indices.
- Mask values selected in ``[0, 1]``:
- ``1`` for tokens that are NOT MASKED, ``0`` for MASKED tokens.
-
- `What are attention masks? <../glossary.html#attention-mask>`__
- token_type_ids (:obj:`Numpy array` or :obj:`tf.Tensor` of shape :obj:`(batch_size, sequence_length)`, `optional`, defaults to :obj:`None`):
- Segment token indices to indicate first and second portions of the inputs.
- Indices are selected in ``[0, 1]``: ``0`` corresponds to a `sentence A` token, ``1``
- corresponds to a `sentence B` token
-
- `What are token type IDs? <../glossary.html#token-type-ids>`__
- position_ids (:obj:`Numpy array` or :obj:`tf.Tensor` of shape :obj:`(batch_size, sequence_length)`, `optional`, defaults to :obj:`None`):
- Indices of positions of each input sequence tokens in the position embeddings.
- Selected in the range ``[0, config.max_position_embeddings - 1]``.
-
- `What are position IDs? <../glossary.html#position-ids>`__
- head_mask (:obj:`Numpy array` or :obj:`tf.Tensor` of shape :obj:`(num_heads,)` or :obj:`(num_layers, num_heads)`, `optional`, defaults to :obj:`None`):
- Mask to nullify selected heads of the self-attention modules.
- Mask values selected in ``[0, 1]``:
- :obj:`1` indicates the head is **not masked**, :obj:`0` indicates the head is **masked**.
- inputs_embeds (:obj:`Numpy array` or :obj:`tf.Tensor` of shape :obj:`(batch_size, sequence_length, embedding_dim)`, `optional`, defaults to :obj:`None`):
- Optionally, instead of passing :obj:`input_ids` you can choose to directly pass an embedded representation.
- This is useful if you want more control over how to convert `input_ids` indices into associated vectors
- than the model's internal embedding lookup matrix.
- training (:obj:`boolean`, `optional`, defaults to :obj:`False`):
- Whether to activate dropout modules (if set to :obj:`True`) during training or to de-activate them
- (if set to :obj:`False`) for evaluation.
-"""
-
-
-@add_start_docstrings(
- "The bare RoBERTa Model transformer outputing raw hidden-states without any specific head on top.",
- ROBERTA_START_DOCSTRING,
-)
-class TFRobertaModel(TFRobertaPreTrainedModel):
- def __init__(self, config, *inputs, **kwargs):
- super().__init__(config, *inputs, **kwargs)
- self.roberta = TFRobertaMainLayer(config, name="roberta")
-
- @add_start_docstrings_to_callable(ROBERTA_INPUTS_DOCSTRING)
- def call(self, inputs, **kwargs):
- r"""
- Returns:
- :obj:`tuple(torch.FloatTensor)` comprising various elements depending on the configuration (:class:`~transformers.RobertaConfig`) and inputs:
- last_hidden_state (:obj:`tf.Tensor` of shape :obj:`(batch_size, sequence_length, hidden_size)`):
- Sequence of hidden-states at the output of the last layer of the model.
- pooler_output (:obj:`tf.Tensor` of shape :obj:`(batch_size, hidden_size)`):
- Last layer hidden-state of the first token of the sequence (classification token)
- further processed by a Linear layer and a Tanh activation function. The Linear
- layer weights are trained from the next sentence prediction (classification)
- objective during Bert pretraining. This output is usually *not* a good summary
- of the semantic content of the input, you're often better with averaging or pooling
- the sequence of hidden-states for the whole input sequence.
- hidden_states (:obj:`tuple(tf.Tensor)`, `optional`, returned when :obj:`config.output_hidden_states=True`):
- tuple of :obj:`tf.Tensor` (one for the output of the embeddings + one for the output of each layer)
- of shape :obj:`(batch_size, sequence_length, hidden_size)`.
-
- Hidden-states of the model at the output of each layer plus the initial embedding outputs.
- attentions (:obj:`tuple(tf.Tensor)`, `optional`, returned when ``config.output_attentions=True``):
- tuple of :obj:`tf.Tensor` (one for each layer) of shape
- :obj:`(batch_size, num_heads, sequence_length, sequence_length)`:
-
- Attentions weights after the attention softmax, used to compute the weighted average in the self-attention heads.
-
- Examples::
-
- import tensorflow as tf
- from transformers import RobertaTokenizer, TFRobertaModel
-
- tokenizer = RobertaTokenizer.from_pretrained('roberta-base')
- model = TFRobertaModel.from_pretrained('roberta-base')
- input_ids = tf.constant(tokenizer.encode("Hello, my dog is cute", add_special_tokens=True))[None, :] # Batch size 1
- outputs = model(input_ids)
- last_hidden_states = outputs[0] # The last hidden-state is the first element of the output tuple
-
- """
- outputs = self.roberta(inputs, **kwargs)
- return outputs
-
-
-class TFRobertaLMHead(tf.keras.layers.Layer):
- """Roberta Head for masked language modeling."""
-
- def __init__(self, config, input_embeddings, **kwargs):
- super().__init__(**kwargs)
- self.vocab_size = config.vocab_size
- self.dense = tf.keras.layers.Dense(
- config.hidden_size, kernel_initializer=get_initializer(config.initializer_range), name="dense"
- )
- self.layer_norm = tf.keras.layers.LayerNormalization(epsilon=config.layer_norm_eps, name="layer_norm")
- self.act = tf.keras.layers.Activation(gelu)
-
- # The output weights are the same as the input embeddings, but there is
- # an output-only bias for each token.
- self.decoder = input_embeddings
-
- def build(self, input_shape):
- self.bias = self.add_weight(shape=(self.vocab_size,), initializer="zeros", trainable=True, name="bias")
- super().build(input_shape)
-
- def call(self, features):
- x = self.dense(features)
- x = self.act(x)
- x = self.layer_norm(x)
-
- # project back to size of vocabulary with bias
- x = self.decoder(x, mode="linear") + self.bias
-
- return x
-
-
-@add_start_docstrings("""RoBERTa Model with a `language modeling` head on top. """, ROBERTA_START_DOCSTRING)
-class TFRobertaForMaskedLM(TFRobertaPreTrainedModel):
- def __init__(self, config, *inputs, **kwargs):
- super().__init__(config, *inputs, **kwargs)
-
- self.roberta = TFRobertaMainLayer(config, name="roberta")
- self.lm_head = TFRobertaLMHead(config, self.roberta.embeddings, name="lm_head")
-
- def get_output_embeddings(self):
- return self.lm_head.decoder
-
- @add_start_docstrings_to_callable(ROBERTA_INPUTS_DOCSTRING)
- def call(self, inputs, **kwargs):
- r"""
- Return:
- :obj:`tuple(torch.FloatTensor)` comprising various elements depending on the configuration (:class:`~transformers.RobertaConfig`) and inputs:
- prediction_scores (:obj:`Numpy array` or :obj:`tf.Tensor` of shape :obj:`(batch_size, sequence_length, config.vocab_size)`):
- Prediction scores of the language modeling head (scores for each vocabulary token before SoftMax).
- hidden_states (:obj:`tuple(tf.Tensor)`, `optional`, returned when :obj:`config.output_hidden_states=True`):
- tuple of :obj:`tf.Tensor` (one for the output of the embeddings + one for the output of each layer)
- of shape :obj:`(batch_size, sequence_length, hidden_size)`.
-
- Hidden-states of the model at the output of each layer plus the initial embedding outputs.
- attentions (:obj:`tuple(tf.Tensor)`, `optional`, returned when ``config.output_attentions=True``):
- tuple of :obj:`tf.Tensor` (one for each layer) of shape
- :obj:`(batch_size, num_heads, sequence_length, sequence_length)`:
-
- Attentions weights after the attention softmax, used to compute the weighted average in the self-attention heads.
-
- Examples::
-
- import tensorflow as tf
- from transformers import RobertaTokenizer, TFRobertaForMaskedLM
-
- tokenizer = RobertaTokenizer.from_pretrained('roberta-base')
- model = TFRobertaForMaskedLM.from_pretrained('roberta-base')
- input_ids = tf.constant(tokenizer.encode("Hello, my dog is cute", add_special_tokens=True))[None, :] # Batch size 1
- outputs = model(input_ids)
- prediction_scores = outputs[0]
-
- """
- outputs = self.roberta(inputs, **kwargs)
-
- sequence_output = outputs[0]
- prediction_scores = self.lm_head(sequence_output)
-
- outputs = (prediction_scores,) + outputs[2:] # Add hidden states and attention if they are here
-
- return outputs # prediction_scores, (hidden_states), (attentions)
-
-
-class TFRobertaClassificationHead(tf.keras.layers.Layer):
- """Head for sentence-level classification tasks."""
-
- def __init__(self, config, **kwargs):
- super().__init__(config, **kwargs)
- self.dense = tf.keras.layers.Dense(
- config.hidden_size,
- kernel_initializer=get_initializer(config.initializer_range),
- activation="tanh",
- name="dense",
- )
- self.dropout = tf.keras.layers.Dropout(config.hidden_dropout_prob)
- self.out_proj = tf.keras.layers.Dense(
- config.num_labels, kernel_initializer=get_initializer(config.initializer_range), name="out_proj"
- )
-
- def call(self, features, training=False):
- x = features[:, 0, :] # take token (equiv. to [CLS])
- x = self.dropout(x, training=training)
- x = self.dense(x)
- x = self.dropout(x, training=training)
- x = self.out_proj(x)
- return x
-
-
-@add_start_docstrings(
- """RoBERTa Model transformer with a sequence classification/regression head on top (a linear layer
- on top of the pooled output) e.g. for GLUE tasks. """,
- ROBERTA_START_DOCSTRING,
-)
-class TFRobertaForSequenceClassification(TFRobertaPreTrainedModel):
- def __init__(self, config, *inputs, **kwargs):
- super().__init__(config, *inputs, **kwargs)
- self.num_labels = config.num_labels
-
- self.roberta = TFRobertaMainLayer(config, name="roberta")
- self.classifier = TFRobertaClassificationHead(config, name="classifier")
-
- @add_start_docstrings_to_callable(ROBERTA_INPUTS_DOCSTRING)
- def call(self, inputs, **kwargs):
- r"""
- Return:
- :obj:`tuple(torch.FloatTensor)` comprising various elements depending on the configuration (:class:`~transformers.RobertaConfig`) and inputs:
- logits (:obj:`Numpy array` or :obj:`tf.Tensor` of shape :obj:`(batch_size, config.num_labels)`):
- Classification (or regression if config.num_labels==1) scores (before SoftMax).
- hidden_states (:obj:`tuple(tf.Tensor)`, `optional`, returned when :obj:`config.output_hidden_states=True`):
- tuple of :obj:`tf.Tensor` (one for the output of the embeddings + one for the output of each layer)
- of shape :obj:`(batch_size, sequence_length, hidden_size)`.
-
- Hidden-states of the model at the output of each layer plus the initial embedding outputs.
- attentions (:obj:`tuple(tf.Tensor)`, `optional`, returned when ``config.output_attentions=True``):
- tuple of :obj:`tf.Tensor` (one for each layer) of shape
- :obj:`(batch_size, num_heads, sequence_length, sequence_length)`:
-
- Attentions weights after the attention softmax, used to compute the weighted average in the self-attention heads.
-
- Examples::
-
- import tensorflow as tf
- from transformers import RobertaTokenizer, TFRobertaForSequenceClassification
-
- tokenizer = RobertaTokenizer.from_pretrained('roberta-base')
- model = TFRobertaForSequenceClassification.from_pretrained('roberta-base')
- input_ids = tf.constant(tokenizer.encode("Hello, my dog is cute", add_special_tokens=True))[None, :] # Batch size 1
- labels = tf.constant([1])[None, :] # Batch size 1
- outputs = model(input_ids)
- logits = outputs[0]
-
- """
- outputs = self.roberta(inputs, **kwargs)
-
- sequence_output = outputs[0]
- logits = self.classifier(sequence_output, training=kwargs.get("training", False))
-
- outputs = (logits,) + outputs[2:]
-
- return outputs # logits, (hidden_states), (attentions)
-
-
-@add_start_docstrings(
- """RoBERTa Model with a token classification head on top (a linear layer on top of
- the hidden-states output) e.g. for Named-Entity-Recognition (NER) tasks. """,
- ROBERTA_START_DOCSTRING,
-)
-class TFRobertaForTokenClassification(TFRobertaPreTrainedModel):
- def __init__(self, config, *inputs, **kwargs):
- super().__init__(config, *inputs, **kwargs)
- self.num_labels = config.num_labels
-
- self.roberta = TFRobertaMainLayer(config, name="roberta")
- self.dropout = tf.keras.layers.Dropout(config.hidden_dropout_prob)
- self.classifier = tf.keras.layers.Dense(
- config.num_labels, kernel_initializer=get_initializer(config.initializer_range), name="classifier"
- )
-
- @add_start_docstrings_to_callable(ROBERTA_INPUTS_DOCSTRING)
- def call(self, inputs, **kwargs):
- r"""
- Return:
- :obj:`tuple(torch.FloatTensor)` comprising various elements depending on the configuration (:class:`~transformers.RobertaConfig`) and inputs:
- scores (:obj:`Numpy array` or :obj:`tf.Tensor` of shape :obj:`(batch_size, sequence_length, config.num_labels)`):
- Classification scores (before SoftMax).
- hidden_states (:obj:`tuple(tf.Tensor)`, `optional`, returned when :obj:`config.output_hidden_states=True`):
- tuple of :obj:`tf.Tensor` (one for the output of the embeddings + one for the output of each layer)
- of shape :obj:`(batch_size, sequence_length, hidden_size)`.
-
- Hidden-states of the model at the output of each layer plus the initial embedding outputs.
- attentions (:obj:`tuple(tf.Tensor)`, `optional`, returned when ``config.output_attentions=True``):
- tuple of :obj:`tf.Tensor` (one for each layer) of shape
- :obj:`(batch_size, num_heads, sequence_length, sequence_length)`:
-
- Attentions weights after the attention softmax, used to compute the weighted average in the self-attention heads.
-
- Examples::
-
- import tensorflow as tf
- from transformers import RobertaTokenizer, TFRobertaForTokenClassification
-
- tokenizer = RobertaTokenizer.from_pretrained('roberta-base')
- model = TFRobertaForTokenClassification.from_pretrained('roberta-base')
- input_ids = tf.constant(tokenizer.encode("Hello, my dog is cute", add_special_tokens=True))[None, :] # Batch size 1
- outputs = model(input_ids)
- scores = outputs[0]
-
- """
- outputs = self.roberta(inputs, **kwargs)
-
- sequence_output = outputs[0]
-
- sequence_output = self.dropout(sequence_output, training=kwargs.get("training", False))
- logits = self.classifier(sequence_output)
-
- outputs = (logits,) + outputs[2:] # add hidden states and attention if they are here
-
- return outputs # scores, (hidden_states), (attentions)
diff --git a/server/transformers/src/transformers/modeling_tf_t5.py b/server/transformers/src/transformers/modeling_tf_t5.py
deleted file mode 100644
index db62e784b10d6e771cd3fe1788535313f9367ea5..0000000000000000000000000000000000000000
--- a/server/transformers/src/transformers/modeling_tf_t5.py
+++ /dev/null
@@ -1,793 +0,0 @@
-# coding=utf-8
-# Copyright 2018 T5 Authors and The HuggingFace Inc. team.
-# Copyright (c) 2018, NVIDIA CORPORATION. All rights reserved.
-#
-# Licensed under the Apache License, Version 2.0 (the "License");
-# you may not use this file except in compliance with the License.
-# You may obtain a copy of the License at
-#
-# http://www.apache.org/licenses/LICENSE-2.0
-#
-# Unless required by applicable law or agreed to in writing, software
-# distributed under the License is distributed on an "AS IS" BASIS,
-# WITHOUT WARRANTIES OR CONDITIONS OF ANY KIND, either express or implied.
-# See the License for the specific language governing permissions and
-# limitations under the License.
-""" TF 2.0 T5 model. """
-
-
-import copy
-import itertools
-import logging
-import math
-
-import tensorflow as tf
-
-from .configuration_t5 import T5Config
-from .file_utils import DUMMY_INPUTS, DUMMY_MASK, add_start_docstrings
-from .modeling_tf_utils import TFPreTrainedModel, TFSharedEmbeddings, shape_list
-
-
-logger = logging.getLogger(__name__)
-
-TF_T5_PRETRAINED_MODEL_ARCHIVE_MAP = {
- "t5-small": "https://s3.amazonaws.com/models.huggingface.co/bert/t5-small-tf_model.h5",
- "t5-base": "https://s3.amazonaws.com/models.huggingface.co/bert/t5-base-tf_model.h5",
- "t5-large": "https://s3.amazonaws.com/models.huggingface.co/bert/t5-large-tf_model.h5",
- "t5-3b": "https://s3.amazonaws.com/models.huggingface.co/bert/t5-3b-tf_model.h5",
- "t5-11b": "https://s3.amazonaws.com/models.huggingface.co/bert/t5-11b-tf_model.h5",
-}
-
-####################################################
-# TF 2.0 Models are constructed using Keras imperative API by sub-classing
-# - tf.keras.layers.Layer for the layers and
-# - TFPreTrainedModel for the models (it-self a sub-class of tf.keras.Model)
-####################################################
-
-
-class TFT5LayerNorm(tf.keras.layers.Layer):
- def __init__(self, epsilon=1e-6, **kwargs):
- """ Construct a layernorm module in the T5 style
- No bias and no substraction of mean.
- """
- super().__init__(**kwargs)
- self.variance_epsilon = epsilon
-
- def build(self, input_shape):
- """Build shared word embedding layer """
- self.weight = self.add_weight("weight", shape=(input_shape[-1],), initializer="ones")
- super().build(input_shape)
-
- def call(self, x):
- variance = tf.math.reduce_mean(tf.math.square(x), axis=-1, keepdims=True)
- x = x * tf.math.rsqrt(variance + self.variance_epsilon)
- return self.weight * x
-
-
-class TFT5DenseReluDense(tf.keras.layers.Layer):
- def __init__(self, config, **kwargs):
- super().__init__(**kwargs)
- self.wi = tf.keras.layers.Dense(config.d_ff, use_bias=False, name="wi")
- self.wo = tf.keras.layers.Dense(config.d_model, use_bias=False, name="wo")
- self.dropout = tf.keras.layers.Dropout(config.dropout_rate)
- self.act = tf.keras.activations.relu
-
- def call(self, hidden_states, training=False):
- h = self.wi(hidden_states)
- h = self.act(h)
- h = self.dropout(h, training=training)
- h = self.wo(h)
- return h
-
-
-class TFT5LayerFF(tf.keras.layers.Layer):
- def __init__(self, config, **kwargs):
- super().__init__(**kwargs)
- self.DenseReluDense = TFT5DenseReluDense(config, name="DenseReluDense")
- self.layer_norm = TFT5LayerNorm(epsilon=config.layer_norm_epsilon, name="layer_norm")
- self.dropout = tf.keras.layers.Dropout(config.dropout_rate)
-
- def call(self, hidden_states, training=False):
- norm_x = self.layer_norm(hidden_states)
- y = self.DenseReluDense(norm_x, training=training)
- layer_output = hidden_states + self.dropout(y, training=training)
- return layer_output
-
-
-class TFT5Attention(tf.keras.layers.Layer):
- NEW_ID = itertools.count()
-
- def __init__(self, config, has_relative_attention_bias=False, **kwargs):
- super().__init__(**kwargs)
- self.layer_id = next(TFT5Attention.NEW_ID)
- self.is_decoder = config.is_decoder
- self.has_relative_attention_bias = has_relative_attention_bias
-
- self.output_attentions = config.output_attentions
- self.relative_attention_num_buckets = config.relative_attention_num_buckets
- self.d_model = config.d_model
- self.d_kv = config.d_kv
- self.n_heads = config.num_heads
- self.inner_dim = self.n_heads * self.d_kv
-
- # Mesh TensorFlow initialization to avoid scaling before softmax
- self.q = tf.keras.layers.Dense(self.inner_dim, use_bias=False, name="q")
- self.k = tf.keras.layers.Dense(self.inner_dim, use_bias=False, name="k")
- self.v = tf.keras.layers.Dense(self.inner_dim, use_bias=False, name="v")
- self.o = tf.keras.layers.Dense(self.d_model, use_bias=False, name="o")
- self.dropout = tf.keras.layers.Dropout(config.dropout_rate)
-
- if self.has_relative_attention_bias:
- self.relative_attention_bias = tf.keras.layers.Embedding(
- self.relative_attention_num_buckets, self.n_heads, name="relative_attention_bias"
- )
- self.pruned_heads = set()
-
- def prune_heads(self, heads):
- raise NotImplementedError
-
- @staticmethod
- def _relative_position_bucket(relative_position, bidirectional=True, num_buckets=32, max_distance=128):
- """
- Adapted from Mesh Tensorflow:
- https://github.com/tensorflow/mesh/blob/0cb87fe07da627bf0b7e60475d59f95ed6b5be3d/mesh_tensorflow/transformer/transformer_layers.py#L593
-
- Translate relative position to a bucket number for relative attention.
- The relative position is defined as memory_position - query_position, i.e.
- the distance in tokens from the attending position to the attended-to
- position. If bidirectional=False, then positive relative positions are
- invalid.
- We use smaller buckets for small absolute relative_position and larger buckets
- for larger absolute relative_positions. All relative positions >=max_distance
- map to the same bucket. All relative positions <=-max_distance map to the
- same bucket. This should allow for more graceful generalization to longer
- sequences than the model has been trained on.
- Args:
- relative_position: an int32 Tensor
- bidirectional: a boolean - whether the attention is bidirectional
- num_buckets: an integer
- max_distance: an integer
- Returns:
- a Tensor with the same shape as relative_position, containing int32
- values in the range [0, num_buckets)
- """
- ret = 0
- n = -relative_position
- if bidirectional:
- num_buckets //= 2
- ret += tf.dtypes.cast(tf.math.less(n, 0), tf.int32) * num_buckets
- n = tf.math.abs(n)
- else:
- n = tf.math.maximum(n, 0)
- # now n is in the range [0, inf)
- max_exact = num_buckets // 2
- is_small = tf.math.less(n, max_exact)
- val_if_large = max_exact + tf.dtypes.cast(
- tf.math.log(tf.dtypes.cast(n, tf.float32) / max_exact)
- / math.log(max_distance / max_exact)
- * (num_buckets - max_exact),
- tf.int32,
- )
- val_if_large = tf.math.minimum(val_if_large, num_buckets - 1)
- ret += tf.where(is_small, n, val_if_large)
- return ret
-
- def compute_bias(self, qlen, klen):
- """ Compute binned relative position bias """
- context_position = tf.range(qlen)[:, None]
- memory_position = tf.range(klen)[None, :]
- relative_position = memory_position - context_position # shape (qlen, klen)
- rp_bucket = self._relative_position_bucket(
- relative_position, bidirectional=not self.is_decoder, num_buckets=self.relative_attention_num_buckets
- )
- values = self.relative_attention_bias(rp_bucket) # shape (qlen, klen, num_heads)
- values = tf.expand_dims(tf.transpose(values, [2, 0, 1]), axis=0) # shape (1, num_heads, qlen, klen)
- return values
-
- def call(self, input, mask=None, kv=None, position_bias=None, cache=None, head_mask=None, training=False):
- """
- Self-attention (if kv is None) or attention over source sentence (provided by kv).
- """
- # Input is (bs, qlen, dim)
- # Mask is (bs, klen) (non-causal) or (bs, klen, klen)
- bs, qlen, dim = shape_list(input)
- if kv is None:
- klen = qlen if cache is None else cache["slen"] + qlen
- else:
- klen = shape_list(kv)[1]
-
- def shape(x):
- """ projection """
- return tf.transpose(tf.reshape(x, (bs, -1, self.n_heads, self.d_kv)), perm=(0, 2, 1, 3))
-
- def unshape(x):
- """ compute context """
- return tf.reshape(tf.transpose(x, perm=(0, 2, 1, 3)), (bs, -1, self.inner_dim))
-
- q = shape(self.q(input)) # (bs, n_heads, qlen, dim_per_head)
- if kv is None:
- k = shape(self.k(input)) # (bs, n_heads, qlen, dim_per_head)
- v = shape(self.v(input)) # (bs, n_heads, qlen, dim_per_head)
- elif cache is None or self.layer_id not in cache:
- k = v = kv
- k = shape(self.k(k)) # (bs, n_heads, qlen, dim_per_head)
- v = shape(self.v(v)) # (bs, n_heads, qlen, dim_per_head)
-
- if cache is not None:
- if self.layer_id in cache:
- if kv is None:
- k_, v_ = cache[self.layer_id]
- k = tf.concat([k_, k], axis=2) # (bs, n_heads, klen, dim_per_head)
- v = tf.concat([v_, v], axis=2) # (bs, n_heads, klen, dim_per_head)
- else:
- k, v = cache[self.layer_id]
- cache[self.layer_id] = (k, v)
-
- # q = q / math.sqrt(dim_per_head) # No scaling in T5
- # scores = tf.matmul(q, k, transpose_b=True) # (bs, n_heads, qlen, klen)
- scores = tf.einsum("bnqd,bnkd->bnqk", q, k) # (bs, n_heads, qlen, klen)
-
- if position_bias is None:
- if not self.has_relative_attention_bias:
- raise ValueError("No position_bias provided and no weights to compute position_bias")
- position_bias = self.compute_bias(qlen, klen)
- if mask is not None:
- position_bias = position_bias + mask
- # mask = (mask == 0).expand_as(scores) # (bs, n_heads, qlen, klen)
- # scores.masked_fill_(mask, -float('inf')) # (bs, n_heads, qlen, klen)
-
- scores += position_bias
- weights = tf.nn.softmax(scores, axis=-1) # (bs, n_heads, qlen, klen)
- weights = self.dropout(weights, training=training) # (bs, n_heads, qlen, klen)
-
- # Mask heads if we want to
- if head_mask is not None:
- weights = weights * head_mask
-
- context = tf.matmul(weights, v) # (bs, n_heads, qlen, dim_per_head)
- context = unshape(context) # (bs, qlen, dim)
-
- context = self.o(context)
-
- outputs = (context,)
- if self.output_attentions:
- outputs = outputs + (weights,)
- if self.has_relative_attention_bias:
- outputs = outputs + (position_bias,)
- return outputs
-
-
-class TFT5LayerSelfAttention(tf.keras.layers.Layer):
- def __init__(self, config, has_relative_attention_bias=False, **kwargs):
- super().__init__(**kwargs)
- self.SelfAttention = TFT5Attention(
- config, has_relative_attention_bias=has_relative_attention_bias, name="SelfAttention"
- )
- self.layer_norm = TFT5LayerNorm(epsilon=config.layer_norm_epsilon, name="layer_norm")
- self.dropout = tf.keras.layers.Dropout(config.dropout_rate)
-
- def call(self, hidden_states, attention_mask=None, position_bias=None, head_mask=None, training=False):
- norm_x = self.layer_norm(hidden_states)
- attention_output = self.SelfAttention(
- norm_x, mask=attention_mask, position_bias=position_bias, head_mask=head_mask, training=training
- )
- y = attention_output[0]
- layer_output = hidden_states + self.dropout(y, training=training)
- outputs = (layer_output,) + attention_output[1:] # add attentions if we output them
- return outputs
-
-
-class TFT5LayerCrossAttention(tf.keras.layers.Layer):
- def __init__(self, config, has_relative_attention_bias=False, **kwargs):
- super().__init__(**kwargs)
- self.EncDecAttention = TFT5Attention(
- config, has_relative_attention_bias=has_relative_attention_bias, name="EncDecAttention"
- )
- self.layer_norm = TFT5LayerNorm(epsilon=config.layer_norm_epsilon, name="layer_norm")
- self.dropout = tf.keras.layers.Dropout(config.dropout_rate)
-
- def call(self, hidden_states, kv, attention_mask=None, position_bias=None, head_mask=None, training=False):
- norm_x = self.layer_norm(hidden_states)
- attention_output = self.EncDecAttention(
- norm_x, mask=attention_mask, kv=kv, position_bias=position_bias, head_mask=head_mask, training=training
- )
- y = attention_output[0]
- layer_output = hidden_states + self.dropout(y, training=training)
- outputs = (layer_output,) + attention_output[1:] # add attentions if we output them
- return outputs
-
-
-class TFT5Block(tf.keras.layers.Layer):
- def __init__(self, config, has_relative_attention_bias=False, **kwargs):
- super().__init__(**kwargs)
- self.is_decoder = config.is_decoder
- self.layer = []
- self.layer.append(
- TFT5LayerSelfAttention(config, has_relative_attention_bias=has_relative_attention_bias, name="layer_._0")
- )
- if self.is_decoder:
- self.layer.append(
- TFT5LayerCrossAttention(
- config, has_relative_attention_bias=has_relative_attention_bias, name="layer_._1"
- )
- )
- self.layer.append(TFT5LayerFF(config, name="layer_._2"))
- else:
- self.layer.append(TFT5LayerFF(config, name="layer_._1"))
-
- def call(
- self,
- hidden_states,
- attention_mask=None,
- position_bias=None,
- encoder_hidden_states=None,
- encoder_attention_mask=None,
- encoder_decoder_position_bias=None,
- head_mask=None,
- training=False,
- ):
- self_attention_outputs = self.layer[0](
- hidden_states,
- attention_mask=attention_mask,
- position_bias=position_bias,
- head_mask=head_mask,
- training=training,
- )
- hidden_states = self_attention_outputs[0]
- outputs = self_attention_outputs[1:]
-
- if not self.is_decoder:
- hidden_states = self.layer[1](hidden_states, training=training)
- else:
- cross_attention_outputs = self.layer[1](
- hidden_states,
- kv=encoder_hidden_states,
- attention_mask=encoder_attention_mask,
- position_bias=encoder_decoder_position_bias,
- head_mask=head_mask,
- training=training,
- )
- hidden_states = cross_attention_outputs[0]
- outputs = outputs + cross_attention_outputs[1:]
- hidden_states = self.layer[2](hidden_states, training=training)
-
- outputs = (hidden_states,) + outputs # add attentions if we output them
- return outputs # hidden-states, (self-attention weights), (self-attention position bias), (cross-attention weights), (cross-attention position bias)
-
-
-####################################################
-# The full model without a specific pretrained or finetuning head is
-# provided as a tf.keras.layers.Layer usually called "TFT5MainLayer"
-####################################################
-class TFT5MainLayer(tf.keras.layers.Layer):
- def __init__(self, config, **kwargs):
- super().__init__(**kwargs)
- self.output_attentions = config.output_attentions
- self.output_hidden_states = config.output_hidden_states
- self.is_decoder = config.is_decoder
- self.config = config
- self.num_hidden_layers = config.num_layers
-
- self.block = [
- TFT5Block(config, has_relative_attention_bias=bool(i == 0), name="block_._{}".format(i))
- for i in range(config.num_layers)
- ]
- self.final_layer_norm = TFT5LayerNorm(epsilon=config.layer_norm_epsilon, name="final_layer_norm")
- self.dropout = tf.keras.layers.Dropout(config.dropout_rate)
-
- def _resize_token_embeddings(self, new_num_tokens):
- raise NotImplementedError # Not implemented yet in the library fr TF 2.0 models
-
- def _prune_heads(self, heads_to_prune):
- raise NotImplementedError # Not implemented yet in the library fr TF 2.0 models
-
- def call(
- self,
- hidden_states,
- attention_mask=None,
- encoder_hidden_states=None,
- encoder_attention_mask=None,
- head_mask=None,
- training=False,
- ):
-
- batch_size, seq_length = shape_list(hidden_states)[:2]
- if attention_mask is None:
- attention_mask = tf.fill((batch_size, seq_length), 1)
- if self.is_decoder and encoder_attention_mask is None:
- encoder_seq_length = encoder_hidden_states.shape[1]
- encoder_attention_mask = tf.fill((batch_size, encoder_seq_length), 1)
-
- # We can provide a self-attention mask of dimensions [batch_size, from_seq_length, to_seq_length]
- # ourselves in which case we just need to make it broadcastable to all heads.
- attention_mask = tf.cast(attention_mask, dtype=tf.float32)
- num_dims_attention_mask = len(shape_list(attention_mask))
- if num_dims_attention_mask == 3:
- extended_attention_mask = attention_mask[:, None, :, :]
- elif num_dims_attention_mask == 2:
- # Provided a padding mask of dimensions [batch_size, seq_length]
- # - if the model is a decoder, apply a causal mask in addition to the padding mask
- # - if the model is an encoder, make the mask broadcastable to [batch_size, num_heads, seq_length, seq_length]
- if self.config.is_decoder:
- seq_ids = tf.range(seq_length)
- causal_mask = tf.less_equal(
- tf.tile(seq_ids[None, None, :], (batch_size, seq_length, 1)), seq_ids[None, :, None]
- )
- causal_mask = tf.cast(causal_mask, dtype=tf.float32)
- extended_attention_mask = causal_mask[:, None, :, :] * attention_mask[:, None, None, :]
- else:
- extended_attention_mask = attention_mask[:, None, None, :]
-
- # Since attention_mask is 1.0 for positions we want to attend and 0.0 for
- # masked positions, this operation will create a tensor which is 0.0 for
- # positions we want to attend and -10000.0 for masked positions.
- # Since we are adding it to the raw scores before the softmax, this is
- # effectively the same as removing these entirely.
-
- # T5 has a mask that can compare sequence ids, we can simulate this here with this transposistion
- # Cf. https://github.com/tensorflow/mesh/blob/8d2465e9bc93129b913b5ccc6a59aa97abd96ec6/mesh_tensorflow/transformer/transformer_layers.py#L270
- # extended_attention_mask = tf.math.equal(extended_attention_mask,
- # tf.transpose(extended_attention_mask, perm=(-1, -2)))
-
- extended_attention_mask = (1.0 - extended_attention_mask) * -1e9
-
- if self.is_decoder:
- # If a 2D ou 3D attention mask is provided for the cross-attention
- # we need to make broadcastabe to [batch_size, num_heads, seq_length, seq_length]
- encoder_attention_mask = tf.cast(encoder_attention_mask, dtype=tf.float32)
- num_dims_encoder_attention_mask = len(shape_list(encoder_attention_mask))
- if num_dims_encoder_attention_mask == 3:
- encoder_extended_attention_mask = encoder_attention_mask[:, None, :, :]
- if num_dims_encoder_attention_mask == 2:
- encoder_extended_attention_mask = encoder_attention_mask[:, None, None, :]
-
- # T5 has a mask that can compare sequence ids, we can simulate this here with this transposistion
- # Cf. https://github.com/tensorflow/mesh/blob/8d2465e9bc93129b913b5ccc6a59aa97abd96ec6/mesh_tensorflow/transformer/transformer_layers.py#L270
- # encoder_extended_attention_mask = tf.math.equal(encoder_extended_attention_mask,
- # tf.transpose(encoder_extended_attention_mask, perm=(-1, -2)))
-
- encoder_extended_attention_mask = (1.0 - encoder_extended_attention_mask) * -1e9
- else:
- encoder_extended_attention_mask = None
-
- # Prepare head mask if needed
- # 1.0 in head_mask indicate we keep the head
- # attention_probs has shape bsz x n_heads x N x N
- # input head_mask has shape [num_heads] or [num_hidden_layers x num_heads]
- # and head_mask is converted to shape [num_hidden_layers x batch x num_heads x seq_length x seq_length]
- if head_mask is not None:
- raise NotImplementedError
- else:
- head_mask = [None] * self.num_hidden_layers
- # head_mask = tf.constant([0] * self.num_hidden_layers)
-
- all_hidden_states = ()
- all_attentions = ()
- position_bias = None
- encoder_decoder_position_bias = None
- for i, layer_module in enumerate(self.block):
- if self.output_hidden_states:
- all_hidden_states = all_hidden_states + (hidden_states,)
-
- layer_outputs = layer_module(
- hidden_states,
- attention_mask=extended_attention_mask,
- position_bias=position_bias,
- encoder_hidden_states=encoder_hidden_states,
- encoder_attention_mask=encoder_extended_attention_mask,
- encoder_decoder_position_bias=encoder_decoder_position_bias,
- head_mask=head_mask[i],
- training=training,
- )
- hidden_states = layer_outputs[0]
- if i == 0:
- # We share the position biases between the layers - the first layer store them
- # layer_outputs = hidden-states, (self-attention weights), (self-attention position bias), (cross-attention weights), (cross-attention position bias)
- position_bias = layer_outputs[2 if self.output_attentions else 1]
- if self.is_decoder:
- encoder_decoder_position_bias = layer_outputs[4 if self.output_attentions else 2]
-
- if self.output_attentions:
- all_attentions = all_attentions + (layer_outputs[1],)
-
- hidden_states = self.final_layer_norm(hidden_states)
- hidden_states = self.dropout(hidden_states, training=training)
-
- # Add last layer
- if self.output_hidden_states:
- all_hidden_states = all_hidden_states + (hidden_states,)
-
- outputs = (hidden_states,)
- if self.output_hidden_states:
- outputs = outputs + (all_hidden_states,)
- if self.output_attentions:
- outputs = outputs + (all_attentions,)
- return outputs # last-layer hidden state, (all hidden states), (all attentions)
-
-
-####################################################
-# TFT5PreTrainedModel is a sub-class of tf.keras.Model
-# which take care of loading and saving pretrained weights
-# and various common utilities.
-# Here you just need to specify a few (self-explanatory)
-# pointers for your model.
-####################################################
-class TFT5PreTrainedModel(TFPreTrainedModel):
- """ An abstract class to handle weights initialization and
- a simple interface for downloading and loading pretrained models.
- """
-
- config_class = T5Config
- pretrained_model_archive_map = TF_T5_PRETRAINED_MODEL_ARCHIVE_MAP
- base_model_prefix = "transformer"
-
- @property
- def dummy_inputs(self):
- input_ids = tf.constant(DUMMY_INPUTS)
- input_mask = tf.constant(DUMMY_MASK)
- dummy_inputs = {
- "decoder_input_ids": input_ids,
- "encoder_input_ids": input_ids,
- "decoder_attention_mask": input_mask,
- }
- return dummy_inputs
-
-
-T5_START_DOCSTRING = r""" The T5 model was proposed in
- `Exploring the Limits of Transfer Learning with a Unified Text-to-Text Transformer`_
- by Colin Raffel, Noam Shazeer, Adam Roberts, Katherine Lee, Sharan Narang, Michael Matena, Yanqi Zhou, Wei Li, Peter J. Liu.
- It's an encoder decoder transformer pre-trained in a text-to-text denoising generative setting.
-
- This model is a tf.keras.Model `tf.keras.Model`_ sub-class. Use it as a regular TF 2.0 Keras Model and
- refer to the TF 2.0 documentation for all matter related to general usage and behavior.
-
- .. _`Exploring the Limits of Transfer Learning with a Unified Text-to-Text Transformer`:
- https://arxiv.org/abs/1910.10683
-
- .. _`tf.keras.Model`:
- https://www.tensorflow.org/versions/r2.0/api_docs/python/tf/keras/Model
-
- Note on the model inputs:
- TF 2.0 models accepts two formats as inputs:
-
- - having all inputs as keyword arguments (like PyTorch models), or
- - having all inputs as a list, tuple or dict in the first positional arguments.
-
- This second option is usefull when using `tf.keras.Model.fit()` method which currently requires having all the tensors in the first argument of the model call function: `model(inputs)`.
-
- If you choose this second option, there are three possibilities you can use to gather all the input Tensors in the first positional argument :
-
- - a single Tensor with input_ids only and nothing else: `model(inputs_ids)
- - a list of varying length with one or several input Tensors IN THE ORDER given in the docstring:
- `model([input_ids, attention_mask])` or `model([input_ids, attention_mask, token_type_ids])`
- - a dictionary with one or several input Tensors associaed to the input names given in the docstring:
- `model({'input_ids': input_ids, 'token_type_ids': token_type_ids})`
-
- Parameters:
- config (:class:`~transformers.T5Config`): Model configuration class with all the parameters of the model.
- Initializing with a config file does not load the weights associated with the model, only the configuration.
- Check out the :meth:`~transformers.PreTrainedModel.from_pretrained` method to load the model weights.
-"""
-
-T5_INPUTS_DOCSTRING = r"""
- Inputs:
- **input_ids**: ``Numpy array`` or ``tf.Tensor`` of shape ``(batch_size, sequence_length)``:
- Indices of input sequence tokens in the vocabulary.
- To match pre-training, T5 input sequence should be formatted with [CLS] and [SEP] tokens as follows:
-
- (a) For sequence pairs:
-
- ``tokens: [CLS] is this jack ##son ##ville ? [SEP] no it is not . [SEP]``
-
- (b) For single sequences:
-
- ``tokens: [CLS] the dog is hairy . [SEP]``
-
-
- T5 is a model with relative position embeddings so you should be able to pad the inputs on
- the right or the left.
-
- Indices can be obtained using :class:`transformers.T5Tokenizer`.
- See :func:`transformers.PreTrainedTokenizer.encode` and
- :func:`transformers.PreTrainedTokenizer.convert_tokens_to_ids` for details.
- **attention_mask**: (`optional`) ``Numpy array`` or ``tf.Tensor`` of shape ``(batch_size, sequence_length)``:
- Mask to avoid performing attention on padding token indices.
- Mask values selected in ``[0, 1]``:
- ``1`` for tokens that are NOT MASKED, ``0`` for MASKED tokens.
- **head_mask**: (`optional`) ``Numpy array`` or ``tf.Tensor`` of shape ``(num_heads,)`` or ``(num_layers, num_heads)``:
- Mask to nullify selected heads of the self-attention modules.
- Mask values selected in ``[0, 1]``:
- ``1`` indicates the head is **not masked**, ``0`` indicates the head is **masked**.
-"""
-
-
-@add_start_docstrings(
- "The bare T5 Model transformer outputting raw hidden-states" "without any specific head on top.",
- T5_START_DOCSTRING,
- T5_INPUTS_DOCSTRING,
-)
-class TFT5Model(TFT5PreTrainedModel):
- r"""
- Outputs: `Tuple` comprising various elements depending on the configuration (config) and inputs:
- **last_hidden_state**: ``tf.Tensor`` of shape ``(batch_size, sequence_length, hidden_size)``
- Sequence of hidden-states at the output of the last layer of the model.
- **hidden_states**: (`optional`, returned when ``config.output_hidden_states=True``)
- list of ``tf.Tensor`` (one for the output of each layer + the output of the embeddings)
- of shape ``(batch_size, sequence_length, hidden_size)``:
- Hidden-states of the model at the output of each layer plus the initial embedding outputs.
- **attentions**: (`optional`, returned when ``config.output_attentions=True``)
- list of ``tf.Tensor`` (one for each layer) of shape ``(batch_size, num_heads, sequence_length, sequence_length)``:
- Attentions weights after the attention softmax, used to compute the weighted average in the self-attention heads.
-
- Examples::
-
- import tensorflow as tf
- from transformers import T5Tokenizer, TFT5Model
-
- tokenizer = T5Tokenizer.from_pretrained('t5-small')
- model = TFT5Model.from_pretrained('t5-small')
- input_ids = tf.constant(tokenizer.encode("Hello, my dog is cute"))[None, :] # Batch size 1
- outputs = model(input_ids=input_ids)
- last_hidden_states = outputs[0] # The last hidden-state is the first element of the output tuple
-
- """
-
- def __init__(self, config, *inputs, **kwargs):
- super().__init__(config, *inputs, **kwargs)
- self.shared = TFSharedEmbeddings(config.vocab_size, config.d_model, name="shared")
-
- encoder_config = copy.deepcopy(config)
- self.encoder = TFT5MainLayer(encoder_config, name="encoder")
-
- decoder_config = copy.deepcopy(config)
- decoder_config.is_decoder = True
- self.decoder = TFT5MainLayer(decoder_config, name="decoder")
-
- def get_input_embeddings(self):
- return self.shared
-
- def get_output_embeddings(self):
- return self.shared
-
- def call(self, decoder_input_ids, **kwargs):
- # We allow two types of multi-inputs:
- # - traditional keyword arguments in the call method
- # - all the arguments provided as a dict in the first positional argument of call
- # The last option is useful to use the tf.keras fit() method.
-
- if isinstance(decoder_input_ids, dict):
- kwargs.update(decoder_input_ids)
- else:
- kwargs["decoder_input_ids"] = decoder_input_ids
-
- kwargs_common = dict(
- (k, v) for k, v in kwargs.items() if not k.startswith("encoder_") and not k.startswith("decoder_")
- )
- kwargs_encoder = kwargs_common.copy()
- kwargs_decoder = kwargs_common.copy()
- kwargs_encoder.update(dict((k[len("encoder_") :], v) for k, v in kwargs.items() if k.startswith("encoder_")))
- kwargs_decoder.update(dict((k[len("decoder_") :], v) for k, v in kwargs.items() if k.startswith("decoder_")))
-
- # Encode if needed (training, first prediction pass)
- encoder_hidden_states = kwargs_encoder.pop("hidden_states", None)
- if encoder_hidden_states is None:
- # Convert encoder inputs in embeddings if needed
- hidden_states = kwargs_encoder.pop("inputs_embeds", None)
- if hidden_states is None:
- encoder_inputs_ids = kwargs_encoder.pop("input_ids")
- hidden_states = self.shared(encoder_inputs_ids) # Convert inputs in embeddings
-
- encoder_outputs = self.encoder(hidden_states, **kwargs_encoder)
- encoder_hidden_states = encoder_outputs[0]
- else:
- encoder_outputs = ()
-
- # Decode
- # Convert decoder inputs in embeddings if needed
- hidden_states = kwargs_decoder.pop("inputs_embeds", None)
- if hidden_states is None:
- decoder_inputs_ids = kwargs_decoder.pop("input_ids")
- hidden_states = self.shared(decoder_inputs_ids)
-
- kwargs_decoder["encoder_hidden_states"] = encoder_hidden_states
- kwargs_decoder["encoder_attention_mask"] = kwargs_encoder.get("attention_mask", None)
- decoder_outputs = self.decoder(hidden_states, **kwargs_decoder)
-
- return decoder_outputs + encoder_outputs
-
-
-@add_start_docstrings("""T5 Model with a `language modeling` head on top. """, T5_START_DOCSTRING, T5_INPUTS_DOCSTRING)
-class TFT5WithLMHeadModel(TFT5PreTrainedModel):
- r"""
- Outputs: `Tuple` comprising various elements depending on the configuration (config) and inputs:
- **prediction_scores**: ``Numpy array`` or ``tf.Tensor`` of shape ``(batch_size, sequence_length, config.vocab_size)``
- Prediction scores of the language modeling head (scores for each vocabulary token before SoftMax).
- **hidden_states**: (`optional`, returned when ``config.output_hidden_states=True``)
- list of ``Numpy array`` or ``tf.Tensor`` (one for the output of each layer + the output of the embeddings)
- of shape ``(batch_size, sequence_length, hidden_size)``:
- Hidden-states of the model at the output of each layer plus the initial embedding outputs.
- **attentions**: (`optional`, returned when ``config.output_attentions=True``)
- list of ``Numpy array`` or ``tf.Tensor`` (one for each layer) of shape ``(batch_size, num_heads, sequence_length, sequence_length)``:
- Attentions weights after the attention softmax, used to compute the weighted average in the self-attention heads.
-
- Examples::
-
- import tensorflow as tf
- from transformers import T5Tokenizer, TFT5WithLMHeadModel
-
- tokenizer = T5Tokenizer.from_pretrained('t5-small')
- model = TFT5WithLMHeadModel.from_pretrained('t5-small')
- input_ids = tf.constant(tokenizer.encode("Hello, my dog is cute"))[None, :] # Batch size 1
- outputs = model(input_ids=input_ids)
- prediction_scores = outputs[0]
-
- """
-
- def __init__(self, config, *inputs, **kwargs):
- super().__init__(config, *inputs, **kwargs)
- self.model_dim = config.d_model
-
- self.shared = TFSharedEmbeddings(config.vocab_size, config.d_model, name="shared")
-
- encoder_config = copy.deepcopy(config)
- self.encoder = TFT5MainLayer(encoder_config, name="encoder")
-
- decoder_config = copy.deepcopy(config)
- decoder_config.is_decoder = True
- self.decoder = TFT5MainLayer(decoder_config, name="decoder")
-
- def get_input_embeddings(self):
- return self.shared
-
- def get_output_embeddings(self):
- return self.shared
-
- def call(self, decoder_input_ids, **kwargs):
- # We allow two types of multi-inputs:
- # - traditional keyword arguments in the call method
- # - all the arguments provided as a dict in the first positional argument of call
- # The last option is useful to use the tf.keras fit() method.
-
- if isinstance(decoder_input_ids, dict):
- kwargs.update(decoder_input_ids)
- else:
- kwargs["decoder_input_ids"] = decoder_input_ids
-
- kwargs_common = dict(
- (k, v) for k, v in kwargs.items() if not k.startswith("encoder_") and not k.startswith("decoder_")
- )
- kwargs_encoder = kwargs_common.copy()
- kwargs_decoder = kwargs_common.copy()
- kwargs_encoder.update(dict((k[len("encoder_") :], v) for k, v in kwargs.items() if k.startswith("encoder_")))
- kwargs_decoder.update(dict((k[len("decoder_") :], v) for k, v in kwargs.items() if k.startswith("decoder_")))
-
- # Encode if needed (training, first prediction pass)
- encoder_hidden_states = kwargs_encoder.pop("hidden_states", None)
- if encoder_hidden_states is None:
- # Convert encoder inputs in embeddings if needed
- hidden_states = kwargs_encoder.pop("inputs_embeds", None)
- if hidden_states is None:
- encoder_inputs_ids = kwargs_encoder.pop("input_ids")
- hidden_states = self.shared(encoder_inputs_ids) # Convert inputs in embeddings
-
- encoder_outputs = self.encoder(hidden_states, **kwargs_encoder)
- encoder_hidden_states = encoder_outputs[0]
- else:
- encoder_outputs = ()
-
- # Decode
- # Convert decoder inputs in embeddings if needed
- hidden_states = kwargs_decoder.pop("inputs_embeds", None)
- if hidden_states is None:
- decoder_inputs_ids = kwargs_decoder.pop("input_ids")
- hidden_states = self.shared(decoder_inputs_ids)
-
- kwargs_decoder["encoder_hidden_states"] = encoder_hidden_states
- kwargs_decoder["encoder_attention_mask"] = kwargs_encoder.get("attention_mask", None)
- decoder_outputs = self.decoder(hidden_states, **kwargs_decoder)
-
- sequence_output = decoder_outputs[0] * (self.model_dim ** -0.5)
- lm_logits = self.shared(sequence_output, mode="linear")
- decoder_outputs = (lm_logits,) + decoder_outputs[1:]
-
- return decoder_outputs + encoder_outputs
diff --git a/server/transformers/src/transformers/modeling_tf_transfo_xl.py b/server/transformers/src/transformers/modeling_tf_transfo_xl.py
deleted file mode 100644
index 659685388e5d5ad4c5b252e77ede314897b6c83c..0000000000000000000000000000000000000000
--- a/server/transformers/src/transformers/modeling_tf_transfo_xl.py
+++ /dev/null
@@ -1,828 +0,0 @@
-# coding=utf-8
-# Copyright 2018 Google AI, Google Brain and Carnegie Mellon University Authors and the HuggingFace Inc. team.
-# Copyright (c) 2018, NVIDIA CORPORATION. All rights reserved.
-#
-# Licensed under the Apache License, Version 2.0 (the "License");
-# you may not use this file except in compliance with the License.
-# You may obtain a copy of the License at
-#
-# http://www.apache.org/licenses/LICENSE-2.0
-#
-# Unless required by applicable law or agreed to in writing, software
-# distributed under the License is distributed on an "AS IS" BASIS,
-# WITHOUT WARRANTIES OR CONDITIONS OF ANY KIND, either express or implied.
-# See the License for the specific language governing permissions and
-# limitations under the License.
-""" TF 2.0 Transformer XL model.
-"""
-
-
-import logging
-
-import tensorflow as tf
-
-from .configuration_transfo_xl import TransfoXLConfig
-from .file_utils import add_start_docstrings, add_start_docstrings_to_callable
-from .modeling_tf_transfo_xl_utilities import TFAdaptiveSoftmaxMask
-from .modeling_tf_utils import TFPreTrainedModel, get_initializer, shape_list
-
-
-logger = logging.getLogger(__name__)
-
-TF_TRANSFO_XL_PRETRAINED_MODEL_ARCHIVE_MAP = {
- "transfo-xl-wt103": "https://s3.amazonaws.com/models.huggingface.co/bert/transfo-xl-wt103-tf_model.h5",
-}
-
-
-class TFPositionalEmbedding(tf.keras.layers.Layer):
- def __init__(self, demb, **kwargs):
- super().__init__(**kwargs)
-
- self.inv_freq = 1 / (10000 ** (tf.range(0, demb, 2.0) / demb))
-
- def call(self, pos_seq, bsz=None):
- sinusoid_inp = tf.einsum("i,j->ij", pos_seq, self.inv_freq)
- pos_emb = tf.concat([tf.sin(sinusoid_inp), tf.cos(sinusoid_inp)], -1)
-
- if bsz is not None:
- return tf.tile(pos_emb[:, None, :], [1, bsz, 1])
- else:
- return pos_emb[:, None, :]
-
-
-class TFPositionwiseFF(tf.keras.layers.Layer):
- def __init__(self, d_model, d_inner, dropout, pre_lnorm=False, layer_norm_epsilon=1e-5, init_std=0.02, **kwargs):
- super().__init__(**kwargs)
-
- self.d_model = d_model
- self.d_inner = d_inner
- self.dropout = dropout
-
- self.layer_1 = tf.keras.layers.Dense(
- d_inner, kernel_initializer=get_initializer(init_std), activation=tf.nn.relu, name="CoreNet_._0"
- )
- self.drop_1 = tf.keras.layers.Dropout(dropout)
- self.layer_2 = tf.keras.layers.Dense(d_model, kernel_initializer=get_initializer(init_std), name="CoreNet_._3")
- self.drop_2 = tf.keras.layers.Dropout(dropout)
-
- self.layer_norm = tf.keras.layers.LayerNormalization(epsilon=layer_norm_epsilon, name="layer_norm")
-
- self.pre_lnorm = pre_lnorm
-
- def call(self, inp, training=False):
- if self.pre_lnorm:
- # layer normalization + positionwise feed-forward
- core_out = self.layer_norm(inp)
- core_out = self.layer_1(core_out)
- core_out = self.drop_1(core_out, training=training)
- core_out = self.layer_2(core_out)
- core_out = self.drop_2(core_out, training=training)
-
- # residual connection
- output = core_out + inp
- else:
- # positionwise feed-forward
- core_out = self.layer_1(inp)
- core_out = self.drop_1(core_out, training=training)
- core_out = self.layer_2(core_out)
- core_out = self.drop_2(core_out, training=training)
-
- # residual connection + layer normalization
- output = self.layer_norm(inp + core_out)
-
- return output
-
-
-class TFRelPartialLearnableMultiHeadAttn(tf.keras.layers.Layer):
- def __init__(
- self,
- n_head,
- d_model,
- d_head,
- dropout,
- dropatt=0,
- tgt_len=None,
- ext_len=None,
- mem_len=None,
- pre_lnorm=False,
- r_r_bias=None,
- r_w_bias=None,
- output_attentions=False,
- layer_norm_epsilon=1e-5,
- init_std=0.02,
- **kwargs
- ):
- super().__init__(**kwargs)
-
- self.output_attentions = output_attentions
- self.n_head = n_head
- self.d_model = d_model
- self.d_head = d_head
- self.dropout = dropout
-
- self.qkv_net = tf.keras.layers.Dense(
- 3 * n_head * d_head, kernel_initializer=get_initializer(init_std), use_bias=False, name="qkv_net"
- )
-
- self.drop = tf.keras.layers.Dropout(dropout)
- self.dropatt = tf.keras.layers.Dropout(dropatt)
- self.o_net = tf.keras.layers.Dense(
- d_model, kernel_initializer=get_initializer(init_std), use_bias=False, name="o_net"
- )
-
- self.layer_norm = tf.keras.layers.LayerNormalization(epsilon=layer_norm_epsilon, name="layer_norm")
-
- self.scale = 1 / (d_head ** 0.5)
-
- self.pre_lnorm = pre_lnorm
-
- if r_r_bias is not None and r_w_bias is not None: # Biases are shared
- self.r_r_bias = r_r_bias
- self.r_w_bias = r_w_bias
- else:
- self.r_r_bias = None
- self.r_w_bias = None
-
- self.r_net = tf.keras.layers.Dense(
- self.n_head * self.d_head, kernel_initializer=get_initializer(init_std), use_bias=False, name="r_net"
- )
-
- def build(self, input_shape):
- if self.r_r_bias is None or self.r_w_bias is None: # Biases are not shared
- self.r_r_bias = self.add_weight(
- shape=(self.n_head, self.d_head), initializer="zeros", trainable=True, name="r_r_bias"
- )
- self.r_w_bias = self.add_weight(
- shape=(self.n_head, self.d_head), initializer="zeros", trainable=True, name="r_w_bias"
- )
- super().build(input_shape)
-
- def _rel_shift(self, x):
- x_size = shape_list(x)
-
- x = tf.pad(x, [[0, 0], [1, 0], [0, 0], [0, 0]])
- x = tf.reshape(x, [x_size[1] + 1, x_size[0], x_size[2], x_size[3]])
- x = tf.slice(x, [1, 0, 0, 0], [-1, -1, -1, -1])
- x = tf.reshape(x, x_size)
-
- return x
-
- def call(self, inputs, training=False):
- w, r, attn_mask, mems, head_mask = inputs
- qlen, rlen, bsz = shape_list(w)[0], shape_list(r)[0], shape_list(w)[1]
-
- if mems is not None:
- cat = tf.concat([mems, w], 0)
- if self.pre_lnorm:
- w_heads = self.qkv_net(self.layer_norm(cat))
- else:
- w_heads = self.qkv_net(cat)
- r_head_k = self.r_net(r)
-
- w_head_q, w_head_k, w_head_v = tf.split(w_heads, 3, axis=-1)
- w_head_q = w_head_q[-qlen:]
- else:
- if self.pre_lnorm:
- w_heads = self.qkv_net(self.layer_norm(w))
- else:
- w_heads = self.qkv_net(w)
- r_head_k = self.r_net(r)
-
- w_head_q, w_head_k, w_head_v = tf.split(w_heads, 3, axis=-1)
-
- klen = shape_list(w_head_k)[0]
-
- w_head_q = tf.reshape(w_head_q, (qlen, bsz, self.n_head, self.d_head)) # qlen x bsz x n_head x d_head
- w_head_k = tf.reshape(w_head_k, (klen, bsz, self.n_head, self.d_head)) # qlen x bsz x n_head x d_head
- w_head_v = tf.reshape(w_head_v, (klen, bsz, self.n_head, self.d_head)) # qlen x bsz x n_head x d_head
-
- r_head_k = tf.reshape(r_head_k, (rlen, self.n_head, self.d_head)) # qlen x n_head x d_head
-
- # compute attention score
- rw_head_q = w_head_q + self.r_w_bias # qlen x bsz x n_head x d_head
- AC = tf.einsum("ibnd,jbnd->ijbn", rw_head_q, w_head_k) # qlen x klen x bsz x n_head
-
- rr_head_q = w_head_q + self.r_r_bias
- BD = tf.einsum("ibnd,jnd->ijbn", rr_head_q, r_head_k) # qlen x klen x bsz x n_head
- BD = self._rel_shift(BD)
-
- # [qlen x klen x bsz x n_head]
- attn_score = AC + BD
- attn_score = attn_score * self.scale
-
- # compute attention probability
- if attn_mask is not None:
- attn_mask_t = attn_mask[:, :, None, None]
- attn_score = attn_score * (1 - attn_mask_t) - 1e30 * attn_mask_t
-
- # [qlen x klen x bsz x n_head]
- attn_prob = tf.nn.softmax(attn_score, axis=1)
- attn_prob = self.dropatt(attn_prob, training=training)
-
- # Mask heads if we want to
- if head_mask is not None:
- attn_prob = attn_prob * head_mask
-
- # compute attention vector
- attn_vec = tf.einsum("ijbn,jbnd->ibnd", attn_prob, w_head_v)
-
- # [qlen x bsz x n_head x d_head]
- attn_vec_sizes = shape_list(attn_vec)
- attn_vec = tf.reshape(attn_vec, (attn_vec_sizes[0], attn_vec_sizes[1], self.n_head * self.d_head))
-
- # linear projection
- attn_out = self.o_net(attn_vec)
- attn_out = self.drop(attn_out, training=training)
-
- if self.pre_lnorm:
- # residual connection
- outputs = [w + attn_out]
- else:
- # residual connection + layer normalization
- outputs = [self.layer_norm(w + attn_out)]
-
- if self.output_attentions:
- outputs.append(attn_prob)
-
- return outputs
-
-
-class TFRelPartialLearnableDecoderLayer(tf.keras.layers.Layer):
- def __init__(
- self,
- n_head,
- d_model,
- d_head,
- d_inner,
- dropout,
- tgt_len=None,
- ext_len=None,
- mem_len=None,
- dropatt=0.0,
- pre_lnorm=False,
- r_w_bias=None,
- r_r_bias=None,
- output_attentions=False,
- layer_norm_epsilon=1e-5,
- init_std=0.02,
- **kwargs
- ):
- super().__init__(**kwargs)
-
- self.dec_attn = TFRelPartialLearnableMultiHeadAttn(
- n_head,
- d_model,
- d_head,
- dropout,
- tgt_len=tgt_len,
- ext_len=ext_len,
- mem_len=mem_len,
- dropatt=dropatt,
- pre_lnorm=pre_lnorm,
- r_w_bias=r_w_bias,
- r_r_bias=r_r_bias,
- init_std=init_std,
- output_attentions=output_attentions,
- layer_norm_epsilon=layer_norm_epsilon,
- name="dec_attn",
- )
- self.pos_ff = TFPositionwiseFF(
- d_model,
- d_inner,
- dropout,
- pre_lnorm=pre_lnorm,
- init_std=init_std,
- layer_norm_epsilon=layer_norm_epsilon,
- name="pos_ff",
- )
-
- def call(self, inputs, training=False):
- dec_inp, r, dec_attn_mask, mems, head_mask = inputs
- attn_outputs = self.dec_attn([dec_inp, r, dec_attn_mask, mems, head_mask], training=training)
- ff_output = self.pos_ff(attn_outputs[0], training=training)
-
- outputs = [ff_output] + attn_outputs[1:]
-
- return outputs
-
-
-class TFAdaptiveEmbedding(tf.keras.layers.Layer):
- def __init__(self, n_token, d_embed, d_proj, cutoffs, div_val=1, init_std=0.02, sample_softmax=False, **kwargs):
- super().__init__(**kwargs)
-
- self.n_token = n_token
- self.d_embed = d_embed
- self.init_std = init_std
-
- self.cutoffs = cutoffs + [n_token]
- self.div_val = div_val
- self.d_proj = d_proj
-
- self.emb_scale = d_proj ** 0.5
-
- self.cutoff_ends = [0] + self.cutoffs
-
- self.emb_layers = []
- self.emb_projs = []
- if div_val == 1:
- raise NotImplementedError # Removed these to avoid maintaining dead code - They are not used in our pretrained checkpoint
- else:
- for i in range(len(self.cutoffs)):
- l_idx, r_idx = self.cutoff_ends[i], self.cutoff_ends[i + 1]
- d_emb_i = d_embed // (div_val ** i)
- self.emb_layers.append(
- tf.keras.layers.Embedding(
- r_idx - l_idx,
- d_emb_i,
- embeddings_initializer=get_initializer(init_std),
- name="emb_layers_._{}".format(i),
- )
- )
-
- def build(self, input_shape):
- for i in range(len(self.cutoffs)):
- d_emb_i = self.d_embed // (self.div_val ** i)
- self.emb_projs.append(
- self.add_weight(
- shape=(d_emb_i, self.d_proj),
- initializer=get_initializer(self.init_std),
- trainable=True,
- name="emb_projs_._{}".format(i),
- )
- )
- super().build(input_shape)
-
- def call(self, inp):
- if self.div_val == 1:
- raise NotImplementedError # Removed these to avoid maintaining dead code - They are not used in our pretrained checkpoint
- else:
- inp_flat = tf.reshape(inp, (-1,))
- emb_flat = tf.zeros([shape_list(inp_flat)[0], self.d_proj])
- for i in range(len(self.cutoffs)):
- l_idx, r_idx = self.cutoff_ends[i], self.cutoff_ends[i + 1]
-
- mask_i = (inp_flat >= l_idx) & (inp_flat < r_idx)
-
- inp_i = tf.boolean_mask(inp_flat, mask_i) - l_idx
- emb_i = self.emb_layers[i](inp_i)
- emb_i = tf.einsum("id,de->ie", emb_i, self.emb_projs[i])
-
- mask_idx = tf.cast(tf.where(mask_i), dtype=tf.int64)
- emb_flat += tf.scatter_nd(mask_idx, emb_i, tf.cast(shape_list(emb_flat), dtype=tf.int64))
-
- embed_shape = shape_list(inp) + [self.d_proj]
- embed = tf.reshape(emb_flat, embed_shape)
-
- embed *= self.emb_scale
-
- return embed
-
-
-class TFTransfoXLMainLayer(tf.keras.layers.Layer):
- def __init__(self, config, **kwargs):
- super().__init__(**kwargs)
- self.output_attentions = config.output_attentions
- self.output_hidden_states = config.output_hidden_states
-
- self.n_token = config.vocab_size
-
- self.d_embed = config.d_embed
- self.d_model = config.d_model
- self.n_head = config.n_head
- self.d_head = config.d_head
- self.untie_r = config.untie_r
-
- self.word_emb = TFAdaptiveEmbedding(
- config.vocab_size,
- config.d_embed,
- config.d_model,
- config.cutoffs,
- div_val=config.div_val,
- init_std=config.init_std,
- name="word_emb",
- )
-
- self.drop = tf.keras.layers.Dropout(config.dropout)
-
- self.n_layer = config.n_layer
-
- self.tgt_len = config.tgt_len
- self.mem_len = config.mem_len
- self.ext_len = config.ext_len
- self.max_klen = config.tgt_len + config.ext_len + config.mem_len
-
- self.attn_type = config.attn_type
-
- self.layers = []
- if config.attn_type == 0: # the default attention
- for i in range(config.n_layer):
- self.layers.append(
- TFRelPartialLearnableDecoderLayer(
- config.n_head,
- config.d_model,
- config.d_head,
- config.d_inner,
- config.dropout,
- tgt_len=config.tgt_len,
- ext_len=config.ext_len,
- mem_len=config.mem_len,
- dropatt=config.dropatt,
- pre_lnorm=config.pre_lnorm,
- r_w_bias=None if self.untie_r else self.r_w_bias,
- r_r_bias=None if self.untie_r else self.r_r_bias,
- output_attentions=self.output_attentions,
- layer_norm_epsilon=config.layer_norm_epsilon,
- init_std=config.init_std,
- name="layers_._{}".format(i),
- )
- )
- else: # learnable embeddings and absolute embeddings
- raise NotImplementedError # Removed these to avoid maintaining dead code - They are not used in our pretrained checkpoint
-
- self.same_length = config.same_length
- self.clamp_len = config.clamp_len
-
- if self.attn_type == 0: # default attention
- self.pos_emb = TFPositionalEmbedding(self.d_model, name="pos_emb")
- else: # learnable embeddings and absolute embeddings
- raise NotImplementedError # Removed these to avoid maintaining dead code - They are not used in our pretrained checkpoint
-
- def build(self, input_shape):
- if not self.untie_r:
- self.r_w_bias = self.add_weight(
- shape=(self.n_head, self.d_head), initializer="zeros", trainable=True, name="r_w_bias"
- )
- self.r_r_bias = self.add_weight(
- shape=(self.n_head, self.d_head), initializer="zeros", trainable=True, name="r_r_bias"
- )
- super().build(input_shape)
-
- def get_input_embeddings(self):
- return self.word_emb
-
- def _resize_token_embeddings(self, new_num_tokens):
- return self.word_emb
-
- def backward_compatible(self):
- self.sample_softmax = -1
-
- def reset_length(self, tgt_len, ext_len, mem_len):
- self.tgt_len = tgt_len
- self.mem_len = mem_len
- self.ext_len = ext_len
-
- def _prune_heads(self, heads):
- raise NotImplementedError
-
- def init_mems(self, bsz):
- if self.mem_len > 0:
- mems = []
- for i in range(self.n_layer):
- empty = tf.zeros([self.mem_len, bsz, self.d_model])
- mems.append(empty)
-
- return mems
- else:
- return None
-
- def _update_mems(self, hids, mems, qlen, mlen):
- # does not deal with None
- if mems is None:
- return None
-
- # mems is not None
- assert len(hids) == len(mems), "len(hids) != len(mems)"
-
- # There are `mlen + qlen` steps that can be cached into mems
- # For the next step, the last `ext_len` of the `qlen` tokens
- # will be used as the extended context. Hence, we only cache
- # the tokens from `mlen + qlen - self.ext_len - self.mem_len`
- # to `mlen + qlen - self.ext_len`.
- new_mems = []
- end_idx = mlen + max(0, qlen - 0 - self.ext_len)
- beg_idx = max(0, end_idx - self.mem_len)
- for i in range(len(hids)):
-
- cat = tf.concat([mems[i], hids[i]], axis=0)
- tf.stop_gradient(cat)
- new_mems.append(cat[beg_idx:end_idx])
-
- return new_mems
-
- def call(self, inputs, mems=None, head_mask=None, inputs_embeds=None, training=False):
- if isinstance(inputs, (tuple, list)):
- input_ids = inputs[0]
- mems = inputs[1] if len(inputs) > 1 else mems
- head_mask = inputs[2] if len(inputs) > 2 else head_mask
- inputs_embeds = inputs[3] if len(inputs) > 3 else inputs_embeds
- assert len(inputs) <= 4, "Too many inputs."
- elif isinstance(inputs, dict):
- input_ids = inputs.get("input_ids")
- mems = inputs.get("mems", mems)
- head_mask = inputs.get("head_mask", head_mask)
- inputs_embeds = inputs.get("inputs_embeds", inputs_embeds)
- assert len(inputs) <= 4, "Too many inputs."
- else:
- input_ids = inputs
-
- # the original code for Transformer-XL used shapes [len, bsz] but we want a unified interface in the library
- # so we transpose here from shape [bsz, len] to shape [len, bsz]
- if input_ids is not None and inputs_embeds is not None:
- raise ValueError("You cannot specify both input_ids and inputs_embeds at the same time")
- elif input_ids is not None:
- input_ids = tf.transpose(input_ids, perm=(1, 0))
- qlen, bsz = shape_list(input_ids)
- elif inputs_embeds is not None:
- inputs_embeds = tf.transpose(inputs_embeds, perm=(1, 0, 2))
- qlen, bsz = shape_list(inputs_embeds)[:2]
- else:
- raise ValueError("You have to specify either input_ids or inputs_embeds")
-
- if mems is None:
- mems = self.init_mems(bsz)
-
- # Prepare head mask if needed
- # 1.0 in head_mask indicate we keep the head
- # attention_probs has shape bsz x n_heads x N x N
- # input head_mask has shape [num_heads] or [num_hidden_layers x num_heads] (a head_mask for each layer)
- # and head_mask is converted to shape [num_hidden_layers x qlen x klen x bsz x n_head]
- if head_mask is not None:
- raise NotImplementedError
- else:
- head_mask = [None] * self.n_layer
-
- if inputs_embeds is not None:
- word_emb = inputs_embeds
- else:
- word_emb = self.word_emb(input_ids)
-
- mlen = shape_list(mems[0])[0] if mems is not None else 0
- klen = mlen + qlen
-
- attn_mask = tf.ones([qlen, qlen])
- mask_u = tf.linalg.band_part(attn_mask, 0, -1)
- mask_dia = tf.linalg.band_part(attn_mask, 0, 0)
- attn_mask_pad = tf.zeros([qlen, mlen])
- dec_attn_mask = tf.concat([attn_mask_pad, mask_u - mask_dia], 1)
- if self.same_length:
- mask_l = tf.linalg.band_part(attn_mask, -1, 0)
- dec_attn_mask = tf.concat([dec_attn_mask[:, :qlen] + mask_l - mask_dia, dec_attn_mask[:, qlen:]], 1)
- # ::: PyTorch masking code for reference :::
- # if self.same_length:
- # all_ones = word_emb.new_ones((qlen, klen), dtype=torch.uint8)
- # mask_len = klen - self.mem_len
- # if mask_len > 0:
- # mask_shift_len = qlen - mask_len
- # else:
- # mask_shift_len = qlen
- # dec_attn_mask = (torch.triu(all_ones, 1+mlen)
- # + torch.tril(all_ones, -mask_shift_len))[:, :, None] # -1
- # else:
- # dec_attn_mask = torch.triu(
- # word_emb.new_ones((qlen, klen), dtype=torch.uint8), diagonal=1+mlen)[:,:,None]
-
- hids = []
- attentions = []
- if self.attn_type == 0: # default
- pos_seq = tf.range(klen - 1, -1, -1.0)
- if self.clamp_len > 0:
- pos_seq = tf.minimum(pos_seq, self.clamp_len)
- pos_emb = self.pos_emb(pos_seq)
-
- core_out = self.drop(word_emb, training=training)
- pos_emb = self.drop(pos_emb, training=training)
-
- for i, layer in enumerate(self.layers):
- hids.append(core_out)
- mems_i = None if mems is None else mems[i]
- layer_outputs = layer([core_out, pos_emb, dec_attn_mask, mems_i, head_mask[i]], training=training)
- core_out = layer_outputs[0]
- if self.output_attentions:
- attentions.append(layer_outputs[1])
- else: # learnable embeddings and absolute embeddings
- raise NotImplementedError # Removed these to avoid maintaining dead code - They are not used in our pretrained checkpoint
-
- core_out = self.drop(core_out, training=training)
-
- new_mems = self._update_mems(hids, mems, mlen, qlen)
-
- # We transpose back here to shape [bsz, len, hidden_dim]
- outputs = [tf.transpose(core_out, perm=(1, 0, 2)), new_mems]
- if self.output_hidden_states:
- # Add last layer and transpose to library standard shape [bsz, len, hidden_dim]
- hids.append(core_out)
- hids = list(tf.transpose(t, perm=(1, 0, 2)) for t in hids)
- outputs.append(hids)
- if self.output_attentions:
- # Transpose to library standard shape [bsz, n_heads, query_seq_len, key_seq_len]
- attentions = list(tf.transpose(t, perm=(2, 3, 0, 1)) for t in attentions)
- outputs.append(attentions)
- return outputs # last hidden state, new_mems, (all hidden states), (all attentions)
-
-
-class TFTransfoXLPreTrainedModel(TFPreTrainedModel):
- """ An abstract class to handle weights initialization and
- a simple interface for downloading and loading pretrained models.
- """
-
- config_class = TransfoXLConfig
- pretrained_model_archive_map = TF_TRANSFO_XL_PRETRAINED_MODEL_ARCHIVE_MAP
- base_model_prefix = "transformer"
-
-
-TRANSFO_XL_START_DOCSTRING = r"""
-
- .. note::
-
- TF 2.0 models accepts two formats as inputs:
-
- - having all inputs as keyword arguments (like PyTorch models), or
- - having all inputs as a list, tuple or dict in the first positional arguments.
-
- This second option is useful when using :obj:`tf.keras.Model.fit()` method which currently requires having
- all the tensors in the first argument of the model call function: :obj:`model(inputs)`.
-
- If you choose this second option, there are three possibilities you can use to gather all the input Tensors
- in the first positional argument :
-
- - a single Tensor with input_ids only and nothing else: :obj:`model(inputs_ids)`
- - a list of varying length with one or several input Tensors IN THE ORDER given in the docstring:
- :obj:`model([input_ids, attention_mask])` or :obj:`model([input_ids, attention_mask, token_type_ids])`
- - a dictionary with one or several input Tensors associated to the input names given in the docstring:
- :obj:`model({'input_ids': input_ids, 'token_type_ids': token_type_ids})`
-
- Parameters:
- config (:class:`~transformers.TransfoXLConfig`): Model configuration class with all the parameters of the model.
- Initializing with a config file does not load the weights associated with the model, only the configuration.
- Check out the :meth:`~transformers.PreTrainedModel.from_pretrained` method to load the model weights.
-"""
-
-TRANSFO_XL_INPUTS_DOCSTRING = r"""
- Args:
- input_ids (:obj:`tf.Tensor` or :obj:`Numpy array` of shape :obj:`(batch_size, sequence_length)`):
- Indices of input sequence tokens in the vocabulary.
-
- Indices can be obtained using :class:`transformers.TransfoXLTokenizer`.
- See :func:`transformers.PreTrainedTokenizer.encode` and
- :func:`transformers.PreTrainedTokenizer.encode_plus` for details.
-
- `What are input IDs? <../glossary.html#input-ids>`__
- mems (:obj:`List[torch.FloatTensor]` of length :obj:`config.n_layers`):
- Contains pre-computed hidden-states (key and values in the attention blocks) as computed by the model
- (see `mems` output below). Can be used to speed up sequential decoding. The token ids which have their mems
- given to this model should not be passed as input ids as they have already been computed.
- head_mask (:obj:`tf.Tensor` or :obj:`Numpy array` of shape :obj:`(num_heads,)` or :obj:`(num_layers, num_heads)`, `optional`, defaults to :obj:`None`):
- Mask to nullify selected heads of the self-attention modules.
- Mask values selected in ``[0, 1]``:
- :obj:`1` indicates the head is **not masked**, :obj:`0` indicates the head is **masked**.
- input_embeds (:obj:`tf.Tensor` or :obj:`Numpy array` of shape :obj:`(batch_size, sequence_length, hidden_size)`, `optional`, defaults to :obj:`None`):
- Optionally, instead of passing :obj:`input_ids` you can choose to directly pass an embedded representation.
- This is useful if you want more control over how to convert `input_ids` indices into associated vectors
- than the model's internal embedding lookup matrix.
-"""
-
-
-@add_start_docstrings(
- "The bare Bert Model transformer outputing raw hidden-states without any specific head on top.",
- TRANSFO_XL_START_DOCSTRING,
-)
-class TFTransfoXLModel(TFTransfoXLPreTrainedModel):
- def __init__(self, config, *inputs, **kwargs):
- super().__init__(config, *inputs, **kwargs)
- self.transformer = TFTransfoXLMainLayer(config, name="transformer")
-
- @add_start_docstrings_to_callable(TRANSFO_XL_INPUTS_DOCSTRING)
- def call(self, inputs, **kwargs):
- r"""
- Return:
- :obj:`tuple(torch.FloatTensor)` comprising various elements depending on the configuration (:class:`~transformers.TransfoXLConfig`) and inputs:
- last_hidden_state (:obj:`torch.FloatTensor` of shape :obj:`(batch_size, sequence_length, hidden_size)`):
- Sequence of hidden-states at the last layer of the model.
- mems (:obj:`List[torch.FloatTensor]` of length :obj:`config.n_layers`):
- Contains pre-computed hidden-states (key and values in the attention blocks).
- Can be used (see `mems` input) to speed up sequential decoding. The token ids which have their past given to this model
- should not be passed as input ids as they have already been computed.
- hidden_states (:obj:`tuple(torch.FloatTensor)`, `optional`, returned when ``config.output_hidden_states=True``):
- Tuple of :obj:`torch.FloatTensor` (one for the output of the embeddings + one for the output of each layer)
- of shape :obj:`(batch_size, sequence_length, hidden_size)`.
-
- Hidden-states of the model at the output of each layer plus the initial embedding outputs.
- attentions (:obj:`tuple(torch.FloatTensor)`, `optional`, returned when ``config.output_attentions=True``):
- Tuple of :obj:`torch.FloatTensor` (one for each layer) of shape
- :obj:`(batch_size, num_heads, sequence_length, sequence_length)`.
-
- Attentions weights after the attention softmax, used to compute the weighted average in the self-attention
- heads.
-
- Examples::
-
- import tensorflow as tf
- from transformers import TransfoXLTokenizer, TFTransfoXLModel
-
- tokenizer = TransfoXLTokenizer.from_pretrained('transfo-xl-wt103')
- model = TFTransfoXLModel.from_pretrained('transfo-xl-wt103')
- input_ids = tf.constant(tokenizer.encode("Hello, my dog is cute", add_special_tokens=True))[None, :] # Batch size 1
- outputs = model(input_ids)
- last_hidden_states, mems = outputs[:2]
-
- """
- outputs = self.transformer(inputs, **kwargs)
- return outputs
-
-
-@add_start_docstrings(
- """The Transformer-XL Model with a language modeling head on top
- (adaptive softmax with weights tied to the adaptive input embeddings)""",
- TRANSFO_XL_START_DOCSTRING,
-)
-class TFTransfoXLLMHeadModel(TFTransfoXLPreTrainedModel):
- def __init__(self, config):
- super().__init__(config)
- self.transformer = TFTransfoXLMainLayer(config, name="transformer")
- self.sample_softmax = config.sample_softmax
- # use sampled softmax
- if config.sample_softmax > 0:
- raise NotImplementedError
- # use adaptive softmax (including standard softmax)
- else:
- self.crit = TFAdaptiveSoftmaxMask(
- config.vocab_size, config.d_embed, config.d_model, config.cutoffs, div_val=config.div_val, name="crit"
- )
-
- def reset_length(self, tgt_len, ext_len, mem_len):
- self.transformer.reset_length(tgt_len, ext_len, mem_len)
-
- def init_mems(self, bsz):
- return self.transformer.init_mems(bsz)
-
- @add_start_docstrings_to_callable(TRANSFO_XL_INPUTS_DOCSTRING)
- def call(self, inputs, mems=None, head_mask=None, inputs_embeds=None, labels=None, training=False):
- r"""
- Return:
- :obj:`tuple(torch.FloatTensor)` comprising various elements depending on the configuration (:class:`~transformers.TransfoXLConfig`) and inputs:
- prediction_scores (:obj:`torch.FloatTensor` of shape :obj:`(batch_size, sequence_length, config.vocab_size)`):
- Prediction scores of the language modeling head (scores for each vocabulary token before SoftMax).
- mems (:obj:`List[torch.FloatTensor]` of length :obj:`config.n_layers`):
- Contains pre-computed hidden-states (key and values in the attention blocks).
- Can be used (see `past` input) to speed up sequential decoding. The token ids which have their past given to this model
- should not be passed as input ids as they have already been computed.
- hidden_states (:obj:`tuple(torch.FloatTensor)`, `optional`, returned when ``config.output_hidden_states=True``):
- Tuple of :obj:`torch.FloatTensor` (one for the output of the embeddings + one for the output of each layer)
- of shape :obj:`(batch_size, sequence_length, hidden_size)`.
-
- Hidden-states of the model at the output of each layer plus the initial embedding outputs.
- attentions (:obj:`tuple(torch.FloatTensor)`, `optional`, returned when ``config.output_attentions=True``):
- Tuple of :obj:`torch.FloatTensor` (one for each layer) of shape
- :obj:`(batch_size, num_heads, sequence_length, sequence_length)`.
-
- Attentions weights after the attention softmax, used to compute the weighted average in the self-attention
- heads.
-
- Examples::
-
- import tensorflow as tf
- from transformers import TransfoXLTokenizer, TFTransfoXLLMHeadModel
-
- tokenizer = TransfoXLTokenizer.from_pretrained('transfo-xl-wt103')
- model = TFTransfoXLLMHeadModel.from_pretrained('transfo-xl-wt103')
- input_ids = tf.constant(tokenizer.encode("Hello, my dog is cute", add_special_tokens=True))[None, :] # Batch size 1
- outputs = model(input_ids)
- prediction_scores, mems = outputs[:2]
-
- """
- if isinstance(inputs, (tuple, list)):
- input_ids = inputs[0]
- mems = inputs[1] if len(inputs) > 1 else mems
- head_mask = inputs[2] if len(inputs) > 2 else head_mask
- inputs_embeds = inputs[3] if len(inputs) > 3 else inputs_embeds
- labels = inputs[4] if len(inputs) > 4 else labels
- assert len(inputs) <= 5, "Too many inputs."
- elif isinstance(inputs, dict):
- input_ids = inputs.get("input_ids")
- mems = inputs.get("mems", mems)
- head_mask = inputs.get("head_mask", head_mask)
- inputs_embeds = inputs.get("inputs_embeds", inputs_embeds)
- labels = inputs.get("labels", labels)
- assert len(inputs) <= 5, "Too many inputs."
- else:
- input_ids = inputs
-
- if input_ids is not None:
- bsz, tgt_len = shape_list(input_ids)[:2]
- else:
- bsz, tgt_len = shape_list(inputs_embeds)[:2]
-
- transformer_outputs = self.transformer([input_ids, mems, head_mask, inputs_embeds], training=training)
-
- last_hidden = transformer_outputs[0]
- pred_hid = last_hidden[:, -tgt_len:]
- outputs = transformer_outputs[1:]
- if self.sample_softmax > 0 and training:
- raise NotImplementedError
- else:
- # pred_hid = tf.reshape(pred_hid, (-1, shape_list(pred_hid)[-1]))
- softmax_output = self.crit([pred_hid, labels], training=training)
- # softmax_output = tf.reshape(softmax_output, (bsz, tgt_len, -1))
- outputs = [softmax_output] + outputs
-
- return outputs # logits, new_mems, (all hidden states), (all attentions)
diff --git a/server/transformers/src/transformers/modeling_tf_transfo_xl_utilities.py b/server/transformers/src/transformers/modeling_tf_transfo_xl_utilities.py
deleted file mode 100644
index 1f6edf3a9b98d142bdb15788b9318d44a4727bed..0000000000000000000000000000000000000000
--- a/server/transformers/src/transformers/modeling_tf_transfo_xl_utilities.py
+++ /dev/null
@@ -1,178 +0,0 @@
-# coding=utf-8
-# Copyright 2018 Google AI, Google Brain and Carnegie Mellon University Authors and the HuggingFace Inc. team.
-# Copyright (c) 2018, NVIDIA CORPORATION. All rights reserved.
-#
-# Licensed under the Apache License, Version 2.0 (the "License");
-# you may not use this file except in compliance with the License.
-# You may obtain a copy of the License at
-#
-# http://www.apache.org/licenses/LICENSE-2.0
-#
-# Unless required by applicable law or agreed to in writing, software
-# distributed under the License is distributed on an "AS IS" BASIS,
-# WITHOUT WARRANTIES OR CONDITIONS OF ANY KIND, either express or implied.
-# See the License for the specific language governing permissions and
-# limitations under the License.
-""" A TF 2.0 Adaptive Softmax for Transformer XL model.
-"""
-
-
-import tensorflow as tf
-
-from .modeling_tf_utils import shape_list
-
-
-class TFAdaptiveSoftmaxMask(tf.keras.layers.Layer):
- def __init__(self, vocab_size, d_embed, d_proj, cutoffs, div_val=1, keep_order=False, **kwargs):
- super().__init__(**kwargs)
-
- self.vocab_size = vocab_size
- self.d_embed = d_embed
- self.d_proj = d_proj
-
- self.cutoffs = cutoffs + [vocab_size]
- self.cutoff_ends = [0] + self.cutoffs
- self.div_val = div_val
-
- self.shortlist_size = self.cutoffs[0]
- self.n_clusters = len(self.cutoffs) - 1
- self.head_size = self.shortlist_size + self.n_clusters
- self.keep_order = keep_order
-
- self.out_layers = []
- self.out_projs = []
-
- def build(self, input_shape):
- if self.n_clusters > 0:
- self.cluster_weight = self.add_weight(
- shape=(self.n_clusters, self.d_embed), initializer="zeros", trainable=True, name="cluster_weight"
- )
- self.cluster_bias = self.add_weight(
- shape=(self.n_clusters,), initializer="zeros", trainable=True, name="cluster_bias"
- )
-
- if self.div_val == 1:
- for i in range(len(self.cutoffs)):
- if self.d_proj != self.d_embed:
- weight = self.add_weight(
- shape=(self.d_embed, self.d_proj),
- initializer="zeros",
- trainable=True,
- name="out_projs_._{}".format(i),
- )
- self.out_projs.append(weight)
- else:
- self.out_projs.append(None)
- weight = self.add_weight(
- shape=(self.vocab_size, self.d_embed,),
- initializer="zeros",
- trainable=True,
- name="out_layers_._{}_._weight".format(i),
- )
- bias = self.add_weight(
- shape=(self.vocab_size,),
- initializer="zeros",
- trainable=True,
- name="out_layers_._{}_._bias".format(i),
- )
- self.out_layers.append((weight, bias))
- else:
- for i in range(len(self.cutoffs)):
- l_idx, r_idx = self.cutoff_ends[i], self.cutoff_ends[i + 1]
- d_emb_i = self.d_embed // (self.div_val ** i)
-
- weight = self.add_weight(
- shape=(d_emb_i, self.d_proj), initializer="zeros", trainable=True, name="out_projs_._{}".format(i)
- )
- self.out_projs.append(weight)
- weight = self.add_weight(
- shape=(r_idx - l_idx, d_emb_i,),
- initializer="zeros",
- trainable=True,
- name="out_layers_._{}_._weight".format(i),
- )
- bias = self.add_weight(
- shape=(r_idx - l_idx,),
- initializer="zeros",
- trainable=True,
- name="out_layers_._{}_._bias".format(i),
- )
- self.out_layers.append((weight, bias))
- super().build(input_shape)
-
- @staticmethod
- def _logit(x, W, b, proj=None):
- y = x
- if proj is not None:
- y = tf.einsum("ibd,ed->ibe", y, proj)
- return tf.einsum("ibd,nd->ibn", y, W) + b
-
- @staticmethod
- def _gather_logprob(logprob, target):
- lp_size = shape_list(logprob)
- r = tf.range(lp_size[0])
- idx = tf.stack([r, target], 1)
- return tf.gather_nd(logprob, idx)
-
- def call(self, inputs, return_mean=True, training=False):
- hidden, target = inputs
- head_logprob = 0
- if self.n_clusters == 0:
- output = self._logit(hidden, self.out_layers[0][0], self.out_layers[0][1], self.out_projs[0])
- if target is not None:
- loss = tf.nn.sparse_softmax_cross_entropy_with_logits(labels=target, logits=output)
- out = tf.nn.log_softmax(output, axis=-1)
- else:
- hidden_sizes = shape_list(hidden)
- out = []
- loss = tf.zeros(hidden_sizes[:2], dtype=tf.float32)
- for i in range(len(self.cutoffs)):
- l_idx, r_idx = self.cutoff_ends[i], self.cutoff_ends[i + 1]
- if target is not None:
- mask = (target >= l_idx) & (target < r_idx)
- mask_idx = tf.where(mask)
- cur_target = tf.boolean_mask(target, mask) - l_idx
-
- if self.div_val == 1:
- cur_W = self.out_layers[0][0][l_idx:r_idx]
- cur_b = self.out_layers[0][1][l_idx:r_idx]
- else:
- cur_W = self.out_layers[i][0]
- cur_b = self.out_layers[i][1]
-
- if i == 0:
- cur_W = tf.concat([cur_W, self.cluster_weight], 0)
- cur_b = tf.concat([cur_b, self.cluster_bias], 0)
-
- head_logit = self._logit(hidden, cur_W, cur_b, self.out_projs[0])
- head_logprob = tf.nn.log_softmax(head_logit)
- out.append(head_logprob[..., : self.cutoffs[0]])
- if target is not None:
- cur_head_logprob = tf.boolean_mask(head_logprob, mask)
- cur_logprob = self._gather_logprob(cur_head_logprob, cur_target)
- else:
- tail_logit = self._logit(hidden, cur_W, cur_b, self.out_projs[i])
- tail_logprob = tf.nn.log_softmax(tail_logit)
- cluster_prob_idx = self.cutoffs[0] + i - 1 # No probability for the head cluster
- logprob_i = head_logprob[..., cluster_prob_idx, None] + tail_logprob
- out.append(logprob_i)
- if target is not None:
- cur_head_logprob = tf.boolean_mask(head_logprob, mask)
- cur_tail_logprob = tf.boolean_mask(tail_logprob, mask)
- cur_logprob = self._gather_logprob(cur_tail_logprob, cur_target)
- cur_logprob += cur_head_logprob[:, self.cutoff_ends[1] + i - 1]
- if target is not None:
- loss += tf.scatter_nd(mask_idx, -cur_logprob, tf.cast(shape_list(loss), dtype=tf.int64))
- out = tf.concat(out, axis=-1)
-
- if target is not None:
- if return_mean:
- loss = tf.reduce_mean(loss)
- # Add the training-time loss value to the layer using `self.add_loss()`.
- self.add_loss(loss)
-
- # Log the loss as a metric (we could log arbitrary metrics,
- # including different metrics for training and inference.
- self.add_metric(loss, name=self.name, aggregation="mean" if return_mean else "")
-
- return out
diff --git a/server/transformers/src/transformers/modeling_tf_utils.py b/server/transformers/src/transformers/modeling_tf_utils.py
deleted file mode 100644
index 4b64f9364ce4e97d66ad8cb49ebb19a9051ab5d1..0000000000000000000000000000000000000000
--- a/server/transformers/src/transformers/modeling_tf_utils.py
+++ /dev/null
@@ -1,602 +0,0 @@
-# coding=utf-8
-# Copyright 2018 The Google AI Language Team Authors and The HuggingFace Inc. team.
-# Copyright (c) 2018, NVIDIA CORPORATION. All rights reserved.
-#
-# Licensed under the Apache License, Version 2.0 (the "License");
-# you may not use this file except in compliance with the License.
-# You may obtain a copy of the License at
-#
-# http://www.apache.org/licenses/LICENSE-2.0
-#
-# Unless required by applicable law or agreed to in writing, software
-# distributed under the License is distributed on an "AS IS" BASIS,
-# WITHOUT WARRANTIES OR CONDITIONS OF ANY KIND, either express or implied.
-# See the License for the specific language governing permissions and
-# limitations under the License.
-"""TF general model utils."""
-
-
-import logging
-import os
-
-import h5py
-import numpy as np
-import tensorflow as tf
-from tensorflow.python.keras.saving import hdf5_format
-
-from .configuration_utils import PretrainedConfig
-from .file_utils import DUMMY_INPUTS, TF2_WEIGHTS_NAME, WEIGHTS_NAME, cached_path, hf_bucket_url, is_remote_url
-from .modeling_tf_pytorch_utils import load_pytorch_checkpoint_in_tf2_model
-
-
-logger = logging.getLogger(__name__)
-
-
-class TFModelUtilsMixin:
- """
- A few utilities for `tf.keras.Model`s, to be used as a mixin.
- """
-
- def num_parameters(self, only_trainable: bool = False) -> int:
- """
- Get number of (optionally, trainable) parameters in the model.
- """
- if only_trainable:
- return int(sum(np.prod(w.shape.as_list()) for w in self.trainable_variables))
- else:
- return self.count_params()
-
-
-class TFPreTrainedModel(tf.keras.Model, TFModelUtilsMixin):
- r""" Base class for all TF models.
-
- :class:`~transformers.TFPreTrainedModel` takes care of storing the configuration of the models and handles methods for loading/downloading/saving models
- as well as a few methods common to all models to (i) resize the input embeddings and (ii) prune heads in the self-attention heads.
-
- Class attributes (overridden by derived classes):
- - ``config_class``: a class derived from :class:`~transformers.PretrainedConfig` to use as configuration class for this model architecture.
- - ``pretrained_model_archive_map``: a python ``dict`` of with `short-cut-names` (string) as keys and `url` (string) of associated pretrained weights as values.
- - ``load_tf_weights``: a python ``method`` for loading a TensorFlow checkpoint in a PyTorch model, taking as arguments:
-
- - ``model``: an instance of the relevant subclass of :class:`~transformers.PreTrainedModel`,
- - ``config``: an instance of the relevant subclass of :class:`~transformers.PretrainedConfig`,
- - ``path``: a path (string) to the TensorFlow checkpoint.
-
- - ``base_model_prefix``: a string indicating the attribute associated to the base model in derived classes of the same architecture adding modules on top of the base model.
- """
- config_class = None
- pretrained_model_archive_map = {}
- base_model_prefix = ""
-
- @property
- def dummy_inputs(self):
- """ Dummy inputs to build the network.
-
- Returns:
- tf.Tensor with dummy inputs
- """
- return {"input_ids": tf.constant(DUMMY_INPUTS)}
-
- def __init__(self, config, *inputs, **kwargs):
- super().__init__(*inputs, **kwargs)
- if not isinstance(config, PretrainedConfig):
- raise ValueError(
- "Parameter config in `{}(config)` should be an instance of class `PretrainedConfig`. "
- "To create a model from a pretrained model use "
- "`model = {}.from_pretrained(PRETRAINED_MODEL_NAME)`".format(
- self.__class__.__name__, self.__class__.__name__
- )
- )
- # Save config in model
- self.config = config
-
- def get_input_embeddings(self):
- """
- Returns the model's input embeddings.
-
- Returns:
- :obj:`tf.keras.layers.Layer`:
- A torch module mapping vocabulary to hidden states.
- """
- base_model = getattr(self, self.base_model_prefix, self)
- if base_model is not self:
- return base_model.get_input_embeddings()
- else:
- raise NotImplementedError
-
- def get_output_embeddings(self):
- """
- Returns the model's output embeddings.
-
- Returns:
- :obj:`tf.keras.layers.Layer`:
- A torch module mapping hidden states to vocabulary.
- """
- return None # Overwrite for models with output embeddings
-
- def _get_resized_embeddings(self, old_embeddings, new_num_tokens=None):
- """ Build a resized Embedding Variable from a provided token Embedding Module.
- Increasing the size will add newly initialized vectors at the end
- Reducing the size will remove vectors from the end
-
- Args:
- new_num_tokens: (`optional`) int
- New number of tokens in the embedding matrix.
- Increasing the size will add newly initialized vectors at the end
- Reducing the size will remove vectors from the end
- If not provided or None: return the provided token Embedding Module.
- Return: ``tf.Variable``
- Pointer to the resized Embedding Module or the old Embedding Module if new_num_tokens is None
- """
- # if new_num_tokens is None:
- # return old_embeddings
-
- # old_num_tokens, old_embedding_dim = old_embeddings.weight.size()
- # if old_num_tokens == new_num_tokens:
- # return old_embeddings
-
- # # Build new embeddings
- # new_embeddings = nn.Embedding(new_num_tokens, old_embedding_dim)
- # new_embeddings.to(old_embeddings.weight.device)
-
- # # initialize all new embeddings (in particular added tokens)
- # self._init_weights(new_embeddings)
-
- # # Copy word embeddings from the previous weights
- # num_tokens_to_copy = min(old_num_tokens, new_num_tokens)
- # new_embeddings.weight.data[:num_tokens_to_copy, :] = old_embeddings.weight.data[:num_tokens_to_copy, :]
-
- # return new_embeddings
-
- def resize_token_embeddings(self, new_num_tokens=None):
- """ Resize input token embeddings matrix of the model if new_num_tokens != config.vocab_size.
- Take care of tying weights embeddings afterwards if the model class has a `tie_weights()` method.
-
- Arguments:
-
- new_num_tokens: (`optional`) int:
- New number of tokens in the embedding matrix. Increasing the size will add newly initialized vectors at the end. Reducing the size will remove vectors from the end.
- If not provided or None: does nothing and just returns a pointer to the input tokens ``tf.Variable`` Module of the model.
-
- Return: ``tf.Variable``
- Pointer to the input tokens Embeddings Module of the model
- """
- raise NotImplementedError
-
- def prune_heads(self, heads_to_prune):
- """ Prunes heads of the base model.
-
- Arguments:
-
- heads_to_prune: dict with keys being selected layer indices (`int`) and associated values being the list of heads to prune in said layer (list of `int`).
- """
- raise NotImplementedError
-
- def save_pretrained(self, save_directory):
- """ Save a model and its configuration file to a directory, so that it
- can be re-loaded using the `:func:`~transformers.PreTrainedModel.from_pretrained`` class method.
- """
- assert os.path.isdir(
- save_directory
- ), "Saving path should be a directory where the model and configuration can be saved"
-
- # Save configuration file
- self.config.save_pretrained(save_directory)
-
- # If we save using the predefined names, we can load using `from_pretrained`
- output_model_file = os.path.join(save_directory, TF2_WEIGHTS_NAME)
- self.save_weights(output_model_file)
- logger.info("Model weights saved in {}".format(output_model_file))
-
- @classmethod
- def from_pretrained(cls, pretrained_model_name_or_path, *model_args, **kwargs):
- r"""Instantiate a pretrained TF 2.0 model from a pre-trained model configuration.
-
- The model is set in evaluation mode by default using ``model.eval()`` (Dropout modules are deactivated)
- To train the model, you should first set it back in training mode with ``model.train()``
-
- The warning ``Weights from XXX not initialized from pretrained model`` means that the weights of XXX do not come pre-trained with the rest of the model.
- It is up to you to train those weights with a downstream fine-tuning task.
-
- The warning ``Weights from XXX not used in YYY`` means that the layer XXX is not used by YYY, therefore those weights are discarded.
-
- Parameters:
- pretrained_model_name_or_path: either:
-
- - a string with the `shortcut name` of a pre-trained model to load from cache or download, e.g.: ``bert-base-uncased``.
- - a string with the `identifier name` of a pre-trained model that was user-uploaded to our S3, e.g.: ``dbmdz/bert-base-german-cased``.
- - a path to a `directory` containing model weights saved using :func:`~transformers.PreTrainedModel.save_pretrained`, e.g.: ``./my_model_directory/``.
- - a path or url to a `PyTorch state_dict save file` (e.g. `./pt_model/pytorch_model.bin`). In this case, ``from_pt`` should be set to True and a configuration object should be provided as ``config`` argument. This loading path is slower than converting the PyTorch checkpoint in a TensorFlow model using the provided conversion scripts and loading the TensorFlow model afterwards.
-
- model_args: (`optional`) Sequence of positional arguments:
- All remaning positional arguments will be passed to the underlying model's ``__init__`` method
-
- config: (`optional`) one of:
- - an instance of a class derived from :class:`~transformers.PretrainedConfig`, or
- - a string valid as input to :func:`~transformers.PretrainedConfig.from_pretrained()`
- Configuration for the model to use instead of an automatically loaded configuation. Configuration can be automatically loaded when:
-
- - the model is a model provided by the library (loaded with the ``shortcut-name`` string of a pretrained model), or
- - the model was saved using :func:`~transformers.PreTrainedModel.save_pretrained` and is reloaded by suppling the save directory.
- - the model is loaded by suppling a local directory as ``pretrained_model_name_or_path`` and a configuration JSON file named `config.json` is found in the directory.
-
- from_pt: (`optional`) boolean, default False:
- Load the model weights from a PyTorch state_dict save file (see docstring of pretrained_model_name_or_path argument).
-
- cache_dir: (`optional`) string:
- Path to a directory in which a downloaded pre-trained model
- configuration should be cached if the standard cache should not be used.
-
- force_download: (`optional`) boolean, default False:
- Force to (re-)download the model weights and configuration files and override the cached versions if they exists.
-
- resume_download: (`optional`) boolean, default False:
- Do not delete incompletely recieved file. Attempt to resume the download if such a file exists.
-
- proxies: (`optional`) dict, default None:
- A dictionary of proxy servers to use by protocol or endpoint, e.g.: {'http': 'foo.bar:3128', 'http://hostname': 'foo.bar:4012'}.
- The proxies are used on each request.
-
- output_loading_info: (`optional`) boolean:
- Set to ``True`` to also return a dictionnary containing missing keys, unexpected keys and error messages.
-
- kwargs: (`optional`) Remaining dictionary of keyword arguments:
- Can be used to update the configuration object (after it being loaded) and initiate the model. (e.g. ``output_attention=True``). Behave differently depending on whether a `config` is provided or automatically loaded:
-
- - If a configuration is provided with ``config``, ``**kwargs`` will be directly passed to the underlying model's ``__init__`` method (we assume all relevant updates to the configuration have already been done)
- - If a configuration is not provided, ``kwargs`` will be first passed to the configuration class initialization function (:func:`~transformers.PretrainedConfig.from_pretrained`). Each key of ``kwargs`` that corresponds to a configuration attribute will be used to override said attribute with the supplied ``kwargs`` value. Remaining keys that do not correspond to any configuration attribute will be passed to the underlying model's ``__init__`` function.
-
- Examples::
-
- # For example purposes. Not runnable.
- model = BertModel.from_pretrained('bert-base-uncased') # Download model and configuration from S3 and cache.
- model = BertModel.from_pretrained('./test/saved_model/') # E.g. model was saved using `save_pretrained('./test/saved_model/')`
- model = BertModel.from_pretrained('bert-base-uncased', output_attention=True) # Update configuration during loading
- assert model.config.output_attention == True
- # Loading from a TF checkpoint file instead of a PyTorch model (slower)
- config = BertConfig.from_json_file('./tf_model/my_tf_model_config.json')
- model = BertModel.from_pretrained('./tf_model/my_tf_checkpoint.ckpt.index', from_pt=True, config=config)
-
- """
- config = kwargs.pop("config", None)
- cache_dir = kwargs.pop("cache_dir", None)
- from_pt = kwargs.pop("from_pt", False)
- force_download = kwargs.pop("force_download", False)
- resume_download = kwargs.pop("resume_download", False)
- proxies = kwargs.pop("proxies", None)
- output_loading_info = kwargs.pop("output_loading_info", False)
-
- # Load config if we don't provide a configuration
- if not isinstance(config, PretrainedConfig):
- config_path = config if config is not None else pretrained_model_name_or_path
- config, model_kwargs = cls.config_class.from_pretrained(
- config_path,
- *model_args,
- cache_dir=cache_dir,
- return_unused_kwargs=True,
- force_download=force_download,
- resume_download=resume_download,
- **kwargs,
- )
- else:
- model_kwargs = kwargs
-
- # Load model
- if pretrained_model_name_or_path is not None:
- if pretrained_model_name_or_path in cls.pretrained_model_archive_map:
- archive_file = cls.pretrained_model_archive_map[pretrained_model_name_or_path]
- elif os.path.isdir(pretrained_model_name_or_path):
- if os.path.isfile(os.path.join(pretrained_model_name_or_path, TF2_WEIGHTS_NAME)):
- # Load from a TF 2.0 checkpoint
- archive_file = os.path.join(pretrained_model_name_or_path, TF2_WEIGHTS_NAME)
- elif from_pt and os.path.isfile(os.path.join(pretrained_model_name_or_path, WEIGHTS_NAME)):
- # Load from a PyTorch checkpoint
- archive_file = os.path.join(pretrained_model_name_or_path, WEIGHTS_NAME)
- else:
- raise EnvironmentError(
- "Error no file named {} found in directory {} or `from_pt` set to False".format(
- [WEIGHTS_NAME, TF2_WEIGHTS_NAME], pretrained_model_name_or_path
- )
- )
- elif os.path.isfile(pretrained_model_name_or_path) or is_remote_url(pretrained_model_name_or_path):
- archive_file = pretrained_model_name_or_path
- elif os.path.isfile(pretrained_model_name_or_path + ".index"):
- archive_file = pretrained_model_name_or_path + ".index"
- else:
- archive_file = hf_bucket_url(pretrained_model_name_or_path, postfix=TF2_WEIGHTS_NAME)
- if from_pt:
- raise EnvironmentError(
- "Loading a TF model from a PyTorch checkpoint is not supported when using a model identifier name."
- )
-
- # redirect to the cache, if necessary
- try:
- resolved_archive_file = cached_path(
- archive_file,
- cache_dir=cache_dir,
- force_download=force_download,
- resume_download=resume_download,
- proxies=proxies,
- )
- except EnvironmentError as e:
- if pretrained_model_name_or_path in cls.pretrained_model_archive_map:
- logger.error("Couldn't reach server at '{}' to download pretrained weights.".format(archive_file))
- else:
- logger.error(
- "Model name '{}' was not found in model name list ({}). "
- "We assumed '{}' was a path or url but couldn't find any file "
- "associated to this path or url.".format(
- pretrained_model_name_or_path,
- ", ".join(cls.pretrained_model_archive_map.keys()),
- archive_file,
- )
- )
- raise e
- if resolved_archive_file == archive_file:
- logger.info("loading weights file {}".format(archive_file))
- else:
- logger.info("loading weights file {} from cache at {}".format(archive_file, resolved_archive_file))
- else:
- resolved_archive_file = None
-
- # Instantiate model.
- model = cls(config, *model_args, **model_kwargs)
-
- if from_pt:
- # Load from a PyTorch checkpoint
- return load_pytorch_checkpoint_in_tf2_model(model, resolved_archive_file, allow_missing_keys=True)
-
- model(model.dummy_inputs, training=False) # build the network with dummy inputs
-
- assert os.path.isfile(resolved_archive_file), "Error retrieving file {}".format(resolved_archive_file)
- # 'by_name' allow us to do transfer learning by skipping/adding layers
- # see https://github.com/tensorflow/tensorflow/blob/00fad90125b18b80fe054de1055770cfb8fe4ba3/tensorflow/python/keras/engine/network.py#L1339-L1357
- try:
- model.load_weights(resolved_archive_file, by_name=True)
- except OSError:
- raise OSError(
- "Unable to load weights from h5 file. "
- "If you tried to load a TF 2.0 model from a PyTorch checkpoint, please set from_pt=True. "
- )
-
- model(model.dummy_inputs, training=False) # Make sure restore ops are run
-
- # Check if the models are the same to output loading informations
- with h5py.File(resolved_archive_file, "r") as f:
- if "layer_names" not in f.attrs and "model_weights" in f:
- f = f["model_weights"]
- hdf5_layer_names = set(hdf5_format.load_attributes_from_hdf5_group(f, "layer_names"))
- model_layer_names = set(layer.name for layer in model.layers)
- missing_keys = list(model_layer_names - hdf5_layer_names)
- unexpected_keys = list(hdf5_layer_names - model_layer_names)
- error_msgs = []
-
- if len(missing_keys) > 0:
- logger.info(
- "Layers of {} not initialized from pretrained model: {}".format(model.__class__.__name__, missing_keys)
- )
- if len(unexpected_keys) > 0:
- logger.info(
- "Layers from pretrained model not used in {}: {}".format(model.__class__.__name__, unexpected_keys)
- )
- if len(error_msgs) > 0:
- raise RuntimeError(
- "Error(s) in loading weights for {}:\n\t{}".format(model.__class__.__name__, "\n\t".join(error_msgs))
- )
- if output_loading_info:
- loading_info = {"missing_keys": missing_keys, "unexpected_keys": unexpected_keys, "error_msgs": error_msgs}
- return model, loading_info
-
- return model
-
-
-class TFConv1D(tf.keras.layers.Layer):
- def __init__(self, nf, nx, initializer_range=0.02, **kwargs):
- """ TFConv1D layer as defined by Radford et al. for OpenAI GPT (and also used in GPT-2)
- Basically works like a Linear layer but the weights are transposed
- """
- super().__init__(**kwargs)
- self.nf = nf
- self.nx = nx
- self.initializer_range = initializer_range
-
- def build(self, input_shape):
- self.weight = self.add_weight(
- "weight", shape=[self.nx, self.nf], initializer=get_initializer(self.initializer_range)
- )
- self.bias = self.add_weight("bias", shape=[1, self.nf], initializer=tf.zeros_initializer())
-
- def call(self, x):
- bz, sl = shape_list(x)[:2]
-
- x = tf.reshape(x, [-1, self.nx])
- x = tf.matmul(x, self.weight) + self.bias
-
- x = tf.reshape(x, [bz, sl, self.nf])
-
- return x
-
-
-class TFSharedEmbeddings(tf.keras.layers.Layer):
- """Construct shared token embeddings.
- """
-
- def __init__(self, vocab_size, hidden_size, initializer_range=None, **kwargs):
- super().__init__(**kwargs)
- self.vocab_size = vocab_size
- self.hidden_size = hidden_size
- self.initializer_range = hidden_size ** -0.5 if initializer_range is None else initializer_range
-
- def build(self, input_shape):
- """Build shared word embedding layer
- Shared weights logic adapted from
- https://github.com/tensorflow/models/blob/a009f4fb9d2fc4949e32192a944688925ef78659/official/transformer/v2/embedding_layer.py#L24
- """
- self.weight = self.add_weight(
- "weight", shape=[self.vocab_size, self.hidden_size], initializer=get_initializer(self.initializer_range)
- )
- super().build(input_shape)
-
- def call(self, inputs, mode="embedding"):
- """Get token embeddings of inputs.
- Args:
- inputs: list of three int64 tensors with shape [batch_size, length]: (input_ids, position_ids, token_type_ids)
- mode: string, a valid value is one of "embedding" and "linear".
- Returns:
- outputs: (1) If mode == "embedding", output embedding tensor, float32 with
- shape [batch_size, length, embedding_size]; (2) mode == "linear", output
- linear tensor, float32 with shape [batch_size, length, vocab_size].
- Raises:
- ValueError: if mode is not valid.
-
- Shared weights logic adapted from
- https://github.com/tensorflow/models/blob/a009f4fb9d2fc4949e32192a944688925ef78659/official/transformer/v2/embedding_layer.py#L24
- """
- if mode == "embedding":
- return self._embedding(inputs)
- elif mode == "linear":
- return self._linear(inputs)
- else:
- raise ValueError("mode {} is not valid.".format(mode))
-
- def _embedding(self, input_ids):
- """Applies embedding based on inputs tensor."""
- return tf.gather(self.weight, input_ids)
-
- def _linear(self, inputs):
- """Computes logits by running inputs through a linear layer.
- Args:
- inputs: A float32 tensor with shape [..., hidden_size]
- Returns:
- float32 tensor with shape [..., vocab_size].
- """
- first_dims = shape_list(inputs)[:-1]
-
- x = tf.reshape(inputs, [-1, self.hidden_size])
- logits = tf.matmul(x, self.weight, transpose_b=True)
-
- return tf.reshape(logits, first_dims + [self.vocab_size])
-
-
-class TFSequenceSummary(tf.keras.layers.Layer):
- r""" Compute a single vector summary of a sequence hidden states according to various possibilities:
- Args of the config class:
- summary_type:
- - 'last' => [default] take the last token hidden state (like XLNet)
- - 'first' => take the first token hidden state (like Bert)
- - 'mean' => take the mean of all tokens hidden states
- - 'cls_index' => supply a Tensor of classification token position (GPT/GPT-2)
- - 'attn' => Not implemented now, use multi-head attention
- summary_use_proj: Add a projection after the vector extraction
- summary_proj_to_labels: If True, the projection outputs to config.num_labels classes (otherwise to hidden_size). Default: False.
- summary_activation: 'tanh' => add a tanh activation to the output, Other => no activation. Default
- summary_first_dropout: Add a dropout before the projection and activation
- summary_last_dropout: Add a dropout after the projection and activation
- """
-
- def __init__(self, config, initializer_range=0.02, **kwargs):
- super().__init__(**kwargs)
-
- self.summary_type = config.summary_type if hasattr(config, "summary_use_proj") else "last"
- if self.summary_type == "attn":
- # We should use a standard multi-head attention module with absolute positional embedding for that.
- # Cf. https://github.com/zihangdai/xlnet/blob/master/modeling.py#L253-L276
- # We can probably just use the multi-head attention module of PyTorch >=1.1.0
- raise NotImplementedError
-
- self.has_summary = hasattr(config, "summary_use_proj") and config.summary_use_proj
- if self.has_summary:
- if hasattr(config, "summary_proj_to_labels") and config.summary_proj_to_labels and config.num_labels > 0:
- num_classes = config.num_labels
- else:
- num_classes = config.hidden_size
- self.summary = tf.keras.layers.Dense(
- num_classes, kernel_initializer=get_initializer(initializer_range), name="summary"
- )
-
- self.has_activation = hasattr(config, "summary_activation") and config.summary_activation == "tanh"
- if self.has_activation:
- self.activation = tf.keras.activations.tanh
-
- self.has_first_dropout = hasattr(config, "summary_first_dropout") and config.summary_first_dropout > 0
- if self.has_first_dropout:
- self.first_dropout = tf.keras.layers.Dropout(config.summary_first_dropout)
-
- self.has_last_dropout = hasattr(config, "summary_last_dropout") and config.summary_last_dropout > 0
- if self.has_last_dropout:
- self.last_dropout = tf.keras.layers.Dropout(config.summary_last_dropout)
-
- def call(self, inputs, training=False):
- """ hidden_states: float Tensor in shape [bsz, seq_len, hidden_size], the hidden-states of the last layer.
- cls_index: [optional] position of the classification token if summary_type == 'cls_index',
- shape (bsz,) or more generally (bsz, ...) where ... are optional leading dimensions of hidden_states.
- if summary_type == 'cls_index' and cls_index is None:
- we take the last token of the sequence as classification token
- """
- if not isinstance(inputs, (dict, tuple, list)):
- hidden_states = inputs
- cls_index = None
- elif isinstance(inputs, (tuple, list)):
- hidden_states = inputs[0]
- cls_index = inputs[1] if len(inputs) > 1 else None
- assert len(inputs) <= 2, "Too many inputs."
- else:
- hidden_states = inputs.get("hidden_states")
- cls_index = inputs.get("cls_index", None)
-
- if self.summary_type == "last":
- output = hidden_states[:, -1]
- elif self.summary_type == "first":
- output = hidden_states[:, 0]
- elif self.summary_type == "mean":
- output = tf.reduce_mean(hidden_states, axis=1)
- elif self.summary_type == "cls_index":
- hidden_shape = shape_list(hidden_states) # e.g. [batch, num choices, seq length, hidden dims]
- if cls_index is None:
- cls_index = tf.fill(
- hidden_shape[:-2], hidden_shape[-2] - 1
- ) # A tensor full of shape [batch] or [batch, num choices] full of sequence length
- cls_shape = shape_list(cls_index)
- if len(cls_shape) <= len(hidden_shape) - 2:
- cls_index = cls_index[..., tf.newaxis]
- # else:
- # cls_index = cls_index[..., tf.newaxis]
- # cls_index = cls_index.expand((-1,) * (cls_index.dim()-1) + (hidden_states.size(-1),))
- # shape of cls_index: (bsz, XX, 1, hidden_size) where XX are optional leading dim of hidden_states
- output = tf.gather(hidden_states, cls_index, batch_dims=len(hidden_shape) - 2)
- output = tf.squeeze(
- output, axis=len(hidden_shape) - 2
- ) # shape of output: (batch, num choices, hidden_size)
- elif self.summary_type == "attn":
- raise NotImplementedError
-
- if self.has_first_dropout:
- output = self.first_dropout(output, training=training)
-
- if self.has_summary:
- output = self.summary(output)
-
- if self.has_activation:
- output = self.activation(output)
-
- if self.has_last_dropout:
- output = self.last_dropout(output, training=training)
-
- return output
-
-
-def shape_list(x):
- """Deal with dynamic shape in tensorflow cleanly."""
- static = x.shape.as_list()
- dynamic = tf.shape(x)
- return [dynamic[i] if s is None else s for i, s in enumerate(static)]
-
-
-def get_initializer(initializer_range=0.02):
- """Creates a `tf.initializers.truncated_normal` with the given range.
- Args:
- initializer_range: float, initializer range for stddev.
- Returns:
- TruncatedNormal initializer with stddev = `initializer_range`.
- """
- return tf.keras.initializers.TruncatedNormal(stddev=initializer_range)
diff --git a/server/transformers/src/transformers/modeling_tf_xlm.py b/server/transformers/src/transformers/modeling_tf_xlm.py
deleted file mode 100644
index 44b991d08cb2fafe0965fd3a4832dc5b57b723e8..0000000000000000000000000000000000000000
--- a/server/transformers/src/transformers/modeling_tf_xlm.py
+++ /dev/null
@@ -1,813 +0,0 @@
-# coding=utf-8
-# Copyright 2019-present, Facebook, Inc and the HuggingFace Inc. team.
-#
-# Licensed under the Apache License, Version 2.0 (the "License");
-# you may not use this file except in compliance with the License.
-# You may obtain a copy of the License at
-#
-# http://www.apache.org/licenses/LICENSE-2.0
-#
-# Unless required by applicable law or agreed to in writing, software
-# distributed under the License is distributed on an "AS IS" BASIS,
-# WITHOUT WARRANTIES OR CONDITIONS OF ANY KIND, either express or implied.
-# See the License for the specific language governing permissions and
-# limitations under the License.
-""" TF 2.0 XLM model.
-"""
-
-
-import itertools
-import logging
-import math
-
-import numpy as np
-import tensorflow as tf
-
-from .configuration_xlm import XLMConfig
-from .file_utils import add_start_docstrings, add_start_docstrings_to_callable
-from .modeling_tf_utils import TFPreTrainedModel, TFSequenceSummary, TFSharedEmbeddings, get_initializer, shape_list
-
-
-logger = logging.getLogger(__name__)
-
-TF_XLM_PRETRAINED_MODEL_ARCHIVE_MAP = {
- "xlm-mlm-en-2048": "https://s3.amazonaws.com/models.huggingface.co/bert/xlm-mlm-en-2048-tf_model.h5",
- "xlm-mlm-ende-1024": "https://s3.amazonaws.com/models.huggingface.co/bert/xlm-mlm-ende-1024-tf_model.h5",
- "xlm-mlm-enfr-1024": "https://s3.amazonaws.com/models.huggingface.co/bert/xlm-mlm-enfr-1024-tf_model.h5",
- "xlm-mlm-enro-1024": "https://s3.amazonaws.com/models.huggingface.co/bert/xlm-mlm-enro-1024-tf_model.h5",
- "xlm-mlm-tlm-xnli15-1024": "https://s3.amazonaws.com/models.huggingface.co/bert/xlm-mlm-tlm-xnli15-1024-tf_model.h5",
- "xlm-mlm-xnli15-1024": "https://s3.amazonaws.com/models.huggingface.co/bert/xlm-mlm-xnli15-1024-tf_model.h5",
- "xlm-clm-enfr-1024": "https://s3.amazonaws.com/models.huggingface.co/bert/xlm-clm-enfr-1024-tf_model.h5",
- "xlm-clm-ende-1024": "https://s3.amazonaws.com/models.huggingface.co/bert/xlm-clm-ende-1024-tf_model.h5",
- "xlm-mlm-17-1280": "https://s3.amazonaws.com/models.huggingface.co/bert/xlm-mlm-17-1280-tf_model.h5",
- "xlm-mlm-100-1280": "https://s3.amazonaws.com/models.huggingface.co/bert/xlm-mlm-100-1280-tf_model.h5",
-}
-
-
-def create_sinusoidal_embeddings(n_pos, dim, out):
- position_enc = np.array([[pos / np.power(10000, 2 * (j // 2) / dim) for j in range(dim)] for pos in range(n_pos)])
- out[:, 0::2] = tf.constant(np.sin(position_enc[:, 0::2]))
- out[:, 1::2] = tf.constant(np.cos(position_enc[:, 1::2]))
-
-
-def gelu(x):
- """ Gaussian Error Linear Unit.
- Original Implementation of the gelu activation function in Google Bert repo when initially created.
- For information: OpenAI GPT's gelu is slightly different (and gives slightly different results):
- 0.5 * x * (1 + torch.tanh(math.sqrt(2 / math.pi) * (x + 0.044715 * torch.pow(x, 3))))
- Also see https://arxiv.org/abs/1606.08415
- """
- cdf = 0.5 * (1.0 + tf.math.erf(x / tf.math.sqrt(2.0)))
- return x * cdf
-
-
-def get_masks(slen, lengths, causal, padding_mask=None, dtype=tf.float32):
- """
- Generate hidden states mask, and optionally an attention mask.
- """
- bs = shape_list(lengths)[0]
- if padding_mask is not None:
- mask = padding_mask
- else:
- # assert lengths.max().item() <= slen
- alen = tf.range(slen)
- mask = tf.math.less(alen, lengths[:, tf.newaxis])
-
- # attention mask is the same as mask, or triangular inferior attention (causal)
- if causal:
- attn_mask = tf.less_equal(
- tf.tile(alen[tf.newaxis, tf.newaxis, :], (bs, slen, 1)), alen[tf.newaxis, :, tf.newaxis]
- )
- else:
- attn_mask = mask
-
- # sanity check
- # assert shape_list(mask) == [bs, slen]
- tf.debugging.assert_equal(shape_list(mask), [bs, slen])
- assert causal is False or shape_list(attn_mask) == [bs, slen, slen]
-
- mask = tf.cast(mask, dtype=dtype)
- attn_mask = tf.cast(attn_mask, dtype=dtype)
-
- return mask, attn_mask
-
-
-class TFMultiHeadAttention(tf.keras.layers.Layer):
-
- NEW_ID = itertools.count()
-
- def __init__(self, n_heads, dim, config, **kwargs):
- super().__init__(**kwargs)
- self.layer_id = next(TFMultiHeadAttention.NEW_ID)
- self.output_attentions = config.output_attentions
- self.dim = dim
- self.n_heads = n_heads
- assert self.dim % self.n_heads == 0
-
- self.q_lin = tf.keras.layers.Dense(dim, kernel_initializer=get_initializer(config.init_std), name="q_lin")
- self.k_lin = tf.keras.layers.Dense(dim, kernel_initializer=get_initializer(config.init_std), name="k_lin")
- self.v_lin = tf.keras.layers.Dense(dim, kernel_initializer=get_initializer(config.init_std), name="v_lin")
- self.out_lin = tf.keras.layers.Dense(dim, kernel_initializer=get_initializer(config.init_std), name="out_lin")
- self.dropout = tf.keras.layers.Dropout(config.attention_dropout)
- self.pruned_heads = set()
-
- def prune_heads(self, heads):
- raise NotImplementedError
-
- def call(self, inputs, training=False):
- """
- Self-attention (if kv is None) or attention over source sentence (provided by kv).
- """
- input, mask, kv, cache, head_mask = inputs
- # Input is (bs, qlen, dim)
- # Mask is (bs, klen) (non-causal) or (bs, klen, klen)
- bs, qlen, dim = shape_list(input)
- if kv is None:
- klen = qlen if cache is None else cache["slen"] + qlen
- else:
- klen = shape_list(kv)[1]
- # assert dim == self.dim, 'Dimensions do not match: %s input vs %s configured' % (dim, self.dim)
- n_heads = self.n_heads
- dim_per_head = self.dim // n_heads
- mask_reshape = (bs, 1, qlen, klen) if len(shape_list(mask)) == 3 else (bs, 1, 1, klen)
-
- def shape(x):
- """ projection """
- return tf.transpose(tf.reshape(x, (bs, -1, self.n_heads, dim_per_head)), perm=(0, 2, 1, 3))
-
- def unshape(x):
- """ compute context """
- return tf.reshape(tf.transpose(x, perm=(0, 2, 1, 3)), (bs, -1, self.n_heads * dim_per_head))
-
- q = shape(self.q_lin(input)) # (bs, n_heads, qlen, dim_per_head)
- if kv is None:
- k = shape(self.k_lin(input)) # (bs, n_heads, qlen, dim_per_head)
- v = shape(self.v_lin(input)) # (bs, n_heads, qlen, dim_per_head)
- elif cache is None or self.layer_id not in cache:
- k = v = kv
- k = shape(self.k_lin(k)) # (bs, n_heads, qlen, dim_per_head)
- v = shape(self.v_lin(v)) # (bs, n_heads, qlen, dim_per_head)
-
- if cache is not None:
- if self.layer_id in cache:
- if kv is None:
- k_, v_ = cache[self.layer_id]
- k = tf.concat([k_, k], axis=2) # (bs, n_heads, klen, dim_per_head)
- v = tf.concat([v_, v], axis=2) # (bs, n_heads, klen, dim_per_head)
- else:
- k, v = cache[self.layer_id]
- cache[self.layer_id] = (k, v)
-
- q = q / math.sqrt(dim_per_head) # (bs, n_heads, qlen, dim_per_head)
- scores = tf.matmul(q, k, transpose_b=True) # (bs, n_heads, qlen, klen)
- mask = tf.reshape(mask, mask_reshape) # (bs, n_heads, qlen, klen)
- # scores.masked_fill_(mask, -float('inf')) # (bs, n_heads, qlen, klen)
- scores = scores - 1e30 * (1.0 - mask)
-
- weights = tf.nn.softmax(scores, axis=-1) # (bs, n_heads, qlen, klen)
- weights = self.dropout(weights, training=training) # (bs, n_heads, qlen, klen)
-
- # Mask heads if we want to
- if head_mask is not None:
- weights = weights * head_mask
-
- context = tf.matmul(weights, v) # (bs, n_heads, qlen, dim_per_head)
- context = unshape(context) # (bs, qlen, dim)
-
- outputs = (self.out_lin(context),)
- if self.output_attentions:
- outputs = outputs + (weights,)
- return outputs
-
-
-class TFTransformerFFN(tf.keras.layers.Layer):
- def __init__(self, in_dim, dim_hidden, out_dim, config, **kwargs):
- super().__init__(**kwargs)
- self.lin1 = tf.keras.layers.Dense(dim_hidden, kernel_initializer=get_initializer(config.init_std), name="lin1")
- self.lin2 = tf.keras.layers.Dense(out_dim, kernel_initializer=get_initializer(config.init_std), name="lin2")
- self.act = tf.keras.layers.Activation(gelu) if config.gelu_activation else tf.keras.activations.relu
- self.dropout = tf.keras.layers.Dropout(config.dropout)
-
- def call(self, input, training=False):
- x = self.lin1(input)
- x = self.act(x)
- x = self.lin2(x)
- x = self.dropout(x, training=training)
- return x
-
-
-class TFXLMMainLayer(tf.keras.layers.Layer):
- def __init__(self, config, **kwargs):
- super().__init__(**kwargs)
- self.output_attentions = config.output_attentions
- self.output_hidden_states = config.output_hidden_states
-
- # encoder / decoder, output layer
- self.is_encoder = config.is_encoder
- self.is_decoder = not config.is_encoder
- if self.is_decoder:
- raise NotImplementedError("Currently XLM can only be used as an encoder")
- # self.with_output = with_output
- self.causal = config.causal
-
- # dictionary / languages
- self.n_langs = config.n_langs
- self.use_lang_emb = config.use_lang_emb
- self.n_words = config.n_words
- self.eos_index = config.eos_index
- self.pad_index = config.pad_index
- # self.dico = dico
- # self.id2lang = config.id2lang
- # self.lang2id = config.lang2id
- # assert len(self.dico) == self.n_words
- # assert len(self.id2lang) == len(self.lang2id) == self.n_langs
-
- # model parameters
- self.dim = config.emb_dim # 512 by default
- self.hidden_dim = self.dim * 4 # 2048 by default
- self.n_heads = config.n_heads # 8 by default
- self.n_layers = config.n_layers
- assert self.dim % self.n_heads == 0, "transformer dim must be a multiple of n_heads"
-
- # embeddings
- self.dropout = tf.keras.layers.Dropout(config.dropout)
- self.attention_dropout = tf.keras.layers.Dropout(config.attention_dropout)
-
- self.position_embeddings = tf.keras.layers.Embedding(
- config.max_position_embeddings,
- self.dim,
- embeddings_initializer=get_initializer(config.embed_init_std),
- name="position_embeddings",
- )
- if config.sinusoidal_embeddings:
- raise NotImplementedError
- # create_sinusoidal_embeddings(config.max_position_embeddings, self.dim, out=self.position_embeddings.weight)
- if config.n_langs > 1 and config.use_lang_emb:
- self.lang_embeddings = tf.keras.layers.Embedding(
- self.n_langs,
- self.dim,
- embeddings_initializer=get_initializer(config.embed_init_std),
- name="lang_embeddings",
- )
- self.embeddings = TFSharedEmbeddings(
- self.n_words, self.dim, initializer_range=config.embed_init_std, name="embeddings"
- ) # padding_idx=self.pad_index)
- self.layer_norm_emb = tf.keras.layers.LayerNormalization(epsilon=config.layer_norm_eps, name="layer_norm_emb")
-
- # transformer layers
- self.attentions = []
- self.layer_norm1 = []
- self.ffns = []
- self.layer_norm2 = []
- # if self.is_decoder:
- # self.layer_norm15 = []
- # self.encoder_attn = []
-
- for i in range(self.n_layers):
- self.attentions.append(
- TFMultiHeadAttention(self.n_heads, self.dim, config=config, name="attentions_._{}".format(i))
- )
- self.layer_norm1.append(
- tf.keras.layers.LayerNormalization(epsilon=config.layer_norm_eps, name="layer_norm1_._{}".format(i))
- )
- # if self.is_decoder:
- # self.layer_norm15.append(nn.LayerNorm(self.dim, eps=config.layer_norm_eps))
- # self.encoder_attn.append(MultiHeadAttention(self.n_heads, self.dim, dropout=self.attention_dropout))
- self.ffns.append(
- TFTransformerFFN(self.dim, self.hidden_dim, self.dim, config=config, name="ffns_._{}".format(i))
- )
- self.layer_norm2.append(
- tf.keras.layers.LayerNormalization(epsilon=config.layer_norm_eps, name="layer_norm2_._{}".format(i))
- )
-
- if hasattr(config, "pruned_heads"):
- pruned_heads = config.pruned_heads.copy().items()
- config.pruned_heads = {}
- for layer, heads in pruned_heads:
- if self.attentions[int(layer)].n_heads == config.n_heads:
- self.prune_heads({int(layer): list(map(int, heads))})
-
- def get_input_embeddings(self):
- return self.embeddings
-
- def _resize_token_embeddings(self, new_num_tokens):
- raise NotImplementedError
-
- def _prune_heads(self, heads_to_prune):
- """ Prunes heads of the model.
- heads_to_prune: dict of {layer_num: list of heads to prune in this layer}
- See base class PreTrainedModel
- """
- raise NotImplementedError
-
- def call(
- self,
- inputs,
- attention_mask=None,
- langs=None,
- token_type_ids=None,
- position_ids=None,
- lengths=None,
- cache=None,
- head_mask=None,
- inputs_embeds=None,
- training=False,
- ): # removed: src_enc=None, src_len=None
- if isinstance(inputs, (tuple, list)):
- input_ids = inputs[0]
- attention_mask = inputs[1] if len(inputs) > 1 else attention_mask
- langs = inputs[2] if len(inputs) > 2 else langs
- token_type_ids = inputs[3] if len(inputs) > 3 else token_type_ids
- position_ids = inputs[4] if len(inputs) > 4 else position_ids
- lengths = inputs[5] if len(inputs) > 5 else lengths
- cache = inputs[6] if len(inputs) > 6 else cache
- head_mask = inputs[7] if len(inputs) > 7 else head_mask
- inputs_embeds = inputs[8] if len(inputs) > 8 else inputs_embeds
- assert len(inputs) <= 9, "Too many inputs."
- elif isinstance(inputs, dict):
- input_ids = inputs.get("input_ids")
- attention_mask = inputs.get("attention_mask", attention_mask)
- langs = inputs.get("langs", langs)
- token_type_ids = inputs.get("token_type_ids", token_type_ids)
- position_ids = inputs.get("position_ids", position_ids)
- lengths = inputs.get("lengths", lengths)
- cache = inputs.get("cache", cache)
- head_mask = inputs.get("head_mask", head_mask)
- inputs_embeds = inputs.get("inputs_embeds", inputs_embeds)
- assert len(inputs) <= 9, "Too many inputs."
- else:
- input_ids = inputs
-
- if input_ids is not None and inputs_embeds is not None:
- raise ValueError("You cannot specify both input_ids and inputs_embeds at the same time")
- elif input_ids is not None:
- bs, slen = shape_list(input_ids)
- elif inputs_embeds is not None:
- bs, slen = shape_list(inputs_embeds)[:2]
- else:
- raise ValueError("You have to specify either input_ids or inputs_embeds")
-
- if lengths is None:
- if input_ids is not None:
- lengths = tf.reduce_sum(tf.cast(tf.not_equal(input_ids, self.pad_index), dtype=tf.int32), axis=1)
- else:
- lengths = tf.convert_to_tensor([slen] * bs, tf.int32)
- # mask = input_ids != self.pad_index
-
- # check inputs
- # assert shape_list(lengths)[0] == bs
- tf.debugging.assert_equal(shape_list(lengths)[0], bs)
- # assert lengths.max().item() <= slen
- # input_ids = input_ids.transpose(0, 1) # batch size as dimension 0
- # assert (src_enc is None) == (src_len is None)
- # if src_enc is not None:
- # assert self.is_decoder
- # assert src_enc.size(0) == bs
-
- # generate masks
- mask, attn_mask = get_masks(slen, lengths, self.causal, padding_mask=attention_mask)
- # if self.is_decoder and src_enc is not None:
- # src_mask = torch.arange(src_len.max(), dtype=torch.long, device=lengths.device) < src_len[:, None]
-
- # position_ids
- if position_ids is None:
- position_ids = tf.expand_dims(tf.range(slen), axis=0)
- else:
- # assert shape_list(position_ids) == [bs, slen] # (slen, bs)
- tf.debugging.assert_equal(shape_list(position_ids), [bs, slen])
- # position_ids = position_ids.transpose(0, 1)
-
- # langs
- if langs is not None:
- # assert shape_list(langs) == [bs, slen] # (slen, bs)
- tf.debugging.assert_equal(shape_list(langs), [bs, slen])
- # langs = langs.transpose(0, 1)
-
- # Prepare head mask if needed
- # 1.0 in head_mask indicate we keep the head
- # attention_probs has shape bsz x n_heads x N x N
- # input head_mask has shape [num_heads] or [num_hidden_layers x num_heads]
- # and head_mask is converted to shape [num_hidden_layers x batch x num_heads x qlen x klen]
- if head_mask is not None:
- raise NotImplementedError
- else:
- head_mask = [None] * self.n_layers
-
- # do not recompute cached elements
- if cache is not None and input_ids is not None:
- _slen = slen - cache["slen"]
- input_ids = input_ids[:, -_slen:]
- position_ids = position_ids[:, -_slen:]
- if langs is not None:
- langs = langs[:, -_slen:]
- mask = mask[:, -_slen:]
- attn_mask = attn_mask[:, -_slen:]
-
- # embeddings
- if inputs_embeds is None:
- inputs_embeds = self.embeddings(input_ids)
-
- tensor = inputs_embeds + self.position_embeddings(position_ids)
- if langs is not None and self.use_lang_emb:
- tensor = tensor + self.lang_embeddings(langs)
- if token_type_ids is not None:
- tensor = tensor + self.embeddings(token_type_ids)
- tensor = self.layer_norm_emb(tensor)
- tensor = self.dropout(tensor, training=training)
- tensor = tensor * mask[..., tf.newaxis]
-
- # transformer layers
- hidden_states = ()
- attentions = ()
- for i in range(self.n_layers):
- if self.output_hidden_states:
- hidden_states = hidden_states + (tensor,)
-
- # self attention
- attn_outputs = self.attentions[i]([tensor, attn_mask, None, cache, head_mask[i]], training=training)
- attn = attn_outputs[0]
- if self.output_attentions:
- attentions = attentions + (attn_outputs[1],)
- attn = self.dropout(attn, training=training)
- tensor = tensor + attn
- tensor = self.layer_norm1[i](tensor)
-
- # encoder attention (for decoder only)
- # if self.is_decoder and src_enc is not None:
- # attn = self.encoder_attn[i](tensor, src_mask, kv=src_enc, cache=cache)
- # attn = F.dropout(attn, p=self.dropout, training=self.training)
- # tensor = tensor + attn
- # tensor = self.layer_norm15[i](tensor)
-
- # FFN
- tensor = tensor + self.ffns[i](tensor)
- tensor = self.layer_norm2[i](tensor)
- tensor = tensor * mask[..., tf.newaxis]
-
- # Add last hidden state
- if self.output_hidden_states:
- hidden_states = hidden_states + (tensor,)
-
- # update cache length
- if cache is not None:
- cache["slen"] += tensor.size(1)
-
- # move back sequence length to dimension 0
- # tensor = tensor.transpose(0, 1)
-
- outputs = (tensor,)
- if self.output_hidden_states:
- outputs = outputs + (hidden_states,)
- if self.output_attentions:
- outputs = outputs + (attentions,)
- return outputs # outputs, (hidden_states), (attentions)
-
-
-class TFXLMPreTrainedModel(TFPreTrainedModel):
- """ An abstract class to handle weights initialization and
- a simple interface for downloading and loading pretrained models.
- """
-
- config_class = XLMConfig
- pretrained_model_archive_map = TF_XLM_PRETRAINED_MODEL_ARCHIVE_MAP
- base_model_prefix = "transformer"
-
- @property
- def dummy_inputs(self):
- # Sometimes XLM has language embeddings so don't forget to build them as well if needed
- inputs_list = tf.constant([[7, 6, 0, 0, 1], [1, 2, 3, 0, 0], [0, 0, 0, 4, 5]])
- attns_list = tf.constant([[1, 1, 0, 0, 1], [1, 1, 1, 0, 0], [1, 0, 0, 1, 1]])
- if self.config.use_lang_emb and self.config.n_langs > 1:
- langs_list = tf.constant([[1, 1, 0, 0, 1], [1, 1, 1, 0, 0], [1, 0, 0, 1, 1]])
- else:
- langs_list = None
- return {"input_ids": inputs_list, "attention_mask": attns_list, "langs": langs_list}
-
-
-XLM_START_DOCSTRING = r"""
-
- .. note::
-
- TF 2.0 models accepts two formats as inputs:
-
- - having all inputs as keyword arguments (like PyTorch models), or
- - having all inputs as a list, tuple or dict in the first positional arguments.
-
- This second option is useful when using :obj:`tf.keras.Model.fit()` method which currently requires having
- all the tensors in the first argument of the model call function: :obj:`model(inputs)`.
-
- If you choose this second option, there are three possibilities you can use to gather all the input Tensors
- in the first positional argument :
-
- - a single Tensor with input_ids only and nothing else: :obj:`model(inputs_ids)`
- - a list of varying length with one or several input Tensors IN THE ORDER given in the docstring:
- :obj:`model([input_ids, attention_mask])` or :obj:`model([input_ids, attention_mask, token_type_ids])`
- - a dictionary with one or several input Tensors associated to the input names given in the docstring:
- :obj:`model({'input_ids': input_ids, 'token_type_ids': token_type_ids})`
-
- Parameters:
- config (:class:`~transformers.XLMConfig`): Model configuration class with all the parameters of the model.
- Initializing with a config file does not load the weights associated with the model, only the configuration.
- Check out the :meth:`~transformers.PreTrainedModel.from_pretrained` method to load the model weights.
-"""
-
-XLM_INPUTS_DOCSTRING = r"""
- Args:
- input_ids (:obj:`tf.Tensor` or :obj:`Numpy array` of shape :obj:`(batch_size, sequence_length)`):
- Indices of input sequence tokens in the vocabulary.
-
- Indices can be obtained using :class:`transformers.BertTokenizer`.
- See :func:`transformers.PreTrainedTokenizer.encode` and
- :func:`transformers.PreTrainedTokenizer.encode_plus` for details.
-
- `What are input IDs? <../glossary.html#input-ids>`__
- attention_mask (:obj:`tf.Tensor` or :obj:`Numpy array` of shape :obj:`(batch_size, sequence_length)`, `optional`, defaults to :obj:`None`):
- Mask to avoid performing attention on padding token indices.
- Mask values selected in ``[0, 1]``:
- ``1`` for tokens that are NOT MASKED, ``0`` for MASKED tokens.
-
- `What are attention masks? <../glossary.html#attention-mask>`__
- langs (:obj:`tf.Tensor` or :obj:`Numpy array` of shape :obj:`(batch_size, sequence_length)`, `optional`, defaults to :obj:`None`):
- A parallel sequence of tokens to be used to indicate the language of each token in the input.
- Indices are languages ids which can be obtained from the language names by using two conversion mappings
- provided in the configuration of the model (only provided for multilingual models).
- More precisely, the `language name -> language id` mapping is in `model.config.lang2id` (dict str -> int) and
- the `language id -> language name` mapping is `model.config.id2lang` (dict int -> str).
-
- See usage examples detailed in the `multilingual documentation `__.
- token_type_ids (:obj:`tf.Tensor` or :obj:`Numpy array` of shape :obj:`(batch_size, sequence_length)`, `optional`, defaults to :obj:`None`):
- Segment token indices to indicate first and second portions of the inputs.
- Indices are selected in ``[0, 1]``: ``0`` corresponds to a `sentence A` token, ``1``
- corresponds to a `sentence B` token
-
- `What are token type IDs? <../glossary.html#token-type-ids>`_
- position_ids (:obj:`tf.Tensor` or :obj:`Numpy array` of shape :obj:`(batch_size, sequence_length)`, `optional`, defaults to :obj:`None`):
- Indices of positions of each input sequence tokens in the position embeddings.
- Selected in the range ``[0, config.max_position_embeddings - 1]``.
-
- `What are position IDs? <../glossary.html#position-ids>`_
- lengths (:obj:`tf.Tensor` or :obj:`Numpy array` of shape :obj:`(batch_size,)`, `optional`, defaults to :obj:`None`):
- Length of each sentence that can be used to avoid performing attention on padding token indices.
- You can also use `attention_mask` for the same result (see above), kept here for compatbility.
- Indices selected in ``[0, ..., input_ids.size(-1)]``:
- cache (:obj:`Dict[str, tf.Tensor]`, `optional`, defaults to :obj:`None`):
- dictionary with ``tf.Tensor`` that contains pre-computed
- hidden-states (key and values in the attention blocks) as computed by the model
- (see `cache` output below). Can be used to speed up sequential decoding.
- The dictionary object will be modified in-place during the forward pass to add newly computed hidden-states.
- head_mask (:obj:`tf.Tensor` or :obj:`Numpy array` of shape :obj:`(num_heads,)` or :obj:`(num_layers, num_heads)`, `optional`, defaults to :obj:`None`):
- Mask to nullify selected heads of the self-attention modules.
- Mask values selected in ``[0, 1]``:
- :obj:`1` indicates the head is **not masked**, :obj:`0` indicates the head is **masked**.
- input_embeds (:obj:`tf.Tensor` or :obj:`Numpy array` of shape :obj:`(batch_size, sequence_length, hidden_size)`, `optional`, defaults to :obj:`None`):
- Optionally, instead of passing :obj:`input_ids` you can choose to directly pass an embedded representation.
- This is useful if you want more control over how to convert `input_ids` indices into associated vectors
- than the model's internal embedding lookup matrix.
-"""
-
-
-@add_start_docstrings(
- "The bare XLM Model transformer outputing raw hidden-states without any specific head on top.",
- XLM_START_DOCSTRING,
-)
-class TFXLMModel(TFXLMPreTrainedModel):
- def __init__(self, config, *inputs, **kwargs):
- super().__init__(config, *inputs, **kwargs)
- self.transformer = TFXLMMainLayer(config, name="transformer")
-
- @add_start_docstrings_to_callable(XLM_INPUTS_DOCSTRING)
- def call(self, inputs, **kwargs):
- r"""
- Return:
- :obj:`tuple(tf.Tensor)` comprising various elements depending on the configuration (:class:`~transformers.XLMConfig`) and inputs:
- last_hidden_state (:obj:`tf.Tensor` or :obj:`Numpy array` of shape :obj:`(batch_size, sequence_length, hidden_size)`):
- Sequence of hidden-states at the output of the last layer of the model.
- hidden_states (:obj:`tuple(tf.Tensor)`, `optional`, returned when ``config.output_hidden_states=True``):
- Tuple of :obj:`tf.Tensor` or :obj:`Numpy array` (one for the output of the embeddings + one for the output of each layer)
- of shape :obj:`(batch_size, sequence_length, hidden_size)`.
-
- Hidden-states of the model at the output of each layer plus the initial embedding outputs.
- attentions (:obj:`tuple(tf.Tensor)`, `optional`, returned when ``config.output_attentions=True``):
- Tuple of :obj:`tf.Tensor` or :obj:`Numpy array` (one for each layer) of shape
- :obj:`(batch_size, num_heads, sequence_length, sequence_length)`.
-
- Attentions weights after the attention softmax, used to compute the weighted average in the self-attention
- heads.
-
- Examples::
-
- import tensorflow as tf
- from transformers import XLMTokenizer, TFXLMModel
-
- tokenizer = XLMTokenizer.from_pretrained('xlm-mlm-en-2048')
- model = TFXLMModel.from_pretrained('xlm-mlm-en-2048')
- input_ids = tf.constant(tokenizer.encode("Hello, my dog is cute", add_special_tokens=True))[None, :] # Batch size 1
- outputs = model(input_ids)
- last_hidden_states = outputs[0] # The last hidden-state is the first element of the output tuple
-
- """
- outputs = self.transformer(inputs, **kwargs)
- return outputs
-
-
-class TFXLMPredLayer(tf.keras.layers.Layer):
- """
- Prediction layer (cross_entropy or adaptive_softmax).
- """
-
- def __init__(self, config, input_embeddings, **kwargs):
- super().__init__(**kwargs)
- self.asm = config.asm
- self.n_words = config.n_words
- self.pad_index = config.pad_index
- if config.asm is False:
- self.input_embeddings = input_embeddings
- else:
- raise NotImplementedError
- # self.proj = nn.AdaptiveLogSoftmaxWithLoss(
- # in_features=dim,
- # n_classes=config.n_words,
- # cutoffs=config.asm_cutoffs,
- # div_value=config.asm_div_value,
- # head_bias=True, # default is False
- # )
-
- def build(self, input_shape):
- # The output weights are the same as the input embeddings, but there is an output-only bias for each token.
- self.bias = self.add_weight(shape=(self.n_words,), initializer="zeros", trainable=True, name="bias")
- super().build(input_shape)
-
- def call(self, hidden_states):
- hidden_states = self.input_embeddings(hidden_states, mode="linear")
- hidden_states = hidden_states + self.bias
- return hidden_states
-
-
-@add_start_docstrings(
- """The XLM Model transformer with a language modeling head on top
- (linear layer with weights tied to the input embeddings). """,
- XLM_START_DOCSTRING,
-)
-class TFXLMWithLMHeadModel(TFXLMPreTrainedModel):
- def __init__(self, config, *inputs, **kwargs):
- super().__init__(config, *inputs, **kwargs)
- self.transformer = TFXLMMainLayer(config, name="transformer")
- self.pred_layer = TFXLMPredLayer(config, self.transformer.embeddings, name="pred_layer_._proj")
-
- def get_output_embeddings(self):
- return self.pred_layer.input_embeddings
-
- @add_start_docstrings_to_callable(XLM_INPUTS_DOCSTRING)
- def call(self, inputs, **kwargs):
- r"""
- Return:
- :obj:`tuple(tf.Tensor)` comprising various elements depending on the configuration (:class:`~transformers.XLMConfig`) and inputs:
- prediction_scores (:obj:`tf.Tensor` or :obj:`Numpy array` of shape :obj:`(batch_size, sequence_length, config.vocab_size)`):
- Prediction scores of the language modeling head (scores for each vocabulary token before SoftMax).
- hidden_states (:obj:`tuple(tf.Tensor)`, `optional`, returned when ``config.output_hidden_states=True``):
- Tuple of :obj:`tf.Tensor` or :obj:`Numpy array` (one for the output of the embeddings + one for the output of each layer)
- of shape :obj:`(batch_size, sequence_length, hidden_size)`.
-
- Hidden-states of the model at the output of each layer plus the initial embedding outputs.
- attentions (:obj:`tuple(tf.Tensor)`, `optional`, returned when ``config.output_attentions=True``):
- Tuple of :obj:`tf.Tensor` or :obj:`Numpy array` (one for each layer) of shape
- :obj:`(batch_size, num_heads, sequence_length, sequence_length)`.
-
- Attentions weights after the attention softmax, used to compute the weighted average in the self-attention
- heads.
-
- Examples::
-
- import tensorflow as tf
- from transformers import XLMTokenizer, TFXLMWithLMHeadModel
-
- tokenizer = XLMTokenizer.from_pretrained('xlm-mlm-en-2048')
- model = TFXLMWithLMHeadModel.from_pretrained('xlm-mlm-en-2048')
- input_ids = tf.constant(tokenizer.encode("Hello, my dog is cute", add_special_tokens=True))[None, :] # Batch size 1
- outputs = model(input_ids)
- last_hidden_states = outputs[0] # The last hidden-state is the first element of the output tuple
-
- """
- transformer_outputs = self.transformer(inputs, **kwargs)
-
- output = transformer_outputs[0]
- outputs = self.pred_layer(output)
- outputs = (outputs,) + transformer_outputs[1:] # Keep new_mems and attention/hidden states if they are here
-
- return outputs
-
-
-@add_start_docstrings(
- """XLM Model with a sequence classification/regression head on top (a linear layer on top of
- the pooled output) e.g. for GLUE tasks. """,
- XLM_START_DOCSTRING,
-)
-class TFXLMForSequenceClassification(TFXLMPreTrainedModel):
- def __init__(self, config, *inputs, **kwargs):
- super().__init__(config, *inputs, **kwargs)
- self.num_labels = config.num_labels
-
- self.transformer = TFXLMMainLayer(config, name="transformer")
- self.sequence_summary = TFSequenceSummary(config, initializer_range=config.init_std, name="sequence_summary")
-
- @add_start_docstrings_to_callable(XLM_INPUTS_DOCSTRING)
- def call(self, inputs, **kwargs):
- r"""
- Returns:
- :obj:`tuple(tf.Tensor)` comprising various elements depending on the configuration (:class:`~transformers.XLMConfig`) and inputs:
- logits (:obj:`tf.Tensor` or :obj:`Numpy array` of shape :obj:`(batch_size, config.num_labels)`):
- Classification (or regression if config.num_labels==1) scores (before SoftMax).
- hidden_states (:obj:`tuple(tf.Tensor)`, `optional`, returned when ``config.output_hidden_states=True``):
- Tuple of :obj:`tf.Tensor` or :obj:`Numpy array` (one for the output of the embeddings + one for the output of each layer)
- of shape :obj:`(batch_size, sequence_length, hidden_size)`.
-
- Hidden-states of the model at the output of each layer plus the initial embedding outputs.
- attentions (:obj:`tuple(tf.Tensor)`, `optional`, returned when ``config.output_attentions=True``):
- Tuple of :obj:`tf.Tensor` or :obj:`Numpy array` (one for each layer) of shape
- :obj:`(batch_size, num_heads, sequence_length, sequence_length)`.
-
- Attentions weights after the attention softmax, used to compute the weighted average in the self-attention
- heads.
-
- Examples::
-
- import tensorflow as tf
- from transformers import XLMTokenizer, TFXLMForSequenceClassification
-
- tokenizer = XLMTokenizer.from_pretrained('xlm-mlm-en-2048')
- model = TFXLMForSequenceClassification.from_pretrained('xlm-mlm-en-2048')
- input_ids = tf.constant(tokenizer.encode("Hello, my dog is cute", add_special_tokens=True))[None, :] # Batch size 1
- labels = tf.constant([1])[None, :] # Batch size 1
- outputs = model(input_ids)
- logits = outputs[0]
-
- """
- transformer_outputs = self.transformer(inputs, **kwargs)
- output = transformer_outputs[0]
-
- logits = self.sequence_summary(output)
-
- outputs = (logits,) + transformer_outputs[1:] # Keep new_mems and attention/hidden states if they are here
- return outputs
-
-
-@add_start_docstrings(
- """XLM Model with a span classification head on top for extractive question-answering tasks like SQuAD (a linear layers on top of
- the hidden-states output to compute `span start logits` and `span end logits`). """,
- XLM_START_DOCSTRING,
-)
-class TFXLMForQuestionAnsweringSimple(TFXLMPreTrainedModel):
- def __init__(self, config, *inputs, **kwargs):
- super().__init__(config, *inputs, **kwargs)
- self.transformer = TFXLMMainLayer(config, name="transformer")
- self.qa_outputs = tf.keras.layers.Dense(
- config.num_labels, kernel_initializer=get_initializer(config.init_std), name="qa_outputs"
- )
-
- @add_start_docstrings_to_callable(XLM_INPUTS_DOCSTRING)
- def call(self, inputs, **kwargs):
- r"""
- Returns:
- :obj:`tuple(tf.Tensor)` comprising various elements depending on the configuration (:class:`~transformers.XLMConfig`) and inputs:
- start_scores (:obj:`tf.Tensor` or :obj:`Numpy array` of shape :obj:`(batch_size, sequence_length,)`):
- Span-start scores (before SoftMax).
- end_scores (:obj:`tf.Tensor` or :obj:`Numpy array` of shape :obj:`(batch_size, sequence_length,)`):
- Span-end scores (before SoftMax).
- hidden_states (:obj:`tuple(tf.Tensor)`, `optional`, returned when ``config.output_hidden_states=True``):
- Tuple of :obj:`tf.Tensor` or :obj:`Numpy array` (one for the output of the embeddings + one for the output of each layer)
- of shape :obj:`(batch_size, sequence_length, hidden_size)`.
-
- Hidden-states of the model at the output of each layer plus the initial embedding outputs.
- attentions (:obj:`tuple(tf.Tensor)`, `optional`, returned when ``config.output_attentions=True``):
- Tuple of :obj:`tf.Tensor` or :obj:`Numpy array` (one for each layer) of shape
- :obj:`(batch_size, num_heads, sequence_length, sequence_length)`.
-
- Attentions weights after the attention softmax, used to compute the weighted average in the self-attention
- heads.
-
- Examples::
-
- import tensorflow as tf
- from transformers import XLMTokenizer, TFXLMForQuestionAnsweringSimple
-
- tokenizer = XLMTokenizer.from_pretrained('xlm-mlm-en-2048')
- model = TFXLMForQuestionAnsweringSimple.from_pretrained('xlm-mlm-en-2048')
- input_ids = tf.constant(tokenizer.encode("Hello, my dog is cute", add_special_tokens=True))[None, :] # Batch size 1
- outputs = model(input_ids)
- start_scores, end_scores = outputs[:2]
-
- """
- transformer_outputs = self.transformer(inputs, **kwargs)
-
- sequence_output = transformer_outputs[0]
-
- logits = self.qa_outputs(sequence_output)
- start_logits, end_logits = tf.split(logits, 2, axis=-1)
- start_logits = tf.squeeze(start_logits, axis=-1)
- end_logits = tf.squeeze(end_logits, axis=-1)
-
- outputs = (start_logits, end_logits,) + transformer_outputs[
- 1:
- ] # Keep mems, hidden states, attentions if there are in it
-
- return outputs # start_logits, end_logits, (hidden_states), (attentions)
diff --git a/server/transformers/src/transformers/modeling_tf_xlm_roberta.py b/server/transformers/src/transformers/modeling_tf_xlm_roberta.py
deleted file mode 100644
index 8b1efdb65df064a788105d045966020227dbb5ae..0000000000000000000000000000000000000000
--- a/server/transformers/src/transformers/modeling_tf_xlm_roberta.py
+++ /dev/null
@@ -1,118 +0,0 @@
-# coding=utf-8
-# Copyright 2019 Facebook AI Research and the HuggingFace Inc. team.
-# Copyright (c) 2018, NVIDIA CORPORATION. All rights reserved.
-#
-# Licensed under the Apache License, Version 2.0 (the "License");
-# you may not use this file except in compliance with the License.
-# You may obtain a copy of the License at
-#
-# http://www.apache.org/licenses/LICENSE-2.0
-#
-# Unless required by applicable law or agreed to in writing, software
-# distributed under the License is distributed on an "AS IS" BASIS,
-# WITHOUT WARRANTIES OR CONDITIONS OF ANY KIND, either express or implied.
-# See the License for the specific language governing permissions and
-# limitations under the License.
-""" TF 2.0 XLM-RoBERTa model. """
-
-
-import logging
-
-from .configuration_xlm_roberta import XLMRobertaConfig
-from .file_utils import add_start_docstrings
-from .modeling_tf_roberta import (
- TFRobertaForMaskedLM,
- TFRobertaForSequenceClassification,
- TFRobertaForTokenClassification,
- TFRobertaModel,
-)
-
-
-logger = logging.getLogger(__name__)
-
-TF_XLM_ROBERTA_PRETRAINED_MODEL_ARCHIVE_MAP = {}
-
-
-XLM_ROBERTA_START_DOCSTRING = r"""
-
- .. note::
-
- TF 2.0 models accepts two formats as inputs:
-
- - having all inputs as keyword arguments (like PyTorch models), or
- - having all inputs as a list, tuple or dict in the first positional arguments.
-
- This second option is useful when using :obj:`tf.keras.Model.fit()` method which currently requires having
- all the tensors in the first argument of the model call function: :obj:`model(inputs)`.
-
- If you choose this second option, there are three possibilities you can use to gather all the input Tensors
- in the first positional argument :
-
- - a single Tensor with input_ids only and nothing else: :obj:`model(inputs_ids)`
- - a list of varying length with one or several input Tensors IN THE ORDER given in the docstring:
- :obj:`model([input_ids, attention_mask])` or :obj:`model([input_ids, attention_mask, token_type_ids])`
- - a dictionary with one or several input Tensors associated to the input names given in the docstring:
- :obj:`model({'input_ids': input_ids, 'token_type_ids': token_type_ids})`
-
- Parameters:
- config (:class:`~transformers.XLMRobertaConfig`): Model configuration class with all the parameters of the
- model. Initializing with a config file does not load the weights associated with the model, only the configuration.
- Check out the :meth:`~transformers.PreTrainedModel.from_pretrained` method to load the model weights.
-"""
-
-
-@add_start_docstrings(
- "The bare XLM-RoBERTa Model transformer outputting raw hidden-states without any specific head on top.",
- XLM_ROBERTA_START_DOCSTRING,
-)
-class TFXLMRobertaModel(TFRobertaModel):
- """
- This class overrides :class:`~transformers.TFRobertaModel`. Please check the
- superclass for the appropriate documentation alongside usage examples.
- """
-
- config_class = XLMRobertaConfig
- pretrained_model_archive_map = TF_XLM_ROBERTA_PRETRAINED_MODEL_ARCHIVE_MAP
-
-
-@add_start_docstrings(
- """XLM-RoBERTa Model with a `language modeling` head on top. """, XLM_ROBERTA_START_DOCSTRING,
-)
-class TFXLMRobertaForMaskedLM(TFRobertaForMaskedLM):
- """
- This class overrides :class:`~transformers.TFRobertaForMaskedLM`. Please check the
- superclass for the appropriate documentation alongside usage examples.
- """
-
- config_class = XLMRobertaConfig
- pretrained_model_archive_map = TF_XLM_ROBERTA_PRETRAINED_MODEL_ARCHIVE_MAP
-
-
-@add_start_docstrings(
- """XLM-RoBERTa Model transformer with a sequence classification/regression head on top (a linear layer
- on top of the pooled output) e.g. for GLUE tasks. """,
- XLM_ROBERTA_START_DOCSTRING,
-)
-class TFXLMRobertaForSequenceClassification(TFRobertaForSequenceClassification):
- """
- This class overrides :class:`~transformers.TFRobertaForSequenceClassification`. Please check the
- superclass for the appropriate documentation alongside usage examples.
- """
-
- config_class = XLMRobertaConfig
- pretrained_model_archive_map = TF_XLM_ROBERTA_PRETRAINED_MODEL_ARCHIVE_MAP
-
-
-@add_start_docstrings(
- """XLM-RoBERTa Model with a token classification head on top (a linear layer on top of
- the hidden-states output) e.g. for Named-Entity-Recognition (NER) tasks. """,
- XLM_ROBERTA_START_DOCSTRING,
-)
-class TFXLMRobertaForTokenClassification(TFRobertaForTokenClassification):
- """
- This class overrides :class:`~transformers.TFRobertaForTokenClassification`. Please check the
- superclass for the appropriate documentation alongside usage examples.
- """
-
- config_class = XLMRobertaConfig
- pretrained_model_archive_map = TF_XLM_ROBERTA_PRETRAINED_MODEL_ARCHIVE_MAP
diff --git a/server/transformers/src/transformers/modeling_tf_xlnet.py b/server/transformers/src/transformers/modeling_tf_xlnet.py
deleted file mode 100644
index d9ced75384c18de6508fbff5b7d3f6e13779404f..0000000000000000000000000000000000000000
--- a/server/transformers/src/transformers/modeling_tf_xlnet.py
+++ /dev/null
@@ -1,1197 +0,0 @@
-# coding=utf-8
-# Copyright 2018 Google AI, Google Brain and Carnegie Mellon University Authors and the HuggingFace Inc. team.
-# Copyright (c) 2018, NVIDIA CORPORATION. All rights reserved.
-#
-# Licensed under the Apache License, Version 2.0 (the "License");
-# you may not use this file except in compliance with the License.
-# You may obtain a copy of the License at
-#
-# http://www.apache.org/licenses/LICENSE-2.0
-#
-# Unless required by applicable law or agreed to in writing, software
-# distributed under the License is distributed on an "AS IS" BASIS,
-# WITHOUT WARRANTIES OR CONDITIONS OF ANY KIND, either express or implied.
-# See the License for the specific language governing permissions and
-# limitations under the License.
-""" TF 2.0 XLNet model.
-"""
-
-
-import logging
-
-import numpy as np
-import tensorflow as tf
-
-from .configuration_xlnet import XLNetConfig
-from .file_utils import add_start_docstrings, add_start_docstrings_to_callable
-from .modeling_tf_utils import TFPreTrainedModel, TFSequenceSummary, TFSharedEmbeddings, get_initializer, shape_list
-
-
-logger = logging.getLogger(__name__)
-
-TF_XLNET_PRETRAINED_MODEL_ARCHIVE_MAP = {
- "xlnet-base-cased": "https://s3.amazonaws.com/models.huggingface.co/bert/xlnet-base-cased-tf_model.h5",
- "xlnet-large-cased": "https://s3.amazonaws.com/models.huggingface.co/bert/xlnet-large-cased-tf_model.h5",
-}
-
-
-def gelu(x):
- """ Implementation of the gelu activation function.
- XLNet is using OpenAI GPT's gelu
- Also see https://arxiv.org/abs/1606.08415
- """
- cdf = 0.5 * (1.0 + tf.tanh((np.sqrt(2 / np.pi) * (x + 0.044715 * tf.pow(x, 3)))))
- return x * cdf
-
-
-def swish(x):
- return x * tf.sigmoid(x)
-
-
-ACT2FN = {
- "gelu": tf.keras.layers.Activation(gelu),
- "relu": tf.keras.activations.relu,
- "swish": tf.keras.layers.Activation(swish),
-}
-
-
-class TFXLNetRelativeAttention(tf.keras.layers.Layer):
- def __init__(self, config, **kwargs):
- super().__init__(**kwargs)
- self.output_attentions = config.output_attentions
-
- if config.d_model % config.n_head != 0:
- raise ValueError(
- "The hidden size (%d) is not a multiple of the number of attention "
- "heads (%d)" % (config.d_model, config.n_head)
- )
-
- self.n_head = config.n_head
- self.d_head = config.d_head
- self.d_model = config.d_model
- self.scale = 1 / (config.d_head ** 0.5)
- self.initializer_range = config.initializer_range
-
- self.layer_norm = tf.keras.layers.LayerNormalization(epsilon=config.layer_norm_eps, name="layer_norm")
- self.dropout = tf.keras.layers.Dropout(config.dropout)
-
- def build(self, input_shape):
- initializer = get_initializer(self.initializer_range)
- self.q = self.add_weight(
- shape=(self.d_model, self.n_head, self.d_head), initializer=initializer, trainable=True, name="q"
- )
- self.k = self.add_weight(
- shape=(self.d_model, self.n_head, self.d_head), initializer=initializer, trainable=True, name="k"
- )
- self.v = self.add_weight(
- shape=(self.d_model, self.n_head, self.d_head), initializer=initializer, trainable=True, name="v"
- )
- self.o = self.add_weight(
- shape=(self.d_model, self.n_head, self.d_head), initializer=initializer, trainable=True, name="o"
- )
- self.r = self.add_weight(
- shape=(self.d_model, self.n_head, self.d_head), initializer=initializer, trainable=True, name="r"
- )
- self.r_r_bias = self.add_weight(
- shape=(self.n_head, self.d_head), initializer="zeros", trainable=True, name="r_r_bias"
- )
- self.r_s_bias = self.add_weight(
- shape=(self.n_head, self.d_head), initializer="zeros", trainable=True, name="r_s_bias"
- )
- self.r_w_bias = self.add_weight(
- shape=(self.n_head, self.d_head), initializer="zeros", trainable=True, name="r_w_bias"
- )
- self.seg_embed = self.add_weight(
- shape=(2, self.n_head, self.d_head), initializer=initializer, trainable=True, name="seg_embed"
- )
- super().build(input_shape)
-
- def prune_heads(self, heads):
- raise NotImplementedError
-
- def rel_shift(self, x, klen=-1):
- """perform relative shift to form the relative attention score."""
- x_size = shape_list(x)
-
- x = tf.reshape(x, (x_size[1], x_size[0], x_size[2], x_size[3]))
- x = x[1:, ...]
- x = tf.reshape(x, (x_size[0], x_size[1] - 1, x_size[2], x_size[3]))
- x = x[:, 0:klen, :, :]
- # x = torch.index_select(x, 1, torch.arange(klen, device=x.device, dtype=torch.long))
-
- return x
-
- def rel_attn_core(self, inputs, training=False):
- """Core relative positional attention operations."""
-
- q_head, k_head_h, v_head_h, k_head_r, seg_mat, attn_mask, head_mask = inputs
-
- # content based attention score
- ac = tf.einsum("ibnd,jbnd->ijbn", q_head + self.r_w_bias, k_head_h)
-
- # position based attention score
- bd = tf.einsum("ibnd,jbnd->ijbn", q_head + self.r_r_bias, k_head_r)
- bd = self.rel_shift(bd, klen=shape_list(ac)[1])
-
- # segment based attention score
- if seg_mat is None:
- ef = 0
- else:
- ef = tf.einsum("ibnd,snd->ibns", q_head + self.r_s_bias, self.seg_embed)
- ef = tf.einsum("ijbs,ibns->ijbn", seg_mat, ef)
-
- # merge attention scores and perform masking
- attn_score = (ac + bd + ef) * self.scale
- if attn_mask is not None:
- # attn_score = attn_score * (1 - attn_mask) - 1e30 * attn_mask
- if attn_mask.dtype == tf.float16:
- attn_score = attn_score - 65500 * attn_mask
- else:
- attn_score = attn_score - 1e30 * attn_mask
-
- # attention probability
- attn_prob = tf.nn.softmax(attn_score, axis=1)
-
- attn_prob = self.dropout(attn_prob, training=training)
-
- # Mask heads if we want to
- if head_mask is not None:
- attn_prob = attn_prob * head_mask
-
- # attention output
- attn_vec = tf.einsum("ijbn,jbnd->ibnd", attn_prob, v_head_h)
-
- if self.output_attentions:
- return attn_vec, attn_prob
-
- return attn_vec
-
- def post_attention(self, inputs, residual=True, training=False):
- """Post-attention processing."""
- # post-attention projection (back to `d_model`)
- h, attn_vec = inputs
-
- attn_out = tf.einsum("ibnd,hnd->ibh", attn_vec, self.o)
-
- attn_out = self.dropout(attn_out, training=training)
-
- if residual:
- attn_out = attn_out + h
- output = self.layer_norm(attn_out)
-
- return output
-
- def call(self, inputs, training=False):
- (h, g, attn_mask_h, attn_mask_g, r, seg_mat, mems, target_mapping, head_mask) = inputs
-
- if g is not None:
- # Two-stream attention with relative positional encoding.
- # content based attention score
- if mems is not None and len(shape_list(mems)) > 1:
- cat = tf.concat([mems, h], axis=0)
- else:
- cat = h
-
- # content-based key head
- k_head_h = tf.einsum("ibh,hnd->ibnd", cat, self.k)
-
- # content-based value head
- v_head_h = tf.einsum("ibh,hnd->ibnd", cat, self.v)
-
- # position-based key head
- k_head_r = tf.einsum("ibh,hnd->ibnd", r, self.r)
-
- # h-stream
- # content-stream query head
- q_head_h = tf.einsum("ibh,hnd->ibnd", h, self.q)
-
- # core attention ops
- attn_vec_h = self.rel_attn_core(
- [q_head_h, k_head_h, v_head_h, k_head_r, seg_mat, attn_mask_h, head_mask], training=training
- )
-
- if self.output_attentions:
- attn_vec_h, attn_prob_h = attn_vec_h
-
- # post processing
- output_h = self.post_attention([h, attn_vec_h], training=training)
-
- # g-stream
- # query-stream query head
- q_head_g = tf.einsum("ibh,hnd->ibnd", g, self.q)
-
- # core attention ops
- if target_mapping is not None:
- q_head_g = tf.einsum("mbnd,mlb->lbnd", q_head_g, target_mapping)
- attn_vec_g = self.rel_attn_core(
- [q_head_g, k_head_h, v_head_h, k_head_r, seg_mat, attn_mask_g, head_mask], training=training
- )
-
- if self.output_attentions:
- attn_vec_g, attn_prob_g = attn_vec_g
-
- attn_vec_g = tf.einsum("lbnd,mlb->mbnd", attn_vec_g, target_mapping)
- else:
- attn_vec_g = self.rel_attn_core(
- [q_head_g, k_head_h, v_head_h, k_head_r, seg_mat, attn_mask_g, head_mask], training=training
- )
-
- if self.output_attentions:
- attn_vec_g, attn_prob_g = attn_vec_g
-
- # post processing
- output_g = self.post_attention([g, attn_vec_g], training=training)
-
- if self.output_attentions:
- attn_prob = attn_prob_h, attn_prob_g
-
- else:
- # Multi-head attention with relative positional encoding
- if mems is not None and len(shape_list(mems)) > 1:
- cat = tf.concat([mems, h], axis=0)
- else:
- cat = h
-
- # content heads
- q_head_h = tf.einsum("ibh,hnd->ibnd", h, self.q)
- k_head_h = tf.einsum("ibh,hnd->ibnd", cat, self.k)
- v_head_h = tf.einsum("ibh,hnd->ibnd", cat, self.v)
-
- # positional heads
- k_head_r = tf.einsum("ibh,hnd->ibnd", r, self.r)
-
- # core attention ops
- attn_vec = self.rel_attn_core(
- [q_head_h, k_head_h, v_head_h, k_head_r, seg_mat, attn_mask_h, head_mask], training=training
- )
-
- if self.output_attentions:
- attn_vec, attn_prob = attn_vec
-
- # post processing
- output_h = self.post_attention([h, attn_vec], training=training)
- output_g = None
-
- outputs = (output_h, output_g)
- if self.output_attentions:
- outputs = outputs + (attn_prob,)
- return outputs
-
-
-class TFXLNetFeedForward(tf.keras.layers.Layer):
- def __init__(self, config, **kwargs):
- super().__init__(**kwargs)
- self.layer_norm = tf.keras.layers.LayerNormalization(epsilon=config.layer_norm_eps, name="layer_norm")
- self.layer_1 = tf.keras.layers.Dense(
- config.d_inner, kernel_initializer=get_initializer(config.initializer_range), name="layer_1"
- )
- self.layer_2 = tf.keras.layers.Dense(
- config.d_model, kernel_initializer=get_initializer(config.initializer_range), name="layer_2"
- )
- self.dropout = tf.keras.layers.Dropout(config.dropout)
- if isinstance(config.ff_activation, str):
- self.activation_function = ACT2FN[config.ff_activation]
- else:
- self.activation_function = config.ff_activation
-
- def call(self, inp, training=False):
- output = inp
- output = self.layer_1(output)
- output = self.activation_function(output)
- output = self.dropout(output, training=training)
- output = self.layer_2(output)
- output = self.dropout(output, training=training)
- output = self.layer_norm(output + inp)
- return output
-
-
-class TFXLNetLayer(tf.keras.layers.Layer):
- def __init__(self, config, **kwargs):
- super().__init__(**kwargs)
- self.rel_attn = TFXLNetRelativeAttention(config, name="rel_attn")
- self.ff = TFXLNetFeedForward(config, name="ff")
- self.dropout = tf.keras.layers.Dropout(config.dropout)
-
- def call(self, inputs, training=False):
- outputs = self.rel_attn(inputs, training=training)
- output_h, output_g = outputs[:2]
-
- if output_g is not None:
- output_g = self.ff(output_g, training=training)
- output_h = self.ff(output_h, training=training)
-
- outputs = (output_h, output_g) + outputs[2:] # Add again attentions if there are there
- return outputs
-
-
-class TFXLNetLMHead(tf.keras.layers.Layer):
- def __init__(self, config, input_embeddings, **kwargs):
- super().__init__(**kwargs)
- self.vocab_size = config.vocab_size
- # The output weights are the same as the input embeddings, but there is
- # an output-only bias for each token.
- self.input_embeddings = input_embeddings
-
- def build(self, input_shape):
- self.bias = self.add_weight(shape=(self.vocab_size,), initializer="zeros", trainable=True, name="bias")
- super().build(input_shape)
-
- def call(self, hidden_states):
- hidden_states = self.input_embeddings(hidden_states, mode="linear")
- hidden_states = hidden_states + self.bias
- return hidden_states
-
-
-class TFXLNetMainLayer(tf.keras.layers.Layer):
- def __init__(self, config, **kwargs):
- super().__init__(**kwargs)
- self.output_attentions = config.output_attentions
- self.output_hidden_states = config.output_hidden_states
- self.output_past = config.output_past
-
- self.mem_len = config.mem_len
- self.reuse_len = config.reuse_len
- self.d_model = config.d_model
- self.same_length = config.same_length
- self.attn_type = config.attn_type
- self.bi_data = config.bi_data
- self.clamp_len = config.clamp_len
- self.n_layer = config.n_layer
- self.use_bfloat16 = config.use_bfloat16
- self.initializer_range = config.initializer_range
-
- self.word_embedding = TFSharedEmbeddings(
- config.vocab_size, config.d_model, initializer_range=config.initializer_range, name="word_embedding"
- )
- self.layer = [TFXLNetLayer(config, name="layer_._{}".format(i)) for i in range(config.n_layer)]
- self.dropout = tf.keras.layers.Dropout(config.dropout)
-
- def get_input_embeddings(self):
- return self.word_embedding
-
- def build(self, input_shape):
- initializer = get_initializer(self.initializer_range)
- self.mask_emb = self.add_weight(
- shape=(1, 1, self.d_model), initializer=initializer, trainable=True, name="mask_emb"
- )
-
- def _resize_token_embeddings(self, new_num_tokens):
- raise NotImplementedError
-
- def _prune_heads(self, heads_to_prune):
- raise NotImplementedError
-
- def create_mask(self, qlen, mlen, dtype=tf.float32):
- """
- Creates causal attention mask. Float mask where 1.0 indicates masked, 0.0 indicates not-masked.
-
- Args:
- qlen: TODO Lysandre didn't fill
- mlen: TODO Lysandre didn't fill
-
- ::
-
- same_length=False: same_length=True:
- < qlen > < qlen >
- ^ [0 0 0 0 0 1 1 1 1] [0 0 0 0 0 1 1 1 1]
- [0 0 0 0 0 0 1 1 1] [1 0 0 0 0 0 1 1 1]
- qlen [0 0 0 0 0 0 0 1 1] [1 1 0 0 0 0 0 1 1]
- [0 0 0 0 0 0 0 0 1] [1 1 1 0 0 0 0 0 1]
- v [0 0 0 0 0 0 0 0 0] [1 1 1 1 0 0 0 0 0]
-
- """
- attn_mask = tf.ones([qlen, qlen], dtype=dtype)
- mask_u = tf.matrix_band_part(attn_mask, 0, -1)
- mask_dia = tf.matrix_band_part(attn_mask, 0, 0)
- attn_mask_pad = tf.zeros([qlen, mlen], dtype=dtype)
- ret = tf.concat([attn_mask_pad, mask_u - mask_dia], 1)
- if self.same_length:
- mask_l = tf.matrix_band_part(attn_mask, -1, 0)
- ret = tf.concat([ret[:, :qlen] + mask_l - mask_dia, ret[:, qlen:]], 1)
- return ret
-
- def cache_mem(self, curr_out, prev_mem):
- """cache hidden states into memory."""
- if self.reuse_len is not None and self.reuse_len > 0:
- curr_out = curr_out[: self.reuse_len]
-
- if prev_mem is None:
- new_mem = curr_out[-self.mem_len :]
- else:
- new_mem = tf.concat([prev_mem, curr_out], 0)[-self.mem_len :]
-
- return tf.stop_gradient(new_mem)
-
- @staticmethod
- def positional_embedding(pos_seq, inv_freq, bsz=None):
- sinusoid_inp = tf.einsum("i,d->id", pos_seq, inv_freq)
- pos_emb = tf.concat([tf.sin(sinusoid_inp), tf.cos(sinusoid_inp)], axis=-1)
- pos_emb = pos_emb[:, None, :]
-
- if bsz is not None:
- pos_emb = tf.tile(pos_emb, [1, bsz, 1])
-
- return pos_emb
-
- def relative_positional_encoding(self, qlen, klen, bsz=None, dtype=None):
- """create relative positional encoding."""
- freq_seq = tf.range(0, self.d_model, 2.0)
- if dtype is not None and dtype != tf.float32:
- freq_seq = tf.cast(freq_seq, dtype=dtype)
- inv_freq = 1 / (10000 ** (freq_seq / self.d_model))
-
- if self.attn_type == "bi":
- # beg, end = klen - 1, -qlen
- beg, end = klen, -qlen
- elif self.attn_type == "uni":
- # beg, end = klen - 1, -1
- beg, end = klen, -1
- else:
- raise ValueError("Unknown `attn_type` {}.".format(self.attn_type))
-
- if self.bi_data:
- fwd_pos_seq = tf.range(beg, end, -1.0)
- bwd_pos_seq = tf.range(-beg, -end, 1.0)
-
- if dtype is not None and dtype != tf.float32:
- fwd_pos_seq = tf.cast(fwd_pos_seq, dtype=dtype)
- bwd_pos_seq = tf.cast(bwd_pos_seq, dtype=dtype)
-
- if self.clamp_len > 0:
- fwd_pos_seq = tf.clip_by_value(fwd_pos_seq, -self.clamp_len, self.clamp_len)
- bwd_pos_seq = tf.clip_by_value(bwd_pos_seq, -self.clamp_len, self.clamp_len)
-
- if bsz is not None:
- # With bi_data, the batch size should be divisible by 2.
- assert bsz % 2 == 0
- fwd_pos_emb = self.positional_embedding(fwd_pos_seq, inv_freq, bsz // 2)
- bwd_pos_emb = self.positional_embedding(bwd_pos_seq, inv_freq, bsz // 2)
- else:
- fwd_pos_emb = self.positional_embedding(fwd_pos_seq, inv_freq)
- bwd_pos_emb = self.positional_embedding(bwd_pos_seq, inv_freq)
-
- pos_emb = tf.concat([fwd_pos_emb, bwd_pos_emb], axis=1)
- else:
- fwd_pos_seq = tf.range(beg, end, -1.0)
- if dtype is not None and dtype != tf.float32:
- fwd_pos_seq = tf.cast(fwd_pos_seq, dtype=dtype)
- if self.clamp_len > 0:
- fwd_pos_seq = tf.clip_by_value(fwd_pos_seq, -self.clamp_len, self.clamp_len)
- pos_emb = self.positional_embedding(fwd_pos_seq, inv_freq, bsz)
-
- return pos_emb
-
- def call(
- self,
- inputs,
- attention_mask=None,
- mems=None,
- perm_mask=None,
- target_mapping=None,
- token_type_ids=None,
- input_mask=None,
- head_mask=None,
- inputs_embeds=None,
- training=False,
- ):
- if isinstance(inputs, (tuple, list)):
- input_ids = inputs[0]
- attention_mask = inputs[1] if len(inputs) > 1 else attention_mask
- mems = inputs[2] if len(inputs) > 2 else mems
- perm_mask = inputs[3] if len(inputs) > 3 else perm_mask
- target_mapping = inputs[4] if len(inputs) > 4 else target_mapping
- token_type_ids = inputs[5] if len(inputs) > 5 else token_type_ids
- input_mask = inputs[6] if len(inputs) > 6 else input_mask
- head_mask = inputs[7] if len(inputs) > 7 else head_mask
- inputs_embeds = inputs[8] if len(inputs) > 8 else inputs_embeds
- assert len(inputs) <= 9, "Too many inputs."
- elif isinstance(inputs, dict):
- input_ids = inputs.get("input_ids")
- attention_mask = inputs.get("attention_mask", attention_mask)
- mems = inputs.get("mems", mems)
- perm_mask = inputs.get("perm_mask", perm_mask)
- target_mapping = inputs.get("target_mapping", target_mapping)
- token_type_ids = inputs.get("token_type_ids", token_type_ids)
- input_mask = inputs.get("input_mask", input_mask)
- head_mask = inputs.get("head_mask", head_mask)
- inputs_embeds = inputs.get("inputs_embeds", inputs_embeds)
- assert len(inputs) <= 9, "Too many inputs."
- else:
- input_ids = inputs
-
- # the original code for XLNet uses shapes [len, bsz] with the batch dimension at the end
- # but we want a unified interface in the library with the batch size on the first dimension
- # so we move here the first dimension (batch) to the end
-
- if input_ids is not None and inputs_embeds is not None:
- raise ValueError("You cannot specify both input_ids and inputs_embeds at the same time")
- elif input_ids is not None:
- input_ids = tf.transpose(input_ids, perm=(1, 0))
- qlen, bsz = shape_list(input_ids)[:2]
- elif inputs_embeds is not None:
- inputs_embeds = tf.transpose(inputs_embeds, perm=(1, 0, 2))
- qlen, bsz = shape_list(inputs_embeds)[:2]
- else:
- raise ValueError("You have to specify either input_ids or inputs_embeds")
-
- token_type_ids = tf.transpose(token_type_ids, perm=(1, 0)) if token_type_ids is not None else None
- input_mask = tf.transpose(input_mask, perm=(1, 0)) if input_mask is not None else None
- attention_mask = tf.transpose(attention_mask, perm=(1, 0)) if attention_mask is not None else None
- perm_mask = tf.transpose(perm_mask, perm=(1, 2, 0)) if perm_mask is not None else None
- target_mapping = tf.transpose(target_mapping, perm=(1, 2, 0)) if target_mapping is not None else None
-
- mlen = shape_list(mems[0])[0] if mems is not None and mems[0] is not None else 0
- klen = mlen + qlen
-
- dtype_float = tf.bfloat16 if self.use_bfloat16 else tf.float32
-
- # Attention mask
- # causal attention mask
- if self.attn_type == "uni":
- attn_mask = self.create_mask(qlen, mlen)
- attn_mask = attn_mask[:, :, None, None]
- elif self.attn_type == "bi":
- attn_mask = None
- else:
- raise ValueError("Unsupported attention type: {}".format(self.attn_type))
-
- # data mask: input mask & perm mask
- assert input_mask is None or attention_mask is None, (
- "You can only use one of input_mask (uses 1 for padding) "
- "or attention_mask (uses 0 for padding, added for compatbility with BERT). Please choose one."
- )
- if input_mask is None and attention_mask is not None:
- input_mask = 1.0 - tf.cast(attention_mask, dtype=dtype_float)
- if input_mask is not None and perm_mask is not None:
- data_mask = input_mask[None] + perm_mask
- elif input_mask is not None and perm_mask is None:
- data_mask = input_mask[None]
- elif input_mask is None and perm_mask is not None:
- data_mask = perm_mask
- else:
- data_mask = None
-
- if data_mask is not None:
- # all mems can be attended to
- mems_mask = tf.zeros([shape_list(data_mask)[0], mlen, bsz], dtype=dtype_float)
- data_mask = tf.concat([mems_mask, data_mask], axis=1)
- if attn_mask is None:
- attn_mask = data_mask[:, :, :, None]
- else:
- attn_mask += data_mask[:, :, :, None]
-
- if attn_mask is not None:
- attn_mask = tf.cast(attn_mask > 0, dtype=dtype_float)
-
- if attn_mask is not None:
- non_tgt_mask = -tf.eye(qlen, dtype=dtype_float)
- non_tgt_mask = tf.concat([tf.zeros([qlen, mlen], dtype=dtype_float), non_tgt_mask], axis=-1)
- non_tgt_mask = tf.cast((attn_mask + non_tgt_mask[:, :, None, None]) > 0, dtype=dtype_float)
- else:
- non_tgt_mask = None
-
- # Word embeddings and prepare h & g hidden states
- if inputs_embeds is not None:
- word_emb_k = inputs_embeds
- else:
- word_emb_k = self.word_embedding(input_ids)
- output_h = self.dropout(word_emb_k, training=training)
- if target_mapping is not None:
- word_emb_q = tf.tile(self.mask_emb, [shape_list(target_mapping)[0], bsz, 1])
- # else: # We removed the inp_q input which was same as target mapping
- # inp_q_ext = inp_q[:, :, None]
- # word_emb_q = inp_q_ext * self.mask_emb + (1 - inp_q_ext) * word_emb_k
- output_g = self.dropout(word_emb_q, training=training)
- else:
- output_g = None
-
- # Segment embedding
- if token_type_ids is not None:
- # Convert `token_type_ids` to one-hot `seg_mat`
- mem_pad = tf.zeros([mlen, bsz], dtype=tf.int32)
- cat_ids = tf.concat([mem_pad, token_type_ids], 0)
-
- # `1` indicates not in the same segment [qlen x klen x bsz]
- seg_mat = tf.cast(tf.logical_not(tf.equal(token_type_ids[:, None], cat_ids[None, :])), tf.int32)
- seg_mat = tf.one_hot(seg_mat, 2, dtype=dtype_float)
- else:
- seg_mat = None
-
- # Positional encoding
- pos_emb = self.relative_positional_encoding(qlen, klen, bsz=bsz, dtype=dtype_float)
- pos_emb = self.dropout(pos_emb, training=training)
-
- # Prepare head mask if needed
- # 1.0 in head_mask indicate we keep the head
- # attention_probs has shape bsz x n_heads x N x N
- # input head_mask has shape [num_heads] or [num_hidden_layers x num_heads] (a head_mask for each layer)
- # and head_mask is converted to shape [num_hidden_layers x qlen x klen x bsz x n_head]
- if head_mask is not None:
- if head_mask.dim() == 1:
- head_mask = head_mask.unsqueeze(0).unsqueeze(0).unsqueeze(0).unsqueeze(0)
- head_mask = head_mask.expand(self.n_layer, -1, -1, -1, -1)
- elif head_mask.dim() == 2:
- head_mask = head_mask.unsqueeze(1).unsqueeze(1).unsqueeze(1)
- head_mask = head_mask.to(
- dtype=next(self.parameters()).dtype
- ) # switch to fload if need + fp16 compatibility
- else:
- head_mask = [None] * self.n_layer
-
- new_mems = ()
- if mems is None:
- mems = [None] * len(self.layer)
-
- attentions = []
- hidden_states = []
- for i, layer_module in enumerate(self.layer):
- # cache new mems
- if self.mem_len is not None and self.mem_len > 0 and self.output_past:
- new_mems = new_mems + (self.cache_mem(output_h, mems[i]),)
- if self.output_hidden_states:
- hidden_states.append((output_h, output_g) if output_g is not None else output_h)
-
- outputs = layer_module(
- [output_h, output_g, non_tgt_mask, attn_mask, pos_emb, seg_mat, mems[i], target_mapping, head_mask[i]],
- training=training,
- )
- output_h, output_g = outputs[:2]
- if self.output_attentions:
- attentions.append(outputs[2])
-
- # Add last hidden state
- if self.output_hidden_states:
- hidden_states.append((output_h, output_g) if output_g is not None else output_h)
-
- output = self.dropout(output_g if output_g is not None else output_h, training=training)
-
- # Prepare outputs, we transpose back here to shape [bsz, len, hidden_dim] (cf. beginning of forward() method)
- outputs = (tf.transpose(output, perm=(1, 0, 2)),)
-
- if self.mem_len is not None and self.mem_len > 0 and self.output_past:
- outputs = outputs + (new_mems,)
-
- if self.output_hidden_states:
- if output_g is not None:
- hidden_states = tuple(tf.transpose(h, perm=(1, 0, 2)) for hs in hidden_states for h in hs)
- else:
- hidden_states = tuple(tf.transpose(hs, perm=(1, 0, 2)) for hs in hidden_states)
- outputs = outputs + (hidden_states,)
- if self.output_attentions:
- attentions = tuple(tf.transpose(t, perm=(2, 3, 0, 1)) for t in attentions)
- outputs = outputs + (attentions,)
-
- return outputs # outputs, (new_mems), (hidden_states), (attentions)
-
-
-class TFXLNetPreTrainedModel(TFPreTrainedModel):
- """ An abstract class to handle weights initialization and
- a simple interface for downloading and loading pretrained models.
- """
-
- config_class = XLNetConfig
- pretrained_model_archive_map = TF_XLNET_PRETRAINED_MODEL_ARCHIVE_MAP
- base_model_prefix = "transformer"
-
-
-XLNET_START_DOCSTRING = r"""
-
- .. note::
-
- TF 2.0 models accepts two formats as inputs:
-
- - having all inputs as keyword arguments (like PyTorch models), or
- - having all inputs as a list, tuple or dict in the first positional arguments.
-
- This second option is useful when using :obj:`tf.keras.Model.fit()` method which currently requires having
- all the tensors in the first argument of the model call function: :obj:`model(inputs)`.
-
- If you choose this second option, there are three possibilities you can use to gather all the input Tensors
- in the first positional argument :
-
- - a single Tensor with input_ids only and nothing else: :obj:`model(inputs_ids)`
- - a list of varying length with one or several input Tensors IN THE ORDER given in the docstring:
- :obj:`model([input_ids, attention_mask])` or :obj:`model([input_ids, attention_mask, token_type_ids])`
- - a dictionary with one or several input Tensors associated to the input names given in the docstring:
- :obj:`model({'input_ids': input_ids, 'token_type_ids': token_type_ids})`
-
- Parameters:
- config (:class:`~transformers.XLNetConfig`): Model configuration class with all the parameters of the model.
- Initializing with a config file does not load the weights associated with the model, only the configuration.
- Check out the :meth:`~transformers.PreTrainedModel.from_pretrained` method to load the model weights.
-"""
-
-XLNET_INPUTS_DOCSTRING = r"""
- Args:
- input_ids (:obj:`tf.Tensor` or :obj:`Numpy array` of shape :obj:`(batch_size, sequence_length)`):
- Indices of input sequence tokens in the vocabulary.
-
- Indices can be obtained using :class:`transformers.XLNetTokenizer`.
- See :func:`transformers.PreTrainedTokenizer.encode` and
- :func:`transformers.PreTrainedTokenizer.encode_plus` for details.
-
- `What are input IDs? <../glossary.html#input-ids>`__
- attention_mask (:obj:`tf.Tensor` or :obj:`Numpy array` of shape :obj:`(batch_size, sequence_length)`, `optional`, defaults to :obj:`None`):
- Mask to avoid performing attention on padding token indices.
- Mask values selected in ``[0, 1]``:
- ``1`` for tokens that are NOT MASKED, ``0`` for MASKED tokens.
-
- `What are attention masks? <../glossary.html#attention-mask>`__
- mems (:obj:`List[tf.Tensor]` of length :obj:`config.n_layers`):
- Contains pre-computed hidden-states (key and values in the attention blocks) as computed by the model
- (see `mems` output below). Can be used to speed up sequential decoding. The token ids which have their mems
- given to this model should not be passed as input ids as they have already been computed.
- perm_mask (:obj:`tf.Tensor` or :obj:`Numpy array` of shape :obj:`(batch_size, sequence_length, sequence_length)`, `optional`, defaults to :obj:`None`):
- Mask to indicate the attention pattern for each input token with values selected in ``[0, 1]``:
- If ``perm_mask[k, i, j] = 0``, i attend to j in batch k;
- if ``perm_mask[k, i, j] = 1``, i does not attend to j in batch k.
- If None, each token attends to all the others (full bidirectional attention).
- Only used during pretraining (to define factorization order) or for sequential decoding (generation).
- target_mapping (:obj:`tf.Tensor` or :obj:`Numpy array` of shape :obj:`(batch_size, num_predict, sequence_length)`, `optional`, defaults to :obj:`None`):
- Mask to indicate the output tokens to use.
- If ``target_mapping[k, i, j] = 1``, the i-th predict in batch k is on the j-th token.
- Only used during pretraining for partial prediction or for sequential decoding (generation).
- token_type_ids (:obj:`tf.Tensor` or :obj:`Numpy array` of shape :obj:`(batch_size, sequence_length)`, `optional`, defaults to :obj:`None`):
- Segment token indices to indicate first and second portions of the inputs.
- Indices are selected in ``[0, 1]``: ``0`` corresponds to a `sentence A` token, ``1``
- corresponds to a `sentence B` token
-
- `What are token type IDs? <../glossary.html#token-type-ids>`_
- input_mask (:obj:`tf.Tensor` or :obj:`Numpy array` of shape :obj:`(batch_size, sequence_length)`, `optional`, defaults to :obj:`None`):
- Mask to avoid performing attention on padding token indices.
- Negative of `attention_mask`, i.e. with 0 for real tokens and 1 for padding.
- Kept for compatibility with the original code base.
- You can only uses one of `input_mask` and `attention_mask`
- Mask values selected in ``[0, 1]``:
- ``1`` for tokens that are MASKED, ``0`` for tokens that are NOT MASKED.
- head_mask (:obj:`tf.Tensor` or :obj:`Numpy array` of shape :obj:`(num_heads,)` or :obj:`(num_layers, num_heads)`, `optional`, defaults to :obj:`None`):
- Mask to nullify selected heads of the self-attention modules.
- Mask values selected in ``[0, 1]``:
- :obj:`1` indicates the head is **not masked**, :obj:`0` indicates the head is **masked**.
- input_embeds (:obj:`tf.Tensor` or :obj:`Numpy array` of shape :obj:`(batch_size, sequence_length, hidden_size)`, `optional`, defaults to :obj:`None`):
- Optionally, instead of passing :obj:`input_ids` you can choose to directly pass an embedded representation.
- This is useful if you want more control over how to convert `input_ids` indices into associated vectors
- than the model's internal embedding lookup matrix.
-"""
-
-
-@add_start_docstrings(
- "The bare XLNet Model transformer outputing raw hidden-states without any specific head on top.",
- XLNET_START_DOCSTRING,
-)
-class TFXLNetModel(TFXLNetPreTrainedModel):
- def __init__(self, config, *inputs, **kwargs):
- super().__init__(config, *inputs, **kwargs)
- self.transformer = TFXLNetMainLayer(config, name="transformer")
-
- @add_start_docstrings_to_callable(XLNET_INPUTS_DOCSTRING)
- def call(self, inputs, **kwargs):
- r"""
- Return:
- :obj:`tuple(tf.Tensor)` comprising various elements depending on the configuration (:class:`~transformers.XLNetConfig`) and inputs:
- last_hidden_state (:obj:`tf.Tensor` or :obj:`Numpy array` of shape :obj:`(batch_size, sequence_length, hidden_size)`):
- Sequence of hidden-states at the last layer of the model.
- mems (:obj:`List[tf.Tensor]` of length :obj:`config.n_layers`):
- Contains pre-computed hidden-states (key and values in the attention blocks).
- Can be used (see `mems` input) to speed up sequential decoding. The token ids which have their past given to this model
- should not be passed as input ids as they have already been computed.
- hidden_states (:obj:`tuple(tf.Tensor)`, `optional`, returned when ``config.output_hidden_states=True``):
- Tuple of :obj:`tf.Tensor` or :obj:`Numpy array` (one for the output of the embeddings + one for the output of each layer)
- of shape :obj:`(batch_size, sequence_length, hidden_size)`.
-
- Hidden-states of the model at the output of each layer plus the initial embedding outputs.
- attentions (:obj:`tuple(tf.Tensor)`, `optional`, returned when ``config.output_attentions=True``):
- Tuple of :obj:`tf.Tensor` or :obj:`Numpy array` (one for each layer) of shape
- :obj:`(batch_size, num_heads, sequence_length, sequence_length)`.
-
- Attentions weights after the attention softmax, used to compute the weighted average in the self-attention
- heads.
-
- Examples::
-
- import tensorflow as tf
- from transformers import XLNetTokenizer, TFXLNetModel
-
- tokenizer = XLNetTokenizer.from_pretrained('xlnet-large-cased')
- model = TFXLNetModel.from_pretrained('xlnet-large-cased')
- input_ids = tf.constant(tokenizer.encode("Hello, my dog is cute", add_special_tokens=True))[None, :] # Batch size 1
- outputs = model(input_ids)
- last_hidden_states = outputs[0] # The last hidden-state is the first element of the output tuple
-
- """
- outputs = self.transformer(inputs, **kwargs)
- return outputs
-
-
-@add_start_docstrings(
- """XLNet Model with a language modeling head on top
- (linear layer with weights tied to the input embeddings). """,
- XLNET_START_DOCSTRING,
-)
-class TFXLNetLMHeadModel(TFXLNetPreTrainedModel):
- def __init__(self, config, *inputs, **kwargs):
- super().__init__(config, *inputs, **kwargs)
- self.transformer = TFXLNetMainLayer(config, name="transformer")
- self.lm_loss = TFXLNetLMHead(config, self.transformer.word_embedding, name="lm_loss")
-
- def get_output_embeddings(self):
- return self.lm_loss.input_embeddings
-
- @add_start_docstrings_to_callable(XLNET_INPUTS_DOCSTRING)
- def call(self, inputs, **kwargs):
- r"""
- Return:
- :obj:`tuple(tf.Tensor)` comprising various elements depending on the configuration (:class:`~transformers.XLNetConfig`) and inputs:
- prediction_scores (:obj:`tf.Tensor` or :obj:`Numpy array` of shape :obj:`(batch_size, sequence_length, config.vocab_size)`):
- Prediction scores of the language modeling head (scores for each vocabulary token before SoftMax).
- mems (:obj:`List[tf.Tensor]` of length :obj:`config.n_layers`):
- Contains pre-computed hidden-states (key and values in the attention blocks).
- Can be used (see `past` input) to speed up sequential decoding. The token ids which have their past given to this model
- should not be passed as input ids as they have already been computed.
- hidden_states (:obj:`tuple(tf.Tensor)`, `optional`, returned when ``config.output_hidden_states=True``):
- Tuple of :obj:`tf.Tensor` or :obj:`Numpy array` (one for the output of the embeddings + one for the output of each layer)
- of shape :obj:`(batch_size, sequence_length, hidden_size)`.
-
- Hidden-states of the model at the output of each layer plus the initial embedding outputs.
- attentions (:obj:`tuple(tf.Tensor)`, `optional`, returned when ``config.output_attentions=True``):
- Tuple of :obj:`tf.Tensor` or :obj:`Numpy array` (one for each layer) of shape
- :obj:`(batch_size, num_heads, sequence_length, sequence_length)`.
-
- Attentions weights after the attention softmax, used to compute the weighted average in the self-attention
- heads.
-
- Examples::
-
- import tensorflow as tf
- import numpy as np
- from transformers import XLNetTokenizer, TFXLNetLMHeadModel
-
- tokenizer = XLNetTokenizer.from_pretrained('xlnet-large-cased')
- model = TFXLNetLMHeadModel.from_pretrained('xlnet-large-cased')
-
- # We show how to setup inputs to predict a next token using a bi-directional context.
- input_ids = tf.constant(tokenizer.encode("Hello, my dog is very ", add_special_tokens=True))[None, :] # We will predict the masked token
- perm_mask = np.zeros((1, input_ids.shape[1], input_ids.shape[1]))
- perm_mask[:, :, -1] = 1.0 # Previous tokens don't see last token
- target_mapping = np.zeros((1, 1, input_ids.shape[1])) # Shape [1, 1, seq_length] => let's predict one token
- target_mapping[0, 0, -1] = 1.0 # Our first (and only) prediction will be the last token of the sequence (the masked token)
- outputs = model(input_ids, perm_mask=tf.constant(perm_mask, dtype=tf.float32), target_mapping=tf.constant(target_mapping, dtype=tf.float32))
-
- next_token_logits = outputs[0] # Output has shape [target_mapping.size(0), target_mapping.size(1), config.vocab_size]
-
- """
- transformer_outputs = self.transformer(inputs, **kwargs)
- hidden_state = transformer_outputs[0]
- logits = self.lm_loss(hidden_state)
-
- outputs = (logits,) + transformer_outputs[1:] # Keep mems, hidden states, attentions if there are in it
-
- return outputs # return logits, (mems), (hidden states), (attentions)
-
-
-@add_start_docstrings(
- """XLNet Model with a sequence classification/regression head on top (a linear layer on top of
- the pooled output) e.g. for GLUE tasks. """,
- XLNET_START_DOCSTRING,
-)
-class TFXLNetForSequenceClassification(TFXLNetPreTrainedModel):
- def __init__(self, config, *inputs, **kwargs):
- super().__init__(config, *inputs, **kwargs)
- self.num_labels = config.num_labels
-
- self.transformer = TFXLNetMainLayer(config, name="transformer")
- self.sequence_summary = TFSequenceSummary(
- config, initializer_range=config.initializer_range, name="sequence_summary"
- )
- self.logits_proj = tf.keras.layers.Dense(
- config.num_labels, kernel_initializer=get_initializer(config.initializer_range), name="logits_proj"
- )
-
- @add_start_docstrings_to_callable(XLNET_INPUTS_DOCSTRING)
- def call(self, inputs, **kwargs):
- r"""
- Return:
- :obj:`tuple(tf.Tensor)` comprising various elements depending on the configuration (:class:`~transformers.XLNetConfig`) and inputs:
- logits (:obj:`tf.Tensor` or :obj:`Numpy array` of shape :obj:(batch_size, config.num_labels)`):
- Classification (or regression if config.num_labels==1) scores (before SoftMax).
- mems (:obj:`List[tf.Tensor]` of length :obj:`config.n_layers`):
- Contains pre-computed hidden-states (key and values in the attention blocks).
- Can be used (see `past` input) to speed up sequential decoding. The token ids which have their past given to this model
- should not be passed as input ids as they have already been computed.
- hidden_states (:obj:`tuple(tf.Tensor)`, `optional`, returned when ``config.output_hidden_states=True``):
- Tuple of :obj:`tf.Tensor` or :obj:`Numpy array` (one for the output of the embeddings + one for the output of each layer)
- of shape :obj:`(batch_size, sequence_length, hidden_size)`.
-
- Hidden-states of the model at the output of each layer plus the initial embedding outputs.
- attentions (:obj:`tuple(tf.Tensor)`, `optional`, returned when ``config.output_attentions=True``):
- Tuple of :obj:`tf.Tensor` or :obj:`Numpy array` (one for each layer) of shape
- :obj:`(batch_size, num_heads, sequence_length, sequence_length)`.
-
- Attentions weights after the attention softmax, used to compute the weighted average in the self-attention
- heads.
-
- Examples::
-
- import tensorflow as tf
- from transformers import XLNetTokenizer, TFXLNetForSequenceClassification
-
- tokenizer = XLNetTokenizer.from_pretrained('xlnet-large-cased')
- model = TFXLNetForSequenceClassification.from_pretrained('xlnet-large-cased')
- input_ids = tf.constant(tokenizer.encode("Hello, my dog is cute", add_special_tokens=True))[None, :] # Batch size 1
- outputs = model(input_ids)
- logits = outputs[0]
-
- """
- transformer_outputs = self.transformer(inputs, **kwargs)
- output = transformer_outputs[0]
-
- output = self.sequence_summary(output)
- logits = self.logits_proj(output)
-
- outputs = (logits,) + transformer_outputs[1:] # Keep mems, hidden states, attentions if there are in it
-
- return outputs # return logits, (mems), (hidden states), (attentions)
-
-
-@add_start_docstrings(
- """XLNet Model with a token classification head on top (a linear layer on top of
- the hidden-states output) e.g. for Named-Entity-Recognition (NER) tasks. """,
- XLNET_START_DOCSTRING,
-)
-class TFXLNetForTokenClassification(TFXLNetPreTrainedModel):
- def __init__(self, config, *inputs, **kwargs):
- super().__init__(config, *inputs, **kwargs)
- self.num_labels = config.num_labels
-
- self.transformer = TFXLNetMainLayer(config, name="transformer")
- self.classifier = tf.keras.layers.Dense(
- config.num_labels, kernel_initializer=get_initializer(config.initializer_range), name="classifier"
- )
-
- def call(self, inputs, **kwargs):
- r"""
- Return:
- :obj:`tuple(tf.Tensor)` comprising various elements depending on the configuration (:class:`~transformers.XLNetConfig`) and inputs:
- logits (:obj:`tf.Tensor` or :obj:`Numpy array` of shape :obj:(batch_size, config.num_labels)`):
- Classification scores (before SoftMax).
- mems (:obj:`List[tf.Tensor]` of length :obj:`config.n_layers`):
- Contains pre-computed hidden-states (key and values in the attention blocks).
- Can be used (see `past` input) to speed up sequential decoding. The token ids which have their past given to this model
- should not be passed as input ids as they have already been computed.
- hidden_states (:obj:`tuple(tf.Tensor)`, `optional`, returned when ``config.output_hidden_states=True``):
- Tuple of :obj:`tf.Tensor` or :obj:`Numpy array` (one for the output of the embeddings + one for the output of each layer)
- of shape :obj:`(batch_size, sequence_length, hidden_size)`.
-
- Hidden-states of the model at the output of each layer plus the initial embedding outputs.
- attentions (:obj:`tuple(tf.Tensor)`, `optional`, returned when ``config.output_attentions=True``):
- Tuple of :obj:`tf.Tensor` or :obj:`Numpy array` (one for each layer) of shape
- :obj:`(batch_size, num_heads, sequence_length, sequence_length)`.
-
- Attentions weights after the attention softmax, used to compute the weighted average in the self-attention
- heads.
-
- Examples::
-
- import tensorflow as tf
- from transformers import XLNetTokenizer, TFXLNetForTokenClassification
-
- tokenizer = XLNetTokenizer.from_pretrained('xlnet-large-cased')
- model = TFXLNetForTokenClassification.from_pretrained('xlnet-large-cased')
- input_ids = tf.constant(tokenizer.encode("Hello, my dog is cute"))[None, :] # Batch size 1
- outputs = model(input_ids)
- scores = outputs[0]
-
- """
- transformer_outputs = self.transformer(inputs, **kwargs)
- output = transformer_outputs[0]
-
- logits = self.classifier(output)
-
- outputs = (logits,) + transformer_outputs[1:] # Keep mems, hidden states, attentions if there are in it
-
- return outputs # return logits, (mems), (hidden states), (attentions)
-
-
-@add_start_docstrings(
- """XLNet Model with a span classification head on top for extractive question-answering tasks like SQuAD (a linear layers on top of
- the hidden-states output to compute `span start logits` and `span end logits`). """,
- XLNET_START_DOCSTRING,
-)
-class TFXLNetForQuestionAnsweringSimple(TFXLNetPreTrainedModel):
- def __init__(self, config, *inputs, **kwargs):
- super().__init__(config, *inputs, **kwargs)
- self.transformer = TFXLNetMainLayer(config, name="transformer")
- self.qa_outputs = tf.keras.layers.Dense(
- config.num_labels, kernel_initializer=get_initializer(config.initializer_range), name="qa_outputs"
- )
-
- @add_start_docstrings_to_callable(XLNET_INPUTS_DOCSTRING)
- def call(self, inputs, **kwargs):
- r"""
- Returns:
- :obj:`tuple(tf.Tensor)` comprising various elements depending on the configuration (:class:`~transformers.XLNetConfig`) and inputs:
- loss (:obj:`tf.Tensor` or :obj:`Numpy array` of shape :obj:`(1,)`, `optional`, returned when :obj:`labels` is provided):
- Total span extraction loss is the sum of a Cross-Entropy for the start and end positions.
- start_scores (:obj:`tf.Tensor` or :obj:`Numpy array` of shape :obj:`(batch_size, sequence_length,)`):
- Span-start scores (before SoftMax).
- end_scores (:obj:`tf.Tensor` or :obj:`Numpy array` of shape :obj:`(batch_size, sequence_length,)`):
- Span-end scores (before SoftMax).
- mems (:obj:`List[tf.Tensor]` of length :obj:`config.n_layers`):
- Contains pre-computed hidden-states (key and values in the attention blocks).
- Can be used (see `past` input) to speed up sequential decoding. The token ids which have their past given to this model
- should not be passed as input ids as they have already been computed.
- hidden_states (:obj:`tuple(tf.Tensor)`, `optional`, returned when ``config.output_hidden_states=True``):
- Tuple of :obj:`tf.Tensor` or :obj:`Numpy array` (one for the output of the embeddings + one for the output of each layer)
- of shape :obj:`(batch_size, sequence_length, hidden_size)`.
-
- Hidden-states of the model at the output of each layer plus the initial embedding outputs.
- attentions (:obj:`tuple(tf.Tensor)`, `optional`, returned when ``config.output_attentions=True``):
- Tuple of :obj:`tf.Tensor` or :obj:`Numpy array` (one for each layer) of shape
- :obj:`(batch_size, num_heads, sequence_length, sequence_length)`.
-
- Attentions weights after the attention softmax, used to compute the weighted average in the self-attention
- heads.
-
- Examples::
-
- import tensorflow as tf
- from transformers import XLNetTokenizer, TFXLNetForQuestionAnsweringSimple
-
- tokenizer = XLNetTokenizer.from_pretrained('xlnet-base-cased')
- model = TFXLNetForQuestionAnsweringSimple.from_pretrained('xlnet-base-cased')
- input_ids = tf.constant(tokenizer.encode("Hello, my dog is cute", add_special_tokens=True))[None, :] # Batch size 1
- outputs = model(input_ids)
- start_scores, end_scores = outputs[:2]
-
- """
- transformer_outputs = self.transformer(inputs, **kwargs)
-
- sequence_output = transformer_outputs[0]
-
- logits = self.qa_outputs(sequence_output)
- start_logits, end_logits = tf.split(logits, 2, axis=-1)
- start_logits = tf.squeeze(start_logits, axis=-1)
- end_logits = tf.squeeze(end_logits, axis=-1)
-
- outputs = (start_logits, end_logits,) + transformer_outputs[
- 1:
- ] # Keep mems, hidden states, attentions if there are in it
-
- return outputs # start_logits, end_logits, (mems), (hidden_states), (attentions)
-
-
-# @add_start_docstrings("""XLNet Model with a span classification head on top for extractive question-answering tasks like SQuAD (a linear layers on top of
-# the hidden-states output to compute `span start logits` and `span end logits`). """,
-# XLNET_START_DOCSTRING, XLNET_INPUTS_DOCSTRING)
-# class TFXLNetForQuestionAnswering(TFXLNetPreTrainedModel):
-# r"""
-# Outputs: `Tuple` comprising various elements depending on the configuration (config) and inputs:
-# **start_top_log_probs**: (`optional`, returned if ``start_positions`` or ``end_positions`` is not provided)
-# ``tf.Tensor`` of shape ``(batch_size, config.start_n_top)``
-# Log probabilities for the top config.start_n_top start token possibilities (beam-search).
-# **start_top_index**: (`optional`, returned if ``start_positions`` or ``end_positions`` is not provided)
-# ``tf.Tensor`` of shape ``(batch_size, config.start_n_top)``
-# Indices for the top config.start_n_top start token possibilities (beam-search).
-# **end_top_log_probs**: (`optional`, returned if ``start_positions`` or ``end_positions`` is not provided)
-# ``tf.Tensor`` of shape ``(batch_size, config.start_n_top * config.end_n_top)``
-# Log probabilities for the top ``config.start_n_top * config.end_n_top`` end token possibilities (beam-search).
-# **end_top_index**: (`optional`, returned if ``start_positions`` or ``end_positions`` is not provided)
-# ``tf.Tensor`` of shape ``(batch_size, config.start_n_top * config.end_n_top)``
-# Indices for the top ``config.start_n_top * config.end_n_top`` end token possibilities (beam-search).
-# **cls_logits**: (`optional`, returned if ``start_positions`` or ``end_positions`` is not provided)
-# ``tf.Tensor`` of shape ``(batch_size,)``
-# Log probabilities for the ``is_impossible`` label of the answers.
-# **mems**:
-# list of ``tf.Tensor`` (one for each layer):
-# that contains pre-computed hidden-states (key and values in the attention blocks) as computed by the model
-# if config.mem_len > 0 else tuple of None. Can be used to speed up sequential decoding and attend to longer context.
-# See details in the docstring of the `mems` input above.
-# **hidden_states**: (`optional`, returned when ``config.output_hidden_states=True``)
-# list of ``tf.Tensor`` (one for the output of each layer + the output of the embeddings)
-# of shape ``(batch_size, sequence_length, hidden_size)``:
-# Hidden-states of the model at the output of each layer plus the initial embedding outputs.
-# **attentions**: (`optional`, returned when ``config.output_attentions=True``)
-# list of ``tf.Tensor`` (one for each layer) of shape ``(batch_size, num_heads, sequence_length, sequence_length)``:
-# Attentions weights after the attention softmax, used to compute the weighted average in the self-attention heads.
-
-# Examples::
-
-# # For example purposes. Not runnable.
-# tokenizer = XLMTokenizer.from_pretrained('xlm-mlm-en-2048')
-# model = XLMForQuestionAnswering.from_pretrained('xlnet-large-cased')
-# input_ids = tf.constant(tokenizer.encode("Hello, my dog is cute", add_special_tokens=True))[None, :] # Batch size 1
-# start_positions = tf.constant([1])
-# end_positions = tf.constant([3])
-# outputs = model(input_ids, start_positions=start_positions, end_positions=end_positions)
-# loss, start_scores, end_scores = outputs[:2]
-
-# """
-# def __init__(self, config, *inputs, **kwargs):
-# super().__init__(config, *inputs, **kwargs)
-# self.start_n_top = config.start_n_top
-# self.end_n_top = config.end_n_top
-
-# self.transformer = TFXLNetMainLayer(config, name='transformer')
-# self.start_logits = TFPoolerStartLogits(config, name='start_logits')
-# self.end_logits = TFPoolerEndLogits(config, name='end_logits')
-# self.answer_class = TFPoolerAnswerClass(config, name='answer_class')
-
-# def call(self, inputs, training=False):
-# transformer_outputs = self.transformer(inputs, training=training)
-# hidden_states = transformer_outputs[0]
-# start_logits = self.start_logits(hidden_states, p_mask=p_mask)
-
-# outputs = transformer_outputs[1:] # Keep mems, hidden states, attentions if there are in it
-
-# if start_positions is not None and end_positions is not None:
-# # If we are on multi-GPU, let's remove the dimension added by batch splitting
-# for x in (start_positions, end_positions, cls_index, is_impossible):
-# if x is not None and x.dim() > 1:
-# x.squeeze_(-1)
-
-# # during training, compute the end logits based on the ground truth of the start position
-# end_logits = self.end_logits(hidden_states, start_positions=start_positions, p_mask=p_mask)
-
-# loss_fct = CrossEntropyLoss()
-# start_loss = loss_fct(start_logits, start_positions)
-# end_loss = loss_fct(end_logits, end_positions)
-# total_loss = (start_loss + end_loss) / 2
-
-# if cls_index is not None and is_impossible is not None:
-# # Predict answerability from the representation of CLS and START
-# cls_logits = self.answer_class(hidden_states, start_positions=start_positions, cls_index=cls_index)
-# loss_fct_cls = nn.BCEWithLogitsLoss()
-# cls_loss = loss_fct_cls(cls_logits, is_impossible)
-
-# # note(zhiliny): by default multiply the loss by 0.5 so that the scale is comparable to start_loss and end_loss
-# total_loss += cls_loss * 0.5
-
-# outputs = (total_loss,) + outputs
-
-# else:
-# # during inference, compute the end logits based on beam search
-# bsz, slen, hsz = hidden_states.size()
-# start_log_probs = F.softmax(start_logits, dim=-1) # shape (bsz, slen)
-
-# start_top_log_probs, start_top_index = torch.topk(start_log_probs, self.start_n_top, dim=-1) # shape (bsz, start_n_top)
-# start_top_index_exp = start_top_index.unsqueeze(-1).expand(-1, -1, hsz) # shape (bsz, start_n_top, hsz)
-# start_states = torch.gather(hidden_states, -2, start_top_index_exp) # shape (bsz, start_n_top, hsz)
-# start_states = start_states.unsqueeze(1).expand(-1, slen, -1, -1) # shape (bsz, slen, start_n_top, hsz)
-
-# hidden_states_expanded = hidden_states.unsqueeze(2).expand_as(start_states) # shape (bsz, slen, start_n_top, hsz)
-# p_mask = p_mask.unsqueeze(-1) if p_mask is not None else None
-# end_logits = self.end_logits(hidden_states_expanded, start_states=start_states, p_mask=p_mask)
-# end_log_probs = F.softmax(end_logits, dim=1) # shape (bsz, slen, start_n_top)
-
-# end_top_log_probs, end_top_index = torch.topk(end_log_probs, self.end_n_top, dim=1) # shape (bsz, end_n_top, start_n_top)
-# end_top_log_probs = end_top_log_probs.view(-1, self.start_n_top * self.end_n_top)
-# end_top_index = end_top_index.view(-1, self.start_n_top * self.end_n_top)
-
-# start_states = torch.einsum("blh,bl->bh", hidden_states, start_log_probs) # get the representation of START as weighted sum of hidden states
-# cls_logits = self.answer_class(hidden_states, start_states=start_states, cls_index=cls_index) # Shape (batch size,): one single `cls_logits` for each sample
-
-# outputs = (start_top_log_probs, start_top_index, end_top_log_probs, end_top_index, cls_logits) + outputs
-
-# # return start_top_log_probs, start_top_index, end_top_log_probs, end_top_index, cls_logits
-# # or (if labels are provided) (total_loss,)
-# return outputs
diff --git a/server/transformers/src/transformers/modeling_transfo_xl.py b/server/transformers/src/transformers/modeling_transfo_xl.py
deleted file mode 100644
index 05bb5f7e3eb29c23d9fc1d03c352491177afa3e3..0000000000000000000000000000000000000000
--- a/server/transformers/src/transformers/modeling_transfo_xl.py
+++ /dev/null
@@ -1,945 +0,0 @@
-# coding=utf-8
-# Copyright 2018 Google AI, Google Brain and Carnegie Mellon University Authors and the HuggingFace Inc. team.
-# Copyright (c) 2018, NVIDIA CORPORATION. All rights reserved.
-#
-# Licensed under the Apache License, Version 2.0 (the "License");
-# you may not use this file except in compliance with the License.
-# You may obtain a copy of the License at
-#
-# http://www.apache.org/licenses/LICENSE-2.0
-#
-# Unless required by applicable law or agreed to in writing, software
-# distributed under the License is distributed on an "AS IS" BASIS,
-# WITHOUT WARRANTIES OR CONDITIONS OF ANY KIND, either express or implied.
-# See the License for the specific language governing permissions and
-# limitations under the License.
-""" PyTorch Transformer XL model.
- Adapted from https://github.com/kimiyoung/transformer-xl.
- In particular https://github.com/kimiyoung/transformer-xl/blob/master/pytorch/mem_transformer.py
-"""
-
-
-import logging
-
-import torch
-import torch.nn as nn
-import torch.nn.functional as F
-
-from .configuration_transfo_xl import TransfoXLConfig
-from .file_utils import add_start_docstrings, add_start_docstrings_to_callable
-from .modeling_transfo_xl_utilities import LogUniformSampler, ProjectedAdaptiveLogSoftmax, sample_logits
-from .modeling_utils import PreTrainedModel
-
-
-logger = logging.getLogger(__name__)
-
-TRANSFO_XL_PRETRAINED_MODEL_ARCHIVE_MAP = {
- "transfo-xl-wt103": "https://s3.amazonaws.com/models.huggingface.co/bert/transfo-xl-wt103-pytorch_model.bin",
-}
-
-
-def build_tf_to_pytorch_map(model, config):
- """ A map of modules from TF to PyTorch.
- This time I use a map to keep the PyTorch model as identical to the original PyTorch model as possible.
- """
- tf_to_pt_map = {}
-
- if hasattr(model, "transformer"):
- # We are loading in a TransfoXLLMHeadModel => we will load also the Adaptive Softmax
- tf_to_pt_map.update(
- {
- "transformer/adaptive_softmax/cutoff_0/cluster_W": model.crit.cluster_weight,
- "transformer/adaptive_softmax/cutoff_0/cluster_b": model.crit.cluster_bias,
- }
- )
- for i, (out_l, proj_l, tie_proj) in enumerate(
- zip(model.crit.out_layers, model.crit.out_projs, config.tie_projs)
- ):
- layer_str = "transformer/adaptive_softmax/cutoff_%d/" % i
- if config.tie_weight:
- tf_to_pt_map.update({layer_str + "b": out_l.bias})
- else:
- raise NotImplementedError
- # I don't think this is implemented in the TF code
- tf_to_pt_map.update({layer_str + "lookup_table": out_l.weight, layer_str + "b": out_l.bias})
- if not tie_proj:
- tf_to_pt_map.update({layer_str + "proj": proj_l})
- # Now load the rest of the transformer
- model = model.transformer
-
- # Embeddings
- for i, (embed_l, proj_l) in enumerate(zip(model.word_emb.emb_layers, model.word_emb.emb_projs)):
- layer_str = "transformer/adaptive_embed/cutoff_%d/" % i
- tf_to_pt_map.update({layer_str + "lookup_table": embed_l.weight, layer_str + "proj_W": proj_l})
-
- # Transformer blocks
- for i, b in enumerate(model.layers):
- layer_str = "transformer/layer_%d/" % i
- tf_to_pt_map.update(
- {
- layer_str + "rel_attn/LayerNorm/gamma": b.dec_attn.layer_norm.weight,
- layer_str + "rel_attn/LayerNorm/beta": b.dec_attn.layer_norm.bias,
- layer_str + "rel_attn/o/kernel": b.dec_attn.o_net.weight,
- layer_str + "rel_attn/qkv/kernel": b.dec_attn.qkv_net.weight,
- layer_str + "rel_attn/r/kernel": b.dec_attn.r_net.weight,
- layer_str + "ff/LayerNorm/gamma": b.pos_ff.layer_norm.weight,
- layer_str + "ff/LayerNorm/beta": b.pos_ff.layer_norm.bias,
- layer_str + "ff/layer_1/kernel": b.pos_ff.CoreNet[0].weight,
- layer_str + "ff/layer_1/bias": b.pos_ff.CoreNet[0].bias,
- layer_str + "ff/layer_2/kernel": b.pos_ff.CoreNet[3].weight,
- layer_str + "ff/layer_2/bias": b.pos_ff.CoreNet[3].bias,
- }
- )
-
- # Relative positioning biases
- if config.untie_r:
- r_r_list = []
- r_w_list = []
- for b in model.layers:
- r_r_list.append(b.dec_attn.r_r_bias)
- r_w_list.append(b.dec_attn.r_w_bias)
- else:
- r_r_list = [model.r_r_bias]
- r_w_list = [model.r_w_bias]
- tf_to_pt_map.update({"transformer/r_r_bias": r_r_list, "transformer/r_w_bias": r_w_list})
- return tf_to_pt_map
-
-
-def load_tf_weights_in_transfo_xl(model, config, tf_path):
- """ Load tf checkpoints in a pytorch model
- """
- try:
- import numpy as np
- import tensorflow as tf
- except ImportError:
- logger.error(
- "Loading a TensorFlow models in PyTorch, requires TensorFlow to be installed. Please see "
- "https://www.tensorflow.org/install/ for installation instructions."
- )
- raise
- # Build TF to PyTorch weights loading map
- tf_to_pt_map = build_tf_to_pytorch_map(model, config)
-
- # Load weights from TF model
- init_vars = tf.train.list_variables(tf_path)
- tf_weights = {}
- for name, shape in init_vars:
- logger.info("Loading TF weight {} with shape {}".format(name, shape))
- array = tf.train.load_variable(tf_path, name)
- tf_weights[name] = array
-
- for name, pointer in tf_to_pt_map.items():
- assert name in tf_weights
- array = tf_weights[name]
- # adam_v and adam_m are variables used in AdamWeightDecayOptimizer to calculated m and v
- # which are not required for using pretrained model
- if "kernel" in name or "proj" in name:
- array = np.transpose(array)
- if ("r_r_bias" in name or "r_w_bias" in name) and len(pointer) > 1:
- # Here we will split the TF weigths
- assert len(pointer) == array.shape[0]
- for i, p_i in enumerate(pointer):
- arr_i = array[i, ...]
- try:
- assert p_i.shape == arr_i.shape
- except AssertionError as e:
- e.args += (p_i.shape, arr_i.shape)
- raise
- logger.info("Initialize PyTorch weight {} for layer {}".format(name, i))
- p_i.data = torch.from_numpy(arr_i)
- else:
- try:
- assert pointer.shape == array.shape
- except AssertionError as e:
- e.args += (pointer.shape, array.shape)
- raise
- logger.info("Initialize PyTorch weight {}".format(name))
- pointer.data = torch.from_numpy(array)
- tf_weights.pop(name, None)
- tf_weights.pop(name + "/Adam", None)
- tf_weights.pop(name + "/Adam_1", None)
-
- logger.info("Weights not copied to PyTorch model: {}".format(", ".join(tf_weights.keys())))
- return model
-
-
-class PositionalEmbedding(nn.Module):
- def __init__(self, demb):
- super().__init__()
-
- self.demb = demb
-
- inv_freq = 1 / (10000 ** (torch.arange(0.0, demb, 2.0) / demb))
- self.register_buffer("inv_freq", inv_freq)
-
- def forward(self, pos_seq, bsz=None):
- sinusoid_inp = torch.ger(pos_seq, self.inv_freq)
- pos_emb = torch.cat([sinusoid_inp.sin(), sinusoid_inp.cos()], dim=-1)
-
- if bsz is not None:
- return pos_emb[:, None, :].expand(-1, bsz, -1)
- else:
- return pos_emb[:, None, :]
-
-
-class PositionwiseFF(nn.Module):
- def __init__(self, d_model, d_inner, dropout, pre_lnorm=False, layer_norm_epsilon=1e-5):
- super().__init__()
-
- self.d_model = d_model
- self.d_inner = d_inner
- self.dropout = dropout
-
- self.CoreNet = nn.Sequential(
- nn.Linear(d_model, d_inner),
- nn.ReLU(inplace=True),
- nn.Dropout(dropout),
- nn.Linear(d_inner, d_model),
- nn.Dropout(dropout),
- )
-
- self.layer_norm = nn.LayerNorm(d_model, eps=layer_norm_epsilon)
-
- self.pre_lnorm = pre_lnorm
-
- def forward(self, inp):
- if self.pre_lnorm:
- # layer normalization + positionwise feed-forward
- core_out = self.CoreNet(self.layer_norm(inp))
-
- # residual connection
- output = core_out + inp
- else:
- # positionwise feed-forward
- core_out = self.CoreNet(inp)
-
- # residual connection + layer normalization
- output = self.layer_norm(inp + core_out)
-
- return output
-
-
-class RelPartialLearnableMultiHeadAttn(nn.Module):
- def __init__(
- self,
- n_head,
- d_model,
- d_head,
- dropout,
- dropatt=0,
- tgt_len=None,
- ext_len=None,
- mem_len=None,
- pre_lnorm=False,
- r_r_bias=None,
- r_w_bias=None,
- output_attentions=False,
- layer_norm_epsilon=1e-5,
- ):
- super().__init__()
-
- self.output_attentions = output_attentions
- self.n_head = n_head
- self.d_model = d_model
- self.d_head = d_head
- self.dropout = dropout
-
- self.qkv_net = nn.Linear(d_model, 3 * n_head * d_head, bias=False)
-
- self.drop = nn.Dropout(dropout)
- self.dropatt = nn.Dropout(dropatt)
- self.o_net = nn.Linear(n_head * d_head, d_model, bias=False)
-
- self.layer_norm = nn.LayerNorm(d_model, eps=layer_norm_epsilon)
-
- self.scale = 1 / (d_head ** 0.5)
-
- self.pre_lnorm = pre_lnorm
-
- if r_r_bias is None or r_w_bias is None: # Biases are not shared
- self.r_r_bias = nn.Parameter(torch.FloatTensor(self.n_head, self.d_head))
- self.r_w_bias = nn.Parameter(torch.FloatTensor(self.n_head, self.d_head))
- else:
- self.r_r_bias = r_r_bias
- self.r_w_bias = r_w_bias
-
- self.r_net = nn.Linear(self.d_model, self.n_head * self.d_head, bias=False)
-
- def _rel_shift(self, x):
- zero_pad_shape = (x.size(0), 1) + x.size()[2:]
- zero_pad = torch.zeros(zero_pad_shape, device=x.device, dtype=x.dtype)
- x_padded = torch.cat([zero_pad, x], dim=1)
-
- x_padded_shape = (x.size(1) + 1, x.size(0)) + x.size()[2:]
- x_padded = x_padded.view(*x_padded_shape)
-
- x = x_padded[1:].view_as(x)
-
- return x
-
- def forward(self, w, r, attn_mask=None, mems=None, head_mask=None):
- qlen, rlen, bsz = w.size(0), r.size(0), w.size(1)
-
- if mems is not None:
- cat = torch.cat([mems, w], 0)
- if self.pre_lnorm:
- w_heads = self.qkv_net(self.layer_norm(cat))
- else:
- w_heads = self.qkv_net(cat)
- r_head_k = self.r_net(r)
-
- w_head_q, w_head_k, w_head_v = torch.chunk(w_heads, 3, dim=-1)
- w_head_q = w_head_q[-qlen:]
- else:
- if self.pre_lnorm:
- w_heads = self.qkv_net(self.layer_norm(w))
- else:
- w_heads = self.qkv_net(w)
- r_head_k = self.r_net(r)
-
- w_head_q, w_head_k, w_head_v = torch.chunk(w_heads, 3, dim=-1)
-
- klen = w_head_k.size(0)
-
- w_head_q = w_head_q.view(qlen, bsz, self.n_head, self.d_head) # qlen x bsz x n_head x d_head
- w_head_k = w_head_k.view(klen, bsz, self.n_head, self.d_head) # qlen x bsz x n_head x d_head
- w_head_v = w_head_v.view(klen, bsz, self.n_head, self.d_head) # qlen x bsz x n_head x d_head
-
- r_head_k = r_head_k.view(rlen, self.n_head, self.d_head) # qlen x n_head x d_head
-
- # compute attention score
- rw_head_q = w_head_q + self.r_w_bias # qlen x bsz x n_head x d_head
- AC = torch.einsum("ibnd,jbnd->ijbn", (rw_head_q, w_head_k)) # qlen x klen x bsz x n_head
-
- rr_head_q = w_head_q + self.r_r_bias
- BD = torch.einsum("ibnd,jnd->ijbn", (rr_head_q, r_head_k)) # qlen x klen x bsz x n_head
- BD = self._rel_shift(BD)
-
- # [qlen x klen x bsz x n_head]
- attn_score = AC + BD
- attn_score.mul_(self.scale)
-
- # compute attention probability
- if attn_mask is not None and torch.sum(attn_mask).item():
- attn_mask = attn_mask == 1 # Switch to bool
- if attn_mask.dim() == 2:
- if next(self.parameters()).dtype == torch.float16:
- attn_score = (
- attn_score.float().masked_fill(attn_mask[None, :, :, None], -65000).type_as(attn_score)
- )
- else:
- attn_score = attn_score.float().masked_fill(attn_mask[None, :, :, None], -1e30).type_as(attn_score)
- elif attn_mask.dim() == 3:
- if next(self.parameters()).dtype == torch.float16:
- attn_score = attn_score.float().masked_fill(attn_mask[:, :, :, None], -65000).type_as(attn_score)
- else:
- attn_score = attn_score.float().masked_fill(attn_mask[:, :, :, None], -1e30).type_as(attn_score)
-
- # [qlen x klen x bsz x n_head]
- attn_prob = F.softmax(attn_score, dim=1)
- attn_prob = self.dropatt(attn_prob)
-
- # Mask heads if we want to
- if head_mask is not None:
- attn_prob = attn_prob * head_mask
-
- # compute attention vector
- attn_vec = torch.einsum("ijbn,jbnd->ibnd", (attn_prob, w_head_v))
-
- # [qlen x bsz x n_head x d_head]
- attn_vec = attn_vec.contiguous().view(attn_vec.size(0), attn_vec.size(1), self.n_head * self.d_head)
-
- # linear projection
- attn_out = self.o_net(attn_vec)
- attn_out = self.drop(attn_out)
-
- if self.pre_lnorm:
- # residual connection
- outputs = [w + attn_out]
- else:
- # residual connection + layer normalization
- outputs = [self.layer_norm(w + attn_out)]
-
- if self.output_attentions:
- outputs.append(attn_prob)
-
- return outputs
-
-
-class RelPartialLearnableDecoderLayer(nn.Module):
- def __init__(self, n_head, d_model, d_head, d_inner, dropout, layer_norm_epsilon=1e-5, **kwargs):
- super().__init__()
-
- self.dec_attn = RelPartialLearnableMultiHeadAttn(
- n_head, d_model, d_head, dropout, layer_norm_epsilon=layer_norm_epsilon, **kwargs
- )
- self.pos_ff = PositionwiseFF(
- d_model, d_inner, dropout, pre_lnorm=kwargs.get("pre_lnorm"), layer_norm_epsilon=layer_norm_epsilon
- )
-
- def forward(self, dec_inp, r, dec_attn_mask=None, mems=None, head_mask=None):
-
- attn_outputs = self.dec_attn(dec_inp, r, attn_mask=dec_attn_mask, mems=mems, head_mask=head_mask)
- ff_output = self.pos_ff(attn_outputs[0])
-
- outputs = [ff_output] + attn_outputs[1:]
-
- return outputs
-
-
-class AdaptiveEmbedding(nn.Module):
- def __init__(self, n_token, d_embed, d_proj, cutoffs, div_val=1, sample_softmax=False):
- super().__init__()
-
- self.n_token = n_token
- self.d_embed = d_embed
-
- self.cutoffs = cutoffs + [n_token]
- self.div_val = div_val
- self.d_proj = d_proj
-
- self.emb_scale = d_proj ** 0.5
-
- self.cutoff_ends = [0] + self.cutoffs
-
- self.emb_layers = nn.ModuleList()
- self.emb_projs = nn.ParameterList()
- if div_val == 1:
- self.emb_layers.append(nn.Embedding(n_token, d_embed, sparse=sample_softmax > 0))
- if d_proj != d_embed:
- self.emb_projs.append(nn.Parameter(torch.FloatTensor(d_proj, d_embed)))
- else:
- for i in range(len(self.cutoffs)):
- l_idx, r_idx = self.cutoff_ends[i], self.cutoff_ends[i + 1]
- d_emb_i = d_embed // (div_val ** i)
- self.emb_layers.append(nn.Embedding(r_idx - l_idx, d_emb_i))
- self.emb_projs.append(nn.Parameter(torch.FloatTensor(d_proj, d_emb_i)))
-
- def forward(self, inp):
- if self.div_val == 1:
- embed = self.emb_layers[0](inp)
- if self.d_proj != self.d_embed:
- embed = F.linear(embed, self.emb_projs[0])
- else:
- param = next(self.parameters())
- inp_flat = inp.view(-1)
- emb_flat = torch.zeros([inp_flat.size(0), self.d_proj], dtype=param.dtype, device=param.device)
- for i in range(len(self.cutoffs)):
- l_idx, r_idx = self.cutoff_ends[i], self.cutoff_ends[i + 1]
-
- mask_i = (inp_flat >= l_idx) & (inp_flat < r_idx)
- indices_i = mask_i.nonzero().squeeze()
-
- if indices_i.numel() == 0:
- continue
-
- inp_i = inp_flat.index_select(0, indices_i) - l_idx
- emb_i = self.emb_layers[i](inp_i)
- emb_i = F.linear(emb_i, self.emb_projs[i])
-
- emb_flat.index_copy_(0, indices_i, emb_i)
-
- embed_shape = inp.size() + (self.d_proj,)
- embed = emb_flat.view(embed_shape)
-
- embed.mul_(self.emb_scale)
-
- return embed
-
-
-class TransfoXLPreTrainedModel(PreTrainedModel):
- """ An abstract class to handle weights initialization and
- a simple interface for downloading and loading pretrained models.
- """
-
- config_class = TransfoXLConfig
- pretrained_model_archive_map = TRANSFO_XL_PRETRAINED_MODEL_ARCHIVE_MAP
- load_tf_weights = load_tf_weights_in_transfo_xl
- base_model_prefix = "transformer"
-
- def _init_weight(self, weight):
- if self.config.init == "uniform":
- nn.init.uniform_(weight, -self.config.init_range, self.config.init_range)
- elif self.config.init == "normal":
- nn.init.normal_(weight, 0.0, self.config.init_std)
-
- def _init_bias(self, bias):
- nn.init.constant_(bias, 0.0)
-
- def _init_weights(self, m):
- """ Initialize the weights.
- """
- classname = m.__class__.__name__
- if classname.find("Linear") != -1:
- if hasattr(m, "weight") and m.weight is not None:
- self._init_weight(m.weight)
- if hasattr(m, "bias") and m.bias is not None:
- self._init_bias(m.bias)
- elif classname.find("AdaptiveEmbedding") != -1:
- if hasattr(m, "emb_projs"):
- for i in range(len(m.emb_projs)):
- if m.emb_projs[i] is not None:
- nn.init.normal_(m.emb_projs[i], 0.0, self.config.proj_init_std)
- elif classname.find("Embedding") != -1:
- if hasattr(m, "weight"):
- self._init_weight(m.weight)
- elif classname.find("ProjectedAdaptiveLogSoftmax") != -1:
- if hasattr(m, "cluster_weight") and m.cluster_weight is not None:
- self._init_weight(m.cluster_weight)
- if hasattr(m, "cluster_bias") and m.cluster_bias is not None:
- self._init_bias(m.cluster_bias)
- if hasattr(m, "out_projs"):
- for i in range(len(m.out_projs)):
- if m.out_projs[i] is not None:
- nn.init.normal_(m.out_projs[i], 0.0, self.config.proj_init_std)
- elif classname.find("LayerNorm") != -1:
- if hasattr(m, "weight"):
- nn.init.normal_(m.weight, 1.0, self.config.init_std)
- if hasattr(m, "bias") and m.bias is not None:
- self._init_bias(m.bias)
- else:
- if hasattr(m, "r_emb"):
- self._init_weight(m.r_emb)
- if hasattr(m, "r_w_bias"):
- self._init_weight(m.r_w_bias)
- if hasattr(m, "r_r_bias"):
- self._init_weight(m.r_r_bias)
- if hasattr(m, "r_bias"):
- self._init_bias(m.r_bias)
-
-
-TRANSFO_XL_START_DOCSTRING = r"""
-
- This model is a PyTorch `torch.nn.Module `_ sub-class.
- Use it as a regular PyTorch Module and refer to the PyTorch documentation for all matter related to general
- usage and behavior.
-
- Parameters:
- config (:class:`~transformers.TransfoXLConfig`): Model configuration class with all the parameters of the model.
- Initializing with a config file does not load the weights associated with the model, only the configuration.
- Check out the :meth:`~transformers.PreTrainedModel.from_pretrained` method to load the model weights.
-"""
-
-TRANSFO_XL_INPUTS_DOCSTRING = r"""
- Args:
- input_ids (:obj:`torch.LongTensor` of shape :obj:`(batch_size, sequence_length)`):
- Indices of input sequence tokens in the vocabulary.
-
- Indices can be obtained using :class:`transformers.TransfoXLTokenizer`.
- See :func:`transformers.PreTrainedTokenizer.encode` and
- :func:`transformers.PreTrainedTokenizer.encode_plus` for details.
-
- `What are input IDs? <../glossary.html#input-ids>`__
- mems (:obj:`List[torch.FloatTensor]` of length :obj:`config.n_layers`):
- Contains pre-computed hidden-states (key and values in the attention blocks) as computed by the model
- (see `mems` output below). Can be used to speed up sequential decoding. The token ids which have their mems
- given to this model should not be passed as input ids as they have already been computed.
- head_mask (:obj:`torch.FloatTensor` of shape :obj:`(num_heads,)` or :obj:`(num_layers, num_heads)`, `optional`, defaults to :obj:`None`):
- Mask to nullify selected heads of the self-attention modules.
- Mask values selected in ``[0, 1]``:
- :obj:`1` indicates the head is **not masked**, :obj:`0` indicates the head is **masked**.
- input_embeds (:obj:`torch.FloatTensor` of shape :obj:`(batch_size, sequence_length, hidden_size)`, `optional`, defaults to :obj:`None`):
- Optionally, instead of passing :obj:`input_ids` you can choose to directly pass an embedded representation.
- This is useful if you want more control over how to convert `input_ids` indices into associated vectors
- than the model's internal embedding lookup matrix.
-"""
-
-
-@add_start_docstrings(
- "The bare Bert Model transformer outputting raw hidden-states without any specific head on top.",
- TRANSFO_XL_START_DOCSTRING,
-)
-class TransfoXLModel(TransfoXLPreTrainedModel):
- def __init__(self, config):
- super().__init__(config)
- self.output_attentions = config.output_attentions
- self.output_hidden_states = config.output_hidden_states
-
- self.n_token = config.vocab_size
-
- self.d_embed = config.d_embed
- self.d_model = config.d_model
- self.n_head = config.n_head
- self.d_head = config.d_head
-
- self.word_emb = AdaptiveEmbedding(
- config.vocab_size, config.d_embed, config.d_model, config.cutoffs, div_val=config.div_val
- )
-
- self.drop = nn.Dropout(config.dropout)
-
- self.n_layer = config.n_layer
-
- self.tgt_len = config.tgt_len
- self.mem_len = config.mem_len
- self.ext_len = config.ext_len
- self.max_klen = config.tgt_len + config.ext_len + config.mem_len
-
- self.attn_type = config.attn_type
-
- if not config.untie_r:
- self.r_w_bias = nn.Parameter(torch.FloatTensor(self.n_head, self.d_head))
- self.r_r_bias = nn.Parameter(torch.FloatTensor(self.n_head, self.d_head))
-
- self.layers = nn.ModuleList()
- if config.attn_type == 0: # the default attention
- for i in range(config.n_layer):
- self.layers.append(
- RelPartialLearnableDecoderLayer(
- config.n_head,
- config.d_model,
- config.d_head,
- config.d_inner,
- config.dropout,
- tgt_len=config.tgt_len,
- ext_len=config.ext_len,
- mem_len=config.mem_len,
- dropatt=config.dropatt,
- pre_lnorm=config.pre_lnorm,
- r_w_bias=None if config.untie_r else self.r_w_bias,
- r_r_bias=None if config.untie_r else self.r_r_bias,
- output_attentions=self.output_attentions,
- layer_norm_epsilon=config.layer_norm_epsilon,
- )
- )
- else: # learnable embeddings and absolute embeddings are not used in our pretrained checkpoints
- raise NotImplementedError # Removed them to avoid maintaining dead code
-
- self.same_length = config.same_length
- self.clamp_len = config.clamp_len
-
- if self.attn_type == 0: # default attention
- self.pos_emb = PositionalEmbedding(self.d_model)
- else: # learnable embeddings and absolute embeddings
- raise NotImplementedError # Removed these to avoid maintaining dead code - They are not used in our pretrained checkpoint
-
- self.init_weights()
-
- def get_input_embeddings(self):
- return self.word_emb
-
- def set_input_embeddings(self, new_embeddings):
- self.word_emb = new_embeddings
-
- def backward_compatible(self):
- self.sample_softmax = -1
-
- def reset_length(self, tgt_len, ext_len, mem_len):
- self.tgt_len = tgt_len
- self.mem_len = mem_len
- self.ext_len = ext_len
-
- def _prune_heads(self, heads):
- logger.info("Head pruning is not implemented for Transformer-XL model")
- pass
-
- def init_mems(self, bsz):
- if self.mem_len > 0:
- mems = []
- param = next(self.parameters())
- for i in range(self.n_layer):
- empty = torch.zeros(self.mem_len, bsz, self.config.d_model, dtype=param.dtype, device=param.device)
- mems.append(empty)
-
- return mems
- else:
- return None
-
- def _update_mems(self, hids, mems, qlen, mlen):
- # does not deal with None
- if mems is None:
- return None
-
- # mems is not None
- assert len(hids) == len(mems), "len(hids) != len(mems)"
-
- # There are `mlen + qlen` steps that can be cached into mems
- # For the next step, the last `ext_len` of the `qlen` tokens
- # will be used as the extended context. Hence, we only cache
- # the tokens from `mlen + qlen - self.ext_len - self.mem_len`
- # to `mlen + qlen - self.ext_len`.
- with torch.no_grad():
- new_mems = []
- end_idx = mlen + max(0, qlen - 0 - self.ext_len)
- beg_idx = max(0, end_idx - self.mem_len)
- for i in range(len(hids)):
-
- cat = torch.cat([mems[i], hids[i]], dim=0)
- new_mems.append(cat[beg_idx:end_idx].detach())
-
- return new_mems
-
- @add_start_docstrings_to_callable(TRANSFO_XL_INPUTS_DOCSTRING)
- def forward(self, input_ids=None, mems=None, head_mask=None, inputs_embeds=None):
- r"""
- Return:
- :obj:`tuple(torch.FloatTensor)` comprising various elements depending on the configuration (:class:`~transformers.TransfoXLConfig`) and inputs:
- last_hidden_state (:obj:`torch.FloatTensor` of shape :obj:`(batch_size, sequence_length, hidden_size)`):
- Sequence of hidden-states at the last layer of the model.
- mems (:obj:`List[torch.FloatTensor]` of length :obj:`config.n_layers`):
- Contains pre-computed hidden-states (key and values in the attention blocks).
- Can be used (see `mems` input) to speed up sequential decoding. The token ids which have their past given to this model
- should not be passed as input ids as they have already been computed.
- hidden_states (:obj:`tuple(torch.FloatTensor)`, `optional`, returned when ``config.output_hidden_states=True``):
- Tuple of :obj:`torch.FloatTensor` (one for the output of the embeddings + one for the output of each layer)
- of shape :obj:`(batch_size, sequence_length, hidden_size)`.
-
- Hidden-states of the model at the output of each layer plus the initial embedding outputs.
- attentions (:obj:`tuple(torch.FloatTensor)`, `optional`, returned when ``config.output_attentions=True``):
- Tuple of :obj:`torch.FloatTensor` (one for each layer) of shape
- :obj:`(batch_size, num_heads, sequence_length, sequence_length)`.
-
- Attentions weights after the attention softmax, used to compute the weighted average in the self-attention
- heads.
-
- Examples::
-
- from transformers import TransfoXLTokenizer, TransfoXLModel
- import torch
-
- tokenizer = TransfoXLTokenizer.from_pretrained('transfo-xl-wt103')
- model = TransfoXLModel.from_pretrained('transfo-xl-wt103')
- input_ids = torch.tensor(tokenizer.encode("Hello, my dog is cute", add_special_tokens=True)).unsqueeze(0) # Batch size 1
- outputs = model(input_ids)
- last_hidden_states, mems = outputs[:2]
-
- """
- # the original code for Transformer-XL used shapes [len, bsz] but we want a unified interface in the library
- # so we transpose here from shape [bsz, len] to shape [len, bsz]
- if input_ids is not None and inputs_embeds is not None:
- raise ValueError("You cannot specify both input_ids and inputs_embeds at the same time")
- elif input_ids is not None:
- input_ids = input_ids.transpose(0, 1).contiguous()
- qlen, bsz = input_ids.size()
- elif inputs_embeds is not None:
- inputs_embeds = inputs_embeds.transpose(0, 1).contiguous()
- qlen, bsz = inputs_embeds.shape[0], inputs_embeds.shape[1]
- else:
- raise ValueError("You have to specify either input_ids or inputs_embeds")
-
- if mems is None:
- mems = self.init_mems(bsz)
-
- # Prepare head mask if needed
- # 1.0 in head_mask indicate we keep the head
- # attention_probs has shape bsz x n_heads x N x N
- # input head_mask has shape [num_heads] or [num_hidden_layers x num_heads] (a head_mask for each layer)
- # and head_mask is converted to shape [num_hidden_layers x qlen x klen x bsz x n_head]
- if head_mask is not None:
- if head_mask.dim() == 1:
- head_mask = head_mask.unsqueeze(0).unsqueeze(0).unsqueeze(0).unsqueeze(0)
- head_mask = head_mask.expand(self.n_layer, -1, -1, -1, -1)
- elif head_mask.dim() == 2:
- head_mask = head_mask.unsqueeze(1).unsqueeze(1).unsqueeze(1)
- head_mask = head_mask.to(
- dtype=next(self.parameters()).dtype
- ) # switch to fload if need + fp16 compatibility
- else:
- head_mask = [None] * self.n_layer
-
- if inputs_embeds is not None:
- word_emb = inputs_embeds
- else:
- word_emb = self.word_emb(input_ids)
-
- mlen = mems[0].size(0) if mems is not None else 0
- klen = mlen + qlen
- if self.same_length:
- all_ones = word_emb.new_ones((qlen, klen), dtype=torch.uint8)
- mask_len = klen - self.mem_len
- if mask_len > 0:
- mask_shift_len = qlen - mask_len
- else:
- mask_shift_len = qlen
- dec_attn_mask = (torch.triu(all_ones, 1 + mlen) + torch.tril(all_ones, -mask_shift_len))[:, :, None] # -1
- else:
- dec_attn_mask = torch.triu(word_emb.new_ones((qlen, klen), dtype=torch.uint8), diagonal=1 + mlen)[
- :, :, None
- ]
-
- hids = []
- attentions = []
- if self.attn_type == 0: # default
- pos_seq = torch.arange(klen - 1, -1, -1.0, device=word_emb.device, dtype=word_emb.dtype)
- if self.clamp_len > 0:
- pos_seq.clamp_(max=self.clamp_len)
- pos_emb = self.pos_emb(pos_seq)
-
- core_out = self.drop(word_emb)
- pos_emb = self.drop(pos_emb)
-
- for i, layer in enumerate(self.layers):
- hids.append(core_out)
- mems_i = None if mems is None else mems[i]
- layer_outputs = layer(
- core_out, pos_emb, dec_attn_mask=dec_attn_mask, mems=mems_i, head_mask=head_mask[i]
- )
- core_out = layer_outputs[0]
- if self.output_attentions:
- attentions.append(layer_outputs[1])
- else: # learnable embeddings and absolute embeddings
- raise NotImplementedError # Removed these to avoid maintaining dead code - They are not used in our pretrained checkpoint
-
- core_out = self.drop(core_out)
-
- new_mems = self._update_mems(hids, mems, mlen, qlen)
-
- # We transpose back here to shape [bsz, len, hidden_dim]
- outputs = [core_out.transpose(0, 1).contiguous(), new_mems]
- if self.output_hidden_states:
- # Add last layer and transpose to library standard shape [bsz, len, hidden_dim]
- hids.append(core_out)
- hids = list(t.transpose(0, 1).contiguous() for t in hids)
- outputs.append(hids)
- if self.output_attentions:
- # Transpose to library standard shape [bsz, n_heads, query_seq_len, key_seq_len]
- attentions = list(t.permute(2, 3, 0, 1).contiguous() for t in attentions)
- outputs.append(attentions)
-
- return outputs # last hidden state, new_mems, (all hidden states), (all attentions)
-
-
-@add_start_docstrings(
- """The Transformer-XL Model with a language modeling head on top
- (adaptive softmax with weights tied to the adaptive input embeddings)""",
- TRANSFO_XL_START_DOCSTRING,
-)
-class TransfoXLLMHeadModel(TransfoXLPreTrainedModel):
- def __init__(self, config):
- super().__init__(config)
- self.transformer = TransfoXLModel(config)
- self.sample_softmax = config.sample_softmax
- # use sampled softmax
- if config.sample_softmax > 0:
- self.out_layer = nn.Linear(config.d_model, config.vocab_size)
- self.sampler = LogUniformSampler(config.vocab_size, config.sample_softmax)
- # use adaptive softmax (including standard softmax)
- else:
- self.crit = ProjectedAdaptiveLogSoftmax(
- config.vocab_size, config.d_embed, config.d_model, config.cutoffs, div_val=config.div_val
- )
- self.init_weights()
-
- def tie_weights(self):
- """
- Run this to be sure output and input (adaptive) softmax weights are tied
- """
- # sampled softmax
- if self.sample_softmax > 0:
- if self.config.tie_weight:
- self.out_layer.weight = self.transformer.word_emb.weight
- # adaptive softmax (including standard softmax)
- else:
- if self.config.tie_weight:
- for i in range(len(self.crit.out_layers)):
- self._tie_or_clone_weights(self.crit.out_layers[i], self.transformer.word_emb.emb_layers[i])
- if self.config.tie_projs:
- for i, tie_proj in enumerate(self.config.tie_projs):
- if tie_proj and self.config.div_val == 1 and self.config.d_model != self.config.d_embed:
- if self.config.torchscript:
- self.crit.out_projs[i] = nn.Parameter(self.transformer.word_emb.emb_projs[0].clone())
- else:
- self.crit.out_projs[i] = self.transformer.word_emb.emb_projs[0]
- elif tie_proj and self.config.div_val != 1:
- if self.config.torchscript:
- self.crit.out_projs[i] = nn.Parameter(self.transformer.word_emb.emb_projs[i].clone())
- else:
- self.crit.out_projs[i] = self.transformer.word_emb.emb_projs[i]
-
- def reset_length(self, tgt_len, ext_len, mem_len):
- self.transformer.reset_length(tgt_len, ext_len, mem_len)
-
- def init_mems(self, bsz):
- return self.transformer.init_mems(bsz)
-
- @add_start_docstrings_to_callable(TRANSFO_XL_INPUTS_DOCSTRING)
- def forward(self, input_ids=None, mems=None, head_mask=None, inputs_embeds=None, labels=None):
- r"""
- labels (:obj:`torch.LongTensor` of shape :obj:`(batch_size, sequence_length)`, `optional`, defaults to :obj:`None`):
- Labels for language modeling.
- Note that the labels **are shifted** inside the model, i.e. you can set ``lm_labels = input_ids``
- Indices are selected in ``[-100, 0, ..., config.vocab_size]``
- All labels set to ``-100`` are ignored (masked), the loss is only
- computed for labels in ``[0, ..., config.vocab_size]``
-
- Return:
- :obj:`tuple(torch.FloatTensor)` comprising various elements depending on the configuration (:class:`~transformers.TransfoXLConfig`) and inputs:
- loss (:obj:`torch.FloatTensor` of shape `(1,)`, `optional`, returned when ``labels`` is provided)
- Language modeling loss.
- prediction_scores (:obj:`torch.FloatTensor` of shape :obj:`(batch_size, sequence_length, config.vocab_size)`):
- Prediction scores of the language modeling head (scores for each vocabulary token before SoftMax).
- mems (:obj:`List[torch.FloatTensor]` of length :obj:`config.n_layers`):
- Contains pre-computed hidden-states (key and values in the attention blocks).
- Can be used (see `past` input) to speed up sequential decoding. The token ids which have their past given to this model
- should not be passed as input ids as they have already been computed.
- hidden_states (:obj:`tuple(torch.FloatTensor)`, `optional`, returned when ``config.output_hidden_states=True``):
- Tuple of :obj:`torch.FloatTensor` (one for the output of the embeddings + one for the output of each layer)
- of shape :obj:`(batch_size, sequence_length, hidden_size)`.
-
- Hidden-states of the model at the output of each layer plus the initial embedding outputs.
- attentions (:obj:`tuple(torch.FloatTensor)`, `optional`, returned when ``config.output_attentions=True``):
- Tuple of :obj:`torch.FloatTensor` (one for each layer) of shape
- :obj:`(batch_size, num_heads, sequence_length, sequence_length)`.
-
- Attentions weights after the attention softmax, used to compute the weighted average in the self-attention
- heads.
-
- Examples::
-
- from transformers import TransfoXLTokenizer, TransfoXLLMHeadModel
- import torch
-
- tokenizer = TransfoXLTokenizer.from_pretrained('transfo-xl-wt103')
- model = TransfoXLLMHeadModel.from_pretrained('transfo-xl-wt103')
- input_ids = torch.tensor(tokenizer.encode("Hello, my dog is cute", add_special_tokens=True)).unsqueeze(0) # Batch size 1
- outputs = model(input_ids)
- prediction_scores, mems = outputs[:2]
-
- """
- if input_ids is not None:
- bsz, tgt_len = input_ids.size(0), input_ids.size(1)
- elif inputs_embeds is not None:
- bsz, tgt_len = inputs_embeds.size(0), inputs_embeds.size(1)
- else:
- raise ValueError("You have to specify either input_ids or inputs_embeds")
-
- transformer_outputs = self.transformer(input_ids, mems=mems, head_mask=head_mask, inputs_embeds=inputs_embeds)
-
- last_hidden = transformer_outputs[0]
- pred_hid = last_hidden[:, -tgt_len:]
- outputs = transformer_outputs[1:]
- if self.sample_softmax > 0 and self.training:
- assert self.config.tie_weight
- logit = sample_logits(self.transformer.word_emb, self.out_layer.bias, labels, pred_hid, self.sampler)
- softmax_output = -F.log_softmax(logit, -1)[:, :, 0]
- outputs = [softmax_output] + outputs
- if labels is not None:
- # TODO: This is not implemented
- raise NotImplementedError
- else:
- softmax_output = self.crit(pred_hid.view(-1, pred_hid.size(-1)), labels)
- if labels is None:
- softmax_output = softmax_output.view(bsz, tgt_len, -1)
- outputs = [softmax_output] + outputs
- else:
- softmax_output = softmax_output.view(bsz, tgt_len)
- outputs = [softmax_output, None] + outputs
-
- return outputs # (loss), logits or None if labels is not None (speed up adaptive softmax), new_mems, (all hidden states), (all attentions)
-
- def get_output_embeddings(self):
- """ Double-check if you are using adaptive softmax.
- """
- if self.sample_softmax > 0:
- return self.out_layer
- else:
- return self.crit.out_layers[-1]
-
- def prepare_inputs_for_generation(self, input_ids, **model_kwargs):
- inputs = {"input_ids": input_ids}
-
- # if past is defined in model kwargs then use it for faster decoding
- if "past" in model_kwargs and model_kwargs["past"]:
- inputs["mems"] = model_kwargs["past"]
-
- return inputs
diff --git a/server/transformers/src/transformers/modeling_transfo_xl_utilities.py b/server/transformers/src/transformers/modeling_transfo_xl_utilities.py
deleted file mode 100644
index ef12316673bdb437ea9ac5062a5c48a99748ee11..0000000000000000000000000000000000000000
--- a/server/transformers/src/transformers/modeling_transfo_xl_utilities.py
+++ /dev/null
@@ -1,317 +0,0 @@
-# coding=utf-8
-# Copyright 2018 Google AI, Google Brain and Carnegie Mellon University Authors and the HuggingFace Inc. team.
-# Copyright (c) 2018, NVIDIA CORPORATION. All rights reserved.
-#
-# Licensed under the Apache License, Version 2.0 (the "License");
-# you may not use this file except in compliance with the License.
-# You may obtain a copy of the License at
-#
-# http://www.apache.org/licenses/LICENSE-2.0
-#
-# Unless required by applicable law or agreed to in writing, software
-# distributed under the License is distributed on an "AS IS" BASIS,
-# WITHOUT WARRANTIES OR CONDITIONS OF ANY KIND, either express or implied.
-# See the License for the specific language governing permissions and
-# limitations under the License.
-""" Utilities for PyTorch Transformer XL model.
- Directly adapted from https://github.com/kimiyoung/transformer-xl.
-"""
-
-
-import torch
-import torch.nn as nn
-import torch.nn.functional as F
-
-
-# CUDA_MAJOR = int(torch.version.cuda.split('.')[0])
-# CUDA_MINOR = int(torch.version.cuda.split('.')[1])
-
-
-class ProjectedAdaptiveLogSoftmax(nn.Module):
- def __init__(self, n_token, d_embed, d_proj, cutoffs, div_val=1, keep_order=False):
- super().__init__()
-
- self.n_token = n_token
- self.d_embed = d_embed
- self.d_proj = d_proj
-
- self.cutoffs = cutoffs + [n_token]
- self.cutoff_ends = [0] + self.cutoffs
- self.div_val = div_val
-
- self.shortlist_size = self.cutoffs[0]
- self.n_clusters = len(self.cutoffs) - 1
- self.head_size = self.shortlist_size + self.n_clusters
-
- if self.n_clusters > 0:
- self.cluster_weight = nn.Parameter(torch.zeros(self.n_clusters, self.d_embed))
- self.cluster_bias = nn.Parameter(torch.zeros(self.n_clusters))
-
- self.out_layers = nn.ModuleList()
- self.out_projs = nn.ParameterList()
-
- if div_val == 1:
- for i in range(len(self.cutoffs)):
- if d_proj != d_embed:
- self.out_projs.append(nn.Parameter(torch.FloatTensor(d_proj, d_embed)))
- else:
- self.out_projs.append(None)
-
- self.out_layers.append(nn.Linear(d_embed, n_token))
- else:
- for i in range(len(self.cutoffs)):
- l_idx, r_idx = self.cutoff_ends[i], self.cutoff_ends[i + 1]
- d_emb_i = d_embed // (div_val ** i)
-
- self.out_projs.append(nn.Parameter(torch.FloatTensor(d_proj, d_emb_i)))
-
- self.out_layers.append(nn.Linear(d_emb_i, r_idx - l_idx))
-
- self.keep_order = keep_order
-
- def _compute_logit(self, hidden, weight, bias, proj):
- if proj is None:
- logit = F.linear(hidden, weight, bias=bias)
- else:
- # if CUDA_MAJOR <= 9 and CUDA_MINOR <= 1:
- proj_hid = F.linear(hidden, proj.t().contiguous())
- logit = F.linear(proj_hid, weight, bias=bias)
- # else:
- # logit = torch.einsum('bd,de,ev->bv', (hidden, proj, weight.t()))
- # if bias is not None:
- # logit = logit + bias
-
- return logit
-
- def forward(self, hidden, labels=None, keep_order=False):
- """
- Params:
- hidden :: [len*bsz x d_proj]
- labels :: [len*bsz]
- Return:
- if labels is None:
- out :: [len*bsz] Negative log likelihood
- else:
- out :: [len*bsz x n_tokens] log probabilities of tokens over the vocabulary
- We could replace this implementation by the native PyTorch one
- if their's had an option to set bias on all clusters in the native one.
- here: https://github.com/pytorch/pytorch/blob/dbe6a7a9ff1a364a8706bf5df58a1ca96d2fd9da/torch/nn/modules/adaptive.py#L138
- """
-
- if labels is not None:
- labels = labels.view(-1)
- if hidden.size(0) != labels.size(0):
- raise RuntimeError("Input and labels should have the same size " "in the batch dimension.")
-
- if self.n_clusters == 0:
- logit = self._compute_logit(hidden, self.out_layers[0].weight, self.out_layers[0].bias, self.out_projs[0])
- if labels is not None:
- out = -F.log_softmax(logit, dim=-1).gather(1, labels.unsqueeze(1)).squeeze(1)
- else:
- out = F.log_softmax(logit, dim=-1)
- else:
- # construct weights and biases
- weights, biases = [], []
- for i in range(len(self.cutoffs)):
- if self.div_val == 1:
- l_idx, r_idx = self.cutoff_ends[i], self.cutoff_ends[i + 1]
- weight_i = self.out_layers[0].weight[l_idx:r_idx]
- bias_i = self.out_layers[0].bias[l_idx:r_idx]
- else:
- weight_i = self.out_layers[i].weight
- bias_i = self.out_layers[i].bias
-
- if i == 0:
- weight_i = torch.cat([weight_i, self.cluster_weight], dim=0)
- bias_i = torch.cat([bias_i, self.cluster_bias], dim=0)
-
- weights.append(weight_i)
- biases.append(bias_i)
-
- head_weight, head_bias, head_proj = weights[0], biases[0], self.out_projs[0]
-
- head_logit = self._compute_logit(hidden, head_weight, head_bias, head_proj)
- head_logprob = F.log_softmax(head_logit, dim=1)
-
- if labels is None:
- out = hidden.new_empty((head_logit.size(0), self.n_token))
- else:
- out = torch.zeros_like(labels, dtype=hidden.dtype, device=hidden.device)
-
- offset = 0
- cutoff_values = [0] + self.cutoffs
- for i in range(len(cutoff_values) - 1):
- l_idx, r_idx = cutoff_values[i], cutoff_values[i + 1]
-
- if labels is not None:
- mask_i = (labels >= l_idx) & (labels < r_idx)
- indices_i = mask_i.nonzero().squeeze()
-
- if indices_i.numel() == 0:
- continue
-
- target_i = labels.index_select(0, indices_i) - l_idx
- head_logprob_i = head_logprob.index_select(0, indices_i)
- hidden_i = hidden.index_select(0, indices_i)
- else:
- hidden_i = hidden
-
- if i == 0:
- if labels is not None:
- logprob_i = head_logprob_i.gather(1, target_i[:, None]).squeeze(1)
- else:
- out[:, : self.cutoffs[0]] = head_logprob[:, : self.cutoffs[0]]
- else:
- weight_i, bias_i, proj_i = weights[i], biases[i], self.out_projs[i]
-
- tail_logit_i = self._compute_logit(hidden_i, weight_i, bias_i, proj_i)
- tail_logprob_i = F.log_softmax(tail_logit_i, dim=1)
- cluster_prob_idx = self.cutoffs[0] + i - 1 # No probability for the head cluster
- if labels is not None:
- logprob_i = head_logprob_i[:, cluster_prob_idx] + tail_logprob_i.gather(
- 1, target_i[:, None]
- ).squeeze(1)
- else:
- logprob_i = head_logprob[:, cluster_prob_idx, None] + tail_logprob_i
- out[:, l_idx:r_idx] = logprob_i
-
- if labels is not None:
- if (hasattr(self, "keep_order") and self.keep_order) or keep_order:
- out.index_copy_(0, indices_i, -logprob_i)
- else:
- out[offset : offset + logprob_i.size(0)].copy_(-logprob_i)
- offset += logprob_i.size(0)
-
- return out
-
- def log_prob(self, hidden):
- r""" Computes log probabilities for all :math:`n\_classes`
- From: https://github.com/pytorch/pytorch/blob/master/torch/nn/modules/adaptive.py
- Args:
- hidden (Tensor): a minibatch of examples
- Returns:
- log-probabilities of for each class :math:`c`
- in range :math:`0 <= c <= n\_classes`, where :math:`n\_classes` is a
- parameter passed to ``AdaptiveLogSoftmaxWithLoss`` constructor.
- Shape:
- - Input: :math:`(N, in\_features)`
- - Output: :math:`(N, n\_classes)`
- """
- if self.n_clusters == 0:
- logit = self._compute_logit(hidden, self.out_layers[0].weight, self.out_layers[0].bias, self.out_projs[0])
- return F.log_softmax(logit, dim=-1)
- else:
- # construct weights and biases
- weights, biases = [], []
- for i in range(len(self.cutoffs)):
- if self.div_val == 1:
- l_idx, r_idx = self.cutoff_ends[i], self.cutoff_ends[i + 1]
- weight_i = self.out_layers[0].weight[l_idx:r_idx]
- bias_i = self.out_layers[0].bias[l_idx:r_idx]
- else:
- weight_i = self.out_layers[i].weight
- bias_i = self.out_layers[i].bias
-
- if i == 0:
- weight_i = torch.cat([weight_i, self.cluster_weight], dim=0)
- bias_i = torch.cat([bias_i, self.cluster_bias], dim=0)
-
- weights.append(weight_i)
- biases.append(bias_i)
-
- head_weight, head_bias, head_proj = weights[0], biases[0], self.out_projs[0]
- head_logit = self._compute_logit(hidden, head_weight, head_bias, head_proj)
-
- out = hidden.new_empty((head_logit.size(0), self.n_token))
- head_logprob = F.log_softmax(head_logit, dim=1)
-
- cutoff_values = [0] + self.cutoffs
- for i in range(len(cutoff_values) - 1):
- start_idx, stop_idx = cutoff_values[i], cutoff_values[i + 1]
-
- if i == 0:
- out[:, : self.cutoffs[0]] = head_logprob[:, : self.cutoffs[0]]
- else:
- weight_i, bias_i, proj_i = weights[i], biases[i], self.out_projs[i]
-
- tail_logit_i = self._compute_logit(hidden, weight_i, bias_i, proj_i)
- tail_logprob_i = F.log_softmax(tail_logit_i, dim=1)
-
- logprob_i = head_logprob[:, -i] + tail_logprob_i
- out[:, start_idx, stop_idx] = logprob_i
-
- return out
-
-
-class LogUniformSampler(object):
- def __init__(self, range_max, n_sample):
- """
- Reference : https://github.com/tensorflow/tensorflow/blob/r1.10/tensorflow/python/ops/candidate_sampling_ops.py
- `P(class) = (log(class + 2) - log(class + 1)) / log(range_max + 1)`
-
- expected count can be approximated by 1 - (1 - p)^n
- and we use a numerically stable version -expm1(num_tries * log1p(-p))
-
- Our implementation fixes num_tries at 2 * n_sample, and the actual #samples will vary from run to run
- """
- with torch.no_grad():
- self.range_max = range_max
- log_indices = torch.arange(1.0, range_max + 2.0, 1.0).log_()
- self.dist = (log_indices[1:] - log_indices[:-1]) / log_indices[-1]
-
- self.log_q = (-(-self.dist.double().log1p_() * 2 * n_sample).expm1_()).log_().float()
-
- self.n_sample = n_sample
-
- def sample(self, labels):
- """
- labels: [b1, b2]
- Return
- true_log_probs: [b1, b2]
- samp_log_probs: [n_sample]
- neg_samples: [n_sample]
- """
-
- # neg_samples = torch.empty(0).long()
- n_sample = self.n_sample
- n_tries = 2 * n_sample
-
- with torch.no_grad():
- neg_samples = torch.multinomial(self.dist, n_tries, replacement=True).unique()
- device = labels.device
- neg_samples = neg_samples.to(device)
- true_log_probs = self.log_q[labels].to(device)
- samp_log_probs = self.log_q[neg_samples].to(device)
- return true_log_probs, samp_log_probs, neg_samples
-
-
-def sample_logits(embedding, bias, labels, inputs, sampler):
- """
- embedding: an nn.Embedding layer
- bias: [n_vocab]
- labels: [b1, b2]
- inputs: [b1, b2, n_emb]
- sampler: you may use a LogUniformSampler
- Return
- logits: [b1, b2, 1 + n_sample]
- """
- true_log_probs, samp_log_probs, neg_samples = sampler.sample(labels)
- n_sample = neg_samples.size(0)
- b1, b2 = labels.size(0), labels.size(1)
- all_ids = torch.cat([labels.view(-1), neg_samples])
- all_w = embedding(all_ids)
- true_w = all_w[:-n_sample].view(b1, b2, -1)
- sample_w = all_w[-n_sample:].view(n_sample, -1)
-
- all_b = bias[all_ids]
- true_b = all_b[:-n_sample].view(b1, b2)
- sample_b = all_b[-n_sample:]
-
- hit = (labels[:, :, None] == neg_samples).detach()
-
- true_logits = torch.einsum("ijk,ijk->ij", [true_w, inputs]) + true_b - true_log_probs
- sample_logits = torch.einsum("lk,ijk->ijl", [sample_w, inputs]) + sample_b - samp_log_probs
- sample_logits.masked_fill_(hit, -1e30)
- logits = torch.cat([true_logits[:, :, None], sample_logits], -1)
-
- return logits
diff --git a/server/transformers/src/transformers/modeling_utils.py b/server/transformers/src/transformers/modeling_utils.py
deleted file mode 100644
index 7edfa7f0b3b82959b9205e2b6cd6b9274a380512..0000000000000000000000000000000000000000
--- a/server/transformers/src/transformers/modeling_utils.py
+++ /dev/null
@@ -1,1517 +0,0 @@
-# coding=utf-8
-# Copyright 2018 The Google AI Language Team Authors, Facebook AI Research authors and The HuggingFace Inc. team.
-# Copyright (c) 2018, NVIDIA CORPORATION. All rights reserved.
-#
-# Licensed under the Apache License, Version 2.0 (the "License");
-# you may not use this file except in compliance with the License.
-# You may obtain a copy of the License at
-#
-# http://www.apache.org/licenses/LICENSE-2.0
-#
-# Unless required by applicable law or agreed to in writing, software
-# distributed under the License is distributed on an "AS IS" BASIS,
-# WITHOUT WARRANTIES OR CONDITIONS OF ANY KIND, either express or implied.
-# See the License for the specific language governing permissions and
-# limitations under the License.
-"""PyTorch BERT model."""
-
-
-import logging
-import os
-from itertools import zip_longest
-
-import torch
-from torch import nn
-from torch.nn import CrossEntropyLoss
-from torch.nn import functional as F
-
-from .configuration_utils import PretrainedConfig
-from .file_utils import (
- DUMMY_INPUTS,
- TF2_WEIGHTS_NAME,
- TF_WEIGHTS_NAME,
- WEIGHTS_NAME,
- cached_path,
- hf_bucket_url,
- is_remote_url,
-)
-
-
-logger = logging.getLogger(__name__)
-
-try:
- from torch.nn import Identity
-except ImportError:
- # Older PyTorch compatibility
- class Identity(nn.Module):
- r"""A placeholder identity operator that is argument-insensitive.
- """
-
- def __init__(self, *args, **kwargs):
- super().__init__()
-
- def forward(self, input):
- return input
-
-
-class ModuleUtilsMixin:
- """
- A few utilities for torch.nn.Modules, to be used as a mixin.
- """
-
- def num_parameters(self, only_trainable: bool = False) -> int:
- """
- Get number of (optionally, trainable) parameters in the module.
- """
- params = filter(lambda x: x.requires_grad, self.parameters()) if only_trainable else self.parameters()
- return sum(p.numel() for p in params)
-
-
-class PreTrainedModel(nn.Module, ModuleUtilsMixin):
- r""" Base class for all models.
-
- :class:`~transformers.PreTrainedModel` takes care of storing the configuration of the models and handles methods for loading/downloading/saving models
- as well as a few methods common to all models to (i) resize the input embeddings and (ii) prune heads in the self-attention heads.
-
- Class attributes (overridden by derived classes):
- - ``config_class``: a class derived from :class:`~transformers.PretrainedConfig` to use as configuration class for this model architecture.
- - ``pretrained_model_archive_map``: a python ``dict`` of with `short-cut-names` (string) as keys and `url` (string) of associated pretrained weights as values.
- - ``load_tf_weights``: a python ``method`` for loading a TensorFlow checkpoint in a PyTorch model, taking as arguments:
-
- - ``model``: an instance of the relevant subclass of :class:`~transformers.PreTrainedModel`,
- - ``config``: an instance of the relevant subclass of :class:`~transformers.PretrainedConfig`,
- - ``path``: a path (string) to the TensorFlow checkpoint.
-
- - ``base_model_prefix``: a string indicating the attribute associated to the base model in derived classes of the same architecture adding modules on top of the base model.
- """
- config_class = None
- pretrained_model_archive_map = {}
- base_model_prefix = ""
-
- @property
- def dummy_inputs(self):
- """ Dummy inputs to do a forward pass in the network.
-
- Returns:
- torch.Tensor with dummy inputs
- """
- return {"input_ids": torch.tensor(DUMMY_INPUTS)}
-
- def __init__(self, config, *inputs, **kwargs):
- super().__init__()
- if not isinstance(config, PretrainedConfig):
- raise ValueError(
- "Parameter config in `{}(config)` should be an instance of class `PretrainedConfig`. "
- "To create a model from a pretrained model use "
- "`model = {}.from_pretrained(PRETRAINED_MODEL_NAME)`".format(
- self.__class__.__name__, self.__class__.__name__
- )
- )
- # Save config in model
- self.config = config
-
- @property
- def base_model(self):
- return getattr(self, self.base_model_prefix, self)
-
- def get_input_embeddings(self):
- """
- Returns the model's input embeddings.
-
- Returns:
- :obj:`nn.Module`:
- A torch module mapping vocabulary to hidden states.
- """
- base_model = getattr(self, self.base_model_prefix, self)
- if base_model is not self:
- return base_model.get_input_embeddings()
- else:
- raise NotImplementedError
-
- def set_input_embeddings(self, value):
- """
- Set model's input embeddings
-
- Args:
- value (:obj:`nn.Module`):
- A module mapping vocabulary to hidden states.
- """
- base_model = getattr(self, self.base_model_prefix, self)
- if base_model is not self:
- base_model.set_input_embeddings(value)
- else:
- raise NotImplementedError
-
- def get_output_embeddings(self):
- """
- Returns the model's output embeddings.
-
- Returns:
- :obj:`nn.Module`:
- A torch module mapping hidden states to vocabulary.
- """
- return None # Overwrite for models with output embeddings
-
- def tie_weights(self):
- """
- Tie the weights between the input embeddings and the output embeddings.
- If the `torchscript` flag is set in the configuration, can't handle parameter sharing so we are cloning
- the weights instead.
- """
- output_embeddings = self.get_output_embeddings()
- if output_embeddings is not None:
- self._tie_or_clone_weights(output_embeddings, self.get_input_embeddings())
-
- def _tie_or_clone_weights(self, output_embeddings, input_embeddings):
- """ Tie or clone module weights depending of weither we are using TorchScript or not
- """
- if self.config.torchscript:
- output_embeddings.weight = nn.Parameter(input_embeddings.weight.clone())
- else:
- output_embeddings.weight = input_embeddings.weight
-
- if hasattr(output_embeddings, "bias") and output_embeddings.bias is not None:
- output_embeddings.bias.data = torch.nn.functional.pad(
- output_embeddings.bias.data,
- (0, output_embeddings.weight.shape[0] - output_embeddings.bias.shape[0]),
- "constant",
- 0,
- )
- if hasattr(output_embeddings, "out_features") and hasattr(input_embeddings, "num_embeddings"):
- output_embeddings.out_features = input_embeddings.num_embeddings
-
- def resize_token_embeddings(self, new_num_tokens=None):
- """ Resize input token embeddings matrix of the model if new_num_tokens != config.vocab_size.
- Take care of tying weights embeddings afterwards if the model class has a `tie_weights()` method.
-
- Arguments:
-
- new_num_tokens: (`optional`) int:
- New number of tokens in the embedding matrix. Increasing the size will add newly initialized vectors at the end. Reducing the size will remove vectors from the end.
- If not provided or None: does nothing and just returns a pointer to the input tokens ``torch.nn.Embeddings`` Module of the model.
-
- Return: ``torch.nn.Embeddings``
- Pointer to the input tokens Embeddings Module of the model
- """
- base_model = getattr(self, self.base_model_prefix, self) # get the base model if needed
- model_embeds = base_model._resize_token_embeddings(new_num_tokens)
- if new_num_tokens is None:
- return model_embeds
-
- # Update base model and current model config
- self.config.vocab_size = new_num_tokens
- base_model.vocab_size = new_num_tokens
-
- # Tie weights again if needed
- self.tie_weights()
-
- return model_embeds
-
- def _resize_token_embeddings(self, new_num_tokens):
- old_embeddings = self.get_input_embeddings()
- new_embeddings = self._get_resized_embeddings(old_embeddings, new_num_tokens)
- self.set_input_embeddings(new_embeddings)
- return self.get_input_embeddings()
-
- def _get_resized_embeddings(self, old_embeddings, new_num_tokens=None):
- """ Build a resized Embedding Module from a provided token Embedding Module.
- Increasing the size will add newly initialized vectors at the end
- Reducing the size will remove vectors from the end
-
- Args:
- new_num_tokens: (`optional`) int
- New number of tokens in the embedding matrix.
- Increasing the size will add newly initialized vectors at the end
- Reducing the size will remove vectors from the end
- If not provided or None: return the provided token Embedding Module.
- Return: ``torch.nn.Embeddings``
- Pointer to the resized Embedding Module or the old Embedding Module if new_num_tokens is None
- """
- if new_num_tokens is None:
- return old_embeddings
-
- old_num_tokens, old_embedding_dim = old_embeddings.weight.size()
- if old_num_tokens == new_num_tokens:
- return old_embeddings
-
- # Build new embeddings
- new_embeddings = nn.Embedding(new_num_tokens, old_embedding_dim)
- new_embeddings.to(old_embeddings.weight.device)
-
- # initialize all new embeddings (in particular added tokens)
- self._init_weights(new_embeddings)
-
- # Copy word embeddings from the previous weights
- num_tokens_to_copy = min(old_num_tokens, new_num_tokens)
- new_embeddings.weight.data[:num_tokens_to_copy, :] = old_embeddings.weight.data[:num_tokens_to_copy, :]
-
- return new_embeddings
-
- def init_weights(self):
- """ Initialize and prunes weights if needed. """
- # Initialize weights
- self.apply(self._init_weights)
-
- # Prune heads if needed
- if self.config.pruned_heads:
- self.prune_heads(self.config.pruned_heads)
-
- # Tie weights if needed
- self.tie_weights()
-
- def prune_heads(self, heads_to_prune):
- """ Prunes heads of the base model.
-
- Arguments:
-
- heads_to_prune: dict with keys being selected layer indices (`int`) and associated values being the list of heads to prune in said layer (list of `int`).
- E.g. {1: [0, 2], 2: [2, 3]} will prune heads 0 and 2 on layer 1 and heads 2 and 3 on layer 2.
- """
- # save new sets of pruned heads as union of previously stored pruned heads and newly pruned heads
- for layer, heads in heads_to_prune.items():
- union_heads = set(self.config.pruned_heads.get(layer, [])) | set(heads)
- self.config.pruned_heads[layer] = list(union_heads) # Unfortunately we have to store it as list for JSON
-
- self.base_model._prune_heads(heads_to_prune)
-
- def save_pretrained(self, save_directory):
- """ Save a model and its configuration file to a directory, so that it
- can be re-loaded using the `:func:`~transformers.PreTrainedModel.from_pretrained`` class method.
- """
- assert os.path.isdir(
- save_directory
- ), "Saving path should be a directory where the model and configuration can be saved"
-
- # Only save the model itself if we are using distributed training
- model_to_save = self.module if hasattr(self, "module") else self
-
- # Attach architecture to the config
- model_to_save.config.architectures = [model_to_save.__class__.__name__]
-
- # Save configuration file
- model_to_save.config.save_pretrained(save_directory)
-
- # If we save using the predefined names, we can load using `from_pretrained`
- output_model_file = os.path.join(save_directory, WEIGHTS_NAME)
- torch.save(model_to_save.state_dict(), output_model_file)
- logger.info("Model weights saved in {}".format(output_model_file))
-
- @classmethod
- def from_pretrained(cls, pretrained_model_name_or_path, *model_args, **kwargs):
- r"""Instantiate a pretrained pytorch model from a pre-trained model configuration.
-
- The model is set in evaluation mode by default using ``model.eval()`` (Dropout modules are deactivated)
- To train the model, you should first set it back in training mode with ``model.train()``
-
- The warning ``Weights from XXX not initialized from pretrained model`` means that the weights of XXX do not come pre-trained with the rest of the model.
- It is up to you to train those weights with a downstream fine-tuning task.
-
- The warning ``Weights from XXX not used in YYY`` means that the layer XXX is not used by YYY, therefore those weights are discarded.
-
- Parameters:
- pretrained_model_name_or_path: either:
- - a string with the `shortcut name` of a pre-trained model to load from cache or download, e.g.: ``bert-base-uncased``.
- - a string with the `identifier name` of a pre-trained model that was user-uploaded to our S3, e.g.: ``dbmdz/bert-base-german-cased``.
- - a path to a `directory` containing model weights saved using :func:`~transformers.PreTrainedModel.save_pretrained`, e.g.: ``./my_model_directory/``.
- - a path or url to a `tensorflow index checkpoint file` (e.g. `./tf_model/model.ckpt.index`). In this case, ``from_tf`` should be set to True and a configuration object should be provided as ``config`` argument. This loading path is slower than converting the TensorFlow checkpoint in a PyTorch model using the provided conversion scripts and loading the PyTorch model afterwards.
- - None if you are both providing the configuration and state dictionary (resp. with keyword arguments ``config`` and ``state_dict``)
-
- model_args: (`optional`) Sequence of positional arguments:
- All remaning positional arguments will be passed to the underlying model's ``__init__`` method
-
- config: (`optional`) one of:
- - an instance of a class derived from :class:`~transformers.PretrainedConfig`, or
- - a string valid as input to :func:`~transformers.PretrainedConfig.from_pretrained()`
- Configuration for the model to use instead of an automatically loaded configuation. Configuration can be automatically loaded when:
- - the model is a model provided by the library (loaded with the ``shortcut-name`` string of a pretrained model), or
- - the model was saved using :func:`~transformers.PreTrainedModel.save_pretrained` and is reloaded by suppling the save directory.
- - the model is loaded by suppling a local directory as ``pretrained_model_name_or_path`` and a configuration JSON file named `config.json` is found in the directory.
-
- state_dict: (`optional`) dict:
- an optional state dictionnary for the model to use instead of a state dictionary loaded from saved weights file.
- This option can be used if you want to create a model from a pretrained configuration but load your own weights.
- In this case though, you should check if using :func:`~transformers.PreTrainedModel.save_pretrained` and :func:`~transformers.PreTrainedModel.from_pretrained` is not a simpler option.
-
- cache_dir: (`optional`) string:
- Path to a directory in which a downloaded pre-trained model
- configuration should be cached if the standard cache should not be used.
-
- force_download: (`optional`) boolean, default False:
- Force to (re-)download the model weights and configuration files and override the cached versions if they exists.
-
- resume_download: (`optional`) boolean, default False:
- Do not delete incompletely recieved file. Attempt to resume the download if such a file exists.
-
- proxies: (`optional`) dict, default None:
- A dictionary of proxy servers to use by protocol or endpoint, e.g.: {'http': 'foo.bar:3128', 'http://hostname': 'foo.bar:4012'}.
- The proxies are used on each request.
-
- output_loading_info: (`optional`) boolean:
- Set to ``True`` to also return a dictionnary containing missing keys, unexpected keys and error messages.
-
- kwargs: (`optional`) Remaining dictionary of keyword arguments:
- Can be used to update the configuration object (after it being loaded) and initiate the model. (e.g. ``output_attention=True``). Behave differently depending on whether a `config` is provided or automatically loaded:
-
- - If a configuration is provided with ``config``, ``**kwargs`` will be directly passed to the underlying model's ``__init__`` method (we assume all relevant updates to the configuration have already been done)
- - If a configuration is not provided, ``kwargs`` will be first passed to the configuration class initialization function (:func:`~transformers.PretrainedConfig.from_pretrained`). Each key of ``kwargs`` that corresponds to a configuration attribute will be used to override said attribute with the supplied ``kwargs`` value. Remaining keys that do not correspond to any configuration attribute will be passed to the underlying model's ``__init__`` function.
-
- Examples::
-
- # For example purposes. Not runnable.
- model = BertModel.from_pretrained('bert-base-uncased') # Download model and configuration from S3 and cache.
- model = BertModel.from_pretrained('./test/saved_model/') # E.g. model was saved using `save_pretrained('./test/saved_model/')`
- model = BertModel.from_pretrained('bert-base-uncased', output_attention=True) # Update configuration during loading
- assert model.config.output_attention == True
- # Loading from a TF checkpoint file instead of a PyTorch model (slower)
- config = BertConfig.from_json_file('./tf_model/my_tf_model_config.json')
- model = BertModel.from_pretrained('./tf_model/my_tf_checkpoint.ckpt.index', from_tf=True, config=config)
-
- """
- config = kwargs.pop("config", None)
- state_dict = kwargs.pop("state_dict", None)
- cache_dir = kwargs.pop("cache_dir", None)
- from_tf = kwargs.pop("from_tf", False)
- force_download = kwargs.pop("force_download", False)
- resume_download = kwargs.pop("resume_download", False)
- proxies = kwargs.pop("proxies", None)
- output_loading_info = kwargs.pop("output_loading_info", False)
-
- # Load config if we don't provide a configuration
- if not isinstance(config, PretrainedConfig):
- config_path = config if config is not None else pretrained_model_name_or_path
- config, model_kwargs = cls.config_class.from_pretrained(
- config_path,
- *model_args,
- cache_dir=cache_dir,
- return_unused_kwargs=True,
- force_download=force_download,
- resume_download=resume_download,
- proxies=proxies,
- **kwargs,
- )
- else:
- model_kwargs = kwargs
-
- # Load model
- if pretrained_model_name_or_path is not None:
- if pretrained_model_name_or_path in cls.pretrained_model_archive_map:
- archive_file = cls.pretrained_model_archive_map[pretrained_model_name_or_path]
- elif os.path.isdir(pretrained_model_name_or_path):
- if from_tf and os.path.isfile(os.path.join(pretrained_model_name_or_path, TF_WEIGHTS_NAME + ".index")):
- # Load from a TF 1.0 checkpoint
- archive_file = os.path.join(pretrained_model_name_or_path, TF_WEIGHTS_NAME + ".index")
- elif from_tf and os.path.isfile(os.path.join(pretrained_model_name_or_path, TF2_WEIGHTS_NAME)):
- # Load from a TF 2.0 checkpoint
- archive_file = os.path.join(pretrained_model_name_or_path, TF2_WEIGHTS_NAME)
- elif os.path.isfile(os.path.join(pretrained_model_name_or_path, WEIGHTS_NAME)):
- # Load from a PyTorch checkpoint
- archive_file = os.path.join(pretrained_model_name_or_path, WEIGHTS_NAME)
- else:
- raise EnvironmentError(
- "Error no file named {} found in directory {} or `from_tf` set to False".format(
- [WEIGHTS_NAME, TF2_WEIGHTS_NAME, TF_WEIGHTS_NAME + ".index"], pretrained_model_name_or_path
- )
- )
- elif os.path.isfile(pretrained_model_name_or_path) or is_remote_url(pretrained_model_name_or_path):
- archive_file = pretrained_model_name_or_path
- elif os.path.isfile(pretrained_model_name_or_path + ".index"):
- assert (
- from_tf
- ), "We found a TensorFlow checkpoint at {}, please set from_tf to True to load from this checkpoint".format(
- pretrained_model_name_or_path + ".index"
- )
- archive_file = pretrained_model_name_or_path + ".index"
- else:
- archive_file = hf_bucket_url(pretrained_model_name_or_path, postfix=WEIGHTS_NAME)
- if from_tf:
- raise EnvironmentError(
- "Loading a PyTorch model from a TF checkpoint is not supported when using a model identifier name."
- )
-
- # redirect to the cache, if necessary
- try:
- resolved_archive_file = cached_path(
- archive_file,
- cache_dir=cache_dir,
- force_download=force_download,
- proxies=proxies,
- resume_download=resume_download,
- )
- except EnvironmentError:
- if pretrained_model_name_or_path in cls.pretrained_model_archive_map:
- msg = "Couldn't reach server at '{}' to download pretrained weights.".format(archive_file)
- else:
- msg = (
- "Model name '{}' was not found in model name list ({}). "
- "We assumed '{}' was a path or url to model weight files named one of {} but "
- "couldn't find any such file at this path or url.".format(
- pretrained_model_name_or_path,
- ", ".join(cls.pretrained_model_archive_map.keys()),
- archive_file,
- [WEIGHTS_NAME, TF2_WEIGHTS_NAME, TF_WEIGHTS_NAME],
- )
- )
- raise EnvironmentError(msg)
-
- if resolved_archive_file == archive_file:
- logger.info("loading weights file {}".format(archive_file))
- else:
- logger.info("loading weights file {} from cache at {}".format(archive_file, resolved_archive_file))
- else:
- resolved_archive_file = None
-
- # Instantiate model.
- model = cls(config, *model_args, **model_kwargs)
-
- if state_dict is None and not from_tf:
- try:
- state_dict = torch.load(resolved_archive_file, map_location="cpu")
- except Exception:
- raise OSError(
- "Unable to load weights from pytorch checkpoint file. "
- "If you tried to load a PyTorch model from a TF 2.0 checkpoint, please set from_tf=True. "
- )
-
- missing_keys = []
- unexpected_keys = []
- error_msgs = []
-
- if from_tf:
- if resolved_archive_file.endswith(".index"):
- # Load from a TensorFlow 1.X checkpoint - provided by original authors
- model = cls.load_tf_weights(model, config, resolved_archive_file[:-6]) # Remove the '.index'
- else:
- # Load from our TensorFlow 2.0 checkpoints
- try:
- from transformers import load_tf2_checkpoint_in_pytorch_model
-
- model = load_tf2_checkpoint_in_pytorch_model(model, resolved_archive_file, allow_missing_keys=True)
- except ImportError:
- logger.error(
- "Loading a TensorFlow model in PyTorch, requires both PyTorch and TensorFlow to be installed. Please see "
- "https://pytorch.org/ and https://www.tensorflow.org/install/ for installation instructions."
- )
- raise
- else:
- # Convert old format to new format if needed from a PyTorch state_dict
- old_keys = []
- new_keys = []
- for key in state_dict.keys():
- new_key = None
- if "gamma" in key:
- new_key = key.replace("gamma", "weight")
- if "beta" in key:
- new_key = key.replace("beta", "bias")
- if new_key:
- old_keys.append(key)
- new_keys.append(new_key)
- for old_key, new_key in zip(old_keys, new_keys):
- state_dict[new_key] = state_dict.pop(old_key)
-
- # copy state_dict so _load_from_state_dict can modify it
- metadata = getattr(state_dict, "_metadata", None)
- state_dict = state_dict.copy()
- if metadata is not None:
- state_dict._metadata = metadata
-
- # PyTorch's `_load_from_state_dict` does not copy parameters in a module's descendants
- # so we need to apply the function recursively.
- def load(module: nn.Module, prefix=""):
- local_metadata = {} if metadata is None else metadata.get(prefix[:-1], {})
- module._load_from_state_dict(
- state_dict, prefix, local_metadata, True, missing_keys, unexpected_keys, error_msgs
- )
- for name, child in module._modules.items():
- if child is not None:
- load(child, prefix + name + ".")
-
- # Make sure we are able to load base models as well as derived models (with heads)
- start_prefix = ""
- model_to_load = model
- if not hasattr(model, cls.base_model_prefix) and any(
- s.startswith(cls.base_model_prefix) for s in state_dict.keys()
- ):
- start_prefix = cls.base_model_prefix + "."
- if hasattr(model, cls.base_model_prefix) and not any(
- s.startswith(cls.base_model_prefix) for s in state_dict.keys()
- ):
- model_to_load = getattr(model, cls.base_model_prefix)
-
- load(model_to_load, prefix=start_prefix)
- if len(missing_keys) > 0:
- logger.info(
- "Weights of {} not initialized from pretrained model: {}".format(
- model.__class__.__name__, missing_keys
- )
- )
- if len(unexpected_keys) > 0:
- logger.info(
- "Weights from pretrained model not used in {}: {}".format(
- model.__class__.__name__, unexpected_keys
- )
- )
- if len(error_msgs) > 0:
- raise RuntimeError(
- "Error(s) in loading state_dict for {}:\n\t{}".format(
- model.__class__.__name__, "\n\t".join(error_msgs)
- )
- )
-
- model.tie_weights() # make sure word embedding weights are still tied if needed
-
- # Set model in evaluation mode to desactivate DropOut modules by default
- model.eval()
-
- if output_loading_info:
- loading_info = {"missing_keys": missing_keys, "unexpected_keys": unexpected_keys, "error_msgs": error_msgs}
- return model, loading_info
-
- return model
-
- def prepare_inputs_for_generation(self, input_ids, **kwargs):
- return {"input_ids": input_ids}
-
- def _do_output_past(self, outputs):
- has_output_past = hasattr(self.config, "output_past") and self.config.output_past
- has_mem_len = hasattr(self.config, "mem_len") and self.config.mem_len
-
- if has_output_past and not has_mem_len and len(outputs) > 1:
- return True
- elif has_mem_len and self.config.mem_len > 0 and len(outputs) > 1:
- return True
-
- return False
-
- @torch.no_grad()
- def generate(
- self,
- input_ids=None,
- max_length=None,
- do_sample=None,
- num_beams=None,
- temperature=None,
- top_k=None,
- top_p=None,
- repetition_penalty=None,
- bos_token_id=None,
- pad_token_id=None,
- eos_token_ids=None,
- length_penalty=None,
- num_return_sequences=None,
- ):
- r""" Generates sequences for models with a LM head. The method currently supports greedy or penalized greedy decoding, sampling with top-k or nucleus sampling
- and beam-search.
-
- Adapted in part from `Facebook's XLM beam search code`_.
-
- .. _`Facebook's XLM beam search code`:
- https://github.com/facebookresearch/XLM/blob/9e6f6814d17be4fe5b15f2e6c43eb2b2d76daeb4/src/model/transformer.py#L529
-
-
- Parameters:
-
- input_ids: (`optional`) `torch.LongTensor` of shape `(batch_size, sequence_length)`
- The sequence used as a prompt for the generation. If `None` the method initializes
- it as an empty `torch.LongTensor` of shape `(1,)`.
-
- max_length: (`optional`) int
- The max length of the sequence to be generated. Between 1 and infinity. Default to 20.
-
- do_sample: (`optional`) bool
- If set to `False` greedy decoding is used. Otherwise sampling is used. Default to greedy sampling.
-
- num_beams: (`optional`) int
- Number of beams for beam search. Must be between 1 and infinity. 1 means no beam search. Default to 1.
-
- temperature: (`optional`) float
- The value used to module the next token probabilities. Must be strictely positive. Default to 1.0.
-
- top_k: (`optional`) int
- The number of highest probability vocabulary tokens to keep for top-k-filtering. Between 1 and infinity. Default to 50.
-
- top_p: (`optional`) float
- The cumulative probability of parameter highest probability vocabulary tokens to keep for nucleus sampling. Must be between 0 and 1. Default to 1.
-
- repetition_penalty: (`optional`) float
- The parameter for repetition penalty. Between 1.0 and infinity. 1.0 means no penalty. Default to 1.0.
-
- bos_token_id: (`optional`) int
- Beginning of sentence token if no prompt is provided. Default to 0.
-
- eos_token_ids: (`optional`) int or list of int
- End of sequence token or list of tokens to stop the generation. Default to 0.
- length_penalty: (`optional`) float
- Exponential penalty to the length. Default to 1.
-
- num_return_sequences: (`optional`) int
- The number of independently computed returned sequences for each element in the batch. Default to 1.
-
- Examples::
-
- tokenizer = AutoTokenizer.from_pretrained('distilgpt2') # Initialize tokenizer
- model = AutoModelWithLMHead.from_pretrained('distilgpt2') # Download model and configuration from S3 and cache.
- outputs = model.generate(max_length=40, bos_token_id=tokenizer.bos_token_id, eos_token_ids=tokenizer.eos_token_id) # do greedy decoding without beam search
- print('Generated: {}'.format(tokenizer.decode(outputs[0], skip_special_tokens=True)))
-
- tokenizer = AutoTokenizer.from_pretrained('openai-gpt') # Initialize tokenizer
- model = AutoModelWithLMHead.from_pretrained('openai-gpt') # Download model and configuration from S3 and cache.
- input_context = 'The dog'
- input_ids = torch.tensor(tokenizer.encode(input_context)).unsqueeze(0) # encode input context
- outputs = model.generate(input_ids=input_ids, do_sample=True, num_beams=5, num_return_sequences=3, temperature=1.5) # generate 3 independent sequences using beam search decoding (5 beams) with sampling from initial context 'The dog'
- for i in range(3): # 3 output sequences were generated
- print('Generated {}: {}'.format(i, tokenizer.decode(outputs[0][i], skip_special_tokens=True)))
-
- tokenizer = AutoTokenizer.from_pretrained('distilgpt2') # Initialize tokenizer
- model = AutoModelWithLMHead.from_pretrained('distilgpt2') # Download model and configuration from S3 and cache.
- input_context = 'The dog'
- input_ids = torch.tensor(tokenizer.encode(input_context)).unsqueeze(0) # encode input context
- outputs = model.generate(input_ids=input_ids, max_length=40, temperature=0.7, bos_token_id=tokenizer.bos_token_id, eos_token_ids=tokenizer.eos_token_id, num_beams=3) # generate sequences using greedy beam search decoding (3 beams)
- print('Generated: {}'.format(tokenizer.decode(outputs[0], skip_special_tokens=True)))
-
- tokenizer = AutoTokenizer.from_pretrained('ctrl') # Initialize tokenizer
- model = AutoModelWithLMHead.from_pretrained('ctrl') # Download model and configuration from S3 and cache.
- input_context = 'Legal My neighbor is' # "Legal" is one of the control codes for ctrl
- input_ids = torch.tensor(tokenizer.encode(input_context)).unsqueeze(0) # encode input context
- outputs = model.generate(input_ids=input_ids, max_length=50, temperature=0.7, repetition_penalty=1.2) # generate sequences using using greedy search
- print('Generated: {}'.format(tokenizer.decode(outputs[0], skip_special_tokens=True)))
-
- """
-
- # We cannot generate if the model does not have a LM head
- if self.get_output_embeddings() is None:
- raise AttributeError(
- "You tried to generate sequences with a model that does not have a LM Head."
- "Please use another model class (e.g. `OpenAIGPTLMHeadModel`, `XLNetLMHeadModel`, `GPT2LMHeadModel`, `CTRLLMHeadModel`, `T5WithLMHeadModel`, `TransfoXLLMHeadModel`)"
- )
-
- max_length = max_length if max_length is not None else self.config.max_length
- do_sample = do_sample if do_sample is not None else self.config.do_sample
- num_beams = num_beams if num_beams is not None else self.config.num_beams
- temperature = temperature if temperature is not None else self.config.temperature
- top_k = top_k if top_k is not None else self.config.top_k
- top_p = top_p if top_p is not None else self.config.top_p
- repetition_penalty = repetition_penalty if repetition_penalty is not None else self.config.repetition_penalty
- bos_token_id = bos_token_id if bos_token_id is not None else self.config.bos_token_id
- pad_token_id = pad_token_id if pad_token_id is not None else self.config.pad_token_id
- eos_token_ids = eos_token_ids if eos_token_ids is not None else self.config.eos_token_ids
- length_penalty = length_penalty if length_penalty is not None else self.config.length_penalty
- num_return_sequences = (
- num_return_sequences if num_return_sequences is not None else self.config.num_return_sequences
- )
-
- if input_ids is not None:
- batch_size = input_ids.shape[0] # overriden by the input batch_size
- else:
- batch_size = 1
- if isinstance(eos_token_ids, int):
- eos_token_ids = [eos_token_ids]
-
- assert isinstance(max_length, int) and max_length > 0, "`max_length` should be a strictely positive integer."
- assert isinstance(do_sample, bool), "`do_sample` should be a boolean."
- assert isinstance(num_beams, int) and num_beams > 0, "`num_beams` should be a strictely positive integer."
- assert temperature > 0, "`temperature` should be strictely positive."
- assert isinstance(top_k, int) and top_k >= 0, "`top_k` should be a positive integer."
- assert 0 <= top_p <= 1, "`top_p` should be between 0 and 1."
- assert repetition_penalty >= 1.0, "`repetition_penalty` should be >= 1."
- assert isinstance(bos_token_id, int) and bos_token_id >= 0, "`bos_token_id` should be a positive integer."
- assert isinstance(pad_token_id, int) and pad_token_id >= 0, "`pad_token_id` should be a positive integer."
- assert isinstance(eos_token_ids, (list, tuple)) and (
- e >= 0 for e in eos_token_ids
- ), "`eos_token_ids` should be a positive integer or a list/tuple of positive integers."
- assert length_penalty > 0, "`length_penalty` should be strictely positive."
- assert (
- isinstance(num_return_sequences, int) and num_return_sequences > 0
- ), "`num_return_sequences` should be a strictely positive integer."
-
- if input_ids is None:
- input_ids = torch.full(
- (batch_size, 1), bos_token_id, dtype=torch.long, device=next(self.parameters()).device
- )
- else:
- assert input_ids.dim() == 2, "Input prompt should be of shape (batch_size, sequence length)."
-
- # current position and vocab size
- cur_len = input_ids.shape[1]
- vocab_size = self.config.vocab_size
-
- if num_return_sequences != 1:
- # Expand input to num return sequences
- input_ids = input_ids.unsqueeze(1).expand(batch_size, num_return_sequences, cur_len)
- input_ids = input_ids.contiguous().view(
- batch_size * num_return_sequences, cur_len
- ) # (batch_size * num_return_sequences, cur_len)
- effective_batch_size = batch_size * num_return_sequences
- else:
- effective_batch_size = batch_size
-
- if num_beams > 1:
- output = self._generate_beam_search(
- input_ids,
- cur_len,
- max_length,
- do_sample,
- temperature,
- top_k,
- top_p,
- repetition_penalty,
- pad_token_id,
- eos_token_ids,
- effective_batch_size,
- length_penalty,
- num_beams,
- vocab_size,
- )
- else:
- output = self._generate_no_beam_search(
- input_ids,
- cur_len,
- max_length,
- do_sample,
- temperature,
- top_k,
- top_p,
- repetition_penalty,
- pad_token_id,
- eos_token_ids,
- effective_batch_size,
- )
-
- if num_return_sequences != 1:
- output = output.view(batch_size, num_return_sequences, -1)
- return output
-
- def _generate_no_beam_search(
- self,
- input_ids,
- cur_len,
- max_length,
- do_sample,
- temperature,
- top_k,
- top_p,
- repetition_penalty,
- pad_token_id,
- eos_token_ids,
- batch_size,
- ):
- """ Generate sequences for each example without beam search (num_beams == 1).
- All returned sequence are generated independantly.
- """
- # current position / max lengths / length of generated sentences / unfinished sentences
- unfinished_sents = input_ids.new(batch_size).fill_(1)
-
- past = None
-
- while cur_len < max_length:
- model_inputs = self.prepare_inputs_for_generation(input_ids, past=past)
- outputs = self(**model_inputs)
- next_token_logits = outputs[0][:, -1, :]
-
- # if model has past, then set the past variable to speed up decoding
- if self._do_output_past(outputs):
- past = outputs[1]
-
- # repetition penalty from CTRL paper (https://arxiv.org/abs/1909.05858)
- if repetition_penalty != 1.0:
- for i in range(batch_size):
- for previous_token in set(input_ids[i].tolist()):
- # if score < 0 then repetition penalty has to multiplied to reduce the previous token probability
- if next_token_logits[i, previous_token] < 0:
- next_token_logits[i, previous_token] *= repetition_penalty
- else:
- next_token_logits[i, previous_token] /= repetition_penalty
-
- if do_sample:
- # Temperature (higher temperature => more likely to sample low probability tokens)
- if temperature != 1.0:
- next_token_logits = next_token_logits / temperature
- # Top-p/top-k filtering
- next_token_logits = top_k_top_p_filtering(next_token_logits, top_k=top_k, top_p=top_p)
- # Sample
- next_token = torch.multinomial(F.softmax(next_token_logits, dim=-1), num_samples=1).squeeze(1)
- else:
- # Greedy decoding
- next_token = torch.argmax(next_token_logits, dim=-1)
-
- # update generations and finished sentences
- tokens_to_add = next_token * unfinished_sents + pad_token_id * (1 - unfinished_sents)
- input_ids = torch.cat([input_ids, tokens_to_add.unsqueeze(-1)], dim=-1)
- for eos_token_id in eos_token_ids:
- unfinished_sents.mul_(tokens_to_add.ne(eos_token_id).long())
- cur_len = cur_len + 1
-
- # stop when there is a in each sentence, or if we exceed the maximul length
- if unfinished_sents.max() == 0:
- break
-
- # add eos_token_ids to unfinished sentences
- if cur_len == max_length:
- input_ids[:, -1].masked_fill_(unfinished_sents.to(dtype=torch.bool), eos_token_ids[0])
-
- return input_ids
-
- def _generate_beam_search(
- self,
- input_ids,
- cur_len,
- max_length,
- do_sample,
- temperature,
- top_k,
- top_p,
- repetition_penalty,
- pad_token_id,
- eos_token_ids,
- batch_size,
- length_penalty,
- num_beams,
- vocab_size,
- ):
- """ Generate sequences for each example with beam search.
- """
- # Expand input to num beams
- input_ids = input_ids.unsqueeze(1).expand(batch_size, num_beams, cur_len)
- input_ids = input_ids.contiguous().view(batch_size * num_beams, cur_len) # (batch_size * num_beams, cur_len)
-
- # generated hypotheses
- generated_hyps = [
- BeamHypotheses(num_beams, max_length, length_penalty, early_stopping=False) for _ in range(batch_size)
- ]
-
- # scores for each sentence in the beam
- beam_scores = torch.zeros((batch_size, num_beams), dtype=torch.float, device=input_ids.device)
- beam_scores[:, 1:] = -1e9
- beam_scores = beam_scores.view(-1) # shape (batch_size * num_beams,)
-
- # cache compute states
- past = None
-
- # done sentences
- done = [False for _ in range(batch_size)]
-
- while cur_len < max_length:
- model_inputs = self.prepare_inputs_for_generation(input_ids, past=past)
- outputs = self(**model_inputs) # (batch_size * num_beams, cur_len, vocab_size)
- scores = outputs[0][:, -1, :] # (batch_size * num_beams, vocab_size)
-
- # if model has past, then set the past variable to speed up decoding
- if self._do_output_past(outputs):
- past = outputs[1]
-
- # repetition penalty (from CTRL paper https://arxiv.org/abs/1909.05858)
- if repetition_penalty != 1.0:
- for i in range(batch_size * num_beams):
- for previous_token in set(input_ids[i].tolist()):
- # if score < 0 then repetition penalty has to multiplied to reduce the previous token probability
- if scores[i, previous_token] < 0:
- scores[i, previous_token] *= repetition_penalty
- else:
- scores[i, previous_token] /= repetition_penalty
-
- if do_sample:
- # Temperature (higher temperature => more likely to sample low probability tokens)
- if temperature != 1.0:
- scores = scores / temperature
- # Top-p/top-k filtering
- scores = top_k_top_p_filtering(
- scores, top_k=top_k, top_p=top_p, min_tokens_to_keep=2
- ) # (batch_size * num_beams, vocab_size)
- # Sample 2 next words for each beam (so we have some spare tokens and match output of greedy beam search)
- next_words = torch.multinomial(F.softmax(scores, dim=-1), num_samples=2) # (batch_size * num_beams, 2)
- # Compute next scores
- _scores = F.log_softmax(scores, dim=-1) # (batch_size * num_beams, vocab_size)
- _scores = torch.gather(_scores, -1, next_words) # (batch_size * num_beams, 2)
- next_scores = _scores + beam_scores[:, None].expand_as(_scores) # (batch_size * num_beams, 2)
- # Match shape of greedy beam search
- next_words = next_words.view(batch_size, 2 * num_beams) # (batch_size, 2 * num_beams)
- next_scores = next_scores.view(batch_size, 2 * num_beams) # (batch_size, 2 * num_beams)
- else:
- # do greedy beam search
- scores = F.log_softmax(scores, dim=-1) # (batch_size * num_beams, vocab_size)
- assert scores.size() == (batch_size * num_beams, vocab_size)
- # Add the log prob of the new beams to the log prob of the beginning of the sequence (sum of logs == log of the product)
- _scores = scores + beam_scores[:, None].expand_as(scores) # (batch_size * num_beams, vocab_size)
- # re-organize to group the beam together (we are keeping top hypothesis accross beams)
- _scores = _scores.view(batch_size, num_beams * vocab_size) # (batch_size, num_beams * vocab_size)
- next_scores, next_words = torch.topk(_scores, 2 * num_beams, dim=1, largest=True, sorted=True)
-
- assert next_scores.size() == next_words.size() == (batch_size, 2 * num_beams)
-
- # next batch beam content
- # list of (batch_size * num_beams) tuple(next hypothesis score, next word, current position in the batch)
- next_batch_beam = []
-
- # for each sentence
- for batch_ex in range(batch_size):
-
- # if we are done with this sentence
- done[batch_ex] = done[batch_ex] or generated_hyps[batch_ex].is_done(next_scores[batch_ex].max().item())
- if done[batch_ex]:
- next_batch_beam.extend([(0, pad_token_id, 0)] * num_beams) # pad the batch
- continue
-
- # next sentence beam content
- next_sent_beam = []
-
- # next words for this sentence
- for idx, score in zip(next_words[batch_ex], next_scores[batch_ex]):
-
- # get beam and word IDs
- beam_id = idx // vocab_size
- word_id = idx % vocab_size
-
- # end of sentence, or next word
- if word_id.item() in eos_token_ids or cur_len + 1 == max_length:
- generated_hyps[batch_ex].add(
- input_ids[batch_ex * num_beams + beam_id, :cur_len].clone(), score.item()
- )
- else:
- next_sent_beam.append((score, word_id, batch_ex * num_beams + beam_id))
-
- # the beam for next step is full
- if len(next_sent_beam) == num_beams:
- break
-
- # update next beam content
- assert len(next_sent_beam) == 0 if cur_len + 1 == max_length else num_beams
- if len(next_sent_beam) == 0:
- next_sent_beam = [(0, pad_token_id, 0)] * num_beams # pad the batch
- next_batch_beam.extend(next_sent_beam)
- assert len(next_batch_beam) == num_beams * (batch_ex + 1)
-
- # sanity check / prepare next batch
- assert len(next_batch_beam) == batch_size * num_beams
- beam_scores = beam_scores.new([x[0] for x in next_batch_beam])
- beam_words = input_ids.new([x[1] for x in next_batch_beam])
- beam_idx = input_ids.new([x[2] for x in next_batch_beam])
-
- # re-order batch
- input_ids = input_ids[beam_idx, :]
- input_ids = torch.cat([input_ids, beam_words.unsqueeze(1)], dim=-1)
-
- # re-order internal states
- if past:
- reordered_past = []
- for layer_past in past:
- # get the correct batch idx from layer past batch dim
- # batch dim of `past` and `mems` is at 2nd position
- reordered_layer_past = [layer_past[:, i].unsqueeze(1).clone().detach() for i in beam_idx]
- reordered_layer_past = torch.cat(reordered_layer_past, dim=1)
- # check that shape matches
- assert reordered_layer_past.shape == layer_past.shape
- reordered_past.append(reordered_layer_past)
- past = tuple(reordered_past)
-
- # update current length
- cur_len = cur_len + 1
-
- # stop when we are done with each sentence
- if all(done):
- break
-
- # visualize hypotheses
- # print([len(x) for x in generated_hyps], cur_len)
- # globals().update( locals() );
- # !import code; code.interact(local=vars())
- # for ii in range(batch_size):
- # for ss, ww in sorted(generated_hyps[ii].hyp, key=lambda x: x[0], reverse=True):
- # print("%.3f " % ss + " ".join(self.dico[x] for x in ww.tolist()))
- # print("")
-
- # select the best hypotheses
- tgt_len = input_ids.new(batch_size)
- best = []
-
- for i, hypotheses in enumerate(generated_hyps):
- best_hyp = max(hypotheses.hyp, key=lambda x: x[0])[1]
- tgt_len[i] = len(best_hyp) + 1 # +1 for the symbol
- best.append(best_hyp)
-
- # generate target batch
- decoded = input_ids.new(batch_size, tgt_len.max().item()).fill_(pad_token_id)
- for i, hypo in enumerate(best):
- decoded[i, : tgt_len[i] - 1] = hypo
- decoded[i, tgt_len[i] - 1] = eos_token_ids[0]
-
- return decoded
-
-
-def top_k_top_p_filtering(logits, top_k=0, top_p=1.0, filter_value=-float("Inf"), min_tokens_to_keep=1):
- """ Filter a distribution of logits using top-k and/or nucleus (top-p) filtering
- Args:
- logits: logits distribution shape (batch size, vocabulary size)
- if top_k > 0: keep only top k tokens with highest probability (top-k filtering).
- if top_p < 1.0: keep the top tokens with cumulative probability >= top_p (nucleus filtering).
- Nucleus filtering is described in Holtzman et al. (http://arxiv.org/abs/1904.09751)
- Make sure we keep at least min_tokens_to_keep per batch example in the output
- From: https://gist.github.com/thomwolf/1a5a29f6962089e871b94cbd09daf317
- """
- if top_k > 0:
- top_k = min(max(top_k, min_tokens_to_keep), logits.size(-1)) # Safety check
- # Remove all tokens with a probability less than the last token of the top-k
- indices_to_remove = logits < torch.topk(logits, top_k)[0][..., -1, None]
- logits[indices_to_remove] = filter_value
-
- if top_p < 1.0:
- sorted_logits, sorted_indices = torch.sort(logits, descending=True)
- cumulative_probs = torch.cumsum(F.softmax(sorted_logits, dim=-1), dim=-1)
-
- # Remove tokens with cumulative probability above the threshold (token with 0 are kept)
- sorted_indices_to_remove = cumulative_probs > top_p
- if min_tokens_to_keep > 1:
- # Keep at least min_tokens_to_keep (set to min_tokens_to_keep-1 because we add the first one below)
- sorted_indices_to_remove[..., :min_tokens_to_keep] = 0
- # Shift the indices to the right to keep also the first token above the threshold
- sorted_indices_to_remove[..., 1:] = sorted_indices_to_remove[..., :-1].clone()
- sorted_indices_to_remove[..., 0] = 0
-
- # scatter sorted tensors to original indexing
- indices_to_remove = sorted_indices_to_remove.scatter(1, sorted_indices, sorted_indices_to_remove)
- logits[indices_to_remove] = filter_value
- return logits
-
-
-class BeamHypotheses(object):
- def __init__(self, n_hyp, max_length, length_penalty, early_stopping):
- """
- Initialize n-best list of hypotheses.
- """
- self.max_length = max_length - 1 # ignoring bos_token
- self.length_penalty = length_penalty
- self.early_stopping = early_stopping
- self.n_hyp = n_hyp
- self.hyp = []
- self.worst_score = 1e9
-
- def __len__(self):
- """
- Number of hypotheses in the list.
- """
- return len(self.hyp)
-
- def add(self, hyp, sum_logprobs):
- """
- Add a new hypothesis to the list.
- """
- score = sum_logprobs / len(hyp) ** self.length_penalty
- if len(self) < self.n_hyp or score > self.worst_score:
- self.hyp.append((score, hyp))
- if len(self) > self.n_hyp:
- sorted_scores = sorted([(s, idx) for idx, (s, _) in enumerate(self.hyp)])
- del self.hyp[sorted_scores[0][1]]
- self.worst_score = sorted_scores[1][0]
- else:
- self.worst_score = min(score, self.worst_score)
-
- def is_done(self, best_sum_logprobs):
- """
- If there are enough hypotheses and that none of the hypotheses being generated
- can become better than the worst one in the heap, then we are done with this sentence.
- """
- if len(self) < self.n_hyp:
- return False
- elif self.early_stopping:
- return True
- else:
- return self.worst_score >= best_sum_logprobs / self.max_length ** self.length_penalty
-
-
-class Conv1D(nn.Module):
- def __init__(self, nf, nx):
- """ Conv1D layer as defined by Radford et al. for OpenAI GPT (and also used in GPT-2)
- Basically works like a Linear layer but the weights are transposed
- """
- super().__init__()
- self.nf = nf
- w = torch.empty(nx, nf)
- nn.init.normal_(w, std=0.02)
- self.weight = nn.Parameter(w)
- self.bias = nn.Parameter(torch.zeros(nf))
-
- def forward(self, x):
- size_out = x.size()[:-1] + (self.nf,)
- x = torch.addmm(self.bias, x.view(-1, x.size(-1)), self.weight)
- x = x.view(*size_out)
- return x
-
-
-class PoolerStartLogits(nn.Module):
- """ Compute SQuAD start_logits from sequence hidden states. """
-
- def __init__(self, config):
- super().__init__()
- self.dense = nn.Linear(config.hidden_size, 1)
-
- def forward(self, hidden_states, p_mask=None):
- """ Args:
- **p_mask**: (`optional`) ``torch.FloatTensor`` of shape `(batch_size, seq_len)`
- invalid position mask such as query and special symbols (PAD, SEP, CLS)
- 1.0 means token should be masked.
- """
- x = self.dense(hidden_states).squeeze(-1)
-
- if p_mask is not None:
- if next(self.parameters()).dtype == torch.float16:
- x = x * (1 - p_mask) - 65500 * p_mask
- else:
- x = x * (1 - p_mask) - 1e30 * p_mask
-
- return x
-
-
-class PoolerEndLogits(nn.Module):
- """ Compute SQuAD end_logits from sequence hidden states and start token hidden state.
- """
-
- def __init__(self, config):
- super().__init__()
- self.dense_0 = nn.Linear(config.hidden_size * 2, config.hidden_size)
- self.activation = nn.Tanh()
- self.LayerNorm = nn.LayerNorm(config.hidden_size, eps=config.layer_norm_eps)
- self.dense_1 = nn.Linear(config.hidden_size, 1)
-
- def forward(self, hidden_states, start_states=None, start_positions=None, p_mask=None):
- """ Args:
- One of ``start_states``, ``start_positions`` should be not None.
- If both are set, ``start_positions`` overrides ``start_states``.
-
- **start_states**: ``torch.LongTensor`` of shape identical to hidden_states
- hidden states of the first tokens for the labeled span.
- **start_positions**: ``torch.LongTensor`` of shape ``(batch_size,)``
- position of the first token for the labeled span:
- **p_mask**: (`optional`) ``torch.FloatTensor`` of shape ``(batch_size, seq_len)``
- Mask of invalid position such as query and special symbols (PAD, SEP, CLS)
- 1.0 means token should be masked.
- """
- assert (
- start_states is not None or start_positions is not None
- ), "One of start_states, start_positions should be not None"
- if start_positions is not None:
- slen, hsz = hidden_states.shape[-2:]
- start_positions = start_positions[:, None, None].expand(-1, -1, hsz) # shape (bsz, 1, hsz)
- start_states = hidden_states.gather(-2, start_positions) # shape (bsz, 1, hsz)
- start_states = start_states.expand(-1, slen, -1) # shape (bsz, slen, hsz)
-
- x = self.dense_0(torch.cat([hidden_states, start_states], dim=-1))
- x = self.activation(x)
- x = self.LayerNorm(x)
- x = self.dense_1(x).squeeze(-1)
-
- if p_mask is not None:
- if next(self.parameters()).dtype == torch.float16:
- x = x * (1 - p_mask) - 65500 * p_mask
- else:
- x = x * (1 - p_mask) - 1e30 * p_mask
-
- return x
-
-
-class PoolerAnswerClass(nn.Module):
- """ Compute SQuAD 2.0 answer class from classification and start tokens hidden states. """
-
- def __init__(self, config):
- super().__init__()
- self.dense_0 = nn.Linear(config.hidden_size * 2, config.hidden_size)
- self.activation = nn.Tanh()
- self.dense_1 = nn.Linear(config.hidden_size, 1, bias=False)
-
- def forward(self, hidden_states, start_states=None, start_positions=None, cls_index=None):
- """
- Args:
- One of ``start_states``, ``start_positions`` should be not None.
- If both are set, ``start_positions`` overrides ``start_states``.
-
- **start_states**: ``torch.LongTensor`` of shape identical to ``hidden_states``.
- hidden states of the first tokens for the labeled span.
- **start_positions**: ``torch.LongTensor`` of shape ``(batch_size,)``
- position of the first token for the labeled span.
- **cls_index**: torch.LongTensor of shape ``(batch_size,)``
- position of the CLS token. If None, take the last token.
-
- note(Original repo):
- no dependency on end_feature so that we can obtain one single `cls_logits`
- for each sample
- """
- hsz = hidden_states.shape[-1]
- assert (
- start_states is not None or start_positions is not None
- ), "One of start_states, start_positions should be not None"
- if start_positions is not None:
- start_positions = start_positions[:, None, None].expand(-1, -1, hsz) # shape (bsz, 1, hsz)
- start_states = hidden_states.gather(-2, start_positions).squeeze(-2) # shape (bsz, hsz)
-
- if cls_index is not None:
- cls_index = cls_index[:, None, None].expand(-1, -1, hsz) # shape (bsz, 1, hsz)
- cls_token_state = hidden_states.gather(-2, cls_index).squeeze(-2) # shape (bsz, hsz)
- else:
- cls_token_state = hidden_states[:, -1, :] # shape (bsz, hsz)
-
- x = self.dense_0(torch.cat([start_states, cls_token_state], dim=-1))
- x = self.activation(x)
- x = self.dense_1(x).squeeze(-1)
-
- return x
-
-
-class SQuADHead(nn.Module):
- r""" A SQuAD head inspired by XLNet.
-
- Parameters:
- config (:class:`~transformers.XLNetConfig`): Model configuration class with all the parameters of the model.
-
- Inputs:
- **hidden_states**: ``torch.FloatTensor`` of shape ``(batch_size, seq_len, hidden_size)``
- hidden states of sequence tokens
- **start_positions**: ``torch.LongTensor`` of shape ``(batch_size,)``
- position of the first token for the labeled span.
- **end_positions**: ``torch.LongTensor`` of shape ``(batch_size,)``
- position of the last token for the labeled span.
- **cls_index**: torch.LongTensor of shape ``(batch_size,)``
- position of the CLS token. If None, take the last token.
- **is_impossible**: ``torch.LongTensor`` of shape ``(batch_size,)``
- Whether the question has a possible answer in the paragraph or not.
- **p_mask**: (`optional`) ``torch.FloatTensor`` of shape ``(batch_size, seq_len)``
- Mask of invalid position such as query and special symbols (PAD, SEP, CLS)
- 1.0 means token should be masked.
-
- Outputs: `Tuple` comprising various elements depending on the configuration (config) and inputs:
- **loss**: (`optional`, returned if both ``start_positions`` and ``end_positions`` are provided) ``torch.FloatTensor`` of shape ``(1,)``:
- Classification loss as the sum of start token, end token (and is_impossible if provided) classification losses.
- **start_top_log_probs**: (`optional`, returned if ``start_positions`` or ``end_positions`` is not provided)
- ``torch.FloatTensor`` of shape ``(batch_size, config.start_n_top)``
- Log probabilities for the top config.start_n_top start token possibilities (beam-search).
- **start_top_index**: (`optional`, returned if ``start_positions`` or ``end_positions`` is not provided)
- ``torch.LongTensor`` of shape ``(batch_size, config.start_n_top)``
- Indices for the top config.start_n_top start token possibilities (beam-search).
- **end_top_log_probs**: (`optional`, returned if ``start_positions`` or ``end_positions`` is not provided)
- ``torch.FloatTensor`` of shape ``(batch_size, config.start_n_top * config.end_n_top)``
- Log probabilities for the top ``config.start_n_top * config.end_n_top`` end token possibilities (beam-search).
- **end_top_index**: (`optional`, returned if ``start_positions`` or ``end_positions`` is not provided)
- ``torch.LongTensor`` of shape ``(batch_size, config.start_n_top * config.end_n_top)``
- Indices for the top ``config.start_n_top * config.end_n_top`` end token possibilities (beam-search).
- **cls_logits**: (`optional`, returned if ``start_positions`` or ``end_positions`` is not provided)
- ``torch.FloatTensor`` of shape ``(batch_size,)``
- Log probabilities for the ``is_impossible`` label of the answers.
- """
-
- def __init__(self, config):
- super().__init__()
- self.start_n_top = config.start_n_top
- self.end_n_top = config.end_n_top
-
- self.start_logits = PoolerStartLogits(config)
- self.end_logits = PoolerEndLogits(config)
- self.answer_class = PoolerAnswerClass(config)
-
- def forward(
- self, hidden_states, start_positions=None, end_positions=None, cls_index=None, is_impossible=None, p_mask=None
- ):
- outputs = ()
-
- start_logits = self.start_logits(hidden_states, p_mask=p_mask)
-
- if start_positions is not None and end_positions is not None:
- # If we are on multi-GPU, let's remove the dimension added by batch splitting
- for x in (start_positions, end_positions, cls_index, is_impossible):
- if x is not None and x.dim() > 1:
- x.squeeze_(-1)
-
- # during training, compute the end logits based on the ground truth of the start position
- end_logits = self.end_logits(hidden_states, start_positions=start_positions, p_mask=p_mask)
-
- loss_fct = CrossEntropyLoss()
- start_loss = loss_fct(start_logits, start_positions)
- end_loss = loss_fct(end_logits, end_positions)
- total_loss = (start_loss + end_loss) / 2
-
- if cls_index is not None and is_impossible is not None:
- # Predict answerability from the representation of CLS and START
- cls_logits = self.answer_class(hidden_states, start_positions=start_positions, cls_index=cls_index)
- loss_fct_cls = nn.BCEWithLogitsLoss()
- cls_loss = loss_fct_cls(cls_logits, is_impossible)
-
- # note(zhiliny): by default multiply the loss by 0.5 so that the scale is comparable to start_loss and end_loss
- total_loss += cls_loss * 0.5
-
- outputs = (total_loss,) + outputs
-
- else:
- # during inference, compute the end logits based on beam search
- bsz, slen, hsz = hidden_states.size()
- start_log_probs = F.softmax(start_logits, dim=-1) # shape (bsz, slen)
-
- start_top_log_probs, start_top_index = torch.topk(
- start_log_probs, self.start_n_top, dim=-1
- ) # shape (bsz, start_n_top)
- start_top_index_exp = start_top_index.unsqueeze(-1).expand(-1, -1, hsz) # shape (bsz, start_n_top, hsz)
- start_states = torch.gather(hidden_states, -2, start_top_index_exp) # shape (bsz, start_n_top, hsz)
- start_states = start_states.unsqueeze(1).expand(-1, slen, -1, -1) # shape (bsz, slen, start_n_top, hsz)
-
- hidden_states_expanded = hidden_states.unsqueeze(2).expand_as(
- start_states
- ) # shape (bsz, slen, start_n_top, hsz)
- p_mask = p_mask.unsqueeze(-1) if p_mask is not None else None
- end_logits = self.end_logits(hidden_states_expanded, start_states=start_states, p_mask=p_mask)
- end_log_probs = F.softmax(end_logits, dim=1) # shape (bsz, slen, start_n_top)
-
- end_top_log_probs, end_top_index = torch.topk(
- end_log_probs, self.end_n_top, dim=1
- ) # shape (bsz, end_n_top, start_n_top)
- end_top_log_probs = end_top_log_probs.view(-1, self.start_n_top * self.end_n_top)
- end_top_index = end_top_index.view(-1, self.start_n_top * self.end_n_top)
-
- start_states = torch.einsum("blh,bl->bh", hidden_states, start_log_probs)
- cls_logits = self.answer_class(hidden_states, start_states=start_states, cls_index=cls_index)
-
- outputs = (start_top_log_probs, start_top_index, end_top_log_probs, end_top_index, cls_logits) + outputs
-
- # return start_top_log_probs, start_top_index, end_top_log_probs, end_top_index, cls_logits
- # or (if labels are provided) (total_loss,)
- return outputs
-
-
-class SequenceSummary(nn.Module):
- r""" Compute a single vector summary of a sequence hidden states according to various possibilities:
- Args of the config class:
- summary_type:
- - 'last' => [default] take the last token hidden state (like XLNet)
- - 'first' => take the first token hidden state (like Bert)
- - 'mean' => take the mean of all tokens hidden states
- - 'cls_index' => supply a Tensor of classification token position (GPT/GPT-2)
- - 'attn' => Not implemented now, use multi-head attention
- summary_use_proj: Add a projection after the vector extraction
- summary_proj_to_labels: If True, the projection outputs to config.num_labels classes (otherwise to hidden_size). Default: False.
- summary_activation: 'tanh' => add a tanh activation to the output, Other => no activation. Default
- summary_first_dropout: Add a dropout before the projection and activation
- summary_last_dropout: Add a dropout after the projection and activation
- """
-
- def __init__(self, config):
- super().__init__()
-
- self.summary_type = config.summary_type if hasattr(config, "summary_type") else "last"
- if self.summary_type == "attn":
- # We should use a standard multi-head attention module with absolute positional embedding for that.
- # Cf. https://github.com/zihangdai/xlnet/blob/master/modeling.py#L253-L276
- # We can probably just use the multi-head attention module of PyTorch >=1.1.0
- raise NotImplementedError
-
- self.summary = Identity()
- if hasattr(config, "summary_use_proj") and config.summary_use_proj:
- if hasattr(config, "summary_proj_to_labels") and config.summary_proj_to_labels and config.num_labels > 0:
- num_classes = config.num_labels
- else:
- num_classes = config.hidden_size
- self.summary = nn.Linear(config.hidden_size, num_classes)
-
- self.activation = Identity()
- if hasattr(config, "summary_activation") and config.summary_activation == "tanh":
- self.activation = nn.Tanh()
-
- self.first_dropout = Identity()
- if hasattr(config, "summary_first_dropout") and config.summary_first_dropout > 0:
- self.first_dropout = nn.Dropout(config.summary_first_dropout)
-
- self.last_dropout = Identity()
- if hasattr(config, "summary_last_dropout") and config.summary_last_dropout > 0:
- self.last_dropout = nn.Dropout(config.summary_last_dropout)
-
- def forward(self, hidden_states, cls_index=None):
- """ hidden_states: float Tensor in shape [bsz, ..., seq_len, hidden_size], the hidden-states of the last layer.
- cls_index: [optional] position of the classification token if summary_type == 'cls_index',
- shape (bsz,) or more generally (bsz, ...) where ... are optional leading dimensions of hidden_states.
- if summary_type == 'cls_index' and cls_index is None:
- we take the last token of the sequence as classification token
- """
- if self.summary_type == "last":
- output = hidden_states[:, -1]
- elif self.summary_type == "first":
- output = hidden_states[:, 0]
- elif self.summary_type == "mean":
- output = hidden_states.mean(dim=1)
- elif self.summary_type == "cls_index":
- if cls_index is None:
- cls_index = torch.full_like(hidden_states[..., :1, :], hidden_states.shape[-2] - 1, dtype=torch.long)
- else:
- cls_index = cls_index.unsqueeze(-1).unsqueeze(-1)
- cls_index = cls_index.expand((-1,) * (cls_index.dim() - 1) + (hidden_states.size(-1),))
- # shape of cls_index: (bsz, XX, 1, hidden_size) where XX are optional leading dim of hidden_states
- output = hidden_states.gather(-2, cls_index).squeeze(-2) # shape (bsz, XX, hidden_size)
- elif self.summary_type == "attn":
- raise NotImplementedError
-
- output = self.first_dropout(output)
- output = self.summary(output)
- output = self.activation(output)
- output = self.last_dropout(output)
-
- return output
-
-
-def prune_linear_layer(layer, index, dim=0):
- """ Prune a linear layer (a model parameters) to keep only entries in index.
- Return the pruned layer as a new layer with requires_grad=True.
- Used to remove heads.
- """
- index = index.to(layer.weight.device)
- W = layer.weight.index_select(dim, index).clone().detach()
- if layer.bias is not None:
- if dim == 1:
- b = layer.bias.clone().detach()
- else:
- b = layer.bias[index].clone().detach()
- new_size = list(layer.weight.size())
- new_size[dim] = len(index)
- new_layer = nn.Linear(new_size[1], new_size[0], bias=layer.bias is not None).to(layer.weight.device)
- new_layer.weight.requires_grad = False
- new_layer.weight.copy_(W.contiguous())
- new_layer.weight.requires_grad = True
- if layer.bias is not None:
- new_layer.bias.requires_grad = False
- new_layer.bias.copy_(b.contiguous())
- new_layer.bias.requires_grad = True
- return new_layer
-
-
-def prune_conv1d_layer(layer, index, dim=1):
- """ Prune a Conv1D layer (a model parameters) to keep only entries in index.
- A Conv1D work as a Linear layer (see e.g. BERT) but the weights are transposed.
- Return the pruned layer as a new layer with requires_grad=True.
- Used to remove heads.
- """
- index = index.to(layer.weight.device)
- W = layer.weight.index_select(dim, index).clone().detach()
- if dim == 0:
- b = layer.bias.clone().detach()
- else:
- b = layer.bias[index].clone().detach()
- new_size = list(layer.weight.size())
- new_size[dim] = len(index)
- new_layer = Conv1D(new_size[1], new_size[0]).to(layer.weight.device)
- new_layer.weight.requires_grad = False
- new_layer.weight.copy_(W.contiguous())
- new_layer.weight.requires_grad = True
- new_layer.bias.requires_grad = False
- new_layer.bias.copy_(b.contiguous())
- new_layer.bias.requires_grad = True
- return new_layer
-
-
-def prune_layer(layer, index, dim=None):
- """ Prune a Conv1D or nn.Linear layer (a model parameters) to keep only entries in index.
- Return the pruned layer as a new layer with requires_grad=True.
- Used to remove heads.
- """
- if isinstance(layer, nn.Linear):
- return prune_linear_layer(layer, index, dim=0 if dim is None else dim)
- elif isinstance(layer, Conv1D):
- return prune_conv1d_layer(layer, index, dim=1 if dim is None else dim)
- else:
- raise ValueError("Can't prune layer of class {}".format(layer.__class__))
-
-def transpose_iterable(ls):
- """Transpose a list of lists (or tuple of identically lengthed tuples)"""
- tp = type(ls)
- if len(ls) > 0: assert type(ls[0]) == tp, f"Expected type {tp}, instead got type {type(ls[0])} inside outer list"
-
- return tp(map(tp, zip_longest(*ls)))
\ No newline at end of file
diff --git a/server/transformers/src/transformers/modeling_xlm.py b/server/transformers/src/transformers/modeling_xlm.py
deleted file mode 100644
index 9ba5540f9c8ea98af1d248e2708ebfd12094d576..0000000000000000000000000000000000000000
--- a/server/transformers/src/transformers/modeling_xlm.py
+++ /dev/null
@@ -1,1052 +0,0 @@
-# coding=utf-8
-# Copyright 2019-present, Facebook, Inc and the HuggingFace Inc. team.
-#
-# Licensed under the Apache License, Version 2.0 (the "License");
-# you may not use this file except in compliance with the License.
-# You may obtain a copy of the License at
-#
-# http://www.apache.org/licenses/LICENSE-2.0
-#
-# Unless required by applicable law or agreed to in writing, software
-# distributed under the License is distributed on an "AS IS" BASIS,
-# WITHOUT WARRANTIES OR CONDITIONS OF ANY KIND, either express or implied.
-# See the License for the specific language governing permissions and
-# limitations under the License.
-""" PyTorch XLM model.
-"""
-
-
-import itertools
-import logging
-import math
-
-import numpy as np
-import torch
-from torch import nn
-from torch.nn import CrossEntropyLoss, MSELoss
-from torch.nn import functional as F
-
-from .configuration_xlm import XLMConfig
-from .file_utils import add_start_docstrings, add_start_docstrings_to_callable
-from .modeling_utils import PreTrainedModel, SequenceSummary, SQuADHead, prune_linear_layer
-
-
-logger = logging.getLogger(__name__)
-
-XLM_PRETRAINED_MODEL_ARCHIVE_MAP = {
- "xlm-mlm-en-2048": "https://s3.amazonaws.com/models.huggingface.co/bert/xlm-mlm-en-2048-pytorch_model.bin",
- "xlm-mlm-ende-1024": "https://s3.amazonaws.com/models.huggingface.co/bert/xlm-mlm-ende-1024-pytorch_model.bin",
- "xlm-mlm-enfr-1024": "https://s3.amazonaws.com/models.huggingface.co/bert/xlm-mlm-enfr-1024-pytorch_model.bin",
- "xlm-mlm-enro-1024": "https://s3.amazonaws.com/models.huggingface.co/bert/xlm-mlm-enro-1024-pytorch_model.bin",
- "xlm-mlm-tlm-xnli15-1024": "https://s3.amazonaws.com/models.huggingface.co/bert/xlm-mlm-tlm-xnli15-1024-pytorch_model.bin",
- "xlm-mlm-xnli15-1024": "https://s3.amazonaws.com/models.huggingface.co/bert/xlm-mlm-xnli15-1024-pytorch_model.bin",
- "xlm-clm-enfr-1024": "https://s3.amazonaws.com/models.huggingface.co/bert/xlm-clm-enfr-1024-pytorch_model.bin",
- "xlm-clm-ende-1024": "https://s3.amazonaws.com/models.huggingface.co/bert/xlm-clm-ende-1024-pytorch_model.bin",
- "xlm-mlm-17-1280": "https://s3.amazonaws.com/models.huggingface.co/bert/xlm-mlm-17-1280-pytorch_model.bin",
- "xlm-mlm-100-1280": "https://s3.amazonaws.com/models.huggingface.co/bert/xlm-mlm-100-1280-pytorch_model.bin",
-}
-
-
-def create_sinusoidal_embeddings(n_pos, dim, out):
- position_enc = np.array([[pos / np.power(10000, 2 * (j // 2) / dim) for j in range(dim)] for pos in range(n_pos)])
- out[:, 0::2] = torch.FloatTensor(np.sin(position_enc[:, 0::2]))
- out[:, 1::2] = torch.FloatTensor(np.cos(position_enc[:, 1::2]))
- out.detach_()
- out.requires_grad = False
-
-
-def gelu(x):
- """
- GELU activation
- https://arxiv.org/abs/1606.08415
- https://github.com/huggingface/pytorch-openai-transformer-lm/blob/master/model_pytorch.py#L14
- https://github.com/huggingface/transformers/blob/master/modeling.py
- """
- # return 0.5 * x * (1 + torch.tanh(math.sqrt(2 / math.pi) * (x + 0.044715 * torch.pow(x, 3))))
- return 0.5 * x * (1.0 + torch.erf(x / math.sqrt(2.0)))
-
-
-def get_masks(slen, lengths, causal, padding_mask=None):
- """
- Generate hidden states mask, and optionally an attention mask.
- """
- alen = torch.arange(slen, dtype=torch.long, device=lengths.device)
- if padding_mask is not None:
- mask = padding_mask
- else:
- assert lengths.max().item() <= slen
- mask = alen < lengths[:, None]
-
- # attention mask is the same as mask, or triangular inferior attention (causal)
- bs = lengths.size(0)
- if causal:
- attn_mask = alen[None, None, :].repeat(bs, slen, 1) <= alen[None, :, None]
- else:
- attn_mask = mask
-
- # sanity check
- assert mask.size() == (bs, slen)
- assert causal is False or attn_mask.size() == (bs, slen, slen)
-
- return mask, attn_mask
-
-
-class MultiHeadAttention(nn.Module):
-
- NEW_ID = itertools.count()
-
- def __init__(self, n_heads, dim, config):
- super().__init__()
- self.layer_id = next(MultiHeadAttention.NEW_ID)
- self.output_attentions = config.output_attentions
- self.dim = dim
- self.n_heads = n_heads
- self.dropout = config.attention_dropout
- assert self.dim % self.n_heads == 0
-
- self.q_lin = nn.Linear(dim, dim)
- self.k_lin = nn.Linear(dim, dim)
- self.v_lin = nn.Linear(dim, dim)
- self.out_lin = nn.Linear(dim, dim)
- self.pruned_heads = set()
-
- def prune_heads(self, heads):
- attention_head_size = self.dim // self.n_heads
- if len(heads) == 0:
- return
- mask = torch.ones(self.n_heads, attention_head_size)
- heads = set(heads) - self.pruned_heads
- for head in heads:
- head -= sum(1 if h < head else 0 for h in self.pruned_heads)
- mask[head] = 0
- mask = mask.view(-1).contiguous().eq(1)
- index = torch.arange(len(mask))[mask].long()
- # Prune linear layers
- self.q_lin = prune_linear_layer(self.q_lin, index)
- self.k_lin = prune_linear_layer(self.k_lin, index)
- self.v_lin = prune_linear_layer(self.v_lin, index)
- self.out_lin = prune_linear_layer(self.out_lin, index, dim=1)
- # Update hyper params
- self.n_heads = self.n_heads - len(heads)
- self.dim = attention_head_size * self.n_heads
- self.pruned_heads = self.pruned_heads.union(heads)
-
- def forward(self, input, mask, kv=None, cache=None, head_mask=None):
- """
- Self-attention (if kv is None) or attention over source sentence (provided by kv).
- """
- # Input is (bs, qlen, dim)
- # Mask is (bs, klen) (non-causal) or (bs, klen, klen)
- bs, qlen, dim = input.size()
- if kv is None:
- klen = qlen if cache is None else cache["slen"] + qlen
- else:
- klen = kv.size(1)
- # assert dim == self.dim, 'Dimensions do not match: %s input vs %s configured' % (dim, self.dim)
- n_heads = self.n_heads
- dim_per_head = self.dim // n_heads
- mask_reshape = (bs, 1, qlen, klen) if mask.dim() == 3 else (bs, 1, 1, klen)
-
- def shape(x):
- """ projection """
- return x.view(bs, -1, self.n_heads, dim_per_head).transpose(1, 2)
-
- def unshape(x):
- """ compute context """
- return x.transpose(1, 2).contiguous().view(bs, -1, self.n_heads * dim_per_head)
-
- q = shape(self.q_lin(input)) # (bs, n_heads, qlen, dim_per_head)
- if kv is None:
- k = shape(self.k_lin(input)) # (bs, n_heads, qlen, dim_per_head)
- v = shape(self.v_lin(input)) # (bs, n_heads, qlen, dim_per_head)
- elif cache is None or self.layer_id not in cache:
- k = v = kv
- k = shape(self.k_lin(k)) # (bs, n_heads, qlen, dim_per_head)
- v = shape(self.v_lin(v)) # (bs, n_heads, qlen, dim_per_head)
-
- if cache is not None:
- if self.layer_id in cache:
- if kv is None:
- k_, v_ = cache[self.layer_id]
- k = torch.cat([k_, k], dim=2) # (bs, n_heads, klen, dim_per_head)
- v = torch.cat([v_, v], dim=2) # (bs, n_heads, klen, dim_per_head)
- else:
- k, v = cache[self.layer_id]
- cache[self.layer_id] = (k, v)
-
- q = q / math.sqrt(dim_per_head) # (bs, n_heads, qlen, dim_per_head)
- scores = torch.matmul(q, k.transpose(2, 3)) # (bs, n_heads, qlen, klen)
- mask = (mask == 0).view(mask_reshape).expand_as(scores) # (bs, n_heads, qlen, klen)
- scores.masked_fill_(mask, -float("inf")) # (bs, n_heads, qlen, klen)
-
- weights = F.softmax(scores.float(), dim=-1).type_as(scores) # (bs, n_heads, qlen, klen)
- weights = F.dropout(weights, p=self.dropout, training=self.training) # (bs, n_heads, qlen, klen)
-
- # Mask heads if we want to
- if head_mask is not None:
- weights = weights * head_mask
-
- context = torch.matmul(weights, v) # (bs, n_heads, qlen, dim_per_head)
- context = unshape(context) # (bs, qlen, dim)
-
- outputs = (self.out_lin(context),)
- if self.output_attentions:
- outputs = outputs + (weights,)
- return outputs
-
-
-class TransformerFFN(nn.Module):
- def __init__(self, in_dim, dim_hidden, out_dim, config):
- super().__init__()
- self.dropout = config.dropout
- self.lin1 = nn.Linear(in_dim, dim_hidden)
- self.lin2 = nn.Linear(dim_hidden, out_dim)
- self.act = gelu if config.gelu_activation else F.relu
-
- def forward(self, input):
- x = self.lin1(input)
- x = self.act(x)
- x = self.lin2(x)
- x = F.dropout(x, p=self.dropout, training=self.training)
- return x
-
-
-class XLMPreTrainedModel(PreTrainedModel):
- """ An abstract class to handle weights initialization and
- a simple interface for downloading and loading pretrained models.
- """
-
- config_class = XLMConfig
- pretrained_model_archive_map = XLM_PRETRAINED_MODEL_ARCHIVE_MAP
- load_tf_weights = None
- base_model_prefix = "transformer"
-
- def __init__(self, *inputs, **kwargs):
- super().__init__(*inputs, **kwargs)
-
- @property
- def dummy_inputs(self):
- inputs_list = torch.tensor([[7, 6, 0, 0, 1], [1, 2, 3, 0, 0], [0, 0, 0, 4, 5]])
- attns_list = torch.tensor([[1, 1, 0, 0, 1], [1, 1, 1, 0, 0], [1, 0, 0, 1, 1]])
- if self.config.use_lang_emb and self.config.n_langs > 1:
- langs_list = torch.tensor([[1, 1, 0, 0, 1], [1, 1, 1, 0, 0], [1, 0, 0, 1, 1]])
- else:
- langs_list = None
- return {"input_ids": inputs_list, "attention_mask": attns_list, "langs": langs_list}
-
- def _init_weights(self, module):
- """ Initialize the weights. """
- if isinstance(module, nn.Embedding):
- if self.config is not None and self.config.embed_init_std is not None:
- nn.init.normal_(module.weight, mean=0, std=self.config.embed_init_std)
- if isinstance(module, nn.Linear):
- if self.config is not None and self.config.init_std is not None:
- nn.init.normal_(module.weight, mean=0, std=self.config.init_std)
- if hasattr(module, "bias") and module.bias is not None:
- nn.init.constant_(module.bias, 0.0)
- if isinstance(module, nn.LayerNorm):
- module.bias.data.zero_()
- module.weight.data.fill_(1.0)
-
-
-XLM_START_DOCSTRING = r"""
-
- This model is a PyTorch `torch.nn.Module `_ sub-class.
- Use it as a regular PyTorch Module and refer to the PyTorch documentation for all matter related to general
- usage and behavior.
-
- Parameters:
- config (:class:`~transformers.XLMConfig`): Model configuration class with all the parameters of the model.
- Initializing with a config file does not load the weights associated with the model, only the configuration.
- Check out the :meth:`~transformers.PreTrainedModel.from_pretrained` method to load the model weights.
-"""
-
-XLM_INPUTS_DOCSTRING = r"""
- Args:
- input_ids (:obj:`torch.LongTensor` of shape :obj:`(batch_size, sequence_length)`):
- Indices of input sequence tokens in the vocabulary.
-
- Indices can be obtained using :class:`transformers.BertTokenizer`.
- See :func:`transformers.PreTrainedTokenizer.encode` and
- :func:`transformers.PreTrainedTokenizer.encode_plus` for details.
-
- `What are input IDs? <../glossary.html#input-ids>`__
- attention_mask (:obj:`torch.FloatTensor` of shape :obj:`(batch_size, sequence_length)`, `optional`, defaults to :obj:`None`):
- Mask to avoid performing attention on padding token indices.
- Mask values selected in ``[0, 1]``:
- ``1`` for tokens that are NOT MASKED, ``0`` for MASKED tokens.
-
- `What are attention masks? <../glossary.html#attention-mask>`__
- langs (:obj:`torch.LongTensor` of shape :obj:`(batch_size, sequence_length)`, `optional`, defaults to :obj:`None`):
- A parallel sequence of tokens to be used to indicate the language of each token in the input.
- Indices are languages ids which can be obtained from the language names by using two conversion mappings
- provided in the configuration of the model (only provided for multilingual models).
- More precisely, the `language name -> language id` mapping is in `model.config.lang2id` (dict str -> int) and
- the `language id -> language name` mapping is `model.config.id2lang` (dict int -> str).
-
- See usage examples detailed in the `multilingual documentation `__.
- token_type_ids (:obj:`torch.LongTensor` of shape :obj:`(batch_size, sequence_length)`, `optional`, defaults to :obj:`None`):
- Segment token indices to indicate first and second portions of the inputs.
- Indices are selected in ``[0, 1]``: ``0`` corresponds to a `sentence A` token, ``1``
- corresponds to a `sentence B` token
-
- `What are token type IDs? <../glossary.html#token-type-ids>`_
- position_ids (:obj:`torch.LongTensor` of shape :obj:`(batch_size, sequence_length)`, `optional`, defaults to :obj:`None`):
- Indices of positions of each input sequence tokens in the position embeddings.
- Selected in the range ``[0, config.max_position_embeddings - 1]``.
-
- `What are position IDs? <../glossary.html#position-ids>`_
- lengths (:obj:`torch.LongTensor` of shape :obj:`(batch_size,)`, `optional`, defaults to :obj:`None`):
- Length of each sentence that can be used to avoid performing attention on padding token indices.
- You can also use `attention_mask` for the same result (see above), kept here for compatbility.
- Indices selected in ``[0, ..., input_ids.size(-1)]``:
- cache (:obj:`Dict[str, torch.FloatTensor]`, `optional`, defaults to :obj:`None`):
- dictionary with ``torch.FloatTensor`` that contains pre-computed
- hidden-states (key and values in the attention blocks) as computed by the model
- (see `cache` output below). Can be used to speed up sequential decoding.
- The dictionary object will be modified in-place during the forward pass to add newly computed hidden-states.
- head_mask (:obj:`torch.FloatTensor` of shape :obj:`(num_heads,)` or :obj:`(num_layers, num_heads)`, `optional`, defaults to :obj:`None`):
- Mask to nullify selected heads of the self-attention modules.
- Mask values selected in ``[0, 1]``:
- :obj:`1` indicates the head is **not masked**, :obj:`0` indicates the head is **masked**.
- input_embeds (:obj:`torch.FloatTensor` of shape :obj:`(batch_size, sequence_length, hidden_size)`, `optional`, defaults to :obj:`None`):
- Optionally, instead of passing :obj:`input_ids` you can choose to directly pass an embedded representation.
- This is useful if you want more control over how to convert `input_ids` indices into associated vectors
- than the model's internal embedding lookup matrix.
-"""
-
-
-@add_start_docstrings(
- "The bare XLM Model transformer outputting raw hidden-states without any specific head on top.",
- XLM_START_DOCSTRING,
-)
-class XLMModel(XLMPreTrainedModel):
- def __init__(self, config): # , dico, is_encoder, with_output):
- super().__init__(config)
- self.output_attentions = config.output_attentions
- self.output_hidden_states = config.output_hidden_states
-
- # encoder / decoder, output layer
- self.is_encoder = config.is_encoder
- self.is_decoder = not config.is_encoder
- if self.is_decoder:
- raise NotImplementedError("Currently XLM can only be used as an encoder")
- # self.with_output = with_output
- self.causal = config.causal
-
- # dictionary / languages
- self.n_langs = config.n_langs
- self.use_lang_emb = config.use_lang_emb
- self.n_words = config.n_words
- self.eos_index = config.eos_index
- self.pad_index = config.pad_index
- # self.dico = dico
- # self.id2lang = config.id2lang
- # self.lang2id = config.lang2id
- # assert len(self.dico) == self.n_words
- # assert len(self.id2lang) == len(self.lang2id) == self.n_langs
-
- # model parameters
- self.dim = config.emb_dim # 512 by default
- self.hidden_dim = self.dim * 4 # 2048 by default
- self.n_heads = config.n_heads # 8 by default
- self.n_layers = config.n_layers
- self.dropout = config.dropout
- self.attention_dropout = config.attention_dropout
- assert self.dim % self.n_heads == 0, "transformer dim must be a multiple of n_heads"
-
- # embeddings
- self.position_embeddings = nn.Embedding(config.max_position_embeddings, self.dim)
- if config.sinusoidal_embeddings:
- create_sinusoidal_embeddings(config.max_position_embeddings, self.dim, out=self.position_embeddings.weight)
- if config.n_langs > 1 and config.use_lang_emb:
- self.lang_embeddings = nn.Embedding(self.n_langs, self.dim)
- self.embeddings = nn.Embedding(self.n_words, self.dim, padding_idx=self.pad_index)
- self.layer_norm_emb = nn.LayerNorm(self.dim, eps=config.layer_norm_eps)
-
- # transformer layers
- self.attentions = nn.ModuleList()
- self.layer_norm1 = nn.ModuleList()
- self.ffns = nn.ModuleList()
- self.layer_norm2 = nn.ModuleList()
- # if self.is_decoder:
- # self.layer_norm15 = nn.ModuleList()
- # self.encoder_attn = nn.ModuleList()
-
- for _ in range(self.n_layers):
- self.attentions.append(MultiHeadAttention(self.n_heads, self.dim, config=config))
- self.layer_norm1.append(nn.LayerNorm(self.dim, eps=config.layer_norm_eps))
- # if self.is_decoder:
- # self.layer_norm15.append(nn.LayerNorm(self.dim, eps=config.layer_norm_eps))
- # self.encoder_attn.append(MultiHeadAttention(self.n_heads, self.dim, dropout=self.attention_dropout))
- self.ffns.append(TransformerFFN(self.dim, self.hidden_dim, self.dim, config=config))
- self.layer_norm2.append(nn.LayerNorm(self.dim, eps=config.layer_norm_eps))
-
- if hasattr(config, "pruned_heads"):
- pruned_heads = config.pruned_heads.copy().items()
- config.pruned_heads = {}
- for layer, heads in pruned_heads:
- if self.attentions[int(layer)].n_heads == config.n_heads:
- self.prune_heads({int(layer): list(map(int, heads))})
-
- self.init_weights()
-
- def get_input_embeddings(self):
- return self.embeddings
-
- def set_input_embeddings(self, new_embeddings):
- self.embeddings = new_embeddings
-
- def _prune_heads(self, heads_to_prune):
- """ Prunes heads of the model.
- heads_to_prune: dict of {layer_num: list of heads to prune in this layer}
- See base class PreTrainedModel
- """
- for layer, heads in heads_to_prune.items():
- self.attentions[layer].prune_heads(heads)
-
- @add_start_docstrings_to_callable(XLM_INPUTS_DOCSTRING)
- def forward(
- self,
- input_ids=None,
- attention_mask=None,
- langs=None,
- token_type_ids=None,
- position_ids=None,
- lengths=None,
- cache=None,
- head_mask=None,
- inputs_embeds=None,
- ):
- r"""
- Return:
- :obj:`tuple(torch.FloatTensor)` comprising various elements depending on the configuration (:class:`~transformers.XLMConfig`) and inputs:
- last_hidden_state (:obj:`torch.FloatTensor` of shape :obj:`(batch_size, sequence_length, hidden_size)`):
- Sequence of hidden-states at the output of the last layer of the model.
- hidden_states (:obj:`tuple(torch.FloatTensor)`, `optional`, returned when ``config.output_hidden_states=True``):
- Tuple of :obj:`torch.FloatTensor` (one for the output of the embeddings + one for the output of each layer)
- of shape :obj:`(batch_size, sequence_length, hidden_size)`.
-
- Hidden-states of the model at the output of each layer plus the initial embedding outputs.
- attentions (:obj:`tuple(torch.FloatTensor)`, `optional`, returned when ``config.output_attentions=True``):
- Tuple of :obj:`torch.FloatTensor` (one for each layer) of shape
- :obj:`(batch_size, num_heads, sequence_length, sequence_length)`.
-
- Attentions weights after the attention softmax, used to compute the weighted average in the self-attention
- heads.
-
- Examples::
-
- from transformers import XLMTokenizer, XLMModel
- import torch
-
- tokenizer = XLMTokenizer.from_pretrained('xlm-mlm-en-2048')
- model = XLMModel.from_pretrained('xlm-mlm-en-2048')
- input_ids = torch.tensor(tokenizer.encode("Hello, my dog is cute", add_special_tokens=True)).unsqueeze(0) # Batch size 1
- outputs = model(input_ids)
- last_hidden_states = outputs[0] # The last hidden-state is the first element of the output tuple
-
- """
- if input_ids is not None:
- bs, slen = input_ids.size()
- else:
- bs, slen = inputs_embeds.size()[:-1]
-
- if lengths is None:
- if input_ids is not None:
- lengths = (input_ids != self.pad_index).sum(dim=1).long()
- else:
- lengths = torch.LongTensor([slen] * bs)
- # mask = input_ids != self.pad_index
-
- # check inputs
- assert lengths.size(0) == bs
- assert lengths.max().item() <= slen
- # input_ids = input_ids.transpose(0, 1) # batch size as dimension 0
- # assert (src_enc is None) == (src_len is None)
- # if src_enc is not None:
- # assert self.is_decoder
- # assert src_enc.size(0) == bs
-
- # generate masks
- mask, attn_mask = get_masks(slen, lengths, self.causal, padding_mask=attention_mask)
- # if self.is_decoder and src_enc is not None:
- # src_mask = torch.arange(src_len.max(), dtype=torch.long, device=lengths.device) < src_len[:, None]
-
- device = input_ids.device if input_ids is not None else inputs_embeds.device
-
- # position_ids
- if position_ids is None:
- position_ids = torch.arange(slen, dtype=torch.long, device=device)
- position_ids = position_ids.unsqueeze(0).expand((bs, slen))
- else:
- assert position_ids.size() == (bs, slen) # (slen, bs)
- # position_ids = position_ids.transpose(0, 1)
-
- # langs
- if langs is not None:
- assert langs.size() == (bs, slen) # (slen, bs)
- # langs = langs.transpose(0, 1)
-
- # Prepare head mask if needed
- # 1.0 in head_mask indicate we keep the head
- # attention_probs has shape bsz x n_heads x N x N
- # input head_mask has shape [num_heads] or [num_hidden_layers x num_heads]
- # and head_mask is converted to shape [num_hidden_layers x batch x num_heads x qlen x klen]
- if head_mask is not None:
- if head_mask.dim() == 1:
- head_mask = head_mask.unsqueeze(0).unsqueeze(0).unsqueeze(-1).unsqueeze(-1)
- head_mask = head_mask.expand(self.n_layers, -1, -1, -1, -1)
- elif head_mask.dim() == 2:
- head_mask = (
- head_mask.unsqueeze(1).unsqueeze(-1).unsqueeze(-1)
- ) # We can specify head_mask for each layer
- head_mask = head_mask.to(
- dtype=next(self.parameters()).dtype
- ) # switch to fload if need + fp16 compatibility
- else:
- head_mask = [None] * self.n_layers
-
- # do not recompute cached elements
- if cache is not None and input_ids is not None:
- _slen = slen - cache["slen"]
- input_ids = input_ids[:, -_slen:]
- position_ids = position_ids[:, -_slen:]
- if langs is not None:
- langs = langs[:, -_slen:]
- mask = mask[:, -_slen:]
- attn_mask = attn_mask[:, -_slen:]
-
- # embeddings
- if inputs_embeds is None:
- inputs_embeds = self.embeddings(input_ids)
-
- tensor = inputs_embeds + self.position_embeddings(position_ids).expand_as(inputs_embeds)
- if langs is not None and self.use_lang_emb:
- tensor = tensor + self.lang_embeddings(langs)
- if token_type_ids is not None:
- tensor = tensor + self.embeddings(token_type_ids)
- tensor = self.layer_norm_emb(tensor)
- tensor = F.dropout(tensor, p=self.dropout, training=self.training)
- tensor *= mask.unsqueeze(-1).to(tensor.dtype)
-
- # transformer layers
- hidden_states = ()
- attentions = ()
- for i in range(self.n_layers):
- if self.output_hidden_states:
- hidden_states = hidden_states + (tensor,)
-
- # self attention
- attn_outputs = self.attentions[i](tensor, attn_mask, cache=cache, head_mask=head_mask[i])
- attn = attn_outputs[0]
- if self.output_attentions:
- attentions = attentions + (attn_outputs[1],)
- attn = F.dropout(attn, p=self.dropout, training=self.training)
- tensor = tensor + attn
- tensor = self.layer_norm1[i](tensor)
-
- # encoder attention (for decoder only)
- # if self.is_decoder and src_enc is not None:
- # attn = self.encoder_attn[i](tensor, src_mask, kv=src_enc, cache=cache)
- # attn = F.dropout(attn, p=self.dropout, training=self.training)
- # tensor = tensor + attn
- # tensor = self.layer_norm15[i](tensor)
-
- # FFN
- tensor = tensor + self.ffns[i](tensor)
- tensor = self.layer_norm2[i](tensor)
- tensor *= mask.unsqueeze(-1).to(tensor.dtype)
-
- # Add last hidden state
- if self.output_hidden_states:
- hidden_states = hidden_states + (tensor,)
-
- # update cache length
- if cache is not None:
- cache["slen"] += tensor.size(1)
-
- # move back sequence length to dimension 0
- # tensor = tensor.transpose(0, 1)
-
- outputs = (tensor,)
- if self.output_hidden_states:
- outputs = outputs + (hidden_states,)
- if self.output_attentions:
- outputs = outputs + (attentions,)
- return outputs # outputs, (hidden_states), (attentions)
-
-
-class XLMPredLayer(nn.Module):
- """
- Prediction layer (cross_entropy or adaptive_softmax).
- """
-
- def __init__(self, config):
- super().__init__()
- self.asm = config.asm
- self.n_words = config.n_words
- self.pad_index = config.pad_index
- dim = config.emb_dim
-
- if config.asm is False:
- self.proj = nn.Linear(dim, config.n_words, bias=True)
- else:
- self.proj = nn.AdaptiveLogSoftmaxWithLoss(
- in_features=dim,
- n_classes=config.n_words,
- cutoffs=config.asm_cutoffs,
- div_value=config.asm_div_value,
- head_bias=True, # default is False
- )
-
- def forward(self, x, y=None):
- """ Compute the loss, and optionally the scores.
- """
- outputs = ()
- if self.asm is False:
- scores = self.proj(x)
- outputs = (scores,) + outputs
- if y is not None:
- loss = F.cross_entropy(scores.view(-1, self.n_words), y.view(-1), reduction="elementwise_mean")
- outputs = (loss,) + outputs
- else:
- scores = self.proj.log_prob(x)
- outputs = (scores,) + outputs
- if y is not None:
- _, loss = self.proj(x, y)
- outputs = (loss,) + outputs
-
- return outputs
-
-
-@add_start_docstrings(
- """The XLM Model transformer with a language modeling head on top
- (linear layer with weights tied to the input embeddings). """,
- XLM_START_DOCSTRING,
-)
-class XLMWithLMHeadModel(XLMPreTrainedModel):
- def __init__(self, config):
- super().__init__(config)
- self.transformer = XLMModel(config)
- self.pred_layer = XLMPredLayer(config)
-
- self.init_weights()
-
- def get_output_embeddings(self):
- return self.pred_layer.proj
-
- def prepare_inputs_for_generation(self, input_ids, **kwargs):
- mask_token_id = self.config.mask_token_id
- lang_id = self.config.lang_id
-
- effective_batch_size = input_ids.shape[0]
- mask_token = torch.full((effective_batch_size, 1), mask_token_id, dtype=torch.long, device=input_ids.device)
- input_ids = torch.cat([input_ids, mask_token], dim=1)
- if lang_id is not None:
- langs = torch.full_like(input_ids, lang_id)
- else:
- langs = None
- return {"input_ids": input_ids, "langs": langs}
-
- @add_start_docstrings_to_callable(XLM_INPUTS_DOCSTRING)
- def forward(
- self,
- input_ids=None,
- attention_mask=None,
- langs=None,
- token_type_ids=None,
- position_ids=None,
- lengths=None,
- cache=None,
- head_mask=None,
- inputs_embeds=None,
- labels=None,
- ):
- r"""
- labels (:obj:`torch.LongTensor` of shape :obj:`(batch_size, sequence_length)`, `optional`, defaults to :obj:`None`):
- Labels for language modeling.
- Note that the labels **are shifted** inside the model, i.e. you can set ``lm_labels = input_ids``
- Indices are selected in ``[-100, 0, ..., config.vocab_size]``
- All labels set to ``-100`` are ignored (masked), the loss is only
- computed for labels in ``[0, ..., config.vocab_size]``
-
- Return:
- :obj:`tuple(torch.FloatTensor)` comprising various elements depending on the configuration (:class:`~transformers.XLMConfig`) and inputs:
- loss (:obj:`torch.FloatTensor` of shape `(1,)`, `optional`, returned when ``labels`` is provided)
- Language modeling loss.
- prediction_scores (:obj:`torch.FloatTensor` of shape :obj:`(batch_size, sequence_length, config.vocab_size)`):
- Prediction scores of the language modeling head (scores for each vocabulary token before SoftMax).
- hidden_states (:obj:`tuple(torch.FloatTensor)`, `optional`, returned when ``config.output_hidden_states=True``):
- Tuple of :obj:`torch.FloatTensor` (one for the output of the embeddings + one for the output of each layer)
- of shape :obj:`(batch_size, sequence_length, hidden_size)`.
-
- Hidden-states of the model at the output of each layer plus the initial embedding outputs.
- attentions (:obj:`tuple(torch.FloatTensor)`, `optional`, returned when ``config.output_attentions=True``):
- Tuple of :obj:`torch.FloatTensor` (one for each layer) of shape
- :obj:`(batch_size, num_heads, sequence_length, sequence_length)`.
-
- Attentions weights after the attention softmax, used to compute the weighted average in the self-attention
- heads.
-
- Examples::
-
- from transformers import XLMTokenizer, XLMWithLMHeadModel
- import torch
-
- tokenizer = XLMTokenizer.from_pretrained('xlm-mlm-en-2048')
- model = XLMWithLMHeadModel.from_pretrained('xlm-mlm-en-2048')
- input_ids = torch.tensor(tokenizer.encode("Hello, my dog is cute", add_special_tokens=True)).unsqueeze(0) # Batch size 1
- outputs = model(input_ids)
- last_hidden_states = outputs[0] # The last hidden-state is the first element of the output tuple
-
- """
- transformer_outputs = self.transformer(
- input_ids,
- attention_mask=attention_mask,
- langs=langs,
- token_type_ids=token_type_ids,
- position_ids=position_ids,
- lengths=lengths,
- cache=cache,
- head_mask=head_mask,
- inputs_embeds=inputs_embeds,
- )
-
- output = transformer_outputs[0]
- outputs = self.pred_layer(output, labels)
- outputs = outputs + transformer_outputs[1:] # Keep new_mems and attention/hidden states if they are here
-
- return outputs
-
-
-@add_start_docstrings(
- """XLM Model with a sequence classification/regression head on top (a linear layer on top of
- the pooled output) e.g. for GLUE tasks. """,
- XLM_START_DOCSTRING,
-)
-class XLMForSequenceClassification(XLMPreTrainedModel):
- def __init__(self, config):
- super().__init__(config)
- self.num_labels = config.num_labels
-
- self.transformer = XLMModel(config)
- self.sequence_summary = SequenceSummary(config)
-
- self.init_weights()
-
- @add_start_docstrings_to_callable(XLM_INPUTS_DOCSTRING)
- def forward(
- self,
- input_ids=None,
- attention_mask=None,
- langs=None,
- token_type_ids=None,
- position_ids=None,
- lengths=None,
- cache=None,
- head_mask=None,
- inputs_embeds=None,
- labels=None,
- ):
- r"""
- labels (:obj:`torch.LongTensor` of shape :obj:`(batch_size,)`, `optional`, defaults to :obj:`None`):
- Labels for computing the sequence classification/regression loss.
- Indices should be in :obj:`[0, ..., config.num_labels - 1]`.
- If :obj:`config.num_labels == 1` a regression loss is computed (Mean-Square loss),
- If :obj:`config.num_labels > 1` a classification loss is computed (Cross-Entropy).
-
- Returns:
- :obj:`tuple(torch.FloatTensor)` comprising various elements depending on the configuration (:class:`~transformers.XLMConfig`) and inputs:
- loss (:obj:`torch.FloatTensor` of shape :obj:`(1,)`, `optional`, returned when :obj:`label` is provided):
- Classification (or regression if config.num_labels==1) loss.
- logits (:obj:`torch.FloatTensor` of shape :obj:`(batch_size, config.num_labels)`):
- Classification (or regression if config.num_labels==1) scores (before SoftMax).
- hidden_states (:obj:`tuple(torch.FloatTensor)`, `optional`, returned when ``config.output_hidden_states=True``):
- Tuple of :obj:`torch.FloatTensor` (one for the output of the embeddings + one for the output of each layer)
- of shape :obj:`(batch_size, sequence_length, hidden_size)`.
-
- Hidden-states of the model at the output of each layer plus the initial embedding outputs.
- attentions (:obj:`tuple(torch.FloatTensor)`, `optional`, returned when ``config.output_attentions=True``):
- Tuple of :obj:`torch.FloatTensor` (one for each layer) of shape
- :obj:`(batch_size, num_heads, sequence_length, sequence_length)`.
-
- Attentions weights after the attention softmax, used to compute the weighted average in the self-attention
- heads.
-
- Examples::
-
- from transformers import XLMTokenizer, XLMForSequenceClassification
- import torch
-
- tokenizer = XLMTokenizer.from_pretrained('xlm-mlm-en-2048')
- model = XLMForSequenceClassification.from_pretrained('xlm-mlm-en-2048')
- input_ids = torch.tensor(tokenizer.encode("Hello, my dog is cute", add_special_tokens=True)).unsqueeze(0) # Batch size 1
- labels = torch.tensor([1]).unsqueeze(0) # Batch size 1
- outputs = model(input_ids, labels=labels)
- loss, logits = outputs[:2]
-
- """
- transformer_outputs = self.transformer(
- input_ids,
- attention_mask=attention_mask,
- langs=langs,
- token_type_ids=token_type_ids,
- position_ids=position_ids,
- lengths=lengths,
- cache=cache,
- head_mask=head_mask,
- inputs_embeds=inputs_embeds,
- )
-
- output = transformer_outputs[0]
- logits = self.sequence_summary(output)
-
- outputs = (logits,) + transformer_outputs[1:] # Keep new_mems and attention/hidden states if they are here
-
- if labels is not None:
- if self.num_labels == 1:
- # We are doing regression
- loss_fct = MSELoss()
- loss = loss_fct(logits.view(-1), labels.view(-1))
- else:
- loss_fct = CrossEntropyLoss()
- loss = loss_fct(logits.view(-1, self.num_labels), labels.view(-1))
- outputs = (loss,) + outputs
-
- return outputs
-
-
-@add_start_docstrings(
- """XLM Model with a span classification head on top for extractive question-answering tasks like SQuAD (a linear layers on top of
- the hidden-states output to compute `span start logits` and `span end logits`). """,
- XLM_START_DOCSTRING,
-)
-class XLMForQuestionAnsweringSimple(XLMPreTrainedModel):
- def __init__(self, config):
- super().__init__(config)
-
- self.transformer = XLMModel(config)
- self.qa_outputs = nn.Linear(config.hidden_size, config.num_labels)
-
- self.init_weights()
-
- @add_start_docstrings_to_callable(XLM_INPUTS_DOCSTRING)
- def forward(
- self,
- input_ids=None,
- attention_mask=None,
- langs=None,
- token_type_ids=None,
- position_ids=None,
- lengths=None,
- cache=None,
- head_mask=None,
- inputs_embeds=None,
- start_positions=None,
- end_positions=None,
- ):
- r"""
- start_positions (:obj:`torch.LongTensor` of shape :obj:`(batch_size,)`, `optional`, defaults to :obj:`None`):
- Labels for position (index) of the start of the labelled span for computing the token classification loss.
- Positions are clamped to the length of the sequence (`sequence_length`).
- Position outside of the sequence are not taken into account for computing the loss.
- end_positions (:obj:`torch.LongTensor` of shape :obj:`(batch_size,)`, `optional`, defaults to :obj:`None`):
- Labels for position (index) of the end of the labelled span for computing the token classification loss.
- Positions are clamped to the length of the sequence (`sequence_length`).
- Position outside of the sequence are not taken into account for computing the loss.
-
- Returns:
- :obj:`tuple(torch.FloatTensor)` comprising various elements depending on the configuration (:class:`~transformers.XLMConfig`) and inputs:
- loss (:obj:`torch.FloatTensor` of shape :obj:`(1,)`, `optional`, returned when :obj:`labels` is provided):
- Total span extraction loss is the sum of a Cross-Entropy for the start and end positions.
- start_scores (:obj:`torch.FloatTensor` of shape :obj:`(batch_size, sequence_length,)`):
- Span-start scores (before SoftMax).
- end_scores (:obj:`torch.FloatTensor` of shape :obj:`(batch_size, sequence_length,)`):
- Span-end scores (before SoftMax).
- hidden_states (:obj:`tuple(torch.FloatTensor)`, `optional`, returned when ``config.output_hidden_states=True``):
- Tuple of :obj:`torch.FloatTensor` (one for the output of the embeddings + one for the output of each layer)
- of shape :obj:`(batch_size, sequence_length, hidden_size)`.
-
- Hidden-states of the model at the output of each layer plus the initial embedding outputs.
- attentions (:obj:`tuple(torch.FloatTensor)`, `optional`, returned when ``config.output_attentions=True``):
- Tuple of :obj:`torch.FloatTensor` (one for each layer) of shape
- :obj:`(batch_size, num_heads, sequence_length, sequence_length)`.
-
- Attentions weights after the attention softmax, used to compute the weighted average in the self-attention
- heads.
-
- Examples::
-
- from transformers import XLMTokenizer, XLMForQuestionAnsweringSimple
- import torch
-
- tokenizer = XLMTokenizer.from_pretrained('xlm-mlm-en-2048')
- model = XLMForQuestionAnsweringSimple.from_pretrained('xlm-mlm-en-2048')
- input_ids = torch.tensor(tokenizer.encode("Hello, my dog is cute", add_special_tokens=True)).unsqueeze(0) # Batch size 1
- start_positions = torch.tensor([1])
- end_positions = torch.tensor([3])
- outputs = model(input_ids, start_positions=start_positions, end_positions=end_positions)
- loss = outputs[0]
-
- """
- transformer_outputs = self.transformer(
- input_ids,
- attention_mask=attention_mask,
- langs=langs,
- token_type_ids=token_type_ids,
- position_ids=position_ids,
- lengths=lengths,
- cache=cache,
- head_mask=head_mask,
- inputs_embeds=inputs_embeds,
- )
-
- sequence_output = transformer_outputs[0]
-
- logits = self.qa_outputs(sequence_output)
- start_logits, end_logits = logits.split(1, dim=-1)
- start_logits = start_logits.squeeze(-1)
- end_logits = end_logits.squeeze(-1)
-
- outputs = (
- start_logits,
- end_logits,
- )
- if start_positions is not None and end_positions is not None:
- # If we are on multi-GPU, split add a dimension
- if len(start_positions.size()) > 1:
- start_positions = start_positions.squeeze(-1)
- if len(end_positions.size()) > 1:
- end_positions = end_positions.squeeze(-1)
- # sometimes the start/end positions are outside our model inputs, we ignore these terms
- ignored_index = start_logits.size(1)
- start_positions.clamp_(0, ignored_index)
- end_positions.clamp_(0, ignored_index)
-
- loss_fct = CrossEntropyLoss(ignore_index=ignored_index)
- start_loss = loss_fct(start_logits, start_positions)
- end_loss = loss_fct(end_logits, end_positions)
- total_loss = (start_loss + end_loss) / 2
- outputs = (total_loss,) + outputs
-
- outputs = outputs + transformer_outputs[1:] # Keep new_mems and attention/hidden states if they are here
-
- return outputs
-
-
-@add_start_docstrings(
- """XLM Model with a beam-search span classification head on top for extractive question-answering tasks like SQuAD (a linear layers on top of
- the hidden-states output to compute `span start logits` and `span end logits`). """,
- XLM_START_DOCSTRING,
-)
-class XLMForQuestionAnswering(XLMPreTrainedModel):
- def __init__(self, config):
- super().__init__(config)
-
- self.transformer = XLMModel(config)
- self.qa_outputs = SQuADHead(config)
-
- self.init_weights()
-
- @add_start_docstrings_to_callable(XLM_INPUTS_DOCSTRING)
- def forward(
- self,
- input_ids=None,
- attention_mask=None,
- langs=None,
- token_type_ids=None,
- position_ids=None,
- lengths=None,
- cache=None,
- head_mask=None,
- inputs_embeds=None,
- start_positions=None,
- end_positions=None,
- is_impossible=None,
- cls_index=None,
- p_mask=None,
- ):
- r"""
- start_positions (:obj:`torch.LongTensor` of shape :obj:`(batch_size,)`, `optional`, defaults to :obj:`None`):
- Labels for position (index) of the start of the labelled span for computing the token classification loss.
- Positions are clamped to the length of the sequence (`sequence_length`).
- Position outside of the sequence are not taken into account for computing the loss.
- end_positions (:obj:`torch.LongTensor` of shape :obj:`(batch_size,)`, `optional`, defaults to :obj:`None`):
- Labels for position (index) of the end of the labelled span for computing the token classification loss.
- Positions are clamped to the length of the sequence (`sequence_length`).
- Position outside of the sequence are not taken into account for computing the loss.
- is_impossible (``torch.LongTensor`` of shape ``(batch_size,)``, `optional`, defaults to :obj:`None`):
- Labels whether a question has an answer or no answer (SQuAD 2.0)
- cls_index (``torch.LongTensor`` of shape ``(batch_size,)``, `optional`, defaults to :obj:`None`):
- Labels for position (index) of the classification token to use as input for computing plausibility of the answer.
- p_mask (``torch.FloatTensor`` of shape ``(batch_size, sequence_length)``, `optional`, defaults to :obj:`None`):
- Optional mask of tokens which can't be in answers (e.g. [CLS], [PAD], ...).
- 1.0 means token should be masked. 0.0 mean token is not masked.
-
- Returns:
- :obj:`tuple(torch.FloatTensor)` comprising various elements depending on the configuration (:class:`~transformers.XLMConfig`) and inputs:
- loss (:obj:`torch.FloatTensor` of shape :obj:`(1,)`, `optional`, returned if both :obj:`start_positions` and :obj:`end_positions` are provided):
- Classification loss as the sum of start token, end token (and is_impossible if provided) classification losses.
- start_top_log_probs (``torch.FloatTensor`` of shape ``(batch_size, config.start_n_top)``, `optional`, returned if ``start_positions`` or ``end_positions`` is not provided):
- Log probabilities for the top config.start_n_top start token possibilities (beam-search).
- start_top_index (``torch.LongTensor`` of shape ``(batch_size, config.start_n_top)``, `optional`, returned if ``start_positions`` or ``end_positions`` is not provided):
- Indices for the top config.start_n_top start token possibilities (beam-search).
- end_top_log_probs (``torch.FloatTensor`` of shape ``(batch_size, config.start_n_top * config.end_n_top)``, `optional`, returned if ``start_positions`` or ``end_positions`` is not provided):
- Log probabilities for the top ``config.start_n_top * config.end_n_top`` end token possibilities (beam-search).
- end_top_index (``torch.LongTensor`` of shape ``(batch_size, config.start_n_top * config.end_n_top)``, `optional`, returned if ``start_positions`` or ``end_positions`` is not provided):
- Indices for the top ``config.start_n_top * config.end_n_top`` end token possibilities (beam-search).
- cls_logits (``torch.FloatTensor`` of shape ``(batch_size,)``, `optional`, returned if ``start_positions`` or ``end_positions`` is not provided):
- Log probabilities for the ``is_impossible`` label of the answers.
- hidden_states (:obj:`tuple(torch.FloatTensor)`, `optional`, returned when ``config.output_hidden_states=True``):
- Tuple of :obj:`torch.FloatTensor` (one for the output of the embeddings + one for the output of each layer)
- of shape :obj:`(batch_size, sequence_length, hidden_size)`.
-
- Hidden-states of the model at the output of each layer plus the initial embedding outputs.
- attentions (:obj:`tuple(torch.FloatTensor)`, `optional`, returned when ``config.output_attentions=True``):
- Tuple of :obj:`torch.FloatTensor` (one for each layer) of shape
- :obj:`(batch_size, num_heads, sequence_length, sequence_length)`.
-
- Attentions weights after the attention softmax, used to compute the weighted average in the self-attention
- heads.
-
- Examples::
-
- from transformers import XLMTokenizer, XLMForQuestionAnswering
- import torch
-
- tokenizer = XLMTokenizer.from_pretrained('xlm-mlm-en-2048')
- model = XLMForQuestionAnswering.from_pretrained('xlm-mlm-en-2048')
- input_ids = torch.tensor(tokenizer.encode("Hello, my dog is cute", add_special_tokens=True)).unsqueeze(0) # Batch size 1
- start_positions = torch.tensor([1])
- end_positions = torch.tensor([3])
- outputs = model(input_ids, start_positions=start_positions, end_positions=end_positions)
- loss = outputs[0]
-
- """
- transformer_outputs = self.transformer(
- input_ids,
- attention_mask=attention_mask,
- langs=langs,
- token_type_ids=token_type_ids,
- position_ids=position_ids,
- lengths=lengths,
- cache=cache,
- head_mask=head_mask,
- inputs_embeds=inputs_embeds,
- )
-
- output = transformer_outputs[0]
-
- outputs = self.qa_outputs(
- output,
- start_positions=start_positions,
- end_positions=end_positions,
- cls_index=cls_index,
- is_impossible=is_impossible,
- p_mask=p_mask,
- )
-
- outputs = outputs + transformer_outputs[1:] # Keep new_mems and attention/hidden states if they are here
-
- return outputs
diff --git a/server/transformers/src/transformers/modeling_xlm_roberta.py b/server/transformers/src/transformers/modeling_xlm_roberta.py
deleted file mode 100644
index c00a2eb4f5dc283315bd32fe1913f1c84405d089..0000000000000000000000000000000000000000
--- a/server/transformers/src/transformers/modeling_xlm_roberta.py
+++ /dev/null
@@ -1,126 +0,0 @@
-# coding=utf-8
-# Copyright 2019 Facebook AI Research and the HuggingFace Inc. team.
-# Copyright (c) 2018, NVIDIA CORPORATION. All rights reserved.
-#
-# Licensed under the Apache License, Version 2.0 (the "License");
-# you may not use this file except in compliance with the License.
-# You may obtain a copy of the License at
-#
-# http://www.apache.org/licenses/LICENSE-2.0
-#
-# Unless required by applicable law or agreed to in writing, software
-# distributed under the License is distributed on an "AS IS" BASIS,
-# WITHOUT WARRANTIES OR CONDITIONS OF ANY KIND, either express or implied.
-# See the License for the specific language governing permissions and
-# limitations under the License.
-"""PyTorch XLM-RoBERTa model. """
-
-
-import logging
-
-from .configuration_xlm_roberta import XLMRobertaConfig
-from .file_utils import add_start_docstrings
-from .modeling_roberta import (
- RobertaForMaskedLM,
- RobertaForMultipleChoice,
- RobertaForSequenceClassification,
- RobertaForTokenClassification,
- RobertaModel,
-)
-
-
-logger = logging.getLogger(__name__)
-
-XLM_ROBERTA_PRETRAINED_MODEL_ARCHIVE_MAP = {
- "xlm-roberta-base": "https://s3.amazonaws.com/models.huggingface.co/bert/xlm-roberta-base-pytorch_model.bin",
- "xlm-roberta-large": "https://s3.amazonaws.com/models.huggingface.co/bert/xlm-roberta-large-pytorch_model.bin",
- "xlm-roberta-large-finetuned-conll02-dutch": "https://s3.amazonaws.com/models.huggingface.co/bert/xlm-roberta-large-finetuned-conll02-dutch-pytorch_model.bin",
- "xlm-roberta-large-finetuned-conll02-spanish": "https://s3.amazonaws.com/models.huggingface.co/bert/xlm-roberta-large-finetuned-conll02-spanish-pytorch_model.bin",
- "xlm-roberta-large-finetuned-conll03-english": "https://s3.amazonaws.com/models.huggingface.co/bert/xlm-roberta-large-finetuned-conll03-english-pytorch_model.bin",
- "xlm-roberta-large-finetuned-conll03-german": "https://s3.amazonaws.com/models.huggingface.co/bert/xlm-roberta-large-finetuned-conll03-german-pytorch_model.bin",
-}
-
-
-XLM_ROBERTA_START_DOCSTRING = r"""
-
- This model is a PyTorch `torch.nn.Module `_ sub-class.
- Use it as a regular PyTorch Module and refer to the PyTorch documentation for all matter related to general
- usage and behavior.
-
- Parameters:
- config (:class:`~transformers.XLMRobertaConfig`): Model configuration class with all the parameters of the
- model. Initializing with a config file does not load the weights associated with the model, only the configuration.
- Check out the :meth:`~transformers.PreTrainedModel.from_pretrained` method to load the model weights.
-"""
-
-
-@add_start_docstrings(
- "The bare XLM-RoBERTa Model transformer outputting raw hidden-states without any specific head on top.",
- XLM_ROBERTA_START_DOCSTRING,
-)
-class XLMRobertaModel(RobertaModel):
- """
- This class overrides :class:`~transformers.RobertaModel`. Please check the
- superclass for the appropriate documentation alongside usage examples.
- """
-
- config_class = XLMRobertaConfig
- pretrained_model_archive_map = XLM_ROBERTA_PRETRAINED_MODEL_ARCHIVE_MAP
-
-
-@add_start_docstrings(
- """XLM-RoBERTa Model with a `language modeling` head on top. """, XLM_ROBERTA_START_DOCSTRING,
-)
-class XLMRobertaForMaskedLM(RobertaForMaskedLM):
- """
- This class overrides :class:`~transformers.RobertaForMaskedLM`. Please check the
- superclass for the appropriate documentation alongside usage examples.
- """
-
- config_class = XLMRobertaConfig
- pretrained_model_archive_map = XLM_ROBERTA_PRETRAINED_MODEL_ARCHIVE_MAP
-
-
-@add_start_docstrings(
- """XLM-RoBERTa Model transformer with a sequence classification/regression head on top (a linear layer
- on top of the pooled output) e.g. for GLUE tasks. """,
- XLM_ROBERTA_START_DOCSTRING,
-)
-class XLMRobertaForSequenceClassification(RobertaForSequenceClassification):
- """
- This class overrides :class:`~transformers.RobertaForSequenceClassification`. Please check the
- superclass for the appropriate documentation alongside usage examples.
- """
-
- config_class = XLMRobertaConfig
- pretrained_model_archive_map = XLM_ROBERTA_PRETRAINED_MODEL_ARCHIVE_MAP
-
-
-@add_start_docstrings(
- """XLM-RoBERTa Model with a multiple choice classification head on top (a linear layer on top of
- the pooled output and a softmax) e.g. for RocStories/SWAG tasks. """,
- XLM_ROBERTA_START_DOCSTRING,
-)
-class XLMRobertaForMultipleChoice(RobertaForMultipleChoice):
- """
- This class overrides :class:`~transformers.RobertaForMultipleChoice`. Please check the
- superclass for the appropriate documentation alongside usage examples.
- """
-
- config_class = XLMRobertaConfig
- pretrained_model_archive_map = XLM_ROBERTA_PRETRAINED_MODEL_ARCHIVE_MAP
-
-
-@add_start_docstrings(
- """XLM-RoBERTa Model with a token classification head on top (a linear layer on top of
- the hidden-states output) e.g. for Named-Entity-Recognition (NER) tasks. """,
- XLM_ROBERTA_START_DOCSTRING,
-)
-class XLMRobertaForTokenClassification(RobertaForTokenClassification):
- """
- This class overrides :class:`~transformers.RobertaForTokenClassification`. Please check the
- superclass for the appropriate documentation alongside usage examples.
- """
-
- config_class = XLMRobertaConfig
- pretrained_model_archive_map = XLM_ROBERTA_PRETRAINED_MODEL_ARCHIVE_MAP
diff --git a/server/transformers/src/transformers/modeling_xlnet.py b/server/transformers/src/transformers/modeling_xlnet.py
deleted file mode 100644
index 2720c848914faace9b4700ca8d47a83e4451c8f9..0000000000000000000000000000000000000000
--- a/server/transformers/src/transformers/modeling_xlnet.py
+++ /dev/null
@@ -1,1682 +0,0 @@
-# coding=utf-8
-# Copyright 2018 Google AI, Google Brain and Carnegie Mellon University Authors and the HuggingFace Inc. team.
-# Copyright (c) 2018, NVIDIA CORPORATION. All rights reserved.
-#
-# Licensed under the Apache License, Version 2.0 (the "License");
-# you may not use this file except in compliance with the License.
-# You may obtain a copy of the License at
-#
-# http://www.apache.org/licenses/LICENSE-2.0
-#
-# Unless required by applicable law or agreed to in writing, software
-# distributed under the License is distributed on an "AS IS" BASIS,
-# WITHOUT WARRANTIES OR CONDITIONS OF ANY KIND, either express or implied.
-# See the License for the specific language governing permissions and
-# limitations under the License.
-""" PyTorch XLNet model.
-"""
-
-
-import logging
-import math
-
-import torch
-from torch import nn
-from torch.nn import CrossEntropyLoss, MSELoss
-from torch.nn import functional as F
-
-from .configuration_xlnet import XLNetConfig
-from .file_utils import add_start_docstrings, add_start_docstrings_to_callable
-from .modeling_utils import PoolerAnswerClass, PoolerEndLogits, PoolerStartLogits, PreTrainedModel, SequenceSummary
-
-
-logger = logging.getLogger(__name__)
-
-XLNET_PRETRAINED_MODEL_ARCHIVE_MAP = {
- "xlnet-base-cased": "https://s3.amazonaws.com/models.huggingface.co/bert/xlnet-base-cased-pytorch_model.bin",
- "xlnet-large-cased": "https://s3.amazonaws.com/models.huggingface.co/bert/xlnet-large-cased-pytorch_model.bin",
-}
-
-
-def build_tf_xlnet_to_pytorch_map(model, config, tf_weights=None):
- """ A map of modules from TF to PyTorch.
- I use a map to keep the PyTorch model as
- identical to the original PyTorch model as possible.
- """
-
- tf_to_pt_map = {}
-
- if hasattr(model, "transformer"):
- if hasattr(model, "lm_loss"):
- # We will load also the output bias
- tf_to_pt_map["model/lm_loss/bias"] = model.lm_loss.bias
- if hasattr(model, "sequence_summary") and "model/sequnece_summary/summary/kernel" in tf_weights:
- # We will load also the sequence summary
- tf_to_pt_map["model/sequnece_summary/summary/kernel"] = model.sequence_summary.summary.weight
- tf_to_pt_map["model/sequnece_summary/summary/bias"] = model.sequence_summary.summary.bias
- if (
- hasattr(model, "logits_proj")
- and config.finetuning_task is not None
- and "model/regression_{}/logit/kernel".format(config.finetuning_task) in tf_weights
- ):
- tf_to_pt_map["model/regression_{}/logit/kernel".format(config.finetuning_task)] = model.logits_proj.weight
- tf_to_pt_map["model/regression_{}/logit/bias".format(config.finetuning_task)] = model.logits_proj.bias
-
- # Now load the rest of the transformer
- model = model.transformer
-
- # Embeddings and output
- tf_to_pt_map.update(
- {
- "model/transformer/word_embedding/lookup_table": model.word_embedding.weight,
- "model/transformer/mask_emb/mask_emb": model.mask_emb,
- }
- )
-
- # Transformer blocks
- for i, b in enumerate(model.layer):
- layer_str = "model/transformer/layer_%d/" % i
- tf_to_pt_map.update(
- {
- layer_str + "rel_attn/LayerNorm/gamma": b.rel_attn.layer_norm.weight,
- layer_str + "rel_attn/LayerNorm/beta": b.rel_attn.layer_norm.bias,
- layer_str + "rel_attn/o/kernel": b.rel_attn.o,
- layer_str + "rel_attn/q/kernel": b.rel_attn.q,
- layer_str + "rel_attn/k/kernel": b.rel_attn.k,
- layer_str + "rel_attn/r/kernel": b.rel_attn.r,
- layer_str + "rel_attn/v/kernel": b.rel_attn.v,
- layer_str + "ff/LayerNorm/gamma": b.ff.layer_norm.weight,
- layer_str + "ff/LayerNorm/beta": b.ff.layer_norm.bias,
- layer_str + "ff/layer_1/kernel": b.ff.layer_1.weight,
- layer_str + "ff/layer_1/bias": b.ff.layer_1.bias,
- layer_str + "ff/layer_2/kernel": b.ff.layer_2.weight,
- layer_str + "ff/layer_2/bias": b.ff.layer_2.bias,
- }
- )
-
- # Relative positioning biases
- if config.untie_r:
- r_r_list = []
- r_w_list = []
- r_s_list = []
- seg_embed_list = []
- for b in model.layer:
- r_r_list.append(b.rel_attn.r_r_bias)
- r_w_list.append(b.rel_attn.r_w_bias)
- r_s_list.append(b.rel_attn.r_s_bias)
- seg_embed_list.append(b.rel_attn.seg_embed)
- else:
- r_r_list = [model.r_r_bias]
- r_w_list = [model.r_w_bias]
- r_s_list = [model.r_s_bias]
- seg_embed_list = [model.seg_embed]
- tf_to_pt_map.update(
- {
- "model/transformer/r_r_bias": r_r_list,
- "model/transformer/r_w_bias": r_w_list,
- "model/transformer/r_s_bias": r_s_list,
- "model/transformer/seg_embed": seg_embed_list,
- }
- )
- return tf_to_pt_map
-
-
-def load_tf_weights_in_xlnet(model, config, tf_path):
- """ Load tf checkpoints in a pytorch model
- """
- try:
- import numpy as np
- import tensorflow as tf
- except ImportError:
- logger.error(
- "Loading a TensorFlow models in PyTorch, requires TensorFlow to be installed. Please see "
- "https://www.tensorflow.org/install/ for installation instructions."
- )
- raise
- # Load weights from TF model
- init_vars = tf.train.list_variables(tf_path)
- tf_weights = {}
- for name, shape in init_vars:
- logger.info("Loading TF weight {} with shape {}".format(name, shape))
- array = tf.train.load_variable(tf_path, name)
- tf_weights[name] = array
-
- # Build TF to PyTorch weights loading map
- tf_to_pt_map = build_tf_xlnet_to_pytorch_map(model, config, tf_weights)
-
- for name, pointer in tf_to_pt_map.items():
- logger.info("Importing {}".format(name))
- if name not in tf_weights:
- logger.info("{} not in tf pre-trained weights, skipping".format(name))
- continue
- array = tf_weights[name]
- # adam_v and adam_m are variables used in AdamWeightDecayOptimizer to calculated m and v
- # which are not required for using pretrained model
- if "kernel" in name and ("ff" in name or "summary" in name or "logit" in name):
- logger.info("Transposing")
- array = np.transpose(array)
- if isinstance(pointer, list):
- # Here we will split the TF weigths
- assert len(pointer) == array.shape[0]
- for i, p_i in enumerate(pointer):
- arr_i = array[i, ...]
- try:
- assert p_i.shape == arr_i.shape
- except AssertionError as e:
- e.args += (p_i.shape, arr_i.shape)
- raise
- logger.info("Initialize PyTorch weight {} for layer {}".format(name, i))
- p_i.data = torch.from_numpy(arr_i)
- else:
- try:
- assert pointer.shape == array.shape
- except AssertionError as e:
- e.args += (pointer.shape, array.shape)
- raise
- logger.info("Initialize PyTorch weight {}".format(name))
- pointer.data = torch.from_numpy(array)
- tf_weights.pop(name, None)
- tf_weights.pop(name + "/Adam", None)
- tf_weights.pop(name + "/Adam_1", None)
-
- logger.info("Weights not copied to PyTorch model: {}".format(", ".join(tf_weights.keys())))
- return model
-
-
-def gelu(x):
- """ Implementation of the gelu activation function.
- XLNet is using OpenAI GPT's gelu (not exactly the same as BERT)
- Also see https://arxiv.org/abs/1606.08415
- """
- cdf = 0.5 * (1.0 + torch.tanh(math.sqrt(2 / math.pi) * (x + 0.044715 * torch.pow(x, 3))))
- return x * cdf
-
-
-def swish(x):
- return x * torch.sigmoid(x)
-
-
-ACT2FN = {"gelu": gelu, "relu": torch.nn.functional.relu, "swish": swish}
-
-
-XLNetLayerNorm = nn.LayerNorm
-
-
-class XLNetRelativeAttention(nn.Module):
- def __init__(self, config):
- super().__init__()
- self.output_attentions = config.output_attentions
-
- if config.d_model % config.n_head != 0:
- raise ValueError(
- "The hidden size (%d) is not a multiple of the number of attention "
- "heads (%d)" % (config.d_model, config.n_head)
- )
-
- self.n_head = config.n_head
- self.d_head = config.d_head
- self.d_model = config.d_model
- self.scale = 1 / (config.d_head ** 0.5)
-
- self.q = nn.Parameter(torch.FloatTensor(config.d_model, self.n_head, self.d_head))
- self.k = nn.Parameter(torch.FloatTensor(config.d_model, self.n_head, self.d_head))
- self.v = nn.Parameter(torch.FloatTensor(config.d_model, self.n_head, self.d_head))
- self.o = nn.Parameter(torch.FloatTensor(config.d_model, self.n_head, self.d_head))
- self.r = nn.Parameter(torch.FloatTensor(config.d_model, self.n_head, self.d_head))
-
- self.r_r_bias = nn.Parameter(torch.FloatTensor(self.n_head, self.d_head))
- self.r_s_bias = nn.Parameter(torch.FloatTensor(self.n_head, self.d_head))
- self.r_w_bias = nn.Parameter(torch.FloatTensor(self.n_head, self.d_head))
- self.seg_embed = nn.Parameter(torch.FloatTensor(2, self.n_head, self.d_head))
-
- self.layer_norm = XLNetLayerNorm(config.d_model, eps=config.layer_norm_eps)
- self.dropout = nn.Dropout(config.dropout)
-
- def prune_heads(self, heads):
- raise NotImplementedError
-
- @staticmethod
- def rel_shift(x, klen=-1):
- """perform relative shift to form the relative attention score."""
- x_size = x.shape
-
- x = x.reshape(x_size[1], x_size[0], x_size[2], x_size[3])
- x = x[1:, ...]
- x = x.reshape(x_size[0], x_size[1] - 1, x_size[2], x_size[3])
- # x = x[:, 0:klen, :, :]
- x = torch.index_select(x, 1, torch.arange(klen, device=x.device, dtype=torch.long))
-
- return x
-
- @staticmethod
- def rel_shift_bnij(x, klen=-1):
- x_size = x.shape
-
- x = x.reshape(x_size[0], x_size[1], x_size[3], x_size[2])
- x = x[:, :, 1:, :]
- x = x.reshape(x_size[0], x_size[1], x_size[2], x_size[3] - 1)
- # Note: the tensor-slice form was faster in my testing than torch.index_select
- # However, tracing doesn't like the nature of the slice, and if klen changes
- # during the run then it'll fail, whereas index_select will be fine.
- x = torch.index_select(x, 3, torch.arange(klen, device=x.device, dtype=torch.long))
- # x = x[:, :, :, :klen]
-
- return x
-
- def rel_attn_core(self, q_head, k_head_h, v_head_h, k_head_r, seg_mat=None, attn_mask=None, head_mask=None):
- """Core relative positional attention operations."""
-
- # content based attention score
- ac = torch.einsum("ibnd,jbnd->bnij", q_head + self.r_w_bias, k_head_h)
-
- # position based attention score
- bd = torch.einsum("ibnd,jbnd->bnij", q_head + self.r_r_bias, k_head_r)
- bd = self.rel_shift_bnij(bd, klen=ac.shape[3])
-
- # segment based attention score
- if seg_mat is None:
- ef = 0
- else:
- ef = torch.einsum("ibnd,snd->ibns", q_head + self.r_s_bias, self.seg_embed)
- ef = torch.einsum("ijbs,ibns->bnij", seg_mat, ef)
-
- # merge attention scores and perform masking
- attn_score = (ac + bd + ef) * self.scale
- if attn_mask is not None:
- # attn_score = attn_score * (1 - attn_mask) - 1e30 * attn_mask
- if attn_mask.dtype == torch.float16:
- attn_score = attn_score - 65500 * torch.einsum("ijbn->bnij", attn_mask)
- else:
- attn_score = attn_score - 1e30 * torch.einsum("ijbn->bnij", attn_mask)
-
- # attention probability
- attn_prob = F.softmax(attn_score, dim=3)
- attn_prob = self.dropout(attn_prob)
-
- # Mask heads if we want to
- if head_mask is not None:
- attn_prob = attn_prob * torch.einsum("ijbn->bnij", head_mask)
-
- # attention output
- attn_vec = torch.einsum("bnij,jbnd->ibnd", attn_prob, v_head_h)
-
- if self.output_attentions:
- return attn_vec, torch.einsum("bnij->ijbn", attn_prob)
-
- return attn_vec
-
- def post_attention(self, h, attn_vec, residual=True):
- """Post-attention processing."""
- # post-attention projection (back to `d_model`)
- attn_out = torch.einsum("ibnd,hnd->ibh", attn_vec, self.o)
-
- attn_out = self.dropout(attn_out)
- if residual:
- attn_out = attn_out + h
- output = self.layer_norm(attn_out)
-
- return output
-
- def forward(self, h, g, attn_mask_h, attn_mask_g, r, seg_mat, mems=None, target_mapping=None, head_mask=None):
- if g is not None:
- # Two-stream attention with relative positional encoding.
- # content based attention score
- if mems is not None and mems.dim() > 1:
- cat = torch.cat([mems, h], dim=0)
- else:
- cat = h
-
- # content-based key head
- k_head_h = torch.einsum("ibh,hnd->ibnd", cat, self.k)
-
- # content-based value head
- v_head_h = torch.einsum("ibh,hnd->ibnd", cat, self.v)
-
- # position-based key head
- k_head_r = torch.einsum("ibh,hnd->ibnd", r, self.r)
-
- # h-stream
- # content-stream query head
- q_head_h = torch.einsum("ibh,hnd->ibnd", h, self.q)
-
- # core attention ops
- attn_vec_h = self.rel_attn_core(
- q_head_h, k_head_h, v_head_h, k_head_r, seg_mat=seg_mat, attn_mask=attn_mask_h, head_mask=head_mask
- )
-
- if self.output_attentions:
- attn_vec_h, attn_prob_h = attn_vec_h
-
- # post processing
- output_h = self.post_attention(h, attn_vec_h)
-
- # g-stream
- # query-stream query head
- q_head_g = torch.einsum("ibh,hnd->ibnd", g, self.q)
-
- # core attention ops
- if target_mapping is not None:
- q_head_g = torch.einsum("mbnd,mlb->lbnd", q_head_g, target_mapping)
- attn_vec_g = self.rel_attn_core(
- q_head_g, k_head_h, v_head_h, k_head_r, seg_mat=seg_mat, attn_mask=attn_mask_g, head_mask=head_mask
- )
-
- if self.output_attentions:
- attn_vec_g, attn_prob_g = attn_vec_g
-
- attn_vec_g = torch.einsum("lbnd,mlb->mbnd", attn_vec_g, target_mapping)
- else:
- attn_vec_g = self.rel_attn_core(
- q_head_g, k_head_h, v_head_h, k_head_r, seg_mat=seg_mat, attn_mask=attn_mask_g, head_mask=head_mask
- )
-
- if self.output_attentions:
- attn_vec_g, attn_prob_g = attn_vec_g
-
- # post processing
- output_g = self.post_attention(g, attn_vec_g)
-
- if self.output_attentions:
- attn_prob = attn_prob_h, attn_prob_g
-
- else:
- # Multi-head attention with relative positional encoding
- if mems is not None and mems.dim() > 1:
- cat = torch.cat([mems, h], dim=0)
- else:
- cat = h
-
- # content heads
- q_head_h = torch.einsum("ibh,hnd->ibnd", h, self.q)
- k_head_h = torch.einsum("ibh,hnd->ibnd", cat, self.k)
- v_head_h = torch.einsum("ibh,hnd->ibnd", cat, self.v)
-
- # positional heads
- k_head_r = torch.einsum("ibh,hnd->ibnd", r, self.r)
-
- # core attention ops
- attn_vec = self.rel_attn_core(
- q_head_h, k_head_h, v_head_h, k_head_r, seg_mat=seg_mat, attn_mask=attn_mask_h, head_mask=head_mask
- )
-
- if self.output_attentions:
- attn_vec, attn_prob = attn_vec
-
- # post processing
- output_h = self.post_attention(h, attn_vec)
- output_g = None
-
- outputs = (output_h, output_g)
- if self.output_attentions:
- outputs = outputs + (attn_prob,)
- return outputs
-
-
-class XLNetFeedForward(nn.Module):
- def __init__(self, config):
- super().__init__()
- self.layer_norm = XLNetLayerNorm(config.d_model, eps=config.layer_norm_eps)
- self.layer_1 = nn.Linear(config.d_model, config.d_inner)
- self.layer_2 = nn.Linear(config.d_inner, config.d_model)
- self.dropout = nn.Dropout(config.dropout)
- if isinstance(config.ff_activation, str):
- self.activation_function = ACT2FN[config.ff_activation]
- else:
- self.activation_function = config.ff_activation
-
- def forward(self, inp):
- output = inp
- output = self.layer_1(output)
- output = self.activation_function(output)
- output = self.dropout(output)
- output = self.layer_2(output)
- output = self.dropout(output)
- output = self.layer_norm(output + inp)
- return output
-
-
-class XLNetLayer(nn.Module):
- def __init__(self, config):
- super().__init__()
- self.rel_attn = XLNetRelativeAttention(config)
- self.ff = XLNetFeedForward(config)
- self.dropout = nn.Dropout(config.dropout)
-
- def forward(
- self, output_h, output_g, attn_mask_h, attn_mask_g, r, seg_mat, mems=None, target_mapping=None, head_mask=None
- ):
- outputs = self.rel_attn(
- output_h,
- output_g,
- attn_mask_h,
- attn_mask_g,
- r,
- seg_mat,
- mems=mems,
- target_mapping=target_mapping,
- head_mask=head_mask,
- )
- output_h, output_g = outputs[:2]
-
- if output_g is not None:
- output_g = self.ff(output_g)
- output_h = self.ff(output_h)
-
- outputs = (output_h, output_g) + outputs[2:] # Add again attentions if there are there
- return outputs
-
-
-class XLNetPreTrainedModel(PreTrainedModel):
- """ An abstract class to handle weights initialization and
- a simple interface for downloading and loading pretrained models.
- """
-
- config_class = XLNetConfig
- pretrained_model_archive_map = XLNET_PRETRAINED_MODEL_ARCHIVE_MAP
- load_tf_weights = load_tf_weights_in_xlnet
- base_model_prefix = "transformer"
-
- def _init_weights(self, module):
- """ Initialize the weights.
- """
- if isinstance(module, (nn.Linear, nn.Embedding)):
- # Slightly different from the TF version which uses truncated_normal for initialization
- # cf https://github.com/pytorch/pytorch/pull/5617
- module.weight.data.normal_(mean=0.0, std=self.config.initializer_range)
- if isinstance(module, nn.Linear) and module.bias is not None:
- module.bias.data.zero_()
- elif isinstance(module, XLNetLayerNorm):
- module.bias.data.zero_()
- module.weight.data.fill_(1.0)
- elif isinstance(module, XLNetRelativeAttention):
- for param in [
- module.q,
- module.k,
- module.v,
- module.o,
- module.r,
- module.r_r_bias,
- module.r_s_bias,
- module.r_w_bias,
- module.seg_embed,
- ]:
- param.data.normal_(mean=0.0, std=self.config.initializer_range)
- elif isinstance(module, XLNetModel):
- module.mask_emb.data.normal_(mean=0.0, std=self.config.initializer_range)
-
-
-XLNET_START_DOCSTRING = r"""
-
- This model is a PyTorch `torch.nn.Module `_ sub-class.
- Use it as a regular PyTorch Module and refer to the PyTorch documentation for all matter related to general
- usage and behavior.
-
- Parameters:
- config (:class:`~transformers.XLNetConfig`): Model configuration class with all the parameters of the model.
- Initializing with a config file does not load the weights associated with the model, only the configuration.
- Check out the :meth:`~transformers.PreTrainedModel.from_pretrained` method to load the model weights.
-"""
-
-XLNET_INPUTS_DOCSTRING = r"""
- Args:
- input_ids (:obj:`torch.LongTensor` of shape :obj:`(batch_size, sequence_length)`):
- Indices of input sequence tokens in the vocabulary.
-
- Indices can be obtained using :class:`transformers.BertTokenizer`.
- See :func:`transformers.PreTrainedTokenizer.encode` and
- :func:`transformers.PreTrainedTokenizer.encode_plus` for details.
-
- `What are input IDs? <../glossary.html#input-ids>`__
- attention_mask (:obj:`torch.FloatTensor` of shape :obj:`(batch_size, sequence_length)`, `optional`, defaults to :obj:`None`):
- Mask to avoid performing attention on padding token indices.
- Mask values selected in ``[0, 1]``:
- ``1`` for tokens that are NOT MASKED, ``0`` for MASKED tokens.
-
- `What are attention masks? <../glossary.html#attention-mask>`__
- mems (:obj:`List[torch.FloatTensor]` of length :obj:`config.n_layers`):
- Contains pre-computed hidden-states (key and values in the attention blocks) as computed by the model
- (see `mems` output below). Can be used to speed up sequential decoding. The token ids which have their mems
- given to this model should not be passed as input ids as they have already been computed.
- perm_mask (:obj:`torch.FloatTensor` of shape :obj:`(batch_size, sequence_length, sequence_length)`, `optional`, defaults to :obj:`None`):
- Mask to indicate the attention pattern for each input token with values selected in ``[0, 1]``:
- If ``perm_mask[k, i, j] = 0``, i attend to j in batch k;
- if ``perm_mask[k, i, j] = 1``, i does not attend to j in batch k.
- If None, each token attends to all the others (full bidirectional attention).
- Only used during pretraining (to define factorization order) or for sequential decoding (generation).
- target_mapping (:obj:`torch.FloatTensor` of shape :obj:`(batch_size, num_predict, sequence_length)`, `optional`, defaults to :obj:`None`):
- Mask to indicate the output tokens to use.
- If ``target_mapping[k, i, j] = 1``, the i-th predict in batch k is on the j-th token.
- Only used during pretraining for partial prediction or for sequential decoding (generation).
- token_type_ids (:obj:`torch.LongTensor` of shape :obj:`(batch_size, sequence_length)`, `optional`, defaults to :obj:`None`):
- Segment token indices to indicate first and second portions of the inputs.
- Indices are selected in ``[0, 1]``: ``0`` corresponds to a `sentence A` token, ``1``
- corresponds to a `sentence B` token
-
- `What are token type IDs? <../glossary.html#token-type-ids>`_
- input_mask (:obj:`torch.FloatTensor` of shape :obj:`(batch_size, sequence_length)`, `optional`, defaults to :obj:`None`):
- Mask to avoid performing attention on padding token indices.
- Negative of `attention_mask`, i.e. with 0 for real tokens and 1 for padding.
- Kept for compatibility with the original code base.
- You can only uses one of `input_mask` and `attention_mask`
- Mask values selected in ``[0, 1]``:
- ``1`` for tokens that are MASKED, ``0`` for tokens that are NOT MASKED.
- head_mask (:obj:`torch.FloatTensor` of shape :obj:`(num_heads,)` or :obj:`(num_layers, num_heads)`, `optional`, defaults to :obj:`None`):
- Mask to nullify selected heads of the self-attention modules.
- Mask values selected in ``[0, 1]``:
- :obj:`1` indicates the head is **not masked**, :obj:`0` indicates the head is **masked**.
- input_embeds (:obj:`torch.FloatTensor` of shape :obj:`(batch_size, sequence_length, hidden_size)`, `optional`, defaults to :obj:`None`):
- Optionally, instead of passing :obj:`input_ids` you can choose to directly pass an embedded representation.
- This is useful if you want more control over how to convert `input_ids` indices into associated vectors
- than the model's internal embedding lookup matrix.
-"""
-
-
-@add_start_docstrings(
- "The bare XLNet Model transformer outputting raw hidden-states without any specific head on top.",
- XLNET_START_DOCSTRING,
-)
-class XLNetModel(XLNetPreTrainedModel):
- def __init__(self, config):
- super().__init__(config)
- self.output_attentions = config.output_attentions
- self.output_hidden_states = config.output_hidden_states
- self.output_past = config.output_past
-
- self.mem_len = config.mem_len
- self.reuse_len = config.reuse_len
- self.d_model = config.d_model
- self.same_length = config.same_length
- self.attn_type = config.attn_type
- self.bi_data = config.bi_data
- self.clamp_len = config.clamp_len
- self.n_layer = config.n_layer
-
- self.word_embedding = nn.Embedding(config.vocab_size, config.d_model)
- self.mask_emb = nn.Parameter(torch.FloatTensor(1, 1, config.d_model))
- self.layer = nn.ModuleList([XLNetLayer(config) for _ in range(config.n_layer)])
- self.dropout = nn.Dropout(config.dropout)
-
- self.init_weights()
-
- def get_input_embeddings(self):
- return self.word_embedding
-
- def set_input_embeddings(self, new_embeddings):
- self.word_embedding = new_embeddings
-
- def _prune_heads(self, heads_to_prune):
- raise NotImplementedError
-
- def create_mask(self, qlen, mlen):
- """
- Creates causal attention mask. Float mask where 1.0 indicates masked, 0.0 indicates not-masked.
-
- Args:
- qlen: Sequence length
- mlen: Mask length
-
- ::
-
- same_length=False: same_length=True:
- < qlen > < qlen >
- ^ [0 0 0 0 0 1 1 1 1] [0 0 0 0 0 1 1 1 1]
- [0 0 0 0 0 0 1 1 1] [1 0 0 0 0 0 1 1 1]
- qlen [0 0 0 0 0 0 0 1 1] [1 1 0 0 0 0 0 1 1]
- [0 0 0 0 0 0 0 0 1] [1 1 1 0 0 0 0 0 1]
- v [0 0 0 0 0 0 0 0 0] [1 1 1 1 0 0 0 0 0]
-
- """
- attn_mask = torch.ones([qlen, qlen])
- mask_up = torch.triu(attn_mask, diagonal=1)
- attn_mask_pad = torch.zeros([qlen, mlen])
- ret = torch.cat([attn_mask_pad, mask_up], dim=1)
- if self.same_length:
- mask_lo = torch.tril(attn_mask, diagonal=-1)
- ret = torch.cat([ret[:, :qlen] + mask_lo, ret[:, qlen:]], dim=1)
-
- ret = ret.to(next(self.parameters()))
- return ret
-
- def cache_mem(self, curr_out, prev_mem):
- # cache hidden states into memory.
- if self.reuse_len is not None and self.reuse_len > 0:
- curr_out = curr_out[: self.reuse_len]
-
- if prev_mem is None:
- new_mem = curr_out[-self.mem_len :]
- else:
- new_mem = torch.cat([prev_mem, curr_out], dim=0)[-self.mem_len :]
-
- return new_mem.detach()
-
- @staticmethod
- def positional_embedding(pos_seq, inv_freq, bsz=None):
- sinusoid_inp = torch.einsum("i,d->id", pos_seq, inv_freq)
- pos_emb = torch.cat([torch.sin(sinusoid_inp), torch.cos(sinusoid_inp)], dim=-1)
- pos_emb = pos_emb[:, None, :]
-
- if bsz is not None:
- pos_emb = pos_emb.expand(-1, bsz, -1)
-
- return pos_emb
-
- def relative_positional_encoding(self, qlen, klen, bsz=None):
- # create relative positional encoding.
- freq_seq = torch.arange(0, self.d_model, 2.0, dtype=torch.float)
- inv_freq = 1 / torch.pow(10000, (freq_seq / self.d_model))
-
- if self.attn_type == "bi":
- # beg, end = klen - 1, -qlen
- beg, end = klen, -qlen
- elif self.attn_type == "uni":
- # beg, end = klen - 1, -1
- beg, end = klen, -1
- else:
- raise ValueError("Unknown `attn_type` {}.".format(self.attn_type))
-
- if self.bi_data:
- fwd_pos_seq = torch.arange(beg, end, -1.0, dtype=torch.float)
- bwd_pos_seq = torch.arange(-beg, -end, 1.0, dtype=torch.float)
-
- if self.clamp_len > 0:
- fwd_pos_seq = fwd_pos_seq.clamp(-self.clamp_len, self.clamp_len)
- bwd_pos_seq = bwd_pos_seq.clamp(-self.clamp_len, self.clamp_len)
-
- if bsz is not None:
- fwd_pos_emb = self.positional_embedding(fwd_pos_seq, inv_freq, bsz // 2)
- bwd_pos_emb = self.positional_embedding(bwd_pos_seq, inv_freq, bsz // 2)
- else:
- fwd_pos_emb = self.positional_embedding(fwd_pos_seq, inv_freq)
- bwd_pos_emb = self.positional_embedding(bwd_pos_seq, inv_freq)
-
- pos_emb = torch.cat([fwd_pos_emb, bwd_pos_emb], dim=1)
- else:
- fwd_pos_seq = torch.arange(beg, end, -1.0)
- if self.clamp_len > 0:
- fwd_pos_seq = fwd_pos_seq.clamp(-self.clamp_len, self.clamp_len)
- pos_emb = self.positional_embedding(fwd_pos_seq, inv_freq, bsz)
-
- pos_emb = pos_emb.to(next(self.parameters()))
- return pos_emb
-
- @add_start_docstrings_to_callable(XLNET_INPUTS_DOCSTRING)
- def forward(
- self,
- input_ids=None,
- attention_mask=None,
- mems=None,
- perm_mask=None,
- target_mapping=None,
- token_type_ids=None,
- input_mask=None,
- head_mask=None,
- inputs_embeds=None,
- ):
- r"""
- Return:
- :obj:`tuple(torch.FloatTensor)` comprising various elements depending on the configuration (:class:`~transformers.XLNetConfig`) and inputs:
- last_hidden_state (:obj:`torch.FloatTensor` of shape :obj:`(batch_size, sequence_length, hidden_size)`):
- Sequence of hidden-states at the last layer of the model.
- mems (:obj:`List[torch.FloatTensor]` of length :obj:`config.n_layers`):
- Contains pre-computed hidden-states (key and values in the attention blocks).
- Can be used (see `mems` input) to speed up sequential decoding. The token ids which have their past given to this model
- should not be passed as input ids as they have already been computed.
- hidden_states (:obj:`tuple(torch.FloatTensor)`, `optional`, returned when ``config.output_hidden_states=True``):
- Tuple of :obj:`torch.FloatTensor` (one for the output of the embeddings + one for the output of each layer)
- of shape :obj:`(batch_size, sequence_length, hidden_size)`.
-
- Hidden-states of the model at the output of each layer plus the initial embedding outputs.
- attentions (:obj:`tuple(torch.FloatTensor)`, `optional`, returned when ``config.output_attentions=True``):
- Tuple of :obj:`torch.FloatTensor` (one for each layer) of shape
- :obj:`(batch_size, num_heads, sequence_length, sequence_length)`.
-
- Attentions weights after the attention softmax, used to compute the weighted average in the self-attention
- heads.
-
- Examples::
-
- from transformers import XLNetTokenizer, XLNetModel
- import torch
-
- tokenizer = XLNetTokenizer.from_pretrained('xlnet-large-cased')
- model = XLNetModel.from_pretrained('xlnet-large-cased')
-
- input_ids = torch.tensor(tokenizer.encode("Hello, my dog is cute", add_special_tokens=True)).unsqueeze(0) # Batch size 1
-
- outputs = model(input_ids)
- last_hidden_states = outputs[0] # The last hidden-state is the first element of the output tuple
-
- """
- # the original code for XLNet uses shapes [len, bsz] with the batch dimension at the end
- # but we want a unified interface in the library with the batch size on the first dimension
- # so we move here the first dimension (batch) to the end
- if input_ids is not None and inputs_embeds is not None:
- raise ValueError("You cannot specify both input_ids and inputs_embeds at the same time")
- elif input_ids is not None:
- input_ids = input_ids.transpose(0, 1).contiguous()
- qlen, bsz = input_ids.shape[0], input_ids.shape[1]
- elif inputs_embeds is not None:
- inputs_embeds = inputs_embeds.transpose(0, 1).contiguous()
- qlen, bsz = inputs_embeds.shape[0], inputs_embeds.shape[1]
- else:
- raise ValueError("You have to specify either input_ids or inputs_embeds")
-
- token_type_ids = token_type_ids.transpose(0, 1).contiguous() if token_type_ids is not None else None
- input_mask = input_mask.transpose(0, 1).contiguous() if input_mask is not None else None
- attention_mask = attention_mask.transpose(0, 1).contiguous() if attention_mask is not None else None
- perm_mask = perm_mask.permute(1, 2, 0).contiguous() if perm_mask is not None else None
- target_mapping = target_mapping.permute(1, 2, 0).contiguous() if target_mapping is not None else None
-
- mlen = mems[0].shape[0] if mems is not None and mems[0] is not None else 0
- klen = mlen + qlen
-
- dtype_float = next(self.parameters()).dtype
- device = next(self.parameters()).device
-
- # Attention mask
- # causal attention mask
- if self.attn_type == "uni":
- attn_mask = self.create_mask(qlen, mlen)
- attn_mask = attn_mask[:, :, None, None]
- elif self.attn_type == "bi":
- attn_mask = None
- else:
- raise ValueError("Unsupported attention type: {}".format(self.attn_type))
-
- # data mask: input mask & perm mask
- assert input_mask is None or attention_mask is None, "You can only use one of input_mask (uses 1 for padding) "
- "or attention_mask (uses 0 for padding, added for compatbility with BERT). Please choose one."
- if input_mask is None and attention_mask is not None:
- input_mask = 1.0 - attention_mask
- if input_mask is not None and perm_mask is not None:
- data_mask = input_mask[None] + perm_mask
- elif input_mask is not None and perm_mask is None:
- data_mask = input_mask[None]
- elif input_mask is None and perm_mask is not None:
- data_mask = perm_mask
- else:
- data_mask = None
-
- if data_mask is not None:
- # all mems can be attended to
- if mlen > 0:
- mems_mask = torch.zeros([data_mask.shape[0], mlen, bsz]).to(data_mask)
- data_mask = torch.cat([mems_mask, data_mask], dim=1)
- if attn_mask is None:
- attn_mask = data_mask[:, :, :, None]
- else:
- attn_mask += data_mask[:, :, :, None]
-
- if attn_mask is not None:
- attn_mask = (attn_mask > 0).to(dtype_float)
-
- if attn_mask is not None:
- non_tgt_mask = -torch.eye(qlen).to(attn_mask)
- if mlen > 0:
- non_tgt_mask = torch.cat([torch.zeros([qlen, mlen]).to(attn_mask), non_tgt_mask], dim=-1)
- non_tgt_mask = ((attn_mask + non_tgt_mask[:, :, None, None]) > 0).to(attn_mask)
- else:
- non_tgt_mask = None
-
- # Word embeddings and prepare h & g hidden states
- if inputs_embeds is not None:
- word_emb_k = inputs_embeds
- else:
- word_emb_k = self.word_embedding(input_ids)
- output_h = self.dropout(word_emb_k)
- if target_mapping is not None:
- word_emb_q = self.mask_emb.expand(target_mapping.shape[0], bsz, -1)
- # else: # We removed the inp_q input which was same as target mapping
- # inp_q_ext = inp_q[:, :, None]
- # word_emb_q = inp_q_ext * self.mask_emb + (1 - inp_q_ext) * word_emb_k
- output_g = self.dropout(word_emb_q)
- else:
- output_g = None
-
- # Segment embedding
- if token_type_ids is not None:
- # Convert `token_type_ids` to one-hot `seg_mat`
- if mlen > 0:
- mem_pad = torch.zeros([mlen, bsz], dtype=torch.long, device=device)
- cat_ids = torch.cat([mem_pad, token_type_ids], dim=0)
- else:
- cat_ids = token_type_ids
-
- # `1` indicates not in the same segment [qlen x klen x bsz]
- seg_mat = (token_type_ids[:, None] != cat_ids[None, :]).long()
- seg_mat = F.one_hot(seg_mat, num_classes=2).to(dtype_float)
- else:
- seg_mat = None
-
- # Positional encoding
- pos_emb = self.relative_positional_encoding(qlen, klen, bsz=bsz)
- pos_emb = self.dropout(pos_emb)
-
- # Prepare head mask if needed
- # 1.0 in head_mask indicate we keep the head
- # attention_probs has shape bsz x n_heads x N x N
- # input head_mask has shape [num_heads] or [num_hidden_layers x num_heads] (a head_mask for each layer)
- # and head_mask is converted to shape [num_hidden_layers x qlen x klen x bsz x n_head]
- if head_mask is not None:
- if head_mask.dim() == 1:
- head_mask = head_mask.unsqueeze(0).unsqueeze(0).unsqueeze(0).unsqueeze(0)
- head_mask = head_mask.expand(self.n_layer, -1, -1, -1, -1)
- elif head_mask.dim() == 2:
- head_mask = head_mask.unsqueeze(1).unsqueeze(1).unsqueeze(1)
- head_mask = head_mask.to(
- dtype=next(self.parameters()).dtype
- ) # switch to fload if need + fp16 compatibility
- else:
- head_mask = [None] * self.n_layer
-
- new_mems = ()
- if mems is None:
- mems = [None] * len(self.layer)
-
- attentions = []
- hidden_states = []
- for i, layer_module in enumerate(self.layer):
- if self.mem_len is not None and self.mem_len > 0 and self.output_past:
- # cache new mems
- new_mems = new_mems + (self.cache_mem(output_h, mems[i]),)
- if self.output_hidden_states:
- hidden_states.append((output_h, output_g) if output_g is not None else output_h)
-
- outputs = layer_module(
- output_h,
- output_g,
- attn_mask_h=non_tgt_mask,
- attn_mask_g=attn_mask,
- r=pos_emb,
- seg_mat=seg_mat,
- mems=mems[i],
- target_mapping=target_mapping,
- head_mask=head_mask[i],
- )
- output_h, output_g = outputs[:2]
- if self.output_attentions:
- attentions.append(outputs[2])
-
- # Add last hidden state
- if self.output_hidden_states:
- hidden_states.append((output_h, output_g) if output_g is not None else output_h)
-
- output = self.dropout(output_g if output_g is not None else output_h)
-
- # Prepare outputs, we transpose back here to shape [bsz, len, hidden_dim] (cf. beginning of forward() method)
- outputs = (output.permute(1, 0, 2).contiguous(),)
-
- if self.mem_len is not None and self.mem_len > 0 and self.output_past:
- outputs = outputs + (new_mems,)
-
- if self.output_hidden_states:
- if output_g is not None:
- hidden_states = tuple(h.permute(1, 0, 2).contiguous() for hs in hidden_states for h in hs)
- else:
- hidden_states = tuple(hs.permute(1, 0, 2).contiguous() for hs in hidden_states)
- outputs = outputs + (hidden_states,)
- if self.output_attentions:
- if target_mapping is not None:
- # when target_mapping is provided, there are 2-tuple of attentions
- attentions = tuple(
- tuple(att_stream.permute(2, 3, 0, 1).contiguous() for att_stream in t) for t in attentions
- )
- else:
- attentions = tuple(t.permute(2, 3, 0, 1).contiguous() for t in attentions)
- outputs = outputs + (attentions,)
-
- return outputs # outputs, (new_mems), (hidden_states), (attentions)
-
-
-@add_start_docstrings(
- """XLNet Model with a language modeling head on top
- (linear layer with weights tied to the input embeddings). """,
- XLNET_START_DOCSTRING,
-)
-class XLNetLMHeadModel(XLNetPreTrainedModel):
- def __init__(self, config):
- super().__init__(config)
- self.attn_type = config.attn_type
- self.same_length = config.same_length
-
- self.transformer = XLNetModel(config)
- self.lm_loss = nn.Linear(config.d_model, config.vocab_size, bias=True)
-
- self.init_weights()
-
- def get_output_embeddings(self):
- return self.lm_loss
-
- def prepare_inputs_for_generation(self, input_ids, **model_kwargs):
- # Add dummy token at the end (no attention on this one)
-
- effective_batch_size = input_ids.shape[0]
- dummy_token = torch.zeros((effective_batch_size, 1), dtype=torch.long, device=input_ids.device)
- input_ids = torch.cat([input_ids, dummy_token], dim=1)
-
- # Build permutation mask so that previous tokens don't see last token
- sequence_length = input_ids.shape[1]
- perm_mask = torch.zeros(
- (effective_batch_size, sequence_length, sequence_length), dtype=torch.float, device=input_ids.device
- )
- perm_mask[:, :, -1] = 1.0
-
- # We'll only predict the last token
- target_mapping = torch.zeros(
- (effective_batch_size, 1, sequence_length), dtype=torch.float, device=input_ids.device
- )
- target_mapping[0, 0, -1] = 1.0
-
- inputs = {"input_ids": input_ids, "perm_mask": perm_mask, "target_mapping": target_mapping}
-
- # if past is defined in model kwargs then use it for faster decoding
- if "past" in model_kwargs and model_kwargs["past"]:
- inputs["mems"] = model_kwargs["past"]
-
- return inputs
-
- @add_start_docstrings_to_callable(XLNET_INPUTS_DOCSTRING)
- def forward(
- self,
- input_ids=None,
- attention_mask=None,
- mems=None,
- perm_mask=None,
- target_mapping=None,
- token_type_ids=None,
- input_mask=None,
- head_mask=None,
- inputs_embeds=None,
- labels=None,
- ):
- r"""
- labels (:obj:`torch.LongTensor` of shape :obj:`(batch_size, sequence_length)`, `optional`, defaults to :obj:`None`):
- Labels for language modeling.
- Note that the labels **are shifted** inside the model, i.e. you can set ``lm_labels = input_ids``
- Indices are selected in ``[-100, 0, ..., config.vocab_size]``
- All labels set to ``-100`` are ignored (masked), the loss is only
- computed for labels in ``[0, ..., config.vocab_size]``
-
- Return:
- :obj:`tuple(torch.FloatTensor)` comprising various elements depending on the configuration (:class:`~transformers.XLNetConfig`) and inputs:
- loss (:obj:`torch.FloatTensor` of shape `(1,)`, `optional`, returned when ``labels`` is provided)
- Language modeling loss.
- prediction_scores (:obj:`torch.FloatTensor` of shape :obj:`(batch_size, sequence_length, config.vocab_size)`):
- Prediction scores of the language modeling head (scores for each vocabulary token before SoftMax).
- mems (:obj:`List[torch.FloatTensor]` of length :obj:`config.n_layers`):
- Contains pre-computed hidden-states (key and values in the attention blocks).
- Can be used (see `past` input) to speed up sequential decoding. The token ids which have their past given to this model
- should not be passed as input ids as they have already been computed.
- hidden_states (:obj:`tuple(torch.FloatTensor)`, `optional`, returned when ``config.output_hidden_states=True``):
- Tuple of :obj:`torch.FloatTensor` (one for the output of the embeddings + one for the output of each layer)
- of shape :obj:`(batch_size, sequence_length, hidden_size)`.
-
- Hidden-states of the model at the output of each layer plus the initial embedding outputs.
- attentions (:obj:`tuple(torch.FloatTensor)`, `optional`, returned when ``config.output_attentions=True``):
- Tuple of :obj:`torch.FloatTensor` (one for each layer) of shape
- :obj:`(batch_size, num_heads, sequence_length, sequence_length)`.
-
- Attentions weights after the attention softmax, used to compute the weighted average in the self-attention
- heads.
-
- Examples::
-
- from transformers import XLNetTokenizer, XLNetLMHeadModel
- import torch
-
- tokenizer = XLNetTokenizer.from_pretrained('xlnet-large-cased')
- model = XLNetLMHeadModel.from_pretrained('xlnet-large-cased')
-
- # We show how to setup inputs to predict a next token using a bi-directional context.
- input_ids = torch.tensor(tokenizer.encode("Hello, my dog is very ", add_special_tokens=True)).unsqueeze(0) # We will predict the masked token
- perm_mask = torch.zeros((1, input_ids.shape[1], input_ids.shape[1]), dtype=torch.float)
- perm_mask[:, :, -1] = 1.0 # Previous tokens don't see last token
- target_mapping = torch.zeros((1, 1, input_ids.shape[1]), dtype=torch.float) # Shape [1, 1, seq_length] => let's predict one token
- target_mapping[0, 0, -1] = 1.0 # Our first (and only) prediction will be the last token of the sequence (the masked token)
-
- outputs = model(input_ids, perm_mask=perm_mask, target_mapping=target_mapping)
- next_token_logits = outputs[0] # Output has shape [target_mapping.size(0), target_mapping.size(1), config.vocab_size]
-
- """
- transformer_outputs = self.transformer(
- input_ids,
- attention_mask=attention_mask,
- mems=mems,
- perm_mask=perm_mask,
- target_mapping=target_mapping,
- token_type_ids=token_type_ids,
- input_mask=input_mask,
- head_mask=head_mask,
- inputs_embeds=inputs_embeds,
- )
-
- logits = self.lm_loss(transformer_outputs[0])
-
- outputs = (logits,) + transformer_outputs[1:] # Keep mems, hidden states, attentions if there are in it
-
- if labels is not None:
- # Flatten the tokens
- loss_fct = CrossEntropyLoss()
- loss = loss_fct(logits.view(-1, logits.size(-1)), labels.view(-1))
- outputs = (loss,) + outputs
-
- return outputs # return (loss), logits, (mems), (hidden states), (attentions)
-
-
-@add_start_docstrings(
- """XLNet Model with a sequence classification/regression head on top (a linear layer on top of
- the pooled output) e.g. for GLUE tasks. """,
- XLNET_START_DOCSTRING,
-)
-class XLNetForSequenceClassification(XLNetPreTrainedModel):
- def __init__(self, config):
- super().__init__(config)
- self.num_labels = config.num_labels
-
- self.transformer = XLNetModel(config)
- self.sequence_summary = SequenceSummary(config)
- self.logits_proj = nn.Linear(config.d_model, config.num_labels)
-
- self.init_weights()
-
- @add_start_docstrings_to_callable(XLNET_INPUTS_DOCSTRING)
- def forward(
- self,
- input_ids=None,
- attention_mask=None,
- mems=None,
- perm_mask=None,
- target_mapping=None,
- token_type_ids=None,
- input_mask=None,
- head_mask=None,
- inputs_embeds=None,
- labels=None,
- ):
- r"""
- labels (:obj:`torch.LongTensor` of shape :obj:`(batch_size,)`, `optional`, defaults to :obj:`None`)
- Labels for computing the sequence classification/regression loss.
- Indices should be in ``[0, ..., config.num_labels - 1]``.
- If ``config.num_labels == 1`` a regression loss is computed (Mean-Square loss),
- If ``config.num_labels > 1`` a classification loss is computed (Cross-Entropy).
-
- Return:
- :obj:`tuple(torch.FloatTensor)` comprising various elements depending on the configuration (:class:`~transformers.XLNetConfig`) and inputs:
- loss (:obj:`torch.FloatTensor` of shape :obj:`(1,)`, `optional`, returned when :obj:`labels` is provided):
- Classification (or regression if config.num_labels==1) loss.
- logits (:obj:`torch.FloatTensor` of shape :obj:(batch_size, config.num_labels)`):
- Classification (or regression if config.num_labels==1) scores (before SoftMax).
- mems (:obj:`List[torch.FloatTensor]` of length :obj:`config.n_layers`):
- Contains pre-computed hidden-states (key and values in the attention blocks).
- Can be used (see `past` input) to speed up sequential decoding. The token ids which have their past given to this model
- should not be passed as input ids as they have already been computed.
- hidden_states (:obj:`tuple(torch.FloatTensor)`, `optional`, returned when ``config.output_hidden_states=True``):
- Tuple of :obj:`torch.FloatTensor` (one for the output of the embeddings + one for the output of each layer)
- of shape :obj:`(batch_size, sequence_length, hidden_size)`.
-
- Hidden-states of the model at the output of each layer plus the initial embedding outputs.
- attentions (:obj:`tuple(torch.FloatTensor)`, `optional`, returned when ``config.output_attentions=True``):
- Tuple of :obj:`torch.FloatTensor` (one for each layer) of shape
- :obj:`(batch_size, num_heads, sequence_length, sequence_length)`.
-
- Attentions weights after the attention softmax, used to compute the weighted average in the self-attention
- heads.
-
- Examples::
-
- from transformers import XLNetTokenizer, XLNetForSequenceClassification
- import torch
-
- tokenizer = XLNetTokenizer.from_pretrained('xlnet-large-cased')
- model = XLNetForSequenceClassification.from_pretrained('xlnet-large-cased')
-
- input_ids = torch.tensor(tokenizer.encode("Hello, my dog is cute", add_special_tokens=True)).unsqueeze(0) # Batch size 1
- labels = torch.tensor([1]).unsqueeze(0) # Batch size 1
- outputs = model(input_ids, labels=labels)
- loss, logits = outputs[:2]
-
- """
- transformer_outputs = self.transformer(
- input_ids,
- attention_mask=attention_mask,
- mems=mems,
- perm_mask=perm_mask,
- target_mapping=target_mapping,
- token_type_ids=token_type_ids,
- input_mask=input_mask,
- head_mask=head_mask,
- inputs_embeds=inputs_embeds,
- )
- output = transformer_outputs[0]
-
- output = self.sequence_summary(output)
- logits = self.logits_proj(output)
-
- outputs = (logits,) + transformer_outputs[1:] # Keep mems, hidden states, attentions if there are in it
-
- if labels is not None:
- if self.num_labels == 1:
- # We are doing regression
- loss_fct = MSELoss()
- loss = loss_fct(logits.view(-1), labels.view(-1))
- else:
- loss_fct = CrossEntropyLoss()
- loss = loss_fct(logits.view(-1, self.num_labels), labels.view(-1))
- outputs = (loss,) + outputs
-
- return outputs # return (loss), logits, (mems), (hidden states), (attentions)
-
-
-@add_start_docstrings(
- """XLNet Model with a token classification head on top (a linear layer on top of
- the hidden-states output) e.g. for Named-Entity-Recognition (NER) tasks. """,
- XLNET_START_DOCSTRING,
-)
-class XLNetForTokenClassification(XLNetPreTrainedModel):
- def __init__(self, config):
- super().__init__(config)
- self.num_labels = config.num_labels
-
- self.transformer = XLNetModel(config)
- self.classifier = nn.Linear(config.hidden_size, config.num_labels)
-
- self.init_weights()
-
- @add_start_docstrings_to_callable(XLNET_INPUTS_DOCSTRING)
- def forward(
- self,
- input_ids=None,
- attention_mask=None,
- mems=None,
- perm_mask=None,
- target_mapping=None,
- token_type_ids=None,
- input_mask=None,
- head_mask=None,
- inputs_embeds=None,
- labels=None,
- ):
- r"""
- labels (:obj:`torch.LongTensor` of shape :obj:`(batch_size,)`, `optional`, defaults to :obj:`None`):
- Labels for computing the multiple choice classification loss.
- Indices should be in ``[0, ..., num_choices]`` where `num_choices` is the size of the second dimension
- of the input tensors. (see `input_ids` above)
-
- Return:
- :obj:`tuple(torch.FloatTensor)` comprising various elements depending on the configuration (:class:`~transformers.XLNetConfig`) and inputs:
- loss (:obj:`torch.FloatTensor` of shape :obj:`(1,)`, `optional`, returned when :obj:`labels` is provided):
- Classification loss.
- logits (:obj:`torch.FloatTensor` of shape :obj:(batch_size, config.num_labels)`):
- Classification scores (before SoftMax).
- mems (:obj:`List[torch.FloatTensor]` of length :obj:`config.n_layers`):
- Contains pre-computed hidden-states (key and values in the attention blocks).
- Can be used (see `past` input) to speed up sequential decoding. The token ids which have their past given to this model
- should not be passed as input ids as they have already been computed.
- hidden_states (:obj:`tuple(torch.FloatTensor)`, `optional`, returned when ``config.output_hidden_states=True``):
- Tuple of :obj:`torch.FloatTensor` (one for the output of the embeddings + one for the output of each layer)
- of shape :obj:`(batch_size, sequence_length, hidden_size)`.
-
- Hidden-states of the model at the output of each layer plus the initial embedding outputs.
- attentions (:obj:`tuple(torch.FloatTensor)`, `optional`, returned when ``config.output_attentions=True``):
- Tuple of :obj:`torch.FloatTensor` (one for each layer) of shape
- :obj:`(batch_size, num_heads, sequence_length, sequence_length)`.
-
- Attentions weights after the attention softmax, used to compute the weighted average in the self-attention
- heads.
-
- Examples::
-
- from transformers import XLNetTokenizer, XLNetForTokenClassification
- import torch
-
- tokenizer = XLNetTokenizer.from_pretrained('xlnet-large-cased')
- model = XLNetForTokenClassification.from_pretrained('xlnet-large-cased')
-
- input_ids = torch.tensor(tokenizer.encode("Hello, my dog is cute")).unsqueeze(0) # Batch size 1
- labels = torch.tensor([1] * input_ids.size(1)).unsqueeze(0) # Batch size 1
- outputs = model(input_ids, labels=labels)
-
- scores = outputs[0]
-
- """
-
- outputs = self.transformer(
- input_ids,
- attention_mask=attention_mask,
- mems=mems,
- perm_mask=perm_mask,
- target_mapping=target_mapping,
- token_type_ids=token_type_ids,
- input_mask=input_mask,
- head_mask=head_mask,
- inputs_embeds=inputs_embeds,
- )
-
- sequence_output = outputs[0]
-
- logits = self.classifier(sequence_output)
-
- outputs = (logits,) + outputs[1:] # Keep mems, hidden states, attentions if there are in it
- if labels is not None:
- loss_fct = CrossEntropyLoss()
- # Only keep active parts of the loss
- if attention_mask is not None:
- active_loss = attention_mask.view(-1) == 1
- active_logits = logits.view(-1, self.num_labels)[active_loss]
- active_labels = labels.view(-1)[active_loss]
- loss = loss_fct(active_logits, active_labels)
- else:
- loss = loss_fct(logits.view(-1, self.num_labels), labels.view(-1))
- outputs = (loss,) + outputs
-
- return outputs # return (loss), logits, (mems), (hidden states), (attentions)
-
-
-@add_start_docstrings(
- """XLNet Model with a multiple choice classification head on top (a linear layer on top of
- the pooled output and a softmax) e.g. for RACE/SWAG tasks. """,
- XLNET_START_DOCSTRING,
-)
-class XLNetForMultipleChoice(XLNetPreTrainedModel):
- def __init__(self, config):
- super().__init__(config)
-
- self.transformer = XLNetModel(config)
- self.sequence_summary = SequenceSummary(config)
- self.logits_proj = nn.Linear(config.d_model, 1)
-
- self.init_weights()
-
- @add_start_docstrings_to_callable(XLNET_INPUTS_DOCSTRING)
- def forward(
- self,
- input_ids=None,
- token_type_ids=None,
- input_mask=None,
- attention_mask=None,
- mems=None,
- perm_mask=None,
- target_mapping=None,
- labels=None,
- head_mask=None,
- inputs_embeds=None,
- ):
- r"""
- labels (:obj:`torch.LongTensor` of shape :obj:`(batch_size,)`, `optional`, defaults to :obj:`None`):
- Labels for computing the multiple choice classification loss.
- Indices should be in ``[0, ..., num_choices]`` where `num_choices` is the size of the second dimension
- of the input tensors. (see `input_ids` above)
-
- Returns:
- :obj:`tuple(torch.FloatTensor)` comprising various elements depending on the configuration (:class:`~transformers.XLNetConfig`) and inputs:
- loss (:obj:`torch.FloatTensor`` of shape ``(1,)`, `optional`, returned when :obj:`labels` is provided):
- Classification loss.
- classification_scores (:obj:`torch.FloatTensor` of shape :obj:`(batch_size, num_choices)`):
- `num_choices` is the second dimension of the input tensors. (see `input_ids` above).
-
- Classification scores (before SoftMax).
- mems (:obj:`List[torch.FloatTensor]` of length :obj:`config.n_layers`):
- Contains pre-computed hidden-states (key and values in the attention blocks).
- Can be used (see `past` input) to speed up sequential decoding. The token ids which have their past given to this model
- should not be passed as input ids as they have already been computed.
- hidden_states (:obj:`tuple(torch.FloatTensor)`, `optional`, returned when ``config.output_hidden_states=True``):
- Tuple of :obj:`torch.FloatTensor` (one for the output of the embeddings + one for the output of each layer)
- of shape :obj:`(batch_size, sequence_length, hidden_size)`.
-
- Hidden-states of the model at the output of each layer plus the initial embedding outputs.
- attentions (:obj:`tuple(torch.FloatTensor)`, `optional`, returned when ``config.output_attentions=True``):
- Tuple of :obj:`torch.FloatTensor` (one for each layer) of shape
- :obj:`(batch_size, num_heads, sequence_length, sequence_length)`.
-
- Attentions weights after the attention softmax, used to compute the weighted average in the self-attention
- heads.
-
- Examples::
-
- from transformers import XLNetTokenizer, XLNetForMultipleChoice
- import torch
-
- tokenizer = XLNetTokenizer.from_pretrained('xlnet-base-cased')
- model = XLNetForMultipleChoice.from_pretrained('xlnet-base-cased')
-
- choices = ["Hello, my dog is cute", "Hello, my cat is amazing"]
- input_ids = torch.tensor([tokenizer.encode(s) for s in choices]).unsqueeze(0) # Batch size 1, 2 choices
- labels = torch.tensor(1).unsqueeze(0) # Batch size 1
-
- outputs = model(input_ids, labels=labels)
- loss, classification_scores = outputs[:2]
-
- """
- num_choices = input_ids.shape[1]
-
- flat_input_ids = input_ids.view(-1, input_ids.size(-1))
- flat_token_type_ids = token_type_ids.view(-1, token_type_ids.size(-1)) if token_type_ids is not None else None
- flat_attention_mask = attention_mask.view(-1, attention_mask.size(-1)) if attention_mask is not None else None
- flat_input_mask = input_mask.view(-1, input_mask.size(-1)) if input_mask is not None else None
-
- transformer_outputs = self.transformer(
- flat_input_ids,
- token_type_ids=flat_token_type_ids,
- input_mask=flat_input_mask,
- attention_mask=flat_attention_mask,
- mems=mems,
- perm_mask=perm_mask,
- target_mapping=target_mapping,
- head_mask=head_mask,
- inputs_embeds=inputs_embeds,
- )
-
- output = transformer_outputs[0]
-
- output = self.sequence_summary(output)
- logits = self.logits_proj(output)
- reshaped_logits = logits.view(-1, num_choices)
- outputs = (reshaped_logits,) + transformer_outputs[
- 1:
- ] # Keep mems, hidden states, attentions if there are in it
-
- if labels is not None:
- loss_fct = CrossEntropyLoss()
- loss = loss_fct(reshaped_logits, labels.view(-1))
- outputs = (loss,) + outputs
-
- return outputs # return (loss), logits, (mems), (hidden states), (attentions)
-
-
-@add_start_docstrings(
- """XLNet Model with a span classification head on top for extractive question-answering tasks like SQuAD (a linear layers on top of
- the hidden-states output to compute `span start logits` and `span end logits`). """,
- XLNET_START_DOCSTRING,
-)
-class XLNetForQuestionAnsweringSimple(XLNetPreTrainedModel):
- def __init__(self, config):
- super().__init__(config)
- self.num_labels = config.num_labels
-
- self.transformer = XLNetModel(config)
- self.qa_outputs = nn.Linear(config.hidden_size, config.num_labels)
-
- self.init_weights()
-
- @add_start_docstrings_to_callable(XLNET_INPUTS_DOCSTRING)
- def forward(
- self,
- input_ids=None,
- attention_mask=None,
- mems=None,
- perm_mask=None,
- target_mapping=None,
- token_type_ids=None,
- input_mask=None,
- head_mask=None,
- inputs_embeds=None,
- start_positions=None,
- end_positions=None,
- ):
- r"""
- start_positions (:obj:`torch.LongTensor` of shape :obj:`(batch_size,)`, `optional`, defaults to :obj:`None`):
- Labels for position (index) of the start of the labelled span for computing the token classification loss.
- Positions are clamped to the length of the sequence (`sequence_length`).
- Position outside of the sequence are not taken into account for computing the loss.
- end_positions (:obj:`torch.LongTensor` of shape :obj:`(batch_size,)`, `optional`, defaults to :obj:`None`):
- Labels for position (index) of the end of the labelled span for computing the token classification loss.
- Positions are clamped to the length of the sequence (`sequence_length`).
- Position outside of the sequence are not taken into account for computing the loss.
-
- Returns:
- :obj:`tuple(torch.FloatTensor)` comprising various elements depending on the configuration (:class:`~transformers.XLNetConfig`) and inputs:
- loss (:obj:`torch.FloatTensor` of shape :obj:`(1,)`, `optional`, returned when :obj:`labels` is provided):
- Total span extraction loss is the sum of a Cross-Entropy for the start and end positions.
- start_scores (:obj:`torch.FloatTensor` of shape :obj:`(batch_size, sequence_length,)`):
- Span-start scores (before SoftMax).
- end_scores (:obj:`torch.FloatTensor` of shape :obj:`(batch_size, sequence_length,)`):
- Span-end scores (before SoftMax).
- mems (:obj:`List[torch.FloatTensor]` of length :obj:`config.n_layers`):
- Contains pre-computed hidden-states (key and values in the attention blocks).
- Can be used (see `past` input) to speed up sequential decoding. The token ids which have their past given to this model
- should not be passed as input ids as they have already been computed.
- hidden_states (:obj:`tuple(torch.FloatTensor)`, `optional`, returned when ``config.output_hidden_states=True``):
- Tuple of :obj:`torch.FloatTensor` (one for the output of the embeddings + one for the output of each layer)
- of shape :obj:`(batch_size, sequence_length, hidden_size)`.
-
- Hidden-states of the model at the output of each layer plus the initial embedding outputs.
- attentions (:obj:`tuple(torch.FloatTensor)`, `optional`, returned when ``config.output_attentions=True``):
- Tuple of :obj:`torch.FloatTensor` (one for each layer) of shape
- :obj:`(batch_size, num_heads, sequence_length, sequence_length)`.
-
- Attentions weights after the attention softmax, used to compute the weighted average in the self-attention
- heads.
-
- Examples::
-
- from transformers import XLNetTokenizer, XLNetForQuestionAnsweringSimple
- import torch
-
- tokenizer = XLNetTokenizer.from_pretrained('xlnet-base-cased')
- model = XLNetForQuestionAnsweringSimple.from_pretrained('xlnet-base-cased')
-
- input_ids = torch.tensor(tokenizer.encode("Hello, my dog is cute", add_special_tokens=True)).unsqueeze(0) # Batch size 1
- start_positions = torch.tensor([1])
- end_positions = torch.tensor([3])
-
- outputs = model(input_ids, start_positions=start_positions, end_positions=end_positions)
- loss = outputs[0]
-
- """
-
- outputs = self.transformer(
- input_ids,
- attention_mask=attention_mask,
- mems=mems,
- perm_mask=perm_mask,
- target_mapping=target_mapping,
- token_type_ids=token_type_ids,
- input_mask=input_mask,
- head_mask=head_mask,
- inputs_embeds=inputs_embeds,
- )
-
- sequence_output = outputs[0]
-
- logits = self.qa_outputs(sequence_output)
- start_logits, end_logits = logits.split(1, dim=-1)
- start_logits = start_logits.squeeze(-1)
- end_logits = end_logits.squeeze(-1)
-
- outputs = (start_logits, end_logits,) + outputs[2:]
- if start_positions is not None and end_positions is not None:
- # If we are on multi-GPU, split add a dimension
- if len(start_positions.size()) > 1:
- start_positions = start_positions.squeeze(-1)
- if len(end_positions.size()) > 1:
- end_positions = end_positions.squeeze(-1)
- # sometimes the start/end positions are outside our model inputs, we ignore these terms
- ignored_index = start_logits.size(1)
- start_positions.clamp_(0, ignored_index)
- end_positions.clamp_(0, ignored_index)
-
- loss_fct = CrossEntropyLoss(ignore_index=ignored_index)
- start_loss = loss_fct(start_logits, start_positions)
- end_loss = loss_fct(end_logits, end_positions)
- total_loss = (start_loss + end_loss) / 2
- outputs = (total_loss,) + outputs
-
- return outputs # (loss), start_logits, end_logits, (mems), (hidden_states), (attentions)
-
-
-@add_start_docstrings(
- """XLNet Model with a span classification head on top for extractive question-answering tasks like SQuAD (a linear layers on top of
- the hidden-states output to compute `span start logits` and `span end logits`). """,
- XLNET_START_DOCSTRING,
-)
-class XLNetForQuestionAnswering(XLNetPreTrainedModel):
- def __init__(self, config):
- super().__init__(config)
- self.start_n_top = config.start_n_top
- self.end_n_top = config.end_n_top
-
- self.transformer = XLNetModel(config)
- self.start_logits = PoolerStartLogits(config)
- self.end_logits = PoolerEndLogits(config)
- self.answer_class = PoolerAnswerClass(config)
-
- self.init_weights()
-
- @add_start_docstrings_to_callable(XLNET_INPUTS_DOCSTRING)
- def forward(
- self,
- input_ids=None,
- attention_mask=None,
- mems=None,
- perm_mask=None,
- target_mapping=None,
- token_type_ids=None,
- input_mask=None,
- head_mask=None,
- inputs_embeds=None,
- start_positions=None,
- end_positions=None,
- is_impossible=None,
- cls_index=None,
- p_mask=None,
- ):
- r"""
- start_positions (:obj:`torch.LongTensor` of shape :obj:`(batch_size,)`, `optional`, defaults to :obj:`None`):
- Labels for position (index) of the start of the labelled span for computing the token classification loss.
- Positions are clamped to the length of the sequence (`sequence_length`).
- Position outside of the sequence are not taken into account for computing the loss.
- end_positions (:obj:`torch.LongTensor` of shape :obj:`(batch_size,)`, `optional`, defaults to :obj:`None`):
- Labels for position (index) of the end of the labelled span for computing the token classification loss.
- Positions are clamped to the length of the sequence (`sequence_length`).
- Position outside of the sequence are not taken into account for computing the loss.
- is_impossible (``torch.LongTensor`` of shape ``(batch_size,)``, `optional`, defaults to :obj:`None`):
- Labels whether a question has an answer or no answer (SQuAD 2.0)
- cls_index (``torch.LongTensor`` of shape ``(batch_size,)``, `optional`, defaults to :obj:`None`):
- Labels for position (index) of the classification token to use as input for computing plausibility of the answer.
- p_mask (``torch.FloatTensor`` of shape ``(batch_size, sequence_length)``, `optional`, defaults to :obj:`None`):
- Optional mask of tokens which can't be in answers (e.g. [CLS], [PAD], ...).
- 1.0 means token should be masked. 0.0 mean token is not masked.
-
- Returns:
- :obj:`tuple(torch.FloatTensor)` comprising various elements depending on the configuration (:class:`~transformers.XLNetConfig`) and inputs:
- loss (:obj:`torch.FloatTensor` of shape :obj:`(1,)`, `optional`, returned if both :obj:`start_positions` and :obj:`end_positions` are provided):
- Classification loss as the sum of start token, end token (and is_impossible if provided) classification losses.
- start_top_log_probs (``torch.FloatTensor`` of shape ``(batch_size, config.start_n_top)``, `optional`, returned if ``start_positions`` or ``end_positions`` is not provided):
- Log probabilities for the top config.start_n_top start token possibilities (beam-search).
- start_top_index (``torch.LongTensor`` of shape ``(batch_size, config.start_n_top)``, `optional`, returned if ``start_positions`` or ``end_positions`` is not provided):
- Indices for the top config.start_n_top start token possibilities (beam-search).
- end_top_log_probs (``torch.FloatTensor`` of shape ``(batch_size, config.start_n_top * config.end_n_top)``, `optional`, returned if ``start_positions`` or ``end_positions`` is not provided):
- Log probabilities for the top ``config.start_n_top * config.end_n_top`` end token possibilities (beam-search).
- end_top_index (``torch.LongTensor`` of shape ``(batch_size, config.start_n_top * config.end_n_top)``, `optional`, returned if ``start_positions`` or ``end_positions`` is not provided):
- Indices for the top ``config.start_n_top * config.end_n_top`` end token possibilities (beam-search).
- cls_logits (``torch.FloatTensor`` of shape ``(batch_size,)``, `optional`, returned if ``start_positions`` or ``end_positions`` is not provided):
- Log probabilities for the ``is_impossible`` label of the answers.
- mems (:obj:`List[torch.FloatTensor]` of length :obj:`config.n_layers`):
- Contains pre-computed hidden-states (key and values in the attention blocks).
- Can be used (see `past` input) to speed up sequential decoding. The token ids which have their past given to this model
- should not be passed as input ids as they have already been computed.
- hidden_states (:obj:`tuple(torch.FloatTensor)`, `optional`, returned when ``config.output_hidden_states=True``):
- Tuple of :obj:`torch.FloatTensor` (one for the output of the embeddings + one for the output of each layer)
- of shape :obj:`(batch_size, sequence_length, hidden_size)`.
-
- Hidden-states of the model at the output of each layer plus the initial embedding outputs.
- attentions (:obj:`tuple(torch.FloatTensor)`, `optional`, returned when ``config.output_attentions=True``):
- Tuple of :obj:`torch.FloatTensor` (one for each layer) of shape
- :obj:`(batch_size, num_heads, sequence_length, sequence_length)`.
-
- Attentions weights after the attention softmax, used to compute the weighted average in the self-attention
- heads.
-
- Examples::
-
- from transformers import XLNetTokenizer, XLNetForQuestionAnswering
- import torch
-
- tokenizer = XLNetTokenizer.from_pretrained('xlnet-base-cased')
- model = XLNetForQuestionAnswering.from_pretrained('xlnet-base-cased')
-
- input_ids = torch.tensor(tokenizer.encode("Hello, my dog is cute", add_special_tokens=True)).unsqueeze(0) # Batch size 1
- start_positions = torch.tensor([1])
- end_positions = torch.tensor([3])
- outputs = model(input_ids, start_positions=start_positions, end_positions=end_positions)
- loss = outputs[0]
-
- """
- transformer_outputs = self.transformer(
- input_ids,
- attention_mask=attention_mask,
- mems=mems,
- perm_mask=perm_mask,
- target_mapping=target_mapping,
- token_type_ids=token_type_ids,
- input_mask=input_mask,
- head_mask=head_mask,
- inputs_embeds=inputs_embeds,
- )
- hidden_states = transformer_outputs[0]
- start_logits = self.start_logits(hidden_states, p_mask=p_mask)
-
- outputs = transformer_outputs[1:] # Keep mems, hidden states, attentions if there are in it
-
- if start_positions is not None and end_positions is not None:
- # If we are on multi-GPU, let's remove the dimension added by batch splitting
- for x in (start_positions, end_positions, cls_index, is_impossible):
- if x is not None and x.dim() > 1:
- x.squeeze_(-1)
-
- # during training, compute the end logits based on the ground truth of the start position
- end_logits = self.end_logits(hidden_states, start_positions=start_positions, p_mask=p_mask)
-
- loss_fct = CrossEntropyLoss()
- start_loss = loss_fct(start_logits, start_positions)
- end_loss = loss_fct(end_logits, end_positions)
- total_loss = (start_loss + end_loss) / 2
-
- if cls_index is not None and is_impossible is not None:
- # Predict answerability from the representation of CLS and START
- cls_logits = self.answer_class(hidden_states, start_positions=start_positions, cls_index=cls_index)
- loss_fct_cls = nn.BCEWithLogitsLoss()
- cls_loss = loss_fct_cls(cls_logits, is_impossible)
-
- # note(zhiliny): by default multiply the loss by 0.5 so that the scale is comparable to start_loss and end_loss
- total_loss += cls_loss * 0.5
-
- outputs = (total_loss,) + outputs
-
- else:
- # during inference, compute the end logits based on beam search
- bsz, slen, hsz = hidden_states.size()
- start_log_probs = F.softmax(start_logits, dim=-1) # shape (bsz, slen)
-
- start_top_log_probs, start_top_index = torch.topk(
- start_log_probs, self.start_n_top, dim=-1
- ) # shape (bsz, start_n_top)
- start_top_index_exp = start_top_index.unsqueeze(-1).expand(-1, -1, hsz) # shape (bsz, start_n_top, hsz)
- start_states = torch.gather(hidden_states, -2, start_top_index_exp) # shape (bsz, start_n_top, hsz)
- start_states = start_states.unsqueeze(1).expand(-1, slen, -1, -1) # shape (bsz, slen, start_n_top, hsz)
-
- hidden_states_expanded = hidden_states.unsqueeze(2).expand_as(
- start_states
- ) # shape (bsz, slen, start_n_top, hsz)
- p_mask = p_mask.unsqueeze(-1) if p_mask is not None else None
- end_logits = self.end_logits(hidden_states_expanded, start_states=start_states, p_mask=p_mask)
- end_log_probs = F.softmax(end_logits, dim=1) # shape (bsz, slen, start_n_top)
-
- end_top_log_probs, end_top_index = torch.topk(
- end_log_probs, self.end_n_top, dim=1
- ) # shape (bsz, end_n_top, start_n_top)
- end_top_log_probs = end_top_log_probs.view(-1, self.start_n_top * self.end_n_top)
- end_top_index = end_top_index.view(-1, self.start_n_top * self.end_n_top)
-
- start_states = torch.einsum(
- "blh,bl->bh", hidden_states, start_log_probs
- ) # get the representation of START as weighted sum of hidden states
- cls_logits = self.answer_class(
- hidden_states, start_states=start_states, cls_index=cls_index
- ) # Shape (batch size,): one single `cls_logits` for each sample
-
- outputs = (start_top_log_probs, start_top_index, end_top_log_probs, end_top_index, cls_logits) + outputs
-
- # return start_top_log_probs, start_top_index, end_top_log_probs, end_top_index, cls_logits
- # or (if labels are provided) (total_loss,)
- return outputs
diff --git a/server/transformers/src/transformers/optimization.py b/server/transformers/src/transformers/optimization.py
deleted file mode 100644
index 5ab7647638e054192b1a122b2121b5c5059ca85d..0000000000000000000000000000000000000000
--- a/server/transformers/src/transformers/optimization.py
+++ /dev/null
@@ -1,178 +0,0 @@
-# coding=utf-8
-# Copyright 2018 The Google AI Language Team Authors and The HuggingFace Inc. team.
-#
-# Licensed under the Apache License, Version 2.0 (the "License");
-# you may not use this file except in compliance with the License.
-# You may obtain a copy of the License at
-#
-# http://www.apache.org/licenses/LICENSE-2.0
-#
-# Unless required by applicable law or agreed to in writing, software
-# distributed under the License is distributed on an "AS IS" BASIS,
-# WITHOUT WARRANTIES OR CONDITIONS OF ANY KIND, either express or implied.
-# See the License for the specific language governing permissions and
-# limitations under the License.
-"""PyTorch optimization for BERT model."""
-
-import logging
-import math
-
-import torch
-from torch.optim import Optimizer
-from torch.optim.lr_scheduler import LambdaLR
-
-
-logger = logging.getLogger(__name__)
-
-
-def get_constant_schedule(optimizer, last_epoch=-1):
- """ Create a schedule with a constant learning rate.
- """
- return LambdaLR(optimizer, lambda _: 1, last_epoch=last_epoch)
-
-
-def get_constant_schedule_with_warmup(optimizer, num_warmup_steps, last_epoch=-1):
- """ Create a schedule with a constant learning rate preceded by a warmup
- period during which the learning rate increases linearly between 0 and 1.
- """
-
- def lr_lambda(current_step):
- if current_step < num_warmup_steps:
- return float(current_step) / float(max(1.0, num_warmup_steps))
- return 1.0
-
- return LambdaLR(optimizer, lr_lambda, last_epoch=last_epoch)
-
-
-def get_linear_schedule_with_warmup(optimizer, num_warmup_steps, num_training_steps, last_epoch=-1):
- """ Create a schedule with a learning rate that decreases linearly after
- linearly increasing during a warmup period.
- """
-
- def lr_lambda(current_step):
- if current_step < num_warmup_steps:
- return float(current_step) / float(max(1, num_warmup_steps))
- return max(
- 0.0, float(num_training_steps - current_step) / float(max(1, num_training_steps - num_warmup_steps))
- )
-
- return LambdaLR(optimizer, lr_lambda, last_epoch)
-
-
-def get_cosine_schedule_with_warmup(optimizer, num_warmup_steps, num_training_steps, num_cycles=0.5, last_epoch=-1):
- """ Create a schedule with a learning rate that decreases following the
- values of the cosine function between 0 and `pi * cycles` after a warmup
- period during which it increases linearly between 0 and 1.
- """
-
- def lr_lambda(current_step):
- if current_step < num_warmup_steps:
- return float(current_step) / float(max(1, num_warmup_steps))
- progress = float(current_step - num_warmup_steps) / float(max(1, num_training_steps - num_warmup_steps))
- return max(0.0, 0.5 * (1.0 + math.cos(math.pi * float(num_cycles) * 2.0 * progress)))
-
- return LambdaLR(optimizer, lr_lambda, last_epoch)
-
-
-def get_cosine_with_hard_restarts_schedule_with_warmup(
- optimizer, num_warmup_steps, num_training_steps, num_cycles=1.0, last_epoch=-1
-):
- """ Create a schedule with a learning rate that decreases following the
- values of the cosine function with several hard restarts, after a warmup
- period during which it increases linearly between 0 and 1.
- """
-
- def lr_lambda(current_step):
- if current_step < num_warmup_steps:
- return float(current_step) / float(max(1, num_warmup_steps))
- progress = float(current_step - num_warmup_steps) / float(max(1, num_training_steps - num_warmup_steps))
- if progress >= 1.0:
- return 0.0
- return max(0.0, 0.5 * (1.0 + math.cos(math.pi * ((float(num_cycles) * progress) % 1.0))))
-
- return LambdaLR(optimizer, lr_lambda, last_epoch)
-
-
-class AdamW(Optimizer):
- """ Implements Adam algorithm with weight decay fix.
-
- Parameters:
- lr (float): learning rate. Default 1e-3.
- betas (tuple of 2 floats): Adams beta parameters (b1, b2). Default: (0.9, 0.999)
- eps (float): Adams epsilon. Default: 1e-6
- weight_decay (float): Weight decay. Default: 0.0
- correct_bias (bool): can be set to False to avoid correcting bias in Adam (e.g. like in Bert TF repository). Default True.
- """
-
- def __init__(self, params, lr=1e-3, betas=(0.9, 0.999), eps=1e-6, weight_decay=0.0, correct_bias=True):
- if lr < 0.0:
- raise ValueError("Invalid learning rate: {} - should be >= 0.0".format(lr))
- if not 0.0 <= betas[0] < 1.0:
- raise ValueError("Invalid beta parameter: {} - should be in [0.0, 1.0[".format(betas[0]))
- if not 0.0 <= betas[1] < 1.0:
- raise ValueError("Invalid beta parameter: {} - should be in [0.0, 1.0[".format(betas[1]))
- if not 0.0 <= eps:
- raise ValueError("Invalid epsilon value: {} - should be >= 0.0".format(eps))
- defaults = dict(lr=lr, betas=betas, eps=eps, weight_decay=weight_decay, correct_bias=correct_bias)
- super().__init__(params, defaults)
-
- def step(self, closure=None):
- """Performs a single optimization step.
-
- Arguments:
- closure (callable, optional): A closure that reevaluates the model
- and returns the loss.
- """
- loss = None
- if closure is not None:
- loss = closure()
-
- for group in self.param_groups:
- for p in group["params"]:
- if p.grad is None:
- continue
- grad = p.grad.data
- if grad.is_sparse:
- raise RuntimeError("Adam does not support sparse gradients, please consider SparseAdam instead")
-
- state = self.state[p]
-
- # State initialization
- if len(state) == 0:
- state["step"] = 0
- # Exponential moving average of gradient values
- state["exp_avg"] = torch.zeros_like(p.data)
- # Exponential moving average of squared gradient values
- state["exp_avg_sq"] = torch.zeros_like(p.data)
-
- exp_avg, exp_avg_sq = state["exp_avg"], state["exp_avg_sq"]
- beta1, beta2 = group["betas"]
-
- state["step"] += 1
-
- # Decay the first and second moment running average coefficient
- # In-place operations to update the averages at the same time
- exp_avg.mul_(beta1).add_(1.0 - beta1, grad)
- exp_avg_sq.mul_(beta2).addcmul_(1.0 - beta2, grad, grad)
- denom = exp_avg_sq.sqrt().add_(group["eps"])
-
- step_size = group["lr"]
- if group["correct_bias"]: # No bias correction for Bert
- bias_correction1 = 1.0 - beta1 ** state["step"]
- bias_correction2 = 1.0 - beta2 ** state["step"]
- step_size = step_size * math.sqrt(bias_correction2) / bias_correction1
-
- p.data.addcdiv_(-step_size, exp_avg, denom)
-
- # Just adding the square of the weights to the loss function is *not*
- # the correct way of using L2 regularization/weight decay with Adam,
- # since that will interact with the m and v parameters in strange ways.
- #
- # Instead we want to decay the weights in a manner that doesn't interact
- # with the m/v parameters. This is equivalent to adding the square
- # of the weights to the loss with plain (non-momentum) SGD.
- # Add weight decay at the end (fixed version)
- if group["weight_decay"] > 0.0:
- p.data.add_(-group["lr"] * group["weight_decay"], p.data)
-
- return loss
diff --git a/server/transformers/src/transformers/optimization_tf.py b/server/transformers/src/transformers/optimization_tf.py
deleted file mode 100644
index d232370905e241a5029200f6f4229f6000368623..0000000000000000000000000000000000000000
--- a/server/transformers/src/transformers/optimization_tf.py
+++ /dev/null
@@ -1,246 +0,0 @@
-# Copyright 2019 The TensorFlow Authors. All Rights Reserved.
-#
-# Licensed under the Apache License, Version 2.0 (the "License");
-# you may not use this file except in compliance with the License.
-# You may obtain a copy of the License at
-#
-# http://www.apache.org/licenses/LICENSE-2.0
-#
-# Unless required by applicable law or agreed to in writing, software
-# distributed under the License is distributed on an "AS IS" BASIS,
-# WITHOUT WARRANTIES OR CONDITIONS OF ANY KIND, either express or implied.
-# See the License for the specific language governing permissions and
-# limitations under the License.
-# ==============================================================================
-"""Functions and classes related to optimization (weight updates)."""
-
-
-import re
-
-import tensorflow as tf
-
-
-class WarmUp(tf.keras.optimizers.schedules.LearningRateSchedule):
- """Applys a warmup schedule on a given learning rate decay schedule."""
-
- def __init__(self, initial_learning_rate, decay_schedule_fn, warmup_steps, power=1.0, name=None):
- super().__init__()
- self.initial_learning_rate = initial_learning_rate
- self.warmup_steps = warmup_steps
- self.power = power
- self.decay_schedule_fn = decay_schedule_fn
- self.name = name
-
- def __call__(self, step):
- with tf.name_scope(self.name or "WarmUp") as name:
- # Implements polynomial warmup. i.e., if global_step < warmup_steps, the
- # learning rate will be `global_step/num_warmup_steps * init_lr`.
- global_step_float = tf.cast(step, tf.float32)
- warmup_steps_float = tf.cast(self.warmup_steps, tf.float32)
- warmup_percent_done = global_step_float / warmup_steps_float
- warmup_learning_rate = self.initial_learning_rate * tf.math.pow(warmup_percent_done, self.power)
- return tf.cond(
- global_step_float < warmup_steps_float,
- lambda: warmup_learning_rate,
- lambda: self.decay_schedule_fn(step),
- name=name,
- )
-
- def get_config(self):
- return {
- "initial_learning_rate": self.initial_learning_rate,
- "decay_schedule_fn": self.decay_schedule_fn,
- "warmup_steps": self.warmup_steps,
- "power": self.power,
- "name": self.name,
- }
-
-
-def create_optimizer(init_lr, num_train_steps, num_warmup_steps):
- """Creates an optimizer with learning rate schedule."""
- # Implements linear decay of the learning rate.
- learning_rate_fn = tf.keras.optimizers.schedules.PolynomialDecay(
- initial_learning_rate=init_lr, decay_steps=num_train_steps, end_learning_rate=0.0
- )
- if num_warmup_steps:
- learning_rate_fn = WarmUp(
- initial_learning_rate=init_lr, decay_schedule_fn=learning_rate_fn, warmup_steps=num_warmup_steps
- )
- optimizer = AdamWeightDecay(
- learning_rate=learning_rate_fn,
- weight_decay_rate=0.01,
- beta_1=0.9,
- beta_2=0.999,
- epsilon=1e-6,
- exclude_from_weight_decay=["layer_norm", "bias"],
- )
- return optimizer
-
-
-class AdamWeightDecay(tf.keras.optimizers.Adam):
- """Adam enables L2 weight decay and clip_by_global_norm on gradients.
-
- Just adding the square of the weights to the loss function is *not* the
- correct way of using L2 regularization/weight decay with Adam, since that will
- interact with the m and v parameters in strange ways.
-
- Instead we want ot decay the weights in a manner that doesn't interact with
- the m/v parameters. This is equivalent to adding the square of the weights to
- the loss with plain (non-momentum) SGD.
- """
-
- def __init__(
- self,
- learning_rate=0.001,
- beta_1=0.9,
- beta_2=0.999,
- epsilon=1e-7,
- amsgrad=False,
- weight_decay_rate=0.0,
- include_in_weight_decay=None,
- exclude_from_weight_decay=None,
- name="AdamWeightDecay",
- **kwargs
- ):
- super().__init__(learning_rate, beta_1, beta_2, epsilon, amsgrad, name, **kwargs)
- self.weight_decay_rate = weight_decay_rate
- self._include_in_weight_decay = include_in_weight_decay
- self._exclude_from_weight_decay = exclude_from_weight_decay
-
- @classmethod
- def from_config(cls, config):
- """Creates an optimizer from its config with WarmUp custom object."""
- custom_objects = {"WarmUp": WarmUp}
- return super().from_config(config, custom_objects=custom_objects)
-
- def _prepare_local(self, var_device, var_dtype, apply_state):
- super()._prepare_local(var_device, var_dtype, apply_state)
- apply_state["weight_decay_rate"] = tf.constant(self.weight_decay_rate, name="adam_weight_decay_rate")
-
- def _decay_weights_op(self, var, learning_rate, apply_state):
- do_decay = self._do_use_weight_decay(var.name)
- if do_decay:
- return var.assign_sub(
- learning_rate * var * apply_state["weight_decay_rate"], use_locking=self._use_locking
- )
- return tf.no_op()
-
- def apply_gradients(self, grads_and_vars, clip_norm, name=None):
- grads, tvars = list(zip(*grads_and_vars))
- (grads, _) = tf.clip_by_global_norm(grads, clip_norm=clip_norm)
- return super().apply_gradients(zip(grads, tvars))
-
- def _get_lr(self, var_device, var_dtype, apply_state):
- """Retrieves the learning rate with the given state."""
- if apply_state is None:
- return self._decayed_lr_t[var_dtype], {}
-
- apply_state = apply_state or {}
- coefficients = apply_state.get((var_device, var_dtype))
- if coefficients is None:
- coefficients = self._fallback_apply_state(var_device, var_dtype)
- apply_state[(var_device, var_dtype)] = coefficients
-
- return coefficients["lr_t"], dict(apply_state=apply_state)
-
- def _resource_apply_dense(self, grad, var, apply_state=None):
- lr_t, kwargs = self._get_lr(var.device, var.dtype.base_dtype, apply_state)
- decay = self._decay_weights_op(var, lr_t, apply_state)
- with tf.control_dependencies([decay]):
- return super()._resource_apply_dense(grad, var, **kwargs)
-
- def _resource_apply_sparse(self, grad, var, indices, apply_state=None):
- lr_t, kwargs = self._get_lr(var.device, var.dtype.base_dtype, apply_state)
- decay = self._decay_weights_op(var, lr_t, apply_state)
- with tf.control_dependencies([decay]):
- return super()._resource_apply_sparse(grad, var, indices, **kwargs)
-
- def get_config(self):
- config = super().get_config()
- config.update({"weight_decay_rate": self.weight_decay_rate})
- return config
-
- def _do_use_weight_decay(self, param_name):
- """Whether to use L2 weight decay for `param_name`."""
- if self.weight_decay_rate == 0:
- return False
-
- if self._include_in_weight_decay:
- for r in self._include_in_weight_decay:
- if re.search(r, param_name) is not None:
- return True
-
- if self._exclude_from_weight_decay:
- for r in self._exclude_from_weight_decay:
- if re.search(r, param_name) is not None:
- return False
- return True
-
-
-# Inspired from https://github.com/OpenNMT/OpenNMT-tf/blob/master/opennmt/optimizers/utils.py
-class GradientAccumulator(object):
- """Distribution strategies-aware gradient accumulation utility."""
-
- def __init__(self):
- """Initializes the accumulator."""
- self._gradients = []
- self._accum_steps = tf.Variable(
- initial_value=0, dtype=tf.int64, trainable=False, aggregation=tf.VariableAggregation.ONLY_FIRST_REPLICA
- )
-
- @property
- def step(self):
- """Number of accumulated steps."""
- return self._accum_steps.value()
-
- @property
- def gradients(self):
- """The accumulated gradients."""
- return list(
- gradient.value() if gradient is not None else gradient for gradient in self._get_replica_gradients()
- )
-
- def __call__(self, gradients):
- """Accumulates :obj:`gradients`."""
- if not self._gradients:
- self._gradients.extend(
- [
- tf.Variable(tf.zeros_like(gradient), trainable=False) if gradient is not None else gradient
- for gradient in gradients
- ]
- )
-
- if len(gradients) != len(self._gradients):
- raise ValueError("Expected %s gradients, but got %d" % (len(self._gradients), len(gradients)))
-
- for accum_gradient, gradient in zip(self._get_replica_gradients(), gradients):
- if accum_gradient is not None:
- accum_gradient.assign_add(gradient)
-
- self._accum_steps.assign_add(1)
-
- def reset(self):
- """Resets the accumulated gradients."""
- if self._gradients:
- self._accum_steps.assign(0)
-
- for gradient in self._get_replica_gradients():
- if gradient is not None:
- gradient.assign(tf.zeros_like(gradient))
-
- def _get_replica_gradients(self):
- if tf.distribute.has_strategy():
- # In a replica context, we want to accumulate gradients on each replica
- # without synchronization, so we directly assign the value of the
- # current replica.
- replica_context = tf.distribute.get_replica_context()
-
- if replica_context is None or tf.distribute.get_strategy().num_replicas_in_sync == 1:
- return self._gradients
-
- return (
- gradient.device_map.select_for_current_replica(gradient.values, replica_context)
- for gradient in self._gradients
- )
- else:
- return self._gradients
diff --git a/server/transformers/src/transformers/pipelines.py b/server/transformers/src/transformers/pipelines.py
deleted file mode 100755
index d694afbaa5d9cb7cad87484c510d9dee0c73f5d0..0000000000000000000000000000000000000000
--- a/server/transformers/src/transformers/pipelines.py
+++ /dev/null
@@ -1,1087 +0,0 @@
-# coding=utf-8
-# Copyright 2018 The HuggingFace Inc. team.
-#
-# Licensed under the Apache License, Version 2.0 (the "License");
-# you may not use this file except in compliance with the License.
-# You may obtain a copy of the License at
-#
-# http://www.apache.org/licenses/LICENSE-2.0
-#
-# Unless required by applicable law or agreed to in writing, software
-# distributed under the License is distributed on an "AS IS" BASIS,
-# WITHOUT WARRANTIES OR CONDITIONS OF ANY KIND, either express or implied.
-# See the License for the specific language governing permissions and
-# limitations under the License.
-
-
-import csv
-import json
-import logging
-import os
-import pickle
-import sys
-from abc import ABC, abstractmethod
-from contextlib import contextmanager
-from os.path import abspath, exists
-from typing import Dict, List, Optional, Tuple, Union
-
-import numpy as np
-
-from .configuration_auto import ALL_PRETRAINED_CONFIG_ARCHIVE_MAP, AutoConfig
-from .configuration_distilbert import DistilBertConfig
-from .configuration_roberta import RobertaConfig
-from .configuration_utils import PretrainedConfig
-from .configuration_xlm import XLMConfig
-from .data import SquadExample, squad_convert_examples_to_features
-from .file_utils import is_tf_available, is_torch_available
-from .modelcard import ModelCard
-from .tokenization_auto import AutoTokenizer
-from .tokenization_bert import BasicTokenizer
-from .tokenization_utils import PreTrainedTokenizer
-
-
-if is_tf_available():
- import tensorflow as tf
- from .modeling_tf_auto import (
- TFAutoModel,
- TFAutoModelForSequenceClassification,
- TFAutoModelForQuestionAnswering,
- TFAutoModelForTokenClassification,
- TFAutoModelWithLMHead,
- )
-
-if is_torch_available():
- import torch
- from .modeling_auto import (
- AutoModel,
- AutoModelForSequenceClassification,
- AutoModelForQuestionAnswering,
- AutoModelForTokenClassification,
- AutoModelWithLMHead,
- )
-
-
-logger = logging.getLogger(__name__)
-
-
-def get_framework(model=None):
- """ Select framework (TensorFlow/PyTorch) to use.
- If both frameworks are installed and no specific model is provided, defaults to using PyTorch.
- """
- if is_tf_available() and is_torch_available() and model is not None and not isinstance(model, str):
- # Both framework are available but the user supplied a model class instance.
- # Try to guess which framework to use from the model classname
- framework = "tf" if model.__class__.__name__.startswith("TF") else "pt"
- elif not is_tf_available() and not is_torch_available():
- raise RuntimeError(
- "At least one of TensorFlow 2.0 or PyTorch should be installed. "
- "To install TensorFlow 2.0, read the instructions at https://www.tensorflow.org/install/ "
- "To install PyTorch, read the instructions at https://pytorch.org/."
- )
- else:
- # framework = 'tf' if is_tf_available() else 'pt'
- framework = "pt" if is_torch_available() else "tf"
- return framework
-
-
-class ArgumentHandler(ABC):
- """
- Base interface for handling varargs for each Pipeline
- """
-
- @abstractmethod
- def __call__(self, *args, **kwargs):
- raise NotImplementedError()
-
-
-class DefaultArgumentHandler(ArgumentHandler):
- """
- Default varargs argument parser handling parameters for each Pipeline
- """
-
- def __call__(self, *args, **kwargs):
- if "X" in kwargs:
- return kwargs["X"]
- elif "data" in kwargs:
- return kwargs["data"]
- elif len(args) == 1:
- if isinstance(args[0], list):
- return args[0]
- else:
- return [args[0]]
- elif len(args) > 1:
- return list(args)
- raise ValueError("Unable to infer the format of the provided data (X=, data=, ...)")
-
-
-class PipelineDataFormat:
- """
- Base class for all the pipeline supported data format both for reading and writing.
- Supported data formats currently includes:
- - JSON
- - CSV
- - stdin/stdout (pipe)
-
- PipelineDataFormat also includes some utilities to work with multi-columns like mapping from datasets columns
- to pipelines keyword arguments through the `dataset_kwarg_1=dataset_column_1` format.
- """
-
- SUPPORTED_FORMATS = ["json", "csv", "pipe"]
-
- def __init__(self, output_path: Optional[str], input_path: Optional[str], column: Optional[str], overwrite=False):
- self.output_path = output_path
- self.input_path = input_path
- self.column = column.split(",") if column is not None else [""]
- self.is_multi_columns = len(self.column) > 1
-
- if self.is_multi_columns:
- self.column = [tuple(c.split("=")) if "=" in c else (c, c) for c in self.column]
-
- if output_path is not None and not overwrite:
- if exists(abspath(self.output_path)):
- raise OSError("{} already exists on disk".format(self.output_path))
-
- if input_path is not None:
- if not exists(abspath(self.input_path)):
- raise OSError("{} doesnt exist on disk".format(self.input_path))
-
- @abstractmethod
- def __iter__(self):
- raise NotImplementedError()
-
- @abstractmethod
- def save(self, data: dict):
- """
- Save the provided data object with the representation for the current `DataFormat`.
- :param data: data to store
- :return:
- """
- raise NotImplementedError()
-
- def save_binary(self, data: Union[dict, List[dict]]) -> str:
- """
- Save the provided data object as a pickle-formatted binary data on the disk.
- :param data: data to store
- :return: (str) Path where the data has been saved
- """
- path, _ = os.path.splitext(self.output_path)
- binary_path = os.path.extsep.join((path, "pickle"))
-
- with open(binary_path, "wb+") as f_output:
- pickle.dump(data, f_output)
-
- return binary_path
-
- @staticmethod
- def from_str(
- format: str, output_path: Optional[str], input_path: Optional[str], column: Optional[str], overwrite=False
- ):
- if format == "json":
- return JsonPipelineDataFormat(output_path, input_path, column, overwrite=overwrite)
- elif format == "csv":
- return CsvPipelineDataFormat(output_path, input_path, column, overwrite=overwrite)
- elif format == "pipe":
- return PipedPipelineDataFormat(output_path, input_path, column, overwrite=overwrite)
- else:
- raise KeyError("Unknown reader {} (Available reader are json/csv/pipe)".format(format))
-
-
-class CsvPipelineDataFormat(PipelineDataFormat):
- def __init__(self, output_path: Optional[str], input_path: Optional[str], column: Optional[str], overwrite=False):
- super().__init__(output_path, input_path, column, overwrite=overwrite)
-
- def __iter__(self):
- with open(self.input_path, "r") as f:
- reader = csv.DictReader(f)
- for row in reader:
- if self.is_multi_columns:
- yield {k: row[c] for k, c in self.column}
- else:
- yield row[self.column[0]]
-
- def save(self, data: List[dict]):
- with open(self.output_path, "w") as f:
- if len(data) > 0:
- writer = csv.DictWriter(f, list(data[0].keys()))
- writer.writeheader()
- writer.writerows(data)
-
-
-class JsonPipelineDataFormat(PipelineDataFormat):
- def __init__(self, output_path: Optional[str], input_path: Optional[str], column: Optional[str], overwrite=False):
- super().__init__(output_path, input_path, column, overwrite=overwrite)
-
- with open(input_path, "r") as f:
- self._entries = json.load(f)
-
- def __iter__(self):
- for entry in self._entries:
- if self.is_multi_columns:
- yield {k: entry[c] for k, c in self.column}
- else:
- yield entry[self.column[0]]
-
- def save(self, data: dict):
- with open(self.output_path, "w") as f:
- json.dump(data, f)
-
-
-class PipedPipelineDataFormat(PipelineDataFormat):
- """
- Read data from piped input to the python process.
- For multi columns data, columns should separated by \t
-
- If columns are provided, then the output will be a dictionary with {column_x: value_x}
- """
-
- def __iter__(self):
- for line in sys.stdin:
- # Split for multi-columns
- if "\t" in line:
-
- line = line.split("\t")
- if self.column:
- # Dictionary to map arguments
- yield {kwargs: l for (kwargs, _), l in zip(self.column, line)}
- else:
- yield tuple(line)
-
- # No dictionary to map arguments
- else:
- yield line
-
- def save(self, data: dict):
- print(data)
-
- def save_binary(self, data: Union[dict, List[dict]]) -> str:
- if self.output_path is None:
- raise KeyError(
- "When using piped input on pipeline outputting large object requires an output file path. "
- "Please provide such output path through --output argument."
- )
-
- return super().save_binary(data)
-
-
-class _ScikitCompat(ABC):
- """
- Interface layer for the Scikit and Keras compatibility.
- """
-
- @abstractmethod
- def transform(self, X):
- raise NotImplementedError()
-
- @abstractmethod
- def predict(self, X):
- raise NotImplementedError()
-
-
-class Pipeline(_ScikitCompat):
- """
- Base class implementing pipelined operations.
- Pipeline workflow is defined as a sequence of the following operations:
- Input -> Tokenization -> Model Inference -> Post-Processing (Task dependent) -> Output
-
- Pipeline supports running on CPU or GPU through the device argument. Users can specify
- device argument as an integer, -1 meaning "CPU", >= 0 referring the CUDA device ordinal.
-
- Some pipeline, like for instance FeatureExtractionPipeline ('feature-extraction') outputs large
- tensor object as nested-lists. In order to avoid dumping such large structure as textual data we
- provide the binary_output constructor argument. If set to True, the output will be stored in the
- pickle format.
-
- Arguments:
- **model**: ``(str, PretrainedModel, TFPretrainedModel)``:
- Reference to the model to use through this pipeline.
-
- **tokenizer**: ``(str, PreTrainedTokenizer)``:
- Reference to the tokenizer to use through this pipeline.
-
- **args_parser**: ``ArgumentHandler``:
- Reference to the object in charge of parsing supplied pipeline parameters.
-
- **device**: ``int``:
- Device ordinal for CPU/GPU supports. Setting this to -1 will leverage CPU, >=0 will run the model
- on the associated CUDA device id.
-
- **binary_output** ``bool`` (default: False):
- Flag indicating if the output the pipeline should happen in a binary format (i.e. pickle) or as raw text.
-
- Return:
- Pipeline returns list or dictionary depending on:
- - Does the user provided multiple sample
- - The pipeline expose multiple fields in the output object
-
- Examples:
- nlp = pipeline('ner')
- nlp = pipeline('ner', model='...', config='...', tokenizer='...')
- nlp = NerPipeline(model='...', config='...', tokenizer='...')
- nlp = QuestionAnsweringPipeline(model=AutoModel.from_pretrained('...'), tokenizer='...')
- """
-
- default_input_names = None
-
- def __init__(
- self,
- model,
- tokenizer: PreTrainedTokenizer = None,
- modelcard: ModelCard = None,
- framework: Optional[str] = None,
- args_parser: ArgumentHandler = None,
- device: int = -1,
- binary_output: bool = False,
- ):
-
- if framework is None:
- framework = get_framework()
-
- self.model = model
- self.tokenizer = tokenizer
- self.modelcard = modelcard
- self.framework = framework
- self.device = device if framework == "tf" else torch.device("cpu" if device < 0 else "cuda:{}".format(device))
- self.binary_output = binary_output
- self._args_parser = args_parser or DefaultArgumentHandler()
-
- # Special handling
- if self.framework == "pt" and self.device.type == "cuda":
- self.model = self.model.to(self.device)
-
- def save_pretrained(self, save_directory):
- """
- Save the pipeline's model and tokenizer to the specified save_directory
- """
- if not os.path.isdir(save_directory):
- logger.error("Provided path ({}) should be a directory".format(save_directory))
- return
-
- self.model.save_pretrained(save_directory)
- self.tokenizer.save_pretrained(save_directory)
- self.modelcard.save_pretrained(save_directory)
-
- def transform(self, X):
- """
- Scikit / Keras interface to transformers' pipelines. This method will forward to __call__().
- """
- return self(X=X)
-
- def predict(self, X):
- """
- Scikit / Keras interface to transformers' pipelines. This method will forward to __call__().
- """
- return self(X=X)
-
- @contextmanager
- def device_placement(self):
- """
- Context Manager allowing tensor allocation on the user-specified device in framework agnostic way.
- example:
- # Explicitly ask for tensor allocation on CUDA device :0
- nlp = pipeline(..., device=0)
- with nlp.device_placement():
- # Every framework specific tensor allocation will be done on the request device
- output = nlp(...)
- Returns:
- Context manager
- """
- if self.framework == "tf":
- with tf.device("/CPU:0" if self.device == -1 else "/device:GPU:{}".format(self.device)):
- yield
- else:
- if self.device.type == "cuda":
- torch.cuda.set_device(self.device)
-
- yield
-
- def ensure_tensor_on_device(self, **inputs):
- """
- Ensure PyTorch tensors are on the specified device.
- :param inputs:
- :return:
- """
- return {name: tensor.to(self.device) for name, tensor in inputs.items()}
-
- def inputs_for_model(self, features: Union[dict, List[dict]]) -> Dict:
- """
- Generates the input dictionary with model-specific parameters.
-
- Returns:
- dict holding all the required parameters for model's forward
- """
- args = ["input_ids", "attention_mask"]
-
- if not isinstance(self.model.config, (DistilBertConfig, XLMConfig, RobertaConfig)):
- args += ["token_type_ids"]
-
- # PR #1548 (CLI) There is an issue with attention_mask
- # if 'xlnet' in model_type or 'xlm' in model_type:
- # args += ['cls_index', 'p_mask']
-
- if isinstance(features, dict):
- return {k: features[k] for k in args}
- else:
- return {k: [feature[k] for feature in features] for k in args}
-
- def _parse_and_tokenize(self, *texts, **kwargs):
- """
- Parse arguments and tokenize
- """
- # Parse arguments
- inputs = self._args_parser(*texts, **kwargs)
- inputs = self.tokenizer.batch_encode_plus(
- inputs, add_special_tokens=True, return_tensors=self.framework, max_length=self.tokenizer.max_len
- )
-
- # Filter out features not available on specific models
- inputs = self.inputs_for_model(inputs)
-
- return inputs
-
- def __call__(self, *texts, **kwargs):
- inputs = self._parse_and_tokenize(*texts, **kwargs)
- return self._forward(inputs)
-
- def _forward(self, inputs, return_tensors=False):
- """
- Internal framework specific forward dispatching.
- Args:
- inputs: dict holding all the keyworded arguments for required by the model forward method.
- return_tensors: Whether to return native framework (pt/tf) tensors rather than numpy array.
- Returns:
- Numpy array
- """
- # Encode for forward
- with self.device_placement():
- if self.framework == "tf":
- # TODO trace model
- predictions = self.model(inputs, training=False)[0]
- else:
- with torch.no_grad():
- inputs = self.ensure_tensor_on_device(**inputs)
- predictions = self.model(**inputs)[0].cpu()
-
- if return_tensors:
- return predictions
- else:
- return predictions.numpy()
-
-
-class FeatureExtractionPipeline(Pipeline):
- """
- Feature extraction pipeline using Model head.
- """
-
- def __init__(
- self,
- model,
- tokenizer: PreTrainedTokenizer = None,
- modelcard: ModelCard = None,
- framework: Optional[str] = None,
- args_parser: ArgumentHandler = None,
- device: int = -1,
- ):
- super().__init__(
- model=model,
- tokenizer=tokenizer,
- modelcard=modelcard,
- framework=framework,
- args_parser=args_parser,
- device=device,
- binary_output=True,
- )
-
- def __call__(self, *args, **kwargs):
- return super().__call__(*args, **kwargs).tolist()
-
-
-class TextClassificationPipeline(Pipeline):
- """
- Text classification pipeline using ModelForTextClassification head.
- """
-
- def __call__(self, *args, **kwargs):
- outputs = super().__call__(*args, **kwargs)
- scores = np.exp(outputs) / np.exp(outputs).sum(-1)
- return [{"label": self.model.config.id2label[item.argmax()], "score": item.max()} for item in scores]
-
-
-class FillMaskPipeline(Pipeline):
- """
- Masked language modeling prediction pipeline using ModelWithLMHead head.
- """
-
- def __init__(
- self,
- model,
- tokenizer: PreTrainedTokenizer = None,
- modelcard: ModelCard = None,
- framework: Optional[str] = None,
- args_parser: ArgumentHandler = None,
- device: int = -1,
- topk=5,
- ):
- super().__init__(
- model=model,
- tokenizer=tokenizer,
- modelcard=modelcard,
- framework=framework,
- args_parser=args_parser,
- device=device,
- binary_output=True,
- )
-
- self.topk = topk
-
- def __call__(self, *args, **kwargs):
- inputs = self._parse_and_tokenize(*args, **kwargs)
- outputs = self._forward(inputs, return_tensors=True)
-
- results = []
- batch_size = outputs.shape[0] if self.framework == "tf" else outputs.size(0)
-
- for i in range(batch_size):
- input_ids = inputs["input_ids"][i]
- result = []
-
- if self.framework == "tf":
- masked_index = tf.where(input_ids == self.tokenizer.mask_token_id).numpy().item()
- logits = outputs[i, masked_index, :]
- probs = tf.nn.softmax(logits)
- topk = tf.math.top_k(probs, k=self.topk)
- values, predictions = topk.values.numpy(), topk.indices.numpy()
- else:
- masked_index = (input_ids == self.tokenizer.mask_token_id).nonzero().item()
- logits = outputs[i, masked_index, :]
- probs = logits.softmax(dim=0)
- values, predictions = probs.topk(self.topk)
-
- for v, p in zip(values.tolist(), predictions.tolist()):
- tokens = input_ids.numpy()
- tokens[masked_index] = p
- # Filter padding out:
- tokens = tokens[np.where(tokens != self.tokenizer.pad_token_id)]
- result.append({"sequence": self.tokenizer.decode(tokens), "score": v, "token": p})
-
- # Append
- results += [result]
-
- if len(results) == 1:
- return results[0]
- return results
-
-
-class NerPipeline(Pipeline):
- """
- Named Entity Recognition pipeline using ModelForTokenClassification head.
- """
-
- default_input_names = "sequences"
-
- def __init__(
- self,
- model,
- tokenizer: PreTrainedTokenizer = None,
- modelcard: ModelCard = None,
- framework: Optional[str] = None,
- args_parser: ArgumentHandler = None,
- device: int = -1,
- binary_output: bool = False,
- ignore_labels=["O"],
- ):
- super().__init__(
- model=model,
- tokenizer=tokenizer,
- modelcard=modelcard,
- framework=framework,
- args_parser=args_parser,
- device=device,
- binary_output=binary_output,
- )
-
- self._basic_tokenizer = BasicTokenizer(do_lower_case=False)
- self.ignore_labels = ignore_labels
-
- def __call__(self, *texts, **kwargs):
- inputs = self._args_parser(*texts, **kwargs)
- answers = []
- for sentence in inputs:
-
- # Manage correct placement of the tensors
- with self.device_placement():
-
- tokens = self.tokenizer.encode_plus(
- sentence,
- return_attention_mask=False,
- return_tensors=self.framework,
- max_length=self.tokenizer.max_len,
- )
-
- # Forward
- if self.framework == "tf":
- entities = self.model(tokens)[0][0].numpy()
- input_ids = tokens["input_ids"].numpy()[0]
- else:
- with torch.no_grad():
- tokens = self.ensure_tensor_on_device(**tokens)
- entities = self.model(**tokens)[0][0].cpu().numpy()
- input_ids = tokens["input_ids"].cpu().numpy()[0]
-
- score = np.exp(entities) / np.exp(entities).sum(-1, keepdims=True)
- labels_idx = score.argmax(axis=-1)
-
- answer = []
- for idx, label_idx in enumerate(labels_idx):
- if self.model.config.id2label[label_idx] not in self.ignore_labels:
- answer += [
- {
- "word": self.tokenizer.decode([int(input_ids[idx])]),
- "score": score[idx][label_idx].item(),
- "entity": self.model.config.id2label[label_idx],
- }
- ]
-
- # Append
- answers += [answer]
- if len(answers) == 1:
- return answers[0]
- return answers
-
-
-class QuestionAnsweringArgumentHandler(ArgumentHandler):
- """
- QuestionAnsweringPipeline requires the user to provide multiple arguments (i.e. question & context) to be mapped
- to internal SquadExample / SquadFeature structures.
-
- QuestionAnsweringArgumentHandler manages all the possible to create SquadExample from the command-line supplied
- arguments.
- """
-
- def __call__(self, *args, **kwargs):
- # Position args, handling is sensibly the same as X and data, so forwarding to avoid duplicating
- if args is not None and len(args) > 0:
- if len(args) == 1:
- kwargs["X"] = args[0]
- else:
- kwargs["X"] = list(args)
-
- # Generic compatibility with sklearn and Keras
- # Batched data
- if "X" in kwargs or "data" in kwargs:
- inputs = kwargs["X"] if "X" in kwargs else kwargs["data"]
-
- if isinstance(inputs, dict):
- inputs = [inputs]
- else:
- # Copy to avoid overriding arguments
- inputs = [i for i in inputs]
-
- for i, item in enumerate(inputs):
- if isinstance(item, dict):
- if any(k not in item for k in ["question", "context"]):
- raise KeyError("You need to provide a dictionary with keys {question:..., context:...}")
-
- inputs[i] = QuestionAnsweringPipeline.create_sample(**item)
-
- elif not isinstance(item, SquadExample):
- raise ValueError(
- "{} argument needs to be of type (list[SquadExample | dict], SquadExample, dict)".format(
- "X" if "X" in kwargs else "data"
- )
- )
-
- # Tabular input
- elif "question" in kwargs and "context" in kwargs:
- if isinstance(kwargs["question"], str):
- kwargs["question"] = [kwargs["question"]]
-
- if isinstance(kwargs["context"], str):
- kwargs["context"] = [kwargs["context"]]
-
- inputs = [
- QuestionAnsweringPipeline.create_sample(q, c) for q, c in zip(kwargs["question"], kwargs["context"])
- ]
- else:
- raise ValueError("Unknown arguments {}".format(kwargs))
-
- if not isinstance(inputs, list):
- inputs = [inputs]
-
- return inputs
-
-
-class QuestionAnsweringPipeline(Pipeline):
- """
- Question Answering pipeline using ModelForQuestionAnswering head.
- """
-
- default_input_names = "question,context"
-
- def __init__(
- self,
- model,
- tokenizer: Optional[PreTrainedTokenizer],
- modelcard: Optional[ModelCard],
- framework: Optional[str] = None,
- device: int = -1,
- **kwargs
- ):
- super().__init__(
- model=model,
- tokenizer=tokenizer,
- modelcard=modelcard,
- framework=framework,
- args_parser=QuestionAnsweringArgumentHandler(),
- device=device,
- **kwargs,
- )
-
- @staticmethod
- def create_sample(
- question: Union[str, List[str]], context: Union[str, List[str]]
- ) -> Union[SquadExample, List[SquadExample]]:
- """
- QuestionAnsweringPipeline leverages the SquadExample/SquadFeatures internally.
- This helper method encapsulate all the logic for converting question(s) and context(s) to SquadExample(s).
- We currently support extractive question answering.
- Arguments:
- question: (str, List[str]) The question to be ask for the associated context
- context: (str, List[str]) The context in which we will look for the answer.
-
- Returns:
- SquadExample initialized with the corresponding question and context.
- """
- if isinstance(question, list):
- return [SquadExample(None, q, c, None, None, None) for q, c in zip(question, context)]
- else:
- return SquadExample(None, question, context, None, None, None)
-
- def __call__(self, *texts, **kwargs):
- """
- Args:
- We support multiple use-cases, the following are exclusive:
- X: sequence of SquadExample
- data: sequence of SquadExample
- question: (str, List[str]), batch of question(s) to map along with context
- context: (str, List[str]), batch of context(s) associated with the provided question keyword argument
- Returns:
- dict: {'answer': str, 'score": float, 'start": int, "end": int}
- answer: the textual answer in the intial context
- score: the score the current answer scored for the model
- start: the character index in the original string corresponding to the beginning of the answer' span
- end: the character index in the original string corresponding to the ending of the answer' span
- """
- # Set defaults values
- kwargs.setdefault("topk", 1)
- kwargs.setdefault("doc_stride", 128)
- kwargs.setdefault("max_answer_len", 15)
- kwargs.setdefault("max_seq_len", 384)
- kwargs.setdefault("max_question_len", 64)
-
- if kwargs["topk"] < 1:
- raise ValueError("topk parameter should be >= 1 (got {})".format(kwargs["topk"]))
-
- if kwargs["max_answer_len"] < 1:
- raise ValueError("max_answer_len parameter should be >= 1 (got {})".format(kwargs["max_answer_len"]))
-
- # Convert inputs to features
- examples = self._args_parser(*texts, **kwargs)
- features_list = [
- squad_convert_examples_to_features(
- [example],
- self.tokenizer,
- kwargs["max_seq_len"],
- kwargs["doc_stride"],
- kwargs["max_question_len"],
- False,
- )
- for example in examples
- ]
- all_answers = []
- for features, example in zip(features_list, examples):
- fw_args = self.inputs_for_model([f.__dict__ for f in features])
-
- # Manage tensor allocation on correct device
- with self.device_placement():
- if self.framework == "tf":
- fw_args = {k: tf.constant(v) for (k, v) in fw_args.items()}
- start, end = self.model(fw_args)
- start, end = start.numpy(), end.numpy()
- else:
- with torch.no_grad():
- # Retrieve the score for the context tokens only (removing question tokens)
- fw_args = {k: torch.tensor(v, device=self.device) for (k, v) in fw_args.items()}
- start, end = self.model(**fw_args)
- start, end = start.cpu().numpy(), end.cpu().numpy()
-
- answers = []
- for (feature, start_, end_) in zip(features, start, end):
- # Normalize logits and spans to retrieve the answer
- start_ = np.exp(start_) / np.sum(np.exp(start_))
- end_ = np.exp(end_) / np.sum(np.exp(end_))
-
- # Mask padding and question
- start_, end_ = (
- start_ * np.abs(np.array(feature.p_mask) - 1),
- end_ * np.abs(np.array(feature.p_mask) - 1),
- )
-
- # TODO : What happens if not possible
- # Mask CLS
- start_[0] = end_[0] = 0
-
- starts, ends, scores = self.decode(start_, end_, kwargs["topk"], kwargs["max_answer_len"])
- char_to_word = np.array(example.char_to_word_offset)
-
- # Convert the answer (tokens) back to the original text
- answers += [
- {
- "score": score.item(),
- "start": np.where(char_to_word == feature.token_to_orig_map[s])[0][0].item(),
- "end": np.where(char_to_word == feature.token_to_orig_map[e])[0][-1].item(),
- "answer": " ".join(
- example.doc_tokens[feature.token_to_orig_map[s] : feature.token_to_orig_map[e] + 1]
- ),
- }
- for s, e, score in zip(starts, ends, scores)
- ]
- answers = sorted(answers, key=lambda x: x["score"], reverse=True)[: kwargs["topk"]]
- all_answers += answers
-
- if len(all_answers) == 1:
- return all_answers[0]
- return all_answers
-
- def decode(self, start: np.ndarray, end: np.ndarray, topk: int, max_answer_len: int) -> Tuple:
- """
- Take the output of any QuestionAnswering head and will generate probalities for each span to be
- the actual answer.
- In addition, it filters out some unwanted/impossible cases like answer len being greater than
- max_answer_len or answer end position being before the starting position.
- The method supports output the k-best answer through the topk argument.
-
- Args:
- start: numpy array, holding individual start probabilities for each token
- end: numpy array, holding individual end probabilities for each token
- topk: int, indicates how many possible answer span(s) to extract from the model's output
- max_answer_len: int, maximum size of the answer to extract from the model's output
- """
- # Ensure we have batch axis
- if start.ndim == 1:
- start = start[None]
-
- if end.ndim == 1:
- end = end[None]
-
- # Compute the score of each tuple(start, end) to be the real answer
- outer = np.matmul(np.expand_dims(start, -1), np.expand_dims(end, 1))
-
- # Remove candidate with end < start and end - start > max_answer_len
- candidates = np.tril(np.triu(outer), max_answer_len - 1)
-
- # Inspired by Chen & al. (https://github.com/facebookresearch/DrQA)
- scores_flat = candidates.flatten()
- if topk == 1:
- idx_sort = [np.argmax(scores_flat)]
- elif len(scores_flat) < topk:
- idx_sort = np.argsort(-scores_flat)
- else:
- idx = np.argpartition(-scores_flat, topk)[0:topk]
- idx_sort = idx[np.argsort(-scores_flat[idx])]
-
- start, end = np.unravel_index(idx_sort, candidates.shape)[1:]
- return start, end, candidates[0, start, end]
-
- def span_to_answer(self, text: str, start: int, end: int):
- """
- When decoding from token probalities, this method maps token indexes to actual word in
- the initial context.
-
- Args:
- text: str, the actual context to extract the answer from
- start: int, starting answer token index
- end: int, ending answer token index
-
- Returns:
- dict: {'answer': str, 'start': int, 'end': int}
- """
- words = []
- token_idx = char_start_idx = char_end_idx = chars_idx = 0
-
- for i, word in enumerate(text.split(" ")):
- token = self.tokenizer.tokenize(word)
-
- # Append words if they are in the span
- if start <= token_idx <= end:
- if token_idx == start:
- char_start_idx = chars_idx
-
- if token_idx == end:
- char_end_idx = chars_idx + len(word)
-
- words += [word]
-
- # Stop if we went over the end of the answer
- if token_idx > end:
- break
-
- # Append the subtokenization length to the running index
- token_idx += len(token)
- chars_idx += len(word) + 1
-
- # Join text with spaces
- return {"answer": " ".join(words), "start": max(0, char_start_idx), "end": min(len(text), char_end_idx)}
-
-
-# Register all the supported task here
-SUPPORTED_TASKS = {
- "feature-extraction": {
- "impl": FeatureExtractionPipeline,
- "tf": TFAutoModel if is_tf_available() else None,
- "pt": AutoModel if is_torch_available() else None,
- "default": {
- "model": {"pt": "distilbert-base-uncased", "tf": "distilbert-base-uncased"},
- "config": None,
- "tokenizer": "distilbert-base-uncased",
- },
- },
- "sentiment-analysis": {
- "impl": TextClassificationPipeline,
- "tf": TFAutoModelForSequenceClassification if is_tf_available() else None,
- "pt": AutoModelForSequenceClassification if is_torch_available() else None,
- "default": {
- "model": {
- "pt": "distilbert-base-uncased-finetuned-sst-2-english",
- "tf": "distilbert-base-uncased-finetuned-sst-2-english",
- },
- "config": "distilbert-base-uncased-finetuned-sst-2-english",
- "tokenizer": "distilbert-base-uncased",
- },
- },
- "ner": {
- "impl": NerPipeline,
- "tf": TFAutoModelForTokenClassification if is_tf_available() else None,
- "pt": AutoModelForTokenClassification if is_torch_available() else None,
- "default": {
- "model": {
- "pt": "dbmdz/bert-large-cased-finetuned-conll03-english",
- "tf": "dbmdz/bert-large-cased-finetuned-conll03-english",
- },
- "config": "dbmdz/bert-large-cased-finetuned-conll03-english",
- "tokenizer": "bert-large-cased",
- },
- },
- "question-answering": {
- "impl": QuestionAnsweringPipeline,
- "tf": TFAutoModelForQuestionAnswering if is_tf_available() else None,
- "pt": AutoModelForQuestionAnswering if is_torch_available() else None,
- "default": {
- "model": {
- "pt": "distilbert-base-uncased-distilled-squad",
- "tf": "distilbert-base-uncased-distilled-squad",
- },
- "config": None,
- "tokenizer": "distilbert-base-uncased",
- },
- },
- "fill-mask": {
- "impl": FillMaskPipeline,
- "tf": TFAutoModelWithLMHead if is_tf_available() else None,
- "pt": AutoModelWithLMHead if is_torch_available() else None,
- "default": {
- "model": {"pt": "distilroberta-base", "tf": "distilroberta-base"},
- "config": None,
- "tokenizer": "distilroberta-base",
- },
- },
-}
-
-
-def pipeline(
- task: str,
- model: Optional = None,
- config: Optional[Union[str, PretrainedConfig]] = None,
- tokenizer: Optional[Union[str, PreTrainedTokenizer]] = None,
- modelcard: Optional[Union[str, ModelCard]] = None,
- **kwargs
-) -> Pipeline:
- """
- Utility factory method to build a pipeline.
- Pipeline are made of:
- A Tokenizer instance in charge of mapping raw textual input to token
- A Model instance
- Some (optional) post processing for enhancing model's output
-
- Examples:
- pipeline('sentiment-analysis')
- pipeline('question-answering', model='distilbert-base-uncased-distilled-squad', tokenizer='bert-base-cased')
- pipeline('ner', model=AutoModel.from_pretrained(...), tokenizer=AutoTokenizer.from_pretrained(...)
- pipeline('ner', model='dbmdz/bert-large-cased-finetuned-conll03-english', tokenizer='bert-base-cased')
- pipeline('ner', model='https://...pytorch-model.bin', config='https://...config.json', tokenizer='bert-base-cased')
- """
- # Retrieve the task
- if task not in SUPPORTED_TASKS:
- raise KeyError("Unknown task {}, available tasks are {}".format(task, list(SUPPORTED_TASKS.keys())))
-
- framework = get_framework(model)
-
- targeted_task = SUPPORTED_TASKS[task]
- task, model_class = targeted_task["impl"], targeted_task[framework]
-
- # Use default model/config/tokenizer for the task if no model is provided
- if model is None:
- models, config, tokenizer = tuple(targeted_task["default"].values())
- model = models[framework]
-
- # Try to infer tokenizer from model or config name (if provided as str)
- if tokenizer is None:
- if isinstance(model, str) and model in ALL_PRETRAINED_CONFIG_ARCHIVE_MAP:
- tokenizer = model
- elif isinstance(config, str) and config in ALL_PRETRAINED_CONFIG_ARCHIVE_MAP:
- tokenizer = config
- else:
- # Impossible to guest what is the right tokenizer here
- raise Exception(
- "Impossible to guess which tokenizer to use. "
- "Please provided a PretrainedTokenizer class or a path/url/shortcut name to a pretrained tokenizer."
- )
-
- # Try to infer modelcard from model or config name (if provided as str)
- if modelcard is None:
- # Try to fallback on one of the provided string for model or config (will replace the suffix)
- if isinstance(model, str):
- modelcard = model
- elif isinstance(config, str):
- modelcard = config
-
- # Instantiate tokenizer if needed
- if isinstance(tokenizer, str):
- tokenizer = AutoTokenizer.from_pretrained(tokenizer)
-
- # Instantiate config if needed
- if isinstance(config, str):
- config = AutoConfig.from_pretrained(config)
-
- # Instantiate modelcard if needed
- if isinstance(modelcard, str):
- modelcard = ModelCard.from_pretrained(modelcard)
-
- # Instantiate model if needed
- if isinstance(model, str):
- # Handle transparent TF/PT model conversion
- model_kwargs = {}
- if framework == "pt" and model.endswith(".h5"):
- model_kwargs["from_tf"] = True
- logger.warning(
- "Model might be a TensorFlow model (ending with `.h5`) but TensorFlow is not available. "
- "Trying to load the model with PyTorch."
- )
- elif framework == "tf" and model.endswith(".bin"):
- model_kwargs["from_pt"] = True
- logger.warning(
- "Model might be a PyTorch model (ending with `.bin`) but PyTorch is not available. "
- "Trying to load the model with Tensorflow."
- )
- model = model_class.from_pretrained(model, config=config, **model_kwargs)
-
- return task(model=model, tokenizer=tokenizer, modelcard=modelcard, framework=framework, **kwargs)
diff --git a/server/transformers/src/transformers/tokenization_albert.py b/server/transformers/src/transformers/tokenization_albert.py
deleted file mode 100644
index 985f82c6fda167184f259482829c6d0949e10928..0000000000000000000000000000000000000000
--- a/server/transformers/src/transformers/tokenization_albert.py
+++ /dev/null
@@ -1,257 +0,0 @@
-# coding=utf-8
-# Copyright 2018 Google AI, Google Brain and the HuggingFace Inc. team.
-#
-# Licensed under the Apache License, Version 2.0 (the "License");
-# you may not use this file except in compliance with the License.
-# You may obtain a copy of the License at
-#
-# http://www.apache.org/licenses/LICENSE-2.0
-#
-# Unless required by applicable law or agreed to in writing, software
-# distributed under the License is distributed on an "AS IS" BASIS,
-# WITHOUT WARRANTIES OR CONDITIONS OF ANY KIND, either express or implied.
-# See the License for the specific language governing permissions and
-# limitations under the License.
-""" Tokenization classes for ALBERT model."""
-
-
-import logging
-import os
-import unicodedata
-from shutil import copyfile
-
-from .tokenization_utils import PreTrainedTokenizer
-
-
-logger = logging.getLogger(__name__)
-VOCAB_FILES_NAMES = {"vocab_file": "spiece.model"}
-
-PRETRAINED_VOCAB_FILES_MAP = {
- "vocab_file": {
- "albert-base-v1": "https://s3.amazonaws.com/models.huggingface.co/bert/albert-base-spiece.model",
- "albert-large-v1": "https://s3.amazonaws.com/models.huggingface.co/bert/albert-large-spiece.model",
- "albert-xlarge-v1": "https://s3.amazonaws.com/models.huggingface.co/bert/albert-xlarge-spiece.model",
- "albert-xxlarge-v1": "https://s3.amazonaws.com/models.huggingface.co/bert/albert-xxlarge-spiece.model",
- "albert-base-v2": "https://s3.amazonaws.com/models.huggingface.co/bert/albert-base-v2-spiece.model",
- "albert-large-v2": "https://s3.amazonaws.com/models.huggingface.co/bert/albert-large-v2-spiece.model",
- "albert-xlarge-v2": "https://s3.amazonaws.com/models.huggingface.co/bert/albert-xlarge-v2-spiece.model",
- "albert-xxlarge-v2": "https://s3.amazonaws.com/models.huggingface.co/bert/albert-xxlarge-v2-spiece.model",
- }
-}
-
-PRETRAINED_POSITIONAL_EMBEDDINGS_SIZES = {
- "albert-base-v1": 512,
- "albert-large-v1": 512,
- "albert-xlarge-v1": 512,
- "albert-xxlarge-v1": 512,
- "albert-base-v2": 512,
- "albert-large-v2": 512,
- "albert-xlarge-v2": 512,
- "albert-xxlarge-v2": 512,
-}
-
-SPIECE_UNDERLINE = "▁"
-
-
-class AlbertTokenizer(PreTrainedTokenizer):
- """
- SentencePiece based tokenizer. Peculiarities:
-
- - requires `SentencePiece `_
- """
-
- vocab_files_names = VOCAB_FILES_NAMES
- pretrained_vocab_files_map = PRETRAINED_VOCAB_FILES_MAP
- max_model_input_sizes = PRETRAINED_POSITIONAL_EMBEDDINGS_SIZES
-
- def __init__(
- self,
- vocab_file,
- do_lower_case=True,
- remove_space=True,
- keep_accents=False,
- bos_token="[CLS]",
- eos_token="[SEP]",
- unk_token="",
- sep_token="[SEP]",
- pad_token="",
- cls_token="[CLS]",
- mask_token="[MASK]",
- **kwargs
- ):
- super().__init__(
- bos_token=bos_token,
- eos_token=eos_token,
- unk_token=unk_token,
- sep_token=sep_token,
- pad_token=pad_token,
- cls_token=cls_token,
- mask_token=mask_token,
- **kwargs,
- )
-
- self.max_len_single_sentence = self.max_len - 2 # take into account special tokens
- self.max_len_sentences_pair = self.max_len - 3 # take into account special tokens
-
- try:
- import sentencepiece as spm
- except ImportError:
- logger.warning(
- "You need to install SentencePiece to use AlbertTokenizer: https://github.com/google/sentencepiece"
- "pip install sentencepiece"
- )
- raise
-
- self.do_lower_case = do_lower_case
- self.remove_space = remove_space
- self.keep_accents = keep_accents
- self.vocab_file = vocab_file
-
- self.sp_model = spm.SentencePieceProcessor()
- self.sp_model.Load(vocab_file)
-
- @property
- def vocab_size(self):
- return len(self.sp_model)
-
- def __getstate__(self):
- state = self.__dict__.copy()
- state["sp_model"] = None
- return state
-
- def __setstate__(self, d):
- self.__dict__ = d
- try:
- import sentencepiece as spm
- except ImportError:
- logger.warning(
- "You need to install SentencePiece to use AlbertTokenizer: https://github.com/google/sentencepiece"
- "pip install sentencepiece"
- )
- raise
- self.sp_model = spm.SentencePieceProcessor()
- self.sp_model.Load(self.vocab_file)
-
- def preprocess_text(self, inputs):
- if self.remove_space:
- outputs = " ".join(inputs.strip().split())
- else:
- outputs = inputs
- outputs = outputs.replace("``", '"').replace("''", '"')
-
- if not self.keep_accents:
- outputs = unicodedata.normalize("NFKD", outputs)
- outputs = "".join([c for c in outputs if not unicodedata.combining(c)])
- if self.do_lower_case:
- outputs = outputs.lower()
-
- return outputs
-
- def _tokenize(self, text, sample=False):
- """ Tokenize a string. """
- text = self.preprocess_text(text)
-
- if not sample:
- pieces = self.sp_model.EncodeAsPieces(text)
- else:
- pieces = self.sp_model.SampleEncodeAsPieces(text, 64, 0.1)
- new_pieces = []
- for piece in pieces:
- if len(piece) > 1 and piece[-1] == str(",") and piece[-2].isdigit():
- cur_pieces = self.sp_model.EncodeAsPieces(piece[:-1].replace(SPIECE_UNDERLINE, ""))
- if piece[0] != SPIECE_UNDERLINE and cur_pieces[0][0] == SPIECE_UNDERLINE:
- if len(cur_pieces[0]) == 1:
- cur_pieces = cur_pieces[1:]
- else:
- cur_pieces[0] = cur_pieces[0][1:]
- cur_pieces.append(piece[-1])
- new_pieces.extend(cur_pieces)
- else:
- new_pieces.append(piece)
-
- return new_pieces
-
- def _convert_token_to_id(self, token):
- """ Converts a token (str) in an id using the vocab. """
- return self.sp_model.PieceToId(token)
-
- def _convert_id_to_token(self, index):
- """Converts an index (integer) in a token (str) using the vocab."""
- return self.sp_model.IdToPiece(index)
-
- def convert_tokens_to_string(self, tokens):
- """Converts a sequence of tokens (strings for sub-words) in a single string."""
- out_string = "".join(tokens).replace(SPIECE_UNDERLINE, " ").strip()
- return out_string
-
- def build_inputs_with_special_tokens(self, token_ids_0, token_ids_1=None):
- """
- Build model inputs from a sequence or a pair of sequence for sequence classification tasks
- by concatenating and adding special tokens.
- An ALBERT sequence has the following format:
- single sequence: [CLS] X [SEP]
- pair of sequences: [CLS] A [SEP] B [SEP]
- """
- sep = [self.sep_token_id]
- cls = [self.cls_token_id]
- if token_ids_1 is None:
- return cls + token_ids_0 + sep
- return cls + token_ids_0 + sep + token_ids_1 + sep
-
- def get_special_tokens_mask(self, token_ids_0, token_ids_1=None, already_has_special_tokens=False):
- """
- Retrieves sequence ids from a token list that has no special tokens added. This method is called when adding
- special tokens using the tokenizer ``prepare_for_model`` or ``encode_plus`` methods.
-
- Args:
- token_ids_0: list of ids (must not contain special tokens)
- token_ids_1: Optional list of ids (must not contain special tokens), necessary when fetching sequence ids
- for sequence pairs
- already_has_special_tokens: (default False) Set to True if the token list is already formated with
- special tokens for the model
-
- Returns:
- A list of integers in the range [0, 1]: 0 for a special token, 1 for a sequence token.
- """
-
- if already_has_special_tokens:
- if token_ids_1 is not None:
- raise ValueError(
- "You should not supply a second sequence if the provided sequence of "
- "ids is already formated with special tokens for the model."
- )
- return list(map(lambda x: 1 if x in [self.sep_token_id, self.cls_token_id] else 0, token_ids_0))
-
- if token_ids_1 is not None:
- return [1] + ([0] * len(token_ids_0)) + [1] + ([0] * len(token_ids_1)) + [1]
- return [1] + ([0] * len(token_ids_0)) + [1]
-
- def create_token_type_ids_from_sequences(self, token_ids_0, token_ids_1=None):
- """
- Creates a mask from the two sequences passed to be used in a sequence-pair classification task.
- An ALBERT sequence pair mask has the following format:
- 0 0 0 0 0 0 0 0 0 0 0 1 1 1 1 1 1 1 1 1 1
- | first sequence | second sequence
-
- if token_ids_1 is None, only returns the first portion of the mask (0's).
- """
- sep = [self.sep_token_id]
- cls = [self.cls_token_id]
-
- if token_ids_1 is None:
- return len(cls + token_ids_0 + sep) * [0]
- return len(cls + token_ids_0 + sep) * [0] + len(token_ids_1 + sep) * [1]
-
- def save_vocabulary(self, save_directory):
- """ Save the sentencepiece vocabulary (copy original file) and special tokens file
- to a directory.
- """
- if not os.path.isdir(save_directory):
- logger.error("Vocabulary path ({}) should be a directory".format(save_directory))
- return
- out_vocab_file = os.path.join(save_directory, VOCAB_FILES_NAMES["vocab_file"])
-
- if os.path.abspath(self.vocab_file) != os.path.abspath(out_vocab_file):
- copyfile(self.vocab_file, out_vocab_file)
-
- return (out_vocab_file,)
diff --git a/server/transformers/src/transformers/tokenization_auto.py b/server/transformers/src/transformers/tokenization_auto.py
deleted file mode 100644
index d272b3367b29360f33e9074086f33a6cd9de56fd..0000000000000000000000000000000000000000
--- a/server/transformers/src/transformers/tokenization_auto.py
+++ /dev/null
@@ -1,189 +0,0 @@
-# coding=utf-8
-# Copyright 2018 The HuggingFace Inc. team.
-#
-# Licensed under the Apache License, Version 2.0 (the "License");
-# you may not use this file except in compliance with the License.
-# You may obtain a copy of the License at
-#
-# http://www.apache.org/licenses/LICENSE-2.0
-#
-# Unless required by applicable law or agreed to in writing, software
-# distributed under the License is distributed on an "AS IS" BASIS,
-# WITHOUT WARRANTIES OR CONDITIONS OF ANY KIND, either express or implied.
-# See the License for the specific language governing permissions and
-# limitations under the License.
-""" Auto Model class. """
-
-
-import logging
-from collections import OrderedDict
-
-from .configuration_auto import (
- AlbertConfig,
- AutoConfig,
- BertConfig,
- CamembertConfig,
- CTRLConfig,
- DistilBertConfig,
- FlaubertConfig,
- GPT2Config,
- OpenAIGPTConfig,
- RobertaConfig,
- T5Config,
- TransfoXLConfig,
- XLMConfig,
- XLMRobertaConfig,
- XLNetConfig,
-)
-from .configuration_utils import PretrainedConfig
-from .tokenization_albert import AlbertTokenizer
-from .tokenization_bert import BertTokenizer
-from .tokenization_bert_japanese import BertJapaneseTokenizer
-from .tokenization_camembert import CamembertTokenizer
-from .tokenization_ctrl import CTRLTokenizer
-from .tokenization_distilbert import DistilBertTokenizer
-from .tokenization_flaubert import FlaubertTokenizer
-from .tokenization_gpt2 import GPT2Tokenizer
-from .tokenization_openai import OpenAIGPTTokenizer
-from .tokenization_roberta import RobertaTokenizer
-from .tokenization_t5 import T5Tokenizer
-from .tokenization_transfo_xl import TransfoXLTokenizer
-from .tokenization_xlm import XLMTokenizer
-from .tokenization_xlm_roberta import XLMRobertaTokenizer
-from .tokenization_xlnet import XLNetTokenizer
-
-
-logger = logging.getLogger(__name__)
-
-
-TOKENIZER_MAPPING = OrderedDict(
- [
- (T5Config, T5Tokenizer),
- (DistilBertConfig, DistilBertTokenizer),
- (AlbertConfig, AlbertTokenizer),
- (CamembertConfig, CamembertTokenizer),
- (XLMRobertaConfig, XLMRobertaTokenizer),
- (RobertaConfig, RobertaTokenizer),
- (BertConfig, BertTokenizer),
- (OpenAIGPTConfig, OpenAIGPTTokenizer),
- (GPT2Config, GPT2Tokenizer),
- (TransfoXLConfig, TransfoXLTokenizer),
- (XLNetConfig, XLNetTokenizer),
- (FlaubertConfig, FlaubertTokenizer),
- (XLMConfig, XLMTokenizer),
- (CTRLConfig, CTRLTokenizer),
- ]
-)
-
-
-class AutoTokenizer:
- r""":class:`~transformers.AutoTokenizer` is a generic tokenizer class
- that will be instantiated as one of the tokenizer classes of the library
- when created with the `AutoTokenizer.from_pretrained(pretrained_model_name_or_path)`
- class method.
-
- The `from_pretrained()` method take care of returning the correct tokenizer class instance
- based on the `model_type` property of the config object, or when it's missing,
- falling back to using pattern matching on the `pretrained_model_name_or_path` string.
-
- The tokenizer class to instantiate is selected as the first pattern matching
- in the `pretrained_model_name_or_path` string (in the following order):
- - contains `t5`: T5Tokenizer (T5 model)
- - contains `distilbert`: DistilBertTokenizer (DistilBert model)
- - contains `albert`: AlbertTokenizer (ALBERT model)
- - contains `camembert`: CamembertTokenizer (CamemBERT model)
- - contains `xlm-roberta`: XLMRobertaTokenizer (XLM-RoBERTa model)
- - contains `roberta`: RobertaTokenizer (RoBERTa model)
- - contains `bert`: BertTokenizer (Bert model)
- - contains `openai-gpt`: OpenAIGPTTokenizer (OpenAI GPT model)
- - contains `gpt2`: GPT2Tokenizer (OpenAI GPT-2 model)
- - contains `transfo-xl`: TransfoXLTokenizer (Transformer-XL model)
- - contains `xlnet`: XLNetTokenizer (XLNet model)
- - contains `xlm`: XLMTokenizer (XLM model)
- - contains `ctrl`: CTRLTokenizer (Salesforce CTRL model)
-
- This class cannot be instantiated using `__init__()` (throw an error).
- """
-
- def __init__(self):
- raise EnvironmentError(
- "AutoTokenizer is designed to be instantiated "
- "using the `AutoTokenizer.from_pretrained(pretrained_model_name_or_path)` method."
- )
-
- @classmethod
- def from_pretrained(cls, pretrained_model_name_or_path, *inputs, **kwargs):
- r""" Instantiate one of the tokenizer classes of the library
- from a pre-trained model vocabulary.
-
- The tokenizer class to instantiate is selected as the first pattern matching
- in the `pretrained_model_name_or_path` string (in the following order):
- - contains `t5`: T5Tokenizer (T5 model)
- - contains `distilbert`: DistilBertTokenizer (DistilBert model)
- - contains `albert`: AlbertTokenizer (ALBERT model)
- - contains `camembert`: CamembertTokenizer (CamemBERT model)
- - contains `xlm-roberta`: XLMRobertaTokenizer (XLM-RoBERTa model)
- - contains `roberta`: RobertaTokenizer (RoBERTa model)
- - contains `bert-base-japanese`: BertJapaneseTokenizer (Bert model)
- - contains `bert`: BertTokenizer (Bert model)
- - contains `openai-gpt`: OpenAIGPTTokenizer (OpenAI GPT model)
- - contains `gpt2`: GPT2Tokenizer (OpenAI GPT-2 model)
- - contains `transfo-xl`: TransfoXLTokenizer (Transformer-XL model)
- - contains `xlnet`: XLNetTokenizer (XLNet model)
- - contains `xlm`: XLMTokenizer (XLM model)
- - contains `ctrl`: CTRLTokenizer (Salesforce CTRL model)
-
- Params:
- pretrained_model_name_or_path: either:
-
- - a string with the `shortcut name` of a predefined tokenizer to load from cache or download, e.g.: ``bert-base-uncased``.
- - a string with the `identifier name` of a predefined tokenizer that was user-uploaded to our S3, e.g.: ``dbmdz/bert-base-german-cased``.
- - a path to a `directory` containing vocabulary files required by the tokenizer, for instance saved using the :func:`~transformers.PreTrainedTokenizer.save_pretrained` method, e.g.: ``./my_model_directory/``.
- - (not applicable to all derived classes) a path or url to a single saved vocabulary file if and only if the tokenizer only requires a single vocabulary file (e.g. Bert, XLNet), e.g.: ``./my_model_directory/vocab.txt``.
-
- cache_dir: (`optional`) string:
- Path to a directory in which a downloaded predefined tokenizer vocabulary files should be cached if the standard cache should not be used.
-
- force_download: (`optional`) boolean, default False:
- Force to (re-)download the vocabulary files and override the cached versions if they exists.
-
- resume_download: (`optional`) boolean, default False:
- Do not delete incompletely recieved file. Attempt to resume the download if such a file exists.
-
- proxies: (`optional`) dict, default None:
- A dictionary of proxy servers to use by protocol or endpoint, e.g.: {'http': 'foo.bar:3128', 'http://hostname': 'foo.bar:4012'}.
- The proxies are used on each request.
-
- inputs: (`optional`) positional arguments: will be passed to the Tokenizer ``__init__`` method.
-
- kwargs: (`optional`) keyword arguments: will be passed to the Tokenizer ``__init__`` method. Can be used to set special tokens like ``bos_token``, ``eos_token``, ``unk_token``, ``sep_token``, ``pad_token``, ``cls_token``, ``mask_token``, ``additional_special_tokens``. See parameters in the doc string of :class:`~transformers.PreTrainedTokenizer` for details.
-
- Examples::
-
- # Download vocabulary from S3 and cache.
- tokenizer = AutoTokenizer.from_pretrained('bert-base-uncased')
-
- # Download vocabulary from S3 (user-uploaded) and cache.
- tokenizer = AutoTokenizer.from_pretrained('dbmdz/bert-base-german-cased')
-
- # If vocabulary files are in a directory (e.g. tokenizer was saved using `save_pretrained('./test/saved_model/')`)
- tokenizer = AutoTokenizer.from_pretrained('./test/bert_saved_model/')
-
- """
- config = kwargs.pop("config", None)
- if not isinstance(config, PretrainedConfig):
- config = AutoConfig.from_pretrained(pretrained_model_name_or_path, **kwargs)
-
- if "bert-base-japanese" in pretrained_model_name_or_path:
- return BertJapaneseTokenizer.from_pretrained(pretrained_model_name_or_path, *inputs, **kwargs)
-
- for config_class, tokenizer_class in TOKENIZER_MAPPING.items():
- if isinstance(config, config_class):
- return tokenizer_class.from_pretrained(pretrained_model_name_or_path, *inputs, **kwargs)
-
- raise ValueError(
- "Unrecognized configuration class {} to build an AutoTokenizer.\n"
- "Model type should be one of {}.".format(
- config.__class__, ", ".join(c.__name__ for c in TOKENIZER_MAPPING.keys())
- )
- )
diff --git a/server/transformers/src/transformers/tokenization_bert.py b/server/transformers/src/transformers/tokenization_bert.py
deleted file mode 100644
index e3f8d00c6fefc352f2bbb106adb4e77a605bbac9..0000000000000000000000000000000000000000
--- a/server/transformers/src/transformers/tokenization_bert.py
+++ /dev/null
@@ -1,597 +0,0 @@
-# coding=utf-8
-# Copyright 2018 The Google AI Language Team Authors and The HuggingFace Inc. team.
-#
-# Licensed under the Apache License, Version 2.0 (the "License");
-# you may not use this file except in compliance with the License.
-# You may obtain a copy of the License at
-#
-# http://www.apache.org/licenses/LICENSE-2.0
-#
-# Unless required by applicable law or agreed to in writing, software
-# distributed under the License is distributed on an "AS IS" BASIS,
-# WITHOUT WARRANTIES OR CONDITIONS OF ANY KIND, either express or implied.
-# See the License for the specific language governing permissions and
-# limitations under the License.
-"""Tokenization classes."""
-
-
-import collections
-import logging
-import os
-import unicodedata
-
-import tokenizers as tk
-
-from .tokenization_utils import PreTrainedTokenizer, PreTrainedTokenizerFast
-
-
-logger = logging.getLogger(__name__)
-
-VOCAB_FILES_NAMES = {"vocab_file": "vocab.txt"}
-
-PRETRAINED_VOCAB_FILES_MAP = {
- "vocab_file": {
- "bert-base-uncased": "https://s3.amazonaws.com/models.huggingface.co/bert/bert-base-uncased-vocab.txt",
- "bert-large-uncased": "https://s3.amazonaws.com/models.huggingface.co/bert/bert-large-uncased-vocab.txt",
- "bert-base-cased": "https://s3.amazonaws.com/models.huggingface.co/bert/bert-base-cased-vocab.txt",
- "bert-large-cased": "https://s3.amazonaws.com/models.huggingface.co/bert/bert-large-cased-vocab.txt",
- "bert-base-multilingual-uncased": "https://s3.amazonaws.com/models.huggingface.co/bert/bert-base-multilingual-uncased-vocab.txt",
- "bert-base-multilingual-cased": "https://s3.amazonaws.com/models.huggingface.co/bert/bert-base-multilingual-cased-vocab.txt",
- "bert-base-chinese": "https://s3.amazonaws.com/models.huggingface.co/bert/bert-base-chinese-vocab.txt",
- "bert-base-german-cased": "https://int-deepset-models-bert.s3.eu-central-1.amazonaws.com/pytorch/bert-base-german-cased-vocab.txt",
- "bert-large-uncased-whole-word-masking": "https://s3.amazonaws.com/models.huggingface.co/bert/bert-large-uncased-whole-word-masking-vocab.txt",
- "bert-large-cased-whole-word-masking": "https://s3.amazonaws.com/models.huggingface.co/bert/bert-large-cased-whole-word-masking-vocab.txt",
- "bert-large-uncased-whole-word-masking-finetuned-squad": "https://s3.amazonaws.com/models.huggingface.co/bert/bert-large-uncased-whole-word-masking-finetuned-squad-vocab.txt",
- "bert-large-cased-whole-word-masking-finetuned-squad": "https://s3.amazonaws.com/models.huggingface.co/bert/bert-large-cased-whole-word-masking-finetuned-squad-vocab.txt",
- "bert-base-cased-finetuned-mrpc": "https://s3.amazonaws.com/models.huggingface.co/bert/bert-base-cased-finetuned-mrpc-vocab.txt",
- "bert-base-german-dbmdz-cased": "https://s3.amazonaws.com/models.huggingface.co/bert/bert-base-german-dbmdz-cased-vocab.txt",
- "bert-base-german-dbmdz-uncased": "https://s3.amazonaws.com/models.huggingface.co/bert/bert-base-german-dbmdz-uncased-vocab.txt",
- "bert-base-finnish-cased-v1": "https://s3.amazonaws.com/models.huggingface.co/bert/TurkuNLP/bert-base-finnish-cased-v1/vocab.txt",
- "bert-base-finnish-uncased-v1": "https://s3.amazonaws.com/models.huggingface.co/bert/TurkuNLP/bert-base-finnish-uncased-v1/vocab.txt",
- "bert-base-dutch-cased": "https://s3.amazonaws.com/models.huggingface.co/bert/wietsedv/bert-base-dutch-cased/vocab.txt",
- }
-}
-
-PRETRAINED_POSITIONAL_EMBEDDINGS_SIZES = {
- "bert-base-uncased": 512,
- "bert-large-uncased": 512,
- "bert-base-cased": 512,
- "bert-large-cased": 512,
- "bert-base-multilingual-uncased": 512,
- "bert-base-multilingual-cased": 512,
- "bert-base-chinese": 512,
- "bert-base-german-cased": 512,
- "bert-large-uncased-whole-word-masking": 512,
- "bert-large-cased-whole-word-masking": 512,
- "bert-large-uncased-whole-word-masking-finetuned-squad": 512,
- "bert-large-cased-whole-word-masking-finetuned-squad": 512,
- "bert-base-cased-finetuned-mrpc": 512,
- "bert-base-german-dbmdz-cased": 512,
- "bert-base-german-dbmdz-uncased": 512,
- "bert-base-finnish-cased-v1": 512,
- "bert-base-finnish-uncased-v1": 512,
- "bert-base-dutch-cased": 512,
-}
-
-PRETRAINED_INIT_CONFIGURATION = {
- "bert-base-uncased": {"do_lower_case": True},
- "bert-large-uncased": {"do_lower_case": True},
- "bert-base-cased": {"do_lower_case": False},
- "bert-large-cased": {"do_lower_case": False},
- "bert-base-multilingual-uncased": {"do_lower_case": True},
- "bert-base-multilingual-cased": {"do_lower_case": False},
- "bert-base-chinese": {"do_lower_case": False},
- "bert-base-german-cased": {"do_lower_case": False},
- "bert-large-uncased-whole-word-masking": {"do_lower_case": True},
- "bert-large-cased-whole-word-masking": {"do_lower_case": False},
- "bert-large-uncased-whole-word-masking-finetuned-squad": {"do_lower_case": True},
- "bert-large-cased-whole-word-masking-finetuned-squad": {"do_lower_case": False},
- "bert-base-cased-finetuned-mrpc": {"do_lower_case": False},
- "bert-base-german-dbmdz-cased": {"do_lower_case": False},
- "bert-base-german-dbmdz-uncased": {"do_lower_case": True},
- "bert-base-finnish-cased-v1": {"do_lower_case": False},
- "bert-base-finnish-uncased-v1": {"do_lower_case": True},
- "bert-base-dutch-cased": {"do_lower_case": False},
-}
-
-
-def load_vocab(vocab_file):
- """Loads a vocabulary file into a dictionary."""
- vocab = collections.OrderedDict()
- with open(vocab_file, "r", encoding="utf-8") as reader:
- tokens = reader.readlines()
- for index, token in enumerate(tokens):
- token = token.rstrip("\n")
- vocab[token] = index
- return vocab
-
-
-def whitespace_tokenize(text):
- """Runs basic whitespace cleaning and splitting on a piece of text."""
- text = text.strip()
- if not text:
- return []
- tokens = text.split()
- return tokens
-
-
-class BertTokenizer(PreTrainedTokenizer):
- r"""
- Constructs a BertTokenizer.
- :class:`~transformers.BertTokenizer` runs end-to-end tokenization: punctuation splitting + wordpiece
-
- Args:
- vocab_file: Path to a one-wordpiece-per-line vocabulary file
- do_lower_case: Whether to lower case the input. Only has an effect when do_basic_tokenize=True
- do_basic_tokenize: Whether to do basic tokenization before wordpiece.
- max_len: An artificial maximum length to truncate tokenized sequences to; Effective maximum length is always the
- minimum of this value (if specified) and the underlying BERT model's sequence length.
- never_split: List of tokens which will never be split during tokenization. Only has an effect when
- do_basic_tokenize=True
- """
-
- vocab_files_names = VOCAB_FILES_NAMES
- pretrained_vocab_files_map = PRETRAINED_VOCAB_FILES_MAP
- pretrained_init_configuration = PRETRAINED_INIT_CONFIGURATION
- max_model_input_sizes = PRETRAINED_POSITIONAL_EMBEDDINGS_SIZES
-
- def __init__(
- self,
- vocab_file,
- do_lower_case=True,
- do_basic_tokenize=True,
- never_split=None,
- unk_token="[UNK]",
- sep_token="[SEP]",
- pad_token="[PAD]",
- cls_token="[CLS]",
- mask_token="[MASK]",
- tokenize_chinese_chars=True,
- **kwargs
- ):
- """Constructs a BertTokenizer.
-
- Args:
- **vocab_file**: Path to a one-wordpiece-per-line vocabulary file
- **do_lower_case**: (`optional`) boolean (default True)
- Whether to lower case the input
- Only has an effect when do_basic_tokenize=True
- **do_basic_tokenize**: (`optional`) boolean (default True)
- Whether to do basic tokenization before wordpiece.
- **never_split**: (`optional`) list of string
- List of tokens which will never be split during tokenization.
- Only has an effect when do_basic_tokenize=True
- **tokenize_chinese_chars**: (`optional`) boolean (default True)
- Whether to tokenize Chinese characters.
- This should likely be deactivated for Japanese:
- see: https://github.com/huggingface/pytorch-pretrained-BERT/issues/328
- """
- super().__init__(
- unk_token=unk_token,
- sep_token=sep_token,
- pad_token=pad_token,
- cls_token=cls_token,
- mask_token=mask_token,
- **kwargs,
- )
- self.max_len_single_sentence = self.max_len - 2 # take into account special tokens
- self.max_len_sentences_pair = self.max_len - 3 # take into account special tokens
-
- if not os.path.isfile(vocab_file):
- raise ValueError(
- "Can't find a vocabulary file at path '{}'. To load the vocabulary from a Google pretrained "
- "model use `tokenizer = BertTokenizer.from_pretrained(PRETRAINED_MODEL_NAME)`".format(vocab_file)
- )
- self.vocab = load_vocab(vocab_file)
- self.ids_to_tokens = collections.OrderedDict([(ids, tok) for tok, ids in self.vocab.items()])
- self.do_basic_tokenize = do_basic_tokenize
- if do_basic_tokenize:
- self.basic_tokenizer = BasicTokenizer(
- do_lower_case=do_lower_case, never_split=never_split, tokenize_chinese_chars=tokenize_chinese_chars
- )
- self.wordpiece_tokenizer = WordpieceTokenizer(vocab=self.vocab, unk_token=self.unk_token)
-
- @property
- def vocab_size(self):
- return len(self.vocab)
-
- def _tokenize(self, text):
- split_tokens = []
- if self.do_basic_tokenize:
- for token in self.basic_tokenizer.tokenize(text, never_split=self.all_special_tokens):
- for sub_token in self.wordpiece_tokenizer.tokenize(token):
- split_tokens.append(sub_token)
- else:
- split_tokens = self.wordpiece_tokenizer.tokenize(text)
- return split_tokens
-
- def _convert_token_to_id(self, token):
- """ Converts a token (str) in an id using the vocab. """
- return self.vocab.get(token, self.vocab.get(self.unk_token))
-
- def _convert_id_to_token(self, index):
- """Converts an index (integer) in a token (str) using the vocab."""
- return self.ids_to_tokens.get(index, self.unk_token)
-
- def convert_tokens_to_string(self, tokens):
- """ Converts a sequence of tokens (string) in a single string. """
- out_string = " ".join(tokens).replace(" ##", "").strip()
- return out_string
-
- def build_inputs_with_special_tokens(self, token_ids_0, token_ids_1=None):
- """
- Build model inputs from a sequence or a pair of sequence for sequence classification tasks
- by concatenating and adding special tokens.
- A BERT sequence has the following format:
- single sequence: [CLS] X [SEP]
- pair of sequences: [CLS] A [SEP] B [SEP]
- """
- if token_ids_1 is None:
- return [self.cls_token_id] + token_ids_0 + [self.sep_token_id]
- cls = [self.cls_token_id]
- sep = [self.sep_token_id]
- return cls + token_ids_0 + sep + token_ids_1 + sep
-
- def get_special_tokens_mask(self, token_ids_0, token_ids_1=None, already_has_special_tokens=False):
- """
- Retrieves sequence ids from a token list that has no special tokens added. This method is called when adding
- special tokens using the tokenizer ``prepare_for_model`` or ``encode_plus`` methods.
-
- Args:
- token_ids_0: list of ids (must not contain special tokens)
- token_ids_1: Optional list of ids (must not contain special tokens), necessary when fetching sequence ids
- for sequence pairs
- already_has_special_tokens: (default False) Set to True if the token list is already formated with
- special tokens for the model
-
- Returns:
- A list of integers in the range [0, 1]: 1 for a special token, 0 for a sequence token.
- """
-
- if already_has_special_tokens:
- if token_ids_1 is not None:
- raise ValueError(
- "You should not supply a second sequence if the provided sequence of "
- "ids is already formated with special tokens for the model."
- )
- return list(map(lambda x: 1 if x in [self.sep_token_id, self.cls_token_id] else 0, token_ids_0))
-
- if token_ids_1 is not None:
- return [1] + ([0] * len(token_ids_0)) + [1] + ([0] * len(token_ids_1)) + [1]
- return [1] + ([0] * len(token_ids_0)) + [1]
-
- def create_token_type_ids_from_sequences(self, token_ids_0, token_ids_1=None):
- """
- Creates a mask from the two sequences passed to be used in a sequence-pair classification task.
- A BERT sequence pair mask has the following format:
- 0 0 0 0 0 0 0 0 0 0 1 1 1 1 1 1 1 1 1 1 1
- | first sequence | second sequence
-
- if token_ids_1 is None, only returns the first portion of the mask (0's).
- """
- sep = [self.sep_token_id]
- cls = [self.cls_token_id]
- if token_ids_1 is None:
- return len(cls + token_ids_0 + sep) * [0]
- return len(cls + token_ids_0 + sep) * [0] + len(token_ids_1 + sep) * [1]
-
- def save_vocabulary(self, vocab_path):
- """Save the tokenizer vocabulary to a directory or file."""
- index = 0
- if os.path.isdir(vocab_path):
- vocab_file = os.path.join(vocab_path, VOCAB_FILES_NAMES["vocab_file"])
- else:
- vocab_file = vocab_path
- with open(vocab_file, "w", encoding="utf-8") as writer:
- for token, token_index in sorted(self.vocab.items(), key=lambda kv: kv[1]):
- if index != token_index:
- logger.warning(
- "Saving vocabulary to {}: vocabulary indices are not consecutive."
- " Please check that the vocabulary is not corrupted!".format(vocab_file)
- )
- index = token_index
- writer.write(token + "\n")
- index += 1
- return (vocab_file,)
-
-
-class BasicTokenizer(object):
- """Runs basic tokenization (punctuation splitting, lower casing, etc.)."""
-
- def __init__(self, do_lower_case=True, never_split=None, tokenize_chinese_chars=True):
- """ Constructs a BasicTokenizer.
-
- Args:
- **do_lower_case**: Whether to lower case the input.
- **never_split**: (`optional`) list of str
- Kept for backward compatibility purposes.
- Now implemented directly at the base class level (see :func:`PreTrainedTokenizer.tokenize`)
- List of token not to split.
- **tokenize_chinese_chars**: (`optional`) boolean (default True)
- Whether to tokenize Chinese characters.
- This should likely be deactivated for Japanese:
- see: https://github.com/huggingface/pytorch-pretrained-BERT/issues/328
- """
- if never_split is None:
- never_split = []
- self.do_lower_case = do_lower_case
- self.never_split = never_split
- self.tokenize_chinese_chars = tokenize_chinese_chars
-
- def tokenize(self, text, never_split=None):
- """ Basic Tokenization of a piece of text.
- Split on "white spaces" only, for sub-word tokenization, see WordPieceTokenizer.
-
- Args:
- **never_split**: (`optional`) list of str
- Kept for backward compatibility purposes.
- Now implemented directly at the base class level (see :func:`PreTrainedTokenizer.tokenize`)
- List of token not to split.
- """
- never_split = self.never_split + (never_split if never_split is not None else [])
- text = self._clean_text(text)
- # This was added on November 1st, 2018 for the multilingual and Chinese
- # models. This is also applied to the English models now, but it doesn't
- # matter since the English models were not trained on any Chinese data
- # and generally don't have any Chinese data in them (there are Chinese
- # characters in the vocabulary because Wikipedia does have some Chinese
- # words in the English Wikipedia.).
- if self.tokenize_chinese_chars:
- text = self._tokenize_chinese_chars(text)
- orig_tokens = whitespace_tokenize(text)
- split_tokens = []
- for token in orig_tokens:
- if self.do_lower_case and token not in never_split:
- token = token.lower()
- token = self._run_strip_accents(token)
- split_tokens.extend(self._run_split_on_punc(token, never_split))
-
- output_tokens = whitespace_tokenize(" ".join(split_tokens))
- return output_tokens
-
- def _run_strip_accents(self, text):
- """Strips accents from a piece of text."""
- text = unicodedata.normalize("NFD", text)
- output = []
- for char in text:
- cat = unicodedata.category(char)
- if cat == "Mn":
- continue
- output.append(char)
- return "".join(output)
-
- def _run_split_on_punc(self, text, never_split=None):
- """Splits punctuation on a piece of text."""
- if never_split is not None and text in never_split:
- return [text]
- chars = list(text)
- i = 0
- start_new_word = True
- output = []
- while i < len(chars):
- char = chars[i]
- if _is_punctuation(char):
- output.append([char])
- start_new_word = True
- else:
- if start_new_word:
- output.append([])
- start_new_word = False
- output[-1].append(char)
- i += 1
-
- return ["".join(x) for x in output]
-
- def _tokenize_chinese_chars(self, text):
- """Adds whitespace around any CJK character."""
- output = []
- for char in text:
- cp = ord(char)
- if self._is_chinese_char(cp):
- output.append(" ")
- output.append(char)
- output.append(" ")
- else:
- output.append(char)
- return "".join(output)
-
- def _is_chinese_char(self, cp):
- """Checks whether CP is the codepoint of a CJK character."""
- # This defines a "chinese character" as anything in the CJK Unicode block:
- # https://en.wikipedia.org/wiki/CJK_Unified_Ideographs_(Unicode_block)
- #
- # Note that the CJK Unicode block is NOT all Japanese and Korean characters,
- # despite its name. The modern Korean Hangul alphabet is a different block,
- # as is Japanese Hiragana and Katakana. Those alphabets are used to write
- # space-separated words, so they are not treated specially and handled
- # like the all of the other languages.
- if (
- (cp >= 0x4E00 and cp <= 0x9FFF)
- or (cp >= 0x3400 and cp <= 0x4DBF) #
- or (cp >= 0x20000 and cp <= 0x2A6DF) #
- or (cp >= 0x2A700 and cp <= 0x2B73F) #
- or (cp >= 0x2B740 and cp <= 0x2B81F) #
- or (cp >= 0x2B820 and cp <= 0x2CEAF) #
- or (cp >= 0xF900 and cp <= 0xFAFF)
- or (cp >= 0x2F800 and cp <= 0x2FA1F) #
- ): #
- return True
-
- return False
-
- def _clean_text(self, text):
- """Performs invalid character removal and whitespace cleanup on text."""
- output = []
- for char in text:
- cp = ord(char)
- if cp == 0 or cp == 0xFFFD or _is_control(char):
- continue
- if _is_whitespace(char):
- output.append(" ")
- else:
- output.append(char)
- return "".join(output)
-
-
-class WordpieceTokenizer(object):
- """Runs WordPiece tokenization."""
-
- def __init__(self, vocab, unk_token, max_input_chars_per_word=100):
- self.vocab = vocab
- self.unk_token = unk_token
- self.max_input_chars_per_word = max_input_chars_per_word
-
- def tokenize(self, text):
- """Tokenizes a piece of text into its word pieces.
-
- This uses a greedy longest-match-first algorithm to perform tokenization
- using the given vocabulary.
-
- For example:
- input = "unaffable"
- output = ["un", "##aff", "##able"]
-
- Args:
- text: A single token or whitespace separated tokens. This should have
- already been passed through `BasicTokenizer`.
-
- Returns:
- A list of wordpiece tokens.
- """
-
- output_tokens = []
- for token in whitespace_tokenize(text):
- chars = list(token)
- if len(chars) > self.max_input_chars_per_word:
- output_tokens.append(self.unk_token)
- continue
-
- is_bad = False
- start = 0
- sub_tokens = []
- while start < len(chars):
- end = len(chars)
- cur_substr = None
- while start < end:
- substr = "".join(chars[start:end])
- if start > 0:
- substr = "##" + substr
- if substr in self.vocab:
- cur_substr = substr
- break
- end -= 1
- if cur_substr is None:
- is_bad = True
- break
- sub_tokens.append(cur_substr)
- start = end
-
- if is_bad:
- output_tokens.append(self.unk_token)
- else:
- output_tokens.extend(sub_tokens)
- return output_tokens
-
-
-def _is_whitespace(char):
- """Checks whether `chars` is a whitespace character."""
- # \t, \n, and \r are technically contorl characters but we treat them
- # as whitespace since they are generally considered as such.
- if char == " " or char == "\t" or char == "\n" or char == "\r":
- return True
- cat = unicodedata.category(char)
- if cat == "Zs":
- return True
- return False
-
-
-def _is_control(char):
- """Checks whether `chars` is a control character."""
- # These are technically control characters but we count them as whitespace
- # characters.
- if char == "\t" or char == "\n" or char == "\r":
- return False
- cat = unicodedata.category(char)
- if cat.startswith("C"):
- return True
- return False
-
-
-def _is_punctuation(char):
- """Checks whether `chars` is a punctuation character."""
- cp = ord(char)
- # We treat all non-letter/number ASCII as punctuation.
- # Characters such as "^", "$", and "`" are not in the Unicode
- # Punctuation class but we treat them as punctuation anyways, for
- # consistency.
- if (cp >= 33 and cp <= 47) or (cp >= 58 and cp <= 64) or (cp >= 91 and cp <= 96) or (cp >= 123 and cp <= 126):
- return True
- cat = unicodedata.category(char)
- if cat.startswith("P"):
- return True
- return False
-
-
-class BertTokenizerFast(PreTrainedTokenizerFast):
- vocab_files_names = VOCAB_FILES_NAMES
- pretrained_vocab_files_map = PRETRAINED_VOCAB_FILES_MAP
- pretrained_init_configuration = PRETRAINED_INIT_CONFIGURATION
- max_model_input_sizes = PRETRAINED_POSITIONAL_EMBEDDINGS_SIZES
-
- def __init__(
- self,
- vocab_file,
- do_lower_case=True,
- do_basic_tokenize=True,
- never_split=None,
- unk_token="[UNK]",
- sep_token="[SEP]",
- pad_token="[PAD]",
- cls_token="[CLS]",
- mask_token="[MASK]",
- tokenize_chinese_chars=True,
- max_length=None,
- pad_to_max_length=False,
- stride=0,
- truncation_strategy="longest_first",
- add_special_tokens=True,
- **kwargs
- ):
- super().__init__(
- unk_token=unk_token,
- sep_token=sep_token,
- pad_token=pad_token,
- cls_token=cls_token,
- mask_token=mask_token,
- **kwargs,
- )
-
- self._tokenizer = tk.Tokenizer(tk.models.WordPiece.from_files(vocab_file, unk_token=unk_token))
- self._update_special_tokens()
- self._tokenizer.with_pre_tokenizer(
- tk.pre_tokenizers.BertPreTokenizer.new(
- do_basic_tokenize=do_basic_tokenize,
- do_lower_case=do_lower_case,
- tokenize_chinese_chars=tokenize_chinese_chars,
- never_split=never_split if never_split is not None else [],
- )
- )
- self._tokenizer.with_decoder(tk.decoders.WordPiece.new())
-
- if add_special_tokens:
- self._tokenizer.with_post_processor(
- tk.processors.BertProcessing.new(
- (sep_token, self._tokenizer.token_to_id(sep_token)),
- (cls_token, self._tokenizer.token_to_id(cls_token)),
- )
- )
- if max_length is not None:
- self._tokenizer.with_truncation(max_length, stride=stride, strategy=truncation_strategy)
- self._tokenizer.with_padding(
- max_length=max_length if pad_to_max_length else None,
- direction=self.padding_side,
- pad_id=self.pad_token_id,
- pad_type_id=self.pad_token_type_id,
- pad_token=self.pad_token,
- )
- self._decoder = tk.decoders.WordPiece.new()
diff --git a/server/transformers/src/transformers/tokenization_bert_japanese.py b/server/transformers/src/transformers/tokenization_bert_japanese.py
deleted file mode 100644
index aaf82c54b3209b6c6d84202dd24ca257d49ba13f..0000000000000000000000000000000000000000
--- a/server/transformers/src/transformers/tokenization_bert_japanese.py
+++ /dev/null
@@ -1,256 +0,0 @@
-# coding=utf-8
-# Copyright 2018 The Google AI Language Team Authors and The HuggingFace Inc. team.
-#
-# Licensed under the Apache License, Version 2.0 (the "License");
-# you may not use this file except in compliance with the License.
-# You may obtain a copy of the License at
-#
-# http://www.apache.org/licenses/LICENSE-2.0
-#
-# Unless required by applicable law or agreed to in writing, software
-# distributed under the License is distributed on an "AS IS" BASIS,
-# WITHOUT WARRANTIES OR CONDITIONS OF ANY KIND, either express or implied.
-# See the License for the specific language governing permissions and
-# limitations under the License.
-"""Tokenization classes."""
-
-
-import collections
-import logging
-import os
-import unicodedata
-
-from .tokenization_bert import BasicTokenizer, BertTokenizer, WordpieceTokenizer, load_vocab
-
-
-logger = logging.getLogger(__name__)
-
-VOCAB_FILES_NAMES = {"vocab_file": "vocab.txt"}
-
-PRETRAINED_VOCAB_FILES_MAP = {
- "vocab_file": {
- "bert-base-japanese": "https://s3.amazonaws.com/models.huggingface.co/bert/cl-tohoku/bert-base-japanese-vocab.txt",
- "bert-base-japanese-whole-word-masking": "https://s3.amazonaws.com/models.huggingface.co/bert/cl-tohoku/bert-base-japanese-whole-word-masking-vocab.txt",
- "bert-base-japanese-char": "https://s3.amazonaws.com/models.huggingface.co/bert/cl-tohoku/bert-base-japanese-char-vocab.txt",
- "bert-base-japanese-char-whole-word-masking": "https://s3.amazonaws.com/models.huggingface.co/bert/cl-tohoku/bert-base-japanese-char-whole-word-masking-vocab.txt",
- }
-}
-
-PRETRAINED_POSITIONAL_EMBEDDINGS_SIZES = {
- "bert-base-japanese": 512,
- "bert-base-japanese-whole-word-masking": 512,
- "bert-base-japanese-char": 512,
- "bert-base-japanese-char-whole-word-masking": 512,
-}
-
-PRETRAINED_INIT_CONFIGURATION = {
- "bert-base-japanese": {
- "do_lower_case": False,
- "word_tokenizer_type": "mecab",
- "subword_tokenizer_type": "wordpiece",
- },
- "bert-base-japanese-whole-word-masking": {
- "do_lower_case": False,
- "word_tokenizer_type": "mecab",
- "subword_tokenizer_type": "wordpiece",
- },
- "bert-base-japanese-char": {
- "do_lower_case": False,
- "word_tokenizer_type": "mecab",
- "subword_tokenizer_type": "character",
- },
- "bert-base-japanese-char-whole-word-masking": {
- "do_lower_case": False,
- "word_tokenizer_type": "mecab",
- "subword_tokenizer_type": "character",
- },
-}
-
-
-class BertJapaneseTokenizer(BertTokenizer):
- """BERT tokenizer for Japanese text"""
-
- vocab_files_names = VOCAB_FILES_NAMES
- pretrained_vocab_files_map = PRETRAINED_VOCAB_FILES_MAP
- pretrained_init_configuration = PRETRAINED_INIT_CONFIGURATION
- max_model_input_sizes = PRETRAINED_POSITIONAL_EMBEDDINGS_SIZES
-
- def __init__(
- self,
- vocab_file,
- do_lower_case=False,
- do_word_tokenize=True,
- do_subword_tokenize=True,
- word_tokenizer_type="basic",
- subword_tokenizer_type="wordpiece",
- never_split=None,
- unk_token="[UNK]",
- sep_token="[SEP]",
- pad_token="[PAD]",
- cls_token="[CLS]",
- mask_token="[MASK]",
- **kwargs
- ):
- """Constructs a MecabBertTokenizer.
-
- Args:
- **vocab_file**: Path to a one-wordpiece-per-line vocabulary file.
- **do_lower_case**: (`optional`) boolean (default True)
- Whether to lower case the input.
- Only has an effect when do_basic_tokenize=True.
- **do_word_tokenize**: (`optional`) boolean (default True)
- Whether to do word tokenization.
- **do_subword_tokenize**: (`optional`) boolean (default True)
- Whether to do subword tokenization.
- **word_tokenizer_type**: (`optional`) string (default "basic")
- Type of word tokenizer.
- **subword_tokenizer_type**: (`optional`) string (default "wordpiece")
- Type of subword tokenizer.
- """
- super(BertTokenizer, self).__init__(
- unk_token=unk_token,
- sep_token=sep_token,
- pad_token=pad_token,
- cls_token=cls_token,
- mask_token=mask_token,
- **kwargs,
- )
- # ^^ We call the grandparent's init, not the parent's.
- self.max_len_single_sentence = self.max_len - 2 # take into account special tokens
- self.max_len_sentences_pair = self.max_len - 3 # take into account special tokens
-
- if not os.path.isfile(vocab_file):
- raise ValueError(
- "Can't find a vocabulary file at path '{}'. To load the vocabulary from a Google pretrained "
- "model use `tokenizer = BertTokenizer.from_pretrained(PRETRAINED_MODEL_NAME)`".format(vocab_file)
- )
- self.vocab = load_vocab(vocab_file)
- self.ids_to_tokens = collections.OrderedDict([(ids, tok) for tok, ids in self.vocab.items()])
-
- self.do_word_tokenize = do_word_tokenize
- if do_word_tokenize:
- if word_tokenizer_type == "basic":
- self.word_tokenizer = BasicTokenizer(
- do_lower_case=do_lower_case, never_split=never_split, tokenize_chinese_chars=False
- )
- elif word_tokenizer_type == "mecab":
- self.word_tokenizer = MecabTokenizer(do_lower_case=do_lower_case, never_split=never_split)
- else:
- raise ValueError("Invalid word_tokenizer_type '{}' is specified.".format(word_tokenizer_type))
-
- self.do_subword_tokenize = do_subword_tokenize
- if do_subword_tokenize:
- if subword_tokenizer_type == "wordpiece":
- self.subword_tokenizer = WordpieceTokenizer(vocab=self.vocab, unk_token=self.unk_token)
- elif subword_tokenizer_type == "character":
- self.subword_tokenizer = CharacterTokenizer(vocab=self.vocab, unk_token=self.unk_token)
- else:
- raise ValueError("Invalid subword_tokenizer_type '{}' is specified.".format(subword_tokenizer_type))
-
- def _tokenize(self, text):
- if self.do_word_tokenize:
- tokens = self.word_tokenizer.tokenize(text, never_split=self.all_special_tokens)
- else:
- tokens = [text]
-
- if self.do_subword_tokenize:
- split_tokens = [sub_token for token in tokens for sub_token in self.subword_tokenizer.tokenize(token)]
- else:
- split_tokens = tokens
-
- return split_tokens
-
-
-class MecabTokenizer(object):
- """Runs basic tokenization with MeCab morphological parser."""
-
- def __init__(self, do_lower_case=False, never_split=None, normalize_text=True):
- """Constructs a MecabTokenizer.
-
- Args:
- **do_lower_case**: (`optional`) boolean (default True)
- Whether to lower case the input.
- **never_split**: (`optional`) list of str
- Kept for backward compatibility purposes.
- Now implemented directly at the base class level (see :func:`PreTrainedTokenizer.tokenize`)
- List of token not to split.
- **normalize_text**: (`optional`) boolean (default True)
- Whether to apply unicode normalization to text before tokenization.
- """
- self.do_lower_case = do_lower_case
- self.never_split = never_split if never_split is not None else []
- self.normalize_text = normalize_text
-
- import MeCab
-
- self.mecab = MeCab.Tagger()
-
- def tokenize(self, text, never_split=None, **kwargs):
- """Tokenizes a piece of text."""
- if self.normalize_text:
- text = unicodedata.normalize("NFKC", text)
-
- never_split = self.never_split + (never_split if never_split is not None else [])
- tokens = []
-
- mecab_output = self.mecab.parse(text)
-
- cursor = 0
- for line in mecab_output.split("\n"):
- if line == "EOS":
- break
-
- token, _ = line.split("\t")
- token_start = text.index(token, cursor)
- token_end = token_start + len(token)
- if self.do_lower_case and token not in never_split:
- token = token.lower()
-
- tokens.append(token)
- cursor = token_end
-
- return tokens
-
-
-class CharacterTokenizer(object):
- """Runs Character tokenziation."""
-
- def __init__(self, vocab, unk_token, normalize_text=True):
- """Constructs a CharacterTokenizer.
-
- Args:
- **vocab**:
- Vocabulary object.
- **unk_token**: str
- A special symbol for out-of-vocabulary token.
- **normalize_text**: (`optional`) boolean (default True)
- Whether to apply unicode normalization to text before tokenization.
- """
- self.vocab = vocab
- self.unk_token = unk_token
- self.normalize_text = normalize_text
-
- def tokenize(self, text):
- """Tokenizes a piece of text into characters.
-
- For example:
- input = "apple"
- output = ["a", "p", "p", "l", "e"]
- Args:
- text: A single token or whitespace separated tokens.
- This should have already been passed through `BasicTokenizer`.
- Returns:
- A list of characters.
- """
- if self.normalize_text:
- text = unicodedata.normalize("NFKC", text)
-
- output_tokens = []
- for i, char in enumerate(text):
- if char not in self.vocab:
- output_tokens.append(self.unk_token)
- continue
-
- output_tokens.append(char)
-
- return output_tokens
diff --git a/server/transformers/src/transformers/tokenization_camembert.py b/server/transformers/src/transformers/tokenization_camembert.py
deleted file mode 100644
index a158419470fb31d37b0e2e14a87bb9b219365640..0000000000000000000000000000000000000000
--- a/server/transformers/src/transformers/tokenization_camembert.py
+++ /dev/null
@@ -1,214 +0,0 @@
-# coding=utf-8
-# Copyright 2018 Google AI, Google Brain and Carnegie Mellon University Authors and the HuggingFace Inc. team.
-#
-# Licensed under the Apache License, Version 2.0 (the "License");
-# you may not use this file except in compliance with the License.
-# You may obtain a copy of the License at
-#
-# http://www.apache.org/licenses/LICENSE-2.0
-#
-# Unless required by applicable law or agreed to in writing, software
-# distributed under the License is distributed on an "AS IS" BASIS,
-# WITHOUT WARRANTIES OR CONDITIONS OF ANY KIND, either express or implied.
-# See the License for the specific language governing permissions and
-# limitations under the License
-""" Tokenization classes for Camembert model."""
-
-
-import logging
-import os
-from shutil import copyfile
-
-import sentencepiece as spm
-
-from transformers.tokenization_utils import PreTrainedTokenizer
-
-from .tokenization_xlnet import SPIECE_UNDERLINE
-
-
-logger = logging.getLogger(__name__)
-
-VOCAB_FILES_NAMES = {"vocab_file": "sentencepiece.bpe.model"}
-
-PRETRAINED_VOCAB_FILES_MAP = {
- "vocab_file": {
- "camembert-base": "https://s3.amazonaws.com/models.huggingface.co/bert/camembert-base-sentencepiece.bpe.model",
- }
-}
-
-PRETRAINED_POSITIONAL_EMBEDDINGS_SIZES = {
- "camembert-base": None,
-}
-
-SHARED_MODEL_IDENTIFIERS = [
- # Load with
- # `tokenizer = AutoTokenizer.from_pretrained("username/pretrained_model")`
- "Musixmatch/umberto-commoncrawl-cased-v1",
- "Musixmatch/umberto-wikipedia-uncased-v1",
-]
-
-
-class CamembertTokenizer(PreTrainedTokenizer):
- """
- Adapted from RobertaTokenizer and XLNetTokenizer
- SentencePiece based tokenizer. Peculiarities:
-
- - requires `SentencePiece `_
- """
-
- vocab_files_names = VOCAB_FILES_NAMES
- pretrained_vocab_files_map = PRETRAINED_VOCAB_FILES_MAP
- max_model_input_sizes = PRETRAINED_POSITIONAL_EMBEDDINGS_SIZES
-
- def __init__(
- self,
- vocab_file,
- bos_token="",
- eos_token="",
- sep_token="",
- cls_token="",
- unk_token="",
- pad_token="",
- mask_token="",
- additional_special_tokens=["NOTUSED", "NOTUSED"],
- **kwargs
- ):
- super().__init__(
- max_len=512,
- bos_token=bos_token,
- eos_token=eos_token,
- unk_token=unk_token,
- sep_token=sep_token,
- cls_token=cls_token,
- pad_token=pad_token,
- mask_token=mask_token,
- additional_special_tokens=additional_special_tokens,
- **kwargs,
- )
- self.max_len_single_sentence = self.max_len - 2 # take into account special tokens
- self.max_len_sentences_pair = self.max_len - 4 # take into account special tokens
- self.sp_model = spm.SentencePieceProcessor()
- self.sp_model.Load(str(vocab_file))
- self.vocab_file = vocab_file
- # HACK: These tokens were added by fairseq but don't seem to be actually used when duplicated in the actual
- # sentencepiece vocabulary (this is the case for and
- self.fairseq_tokens_to_ids = {"NOTUSED": 0, "": 1, "NOTUSED": 2, "": 3}
- self.fairseq_offset = len(self.fairseq_tokens_to_ids)
- self.fairseq_tokens_to_ids[""] = len(self.sp_model) + len(self.fairseq_tokens_to_ids)
- self.fairseq_ids_to_tokens = {v: k for k, v in self.fairseq_tokens_to_ids.items()}
-
- def build_inputs_with_special_tokens(self, token_ids_0, token_ids_1=None):
- """
- Build model inputs from a sequence or a pair of sequence for sequence classification tasks
- by concatenating and adding special tokens.
- A RoBERTa sequence has the following format:
- single sequence: X
- pair of sequences: A B
- """
- if token_ids_1 is None:
- return [self.cls_token_id] + token_ids_0 + [self.sep_token_id]
- cls = [self.cls_token_id]
- sep = [self.sep_token_id]
- return cls + token_ids_0 + sep + sep + token_ids_1 + sep
-
- def get_special_tokens_mask(self, token_ids_0, token_ids_1=None, already_has_special_tokens=False):
- """
- Retrieves sequence ids from a token list that has no special tokens added. This method is called when adding
- special tokens using the tokenizer ``prepare_for_model`` or ``encode_plus`` methods.
-
- Args:
- token_ids_0: list of ids (must not contain special tokens)
- token_ids_1: Optional list of ids (must not contain special tokens), necessary when fetching sequence ids
- for sequence pairs
- already_has_special_tokens: (default False) Set to True if the token list is already formated with
- special tokens for the model
-
- Returns:
- A list of integers in the range [0, 1]: 1 for a special token, 0 for a sequence token.
- """
- if already_has_special_tokens:
- if token_ids_1 is not None:
- raise ValueError(
- "You should not supply a second sequence if the provided sequence of "
- "ids is already formated with special tokens for the model."
- )
- return list(map(lambda x: 1 if x in [self.sep_token_id, self.cls_token_id] else 0, token_ids_0))
-
- if token_ids_1 is None:
- return [1] + ([0] * len(token_ids_0)) + [1]
- return [1] + ([0] * len(token_ids_0)) + [1, 1] + ([0] * len(token_ids_1)) + [1]
-
- def create_token_type_ids_from_sequences(self, token_ids_0, token_ids_1=None):
- """
- Creates a mask from the two sequences passed to be used in a sequence-pair classification task.
- A RoBERTa sequence pair mask has the following format:
- 0 0 0 0 0 0 0 0 0 0 1 1 1 1 1 1 1 1 1 1 1
- | first sequence | second sequence
-
- if token_ids_1 is None, only returns the first portion of the mask (0's).
- """
- sep = [self.sep_token_id]
- cls = [self.cls_token_id]
-
- if token_ids_1 is None:
- return len(cls + token_ids_0 + sep) * [0]
- return len(cls + token_ids_0 + sep + sep) * [0] + len(token_ids_1 + sep) * [1]
-
- @property
- def vocab_size(self):
- return len(self.fairseq_tokens_to_ids) + len(self.sp_model)
-
- def _tokenize(self, text):
- return self.sp_model.EncodeAsPieces(text)
-
- def _convert_token_to_id(self, token):
- """ Converts a token (str) in an id using the vocab. """
- if token in self.fairseq_tokens_to_ids:
- return self.fairseq_tokens_to_ids[token]
- elif self.sp_model.PieceToId(token) == 0:
- # Convert sentence piece unk token to fairseq unk token index
- return self.unk_token_id
- return self.fairseq_offset + self.sp_model.PieceToId(token)
-
- def _convert_id_to_token(self, index):
- """Converts an index (integer) in a token (str) using the vocab."""
- if index in self.fairseq_ids_to_tokens:
- return self.fairseq_ids_to_tokens[index]
- return self.sp_model.IdToPiece(index - self.fairseq_offset)
-
- def __getstate__(self):
- state = self.__dict__.copy()
- state["sp_model"] = None
- return state
-
- def __setstate__(self, d):
- self.__dict__ = d
- try:
- import sentencepiece as spm
- except ImportError:
- logger.warning(
- "You need to install SentencePiece to use AlbertTokenizer: https://github.com/google/sentencepiece"
- "pip install sentencepiece"
- )
- raise
- self.sp_model = spm.SentencePieceProcessor()
- self.sp_model.Load(self.vocab_file)
-
- def convert_tokens_to_string(self, tokens):
- """Converts a sequence of tokens (strings for sub-words) in a single string."""
- out_string = "".join(tokens).replace(SPIECE_UNDERLINE, " ").strip()
- return out_string
-
- def save_vocabulary(self, save_directory):
- """ Save the sentencepiece vocabulary (copy original file) and special tokens file
- to a directory.
- """
- if not os.path.isdir(save_directory):
- logger.error("Vocabulary path ({}) should be a directory".format(save_directory))
- return
- out_vocab_file = os.path.join(save_directory, VOCAB_FILES_NAMES["vocab_file"])
-
- if os.path.abspath(self.vocab_file) != os.path.abspath(out_vocab_file):
- copyfile(self.vocab_file, out_vocab_file)
-
- return (out_vocab_file,)
diff --git a/server/transformers/src/transformers/tokenization_ctrl.py b/server/transformers/src/transformers/tokenization_ctrl.py
deleted file mode 100644
index 1f2184f0a12e31f7a5a575758781b47b5294cfd0..0000000000000000000000000000000000000000
--- a/server/transformers/src/transformers/tokenization_ctrl.py
+++ /dev/null
@@ -1,248 +0,0 @@
-# coding=utf-8
-# Copyright 2018 Salesforce and The HuggingFace Inc. team.
-#
-# Licensed under the Apache License, Version 2.0 (the "License");
-# you may not use this file except in compliance with the License.
-# You may obtain a copy of the License at
-#
-# http://www.apache.org/licenses/LICENSE-2.0
-#
-# Unless required by applicable law or agreed to in writing, software
-# distributed under the License is distributed on an "AS IS" BASIS,
-# WITHOUT WARRANTIES OR CONDITIONS OF ANY KIND, either express or implied.
-# See the License for the specific language governing permissions and
-# limitations under the License.
-"""Tokenization classes for Salesforce CTRL."""
-
-
-import json
-import logging
-import os
-
-import regex as re
-
-from .tokenization_utils import PreTrainedTokenizer
-
-
-logger = logging.getLogger(__name__)
-
-VOCAB_FILES_NAMES = {
- "vocab_file": "vocab.json",
- "merges_file": "merges.txt",
-}
-
-PRETRAINED_VOCAB_FILES_MAP = {
- "vocab_file": {"ctrl": "https://raw.githubusercontent.com/salesforce/ctrl/master/ctrl-vocab.json"},
- "merges_file": {"ctrl": "https://raw.githubusercontent.com/salesforce/ctrl/master/ctrl-merges.txt"},
-}
-
-PRETRAINED_POSITIONAL_EMBEDDINGS_SIZES = {
- "ctrl": 256,
-}
-
-CONTROL_CODES = {
- "Pregnancy": 168629,
- "Christianity": 7675,
- "Explain": 106423,
- "Fitness": 63440,
- "Saving": 63163,
- "Ask": 27171,
- "Ass": 95985,
- "Joke": 163509,
- "Questions": 45622,
- "Thoughts": 49605,
- "Retail": 52342,
- "Feminism": 164338,
- "Writing": 11992,
- "Atheism": 192263,
- "Netflix": 48616,
- "Computing": 39639,
- "Opinion": 43213,
- "Alone": 44967,
- "Funny": 58917,
- "Gaming": 40358,
- "Human": 4088,
- "India": 1331,
- "Joker": 77138,
- "Diet": 36206,
- "Legal": 11859,
- "Norman": 4939,
- "Tip": 72689,
- "Weight": 52343,
- "Movies": 46273,
- "Running": 23425,
- "Science": 2090,
- "Horror": 37793,
- "Confession": 60572,
- "Finance": 12250,
- "Politics": 16360,
- "Scary": 191985,
- "Support": 12654,
- "Technologies": 32516,
- "Teenage": 66160,
- "Event": 32769,
- "Learned": 67460,
- "Notion": 182770,
- "Wikipedia": 37583,
- "Books": 6665,
- "Extract": 76050,
- "Confessions": 102701,
- "Conspiracy": 75932,
- "Links": 63674,
- "Narcissus": 150425,
- "Relationship": 54766,
- "Relationships": 134796,
- "Reviews": 41671,
- "News": 4256,
- "Translation": 26820,
- "multilingual": 128406,
-}
-
-
-def get_pairs(word):
- """Return set of symbol pairs in a word.
-
- Word is represented as tuple of symbols (symbols being variable-length strings).
- """
- pairs = set()
- prev_char = word[0]
- for char in word[1:]:
- pairs.add((prev_char, char))
- prev_char = char
-
- pairs = set(pairs)
- return pairs
-
-
-class CTRLTokenizer(PreTrainedTokenizer):
- """
- CTRL BPE tokenizer. Peculiarities:
- - Byte-Pair-Encoding
- """
-
- vocab_files_names = VOCAB_FILES_NAMES
- pretrained_vocab_files_map = PRETRAINED_VOCAB_FILES_MAP
- max_model_input_sizes = PRETRAINED_POSITIONAL_EMBEDDINGS_SIZES
- control_codes = CONTROL_CODES
-
- def __init__(self, vocab_file, merges_file, unk_token="", **kwargs):
- super().__init__(unk_token=unk_token, **kwargs)
- self.max_len_single_sentence = (
- self.max_len
- ) # no default special tokens - you can update this value if you add special tokens
- self.max_len_sentences_pair = (
- self.max_len
- ) # no default special tokens - you can update this value if you add special tokens
-
- with open(vocab_file, encoding="utf-8") as vocab_handle:
- self.encoder = json.load(vocab_handle)
- self.decoder = {v: k for k, v in self.encoder.items()}
- with open(merges_file, encoding="utf-8") as merges_handle:
- merges = merges_handle.read().split("\n")[1:-1]
- merges = [tuple(merge.split()) for merge in merges]
- self.bpe_ranks = dict(zip(merges, range(len(merges))))
- self.cache = {}
-
- @property
- def vocab_size(self):
- return len(self.encoder)
-
- def bpe(self, token):
- if token in self.cache:
- return self.cache[token]
- word = tuple(token)
- word = tuple(list(word[:-1]) + [word[-1] + ""])
- pairs = get_pairs(word)
-
- if not pairs:
- return token
-
- while True:
- bigram = min(pairs, key=lambda pair: self.bpe_ranks.get(pair, float("inf")))
- if bigram not in self.bpe_ranks:
- break
- first, second = bigram
- new_word = []
- i = 0
- while i < len(word):
- try:
- j = word.index(first, i)
- except ValueError:
- new_word.extend(word[i:])
- break
- else:
- new_word.extend(word[i:j])
- i = j
-
- if word[i] == first and i < len(word) - 1 and word[i + 1] == second:
- new_word.append(first + second)
- i += 2
- else:
- new_word.append(word[i])
- i += 1
- new_word = tuple(new_word)
- word = new_word
- if len(word) == 1:
- break
- else:
- pairs = get_pairs(word)
- word = "@@ ".join(word)
- word = word[:-4]
- self.cache[token] = word
- return word
-
- def _tokenize(self, text):
- """ Tokenize a string.
- """
- split_tokens = []
-
- words = re.findall(r"\S+\n?", text)
-
- for token in words:
- split_tokens.extend([t for t in self.bpe(token).split(" ")])
- return split_tokens
-
- def _convert_token_to_id(self, token):
- """ Converts a token (str) in an id using the vocab. """
- return self.encoder.get(token, self.encoder.get(self.unk_token))
-
- def _convert_id_to_token(self, index):
- """Converts an index (integer) in a token (str) using the vocab."""
- return self.decoder.get(index, self.unk_token)
-
- def convert_tokens_to_string(self, tokens):
- """ Converts a sequence of tokens (string) in a single string. """
- out_string = " ".join(tokens).replace("@@ ", "").strip()
- return out_string
-
- def save_vocabulary(self, save_directory):
- """Save the tokenizer vocabulary and merge files to a directory."""
- if not os.path.isdir(save_directory):
- logger.error("Vocabulary path ({}) should be a directory".format(save_directory))
- return
- vocab_file = os.path.join(save_directory, VOCAB_FILES_NAMES["vocab_file"])
- merge_file = os.path.join(save_directory, VOCAB_FILES_NAMES["merges_file"])
-
- with open(vocab_file, "w", encoding="utf-8") as f:
- f.write(json.dumps(self.encoder, ensure_ascii=False))
-
- index = 0
- with open(merge_file, "w", encoding="utf-8") as writer:
- writer.write("#version: 0.2\n")
- for bpe_tokens, token_index in sorted(self.bpe_ranks.items(), key=lambda kv: kv[1]):
- if index != token_index:
- logger.warning(
- "Saving vocabulary to {}: BPE merge indices are not consecutive."
- " Please check that the tokenizer is not corrupted!".format(merge_file)
- )
- index = token_index
- writer.write(" ".join(bpe_tokens) + "\n")
- index += 1
-
- return vocab_file, merge_file
-
- # def decode(self, token_ids, skip_special_tokens=False, clean_up_tokenization_spaces=True):
- # filtered_tokens = ' '.join(self.convert_ids_to_tokens(token_ids, skip_special_tokens=skip_special_tokens))
- # tokens_generated_so_far = re.sub('(@@ )', '', string=filtered_tokens)
- # tokens_generated_so_far = re.sub('(@@ ?$)', '', string=tokens_generated_so_far)
- # return ''.join(tokens_generated_so_far)
diff --git a/server/transformers/src/transformers/tokenization_distilbert.py b/server/transformers/src/transformers/tokenization_distilbert.py
deleted file mode 100644
index 82dbfdb414f63cc1fc5606c188298e387ef37376..0000000000000000000000000000000000000000
--- a/server/transformers/src/transformers/tokenization_distilbert.py
+++ /dev/null
@@ -1,70 +0,0 @@
-# coding=utf-8
-# Copyright 2018 The HuggingFace Inc. team.
-#
-# Licensed under the Apache License, Version 2.0 (the "License");
-# you may not use this file except in compliance with the License.
-# You may obtain a copy of the License at
-#
-# http://www.apache.org/licenses/LICENSE-2.0
-#
-# Unless required by applicable law or agreed to in writing, software
-# distributed under the License is distributed on an "AS IS" BASIS,
-# WITHOUT WARRANTIES OR CONDITIONS OF ANY KIND, either express or implied.
-# See the License for the specific language governing permissions and
-# limitations under the License.
-"""Tokenization classes for DistilBERT."""
-
-
-import logging
-
-from .tokenization_bert import BertTokenizer
-
-
-logger = logging.getLogger(__name__)
-
-VOCAB_FILES_NAMES = {"vocab_file": "vocab.txt"}
-
-PRETRAINED_VOCAB_FILES_MAP = {
- "vocab_file": {
- "distilbert-base-uncased": "https://s3.amazonaws.com/models.huggingface.co/bert/bert-base-uncased-vocab.txt",
- "distilbert-base-uncased-distilled-squad": "https://s3.amazonaws.com/models.huggingface.co/bert/bert-large-uncased-vocab.txt",
- "distilbert-base-german-cased": "https://s3.amazonaws.com/models.huggingface.co/bert/distilbert-base-german-cased-vocab.txt",
- "distilbert-base-multilingual-cased": "https://s3.amazonaws.com/models.huggingface.co/bert/bert-base-multilingual-cased-vocab.txt",
- }
-}
-
-PRETRAINED_POSITIONAL_EMBEDDINGS_SIZES = {
- "distilbert-base-uncased": 512,
- "distilbert-base-uncased-distilled-squad": 512,
- "distilbert-base-german-cased": 512,
- "distilbert-base-multilingual-cased": 512,
-}
-
-
-PRETRAINED_INIT_CONFIGURATION = {
- "distilbert-base-uncased": {"do_lower_case": True},
- "distilbert-base-uncased-distilled-squad": {"do_lower_case": True},
- "distilbert-base-german-cased": {"do_lower_case": False},
- "distilbert-base-multilingual-cased": {"do_lower_case": False},
-}
-
-
-class DistilBertTokenizer(BertTokenizer):
- r"""
- Constructs a DistilBertTokenizer.
- :class:`~transformers.DistilBertTokenizer` is identical to BertTokenizer and runs end-to-end tokenization: punctuation splitting + wordpiece
-
- Args:
- vocab_file: Path to a one-wordpiece-per-line vocabulary file
- do_lower_case: Whether to lower case the input. Only has an effect when do_basic_tokenize=True
- do_basic_tokenize: Whether to do basic tokenization before wordpiece.
- max_len: An artificial maximum length to truncate tokenized sequences to; Effective maximum length is always the
- minimum of this value (if specified) and the underlying BERT model's sequence length.
- never_split: List of tokens which will never be split during tokenization. Only has an effect when
- do_basic_tokenize=True
- """
-
- vocab_files_names = VOCAB_FILES_NAMES
- pretrained_vocab_files_map = PRETRAINED_VOCAB_FILES_MAP
- max_model_input_sizes = PRETRAINED_POSITIONAL_EMBEDDINGS_SIZES
- pretrained_init_configuration = PRETRAINED_INIT_CONFIGURATION
diff --git a/server/transformers/src/transformers/tokenization_flaubert.py b/server/transformers/src/transformers/tokenization_flaubert.py
deleted file mode 100644
index e648a61c94f4d6aa3a8ffca9de25b4854edcdbc2..0000000000000000000000000000000000000000
--- a/server/transformers/src/transformers/tokenization_flaubert.py
+++ /dev/null
@@ -1,145 +0,0 @@
-# coding=utf-8
-# Copyright 2019-present CNRS, Facebook Inc. and the HuggingFace Inc. team.
-#
-# Licensed under the Apache License, Version 2.0 (the "License");
-# you may not use this file except in compliance with the License.
-# You may obtain a copy of the License at
-#
-# http://www.apache.org/licenses/LICENSE-2.0
-#
-# Unless required by applicable law or agreed to in writing, software
-# distributed under the License is distributed on an "AS IS" BASIS,
-# WITHOUT WARRANTIES OR CONDITIONS OF ANY KIND, either express or implied.
-# See the License for the specific language governing permissions and
-# limitations under the License.
-"""Tokenization classes for Flaubert, based on XLM."""
-
-
-import logging
-import unicodedata
-
-import six
-
-from .tokenization_xlm import XLMTokenizer
-
-
-logger = logging.getLogger(__name__)
-
-VOCAB_FILES_NAMES = {
- "vocab_file": "vocab.json",
- "merges_file": "merges.txt",
-}
-
-PRETRAINED_VOCAB_FILES_MAP = {
- "vocab_file": {
- "flaubert-small-cased": "https://s3.amazonaws.com/models.huggingface.co/bert/flaubert/flaubert_small_cased/vocab.json",
- "flaubert-base-uncased": "https://s3.amazonaws.com/models.huggingface.co/bert/flaubert/flaubert_base_uncased/vocab.json",
- "flaubert-base-cased": "https://s3.amazonaws.com/models.huggingface.co/bert/flaubert/flaubert_base_cased/vocab.json",
- "flaubert-large-cased": "https://s3.amazonaws.com/models.huggingface.co/bert/flaubert/flaubert_large_cased/vocab.json",
- },
- "merges_file": {
- "flaubert-small-cased": "https://s3.amazonaws.com/models.huggingface.co/bert/flaubert/flaubert_small_cased/merges.txt",
- "flaubert-base-uncased": "https://s3.amazonaws.com/models.huggingface.co/bert/flaubert/flaubert_base_uncased/merges.txt",
- "flaubert-base-cased": "https://s3.amazonaws.com/models.huggingface.co/bert/flaubert/flaubert_base_cased/merges.txt",
- "flaubert-large-cased": "https://s3.amazonaws.com/models.huggingface.co/bert/flaubert/flaubert_large_cased/merges.txt",
- },
-}
-
-PRETRAINED_POSITIONAL_EMBEDDINGS_SIZES = {
- "flaubert-small-cased": 512,
- "flaubert-base-uncased": 512,
- "flaubert-base-cased": 512,
- "flaubert-large-cased": 512,
-}
-
-PRETRAINED_INIT_CONFIGURATION = {
- "flaubert-small-cased": {"do_lowercase": False},
- "flaubert-base-uncased": {"do_lowercase": True},
- "flaubert-base-cased": {"do_lowercase": False},
- "flaubert-large-cased": {"do_lowercase": False},
-}
-
-
-def convert_to_unicode(text):
- """
- Converts `text` to Unicode (if it's not already), assuming UTF-8 input.
- """
- # six_ensure_text is copied from https://github.com/benjaminp/six
- def six_ensure_text(s, encoding="utf-8", errors="strict"):
- if isinstance(s, six.binary_type):
- return s.decode(encoding, errors)
- elif isinstance(s, six.text_type):
- return s
- else:
- raise TypeError("not expecting type '%s'" % type(s))
-
- return six_ensure_text(text, encoding="utf-8", errors="ignore")
-
-
-class FlaubertTokenizer(XLMTokenizer):
- """
- BPE tokenizer for Flaubert
-
- - Moses preprocessing & tokenization
-
- - Normalize all inputs text
-
- - argument ``special_tokens`` and function ``set_special_tokens``, can be used to add additional symbols \
- (ex: "__classify__") to a vocabulary
-
- - `do_lowercase` controle lower casing (automatically set for pretrained vocabularies)
- """
-
- vocab_files_names = VOCAB_FILES_NAMES
- pretrained_vocab_files_map = PRETRAINED_VOCAB_FILES_MAP
- pretrained_init_configuration = PRETRAINED_INIT_CONFIGURATION
- max_model_input_sizes = PRETRAINED_POSITIONAL_EMBEDDINGS_SIZES
-
- def __init__(self, do_lowercase=False, **kwargs):
- super().__init__(**kwargs)
- self.do_lowercase = do_lowercase
- self.do_lowercase_and_remove_accent = False
-
- def preprocess_text(self, text):
- text = text.replace("``", '"').replace("''", '"')
- text = convert_to_unicode(text)
- text = unicodedata.normalize("NFC", text)
-
- if self.do_lowercase:
- text = text.lower()
-
- return text
-
- def _tokenize(self, text, bypass_tokenizer=False):
- """
- Tokenize a string given language code using Moses.
-
- Details of tokenization:
- - [sacremoses](https://github.com/alvations/sacremoses): port of Moses
- - Install with `pip install sacremoses`
-
- Args:
- - bypass_tokenizer: Allow users to preprocess and tokenize the sentences externally (default = False) (bool). If True, we only apply BPE.
-
- Returns:
- List of tokens.
- """
- lang = "fr"
- if lang and self.lang2id and lang not in self.lang2id:
- logger.error(
- "Supplied language code not found in lang2id mapping. Please check that your language is supported by the loaded pretrained model."
- )
-
- if bypass_tokenizer:
- text = text.split()
- else:
- text = self.preprocess_text(text)
- text = self.moses_pipeline(text, lang=lang)
- text = self.moses_tokenize(text, lang=lang)
-
- split_tokens = []
- for token in text:
- if token:
- split_tokens.extend([t for t in self.bpe(token).split(" ")])
-
- return split_tokens
diff --git a/server/transformers/src/transformers/tokenization_gpt2.py b/server/transformers/src/transformers/tokenization_gpt2.py
deleted file mode 100644
index 4f2de845b569bc8f38880fab521607610e4024d8..0000000000000000000000000000000000000000
--- a/server/transformers/src/transformers/tokenization_gpt2.py
+++ /dev/null
@@ -1,286 +0,0 @@
-# coding=utf-8
-# Copyright 2018 The Open AI Team Authors and The HuggingFace Inc. team.
-#
-# Licensed under the Apache License, Version 2.0 (the "License");
-# you may not use this file except in compliance with the License.
-# You may obtain a copy of the License at
-#
-# http://www.apache.org/licenses/LICENSE-2.0
-#
-# Unless required by applicable law or agreed to in writing, software
-# distributed under the License is distributed on an "AS IS" BASIS,
-# WITHOUT WARRANTIES OR CONDITIONS OF ANY KIND, either express or implied.
-# See the License for the specific language governing permissions and
-# limitations under the License.
-"""Tokenization classes for OpenAI GPT."""
-
-
-import json
-import logging
-import os
-from functools import lru_cache
-
-import regex as re
-import tokenizers as tk
-
-from .tokenization_utils import PreTrainedTokenizer, PreTrainedTokenizerFast
-
-
-logger = logging.getLogger(__name__)
-
-VOCAB_FILES_NAMES = {
- "vocab_file": "vocab.json",
- "merges_file": "merges.txt",
-}
-
-PRETRAINED_VOCAB_FILES_MAP = {
- "vocab_file": {
- "gpt2": "https://s3.amazonaws.com/models.huggingface.co/bert/gpt2-vocab.json",
- "gpt2-medium": "https://s3.amazonaws.com/models.huggingface.co/bert/gpt2-medium-vocab.json",
- "gpt2-large": "https://s3.amazonaws.com/models.huggingface.co/bert/gpt2-large-vocab.json",
- "gpt2-xl": "https://s3.amazonaws.com/models.huggingface.co/bert/gpt2-xl-vocab.json",
- "distilgpt2": "https://s3.amazonaws.com/models.huggingface.co/bert/distilgpt2-vocab.json",
- },
- "merges_file": {
- "gpt2": "https://s3.amazonaws.com/models.huggingface.co/bert/gpt2-merges.txt",
- "gpt2-medium": "https://s3.amazonaws.com/models.huggingface.co/bert/gpt2-medium-merges.txt",
- "gpt2-large": "https://s3.amazonaws.com/models.huggingface.co/bert/gpt2-large-merges.txt",
- "gpt2-xl": "https://s3.amazonaws.com/models.huggingface.co/bert/gpt2-xl-merges.txt",
- "distilgpt2": "https://s3.amazonaws.com/models.huggingface.co/bert/distilgpt2-merges.txt",
- },
-}
-
-PRETRAINED_POSITIONAL_EMBEDDINGS_SIZES = {
- "gpt2": 1024,
- "gpt2-medium": 1024,
- "gpt2-large": 1024,
- "gpt2-xl": 1024,
- "distilgpt2": 1024,
-}
-
-
-@lru_cache()
-def bytes_to_unicode():
- """
- Returns list of utf-8 byte and a mapping to unicode strings.
- We specifically avoids mapping to whitespace/control characters the bpe code barfs on.
-
- The reversible bpe codes work on unicode strings.
- This means you need a large # of unicode characters in your vocab if you want to avoid UNKs.
- When you're at something like a 10B token dataset you end up needing around 5K for decent coverage.
- This is a signficant percentage of your normal, say, 32K bpe vocab.
- To avoid that, we want lookup tables between utf-8 bytes and unicode strings.
- """
- bs = (
- list(range(ord("!"), ord("~") + 1)) + list(range(ord("¡"), ord("¬") + 1)) + list(range(ord("®"), ord("ÿ") + 1))
- )
- cs = bs[:]
- n = 0
- for b in range(2 ** 8):
- if b not in bs:
- bs.append(b)
- cs.append(2 ** 8 + n)
- n += 1
- cs = [chr(n) for n in cs]
- return dict(zip(bs, cs))
-
-
-def get_pairs(word):
- """Return set of symbol pairs in a word.
-
- Word is represented as tuple of symbols (symbols being variable-length strings).
- """
- pairs = set()
- prev_char = word[0]
- for char in word[1:]:
- pairs.add((prev_char, char))
- prev_char = char
- return pairs
-
-
-class GPT2Tokenizer(PreTrainedTokenizer):
- """
- GPT-2 BPE tokenizer. Peculiarities:
- - Byte-level Byte-Pair-Encoding
- - Requires a space to start the input string => the encoding and tokenize methods should be called with the
- ``add_prefix_space`` flag set to ``True``.
- Otherwise, this tokenizer's ``encode``, ``decode``, and ``tokenize`` methods will not conserve
- the spaces at the beginning of a string: `tokenizer.decode(tokenizer.encode(" Hello")) = "Hello"`
- """
-
- vocab_files_names = VOCAB_FILES_NAMES
- pretrained_vocab_files_map = PRETRAINED_VOCAB_FILES_MAP
- max_model_input_sizes = PRETRAINED_POSITIONAL_EMBEDDINGS_SIZES
-
- def __init__(
- self,
- vocab_file,
- merges_file,
- errors="replace",
- unk_token="<|endoftext|>",
- bos_token="<|endoftext|>",
- eos_token="<|endoftext|>",
- **kwargs
- ):
- super().__init__(bos_token=bos_token, eos_token=eos_token, unk_token=unk_token, **kwargs)
- self.max_len_single_sentence = (
- self.max_len
- ) # no default special tokens - you can update this value if you add special tokens
- self.max_len_sentences_pair = (
- self.max_len
- ) # no default special tokens - you can update this value if you add special tokens
-
- with open(vocab_file, encoding="utf-8") as vocab_handle:
- self.encoder = json.load(vocab_handle)
- self.decoder = {v: k for k, v in self.encoder.items()}
- self.errors = errors # how to handle errors in decoding
- self.byte_encoder = bytes_to_unicode()
- self.byte_decoder = {v: k for k, v in self.byte_encoder.items()}
- with open(merges_file, encoding="utf-8") as merges_handle:
- bpe_merges = merges_handle.read().split("\n")[1:-1]
- bpe_merges = [tuple(merge.split()) for merge in bpe_merges]
- self.bpe_ranks = dict(zip(bpe_merges, range(len(bpe_merges))))
- self.cache = {}
-
- # Should haved added re.IGNORECASE so BPE merges can happen for capitalized versions of contractions
- self.pat = re.compile(r"""'s|'t|'re|'ve|'m|'ll|'d| ?\p{L}+| ?\p{N}+| ?[^\s\p{L}\p{N}]+|\s+(?!\S)|\s+""")
-
- @property
- def vocab_size(self):
- return len(self.encoder)
-
- def bpe(self, token):
- if token in self.cache:
- return self.cache[token]
- word = tuple(token)
- pairs = get_pairs(word)
-
- if not pairs:
- return token
-
- while True:
- bigram = min(pairs, key=lambda pair: self.bpe_ranks.get(pair, float("inf")))
- if bigram not in self.bpe_ranks:
- break
- first, second = bigram
- new_word = []
- i = 0
- while i < len(word):
- try:
- j = word.index(first, i)
- except ValueError:
- new_word.extend(word[i:])
- break
- else:
- new_word.extend(word[i:j])
- i = j
-
- if word[i] == first and i < len(word) - 1 and word[i + 1] == second:
- new_word.append(first + second)
- i += 2
- else:
- new_word.append(word[i])
- i += 1
- new_word = tuple(new_word)
- word = new_word
- if len(word) == 1:
- break
- else:
- pairs = get_pairs(word)
- word = " ".join(word)
- self.cache[token] = word
- return word
-
- def _tokenize(self, text, add_prefix_space=False):
- """ Tokenize a string.
- Args:
- - add_prefix_space (boolean, default False):
- Begin the sentence with at least one space to get invariance to word order in GPT-2 (and RoBERTa) tokenizers.
- """
- if add_prefix_space:
- text = " " + text
-
- bpe_tokens = []
- for token in re.findall(self.pat, text):
- token = "".join(
- self.byte_encoder[b] for b in token.encode("utf-8")
- ) # Maps all our bytes to unicode strings, avoiding controle tokens of the BPE (spaces in our case)
- bpe_tokens.extend(bpe_token for bpe_token in self.bpe(token).split(" "))
- return bpe_tokens
-
- def _convert_token_to_id(self, token):
- """ Converts a token (str) in an id using the vocab. """
- return self.encoder.get(token, self.encoder.get(self.unk_token))
-
- def _convert_id_to_token(self, index):
- """Converts an index (integer) in a token (str) using the vocab."""
- return self.decoder.get(index)
-
- def convert_tokens_to_string(self, tokens):
- """ Converts a sequence of tokens (string) in a single string. """
- text = "".join(tokens)
- text = bytearray([self.byte_decoder[c] for c in text]).decode("utf-8", errors=self.errors)
- return text
-
- def save_vocabulary(self, save_directory):
- """Save the tokenizer vocabulary and merge files to a directory."""
- if not os.path.isdir(save_directory):
- logger.error("Vocabulary path ({}) should be a directory".format(save_directory))
- return
- vocab_file = os.path.join(save_directory, VOCAB_FILES_NAMES["vocab_file"])
- merge_file = os.path.join(save_directory, VOCAB_FILES_NAMES["merges_file"])
-
- with open(vocab_file, "w", encoding="utf-8") as f:
- f.write(json.dumps(self.encoder, ensure_ascii=False))
-
- index = 0
- with open(merge_file, "w", encoding="utf-8") as writer:
- writer.write("#version: 0.2\n")
- for bpe_tokens, token_index in sorted(self.bpe_ranks.items(), key=lambda kv: kv[1]):
- if index != token_index:
- logger.warning(
- "Saving vocabulary to {}: BPE merge indices are not consecutive."
- " Please check that the tokenizer is not corrupted!".format(merge_file)
- )
- index = token_index
- writer.write(" ".join(bpe_tokens) + "\n")
- index += 1
-
- return vocab_file, merge_file
-
-
-class GPT2TokenizerFast(PreTrainedTokenizerFast):
- vocab_files_names = VOCAB_FILES_NAMES
- pretrained_vocab_files_map = PRETRAINED_VOCAB_FILES_MAP
- max_model_input_sizes = PRETRAINED_POSITIONAL_EMBEDDINGS_SIZES
-
- def __init__(
- self,
- vocab_file,
- merges_file,
- unk_token="<|endoftext|>",
- bos_token="<|endoftext|>",
- eos_token="<|endoftext|>",
- pad_to_max_length=False,
- add_prefix_space=False,
- max_length=None,
- stride=0,
- truncation_strategy="longest_first",
- **kwargs
- ):
- super().__init__(bos_token=bos_token, eos_token=eos_token, unk_token=unk_token, **kwargs)
-
- self._tokenizer = tk.Tokenizer(tk.models.BPE.from_files(vocab_file, merges_file))
- self._update_special_tokens()
- self._tokenizer.with_pre_tokenizer(tk.pre_tokenizers.ByteLevel.new(add_prefix_space=add_prefix_space))
- self._tokenizer.with_decoder(tk.decoders.ByteLevel.new())
- if max_length:
- self._tokenizer.with_truncation(max_length, stride=stride, strategy=truncation_strategy)
- self._tokenizer.with_padding(
- max_length=max_length if pad_to_max_length else None,
- direction=self.padding_side,
- pad_id=self.pad_token_id if self.pad_token_id is not None else 0,
- pad_type_id=self.pad_token_type_id,
- pad_token=self.pad_token if self.pad_token is not None else "",
- )
- self._decoder = tk.decoders.ByteLevel.new()
diff --git a/server/transformers/src/transformers/tokenization_openai.py b/server/transformers/src/transformers/tokenization_openai.py
deleted file mode 100644
index eca9f81c3ef631d6f27f34965eadc5c793c928e1..0000000000000000000000000000000000000000
--- a/server/transformers/src/transformers/tokenization_openai.py
+++ /dev/null
@@ -1,215 +0,0 @@
-# coding=utf-8
-# Copyright 2018 The Open AI Team Authors and The HuggingFace Inc. team.
-#
-# Licensed under the Apache License, Version 2.0 (the "License");
-# you may not use this file except in compliance with the License.
-# You may obtain a copy of the License at
-#
-# http://www.apache.org/licenses/LICENSE-2.0
-#
-# Unless required by applicable law or agreed to in writing, software
-# distributed under the License is distributed on an "AS IS" BASIS,
-# WITHOUT WARRANTIES OR CONDITIONS OF ANY KIND, either express or implied.
-# See the License for the specific language governing permissions and
-# limitations under the License.
-"""Tokenization classes for OpenAI GPT."""
-
-
-import json
-import logging
-import os
-import re
-
-from .tokenization_bert import BasicTokenizer
-from .tokenization_utils import PreTrainedTokenizer
-
-
-logger = logging.getLogger(__name__)
-
-VOCAB_FILES_NAMES = {
- "vocab_file": "vocab.json",
- "merges_file": "merges.txt",
-}
-
-PRETRAINED_VOCAB_FILES_MAP = {
- "vocab_file": {"openai-gpt": "https://s3.amazonaws.com/models.huggingface.co/bert/openai-gpt-vocab.json"},
- "merges_file": {"openai-gpt": "https://s3.amazonaws.com/models.huggingface.co/bert/openai-gpt-merges.txt"},
-}
-
-PRETRAINED_POSITIONAL_EMBEDDINGS_SIZES = {
- "openai-gpt": 512,
-}
-
-
-def get_pairs(word):
- """
- Return set of symbol pairs in a word.
- word is represented as tuple of symbols (symbols being variable-length strings)
- """
- pairs = set()
- prev_char = word[0]
- for char in word[1:]:
- pairs.add((prev_char, char))
- prev_char = char
- return pairs
-
-
-def text_standardize(text):
- """
- fixes some issues the spacy tokenizer had on books corpus
- also does some whitespace standardization
- """
- text = text.replace("—", "-")
- text = text.replace("–", "-")
- text = text.replace("―", "-")
- text = text.replace("…", "...")
- text = text.replace("´", "'")
- text = re.sub(r"""(-+|~+|!+|"+|;+|\?+|\++|,+|\)+|\(+|\\+|\/+|\*+|\[+|\]+|}+|{+|\|+|_+)""", r" \1 ", text)
- text = re.sub(r"\s*\n\s*", " \n ", text)
- text = re.sub(r"[^\S\n]+", " ", text)
- return text.strip()
-
-
-class OpenAIGPTTokenizer(PreTrainedTokenizer):
- """
- BPE tokenizer. Peculiarities:
- - lower case all inputs
- - uses SpaCy tokenizer and ftfy for pre-BPE tokenization if they are installed, fallback to BERT's BasicTokenizer if not.
- """
-
- vocab_files_names = VOCAB_FILES_NAMES
- pretrained_vocab_files_map = PRETRAINED_VOCAB_FILES_MAP
- max_model_input_sizes = PRETRAINED_POSITIONAL_EMBEDDINGS_SIZES
-
- def __init__(self, vocab_file, merges_file, unk_token="", **kwargs):
- super().__init__(unk_token=unk_token, **kwargs)
-
- self.max_len_single_sentence = (
- self.max_len
- ) # no default special tokens - you can update this value if you add special tokens
- self.max_len_sentences_pair = (
- self.max_len
- ) # no default special tokens - you can update this value if you add special tokens
-
- try:
- import ftfy
- from spacy.lang.en import English
-
- _nlp = English()
- self.nlp = _nlp.Defaults.create_tokenizer(_nlp)
- self.fix_text = ftfy.fix_text
- except ImportError:
- logger.warning("ftfy or spacy is not installed using BERT BasicTokenizer instead of SpaCy & ftfy.")
- self.nlp = BasicTokenizer(do_lower_case=True)
- self.fix_text = None
-
- with open(vocab_file, encoding="utf-8") as vocab_handle:
- self.encoder = json.load(vocab_handle)
- self.decoder = {v: k for k, v in self.encoder.items()}
- with open(merges_file, encoding="utf-8") as merges_handle:
- merges = merges_handle.read().split("\n")[1:-1]
- merges = [tuple(merge.split()) for merge in merges]
- self.bpe_ranks = dict(zip(merges, range(len(merges))))
- self.cache = {}
-
- @property
- def vocab_size(self):
- return len(self.encoder)
-
- def bpe(self, token):
- word = tuple(token[:-1]) + (token[-1] + "",)
- if token in self.cache:
- return self.cache[token]
- pairs = get_pairs(word)
-
- if not pairs:
- return token + ""
-
- while True:
- bigram = min(pairs, key=lambda pair: self.bpe_ranks.get(pair, float("inf")))
- if bigram not in self.bpe_ranks:
- break
- first, second = bigram
- new_word = []
- i = 0
- while i < len(word):
- try:
- j = word.index(first, i)
- except ValueError:
- new_word.extend(word[i:])
- break
- else:
- new_word.extend(word[i:j])
- i = j
-
- if word[i] == first and i < len(word) - 1 and word[i + 1] == second:
- new_word.append(first + second)
- i += 2
- else:
- new_word.append(word[i])
- i += 1
- new_word = tuple(new_word)
- word = new_word
- if len(word) == 1:
- break
- else:
- pairs = get_pairs(word)
- word = " ".join(word)
- if word == "\n ":
- word = "\n"
- self.cache[token] = word
- return word
-
- def _tokenize(self, text):
- """ Tokenize a string. """
- split_tokens = []
- if self.fix_text is None:
- # Using BERT's BasicTokenizer
- text = self.nlp.tokenize(text)
- for token in text:
- split_tokens.extend([t for t in self.bpe(token).split(" ")])
- else:
- # Using SpaCy & ftfy (original tokenization process of OpenAI GPT)
- text = self.nlp(text_standardize(self.fix_text(text)))
- for token in text:
- split_tokens.extend([t for t in self.bpe(token.text.lower()).split(" ")])
- return split_tokens
-
- def _convert_token_to_id(self, token):
- """ Converts a token (str) in an id using the vocab. """
- return self.encoder.get(token, self.encoder.get(self.unk_token))
-
- def _convert_id_to_token(self, index):
- """Converts an id in a token (BPE) using the vocab."""
- return self.decoder.get(index, self.unk_token)
-
- def convert_tokens_to_string(self, tokens):
- """ Converts a sequence of tokens (string) in a single string. """
- out_string = "".join(tokens).replace("", " ").strip()
- return out_string
-
- def save_vocabulary(self, save_directory):
- """Save the tokenizer vocabulary and merge files to a directory."""
- if not os.path.isdir(save_directory):
- logger.error("Vocabulary path ({}) should be a directory".format(save_directory))
- return
- vocab_file = os.path.join(save_directory, VOCAB_FILES_NAMES["vocab_file"])
- merge_file = os.path.join(save_directory, VOCAB_FILES_NAMES["merges_file"])
-
- with open(vocab_file, "w", encoding="utf-8") as f:
- f.write(json.dumps(self.encoder, ensure_ascii=False))
-
- index = 0
- with open(merge_file, "w", encoding="utf-8") as writer:
- writer.write("#version: 0.2\n")
- for bpe_tokens, token_index in sorted(self.bpe_ranks.items(), key=lambda kv: kv[1]):
- if index != token_index:
- logger.warning(
- "Saving vocabulary to {}: BPE merge indices are not consecutive."
- " Please check that the tokenizer is not corrupted!".format(merge_file)
- )
- index = token_index
- writer.write(" ".join(bpe_tokens) + "\n")
- index += 1
-
- return vocab_file, merge_file
diff --git a/server/transformers/src/transformers/tokenization_roberta.py b/server/transformers/src/transformers/tokenization_roberta.py
deleted file mode 100644
index caaaf98cd0dbd90f8b944328a96403be5e3ebb6e..0000000000000000000000000000000000000000
--- a/server/transformers/src/transformers/tokenization_roberta.py
+++ /dev/null
@@ -1,156 +0,0 @@
-# coding=utf-8
-# Copyright 2018 The Open AI Team Authors and The HuggingFace Inc. team.
-#
-# Licensed under the Apache License, Version 2.0 (the "License");
-# you may not use this file except in compliance with the License.
-# You may obtain a copy of the License at
-#
-# http://www.apache.org/licenses/LICENSE-2.0
-#
-# Unless required by applicable law or agreed to in writing, software
-# distributed under the License is distributed on an "AS IS" BASIS,
-# WITHOUT WARRANTIES OR CONDITIONS OF ANY KIND, either express or implied.
-# See the License for the specific language governing permissions and
-# limitations under the License.
-"""Tokenization classes for RoBERTa."""
-
-
-import logging
-
-from .tokenization_gpt2 import GPT2Tokenizer
-
-
-logger = logging.getLogger(__name__)
-
-VOCAB_FILES_NAMES = {
- "vocab_file": "vocab.json",
- "merges_file": "merges.txt",
-}
-
-PRETRAINED_VOCAB_FILES_MAP = {
- "vocab_file": {
- "roberta-base": "https://s3.amazonaws.com/models.huggingface.co/bert/roberta-base-vocab.json",
- "roberta-large": "https://s3.amazonaws.com/models.huggingface.co/bert/roberta-large-vocab.json",
- "roberta-large-mnli": "https://s3.amazonaws.com/models.huggingface.co/bert/roberta-large-mnli-vocab.json",
- "distilroberta-base": "https://s3.amazonaws.com/models.huggingface.co/bert/distilroberta-base-vocab.json",
- "roberta-base-openai-detector": "https://s3.amazonaws.com/models.huggingface.co/bert/roberta-base-vocab.json",
- "roberta-large-openai-detector": "https://s3.amazonaws.com/models.huggingface.co/bert/roberta-large-vocab.json",
- },
- "merges_file": {
- "roberta-base": "https://s3.amazonaws.com/models.huggingface.co/bert/roberta-base-merges.txt",
- "roberta-large": "https://s3.amazonaws.com/models.huggingface.co/bert/roberta-large-merges.txt",
- "roberta-large-mnli": "https://s3.amazonaws.com/models.huggingface.co/bert/roberta-large-mnli-merges.txt",
- "distilroberta-base": "https://s3.amazonaws.com/models.huggingface.co/bert/distilroberta-base-merges.txt",
- "roberta-base-openai-detector": "https://s3.amazonaws.com/models.huggingface.co/bert/roberta-base-merges.txt",
- "roberta-large-openai-detector": "https://s3.amazonaws.com/models.huggingface.co/bert/roberta-large-merges.txt",
- },
-}
-
-PRETRAINED_POSITIONAL_EMBEDDINGS_SIZES = {
- "roberta-base": 512,
- "roberta-large": 512,
- "roberta-large-mnli": 512,
- "distilroberta-base": 512,
- "roberta-base-openai-detector": 512,
- "roberta-large-openai-detector": 512,
-}
-
-
-class RobertaTokenizer(GPT2Tokenizer):
- """
- RoBERTa BPE tokenizer, derived from the GPT-2 tokenizer. Peculiarities:
- - Byte-level Byte-Pair-Encoding
- - Requires a space to start the input string => the encoding methods should be called with the
- ``add_prefix_space`` flag set to ``True``.
- Otherwise, this tokenizer ``encode`` and ``decode`` method will not conserve
- the absence of a space at the beginning of a string: `tokenizer.decode(tokenizer.encode("Hello")) = " Hello"`
- """
-
- vocab_files_names = VOCAB_FILES_NAMES
- pretrained_vocab_files_map = PRETRAINED_VOCAB_FILES_MAP
- max_model_input_sizes = PRETRAINED_POSITIONAL_EMBEDDINGS_SIZES
-
- def __init__(
- self,
- vocab_file,
- merges_file,
- errors="replace",
- bos_token="",
- eos_token="",
- sep_token="",
- cls_token="",
- unk_token="",
- pad_token="",
- mask_token="",
- **kwargs
- ):
- super().__init__(
- vocab_file=vocab_file,
- merges_file=merges_file,
- errors=errors,
- bos_token=bos_token,
- eos_token=eos_token,
- unk_token=unk_token,
- sep_token=sep_token,
- cls_token=cls_token,
- pad_token=pad_token,
- mask_token=mask_token,
- **kwargs,
- )
- self.max_len_single_sentence = self.max_len - 2 # take into account special tokens
- self.max_len_sentences_pair = self.max_len - 4 # take into account special tokens
-
- def build_inputs_with_special_tokens(self, token_ids_0, token_ids_1=None):
- """
- Build model inputs from a sequence or a pair of sequence for sequence classification tasks
- by concatenating and adding special tokens.
- A RoBERTa sequence has the following format:
- single sequence: X
- pair of sequences: A B
- """
- if token_ids_1 is None:
- return [self.cls_token_id] + token_ids_0 + [self.sep_token_id]
- cls = [self.cls_token_id]
- sep = [self.sep_token_id]
- return cls + token_ids_0 + sep + sep + token_ids_1 + sep
-
- def get_special_tokens_mask(self, token_ids_0, token_ids_1=None, already_has_special_tokens=False):
- """
- Retrieves sequence ids from a token list that has no special tokens added. This method is called when adding
- special tokens using the tokenizer ``prepare_for_model`` or ``encode_plus`` methods.
-
- Args:
- token_ids_0: list of ids (must not contain special tokens)
- token_ids_1: Optional list of ids (must not contain special tokens), necessary when fetching sequence ids
- for sequence pairs
- already_has_special_tokens: (default False) Set to True if the token list is already formated with
- special tokens for the model
-
- Returns:
- A list of integers in the range [0, 1]: 1 for a special token, 0 for a sequence token.
- """
- if already_has_special_tokens:
- if token_ids_1 is not None:
- raise ValueError(
- "You should not supply a second sequence if the provided sequence of "
- "ids is already formated with special tokens for the model."
- )
- return list(map(lambda x: 1 if x in [self.sep_token_id, self.cls_token_id] else 0, token_ids_0))
-
- if token_ids_1 is None:
- return [1] + ([0] * len(token_ids_0)) + [1]
- return [1] + ([0] * len(token_ids_0)) + [1, 1] + ([0] * len(token_ids_1)) + [1]
-
- def create_token_type_ids_from_sequences(self, token_ids_0, token_ids_1=None):
- """
- Creates a mask from the two sequences passed to be used in a sequence-pair classification task.
- RoBERTa does not make use of token type ids, therefore a list of zeros is returned.
-
- if token_ids_1 is None, only returns the first portion of the mask (0's).
- """
- sep = [self.sep_token_id]
- cls = [self.cls_token_id]
-
- if token_ids_1 is None:
- return len(cls + token_ids_0 + sep) * [0]
- return len(cls + token_ids_0 + sep + sep + token_ids_1 + sep) * [0]
diff --git a/server/transformers/src/transformers/tokenization_t5.py b/server/transformers/src/transformers/tokenization_t5.py
deleted file mode 100644
index 2196cc82e726effbf8d8339626efd9ac38c6faf7..0000000000000000000000000000000000000000
--- a/server/transformers/src/transformers/tokenization_t5.py
+++ /dev/null
@@ -1,182 +0,0 @@
-# coding=utf-8
-# Copyright 2018 T5 Authors and HuggingFace Inc. team.
-#
-# Licensed under the Apache License, Version 2.0 (the "License");
-# you may not use this file except in compliance with the License.
-# You may obtain a copy of the License at
-#
-# http://www.apache.org/licenses/LICENSE-2.0
-#
-# Unless required by applicable law or agreed to in writing, software
-# distributed under the License is distributed on an "AS IS" BASIS,
-# WITHOUT WARRANTIES OR CONDITIONS OF ANY KIND, either express or implied.
-# See the License for the specific language governing permissions and
-# limitations under the License.
-""" Tokenization class for model T5."""
-
-
-import logging
-import os
-import re
-from shutil import copyfile
-
-from .tokenization_utils import PreTrainedTokenizer
-
-
-logger = logging.getLogger(__name__)
-
-SPIECE_UNDERLINE = "▁"
-
-####################################################
-# Mapping from the keyword arguments names of Tokenizer `__init__`
-# to file names for serializing Tokenizer instances
-####################################################
-VOCAB_FILES_NAMES = {"vocab_file": "spiece.model"}
-
-####################################################
-# Mapping from the keyword arguments names of Tokenizer `__init__`
-# to pretrained vocabulary URL for all the model shortcut names.
-####################################################
-PRETRAINED_VOCAB_FILES_MAP = {
- "vocab_file": {
- "t5-small": "https://s3.amazonaws.com/models.huggingface.co/bert/t5-spiece.model",
- "t5-base": "https://s3.amazonaws.com/models.huggingface.co/bert/t5-spiece.model",
- "t5-large": "https://s3.amazonaws.com/models.huggingface.co/bert/t5-spiece.model",
- "t5-3b": "https://s3.amazonaws.com/models.huggingface.co/bert/t5-spiece.model",
- "t5-11b": "https://s3.amazonaws.com/models.huggingface.co/bert/t5-spiece.model",
- }
-}
-
-####################################################
-# Mapping from model shortcut names to max length of inputs
-####################################################
-PRETRAINED_POSITIONAL_EMBEDDINGS_SIZES = {
- "t5-small": 512,
- "t5-base": 512,
- "t5-large": 512,
- "t5-3b": 512,
- "t5-11b": 512,
-}
-
-
-class T5Tokenizer(PreTrainedTokenizer):
- """
- SentencePiece based tokenizer. Peculiarities:
-
- - requires `SentencePiece `_
- - `extra_ids` add a number of extra ids added to the end of the vocabulary for use as sentinels.
- These tokens are accessible as `` where `{%d}` is a number between 0 and extra_ids-1.
- Extra tokens are indexed from the end of the vocabulary up to beginnning ( is the last token in the vocabulary)
- (like in T5 preprocessing
- see: https://github.com/google-research/text-to-text-transfer-transformer/blob/9fd7b14a769417be33bc6c850f9598764913c833/t5/data/preprocessors.py#L2117)
- """
-
- vocab_files_names = VOCAB_FILES_NAMES
- pretrained_vocab_files_map = PRETRAINED_VOCAB_FILES_MAP
- max_model_input_sizes = PRETRAINED_POSITIONAL_EMBEDDINGS_SIZES
-
- def __init__(
- self,
- vocab_file,
- eos_token="",
- unk_token="",
- pad_token="",
- extra_ids=100,
- additional_special_tokens=None,
- **kwargs
- ):
- # Add extra_ids to the special token list
- if extra_ids > 0:
- if additional_special_tokens is None:
- additional_special_tokens = []
- additional_special_tokens.extend(["".format(i) for i in range(extra_ids)])
-
- super().__init__(
- eos_token=eos_token,
- unk_token=unk_token,
- pad_token=pad_token,
- additional_special_tokens=additional_special_tokens,
- **kwargs,
- )
-
- try:
- import sentencepiece as spm
- except ImportError:
- logger.warning(
- "You need to install SentencePiece to use T5Tokenizer:"
- "https://github.com/google/sentencepiece"
- "pip install sentencepiece"
- )
- raise
-
- self.vocab_file = vocab_file
- self._extra_ids = extra_ids
-
- self.sp_model = spm.SentencePieceProcessor()
- self.sp_model.Load(vocab_file)
-
- @property
- def vocab_size(self):
- return self.sp_model.get_piece_size() + self._extra_ids
-
- def __getstate__(self):
- state = self.__dict__.copy()
- state["sp_model"] = None
- return state
-
- def __setstate__(self, d):
- self.__dict__ = d
- try:
- import sentencepiece as spm
- except ImportError:
- logger.warning(
- "You need to install SentencePiece to use XLNetTokenizer: https://github.com/google/sentencepiece"
- "pip install sentencepiece"
- )
- raise
- self.sp_model = spm.SentencePieceProcessor()
- self.sp_model.Load(self.vocab_file)
-
- def _tokenize(self, text, sample=False):
- """ Take as input a string and return a list of strings (tokens) for words/sub-words
- """
- if not sample:
- pieces = self.sp_model.EncodeAsPieces(text)
- else:
- pieces = self.sp_model.SampleEncodeAsPieces(text, 64, 0.1)
- return pieces
-
- def _convert_token_to_id(self, token):
- """ Converts a token (str) in an id using the vocab. """
- if token.startswith("", token)
- num = int(match.group(1))
- return self.vocab_size - num - 1
- return self.sp_model.piece_to_id(token)
-
- def _convert_id_to_token(self, index):
- """Converts an index (integer) in a token (str) using the vocab."""
- if index < self.sp_model.get_piece_size():
- token = self.sp_model.IdToPiece(index)
- else:
- token = "".format(self.vocab_size - 1 - index)
- return token
-
- def convert_tokens_to_string(self, tokens):
- """ Converts a sequence of tokens (string) in a single string. """
- out_string = self.sp_model.decode_pieces(tokens)
- return out_string
-
- def save_vocabulary(self, save_directory):
- """ Save the sentencepiece vocabulary (copy original file) and special tokens file
- to a directory.
- """
- if not os.path.isdir(save_directory):
- logger.error("Vocabulary path ({}) should be a directory".format(save_directory))
- return
- out_vocab_file = os.path.join(save_directory, VOCAB_FILES_NAMES["vocab_file"])
-
- if os.path.abspath(self.vocab_file) != os.path.abspath(out_vocab_file):
- copyfile(self.vocab_file, out_vocab_file)
-
- return (out_vocab_file,)
diff --git a/server/transformers/src/transformers/tokenization_transfo_xl.py b/server/transformers/src/transformers/tokenization_transfo_xl.py
deleted file mode 100644
index 9d847e6f8ca491219d5b96b8a1ec38cdb819bf79..0000000000000000000000000000000000000000
--- a/server/transformers/src/transformers/tokenization_transfo_xl.py
+++ /dev/null
@@ -1,581 +0,0 @@
-# coding=utf-8
-# Copyright 2018 Google AI, Google Brain and Carnegie Mellon University Authors and the HuggingFace Inc. team.
-# Copyright (c) 2018, NVIDIA CORPORATION. All rights reserved.
-#
-# Licensed under the Apache License, Version 2.0 (the "License");
-# you may not use this file except in compliance with the License.
-# You may obtain a copy of the License at
-#
-# http://www.apache.org/licenses/LICENSE-2.0
-#
-# Unless required by applicable law or agreed to in writing, software
-# distributed under the License is distributed on an "AS IS" BASIS,
-# WITHOUT WARRANTIES OR CONDITIONS OF ANY KIND, either express or implied.
-# See the License for the specific language governing permissions and
-# limitations under the License.
-""" Tokenization classes for Transformer XL model.
- Adapted from https://github.com/kimiyoung/transformer-xl.
-"""
-
-
-import glob
-import logging
-import os
-import pickle
-from collections import Counter, OrderedDict
-
-import numpy as np
-
-from .file_utils import cached_path, is_torch_available
-from .tokenization_utils import PreTrainedTokenizer
-
-
-if is_torch_available():
- import torch
-
-
-logger = logging.getLogger(__name__)
-
-VOCAB_FILES_NAMES = {"pretrained_vocab_file": "vocab.bin", "vocab_file": "vocab.txt"}
-
-PRETRAINED_VOCAB_FILES_MAP = {
- "pretrained_vocab_file": {
- "transfo-xl-wt103": "https://s3.amazonaws.com/models.huggingface.co/bert/transfo-xl-wt103-vocab.bin",
- }
-}
-
-PRETRAINED_POSITIONAL_EMBEDDINGS_SIZES = {
- "transfo-xl-wt103": None,
-}
-
-PRETRAINED_CORPUS_ARCHIVE_MAP = {
- "transfo-xl-wt103": "https://s3.amazonaws.com/models.huggingface.co/bert/transfo-xl-wt103-corpus.bin",
-}
-CORPUS_NAME = "corpus.bin"
-
-
-class TransfoXLTokenizer(PreTrainedTokenizer):
- """
- Transformer-XL tokenizer adapted from Vocab class in https://github.com/kimiyoung/transformer-xl
- """
-
- vocab_files_names = VOCAB_FILES_NAMES
- pretrained_vocab_files_map = PRETRAINED_VOCAB_FILES_MAP
- max_model_input_sizes = PRETRAINED_POSITIONAL_EMBEDDINGS_SIZES
-
- def __init__(
- self,
- special=None,
- min_freq=0,
- max_size=None,
- lower_case=False,
- delimiter=None,
- vocab_file=None,
- pretrained_vocab_file=None,
- never_split=None,
- unk_token="",
- eos_token="",
- additional_special_tokens=[""],
- **kwargs
- ):
- super().__init__(
- unk_token=unk_token, eos_token=eos_token, additional_special_tokens=additional_special_tokens, **kwargs
- )
-
- self.max_len_single_sentence = (
- self.max_len
- ) # no default special tokens - you can update this value if you add special tokens
- self.max_len_sentences_pair = (
- self.max_len
- ) # no default special tokens - you can update this value if you add special tokens
-
- if never_split is None:
- never_split = self.all_special_tokens
- if special is None:
- special = []
- self.counter = Counter()
- self.special = special
- self.min_freq = min_freq
- self.max_size = max_size
- self.lower_case = lower_case
- self.delimiter = delimiter
- self.vocab_file = vocab_file
- self.never_split = never_split
-
- if pretrained_vocab_file is not None:
- # Hack because, honestly this tokenizer was not made to be used
- # in a library like ours, at all.
- vocab_dict = torch.load(pretrained_vocab_file)
- for key, value in vocab_dict.items():
- if key not in self.__dict__:
- self.__dict__[key] = value
-
- if vocab_file is not None:
- self.build_vocab()
-
- def count_file(self, path, verbose=False, add_eos=False):
- if verbose:
- logger.info("counting file {} ...".format(path))
- assert os.path.exists(path)
-
- sents = []
- with open(path, "r", encoding="utf-8") as f:
- for idx, line in enumerate(f):
- if verbose and idx > 0 and idx % 500000 == 0:
- logger.info(" line {}".format(idx))
- symbols = self.tokenize(line, add_eos=add_eos)
- self.counter.update(symbols)
- sents.append(symbols)
-
- return sents
-
- def count_sents(self, sents, verbose=False):
- """
- sents : a list of sentences, each a list of tokenized symbols
- """
- if verbose:
- logger.info("counting {} sents ...".format(len(sents)))
- for idx, symbols in enumerate(sents):
- if verbose and idx > 0 and idx % 500000 == 0:
- logger.info(" line {}".format(idx))
- self.counter.update(symbols)
-
- def _build_from_file(self, vocab_file):
- self.idx2sym = []
- self.sym2idx = OrderedDict()
-
- with open(vocab_file, "r", encoding="utf-8") as f:
- for line in f:
- symb = line.strip().split()[0]
- self.add_symbol(symb)
- if "" in self.sym2idx:
- self.unk_idx = self.sym2idx[""]
- elif "" in self.sym2idx:
- self.unk_idx = self.sym2idx[""]
- else:
- raise ValueError("No token in vocabulary")
-
- def save_vocabulary(self, vocab_path):
- """Save the tokenizer vocabulary to a directory or file."""
- if os.path.isdir(vocab_path):
- vocab_file = os.path.join(vocab_path, VOCAB_FILES_NAMES["pretrained_vocab_file"])
- torch.save(self.__dict__, vocab_file)
- return (vocab_file,)
-
- def build_vocab(self):
- if self.vocab_file:
- logger.info("building vocab from {}".format(self.vocab_file))
- self._build_from_file(self.vocab_file)
- logger.info("final vocab size {}".format(len(self)))
- else:
- logger.info("building vocab with min_freq={}, max_size={}".format(self.min_freq, self.max_size))
- self.idx2sym = []
- self.sym2idx = OrderedDict()
-
- for sym in self.special:
- self.add_special(sym)
-
- for sym, cnt in self.counter.most_common(self.max_size):
- if cnt < self.min_freq:
- break
- self.add_symbol(sym)
-
- logger.info("final vocab size {} from {} unique tokens".format(len(self), len(self.counter)))
-
- def encode_file(self, path, ordered=False, verbose=False, add_eos=True, add_double_eos=False):
- if verbose:
- logger.info("encoding file {} ...".format(path))
- assert os.path.exists(path)
- encoded = []
- with open(path, "r", encoding="utf-8") as f:
- for idx, line in enumerate(f):
- if verbose and idx > 0 and idx % 500000 == 0:
- logger.info(" line {}".format(idx))
- symbols = self.tokenize(line, add_eos=add_eos, add_double_eos=add_double_eos)
- encoded.append(self.convert_to_tensor(symbols))
-
- if ordered:
- encoded = torch.cat(encoded)
-
- return encoded
-
- def encode_sents(self, sents, ordered=False, verbose=False):
- if verbose:
- logger.info("encoding {} sents ...".format(len(sents)))
- encoded = []
- for idx, symbols in enumerate(sents):
- if verbose and idx > 0 and idx % 500000 == 0:
- logger.info(" line {}".format(idx))
- encoded.append(self.convert_to_tensor(symbols))
-
- if ordered:
- encoded = torch.cat(encoded)
-
- return encoded
-
- def add_special(self, sym):
- if sym not in self.sym2idx:
- self.idx2sym.append(sym)
- self.sym2idx[sym] = len(self.idx2sym) - 1
- setattr(self, "{}_idx".format(sym.strip("<>")), self.sym2idx[sym])
-
- def add_symbol(self, sym):
- if sym not in self.sym2idx:
- self.idx2sym.append(sym)
- self.sym2idx[sym] = len(self.idx2sym) - 1
-
- def _convert_id_to_token(self, idx):
- """Converts an id in a token (BPE) using the vocab."""
- assert 0 <= idx < len(self), "Index {} out of vocabulary range".format(idx)
- return self.idx2sym[idx]
-
- def _convert_token_to_id(self, sym):
- """ Converts a token (str) in an id using the vocab. """
- if sym in self.sym2idx:
- return self.sym2idx[sym]
- else:
- # logger.info('encounter unk {}'.format(sym))
- # assert '' not in sym
- if hasattr(self, "unk_idx"):
- return self.sym2idx.get(sym, self.unk_idx)
- # Backward compatibility with pre-trained models
- elif "" in self.sym2idx:
- return self.sym2idx[""]
- elif "" in self.sym2idx:
- return self.sym2idx[""]
- else:
- raise ValueError("Token not in vocabulary and no token in vocabulary for replacement")
-
- def convert_tokens_to_string(self, tokens):
- """ Converts a sequence of tokens (string) in a single string. """
- out_string = " ".join(tokens).strip()
- return out_string
-
- def convert_to_tensor(self, symbols):
- return torch.LongTensor(self.convert_tokens_to_ids(symbols))
-
- @property
- def vocab_size(self):
- return len(self.idx2sym)
-
- def _tokenize(self, line, add_eos=False, add_double_eos=False):
- line = line.strip()
- # convert to lower case
- if self.lower_case:
- line = line.lower()
-
- # empty delimiter '' will evaluate False
- if self.delimiter == "":
- symbols = line
- else:
- symbols = line.split(self.delimiter)
-
- if add_double_eos: # lm1b
- return [""] + symbols + [""]
- elif add_eos:
- return symbols + [""]
- else:
- return symbols
-
-
-class LMOrderedIterator(object):
- def __init__(self, data, bsz, bptt, device="cpu", ext_len=None):
- """
- data -- LongTensor -- the LongTensor is strictly ordered
- """
- self.bsz = bsz
- self.bptt = bptt
- self.ext_len = ext_len if ext_len is not None else 0
-
- self.device = device
-
- # Work out how cleanly we can divide the dataset into bsz parts.
- self.n_step = data.size(0) // bsz
-
- # Trim off any extra elements that wouldn't cleanly fit (remainders).
- data = data.narrow(0, 0, self.n_step * bsz)
-
- # Evenly divide the data across the bsz batches.
- self.data = data.view(bsz, -1).t().contiguous().to(device)
-
- # Number of mini-batches
- self.n_batch = (self.n_step + self.bptt - 1) // self.bptt
-
- def get_batch(self, i, bptt=None):
- if bptt is None:
- bptt = self.bptt
- seq_len = min(bptt, self.data.size(0) - 1 - i)
-
- end_idx = i + seq_len
- beg_idx = max(0, i - self.ext_len)
-
- data = self.data[beg_idx:end_idx]
- target = self.data[i + 1 : i + 1 + seq_len]
-
- data_out = data.transpose(0, 1).contiguous().to(self.device)
- target_out = target.transpose(0, 1).contiguous().to(self.device)
-
- return data_out, target_out, seq_len
-
- def get_fixlen_iter(self, start=0):
- for i in range(start, self.data.size(0) - 1, self.bptt):
- yield self.get_batch(i)
-
- def get_varlen_iter(self, start=0, std=5, min_len=5, max_deviation=3):
- max_len = self.bptt + max_deviation * std
- i = start
- while True:
- bptt = self.bptt if np.random.random() < 0.95 else self.bptt / 2.0
- bptt = min(max_len, max(min_len, int(np.random.normal(bptt, std))))
- data, target, seq_len = self.get_batch(i, bptt)
- i += seq_len
- yield data, target, seq_len
- if i >= self.data.size(0) - 2:
- break
-
- def __iter__(self):
- return self.get_fixlen_iter()
-
-
-class LMShuffledIterator(object):
- def __init__(self, data, bsz, bptt, device="cpu", ext_len=None, shuffle=False):
- """
- data -- list[LongTensor] -- there is no order among the LongTensors
- """
- self.data = data
-
- self.bsz = bsz
- self.bptt = bptt
- self.ext_len = ext_len if ext_len is not None else 0
-
- self.device = device
- self.shuffle = shuffle
-
- def get_sent_stream(self):
- # index iterator
- epoch_indices = np.random.permutation(len(self.data)) if self.shuffle else np.array(range(len(self.data)))
-
- # sentence iterator
- for idx in epoch_indices:
- yield self.data[idx]
-
- def stream_iterator(self, sent_stream):
- # streams for each data in the batch
- streams = [None] * self.bsz
-
- data = torch.LongTensor(self.bptt, self.bsz)
- target = torch.LongTensor(self.bptt, self.bsz)
-
- n_retain = 0
-
- while True:
- # data : [n_retain+bptt x bsz]
- # target : [bptt x bsz]
- data[n_retain:].fill_(-1)
- target.fill_(-1)
-
- valid_batch = True
-
- for i in range(self.bsz):
- n_filled = 0
- try:
- while n_filled < self.bptt:
- if streams[i] is None or len(streams[i]) <= 1:
- streams[i] = next(sent_stream)
- # number of new tokens to fill in
- n_new = min(len(streams[i]) - 1, self.bptt - n_filled)
- # first n_retain tokens are retained from last batch
- data[n_retain + n_filled : n_retain + n_filled + n_new, i] = streams[i][:n_new]
- target[n_filled : n_filled + n_new, i] = streams[i][1 : n_new + 1]
- streams[i] = streams[i][n_new:]
- n_filled += n_new
- except StopIteration:
- valid_batch = False
- break
-
- if not valid_batch:
- return
-
- data_out = data.transpose(0, 1).contiguous().to(self.device)
- target_out = target.transpose(0, 1).contiguous().to(self.device)
-
- yield data_out, target_out, self.bptt
-
- n_retain = min(data.size(0), self.ext_len)
- if n_retain > 0:
- data[:n_retain] = data[-n_retain:]
- data.resize_(n_retain + self.bptt, data.size(1))
-
- def __iter__(self):
- # sent_stream is an iterator
- sent_stream = self.get_sent_stream()
-
- for batch in self.stream_iterator(sent_stream):
- yield batch
-
-
-class LMMultiFileIterator(LMShuffledIterator):
- def __init__(self, paths, vocab, bsz, bptt, device="cpu", ext_len=None, shuffle=False):
-
- self.paths = paths
- self.vocab = vocab
-
- self.bsz = bsz
- self.bptt = bptt
- self.ext_len = ext_len if ext_len is not None else 0
-
- self.device = device
- self.shuffle = shuffle
-
- def get_sent_stream(self, path):
- sents = self.vocab.encode_file(path, add_double_eos=True)
- if self.shuffle:
- np.random.shuffle(sents)
- sent_stream = iter(sents)
-
- return sent_stream
-
- def __iter__(self):
- if self.shuffle:
- np.random.shuffle(self.paths)
-
- for path in self.paths:
- # sent_stream is an iterator
- sent_stream = self.get_sent_stream(path)
- for batch in self.stream_iterator(sent_stream):
- yield batch
-
-
-class TransfoXLCorpus(object):
- @classmethod
- def from_pretrained(cls, pretrained_model_name_or_path, cache_dir=None, *inputs, **kwargs):
- """
- Instantiate a pre-processed corpus.
- """
- vocab = TransfoXLTokenizer.from_pretrained(pretrained_model_name_or_path, *inputs, **kwargs)
- if pretrained_model_name_or_path in PRETRAINED_CORPUS_ARCHIVE_MAP:
- corpus_file = PRETRAINED_CORPUS_ARCHIVE_MAP[pretrained_model_name_or_path]
- else:
- corpus_file = os.path.join(pretrained_model_name_or_path, CORPUS_NAME)
- # redirect to the cache, if necessary
- try:
- resolved_corpus_file = cached_path(corpus_file, cache_dir=cache_dir)
- except EnvironmentError:
- logger.error(
- "Corpus '{}' was not found in corpus list ({}). "
- "We assumed '{}' was a path or url but couldn't find files {} "
- "at this path or url.".format(
- pretrained_model_name_or_path,
- ", ".join(PRETRAINED_CORPUS_ARCHIVE_MAP.keys()),
- pretrained_model_name_or_path,
- corpus_file,
- )
- )
- return None
- if resolved_corpus_file == corpus_file:
- logger.info("loading corpus file {}".format(corpus_file))
- else:
- logger.info("loading corpus file {} from cache at {}".format(corpus_file, resolved_corpus_file))
-
- # Instantiate tokenizer.
- corpus = cls(*inputs, **kwargs)
- corpus_dict = torch.load(resolved_corpus_file)
- for key, value in corpus_dict.items():
- corpus.__dict__[key] = value
- corpus.vocab = vocab
- if corpus.train is not None:
- corpus.train = torch.tensor(corpus.train, dtype=torch.long)
- if corpus.valid is not None:
- corpus.valid = torch.tensor(corpus.valid, dtype=torch.long)
- if corpus.test is not None:
- corpus.test = torch.tensor(corpus.test, dtype=torch.long)
- return corpus
-
- def __init__(self, *args, **kwargs):
- self.vocab = TransfoXLTokenizer(*args, **kwargs)
- self.dataset = None
- self.train = None
- self.valid = None
- self.test = None
-
- def build_corpus(self, path, dataset):
- self.dataset = dataset
-
- if self.dataset in ["ptb", "wt2", "enwik8", "text8"]:
- self.vocab.count_file(os.path.join(path, "train.txt"))
- self.vocab.count_file(os.path.join(path, "valid.txt"))
- self.vocab.count_file(os.path.join(path, "test.txt"))
- elif self.dataset == "wt103":
- self.vocab.count_file(os.path.join(path, "train.txt"))
- elif self.dataset == "lm1b":
- train_path_pattern = os.path.join(
- path,
- "1-billion-word-language-modeling-benchmark-r13output",
- "training-monolingual.tokenized.shuffled",
- "news.en-*",
- )
- train_paths = glob.glob(train_path_pattern)
- # the vocab will load from file when build_vocab() is called
-
- self.vocab.build_vocab()
-
- if self.dataset in ["ptb", "wt2", "wt103"]:
- self.train = self.vocab.encode_file(os.path.join(path, "train.txt"), ordered=True)
- self.valid = self.vocab.encode_file(os.path.join(path, "valid.txt"), ordered=True)
- self.test = self.vocab.encode_file(os.path.join(path, "test.txt"), ordered=True)
- elif self.dataset in ["enwik8", "text8"]:
- self.train = self.vocab.encode_file(os.path.join(path, "train.txt"), ordered=True, add_eos=False)
- self.valid = self.vocab.encode_file(os.path.join(path, "valid.txt"), ordered=True, add_eos=False)
- self.test = self.vocab.encode_file(os.path.join(path, "test.txt"), ordered=True, add_eos=False)
- elif self.dataset == "lm1b":
- self.train = train_paths
- self.valid = self.vocab.encode_file(os.path.join(path, "valid.txt"), ordered=False, add_double_eos=True)
- self.test = self.vocab.encode_file(os.path.join(path, "test.txt"), ordered=False, add_double_eos=True)
-
- def get_iterator(self, split, *args, **kwargs):
- if split == "train":
- if self.dataset in ["ptb", "wt2", "wt103", "enwik8", "text8"]:
- data_iter = LMOrderedIterator(self.train, *args, **kwargs)
- elif self.dataset == "lm1b":
- kwargs["shuffle"] = True
- data_iter = LMMultiFileIterator(self.train, self.vocab, *args, **kwargs)
- elif split in ["valid", "test"]:
- data = self.valid if split == "valid" else self.test
- if self.dataset in ["ptb", "wt2", "wt103", "enwik8", "text8"]:
- data_iter = LMOrderedIterator(data, *args, **kwargs)
- elif self.dataset == "lm1b":
- data_iter = LMShuffledIterator(data, *args, **kwargs)
-
- return data_iter
-
-
-def get_lm_corpus(datadir, dataset):
- fn = os.path.join(datadir, "cache.pt")
- fn_pickle = os.path.join(datadir, "cache.pkl")
- if os.path.exists(fn):
- logger.info("Loading cached dataset...")
- corpus = torch.load(fn_pickle)
- elif os.path.exists(fn):
- logger.info("Loading cached dataset from pickle...")
- with open(fn, "rb") as fp:
- corpus = pickle.load(fp)
- else:
- logger.info("Producing dataset {}...".format(dataset))
- kwargs = {}
- if dataset in ["wt103", "wt2"]:
- kwargs["special"] = [""]
- kwargs["lower_case"] = False
- elif dataset == "ptb":
- kwargs["special"] = [""]
- kwargs["lower_case"] = True
- elif dataset == "lm1b":
- kwargs["special"] = []
- kwargs["lower_case"] = False
- kwargs["vocab_file"] = os.path.join(datadir, "1b_word_vocab.txt")
- elif dataset in ["enwik8", "text8"]:
- pass
-
- corpus = TransfoXLCorpus(datadir, dataset, **kwargs)
- torch.save(corpus, fn)
-
- return corpus
diff --git a/server/transformers/src/transformers/tokenization_utils.py b/server/transformers/src/transformers/tokenization_utils.py
deleted file mode 100644
index 469181325aaa9ab582ba462a381b93e7761bdd7a..0000000000000000000000000000000000000000
--- a/server/transformers/src/transformers/tokenization_utils.py
+++ /dev/null
@@ -1,1615 +0,0 @@
-# coding=utf-8
-# Copyright 2018 The Open AI Team Authors and The HuggingFace Inc. team.
-#
-# Licensed under the Apache License, Version 2.0 (the "License");
-# you may not use this file except in compliance with the License.
-# You may obtain a copy of the License at
-#
-# http://www.apache.org/licenses/LICENSE-2.0
-#
-# Unless required by applicable law or agreed to in writing, software
-# distributed under the License is distributed on an "AS IS" BASIS,
-# WITHOUT WARRANTIES OR CONDITIONS OF ANY KIND, either express or implied.
-# See the License for the specific language governing permissions and
-# limitations under the License.
-"""Tokenization classes for OpenAI GPT."""
-
-
-import copy
-import itertools
-import json
-import logging
-import os
-import re
-
-from .file_utils import cached_path, hf_bucket_url, is_remote_url, is_tf_available, is_torch_available
-
-
-if is_tf_available():
- import tensorflow as tf
-if is_torch_available():
- import torch
-
-logger = logging.getLogger(__name__)
-
-SPECIAL_TOKENS_MAP_FILE = "special_tokens_map.json"
-ADDED_TOKENS_FILE = "added_tokens.json"
-TOKENIZER_CONFIG_FILE = "tokenizer_config.json"
-
-
-class PreTrainedTokenizer(object):
- """ Base class for all tokenizers.
- Handle all the shared methods for tokenization and special tokens as well as methods downloading/caching/loading pretrained tokenizers as well as adding tokens to the vocabulary.
-
- This class also contain the added tokens in a unified way on top of all tokenizers so we don't have to handle the specific vocabulary augmentation methods of the various underlying dictionary structures (BPE, sentencepiece...).
-
- Class attributes (overridden by derived classes):
-
- - ``vocab_files_names``: a python ``dict`` with, as keys, the ``__init__`` keyword name of each vocabulary file required by the model, and as associated values, the filename for saving the associated file (string).
- - ``pretrained_vocab_files_map``: a python ``dict of dict`` the high-level keys being the ``__init__`` keyword name of each vocabulary file required by the model, the low-level being the `short-cut-names` (string) of the pretrained models with, as associated values, the `url` (string) to the associated pretrained vocabulary file.
- - ``max_model_input_sizes``: a python ``dict`` with, as keys, the `short-cut-names` (string) of the pretrained models, and as associated values, the maximum length of the sequence inputs of this model, or None if the model has no maximum input size.
- - ``pretrained_init_configuration``: a python ``dict`` with, as keys, the `short-cut-names` (string) of the pretrained models, and as associated values, a dictionnary of specific arguments to pass to the ``__init__``method of the tokenizer class for this pretrained model when loading the tokenizer with the ``from_pretrained()`` method.
-
- Parameters:
-
- - ``bos_token``: (`Optional`) string: a beginning of sentence token. Will be associated to ``self.bos_token`` and ``self.bos_token_id``
-
- - ``eos_token``: (`Optional`) string: an end of sentence token. Will be associated to ``self.eos_token`` and ``self.eos_token_id``
-
- - ``unk_token``: (`Optional`) string: an unknown token. Will be associated to ``self.unk_token`` and ``self.unk_token_id``
-
- - ``sep_token``: (`Optional`) string: a separation token (e.g. to separate context and query in an input sequence). Will be associated to ``self.sep_token`` and ``self.sep_token_id``
-
- - ``pad_token``: (`Optional`) string: a padding token. Will be associated to ``self.pad_token`` and ``self.pad_token_id``
-
- - ``cls_token``: (`Optional`) string: a classification token (e.g. to extract a summary of an input sequence leveraging self-attention along the full depth of the model). Will be associated to ``self.cls_token`` and ``self.cls_token_id``
-
- - ``mask_token``: (`Optional`) string: a masking token (e.g. when training a model with masked-language modeling). Will be associated to ``self.mask_token`` and ``self.mask_token_id``
-
- - ``additional_special_tokens``: (`Optional`) list: a list of additional special tokens. Adding all special tokens here ensure they won't be split by the tokenization process. Will be associated to ``self.additional_special_tokens`` and ``self.additional_special_tokens_ids``
- """
-
- vocab_files_names = {}
- pretrained_vocab_files_map = {}
- pretrained_init_configuration = {}
- max_model_input_sizes = {}
-
- SPECIAL_TOKENS_ATTRIBUTES = [
- "bos_token",
- "eos_token",
- "unk_token",
- "sep_token",
- "pad_token",
- "cls_token",
- "mask_token",
- "additional_special_tokens",
- ]
-
- padding_side = "right"
-
- @property
- def bos_token(self):
- """ Beginning of sentence token (string). Log an error if used while not having been set. """
- if self._bos_token is None:
- logger.error("Using bos_token, but it is not set yet.")
- return self._bos_token
-
- @property
- def eos_token(self):
- """ End of sentence token (string). Log an error if used while not having been set. """
- if self._eos_token is None:
- logger.error("Using eos_token, but it is not set yet.")
- return self._eos_token
-
- @property
- def unk_token(self):
- """ Unknown token (string). Log an error if used while not having been set. """
- if self._unk_token is None:
- logger.error("Using unk_token, but it is not set yet.")
- return self._unk_token
-
- @property
- def sep_token(self):
- """ Separation token (string). E.g. separate context and query in an input sequence. Log an error if used while not having been set. """
- if self._sep_token is None:
- logger.error("Using sep_token, but it is not set yet.")
- return self._sep_token
-
- @property
- def pad_token(self):
- """ Padding token (string). Log an error if used while not having been set. """
- if self._pad_token is None:
- logger.error("Using pad_token, but it is not set yet.")
- return self._pad_token
-
- @property
- def cls_token(self):
- """ Classification token (string). E.g. to extract a summary of an input sequence leveraging self-attention along the full depth of the model. Log an error if used while not having been set. """
- if self._cls_token is None:
- logger.error("Using cls_token, but it is not set yet.")
- return self._cls_token
-
- @property
- def mask_token(self):
- """ Mask token (string). E.g. when training a model with masked-language modeling. Log an error if used while not having been set. """
- if self._mask_token is None:
- logger.error("Using mask_token, but it is not set yet.")
- return self._mask_token
-
- @property
- def additional_special_tokens(self):
- """ All the additional special tokens you may want to use (list of strings). Log an error if used while not having been set. """
- if self._additional_special_tokens is None:
- logger.error("Using additional_special_tokens, but it is not set yet.")
- return self._additional_special_tokens
-
- @bos_token.setter
- def bos_token(self, value):
- self._bos_token = value
-
- @eos_token.setter
- def eos_token(self, value):
- self._eos_token = value
-
- @unk_token.setter
- def unk_token(self, value):
- self._unk_token = value
-
- @sep_token.setter
- def sep_token(self, value):
- self._sep_token = value
-
- @pad_token.setter
- def pad_token(self, value):
- self._pad_token = value
-
- @cls_token.setter
- def cls_token(self, value):
- self._cls_token = value
-
- @mask_token.setter
- def mask_token(self, value):
- self._mask_token = value
-
- @additional_special_tokens.setter
- def additional_special_tokens(self, value):
- self._additional_special_tokens = value
-
- @property
- def bos_token_id(self):
- """ Id of the beginning of sentence token in the vocabulary. Log an error if used while not having been set. """
- return self.convert_tokens_to_ids(self.bos_token)
-
- @property
- def eos_token_id(self):
- """ Id of the end of sentence token in the vocabulary. Log an error if used while not having been set. """
- return self.convert_tokens_to_ids(self.eos_token)
-
- @property
- def unk_token_id(self):
- """ Id of the unknown token in the vocabulary. Log an error if used while not having been set. """
- return self.convert_tokens_to_ids(self.unk_token)
-
- @property
- def sep_token_id(self):
- """ Id of the separation token in the vocabulary. E.g. separate context and query in an input sequence. Log an error if used while not having been set. """
- return self.convert_tokens_to_ids(self.sep_token)
-
- @property
- def pad_token_id(self):
- """ Id of the padding token in the vocabulary. Log an error if used while not having been set. """
- return self.convert_tokens_to_ids(self.pad_token)
-
- @property
- def pad_token_type_id(self):
- """ Id of the padding token type in the vocabulary."""
- return self._pad_token_type_id
-
- @property
- def cls_token_id(self):
- """ Id of the classification token in the vocabulary. E.g. to extract a summary of an input sequence leveraging self-attention along the full depth of the model. Log an error if used while not having been set. """
- return self.convert_tokens_to_ids(self.cls_token)
-
- @property
- def mask_token_id(self):
- """ Id of the mask token in the vocabulary. E.g. when training a model with masked-language modeling. Log an error if used while not having been set. """
- return self.convert_tokens_to_ids(self.mask_token)
-
- @property
- def additional_special_tokens_ids(self):
- """ Ids of all the additional special tokens in the vocabulary (list of integers). Log an error if used while not having been set. """
- return self.convert_tokens_to_ids(self.additional_special_tokens)
-
- def __init__(self, max_len=None, **kwargs):
- self._bos_token = None
- self._eos_token = None
- self._unk_token = None
- self._sep_token = None
- self._pad_token = None
- self._cls_token = None
- self._mask_token = None
- self._pad_token_type_id = 0
- self._additional_special_tokens = []
-
- self.max_len = max_len if max_len is not None else int(1e12)
-
- # Padding side is right by default and over-riden in subclasses. If specified in the kwargs, it is changed.
- self.padding_side = kwargs.pop("padding_side", self.padding_side)
-
- # Added tokens
- self.added_tokens_encoder = {}
- self.unique_added_tokens_encoder = set()
- self.added_tokens_decoder = {}
-
- # inputs and kwargs for saving and re-loading (see ``from_pretrained`` and ``save_pretrained``)
- self.init_inputs = ()
- self.init_kwargs = {}
-
- for key, value in kwargs.items():
- if key in self.SPECIAL_TOKENS_ATTRIBUTES:
- if key == "additional_special_tokens":
- assert isinstance(value, (list, tuple)) and all(isinstance(t, str) for t in value)
- else:
- assert isinstance(value, str)
- setattr(self, key, value)
-
- @classmethod
- def from_pretrained(cls, *inputs, **kwargs):
- r"""
- Instantiate a :class:`~transformers.PreTrainedTokenizer` (or a derived class) from a predefined tokenizer.
-
- Args:
- pretrained_model_name_or_path: either:
-
- - a string with the `shortcut name` of a predefined tokenizer to load from cache or download, e.g.: ``bert-base-uncased``.
- - a string with the `identifier name` of a predefined tokenizer that was user-uploaded to our S3, e.g.: ``dbmdz/bert-base-german-cased``.
- - a path to a `directory` containing vocabulary files required by the tokenizer, for instance saved using the :func:`~transformers.PreTrainedTokenizer.save_pretrained` method, e.g.: ``./my_model_directory/``.
- - (not applicable to all derived classes, deprecated) a path or url to a single saved vocabulary file if and only if the tokenizer only requires a single vocabulary file (e.g. Bert, XLNet), e.g.: ``./my_model_directory/vocab.txt``.
-
- cache_dir: (`optional`) string:
- Path to a directory in which a downloaded predefined tokenizer vocabulary files should be cached if the standard cache should not be used.
-
- force_download: (`optional`) boolean, default False:
- Force to (re-)download the vocabulary files and override the cached versions if they exists.
-
- resume_download: (`optional`) boolean, default False:
- Do not delete incompletely recieved file. Attempt to resume the download if such a file exists.
-
- proxies: (`optional`) dict, default None:
- A dictionary of proxy servers to use by protocol or endpoint, e.g.: {'http': 'foo.bar:3128', 'http://hostname': 'foo.bar:4012'}.
- The proxies are used on each request.
-
- inputs: (`optional`) positional arguments: will be passed to the Tokenizer ``__init__`` method.
-
- kwargs: (`optional`) keyword arguments: will be passed to the Tokenizer ``__init__`` method. Can be used to set special tokens like ``bos_token``, ``eos_token``, ``unk_token``, ``sep_token``, ``pad_token``, ``cls_token``, ``mask_token``, ``additional_special_tokens``. See parameters in the doc string of :class:`~transformers.PreTrainedTokenizer` for details.
-
- Examples::
-
- # We can't instantiate directly the base class `PreTrainedTokenizer` so let's show our examples on a derived class: BertTokenizer
-
- # Download vocabulary from S3 and cache.
- tokenizer = BertTokenizer.from_pretrained('bert-base-uncased')
-
- # Download vocabulary from S3 (user-uploaded) and cache.
- tokenizer = BertTokenizer.from_pretrained('dbmdz/bert-base-german-cased')
-
- # If vocabulary files are in a directory (e.g. tokenizer was saved using `save_pretrained('./test/saved_model/')`)
- tokenizer = BertTokenizer.from_pretrained('./test/saved_model/')
-
- # If the tokenizer uses a single vocabulary file, you can point directly to this file
- tokenizer = BertTokenizer.from_pretrained('./test/saved_model/my_vocab.txt')
-
- # You can link tokens to special vocabulary when instantiating
- tokenizer = BertTokenizer.from_pretrained('bert-base-uncased', unk_token='')
- # You should be sure '' is in the vocabulary when doing that.
- # Otherwise use tokenizer.add_special_tokens({'unk_token': ''}) instead)
- assert tokenizer.unk_token == ''
-
- """
- return cls._from_pretrained(*inputs, **kwargs)
-
- @classmethod
- def _from_pretrained(cls, pretrained_model_name_or_path, *init_inputs, **kwargs):
- cache_dir = kwargs.pop("cache_dir", None)
- force_download = kwargs.pop("force_download", False)
- resume_download = kwargs.pop("resume_download", False)
- proxies = kwargs.pop("proxies", None)
-
- s3_models = list(cls.max_model_input_sizes.keys())
- vocab_files = {}
- init_configuration = {}
- if pretrained_model_name_or_path in s3_models:
- # Get the vocabulary from AWS S3 bucket
- for file_id, map_list in cls.pretrained_vocab_files_map.items():
- vocab_files[file_id] = map_list[pretrained_model_name_or_path]
- if (
- cls.pretrained_init_configuration
- and pretrained_model_name_or_path in cls.pretrained_init_configuration
- ):
- init_configuration = cls.pretrained_init_configuration[pretrained_model_name_or_path].copy()
- else:
- # Get the vocabulary from local files
- logger.info(
- "Model name '{}' not found in model shortcut name list ({}). "
- "Assuming '{}' is a path, a model identifier, or url to a directory containing tokenizer files.".format(
- pretrained_model_name_or_path, ", ".join(s3_models), pretrained_model_name_or_path
- )
- )
-
- if os.path.isfile(pretrained_model_name_or_path) or is_remote_url(pretrained_model_name_or_path):
- if len(cls.vocab_files_names) > 1:
- raise ValueError(
- "Calling {}.from_pretrained() with the path to a single file or url is not supported."
- "Use a model identifier or the path to a directory instead.".format(cls.__name__)
- )
- logger.warning(
- "Calling {}.from_pretrained() with the path to a single file or url is deprecated".format(
- cls.__name__
- )
- )
- file_id = list(cls.vocab_files_names.keys())[0]
- vocab_files[file_id] = pretrained_model_name_or_path
- else:
- # At this point pretrained_model_name_or_path is either a directory or a model identifier name
- additional_files_names = {
- "added_tokens_file": ADDED_TOKENS_FILE,
- "special_tokens_map_file": SPECIAL_TOKENS_MAP_FILE,
- "tokenizer_config_file": TOKENIZER_CONFIG_FILE,
- }
- # Look for the tokenizer main vocabulary files + the additional tokens files
- for file_id, file_name in {**cls.vocab_files_names, **additional_files_names}.items():
- if os.path.isdir(pretrained_model_name_or_path):
- full_file_name = os.path.join(pretrained_model_name_or_path, file_name)
- if not os.path.exists(full_file_name):
- logger.info("Didn't find file {}. We won't load it.".format(full_file_name))
- full_file_name = None
- else:
- full_file_name = hf_bucket_url(pretrained_model_name_or_path, postfix=file_name)
-
- vocab_files[file_id] = full_file_name
-
- # Get files from url, cache, or disk depending on the case
- try:
- resolved_vocab_files = {}
- for file_id, file_path in vocab_files.items():
- if file_path is None:
- resolved_vocab_files[file_id] = None
- else:
- resolved_vocab_files[file_id] = cached_path(
- file_path,
- cache_dir=cache_dir,
- force_download=force_download,
- proxies=proxies,
- resume_download=resume_download,
- )
- except EnvironmentError:
- if pretrained_model_name_or_path in s3_models:
- msg = "Couldn't reach server at '{}' to download vocabulary files."
- else:
- msg = (
- "Model name '{}' was not found in tokenizers model name list ({}). "
- "We assumed '{}' was a path or url to a directory containing vocabulary files "
- "named {}, but couldn't find such vocabulary files at this path or url.".format(
- pretrained_model_name_or_path,
- ", ".join(s3_models),
- pretrained_model_name_or_path,
- list(cls.vocab_files_names.values()),
- )
- )
-
- raise EnvironmentError(msg)
-
- if all(full_file_name is None for full_file_name in resolved_vocab_files.values()):
- raise EnvironmentError(
- "Model name '{}' was not found in tokenizers model name list ({}). "
- "We assumed '{}' was a path, a model identifier, or url to a directory containing vocabulary files "
- "named {} but couldn't find such vocabulary files at this path or url.".format(
- pretrained_model_name_or_path,
- ", ".join(s3_models),
- pretrained_model_name_or_path,
- list(cls.vocab_files_names.values()),
- )
- )
-
- for file_id, file_path in vocab_files.items():
- if file_path == resolved_vocab_files[file_id]:
- logger.info("loading file {}".format(file_path))
- else:
- logger.info("loading file {} from cache at {}".format(file_path, resolved_vocab_files[file_id]))
-
- # Prepare tokenizer initialization kwargs
- # Did we saved some inputs and kwargs to reload ?
- tokenizer_config_file = resolved_vocab_files.pop("tokenizer_config_file", None)
- if tokenizer_config_file is not None:
- with open(tokenizer_config_file, encoding="utf-8") as tokenizer_config_handle:
- init_kwargs = json.load(tokenizer_config_handle)
- saved_init_inputs = init_kwargs.pop("init_inputs", ())
- if not init_inputs:
- init_inputs = saved_init_inputs
- else:
- init_kwargs = init_configuration
-
- # Update with newly provided kwargs
- init_kwargs.update(kwargs)
-
- # Set max length if needed
- if pretrained_model_name_or_path in cls.max_model_input_sizes:
- # if we're using a pretrained model, ensure the tokenizer
- # wont index sequences longer than the number of positional embeddings
- max_len = cls.max_model_input_sizes[pretrained_model_name_or_path]
- if max_len is not None and isinstance(max_len, (int, float)):
- init_kwargs["max_len"] = min(init_kwargs.get("max_len", int(1e12)), max_len)
-
- # Merge resolved_vocab_files arguments in init_kwargs.
- added_tokens_file = resolved_vocab_files.pop("added_tokens_file", None)
- special_tokens_map_file = resolved_vocab_files.pop("special_tokens_map_file", None)
- for args_name, file_path in resolved_vocab_files.items():
- if args_name not in init_kwargs:
- init_kwargs[args_name] = file_path
- if special_tokens_map_file is not None:
- with open(special_tokens_map_file, encoding="utf-8") as special_tokens_map_handle:
- special_tokens_map = json.load(special_tokens_map_handle)
- for key, value in special_tokens_map.items():
- if key not in init_kwargs:
- init_kwargs[key] = value
-
- # Instantiate tokenizer.
- try:
- tokenizer = cls(*init_inputs, **init_kwargs)
- except OSError:
- raise OSError(
- "Unable to load vocabulary from file. "
- "Please check that the provided vocabulary is accessible and not corrupted."
- )
-
- # Save inputs and kwargs for saving and re-loading with ``save_pretrained``
- tokenizer.init_inputs = init_inputs
- tokenizer.init_kwargs = init_kwargs
-
- # update unique_added_tokens_encoder with special tokens for correct tokenization
- tokenizer.unique_added_tokens_encoder.update(set(tokenizer.all_special_tokens))
-
- # Add supplementary tokens.
- if added_tokens_file is not None:
- with open(added_tokens_file, encoding="utf-8") as added_tokens_handle:
- added_tok_encoder = json.load(added_tokens_handle)
- added_tok_decoder = {v: k for k, v in added_tok_encoder.items()}
- tokenizer.added_tokens_encoder.update(added_tok_encoder)
- tokenizer.added_tokens_decoder.update(added_tok_decoder)
- tokenizer.unique_added_tokens_encoder.update(set(tokenizer.added_tokens_encoder.keys()))
-
- return tokenizer
-
- def save_pretrained(self, save_directory):
- """ Save the tokenizer vocabulary files together with:
- - added tokens,
- - special-tokens-to-class-attributes-mapping,
- - tokenizer instantiation positional and keywords inputs (e.g. do_lower_case for Bert).
-
- This won't save modifications other than (added tokens and special token mapping) you may have
- applied to the tokenizer after the instantiation (e.g. modifying tokenizer.do_lower_case after creation).
-
- This method make sure the full tokenizer can then be re-loaded using the :func:`~transformers.PreTrainedTokenizer.from_pretrained` class method.
- """
- if not os.path.isdir(save_directory):
- logger.error("Saving directory ({}) should be a directory".format(save_directory))
- return
-
- special_tokens_map_file = os.path.join(save_directory, SPECIAL_TOKENS_MAP_FILE)
- added_tokens_file = os.path.join(save_directory, ADDED_TOKENS_FILE)
- tokenizer_config_file = os.path.join(save_directory, TOKENIZER_CONFIG_FILE)
-
- tokenizer_config = copy.deepcopy(self.init_kwargs)
- if len(self.init_inputs) > 0:
- tokenizer_config["init_inputs"] = copy.deepcopy(self.init_inputs)
- for file_id in self.vocab_files_names.keys():
- tokenizer_config.pop(file_id, None)
-
- with open(tokenizer_config_file, "w", encoding="utf-8") as f:
- f.write(json.dumps(tokenizer_config, ensure_ascii=False))
-
- with open(special_tokens_map_file, "w", encoding="utf-8") as f:
- f.write(json.dumps(self.special_tokens_map, ensure_ascii=False))
-
- if len(self.added_tokens_encoder) > 0:
- with open(added_tokens_file, "w", encoding="utf-8") as f:
- out_str = json.dumps(self.added_tokens_encoder, ensure_ascii=False)
- f.write(out_str)
-
- vocab_files = self.save_vocabulary(save_directory)
-
- return vocab_files + (special_tokens_map_file, added_tokens_file)
-
- def save_vocabulary(self, save_directory):
- """ Save the tokenizer vocabulary to a directory. This method does *NOT* save added tokens
- and special token mappings.
-
- Please use :func:`~transformers.PreTrainedTokenizer.save_pretrained` `()` to save the full Tokenizer state if you want to reload it using the :func:`~transformers.PreTrainedTokenizer.from_pretrained` class method.
- """
- raise NotImplementedError
-
- def vocab_size(self):
- """ Size of the base vocabulary (without the added tokens) """
- raise NotImplementedError
-
- def __len__(self):
- """ Size of the full vocabulary with the added tokens """
- return self.vocab_size + len(self.added_tokens_encoder)
-
- def add_tokens(self, new_tokens):
- """
- Add a list of new tokens to the tokenizer class. If the new tokens are not in the
- vocabulary, they are added to it with indices starting from length of the current vocabulary.
-
- Args:
- new_tokens: list of string. Each string is a token to add. Tokens are only added if they are not already in the vocabulary (tested by checking if the tokenizer assign the index of the ``unk_token`` to them).
-
- Returns:
- Number of tokens added to the vocabulary.
-
- Examples::
-
- # Let's see how to increase the vocabulary of Bert model and tokenizer
- tokenizer = BertTokenizer.from_pretrained('bert-base-uncased')
- model = BertModel.from_pretrained('bert-base-uncased')
-
- num_added_toks = tokenizer.add_tokens(['new_tok1', 'my_new-tok2'])
- print('We have added', num_added_toks, 'tokens')
- model.resize_token_embeddings(len(tokenizer)) # Notice: resize_token_embeddings expect to receive the full size of the new vocabulary, i.e. the length of the tokenizer.
- """
- if not new_tokens:
- return 0
-
- to_add_tokens = []
- for token in new_tokens:
- assert isinstance(token, str)
- if self.init_kwargs.get("do_lower_case", False) and token not in self.all_special_tokens:
- token = token.lower()
- if (
- token != self.unk_token
- and self.convert_tokens_to_ids(token) == self.convert_tokens_to_ids(self.unk_token)
- and token not in to_add_tokens
- ):
- to_add_tokens.append(token)
- logger.info("Adding %s to the vocabulary", token)
-
- added_tok_encoder = dict((tok, len(self) + i) for i, tok in enumerate(to_add_tokens))
- added_tok_decoder = {v: k for k, v in added_tok_encoder.items()}
- self.added_tokens_encoder.update(added_tok_encoder)
- self.unique_added_tokens_encoder = set(self.added_tokens_encoder.keys()).union(set(self.all_special_tokens))
- self.added_tokens_decoder.update(added_tok_decoder)
-
- return len(to_add_tokens)
-
- def num_added_tokens(self, pair=False):
- """
- Returns the number of added tokens when encoding a sequence with special tokens.
-
- Note:
- This encodes inputs and checks the number of added tokens, and is therefore not efficient. Do not put this
- inside your training loop.
-
- Args:
- pair: Returns the number of added tokens in the case of a sequence pair if set to True, returns the
- number of added tokens in the case of a single sequence if set to False.
-
- Returns:
- Number of tokens added to sequences
- """
- token_ids_0 = []
- token_ids_1 = []
- return len(self.build_inputs_with_special_tokens(token_ids_0, token_ids_1 if pair else None))
-
- def add_special_tokens(self, special_tokens_dict):
- """
- Add a dictionary of special tokens (eos, pad, cls...) to the encoder and link them
- to class attributes. If special tokens are NOT in the vocabulary, they are added
- to it (indexed starting from the last index of the current vocabulary).
-
- Using `add_special_tokens` will ensure your special tokens can be used in several ways:
-
- - special tokens are carefully handled by the tokenizer (they are never split)
- - you can easily refer to special tokens using tokenizer class attributes like `tokenizer.cls_token`. This makes it easy to develop model-agnostic training and fine-tuning scripts.
-
- When possible, special tokens are already registered for provided pretrained models (ex: BertTokenizer cls_token is already registered to be '[CLS]' and XLM's one is also registered to be '')
-
- Args:
- special_tokens_dict: dict of string. Keys should be in the list of predefined special attributes:
- [``bos_token``, ``eos_token``, ``unk_token``, ``sep_token``, ``pad_token``, ``cls_token``, ``mask_token``,
- ``additional_special_tokens``].
-
- Tokens are only added if they are not already in the vocabulary (tested by checking if the tokenizer assign the index of the ``unk_token`` to them).
-
- Returns:
- Number of tokens added to the vocabulary.
-
- Examples::
-
- # Let's see how to add a new classification token to GPT-2
- tokenizer = GPT2Tokenizer.from_pretrained('gpt2')
- model = GPT2Model.from_pretrained('gpt2')
-
- special_tokens_dict = {'cls_token': ''}
-
- num_added_toks = tokenizer.add_special_tokens(special_tokens_dict)
- print('We have added', num_added_toks, 'tokens')
- model.resize_token_embeddings(len(tokenizer)) # Notice: resize_token_embeddings expect to receive the full size of the new vocabulary, i.e. the length of the tokenizer.
-
- assert tokenizer.cls_token == ''
- """
- if not special_tokens_dict:
- return 0
-
- added_tokens = 0
- for key, value in special_tokens_dict.items():
- assert key in self.SPECIAL_TOKENS_ATTRIBUTES
- if key == "additional_special_tokens":
- assert isinstance(value, (list, tuple)) and all(isinstance(t, str) for t in value)
- added_tokens += self.add_tokens(value)
- else:
- assert isinstance(value, str)
- added_tokens += self.add_tokens([value])
- logger.info("Assigning %s to the %s key of the tokenizer", value, key)
- setattr(self, key, value)
-
- return added_tokens
-
- def tokenize(self, text, **kwargs):
- """ Converts a string in a sequence of tokens (string), using the tokenizer.
- Split in words for word-based vocabulary or sub-words for sub-word-based
- vocabularies (BPE/SentencePieces/WordPieces).
-
- Take care of added tokens.
-
- text: The sequence to be encoded.
- **kwargs: passed to the child `self.tokenize()` method
- """
- all_special_tokens = self.all_special_tokens
-
- def lowercase_text(t):
- # convert non-special tokens to lowercase
- escaped_special_toks = [re.escape(s_tok) for s_tok in all_special_tokens]
- pattern = r"(" + r"|".join(escaped_special_toks) + r")|" + r"(.+?)"
- return re.sub(pattern, lambda m: m.groups()[0] or m.groups()[1].lower(), t)
-
- if self.init_kwargs.get("do_lower_case", False):
- text = lowercase_text(text)
-
- def split_on_token(tok, text):
- result = []
- split_text = text.split(tok)
- for i, sub_text in enumerate(split_text):
- sub_text = sub_text.strip()
- if i == 0 and not sub_text:
- result += [tok]
- elif i == len(split_text) - 1:
- if sub_text:
- result += [sub_text]
- else:
- pass
- else:
- if sub_text:
- result += [sub_text]
- result += [tok]
- return result
-
- def split_on_tokens(tok_list, text):
- if not text.strip():
- return []
- if not tok_list:
- return self._tokenize(text, **kwargs)
-
- tokenized_text = []
- text_list = [text]
- for tok in tok_list:
- tokenized_text = []
- for sub_text in text_list:
- if sub_text not in self.unique_added_tokens_encoder:
- tokenized_text += split_on_token(tok, sub_text)
- else:
- tokenized_text += [sub_text]
- text_list = tokenized_text
-
- return list(
- itertools.chain.from_iterable(
- (
- self._tokenize(token, **kwargs) if token not in self.unique_added_tokens_encoder else [token]
- for token in tokenized_text
- )
- )
- )
-
- added_tokens = self.unique_added_tokens_encoder
- tokenized_text = split_on_tokens(added_tokens, text)
- return tokenized_text
-
- def _tokenize(self, text, **kwargs):
- """ Converts a string in a sequence of tokens (string), using the tokenizer.
- Split in words for word-based vocabulary or sub-words for sub-word-based
- vocabularies (BPE/SentencePieces/WordPieces).
-
- Do NOT take care of added tokens.
- """
- raise NotImplementedError
-
- def convert_tokens_to_ids(self, tokens):
- """ Converts a single token, or a sequence of tokens, (str) in a single integer id
- (resp. a sequence of ids), using the vocabulary.
- """
- if tokens is None:
- return None
-
- if isinstance(tokens, str):
- return self._convert_token_to_id_with_added_voc(tokens)
-
- ids = []
- for token in tokens:
- ids.append(self._convert_token_to_id_with_added_voc(token))
- return ids
-
- def _convert_token_to_id_with_added_voc(self, token):
- if token is None:
- return None
-
- if token in self.added_tokens_encoder:
- return self.added_tokens_encoder[token]
- return self._convert_token_to_id(token)
-
- def _convert_token_to_id(self, token):
- raise NotImplementedError
-
- def encode(
- self,
- text,
- text_pair=None,
- add_special_tokens=True,
- max_length=None,
- stride=0,
- truncation_strategy="longest_first",
- pad_to_max_length=False,
- return_tensors=None,
- **kwargs
- ):
- """
- Converts a string in a sequence of ids (integer), using the tokenizer and vocabulary.
-
- Same as doing ``self.convert_tokens_to_ids(self.tokenize(text))``.
-
- Args:
- text: The first sequence to be encoded. This can be a string, a list of strings (tokenized string using
- the `tokenize` method) or a list of integers (tokenized string ids using the `convert_tokens_to_ids`
- method)
- text_pair: Optional second sequence to be encoded. This can be a string, a list of strings (tokenized
- string using the `tokenize` method) or a list of integers (tokenized string ids using the
- `convert_tokens_to_ids` method)
- add_special_tokens: if set to ``True``, the sequences will be encoded with the special tokens relative
- to their model.
- max_length: if set to a number, will limit the total sequence returned so that it has a maximum length.
- If there are overflowing tokens, those will be added to the returned dictionary
- stride: if set to a number along with max_length, the overflowing tokens returned will contain some tokens
- from the main sequence returned. The value of this argument defines the number of additional tokens.
- truncation_strategy: string selected in the following options:
- - 'longest_first' (default) Iteratively reduce the inputs sequence until the input is under max_length
- starting from the longest one at each token (when there is a pair of input sequences)
- - 'only_first': Only truncate the first sequence
- - 'only_second': Only truncate the second sequence
- - 'do_not_truncate': Does not truncate (raise an error if the input sequence is longer than max_length)
- pad_to_max_length: if set to True, the returned sequences will be padded according to the model's padding side and
- padding index, up to their max length. If no max length is specified, the padding is done up to the model's max length.
- The tokenizer padding sides are handled by the class attribute `padding_side` which can be set to the following strings:
- - 'left': pads on the left of the sequences
- - 'right': pads on the right of the sequences
- Defaults to False: no padding.
- return_tensors: (optional) can be set to 'tf' or 'pt' to return respectively TensorFlow tf.constant
- or PyTorch torch.Tensor instead of a list of python integers.
- **kwargs: passed to the `self.tokenize()` method
- """
- encoded_inputs = self.encode_plus(
- text,
- text_pair=text_pair,
- max_length=max_length,
- add_special_tokens=add_special_tokens,
- stride=stride,
- truncation_strategy=truncation_strategy,
- pad_to_max_length=pad_to_max_length,
- return_tensors=return_tensors,
- **kwargs,
- )
-
- return encoded_inputs["input_ids"]
-
- def encode_plus(
- self,
- text,
- text_pair=None,
- add_special_tokens=True,
- max_length=None,
- stride=0,
- truncation_strategy="longest_first",
- pad_to_max_length=False,
- return_tensors=None,
- return_token_type_ids=True,
- return_attention_mask=True,
- return_overflowing_tokens=False,
- return_special_tokens_mask=False,
- **kwargs
- ):
- """
- Returns a dictionary containing the encoded sequence or sequence pair and additional informations:
- the mask for sequence classification and the overflowing elements if a ``max_length`` is specified.
-
- Args:
- text: The first sequence to be encoded. This can be a string, a list of strings (tokenized string using
- the `tokenize` method) or a list of integers (tokenized string ids using the `convert_tokens_to_ids`
- method)
- text_pair: Optional second sequence to be encoded. This can be a string, a list of strings (tokenized
- string using the `tokenize` method) or a list of integers (tokenized string ids using the
- `convert_tokens_to_ids` method)
- add_special_tokens: if set to ``True``, the sequences will be encoded with the special tokens relative
- to their model.
- max_length: if set to a number, will limit the total sequence returned so that it has a maximum length.
- If there are overflowing tokens, those will be added to the returned dictionary
- stride: if set to a number along with max_length, the overflowing tokens returned will contain some tokens
- from the main sequence returned. The value of this argument defines the number of additional tokens.
- truncation_strategy: string selected in the following options:
- - 'longest_first' (default) Iteratively reduce the inputs sequence until the input is under max_length
- starting from the longest one at each token (when there is a pair of input sequences)
- - 'only_first': Only truncate the first sequence
- - 'only_second': Only truncate the second sequence
- - 'do_not_truncate': Does not truncate (raise an error if the input sequence is longer than max_length)
- pad_to_max_length: if set to True, the returned sequences will be padded according to the model's padding side and
- padding index, up to their max length. If no max length is specified, the padding is done up to the model's max length.
- The tokenizer padding sides are handled by the class attribute `padding_side` which can be set to the following strings:
- - 'left': pads on the left of the sequences
- - 'right': pads on the right of the sequences
- Defaults to False: no padding.
- return_tensors: (optional) can be set to 'tf' or 'pt' to return respectively TensorFlow tf.constant
- or PyTorch torch.Tensor instead of a list of python integers.
- return_token_type_ids: (optional) Set to False to avoid returning token_type_ids (default True).
- return_attention_mask: (optional) Set to False to avoid returning attention mask (default True)
- return_overflowing_tokens: (optional) Set to True to return overflowing token information (default False).
- return_special_tokens_mask: (optional) Set to True to return special tokens mask information (default False).
- **kwargs: passed to the `self.tokenize()` method
-
- Return:
- A Dictionary of shape::
-
- {
- input_ids: list[int],
- token_type_ids: list[int] if return_token_type_ids is True (default)
- attention_mask: list[int] if return_attention_mask is True (default)
- overflowing_tokens: list[int] if a ``max_length`` is specified and return_overflowing_tokens is True
- num_truncated_tokens: int if a ``max_length`` is specified and return_overflowing_tokens is True
- special_tokens_mask: list[int] if ``add_special_tokens`` if set to ``True`` and return_special_tokens_mask is True
- }
-
- With the fields:
- ``input_ids``: list of token ids to be fed to a model
- ``token_type_ids``: list of token type ids to be fed to a model
- ``attention_mask``: list of indices specifying which tokens should be attended to by the model
- ``overflowing_tokens``: list of overflowing tokens if a max length is specified.
- ``num_truncated_tokens``: number of overflowing tokens a ``max_length`` is specified
- ``special_tokens_mask``: if adding special tokens, this is a list of [0, 1], with 0 specifying special added
- tokens and 1 specifying sequence tokens.
- """
-
- def get_input_ids(text):
- if isinstance(text, str):
- return self.convert_tokens_to_ids(self.tokenize(text, **kwargs))
- elif isinstance(text, (list, tuple)) and len(text) > 0 and isinstance(text[0], str):
- return self.convert_tokens_to_ids(text)
- elif isinstance(text, (list, tuple)) and len(text) > 0 and isinstance(text[0], int):
- return text
- else:
- raise ValueError(
- "Input is not valid. Should be a string, a list/tuple of strings or a list/tuple of integers."
- )
-
- first_ids = get_input_ids(text)
- second_ids = get_input_ids(text_pair) if text_pair is not None else None
-
- return self.prepare_for_model(
- first_ids,
- pair_ids=second_ids,
- max_length=max_length,
- pad_to_max_length=pad_to_max_length,
- add_special_tokens=add_special_tokens,
- stride=stride,
- truncation_strategy=truncation_strategy,
- return_tensors=return_tensors,
- return_attention_mask=return_attention_mask,
- return_token_type_ids=return_token_type_ids,
- return_overflowing_tokens=return_overflowing_tokens,
- return_special_tokens_mask=return_special_tokens_mask,
- )
-
- def batch_encode_plus(
- self,
- batch_text_or_text_pairs=None,
- add_special_tokens=False,
- max_length=None,
- stride=0,
- truncation_strategy="longest_first",
- return_tensors=None,
- return_input_lengths=False,
- return_attention_masks=False,
- **kwargs
- ):
- """
- Returns a dictionary containing the encoded sequence or sequence pair and additional information:
- the mask for sequence classification and the overflowing elements if a ``max_length`` is specified.
-
- Args:
- batch_text_or_text_pairs: Batch of sequences or pair of sequences to be encoded.
- This can be a list of string/string-sequences/int-sequences or a list of pair of
- string/string-sequences/int-sequence (see details in encode_plus)
- add_special_tokens: if set to ``True``, the sequences will be encoded with the special tokens relative
- to their model.
- max_length: if set to a number, will limit the total sequence returned so that it has a maximum length.
- If there are overflowing tokens, those will be added to the returned dictionary`
- stride: if set to a number along with max_length, the overflowing tokens returned will contain some tokens
- from the main sequence returned. The value of this argument defines the number of additional tokens.
- truncation_strategy: string selected in the following options:
- - 'longest_first' (default) Iteratively reduce the inputs sequence until the input is under max_length
- starting from the longest one at each token (when there is a pair of input sequences)
- - 'only_first': Only truncate the first sequence
- - 'only_second': Only truncate the second sequence
- - 'do_not_truncate': Does not truncate (raise an error if the input sequence is longer than max_length)
- return_tensors: (optional) can be set to 'tf' or 'pt' to return respectively TensorFlow tf.constant
- or PyTorch torch.Tensor instead of a list of python integers.
- **kwargs: passed to the `self.tokenize()` method
- """
- batch_outputs = {}
- for ids_or_pair_ids in batch_text_or_text_pairs:
- if isinstance(ids_or_pair_ids, (list, tuple)):
- assert len(ids_or_pair_ids) == 2
- ids, pair_ids = ids_or_pair_ids
- else:
- ids, pair_ids = ids_or_pair_ids, None
- outputs = self.encode_plus(
- ids,
- pair_ids,
- add_special_tokens=add_special_tokens,
- max_length=max_length,
- stride=stride,
- truncation_strategy=truncation_strategy,
- return_tensors=None,
- )
-
- # Append the non-padded length to the output
- if return_input_lengths:
- outputs["input_len"] = len(outputs["input_ids"])
-
- for key, value in outputs.items():
- if key not in batch_outputs:
- batch_outputs[key] = []
- batch_outputs[key].append(value)
-
- # Compute longest sequence size
- max_seq_len = max(map(len, batch_outputs["input_ids"]))
-
- if return_attention_masks:
- # Allow the model to not give any special attention to padded input
- batch_outputs["attention_mask"] = [[0] * len(v) for v in batch_outputs["input_ids"]]
-
- if return_tensors is not None:
-
- # Do the tensor conversion in batch
- for key, value in batch_outputs.items():
-
- padded_value = value
- # verify that the tokenizer has a pad_token_id
- if key != "input_len" and self._pad_token is not None:
- # Padding handle
- padded_value = [
- v + [self.pad_token_id if key == "input_ids" else 1] * (max_seq_len - len(v))
- for v in padded_value
- ]
-
- if return_tensors == "tf" and is_tf_available():
- batch_outputs[key] = tf.constant(padded_value)
- elif return_tensors == "pt" and is_torch_available():
- batch_outputs[key] = torch.tensor(padded_value)
- elif return_tensors is not None:
- logger.warning(
- "Unable to convert output to tensors format {}, PyTorch or TensorFlow is not available.".format(
- return_tensors
- )
- )
-
- # encoder_attention_mask requires 1 for real token, 0 for padding, just invert value
- if return_attention_masks:
- if is_tf_available():
- batch_outputs["attention_mask"] = tf.abs(batch_outputs["attention_mask"] - 1)
- else:
- batch_outputs["attention_mask"] = torch.abs(batch_outputs["attention_mask"] - 1)
-
- return batch_outputs
-
- def prepare_for_model(
- self,
- ids,
- pair_ids=None,
- max_length=None,
- add_special_tokens=True,
- stride=0,
- truncation_strategy="longest_first",
- pad_to_max_length=False,
- return_tensors=None,
- return_token_type_ids=True,
- return_attention_mask=True,
- return_overflowing_tokens=False,
- return_special_tokens_mask=False,
- ):
- """
- Prepares a sequence of input id, or a pair of sequences of inputs ids so that it can be used by the model.
- It adds special tokens, truncates
- sequences if overflowing while taking into account the special tokens and manages a window stride for
- overflowing tokens
-
- Args:
- ids: list of tokenized input ids. Can be obtained from a string by chaining the
- `tokenize` and `convert_tokens_to_ids` methods.
- pair_ids: Optional second list of input ids. Can be obtained from a string by chaining the
- `tokenize` and `convert_tokens_to_ids` methods.
- max_length: maximum length of the returned list. Will truncate by taking into account the special tokens.
- add_special_tokens: if set to ``True``, the sequences will be encoded with the special tokens relative
- to their model.
- stride: window stride for overflowing tokens. Can be useful for edge effect removal when using sequential
- list of inputs.
- truncation_strategy: string selected in the following options:
- - 'longest_first' (default) Iteratively reduce the inputs sequence until the input is under max_length
- starting from the longest one at each token (when there is a pair of input sequences)
- - 'only_first': Only truncate the first sequence
- - 'only_second': Only truncate the second sequence
- - 'do_not_truncate': Does not truncate (raise an error if the input sequence is longer than max_length)
- pad_to_max_length: if set to True, the returned sequences will be padded according to the model's padding side and
- padding index, up to their max length. If no max length is specified, the padding is done up to the model's max length.
- The tokenizer padding sides are handled by the following strings:
- - 'left': pads on the left of the sequences
- - 'right': pads on the right of the sequences
- Defaults to False: no padding.
- return_tensors: (optional) can be set to 'tf' or 'pt' to return respectively TensorFlow tf.constant
- or PyTorch torch.Tensor instead of a list of python integers.
- return_token_type_ids: (optional) Set to False to avoid returning token_type_ids (default True).
- return_attention_mask: (optional) Set to False to avoid returning attention mask (default True)
- return_overflowing_tokens: (optional) Set to True to return overflowing token information (default False).
- return_special_tokens_mask: (optional) Set to True to return special tokens mask information (default False).
-
- Return:
- A Dictionary of shape::
-
- {
- input_ids: list[int],
- token_type_ids: list[int] if return_token_type_ids is True (default)
- overflowing_tokens: list[int] if a ``max_length`` is specified and return_overflowing_tokens is True
- num_truncated_tokens: int if a ``max_length`` is specified and return_overflowing_tokens is True
- special_tokens_mask: list[int] if ``add_special_tokens`` if set to ``True`` and return_special_tokens_mask is True
- }
-
- With the fields:
- ``input_ids``: list of token ids to be fed to a model
- ``token_type_ids``: list of token type ids to be fed to a model
-
- ``overflowing_tokens``: list of overflowing tokens if a max length is specified.
- ``num_truncated_tokens``: number of overflowing tokens a ``max_length`` is specified
- ``special_tokens_mask``: if adding special tokens, this is a list of [0, 1], with 0 specifying special added
- tokens and 1 specifying sequence tokens.
- """
- pair = bool(pair_ids is not None)
- len_ids = len(ids)
- len_pair_ids = len(pair_ids) if pair else 0
-
- encoded_inputs = {}
-
- # Handle max sequence length
- total_len = len_ids + len_pair_ids + (self.num_added_tokens(pair=pair) if add_special_tokens else 0)
- if max_length and total_len > max_length:
- ids, pair_ids, overflowing_tokens = self.truncate_sequences(
- ids,
- pair_ids=pair_ids,
- num_tokens_to_remove=total_len - max_length,
- truncation_strategy=truncation_strategy,
- stride=stride,
- )
- if return_overflowing_tokens:
- encoded_inputs["overflowing_tokens"] = overflowing_tokens
- encoded_inputs["num_truncated_tokens"] = total_len - max_length
-
- # Handle special_tokens
- if add_special_tokens:
- sequence = self.build_inputs_with_special_tokens(ids, pair_ids)
- token_type_ids = self.create_token_type_ids_from_sequences(ids, pair_ids)
- else:
- sequence = ids + pair_ids if pair else ids
- token_type_ids = [0] * len(ids) + ([1] * len(pair_ids) if pair else [])
-
- if return_special_tokens_mask:
- encoded_inputs["special_tokens_mask"] = self.get_special_tokens_mask(ids, pair_ids)
-
- encoded_inputs["input_ids"] = sequence
- if return_token_type_ids:
- encoded_inputs["token_type_ids"] = token_type_ids
-
- if max_length and len(encoded_inputs["input_ids"]) > max_length:
- encoded_inputs["input_ids"] = encoded_inputs["input_ids"][:max_length]
- if return_token_type_ids:
- encoded_inputs["token_type_ids"] = encoded_inputs["token_type_ids"][:max_length]
- if return_special_tokens_mask:
- encoded_inputs["special_tokens_mask"] = encoded_inputs["special_tokens_mask"][:max_length]
-
- if max_length is None and len(encoded_inputs["input_ids"]) > self.max_len:
- logger.warning(
- "Token indices sequence length is longer than the specified maximum sequence length "
- "for this model ({} > {}). Running this sequence through the model will result in "
- "indexing errors".format(len(ids), self.max_len)
- )
-
- needs_to_be_padded = pad_to_max_length and (
- max_length
- and len(encoded_inputs["input_ids"]) < max_length
- or max_length is None
- and len(encoded_inputs["input_ids"]) < self.max_len
- and self.max_len <= 10000
- )
-
- if pad_to_max_length and max_length is None and self.max_len > 10000:
- logger.warning(
- "Sequence can't be padded as no maximum length is specified and the model maximum length is too high."
- )
-
- if needs_to_be_padded:
- difference = (max_length if max_length is not None else self.max_len) - len(encoded_inputs["input_ids"])
-
- if self.padding_side == "right":
- if return_attention_mask:
- encoded_inputs["attention_mask"] = [1] * len(encoded_inputs["input_ids"]) + [0] * difference
- if return_token_type_ids:
- encoded_inputs["token_type_ids"] = (
- encoded_inputs["token_type_ids"] + [self.pad_token_type_id] * difference
- )
- if return_special_tokens_mask:
- encoded_inputs["special_tokens_mask"] = encoded_inputs["special_tokens_mask"] + [1] * difference
- encoded_inputs["input_ids"] = encoded_inputs["input_ids"] + [self.pad_token_id] * difference
- elif self.padding_side == "left":
- if return_attention_mask:
- encoded_inputs["attention_mask"] = [0] * difference + [1] * len(encoded_inputs["input_ids"])
- if return_token_type_ids:
- encoded_inputs["token_type_ids"] = [self.pad_token_type_id] * difference + encoded_inputs[
- "token_type_ids"
- ]
- if return_special_tokens_mask:
- encoded_inputs["special_tokens_mask"] = [1] * difference + encoded_inputs["special_tokens_mask"]
- encoded_inputs["input_ids"] = [self.pad_token_id] * difference + encoded_inputs["input_ids"]
-
- else:
- raise ValueError("Invalid padding strategy:" + str(self.padding_side))
-
- elif return_attention_mask:
- encoded_inputs["attention_mask"] = [1] * len(encoded_inputs["input_ids"])
-
- # Prepare inputs as tensors if asked
- if return_tensors == "tf" and is_tf_available():
- encoded_inputs["input_ids"] = tf.constant([encoded_inputs["input_ids"]])
-
- if "token_type_ids" in encoded_inputs:
- encoded_inputs["token_type_ids"] = tf.constant([encoded_inputs["token_type_ids"]])
-
- if "attention_mask" in encoded_inputs:
- encoded_inputs["attention_mask"] = tf.constant([encoded_inputs["attention_mask"]])
-
- elif return_tensors == "pt" and is_torch_available():
- encoded_inputs["input_ids"] = torch.tensor([encoded_inputs["input_ids"]])
-
- if "token_type_ids" in encoded_inputs:
- encoded_inputs["token_type_ids"] = torch.tensor([encoded_inputs["token_type_ids"]])
-
- if "attention_mask" in encoded_inputs:
- encoded_inputs["attention_mask"] = torch.tensor([encoded_inputs["attention_mask"]])
- elif return_tensors is not None:
- logger.warning(
- "Unable to convert output to tensors format {}, PyTorch or TensorFlow is not available.".format(
- return_tensors
- )
- )
-
- return encoded_inputs
-
- def truncate_sequences(
- self, ids, pair_ids=None, num_tokens_to_remove=0, truncation_strategy="longest_first", stride=0
- ):
- """Truncates a sequence pair in place to the maximum length.
- truncation_strategy: string selected in the following options:
- - 'longest_first' (default) Iteratively reduce the inputs sequence until the input is under max_length
- starting from the longest one at each token (when there is a pair of input sequences).
- Overflowing tokens only contains overflow from the first sequence.
- - 'only_first': Only truncate the first sequence. raise an error if the first sequence is shorter or equal to than num_tokens_to_remove.
- - 'only_second': Only truncate the second sequence
- - 'do_not_truncate': Does not truncate (raise an error if the input sequence is longer than max_length)
- """
- if num_tokens_to_remove <= 0:
- return ids, pair_ids, []
-
- if truncation_strategy == "longest_first":
- overflowing_tokens = []
- for _ in range(num_tokens_to_remove):
- if pair_ids is None or len(ids) > len(pair_ids):
- overflowing_tokens = [ids[-1]] + overflowing_tokens
- ids = ids[:-1]
- else:
- pair_ids = pair_ids[:-1]
- window_len = min(len(ids), stride)
- if window_len > 0:
- overflowing_tokens = ids[-window_len:] + overflowing_tokens
- elif truncation_strategy == "only_first":
- assert len(ids) > num_tokens_to_remove
- window_len = min(len(ids), stride + num_tokens_to_remove)
- overflowing_tokens = ids[-window_len:]
- ids = ids[:-num_tokens_to_remove]
- elif truncation_strategy == "only_second":
- assert pair_ids is not None and len(pair_ids) > num_tokens_to_remove
- window_len = min(len(pair_ids), stride + num_tokens_to_remove)
- overflowing_tokens = pair_ids[-window_len:]
- pair_ids = pair_ids[:-num_tokens_to_remove]
- elif truncation_strategy == "do_not_truncate":
- raise ValueError("Input sequence are too long for max_length. Please select a truncation strategy.")
- else:
- raise ValueError(
- "Truncation_strategy should be selected in ['longest_first', 'only_first', 'only_second', 'do_not_truncate']"
- )
- return (ids, pair_ids, overflowing_tokens)
-
- def create_token_type_ids_from_sequences(self, token_ids_0, token_ids_1=None):
- if token_ids_1 is None:
- return len(token_ids_0) * [0]
- return [0] * len(token_ids_0) + [1] * len(token_ids_1)
-
- def build_inputs_with_special_tokens(self, token_ids_0, token_ids_1=None):
- """
- Build model inputs from a sequence or a pair of sequence for sequence classification tasks
- by concatenating and adding special tokens.
- A RoBERTa sequence has the following format:
- single sequence: X
- pair of sequences: A B
- """
- if token_ids_1 is None:
- return token_ids_0
- return token_ids_0 + token_ids_1
-
- def get_special_tokens_mask(self, token_ids_0, token_ids_1=None, already_has_special_tokens=False):
- """
- Retrieves sequence ids from a token list that has no special tokens added. This method is called when adding
- special tokens using the tokenizer ``prepare_for_model`` or ``encode_plus`` methods.
-
- Args:
- token_ids_0: list of ids (must not contain special tokens)
- token_ids_1: Optional list of ids (must not contain special tokens), necessary when fetching sequence ids
- for sequence pairs
- already_has_special_tokens: (default False) Set to True if the token list is already formated with
- special tokens for the model
-
- Returns:
- A list of integers in the range [0, 1]: 1 for a special token, 0 for a sequence token.
- """
- return [0] * ((len(token_ids_1) if token_ids_1 else 0) + len(token_ids_0))
-
- def convert_ids_to_tokens(self, ids, skip_special_tokens=False):
- """ Converts a single index or a sequence of indices (integers) in a token "
- (resp.) a sequence of tokens (str), using the vocabulary and added tokens.
-
- Args:
- skip_special_tokens: Don't decode special tokens (self.all_special_tokens). Default: False
- """
- if isinstance(ids, int):
- if ids in self.added_tokens_decoder:
- return self.added_tokens_decoder[ids]
- else:
- return self._convert_id_to_token(ids)
- tokens = []
- for index in ids:
- index = int(index)
- if skip_special_tokens and index in self.all_special_ids:
- continue
- if index in self.added_tokens_decoder:
- tokens.append(self.added_tokens_decoder[index])
- else:
- tokens.append(self._convert_id_to_token(index))
- return tokens
-
- def _convert_id_to_token(self, index):
- raise NotImplementedError
-
- def convert_tokens_to_string(self, tokens):
- """ Converts a sequence of tokens (string) in a single string.
- The most simple way to do it is ' '.join(self.convert_ids_to_tokens(token_ids))
- but we often want to remove sub-word tokenization artifacts at the same time.
- """
- return " ".join(self.convert_ids_to_tokens(tokens))
-
- def decode(self, token_ids, skip_special_tokens=False, clean_up_tokenization_spaces=True):
- """
- Converts a sequence of ids (integer) in a string, using the tokenizer and vocabulary
- with options to remove special tokens and clean up tokenization spaces.
- Similar to doing ``self.convert_tokens_to_string(self.convert_ids_to_tokens(token_ids))``.
-
- Args:
- token_ids: list of tokenized input ids. Can be obtained using the `encode` or `encode_plus` methods.
- skip_special_tokens: if set to True, will replace special tokens.
- clean_up_tokenization_spaces: if set to True, will clean up the tokenization spaces.
- """
- filtered_tokens = self.convert_ids_to_tokens(token_ids, skip_special_tokens=skip_special_tokens)
-
- # To avoid mixing byte-level and unicode for byte-level BPT
- # we need to build string separatly for added tokens and byte-level tokens
- # cf. https://github.com/huggingface/transformers/issues/1133
- sub_texts = []
- current_sub_text = []
- for token in filtered_tokens:
- if skip_special_tokens and token in self.all_special_ids:
- continue
- if token in self.added_tokens_encoder:
- if current_sub_text:
- sub_texts.append(self.convert_tokens_to_string(current_sub_text))
- current_sub_text = []
- sub_texts.append(token)
- else:
- current_sub_text.append(token)
- if current_sub_text:
- sub_texts.append(self.convert_tokens_to_string(current_sub_text))
- text = " ".join(sub_texts)
-
- if clean_up_tokenization_spaces:
- clean_text = self.clean_up_tokenization(text)
- return clean_text
- else:
- return text
-
- @property
- def special_tokens_map(self):
- """ A dictionary mapping special token class attribute (cls_token, unk_token...) to their
- values ('', ''...)
- """
- set_attr = {}
- for attr in self.SPECIAL_TOKENS_ATTRIBUTES:
- attr_value = getattr(self, "_" + attr)
- if attr_value:
- set_attr[attr] = attr_value
- return set_attr
-
- @property
- def all_special_tokens(self):
- """ List all the special tokens ('', ''...) mapped to class attributes
- (cls_token, unk_token...).
- """
- all_toks = []
- set_attr = self.special_tokens_map
- for attr_value in set_attr.values():
- all_toks = all_toks + (list(attr_value) if isinstance(attr_value, (list, tuple)) else [attr_value])
- all_toks = list(set(all_toks))
- return all_toks
-
- @property
- def all_special_ids(self):
- """ List the vocabulary indices of the special tokens ('', ''...) mapped to
- class attributes (cls_token, unk_token...).
- """
- all_toks = self.all_special_tokens
- all_ids = self.convert_tokens_to_ids(all_toks)
- return all_ids
-
- @staticmethod
- def clean_up_tokenization(out_string):
- """ Clean up a list of simple English tokenization artifacts like spaces before punctuations and abreviated forms.
- """
- out_string = (
- out_string.replace(" .", ".")
- .replace(" ?", "?")
- .replace(" !", "!")
- .replace(" ,", ",")
- .replace(" ' ", "'")
- .replace(" n't", "n't")
- .replace(" 'm", "'m")
- .replace(" do not", " don't")
- .replace(" 's", "'s")
- .replace(" 've", "'ve")
- .replace(" 're", "'re")
- )
- return out_string
-
-
-class PreTrainedTokenizerFast(PreTrainedTokenizer):
- _tokenizer = None
- _decoder = None
-
- def __init__(self, **kwargs):
- super().__init__(**kwargs)
-
- @property
- def tokenizer(self):
- if self._tokenizer is None:
- raise NotImplementedError
- return self._tokenizer
-
- @property
- def decoder(self):
- if self._decoder is None:
- raise NotImplementedError
- return self._decoder
-
- @property
- def vocab_size(self):
- return self.tokenizer.get_vocab_size(with_added_tokens=False)
-
- def __len__(self):
- return self.tokenizer.get_vocab_size(with_added_tokens=True)
-
- @PreTrainedTokenizer.bos_token.setter
- def bos_token(self, value):
- self._bos_token = value
- self._update_special_tokens()
-
- @PreTrainedTokenizer.eos_token.setter
- def eos_token(self, value):
- self._eos_token = value
- self._update_special_tokens()
-
- @PreTrainedTokenizer.unk_token.setter
- def unk_token(self, value):
- self._unk_token = value
- self._update_special_tokens()
-
- @PreTrainedTokenizer.sep_token.setter
- def sep_token(self, value):
- self._sep_token = value
- self._update_special_tokens()
-
- @PreTrainedTokenizer.pad_token.setter
- def pad_token(self, value):
- self._pad_token = value
- self._update_special_tokens()
-
- @PreTrainedTokenizer.cls_token.setter
- def cls_token(self, value):
- self._cls_token = value
- self._update_special_tokens()
-
- @PreTrainedTokenizer.mask_token.setter
- def mask_token(self, value):
- self._mask_token = value
- self._update_special_tokens()
-
- @PreTrainedTokenizer.additional_special_tokens.setter
- def additional_special_tokens(self, value):
- self._additional_special_tokens = value
- self._update_special_tokens()
-
- def _update_special_tokens(self):
- if self._tokenizer is not None:
- self._tokenizer.add_special_tokens(self.all_special_tokens)
-
- @staticmethod
- def _convert_encoding(
- encoding,
- return_tensors=None,
- return_token_type_ids=True,
- return_attention_mask=True,
- return_overflowing_tokens=False,
- return_special_tokens_mask=False,
- ):
- encoding_dict = {
- "input_ids": encoding.ids,
- }
- if return_token_type_ids:
- encoding_dict["token_type_ids"] = encoding.type_ids
- if return_attention_mask:
- encoding_dict["attention_mask"] = encoding.attention_mask
- if return_overflowing_tokens:
- overflowing = encoding.overflowing
- encoding_dict["overflowing_tokens"] = overflowing.ids if overflowing is not None else []
- if return_special_tokens_mask:
- encoding_dict["special_tokens_mask"] = encoding.special_tokens_mask
-
- # Prepare inputs as tensors if asked
- if return_tensors == "tf" and is_tf_available():
- encoding_dict["input_ids"] = tf.constant([encoding_dict["input_ids"]])
- if "token_type_ids" in encoding_dict:
- encoding_dict["token_type_ids"] = tf.constant([encoding_dict["token_type_ids"]])
-
- if "attention_mask" in encoding_dict:
- encoding_dict["attention_mask"] = tf.constant([encoding_dict["attention_mask"]])
-
- elif return_tensors == "pt" and is_torch_available():
- encoding_dict["input_ids"] = torch.tensor([encoding_dict["input_ids"]])
- if "token_type_ids" in encoding_dict:
- encoding_dict["token_type_ids"] = torch.tensor([encoding_dict["token_type_ids"]])
-
- if "attention_mask" in encoding_dict:
- encoding_dict["attention_mask"] = torch.tensor([encoding_dict["attention_mask"]])
- elif return_tensors is not None:
- logger.warning(
- "Unable to convert output to tensors format {}, PyTorch or TensorFlow is not available.".format(
- return_tensors
- )
- )
-
- return encoding_dict
-
- def encode_plus(
- self,
- text,
- text_pair=None,
- return_tensors=None,
- return_token_type_ids=True,
- return_attention_mask=True,
- return_overflowing_tokens=False,
- return_special_tokens_mask=False,
- **kwargs
- ):
- encoding = self.tokenizer.encode(text, text_pair)
- return self._convert_encoding(
- encoding,
- return_tensors=return_tensors,
- return_token_type_ids=return_token_type_ids,
- return_attention_mask=return_attention_mask,
- return_overflowing_tokens=return_overflowing_tokens,
- return_special_tokens_mask=return_special_tokens_mask,
- )
-
- def tokenize(self, text):
- return self.tokenizer.encode(text).tokens
-
- def _convert_token_to_id_with_added_voc(self, token):
- id = self.tokenizer.token_to_id(token)
- if id is None:
- return self.unk_token_id
- return id
-
- def _convert_id_to_token(self, index):
- return self.tokenizer.id_to_token(int(index))
-
- def convert_tokens_to_string(self, tokens):
- return self.decoder.decode(tokens)
-
- def add_tokens(self, new_tokens):
- self.tokenizer.add_tokens(new_tokens)
-
- def add_special_tokens(self, special_tokens_dict):
- added = super().add_special_tokens(special_tokens_dict)
- self._update_special_tokens()
- return added
-
- def encode_batch(
- self,
- texts,
- return_tensors=None,
- return_token_type_ids=True,
- return_attention_mask=True,
- return_overflowing_tokens=False,
- return_special_tokens_mask=False,
- ):
- return [
- self._convert_encoding(
- encoding,
- return_tensors=return_tensors,
- return_token_type_ids=return_token_type_ids,
- return_attention_mask=return_attention_mask,
- return_overflowing_tokens=return_overflowing_tokens,
- return_special_tokens_mask=return_special_tokens_mask,
- )
- for encoding in self.tokenizer.encode_batch(texts)
- ]
-
- def decode(self, token_ids, skip_special_tokens=False, clean_up_tokenization_spaces=True):
- text = self.tokenizer.decode(token_ids, skip_special_tokens)
-
- if clean_up_tokenization_spaces:
- clean_text = self.clean_up_tokenization(text)
- return clean_text
- else:
- return text
-
- def decode_batch(self, ids_batch, skip_special_tokens=False, clear_up_tokenization_spaces=True):
- return [
- self.clean_up_tokenization(text) if clear_up_tokenization_spaces else text
- for text in self.tokenizer.decode_batch(ids_batch, skip_special_tokens)
- ]
diff --git a/server/transformers/src/transformers/tokenization_xlm.py b/server/transformers/src/transformers/tokenization_xlm.py
deleted file mode 100644
index 518f3dd7ffbff955830e07be02a561d53e3a060e..0000000000000000000000000000000000000000
--- a/server/transformers/src/transformers/tokenization_xlm.py
+++ /dev/null
@@ -1,892 +0,0 @@
-# coding=utf-8
-# Copyright 2019 The Open AI Team Authors and The HuggingFace Inc. team.
-#
-# Licensed under the Apache License, Version 2.0 (the "License");
-# you may not use this file except in compliance with the License.
-# You may obtain a copy of the License at
-#
-# http://www.apache.org/licenses/LICENSE-2.0
-#
-# Unless required by applicable law or agreed to in writing, software
-# distributed under the License is distributed on an "AS IS" BASIS,
-# WITHOUT WARRANTIES OR CONDITIONS OF ANY KIND, either express or implied.
-# See the License for the specific language governing permissions and
-# limitations under the License.
-"""Tokenization classes for XLM."""
-
-
-import json
-import logging
-import os
-import re
-import sys
-import unicodedata
-
-import sacremoses as sm
-
-from .tokenization_utils import PreTrainedTokenizer
-
-
-logger = logging.getLogger(__name__)
-
-VOCAB_FILES_NAMES = {
- "vocab_file": "vocab.json",
- "merges_file": "merges.txt",
-}
-
-PRETRAINED_VOCAB_FILES_MAP = {
- "vocab_file": {
- "xlm-mlm-en-2048": "https://s3.amazonaws.com/models.huggingface.co/bert/xlm-mlm-en-2048-vocab.json",
- "xlm-mlm-ende-1024": "https://s3.amazonaws.com/models.huggingface.co/bert/xlm-mlm-ende-1024-vocab.json",
- "xlm-mlm-enfr-1024": "https://s3.amazonaws.com/models.huggingface.co/bert/xlm-mlm-enfr-1024-vocab.json",
- "xlm-mlm-enro-1024": "https://s3.amazonaws.com/models.huggingface.co/bert/xlm-mlm-enro-1024-vocab.json",
- "xlm-mlm-tlm-xnli15-1024": "https://s3.amazonaws.com/models.huggingface.co/bert/xlm-mlm-tlm-xnli15-1024-vocab.json",
- "xlm-mlm-xnli15-1024": "https://s3.amazonaws.com/models.huggingface.co/bert/xlm-mlm-xnli15-1024-vocab.json",
- "xlm-clm-enfr-1024": "https://s3.amazonaws.com/models.huggingface.co/bert/xlm-clm-enfr-1024-vocab.json",
- "xlm-clm-ende-1024": "https://s3.amazonaws.com/models.huggingface.co/bert/xlm-clm-ende-1024-vocab.json",
- "xlm-mlm-17-1280": "https://s3.amazonaws.com/models.huggingface.co/bert/xlm-mlm-17-1280-vocab.json",
- "xlm-mlm-100-1280": "https://s3.amazonaws.com/models.huggingface.co/bert/xlm-mlm-100-1280-vocab.json",
- },
- "merges_file": {
- "xlm-mlm-en-2048": "https://s3.amazonaws.com/models.huggingface.co/bert/xlm-mlm-en-2048-merges.txt",
- "xlm-mlm-ende-1024": "https://s3.amazonaws.com/models.huggingface.co/bert/xlm-mlm-ende-1024-merges.txt",
- "xlm-mlm-enfr-1024": "https://s3.amazonaws.com/models.huggingface.co/bert/xlm-mlm-enfr-1024-merges.txt",
- "xlm-mlm-enro-1024": "https://s3.amazonaws.com/models.huggingface.co/bert/xlm-mlm-enro-1024-merges.txt",
- "xlm-mlm-tlm-xnli15-1024": "https://s3.amazonaws.com/models.huggingface.co/bert/xlm-mlm-tlm-xnli15-1024-merges.txt",
- "xlm-mlm-xnli15-1024": "https://s3.amazonaws.com/models.huggingface.co/bert/xlm-mlm-xnli15-1024-merges.txt",
- "xlm-clm-enfr-1024": "https://s3.amazonaws.com/models.huggingface.co/bert/xlm-mlm-enfr-1024-merges.txt",
- "xlm-clm-ende-1024": "https://s3.amazonaws.com/models.huggingface.co/bert/xlm-mlm-ende-1024-merges.txt",
- "xlm-mlm-17-1280": "https://s3.amazonaws.com/models.huggingface.co/bert/xlm-mlm-17-1280-merges.txt",
- "xlm-mlm-100-1280": "https://s3.amazonaws.com/models.huggingface.co/bert/xlm-mlm-100-1280-merges.txt",
- },
-}
-
-PRETRAINED_POSITIONAL_EMBEDDINGS_SIZES = {
- "xlm-mlm-en-2048": 512,
- "xlm-mlm-ende-1024": 512,
- "xlm-mlm-enfr-1024": 512,
- "xlm-mlm-enro-1024": 512,
- "xlm-mlm-tlm-xnli15-1024": 512,
- "xlm-mlm-xnli15-1024": 512,
- "xlm-clm-enfr-1024": 512,
- "xlm-clm-ende-1024": 512,
- "xlm-mlm-17-1280": 512,
- "xlm-mlm-100-1280": 512,
-}
-
-PRETRAINED_INIT_CONFIGURATION = {
- "xlm-mlm-en-2048": {"do_lowercase_and_remove_accent": True},
- "xlm-mlm-ende-1024": {
- "do_lowercase_and_remove_accent": True,
- "id2lang": {"0": "de", "1": "en"},
- "lang2id": {"de": 0, "en": 1},
- },
- "xlm-mlm-enfr-1024": {
- "do_lowercase_and_remove_accent": True,
- "id2lang": {"0": "en", "1": "fr"},
- "lang2id": {"en": 0, "fr": 1},
- },
- "xlm-mlm-enro-1024": {
- "do_lowercase_and_remove_accent": True,
- "id2lang": {"0": "en", "1": "ro"},
- "lang2id": {"en": 0, "ro": 1},
- },
- "xlm-mlm-tlm-xnli15-1024": {
- "do_lowercase_and_remove_accent": True,
- "id2lang": {
- "0": "ar",
- "1": "bg",
- "2": "de",
- "3": "el",
- "4": "en",
- "5": "es",
- "6": "fr",
- "7": "hi",
- "8": "ru",
- "9": "sw",
- "10": "th",
- "11": "tr",
- "12": "ur",
- "13": "vi",
- "14": "zh",
- },
- "lang2id": {
- "ar": 0,
- "bg": 1,
- "de": 2,
- "el": 3,
- "en": 4,
- "es": 5,
- "fr": 6,
- "hi": 7,
- "ru": 8,
- "sw": 9,
- "th": 10,
- "tr": 11,
- "ur": 12,
- "vi": 13,
- "zh": 14,
- },
- },
- "xlm-mlm-xnli15-1024": {
- "do_lowercase_and_remove_accent": True,
- "id2lang": {
- "0": "ar",
- "1": "bg",
- "2": "de",
- "3": "el",
- "4": "en",
- "5": "es",
- "6": "fr",
- "7": "hi",
- "8": "ru",
- "9": "sw",
- "10": "th",
- "11": "tr",
- "12": "ur",
- "13": "vi",
- "14": "zh",
- },
- "lang2id": {
- "ar": 0,
- "bg": 1,
- "de": 2,
- "el": 3,
- "en": 4,
- "es": 5,
- "fr": 6,
- "hi": 7,
- "ru": 8,
- "sw": 9,
- "th": 10,
- "tr": 11,
- "ur": 12,
- "vi": 13,
- "zh": 14,
- },
- },
- "xlm-clm-enfr-1024": {
- "do_lowercase_and_remove_accent": True,
- "id2lang": {"0": "en", "1": "fr"},
- "lang2id": {"en": 0, "fr": 1},
- },
- "xlm-clm-ende-1024": {
- "do_lowercase_and_remove_accent": True,
- "id2lang": {"0": "de", "1": "en"},
- "lang2id": {"de": 0, "en": 1},
- },
- "xlm-mlm-17-1280": {
- "do_lowercase_and_remove_accent": False,
- "id2lang": {
- "0": "ar",
- "1": "de",
- "2": "en",
- "3": "es",
- "4": "fr",
- "5": "hi",
- "6": "it",
- "7": "ja",
- "8": "ko",
- "9": "nl",
- "10": "pl",
- "11": "pt",
- "12": "ru",
- "13": "sv",
- "14": "tr",
- "15": "vi",
- "16": "zh",
- },
- "lang2id": {
- "ar": 0,
- "de": 1,
- "en": 2,
- "es": 3,
- "fr": 4,
- "hi": 5,
- "it": 6,
- "ja": 7,
- "ko": 8,
- "nl": 9,
- "pl": 10,
- "pt": 11,
- "ru": 12,
- "sv": 13,
- "tr": 14,
- "vi": 15,
- "zh": 16,
- },
- },
- "xlm-mlm-100-1280": {
- "do_lowercase_and_remove_accent": False,
- "id2lang": {
- "0": "af",
- "1": "als",
- "2": "am",
- "3": "an",
- "4": "ang",
- "5": "ar",
- "6": "arz",
- "7": "ast",
- "8": "az",
- "9": "bar",
- "10": "be",
- "11": "bg",
- "12": "bn",
- "13": "br",
- "14": "bs",
- "15": "ca",
- "16": "ceb",
- "17": "ckb",
- "18": "cs",
- "19": "cy",
- "20": "da",
- "21": "de",
- "22": "el",
- "23": "en",
- "24": "eo",
- "25": "es",
- "26": "et",
- "27": "eu",
- "28": "fa",
- "29": "fi",
- "30": "fr",
- "31": "fy",
- "32": "ga",
- "33": "gan",
- "34": "gl",
- "35": "gu",
- "36": "he",
- "37": "hi",
- "38": "hr",
- "39": "hu",
- "40": "hy",
- "41": "ia",
- "42": "id",
- "43": "is",
- "44": "it",
- "45": "ja",
- "46": "jv",
- "47": "ka",
- "48": "kk",
- "49": "kn",
- "50": "ko",
- "51": "ku",
- "52": "la",
- "53": "lb",
- "54": "lt",
- "55": "lv",
- "56": "mk",
- "57": "ml",
- "58": "mn",
- "59": "mr",
- "60": "ms",
- "61": "my",
- "62": "nds",
- "63": "ne",
- "64": "nl",
- "65": "nn",
- "66": "no",
- "67": "oc",
- "68": "pl",
- "69": "pt",
- "70": "ro",
- "71": "ru",
- "72": "scn",
- "73": "sco",
- "74": "sh",
- "75": "si",
- "76": "simple",
- "77": "sk",
- "78": "sl",
- "79": "sq",
- "80": "sr",
- "81": "sv",
- "82": "sw",
- "83": "ta",
- "84": "te",
- "85": "th",
- "86": "tl",
- "87": "tr",
- "88": "tt",
- "89": "uk",
- "90": "ur",
- "91": "uz",
- "92": "vi",
- "93": "war",
- "94": "wuu",
- "95": "yi",
- "96": "zh",
- "97": "zh_classical",
- "98": "zh_min_nan",
- "99": "zh_yue",
- },
- "lang2id": {
- "af": 0,
- "als": 1,
- "am": 2,
- "an": 3,
- "ang": 4,
- "ar": 5,
- "arz": 6,
- "ast": 7,
- "az": 8,
- "bar": 9,
- "be": 10,
- "bg": 11,
- "bn": 12,
- "br": 13,
- "bs": 14,
- "ca": 15,
- "ceb": 16,
- "ckb": 17,
- "cs": 18,
- "cy": 19,
- "da": 20,
- "de": 21,
- "el": 22,
- "en": 23,
- "eo": 24,
- "es": 25,
- "et": 26,
- "eu": 27,
- "fa": 28,
- "fi": 29,
- "fr": 30,
- "fy": 31,
- "ga": 32,
- "gan": 33,
- "gl": 34,
- "gu": 35,
- "he": 36,
- "hi": 37,
- "hr": 38,
- "hu": 39,
- "hy": 40,
- "ia": 41,
- "id": 42,
- "is": 43,
- "it": 44,
- "ja": 45,
- "jv": 46,
- "ka": 47,
- "kk": 48,
- "kn": 49,
- "ko": 50,
- "ku": 51,
- "la": 52,
- "lb": 53,
- "lt": 54,
- "lv": 55,
- "mk": 56,
- "ml": 57,
- "mn": 58,
- "mr": 59,
- "ms": 60,
- "my": 61,
- "nds": 62,
- "ne": 63,
- "nl": 64,
- "nn": 65,
- "no": 66,
- "oc": 67,
- "pl": 68,
- "pt": 69,
- "ro": 70,
- "ru": 71,
- "scn": 72,
- "sco": 73,
- "sh": 74,
- "si": 75,
- "simple": 76,
- "sk": 77,
- "sl": 78,
- "sq": 79,
- "sr": 80,
- "sv": 81,
- "sw": 82,
- "ta": 83,
- "te": 84,
- "th": 85,
- "tl": 86,
- "tr": 87,
- "tt": 88,
- "uk": 89,
- "ur": 90,
- "uz": 91,
- "vi": 92,
- "war": 93,
- "wuu": 94,
- "yi": 95,
- "zh": 96,
- "zh_classical": 97,
- "zh_min_nan": 98,
- "zh_yue": 99,
- },
- },
-}
-
-
-def get_pairs(word):
- """
- Return set of symbol pairs in a word.
- word is represented as tuple of symbols (symbols being variable-length strings)
- """
- pairs = set()
- prev_char = word[0]
- for char in word[1:]:
- pairs.add((prev_char, char))
- prev_char = char
- return pairs
-
-
-def lowercase_and_remove_accent(text):
- """
- Lowercase and strips accents from a piece of text based on
- https://github.com/facebookresearch/XLM/blob/master/tools/lowercase_and_remove_accent.py
- """
- text = " ".join(text)
- text = text.lower()
- text = unicodedata.normalize("NFD", text)
- output = []
- for char in text:
- cat = unicodedata.category(char)
- if cat == "Mn":
- continue
- output.append(char)
- return "".join(output).lower().split(" ")
-
-
-def replace_unicode_punct(text):
- """
- Port of https://github.com/moses-smt/mosesdecoder/blob/master/scripts/tokenizer/replace-unicode-punctuation.perl
- """
- text = text.replace(",", ",")
- text = re.sub(r"。\s*", ". ", text)
- text = text.replace("、", ",")
- text = text.replace("”", '"')
- text = text.replace("“", '"')
- text = text.replace("∶", ":")
- text = text.replace(":", ":")
- text = text.replace("?", "?")
- text = text.replace("《", '"')
- text = text.replace("》", '"')
- text = text.replace(")", ")")
- text = text.replace("!", "!")
- text = text.replace("(", "(")
- text = text.replace(";", ";")
- text = text.replace("1", "1")
- text = text.replace("」", '"')
- text = text.replace("「", '"')
- text = text.replace("0", "0")
- text = text.replace("3", "3")
- text = text.replace("2", "2")
- text = text.replace("5", "5")
- text = text.replace("6", "6")
- text = text.replace("9", "9")
- text = text.replace("7", "7")
- text = text.replace("8", "8")
- text = text.replace("4", "4")
- text = re.sub(r".\s*", ". ", text)
- text = text.replace("~", "~")
- text = text.replace("’", "'")
- text = text.replace("…", "...")
- text = text.replace("━", "-")
- text = text.replace("〈", "<")
- text = text.replace("〉", ">")
- text = text.replace("【", "[")
- text = text.replace("】", "]")
- text = text.replace("%", "%")
- return text
-
-
-def remove_non_printing_char(text):
- """
- Port of https://github.com/moses-smt/mosesdecoder/blob/master/scripts/tokenizer/remove-non-printing-char.perl
- """
- output = []
- for char in text:
- cat = unicodedata.category(char)
- if cat.startswith("C"):
- continue
- output.append(char)
- return "".join(output)
-
-
-def romanian_preprocessing(text):
- """Sennrich's WMT16 scripts for Romanian preprocessing, used by model `xlm-mlm-enro-1024`"""
- # https://github.com/rsennrich/wmt16-scripts/blob/master/preprocess/normalise-romanian.py
- text = text.replace("\u015e", "\u0218").replace("\u015f", "\u0219")
- text = text.replace("\u0162", "\u021a").replace("\u0163", "\u021b")
- # https://github.com/rsennrich/wmt16-scripts/blob/master/preprocess/remove-diacritics.py
- text = text.replace("\u0218", "S").replace("\u0219", "s") # s-comma
- text = text.replace("\u021a", "T").replace("\u021b", "t") # t-comma
- text = text.replace("\u0102", "A").replace("\u0103", "a")
- text = text.replace("\u00C2", "A").replace("\u00E2", "a")
- text = text.replace("\u00CE", "I").replace("\u00EE", "i")
- return text
-
-
-class XLMTokenizer(PreTrainedTokenizer):
- """
- BPE tokenizer for XLM
-
- - Moses preprocessing & tokenization for most supported languages
-
- - Language specific tokenization for Chinese (Jieba), Japanese (KyTea) and Thai (PyThaiNLP)
-
- - (optionally) lower case & normalize all inputs text
-
- - argument ``special_tokens`` and function ``set_special_tokens``, can be used to add additional symbols \
- (ex: "__classify__") to a vocabulary
-
- - `lang2id` attribute maps the languages supported by the model with their ids if provided (automatically set for pretrained vocabularies)
-
- - `id2lang` attributes does reverse mapping if provided (automatically set for pretrained vocabularies)
-
- - `do_lowercase_and_remove_accent` controle lower casing and accent (automatically set for pretrained vocabularies)
- """
-
- vocab_files_names = VOCAB_FILES_NAMES
- pretrained_vocab_files_map = PRETRAINED_VOCAB_FILES_MAP
- pretrained_init_configuration = PRETRAINED_INIT_CONFIGURATION
- max_model_input_sizes = PRETRAINED_POSITIONAL_EMBEDDINGS_SIZES
-
- def __init__(
- self,
- vocab_file,
- merges_file,
- unk_token="",
- bos_token="",
- sep_token="",
- pad_token="",
- cls_token="",
- mask_token="",
- additional_special_tokens=[
- "",
- "",
- "",
- "",
- "",
- "",
- "",
- "",
- "",
- "",
- ],
- lang2id=None,
- id2lang=None,
- do_lowercase_and_remove_accent=True,
- **kwargs
- ):
- super().__init__(
- unk_token=unk_token,
- bos_token=bos_token,
- sep_token=sep_token,
- pad_token=pad_token,
- cls_token=cls_token,
- mask_token=mask_token,
- additional_special_tokens=additional_special_tokens,
- **kwargs,
- )
-
- self.max_len_single_sentence = self.max_len - 2 # take into account special tokens
- self.max_len_sentences_pair = self.max_len - 3 # take into account special tokens
-
- # cache of sm.MosesPunctNormalizer instance
- self.cache_moses_punct_normalizer = dict()
- # cache of sm.MosesTokenizer instance
- self.cache_moses_tokenizer = dict()
- self.lang_with_custom_tokenizer = set(["zh", "th", "ja"])
- # True for current supported model (v1.2.0), False for XLM-17 & 100
- self.do_lowercase_and_remove_accent = do_lowercase_and_remove_accent
- self.lang2id = lang2id
- self.id2lang = id2lang
- if lang2id is not None and id2lang is not None:
- assert len(lang2id) == len(id2lang)
-
- self.ja_word_tokenizer = None
- self.zh_word_tokenizer = None
-
- with open(vocab_file, encoding="utf-8") as vocab_handle:
- self.encoder = json.load(vocab_handle)
- self.decoder = {v: k for k, v in self.encoder.items()}
- with open(merges_file, encoding="utf-8") as merges_handle:
- merges = merges_handle.read().split("\n")[:-1]
- merges = [tuple(merge.split()[:2]) for merge in merges]
- self.bpe_ranks = dict(zip(merges, range(len(merges))))
- self.cache = {}
-
- def moses_punct_norm(self, text, lang):
- if lang not in self.cache_moses_punct_normalizer:
- punct_normalizer = sm.MosesPunctNormalizer(lang=lang)
- self.cache_moses_punct_normalizer[lang] = punct_normalizer
- else:
- punct_normalizer = self.cache_moses_punct_normalizer[lang]
- return punct_normalizer.normalize(text)
-
- def moses_tokenize(self, text, lang):
- if lang not in self.cache_moses_tokenizer:
- moses_tokenizer = sm.MosesTokenizer(lang=lang)
- self.cache_moses_tokenizer[lang] = moses_tokenizer
- else:
- moses_tokenizer = self.cache_moses_tokenizer[lang]
- return moses_tokenizer.tokenize(text, return_str=False, escape=False)
-
- def moses_pipeline(self, text, lang):
- text = replace_unicode_punct(text)
- text = self.moses_punct_norm(text, lang)
- text = remove_non_printing_char(text)
- return text
-
- def ja_tokenize(self, text):
- if self.ja_word_tokenizer is None:
- try:
- import Mykytea
-
- self.ja_word_tokenizer = Mykytea.Mykytea(
- "-model %s/local/share/kytea/model.bin" % os.path.expanduser("~")
- )
- except (AttributeError, ImportError):
- logger.error(
- "Make sure you install KyTea (https://github.com/neubig/kytea) and it's python wrapper (https://github.com/chezou/Mykytea-python) with the following steps"
- )
- logger.error("1. git clone git@github.com:neubig/kytea.git && cd kytea")
- logger.error("2. autoreconf -i")
- logger.error("3. ./configure --prefix=$HOME/local")
- logger.error("4. make && make install")
- logger.error("5. pip install kytea")
- raise
- return list(self.ja_word_tokenizer.getWS(text))
-
- @property
- def vocab_size(self):
- return len(self.encoder)
-
- def bpe(self, token):
- word = tuple(token[:-1]) + (token[-1] + "",)
- if token in self.cache:
- return self.cache[token]
- pairs = get_pairs(word)
-
- if not pairs:
- return token + ""
-
- while True:
- bigram = min(pairs, key=lambda pair: self.bpe_ranks.get(pair, float("inf")))
- if bigram not in self.bpe_ranks:
- break
- first, second = bigram
- new_word = []
- i = 0
- while i < len(word):
- try:
- j = word.index(first, i)
- except ValueError:
- new_word.extend(word[i:])
- break
- else:
- new_word.extend(word[i:j])
- i = j
-
- if word[i] == first and i < len(word) - 1 and word[i + 1] == second:
- new_word.append(first + second)
- i += 2
- else:
- new_word.append(word[i])
- i += 1
- new_word = tuple(new_word)
- word = new_word
- if len(word) == 1:
- break
- else:
- pairs = get_pairs(word)
- word = " ".join(word)
- if word == "\n ":
- word = "\n"
- self.cache[token] = word
- return word
-
- def _tokenize(self, text, lang="en", bypass_tokenizer=False):
- """
- Tokenize a string given language code. For Chinese, Japanese and Thai, we use a language specific tokenizerself. Otherwise, we use Moses.
-
- Details of tokenization:
- - [sacremoses](https://github.com/alvations/sacremoses): port of Moses
- - Install with `pip install sacremoses`
- - [pythainlp](https://github.com/PyThaiNLP/pythainlp): Thai tokenizer
- - Install with `pip install pythainlp`
- - [kytea](https://github.com/chezou/Mykytea-python): Japanese tokenizer, wrapper of [KyTea](https://github.com/neubig/kytea)
- - Install with the following steps:
- ```
- git clone git@github.com:neubig/kytea.git && cd kytea
- autoreconf -i
- ./configure --prefix=$HOME/local
- make && make install
- pip install kytea
- ```
- - [jieba](https://github.com/fxsjy/jieba): Chinese tokenizer (*)
- - Install with `pip install jieba`
-
- (*) The original XLM used [Stanford Segmenter](https://nlp.stanford.edu/software/stanford-segmenter-2018-10-16.zip).
- However, the wrapper (`nltk.tokenize.stanford_segmenter`) is slow due to JVM overhead, and it will be deprecated.
- Jieba is a lot faster and pip-installable. Note there is some mismatch with the Stanford Segmenter. It should be fine
- if you fine-tune the model with Chinese supervisionself. If you want the same exact behaviour, use the original XLM
- [preprocessing script](https://github.com/facebookresearch/XLM/tree/master/tools) to tokenize the sentence externally,
- and set `bypass_tokenizer=True` to bypass the tokenizer.
-
- Args:
- - lang: ISO language code (default = 'en') (string). Languages should belong of the model supported languages. However, we don't enforce it.
- - bypass_tokenizer: Allow users to preprocess and tokenize the sentences externally (default = False) (bool). If True, we only apply BPE.
-
- Returns:
- List of tokens.
- """
- if lang and self.lang2id and lang not in self.lang2id:
- logger.error(
- "Supplied language code not found in lang2id mapping. Please check that your language is supported by the loaded pretrained model."
- )
- if bypass_tokenizer:
- text = text.split()
- elif lang not in self.lang_with_custom_tokenizer:
- text = self.moses_pipeline(text, lang=lang)
- # TODO: make sure we are using `xlm-mlm-enro-1024`, since XLM-100 doesn't have this step
- if lang == "ro":
- text = romanian_preprocessing(text)
- text = self.moses_tokenize(text, lang=lang)
- elif lang == "th":
- text = self.moses_pipeline(text, lang=lang)
- try:
- if "pythainlp" not in sys.modules:
- from pythainlp.tokenize import word_tokenize as th_word_tokenize
- else:
- th_word_tokenize = sys.modules["pythainlp"].word_tokenize
- except (AttributeError, ImportError):
- logger.error(
- "Make sure you install PyThaiNLP (https://github.com/PyThaiNLP/pythainlp) with the following steps"
- )
- logger.error("1. pip install pythainlp")
- raise
- text = th_word_tokenize(text)
- elif lang == "zh":
- try:
- if "jieba" not in sys.modules:
- import jieba
- else:
- jieba = sys.modules["jieba"]
- except (AttributeError, ImportError):
- logger.error("Make sure you install Jieba (https://github.com/fxsjy/jieba) with the following steps")
- logger.error("1. pip install jieba")
- raise
- text = " ".join(jieba.cut(text))
- text = self.moses_pipeline(text, lang=lang)
- text = text.split()
- elif lang == "ja":
- text = self.moses_pipeline(text, lang=lang)
- text = self.ja_tokenize(text)
- else:
- raise ValueError("It should not reach here")
-
- if self.do_lowercase_and_remove_accent and not bypass_tokenizer:
- text = lowercase_and_remove_accent(text)
-
- split_tokens = []
- for token in text:
- if token:
- split_tokens.extend([t for t in self.bpe(token).split(" ")])
-
- return split_tokens
-
- def _convert_token_to_id(self, token):
- """ Converts a token (str) in an id using the vocab. """
- return self.encoder.get(token, self.encoder.get(self.unk_token))
-
- def _convert_id_to_token(self, index):
- """Converts an index (integer) in a token (str) using the vocab."""
- return self.decoder.get(index, self.unk_token)
-
- def convert_tokens_to_string(self, tokens):
- """ Converts a sequence of tokens (string) in a single string. """
- out_string = "".join(tokens).replace("", " ").strip()
- return out_string
-
- def build_inputs_with_special_tokens(self, token_ids_0, token_ids_1=None):
- """
- Build model inputs from a sequence or a pair of sequence for sequence classification tasks
- by concatenating and adding special tokens.
- A XLM sequence has the following format:
- single sequence: X
- pair of sequences: A B
- """
- if token_ids_1 is None:
- return [self.cls_token_id] + token_ids_0 + [self.sep_token_id]
- sep = [self.sep_token_id]
- cls = [self.cls_token_id]
- return cls + token_ids_0 + sep + token_ids_1 + sep
-
- def get_special_tokens_mask(self, token_ids_0, token_ids_1=None, already_has_special_tokens=False):
- """
- Retrieves sequence ids from a token list that has no special tokens added. This method is called when adding
- special tokens using the tokenizer ``prepare_for_model`` or ``encode_plus`` methods.
-
- Args:
- token_ids_0: list of ids (must not contain special tokens)
- token_ids_1: Optional list of ids (must not contain special tokens), necessary when fetching sequence ids
- for sequence pairs
- already_has_special_tokens: (default False) Set to True if the token list is already formated with
- special tokens for the model
-
- Returns:
- A list of integers in the range [0, 1]: 1 for a special token, 0 for a sequence token.
- """
-
- if already_has_special_tokens:
- if token_ids_1 is not None:
- raise ValueError(
- "You should not supply a second sequence if the provided sequence of "
- "ids is already formated with special tokens for the model."
- )
- return list(map(lambda x: 1 if x in [self.sep_token_id, self.cls_token_id] else 0, token_ids_0,))
-
- if token_ids_1 is not None:
- return [1] + ([0] * len(token_ids_0)) + [1] + ([0] * len(token_ids_1)) + [1]
- return [1] + ([0] * len(token_ids_0)) + [1]
-
- def create_token_type_ids_from_sequences(self, token_ids_0, token_ids_1=None):
- """
- Creates a mask from the two sequences passed to be used in a sequence-pair classification task.
- An XLM sequence pair mask has the following format:
- 0 0 0 0 0 0 0 0 0 0 1 1 1 1 1 1 1 1 1 1 1
- | first sequence | second sequence
-
- if token_ids_1 is None, only returns the first portion of the mask (0's).
- """
- sep = [self.sep_token_id]
- cls = [self.cls_token_id]
- if token_ids_1 is None:
- return len(cls + token_ids_0 + sep) * [0]
- return len(cls + token_ids_0 + sep) * [0] + len(token_ids_1 + sep) * [1]
-
- def save_vocabulary(self, save_directory):
- """Save the tokenizer vocabulary and merge files to a directory."""
- if not os.path.isdir(save_directory):
- logger.error("Vocabulary path ({}) should be a directory".format(save_directory))
- return
- vocab_file = os.path.join(save_directory, VOCAB_FILES_NAMES["vocab_file"])
- merge_file = os.path.join(save_directory, VOCAB_FILES_NAMES["merges_file"])
-
- with open(vocab_file, "w", encoding="utf-8") as f:
- f.write(json.dumps(self.encoder, ensure_ascii=False))
-
- index = 0
- with open(merge_file, "w", encoding="utf-8") as writer:
- for bpe_tokens, token_index in sorted(self.bpe_ranks.items(), key=lambda kv: kv[1]):
- if index != token_index:
- logger.warning(
- "Saving vocabulary to {}: BPE merge indices are not consecutive."
- " Please check that the tokenizer is not corrupted!".format(merge_file)
- )
- index = token_index
- writer.write(" ".join(bpe_tokens) + "\n")
- index += 1
-
- return vocab_file, merge_file
diff --git a/server/transformers/src/transformers/tokenization_xlm_roberta.py b/server/transformers/src/transformers/tokenization_xlm_roberta.py
deleted file mode 100644
index ea39d945ae78fd703f05392ecdf8910805e10324..0000000000000000000000000000000000000000
--- a/server/transformers/src/transformers/tokenization_xlm_roberta.py
+++ /dev/null
@@ -1,225 +0,0 @@
-# coding=utf-8
-# Copyright 2018 Google AI, Google Brain and Carnegie Mellon University Authors and the HuggingFace Inc. team.
-#
-# Licensed under the Apache License, Version 2.0 (the "License");
-# you may not use this file except in compliance with the License.
-# You may obtain a copy of the License at
-#
-# http://www.apache.org/licenses/LICENSE-2.0
-#
-# Unless required by applicable law or agreed to in writing, software
-# distributed under the License is distributed on an "AS IS" BASIS,
-# WITHOUT WARRANTIES OR CONDITIONS OF ANY KIND, either express or implied.
-# See the License for the specific language governing permissions and
-# limitations under the License
-""" Tokenization classes for XLM-RoBERTa model."""
-
-
-import logging
-import os
-from shutil import copyfile
-
-from transformers.tokenization_utils import PreTrainedTokenizer
-
-from .tokenization_xlnet import SPIECE_UNDERLINE
-
-
-logger = logging.getLogger(__name__)
-
-VOCAB_FILES_NAMES = {"vocab_file": "sentencepiece.bpe.model"}
-
-PRETRAINED_VOCAB_FILES_MAP = {
- "vocab_file": {
- "xlm-roberta-base": "https://s3.amazonaws.com/models.huggingface.co/bert/xlm-roberta-base-sentencepiece.bpe.model",
- "xlm-roberta-large": "https://s3.amazonaws.com/models.huggingface.co/bert/xlm-roberta-large-sentencepiece.bpe.model",
- "xlm-roberta-large-finetuned-conll02-dutch": "https://s3.amazonaws.com/models.huggingface.co/bert/xlm-roberta-large-finetuned-conll02-dutch-sentencepiece.bpe.model",
- "xlm-roberta-large-finetuned-conll02-spanish": "https://s3.amazonaws.com/models.huggingface.co/bert/xlm-roberta-large-finetuned-conll02-spanish-sentencepiece.bpe.model",
- "xlm-roberta-large-finetuned-conll03-english": "https://s3.amazonaws.com/models.huggingface.co/bert/xlm-roberta-large-finetuned-conll03-english-sentencepiece.bpe.model",
- "xlm-roberta-large-finetuned-conll03-german": "https://s3.amazonaws.com/models.huggingface.co/bert/xlm-roberta-large-finetuned-conll03-german-sentencepiece.bpe.model",
- }
-}
-
-PRETRAINED_POSITIONAL_EMBEDDINGS_SIZES = {
- "xlm-roberta-base": 512,
- "xlm-roberta-large": 512,
- "xlm-roberta-large-finetuned-conll02-dutch": 512,
- "xlm-roberta-large-finetuned-conll02-spanish": 512,
- "xlm-roberta-large-finetuned-conll03-english": 512,
- "xlm-roberta-large-finetuned-conll03-german": 512,
-}
-
-
-class XLMRobertaTokenizer(PreTrainedTokenizer):
- """
- Adapted from RobertaTokenizer and XLNetTokenizer
- SentencePiece based tokenizer. Peculiarities:
-
- - requires `SentencePiece `_
- """
-
- vocab_files_names = VOCAB_FILES_NAMES
- pretrained_vocab_files_map = PRETRAINED_VOCAB_FILES_MAP
- max_model_input_sizes = PRETRAINED_POSITIONAL_EMBEDDINGS_SIZES
-
- def __init__(
- self,
- vocab_file,
- bos_token="",
- eos_token="",
- sep_token="",
- cls_token="",
- unk_token="",
- pad_token="",
- mask_token="",
- **kwargs
- ):
- super().__init__(
- bos_token=bos_token,
- eos_token=eos_token,
- unk_token=unk_token,
- sep_token=sep_token,
- cls_token=cls_token,
- pad_token=pad_token,
- mask_token=mask_token,
- **kwargs,
- )
- self.max_len_single_sentence = self.max_len - 2 # take into account special tokens
- self.max_len_sentences_pair = self.max_len - 4 # take into account special tokens
-
- try:
- import sentencepiece as spm
- except ImportError:
- logger.warning(
- "You need to install SentencePiece to use XLMRobertaTokenizer: https://github.com/google/sentencepiece"
- "pip install sentencepiece"
- )
- raise
-
- self.sp_model = spm.SentencePieceProcessor()
- self.sp_model.Load(str(vocab_file))
- self.vocab_file = vocab_file
-
- # Original fairseq vocab and spm vocab must be "aligned":
- # Vocab | 0 | 1 | 2 | 3 | 4 | 5 | 6 | 7 | 8 | 9
- # -------- | ------- | ------- | ------ | ------- | --- | --- | --- | ----- | ----- | ----
- # fairseq | '' | '' | '' | '' | ',' | '.' | '▁' | 's' | '▁de' | '-'
- # spm | '' | '' | '' | ',' | '.' | '▁' | 's' | '▁de' | '-' | '▁a'
-
- # Mimic fairseq token-to-id alignment for the first 4 token
- self.fairseq_tokens_to_ids = {"": 0, "": 1, "": 2, "": 3}
-
- # The first "real" token "," has position 4 in the original fairseq vocab and position 3 in the spm vocab
- self.fairseq_offset = 1
-
- self.fairseq_tokens_to_ids[""] = len(self.sp_model) + len(self.fairseq_tokens_to_ids)
- self.fairseq_ids_to_tokens = {v: k for k, v in self.fairseq_tokens_to_ids.items()}
-
- def __getstate__(self):
- state = self.__dict__.copy()
- state["sp_model"] = None
- return state
-
- def __setstate__(self, d):
- self.__dict__ = d
- try:
- import sentencepiece as spm
- except ImportError:
- logger.warning(
- "You need to install SentencePiece to use XLMRobertaTokenizer: https://github.com/google/sentencepiece"
- "pip install sentencepiece"
- )
- raise
- self.sp_model = spm.SentencePieceProcessor()
- self.sp_model.Load(self.vocab_file)
-
- def build_inputs_with_special_tokens(self, token_ids_0, token_ids_1=None):
- """
- Build model inputs from a sequence or a pair of sequence for sequence classification tasks
- by concatenating and adding special tokens.
- A RoBERTa sequence has the following format:
- single sequence: X
- pair of sequences: A B
- """
- if token_ids_1 is None:
- return [self.cls_token_id] + token_ids_0 + [self.sep_token_id]
- cls = [self.cls_token_id]
- sep = [self.sep_token_id]
- return cls + token_ids_0 + sep + sep + token_ids_1 + sep
-
- def get_special_tokens_mask(self, token_ids_0, token_ids_1=None, already_has_special_tokens=False):
- """
- Retrieves sequence ids from a token list that has no special tokens added. This method is called when adding
- special tokens using the tokenizer ``prepare_for_model`` or ``encode_plus`` methods.
-
- Args:
- token_ids_0: list of ids (must not contain special tokens)
- token_ids_1: Optional list of ids (must not contain special tokens), necessary when fetching sequence ids
- for sequence pairs
- already_has_special_tokens: (default False) Set to True if the token list is already formated with
- special tokens for the model
-
- Returns:
- A list of integers in the range [0, 1]: 1 for a special token, 0 for a sequence token.
- """
- if already_has_special_tokens:
- if token_ids_1 is not None:
- raise ValueError(
- "You should not supply a second sequence if the provided sequence of "
- "ids is already formated with special tokens for the model."
- )
- return list(map(lambda x: 1 if x in [self.sep_token_id, self.cls_token_id] else 0, token_ids_0))
-
- if token_ids_1 is None:
- return [1] + ([0] * len(token_ids_0)) + [1]
- return [1] + ([0] * len(token_ids_0)) + [1, 1] + ([0] * len(token_ids_1)) + [1]
-
- def create_token_type_ids_from_sequences(self, token_ids_0, token_ids_1=None):
- """
- Creates a mask from the two sequences passed to be used in a sequence-pair classification task.
- RoBERTa does not make use of token type ids, therefore a list of zeros is returned.
- if token_ids_1 is None, only returns the first portion of the mask (0's).
- """
- sep = [self.sep_token_id]
- cls = [self.cls_token_id]
-
- if token_ids_1 is None:
- return len(cls + token_ids_0 + sep) * [0]
- return len(cls + token_ids_0 + sep + sep + token_ids_1 + sep) * [0]
-
- @property
- def vocab_size(self):
- return len(self.sp_model) + len(self.fairseq_tokens_to_ids)
-
- def _tokenize(self, text):
- return self.sp_model.EncodeAsPieces(text)
-
- def _convert_token_to_id(self, token):
- """ Converts a token (str) in an id using the vocab. """
- if token in self.fairseq_tokens_to_ids:
- return self.fairseq_tokens_to_ids[token]
- return self.sp_model.PieceToId(token) + self.fairseq_offset
-
- def _convert_id_to_token(self, index):
- """Converts an index (integer) in a token (str) using the vocab."""
- if index in self.fairseq_ids_to_tokens:
- return self.fairseq_ids_to_tokens[index]
- return self.sp_model.IdToPiece(index - self.fairseq_offset)
-
- def convert_tokens_to_string(self, tokens):
- """Converts a sequence of tokens (strings for sub-words) in a single string."""
- out_string = "".join(tokens).replace(SPIECE_UNDERLINE, " ").strip()
- return out_string
-
- def save_vocabulary(self, save_directory):
- """ Save the sentencepiece vocabulary (copy original file) and special tokens file
- to a directory.
- """
- if not os.path.isdir(save_directory):
- logger.error("Vocabulary path ({}) should be a directory".format(save_directory))
- return
- out_vocab_file = os.path.join(save_directory, VOCAB_FILES_NAMES["vocab_file"])
-
- if os.path.abspath(self.vocab_file) != os.path.abspath(out_vocab_file):
- copyfile(self.vocab_file, out_vocab_file)
-
- return (out_vocab_file,)
diff --git a/server/transformers/src/transformers/tokenization_xlnet.py b/server/transformers/src/transformers/tokenization_xlnet.py
deleted file mode 100644
index e3ebc7107244f3c5258f7f59c6227023a1317b65..0000000000000000000000000000000000000000
--- a/server/transformers/src/transformers/tokenization_xlnet.py
+++ /dev/null
@@ -1,257 +0,0 @@
-# coding=utf-8
-# Copyright 2018 Google AI, Google Brain and Carnegie Mellon University Authors and the HuggingFace Inc. team.
-#
-# Licensed under the Apache License, Version 2.0 (the "License");
-# you may not use this file except in compliance with the License.
-# You may obtain a copy of the License at
-#
-# http://www.apache.org/licenses/LICENSE-2.0
-#
-# Unless required by applicable law or agreed to in writing, software
-# distributed under the License is distributed on an "AS IS" BASIS,
-# WITHOUT WARRANTIES OR CONDITIONS OF ANY KIND, either express or implied.
-# See the License for the specific language governing permissions and
-# limitations under the License.
-""" Tokenization classes for XLNet model."""
-
-
-import logging
-import os
-import unicodedata
-from shutil import copyfile
-
-from .tokenization_utils import PreTrainedTokenizer
-
-
-logger = logging.getLogger(__name__)
-
-VOCAB_FILES_NAMES = {"vocab_file": "spiece.model"}
-
-PRETRAINED_VOCAB_FILES_MAP = {
- "vocab_file": {
- "xlnet-base-cased": "https://s3.amazonaws.com/models.huggingface.co/bert/xlnet-base-cased-spiece.model",
- "xlnet-large-cased": "https://s3.amazonaws.com/models.huggingface.co/bert/xlnet-large-cased-spiece.model",
- }
-}
-
-PRETRAINED_POSITIONAL_EMBEDDINGS_SIZES = {
- "xlnet-base-cased": None,
- "xlnet-large-cased": None,
-}
-
-SPIECE_UNDERLINE = "▁"
-
-# Segments (not really needed)
-SEG_ID_A = 0
-SEG_ID_B = 1
-SEG_ID_CLS = 2
-SEG_ID_SEP = 3
-SEG_ID_PAD = 4
-
-
-class XLNetTokenizer(PreTrainedTokenizer):
- """
- SentencePiece based tokenizer. Peculiarities:
-
- - requires `SentencePiece `_
- """
-
- vocab_files_names = VOCAB_FILES_NAMES
- pretrained_vocab_files_map = PRETRAINED_VOCAB_FILES_MAP
- max_model_input_sizes = PRETRAINED_POSITIONAL_EMBEDDINGS_SIZES
- padding_side = "left"
-
- def __init__(
- self,
- vocab_file,
- do_lower_case=False,
- remove_space=True,
- keep_accents=False,
- bos_token="",
- eos_token="",
- unk_token="",
- sep_token="",
- pad_token="",
- cls_token="",
- mask_token="",
- additional_special_tokens=["", ""],
- **kwargs
- ):
- super().__init__(
- bos_token=bos_token,
- eos_token=eos_token,
- unk_token=unk_token,
- sep_token=sep_token,
- pad_token=pad_token,
- cls_token=cls_token,
- mask_token=mask_token,
- additional_special_tokens=additional_special_tokens,
- **kwargs,
- )
-
- self.max_len_single_sentence = self.max_len - 2 # take into account special tokens
- self.max_len_sentences_pair = self.max_len - 3 # take into account special tokens
- self._pad_token_type_id = 3
-
- try:
- import sentencepiece as spm
- except ImportError:
- logger.warning(
- "You need to install SentencePiece to use XLNetTokenizer: https://github.com/google/sentencepiece"
- "pip install sentencepiece"
- )
- raise
-
- self.do_lower_case = do_lower_case
- self.remove_space = remove_space
- self.keep_accents = keep_accents
- self.vocab_file = vocab_file
-
- self.sp_model = spm.SentencePieceProcessor()
- self.sp_model.Load(vocab_file)
-
- @property
- def vocab_size(self):
- return len(self.sp_model)
-
- def __getstate__(self):
- state = self.__dict__.copy()
- state["sp_model"] = None
- return state
-
- def __setstate__(self, d):
- self.__dict__ = d
- try:
- import sentencepiece as spm
- except ImportError:
- logger.warning(
- "You need to install SentencePiece to use XLNetTokenizer: https://github.com/google/sentencepiece"
- "pip install sentencepiece"
- )
- raise
- self.sp_model = spm.SentencePieceProcessor()
- self.sp_model.Load(self.vocab_file)
-
- def preprocess_text(self, inputs):
- if self.remove_space:
- outputs = " ".join(inputs.strip().split())
- else:
- outputs = inputs
- outputs = outputs.replace("``", '"').replace("''", '"')
-
- if not self.keep_accents:
- outputs = unicodedata.normalize("NFKD", outputs)
- outputs = "".join([c for c in outputs if not unicodedata.combining(c)])
- if self.do_lower_case:
- outputs = outputs.lower()
-
- return outputs
-
- def _tokenize(self, text, sample=False):
- """ Tokenize a string. """
- text = self.preprocess_text(text)
-
- if not sample:
- pieces = self.sp_model.EncodeAsPieces(text)
- else:
- pieces = self.sp_model.SampleEncodeAsPieces(text, 64, 0.1)
- new_pieces = []
- for piece in pieces:
- if len(piece) > 1 and piece[-1] == str(",") and piece[-2].isdigit():
- cur_pieces = self.sp_model.EncodeAsPieces(piece[:-1].replace(SPIECE_UNDERLINE, ""))
- if piece[0] != SPIECE_UNDERLINE and cur_pieces[0][0] == SPIECE_UNDERLINE:
- if len(cur_pieces[0]) == 1:
- cur_pieces = cur_pieces[1:]
- else:
- cur_pieces[0] = cur_pieces[0][1:]
- cur_pieces.append(piece[-1])
- new_pieces.extend(cur_pieces)
- else:
- new_pieces.append(piece)
-
- return new_pieces
-
- def _convert_token_to_id(self, token):
- """ Converts a token (str) in an id using the vocab. """
- return self.sp_model.PieceToId(token)
-
- def _convert_id_to_token(self, index):
- """Converts an index (integer) in a token (str) using the vocab."""
- return self.sp_model.IdToPiece(index)
-
- def convert_tokens_to_string(self, tokens):
- """Converts a sequence of tokens (strings for sub-words) in a single string."""
- out_string = "".join(tokens).replace(SPIECE_UNDERLINE, " ").strip()
- return out_string
-
- def build_inputs_with_special_tokens(self, token_ids_0, token_ids_1=None):
- """
- Build model inputs from a sequence or a pair of sequence for sequence classification tasks
- by concatenating and adding special tokens.
- An XLNet sequence has the following format:
- single sequence: X
- pair of sequences: A B
- """
- sep = [self.sep_token_id]
- cls = [self.cls_token_id]
- if token_ids_1 is None:
- return token_ids_0 + sep + cls
- return token_ids_0 + sep + token_ids_1 + sep + cls
-
- def get_special_tokens_mask(self, token_ids_0, token_ids_1=None, already_has_special_tokens=False):
- """
- Retrieves sequence ids from a token list that has no special tokens added. This method is called when adding
- special tokens using the tokenizer ``prepare_for_model`` or ``encode_plus`` methods.
-
- Args:
- token_ids_0: list of ids (must not contain special tokens)
- token_ids_1: Optional list of ids (must not contain special tokens), necessary when fetching sequence ids
- for sequence pairs
- already_has_special_tokens: (default False) Set to True if the token list is already formated with
- special tokens for the model
-
- Returns:
- A list of integers in the range [0, 1]: 1 for a special token, 0 for a sequence token.
- """
-
- if already_has_special_tokens:
- if token_ids_1 is not None:
- raise ValueError(
- "You should not supply a second sequence if the provided sequence of "
- "ids is already formated with special tokens for the model."
- )
- return list(map(lambda x: 1 if x in [self.sep_token_id, self.cls_token_id] else 0, token_ids_0))
-
- if token_ids_1 is not None:
- return ([0] * len(token_ids_0)) + [1] + ([0] * len(token_ids_1)) + [1, 1]
- return ([0] * len(token_ids_0)) + [1, 1]
-
- def create_token_type_ids_from_sequences(self, token_ids_0, token_ids_1=None):
- """
- Creates a mask from the two sequences passed to be used in a sequence-pair classification task.
- An XLNet sequence pair mask has the following format:
- 0 0 0 0 0 0 0 0 0 0 1 1 1 1 1 1 1 1 1 1 1 2
- | first sequence | second sequence | CLS segment ID
-
- if token_ids_1 is None, only returns the first portion of the mask (0's).
- """
- sep = [self.sep_token_id]
- cls_segment_id = [2]
-
- if token_ids_1 is None:
- return len(token_ids_0 + sep) * [0] + cls_segment_id
- return len(token_ids_0 + sep) * [0] + len(token_ids_1 + sep) * [1] + cls_segment_id
-
- def save_vocabulary(self, save_directory):
- """ Save the sentencepiece vocabulary (copy original file) and special tokens file
- to a directory.
- """
- if not os.path.isdir(save_directory):
- logger.error("Vocabulary path ({}) should be a directory".format(save_directory))
- return
- out_vocab_file = os.path.join(save_directory, VOCAB_FILES_NAMES["vocab_file"])
-
- if os.path.abspath(self.vocab_file) != os.path.abspath(out_vocab_file):
- copyfile(self.vocab_file, out_vocab_file)
-
- return (out_vocab_file,)
diff --git a/server/transformers/templates/adding_a_new_example_script/README.md b/server/transformers/templates/adding_a_new_example_script/README.md
deleted file mode 100644
index 2afca08bf8456375c2bca786ce28a5605ada2b31..0000000000000000000000000000000000000000
--- a/server/transformers/templates/adding_a_new_example_script/README.md
+++ /dev/null
@@ -1,5 +0,0 @@
-# How to add a new example script in 🤗Transformers
-
-This folder provide a template for adding a new example script implementing a training or inference task with the models in the 🤗Transformers library.
-
-Currently only examples for PyTorch are provided which are adaptations of the library's SQuAD examples which implement single-GPU and distributed training with gradient accumulation and mixed-precision (using NVIDIA's apex library) to cover a reasonable range of use cases.
diff --git a/server/transformers/templates/adding_a_new_example_script/run_xxx.py b/server/transformers/templates/adding_a_new_example_script/run_xxx.py
deleted file mode 100644
index 6de065ce65ce57729f02cf6fc593a028d27b1dae..0000000000000000000000000000000000000000
--- a/server/transformers/templates/adding_a_new_example_script/run_xxx.py
+++ /dev/null
@@ -1,724 +0,0 @@
-# coding=utf-8
-# Copyright 2018 XXX. All rights reserved.
-#
-# Licensed under the Apache License, Version 2.0 (the "License");
-# you may not use this file except in compliance with the License.
-# You may obtain a copy of the License at
-#
-# http://www.apache.org/licenses/LICENSE-2.0
-#
-# Unless required by applicable law or agreed to in writing, software
-# distributed under the License is distributed on an "AS IS" BASIS,
-# WITHOUT WARRANTIES OR CONDITIONS OF ANY KIND, either express or implied.
-# See the License for the specific language governing permissions and
-# limitations under the License.
-""" Finetuning the library models for task XXX."""
-
-
-import argparse
-import glob
-import logging
-import os
-import random
-
-import numpy as np
-import torch
-from torch.utils.data import DataLoader, RandomSampler, SequentialSampler, TensorDataset
-from torch.utils.data.distributed import DistributedSampler
-from tqdm import tqdm, trange
-
-from transformers import (
- WEIGHTS_NAME,
- AdamW,
- BertConfig,
- BertForQuestionAnswering,
- BertTokenizer,
- DistilBertConfig,
- DistilBertForQuestionAnswering,
- DistilBertTokenizer,
- XLMConfig,
- XLMForQuestionAnswering,
- XLMTokenizer,
- XLNetConfig,
- XLNetForQuestionAnswering,
- XLNetTokenizer,
- get_linear_schedule_with_warmup,
-)
-from utils_squad import (
- RawResult,
- RawResultExtended,
- convert_examples_to_features,
- read_squad_examples,
- write_predictions,
- write_predictions_extended,
-)
-
-# The follwing import is the official SQuAD evaluation script (2.0).
-# You can remove it from the dependencies if you are using this script outside of the library
-# We've added it here for automated tests (see examples/test_examples.py file)
-from utils_squad_evaluate import EVAL_OPTS
-from utils_squad_evaluate import main as evaluate_on_squad
-
-
-try:
- from torch.utils.tensorboard import SummaryWriter
-except ImportError:
- from tensorboardX import SummaryWriter
-
-
-logger = logging.getLogger(__name__)
-
-ALL_MODELS = sum(
- (tuple(conf.pretrained_config_archive_map.keys()) for conf in (BertConfig, XLNetConfig, XLMConfig)), ()
-)
-
-MODEL_CLASSES = {
- "bert": (BertConfig, BertForQuestionAnswering, BertTokenizer),
- "xlnet": (XLNetConfig, XLNetForQuestionAnswering, XLNetTokenizer),
- "xlm": (XLMConfig, XLMForQuestionAnswering, XLMTokenizer),
- "distilbert": (DistilBertConfig, DistilBertForQuestionAnswering, DistilBertTokenizer),
-}
-
-
-def set_seed(args):
- random.seed(args.seed)
- np.random.seed(args.seed)
- torch.manual_seed(args.seed)
- if args.n_gpu > 0:
- torch.cuda.manual_seed_all(args.seed)
-
-
-def to_list(tensor):
- return tensor.detach().cpu().tolist()
-
-
-def train(args, train_dataset, model, tokenizer):
- """ Train the model """
- if args.local_rank in [-1, 0]:
- tb_writer = SummaryWriter()
-
- args.train_batch_size = args.per_gpu_train_batch_size * max(1, args.n_gpu)
- train_sampler = RandomSampler(train_dataset) if args.local_rank == -1 else DistributedSampler(train_dataset)
- train_dataloader = DataLoader(train_dataset, sampler=train_sampler, batch_size=args.train_batch_size)
-
- if args.max_steps > 0:
- t_total = args.max_steps
- args.num_train_epochs = args.max_steps // (len(train_dataloader) // args.gradient_accumulation_steps) + 1
- else:
- t_total = len(train_dataloader) // args.gradient_accumulation_steps * args.num_train_epochs
-
- # Prepare optimizer and schedule (linear warmup and decay)
- no_decay = ["bias", "LayerNorm.weight"]
- optimizer_grouped_parameters = [
- {
- "params": [p for n, p in model.named_parameters() if not any(nd in n for nd in no_decay)],
- "weight_decay": args.weight_decay,
- },
- {"params": [p for n, p in model.named_parameters() if any(nd in n for nd in no_decay)], "weight_decay": 0.0},
- ]
- optimizer = AdamW(optimizer_grouped_parameters, lr=args.learning_rate, eps=args.adam_epsilon)
- scheduler = get_linear_schedule_with_warmup(
- optimizer, num_warmup_steps=args.warmup_steps, num_training_steps=t_total
- )
- if args.fp16:
- try:
- from apex import amp
- except ImportError:
- raise ImportError("Please install apex from https://www.github.com/nvidia/apex to use fp16 training.")
- model, optimizer = amp.initialize(model, optimizer, opt_level=args.fp16_opt_level)
-
- # multi-gpu training (should be after apex fp16 initialization)
- if args.n_gpu > 1:
- model = torch.nn.DataParallel(model)
-
- # Distributed training (should be after apex fp16 initialization)
- if args.local_rank != -1:
- model = torch.nn.parallel.DistributedDataParallel(
- model, device_ids=[args.local_rank], output_device=args.local_rank, find_unused_parameters=True
- )
-
- # Train!
- logger.info("***** Running training *****")
- logger.info(" Num examples = %d", len(train_dataset))
- logger.info(" Num Epochs = %d", args.num_train_epochs)
- logger.info(" Instantaneous batch size per GPU = %d", args.per_gpu_train_batch_size)
- logger.info(
- " Total train batch size (w. parallel, distributed & accumulation) = %d",
- args.train_batch_size
- * args.gradient_accumulation_steps
- * (torch.distributed.get_world_size() if args.local_rank != -1 else 1),
- )
- logger.info(" Gradient Accumulation steps = %d", args.gradient_accumulation_steps)
- logger.info(" Total optimization steps = %d", t_total)
-
- global_step = 0
- tr_loss, logging_loss = 0.0, 0.0
- model.zero_grad()
- train_iterator = trange(int(args.num_train_epochs), desc="Epoch", disable=args.local_rank not in [-1, 0])
- set_seed(args) # Added here for reproductibility
- for _ in train_iterator:
- epoch_iterator = tqdm(train_dataloader, desc="Iteration", disable=args.local_rank not in [-1, 0])
- for step, batch in enumerate(epoch_iterator):
- model.train()
- batch = tuple(t.to(args.device) for t in batch)
- inputs = {
- "input_ids": batch[0],
- "attention_mask": batch[1],
- "start_positions": batch[3],
- "end_positions": batch[4],
- }
- if args.model_type != "distilbert":
- inputs["token_type_ids"] = None if args.model_type == "xlm" else batch[2]
- if args.model_type in ["xlnet", "xlm"]:
- inputs.update({"cls_index": batch[5], "p_mask": batch[6]})
- outputs = model(**inputs)
- loss = outputs[0] # model outputs are always tuple in transformers (see doc)
-
- if args.n_gpu > 1:
- loss = loss.mean() # mean() to average on multi-gpu parallel (not distributed) training
- if args.gradient_accumulation_steps > 1:
- loss = loss / args.gradient_accumulation_steps
-
- if args.fp16:
- with amp.scale_loss(loss, optimizer) as scaled_loss:
- scaled_loss.backward()
- else:
- loss.backward()
-
- tr_loss += loss.item()
- if (step + 1) % args.gradient_accumulation_steps == 0:
- if args.fp16:
- torch.nn.utils.clip_grad_norm_(amp.master_params(optimizer), args.max_grad_norm)
- else:
- torch.nn.utils.clip_grad_norm_(model.parameters(), args.max_grad_norm)
-
- optimizer.step()
- scheduler.step() # Update learning rate schedule
- model.zero_grad()
- global_step += 1
-
- if args.local_rank in [-1, 0] and args.logging_steps > 0 and global_step % args.logging_steps == 0:
- # Log metrics
- if (
- args.local_rank == -1 and args.evaluate_during_training
- ): # Only evaluate when single GPU otherwise metrics may not average well
- results = evaluate(args, model, tokenizer)
- for key, value in results.items():
- tb_writer.add_scalar("eval_{}".format(key), value, global_step)
- tb_writer.add_scalar("lr", scheduler.get_lr()[0], global_step)
- tb_writer.add_scalar("loss", (tr_loss - logging_loss) / args.logging_steps, global_step)
- logging_loss = tr_loss
-
- if args.local_rank in [-1, 0] and args.save_steps > 0 and global_step % args.save_steps == 0:
- # Save model checkpoint
- output_dir = os.path.join(args.output_dir, "checkpoint-{}".format(global_step))
- if not os.path.exists(output_dir):
- os.makedirs(output_dir)
- model_to_save = (
- model.module if hasattr(model, "module") else model
- ) # Take care of distributed/parallel training
- model_to_save.save_pretrained(output_dir)
- torch.save(args, os.path.join(output_dir, "training_args.bin"))
- logger.info("Saving model checkpoint to %s", output_dir)
-
- if args.max_steps > 0 and global_step > args.max_steps:
- epoch_iterator.close()
- break
- if args.max_steps > 0 and global_step > args.max_steps:
- train_iterator.close()
- break
-
- if args.local_rank in [-1, 0]:
- tb_writer.close()
-
- return global_step, tr_loss / global_step
-
-
-def evaluate(args, model, tokenizer, prefix=""):
- dataset, examples, features = load_and_cache_examples(args, tokenizer, evaluate=True, output_examples=True)
-
- if not os.path.exists(args.output_dir) and args.local_rank in [-1, 0]:
- os.makedirs(args.output_dir)
-
- args.eval_batch_size = args.per_gpu_eval_batch_size * max(1, args.n_gpu)
- # Note that DistributedSampler samples randomly
- eval_sampler = SequentialSampler(dataset) if args.local_rank == -1 else DistributedSampler(dataset)
- eval_dataloader = DataLoader(dataset, sampler=eval_sampler, batch_size=args.eval_batch_size)
-
- # Eval!
- logger.info("***** Running evaluation {} *****".format(prefix))
- logger.info(" Num examples = %d", len(dataset))
- logger.info(" Batch size = %d", args.eval_batch_size)
- all_results = []
- for batch in tqdm(eval_dataloader, desc="Evaluating"):
- model.eval()
- batch = tuple(t.to(args.device) for t in batch)
- with torch.no_grad():
- inputs = {"input_ids": batch[0], "attention_mask": batch[1]}
- if args.model_type != "distilbert":
- inputs["token_type_ids"] = None if args.model_type == "xlm" else batch[2] # XLM don't use segment_ids
- example_indices = batch[3]
- if args.model_type in ["xlnet", "xlm"]:
- inputs.update({"cls_index": batch[4], "p_mask": batch[5]})
- outputs = model(**inputs)
-
- for i, example_index in enumerate(example_indices):
- eval_feature = features[example_index.item()]
- unique_id = int(eval_feature.unique_id)
- if args.model_type in ["xlnet", "xlm"]:
- # XLNet uses a more complex post-processing procedure
- result = RawResultExtended(
- unique_id=unique_id,
- start_top_log_probs=to_list(outputs[0][i]),
- start_top_index=to_list(outputs[1][i]),
- end_top_log_probs=to_list(outputs[2][i]),
- end_top_index=to_list(outputs[3][i]),
- cls_logits=to_list(outputs[4][i]),
- )
- else:
- result = RawResult(
- unique_id=unique_id, start_logits=to_list(outputs[0][i]), end_logits=to_list(outputs[1][i])
- )
- all_results.append(result)
-
- # Compute predictions
- output_prediction_file = os.path.join(args.output_dir, "predictions_{}.json".format(prefix))
- output_nbest_file = os.path.join(args.output_dir, "nbest_predictions_{}.json".format(prefix))
- if args.version_2_with_negative:
- output_null_log_odds_file = os.path.join(args.output_dir, "null_odds_{}.json".format(prefix))
- else:
- output_null_log_odds_file = None
-
- if args.model_type in ["xlnet", "xlm"]:
- # XLNet uses a more complex post-processing procedure
- write_predictions_extended(
- examples,
- features,
- all_results,
- args.n_best_size,
- args.max_answer_length,
- output_prediction_file,
- output_nbest_file,
- output_null_log_odds_file,
- args.predict_file,
- model.config.start_n_top,
- model.config.end_n_top,
- args.version_2_with_negative,
- tokenizer,
- args.verbose_logging,
- )
- else:
- write_predictions(
- examples,
- features,
- all_results,
- args.n_best_size,
- args.max_answer_length,
- args.do_lower_case,
- output_prediction_file,
- output_nbest_file,
- output_null_log_odds_file,
- args.verbose_logging,
- args.version_2_with_negative,
- args.null_score_diff_threshold,
- )
-
- # Evaluate with the official SQuAD script
- evaluate_options = EVAL_OPTS(
- data_file=args.predict_file, pred_file=output_prediction_file, na_prob_file=output_null_log_odds_file
- )
- results = evaluate_on_squad(evaluate_options)
- return results
-
-
-def load_and_cache_examples(args, tokenizer, evaluate=False, output_examples=False):
- if args.local_rank not in [-1, 0] and not evaluate:
- torch.distributed.barrier() # Make sure only the first process in distributed training process the dataset,
- # and the others will use the cache
-
- # Load data features from cache or dataset file
- input_file = args.predict_file if evaluate else args.train_file
- cached_features_file = os.path.join(
- os.path.dirname(input_file),
- "cached_{}_{}_{}".format(
- "dev" if evaluate else "train",
- list(filter(None, args.model_name_or_path.split("/"))).pop(),
- str(args.max_seq_length),
- ),
- )
- if os.path.exists(cached_features_file) and not args.overwrite_cache and not output_examples:
- logger.info("Loading features from cached file %s", cached_features_file)
- features = torch.load(cached_features_file)
- else:
- logger.info("Creating features from dataset file at %s", input_file)
- examples = read_squad_examples(
- input_file=input_file, is_training=not evaluate, version_2_with_negative=args.version_2_with_negative
- )
- features = convert_examples_to_features(
- examples=examples,
- tokenizer=tokenizer,
- max_seq_length=args.max_seq_length,
- doc_stride=args.doc_stride,
- max_query_length=args.max_query_length,
- is_training=not evaluate,
- )
- if args.local_rank in [-1, 0]:
- logger.info("Saving features into cached file %s", cached_features_file)
- torch.save(features, cached_features_file)
-
- if args.local_rank == 0 and not evaluate:
- torch.distributed.barrier() # Make sure only the first process in distributed training process the dataset,
- # and the others will use the cache
-
- # Convert to Tensors and build dataset
- all_input_ids = torch.tensor([f.input_ids for f in features], dtype=torch.long)
- all_input_mask = torch.tensor([f.input_mask for f in features], dtype=torch.long)
- all_segment_ids = torch.tensor([f.segment_ids for f in features], dtype=torch.long)
- all_cls_index = torch.tensor([f.cls_index for f in features], dtype=torch.long)
- all_p_mask = torch.tensor([f.p_mask for f in features], dtype=torch.float)
- if evaluate:
- all_example_index = torch.arange(all_input_ids.size(0), dtype=torch.long)
- dataset = TensorDataset(
- all_input_ids, all_input_mask, all_segment_ids, all_example_index, all_cls_index, all_p_mask
- )
- else:
- all_start_positions = torch.tensor([f.start_position for f in features], dtype=torch.long)
- all_end_positions = torch.tensor([f.end_position for f in features], dtype=torch.long)
- dataset = TensorDataset(
- all_input_ids,
- all_input_mask,
- all_segment_ids,
- all_start_positions,
- all_end_positions,
- all_cls_index,
- all_p_mask,
- )
-
- if output_examples:
- return dataset, examples, features
- return dataset
-
-
-def main():
- parser = argparse.ArgumentParser()
-
- # Required parameters
- parser.add_argument(
- "--train_file", default=None, type=str, required=True, help="SQuAD json for training. E.g., train-v1.1.json"
- )
- parser.add_argument(
- "--predict_file",
- default=None,
- type=str,
- required=True,
- help="SQuAD json for predictions. E.g., dev-v1.1.json or test-v1.1.json",
- )
- parser.add_argument(
- "--model_type",
- default=None,
- type=str,
- required=True,
- help="Model type selected in the list: " + ", ".join(MODEL_CLASSES.keys()),
- )
- parser.add_argument(
- "--model_name_or_path",
- default=None,
- type=str,
- required=True,
- help="Path to pre-trained model or shortcut name selected in the list: " + ", ".join(ALL_MODELS),
- )
- parser.add_argument(
- "--output_dir",
- default=None,
- type=str,
- required=True,
- help="The output directory where the model checkpoints and predictions will be written.",
- )
-
- # Other parameters
- parser.add_argument(
- "--config_name", default="", type=str, help="Pretrained config name or path if not the same as model_name"
- )
- parser.add_argument(
- "--tokenizer_name",
- default="",
- type=str,
- help="Pretrained tokenizer name or path if not the same as model_name",
- )
- parser.add_argument(
- "--cache_dir",
- default="",
- type=str,
- help="Where do you want to store the pre-trained models downloaded from s3",
- )
-
- parser.add_argument(
- "--version_2_with_negative",
- action="store_true",
- help="If true, the SQuAD examples contain some that do not have an answer.",
- )
- parser.add_argument(
- "--null_score_diff_threshold",
- type=float,
- default=0.0,
- help="If null_score - best_non_null is greater than the threshold predict null.",
- )
-
- parser.add_argument(
- "--max_seq_length",
- default=384,
- type=int,
- help="The maximum total input sequence length after WordPiece tokenization. Sequences "
- "longer than this will be truncated, and sequences shorter than this will be padded.",
- )
- parser.add_argument(
- "--doc_stride",
- default=128,
- type=int,
- help="When splitting up a long document into chunks, how much stride to take between chunks.",
- )
- parser.add_argument(
- "--max_query_length",
- default=64,
- type=int,
- help="The maximum number of tokens for the question. Questions longer than this will "
- "be truncated to this length.",
- )
- parser.add_argument("--do_train", action="store_true", help="Whether to run training.")
- parser.add_argument("--do_eval", action="store_true", help="Whether to run eval on the dev set.")
- parser.add_argument(
- "--evaluate_during_training", action="store_true", help="Rul evaluation during training at each logging step."
- )
- parser.add_argument(
- "--do_lower_case", action="store_true", help="Set this flag if you are using an uncased model."
- )
-
- parser.add_argument("--per_gpu_train_batch_size", default=8, type=int, help="Batch size per GPU/CPU for training.")
- parser.add_argument(
- "--per_gpu_eval_batch_size", default=8, type=int, help="Batch size per GPU/CPU for evaluation."
- )
- parser.add_argument("--learning_rate", default=5e-5, type=float, help="The initial learning rate for Adam.")
- parser.add_argument(
- "--gradient_accumulation_steps",
- type=int,
- default=1,
- help="Number of updates steps to accumulate before performing a backward/update pass.",
- )
- parser.add_argument("--weight_decay", default=0.0, type=float, help="Weight deay if we apply some.")
- parser.add_argument("--adam_epsilon", default=1e-8, type=float, help="Epsilon for Adam optimizer.")
- parser.add_argument("--max_grad_norm", default=1.0, type=float, help="Max gradient norm.")
- parser.add_argument(
- "--num_train_epochs", default=3.0, type=float, help="Total number of training epochs to perform."
- )
- parser.add_argument(
- "--max_steps",
- default=-1,
- type=int,
- help="If > 0: set total number of training steps to perform. Override num_train_epochs.",
- )
- parser.add_argument("--warmup_steps", default=0, type=int, help="Linear warmup over warmup_steps.")
- parser.add_argument(
- "--n_best_size",
- default=20,
- type=int,
- help="The total number of n-best predictions to generate in the nbest_predictions.json output file.",
- )
- parser.add_argument(
- "--max_answer_length",
- default=30,
- type=int,
- help="The maximum length of an answer that can be generated. This is needed because the start "
- "and end predictions are not conditioned on one another.",
- )
- parser.add_argument(
- "--verbose_logging",
- action="store_true",
- help="If true, all of the warnings related to data processing will be printed. "
- "A number of warnings are expected for a normal SQuAD evaluation.",
- )
-
- parser.add_argument("--logging_steps", type=int, default=50, help="Log every X updates steps.")
- parser.add_argument("--save_steps", type=int, default=50, help="Save checkpoint every X updates steps.")
- parser.add_argument(
- "--eval_all_checkpoints",
- action="store_true",
- help="Evaluate all checkpoints starting with the same prefix as model_name ending and ending with step number",
- )
- parser.add_argument("--no_cuda", action="store_true", help="Whether not to use CUDA when available")
- parser.add_argument(
- "--overwrite_output_dir", action="store_true", help="Overwrite the content of the output directory"
- )
- parser.add_argument(
- "--overwrite_cache", action="store_true", help="Overwrite the cached training and evaluation sets"
- )
- parser.add_argument("--seed", type=int, default=42, help="random seed for initialization")
-
- parser.add_argument("--local_rank", type=int, default=-1, help="local_rank for distributed training on gpus")
- parser.add_argument(
- "--fp16",
- action="store_true",
- help="Whether to use 16-bit (mixed) precision (through NVIDIA apex) instead of 32-bit",
- )
- parser.add_argument(
- "--fp16_opt_level",
- type=str,
- default="O1",
- help="For fp16: Apex AMP optimization level selected in ['O0', 'O1', 'O2', and 'O3']."
- "See details at https://nvidia.github.io/apex/amp.html",
- )
- parser.add_argument("--server_ip", type=str, default="", help="Can be used for distant debugging.")
- parser.add_argument("--server_port", type=str, default="", help="Can be used for distant debugging.")
- args = parser.parse_args()
-
- if (
- os.path.exists(args.output_dir)
- and os.listdir(args.output_dir)
- and args.do_train
- and not args.overwrite_output_dir
- ):
- raise ValueError(
- "Output directory ({}) already exists and is not empty. Use --overwrite_output_dir to overcome.".format(
- args.output_dir
- )
- )
-
- # Setup distant debugging if needed
- if args.server_ip and args.server_port:
- # Distant debugging - see https://code.visualstudio.com/docs/python/debugging#_attach-to-a-local-script
- import ptvsd
-
- print("Waiting for debugger attach")
- ptvsd.enable_attach(address=(args.server_ip, args.server_port), redirect_output=True)
- ptvsd.wait_for_attach()
-
- # Setup CUDA, GPU & distributed training
- if args.local_rank == -1 or args.no_cuda:
- device = torch.device("cuda" if torch.cuda.is_available() and not args.no_cuda else "cpu")
- args.n_gpu = torch.cuda.device_count()
- else: # Initializes the distributed backend which will take care of sychronizing nodes/GPUs
- torch.cuda.set_device(args.local_rank)
- device = torch.device("cuda", args.local_rank)
- torch.distributed.init_process_group(backend="nccl")
- args.n_gpu = 1
- args.device = device
-
- # Setup logging
- logging.basicConfig(
- format="%(asctime)s - %(levelname)s - %(name)s - %(message)s",
- datefmt="%m/%d/%Y %H:%M:%S",
- level=logging.INFO if args.local_rank in [-1, 0] else logging.WARN,
- )
- logger.warning(
- "Process rank: %s, device: %s, n_gpu: %s, distributed training: %s, 16-bits training: %s",
- args.local_rank,
- device,
- args.n_gpu,
- bool(args.local_rank != -1),
- args.fp16,
- )
-
- # Set seed
- set_seed(args)
-
- # Load pretrained model and tokenizer
- if args.local_rank not in [-1, 0]:
- torch.distributed.barrier() # Make sure only the first process in distributed training will
- # download model & vocab
-
- args.model_type = args.model_type.lower()
- config_class, model_class, tokenizer_class = MODEL_CLASSES[args.model_type]
- config = config_class.from_pretrained(
- args.config_name if args.config_name else args.model_name_or_path,
- cache_dir=args.cache_dir if args.cache_dir else None,
- )
- tokenizer = tokenizer_class.from_pretrained(
- args.tokenizer_name if args.tokenizer_name else args.model_name_or_path,
- do_lower_case=args.do_lower_case,
- cache_dir=args.cache_dir if args.cache_dir else None,
- )
- model = model_class.from_pretrained(
- args.model_name_or_path,
- from_tf=bool(".ckpt" in args.model_name_or_path),
- config=config,
- cache_dir=args.cache_dir if args.cache_dir else None,
- )
-
- if args.local_rank == 0:
- torch.distributed.barrier() # Make sure only the first process in distributed training will
- # download model & vocab
-
- model.to(args.device)
-
- logger.info("Training/evaluation parameters %s", args)
-
- # Before we do anything with models, we want to ensure that we get fp16 execution of torch.einsum
- # if args.fp16 is set. Otherwise it'll default to "promote" mode, and we'll get fp32 operations.
- # Note that running `--fp16_opt_level="O2"` will remove the need for this code, but it is still valid.
- if args.fp16:
- try:
- import apex
-
- apex.amp.register_half_function(torch, "einsum")
- except ImportError:
- raise ImportError("Please install apex from https://www.github.com/nvidia/apex to use fp16 training.")
-
- # Training
- if args.do_train:
- train_dataset = load_and_cache_examples(args, tokenizer, evaluate=False, output_examples=False)
- global_step, tr_loss = train(args, train_dataset, model, tokenizer)
- logger.info(" global_step = %s, average loss = %s", global_step, tr_loss)
-
- # Save the trained model and the tokenizer
- if args.do_train and (args.local_rank == -1 or torch.distributed.get_rank() == 0):
- # Create output directory if needed
- if not os.path.exists(args.output_dir) and args.local_rank in [-1, 0]:
- os.makedirs(args.output_dir)
-
- logger.info("Saving model checkpoint to %s", args.output_dir)
- # Save a trained model, configuration and tokenizer using `save_pretrained()`.
- # They can then be reloaded using `from_pretrained()`
- model_to_save = (
- model.module if hasattr(model, "module") else model
- ) # Take care of distributed/parallel training
- model_to_save.save_pretrained(args.output_dir)
- tokenizer.save_pretrained(args.output_dir)
-
- # Good practice: save your training arguments together with the trained model
- torch.save(args, os.path.join(args.output_dir, "training_args.bin"))
-
- # Load a trained model and vocabulary that you have fine-tuned
- model = model_class.from_pretrained(args.output_dir)
- tokenizer = tokenizer_class.from_pretrained(args.output_dir, do_lower_case=args.do_lower_case)
- model.to(args.device)
-
- # Evaluation - we can ask to evaluate all the checkpoints (sub-directories) in a directory
- results = {}
- if args.do_eval and args.local_rank in [-1, 0]:
- checkpoints = [args.output_dir]
- if args.eval_all_checkpoints:
- checkpoints = list(
- os.path.dirname(c) for c in sorted(glob.glob(args.output_dir + "/**/" + WEIGHTS_NAME, recursive=True))
- )
- logging.getLogger("transformers.modeling_utils").setLevel(logging.WARN) # Reduce model loading logs
-
- logger.info("Evaluate the following checkpoints: %s", checkpoints)
-
- for checkpoint in checkpoints:
- # Reload the model
- global_step = checkpoint.split("-")[-1] if len(checkpoints) > 1 else ""
- model = model_class.from_pretrained(checkpoint)
- model.to(args.device)
-
- # Evaluate
- result = evaluate(args, model, tokenizer, prefix=global_step)
-
- result = dict((k + ("_{}".format(global_step) if global_step else ""), v) for k, v in result.items())
- results.update(result)
-
- logger.info("Results: {}".format(results))
-
- return results
-
-
-if __name__ == "__main__":
- main()
diff --git a/server/transformers/templates/adding_a_new_example_script/utils_xxx.py b/server/transformers/templates/adding_a_new_example_script/utils_xxx.py
deleted file mode 100644
index b8f8cdf2b962c061722aadaad0a7ae3dab88ce8b..0000000000000000000000000000000000000000
--- a/server/transformers/templates/adding_a_new_example_script/utils_xxx.py
+++ /dev/null
@@ -1,1005 +0,0 @@
-# coding=utf-8
-# Copyright 2018 XXX. All rights reserved.
-#
-# Licensed under the Apache License, Version 2.0 (the "License");
-# you may not use this file except in compliance with the License.
-# You may obtain a copy of the License at
-#
-# http://www.apache.org/licenses/LICENSE-2.0
-#
-# Unless required by applicable law or agreed to in writing, software
-# distributed under the License is distributed on an "AS IS" BASIS,
-# WITHOUT WARRANTIES OR CONDITIONS OF ANY KIND, either express or implied.
-# See the License for the specific language governing permissions and
-# limitations under the License.
-""" Load XXX dataset. """
-
-
-import collections
-import json
-import logging
-import math
-
-from transformers.tokenization_bert import BasicTokenizer, whitespace_tokenize
-
-# Required by XLNet evaluation method to compute optimal threshold (see write_predictions_extended() method)
-from utils_squad_evaluate import find_all_best_thresh_v2, get_raw_scores, make_qid_to_has_ans
-
-
-logger = logging.getLogger(__name__)
-
-
-class SquadExample(object):
- """
- A single training/test example for the Squad dataset.
- For examples without an answer, the start and end position are -1.
- """
-
- def __init__(
- self,
- qas_id,
- question_text,
- doc_tokens,
- orig_answer_text=None,
- start_position=None,
- end_position=None,
- is_impossible=None,
- ):
- self.qas_id = qas_id
- self.question_text = question_text
- self.doc_tokens = doc_tokens
- self.orig_answer_text = orig_answer_text
- self.start_position = start_position
- self.end_position = end_position
- self.is_impossible = is_impossible
-
- def __str__(self):
- return self.__repr__()
-
- def __repr__(self):
- s = ""
- s += "qas_id: %s" % (self.qas_id)
- s += ", question_text: %s" % (self.question_text)
- s += ", doc_tokens: [%s]" % (" ".join(self.doc_tokens))
- if self.start_position:
- s += ", start_position: %d" % (self.start_position)
- if self.end_position:
- s += ", end_position: %d" % (self.end_position)
- if self.is_impossible:
- s += ", is_impossible: %r" % (self.is_impossible)
- return s
-
-
-class InputFeatures(object):
- """A single set of features of data."""
-
- def __init__(
- self,
- unique_id,
- example_index,
- doc_span_index,
- tokens,
- token_to_orig_map,
- token_is_max_context,
- input_ids,
- input_mask,
- segment_ids,
- cls_index,
- p_mask,
- paragraph_len,
- start_position=None,
- end_position=None,
- is_impossible=None,
- ):
- self.unique_id = unique_id
- self.example_index = example_index
- self.doc_span_index = doc_span_index
- self.tokens = tokens
- self.token_to_orig_map = token_to_orig_map
- self.token_is_max_context = token_is_max_context
- self.input_ids = input_ids
- self.input_mask = input_mask
- self.segment_ids = segment_ids
- self.cls_index = cls_index
- self.p_mask = p_mask
- self.paragraph_len = paragraph_len
- self.start_position = start_position
- self.end_position = end_position
- self.is_impossible = is_impossible
-
-
-def read_squad_examples(input_file, is_training, version_2_with_negative):
- """Read a SQuAD json file into a list of SquadExample."""
- with open(input_file, "r", encoding="utf-8") as reader:
- input_data = json.load(reader)["data"]
-
- def is_whitespace(c):
- if c == " " or c == "\t" or c == "\r" or c == "\n" or ord(c) == 0x202F:
- return True
- return False
-
- examples = []
- for entry in input_data:
- for paragraph in entry["paragraphs"]:
- paragraph_text = paragraph["context"]
- doc_tokens = []
- char_to_word_offset = []
- prev_is_whitespace = True
- for c in paragraph_text:
- if is_whitespace(c):
- prev_is_whitespace = True
- else:
- if prev_is_whitespace:
- doc_tokens.append(c)
- else:
- doc_tokens[-1] += c
- prev_is_whitespace = False
- char_to_word_offset.append(len(doc_tokens) - 1)
-
- for qa in paragraph["qas"]:
- qas_id = qa["id"]
- question_text = qa["question"]
- start_position = None
- end_position = None
- orig_answer_text = None
- is_impossible = False
- if is_training:
- if version_2_with_negative:
- is_impossible = qa["is_impossible"]
- if (len(qa["answers"]) != 1) and (not is_impossible):
- raise ValueError("For training, each question should have exactly 1 answer.")
- if not is_impossible:
- answer = qa["answers"][0]
- orig_answer_text = answer["text"]
- answer_offset = answer["answer_start"]
- answer_length = len(orig_answer_text)
- start_position = char_to_word_offset[answer_offset]
- end_position = char_to_word_offset[answer_offset + answer_length - 1]
- # Only add answers where the text can be exactly recovered from the
- # document. If this CAN'T happen it's likely due to weird Unicode
- # stuff so we will just skip the example.
- #
- # Note that this means for training mode, every example is NOT
- # guaranteed to be preserved.
- actual_text = " ".join(doc_tokens[start_position : (end_position + 1)])
- cleaned_answer_text = " ".join(whitespace_tokenize(orig_answer_text))
- if actual_text.find(cleaned_answer_text) == -1:
- logger.warning("Could not find answer: '%s' vs. '%s'", actual_text, cleaned_answer_text)
- continue
- else:
- start_position = -1
- end_position = -1
- orig_answer_text = ""
-
- example = SquadExample(
- qas_id=qas_id,
- question_text=question_text,
- doc_tokens=doc_tokens,
- orig_answer_text=orig_answer_text,
- start_position=start_position,
- end_position=end_position,
- is_impossible=is_impossible,
- )
- examples.append(example)
- return examples
-
-
-def convert_examples_to_features(
- examples,
- tokenizer,
- max_seq_length,
- doc_stride,
- max_query_length,
- is_training,
- cls_token_at_end=False,
- cls_token="[CLS]",
- sep_token="[SEP]",
- pad_token=0,
- sequence_a_segment_id=0,
- sequence_b_segment_id=1,
- cls_token_segment_id=0,
- pad_token_segment_id=0,
- mask_padding_with_zero=True,
-):
- """Loads a data file into a list of `InputBatch`s."""
-
- unique_id = 1000000000
- # cnt_pos, cnt_neg = 0, 0
- # max_N, max_M = 1024, 1024
- # f = np.zeros((max_N, max_M), dtype=np.float32)
-
- features = []
- for (example_index, example) in enumerate(examples):
-
- # if example_index % 100 == 0:
- # logger.info('Converting %s/%s pos %s neg %s', example_index, len(examples), cnt_pos, cnt_neg)
-
- query_tokens = tokenizer.tokenize(example.question_text)
-
- if len(query_tokens) > max_query_length:
- query_tokens = query_tokens[0:max_query_length]
-
- tok_to_orig_index = []
- orig_to_tok_index = []
- all_doc_tokens = []
- for (i, token) in enumerate(example.doc_tokens):
- orig_to_tok_index.append(len(all_doc_tokens))
- sub_tokens = tokenizer.tokenize(token)
- for sub_token in sub_tokens:
- tok_to_orig_index.append(i)
- all_doc_tokens.append(sub_token)
-
- tok_start_position = None
- tok_end_position = None
- if is_training and example.is_impossible:
- tok_start_position = -1
- tok_end_position = -1
- if is_training and not example.is_impossible:
- tok_start_position = orig_to_tok_index[example.start_position]
- if example.end_position < len(example.doc_tokens) - 1:
- tok_end_position = orig_to_tok_index[example.end_position + 1] - 1
- else:
- tok_end_position = len(all_doc_tokens) - 1
- (tok_start_position, tok_end_position) = _improve_answer_span(
- all_doc_tokens, tok_start_position, tok_end_position, tokenizer, example.orig_answer_text
- )
-
- # The -3 accounts for [CLS], [SEP] and [SEP]
- max_tokens_for_doc = max_seq_length - len(query_tokens) - 3
-
- # We can have documents that are longer than the maximum sequence length.
- # To deal with this we do a sliding window approach, where we take chunks
- # of the up to our max length with a stride of `doc_stride`.
- _DocSpan = collections.namedtuple("DocSpan", ["start", "length"]) # pylint: disable=invalid-name
- doc_spans = []
- start_offset = 0
- while start_offset < len(all_doc_tokens):
- length = len(all_doc_tokens) - start_offset
- if length > max_tokens_for_doc:
- length = max_tokens_for_doc
- doc_spans.append(_DocSpan(start=start_offset, length=length))
- if start_offset + length == len(all_doc_tokens):
- break
- start_offset += min(length, doc_stride)
-
- for (doc_span_index, doc_span) in enumerate(doc_spans):
- tokens = []
- token_to_orig_map = {}
- token_is_max_context = {}
- segment_ids = []
-
- # p_mask: mask with 1 for token than cannot be in the answer (0 for token which can be in an answer)
- # Original TF implem also keep the classification token (set to 0) (not sure why...)
- p_mask = []
-
- # CLS token at the beginning
- if not cls_token_at_end:
- tokens.append(cls_token)
- segment_ids.append(cls_token_segment_id)
- p_mask.append(0)
- cls_index = 0
-
- # Query
- for token in query_tokens:
- tokens.append(token)
- segment_ids.append(sequence_a_segment_id)
- p_mask.append(1)
-
- # SEP token
- tokens.append(sep_token)
- segment_ids.append(sequence_a_segment_id)
- p_mask.append(1)
-
- # Paragraph
- for i in range(doc_span.length):
- split_token_index = doc_span.start + i
- token_to_orig_map[len(tokens)] = tok_to_orig_index[split_token_index]
-
- is_max_context = _check_is_max_context(doc_spans, doc_span_index, split_token_index)
- token_is_max_context[len(tokens)] = is_max_context
- tokens.append(all_doc_tokens[split_token_index])
- segment_ids.append(sequence_b_segment_id)
- p_mask.append(0)
- paragraph_len = doc_span.length
-
- # SEP token
- tokens.append(sep_token)
- segment_ids.append(sequence_b_segment_id)
- p_mask.append(1)
-
- # CLS token at the end
- if cls_token_at_end:
- tokens.append(cls_token)
- segment_ids.append(cls_token_segment_id)
- p_mask.append(0)
- cls_index = len(tokens) - 1 # Index of classification token
-
- input_ids = tokenizer.convert_tokens_to_ids(tokens)
-
- # The mask has 1 for real tokens and 0 for padding tokens. Only real
- # tokens are attended to.
- input_mask = [1 if mask_padding_with_zero else 0] * len(input_ids)
-
- # Zero-pad up to the sequence length.
- while len(input_ids) < max_seq_length:
- input_ids.append(pad_token)
- input_mask.append(0 if mask_padding_with_zero else 1)
- segment_ids.append(pad_token_segment_id)
- p_mask.append(1)
-
- assert len(input_ids) == max_seq_length
- assert len(input_mask) == max_seq_length
- assert len(segment_ids) == max_seq_length
-
- span_is_impossible = example.is_impossible
- start_position = None
- end_position = None
- if is_training and not span_is_impossible:
- # For training, if our document chunk does not contain an annotation
- # we throw it out, since there is nothing to predict.
- doc_start = doc_span.start
- doc_end = doc_span.start + doc_span.length - 1
- out_of_span = False
- if not (tok_start_position >= doc_start and tok_end_position <= doc_end):
- out_of_span = True
- if out_of_span:
- start_position = 0
- end_position = 0
- span_is_impossible = True
- else:
- doc_offset = len(query_tokens) + 2
- start_position = tok_start_position - doc_start + doc_offset
- end_position = tok_end_position - doc_start + doc_offset
-
- if is_training and span_is_impossible:
- start_position = cls_index
- end_position = cls_index
-
- if example_index < 20:
- logger.info("*** Example ***")
- logger.info("unique_id: %s" % (unique_id))
- logger.info("example_index: %s" % (example_index))
- logger.info("doc_span_index: %s" % (doc_span_index))
- logger.info("tokens: %s" % " ".join(tokens))
- logger.info(
- "token_to_orig_map: %s" % " ".join(["%d:%d" % (x, y) for (x, y) in token_to_orig_map.items()])
- )
- logger.info(
- "token_is_max_context: %s"
- % " ".join(["%d:%s" % (x, y) for (x, y) in token_is_max_context.items()])
- )
- logger.info("input_ids: %s" % " ".join([str(x) for x in input_ids]))
- logger.info("input_mask: %s" % " ".join([str(x) for x in input_mask]))
- logger.info("segment_ids: %s" % " ".join([str(x) for x in segment_ids]))
- if is_training and span_is_impossible:
- logger.info("impossible example")
- if is_training and not span_is_impossible:
- answer_text = " ".join(tokens[start_position : (end_position + 1)])
- logger.info("start_position: %d" % (start_position))
- logger.info("end_position: %d" % (end_position))
- logger.info("answer: %s" % (answer_text))
-
- features.append(
- InputFeatures(
- unique_id=unique_id,
- example_index=example_index,
- doc_span_index=doc_span_index,
- tokens=tokens,
- token_to_orig_map=token_to_orig_map,
- token_is_max_context=token_is_max_context,
- input_ids=input_ids,
- input_mask=input_mask,
- segment_ids=segment_ids,
- cls_index=cls_index,
- p_mask=p_mask,
- paragraph_len=paragraph_len,
- start_position=start_position,
- end_position=end_position,
- is_impossible=span_is_impossible,
- )
- )
- unique_id += 1
-
- return features
-
-
-def _improve_answer_span(doc_tokens, input_start, input_end, tokenizer, orig_answer_text):
- """Returns tokenized answer spans that better match the annotated answer."""
-
- # The SQuAD annotations are character based. We first project them to
- # whitespace-tokenized words. But then after WordPiece tokenization, we can
- # often find a "better match". For example:
- #
- # Question: What year was John Smith born?
- # Context: The leader was John Smith (1895-1943).
- # Answer: 1895
- #
- # The original whitespace-tokenized answer will be "(1895-1943).". However
- # after tokenization, our tokens will be "( 1895 - 1943 ) .". So we can match
- # the exact answer, 1895.
- #
- # However, this is not always possible. Consider the following:
- #
- # Question: What country is the top exporter of electornics?
- # Context: The Japanese electronics industry is the lagest in the world.
- # Answer: Japan
- #
- # In this case, the annotator chose "Japan" as a character sub-span of
- # the word "Japanese". Since our WordPiece tokenizer does not split
- # "Japanese", we just use "Japanese" as the annotation. This is fairly rare
- # in SQuAD, but does happen.
- tok_answer_text = " ".join(tokenizer.tokenize(orig_answer_text))
-
- for new_start in range(input_start, input_end + 1):
- for new_end in range(input_end, new_start - 1, -1):
- text_span = " ".join(doc_tokens[new_start : (new_end + 1)])
- if text_span == tok_answer_text:
- return (new_start, new_end)
-
- return (input_start, input_end)
-
-
-def _check_is_max_context(doc_spans, cur_span_index, position):
- """Check if this is the 'max context' doc span for the token."""
-
- # Because of the sliding window approach taken to scoring documents, a single
- # token can appear in multiple documents. E.g.
- # Doc: the man went to the store and bought a gallon of milk
- # Span A: the man went to the
- # Span B: to the store and bought
- # Span C: and bought a gallon of
- # ...
- #
- # Now the word 'bought' will have two scores from spans B and C. We only
- # want to consider the score with "maximum context", which we define as
- # the *minimum* of its left and right context (the *sum* of left and
- # right context will always be the same, of course).
- #
- # In the example the maximum context for 'bought' would be span C since
- # it has 1 left context and 3 right context, while span B has 4 left context
- # and 0 right context.
- best_score = None
- best_span_index = None
- for (span_index, doc_span) in enumerate(doc_spans):
- end = doc_span.start + doc_span.length - 1
- if position < doc_span.start:
- continue
- if position > end:
- continue
- num_left_context = position - doc_span.start
- num_right_context = end - position
- score = min(num_left_context, num_right_context) + 0.01 * doc_span.length
- if best_score is None or score > best_score:
- best_score = score
- best_span_index = span_index
-
- return cur_span_index == best_span_index
-
-
-RawResult = collections.namedtuple("RawResult", ["unique_id", "start_logits", "end_logits"])
-
-
-def write_predictions(
- all_examples,
- all_features,
- all_results,
- n_best_size,
- max_answer_length,
- do_lower_case,
- output_prediction_file,
- output_nbest_file,
- output_null_log_odds_file,
- verbose_logging,
- version_2_with_negative,
- null_score_diff_threshold,
-):
- """Write final predictions to the json file and log-odds of null if needed."""
- logger.info("Writing predictions to: %s" % (output_prediction_file))
- logger.info("Writing nbest to: %s" % (output_nbest_file))
-
- example_index_to_features = collections.defaultdict(list)
- for feature in all_features:
- example_index_to_features[feature.example_index].append(feature)
-
- unique_id_to_result = {}
- for result in all_results:
- unique_id_to_result[result.unique_id] = result
-
- _PrelimPrediction = collections.namedtuple( # pylint: disable=invalid-name
- "PrelimPrediction", ["feature_index", "start_index", "end_index", "start_logit", "end_logit"]
- )
-
- all_predictions = collections.OrderedDict()
- all_nbest_json = collections.OrderedDict()
- scores_diff_json = collections.OrderedDict()
-
- for (example_index, example) in enumerate(all_examples):
- features = example_index_to_features[example_index]
-
- prelim_predictions = []
- # keep track of the minimum score of null start+end of position 0
- score_null = 1000000 # large and positive
- min_null_feature_index = 0 # the paragraph slice with min null score
- null_start_logit = 0 # the start logit at the slice with min null score
- null_end_logit = 0 # the end logit at the slice with min null score
- for (feature_index, feature) in enumerate(features):
- result = unique_id_to_result[feature.unique_id]
- start_indexes = _get_best_indexes(result.start_logits, n_best_size)
- end_indexes = _get_best_indexes(result.end_logits, n_best_size)
- # if we could have irrelevant answers, get the min score of irrelevant
- if version_2_with_negative:
- feature_null_score = result.start_logits[0] + result.end_logits[0]
- if feature_null_score < score_null:
- score_null = feature_null_score
- min_null_feature_index = feature_index
- null_start_logit = result.start_logits[0]
- null_end_logit = result.end_logits[0]
- for start_index in start_indexes:
- for end_index in end_indexes:
- # We could hypothetically create invalid predictions, e.g., predict
- # that the start of the span is in the question. We throw out all
- # invalid predictions.
- if start_index >= len(feature.tokens):
- continue
- if end_index >= len(feature.tokens):
- continue
- if start_index not in feature.token_to_orig_map:
- continue
- if end_index not in feature.token_to_orig_map:
- continue
- if not feature.token_is_max_context.get(start_index, False):
- continue
- if end_index < start_index:
- continue
- length = end_index - start_index + 1
- if length > max_answer_length:
- continue
- prelim_predictions.append(
- _PrelimPrediction(
- feature_index=feature_index,
- start_index=start_index,
- end_index=end_index,
- start_logit=result.start_logits[start_index],
- end_logit=result.end_logits[end_index],
- )
- )
- if version_2_with_negative:
- prelim_predictions.append(
- _PrelimPrediction(
- feature_index=min_null_feature_index,
- start_index=0,
- end_index=0,
- start_logit=null_start_logit,
- end_logit=null_end_logit,
- )
- )
- prelim_predictions = sorted(prelim_predictions, key=lambda x: (x.start_logit + x.end_logit), reverse=True)
-
- _NbestPrediction = collections.namedtuple( # pylint: disable=invalid-name
- "NbestPrediction", ["text", "start_logit", "end_logit"]
- )
-
- seen_predictions = {}
- nbest = []
- for pred in prelim_predictions:
- if len(nbest) >= n_best_size:
- break
- feature = features[pred.feature_index]
- if pred.start_index > 0: # this is a non-null prediction
- tok_tokens = feature.tokens[pred.start_index : (pred.end_index + 1)]
- orig_doc_start = feature.token_to_orig_map[pred.start_index]
- orig_doc_end = feature.token_to_orig_map[pred.end_index]
- orig_tokens = example.doc_tokens[orig_doc_start : (orig_doc_end + 1)]
- tok_text = " ".join(tok_tokens)
-
- # De-tokenize WordPieces that have been split off.
- tok_text = tok_text.replace(" ##", "")
- tok_text = tok_text.replace("##", "")
-
- # Clean whitespace
- tok_text = tok_text.strip()
- tok_text = " ".join(tok_text.split())
- orig_text = " ".join(orig_tokens)
-
- final_text = get_final_text(tok_text, orig_text, do_lower_case, verbose_logging)
- if final_text in seen_predictions:
- continue
-
- seen_predictions[final_text] = True
- else:
- final_text = ""
- seen_predictions[final_text] = True
-
- nbest.append(_NbestPrediction(text=final_text, start_logit=pred.start_logit, end_logit=pred.end_logit))
- # if we didn't include the empty option in the n-best, include it
- if version_2_with_negative:
- if "" not in seen_predictions:
- nbest.append(_NbestPrediction(text="", start_logit=null_start_logit, end_logit=null_end_logit))
-
- # In very rare edge cases we could only have single null prediction.
- # So we just create a nonce prediction in this case to avoid failure.
- if len(nbest) == 1:
- nbest.insert(0, _NbestPrediction(text="empty", start_logit=0.0, end_logit=0.0))
-
- # In very rare edge cases we could have no valid predictions. So we
- # just create a nonce prediction in this case to avoid failure.
- if not nbest:
- nbest.append(_NbestPrediction(text="empty", start_logit=0.0, end_logit=0.0))
-
- assert len(nbest) >= 1
-
- total_scores = []
- best_non_null_entry = None
- for entry in nbest:
- total_scores.append(entry.start_logit + entry.end_logit)
- if not best_non_null_entry:
- if entry.text:
- best_non_null_entry = entry
-
- probs = _compute_softmax(total_scores)
-
- nbest_json = []
- for (i, entry) in enumerate(nbest):
- output = collections.OrderedDict()
- output["text"] = entry.text
- output["probability"] = probs[i]
- output["start_logit"] = entry.start_logit
- output["end_logit"] = entry.end_logit
- nbest_json.append(output)
-
- assert len(nbest_json) >= 1
-
- if not version_2_with_negative:
- all_predictions[example.qas_id] = nbest_json[0]["text"]
- else:
- # predict "" iff the null score - the score of best non-null > threshold
- score_diff = score_null - best_non_null_entry.start_logit - (best_non_null_entry.end_logit)
- scores_diff_json[example.qas_id] = score_diff
- if score_diff > null_score_diff_threshold:
- all_predictions[example.qas_id] = ""
- else:
- all_predictions[example.qas_id] = best_non_null_entry.text
- all_nbest_json[example.qas_id] = nbest_json
-
- with open(output_prediction_file, "w") as writer:
- writer.write(json.dumps(all_predictions, indent=4) + "\n")
-
- with open(output_nbest_file, "w") as writer:
- writer.write(json.dumps(all_nbest_json, indent=4) + "\n")
-
- if version_2_with_negative:
- with open(output_null_log_odds_file, "w") as writer:
- writer.write(json.dumps(scores_diff_json, indent=4) + "\n")
-
- return all_predictions
-
-
-# For XLNet (and XLM which uses the same head)
-RawResultExtended = collections.namedtuple(
- "RawResultExtended",
- ["unique_id", "start_top_log_probs", "start_top_index", "end_top_log_probs", "end_top_index", "cls_logits"],
-)
-
-
-def write_predictions_extended(
- all_examples,
- all_features,
- all_results,
- n_best_size,
- max_answer_length,
- output_prediction_file,
- output_nbest_file,
- output_null_log_odds_file,
- orig_data_file,
- start_n_top,
- end_n_top,
- version_2_with_negative,
- tokenizer,
- verbose_logging,
-):
- """ XLNet write prediction logic (more complex than Bert's).
- Write final predictions to the json file and log-odds of null if needed.
-
- Requires utils_squad_evaluate.py
- """
- _PrelimPrediction = collections.namedtuple( # pylint: disable=invalid-name
- "PrelimPrediction", ["feature_index", "start_index", "end_index", "start_log_prob", "end_log_prob"]
- )
-
- _NbestPrediction = collections.namedtuple( # pylint: disable=invalid-name
- "NbestPrediction", ["text", "start_log_prob", "end_log_prob"]
- )
-
- logger.info("Writing predictions to: %s", output_prediction_file)
- # logger.info("Writing nbest to: %s" % (output_nbest_file))
-
- example_index_to_features = collections.defaultdict(list)
- for feature in all_features:
- example_index_to_features[feature.example_index].append(feature)
-
- unique_id_to_result = {}
- for result in all_results:
- unique_id_to_result[result.unique_id] = result
-
- all_predictions = collections.OrderedDict()
- all_nbest_json = collections.OrderedDict()
- scores_diff_json = collections.OrderedDict()
-
- for (example_index, example) in enumerate(all_examples):
- features = example_index_to_features[example_index]
-
- prelim_predictions = []
- # keep track of the minimum score of null start+end of position 0
- score_null = 1000000 # large and positive
-
- for (feature_index, feature) in enumerate(features):
- result = unique_id_to_result[feature.unique_id]
-
- cur_null_score = result.cls_logits
-
- # if we could have irrelevant answers, get the min score of irrelevant
- score_null = min(score_null, cur_null_score)
-
- for i in range(start_n_top):
- for j in range(end_n_top):
- start_log_prob = result.start_top_log_probs[i]
- start_index = result.start_top_index[i]
-
- j_index = i * end_n_top + j
-
- end_log_prob = result.end_top_log_probs[j_index]
- end_index = result.end_top_index[j_index]
-
- # We could hypothetically create invalid predictions, e.g., predict
- # that the start of the span is in the question. We throw out all
- # invalid predictions.
- if start_index >= feature.paragraph_len - 1:
- continue
- if end_index >= feature.paragraph_len - 1:
- continue
-
- if not feature.token_is_max_context.get(start_index, False):
- continue
- if end_index < start_index:
- continue
- length = end_index - start_index + 1
- if length > max_answer_length:
- continue
-
- prelim_predictions.append(
- _PrelimPrediction(
- feature_index=feature_index,
- start_index=start_index,
- end_index=end_index,
- start_log_prob=start_log_prob,
- end_log_prob=end_log_prob,
- )
- )
-
- prelim_predictions = sorted(
- prelim_predictions, key=lambda x: (x.start_log_prob + x.end_log_prob), reverse=True
- )
-
- seen_predictions = {}
- nbest = []
- for pred in prelim_predictions:
- if len(nbest) >= n_best_size:
- break
- feature = features[pred.feature_index]
-
- # XLNet un-tokenizer
- # Let's keep it simple for now and see if we need all this later.
- #
- # tok_start_to_orig_index = feature.tok_start_to_orig_index
- # tok_end_to_orig_index = feature.tok_end_to_orig_index
- # start_orig_pos = tok_start_to_orig_index[pred.start_index]
- # end_orig_pos = tok_end_to_orig_index[pred.end_index]
- # paragraph_text = example.paragraph_text
- # final_text = paragraph_text[start_orig_pos: end_orig_pos + 1].strip()
-
- # Previously used Bert untokenizer
- tok_tokens = feature.tokens[pred.start_index : (pred.end_index + 1)]
- orig_doc_start = feature.token_to_orig_map[pred.start_index]
- orig_doc_end = feature.token_to_orig_map[pred.end_index]
- orig_tokens = example.doc_tokens[orig_doc_start : (orig_doc_end + 1)]
- tok_text = tokenizer.convert_tokens_to_string(tok_tokens)
-
- # Clean whitespace
- tok_text = tok_text.strip()
- tok_text = " ".join(tok_text.split())
- orig_text = " ".join(orig_tokens)
-
- final_text = get_final_text(tok_text, orig_text, tokenizer.do_lower_case, verbose_logging)
-
- if final_text in seen_predictions:
- continue
-
- seen_predictions[final_text] = True
-
- nbest.append(
- _NbestPrediction(text=final_text, start_log_prob=pred.start_log_prob, end_log_prob=pred.end_log_prob)
- )
-
- # In very rare edge cases we could have no valid predictions. So we
- # just create a nonce prediction in this case to avoid failure.
- if not nbest:
- nbest.append(_NbestPrediction(text="", start_log_prob=-1e6, end_log_prob=-1e6))
-
- total_scores = []
- best_non_null_entry = None
- for entry in nbest:
- total_scores.append(entry.start_log_prob + entry.end_log_prob)
- if not best_non_null_entry:
- best_non_null_entry = entry
-
- probs = _compute_softmax(total_scores)
-
- nbest_json = []
- for (i, entry) in enumerate(nbest):
- output = collections.OrderedDict()
- output["text"] = entry.text
- output["probability"] = probs[i]
- output["start_log_prob"] = entry.start_log_prob
- output["end_log_prob"] = entry.end_log_prob
- nbest_json.append(output)
-
- assert len(nbest_json) >= 1
- assert best_non_null_entry is not None
-
- score_diff = score_null
- scores_diff_json[example.qas_id] = score_diff
- # note(zhiliny): always predict best_non_null_entry
- # and the evaluation script will search for the best threshold
- all_predictions[example.qas_id] = best_non_null_entry.text
-
- all_nbest_json[example.qas_id] = nbest_json
-
- with open(output_prediction_file, "w") as writer:
- writer.write(json.dumps(all_predictions, indent=4) + "\n")
-
- with open(output_nbest_file, "w") as writer:
- writer.write(json.dumps(all_nbest_json, indent=4) + "\n")
-
- if version_2_with_negative:
- with open(output_null_log_odds_file, "w") as writer:
- writer.write(json.dumps(scores_diff_json, indent=4) + "\n")
-
- with open(orig_data_file, "r", encoding="utf-8") as reader:
- orig_data = json.load(reader)["data"]
-
- qid_to_has_ans = make_qid_to_has_ans(orig_data)
- exact_raw, f1_raw = get_raw_scores(orig_data, all_predictions)
- out_eval = {}
-
- find_all_best_thresh_v2(out_eval, all_predictions, exact_raw, f1_raw, scores_diff_json, qid_to_has_ans)
-
- return out_eval
-
-
-def get_final_text(pred_text, orig_text, do_lower_case, verbose_logging=False):
- """Project the tokenized prediction back to the original text."""
-
- # When we created the data, we kept track of the alignment between original
- # (whitespace tokenized) tokens and our WordPiece tokenized tokens. So
- # now `orig_text` contains the span of our original text corresponding to the
- # span that we predicted.
- #
- # However, `orig_text` may contain extra characters that we don't want in
- # our prediction.
- #
- # For example, let's say:
- # pred_text = steve smith
- # orig_text = Steve Smith's
- #
- # We don't want to return `orig_text` because it contains the extra "'s".
- #
- # We don't want to return `pred_text` because it's already been normalized
- # (the SQuAD eval script also does punctuation stripping/lower casing but
- # our tokenizer does additional normalization like stripping accent
- # characters).
- #
- # What we really want to return is "Steve Smith".
- #
- # Therefore, we have to apply a semi-complicated alignment heuristic between
- # `pred_text` and `orig_text` to get a character-to-character alignment. This
- # can fail in certain cases in which case we just return `orig_text`.
-
- def _strip_spaces(text):
- ns_chars = []
- ns_to_s_map = collections.OrderedDict()
- for (i, c) in enumerate(text):
- if c == " ":
- continue
- ns_to_s_map[len(ns_chars)] = i
- ns_chars.append(c)
- ns_text = "".join(ns_chars)
- return (ns_text, ns_to_s_map)
-
- # We first tokenize `orig_text`, strip whitespace from the result
- # and `pred_text`, and check if they are the same length. If they are
- # NOT the same length, the heuristic has failed. If they are the same
- # length, we assume the characters are one-to-one aligned.
- tokenizer = BasicTokenizer(do_lower_case=do_lower_case)
-
- tok_text = " ".join(tokenizer.tokenize(orig_text))
-
- start_position = tok_text.find(pred_text)
- if start_position == -1:
- if verbose_logging:
- logger.info("Unable to find text: '%s' in '%s'" % (pred_text, orig_text))
- return orig_text
- end_position = start_position + len(pred_text) - 1
-
- (orig_ns_text, orig_ns_to_s_map) = _strip_spaces(orig_text)
- (tok_ns_text, tok_ns_to_s_map) = _strip_spaces(tok_text)
-
- if len(orig_ns_text) != len(tok_ns_text):
- if verbose_logging:
- logger.info("Length not equal after stripping spaces: '%s' vs '%s'", orig_ns_text, tok_ns_text)
- return orig_text
-
- # We then project the characters in `pred_text` back to `orig_text` using
- # the character-to-character alignment.
- tok_s_to_ns_map = {}
- for (i, tok_index) in tok_ns_to_s_map.items():
- tok_s_to_ns_map[tok_index] = i
-
- orig_start_position = None
- if start_position in tok_s_to_ns_map:
- ns_start_position = tok_s_to_ns_map[start_position]
- if ns_start_position in orig_ns_to_s_map:
- orig_start_position = orig_ns_to_s_map[ns_start_position]
-
- if orig_start_position is None:
- if verbose_logging:
- logger.info("Couldn't map start position")
- return orig_text
-
- orig_end_position = None
- if end_position in tok_s_to_ns_map:
- ns_end_position = tok_s_to_ns_map[end_position]
- if ns_end_position in orig_ns_to_s_map:
- orig_end_position = orig_ns_to_s_map[ns_end_position]
-
- if orig_end_position is None:
- if verbose_logging:
- logger.info("Couldn't map end position")
- return orig_text
-
- output_text = orig_text[orig_start_position : (orig_end_position + 1)]
- return output_text
-
-
-def _get_best_indexes(logits, n_best_size):
- """Get the n-best logits from a list."""
- index_and_score = sorted(enumerate(logits), key=lambda x: x[1], reverse=True)
-
- best_indexes = []
- for i in range(len(index_and_score)):
- if i >= n_best_size:
- break
- best_indexes.append(index_and_score[i][0])
- return best_indexes
-
-
-def _compute_softmax(scores):
- """Compute softmax probability over raw logits."""
- if not scores:
- return []
-
- max_score = None
- for score in scores:
- if max_score is None or score > max_score:
- max_score = score
-
- exp_scores = []
- total_sum = 0.0
- for score in scores:
- x = math.exp(score - max_score)
- exp_scores.append(x)
- total_sum += x
-
- probs = []
- for score in exp_scores:
- probs.append(score / total_sum)
- return probs
diff --git a/server/transformers/templates/adding_a_new_model/README.md b/server/transformers/templates/adding_a_new_model/README.md
deleted file mode 100644
index 5397ca4c789817bbb244bcfbd7679adc9381f8d2..0000000000000000000000000000000000000000
--- a/server/transformers/templates/adding_a_new_model/README.md
+++ /dev/null
@@ -1,62 +0,0 @@
-# How to add a new model in 🤗Transformers
-
-This folder describes the process to add a new model in 🤗Transformers and provide templates for the required files.
-
-The library is designed to incorporate a variety of models and code bases. As such the process for adding a new model usually mostly consists in copy-pasting to relevant original code in the various sections of the templates included in the present repository.
-
-One important point though is that the library has the following goals impacting the way models are incorporated:
-
-- one specific feature of the API is the capability to run the model and tokenizer inline. The tokenization code thus often have to be slightly adapted to allow for running in the python interpreter.
-- the package is also designed to be as self-consistent and with a small and reliable set of packages dependencies. In consequence, additional dependencies are usually not allowed when adding a model but can be allowed for the inclusion of a new tokenizer (recent examples of dependencies added for tokenizer specificities include `sentencepiece` and `sacremoses`). Please make sure to check the existing dependencies when possible before adding a new one.
-
-For a quick overview of the library organization, please check the [QuickStart section of the documentation](https://huggingface.co/transformers/quickstart.html).
-
-# Typical workflow for including a model
-
-Here an overview of the general workflow:
-
-- [ ] add model/configuration/tokenization classes
-- [ ] add conversion scripts
-- [ ] add tests
-- [ ] finalize
-
-Let's detail what should be done at each step
-
-## Adding model/configuration/tokenization classes
-
-Here is the workflow for adding model/configuration/tokenization classes:
-
-- [ ] copy the python files from the present folder to the main folder and rename them, replacing `xxx` with your model name,
-- [ ] edit the files to replace `XXX` (with various casing) with your model name
-- [ ] copy-paste or create a simple configuration class for your model in the `configuration_...` file
-- [ ] copy-paste or create the code for your model in the `modeling_...` files (PyTorch and TF 2.0)
-- [ ] copy-paste or create a tokenizer class for your model in the `tokenization_...` file
-
-# Adding conversion scripts
-
-Here is the workflow for the conversion scripts:
-
-- [ ] copy the conversion script (`convert_...`) from the present folder to the main folder.
-- [ ] edit this script to convert your original checkpoint weights to the current pytorch ones.
-
-# Adding tests:
-
-Here is the workflow for the adding tests:
-
-- [ ] copy the python files from the `tests` sub-folder of the present folder to the `tests` subfolder of the main folder and rename them, replacing `xxx` with your model name,
-- [ ] edit the tests files to replace `XXX` (with various casing) with your model name
-- [ ] edit the tests code as needed
-
-# Final steps
-
-You can then finish the addition step by adding imports for your classes in the common files:
-
-- [ ] add import for all the relevant classes in `__init__.py`
-- [ ] add your configuration in `configuration_auto.py`
-- [ ] add your PyTorch and TF 2.0 model respectively in `modeling_auto.py` and `modeling_tf_auto.py`
-- [ ] add your tokenizer in `tokenization_auto.py`
-- [ ] add your models and tokenizer to `pipeline.py`
-- [ ] add a link to your conversion script in the main conversion utility (in `commands/convert.py`)
-- [ ] edit the PyTorch to TF 2.0 conversion script to add your model in the `convert_pytorch_checkpoint_to_tf2.py` file
-- [ ] add a mention of your model in the doc: `README.md` and the documentation itself at `docs/source/pretrained_models.rst`.
-- [ ] upload the pretrained weigths, configurations and vocabulary files.
diff --git a/server/transformers/templates/adding_a_new_model/configuration_xxx.py b/server/transformers/templates/adding_a_new_model/configuration_xxx.py
deleted file mode 100644
index d23bce43d2f43bf6cda25feea3197a9ddfc56f01..0000000000000000000000000000000000000000
--- a/server/transformers/templates/adding_a_new_model/configuration_xxx.py
+++ /dev/null
@@ -1,115 +0,0 @@
-# coding=utf-8
-# Copyright 2010, XXX authors
-#
-# Licensed under the Apache License, Version 2.0 (the "License");
-# you may not use this file except in compliance with the License.
-# You may obtain a copy of the License at
-#
-# http://www.apache.org/licenses/LICENSE-2.0
-#
-# Unless required by applicable law or agreed to in writing, software
-# distributed under the License is distributed on an "AS IS" BASIS,
-# WITHOUT WARRANTIES OR CONDITIONS OF ANY KIND, either express or implied.
-# See the License for the specific language governing permissions and
-# limitations under the License.
-""" XXX model configuration """
-
-
-import logging
-
-from .configuration_utils import PretrainedConfig
-
-
-logger = logging.getLogger(__name__)
-
-XXX_PRETRAINED_CONFIG_ARCHIVE_MAP = {
- "xxx-base-uncased": "https://s3.amazonaws.com/models.huggingface.co/bert/xxx-base-uncased-config.json",
- "xxx-large-uncased": "https://s3.amazonaws.com/models.huggingface.co/bert/xxx-large-uncased-config.json",
-}
-
-
-class XxxConfig(PretrainedConfig):
- r"""
- :class:`~transformers.XxxConfig` is the configuration class to store the configuration of a
- `XxxModel`.
-
-
- Arguments:
- vocab_size: Vocabulary size of `inputs_ids` in `XxxModel`.
- hidden_size: Size of the encoder layers and the pooler layer.
- num_hidden_layers: Number of hidden layers in the Transformer encoder.
- num_attention_heads: Number of attention heads for each attention layer in
- the Transformer encoder.
- intermediate_size: The size of the "intermediate" (i.e., feed-forward)
- layer in the Transformer encoder.
- hidden_act: The non-linear activation function (function or string) in the
- encoder and pooler. If string, "gelu", "relu", "swish" and "gelu_new" are supported.
- hidden_dropout_prob: The dropout probabilitiy for all fully connected
- layers in the embeddings, encoder, and pooler.
- attention_probs_dropout_prob: The dropout ratio for the attention
- probabilities.
- max_position_embeddings: The maximum sequence length that this model might
- ever be used with. Typically set this to something large just in case
- (e.g., 512 or 1024 or 2048).
- type_vocab_size: The vocabulary size of the `token_type_ids` passed into
- `XxxModel`.
- initializer_range: The sttdev of the truncated_normal_initializer for
- initializing all weight matrices.
- layer_norm_eps: The epsilon used by LayerNorm.
- """
- pretrained_config_archive_map = XXX_PRETRAINED_CONFIG_ARCHIVE_MAP
- model_type = "xxx"
-
- def __init__(
- self,
- vocab_size=50257,
- n_positions=1024,
- n_ctx=1024,
- n_embd=768,
- n_layer=12,
- n_head=12,
- resid_pdrop=0.1,
- embd_pdrop=0.1,
- attn_pdrop=0.1,
- layer_norm_epsilon=1e-5,
- initializer_range=0.02,
- summary_type="cls_index",
- summary_use_proj=True,
- summary_activation=None,
- summary_proj_to_labels=True,
- summary_first_dropout=0.1,
- **kwargs
- ):
- super().__init__(**kwargs)
- self.vocab_size = vocab_size
- self.n_ctx = n_ctx
- self.n_positions = n_positions
- self.n_embd = n_embd
- self.n_layer = n_layer
- self.n_head = n_head
- self.resid_pdrop = resid_pdrop
- self.embd_pdrop = embd_pdrop
- self.attn_pdrop = attn_pdrop
- self.layer_norm_epsilon = layer_norm_epsilon
- self.initializer_range = initializer_range
- self.summary_type = summary_type
- self.summary_use_proj = summary_use_proj
- self.summary_activation = summary_activation
- self.summary_first_dropout = summary_first_dropout
- self.summary_proj_to_labels = summary_proj_to_labels
-
- @property
- def max_position_embeddings(self):
- return self.n_positions
-
- @property
- def hidden_size(self):
- return self.n_embd
-
- @property
- def num_attention_heads(self):
- return self.n_head
-
- @property
- def num_hidden_layers(self):
- return self.n_layer
diff --git a/server/transformers/templates/adding_a_new_model/convert_xxx_original_tf_checkpoint_to_pytorch.py b/server/transformers/templates/adding_a_new_model/convert_xxx_original_tf_checkpoint_to_pytorch.py
deleted file mode 100755
index b57d3bbdcaeacce796833750f259f5f809beca58..0000000000000000000000000000000000000000
--- a/server/transformers/templates/adding_a_new_model/convert_xxx_original_tf_checkpoint_to_pytorch.py
+++ /dev/null
@@ -1,61 +0,0 @@
-# coding=utf-8
-# Copyright 2018 The HuggingFace Inc. team.
-#
-# Licensed under the Apache License, Version 2.0 (the "License");
-# you may not use this file except in compliance with the License.
-# You may obtain a copy of the License at
-#
-# http://www.apache.org/licenses/LICENSE-2.0
-#
-# Unless required by applicable law or agreed to in writing, software
-# distributed under the License is distributed on an "AS IS" BASIS,
-# WITHOUT WARRANTIES OR CONDITIONS OF ANY KIND, either express or implied.
-# See the License for the specific language governing permissions and
-# limitations under the License.
-"""Convert XXX checkpoint."""
-
-
-import argparse
-import logging
-
-import torch
-
-from transformers import XxxConfig, XxxForPreTraining, load_tf_weights_in_xxx
-
-
-logging.basicConfig(level=logging.INFO)
-
-
-def convert_tf_checkpoint_to_pytorch(tf_checkpoint_path, config_file, pytorch_dump_path):
- # Initialise PyTorch model
- config = XxxConfig.from_json_file(config_file)
- print("Building PyTorch model from configuration: {}".format(str(config)))
- model = XxxForPreTraining(config)
-
- # Load weights from tf checkpoint
- load_tf_weights_in_xxx(model, config, tf_checkpoint_path)
-
- # Save pytorch-model
- print("Save PyTorch model to {}".format(pytorch_dump_path))
- torch.save(model.state_dict(), pytorch_dump_path)
-
-
-if __name__ == "__main__":
- parser = argparse.ArgumentParser()
- # Required parameters
- parser.add_argument(
- "--tf_checkpoint_path", default=None, type=str, required=True, help="Path to the TensorFlow checkpoint path."
- )
- parser.add_argument(
- "--config_file",
- default=None,
- type=str,
- required=True,
- help="The config json file corresponding to the pre-trained model. \n"
- "This specifies the model architecture.",
- )
- parser.add_argument(
- "--pytorch_dump_path", default=None, type=str, required=True, help="Path to the output PyTorch model."
- )
- args = parser.parse_args()
- convert_tf_checkpoint_to_pytorch(args.tf_checkpoint_path, args.config_file, args.pytorch_dump_path)
diff --git a/server/transformers/templates/adding_a_new_model/modeling_tf_xxx.py b/server/transformers/templates/adding_a_new_model/modeling_tf_xxx.py
deleted file mode 100644
index 4e3791e481d9900bbe5d6454a7483440de642885..0000000000000000000000000000000000000000
--- a/server/transformers/templates/adding_a_new_model/modeling_tf_xxx.py
+++ /dev/null
@@ -1,532 +0,0 @@
-# coding=utf-8
-# Copyright 2018 The Google AI Language Team Authors and The HuggingFace Inc. team.
-# Copyright (c) 2018, NVIDIA CORPORATION. All rights reserved.
-#
-# Licensed under the Apache License, Version 2.0 (the "License");
-# you may not use this file except in compliance with the License.
-# You may obtain a copy of the License at
-#
-# http://www.apache.org/licenses/LICENSE-2.0
-#
-# Unless required by applicable law or agreed to in writing, software
-# distributed under the License is distributed on an "AS IS" BASIS,
-# WITHOUT WARRANTIES OR CONDITIONS OF ANY KIND, either express or implied.
-# See the License for the specific language governing permissions and
-# limitations under the License.
-""" TF 2.0 XXX model. """
-
-####################################################
-# In this template, replace all the XXX (various casings) with your model name
-####################################################
-
-
-import logging
-
-import tensorflow as tf
-
-from .configuration_xxx import XxxConfig
-from .file_utils import add_start_docstrings
-from .modeling_tf_utils import TFPreTrainedModel, get_initializer, shape_list
-
-
-logger = logging.getLogger(__name__)
-
-####################################################
-# This dict contrains shortcut names and associated url
-# for the pretrained weights provided with the models
-####################################################
-TF_XXX_PRETRAINED_MODEL_ARCHIVE_MAP = {
- "xxx-base-uncased": "https://s3.amazonaws.com/models.huggingface.co/bert/xxx-base-uncased-tf_model.h5",
- "xxx-large-uncased": "https://s3.amazonaws.com/models.huggingface.co/bert/xxx-large-uncased-tf_model.h5",
-}
-
-
-####################################################
-# TF 2.0 Models are constructed using Keras imperative API by sub-classing
-# - tf.keras.layers.Layer for the layers and
-# - TFPreTrainedModel for the models (itself a sub-class of tf.keras.Model)
-####################################################
-
-####################################################
-# Here is an example of typical layer in a TF 2.0 model of the library
-# The classes are usually identical to the PyTorch ones and prefixed with 'TF'.
-#
-# Note that class __init__ parameters includes **kwargs (send to 'super').
-# This let us have a control on class scope and variable names:
-# More precisely, we set the names of the class attributes (lower level layers) to
-# to the equivalent attributes names in the PyTorch model so we can have equivalent
-# class and scope structure between PyTorch and TF 2.0 models and easily load one in the other.
-#
-# See the conversion methods in modeling_tf_pytorch_utils.py for more details
-####################################################
-
-TFXxxAttention = tf.keras.layers.Layer
-
-TFXxxIntermediate = tf.keras.layers.Layer
-
-TFXxxOutput = tf.keras.layers.Layer
-
-
-class TFXxxLayer(tf.keras.layers.Layer):
- def __init__(self, config, **kwargs):
- super().__init__(**kwargs)
- self.attention = TFXxxAttention(config, name="attention")
- self.intermediate = TFXxxIntermediate(config, name="intermediate")
- self.transformer_output = TFXxxOutput(config, name="output")
-
- def call(self, inputs, training=False):
- hidden_states, attention_mask, head_mask = inputs
-
- attention_outputs = self.attention([hidden_states, attention_mask, head_mask], training=training)
- attention_output = attention_outputs[0]
- intermediate_output = self.intermediate(attention_output)
- layer_output = self.transformer_output([intermediate_output, attention_output], training=training)
- outputs = (layer_output,) + attention_outputs[1:] # add attentions if we output them
- return outputs
-
-
-####################################################
-# The full model without a specific pretrained or finetuning head is
-# provided as a tf.keras.layers.Layer usually called "TFXxxMainLayer"
-####################################################
-class TFXxxMainLayer(tf.keras.layers.Layer):
- def __init__(self, config, **kwargs):
- super().__init__(**kwargs)
-
- def _resize_token_embeddings(self, new_num_tokens):
- raise NotImplementedError # Not implemented yet in the library fr TF 2.0 models
-
- def _prune_heads(self, heads_to_prune):
- raise NotImplementedError # Not implemented yet in the library fr TF 2.0 models
-
- def call(
- self, inputs, attention_mask=None, token_type_ids=None, position_ids=None, head_mask=None, training=False
- ):
- # We allow three types of multi-inputs:
- # - traditional keyword arguments in the call method
- # - all the arguments provided as a dict in the first positional argument of call
- # - all the arguments provided as a list/tuple (ordered) in the first positional argument of call
- # The last two options are useful to use the tf.keras fit() method.
-
- if isinstance(inputs, (tuple, list)):
- input_ids = inputs[0]
- attention_mask = inputs[1] if len(inputs) > 1 else attention_mask
- token_type_ids = inputs[2] if len(inputs) > 2 else token_type_ids
- position_ids = inputs[3] if len(inputs) > 3 else position_ids
- head_mask = inputs[4] if len(inputs) > 4 else head_mask
- assert len(inputs) <= 5, "Too many inputs."
- elif isinstance(inputs, dict):
- input_ids = inputs.get("input_ids")
- attention_mask = inputs.get("attention_mask", attention_mask)
- token_type_ids = inputs.get("token_type_ids", token_type_ids)
- position_ids = inputs.get("position_ids", position_ids)
- head_mask = inputs.get("head_mask", head_mask)
- assert len(inputs) <= 5, "Too many inputs."
- else:
- input_ids = inputs
-
- if attention_mask is None:
- attention_mask = tf.fill(shape_list(input_ids), 1)
- if token_type_ids is None:
- token_type_ids = tf.fill(shape_list(input_ids), 0)
-
- # We create a 3D attention mask from a 2D tensor mask.
- # Sizes are [batch_size, 1, 1, to_seq_length]
- # So we can broadcast to [batch_size, num_heads, from_seq_length, to_seq_length]
- # this attention mask is more simple than the triangular masking of causal attention
- # used in OpenAI GPT, we just need to prepare the broadcast dimension here.
- extended_attention_mask = attention_mask[:, tf.newaxis, tf.newaxis, :]
-
- # Since attention_mask is 1.0 for positions we want to attend and 0.0 for
- # masked positions, this operation will create a tensor which is 0.0 for
- # positions we want to attend and -10000.0 for masked positions.
- # Since we are adding it to the raw scores before the softmax, this is
- # effectively the same as removing these entirely.
-
- extended_attention_mask = tf.cast(extended_attention_mask, tf.float32)
- extended_attention_mask = (1.0 - extended_attention_mask) * -10000.0
-
- # Prepare head mask if needed
- # 1.0 in head_mask indicate we keep the head
- # attention_probs has shape bsz x n_heads x N x N
- # input head_mask has shape [num_heads] or [num_hidden_layers x num_heads]
- # and head_mask is converted to shape [num_hidden_layers x batch x num_heads x seq_length x seq_length]
- if head_mask is not None:
- raise NotImplementedError
- else:
- head_mask = [None] * self.num_hidden_layers
- # head_mask = tf.constant([0] * self.num_hidden_layers)
-
- ##################################
- # Replace this with your model code
- embedding_output = self.embeddings(input_ids, position_ids=position_ids, token_type_ids=token_type_ids)
- encoder_outputs = self.encoder([embedding_output, extended_attention_mask, head_mask], training=training)
- sequence_output = encoder_outputs[0]
- outputs = (sequence_output,) + encoder_outputs[1:] # add hidden_states and attentions if they are here
-
- return outputs # sequence_output, (hidden_states), (attentions)
-
-
-####################################################
-# TFXxxPreTrainedModel is a sub-class of tf.keras.Model
-# which take care of loading and saving pretrained weights
-# and various common utilities.
-# Here you just need to specify a few (self-explanatory)
-# pointers for your model.
-####################################################
-class TFXxxPreTrainedModel(TFPreTrainedModel):
- """ An abstract class to handle weights initialization and
- a simple interface for downloading and loading pretrained models.
- """
-
- config_class = XxxConfig
- pretrained_model_archive_map = TF_XXX_PRETRAINED_MODEL_ARCHIVE_MAP
- base_model_prefix = "transformer"
-
-
-XXX_START_DOCSTRING = r""" The XXX model was proposed in
- `XXX: Pre-training of Deep Bidirectional Transformers for Language Understanding`_
- by Jacob Devlin, Ming-Wei Chang, Kenton Lee and Kristina Toutanova. It's a bidirectional transformer
- pre-trained using a combination of masked language modeling objective and next sentence prediction
- on a large corpus comprising the Toronto Book Corpus and Wikipedia.
-
- This model is a tf.keras.Model `tf.keras.Model`_ sub-class. Use it as a regular TF 2.0 Keras Model and
- refer to the TF 2.0 documentation for all matter related to general usage and behavior.
-
- .. _`XXX: Pre-training of Deep Bidirectional Transformers for Language Understanding`:
- https://arxiv.org/abs/1810.04805
-
- .. _`tf.keras.Model`:
- https://www.tensorflow.org/versions/r2.0/api_docs/python/tf/keras/Model
-
- Note on the model inputs:
- TF 2.0 models accepts two formats as inputs:
-
- - having all inputs as keyword arguments (like PyTorch models), or
- - having all inputs as a list, tuple or dict in the first positional arguments.
-
- This second option is usefull when using `tf.keras.Model.fit()` method which currently requires having all the tensors in the first argument of the model call function: `model(inputs)`.
-
- If you choose this second option, there are three possibilities you can use to gather all the input Tensors in the first positional argument :
-
- - a single Tensor with input_ids only and nothing else: `model(inputs_ids)
- - a list of varying length with one or several input Tensors IN THE ORDER given in the docstring:
- `model([input_ids, attention_mask])` or `model([input_ids, attention_mask, token_type_ids])`
- - a dictionary with one or several input Tensors associaed to the input names given in the docstring:
- `model({'input_ids': input_ids, 'token_type_ids': token_type_ids})`
-
- Parameters:
- config (:class:`~transformers.XxxConfig`): Model configuration class with all the parameters of the model.
- Initializing with a config file does not load the weights associated with the model, only the configuration.
- Check out the :meth:`~transformers.PreTrainedModel.from_pretrained` method to load the model weights.
-"""
-
-XXX_INPUTS_DOCSTRING = r"""
- Inputs:
- **input_ids**: ``Numpy array`` or ``tf.Tensor`` of shape ``(batch_size, sequence_length)``:
- Indices of input sequence tokens in the vocabulary.
- To match pre-training, XXX input sequence should be formatted with [CLS] and [SEP] tokens as follows:
-
- (a) For sequence pairs:
-
- ``tokens: [CLS] is this jack ##son ##ville ? [SEP] no it is not . [SEP]``
-
- ``token_type_ids: 0 0 0 0 0 0 0 0 1 1 1 1 1 1``
-
- (b) For single sequences:
-
- ``tokens: [CLS] the dog is hairy . [SEP]``
-
- ``token_type_ids: 0 0 0 0 0 0 0``
-
- Xxx is a model with absolute position embeddings so it's usually advised to pad the inputs on
- the right rather than the left.
-
- Indices can be obtained using :class:`transformers.XxxTokenizer`.
- See :func:`transformers.PreTrainedTokenizer.encode` and
- :func:`transformers.PreTrainedTokenizer.convert_tokens_to_ids` for details.
- **attention_mask**: (`optional`) ``Numpy array`` or ``tf.Tensor`` of shape ``(batch_size, sequence_length)``:
- Mask to avoid performing attention on padding token indices.
- Mask values selected in ``[0, 1]``:
- ``1`` for tokens that are NOT MASKED, ``0`` for MASKED tokens.
- **token_type_ids**: (`optional`) ``Numpy array`` or ``tf.Tensor`` of shape ``(batch_size, sequence_length)``:
- Segment token indices to indicate first and second portions of the inputs.
- Indices are selected in ``[0, 1]``: ``0`` corresponds to a `sentence A` token, ``1``
- corresponds to a `sentence B` token
- (see `XXX: Pre-training of Deep Bidirectional Transformers for Language Understanding`_ for more details).
- **position_ids**: (`optional`) ``Numpy array`` or ``tf.Tensor`` of shape ``(batch_size, sequence_length)``:
- Indices of positions of each input sequence tokens in the position embeddings.
- Selected in the range ``[0, config.max_position_embeddings - 1]``.
- **head_mask**: (`optional`) ``Numpy array`` or ``tf.Tensor`` of shape ``(num_heads,)`` or ``(num_layers, num_heads)``:
- Mask to nullify selected heads of the self-attention modules.
- Mask values selected in ``[0, 1]``:
- ``1`` indicates the head is **not masked**, ``0`` indicates the head is **masked**.
- **inputs_embeds**: (`optional`) ``Numpy array`` or ``tf.Tensor`` of shape ``(batch_size, sequence_length, embedding_dim)``:
- Optionally, instead of passing ``input_ids`` you can choose to directly pass an embedded representation.
- This is useful if you want more control over how to convert `input_ids` indices into associated vectors
- than the model's internal embedding lookup matrix.
-"""
-
-
-@add_start_docstrings(
- "The bare Xxx Model transformer outputing raw hidden-states without any specific head on top.",
- XXX_START_DOCSTRING,
- XXX_INPUTS_DOCSTRING,
-)
-class TFXxxModel(TFXxxPreTrainedModel):
- r"""
- Outputs: `Tuple` comprising various elements depending on the configuration (config) and inputs:
- **last_hidden_state**: ``tf.Tensor`` of shape ``(batch_size, sequence_length, hidden_size)``
- Sequence of hidden-states at the output of the last layer of the model.
- **pooler_output**: ``tf.Tensor`` of shape ``(batch_size, hidden_size)``
- Last layer hidden-state of the first token of the sequence (classification token)
- further processed by a Linear layer and a Tanh activation function. The Linear
- layer weights are trained from the next sentence prediction (classification)
- objective during Xxx pretraining. This output is usually *not* a good summary
- of the semantic content of the input, you're often better with averaging or pooling
- the sequence of hidden-states for the whole input sequence.
- **hidden_states**: (`optional`, returned when ``config.output_hidden_states=True``)
- list of ``tf.Tensor`` (one for the output of each layer + the output of the embeddings)
- of shape ``(batch_size, sequence_length, hidden_size)``:
- Hidden-states of the model at the output of each layer plus the initial embedding outputs.
- **attentions**: (`optional`, returned when ``config.output_attentions=True``)
- list of ``tf.Tensor`` (one for each layer) of shape ``(batch_size, num_heads, sequence_length, sequence_length)``:
- Attentions weights after the attention softmax, used to compute the weighted average in the self-attention heads.
-
- Examples::
-
- import tensorflow as tf
- from transformers import XxxTokenizer, TFXxxModel
-
- tokenizer = XxxTokenizer.from_pretrained('xxx-base-uncased')
- model = TFXxxModel.from_pretrained('xxx-base-uncased')
- input_ids = tf.constant(tokenizer.encode("Hello, my dog is cute"))[None, :] # Batch size 1
- outputs = model(input_ids)
- last_hidden_states = outputs[0] # The last hidden-state is the first element of the output tuple
-
- """
-
- def __init__(self, config, *inputs, **kwargs):
- super().__init__(config, *inputs, **kwargs)
- self.transformer = TFXxxMainLayer(config, name="transformer")
-
- def call(self, inputs, **kwargs):
- outputs = self.transformer(inputs, **kwargs)
- return outputs
-
-
-TFXxxMLMHead = tf.keras.layers.Layer
-
-
-@add_start_docstrings(
- """Xxx Model with a `language modeling` head on top. """, XXX_START_DOCSTRING, XXX_INPUTS_DOCSTRING
-)
-class TFXxxForMaskedLM(TFXxxPreTrainedModel):
- r"""
- Outputs: `Tuple` comprising various elements depending on the configuration (config) and inputs:
- **prediction_scores**: ``Numpy array`` or ``tf.Tensor`` of shape ``(batch_size, sequence_length, config.vocab_size)``
- Prediction scores of the language modeling head (scores for each vocabulary token before SoftMax).
- **hidden_states**: (`optional`, returned when ``config.output_hidden_states=True``)
- list of ``Numpy array`` or ``tf.Tensor`` (one for the output of each layer + the output of the embeddings)
- of shape ``(batch_size, sequence_length, hidden_size)``:
- Hidden-states of the model at the output of each layer plus the initial embedding outputs.
- **attentions**: (`optional`, returned when ``config.output_attentions=True``)
- list of ``Numpy array`` or ``tf.Tensor`` (one for each layer) of shape ``(batch_size, num_heads, sequence_length, sequence_length)``:
- Attentions weights after the attention softmax, used to compute the weighted average in the self-attention heads.
-
- Examples::
-
- import tensorflow as tf
- from transformers import XxxTokenizer, TFXxxForMaskedLM
-
- tokenizer = XxxTokenizer.from_pretrained('xxx-base-uncased')
- model = TFXxxForMaskedLM.from_pretrained('xxx-base-uncased')
- input_ids = tf.constant(tokenizer.encode("Hello, my dog is cute"))[None, :] # Batch size 1
- outputs = model(input_ids)
- prediction_scores = outputs[0]
-
- """
-
- def __init__(self, config, *inputs, **kwargs):
- super().__init__(config, *inputs, **kwargs)
-
- self.transformer = TFXxxMainLayer(config, name="transformer")
- self.mlm = TFXxxMLMHead(config, self.transformer.embeddings, name="mlm")
-
- def call(self, inputs, **kwargs):
- outputs = self.transformer(inputs, **kwargs)
-
- sequence_output = outputs[0]
- prediction_scores = self.mlm(sequence_output, training=kwargs.get("training", False))
-
- outputs = (prediction_scores,) + outputs[2:] # Add hidden states and attention if they are here
-
- return outputs # prediction_scores, (hidden_states), (attentions)
-
-
-@add_start_docstrings(
- """Xxx Model transformer with a sequence classification/regression head on top (a linear layer on top of
- the pooled output) e.g. for GLUE tasks. """,
- XXX_START_DOCSTRING,
- XXX_INPUTS_DOCSTRING,
-)
-class TFXxxForSequenceClassification(TFXxxPreTrainedModel):
- r"""
- Outputs: `Tuple` comprising various elements depending on the configuration (config) and inputs:
- **logits**: ``Numpy array`` or ``tf.Tensor`` of shape ``(batch_size, config.num_labels)``
- Classification (or regression if config.num_labels==1) scores (before SoftMax).
- **hidden_states**: (`optional`, returned when ``config.output_hidden_states=True``)
- list of ``Numpy array`` or ``tf.Tensor`` (one for the output of each layer + the output of the embeddings)
- of shape ``(batch_size, sequence_length, hidden_size)``:
- Hidden-states of the model at the output of each layer plus the initial embedding outputs.
- **attentions**: (`optional`, returned when ``config.output_attentions=True``)
- list of ``Numpy array`` or ``tf.Tensor`` (one for each layer) of shape ``(batch_size, num_heads, sequence_length, sequence_length)``:
- Attentions weights after the attention softmax, used to compute the weighted average in the self-attention heads.
-
- Examples::
-
- import tensorflow as tf
- from transformers import XxxTokenizer, TFXxxForSequenceClassification
-
- tokenizer = XxxTokenizer.from_pretrained('xxx-base-uncased')
- model = TFXxxForSequenceClassification.from_pretrained('xxx-base-uncased')
- input_ids = tf.constant(tokenizer.encode("Hello, my dog is cute"))[None, :] # Batch size 1
- outputs = model(input_ids)
- logits = outputs[0]
-
- """
-
- def __init__(self, config, *inputs, **kwargs):
- super().__init__(config, *inputs, **kwargs)
- self.num_labels = config.num_labels
-
- self.transformer = TFXxxMainLayer(config, name="transformer")
- self.dropout = tf.keras.layers.Dropout(config.hidden_dropout_prob)
- self.classifier = tf.keras.layers.Dense(
- config.num_labels, kernel_initializer=get_initializer(config.initializer_range), name="classifier"
- )
-
- def call(self, inputs, **kwargs):
- outputs = self.transformer(inputs, **kwargs)
-
- pooled_output = outputs[1]
-
- pooled_output = self.dropout(pooled_output, training=kwargs.get("training", False))
- logits = self.classifier(pooled_output)
-
- outputs = (logits,) + outputs[2:] # add hidden states and attention if they are here
-
- return outputs # logits, (hidden_states), (attentions)
-
-
-@add_start_docstrings(
- """Xxx Model with a token classification head on top (a linear layer on top of
- the hidden-states output) e.g. for Named-Entity-Recognition (NER) tasks. """,
- XXX_START_DOCSTRING,
- XXX_INPUTS_DOCSTRING,
-)
-class TFXxxForTokenClassification(TFXxxPreTrainedModel):
- r"""
- Outputs: `Tuple` comprising various elements depending on the configuration (config) and inputs:
- **scores**: ``Numpy array`` or ``tf.Tensor`` of shape ``(batch_size, sequence_length, config.num_labels)``
- Classification scores (before SoftMax).
- **hidden_states**: (`optional`, returned when ``config.output_hidden_states=True``)
- list of ``Numpy array`` or ``tf.Tensor`` (one for the output of each layer + the output of the embeddings)
- of shape ``(batch_size, sequence_length, hidden_size)``:
- Hidden-states of the model at the output of each layer plus the initial embedding outputs.
- **attentions**: (`optional`, returned when ``config.output_attentions=True``)
- list of ``Numpy array`` or ``tf.Tensor`` (one for each layer) of shape ``(batch_size, num_heads, sequence_length, sequence_length)``:
- Attentions weights after the attention softmax, used to compute the weighted average in the self-attention heads.
-
- Examples::
-
- import tensorflow as tf
- from transformers import XxxTokenizer, TFXxxForTokenClassification
-
- tokenizer = XxxTokenizer.from_pretrained('xxx-base-uncased')
- model = TFXxxForTokenClassification.from_pretrained('xxx-base-uncased')
- input_ids = tf.constant(tokenizer.encode("Hello, my dog is cute"))[None, :] # Batch size 1
- outputs = model(input_ids)
- scores = outputs[0]
-
- """
-
- def __init__(self, config, *inputs, **kwargs):
- super().__init__(config, *inputs, **kwargs)
- self.num_labels = config.num_labels
-
- self.transformer = TFXxxMainLayer(config, name="transformer")
- self.dropout = tf.keras.layers.Dropout(config.hidden_dropout_prob)
- self.classifier = tf.keras.layers.Dense(
- config.num_labels, kernel_initializer=get_initializer(config.initializer_range), name="classifier"
- )
-
- def call(self, inputs, **kwargs):
- outputs = self.transformer(inputs, **kwargs)
-
- sequence_output = outputs[0]
-
- sequence_output = self.dropout(sequence_output, training=kwargs.get("training", False))
- logits = self.classifier(sequence_output)
-
- outputs = (logits,) + outputs[2:] # add hidden states and attention if they are here
-
- return outputs # scores, (hidden_states), (attentions)
-
-
-@add_start_docstrings(
- """Xxx Model with a span classification head on top for extractive question-answering tasks like SQuAD (a linear layers on top of
- the hidden-states output to compute `span start logits` and `span end logits`). """,
- XXX_START_DOCSTRING,
- XXX_INPUTS_DOCSTRING,
-)
-class TFXxxForQuestionAnswering(TFXxxPreTrainedModel):
- r"""
- Outputs: `Tuple` comprising various elements depending on the configuration (config) and inputs:
- **start_scores**: ``Numpy array`` or ``tf.Tensor`` of shape ``(batch_size, sequence_length,)``
- Span-start scores (before SoftMax).
- **end_scores**: ``Numpy array`` or ``tf.Tensor`` of shape ``(batch_size, sequence_length,)``
- Span-end scores (before SoftMax).
- **hidden_states**: (`optional`, returned when ``config.output_hidden_states=True``)
- list of ``Numpy array`` or ``tf.Tensor`` (one for the output of each layer + the output of the embeddings)
- of shape ``(batch_size, sequence_length, hidden_size)``:
- Hidden-states of the model at the output of each layer plus the initial embedding outputs.
- **attentions**: (`optional`, returned when ``config.output_attentions=True``)
- list of ``Numpy array`` or ``tf.Tensor`` (one for each layer) of shape ``(batch_size, num_heads, sequence_length, sequence_length)``:
- Attentions weights after the attention softmax, used to compute the weighted average in the self-attention heads.
-
- Examples::
-
- import tensorflow as tf
- from transformers import XxxTokenizer, TFXxxForQuestionAnswering
-
- tokenizer = XxxTokenizer.from_pretrained('xxx-base-uncased')
- model = TFXxxForQuestionAnswering.from_pretrained('xxx-base-uncased')
- input_ids = tf.constant(tokenizer.encode("Hello, my dog is cute"))[None, :] # Batch size 1
- outputs = model(input_ids)
- start_scores, end_scores = outputs[:2]
-
- """
-
- def __init__(self, config, *inputs, **kwargs):
- super().__init__(config, *inputs, **kwargs)
- self.num_labels = config.num_labels
-
- self.transformer = TFXxxMainLayer(config, name="transformer")
- self.qa_outputs = tf.keras.layers.Dense(
- config.num_labels, kernel_initializer=get_initializer(config.initializer_range), name="qa_outputs"
- )
-
- def call(self, inputs, **kwargs):
- outputs = self.transformer(inputs, **kwargs)
-
- sequence_output = outputs[0]
-
- logits = self.qa_outputs(sequence_output)
- start_logits, end_logits = tf.split(logits, 2, axis=-1)
- start_logits = tf.squeeze(start_logits, axis=-1)
- end_logits = tf.squeeze(end_logits, axis=-1)
-
- outputs = (start_logits, end_logits,) + outputs[2:]
-
- return outputs # start_logits, end_logits, (hidden_states), (attentions)
diff --git a/server/transformers/templates/adding_a_new_model/modeling_xxx.py b/server/transformers/templates/adding_a_new_model/modeling_xxx.py
deleted file mode 100644
index f9f4daa9506fc9731b03b326444d63aa45a27be5..0000000000000000000000000000000000000000
--- a/server/transformers/templates/adding_a_new_model/modeling_xxx.py
+++ /dev/null
@@ -1,749 +0,0 @@
-# coding=utf-8
-# Copyright 2018 XXX Authors
-#
-# Licensed under the Apache License, Version 2.0 (the "License");
-# you may not use this file except in compliance with the License.
-# You may obtain a copy of the License at
-#
-# http://www.apache.org/licenses/LICENSE-2.0
-#
-# Unless required by applicable law or agreed to in writing, software
-# distributed under the License is distributed on an "AS IS" BASIS,
-# WITHOUT WARRANTIES OR CONDITIONS OF ANY KIND, either express or implied.
-# See the License for the specific language governing permissions and
-# limitations under the License.
-""" PyTorch XXX model. """
-
-####################################################
-# In this template, replace all the XXX (various casings) with your model name
-####################################################
-
-
-import logging
-import os
-
-import torch
-from torch import nn
-from torch.nn import CrossEntropyLoss, MSELoss
-
-from .configuration_xxx import XxxConfig
-from .file_utils import add_start_docstrings
-from .modeling_utils import PreTrainedModel
-
-
-logger = logging.getLogger(__name__)
-
-####################################################
-# This dict contrains shortcut names and associated url
-# for the pretrained weights provided with the models
-####################################################
-XXX_PRETRAINED_MODEL_ARCHIVE_MAP = {
- "xxx-base-uncased": "https://s3.amazonaws.com/models.huggingface.co/bert/xxx-base-uncased-pytorch_model.bin",
- "xxx-large-uncased": "https://s3.amazonaws.com/models.huggingface.co/bert/xxx-large-uncased-pytorch_model.bin",
-}
-
-
-####################################################
-# This is a conversion method from TF 1.0 to PyTorch
-# More details: https://medium.com/huggingface/from-tensorflow-to-pytorch-265f40ef2a28
-####################################################
-def load_tf_weights_in_xxx(model, config, tf_checkpoint_path):
- """ Load tf checkpoints in a pytorch model.
- """
- try:
- import re
- import numpy as np
- import tensorflow as tf
- except ImportError:
- logger.error(
- "Loading a TensorFlow model in PyTorch, requires TensorFlow to be installed. Please see "
- "https://www.tensorflow.org/install/ for installation instructions."
- )
- raise
- tf_path = os.path.abspath(tf_checkpoint_path)
- logger.info("Converting TensorFlow checkpoint from {}".format(tf_path))
- # Load weights from TF model
- init_vars = tf.train.list_variables(tf_path)
- names = []
- arrays = []
- for name, shape in init_vars:
- logger.info("Loading TF weight {} with shape {}".format(name, shape))
- array = tf.train.load_variable(tf_path, name)
- names.append(name)
- arrays.append(array)
-
- for name, array in zip(names, arrays):
- name = name.split("/")
- # adam_v and adam_m are variables used in AdamWeightDecayOptimizer to calculated m and v
- # which are not required for using pretrained model
- if any(n in ["adam_v", "adam_m", "global_step"] for n in name):
- logger.info("Skipping {}".format("/".join(name)))
- continue
- pointer = model
- for m_name in name:
- if re.fullmatch(r"[A-Za-z]+_\d+", m_name):
- scope_names = re.split(r"_(\d+)", m_name)
- else:
- scope_names = [m_name]
- if scope_names[0] == "kernel" or scope_names[0] == "gamma":
- pointer = getattr(pointer, "weight")
- elif scope_names[0] == "output_bias" or scope_names[0] == "beta":
- pointer = getattr(pointer, "bias")
- elif scope_names[0] == "output_weights":
- pointer = getattr(pointer, "weight")
- elif scope_names[0] == "squad":
- pointer = getattr(pointer, "classifier")
- else:
- try:
- pointer = getattr(pointer, scope_names[0])
- except AttributeError:
- logger.info("Skipping {}".format("/".join(name)))
- continue
- if len(scope_names) >= 2:
- num = int(scope_names[1])
- pointer = pointer[num]
- if m_name[-11:] == "_embeddings":
- pointer = getattr(pointer, "weight")
- elif m_name == "kernel":
- array = np.transpose(array)
- try:
- assert pointer.shape == array.shape
- except AssertionError as e:
- e.args += (pointer.shape, array.shape)
- raise
- logger.info("Initialize PyTorch weight {}".format(name))
- pointer.data = torch.from_numpy(array)
- return model
-
-
-####################################################
-# PyTorch Models are constructed by sub-classing
-# - torch.nn.Module for the layers and
-# - PreTrainedModel for the models (itself a sub-class of torch.nn.Module)
-####################################################
-
-####################################################
-# Here is an example of typical layer in a PyTorch model of the library
-# The classes are usually identical to the TF 2.0 ones without the 'TF' prefix.
-#
-# See the conversion methods in modeling_tf_pytorch_utils.py for more details
-####################################################
-
-XxxAttention = nn.Module
-
-XxxIntermediate = nn.Module
-
-XxxOutput = nn.Module
-
-
-class XxxLayer(nn.Module):
- def __init__(self, config):
- super().__init__()
- self.attention = XxxAttention(config)
- self.intermediate = XxxIntermediate(config)
- self.output = XxxOutput(config)
-
- def forward(self, hidden_states, attention_mask=None, head_mask=None):
- attention_outputs = self.attention(hidden_states, attention_mask, head_mask)
- attention_output = attention_outputs[0]
- intermediate_output = self.intermediate(attention_output)
- layer_output = self.output(intermediate_output, attention_output)
- outputs = (layer_output,) + attention_outputs[1:] # add attentions if we output them
- return outputs
-
-
-####################################################
-# PreTrainedModel is a sub-class of torch.nn.Module
-# which take care of loading and saving pretrained weights
-# and various common utilities.
-#
-# Here you just need to specify a few (self-explanatory)
-# pointers for your model and the weights initialization
-# method if its not fully covered by PreTrainedModel's default method
-####################################################
-
-XxxLayerNorm = torch.nn.LayerNorm
-
-XxxEmbeddings = nn.Module
-
-XxxEncoder = nn.Module
-
-XxxPooler = nn.Module
-
-
-class XxxPreTrainedModel(PreTrainedModel):
- """ An abstract class to handle weights initialization and
- a simple interface for downloading and loading pretrained models.
- """
-
- config_class = XxxConfig
- pretrained_model_archive_map = XXX_PRETRAINED_MODEL_ARCHIVE_MAP
- load_tf_weights = load_tf_weights_in_xxx
- base_model_prefix = "transformer"
-
- def _init_weights(self, module):
- """ Initialize the weights """
- if isinstance(module, (nn.Linear, nn.Embedding)):
- # Slightly different from the TF version which uses truncated_normal for initialization
- # cf https://github.com/pytorch/pytorch/pull/5617
- module.weight.data.normal_(mean=0.0, std=self.config.initializer_range)
- elif isinstance(module, XxxLayerNorm):
- module.bias.data.zero_()
- module.weight.data.fill_(1.0)
- if isinstance(module, nn.Linear) and module.bias is not None:
- module.bias.data.zero_()
-
-
-XXX_START_DOCSTRING = r""" The XXX model was proposed in
- `XXX: Pre-training of Deep Bidirectional Transformers for Language Understanding`_
- by Jacob Devlin, Ming-Wei Chang, Kenton Lee and Kristina Toutanova. It's a bidirectional transformer
- pre-trained using a combination of masked language modeling objective and next sentence prediction
- on a large corpus comprising the Toronto Book Corpus and Wikipedia.
-
- This model is a PyTorch `torch.nn.Module`_ sub-class. Use it as a regular PyTorch Module and
- refer to the PyTorch documentation for all matter related to general usage and behavior.
-
- .. _`XXX: Pre-training of Deep Bidirectional Transformers for Language Understanding`:
- https://arxiv.org/abs/1810.04805
-
- .. _`torch.nn.Module`:
- https://pytorch.org/docs/stable/nn.html#module
-
- Parameters:
- config (:class:`~transformers.XxxConfig`): Model configuration class with all the parameters of the model.
- Initializing with a config file does not load the weights associated with the model, only the configuration.
- Check out the :meth:`~transformers.PreTrainedModel.from_pretrained` method to load the model weights.
-"""
-
-XXX_INPUTS_DOCSTRING = r"""
- Inputs:
- **input_ids**: ``torch.LongTensor`` of shape ``(batch_size, sequence_length)``:
- Indices of input sequence tokens in the vocabulary.
- To match pre-training, XXX input sequence should be formatted with [CLS] and [SEP] tokens as follows:
-
- (a) For sequence pairs:
-
- ``tokens: [CLS] is this jack ##son ##ville ? [SEP] no it is not . [SEP]``
-
- ``token_type_ids: 0 0 0 0 0 0 0 0 1 1 1 1 1 1``
-
- (b) For single sequences:
-
- ``tokens: [CLS] the dog is hairy . [SEP]``
-
- ``token_type_ids: 0 0 0 0 0 0 0``
-
- Xxx is a model with absolute position embeddings so it's usually advised to pad the inputs on
- the right rather than the left.
-
- Indices can be obtained using :class:`transformers.XxxTokenizer`.
- See :func:`transformers.PreTrainedTokenizer.encode` and
- :func:`transformers.PreTrainedTokenizer.convert_tokens_to_ids` for details.
- **attention_mask**: (`optional`) ``torch.FloatTensor`` of shape ``(batch_size, sequence_length)``:
- Mask to avoid performing attention on padding token indices.
- Mask values selected in ``[0, 1]``:
- ``1`` for tokens that are NOT MASKED, ``0`` for MASKED tokens.
- **token_type_ids**: (`optional`) ``torch.LongTensor`` of shape ``(batch_size, sequence_length)``:
- Segment token indices to indicate first and second portions of the inputs.
- Indices are selected in ``[0, 1]``: ``0`` corresponds to a `sentence A` token, ``1``
- corresponds to a `sentence B` token
- (see `XXX: Pre-training of Deep Bidirectional Transformers for Language Understanding`_ for more details).
- **position_ids**: (`optional`) ``torch.LongTensor`` of shape ``(batch_size, sequence_length)``:
- Indices of positions of each input sequence tokens in the position embeddings.
- Selected in the range ``[0, config.max_position_embeddings - 1]``.
- **head_mask**: (`optional`) ``torch.FloatTensor`` of shape ``(num_heads,)`` or ``(num_layers, num_heads)``:
- Mask to nullify selected heads of the self-attention modules.
- Mask values selected in ``[0, 1]``:
- ``1`` indicates the head is **not masked**, ``0`` indicates the head is **masked**.
- **inputs_embeds**: (`optional`) ``torch.FloatTensor`` of shape ``(batch_size, sequence_length, embedding_dim)``:
- Optionally, instead of passing ``input_ids`` you can choose to directly pass an embedded representation.
- This is useful if you want more control over how to convert `input_ids` indices into associated vectors
- than the model's internal embedding lookup matrix.
-"""
-
-
-@add_start_docstrings(
- "The bare Xxx Model transformer outputting raw hidden-states without any specific head on top.",
- XXX_START_DOCSTRING,
- XXX_INPUTS_DOCSTRING,
-)
-class XxxModel(XxxPreTrainedModel):
- r"""
- Outputs: `Tuple` comprising various elements depending on the configuration (config) and inputs:
- **last_hidden_state**: ``torch.FloatTensor`` of shape ``(batch_size, sequence_length, hidden_size)``
- Sequence of hidden-states at the output of the last layer of the model.
- **pooler_output**: ``torch.FloatTensor`` of shape ``(batch_size, hidden_size)``
- Last layer hidden-state of the first token of the sequence (classification token)
- further processed by a Linear layer and a Tanh activation function. The Linear
- layer weights are trained from the next sentence prediction (classification)
- objective during Xxx pretraining. This output is usually *not* a good summary
- of the semantic content of the input, you're often better with averaging or pooling
- the sequence of hidden-states for the whole input sequence.
- **hidden_states**: (`optional`, returned when ``config.output_hidden_states=True``)
- list of ``torch.FloatTensor`` (one for the output of each layer + the output of the embeddings)
- of shape ``(batch_size, sequence_length, hidden_size)``:
- Hidden-states of the model at the output of each layer plus the initial embedding outputs.
- **attentions**: (`optional`, returned when ``config.output_attentions=True``)
- list of ``torch.FloatTensor`` (one for each layer) of shape ``(batch_size, num_heads, sequence_length, sequence_length)``:
- Attentions weights after the attention softmax, used to compute the weighted average in the self-attention heads.
-
- Examples::
-
- tokenizer = XxxTokenizer.from_pretrained('xxx-base-uncased')
- model = XxxModel.from_pretrained('xxx-base-uncased')
- input_ids = torch.tensor(tokenizer.encode("Hello, my dog is cute")).unsqueeze(0) # Batch size 1
- outputs = model(input_ids)
- last_hidden_states = outputs[0] # The last hidden-state is the first element of the output tuple
-
- """
-
- def __init__(self, config):
- super().__init__(config)
-
- self.embeddings = XxxEmbeddings(config)
- self.encoder = XxxEncoder(config)
- self.pooler = XxxPooler(config)
-
- self.init_weights()
-
- def get_input_embeddings(self):
- return self.embeddings.word_embeddings
-
- def set_input_embeddings(self, new_embeddings):
- self.embeddings.word_embeddings = new_embeddings
-
- def _prune_heads(self, heads_to_prune):
- """ Prunes heads of the model.
- heads_to_prune: dict of {layer_num: list of heads to prune in this layer}
- See base class PreTrainedModel
- """
- for layer, heads in heads_to_prune.items():
- self.encoder.layer[layer].attention.prune_heads(heads)
-
- def forward(
- self,
- input_ids=None,
- attention_mask=None,
- token_type_ids=None,
- position_ids=None,
- head_mask=None,
- inputs_embeds=None,
- ):
- if input_ids is not None and inputs_embeds is not None:
- raise ValueError("You cannot specify both input_ids and inputs_embeds at the same time")
- elif input_ids is not None:
- input_shape = input_ids.size()
- elif inputs_embeds is not None:
- input_shape = inputs_embeds.size()[:-1]
- else:
- raise ValueError("You have to specify either input_ids or inputs_embeds")
-
- device = input_ids.device if input_ids is not None else inputs_embeds.device
-
- if attention_mask is None:
- attention_mask = torch.ones(input_shape, device=device)
- if token_type_ids is None:
- token_type_ids = torch.zeros(input_shape, dtype=torch.long, device=device)
-
- # We create a 3D attention mask from a 2D tensor mask.
- # Sizes are [batch_size, 1, 1, to_seq_length]
- # So we can broadcast to [batch_size, num_heads, from_seq_length, to_seq_length]
- # this attention mask is more simple than the triangular masking of causal attention
- # used in OpenAI GPT, we just need to prepare the broadcast dimension here.
- extended_attention_mask = attention_mask.unsqueeze(1).unsqueeze(2)
-
- # Since attention_mask is 1.0 for positions we want to attend and 0.0 for
- # masked positions, this operation will create a tensor which is 0.0 for
- # positions we want to attend and -10000.0 for masked positions.
- # Since we are adding it to the raw scores before the softmax, this is
- # effectively the same as removing these entirely.
- extended_attention_mask = extended_attention_mask.to(dtype=next(self.parameters()).dtype) # fp16 compatibility
- extended_attention_mask = (1.0 - extended_attention_mask) * -10000.0
-
- # Prepare head mask if needed
- # 1.0 in head_mask indicate we keep the head
- # attention_probs has shape bsz x n_heads x N x N
- # input head_mask has shape [num_heads] or [num_hidden_layers x num_heads]
- # and head_mask is converted to shape [num_hidden_layers x batch x num_heads x seq_length x seq_length]
- if head_mask is not None:
- if head_mask.dim() == 1:
- head_mask = head_mask.unsqueeze(0).unsqueeze(0).unsqueeze(-1).unsqueeze(-1)
- head_mask = head_mask.expand(self.config.num_hidden_layers, -1, -1, -1, -1)
- elif head_mask.dim() == 2:
- head_mask = (
- head_mask.unsqueeze(1).unsqueeze(-1).unsqueeze(-1)
- ) # We can specify head_mask for each layer
- head_mask = head_mask.to(
- dtype=next(self.parameters()).dtype
- ) # switch to fload if need + fp16 compatibility
- else:
- head_mask = [None] * self.config.num_hidden_layers
-
- ##################################
- # Replace this with your model code
- embedding_output = self.embeddings(
- input_ids=input_ids, position_ids=position_ids, token_type_ids=token_type_ids, inputs_embeds=inputs_embeds
- )
- encoder_outputs = self.encoder(embedding_output, extended_attention_mask, head_mask=head_mask)
- sequence_output = encoder_outputs[0]
- outputs = (sequence_output,) + encoder_outputs[1:] # add hidden_states and attentions if they are here
-
- return outputs # sequence_output, (hidden_states), (attentions)
-
-
-@add_start_docstrings(
- """Xxx Model with a `language modeling` head on top. """, XXX_START_DOCSTRING, XXX_INPUTS_DOCSTRING
-)
-class XxxForMaskedLM(XxxPreTrainedModel):
- r"""
- **masked_lm_labels**: (`optional`) ``torch.LongTensor`` of shape ``(batch_size, sequence_length)``:
- Labels for computing the masked language modeling loss.
- Indices should be in ``[-1, 0, ..., config.vocab_size]`` (see ``input_ids`` docstring)
- Tokens with indices set to ``-100`` are ignored (masked), the loss is only computed for the tokens with labels
- in ``[0, ..., config.vocab_size]``
-
- Outputs: `Tuple` comprising various elements depending on the configuration (config) and inputs:
- **loss**: (`optional`, returned when ``masked_lm_labels`` is provided) ``torch.FloatTensor`` of shape ``(1,)``:
- Masked language modeling loss.
- **prediction_scores**: ``torch.FloatTensor`` of shape ``(batch_size, sequence_length, config.vocab_size)``
- Prediction scores of the language modeling head (scores for each vocabulary token before SoftMax).
- **hidden_states**: (`optional`, returned when ``config.output_hidden_states=True``)
- list of ``torch.FloatTensor`` (one for the output of each layer + the output of the embeddings)
- of shape ``(batch_size, sequence_length, hidden_size)``:
- Hidden-states of the model at the output of each layer plus the initial embedding outputs.
- **attentions**: (`optional`, returned when ``config.output_attentions=True``)
- list of ``torch.FloatTensor`` (one for each layer) of shape ``(batch_size, num_heads, sequence_length, sequence_length)``:
- Attentions weights after the attention softmax, used to compute the weighted average in the self-attention heads.
-
- Examples::
-
- tokenizer = XxxTokenizer.from_pretrained('xxx-base-uncased')
- model = XxxForMaskedLM.from_pretrained('xxx-base-uncased')
- input_ids = torch.tensor(tokenizer.encode("Hello, my dog is cute")).unsqueeze(0) # Batch size 1
- outputs = model(input_ids, masked_lm_labels=input_ids)
- loss, prediction_scores = outputs[:2]
-
- """
-
- def __init__(self, config):
- super().__init__(config)
-
- self.transformer = XxxModel(config)
- self.lm_head = nn.Linear(config.n_embd, config.vocab_size)
-
- self.init_weights()
-
- def get_output_embeddings(self):
- return self.lm_head
-
- def forward(
- self,
- input_ids=None,
- attention_mask=None,
- token_type_ids=None,
- position_ids=None,
- head_mask=None,
- inputs_embeds=None,
- masked_lm_labels=None,
- ):
-
- outputs = self.transformer(
- input_ids,
- attention_mask=attention_mask,
- token_type_ids=token_type_ids,
- position_ids=position_ids,
- head_mask=head_mask,
- inputs_embeds=inputs_embeds,
- )
-
- sequence_output = outputs[0]
- prediction_scores = self.cls(sequence_output)
-
- outputs = (prediction_scores,) + outputs[2:] # Add hidden states and attention if they are here
- if masked_lm_labels is not None:
- loss_fct = CrossEntropyLoss()
- masked_lm_loss = loss_fct(prediction_scores.view(-1, self.config.vocab_size), masked_lm_labels.view(-1))
- outputs = (masked_lm_loss,) + outputs
-
- return outputs # (masked_lm_loss), prediction_scores, (hidden_states), (attentions)
-
-
-@add_start_docstrings(
- """Xxx Model transformer with a sequence classification/regression head on top (a linear layer on top of
- the pooled output) e.g. for GLUE tasks. """,
- XXX_START_DOCSTRING,
- XXX_INPUTS_DOCSTRING,
-)
-class XxxForSequenceClassification(XxxPreTrainedModel):
- r"""
- **labels**: (`optional`) ``torch.LongTensor`` of shape ``(batch_size,)``:
- Labels for computing the sequence classification/regression loss.
- Indices should be in ``[0, ..., config.num_labels - 1]``.
- If ``config.num_labels == 1`` a regression loss is computed (Mean-Square loss),
- If ``config.num_labels > 1`` a classification loss is computed (Cross-Entropy).
-
- Outputs: `Tuple` comprising various elements depending on the configuration (config) and inputs:
- **loss**: (`optional`, returned when ``labels`` is provided) ``torch.FloatTensor`` of shape ``(1,)``:
- Classification (or regression if config.num_labels==1) loss.
- **logits**: ``torch.FloatTensor`` of shape ``(batch_size, config.num_labels)``
- Classification (or regression if config.num_labels==1) scores (before SoftMax).
- **hidden_states**: (`optional`, returned when ``config.output_hidden_states=True``)
- list of ``torch.FloatTensor`` (one for the output of each layer + the output of the embeddings)
- of shape ``(batch_size, sequence_length, hidden_size)``:
- Hidden-states of the model at the output of each layer plus the initial embedding outputs.
- **attentions**: (`optional`, returned when ``config.output_attentions=True``)
- list of ``torch.FloatTensor`` (one for each layer) of shape ``(batch_size, num_heads, sequence_length, sequence_length)``:
- Attentions weights after the attention softmax, used to compute the weighted average in the self-attention heads.
-
- Examples::
-
- tokenizer = XxxTokenizer.from_pretrained('xxx-base-uncased')
- model = XxxForSequenceClassification.from_pretrained('xxx-base-uncased')
- input_ids = torch.tensor(tokenizer.encode("Hello, my dog is cute")).unsqueeze(0) # Batch size 1
- labels = torch.tensor([1]).unsqueeze(0) # Batch size 1
- outputs = model(input_ids, labels=labels)
- loss, logits = outputs[:2]
-
- """
-
- def __init__(self, config):
- super().__init__(config)
- self.num_labels = config.num_labels
-
- self.transformer = XxxModel(config)
- self.dropout = nn.Dropout(config.hidden_dropout_prob)
- self.classifier = nn.Linear(config.hidden_size, self.config.num_labels)
-
- self.init_weights()
-
- def forward(
- self,
- input_ids=None,
- attention_mask=None,
- token_type_ids=None,
- position_ids=None,
- head_mask=None,
- inputs_embeds=None,
- labels=None,
- ):
-
- outputs = self.transformer(
- input_ids,
- attention_mask=attention_mask,
- token_type_ids=token_type_ids,
- position_ids=position_ids,
- head_mask=head_mask,
- inputs_embeds=inputs_embeds,
- )
-
- pooled_output = outputs[1]
-
- pooled_output = self.dropout(pooled_output)
- logits = self.classifier(pooled_output)
-
- outputs = (logits,) + outputs[2:] # add hidden states and attention if they are here
-
- if labels is not None:
- if self.num_labels == 1:
- # We are doing regression
- loss_fct = MSELoss()
- loss = loss_fct(logits.view(-1), labels.view(-1))
- else:
- loss_fct = CrossEntropyLoss()
- loss = loss_fct(logits.view(-1, self.num_labels), labels.view(-1))
- outputs = (loss,) + outputs
-
- return outputs # (loss), logits, (hidden_states), (attentions)
-
-
-@add_start_docstrings(
- """Xxx Model with a token classification head on top (a linear layer on top of
- the hidden-states output) e.g. for Named-Entity-Recognition (NER) tasks. """,
- XXX_START_DOCSTRING,
- XXX_INPUTS_DOCSTRING,
-)
-class XxxForTokenClassification(XxxPreTrainedModel):
- r"""
- **labels**: (`optional`) ``torch.LongTensor`` of shape ``(batch_size, sequence_length)``:
- Labels for computing the token classification loss.
- Indices should be in ``[0, ..., config.num_labels - 1]``.
-
- Outputs: `Tuple` comprising various elements depending on the configuration (config) and inputs:
- **loss**: (`optional`, returned when ``labels`` is provided) ``torch.FloatTensor`` of shape ``(1,)``:
- Classification loss.
- **scores**: ``torch.FloatTensor`` of shape ``(batch_size, sequence_length, config.num_labels)``
- Classification scores (before SoftMax).
- **hidden_states**: (`optional`, returned when ``config.output_hidden_states=True``)
- list of ``torch.FloatTensor`` (one for the output of each layer + the output of the embeddings)
- of shape ``(batch_size, sequence_length, hidden_size)``:
- Hidden-states of the model at the output of each layer plus the initial embedding outputs.
- **attentions**: (`optional`, returned when ``config.output_attentions=True``)
- list of ``torch.FloatTensor`` (one for each layer) of shape ``(batch_size, num_heads, sequence_length, sequence_length)``:
- Attentions weights after the attention softmax, used to compute the weighted average in the self-attention heads.
-
- Examples::
-
- tokenizer = XxxTokenizer.from_pretrained('xxx-base-uncased')
- model = XxxForTokenClassification.from_pretrained('xxx-base-uncased')
- input_ids = torch.tensor(tokenizer.encode("Hello, my dog is cute")).unsqueeze(0) # Batch size 1
- labels = torch.tensor([1] * input_ids.size(1)).unsqueeze(0) # Batch size 1
- outputs = model(input_ids, labels=labels)
- loss, scores = outputs[:2]
-
- """
-
- def __init__(self, config):
- super().__init__(config)
- self.num_labels = config.num_labels
-
- self.transformer = XxxModel(config)
- self.dropout = nn.Dropout(config.hidden_dropout_prob)
- self.classifier = nn.Linear(config.hidden_size, config.num_labels)
-
- self.init_weights()
-
- def forward(
- self,
- input_ids=None,
- attention_mask=None,
- token_type_ids=None,
- position_ids=None,
- head_mask=None,
- inputs_embeds=None,
- labels=None,
- ):
-
- outputs = self.transformer(
- input_ids,
- attention_mask=attention_mask,
- token_type_ids=token_type_ids,
- position_ids=position_ids,
- head_mask=head_mask,
- inputs_embeds=inputs_embeds,
- )
-
- sequence_output = outputs[0]
-
- sequence_output = self.dropout(sequence_output)
- logits = self.classifier(sequence_output)
-
- outputs = (logits,) + outputs[2:] # add hidden states and attention if they are here
- if labels is not None:
- loss_fct = CrossEntropyLoss()
- # Only keep active parts of the loss
- if attention_mask is not None:
- active_loss = attention_mask.view(-1) == 1
- active_logits = logits.view(-1, self.num_labels)[active_loss]
- active_labels = labels.view(-1)[active_loss]
- loss = loss_fct(active_logits, active_labels)
- else:
- loss = loss_fct(logits.view(-1, self.num_labels), labels.view(-1))
- outputs = (loss,) + outputs
-
- return outputs # (loss), scores, (hidden_states), (attentions)
-
-
-@add_start_docstrings(
- """Xxx Model with a span classification head on top for extractive question-answering tasks like SQuAD (a linear layers on top of
- the hidden-states output to compute `span start logits` and `span end logits`). """,
- XXX_START_DOCSTRING,
- XXX_INPUTS_DOCSTRING,
-)
-class XxxForQuestionAnswering(XxxPreTrainedModel):
- r"""
- **start_positions**: (`optional`) ``torch.LongTensor`` of shape ``(batch_size,)``:
- Labels for position (index) of the start of the labelled span for computing the token classification loss.
- Positions are clamped to the length of the sequence (`sequence_length`).
- Position outside of the sequence are not taken into account for computing the loss.
- **end_positions**: (`optional`) ``torch.LongTensor`` of shape ``(batch_size,)``:
- Labels for position (index) of the end of the labelled span for computing the token classification loss.
- Positions are clamped to the length of the sequence (`sequence_length`).
- Position outside of the sequence are not taken into account for computing the loss.
-
- Outputs: `Tuple` comprising various elements depending on the configuration (config) and inputs:
- **loss**: (`optional`, returned when ``labels`` is provided) ``torch.FloatTensor`` of shape ``(1,)``:
- Total span extraction loss is the sum of a Cross-Entropy for the start and end positions.
- **start_scores**: ``torch.FloatTensor`` of shape ``(batch_size, sequence_length,)``
- Span-start scores (before SoftMax).
- **end_scores**: ``torch.FloatTensor`` of shape ``(batch_size, sequence_length,)``
- Span-end scores (before SoftMax).
- **hidden_states**: (`optional`, returned when ``config.output_hidden_states=True``)
- list of ``torch.FloatTensor`` (one for the output of each layer + the output of the embeddings)
- of shape ``(batch_size, sequence_length, hidden_size)``:
- Hidden-states of the model at the output of each layer plus the initial embedding outputs.
- **attentions**: (`optional`, returned when ``config.output_attentions=True``)
- list of ``torch.FloatTensor`` (one for each layer) of shape ``(batch_size, num_heads, sequence_length, sequence_length)``:
- Attentions weights after the attention softmax, used to compute the weighted average in the self-attention heads.
-
- Examples::
-
- tokenizer = XxxTokenizer.from_pretrained('xxx-base-uncased')
- model = XxxForQuestionAnswering.from_pretrained('xxx-large-uncased-whole-word-masking-finetuned-squad')
- question, text = "Who was Jim Henson?", "Jim Henson was a nice puppet"
- input_text = "[CLS] " + question + " [SEP] " + text + " [SEP]"
- input_ids = tokenizer.encode(input_text)
- token_type_ids = [0 if i <= input_ids.index(102) else 1 for i in range(len(input_ids))]
- start_scores, end_scores = model(torch.tensor([input_ids]), token_type_ids=torch.tensor([token_type_ids]))
- all_tokens = tokenizer.convert_ids_to_tokens(input_ids)
- print(' '.join(all_tokens[torch.argmax(start_scores) : torch.argmax(end_scores)+1]))
- # a nice puppet
-
-
- """
-
- def __init__(self, config):
- super().__init__(config)
- self.num_labels = config.num_labels
-
- self.transformer = XxxModel(config)
- self.qa_outputs = nn.Linear(config.hidden_size, config.num_labels)
-
- self.init_weights()
-
- def forward(
- self,
- input_ids=None,
- attention_mask=None,
- token_type_ids=None,
- position_ids=None,
- head_mask=None,
- inputs_embeds=None,
- start_positions=None,
- end_positions=None,
- ):
-
- outputs = self.transformer(
- input_ids,
- attention_mask=attention_mask,
- token_type_ids=token_type_ids,
- position_ids=position_ids,
- head_mask=head_mask,
- inputs_embeds=inputs_embeds,
- )
-
- sequence_output = outputs[0]
-
- logits = self.qa_outputs(sequence_output)
- start_logits, end_logits = logits.split(1, dim=-1)
- start_logits = start_logits.squeeze(-1)
- end_logits = end_logits.squeeze(-1)
-
- outputs = (start_logits, end_logits,) + outputs[2:]
- if start_positions is not None and end_positions is not None:
- # If we are on multi-GPU, split add a dimension
- if len(start_positions.size()) > 1:
- start_positions = start_positions.squeeze(-1)
- if len(end_positions.size()) > 1:
- end_positions = end_positions.squeeze(-1)
- # sometimes the start/end positions are outside our model inputs, we ignore these terms
- ignored_index = start_logits.size(1)
- start_positions.clamp_(0, ignored_index)
- end_positions.clamp_(0, ignored_index)
-
- loss_fct = CrossEntropyLoss(ignore_index=ignored_index)
- start_loss = loss_fct(start_logits, start_positions)
- end_loss = loss_fct(end_logits, end_positions)
- total_loss = (start_loss + end_loss) / 2
- outputs = (total_loss,) + outputs
-
- return outputs # (loss), start_logits, end_logits, (hidden_states), (attentions)
diff --git a/server/transformers/templates/adding_a_new_model/tests/test_modeling_tf_xxx.py b/server/transformers/templates/adding_a_new_model/tests/test_modeling_tf_xxx.py
deleted file mode 100644
index 3e12b3f745997f149d7c635e07670ecf234e05d9..0000000000000000000000000000000000000000
--- a/server/transformers/templates/adding_a_new_model/tests/test_modeling_tf_xxx.py
+++ /dev/null
@@ -1,253 +0,0 @@
-# coding=utf-8
-# Copyright 2018 XXX Authors.
-#
-# Licensed under the Apache License, Version 2.0 (the "License");
-# you may not use this file except in compliance with the License.
-# You may obtain a copy of the License at
-#
-# http://www.apache.org/licenses/LICENSE-2.0
-#
-# Unless required by applicable law or agreed to in writing, software
-# distributed under the License is distributed on an "AS IS" BASIS,
-# WITHOUT WARRANTIES OR CONDITIONS OF ANY KIND, either express or implied.
-# See the License for the specific language governing permissions and
-# limitations under the License.
-
-
-import unittest
-
-from transformers import XxxConfig, is_tf_available
-
-from .test_configuration_common import ConfigTester
-from .test_modeling_tf_common import TFModelTesterMixin, ids_tensor
-from .utils import CACHE_DIR, require_tf, slow
-
-
-if is_tf_available():
- from transformers.modeling_tf_xxx import (
- TFXxxModel,
- TFXxxForMaskedLM,
- TFXxxForSequenceClassification,
- TFXxxForTokenClassification,
- TFXxxForQuestionAnswering,
- )
-
-
-@require_tf
-class TFXxxModelTest(TFModelTesterMixin, unittest.TestCase):
-
- all_model_classes = (
- (
- TFXxxModel,
- TFXxxForMaskedLM,
- TFXxxForQuestionAnswering,
- TFXxxForSequenceClassification,
- TFXxxForTokenClassification,
- )
- if is_tf_available()
- else ()
- )
-
- class TFXxxModelTester(object):
- def __init__(
- self,
- parent,
- batch_size=13,
- seq_length=7,
- is_training=True,
- use_input_mask=True,
- use_token_type_ids=True,
- use_labels=True,
- vocab_size=99,
- hidden_size=32,
- num_hidden_layers=5,
- num_attention_heads=4,
- intermediate_size=37,
- hidden_act="gelu",
- hidden_dropout_prob=0.1,
- attention_probs_dropout_prob=0.1,
- max_position_embeddings=512,
- type_vocab_size=16,
- type_sequence_label_size=2,
- initializer_range=0.02,
- num_labels=3,
- num_choices=4,
- scope=None,
- ):
- self.parent = parent
- self.batch_size = batch_size
- self.seq_length = seq_length
- self.is_training = is_training
- self.use_input_mask = use_input_mask
- self.use_token_type_ids = use_token_type_ids
- self.use_labels = use_labels
- self.vocab_size = vocab_size
- self.hidden_size = hidden_size
- self.num_hidden_layers = num_hidden_layers
- self.num_attention_heads = num_attention_heads
- self.intermediate_size = intermediate_size
- self.hidden_act = hidden_act
- self.hidden_dropout_prob = hidden_dropout_prob
- self.attention_probs_dropout_prob = attention_probs_dropout_prob
- self.max_position_embeddings = max_position_embeddings
- self.type_vocab_size = type_vocab_size
- self.type_sequence_label_size = type_sequence_label_size
- self.initializer_range = initializer_range
- self.num_labels = num_labels
- self.num_choices = num_choices
- self.scope = scope
-
- def prepare_config_and_inputs(self):
- input_ids = ids_tensor([self.batch_size, self.seq_length], self.vocab_size)
-
- input_mask = None
- if self.use_input_mask:
- input_mask = ids_tensor([self.batch_size, self.seq_length], vocab_size=2)
-
- token_type_ids = None
- if self.use_token_type_ids:
- token_type_ids = ids_tensor([self.batch_size, self.seq_length], self.type_vocab_size)
-
- sequence_labels = None
- token_labels = None
- choice_labels = None
- if self.use_labels:
- sequence_labels = ids_tensor([self.batch_size], self.type_sequence_label_size)
- token_labels = ids_tensor([self.batch_size, self.seq_length], self.num_labels)
- choice_labels = ids_tensor([self.batch_size], self.num_choices)
-
- config = XxxConfig(
- vocab_size=self.vocab_size,
- hidden_size=self.hidden_size,
- num_hidden_layers=self.num_hidden_layers,
- num_attention_heads=self.num_attention_heads,
- intermediate_size=self.intermediate_size,
- hidden_act=self.hidden_act,
- hidden_dropout_prob=self.hidden_dropout_prob,
- attention_probs_dropout_prob=self.attention_probs_dropout_prob,
- max_position_embeddings=self.max_position_embeddings,
- type_vocab_size=self.type_vocab_size,
- initializer_range=self.initializer_range,
- )
-
- return config, input_ids, token_type_ids, input_mask, sequence_labels, token_labels, choice_labels
-
- def create_and_check_xxx_model(
- self, config, input_ids, token_type_ids, input_mask, sequence_labels, token_labels, choice_labels
- ):
- model = TFXxxModel(config=config)
- inputs = {"input_ids": input_ids, "attention_mask": input_mask, "token_type_ids": token_type_ids}
- sequence_output, pooled_output = model(inputs)
-
- inputs = [input_ids, input_mask]
- sequence_output, pooled_output = model(inputs)
-
- sequence_output, pooled_output = model(input_ids)
-
- result = {
- "sequence_output": sequence_output.numpy(),
- "pooled_output": pooled_output.numpy(),
- }
- self.parent.assertListEqual(
- list(result["sequence_output"].shape), [self.batch_size, self.seq_length, self.hidden_size]
- )
- self.parent.assertListEqual(list(result["pooled_output"].shape), [self.batch_size, self.hidden_size])
-
- def create_and_check_xxx_for_masked_lm(
- self, config, input_ids, token_type_ids, input_mask, sequence_labels, token_labels, choice_labels
- ):
- model = TFXxxForMaskedLM(config=config)
- inputs = {"input_ids": input_ids, "attention_mask": input_mask, "token_type_ids": token_type_ids}
- (prediction_scores,) = model(inputs)
- result = {
- "prediction_scores": prediction_scores.numpy(),
- }
- self.parent.assertListEqual(
- list(result["prediction_scores"].shape), [self.batch_size, self.seq_length, self.vocab_size]
- )
-
- def create_and_check_xxx_for_sequence_classification(
- self, config, input_ids, token_type_ids, input_mask, sequence_labels, token_labels, choice_labels
- ):
- config.num_labels = self.num_labels
- model = TFXxxForSequenceClassification(config=config)
- inputs = {"input_ids": input_ids, "attention_mask": input_mask, "token_type_ids": token_type_ids}
- (logits,) = model(inputs)
- result = {
- "logits": logits.numpy(),
- }
- self.parent.assertListEqual(list(result["logits"].shape), [self.batch_size, self.num_labels])
-
- def create_and_check_xxx_for_token_classification(
- self, config, input_ids, token_type_ids, input_mask, sequence_labels, token_labels, choice_labels
- ):
- config.num_labels = self.num_labels
- model = TFXxxForTokenClassification(config=config)
- inputs = {"input_ids": input_ids, "attention_mask": input_mask, "token_type_ids": token_type_ids}
- (logits,) = model(inputs)
- result = {
- "logits": logits.numpy(),
- }
- self.parent.assertListEqual(
- list(result["logits"].shape), [self.batch_size, self.seq_length, self.num_labels]
- )
-
- def create_and_check_xxx_for_question_answering(
- self, config, input_ids, token_type_ids, input_mask, sequence_labels, token_labels, choice_labels
- ):
- model = TFXxxForQuestionAnswering(config=config)
- inputs = {"input_ids": input_ids, "attention_mask": input_mask, "token_type_ids": token_type_ids}
- start_logits, end_logits = model(inputs)
- result = {
- "start_logits": start_logits.numpy(),
- "end_logits": end_logits.numpy(),
- }
- self.parent.assertListEqual(list(result["start_logits"].shape), [self.batch_size, self.seq_length])
- self.parent.assertListEqual(list(result["end_logits"].shape), [self.batch_size, self.seq_length])
-
- def prepare_config_and_inputs_for_common(self):
- config_and_inputs = self.prepare_config_and_inputs()
- (
- config,
- input_ids,
- token_type_ids,
- input_mask,
- sequence_labels,
- token_labels,
- choice_labels,
- ) = config_and_inputs
- inputs_dict = {"input_ids": input_ids, "token_type_ids": token_type_ids, "attention_mask": input_mask}
- return config, inputs_dict
-
- def setUp(self):
- self.model_tester = TFXxxModelTest.TFXxxModelTester(self)
- self.config_tester = ConfigTester(self, config_class=XxxConfig, hidden_size=37)
-
- def test_config(self):
- self.config_tester.run_common_tests()
-
- def test_xxx_model(self):
- config_and_inputs = self.model_tester.prepare_config_and_inputs()
- self.model_tester.create_and_check_xxx_model(*config_and_inputs)
-
- def test_for_masked_lm(self):
- config_and_inputs = self.model_tester.prepare_config_and_inputs()
- self.model_tester.create_and_check_xxx_for_masked_lm(*config_and_inputs)
-
- def test_for_question_answering(self):
- config_and_inputs = self.model_tester.prepare_config_and_inputs()
- self.model_tester.create_and_check_xxx_for_question_answering(*config_and_inputs)
-
- def test_for_sequence_classification(self):
- config_and_inputs = self.model_tester.prepare_config_and_inputs()
- self.model_tester.create_and_check_xxx_for_sequence_classification(*config_and_inputs)
-
- def test_for_token_classification(self):
- config_and_inputs = self.model_tester.prepare_config_and_inputs()
- self.model_tester.create_and_check_xxx_for_token_classification(*config_and_inputs)
-
- @slow
- def test_model_from_pretrained(self):
- for model_name in ["xxx-base-uncased"]:
- model = TFXxxModel.from_pretrained(model_name, cache_dir=CACHE_DIR)
- self.assertIsNotNone(model)
diff --git a/server/transformers/templates/adding_a_new_model/tests/test_modeling_xxx.py b/server/transformers/templates/adding_a_new_model/tests/test_modeling_xxx.py
deleted file mode 100644
index 281a9226fc25490aba3030fcf18dc8d417b4958a..0000000000000000000000000000000000000000
--- a/server/transformers/templates/adding_a_new_model/tests/test_modeling_xxx.py
+++ /dev/null
@@ -1,274 +0,0 @@
-# coding=utf-8
-# Copyright 2018 XXX Authors.
-#
-# Licensed under the Apache License, Version 2.0 (the "License");
-# you may not use this file except in compliance with the License.
-# You may obtain a copy of the License at
-#
-# http://www.apache.org/licenses/LICENSE-2.0
-#
-# Unless required by applicable law or agreed to in writing, software
-# distributed under the License is distributed on an "AS IS" BASIS,
-# WITHOUT WARRANTIES OR CONDITIONS OF ANY KIND, either express or implied.
-# See the License for the specific language governing permissions and
-# limitations under the License.
-
-
-import unittest
-
-from transformers import is_torch_available
-
-from .test_configuration_common import ConfigTester
-from .test_modeling_common import ModelTesterMixin, ids_tensor
-from .utils import CACHE_DIR, require_torch, slow, torch_device
-
-
-if is_torch_available():
- from transformers import (
- XxxConfig,
- XxxModel,
- XxxForMaskedLM,
- XxxForQuestionAnswering,
- XxxForSequenceClassification,
- XxxForTokenClassification,
- )
- from transformers.modeling_xxx import XXX_PRETRAINED_MODEL_ARCHIVE_MAP
-
-
-@require_torch
-class XxxModelTest(ModelTesterMixin, unittest.TestCase):
-
- all_model_classes = (
- (XxxModel, XxxForMaskedLM, XxxForQuestionAnswering, XxxForSequenceClassification, XxxForTokenClassification)
- if is_torch_available()
- else ()
- )
-
- class XxxModelTester(object):
- def __init__(
- self,
- parent,
- batch_size=13,
- seq_length=7,
- is_training=True,
- use_input_mask=True,
- use_token_type_ids=True,
- use_labels=True,
- vocab_size=99,
- hidden_size=32,
- num_hidden_layers=5,
- num_attention_heads=4,
- intermediate_size=37,
- hidden_act="gelu",
- hidden_dropout_prob=0.1,
- attention_probs_dropout_prob=0.1,
- max_position_embeddings=512,
- type_vocab_size=16,
- type_sequence_label_size=2,
- initializer_range=0.02,
- num_labels=3,
- num_choices=4,
- scope=None,
- ):
- self.parent = parent
- self.batch_size = batch_size
- self.seq_length = seq_length
- self.is_training = is_training
- self.use_input_mask = use_input_mask
- self.use_token_type_ids = use_token_type_ids
- self.use_labels = use_labels
- self.vocab_size = vocab_size
- self.hidden_size = hidden_size
- self.num_hidden_layers = num_hidden_layers
- self.num_attention_heads = num_attention_heads
- self.intermediate_size = intermediate_size
- self.hidden_act = hidden_act
- self.hidden_dropout_prob = hidden_dropout_prob
- self.attention_probs_dropout_prob = attention_probs_dropout_prob
- self.max_position_embeddings = max_position_embeddings
- self.type_vocab_size = type_vocab_size
- self.type_sequence_label_size = type_sequence_label_size
- self.initializer_range = initializer_range
- self.num_labels = num_labels
- self.num_choices = num_choices
- self.scope = scope
-
- def prepare_config_and_inputs(self):
- input_ids = ids_tensor([self.batch_size, self.seq_length], self.vocab_size)
-
- input_mask = None
- if self.use_input_mask:
- input_mask = ids_tensor([self.batch_size, self.seq_length], vocab_size=2)
-
- token_type_ids = None
- if self.use_token_type_ids:
- token_type_ids = ids_tensor([self.batch_size, self.seq_length], self.type_vocab_size)
-
- sequence_labels = None
- token_labels = None
- choice_labels = None
- if self.use_labels:
- sequence_labels = ids_tensor([self.batch_size], self.type_sequence_label_size)
- token_labels = ids_tensor([self.batch_size, self.seq_length], self.num_labels)
- choice_labels = ids_tensor([self.batch_size], self.num_choices)
-
- config = XxxConfig(
- vocab_size=self.vocab_size,
- hidden_size=self.hidden_size,
- num_hidden_layers=self.num_hidden_layers,
- num_attention_heads=self.num_attention_heads,
- intermediate_size=self.intermediate_size,
- hidden_act=self.hidden_act,
- hidden_dropout_prob=self.hidden_dropout_prob,
- attention_probs_dropout_prob=self.attention_probs_dropout_prob,
- max_position_embeddings=self.max_position_embeddings,
- type_vocab_size=self.type_vocab_size,
- initializer_range=self.initializer_range,
- )
-
- return config, input_ids, token_type_ids, input_mask, sequence_labels, token_labels, choice_labels
-
- def check_loss_output(self, result):
- self.parent.assertListEqual(list(result["loss"].size()), [])
-
- def create_and_check_xxx_model(
- self, config, input_ids, token_type_ids, input_mask, sequence_labels, token_labels, choice_labels
- ):
- model = XxxModel(config=config)
- model.to(torch_device)
- model.eval()
- sequence_output, pooled_output = model(input_ids, attention_mask=input_mask, token_type_ids=token_type_ids)
- sequence_output, pooled_output = model(input_ids, token_type_ids=token_type_ids)
- sequence_output, pooled_output = model(input_ids)
-
- result = {
- "sequence_output": sequence_output,
- "pooled_output": pooled_output,
- }
- self.parent.assertListEqual(
- list(result["sequence_output"].size()), [self.batch_size, self.seq_length, self.hidden_size]
- )
- self.parent.assertListEqual(list(result["pooled_output"].size()), [self.batch_size, self.hidden_size])
-
- def create_and_check_xxx_for_masked_lm(
- self, config, input_ids, token_type_ids, input_mask, sequence_labels, token_labels, choice_labels
- ):
- model = XxxForMaskedLM(config=config)
- model.to(torch_device)
- model.eval()
- loss, prediction_scores = model(
- input_ids, attention_mask=input_mask, token_type_ids=token_type_ids, masked_lm_labels=token_labels
- )
- result = {
- "loss": loss,
- "prediction_scores": prediction_scores,
- }
- self.parent.assertListEqual(
- list(result["prediction_scores"].size()), [self.batch_size, self.seq_length, self.vocab_size]
- )
- self.check_loss_output(result)
-
- def create_and_check_xxx_for_question_answering(
- self, config, input_ids, token_type_ids, input_mask, sequence_labels, token_labels, choice_labels
- ):
- model = XxxForQuestionAnswering(config=config)
- model.to(torch_device)
- model.eval()
- loss, start_logits, end_logits = model(
- input_ids,
- attention_mask=input_mask,
- token_type_ids=token_type_ids,
- start_positions=sequence_labels,
- end_positions=sequence_labels,
- )
- result = {
- "loss": loss,
- "start_logits": start_logits,
- "end_logits": end_logits,
- }
- self.parent.assertListEqual(list(result["start_logits"].size()), [self.batch_size, self.seq_length])
- self.parent.assertListEqual(list(result["end_logits"].size()), [self.batch_size, self.seq_length])
- self.check_loss_output(result)
-
- def create_and_check_xxx_for_sequence_classification(
- self, config, input_ids, token_type_ids, input_mask, sequence_labels, token_labels, choice_labels
- ):
- config.num_labels = self.num_labels
- model = XxxForSequenceClassification(config)
- model.to(torch_device)
- model.eval()
- loss, logits = model(
- input_ids, attention_mask=input_mask, token_type_ids=token_type_ids, labels=sequence_labels
- )
- result = {
- "loss": loss,
- "logits": logits,
- }
- self.parent.assertListEqual(list(result["logits"].size()), [self.batch_size, self.num_labels])
- self.check_loss_output(result)
-
- def create_and_check_xxx_for_token_classification(
- self, config, input_ids, token_type_ids, input_mask, sequence_labels, token_labels, choice_labels
- ):
- config.num_labels = self.num_labels
- model = XxxForTokenClassification(config=config)
- model.to(torch_device)
- model.eval()
- loss, logits = model(
- input_ids, attention_mask=input_mask, token_type_ids=token_type_ids, labels=token_labels
- )
- result = {
- "loss": loss,
- "logits": logits,
- }
- self.parent.assertListEqual(
- list(result["logits"].size()), [self.batch_size, self.seq_length, self.num_labels]
- )
- self.check_loss_output(result)
-
- def prepare_config_and_inputs_for_common(self):
- config_and_inputs = self.prepare_config_and_inputs()
- (
- config,
- input_ids,
- token_type_ids,
- input_mask,
- sequence_labels,
- token_labels,
- choice_labels,
- ) = config_and_inputs
- inputs_dict = {"input_ids": input_ids, "token_type_ids": token_type_ids, "attention_mask": input_mask}
- return config, inputs_dict
-
- def setUp(self):
- self.model_tester = XxxModelTest.XxxModelTester(self)
- self.config_tester = ConfigTester(self, config_class=XxxConfig, hidden_size=37)
-
- def test_config(self):
- self.config_tester.run_common_tests()
-
- def test_xxx_model(self):
- config_and_inputs = self.model_tester.prepare_config_and_inputs()
- self.model_tester.create_and_check_xxx_model(*config_and_inputs)
-
- def test_for_masked_lm(self):
- config_and_inputs = self.model_tester.prepare_config_and_inputs()
- self.model_tester.create_and_check_xxx_for_masked_lm(*config_and_inputs)
-
- def test_for_question_answering(self):
- config_and_inputs = self.model_tester.prepare_config_and_inputs()
- self.model_tester.create_and_check_xxx_for_question_answering(*config_and_inputs)
-
- def test_for_sequence_classification(self):
- config_and_inputs = self.model_tester.prepare_config_and_inputs()
- self.model_tester.create_and_check_xxx_for_sequence_classification(*config_and_inputs)
-
- def test_for_token_classification(self):
- config_and_inputs = self.model_tester.prepare_config_and_inputs()
- self.model_tester.create_and_check_xxx_for_token_classification(*config_and_inputs)
-
- @slow
- def test_model_from_pretrained(self):
- for model_name in list(XXX_PRETRAINED_MODEL_ARCHIVE_MAP.keys())[:1]:
- model = XxxModel.from_pretrained(model_name, cache_dir=CACHE_DIR)
- self.assertIsNotNone(model)
diff --git a/server/transformers/templates/adding_a_new_model/tests/test_tokenization_xxx.py b/server/transformers/templates/adding_a_new_model/tests/test_tokenization_xxx.py
deleted file mode 100644
index 1a24f76b0fb1327c41be50117db59b8c572ef74f..0000000000000000000000000000000000000000
--- a/server/transformers/templates/adding_a_new_model/tests/test_tokenization_xxx.py
+++ /dev/null
@@ -1,64 +0,0 @@
-# coding=utf-8
-# Copyright 2018 XXX Authors.
-#
-# Licensed under the Apache License, Version 2.0 (the "License");
-# you may not use this file except in compliance with the License.
-# You may obtain a copy of the License at
-#
-# http://www.apache.org/licenses/LICENSE-2.0
-#
-# Unless required by applicable law or agreed to in writing, software
-# distributed under the License is distributed on an "AS IS" BASIS,
-# WITHOUT WARRANTIES OR CONDITIONS OF ANY KIND, either express or implied.
-# See the License for the specific language governing permissions and
-# limitations under the License.
-
-
-import os
-import unittest
-
-from transformers.tokenization_bert import VOCAB_FILES_NAMES, XxxTokenizer
-
-from .test_tokenization_common import TokenizerTesterMixin
-
-
-class XxxTokenizationTest(TokenizerTesterMixin, unittest.TestCase):
-
- tokenizer_class = XxxTokenizer
-
- def setUp(self):
- super().setUp()
-
- vocab_tokens = [
- "[UNK]",
- "[CLS]",
- "[SEP]",
- "want",
- "##want",
- "##ed",
- "wa",
- "un",
- "runn",
- "##ing",
- ",",
- "low",
- "lowest",
- ]
- self.vocab_file = os.path.join(self.tmpdirname, VOCAB_FILES_NAMES["vocab_file"])
- with open(self.vocab_file, "w", encoding="utf-8") as vocab_writer:
- vocab_writer.write("".join([x + "\n" for x in vocab_tokens]))
-
- def get_tokenizer(self, **kwargs):
- return XxxTokenizer.from_pretrained(self.tmpdirname, **kwargs)
-
- def get_input_output_texts(self):
- input_text = "UNwant\u00E9d,running"
- output_text = "unwanted, running"
- return input_text, output_text
-
- def test_full_tokenizer(self):
- tokenizer = self.tokenizer_class(self.vocab_file)
-
- tokens = tokenizer.tokenize("UNwant\u00E9d,running")
- self.assertListEqual(tokens, ["un", "##want", "##ed", ",", "runn", "##ing"])
- self.assertListEqual(tokenizer.convert_tokens_to_ids(tokens), [7, 4, 5, 10, 8, 9])
diff --git a/server/transformers/templates/adding_a_new_model/tokenization_xxx.py b/server/transformers/templates/adding_a_new_model/tokenization_xxx.py
deleted file mode 100644
index 667a130a9bf5a7d99c8bae4ec52b739d39377092..0000000000000000000000000000000000000000
--- a/server/transformers/templates/adding_a_new_model/tokenization_xxx.py
+++ /dev/null
@@ -1,233 +0,0 @@
-# coding=utf-8
-# Copyright 2018 XXX Authors.
-#
-# Licensed under the Apache License, Version 2.0 (the "License");
-# you may not use this file except in compliance with the License.
-# You may obtain a copy of the License at
-#
-# http://www.apache.org/licenses/LICENSE-2.0
-#
-# Unless required by applicable law or agreed to in writing, software
-# distributed under the License is distributed on an "AS IS" BASIS,
-# WITHOUT WARRANTIES OR CONDITIONS OF ANY KIND, either express or implied.
-# See the License for the specific language governing permissions and
-# limitations under the License.
-""" Tokenization class for model XXX."""
-
-
-import collections
-import logging
-import os
-
-from .tokenization_utils import PreTrainedTokenizer
-
-
-logger = logging.getLogger(__name__)
-
-####################################################
-# In this template, replace all the XXX (various casings) with your model name
-####################################################
-
-####################################################
-# Mapping from the keyword arguments names of Tokenizer `__init__`
-# to file names for serializing Tokenizer instances
-####################################################
-VOCAB_FILES_NAMES = {"vocab_file": "vocab.txt"}
-
-####################################################
-# Mapping from the keyword arguments names of Tokenizer `__init__`
-# to pretrained vocabulary URL for all the model shortcut names.
-####################################################
-PRETRAINED_VOCAB_FILES_MAP = {
- "vocab_file": {
- "xxx-base-uncased": "https://s3.amazonaws.com/models.huggingface.co/bert/xxx-base-uncased-vocab.txt",
- "xxx-large-uncased": "https://s3.amazonaws.com/models.huggingface.co/bert/xxx-large-uncased-vocab.txt",
- }
-}
-
-####################################################
-# Mapping from model shortcut names to max length of inputs
-####################################################
-PRETRAINED_POSITIONAL_EMBEDDINGS_SIZES = {
- "xxx-base-uncased": 512,
- "xxx-large-uncased": 512,
-}
-
-####################################################
-# Mapping from model shortcut names to a dictionary of additional
-# keyword arguments for Tokenizer `__init__`.
-# To be used for checkpoint specific configurations.
-####################################################
-PRETRAINED_INIT_CONFIGURATION = {
- "xxx-base-uncased": {"do_lower_case": True},
- "xxx-large-uncased": {"do_lower_case": True},
-}
-
-
-def load_vocab(vocab_file):
- """Loads a vocabulary file into a dictionary."""
- vocab = collections.OrderedDict()
- with open(vocab_file, "r", encoding="utf-8") as reader:
- tokens = reader.readlines()
- for index, token in enumerate(tokens):
- token = token.rstrip("\n")
- vocab[token] = index
- return vocab
-
-
-class XxxTokenizer(PreTrainedTokenizer):
- r"""
- Constructs a XxxTokenizer.
- :class:`~transformers.XxxTokenizer` runs end-to-end tokenization: punctuation splitting + wordpiece
-
- Args:
- vocab_file: Path to a one-wordpiece-per-line vocabulary file
- do_lower_case: Whether to lower case the input. Only has an effect when do_basic_tokenize=True
- """
-
- vocab_files_names = VOCAB_FILES_NAMES
- pretrained_vocab_files_map = PRETRAINED_VOCAB_FILES_MAP
- pretrained_init_configuration = PRETRAINED_INIT_CONFIGURATION
- max_model_input_sizes = PRETRAINED_POSITIONAL_EMBEDDINGS_SIZES
-
- def __init__(
- self,
- vocab_file,
- do_lower_case=True,
- unk_token="[UNK]",
- sep_token="[SEP]",
- pad_token="[PAD]",
- cls_token="[CLS]",
- mask_token="[MASK]",
- **kwargs
- ):
- """Constructs a XxxTokenizer.
-
- Args:
- **vocab_file**: Path to a one-wordpiece-per-line vocabulary file
- **do_lower_case**: (`optional`) boolean (default True)
- Whether to lower case the input
- Only has an effect when do_basic_tokenize=True
- """
- super().__init__(
- unk_token=unk_token,
- sep_token=sep_token,
- pad_token=pad_token,
- cls_token=cls_token,
- mask_token=mask_token,
- **kwargs,
- )
- self.max_len_single_sentence = self.max_len - 2 # take into account special tokens
- self.max_len_sentences_pair = self.max_len - 3 # take into account special tokens
-
- if not os.path.isfile(vocab_file):
- raise ValueError(
- "Can't find a vocabulary file at path '{}'. To load the vocabulary from a Google pretrained "
- "model use `tokenizer = XxxTokenizer.from_pretrained(PRETRAINED_MODEL_NAME)`".format(vocab_file)
- )
- self.vocab = load_vocab(vocab_file)
-
- @property
- def vocab_size(self):
- return len(self.vocab)
-
- def _tokenize(self, text):
- """ Take as input a string and return a list of strings (tokens) for words/sub-words
- """
- split_tokens = []
- if self.do_basic_tokenize:
- for token in self.basic_tokenizer.tokenize(text, never_split=self.all_special_tokens):
- for sub_token in self.wordpiece_tokenizer.tokenize(token):
- split_tokens.append(sub_token)
- else:
- split_tokens = self.wordpiece_tokenizer.tokenize(text)
- return split_tokens
-
- def _convert_token_to_id(self, token):
- """ Converts a token (str) in an id using the vocab. """
- return self.vocab.get(token, self.vocab.get(self.unk_token))
-
- def _convert_id_to_token(self, index):
- """Converts an index (integer) in a token (str) using the vocab."""
- return self.ids_to_tokens.get(index, self.unk_token)
-
- def convert_tokens_to_string(self, tokens):
- """ Converts a sequence of tokens (string) in a single string. """
- out_string = " ".join(tokens).replace(" ##", "").strip()
- return out_string
-
- def build_inputs_with_special_tokens(self, token_ids_0, token_ids_1=None):
- """
- Build model inputs from a sequence or a pair of sequence for sequence classification tasks
- by concatenating and adding special tokens.
- A BERT sequence has the following format:
- single sequence: [CLS] X [SEP]
- pair of sequences: [CLS] A [SEP] B [SEP]
- """
- if token_ids_1 is None:
- return [self.cls_token_id] + token_ids_0 + [self.sep_token_id]
- cls = [self.cls_token_id]
- sep = [self.sep_token_id]
- return cls + token_ids_0 + sep + token_ids_1 + sep
-
- def get_special_tokens_mask(self, token_ids_0, token_ids_1=None, already_has_special_tokens=False):
- """
- Retrieves sequence ids from a token list that has no special tokens added. This method is called when adding
- special tokens using the tokenizer ``prepare_for_model`` or ``encode_plus`` methods.
-
- Args:
- token_ids_0: list of ids (must not contain special tokens)
- token_ids_1: Optional list of ids (must not contain special tokens), necessary when fetching sequence ids
- for sequence pairs
- already_has_special_tokens: (default False) Set to True if the token list is already formated with
- special tokens for the model
-
- Returns:
- A list of integers in the range [0, 1]: 1 for a special token, 0 for a sequence token.
- """
-
- if already_has_special_tokens:
- if token_ids_1 is not None:
- raise ValueError(
- "You should not supply a second sequence if the provided sequence of "
- "ids is already formated with special tokens for the model."
- )
- return list(map(lambda x: 1 if x in [self.sep_token_id, self.cls_token_id] else 0, token_ids_0))
-
- if token_ids_1 is not None:
- return [1] + ([0] * len(token_ids_0)) + [1] + ([0] * len(token_ids_1)) + [1]
- return [1] + ([0] * len(token_ids_0)) + [1]
-
- def create_token_type_ids_from_sequences(self, token_ids_0, token_ids_1=None):
- """
- Creates a mask from the two sequences passed to be used in a sequence-pair classification task.
- A BERT sequence pair mask has the following format:
- 0 0 0 0 0 0 0 0 0 0 1 1 1 1 1 1 1 1 1 1 1
- | first sequence | second sequence
-
- if token_ids_1 is None, only returns the first portion of the mask (0's).
- """
- sep = [self.sep_token_id]
- cls = [self.cls_token_id]
- if token_ids_1 is None:
- return len(cls + token_ids_0 + sep) * [0]
- return len(cls + token_ids_0 + sep) * [0] + len(token_ids_1 + sep) * [1]
-
- def save_vocabulary(self, vocab_path):
- """Save the tokenizer vocabulary to a directory or file."""
- index = 0
- if os.path.isdir(vocab_path):
- vocab_file = os.path.join(vocab_path, VOCAB_FILES_NAMES["vocab_file"])
- else:
- vocab_file = vocab_path
- with open(vocab_file, "w", encoding="utf-8") as writer:
- for token, token_index in sorted(self.vocab.items(), key=lambda kv: kv[1]):
- if index != token_index:
- logger.warning(
- "Saving vocabulary to {}: vocabulary indices are not consecutive."
- " Please check that the vocabulary is not corrupted!".format(vocab_file)
- )
- index = token_index
- writer.write(token + "\n")
- index += 1
- return (vocab_file,)
diff --git a/server/transformers/tests/__init__.py b/server/transformers/tests/__init__.py
deleted file mode 100644
index e69de29bb2d1d6434b8b29ae775ad8c2e48c5391..0000000000000000000000000000000000000000
diff --git a/server/transformers/tests/fixtures/dummy-config.json b/server/transformers/tests/fixtures/dummy-config.json
deleted file mode 100644
index e388bdf71151db7c014ae6e0174dd07c1a6acbee..0000000000000000000000000000000000000000
--- a/server/transformers/tests/fixtures/dummy-config.json
+++ /dev/null
@@ -1,3 +0,0 @@
-{
- "model_type": "roberta"
-}
\ No newline at end of file
diff --git a/server/transformers/tests/fixtures/empty.txt b/server/transformers/tests/fixtures/empty.txt
deleted file mode 100644
index e69de29bb2d1d6434b8b29ae775ad8c2e48c5391..0000000000000000000000000000000000000000
diff --git a/server/transformers/tests/fixtures/input.txt b/server/transformers/tests/fixtures/input.txt
deleted file mode 100644
index d1e3f410d07833e4c5c233ffd54f8d2b54ebb7cf..0000000000000000000000000000000000000000
--- a/server/transformers/tests/fixtures/input.txt
+++ /dev/null
@@ -1 +0,0 @@
-Who was Jim Henson ? ||| Jim Henson was a puppeteer
diff --git a/server/transformers/tests/fixtures/sample_text.txt b/server/transformers/tests/fixtures/sample_text.txt
deleted file mode 100644
index a42812060c576bae870eb29b1ac083fda0d239d3..0000000000000000000000000000000000000000
--- a/server/transformers/tests/fixtures/sample_text.txt
+++ /dev/null
@@ -1,33 +0,0 @@
-This text is included to make sure Unicode is handled properly: 力加勝北区ᴵᴺᵀᵃছজটডণত
-Text should be one-sentence-per-line, with empty lines between documents.
-This sample text is public domain and was randomly selected from Project Guttenberg.
-
-The rain had only ceased with the gray streaks of morning at Blazing Star, and the settlement awoke to a moral sense of cleanliness, and the finding of forgotten knives, tin cups, and smaller camp utensils, where the heavy showers had washed away the debris and dust heaps before the cabin doors.
-Indeed, it was recorded in Blazing Star that a fortunate early riser had once picked up on the highway a solid chunk of gold quartz which the rain had freed from its incumbering soil, and washed into immediate and glittering popularity.
-Possibly this may have been the reason why early risers in that locality, during the rainy season, adopted a thoughtful habit of body, and seldom lifted their eyes to the rifted or india-ink washed skies above them.
-"Cass" Beard had risen early that morning, but not with a view to discovery.
-A leak in his cabin roof,--quite consistent with his careless, improvident habits,--had roused him at 4 A. M., with a flooded "bunk" and wet blankets.
-The chips from his wood pile refused to kindle a fire to dry his bed-clothes, and he had recourse to a more provident neighbor's to supply the deficiency.
-This was nearly opposite.
-Mr. Cassius crossed the highway, and stopped suddenly.
-Something glittered in the nearest red pool before him.
-Gold, surely!
-But, wonderful to relate, not an irregular, shapeless fragment of crude ore, fresh from Nature's crucible, but a bit of jeweler's handicraft in the form of a plain gold ring.
-Looking at it more attentively, he saw that it bore the inscription, "May to Cass."
-Like most of his fellow gold-seekers, Cass was superstitious.
-
-The fountain of classic wisdom, Hypatia herself.
-As the ancient sage--the name is unimportant to a monk--pumped water nightly that he might study by day, so I, the guardian of cloaks and parasols, at the sacred doors of her lecture-room, imbibe celestial knowledge.
-From my youth I felt in me a soul above the matter-entangled herd.
-She revealed to me the glorious fact, that I am a spark of Divinity itself.
-A fallen star, I am, sir!' continued he, pensively, stroking his lean stomach--'a fallen star!--fallen, if the dignity of philosophy will allow of the simile, among the hogs of the lower world--indeed, even into the hog-bucket itself. Well, after all, I will show you the way to the Archbishop's.
-There is a philosophic pleasure in opening one's treasures to the modest young.
-Perhaps you will assist me by carrying this basket of fruit?' And the little man jumped up, put his basket on Philammon's head, and trotted off up a neighbouring street.
-Philammon followed, half contemptuous, half wondering at what this philosophy might be, which could feed the self-conceit of anything so abject as his ragged little apish guide;
-but the novel roar and whirl of the street, the perpetual stream of busy faces, the line of curricles, palanquins, laden asses, camels, elephants, which met and passed him, and squeezed him up steps and into doorways, as they threaded their way through the great Moon-gate into the ample street beyond, drove everything from his mind but wondering curiosity, and a vague, helpless dread of that great living wilderness, more terrible than any dead wilderness of sand which he had left behind.
-Already he longed for the repose, the silence of the Laura--for faces which knew him and smiled upon him; but it was too late to turn back now.
-His guide held on for more than a mile up the great main street, crossed in the centre of the city, at right angles, by one equally magnificent, at each end of which, miles away, appeared, dim and distant over the heads of the living stream of passengers, the yellow sand-hills of the desert;
-while at the end of the vista in front of them gleamed the blue harbour, through a network of countless masts.
-At last they reached the quay at the opposite end of the street;
-and there burst on Philammon's astonished eyes a vast semicircle of blue sea, ringed with palaces and towers.
-He stopped involuntarily; and his little guide stopped also, and looked askance at the young monk, to watch the effect which that grand panorama should produce on him.
diff --git a/server/transformers/tests/fixtures/spiece.model b/server/transformers/tests/fixtures/spiece.model
deleted file mode 100644
index c91b8acfa56ccfc80e1cdd854ddcaf9b6c44ab2a..0000000000000000000000000000000000000000
Binary files a/server/transformers/tests/fixtures/spiece.model and /dev/null differ
diff --git a/server/transformers/tests/fixtures/test_sentencepiece.model b/server/transformers/tests/fixtures/test_sentencepiece.model
deleted file mode 100644
index 376dda73010c6f93acfa3b974bea81a9ac9e1740..0000000000000000000000000000000000000000
Binary files a/server/transformers/tests/fixtures/test_sentencepiece.model and /dev/null differ
diff --git a/server/transformers/tests/test_configuration_auto.py b/server/transformers/tests/test_configuration_auto.py
deleted file mode 100644
index 5262be2e7cccd5ee1143dc0388e8ea0ff0eedb11..0000000000000000000000000000000000000000
--- a/server/transformers/tests/test_configuration_auto.py
+++ /dev/null
@@ -1,54 +0,0 @@
-# coding=utf-8
-# Copyright 2019-present, the HuggingFace Inc. team.
-#
-# Licensed under the Apache License, Version 2.0 (the "License");
-# you may not use this file except in compliance with the License.
-# You may obtain a copy of the License at
-#
-# http://www.apache.org/licenses/LICENSE-2.0
-#
-# Unless required by applicable law or agreed to in writing, software
-# distributed under the License is distributed on an "AS IS" BASIS,
-# WITHOUT WARRANTIES OR CONDITIONS OF ANY KIND, either express or implied.
-# See the License for the specific language governing permissions and
-# limitations under the License.
-
-import os
-import unittest
-
-from transformers.configuration_auto import CONFIG_MAPPING, AutoConfig
-from transformers.configuration_bert import BertConfig
-from transformers.configuration_roberta import RobertaConfig
-
-from .utils import DUMMY_UNKWOWN_IDENTIFIER
-
-
-SAMPLE_ROBERTA_CONFIG = os.path.join(os.path.dirname(os.path.abspath(__file__)), "fixtures/dummy-config.json")
-
-
-class AutoConfigTest(unittest.TestCase):
- def test_config_from_model_shortcut(self):
- config = AutoConfig.from_pretrained("bert-base-uncased")
- self.assertIsInstance(config, BertConfig)
-
- def test_config_model_type_from_local_file(self):
- config = AutoConfig.from_pretrained(SAMPLE_ROBERTA_CONFIG)
- self.assertIsInstance(config, RobertaConfig)
-
- def test_config_model_type_from_model_identifier(self):
- config = AutoConfig.from_pretrained(DUMMY_UNKWOWN_IDENTIFIER)
- self.assertIsInstance(config, RobertaConfig)
-
- def test_config_for_model_str(self):
- config = AutoConfig.for_model("roberta")
- self.assertIsInstance(config, RobertaConfig)
-
- def test_pattern_matching_fallback(self):
- """
- In cases where config.json doesn't include a model_type,
- perform a few safety checks on the config mapping's order.
- """
- # no key string should be included in a later key string (typical failure case)
- keys = list(CONFIG_MAPPING.keys())
- for i, key in enumerate(keys):
- self.assertFalse(any(key in later_key for later_key in keys[i + 1 :]))
diff --git a/server/transformers/tests/test_configuration_common.py b/server/transformers/tests/test_configuration_common.py
deleted file mode 100644
index 471f0f012d549ae17bf4cefd12d0d91ea230857e..0000000000000000000000000000000000000000
--- a/server/transformers/tests/test_configuration_common.py
+++ /dev/null
@@ -1,64 +0,0 @@
-# coding=utf-8
-# Copyright 2019 HuggingFace Inc.
-#
-# Licensed under the Apache License, Version 2.0 (the "License");
-# you may not use this file except in compliance with the License.
-# You may obtain a copy of the License at
-#
-# http://www.apache.org/licenses/LICENSE-2.0
-#
-# Unless required by applicable law or agreed to in writing, software
-# distributed under the License is distributed on an "AS IS" BASIS,
-# WITHOUT WARRANTIES OR CONDITIONS OF ANY KIND, either express or implied.
-# See the License for the specific language governing permissions and
-# limitations under the License.
-
-
-import json
-import os
-import tempfile
-
-
-class ConfigTester(object):
- def __init__(self, parent, config_class=None, **kwargs):
- self.parent = parent
- self.config_class = config_class
- self.inputs_dict = kwargs
-
- def create_and_test_config_common_properties(self):
- config = self.config_class(**self.inputs_dict)
- self.parent.assertTrue(hasattr(config, "vocab_size"))
- self.parent.assertTrue(hasattr(config, "hidden_size"))
- self.parent.assertTrue(hasattr(config, "num_attention_heads"))
- self.parent.assertTrue(hasattr(config, "num_hidden_layers"))
-
- def create_and_test_config_to_json_string(self):
- config = self.config_class(**self.inputs_dict)
- obj = json.loads(config.to_json_string())
- for key, value in self.inputs_dict.items():
- self.parent.assertEqual(obj[key], value)
-
- def create_and_test_config_to_json_file(self):
- config_first = self.config_class(**self.inputs_dict)
-
- with tempfile.TemporaryDirectory() as tmpdirname:
- json_file_path = os.path.join(tmpdirname, "config.json")
- config_first.to_json_file(json_file_path)
- config_second = self.config_class.from_json_file(json_file_path)
-
- self.parent.assertEqual(config_second.to_dict(), config_first.to_dict())
-
- def create_and_test_config_from_and_save_pretrained(self):
- config_first = self.config_class(**self.inputs_dict)
-
- with tempfile.TemporaryDirectory() as tmpdirname:
- config_first.save_pretrained(tmpdirname)
- config_second = self.config_class.from_pretrained(tmpdirname)
-
- self.parent.assertEqual(config_second.to_dict(), config_first.to_dict())
-
- def run_common_tests(self):
- self.create_and_test_config_common_properties()
- self.create_and_test_config_to_json_string()
- self.create_and_test_config_to_json_file()
- self.create_and_test_config_from_and_save_pretrained()
diff --git a/server/transformers/tests/test_doc_samples.py b/server/transformers/tests/test_doc_samples.py
deleted file mode 100644
index c97af35200ac4b38875d4be4e33b379221b92b99..0000000000000000000000000000000000000000
--- a/server/transformers/tests/test_doc_samples.py
+++ /dev/null
@@ -1,129 +0,0 @@
-# coding=utf-8
-# Copyright 2019-present, the HuggingFace Inc. team.
-#
-# Licensed under the Apache License, Version 2.0 (the "License");
-# you may not use this file except in compliance with the License.
-# You may obtain a copy of the License at
-#
-# http://www.apache.org/licenses/LICENSE-2.0
-#
-# Unless required by applicable law or agreed to in writing, software
-# distributed under the License is distributed on an "AS IS" BASIS,
-# WITHOUT WARRANTIES OR CONDITIONS OF ANY KIND, either express or implied.
-# See the License for the specific language governing permissions and
-# limitations under the License.
-
-import os
-import unittest
-from typing import List, Union
-
-from .utils import require_tf, require_torch, slow
-
-
-def get_examples_from_file(file):
- examples = []
- example = []
- example_mode = False
- example_indentation = None
- for i, line in enumerate(file):
- if example_mode:
- current_indentation = len(line) - len(line.strip()) - 1
-
- # Check if the indentation is 0 for the example, so that we don't exit as soon as there's a line return.
- empty_line = example_indentation == 0 and len(line) == 1
-
- # If we're back to the example indentation or if it's the end of the docstring.
- if (current_indentation == example_indentation and not empty_line) or '"""' in line:
- # Exit the example mode and add the example to the examples list
- example_mode = False
- example_indentation = None
- examples.append(example)
- example = []
- else:
- # If line is not empty, add it to the current example
- if line != "\n":
- example.append(line[example_indentation + 4 : -1])
-
- # Detect the example from '::' or 'example::'
- if "example::" in line.lower():
- example_mode = True
- example_indentation = line.lower().find("example::")
- elif "examples::" in line.lower():
- example_mode = True
- example_indentation = line.lower().find("examples::")
- # elif "::" in line.lower() and len(line.strip()) == 2:
- # example_mode = True
- # example_indentation = line.lower().find("::")
-
- examples = ["\n".join(example) for example in examples]
- examples = [example for example in examples if "not runnable" not in example.lower()]
-
- return examples
-
-
-@require_torch
-@require_tf
-@slow
-class TestCodeExamples(unittest.TestCase):
- def analyze_directory(
- self, directory: str, identifier: Union[str, None] = None, ignore_files: Union[List[str], None] = None
- ):
- files = [file for file in os.listdir(directory) if os.path.isfile(os.path.join(directory, file))]
-
- if identifier is not None:
- files = [file for file in files if identifier in file]
-
- if ignore_files is not None:
- files = [file for file in files if file not in ignore_files]
-
- for file in files:
- # Open all files
- with open(os.path.join(directory, file)) as f:
- # Retrieve examples
- examples = get_examples_from_file(f)
- joined_examples = []
-
- def execute_example(code_example):
- exec(code_example, {})
-
- # Some examples are the continuation of others.
- if len(examples) > 0:
- joined_examples.append(examples[0])
- joined_examples_index = 0
- for example in examples[1:]:
- # If they contain this line, then they're a continuation of the previous script
- if "# Continuation of the previous script" in example:
- joined_examples[joined_examples_index] += "\n" + example
- # If not, create a new example and increment the index
- else:
- joined_examples.append(example)
- joined_examples_index += 1
-
- print("Testing", file, str(len(joined_examples)) + "/" + str(len(joined_examples)))
-
- # Execute sub tests with every example.
- for index, code_example in enumerate(joined_examples):
- with self.subTest(msg=file + " " + str(index) + "/" + str(len(joined_examples)) + code_example):
- execute_example(code_example)
-
- def test_configuration_examples(self):
- transformers_directory = "src/transformers"
- configuration_files = "configuration"
- ignore_files = ["configuration_auto.py", "configuration_utils.py"]
- self.analyze_directory(transformers_directory, identifier=configuration_files, ignore_files=ignore_files)
-
- def test_main_doc_examples(self):
- doc_directory = "docs/source"
- self.analyze_directory(doc_directory)
-
- def test_modeling_examples(self):
- transformers_directory = "src/transformers"
- modeling_files = "modeling"
- ignore_files = [
- "modeling_auto.py",
- "modeling_t5.py",
- "modeling_tf_auto.py",
- "modeling_utils.py",
- "modeling_tf_t5.py",
- ]
- self.analyze_directory(transformers_directory, identifier=modeling_files, ignore_files=ignore_files)
diff --git a/server/transformers/tests/test_hf_api.py b/server/transformers/tests/test_hf_api.py
deleted file mode 100644
index c791390959cd3599d148fec0a13205591decda28..0000000000000000000000000000000000000000
--- a/server/transformers/tests/test_hf_api.py
+++ /dev/null
@@ -1,108 +0,0 @@
-# coding=utf-8
-# Copyright 2019-present, the HuggingFace Inc. team.
-#
-# Licensed under the Apache License, Version 2.0 (the "License");
-# you may not use this file except in compliance with the License.
-# You may obtain a copy of the License at
-#
-# http://www.apache.org/licenses/LICENSE-2.0
-#
-# Unless required by applicable law or agreed to in writing, software
-# distributed under the License is distributed on an "AS IS" BASIS,
-# WITHOUT WARRANTIES OR CONDITIONS OF ANY KIND, either express or implied.
-# See the License for the specific language governing permissions and
-# limitations under the License.
-
-
-import os
-import time
-import unittest
-
-import requests
-from requests.exceptions import HTTPError
-
-from transformers.hf_api import HfApi, HfFolder, PresignedUrl, S3Obj
-
-
-USER = "__DUMMY_TRANSFORMERS_USER__"
-PASS = "__DUMMY_TRANSFORMERS_PASS__"
-FILES = [
- (
- "Test-{}.txt".format(int(time.time())),
- os.path.join(os.path.dirname(os.path.abspath(__file__)), "fixtures/input.txt"),
- ),
- (
- "yoyo {}.txt".format(int(time.time())), # space is intentional
- os.path.join(os.path.dirname(os.path.abspath(__file__)), "fixtures/empty.txt"),
- ),
-]
-
-
-class HfApiCommonTest(unittest.TestCase):
- _api = HfApi(endpoint="https://moon-staging.huggingface.co")
-
-
-class HfApiLoginTest(HfApiCommonTest):
- def test_login_invalid(self):
- with self.assertRaises(HTTPError):
- self._api.login(username=USER, password="fake")
-
- def test_login_valid(self):
- token = self._api.login(username=USER, password=PASS)
- self.assertIsInstance(token, str)
-
-
-class HfApiEndpointsTest(HfApiCommonTest):
- @classmethod
- def setUpClass(cls):
- """
- Share this valid token in all tests below.
- """
- cls._token = cls._api.login(username=USER, password=PASS)
-
- @classmethod
- def tearDownClass(cls):
- for FILE_KEY, FILE_PATH in FILES:
- cls._api.delete_obj(token=cls._token, filename=FILE_KEY)
-
- def test_whoami(self):
- user = self._api.whoami(token=self._token)
- self.assertEqual(user, USER)
-
- def test_presign(self):
- for FILE_KEY, FILE_PATH in FILES:
- urls = self._api.presign(token=self._token, filename=FILE_KEY)
- self.assertIsInstance(urls, PresignedUrl)
- self.assertEqual(urls.type, "text/plain")
-
- def test_presign_and_upload(self):
- for FILE_KEY, FILE_PATH in FILES:
- access_url = self._api.presign_and_upload(token=self._token, filename=FILE_KEY, filepath=FILE_PATH)
- self.assertIsInstance(access_url, str)
- with open(FILE_PATH, "r") as f:
- body = f.read()
- r = requests.get(access_url)
- self.assertEqual(r.text, body)
-
- def test_list_objs(self):
- objs = self._api.list_objs(token=self._token)
- self.assertIsInstance(objs, list)
- if len(objs) > 0:
- o = objs[-1]
- self.assertIsInstance(o, S3Obj)
-
-
-class HfFolderTest(unittest.TestCase):
- def test_token_workflow(self):
- """
- Test the whole token save/get/delete workflow,
- with the desired behavior with respect to non-existent tokens.
- """
- token = "token-{}".format(int(time.time()))
- HfFolder.save_token(token)
- self.assertEqual(HfFolder.get_token(), token)
- HfFolder.delete_token()
- HfFolder.delete_token()
- # ^^ not an error, we test that the
- # second call does not fail.
- self.assertEqual(HfFolder.get_token(), None)
diff --git a/server/transformers/tests/test_model_card.py b/server/transformers/tests/test_model_card.py
deleted file mode 100644
index 1004642a92a2a6253da5cc91a05ac5c3545ffed9..0000000000000000000000000000000000000000
--- a/server/transformers/tests/test_model_card.py
+++ /dev/null
@@ -1,81 +0,0 @@
-# coding=utf-8
-# Copyright 2019 HuggingFace Inc.
-#
-# Licensed under the Apache License, Version 2.0 (the "License");
-# you may not use this file except in compliance with the License.
-# You may obtain a copy of the License at
-#
-# http://www.apache.org/licenses/LICENSE-2.0
-#
-# Unless required by applicable law or agreed to in writing, software
-# distributed under the License is distributed on an "AS IS" BASIS,
-# WITHOUT WARRANTIES OR CONDITIONS OF ANY KIND, either express or implied.
-# See the License for the specific language governing permissions and
-# limitations under the License.
-
-
-import json
-import os
-import tempfile
-import unittest
-
-from transformers.modelcard import ModelCard
-
-
-class ModelCardTester(unittest.TestCase):
- def setUp(self):
- self.inputs_dict = {
- "model_details": {
- "Organization": "testing",
- "Model date": "today",
- "Model version": "v2.1, Developed by Test Corp in 2019.",
- "Architecture": "Convolutional Neural Network.",
- },
- "metrics": "BLEU and ROUGE-1",
- "evaluation_data": {
- "Datasets": {"BLEU": "My-great-dataset-v1", "ROUGE-1": "My-short-dataset-v2.1"},
- "Preprocessing": "See details on https://arxiv.org/pdf/1810.03993.pdf",
- },
- "training_data": {
- "Dataset": "English Wikipedia dump dated 2018-12-01",
- "Preprocessing": "Using SentencePiece vocabulary of size 52k tokens. See details on https://arxiv.org/pdf/1810.03993.pdf",
- },
- "quantitative_analyses": {"BLEU": 55.1, "ROUGE-1": 76},
- }
-
- def test_model_card_common_properties(self):
- modelcard = ModelCard.from_dict(self.inputs_dict)
- self.assertTrue(hasattr(modelcard, "model_details"))
- self.assertTrue(hasattr(modelcard, "intended_use"))
- self.assertTrue(hasattr(modelcard, "factors"))
- self.assertTrue(hasattr(modelcard, "metrics"))
- self.assertTrue(hasattr(modelcard, "evaluation_data"))
- self.assertTrue(hasattr(modelcard, "training_data"))
- self.assertTrue(hasattr(modelcard, "quantitative_analyses"))
- self.assertTrue(hasattr(modelcard, "ethical_considerations"))
- self.assertTrue(hasattr(modelcard, "caveats_and_recommendations"))
-
- def test_model_card_to_json_string(self):
- modelcard = ModelCard.from_dict(self.inputs_dict)
- obj = json.loads(modelcard.to_json_string())
- for key, value in self.inputs_dict.items():
- self.assertEqual(obj[key], value)
-
- def test_model_card_to_json_file(self):
- model_card_first = ModelCard.from_dict(self.inputs_dict)
-
- with tempfile.TemporaryDirectory() as tmpdirname:
- filename = os.path.join(tmpdirname, "modelcard.json")
- model_card_first.to_json_file(filename)
- model_card_second = ModelCard.from_json_file(filename)
-
- self.assertEqual(model_card_second.to_dict(), model_card_first.to_dict())
-
- def test_model_card_from_and_save_pretrained(self):
- model_card_first = ModelCard.from_dict(self.inputs_dict)
-
- with tempfile.TemporaryDirectory() as tmpdirname:
- model_card_first.save_pretrained(tmpdirname)
- model_card_second = ModelCard.from_pretrained(tmpdirname)
-
- self.assertEqual(model_card_second.to_dict(), model_card_first.to_dict())
diff --git a/server/transformers/tests/test_modeling_albert.py b/server/transformers/tests/test_modeling_albert.py
deleted file mode 100644
index 05d7aaefb5014a16b5908ddff8e1194011f49d46..0000000000000000000000000000000000000000
--- a/server/transformers/tests/test_modeling_albert.py
+++ /dev/null
@@ -1,251 +0,0 @@
-# coding=utf-8
-# Copyright 2018 The Google AI Language Team Authors.
-#
-# Licensed under the Apache License, Version 2.0 (the "License");
-# you may not use this file except in compliance with the License.
-# You may obtain a copy of the License at
-#
-# http://www.apache.org/licenses/LICENSE-2.0
-#
-# Unless required by applicable law or agreed to in writing, software
-# distributed under the License is distributed on an "AS IS" BASIS,
-# WITHOUT WARRANTIES OR CONDITIONS OF ANY KIND, either express or implied.
-# See the License for the specific language governing permissions and
-# limitations under the License.
-
-
-import unittest
-
-from transformers import is_torch_available
-
-from .test_configuration_common import ConfigTester
-from .test_modeling_common import ModelTesterMixin, ids_tensor
-from .utils import CACHE_DIR, require_torch, slow, torch_device
-
-
-if is_torch_available():
- from transformers import (
- AlbertConfig,
- AlbertModel,
- AlbertForMaskedLM,
- AlbertForSequenceClassification,
- AlbertForQuestionAnswering,
- )
- from transformers.modeling_albert import ALBERT_PRETRAINED_MODEL_ARCHIVE_MAP
-
-
-@require_torch
-class AlbertModelTest(ModelTesterMixin, unittest.TestCase):
-
- all_model_classes = (AlbertModel, AlbertForMaskedLM) if is_torch_available() else ()
-
- class AlbertModelTester(object):
- def __init__(
- self,
- parent,
- batch_size=13,
- seq_length=7,
- is_training=True,
- use_input_mask=True,
- use_token_type_ids=True,
- use_labels=True,
- vocab_size=99,
- embedding_size=16,
- hidden_size=36,
- num_hidden_layers=6,
- num_hidden_groups=6,
- num_attention_heads=6,
- intermediate_size=37,
- hidden_act="gelu",
- hidden_dropout_prob=0.1,
- attention_probs_dropout_prob=0.1,
- max_position_embeddings=512,
- type_vocab_size=16,
- type_sequence_label_size=2,
- initializer_range=0.02,
- num_labels=3,
- num_choices=4,
- scope=None,
- ):
- self.parent = parent
- self.batch_size = batch_size
- self.seq_length = seq_length
- self.is_training = is_training
- self.use_input_mask = use_input_mask
- self.use_token_type_ids = use_token_type_ids
- self.use_labels = use_labels
- self.vocab_size = vocab_size
- self.embedding_size = embedding_size
- self.hidden_size = hidden_size
- self.num_hidden_layers = num_hidden_layers
- self.num_attention_heads = num_attention_heads
- self.intermediate_size = intermediate_size
- self.hidden_act = hidden_act
- self.hidden_dropout_prob = hidden_dropout_prob
- self.attention_probs_dropout_prob = attention_probs_dropout_prob
- self.max_position_embeddings = max_position_embeddings
- self.type_vocab_size = type_vocab_size
- self.type_sequence_label_size = type_sequence_label_size
- self.initializer_range = initializer_range
- self.num_labels = num_labels
- self.num_choices = num_choices
- self.scope = scope
- self.num_hidden_groups = num_hidden_groups
-
- def prepare_config_and_inputs(self):
- input_ids = ids_tensor([self.batch_size, self.seq_length], self.vocab_size)
-
- input_mask = None
- if self.use_input_mask:
- input_mask = ids_tensor([self.batch_size, self.seq_length], vocab_size=2)
-
- token_type_ids = None
- if self.use_token_type_ids:
- token_type_ids = ids_tensor([self.batch_size, self.seq_length], self.type_vocab_size)
-
- sequence_labels = None
- token_labels = None
- choice_labels = None
- if self.use_labels:
- sequence_labels = ids_tensor([self.batch_size], self.type_sequence_label_size)
- token_labels = ids_tensor([self.batch_size, self.seq_length], self.num_labels)
- choice_labels = ids_tensor([self.batch_size], self.num_choices)
-
- config = AlbertConfig(
- vocab_size=self.vocab_size,
- hidden_size=self.hidden_size,
- num_hidden_layers=self.num_hidden_layers,
- num_attention_heads=self.num_attention_heads,
- intermediate_size=self.intermediate_size,
- hidden_act=self.hidden_act,
- hidden_dropout_prob=self.hidden_dropout_prob,
- attention_probs_dropout_prob=self.attention_probs_dropout_prob,
- max_position_embeddings=self.max_position_embeddings,
- type_vocab_size=self.type_vocab_size,
- initializer_range=self.initializer_range,
- num_hidden_groups=self.num_hidden_groups,
- )
-
- return config, input_ids, token_type_ids, input_mask, sequence_labels, token_labels, choice_labels
-
- def check_loss_output(self, result):
- self.parent.assertListEqual(list(result["loss"].size()), [])
-
- def create_and_check_albert_model(
- self, config, input_ids, token_type_ids, input_mask, sequence_labels, token_labels, choice_labels
- ):
- model = AlbertModel(config=config)
- model.to(torch_device)
- model.eval()
- sequence_output, pooled_output = model(input_ids, attention_mask=input_mask, token_type_ids=token_type_ids)
- sequence_output, pooled_output = model(input_ids, token_type_ids=token_type_ids)
- sequence_output, pooled_output = model(input_ids)
-
- result = {
- "sequence_output": sequence_output,
- "pooled_output": pooled_output,
- }
- self.parent.assertListEqual(
- list(result["sequence_output"].size()), [self.batch_size, self.seq_length, self.hidden_size]
- )
- self.parent.assertListEqual(list(result["pooled_output"].size()), [self.batch_size, self.hidden_size])
-
- def create_and_check_albert_for_masked_lm(
- self, config, input_ids, token_type_ids, input_mask, sequence_labels, token_labels, choice_labels
- ):
- model = AlbertForMaskedLM(config=config)
- model.to(torch_device)
- model.eval()
- loss, prediction_scores = model(
- input_ids, attention_mask=input_mask, token_type_ids=token_type_ids, masked_lm_labels=token_labels
- )
- result = {
- "loss": loss,
- "prediction_scores": prediction_scores,
- }
- self.parent.assertListEqual(
- list(result["prediction_scores"].size()), [self.batch_size, self.seq_length, self.vocab_size]
- )
- self.check_loss_output(result)
-
- def create_and_check_albert_for_question_answering(
- self, config, input_ids, token_type_ids, input_mask, sequence_labels, token_labels, choice_labels
- ):
- model = AlbertForQuestionAnswering(config=config)
- model.to(torch_device)
- model.eval()
- loss, start_logits, end_logits = model(
- input_ids,
- attention_mask=input_mask,
- token_type_ids=token_type_ids,
- start_positions=sequence_labels,
- end_positions=sequence_labels,
- )
- result = {
- "loss": loss,
- "start_logits": start_logits,
- "end_logits": end_logits,
- }
- self.parent.assertListEqual(list(result["start_logits"].size()), [self.batch_size, self.seq_length])
- self.parent.assertListEqual(list(result["end_logits"].size()), [self.batch_size, self.seq_length])
- self.check_loss_output(result)
-
- def create_and_check_albert_for_sequence_classification(
- self, config, input_ids, token_type_ids, input_mask, sequence_labels, token_labels, choice_labels
- ):
- config.num_labels = self.num_labels
- model = AlbertForSequenceClassification(config)
- model.to(torch_device)
- model.eval()
- loss, logits = model(
- input_ids, attention_mask=input_mask, token_type_ids=token_type_ids, labels=sequence_labels
- )
- result = {
- "loss": loss,
- "logits": logits,
- }
- self.parent.assertListEqual(list(result["logits"].size()), [self.batch_size, self.num_labels])
- self.check_loss_output(result)
-
- def prepare_config_and_inputs_for_common(self):
- config_and_inputs = self.prepare_config_and_inputs()
- (
- config,
- input_ids,
- token_type_ids,
- input_mask,
- sequence_labels,
- token_labels,
- choice_labels,
- ) = config_and_inputs
- inputs_dict = {"input_ids": input_ids, "token_type_ids": token_type_ids, "attention_mask": input_mask}
- return config, inputs_dict
-
- def setUp(self):
- self.model_tester = AlbertModelTest.AlbertModelTester(self)
- self.config_tester = ConfigTester(self, config_class=AlbertConfig, hidden_size=37)
-
- def test_config(self):
- self.config_tester.run_common_tests()
-
- def test_albert_model(self):
- config_and_inputs = self.model_tester.prepare_config_and_inputs()
- self.model_tester.create_and_check_albert_model(*config_and_inputs)
-
- def test_for_masked_lm(self):
- config_and_inputs = self.model_tester.prepare_config_and_inputs()
- self.model_tester.create_and_check_albert_for_masked_lm(*config_and_inputs)
-
- def test_for_question_answering(self):
- config_and_inputs = self.model_tester.prepare_config_and_inputs()
- self.model_tester.create_and_check_albert_for_question_answering(*config_and_inputs)
-
- def test_for_sequence_classification(self):
- config_and_inputs = self.model_tester.prepare_config_and_inputs()
- self.model_tester.create_and_check_albert_for_sequence_classification(*config_and_inputs)
-
- @slow
- def test_model_from_pretrained(self):
- for model_name in list(ALBERT_PRETRAINED_MODEL_ARCHIVE_MAP.keys())[:1]:
- model = AlbertModel.from_pretrained(model_name, cache_dir=CACHE_DIR)
- self.assertIsNotNone(model)
diff --git a/server/transformers/tests/test_modeling_auto.py b/server/transformers/tests/test_modeling_auto.py
deleted file mode 100644
index b39c9de5228df75c9ee1eca08592fe917a380500..0000000000000000000000000000000000000000
--- a/server/transformers/tests/test_modeling_auto.py
+++ /dev/null
@@ -1,160 +0,0 @@
-# coding=utf-8
-# Copyright 2018 The Google AI Language Team Authors.
-#
-# Licensed under the Apache License, Version 2.0 (the "License");
-# you may not use this file except in compliance with the License.
-# You may obtain a copy of the License at
-#
-# http://www.apache.org/licenses/LICENSE-2.0
-#
-# Unless required by applicable law or agreed to in writing, software
-# distributed under the License is distributed on an "AS IS" BASIS,
-# WITHOUT WARRANTIES OR CONDITIONS OF ANY KIND, either express or implied.
-# See the License for the specific language governing permissions and
-# limitations under the License.
-
-
-import logging
-import unittest
-
-from transformers import is_torch_available
-
-from .utils import DUMMY_UNKWOWN_IDENTIFIER, SMALL_MODEL_IDENTIFIER, require_torch, slow
-
-
-if is_torch_available():
- from transformers import (
- AutoConfig,
- BertConfig,
- AutoModel,
- BertModel,
- AutoModelForPreTraining,
- BertForPreTraining,
- AutoModelWithLMHead,
- BertForMaskedLM,
- RobertaForMaskedLM,
- AutoModelForSequenceClassification,
- BertForSequenceClassification,
- AutoModelForQuestionAnswering,
- BertForQuestionAnswering,
- )
- from transformers.modeling_bert import BERT_PRETRAINED_MODEL_ARCHIVE_MAP
- from transformers.modeling_auto import (
- MODEL_MAPPING,
- MODEL_FOR_PRETRAINING_MAPPING,
- MODEL_FOR_QUESTION_ANSWERING_MAPPING,
- MODEL_FOR_SEQUENCE_CLASSIFICATION_MAPPING,
- MODEL_FOR_TOKEN_CLASSIFICATION_MAPPING,
- MODEL_WITH_LM_HEAD_MAPPING,
- )
-
-
-@require_torch
-class AutoModelTest(unittest.TestCase):
- @slow
- def test_model_from_pretrained(self):
- logging.basicConfig(level=logging.INFO)
- for model_name in list(BERT_PRETRAINED_MODEL_ARCHIVE_MAP.keys())[:1]:
- config = AutoConfig.from_pretrained(model_name)
- self.assertIsNotNone(config)
- self.assertIsInstance(config, BertConfig)
-
- model = AutoModel.from_pretrained(model_name)
- model, loading_info = AutoModel.from_pretrained(model_name, output_loading_info=True)
- self.assertIsNotNone(model)
- self.assertIsInstance(model, BertModel)
- for value in loading_info.values():
- self.assertEqual(len(value), 0)
-
- @slow
- def test_model_for_pretraining_from_pretrained(self):
- logging.basicConfig(level=logging.INFO)
- for model_name in list(BERT_PRETRAINED_MODEL_ARCHIVE_MAP.keys())[:1]:
- config = AutoConfig.from_pretrained(model_name)
- self.assertIsNotNone(config)
- self.assertIsInstance(config, BertConfig)
-
- model = AutoModelForPreTraining.from_pretrained(model_name)
- model, loading_info = AutoModelForPreTraining.from_pretrained(model_name, output_loading_info=True)
- self.assertIsNotNone(model)
- self.assertIsInstance(model, BertForPreTraining)
- for value in loading_info.values():
- self.assertEqual(len(value), 0)
-
- @slow
- def test_lmhead_model_from_pretrained(self):
- logging.basicConfig(level=logging.INFO)
- for model_name in list(BERT_PRETRAINED_MODEL_ARCHIVE_MAP.keys())[:1]:
- config = AutoConfig.from_pretrained(model_name)
- self.assertIsNotNone(config)
- self.assertIsInstance(config, BertConfig)
-
- model = AutoModelWithLMHead.from_pretrained(model_name)
- model, loading_info = AutoModelWithLMHead.from_pretrained(model_name, output_loading_info=True)
- self.assertIsNotNone(model)
- self.assertIsInstance(model, BertForMaskedLM)
-
- @slow
- def test_sequence_classification_model_from_pretrained(self):
- logging.basicConfig(level=logging.INFO)
- for model_name in list(BERT_PRETRAINED_MODEL_ARCHIVE_MAP.keys())[:1]:
- config = AutoConfig.from_pretrained(model_name)
- self.assertIsNotNone(config)
- self.assertIsInstance(config, BertConfig)
-
- model = AutoModelForSequenceClassification.from_pretrained(model_name)
- model, loading_info = AutoModelForSequenceClassification.from_pretrained(
- model_name, output_loading_info=True
- )
- self.assertIsNotNone(model)
- self.assertIsInstance(model, BertForSequenceClassification)
-
- # @slow
- def test_question_answering_model_from_pretrained(self):
- logging.basicConfig(level=logging.INFO)
- for model_name in list(BERT_PRETRAINED_MODEL_ARCHIVE_MAP.keys())[:1]:
- config = AutoConfig.from_pretrained(model_name)
- self.assertIsNotNone(config)
- self.assertIsInstance(config, BertConfig)
-
- model = AutoModelForQuestionAnswering.from_pretrained(model_name)
- model, loading_info = AutoModelForQuestionAnswering.from_pretrained(model_name, output_loading_info=True)
- self.assertIsNotNone(model)
- self.assertIsInstance(model, BertForQuestionAnswering)
-
- def test_from_pretrained_identifier(self):
- logging.basicConfig(level=logging.INFO)
- model = AutoModelWithLMHead.from_pretrained(SMALL_MODEL_IDENTIFIER)
- self.assertIsInstance(model, BertForMaskedLM)
- self.assertEqual(model.num_parameters(), 14830)
- self.assertEqual(model.num_parameters(only_trainable=True), 14830)
-
- def test_from_identifier_from_model_type(self):
- logging.basicConfig(level=logging.INFO)
- model = AutoModelWithLMHead.from_pretrained(DUMMY_UNKWOWN_IDENTIFIER)
- self.assertIsInstance(model, RobertaForMaskedLM)
- self.assertEqual(model.num_parameters(), 14830)
- self.assertEqual(model.num_parameters(only_trainable=True), 14830)
-
- def test_parents_and_children_in_mappings(self):
- # Test that the children are placed before the parents in the mappings, as the `instanceof` will be triggered
- # by the parents and will return the wrong configuration type when using auto models
-
- mappings = (
- MODEL_MAPPING,
- MODEL_FOR_PRETRAINING_MAPPING,
- MODEL_FOR_QUESTION_ANSWERING_MAPPING,
- MODEL_FOR_SEQUENCE_CLASSIFICATION_MAPPING,
- MODEL_FOR_TOKEN_CLASSIFICATION_MAPPING,
- MODEL_WITH_LM_HEAD_MAPPING,
- )
-
- for mapping in mappings:
- mapping = tuple(mapping.items())
- for index, (child_config, child_model) in enumerate(mapping[1:]):
- for parent_config, parent_model in mapping[: index + 1]:
- with self.subTest(
- msg="Testing if {} is child of {}".format(child_config.__name__, parent_config.__name__)
- ):
- self.assertFalse(issubclass(child_config, parent_config))
- self.assertFalse(issubclass(child_model, parent_model))
diff --git a/server/transformers/tests/test_modeling_bert.py b/server/transformers/tests/test_modeling_bert.py
deleted file mode 100644
index 946246ea2e32c0d4ad35d417df35682b522a5e74..0000000000000000000000000000000000000000
--- a/server/transformers/tests/test_modeling_bert.py
+++ /dev/null
@@ -1,477 +0,0 @@
-# coding=utf-8
-# Copyright 2018 The Google AI Language Team Authors.
-#
-# Licensed under the Apache License, Version 2.0 (the "License");
-# you may not use this file except in compliance with the License.
-# You may obtain a copy of the License at
-#
-# http://www.apache.org/licenses/LICENSE-2.0
-#
-# Unless required by applicable law or agreed to in writing, software
-# distributed under the License is distributed on an "AS IS" BASIS,
-# WITHOUT WARRANTIES OR CONDITIONS OF ANY KIND, either express or implied.
-# See the License for the specific language governing permissions and
-# limitations under the License.
-
-
-import unittest
-
-from transformers import is_torch_available
-
-from .test_configuration_common import ConfigTester
-from .test_modeling_common import ModelTesterMixin, floats_tensor, ids_tensor
-from .utils import CACHE_DIR, require_torch, slow, torch_device
-
-
-if is_torch_available():
- from transformers import (
- BertConfig,
- BertModel,
- BertForMaskedLM,
- BertForNextSentencePrediction,
- BertForPreTraining,
- BertForQuestionAnswering,
- BertForSequenceClassification,
- BertForTokenClassification,
- BertForMultipleChoice,
- )
- from transformers.modeling_bert import BERT_PRETRAINED_MODEL_ARCHIVE_MAP
-
-
-@require_torch
-class BertModelTest(ModelTesterMixin, unittest.TestCase):
-
- all_model_classes = (
- (
- BertModel,
- BertForMaskedLM,
- BertForNextSentencePrediction,
- BertForPreTraining,
- BertForQuestionAnswering,
- BertForSequenceClassification,
- BertForTokenClassification,
- )
- if is_torch_available()
- else ()
- )
-
- class BertModelTester(object):
- def __init__(
- self,
- parent,
- batch_size=13,
- seq_length=7,
- is_training=True,
- use_input_mask=True,
- use_token_type_ids=True,
- use_labels=True,
- vocab_size=99,
- hidden_size=32,
- num_hidden_layers=5,
- num_attention_heads=4,
- intermediate_size=37,
- hidden_act="gelu",
- hidden_dropout_prob=0.1,
- attention_probs_dropout_prob=0.1,
- max_position_embeddings=512,
- type_vocab_size=16,
- type_sequence_label_size=2,
- initializer_range=0.02,
- num_labels=3,
- num_choices=4,
- scope=None,
- ):
- self.parent = parent
- self.batch_size = batch_size
- self.seq_length = seq_length
- self.is_training = is_training
- self.use_input_mask = use_input_mask
- self.use_token_type_ids = use_token_type_ids
- self.use_labels = use_labels
- self.vocab_size = vocab_size
- self.hidden_size = hidden_size
- self.num_hidden_layers = num_hidden_layers
- self.num_attention_heads = num_attention_heads
- self.intermediate_size = intermediate_size
- self.hidden_act = hidden_act
- self.hidden_dropout_prob = hidden_dropout_prob
- self.attention_probs_dropout_prob = attention_probs_dropout_prob
- self.max_position_embeddings = max_position_embeddings
- self.type_vocab_size = type_vocab_size
- self.type_sequence_label_size = type_sequence_label_size
- self.initializer_range = initializer_range
- self.num_labels = num_labels
- self.num_choices = num_choices
- self.scope = scope
-
- def prepare_config_and_inputs(self):
- input_ids = ids_tensor([self.batch_size, self.seq_length], self.vocab_size)
-
- input_mask = None
- if self.use_input_mask:
- input_mask = ids_tensor([self.batch_size, self.seq_length], vocab_size=2)
-
- token_type_ids = None
- if self.use_token_type_ids:
- token_type_ids = ids_tensor([self.batch_size, self.seq_length], self.type_vocab_size)
-
- sequence_labels = None
- token_labels = None
- choice_labels = None
- if self.use_labels:
- sequence_labels = ids_tensor([self.batch_size], self.type_sequence_label_size)
- token_labels = ids_tensor([self.batch_size, self.seq_length], self.num_labels)
- choice_labels = ids_tensor([self.batch_size], self.num_choices)
-
- config = BertConfig(
- vocab_size=self.vocab_size,
- hidden_size=self.hidden_size,
- num_hidden_layers=self.num_hidden_layers,
- num_attention_heads=self.num_attention_heads,
- intermediate_size=self.intermediate_size,
- hidden_act=self.hidden_act,
- hidden_dropout_prob=self.hidden_dropout_prob,
- attention_probs_dropout_prob=self.attention_probs_dropout_prob,
- max_position_embeddings=self.max_position_embeddings,
- type_vocab_size=self.type_vocab_size,
- is_decoder=False,
- initializer_range=self.initializer_range,
- )
-
- return config, input_ids, token_type_ids, input_mask, sequence_labels, token_labels, choice_labels
-
- def prepare_config_and_inputs_for_decoder(self):
- (
- config,
- input_ids,
- token_type_ids,
- input_mask,
- sequence_labels,
- token_labels,
- choice_labels,
- ) = self.prepare_config_and_inputs()
-
- config.is_decoder = True
- encoder_hidden_states = floats_tensor([self.batch_size, self.seq_length, self.hidden_size])
- encoder_attention_mask = ids_tensor([self.batch_size, self.seq_length], vocab_size=2)
-
- return (
- config,
- input_ids,
- token_type_ids,
- input_mask,
- sequence_labels,
- token_labels,
- choice_labels,
- encoder_hidden_states,
- encoder_attention_mask,
- )
-
- def check_loss_output(self, result):
- self.parent.assertListEqual(list(result["loss"].size()), [])
-
- def create_and_check_bert_model(
- self, config, input_ids, token_type_ids, input_mask, sequence_labels, token_labels, choice_labels
- ):
- model = BertModel(config=config)
- model.to(torch_device)
- model.eval()
- sequence_output, pooled_output = model(input_ids, attention_mask=input_mask, token_type_ids=token_type_ids)
- sequence_output, pooled_output = model(input_ids, token_type_ids=token_type_ids)
- sequence_output, pooled_output = model(input_ids)
-
- result = {
- "sequence_output": sequence_output,
- "pooled_output": pooled_output,
- }
- self.parent.assertListEqual(
- list(result["sequence_output"].size()), [self.batch_size, self.seq_length, self.hidden_size]
- )
- self.parent.assertListEqual(list(result["pooled_output"].size()), [self.batch_size, self.hidden_size])
-
- def create_and_check_bert_model_as_decoder(
- self,
- config,
- input_ids,
- token_type_ids,
- input_mask,
- sequence_labels,
- token_labels,
- choice_labels,
- encoder_hidden_states,
- encoder_attention_mask,
- ):
- model = BertModel(config)
- model.to(torch_device)
- model.eval()
- sequence_output, pooled_output = model(
- input_ids,
- attention_mask=input_mask,
- token_type_ids=token_type_ids,
- encoder_hidden_states=encoder_hidden_states,
- encoder_attention_mask=encoder_attention_mask,
- )
- sequence_output, pooled_output = model(
- input_ids,
- attention_mask=input_mask,
- token_type_ids=token_type_ids,
- encoder_hidden_states=encoder_hidden_states,
- )
- sequence_output, pooled_output = model(input_ids, attention_mask=input_mask, token_type_ids=token_type_ids)
-
- result = {
- "sequence_output": sequence_output,
- "pooled_output": pooled_output,
- }
- self.parent.assertListEqual(
- list(result["sequence_output"].size()), [self.batch_size, self.seq_length, self.hidden_size]
- )
- self.parent.assertListEqual(list(result["pooled_output"].size()), [self.batch_size, self.hidden_size])
-
- def create_and_check_bert_for_masked_lm(
- self, config, input_ids, token_type_ids, input_mask, sequence_labels, token_labels, choice_labels
- ):
- model = BertForMaskedLM(config=config)
- model.to(torch_device)
- model.eval()
- loss, prediction_scores = model(
- input_ids, attention_mask=input_mask, token_type_ids=token_type_ids, masked_lm_labels=token_labels
- )
- result = {
- "loss": loss,
- "prediction_scores": prediction_scores,
- }
- self.parent.assertListEqual(
- list(result["prediction_scores"].size()), [self.batch_size, self.seq_length, self.vocab_size]
- )
- self.check_loss_output(result)
-
- def create_and_check_bert_model_for_masked_lm_as_decoder(
- self,
- config,
- input_ids,
- token_type_ids,
- input_mask,
- sequence_labels,
- token_labels,
- choice_labels,
- encoder_hidden_states,
- encoder_attention_mask,
- ):
- model = BertForMaskedLM(config=config)
- model.to(torch_device)
- model.eval()
- loss, prediction_scores = model(
- input_ids,
- attention_mask=input_mask,
- token_type_ids=token_type_ids,
- masked_lm_labels=token_labels,
- encoder_hidden_states=encoder_hidden_states,
- encoder_attention_mask=encoder_attention_mask,
- )
- loss, prediction_scores = model(
- input_ids,
- attention_mask=input_mask,
- token_type_ids=token_type_ids,
- masked_lm_labels=token_labels,
- encoder_hidden_states=encoder_hidden_states,
- )
- result = {
- "loss": loss,
- "prediction_scores": prediction_scores,
- }
- self.parent.assertListEqual(
- list(result["prediction_scores"].size()), [self.batch_size, self.seq_length, self.vocab_size]
- )
- self.check_loss_output(result)
-
- def create_and_check_bert_for_next_sequence_prediction(
- self, config, input_ids, token_type_ids, input_mask, sequence_labels, token_labels, choice_labels
- ):
- model = BertForNextSentencePrediction(config=config)
- model.to(torch_device)
- model.eval()
- loss, seq_relationship_score = model(
- input_ids,
- attention_mask=input_mask,
- token_type_ids=token_type_ids,
- next_sentence_label=sequence_labels,
- )
- result = {
- "loss": loss,
- "seq_relationship_score": seq_relationship_score,
- }
- self.parent.assertListEqual(list(result["seq_relationship_score"].size()), [self.batch_size, 2])
- self.check_loss_output(result)
-
- def create_and_check_bert_for_pretraining(
- self, config, input_ids, token_type_ids, input_mask, sequence_labels, token_labels, choice_labels
- ):
- model = BertForPreTraining(config=config)
- model.to(torch_device)
- model.eval()
- loss, prediction_scores, seq_relationship_score = model(
- input_ids,
- attention_mask=input_mask,
- token_type_ids=token_type_ids,
- masked_lm_labels=token_labels,
- next_sentence_label=sequence_labels,
- )
- result = {
- "loss": loss,
- "prediction_scores": prediction_scores,
- "seq_relationship_score": seq_relationship_score,
- }
- self.parent.assertListEqual(
- list(result["prediction_scores"].size()), [self.batch_size, self.seq_length, self.vocab_size]
- )
- self.parent.assertListEqual(list(result["seq_relationship_score"].size()), [self.batch_size, 2])
- self.check_loss_output(result)
-
- def create_and_check_bert_for_question_answering(
- self, config, input_ids, token_type_ids, input_mask, sequence_labels, token_labels, choice_labels
- ):
- model = BertForQuestionAnswering(config=config)
- model.to(torch_device)
- model.eval()
- loss, start_logits, end_logits = model(
- input_ids,
- attention_mask=input_mask,
- token_type_ids=token_type_ids,
- start_positions=sequence_labels,
- end_positions=sequence_labels,
- )
- result = {
- "loss": loss,
- "start_logits": start_logits,
- "end_logits": end_logits,
- }
- self.parent.assertListEqual(list(result["start_logits"].size()), [self.batch_size, self.seq_length])
- self.parent.assertListEqual(list(result["end_logits"].size()), [self.batch_size, self.seq_length])
- self.check_loss_output(result)
-
- def create_and_check_bert_for_sequence_classification(
- self, config, input_ids, token_type_ids, input_mask, sequence_labels, token_labels, choice_labels
- ):
- config.num_labels = self.num_labels
- model = BertForSequenceClassification(config)
- model.to(torch_device)
- model.eval()
- loss, logits = model(
- input_ids, attention_mask=input_mask, token_type_ids=token_type_ids, labels=sequence_labels
- )
- result = {
- "loss": loss,
- "logits": logits,
- }
- self.parent.assertListEqual(list(result["logits"].size()), [self.batch_size, self.num_labels])
- self.check_loss_output(result)
-
- def create_and_check_bert_for_token_classification(
- self, config, input_ids, token_type_ids, input_mask, sequence_labels, token_labels, choice_labels
- ):
- config.num_labels = self.num_labels
- model = BertForTokenClassification(config=config)
- model.to(torch_device)
- model.eval()
- loss, logits = model(
- input_ids, attention_mask=input_mask, token_type_ids=token_type_ids, labels=token_labels
- )
- result = {
- "loss": loss,
- "logits": logits,
- }
- self.parent.assertListEqual(
- list(result["logits"].size()), [self.batch_size, self.seq_length, self.num_labels]
- )
- self.check_loss_output(result)
-
- def create_and_check_bert_for_multiple_choice(
- self, config, input_ids, token_type_ids, input_mask, sequence_labels, token_labels, choice_labels
- ):
- config.num_choices = self.num_choices
- model = BertForMultipleChoice(config=config)
- model.to(torch_device)
- model.eval()
- multiple_choice_inputs_ids = input_ids.unsqueeze(1).expand(-1, self.num_choices, -1).contiguous()
- multiple_choice_token_type_ids = token_type_ids.unsqueeze(1).expand(-1, self.num_choices, -1).contiguous()
- multiple_choice_input_mask = input_mask.unsqueeze(1).expand(-1, self.num_choices, -1).contiguous()
- loss, logits = model(
- multiple_choice_inputs_ids,
- attention_mask=multiple_choice_input_mask,
- token_type_ids=multiple_choice_token_type_ids,
- labels=choice_labels,
- )
- result = {
- "loss": loss,
- "logits": logits,
- }
- self.parent.assertListEqual(list(result["logits"].size()), [self.batch_size, self.num_choices])
- self.check_loss_output(result)
-
- def prepare_config_and_inputs_for_common(self):
- config_and_inputs = self.prepare_config_and_inputs()
- (
- config,
- input_ids,
- token_type_ids,
- input_mask,
- sequence_labels,
- token_labels,
- choice_labels,
- ) = config_and_inputs
- inputs_dict = {"input_ids": input_ids, "token_type_ids": token_type_ids, "attention_mask": input_mask}
- return config, inputs_dict
-
- def setUp(self):
- self.model_tester = BertModelTest.BertModelTester(self)
- self.config_tester = ConfigTester(self, config_class=BertConfig, hidden_size=37)
-
- def test_config(self):
- self.config_tester.run_common_tests()
-
- def test_bert_model(self):
- config_and_inputs = self.model_tester.prepare_config_and_inputs()
- self.model_tester.create_and_check_bert_model(*config_and_inputs)
-
- def test_bert_model_as_decoder(self):
- config_and_inputs = self.model_tester.prepare_config_and_inputs_for_decoder()
- self.model_tester.create_and_check_bert_model_as_decoder(*config_and_inputs)
-
- def test_for_masked_lm(self):
- config_and_inputs = self.model_tester.prepare_config_and_inputs()
- self.model_tester.create_and_check_bert_for_masked_lm(*config_and_inputs)
-
- def test_for_masked_lm_decoder(self):
- config_and_inputs = self.model_tester.prepare_config_and_inputs_for_decoder()
- self.model_tester.create_and_check_bert_model_for_masked_lm_as_decoder(*config_and_inputs)
-
- def test_for_multiple_choice(self):
- config_and_inputs = self.model_tester.prepare_config_and_inputs()
- self.model_tester.create_and_check_bert_for_multiple_choice(*config_and_inputs)
-
- def test_for_next_sequence_prediction(self):
- config_and_inputs = self.model_tester.prepare_config_and_inputs()
- self.model_tester.create_and_check_bert_for_next_sequence_prediction(*config_and_inputs)
-
- def test_for_pretraining(self):
- config_and_inputs = self.model_tester.prepare_config_and_inputs()
- self.model_tester.create_and_check_bert_for_pretraining(*config_and_inputs)
-
- def test_for_question_answering(self):
- config_and_inputs = self.model_tester.prepare_config_and_inputs()
- self.model_tester.create_and_check_bert_for_question_answering(*config_and_inputs)
-
- def test_for_sequence_classification(self):
- config_and_inputs = self.model_tester.prepare_config_and_inputs()
- self.model_tester.create_and_check_bert_for_sequence_classification(*config_and_inputs)
-
- def test_for_token_classification(self):
- config_and_inputs = self.model_tester.prepare_config_and_inputs()
- self.model_tester.create_and_check_bert_for_token_classification(*config_and_inputs)
-
- @slow
- def test_model_from_pretrained(self):
- for model_name in list(BERT_PRETRAINED_MODEL_ARCHIVE_MAP.keys())[:1]:
- model = BertModel.from_pretrained(model_name, cache_dir=CACHE_DIR)
- self.assertIsNotNone(model)
diff --git a/server/transformers/tests/test_modeling_common.py b/server/transformers/tests/test_modeling_common.py
deleted file mode 100644
index a5d69fbd6c196096b55b28afdbeb6a4404c02a97..0000000000000000000000000000000000000000
--- a/server/transformers/tests/test_modeling_common.py
+++ /dev/null
@@ -1,659 +0,0 @@
-# coding=utf-8
-# Copyright 2019 HuggingFace Inc.
-#
-# Licensed under the Apache License, Version 2.0 (the "License");
-# you may not use this file except in compliance with the License.
-# You may obtain a copy of the License at
-#
-# http://www.apache.org/licenses/LICENSE-2.0
-#
-# Unless required by applicable law or agreed to in writing, software
-# distributed under the License is distributed on an "AS IS" BASIS,
-# WITHOUT WARRANTIES OR CONDITIONS OF ANY KIND, either express or implied.
-# See the License for the specific language governing permissions and
-# limitations under the License.
-
-
-import copy
-import logging
-import os.path
-import random
-import tempfile
-import unittest
-
-from transformers import is_torch_available
-
-from .utils import require_torch, slow, torch_device
-
-
-if is_torch_available():
- import torch
- import numpy as np
-
- from transformers import (
- AdaptiveEmbedding,
- PretrainedConfig,
- PreTrainedModel,
- BertModel,
- BertConfig,
- BERT_PRETRAINED_MODEL_ARCHIVE_MAP,
- )
-
-
-def _config_zero_init(config):
- configs_no_init = copy.deepcopy(config)
- for key in configs_no_init.__dict__.keys():
- if "_range" in key or "_std" in key or "initializer_factor" in key:
- setattr(configs_no_init, key, 0.0)
- return configs_no_init
-
-
-@require_torch
-class ModelTesterMixin:
-
- model_tester = None
- all_model_classes = ()
- test_torchscript = True
- test_pruning = True
- test_resize_embeddings = True
- test_head_masking = True
- is_encoder_decoder = False
-
- def test_save_load(self):
- config, inputs_dict = self.model_tester.prepare_config_and_inputs_for_common()
-
- for model_class in self.all_model_classes:
- model = model_class(config)
- model.to(torch_device)
- model.eval()
- with torch.no_grad():
- outputs = model(**inputs_dict)
- out_2 = outputs[0].numpy()
- out_2[np.isnan(out_2)] = 0
-
- with tempfile.TemporaryDirectory() as tmpdirname:
- model.save_pretrained(tmpdirname)
- model = model_class.from_pretrained(tmpdirname)
- model.to(torch_device)
- with torch.no_grad():
- after_outputs = model(**inputs_dict)
-
- # Make sure we don't have nans
- out_1 = after_outputs[0].cpu().numpy()
- out_1[np.isnan(out_1)] = 0
- max_diff = np.amax(np.abs(out_1 - out_2))
- self.assertLessEqual(max_diff, 1e-5)
-
- def test_initialization(self):
- config, inputs_dict = self.model_tester.prepare_config_and_inputs_for_common()
-
- configs_no_init = _config_zero_init(config)
- for model_class in self.all_model_classes:
- model = model_class(config=configs_no_init)
- for name, param in model.named_parameters():
- if param.requires_grad:
- self.assertIn(
- param.data.mean().item(),
- [0.0, 1.0],
- msg="Parameter {} of model {} seems not properly initialized".format(name, model_class),
- )
-
- def test_determinism(self):
- config, inputs_dict = self.model_tester.prepare_config_and_inputs_for_common()
-
- for model_class in self.all_model_classes:
- model = model_class(config)
- model.to(torch_device)
- model.eval()
- with torch.no_grad():
- first = model(**inputs_dict)[0]
- second = model(**inputs_dict)[0]
- out_1 = first.cpu().numpy()
- out_2 = second.cpu().numpy()
- out_1 = out_1[~np.isnan(out_1)]
- out_2 = out_2[~np.isnan(out_2)]
- max_diff = np.amax(np.abs(out_1 - out_2))
- self.assertLessEqual(max_diff, 1e-5)
-
- def test_attention_outputs(self):
- config, inputs_dict = self.model_tester.prepare_config_and_inputs_for_common()
-
- decoder_seq_length = (
- self.model_tester.decoder_seq_length
- if hasattr(self.model_tester, "decoder_seq_length")
- else self.model_tester.seq_length
- )
- encoder_seq_length = (
- self.model_tester.encoder_seq_length
- if hasattr(self.model_tester, "encoder_seq_length")
- else self.model_tester.seq_length
- )
- decoder_key_length = (
- self.model_tester.key_length if hasattr(self.model_tester, "key_length") else decoder_seq_length
- )
- encoder_key_length = (
- self.model_tester.key_length if hasattr(self.model_tester, "key_length") else encoder_seq_length
- )
-
- for model_class in self.all_model_classes:
- config.output_attentions = True
- config.output_hidden_states = False
- model = model_class(config)
- model.to(torch_device)
- model.eval()
- with torch.no_grad():
- outputs = model(**inputs_dict)
- attentions = outputs[-1]
- self.assertEqual(model.config.output_attentions, True)
- self.assertEqual(model.config.output_hidden_states, False)
- self.assertEqual(len(attentions), self.model_tester.num_hidden_layers)
- self.assertListEqual(
- list(attentions[0].shape[-3:]),
- [self.model_tester.num_attention_heads, encoder_seq_length, encoder_key_length],
- )
- out_len = len(outputs)
-
- if self.is_encoder_decoder:
- self.assertEqual(out_len % 2, 0)
- decoder_attentions = outputs[(out_len // 2) - 1]
- self.assertEqual(model.config.output_attentions, True)
- self.assertEqual(model.config.output_hidden_states, False)
- self.assertEqual(len(decoder_attentions), self.model_tester.num_hidden_layers)
- self.assertListEqual(
- list(decoder_attentions[0].shape[-3:]),
- [self.model_tester.num_attention_heads, decoder_seq_length, decoder_key_length],
- )
-
- # Check attention is always last and order is fine
- config.output_attentions = True
- config.output_hidden_states = True
- model = model_class(config)
- model.to(torch_device)
- model.eval()
- with torch.no_grad():
- outputs = model(**inputs_dict)
- self.assertEqual(out_len + (2 if self.is_encoder_decoder else 1), len(outputs))
- self.assertEqual(model.config.output_attentions, True)
- self.assertEqual(model.config.output_hidden_states, True)
-
- self_attentions = outputs[-1]
- self.assertEqual(len(self_attentions), self.model_tester.num_hidden_layers)
- self.assertListEqual(
- list(self_attentions[0].shape[-3:]),
- [self.model_tester.num_attention_heads, encoder_seq_length, encoder_key_length],
- )
-
- def test_torchscript(self):
- config, inputs_dict = self.model_tester.prepare_config_and_inputs_for_common()
-
- self._create_and_check_torchscript(config, inputs_dict)
-
- def test_torchscript_output_attentions(self):
- config, inputs_dict = self.model_tester.prepare_config_and_inputs_for_common()
-
- config.output_attentions = True
- self._create_and_check_torchscript(config, inputs_dict)
-
- def test_torchscript_output_hidden_state(self):
- config, inputs_dict = self.model_tester.prepare_config_and_inputs_for_common()
-
- config.output_hidden_states = True
- self._create_and_check_torchscript(config, inputs_dict)
-
- def _create_and_check_torchscript(self, config, inputs_dict):
- if not self.test_torchscript:
- return
-
- configs_no_init = _config_zero_init(config) # To be sure we have no Nan
- configs_no_init.torchscript = True
- for model_class in self.all_model_classes:
- model = model_class(config=configs_no_init)
- model.to(torch_device)
- model.eval()
- inputs = inputs_dict["input_ids"] # Let's keep only input_ids
-
- try:
- traced_gpt2 = torch.jit.trace(model, inputs)
- except RuntimeError:
- self.fail("Couldn't trace module.")
-
- with tempfile.TemporaryDirectory() as tmp_dir_name:
- pt_file_name = os.path.join(tmp_dir_name, "traced_model.pt")
-
- try:
- torch.jit.save(traced_gpt2, pt_file_name)
- except Exception:
- self.fail("Couldn't save module.")
-
- try:
- loaded_model = torch.jit.load(pt_file_name)
- except Exception:
- self.fail("Couldn't load module.")
-
- model.to(torch_device)
- model.eval()
-
- loaded_model.to(torch_device)
- loaded_model.eval()
-
- model_state_dict = model.state_dict()
- loaded_model_state_dict = loaded_model.state_dict()
-
- self.assertEqual(set(model_state_dict.keys()), set(loaded_model_state_dict.keys()))
-
- models_equal = True
- for layer_name, p1 in model_state_dict.items():
- p2 = loaded_model_state_dict[layer_name]
- if p1.data.ne(p2.data).sum() > 0:
- models_equal = False
-
- self.assertTrue(models_equal)
-
- def test_headmasking(self):
- if not self.test_head_masking:
- return
-
- global_rng.seed(42)
- config, inputs_dict = self.model_tester.prepare_config_and_inputs_for_common()
- global_rng.seed()
-
- config.output_attentions = True
- config.output_hidden_states = True
- configs_no_init = _config_zero_init(config) # To be sure we have no Nan
- for model_class in self.all_model_classes:
- model = model_class(config=configs_no_init)
- model.to(torch_device)
- model.eval()
-
- # Prepare head_mask
- # Set require_grad after having prepared the tensor to avoid error (leaf variable has been moved into the graph interior)
- head_mask = torch.ones(
- self.model_tester.num_hidden_layers, self.model_tester.num_attention_heads, device=torch_device
- )
- head_mask[0, 0] = 0
- head_mask[-1, :-1] = 0
- head_mask.requires_grad_(requires_grad=True)
- inputs = inputs_dict.copy()
- inputs["head_mask"] = head_mask
-
- outputs = model(**inputs)
-
- # Test that we can get a gradient back for importance score computation
- output = sum(t.sum() for t in outputs[0])
- output = output.sum()
- output.backward()
- multihead_outputs = head_mask.grad
-
- attentions = outputs[-1]
-
- # Remove Nan
- for t in attentions:
- self.assertLess(
- torch.sum(torch.isnan(t)), t.numel() / 4
- ) # Check we don't have more than 25% nans (arbitrary)
- attentions = [
- t.masked_fill(torch.isnan(t), 0.0) for t in attentions
- ] # remove them (the test is less complete)
-
- self.assertIsNotNone(multihead_outputs)
- self.assertEqual(len(multihead_outputs), self.model_tester.num_hidden_layers)
- self.assertAlmostEqual(attentions[0][..., 0, :, :].flatten().sum().item(), 0.0)
- self.assertNotEqual(attentions[0][..., -1, :, :].flatten().sum().item(), 0.0)
- self.assertNotEqual(attentions[1][..., 0, :, :].flatten().sum().item(), 0.0)
- self.assertAlmostEqual(attentions[-1][..., -2, :, :].flatten().sum().item(), 0.0)
- self.assertNotEqual(attentions[-1][..., -1, :, :].flatten().sum().item(), 0.0)
-
- def test_head_pruning(self):
- if not self.test_pruning:
- return
-
- for model_class in self.all_model_classes:
- config, inputs_dict = self.model_tester.prepare_config_and_inputs_for_common()
-
- if "head_mask" in inputs_dict:
- del inputs_dict["head_mask"]
-
- config.output_attentions = True
- config.output_hidden_states = False
- model = model_class(config=config)
- model.to(torch_device)
- model.eval()
- heads_to_prune = {0: list(range(1, self.model_tester.num_attention_heads)), -1: [0]}
- model.prune_heads(heads_to_prune)
- with torch.no_grad():
- outputs = model(**inputs_dict)
-
- attentions = outputs[-1]
-
- self.assertEqual(attentions[0].shape[-3], 1)
- self.assertEqual(attentions[1].shape[-3], self.model_tester.num_attention_heads)
- self.assertEqual(attentions[-1].shape[-3], self.model_tester.num_attention_heads - 1)
-
- def test_head_pruning_save_load_from_pretrained(self):
- if not self.test_pruning:
- return
-
- for model_class in self.all_model_classes:
- config, inputs_dict = self.model_tester.prepare_config_and_inputs_for_common()
-
- if "head_mask" in inputs_dict:
- del inputs_dict["head_mask"]
-
- config.output_attentions = True
- config.output_hidden_states = False
- model = model_class(config=config)
- model.to(torch_device)
- model.eval()
- heads_to_prune = {0: list(range(1, self.model_tester.num_attention_heads)), -1: [0]}
- model.prune_heads(heads_to_prune)
-
- with tempfile.TemporaryDirectory() as temp_dir_name:
- model.save_pretrained(temp_dir_name)
- model = model_class.from_pretrained(temp_dir_name)
- model.to(torch_device)
-
- with torch.no_grad():
- outputs = model(**inputs_dict)
- attentions = outputs[-1]
- self.assertEqual(attentions[0].shape[-3], 1)
- self.assertEqual(attentions[1].shape[-3], self.model_tester.num_attention_heads)
- self.assertEqual(attentions[-1].shape[-3], self.model_tester.num_attention_heads - 1)
-
- def test_head_pruning_save_load_from_config_init(self):
- if not self.test_pruning:
- return
-
- for model_class in self.all_model_classes:
- config, inputs_dict = self.model_tester.prepare_config_and_inputs_for_common()
-
- if "head_mask" in inputs_dict:
- del inputs_dict["head_mask"]
-
- config.output_attentions = True
- config.output_hidden_states = False
-
- heads_to_prune = {0: list(range(1, self.model_tester.num_attention_heads)), -1: [0]}
- config.pruned_heads = heads_to_prune
-
- model = model_class(config=config)
- model.to(torch_device)
- model.eval()
-
- with torch.no_grad():
- outputs = model(**inputs_dict)
- attentions = outputs[-1]
-
- self.assertEqual(attentions[0].shape[-3], 1)
- self.assertEqual(attentions[1].shape[-3], self.model_tester.num_attention_heads)
- self.assertEqual(attentions[-1].shape[-3], self.model_tester.num_attention_heads - 1)
-
- def test_head_pruning_integration(self):
- if not self.test_pruning:
- return
-
- for model_class in self.all_model_classes:
- config, inputs_dict = self.model_tester.prepare_config_and_inputs_for_common()
-
- if "head_mask" in inputs_dict:
- del inputs_dict["head_mask"]
-
- config.output_attentions = True
- config.output_hidden_states = False
-
- heads_to_prune = {0: [0], 1: [1, 2]}
- config.pruned_heads = heads_to_prune
-
- model = model_class(config=config)
- model.to(torch_device)
- model.eval()
-
- with torch.no_grad():
- outputs = model(**inputs_dict)
- attentions = outputs[-1]
-
- self.assertEqual(attentions[0].shape[-3], self.model_tester.num_attention_heads - 1)
- self.assertEqual(attentions[1].shape[-3], self.model_tester.num_attention_heads - 2)
- self.assertEqual(attentions[2].shape[-3], self.model_tester.num_attention_heads)
- self.assertEqual(attentions[3].shape[-3], self.model_tester.num_attention_heads)
-
- with tempfile.TemporaryDirectory() as temp_dir_name:
- model.save_pretrained(temp_dir_name)
- model = model_class.from_pretrained(temp_dir_name)
- model.to(torch_device)
-
- with torch.no_grad():
- outputs = model(**inputs_dict)
- attentions = outputs[-1]
-
- self.assertEqual(attentions[0].shape[-3], self.model_tester.num_attention_heads - 1)
- self.assertEqual(attentions[1].shape[-3], self.model_tester.num_attention_heads - 2)
- self.assertEqual(attentions[2].shape[-3], self.model_tester.num_attention_heads)
- self.assertEqual(attentions[3].shape[-3], self.model_tester.num_attention_heads)
-
- heads_to_prune = {0: [0], 2: [1, 2]}
- model.prune_heads(heads_to_prune)
-
- with torch.no_grad():
- outputs = model(**inputs_dict)
- attentions = outputs[-1]
-
- self.assertEqual(attentions[0].shape[-3], self.model_tester.num_attention_heads - 1)
- self.assertEqual(attentions[1].shape[-3], self.model_tester.num_attention_heads - 2)
- self.assertEqual(attentions[2].shape[-3], self.model_tester.num_attention_heads - 2)
- self.assertEqual(attentions[3].shape[-3], self.model_tester.num_attention_heads)
-
- self.assertDictEqual(model.config.pruned_heads, {0: [0], 1: [1, 2], 2: [1, 2]})
-
- def test_hidden_states_output(self):
- config, inputs_dict = self.model_tester.prepare_config_and_inputs_for_common()
-
- for model_class in self.all_model_classes:
- config.output_hidden_states = True
- config.output_attentions = False
- model = model_class(config)
- model.to(torch_device)
- model.eval()
- with torch.no_grad():
- outputs = model(**inputs_dict)
- hidden_states = outputs[-1]
- self.assertEqual(model.config.output_attentions, False)
- self.assertEqual(model.config.output_hidden_states, True)
- self.assertEqual(len(hidden_states), self.model_tester.num_hidden_layers + 1)
- self.assertListEqual(
- list(hidden_states[0].shape[-2:]),
- [
- self.model_tester.encoder_seq_length
- if hasattr(self.model_tester, "encoder_seq_length")
- else self.model_tester.seq_length,
- self.model_tester.hidden_size,
- ],
- )
-
- def test_resize_tokens_embeddings(self):
- original_config, inputs_dict = self.model_tester.prepare_config_and_inputs_for_common()
- if not self.test_resize_embeddings:
- return
-
- for model_class in self.all_model_classes:
- config = copy.deepcopy(original_config)
- model = model_class(config)
-
- model_vocab_size = config.vocab_size
- # Retrieve the embeddings and clone theme
- model_embed = model.resize_token_embeddings(model_vocab_size)
- cloned_embeddings = model_embed.weight.clone()
-
- # Check that resizing the token embeddings with a larger vocab size increases the model's vocab size
- model_embed = model.resize_token_embeddings(model_vocab_size + 10)
- self.assertEqual(model.config.vocab_size, model_vocab_size + 10)
- # Check that it actually resizes the embeddings matrix
- self.assertEqual(model_embed.weight.shape[0], cloned_embeddings.shape[0] + 10)
- # Check that the model can still do a forward pass successfully (every parameter should be resized)
- model(**inputs_dict)
-
- # Check that resizing the token embeddings with a smaller vocab size decreases the model's vocab size
- model_embed = model.resize_token_embeddings(model_vocab_size - 15)
- self.assertEqual(model.config.vocab_size, model_vocab_size - 15)
- # Check that it actually resizes the embeddings matrix
- self.assertEqual(model_embed.weight.shape[0], cloned_embeddings.shape[0] - 15)
-
- # Check that the model can still do a forward pass successfully (every parameter should be resized)
- # Input ids should be clamped to the maximum size of the vocabulary
- inputs_dict["input_ids"].clamp_(max=model_vocab_size - 15 - 1)
- model(**inputs_dict)
-
- # Check that adding and removing tokens has not modified the first part of the embedding matrix.
- models_equal = True
- for p1, p2 in zip(cloned_embeddings, model_embed.weight):
- if p1.data.ne(p2.data).sum() > 0:
- models_equal = False
-
- self.assertTrue(models_equal)
-
- def test_model_common_attributes(self):
- config, inputs_dict = self.model_tester.prepare_config_and_inputs_for_common()
-
- for model_class in self.all_model_classes:
- model = model_class(config)
- self.assertIsInstance(model.get_input_embeddings(), (torch.nn.Embedding, AdaptiveEmbedding))
- model.set_input_embeddings(torch.nn.Embedding(10, 10))
- x = model.get_output_embeddings()
- self.assertTrue(x is None or isinstance(x, torch.nn.Linear))
-
- def test_tie_model_weights(self):
- if not self.test_torchscript:
- return
-
- config, inputs_dict = self.model_tester.prepare_config_and_inputs_for_common()
-
- def check_same_values(layer_1, layer_2):
- equal = True
- for p1, p2 in zip(layer_1.weight, layer_2.weight):
- if p1.data.ne(p2.data).sum() > 0:
- equal = False
- return equal
-
- for model_class in self.all_model_classes:
- config.torchscript = True
- model_not_tied = model_class(config)
- if model_not_tied.get_output_embeddings() is None:
- continue
-
- params_not_tied = list(model_not_tied.parameters())
-
- config_tied = copy.deepcopy(config)
- config_tied.torchscript = False
- model_tied = model_class(config_tied)
- params_tied = list(model_tied.parameters())
-
- # Check that the embedding layer and decoding layer are the same in size and in value
- self.assertGreater(len(params_not_tied), len(params_tied))
- # self.assertTrue(check_same_values(embeddings, decoding))
-
- # # Check that after modification, they remain the same.
- # embeddings.weight.data.div_(2)
- # # Check that the embedding layer and decoding layer are the same in size and in value
- # self.assertTrue(embeddings.weight.shape, decoding.weight.shape)
- # self.assertTrue(check_same_values(embeddings, decoding))
-
- # # Check that after modification, they remain the same.
- # decoding.weight.data.div_(4)
- # # Check that the embedding layer and decoding layer are the same in size and in value
- # self.assertTrue(embeddings.weight.shape, decoding.weight.shape)
- # self.assertTrue(check_same_values(embeddings, decoding))
-
- # Check that after resize they remain tied.
- model_tied.resize_token_embeddings(config.vocab_size + 10)
- params_tied_2 = list(model_tied.parameters())
- self.assertGreater(len(params_not_tied), len(params_tied))
- self.assertEqual(len(params_tied_2), len(params_tied))
-
- # decoding.weight.data.mul_(20)
- # # Check that the embedding layer and decoding layer are the same in size and in value
- # self.assertTrue(model.transformer.wte.weight.shape, model.lm_head.weight.shape)
- # self.assertTrue(check_same_values(model.transformer.wte, model.lm_head))
-
- def test_inputs_embeds(self):
- config, inputs_dict = self.model_tester.prepare_config_and_inputs_for_common()
- if not self.is_encoder_decoder:
- input_ids = inputs_dict["input_ids"]
- del inputs_dict["input_ids"]
- else:
- encoder_input_ids = inputs_dict["encoder_input_ids"]
- decoder_input_ids = inputs_dict["decoder_input_ids"]
- del inputs_dict["encoder_input_ids"]
- del inputs_dict["decoder_input_ids"]
-
- for model_class in self.all_model_classes:
- model = model_class(config)
- model.to(torch_device)
- model.eval()
-
- wte = model.get_input_embeddings()
- if not self.is_encoder_decoder:
- inputs_dict["inputs_embeds"] = wte(input_ids)
- else:
- inputs_dict["encoder_inputs_embeds"] = wte(encoder_input_ids)
- inputs_dict["decoder_inputs_embeds"] = wte(decoder_input_ids)
-
- with torch.no_grad():
- model(**inputs_dict)
-
-
-global_rng = random.Random()
-
-
-def ids_tensor(shape, vocab_size, rng=None, name=None):
- """Creates a random int32 tensor of the shape within the vocab size."""
- if rng is None:
- rng = global_rng
-
- total_dims = 1
- for dim in shape:
- total_dims *= dim
-
- values = []
- for _ in range(total_dims):
- values.append(rng.randint(0, vocab_size - 1))
-
- return torch.tensor(data=values, dtype=torch.long, device=torch_device).view(shape).contiguous()
-
-
-def floats_tensor(shape, scale=1.0, rng=None, name=None):
- """Creates a random float32 tensor of the shape within the vocab size."""
- if rng is None:
- rng = global_rng
-
- total_dims = 1
- for dim in shape:
- total_dims *= dim
-
- values = []
- for _ in range(total_dims):
- values.append(rng.random() * scale)
-
- return torch.tensor(data=values, dtype=torch.float, device=torch_device).view(shape).contiguous()
-
-
-@require_torch
-class ModelUtilsTest(unittest.TestCase):
- @slow
- def test_model_from_pretrained(self):
- logging.basicConfig(level=logging.INFO)
- for model_name in list(BERT_PRETRAINED_MODEL_ARCHIVE_MAP.keys())[:1]:
- config = BertConfig.from_pretrained(model_name)
- self.assertIsNotNone(config)
- self.assertIsInstance(config, PretrainedConfig)
-
- model = BertModel.from_pretrained(model_name)
- model, loading_info = BertModel.from_pretrained(model_name, output_loading_info=True)
- self.assertIsNotNone(model)
- self.assertIsInstance(model, PreTrainedModel)
- for value in loading_info.values():
- self.assertEqual(len(value), 0)
-
- config = BertConfig.from_pretrained(model_name, output_attentions=True, output_hidden_states=True)
- model = BertModel.from_pretrained(model_name, output_attentions=True, output_hidden_states=True)
- self.assertEqual(model.config.output_attentions, True)
- self.assertEqual(model.config.output_hidden_states, True)
- self.assertEqual(model.config, config)
diff --git a/server/transformers/tests/test_modeling_ctrl.py b/server/transformers/tests/test_modeling_ctrl.py
deleted file mode 100644
index 3d1a1cb2dc728952f1ef36f667b59ccf7af1a48b..0000000000000000000000000000000000000000
--- a/server/transformers/tests/test_modeling_ctrl.py
+++ /dev/null
@@ -1,213 +0,0 @@
-# coding=utf-8
-# Copyright 2018 Salesforce and HuggingFace Inc. team.
-# Licensed under the Apache License, Version 2.0 (the "License");
-# you may not use this file except in compliance with the License.
-# You may obtain a copy of the License at
-#
-# http://www.apache.org/licenses/LICENSE-2.0
-#
-# Unless required by applicable law or agreed to in writing, software
-# distributed under the License is distributed on an "AS IS" BASIS,
-# WITHOUT WARRANTIES OR CONDITIONS OF ANY KIND, either express or implied.
-# See the License for the specific language governing permissions and
-# limitations under the License.
-
-
-import unittest
-
-from transformers import is_torch_available
-
-from .test_configuration_common import ConfigTester
-from .test_modeling_common import ModelTesterMixin, ids_tensor
-from .utils import CACHE_DIR, require_torch, slow, torch_device
-
-
-if is_torch_available():
- from transformers import CTRLConfig, CTRLModel, CTRL_PRETRAINED_MODEL_ARCHIVE_MAP, CTRLLMHeadModel
-
-
-@require_torch
-class CTRLModelTest(ModelTesterMixin, unittest.TestCase):
-
- all_model_classes = (CTRLModel, CTRLLMHeadModel) if is_torch_available() else ()
- test_pruning = False
- test_torchscript = False
- test_resize_embeddings = False
- test_head_masking = False
-
- class CTRLModelTester(object):
- def __init__(
- self,
- parent,
- batch_size=13,
- seq_length=7,
- is_training=True,
- use_token_type_ids=True,
- use_input_mask=True,
- use_labels=True,
- use_mc_token_ids=True,
- vocab_size=99,
- hidden_size=32,
- num_hidden_layers=5,
- num_attention_heads=4,
- intermediate_size=37,
- hidden_act="gelu",
- hidden_dropout_prob=0.1,
- attention_probs_dropout_prob=0.1,
- max_position_embeddings=512,
- type_vocab_size=16,
- type_sequence_label_size=2,
- initializer_range=0.02,
- num_labels=3,
- num_choices=4,
- scope=None,
- ):
- self.parent = parent
- self.batch_size = batch_size
- self.seq_length = seq_length
- self.is_training = is_training
- self.use_token_type_ids = use_token_type_ids
- self.use_input_mask = use_input_mask
- self.use_labels = use_labels
- self.use_mc_token_ids = use_mc_token_ids
- self.vocab_size = vocab_size
- self.hidden_size = hidden_size
- self.num_hidden_layers = num_hidden_layers
- self.num_attention_heads = num_attention_heads
- self.intermediate_size = intermediate_size
- self.hidden_act = hidden_act
- self.hidden_dropout_prob = hidden_dropout_prob
- self.attention_probs_dropout_prob = attention_probs_dropout_prob
- self.max_position_embeddings = max_position_embeddings
- self.type_vocab_size = type_vocab_size
- self.type_sequence_label_size = type_sequence_label_size
- self.initializer_range = initializer_range
- self.num_labels = num_labels
- self.num_choices = num_choices
- self.scope = scope
-
- def prepare_config_and_inputs(self):
- input_ids = ids_tensor([self.batch_size, self.seq_length], self.vocab_size)
-
- input_mask = None
- if self.use_input_mask:
- input_mask = ids_tensor([self.batch_size, self.seq_length], vocab_size=2)
-
- token_type_ids = None
- if self.use_token_type_ids:
- token_type_ids = ids_tensor([self.batch_size, self.seq_length], self.type_vocab_size)
-
- mc_token_ids = None
- if self.use_mc_token_ids:
- mc_token_ids = ids_tensor([self.batch_size, self.num_choices], self.seq_length)
-
- sequence_labels = None
- token_labels = None
- choice_labels = None
- if self.use_labels:
- sequence_labels = ids_tensor([self.batch_size], self.type_sequence_label_size)
- token_labels = ids_tensor([self.batch_size, self.seq_length], self.num_labels)
- choice_labels = ids_tensor([self.batch_size], self.num_choices)
-
- config = CTRLConfig(
- vocab_size=self.vocab_size,
- n_embd=self.hidden_size,
- n_layer=self.num_hidden_layers,
- n_head=self.num_attention_heads,
- # intermediate_size=self.intermediate_size,
- # hidden_act=self.hidden_act,
- # hidden_dropout_prob=self.hidden_dropout_prob,
- # attention_probs_dropout_prob=self.attention_probs_dropout_prob,
- n_positions=self.max_position_embeddings,
- n_ctx=self.max_position_embeddings
- # type_vocab_size=self.type_vocab_size,
- # initializer_range=self.initializer_range
- )
-
- head_mask = ids_tensor([self.num_hidden_layers, self.num_attention_heads], 2)
-
- return (
- config,
- input_ids,
- input_mask,
- head_mask,
- token_type_ids,
- mc_token_ids,
- sequence_labels,
- token_labels,
- choice_labels,
- )
-
- def check_loss_output(self, result):
- self.parent.assertListEqual(list(result["loss"].size()), [])
-
- def create_and_check_ctrl_model(self, config, input_ids, input_mask, head_mask, token_type_ids, *args):
- model = CTRLModel(config=config)
- model.to(torch_device)
- model.eval()
-
- model(input_ids, token_type_ids=token_type_ids, head_mask=head_mask)
- model(input_ids, token_type_ids=token_type_ids)
- sequence_output, presents = model(input_ids)
-
- result = {
- "sequence_output": sequence_output,
- "presents": presents,
- }
- self.parent.assertListEqual(
- list(result["sequence_output"].size()), [self.batch_size, self.seq_length, self.hidden_size]
- )
- self.parent.assertEqual(len(result["presents"]), config.n_layer)
-
- def create_and_check_lm_head_model(self, config, input_ids, input_mask, head_mask, token_type_ids, *args):
- model = CTRLLMHeadModel(config)
- model.to(torch_device)
- model.eval()
-
- loss, lm_logits, _ = model(input_ids, token_type_ids=token_type_ids, labels=input_ids)
-
- result = {"loss": loss, "lm_logits": lm_logits}
- self.parent.assertListEqual(list(result["loss"].size()), [])
- self.parent.assertListEqual(
- list(result["lm_logits"].size()), [self.batch_size, self.seq_length, self.vocab_size]
- )
-
- def prepare_config_and_inputs_for_common(self):
- config_and_inputs = self.prepare_config_and_inputs()
-
- (
- config,
- input_ids,
- input_mask,
- head_mask,
- token_type_ids,
- mc_token_ids,
- sequence_labels,
- token_labels,
- choice_labels,
- ) = config_and_inputs
-
- inputs_dict = {"input_ids": input_ids, "token_type_ids": token_type_ids, "head_mask": head_mask}
-
- return config, inputs_dict
-
- def setUp(self):
- self.model_tester = CTRLModelTest.CTRLModelTester(self)
- self.config_tester = ConfigTester(self, config_class=CTRLConfig, n_embd=37)
-
- def test_config(self):
- self.config_tester.run_common_tests()
-
- def test_ctrl_model(self):
- config_and_inputs = self.model_tester.prepare_config_and_inputs()
- self.model_tester.create_and_check_ctrl_model(*config_and_inputs)
-
- def test_ctrl_lm_head_model(self):
- config_and_inputs = self.model_tester.prepare_config_and_inputs()
- self.model_tester.create_and_check_lm_head_model(*config_and_inputs)
-
- @slow
- def test_model_from_pretrained(self):
- for model_name in list(CTRL_PRETRAINED_MODEL_ARCHIVE_MAP.keys())[:1]:
- model = CTRLModel.from_pretrained(model_name, cache_dir=CACHE_DIR)
- self.assertIsNotNone(model)
diff --git a/server/transformers/tests/test_modeling_distilbert.py b/server/transformers/tests/test_modeling_distilbert.py
deleted file mode 100644
index 96f487916660c5aacbce6eb82f1f8f1a0a8b9be3..0000000000000000000000000000000000000000
--- a/server/transformers/tests/test_modeling_distilbert.py
+++ /dev/null
@@ -1,252 +0,0 @@
-# coding=utf-8
-# Copyright 2018 The Google AI Language Team Authors.
-#
-# Licensed under the Apache License, Version 2.0 (the "License");
-# you may not use this file except in compliance with the License.
-# You may obtain a copy of the License at
-#
-# http://www.apache.org/licenses/LICENSE-2.0
-#
-# Unless required by applicable law or agreed to in writing, software
-# distributed under the License is distributed on an "AS IS" BASIS,
-# WITHOUT WARRANTIES OR CONDITIONS OF ANY KIND, either express or implied.
-# See the License for the specific language governing permissions and
-# limitations under the License.
-
-
-import unittest
-
-from transformers import is_torch_available
-
-from .test_configuration_common import ConfigTester
-from .test_modeling_common import ModelTesterMixin, ids_tensor
-from .utils import require_torch, torch_device
-
-
-if is_torch_available():
- from transformers import (
- DistilBertConfig,
- DistilBertModel,
- DistilBertForMaskedLM,
- DistilBertForTokenClassification,
- DistilBertForQuestionAnswering,
- DistilBertForSequenceClassification,
- )
-
-
-@require_torch
-class DistilBertModelTest(ModelTesterMixin, unittest.TestCase):
-
- all_model_classes = (
- (DistilBertModel, DistilBertForMaskedLM, DistilBertForQuestionAnswering, DistilBertForSequenceClassification)
- if is_torch_available()
- else None
- )
- test_pruning = True
- test_torchscript = True
- test_resize_embeddings = True
- test_head_masking = True
-
- class DistilBertModelTester(object):
- def __init__(
- self,
- parent,
- batch_size=13,
- seq_length=7,
- is_training=True,
- use_input_mask=True,
- use_token_type_ids=False,
- use_labels=True,
- vocab_size=99,
- hidden_size=32,
- num_hidden_layers=5,
- num_attention_heads=4,
- intermediate_size=37,
- hidden_act="gelu",
- hidden_dropout_prob=0.1,
- attention_probs_dropout_prob=0.1,
- max_position_embeddings=512,
- type_vocab_size=16,
- type_sequence_label_size=2,
- initializer_range=0.02,
- num_labels=3,
- num_choices=4,
- scope=None,
- ):
- self.parent = parent
- self.batch_size = batch_size
- self.seq_length = seq_length
- self.is_training = is_training
- self.use_input_mask = use_input_mask
- self.use_token_type_ids = use_token_type_ids
- self.use_labels = use_labels
- self.vocab_size = vocab_size
- self.hidden_size = hidden_size
- self.num_hidden_layers = num_hidden_layers
- self.num_attention_heads = num_attention_heads
- self.intermediate_size = intermediate_size
- self.hidden_act = hidden_act
- self.hidden_dropout_prob = hidden_dropout_prob
- self.attention_probs_dropout_prob = attention_probs_dropout_prob
- self.max_position_embeddings = max_position_embeddings
- self.type_vocab_size = type_vocab_size
- self.type_sequence_label_size = type_sequence_label_size
- self.initializer_range = initializer_range
- self.num_labels = num_labels
- self.num_choices = num_choices
- self.scope = scope
-
- def prepare_config_and_inputs(self):
- input_ids = ids_tensor([self.batch_size, self.seq_length], self.vocab_size)
-
- input_mask = None
- if self.use_input_mask:
- input_mask = ids_tensor([self.batch_size, self.seq_length], vocab_size=2)
-
- sequence_labels = None
- token_labels = None
- choice_labels = None
- if self.use_labels:
- sequence_labels = ids_tensor([self.batch_size], self.type_sequence_label_size)
- token_labels = ids_tensor([self.batch_size, self.seq_length], self.num_labels)
- choice_labels = ids_tensor([self.batch_size], self.num_choices)
-
- config = DistilBertConfig(
- vocab_size=self.vocab_size,
- dim=self.hidden_size,
- n_layers=self.num_hidden_layers,
- n_heads=self.num_attention_heads,
- hidden_dim=self.intermediate_size,
- hidden_act=self.hidden_act,
- dropout=self.hidden_dropout_prob,
- attention_dropout=self.attention_probs_dropout_prob,
- max_position_embeddings=self.max_position_embeddings,
- initializer_range=self.initializer_range,
- )
-
- return config, input_ids, input_mask, sequence_labels, token_labels, choice_labels
-
- def check_loss_output(self, result):
- self.parent.assertListEqual(list(result["loss"].size()), [])
-
- def create_and_check_distilbert_model(
- self, config, input_ids, input_mask, sequence_labels, token_labels, choice_labels
- ):
- model = DistilBertModel(config=config)
- model.to(torch_device)
- model.eval()
- (sequence_output,) = model(input_ids, input_mask)
- (sequence_output,) = model(input_ids)
-
- result = {
- "sequence_output": sequence_output,
- }
- self.parent.assertListEqual(
- list(result["sequence_output"].size()), [self.batch_size, self.seq_length, self.hidden_size]
- )
-
- def create_and_check_distilbert_for_masked_lm(
- self, config, input_ids, input_mask, sequence_labels, token_labels, choice_labels
- ):
- model = DistilBertForMaskedLM(config=config)
- model.to(torch_device)
- model.eval()
- loss, prediction_scores = model(input_ids, attention_mask=input_mask, masked_lm_labels=token_labels)
- result = {
- "loss": loss,
- "prediction_scores": prediction_scores,
- }
- self.parent.assertListEqual(
- list(result["prediction_scores"].size()), [self.batch_size, self.seq_length, self.vocab_size]
- )
- self.check_loss_output(result)
-
- def create_and_check_distilbert_for_question_answering(
- self, config, input_ids, input_mask, sequence_labels, token_labels, choice_labels
- ):
- model = DistilBertForQuestionAnswering(config=config)
- model.to(torch_device)
- model.eval()
- loss, start_logits, end_logits = model(
- input_ids, attention_mask=input_mask, start_positions=sequence_labels, end_positions=sequence_labels
- )
- result = {
- "loss": loss,
- "start_logits": start_logits,
- "end_logits": end_logits,
- }
- self.parent.assertListEqual(list(result["start_logits"].size()), [self.batch_size, self.seq_length])
- self.parent.assertListEqual(list(result["end_logits"].size()), [self.batch_size, self.seq_length])
- self.check_loss_output(result)
-
- def create_and_check_distilbert_for_sequence_classification(
- self, config, input_ids, input_mask, sequence_labels, token_labels, choice_labels
- ):
- config.num_labels = self.num_labels
- model = DistilBertForSequenceClassification(config)
- model.to(torch_device)
- model.eval()
- loss, logits = model(input_ids, attention_mask=input_mask, labels=sequence_labels)
- result = {
- "loss": loss,
- "logits": logits,
- }
- self.parent.assertListEqual(list(result["logits"].size()), [self.batch_size, self.num_labels])
- self.check_loss_output(result)
-
- def create_and_check_distilbert_for_token_classification(
- self, config, input_ids, input_mask, sequence_labels, token_labels, choice_labels
- ):
- config.num_labels = self.num_labels
- model = DistilBertForTokenClassification(config=config)
- model.to(torch_device)
- model.eval()
-
- loss, logits = model(input_ids, attention_mask=input_mask, labels=token_labels)
- result = {
- "loss": loss,
- "logits": logits,
- }
- self.parent.assertListEqual(
- list(result["logits"].size()), [self.batch_size, self.seq_length, self.num_labels]
- )
- self.check_loss_output(result)
-
- def prepare_config_and_inputs_for_common(self):
- config_and_inputs = self.prepare_config_and_inputs()
- (config, input_ids, input_mask, sequence_labels, token_labels, choice_labels) = config_and_inputs
- inputs_dict = {"input_ids": input_ids, "attention_mask": input_mask}
- return config, inputs_dict
-
- def setUp(self):
- self.model_tester = DistilBertModelTest.DistilBertModelTester(self)
- self.config_tester = ConfigTester(self, config_class=DistilBertConfig, dim=37)
-
- def test_config(self):
- self.config_tester.run_common_tests()
-
- def test_distilbert_model(self):
- config_and_inputs = self.model_tester.prepare_config_and_inputs()
- self.model_tester.create_and_check_distilbert_model(*config_and_inputs)
-
- def test_for_masked_lm(self):
- config_and_inputs = self.model_tester.prepare_config_and_inputs()
- self.model_tester.create_and_check_distilbert_for_masked_lm(*config_and_inputs)
-
- def test_for_question_answering(self):
- config_and_inputs = self.model_tester.prepare_config_and_inputs()
- self.model_tester.create_and_check_distilbert_for_question_answering(*config_and_inputs)
-
- def test_for_sequence_classification(self):
- config_and_inputs = self.model_tester.prepare_config_and_inputs()
- self.model_tester.create_and_check_distilbert_for_sequence_classification(*config_and_inputs)
-
- def test_for_token_classification(self):
- config_and_inputs = self.model_tester.prepare_config_and_inputs()
- self.model_tester.create_and_check_distilbert_for_token_classification(*config_and_inputs)
-
- # @slow
- # def test_model_from_pretrained(self):
- # for model_name in list(DISTILBERT_PRETRAINED_MODEL_ARCHIVE_MAP.keys())[:1]:
- # model = DistilBertModel.from_pretrained(model_name, cache_dir=CACHE_DIR)
- # self.assertIsNotNone(model)
diff --git a/server/transformers/tests/test_modeling_encoder_decoder.py b/server/transformers/tests/test_modeling_encoder_decoder.py
deleted file mode 100644
index ac01e7b5615f5bcc5d827e0f5bf6aa9d3337a73b..0000000000000000000000000000000000000000
--- a/server/transformers/tests/test_modeling_encoder_decoder.py
+++ /dev/null
@@ -1,50 +0,0 @@
-# coding=utf-8
-# Copyright 2018 The Hugging Face Inc. Team
-#
-# Licensed under the Apache License, Version 2.0 (the "License");
-# you may not use this file except in compliance with the License.
-# You may obtain a copy of the License at
-#
-# http://www.apache.org/licenses/LICENSE-2.0
-#
-# Unless required by applicable law or agreed to in writing, software
-# distributed under the License is distributed on an "AS IS" BASIS,
-# WITHOUT WARRANTIES OR CONDITIONS OF ANY KIND, either express or implied.
-# See the License for the specific language governing permissions and
-# limitations under the License.
-
-import logging
-import unittest
-
-from transformers import is_torch_available
-
-from .utils import require_torch, slow
-
-
-if is_torch_available():
- from transformers import BertModel, BertForMaskedLM, Model2Model
- from transformers.modeling_bert import BERT_PRETRAINED_MODEL_ARCHIVE_MAP
-
-
-@require_torch
-class EncoderDecoderModelTest(unittest.TestCase):
- @slow
- def test_model2model_from_pretrained(self):
- logging.basicConfig(level=logging.INFO)
- for model_name in list(BERT_PRETRAINED_MODEL_ARCHIVE_MAP.keys())[:1]:
- model = Model2Model.from_pretrained(model_name)
- self.assertIsInstance(model.encoder, BertModel)
- self.assertIsInstance(model.decoder, BertForMaskedLM)
- self.assertEqual(model.decoder.config.is_decoder, True)
- self.assertEqual(model.encoder.config.is_decoder, False)
-
- def test_model2model_from_pretrained_not_bert(self):
- logging.basicConfig(level=logging.INFO)
- with self.assertRaises(ValueError):
- _ = Model2Model.from_pretrained("roberta")
-
- with self.assertRaises(ValueError):
- _ = Model2Model.from_pretrained("distilbert")
-
- with self.assertRaises(ValueError):
- _ = Model2Model.from_pretrained("does-not-exist")
diff --git a/server/transformers/tests/test_modeling_gpt2.py b/server/transformers/tests/test_modeling_gpt2.py
deleted file mode 100644
index 3976c7d452a9d96281bdbd6da55c8eab824c99da..0000000000000000000000000000000000000000
--- a/server/transformers/tests/test_modeling_gpt2.py
+++ /dev/null
@@ -1,250 +0,0 @@
-# coding=utf-8
-# Copyright 2018 The Google AI Language Team Authors.
-#
-# Licensed under the Apache License, Version 2.0 (the "License");
-# you may not use this file except in compliance with the License.
-# You may obtain a copy of the License at
-#
-# http://www.apache.org/licenses/LICENSE-2.0
-#
-# Unless required by applicable law or agreed to in writing, software
-# distributed under the License is distributed on an "AS IS" BASIS,
-# WITHOUT WARRANTIES OR CONDITIONS OF ANY KIND, either express or implied.
-# See the License for the specific language governing permissions and
-# limitations under the License.
-
-
-import unittest
-
-from transformers import is_torch_available
-
-from .test_configuration_common import ConfigTester
-from .test_modeling_common import ModelTesterMixin, ids_tensor
-from .utils import CACHE_DIR, require_torch, slow, torch_device
-
-
-if is_torch_available():
- from transformers import (
- GPT2Config,
- GPT2Model,
- GPT2_PRETRAINED_MODEL_ARCHIVE_MAP,
- GPT2LMHeadModel,
- GPT2DoubleHeadsModel,
- )
-
-
-@require_torch
-class GPT2ModelTest(ModelTesterMixin, unittest.TestCase):
-
- all_model_classes = (GPT2Model, GPT2LMHeadModel, GPT2DoubleHeadsModel) if is_torch_available() else ()
-
- class GPT2ModelTester(object):
- def __init__(
- self,
- parent,
- batch_size=13,
- seq_length=7,
- is_training=True,
- use_token_type_ids=True,
- use_input_mask=True,
- use_labels=True,
- use_mc_token_ids=True,
- vocab_size=99,
- hidden_size=32,
- num_hidden_layers=5,
- num_attention_heads=4,
- intermediate_size=37,
- hidden_act="gelu",
- hidden_dropout_prob=0.1,
- attention_probs_dropout_prob=0.1,
- max_position_embeddings=512,
- type_vocab_size=16,
- type_sequence_label_size=2,
- initializer_range=0.02,
- num_labels=3,
- num_choices=4,
- scope=None,
- ):
- self.parent = parent
- self.batch_size = batch_size
- self.seq_length = seq_length
- self.is_training = is_training
- self.use_token_type_ids = use_token_type_ids
- self.use_input_mask = use_input_mask
- self.use_labels = use_labels
- self.use_mc_token_ids = use_mc_token_ids
- self.vocab_size = vocab_size
- self.hidden_size = hidden_size
- self.num_hidden_layers = num_hidden_layers
- self.num_attention_heads = num_attention_heads
- self.intermediate_size = intermediate_size
- self.hidden_act = hidden_act
- self.hidden_dropout_prob = hidden_dropout_prob
- self.attention_probs_dropout_prob = attention_probs_dropout_prob
- self.max_position_embeddings = max_position_embeddings
- self.type_vocab_size = type_vocab_size
- self.type_sequence_label_size = type_sequence_label_size
- self.initializer_range = initializer_range
- self.num_labels = num_labels
- self.num_choices = num_choices
- self.scope = scope
-
- def prepare_config_and_inputs(self):
- input_ids = ids_tensor([self.batch_size, self.seq_length], self.vocab_size)
-
- input_mask = None
- if self.use_input_mask:
- input_mask = ids_tensor([self.batch_size, self.seq_length], vocab_size=2)
-
- token_type_ids = None
- if self.use_token_type_ids:
- token_type_ids = ids_tensor([self.batch_size, self.seq_length], self.type_vocab_size)
-
- mc_token_ids = None
- if self.use_mc_token_ids:
- mc_token_ids = ids_tensor([self.batch_size, self.num_choices], self.seq_length)
-
- sequence_labels = None
- token_labels = None
- choice_labels = None
- if self.use_labels:
- sequence_labels = ids_tensor([self.batch_size], self.type_sequence_label_size)
- token_labels = ids_tensor([self.batch_size, self.seq_length], self.num_labels)
- choice_labels = ids_tensor([self.batch_size], self.num_choices)
-
- config = GPT2Config(
- vocab_size=self.vocab_size,
- n_embd=self.hidden_size,
- n_layer=self.num_hidden_layers,
- n_head=self.num_attention_heads,
- # intermediate_size=self.intermediate_size,
- # hidden_act=self.hidden_act,
- # hidden_dropout_prob=self.hidden_dropout_prob,
- # attention_probs_dropout_prob=self.attention_probs_dropout_prob,
- n_positions=self.max_position_embeddings,
- n_ctx=self.max_position_embeddings
- # type_vocab_size=self.type_vocab_size,
- # initializer_range=self.initializer_range
- )
-
- head_mask = ids_tensor([self.num_hidden_layers, self.num_attention_heads], 2)
-
- return (
- config,
- input_ids,
- input_mask,
- head_mask,
- token_type_ids,
- mc_token_ids,
- sequence_labels,
- token_labels,
- choice_labels,
- )
-
- def check_loss_output(self, result):
- self.parent.assertListEqual(list(result["loss"].size()), [])
-
- def create_and_check_gpt2_model(self, config, input_ids, input_mask, head_mask, token_type_ids, *args):
- model = GPT2Model(config=config)
- model.to(torch_device)
- model.eval()
-
- model(input_ids, token_type_ids=token_type_ids, head_mask=head_mask)
- model(input_ids, token_type_ids=token_type_ids)
- sequence_output, presents = model(input_ids)
-
- result = {
- "sequence_output": sequence_output,
- "presents": presents,
- }
- self.parent.assertListEqual(
- list(result["sequence_output"].size()), [self.batch_size, self.seq_length, self.hidden_size]
- )
- self.parent.assertEqual(len(result["presents"]), config.n_layer)
-
- def create_and_check_lm_head_model(self, config, input_ids, input_mask, head_mask, token_type_ids, *args):
- model = GPT2LMHeadModel(config)
- model.to(torch_device)
- model.eval()
-
- loss, lm_logits, _ = model(input_ids, token_type_ids=token_type_ids, labels=input_ids)
-
- result = {"loss": loss, "lm_logits": lm_logits}
-
- self.parent.assertListEqual(list(result["loss"].size()), [])
- self.parent.assertListEqual(
- list(result["lm_logits"].size()), [self.batch_size, self.seq_length, self.vocab_size]
- )
-
- def create_and_check_double_lm_head_model(
- self, config, input_ids, input_mask, head_mask, token_type_ids, mc_token_ids, *args
- ):
- model = GPT2DoubleHeadsModel(config)
- model.to(torch_device)
- model.eval()
-
- multiple_choice_inputs_ids = input_ids.unsqueeze(1).expand(-1, self.num_choices, -1).contiguous()
- multiple_choice_input_mask = input_mask.unsqueeze(1).expand(-1, self.num_choices, -1).contiguous()
- multiple_choice_token_type_ids = token_type_ids.unsqueeze(1).expand(-1, self.num_choices, -1).contiguous()
-
- inputs = {
- "input_ids": multiple_choice_inputs_ids,
- "mc_token_ids": mc_token_ids,
- "attention_mask": multiple_choice_input_mask,
- "token_type_ids": multiple_choice_token_type_ids,
- "lm_labels": multiple_choice_inputs_ids,
- }
-
- loss, lm_logits, mc_logits, _ = model(**inputs)
-
- result = {"loss": loss, "lm_logits": lm_logits, "mc_logits": mc_logits}
-
- self.parent.assertListEqual(list(result["loss"].size()), [])
- self.parent.assertListEqual(
- list(result["lm_logits"].size()), [self.batch_size, self.num_choices, self.seq_length, self.vocab_size]
- )
- self.parent.assertListEqual(list(result["mc_logits"].size()), [self.batch_size, self.num_choices])
-
- def prepare_config_and_inputs_for_common(self):
- config_and_inputs = self.prepare_config_and_inputs()
-
- (
- config,
- input_ids,
- input_mask,
- head_mask,
- token_type_ids,
- mc_token_ids,
- sequence_labels,
- token_labels,
- choice_labels,
- ) = config_and_inputs
-
- inputs_dict = {"input_ids": input_ids, "token_type_ids": token_type_ids, "head_mask": head_mask}
-
- return config, inputs_dict
-
- def setUp(self):
- self.model_tester = GPT2ModelTest.GPT2ModelTester(self)
- self.config_tester = ConfigTester(self, config_class=GPT2Config, n_embd=37)
-
- def test_config(self):
- self.config_tester.run_common_tests()
-
- def test_gpt2_model(self):
- config_and_inputs = self.model_tester.prepare_config_and_inputs()
- self.model_tester.create_and_check_gpt2_model(*config_and_inputs)
-
- def test_gpt2_lm_head_model(self):
- config_and_inputs = self.model_tester.prepare_config_and_inputs()
- self.model_tester.create_and_check_lm_head_model(*config_and_inputs)
-
- def test_gpt2_double_lm_head_model(self):
- config_and_inputs = self.model_tester.prepare_config_and_inputs()
- self.model_tester.create_and_check_double_lm_head_model(*config_and_inputs)
-
- @slow
- def test_model_from_pretrained(self):
- for model_name in list(GPT2_PRETRAINED_MODEL_ARCHIVE_MAP.keys())[:1]:
- model = GPT2Model.from_pretrained(model_name, cache_dir=CACHE_DIR)
- self.assertIsNotNone(model)
diff --git a/server/transformers/tests/test_modeling_openai.py b/server/transformers/tests/test_modeling_openai.py
deleted file mode 100644
index a2aaabb645db62fe7544fec7a1e9bb0aa608f1ef..0000000000000000000000000000000000000000
--- a/server/transformers/tests/test_modeling_openai.py
+++ /dev/null
@@ -1,207 +0,0 @@
-# coding=utf-8
-# Copyright 2018 The Google AI Language Team Authors.
-#
-# Licensed under the Apache License, Version 2.0 (the "License");
-# you may not use this file except in compliance with the License.
-# You may obtain a copy of the License at
-#
-# http://www.apache.org/licenses/LICENSE-2.0
-#
-# Unless required by applicable law or agreed to in writing, software
-# distributed under the License is distributed on an "AS IS" BASIS,
-# WITHOUT WARRANTIES OR CONDITIONS OF ANY KIND, either express or implied.
-# See the License for the specific language governing permissions and
-# limitations under the License.
-
-
-import unittest
-
-from transformers import is_torch_available
-
-from .test_configuration_common import ConfigTester
-from .test_modeling_common import ModelTesterMixin, ids_tensor
-from .utils import CACHE_DIR, require_torch, slow, torch_device
-
-
-if is_torch_available():
- from transformers import (
- OpenAIGPTConfig,
- OpenAIGPTModel,
- OPENAI_GPT_PRETRAINED_MODEL_ARCHIVE_MAP,
- OpenAIGPTLMHeadModel,
- OpenAIGPTDoubleHeadsModel,
- )
-
-
-@require_torch
-class OpenAIGPTModelTest(ModelTesterMixin, unittest.TestCase):
-
- all_model_classes = (
- (OpenAIGPTModel, OpenAIGPTLMHeadModel, OpenAIGPTDoubleHeadsModel) if is_torch_available() else ()
- )
-
- class OpenAIGPTModelTester(object):
- def __init__(
- self,
- parent,
- batch_size=13,
- seq_length=7,
- is_training=True,
- use_token_type_ids=True,
- use_labels=True,
- vocab_size=99,
- hidden_size=32,
- num_hidden_layers=5,
- num_attention_heads=4,
- intermediate_size=37,
- hidden_act="gelu",
- hidden_dropout_prob=0.1,
- attention_probs_dropout_prob=0.1,
- max_position_embeddings=512,
- type_vocab_size=16,
- type_sequence_label_size=2,
- initializer_range=0.02,
- num_labels=3,
- num_choices=4,
- scope=None,
- ):
- self.parent = parent
- self.batch_size = batch_size
- self.seq_length = seq_length
- self.is_training = is_training
- self.use_token_type_ids = use_token_type_ids
- self.use_labels = use_labels
- self.vocab_size = vocab_size
- self.hidden_size = hidden_size
- self.num_hidden_layers = num_hidden_layers
- self.num_attention_heads = num_attention_heads
- self.intermediate_size = intermediate_size
- self.hidden_act = hidden_act
- self.hidden_dropout_prob = hidden_dropout_prob
- self.attention_probs_dropout_prob = attention_probs_dropout_prob
- self.max_position_embeddings = max_position_embeddings
- self.type_vocab_size = type_vocab_size
- self.type_sequence_label_size = type_sequence_label_size
- self.initializer_range = initializer_range
- self.num_labels = num_labels
- self.num_choices = num_choices
- self.scope = scope
-
- def prepare_config_and_inputs(self):
- input_ids = ids_tensor([self.batch_size, self.seq_length], self.vocab_size)
-
- token_type_ids = None
- if self.use_token_type_ids:
- token_type_ids = ids_tensor([self.batch_size, self.seq_length], self.type_vocab_size)
-
- sequence_labels = None
- token_labels = None
- choice_labels = None
- if self.use_labels:
- sequence_labels = ids_tensor([self.batch_size], self.type_sequence_label_size)
- token_labels = ids_tensor([self.batch_size, self.seq_length], self.num_labels)
- choice_labels = ids_tensor([self.batch_size], self.num_choices)
-
- config = OpenAIGPTConfig(
- vocab_size=self.vocab_size,
- n_embd=self.hidden_size,
- n_layer=self.num_hidden_layers,
- n_head=self.num_attention_heads,
- # intermediate_size=self.intermediate_size,
- # hidden_act=self.hidden_act,
- # hidden_dropout_prob=self.hidden_dropout_prob,
- # attention_probs_dropout_prob=self.attention_probs_dropout_prob,
- n_positions=self.max_position_embeddings,
- n_ctx=self.max_position_embeddings
- # type_vocab_size=self.type_vocab_size,
- # initializer_range=self.initializer_range
- )
-
- head_mask = ids_tensor([self.num_hidden_layers, self.num_attention_heads], 2)
-
- return config, input_ids, head_mask, token_type_ids, sequence_labels, token_labels, choice_labels
-
- def check_loss_output(self, result):
- self.parent.assertListEqual(list(result["loss"].size()), [])
-
- def create_and_check_openai_gpt_model(self, config, input_ids, head_mask, token_type_ids, *args):
- model = OpenAIGPTModel(config=config)
- model.to(torch_device)
- model.eval()
-
- model(input_ids, token_type_ids=token_type_ids, head_mask=head_mask)
- model(input_ids, token_type_ids=token_type_ids)
- (sequence_output,) = model(input_ids)
-
- result = {"sequence_output": sequence_output}
- self.parent.assertListEqual(
- list(result["sequence_output"].size()), [self.batch_size, self.seq_length, self.hidden_size]
- )
-
- def create_and_check_lm_head_model(self, config, input_ids, head_mask, token_type_ids, *args):
- model = OpenAIGPTLMHeadModel(config)
- model.to(torch_device)
- model.eval()
-
- loss, lm_logits = model(input_ids, token_type_ids=token_type_ids, labels=input_ids)
-
- result = {"loss": loss, "lm_logits": lm_logits}
-
- self.parent.assertListEqual(list(result["loss"].size()), [])
- self.parent.assertListEqual(
- list(result["lm_logits"].size()), [self.batch_size, self.seq_length, self.vocab_size]
- )
-
- def create_and_check_double_lm_head_model(self, config, input_ids, head_mask, token_type_ids, *args):
- model = OpenAIGPTDoubleHeadsModel(config)
- model.to(torch_device)
- model.eval()
-
- loss, lm_logits, mc_logits = model(input_ids, token_type_ids=token_type_ids, lm_labels=input_ids)
-
- result = {"loss": loss, "lm_logits": lm_logits}
-
- self.parent.assertListEqual(list(result["loss"].size()), [])
- self.parent.assertListEqual(
- list(result["lm_logits"].size()), [self.batch_size, self.seq_length, self.vocab_size]
- )
-
- def prepare_config_and_inputs_for_common(self):
- config_and_inputs = self.prepare_config_and_inputs()
- (
- config,
- input_ids,
- head_mask,
- token_type_ids,
- sequence_labels,
- token_labels,
- choice_labels,
- ) = config_and_inputs
- inputs_dict = {"input_ids": input_ids, "token_type_ids": token_type_ids, "head_mask": head_mask}
-
- return config, inputs_dict
-
- def setUp(self):
- self.model_tester = OpenAIGPTModelTest.OpenAIGPTModelTester(self)
- self.config_tester = ConfigTester(self, config_class=OpenAIGPTConfig, n_embd=37)
-
- def test_config(self):
- self.config_tester.run_common_tests()
-
- def test_openai_gpt_model(self):
- config_and_inputs = self.model_tester.prepare_config_and_inputs()
- self.model_tester.create_and_check_openai_gpt_model(*config_and_inputs)
-
- def test_openai_gpt_lm_head_model(self):
- config_and_inputs = self.model_tester.prepare_config_and_inputs()
- self.model_tester.create_and_check_lm_head_model(*config_and_inputs)
-
- def test_openai_gpt_double_lm_head_model(self):
- config_and_inputs = self.model_tester.prepare_config_and_inputs()
- self.model_tester.create_and_check_double_lm_head_model(*config_and_inputs)
-
- @slow
- def test_model_from_pretrained(self):
- for model_name in list(OPENAI_GPT_PRETRAINED_MODEL_ARCHIVE_MAP.keys())[:1]:
- model = OpenAIGPTModel.from_pretrained(model_name, cache_dir=CACHE_DIR)
- self.assertIsNotNone(model)
diff --git a/server/transformers/tests/test_modeling_roberta.py b/server/transformers/tests/test_modeling_roberta.py
deleted file mode 100644
index 2a63ac232a70943937de03f2daa90667e5da6a28..0000000000000000000000000000000000000000
--- a/server/transformers/tests/test_modeling_roberta.py
+++ /dev/null
@@ -1,300 +0,0 @@
-# coding=utf-8
-# Copyright 2018 The Google AI Language Team Authors.
-#
-# Licensed under the Apache License, Version 2.0 (the "License");
-# you may not use this file except in compliance with the License.
-# You may obtain a copy of the License at
-#
-# http://www.apache.org/licenses/LICENSE-2.0
-#
-# Unless required by applicable law or agreed to in writing, software
-# distributed under the License is distributed on an "AS IS" BASIS,
-# WITHOUT WARRANTIES OR CONDITIONS OF ANY KIND, either express or implied.
-# See the License for the specific language governing permissions and
-# limitations under the License.
-
-
-import unittest
-
-from transformers import is_torch_available
-
-from .test_configuration_common import ConfigTester
-from .test_modeling_common import ModelTesterMixin, ids_tensor
-from .utils import CACHE_DIR, require_torch, slow, torch_device
-
-
-if is_torch_available():
- import torch
- from transformers import (
- RobertaConfig,
- RobertaModel,
- RobertaForMaskedLM,
- RobertaForSequenceClassification,
- RobertaForTokenClassification,
- )
- from transformers.modeling_roberta import RobertaEmbeddings
- from transformers.modeling_roberta import ROBERTA_PRETRAINED_MODEL_ARCHIVE_MAP
-
-
-@require_torch
-class RobertaModelTest(ModelTesterMixin, unittest.TestCase):
-
- all_model_classes = (RobertaForMaskedLM, RobertaModel) if is_torch_available() else ()
-
- class RobertaModelTester(object):
- def __init__(
- self,
- parent,
- batch_size=13,
- seq_length=7,
- is_training=True,
- use_input_mask=True,
- use_token_type_ids=True,
- use_labels=True,
- vocab_size=99,
- hidden_size=32,
- num_hidden_layers=5,
- num_attention_heads=4,
- intermediate_size=37,
- hidden_act="gelu",
- hidden_dropout_prob=0.1,
- attention_probs_dropout_prob=0.1,
- max_position_embeddings=512,
- type_vocab_size=16,
- type_sequence_label_size=2,
- initializer_range=0.02,
- num_labels=3,
- num_choices=4,
- scope=None,
- ):
- self.parent = parent
- self.batch_size = batch_size
- self.seq_length = seq_length
- self.is_training = is_training
- self.use_input_mask = use_input_mask
- self.use_token_type_ids = use_token_type_ids
- self.use_labels = use_labels
- self.vocab_size = vocab_size
- self.hidden_size = hidden_size
- self.num_hidden_layers = num_hidden_layers
- self.num_attention_heads = num_attention_heads
- self.intermediate_size = intermediate_size
- self.hidden_act = hidden_act
- self.hidden_dropout_prob = hidden_dropout_prob
- self.attention_probs_dropout_prob = attention_probs_dropout_prob
- self.max_position_embeddings = max_position_embeddings
- self.type_vocab_size = type_vocab_size
- self.type_sequence_label_size = type_sequence_label_size
- self.initializer_range = initializer_range
- self.num_labels = num_labels
- self.num_choices = num_choices
- self.scope = scope
-
- def prepare_config_and_inputs(self):
- input_ids = ids_tensor([self.batch_size, self.seq_length], self.vocab_size)
-
- input_mask = None
- if self.use_input_mask:
- input_mask = ids_tensor([self.batch_size, self.seq_length], vocab_size=2)
-
- token_type_ids = None
- if self.use_token_type_ids:
- token_type_ids = ids_tensor([self.batch_size, self.seq_length], self.type_vocab_size)
-
- sequence_labels = None
- token_labels = None
- choice_labels = None
- if self.use_labels:
- sequence_labels = ids_tensor([self.batch_size], self.type_sequence_label_size)
- token_labels = ids_tensor([self.batch_size, self.seq_length], self.num_labels)
- choice_labels = ids_tensor([self.batch_size], self.num_choices)
-
- config = RobertaConfig(
- vocab_size=self.vocab_size,
- hidden_size=self.hidden_size,
- num_hidden_layers=self.num_hidden_layers,
- num_attention_heads=self.num_attention_heads,
- intermediate_size=self.intermediate_size,
- hidden_act=self.hidden_act,
- hidden_dropout_prob=self.hidden_dropout_prob,
- attention_probs_dropout_prob=self.attention_probs_dropout_prob,
- max_position_embeddings=self.max_position_embeddings,
- type_vocab_size=self.type_vocab_size,
- initializer_range=self.initializer_range,
- )
-
- return config, input_ids, token_type_ids, input_mask, sequence_labels, token_labels, choice_labels
-
- def check_loss_output(self, result):
- self.parent.assertListEqual(list(result["loss"].size()), [])
-
- def create_and_check_roberta_model(
- self, config, input_ids, token_type_ids, input_mask, sequence_labels, token_labels, choice_labels
- ):
- model = RobertaModel(config=config)
- model.to(torch_device)
- model.eval()
- sequence_output, pooled_output = model(input_ids, attention_mask=input_mask, token_type_ids=token_type_ids)
- sequence_output, pooled_output = model(input_ids, token_type_ids=token_type_ids)
- sequence_output, pooled_output = model(input_ids)
-
- result = {
- "sequence_output": sequence_output,
- "pooled_output": pooled_output,
- }
- self.parent.assertListEqual(
- list(result["sequence_output"].size()), [self.batch_size, self.seq_length, self.hidden_size]
- )
- self.parent.assertListEqual(list(result["pooled_output"].size()), [self.batch_size, self.hidden_size])
-
- def create_and_check_roberta_for_masked_lm(
- self, config, input_ids, token_type_ids, input_mask, sequence_labels, token_labels, choice_labels
- ):
- model = RobertaForMaskedLM(config=config)
- model.to(torch_device)
- model.eval()
- loss, prediction_scores = model(
- input_ids, attention_mask=input_mask, token_type_ids=token_type_ids, masked_lm_labels=token_labels
- )
- result = {
- "loss": loss,
- "prediction_scores": prediction_scores,
- }
- self.parent.assertListEqual(
- list(result["prediction_scores"].size()), [self.batch_size, self.seq_length, self.vocab_size]
- )
- self.check_loss_output(result)
-
- def create_and_check_roberta_for_token_classification(
- self, config, input_ids, token_type_ids, input_mask, sequence_labels, token_labels, choice_labels
- ):
- config.num_labels = self.num_labels
- model = RobertaForTokenClassification(config=config)
- model.to(torch_device)
- model.eval()
- loss, logits = model(
- input_ids, attention_mask=input_mask, token_type_ids=token_type_ids, labels=token_labels
- )
- result = {
- "loss": loss,
- "logits": logits,
- }
- self.parent.assertListEqual(
- list(result["logits"].size()), [self.batch_size, self.seq_length, self.num_labels]
- )
- self.check_loss_output(result)
-
- def prepare_config_and_inputs_for_common(self):
- config_and_inputs = self.prepare_config_and_inputs()
- (
- config,
- input_ids,
- token_type_ids,
- input_mask,
- sequence_labels,
- token_labels,
- choice_labels,
- ) = config_and_inputs
- inputs_dict = {"input_ids": input_ids, "token_type_ids": token_type_ids, "attention_mask": input_mask}
- return config, inputs_dict
-
- def setUp(self):
- self.model_tester = RobertaModelTest.RobertaModelTester(self)
- self.config_tester = ConfigTester(self, config_class=RobertaConfig, hidden_size=37)
-
- def test_config(self):
- self.config_tester.run_common_tests()
-
- def test_roberta_model(self):
- config_and_inputs = self.model_tester.prepare_config_and_inputs()
- self.model_tester.create_and_check_roberta_model(*config_and_inputs)
-
- def test_for_masked_lm(self):
- config_and_inputs = self.model_tester.prepare_config_and_inputs()
- self.model_tester.create_and_check_roberta_for_masked_lm(*config_and_inputs)
-
- @slow
- def test_model_from_pretrained(self):
- for model_name in list(ROBERTA_PRETRAINED_MODEL_ARCHIVE_MAP.keys())[:1]:
- model = RobertaModel.from_pretrained(model_name, cache_dir=CACHE_DIR)
- self.assertIsNotNone(model)
-
- def test_create_position_ids_respects_padding_index(self):
- """ Ensure that the default position ids only assign a sequential . This is a regression
- test for https://github.com/huggingface/transformers/issues/1761
-
- The position ids should be masked with the embedding object's padding index. Therefore, the
- first available non-padding position index is RobertaEmbeddings.padding_idx + 1
- """
- config = self.model_tester.prepare_config_and_inputs()[0]
- model = RobertaEmbeddings(config=config)
-
- input_ids = torch.as_tensor([[12, 31, 13, model.padding_idx]])
- expected_positions = torch.as_tensor(
- [[0 + model.padding_idx + 1, 1 + model.padding_idx + 1, 2 + model.padding_idx + 1, model.padding_idx]]
- )
-
- position_ids = model.create_position_ids_from_input_ids(input_ids)
- self.assertEqual(position_ids.shape, expected_positions.shape)
- self.assertTrue(torch.all(torch.eq(position_ids, expected_positions)))
-
- def test_create_position_ids_from_inputs_embeds(self):
- """ Ensure that the default position ids only assign a sequential . This is a regression
- test for https://github.com/huggingface/transformers/issues/1761
-
- The position ids should be masked with the embedding object's padding index. Therefore, the
- first available non-padding position index is RobertaEmbeddings.padding_idx + 1
- """
- config = self.model_tester.prepare_config_and_inputs()[0]
- embeddings = RobertaEmbeddings(config=config)
-
- inputs_embeds = torch.Tensor(2, 4, 30)
- expected_single_positions = [
- 0 + embeddings.padding_idx + 1,
- 1 + embeddings.padding_idx + 1,
- 2 + embeddings.padding_idx + 1,
- 3 + embeddings.padding_idx + 1,
- ]
- expected_positions = torch.as_tensor([expected_single_positions, expected_single_positions])
- position_ids = embeddings.create_position_ids_from_inputs_embeds(inputs_embeds)
- self.assertEqual(position_ids.shape, expected_positions.shape)
- self.assertTrue(torch.all(torch.eq(position_ids, expected_positions)))
-
-
-class RobertaModelIntegrationTest(unittest.TestCase):
- @slow
- def test_inference_masked_lm(self):
- model = RobertaForMaskedLM.from_pretrained("roberta-base")
-
- input_ids = torch.tensor([[0, 31414, 232, 328, 740, 1140, 12695, 69, 46078, 1588, 2]])
- output = model(input_ids)[0]
- expected_shape = torch.Size((1, 11, 50265))
- self.assertEqual(output.shape, expected_shape)
- # compare the actual values for a slice.
- expected_slice = torch.Tensor(
- [[[33.8843, -4.3107, 22.7779], [4.6533, -2.8099, 13.6252], [1.8222, -3.6898, 8.8600]]]
- )
- self.assertTrue(torch.allclose(output[:, :3, :3], expected_slice, atol=1e-3))
-
- @slow
- def test_inference_no_head(self):
- model = RobertaModel.from_pretrained("roberta-base")
-
- input_ids = torch.tensor([[0, 31414, 232, 328, 740, 1140, 12695, 69, 46078, 1588, 2]])
- output = model(input_ids)[0]
- # compare the actual values for a slice.
- expected_slice = torch.Tensor(
- [[[-0.0231, 0.0782, 0.0074], [-0.1854, 0.0539, -0.0174], [0.0548, 0.0799, 0.1687]]]
- )
- self.assertTrue(torch.allclose(output[:, :3, :3], expected_slice, atol=1e-3))
-
- @slow
- def test_inference_classification_head(self):
- model = RobertaForSequenceClassification.from_pretrained("roberta-large-mnli")
-
- input_ids = torch.tensor([[0, 31414, 232, 328, 740, 1140, 12695, 69, 46078, 1588, 2]])
- output = model(input_ids)[0]
- expected_shape = torch.Size((1, 3))
- self.assertEqual(output.shape, expected_shape)
- expected_tensor = torch.Tensor([[-0.9469, 0.3913, 0.5118]])
- self.assertTrue(torch.allclose(output, expected_tensor, atol=1e-3))
diff --git a/server/transformers/tests/test_modeling_t5.py b/server/transformers/tests/test_modeling_t5.py
deleted file mode 100644
index 964d5d4afee1f524c4f820710aa7d22b772fd9c1..0000000000000000000000000000000000000000
--- a/server/transformers/tests/test_modeling_t5.py
+++ /dev/null
@@ -1,214 +0,0 @@
-# coding=utf-8
-# Copyright 2018 Google T5 Authors and HuggingFace Inc. team.
-#
-# Licensed under the Apache License, Version 2.0 (the "License");
-# you may not use this file except in compliance with the License.
-# You may obtain a copy of the License at
-#
-# http://www.apache.org/licenses/LICENSE-2.0
-#
-# Unless required by applicable law or agreed to in writing, software
-# distributed under the License is distributed on an "AS IS" BASIS,
-# WITHOUT WARRANTIES OR CONDITIONS OF ANY KIND, either express or implied.
-# See the License for the specific language governing permissions and
-# limitations under the License.
-
-
-import unittest
-
-from transformers import is_torch_available
-
-from .test_configuration_common import ConfigTester
-from .test_modeling_common import ModelTesterMixin, ids_tensor
-from .utils import CACHE_DIR, require_torch, slow
-
-
-if is_torch_available():
- from transformers import T5Config, T5Model, T5WithLMHeadModel
- from transformers.modeling_t5 import T5_PRETRAINED_MODEL_ARCHIVE_MAP
-
-
-@require_torch
-class T5ModelTest(ModelTesterMixin, unittest.TestCase):
-
- all_model_classes = (T5Model, T5WithLMHeadModel) if is_torch_available() else ()
- test_pruning = False
- test_torchscript = False
- test_resize_embeddings = False
- is_encoder_decoder = True
-
- class T5ModelTester(object):
- def __init__(
- self,
- parent,
- batch_size=13,
- encoder_seq_length=7,
- decoder_seq_length=9,
- is_training=True,
- use_attention_mask=True,
- use_labels=True,
- vocab_size=99,
- n_positions=14,
- hidden_size=32,
- num_hidden_layers=5,
- num_attention_heads=4,
- d_ff=37,
- relative_attention_num_buckets=8,
- dropout_rate=0.1,
- initializer_factor=0.002,
- scope=None,
- ):
- self.parent = parent
- self.batch_size = batch_size
- self.encoder_seq_length = encoder_seq_length
- self.decoder_seq_length = decoder_seq_length
- self.is_training = is_training
- self.use_attention_mask = use_attention_mask
- self.use_labels = use_labels
- self.vocab_size = vocab_size
- self.n_positions = n_positions
- self.hidden_size = hidden_size
- self.num_hidden_layers = num_hidden_layers
- self.num_attention_heads = num_attention_heads
- self.d_ff = d_ff
- self.relative_attention_num_buckets = relative_attention_num_buckets
- self.dropout_rate = dropout_rate
- self.initializer_factor = initializer_factor
- self.scope = scope
-
- def prepare_config_and_inputs(self):
- encoder_input_ids = ids_tensor([self.batch_size, self.encoder_seq_length], self.vocab_size)
- decoder_input_ids = ids_tensor([self.batch_size, self.decoder_seq_length], self.vocab_size)
-
- encoder_attention_mask = None
- decoder_attention_mask = None
- if self.use_attention_mask:
- encoder_attention_mask = ids_tensor([self.batch_size, self.encoder_seq_length], vocab_size=2)
- decoder_attention_mask = ids_tensor([self.batch_size, self.decoder_seq_length], vocab_size=2)
-
- decoder_lm_labels = None
- if self.use_labels:
- decoder_lm_labels = ids_tensor([self.batch_size, self.decoder_seq_length], self.vocab_size)
-
- config = T5Config(
- vocab_size=self.vocab_size,
- n_positions=self.n_positions,
- d_model=self.hidden_size,
- d_ff=self.d_ff,
- d_kv=self.hidden_size // self.num_attention_heads,
- num_layers=self.num_hidden_layers,
- num_heads=self.num_attention_heads,
- relative_attention_num_buckets=self.relative_attention_num_buckets,
- dropout_rate=self.dropout_rate,
- initializer_factor=self.initializer_factor,
- )
-
- return (
- config,
- encoder_input_ids,
- decoder_input_ids,
- encoder_attention_mask,
- decoder_attention_mask,
- decoder_lm_labels,
- )
-
- def check_loss_output(self, result):
- self.parent.assertListEqual(list(result["loss"].size()), [])
-
- def create_and_check_t5_model(
- self,
- config,
- encoder_input_ids,
- decoder_input_ids,
- encoder_attention_mask,
- decoder_attention_mask,
- decoder_lm_labels,
- ):
- model = T5Model(config=config)
- model.eval()
- decoder_output, encoder_output = model(
- encoder_input_ids=encoder_input_ids,
- decoder_input_ids=decoder_input_ids,
- encoder_attention_mask=encoder_attention_mask,
- decoder_attention_mask=decoder_attention_mask,
- )
- decoder_output, encoder_output = model(
- encoder_input_ids=encoder_input_ids, decoder_input_ids=decoder_input_ids
- )
-
- result = {
- "encoder_output": encoder_output,
- "decoder_output": decoder_output,
- }
- self.parent.assertListEqual(
- list(result["encoder_output"].size()), [self.batch_size, self.encoder_seq_length, self.hidden_size]
- )
- self.parent.assertListEqual(
- list(result["decoder_output"].size()), [self.batch_size, self.decoder_seq_length, self.hidden_size]
- )
-
- def create_and_check_t5_with_lm_head(
- self,
- config,
- encoder_input_ids,
- decoder_input_ids,
- encoder_attention_mask,
- decoder_attention_mask,
- decoder_lm_labels,
- ):
- model = T5WithLMHeadModel(config=config)
- model.eval()
- outputs = model(
- encoder_input_ids=encoder_input_ids,
- decoder_input_ids=decoder_input_ids,
- decoder_attention_mask=decoder_attention_mask,
- decoder_lm_labels=decoder_lm_labels,
- )
- loss, prediction_scores = outputs[0], outputs[1]
- result = {
- "loss": loss,
- "prediction_scores": prediction_scores,
- }
- self.parent.assertListEqual(
- list(result["prediction_scores"].size()), [self.batch_size, self.decoder_seq_length, self.vocab_size]
- )
- self.check_loss_output(result)
-
- def prepare_config_and_inputs_for_common(self):
- config_and_inputs = self.prepare_config_and_inputs()
- (
- config,
- encoder_input_ids,
- decoder_input_ids,
- encoder_attention_mask,
- decoder_attention_mask,
- decoder_lm_labels,
- ) = config_and_inputs
- inputs_dict = {
- "encoder_input_ids": encoder_input_ids,
- "decoder_input_ids": decoder_input_ids,
- "decoder_attention_mask": decoder_attention_mask,
- "encoder_attention_mask": encoder_attention_mask,
- }
- return config, inputs_dict
-
- def setUp(self):
- self.model_tester = T5ModelTest.T5ModelTester(self)
- self.config_tester = ConfigTester(self, config_class=T5Config, d_model=37)
-
- def test_config(self):
- self.config_tester.run_common_tests()
-
- def test_t5_model(self):
- config_and_inputs = self.model_tester.prepare_config_and_inputs()
- self.model_tester.create_and_check_t5_model(*config_and_inputs)
-
- def test_with_lm_head(self):
- config_and_inputs = self.model_tester.prepare_config_and_inputs()
- self.model_tester.create_and_check_t5_with_lm_head(*config_and_inputs)
-
- @slow
- def test_model_from_pretrained(self):
- for model_name in list(T5_PRETRAINED_MODEL_ARCHIVE_MAP.keys())[:1]:
- model = T5Model.from_pretrained(model_name, cache_dir=CACHE_DIR)
- self.assertIsNotNone(model)
diff --git a/server/transformers/tests/test_modeling_tf_albert.py b/server/transformers/tests/test_modeling_tf_albert.py
deleted file mode 100644
index fb7b269cdcabde5993e916c7ac8818e95b2932ff..0000000000000000000000000000000000000000
--- a/server/transformers/tests/test_modeling_tf_albert.py
+++ /dev/null
@@ -1,215 +0,0 @@
-# coding=utf-8
-# Copyright 2018 The Google AI Language Team Authors.
-#
-# Licensed under the Apache License, Version 2.0 (the "License");
-# you may not use this file except in compliance with the License.
-# You may obtain a copy of the License at
-#
-# http://www.apache.org/licenses/LICENSE-2.0
-#
-# Unless required by applicable law or agreed to in writing, software
-# distributed under the License is distributed on an "AS IS" BASIS,
-# WITHOUT WARRANTIES OR CONDITIONS OF ANY KIND, either express or implied.
-# See the License for the specific language governing permissions and
-# limitations under the License.
-
-
-import unittest
-
-from transformers import AlbertConfig, is_tf_available
-
-from .test_configuration_common import ConfigTester
-from .test_modeling_tf_common import TFModelTesterMixin, ids_tensor
-from .utils import CACHE_DIR, require_tf, slow
-
-
-if is_tf_available():
- from transformers.modeling_tf_albert import (
- TFAlbertModel,
- TFAlbertForMaskedLM,
- TFAlbertForSequenceClassification,
- TF_ALBERT_PRETRAINED_MODEL_ARCHIVE_MAP,
- )
-
-
-@require_tf
-class TFAlbertModelTest(TFModelTesterMixin, unittest.TestCase):
-
- all_model_classes = (
- (TFAlbertModel, TFAlbertForMaskedLM, TFAlbertForSequenceClassification) if is_tf_available() else ()
- )
-
- class TFAlbertModelTester(object):
- def __init__(
- self,
- parent,
- batch_size=13,
- seq_length=7,
- is_training=True,
- use_input_mask=True,
- use_token_type_ids=True,
- use_labels=True,
- vocab_size=99,
- embedding_size=16,
- hidden_size=32,
- num_hidden_layers=5,
- num_attention_heads=4,
- intermediate_size=37,
- hidden_act="gelu",
- hidden_dropout_prob=0.1,
- attention_probs_dropout_prob=0.1,
- max_position_embeddings=512,
- type_vocab_size=16,
- type_sequence_label_size=2,
- initializer_range=0.02,
- num_labels=3,
- num_choices=4,
- scope=None,
- ):
- self.parent = parent
- self.batch_size = batch_size
- self.seq_length = seq_length
- self.is_training = is_training
- self.use_input_mask = use_input_mask
- self.use_token_type_ids = use_token_type_ids
- self.use_labels = use_labels
- self.vocab_size = vocab_size
- self.embedding_size = embedding_size
- self.hidden_size = hidden_size
- self.num_hidden_layers = num_hidden_layers
- self.num_attention_heads = num_attention_heads
- self.intermediate_size = intermediate_size
- self.hidden_act = hidden_act
- self.hidden_dropout_prob = hidden_dropout_prob
- self.attention_probs_dropout_prob = attention_probs_dropout_prob
- self.max_position_embeddings = max_position_embeddings
- self.type_vocab_size = type_vocab_size
- self.type_sequence_label_size = type_sequence_label_size
- self.initializer_range = initializer_range
- self.num_labels = num_labels
- self.num_choices = num_choices
- self.scope = scope
-
- def prepare_config_and_inputs(self):
- input_ids = ids_tensor([self.batch_size, self.seq_length], self.vocab_size)
-
- input_mask = None
- if self.use_input_mask:
- input_mask = ids_tensor([self.batch_size, self.seq_length], vocab_size=2)
-
- token_type_ids = None
- if self.use_token_type_ids:
- token_type_ids = ids_tensor([self.batch_size, self.seq_length], self.type_vocab_size)
-
- sequence_labels = None
- token_labels = None
- choice_labels = None
- if self.use_labels:
- sequence_labels = ids_tensor([self.batch_size], self.type_sequence_label_size)
- token_labels = ids_tensor([self.batch_size, self.seq_length], self.num_labels)
- choice_labels = ids_tensor([self.batch_size], self.num_choices)
-
- config = AlbertConfig(
- vocab_size=self.vocab_size,
- hidden_size=self.hidden_size,
- num_hidden_layers=self.num_hidden_layers,
- num_attention_heads=self.num_attention_heads,
- intermediate_size=self.intermediate_size,
- hidden_act=self.hidden_act,
- hidden_dropout_prob=self.hidden_dropout_prob,
- attention_probs_dropout_prob=self.attention_probs_dropout_prob,
- max_position_embeddings=self.max_position_embeddings,
- type_vocab_size=self.type_vocab_size,
- initializer_range=self.initializer_range,
- )
-
- return config, input_ids, token_type_ids, input_mask, sequence_labels, token_labels, choice_labels
-
- def create_and_check_albert_model(
- self, config, input_ids, token_type_ids, input_mask, sequence_labels, token_labels, choice_labels
- ):
- model = TFAlbertModel(config=config)
- # inputs = {'input_ids': input_ids,
- # 'attention_mask': input_mask,
- # 'token_type_ids': token_type_ids}
- # sequence_output, pooled_output = model(**inputs)
- inputs = {"input_ids": input_ids, "attention_mask": input_mask, "token_type_ids": token_type_ids}
- sequence_output, pooled_output = model(inputs)
-
- inputs = [input_ids, input_mask]
- sequence_output, pooled_output = model(inputs)
-
- sequence_output, pooled_output = model(input_ids)
-
- result = {
- "sequence_output": sequence_output.numpy(),
- "pooled_output": pooled_output.numpy(),
- }
- self.parent.assertListEqual(
- list(result["sequence_output"].shape), [self.batch_size, self.seq_length, self.hidden_size]
- )
- self.parent.assertListEqual(list(result["pooled_output"].shape), [self.batch_size, self.hidden_size])
-
- def create_and_check_albert_for_masked_lm(
- self, config, input_ids, token_type_ids, input_mask, sequence_labels, token_labels, choice_labels
- ):
- model = TFAlbertForMaskedLM(config=config)
- inputs = {"input_ids": input_ids, "attention_mask": input_mask, "token_type_ids": token_type_ids}
- (prediction_scores,) = model(inputs)
- result = {
- "prediction_scores": prediction_scores.numpy(),
- }
- self.parent.assertListEqual(
- list(result["prediction_scores"].shape), [self.batch_size, self.seq_length, self.vocab_size]
- )
-
- def create_and_check_albert_for_sequence_classification(
- self, config, input_ids, token_type_ids, input_mask, sequence_labels, token_labels, choice_labels
- ):
- config.num_labels = self.num_labels
- model = TFAlbertForSequenceClassification(config=config)
- inputs = {"input_ids": input_ids, "attention_mask": input_mask, "token_type_ids": token_type_ids}
- (logits,) = model(inputs)
- result = {
- "logits": logits.numpy(),
- }
- self.parent.assertListEqual(list(result["logits"].shape), [self.batch_size, self.num_labels])
-
- def prepare_config_and_inputs_for_common(self):
- config_and_inputs = self.prepare_config_and_inputs()
- (
- config,
- input_ids,
- token_type_ids,
- input_mask,
- sequence_labels,
- token_labels,
- choice_labels,
- ) = config_and_inputs
- inputs_dict = {"input_ids": input_ids, "token_type_ids": token_type_ids, "attention_mask": input_mask}
- return config, inputs_dict
-
- def setUp(self):
- self.model_tester = TFAlbertModelTest.TFAlbertModelTester(self)
- self.config_tester = ConfigTester(self, config_class=AlbertConfig, hidden_size=37)
-
- def test_config(self):
- self.config_tester.run_common_tests()
-
- def test_albert_model(self):
- config_and_inputs = self.model_tester.prepare_config_and_inputs()
- self.model_tester.create_and_check_albert_model(*config_and_inputs)
-
- def test_for_masked_lm(self):
- config_and_inputs = self.model_tester.prepare_config_and_inputs()
- self.model_tester.create_and_check_albert_for_masked_lm(*config_and_inputs)
-
- def test_for_sequence_classification(self):
- config_and_inputs = self.model_tester.prepare_config_and_inputs()
- self.model_tester.create_and_check_albert_for_sequence_classification(*config_and_inputs)
-
- @slow
- def test_model_from_pretrained(self):
- for model_name in list(TF_ALBERT_PRETRAINED_MODEL_ARCHIVE_MAP.keys())[:1]:
- model = TFAlbertModel.from_pretrained(model_name, cache_dir=CACHE_DIR)
- self.assertIsNotNone(model)
diff --git a/server/transformers/tests/test_modeling_tf_auto.py b/server/transformers/tests/test_modeling_tf_auto.py
deleted file mode 100644
index 6994f6eaa949c43be73219df544867d3d57a5bfd..0000000000000000000000000000000000000000
--- a/server/transformers/tests/test_modeling_tf_auto.py
+++ /dev/null
@@ -1,130 +0,0 @@
-# coding=utf-8
-# Copyright 2018 The Google AI Language Team Authors.
-#
-# Licensed under the Apache License, Version 2.0 (the "License");
-# you may not use this file except in compliance with the License.
-# You may obtain a copy of the License at
-#
-# http://www.apache.org/licenses/LICENSE-2.0
-#
-# Unless required by applicable law or agreed to in writing, software
-# distributed under the License is distributed on an "AS IS" BASIS,
-# WITHOUT WARRANTIES OR CONDITIONS OF ANY KIND, either express or implied.
-# See the License for the specific language governing permissions and
-# limitations under the License.
-
-
-import logging
-import unittest
-
-from transformers import is_tf_available
-
-from .utils import DUMMY_UNKWOWN_IDENTIFIER, SMALL_MODEL_IDENTIFIER, require_tf, slow
-
-
-if is_tf_available():
- from transformers import (
- AutoConfig,
- BertConfig,
- TFAutoModel,
- TFBertModel,
- TFAutoModelForPreTraining,
- TFBertForPreTraining,
- TFAutoModelWithLMHead,
- TFBertForMaskedLM,
- TFRobertaForMaskedLM,
- TFAutoModelForSequenceClassification,
- TFBertForSequenceClassification,
- TFAutoModelForQuestionAnswering,
- TFBertForQuestionAnswering,
- )
-
-
-@require_tf
-class TFAutoModelTest(unittest.TestCase):
- @slow
- def test_model_from_pretrained(self):
- import h5py
-
- self.assertTrue(h5py.version.hdf5_version.startswith("1.10"))
-
- logging.basicConfig(level=logging.INFO)
- # for model_name in list(TF_BERT_PRETRAINED_MODEL_ARCHIVE_MAP.keys())[:1]:
- for model_name in ["bert-base-uncased"]:
- config = AutoConfig.from_pretrained(model_name)
- self.assertIsNotNone(config)
- self.assertIsInstance(config, BertConfig)
-
- model = TFAutoModel.from_pretrained(model_name)
- self.assertIsNotNone(model)
- self.assertIsInstance(model, TFBertModel)
-
- @slow
- def test_model_for_pretraining_from_pretrained(self):
- import h5py
-
- self.assertTrue(h5py.version.hdf5_version.startswith("1.10"))
-
- logging.basicConfig(level=logging.INFO)
- # for model_name in list(TF_BERT_PRETRAINED_MODEL_ARCHIVE_MAP.keys())[:1]:
- for model_name in ["bert-base-uncased"]:
- config = AutoConfig.from_pretrained(model_name)
- self.assertIsNotNone(config)
- self.assertIsInstance(config, BertConfig)
-
- model = TFAutoModelForPreTraining.from_pretrained(model_name)
- self.assertIsNotNone(model)
- self.assertIsInstance(model, TFBertForPreTraining)
-
- @slow
- def test_lmhead_model_from_pretrained(self):
- logging.basicConfig(level=logging.INFO)
- # for model_name in list(TF_BERT_PRETRAINED_MODEL_ARCHIVE_MAP.keys())[:1]:
- for model_name in ["bert-base-uncased"]:
- config = AutoConfig.from_pretrained(model_name)
- self.assertIsNotNone(config)
- self.assertIsInstance(config, BertConfig)
-
- model = TFAutoModelWithLMHead.from_pretrained(model_name)
- self.assertIsNotNone(model)
- self.assertIsInstance(model, TFBertForMaskedLM)
-
- @slow
- def test_sequence_classification_model_from_pretrained(self):
- logging.basicConfig(level=logging.INFO)
- # for model_name in list(TF_BERT_PRETRAINED_MODEL_ARCHIVE_MAP.keys())[:1]:
- for model_name in ["bert-base-uncased"]:
- config = AutoConfig.from_pretrained(model_name)
- self.assertIsNotNone(config)
- self.assertIsInstance(config, BertConfig)
-
- model = TFAutoModelForSequenceClassification.from_pretrained(model_name)
- self.assertIsNotNone(model)
- self.assertIsInstance(model, TFBertForSequenceClassification)
-
- @slow
- def test_question_answering_model_from_pretrained(self):
- logging.basicConfig(level=logging.INFO)
- # for model_name in list(TF_BERT_PRETRAINED_MODEL_ARCHIVE_MAP.keys())[:1]:
- for model_name in ["bert-base-uncased"]:
- config = AutoConfig.from_pretrained(model_name)
- self.assertIsNotNone(config)
- self.assertIsInstance(config, BertConfig)
-
- model = TFAutoModelForQuestionAnswering.from_pretrained(model_name)
- self.assertIsNotNone(model)
- self.assertIsInstance(model, TFBertForQuestionAnswering)
-
- def test_from_pretrained_identifier(self):
- logging.basicConfig(level=logging.INFO)
- model = TFAutoModelWithLMHead.from_pretrained(SMALL_MODEL_IDENTIFIER)
- self.assertIsInstance(model, TFBertForMaskedLM)
- self.assertEqual(model.num_parameters(), 14830)
- self.assertEqual(model.num_parameters(only_trainable=True), 14830)
-
- def test_from_identifier_from_model_type(self):
- logging.basicConfig(level=logging.INFO)
- model = TFAutoModelWithLMHead.from_pretrained(DUMMY_UNKWOWN_IDENTIFIER)
- self.assertIsInstance(model, TFRobertaForMaskedLM)
- self.assertEqual(model.num_parameters(), 14830)
- self.assertEqual(model.num_parameters(only_trainable=True), 14830)
diff --git a/server/transformers/tests/test_modeling_tf_bert.py b/server/transformers/tests/test_modeling_tf_bert.py
deleted file mode 100644
index d91d4863afe42543d071eecc09864f1a0913ec80..0000000000000000000000000000000000000000
--- a/server/transformers/tests/test_modeling_tf_bert.py
+++ /dev/null
@@ -1,317 +0,0 @@
-# coding=utf-8
-# Copyright 2018 The Google AI Language Team Authors.
-#
-# Licensed under the Apache License, Version 2.0 (the "License");
-# you may not use this file except in compliance with the License.
-# You may obtain a copy of the License at
-#
-# http://www.apache.org/licenses/LICENSE-2.0
-#
-# Unless required by applicable law or agreed to in writing, software
-# distributed under the License is distributed on an "AS IS" BASIS,
-# WITHOUT WARRANTIES OR CONDITIONS OF ANY KIND, either express or implied.
-# See the License for the specific language governing permissions and
-# limitations under the License.
-
-
-import unittest
-
-from transformers import BertConfig, is_tf_available
-
-from .test_configuration_common import ConfigTester
-from .test_modeling_tf_common import TFModelTesterMixin, ids_tensor
-from .utils import CACHE_DIR, require_tf, slow
-
-
-if is_tf_available():
- import tensorflow as tf
- from transformers.modeling_tf_bert import (
- TFBertModel,
- TFBertForMaskedLM,
- TFBertForNextSentencePrediction,
- TFBertForPreTraining,
- TFBertForSequenceClassification,
- TFBertForMultipleChoice,
- TFBertForTokenClassification,
- TFBertForQuestionAnswering,
- )
-
-
-@require_tf
-class TFBertModelTest(TFModelTesterMixin, unittest.TestCase):
-
- all_model_classes = (
- (
- TFBertModel,
- TFBertForMaskedLM,
- TFBertForNextSentencePrediction,
- TFBertForPreTraining,
- TFBertForQuestionAnswering,
- TFBertForSequenceClassification,
- TFBertForTokenClassification,
- )
- if is_tf_available()
- else ()
- )
-
- class TFBertModelTester(object):
- def __init__(
- self,
- parent,
- batch_size=13,
- seq_length=7,
- is_training=True,
- use_input_mask=True,
- use_token_type_ids=True,
- use_labels=True,
- vocab_size=99,
- hidden_size=32,
- num_hidden_layers=5,
- num_attention_heads=4,
- intermediate_size=37,
- hidden_act="gelu",
- hidden_dropout_prob=0.1,
- attention_probs_dropout_prob=0.1,
- max_position_embeddings=512,
- type_vocab_size=16,
- type_sequence_label_size=2,
- initializer_range=0.02,
- num_labels=3,
- num_choices=4,
- scope=None,
- ):
- self.parent = parent
- self.batch_size = batch_size
- self.seq_length = seq_length
- self.is_training = is_training
- self.use_input_mask = use_input_mask
- self.use_token_type_ids = use_token_type_ids
- self.use_labels = use_labels
- self.vocab_size = vocab_size
- self.hidden_size = hidden_size
- self.num_hidden_layers = num_hidden_layers
- self.num_attention_heads = num_attention_heads
- self.intermediate_size = intermediate_size
- self.hidden_act = hidden_act
- self.hidden_dropout_prob = hidden_dropout_prob
- self.attention_probs_dropout_prob = attention_probs_dropout_prob
- self.max_position_embeddings = max_position_embeddings
- self.type_vocab_size = type_vocab_size
- self.type_sequence_label_size = type_sequence_label_size
- self.initializer_range = initializer_range
- self.num_labels = num_labels
- self.num_choices = num_choices
- self.scope = scope
-
- def prepare_config_and_inputs(self):
- input_ids = ids_tensor([self.batch_size, self.seq_length], self.vocab_size)
-
- input_mask = None
- if self.use_input_mask:
- input_mask = ids_tensor([self.batch_size, self.seq_length], vocab_size=2)
-
- token_type_ids = None
- if self.use_token_type_ids:
- token_type_ids = ids_tensor([self.batch_size, self.seq_length], self.type_vocab_size)
-
- sequence_labels = None
- token_labels = None
- choice_labels = None
- if self.use_labels:
- sequence_labels = ids_tensor([self.batch_size], self.type_sequence_label_size)
- token_labels = ids_tensor([self.batch_size, self.seq_length], self.num_labels)
- choice_labels = ids_tensor([self.batch_size], self.num_choices)
-
- config = BertConfig(
- vocab_size=self.vocab_size,
- hidden_size=self.hidden_size,
- num_hidden_layers=self.num_hidden_layers,
- num_attention_heads=self.num_attention_heads,
- intermediate_size=self.intermediate_size,
- hidden_act=self.hidden_act,
- hidden_dropout_prob=self.hidden_dropout_prob,
- attention_probs_dropout_prob=self.attention_probs_dropout_prob,
- max_position_embeddings=self.max_position_embeddings,
- type_vocab_size=self.type_vocab_size,
- initializer_range=self.initializer_range,
- )
-
- return config, input_ids, token_type_ids, input_mask, sequence_labels, token_labels, choice_labels
-
- def create_and_check_bert_model(
- self, config, input_ids, token_type_ids, input_mask, sequence_labels, token_labels, choice_labels
- ):
- model = TFBertModel(config=config)
- inputs = {"input_ids": input_ids, "attention_mask": input_mask, "token_type_ids": token_type_ids}
- sequence_output, pooled_output = model(inputs)
-
- inputs = [input_ids, input_mask]
- sequence_output, pooled_output = model(inputs)
-
- sequence_output, pooled_output = model(input_ids)
-
- result = {
- "sequence_output": sequence_output.numpy(),
- "pooled_output": pooled_output.numpy(),
- }
- self.parent.assertListEqual(
- list(result["sequence_output"].shape), [self.batch_size, self.seq_length, self.hidden_size]
- )
- self.parent.assertListEqual(list(result["pooled_output"].shape), [self.batch_size, self.hidden_size])
-
- def create_and_check_bert_for_masked_lm(
- self, config, input_ids, token_type_ids, input_mask, sequence_labels, token_labels, choice_labels
- ):
- model = TFBertForMaskedLM(config=config)
- inputs = {"input_ids": input_ids, "attention_mask": input_mask, "token_type_ids": token_type_ids}
- (prediction_scores,) = model(inputs)
- result = {
- "prediction_scores": prediction_scores.numpy(),
- }
- self.parent.assertListEqual(
- list(result["prediction_scores"].shape), [self.batch_size, self.seq_length, self.vocab_size]
- )
-
- def create_and_check_bert_for_next_sequence_prediction(
- self, config, input_ids, token_type_ids, input_mask, sequence_labels, token_labels, choice_labels
- ):
- model = TFBertForNextSentencePrediction(config=config)
- inputs = {"input_ids": input_ids, "attention_mask": input_mask, "token_type_ids": token_type_ids}
- (seq_relationship_score,) = model(inputs)
- result = {
- "seq_relationship_score": seq_relationship_score.numpy(),
- }
- self.parent.assertListEqual(list(result["seq_relationship_score"].shape), [self.batch_size, 2])
-
- def create_and_check_bert_for_pretraining(
- self, config, input_ids, token_type_ids, input_mask, sequence_labels, token_labels, choice_labels
- ):
- model = TFBertForPreTraining(config=config)
- inputs = {"input_ids": input_ids, "attention_mask": input_mask, "token_type_ids": token_type_ids}
- prediction_scores, seq_relationship_score = model(inputs)
- result = {
- "prediction_scores": prediction_scores.numpy(),
- "seq_relationship_score": seq_relationship_score.numpy(),
- }
- self.parent.assertListEqual(
- list(result["prediction_scores"].shape), [self.batch_size, self.seq_length, self.vocab_size]
- )
- self.parent.assertListEqual(list(result["seq_relationship_score"].shape), [self.batch_size, 2])
-
- def create_and_check_bert_for_sequence_classification(
- self, config, input_ids, token_type_ids, input_mask, sequence_labels, token_labels, choice_labels
- ):
- config.num_labels = self.num_labels
- model = TFBertForSequenceClassification(config=config)
- inputs = {"input_ids": input_ids, "attention_mask": input_mask, "token_type_ids": token_type_ids}
- (logits,) = model(inputs)
- result = {
- "logits": logits.numpy(),
- }
- self.parent.assertListEqual(list(result["logits"].shape), [self.batch_size, self.num_labels])
-
- def create_and_check_bert_for_multiple_choice(
- self, config, input_ids, token_type_ids, input_mask, sequence_labels, token_labels, choice_labels
- ):
- config.num_choices = self.num_choices
- model = TFBertForMultipleChoice(config=config)
- multiple_choice_inputs_ids = tf.tile(tf.expand_dims(input_ids, 1), (1, self.num_choices, 1))
- multiple_choice_input_mask = tf.tile(tf.expand_dims(input_mask, 1), (1, self.num_choices, 1))
- multiple_choice_token_type_ids = tf.tile(tf.expand_dims(token_type_ids, 1), (1, self.num_choices, 1))
- inputs = {
- "input_ids": multiple_choice_inputs_ids,
- "attention_mask": multiple_choice_input_mask,
- "token_type_ids": multiple_choice_token_type_ids,
- }
- (logits,) = model(inputs)
- result = {
- "logits": logits.numpy(),
- }
- self.parent.assertListEqual(list(result["logits"].shape), [self.batch_size, self.num_choices])
-
- def create_and_check_bert_for_token_classification(
- self, config, input_ids, token_type_ids, input_mask, sequence_labels, token_labels, choice_labels
- ):
- config.num_labels = self.num_labels
- model = TFBertForTokenClassification(config=config)
- inputs = {"input_ids": input_ids, "attention_mask": input_mask, "token_type_ids": token_type_ids}
- (logits,) = model(inputs)
- result = {
- "logits": logits.numpy(),
- }
- self.parent.assertListEqual(
- list(result["logits"].shape), [self.batch_size, self.seq_length, self.num_labels]
- )
-
- def create_and_check_bert_for_question_answering(
- self, config, input_ids, token_type_ids, input_mask, sequence_labels, token_labels, choice_labels
- ):
- model = TFBertForQuestionAnswering(config=config)
- inputs = {"input_ids": input_ids, "attention_mask": input_mask, "token_type_ids": token_type_ids}
- start_logits, end_logits = model(inputs)
- result = {
- "start_logits": start_logits.numpy(),
- "end_logits": end_logits.numpy(),
- }
- self.parent.assertListEqual(list(result["start_logits"].shape), [self.batch_size, self.seq_length])
- self.parent.assertListEqual(list(result["end_logits"].shape), [self.batch_size, self.seq_length])
-
- def prepare_config_and_inputs_for_common(self):
- config_and_inputs = self.prepare_config_and_inputs()
- (
- config,
- input_ids,
- token_type_ids,
- input_mask,
- sequence_labels,
- token_labels,
- choice_labels,
- ) = config_and_inputs
- inputs_dict = {"input_ids": input_ids, "token_type_ids": token_type_ids, "attention_mask": input_mask}
- return config, inputs_dict
-
- def setUp(self):
- self.model_tester = TFBertModelTest.TFBertModelTester(self)
- self.config_tester = ConfigTester(self, config_class=BertConfig, hidden_size=37)
-
- def test_config(self):
- self.config_tester.run_common_tests()
-
- def test_bert_model(self):
- config_and_inputs = self.model_tester.prepare_config_and_inputs()
- self.model_tester.create_and_check_bert_model(*config_and_inputs)
-
- def test_for_masked_lm(self):
- config_and_inputs = self.model_tester.prepare_config_and_inputs()
- self.model_tester.create_and_check_bert_for_masked_lm(*config_and_inputs)
-
- def test_for_multiple_choice(self):
- config_and_inputs = self.model_tester.prepare_config_and_inputs()
- self.model_tester.create_and_check_bert_for_multiple_choice(*config_and_inputs)
-
- def test_for_next_sequence_prediction(self):
- config_and_inputs = self.model_tester.prepare_config_and_inputs()
- self.model_tester.create_and_check_bert_for_next_sequence_prediction(*config_and_inputs)
-
- def test_for_pretraining(self):
- config_and_inputs = self.model_tester.prepare_config_and_inputs()
- self.model_tester.create_and_check_bert_for_pretraining(*config_and_inputs)
-
- def test_for_question_answering(self):
- config_and_inputs = self.model_tester.prepare_config_and_inputs()
- self.model_tester.create_and_check_bert_for_question_answering(*config_and_inputs)
-
- def test_for_sequence_classification(self):
- config_and_inputs = self.model_tester.prepare_config_and_inputs()
- self.model_tester.create_and_check_bert_for_sequence_classification(*config_and_inputs)
-
- def test_for_token_classification(self):
- config_and_inputs = self.model_tester.prepare_config_and_inputs()
- self.model_tester.create_and_check_bert_for_token_classification(*config_and_inputs)
-
- @slow
- def test_model_from_pretrained(self):
- # for model_name in list(TF_BERT_PRETRAINED_MODEL_ARCHIVE_MAP.keys())[:1]:
- for model_name in ["bert-base-uncased"]:
- model = TFBertModel.from_pretrained(model_name, cache_dir=CACHE_DIR)
- self.assertIsNotNone(model)
diff --git a/server/transformers/tests/test_modeling_tf_common.py b/server/transformers/tests/test_modeling_tf_common.py
deleted file mode 100644
index bcfb6bfe5d457e7dece90b8a6aada8a670bd9b58..0000000000000000000000000000000000000000
--- a/server/transformers/tests/test_modeling_tf_common.py
+++ /dev/null
@@ -1,373 +0,0 @@
-# coding=utf-8
-# Copyright 2019 HuggingFace Inc.
-#
-# Licensed under the Apache License, Version 2.0 (the "License");
-# you may not use this file except in compliance with the License.
-# You may obtain a copy of the License at
-#
-# http://www.apache.org/licenses/LICENSE-2.0
-#
-# Unless required by applicable law or agreed to in writing, software
-# distributed under the License is distributed on an "AS IS" BASIS,
-# WITHOUT WARRANTIES OR CONDITIONS OF ANY KIND, either express or implied.
-# See the License for the specific language governing permissions and
-# limitations under the License.
-
-
-import copy
-import os
-import random
-import tempfile
-
-from transformers import is_tf_available, is_torch_available
-
-from .utils import require_tf
-
-
-if is_tf_available():
- import tensorflow as tf
- import numpy as np
-
- # from transformers.modeling_bert import BertModel, BertConfig, BERT_PRETRAINED_MODEL_ARCHIVE_MAP
-
-
-def _config_zero_init(config):
- configs_no_init = copy.deepcopy(config)
- for key in configs_no_init.__dict__.keys():
- if "_range" in key or "_std" in key:
- setattr(configs_no_init, key, 0.0)
- return configs_no_init
-
-
-@require_tf
-class TFModelTesterMixin:
-
- model_tester = None
- all_model_classes = ()
- test_torchscript = True
- test_pruning = True
- test_resize_embeddings = True
- is_encoder_decoder = False
-
- def test_initialization(self):
- pass
- # config, inputs_dict = self.model_tester.prepare_config_and_inputs_for_common()
-
- # configs_no_init = _config_zero_init(config)
- # for model_class in self.all_model_classes:
- # model = model_class(config=configs_no_init)
- # for name, param in model.named_parameters():
- # if param.requires_grad:
- # self.assertIn(param.data.mean().item(), [0.0, 1.0],
- # msg="Parameter {} of model {} seems not properly initialized".format(name, model_class))
-
- def test_save_load(self):
- config, inputs_dict = self.model_tester.prepare_config_and_inputs_for_common()
-
- for model_class in self.all_model_classes:
- model = model_class(config)
- outputs = model(inputs_dict)
-
- with tempfile.TemporaryDirectory() as tmpdirname:
- model.save_pretrained(tmpdirname)
- model = model_class.from_pretrained(tmpdirname)
- after_outputs = model(inputs_dict)
-
- # Make sure we don't have nans
- out_1 = after_outputs[0].numpy()
- out_2 = outputs[0].numpy()
- out_1 = out_1[~np.isnan(out_1)]
- out_2 = out_2[~np.isnan(out_2)]
- max_diff = np.amax(np.abs(out_1 - out_2))
- self.assertLessEqual(max_diff, 1e-5)
-
- def test_pt_tf_model_equivalence(self):
- if not is_torch_available():
- return
-
- import torch
- import transformers
-
- config, inputs_dict = self.model_tester.prepare_config_and_inputs_for_common()
-
- for model_class in self.all_model_classes:
- pt_model_class_name = model_class.__name__[2:] # Skip the "TF" at the beggining
- pt_model_class = getattr(transformers, pt_model_class_name)
-
- config.output_hidden_states = True
- tf_model = model_class(config)
- pt_model = pt_model_class(config)
-
- # Check we can load pt model in tf and vice-versa with model => model functions
- tf_model = transformers.load_pytorch_model_in_tf2_model(tf_model, pt_model, tf_inputs=inputs_dict)
- pt_model = transformers.load_tf2_model_in_pytorch_model(pt_model, tf_model)
-
- # Check predictions on first output (logits/hidden-states) are close enought given low-level computational differences
- pt_model.eval()
- pt_inputs_dict = dict(
- (name, torch.from_numpy(key.numpy()).to(torch.long)) for name, key in inputs_dict.items()
- )
- with torch.no_grad():
- pto = pt_model(**pt_inputs_dict)
- tfo = tf_model(inputs_dict, training=False)
- tf_hidden_states = tfo[0].numpy()
- pt_hidden_states = pto[0].numpy()
-
- tf_nans = np.copy(np.isnan(tf_hidden_states))
- pt_nans = np.copy(np.isnan(pt_hidden_states))
-
- pt_hidden_states[tf_nans] = 0
- tf_hidden_states[tf_nans] = 0
- pt_hidden_states[pt_nans] = 0
- tf_hidden_states[pt_nans] = 0
-
- max_diff = np.amax(np.abs(tf_hidden_states - pt_hidden_states))
- # Debug info (remove when fixed)
- if max_diff >= 2e-2:
- print("===")
- print(model_class)
- print(config)
- print(inputs_dict)
- print(pt_inputs_dict)
- self.assertLessEqual(max_diff, 2e-2)
-
- # Check we can load pt model in tf and vice-versa with checkpoint => model functions
- with tempfile.TemporaryDirectory() as tmpdirname:
- pt_checkpoint_path = os.path.join(tmpdirname, "pt_model.bin")
- torch.save(pt_model.state_dict(), pt_checkpoint_path)
- tf_model = transformers.load_pytorch_checkpoint_in_tf2_model(tf_model, pt_checkpoint_path)
-
- tf_checkpoint_path = os.path.join(tmpdirname, "tf_model.h5")
- tf_model.save_weights(tf_checkpoint_path)
- pt_model = transformers.load_tf2_checkpoint_in_pytorch_model(pt_model, tf_checkpoint_path)
-
- # Check predictions on first output (logits/hidden-states) are close enought given low-level computational differences
- pt_model.eval()
- pt_inputs_dict = dict(
- (name, torch.from_numpy(key.numpy()).to(torch.long)) for name, key in inputs_dict.items()
- )
- with torch.no_grad():
- pto = pt_model(**pt_inputs_dict)
- tfo = tf_model(inputs_dict)
- tfo = tfo[0].numpy()
- pto = pto[0].numpy()
- tf_nans = np.copy(np.isnan(tfo))
- pt_nans = np.copy(np.isnan(pto))
-
- pto[tf_nans] = 0
- tfo[tf_nans] = 0
- pto[pt_nans] = 0
- tfo[pt_nans] = 0
-
- max_diff = np.amax(np.abs(tfo - pto))
- self.assertLessEqual(max_diff, 2e-2)
-
- def test_compile_tf_model(self):
- config, inputs_dict = self.model_tester.prepare_config_and_inputs_for_common()
-
- if self.is_encoder_decoder:
- input_ids = {
- "decoder_input_ids": tf.keras.Input(batch_shape=(2, 2000), name="decoder_input_ids", dtype="int32"),
- "encoder_input_ids": tf.keras.Input(batch_shape=(2, 2000), name="encoder_input_ids", dtype="int32"),
- }
- else:
- input_ids = tf.keras.Input(batch_shape=(2, 2000), name="input_ids", dtype="int32")
- optimizer = tf.keras.optimizers.Adam(learning_rate=3e-5, epsilon=1e-08, clipnorm=1.0)
- loss = tf.keras.losses.SparseCategoricalCrossentropy(from_logits=True)
- metric = tf.keras.metrics.SparseCategoricalAccuracy("accuracy")
-
- for model_class in self.all_model_classes:
- # Prepare our model
- model = model_class(config)
-
- # Let's load it from the disk to be sure we can use pretrained weights
- with tempfile.TemporaryDirectory() as tmpdirname:
- outputs = model(inputs_dict) # build the model
- model.save_pretrained(tmpdirname)
- model = model_class.from_pretrained(tmpdirname)
-
- outputs_dict = model(input_ids)
- hidden_states = outputs_dict[0]
-
- # Add a dense layer on top to test intetgration with other keras modules
- outputs = tf.keras.layers.Dense(2, activation="softmax", name="outputs")(hidden_states)
-
- # Compile extended model
- extended_model = tf.keras.Model(inputs=[input_ids], outputs=[outputs])
- extended_model.compile(optimizer=optimizer, loss=loss, metrics=[metric])
-
- def test_keyword_and_dict_args(self):
- config, inputs_dict = self.model_tester.prepare_config_and_inputs_for_common()
-
- for model_class in self.all_model_classes:
- model = model_class(config)
- outputs_dict = model(inputs_dict)
-
- inputs_keywords = copy.deepcopy(inputs_dict)
- input_ids = inputs_keywords.pop("input_ids" if not self.is_encoder_decoder else "decoder_input_ids", None)
- outputs_keywords = model(input_ids, **inputs_keywords)
-
- output_dict = outputs_dict[0].numpy()
- output_keywords = outputs_keywords[0].numpy()
-
- self.assertLess(np.sum(np.abs(output_dict - output_keywords)), 1e-6)
-
- def test_attention_outputs(self):
- config, inputs_dict = self.model_tester.prepare_config_and_inputs_for_common()
-
- decoder_seq_length = (
- self.model_tester.decoder_seq_length
- if hasattr(self.model_tester, "decoder_seq_length")
- else self.model_tester.seq_length
- )
- encoder_seq_length = (
- self.model_tester.encoder_seq_length
- if hasattr(self.model_tester, "encoder_seq_length")
- else self.model_tester.seq_length
- )
- decoder_key_length = (
- self.model_tester.key_length if hasattr(self.model_tester, "key_length") else decoder_seq_length
- )
- encoder_key_length = (
- self.model_tester.key_length if hasattr(self.model_tester, "key_length") else encoder_seq_length
- )
-
- for model_class in self.all_model_classes:
- config.output_attentions = True
- config.output_hidden_states = False
- model = model_class(config)
- outputs = model(inputs_dict)
- attentions = [t.numpy() for t in outputs[-1]]
- self.assertEqual(model.config.output_attentions, True)
- self.assertEqual(model.config.output_hidden_states, False)
- self.assertEqual(len(attentions), self.model_tester.num_hidden_layers)
- self.assertListEqual(
- list(attentions[0].shape[-3:]),
- [self.model_tester.num_attention_heads, encoder_seq_length, encoder_key_length],
- )
- out_len = len(outputs)
-
- if self.is_encoder_decoder:
- self.assertEqual(out_len % 2, 0)
- decoder_attentions = outputs[(out_len // 2) - 1]
- self.assertEqual(model.config.output_attentions, True)
- self.assertEqual(model.config.output_hidden_states, False)
- self.assertEqual(len(decoder_attentions), self.model_tester.num_hidden_layers)
- self.assertListEqual(
- list(decoder_attentions[0].shape[-3:]),
- [self.model_tester.num_attention_heads, decoder_seq_length, decoder_key_length],
- )
-
- # Check attention is always last and order is fine
- config.output_attentions = True
- config.output_hidden_states = True
- model = model_class(config)
- outputs = model(inputs_dict)
- self.assertEqual(out_len + (2 if self.is_encoder_decoder else 1), len(outputs))
- self.assertEqual(model.config.output_attentions, True)
- self.assertEqual(model.config.output_hidden_states, True)
-
- attentions = [t.numpy() for t in outputs[-1]]
- self.assertEqual(len(attentions), self.model_tester.num_hidden_layers)
- self.assertListEqual(
- list(attentions[0].shape[-3:]),
- [self.model_tester.num_attention_heads, encoder_seq_length, encoder_key_length],
- )
-
- def test_hidden_states_output(self):
- config, inputs_dict = self.model_tester.prepare_config_and_inputs_for_common()
-
- for model_class in self.all_model_classes:
- config.output_hidden_states = True
- config.output_attentions = False
- model = model_class(config)
- outputs = model(inputs_dict)
- hidden_states = [t.numpy() for t in outputs[-1]]
- self.assertEqual(model.config.output_attentions, False)
- self.assertEqual(model.config.output_hidden_states, True)
- self.assertEqual(len(hidden_states), self.model_tester.num_hidden_layers + 1)
- self.assertListEqual(
- list(hidden_states[0].shape[-2:]), [self.model_tester.seq_length, self.model_tester.hidden_size]
- )
-
- def test_model_common_attributes(self):
- config, inputs_dict = self.model_tester.prepare_config_and_inputs_for_common()
-
- for model_class in self.all_model_classes:
- model = model_class(config)
- assert isinstance(model.get_input_embeddings(), tf.keras.layers.Layer)
- x = model.get_output_embeddings()
- assert x is None or isinstance(x, tf.keras.layers.Layer)
-
- def test_determinism(self):
- config, inputs_dict = self.model_tester.prepare_config_and_inputs_for_common()
-
- for model_class in self.all_model_classes:
- model = model_class(config)
- first, second = model(inputs_dict, training=False)[0], model(inputs_dict, training=False)[0]
- out_1 = first.numpy()
- out_2 = second.numpy()
- out_1 = out_1[~np.isnan(out_1)]
- out_2 = out_2[~np.isnan(out_2)]
- max_diff = np.amax(np.abs(out_1 - out_2))
- self.assertLessEqual(max_diff, 1e-5)
-
- def _get_embeds(self, wte, input_ids):
- # ^^ In our TF models, the input_embeddings can take slightly different forms,
- # so we try a few of them.
- # We used to fall back to just synthetically creating a dummy tensor of ones:
- try:
- x = wte(input_ids, mode="embedding")
- except Exception:
- try:
- x = wte([input_ids], mode="embedding")
- except Exception:
- try:
- x = wte([input_ids, None, None, None], mode="embedding")
- except Exception:
- if hasattr(self.model_tester, "embedding_size"):
- x = tf.ones(input_ids.shape + [self.model_tester.embedding_size], dtype=tf.dtypes.float32)
- else:
- x = tf.ones(input_ids.shape + [self.model_tester.hidden_size], dtype=tf.dtypes.float32)
- return x
-
- def test_inputs_embeds(self):
- config, inputs_dict = self.model_tester.prepare_config_and_inputs_for_common()
- if not self.is_encoder_decoder:
- input_ids = inputs_dict["input_ids"]
- del inputs_dict["input_ids"]
- else:
- encoder_input_ids = inputs_dict["encoder_input_ids"]
- decoder_input_ids = inputs_dict["decoder_input_ids"]
- del inputs_dict["encoder_input_ids"]
- del inputs_dict["decoder_input_ids"]
-
- for model_class in self.all_model_classes:
- model = model_class(config)
-
- wte = model.get_input_embeddings()
- if not self.is_encoder_decoder:
- inputs_dict["inputs_embeds"] = self._get_embeds(wte, input_ids)
- else:
- inputs_dict["encoder_inputs_embeds"] = self._get_embeds(wte, encoder_input_ids)
- inputs_dict["decoder_inputs_embeds"] = self._get_embeds(wte, decoder_input_ids)
-
- model(inputs_dict)
-
-
-def ids_tensor(shape, vocab_size, rng=None, name=None, dtype=None):
- """Creates a random int32 tensor of the shape within the vocab size."""
- if rng is None:
- rng = random.Random()
-
- total_dims = 1
- for dim in shape:
- total_dims *= dim
-
- values = []
- for _ in range(total_dims):
- values.append(rng.randint(0, vocab_size - 1))
-
- output = tf.constant(values, shape=shape, dtype=dtype if dtype is not None else tf.int32)
-
- return output
diff --git a/server/transformers/tests/test_modeling_tf_ctrl.py b/server/transformers/tests/test_modeling_tf_ctrl.py
deleted file mode 100644
index 4997c2a573a12c87071ae4fbdf6aeec1c6ac9646..0000000000000000000000000000000000000000
--- a/server/transformers/tests/test_modeling_tf_ctrl.py
+++ /dev/null
@@ -1,203 +0,0 @@
-# coding=utf-8
-# Copyright 2018 The Google AI Language Team Authors.
-#
-# Licensed under the Apache License, Version 2.0 (the "License");
-# you may not use this file except in compliance with the License.
-# You may obtain a copy of the License at
-#
-# http://www.apache.org/licenses/LICENSE-2.0
-#
-# Unless required by applicable law or agreed to in writing, software
-# distributed under the License is distributed on an "AS IS" BASIS,
-# WITHOUT WARRANTIES OR CONDITIONS OF ANY KIND, either express or implied.
-# See the License for the specific language governing permissions and
-# limitations under the License.
-
-
-import unittest
-
-from transformers import CTRLConfig, is_tf_available
-
-from .test_configuration_common import ConfigTester
-from .test_modeling_tf_common import TFModelTesterMixin, ids_tensor
-from .utils import CACHE_DIR, require_tf, slow
-
-
-if is_tf_available():
- from transformers.modeling_tf_ctrl import TFCTRLModel, TFCTRLLMHeadModel, TF_CTRL_PRETRAINED_MODEL_ARCHIVE_MAP
-
-
-@require_tf
-class TFCTRLModelTest(TFModelTesterMixin, unittest.TestCase):
-
- all_model_classes = (TFCTRLModel, TFCTRLLMHeadModel) if is_tf_available() else ()
-
- class TFCTRLModelTester(object):
- def __init__(
- self,
- parent,
- batch_size=13,
- seq_length=7,
- is_training=True,
- use_token_type_ids=True,
- use_input_mask=True,
- use_labels=True,
- use_mc_token_ids=True,
- vocab_size=99,
- hidden_size=32,
- num_hidden_layers=5,
- num_attention_heads=4,
- intermediate_size=37,
- hidden_act="gelu",
- hidden_dropout_prob=0.1,
- attention_probs_dropout_prob=0.1,
- max_position_embeddings=512,
- type_vocab_size=16,
- type_sequence_label_size=2,
- initializer_range=0.02,
- num_labels=3,
- num_choices=4,
- scope=None,
- ):
- self.parent = parent
- self.batch_size = batch_size
- self.seq_length = seq_length
- self.is_training = is_training
- self.use_token_type_ids = use_token_type_ids
- self.use_input_mask = use_input_mask
- self.use_labels = use_labels
- self.use_mc_token_ids = use_mc_token_ids
- self.vocab_size = vocab_size
- self.hidden_size = hidden_size
- self.num_hidden_layers = num_hidden_layers
- self.num_attention_heads = num_attention_heads
- self.intermediate_size = intermediate_size
- self.hidden_act = hidden_act
- self.hidden_dropout_prob = hidden_dropout_prob
- self.attention_probs_dropout_prob = attention_probs_dropout_prob
- self.max_position_embeddings = max_position_embeddings
- self.type_vocab_size = type_vocab_size
- self.type_sequence_label_size = type_sequence_label_size
- self.initializer_range = initializer_range
- self.num_labels = num_labels
- self.num_choices = num_choices
- self.scope = scope
-
- def prepare_config_and_inputs(self):
- input_ids = ids_tensor([self.batch_size, self.seq_length], self.vocab_size)
-
- input_mask = None
- if self.use_input_mask:
- input_mask = ids_tensor([self.batch_size, self.seq_length], vocab_size=2)
-
- token_type_ids = None
- if self.use_token_type_ids:
- token_type_ids = ids_tensor([self.batch_size, self.seq_length], self.type_vocab_size)
-
- mc_token_ids = None
- if self.use_mc_token_ids:
- mc_token_ids = ids_tensor([self.batch_size, self.num_choices], self.seq_length)
-
- sequence_labels = None
- token_labels = None
- choice_labels = None
- if self.use_labels:
- sequence_labels = ids_tensor([self.batch_size], self.type_sequence_label_size)
- token_labels = ids_tensor([self.batch_size, self.seq_length], self.num_labels)
- choice_labels = ids_tensor([self.batch_size], self.num_choices)
-
- config = CTRLConfig(
- vocab_size=self.vocab_size,
- n_embd=self.hidden_size,
- n_layer=self.num_hidden_layers,
- n_head=self.num_attention_heads,
- # intermediate_size=self.intermediate_size,
- # hidden_act=self.hidden_act,
- # hidden_dropout_prob=self.hidden_dropout_prob,
- # attention_probs_dropout_prob=self.attention_probs_dropout_prob,
- n_positions=self.max_position_embeddings,
- n_ctx=self.max_position_embeddings
- # type_vocab_size=self.type_vocab_size,
- # initializer_range=self.initializer_range
- )
-
- head_mask = ids_tensor([self.num_hidden_layers, self.num_attention_heads], 2)
-
- return (
- config,
- input_ids,
- input_mask,
- head_mask,
- token_type_ids,
- mc_token_ids,
- sequence_labels,
- token_labels,
- choice_labels,
- )
-
- def create_and_check_ctrl_model(self, config, input_ids, input_mask, head_mask, token_type_ids, *args):
- model = TFCTRLModel(config=config)
- inputs = {"input_ids": input_ids, "attention_mask": input_mask, "token_type_ids": token_type_ids}
- sequence_output = model(inputs)[0]
-
- inputs = [input_ids, None, input_mask] # None is the input for 'past'
- sequence_output = model(inputs)[0]
-
- sequence_output = model(input_ids)[0]
-
- result = {
- "sequence_output": sequence_output.numpy(),
- }
- self.parent.assertListEqual(
- list(result["sequence_output"].shape), [self.batch_size, self.seq_length, self.hidden_size]
- )
-
- def create_and_check_ctrl_lm_head(self, config, input_ids, input_mask, head_mask, token_type_ids, *args):
- model = TFCTRLLMHeadModel(config=config)
- inputs = {"input_ids": input_ids, "attention_mask": input_mask, "token_type_ids": token_type_ids}
- prediction_scores = model(inputs)[0]
- result = {
- "prediction_scores": prediction_scores.numpy(),
- }
- self.parent.assertListEqual(
- list(result["prediction_scores"].shape), [self.batch_size, self.seq_length, self.vocab_size]
- )
-
- def prepare_config_and_inputs_for_common(self):
- config_and_inputs = self.prepare_config_and_inputs()
-
- (
- config,
- input_ids,
- input_mask,
- head_mask,
- token_type_ids,
- mc_token_ids,
- sequence_labels,
- token_labels,
- choice_labels,
- ) = config_and_inputs
-
- inputs_dict = {"input_ids": input_ids, "token_type_ids": token_type_ids, "attention_mask": input_mask}
- return config, inputs_dict
-
- def setUp(self):
- self.model_tester = TFCTRLModelTest.TFCTRLModelTester(self)
- self.config_tester = ConfigTester(self, config_class=CTRLConfig, n_embd=37)
-
- def test_config(self):
- self.config_tester.run_common_tests()
-
- def test_ctrl_model(self):
- config_and_inputs = self.model_tester.prepare_config_and_inputs()
- self.model_tester.create_and_check_ctrl_model(*config_and_inputs)
-
- def test_ctrl_lm_head(self):
- config_and_inputs = self.model_tester.prepare_config_and_inputs()
- self.model_tester.create_and_check_ctrl_lm_head(*config_and_inputs)
-
- @slow
- def test_model_from_pretrained(self):
- for model_name in list(TF_CTRL_PRETRAINED_MODEL_ARCHIVE_MAP.keys())[:1]:
- model = TFCTRLModel.from_pretrained(model_name, cache_dir=CACHE_DIR)
- self.assertIsNotNone(model)
diff --git a/server/transformers/tests/test_modeling_tf_distilbert.py b/server/transformers/tests/test_modeling_tf_distilbert.py
deleted file mode 100644
index 5546e7a5b850412a82c14332da37b041b1e3adac..0000000000000000000000000000000000000000
--- a/server/transformers/tests/test_modeling_tf_distilbert.py
+++ /dev/null
@@ -1,223 +0,0 @@
-# coding=utf-8
-# Copyright 2018 The Google AI Language Team Authors.
-#
-# Licensed under the Apache License, Version 2.0 (the "License");
-# you may not use this file except in compliance with the License.
-# You may obtain a copy of the License at
-#
-# http://www.apache.org/licenses/LICENSE-2.0
-#
-# Unless required by applicable law or agreed to in writing, software
-# distributed under the License is distributed on an "AS IS" BASIS,
-# WITHOUT WARRANTIES OR CONDITIONS OF ANY KIND, either express or implied.
-# See the License for the specific language governing permissions and
-# limitations under the License.
-
-
-import unittest
-
-from transformers import DistilBertConfig, is_tf_available
-
-from .test_configuration_common import ConfigTester
-from .test_modeling_tf_common import TFModelTesterMixin, ids_tensor
-from .utils import require_tf
-
-
-if is_tf_available():
- from transformers.modeling_tf_distilbert import (
- TFDistilBertModel,
- TFDistilBertForMaskedLM,
- TFDistilBertForQuestionAnswering,
- TFDistilBertForSequenceClassification,
- )
-
-
-@require_tf
-class TFDistilBertModelTest(TFModelTesterMixin, unittest.TestCase):
-
- all_model_classes = (
- (
- TFDistilBertModel,
- TFDistilBertForMaskedLM,
- TFDistilBertForQuestionAnswering,
- TFDistilBertForSequenceClassification,
- )
- if is_tf_available()
- else None
- )
- test_pruning = True
- test_torchscript = True
- test_resize_embeddings = True
- test_head_masking = True
-
- class TFDistilBertModelTester(object):
- def __init__(
- self,
- parent,
- batch_size=13,
- seq_length=7,
- is_training=True,
- use_input_mask=True,
- use_token_type_ids=False,
- use_labels=True,
- vocab_size=99,
- hidden_size=32,
- num_hidden_layers=5,
- num_attention_heads=4,
- intermediate_size=37,
- hidden_act="gelu",
- hidden_dropout_prob=0.1,
- attention_probs_dropout_prob=0.1,
- max_position_embeddings=512,
- type_vocab_size=16,
- type_sequence_label_size=2,
- initializer_range=0.02,
- num_labels=3,
- num_choices=4,
- scope=None,
- ):
- self.parent = parent
- self.batch_size = batch_size
- self.seq_length = seq_length
- self.is_training = is_training
- self.use_input_mask = use_input_mask
- self.use_token_type_ids = use_token_type_ids
- self.use_labels = use_labels
- self.vocab_size = vocab_size
- self.hidden_size = hidden_size
- self.num_hidden_layers = num_hidden_layers
- self.num_attention_heads = num_attention_heads
- self.intermediate_size = intermediate_size
- self.hidden_act = hidden_act
- self.hidden_dropout_prob = hidden_dropout_prob
- self.attention_probs_dropout_prob = attention_probs_dropout_prob
- self.max_position_embeddings = max_position_embeddings
- self.type_vocab_size = type_vocab_size
- self.type_sequence_label_size = type_sequence_label_size
- self.initializer_range = initializer_range
- self.num_labels = num_labels
- self.num_choices = num_choices
- self.scope = scope
-
- def prepare_config_and_inputs(self):
- input_ids = ids_tensor([self.batch_size, self.seq_length], self.vocab_size)
-
- input_mask = None
- if self.use_input_mask:
- input_mask = ids_tensor([self.batch_size, self.seq_length], vocab_size=2)
-
- sequence_labels = None
- token_labels = None
- choice_labels = None
- if self.use_labels:
- sequence_labels = ids_tensor([self.batch_size], self.type_sequence_label_size)
- token_labels = ids_tensor([self.batch_size, self.seq_length], self.num_labels)
- choice_labels = ids_tensor([self.batch_size], self.num_choices)
-
- config = DistilBertConfig(
- vocab_size=self.vocab_size,
- dim=self.hidden_size,
- n_layers=self.num_hidden_layers,
- n_heads=self.num_attention_heads,
- hidden_dim=self.intermediate_size,
- hidden_act=self.hidden_act,
- dropout=self.hidden_dropout_prob,
- attention_dropout=self.attention_probs_dropout_prob,
- max_position_embeddings=self.max_position_embeddings,
- initializer_range=self.initializer_range,
- )
-
- return config, input_ids, input_mask, sequence_labels, token_labels, choice_labels
-
- def create_and_check_distilbert_model(
- self, config, input_ids, input_mask, sequence_labels, token_labels, choice_labels
- ):
- model = TFDistilBertModel(config=config)
- inputs = {"input_ids": input_ids, "attention_mask": input_mask}
-
- outputs = model(inputs)
- sequence_output = outputs[0]
-
- inputs = [input_ids, input_mask]
-
- (sequence_output,) = model(inputs)
-
- result = {
- "sequence_output": sequence_output.numpy(),
- }
- self.parent.assertListEqual(
- list(result["sequence_output"].shape), [self.batch_size, self.seq_length, self.hidden_size]
- )
-
- def create_and_check_distilbert_for_masked_lm(
- self, config, input_ids, input_mask, sequence_labels, token_labels, choice_labels
- ):
- model = TFDistilBertForMaskedLM(config=config)
- inputs = {"input_ids": input_ids, "attention_mask": input_mask}
- (prediction_scores,) = model(inputs)
- result = {
- "prediction_scores": prediction_scores.numpy(),
- }
- self.parent.assertListEqual(
- list(result["prediction_scores"].shape), [self.batch_size, self.seq_length, self.vocab_size]
- )
-
- def create_and_check_distilbert_for_question_answering(
- self, config, input_ids, input_mask, sequence_labels, token_labels, choice_labels
- ):
- model = TFDistilBertForQuestionAnswering(config=config)
- inputs = {"input_ids": input_ids, "attention_mask": input_mask}
- start_logits, end_logits = model(inputs)
- result = {
- "start_logits": start_logits.numpy(),
- "end_logits": end_logits.numpy(),
- }
- self.parent.assertListEqual(list(result["start_logits"].shape), [self.batch_size, self.seq_length])
- self.parent.assertListEqual(list(result["end_logits"].shape), [self.batch_size, self.seq_length])
-
- def create_and_check_distilbert_for_sequence_classification(
- self, config, input_ids, input_mask, sequence_labels, token_labels, choice_labels
- ):
- config.num_labels = self.num_labels
- model = TFDistilBertForSequenceClassification(config)
- inputs = {"input_ids": input_ids, "attention_mask": input_mask}
- (logits,) = model(inputs)
- result = {
- "logits": logits.numpy(),
- }
- self.parent.assertListEqual(list(result["logits"].shape), [self.batch_size, self.num_labels])
-
- def prepare_config_and_inputs_for_common(self):
- config_and_inputs = self.prepare_config_and_inputs()
- (config, input_ids, input_mask, sequence_labels, token_labels, choice_labels) = config_and_inputs
- inputs_dict = {"input_ids": input_ids, "attention_mask": input_mask}
- return config, inputs_dict
-
- def setUp(self):
- self.model_tester = TFDistilBertModelTest.TFDistilBertModelTester(self)
- self.config_tester = ConfigTester(self, config_class=DistilBertConfig, dim=37)
-
- def test_config(self):
- self.config_tester.run_common_tests()
-
- def test_distilbert_model(self):
- config_and_inputs = self.model_tester.prepare_config_and_inputs()
- self.model_tester.create_and_check_distilbert_model(*config_and_inputs)
-
- def test_for_masked_lm(self):
- config_and_inputs = self.model_tester.prepare_config_and_inputs()
- self.model_tester.create_and_check_distilbert_for_masked_lm(*config_and_inputs)
-
- def test_for_question_answering(self):
- config_and_inputs = self.model_tester.prepare_config_and_inputs()
- self.model_tester.create_and_check_distilbert_for_question_answering(*config_and_inputs)
-
- def test_for_sequence_classification(self):
- config_and_inputs = self.model_tester.prepare_config_and_inputs()
- self.model_tester.create_and_check_distilbert_for_sequence_classification(*config_and_inputs)
-
- # @slow
- # def test_model_from_pretrained(self):
- # for model_name in list(DISTILBERT_PRETRAINED_MODEL_ARCHIVE_MAP.keys())[:1]:
- # model = DistilBertModesss.from_pretrained(model_name, cache_dir=CACHE_DIR)
- # self.assertIsNotNone(model)
diff --git a/server/transformers/tests/test_modeling_tf_gpt2.py b/server/transformers/tests/test_modeling_tf_gpt2.py
deleted file mode 100644
index d7b0809964799f35eebe87144dddf4d7e01b0960..0000000000000000000000000000000000000000
--- a/server/transformers/tests/test_modeling_tf_gpt2.py
+++ /dev/null
@@ -1,236 +0,0 @@
-# coding=utf-8
-# Copyright 2018 The Google AI Language Team Authors.
-#
-# Licensed under the Apache License, Version 2.0 (the "License");
-# you may not use this file except in compliance with the License.
-# You may obtain a copy of the License at
-#
-# http://www.apache.org/licenses/LICENSE-2.0
-#
-# Unless required by applicable law or agreed to in writing, software
-# distributed under the License is distributed on an "AS IS" BASIS,
-# WITHOUT WARRANTIES OR CONDITIONS OF ANY KIND, either express or implied.
-# See the License for the specific language governing permissions and
-# limitations under the License.
-
-
-import unittest
-
-from transformers import GPT2Config, is_tf_available
-
-from .test_configuration_common import ConfigTester
-from .test_modeling_tf_common import TFModelTesterMixin, ids_tensor
-from .utils import CACHE_DIR, require_tf, slow
-
-
-if is_tf_available():
- import tensorflow as tf
- from transformers.modeling_tf_gpt2 import (
- TFGPT2Model,
- TFGPT2LMHeadModel,
- TFGPT2DoubleHeadsModel,
- TF_GPT2_PRETRAINED_MODEL_ARCHIVE_MAP,
- )
-
-
-@require_tf
-class TFGPT2ModelTest(TFModelTesterMixin, unittest.TestCase):
-
- all_model_classes = (TFGPT2Model, TFGPT2LMHeadModel, TFGPT2DoubleHeadsModel) if is_tf_available() else ()
- # all_model_classes = (TFGPT2Model, TFGPT2LMHeadModel) if is_tf_available() else ()
-
- class TFGPT2ModelTester(object):
- def __init__(
- self,
- parent,
- batch_size=13,
- seq_length=7,
- is_training=True,
- use_token_type_ids=True,
- use_input_mask=True,
- use_labels=True,
- use_mc_token_ids=True,
- vocab_size=99,
- hidden_size=32,
- num_hidden_layers=5,
- num_attention_heads=4,
- intermediate_size=37,
- hidden_act="gelu",
- hidden_dropout_prob=0.1,
- attention_probs_dropout_prob=0.1,
- max_position_embeddings=512,
- type_vocab_size=16,
- type_sequence_label_size=2,
- initializer_range=0.02,
- num_labels=3,
- num_choices=4,
- scope=None,
- ):
- self.parent = parent
- self.batch_size = batch_size
- self.seq_length = seq_length
- self.is_training = is_training
- self.use_token_type_ids = use_token_type_ids
- self.use_input_mask = use_input_mask
- self.use_labels = use_labels
- self.use_mc_token_ids = use_mc_token_ids
- self.vocab_size = vocab_size
- self.hidden_size = hidden_size
- self.num_hidden_layers = num_hidden_layers
- self.num_attention_heads = num_attention_heads
- self.intermediate_size = intermediate_size
- self.hidden_act = hidden_act
- self.hidden_dropout_prob = hidden_dropout_prob
- self.attention_probs_dropout_prob = attention_probs_dropout_prob
- self.max_position_embeddings = max_position_embeddings
- self.type_vocab_size = type_vocab_size
- self.type_sequence_label_size = type_sequence_label_size
- self.initializer_range = initializer_range
- self.num_labels = num_labels
- self.num_choices = num_choices
- self.scope = scope
-
- def prepare_config_and_inputs(self):
- input_ids = ids_tensor([self.batch_size, self.seq_length], self.vocab_size)
-
- input_mask = None
- if self.use_input_mask:
- input_mask = ids_tensor([self.batch_size, self.seq_length], vocab_size=2)
-
- token_type_ids = None
- if self.use_token_type_ids:
- token_type_ids = ids_tensor([self.batch_size, self.seq_length], self.type_vocab_size)
-
- mc_token_ids = None
- if self.use_mc_token_ids:
- mc_token_ids = ids_tensor([self.batch_size, self.num_choices], self.seq_length)
-
- sequence_labels = None
- token_labels = None
- choice_labels = None
- if self.use_labels:
- sequence_labels = ids_tensor([self.batch_size], self.type_sequence_label_size)
- token_labels = ids_tensor([self.batch_size, self.seq_length], self.num_labels)
- choice_labels = ids_tensor([self.batch_size], self.num_choices)
-
- config = GPT2Config(
- vocab_size=self.vocab_size,
- n_embd=self.hidden_size,
- n_layer=self.num_hidden_layers,
- n_head=self.num_attention_heads,
- # intermediate_size=self.intermediate_size,
- # hidden_act=self.hidden_act,
- # hidden_dropout_prob=self.hidden_dropout_prob,
- # attention_probs_dropout_prob=self.attention_probs_dropout_prob,
- n_positions=self.max_position_embeddings,
- n_ctx=self.max_position_embeddings
- # type_vocab_size=self.type_vocab_size,
- # initializer_range=self.initializer_range
- )
-
- head_mask = ids_tensor([self.num_hidden_layers, self.num_attention_heads], 2)
-
- return (
- config,
- input_ids,
- input_mask,
- head_mask,
- token_type_ids,
- mc_token_ids,
- sequence_labels,
- token_labels,
- choice_labels,
- )
-
- def create_and_check_gpt2_model(self, config, input_ids, input_mask, head_mask, token_type_ids, *args):
- model = TFGPT2Model(config=config)
- inputs = {"input_ids": input_ids, "attention_mask": input_mask, "token_type_ids": token_type_ids}
- sequence_output = model(inputs)[0]
-
- inputs = [input_ids, None, input_mask] # None is the input for 'past'
- sequence_output = model(inputs)[0]
-
- sequence_output = model(input_ids)[0]
-
- result = {
- "sequence_output": sequence_output.numpy(),
- }
- self.parent.assertListEqual(
- list(result["sequence_output"].shape), [self.batch_size, self.seq_length, self.hidden_size]
- )
-
- def create_and_check_gpt2_lm_head(self, config, input_ids, input_mask, head_mask, token_type_ids, *args):
- model = TFGPT2LMHeadModel(config=config)
- inputs = {"input_ids": input_ids, "attention_mask": input_mask, "token_type_ids": token_type_ids}
- prediction_scores = model(inputs)[0]
- result = {
- "prediction_scores": prediction_scores.numpy(),
- }
- self.parent.assertListEqual(
- list(result["prediction_scores"].shape), [self.batch_size, self.seq_length, self.vocab_size]
- )
-
- def create_and_check_gpt2_double_head(
- self, config, input_ids, input_mask, head_mask, token_type_ids, mc_token_ids, *args
- ):
- model = TFGPT2DoubleHeadsModel(config=config)
-
- multiple_choice_inputs_ids = tf.tile(tf.expand_dims(input_ids, 1), (1, self.num_choices, 1))
- multiple_choice_input_mask = tf.tile(tf.expand_dims(input_mask, 1), (1, self.num_choices, 1))
- multiple_choice_token_type_ids = tf.tile(tf.expand_dims(token_type_ids, 1), (1, self.num_choices, 1))
-
- inputs = {
- "input_ids": multiple_choice_inputs_ids,
- "mc_token_ids": mc_token_ids,
- "attention_mask": multiple_choice_input_mask,
- "token_type_ids": multiple_choice_token_type_ids,
- }
- lm_logits, mc_logits = model(inputs)[:2]
- result = {"lm_logits": lm_logits.numpy(), "mc_logits": mc_logits.numpy()}
- self.parent.assertListEqual(
- list(result["lm_logits"].shape), [self.batch_size, self.num_choices, self.seq_length, self.vocab_size]
- )
- self.parent.assertListEqual(list(result["mc_logits"].shape), [self.batch_size, self.num_choices])
-
- def prepare_config_and_inputs_for_common(self):
- config_and_inputs = self.prepare_config_and_inputs()
-
- (
- config,
- input_ids,
- input_mask,
- head_mask,
- token_type_ids,
- mc_token_ids,
- sequence_labels,
- token_labels,
- choice_labels,
- ) = config_and_inputs
-
- inputs_dict = {"input_ids": input_ids, "token_type_ids": token_type_ids, "attention_mask": input_mask}
- return config, inputs_dict
-
- def setUp(self):
- self.model_tester = TFGPT2ModelTest.TFGPT2ModelTester(self)
- self.config_tester = ConfigTester(self, config_class=GPT2Config, n_embd=37)
-
- def test_config(self):
- self.config_tester.run_common_tests()
-
- def test_gpt2_model(self):
- config_and_inputs = self.model_tester.prepare_config_and_inputs()
- self.model_tester.create_and_check_gpt2_model(*config_and_inputs)
-
- def test_gpt2_lm_head(self):
- config_and_inputs = self.model_tester.prepare_config_and_inputs()
- self.model_tester.create_and_check_gpt2_lm_head(*config_and_inputs)
-
- def test_gpt2_double_head(self):
- config_and_inputs = self.model_tester.prepare_config_and_inputs()
- self.model_tester.create_and_check_gpt2_double_head(*config_and_inputs)
-
- @slow
- def test_model_from_pretrained(self):
- for model_name in list(TF_GPT2_PRETRAINED_MODEL_ARCHIVE_MAP.keys())[:1]:
- model = TFGPT2Model.from_pretrained(model_name, cache_dir=CACHE_DIR)
- self.assertIsNotNone(model)
diff --git a/server/transformers/tests/test_modeling_tf_openai_gpt.py b/server/transformers/tests/test_modeling_tf_openai_gpt.py
deleted file mode 100644
index b825c94fca27aeb4c598ba1ea08bb55bb6cfef96..0000000000000000000000000000000000000000
--- a/server/transformers/tests/test_modeling_tf_openai_gpt.py
+++ /dev/null
@@ -1,237 +0,0 @@
-# coding=utf-8
-# Copyright 2018 The Google AI Language Team Authors.
-#
-# Licensed under the Apache License, Version 2.0 (the "License");
-# you may not use this file except in compliance with the License.
-# You may obtain a copy of the License at
-#
-# http://www.apache.org/licenses/LICENSE-2.0
-#
-# Unless required by applicable law or agreed to in writing, software
-# distributed under the License is distributed on an "AS IS" BASIS,
-# WITHOUT WARRANTIES OR CONDITIONS OF ANY KIND, either express or implied.
-# See the License for the specific language governing permissions and
-# limitations under the License.
-
-
-import unittest
-
-from transformers import OpenAIGPTConfig, is_tf_available
-
-from .test_configuration_common import ConfigTester
-from .test_modeling_tf_common import TFModelTesterMixin, ids_tensor
-from .utils import CACHE_DIR, require_tf, slow
-
-
-if is_tf_available():
- import tensorflow as tf
- from transformers.modeling_tf_openai import (
- TFOpenAIGPTModel,
- TFOpenAIGPTLMHeadModel,
- TFOpenAIGPTDoubleHeadsModel,
- TF_OPENAI_GPT_PRETRAINED_MODEL_ARCHIVE_MAP,
- )
-
-
-@require_tf
-class TFOpenAIGPTModelTest(TFModelTesterMixin, unittest.TestCase):
-
- all_model_classes = (
- (TFOpenAIGPTModel, TFOpenAIGPTLMHeadModel, TFOpenAIGPTDoubleHeadsModel) if is_tf_available() else ()
- )
-
- class TFOpenAIGPTModelTester(object):
- def __init__(
- self,
- parent,
- batch_size=13,
- seq_length=7,
- is_training=True,
- use_token_type_ids=True,
- use_input_mask=True,
- use_labels=True,
- use_mc_token_ids=True,
- vocab_size=99,
- hidden_size=32,
- num_hidden_layers=5,
- num_attention_heads=4,
- intermediate_size=37,
- hidden_act="gelu",
- hidden_dropout_prob=0.1,
- attention_probs_dropout_prob=0.1,
- max_position_embeddings=512,
- type_vocab_size=16,
- type_sequence_label_size=2,
- initializer_range=0.02,
- num_labels=3,
- num_choices=4,
- scope=None,
- ):
- self.parent = parent
- self.batch_size = batch_size
- self.seq_length = seq_length
- self.is_training = is_training
- self.use_token_type_ids = use_token_type_ids
- self.use_input_mask = use_input_mask
- self.use_labels = use_labels
- self.use_mc_token_ids = use_mc_token_ids
- self.vocab_size = vocab_size
- self.hidden_size = hidden_size
- self.num_hidden_layers = num_hidden_layers
- self.num_attention_heads = num_attention_heads
- self.intermediate_size = intermediate_size
- self.hidden_act = hidden_act
- self.hidden_dropout_prob = hidden_dropout_prob
- self.attention_probs_dropout_prob = attention_probs_dropout_prob
- self.max_position_embeddings = max_position_embeddings
- self.type_vocab_size = type_vocab_size
- self.type_sequence_label_size = type_sequence_label_size
- self.initializer_range = initializer_range
- self.num_labels = num_labels
- self.num_choices = num_choices
- self.scope = scope
-
- def prepare_config_and_inputs(self):
- input_ids = ids_tensor([self.batch_size, self.seq_length], self.vocab_size)
-
- input_mask = None
- if self.use_input_mask:
- input_mask = ids_tensor([self.batch_size, self.seq_length], vocab_size=2)
-
- token_type_ids = None
- if self.use_token_type_ids:
- token_type_ids = ids_tensor([self.batch_size, self.seq_length], self.type_vocab_size)
-
- mc_token_ids = None
- if self.use_mc_token_ids:
- mc_token_ids = ids_tensor([self.batch_size, self.num_choices], self.seq_length)
-
- sequence_labels = None
- token_labels = None
- choice_labels = None
- if self.use_labels:
- sequence_labels = ids_tensor([self.batch_size], self.type_sequence_label_size)
- token_labels = ids_tensor([self.batch_size, self.seq_length], self.num_labels)
- choice_labels = ids_tensor([self.batch_size], self.num_choices)
-
- config = OpenAIGPTConfig(
- vocab_size=self.vocab_size,
- n_embd=self.hidden_size,
- n_layer=self.num_hidden_layers,
- n_head=self.num_attention_heads,
- # intermediate_size=self.intermediate_size,
- # hidden_act=self.hidden_act,
- # hidden_dropout_prob=self.hidden_dropout_prob,
- # attention_probs_dropout_prob=self.attention_probs_dropout_prob,
- n_positions=self.max_position_embeddings,
- n_ctx=self.max_position_embeddings
- # type_vocab_size=self.type_vocab_size,
- # initializer_range=self.initializer_range
- )
-
- head_mask = ids_tensor([self.num_hidden_layers, self.num_attention_heads], 2)
-
- return (
- config,
- input_ids,
- input_mask,
- head_mask,
- token_type_ids,
- mc_token_ids,
- sequence_labels,
- token_labels,
- choice_labels,
- )
-
- def create_and_check_openai_gpt_model(self, config, input_ids, input_mask, head_mask, token_type_ids, *args):
- model = TFOpenAIGPTModel(config=config)
- inputs = {"input_ids": input_ids, "attention_mask": input_mask, "token_type_ids": token_type_ids}
- sequence_output = model(inputs)[0]
-
- inputs = [input_ids, input_mask]
- sequence_output = model(inputs)[0]
-
- sequence_output = model(input_ids)[0]
-
- result = {
- "sequence_output": sequence_output.numpy(),
- }
- self.parent.assertListEqual(
- list(result["sequence_output"].shape), [self.batch_size, self.seq_length, self.hidden_size]
- )
-
- def create_and_check_openai_gpt_lm_head(self, config, input_ids, input_mask, head_mask, token_type_ids, *args):
- model = TFOpenAIGPTLMHeadModel(config=config)
- inputs = {"input_ids": input_ids, "attention_mask": input_mask, "token_type_ids": token_type_ids}
- prediction_scores = model(inputs)[0]
- result = {
- "prediction_scores": prediction_scores.numpy(),
- }
- self.parent.assertListEqual(
- list(result["prediction_scores"].shape), [self.batch_size, self.seq_length, self.vocab_size]
- )
-
- def create_and_check_openai_gpt_double_head(
- self, config, input_ids, input_mask, head_mask, token_type_ids, mc_token_ids, *args
- ):
- model = TFOpenAIGPTDoubleHeadsModel(config=config)
-
- multiple_choice_inputs_ids = tf.tile(tf.expand_dims(input_ids, 1), (1, self.num_choices, 1))
- multiple_choice_input_mask = tf.tile(tf.expand_dims(input_mask, 1), (1, self.num_choices, 1))
- multiple_choice_token_type_ids = tf.tile(tf.expand_dims(token_type_ids, 1), (1, self.num_choices, 1))
-
- inputs = {
- "input_ids": multiple_choice_inputs_ids,
- "mc_token_ids": mc_token_ids,
- "attention_mask": multiple_choice_input_mask,
- "token_type_ids": multiple_choice_token_type_ids,
- }
- lm_logits, mc_logits = model(inputs)[:2]
- result = {"lm_logits": lm_logits.numpy(), "mc_logits": mc_logits.numpy()}
- self.parent.assertListEqual(
- list(result["lm_logits"].shape), [self.batch_size, self.num_choices, self.seq_length, self.vocab_size]
- )
- self.parent.assertListEqual(list(result["mc_logits"].shape), [self.batch_size, self.num_choices])
-
- def prepare_config_and_inputs_for_common(self):
- config_and_inputs = self.prepare_config_and_inputs()
-
- (
- config,
- input_ids,
- input_mask,
- head_mask,
- token_type_ids,
- mc_token_ids,
- sequence_labels,
- token_labels,
- choice_labels,
- ) = config_and_inputs
-
- inputs_dict = {"input_ids": input_ids, "token_type_ids": token_type_ids, "attention_mask": input_mask}
- return config, inputs_dict
-
- def setUp(self):
- self.model_tester = TFOpenAIGPTModelTest.TFOpenAIGPTModelTester(self)
- self.config_tester = ConfigTester(self, config_class=OpenAIGPTConfig, n_embd=37)
-
- def test_config(self):
- self.config_tester.run_common_tests()
-
- def test_openai_gpt_model(self):
- config_and_inputs = self.model_tester.prepare_config_and_inputs()
- self.model_tester.create_and_check_openai_gpt_model(*config_and_inputs)
-
- def test_openai_gpt_lm_head(self):
- config_and_inputs = self.model_tester.prepare_config_and_inputs()
- self.model_tester.create_and_check_openai_gpt_lm_head(*config_and_inputs)
-
- def test_openai_gpt_double_head(self):
- config_and_inputs = self.model_tester.prepare_config_and_inputs()
- self.model_tester.create_and_check_openai_gpt_double_head(*config_and_inputs)
-
- @slow
- def test_model_from_pretrained(self):
- for model_name in list(TF_OPENAI_GPT_PRETRAINED_MODEL_ARCHIVE_MAP.keys())[:1]:
- model = TFOpenAIGPTModel.from_pretrained(model_name, cache_dir=CACHE_DIR)
- self.assertIsNotNone(model)
diff --git a/server/transformers/tests/test_modeling_tf_roberta.py b/server/transformers/tests/test_modeling_tf_roberta.py
deleted file mode 100644
index 21b0ffee0e8069b9aa56afad5678d870445aea41..0000000000000000000000000000000000000000
--- a/server/transformers/tests/test_modeling_tf_roberta.py
+++ /dev/null
@@ -1,246 +0,0 @@
-# coding=utf-8
-# Copyright 2018 The Google AI Language Team Authors.
-#
-# Licensed under the Apache License, Version 2.0 (the "License");
-# you may not use this file except in compliance with the License.
-# You may obtain a copy of the License at
-#
-# http://www.apache.org/licenses/LICENSE-2.0
-#
-# Unless required by applicable law or agreed to in writing, software
-# distributed under the License is distributed on an "AS IS" BASIS,
-# WITHOUT WARRANTIES OR CONDITIONS OF ANY KIND, either express or implied.
-# See the License for the specific language governing permissions and
-# limitations under the License.
-
-
-import unittest
-
-from transformers import RobertaConfig, is_tf_available
-
-from .test_configuration_common import ConfigTester
-from .test_modeling_tf_common import TFModelTesterMixin, ids_tensor
-from .utils import CACHE_DIR, require_tf, slow
-
-
-if is_tf_available():
- import tensorflow as tf
- import numpy
- from transformers.modeling_tf_roberta import (
- TFRobertaModel,
- TFRobertaForMaskedLM,
- TFRobertaForSequenceClassification,
- TFRobertaForTokenClassification,
- TF_ROBERTA_PRETRAINED_MODEL_ARCHIVE_MAP,
- )
-
-
-@require_tf
-class TFRobertaModelTest(TFModelTesterMixin, unittest.TestCase):
-
- all_model_classes = (
- (TFRobertaModel, TFRobertaForMaskedLM, TFRobertaForSequenceClassification) if is_tf_available() else ()
- )
-
- class TFRobertaModelTester(object):
- def __init__(
- self,
- parent,
- batch_size=13,
- seq_length=7,
- is_training=True,
- use_input_mask=True,
- use_token_type_ids=True,
- use_labels=True,
- vocab_size=99,
- hidden_size=32,
- num_hidden_layers=5,
- num_attention_heads=4,
- intermediate_size=37,
- hidden_act="gelu",
- hidden_dropout_prob=0.1,
- attention_probs_dropout_prob=0.1,
- max_position_embeddings=512,
- type_vocab_size=16,
- type_sequence_label_size=2,
- initializer_range=0.02,
- num_labels=3,
- num_choices=4,
- scope=None,
- ):
- self.parent = parent
- self.batch_size = batch_size
- self.seq_length = seq_length
- self.is_training = is_training
- self.use_input_mask = use_input_mask
- self.use_token_type_ids = use_token_type_ids
- self.use_labels = use_labels
- self.vocab_size = vocab_size
- self.hidden_size = hidden_size
- self.num_hidden_layers = num_hidden_layers
- self.num_attention_heads = num_attention_heads
- self.intermediate_size = intermediate_size
- self.hidden_act = hidden_act
- self.hidden_dropout_prob = hidden_dropout_prob
- self.attention_probs_dropout_prob = attention_probs_dropout_prob
- self.max_position_embeddings = max_position_embeddings
- self.type_vocab_size = type_vocab_size
- self.type_sequence_label_size = type_sequence_label_size
- self.initializer_range = initializer_range
- self.num_labels = num_labels
- self.num_choices = num_choices
- self.scope = scope
-
- def prepare_config_and_inputs(self):
- input_ids = ids_tensor([self.batch_size, self.seq_length], self.vocab_size)
-
- input_mask = None
- if self.use_input_mask:
- input_mask = ids_tensor([self.batch_size, self.seq_length], vocab_size=2)
-
- token_type_ids = None
- if self.use_token_type_ids:
- token_type_ids = ids_tensor([self.batch_size, self.seq_length], self.type_vocab_size)
-
- sequence_labels = None
- token_labels = None
- choice_labels = None
- if self.use_labels:
- sequence_labels = ids_tensor([self.batch_size], self.type_sequence_label_size)
- token_labels = ids_tensor([self.batch_size, self.seq_length], self.num_labels)
- choice_labels = ids_tensor([self.batch_size], self.num_choices)
-
- config = RobertaConfig(
- vocab_size=self.vocab_size,
- hidden_size=self.hidden_size,
- num_hidden_layers=self.num_hidden_layers,
- num_attention_heads=self.num_attention_heads,
- intermediate_size=self.intermediate_size,
- hidden_act=self.hidden_act,
- hidden_dropout_prob=self.hidden_dropout_prob,
- attention_probs_dropout_prob=self.attention_probs_dropout_prob,
- max_position_embeddings=self.max_position_embeddings,
- type_vocab_size=self.type_vocab_size,
- initializer_range=self.initializer_range,
- )
-
- return config, input_ids, token_type_ids, input_mask, sequence_labels, token_labels, choice_labels
-
- def create_and_check_roberta_model(
- self, config, input_ids, token_type_ids, input_mask, sequence_labels, token_labels, choice_labels
- ):
- model = TFRobertaModel(config=config)
- inputs = {"input_ids": input_ids, "attention_mask": input_mask, "token_type_ids": token_type_ids}
- sequence_output = model(inputs)[0]
-
- inputs = [input_ids, input_mask]
- sequence_output = model(inputs)[0]
-
- sequence_output = model(input_ids)[0]
-
- result = {
- "sequence_output": sequence_output.numpy(),
- }
- self.parent.assertListEqual(
- list(result["sequence_output"].shape), [self.batch_size, self.seq_length, self.hidden_size]
- )
-
- def create_and_check_roberta_for_masked_lm(
- self, config, input_ids, token_type_ids, input_mask, sequence_labels, token_labels, choice_labels
- ):
- model = TFRobertaForMaskedLM(config=config)
- prediction_scores = model([input_ids, input_mask, token_type_ids])[0]
- result = {
- "prediction_scores": prediction_scores.numpy(),
- }
- self.parent.assertListEqual(
- list(result["prediction_scores"].shape), [self.batch_size, self.seq_length, self.vocab_size]
- )
-
- def create_and_check_roberta_for_token_classification(
- self, config, input_ids, token_type_ids, input_mask, sequence_labels, token_labels, choice_labels
- ):
- config.num_labels = self.num_labels
- model = TFRobertaForTokenClassification(config=config)
- inputs = {"input_ids": input_ids, "attention_mask": input_mask, "token_type_ids": token_type_ids}
- (logits,) = model(inputs)
- result = {
- "logits": logits.numpy(),
- }
- self.parent.assertListEqual(
- list(result["logits"].shape), [self.batch_size, self.seq_length, self.num_labels]
- )
-
- def prepare_config_and_inputs_for_common(self):
- config_and_inputs = self.prepare_config_and_inputs()
- (
- config,
- input_ids,
- token_type_ids,
- input_mask,
- sequence_labels,
- token_labels,
- choice_labels,
- ) = config_and_inputs
- inputs_dict = {"input_ids": input_ids, "token_type_ids": token_type_ids, "attention_mask": input_mask}
- return config, inputs_dict
-
- def setUp(self):
- self.model_tester = TFRobertaModelTest.TFRobertaModelTester(self)
- self.config_tester = ConfigTester(self, config_class=RobertaConfig, hidden_size=37)
-
- def test_config(self):
- self.config_tester.run_common_tests()
-
- def test_roberta_model(self):
- config_and_inputs = self.model_tester.prepare_config_and_inputs()
- self.model_tester.create_and_check_roberta_model(*config_and_inputs)
-
- def test_for_masked_lm(self):
- config_and_inputs = self.model_tester.prepare_config_and_inputs()
- self.model_tester.create_and_check_roberta_for_masked_lm(*config_and_inputs)
-
- @slow
- def test_model_from_pretrained(self):
- for model_name in list(TF_ROBERTA_PRETRAINED_MODEL_ARCHIVE_MAP.keys())[:1]:
- model = TFRobertaModel.from_pretrained(model_name, cache_dir=CACHE_DIR)
- self.assertIsNotNone(model)
-
-
-class TFRobertaModelIntegrationTest(unittest.TestCase):
- @slow
- def test_inference_masked_lm(self):
- model = TFRobertaForMaskedLM.from_pretrained("roberta-base")
-
- input_ids = tf.constant([[0, 31414, 232, 328, 740, 1140, 12695, 69, 46078, 1588, 2]])
- output = model(input_ids)[0]
- expected_shape = [1, 11, 50265]
- self.assertEqual(list(output.numpy().shape), expected_shape)
- # compare the actual values for a slice.
- expected_slice = tf.constant(
- [[[33.8843, -4.3107, 22.7779], [4.6533, -2.8099, 13.6252], [1.8222, -3.6898, 8.8600]]]
- )
- self.assertTrue(numpy.allclose(output[:, :3, :3].numpy(), expected_slice.numpy(), atol=1e-3))
-
- @slow
- def test_inference_no_head(self):
- model = TFRobertaModel.from_pretrained("roberta-base")
-
- input_ids = tf.constant([[0, 31414, 232, 328, 740, 1140, 12695, 69, 46078, 1588, 2]])
- output = model(input_ids)[0]
- # compare the actual values for a slice.
- expected_slice = tf.constant(
- [[[-0.0231, 0.0782, 0.0074], [-0.1854, 0.0539, -0.0174], [0.0548, 0.0799, 0.1687]]]
- )
- self.assertTrue(numpy.allclose(output[:, :3, :3].numpy(), expected_slice.numpy(), atol=1e-3))
-
- @slow
- def test_inference_classification_head(self):
- model = TFRobertaForSequenceClassification.from_pretrained("roberta-large-mnli")
-
- input_ids = tf.constant([[0, 31414, 232, 328, 740, 1140, 12695, 69, 46078, 1588, 2]])
- output = model(input_ids)[0]
- expected_shape = [1, 3]
- self.assertEqual(list(output.numpy().shape), expected_shape)
- expected_tensor = tf.constant([[-0.9469, 0.3913, 0.5118]])
- self.assertTrue(numpy.allclose(output.numpy(), expected_tensor.numpy(), atol=1e-3))
diff --git a/server/transformers/tests/test_modeling_tf_t5.py b/server/transformers/tests/test_modeling_tf_t5.py
deleted file mode 100644
index d5589eaf165cbdcb42c8a60c77eb9be4f9493930..0000000000000000000000000000000000000000
--- a/server/transformers/tests/test_modeling_tf_t5.py
+++ /dev/null
@@ -1,167 +0,0 @@
-# coding=utf-8
-# Copyright 2018 Google T5 Authors and HuggingFace Inc. team.
-#
-# Licensed under the Apache License, Version 2.0 (the "License");
-# you may not use this file except in compliance with the License.
-# You may obtain a copy of the License at
-#
-# http://www.apache.org/licenses/LICENSE-2.0
-#
-# Unless required by applicable law or agreed to in writing, software
-# distributed under the License is distributed on an "AS IS" BASIS,
-# WITHOUT WARRANTIES OR CONDITIONS OF ANY KIND, either express or implied.
-# See the License for the specific language governing permissions and
-# limitations under the License.
-
-
-import unittest
-
-from transformers import T5Config, is_tf_available
-
-from .test_configuration_common import ConfigTester
-from .test_modeling_tf_common import TFModelTesterMixin, ids_tensor
-from .utils import CACHE_DIR, require_tf, slow
-
-
-if is_tf_available():
- from transformers.modeling_tf_t5 import TFT5Model, TFT5WithLMHeadModel
-
-
-@require_tf
-class TFT5ModelTest(TFModelTesterMixin, unittest.TestCase):
-
- is_encoder_decoder = True
- all_model_classes = (TFT5Model, TFT5WithLMHeadModel) if is_tf_available() else ()
-
- class TFT5ModelTester(object):
- def __init__(
- self,
- parent,
- batch_size=13,
- seq_length=7,
- is_training=True,
- use_input_mask=True,
- use_labels=True,
- vocab_size=99,
- n_positions=14,
- hidden_size=32,
- num_hidden_layers=5,
- num_attention_heads=4,
- d_ff=37,
- relative_attention_num_buckets=8,
- dropout_rate=0.1,
- initializer_factor=0.002,
- scope=None,
- ):
- self.parent = parent
- self.batch_size = batch_size
- self.seq_length = seq_length
- self.is_training = is_training
- self.use_input_mask = use_input_mask
- self.use_labels = use_labels
- self.vocab_size = vocab_size
- self.n_positions = n_positions
- self.hidden_size = hidden_size
- self.num_hidden_layers = num_hidden_layers
- self.num_attention_heads = num_attention_heads
- self.d_ff = d_ff
- self.relative_attention_num_buckets = relative_attention_num_buckets
- self.dropout_rate = dropout_rate
- self.initializer_factor = initializer_factor
- self.scope = scope
-
- def prepare_config_and_inputs(self):
- input_ids = ids_tensor([self.batch_size, self.seq_length], self.vocab_size)
-
- input_mask = None
- if self.use_input_mask:
- input_mask = ids_tensor([self.batch_size, self.seq_length], vocab_size=2)
-
- token_labels = None
- if self.use_labels:
- token_labels = ids_tensor([self.batch_size, self.seq_length], self.vocab_size)
-
- config = T5Config(
- vocab_size=self.vocab_size,
- n_positions=self.n_positions,
- d_model=self.hidden_size,
- d_ff=self.d_ff,
- d_kv=self.hidden_size // self.num_attention_heads,
- num_layers=self.num_hidden_layers,
- num_heads=self.num_attention_heads,
- relative_attention_num_buckets=self.relative_attention_num_buckets,
- dropout_rate=self.dropout_rate,
- initializer_factor=self.initializer_factor,
- )
-
- return (config, input_ids, input_mask, token_labels)
-
- def create_and_check_t5_model(self, config, input_ids, input_mask, token_labels):
- model = TFT5Model(config=config)
- inputs = {
- "encoder_input_ids": input_ids,
- "decoder_input_ids": input_ids,
- "decoder_attention_mask": input_mask,
- }
- encoder_output, decoder_output = model(inputs)
-
- encoder_output, decoder_output = model(
- input_ids, decoder_attention_mask=input_mask, encoder_input_ids=input_ids
- )
-
- result = {
- "encoder_output": encoder_output.numpy(),
- "decoder_output": decoder_output.numpy(),
- }
- self.parent.assertListEqual(
- list(result["encoder_output"].shape), [self.batch_size, self.seq_length, self.hidden_size]
- )
- self.parent.assertListEqual(
- list(result["decoder_output"].shape), [self.batch_size, self.seq_length, self.hidden_size]
- )
-
- def create_and_check_t5_with_lm_head(self, config, input_ids, input_mask, token_labels):
- model = TFT5WithLMHeadModel(config=config)
- inputs = {
- "encoder_input_ids": input_ids,
- "decoder_input_ids": input_ids,
- "decoder_attention_mask": input_mask,
- }
- prediction_scores, decoder_output = model(inputs)
- result = {
- "prediction_scores": prediction_scores.numpy(),
- }
- self.parent.assertListEqual(
- list(result["prediction_scores"].shape), [self.batch_size, self.seq_length, self.vocab_size]
- )
-
- def prepare_config_and_inputs_for_common(self):
- config_and_inputs = self.prepare_config_and_inputs()
- (config, input_ids, input_mask, token_labels) = config_and_inputs
- inputs_dict = {
- "encoder_input_ids": input_ids,
- "decoder_input_ids": input_ids,
- "decoder_attention_mask": input_mask,
- }
- return config, inputs_dict
-
- def setUp(self):
- self.model_tester = TFT5ModelTest.TFT5ModelTester(self)
- self.config_tester = ConfigTester(self, config_class=T5Config, d_model=37)
-
- def test_config(self):
- self.config_tester.run_common_tests()
-
- def test_t5_model(self):
- config_and_inputs = self.model_tester.prepare_config_and_inputs()
- self.model_tester.create_and_check_t5_model(*config_and_inputs)
-
- def test_with_lm_head(self):
- config_and_inputs = self.model_tester.prepare_config_and_inputs()
- self.model_tester.create_and_check_t5_with_lm_head(*config_and_inputs)
-
- @slow
- def test_model_from_pretrained(self):
- for model_name in ["t5-small"]:
- model = TFT5Model.from_pretrained(model_name, cache_dir=CACHE_DIR)
- self.assertIsNotNone(model)
diff --git a/server/transformers/tests/test_modeling_tf_transfo_xl.py b/server/transformers/tests/test_modeling_tf_transfo_xl.py
deleted file mode 100644
index f94f2032a26b753f6003372499e0fccdbab4d864..0000000000000000000000000000000000000000
--- a/server/transformers/tests/test_modeling_tf_transfo_xl.py
+++ /dev/null
@@ -1,209 +0,0 @@
-# coding=utf-8
-# Copyright 2018 The Google AI Language Team Authors.
-#
-# Licensed under the Apache License, Version 2.0 (the "License");
-# you may not use this file except in compliance with the License.
-# You may obtain a copy of the License at
-#
-# http://www.apache.org/licenses/LICENSE-2.0
-#
-# Unless required by applicable law or agreed to in writing, software
-# distributed under the License is distributed on an "AS IS" BASIS,
-# WITHOUT WARRANTIES OR CONDITIONS OF ANY KIND, either express or implied.
-# See the License for the specific language governing permissions and
-# limitations under the License.
-
-
-import random
-import unittest
-
-from transformers import TransfoXLConfig, is_tf_available
-
-from .test_configuration_common import ConfigTester
-from .test_modeling_tf_common import TFModelTesterMixin, ids_tensor
-from .utils import CACHE_DIR, require_tf, slow
-
-
-if is_tf_available():
- import tensorflow as tf
- from transformers.modeling_tf_transfo_xl import (
- TFTransfoXLModel,
- TFTransfoXLLMHeadModel,
- TF_TRANSFO_XL_PRETRAINED_MODEL_ARCHIVE_MAP,
- )
-
-
-@require_tf
-class TFTransfoXLModelTest(TFModelTesterMixin, unittest.TestCase):
-
- all_model_classes = (TFTransfoXLModel, TFTransfoXLLMHeadModel) if is_tf_available() else ()
- test_pruning = False
- test_torchscript = False
- test_resize_embeddings = False
-
- class TFTransfoXLModelTester(object):
- def __init__(
- self,
- parent,
- batch_size=13,
- seq_length=7,
- mem_len=30,
- clamp_len=15,
- is_training=True,
- use_labels=True,
- vocab_size=99,
- cutoffs=[10, 50, 80],
- hidden_size=32,
- d_embed=32,
- num_attention_heads=4,
- d_head=8,
- d_inner=128,
- div_val=2,
- num_hidden_layers=5,
- scope=None,
- seed=1,
- ):
- self.parent = parent
- self.batch_size = batch_size
- self.seq_length = seq_length
- self.mem_len = mem_len
- self.key_length = seq_length + mem_len
- self.clamp_len = clamp_len
- self.is_training = is_training
- self.use_labels = use_labels
- self.vocab_size = vocab_size
- self.cutoffs = cutoffs
- self.hidden_size = hidden_size
- self.d_embed = d_embed
- self.num_attention_heads = num_attention_heads
- self.d_head = d_head
- self.d_inner = d_inner
- self.div_val = div_val
- self.num_hidden_layers = num_hidden_layers
- self.scope = scope
- self.seed = seed
-
- def prepare_config_and_inputs(self):
- input_ids_1 = ids_tensor([self.batch_size, self.seq_length], self.vocab_size)
- input_ids_2 = ids_tensor([self.batch_size, self.seq_length], self.vocab_size)
-
- lm_labels = None
- if self.use_labels:
- lm_labels = ids_tensor([self.batch_size, self.seq_length], self.vocab_size)
-
- config = TransfoXLConfig(
- vocab_size=self.vocab_size,
- mem_len=self.mem_len,
- clamp_len=self.clamp_len,
- cutoffs=self.cutoffs,
- d_model=self.hidden_size,
- d_embed=self.d_embed,
- n_head=self.num_attention_heads,
- d_head=self.d_head,
- d_inner=self.d_inner,
- div_val=self.div_val,
- n_layer=self.num_hidden_layers,
- )
-
- return (config, input_ids_1, input_ids_2, lm_labels)
-
- def set_seed(self):
- random.seed(self.seed)
- tf.random.set_seed(self.seed)
-
- def create_and_check_transfo_xl_model(self, config, input_ids_1, input_ids_2, lm_labels):
- model = TFTransfoXLModel(config)
-
- hidden_states_1, mems_1 = model(input_ids_1)
-
- inputs = {"input_ids": input_ids_2, "mems": mems_1}
-
- hidden_states_2, mems_2 = model(inputs)
-
- result = {
- "hidden_states_1": hidden_states_1.numpy(),
- "mems_1": [mem.numpy() for mem in mems_1],
- "hidden_states_2": hidden_states_2.numpy(),
- "mems_2": [mem.numpy() for mem in mems_2],
- }
-
- self.parent.assertListEqual(
- list(result["hidden_states_1"].shape), [self.batch_size, self.seq_length, self.hidden_size]
- )
- self.parent.assertListEqual(
- list(result["hidden_states_2"].shape), [self.batch_size, self.seq_length, self.hidden_size]
- )
- self.parent.assertListEqual(
- list(list(mem.shape) for mem in result["mems_1"]),
- [[self.mem_len, self.batch_size, self.hidden_size]] * self.num_hidden_layers,
- )
- self.parent.assertListEqual(
- list(list(mem.shape) for mem in result["mems_2"]),
- [[self.mem_len, self.batch_size, self.hidden_size]] * self.num_hidden_layers,
- )
-
- def create_and_check_transfo_xl_lm_head(self, config, input_ids_1, input_ids_2, lm_labels):
- model = TFTransfoXLLMHeadModel(config)
-
- lm_logits_1, mems_1 = model(input_ids_1)
-
- inputs = {"input_ids": input_ids_1, "labels": lm_labels}
- _, mems_1 = model(inputs)
-
- lm_logits_2, mems_2 = model([input_ids_2, mems_1])
-
- inputs = {"input_ids": input_ids_1, "mems": mems_1, "labels": lm_labels}
-
- _, mems_2 = model(inputs)
-
- result = {
- "mems_1": [mem.numpy() for mem in mems_1],
- "lm_logits_1": lm_logits_1.numpy(),
- "mems_2": [mem.numpy() for mem in mems_2],
- "lm_logits_2": lm_logits_2.numpy(),
- }
-
- self.parent.assertListEqual(
- list(result["lm_logits_1"].shape), [self.batch_size, self.seq_length, self.vocab_size]
- )
- self.parent.assertListEqual(
- list(list(mem.shape) for mem in result["mems_1"]),
- [[self.mem_len, self.batch_size, self.hidden_size]] * self.num_hidden_layers,
- )
-
- self.parent.assertListEqual(
- list(result["lm_logits_2"].shape), [self.batch_size, self.seq_length, self.vocab_size]
- )
- self.parent.assertListEqual(
- list(list(mem.shape) for mem in result["mems_2"]),
- [[self.mem_len, self.batch_size, self.hidden_size]] * self.num_hidden_layers,
- )
-
- def prepare_config_and_inputs_for_common(self):
- config_and_inputs = self.prepare_config_and_inputs()
- (config, input_ids_1, input_ids_2, lm_labels) = config_and_inputs
- inputs_dict = {"input_ids": input_ids_1}
- return config, inputs_dict
-
- def setUp(self):
- self.model_tester = TFTransfoXLModelTest.TFTransfoXLModelTester(self)
- self.config_tester = ConfigTester(self, config_class=TransfoXLConfig, d_embed=37)
-
- def test_config(self):
- self.config_tester.run_common_tests()
-
- def test_transfo_xl_model(self):
- self.model_tester.set_seed()
- config_and_inputs = self.model_tester.prepare_config_and_inputs()
- self.model_tester.create_and_check_transfo_xl_model(*config_and_inputs)
-
- def test_transfo_xl_lm_head(self):
- self.model_tester.set_seed()
- config_and_inputs = self.model_tester.prepare_config_and_inputs()
- self.model_tester.create_and_check_transfo_xl_lm_head(*config_and_inputs)
-
- @slow
- def test_model_from_pretrained(self):
- for model_name in list(TF_TRANSFO_XL_PRETRAINED_MODEL_ARCHIVE_MAP.keys())[:1]:
- model = TFTransfoXLModel.from_pretrained(model_name, cache_dir=CACHE_DIR)
- self.assertIsNotNone(model)
diff --git a/server/transformers/tests/test_modeling_tf_xlm.py b/server/transformers/tests/test_modeling_tf_xlm.py
deleted file mode 100644
index 53719f63f4bda65d759df84e14039a329872402e..0000000000000000000000000000000000000000
--- a/server/transformers/tests/test_modeling_tf_xlm.py
+++ /dev/null
@@ -1,307 +0,0 @@
-# coding=utf-8
-# Copyright 2018 The Google AI Language Team Authors.
-#
-# Licensed under the Apache License, Version 2.0 (the "License");
-# you may not use this file except in compliance with the License.
-# You may obtain a copy of the License at
-#
-# http://www.apache.org/licenses/LICENSE-2.0
-#
-# Unless required by applicable law or agreed to in writing, software
-# distributed under the License is distributed on an "AS IS" BASIS,
-# WITHOUT WARRANTIES OR CONDITIONS OF ANY KIND, either express or implied.
-# See the License for the specific language governing permissions and
-# limitations under the License.
-
-
-import unittest
-
-from transformers import is_tf_available
-
-from .test_configuration_common import ConfigTester
-from .test_modeling_tf_common import TFModelTesterMixin, ids_tensor
-from .utils import CACHE_DIR, require_tf, slow
-
-
-if is_tf_available():
- import tensorflow as tf
- from transformers import (
- XLMConfig,
- TFXLMModel,
- TFXLMWithLMHeadModel,
- TFXLMForSequenceClassification,
- TFXLMForQuestionAnsweringSimple,
- TF_XLM_PRETRAINED_MODEL_ARCHIVE_MAP,
- )
-
-
-@require_tf
-class TFXLMModelTest(TFModelTesterMixin, unittest.TestCase):
-
- all_model_classes = (
- (TFXLMModel, TFXLMWithLMHeadModel, TFXLMForSequenceClassification, TFXLMForQuestionAnsweringSimple)
- if is_tf_available()
- else ()
- )
-
- class TFXLMModelTester(object):
- def __init__(
- self,
- parent,
- batch_size=13,
- seq_length=7,
- is_training=True,
- use_input_lengths=True,
- use_token_type_ids=True,
- use_labels=True,
- gelu_activation=True,
- sinusoidal_embeddings=False,
- causal=False,
- asm=False,
- n_langs=2,
- vocab_size=99,
- n_special=0,
- hidden_size=32,
- num_hidden_layers=5,
- num_attention_heads=4,
- hidden_dropout_prob=0.1,
- attention_probs_dropout_prob=0.1,
- max_position_embeddings=512,
- type_vocab_size=16,
- type_sequence_label_size=2,
- initializer_range=0.02,
- num_labels=3,
- num_choices=4,
- summary_type="last",
- use_proj=True,
- scope=None,
- ):
- self.parent = parent
- self.batch_size = batch_size
- self.seq_length = seq_length
- self.is_training = is_training
- self.use_input_lengths = use_input_lengths
- self.use_token_type_ids = use_token_type_ids
- self.use_labels = use_labels
- self.gelu_activation = gelu_activation
- self.sinusoidal_embeddings = sinusoidal_embeddings
- self.asm = asm
- self.n_langs = n_langs
- self.vocab_size = vocab_size
- self.n_special = n_special
- self.summary_type = summary_type
- self.causal = causal
- self.use_proj = use_proj
- self.hidden_size = hidden_size
- self.num_hidden_layers = num_hidden_layers
- self.num_attention_heads = num_attention_heads
- self.hidden_dropout_prob = hidden_dropout_prob
- self.attention_probs_dropout_prob = attention_probs_dropout_prob
- self.max_position_embeddings = max_position_embeddings
- self.n_langs = n_langs
- self.type_sequence_label_size = type_sequence_label_size
- self.initializer_range = initializer_range
- self.summary_type = summary_type
- self.num_labels = num_labels
- self.num_choices = num_choices
- self.scope = scope
-
- def prepare_config_and_inputs(self):
- input_ids = ids_tensor([self.batch_size, self.seq_length], self.vocab_size)
- input_mask = ids_tensor([self.batch_size, self.seq_length], 2, dtype=tf.float32)
-
- input_lengths = None
- if self.use_input_lengths:
- input_lengths = (
- ids_tensor([self.batch_size], vocab_size=2) + self.seq_length - 2
- ) # small variation of seq_length
-
- token_type_ids = None
- if self.use_token_type_ids:
- token_type_ids = ids_tensor([self.batch_size, self.seq_length], self.n_langs)
-
- sequence_labels = None
- token_labels = None
- is_impossible_labels = None
- if self.use_labels:
- sequence_labels = ids_tensor([self.batch_size], self.type_sequence_label_size)
- token_labels = ids_tensor([self.batch_size, self.seq_length], self.num_labels)
- is_impossible_labels = ids_tensor([self.batch_size], 2, dtype=tf.float32)
-
- config = XLMConfig(
- vocab_size=self.vocab_size,
- n_special=self.n_special,
- emb_dim=self.hidden_size,
- n_layers=self.num_hidden_layers,
- n_heads=self.num_attention_heads,
- dropout=self.hidden_dropout_prob,
- attention_dropout=self.attention_probs_dropout_prob,
- gelu_activation=self.gelu_activation,
- sinusoidal_embeddings=self.sinusoidal_embeddings,
- asm=self.asm,
- causal=self.causal,
- n_langs=self.n_langs,
- max_position_embeddings=self.max_position_embeddings,
- initializer_range=self.initializer_range,
- summary_type=self.summary_type,
- use_proj=self.use_proj,
- )
-
- return (
- config,
- input_ids,
- token_type_ids,
- input_lengths,
- sequence_labels,
- token_labels,
- is_impossible_labels,
- input_mask,
- )
-
- def create_and_check_xlm_model(
- self,
- config,
- input_ids,
- token_type_ids,
- input_lengths,
- sequence_labels,
- token_labels,
- is_impossible_labels,
- input_mask,
- ):
- model = TFXLMModel(config=config)
- inputs = {"input_ids": input_ids, "lengths": input_lengths, "langs": token_type_ids}
- outputs = model(inputs)
-
- inputs = [input_ids, input_mask]
- outputs = model(inputs)
- sequence_output = outputs[0]
- result = {
- "sequence_output": sequence_output.numpy(),
- }
- self.parent.assertListEqual(
- list(result["sequence_output"].shape), [self.batch_size, self.seq_length, self.hidden_size]
- )
-
- def create_and_check_xlm_lm_head(
- self,
- config,
- input_ids,
- token_type_ids,
- input_lengths,
- sequence_labels,
- token_labels,
- is_impossible_labels,
- input_mask,
- ):
- model = TFXLMWithLMHeadModel(config)
-
- inputs = {"input_ids": input_ids, "lengths": input_lengths, "langs": token_type_ids}
- outputs = model(inputs)
-
- logits = outputs[0]
-
- result = {
- "logits": logits.numpy(),
- }
-
- self.parent.assertListEqual(
- list(result["logits"].shape), [self.batch_size, self.seq_length, self.vocab_size]
- )
-
- def create_and_check_xlm_qa(
- self,
- config,
- input_ids,
- token_type_ids,
- input_lengths,
- sequence_labels,
- token_labels,
- is_impossible_labels,
- input_mask,
- ):
- model = TFXLMForQuestionAnsweringSimple(config)
-
- inputs = {"input_ids": input_ids, "lengths": input_lengths}
-
- start_logits, end_logits = model(inputs)
-
- result = {
- "start_logits": start_logits.numpy(),
- "end_logits": end_logits.numpy(),
- }
-
- self.parent.assertListEqual(list(result["start_logits"].shape), [self.batch_size, self.seq_length])
- self.parent.assertListEqual(list(result["end_logits"].shape), [self.batch_size, self.seq_length])
-
- def create_and_check_xlm_sequence_classif(
- self,
- config,
- input_ids,
- token_type_ids,
- input_lengths,
- sequence_labels,
- token_labels,
- is_impossible_labels,
- input_mask,
- ):
- model = TFXLMForSequenceClassification(config)
-
- inputs = {"input_ids": input_ids, "lengths": input_lengths}
-
- (logits,) = model(inputs)
-
- result = {
- "logits": logits.numpy(),
- }
-
- self.parent.assertListEqual(list(result["logits"].shape), [self.batch_size, self.type_sequence_label_size])
-
- def prepare_config_and_inputs_for_common(self):
- config_and_inputs = self.prepare_config_and_inputs()
- (
- config,
- input_ids,
- token_type_ids,
- input_lengths,
- sequence_labels,
- token_labels,
- is_impossible_labels,
- input_mask,
- ) = config_and_inputs
- inputs_dict = {
- "input_ids": input_ids,
- "token_type_ids": token_type_ids,
- "langs": token_type_ids,
- "lengths": input_lengths,
- }
- return config, inputs_dict
-
- def setUp(self):
- self.model_tester = TFXLMModelTest.TFXLMModelTester(self)
- self.config_tester = ConfigTester(self, config_class=XLMConfig, emb_dim=37)
-
- def test_config(self):
- self.config_tester.run_common_tests()
-
- def test_xlm_model(self):
- config_and_inputs = self.model_tester.prepare_config_and_inputs()
- self.model_tester.create_and_check_xlm_model(*config_and_inputs)
-
- def test_xlm_lm_head(self):
- config_and_inputs = self.model_tester.prepare_config_and_inputs()
- self.model_tester.create_and_check_xlm_lm_head(*config_and_inputs)
-
- def test_xlm_qa(self):
- config_and_inputs = self.model_tester.prepare_config_and_inputs()
- self.model_tester.create_and_check_xlm_qa(*config_and_inputs)
-
- def test_xlm_sequence_classif(self):
- config_and_inputs = self.model_tester.prepare_config_and_inputs()
- self.model_tester.create_and_check_xlm_sequence_classif(*config_and_inputs)
-
- @slow
- def test_model_from_pretrained(self):
- for model_name in list(TF_XLM_PRETRAINED_MODEL_ARCHIVE_MAP.keys())[:1]:
- model = TFXLMModel.from_pretrained(model_name, cache_dir=CACHE_DIR)
- self.assertIsNotNone(model)
diff --git a/server/transformers/tests/test_modeling_tf_xlnet.py b/server/transformers/tests/test_modeling_tf_xlnet.py
deleted file mode 100644
index 65c83395e542e90bd70117e3ab819b0d70e60183..0000000000000000000000000000000000000000
--- a/server/transformers/tests/test_modeling_tf_xlnet.py
+++ /dev/null
@@ -1,403 +0,0 @@
-# coding=utf-8
-# Copyright 2018 The Google AI Language Team Authors.
-#
-# Licensed under the Apache License, Version 2.0 (the "License");
-# you may not use this file except in compliance with the License.
-# You may obtain a copy of the License at
-#
-# http://www.apache.org/licenses/LICENSE-2.0
-#
-# Unless required by applicable law or agreed to in writing, software
-# distributed under the License is distributed on an "AS IS" BASIS,
-# WITHOUT WARRANTIES OR CONDITIONS OF ANY KIND, either express or implied.
-# See the License for the specific language governing permissions and
-# limitations under the License.
-
-
-import random
-import unittest
-
-from transformers import XLNetConfig, is_tf_available
-
-from .test_configuration_common import ConfigTester
-from .test_modeling_tf_common import TFModelTesterMixin, ids_tensor
-from .utils import CACHE_DIR, require_tf, slow
-
-
-if is_tf_available():
- import tensorflow as tf
-
- from transformers.modeling_tf_xlnet import (
- TFXLNetModel,
- TFXLNetLMHeadModel,
- TFXLNetForSequenceClassification,
- TFXLNetForTokenClassification,
- TFXLNetForQuestionAnsweringSimple,
- TF_XLNET_PRETRAINED_MODEL_ARCHIVE_MAP,
- )
-
-
-@require_tf
-class TFXLNetModelTest(TFModelTesterMixin, unittest.TestCase):
-
- all_model_classes = (
- (
- TFXLNetModel,
- TFXLNetLMHeadModel,
- TFXLNetForSequenceClassification,
- TFXLNetForTokenClassification,
- TFXLNetForQuestionAnsweringSimple,
- )
- if is_tf_available()
- else ()
- )
- test_pruning = False
-
- class TFXLNetModelTester(object):
- def __init__(
- self,
- parent,
- batch_size=13,
- seq_length=7,
- mem_len=10,
- clamp_len=-1,
- reuse_len=15,
- is_training=True,
- use_labels=True,
- vocab_size=99,
- cutoffs=[10, 50, 80],
- hidden_size=32,
- num_attention_heads=4,
- d_inner=128,
- num_hidden_layers=5,
- type_sequence_label_size=2,
- untie_r=True,
- bi_data=False,
- same_length=False,
- initializer_range=0.05,
- seed=1,
- type_vocab_size=2,
- ):
- self.parent = parent
- self.batch_size = batch_size
- self.seq_length = seq_length
- self.mem_len = mem_len
- # self.key_len = seq_length + mem_len
- self.clamp_len = clamp_len
- self.reuse_len = reuse_len
- self.is_training = is_training
- self.use_labels = use_labels
- self.vocab_size = vocab_size
- self.cutoffs = cutoffs
- self.hidden_size = hidden_size
- self.num_attention_heads = num_attention_heads
- self.d_inner = d_inner
- self.num_hidden_layers = num_hidden_layers
- self.bi_data = bi_data
- self.untie_r = untie_r
- self.same_length = same_length
- self.initializer_range = initializer_range
- self.seed = seed
- self.type_vocab_size = type_vocab_size
- self.type_sequence_label_size = type_sequence_label_size
-
- def prepare_config_and_inputs(self):
- input_ids_1 = ids_tensor([self.batch_size, self.seq_length], self.vocab_size)
- input_ids_2 = ids_tensor([self.batch_size, self.seq_length], self.vocab_size)
- segment_ids = ids_tensor([self.batch_size, self.seq_length], self.type_vocab_size)
- input_mask = ids_tensor([self.batch_size, self.seq_length], 2, dtype=tf.float32)
-
- input_ids_q = ids_tensor([self.batch_size, self.seq_length + 1], self.vocab_size)
- perm_mask = tf.zeros((self.batch_size, self.seq_length + 1, self.seq_length), dtype=tf.float32)
- perm_mask_last = tf.ones((self.batch_size, self.seq_length + 1, 1), dtype=tf.float32)
- perm_mask = tf.concat([perm_mask, perm_mask_last], axis=-1)
- # perm_mask[:, :, -1] = 1.0 # Previous tokens don't see last token
- target_mapping = tf.zeros((self.batch_size, 1, self.seq_length), dtype=tf.float32)
- target_mapping_last = tf.ones((self.batch_size, 1, 1), dtype=tf.float32)
- target_mapping = tf.concat([target_mapping, target_mapping_last], axis=-1)
- # target_mapping[:, 0, -1] = 1.0 # predict last token
-
- sequence_labels = None
- lm_labels = None
- is_impossible_labels = None
- if self.use_labels:
- lm_labels = ids_tensor([self.batch_size, self.seq_length], self.vocab_size)
- sequence_labels = ids_tensor([self.batch_size], self.type_sequence_label_size)
- is_impossible_labels = ids_tensor([self.batch_size], 2, dtype=tf.float32)
-
- config = XLNetConfig(
- vocab_size=self.vocab_size,
- d_model=self.hidden_size,
- n_head=self.num_attention_heads,
- d_inner=self.d_inner,
- n_layer=self.num_hidden_layers,
- untie_r=self.untie_r,
- mem_len=self.mem_len,
- clamp_len=self.clamp_len,
- same_length=self.same_length,
- reuse_len=self.reuse_len,
- bi_data=self.bi_data,
- initializer_range=self.initializer_range,
- num_labels=self.type_sequence_label_size,
- )
-
- return (
- config,
- input_ids_1,
- input_ids_2,
- input_ids_q,
- perm_mask,
- input_mask,
- target_mapping,
- segment_ids,
- lm_labels,
- sequence_labels,
- is_impossible_labels,
- )
-
- def set_seed(self):
- random.seed(self.seed)
- tf.random.set_seed(self.seed)
-
- def create_and_check_xlnet_base_model(
- self,
- config,
- input_ids_1,
- input_ids_2,
- input_ids_q,
- perm_mask,
- input_mask,
- target_mapping,
- segment_ids,
- lm_labels,
- sequence_labels,
- is_impossible_labels,
- ):
- model = TFXLNetModel(config)
-
- inputs = {"input_ids": input_ids_1, "input_mask": input_mask, "token_type_ids": segment_ids}
-
- _, _ = model(inputs)
-
- inputs = [input_ids_1, input_mask]
-
- outputs, mems_1 = model(inputs)
-
- result = {
- "mems_1": [mem.numpy() for mem in mems_1],
- "outputs": outputs.numpy(),
- }
-
- config.mem_len = 0
- model = TFXLNetModel(config)
- no_mems_outputs = model(inputs)
- self.parent.assertEqual(len(no_mems_outputs), 1)
-
- self.parent.assertListEqual(
- list(result["outputs"].shape), [self.batch_size, self.seq_length, self.hidden_size]
- )
- self.parent.assertListEqual(
- list(list(mem.shape) for mem in result["mems_1"]),
- [[self.seq_length, self.batch_size, self.hidden_size]] * self.num_hidden_layers,
- )
-
- def create_and_check_xlnet_lm_head(
- self,
- config,
- input_ids_1,
- input_ids_2,
- input_ids_q,
- perm_mask,
- input_mask,
- target_mapping,
- segment_ids,
- lm_labels,
- sequence_labels,
- is_impossible_labels,
- ):
- model = TFXLNetLMHeadModel(config)
-
- inputs_1 = {"input_ids": input_ids_1, "token_type_ids": segment_ids}
-
- all_logits_1, mems_1 = model(inputs_1)
-
- inputs_2 = {"input_ids": input_ids_2, "mems": mems_1, "token_type_ids": segment_ids}
-
- all_logits_2, mems_2 = model(inputs_2)
-
- inputs_3 = {"input_ids": input_ids_q, "perm_mask": perm_mask, "target_mapping": target_mapping}
-
- logits, _ = model(inputs_3)
-
- result = {
- "mems_1": [mem.numpy() for mem in mems_1],
- "all_logits_1": all_logits_1.numpy(),
- "mems_2": [mem.numpy() for mem in mems_2],
- "all_logits_2": all_logits_2.numpy(),
- }
-
- self.parent.assertListEqual(
- list(result["all_logits_1"].shape), [self.batch_size, self.seq_length, self.vocab_size]
- )
- self.parent.assertListEqual(
- list(list(mem.shape) for mem in result["mems_1"]),
- [[self.seq_length, self.batch_size, self.hidden_size]] * self.num_hidden_layers,
- )
-
- self.parent.assertListEqual(
- list(result["all_logits_2"].shape), [self.batch_size, self.seq_length, self.vocab_size]
- )
- self.parent.assertListEqual(
- list(list(mem.shape) for mem in result["mems_2"]),
- [[self.mem_len, self.batch_size, self.hidden_size]] * self.num_hidden_layers,
- )
-
- def create_and_check_xlnet_qa(
- self,
- config,
- input_ids_1,
- input_ids_2,
- input_ids_q,
- perm_mask,
- input_mask,
- target_mapping,
- segment_ids,
- lm_labels,
- sequence_labels,
- is_impossible_labels,
- ):
- model = TFXLNetForQuestionAnsweringSimple(config)
-
- inputs = {"input_ids": input_ids_1, "attention_mask": input_mask, "token_type_ids": segment_ids}
- start_logits, end_logits, mems = model(inputs)
-
- result = {
- "start_logits": start_logits.numpy(),
- "end_logits": end_logits.numpy(),
- "mems": [m.numpy() for m in mems],
- }
-
- self.parent.assertListEqual(list(result["start_logits"].shape), [self.batch_size, self.seq_length])
- self.parent.assertListEqual(list(result["end_logits"].shape), [self.batch_size, self.seq_length])
- self.parent.assertListEqual(
- list(list(mem.shape) for mem in result["mems"]),
- [[self.seq_length, self.batch_size, self.hidden_size]] * self.num_hidden_layers,
- )
-
- def create_and_check_xlnet_sequence_classif(
- self,
- config,
- input_ids_1,
- input_ids_2,
- input_ids_q,
- perm_mask,
- input_mask,
- target_mapping,
- segment_ids,
- lm_labels,
- sequence_labels,
- is_impossible_labels,
- ):
- model = TFXLNetForSequenceClassification(config)
-
- logits, mems_1 = model(input_ids_1)
-
- result = {
- "mems_1": [mem.numpy() for mem in mems_1],
- "logits": logits.numpy(),
- }
-
- self.parent.assertListEqual(list(result["logits"].shape), [self.batch_size, self.type_sequence_label_size])
- self.parent.assertListEqual(
- list(list(mem.shape) for mem in result["mems_1"]),
- [[self.seq_length, self.batch_size, self.hidden_size]] * self.num_hidden_layers,
- )
-
- def create_and_check_xlnet_for_token_classification(
- self,
- config,
- input_ids_1,
- input_ids_2,
- input_ids_q,
- perm_mask,
- input_mask,
- target_mapping,
- segment_ids,
- lm_labels,
- sequence_labels,
- is_impossible_labels,
- ):
- config.num_labels = input_ids_1.shape[1]
- model = TFXLNetForTokenClassification(config)
- inputs = {
- "input_ids": input_ids_1,
- "attention_mask": input_mask,
- # 'token_type_ids': token_type_ids
- }
- logits, mems_1 = model(inputs)
- result = {
- "mems_1": [mem.numpy() for mem in mems_1],
- "logits": logits.numpy(),
- }
- self.parent.assertListEqual(
- list(result["logits"].shape), [self.batch_size, self.seq_length, config.num_labels]
- )
- self.parent.assertListEqual(
- list(list(mem.shape) for mem in result["mems_1"]),
- [[self.seq_length, self.batch_size, self.hidden_size]] * self.num_hidden_layers,
- )
-
- def prepare_config_and_inputs_for_common(self):
- config_and_inputs = self.prepare_config_and_inputs()
- (
- config,
- input_ids_1,
- input_ids_2,
- input_ids_q,
- perm_mask,
- input_mask,
- target_mapping,
- segment_ids,
- lm_labels,
- sequence_labels,
- is_impossible_labels,
- ) = config_and_inputs
- inputs_dict = {"input_ids": input_ids_1}
- return config, inputs_dict
-
- def setUp(self):
- self.model_tester = TFXLNetModelTest.TFXLNetModelTester(self)
- self.config_tester = ConfigTester(self, config_class=XLNetConfig, d_inner=37)
-
- def test_config(self):
- self.config_tester.run_common_tests()
-
- def test_xlnet_base_model(self):
- self.model_tester.set_seed()
- config_and_inputs = self.model_tester.prepare_config_and_inputs()
- self.model_tester.create_and_check_xlnet_base_model(*config_and_inputs)
-
- def test_xlnet_lm_head(self):
- self.model_tester.set_seed()
- config_and_inputs = self.model_tester.prepare_config_and_inputs()
- self.model_tester.create_and_check_xlnet_lm_head(*config_and_inputs)
-
- def test_xlnet_sequence_classif(self):
- self.model_tester.set_seed()
- config_and_inputs = self.model_tester.prepare_config_and_inputs()
- self.model_tester.create_and_check_xlnet_sequence_classif(*config_and_inputs)
-
- def test_xlnet_token_classification(self):
- config_and_inputs = self.model_tester.prepare_config_and_inputs()
- self.model_tester.create_and_check_xlnet_for_token_classification(*config_and_inputs)
-
- def test_xlnet_qa(self):
- self.model_tester.set_seed()
- config_and_inputs = self.model_tester.prepare_config_and_inputs()
- self.model_tester.create_and_check_xlnet_qa(*config_and_inputs)
-
- @slow
- def test_model_from_pretrained(self):
- for model_name in list(TF_XLNET_PRETRAINED_MODEL_ARCHIVE_MAP.keys())[:1]:
- model = TFXLNetModel.from_pretrained(model_name, cache_dir=CACHE_DIR)
- self.assertIsNotNone(model)
diff --git a/server/transformers/tests/test_modeling_transfo_xl.py b/server/transformers/tests/test_modeling_transfo_xl.py
deleted file mode 100644
index b06bd8510673a84a97c44e82e8b0e14f0db42144..0000000000000000000000000000000000000000
--- a/server/transformers/tests/test_modeling_transfo_xl.py
+++ /dev/null
@@ -1,210 +0,0 @@
-# coding=utf-8
-# Copyright 2018 The Google AI Language Team Authors.
-#
-# Licensed under the Apache License, Version 2.0 (the "License");
-# you may not use this file except in compliance with the License.
-# You may obtain a copy of the License at
-#
-# http://www.apache.org/licenses/LICENSE-2.0
-#
-# Unless required by applicable law or agreed to in writing, software
-# distributed under the License is distributed on an "AS IS" BASIS,
-# WITHOUT WARRANTIES OR CONDITIONS OF ANY KIND, either express or implied.
-# See the License for the specific language governing permissions and
-# limitations under the License.
-
-
-import random
-import unittest
-
-from transformers import is_torch_available
-
-from .test_configuration_common import ConfigTester
-from .test_modeling_common import ModelTesterMixin, ids_tensor
-from .utils import CACHE_DIR, require_torch, slow, torch_device
-
-
-if is_torch_available():
- import torch
- from transformers import TransfoXLConfig, TransfoXLModel, TransfoXLLMHeadModel
- from transformers.modeling_transfo_xl import TRANSFO_XL_PRETRAINED_MODEL_ARCHIVE_MAP
-
-
-@require_torch
-class TransfoXLModelTest(ModelTesterMixin, unittest.TestCase):
-
- all_model_classes = (TransfoXLModel, TransfoXLLMHeadModel) if is_torch_available() else ()
- test_pruning = False
- test_torchscript = False
- test_resize_embeddings = False
-
- class TransfoXLModelTester(object):
- def __init__(
- self,
- parent,
- batch_size=13,
- seq_length=7,
- mem_len=30,
- clamp_len=15,
- is_training=True,
- use_labels=True,
- vocab_size=99,
- cutoffs=[10, 50, 80],
- hidden_size=32,
- d_embed=32,
- num_attention_heads=4,
- d_head=8,
- d_inner=128,
- div_val=2,
- num_hidden_layers=5,
- scope=None,
- seed=1,
- ):
- self.parent = parent
- self.batch_size = batch_size
- self.seq_length = seq_length
- self.mem_len = mem_len
- self.key_length = seq_length + mem_len
- self.clamp_len = clamp_len
- self.is_training = is_training
- self.use_labels = use_labels
- self.vocab_size = vocab_size
- self.cutoffs = cutoffs
- self.hidden_size = hidden_size
- self.d_embed = d_embed
- self.num_attention_heads = num_attention_heads
- self.d_head = d_head
- self.d_inner = d_inner
- self.div_val = div_val
- self.num_hidden_layers = num_hidden_layers
- self.scope = scope
- self.seed = seed
-
- def prepare_config_and_inputs(self):
- input_ids_1 = ids_tensor([self.batch_size, self.seq_length], self.vocab_size)
- input_ids_2 = ids_tensor([self.batch_size, self.seq_length], self.vocab_size)
-
- lm_labels = None
- if self.use_labels:
- lm_labels = ids_tensor([self.batch_size, self.seq_length], self.vocab_size)
-
- config = TransfoXLConfig(
- vocab_size=self.vocab_size,
- mem_len=self.mem_len,
- clamp_len=self.clamp_len,
- cutoffs=self.cutoffs,
- d_model=self.hidden_size,
- d_embed=self.d_embed,
- n_head=self.num_attention_heads,
- d_head=self.d_head,
- d_inner=self.d_inner,
- div_val=self.div_val,
- n_layer=self.num_hidden_layers,
- )
-
- return (config, input_ids_1, input_ids_2, lm_labels)
-
- def set_seed(self):
- random.seed(self.seed)
- torch.manual_seed(self.seed)
-
- def create_transfo_xl_model(self, config, input_ids_1, input_ids_2, lm_labels):
- model = TransfoXLModel(config)
- model.to(torch_device)
- model.eval()
-
- hidden_states_1, mems_1 = model(input_ids_1)
- hidden_states_2, mems_2 = model(input_ids_2, mems_1)
- outputs = {
- "hidden_states_1": hidden_states_1,
- "mems_1": mems_1,
- "hidden_states_2": hidden_states_2,
- "mems_2": mems_2,
- }
- return outputs
-
- def check_transfo_xl_model_output(self, result):
- self.parent.assertListEqual(
- list(result["hidden_states_1"].size()), [self.batch_size, self.seq_length, self.hidden_size]
- )
- self.parent.assertListEqual(
- list(result["hidden_states_2"].size()), [self.batch_size, self.seq_length, self.hidden_size]
- )
- self.parent.assertListEqual(
- list(list(mem.size()) for mem in result["mems_1"]),
- [[self.mem_len, self.batch_size, self.hidden_size]] * self.num_hidden_layers,
- )
- self.parent.assertListEqual(
- list(list(mem.size()) for mem in result["mems_2"]),
- [[self.mem_len, self.batch_size, self.hidden_size]] * self.num_hidden_layers,
- )
-
- def create_transfo_xl_lm_head(self, config, input_ids_1, input_ids_2, lm_labels):
- model = TransfoXLLMHeadModel(config)
- model.to(torch_device)
- model.eval()
-
- lm_logits_1, mems_1 = model(input_ids_1)
- loss_1, _, mems_1 = model(input_ids_1, labels=lm_labels)
- lm_logits_2, mems_2 = model(input_ids_2, mems=mems_1)
- loss_2, _, mems_2 = model(input_ids_2, labels=lm_labels, mems=mems_1)
-
- outputs = {
- "loss_1": loss_1,
- "mems_1": mems_1,
- "lm_logits_1": lm_logits_1,
- "loss_2": loss_2,
- "mems_2": mems_2,
- "lm_logits_2": lm_logits_2,
- }
- return outputs
-
- def check_transfo_xl_lm_head_output(self, result):
- self.parent.assertListEqual(list(result["loss_1"].size()), [self.batch_size, self.seq_length])
- self.parent.assertListEqual(
- list(result["lm_logits_1"].size()), [self.batch_size, self.seq_length, self.vocab_size]
- )
- self.parent.assertListEqual(
- list(list(mem.size()) for mem in result["mems_1"]),
- [[self.mem_len, self.batch_size, self.hidden_size]] * self.num_hidden_layers,
- )
-
- self.parent.assertListEqual(list(result["loss_2"].size()), [self.batch_size, self.seq_length])
- self.parent.assertListEqual(
- list(result["lm_logits_2"].size()), [self.batch_size, self.seq_length, self.vocab_size]
- )
- self.parent.assertListEqual(
- list(list(mem.size()) for mem in result["mems_2"]),
- [[self.mem_len, self.batch_size, self.hidden_size]] * self.num_hidden_layers,
- )
-
- def prepare_config_and_inputs_for_common(self):
- config_and_inputs = self.prepare_config_and_inputs()
- (config, input_ids_1, input_ids_2, lm_labels) = config_and_inputs
- inputs_dict = {"input_ids": input_ids_1}
- return config, inputs_dict
-
- def setUp(self):
- self.model_tester = TransfoXLModelTest.TransfoXLModelTester(self)
- self.config_tester = ConfigTester(self, config_class=TransfoXLConfig, d_embed=37)
-
- def test_config(self):
- self.config_tester.run_common_tests()
-
- def test_transfo_xl_model(self):
- self.model_tester.set_seed()
- config_and_inputs = self.model_tester.prepare_config_and_inputs()
- output_result = self.model_tester.create_transfo_xl_model(*config_and_inputs)
- self.model_tester.check_transfo_xl_model_output(output_result)
-
- def test_transfo_xl_lm_head(self):
- self.model_tester.set_seed()
- config_and_inputs = self.model_tester.prepare_config_and_inputs()
- output_result = self.model_tester.create_transfo_xl_lm_head(*config_and_inputs)
- self.model_tester.check_transfo_xl_lm_head_output(output_result)
-
- @slow
- def test_model_from_pretrained(self):
- for model_name in list(TRANSFO_XL_PRETRAINED_MODEL_ARCHIVE_MAP.keys())[:1]:
- model = TransfoXLModel.from_pretrained(model_name, cache_dir=CACHE_DIR)
- self.assertIsNotNone(model)
diff --git a/server/transformers/tests/test_modeling_xlm.py b/server/transformers/tests/test_modeling_xlm.py
deleted file mode 100644
index df5ac260fabdd0018657b99b7ccfbe4994aa44db..0000000000000000000000000000000000000000
--- a/server/transformers/tests/test_modeling_xlm.py
+++ /dev/null
@@ -1,392 +0,0 @@
-# coding=utf-8
-# Copyright 2018 The Google AI Language Team Authors.
-#
-# Licensed under the Apache License, Version 2.0 (the "License");
-# you may not use this file except in compliance with the License.
-# You may obtain a copy of the License at
-#
-# http://www.apache.org/licenses/LICENSE-2.0
-#
-# Unless required by applicable law or agreed to in writing, software
-# distributed under the License is distributed on an "AS IS" BASIS,
-# WITHOUT WARRANTIES OR CONDITIONS OF ANY KIND, either express or implied.
-# See the License for the specific language governing permissions and
-# limitations under the License.
-
-
-import unittest
-
-from transformers import is_torch_available
-
-from .test_configuration_common import ConfigTester
-from .test_modeling_common import ModelTesterMixin, ids_tensor
-from .utils import CACHE_DIR, require_torch, slow, torch_device
-
-
-if is_torch_available():
- from transformers import (
- XLMConfig,
- XLMModel,
- XLMWithLMHeadModel,
- XLMForQuestionAnswering,
- XLMForSequenceClassification,
- XLMForQuestionAnsweringSimple,
- )
- from transformers.modeling_xlm import XLM_PRETRAINED_MODEL_ARCHIVE_MAP
-
-
-@require_torch
-class XLMModelTest(ModelTesterMixin, unittest.TestCase):
-
- all_model_classes = (
- (
- XLMModel,
- XLMWithLMHeadModel,
- XLMForQuestionAnswering,
- XLMForSequenceClassification,
- XLMForQuestionAnsweringSimple,
- )
- if is_torch_available()
- else ()
- )
-
- class XLMModelTester(object):
- def __init__(
- self,
- parent,
- batch_size=13,
- seq_length=7,
- is_training=True,
- use_input_lengths=True,
- use_token_type_ids=True,
- use_labels=True,
- gelu_activation=True,
- sinusoidal_embeddings=False,
- causal=False,
- asm=False,
- n_langs=2,
- vocab_size=99,
- n_special=0,
- hidden_size=32,
- num_hidden_layers=5,
- num_attention_heads=4,
- hidden_dropout_prob=0.1,
- attention_probs_dropout_prob=0.1,
- max_position_embeddings=512,
- type_vocab_size=16,
- type_sequence_label_size=2,
- initializer_range=0.02,
- num_labels=3,
- num_choices=4,
- summary_type="last",
- use_proj=True,
- scope=None,
- ):
- self.parent = parent
- self.batch_size = batch_size
- self.seq_length = seq_length
- self.is_training = is_training
- self.use_input_lengths = use_input_lengths
- self.use_token_type_ids = use_token_type_ids
- self.use_labels = use_labels
- self.gelu_activation = gelu_activation
- self.sinusoidal_embeddings = sinusoidal_embeddings
- self.asm = asm
- self.n_langs = n_langs
- self.vocab_size = vocab_size
- self.n_special = n_special
- self.summary_type = summary_type
- self.causal = causal
- self.use_proj = use_proj
- self.hidden_size = hidden_size
- self.num_hidden_layers = num_hidden_layers
- self.num_attention_heads = num_attention_heads
- self.hidden_dropout_prob = hidden_dropout_prob
- self.attention_probs_dropout_prob = attention_probs_dropout_prob
- self.max_position_embeddings = max_position_embeddings
- self.n_langs = n_langs
- self.type_sequence_label_size = type_sequence_label_size
- self.initializer_range = initializer_range
- self.summary_type = summary_type
- self.num_labels = num_labels
- self.num_choices = num_choices
- self.scope = scope
-
- def prepare_config_and_inputs(self):
- input_ids = ids_tensor([self.batch_size, self.seq_length], self.vocab_size)
- input_mask = ids_tensor([self.batch_size, self.seq_length], 2).float()
-
- input_lengths = None
- if self.use_input_lengths:
- input_lengths = (
- ids_tensor([self.batch_size], vocab_size=2) + self.seq_length - 2
- ) # small variation of seq_length
-
- token_type_ids = None
- if self.use_token_type_ids:
- token_type_ids = ids_tensor([self.batch_size, self.seq_length], self.n_langs)
-
- sequence_labels = None
- token_labels = None
- is_impossible_labels = None
- if self.use_labels:
- sequence_labels = ids_tensor([self.batch_size], self.type_sequence_label_size)
- token_labels = ids_tensor([self.batch_size, self.seq_length], self.num_labels)
- is_impossible_labels = ids_tensor([self.batch_size], 2).float()
-
- config = XLMConfig(
- vocab_size=self.vocab_size,
- n_special=self.n_special,
- emb_dim=self.hidden_size,
- n_layers=self.num_hidden_layers,
- n_heads=self.num_attention_heads,
- dropout=self.hidden_dropout_prob,
- attention_dropout=self.attention_probs_dropout_prob,
- gelu_activation=self.gelu_activation,
- sinusoidal_embeddings=self.sinusoidal_embeddings,
- asm=self.asm,
- causal=self.causal,
- n_langs=self.n_langs,
- max_position_embeddings=self.max_position_embeddings,
- initializer_range=self.initializer_range,
- summary_type=self.summary_type,
- use_proj=self.use_proj,
- )
-
- return (
- config,
- input_ids,
- token_type_ids,
- input_lengths,
- sequence_labels,
- token_labels,
- is_impossible_labels,
- input_mask,
- )
-
- def check_loss_output(self, result):
- self.parent.assertListEqual(list(result["loss"].size()), [])
-
- def create_and_check_xlm_model(
- self,
- config,
- input_ids,
- token_type_ids,
- input_lengths,
- sequence_labels,
- token_labels,
- is_impossible_labels,
- input_mask,
- ):
- model = XLMModel(config=config)
- model.to(torch_device)
- model.eval()
- outputs = model(input_ids, lengths=input_lengths, langs=token_type_ids)
- outputs = model(input_ids, langs=token_type_ids)
- outputs = model(input_ids)
- sequence_output = outputs[0]
- result = {
- "sequence_output": sequence_output,
- }
- self.parent.assertListEqual(
- list(result["sequence_output"].size()), [self.batch_size, self.seq_length, self.hidden_size]
- )
-
- def create_and_check_xlm_lm_head(
- self,
- config,
- input_ids,
- token_type_ids,
- input_lengths,
- sequence_labels,
- token_labels,
- is_impossible_labels,
- input_mask,
- ):
- model = XLMWithLMHeadModel(config)
- model.to(torch_device)
- model.eval()
-
- loss, logits = model(input_ids, token_type_ids=token_type_ids, labels=token_labels)
-
- result = {
- "loss": loss,
- "logits": logits,
- }
-
- self.parent.assertListEqual(list(result["loss"].size()), [])
- self.parent.assertListEqual(
- list(result["logits"].size()), [self.batch_size, self.seq_length, self.vocab_size]
- )
-
- def create_and_check_xlm_simple_qa(
- self,
- config,
- input_ids,
- token_type_ids,
- input_lengths,
- sequence_labels,
- token_labels,
- is_impossible_labels,
- input_mask,
- ):
- model = XLMForQuestionAnsweringSimple(config)
- model.to(torch_device)
- model.eval()
-
- outputs = model(input_ids)
-
- outputs = model(input_ids, start_positions=sequence_labels, end_positions=sequence_labels)
- loss, start_logits, end_logits = outputs
-
- result = {
- "loss": loss,
- "start_logits": start_logits,
- "end_logits": end_logits,
- }
- self.parent.assertListEqual(list(result["start_logits"].size()), [self.batch_size, self.seq_length])
- self.parent.assertListEqual(list(result["end_logits"].size()), [self.batch_size, self.seq_length])
- self.check_loss_output(result)
-
- def create_and_check_xlm_qa(
- self,
- config,
- input_ids,
- token_type_ids,
- input_lengths,
- sequence_labels,
- token_labels,
- is_impossible_labels,
- input_mask,
- ):
- model = XLMForQuestionAnswering(config)
- model.to(torch_device)
- model.eval()
-
- outputs = model(input_ids)
- start_top_log_probs, start_top_index, end_top_log_probs, end_top_index, cls_logits = outputs
-
- outputs = model(
- input_ids,
- start_positions=sequence_labels,
- end_positions=sequence_labels,
- cls_index=sequence_labels,
- is_impossible=is_impossible_labels,
- p_mask=input_mask,
- )
-
- outputs = model(
- input_ids,
- start_positions=sequence_labels,
- end_positions=sequence_labels,
- cls_index=sequence_labels,
- is_impossible=is_impossible_labels,
- )
-
- (total_loss,) = outputs
-
- outputs = model(input_ids, start_positions=sequence_labels, end_positions=sequence_labels)
-
- (total_loss,) = outputs
-
- result = {
- "loss": total_loss,
- "start_top_log_probs": start_top_log_probs,
- "start_top_index": start_top_index,
- "end_top_log_probs": end_top_log_probs,
- "end_top_index": end_top_index,
- "cls_logits": cls_logits,
- }
-
- self.parent.assertListEqual(list(result["loss"].size()), [])
- self.parent.assertListEqual(
- list(result["start_top_log_probs"].size()), [self.batch_size, model.config.start_n_top]
- )
- self.parent.assertListEqual(
- list(result["start_top_index"].size()), [self.batch_size, model.config.start_n_top]
- )
- self.parent.assertListEqual(
- list(result["end_top_log_probs"].size()),
- [self.batch_size, model.config.start_n_top * model.config.end_n_top],
- )
- self.parent.assertListEqual(
- list(result["end_top_index"].size()),
- [self.batch_size, model.config.start_n_top * model.config.end_n_top],
- )
- self.parent.assertListEqual(list(result["cls_logits"].size()), [self.batch_size])
-
- def create_and_check_xlm_sequence_classif(
- self,
- config,
- input_ids,
- token_type_ids,
- input_lengths,
- sequence_labels,
- token_labels,
- is_impossible_labels,
- input_mask,
- ):
- model = XLMForSequenceClassification(config)
- model.to(torch_device)
- model.eval()
-
- (logits,) = model(input_ids)
- loss, logits = model(input_ids, labels=sequence_labels)
-
- result = {
- "loss": loss,
- "logits": logits,
- }
-
- self.parent.assertListEqual(list(result["loss"].size()), [])
- self.parent.assertListEqual(
- list(result["logits"].size()), [self.batch_size, self.type_sequence_label_size]
- )
-
- def prepare_config_and_inputs_for_common(self):
- config_and_inputs = self.prepare_config_and_inputs()
- (
- config,
- input_ids,
- token_type_ids,
- input_lengths,
- sequence_labels,
- token_labels,
- is_impossible_labels,
- input_mask,
- ) = config_and_inputs
- inputs_dict = {"input_ids": input_ids, "token_type_ids": token_type_ids, "lengths": input_lengths}
- return config, inputs_dict
-
- def setUp(self):
- self.model_tester = XLMModelTest.XLMModelTester(self)
- self.config_tester = ConfigTester(self, config_class=XLMConfig, emb_dim=37)
-
- def test_config(self):
- self.config_tester.run_common_tests()
-
- def test_xlm_model(self):
- config_and_inputs = self.model_tester.prepare_config_and_inputs()
- self.model_tester.create_and_check_xlm_model(*config_and_inputs)
-
- def test_xlm_lm_head(self):
- config_and_inputs = self.model_tester.prepare_config_and_inputs()
- self.model_tester.create_and_check_xlm_lm_head(*config_and_inputs)
-
- def test_xlm_simple_qa(self):
- config_and_inputs = self.model_tester.prepare_config_and_inputs()
- self.model_tester.create_and_check_xlm_simple_qa(*config_and_inputs)
-
- def test_xlm_qa(self):
- config_and_inputs = self.model_tester.prepare_config_and_inputs()
- self.model_tester.create_and_check_xlm_qa(*config_and_inputs)
-
- def test_xlm_sequence_classif(self):
- config_and_inputs = self.model_tester.prepare_config_and_inputs()
- self.model_tester.create_and_check_xlm_sequence_classif(*config_and_inputs)
-
- @slow
- def test_model_from_pretrained(self):
- for model_name in list(XLM_PRETRAINED_MODEL_ARCHIVE_MAP.keys())[:1]:
- model = XLMModel.from_pretrained(model_name, cache_dir=CACHE_DIR)
- self.assertIsNotNone(model)
diff --git a/server/transformers/tests/test_modeling_xlnet.py b/server/transformers/tests/test_modeling_xlnet.py
deleted file mode 100644
index 8b57e4ae82a26e44af82a14b3024009073d213ba..0000000000000000000000000000000000000000
--- a/server/transformers/tests/test_modeling_xlnet.py
+++ /dev/null
@@ -1,501 +0,0 @@
-# coding=utf-8
-# Copyright 2018 The Google AI Language Team Authors.
-#
-# Licensed under the Apache License, Version 2.0 (the "License");
-# you may not use this file except in compliance with the License.
-# You may obtain a copy of the License at
-#
-# http://www.apache.org/licenses/LICENSE-2.0
-#
-# Unless required by applicable law or agreed to in writing, software
-# distributed under the License is distributed on an "AS IS" BASIS,
-# WITHOUT WARRANTIES OR CONDITIONS OF ANY KIND, either express or implied.
-# See the License for the specific language governing permissions and
-# limitations under the License.
-
-
-import random
-import unittest
-
-from transformers import is_torch_available
-
-from .test_configuration_common import ConfigTester
-from .test_modeling_common import ModelTesterMixin, ids_tensor
-from .utils import CACHE_DIR, require_torch, slow, torch_device
-
-
-if is_torch_available():
- import torch
-
- from transformers import (
- XLNetConfig,
- XLNetModel,
- XLNetLMHeadModel,
- XLNetForSequenceClassification,
- XLNetForTokenClassification,
- XLNetForQuestionAnswering,
- )
- from transformers.modeling_xlnet import XLNET_PRETRAINED_MODEL_ARCHIVE_MAP
-
-
-@require_torch
-class XLNetModelTest(ModelTesterMixin, unittest.TestCase):
-
- all_model_classes = (
- (
- XLNetModel,
- XLNetLMHeadModel,
- XLNetForTokenClassification,
- XLNetForSequenceClassification,
- XLNetForQuestionAnswering,
- )
- if is_torch_available()
- else ()
- )
- test_pruning = False
-
- class XLNetModelTester(object):
- def __init__(
- self,
- parent,
- batch_size=13,
- seq_length=7,
- mem_len=10,
- clamp_len=-1,
- reuse_len=15,
- is_training=True,
- use_labels=True,
- vocab_size=99,
- cutoffs=[10, 50, 80],
- hidden_size=32,
- num_attention_heads=4,
- d_inner=128,
- num_hidden_layers=5,
- type_sequence_label_size=2,
- untie_r=True,
- bi_data=False,
- same_length=False,
- initializer_range=0.05,
- seed=1,
- type_vocab_size=2,
- ):
- self.parent = parent
- self.batch_size = batch_size
- self.seq_length = seq_length
- self.mem_len = mem_len
- # self.key_len = seq_length + mem_len
- self.clamp_len = clamp_len
- self.reuse_len = reuse_len
- self.is_training = is_training
- self.use_labels = use_labels
- self.vocab_size = vocab_size
- self.cutoffs = cutoffs
- self.hidden_size = hidden_size
- self.num_attention_heads = num_attention_heads
- self.d_inner = d_inner
- self.num_hidden_layers = num_hidden_layers
- self.bi_data = bi_data
- self.untie_r = untie_r
- self.same_length = same_length
- self.initializer_range = initializer_range
- self.seed = seed
- self.type_vocab_size = type_vocab_size
- self.type_sequence_label_size = type_sequence_label_size
-
- def prepare_config_and_inputs(self):
- input_ids_1 = ids_tensor([self.batch_size, self.seq_length], self.vocab_size)
- input_ids_2 = ids_tensor([self.batch_size, self.seq_length], self.vocab_size)
- segment_ids = ids_tensor([self.batch_size, self.seq_length], self.type_vocab_size)
- input_mask = ids_tensor([self.batch_size, self.seq_length], 2).float()
-
- input_ids_q = ids_tensor([self.batch_size, self.seq_length + 1], self.vocab_size)
- perm_mask = torch.zeros(
- self.batch_size, self.seq_length + 1, self.seq_length + 1, dtype=torch.float, device=torch_device
- )
- perm_mask[:, :, -1] = 1.0 # Previous tokens don't see last token
- target_mapping = torch.zeros(
- self.batch_size, 1, self.seq_length + 1, dtype=torch.float, device=torch_device
- )
- target_mapping[:, 0, -1] = 1.0 # predict last token
-
- sequence_labels = None
- lm_labels = None
- is_impossible_labels = None
- token_labels = None
- if self.use_labels:
- lm_labels = ids_tensor([self.batch_size, self.seq_length], self.vocab_size)
- sequence_labels = ids_tensor([self.batch_size], self.type_sequence_label_size)
- is_impossible_labels = ids_tensor([self.batch_size], 2).float()
- token_labels = ids_tensor([self.batch_size, self.seq_length], self.type_vocab_size)
-
- config = XLNetConfig(
- vocab_size=self.vocab_size,
- d_model=self.hidden_size,
- n_head=self.num_attention_heads,
- d_inner=self.d_inner,
- n_layer=self.num_hidden_layers,
- untie_r=self.untie_r,
- mem_len=self.mem_len,
- clamp_len=self.clamp_len,
- same_length=self.same_length,
- reuse_len=self.reuse_len,
- bi_data=self.bi_data,
- initializer_range=self.initializer_range,
- num_labels=self.type_sequence_label_size,
- )
-
- return (
- config,
- input_ids_1,
- input_ids_2,
- input_ids_q,
- perm_mask,
- input_mask,
- target_mapping,
- segment_ids,
- lm_labels,
- sequence_labels,
- is_impossible_labels,
- token_labels,
- )
-
- def set_seed(self):
- random.seed(self.seed)
- torch.manual_seed(self.seed)
-
- def create_and_check_xlnet_base_model(
- self,
- config,
- input_ids_1,
- input_ids_2,
- input_ids_q,
- perm_mask,
- input_mask,
- target_mapping,
- segment_ids,
- lm_labels,
- sequence_labels,
- is_impossible_labels,
- token_labels,
- ):
- model = XLNetModel(config)
- model.to(torch_device)
- model.eval()
-
- _, _ = model(input_ids_1, input_mask=input_mask)
- _, _ = model(input_ids_1, attention_mask=input_mask)
- _, _ = model(input_ids_1, token_type_ids=segment_ids)
- outputs, mems_1 = model(input_ids_1)
-
- result = {
- "mems_1": mems_1,
- "outputs": outputs,
- }
-
- config.mem_len = 0
- model = XLNetModel(config)
- model.to(torch_device)
- model.eval()
- no_mems_outputs = model(input_ids_1)
- self.parent.assertEqual(len(no_mems_outputs), 1)
-
- self.parent.assertListEqual(
- list(result["outputs"].size()), [self.batch_size, self.seq_length, self.hidden_size]
- )
- self.parent.assertListEqual(
- list(list(mem.size()) for mem in result["mems_1"]),
- [[self.seq_length, self.batch_size, self.hidden_size]] * self.num_hidden_layers,
- )
-
- def create_and_check_xlnet_base_model_with_att_output(
- self,
- config,
- input_ids_1,
- input_ids_2,
- input_ids_q,
- perm_mask,
- input_mask,
- target_mapping,
- segment_ids,
- lm_labels,
- sequence_labels,
- is_impossible_labels,
- token_labels,
- ):
- model = XLNetModel(config)
- model.to(torch_device)
- model.eval()
-
- _, _, attentions = model(input_ids_1, target_mapping=target_mapping)
-
- self.parent.assertEqual(len(attentions), config.n_layer)
- self.parent.assertIsInstance(attentions[0], tuple)
- self.parent.assertEqual(len(attentions[0]), 2)
- self.parent.assertTrue(attentions[0][0].shape, attentions[0][0].shape)
-
- def create_and_check_xlnet_lm_head(
- self,
- config,
- input_ids_1,
- input_ids_2,
- input_ids_q,
- perm_mask,
- input_mask,
- target_mapping,
- segment_ids,
- lm_labels,
- sequence_labels,
- is_impossible_labels,
- token_labels,
- ):
- model = XLNetLMHeadModel(config)
- model.to(torch_device)
- model.eval()
-
- loss_1, all_logits_1, mems_1 = model(input_ids_1, token_type_ids=segment_ids, labels=lm_labels)
-
- loss_2, all_logits_2, mems_2 = model(
- input_ids_2, token_type_ids=segment_ids, labels=lm_labels, mems=mems_1
- )
-
- logits, _ = model(input_ids_q, perm_mask=perm_mask, target_mapping=target_mapping)
-
- result = {
- "loss_1": loss_1,
- "mems_1": mems_1,
- "all_logits_1": all_logits_1,
- "loss_2": loss_2,
- "mems_2": mems_2,
- "all_logits_2": all_logits_2,
- }
-
- self.parent.assertListEqual(list(result["loss_1"].size()), [])
- self.parent.assertListEqual(
- list(result["all_logits_1"].size()), [self.batch_size, self.seq_length, self.vocab_size]
- )
- self.parent.assertListEqual(
- list(list(mem.size()) for mem in result["mems_1"]),
- [[self.seq_length, self.batch_size, self.hidden_size]] * self.num_hidden_layers,
- )
-
- self.parent.assertListEqual(list(result["loss_2"].size()), [])
- self.parent.assertListEqual(
- list(result["all_logits_2"].size()), [self.batch_size, self.seq_length, self.vocab_size]
- )
- self.parent.assertListEqual(
- list(list(mem.size()) for mem in result["mems_2"]),
- [[self.mem_len, self.batch_size, self.hidden_size]] * self.num_hidden_layers,
- )
-
- def create_and_check_xlnet_qa(
- self,
- config,
- input_ids_1,
- input_ids_2,
- input_ids_q,
- perm_mask,
- input_mask,
- target_mapping,
- segment_ids,
- lm_labels,
- sequence_labels,
- is_impossible_labels,
- token_labels,
- ):
- model = XLNetForQuestionAnswering(config)
- model.to(torch_device)
- model.eval()
-
- outputs = model(input_ids_1)
- start_top_log_probs, start_top_index, end_top_log_probs, end_top_index, cls_logits, mems = outputs
-
- outputs = model(
- input_ids_1,
- start_positions=sequence_labels,
- end_positions=sequence_labels,
- cls_index=sequence_labels,
- is_impossible=is_impossible_labels,
- p_mask=input_mask,
- )
-
- outputs = model(
- input_ids_1,
- start_positions=sequence_labels,
- end_positions=sequence_labels,
- cls_index=sequence_labels,
- is_impossible=is_impossible_labels,
- )
-
- total_loss, mems = outputs
-
- outputs = model(input_ids_1, start_positions=sequence_labels, end_positions=sequence_labels)
-
- total_loss, mems = outputs
-
- result = {
- "loss": total_loss,
- "start_top_log_probs": start_top_log_probs,
- "start_top_index": start_top_index,
- "end_top_log_probs": end_top_log_probs,
- "end_top_index": end_top_index,
- "cls_logits": cls_logits,
- "mems": mems,
- }
-
- self.parent.assertListEqual(list(result["loss"].size()), [])
- self.parent.assertListEqual(
- list(result["start_top_log_probs"].size()), [self.batch_size, model.config.start_n_top]
- )
- self.parent.assertListEqual(
- list(result["start_top_index"].size()), [self.batch_size, model.config.start_n_top]
- )
- self.parent.assertListEqual(
- list(result["end_top_log_probs"].size()),
- [self.batch_size, model.config.start_n_top * model.config.end_n_top],
- )
- self.parent.assertListEqual(
- list(result["end_top_index"].size()),
- [self.batch_size, model.config.start_n_top * model.config.end_n_top],
- )
- self.parent.assertListEqual(list(result["cls_logits"].size()), [self.batch_size])
- self.parent.assertListEqual(
- list(list(mem.size()) for mem in result["mems"]),
- [[self.seq_length, self.batch_size, self.hidden_size]] * self.num_hidden_layers,
- )
-
- def create_and_check_xlnet_token_classif(
- self,
- config,
- input_ids_1,
- input_ids_2,
- input_ids_q,
- perm_mask,
- input_mask,
- target_mapping,
- segment_ids,
- lm_labels,
- sequence_labels,
- is_impossible_labels,
- token_labels,
- ):
- model = XLNetForTokenClassification(config)
- model.to(torch_device)
- model.eval()
-
- logits, mems_1 = model(input_ids_1)
- loss, logits, mems_1 = model(input_ids_1, labels=token_labels)
-
- result = {
- "loss": loss,
- "mems_1": mems_1,
- "logits": logits,
- }
-
- self.parent.assertListEqual(list(result["loss"].size()), [])
- self.parent.assertListEqual(
- list(result["logits"].size()), [self.batch_size, self.seq_length, self.type_sequence_label_size]
- )
- self.parent.assertListEqual(
- list(list(mem.size()) for mem in result["mems_1"]),
- [[self.seq_length, self.batch_size, self.hidden_size]] * self.num_hidden_layers,
- )
-
- def create_and_check_xlnet_sequence_classif(
- self,
- config,
- input_ids_1,
- input_ids_2,
- input_ids_q,
- perm_mask,
- input_mask,
- target_mapping,
- segment_ids,
- lm_labels,
- sequence_labels,
- is_impossible_labels,
- token_labels,
- ):
- model = XLNetForSequenceClassification(config)
- model.to(torch_device)
- model.eval()
-
- logits, mems_1 = model(input_ids_1)
- loss, logits, mems_1 = model(input_ids_1, labels=sequence_labels)
-
- result = {
- "loss": loss,
- "mems_1": mems_1,
- "logits": logits,
- }
-
- self.parent.assertListEqual(list(result["loss"].size()), [])
- self.parent.assertListEqual(
- list(result["logits"].size()), [self.batch_size, self.type_sequence_label_size]
- )
- self.parent.assertListEqual(
- list(list(mem.size()) for mem in result["mems_1"]),
- [[self.seq_length, self.batch_size, self.hidden_size]] * self.num_hidden_layers,
- )
-
- def prepare_config_and_inputs_for_common(self):
- config_and_inputs = self.prepare_config_and_inputs()
- (
- config,
- input_ids_1,
- input_ids_2,
- input_ids_q,
- perm_mask,
- input_mask,
- target_mapping,
- segment_ids,
- lm_labels,
- sequence_labels,
- is_impossible_labels,
- token_labels,
- ) = config_and_inputs
- inputs_dict = {"input_ids": input_ids_1}
- return config, inputs_dict
-
- def setUp(self):
- self.model_tester = XLNetModelTest.XLNetModelTester(self)
- self.config_tester = ConfigTester(self, config_class=XLNetConfig, d_inner=37)
-
- def test_config(self):
- self.config_tester.run_common_tests()
-
- def test_xlnet_base_model(self):
- self.model_tester.set_seed()
- config_and_inputs = self.model_tester.prepare_config_and_inputs()
- self.model_tester.create_and_check_xlnet_base_model(*config_and_inputs)
-
- def test_xlnet_base_model_with_att_output(self):
- self.model_tester.set_seed()
- config_and_inputs = self.model_tester.prepare_config_and_inputs()
- config_and_inputs[0].output_attentions = True
- self.model_tester.create_and_check_xlnet_base_model_with_att_output(*config_and_inputs)
-
- def test_xlnet_lm_head(self):
- self.model_tester.set_seed()
- config_and_inputs = self.model_tester.prepare_config_and_inputs()
- self.model_tester.create_and_check_xlnet_lm_head(*config_and_inputs)
-
- def test_xlnet_sequence_classif(self):
- self.model_tester.set_seed()
- config_and_inputs = self.model_tester.prepare_config_and_inputs()
- self.model_tester.create_and_check_xlnet_sequence_classif(*config_and_inputs)
-
- def test_xlnet_token_classif(self):
- self.model_tester.set_seed()
- config_and_inputs = self.model_tester.prepare_config_and_inputs()
- self.model_tester.create_and_check_xlnet_token_classif(*config_and_inputs)
-
- def test_xlnet_qa(self):
- self.model_tester.set_seed()
- config_and_inputs = self.model_tester.prepare_config_and_inputs()
- self.model_tester.create_and_check_xlnet_qa(*config_and_inputs)
-
- @slow
- def test_model_from_pretrained(self):
- for model_name in list(XLNET_PRETRAINED_MODEL_ARCHIVE_MAP.keys())[:1]:
- model = XLNetModel.from_pretrained(model_name, cache_dir=CACHE_DIR)
- self.assertIsNotNone(model)
diff --git a/server/transformers/tests/test_optimization.py b/server/transformers/tests/test_optimization.py
deleted file mode 100644
index 8c9ebb2dd27a96cb9f60b1e8d4068af54a9ba8b7..0000000000000000000000000000000000000000
--- a/server/transformers/tests/test_optimization.py
+++ /dev/null
@@ -1,152 +0,0 @@
-# coding=utf-8
-# Copyright 2018 The Google AI Language Team Authors.
-#
-# Licensed under the Apache License, Version 2.0 (the "License");
-# you may not use this file except in compliance with the License.
-# You may obtain a copy of the License at
-#
-# http://www.apache.org/licenses/LICENSE-2.0
-#
-# Unless required by applicable law or agreed to in writing, software
-# distributed under the License is distributed on an "AS IS" BASIS,
-# WITHOUT WARRANTIES OR CONDITIONS OF ANY KIND, either express or implied.
-# See the License for the specific language governing permissions and
-# limitations under the License.
-
-
-import os
-import tempfile
-import unittest
-
-from transformers import is_torch_available
-
-from .utils import require_torch
-
-
-if is_torch_available():
- import torch
-
- from transformers import (
- AdamW,
- get_constant_schedule,
- get_constant_schedule_with_warmup,
- get_cosine_schedule_with_warmup,
- get_cosine_with_hard_restarts_schedule_with_warmup,
- get_linear_schedule_with_warmup,
- )
-
-
-def unwrap_schedule(scheduler, num_steps=10):
- lrs = []
- for _ in range(num_steps):
- scheduler.step()
- lrs.append(scheduler.get_lr())
- return lrs
-
-
-def unwrap_and_save_reload_schedule(scheduler, num_steps=10):
- lrs = []
- for step in range(num_steps):
- scheduler.step()
- lrs.append(scheduler.get_lr())
- if step == num_steps // 2:
- with tempfile.TemporaryDirectory() as tmpdirname:
- file_name = os.path.join(tmpdirname, "schedule.bin")
- torch.save(scheduler.state_dict(), file_name)
-
- state_dict = torch.load(file_name)
- scheduler.load_state_dict(state_dict)
- return lrs
-
-
-@require_torch
-class OptimizationTest(unittest.TestCase):
- def assertListAlmostEqual(self, list1, list2, tol):
- self.assertEqual(len(list1), len(list2))
- for a, b in zip(list1, list2):
- self.assertAlmostEqual(a, b, delta=tol)
-
- def test_adam_w(self):
- w = torch.tensor([0.1, -0.2, -0.1], requires_grad=True)
- target = torch.tensor([0.4, 0.2, -0.5])
- criterion = torch.nn.MSELoss()
- # No warmup, constant schedule, no gradient clipping
- optimizer = AdamW(params=[w], lr=2e-1, weight_decay=0.0)
- for _ in range(100):
- loss = criterion(w, target)
- loss.backward()
- optimizer.step()
- w.grad.detach_() # No zero_grad() function on simple tensors. we do it ourselves.
- w.grad.zero_()
- self.assertListAlmostEqual(w.tolist(), [0.4, 0.2, -0.5], tol=1e-2)
-
-
-@require_torch
-class ScheduleInitTest(unittest.TestCase):
- m = torch.nn.Linear(50, 50) if is_torch_available() else None
- optimizer = AdamW(m.parameters(), lr=10.0) if is_torch_available() else None
- num_steps = 10
-
- def assertListAlmostEqual(self, list1, list2, tol):
- self.assertEqual(len(list1), len(list2))
- for a, b in zip(list1, list2):
- self.assertAlmostEqual(a, b, delta=tol)
-
- def test_constant_scheduler(self):
- scheduler = get_constant_schedule(self.optimizer)
- lrs = unwrap_schedule(scheduler, self.num_steps)
- expected_learning_rates = [10.0] * self.num_steps
- self.assertEqual(len(lrs[0]), 1)
- self.assertListEqual([l[0] for l in lrs], expected_learning_rates)
-
- scheduler = get_constant_schedule(self.optimizer)
- lrs_2 = unwrap_and_save_reload_schedule(scheduler, self.num_steps)
- self.assertListEqual([l[0] for l in lrs], [l[0] for l in lrs_2])
-
- def test_warmup_constant_scheduler(self):
- scheduler = get_constant_schedule_with_warmup(self.optimizer, num_warmup_steps=4)
- lrs = unwrap_schedule(scheduler, self.num_steps)
- expected_learning_rates = [2.5, 5.0, 7.5, 10.0, 10.0, 10.0, 10.0, 10.0, 10.0, 10.0]
- self.assertEqual(len(lrs[0]), 1)
- self.assertListEqual([l[0] for l in lrs], expected_learning_rates)
-
- scheduler = get_constant_schedule_with_warmup(self.optimizer, num_warmup_steps=4)
- lrs_2 = unwrap_and_save_reload_schedule(scheduler, self.num_steps)
- self.assertListEqual([l[0] for l in lrs], [l[0] for l in lrs_2])
-
- def test_warmup_linear_scheduler(self):
- scheduler = get_linear_schedule_with_warmup(self.optimizer, num_warmup_steps=2, num_training_steps=10)
- lrs = unwrap_schedule(scheduler, self.num_steps)
- expected_learning_rates = [5.0, 10.0, 8.75, 7.5, 6.25, 5.0, 3.75, 2.5, 1.25, 0.0]
- self.assertEqual(len(lrs[0]), 1)
- self.assertListEqual([l[0] for l in lrs], expected_learning_rates)
-
- scheduler = get_linear_schedule_with_warmup(self.optimizer, num_warmup_steps=2, num_training_steps=10)
- lrs_2 = unwrap_and_save_reload_schedule(scheduler, self.num_steps)
- self.assertListEqual([l[0] for l in lrs], [l[0] for l in lrs_2])
-
- def test_warmup_cosine_scheduler(self):
- scheduler = get_cosine_schedule_with_warmup(self.optimizer, num_warmup_steps=2, num_training_steps=10)
- lrs = unwrap_schedule(scheduler, self.num_steps)
- expected_learning_rates = [5.0, 10.0, 9.61, 8.53, 6.91, 5.0, 3.08, 1.46, 0.38, 0.0]
- self.assertEqual(len(lrs[0]), 1)
- self.assertListAlmostEqual([l[0] for l in lrs], expected_learning_rates, tol=1e-2)
-
- scheduler = get_cosine_schedule_with_warmup(self.optimizer, num_warmup_steps=2, num_training_steps=10)
- lrs_2 = unwrap_and_save_reload_schedule(scheduler, self.num_steps)
- self.assertListEqual([l[0] for l in lrs], [l[0] for l in lrs_2])
-
- def test_warmup_cosine_hard_restart_scheduler(self):
- scheduler = get_cosine_with_hard_restarts_schedule_with_warmup(
- self.optimizer, num_warmup_steps=2, num_cycles=2, num_training_steps=10
- )
- lrs = unwrap_schedule(scheduler, self.num_steps)
- expected_learning_rates = [5.0, 10.0, 8.53, 5.0, 1.46, 10.0, 8.53, 5.0, 1.46, 0.0]
- self.assertEqual(len(lrs[0]), 1)
- self.assertListAlmostEqual([l[0] for l in lrs], expected_learning_rates, tol=1e-2)
-
- scheduler = get_cosine_with_hard_restarts_schedule_with_warmup(
- self.optimizer, num_warmup_steps=2, num_cycles=2, num_training_steps=10
- )
- lrs_2 = unwrap_and_save_reload_schedule(scheduler, self.num_steps)
- self.assertListEqual([l[0] for l in lrs], [l[0] for l in lrs_2])
diff --git a/server/transformers/tests/test_optimization_tf.py b/server/transformers/tests/test_optimization_tf.py
deleted file mode 100644
index 6236c312967c04f311ff721b224d9a005ba8e98b..0000000000000000000000000000000000000000
--- a/server/transformers/tests/test_optimization_tf.py
+++ /dev/null
@@ -1,83 +0,0 @@
-import unittest
-
-from transformers import is_tf_available
-
-from .utils import require_tf
-
-
-if is_tf_available():
- import tensorflow as tf
- from tensorflow.python.eager import context
- from tensorflow.python.framework import ops
- from transformers import create_optimizer, GradientAccumulator
-
-
-@require_tf
-class OptimizationFTest(unittest.TestCase):
- def assertListAlmostEqual(self, list1, list2, tol):
- self.assertEqual(len(list1), len(list2))
- for a, b in zip(list1, list2):
- self.assertAlmostEqual(a, b, delta=tol)
-
- def testGradientAccumulator(self):
- accumulator = GradientAccumulator()
- accumulator([tf.constant([1.0, 2.0])])
- accumulator([tf.constant([-2.0, 1.0])])
- accumulator([tf.constant([-1.0, 2.0])])
- with self.assertRaises(ValueError):
- accumulator([tf.constant([1.0, 1.0]), tf.constant([2.0, 2.0])])
- self.assertEqual(accumulator.step, 3)
- self.assertEqual(len(accumulator.gradients), 1)
- self.assertListAlmostEqual(accumulator.gradients[0].numpy().tolist(), [-2.0, 5.0], tol=1e-2)
- accumulator.reset()
- self.assertEqual(accumulator.step, 0)
- self.assertListAlmostEqual(accumulator.gradients[0].numpy().tolist(), [0.0, 0.0], tol=1e-2)
-
- def testGradientAccumulatorDistributionStrategy(self):
- context._context = None
- ops.enable_eager_execution_internal()
- physical_devices = tf.config.experimental.list_physical_devices("CPU")
- tf.config.experimental.set_virtual_device_configuration(
- physical_devices[0],
- [tf.config.experimental.VirtualDeviceConfiguration(), tf.config.experimental.VirtualDeviceConfiguration()],
- )
-
- devices = tf.config.experimental.list_logical_devices(device_type="CPU")
- strategy = tf.distribute.MirroredStrategy(devices=[device.name for device in devices])
-
- with strategy.scope():
- accumulator = GradientAccumulator()
- variable = tf.Variable([4.0, 3.0])
- optimizer = create_optimizer(5e-5, 10, 5)
- gradient_placeholder = tf.Variable([0.0, 0.0], trainable=False)
-
- def accumulate_on_replica(gradient):
- accumulator([gradient])
-
- def apply_on_replica():
- optimizer.apply_gradients(list(zip(accumulator.gradients, [variable])), 1.0)
-
- @tf.function
- def accumulate(grad1, grad2):
- with strategy.scope():
- gradient_placeholder.values[0].assign(grad1)
- gradient_placeholder.values[1].assign(grad2)
- strategy.experimental_run_v2(accumulate_on_replica, args=(gradient_placeholder,))
-
- @tf.function
- def apply_grad():
- with strategy.scope():
- strategy.experimental_run_v2(apply_on_replica)
-
- accumulate([1.0, 2.0], [-1.0, 1.0])
- accumulate([3.0, -1.0], [-1.0, -1.0])
- accumulate([-2.0, 2.0], [3.0, -2.0])
- self.assertEqual(accumulator.step, 3)
- self.assertListAlmostEqual(accumulator._gradients[0].values[0].value().numpy().tolist(), [2.0, 3.0], tol=1e-2)
- self.assertListAlmostEqual(accumulator._gradients[0].values[1].value().numpy().tolist(), [1.0, -2.0], tol=1e-2)
- apply_grad()
- self.assertListAlmostEqual(variable.value().numpy().tolist(), [4.0, 3.0], tol=1e-2)
- accumulator.reset()
- self.assertEqual(accumulator.step, 0)
- self.assertListAlmostEqual(accumulator._gradients[0].values[0].value().numpy().tolist(), [0.0, 0.0], tol=1e-2)
- self.assertListAlmostEqual(accumulator._gradients[0].values[1].value().numpy().tolist(), [0.0, 0.0], tol=1e-2)
diff --git a/server/transformers/tests/test_pipelines.py b/server/transformers/tests/test_pipelines.py
deleted file mode 100644
index 3a4535d153828820d8973af36c487750ff95a13f..0000000000000000000000000000000000000000
--- a/server/transformers/tests/test_pipelines.py
+++ /dev/null
@@ -1,297 +0,0 @@
-import unittest
-from typing import Iterable, List, Optional
-
-from transformers import pipeline
-from transformers.pipelines import Pipeline
-
-from .utils import require_tf, require_torch
-
-
-QA_FINETUNED_MODELS = {
- ("bert-base-uncased", "bert-large-uncased-whole-word-masking-finetuned-squad", None),
- ("bert-base-cased", "bert-large-cased-whole-word-masking-finetuned-squad", None),
- ("bert-base-uncased", "distilbert-base-uncased-distilled-squad", None),
-}
-
-TF_QA_FINETUNED_MODELS = {
- ("bert-base-uncased", "bert-large-uncased-whole-word-masking-finetuned-squad", None),
- ("bert-base-cased", "bert-large-cased-whole-word-masking-finetuned-squad", None),
- ("bert-base-uncased", "distilbert-base-uncased-distilled-squad", None),
-}
-
-TF_NER_FINETUNED_MODELS = {
- (
- "bert-base-cased",
- "dbmdz/bert-large-cased-finetuned-conll03-english",
- "dbmdz/bert-large-cased-finetuned-conll03-english",
- )
-}
-
-NER_FINETUNED_MODELS = {
- (
- "bert-base-cased",
- "dbmdz/bert-large-cased-finetuned-conll03-english",
- "dbmdz/bert-large-cased-finetuned-conll03-english",
- )
-}
-
-FEATURE_EXTRACT_FINETUNED_MODELS = {
- ("bert-base-cased", "bert-base-cased", None),
- # ('xlnet-base-cased', 'xlnet-base-cased', None), # Disabled for now as it crash for TF2
- ("distilbert-base-uncased", "distilbert-base-uncased", None),
-}
-
-TF_FEATURE_EXTRACT_FINETUNED_MODELS = {
- ("bert-base-cased", "bert-base-cased", None),
- # ('xlnet-base-cased', 'xlnet-base-cased', None), # Disabled for now as it crash for TF2
- ("distilbert-base-uncased", "distilbert-base-uncased", None),
-}
-
-TF_TEXT_CLASSIF_FINETUNED_MODELS = {
- (
- "bert-base-uncased",
- "distilbert-base-uncased-finetuned-sst-2-english",
- "distilbert-base-uncased-finetuned-sst-2-english",
- )
-}
-
-TEXT_CLASSIF_FINETUNED_MODELS = {
- (
- "bert-base-uncased",
- "distilbert-base-uncased-finetuned-sst-2-english",
- "distilbert-base-uncased-finetuned-sst-2-english",
- )
-}
-
-FILL_MASK_FINETUNED_MODELS = {
- ("distilroberta-base", "distilroberta-base", None),
-}
-
-TF_FILL_MASK_FINETUNED_MODELS = {
- ("distilroberta-base", "distilroberta-base", None),
-}
-
-
-class MonoColumnInputTestCase(unittest.TestCase):
- def _test_mono_column_pipeline(
- self,
- nlp: Pipeline,
- valid_inputs: List,
- invalid_inputs: List,
- output_keys: Iterable[str],
- expected_multi_result: Optional[List] = None,
- expected_check_keys: Optional[List[str]] = None,
- ):
- self.assertIsNotNone(nlp)
-
- mono_result = nlp(valid_inputs[0])
- self.assertIsInstance(mono_result, list)
- self.assertIsInstance(mono_result[0], (dict, list))
-
- if isinstance(mono_result[0], list):
- mono_result = mono_result[0]
-
- for key in output_keys:
- self.assertIn(key, mono_result[0])
-
- multi_result = nlp(valid_inputs)
- self.assertIsInstance(multi_result, list)
- self.assertIsInstance(multi_result[0], (dict, list))
-
- if expected_multi_result is not None:
- for result, expect in zip(multi_result, expected_multi_result):
- for key in expected_check_keys or []:
- self.assertEqual(
- set([o[key] for o in result]), set([o[key] for o in expect]),
- )
-
- if isinstance(multi_result[0], list):
- multi_result = multi_result[0]
-
- for result in multi_result:
- for key in output_keys:
- self.assertIn(key, result)
-
- self.assertRaises(Exception, nlp, invalid_inputs)
-
- @require_torch
- def test_ner(self):
- mandatory_keys = {"entity", "word", "score"}
- valid_inputs = ["HuggingFace is solving NLP one commit at a time.", "HuggingFace is based in New-York & Paris"]
- invalid_inputs = [None]
- for tokenizer, model, config in NER_FINETUNED_MODELS:
- nlp = pipeline(task="ner", model=model, config=config, tokenizer=tokenizer)
- self._test_mono_column_pipeline(nlp, valid_inputs, invalid_inputs, mandatory_keys)
-
- @require_tf
- def test_tf_ner(self):
- mandatory_keys = {"entity", "word", "score"}
- valid_inputs = ["HuggingFace is solving NLP one commit at a time.", "HuggingFace is based in New-York & Paris"]
- invalid_inputs = [None]
- for tokenizer, model, config in TF_NER_FINETUNED_MODELS:
- nlp = pipeline(task="ner", model=model, config=config, tokenizer=tokenizer)
- self._test_mono_column_pipeline(nlp, valid_inputs, invalid_inputs, mandatory_keys)
-
- @require_torch
- def test_sentiment_analysis(self):
- mandatory_keys = {"label", "score"}
- valid_inputs = ["HuggingFace is solving NLP one commit at a time.", "HuggingFace is based in New-York & Paris"]
- invalid_inputs = [None]
- for tokenizer, model, config in TEXT_CLASSIF_FINETUNED_MODELS:
- nlp = pipeline(task="sentiment-analysis", model=model, config=config, tokenizer=tokenizer)
- self._test_mono_column_pipeline(nlp, valid_inputs, invalid_inputs, mandatory_keys)
-
- @require_tf
- def test_tf_sentiment_analysis(self):
- mandatory_keys = {"label", "score"}
- valid_inputs = ["HuggingFace is solving NLP one commit at a time.", "HuggingFace is based in New-York & Paris"]
- invalid_inputs = [None]
- for tokenizer, model, config in TF_TEXT_CLASSIF_FINETUNED_MODELS:
- nlp = pipeline(task="sentiment-analysis", model=model, config=config, tokenizer=tokenizer)
- self._test_mono_column_pipeline(nlp, valid_inputs, invalid_inputs, mandatory_keys)
-
- @require_torch
- def test_feature_extraction(self):
- valid_inputs = ["HuggingFace is solving NLP one commit at a time.", "HuggingFace is based in New-York & Paris"]
- invalid_inputs = [None]
- for tokenizer, model, config in FEATURE_EXTRACT_FINETUNED_MODELS:
- nlp = pipeline(task="feature-extraction", model=model, config=config, tokenizer=tokenizer)
- self._test_mono_column_pipeline(nlp, valid_inputs, invalid_inputs, {})
-
- @require_tf
- def test_tf_feature_extraction(self):
- valid_inputs = ["HuggingFace is solving NLP one commit at a time.", "HuggingFace is based in New-York & Paris"]
- invalid_inputs = [None]
- for tokenizer, model, config in TF_FEATURE_EXTRACT_FINETUNED_MODELS:
- nlp = pipeline(task="feature-extraction", model=model, config=config, tokenizer=tokenizer)
- self._test_mono_column_pipeline(nlp, valid_inputs, invalid_inputs, {})
-
- @require_torch
- def test_fill_mask(self):
- mandatory_keys = {"sequence", "score", "token"}
- valid_inputs = [
- "My name is ",
- "The largest city in France is ",
- ]
- invalid_inputs = [None]
- expected_multi_result = [
- [
- {"score": 0.008698059245944023, "sequence": "My name is John", "token": 610},
- {"score": 0.007750614080578089, "sequence": "My name is Chris", "token": 1573},
- ],
- [
- {"score": 0.2721288502216339, "sequence": "The largest city in France is Paris", "token": 2201},
- {
- "score": 0.19764970242977142,
- "sequence": "The largest city in France is Lyon",
- "token": 12790,
- },
- ],
- ]
- for tokenizer, model, config in FILL_MASK_FINETUNED_MODELS:
- nlp = pipeline(task="fill-mask", model=model, config=config, tokenizer=tokenizer, topk=2)
- self._test_mono_column_pipeline(
- nlp,
- valid_inputs,
- invalid_inputs,
- mandatory_keys,
- expected_multi_result=expected_multi_result,
- expected_check_keys=["sequence"],
- )
-
- @require_tf
- def test_tf_fill_mask(self):
- mandatory_keys = {"sequence", "score", "token"}
- valid_inputs = [
- "My name is ",
- "The largest city in France is ",
- ]
- invalid_inputs = [None]
- expected_multi_result = [
- [
- {"score": 0.008698059245944023, "sequence": "My name is John", "token": 610},
- {"score": 0.007750614080578089, "sequence": "My name is Chris", "token": 1573},
- ],
- [
- {"score": 0.2721288502216339, "sequence": "The largest city in France is Paris", "token": 2201},
- {
- "score": 0.19764970242977142,
- "sequence": "The largest city in France is Lyon",
- "token": 12790,
- },
- ],
- ]
- for tokenizer, model, config in TF_FILL_MASK_FINETUNED_MODELS:
- nlp = pipeline(task="fill-mask", model=model, config=config, tokenizer=tokenizer, topk=2)
- self._test_mono_column_pipeline(
- nlp,
- valid_inputs,
- invalid_inputs,
- mandatory_keys,
- expected_multi_result=expected_multi_result,
- expected_check_keys=["sequence"],
- )
-
-
-class MultiColumnInputTestCase(unittest.TestCase):
- def _test_multicolumn_pipeline(self, nlp, valid_inputs: list, invalid_inputs: list, output_keys: Iterable[str]):
- self.assertIsNotNone(nlp)
-
- mono_result = nlp(valid_inputs[0])
- self.assertIsInstance(mono_result, dict)
-
- for key in output_keys:
- self.assertIn(key, mono_result)
-
- multi_result = nlp(valid_inputs)
- self.assertIsInstance(multi_result, list)
- self.assertIsInstance(multi_result[0], dict)
-
- for result in multi_result:
- for key in output_keys:
- self.assertIn(key, result)
-
- self.assertRaises(Exception, nlp, invalid_inputs[0])
- self.assertRaises(Exception, nlp, invalid_inputs)
-
- @require_torch
- def test_question_answering(self):
- mandatory_output_keys = {"score", "answer", "start", "end"}
- valid_samples = [
- {"question": "Where was HuggingFace founded ?", "context": "HuggingFace was founded in Paris."},
- {
- "question": "In what field is HuggingFace working ?",
- "context": "HuggingFace is a startup based in New-York founded in Paris which is trying to solve NLP.",
- },
- ]
- invalid_samples = [
- {"question": "", "context": "This is a test to try empty question edge case"},
- {"question": None, "context": "This is a test to try empty question edge case"},
- {"question": "What is does with empty context ?", "context": ""},
- {"question": "What is does with empty context ?", "context": None},
- ]
-
- for tokenizer, model, config in QA_FINETUNED_MODELS:
- nlp = pipeline(task="question-answering", model=model, config=config, tokenizer=tokenizer)
- self._test_multicolumn_pipeline(nlp, valid_samples, invalid_samples, mandatory_output_keys)
-
- @require_tf
- def test_tf_question_answering(self):
- mandatory_output_keys = {"score", "answer", "start", "end"}
- valid_samples = [
- {"question": "Where was HuggingFace founded ?", "context": "HuggingFace was founded in Paris."},
- {
- "question": "In what field is HuggingFace working ?",
- "context": "HuggingFace is a startup based in New-York founded in Paris which is trying to solve NLP.",
- },
- ]
- invalid_samples = [
- {"question": "", "context": "This is a test to try empty question edge case"},
- {"question": None, "context": "This is a test to try empty question edge case"},
- {"question": "What is does with empty context ?", "context": ""},
- {"question": "What is does with empty context ?", "context": None},
- ]
-
- for tokenizer, model, config in TF_QA_FINETUNED_MODELS:
- nlp = pipeline(task="question-answering", model=model, config=config, tokenizer=tokenizer)
- self._test_multicolumn_pipeline(nlp, valid_samples, invalid_samples, mandatory_output_keys)
diff --git a/server/transformers/tests/test_tokenization_albert.py b/server/transformers/tests/test_tokenization_albert.py
deleted file mode 100644
index c190d8ed826330e5c88d9be09c25a8a406b86b3e..0000000000000000000000000000000000000000
--- a/server/transformers/tests/test_tokenization_albert.py
+++ /dev/null
@@ -1,80 +0,0 @@
-# coding=utf-8
-# Copyright 2019 Hugging Face inc.
-#
-# Licensed under the Apache License, Version 2.0 (the "License");
-# you may not use this file except in compliance with the License.
-# You may obtain a copy of the License at
-#
-# http://www.apache.org/licenses/LICENSE-2.0
-#
-# Unless required by applicable law or agreed to in writing, software
-# distributed under the License is distributed on an "AS IS" BASIS,
-# WITHOUT WARRANTIES OR CONDITIONS OF ANY KIND, either express or implied.
-# See the License for the specific language governing permissions and
-# limitations under the License.
-
-
-import os
-import unittest
-
-from transformers.tokenization_albert import AlbertTokenizer
-
-from .test_tokenization_common import TokenizerTesterMixin
-
-
-SAMPLE_VOCAB = os.path.join(os.path.dirname(os.path.abspath(__file__)), "fixtures/spiece.model")
-
-
-class AlbertTokenizationTest(TokenizerTesterMixin, unittest.TestCase):
-
- tokenizer_class = AlbertTokenizer
-
- def setUp(self):
- super().setUp()
-
- # We have a SentencePiece fixture for testing
- tokenizer = AlbertTokenizer(SAMPLE_VOCAB)
- tokenizer.save_pretrained(self.tmpdirname)
-
- def get_tokenizer(self, **kwargs):
- return AlbertTokenizer.from_pretrained(self.tmpdirname, **kwargs)
-
- def get_input_output_texts(self):
- input_text = "this is a test"
- output_text = "this is a test"
- return input_text, output_text
-
- def test_full_tokenizer(self):
- tokenizer = AlbertTokenizer(SAMPLE_VOCAB, keep_accents=True)
-
- tokens = tokenizer.tokenize("This is a test")
- self.assertListEqual(tokens, ["▁this", "▁is", "▁a", "▁test"])
-
- self.assertListEqual(tokenizer.convert_tokens_to_ids(tokens), [48, 25, 21, 1289])
-
- tokens = tokenizer.tokenize("I was born in 92000, and this is falsé.")
- self.assertListEqual(
- tokens, ["▁i", "▁was", "▁born", "▁in", "▁9", "2000", ",", "▁and", "▁this", "▁is", "▁fal", "s", "é", "."]
- )
- ids = tokenizer.convert_tokens_to_ids(tokens)
- self.assertListEqual(ids, [31, 23, 386, 19, 561, 3050, 15, 17, 48, 25, 8256, 18, 1, 9])
-
- back_tokens = tokenizer.convert_ids_to_tokens(ids)
- self.assertListEqual(
- back_tokens,
- ["▁i", "▁was", "▁born", "▁in", "▁9", "2000", ",", "▁and", "▁this", "▁is", "▁fal", "s", "", "."],
- )
-
- def test_sequence_builders(self):
- tokenizer = AlbertTokenizer(SAMPLE_VOCAB)
-
- text = tokenizer.encode("sequence builders")
- text_2 = tokenizer.encode("multi-sequence build")
-
- encoded_sentence = tokenizer.build_inputs_with_special_tokens(text)
- encoded_pair = tokenizer.build_inputs_with_special_tokens(text, text_2)
-
- assert encoded_sentence == [tokenizer.cls_token_id] + text + [tokenizer.sep_token_id]
- assert encoded_pair == [tokenizer.cls_token_id] + text + [tokenizer.sep_token_id] + text_2 + [
- tokenizer.sep_token_id
- ]
diff --git a/server/transformers/tests/test_tokenization_auto.py b/server/transformers/tests/test_tokenization_auto.py
deleted file mode 100644
index 5ce9228287046e066172eba3c91d0788fda63918..0000000000000000000000000000000000000000
--- a/server/transformers/tests/test_tokenization_auto.py
+++ /dev/null
@@ -1,89 +0,0 @@
-# coding=utf-8
-# Copyright 2018 The Google AI Language Team Authors.
-#
-# Licensed under the Apache License, Version 2.0 (the "License");
-# you may not use this file except in compliance with the License.
-# You may obtain a copy of the License at
-#
-# http://www.apache.org/licenses/LICENSE-2.0
-#
-# Unless required by applicable law or agreed to in writing, software
-# distributed under the License is distributed on an "AS IS" BASIS,
-# WITHOUT WARRANTIES OR CONDITIONS OF ANY KIND, either express or implied.
-# See the License for the specific language governing permissions and
-# limitations under the License.
-
-
-import logging
-import unittest
-
-from transformers import (
- BERT_PRETRAINED_CONFIG_ARCHIVE_MAP,
- GPT2_PRETRAINED_CONFIG_ARCHIVE_MAP,
- AutoTokenizer,
- BertTokenizer,
- GPT2Tokenizer,
- RobertaTokenizer,
-)
-from transformers.tokenization_auto import TOKENIZER_MAPPING
-
-from .utils import DUMMY_UNKWOWN_IDENTIFIER, SMALL_MODEL_IDENTIFIER, slow # noqa: F401
-
-
-class AutoTokenizerTest(unittest.TestCase):
- # @slow
- def test_tokenizer_from_pretrained(self):
- logging.basicConfig(level=logging.INFO)
- for model_name in (x for x in BERT_PRETRAINED_CONFIG_ARCHIVE_MAP.keys() if "japanese" not in x):
- tokenizer = AutoTokenizer.from_pretrained(model_name)
- self.assertIsNotNone(tokenizer)
- self.assertIsInstance(tokenizer, BertTokenizer)
- self.assertGreater(len(tokenizer), 0)
-
- for model_name in GPT2_PRETRAINED_CONFIG_ARCHIVE_MAP.keys():
- tokenizer = AutoTokenizer.from_pretrained(model_name)
- self.assertIsNotNone(tokenizer)
- self.assertIsInstance(tokenizer, GPT2Tokenizer)
- self.assertGreater(len(tokenizer), 0)
-
- def test_tokenizer_from_pretrained_identifier(self):
- logging.basicConfig(level=logging.INFO)
- tokenizer = AutoTokenizer.from_pretrained(SMALL_MODEL_IDENTIFIER)
- self.assertIsInstance(tokenizer, BertTokenizer)
- self.assertEqual(len(tokenizer), 12)
-
- def test_tokenizer_from_model_type(self):
- logging.basicConfig(level=logging.INFO)
- tokenizer = AutoTokenizer.from_pretrained(DUMMY_UNKWOWN_IDENTIFIER)
- self.assertIsInstance(tokenizer, RobertaTokenizer)
- self.assertEqual(len(tokenizer), 20)
-
- def test_tokenizer_identifier_with_correct_config(self):
- logging.basicConfig(level=logging.INFO)
- for tokenizer_class in [BertTokenizer, AutoTokenizer]:
- tokenizer = tokenizer_class.from_pretrained("wietsedv/bert-base-dutch-cased")
- self.assertIsInstance(tokenizer, BertTokenizer)
- self.assertEqual(tokenizer.basic_tokenizer.do_lower_case, False)
- self.assertEqual(tokenizer.max_len, 512)
-
- def test_tokenizer_identifier_non_existent(self):
- logging.basicConfig(level=logging.INFO)
- for tokenizer_class in [BertTokenizer, AutoTokenizer]:
- with self.assertRaises(EnvironmentError):
- _ = tokenizer_class.from_pretrained("julien-c/herlolip-not-exists")
-
- def test_parents_and_children_in_mappings(self):
- # Test that the children are placed before the parents in the mappings, as the `instanceof` will be triggered
- # by the parents and will return the wrong configuration type when using auto models
-
- mappings = (TOKENIZER_MAPPING,)
-
- for mapping in mappings:
- mapping = tuple(mapping.items())
- for index, (child_config, child_model) in enumerate(mapping[1:]):
- for parent_config, parent_model in mapping[: index + 1]:
- with self.subTest(
- msg="Testing if {} is child of {}".format(child_config.__name__, parent_config.__name__)
- ):
- self.assertFalse(issubclass(child_config, parent_config))
- self.assertFalse(issubclass(child_model, parent_model))
diff --git a/server/transformers/tests/test_tokenization_bert.py b/server/transformers/tests/test_tokenization_bert.py
deleted file mode 100644
index 49bb073351d150ee2737783defe61c35814ccc22..0000000000000000000000000000000000000000
--- a/server/transformers/tests/test_tokenization_bert.py
+++ /dev/null
@@ -1,181 +0,0 @@
-# coding=utf-8
-# Copyright 2018 The Google AI Language Team Authors.
-#
-# Licensed under the Apache License, Version 2.0 (the "License");
-# you may not use this file except in compliance with the License.
-# You may obtain a copy of the License at
-#
-# http://www.apache.org/licenses/LICENSE-2.0
-#
-# Unless required by applicable law or agreed to in writing, software
-# distributed under the License is distributed on an "AS IS" BASIS,
-# WITHOUT WARRANTIES OR CONDITIONS OF ANY KIND, either express or implied.
-# See the License for the specific language governing permissions and
-# limitations under the License.
-
-
-import os
-import unittest
-
-from transformers.tokenization_bert import (
- VOCAB_FILES_NAMES,
- BasicTokenizer,
- BertTokenizer,
- BertTokenizerFast,
- WordpieceTokenizer,
- _is_control,
- _is_punctuation,
- _is_whitespace,
-)
-
-from .test_tokenization_common import TokenizerTesterMixin
-from .utils import slow
-
-
-class BertTokenizationTest(TokenizerTesterMixin, unittest.TestCase):
-
- tokenizer_class = BertTokenizer
- test_rust_tokenizer = True
-
- def setUp(self):
- super().setUp()
-
- vocab_tokens = [
- "[UNK]",
- "[CLS]",
- "[SEP]",
- "want",
- "##want",
- "##ed",
- "wa",
- "un",
- "runn",
- "##ing",
- ",",
- "low",
- "lowest",
- ]
- self.vocab_file = os.path.join(self.tmpdirname, VOCAB_FILES_NAMES["vocab_file"])
- with open(self.vocab_file, "w", encoding="utf-8") as vocab_writer:
- vocab_writer.write("".join([x + "\n" for x in vocab_tokens]))
-
- def get_tokenizer(self, **kwargs):
- return BertTokenizer.from_pretrained(self.tmpdirname, **kwargs)
-
- def get_rust_tokenizer(self, **kwargs):
- return BertTokenizerFast.from_pretrained(self.tmpdirname, **kwargs)
-
- def get_input_output_texts(self):
- input_text = "UNwant\u00E9d,running"
- output_text = "unwanted, running"
- return input_text, output_text
-
- def test_full_tokenizer(self):
- tokenizer = self.tokenizer_class(self.vocab_file)
-
- tokens = tokenizer.tokenize("UNwant\u00E9d,running")
- self.assertListEqual(tokens, ["un", "##want", "##ed", ",", "runn", "##ing"])
- self.assertListEqual(tokenizer.convert_tokens_to_ids(tokens), [7, 4, 5, 10, 8, 9])
-
- def test_rust_and_python_full_tokenizers(self):
- if not self.test_rust_tokenizer:
- return
-
- tokenizer = self.get_tokenizer()
- rust_tokenizer = self.get_rust_tokenizer(add_special_tokens=False)
-
- sequence = "UNwant\u00E9d,running"
-
- tokens = tokenizer.tokenize(sequence)
- rust_tokens = rust_tokenizer.tokenize(sequence)
- self.assertListEqual(tokens, rust_tokens)
-
- ids = tokenizer.encode(sequence, add_special_tokens=False)
- rust_ids = rust_tokenizer.encode(sequence)
- self.assertListEqual(ids, rust_ids)
-
- rust_tokenizer = self.get_rust_tokenizer()
- ids = tokenizer.encode(sequence)
- rust_ids = rust_tokenizer.encode(sequence)
- self.assertListEqual(ids, rust_ids)
-
- def test_chinese(self):
- tokenizer = BasicTokenizer()
-
- self.assertListEqual(tokenizer.tokenize("ah\u535A\u63A8zz"), ["ah", "\u535A", "\u63A8", "zz"])
-
- def test_basic_tokenizer_lower(self):
- tokenizer = BasicTokenizer(do_lower_case=True)
-
- self.assertListEqual(
- tokenizer.tokenize(" \tHeLLo!how \n Are yoU? "), ["hello", "!", "how", "are", "you", "?"]
- )
- self.assertListEqual(tokenizer.tokenize("H\u00E9llo"), ["hello"])
-
- def test_basic_tokenizer_no_lower(self):
- tokenizer = BasicTokenizer(do_lower_case=False)
-
- self.assertListEqual(
- tokenizer.tokenize(" \tHeLLo!how \n Are yoU? "), ["HeLLo", "!", "how", "Are", "yoU", "?"]
- )
-
- def test_basic_tokenizer_respects_never_split_tokens(self):
- tokenizer = BasicTokenizer(do_lower_case=False, never_split=["[UNK]"])
-
- self.assertListEqual(
- tokenizer.tokenize(" \tHeLLo!how \n Are yoU? [UNK]"), ["HeLLo", "!", "how", "Are", "yoU", "?", "[UNK]"]
- )
-
- def test_wordpiece_tokenizer(self):
- vocab_tokens = ["[UNK]", "[CLS]", "[SEP]", "want", "##want", "##ed", "wa", "un", "runn", "##ing"]
-
- vocab = {}
- for (i, token) in enumerate(vocab_tokens):
- vocab[token] = i
- tokenizer = WordpieceTokenizer(vocab=vocab, unk_token="[UNK]")
-
- self.assertListEqual(tokenizer.tokenize(""), [])
-
- self.assertListEqual(tokenizer.tokenize("unwanted running"), ["un", "##want", "##ed", "runn", "##ing"])
-
- self.assertListEqual(tokenizer.tokenize("unwantedX running"), ["[UNK]", "runn", "##ing"])
-
- def test_is_whitespace(self):
- self.assertTrue(_is_whitespace(" "))
- self.assertTrue(_is_whitespace("\t"))
- self.assertTrue(_is_whitespace("\r"))
- self.assertTrue(_is_whitespace("\n"))
- self.assertTrue(_is_whitespace("\u00A0"))
-
- self.assertFalse(_is_whitespace("A"))
- self.assertFalse(_is_whitespace("-"))
-
- def test_is_control(self):
- self.assertTrue(_is_control("\u0005"))
-
- self.assertFalse(_is_control("A"))
- self.assertFalse(_is_control(" "))
- self.assertFalse(_is_control("\t"))
- self.assertFalse(_is_control("\r"))
-
- def test_is_punctuation(self):
- self.assertTrue(_is_punctuation("-"))
- self.assertTrue(_is_punctuation("$"))
- self.assertTrue(_is_punctuation("`"))
- self.assertTrue(_is_punctuation("."))
-
- self.assertFalse(_is_punctuation("A"))
- self.assertFalse(_is_punctuation(" "))
-
- @slow
- def test_sequence_builders(self):
- tokenizer = self.tokenizer_class.from_pretrained("bert-base-uncased")
-
- text = tokenizer.encode("sequence builders", add_special_tokens=False)
- text_2 = tokenizer.encode("multi-sequence build", add_special_tokens=False)
-
- encoded_sentence = tokenizer.build_inputs_with_special_tokens(text)
- encoded_pair = tokenizer.build_inputs_with_special_tokens(text, text_2)
-
- assert encoded_sentence == [101] + text + [102]
- assert encoded_pair == [101] + text + [102] + text_2 + [102]
diff --git a/server/transformers/tests/test_tokenization_bert_japanese.py b/server/transformers/tests/test_tokenization_bert_japanese.py
deleted file mode 100644
index 4900ff49da50690e129038716a03d558ba614b9e..0000000000000000000000000000000000000000
--- a/server/transformers/tests/test_tokenization_bert_japanese.py
+++ /dev/null
@@ -1,191 +0,0 @@
-# coding=utf-8
-# Copyright 2018 The Google AI Language Team Authors.
-#
-# Licensed under the Apache License, Version 2.0 (the "License");
-# you may not use this file except in compliance with the License.
-# You may obtain a copy of the License at
-#
-# http://www.apache.org/licenses/LICENSE-2.0
-#
-# Unless required by applicable law or agreed to in writing, software
-# distributed under the License is distributed on an "AS IS" BASIS,
-# WITHOUT WARRANTIES OR CONDITIONS OF ANY KIND, either express or implied.
-# See the License for the specific language governing permissions and
-# limitations under the License.
-
-
-import os
-import unittest
-
-from transformers.tokenization_bert import WordpieceTokenizer
-from transformers.tokenization_bert_japanese import (
- VOCAB_FILES_NAMES,
- BertJapaneseTokenizer,
- CharacterTokenizer,
- MecabTokenizer,
-)
-
-from .test_tokenization_common import TokenizerTesterMixin
-from .utils import custom_tokenizers, slow
-
-
-@custom_tokenizers
-class BertJapaneseTokenizationTest(TokenizerTesterMixin, unittest.TestCase):
-
- tokenizer_class = BertJapaneseTokenizer
-
- def setUp(self):
- super().setUp()
-
- vocab_tokens = [
- "[UNK]",
- "[CLS]",
- "[SEP]",
- "こんにちは",
- "こん",
- "にちは",
- "ばんは",
- "##こん",
- "##にちは",
- "##ばんは",
- "世界",
- "##世界",
- "、",
- "##、",
- "。",
- "##。",
- ]
-
- self.vocab_file = os.path.join(self.tmpdirname, VOCAB_FILES_NAMES["vocab_file"])
- with open(self.vocab_file, "w", encoding="utf-8") as vocab_writer:
- vocab_writer.write("".join([x + "\n" for x in vocab_tokens]))
-
- def get_tokenizer(self, **kwargs):
- return BertJapaneseTokenizer.from_pretrained(self.tmpdirname, **kwargs)
-
- def get_input_output_texts(self):
- input_text = "こんにちは、世界。 \nこんばんは、世界。"
- output_text = "こんにちは 、 世界 。 こんばんは 、 世界 。"
- return input_text, output_text
-
- def test_full_tokenizer(self):
- tokenizer = self.tokenizer_class(self.vocab_file)
-
- tokens = tokenizer.tokenize("こんにちは、世界。\nこんばんは、世界。")
- self.assertListEqual(tokens, ["こんにちは", "、", "世界", "。", "こん", "##ばんは", "、", "世界", "。"])
- self.assertListEqual(tokenizer.convert_tokens_to_ids(tokens), [3, 12, 10, 14, 4, 9, 12, 10, 14])
-
- def test_mecab_tokenizer(self):
- tokenizer = MecabTokenizer()
-
- self.assertListEqual(
- tokenizer.tokenize(" \tアップルストアでiPhone8 が \n 発売された 。 "),
- ["アップルストア", "で", "iPhone", "8", "が", "発売", "さ", "れ", "た", "。"],
- )
-
- def test_mecab_tokenizer_lower(self):
- tokenizer = MecabTokenizer(do_lower_case=True)
-
- self.assertListEqual(
- tokenizer.tokenize(" \tアップルストアでiPhone8 が \n 発売された 。 "),
- ["アップルストア", "で", "iphone", "8", "が", "発売", "さ", "れ", "た", "。"],
- )
-
- def test_mecab_tokenizer_no_normalize(self):
- tokenizer = MecabTokenizer(normalize_text=False)
-
- self.assertListEqual(
- tokenizer.tokenize(" \tアップルストアでiPhone8 が \n 発売された 。 "),
- ["アップルストア", "で", "iPhone", "8", "が", "発売", "さ", "れ", "た", " ", "。"],
- )
-
- def test_wordpiece_tokenizer(self):
- vocab_tokens = ["[UNK]", "[CLS]", "[SEP]", "こんにちは", "こん", "にちは" "ばんは", "##こん", "##にちは", "##ばんは"]
-
- vocab = {}
- for (i, token) in enumerate(vocab_tokens):
- vocab[token] = i
- tokenizer = WordpieceTokenizer(vocab=vocab, unk_token="[UNK]")
-
- self.assertListEqual(tokenizer.tokenize(""), [])
-
- self.assertListEqual(tokenizer.tokenize("こんにちは"), ["こんにちは"])
-
- self.assertListEqual(tokenizer.tokenize("こんばんは"), ["こん", "##ばんは"])
-
- self.assertListEqual(tokenizer.tokenize("こんばんは こんばんにちは こんにちは"), ["こん", "##ばんは", "[UNK]", "こんにちは"])
-
- @slow
- def test_sequence_builders(self):
- tokenizer = self.tokenizer_class.from_pretrained("bert-base-japanese")
-
- text = tokenizer.encode("ありがとう。", add_special_tokens=False)
- text_2 = tokenizer.encode("どういたしまして。", add_special_tokens=False)
-
- encoded_sentence = tokenizer.build_inputs_with_special_tokens(text)
- encoded_pair = tokenizer.build_inputs_with_special_tokens(text, text_2)
-
- # 2 is for "[CLS]", 3 is for "[SEP]"
- assert encoded_sentence == [2] + text + [3]
- assert encoded_pair == [2] + text + [3] + text_2 + [3]
-
-
-class BertJapaneseCharacterTokenizationTest(TokenizerTesterMixin, unittest.TestCase):
-
- tokenizer_class = BertJapaneseTokenizer
-
- def setUp(self):
- super().setUp()
-
- vocab_tokens = ["[UNK]", "[CLS]", "[SEP]", "こ", "ん", "に", "ち", "は", "ば", "世", "界", "、", "。"]
-
- self.vocab_file = os.path.join(self.tmpdirname, VOCAB_FILES_NAMES["vocab_file"])
- with open(self.vocab_file, "w", encoding="utf-8") as vocab_writer:
- vocab_writer.write("".join([x + "\n" for x in vocab_tokens]))
-
- def get_tokenizer(self, **kwargs):
- return BertJapaneseTokenizer.from_pretrained(self.tmpdirname, subword_tokenizer_type="character", **kwargs)
-
- def get_input_output_texts(self):
- input_text = "こんにちは、世界。 \nこんばんは、世界。"
- output_text = "こ ん に ち は 、 世 界 。 こ ん ば ん は 、 世 界 。"
- return input_text, output_text
-
- def test_full_tokenizer(self):
- tokenizer = self.tokenizer_class(self.vocab_file, subword_tokenizer_type="character")
-
- tokens = tokenizer.tokenize("こんにちは、世界。 \nこんばんは、世界。")
- self.assertListEqual(
- tokens, ["こ", "ん", "に", "ち", "は", "、", "世", "界", "。", "こ", "ん", "ば", "ん", "は", "、", "世", "界", "。"]
- )
- self.assertListEqual(
- tokenizer.convert_tokens_to_ids(tokens), [3, 4, 5, 6, 7, 11, 9, 10, 12, 3, 4, 8, 4, 7, 11, 9, 10, 12]
- )
-
- def test_character_tokenizer(self):
- vocab_tokens = ["[UNK]", "[CLS]", "[SEP]", "こ", "ん", "に", "ち", "は", "ば", "世", "界" "、", "。"]
-
- vocab = {}
- for (i, token) in enumerate(vocab_tokens):
- vocab[token] = i
- tokenizer = CharacterTokenizer(vocab=vocab, unk_token="[UNK]")
-
- self.assertListEqual(tokenizer.tokenize(""), [])
-
- self.assertListEqual(tokenizer.tokenize("こんにちは"), ["こ", "ん", "に", "ち", "は"])
-
- self.assertListEqual(tokenizer.tokenize("こんにちほ"), ["こ", "ん", "に", "ち", "[UNK]"])
-
- @slow
- def test_sequence_builders(self):
- tokenizer = self.tokenizer_class.from_pretrained("bert-base-japanese-char")
-
- text = tokenizer.encode("ありがとう。", add_special_tokens=False)
- text_2 = tokenizer.encode("どういたしまして。", add_special_tokens=False)
-
- encoded_sentence = tokenizer.build_inputs_with_special_tokens(text)
- encoded_pair = tokenizer.build_inputs_with_special_tokens(text, text_2)
-
- # 2 is for "[CLS]", 3 is for "[SEP]"
- assert encoded_sentence == [2] + text + [3]
- assert encoded_pair == [2] + text + [3] + text_2 + [3]
diff --git a/server/transformers/tests/test_tokenization_common.py b/server/transformers/tests/test_tokenization_common.py
deleted file mode 100644
index 9867b189915fb4d56fa61c63a833f275e2c99b02..0000000000000000000000000000000000000000
--- a/server/transformers/tests/test_tokenization_common.py
+++ /dev/null
@@ -1,510 +0,0 @@
-# coding=utf-8
-# Copyright 2019 HuggingFace Inc.
-#
-# Licensed under the Apache License, Version 2.0 (the "License");
-# you may not use this file except in compliance with the License.
-# You may obtain a copy of the License at
-#
-# http://www.apache.org/licenses/LICENSE-2.0
-#
-# Unless required by applicable law or agreed to in writing, software
-# distributed under the License is distributed on an "AS IS" BASIS,
-# WITHOUT WARRANTIES OR CONDITIONS OF ANY KIND, either express or implied.
-# See the License for the specific language governing permissions and
-# limitations under the License.
-
-
-import os
-import pickle
-import shutil
-import tempfile
-
-
-class TokenizerTesterMixin:
-
- tokenizer_class = None
- test_rust_tokenizer = False
-
- def setUp(self):
- self.tmpdirname = tempfile.mkdtemp()
-
- def tearDown(self):
- shutil.rmtree(self.tmpdirname)
-
- def get_tokenizer(self, **kwargs):
- raise NotImplementedError
-
- def get_rust_tokenizer(self, **kwargs):
- raise NotImplementedError
-
- def get_input_output_texts(self):
- raise NotImplementedError
-
- def test_tokenizers_common_properties(self):
- tokenizer = self.get_tokenizer()
- attributes_list = [
- "bos_token",
- "eos_token",
- "unk_token",
- "sep_token",
- "pad_token",
- "cls_token",
- "mask_token",
- ]
- for attr in attributes_list:
- self.assertTrue(hasattr(tokenizer, attr))
- self.assertTrue(hasattr(tokenizer, attr + "_id"))
-
- self.assertTrue(hasattr(tokenizer, "additional_special_tokens"))
- self.assertTrue(hasattr(tokenizer, "additional_special_tokens_ids"))
-
- attributes_list = ["max_len", "init_inputs", "init_kwargs", "added_tokens_encoder", "added_tokens_decoder"]
- for attr in attributes_list:
- self.assertTrue(hasattr(tokenizer, attr))
-
- def test_save_and_load_tokenizer(self):
- # safety check on max_len default value so we are sure the test works
- tokenizer = self.get_tokenizer()
- self.assertNotEqual(tokenizer.max_len, 42)
-
- # Now let's start the test
- tokenizer = self.get_tokenizer(max_len=42)
-
- before_tokens = tokenizer.encode("He is very happy, UNwant\u00E9d,running", add_special_tokens=False)
-
- with tempfile.TemporaryDirectory() as tmpdirname:
- tokenizer.save_pretrained(tmpdirname)
- tokenizer = self.tokenizer_class.from_pretrained(tmpdirname)
-
- after_tokens = tokenizer.encode("He is very happy, UNwant\u00E9d,running", add_special_tokens=False)
- self.assertListEqual(before_tokens, after_tokens)
-
- self.assertEqual(tokenizer.max_len, 42)
- tokenizer = self.tokenizer_class.from_pretrained(tmpdirname, max_len=43)
- self.assertEqual(tokenizer.max_len, 43)
-
- def test_pickle_tokenizer(self):
- tokenizer = self.get_tokenizer()
- self.assertIsNotNone(tokenizer)
-
- text = "Munich and Berlin are nice cities"
- subwords = tokenizer.tokenize(text)
-
- with tempfile.TemporaryDirectory() as tmpdirname:
-
- filename = os.path.join(tmpdirname, "tokenizer.bin")
- with open(filename, "wb") as handle:
- pickle.dump(tokenizer, handle)
-
- with open(filename, "rb") as handle:
- tokenizer_new = pickle.load(handle)
-
- subwords_loaded = tokenizer_new.tokenize(text)
-
- self.assertListEqual(subwords, subwords_loaded)
-
- def test_added_tokens_do_lower_case(self):
- tokenizer = self.get_tokenizer(do_lower_case=True)
-
- special_token = tokenizer.all_special_tokens[0]
-
- text = special_token + " aaaaa bbbbbb low cccccccccdddddddd l " + special_token
- text2 = special_token + " AAAAA BBBBBB low CCCCCCCCCDDDDDDDD l " + special_token
-
- toks0 = tokenizer.tokenize(text) # toks before adding new_toks
-
- new_toks = ["aaaaa bbbbbb", "cccccccccdddddddd", "AAAAA BBBBBB", "CCCCCCCCCDDDDDDDD"]
- added = tokenizer.add_tokens(new_toks)
- self.assertEqual(added, 2)
-
- toks = tokenizer.tokenize(text)
- toks2 = tokenizer.tokenize(text2)
-
- self.assertEqual(len(toks), len(toks2))
- self.assertNotEqual(len(toks), len(toks0)) # toks0 should be longer
- self.assertListEqual(toks, toks2)
-
- # Check that none of the special tokens are lowercased
- sequence_with_special_tokens = "A " + " yEs ".join(tokenizer.all_special_tokens) + " B"
- tokenized_sequence = tokenizer.tokenize(sequence_with_special_tokens)
-
- for special_token in tokenizer.all_special_tokens:
- self.assertTrue(special_token in tokenized_sequence)
-
- tokenizer = self.get_tokenizer(do_lower_case=False)
-
- added = tokenizer.add_tokens(new_toks)
- self.assertEqual(added, 4)
-
- toks = tokenizer.tokenize(text)
- toks2 = tokenizer.tokenize(text2)
-
- self.assertEqual(len(toks), len(toks2)) # Length should still be the same
- self.assertNotEqual(len(toks), len(toks0))
- self.assertNotEqual(toks[1], toks2[1]) # But at least the first non-special tokens should differ
-
- def test_add_tokens_tokenizer(self):
- tokenizer = self.get_tokenizer()
-
- vocab_size = tokenizer.vocab_size
- all_size = len(tokenizer)
-
- self.assertNotEqual(vocab_size, 0)
- self.assertEqual(vocab_size, all_size)
-
- new_toks = ["aaaaa bbbbbb", "cccccccccdddddddd"]
- added_toks = tokenizer.add_tokens(new_toks)
- vocab_size_2 = tokenizer.vocab_size
- all_size_2 = len(tokenizer)
-
- self.assertNotEqual(vocab_size_2, 0)
- self.assertEqual(vocab_size, vocab_size_2)
- self.assertEqual(added_toks, len(new_toks))
- self.assertEqual(all_size_2, all_size + len(new_toks))
-
- tokens = tokenizer.encode("aaaaa bbbbbb low cccccccccdddddddd l", add_special_tokens=False)
-
- self.assertGreaterEqual(len(tokens), 4)
- self.assertGreater(tokens[0], tokenizer.vocab_size - 1)
- self.assertGreater(tokens[-2], tokenizer.vocab_size - 1)
-
- new_toks_2 = {"eos_token": ">>>>|||<||<<|<<", "pad_token": "<<<<<|||>|>>>>|>"}
- added_toks_2 = tokenizer.add_special_tokens(new_toks_2)
- vocab_size_3 = tokenizer.vocab_size
- all_size_3 = len(tokenizer)
-
- self.assertNotEqual(vocab_size_3, 0)
- self.assertEqual(vocab_size, vocab_size_3)
- self.assertEqual(added_toks_2, len(new_toks_2))
- self.assertEqual(all_size_3, all_size_2 + len(new_toks_2))
-
- tokens = tokenizer.encode(
- ">>>>|||<||<<|<< aaaaabbbbbb low cccccccccdddddddd <<<<<|||>|>>>>|> l", add_special_tokens=False
- )
-
- self.assertGreaterEqual(len(tokens), 6)
- self.assertGreater(tokens[0], tokenizer.vocab_size - 1)
- self.assertGreater(tokens[0], tokens[1])
- self.assertGreater(tokens[-2], tokenizer.vocab_size - 1)
- self.assertGreater(tokens[-2], tokens[-3])
- self.assertEqual(tokens[0], tokenizer.eos_token_id)
- self.assertEqual(tokens[-2], tokenizer.pad_token_id)
-
- def test_add_special_tokens(self):
- tokenizer = self.get_tokenizer()
- input_text, output_text = self.get_input_output_texts()
-
- special_token = "[SPECIAL TOKEN]"
-
- tokenizer.add_special_tokens({"cls_token": special_token})
- encoded_special_token = tokenizer.encode(special_token, add_special_tokens=False)
- assert len(encoded_special_token) == 1
-
- text = " ".join([input_text, special_token, output_text])
- encoded = tokenizer.encode(text, add_special_tokens=False)
-
- input_encoded = tokenizer.encode(input_text, add_special_tokens=False)
- output_encoded = tokenizer.encode(output_text, add_special_tokens=False)
- special_token_id = tokenizer.encode(special_token, add_special_tokens=False)
- assert encoded == input_encoded + special_token_id + output_encoded
-
- decoded = tokenizer.decode(encoded, skip_special_tokens=True)
- assert special_token not in decoded
-
- def test_required_methods_tokenizer(self):
- tokenizer = self.get_tokenizer()
- input_text, output_text = self.get_input_output_texts()
-
- tokens = tokenizer.tokenize(input_text)
- ids = tokenizer.convert_tokens_to_ids(tokens)
- ids_2 = tokenizer.encode(input_text, add_special_tokens=False)
- self.assertListEqual(ids, ids_2)
-
- tokens_2 = tokenizer.convert_ids_to_tokens(ids)
- text_2 = tokenizer.decode(ids)
-
- self.assertEqual(text_2, output_text)
-
- self.assertNotEqual(len(tokens_2), 0)
- self.assertIsInstance(text_2, str)
-
- def test_encode_decode_with_spaces(self):
- tokenizer = self.get_tokenizer()
-
- new_toks = ["[ABC]", "[DEF]", "GHI IHG"]
- tokenizer.add_tokens(new_toks)
- input = "[ABC] [DEF] [ABC] GHI IHG [DEF]"
- encoded = tokenizer.encode(input, add_special_tokens=False)
- decoded = tokenizer.decode(encoded)
- self.assertEqual(decoded, input)
-
- def test_pretrained_model_lists(self):
- weights_list = list(self.tokenizer_class.max_model_input_sizes.keys())
- weights_lists_2 = []
- for file_id, map_list in self.tokenizer_class.pretrained_vocab_files_map.items():
- weights_lists_2.append(list(map_list.keys()))
-
- for weights_list_2 in weights_lists_2:
- self.assertListEqual(weights_list, weights_list_2)
-
- def test_mask_output(self):
- tokenizer = self.get_tokenizer()
-
- if tokenizer.build_inputs_with_special_tokens.__qualname__.split(".")[0] != "PreTrainedTokenizer":
- seq_0 = "Test this method."
- seq_1 = "With these inputs."
- information = tokenizer.encode_plus(seq_0, seq_1, add_special_tokens=True)
- sequences, mask = information["input_ids"], information["token_type_ids"]
- self.assertEqual(len(sequences), len(mask))
-
- def test_number_of_added_tokens(self):
- tokenizer = self.get_tokenizer()
-
- seq_0 = "Test this method."
- seq_1 = "With these inputs."
-
- sequences = tokenizer.encode(seq_0, seq_1, add_special_tokens=False)
- attached_sequences = tokenizer.encode(seq_0, seq_1, add_special_tokens=True)
-
- # Method is implemented (e.g. not GPT-2)
- if len(attached_sequences) != 2:
- self.assertEqual(tokenizer.num_added_tokens(pair=True), len(attached_sequences) - len(sequences))
-
- def test_maximum_encoding_length_single_input(self):
- tokenizer = self.get_tokenizer()
-
- seq_0 = "This is a sentence to be encoded."
- stride = 2
-
- sequence = tokenizer.encode(seq_0, add_special_tokens=False)
- num_added_tokens = tokenizer.num_added_tokens()
- total_length = len(sequence) + num_added_tokens
- information = tokenizer.encode_plus(
- seq_0, max_length=total_length - 2, add_special_tokens=True, stride=stride, return_overflowing_tokens=True,
- )
-
- truncated_sequence = information["input_ids"]
- overflowing_tokens = information["overflowing_tokens"]
-
- self.assertEqual(len(overflowing_tokens), 2 + stride)
- self.assertEqual(overflowing_tokens, sequence[-(2 + stride) :])
- self.assertEqual(len(truncated_sequence), total_length - 2)
- self.assertEqual(truncated_sequence, tokenizer.build_inputs_with_special_tokens(sequence[:-2]))
-
- def test_maximum_encoding_length_pair_input(self):
- tokenizer = self.get_tokenizer()
-
- seq_0 = "This is a sentence to be encoded."
- seq_1 = "This is another sentence to be encoded."
- stride = 2
-
- sequence_0_no_special_tokens = tokenizer.encode(seq_0, add_special_tokens=False)
- sequence_1_no_special_tokens = tokenizer.encode(seq_1, add_special_tokens=False)
-
- sequence = tokenizer.encode(seq_0, seq_1, add_special_tokens=True)
- truncated_second_sequence = tokenizer.build_inputs_with_special_tokens(
- tokenizer.encode(seq_0, add_special_tokens=False), tokenizer.encode(seq_1, add_special_tokens=False)[:-2],
- )
-
- information = tokenizer.encode_plus(
- seq_0,
- seq_1,
- max_length=len(sequence) - 2,
- add_special_tokens=True,
- stride=stride,
- truncation_strategy="only_second",
- return_overflowing_tokens=True,
- )
- information_first_truncated = tokenizer.encode_plus(
- seq_0,
- seq_1,
- max_length=len(sequence) - 2,
- add_special_tokens=True,
- stride=stride,
- truncation_strategy="only_first",
- return_overflowing_tokens=True,
- )
-
- truncated_sequence = information["input_ids"]
- overflowing_tokens = information["overflowing_tokens"]
- overflowing_tokens_first_truncated = information_first_truncated["overflowing_tokens"]
-
- self.assertEqual(len(overflowing_tokens), 2 + stride)
- self.assertEqual(overflowing_tokens, sequence_1_no_special_tokens[-(2 + stride) :])
- self.assertEqual(overflowing_tokens_first_truncated, sequence_0_no_special_tokens[-(2 + stride) :])
- self.assertEqual(len(truncated_sequence), len(sequence) - 2)
- self.assertEqual(truncated_sequence, truncated_second_sequence)
-
- def test_encode_input_type(self):
- tokenizer = self.get_tokenizer()
-
- sequence = "Let's encode this sequence"
-
- tokens = tokenizer.tokenize(sequence)
- input_ids = tokenizer.convert_tokens_to_ids(tokens)
- formatted_input = tokenizer.encode(sequence, add_special_tokens=True)
-
- self.assertEqual(tokenizer.encode(tokens, add_special_tokens=True), formatted_input)
- self.assertEqual(tokenizer.encode(input_ids, add_special_tokens=True), formatted_input)
-
- def test_special_tokens_mask(self):
- tokenizer = self.get_tokenizer()
-
- sequence_0 = "Encode this."
- sequence_1 = "This one too please."
-
- # Testing single inputs
- encoded_sequence = tokenizer.encode(sequence_0, add_special_tokens=False)
- encoded_sequence_dict = tokenizer.encode_plus(
- sequence_0, add_special_tokens=True, return_special_tokens_mask=True
- )
- encoded_sequence_w_special = encoded_sequence_dict["input_ids"]
- special_tokens_mask = encoded_sequence_dict["special_tokens_mask"]
- self.assertEqual(len(special_tokens_mask), len(encoded_sequence_w_special))
-
- filtered_sequence = [
- (x if not special_tokens_mask[i] else None) for i, x in enumerate(encoded_sequence_w_special)
- ]
- filtered_sequence = [x for x in filtered_sequence if x is not None]
- self.assertEqual(encoded_sequence, filtered_sequence)
-
- # Testing inputs pairs
- encoded_sequence = tokenizer.encode(sequence_0, add_special_tokens=False) + tokenizer.encode(
- sequence_1, add_special_tokens=False
- )
- encoded_sequence_dict = tokenizer.encode_plus(
- sequence_0, sequence_1, add_special_tokens=True, return_special_tokens_mask=True
- )
- encoded_sequence_w_special = encoded_sequence_dict["input_ids"]
- special_tokens_mask = encoded_sequence_dict["special_tokens_mask"]
- self.assertEqual(len(special_tokens_mask), len(encoded_sequence_w_special))
-
- filtered_sequence = [
- (x if not special_tokens_mask[i] else None) for i, x in enumerate(encoded_sequence_w_special)
- ]
- filtered_sequence = [x for x in filtered_sequence if x is not None]
- self.assertEqual(encoded_sequence, filtered_sequence)
-
- # Testing with already existing special tokens
- if tokenizer.cls_token_id == tokenizer.unk_token_id and tokenizer.cls_token_id == tokenizer.unk_token_id:
- tokenizer.add_special_tokens({"cls_token": "", "sep_token": ""})
- encoded_sequence_dict = tokenizer.encode_plus(
- sequence_0, add_special_tokens=True, return_special_tokens_mask=True
- )
- encoded_sequence_w_special = encoded_sequence_dict["input_ids"]
- special_tokens_mask_orig = encoded_sequence_dict["special_tokens_mask"]
- special_tokens_mask = tokenizer.get_special_tokens_mask(
- encoded_sequence_w_special, already_has_special_tokens=True
- )
- self.assertEqual(len(special_tokens_mask), len(encoded_sequence_w_special))
- self.assertEqual(special_tokens_mask_orig, special_tokens_mask)
-
- def test_padding_to_max_length(self):
- tokenizer = self.get_tokenizer()
-
- sequence = "Sequence"
- padding_size = 10
- padding_idx = tokenizer.pad_token_id
-
- # RIGHT PADDING - Check that it correctly pads when a maximum length is specified along with the padding flag set to True
- tokenizer.padding_side = "right"
- encoded_sequence = tokenizer.encode(sequence)
- sequence_length = len(encoded_sequence)
- padded_sequence = tokenizer.encode(sequence, max_length=sequence_length + padding_size, pad_to_max_length=True)
- padded_sequence_length = len(padded_sequence)
- assert sequence_length + padding_size == padded_sequence_length
- assert encoded_sequence + [padding_idx] * padding_size == padded_sequence
-
- # LEFT PADDING - Check that it correctly pads when a maximum length is specified along with the padding flag set to True
- tokenizer.padding_side = "left"
- encoded_sequence = tokenizer.encode(sequence)
- sequence_length = len(encoded_sequence)
- padded_sequence = tokenizer.encode(sequence, max_length=sequence_length + padding_size, pad_to_max_length=True)
- padded_sequence_length = len(padded_sequence)
- assert sequence_length + padding_size == padded_sequence_length
- assert [padding_idx] * padding_size + encoded_sequence == padded_sequence
-
- # RIGHT & LEFT PADDING - Check that nothing is done when a maximum length is not specified
- encoded_sequence = tokenizer.encode(sequence)
- sequence_length = len(encoded_sequence)
-
- tokenizer.padding_side = "right"
- padded_sequence_right = tokenizer.encode(sequence, pad_to_max_length=True)
- padded_sequence_right_length = len(padded_sequence_right)
-
- tokenizer.padding_side = "left"
- padded_sequence_left = tokenizer.encode(sequence, pad_to_max_length=True)
- padded_sequence_left_length = len(padded_sequence_left)
-
- assert sequence_length == padded_sequence_right_length
- assert encoded_sequence == padded_sequence_right
- assert sequence_length == padded_sequence_left_length
- assert encoded_sequence == padded_sequence_left
-
- def test_encode_plus_with_padding(self):
- tokenizer = self.get_tokenizer()
-
- sequence = "Sequence"
- padding_size = 10
- padding_idx = tokenizer.pad_token_id
- token_type_padding_idx = tokenizer.pad_token_type_id
-
- encoded_sequence = tokenizer.encode_plus(sequence, return_special_tokens_mask=True)
- input_ids = encoded_sequence["input_ids"]
- token_type_ids = encoded_sequence["token_type_ids"]
- attention_mask = encoded_sequence["attention_mask"]
- special_tokens_mask = encoded_sequence["special_tokens_mask"]
- sequence_length = len(input_ids)
-
- # Test right padding
- tokenizer.padding_side = "right"
- padded_sequence = tokenizer.encode_plus(
- sequence,
- max_length=sequence_length + padding_size,
- pad_to_max_length=True,
- return_special_tokens_mask=True,
- )
- padded_input_ids = padded_sequence["input_ids"]
- padded_token_type_ids = padded_sequence["token_type_ids"]
- padded_attention_mask = padded_sequence["attention_mask"]
- padded_special_tokens_mask = padded_sequence["special_tokens_mask"]
- padded_sequence_length = len(padded_input_ids)
-
- assert sequence_length + padding_size == padded_sequence_length
- assert input_ids + [padding_idx] * padding_size == padded_input_ids
- assert token_type_ids + [token_type_padding_idx] * padding_size == padded_token_type_ids
- assert attention_mask + [0] * padding_size == padded_attention_mask
- assert special_tokens_mask + [1] * padding_size == padded_special_tokens_mask
-
- # Test left padding
- tokenizer.padding_side = "left"
- padded_sequence = tokenizer.encode_plus(
- sequence,
- max_length=sequence_length + padding_size,
- pad_to_max_length=True,
- return_special_tokens_mask=True,
- )
- padded_input_ids = padded_sequence["input_ids"]
- padded_token_type_ids = padded_sequence["token_type_ids"]
- padded_attention_mask = padded_sequence["attention_mask"]
- padded_special_tokens_mask = padded_sequence["special_tokens_mask"]
- padded_sequence_length = len(padded_input_ids)
-
- assert sequence_length + padding_size == padded_sequence_length
- assert [padding_idx] * padding_size + input_ids == padded_input_ids
- assert [token_type_padding_idx] * padding_size + token_type_ids == padded_token_type_ids
- assert [0] * padding_size + attention_mask == padded_attention_mask
- assert [1] * padding_size + special_tokens_mask == padded_special_tokens_mask
-
- def test_separate_tokenizers(self):
- # This tests that tokenizers don't impact others. Unfortunately the case where it fails is when
- # we're loading an S3 configuration from a pre-trained identifier, and we have no way of testing those today.
-
- tokenizer = self.get_tokenizer(random_argument=True)
- print(tokenizer.init_kwargs)
- assert tokenizer.init_kwargs["random_argument"] is True
- new_tokenizer = self.get_tokenizer(random_argument=False)
- print(tokenizer.init_kwargs)
- print(new_tokenizer.init_kwargs)
- assert tokenizer.init_kwargs["random_argument"] is True
- assert new_tokenizer.init_kwargs["random_argument"] is False
diff --git a/server/transformers/tests/test_tokenization_ctrl.py b/server/transformers/tests/test_tokenization_ctrl.py
deleted file mode 100644
index 8b57dc49d347c3515e9c30804c660640c20ccf0c..0000000000000000000000000000000000000000
--- a/server/transformers/tests/test_tokenization_ctrl.py
+++ /dev/null
@@ -1,64 +0,0 @@
-# coding=utf-8
-# Copyright 2018 Salesforce and HuggingFace Inc. team.
-# Licensed under the Apache License, Version 2.0 (the "License");
-# you may not use this file except in compliance with the License.
-# You may obtain a copy of the License at
-#
-# http://www.apache.org/licenses/LICENSE-2.0
-#
-# Unless required by applicable law or agreed to in writing, software
-# distributed under the License is distributed on an "AS IS" BASIS,
-# WITHOUT WARRANTIES OR CONDITIONS OF ANY KIND, either express or implied.
-# See the License for the specific language governing permissions and
-# limitations under the License.
-
-
-import json
-import os
-import unittest
-
-from transformers.tokenization_ctrl import VOCAB_FILES_NAMES, CTRLTokenizer
-
-from .test_tokenization_common import TokenizerTesterMixin
-
-
-class CTRLTokenizationTest(TokenizerTesterMixin, unittest.TestCase):
-
- tokenizer_class = CTRLTokenizer
-
- def setUp(self):
- super().setUp()
-
- # Adapted from Sennrich et al. 2015 and https://github.com/rsennrich/subword-nmt
- vocab = ["adapt", "re@@", "a@@", "apt", "c@@", "t", ""]
- vocab_tokens = dict(zip(vocab, range(len(vocab))))
- merges = ["#version: 0.2", "a p", "ap t", "r e", "a d", "ad apt", ""]
- self.special_tokens_map = {"unk_token": ""}
-
- self.vocab_file = os.path.join(self.tmpdirname, VOCAB_FILES_NAMES["vocab_file"])
- self.merges_file = os.path.join(self.tmpdirname, VOCAB_FILES_NAMES["merges_file"])
- with open(self.vocab_file, "w", encoding="utf-8") as fp:
- fp.write(json.dumps(vocab_tokens) + "\n")
- with open(self.merges_file, "w", encoding="utf-8") as fp:
- fp.write("\n".join(merges))
-
- def get_tokenizer(self, **kwargs):
- kwargs.update(self.special_tokens_map)
- return CTRLTokenizer.from_pretrained(self.tmpdirname, **kwargs)
-
- def get_input_output_texts(self):
- input_text = "adapt react readapt apt"
- output_text = "adapt react readapt apt"
- return input_text, output_text
-
- def test_full_tokenizer(self):
- tokenizer = CTRLTokenizer(self.vocab_file, self.merges_file, **self.special_tokens_map)
- text = "adapt react readapt apt"
- bpe_tokens = "adapt re@@ a@@ c@@ t re@@ adapt apt".split()
- tokens = tokenizer.tokenize(text)
- self.assertListEqual(tokens, bpe_tokens)
-
- input_tokens = tokens + [tokenizer.unk_token]
-
- input_bpe_tokens = [0, 1, 2, 4, 5, 1, 0, 3, 6]
- self.assertListEqual(tokenizer.convert_tokens_to_ids(input_tokens), input_bpe_tokens)
diff --git a/server/transformers/tests/test_tokenization_distilbert.py b/server/transformers/tests/test_tokenization_distilbert.py
deleted file mode 100644
index a142b8d8f92f0dee5bc747929f78895fb6a3f9ad..0000000000000000000000000000000000000000
--- a/server/transformers/tests/test_tokenization_distilbert.py
+++ /dev/null
@@ -1,43 +0,0 @@
-# coding=utf-8
-# Copyright 2018 The Google AI Language Team Authors.
-#
-# Licensed under the Apache License, Version 2.0 (the "License");
-# you may not use this file except in compliance with the License.
-# You may obtain a copy of the License at
-#
-# http://www.apache.org/licenses/LICENSE-2.0
-#
-# Unless required by applicable law or agreed to in writing, software
-# distributed under the License is distributed on an "AS IS" BASIS,
-# WITHOUT WARRANTIES OR CONDITIONS OF ANY KIND, either express or implied.
-# See the License for the specific language governing permissions and
-# limitations under the License.
-
-
-from transformers.tokenization_distilbert import DistilBertTokenizer
-
-from .test_tokenization_bert import BertTokenizationTest
-from .utils import slow
-
-
-class DistilBertTokenizationTest(BertTokenizationTest):
-
- tokenizer_class = DistilBertTokenizer
-
- def get_tokenizer(self, **kwargs):
- return DistilBertTokenizer.from_pretrained(self.tmpdirname, **kwargs)
-
- @slow
- def test_sequence_builders(self):
- tokenizer = DistilBertTokenizer.from_pretrained("distilbert-base-uncased")
-
- text = tokenizer.encode("sequence builders", add_special_tokens=False)
- text_2 = tokenizer.encode("multi-sequence build", add_special_tokens=False)
-
- encoded_sentence = tokenizer.build_inputs_with_special_tokens(text)
- encoded_pair = tokenizer.build_inputs_with_special_tokens(text, text_2)
-
- assert encoded_sentence == [tokenizer.cls_token_id] + text + [tokenizer.sep_token_id]
- assert encoded_pair == [tokenizer.cls_token_id] + text + [tokenizer.sep_token_id] + text_2 + [
- tokenizer.sep_token_id
- ]
diff --git a/server/transformers/tests/test_tokenization_gpt2.py b/server/transformers/tests/test_tokenization_gpt2.py
deleted file mode 100644
index 12b7b0eeb1674f4719246ecadeea3c5fc823a5dc..0000000000000000000000000000000000000000
--- a/server/transformers/tests/test_tokenization_gpt2.py
+++ /dev/null
@@ -1,120 +0,0 @@
-# coding=utf-8
-# Copyright 2018 The Google AI Language Team Authors.
-#
-# Licensed under the Apache License, Version 2.0 (the "License");
-# you may not use this file except in compliance with the License.
-# You may obtain a copy of the License at
-#
-# http://www.apache.org/licenses/LICENSE-2.0
-#
-# Unless required by applicable law or agreed to in writing, software
-# distributed under the License is distributed on an "AS IS" BASIS,
-# WITHOUT WARRANTIES OR CONDITIONS OF ANY KIND, either express or implied.
-# See the License for the specific language governing permissions and
-# limitations under the License.
-
-
-import json
-import os
-import unittest
-
-from transformers.tokenization_gpt2 import VOCAB_FILES_NAMES, GPT2Tokenizer, GPT2TokenizerFast
-
-from .test_tokenization_common import TokenizerTesterMixin
-
-
-class GPT2TokenizationTest(TokenizerTesterMixin, unittest.TestCase):
-
- tokenizer_class = GPT2Tokenizer
- test_rust_tokenizer = True
-
- def setUp(self):
- super().setUp()
-
- # Adapted from Sennrich et al. 2015 and https://github.com/rsennrich/subword-nmt
- vocab = [
- "l",
- "o",
- "w",
- "e",
- "r",
- "s",
- "t",
- "i",
- "d",
- "n",
- "\u0120",
- "\u0120l",
- "\u0120n",
- "\u0120lo",
- "\u0120low",
- "er",
- "\u0120lowest",
- "\u0120newer",
- "\u0120wider",
- "",
- ]
- vocab_tokens = dict(zip(vocab, range(len(vocab))))
- merges = ["#version: 0.2", "\u0120 l", "\u0120l o", "\u0120lo w", "e r", ""]
- self.special_tokens_map = {"unk_token": ""}
-
- self.vocab_file = os.path.join(self.tmpdirname, VOCAB_FILES_NAMES["vocab_file"])
- self.merges_file = os.path.join(self.tmpdirname, VOCAB_FILES_NAMES["merges_file"])
- with open(self.vocab_file, "w", encoding="utf-8") as fp:
- fp.write(json.dumps(vocab_tokens) + "\n")
- with open(self.merges_file, "w", encoding="utf-8") as fp:
- fp.write("\n".join(merges))
-
- def get_tokenizer(self, **kwargs):
- kwargs.update(self.special_tokens_map)
- return GPT2Tokenizer.from_pretrained(self.tmpdirname, **kwargs)
-
- def get_rust_tokenizer(self, **kwargs):
- kwargs.update(self.special_tokens_map)
- return GPT2TokenizerFast.from_pretrained(self.tmpdirname, **kwargs)
-
- def get_input_output_texts(self):
- input_text = "lower newer"
- output_text = "lower newer"
- return input_text, output_text
-
- def test_full_tokenizer(self):
- tokenizer = GPT2Tokenizer(self.vocab_file, self.merges_file, **self.special_tokens_map)
- text = "lower newer"
- bpe_tokens = ["\u0120low", "er", "\u0120", "n", "e", "w", "er"]
- tokens = tokenizer.tokenize(text, add_prefix_space=True)
- self.assertListEqual(tokens, bpe_tokens)
-
- input_tokens = tokens + [tokenizer.unk_token]
- input_bpe_tokens = [14, 15, 10, 9, 3, 2, 15, 19]
- self.assertListEqual(tokenizer.convert_tokens_to_ids(input_tokens), input_bpe_tokens)
-
- def test_rust_and_python_full_tokenizers(self):
- if not self.test_rust_tokenizer:
- return
-
- tokenizer = self.get_tokenizer()
- rust_tokenizer = self.get_rust_tokenizer(add_special_tokens=False, add_prefix_space=True)
-
- sequence = "lower newer"
-
- # Testing tokenization
- tokens = tokenizer.tokenize(sequence, add_prefix_space=True)
- rust_tokens = rust_tokenizer.tokenize(sequence)
- self.assertListEqual(tokens, rust_tokens)
-
- # Testing conversion to ids without special tokens
- ids = tokenizer.encode(sequence, add_special_tokens=False, add_prefix_space=True)
- rust_ids = rust_tokenizer.encode(sequence)
- self.assertListEqual(ids, rust_ids)
-
- # Testing conversion to ids with special tokens
- rust_tokenizer = self.get_rust_tokenizer(add_prefix_space=True)
- ids = tokenizer.encode(sequence, add_prefix_space=True)
- rust_ids = rust_tokenizer.encode(sequence)
- self.assertListEqual(ids, rust_ids)
-
- # Testing the unknown token
- input_tokens = tokens + [rust_tokenizer.unk_token]
- input_bpe_tokens = [14, 15, 10, 9, 3, 2, 15, 19]
- self.assertListEqual(rust_tokenizer.convert_tokens_to_ids(input_tokens), input_bpe_tokens)
diff --git a/server/transformers/tests/test_tokenization_openai.py b/server/transformers/tests/test_tokenization_openai.py
deleted file mode 100644
index f89ec61ff61153f244adc47ea8c777cd404593d8..0000000000000000000000000000000000000000
--- a/server/transformers/tests/test_tokenization_openai.py
+++ /dev/null
@@ -1,85 +0,0 @@
-# coding=utf-8
-# Copyright 2018 The Google AI Language Team Authors.
-#
-# Licensed under the Apache License, Version 2.0 (the "License");
-# you may not use this file except in compliance with the License.
-# You may obtain a copy of the License at
-#
-# http://www.apache.org/licenses/LICENSE-2.0
-#
-# Unless required by applicable law or agreed to in writing, software
-# distributed under the License is distributed on an "AS IS" BASIS,
-# WITHOUT WARRANTIES OR CONDITIONS OF ANY KIND, either express or implied.
-# See the License for the specific language governing permissions and
-# limitations under the License.
-
-
-import json
-import os
-import unittest
-
-from transformers.tokenization_openai import VOCAB_FILES_NAMES, OpenAIGPTTokenizer
-
-from .test_tokenization_common import TokenizerTesterMixin
-
-
-class OpenAIGPTTokenizationTest(TokenizerTesterMixin, unittest.TestCase):
-
- tokenizer_class = OpenAIGPTTokenizer
-
- def setUp(self):
- super().setUp()
-
- # Adapted from Sennrich et al. 2015 and https://github.com/rsennrich/subword-nmt
- vocab = [
- "l",
- "o",
- "w",
- "e",
- "r",
- "s",
- "t",
- "i",
- "d",
- "n",
- "w",
- "r",
- "t",
- "lo",
- "low",
- "er",
- "low",
- "lowest",
- "newer",
- "wider",
- "",
- ]
- vocab_tokens = dict(zip(vocab, range(len(vocab))))
- merges = ["#version: 0.2", "l o", "lo w", "e r", ""]
-
- self.vocab_file = os.path.join(self.tmpdirname, VOCAB_FILES_NAMES["vocab_file"])
- self.merges_file = os.path.join(self.tmpdirname, VOCAB_FILES_NAMES["merges_file"])
- with open(self.vocab_file, "w") as fp:
- fp.write(json.dumps(vocab_tokens))
- with open(self.merges_file, "w") as fp:
- fp.write("\n".join(merges))
-
- def get_tokenizer(self, **kwargs):
- return OpenAIGPTTokenizer.from_pretrained(self.tmpdirname, **kwargs)
-
- def get_input_output_texts(self):
- input_text = "lower newer"
- output_text = "lower newer"
- return input_text, output_text
-
- def test_full_tokenizer(self):
- tokenizer = OpenAIGPTTokenizer(self.vocab_file, self.merges_file)
-
- text = "lower"
- bpe_tokens = ["low", "er"]
- tokens = tokenizer.tokenize(text)
- self.assertListEqual(tokens, bpe_tokens)
-
- input_tokens = tokens + [""]
- input_bpe_tokens = [14, 15, 20]
- self.assertListEqual(tokenizer.convert_tokens_to_ids(input_tokens), input_bpe_tokens)
diff --git a/server/transformers/tests/test_tokenization_roberta.py b/server/transformers/tests/test_tokenization_roberta.py
deleted file mode 100644
index f9abdea66623af2b9aa2aeca27d18dfdd7b9d5e2..0000000000000000000000000000000000000000
--- a/server/transformers/tests/test_tokenization_roberta.py
+++ /dev/null
@@ -1,112 +0,0 @@
-# coding=utf-8
-# Copyright 2018 The Google AI Language Team Authors.
-#
-# Licensed under the Apache License, Version 2.0 (the "License");
-# you may not use this file except in compliance with the License.
-# You may obtain a copy of the License at
-#
-# http://www.apache.org/licenses/LICENSE-2.0
-#
-# Unless required by applicable law or agreed to in writing, software
-# distributed under the License is distributed on an "AS IS" BASIS,
-# WITHOUT WARRANTIES OR CONDITIONS OF ANY KIND, either express or implied.
-# See the License for the specific language governing permissions and
-# limitations under the License.
-
-
-import json
-import os
-import unittest
-
-from transformers.tokenization_roberta import VOCAB_FILES_NAMES, RobertaTokenizer
-
-from .test_tokenization_common import TokenizerTesterMixin
-from .utils import slow
-
-
-class RobertaTokenizationTest(TokenizerTesterMixin, unittest.TestCase):
- tokenizer_class = RobertaTokenizer
-
- def setUp(self):
- super().setUp()
-
- # Adapted from Sennrich et al. 2015 and https://github.com/rsennrich/subword-nmt
- vocab = [
- "l",
- "o",
- "w",
- "e",
- "r",
- "s",
- "t",
- "i",
- "d",
- "n",
- "\u0120",
- "\u0120l",
- "\u0120n",
- "\u0120lo",
- "\u0120low",
- "er",
- "\u0120lowest",
- "\u0120newer",
- "\u0120wider",
- "",
- ]
- vocab_tokens = dict(zip(vocab, range(len(vocab))))
- merges = ["#version: 0.2", "\u0120 l", "\u0120l o", "\u0120lo w", "e r", ""]
- self.special_tokens_map = {"unk_token": ""}
-
- self.vocab_file = os.path.join(self.tmpdirname, VOCAB_FILES_NAMES["vocab_file"])
- self.merges_file = os.path.join(self.tmpdirname, VOCAB_FILES_NAMES["merges_file"])
- with open(self.vocab_file, "w", encoding="utf-8") as fp:
- fp.write(json.dumps(vocab_tokens) + "\n")
- with open(self.merges_file, "w", encoding="utf-8") as fp:
- fp.write("\n".join(merges))
-
- def get_tokenizer(self, **kwargs):
- kwargs.update(self.special_tokens_map)
- return RobertaTokenizer.from_pretrained(self.tmpdirname, **kwargs)
-
- def get_input_output_texts(self):
- input_text = "lower newer"
- output_text = "lower newer"
- return input_text, output_text
-
- def test_full_tokenizer(self):
- tokenizer = RobertaTokenizer(self.vocab_file, self.merges_file, **self.special_tokens_map)
- text = "lower newer"
- bpe_tokens = ["\u0120low", "er", "\u0120", "n", "e", "w", "er"]
- tokens = tokenizer.tokenize(text, add_prefix_space=True)
- self.assertListEqual(tokens, bpe_tokens)
-
- input_tokens = tokens + [tokenizer.unk_token]
- input_bpe_tokens = [14, 15, 10, 9, 3, 2, 15, 19]
- self.assertListEqual(tokenizer.convert_tokens_to_ids(input_tokens), input_bpe_tokens)
-
- def roberta_dict_integration_testing(self):
- tokenizer = self.get_tokenizer()
-
- self.assertListEqual(tokenizer.encode("Hello world!", add_special_tokens=False), [0, 31414, 232, 328, 2])
- self.assertListEqual(
- tokenizer.encode("Hello world! cécé herlolip 418", add_special_tokens=False),
- [0, 31414, 232, 328, 740, 1140, 12695, 69, 46078, 1588, 2],
- )
-
- @slow
- def test_sequence_builders(self):
- tokenizer = RobertaTokenizer.from_pretrained("roberta-base")
-
- text = tokenizer.encode("sequence builders", add_special_tokens=False)
- text_2 = tokenizer.encode("multi-sequence build", add_special_tokens=False)
-
- encoded_text_from_decode = tokenizer.encode("sequence builders", add_special_tokens=True)
- encoded_pair_from_decode = tokenizer.encode(
- "sequence builders", "multi-sequence build", add_special_tokens=True
- )
-
- encoded_sentence = tokenizer.build_inputs_with_special_tokens(text)
- encoded_pair = tokenizer.build_inputs_with_special_tokens(text, text_2)
-
- assert encoded_sentence == encoded_text_from_decode
- assert encoded_pair == encoded_pair_from_decode
diff --git a/server/transformers/tests/test_tokenization_t5.py b/server/transformers/tests/test_tokenization_t5.py
deleted file mode 100644
index 793d80ac646ac23718d50917d40a12a8408c0b8c..0000000000000000000000000000000000000000
--- a/server/transformers/tests/test_tokenization_t5.py
+++ /dev/null
@@ -1,112 +0,0 @@
-# coding=utf-8
-# Copyright 2018 Google T5 Authors and HuggingFace Inc. team.
-#
-# Licensed under the Apache License, Version 2.0 (the "License");
-# you may not use this file except in compliance with the License.
-# You may obtain a copy of the License at
-#
-# http://www.apache.org/licenses/LICENSE-2.0
-#
-# Unless required by applicable law or agreed to in writing, software
-# distributed under the License is distributed on an "AS IS" BASIS,
-# WITHOUT WARRANTIES OR CONDITIONS OF ANY KIND, either express or implied.
-# See the License for the specific language governing permissions and
-# limitations under the License.
-
-
-import os
-import unittest
-
-from transformers.tokenization_t5 import T5Tokenizer
-from transformers.tokenization_xlnet import SPIECE_UNDERLINE
-
-from .test_tokenization_common import TokenizerTesterMixin
-
-
-SAMPLE_VOCAB = os.path.join(os.path.dirname(os.path.abspath(__file__)), "fixtures/test_sentencepiece.model")
-
-
-class T5TokenizationTest(TokenizerTesterMixin, unittest.TestCase):
-
- tokenizer_class = T5Tokenizer
-
- def setUp(self):
- super().setUp()
-
- # We have a SentencePiece fixture for testing
- tokenizer = T5Tokenizer(SAMPLE_VOCAB)
- tokenizer.save_pretrained(self.tmpdirname)
-
- def get_tokenizer(self, **kwargs):
- return T5Tokenizer.from_pretrained(self.tmpdirname, **kwargs)
-
- def get_input_output_texts(self):
- input_text = "This is a test"
- output_text = "This is a test"
- return input_text, output_text
-
- def test_full_tokenizer(self):
- tokenizer = T5Tokenizer(SAMPLE_VOCAB)
-
- tokens = tokenizer.tokenize("This is a test")
- self.assertListEqual(tokens, ["▁This", "▁is", "▁a", "▁t", "est"])
-
- self.assertListEqual(tokenizer.convert_tokens_to_ids(tokens), [285, 46, 10, 170, 382])
-
- tokens = tokenizer.tokenize("I was born in 92000, and this is falsé.")
- self.assertListEqual(
- tokens,
- [
- SPIECE_UNDERLINE + "I",
- SPIECE_UNDERLINE + "was",
- SPIECE_UNDERLINE + "b",
- "or",
- "n",
- SPIECE_UNDERLINE + "in",
- SPIECE_UNDERLINE + "",
- "9",
- "2",
- "0",
- "0",
- "0",
- ",",
- SPIECE_UNDERLINE + "and",
- SPIECE_UNDERLINE + "this",
- SPIECE_UNDERLINE + "is",
- SPIECE_UNDERLINE + "f",
- "al",
- "s",
- "é",
- ".",
- ],
- )
- ids = tokenizer.convert_tokens_to_ids(tokens)
- self.assertListEqual(ids, [8, 21, 84, 55, 24, 19, 7, 0, 602, 347, 347, 347, 3, 12, 66, 46, 72, 80, 6, 0, 4])
-
- back_tokens = tokenizer.convert_ids_to_tokens(ids)
- self.assertListEqual(
- back_tokens,
- [
- SPIECE_UNDERLINE + "I",
- SPIECE_UNDERLINE + "was",
- SPIECE_UNDERLINE + "b",
- "or",
- "n",
- SPIECE_UNDERLINE + "in",
- SPIECE_UNDERLINE + "",
- "",
- "2",
- "0",
- "0",
- "0",
- ",",
- SPIECE_UNDERLINE + "and",
- SPIECE_UNDERLINE + "this",
- SPIECE_UNDERLINE + "is",
- SPIECE_UNDERLINE + "f",
- "al",
- "s",
- "",
- ".",
- ],
- )
diff --git a/server/transformers/tests/test_tokenization_transfo_xl.py b/server/transformers/tests/test_tokenization_transfo_xl.py
deleted file mode 100644
index 8d4814699e086a8363c003fcf475bdba53734602..0000000000000000000000000000000000000000
--- a/server/transformers/tests/test_tokenization_transfo_xl.py
+++ /dev/null
@@ -1,84 +0,0 @@
-# coding=utf-8
-# Copyright 2018 The Google AI Language Team Authors.
-#
-# Licensed under the Apache License, Version 2.0 (the "License");
-# you may not use this file except in compliance with the License.
-# You may obtain a copy of the License at
-#
-# http://www.apache.org/licenses/LICENSE-2.0
-#
-# Unless required by applicable law or agreed to in writing, software
-# distributed under the License is distributed on an "AS IS" BASIS,
-# WITHOUT WARRANTIES OR CONDITIONS OF ANY KIND, either express or implied.
-# See the License for the specific language governing permissions and
-# limitations under the License.
-
-
-import os
-import unittest
-
-from transformers import is_torch_available
-
-from .test_tokenization_common import TokenizerTesterMixin
-from .utils import require_torch
-
-
-if is_torch_available():
- from transformers.tokenization_transfo_xl import TransfoXLTokenizer, VOCAB_FILES_NAMES
-
-
-@require_torch
-class TransfoXLTokenizationTest(TokenizerTesterMixin, unittest.TestCase):
-
- tokenizer_class = TransfoXLTokenizer if is_torch_available() else None
-
- def setUp(self):
- super().setUp()
-
- vocab_tokens = [
- "",
- "[CLS]",
- "[SEP]",
- "want",
- "unwanted",
- "wa",
- "un",
- "running",
- ",",
- "low",
- "l",
- ]
- self.vocab_file = os.path.join(self.tmpdirname, VOCAB_FILES_NAMES["vocab_file"])
- with open(self.vocab_file, "w", encoding="utf-8") as vocab_writer:
- vocab_writer.write("".join([x + "\n" for x in vocab_tokens]))
-
- def get_tokenizer(self, **kwargs):
- kwargs["lower_case"] = True
- return TransfoXLTokenizer.from_pretrained(self.tmpdirname, **kwargs)
-
- def get_input_output_texts(self):
- input_text = " UNwanted , running"
- output_text = " unwanted, running"
- return input_text, output_text
-
- def test_full_tokenizer(self):
- tokenizer = TransfoXLTokenizer(vocab_file=self.vocab_file, lower_case=True)
-
- tokens = tokenizer.tokenize(" UNwanted , running")
- self.assertListEqual(tokens, ["", "unwanted", ",", "running"])
-
- self.assertListEqual(tokenizer.convert_tokens_to_ids(tokens), [0, 4, 8, 7])
-
- def test_full_tokenizer_lower(self):
- tokenizer = TransfoXLTokenizer(lower_case=True)
-
- self.assertListEqual(
- tokenizer.tokenize(" \tHeLLo ! how \n Are yoU ? "), ["hello", "!", "how", "are", "you", "?"]
- )
-
- def test_full_tokenizer_no_lower(self):
- tokenizer = TransfoXLTokenizer(lower_case=False)
-
- self.assertListEqual(
- tokenizer.tokenize(" \tHeLLo ! how \n Are yoU ? "), ["HeLLo", "!", "how", "Are", "yoU", "?"]
- )
diff --git a/server/transformers/tests/test_tokenization_utils.py b/server/transformers/tests/test_tokenization_utils.py
deleted file mode 100644
index 2909b4f9daa4bf2f80e01ef6966585f46beace23..0000000000000000000000000000000000000000
--- a/server/transformers/tests/test_tokenization_utils.py
+++ /dev/null
@@ -1,41 +0,0 @@
-# coding=utf-8
-# Copyright 2018 HuggingFace Inc..
-#
-# Licensed under the Apache License, Version 2.0 (the "License");
-# you may not use this file except in compliance with the License.
-# You may obtain a copy of the License at
-#
-# http://www.apache.org/licenses/LICENSE-2.0
-#
-# Unless required by applicable law or agreed to in writing, software
-# distributed under the License is distributed on an "AS IS" BASIS,
-# WITHOUT WARRANTIES OR CONDITIONS OF ANY KIND, either express or implied.
-# See the License for the specific language governing permissions and
-# limitations under the License.
-
-
-import unittest
-
-from transformers import PreTrainedTokenizer
-from transformers.tokenization_gpt2 import GPT2Tokenizer
-
-from .utils import slow
-
-
-class TokenizerUtilsTest(unittest.TestCase):
- def check_tokenizer_from_pretrained(self, tokenizer_class):
- s3_models = list(tokenizer_class.max_model_input_sizes.keys())
- for model_name in s3_models[:1]:
- tokenizer = tokenizer_class.from_pretrained(model_name)
- self.assertIsNotNone(tokenizer)
- self.assertIsInstance(tokenizer, tokenizer_class)
- self.assertIsInstance(tokenizer, PreTrainedTokenizer)
-
- for special_tok in tokenizer.all_special_tokens:
- self.assertIsInstance(special_tok, str)
- special_tok_id = tokenizer.convert_tokens_to_ids(special_tok)
- self.assertIsInstance(special_tok_id, int)
-
- @slow
- def test_pretrained_tokenizers(self):
- self.check_tokenizer_from_pretrained(GPT2Tokenizer)
diff --git a/server/transformers/tests/test_tokenization_xlm.py b/server/transformers/tests/test_tokenization_xlm.py
deleted file mode 100644
index 5fd7379388b54abc299d2527809b71a0bb2f7d47..0000000000000000000000000000000000000000
--- a/server/transformers/tests/test_tokenization_xlm.py
+++ /dev/null
@@ -1,100 +0,0 @@
-# coding=utf-8
-# Copyright 2018 The Google AI Language Team Authors.
-#
-# Licensed under the Apache License, Version 2.0 (the "License");
-# you may not use this file except in compliance with the License.
-# You may obtain a copy of the License at
-#
-# http://www.apache.org/licenses/LICENSE-2.0
-#
-# Unless required by applicable law or agreed to in writing, software
-# distributed under the License is distributed on an "AS IS" BASIS,
-# WITHOUT WARRANTIES OR CONDITIONS OF ANY KIND, either express or implied.
-# See the License for the specific language governing permissions and
-# limitations under the License.
-
-
-import json
-import os
-import unittest
-
-from transformers.tokenization_xlm import VOCAB_FILES_NAMES, XLMTokenizer
-
-from .test_tokenization_common import TokenizerTesterMixin
-from .utils import slow
-
-
-class XLMTokenizationTest(TokenizerTesterMixin, unittest.TestCase):
-
- tokenizer_class = XLMTokenizer
-
- def setUp(self):
- super().setUp()
-
- # Adapted from Sennrich et al. 2015 and https://github.com/rsennrich/subword-nmt
- vocab = [
- "l",
- "o",
- "w",
- "e",
- "r",
- "s",
- "t",
- "i",
- "d",
- "n",
- "w",
- "r",
- "t",
- "lo",
- "low",
- "er",
- "low",
- "lowest",
- "newer",
- "wider",
- "",
- ]
- vocab_tokens = dict(zip(vocab, range(len(vocab))))
- merges = ["l o 123", "lo w 1456", "e r 1789", ""]
-
- self.vocab_file = os.path.join(self.tmpdirname, VOCAB_FILES_NAMES["vocab_file"])
- self.merges_file = os.path.join(self.tmpdirname, VOCAB_FILES_NAMES["merges_file"])
- with open(self.vocab_file, "w") as fp:
- fp.write(json.dumps(vocab_tokens))
- with open(self.merges_file, "w") as fp:
- fp.write("\n".join(merges))
-
- def get_tokenizer(self, **kwargs):
- return XLMTokenizer.from_pretrained(self.tmpdirname, **kwargs)
-
- def get_input_output_texts(self):
- input_text = "lower newer"
- output_text = "lower newer"
- return input_text, output_text
-
- def test_full_tokenizer(self):
- """ Adapted from Sennrich et al. 2015 and https://github.com/rsennrich/subword-nmt """
- tokenizer = XLMTokenizer(self.vocab_file, self.merges_file)
-
- text = "lower"
- bpe_tokens = ["low", "er"]
- tokens = tokenizer.tokenize(text)
- self.assertListEqual(tokens, bpe_tokens)
-
- input_tokens = tokens + [""]
- input_bpe_tokens = [14, 15, 20]
- self.assertListEqual(tokenizer.convert_tokens_to_ids(input_tokens), input_bpe_tokens)
-
- @slow
- def test_sequence_builders(self):
- tokenizer = XLMTokenizer.from_pretrained("xlm-mlm-en-2048")
-
- text = tokenizer.encode("sequence builders", add_special_tokens=False)
- text_2 = tokenizer.encode("multi-sequence build", add_special_tokens=False)
-
- encoded_sentence = tokenizer.build_inputs_with_special_tokens(text)
- encoded_pair = tokenizer.build_inputs_with_special_tokens(text, text_2)
-
- assert encoded_sentence == [1] + text + [1]
- assert encoded_pair == [1] + text + [1] + text_2 + [1]
diff --git a/server/transformers/tests/test_tokenization_xlnet.py b/server/transformers/tests/test_tokenization_xlnet.py
deleted file mode 100644
index 2fa94bfbc928dbad0ae1c2f6c6ed2f5dc6ab1326..0000000000000000000000000000000000000000
--- a/server/transformers/tests/test_tokenization_xlnet.py
+++ /dev/null
@@ -1,185 +0,0 @@
-# coding=utf-8
-# Copyright 2018 The Google AI Language Team Authors.
-#
-# Licensed under the Apache License, Version 2.0 (the "License");
-# you may not use this file except in compliance with the License.
-# You may obtain a copy of the License at
-#
-# http://www.apache.org/licenses/LICENSE-2.0
-#
-# Unless required by applicable law or agreed to in writing, software
-# distributed under the License is distributed on an "AS IS" BASIS,
-# WITHOUT WARRANTIES OR CONDITIONS OF ANY KIND, either express or implied.
-# See the License for the specific language governing permissions and
-# limitations under the License.
-
-
-import os
-import unittest
-
-from transformers.tokenization_xlnet import SPIECE_UNDERLINE, XLNetTokenizer
-
-from .test_tokenization_common import TokenizerTesterMixin
-from .utils import slow
-
-
-SAMPLE_VOCAB = os.path.join(os.path.dirname(os.path.abspath(__file__)), "fixtures/test_sentencepiece.model")
-
-
-class XLNetTokenizationTest(TokenizerTesterMixin, unittest.TestCase):
-
- tokenizer_class = XLNetTokenizer
-
- def setUp(self):
- super().setUp()
-
- # We have a SentencePiece fixture for testing
- tokenizer = XLNetTokenizer(SAMPLE_VOCAB, keep_accents=True)
- tokenizer.save_pretrained(self.tmpdirname)
-
- def get_tokenizer(self, **kwargs):
- return XLNetTokenizer.from_pretrained(self.tmpdirname, **kwargs)
-
- def get_input_output_texts(self):
- input_text = "This is a test"
- output_text = "This is a test"
- return input_text, output_text
-
- def test_full_tokenizer(self):
- tokenizer = XLNetTokenizer(SAMPLE_VOCAB, keep_accents=True)
-
- tokens = tokenizer.tokenize("This is a test")
- self.assertListEqual(tokens, ["▁This", "▁is", "▁a", "▁t", "est"])
-
- self.assertListEqual(tokenizer.convert_tokens_to_ids(tokens), [285, 46, 10, 170, 382])
-
- tokens = tokenizer.tokenize("I was born in 92000, and this is falsé.")
- self.assertListEqual(
- tokens,
- [
- SPIECE_UNDERLINE + "I",
- SPIECE_UNDERLINE + "was",
- SPIECE_UNDERLINE + "b",
- "or",
- "n",
- SPIECE_UNDERLINE + "in",
- SPIECE_UNDERLINE + "",
- "9",
- "2",
- "0",
- "0",
- "0",
- ",",
- SPIECE_UNDERLINE + "and",
- SPIECE_UNDERLINE + "this",
- SPIECE_UNDERLINE + "is",
- SPIECE_UNDERLINE + "f",
- "al",
- "s",
- "é",
- ".",
- ],
- )
- ids = tokenizer.convert_tokens_to_ids(tokens)
- self.assertListEqual(ids, [8, 21, 84, 55, 24, 19, 7, 0, 602, 347, 347, 347, 3, 12, 66, 46, 72, 80, 6, 0, 4])
-
- back_tokens = tokenizer.convert_ids_to_tokens(ids)
- self.assertListEqual(
- back_tokens,
- [
- SPIECE_UNDERLINE + "I",
- SPIECE_UNDERLINE + "was",
- SPIECE_UNDERLINE + "b",
- "or",
- "n",
- SPIECE_UNDERLINE + "in",
- SPIECE_UNDERLINE + "",
- "",
- "2",
- "0",
- "0",
- "0",
- ",",
- SPIECE_UNDERLINE + "and",
- SPIECE_UNDERLINE + "this",
- SPIECE_UNDERLINE + "is",
- SPIECE_UNDERLINE + "f",
- "al",
- "s",
- "",
- ".",
- ],
- )
-
- def test_tokenizer_lower(self):
- tokenizer = XLNetTokenizer(SAMPLE_VOCAB, do_lower_case=True)
- tokens = tokenizer.tokenize("I was born in 92000, and this is falsé.")
- self.assertListEqual(
- tokens,
- [
- SPIECE_UNDERLINE + "",
- "i",
- SPIECE_UNDERLINE + "was",
- SPIECE_UNDERLINE + "b",
- "or",
- "n",
- SPIECE_UNDERLINE + "in",
- SPIECE_UNDERLINE + "",
- "9",
- "2",
- "0",
- "0",
- "0",
- ",",
- SPIECE_UNDERLINE + "and",
- SPIECE_UNDERLINE + "this",
- SPIECE_UNDERLINE + "is",
- SPIECE_UNDERLINE + "f",
- "al",
- "se",
- ".",
- ],
- )
- self.assertListEqual(tokenizer.tokenize("H\u00E9llo"), ["▁he", "ll", "o"])
-
- def test_tokenizer_no_lower(self):
- tokenizer = XLNetTokenizer(SAMPLE_VOCAB, do_lower_case=False)
- tokens = tokenizer.tokenize("I was born in 92000, and this is falsé.")
- self.assertListEqual(
- tokens,
- [
- SPIECE_UNDERLINE + "I",
- SPIECE_UNDERLINE + "was",
- SPIECE_UNDERLINE + "b",
- "or",
- "n",
- SPIECE_UNDERLINE + "in",
- SPIECE_UNDERLINE + "",
- "9",
- "2",
- "0",
- "0",
- "0",
- ",",
- SPIECE_UNDERLINE + "and",
- SPIECE_UNDERLINE + "this",
- SPIECE_UNDERLINE + "is",
- SPIECE_UNDERLINE + "f",
- "al",
- "se",
- ".",
- ],
- )
-
- @slow
- def test_sequence_builders(self):
- tokenizer = XLNetTokenizer.from_pretrained("xlnet-base-cased")
-
- text = tokenizer.encode("sequence builders", add_special_tokens=False)
- text_2 = tokenizer.encode("multi-sequence build", add_special_tokens=False)
-
- encoded_sentence = tokenizer.build_inputs_with_special_tokens(text)
- encoded_pair = tokenizer.build_inputs_with_special_tokens(text, text_2)
-
- assert encoded_sentence == text + [4, 3]
- assert encoded_pair == text + [4] + text_2 + [4, 3]
diff --git a/server/transformers/tests/utils.py b/server/transformers/tests/utils.py
deleted file mode 100644
index 163628d3a7d682d59f66e8fe038e360daa602308..0000000000000000000000000000000000000000
--- a/server/transformers/tests/utils.py
+++ /dev/null
@@ -1,90 +0,0 @@
-import os
-import tempfile
-import unittest
-from distutils.util import strtobool
-
-from transformers.file_utils import _tf_available, _torch_available
-
-
-CACHE_DIR = os.path.join(tempfile.gettempdir(), "transformers_test")
-
-SMALL_MODEL_IDENTIFIER = "julien-c/bert-xsmall-dummy"
-DUMMY_UNKWOWN_IDENTIFIER = "julien-c/dummy-unknown"
-# Used to test Auto{Config, Model, Tokenizer} model_type detection.
-
-
-def parse_flag_from_env(key, default=False):
- try:
- value = os.environ[key]
- except KeyError:
- # KEY isn't set, default to `default`.
- _value = default
- else:
- # KEY is set, convert it to True or False.
- try:
- _value = strtobool(value)
- except ValueError:
- # More values are supported, but let's keep the message simple.
- raise ValueError("If set, {} must be yes or no.".format(key))
- return _value
-
-
-_run_slow_tests = parse_flag_from_env("RUN_SLOW", default=False)
-_run_custom_tokenizers = parse_flag_from_env("RUN_CUSTOM_TOKENIZERS", default=False)
-
-
-def slow(test_case):
- """
- Decorator marking a test as slow.
-
- Slow tests are skipped by default. Set the RUN_SLOW environment variable
- to a truthy value to run them.
-
- """
- if not _run_slow_tests:
- test_case = unittest.skip("test is slow")(test_case)
- return test_case
-
-
-def custom_tokenizers(test_case):
- """
- Decorator marking a test for a custom tokenizer.
-
- Custom tokenizers require additional dependencies, and are skipped
- by default. Set the RUN_CUSTOM_TOKENIZERS environment variable
- to a truthy value to run them.
- """
- if not _run_custom_tokenizers:
- test_case = unittest.skip("test of custom tokenizers")(test_case)
- return test_case
-
-
-def require_torch(test_case):
- """
- Decorator marking a test that requires PyTorch.
-
- These tests are skipped when PyTorch isn't installed.
-
- """
- if not _torch_available:
- test_case = unittest.skip("test requires PyTorch")(test_case)
- return test_case
-
-
-def require_tf(test_case):
- """
- Decorator marking a test that requires TensorFlow.
-
- These tests are skipped when TensorFlow isn't installed.
-
- """
- if not _tf_available:
- test_case = unittest.skip("test requires TensorFlow")(test_case)
- return test_case
-
-
-if _torch_available:
- # Set the USE_CUDA environment variable to select a GPU.
- torch_device = "cuda" if parse_flag_from_env("USE_CUDA") else "cpu"
-else:
- torch_device = None
diff --git a/server/transformers/transformers-cli b/server/transformers/transformers-cli
deleted file mode 100755
index 9813b838433252821ec44e726275326e55bbc3c8..0000000000000000000000000000000000000000
--- a/server/transformers/transformers-cli
+++ /dev/null
@@ -1,32 +0,0 @@
-#!/usr/bin/env python
-from argparse import ArgumentParser
-
-from transformers.commands.convert import ConvertCommand
-from transformers.commands.download import DownloadCommand
-from transformers.commands.env import EnvironmentCommand
-from transformers.commands.run import RunCommand
-from transformers.commands.serving import ServeCommand
-from transformers.commands.user import UserCommands
-
-if __name__ == '__main__':
- parser = ArgumentParser('Transformers CLI tool', usage='transformers-cli []')
- commands_parser = parser.add_subparsers(help='transformers-cli command helpers')
-
- # Register commands
- ConvertCommand.register_subcommand(commands_parser)
- DownloadCommand.register_subcommand(commands_parser)
- EnvironmentCommand.register_subcommand(commands_parser)
- RunCommand.register_subcommand(commands_parser)
- ServeCommand.register_subcommand(commands_parser)
- UserCommands.register_subcommand(commands_parser)
-
- # Let's go
- args = parser.parse_args()
-
- if not hasattr(args, 'func'):
- parser.print_help()
- exit(1)
-
- # Run
- service = args.func(args)
- service.run()
diff --git a/server/transformers/utils/download_glue_data.py b/server/transformers/utils/download_glue_data.py
deleted file mode 100644
index b46cbcd7b22f00547e93f98be035f98aaf59e18a..0000000000000000000000000000000000000000
--- a/server/transformers/utils/download_glue_data.py
+++ /dev/null
@@ -1,154 +0,0 @@
-""" Script for downloading all GLUE data.
-Original source: https://gist.github.com/W4ngatang/60c2bdb54d156a41194446737ce03e2e
-
-Note: for legal reasons, we are unable to host MRPC.
-You can either use the version hosted by the SentEval team, which is already tokenized,
-or you can download the original data from (https://download.microsoft.com/download/D/4/6/D46FF87A-F6B9-4252-AA8B-3604ED519838/MSRParaphraseCorpus.msi) and extract the data from it manually.
-For Windows users, you can run the .msi file. For Mac and Linux users, consider an external library such as 'cabextract' (see below for an example).
-You should then rename and place specific files in a folder (see below for an example).
-
-mkdir MRPC
-cabextract MSRParaphraseCorpus.msi -d MRPC
-cat MRPC/_2DEC3DBE877E4DB192D17C0256E90F1D | tr -d $'\r' > MRPC/msr_paraphrase_train.txt
-cat MRPC/_D7B391F9EAFF4B1B8BCE8F21B20B1B61 | tr -d $'\r' > MRPC/msr_paraphrase_test.txt
-rm MRPC/_*
-rm MSRParaphraseCorpus.msi
-
-1/30/19: It looks like SentEval is no longer hosting their extracted and tokenized MRPC data, so you'll need to download the data from the original source for now.
-2/11/19: It looks like SentEval actually *is* hosting the extracted data. Hooray!
-"""
-
-import argparse
-import os
-import sys
-import urllib.request
-import zipfile
-
-
-TASKS = ["CoLA", "SST", "MRPC", "QQP", "STS", "MNLI", "SNLI", "QNLI", "RTE", "WNLI", "diagnostic"]
-TASK2PATH = {
- "CoLA": "https://firebasestorage.googleapis.com/v0/b/mtl-sentence-representations.appspot.com/o/data%2FCoLA.zip?alt=media&token=46d5e637-3411-4188-bc44-5809b5bfb5f4",
- "SST": "https://firebasestorage.googleapis.com/v0/b/mtl-sentence-representations.appspot.com/o/data%2FSST-2.zip?alt=media&token=aabc5f6b-e466-44a2-b9b4-cf6337f84ac8",
- "MRPC": "https://firebasestorage.googleapis.com/v0/b/mtl-sentence-representations.appspot.com/o/data%2Fmrpc_dev_ids.tsv?alt=media&token=ec5c0836-31d5-48f4-b431-7480817f1adc",
- "QQP": "https://firebasestorage.googleapis.com/v0/b/mtl-sentence-representations.appspot.com/o/data%2FQQP.zip?alt=media&token=700c6acf-160d-4d89-81d1-de4191d02cb5",
- "STS": "https://firebasestorage.googleapis.com/v0/b/mtl-sentence-representations.appspot.com/o/data%2FSTS-B.zip?alt=media&token=bddb94a7-8706-4e0d-a694-1109e12273b5",
- "MNLI": "https://firebasestorage.googleapis.com/v0/b/mtl-sentence-representations.appspot.com/o/data%2FMNLI.zip?alt=media&token=50329ea1-e339-40e2-809c-10c40afff3ce",
- "SNLI": "https://firebasestorage.googleapis.com/v0/b/mtl-sentence-representations.appspot.com/o/data%2FSNLI.zip?alt=media&token=4afcfbb2-ff0c-4b2d-a09a-dbf07926f4df",
- "QNLI": "https://firebasestorage.googleapis.com/v0/b/mtl-sentence-representations.appspot.com/o/data%2FQNLIv2.zip?alt=media&token=6fdcf570-0fc5-4631-8456-9505272d1601",
- "RTE": "https://firebasestorage.googleapis.com/v0/b/mtl-sentence-representations.appspot.com/o/data%2FRTE.zip?alt=media&token=5efa7e85-a0bb-4f19-8ea2-9e1840f077fb",
- "WNLI": "https://firebasestorage.googleapis.com/v0/b/mtl-sentence-representations.appspot.com/o/data%2FWNLI.zip?alt=media&token=068ad0a0-ded7-4bd7-99a5-5e00222e0faf",
- "diagnostic": "https://storage.googleapis.com/mtl-sentence-representations.appspot.com/tsvsWithoutLabels%2FAX.tsv?GoogleAccessId=firebase-adminsdk-0khhl@mtl-sentence-representations.iam.gserviceaccount.com&Expires=2498860800&Signature=DuQ2CSPt2Yfre0C%2BiISrVYrIFaZH1Lc7hBVZDD4ZyR7fZYOMNOUGpi8QxBmTNOrNPjR3z1cggo7WXFfrgECP6FBJSsURv8Ybrue8Ypt%2FTPxbuJ0Xc2FhDi%2BarnecCBFO77RSbfuz%2Bs95hRrYhTnByqu3U%2FYZPaj3tZt5QdfpH2IUROY8LiBXoXS46LE%2FgOQc%2FKN%2BA9SoscRDYsnxHfG0IjXGwHN%2Bf88q6hOmAxeNPx6moDulUF6XMUAaXCSFU%2BnRO2RDL9CapWxj%2BDl7syNyHhB7987hZ80B%2FwFkQ3MEs8auvt5XW1%2Bd4aCU7ytgM69r8JDCwibfhZxpaa4gd50QXQ%3D%3D",
-}
-
-MRPC_TRAIN = "https://dl.fbaipublicfiles.com/senteval/senteval_data/msr_paraphrase_train.txt"
-MRPC_TEST = "https://dl.fbaipublicfiles.com/senteval/senteval_data/msr_paraphrase_test.txt"
-
-
-def download_and_extract(task, data_dir):
- print("Downloading and extracting %s..." % task)
- data_file = "%s.zip" % task
- urllib.request.urlretrieve(TASK2PATH[task], data_file)
- with zipfile.ZipFile(data_file) as zip_ref:
- zip_ref.extractall(data_dir)
- os.remove(data_file)
- print("\tCompleted!")
-
-
-def format_mrpc(data_dir, path_to_data):
- print("Processing MRPC...")
- mrpc_dir = os.path.join(data_dir, "MRPC")
- if not os.path.isdir(mrpc_dir):
- os.mkdir(mrpc_dir)
- if path_to_data:
- mrpc_train_file = os.path.join(path_to_data, "msr_paraphrase_train.txt")
- mrpc_test_file = os.path.join(path_to_data, "msr_paraphrase_test.txt")
- else:
- print("Local MRPC data not specified, downloading data from %s" % MRPC_TRAIN)
- mrpc_train_file = os.path.join(mrpc_dir, "msr_paraphrase_train.txt")
- mrpc_test_file = os.path.join(mrpc_dir, "msr_paraphrase_test.txt")
- urllib.request.urlretrieve(MRPC_TRAIN, mrpc_train_file)
- urllib.request.urlretrieve(MRPC_TEST, mrpc_test_file)
- assert os.path.isfile(mrpc_train_file), "Train data not found at %s" % mrpc_train_file
- assert os.path.isfile(mrpc_test_file), "Test data not found at %s" % mrpc_test_file
- urllib.request.urlretrieve(TASK2PATH["MRPC"], os.path.join(mrpc_dir, "dev_ids.tsv"))
-
- dev_ids = []
- with open(os.path.join(mrpc_dir, "dev_ids.tsv"), encoding="utf8") as ids_fh:
- for row in ids_fh:
- dev_ids.append(row.strip().split("\t"))
-
- with open(mrpc_train_file, encoding="utf8") as data_fh, open(
- os.path.join(mrpc_dir, "train.tsv"), "w", encoding="utf8"
- ) as train_fh, open(os.path.join(mrpc_dir, "dev.tsv"), "w", encoding="utf8") as dev_fh:
- header = data_fh.readline()
- train_fh.write(header)
- dev_fh.write(header)
- for row in data_fh:
- label, id1, id2, s1, s2 = row.strip().split("\t")
- if [id1, id2] in dev_ids:
- dev_fh.write("%s\t%s\t%s\t%s\t%s\n" % (label, id1, id2, s1, s2))
- else:
- train_fh.write("%s\t%s\t%s\t%s\t%s\n" % (label, id1, id2, s1, s2))
-
- with open(mrpc_test_file, encoding="utf8") as data_fh, open(
- os.path.join(mrpc_dir, "test.tsv"), "w", encoding="utf8"
- ) as test_fh:
- header = data_fh.readline()
- test_fh.write("index\t#1 ID\t#2 ID\t#1 String\t#2 String\n")
- for idx, row in enumerate(data_fh):
- label, id1, id2, s1, s2 = row.strip().split("\t")
- test_fh.write("%d\t%s\t%s\t%s\t%s\n" % (idx, id1, id2, s1, s2))
- print("\tCompleted!")
-
-
-def download_diagnostic(data_dir):
- print("Downloading and extracting diagnostic...")
- if not os.path.isdir(os.path.join(data_dir, "diagnostic")):
- os.mkdir(os.path.join(data_dir, "diagnostic"))
- data_file = os.path.join(data_dir, "diagnostic", "diagnostic.tsv")
- urllib.request.urlretrieve(TASK2PATH["diagnostic"], data_file)
- print("\tCompleted!")
- return
-
-
-def get_tasks(task_names):
- task_names = task_names.split(",")
- if "all" in task_names:
- tasks = TASKS
- else:
- tasks = []
- for task_name in task_names:
- assert task_name in TASKS, "Task %s not found!" % task_name
- tasks.append(task_name)
- return tasks
-
-
-def main(arguments):
- parser = argparse.ArgumentParser()
- parser.add_argument("--data_dir", help="directory to save data to", type=str, default="glue_data")
- parser.add_argument(
- "--tasks", help="tasks to download data for as a comma separated string", type=str, default="all"
- )
- parser.add_argument(
- "--path_to_mrpc",
- help="path to directory containing extracted MRPC data, msr_paraphrase_train.txt and msr_paraphrase_text.txt",
- type=str,
- default="",
- )
- args = parser.parse_args(arguments)
-
- if not os.path.isdir(args.data_dir):
- os.mkdir(args.data_dir)
- tasks = get_tasks(args.tasks)
-
- for task in tasks:
- if task == "MRPC":
- format_mrpc(args.data_dir, args.path_to_mrpc)
- elif task == "diagnostic":
- download_diagnostic(args.data_dir)
- else:
- download_and_extract(task, args.data_dir)
-
-
-if __name__ == "__main__":
- sys.exit(main(sys.argv[1:]))
diff --git a/server/transformers/utils/link_tester.py b/server/transformers/utils/link_tester.py
deleted file mode 100644
index 0ef165c401b84f8b15ac9a7eea1e699a888b77fd..0000000000000000000000000000000000000000
--- a/server/transformers/utils/link_tester.py
+++ /dev/null
@@ -1,79 +0,0 @@
-""" Link tester.
-
-This little utility reads all the python files in the repository,
-scans for links pointing to S3 and tests the links one by one. Raises an error
-at the end of the scan if at least one link was reported broken.
-"""
-import os
-import re
-import sys
-
-import requests
-
-
-REGEXP_FIND_S3_LINKS = r"""([\"'])(https:\/\/s3)(.*)?\1"""
-
-
-def list_python_files_in_repository():
- """ List all python files in the repository.
-
- This function assumes that the script is executed in the root folder.
- """
- source_code_files = []
- for path, subdirs, files in os.walk("."):
- if "templates" in path:
- continue
- for name in files:
- if ".py" in name and ".pyc" not in name:
- path_to_files = os.path.join(path, name)
- source_code_files.append(path_to_files)
-
- return source_code_files
-
-
-def find_all_links(file_paths):
- links = []
- for path in file_paths:
- links += scan_code_for_links(path)
-
- return links
-
-
-def scan_code_for_links(source):
- """ Scans the file to find links using a regular expression.
- Returns a list of links.
- """
- with open(source, "r") as content:
- content = content.read()
- raw_links = re.findall(REGEXP_FIND_S3_LINKS, content)
- links = [prefix + suffix for _, prefix, suffix in raw_links]
-
- return links
-
-
-def check_all_links(links):
- """ Check that the provided links are valid.
-
- Links are considered valid if a HEAD request to the server
- returns a 200 status code.
- """
- broken_links = []
- for link in links:
- head = requests.head(link)
- if head.status_code != 200:
- broken_links.append(link)
-
- return broken_links
-
-
-if __name__ == "__main__":
- file_paths = list_python_files_in_repository()
- links = find_all_links(file_paths)
- broken_links = check_all_links(links)
- print("Looking for broken links to pre-trained models/configs/tokenizers...")
- if broken_links:
- print("The following links did not respond:")
- for link in broken_links:
- print("- {}".format(link))
- sys.exit(1)
- print("All links are ok.")
diff --git a/server/transformers/valohai.yaml b/server/transformers/valohai.yaml
deleted file mode 100644
index 2573551b4e23d6f2243f4584f2c20007fed155f2..0000000000000000000000000000000000000000
--- a/server/transformers/valohai.yaml
+++ /dev/null
@@ -1,94 +0,0 @@
----
-
-- step:
- name: Execute python examples/run_glue.py
- image: pytorch/pytorch:nightly-devel-cuda10.0-cudnn7
- command:
- - python /valohai/repository/utils/download_glue_data.py --data_dir=/glue_data
- - pip install -e .
- - pip install -r examples/requirements.txt
- - python examples/run_glue.py --do_train --data_dir=/glue_data/{parameter-value:task_name} {parameters}
- parameters:
- - name: model_type
- pass-as: --model_type={v}
- type: string
- default: bert
- - name: model_name_or_path
- pass-as: --model_name_or_path={v}
- type: string
- default: bert-base-uncased
- - name: task_name
- pass-as: --task_name={v}
- type: string
- default: MRPC
- - name: max_seq_length
- pass-as: --max_seq_length={v}
- description: The maximum total input sequence length after tokenization. Sequences longer than this will be truncated, sequences shorter will be padded.
- type: integer
- default: 128
- - name: per_gpu_train_batch_size
- pass-as: --per_gpu_train_batch_size={v}
- description: Batch size per GPU/CPU for training.
- type: integer
- default: 8
- - name: per_gpu_eval_batch_size
- pass-as: --per_gpu_eval_batch_size={v}
- description: Batch size per GPU/CPU for evaluation.
- type: integer
- default: 8
- - name: gradient_accumulation_steps
- pass-as: --gradient_accumulation_steps={v}
- description: Number of updates steps to accumulate before performing a backward/update pass.
- type: integer
- default: 1
- - name: learning_rate
- pass-as: --learning_rate={v}
- description: The initial learning rate for Adam.
- type: float
- default: 0.00005
- - name: adam_epsilon
- pass-as: --adam_epsilon={v}
- description: Epsilon for Adam optimizer.
- type: float
- default: 0.00000001
- - name: max_grad_norm
- pass-as: --max_grad_norm={v}
- description: Max gradient norm.
- type: float
- default: 1.0
- - name: num_train_epochs
- pass-as: --num_train_epochs={v}
- description: Total number of training epochs to perform.
- type: integer
- default: 3
- - name: max_steps
- pass-as: --max_steps={v}
- description: If > 0, set total number of training steps to perform. Override num_train_epochs.
- type: integer
- default: -1
- - name: warmup_steps
- pass-as: --warmup_steps={v}
- description: Linear warmup over warmup_steps.
- type: integer
- default: -1
- - name: logging_steps
- pass-as: --logging_steps={v}
- description: Log every X updates steps.
- type: integer
- default: 25
- - name: save_steps
- pass-as: --save_steps={v}
- description: Save checkpoint every X updates steps.
- type: integer
- default: -1
- - name: output_dir
- pass-as: --output_dir={v}
- type: string
- default: /valohai/outputs
- - name: evaluate_during_training
- description: Run evaluation during training at each logging step.
- type: flag
- default: true
- - name: do_lower_case
- description: Set this flag if you are using an uncased model.
- type: flag