Spaces:
Sleeping
Sleeping
File size: 9,869 Bytes
7370e5c |
1 2 3 4 5 6 7 8 9 10 11 12 13 14 15 16 17 18 19 20 21 22 23 24 25 26 27 28 29 30 31 32 33 34 35 36 37 38 39 40 41 42 43 44 45 46 47 48 49 50 51 52 53 54 55 56 57 58 59 60 61 62 63 64 65 66 67 68 69 70 71 72 73 74 75 76 77 78 79 80 81 82 83 84 85 86 87 88 89 90 91 92 93 94 95 96 97 98 99 100 101 102 103 104 105 106 107 108 109 110 111 112 113 114 115 116 117 118 119 120 121 122 123 124 125 126 127 128 129 130 131 132 133 134 135 136 137 138 139 140 141 142 143 144 145 146 147 148 149 150 151 152 153 154 155 156 157 158 159 160 161 162 163 |
---
comments: true
description: Explore Meituan YOLOv6, a top-tier object detector balancing speed and accuracy. Learn about its unique features and performance metrics on Ultralytics Docs.
keywords: Meituan YOLOv6, object detection, real-time applications, BiC module, Anchor-Aided Training, COCO dataset, high-performance models, Ultralytics Docs
---
# Meituan YOLOv6
## Overview
[Meituan](https://about.meituan.com/) YOLOv6 is a cutting-edge object detector that offers remarkable balance between speed and accuracy, making it a popular choice for real-time applications. This model introduces several notable enhancements on its architecture and training scheme, including the implementation of a Bi-directional Concatenation (BiC) module, an anchor-aided training (AAT) strategy, and an improved backbone and neck design for state-of-the-art accuracy on the COCO dataset.
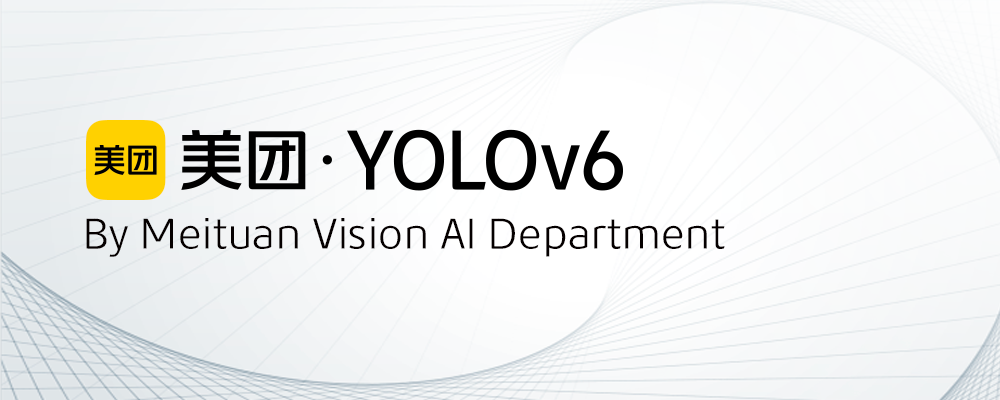
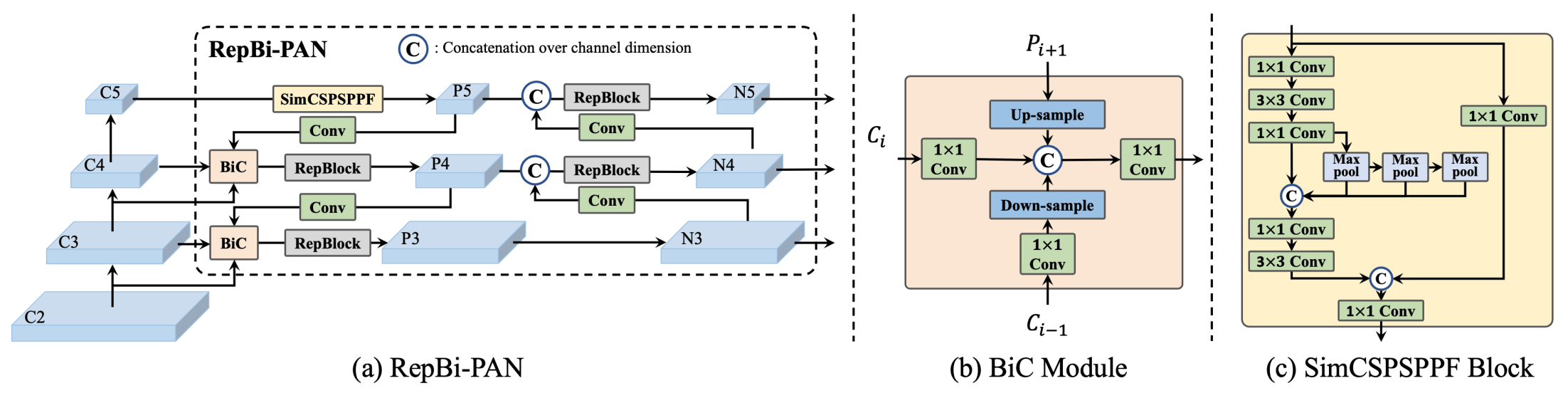 **Overview of YOLOv6.** Model architecture diagram showing the redesigned network components and training strategies that have led to significant performance improvements. (a) The neck of YOLOv6 (N and S are shown). Note for M/L, RepBlocks is replaced with CSPStackRep. (b) The structure of a BiC module. (c) A SimCSPSPPF block. ([source](https://arxiv.org/pdf/2301.05586.pdf)).
### Key Features
- **Bidirectional Concatenation (BiC) Module:** YOLOv6 introduces a BiC module in the neck of the detector, enhancing localization signals and delivering performance gains with negligible speed degradation.
- **Anchor-Aided Training (AAT) Strategy:** This model proposes AAT to enjoy the benefits of both anchor-based and anchor-free paradigms without compromising inference efficiency.
- **Enhanced Backbone and Neck Design:** By deepening YOLOv6 to include another stage in the backbone and neck, this model achieves state-of-the-art performance on the COCO dataset at high-resolution input.
- **Self-Distillation Strategy:** A new self-distillation strategy is implemented to boost the performance of smaller models of YOLOv6, enhancing the auxiliary regression branch during training and removing it at inference to avoid a marked speed decline.
## Performance Metrics
YOLOv6 provides various pre-trained models with different scales:
- YOLOv6-N: 37.5% AP on COCO val2017 at 1187 FPS with NVIDIA Tesla T4 GPU.
- YOLOv6-S: 45.0% AP at 484 FPS.
- YOLOv6-M: 50.0% AP at 226 FPS.
- YOLOv6-L: 52.8% AP at 116 FPS.
- YOLOv6-L6: State-of-the-art accuracy in real-time.
YOLOv6 also provides quantized models for different precisions and models optimized for mobile platforms.
## Usage Examples
This example provides simple YOLOv6 training and inference examples. For full documentation on these and other [modes](../modes/index.md) see the [Predict](../modes/predict.md), [Train](../modes/train.md), [Val](../modes/val.md) and [Export](../modes/export.md) docs pages.
!!! Example
=== "Python"
PyTorch pretrained `*.pt` models as well as configuration `*.yaml` files can be passed to the `YOLO()` class to create a model instance in python:
```python
from ultralytics import YOLO
# Build a YOLOv6n model from scratch
model = YOLO("yolov6n.yaml")
# Display model information (optional)
model.info()
# Train the model on the COCO8 example dataset for 100 epochs
results = model.train(data="coco8.yaml", epochs=100, imgsz=640)
# Run inference with the YOLOv6n model on the 'bus.jpg' image
results = model("path/to/bus.jpg")
```
=== "CLI"
CLI commands are available to directly run the models:
```bash
# Build a YOLOv6n model from scratch and train it on the COCO8 example dataset for 100 epochs
yolo train model=yolov6n.yaml data=coco8.yaml epochs=100 imgsz=640
# Build a YOLOv6n model from scratch and run inference on the 'bus.jpg' image
yolo predict model=yolov6n.yaml source=path/to/bus.jpg
```
## Supported Tasks and Modes
The YOLOv6 series offers a range of models, each optimized for high-performance [Object Detection](../tasks/detect.md). These models cater to varying computational needs and accuracy requirements, making them versatile for a wide array of applications.
| Model Type | Pre-trained Weights | Tasks Supported | Inference | Validation | Training | Export |
| ---------- | ------------------- | -------------------------------------- | --------- | ---------- | -------- | ------ |
| YOLOv6-N | `yolov6-n.pt` | [Object Detection](../tasks/detect.md) | β
| β
| β
| β
|
| YOLOv6-S | `yolov6-s.pt` | [Object Detection](../tasks/detect.md) | β
| β
| β
| β
|
| YOLOv6-M | `yolov6-m.pt` | [Object Detection](../tasks/detect.md) | β
| β
| β
| β
|
| YOLOv6-L | `yolov6-l.pt` | [Object Detection](../tasks/detect.md) | β
| β
| β
| β
|
| YOLOv6-L6 | `yolov6-l6.pt` | [Object Detection](../tasks/detect.md) | β
| β
| β
| β
|
This table provides a detailed overview of the YOLOv6 model variants, highlighting their capabilities in object detection tasks and their compatibility with various operational modes such as [Inference](../modes/predict.md), [Validation](../modes/val.md), [Training](../modes/train.md), and [Export](../modes/export.md). This comprehensive support ensures that users can fully leverage the capabilities of YOLOv6 models in a broad range of object detection scenarios.
## Citations and Acknowledgements
We would like to acknowledge the authors for their significant contributions in the field of real-time object detection:
!!! Quote ""
=== "BibTeX"
```bibtex
@misc{li2023yolov6,
title={YOLOv6 v3.0: A Full-Scale Reloading},
author={Chuyi Li and Lulu Li and Yifei Geng and Hongliang Jiang and Meng Cheng and Bo Zhang and Zaidan Ke and Xiaoming Xu and Xiangxiang Chu},
year={2023},
eprint={2301.05586},
archivePrefix={arXiv},
primaryClass={cs.CV}
}
```
The original YOLOv6 paper can be found on [arXiv](https://arxiv.org/abs/2301.05586). The authors have made their work publicly available, and the codebase can be accessed on [GitHub](https://github.com/meituan/YOLOv6). We appreciate their efforts in advancing the field and making their work accessible to the broader community.
## FAQ
### What is Meituan YOLOv6 and what makes it unique?
Meituan YOLOv6 is a state-of-the-art object detector that balances speed and accuracy, ideal for real-time applications. It features notable architectural enhancements like the Bi-directional Concatenation (BiC) module and an Anchor-Aided Training (AAT) strategy. These innovations provide substantial performance gains with minimal speed degradation, making YOLOv6 a competitive choice for object detection tasks.
### How does the Bi-directional Concatenation (BiC) Module in YOLOv6 improve performance?
The Bi-directional Concatenation (BiC) module in YOLOv6 enhances localization signals in the detector's neck, delivering performance improvements with negligible speed impact. This module effectively combines different feature maps, increasing the model's ability to detect objects accurately. For more details on YOLOv6's features, refer to the [Key Features](#key-features) section.
### How can I train a YOLOv6 model using Ultralytics?
You can train a YOLOv6 model using Ultralytics with simple Python or CLI commands. For instance:
!!! Example
=== "Python"
```python
from ultralytics import YOLO
# Build a YOLOv6n model from scratch
model = YOLO("yolov6n.yaml")
# Train the model on the COCO8 example dataset for 100 epochs
results = model.train(data="coco8.yaml", epochs=100, imgsz=640)
```
=== "CLI"
```bash
yolo train model=yolov6n.yaml data=coco8.yaml epochs=100 imgsz=640
```
For more information, visit the [Train](../modes/train.md) page.
### What are the different versions of YOLOv6 and their performance metrics?
YOLOv6 offers multiple versions, each optimized for different performance requirements:
- YOLOv6-N: 37.5% AP at 1187 FPS
- YOLOv6-S: 45.0% AP at 484 FPS
- YOLOv6-M: 50.0% AP at 226 FPS
- YOLOv6-L: 52.8% AP at 116 FPS
- YOLOv6-L6: State-of-the-art accuracy in real-time scenarios
These models are evaluated on the COCO dataset using an NVIDIA Tesla T4 GPU. For more on performance metrics, see the [Performance Metrics](#performance-metrics) section.
### How does the Anchor-Aided Training (AAT) strategy benefit YOLOv6?
Anchor-Aided Training (AAT) in YOLOv6 combines elements of anchor-based and anchor-free approaches, enhancing the model's detection capabilities without compromising inference efficiency. This strategy leverages anchors during training to improve bounding box predictions, making YOLOv6 effective in diverse object detection tasks.
### Which operational modes are supported by YOLOv6 models in Ultralytics?
YOLOv6 supports various operational modes including Inference, Validation, Training, and Export. This flexibility allows users to fully exploit the model's capabilities in different scenarios. Check out the [Supported Tasks and Modes](#supported-tasks-and-modes) section for a detailed overview of each mode.
|