Spaces:
Running
on
CPU Upgrade
Running
on
CPU Upgrade
Remove old files
Browse filesThis view is limited to 50 files because it contains too many changes.
See raw diff
- server/transformers/CONTRIBUTING.md +0 -262
- server/transformers/LICENSE +0 -202
- server/transformers/MANIFEST.in +0 -1
- server/transformers/Makefile +0 -24
- server/transformers/README.md +0 -676
- server/transformers/deploy_multi_version_doc.sh +0 -23
- server/transformers/docker/Dockerfile +0 -7
- server/transformers/docs/Makefile +0 -19
- server/transformers/docs/README.md +0 -67
- server/transformers/docs/source/_static/css/Calibre-Light.ttf +0 -0
- server/transformers/docs/source/_static/css/Calibre-Medium.otf +0 -0
- server/transformers/docs/source/_static/css/Calibre-Regular.otf +0 -0
- server/transformers/docs/source/_static/css/Calibre-Thin.otf +0 -0
- server/transformers/docs/source/_static/css/code-snippets.css +0 -12
- server/transformers/docs/source/_static/css/huggingface.css +0 -196
- server/transformers/docs/source/_static/js/custom.js +0 -79
- server/transformers/docs/source/_static/js/huggingface_logo.svg +0 -47
- server/transformers/docs/source/benchmarks.md +0 -54
- server/transformers/docs/source/bertology.rst +0 -18
- server/transformers/docs/source/conf.py +0 -188
- server/transformers/docs/source/converting_tensorflow_models.rst +0 -137
- server/transformers/docs/source/examples.md +0 -801
- server/transformers/docs/source/imgs/transformers_logo_name.png +0 -0
- server/transformers/docs/source/imgs/warmup_constant_schedule.png +0 -0
- server/transformers/docs/source/imgs/warmup_cosine_hard_restarts_schedule.png +0 -0
- server/transformers/docs/source/imgs/warmup_cosine_schedule.png +0 -0
- server/transformers/docs/source/imgs/warmup_cosine_warm_restarts_schedule.png +0 -0
- server/transformers/docs/source/imgs/warmup_linear_schedule.png +0 -0
- server/transformers/docs/source/index.rst +0 -98
- server/transformers/docs/source/installation.md +0 -51
- server/transformers/docs/source/main_classes/configuration.rst +0 -10
- server/transformers/docs/source/main_classes/model.rst +0 -21
- server/transformers/docs/source/main_classes/optimizer_schedules.rst +0 -75
- server/transformers/docs/source/main_classes/processors.rst +0 -153
- server/transformers/docs/source/main_classes/tokenizer.rst +0 -16
- server/transformers/docs/source/migration.md +0 -109
- server/transformers/docs/source/model_doc/albert.rst +0 -64
- server/transformers/docs/source/model_doc/auto.rst +0 -29
- server/transformers/docs/source/model_doc/bert.rst +0 -128
- server/transformers/docs/source/model_doc/camembert.rst +0 -50
- server/transformers/docs/source/model_doc/ctrl.rst +0 -49
- server/transformers/docs/source/model_doc/distilbert.rst +0 -70
- server/transformers/docs/source/model_doc/gpt.rst +0 -57
- server/transformers/docs/source/model_doc/gpt2.rst +0 -57
- server/transformers/docs/source/model_doc/roberta.rst +0 -57
- server/transformers/docs/source/model_doc/transformerxl.rst +0 -44
- server/transformers/docs/source/model_doc/xlm.rst +0 -76
- server/transformers/docs/source/model_doc/xlnet.rst +0 -92
- server/transformers/docs/source/model_sharing.md +0 -40
- server/transformers/docs/source/multilingual.rst +0 -103
server/transformers/CONTRIBUTING.md
DELETED
@@ -1,262 +0,0 @@
|
|
1 |
-
# How to contribute to transformers?
|
2 |
-
|
3 |
-
Everyone is welcome to contribute, and we value everybody's contribution. Code
|
4 |
-
is thus not the only way to help the community. Answering questions, helping
|
5 |
-
others, reaching out and improving the documentations are immensely valuable to
|
6 |
-
the community.
|
7 |
-
|
8 |
-
It also helps us if you spread the word: reference the library from blog posts
|
9 |
-
on the awesome projects it made possible, shout out on Twitter every time it has
|
10 |
-
helped you, or simply star the repo to say "thank you".
|
11 |
-
|
12 |
-
## You can contribute in so many ways!
|
13 |
-
|
14 |
-
There are 4 ways you can contribute to transformers:
|
15 |
-
* Fixing outstanding issues with the existing code;
|
16 |
-
* Implementing new models;
|
17 |
-
* Contributing to the examples or to the documentation;
|
18 |
-
* Submitting issues related to bugs or desired new features.
|
19 |
-
|
20 |
-
*All are equally valuable to the community.*
|
21 |
-
|
22 |
-
## Submitting a new issue or feature request
|
23 |
-
|
24 |
-
Do your best to follow these guidelines when submitting an issue or a feature
|
25 |
-
request. It will make it easier for us to come back to you quickly and with good
|
26 |
-
feedback.
|
27 |
-
|
28 |
-
### Did you find a bug?
|
29 |
-
|
30 |
-
The transformers are robust and reliable thanks to the users who notify us of
|
31 |
-
the problems they encounter. So thank you for reporting an issue.
|
32 |
-
|
33 |
-
First, we would really appreciate it if you could **make sure the bug was not
|
34 |
-
already reported** (use the search bar on Github under Issues).
|
35 |
-
|
36 |
-
Did not find it? :( So we can act quickly on it, please follow these steps:
|
37 |
-
|
38 |
-
* Include your **OS type and version**, the versions of **Python**, **PyTorch** and
|
39 |
-
**Tensorflow** when applicable;
|
40 |
-
* A short, self-contained, code snippet that allows us to reproduce the bug in
|
41 |
-
less than 30s;
|
42 |
-
* Provide the *full* traceback if an exception is raised.
|
43 |
-
|
44 |
-
To get the OS and software versions, execute the following code and copy-paste
|
45 |
-
the output:
|
46 |
-
|
47 |
-
```
|
48 |
-
import platform; print("Platform", platform.platform())
|
49 |
-
import sys; print("Python", sys.version)
|
50 |
-
import torch; print("PyTorch", torch.__version__)
|
51 |
-
import tensorflow; print("Tensorflow", tensorflow.__version__)
|
52 |
-
```
|
53 |
-
|
54 |
-
### Do you want to implement a new model?
|
55 |
-
|
56 |
-
Awesome! Please provide the following information:
|
57 |
-
|
58 |
-
* Short description of the model and link to the paper;
|
59 |
-
* Link to the implementation if it is open-source;
|
60 |
-
* Link to the model weights if they are available.
|
61 |
-
|
62 |
-
If you are willing to contribute the model yourself, let us know so we can best
|
63 |
-
guide you.
|
64 |
-
|
65 |
-
We have added a **detailed guide and templates** to guide you in the process of adding a new model. You can find them in the [`templates`](./templates) folder.
|
66 |
-
|
67 |
-
### Do you want a new feature (that is not a model)?
|
68 |
-
|
69 |
-
A world-class feature request addresses the following points:
|
70 |
-
|
71 |
-
1. Motivation first:
|
72 |
-
* Is it related to a problem/frustration with the library? If so, please explain
|
73 |
-
why. Providing a code snippet that demonstrates the problem is best.
|
74 |
-
* Is it related to something you would need for a project? We'd love to hear
|
75 |
-
about it!
|
76 |
-
* Is it something you worked on and think could benefit the community?
|
77 |
-
Awesome! Tell us what problem it solved for you.
|
78 |
-
2. Write a *full paragraph* describing the feature;
|
79 |
-
3. Provide a **code snippet** that demonstrates its future use;
|
80 |
-
4. In case this is related to a paper, please attach a link;
|
81 |
-
5. Attach any additional information (drawings, screenshots, etc.) you think may help.
|
82 |
-
|
83 |
-
If your issue is well written we're already 80% of the way there by the time you
|
84 |
-
post it.
|
85 |
-
|
86 |
-
We have added **templates** to guide you in the process of adding a new example script for training or testing the models in the library. You can find them in the [`templates`](./templates) folder.
|
87 |
-
|
88 |
-
## Start contributing! (Pull Requests)
|
89 |
-
|
90 |
-
Before writing code, we strongly advise you to search through the exising PRs or
|
91 |
-
issues to make sure that nobody is already working on the same thing. If you are
|
92 |
-
unsure, it is always a good idea to open an issue to get some feedback.
|
93 |
-
|
94 |
-
You will need basic `git` proficiency to be able to contribute to
|
95 |
-
`transformers`. `git` is not the easiest tool to use but it has the greatest
|
96 |
-
manual. Type `git --help` in a shell and enjoy. If you prefer books, [Pro
|
97 |
-
Git](https://git-scm.com/book/en/v2) is a very good reference.
|
98 |
-
|
99 |
-
Follow these steps to start contributing:
|
100 |
-
|
101 |
-
1. Fork the [repository](https://github.com/huggingface/transformers) by
|
102 |
-
clicking on the 'Fork' button on the repository's page. This creates a copy of the code
|
103 |
-
under your GitHub user account.
|
104 |
-
|
105 |
-
2. Clone your fork to your local disk, and add the base repository as a remote:
|
106 |
-
|
107 |
-
```bash
|
108 |
-
$ git clone [email protected]:<your Github handle>/transformers.git
|
109 |
-
$ cd transformers
|
110 |
-
$ git remote add upstream https://github.com/huggingface/transformers.git
|
111 |
-
```
|
112 |
-
|
113 |
-
3. Create a new branch to hold your development changes:
|
114 |
-
|
115 |
-
```bash
|
116 |
-
$ git checkout -b a-descriptive-name-for-my-changes
|
117 |
-
```
|
118 |
-
|
119 |
-
**do not** work on the `master` branch.
|
120 |
-
|
121 |
-
4. Set up a development environment by running the following command in a virtual environment:
|
122 |
-
|
123 |
-
```bash
|
124 |
-
$ pip install -e ".[dev]"
|
125 |
-
```
|
126 |
-
|
127 |
-
(If transformers was already installed in the virtual environment, remove
|
128 |
-
it with `pip uninstall transformers` before reinstalling it in editable
|
129 |
-
mode with the `-e` flag.)
|
130 |
-
|
131 |
-
Right now, we need an unreleased version of `isort` to avoid a
|
132 |
-
[bug](https://github.com/timothycrosley/isort/pull/1000):
|
133 |
-
|
134 |
-
```bash
|
135 |
-
$ pip install -U git+git://github.com/timothycrosley/isort.git@e63ae06ec7d70b06df9e528357650281a3d3ec22#egg=isort
|
136 |
-
```
|
137 |
-
|
138 |
-
5. Develop the features on your branch.
|
139 |
-
|
140 |
-
As you work on the features, you should make sure that the test suite
|
141 |
-
passes:
|
142 |
-
|
143 |
-
```bash
|
144 |
-
$ make test
|
145 |
-
```
|
146 |
-
|
147 |
-
`transformers` relies on `black` and `isort` to format its source code
|
148 |
-
consistently. After you make changes, format them with:
|
149 |
-
|
150 |
-
```bash
|
151 |
-
$ make style
|
152 |
-
```
|
153 |
-
|
154 |
-
`transformers` also uses `flake8` to check for coding mistakes. Quality
|
155 |
-
control runs in CI, however you can also run the same checks with:
|
156 |
-
|
157 |
-
```bash
|
158 |
-
$ make quality
|
159 |
-
```
|
160 |
-
|
161 |
-
Once you're happy with your changes, add changed files using `git add` and
|
162 |
-
make a commit with `git commit` to record your changes locally:
|
163 |
-
|
164 |
-
```bash
|
165 |
-
$ git add modified_file.py
|
166 |
-
$ git commit
|
167 |
-
```
|
168 |
-
|
169 |
-
Please write [good commit
|
170 |
-
messages](https://chris.beams.io/posts/git-commit/).
|
171 |
-
|
172 |
-
It is a good idea to sync your copy of the code with the original
|
173 |
-
repository regularly. This way you can quickly account for changes:
|
174 |
-
|
175 |
-
```bash
|
176 |
-
$ git fetch upstream
|
177 |
-
$ git rebase upstream/master
|
178 |
-
```
|
179 |
-
|
180 |
-
Push the changes to your account using:
|
181 |
-
|
182 |
-
```bash
|
183 |
-
$ git push -u origin a-descriptive-name-for-my-changes
|
184 |
-
```
|
185 |
-
|
186 |
-
6. Once you are satisfied (**and the checklist below is happy too**), go to the
|
187 |
-
webpage of your fork on GitHub. Click on 'Pull request' to send your changes
|
188 |
-
to the project maintainers for review.
|
189 |
-
|
190 |
-
7. It's ok if maintainers ask you for changes. It happens to core contributors
|
191 |
-
too! So everyone can see the changes in the Pull request, work in your local
|
192 |
-
branch and push the changes to your fork. They will automatically appear in
|
193 |
-
the pull request.
|
194 |
-
|
195 |
-
|
196 |
-
### Checklist
|
197 |
-
|
198 |
-
1. The title of your pull request should be a summary of its contribution;
|
199 |
-
2. If your pull request adresses an issue, please mention the issue number in
|
200 |
-
the pull request description to make sure they are linked (and people
|
201 |
-
consulting the issue know you are working on it);
|
202 |
-
3. To indicate a work in progress please prefix the title with `[WIP]`. These
|
203 |
-
are useful to avoid duplicated work, and to differentiate it from PRs ready
|
204 |
-
to be merged;
|
205 |
-
4. Make sure pre-existing tests still pass;
|
206 |
-
5. Add high-coverage tests. No quality test, no merge;
|
207 |
-
6. All public methods must have informative docstrings;
|
208 |
-
|
209 |
-
|
210 |
-
### Tests
|
211 |
-
|
212 |
-
You can run 🤗 Transformers tests with `unittest` or `pytest`.
|
213 |
-
|
214 |
-
We like `pytest` and `pytest-xdist` because it's faster. From the root of the
|
215 |
-
repository, here's how to run tests with `pytest` for the library:
|
216 |
-
|
217 |
-
```bash
|
218 |
-
$ python -m pytest -n auto --dist=loadfile -s -v ./tests/
|
219 |
-
```
|
220 |
-
|
221 |
-
and for the examples:
|
222 |
-
|
223 |
-
```bash
|
224 |
-
$ pip install -r examples/requirements.txt # only needed the first time
|
225 |
-
$ python -m pytest -n auto --dist=loadfile -s -v ./examples/
|
226 |
-
```
|
227 |
-
|
228 |
-
In fact, that's how `make test` and `make test-examples` are implemented!
|
229 |
-
|
230 |
-
You can specify a smaller set of tests in order to test only the feature
|
231 |
-
you're working on.
|
232 |
-
|
233 |
-
By default, slow tests are skipped. Set the `RUN_SLOW` environment variable to
|
234 |
-
`yes` to run them. This will download many gigabytes of models — make sure you
|
235 |
-
have enough disk space and a good Internet connection, or a lot of patience!
|
236 |
-
|
237 |
-
```bash
|
238 |
-
$ RUN_SLOW=yes python -m pytest -n auto --dist=loadfile -s -v ./tests/
|
239 |
-
$ RUN_SLOW=yes python -m pytest -n auto --dist=loadfile -s -v ./examples/
|
240 |
-
```
|
241 |
-
|
242 |
-
Likewise, set the `RUN_CUSTOM_TOKENIZERS` environment variable to `yes` to run
|
243 |
-
tests for custom tokenizers, which don't run by default either.
|
244 |
-
|
245 |
-
🤗 Transformers uses `pytest` as a test runner only. It doesn't use any
|
246 |
-
`pytest`-specific features in the test suite itself.
|
247 |
-
|
248 |
-
This means `unittest` is fully supported. Here's how to run tests with
|
249 |
-
`unittest`:
|
250 |
-
|
251 |
-
```bash
|
252 |
-
$ python -m unittest discover -s tests -t . -v
|
253 |
-
$ python -m unittest discover -s examples -t examples -v
|
254 |
-
```
|
255 |
-
|
256 |
-
|
257 |
-
### Style guide
|
258 |
-
|
259 |
-
For documentation strings, `transformers` follows the [google
|
260 |
-
style](https://google.github.io/styleguide/pyguide.html).
|
261 |
-
|
262 |
-
#### This guide was heavily inspired by the awesome [scikit-learn guide to contributing](https://github.com/scikit-learn/scikit-learn/blob/master/CONTRIBUTING.md)
|
|
|
|
|
|
|
|
|
|
|
|
|
|
|
|
|
|
|
|
|
|
|
|
|
|
|
|
|
|
|
|
|
|
|
|
|
|
|
|
|
|
|
|
|
|
|
|
|
|
|
|
|
|
|
|
|
|
|
|
|
|
|
|
|
|
|
|
|
|
|
|
|
|
|
|
|
|
|
|
|
|
|
|
|
|
|
|
|
|
|
|
|
|
|
|
|
|
|
|
|
|
|
|
|
|
|
|
|
|
|
|
|
|
|
|
|
|
|
|
|
|
|
|
|
|
|
|
|
|
|
|
|
|
|
|
|
|
|
|
|
|
|
|
|
|
|
|
|
|
|
|
|
|
|
|
|
|
|
|
|
|
|
|
|
|
|
|
|
|
|
|
|
|
|
|
|
|
|
|
|
|
|
|
|
|
|
|
|
|
|
|
|
|
|
|
|
|
|
|
|
|
|
|
|
|
|
|
|
|
|
|
|
|
|
|
|
|
|
|
|
|
|
|
|
|
|
|
|
|
|
|
|
|
|
|
|
|
|
|
|
|
|
|
|
|
|
|
|
|
|
|
|
|
|
|
|
|
|
|
|
|
|
|
|
|
|
|
|
|
|
|
|
|
|
|
|
|
|
|
|
|
|
|
|
|
|
|
|
|
|
|
|
|
|
|
|
|
|
|
|
|
|
|
|
|
|
|
|
|
|
|
|
|
|
|
|
|
|
|
|
|
|
|
|
|
|
|
|
|
|
|
|
|
|
|
|
|
|
|
|
|
|
|
|
|
|
|
|
|
|
|
|
|
|
|
|
|
|
|
|
|
|
|
|
|
|
|
|
|
|
|
|
|
|
|
|
|
|
|
|
|
|
|
|
|
|
|
|
|
|
|
|
|
|
|
|
|
|
|
|
|
|
|
|
|
|
|
|
|
|
|
|
|
|
|
|
|
|
|
|
|
|
|
|
|
|
|
|
|
|
|
|
|
|
|
|
|
|
|
|
|
|
|
|
|
|
|
|
|
|
|
|
|
|
|
|
|
|
|
|
|
|
|
|
|
|
|
|
|
|
|
|
|
|
|
|
|
|
|
|
|
|
|
|
|
|
|
|
|
|
|
|
|
|
|
|
|
|
|
|
|
|
|
|
|
|
|
|
|
|
|
|
|
|
|
|
|
|
|
|
|
|
|
|
|
server/transformers/LICENSE
DELETED
@@ -1,202 +0,0 @@
|
|
1 |
-
|
2 |
-
Apache License
|
3 |
-
Version 2.0, January 2004
|
4 |
-
http://www.apache.org/licenses/
|
5 |
-
|
6 |
-
TERMS AND CONDITIONS FOR USE, REPRODUCTION, AND DISTRIBUTION
|
7 |
-
|
8 |
-
1. Definitions.
|
9 |
-
|
10 |
-
"License" shall mean the terms and conditions for use, reproduction,
|
11 |
-
and distribution as defined by Sections 1 through 9 of this document.
|
12 |
-
|
13 |
-
"Licensor" shall mean the copyright owner or entity authorized by
|
14 |
-
the copyright owner that is granting the License.
|
15 |
-
|
16 |
-
"Legal Entity" shall mean the union of the acting entity and all
|
17 |
-
other entities that control, are controlled by, or are under common
|
18 |
-
control with that entity. For the purposes of this definition,
|
19 |
-
"control" means (i) the power, direct or indirect, to cause the
|
20 |
-
direction or management of such entity, whether by contract or
|
21 |
-
otherwise, or (ii) ownership of fifty percent (50%) or more of the
|
22 |
-
outstanding shares, or (iii) beneficial ownership of such entity.
|
23 |
-
|
24 |
-
"You" (or "Your") shall mean an individual or Legal Entity
|
25 |
-
exercising permissions granted by this License.
|
26 |
-
|
27 |
-
"Source" form shall mean the preferred form for making modifications,
|
28 |
-
including but not limited to software source code, documentation
|
29 |
-
source, and configuration files.
|
30 |
-
|
31 |
-
"Object" form shall mean any form resulting from mechanical
|
32 |
-
transformation or translation of a Source form, including but
|
33 |
-
not limited to compiled object code, generated documentation,
|
34 |
-
and conversions to other media types.
|
35 |
-
|
36 |
-
"Work" shall mean the work of authorship, whether in Source or
|
37 |
-
Object form, made available under the License, as indicated by a
|
38 |
-
copyright notice that is included in or attached to the work
|
39 |
-
(an example is provided in the Appendix below).
|
40 |
-
|
41 |
-
"Derivative Works" shall mean any work, whether in Source or Object
|
42 |
-
form, that is based on (or derived from) the Work and for which the
|
43 |
-
editorial revisions, annotations, elaborations, or other modifications
|
44 |
-
represent, as a whole, an original work of authorship. For the purposes
|
45 |
-
of this License, Derivative Works shall not include works that remain
|
46 |
-
separable from, or merely link (or bind by name) to the interfaces of,
|
47 |
-
the Work and Derivative Works thereof.
|
48 |
-
|
49 |
-
"Contribution" shall mean any work of authorship, including
|
50 |
-
the original version of the Work and any modifications or additions
|
51 |
-
to that Work or Derivative Works thereof, that is intentionally
|
52 |
-
submitted to Licensor for inclusion in the Work by the copyright owner
|
53 |
-
or by an individual or Legal Entity authorized to submit on behalf of
|
54 |
-
the copyright owner. For the purposes of this definition, "submitted"
|
55 |
-
means any form of electronic, verbal, or written communication sent
|
56 |
-
to the Licensor or its representatives, including but not limited to
|
57 |
-
communication on electronic mailing lists, source code control systems,
|
58 |
-
and issue tracking systems that are managed by, or on behalf of, the
|
59 |
-
Licensor for the purpose of discussing and improving the Work, but
|
60 |
-
excluding communication that is conspicuously marked or otherwise
|
61 |
-
designated in writing by the copyright owner as "Not a Contribution."
|
62 |
-
|
63 |
-
"Contributor" shall mean Licensor and any individual or Legal Entity
|
64 |
-
on behalf of whom a Contribution has been received by Licensor and
|
65 |
-
subsequently incorporated within the Work.
|
66 |
-
|
67 |
-
2. Grant of Copyright License. Subject to the terms and conditions of
|
68 |
-
this License, each Contributor hereby grants to You a perpetual,
|
69 |
-
worldwide, non-exclusive, no-charge, royalty-free, irrevocable
|
70 |
-
copyright license to reproduce, prepare Derivative Works of,
|
71 |
-
publicly display, publicly perform, sublicense, and distribute the
|
72 |
-
Work and such Derivative Works in Source or Object form.
|
73 |
-
|
74 |
-
3. Grant of Patent License. Subject to the terms and conditions of
|
75 |
-
this License, each Contributor hereby grants to You a perpetual,
|
76 |
-
worldwide, non-exclusive, no-charge, royalty-free, irrevocable
|
77 |
-
(except as stated in this section) patent license to make, have made,
|
78 |
-
use, offer to sell, sell, import, and otherwise transfer the Work,
|
79 |
-
where such license applies only to those patent claims licensable
|
80 |
-
by such Contributor that are necessarily infringed by their
|
81 |
-
Contribution(s) alone or by combination of their Contribution(s)
|
82 |
-
with the Work to which such Contribution(s) was submitted. If You
|
83 |
-
institute patent litigation against any entity (including a
|
84 |
-
cross-claim or counterclaim in a lawsuit) alleging that the Work
|
85 |
-
or a Contribution incorporated within the Work constitutes direct
|
86 |
-
or contributory patent infringement, then any patent licenses
|
87 |
-
granted to You under this License for that Work shall terminate
|
88 |
-
as of the date such litigation is filed.
|
89 |
-
|
90 |
-
4. Redistribution. You may reproduce and distribute copies of the
|
91 |
-
Work or Derivative Works thereof in any medium, with or without
|
92 |
-
modifications, and in Source or Object form, provided that You
|
93 |
-
meet the following conditions:
|
94 |
-
|
95 |
-
(a) You must give any other recipients of the Work or
|
96 |
-
Derivative Works a copy of this License; and
|
97 |
-
|
98 |
-
(b) You must cause any modified files to carry prominent notices
|
99 |
-
stating that You changed the files; and
|
100 |
-
|
101 |
-
(c) You must retain, in the Source form of any Derivative Works
|
102 |
-
that You distribute, all copyright, patent, trademark, and
|
103 |
-
attribution notices from the Source form of the Work,
|
104 |
-
excluding those notices that do not pertain to any part of
|
105 |
-
the Derivative Works; and
|
106 |
-
|
107 |
-
(d) If the Work includes a "NOTICE" text file as part of its
|
108 |
-
distribution, then any Derivative Works that You distribute must
|
109 |
-
include a readable copy of the attribution notices contained
|
110 |
-
within such NOTICE file, excluding those notices that do not
|
111 |
-
pertain to any part of the Derivative Works, in at least one
|
112 |
-
of the following places: within a NOTICE text file distributed
|
113 |
-
as part of the Derivative Works; within the Source form or
|
114 |
-
documentation, if provided along with the Derivative Works; or,
|
115 |
-
within a display generated by the Derivative Works, if and
|
116 |
-
wherever such third-party notices normally appear. The contents
|
117 |
-
of the NOTICE file are for informational purposes only and
|
118 |
-
do not modify the License. You may add Your own attribution
|
119 |
-
notices within Derivative Works that You distribute, alongside
|
120 |
-
or as an addendum to the NOTICE text from the Work, provided
|
121 |
-
that such additional attribution notices cannot be construed
|
122 |
-
as modifying the License.
|
123 |
-
|
124 |
-
You may add Your own copyright statement to Your modifications and
|
125 |
-
may provide additional or different license terms and conditions
|
126 |
-
for use, reproduction, or distribution of Your modifications, or
|
127 |
-
for any such Derivative Works as a whole, provided Your use,
|
128 |
-
reproduction, and distribution of the Work otherwise complies with
|
129 |
-
the conditions stated in this License.
|
130 |
-
|
131 |
-
5. Submission of Contributions. Unless You explicitly state otherwise,
|
132 |
-
any Contribution intentionally submitted for inclusion in the Work
|
133 |
-
by You to the Licensor shall be under the terms and conditions of
|
134 |
-
this License, without any additional terms or conditions.
|
135 |
-
Notwithstanding the above, nothing herein shall supersede or modify
|
136 |
-
the terms of any separate license agreement you may have executed
|
137 |
-
with Licensor regarding such Contributions.
|
138 |
-
|
139 |
-
6. Trademarks. This License does not grant permission to use the trade
|
140 |
-
names, trademarks, service marks, or product names of the Licensor,
|
141 |
-
except as required for reasonable and customary use in describing the
|
142 |
-
origin of the Work and reproducing the content of the NOTICE file.
|
143 |
-
|
144 |
-
7. Disclaimer of Warranty. Unless required by applicable law or
|
145 |
-
agreed to in writing, Licensor provides the Work (and each
|
146 |
-
Contributor provides its Contributions) on an "AS IS" BASIS,
|
147 |
-
WITHOUT WARRANTIES OR CONDITIONS OF ANY KIND, either express or
|
148 |
-
implied, including, without limitation, any warranties or conditions
|
149 |
-
of TITLE, NON-INFRINGEMENT, MERCHANTABILITY, or FITNESS FOR A
|
150 |
-
PARTICULAR PURPOSE. You are solely responsible for determining the
|
151 |
-
appropriateness of using or redistributing the Work and assume any
|
152 |
-
risks associated with Your exercise of permissions under this License.
|
153 |
-
|
154 |
-
8. Limitation of Liability. In no event and under no legal theory,
|
155 |
-
whether in tort (including negligence), contract, or otherwise,
|
156 |
-
unless required by applicable law (such as deliberate and grossly
|
157 |
-
negligent acts) or agreed to in writing, shall any Contributor be
|
158 |
-
liable to You for damages, including any direct, indirect, special,
|
159 |
-
incidental, or consequential damages of any character arising as a
|
160 |
-
result of this License or out of the use or inability to use the
|
161 |
-
Work (including but not limited to damages for loss of goodwill,
|
162 |
-
work stoppage, computer failure or malfunction, or any and all
|
163 |
-
other commercial damages or losses), even if such Contributor
|
164 |
-
has been advised of the possibility of such damages.
|
165 |
-
|
166 |
-
9. Accepting Warranty or Additional Liability. While redistributing
|
167 |
-
the Work or Derivative Works thereof, You may choose to offer,
|
168 |
-
and charge a fee for, acceptance of support, warranty, indemnity,
|
169 |
-
or other liability obligations and/or rights consistent with this
|
170 |
-
License. However, in accepting such obligations, You may act only
|
171 |
-
on Your own behalf and on Your sole responsibility, not on behalf
|
172 |
-
of any other Contributor, and only if You agree to indemnify,
|
173 |
-
defend, and hold each Contributor harmless for any liability
|
174 |
-
incurred by, or claims asserted against, such Contributor by reason
|
175 |
-
of your accepting any such warranty or additional liability.
|
176 |
-
|
177 |
-
END OF TERMS AND CONDITIONS
|
178 |
-
|
179 |
-
APPENDIX: How to apply the Apache License to your work.
|
180 |
-
|
181 |
-
To apply the Apache License to your work, attach the following
|
182 |
-
boilerplate notice, with the fields enclosed by brackets "[]"
|
183 |
-
replaced with your own identifying information. (Don't include
|
184 |
-
the brackets!) The text should be enclosed in the appropriate
|
185 |
-
comment syntax for the file format. We also recommend that a
|
186 |
-
file or class name and description of purpose be included on the
|
187 |
-
same "printed page" as the copyright notice for easier
|
188 |
-
identification within third-party archives.
|
189 |
-
|
190 |
-
Copyright [yyyy] [name of copyright owner]
|
191 |
-
|
192 |
-
Licensed under the Apache License, Version 2.0 (the "License");
|
193 |
-
you may not use this file except in compliance with the License.
|
194 |
-
You may obtain a copy of the License at
|
195 |
-
|
196 |
-
http://www.apache.org/licenses/LICENSE-2.0
|
197 |
-
|
198 |
-
Unless required by applicable law or agreed to in writing, software
|
199 |
-
distributed under the License is distributed on an "AS IS" BASIS,
|
200 |
-
WITHOUT WARRANTIES OR CONDITIONS OF ANY KIND, either express or implied.
|
201 |
-
See the License for the specific language governing permissions and
|
202 |
-
limitations under the License.
|
|
|
|
|
|
|
|
|
|
|
|
|
|
|
|
|
|
|
|
|
|
|
|
|
|
|
|
|
|
|
|
|
|
|
|
|
|
|
|
|
|
|
|
|
|
|
|
|
|
|
|
|
|
|
|
|
|
|
|
|
|
|
|
|
|
|
|
|
|
|
|
|
|
|
|
|
|
|
|
|
|
|
|
|
|
|
|
|
|
|
|
|
|
|
|
|
|
|
|
|
|
|
|
|
|
|
|
|
|
|
|
|
|
|
|
|
|
|
|
|
|
|
|
|
|
|
|
|
|
|
|
|
|
|
|
|
|
|
|
|
|
|
|
|
|
|
|
|
|
|
|
|
|
|
|
|
|
|
|
|
|
|
|
|
|
|
|
|
|
|
|
|
|
|
|
|
|
|
|
|
|
|
|
|
|
|
|
|
|
|
|
|
|
|
|
|
|
|
|
|
|
|
|
|
|
|
|
|
|
|
|
|
|
|
|
|
|
|
|
|
|
|
|
|
|
|
|
|
|
|
|
|
|
|
|
|
|
|
|
|
|
|
|
|
|
|
|
|
|
|
|
|
|
|
|
|
|
|
|
|
|
|
|
|
|
|
|
|
|
|
|
|
|
|
|
|
|
|
|
|
|
|
|
|
|
|
|
|
|
|
|
|
|
|
|
|
|
|
|
|
|
|
|
|
|
|
|
|
|
|
|
|
|
|
|
|
|
|
|
|
|
|
|
|
|
|
|
|
|
|
|
|
|
|
|
|
|
|
|
|
|
|
|
|
|
|
|
|
|
|
|
|
|
|
|
|
|
|
|
|
|
|
|
|
|
|
|
|
|
|
|
|
|
|
|
|
|
|
|
|
|
|
|
|
|
|
|
|
|
|
|
|
|
|
|
|
|
|
|
|
|
|
|
|
|
server/transformers/MANIFEST.in
DELETED
@@ -1 +0,0 @@
|
|
1 |
-
include LICENSE
|
|
|
|
server/transformers/Makefile
DELETED
@@ -1,24 +0,0 @@
|
|
1 |
-
.PHONY: quality style test test-examples
|
2 |
-
|
3 |
-
# Check that source code meets quality standards
|
4 |
-
|
5 |
-
quality:
|
6 |
-
black --check --line-length 119 --target-version py35 examples templates tests src utils
|
7 |
-
isort --check-only --recursive examples templates tests src utils
|
8 |
-
flake8 examples templates tests src utils
|
9 |
-
|
10 |
-
# Format source code automatically
|
11 |
-
|
12 |
-
style:
|
13 |
-
black --line-length 119 --target-version py35 examples templates tests src utils
|
14 |
-
isort --recursive examples templates tests src utils
|
15 |
-
|
16 |
-
# Run tests for the library
|
17 |
-
|
18 |
-
test:
|
19 |
-
python -m pytest -n auto --dist=loadfile -s -v ./tests/
|
20 |
-
|
21 |
-
# Run tests for examples
|
22 |
-
|
23 |
-
test-examples:
|
24 |
-
python -m pytest -n auto --dist=loadfile -s -v ./examples/
|
|
|
|
|
|
|
|
|
|
|
|
|
|
|
|
|
|
|
|
|
|
|
|
|
|
|
|
|
|
|
|
|
|
|
|
|
|
|
|
|
|
|
|
|
|
|
|
|
|
server/transformers/README.md
DELETED
@@ -1,676 +0,0 @@
|
|
1 |
-
<p align="center">
|
2 |
-
<br>
|
3 |
-
<img src="https://raw.githubusercontent.com/huggingface/transformers/master/docs/source/imgs/transformers_logo_name.png" width="400"/>
|
4 |
-
<br>
|
5 |
-
<p>
|
6 |
-
<p align="center">
|
7 |
-
<a href="https://circleci.com/gh/huggingface/transformers">
|
8 |
-
<img alt="Build" src="https://img.shields.io/circleci/build/github/huggingface/transformers/master">
|
9 |
-
</a>
|
10 |
-
<a href="https://github.com/huggingface/transformers/blob/master/LICENSE">
|
11 |
-
<img alt="GitHub" src="https://img.shields.io/github/license/huggingface/transformers.svg?color=blue">
|
12 |
-
</a>
|
13 |
-
<a href="https://huggingface.co/transformers/index.html">
|
14 |
-
<img alt="Documentation" src="https://img.shields.io/website/http/huggingface.co/transformers/index.html.svg?down_color=red&down_message=offline&up_message=online">
|
15 |
-
</a>
|
16 |
-
<a href="https://github.com/huggingface/transformers/releases">
|
17 |
-
<img alt="GitHub release" src="https://img.shields.io/github/release/huggingface/transformers.svg">
|
18 |
-
</a>
|
19 |
-
</p>
|
20 |
-
|
21 |
-
<h3 align="center">
|
22 |
-
<p>State-of-the-art Natural Language Processing for TensorFlow 2.0 and PyTorch
|
23 |
-
</h3>
|
24 |
-
|
25 |
-
🤗 Transformers (formerly known as `pytorch-transformers` and `pytorch-pretrained-bert`) provides state-of-the-art general-purpose architectures (BERT, GPT-2, RoBERTa, XLM, DistilBert, XLNet, CTRL...) for Natural Language Understanding (NLU) and Natural Language Generation (NLG) with over 32+ pretrained models in 100+ languages and deep interoperability between TensorFlow 2.0 and PyTorch.
|
26 |
-
|
27 |
-
### Features
|
28 |
-
|
29 |
-
- As easy to use as pytorch-transformers
|
30 |
-
- As powerful and concise as Keras
|
31 |
-
- High performance on NLU and NLG tasks
|
32 |
-
- Low barrier to entry for educators and practitioners
|
33 |
-
|
34 |
-
State-of-the-art NLP for everyone
|
35 |
-
- Deep learning researchers
|
36 |
-
- Hands-on practitioners
|
37 |
-
- AI/ML/NLP teachers and educators
|
38 |
-
|
39 |
-
Lower compute costs, smaller carbon footprint
|
40 |
-
- Researchers can share trained models instead of always retraining
|
41 |
-
- Practitioners can reduce compute time and production costs
|
42 |
-
- 10 architectures with over 30 pretrained models, some in more than 100 languages
|
43 |
-
|
44 |
-
Choose the right framework for every part of a model's lifetime
|
45 |
-
- Train state-of-the-art models in 3 lines of code
|
46 |
-
- Deep interoperability between TensorFlow 2.0 and PyTorch models
|
47 |
-
- Move a single model between TF2.0/PyTorch frameworks at will
|
48 |
-
- Seamlessly pick the right framework for training, evaluation, production
|
49 |
-
|
50 |
-
|
51 |
-
| Section | Description |
|
52 |
-
|-|-|
|
53 |
-
| [Installation](#installation) | How to install the package |
|
54 |
-
| [Model architectures](#model-architectures) | Architectures (with pretrained weights) |
|
55 |
-
| [Online demo](#online-demo) | Experimenting with this repo’s text generation capabilities |
|
56 |
-
| [Quick tour: Usage](#quick-tour) | Tokenizers & models usage: Bert and GPT-2 |
|
57 |
-
| [Quick tour: TF 2.0 and PyTorch ](#Quick-tour-TF-20-training-and-PyTorch-interoperability) | Train a TF 2.0 model in 10 lines of code, load it in PyTorch |
|
58 |
-
| [Quick tour: pipelines](#quick-tour-of-pipelines) | Using Pipelines: Wrapper around tokenizer and models to use finetuned models |
|
59 |
-
| [Quick tour: Fine-tuning/usage scripts](#quick-tour-of-the-fine-tuningusage-scripts) | Using provided scripts: GLUE, SQuAD and Text generation |
|
60 |
-
| [Quick tour: Share your models ](#Quick-tour-of-model-sharing) | Upload and share your fine-tuned models with the community |
|
61 |
-
| [Migrating from pytorch-transformers to transformers](#Migrating-from-pytorch-transformers-to-transformers) | Migrating your code from pytorch-transformers to transformers |
|
62 |
-
| [Migrating from pytorch-pretrained-bert to pytorch-transformers](#Migrating-from-pytorch-pretrained-bert-to-transformers) | Migrating your code from pytorch-pretrained-bert to transformers |
|
63 |
-
| [Documentation][(v2.3.0)](https://huggingface.co/transformers/v2.3.0)[(v2.2.0/v2.2.1/v2.2.2)](https://huggingface.co/transformers/v2.2.0) [(v2.1.1)](https://huggingface.co/transformers/v2.1.1) [(v2.0.0)](https://huggingface.co/transformers/v2.0.0) [(v1.2.0)](https://huggingface.co/transformers/v1.2.0) [(v1.1.0)](https://huggingface.co/transformers/v1.1.0) [(v1.0.0)](https://huggingface.co/transformers/v1.0.0) [(master)](https://huggingface.co/transformers) | Full API documentation and more |
|
64 |
-
|
65 |
-
## Installation
|
66 |
-
|
67 |
-
This repo is tested on Python 3.5+, PyTorch 1.0.0+ and TensorFlow 2.0.0-rc1
|
68 |
-
|
69 |
-
You should install 🤗 Transformers in a [virtual environment](https://docs.python.org/3/library/venv.html). If you're unfamiliar with Python virtual environments, check out the [user guide](https://packaging.python.org/guides/installing-using-pip-and-virtual-environments/).
|
70 |
-
|
71 |
-
Create a virtual environment with the version of Python you're going to use and activate it.
|
72 |
-
|
73 |
-
Now, if you want to use 🤗 Transformers, you can install it with pip. If you'd like to play with the examples, you must install it from source.
|
74 |
-
|
75 |
-
### With pip
|
76 |
-
|
77 |
-
First you need to install one of, or both, TensorFlow 2.0 and PyTorch.
|
78 |
-
Please refer to [TensorFlow installation page](https://www.tensorflow.org/install/pip#tensorflow-2.0-rc-is-available) and/or [PyTorch installation page](https://pytorch.org/get-started/locally/#start-locally) regarding the specific install command for your platform.
|
79 |
-
|
80 |
-
When TensorFlow 2.0 and/or PyTorch has been installed, 🤗 Transformers can be installed using pip as follows:
|
81 |
-
|
82 |
-
```bash
|
83 |
-
pip install transformers
|
84 |
-
```
|
85 |
-
|
86 |
-
### From source
|
87 |
-
|
88 |
-
Here also, you first need to install one of, or both, TensorFlow 2.0 and PyTorch.
|
89 |
-
Please refer to [TensorFlow installation page](https://www.tensorflow.org/install/pip#tensorflow-2.0-rc-is-available) and/or [PyTorch installation page](https://pytorch.org/get-started/locally/#start-locally) regarding the specific install command for your platform.
|
90 |
-
|
91 |
-
When TensorFlow 2.0 and/or PyTorch has been installed, you can install from source by cloning the repository and running:
|
92 |
-
|
93 |
-
```bash
|
94 |
-
git clone https://github.com/huggingface/transformers
|
95 |
-
cd transformers
|
96 |
-
pip install .
|
97 |
-
```
|
98 |
-
|
99 |
-
When you update the repository, you should upgrade the transformers installation and its dependencies as follows:
|
100 |
-
|
101 |
-
```bash
|
102 |
-
git pull
|
103 |
-
pip install --upgrade .
|
104 |
-
```
|
105 |
-
|
106 |
-
### Run the examples
|
107 |
-
|
108 |
-
Examples are included in the repository but are not shipped with the library.
|
109 |
-
|
110 |
-
Therefore, in order to run the latest versions of the examples, you need to install from source, as described above.
|
111 |
-
|
112 |
-
Look at the [README](https://github.com/huggingface/transformers/blob/master/examples/README.md) for how to run examples.
|
113 |
-
|
114 |
-
### Tests
|
115 |
-
|
116 |
-
A series of tests are included for the library and for some example scripts. Library tests can be found in the [tests folder](https://github.com/huggingface/transformers/tree/master/tests) and examples tests in the [examples folder](https://github.com/huggingface/transformers/tree/master/examples).
|
117 |
-
|
118 |
-
Depending on which framework is installed (TensorFlow 2.0 and/or PyTorch), the irrelevant tests will be skipped. Ensure that both frameworks are installed if you want to execute all tests.
|
119 |
-
|
120 |
-
Here's the easiest way to run tests for the library:
|
121 |
-
|
122 |
-
```bash
|
123 |
-
pip install -e ".[testing]"
|
124 |
-
make test
|
125 |
-
```
|
126 |
-
|
127 |
-
and for the examples:
|
128 |
-
|
129 |
-
```bash
|
130 |
-
pip install -e ".[testing]"
|
131 |
-
pip install -r examples/requirements.txt
|
132 |
-
make test-examples
|
133 |
-
```
|
134 |
-
|
135 |
-
For details, refer to the [contributing guide](https://github.com/huggingface/transformers/blob/master/CONTRIBUTING.md#tests).
|
136 |
-
|
137 |
-
### Do you want to run a Transformer model on a mobile device?
|
138 |
-
|
139 |
-
You should check out our [`swift-coreml-transformers`](https://github.com/huggingface/swift-coreml-transformers) repo.
|
140 |
-
|
141 |
-
It contains a set of tools to convert PyTorch or TensorFlow 2.0 trained Transformer models (currently contains `GPT-2`, `DistilGPT-2`, `BERT`, and `DistilBERT`) to CoreML models that run on iOS devices.
|
142 |
-
|
143 |
-
At some point in the future, you'll be able to seamlessly move from pre-training or fine-tuning models to productizing them in CoreML, or prototype a model or an app in CoreML then research its hyperparameters or architecture from TensorFlow 2.0 and/or PyTorch. Super exciting!
|
144 |
-
|
145 |
-
## Model architectures
|
146 |
-
|
147 |
-
🤗 Transformers currently provides the following NLU/NLG architectures:
|
148 |
-
|
149 |
-
1. **[BERT](https://github.com/google-research/bert)** (from Google) released with the paper [BERT: Pre-training of Deep Bidirectional Transformers for Language Understanding](https://arxiv.org/abs/1810.04805) by Jacob Devlin, Ming-Wei Chang, Kenton Lee and Kristina Toutanova.
|
150 |
-
2. **[GPT](https://github.com/openai/finetune-transformer-lm)** (from OpenAI) released with the paper [Improving Language Understanding by Generative Pre-Training](https://blog.openai.com/language-unsupervised/) by Alec Radford, Karthik Narasimhan, Tim Salimans and Ilya Sutskever.
|
151 |
-
3. **[GPT-2](https://blog.openai.com/better-language-models/)** (from OpenAI) released with the paper [Language Models are Unsupervised Multitask Learners](https://blog.openai.com/better-language-models/) by Alec Radford*, Jeffrey Wu*, Rewon Child, David Luan, Dario Amodei** and Ilya Sutskever**.
|
152 |
-
4. **[Transformer-XL](https://github.com/kimiyoung/transformer-xl)** (from Google/CMU) released with the paper [Transformer-XL: Attentive Language Models Beyond a Fixed-Length Context](https://arxiv.org/abs/1901.02860) by Zihang Dai*, Zhilin Yang*, Yiming Yang, Jaime Carbonell, Quoc V. Le, Ruslan Salakhutdinov.
|
153 |
-
5. **[XLNet](https://github.com/zihangdai/xlnet/)** (from Google/CMU) released with the paper [XLNet: Generalized Autoregressive Pretraining for Language Understanding](https://arxiv.org/abs/1906.08237) by Zhilin Yang*, Zihang Dai*, Yiming Yang, Jaime Carbonell, Ruslan Salakhutdinov, Quoc V. Le.
|
154 |
-
6. **[XLM](https://github.com/facebookresearch/XLM/)** (from Facebook) released together with the paper [Cross-lingual Language Model Pretraining](https://arxiv.org/abs/1901.07291) by Guillaume Lample and Alexis Conneau.
|
155 |
-
7. **[RoBERTa](https://github.com/pytorch/fairseq/tree/master/examples/roberta)** (from Facebook), released together with the paper a [Robustly Optimized BERT Pretraining Approach](https://arxiv.org/abs/1907.11692) by Yinhan Liu, Myle Ott, Naman Goyal, Jingfei Du, Mandar Joshi, Danqi Chen, Omer Levy, Mike Lewis, Luke Zettlemoyer, Veselin Stoyanov.
|
156 |
-
8. **[DistilBERT](https://github.com/huggingface/transformers/tree/master/examples/distillation)** (from HuggingFace), released together with the paper [DistilBERT, a distilled version of BERT: smaller, faster, cheaper and lighter](https://arxiv.org/abs/1910.01108) by Victor Sanh, Lysandre Debut and Thomas Wolf. The same method has been applied to compress GPT2 into [DistilGPT2](https://github.com/huggingface/transformers/tree/master/examples/distillation), RoBERTa into [DistilRoBERTa](https://github.com/huggingface/transformers/tree/master/examples/distillation), Multilingual BERT into [DistilmBERT](https://github.com/huggingface/transformers/tree/master/examples/distillation) and a German version of DistilBERT.
|
157 |
-
9. **[CTRL](https://github.com/salesforce/ctrl/)** (from Salesforce) released with the paper [CTRL: A Conditional Transformer Language Model for Controllable Generation](https://arxiv.org/abs/1909.05858) by Nitish Shirish Keskar*, Bryan McCann*, Lav R. Varshney, Caiming Xiong and Richard Socher.
|
158 |
-
10. **[CamemBERT](https://camembert-model.fr)** (from Inria/Facebook/Sorbonne) released with the paper [CamemBERT: a Tasty French Language Model](https://arxiv.org/abs/1911.03894) by Louis Martin*, Benjamin Muller*, Pedro Javier Ortiz Suárez*, Yoann Dupont, Laurent Romary, Éric Villemonte de la Clergerie, Djamé Seddah and Benoît Sagot.
|
159 |
-
11. **[ALBERT](https://github.com/google-research/ALBERT)** (from Google Research and the Toyota Technological Institute at Chicago) released with the paper [ALBERT: A Lite BERT for Self-supervised Learning of Language Representations](https://arxiv.org/abs/1909.11942), by Zhenzhong Lan, Mingda Chen, Sebastian Goodman, Kevin Gimpel, Piyush Sharma, Radu Soricut.
|
160 |
-
12. **[T5](https://github.com/google-research/text-to-text-transfer-transformer)** (from Google AI) released with the paper [Exploring the Limits of Transfer Learning with a Unified Text-to-Text Transformer](https://arxiv.org/abs/1910.10683) by Colin Raffel and Noam Shazeer and Adam Roberts and Katherine Lee and Sharan Narang and Michael Matena and Yanqi Zhou and Wei Li and Peter J. Liu.
|
161 |
-
13. **[XLM-RoBERTa](https://github.com/pytorch/fairseq/tree/master/examples/xlmr)** (from Facebook AI), released together with the paper [Unsupervised Cross-lingual Representation Learning at Scale](https://arxiv.org/abs/1911.02116) by Alexis Conneau*, Kartikay Khandelwal*, Naman Goyal, Vishrav Chaudhary, Guillaume Wenzek, Francisco Guzmán, Edouard Grave, Myle Ott, Luke Zettlemoyer and Veselin Stoyanov.
|
162 |
-
14. **[MMBT](https://github.com/facebookresearch/mmbt/)** (from Facebook), released together with the paper a [Supervised Multimodal Bitransformers for Classifying Images and Text](https://arxiv.org/pdf/1909.02950.pdf) by Douwe Kiela, Suvrat Bhooshan, Hamed Firooz, Davide Testuggine.
|
163 |
-
15. **[Other community models](https://huggingface.co/models)**, contributed by the [community](https://huggingface.co/users).
|
164 |
-
16. Want to contribute a new model? We have added a **detailed guide and templates** to guide you in the process of adding a new model. You can find them in the [`templates`](./templates) folder of the repository. Be sure to check the [contributing guidelines](./CONTRIBUTING.md) and contact the maintainers or open an issue to collect feedbacks before starting your PR.
|
165 |
-
|
166 |
-
These implementations have been tested on several datasets (see the example scripts) and should match the performances of the original implementations (e.g. ~93 F1 on SQuAD for BERT Whole-Word-Masking, ~88 F1 on RocStories for OpenAI GPT, ~18.3 perplexity on WikiText 103 for Transformer-XL, ~0.916 Peason R coefficient on STS-B for XLNet). You can find more details on the performances in the Examples section of the [documentation](https://huggingface.co/transformers/examples.html).
|
167 |
-
|
168 |
-
## Online demo
|
169 |
-
|
170 |
-
**[Write With Transformer](https://transformer.huggingface.co)**, built by the Hugging Face team at transformer.huggingface.co, is the official demo of this repo’s text generation capabilities.
|
171 |
-
You can use it to experiment with completions generated by `GPT2Model`, `TransfoXLModel`, and `XLNetModel`.
|
172 |
-
|
173 |
-
> “🦄 Write with transformer is to writing what calculators are to calculus.”
|
174 |
-
|
175 |
-
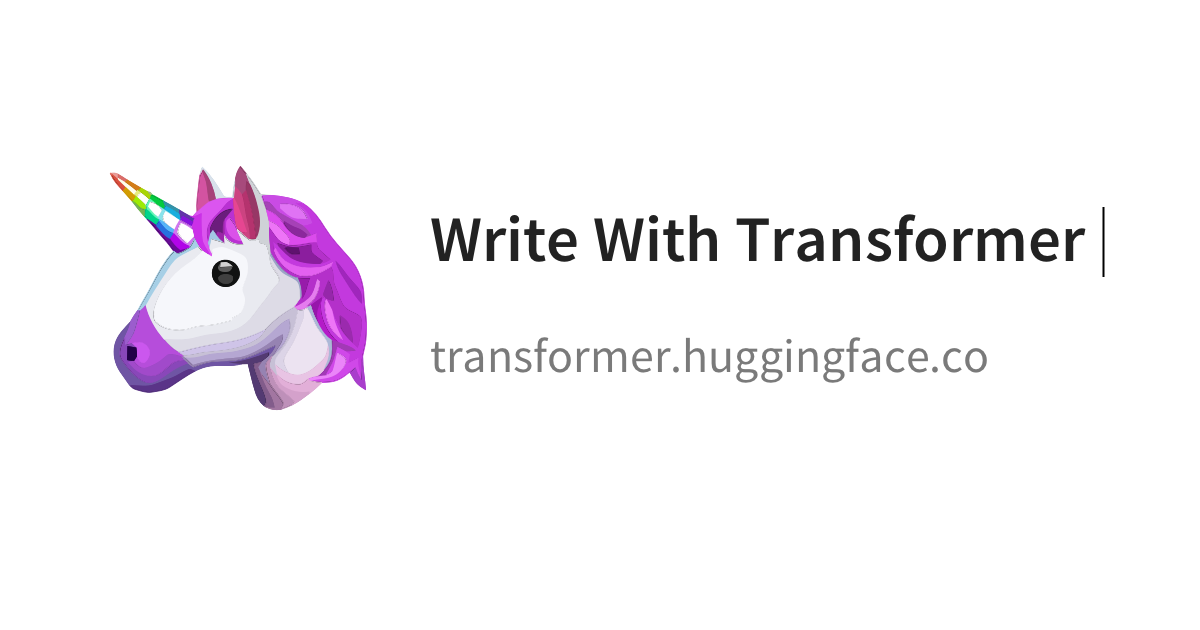
|
176 |
-
|
177 |
-
## Quick tour
|
178 |
-
|
179 |
-
Let's do a very quick overview of the model architectures in 🤗 Transformers. Detailed examples for each model architecture (Bert, GPT, GPT-2, Transformer-XL, XLNet and XLM) can be found in the [full documentation](https://huggingface.co/transformers/).
|
180 |
-
|
181 |
-
```python
|
182 |
-
import torch
|
183 |
-
from transformers import *
|
184 |
-
|
185 |
-
# Transformers has a unified API
|
186 |
-
# for 10 transformer architectures and 30 pretrained weights.
|
187 |
-
# Model | Tokenizer | Pretrained weights shortcut
|
188 |
-
MODELS = [(BertModel, BertTokenizer, 'bert-base-uncased'),
|
189 |
-
(OpenAIGPTModel, OpenAIGPTTokenizer, 'openai-gpt'),
|
190 |
-
(GPT2Model, GPT2Tokenizer, 'gpt2'),
|
191 |
-
(CTRLModel, CTRLTokenizer, 'ctrl'),
|
192 |
-
(TransfoXLModel, TransfoXLTokenizer, 'transfo-xl-wt103'),
|
193 |
-
(XLNetModel, XLNetTokenizer, 'xlnet-base-cased'),
|
194 |
-
(XLMModel, XLMTokenizer, 'xlm-mlm-enfr-1024'),
|
195 |
-
(DistilBertModel, DistilBertTokenizer, 'distilbert-base-uncased'),
|
196 |
-
(RobertaModel, RobertaTokenizer, 'roberta-base'),
|
197 |
-
(XLMRobertaModel, XLMRobertaTokenizer, 'xlm-roberta-base'),
|
198 |
-
]
|
199 |
-
|
200 |
-
# To use TensorFlow 2.0 versions of the models, simply prefix the class names with 'TF', e.g. `TFRobertaModel` is the TF 2.0 counterpart of the PyTorch model `RobertaModel`
|
201 |
-
|
202 |
-
# Let's encode some text in a sequence of hidden-states using each model:
|
203 |
-
for model_class, tokenizer_class, pretrained_weights in MODELS:
|
204 |
-
# Load pretrained model/tokenizer
|
205 |
-
tokenizer = tokenizer_class.from_pretrained(pretrained_weights)
|
206 |
-
model = model_class.from_pretrained(pretrained_weights)
|
207 |
-
|
208 |
-
# Encode text
|
209 |
-
input_ids = torch.tensor([tokenizer.encode("Here is some text to encode", add_special_tokens=True)]) # Add special tokens takes care of adding [CLS], [SEP], <s>... tokens in the right way for each model.
|
210 |
-
with torch.no_grad():
|
211 |
-
last_hidden_states = model(input_ids)[0] # Models outputs are now tuples
|
212 |
-
|
213 |
-
# Each architecture is provided with several class for fine-tuning on down-stream tasks, e.g.
|
214 |
-
BERT_MODEL_CLASSES = [BertModel, BertForPreTraining, BertForMaskedLM, BertForNextSentencePrediction,
|
215 |
-
BertForSequenceClassification, BertForTokenClassification, BertForQuestionAnswering]
|
216 |
-
|
217 |
-
# All the classes for an architecture can be initiated from pretrained weights for this architecture
|
218 |
-
# Note that additional weights added for fine-tuning are only initialized
|
219 |
-
# and need to be trained on the down-stream task
|
220 |
-
pretrained_weights = 'bert-base-uncased'
|
221 |
-
tokenizer = BertTokenizer.from_pretrained(pretrained_weights)
|
222 |
-
for model_class in BERT_MODEL_CLASSES:
|
223 |
-
# Load pretrained model/tokenizer
|
224 |
-
model = model_class.from_pretrained(pretrained_weights)
|
225 |
-
|
226 |
-
# Models can return full list of hidden-states & attentions weights at each layer
|
227 |
-
model = model_class.from_pretrained(pretrained_weights,
|
228 |
-
output_hidden_states=True,
|
229 |
-
output_attentions=True)
|
230 |
-
input_ids = torch.tensor([tokenizer.encode("Let's see all hidden-states and attentions on this text")])
|
231 |
-
all_hidden_states, all_attentions = model(input_ids)[-2:]
|
232 |
-
|
233 |
-
# Models are compatible with Torchscript
|
234 |
-
model = model_class.from_pretrained(pretrained_weights, torchscript=True)
|
235 |
-
traced_model = torch.jit.trace(model, (input_ids,))
|
236 |
-
|
237 |
-
# Simple serialization for models and tokenizers
|
238 |
-
model.save_pretrained('./directory/to/save/') # save
|
239 |
-
model = model_class.from_pretrained('./directory/to/save/') # re-load
|
240 |
-
tokenizer.save_pretrained('./directory/to/save/') # save
|
241 |
-
tokenizer = BertTokenizer.from_pretrained('./directory/to/save/') # re-load
|
242 |
-
|
243 |
-
# SOTA examples for GLUE, SQUAD, text generation...
|
244 |
-
```
|
245 |
-
|
246 |
-
## Quick tour TF 2.0 training and PyTorch interoperability
|
247 |
-
|
248 |
-
Let's do a quick example of how a TensorFlow 2.0 model can be trained in 12 lines of code with 🤗 Transformers and then loaded in PyTorch for fast inspection/tests.
|
249 |
-
|
250 |
-
```python
|
251 |
-
import tensorflow as tf
|
252 |
-
import tensorflow_datasets
|
253 |
-
from transformers import *
|
254 |
-
|
255 |
-
# Load dataset, tokenizer, model from pretrained model/vocabulary
|
256 |
-
tokenizer = BertTokenizer.from_pretrained('bert-base-cased')
|
257 |
-
model = TFBertForSequenceClassification.from_pretrained('bert-base-cased')
|
258 |
-
data = tensorflow_datasets.load('glue/mrpc')
|
259 |
-
|
260 |
-
# Prepare dataset for GLUE as a tf.data.Dataset instance
|
261 |
-
train_dataset = glue_convert_examples_to_features(data['train'], tokenizer, max_length=128, task='mrpc')
|
262 |
-
valid_dataset = glue_convert_examples_to_features(data['validation'], tokenizer, max_length=128, task='mrpc')
|
263 |
-
train_dataset = train_dataset.shuffle(100).batch(32).repeat(2)
|
264 |
-
valid_dataset = valid_dataset.batch(64)
|
265 |
-
|
266 |
-
# Prepare training: Compile tf.keras model with optimizer, loss and learning rate schedule
|
267 |
-
optimizer = tf.keras.optimizers.Adam(learning_rate=3e-5, epsilon=1e-08, clipnorm=1.0)
|
268 |
-
loss = tf.keras.losses.SparseCategoricalCrossentropy(from_logits=True)
|
269 |
-
metric = tf.keras.metrics.SparseCategoricalAccuracy('accuracy')
|
270 |
-
model.compile(optimizer=optimizer, loss=loss, metrics=[metric])
|
271 |
-
|
272 |
-
# Train and evaluate using tf.keras.Model.fit()
|
273 |
-
history = model.fit(train_dataset, epochs=2, steps_per_epoch=115,
|
274 |
-
validation_data=valid_dataset, validation_steps=7)
|
275 |
-
|
276 |
-
# Load the TensorFlow model in PyTorch for inspection
|
277 |
-
model.save_pretrained('./save/')
|
278 |
-
pytorch_model = BertForSequenceClassification.from_pretrained('./save/', from_tf=True)
|
279 |
-
|
280 |
-
# Quickly test a few predictions - MRPC is a paraphrasing task, let's see if our model learned the task
|
281 |
-
sentence_0 = "This research was consistent with his findings."
|
282 |
-
sentence_1 = "His findings were compatible with this research."
|
283 |
-
sentence_2 = "His findings were not compatible with this research."
|
284 |
-
inputs_1 = tokenizer.encode_plus(sentence_0, sentence_1, add_special_tokens=True, return_tensors='pt')
|
285 |
-
inputs_2 = tokenizer.encode_plus(sentence_0, sentence_2, add_special_tokens=True, return_tensors='pt')
|
286 |
-
|
287 |
-
pred_1 = pytorch_model(inputs_1['input_ids'], token_type_ids=inputs_1['token_type_ids'])[0].argmax().item()
|
288 |
-
pred_2 = pytorch_model(inputs_2['input_ids'], token_type_ids=inputs_2['token_type_ids'])[0].argmax().item()
|
289 |
-
|
290 |
-
print("sentence_1 is", "a paraphrase" if pred_1 else "not a paraphrase", "of sentence_0")
|
291 |
-
print("sentence_2 is", "a paraphrase" if pred_2 else "not a paraphrase", "of sentence_0")
|
292 |
-
```
|
293 |
-
|
294 |
-
## Quick tour of the fine-tuning/usage scripts
|
295 |
-
|
296 |
-
**Important**
|
297 |
-
Before running the fine-tuning scripts, please read the
|
298 |
-
[instructions](#run-the-examples) on how to
|
299 |
-
setup your environment to run the examples.
|
300 |
-
|
301 |
-
The library comprises several example scripts with SOTA performances for NLU and NLG tasks:
|
302 |
-
|
303 |
-
- `run_glue.py`: an example fine-tuning Bert, XLNet and XLM on nine different GLUE tasks (*sequence-level classification*)
|
304 |
-
- `run_squad.py`: an example fine-tuning Bert, XLNet and XLM on the question answering dataset SQuAD 2.0 (*token-level classification*)
|
305 |
-
- `run_generation.py`: an example using GPT, GPT-2, CTRL, Transformer-XL and XLNet for conditional language generation
|
306 |
-
- other model-specific examples (see the documentation).
|
307 |
-
|
308 |
-
Here are three quick usage examples for these scripts:
|
309 |
-
|
310 |
-
### `run_glue.py`: Fine-tuning on GLUE tasks for sequence classification
|
311 |
-
|
312 |
-
The [General Language Understanding Evaluation (GLUE) benchmark](https://gluebenchmark.com/) is a collection of nine sentence- or sentence-pair language understanding tasks for evaluating and analyzing natural language understanding systems.
|
313 |
-
|
314 |
-
Before running anyone of these GLUE tasks you should download the
|
315 |
-
[GLUE data](https://gluebenchmark.com/tasks) by running
|
316 |
-
[this script](https://gist.github.com/W4ngatang/60c2bdb54d156a41194446737ce03e2e)
|
317 |
-
and unpack it to some directory `$GLUE_DIR`.
|
318 |
-
|
319 |
-
You should also install the additional packages required by the examples:
|
320 |
-
|
321 |
-
```shell
|
322 |
-
pip install -r ./examples/requirements.txt
|
323 |
-
```
|
324 |
-
|
325 |
-
```shell
|
326 |
-
export GLUE_DIR=/path/to/glue
|
327 |
-
export TASK_NAME=MRPC
|
328 |
-
|
329 |
-
python ./examples/run_glue.py \
|
330 |
-
--model_type bert \
|
331 |
-
--model_name_or_path bert-base-uncased \
|
332 |
-
--task_name $TASK_NAME \
|
333 |
-
--do_train \
|
334 |
-
--do_eval \
|
335 |
-
--do_lower_case \
|
336 |
-
--data_dir $GLUE_DIR/$TASK_NAME \
|
337 |
-
--max_seq_length 128 \
|
338 |
-
--per_gpu_eval_batch_size=8 \
|
339 |
-
--per_gpu_train_batch_size=8 \
|
340 |
-
--learning_rate 2e-5 \
|
341 |
-
--num_train_epochs 3.0 \
|
342 |
-
--output_dir /tmp/$TASK_NAME/
|
343 |
-
```
|
344 |
-
|
345 |
-
where task name can be one of CoLA, SST-2, MRPC, STS-B, QQP, MNLI, QNLI, RTE, WNLI.
|
346 |
-
|
347 |
-
The dev set results will be present within the text file 'eval_results.txt' in the specified output_dir. In case of MNLI, since there are two separate dev sets, matched and mismatched, there will be a separate output folder called '/tmp/MNLI-MM/' in addition to '/tmp/MNLI/'.
|
348 |
-
|
349 |
-
#### Fine-tuning XLNet model on the STS-B regression task
|
350 |
-
|
351 |
-
This example code fine-tunes XLNet on the STS-B corpus using parallel training on a server with 4 V100 GPUs.
|
352 |
-
Parallel training is a simple way to use several GPUs (but is slower and less flexible than distributed training, see below).
|
353 |
-
|
354 |
-
```shell
|
355 |
-
export GLUE_DIR=/path/to/glue
|
356 |
-
|
357 |
-
python ./examples/run_glue.py \
|
358 |
-
--model_type xlnet \
|
359 |
-
--model_name_or_path xlnet-large-cased \
|
360 |
-
--do_train \
|
361 |
-
--do_eval \
|
362 |
-
--task_name=sts-b \
|
363 |
-
--data_dir=${GLUE_DIR}/STS-B \
|
364 |
-
--output_dir=./proc_data/sts-b-110 \
|
365 |
-
--max_seq_length=128 \
|
366 |
-
--per_gpu_eval_batch_size=8 \
|
367 |
-
--per_gpu_train_batch_size=8 \
|
368 |
-
--gradient_accumulation_steps=1 \
|
369 |
-
--max_steps=1200 \
|
370 |
-
--model_name=xlnet-large-cased \
|
371 |
-
--overwrite_output_dir \
|
372 |
-
--overwrite_cache \
|
373 |
-
--warmup_steps=120
|
374 |
-
```
|
375 |
-
|
376 |
-
On this machine we thus have a batch size of 32, please increase `gradient_accumulation_steps` to reach the same batch size if you have a smaller machine. These hyper-parameters should result in a Pearson correlation coefficient of `+0.917` on the development set.
|
377 |
-
|
378 |
-
#### Fine-tuning Bert model on the MRPC classification task
|
379 |
-
|
380 |
-
This example code fine-tunes the Bert Whole Word Masking model on the Microsoft Research Paraphrase Corpus (MRPC) corpus using distributed training on 8 V100 GPUs to reach a F1 > 92.
|
381 |
-
|
382 |
-
```bash
|
383 |
-
python -m torch.distributed.launch --nproc_per_node 8 ./examples/run_glue.py \
|
384 |
-
--model_type bert \
|
385 |
-
--model_name_or_path bert-large-uncased-whole-word-masking \
|
386 |
-
--task_name MRPC \
|
387 |
-
--do_train \
|
388 |
-
--do_eval \
|
389 |
-
--do_lower_case \
|
390 |
-
--data_dir $GLUE_DIR/MRPC/ \
|
391 |
-
--max_seq_length 128 \
|
392 |
-
--per_gpu_eval_batch_size=8 \
|
393 |
-
--per_gpu_train_batch_size=8 \
|
394 |
-
--learning_rate 2e-5 \
|
395 |
-
--num_train_epochs 3.0 \
|
396 |
-
--output_dir /tmp/mrpc_output/ \
|
397 |
-
--overwrite_output_dir \
|
398 |
-
--overwrite_cache \
|
399 |
-
```
|
400 |
-
|
401 |
-
Training with these hyper-parameters gave us the following results:
|
402 |
-
|
403 |
-
```bash
|
404 |
-
acc = 0.8823529411764706
|
405 |
-
acc_and_f1 = 0.901702786377709
|
406 |
-
eval_loss = 0.3418912578906332
|
407 |
-
f1 = 0.9210526315789473
|
408 |
-
global_step = 174
|
409 |
-
loss = 0.07231863956341798
|
410 |
-
```
|
411 |
-
|
412 |
-
### `run_squad.py`: Fine-tuning on SQuAD for question-answering
|
413 |
-
|
414 |
-
This example code fine-tunes BERT on the SQuAD dataset using distributed training on 8 V100 GPUs and Bert Whole Word Masking uncased model to reach a F1 > 93 on SQuAD:
|
415 |
-
|
416 |
-
```bash
|
417 |
-
python -m torch.distributed.launch --nproc_per_node=8 ./examples/run_squad.py \
|
418 |
-
--model_type bert \
|
419 |
-
--model_name_or_path bert-large-uncased-whole-word-masking \
|
420 |
-
--do_train \
|
421 |
-
--do_eval \
|
422 |
-
--do_lower_case \
|
423 |
-
--train_file $SQUAD_DIR/train-v1.1.json \
|
424 |
-
--predict_file $SQUAD_DIR/dev-v1.1.json \
|
425 |
-
--learning_rate 3e-5 \
|
426 |
-
--num_train_epochs 2 \
|
427 |
-
--max_seq_length 384 \
|
428 |
-
--doc_stride 128 \
|
429 |
-
--output_dir ../models/wwm_uncased_finetuned_squad/ \
|
430 |
-
--per_gpu_eval_batch_size=3 \
|
431 |
-
--per_gpu_train_batch_size=3 \
|
432 |
-
```
|
433 |
-
|
434 |
-
Training with these hyper-parameters gave us the following results:
|
435 |
-
|
436 |
-
```bash
|
437 |
-
python $SQUAD_DIR/evaluate-v1.1.py $SQUAD_DIR/dev-v1.1.json ../models/wwm_uncased_finetuned_squad/predictions.json
|
438 |
-
{"exact_match": 86.91579943235573, "f1": 93.1532499015869}
|
439 |
-
```
|
440 |
-
|
441 |
-
This is the model provided as `bert-large-uncased-whole-word-masking-finetuned-squad`.
|
442 |
-
|
443 |
-
### `run_generation.py`: Text generation with GPT, GPT-2, CTRL, Transformer-XL and XLNet
|
444 |
-
|
445 |
-
A conditional generation script is also included to generate text from a prompt.
|
446 |
-
The generation script includes the [tricks](https://github.com/rusiaaman/XLNet-gen#methodology) proposed by Aman Rusia to get high-quality generation with memory models like Transformer-XL and XLNet (include a predefined text to make short inputs longer).
|
447 |
-
|
448 |
-
Here is how to run the script with the small version of OpenAI GPT-2 model:
|
449 |
-
|
450 |
-
```shell
|
451 |
-
python ./examples/run_generation.py \
|
452 |
-
--model_type=gpt2 \
|
453 |
-
--length=20 \
|
454 |
-
--model_name_or_path=gpt2 \
|
455 |
-
```
|
456 |
-
|
457 |
-
and from the Salesforce CTRL model:
|
458 |
-
```shell
|
459 |
-
python ./examples/run_generation.py \
|
460 |
-
--model_type=ctrl \
|
461 |
-
--length=20 \
|
462 |
-
--model_name_or_path=ctrl \
|
463 |
-
--temperature=0 \
|
464 |
-
--repetition_penalty=1.2 \
|
465 |
-
```
|
466 |
-
|
467 |
-
## Quick tour of model sharing
|
468 |
-
|
469 |
-
New in `v2.2.2`: you can now upload and share your fine-tuned models with the community, using the <abbr title="Command-line interface">CLI</abbr> that's built-in to the library.
|
470 |
-
|
471 |
-
**First, create an account on [https://huggingface.co/join](https://huggingface.co/join)**. Then:
|
472 |
-
|
473 |
-
```shell
|
474 |
-
transformers-cli login
|
475 |
-
# log in using the same credentials as on huggingface.co
|
476 |
-
```
|
477 |
-
Upload your model:
|
478 |
-
```shell
|
479 |
-
transformers-cli upload ./path/to/pretrained_model/
|
480 |
-
|
481 |
-
# ^^ Upload folder containing weights/tokenizer/config
|
482 |
-
# saved via `.save_pretrained()`
|
483 |
-
|
484 |
-
transformers-cli upload ./config.json [--filename folder/foobar.json]
|
485 |
-
|
486 |
-
# ^^ Upload a single file
|
487 |
-
# (you can optionally override its filename, which can be nested inside a folder)
|
488 |
-
```
|
489 |
-
|
490 |
-
Your model will then be accessible through its identifier, a concatenation of your username and the folder name above:
|
491 |
-
```python
|
492 |
-
"username/model_name"
|
493 |
-
```
|
494 |
-
|
495 |
-
Anyone can load it from code:
|
496 |
-
```python
|
497 |
-
tokenizer = AutoTokenizer.from_pretrained("username/pretrained_model")
|
498 |
-
model = AutoModel.from_pretrained("username/pretrained_model")
|
499 |
-
```
|
500 |
-
|
501 |
-
Finally, list all your files on S3:
|
502 |
-
```shell
|
503 |
-
transformers-cli s3 ls
|
504 |
-
# List all your S3 objects.
|
505 |
-
```
|
506 |
-
|
507 |
-
## Quick tour of pipelines
|
508 |
-
|
509 |
-
New in version `v2.3`: `Pipeline` are high-level objects which automatically handle tokenization, running your data through a transformers model
|
510 |
-
and outputting the result in a structured object.
|
511 |
-
|
512 |
-
You can create `Pipeline` objects for the following down-stream tasks:
|
513 |
-
|
514 |
-
- `feature-extraction`: Generates a tensor representation for the input sequence
|
515 |
-
- `ner`: Generates named entity mapping for each word in the input sequence.
|
516 |
-
- `sentiment-analysis`: Gives the polarity (positive / negative) of the whole input sequence.
|
517 |
-
- `question-answering`: Provided some context and a question refering to the context, it will extract the answer to the question
|
518 |
-
in the context.
|
519 |
-
|
520 |
-
```python
|
521 |
-
from transformers import pipeline
|
522 |
-
|
523 |
-
# Allocate a pipeline for sentiment-analysis
|
524 |
-
nlp = pipeline('sentiment-analysis')
|
525 |
-
nlp('We are very happy to include pipeline into the transformers repository.')
|
526 |
-
>>> {'label': 'POSITIVE', 'score': 0.99893874}
|
527 |
-
|
528 |
-
# Allocate a pipeline for question-answering
|
529 |
-
nlp = pipeline('question-answering')
|
530 |
-
nlp({
|
531 |
-
'question': 'What is the name of the repository ?',
|
532 |
-
'context': 'Pipeline have been included in the huggingface/transformers repository'
|
533 |
-
})
|
534 |
-
>>> {'score': 0.28756016668193496, 'start': 35, 'end': 59, 'answer': 'huggingface/transformers'}
|
535 |
-
```
|
536 |
-
|
537 |
-
## Migrating from pytorch-transformers to transformers
|
538 |
-
|
539 |
-
Here is a quick summary of what you should take care of when migrating from `pytorch-transformers` to `transformers`.
|
540 |
-
|
541 |
-
### Positional order of some models' keywords inputs (`attention_mask`, `token_type_ids`...) changed
|
542 |
-
|
543 |
-
To be able to use Torchscript (see #1010, #1204 and #1195) the specific order of some models **keywords inputs** (`attention_mask`, `token_type_ids`...) has been changed.
|
544 |
-
|
545 |
-
If you used to call the models with keyword names for keyword arguments, e.g. `model(inputs_ids, attention_mask=attention_mask, token_type_ids=token_type_ids)`, this should not cause any change.
|
546 |
-
|
547 |
-
If you used to call the models with positional inputs for keyword arguments, e.g. `model(inputs_ids, attention_mask, token_type_ids)`, you may have to double check the exact order of input arguments.
|
548 |
-
|
549 |
-
|
550 |
-
## Migrating from pytorch-pretrained-bert to transformers
|
551 |
-
|
552 |
-
Here is a quick summary of what you should take care of when migrating from `pytorch-pretrained-bert` to `transformers`.
|
553 |
-
|
554 |
-
### Models always output `tuples`
|
555 |
-
|
556 |
-
The main breaking change when migrating from `pytorch-pretrained-bert` to `transformers` is that every model's forward method always outputs a `tuple` with various elements depending on the model and the configuration parameters.
|
557 |
-
|
558 |
-
The exact content of the tuples for each model is detailed in the models' docstrings and the [documentation](https://huggingface.co/transformers/).
|
559 |
-
|
560 |
-
In pretty much every case, you will be fine by taking the first element of the output as the output you previously used in `pytorch-pretrained-bert`.
|
561 |
-
|
562 |
-
Here is a `pytorch-pretrained-bert` to `transformers` conversion example for a `BertForSequenceClassification` classification model:
|
563 |
-
|
564 |
-
```python
|
565 |
-
# Let's load our model
|
566 |
-
model = BertForSequenceClassification.from_pretrained('bert-base-uncased')
|
567 |
-
|
568 |
-
# If you used to have this line in pytorch-pretrained-bert:
|
569 |
-
loss = model(input_ids, labels=labels)
|
570 |
-
|
571 |
-
# Now just use this line in transformers to extract the loss from the output tuple:
|
572 |
-
outputs = model(input_ids, labels=labels)
|
573 |
-
loss = outputs[0]
|
574 |
-
|
575 |
-
# In transformers you can also have access to the logits:
|
576 |
-
loss, logits = outputs[:2]
|
577 |
-
|
578 |
-
# And even the attention weights if you configure the model to output them (and other outputs too, see the docstrings and documentation)
|
579 |
-
model = BertForSequenceClassification.from_pretrained('bert-base-uncased', output_attentions=True)
|
580 |
-
outputs = model(input_ids, labels=labels)
|
581 |
-
loss, logits, attentions = outputs
|
582 |
-
```
|
583 |
-
|
584 |
-
### Using hidden states
|
585 |
-
|
586 |
-
By enabling the configuration option `output_hidden_states`, it was possible to retrieve the last hidden states of the encoder. In `pytorch-transformers` as well as `transformers` the return value has changed slightly: `all_hidden_states` now also includes the hidden state of the embeddings in addition to those of the encoding layers. This allows users to easily access the embeddings final state.
|
587 |
-
|
588 |
-
### Serialization
|
589 |
-
|
590 |
-
Breaking change in the `from_pretrained()` method:
|
591 |
-
|
592 |
-
1. Models are now set in evaluation mode by default when instantiated with the `from_pretrained()` method. To train them, don't forget to set them back in training mode (`model.train()`) to activate the dropout modules.
|
593 |
-
|
594 |
-
2. The additional `*input` and `**kwargs` arguments supplied to the `from_pretrained()` method used to be directly passed to the underlying model's class `__init__()` method. They are now used to update the model configuration attribute instead, which can break derived model classes built based on the previous `BertForSequenceClassification` examples. We are working on a way to mitigate this breaking change in [#866](https://github.com/huggingface/transformers/pull/866) by forwarding the the model's `__init__()` method (i) the provided positional arguments and (ii) the keyword arguments which do not match any configuration class attributes.
|
595 |
-
|
596 |
-
Also, while not a breaking change, the serialization methods have been standardized and you probably should switch to the new method `save_pretrained(save_directory)` if you were using any other serialization method before.
|
597 |
-
|
598 |
-
Here is an example:
|
599 |
-
|
600 |
-
```python
|
601 |
-
### Let's load a model and tokenizer
|
602 |
-
model = BertForSequenceClassification.from_pretrained('bert-base-uncased')
|
603 |
-
tokenizer = BertTokenizer.from_pretrained('bert-base-uncased')
|
604 |
-
|
605 |
-
### Do some stuff to our model and tokenizer
|
606 |
-
# Ex: add new tokens to the vocabulary and embeddings of our model
|
607 |
-
tokenizer.add_tokens(['[SPECIAL_TOKEN_1]', '[SPECIAL_TOKEN_2]'])
|
608 |
-
model.resize_token_embeddings(len(tokenizer))
|
609 |
-
# Train our model
|
610 |
-
train(model)
|
611 |
-
|
612 |
-
### Now let's save our model and tokenizer to a directory
|
613 |
-
model.save_pretrained('./my_saved_model_directory/')
|
614 |
-
tokenizer.save_pretrained('./my_saved_model_directory/')
|
615 |
-
|
616 |
-
### Reload the model and the tokenizer
|
617 |
-
model = BertForSequenceClassification.from_pretrained('./my_saved_model_directory/')
|
618 |
-
tokenizer = BertTokenizer.from_pretrained('./my_saved_model_directory/')
|
619 |
-
```
|
620 |
-
|
621 |
-
### Optimizers: BertAdam & OpenAIAdam are now AdamW, schedules are standard PyTorch schedules
|
622 |
-
|
623 |
-
The two optimizers previously included, `BertAdam` and `OpenAIAdam`, have been replaced by a single `AdamW` optimizer which has a few differences:
|
624 |
-
|
625 |
-
- it only implements weights decay correction,
|
626 |
-
- schedules are now externals (see below),
|
627 |
-
- gradient clipping is now also external (see below).
|
628 |
-
|
629 |
-
The new optimizer `AdamW` matches PyTorch `Adam` optimizer API and let you use standard PyTorch or apex methods for the schedule and clipping.
|
630 |
-
|
631 |
-
The schedules are now standard [PyTorch learning rate schedulers](https://pytorch.org/docs/stable/optim.html#how-to-adjust-learning-rate) and not part of the optimizer anymore.
|
632 |
-
|
633 |
-
Here is a conversion examples from `BertAdam` with a linear warmup and decay schedule to `AdamW` and the same schedule:
|
634 |
-
|
635 |
-
```python
|
636 |
-
# Parameters:
|
637 |
-
lr = 1e-3
|
638 |
-
max_grad_norm = 1.0
|
639 |
-
num_training_steps = 1000
|
640 |
-
num_warmup_steps = 100
|
641 |
-
warmup_proportion = float(num_warmup_steps) / float(num_training_steps) # 0.1
|
642 |
-
|
643 |
-
### Previously BertAdam optimizer was instantiated like this:
|
644 |
-
optimizer = BertAdam(model.parameters(), lr=lr, schedule='warmup_linear', warmup=warmup_proportion, t_total=num_training_steps)
|
645 |
-
### and used like this:
|
646 |
-
for batch in train_data:
|
647 |
-
loss = model(batch)
|
648 |
-
loss.backward()
|
649 |
-
optimizer.step()
|
650 |
-
|
651 |
-
### In Transformers, optimizer and schedules are splitted and instantiated like this:
|
652 |
-
optimizer = AdamW(model.parameters(), lr=lr, correct_bias=False) # To reproduce BertAdam specific behavior set correct_bias=False
|
653 |
-
scheduler = get_linear_schedule_with_warmup(optimizer, num_warmup_steps=num_warmup_steps, num_training_steps=num_training_steps) # PyTorch scheduler
|
654 |
-
### and used like this:
|
655 |
-
for batch in train_data:
|
656 |
-
model.train()
|
657 |
-
loss = model(batch)
|
658 |
-
loss.backward()
|
659 |
-
torch.nn.utils.clip_grad_norm_(model.parameters(), max_grad_norm) # Gradient clipping is not in AdamW anymore (so you can use amp without issue)
|
660 |
-
optimizer.step()
|
661 |
-
scheduler.step()
|
662 |
-
optimizer.zero_grad()
|
663 |
-
```
|
664 |
-
|
665 |
-
## Citation
|
666 |
-
|
667 |
-
We now have a paper you can cite for the 🤗 Transformers library:
|
668 |
-
```
|
669 |
-
@article{Wolf2019HuggingFacesTS,
|
670 |
-
title={HuggingFace's Transformers: State-of-the-art Natural Language Processing},
|
671 |
-
author={Thomas Wolf and Lysandre Debut and Victor Sanh and Julien Chaumond and Clement Delangue and Anthony Moi and Pierric Cistac and Tim Rault and R'emi Louf and Morgan Funtowicz and Jamie Brew},
|
672 |
-
journal={ArXiv},
|
673 |
-
year={2019},
|
674 |
-
volume={abs/1910.03771}
|
675 |
-
}
|
676 |
-
```
|
|
|
|
|
|
|
|
|
|
|
|
|
|
|
|
|
|
|
|
|
|
|
|
|
|
|
|
|
|
|
|
|
|
|
|
|
|
|
|
|
|
|
|
|
|
|
|
|
|
|
|
|
|
|
|
|
|
|
|
|
|
|
|
|
|
|
|
|
|
|
|
|
|
|
|
|
|
|
|
|
|
|
|
|
|
|
|
|
|
|
|
|
|
|
|
|
|
|
|
|
|
|
|
|
|
|
|
|
|
|
|
|
|
|
|
|
|
|
|
|
|
|
|
|
|
|
|
|
|
|
|
|
|
|
|
|
|
|
|
|
|
|
|
|
|
|
|
|
|
|
|
|
|
|
|
|
|
|
|
|
|
|
|
|
|
|
|
|
|
|
|
|
|
|
|
|
|
|
|
|
|
|
|
|
|
|
|
|
|
|
|
|
|
|
|
|
|
|
|
|
|
|
|
|
|
|
|
|
|
|
|
|
|
|
|
|
|
|
|
|
|
|
|
|
|
|
|
|
|
|
|
|
|
|
|
|
|
|
|
|
|
|
|
|
|
|
|
|
|
|
|
|
|
|
|
|
|
|
|
|
|
|
|
|
|
|
|
|
|
|
|
|
|
|
|
|
|
|
|
|
|
|
|
|
|
|
|
|
|
|
|
|
|
|
|
|
|
|
|
|
|
|
|
|
|
|
|
|
|
|
|
|
|
|
|
|
|
|
|
|
|
|
|
|
|
|
|
|
|
|
|
|
|
|
|
|
|
|
|
|
|
|
|
|
|
|
|
|
|
|
|
|
|
|
|
|
|
|
|
|
|
|
|
|
|
|
|
|
|
|
|
|
|
|
|
|
|
|
|
|
|
|
|
|
|
|
|
|
|
|
|
|
|
|
|
|
|
|
|
|
|
|
|
|
|
|
|
|
|
|
|
|
|
|
|
|
|
|
|
|
|
|
|
|
|
|
|
|
|
|
|
|
|
|
|
|
|
|
|
|
|
|
|
|
|
|
|
|
|
|
|
|
|
|
|
|
|
|
|
|
|
|
|
|
|
|
|
|
|
|
|
|
|
|
|
|
|
|
|
|
|
|
|
|
|
|
|
|
|
|
|
|
|
|
|
|
|
|
|
|
|
|
|
|
|
|
|
|
|
|
|
|
|
|
|
|
|
|
|
|
|
|
|
|
|
|
|
|
|
|
|
|
|
|
|
|
|
|
|
|
|
|
|
|
|
|
|
|
|
|
|
|
|
|
|
|
|
|
|
|
|
|
|
|
|
|
|
|
|
|
|
|
|
|
|
|
|
|
|
|
|
|
|
|
|
|
|
|
|
|
|
|
|
|
|
|
|
|
|
|
|
|
|
|
|
|
|
|
|
|
|
|
|
|
|
|
|
|
|
|
|
|
|
|
|
|
|
|
|
|
|
|
|
|
|
|
|
|
|
|
|
|
|
|
|
|
|
|
|
|
|
|
|
|
|
|
|
|
|
|
|
|
|
|
|
|
|
|
|
|
|
|
|
|
|
|
|
|
|
|
|
|
|
|
|
|
|
|
|
|
|
|
|
|
|
|
|
|
|
|
|
|
|
|
|
|
|
|
|
|
|
|
|
|
|
|
|
|
|
|
|
|
|
|
|
|
|
|
|
|
|
|
|
|
|
|
|
|
|
|
|
|
|
|
|
|
|
|
|
|
|
|
|
|
|
|
|
|
|
|
|
|
|
|
|
|
|
|
|
|
|
|
|
|
|
|
|
|
|
|
|
|
|
|
|
|
|
|
|
|
|
|
|
|
|
|
|
|
|
|
|
|
|
|
|
|
|
|
|
|
|
|
|
|
|
|
|
|
|
|
|
|
|
|
|
|
|
|
|
|
|
|
|
|
|
|
|
|
|
|
|
|
|
|
|
|
|
|
|
|
|
|
|
|
|
|
|
|
|
|
|
|
|
|
|
|
|
|
|
|
|
|
|
|
|
|
|
|
|
|
|
|
|
|
|
|
|
|
|
|
|
|
|
|
|
|
|
|
|
|
|
|
|
|
|
|
|
|
|
|
|
|
|
|
|
|
|
|
|
|
|
|
|
|
|
|
|
|
|
|
|
|
|
|
|
|
|
|
|
|
|
|
|
|
|
|
|
|
|
|
|
|
|
|
|
|
|
|
|
|
|
|
|
|
|
|
|
|
|
|
|
|
|
|
|
|
|
|
|
|
|
|
|
|
|
|
|
|
|
|
|
|
|
|
|
|
|
|
|
|
|
|
|
|
|
|
|
|
|
|
|
|
|
|
|
|
|
|
|
|
|
|
|
|
|
|
|
|
|
|
|
|
|
|
|
|
|
|
|
|
|
|
|
|
|
|
|
|
|
|
|
|
|
|
|
|
|
|
|
|
|
|
|
|
|
|
|
|
|
|
|
|
|
|
|
|
|
|
|
|
|
|
|
|
|
|
|
|
|
|
|
|
|
|
|
|
|
|
|
|
|
|
|
|
|
|
|
|
|
|
|
|
|
|
|
|
|
|
|
|
|
|
|
|
|
|
|
|
|
|
|
|
|
|
|
|
|
|
|
|
|
|
|
|
|
|
|
|
|
|
|
|
|
|
|
|
|
|
|
|
|
|
|
|
|
|
|
|
|
|
|
|
|
|
|
|
|
|
|
|
|
|
|
|
|
|
|
|
|
|
|
|
|
|
|
|
|
|
|
|
|
|
|
|
|
|
|
|
|
|
|
|
|
|
|
|
|
|
|
|
|
|
|
|
|
|
|
|
|
|
|
|
|
|
|
|
|
|
|
|
|
|
|
|
|
|
|
|
|
|
|
|
|
|
|
|
|
|
|
|
|
|
|
|
|
|
|
|
|
|
|
|
|
|
|
|
|
|
|
|
|
|
|
|
|
|
|
|
|
|
|
|
|
|
|
|
|
|
|
|
|
|
|
|
|
|
|
|
|
|
|
|
|
|
|
|
|
|
|
|
|
|
|
|
|
|
|
|
|
|
|
|
|
|
|
|
|
|
|
|
|
|
|
|
|
|
|
|
|
|
|
|
|
server/transformers/deploy_multi_version_doc.sh
DELETED
@@ -1,23 +0,0 @@
|
|
1 |
-
cd docs
|
2 |
-
|
3 |
-
function deploy_doc(){
|
4 |
-
echo "Creating doc at commit $1 and pushing to folder $2"
|
5 |
-
git checkout $1
|
6 |
-
if [ ! -z "$2" ]
|
7 |
-
then
|
8 |
-
echo "Pushing version" $2
|
9 |
-
make clean && make html && scp -r -oStrictHostKeyChecking=no _build/html $doc:$dir/$2
|
10 |
-
else
|
11 |
-
echo "Pushing master"
|
12 |
-
make clean && make html && scp -r -oStrictHostKeyChecking=no _build/html/* $doc:$dir
|
13 |
-
fi
|
14 |
-
}
|
15 |
-
|
16 |
-
deploy_doc "master"
|
17 |
-
deploy_doc "b33a385" v1.0.0
|
18 |
-
deploy_doc "fe02e45" v1.1.0
|
19 |
-
deploy_doc "89fd345" v1.2.0
|
20 |
-
deploy_doc "fc9faa8" v2.0.0
|
21 |
-
deploy_doc "3ddce1d" v2.1.1
|
22 |
-
deploy_doc "f2f3294" v2.2.0
|
23 |
-
deploy_doc "d0f8b9a" v2.3.0
|
|
|
|
|
|
|
|
|
|
|
|
|
|
|
|
|
|
|
|
|
|
|
|
|
|
|
|
|
|
|
|
|
|
|
|
|
|
|
|
|
|
|
|
|
|
|
|
server/transformers/docker/Dockerfile
DELETED
@@ -1,7 +0,0 @@
|
|
1 |
-
FROM pytorch/pytorch:latest
|
2 |
-
|
3 |
-
RUN git clone https://github.com/NVIDIA/apex.git && cd apex && python setup.py install --cuda_ext --cpp_ext
|
4 |
-
|
5 |
-
RUN pip install transformers
|
6 |
-
|
7 |
-
WORKDIR /workspace
|
|
|
|
|
|
|
|
|
|
|
|
|
|
|
|
server/transformers/docs/Makefile
DELETED
@@ -1,19 +0,0 @@
|
|
1 |
-
# Minimal makefile for Sphinx documentation
|
2 |
-
#
|
3 |
-
|
4 |
-
# You can set these variables from the command line.
|
5 |
-
SPHINXOPTS =
|
6 |
-
SPHINXBUILD = sphinx-build
|
7 |
-
SOURCEDIR = source
|
8 |
-
BUILDDIR = _build
|
9 |
-
|
10 |
-
# Put it first so that "make" without argument is like "make help".
|
11 |
-
help:
|
12 |
-
@$(SPHINXBUILD) -M help "$(SOURCEDIR)" "$(BUILDDIR)" $(SPHINXOPTS) $(O)
|
13 |
-
|
14 |
-
.PHONY: help Makefile
|
15 |
-
|
16 |
-
# Catch-all target: route all unknown targets to Sphinx using the new
|
17 |
-
# "make mode" option. $(O) is meant as a shortcut for $(SPHINXOPTS).
|
18 |
-
%: Makefile
|
19 |
-
@$(SPHINXBUILD) -M $@ "$(SOURCEDIR)" "$(BUILDDIR)" $(SPHINXOPTS) $(O)
|
|
|
|
|
|
|
|
|
|
|
|
|
|
|
|
|
|
|
|
|
|
|
|
|
|
|
|
|
|
|
|
|
|
|
|
|
|
|
|
server/transformers/docs/README.md
DELETED
@@ -1,67 +0,0 @@
|
|
1 |
-
# Generating the documentation
|
2 |
-
|
3 |
-
To generate the documentation, you first have to build it. Several packages are necessary to build the doc,
|
4 |
-
you can install them with the following command, at the root of the code repository:
|
5 |
-
|
6 |
-
```bash
|
7 |
-
pip install -e ".[docs]"
|
8 |
-
```
|
9 |
-
|
10 |
-
## Packages installed
|
11 |
-
|
12 |
-
Here's an overview of all the packages installed. If you ran the previous command installing all packages from
|
13 |
-
`requirements.txt`, you do not need to run the following commands.
|
14 |
-
|
15 |
-
Building it requires the package `sphinx` that you can
|
16 |
-
install using:
|
17 |
-
|
18 |
-
```bash
|
19 |
-
pip install -U sphinx
|
20 |
-
```
|
21 |
-
|
22 |
-
You would also need the custom installed [theme](https://github.com/readthedocs/sphinx_rtd_theme) by
|
23 |
-
[Read The Docs](https://readthedocs.org/). You can install it using the following command:
|
24 |
-
|
25 |
-
```bash
|
26 |
-
pip install sphinx_rtd_theme
|
27 |
-
```
|
28 |
-
|
29 |
-
The third necessary package is the `recommonmark` package to accept Markdown as well as Restructured text:
|
30 |
-
|
31 |
-
```bash
|
32 |
-
pip install recommonmark
|
33 |
-
```
|
34 |
-
|
35 |
-
## Building the documentation
|
36 |
-
|
37 |
-
Make sure that there is a symlink from the `example` file (in /examples) inside the source folder. Run the following
|
38 |
-
command to generate it:
|
39 |
-
|
40 |
-
```bash
|
41 |
-
ln -s ../../examples/README.md examples.md
|
42 |
-
```
|
43 |
-
|
44 |
-
Once you have setup `sphinx`, you can build the documentation by running the following command in the `/docs` folder:
|
45 |
-
|
46 |
-
```bash
|
47 |
-
make html
|
48 |
-
```
|
49 |
-
|
50 |
-
---
|
51 |
-
**NOTE**
|
52 |
-
|
53 |
-
If you are adding/removing elements from the toc-tree or from any structural item, it is recommended to clean the build
|
54 |
-
directory before rebuilding. Run the following command to clean and build:
|
55 |
-
|
56 |
-
```bash
|
57 |
-
make clean && make html
|
58 |
-
```
|
59 |
-
|
60 |
-
---
|
61 |
-
|
62 |
-
It should build the static app that will be available under `/docs/_build/html`
|
63 |
-
|
64 |
-
## Adding a new element to the tree (toc-tree)
|
65 |
-
|
66 |
-
Accepted files are reStructuredText (.rst) and Markdown (.md). Create a file with its extension and put it
|
67 |
-
in the source directory. You can then link it to the toc-tree by putting the filename without the extension.
|
|
|
|
|
|
|
|
|
|
|
|
|
|
|
|
|
|
|
|
|
|
|
|
|
|
|
|
|
|
|
|
|
|
|
|
|
|
|
|
|
|
|
|
|
|
|
|
|
|
|
|
|
|
|
|
|
|
|
|
|
|
|
|
|
|
|
|
|
|
|
|
|
|
|
|
|
|
|
|
|
|
|
|
|
|
|
|
|
|
|
|
|
|
|
|
|
|
|
|
|
|
|
|
|
|
|
|
|
|
|
|
|
|
|
|
|
|
|
|
|
|
|
|
|
|
|
|
|
|
|
|
|
|
|
|
server/transformers/docs/source/_static/css/Calibre-Light.ttf
DELETED
Binary file (62.5 kB)
|
|
server/transformers/docs/source/_static/css/Calibre-Medium.otf
DELETED
Binary file (47.9 kB)
|
|
server/transformers/docs/source/_static/css/Calibre-Regular.otf
DELETED
Binary file (49.9 kB)
|
|
server/transformers/docs/source/_static/css/Calibre-Thin.otf
DELETED
Binary file (46.7 kB)
|
|
server/transformers/docs/source/_static/css/code-snippets.css
DELETED
@@ -1,12 +0,0 @@
|
|
1 |
-
|
2 |
-
.highlight .c1, .highlight .sd{
|
3 |
-
color: #999
|
4 |
-
}
|
5 |
-
|
6 |
-
.highlight .nn, .highlight .k, .highlight .s1, .highlight .nb, .highlight .bp, .highlight .kc {
|
7 |
-
color: #FB8D68;
|
8 |
-
}
|
9 |
-
|
10 |
-
.highlight .kn, .highlight .nv, .highlight .s2, .highlight .ow {
|
11 |
-
color: #6670FF;
|
12 |
-
}
|
|
|
|
|
|
|
|
|
|
|
|
|
|
|
|
|
|
|
|
|
|
|
|
|
|
server/transformers/docs/source/_static/css/huggingface.css
DELETED
@@ -1,196 +0,0 @@
|
|
1 |
-
/* The literal code blocks */
|
2 |
-
.rst-content tt.literal, .rst-content tt.literal, .rst-content code.literal {
|
3 |
-
color: #6670FF;
|
4 |
-
}
|
5 |
-
|
6 |
-
/* To keep the logo centered */
|
7 |
-
.wy-side-scroll {
|
8 |
-
width: auto;
|
9 |
-
font-size: 20px;
|
10 |
-
}
|
11 |
-
|
12 |
-
/* The div that holds the Hugging Face logo */
|
13 |
-
.HuggingFaceDiv {
|
14 |
-
width: 100%
|
15 |
-
}
|
16 |
-
|
17 |
-
/* The research field on top of the toc tree */
|
18 |
-
.wy-side-nav-search{
|
19 |
-
background-color: #6670FF;
|
20 |
-
}
|
21 |
-
|
22 |
-
/* The toc tree */
|
23 |
-
.wy-nav-side{
|
24 |
-
background-color: #6670FF;
|
25 |
-
}
|
26 |
-
|
27 |
-
/* The selected items in the toc tree */
|
28 |
-
.wy-menu-vertical li.current{
|
29 |
-
background-color: #A6B0FF;
|
30 |
-
}
|
31 |
-
|
32 |
-
/* When a list item that does belong to the selected block from the toc tree is hovered */
|
33 |
-
.wy-menu-vertical li.current a:hover{
|
34 |
-
background-color: #B6C0FF;
|
35 |
-
}
|
36 |
-
|
37 |
-
/* When a list item that does NOT belong to the selected block from the toc tree is hovered. */
|
38 |
-
.wy-menu-vertical li a:hover{
|
39 |
-
background-color: #A7AFFB;
|
40 |
-
}
|
41 |
-
|
42 |
-
/* The text items on the toc tree */
|
43 |
-
.wy-menu-vertical a {
|
44 |
-
color: #FFFFDD;
|
45 |
-
font-family: Calibre-Light, sans-serif;
|
46 |
-
}
|
47 |
-
.wy-menu-vertical header, .wy-menu-vertical p.caption{
|
48 |
-
color: white;
|
49 |
-
font-family: Calibre-Light, sans-serif;
|
50 |
-
}
|
51 |
-
|
52 |
-
/* The color inside the selected toc tree block */
|
53 |
-
.wy-menu-vertical li.toctree-l2 a, .wy-menu-vertical li.toctree-l3 a, .wy-menu-vertical li.toctree-l4 a {
|
54 |
-
color: black;
|
55 |
-
}
|
56 |
-
|
57 |
-
/* Inside the depth-2 selected toc tree block */
|
58 |
-
.wy-menu-vertical li.toctree-l2.current>a {
|
59 |
-
background-color: #B6C0FF
|
60 |
-
}
|
61 |
-
.wy-menu-vertical li.toctree-l2.current li.toctree-l3>a {
|
62 |
-
background-color: #C6D0FF
|
63 |
-
}
|
64 |
-
|
65 |
-
/* Inside the depth-3 selected toc tree block */
|
66 |
-
.wy-menu-vertical li.toctree-l3.current li.toctree-l4>a{
|
67 |
-
background-color: #D6E0FF
|
68 |
-
}
|
69 |
-
|
70 |
-
/* Inside code snippets */
|
71 |
-
.rst-content dl:not(.docutils) dt{
|
72 |
-
font-size: 15px;
|
73 |
-
}
|
74 |
-
|
75 |
-
/* Links */
|
76 |
-
a {
|
77 |
-
color: #6670FF;
|
78 |
-
}
|
79 |
-
|
80 |
-
/* Content bars */
|
81 |
-
.rst-content dl:not(.docutils) dt {
|
82 |
-
background-color: rgba(251, 141, 104, 0.1);
|
83 |
-
border-right: solid 2px #FB8D68;
|
84 |
-
border-left: solid 2px #FB8D68;
|
85 |
-
color: #FB8D68;
|
86 |
-
font-family: Calibre-Light, sans-serif;
|
87 |
-
border-top: none;
|
88 |
-
font-style: normal !important;
|
89 |
-
}
|
90 |
-
|
91 |
-
/* Expand button */
|
92 |
-
.wy-menu-vertical li.toctree-l2 span.toctree-expand,
|
93 |
-
.wy-menu-vertical li.on a span.toctree-expand, .wy-menu-vertical li.current>a span.toctree-expand,
|
94 |
-
.wy-menu-vertical li.toctree-l3 span.toctree-expand{
|
95 |
-
color: black;
|
96 |
-
}
|
97 |
-
|
98 |
-
/* Max window size */
|
99 |
-
.wy-nav-content{
|
100 |
-
max-width: 1200px;
|
101 |
-
}
|
102 |
-
|
103 |
-
/* Mobile header */
|
104 |
-
.wy-nav-top{
|
105 |
-
background-color: #6670FF;
|
106 |
-
}
|
107 |
-
|
108 |
-
|
109 |
-
/* Source spans */
|
110 |
-
.rst-content .viewcode-link, .rst-content .viewcode-back{
|
111 |
-
color: #6670FF;
|
112 |
-
font-size: 110%;
|
113 |
-
letter-spacing: 2px;
|
114 |
-
text-transform: uppercase;
|
115 |
-
}
|
116 |
-
|
117 |
-
/* It would be better for table to be visible without horizontal scrolling */
|
118 |
-
.wy-table-responsive table td, .wy-table-responsive table th{
|
119 |
-
white-space: normal;
|
120 |
-
}
|
121 |
-
|
122 |
-
.footer {
|
123 |
-
margin-top: 20px;
|
124 |
-
}
|
125 |
-
|
126 |
-
.footer__Social {
|
127 |
-
display: flex;
|
128 |
-
flex-direction: row;
|
129 |
-
}
|
130 |
-
|
131 |
-
.footer__CustomImage {
|
132 |
-
margin: 2px 5px 0 0;
|
133 |
-
}
|
134 |
-
|
135 |
-
/* class and method names in doc */
|
136 |
-
.rst-content dl:not(.docutils) tt.descname, .rst-content dl:not(.docutils) tt.descclassname, .rst-content dl:not(.docutils) tt.descname, .rst-content dl:not(.docutils) code.descname, .rst-content dl:not(.docutils) tt.descclassname, .rst-content dl:not(.docutils) code.descclassname{
|
137 |
-
font-family: Calibre, sans-serif;
|
138 |
-
font-size: 20px !important;
|
139 |
-
}
|
140 |
-
|
141 |
-
/* class name in doc*/
|
142 |
-
.rst-content dl:not(.docutils) tt.descname, .rst-content dl:not(.docutils) tt.descname, .rst-content dl:not(.docutils) code.descname{
|
143 |
-
margin-right: 10px;
|
144 |
-
font-family: Calibre-Medium, sans-serif;
|
145 |
-
}
|
146 |
-
|
147 |
-
/* Method and class parameters */
|
148 |
-
.sig-param{
|
149 |
-
line-height: 23px;
|
150 |
-
}
|
151 |
-
|
152 |
-
/* Class introduction "class" string at beginning */
|
153 |
-
.rst-content dl:not(.docutils) .property{
|
154 |
-
font-size: 18px;
|
155 |
-
color: black;
|
156 |
-
}
|
157 |
-
|
158 |
-
|
159 |
-
/* FONTS */
|
160 |
-
body{
|
161 |
-
font-family: Calibre, sans-serif;
|
162 |
-
font-size: 16px;
|
163 |
-
}
|
164 |
-
|
165 |
-
h1 {
|
166 |
-
font-family: Calibre-Thin, sans-serif;
|
167 |
-
font-size: 70px;
|
168 |
-
}
|
169 |
-
|
170 |
-
h2, .rst-content .toctree-wrapper p.caption, h3, h4, h5, h6, legend{
|
171 |
-
font-family: Calibre-Medium, sans-serif;
|
172 |
-
}
|
173 |
-
|
174 |
-
@font-face {
|
175 |
-
font-family: Calibre-Medium;
|
176 |
-
src: url(./Calibre-Medium.otf);
|
177 |
-
font-weight:400;
|
178 |
-
}
|
179 |
-
|
180 |
-
@font-face {
|
181 |
-
font-family: Calibre;
|
182 |
-
src: url(./Calibre-Regular.otf);
|
183 |
-
font-weight:400;
|
184 |
-
}
|
185 |
-
|
186 |
-
@font-face {
|
187 |
-
font-family: Calibre-Light;
|
188 |
-
src: url(./Calibre-Light.ttf);
|
189 |
-
font-weight:400;
|
190 |
-
}
|
191 |
-
|
192 |
-
@font-face {
|
193 |
-
font-family: Calibre-Thin;
|
194 |
-
src: url(./Calibre-Thin.otf);
|
195 |
-
font-weight:400;
|
196 |
-
}
|
|
|
|
|
|
|
|
|
|
|
|
|
|
|
|
|
|
|
|
|
|
|
|
|
|
|
|
|
|
|
|
|
|
|
|
|
|
|
|
|
|
|
|
|
|
|
|
|
|
|
|
|
|
|
|
|
|
|
|
|
|
|
|
|
|
|
|
|
|
|
|
|
|
|
|
|
|
|
|
|
|
|
|
|
|
|
|
|
|
|
|
|
|
|
|
|
|
|
|
|
|
|
|
|
|
|
|
|
|
|
|
|
|
|
|
|
|
|
|
|
|
|
|
|
|
|
|
|
|
|
|
|
|
|
|
|
|
|
|
|
|
|
|
|
|
|
|
|
|
|
|
|
|
|
|
|
|
|
|
|
|
|
|
|
|
|
|
|
|
|
|
|
|
|
|
|
|
|
|
|
|
|
|
|
|
|
|
|
|
|
|
|
|
|
|
|
|
|
|
|
|
|
|
|
|
|
|
|
|
|
|
|
|
|
|
|
|
|
|
|
|
|
|
|
|
|
|
|
|
|
|
|
|
|
|
|
|
|
|
|
|
|
|
|
|
|
|
|
|
|
|
|
|
|
|
|
|
|
|
|
|
|
|
|
|
|
|
|
|
|
|
|
|
|
|
|
|
|
|
|
|
|
|
|
|
|
|
|
|
|
|
|
|
|
|
|
|
|
|
|
|
|
|
|
|
|
|
|
|
|
|
|
|
|
|
|
|
|
|
|
|
|
|
|
|
|
|
|
|
|
|
|
|
|
|
|
|
|
|
|
|
|
|
|
|
|
|
|
|
|
|
|
|
|
|
|
|
|
|
|
|
|
|
|
|
|
|
|
|
|
|
|
|
|
|
|
|
|
|
|
|
|
|
|
|
|
|
|
|
|
|
|
|
server/transformers/docs/source/_static/js/custom.js
DELETED
@@ -1,79 +0,0 @@
|
|
1 |
-
function addIcon() {
|
2 |
-
const huggingFaceLogo = "https://huggingface.co/landing/assets/transformers-docs/huggingface_logo.svg";
|
3 |
-
const image = document.createElement("img");
|
4 |
-
image.setAttribute("src", huggingFaceLogo);
|
5 |
-
|
6 |
-
const div = document.createElement("div");
|
7 |
-
div.appendChild(image);
|
8 |
-
div.style.textAlign = 'center';
|
9 |
-
div.style.paddingTop = '30px';
|
10 |
-
div.style.backgroundColor = '#6670FF';
|
11 |
-
|
12 |
-
const scrollDiv = document.querySelector(".wy-side-scroll");
|
13 |
-
scrollDiv.prepend(div);
|
14 |
-
}
|
15 |
-
|
16 |
-
function addCustomFooter() {
|
17 |
-
const customFooter = document.createElement("div");
|
18 |
-
const questionOrIssue = document.createElement("div");
|
19 |
-
questionOrIssue.innerHTML = "Stuck? Read our <a href='https://medium.com/huggingface'>Blog posts</a> or <a href='https://github.com/huggingface/transformers'>Create an issue</a>";
|
20 |
-
customFooter.appendChild(questionOrIssue);
|
21 |
-
customFooter.classList.add("footer");
|
22 |
-
|
23 |
-
const social = document.createElement("div");
|
24 |
-
social.classList.add("footer__Social");
|
25 |
-
|
26 |
-
const imageDetails = [
|
27 |
-
{ link: "https://huggingface.co", imageLink: "https://huggingface.co/landing/assets/transformers-docs/website.svg" },
|
28 |
-
{ link: "https://twitter.com/huggingface", imageLink: "https://huggingface.co/landing/assets/transformers-docs/twitter.svg" },
|
29 |
-
{ link: "https://github.com/huggingface", imageLink: "https://huggingface.co/landing/assets/transformers-docs/github.svg" },
|
30 |
-
{ link: "https://www.linkedin.com/company/huggingface/", imageLink: "https://huggingface.co/landing/assets/transformers-docs/linkedin.svg" }
|
31 |
-
];
|
32 |
-
|
33 |
-
imageDetails.forEach(imageLinks => {
|
34 |
-
const link = document.createElement("a");
|
35 |
-
const image = document.createElement("img");
|
36 |
-
image.src = imageLinks.imageLink;
|
37 |
-
link.href = imageLinks.link;
|
38 |
-
image.style.width = "30px";
|
39 |
-
image.classList.add("footer__CustomImage");
|
40 |
-
link.appendChild(image);
|
41 |
-
social.appendChild(link);
|
42 |
-
});
|
43 |
-
|
44 |
-
customFooter.appendChild(social);
|
45 |
-
document.querySelector("footer").appendChild(customFooter);
|
46 |
-
}
|
47 |
-
|
48 |
-
function addGithubButton() {
|
49 |
-
const div = `
|
50 |
-
<div class="github-repo">
|
51 |
-
<a
|
52 |
-
class="github-button"
|
53 |
-
href="https://github.com/huggingface/transformers" data-size="large" data-show-count="true" aria-label="Star huggingface/pytorch-transformers on GitHub">
|
54 |
-
Star
|
55 |
-
</a>
|
56 |
-
</div>
|
57 |
-
`;
|
58 |
-
document.querySelector(".wy-side-nav-search .icon-home").insertAdjacentHTML('afterend', div);
|
59 |
-
}
|
60 |
-
|
61 |
-
/*!
|
62 |
-
* github-buttons v2.2.10
|
63 |
-
* (c) 2019 なつき
|
64 |
-
* @license BSD-2-Clause
|
65 |
-
*/
|
66 |
-
/**
|
67 |
-
* modified to run programmatically
|
68 |
-
*/
|
69 |
-
function parseGithubButtons (){"use strict";var e=window.document,t=e.location,o=window.encodeURIComponent,r=window.decodeURIComponent,n=window.Math,a=window.HTMLElement,i=window.XMLHttpRequest,l="https://unpkg.com/[email protected]/dist/buttons.html",c=i&&i.prototype&&"withCredentials"in i.prototype,d=c&&a&&a.prototype.attachShadow&&!a.prototype.attachShadow.prototype,s=function(e,t,o){e.addEventListener?e.addEventListener(t,o):e.attachEvent("on"+t,o)},u=function(e,t,o){e.removeEventListener?e.removeEventListener(t,o):e.detachEvent("on"+t,o)},h=function(e,t,o){var r=function(n){return u(e,t,r),o(n)};s(e,t,r)},f=function(e,t,o){var r=function(n){if(t.test(e.readyState))return u(e,"readystatechange",r),o(n)};s(e,"readystatechange",r)},p=function(e){return function(t,o,r){var n=e.createElement(t);if(o)for(var a in o){var i=o[a];null!=i&&(null!=n[a]?n[a]=i:n.setAttribute(a,i))}if(r)for(var l=0,c=r.length;l<c;l++){var d=r[l];n.appendChild("string"==typeof d?e.createTextNode(d):d)}return n}},g=p(e),b=function(e){var t;return function(){t||(t=1,e.apply(this,arguments))}},m="body{margin:0}a{color:#24292e;text-decoration:none;outline:0}.octicon{display:inline-block;vertical-align:text-top;fill:currentColor}.widget{ display:inline-block;overflow:hidden;font-family:-apple-system, BlinkMacSystemFont, \"Segoe UI\", Helvetica, Arial, sans-serif;font-size:0;white-space:nowrap;-webkit-user-select:none;-moz-user-select:none;-ms-user-select:none;user-select:none}.btn,.social-count{display:inline-block;height:14px;padding:2px 5px;font-size:11px;font-weight:600;line-height:14px;vertical-align:bottom;cursor:pointer;border:1px solid #c5c9cc;border-radius:0.25em}.btn{background-color:#eff3f6;background-image:-webkit-linear-gradient(top, #fafbfc, #eff3f6 90%);background-image:-moz-linear-gradient(top, #fafbfc, #eff3f6 90%);background-image:linear-gradient(180deg, #fafbfc, #eff3f6 90%);background-position:-1px -1px;background-repeat:repeat-x;background-size:110% 110%;border-color:rgba(27,31,35,0.2);-ms-filter:\"progid:DXImageTransform.Microsoft.Gradient(startColorstr='#FFFAFBFC', endColorstr='#FFEEF2F5')\";*filter:progid:DXImageTransform.Microsoft.Gradient(startColorstr='#FFFAFBFC', endColorstr='#FFEEF2F5')}.btn:active{background-color:#e9ecef;background-image:none;border-color:#a5a9ac;border-color:rgba(27,31,35,0.35);box-shadow:inset 0 0.15em 0.3em rgba(27,31,35,0.15)}.btn:focus,.btn:hover{background-color:#e6ebf1;background-image:-webkit-linear-gradient(top, #f0f3f6, #e6ebf1 90%);background-image:-moz-linear-gradient(top, #f0f3f6, #e6ebf1 90%);background-image:linear-gradient(180deg, #f0f3f6, #e6ebf1 90%);border-color:#a5a9ac;border-color:rgba(27,31,35,0.35);-ms-filter:\"progid:DXImageTransform.Microsoft.Gradient(startColorstr='#FFF0F3F6', endColorstr='#FFE5EAF0')\";*filter:progid:DXImageTransform.Microsoft.Gradient(startColorstr='#FFF0F3F6', endColorstr='#FFE5EAF0')}.social-count{position:relative;margin-left:5px;background-color:#fff}.social-count:focus,.social-count:hover{color:#0366d6}.social-count b,.social-count i{position:absolute;top:50%;left:0;display:block;width:0;height:0;margin:-4px 0 0 -4px;border:solid transparent;border-width:4px 4px 4px 0;_line-height:0;_border-top-color:red !important;_border-bottom-color:red !important;_border-left-color:red !important;_filter:chroma(color=red)}.social-count b{border-right-color:#c5c9cc}.social-count i{margin-left:-3px;border-right-color:#fff}.lg .btn,.lg .social-count{height:16px;padding:5px 10px;font-size:12px;line-height:16px}.lg .social-count{margin-left:6px}.lg .social-count b,.lg .social-count i{margin:-5px 0 0 -5px;border-width:5px 5px 5px 0}.lg .social-count i{margin-left:-4px}\n",v={"mark-github":{width:16,height:16,path:'<path fill-rule="evenodd" d="M8 0C3.58 0 0 3.58 0 8c0 3.54 2.29 6.53 5.47 7.59.4.07.55-.17.55-.38 0-.19-.01-.82-.01-1.49-2.01.37-2.53-.49-2.69-.94-.09-.23-.48-.94-.82-1.13-.28-.15-.68-.52-.01-.53.63-.01 1.08.58 1.23.82.72 1.21 1.87.87 2.33.66.07-.52.28-.87.51-1.07-1.78-.2-3.64-.89-3.64-3.95 0-.87.31-1.59.82-2.15-.08-.2-.36-1.02.08-2.12 0 0 .67-.21 2.2.82.64-.18 1.32-.27 2-.27.68 0 1.36.09 2 .27 1.53-1.04 2.2-.82 2.2-.82.44 1.1.16 1.92.08 2.12.51.56.82 1.27.82 2.15 0 3.07-1.87 3.75-3.65 3.95.29.25.54.73.54 1.48 0 1.07-.01 1.93-.01 2.2 0 .21.15.46.55.38A8.013 8.013 0 0 0 16 8c0-4.42-3.58-8-8-8z"/>'},eye:{width:16,height:16,path:'<path fill-rule="evenodd" d="M8.06 2C3 2 0 8 0 8s3 6 8.06 6C13 14 16 8 16 8s-3-6-7.94-6zM8 12c-2.2 0-4-1.78-4-4 0-2.2 1.8-4 4-4 2.22 0 4 1.8 4 4 0 2.22-1.78 4-4 4zm2-4c0 1.11-.89 2-2 2-1.11 0-2-.89-2-2 0-1.11.89-2 2-2 1.11 0 2 .89 2 2z"/>'},star:{width:14,height:16,path:'<path fill-rule="evenodd" d="M14 6l-4.9-.64L7 1 4.9 5.36 0 6l3.6 3.26L2.67 14 7 11.67 11.33 14l-.93-4.74L14 6z"/>'},"repo-forked":{width:10,height:16,path:'<path fill-rule="evenodd" d="M8 1a1.993 1.993 0 0 0-1 3.72V6L5 8 3 6V4.72A1.993 1.993 0 0 0 2 1a1.993 1.993 0 0 0-1 3.72V6.5l3 3v1.78A1.993 1.993 0 0 0 5 15a1.993 1.993 0 0 0 1-3.72V9.5l3-3V4.72A1.993 1.993 0 0 0 8 1zM2 4.2C1.34 4.2.8 3.65.8 3c0-.65.55-1.2 1.2-1.2.65 0 1.2.55 1.2 1.2 0 .65-.55 1.2-1.2 1.2zm3 10c-.66 0-1.2-.55-1.2-1.2 0-.65.55-1.2 1.2-1.2.65 0 1.2.55 1.2 1.2 0 .65-.55 1.2-1.2 1.2zm3-10c-.66 0-1.2-.55-1.2-1.2 0-.65.55-1.2 1.2-1.2.65 0 1.2.55 1.2 1.2 0 .65-.55 1.2-1.2 1.2z"/>'},"issue-opened":{width:14,height:16,path:'<path fill-rule="evenodd" d="M7 2.3c3.14 0 5.7 2.56 5.7 5.7s-2.56 5.7-5.7 5.7A5.71 5.71 0 0 1 1.3 8c0-3.14 2.56-5.7 5.7-5.7zM7 1C3.14 1 0 4.14 0 8s3.14 7 7 7 7-3.14 7-7-3.14-7-7-7zm1 3H6v5h2V4zm0 6H6v2h2v-2z"/>'},"cloud-download":{width:16,height:16,path:'<path fill-rule="evenodd" d="M9 12h2l-3 3-3-3h2V7h2v5zm3-8c0-.44-.91-3-4.5-3C5.08 1 3 2.92 3 5 1.02 5 0 6.52 0 8c0 1.53 1 3 3 3h3V9.7H3C1.38 9.7 1.3 8.28 1.3 8c0-.17.05-1.7 1.7-1.7h1.3V5c0-1.39 1.56-2.7 3.2-2.7 2.55 0 3.13 1.55 3.2 1.8v1.2H12c.81 0 2.7.22 2.7 2.2 0 2.09-2.25 2.2-2.7 2.2h-2V11h2c2.08 0 4-1.16 4-3.5C16 5.06 14.08 4 12 4z"/>'}},w={},x=function(e,t,o){var r=p(e.ownerDocument),n=e.appendChild(r("style",{type:"text/css"}));n.styleSheet?n.styleSheet.cssText=m:n.appendChild(e.ownerDocument.createTextNode(m));var a,l,d=r("a",{className:"btn",href:t.href,target:"_blank",innerHTML:(a=t["data-icon"],l=/^large$/i.test(t["data-size"])?16:14,a=(""+a).toLowerCase().replace(/^octicon-/,""),{}.hasOwnProperty.call(v,a)||(a="mark-github"),'<svg version="1.1" width="'+l*v[a].width/v[a].height+'" height="'+l+'" viewBox="0 0 '+v[a].width+" "+v[a].height+'" class="octicon octicon-'+a+'" aria-hidden="true">'+v[a].path+"</svg>"),"aria-label":t["aria-label"]||void 0},[" ",r("span",{},[t["data-text"]||""])]);/\.github\.com$/.test("."+d.hostname)?/^https?:\/\/((gist\.)?github\.com\/[^\/?#]+\/[^\/?#]+\/archive\/|github\.com\/[^\/?#]+\/[^\/?#]+\/releases\/download\/|codeload\.github\.com\/)/.test(d.href)&&(d.target="_top"):(d.href="#",d.target="_self");var u,h,g,x,y=e.appendChild(r("div",{className:"widget"+(/^large$/i.test(t["data-size"])?" lg":"")},[d]));/^(true|1)$/i.test(t["data-show-count"])&&"github.com"===d.hostname&&(u=d.pathname.replace(/^(?!\/)/,"/").match(/^\/([^\/?#]+)(?:\/([^\/?#]+)(?:\/(?:(subscription)|(fork)|(issues)|([^\/?#]+)))?)?(?:[\/?#]|$)/))&&!u[6]?(u[2]?(h="/repos/"+u[1]+"/"+u[2],u[3]?(x="subscribers_count",g="watchers"):u[4]?(x="forks_count",g="network"):u[5]?(x="open_issues_count",g="issues"):(x="stargazers_count",g="stargazers")):(h="/users/"+u[1],g=x="followers"),function(e,t){var o=w[e]||(w[e]=[]);if(!(o.push(t)>1)){var r=b(function(){for(delete w[e];t=o.shift();)t.apply(null,arguments)});if(c){var n=new i;s(n,"abort",r),s(n,"error",r),s(n,"load",function(){var e;try{e=JSON.parse(n.responseText)}catch(e){return void r(e)}r(200!==n.status,e)}),n.open("GET",e),n.send()}else{var a=this||window;a._=function(e){a._=null,r(200!==e.meta.status,e.data)};var l=p(a.document)("script",{async:!0,src:e+(/\?/.test(e)?"&":"?")+"callback=_"}),d=function(){a._&&a._({meta:{}})};s(l,"load",d),s(l,"error",d),l.readyState&&f(l,/de|m/,d),a.document.getElementsByTagName("head")[0].appendChild(l)}}}.call(this,"https://api.github.com"+h,function(e,t){if(!e){var n=t[x];y.appendChild(r("a",{className:"social-count",href:t.html_url+"/"+g,target:"_blank","aria-label":n+" "+x.replace(/_count$/,"").replace("_"," ").slice(0,n<2?-1:void 0)+" on GitHub"},[r("b"),r("i"),r("span",{},[(""+n).replace(/\B(?=(\d{3})+(?!\d))/g,",")])]))}o&&o(y)})):o&&o(y)},y=window.devicePixelRatio||1,C=function(e){return(y>1?n.ceil(n.round(e*y)/y*2)/2:n.ceil(e))||0},F=function(e,t){e.style.width=t[0]+"px",e.style.height=t[1]+"px"},k=function(t,r){if(null!=t&&null!=r)if(t.getAttribute&&(t=function(e){for(var t={href:e.href,title:e.title,"aria-label":e.getAttribute("aria-label")},o=["icon","text","size","show-count"],r=0,n=o.length;r<n;r++){var a="data-"+o[r];t[a]=e.getAttribute(a)}return null==t["data-text"]&&(t["data-text"]=e.textContent||e.innerText),t}(t)),d){var a=g("span",{title:t.title||void 0});x(a.attachShadow({mode:"closed"}),t,function(){r(a)})}else{var i=g("iframe",{src:"javascript:0",title:t.title||void 0,allowtransparency:!0,scrolling:"no",frameBorder:0});F(i,[0,0]),i.style.border="none";var c=function(){var a,d=i.contentWindow;try{a=d.document.body}catch(t){return void e.body.appendChild(i.parentNode.removeChild(i))}u(i,"load",c),x.call(d,a,t,function(e){var a=function(e){var t=e.offsetWidth,o=e.offsetHeight;if(e.getBoundingClientRect){var r=e.getBoundingClientRect();t=n.max(t,C(r.width)),o=n.max(o,C(r.height))}return[t,o]}(e);i.parentNode.removeChild(i),h(i,"load",function(){F(i,a)}),i.src=l+"#"+(i.name=function(e){var t=[];for(var r in e){var n=e[r];null!=n&&t.push(o(r)+"="+o(n))}return t.join("&")}(t)),r(i)})};s(i,"load",c),e.body.appendChild(i)}};t.protocol+"//"+t.host+t.pathname===l?x(e.body,function(e){for(var t={},o=e.split("&"),n=0,a=o.length;n<a;n++){var i=o[n];if(""!==i){var l=i.split("=");t[r(l[0])]=null!=l[1]?r(l.slice(1).join("=")):void 0}}return t}(window.name||t.hash.replace(/^#/,""))):function(t){if(/m/.test(e.readyState)||!/g/.test(e.readyState)&&!e.documentElement.doScroll)setTimeout(t);else if(e.addEventListener){var o=b(t);h(e,"DOMContentLoaded",o),h(window,"load",o)}else f(e,/m/,t)}(function(){for(var t=e.querySelectorAll?e.querySelectorAll("a.github-button"):function(){for(var t=[],o=e.getElementsByTagName("a"),r=0,n=o.length;r<n;r++)~(" "+o[r].className+" ").replace(/[ \t\n\f\r]+/g," ").indexOf(" github-button ")&&t.push(o[r]);return t}(),o=0,r=t.length;o<r;o++)!function(e){k(e,function(t){e.parentNode.replaceChild(t,e)})}(t[o])})};
|
70 |
-
|
71 |
-
|
72 |
-
function onLoad() {
|
73 |
-
addIcon();
|
74 |
-
addCustomFooter();
|
75 |
-
addGithubButton();
|
76 |
-
parseGithubButtons();
|
77 |
-
}
|
78 |
-
|
79 |
-
window.addEventListener("load", onLoad);
|
|
|
|
|
|
|
|
|
|
|
|
|
|
|
|
|
|
|
|
|
|
|
|
|
|
|
|
|
|
|
|
|
|
|
|
|
|
|
|
|
|
|
|
|
|
|
|
|
|
|
|
|
|
|
|
|
|
|
|
|
|
|
|
|
|
|
|
|
|
|
|
|
|
|
|
|
|
|
|
|
|
|
|
|
|
|
|
|
|
|
|
|
|
|
|
|
|
|
|
|
|
|
|
|
|
|
|
|
|
|
|
|
|
|
|
|
|
|
|
|
|
|
|
|
|
|
|
|
|
|
|
|
|
|
|
|
|
|
|
|
|
|
|
|
|
|
|
|
|
|
|
|
|
|
|
|
|
|
|
server/transformers/docs/source/_static/js/huggingface_logo.svg
DELETED
server/transformers/docs/source/benchmarks.md
DELETED
@@ -1,54 +0,0 @@
|
|
1 |
-
# Benchmarks
|
2 |
-
|
3 |
-
This section is dedicated to the Benchmarks done by the library, both by maintainers, contributors and users. These
|
4 |
-
benchmark will help keep track of the preformance improvements that are brought to our models across versions.
|
5 |
-
|
6 |
-
## Benchmarking all models for inference
|
7 |
-
|
8 |
-
As of version 2.1 we have benchmarked all models for inference, across many different settings: using PyTorch, with
|
9 |
-
and without TorchScript, using TensorFlow, with and without XLA. All of those tests were done across CPUs (except for
|
10 |
-
TensorFlow XLA) and GPUs.
|
11 |
-
|
12 |
-
The approach is detailed in the [following blogpost](https://medium.com/huggingface/benchmarking-transformers-pytorch-and-tensorflow-e2917fb891c2)
|
13 |
-
|
14 |
-
The results are available [here](https://docs.google.com/spreadsheets/d/1sryqufw2D0XlUH4sq3e9Wnxu5EAQkaohzrJbd5HdQ_w/edit?usp=sharing).
|
15 |
-
|
16 |
-
## TF2 with mixed precision, XLA, Distribution (@tlkh)
|
17 |
-
|
18 |
-
This work was done by [Timothy Liu](https://github.com/tlkh).
|
19 |
-
|
20 |
-
There are very positive results to be gained from the various TensorFlow 2.0 features:
|
21 |
-
|
22 |
-
- Automatic Mixed Precision (AMP)
|
23 |
-
- XLA compiler
|
24 |
-
- Distribution strategies (multi-GPU)
|
25 |
-
|
26 |
-
The benefits are listed here (tested on CoLA, MRPC, SST-2):
|
27 |
-
|
28 |
-
- AMP: Between 1.4x to 1.6x decrease in overall time without change in batch size
|
29 |
-
- AMP+XLA: Up to 2.5x decrease in overall time on SST-2 (larger dataset)
|
30 |
-
- Distribution: Between 1.4x to 3.4x decrease in overall time on 4xV100
|
31 |
-
- Combined: Up to 5.7x decrease in overall training time, or 9.1x training throughput
|
32 |
-
|
33 |
-
The model quality (measured by the validation accuracy) fluctuates slightly. Taking an average of 4 training runs
|
34 |
-
on a single GPU gives the following results:
|
35 |
-
|
36 |
-
- CoLA: AMP results in slighter lower acc (0.820 vs 0.824)
|
37 |
-
- MRPC: AMP results in lower acc (0.823 vs 0.835)
|
38 |
-
- SST-2: AMP results in slighter lower acc (0.918 vs 0.922)
|
39 |
-
|
40 |
-
However, in a distributed setting with 4xV100 (4x batch size), AMP can yield in better results:
|
41 |
-
|
42 |
-
CoLA: AMP results in higher acc (0.828 vs 0.812)
|
43 |
-
MRPC: AMP results in lower acc (0.817 vs 0.827)
|
44 |
-
SST-2: AMP results in slightly lower acc (0.926 vs 0.929)
|
45 |
-
|
46 |
-
The benchmark script is available [here](https://github.com/NVAITC/benchmarking/blob/master/tf2/bert_dist.py).
|
47 |
-
|
48 |
-
Note: on some tasks (e.g. MRPC), the dataset is too small. The overhead due to the model compilation with XLA as well
|
49 |
-
as the distribution strategy setup does not speed things up. The XLA compile time is also the reason why although throughput
|
50 |
-
can increase a lot (e.g. 2.7x for single GPU), overall (end-to-end) training speed-up is not as fast (as low as 1.4x)
|
51 |
-
|
52 |
-
The benefits as seen on SST-2 (larger dataset) is much clear.
|
53 |
-
|
54 |
-
All results can be seen on this [Google Sheet](https://docs.google.com/spreadsheets/d/1538MN224EzjbRL239sqSiUy6YY-rAjHyXhTzz_Zptls/edit#gid=960868445).
|
|
|
|
|
|
|
|
|
|
|
|
|
|
|
|
|
|
|
|
|
|
|
|
|
|
|
|
|
|
|
|
|
|
|
|
|
|
|
|
|
|
|
|
|
|
|
|
|
|
|
|
|
|
|
|
|
|
|
|
|
|
|
|
|
|
|
|
|
|
|
|
|
|
|
|
|
|
|
|
|
|
|
|
|
|
|
|
|
|
|
|
|
|
|
|
|
|
|
|
|
|
|
|
|
|
|
|
|
|
server/transformers/docs/source/bertology.rst
DELETED
@@ -1,18 +0,0 @@
|
|
1 |
-
BERTology
|
2 |
-
---------
|
3 |
-
|
4 |
-
There is a growing field of study concerned with investigating the inner working of large-scale transformers like BERT (that some call "BERTology"). Some good examples of this field are:
|
5 |
-
|
6 |
-
|
7 |
-
* BERT Rediscovers the Classical NLP Pipeline by Ian Tenney, Dipanjan Das, Ellie Pavlick: https://arxiv.org/abs/1905.05950
|
8 |
-
* Are Sixteen Heads Really Better than One? by Paul Michel, Omer Levy, Graham Neubig: https://arxiv.org/abs/1905.10650
|
9 |
-
* What Does BERT Look At? An Analysis of BERT's Attention by Kevin Clark, Urvashi Khandelwal, Omer Levy, Christopher D. Manning: https://arxiv.org/abs/1906.04341
|
10 |
-
|
11 |
-
In order to help this new field develop, we have included a few additional features in the BERT/GPT/GPT-2 models to help people access the inner representations, mainly adapted from the great work of Paul Michel (https://arxiv.org/abs/1905.10650):
|
12 |
-
|
13 |
-
|
14 |
-
* accessing all the hidden-states of BERT/GPT/GPT-2,
|
15 |
-
* accessing all the attention weights for each head of BERT/GPT/GPT-2,
|
16 |
-
* retrieving heads output values and gradients to be able to compute head importance score and prune head as explained in https://arxiv.org/abs/1905.10650.
|
17 |
-
|
18 |
-
To help you understand and use these features, we have added a specific example script: `bertology.py <https://github.com/huggingface/transformers/blob/master/examples/run_bertology.py>`_ while extract information and prune a model pre-trained on GLUE.
|
|
|
|
|
|
|
|
|
|
|
|
|
|
|
|
|
|
|
|
|
|
|
|
|
|
|
|
|
|
|
|
|
|
|
|
|
|
server/transformers/docs/source/conf.py
DELETED
@@ -1,188 +0,0 @@
|
|
1 |
-
# -*- coding: utf-8 -*-
|
2 |
-
#
|
3 |
-
# Configuration file for the Sphinx documentation builder.
|
4 |
-
#
|
5 |
-
# This file does only contain a selection of the most common options. For a
|
6 |
-
# full list see the documentation:
|
7 |
-
# http://www.sphinx-doc.org/en/master/config
|
8 |
-
|
9 |
-
# -- Path setup --------------------------------------------------------------
|
10 |
-
|
11 |
-
# If extensions (or modules to document with autodoc) are in another directory,
|
12 |
-
# add these directories to sys.path here. If the directory is relative to the
|
13 |
-
# documentation root, use os.path.abspath to make it absolute, like shown here.
|
14 |
-
#
|
15 |
-
import os
|
16 |
-
import sys
|
17 |
-
sys.path.insert(0, os.path.abspath('../../src'))
|
18 |
-
|
19 |
-
|
20 |
-
# -- Project information -----------------------------------------------------
|
21 |
-
|
22 |
-
project = u'transformers'
|
23 |
-
copyright = u'2019, huggingface'
|
24 |
-
author = u'huggingface'
|
25 |
-
|
26 |
-
# The short X.Y version
|
27 |
-
version = u''
|
28 |
-
# The full version, including alpha/beta/rc tags
|
29 |
-
release = u'2.3.0'
|
30 |
-
|
31 |
-
|
32 |
-
# -- General configuration ---------------------------------------------------
|
33 |
-
|
34 |
-
# If your documentation needs a minimal Sphinx version, state it here.
|
35 |
-
#
|
36 |
-
# needs_sphinx = '1.0'
|
37 |
-
|
38 |
-
# Add any Sphinx extension module names here, as strings. They can be
|
39 |
-
# extensions coming with Sphinx (named 'sphinx.ext.*') or your custom
|
40 |
-
# ones.
|
41 |
-
extensions = [
|
42 |
-
'sphinx.ext.autodoc',
|
43 |
-
'sphinx.ext.coverage',
|
44 |
-
'sphinx.ext.napoleon',
|
45 |
-
'recommonmark',
|
46 |
-
'sphinx.ext.viewcode',
|
47 |
-
'sphinx_markdown_tables'
|
48 |
-
]
|
49 |
-
|
50 |
-
# Add any paths that contain templates here, relative to this directory.
|
51 |
-
templates_path = ['_templates']
|
52 |
-
|
53 |
-
# The suffix(es) of source filenames.
|
54 |
-
# You can specify multiple suffix as a list of string:
|
55 |
-
#
|
56 |
-
source_suffix = ['.rst', '.md']
|
57 |
-
# source_suffix = '.rst'
|
58 |
-
|
59 |
-
# The master toctree document.
|
60 |
-
master_doc = 'index'
|
61 |
-
|
62 |
-
# The language for content autogenerated by Sphinx. Refer to documentation
|
63 |
-
# for a list of supported languages.
|
64 |
-
#
|
65 |
-
# This is also used if you do content translation via gettext catalogs.
|
66 |
-
# Usually you set "language" from the command line for these cases.
|
67 |
-
language = None
|
68 |
-
|
69 |
-
# List of patterns, relative to source directory, that match files and
|
70 |
-
# directories to ignore when looking for source files.
|
71 |
-
# This pattern also affects html_static_path and html_extra_path.
|
72 |
-
exclude_patterns = [u'_build', 'Thumbs.db', '.DS_Store']
|
73 |
-
|
74 |
-
# The name of the Pygments (syntax highlighting) style to use.
|
75 |
-
pygments_style = None
|
76 |
-
|
77 |
-
|
78 |
-
# -- Options for HTML output -------------------------------------------------
|
79 |
-
|
80 |
-
# The theme to use for HTML and HTML Help pages. See the documentation for
|
81 |
-
# a list of builtin themes.
|
82 |
-
#
|
83 |
-
html_theme = 'sphinx_rtd_theme'
|
84 |
-
|
85 |
-
# Theme options are theme-specific and customize the look and feel of a theme
|
86 |
-
# further. For a list of options available for each theme, see the
|
87 |
-
# documentation.
|
88 |
-
#
|
89 |
-
html_theme_options = {
|
90 |
-
'analytics_id': 'UA-83738774-2'
|
91 |
-
}
|
92 |
-
|
93 |
-
# Add any paths that contain custom static files (such as style sheets) here,
|
94 |
-
# relative to this directory. They are copied after the builtin static files,
|
95 |
-
# so a file named "default.css" will overwrite the builtin "default.css".
|
96 |
-
html_static_path = ['_static']
|
97 |
-
|
98 |
-
# Custom sidebar templates, must be a dictionary that maps document names
|
99 |
-
# to template names.
|
100 |
-
#
|
101 |
-
# The default sidebars (for documents that don't match any pattern) are
|
102 |
-
# defined by theme itself. Builtin themes are using these templates by
|
103 |
-
# default: ``['localtoc.html', 'relations.html', 'sourcelink.html',
|
104 |
-
# 'searchbox.html']``.
|
105 |
-
#
|
106 |
-
# html_sidebars = {}
|
107 |
-
|
108 |
-
|
109 |
-
# -- Options for HTMLHelp output ---------------------------------------------
|
110 |
-
|
111 |
-
# Output file base name for HTML help builder.
|
112 |
-
htmlhelp_basename = 'transformersdoc'
|
113 |
-
|
114 |
-
|
115 |
-
# -- Options for LaTeX output ------------------------------------------------
|
116 |
-
|
117 |
-
latex_elements = {
|
118 |
-
# The paper size ('letterpaper' or 'a4paper').
|
119 |
-
#
|
120 |
-
# 'papersize': 'letterpaper',
|
121 |
-
|
122 |
-
# The font size ('10pt', '11pt' or '12pt').
|
123 |
-
#
|
124 |
-
# 'pointsize': '10pt',
|
125 |
-
|
126 |
-
# Additional stuff for the LaTeX preamble.
|
127 |
-
#
|
128 |
-
# 'preamble': '',
|
129 |
-
|
130 |
-
# Latex figure (float) alignment
|
131 |
-
#
|
132 |
-
# 'figure_align': 'htbp',
|
133 |
-
}
|
134 |
-
|
135 |
-
# Grouping the document tree into LaTeX files. List of tuples
|
136 |
-
# (source start file, target name, title,
|
137 |
-
# author, documentclass [howto, manual, or own class]).
|
138 |
-
latex_documents = [
|
139 |
-
(master_doc, 'transformers.tex', u'transformers Documentation',
|
140 |
-
u'huggingface', 'manual'),
|
141 |
-
]
|
142 |
-
|
143 |
-
|
144 |
-
# -- Options for manual page output ------------------------------------------
|
145 |
-
|
146 |
-
# One entry per manual page. List of tuples
|
147 |
-
# (source start file, name, description, authors, manual section).
|
148 |
-
man_pages = [
|
149 |
-
(master_doc, 'transformers', u'transformers Documentation',
|
150 |
-
[author], 1)
|
151 |
-
]
|
152 |
-
|
153 |
-
|
154 |
-
# -- Options for Texinfo output ----------------------------------------------
|
155 |
-
|
156 |
-
# Grouping the document tree into Texinfo files. List of tuples
|
157 |
-
# (source start file, target name, title, author,
|
158 |
-
# dir menu entry, description, category)
|
159 |
-
texinfo_documents = [
|
160 |
-
(master_doc, 'transformers', u'transformers Documentation',
|
161 |
-
author, 'transformers', 'One line description of project.',
|
162 |
-
'Miscellaneous'),
|
163 |
-
]
|
164 |
-
|
165 |
-
|
166 |
-
# -- Options for Epub output -------------------------------------------------
|
167 |
-
|
168 |
-
# Bibliographic Dublin Core info.
|
169 |
-
epub_title = project
|
170 |
-
|
171 |
-
# The unique identifier of the text. This can be a ISBN number
|
172 |
-
# or the project homepage.
|
173 |
-
#
|
174 |
-
# epub_identifier = ''
|
175 |
-
|
176 |
-
# A unique identification for the text.
|
177 |
-
#
|
178 |
-
# epub_uid = ''
|
179 |
-
|
180 |
-
# A list of files that should not be packed into the epub file.
|
181 |
-
epub_exclude_files = ['search.html']
|
182 |
-
|
183 |
-
def setup(app):
|
184 |
-
app.add_stylesheet('css/huggingface.css')
|
185 |
-
app.add_stylesheet('css/code-snippets.css')
|
186 |
-
app.add_js_file('js/custom.js')
|
187 |
-
|
188 |
-
# -- Extension configuration -------------------------------------------------
|
|
|
|
|
|
|
|
|
|
|
|
|
|
|
|
|
|
|
|
|
|
|
|
|
|
|
|
|
|
|
|
|
|
|
|
|
|
|
|
|
|
|
|
|
|
|
|
|
|
|
|
|
|
|
|
|
|
|
|
|
|
|
|
|
|
|
|
|
|
|
|
|
|
|
|
|
|
|
|
|
|
|
|
|
|
|
|
|
|
|
|
|
|
|
|
|
|
|
|
|
|
|
|
|
|
|
|
|
|
|
|
|
|
|
|
|
|
|
|
|
|
|
|
|
|
|
|
|
|
|
|
|
|
|
|
|
|
|
|
|
|
|
|
|
|
|
|
|
|
|
|
|
|
|
|
|
|
|
|
|
|
|
|
|
|
|
|
|
|
|
|
|
|
|
|
|
|
|
|
|
|
|
|
|
|
|
|
|
|
|
|
|
|
|
|
|
|
|
|
|
|
|
|
|
|
|
|
|
|
|
|
|
|
|
|
|
|
|
|
|
|
|
|
|
|
|
|
|
|
|
|
|
|
|
|
|
|
|
|
|
|
|
|
|
|
|
|
|
|
|
|
|
|
|
|
|
|
|
|
|
|
|
|
|
|
|
|
|
|
|
|
|
|
|
|
|
|
|
|
|
|
|
|
|
|
|
|
|
|
|
|
|
|
|
|
|
|
|
|
|
|
|
|
|
|
|
|
|
|
|
|
|
|
|
|
|
|
|
|
|
|
|
|
|
|
|
|
|
|
|
|
|
|
|
|
|
|
|
|
|
|
|
|
|
|
|
|
|
|
|
|
|
|
|
|
|
|
|
|
|
|
|
|
|
|
|
|
|
|
|
|
|
|
|
|
|
|
server/transformers/docs/source/converting_tensorflow_models.rst
DELETED
@@ -1,137 +0,0 @@
|
|
1 |
-
Converting Tensorflow Checkpoints
|
2 |
-
================================================
|
3 |
-
|
4 |
-
A command-line interface is provided to convert original Bert/GPT/GPT-2/Transformer-XL/XLNet/XLM checkpoints in models than be loaded using the ``from_pretrained`` methods of the library.
|
5 |
-
|
6 |
-
.. note::
|
7 |
-
Since 2.3.0 the conversion script is now part of the transformers CLI (**transformers-cli**)
|
8 |
-
available in any transformers >= 2.3.0 installation.
|
9 |
-
|
10 |
-
The documentation below reflects the **transformers-cli convert** command format.
|
11 |
-
|
12 |
-
BERT
|
13 |
-
^^^^
|
14 |
-
|
15 |
-
You can convert any TensorFlow checkpoint for BERT (in particular `the pre-trained models released by Google <https://github.com/google-research/bert#pre-trained-models>`_\ ) in a PyTorch save file by using the `convert_tf_checkpoint_to_pytorch.py <https://github.com/huggingface/transformers/blob/master/transformers/convert_tf_checkpoint_to_pytorch.py>`_ script.
|
16 |
-
|
17 |
-
This CLI takes as input a TensorFlow checkpoint (three files starting with ``bert_model.ckpt``\ ) and the associated configuration file (\ ``bert_config.json``\ ), and creates a PyTorch model for this configuration, loads the weights from the TensorFlow checkpoint in the PyTorch model and saves the resulting model in a standard PyTorch save file that can be imported using ``torch.load()`` (see examples in `run_bert_extract_features.py <https://github.com/huggingface/pytorch-pretrained-BERT/tree/master/examples/run_bert_extract_features.py>`_\ , `run_bert_classifier.py <https://github.com/huggingface/pytorch-pretrained-BERT/tree/master/examples/run_bert_classifier.py>`_ and `run_bert_squad.py <https://github.com/huggingface/pytorch-pretrained-BERT/tree/master/examples/run_bert_squad.py>`_\ ).
|
18 |
-
|
19 |
-
You only need to run this conversion script **once** to get a PyTorch model. You can then disregard the TensorFlow checkpoint (the three files starting with ``bert_model.ckpt``\ ) but be sure to keep the configuration file (\ ``bert_config.json``\ ) and the vocabulary file (\ ``vocab.txt``\ ) as these are needed for the PyTorch model too.
|
20 |
-
|
21 |
-
To run this specific conversion script you will need to have TensorFlow and PyTorch installed (\ ``pip install tensorflow``\ ). The rest of the repository only requires PyTorch.
|
22 |
-
|
23 |
-
Here is an example of the conversion process for a pre-trained ``BERT-Base Uncased`` model:
|
24 |
-
|
25 |
-
.. code-block:: shell
|
26 |
-
|
27 |
-
export BERT_BASE_DIR=/path/to/bert/uncased_L-12_H-768_A-12
|
28 |
-
|
29 |
-
<<<<<<< HEAD
|
30 |
-
transformers-cli --model_type bert \
|
31 |
-
=======
|
32 |
-
transformers-cli convert --model_type bert \
|
33 |
-
>>>>>>> bfec203d4ed95255619e7e2f28c9040744a16232
|
34 |
-
--tf_checkpoint $BERT_BASE_DIR/bert_model.ckpt \
|
35 |
-
--config $BERT_BASE_DIR/bert_config.json \
|
36 |
-
--pytorch_dump_output $BERT_BASE_DIR/pytorch_model.bin
|
37 |
-
|
38 |
-
You can download Google's pre-trained models for the conversion `here <https://github.com/google-research/bert#pre-trained-models>`__.
|
39 |
-
|
40 |
-
OpenAI GPT
|
41 |
-
^^^^^^^^^^
|
42 |
-
|
43 |
-
Here is an example of the conversion process for a pre-trained OpenAI GPT model, assuming that your NumPy checkpoint save as the same format than OpenAI pretrained model (see `here <https://github.com/openai/finetune-transformer-lm>`__\ )
|
44 |
-
|
45 |
-
.. code-block:: shell
|
46 |
-
|
47 |
-
export OPENAI_GPT_CHECKPOINT_FOLDER_PATH=/path/to/openai/pretrained/numpy/weights
|
48 |
-
|
49 |
-
<<<<<<< HEAD
|
50 |
-
transformers-cli --model_type gpt \
|
51 |
-
=======
|
52 |
-
transformers-cli convert --model_type gpt \
|
53 |
-
>>>>>>> bfec203d4ed95255619e7e2f28c9040744a16232
|
54 |
-
--tf_checkpoint $OPENAI_GPT_CHECKPOINT_FOLDER_PATH \
|
55 |
-
--pytorch_dump_output $PYTORCH_DUMP_OUTPUT \
|
56 |
-
[--config OPENAI_GPT_CONFIG] \
|
57 |
-
[--finetuning_task_name OPENAI_GPT_FINETUNED_TASK] \
|
58 |
-
|
59 |
-
|
60 |
-
OpenAI GPT-2
|
61 |
-
^^^^^^^^^^^^
|
62 |
-
|
63 |
-
Here is an example of the conversion process for a pre-trained OpenAI GPT-2 model (see `here <https://github.com/openai/gpt-2>`__\ )
|
64 |
-
|
65 |
-
.. code-block:: shell
|
66 |
-
|
67 |
-
export OPENAI_GPT2_CHECKPOINT_PATH=/path/to/gpt2/pretrained/weights
|
68 |
-
|
69 |
-
<<<<<<< HEAD
|
70 |
-
transformers-cli --model_type gpt2 \
|
71 |
-
=======
|
72 |
-
transformers-cli convert --model_type gpt2 \
|
73 |
-
>>>>>>> bfec203d4ed95255619e7e2f28c9040744a16232
|
74 |
-
--tf_checkpoint $OPENAI_GPT2_CHECKPOINT_PATH \
|
75 |
-
--pytorch_dump_output $PYTORCH_DUMP_OUTPUT \
|
76 |
-
[--config OPENAI_GPT2_CONFIG] \
|
77 |
-
[--finetuning_task_name OPENAI_GPT2_FINETUNED_TASK]
|
78 |
-
|
79 |
-
Transformer-XL
|
80 |
-
^^^^^^^^^^^^^^
|
81 |
-
|
82 |
-
Here is an example of the conversion process for a pre-trained Transformer-XL model (see `here <https://github.com/kimiyoung/transformer-xl/tree/master/tf#obtain-and-evaluate-pretrained-sota-models>`__\ )
|
83 |
-
|
84 |
-
.. code-block:: shell
|
85 |
-
|
86 |
-
export TRANSFO_XL_CHECKPOINT_FOLDER_PATH=/path/to/transfo/xl/checkpoint
|
87 |
-
|
88 |
-
<<<<<<< HEAD
|
89 |
-
transformers-cli --model_type transfo_xl \
|
90 |
-
=======
|
91 |
-
transformers-cli convert --model_type transfo_xl \
|
92 |
-
>>>>>>> bfec203d4ed95255619e7e2f28c9040744a16232
|
93 |
-
--tf_checkpoint $TRANSFO_XL_CHECKPOINT_FOLDER_PATH \
|
94 |
-
--pytorch_dump_output $PYTORCH_DUMP_OUTPUT \
|
95 |
-
[--config TRANSFO_XL_CONFIG] \
|
96 |
-
[--finetuning_task_name TRANSFO_XL_FINETUNED_TASK]
|
97 |
-
|
98 |
-
|
99 |
-
XLNet
|
100 |
-
^^^^^
|
101 |
-
|
102 |
-
Here is an example of the conversion process for a pre-trained XLNet model:
|
103 |
-
|
104 |
-
.. code-block:: shell
|
105 |
-
|
106 |
-
export TRANSFO_XL_CHECKPOINT_PATH=/path/to/xlnet/checkpoint
|
107 |
-
export TRANSFO_XL_CONFIG_PATH=/path/to/xlnet/config
|
108 |
-
|
109 |
-
<<<<<<< HEAD
|
110 |
-
transformers-cli --model_type xlnet \
|
111 |
-
=======
|
112 |
-
transformers-cli convert --model_type xlnet \
|
113 |
-
>>>>>>> bfec203d4ed95255619e7e2f28c9040744a16232
|
114 |
-
--tf_checkpoint $TRANSFO_XL_CHECKPOINT_PATH \
|
115 |
-
--config $TRANSFO_XL_CONFIG_PATH \
|
116 |
-
--pytorch_dump_output $PYTORCH_DUMP_OUTPUT \
|
117 |
-
[--finetuning_task_name XLNET_FINETUNED_TASK] \
|
118 |
-
|
119 |
-
|
120 |
-
XLM
|
121 |
-
^^^
|
122 |
-
|
123 |
-
Here is an example of the conversion process for a pre-trained XLM model:
|
124 |
-
|
125 |
-
.. code-block:: shell
|
126 |
-
|
127 |
-
export XLM_CHECKPOINT_PATH=/path/to/xlm/checkpoint
|
128 |
-
|
129 |
-
<<<<<<< HEAD
|
130 |
-
transformers-cli --model_type xlm \
|
131 |
-
=======
|
132 |
-
transformers-cli convert --model_type xlm \
|
133 |
-
>>>>>>> bfec203d4ed95255619e7e2f28c9040744a16232
|
134 |
-
--tf_checkpoint $XLM_CHECKPOINT_PATH \
|
135 |
-
--pytorch_dump_output $PYTORCH_DUMP_OUTPUT
|
136 |
-
[--config XML_CONFIG] \
|
137 |
-
[--finetuning_task_name XML_FINETUNED_TASK]
|
|
|
|
|
|
|
|
|
|
|
|
|
|
|
|
|
|
|
|
|
|
|
|
|
|
|
|
|
|
|
|
|
|
|
|
|
|
|
|
|
|
|
|
|
|
|
|
|
|
|
|
|
|
|
|
|
|
|
|
|
|
|
|
|
|
|
|
|
|
|
|
|
|
|
|
|
|
|
|
|
|
|
|
|
|
|
|
|
|
|
|
|
|
|
|
|
|
|
|
|
|
|
|
|
|
|
|
|
|
|
|
|
|
|
|
|
|
|
|
|
|
|
|
|
|
|
|
|
|
|
|
|
|
|
|
|
|
|
|
|
|
|
|
|
|
|
|
|
|
|
|
|
|
|
|
|
|
|
|
|
|
|
|
|
|
|
|
|
|
|
|
|
|
|
|
|
|
|
|
|
|
|
|
|
|
|
|
|
|
|
|
|
|
|
|
|
|
|
|
|
|
|
|
|
|
|
|
|
|
|
|
|
|
|
|
|
|
|
|
|
|
|
|
|
|
|
|
|
|
|
|
|
|
|
|
|
|
|
|
|
|
|
|
|
|
|
|
|
|
|
|
|
|
|
|
|
|
|
|
|
|
|
|
|
|
|
|
|
|
|
|
|
|
|
|
server/transformers/docs/source/examples.md
DELETED
@@ -1,801 +0,0 @@
|
|
1 |
-
# Examples
|
2 |
-
|
3 |
-
In this section a few examples are put together. All of these examples work for several models, making use of the very
|
4 |
-
similar API between the different models.
|
5 |
-
|
6 |
-
**Important**
|
7 |
-
To run the latest versions of the examples, you have to install from source and install some specific requirements for the examples.
|
8 |
-
Execute the following steps in a new virtual environment:
|
9 |
-
|
10 |
-
```bash
|
11 |
-
git clone https://github.com/huggingface/transformers
|
12 |
-
cd transformers
|
13 |
-
pip install .
|
14 |
-
pip install -r ./examples/requirements.txt
|
15 |
-
```
|
16 |
-
|
17 |
-
| Section | Description |
|
18 |
-
|----------------------------|------------------------------------------------------------------------------------------------------------------------------------------------------------|
|
19 |
-
| [TensorFlow 2.0 models on GLUE](#TensorFlow-2.0-Bert-models-on-GLUE) | Examples running BERT TensorFlow 2.0 model on the GLUE tasks.
|
20 |
-
| [Language Model fine-tuning](#language-model-fine-tuning) | Fine-tuning the library models for language modeling on a text dataset. Causal language modeling for GPT/GPT-2, masked language modeling for BERT/RoBERTa. |
|
21 |
-
| [Language Generation](#language-generation) | Conditional text generation using the auto-regressive models of the library: GPT, GPT-2, Transformer-XL and XLNet. |
|
22 |
-
| [GLUE](#glue) | Examples running BERT/XLM/XLNet/RoBERTa on the 9 GLUE tasks. Examples feature distributed training as well as half-precision. |
|
23 |
-
| [SQuAD](#squad) | Using BERT/RoBERTa/XLNet/XLM for question answering, examples with distributed training. |
|
24 |
-
| [Multiple Choice](#multiple-choice) | Examples running BERT/XLNet/RoBERTa on the SWAG/RACE/ARC tasks.
|
25 |
-
| [Named Entity Recognition](#named-entity-recognition) | Using BERT for Named Entity Recognition (NER) on the CoNLL 2003 dataset, examples with distributed training. |
|
26 |
-
| [XNLI](#xnli) | Examples running BERT/XLM on the XNLI benchmark. |
|
27 |
-
| [Adversarial evaluation of model performances](#adversarial-evaluation-of-model-performances) | Testing a model with adversarial evaluation of natural language
|
28 |
-
inference on the Heuristic Analysis for NLI Systems (HANS) dataset (McCoy et al., 2019.) |
|
29 |
-
|
30 |
-
## TensorFlow 2.0 Bert models on GLUE
|
31 |
-
|
32 |
-
Based on the script [`run_tf_glue.py`](https://github.com/huggingface/transformers/blob/master/examples/run_tf_glue.py).
|
33 |
-
|
34 |
-
Fine-tuning the library TensorFlow 2.0 Bert model for sequence classification on the MRPC task of the GLUE benchmark: [General Language Understanding Evaluation](https://gluebenchmark.com/).
|
35 |
-
|
36 |
-
This script has an option for mixed precision (Automatic Mixed Precision / AMP) to run models on Tensor Cores (NVIDIA Volta/Turing GPUs) and future hardware and an option for XLA, which uses the XLA compiler to reduce model runtime.
|
37 |
-
Options are toggled using `USE_XLA` or `USE_AMP` variables in the script.
|
38 |
-
These options and the below benchmark are provided by @tlkh.
|
39 |
-
|
40 |
-
Quick benchmarks from the script (no other modifications):
|
41 |
-
|
42 |
-
| GPU | Mode | Time (2nd epoch) | Val Acc (3 runs) |
|
43 |
-
| --------- | -------- | ----------------------- | ----------------------|
|
44 |
-
| Titan V | FP32 | 41s | 0.8438/0.8281/0.8333 |
|
45 |
-
| Titan V | AMP | 26s | 0.8281/0.8568/0.8411 |
|
46 |
-
| V100 | FP32 | 35s | 0.8646/0.8359/0.8464 |
|
47 |
-
| V100 | AMP | 22s | 0.8646/0.8385/0.8411 |
|
48 |
-
| 1080 Ti | FP32 | 55s | - |
|
49 |
-
|
50 |
-
Mixed precision (AMP) reduces the training time considerably for the same hardware and hyper-parameters (same batch size was used).
|
51 |
-
|
52 |
-
## Language model fine-tuning
|
53 |
-
|
54 |
-
Based on the script [`run_lm_finetuning.py`](https://github.com/huggingface/transformers/blob/master/examples/run_lm_finetuning.py).
|
55 |
-
|
56 |
-
Fine-tuning the library models for language modeling on a text dataset for GPT, GPT-2, BERT and RoBERTa (DistilBERT
|
57 |
-
to be added soon). GPT and GPT-2 are fine-tuned using a causal language modeling (CLM) loss while BERT and RoBERTa
|
58 |
-
are fine-tuned using a masked language modeling (MLM) loss.
|
59 |
-
|
60 |
-
Before running the following example, you should get a file that contains text on which the language model will be
|
61 |
-
fine-tuned. A good example of such text is the [WikiText-2 dataset](https://blog.einstein.ai/the-wikitext-long-term-dependency-language-modeling-dataset/).
|
62 |
-
|
63 |
-
We will refer to two different files: `$TRAIN_FILE`, which contains text for training, and `$TEST_FILE`, which contains
|
64 |
-
text that will be used for evaluation.
|
65 |
-
|
66 |
-
### GPT-2/GPT and causal language modeling
|
67 |
-
|
68 |
-
The following example fine-tunes GPT-2 on WikiText-2. We're using the raw WikiText-2 (no tokens were replaced before
|
69 |
-
the tokenization). The loss here is that of causal language modeling.
|
70 |
-
|
71 |
-
```bash
|
72 |
-
export TRAIN_FILE=/path/to/dataset/wiki.train.raw
|
73 |
-
export TEST_FILE=/path/to/dataset/wiki.test.raw
|
74 |
-
|
75 |
-
python run_lm_finetuning.py \
|
76 |
-
--output_dir=output \
|
77 |
-
--model_type=gpt2 \
|
78 |
-
--model_name_or_path=gpt2 \
|
79 |
-
--do_train \
|
80 |
-
--train_data_file=$TRAIN_FILE \
|
81 |
-
--do_eval \
|
82 |
-
--eval_data_file=$TEST_FILE
|
83 |
-
```
|
84 |
-
|
85 |
-
This takes about half an hour to train on a single K80 GPU and about one minute for the evaluation to run. It reaches
|
86 |
-
a score of ~20 perplexity once fine-tuned on the dataset.
|
87 |
-
|
88 |
-
### RoBERTa/BERT and masked language modeling
|
89 |
-
|
90 |
-
The following example fine-tunes RoBERTa on WikiText-2. Here too, we're using the raw WikiText-2. The loss is different
|
91 |
-
as BERT/RoBERTa have a bidirectional mechanism; we're therefore using the same loss that was used during their
|
92 |
-
pre-training: masked language modeling.
|
93 |
-
|
94 |
-
In accordance to the RoBERTa paper, we use dynamic masking rather than static masking. The model may, therefore, converge
|
95 |
-
slightly slower (over-fitting takes more epochs).
|
96 |
-
|
97 |
-
We use the `--mlm` flag so that the script may change its loss function.
|
98 |
-
|
99 |
-
```bash
|
100 |
-
export TRAIN_FILE=/path/to/dataset/wiki.train.raw
|
101 |
-
export TEST_FILE=/path/to/dataset/wiki.test.raw
|
102 |
-
|
103 |
-
python run_lm_finetuning.py \
|
104 |
-
--output_dir=output \
|
105 |
-
--model_type=roberta \
|
106 |
-
--model_name_or_path=roberta-base \
|
107 |
-
--do_train \
|
108 |
-
--train_data_file=$TRAIN_FILE \
|
109 |
-
--do_eval \
|
110 |
-
--eval_data_file=$TEST_FILE \
|
111 |
-
--mlm
|
112 |
-
```
|
113 |
-
|
114 |
-
## Language generation
|
115 |
-
|
116 |
-
Based on the script [`run_generation.py`](https://github.com/huggingface/transformers/blob/master/examples/run_generation.py).
|
117 |
-
|
118 |
-
Conditional text generation using the auto-regressive models of the library: GPT, GPT-2, Transformer-XL, XLNet, CTRL.
|
119 |
-
A similar script is used for our official demo [Write With Transfomer](https://transformer.huggingface.co), where you
|
120 |
-
can try out the different models available in the library.
|
121 |
-
|
122 |
-
Example usage:
|
123 |
-
|
124 |
-
```bash
|
125 |
-
python run_generation.py \
|
126 |
-
--model_type=gpt2 \
|
127 |
-
--model_name_or_path=gpt2
|
128 |
-
```
|
129 |
-
|
130 |
-
## GLUE
|
131 |
-
|
132 |
-
Based on the script [`run_glue.py`](https://github.com/huggingface/transformers/blob/master/examples/run_glue.py).
|
133 |
-
|
134 |
-
Fine-tuning the library models for sequence classification on the GLUE benchmark: [General Language Understanding
|
135 |
-
Evaluation](https://gluebenchmark.com/). This script can fine-tune the following models: BERT, XLM, XLNet and RoBERTa.
|
136 |
-
|
137 |
-
GLUE is made up of a total of 9 different tasks. We get the following results on the dev set of the benchmark with an
|
138 |
-
uncased BERT base model (the checkpoint `bert-base-uncased`). All experiments ran on 8 V100 GPUs with a total train
|
139 |
-
batch size of 24. Some of these tasks have a small dataset and training can lead to high variance in the results
|
140 |
-
between different runs. We report the median on 5 runs (with different seeds) for each of the metrics.
|
141 |
-
|
142 |
-
| Task | Metric | Result |
|
143 |
-
|-------|------------------------------|-------------|
|
144 |
-
| CoLA | Matthew's corr | 48.87 |
|
145 |
-
| SST-2 | Accuracy | 91.74 |
|
146 |
-
| MRPC | F1/Accuracy | 90.70/86.27 |
|
147 |
-
| STS-B | Person/Spearman corr. | 91.39/91.04 |
|
148 |
-
| QQP | Accuracy/F1 | 90.79/87.66 |
|
149 |
-
| MNLI | Matched acc./Mismatched acc. | 83.70/84.83 |
|
150 |
-
| QNLI | Accuracy | 89.31 |
|
151 |
-
| RTE | Accuracy | 71.43 |
|
152 |
-
| WNLI | Accuracy | 43.66 |
|
153 |
-
|
154 |
-
Some of these results are significantly different from the ones reported on the test set
|
155 |
-
of GLUE benchmark on the website. For QQP and WNLI, please refer to [FAQ #12](https://gluebenchmark.com/faq) on the webite.
|
156 |
-
|
157 |
-
Before running anyone of these GLUE tasks you should download the
|
158 |
-
[GLUE data](https://gluebenchmark.com/tasks) by running
|
159 |
-
[this script](https://gist.github.com/W4ngatang/60c2bdb54d156a41194446737ce03e2e)
|
160 |
-
and unpack it to some directory `$GLUE_DIR`.
|
161 |
-
|
162 |
-
```bash
|
163 |
-
export GLUE_DIR=/path/to/glue
|
164 |
-
export TASK_NAME=MRPC
|
165 |
-
|
166 |
-
python run_glue.py \
|
167 |
-
--model_type bert \
|
168 |
-
--model_name_or_path bert-base-cased \
|
169 |
-
--task_name $TASK_NAME \
|
170 |
-
--do_train \
|
171 |
-
--do_eval \
|
172 |
-
--do_lower_case \
|
173 |
-
--data_dir $GLUE_DIR/$TASK_NAME \
|
174 |
-
--max_seq_length 128 \
|
175 |
-
--per_gpu_train_batch_size 32 \
|
176 |
-
--learning_rate 2e-5 \
|
177 |
-
--num_train_epochs 3.0 \
|
178 |
-
--output_dir /tmp/$TASK_NAME/
|
179 |
-
```
|
180 |
-
|
181 |
-
where task name can be one of CoLA, SST-2, MRPC, STS-B, QQP, MNLI, QNLI, RTE, WNLI.
|
182 |
-
|
183 |
-
The dev set results will be present within the text file `eval_results.txt` in the specified output_dir.
|
184 |
-
In case of MNLI, since there are two separate dev sets (matched and mismatched), there will be a separate
|
185 |
-
output folder called `/tmp/MNLI-MM/` in addition to `/tmp/MNLI/`.
|
186 |
-
|
187 |
-
The code has not been tested with half-precision training with apex on any GLUE task apart from MRPC, MNLI,
|
188 |
-
CoLA, SST-2. The following section provides details on how to run half-precision training with MRPC. With that being
|
189 |
-
said, there shouldn’t be any issues in running half-precision training with the remaining GLUE tasks as well,
|
190 |
-
since the data processor for each task inherits from the base class DataProcessor.
|
191 |
-
|
192 |
-
### MRPC
|
193 |
-
|
194 |
-
#### Fine-tuning example
|
195 |
-
|
196 |
-
The following examples fine-tune BERT on the Microsoft Research Paraphrase Corpus (MRPC) corpus and runs in less
|
197 |
-
than 10 minutes on a single K-80 and in 27 seconds (!) on single tesla V100 16GB with apex installed.
|
198 |
-
|
199 |
-
Before running anyone of these GLUE tasks you should download the
|
200 |
-
[GLUE data](https://gluebenchmark.com/tasks) by running
|
201 |
-
[this script](https://gist.github.com/W4ngatang/60c2bdb54d156a41194446737ce03e2e)
|
202 |
-
and unpack it to some directory `$GLUE_DIR`.
|
203 |
-
|
204 |
-
```bash
|
205 |
-
export GLUE_DIR=/path/to/glue
|
206 |
-
|
207 |
-
python run_glue.py \
|
208 |
-
--model_type bert \
|
209 |
-
--model_name_or_path bert-base-cased \
|
210 |
-
--task_name MRPC \
|
211 |
-
--do_train \
|
212 |
-
--do_eval \
|
213 |
-
--do_lower_case \
|
214 |
-
--data_dir $GLUE_DIR/MRPC/ \
|
215 |
-
--max_seq_length 128 \
|
216 |
-
--per_gpu_train_batch_size 32 \
|
217 |
-
--learning_rate 2e-5 \
|
218 |
-
--num_train_epochs 3.0 \
|
219 |
-
--output_dir /tmp/mrpc_output/
|
220 |
-
```
|
221 |
-
|
222 |
-
Our test ran on a few seeds with [the original implementation hyper-
|
223 |
-
parameters](https://github.com/google-research/bert#sentence-and-sentence-pair-classification-tasks) gave evaluation
|
224 |
-
results between 84% and 88%.
|
225 |
-
|
226 |
-
#### Using Apex and mixed-precision
|
227 |
-
|
228 |
-
Using Apex and 16 bit precision, the fine-tuning on MRPC only takes 27 seconds. First install
|
229 |
-
[apex](https://github.com/NVIDIA/apex), then run the following example:
|
230 |
-
|
231 |
-
```bash
|
232 |
-
export GLUE_DIR=/path/to/glue
|
233 |
-
|
234 |
-
python run_glue.py \
|
235 |
-
--model_type bert \
|
236 |
-
--model_name_or_path bert-base-cased \
|
237 |
-
--task_name MRPC \
|
238 |
-
--do_train \
|
239 |
-
--do_eval \
|
240 |
-
--do_lower_case \
|
241 |
-
--data_dir $GLUE_DIR/MRPC/ \
|
242 |
-
--max_seq_length 128 \
|
243 |
-
--per_gpu_train_batch_size 32 \
|
244 |
-
--learning_rate 2e-5 \
|
245 |
-
--num_train_epochs 3.0 \
|
246 |
-
--output_dir /tmp/mrpc_output/ \
|
247 |
-
--fp16
|
248 |
-
```
|
249 |
-
|
250 |
-
#### Distributed training
|
251 |
-
|
252 |
-
Here is an example using distributed training on 8 V100 GPUs. The model used is the BERT whole-word-masking and it
|
253 |
-
reaches F1 > 92 on MRPC.
|
254 |
-
|
255 |
-
```bash
|
256 |
-
export GLUE_DIR=/path/to/glue
|
257 |
-
|
258 |
-
python -m torch.distributed.launch \
|
259 |
-
--nproc_per_node 8 run_glue.py \
|
260 |
-
--model_type bert \
|
261 |
-
--model_name_or_path bert-base-cased \
|
262 |
-
--task_name MRPC \
|
263 |
-
--do_train \
|
264 |
-
--do_eval \
|
265 |
-
--do_lower_case \
|
266 |
-
--data_dir $GLUE_DIR/MRPC/ \
|
267 |
-
--max_seq_length 128 \
|
268 |
-
--per_gpu_train_batch_size 8 \
|
269 |
-
--learning_rate 2e-5 \
|
270 |
-
--num_train_epochs 3.0 \
|
271 |
-
--output_dir /tmp/mrpc_output/
|
272 |
-
```
|
273 |
-
|
274 |
-
Training with these hyper-parameters gave us the following results:
|
275 |
-
|
276 |
-
```bash
|
277 |
-
acc = 0.8823529411764706
|
278 |
-
acc_and_f1 = 0.901702786377709
|
279 |
-
eval_loss = 0.3418912578906332
|
280 |
-
f1 = 0.9210526315789473
|
281 |
-
global_step = 174
|
282 |
-
loss = 0.07231863956341798
|
283 |
-
```
|
284 |
-
|
285 |
-
### MNLI
|
286 |
-
|
287 |
-
The following example uses the BERT-large, uncased, whole-word-masking model and fine-tunes it on the MNLI task.
|
288 |
-
|
289 |
-
```bash
|
290 |
-
export GLUE_DIR=/path/to/glue
|
291 |
-
|
292 |
-
python -m torch.distributed.launch \
|
293 |
-
--nproc_per_node 8 run_glue.py \
|
294 |
-
--model_type bert \
|
295 |
-
--model_name_or_path bert-base-cased \
|
296 |
-
--task_name mnli \
|
297 |
-
--do_train \
|
298 |
-
--do_eval \
|
299 |
-
--do_lower_case \
|
300 |
-
--data_dir $GLUE_DIR/MNLI/ \
|
301 |
-
--max_seq_length 128 \
|
302 |
-
--per_gpu_train_batch_size 8 \
|
303 |
-
--learning_rate 2e-5 \
|
304 |
-
--num_train_epochs 3.0 \
|
305 |
-
--output_dir output_dir \
|
306 |
-
```
|
307 |
-
|
308 |
-
The results are the following:
|
309 |
-
|
310 |
-
```bash
|
311 |
-
***** Eval results *****
|
312 |
-
acc = 0.8679706601466992
|
313 |
-
eval_loss = 0.4911287787382479
|
314 |
-
global_step = 18408
|
315 |
-
loss = 0.04755385363816904
|
316 |
-
|
317 |
-
***** Eval results *****
|
318 |
-
acc = 0.8747965825874695
|
319 |
-
eval_loss = 0.45516540421714036
|
320 |
-
global_step = 18408
|
321 |
-
loss = 0.04755385363816904
|
322 |
-
```
|
323 |
-
|
324 |
-
## Multiple Choice
|
325 |
-
|
326 |
-
Based on the script [`run_multiple_choice.py`]().
|
327 |
-
|
328 |
-
#### Fine-tuning on SWAG
|
329 |
-
Download [swag](https://github.com/rowanz/swagaf/tree/master/data) data
|
330 |
-
|
331 |
-
```bash
|
332 |
-
#training on 4 tesla V100(16GB) GPUS
|
333 |
-
export SWAG_DIR=/path/to/swag_data_dir
|
334 |
-
python ./examples/run_multiple_choice.py \
|
335 |
-
--model_type roberta \
|
336 |
-
--task_name swag \
|
337 |
-
--model_name_or_path roberta-base \
|
338 |
-
--do_train \
|
339 |
-
--do_eval \
|
340 |
-
--do_lower_case \
|
341 |
-
--data_dir $SWAG_DIR \
|
342 |
-
--learning_rate 5e-5 \
|
343 |
-
--num_train_epochs 3 \
|
344 |
-
--max_seq_length 80 \
|
345 |
-
--output_dir models_bert/swag_base \
|
346 |
-
--per_gpu_eval_batch_size=16 \
|
347 |
-
--per_gpu_train_batch_size=16 \
|
348 |
-
--gradient_accumulation_steps 2 \
|
349 |
-
--overwrite_output
|
350 |
-
```
|
351 |
-
Training with the defined hyper-parameters yields the following results:
|
352 |
-
```
|
353 |
-
***** Eval results *****
|
354 |
-
eval_acc = 0.8338998300509847
|
355 |
-
eval_loss = 0.44457291918821606
|
356 |
-
```
|
357 |
-
|
358 |
-
## SQuAD
|
359 |
-
|
360 |
-
Based on the script [`run_squad.py`](https://github.com/huggingface/transformers/blob/master/examples/run_squad.py).
|
361 |
-
|
362 |
-
#### Fine-tuning BERT on SQuAD1.0
|
363 |
-
|
364 |
-
This example code fine-tunes BERT on the SQuAD1.0 dataset. It runs in 24 min (with BERT-base) or 68 min (with BERT-large)
|
365 |
-
on a single tesla V100 16GB. The data for SQuAD can be downloaded with the following links and should be saved in a
|
366 |
-
$SQUAD_DIR directory.
|
367 |
-
|
368 |
-
* [train-v1.1.json](https://rajpurkar.github.io/SQuAD-explorer/dataset/train-v1.1.json)
|
369 |
-
* [dev-v1.1.json](https://rajpurkar.github.io/SQuAD-explorer/dataset/dev-v1.1.json)
|
370 |
-
* [evaluate-v1.1.py](https://github.com/allenai/bi-att-flow/blob/master/squad/evaluate-v1.1.py)
|
371 |
-
|
372 |
-
And for SQuAD2.0, you need to download:
|
373 |
-
|
374 |
-
- [train-v2.0.json](https://rajpurkar.github.io/SQuAD-explorer/dataset/train-v2.0.json)
|
375 |
-
- [dev-v2.0.json](https://rajpurkar.github.io/SQuAD-explorer/dataset/dev-v2.0.json)
|
376 |
-
- [evaluate-v2.0.py](https://worksheets.codalab.org/rest/bundles/0x6b567e1cf2e041ec80d7098f031c5c9e/contents/blob/)
|
377 |
-
|
378 |
-
```bash
|
379 |
-
export SQUAD_DIR=/path/to/SQUAD
|
380 |
-
|
381 |
-
python run_squad.py \
|
382 |
-
--model_type bert \
|
383 |
-
--model_name_or_path bert-base-cased \
|
384 |
-
--do_train \
|
385 |
-
--do_eval \
|
386 |
-
--do_lower_case \
|
387 |
-
--train_file $SQUAD_DIR/train-v1.1.json \
|
388 |
-
--predict_file $SQUAD_DIR/dev-v1.1.json \
|
389 |
-
--per_gpu_train_batch_size 12 \
|
390 |
-
--learning_rate 3e-5 \
|
391 |
-
--num_train_epochs 2.0 \
|
392 |
-
--max_seq_length 384 \
|
393 |
-
--doc_stride 128 \
|
394 |
-
--output_dir /tmp/debug_squad/
|
395 |
-
```
|
396 |
-
|
397 |
-
Training with the previously defined hyper-parameters yields the following results:
|
398 |
-
|
399 |
-
```bash
|
400 |
-
f1 = 88.52
|
401 |
-
exact_match = 81.22
|
402 |
-
```
|
403 |
-
|
404 |
-
#### Distributed training
|
405 |
-
|
406 |
-
|
407 |
-
Here is an example using distributed training on 8 V100 GPUs and Bert Whole Word Masking uncased model to reach a F1 > 93 on SQuAD1.0:
|
408 |
-
|
409 |
-
```bash
|
410 |
-
python -m torch.distributed.launch --nproc_per_node=8 run_squad.py \
|
411 |
-
--model_type bert \
|
412 |
-
--model_name_or_path bert-base-cased \
|
413 |
-
--do_train \
|
414 |
-
--do_eval \
|
415 |
-
--do_lower_case \
|
416 |
-
--train_file $SQUAD_DIR/train-v1.1.json \
|
417 |
-
--predict_file $SQUAD_DIR/dev-v1.1.json \
|
418 |
-
--learning_rate 3e-5 \
|
419 |
-
--num_train_epochs 2 \
|
420 |
-
--max_seq_length 384 \
|
421 |
-
--doc_stride 128 \
|
422 |
-
--output_dir ../models/wwm_uncased_finetuned_squad/ \
|
423 |
-
--per_gpu_train_batch_size 24 \
|
424 |
-
--gradient_accumulation_steps 12
|
425 |
-
```
|
426 |
-
|
427 |
-
Training with the previously defined hyper-parameters yields the following results:
|
428 |
-
|
429 |
-
```bash
|
430 |
-
f1 = 93.15
|
431 |
-
exact_match = 86.91
|
432 |
-
```
|
433 |
-
|
434 |
-
This fine-tuned model is available as a checkpoint under the reference
|
435 |
-
`bert-large-uncased-whole-word-masking-finetuned-squad`.
|
436 |
-
|
437 |
-
#### Fine-tuning XLNet on SQuAD
|
438 |
-
|
439 |
-
This example code fine-tunes XLNet on both SQuAD1.0 and SQuAD2.0 dataset. See above to download the data for SQuAD .
|
440 |
-
|
441 |
-
##### Command for SQuAD1.0:
|
442 |
-
|
443 |
-
```bash
|
444 |
-
export SQUAD_DIR=/path/to/SQUAD
|
445 |
-
|
446 |
-
python /data/home/hlu/transformers/examples/run_squad.py \
|
447 |
-
--model_type xlnet \
|
448 |
-
--model_name_or_path xlnet-large-cased \
|
449 |
-
--do_train \
|
450 |
-
--do_eval \
|
451 |
-
--do_lower_case \
|
452 |
-
--train_file /data/home/hlu/notebooks/NLP/examples/question_answering/train-v1.1.json \
|
453 |
-
--predict_file /data/home/hlu/notebooks/NLP/examples/question_answering/dev-v1.1.json \
|
454 |
-
--learning_rate 3e-5 \
|
455 |
-
--num_train_epochs 2 \
|
456 |
-
--max_seq_length 384 \
|
457 |
-
--doc_stride 128 \
|
458 |
-
--output_dir ./wwm_cased_finetuned_squad/ \
|
459 |
-
--per_gpu_eval_batch_size=4 \
|
460 |
-
--per_gpu_train_batch_size=4 \
|
461 |
-
--save_steps 5000
|
462 |
-
```
|
463 |
-
|
464 |
-
##### Command for SQuAD2.0:
|
465 |
-
|
466 |
-
```bash
|
467 |
-
export SQUAD_DIR=/path/to/SQUAD
|
468 |
-
|
469 |
-
python run_squad.py \
|
470 |
-
--model_type xlnet \
|
471 |
-
--model_name_or_path xlnet-large-cased \
|
472 |
-
--do_train \
|
473 |
-
--do_eval \
|
474 |
-
--version_2_with_negative \
|
475 |
-
--train_file $SQUAD_DIR/train-v2.0.json \
|
476 |
-
--predict_file $SQUAD_DIR/dev-v2.0.json \
|
477 |
-
--learning_rate 3e-5 \
|
478 |
-
--num_train_epochs 4 \
|
479 |
-
--max_seq_length 384 \
|
480 |
-
--doc_stride 128 \
|
481 |
-
--output_dir ./wwm_cased_finetuned_squad/ \
|
482 |
-
--per_gpu_eval_batch_size=2 \
|
483 |
-
--per_gpu_train_batch_size=2 \
|
484 |
-
--save_steps 5000
|
485 |
-
```
|
486 |
-
|
487 |
-
Larger batch size may improve the performance while costing more memory.
|
488 |
-
|
489 |
-
##### Results for SQuAD1.0 with the previously defined hyper-parameters:
|
490 |
-
|
491 |
-
```python
|
492 |
-
{
|
493 |
-
"exact": 85.45884578997162,
|
494 |
-
"f1": 92.5974600601065,
|
495 |
-
"total": 10570,
|
496 |
-
"HasAns_exact": 85.45884578997162,
|
497 |
-
"HasAns_f1": 92.59746006010651,
|
498 |
-
"HasAns_total": 10570
|
499 |
-
}
|
500 |
-
```
|
501 |
-
|
502 |
-
##### Results for SQuAD2.0 with the previously defined hyper-parameters:
|
503 |
-
|
504 |
-
```python
|
505 |
-
{
|
506 |
-
"exact": 80.4177545691906,
|
507 |
-
"f1": 84.07154997729623,
|
508 |
-
"total": 11873,
|
509 |
-
"HasAns_exact": 76.73751686909581,
|
510 |
-
"HasAns_f1": 84.05558584352873,
|
511 |
-
"HasAns_total": 5928,
|
512 |
-
"NoAns_exact": 84.0874684608915,
|
513 |
-
"NoAns_f1": 84.0874684608915,
|
514 |
-
"NoAns_total": 5945
|
515 |
-
}
|
516 |
-
```
|
517 |
-
|
518 |
-
|
519 |
-
|
520 |
-
## Named Entity Recognition
|
521 |
-
|
522 |
-
Based on the scripts [`run_ner.py`](https://github.com/huggingface/transformers/blob/master/examples/run_ner.py) for Pytorch and
|
523 |
-
[`run_tf_ner.py`](https://github.com/huggingface/transformers/blob/master/examples/run_tf_ner.py) for Tensorflow 2.
|
524 |
-
This example fine-tune Bert Multilingual on GermEval 2014 (German NER).
|
525 |
-
Details and results for the fine-tuning provided by @stefan-it.
|
526 |
-
|
527 |
-
### Data (Download and pre-processing steps)
|
528 |
-
|
529 |
-
Data can be obtained from the [GermEval 2014](https://sites.google.com/site/germeval2014ner/data) shared task page.
|
530 |
-
|
531 |
-
Here are the commands for downloading and pre-processing train, dev and test datasets. The original data format has four (tab-separated) columns, in a pre-processing step only the two relevant columns (token and outer span NER annotation) are extracted:
|
532 |
-
|
533 |
-
```bash
|
534 |
-
curl -L 'https://sites.google.com/site/germeval2014ner/data/NER-de-train.tsv?attredirects=0&d=1' \
|
535 |
-
| grep -v "^#" | cut -f 2,3 | tr '\t' ' ' > train.txt.tmp
|
536 |
-
curl -L 'https://sites.google.com/site/germeval2014ner/data/NER-de-dev.tsv?attredirects=0&d=1' \
|
537 |
-
| grep -v "^#" | cut -f 2,3 | tr '\t' ' ' > dev.txt.tmp
|
538 |
-
curl -L 'https://sites.google.com/site/germeval2014ner/data/NER-de-test.tsv?attredirects=0&d=1' \
|
539 |
-
| grep -v "^#" | cut -f 2,3 | tr '\t' ' ' > test.txt.tmp
|
540 |
-
```
|
541 |
-
|
542 |
-
The GermEval 2014 dataset contains some strange "control character" tokens like `'\x96', '\u200e', '\x95', '\xad' or '\x80'`. One problem with these tokens is, that `BertTokenizer` returns an empty token for them, resulting in misaligned `InputExample`s. I wrote a script that a) filters these tokens and b) splits longer sentences into smaller ones (once the max. subtoken length is reached).
|
543 |
-
|
544 |
-
```bash
|
545 |
-
wget "https://raw.githubusercontent.com/stefan-it/fine-tuned-berts-seq/master/scripts/preprocess.py"
|
546 |
-
```
|
547 |
-
Let's define some variables that we need for further pre-processing steps and training the model:
|
548 |
-
|
549 |
-
```bash
|
550 |
-
export MAX_LENGTH=128
|
551 |
-
export BERT_MODEL=bert-base-multilingual-cased
|
552 |
-
```
|
553 |
-
|
554 |
-
Run the pre-processing script on training, dev and test datasets:
|
555 |
-
|
556 |
-
```bash
|
557 |
-
python3 preprocess.py train.txt.tmp $BERT_MODEL $MAX_LENGTH > train.txt
|
558 |
-
python3 preprocess.py dev.txt.tmp $BERT_MODEL $MAX_LENGTH > dev.txt
|
559 |
-
python3 preprocess.py test.txt.tmp $BERT_MODEL $MAX_LENGTH > test.txt
|
560 |
-
```
|
561 |
-
|
562 |
-
The GermEval 2014 dataset has much more labels than CoNLL-2002/2003 datasets, so an own set of labels must be used:
|
563 |
-
|
564 |
-
```bash
|
565 |
-
cat train.txt dev.txt test.txt | cut -d " " -f 2 | grep -v "^$"| sort | uniq > labels.txt
|
566 |
-
```
|
567 |
-
|
568 |
-
### Prepare the run
|
569 |
-
|
570 |
-
Additional environment variables must be set:
|
571 |
-
|
572 |
-
```bash
|
573 |
-
export OUTPUT_DIR=germeval-model
|
574 |
-
export BATCH_SIZE=32
|
575 |
-
export NUM_EPOCHS=3
|
576 |
-
export SAVE_STEPS=750
|
577 |
-
export SEED=1
|
578 |
-
```
|
579 |
-
|
580 |
-
### Run the Pytorch version
|
581 |
-
|
582 |
-
To start training, just run:
|
583 |
-
|
584 |
-
```bash
|
585 |
-
python3 run_ner.py --data_dir ./ \
|
586 |
-
--model_type bert \
|
587 |
-
--labels ./labels.txt \
|
588 |
-
--model_name_or_path $BERT_MODEL \
|
589 |
-
--output_dir $OUTPUT_DIR \
|
590 |
-
--max_seq_length $MAX_LENGTH \
|
591 |
-
--num_train_epochs $NUM_EPOCHS \
|
592 |
-
--per_gpu_train_batch_size $BATCH_SIZE \
|
593 |
-
--save_steps $SAVE_STEPS \
|
594 |
-
--seed $SEED \
|
595 |
-
--do_train \
|
596 |
-
--do_eval \
|
597 |
-
--do_predict
|
598 |
-
```
|
599 |
-
|
600 |
-
If your GPU supports half-precision training, just add the `--fp16` flag. After training, the model will be both evaluated on development and test datasets.
|
601 |
-
|
602 |
-
#### Evaluation
|
603 |
-
|
604 |
-
Evaluation on development dataset outputs the following for our example:
|
605 |
-
|
606 |
-
```bash
|
607 |
-
10/04/2019 00:42:06 - INFO - __main__ - ***** Eval results *****
|
608 |
-
10/04/2019 00:42:06 - INFO - __main__ - f1 = 0.8623348017621146
|
609 |
-
10/04/2019 00:42:06 - INFO - __main__ - loss = 0.07183869666975543
|
610 |
-
10/04/2019 00:42:06 - INFO - __main__ - precision = 0.8467916366258111
|
611 |
-
10/04/2019 00:42:06 - INFO - __main__ - recall = 0.8784592370979806
|
612 |
-
```
|
613 |
-
|
614 |
-
On the test dataset the following results could be achieved:
|
615 |
-
|
616 |
-
```bash
|
617 |
-
10/04/2019 00:42:42 - INFO - __main__ - ***** Eval results *****
|
618 |
-
10/04/2019 00:42:42 - INFO - __main__ - f1 = 0.8614389652384803
|
619 |
-
10/04/2019 00:42:42 - INFO - __main__ - loss = 0.07064602487454782
|
620 |
-
10/04/2019 00:42:42 - INFO - __main__ - precision = 0.8604651162790697
|
621 |
-
10/04/2019 00:42:42 - INFO - __main__ - recall = 0.8624150210424085
|
622 |
-
```
|
623 |
-
|
624 |
-
#### Comparing BERT (large, cased), RoBERTa (large, cased) and DistilBERT (base, uncased)
|
625 |
-
|
626 |
-
Here is a small comparison between BERT (large, cased), RoBERTa (large, cased) and DistilBERT (base, uncased) with the same hyperparameters as specified in the [example documentation](https://huggingface.co/transformers/examples.html#named-entity-recognition) (one run):
|
627 |
-
|
628 |
-
| Model | F-Score Dev | F-Score Test
|
629 |
-
| --------------------------------- | ------- | --------
|
630 |
-
| `bert-large-cased` | 95.59 | 91.70
|
631 |
-
| `roberta-large` | 95.96 | 91.87
|
632 |
-
| `distilbert-base-uncased` | 94.34 | 90.32
|
633 |
-
|
634 |
-
### Run the Tensorflow 2 version
|
635 |
-
|
636 |
-
To start training, just run:
|
637 |
-
|
638 |
-
```bash
|
639 |
-
python3 run_tf_ner.py --data_dir ./ \
|
640 |
-
--model_type bert \
|
641 |
-
--labels ./labels.txt \
|
642 |
-
--model_name_or_path $BERT_MODEL \
|
643 |
-
--output_dir $OUTPUT_DIR \
|
644 |
-
--max_seq_length $MAX_LENGTH \
|
645 |
-
--num_train_epochs $NUM_EPOCHS \
|
646 |
-
--per_device_train_batch_size $BATCH_SIZE \
|
647 |
-
--save_steps $SAVE_STEPS \
|
648 |
-
--seed $SEED \
|
649 |
-
--do_train \
|
650 |
-
--do_eval \
|
651 |
-
--do_predict
|
652 |
-
```
|
653 |
-
|
654 |
-
Such as the Pytorch version, if your GPU supports half-precision training, just add the `--fp16` flag. After training, the model will be both evaluated on development and test datasets.
|
655 |
-
|
656 |
-
#### Evaluation
|
657 |
-
|
658 |
-
Evaluation on development dataset outputs the following for our example:
|
659 |
-
```bash
|
660 |
-
precision recall f1-score support
|
661 |
-
|
662 |
-
LOCderiv 0.7619 0.6154 0.6809 52
|
663 |
-
PERpart 0.8724 0.8997 0.8858 4057
|
664 |
-
OTHpart 0.9360 0.9466 0.9413 711
|
665 |
-
ORGpart 0.7015 0.6989 0.7002 269
|
666 |
-
LOCpart 0.7668 0.8488 0.8057 496
|
667 |
-
LOC 0.8745 0.9191 0.8963 235
|
668 |
-
ORGderiv 0.7723 0.8571 0.8125 91
|
669 |
-
OTHderiv 0.4800 0.6667 0.5581 18
|
670 |
-
OTH 0.5789 0.6875 0.6286 16
|
671 |
-
PERderiv 0.5385 0.3889 0.4516 18
|
672 |
-
PER 0.5000 0.5000 0.5000 2
|
673 |
-
ORG 0.0000 0.0000 0.0000 3
|
674 |
-
|
675 |
-
micro avg 0.8574 0.8862 0.8715 5968
|
676 |
-
macro avg 0.8575 0.8862 0.8713 5968
|
677 |
-
```
|
678 |
-
|
679 |
-
On the test dataset the following results could be achieved:
|
680 |
-
```bash
|
681 |
-
precision recall f1-score support
|
682 |
-
|
683 |
-
PERpart 0.8847 0.8944 0.8896 9397
|
684 |
-
OTHpart 0.9376 0.9353 0.9365 1639
|
685 |
-
ORGpart 0.7307 0.7044 0.7173 697
|
686 |
-
LOC 0.9133 0.9394 0.9262 561
|
687 |
-
LOCpart 0.8058 0.8157 0.8107 1150
|
688 |
-
ORG 0.0000 0.0000 0.0000 8
|
689 |
-
OTHderiv 0.5882 0.4762 0.5263 42
|
690 |
-
PERderiv 0.6571 0.5227 0.5823 44
|
691 |
-
OTH 0.4906 0.6667 0.5652 39
|
692 |
-
ORGderiv 0.7016 0.7791 0.7383 172
|
693 |
-
LOCderiv 0.8256 0.6514 0.7282 109
|
694 |
-
PER 0.0000 0.0000 0.0000 11
|
695 |
-
|
696 |
-
micro avg 0.8722 0.8774 0.8748 13869
|
697 |
-
macro avg 0.8712 0.8774 0.8740 13869
|
698 |
-
```
|
699 |
-
|
700 |
-
## XNLI
|
701 |
-
|
702 |
-
Based on the script [`run_xnli.py`](https://github.com/huggingface/transformers/blob/master/examples/run_xnli.py).
|
703 |
-
|
704 |
-
[XNLI](https://www.nyu.edu/projects/bowman/xnli/) is crowd-sourced dataset based on [MultiNLI](http://www.nyu.edu/projects/bowman/multinli/). It is an evaluation benchmark for cross-lingual text representations. Pairs of text are labeled with textual entailment annotations for 15 different languages (including both high-ressource language such as English and low-ressource languages such as Swahili).
|
705 |
-
|
706 |
-
#### Fine-tuning on XNLI
|
707 |
-
|
708 |
-
This example code fine-tunes mBERT (multi-lingual BERT) on the XNLI dataset. It runs in 106 mins
|
709 |
-
on a single tesla V100 16GB. The data for XNLI can be downloaded with the following links and should be both saved (and un-zipped) in a
|
710 |
-
`$XNLI_DIR` directory.
|
711 |
-
|
712 |
-
* [XNLI 1.0](https://www.nyu.edu/projects/bowman/xnli/XNLI-1.0.zip)
|
713 |
-
* [XNLI-MT 1.0](https://www.nyu.edu/projects/bowman/xnli/XNLI-MT-1.0.zip)
|
714 |
-
|
715 |
-
```bash
|
716 |
-
export XNLI_DIR=/path/to/XNLI
|
717 |
-
|
718 |
-
python run_xnli.py \
|
719 |
-
--model_type bert \
|
720 |
-
--model_name_or_path bert-base-multilingual-cased \
|
721 |
-
--language de \
|
722 |
-
--train_language en \
|
723 |
-
--do_train \
|
724 |
-
--do_eval \
|
725 |
-
--data_dir $XNLI_DIR \
|
726 |
-
--per_gpu_train_batch_size 32 \
|
727 |
-
--learning_rate 5e-5 \
|
728 |
-
--num_train_epochs 2.0 \
|
729 |
-
--max_seq_length 128 \
|
730 |
-
--output_dir /tmp/debug_xnli/ \
|
731 |
-
--save_steps -1
|
732 |
-
```
|
733 |
-
|
734 |
-
Training with the previously defined hyper-parameters yields the following results on the **test** set:
|
735 |
-
|
736 |
-
```bash
|
737 |
-
acc = 0.7093812375249501
|
738 |
-
```
|
739 |
-
|
740 |
-
## MM-IMDb
|
741 |
-
|
742 |
-
Based on the script [`run_mmimdb.py`](https://github.com/huggingface/transformers/blob/master/examples/mm-imdb/run_mmimdb.py).
|
743 |
-
|
744 |
-
[MM-IMDb](http://lisi1.unal.edu.co/mmimdb/) is a Multimodal dataset with around 26,000 movies including images, plots and other metadata.
|
745 |
-
|
746 |
-
### Training on MM-IMDb
|
747 |
-
|
748 |
-
```
|
749 |
-
python run_mmimdb.py \
|
750 |
-
--data_dir /path/to/mmimdb/dataset/ \
|
751 |
-
--model_type bert \
|
752 |
-
--model_name_or_path bert-base-uncased \
|
753 |
-
--output_dir /path/to/save/dir/ \
|
754 |
-
--do_train \
|
755 |
-
--do_eval \
|
756 |
-
--max_seq_len 512 \
|
757 |
-
--gradient_accumulation_steps 20 \
|
758 |
-
--num_image_embeds 3 \
|
759 |
-
--num_train_epochs 100 \
|
760 |
-
--patience 5
|
761 |
-
```
|
762 |
-
|
763 |
-
## Adversarial evaluation of model performances
|
764 |
-
|
765 |
-
Here is an example on evaluating a model using adversarial evaluation of natural language inference with the Heuristic Analysis for NLI Systems (HANS) dataset [McCoy et al., 2019](https://arxiv.org/abs/1902.01007). The example was gracefully provided by [Nafise Sadat Moosavi](https://github.com/ns-moosavi).
|
766 |
-
|
767 |
-
The HANS dataset can be downloaded from [this location](https://github.com/tommccoy1/hans).
|
768 |
-
|
769 |
-
This is an example of using test_hans.py:
|
770 |
-
|
771 |
-
```bash
|
772 |
-
export HANS_DIR=path-to-hans
|
773 |
-
export MODEL_TYPE=type-of-the-model-e.g.-bert-roberta-xlnet-etc
|
774 |
-
export MODEL_PATH=path-to-the-model-directory-that-is-trained-on-NLI-e.g.-by-using-run_glue.py
|
775 |
-
|
776 |
-
python examples/test_hans.py \
|
777 |
-
--task_name hans \
|
778 |
-
--model_type $MODEL_TYPE \
|
779 |
-
--do_eval \
|
780 |
-
--do_lower_case \
|
781 |
-
--data_dir $HANS_DIR \
|
782 |
-
--model_name_or_path $MODEL_PATH \
|
783 |
-
--max_seq_length 128 \
|
784 |
-
-output_dir $MODEL_PATH \
|
785 |
-
```
|
786 |
-
|
787 |
-
This will create the hans_predictions.txt file in MODEL_PATH, which can then be evaluated using hans/evaluate_heur_output.py from the HANS dataset.
|
788 |
-
|
789 |
-
The results of the BERT-base model that is trained on MNLI using batch size 8 and the random seed 42 on the HANS dataset is as follows:
|
790 |
-
|
791 |
-
```bash
|
792 |
-
Heuristic entailed results:
|
793 |
-
lexical_overlap: 0.9702
|
794 |
-
subsequence: 0.9942
|
795 |
-
constituent: 0.9962
|
796 |
-
|
797 |
-
Heuristic non-entailed results:
|
798 |
-
lexical_overlap: 0.199
|
799 |
-
subsequence: 0.0396
|
800 |
-
constituent: 0.118
|
801 |
-
```
|
|
|
|
|
|
|
|
|
|
|
|
|
|
|
|
|
|
|
|
|
|
|
|
|
|
|
|
|
|
|
|
|
|
|
|
|
|
|
|
|
|
|
|
|
|
|
|
|
|
|
|
|
|
|
|
|
|
|
|
|
|
|
|
|
|
|
|
|
|
|
|
|
|
|
|
|
|
|
|
|
|
|
|
|
|
|
|
|
|
|
|
|
|
|
|
|
|
|
|
|
|
|
|
|
|
|
|
|
|
|
|
|
|
|
|
|
|
|
|
|
|
|
|
|
|
|
|
|
|
|
|
|
|
|
|
|
|
|
|
|
|
|
|
|
|
|
|
|
|
|
|
|
|
|
|
|
|
|
|
|
|
|
|
|
|
|
|
|
|
|
|
|
|
|
|
|
|
|
|
|
|
|
|
|
|
|
|
|
|
|
|
|
|
|
|
|
|
|
|
|
|
|
|
|
|
|
|
|
|
|
|
|
|
|
|
|
|
|
|
|
|
|
|
|
|
|
|
|
|
|
|
|
|
|
|
|
|
|
|
|
|
|
|
|
|
|
|
|
|
|
|
|
|
|
|
|
|
|
|
|
|
|
|
|
|
|
|
|
|
|
|
|
|
|
|
|
|
|
|
|
|
|
|
|
|
|
|
|
|
|
|
|
|
|
|
|
|
|
|
|
|
|
|
|
|
|
|
|
|
|
|
|
|
|
|
|
|
|
|
|
|
|
|
|
|
|
|
|
|
|
|
|
|
|
|
|
|
|
|
|
|
|
|
|
|
|
|
|
|
|
|
|
|
|
|
|
|
|
|
|
|
|
|
|
|
|
|
|
|
|
|
|
|
|
|
|
|
|
|
|
|
|
|
|
|
|
|
|
|
|
|
|
|
|
|
|
|
|
|
|
|
|
|
|
|
|
|
|
|
|
|
|
|
|
|
|
|
|
|
|
|
|
|
|
|
|
|
|
|
|
|
|
|
|
|
|
|
|
|
|
|
|
|
|
|
|
|
|
|
|
|
|
|
|
|
|
|
|
|
|
|
|
|
|
|
|
|
|
|
|
|
|
|
|
|
|
|
|
|
|
|
|
|
|
|
|
|
|
|
|
|
|
|
|
|
|
|
|
|
|
|
|
|
|
|
|
|
|
|
|
|
|
|
|
|
|
|
|
|
|
|
|
|
|
|
|
|
|
|
|
|
|
|
|
|
|
|
|
|
|
|
|
|
|
|
|
|
|
|
|
|
|
|
|
|
|
|
|
|
|
|
|
|
|
|
|
|
|
|
|
|
|
|
|
|
|
|
|
|
|
|
|
|
|
|
|
|
|
|
|
|
|
|
|
|
|
|
|
|
|
|
|
|
|
|
|
|
|
|
|
|
|
|
|
|
|
|
|
|
|
|
|
|
|
|
|
|
|
|
|
|
|
|
|
|
|
|
|
|
|
|
|
|
|
|
|
|
|
|
|
|
|
|
|
|
|
|
|
|
|
|
|
|
|
|
|
|
|
|
|
|
|
|
|
|
|
|
|
|
|
|
|
|
|
|
|
|
|
|
|
|
|
|
|
|
|
|
|
|
|
|
|
|
|
|
|
|
|
|
|
|
|
|
|
|
|
|
|
|
|
|
|
|
|
|
|
|
|
|
|
|
|
|
|
|
|
|
|
|
|
|
|
|
|
|
|
|
|
|
|
|
|
|
|
|
|
|
|
|
|
|
|
|
|
|
|
|
|
|
|
|
|
|
|
|
|
|
|
|
|
|
|
|
|
|
|
|
|
|
|
|
|
|
|
|
|
|
|
|
|
|
|
|
|
|
|
|
|
|
|
|
|
|
|
|
|
|
|
|
|
|
|
|
|
|
|
|
|
|
|
|
|
|
|
|
|
|
|
|
|
|
|
|
|
|
|
|
|
|
|
|
|
|
|
|
|
|
|
|
|
|
|
|
|
|
|
|
|
|
|
|
|
|
|
|
|
|
|
|
|
|
|
|
|
|
|
|
|
|
|
|
|
|
|
|
|
|
|
|
|
|
|
|
|
|
|
|
|
|
|
|
|
|
|
|
|
|
|
|
|
|
|
|
|
|
|
|
|
|
|
|
|
|
|
|
|
|
|
|
|
|
|
|
|
|
|
|
|
|
|
|
|
|
|
|
|
|
|
|
|
|
|
|
|
|
|
|
|
|
|
|
|
|
|
|
|
|
|
|
|
|
|
|
|
|
|
|
|
|
|
|
|
|
|
|
|
|
|
|
|
|
|
|
|
|
|
|
|
|
|
|
|
|
|
|
|
|
|
|
|
|
|
|
|
|
|
|
|
|
|
|
|
|
|
|
|
|
|
|
|
|
|
|
|
|
|
|
|
|
|
|
|
|
|
|
|
|
|
|
|
|
|
|
|
|
|
|
|
|
|
|
|
|
|
|
|
|
|
|
|
|
|
|
|
|
|
|
|
|
|
|
|
|
|
|
|
|
|
|
|
|
|
|
|
|
|
|
|
|
|
|
|
|
|
|
|
|
|
|
|
|
|
|
|
|
|
|
|
|
|
|
|
|
|
|
|
|
|
|
|
|
|
|
|
|
|
|
|
|
|
|
|
|
|
|
|
|
|
|
|
|
|
|
|
|
|
|
|
|
|
|
|
|
|
|
|
|
|
|
|
|
|
|
|
|
|
|
|
|
|
|
|
|
|
|
|
|
|
|
|
|
|
|
|
|
|
|
|
|
|
|
|
|
|
|
|
|
|
|
|
|
|
|
|
|
|
|
|
|
|
|
|
|
|
|
|
|
|
|
|
|
|
|
|
|
|
|
|
|
|
|
|
|
|
|
|
|
|
|
|
|
|
|
|
|
|
|
|
|
|
|
|
|
|
|
|
|
|
|
|
|
|
|
|
|
|
|
|
|
|
|
|
|
|
|
|
|
|
|
|
|
|
|
|
|
|
|
|
|
|
|
|
|
|
|
|
|
|
|
|
|
|
|
|
|
|
|
|
|
|
|
|
|
|
|
|
|
|
|
|
|
|
|
|
|
|
|
|
|
|
|
|
|
|
|
|
|
|
|
|
|
|
|
|
|
|
|
|
|
|
|
|
|
|
|
|
|
|
|
|
|
|
|
|
|
|
|
|
|
|
|
|
|
|
|
|
|
|
|
|
|
|
|
|
|
|
|
|
|
|
|
|
|
|
|
|
|
|
|
|
|
|
|
|
|
|
|
|
|
|
|
|
|
|
|
|
|
|
|
|
|
|
|
|
|
|
|
|
|
|
|
|
|
|
|
|
|
|
|
|
|
|
|
|
|
|
|
|
|
|
|
|
|
|
|
|
|
|
|
|
|
|
|
|
|
|
|
|
|
|
|
|
|
|
|
|
|
|
|
|
|
|
|
|
|
|
|
|
|
|
|
|
|
|
|
|
|
|
|
|
|
|
|
|
|
|
|
|
|
|
|
|
|
|
|
|
|
|
|
|
|
|
|
|
|
|
|
|
|
|
|
|
|
|
|
|
|
|
|
|
|
|
|
|
|
|
|
|
|
|
|
|
|
|
|
|
|
|
|
|
|
|
|
|
|
|
|
|
|
|
|
|
|
|
|
|
|
|
|
|
|
|
|
server/transformers/docs/source/imgs/transformers_logo_name.png
DELETED
Binary file (8.87 kB)
|
|
server/transformers/docs/source/imgs/warmup_constant_schedule.png
DELETED
Binary file (9.98 kB)
|
|
server/transformers/docs/source/imgs/warmup_cosine_hard_restarts_schedule.png
DELETED
Binary file (22.4 kB)
|
|
server/transformers/docs/source/imgs/warmup_cosine_schedule.png
DELETED
Binary file (17.3 kB)
|
|
server/transformers/docs/source/imgs/warmup_cosine_warm_restarts_schedule.png
DELETED
Binary file (22.3 kB)
|
|
server/transformers/docs/source/imgs/warmup_linear_schedule.png
DELETED
Binary file (16.8 kB)
|
|
server/transformers/docs/source/index.rst
DELETED
@@ -1,98 +0,0 @@
|
|
1 |
-
Transformers
|
2 |
-
================================================================================================================================================
|
3 |
-
|
4 |
-
🤗 Transformers (formerly known as `pytorch-transformers` and `pytorch-pretrained-bert`) provides general-purpose architectures
|
5 |
-
(BERT, GPT-2, RoBERTa, XLM, DistilBert, XLNet...) for Natural Language Understanding (NLU) and Natural Language Generation
|
6 |
-
(NLG) with over 32+ pretrained models in 100+ languages and deep interoperability between TensorFlow 2.0 and PyTorch.
|
7 |
-
|
8 |
-
This is the documentation of our repository `transformers <https://github.com/huggingface/transformers>`__.
|
9 |
-
|
10 |
-
Features
|
11 |
-
---------------------------------------------------
|
12 |
-
|
13 |
-
- As easy to use as pytorch-transformers
|
14 |
-
- As powerful and concise as Keras
|
15 |
-
- High performance on NLU and NLG tasks
|
16 |
-
- Low barrier to entry for educators and practitioners
|
17 |
-
|
18 |
-
State-of-the-art NLP for everyone:
|
19 |
-
|
20 |
-
- Deep learning researchers
|
21 |
-
- Hands-on practitioners
|
22 |
-
- AI/ML/NLP teachers and educators
|
23 |
-
|
24 |
-
Lower compute costs, smaller carbon footprint:
|
25 |
-
|
26 |
-
- Researchers can share trained models instead of always retraining
|
27 |
-
- Practitioners can reduce compute time and production costs
|
28 |
-
- 8 architectures with over 30 pretrained models, some in more than 100 languages
|
29 |
-
|
30 |
-
Choose the right framework for every part of a model's lifetime:
|
31 |
-
|
32 |
-
- Train state-of-the-art models in 3 lines of code
|
33 |
-
- Deep interoperability between TensorFlow 2.0 and PyTorch models
|
34 |
-
- Move a single model between TF2.0/PyTorch frameworks at will
|
35 |
-
- Seamlessly pick the right framework for training, evaluation, production
|
36 |
-
|
37 |
-
Contents
|
38 |
-
---------------------------------
|
39 |
-
|
40 |
-
The library currently contains PyTorch and Tensorflow implementations, pre-trained model weights, usage scripts and conversion utilities for the following models:
|
41 |
-
|
42 |
-
1. `BERT <https://github.com/google-research/bert>`_ (from Google) released with the paper `BERT: Pre-training of Deep Bidirectional Transformers for Language Understanding <https://arxiv.org/abs/1810.04805>`_ by Jacob Devlin, Ming-Wei Chang, Kenton Lee and Kristina Toutanova.
|
43 |
-
2. `GPT <https://github.com/openai/finetune-transformer-lm>`_ (from OpenAI) released with the paper `Improving Language Understanding by Generative Pre-Training <https://blog.openai.com/language-unsupervised>`_ by Alec Radford, Karthik Narasimhan, Tim Salimans and Ilya Sutskever.
|
44 |
-
3. `GPT-2 <https://blog.openai.com/better-language-models>`_ (from OpenAI) released with the paper `Language Models are Unsupervised Multitask Learners <https://blog.openai.com/better-language-models>`_ by Alec Radford*, Jeffrey Wu*, Rewon Child, David Luan, Dario Amodei** and Ilya Sutskever**.
|
45 |
-
4. `Transformer-XL <https://github.com/kimiyoung/transformer-xl>`_ (from Google/CMU) released with the paper `Transformer-XL: Attentive Language Models Beyond a Fixed-Length Context <https://arxiv.org/abs/1901.02860>`_ by Zihang Dai*, Zhilin Yang*, Yiming Yang, Jaime Carbonell, Quoc V. Le, Ruslan Salakhutdinov.
|
46 |
-
5. `XLNet <https://github.com/zihangdai/xlnet>`_ (from Google/CMU) released with the paper `XLNet: Generalized Autoregressive Pretraining for Language Understanding <https://arxiv.org/abs/1906.08237>`_ by Zhilin Yang*, Zihang Dai*, Yiming Yang, Jaime Carbonell, Ruslan Salakhutdinov, Quoc V. Le.
|
47 |
-
6. `XLM <https://github.com/facebookresearch/XLM>`_ (from Facebook) released together with the paper `Cross-lingual Language Model Pretraining <https://arxiv.org/abs/1901.07291>`_ by Guillaume Lample and Alexis Conneau.
|
48 |
-
7. `RoBERTa <https://github.com/pytorch/fairseq/tree/master/examples/roberta>`_ (from Facebook), released together with the paper a `Robustly Optimized BERT Pretraining Approach <https://arxiv.org/abs/1907.11692>`_ by Yinhan Liu, Myle Ott, Naman Goyal, Jingfei Du, Mandar Joshi, Danqi Chen, Omer Levy, Mike Lewis, Luke Zettlemoyer, Veselin Stoyanov.
|
49 |
-
8. `DistilBERT <https://huggingface.co/transformers/model_doc/distilbert.html>`_ (from HuggingFace) released together with the paper `DistilBERT, a distilled version of BERT: smaller, faster, cheaper and lighter <https://arxiv.org/abs/1910.01108>`_ by Victor Sanh, Lysandre Debut and Thomas Wolf. The same method has been applied to compress GPT2 into `DistilGPT2 <https://github.com/huggingface/transformers/tree/master/examples/distillation>`_.
|
50 |
-
9. `CTRL <https://github.com/pytorch/fairseq/tree/master/examples/ctrl>`_ (from Salesforce), released together with the paper `CTRL: A Conditional Transformer Language Model for Controllable Generation <https://www.github.com/salesforce/ctrl>`_ by Nitish Shirish Keskar*, Bryan McCann*, Lav R. Varshney, Caiming Xiong and Richard Socher.
|
51 |
-
10. `CamemBERT <https://huggingface.co/transformers/model_doc/camembert.html>`_ (from FAIR, Inria, Sorbonne Université) released together with the paper `CamemBERT: a Tasty French Language Model <https://arxiv.org/abs/1911.03894>`_ by Louis Martin, Benjamin Muller, Pedro Javier Ortiz Suarez, Yoann Dupont, Laurent Romary, Eric Villemonte de la Clergerie, Djame Seddah, and Benoît Sagot.
|
52 |
-
11. `ALBERT <https://github.com/google-research/ALBERT>`_ (from Google Research), released together with the paper a `ALBERT: A Lite BERT for Self-supervised Learning of Language Representations <https://arxiv.org/abs/1909.11942>`_ by Zhenzhong Lan, Mingda Chen, Sebastian Goodman, Kevin Gimpel, Piyush Sharma, Radu Soricut.
|
53 |
-
12. `XLM-RoBERTa <https://github.com/pytorch/fairseq/tree/master/examples/xlmr>`_ (from Facebook AI), released together with the paper `Unsupervised Cross-lingual Representation Learning at Scale <https://arxiv.org/abs/1911.02116>`_ by Alexis Conneau*, Kartikay Khandelwal*, Naman Goyal, Vishrav Chaudhary, Guillaume Wenzek, Francisco Guzmán, Edouard Grave, Myle Ott, Luke Zettlemoyer and Veselin Stoyanov.
|
54 |
-
|
55 |
-
.. toctree::
|
56 |
-
:maxdepth: 2
|
57 |
-
:caption: Notes
|
58 |
-
|
59 |
-
installation
|
60 |
-
quickstart
|
61 |
-
pretrained_models
|
62 |
-
model_sharing
|
63 |
-
examples
|
64 |
-
notebooks
|
65 |
-
serialization
|
66 |
-
converting_tensorflow_models
|
67 |
-
migration
|
68 |
-
bertology
|
69 |
-
torchscript
|
70 |
-
multilingual
|
71 |
-
benchmarks
|
72 |
-
|
73 |
-
.. toctree::
|
74 |
-
:maxdepth: 2
|
75 |
-
:caption: Main classes
|
76 |
-
|
77 |
-
main_classes/configuration
|
78 |
-
main_classes/model
|
79 |
-
main_classes/tokenizer
|
80 |
-
main_classes/optimizer_schedules
|
81 |
-
main_classes/processors
|
82 |
-
|
83 |
-
.. toctree::
|
84 |
-
:maxdepth: 2
|
85 |
-
:caption: Package Reference
|
86 |
-
|
87 |
-
model_doc/auto
|
88 |
-
model_doc/bert
|
89 |
-
model_doc/gpt
|
90 |
-
model_doc/transformerxl
|
91 |
-
model_doc/gpt2
|
92 |
-
model_doc/xlm
|
93 |
-
model_doc/xlnet
|
94 |
-
model_doc/roberta
|
95 |
-
model_doc/distilbert
|
96 |
-
model_doc/ctrl
|
97 |
-
model_doc/camembert
|
98 |
-
model_doc/albert
|
|
|
|
|
|
|
|
|
|
|
|
|
|
|
|
|
|
|
|
|
|
|
|
|
|
|
|
|
|
|
|
|
|
|
|
|
|
|
|
|
|
|
|
|
|
|
|
|
|
|
|
|
|
|
|
|
|
|
|
|
|
|
|
|
|
|
|
|
|
|
|
|
|
|
|
|
|
|
|
|
|
|
|
|
|
|
|
|
|
|
|
|
|
|
|
|
|
|
|
|
|
|
|
|
|
|
|
|
|
|
|
|
|
|
|
|
|
|
|
|
|
|
|
|
|
|
|
|
|
|
|
|
|
|
|
|
|
|
|
|
|
|
|
|
|
|
|
|
|
|
|
|
|
|
|
|
|
|
|
|
|
|
|
|
|
|
|
|
|
|
|
|
|
|
|
|
|
|
|
|
|
|
|
|
|
|
|
|
|
|
|
|
|
|
|
|
|
server/transformers/docs/source/installation.md
DELETED
@@ -1,51 +0,0 @@
|
|
1 |
-
# Installation
|
2 |
-
|
3 |
-
Transformers is tested on Python 3.5+ and PyTorch 1.1.0
|
4 |
-
|
5 |
-
## With pip
|
6 |
-
|
7 |
-
PyTorch Transformers can be installed using pip as follows:
|
8 |
-
|
9 |
-
``` bash
|
10 |
-
pip install transformers
|
11 |
-
```
|
12 |
-
|
13 |
-
## From source
|
14 |
-
|
15 |
-
To install from source, clone the repository and install with:
|
16 |
-
|
17 |
-
``` bash
|
18 |
-
git clone https://github.com/huggingface/transformers.git
|
19 |
-
cd transformers
|
20 |
-
pip install .
|
21 |
-
```
|
22 |
-
|
23 |
-
## Tests
|
24 |
-
|
25 |
-
An extensive test suite is included to test the library behavior and several examples. Library tests can be found in the [tests folder](https://github.com/huggingface/transformers/tree/master/tests) and examples tests in the [examples folder](https://github.com/huggingface/transformers/tree/master/examples).
|
26 |
-
|
27 |
-
Refer to the [contributing guide](https://github.com/huggingface/transformers/blob/master/CONTRIBUTING.md#tests) for details about running tests.
|
28 |
-
|
29 |
-
## OpenAI GPT original tokenization workflow
|
30 |
-
|
31 |
-
If you want to reproduce the original tokenization process of the `OpenAI GPT` paper, you will need to install `ftfy` and `SpaCy`:
|
32 |
-
|
33 |
-
``` bash
|
34 |
-
pip install spacy ftfy==4.4.3
|
35 |
-
python -m spacy download en
|
36 |
-
```
|
37 |
-
|
38 |
-
If you don't install `ftfy` and `SpaCy`, the `OpenAI GPT` tokenizer will default to tokenize using BERT's `BasicTokenizer` followed by Byte-Pair Encoding (which should be fine for most usage, don't worry).
|
39 |
-
|
40 |
-
## Note on model downloads (Continuous Integration or large-scale deployments)
|
41 |
-
|
42 |
-
If you expect to be downloading large volumes of models (more than 1,000) from our hosted bucket (for instance through your CI setup, or a large-scale production deployment), please cache the model files on your end. It will be way faster, and cheaper. Feel free to contact us privately if you need any help.
|
43 |
-
|
44 |
-
## Do you want to run a Transformer model on a mobile device?
|
45 |
-
|
46 |
-
You should check out our [swift-coreml-transformers](https://github.com/huggingface/swift-coreml-transformers) repo.
|
47 |
-
|
48 |
-
It contains a set of tools to convert PyTorch or TensorFlow 2.0 trained Transformer models (currently contains `GPT-2`, `DistilGPT-2`, `BERT`, and `DistilBERT`) to CoreML models that run on iOS devices.
|
49 |
-
|
50 |
-
At some point in the future, you'll be able to seamlessly move from pre-training or fine-tuning models in PyTorch to productizing them in CoreML,
|
51 |
-
or prototype a model or an app in CoreML then research its hyperparameters or architecture from PyTorch. Super exciting!
|
|
|
|
|
|
|
|
|
|
|
|
|
|
|
|
|
|
|
|
|
|
|
|
|
|
|
|
|
|
|
|
|
|
|
|
|
|
|
|
|
|
|
|
|
|
|
|
|
|
|
|
|
|
|
|
|
|
|
|
|
|
|
|
|
|
|
|
|
|
|
|
|
|
|
|
|
|
|
|
|
|
|
|
|
|
|
|
|
|
|
|
|
|
|
|
|
|
|
|
|
|
|
|
server/transformers/docs/source/main_classes/configuration.rst
DELETED
@@ -1,10 +0,0 @@
|
|
1 |
-
Configuration
|
2 |
-
----------------------------------------------------
|
3 |
-
|
4 |
-
The base class ``PretrainedConfig`` implements the common methods for loading/saving a configuration either from a local file or directory, or from a pretrained model configuration provided by the library (downloaded from HuggingFace's AWS S3 repository).
|
5 |
-
|
6 |
-
``PretrainedConfig``
|
7 |
-
~~~~~~~~~~~~~~~~~~~~~
|
8 |
-
|
9 |
-
.. autoclass:: transformers.PretrainedConfig
|
10 |
-
:members:
|
|
|
|
|
|
|
|
|
|
|
|
|
|
|
|
|
|
|
|
|
|
server/transformers/docs/source/main_classes/model.rst
DELETED
@@ -1,21 +0,0 @@
|
|
1 |
-
Models
|
2 |
-
----------------------------------------------------
|
3 |
-
|
4 |
-
The base class ``PreTrainedModel`` implements the common methods for loading/saving a model either from a local file or directory, or from a pretrained model configuration provided by the library (downloaded from HuggingFace's AWS S3 repository).
|
5 |
-
|
6 |
-
``PreTrainedModel`` also implements a few methods which are common among all the models to:
|
7 |
-
|
8 |
-
- resize the input token embeddings when new tokens are added to the vocabulary
|
9 |
-
- prune the attention heads of the model.
|
10 |
-
|
11 |
-
``PreTrainedModel``
|
12 |
-
~~~~~~~~~~~~~~~~~~~~~
|
13 |
-
|
14 |
-
.. autoclass:: transformers.PreTrainedModel
|
15 |
-
:members:
|
16 |
-
|
17 |
-
``TFPreTrainedModel``
|
18 |
-
~~~~~~~~~~~~~~~~~~~~~
|
19 |
-
|
20 |
-
.. autoclass:: transformers.TFPreTrainedModel
|
21 |
-
:members:
|
|
|
|
|
|
|
|
|
|
|
|
|
|
|
|
|
|
|
|
|
|
|
|
|
|
|
|
|
|
|
|
|
|
|
|
|
|
|
|
|
|
|
|
server/transformers/docs/source/main_classes/optimizer_schedules.rst
DELETED
@@ -1,75 +0,0 @@
|
|
1 |
-
Optimizer
|
2 |
-
----------------------------------------------------
|
3 |
-
|
4 |
-
The ``.optimization`` module provides:
|
5 |
-
|
6 |
-
- an optimizer with weight decay fixed that can be used to fine-tuned models, and
|
7 |
-
- several schedules in the form of schedule objects that inherit from ``_LRSchedule``:
|
8 |
-
- a gradient accumulation class to accumulate the gradients of multiple batches
|
9 |
-
|
10 |
-
``AdamW``
|
11 |
-
~~~~~~~~~~~~~~~~
|
12 |
-
|
13 |
-
.. autoclass:: transformers.AdamW
|
14 |
-
:members:
|
15 |
-
|
16 |
-
``AdamWeightDecay``
|
17 |
-
~~~~~~~~~~~~~~~~~~~
|
18 |
-
|
19 |
-
.. autoclass:: transformers.AdamWeightDecay
|
20 |
-
:members:
|
21 |
-
|
22 |
-
.. autofunction:: transformers.create_optimizer
|
23 |
-
:members:
|
24 |
-
|
25 |
-
Schedules
|
26 |
-
----------------------------------------------------
|
27 |
-
|
28 |
-
Learning Rate Schedules
|
29 |
-
^^^^^^^^^^^^^^^^^^^^^^^^^^^^^^^^^^^^^^^^^^^^^^^^^^^^^^^^^^^^^^^^^^
|
30 |
-
|
31 |
-
.. autofunction:: transformers.get_constant_schedule
|
32 |
-
|
33 |
-
|
34 |
-
.. autofunction:: transformers.get_constant_schedule_with_warmup
|
35 |
-
|
36 |
-
.. image:: /imgs/warmup_constant_schedule.png
|
37 |
-
:target: /imgs/warmup_constant_schedule.png
|
38 |
-
:alt:
|
39 |
-
|
40 |
-
|
41 |
-
.. autofunction:: transformers.get_cosine_schedule_with_warmup
|
42 |
-
:members:
|
43 |
-
|
44 |
-
.. image:: /imgs/warmup_cosine_schedule.png
|
45 |
-
:target: /imgs/warmup_cosine_schedule.png
|
46 |
-
:alt:
|
47 |
-
|
48 |
-
|
49 |
-
.. autofunction:: transformers.get_cosine_with_hard_restarts_schedule_with_warmup
|
50 |
-
|
51 |
-
.. image:: /imgs/warmup_cosine_hard_restarts_schedule.png
|
52 |
-
:target: /imgs/warmup_cosine_hard_restarts_schedule.png
|
53 |
-
:alt:
|
54 |
-
|
55 |
-
|
56 |
-
|
57 |
-
.. autofunction:: transformers.get_linear_schedule_with_warmup
|
58 |
-
|
59 |
-
.. image:: /imgs/warmup_linear_schedule.png
|
60 |
-
:target: /imgs/warmup_linear_schedule.png
|
61 |
-
:alt:
|
62 |
-
|
63 |
-
``Warmup``
|
64 |
-
~~~~~~~~~~~~~~~~
|
65 |
-
|
66 |
-
.. autoclass:: transformers.Warmup
|
67 |
-
:members:
|
68 |
-
|
69 |
-
Gradient Strategies
|
70 |
-
----------------------------------------------------
|
71 |
-
|
72 |
-
``GradientAccumulator``
|
73 |
-
~~~~~~~~~~~~~~~~~~~~~~~
|
74 |
-
|
75 |
-
.. autoclass:: transformers.GradientAccumulator
|
|
|
|
|
|
|
|
|
|
|
|
|
|
|
|
|
|
|
|
|
|
|
|
|
|
|
|
|
|
|
|
|
|
|
|
|
|
|
|
|
|
|
|
|
|
|
|
|
|
|
|
|
|
|
|
|
|
|
|
|
|
|
|
|
|
|
|
|
|
|
|
|
|
|
|
|
|
|
|
|
|
|
|
|
|
|
|
|
|
|
|
|
|
|
|
|
|
|
|
|
|
|
|
|
|
|
|
|
|
|
|
|
|
|
|
|
|
|
|
|
|
|
|
|
|
|
|
|
|
|
|
|
|
|
|
|
|
|
|
|
|
|
|
|
|
|
|
|
|
|
|
server/transformers/docs/source/main_classes/processors.rst
DELETED
@@ -1,153 +0,0 @@
|
|
1 |
-
Processors
|
2 |
-
----------------------------------------------------
|
3 |
-
|
4 |
-
This library includes processors for several traditional tasks. These processors can be used to process a dataset into
|
5 |
-
examples that can be fed to a model.
|
6 |
-
|
7 |
-
Processors
|
8 |
-
~~~~~~~~~~~~~~~~~~~~~
|
9 |
-
|
10 |
-
All processors follow the same architecture which is that of the
|
11 |
-
:class:`~transformers.data.processors.utils.DataProcessor`. The processor returns a list
|
12 |
-
of :class:`~transformers.data.processors.utils.InputExample`. These
|
13 |
-
:class:`~transformers.data.processors.utils.InputExample` can be converted to
|
14 |
-
:class:`~transformers.data.processors.utils.InputFeatures` in order to be fed to the model.
|
15 |
-
|
16 |
-
.. autoclass:: transformers.data.processors.utils.DataProcessor
|
17 |
-
:members:
|
18 |
-
|
19 |
-
|
20 |
-
.. autoclass:: transformers.data.processors.utils.InputExample
|
21 |
-
:members:
|
22 |
-
|
23 |
-
|
24 |
-
.. autoclass:: transformers.data.processors.utils.InputFeatures
|
25 |
-
:members:
|
26 |
-
|
27 |
-
|
28 |
-
GLUE
|
29 |
-
~~~~~~~~~~~~~~~~~~~~~
|
30 |
-
|
31 |
-
`General Language Understanding Evaluation (GLUE) <https://gluebenchmark.com/>`__ is a benchmark that evaluates
|
32 |
-
the performance of models across a diverse set of existing NLU tasks. It was released together with the paper
|
33 |
-
`GLUE: A multi-task benchmark and analysis platform for natural language understanding <https://openreview.net/pdf?id=rJ4km2R5t7>`__
|
34 |
-
|
35 |
-
This library hosts a total of 10 processors for the following tasks: MRPC, MNLI, MNLI (mismatched),
|
36 |
-
CoLA, SST2, STSB, QQP, QNLI, RTE and WNLI.
|
37 |
-
|
38 |
-
Those processors are:
|
39 |
-
- :class:`~transformers.data.processors.utils.MrpcProcessor`
|
40 |
-
- :class:`~transformers.data.processors.utils.MnliProcessor`
|
41 |
-
- :class:`~transformers.data.processors.utils.MnliMismatchedProcessor`
|
42 |
-
- :class:`~transformers.data.processors.utils.Sst2Processor`
|
43 |
-
- :class:`~transformers.data.processors.utils.StsbProcessor`
|
44 |
-
- :class:`~transformers.data.processors.utils.QqpProcessor`
|
45 |
-
- :class:`~transformers.data.processors.utils.QnliProcessor`
|
46 |
-
- :class:`~transformers.data.processors.utils.RteProcessor`
|
47 |
-
- :class:`~transformers.data.processors.utils.WnliProcessor`
|
48 |
-
|
49 |
-
Additionally, the following method can be used to load values from a data file and convert them to a list of
|
50 |
-
:class:`~transformers.data.processors.utils.InputExample`.
|
51 |
-
|
52 |
-
.. automethod:: transformers.data.processors.glue.glue_convert_examples_to_features
|
53 |
-
|
54 |
-
Example usage
|
55 |
-
^^^^^^^^^^^^^^^^^^^^^^^^^
|
56 |
-
|
57 |
-
An example using these processors is given in the `run_glue.py <https://github.com/huggingface/pytorch-transformers/blob/master/examples/run_glue.py>`__ script.
|
58 |
-
|
59 |
-
|
60 |
-
XNLI
|
61 |
-
~~~~~~~~~~~~~~~~~~~~~
|
62 |
-
|
63 |
-
`The Cross-Lingual NLI Corpus (XNLI) <https://www.nyu.edu/projects/bowman/xnli/>`__ is a benchmark that evaluates
|
64 |
-
the quality of cross-lingual text representations.
|
65 |
-
XNLI is crowd-sourced dataset based on `MultiNLI <http://www.nyu.edu/projects/bowman/multinli/>`: pairs of text are labeled with textual entailment
|
66 |
-
annotations for 15 different languages (including both high-ressource language such as English and low-ressource languages such as Swahili).
|
67 |
-
|
68 |
-
It was released together with the paper
|
69 |
-
`XNLI: Evaluating Cross-lingual Sentence Representations <https://arxiv.org/abs/1809.05053>`__
|
70 |
-
|
71 |
-
This library hosts the processor to load the XNLI data:
|
72 |
-
- :class:`~transformers.data.processors.utils.XnliProcessor`
|
73 |
-
|
74 |
-
Please note that since the gold labels are available on the test set, evaluation is performed on the test set.
|
75 |
-
|
76 |
-
An example using these processors is given in the
|
77 |
-
`run_xnli.py <https://github.com/huggingface/pytorch-transformers/blob/master/examples/run_xnli.py>`__ script.
|
78 |
-
|
79 |
-
|
80 |
-
SQuAD
|
81 |
-
~~~~~~~~~~~~~~~~~~~~~
|
82 |
-
|
83 |
-
`The Stanford Question Answering Dataset (SQuAD) <https://rajpurkar.github.io/SQuAD-explorer//>`__ is a benchmark that evaluates
|
84 |
-
the performance of models on question answering. Two versions are available, v1.1 and v2.0. The first version (v1.1) was released together with the paper
|
85 |
-
`SQuAD: 100,000+ Questions for Machine Comprehension of Text <https://arxiv.org/abs/1606.05250>`__. The second version (v2.0) was released alongside
|
86 |
-
the paper `Know What You Don't Know: Unanswerable Questions for SQuAD <https://arxiv.org/abs/1806.03822>`__.
|
87 |
-
|
88 |
-
This library hosts a processor for each of the two versions:
|
89 |
-
|
90 |
-
Processors
|
91 |
-
^^^^^^^^^^^^^^^^^^^^^^^^^
|
92 |
-
|
93 |
-
Those processors are:
|
94 |
-
- :class:`~transformers.data.processors.utils.SquadV1Processor`
|
95 |
-
- :class:`~transformers.data.processors.utils.SquadV2Processor`
|
96 |
-
|
97 |
-
They both inherit from the abstract class :class:`~transformers.data.processors.utils.SquadProcessor`
|
98 |
-
|
99 |
-
.. autoclass:: transformers.data.processors.squad.SquadProcessor
|
100 |
-
:members:
|
101 |
-
|
102 |
-
Additionally, the following method can be used to convert SQuAD examples into :class:`~transformers.data.processors.utils.SquadFeatures`
|
103 |
-
that can be used as model inputs.
|
104 |
-
|
105 |
-
.. automethod:: transformers.data.processors.squad.squad_convert_examples_to_features
|
106 |
-
|
107 |
-
These processors as well as the aforementionned method can be used with files containing the data as well as with the `tensorflow_datasets` package.
|
108 |
-
Examples are given below.
|
109 |
-
|
110 |
-
|
111 |
-
Example usage
|
112 |
-
^^^^^^^^^^^^^^^^^^^^^^^^^
|
113 |
-
Here is an example using the processors as well as the conversion method using data files:
|
114 |
-
|
115 |
-
Example::
|
116 |
-
|
117 |
-
# Loading a V2 processor
|
118 |
-
processor = SquadV2Processor()
|
119 |
-
examples = processor.get_dev_examples(squad_v2_data_dir)
|
120 |
-
|
121 |
-
# Loading a V1 processor
|
122 |
-
processor = SquadV1Processor()
|
123 |
-
examples = processor.get_dev_examples(squad_v1_data_dir)
|
124 |
-
|
125 |
-
features = squad_convert_examples_to_features(
|
126 |
-
examples=examples,
|
127 |
-
tokenizer=tokenizer,
|
128 |
-
max_seq_length=max_seq_length,
|
129 |
-
doc_stride=args.doc_stride,
|
130 |
-
max_query_length=max_query_length,
|
131 |
-
is_training=not evaluate,
|
132 |
-
)
|
133 |
-
|
134 |
-
Using `tensorflow_datasets` is as easy as using a data file:
|
135 |
-
|
136 |
-
Example::
|
137 |
-
|
138 |
-
# tensorflow_datasets only handle Squad V1.
|
139 |
-
tfds_examples = tfds.load("squad")
|
140 |
-
examples = SquadV1Processor().get_examples_from_dataset(tfds_examples, evaluate=evaluate)
|
141 |
-
|
142 |
-
features = squad_convert_examples_to_features(
|
143 |
-
examples=examples,
|
144 |
-
tokenizer=tokenizer,
|
145 |
-
max_seq_length=max_seq_length,
|
146 |
-
doc_stride=args.doc_stride,
|
147 |
-
max_query_length=max_query_length,
|
148 |
-
is_training=not evaluate,
|
149 |
-
)
|
150 |
-
|
151 |
-
|
152 |
-
Another example using these processors is given in the
|
153 |
-
`run_squad.py <https://github.com/huggingface/transformers/blob/master/examples/run_squad.py>`__ script.
|
|
|
|
|
|
|
|
|
|
|
|
|
|
|
|
|
|
|
|
|
|
|
|
|
|
|
|
|
|
|
|
|
|
|
|
|
|
|
|
|
|
|
|
|
|
|
|
|
|
|
|
|
|
|
|
|
|
|
|
|
|
|
|
|
|
|
|
|
|
|
|
|
|
|
|
|
|
|
|
|
|
|
|
|
|
|
|
|
|
|
|
|
|
|
|
|
|
|
|
|
|
|
|
|
|
|
|
|
|
|
|
|
|
|
|
|
|
|
|
|
|
|
|
|
|
|
|
|
|
|
|
|
|
|
|
|
|
|
|
|
|
|
|
|
|
|
|
|
|
|
|
|
|
|
|
|
|
|
|
|
|
|
|
|
|
|
|
|
|
|
|
|
|
|
|
|
|
|
|
|
|
|
|
|
|
|
|
|
|
|
|
|
|
|
|
|
|
|
|
|
|
|
|
|
|
|
|
|
|
|
|
|
|
|
|
|
|
|
|
|
|
|
|
|
|
|
|
|
|
|
|
|
|
|
|
|
|
|
|
|
|
|
|
|
|
|
|
|
|
|
|
|
|
|
|
|
|
|
|
|
|
|
|
|
|
|
|
|
|
|
|
|
|
|
|
|
|
|
|
|
|
|
|
|
|
|
|
|
|
|
|
|
|
|
|
|
|
|
|
|
|
|
|
|
|
|
|
server/transformers/docs/source/main_classes/tokenizer.rst
DELETED
@@ -1,16 +0,0 @@
|
|
1 |
-
Tokenizer
|
2 |
-
----------------------------------------------------
|
3 |
-
|
4 |
-
The base class ``PreTrainedTokenizer`` implements the common methods for loading/saving a tokenizer either from a local file or directory, or from a pretrained tokenizer provided by the library (downloaded from HuggingFace's AWS S3 repository).
|
5 |
-
|
6 |
-
``PreTrainedTokenizer`` is the main entry point into tokenizers as it also implements the main methods for using all the tokenizers:
|
7 |
-
|
8 |
-
- tokenizing, converting tokens to ids and back and encoding/decoding,
|
9 |
-
- adding new tokens to the vocabulary in a way that is independant of the underlying structure (BPE, SentencePiece...),
|
10 |
-
- managing special tokens (adding them, assigning them to roles, making sure they are not split during tokenization)
|
11 |
-
|
12 |
-
``PreTrainedTokenizer``
|
13 |
-
~~~~~~~~~~~~~~~~~~~~~~~~
|
14 |
-
|
15 |
-
.. autoclass:: transformers.PreTrainedTokenizer
|
16 |
-
:members:
|
|
|
|
|
|
|
|
|
|
|
|
|
|
|
|
|
|
|
|
|
|
|
|
|
|
|
|
|
|
|
|
|
|
server/transformers/docs/source/migration.md
DELETED
@@ -1,109 +0,0 @@
|
|
1 |
-
# Migrating from pytorch-pretrained-bert
|
2 |
-
|
3 |
-
|
4 |
-
Here is a quick summary of what you should take care of when migrating from `pytorch-pretrained-bert` to `transformers`
|
5 |
-
|
6 |
-
### Models always output `tuples`
|
7 |
-
|
8 |
-
The main breaking change when migrating from `pytorch-pretrained-bert` to `transformers` is that the models forward method always outputs a `tuple` with various elements depending on the model and the configuration parameters.
|
9 |
-
|
10 |
-
The exact content of the tuples for each model are detailled in the models' docstrings and the [documentation](https://huggingface.co/transformers/).
|
11 |
-
|
12 |
-
In pretty much every case, you will be fine by taking the first element of the output as the output you previously used in `pytorch-pretrained-bert`.
|
13 |
-
|
14 |
-
Here is a `pytorch-pretrained-bert` to `transformers` conversion example for a `BertForSequenceClassification` classification model:
|
15 |
-
|
16 |
-
```python
|
17 |
-
# Let's load our model
|
18 |
-
model = BertForSequenceClassification.from_pretrained('bert-base-uncased')
|
19 |
-
|
20 |
-
# If you used to have this line in pytorch-pretrained-bert:
|
21 |
-
loss = model(input_ids, labels=labels)
|
22 |
-
|
23 |
-
# Now just use this line in transformers to extract the loss from the output tuple:
|
24 |
-
outputs = model(input_ids, labels=labels)
|
25 |
-
loss = outputs[0]
|
26 |
-
|
27 |
-
# In transformers you can also have access to the logits:
|
28 |
-
loss, logits = outputs[:2]
|
29 |
-
|
30 |
-
# And even the attention weigths if you configure the model to output them (and other outputs too, see the docstrings and documentation)
|
31 |
-
model = BertForSequenceClassification.from_pretrained('bert-base-uncased', output_attentions=True)
|
32 |
-
outputs = model(input_ids, labels=labels)
|
33 |
-
loss, logits, attentions = outputs
|
34 |
-
```
|
35 |
-
|
36 |
-
### Serialization
|
37 |
-
|
38 |
-
Breaking change in the `from_pretrained()`method:
|
39 |
-
|
40 |
-
1. Models are now set in evaluation mode by default when instantiated with the `from_pretrained()` method. To train them don't forget to set them back in training mode (`model.train()`) to activate the dropout modules.
|
41 |
-
|
42 |
-
2. The additional `*inputs` and `**kwargs` arguments supplied to the `from_pretrained()` method used to be directly passed to the underlying model's class `__init__()` method. They are now used to update the model configuration attribute first which can break derived model classes build based on the previous `BertForSequenceClassification` examples. More precisely, the positional arguments `*inputs` provided to `from_pretrained()` are directly forwarded the model `__init__()` method while the keyword arguments `**kwargs` (i) which match configuration class attributes are used to update said attributes (ii) which don't match any configuration class attributes are forwarded to the model `__init__()` method.
|
43 |
-
|
44 |
-
Also, while not a breaking change, the serialization methods have been standardized and you probably should switch to the new method `save_pretrained(save_directory)` if you were using any other serialization method before.
|
45 |
-
|
46 |
-
Here is an example:
|
47 |
-
|
48 |
-
```python
|
49 |
-
### Let's load a model and tokenizer
|
50 |
-
model = BertForSequenceClassification.from_pretrained('bert-base-uncased')
|
51 |
-
tokenizer = BertTokenizer.from_pretrained('bert-base-uncased')
|
52 |
-
|
53 |
-
### Do some stuff to our model and tokenizer
|
54 |
-
# Ex: add new tokens to the vocabulary and embeddings of our model
|
55 |
-
tokenizer.add_tokens(['[SPECIAL_TOKEN_1]', '[SPECIAL_TOKEN_2]'])
|
56 |
-
model.resize_token_embeddings(len(tokenizer))
|
57 |
-
# Train our model
|
58 |
-
train(model)
|
59 |
-
|
60 |
-
### Now let's save our model and tokenizer to a directory
|
61 |
-
model.save_pretrained('./my_saved_model_directory/')
|
62 |
-
tokenizer.save_pretrained('./my_saved_model_directory/')
|
63 |
-
|
64 |
-
### Reload the model and the tokenizer
|
65 |
-
model = BertForSequenceClassification.from_pretrained('./my_saved_model_directory/')
|
66 |
-
tokenizer = BertTokenizer.from_pretrained('./my_saved_model_directory/')
|
67 |
-
```
|
68 |
-
|
69 |
-
### Optimizers: BertAdam & OpenAIAdam are now AdamW, schedules are standard PyTorch schedules
|
70 |
-
|
71 |
-
The two optimizers previously included, `BertAdam` and `OpenAIAdam`, have been replaced by a single `AdamW` optimizer which has a few differences:
|
72 |
-
|
73 |
-
- it only implements weights decay correction,
|
74 |
-
- schedules are now externals (see below),
|
75 |
-
- gradient clipping is now also external (see below).
|
76 |
-
|
77 |
-
The new optimizer `AdamW` matches PyTorch `Adam` optimizer API and let you use standard PyTorch or apex methods for the schedule and clipping.
|
78 |
-
|
79 |
-
The schedules are now standard [PyTorch learning rate schedulers](https://pytorch.org/docs/stable/optim.html#how-to-adjust-learning-rate) and not part of the optimizer anymore.
|
80 |
-
|
81 |
-
Here is a conversion examples from `BertAdam` with a linear warmup and decay schedule to `AdamW` and the same schedule:
|
82 |
-
|
83 |
-
```python
|
84 |
-
# Parameters:
|
85 |
-
lr = 1e-3
|
86 |
-
max_grad_norm = 1.0
|
87 |
-
num_training_steps = 1000
|
88 |
-
num_warmup_steps = 100
|
89 |
-
warmup_proportion = float(num_warmup_steps) / float(num_training_steps) # 0.1
|
90 |
-
|
91 |
-
### Previously BertAdam optimizer was instantiated like this:
|
92 |
-
optimizer = BertAdam(model.parameters(), lr=lr, schedule='warmup_linear', warmup=warmup_proportion, num_training_steps=num_training_steps)
|
93 |
-
### and used like this:
|
94 |
-
for batch in train_data:
|
95 |
-
loss = model(batch)
|
96 |
-
loss.backward()
|
97 |
-
optimizer.step()
|
98 |
-
|
99 |
-
### In Transformers, optimizer and schedules are splitted and instantiated like this:
|
100 |
-
optimizer = AdamW(model.parameters(), lr=lr, correct_bias=False) # To reproduce BertAdam specific behavior set correct_bias=False
|
101 |
-
scheduler = get_linear_schedule_with_warmup(optimizer, num_warmup_steps=num_warmup_steps, num_training_steps=num_training_steps) # PyTorch scheduler
|
102 |
-
### and used like this:
|
103 |
-
for batch in train_data:
|
104 |
-
loss = model(batch)
|
105 |
-
loss.backward()
|
106 |
-
torch.nn.utils.clip_grad_norm_(model.parameters(), max_grad_norm) # Gradient clipping is not in AdamW anymore (so you can use amp without issue)
|
107 |
-
optimizer.step()
|
108 |
-
scheduler.step()
|
109 |
-
```
|
|
|
|
|
|
|
|
|
|
|
|
|
|
|
|
|
|
|
|
|
|
|
|
|
|
|
|
|
|
|
|
|
|
|
|
|
|
|
|
|
|
|
|
|
|
|
|
|
|
|
|
|
|
|
|
|
|
|
|
|
|
|
|
|
|
|
|
|
|
|
|
|
|
|
|
|
|
|
|
|
|
|
|
|
|
|
|
|
|
|
|
|
|
|
|
|
|
|
|
|
|
|
|
|
|
|
|
|
|
|
|
|
|
|
|
|
|
|
|
|
|
|
|
|
|
|
|
|
|
|
|
|
|
|
|
|
|
|
|
|
|
|
|
|
|
|
|
|
|
|
|
|
|
|
|
|
|
|
|
|
|
|
|
|
|
|
|
|
|
|
|
|
|
|
|
|
|
|
|
|
|
|
|
|
|
|
|
|
|
|
|
|
|
|
|
|
|
|
|
|
|
|
|
|
|
|
|
|
|
|
|
|
|
|
|
|
|
|
|
server/transformers/docs/source/model_doc/albert.rst
DELETED
@@ -1,64 +0,0 @@
|
|
1 |
-
ALBERT
|
2 |
-
----------------------------------------------------
|
3 |
-
|
4 |
-
``AlbertConfig``
|
5 |
-
~~~~~~~~~~~~~~~~~~~~~
|
6 |
-
|
7 |
-
.. autoclass:: transformers.AlbertConfig
|
8 |
-
:members:
|
9 |
-
|
10 |
-
|
11 |
-
``AlbertTokenizer``
|
12 |
-
~~~~~~~~~~~~~~~~~~~~~
|
13 |
-
|
14 |
-
.. autoclass:: transformers.AlbertTokenizer
|
15 |
-
:members:
|
16 |
-
|
17 |
-
|
18 |
-
``AlbertModel``
|
19 |
-
~~~~~~~~~~~~~~~~~~~~
|
20 |
-
|
21 |
-
.. autoclass:: transformers.AlbertModel
|
22 |
-
:members:
|
23 |
-
|
24 |
-
|
25 |
-
``AlbertForMaskedLM``
|
26 |
-
~~~~~~~~~~~~~~~~~~~~~~~~~~~~~
|
27 |
-
|
28 |
-
.. autoclass:: transformers.AlbertForMaskedLM
|
29 |
-
:members:
|
30 |
-
|
31 |
-
|
32 |
-
``AlbertForSequenceClassification``
|
33 |
-
~~~~~~~~~~~~~~~~~~~~~~~~~~
|
34 |
-
|
35 |
-
.. autoclass:: transformers.AlbertForSequenceClassification
|
36 |
-
:members:
|
37 |
-
|
38 |
-
|
39 |
-
``AlbertForQuestionAnswering``
|
40 |
-
~~~~~~~~~~~~~~~~~~~~~~~~~~~~~~~~~~~~~~~~
|
41 |
-
|
42 |
-
.. autoclass:: transformers.AlbertForQuestionAnswering
|
43 |
-
:members:
|
44 |
-
|
45 |
-
|
46 |
-
``TFAlbertModel``
|
47 |
-
~~~~~~~~~~~~~~~~~~~~
|
48 |
-
|
49 |
-
.. autoclass:: transformers.TFAlbertModel
|
50 |
-
:members:
|
51 |
-
|
52 |
-
|
53 |
-
``TFAlbertForMaskedLM``
|
54 |
-
~~~~~~~~~~~~~~~~~~~~~~~~~~
|
55 |
-
|
56 |
-
.. autoclass:: transformers.TFAlbertForMaskedLM
|
57 |
-
:members:
|
58 |
-
|
59 |
-
|
60 |
-
``TFAlbertForSequenceClassification``
|
61 |
-
~~~~~~~~~~~~~~~~~~~~~~~~~~~~~~~~~~~~~~~~
|
62 |
-
|
63 |
-
.. autoclass:: transformers.TFAlbertForSequenceClassification
|
64 |
-
:members:
|
|
|
|
|
|
|
|
|
|
|
|
|
|
|
|
|
|
|
|
|
|
|
|
|
|
|
|
|
|
|
|
|
|
|
|
|
|
|
|
|
|
|
|
|
|
|
|
|
|
|
|
|
|
|
|
|
|
|
|
|
|
|
|
|
|
|
|
|
|
|
|
|
|
|
|
|
|
|
|
|
|
|
|
|
|
|
|
|
|
|
|
|
|
|
|
|
|
|
|
|
|
|
|
|
|
|
|
|
|
|
|
|
|
|
|
|
|
|
|
|
|
|
|
|
|
|
|
|
|
server/transformers/docs/source/model_doc/auto.rst
DELETED
@@ -1,29 +0,0 @@
|
|
1 |
-
AutoModels
|
2 |
-
-----------
|
3 |
-
|
4 |
-
In many cases, the architecture you want to use can be guessed from the name or the path of the pretrained model you are supplying to the ``from_pretrained`` method.
|
5 |
-
|
6 |
-
AutoClasses are here to do this job for you so that you automatically retreive the relevant model given the name/path to the pretrained weights/config/vocabulary:
|
7 |
-
|
8 |
-
Instantiating one of ``AutoModel``, ``AutoConfig`` and ``AutoTokenizer`` will directly create a class of the relevant architecture (ex: ``model = AutoModel.from_pretrained('bert-base-cased')`` will create a instance of ``BertModel``).
|
9 |
-
|
10 |
-
|
11 |
-
``AutoConfig``
|
12 |
-
~~~~~~~~~~~~~~~~~~~~~
|
13 |
-
|
14 |
-
.. autoclass:: transformers.AutoConfig
|
15 |
-
:members:
|
16 |
-
|
17 |
-
|
18 |
-
``AutoModel``
|
19 |
-
~~~~~~~~~~~~~~~~~~~~~
|
20 |
-
|
21 |
-
.. autoclass:: transformers.AutoModel
|
22 |
-
:members:
|
23 |
-
|
24 |
-
|
25 |
-
``AutoTokenizer``
|
26 |
-
~~~~~~~~~~~~~~~~~~~~~~~~~~~~~
|
27 |
-
|
28 |
-
.. autoclass:: transformers.AutoTokenizer
|
29 |
-
:members:
|
|
|
|
|
|
|
|
|
|
|
|
|
|
|
|
|
|
|
|
|
|
|
|
|
|
|
|
|
|
|
|
|
|
|
|
|
|
|
|
|
|
|
|
|
|
|
|
|
|
|
|
|
|
|
|
|
|
|
|
server/transformers/docs/source/model_doc/bert.rst
DELETED
@@ -1,128 +0,0 @@
|
|
1 |
-
BERT
|
2 |
-
----------------------------------------------------
|
3 |
-
|
4 |
-
``BertConfig``
|
5 |
-
~~~~~~~~~~~~~~~~~~~~~
|
6 |
-
|
7 |
-
.. autoclass:: transformers.BertConfig
|
8 |
-
:members:
|
9 |
-
|
10 |
-
|
11 |
-
``BertTokenizer``
|
12 |
-
~~~~~~~~~~~~~~~~~~~~~
|
13 |
-
|
14 |
-
.. autoclass:: transformers.BertTokenizer
|
15 |
-
:members:
|
16 |
-
|
17 |
-
|
18 |
-
``BertModel``
|
19 |
-
~~~~~~~~~~~~~~~~~~~~
|
20 |
-
|
21 |
-
.. autoclass:: transformers.BertModel
|
22 |
-
:members:
|
23 |
-
|
24 |
-
|
25 |
-
``BertForPreTraining``
|
26 |
-
~~~~~~~~~~~~~~~~~~~~~~~~~~~~~
|
27 |
-
|
28 |
-
.. autoclass:: transformers.BertForPreTraining
|
29 |
-
:members:
|
30 |
-
|
31 |
-
|
32 |
-
``BertForMaskedLM``
|
33 |
-
~~~~~~~~~~~~~~~~~~~~~~~~~~
|
34 |
-
|
35 |
-
.. autoclass:: transformers.BertForMaskedLM
|
36 |
-
:members:
|
37 |
-
|
38 |
-
|
39 |
-
``BertForNextSentencePrediction``
|
40 |
-
~~~~~~~~~~~~~~~~~~~~~~~~~~~~~~~~~~~~~~~~
|
41 |
-
|
42 |
-
.. autoclass:: transformers.BertForNextSentencePrediction
|
43 |
-
:members:
|
44 |
-
|
45 |
-
|
46 |
-
``BertForSequenceClassification``
|
47 |
-
~~~~~~~~~~~~~~~~~~~~~~~~~~~~~~~~~~~~~~~~
|
48 |
-
|
49 |
-
.. autoclass:: transformers.BertForSequenceClassification
|
50 |
-
:members:
|
51 |
-
|
52 |
-
|
53 |
-
``BertForMultipleChoice``
|
54 |
-
~~~~~~~~~~~~~~~~~~~~~~~~~~~~~~~~
|
55 |
-
|
56 |
-
.. autoclass:: transformers.BertForMultipleChoice
|
57 |
-
:members:
|
58 |
-
|
59 |
-
|
60 |
-
``BertForTokenClassification``
|
61 |
-
~~~~~~~~~~~~~~~~~~~~~~~~~~~~~~~~~~~~~
|
62 |
-
|
63 |
-
.. autoclass:: transformers.BertForTokenClassification
|
64 |
-
:members:
|
65 |
-
|
66 |
-
|
67 |
-
``BertForQuestionAnswering``
|
68 |
-
~~~~~~~~~~~~~~~~~~~~~~~~~~~~~~~~~~~
|
69 |
-
|
70 |
-
.. autoclass:: transformers.BertForQuestionAnswering
|
71 |
-
:members:
|
72 |
-
|
73 |
-
|
74 |
-
``TFBertModel``
|
75 |
-
~~~~~~~~~~~~~~~~~~~~
|
76 |
-
|
77 |
-
.. autoclass:: transformers.TFBertModel
|
78 |
-
:members:
|
79 |
-
|
80 |
-
|
81 |
-
``TFBertForPreTraining``
|
82 |
-
~~~~~~~~~~~~~~~~~~~~~~~~~~~~~
|
83 |
-
|
84 |
-
.. autoclass:: transformers.TFBertForPreTraining
|
85 |
-
:members:
|
86 |
-
|
87 |
-
|
88 |
-
``TFBertForMaskedLM``
|
89 |
-
~~~~~~~~~~~~~~~~~~~~~~~~~~
|
90 |
-
|
91 |
-
.. autoclass:: transformers.TFBertForMaskedLM
|
92 |
-
:members:
|
93 |
-
|
94 |
-
|
95 |
-
``TFBertForNextSentencePrediction``
|
96 |
-
~~~~~~~~~~~~~~~~~~~~~~~~~~~~~~~~~~~~~~~~
|
97 |
-
|
98 |
-
.. autoclass:: transformers.TFBertForNextSentencePrediction
|
99 |
-
:members:
|
100 |
-
|
101 |
-
|
102 |
-
``TFBertForSequenceClassification``
|
103 |
-
~~~~~~~~~~~~~~~~~~~~~~~~~~~~~~~~~~~~~~~~
|
104 |
-
|
105 |
-
.. autoclass:: transformers.TFBertForSequenceClassification
|
106 |
-
:members:
|
107 |
-
|
108 |
-
|
109 |
-
``TFBertForMultipleChoice``
|
110 |
-
~~~~~~~~~~~~~~~~~~~~~~~~~~~~~~~~
|
111 |
-
|
112 |
-
.. autoclass:: transformers.TFBertForMultipleChoice
|
113 |
-
:members:
|
114 |
-
|
115 |
-
|
116 |
-
``TFBertForTokenClassification``
|
117 |
-
~~~~~~~~~~~~~~~~~~~~~~~~~~~~~~~~~~~~~
|
118 |
-
|
119 |
-
.. autoclass:: transformers.TFBertForTokenClassification
|
120 |
-
:members:
|
121 |
-
|
122 |
-
|
123 |
-
``TFBertForQuestionAnswering``
|
124 |
-
~~~~~~~~~~~~~~~~~~~~~~~~~~~~~~~~~~~
|
125 |
-
|
126 |
-
.. autoclass:: transformers.TFBertForQuestionAnswering
|
127 |
-
:members:
|
128 |
-
|
|
|
|
|
|
|
|
|
|
|
|
|
|
|
|
|
|
|
|
|
|
|
|
|
|
|
|
|
|
|
|
|
|
|
|
|
|
|
|
|
|
|
|
|
|
|
|
|
|
|
|
|
|
|
|
|
|
|
|
|
|
|
|
|
|
|
|
|
|
|
|
|
|
|
|
|
|
|
|
|
|
|
|
|
|
|
|
|
|
|
|
|
|
|
|
|
|
|
|
|
|
|
|
|
|
|
|
|
|
|
|
|
|
|
|
|
|
|
|
|
|
|
|
|
|
|
|
|
|
|
|
|
|
|
|
|
|
|
|
|
|
|
|
|
|
|
|
|
|
|
|
|
|
|
|
|
|
|
|
|
|
|
|
|
|
|
|
|
|
|
|
|
|
|
|
|
|
|
|
|
|
|
|
|
|
|
|
|
|
|
|
|
|
|
|
|
|
|
|
|
|
|
|
|
|
|
|
|
|
|
|
|
|
|
|
|
|
|
|
|
|
|
|
|
|
|
|
|
|
|
|
|
|
|
|
|
|
|
|
|
|
|
|
|
|
|
|
|
|
|
|
|
|
|
|
|
|
server/transformers/docs/source/model_doc/camembert.rst
DELETED
@@ -1,50 +0,0 @@
|
|
1 |
-
CamemBERT
|
2 |
-
----------------------------------------------------
|
3 |
-
|
4 |
-
``CamembertConfig``
|
5 |
-
~~~~~~~~~~~~~~~~~~~~~
|
6 |
-
|
7 |
-
.. autoclass:: transformers.CamembertConfig
|
8 |
-
:members:
|
9 |
-
|
10 |
-
|
11 |
-
``CamembertTokenizer``
|
12 |
-
~~~~~~~~~~~~~~~~~~~~~
|
13 |
-
|
14 |
-
.. autoclass:: transformers.CamembertTokenizer
|
15 |
-
:members:
|
16 |
-
|
17 |
-
|
18 |
-
``CamembertModel``
|
19 |
-
~~~~~~~~~~~~~~~~~~~~
|
20 |
-
|
21 |
-
.. autoclass:: transformers.CamembertModel
|
22 |
-
:members:
|
23 |
-
|
24 |
-
|
25 |
-
``CamembertForMaskedLM``
|
26 |
-
~~~~~~~~~~~~~~~~~~~~~~~~~~~~~
|
27 |
-
|
28 |
-
.. autoclass:: transformers.CamembertForMaskedLM
|
29 |
-
:members:
|
30 |
-
|
31 |
-
|
32 |
-
``CamembertForSequenceClassification``
|
33 |
-
~~~~~~~~~~~~~~~~~~~~~~~~~~
|
34 |
-
|
35 |
-
.. autoclass:: transformers.CamembertForSequenceClassification
|
36 |
-
:members:
|
37 |
-
|
38 |
-
|
39 |
-
``CamembertForMultipleChoice``
|
40 |
-
~~~~~~~~~~~~~~~~~~~~~~~~~~~~~~~~~~~~~~~~
|
41 |
-
|
42 |
-
.. autoclass:: transformers.CamembertForMultipleChoice
|
43 |
-
:members:
|
44 |
-
|
45 |
-
|
46 |
-
``CamembertForTokenClassification``
|
47 |
-
~~~~~~~~~~~~~~~~~~~~~~~~~~~~~~~~~~~~~~~~
|
48 |
-
|
49 |
-
.. autoclass:: transformers.CamembertForTokenClassification
|
50 |
-
:members:
|
|
|
|
|
|
|
|
|
|
|
|
|
|
|
|
|
|
|
|
|
|
|
|
|
|
|
|
|
|
|
|
|
|
|
|
|
|
|
|
|
|
|
|
|
|
|
|
|
|
|
|
|
|
|
|
|
|
|
|
|
|
|
|
|
|
|
|
|
|
|
|
|
|
|
|
|
|
|
|
|
|
|
|
|
|
|
|
|
|
|
|
|
|
|
|
|
|
|
|
|
|
server/transformers/docs/source/model_doc/ctrl.rst
DELETED
@@ -1,49 +0,0 @@
|
|
1 |
-
CTRL
|
2 |
-
----------------------------------------------------
|
3 |
-
|
4 |
-
Note: if you fine-tune a CTRL model using the Salesforce code (https://github.com/salesforce/ctrl),
|
5 |
-
you'll be able to convert from TF to our HuggingFace/Transformers format using the
|
6 |
-
``convert_tf_to_huggingface_pytorch.py`` script (see `issue #1654 <https://github.com/huggingface/transformers/issues/1654>`_).
|
7 |
-
|
8 |
-
|
9 |
-
``CTRLConfig``
|
10 |
-
~~~~~~~~~~~~~~~~~~~~~~~~~~~~~~~~
|
11 |
-
|
12 |
-
.. autoclass:: transformers.CTRLConfig
|
13 |
-
:members:
|
14 |
-
|
15 |
-
|
16 |
-
``CTRLTokenizer``
|
17 |
-
~~~~~~~~~~~~~~~~~~~~~~~~~~~~~~~~
|
18 |
-
|
19 |
-
.. autoclass:: transformers.CTRLTokenizer
|
20 |
-
:members:
|
21 |
-
|
22 |
-
|
23 |
-
``CTRLModel``
|
24 |
-
~~~~~~~~~~~~~~~~~~~~~~~~~~~~~~~
|
25 |
-
|
26 |
-
.. autoclass:: transformers.CTRLModel
|
27 |
-
:members:
|
28 |
-
|
29 |
-
|
30 |
-
``CTRLLMHeadModel``
|
31 |
-
~~~~~~~~~~~~~~~~~~~~~~~~~~~~~~~~~~~~~
|
32 |
-
|
33 |
-
.. autoclass:: transformers.CTRLLMHeadModel
|
34 |
-
:members:
|
35 |
-
|
36 |
-
|
37 |
-
``TFCTRLModel``
|
38 |
-
~~~~~~~~~~~~~~~~~~~~~~~~~~~~~~~
|
39 |
-
|
40 |
-
.. autoclass:: transformers.TFCTRLModel
|
41 |
-
:members:
|
42 |
-
|
43 |
-
|
44 |
-
``TFCTRLLMHeadModel``
|
45 |
-
~~~~~~~~~~~~~~~~~~~~~~~~~~~~~~~~~~~~~
|
46 |
-
|
47 |
-
.. autoclass:: transformers.TFCTRLLMHeadModel
|
48 |
-
:members:
|
49 |
-
|
|
|
|
|
|
|
|
|
|
|
|
|
|
|
|
|
|
|
|
|
|
|
|
|
|
|
|
|
|
|
|
|
|
|
|
|
|
|
|
|
|
|
|
|
|
|
|
|
|
|
|
|
|
|
|
|
|
|
|
|
|
|
|
|
|
|
|
|
|
|
|
|
|
|
|
|
|
|
|
|
|
|
|
|
|
|
|
|
|
|
|
|
|
|
|
|
|
|
|
server/transformers/docs/source/model_doc/distilbert.rst
DELETED
@@ -1,70 +0,0 @@
|
|
1 |
-
DistilBERT
|
2 |
-
----------------------------------------------------
|
3 |
-
|
4 |
-
``DistilBertConfig``
|
5 |
-
~~~~~~~~~~~~~~~~~~~~~~~~~~~~~~~~
|
6 |
-
|
7 |
-
.. autoclass:: transformers.DistilBertConfig
|
8 |
-
:members:
|
9 |
-
|
10 |
-
|
11 |
-
``DistilBertTokenizer``
|
12 |
-
~~~~~~~~~~~~~~~~~~~~~~~~~~~~~~~~
|
13 |
-
|
14 |
-
.. autoclass:: transformers.DistilBertTokenizer
|
15 |
-
:members:
|
16 |
-
|
17 |
-
|
18 |
-
``DistilBertModel``
|
19 |
-
~~~~~~~~~~~~~~~~~~~~~~~~~~~~~~~
|
20 |
-
|
21 |
-
.. autoclass:: transformers.DistilBertModel
|
22 |
-
:members:
|
23 |
-
|
24 |
-
|
25 |
-
``DistilBertForMaskedLM``
|
26 |
-
~~~~~~~~~~~~~~~~~~~~~~~~~~~~~~~~~~~~~
|
27 |
-
|
28 |
-
.. autoclass:: transformers.DistilBertForMaskedLM
|
29 |
-
:members:
|
30 |
-
|
31 |
-
|
32 |
-
``DistilBertForSequenceClassification``
|
33 |
-
~~~~~~~~~~~~~~~~~~~~~~~~~~~~~~~~~~~~~~~~~~~~~~~~~~~
|
34 |
-
|
35 |
-
.. autoclass:: transformers.DistilBertForSequenceClassification
|
36 |
-
:members:
|
37 |
-
|
38 |
-
|
39 |
-
``DistilBertForQuestionAnswering``
|
40 |
-
~~~~~~~~~~~~~~~~~~~~~~~~~~~~~~~~~~~~~~~~
|
41 |
-
|
42 |
-
.. autoclass:: transformers.DistilBertForQuestionAnswering
|
43 |
-
:members:
|
44 |
-
|
45 |
-
``TFDistilBertModel``
|
46 |
-
~~~~~~~~~~~~~~~~~~~~~~~~~~~~~~~
|
47 |
-
|
48 |
-
.. autoclass:: transformers.TFDistilBertModel
|
49 |
-
:members:
|
50 |
-
|
51 |
-
|
52 |
-
``TFDistilBertForMaskedLM``
|
53 |
-
~~~~~~~~~~~~~~~~~~~~~~~~~~~~~~~~~~~~~
|
54 |
-
|
55 |
-
.. autoclass:: transformers.TFDistilBertForMaskedLM
|
56 |
-
:members:
|
57 |
-
|
58 |
-
|
59 |
-
``TFDistilBertForSequenceClassification``
|
60 |
-
~~~~~~~~~~~~~~~~~~~~~~~~~~~~~~~~~~~~~~~~~~~~~~~~~~~
|
61 |
-
|
62 |
-
.. autoclass:: transformers.TFDistilBertForSequenceClassification
|
63 |
-
:members:
|
64 |
-
|
65 |
-
|
66 |
-
``TFDistilBertForQuestionAnswering``
|
67 |
-
~~~~~~~~~~~~~~~~~~~~~~~~~~~~~~~~~~~~~~~~
|
68 |
-
|
69 |
-
.. autoclass:: transformers.TFDistilBertForQuestionAnswering
|
70 |
-
:members:
|
|
|
|
|
|
|
|
|
|
|
|
|
|
|
|
|
|
|
|
|
|
|
|
|
|
|
|
|
|
|
|
|
|
|
|
|
|
|
|
|
|
|
|
|
|
|
|
|
|
|
|
|
|
|
|
|
|
|
|
|
|
|
|
|
|
|
|
|
|
|
|
|
|
|
|
|
|
|
|
|
|
|
|
|
|
|
|
|
|
|
|
|
|
|
|
|
|
|
|
|
|
|
|
|
|
|
|
|
|
|
|
|
|
|
|
|
|
|
|
|
|
|
|
|
|
|
|
|
|
|
|
|
|
|
|
|
|
|
|
|
|
server/transformers/docs/source/model_doc/gpt.rst
DELETED
@@ -1,57 +0,0 @@
|
|
1 |
-
OpenAI GPT
|
2 |
-
----------------------------------------------------
|
3 |
-
|
4 |
-
``OpenAIGPTConfig``
|
5 |
-
~~~~~~~~~~~~~~~~~~~~~
|
6 |
-
|
7 |
-
.. autoclass:: transformers.OpenAIGPTConfig
|
8 |
-
:members:
|
9 |
-
|
10 |
-
|
11 |
-
``OpenAIGPTTokenizer``
|
12 |
-
~~~~~~~~~~~~~~~~~~~~~~~~~~
|
13 |
-
|
14 |
-
.. autoclass:: transformers.OpenAIGPTTokenizer
|
15 |
-
:members:
|
16 |
-
|
17 |
-
|
18 |
-
``OpenAIGPTModel``
|
19 |
-
~~~~~~~~~~~~~~~~~~~~~~~~~
|
20 |
-
|
21 |
-
.. autoclass:: transformers.OpenAIGPTModel
|
22 |
-
:members:
|
23 |
-
|
24 |
-
|
25 |
-
``OpenAIGPTLMHeadModel``
|
26 |
-
~~~~~~~~~~~~~~~~~~~~~~~~~~~~~~~~
|
27 |
-
|
28 |
-
.. autoclass:: transformers.OpenAIGPTLMHeadModel
|
29 |
-
:members:
|
30 |
-
|
31 |
-
|
32 |
-
``OpenAIGPTDoubleHeadsModel``
|
33 |
-
~~~~~~~~~~~~~~~~~~~~~~~~~~~~~~~~~~~~~
|
34 |
-
|
35 |
-
.. autoclass:: transformers.OpenAIGPTDoubleHeadsModel
|
36 |
-
:members:
|
37 |
-
|
38 |
-
|
39 |
-
``TFOpenAIGPTModel``
|
40 |
-
~~~~~~~~~~~~~~~~~~~~~~~~~
|
41 |
-
|
42 |
-
.. autoclass:: transformers.TFOpenAIGPTModel
|
43 |
-
:members:
|
44 |
-
|
45 |
-
|
46 |
-
``TFOpenAIGPTLMHeadModel``
|
47 |
-
~~~~~~~~~~~~~~~~~~~~~~~~~~~~~~~~
|
48 |
-
|
49 |
-
.. autoclass:: transformers.TFOpenAIGPTLMHeadModel
|
50 |
-
:members:
|
51 |
-
|
52 |
-
|
53 |
-
``TFOpenAIGPTDoubleHeadsModel``
|
54 |
-
~~~~~~~~~~~~~~~~~~~~~~~~~~~~~~~~~~~~~
|
55 |
-
|
56 |
-
.. autoclass:: transformers.TFOpenAIGPTDoubleHeadsModel
|
57 |
-
:members:
|
|
|
|
|
|
|
|
|
|
|
|
|
|
|
|
|
|
|
|
|
|
|
|
|
|
|
|
|
|
|
|
|
|
|
|
|
|
|
|
|
|
|
|
|
|
|
|
|
|
|
|
|
|
|
|
|
|
|
|
|
|
|
|
|
|
|
|
|
|
|
|
|
|
|
|
|
|
|
|
|
|
|
|
|
|
|
|
|
|
|
|
|
|
|
|
|
|
|
|
|
|
|
|
|
|
|
|
|
|
|
|
|
|
|
|
server/transformers/docs/source/model_doc/gpt2.rst
DELETED
@@ -1,57 +0,0 @@
|
|
1 |
-
OpenAI GPT2
|
2 |
-
----------------------------------------------------
|
3 |
-
|
4 |
-
``GPT2Config``
|
5 |
-
~~~~~~~~~~~~~~~~~~~~~
|
6 |
-
|
7 |
-
.. autoclass:: transformers.GPT2Config
|
8 |
-
:members:
|
9 |
-
|
10 |
-
|
11 |
-
``GPT2Tokenizer``
|
12 |
-
~~~~~~~~~~~~~~~~~~~~~
|
13 |
-
|
14 |
-
.. autoclass:: transformers.GPT2Tokenizer
|
15 |
-
:members:
|
16 |
-
|
17 |
-
|
18 |
-
``GPT2Model``
|
19 |
-
~~~~~~~~~~~~~~~~~~~~~
|
20 |
-
|
21 |
-
.. autoclass:: transformers.GPT2Model
|
22 |
-
:members:
|
23 |
-
|
24 |
-
|
25 |
-
``GPT2LMHeadModel``
|
26 |
-
~~~~~~~~~~~~~~~~~~~~~~~~~~~
|
27 |
-
|
28 |
-
.. autoclass:: transformers.GPT2LMHeadModel
|
29 |
-
:members:
|
30 |
-
|
31 |
-
|
32 |
-
``GPT2DoubleHeadsModel``
|
33 |
-
~~~~~~~~~~~~~~~~~~~~~~~~~~~~~~~~
|
34 |
-
|
35 |
-
.. autoclass:: transformers.GPT2DoubleHeadsModel
|
36 |
-
:members:
|
37 |
-
|
38 |
-
|
39 |
-
``TFGPT2Model``
|
40 |
-
~~~~~~~~~~~~~~~~~~~~~
|
41 |
-
|
42 |
-
.. autoclass:: transformers.TFGPT2Model
|
43 |
-
:members:
|
44 |
-
|
45 |
-
|
46 |
-
``TFGPT2LMHeadModel``
|
47 |
-
~~~~~~~~~~~~~~~~~~~~~~~~~~~
|
48 |
-
|
49 |
-
.. autoclass:: transformers.TFGPT2LMHeadModel
|
50 |
-
:members:
|
51 |
-
|
52 |
-
|
53 |
-
``TFGPT2DoubleHeadsModel``
|
54 |
-
~~~~~~~~~~~~~~~~~~~~~~~~~~~~~~~~
|
55 |
-
|
56 |
-
.. autoclass:: transformers.TFGPT2DoubleHeadsModel
|
57 |
-
:members:
|
|
|
|
|
|
|
|
|
|
|
|
|
|
|
|
|
|
|
|
|
|
|
|
|
|
|
|
|
|
|
|
|
|
|
|
|
|
|
|
|
|
|
|
|
|
|
|
|
|
|
|
|
|
|
|
|
|
|
|
|
|
|
|
|
|
|
|
|
|
|
|
|
|
|
|
|
|
|
|
|
|
|
|
|
|
|
|
|
|
|
|
|
|
|
|
|
|
|
|
|
|
|
|
|
|
|
|
|
|
|
|
|
|
|
|
server/transformers/docs/source/model_doc/roberta.rst
DELETED
@@ -1,57 +0,0 @@
|
|
1 |
-
RoBERTa
|
2 |
-
----------------------------------------------------
|
3 |
-
|
4 |
-
``RobertaConfig``
|
5 |
-
~~~~~~~~~~~~~~~~~~~~~
|
6 |
-
|
7 |
-
.. autoclass:: transformers.RobertaConfig
|
8 |
-
:members:
|
9 |
-
|
10 |
-
|
11 |
-
``RobertaTokenizer``
|
12 |
-
~~~~~~~~~~~~~~~~~~~~~
|
13 |
-
|
14 |
-
.. autoclass:: transformers.RobertaTokenizer
|
15 |
-
:members:
|
16 |
-
|
17 |
-
|
18 |
-
``RobertaModel``
|
19 |
-
~~~~~~~~~~~~~~~~~~~~
|
20 |
-
|
21 |
-
.. autoclass:: transformers.RobertaModel
|
22 |
-
:members:
|
23 |
-
|
24 |
-
|
25 |
-
``RobertaForMaskedLM``
|
26 |
-
~~~~~~~~~~~~~~~~~~~~~~~~~~
|
27 |
-
|
28 |
-
.. autoclass:: transformers.RobertaForMaskedLM
|
29 |
-
:members:
|
30 |
-
|
31 |
-
|
32 |
-
``RobertaForSequenceClassification``
|
33 |
-
~~~~~~~~~~~~~~~~~~~~~~~~~~~~~~~~~~~~~~~~
|
34 |
-
|
35 |
-
.. autoclass:: transformers.RobertaForSequenceClassification
|
36 |
-
:members:
|
37 |
-
|
38 |
-
|
39 |
-
``TFRobertaModel``
|
40 |
-
~~~~~~~~~~~~~~~~~~~~
|
41 |
-
|
42 |
-
.. autoclass:: transformers.TFRobertaModel
|
43 |
-
:members:
|
44 |
-
|
45 |
-
|
46 |
-
``TFRobertaForMaskedLM``
|
47 |
-
~~~~~~~~~~~~~~~~~~~~~~~~~~
|
48 |
-
|
49 |
-
.. autoclass:: transformers.TFRobertaForMaskedLM
|
50 |
-
:members:
|
51 |
-
|
52 |
-
|
53 |
-
``TFRobertaForSequenceClassification``
|
54 |
-
~~~~~~~~~~~~~~~~~~~~~~~~~~~~~~~~~~~~~~~~
|
55 |
-
|
56 |
-
.. autoclass:: transformers.TFRobertaForSequenceClassification
|
57 |
-
:members:
|
|
|
|
|
|
|
|
|
|
|
|
|
|
|
|
|
|
|
|
|
|
|
|
|
|
|
|
|
|
|
|
|
|
|
|
|
|
|
|
|
|
|
|
|
|
|
|
|
|
|
|
|
|
|
|
|
|
|
|
|
|
|
|
|
|
|
|
|
|
|
|
|
|
|
|
|
|
|
|
|
|
|
|
|
|
|
|
|
|
|
|
|
|
|
|
|
|
|
|
|
|
|
|
|
|
|
|
|
|
|
|
|
|
|
|
server/transformers/docs/source/model_doc/transformerxl.rst
DELETED
@@ -1,44 +0,0 @@
|
|
1 |
-
Transformer XL
|
2 |
-
----------------------------------------------------
|
3 |
-
|
4 |
-
|
5 |
-
``TransfoXLConfig``
|
6 |
-
~~~~~~~~~~~~~~~~~~~~~
|
7 |
-
|
8 |
-
.. autoclass:: transformers.TransfoXLConfig
|
9 |
-
:members:
|
10 |
-
|
11 |
-
|
12 |
-
``TransfoXLTokenizer``
|
13 |
-
~~~~~~~~~~~~~~~~~~~~~~~~~~
|
14 |
-
|
15 |
-
.. autoclass:: transformers.TransfoXLTokenizer
|
16 |
-
:members:
|
17 |
-
|
18 |
-
|
19 |
-
``TransfoXLModel``
|
20 |
-
~~~~~~~~~~~~~~~~~~~~~~~~~~
|
21 |
-
|
22 |
-
.. autoclass:: transformers.TransfoXLModel
|
23 |
-
:members:
|
24 |
-
|
25 |
-
|
26 |
-
``TransfoXLLMHeadModel``
|
27 |
-
~~~~~~~~~~~~~~~~~~~~~~~~~~~~~~~~
|
28 |
-
|
29 |
-
.. autoclass:: transformers.TransfoXLLMHeadModel
|
30 |
-
:members:
|
31 |
-
|
32 |
-
|
33 |
-
``TFTransfoXLModel``
|
34 |
-
~~~~~~~~~~~~~~~~~~~~~~~~~~
|
35 |
-
|
36 |
-
.. autoclass:: transformers.TFTransfoXLModel
|
37 |
-
:members:
|
38 |
-
|
39 |
-
|
40 |
-
``TFTransfoXLLMHeadModel``
|
41 |
-
~~~~~~~~~~~~~~~~~~~~~~~~~~~~~~~~
|
42 |
-
|
43 |
-
.. autoclass:: transformers.TFTransfoXLLMHeadModel
|
44 |
-
:members:
|
|
|
|
|
|
|
|
|
|
|
|
|
|
|
|
|
|
|
|
|
|
|
|
|
|
|
|
|
|
|
|
|
|
|
|
|
|
|
|
|
|
|
|
|
|
|
|
|
|
|
|
|
|
|
|
|
|
|
|
|
|
|
|
|
|
|
|
|
|
|
|
|
|
|
|
|
|
|
|
|
|
|
|
|
|
|
|
|
|
server/transformers/docs/source/model_doc/xlm.rst
DELETED
@@ -1,76 +0,0 @@
|
|
1 |
-
XLM
|
2 |
-
----------------------------------------------------
|
3 |
-
|
4 |
-
``XLMConfig``
|
5 |
-
~~~~~~~~~~~~~~~~~~~~~~~~~~~~~~~~~~~~~~~~~~
|
6 |
-
|
7 |
-
.. autoclass:: transformers.XLMConfig
|
8 |
-
:members:
|
9 |
-
|
10 |
-
``XLMTokenizer``
|
11 |
-
~~~~~~~~~~~~~~~~~~~~~~~~~~~~~~~~~~~~~~~~~~
|
12 |
-
|
13 |
-
.. autoclass:: transformers.XLMTokenizer
|
14 |
-
:members:
|
15 |
-
|
16 |
-
``XLMModel``
|
17 |
-
~~~~~~~~~~~~~~~~~~~~~~~~~~~~~~~~~~~~~~~~~~~~~~~
|
18 |
-
|
19 |
-
.. autoclass:: transformers.XLMModel
|
20 |
-
:members:
|
21 |
-
|
22 |
-
|
23 |
-
``XLMWithLMHeadModel``
|
24 |
-
~~~~~~~~~~~~~~~~~~~~~~~~~~~~~~~~~~~~~~~~~~~~~~~
|
25 |
-
|
26 |
-
.. autoclass:: transformers.XLMWithLMHeadModel
|
27 |
-
:members:
|
28 |
-
|
29 |
-
|
30 |
-
``XLMForSequenceClassification``
|
31 |
-
~~~~~~~~~~~~~~~~~~~~~~~~~~~~~~~~~~~~~~~~~~~~~~~~~~~~~
|
32 |
-
|
33 |
-
.. autoclass:: transformers.XLMForSequenceClassification
|
34 |
-
:members:
|
35 |
-
|
36 |
-
|
37 |
-
``XLMForQuestionAnsweringSimple``
|
38 |
-
~~~~~~~~~~~~~~~~~~~~~~~~~~~~~~~~~~~~~~~~~~~~~~~~~~~~~
|
39 |
-
|
40 |
-
.. autoclass:: transformers.XLMForQuestionAnsweringSimple
|
41 |
-
:members:
|
42 |
-
|
43 |
-
|
44 |
-
``XLMForQuestionAnswering``
|
45 |
-
~~~~~~~~~~~~~~~~~~~~~~~~~~~~~~~~~~~~~~~~~~~~~~~~~~~~~
|
46 |
-
|
47 |
-
.. autoclass:: transformers.XLMForQuestionAnswering
|
48 |
-
:members:
|
49 |
-
|
50 |
-
|
51 |
-
``TFXLMModel``
|
52 |
-
~~~~~~~~~~~~~~~~~~~~~~~~~~~~~~~~~~~~~~~~~~~~~~~
|
53 |
-
|
54 |
-
.. autoclass:: transformers.TFXLMModel
|
55 |
-
:members:
|
56 |
-
|
57 |
-
|
58 |
-
``TFXLMWithLMHeadModel``
|
59 |
-
~~~~~~~~~~~~~~~~~~~~~~~~~~~~~~~~~~~~~~~~~~~~~~~
|
60 |
-
|
61 |
-
.. autoclass:: transformers.TFXLMWithLMHeadModel
|
62 |
-
:members:
|
63 |
-
|
64 |
-
|
65 |
-
``TFXLMForSequenceClassification``
|
66 |
-
~~~~~~~~~~~~~~~~~~~~~~~~~~~~~~~~~~~~~~~~~~~~~~~~~~~~~
|
67 |
-
|
68 |
-
.. autoclass:: transformers.TFXLMForSequenceClassification
|
69 |
-
:members:
|
70 |
-
|
71 |
-
|
72 |
-
``TFXLMForQuestionAnsweringSimple``
|
73 |
-
~~~~~~~~~~~~~~~~~~~~~~~~~~~~~~~~~~~~~~~~~~~~~~~~~~~~~
|
74 |
-
|
75 |
-
.. autoclass:: transformers.TFXLMForQuestionAnsweringSimple
|
76 |
-
:members:
|
|
|
|
|
|
|
|
|
|
|
|
|
|
|
|
|
|
|
|
|
|
|
|
|
|
|
|
|
|
|
|
|
|
|
|
|
|
|
|
|
|
|
|
|
|
|
|
|
|
|
|
|
|
|
|
|
|
|
|
|
|
|
|
|
|
|
|
|
|
|
|
|
|
|
|
|
|
|
|
|
|
|
|
|
|
|
|
|
|
|
|
|
|
|
|
|
|
|
|
|
|
|
|
|
|
|
|
|
|
|
|
|
|
|
|
|
|
|
|
|
|
|
|
|
|
|
|
|
|
|
|
|
|
|
|
|
|
|
|
|
|
|
|
|
|
|
|
|
|
|
|
|
|
server/transformers/docs/source/model_doc/xlnet.rst
DELETED
@@ -1,92 +0,0 @@
|
|
1 |
-
XLNet
|
2 |
-
----------------------------------------------------
|
3 |
-
|
4 |
-
``XLNetConfig``
|
5 |
-
~~~~~~~~~~~~~~~~~~~~~~~~~~~~~~~~~~~~~~~~~~
|
6 |
-
|
7 |
-
.. autoclass:: transformers.XLNetConfig
|
8 |
-
:members:
|
9 |
-
|
10 |
-
|
11 |
-
``XLNetTokenizer``
|
12 |
-
~~~~~~~~~~~~~~~~~~~~~~~~~~~~~~~~~~~~~~~~~~
|
13 |
-
|
14 |
-
.. autoclass:: transformers.XLNetTokenizer
|
15 |
-
:members:
|
16 |
-
|
17 |
-
|
18 |
-
``XLNetModel``
|
19 |
-
~~~~~~~~~~~~~~~~~~~~~~~~~~~~~~~~~~~~~~~~~~~~~~~
|
20 |
-
|
21 |
-
.. autoclass:: transformers.XLNetModel
|
22 |
-
:members:
|
23 |
-
|
24 |
-
|
25 |
-
``XLNetLMHeadModel``
|
26 |
-
~~~~~~~~~~~~~~~~~~~~~~~~~~~~~~~~~~~~~~~~~~~~~~~
|
27 |
-
|
28 |
-
.. autoclass:: transformers.XLNetLMHeadModel
|
29 |
-
:members:
|
30 |
-
|
31 |
-
|
32 |
-
``XLNetForSequenceClassification``
|
33 |
-
~~~~~~~~~~~~~~~~~~~~~~~~~~~~~~~~~~~~~~~~~~~~~~~~~~~~~
|
34 |
-
|
35 |
-
.. autoclass:: transformers.XLNetForSequenceClassification
|
36 |
-
:members:
|
37 |
-
|
38 |
-
|
39 |
-
``XLNetForTokenClassification``
|
40 |
-
~~~~~~~~~~~~~~~~~~~~~~~~~~~~~~~~~~~~~~~~~~~~~~~~~~~~~
|
41 |
-
|
42 |
-
.. autoclass:: transformers.XLNetForTokenClassification
|
43 |
-
:members:
|
44 |
-
|
45 |
-
|
46 |
-
``XLNetForMultipleChoice``
|
47 |
-
~~~~~~~~~~~~~~~~~~~~~~~~~~~~~~~~~~~~~~~~~~~~~~~~~~~~~
|
48 |
-
|
49 |
-
.. autoclass:: transformers.XLNetForMultipleChoice
|
50 |
-
:members:
|
51 |
-
|
52 |
-
|
53 |
-
``XLNetForQuestionAnsweringSimple``
|
54 |
-
~~~~~~~~~~~~~~~~~~~~~~~~~~~~~~~~~~~~~~~~~~~~~~~~~~~~~
|
55 |
-
|
56 |
-
.. autoclass:: transformers.XLNetForQuestionAnsweringSimple
|
57 |
-
:members:
|
58 |
-
|
59 |
-
|
60 |
-
``XLNetForQuestionAnswering``
|
61 |
-
~~~~~~~~~~~~~~~~~~~~~~~~~~~~~~~~~~~~~~~~~~~~~~~~~~~~~
|
62 |
-
|
63 |
-
.. autoclass:: transformers.XLNetForQuestionAnswering
|
64 |
-
:members:
|
65 |
-
|
66 |
-
|
67 |
-
``TFXLNetModel``
|
68 |
-
~~~~~~~~~~~~~~~~~~~~~~~~~~~~~~~~~~~~~~~~~~~~~~~
|
69 |
-
|
70 |
-
.. autoclass:: transformers.TFXLNetModel
|
71 |
-
:members:
|
72 |
-
|
73 |
-
|
74 |
-
``TFXLNetLMHeadModel``
|
75 |
-
~~~~~~~~~~~~~~~~~~~~~~~~~~~~~~~~~~~~~~~~~~~~~~~
|
76 |
-
|
77 |
-
.. autoclass:: transformers.TFXLNetLMHeadModel
|
78 |
-
:members:
|
79 |
-
|
80 |
-
|
81 |
-
``TFXLNetForSequenceClassification``
|
82 |
-
~~~~~~~~~~~~~~~~~~~~~~~~~~~~~~~~~~~~~~~~~~~~~~~~~~~~~
|
83 |
-
|
84 |
-
.. autoclass:: transformers.TFXLNetForSequenceClassification
|
85 |
-
:members:
|
86 |
-
|
87 |
-
|
88 |
-
``TFXLNetForQuestionAnsweringSimple``
|
89 |
-
~~~~~~~~~~~~~~~~~~~~~~~~~~~~~~~~~~~~~~~~~~~~~~~~~~~~~
|
90 |
-
|
91 |
-
.. autoclass:: transformers.TFXLNetForQuestionAnsweringSimple
|
92 |
-
:members:
|
|
|
|
|
|
|
|
|
|
|
|
|
|
|
|
|
|
|
|
|
|
|
|
|
|
|
|
|
|
|
|
|
|
|
|
|
|
|
|
|
|
|
|
|
|
|
|
|
|
|
|
|
|
|
|
|
|
|
|
|
|
|
|
|
|
|
|
|
|
|
|
|
|
|
|
|
|
|
|
|
|
|
|
|
|
|
|
|
|
|
|
|
|
|
|
|
|
|
|
|
|
|
|
|
|
|
|
|
|
|
|
|
|
|
|
|
|
|
|
|
|
|
|
|
|
|
|
|
|
|
|
|
|
|
|
|
|
|
|
|
|
|
|
|
|
|
|
|
|
|
|
|
|
|
|
|
|
|
|
|
|
|
|
|
|
|
|
|
|
|
|
|
|
|
|
|
|
|
|
|
|
|
|
|
|
server/transformers/docs/source/model_sharing.md
DELETED
@@ -1,40 +0,0 @@
|
|
1 |
-
# Model upload and sharing
|
2 |
-
|
3 |
-
Starting with `v2.2.2`, you can now upload and share your fine-tuned models with the community, using the <abbr title="Command-line interface">CLI</abbr> that's built-in to the library.
|
4 |
-
|
5 |
-
**First, create an account on [https://huggingface.co/join](https://huggingface.co/join)**. Then:
|
6 |
-
|
7 |
-
```shell
|
8 |
-
transformers-cli login
|
9 |
-
# log in using the same credentials as on huggingface.co
|
10 |
-
```
|
11 |
-
Upload your model:
|
12 |
-
```shell
|
13 |
-
transformers-cli upload ./path/to/pretrained_model/
|
14 |
-
|
15 |
-
# ^^ Upload folder containing weights/tokenizer/config
|
16 |
-
# saved via `.save_pretrained()`
|
17 |
-
|
18 |
-
transformers-cli upload ./config.json [--filename folder/foobar.json]
|
19 |
-
|
20 |
-
# ^^ Upload a single file
|
21 |
-
# (you can optionally override its filename, which can be nested inside a folder)
|
22 |
-
```
|
23 |
-
|
24 |
-
Your model will then be accessible through its identifier, a concatenation of your username and the folder name above:
|
25 |
-
```python
|
26 |
-
"username/pretrained_model"
|
27 |
-
```
|
28 |
-
|
29 |
-
Anyone can load it from code:
|
30 |
-
```python
|
31 |
-
tokenizer = AutoTokenizer.from_pretrained("username/pretrained_model")
|
32 |
-
model = AutoModel.from_pretrained("username/pretrained_model")
|
33 |
-
```
|
34 |
-
|
35 |
-
Finally, list all your files on S3:
|
36 |
-
```shell
|
37 |
-
transformers-cli s3 ls
|
38 |
-
# List all your S3 objects.
|
39 |
-
```
|
40 |
-
|
|
|
|
|
|
|
|
|
|
|
|
|
|
|
|
|
|
|
|
|
|
|
|
|
|
|
|
|
|
|
|
|
|
|
|
|
|
|
|
|
|
|
|
|
|
|
|
|
|
|
|
|
|
|
|
|
|
|
|
|
|
|
|
|
|
|
|
|
|
|
|
|
|
|
|
|
|
|
|
|
|
server/transformers/docs/source/multilingual.rst
DELETED
@@ -1,103 +0,0 @@
|
|
1 |
-
Multi-lingual models
|
2 |
-
================================================
|
3 |
-
|
4 |
-
Most of the models available in this library are mono-lingual models (English, Chinese and German). A few
|
5 |
-
multi-lingual models are available and have a different mechanisms than mono-lingual models.
|
6 |
-
This page details the usage of these models.
|
7 |
-
|
8 |
-
The two models that currently support multiple languages are BERT and XLM.
|
9 |
-
|
10 |
-
XLM
|
11 |
-
^^^^^^^^^^^^^^^^^^^^^^^^^^^^^^^^^^^^^^^^^^^^
|
12 |
-
|
13 |
-
XLM has a total of 10 different checkpoints, only one of which is mono-lingual. The 9 remaining model checkpoints can
|
14 |
-
be split in two categories: the checkpoints that make use of language embeddings, and those that don't
|
15 |
-
|
16 |
-
XLM & Language Embeddings
|
17 |
-
------------------------------------------------
|
18 |
-
|
19 |
-
This section concerns the following checkpoints:
|
20 |
-
|
21 |
-
- ``xlm-mlm-ende-1024`` (Masked language modeling, English-German)
|
22 |
-
- ``xlm-mlm-enfr-1024`` (Masked language modeling, English-French)
|
23 |
-
- ``xlm-mlm-enro-1024`` (Masked language modeling, English-Romanian)
|
24 |
-
- ``xlm-mlm-xnli15-1024`` (Masked language modeling, XNLI languages)
|
25 |
-
- ``xlm-mlm-tlm-xnli15-1024`` (Masked language modeling + Translation, XNLI languages)
|
26 |
-
- ``xlm-clm-enfr-1024`` (Causal language modeling, English-French)
|
27 |
-
- ``xlm-clm-ende-1024`` (Causal language modeling, English-German)
|
28 |
-
|
29 |
-
These checkpoints require language embeddings that will specify the language used at inference time. These language
|
30 |
-
embeddings are represented as a tensor that is of the same shape as the input ids passed to the model. The values in
|
31 |
-
these tensors depend on the language used and are identifiable using the ``lang2id`` and ``id2lang`` attributes
|
32 |
-
from the tokenizer.
|
33 |
-
|
34 |
-
Here is an example using the ``xlm-clm-enfr-1024`` checkpoint (Causal language modeling, English-French):
|
35 |
-
|
36 |
-
|
37 |
-
.. code-block::
|
38 |
-
|
39 |
-
import torch
|
40 |
-
from transformers import XLMTokenizer, XLMWithLMHeadModel
|
41 |
-
|
42 |
-
tokenizer = XLMTokenizer.from_pretrained("xlm-clm-1024-enfr")
|
43 |
-
|
44 |
-
|
45 |
-
The different languages this model/tokenizer handles, as well as the ids of these languages are visible using the
|
46 |
-
``lang2id`` attribute:
|
47 |
-
|
48 |
-
.. code-block::
|
49 |
-
|
50 |
-
print(tokenizer.lang2id) # {'en': 0, 'fr': 1}
|
51 |
-
|
52 |
-
|
53 |
-
These ids should be used when passing a language parameter during a model pass. Let's define our inputs:
|
54 |
-
|
55 |
-
.. code-block::
|
56 |
-
|
57 |
-
input_ids = torch.tensor([tokenizer.encode("Wikipedia was used to")]) # batch size of 1
|
58 |
-
|
59 |
-
|
60 |
-
We should now define the language embedding by using the previously defined language id. We want to create a tensor
|
61 |
-
filled with the appropriate language ids, of the same size as input_ids. For english, the id is 0:
|
62 |
-
|
63 |
-
.. code-block::
|
64 |
-
|
65 |
-
language_id = tokenizer.lang2id['en'] # 0
|
66 |
-
langs = torch.tensor([language_id] * input_ids.shape[1]) # torch.tensor([0, 0, 0, ..., 0])
|
67 |
-
|
68 |
-
# We reshape it to be of size (batch_size, sequence_length)
|
69 |
-
langs = langs.view(1, -1) # is now of shape [1, sequence_length] (we have a batch size of 1)
|
70 |
-
|
71 |
-
|
72 |
-
You can then feed it all as input to your model:
|
73 |
-
|
74 |
-
.. code-block::
|
75 |
-
|
76 |
-
outputs = model(input_ids, langs=langs)
|
77 |
-
|
78 |
-
|
79 |
-
The example `run_generation.py <https://github.com/huggingface/transformers/blob/master/examples/run_generation.py>`__
|
80 |
-
can generate text using the CLM checkpoints from XLM, using the language embeddings.
|
81 |
-
|
82 |
-
XLM without Language Embeddings
|
83 |
-
------------------------------------------------
|
84 |
-
|
85 |
-
This section concerns the following checkpoints:
|
86 |
-
|
87 |
-
- ``xlm-mlm-17-1280`` (Masked language modeling, 17 languages)
|
88 |
-
- ``xlm-mlm-100-1280`` (Masked language modeling, 100 languages)
|
89 |
-
|
90 |
-
These checkpoints do not require language embeddings at inference time. These models are used to have generic
|
91 |
-
sentence representations, differently from previously-mentioned XLM checkpoints.
|
92 |
-
|
93 |
-
|
94 |
-
BERT
|
95 |
-
^^^^^^^^^^^^^^^^^^^^^^^^^^^^^^^^^^^^^^^^^^^^
|
96 |
-
|
97 |
-
BERT has two checkpoints that can be used for multi-lingual tasks:
|
98 |
-
|
99 |
-
- ``bert-base-multilingual-uncased`` (Masked language modeling + Next sentence prediction, 102 languages)
|
100 |
-
- ``bert-base-multilingual-cased`` (Masked language modeling + Next sentence prediction, 104 languages)
|
101 |
-
|
102 |
-
These checkpoints do not require language embeddings at inference time. They should identify the language
|
103 |
-
used in the context and infer accordingly.
|
|
|
|
|
|
|
|
|
|
|
|
|
|
|
|
|
|
|
|
|
|
|
|
|
|
|
|
|
|
|
|
|
|
|
|
|
|
|
|
|
|
|
|
|
|
|
|
|
|
|
|
|
|
|
|
|
|
|
|
|
|
|
|
|
|
|
|
|
|
|
|
|
|
|
|
|
|
|
|
|
|
|
|
|
|
|
|
|
|
|
|
|
|
|
|
|
|
|
|
|
|
|
|
|
|
|
|
|
|
|
|
|
|
|
|
|
|
|
|
|
|
|
|
|
|
|
|
|
|
|
|
|
|
|
|
|
|
|
|
|
|
|
|
|
|
|
|
|
|
|
|
|
|
|
|
|
|
|
|
|
|
|
|
|
|
|
|
|
|
|
|
|
|
|
|
|
|
|
|
|
|
|
|
|
|
|
|
|
|
|
|
|
|
|
|
|
|
|
|
|
|
|
|
|
|
|
|