diff --git a/.gitattributes b/.gitattributes
index a6344aac8c09253b3b630fb776ae94478aa0275b..7307b16ff423aa171ac31571fa774436a3ed6b9f 100644
--- a/.gitattributes
+++ b/.gitattributes
@@ -33,3 +33,4 @@ saved_model/**/* filter=lfs diff=lfs merge=lfs -text
*.zip filter=lfs diff=lfs merge=lfs -text
*.zst filter=lfs diff=lfs merge=lfs -text
*tfevents* filter=lfs diff=lfs merge=lfs -text
+difpoint/src/utils/dependencies/insightface/data/images/t1.jpg filter=lfs diff=lfs merge=lfs -text
diff --git a/app.py b/app.py
new file mode 100644
index 0000000000000000000000000000000000000000..b6df7955417669042688751f1acf6afa956d3d82
--- /dev/null
+++ b/app.py
@@ -0,0 +1,124 @@
+import os, sys
+import gradio as gr
+from difpoint.inference import Inferencer
+from TTS.api import TTS
+import torch
+import time
+from flask import send_from_directory
+from huggingface_hub import snapshot_download
+import spaces
+import tensorrt
+import multiprocessing as mp
+import pickle
+mp.set_start_method('spawn', force=True)
+
+
+repo_id = "ChaolongYang/KDTalker"
+local_dir = "./downloaded_repo"
+snapshot_download(repo_id=repo_id, local_dir=local_dir)
+print("\nFiles downloaded:")
+for root, dirs, files in os.walk(local_dir):
+ for file in files:
+ file_path = os.path.join(root, file)
+ print(file_path)
+
+result_dir = "results"
+def set_upload():
+ return "upload"
+def set_microphone():
+ return "microphone"
+def set_tts():
+ return "tts"
+def create_kd_talker():
+ return Inferencer()
+
+@spaces.GPU
+def predict(prompt, upload_reference_audio, microphone_reference_audio, reference_audio_type):
+ global result_dir
+ output_file_path = os.path.join('./downloaded_repo/', 'output.wav')
+ if reference_audio_type == 'upload':
+ audio_file_pth = upload_reference_audio
+ elif reference_audio_type == 'microphone':
+ audio_file_pth = microphone_reference_audio
+ tts = TTS('tts_models/multilingual/multi-dataset/your_tts')
+ tts.tts_to_file(
+ text=prompt,
+ file_path=output_file_path,
+ speaker_wav=audio_file_pth,
+ language="en",
+ )
+ return gr.Audio(value=output_file_path, type='filepath')
+
+@spaces.GPU
+def generate(upload_driven_audio, tts_driven_audio, driven_audio_type, source_image, smoothed_pitch, smoothed_yaw, smoothed_roll, smoothed_t):
+ return Inferencer().generate_with_audio_img(upload_driven_audio, tts_driven_audio, driven_audio_type, source_image,
+ smoothed_pitch, smoothed_yaw, smoothed_roll, smoothed_t)
+
+
+def main():
+ if torch.cuda.is_available():
+ device = "cuda"
+ else:
+ device = "cpu"
+
+ with gr.Blocks(analytics_enabled=False) as interface:
+ gr.Markdown(
+ """
+
+
Unlock Pose Diversity: Accurate and Efficient Implicit Keypoint-based Spatiotemporal Diffusion for Audio-driven Talking Portrait
+
+
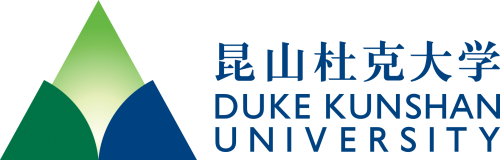
+
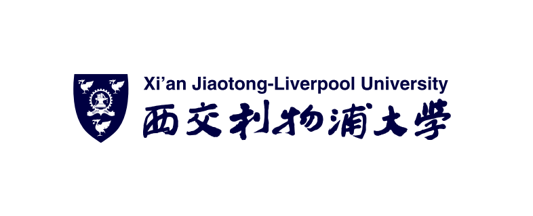
+
+
+ """
+ )
+ driven_audio_type = gr.Textbox(value="upload", visible=False)
+ reference_audio_type = gr.Textbox(value="upload", visible=False)
+
+ with gr.Row():
+ with gr.Column(variant="panel"):
+ with gr.Tabs(elem_id="kdtalker_source_image"):
+ with gr.TabItem("Upload image"):
+ source_image = gr.Image(label="Source image", sources="upload", type="filepath", scale=256)
+
+ with gr.Tabs(elem_id="kdtalker_driven_audio"):
+ with gr.TabItem("Upload"):
+ upload_driven_audio = gr.Audio(label="Upload audio", sources="upload", type="filepath")
+ upload_driven_audio.change(set_upload, outputs=driven_audio_type)
+ with gr.TabItem("TTS"):
+ upload_reference_audio = gr.Audio(label="Upload Reference Audio", sources="upload", type="filepath")
+ upload_reference_audio.change(set_upload, outputs=reference_audio_type)
+ microphone_reference_audio = gr.Audio(label="Recorded Reference Audio", sources="microphone", type="filepath")
+ microphone_reference_audio.change(set_microphone, outputs=reference_audio_type)
+ input_text = gr.Textbox(
+ label="Generating audio from text",
+ lines=5,
+ placeholder="please enter some text here, we generate the audio from text using @Coqui.ai TTS."
+ )
+ tts_button = gr.Button("Generate audio", elem_id="kdtalker_audio_generate", variant="primary")
+ tts_driven_audio = gr.Audio(label="Synthesised Audio", type="filepath")
+ tts_button.click(fn=predict, inputs=[input_text, upload_reference_audio, microphone_reference_audio, reference_audio_type], outputs=[tts_driven_audio])
+ tts_button.click(set_tts, outputs=driven_audio_type)
+ with gr.Column(variant="panel"):
+ gen_video = gr.Video(label="Generated video", format="mp4", width=256)
+ with gr.Tabs(elem_id="talker_checkbox"):
+ with gr.TabItem("KDTalker"):
+ smoothed_pitch = gr.Slider(minimum=0, maximum=1, step=0.1, label="Pitch", value=0.8)
+ smoothed_yaw = gr.Slider(minimum=0, maximum=1, step=0.1, label="Yaw", value=0.8)
+ smoothed_roll = gr.Slider(minimum=0, maximum=1, step=0.1, label="Roll", value=0.8)
+ smoothed_t = gr.Slider(minimum=0, maximum=1, step=0.1, label="T", value=0.8)
+ kd_submit = gr.Button("Generate", elem_id="kdtalker_generate", variant="primary")
+ kd_submit.click(
+ fn=generate,
+ inputs=[
+ upload_driven_audio, tts_driven_audio, driven_audio_type, source_image,
+ smoothed_pitch, smoothed_yaw, smoothed_roll, smoothed_t
+ ],
+ outputs=[gen_video]
+ )
+ return interface
+
+
+demo = main()
+demo.queue().launch()
\ No newline at end of file
diff --git a/difpoint/.DS_Store b/difpoint/.DS_Store
new file mode 100644
index 0000000000000000000000000000000000000000..fc1361d70c469c81f849e2356f86479c34b61857
Binary files /dev/null and b/difpoint/.DS_Store differ
diff --git a/difpoint/configs/onnx_infer.yaml b/difpoint/configs/onnx_infer.yaml
new file mode 100644
index 0000000000000000000000000000000000000000..cac7c83c83acee683db0dd0959959b737fceb5f8
--- /dev/null
+++ b/difpoint/configs/onnx_infer.yaml
@@ -0,0 +1,105 @@
+models:
+ warping_spade:
+ name: "WarpingSpadeModel"
+ predict_type: "ort"
+ model_path: "./downloaded_repo/pretrained_weights/liveportrait_onnx/warping_spade.onnx"
+ motion_extractor:
+ name: "MotionExtractorModel"
+ predict_type: "ort"
+ model_path: "./downloaded_repo/pretrained_weights/liveportrait_onnx/motion_extractor.onnx"
+ landmark:
+ name: "LandmarkModel"
+ predict_type: "ort"
+ model_path: "./downloaded_repo/pretrained_weights/liveportrait_onnx/landmark.onnx"
+ face_analysis:
+ name: "FaceAnalysisModel"
+ predict_type: "ort"
+ model_path:
+ - "./downloaded_repo/pretrained_weights/liveportrait_onnx/retinaface_det_static.onnx"
+ - "./downloaded_repo/pretrained_weights/liveportrait_onnx/face_2dpose_106_static.onnx"
+ app_feat_extractor:
+ name: "AppearanceFeatureExtractorModel"
+ predict_type: "ort"
+ model_path: "./downloaded_repo/pretrained_weights/liveportrait_onnx/appearance_feature_extractor.onnx"
+ stitching:
+ name: "StitchingModel"
+ predict_type: "ort"
+ model_path: "./downloaded_repo/pretrained_weights/liveportrait_onnx/stitching.onnx"
+ stitching_eye_retarget:
+ name: "StitchingModel"
+ predict_type: "ort"
+ model_path: "./downloaded_repo/pretrained_weights/liveportrait_onnx/stitching_eye.onnx"
+ stitching_lip_retarget:
+ name: "StitchingModel"
+ predict_type: "ort"
+ model_path: "./downloaded_repo/pretrained_weights/liveportrait_onnx/stitching_lip.onnx"
+
+animal_models:
+ warping_spade:
+ name: "WarpingSpadeModel"
+ predict_type: "ort"
+ model_path: "./downloaded_repo/pretrained_weights/liveportrait_animal_onnx/warping_spade.onnx"
+ motion_extractor:
+ name: "MotionExtractorModel"
+ predict_type: "ort"
+ model_path: "./downloaded_repo/pretrained_weights/liveportrait_animal_onnx/motion_extractor.onnx"
+ app_feat_extractor:
+ name: "AppearanceFeatureExtractorModel"
+ predict_type: "ort"
+ model_path: "./downloaded_repo/pretrained_weights/liveportrait_animal_onnx/appearance_feature_extractor.onnx"
+ stitching:
+ name: "StitchingModel"
+ predict_type: "ort"
+ model_path: "./downloaded_repo/pretrained_weights/liveportrait_animal_onnx/stitching.onnx"
+ stitching_eye_retarget:
+ name: "StitchingModel"
+ predict_type: "ort"
+ model_path: "./downloaded_repo/pretrained_weights/liveportrait_animal_onnx/stitching_eye.onnx"
+ stitching_lip_retarget:
+ name: "StitchingModel"
+ predict_type: "ort"
+ model_path: "./downloaded_repo/pretrained_weights/liveportrait_animal_onnx/stitching_lip.onnx"
+ landmark:
+ name: "LandmarkModel"
+ predict_type: "ort"
+ model_path: "./downloaded_repo/pretrained_weights/liveportrait_onnx/landmark.onnx"
+ face_analysis:
+ name: "FaceAnalysisModel"
+ predict_type: "ort"
+ model_path:
+ - "./downloaded_repo/pretrained_weights/liveportrait_onnx/retinaface_det_static.onnx"
+ - "./downloaded_repo/pretrained_weights/liveportrait_onnx/face_2dpose_106_static.onnx"
+
+crop_params:
+ src_dsize: 512
+ src_scale: 2.3
+ src_vx_ratio: 0.0
+ src_vy_ratio: -0.125
+ dri_scale: 2.2
+ dri_vx_ratio: 0.0
+ dri_vy_ratio: -0.1
+
+
+infer_params:
+ flag_crop_driving_video: False
+ flag_normalize_lip: True
+ flag_source_video_eye_retargeting: False
+ flag_video_editing_head_rotation: False
+ flag_eye_retargeting: False
+ flag_lip_retargeting: False
+ flag_stitching: True
+ flag_relative_motion: True
+ flag_pasteback: True
+ flag_do_crop: True
+ flag_do_rot: True
+
+ # NOT EXPOERTED PARAMS
+ lip_normalize_threshold: 0.03 # threshold for flag_normalize_lip
+ source_video_eye_retargeting_threshold: 0.18 # threshold for eyes retargeting if the input is a source video
+ driving_smooth_observation_variance: 1e-7 # smooth strength scalar for the animated video when the input is a source video, the larger the number, the smoother the animated video; too much smoothness would result in loss of motion accuracy
+ anchor_frame: 0 # TO IMPLEMENT
+ mask_crop_path: "./assets/mask_template.png"
+ driving_multiplier: 1.0
+
+ source_max_dim: 1280 # the max dim of height and width of source image
+ source_division: 2 # make sure the height and width of source image can be divided by this number
\ No newline at end of file
diff --git a/difpoint/configs/onnx_mp_infer.yaml b/difpoint/configs/onnx_mp_infer.yaml
new file mode 100644
index 0000000000000000000000000000000000000000..b14352c707c26ebe0b87da8c6a157ee1e1921645
--- /dev/null
+++ b/difpoint/configs/onnx_mp_infer.yaml
@@ -0,0 +1,99 @@
+models:
+ warping_spade:
+ name: "WarpingSpadeModel"
+ predict_type: "ort"
+ model_path: "./downloaded_repo/pretrained_weights/liveportrait_onnx/warping_spade.onnx"
+ motion_extractor:
+ name: "MotionExtractorModel"
+ predict_type: "ort"
+ model_path: "./downloaded_repo/pretrained_weights/liveportrait_onnx/motion_extractor.onnx"
+ landmark:
+ name: "LandmarkModel"
+ predict_type: "ort"
+ model_path: "./downloaded_repo/pretrained_weights/liveportrait_onnx/landmark.onnx"
+ face_analysis:
+ name: "MediaPipeFaceModel"
+ predict_type: "mp"
+ app_feat_extractor:
+ name: "AppearanceFeatureExtractorModel"
+ predict_type: "ort"
+ model_path: "./downloaded_repo/pretrained_weights/liveportrait_onnx/appearance_feature_extractor.onnx"
+ stitching:
+ name: "StitchingModel"
+ predict_type: "ort"
+ model_path: "./downloaded_repo/pretrained_weights/liveportrait_onnx/stitching.onnx"
+ stitching_eye_retarget:
+ name: "StitchingModel"
+ predict_type: "ort"
+ model_path: "./downloaded_repo/pretrained_weights/liveportrait_onnx/stitching_eye.onnx"
+ stitching_lip_retarget:
+ name: "StitchingModel"
+ predict_type: "ort"
+ model_path: "./downloaded_repo/pretrained_weights/liveportrait_onnx/stitching_lip.onnx"
+
+animal_models:
+ warping_spade:
+ name: "WarpingSpadeModel"
+ predict_type: "ort"
+ model_path: "./downloaded_repo/pretrained_weights/liveportrait_animal_onnx/warping_spade.onnx"
+ motion_extractor:
+ name: "MotionExtractorModel"
+ predict_type: "ort"
+ model_path: "./downloaded_repo/pretrained_weights/liveportrait_animal_onnx/motion_extractor.onnx"
+ app_feat_extractor:
+ name: "AppearanceFeatureExtractorModel"
+ predict_type: "ort"
+ model_path: "./downloaded_repo/pretrained_weights/liveportrait_animal_onnx/appearance_feature_extractor.onnx"
+ stitching:
+ name: "StitchingModel"
+ predict_type: "ort"
+ model_path: "./downloaded_repo/pretrained_weights/liveportrait_animal_onnx/stitching.onnx"
+ stitching_eye_retarget:
+ name: "StitchingModel"
+ predict_type: "ort"
+ model_path: "./downloaded_repo/pretrained_weights/liveportrait_animal_onnx/stitching_eye.onnx"
+ stitching_lip_retarget:
+ name: "StitchingModel"
+ predict_type: "ort"
+ model_path: "./downloaded_repo/pretrained_weights/liveportrait_animal_onnx/stitching_lip.onnx"
+ landmark:
+ name: "LandmarkModel"
+ predict_type: "ort"
+ model_path: "./downloaded_repo/pretrained_weights/liveportrait_onnx/landmark.onnx"
+ face_analysis:
+ name: "MediaPipeFaceModel"
+ predict_type: "mp"
+
+crop_params:
+ src_dsize: 512
+ src_scale: 2.3
+ src_vx_ratio: 0.0
+ src_vy_ratio: -0.125
+ dri_scale: 2.2
+ dri_vx_ratio: 0.0
+ dri_vy_ratio: -0.1
+
+
+infer_params:
+ flag_crop_driving_video: False
+ flag_normalize_lip: True
+ flag_source_video_eye_retargeting: False
+ flag_video_editing_head_rotation: False
+ flag_eye_retargeting: False
+ flag_lip_retargeting: False
+ flag_stitching: True
+ flag_relative_motion: True
+ flag_pasteback: True
+ flag_do_crop: True
+ flag_do_rot: True
+
+ # NOT EXPOERTED PARAMS
+ lip_normalize_threshold: 0.03 # threshold for flag_normalize_lip
+ source_video_eye_retargeting_threshold: 0.18 # threshold for eyes retargeting if the input is a source video
+ driving_smooth_observation_variance: 1e-7 # smooth strength scalar for the animated video when the input is a source video, the larger the number, the smoother the animated video; too much smoothness would result in loss of motion accuracy
+ anchor_frame: 0 # TO IMPLEMENT
+ mask_crop_path: "./assets/mask_template.png"
+ driving_multiplier: 1.0
+
+ source_max_dim: 1280 # the max dim of height and width of source image
+ source_division: 2 # make sure the height and width of source image can be divided by this number
\ No newline at end of file
diff --git a/difpoint/configs/trt_infer.yaml b/difpoint/configs/trt_infer.yaml
new file mode 100644
index 0000000000000000000000000000000000000000..c6f532d15ec1d47f01f7d1ba9dd259eac5b1eadf
--- /dev/null
+++ b/difpoint/configs/trt_infer.yaml
@@ -0,0 +1,105 @@
+models:
+ warping_spade:
+ name: "WarpingSpadeModel"
+ predict_type: "trt"
+ model_path: "./downloaded_repo/pretrained_weights/liveportrait_onnx/warping_spade-fix.trt"
+ motion_extractor:
+ name: "MotionExtractorModel"
+ predict_type: "trt"
+ model_path: "./downloaded_repo/pretrained_weights/liveportrait_onnx/motion_extractor.trt"
+ landmark:
+ name: "LandmarkModel"
+ predict_type: "trt"
+ model_path: "./downloaded_repo/pretrained_weights/liveportrait_onnx/landmark.trt"
+ face_analysis:
+ name: "FaceAnalysisModel"
+ predict_type: "trt"
+ model_path:
+ - "./downloaded_repo/pretrained_weights/liveportrait_onnx/retinaface_det_static.trt"
+ - "./downloaded_repo/pretrained_weights/liveportrait_onnx/face_2dpose_106_static.trt"
+ app_feat_extractor:
+ name: "AppearanceFeatureExtractorModel"
+ predict_type: "trt"
+ model_path: "./downloaded_repo/pretrained_weights/liveportrait_onnx/appearance_feature_extractor.trt"
+ stitching:
+ name: "StitchingModel"
+ predict_type: "trt"
+ model_path: "./downloaded_repo/pretrained_weights/liveportrait_onnx/stitching.trt"
+ stitching_eye_retarget:
+ name: "StitchingModel"
+ predict_type: "trt"
+ model_path: "./downloaded_repo/pretrained_weights/liveportrait_onnx/stitching_eye.trt"
+ stitching_lip_retarget:
+ name: "StitchingModel"
+ predict_type: "trt"
+ model_path: "./downloaded_repo/pretrained_weights/liveportrait_onnx/stitching_lip.trt"
+
+animal_models:
+ warping_spade:
+ name: "WarpingSpadeModel"
+ predict_type: "trt"
+ model_path: "./downloaded_repo/pretrained_weights/liveportrait_animal_onnx/warping_spade-fix.trt"
+ motion_extractor:
+ name: "MotionExtractorModel"
+ predict_type: "trt"
+ model_path: "./downloaded_repo/pretrained_weights/liveportrait_animal_onnx/motion_extractor.trt"
+ app_feat_extractor:
+ name: "AppearanceFeatureExtractorModel"
+ predict_type: "trt"
+ model_path: "./downloaded_repo/pretrained_weights/liveportrait_animal_onnx/appearance_feature_extractor.trt"
+ stitching:
+ name: "StitchingModel"
+ predict_type: "trt"
+ model_path: "./downloaded_repo/pretrained_weights/liveportrait_animal_onnx/stitching.trt"
+ stitching_eye_retarget:
+ name: "StitchingModel"
+ predict_type: "trt"
+ model_path: "./downloaded_repo/pretrained_weights/liveportrait_animal_onnx/stitching_eye.trt"
+ stitching_lip_retarget:
+ name: "StitchingModel"
+ predict_type: "trt"
+ model_path: "./downloaded_repo/pretrained_weights/liveportrait_animal_onnx/stitching_lip.trt"
+ landmark:
+ name: "LandmarkModel"
+ predict_type: "trt"
+ model_path: "./downloaded_repo/pretrained_weights/liveportrait_onnx/landmark.trt"
+ face_analysis:
+ name: "FaceAnalysisModel"
+ predict_type: "trt"
+ model_path:
+ - "./downloaded_repo/pretrained_weights/liveportrait_onnx/retinaface_det_static.trt"
+ - "./downloaded_repo/pretrained_weights/liveportrait_onnx/face_2dpose_106_static.trt"
+
+crop_params:
+ src_dsize: 512
+ src_scale: 2.3
+ src_vx_ratio: 0.0
+ src_vy_ratio: -0.125
+ dri_scale: 2.2
+ dri_vx_ratio: 0.0
+ dri_vy_ratio: -0.1
+
+
+infer_params:
+ flag_crop_driving_video: False
+ flag_normalize_lip: False
+ flag_source_video_eye_retargeting: False
+ flag_video_editing_head_rotation: False
+ flag_eye_retargeting: False
+ flag_lip_retargeting: False
+ flag_stitching: True
+ flag_relative_motion: True
+ flag_pasteback: True
+ flag_do_crop: True
+ flag_do_rot: True
+
+ # NOT EXPOERTED PARAMS
+ lip_normalize_threshold: 0.03 # threshold for flag_normalize_lip
+ source_video_eye_retargeting_threshold: 0.18 # threshold for eyes retargeting if the input is a source video
+ driving_smooth_observation_variance: 1e-7 # smooth strength scalar for the animated video when the input is a source video, the larger the number, the smoother the animated video; too much smoothness would result in loss of motion accuracy
+ anchor_frame: 0 # TO IMPLEMENT
+ mask_crop_path: "./assets/mask_template.png"
+ driving_multiplier: 1.0
+
+ source_max_dim: 1280 # the max dim of height and width of source image
+ source_division: 2 # make sure the height and width of source image can be divided by this number
\ No newline at end of file
diff --git a/difpoint/configs/trt_mp_infer.yaml b/difpoint/configs/trt_mp_infer.yaml
new file mode 100644
index 0000000000000000000000000000000000000000..76dc69e5c7b8ef9ab6cb61aff3a20c84adb950b2
--- /dev/null
+++ b/difpoint/configs/trt_mp_infer.yaml
@@ -0,0 +1,99 @@
+models:
+ warping_spade:
+ name: "WarpingSpadeModel"
+ predict_type: "trt"
+ model_path: "./downloaded_repo/pretrained_weights/liveportrait_onnx/warping_spade-fix.trt"
+ motion_extractor:
+ name: "MotionExtractorModel"
+ predict_type: "trt"
+ model_path: "./downloaded_repo/pretrained_weights/liveportrait_onnx/motion_extractor.trt"
+ landmark:
+ name: "LandmarkModel"
+ predict_type: "trt"
+ model_path: "./downloaded_repo/pretrained_weights/liveportrait_onnx/landmark.trt"
+ face_analysis:
+ name: "MediaPipeFaceModel"
+ predict_type: "mp"
+ app_feat_extractor:
+ name: "AppearanceFeatureExtractorModel"
+ predict_type: "trt"
+ model_path: "./downloaded_repo/pretrained_weights/liveportrait_onnx/appearance_feature_extractor.trt"
+ stitching:
+ name: "StitchingModel"
+ predict_type: "trt"
+ model_path: "./downloaded_repo/pretrained_weights/liveportrait_onnx/stitching.trt"
+ stitching_eye_retarget:
+ name: "StitchingModel"
+ predict_type: "trt"
+ model_path: "./downloaded_repo/pretrained_weights/liveportrait_onnx/stitching_eye.trt"
+ stitching_lip_retarget:
+ name: "StitchingModel"
+ predict_type: "trt"
+ model_path: "./downloaded_repo/pretrained_weights/liveportrait_onnx/stitching_lip.trt"
+
+animal_models:
+ warping_spade:
+ name: "WarpingSpadeModel"
+ predict_type: "trt"
+ model_path: "./downloaded_repo/pretrained_weights/liveportrait_animal_onnx/warping_spade-fix.trt"
+ motion_extractor:
+ name: "MotionExtractorModel"
+ predict_type: "trt"
+ model_path: "./downloaded_repo/pretrained_weights/liveportrait_animal_onnx/motion_extractor.trt"
+ app_feat_extractor:
+ name: "AppearanceFeatureExtractorModel"
+ predict_type: "trt"
+ model_path: "./downloaded_repo/pretrained_weights/liveportrait_animal_onnx/appearance_feature_extractor.trt"
+ stitching:
+ name: "StitchingModel"
+ predict_type: "trt"
+ model_path: "./downloaded_repo/pretrained_weights/liveportrait_animal_onnx/stitching.trt"
+ stitching_eye_retarget:
+ name: "StitchingModel"
+ predict_type: "trt"
+ model_path: "./downloaded_repo/pretrained_weights/liveportrait_animal_onnx/stitching_eye.trt"
+ stitching_lip_retarget:
+ name: "StitchingModel"
+ predict_type: "trt"
+ model_path: "./downloaded_repo/pretrained_weights/liveportrait_animal_onnx/stitching_lip.trt"
+ landmark:
+ name: "LandmarkModel"
+ predict_type: "trt"
+ model_path: "./downloaded_repo/pretrained_weights/liveportrait_onnx/landmark.trt"
+ face_analysis:
+ name: "MediaPipeFaceModel"
+ predict_type: "mp"
+
+crop_params:
+ src_dsize: 512
+ src_scale: 2.0
+ src_vx_ratio: 0.0
+ src_vy_ratio: -0.125
+ dri_scale: 2.2
+ dri_vx_ratio: 0.0
+ dri_vy_ratio: -0.1
+
+
+infer_params:
+ flag_crop_driving_video: False
+ flag_normalize_lip: False
+ flag_source_video_eye_retargeting: False
+ flag_video_editing_head_rotation: False
+ flag_eye_retargeting: False
+ flag_lip_retargeting: False
+ flag_stitching: False
+ flag_relative_motion: False
+ flag_pasteback: False
+ flag_do_crop: False
+ flag_do_rot: False
+
+ # NOT EXPOERTED PARAMS
+ lip_normalize_threshold: 0.03 # threshold for flag_normalize_lip
+ source_video_eye_retargeting_threshold: 0.18 # threshold for eyes retargeting if the input is a source video
+ driving_smooth_observation_variance: 1e-7 # smooth strength scalar for the animated video when the input is a source video, the larger the number, the smoother the animated video; too much smoothness would result in loss of motion accuracy
+ anchor_frame: 0 # TO IMPLEMENT
+ mask_crop_path: "./assets/mask_template.png"
+ driving_multiplier: 1.0
+
+ source_max_dim: 1280 # the max dim of height and width of source image
+ source_division: 2 # make sure the height and width of source image can be divided by this number
\ No newline at end of file
diff --git a/difpoint/croper.py b/difpoint/croper.py
new file mode 100644
index 0000000000000000000000000000000000000000..4569a3d8b4d98c59adf6c50997cba8be213fc0a8
--- /dev/null
+++ b/difpoint/croper.py
@@ -0,0 +1,269 @@
+import os
+import cv2
+import time
+import glob
+import argparse
+import scipy
+import numpy as np
+from PIL import Image
+from tqdm import tqdm
+from itertools import cycle
+
+
+"""
+brief: face alignment with FFHQ method (https://github.com/NVlabs/ffhq-dataset)
+author: lzhbrian (https://lzhbrian.me)
+date: 2020.1.5
+note: code is heavily borrowed from
+ https://github.com/NVlabs/ffhq-dataset
+ http://dlib.net/face_landmark_detection.py.html
+requirements:
+ apt install cmake
+ conda install Pillow numpy scipy
+ pip install dlib
+ # download face landmark model from:
+ # http://dlib.net/files/shape_predictor_68_face_landmarks.dat.bz2
+"""
+
+import numpy as np
+from PIL import Image
+import dlib
+
+
+class Croper:
+
+ def __init__(self, path_of_lm):
+ # download model from: http://dlib.net/files/shape_predictor_68_face_landmarks.dat.bz2
+ self.predictor = dlib.shape_predictor(path_of_lm)
+
+ def get_landmark(self, img_np):
+ """get landmark with dlib
+ :return: np.array shape=(68, 2)
+ """
+ detector = dlib.get_frontal_face_detector()
+ dets = detector(img_np, 1)
+ # print("Number of faces detected: {}".format(len(dets)))
+ # for k, d in enumerate(dets):
+ if len(dets) == 0:
+ return None
+ d = dets[0]
+ # Get the landmarks/parts for the face in box d.
+ shape = self.predictor(img_np, d)
+ # print("Part 0: {}, Part 1: {} ...".format(shape.part(0), shape.part(1)))
+ t = list(shape.parts())
+ a = []
+ for tt in t:
+ a.append([tt.x, tt.y])
+ lm = np.array(a)
+ # lm is a shape=(68,2) np.array
+ return lm
+
+ def align_face(self, img, lm, output_size=1024):
+ """
+ :param filepath: str
+ :return: PIL Image
+ """
+ lm_chin = lm[0: 17] # left-right
+ lm_eyebrow_left = lm[17: 22] # left-right
+ lm_eyebrow_right = lm[22: 27] # left-right
+ lm_nose = lm[27: 31] # top-down
+ lm_nostrils = lm[31: 36] # top-down
+ lm_eye_left = lm[36: 42] # left-clockwise
+ lm_eye_right = lm[42: 48] # left-clockwise
+ lm_mouth_outer = lm[48: 60] # left-clockwise
+ lm_mouth_inner = lm[60: 68] # left-clockwise
+
+ # Calculate auxiliary vectors.
+ eye_left = np.mean(lm_eye_left, axis=0)
+ eye_right = np.mean(lm_eye_right, axis=0)
+ eye_avg = (eye_left + eye_right) * 0.5
+ eye_to_eye = eye_right - eye_left
+ mouth_left = lm_mouth_outer[0]
+ mouth_right = lm_mouth_outer[6]
+ mouth_avg = (mouth_left + mouth_right) * 0.5
+ eye_to_mouth = mouth_avg - eye_avg
+
+ # Choose oriented crop rectangle.
+ x = eye_to_eye - np.flipud(eye_to_mouth) * [-1, 1] # Addition of binocular difference and double mouth difference
+ x /= np.hypot(*x) # hypot函数计算直角三角形的斜边长,用斜边长对三角形两条直边做归一化
+ x *= max(np.hypot(*eye_to_eye) * 2.0, np.hypot(*eye_to_mouth) * 1.8) # 双眼差和眼嘴差,选较大的作为基准尺度
+ y = np.flipud(x) * [-1, 1]
+ c = eye_avg + eye_to_mouth * 0.1
+ quad = np.stack([c - x - y, c - x + y, c + x + y, c + x - y]) # 定义四边形,以面部基准位置为中心上下左右平移得到四个顶点
+ qsize = np.hypot(*x) * 2 # 定义四边形的大小(边长),为基准尺度的2倍
+
+ # Shrink.
+ # 如果计算出的四边形太大了,就按比例缩小它
+ shrink = int(np.floor(qsize / output_size * 0.5))
+ if shrink > 1:
+ rsize = (int(np.rint(float(img.size[0]) / shrink)), int(np.rint(float(img.size[1]) / shrink)))
+ img = img.resize(rsize, Image.ANTIALIAS)
+ quad /= shrink
+ qsize /= shrink
+ else:
+ rsize = (int(np.rint(float(img.size[0]))), int(np.rint(float(img.size[1]))))
+
+ # Crop.
+ border = max(int(np.rint(qsize * 0.1)), 3)
+ crop = (int(np.floor(min(quad[:, 0]))), int(np.floor(min(quad[:, 1]))), int(np.ceil(max(quad[:, 0]))),
+ int(np.ceil(max(quad[:, 1]))))
+ crop = (max(crop[0] - border, 0), max(crop[1] - border, 0), min(crop[2] + border, img.size[0]),
+ min(crop[3] + border, img.size[1]))
+ if crop[2] - crop[0] < img.size[0] or crop[3] - crop[1] < img.size[1]:
+ # img = img.crop(crop)
+ quad -= crop[0:2]
+
+ # Pad.
+ pad = (int(np.floor(min(quad[:, 0]))), int(np.floor(min(quad[:, 1]))), int(np.ceil(max(quad[:, 0]))),
+ int(np.ceil(max(quad[:, 1]))))
+ pad = (max(-pad[0] + border, 0), max(-pad[1] + border, 0), max(pad[2] - img.size[0] + border, 0),
+ max(pad[3] - img.size[1] + border, 0))
+ # if enable_padding and max(pad) > border - 4:
+ # pad = np.maximum(pad, int(np.rint(qsize * 0.3)))
+ # img = np.pad(np.float32(img), ((pad[1], pad[3]), (pad[0], pad[2]), (0, 0)), 'reflect')
+ # h, w, _ = img.shape
+ # y, x, _ = np.ogrid[:h, :w, :1]
+ # mask = np.maximum(1.0 - np.minimum(np.float32(x) / pad[0], np.float32(w - 1 - x) / pad[2]),
+ # 1.0 - np.minimum(np.float32(y) / pad[1], np.float32(h - 1 - y) / pad[3]))
+ # blur = qsize * 0.02
+ # img += (scipy.ndimage.gaussian_filter(img, [blur, blur, 0]) - img) * np.clip(mask * 3.0 + 1.0, 0.0, 1.0)
+ # img += (np.median(img, axis=(0, 1)) - img) * np.clip(mask, 0.0, 1.0)
+ # img = Image.fromarray(np.uint8(np.clip(np.rint(img), 0, 255)), 'RGB')
+ # quad += pad[:2]
+
+ # Transform.
+ quad = (quad + 0.5).flatten()
+ lx = max(min(quad[0], quad[2]), 0)
+ ly = max(min(quad[1], quad[7]), 0)
+ rx = min(max(quad[4], quad[6]), img.size[0])
+ ry = min(max(quad[3], quad[5]), img.size[0])
+ # img = img.transform((transform_size, transform_size), Image.QUAD, (quad + 0.5).flatten(),
+ # Image.BILINEAR)
+ # if output_size < transform_size:
+ # img = img.resize((output_size, output_size), Image.ANTIALIAS)
+
+ # Save aligned image.
+ return rsize, crop, [lx, ly, rx, ry]
+
+ # def crop(self, img_np_list):
+ # for _i in range(len(img_np_list)):
+ # img_np = img_np_list[_i]
+ # lm = self.get_landmark(img_np)
+ # if lm is None:
+ # return None
+ # crop, quad = self.align_face(img=Image.fromarray(img_np), lm=lm, output_size=512)
+ # clx, cly, crx, cry = crop
+ # lx, ly, rx, ry = quad
+ # lx, ly, rx, ry = int(lx), int(ly), int(rx), int(ry)
+
+ # _inp = img_np_list[_i]
+ # _inp = _inp[cly:cry, clx:crx]
+ # _inp = _inp[ly:ry, lx:rx]
+ # img_np_list[_i] = _inp
+ # return img_np_list
+
+ def crop(self, img_np_list, still=False, xsize=512): # first frame for all video
+ img_np = img_np_list[0]
+ lm = self.get_landmark(img_np)
+ if lm is None:
+ raise 'can not detect the landmark from source image'
+ rsize, crop, quad = self.align_face(img=Image.fromarray(img_np), lm=lm, output_size=xsize)
+ clx, cly, crx, cry = crop
+ lx, ly, rx, ry = quad
+ lx, ly, rx, ry = int(lx), int(ly), int(rx), int(ry)
+ for _i in range(len(img_np_list)):
+ _inp = img_np_list[_i]
+ _inp = cv2.resize(_inp, (rsize[0], rsize[1]))
+ _inp = _inp[cly:cry, clx:crx]
+ # cv2.imwrite('test1.jpg', _inp)
+ if not still:
+ _inp = _inp[ly:ry, lx:rx]
+ # cv2.imwrite('test2.jpg', _inp)
+ img_np_list[_i] = _inp
+ return img_np_list, crop, quad
+
+
+def read_video(filename, uplimit=100):
+ frames = []
+ cap = cv2.VideoCapture(filename)
+ cnt = 0
+ while cap.isOpened():
+ ret, frame = cap.read()
+ if ret:
+ frame = cv2.resize(frame, (512, 512))
+ frames.append(frame)
+ else:
+ break
+ cnt += 1
+ if cnt >= uplimit:
+ break
+ cap.release()
+ assert len(frames) > 0, f'{filename}: video with no frames!'
+ return frames
+
+
+def create_video(video_name, frames, fps=25, video_format='.mp4', resize_ratio=1):
+ # video_name = os.path.dirname(image_folder) + video_format
+ # img_list = glob.glob1(image_folder, 'frame*')
+ # img_list.sort()
+ # frame = cv2.imread(os.path.join(image_folder, img_list[0]))
+ # frame = cv2.resize(frame, (0, 0), fx=resize_ratio, fy=resize_ratio)
+ # height, width, layers = frames[0].shape
+ height, width, layers = 512, 512, 3
+ if video_format == '.mp4':
+ fourcc = cv2.VideoWriter_fourcc(*'mp4v')
+ elif video_format == '.avi':
+ fourcc = cv2.VideoWriter_fourcc(*'XVID')
+ video = cv2.VideoWriter(video_name, fourcc, fps, (width, height))
+ for _frame in frames:
+ _frame = cv2.resize(_frame, (height, width), interpolation=cv2.INTER_LINEAR)
+ video.write(_frame)
+
+def create_images(video_name, frames):
+ height, width, layers = 512, 512, 3
+ images_dir = video_name.split('.')[0]
+ os.makedirs(images_dir, exist_ok=True)
+ for i, _frame in enumerate(frames):
+ _frame = cv2.resize(_frame, (height, width), interpolation=cv2.INTER_LINEAR)
+ _frame_path = os.path.join(images_dir, str(i)+'.jpg')
+ cv2.imwrite(_frame_path, _frame)
+
+def run(data):
+ filename, opt, device = data
+ os.environ['CUDA_VISIBLE_DEVICES'] = device
+ croper = Croper()
+
+ frames = read_video(filename, uplimit=opt.uplimit)
+ name = filename.split('/')[-1] # .split('.')[0]
+ name = os.path.join(opt.output_dir, name)
+
+ frames = croper.crop(frames)
+ if frames is None:
+ print(f'{name}: detect no face. should removed')
+ return
+ # create_video(name, frames)
+ create_images(name, frames)
+
+
+def get_data_path(video_dir):
+ eg_video_files = ['/apdcephfs/share_1290939/quincheng/datasets/HDTF/backup_fps25/WDA_KatieHill_000.mp4']
+ # filenames = list()
+ # VIDEO_EXTENSIONS_LOWERCASE = {'mp4'}
+ # VIDEO_EXTENSIONS = VIDEO_EXTENSIONS_LOWERCASE.union({f.upper() for f in VIDEO_EXTENSIONS_LOWERCASE})
+ # extensions = VIDEO_EXTENSIONS
+ # for ext in extensions:
+ # filenames = sorted(glob.glob(f'{opt.input_dir}/**/*.{ext}'))
+ # print('Total number of videos:', len(filenames))
+ return eg_video_files
+
+
+def get_wra_data_path(video_dir):
+ if opt.option == 'video':
+ videos_path = sorted(glob.glob(f'{video_dir}/*.mp4'))
+ elif opt.option == 'image':
+ videos_path = sorted(glob.glob(f'{video_dir}/*/'))
+ else:
+ raise NotImplementedError
+ print('Example videos: ', videos_path[:2])
+ return videos_path
+
diff --git a/difpoint/dataset_process/.DS_Store b/difpoint/dataset_process/.DS_Store
new file mode 100644
index 0000000000000000000000000000000000000000..5df00aa963635f954ee077a9456757c74db5db74
Binary files /dev/null and b/difpoint/dataset_process/.DS_Store differ
diff --git a/difpoint/dataset_process/__pycache__/audio.cpython-310.pyc b/difpoint/dataset_process/__pycache__/audio.cpython-310.pyc
new file mode 100644
index 0000000000000000000000000000000000000000..8018e0b466008efb242cc4b3e4423afa7044a321
Binary files /dev/null and b/difpoint/dataset_process/__pycache__/audio.cpython-310.pyc differ
diff --git a/difpoint/dataset_process/__pycache__/audio.cpython-38.pyc b/difpoint/dataset_process/__pycache__/audio.cpython-38.pyc
new file mode 100644
index 0000000000000000000000000000000000000000..321cf105f1c19e4716dca62eb652abde953eee02
Binary files /dev/null and b/difpoint/dataset_process/__pycache__/audio.cpython-38.pyc differ
diff --git a/difpoint/dataset_process/audio.py b/difpoint/dataset_process/audio.py
new file mode 100644
index 0000000000000000000000000000000000000000..9cbb30a5700a4df2c393ab6a6c432d7ce03de005
--- /dev/null
+++ b/difpoint/dataset_process/audio.py
@@ -0,0 +1,156 @@
+import librosa
+import librosa.filters
+import numpy as np
+# import tensorflow as tf
+from scipy import signal
+from scipy.io import wavfile
+from difpoint.src.utils.hparams import hparams as hp
+
+
+def load_wav(path, sr):
+ return librosa.core.load(path, sr=sr)[0]
+
+
+def save_wav(wav, path, sr):
+ wav *= 32767 / max(0.01, np.max(np.abs(wav)))
+ # proposed by @dsmiller
+ wavfile.write(path, sr, wav.astype(np.int16))
+
+
+def save_wavenet_wav(wav, path, sr):
+ librosa.output.write_wav(path, wav, sr=sr)
+
+
+def preemphasis(wav, k, preemphasize=True):
+ if preemphasize:
+ return signal.lfilter([1, -k], [1], wav)
+ return wav
+
+
+def inv_preemphasis(wav, k, inv_preemphasize=True):
+ if inv_preemphasize:
+ return signal.lfilter([1], [1, -k], wav)
+ return wav
+
+
+def get_hop_size():
+ hop_size = hp.hop_size
+ if hop_size is None:
+ assert hp.frame_shift_ms is not None
+ hop_size = int(hp.frame_shift_ms / 1000 * hp.sample_rate)
+ return hop_size
+
+
+def linearspectrogram(wav):
+ D = _stft(preemphasis(wav, hp.preemphasis, hp.preemphasize))
+ S = _amp_to_db(np.abs(D)) - hp.ref_level_db
+
+ if hp.signal_normalization:
+ return _normalize(S)
+ return S
+
+
+def melspectrogram(wav):
+ D = _stft(preemphasis(wav, hp.preemphasis, hp.preemphasize))
+ S = _amp_to_db(_linear_to_mel(np.abs(D))) - hp.ref_level_db
+
+ if hp.signal_normalization:
+ return _normalize(S)
+ return S
+
+
+def _lws_processor():
+ import lws
+ return lws.lws(hp.n_fft, get_hop_size(), fftsize=hp.win_size, mode="speech")
+
+
+def _stft(y):
+ if hp.use_lws:
+ return _lws_processor(hp).stft(y).T
+ else:
+ return librosa.stft(y=y, n_fft=hp.n_fft, hop_length=get_hop_size(), win_length=hp.win_size)
+
+
+##########################################################
+# Those are only correct when using lws!!! (This was messing with Wavenet quality for a long time!)
+def num_frames(length, fsize, fshift):
+ """Compute number of time frames of spectrogram
+ """
+ pad = (fsize - fshift)
+ if length % fshift == 0:
+ M = (length + pad * 2 - fsize) // fshift + 1
+ else:
+ M = (length + pad * 2 - fsize) // fshift + 2
+ return M
+
+
+def pad_lr(x, fsize, fshift):
+ """Compute left and right padding
+ """
+ M = num_frames(len(x), fsize, fshift)
+ pad = (fsize - fshift)
+ T = len(x) + 2 * pad
+ r = (M - 1) * fshift + fsize - T
+ return pad, pad + r
+
+
+##########################################################
+# Librosa correct padding
+def librosa_pad_lr(x, fsize, fshift):
+ return 0, (x.shape[0] // fshift + 1) * fshift - x.shape[0]
+
+
+# Conversions
+_mel_basis = None
+
+
+def _linear_to_mel(spectogram):
+ global _mel_basis
+ if _mel_basis is None:
+ _mel_basis = _build_mel_basis()
+ return np.dot(_mel_basis, spectogram)
+
+
+def _build_mel_basis():
+ assert hp.fmax <= hp.sample_rate // 2
+ return librosa.filters.mel(sr=hp.sample_rate, n_fft=hp.n_fft, n_mels=hp.num_mels,
+ fmin=hp.fmin, fmax=hp.fmax)
+
+
+def _amp_to_db(x):
+ min_level = np.exp(hp.min_level_db / 20 * np.log(10))
+ return 20 * np.log10(np.maximum(min_level, x))
+
+
+def _db_to_amp(x):
+ return np.power(10.0, (x) * 0.05)
+
+
+def _normalize(S):
+ if hp.allow_clipping_in_normalization:
+ if hp.symmetric_mels:
+ return np.clip((2 * hp.max_abs_value) * ((S - hp.min_level_db) / (-hp.min_level_db)) - hp.max_abs_value,
+ -hp.max_abs_value, hp.max_abs_value)
+ else:
+ return np.clip(hp.max_abs_value * ((S - hp.min_level_db) / (-hp.min_level_db)), 0, hp.max_abs_value)
+
+ assert S.max() <= 0 and S.min() - hp.min_level_db >= 0
+ if hp.symmetric_mels:
+ return (2 * hp.max_abs_value) * ((S - hp.min_level_db) / (-hp.min_level_db)) - hp.max_abs_value
+ else:
+ return hp.max_abs_value * ((S - hp.min_level_db) / (-hp.min_level_db))
+
+
+def _denormalize(D):
+ if hp.allow_clipping_in_normalization:
+ if hp.symmetric_mels:
+ return (((np.clip(D, -hp.max_abs_value,
+ hp.max_abs_value) + hp.max_abs_value) * -hp.min_level_db / (2 * hp.max_abs_value))
+ + hp.min_level_db)
+ else:
+ return ((np.clip(D, 0, hp.max_abs_value) * -hp.min_level_db / hp.max_abs_value) + hp.min_level_db)
+
+ if hp.symmetric_mels:
+ return (((D + hp.max_abs_value) * -hp.min_level_db / (2 * hp.max_abs_value)) + hp.min_level_db)
+ else:
+ return ((D * -hp.min_level_db / hp.max_abs_value) + hp.min_level_db)
diff --git a/difpoint/dataset_process/wav2lip.py b/difpoint/dataset_process/wav2lip.py
new file mode 100644
index 0000000000000000000000000000000000000000..d4746a03658a7ef4e38967930435fb23e47ea7ee
--- /dev/null
+++ b/difpoint/dataset_process/wav2lip.py
@@ -0,0 +1,75 @@
+import torch
+import torch.nn.functional as F
+from torch import nn
+
+
+class Conv2d(nn.Module):
+ def __init__(self, cin, cout, kernel_size, stride, padding, residual=False, use_act=True, *args, **kwargs):
+ super().__init__(*args, **kwargs)
+ self.conv_block = nn.Sequential(
+ nn.Conv2d(cin, cout, kernel_size, stride, padding),
+ nn.BatchNorm2d(cout)
+ )
+ self.act = nn.ReLU()
+ self.residual = residual
+ self.use_act = use_act
+
+ def forward(self, x):
+ out = self.conv_block(x)
+ if self.residual:
+ out += x
+
+ if self.use_act:
+ return self.act(out)
+ else:
+ return out
+
+class AudioEncoder(nn.Module):
+ def __init__(self, wav2lip_checkpoint, device):
+ super(AudioEncoder, self).__init__()
+
+ self.audio_encoder = nn.Sequential(
+ Conv2d(1, 32, kernel_size=3, stride=1, padding=1),
+ Conv2d(32, 32, kernel_size=3, stride=1, padding=1, residual=True),
+ Conv2d(32, 32, kernel_size=3, stride=1, padding=1, residual=True),
+
+ Conv2d(32, 64, kernel_size=3, stride=(3, 1), padding=1),
+ Conv2d(64, 64, kernel_size=3, stride=1, padding=1, residual=True),
+ Conv2d(64, 64, kernel_size=3, stride=1, padding=1, residual=True),
+
+ Conv2d(64, 128, kernel_size=3, stride=3, padding=1),
+ Conv2d(128, 128, kernel_size=3, stride=1, padding=1, residual=True),
+ Conv2d(128, 128, kernel_size=3, stride=1, padding=1, residual=True),
+
+ Conv2d(128, 256, kernel_size=3, stride=(3, 2), padding=1),
+ Conv2d(256, 256, kernel_size=3, stride=1, padding=1, residual=True),
+
+ Conv2d(256, 512, kernel_size=3, stride=1, padding=0),
+ Conv2d(512, 512, kernel_size=1, stride=1, padding=0),)
+
+ #### load the pre-trained audio_encoder
+ wav2lip_state_dict = torch.load(wav2lip_checkpoint, map_location=torch.device(device))['state_dict']
+ state_dict = self.audio_encoder.state_dict()
+
+ for k,v in wav2lip_state_dict.items():
+ if 'audio_encoder' in k:
+ state_dict[k.replace('module.audio_encoder.', '')] = v
+ self.audio_encoder.load_state_dict(state_dict)
+
+
+ def forward(self, audio_sequences):
+ # audio_sequences = (B, T, 1, 80, 16)
+ B = audio_sequences.size(0)
+
+ audio_sequences = torch.cat([audio_sequences[:, i] for i in range(audio_sequences.size(1))], dim=0)
+
+ audio_embedding = self.audio_encoder(audio_sequences) # B, 512, 1, 1
+ dim = audio_embedding.shape[1]
+ audio_embedding = audio_embedding.reshape((B, -1, dim, 1, 1))
+
+ return audio_embedding.squeeze(-1).squeeze(-1) #B seq_len+1 512
+
+wav2lip_checkpoint='ckpts/wav2lip.pth'
+wav2lip_model = AudioEncoder(wav2lip_checkpoint, 'cuda')
+wav2lip_model.cuda()
+wav2lip_model.eval()
\ No newline at end of file
diff --git a/difpoint/datasets/norm_info_d6.5_c8.5_vox1_train.npz b/difpoint/datasets/norm_info_d6.5_c8.5_vox1_train.npz
new file mode 100644
index 0000000000000000000000000000000000000000..09d73394389ba43100ca7e4355130aac7fe4a4f4
--- /dev/null
+++ b/difpoint/datasets/norm_info_d6.5_c8.5_vox1_train.npz
@@ -0,0 +1,3 @@
+version https://git-lfs.github.com/spec/v1
+oid sha256:9422e503e75df9d1bd455d8e0f9f5e2826b12956cdedbb5566097c0151bddafb
+size 5580
diff --git a/difpoint/inference.py b/difpoint/inference.py
new file mode 100644
index 0000000000000000000000000000000000000000..67e924ebc6c9f84175e34c8caf495c9656ebed79
--- /dev/null
+++ b/difpoint/inference.py
@@ -0,0 +1,474 @@
+# -*- coding: UTF-8 -*-
+'''
+@File :inference.py
+@Author :Chaolong Yang
+@Date :2024/5/29 19:26
+'''
+import glob
+
+import os
+os.environ['HYDRA_FULL_ERROR']='1'
+
+
+import os
+import time
+import shutil
+import uuid
+import os
+import cv2
+import tyro
+
+import numpy as np
+from tqdm import tqdm
+import cv2
+from rich.progress import track
+
+from difpoint.croper import Croper
+from PIL import Image
+import time
+
+
+import torch
+import torch.nn.functional as F
+from torch import nn
+import imageio
+from pydub import AudioSegment
+from pykalman import KalmanFilter
+import scipy
+import matplotlib.pyplot as plt
+import matplotlib
+matplotlib.use('Agg')
+
+from difpoint.dataset_process import audio
+import os
+import argparse
+import pdb
+import ffmpeg
+import cv2
+import time
+import numpy as np
+import os
+import datetime
+import platform
+from omegaconf import OmegaConf
+#from difpoint.src.pipelines.faster_live_portrait_pipeline import FasterLivePortraitPipeline
+from difpoint.src.live_portrait_pipeline import LivePortraitPipeline
+from difpoint.src.config.argument_config import ArgumentConfig
+from difpoint.src.config.inference_config import InferenceConfig
+from difpoint.src.config.crop_config import CropConfig
+from difpoint.src.live_portrait_pipeline import LivePortraitPipeline
+from difpoint.src.utils.retargeting_utils import calc_eye_close_ratio, calc_lip_close_ratio
+from difpoint.src.utils.camera import get_rotation_matrix
+from difpoint.src.utils.video import images2video, concat_frames, get_fps, add_audio_to_video, has_audio_stream
+
+
+FFMPEG = "ffmpeg"
+
+def parse_audio_length(audio_length, sr, fps):
+ bit_per_frames = sr / fps
+ num_frames = int(audio_length / bit_per_frames)
+ audio_length = int(num_frames * bit_per_frames)
+ return audio_length, num_frames
+
+def crop_pad_audio(wav, audio_length):
+ if len(wav) > audio_length:
+ wav = wav[:audio_length]
+ elif len(wav) < audio_length:
+ wav = np.pad(wav, [0, audio_length - len(wav)], mode='constant', constant_values=0)
+ return wav
+
+class Conv2d(nn.Module):
+ def __init__(self, cin, cout, kernel_size, stride, padding, residual=False, use_act=True, *args, **kwargs):
+ super().__init__(*args, **kwargs)
+ self.conv_block = nn.Sequential(
+ nn.Conv2d(cin, cout, kernel_size, stride, padding),
+ nn.BatchNorm2d(cout)
+ )
+ self.act = nn.ReLU()
+ self.residual = residual
+ self.use_act = use_act
+
+ def forward(self, x):
+ out = self.conv_block(x)
+ if self.residual:
+ out += x
+
+ if self.use_act:
+ return self.act(out)
+ else:
+ return out
+
+class AudioEncoder(nn.Module):
+ def __init__(self, wav2lip_checkpoint, device):
+ super(AudioEncoder, self).__init__()
+
+ self.audio_encoder = nn.Sequential(
+ Conv2d(1, 32, kernel_size=3, stride=1, padding=1),
+ Conv2d(32, 32, kernel_size=3, stride=1, padding=1, residual=True),
+ Conv2d(32, 32, kernel_size=3, stride=1, padding=1, residual=True),
+
+ Conv2d(32, 64, kernel_size=3, stride=(3, 1), padding=1),
+ Conv2d(64, 64, kernel_size=3, stride=1, padding=1, residual=True),
+ Conv2d(64, 64, kernel_size=3, stride=1, padding=1, residual=True),
+
+ Conv2d(64, 128, kernel_size=3, stride=3, padding=1),
+ Conv2d(128, 128, kernel_size=3, stride=1, padding=1, residual=True),
+ Conv2d(128, 128, kernel_size=3, stride=1, padding=1, residual=True),
+
+ Conv2d(128, 256, kernel_size=3, stride=(3, 2), padding=1),
+ Conv2d(256, 256, kernel_size=3, stride=1, padding=1, residual=True),
+
+ Conv2d(256, 512, kernel_size=3, stride=1, padding=0),
+ Conv2d(512, 512, kernel_size=1, stride=1, padding=0),)
+
+ #### load the pre-trained audio_encoder
+ wav2lip_state_dict = torch.load(wav2lip_checkpoint, map_location=torch.device(device))['state_dict']
+ state_dict = self.audio_encoder.state_dict()
+
+ for k,v in wav2lip_state_dict.items():
+ if 'audio_encoder' in k:
+ state_dict[k.replace('module.audio_encoder.', '')] = v
+ self.audio_encoder.load_state_dict(state_dict)
+
+ def forward(self, audio_sequences):
+ # audio_sequences = (B, T, 1, 80, 16)
+ B = audio_sequences.size(0)
+
+ audio_sequences = torch.cat([audio_sequences[:, i] for i in range(audio_sequences.size(1))], dim=0)
+
+ audio_embedding = self.audio_encoder(audio_sequences) # B, 512, 1, 1
+ dim = audio_embedding.shape[1]
+ audio_embedding = audio_embedding.reshape((B, -1, dim, 1, 1))
+
+ return audio_embedding.squeeze(-1).squeeze(-1) #B seq_len+1 512
+
+def partial_fields(target_class, kwargs):
+ return target_class(**{k: v for k, v in kwargs.items() if hasattr(target_class, k)})
+
+def dct2device(dct: dict, device):
+ for key in dct:
+ dct[key] = torch.tensor(dct[key]).to(device)
+ return dct
+
+def save_video_with_watermark(video, audio, save_path, watermark=False):
+ temp_file = str(uuid.uuid4())+'.mp4'
+ cmd = r'ffmpeg -y -i "%s" -i "%s" -vcodec copy "%s"' % (video, audio, temp_file)
+ os.system(cmd)
+ shutil.move(temp_file, save_path)
+
+
+
+class Inferencer(object):
+ def __init__(self):
+
+ st=time.time()
+ print('#'*25+'Start initialization'+'#'*25)
+ self.device = 'cuda'
+ from difpoint.model import get_model
+ self.point_diffusion = get_model()
+ ckpt = torch.load('./downloaded_repo/ckpts/KDTalker.pth', weights_only=False)
+
+ self.point_diffusion.load_state_dict(ckpt['model'])
+ print('model', self.point_diffusion.children())
+ self.point_diffusion.eval()
+ self.point_diffusion.to(self.device)
+
+ lm_croper_checkpoint = './downloaded_repo/ckpts/shape_predictor_68_face_landmarks.dat'
+ self.croper = Croper(lm_croper_checkpoint)
+
+ self.norm_info = dict(np.load(r'difpoint/datasets/norm_info_d6.5_c8.5_vox1_train.npz'))
+
+ wav2lip_checkpoint = './downloaded_repo/ckpts/wav2lip.pth'
+ self.wav2lip_model = AudioEncoder(wav2lip_checkpoint, 'cuda')
+ self.wav2lip_model.cuda()
+ self.wav2lip_model.eval()
+
+ args = tyro.cli(ArgumentConfig)
+
+ self.inf_cfg = partial_fields(InferenceConfig, args.__dict__) # use attribute of args to initial InferenceConfig
+ self.crop_cfg = partial_fields(CropConfig, args.__dict__) # use attribute of args to initial CropConfig
+
+ self.live_portrait_pipeline = LivePortraitPipeline(inference_cfg=self.inf_cfg, crop_cfg=self.crop_cfg)
+ print('#'*25+f'End initialization, cost time {time.time()-st}'+'#'*25)
+
+ def _norm(self, data_dict):
+ for k in data_dict.keys():
+ if k in ['yaw', 'pitch', 'roll', 't', 'exp', 'scale', 'kp', 'c_lip', 'c_eye']:
+ v=data_dict[k]
+ data_dict[k] = (v - self.norm_info[k+'_mean'])/self.norm_info[k+'_std']
+ return data_dict
+
+ def _denorm(self, data_dict):
+ for k in data_dict.keys():
+ if k in ['yaw', 'pitch', 'roll', 't', 'exp', 'scale', 'kp', 'c_lip', 'c_eye']:
+ v=data_dict[k]
+ data_dict[k] = v * self.norm_info[k+'_std'] + self.norm_info[k+'_mean']
+ return data_dict
+
+
+ def output_to_dict(self, data):
+ output = {}
+
+ output['scale'] = data[:, 0]
+ output['yaw'] = data[:, 1, None]
+ output['pitch'] = data[:, 2, None]
+ output['roll'] = data[:, 3, None]
+ output['t'] = data[:, 4:7]
+ output['exp'] = data[:, 7:]
+
+ return output
+
+ def extract_mel_from_audio(self, audio_file_path):
+ syncnet_mel_step_size = 16
+ fps = 25
+ wav = audio.load_wav(audio_file_path, 16000)
+ wav_length, num_frames = parse_audio_length(len(wav), 16000, 25)
+ wav = crop_pad_audio(wav, wav_length)
+ orig_mel = audio.melspectrogram(wav).T
+ spec = orig_mel.copy()
+ indiv_mels = []
+
+ for i in tqdm(range(num_frames), 'mel:'):
+ start_frame_num = i - 2
+ start_idx = int(80. * (start_frame_num / float(fps)))
+ end_idx = start_idx + syncnet_mel_step_size
+ seq = list(range(start_idx, end_idx))
+ seq = [min(max(item, 0), orig_mel.shape[0] - 1) for item in seq]
+ m = spec[seq, :]
+ indiv_mels.append(m.T)
+ indiv_mels = np.asarray(indiv_mels) # T 80 16
+ return indiv_mels
+
+ def extract_wav2lip_from_audio(self, audio_file_path):
+ asd_mel = self.extract_mel_from_audio(audio_file_path)
+ asd_mel = torch.FloatTensor(asd_mel).cuda().unsqueeze(0).unsqueeze(2)
+ with torch.no_grad():
+ hidden = self.wav2lip_model(asd_mel)
+ return hidden[0].cpu().detach().numpy()
+
+ def headpose_pred_to_degree(self, pred):
+ device = pred.device
+ idx_tensor = [idx for idx in range(66)]
+ idx_tensor = torch.FloatTensor(idx_tensor).to(device)
+ pred = F.softmax(pred)
+ degree = torch.sum(pred * idx_tensor, 1) * 3 - 99
+ return degree
+
+ def calc_combined_eye_ratio(self, c_d_eyes_i, c_s_eyes):
+ c_s_eyes_tensor = torch.from_numpy(c_s_eyes).float().to(self.device)
+ c_d_eyes_i_tensor = c_d_eyes_i[0].reshape(1, 1).to(self.device)
+ # [c_s,eyes, c_d,eyes,i]
+ combined_eye_ratio_tensor = torch.cat([c_s_eyes_tensor, c_d_eyes_i_tensor], dim=1)
+ return combined_eye_ratio_tensor
+
+ def calc_combined_lip_ratio(self, c_d_lip_i, c_s_lip):
+ c_s_lip_tensor = torch.from_numpy(c_s_lip).float().to(self.device)
+ c_d_lip_i_tensor = c_d_lip_i[0].to(self.device).reshape(1, 1) # 1x1
+ # [c_s,lip, c_d,lip,i]
+ combined_lip_ratio_tensor = torch.cat([c_s_lip_tensor, c_d_lip_i_tensor], dim=1) # 1x2
+ return combined_lip_ratio_tensor
+
+ # 2024.06.26
+ @torch.no_grad()
+ def generate_with_audio_img(self, upload_audio_path, tts_audio_path, audio_type, image_path, smoothed_pitch, smoothed_yaw, smoothed_roll, smoothed_t, save_path='./downloaded_repo/'):
+ print(audio_type)
+ if audio_type == 'upload':
+ audio_path = upload_audio_path
+ elif audio_type == 'tts':
+ audio_path = tts_audio_path
+ save_path = os.path.join(save_path, "output.mp4")
+ image = [np.array(Image.open(image_path).convert('RGB'))]
+ if image[0].shape[0] != 256 or image[0].shape[1] != 256:
+ cropped_image, crop, quad = self.croper.crop(image, still=False, xsize=512)
+ input_image = cv2.resize(cropped_image[0], (256, 256))
+ else:
+ input_image = image[0]
+
+ I_s = torch.FloatTensor(input_image.transpose((2, 0, 1))).unsqueeze(0).cuda() / 255
+
+ x_s_info = self.live_portrait_pipeline.live_portrait_wrapper.get_kp_info(I_s)
+ x_c_s = x_s_info['kp'].reshape(1, 21, -1)
+ R_s = get_rotation_matrix(x_s_info['pitch'], x_s_info['yaw'], x_s_info['roll'])
+ f_s = self.live_portrait_pipeline.live_portrait_wrapper.extract_feature_3d(I_s)
+ x_s = self.live_portrait_pipeline.live_portrait_wrapper.transform_keypoint(x_s_info)
+
+ flag_lip_zero = self.inf_cfg.flag_lip_zero # not overwrite
+
+
+ ######## process driving info ########
+ kp_info = {}
+ for k in x_s_info.keys():
+ kp_info[k] = x_s_info[k].cpu().numpy()
+ # kp_info['c_lip'] = c_s_lip
+ # kp_info['c_eye'] = c_s_eye
+
+ kp_info = self._norm(kp_info)
+
+ ori_kp = torch.cat([torch.zeros([1, 7]), torch.Tensor(kp_info['kp'])], -1).cuda()
+
+ input_x = np.concatenate([kp_info[k] for k in ['scale', 'yaw', 'pitch', 'roll', 't']], 1)
+ input_x = np.concatenate((input_x, kp_info['exp'].reshape(1, 63)), axis=1)
+ input_x = np.expand_dims(input_x, -1)
+ input_x = np.expand_dims(input_x, 0)
+ input_x = np.concatenate([input_x, input_x, input_x], -1)
+
+ aud_feat = self.extract_wav2lip_from_audio(audio_path)
+
+ outputs = [input_x]
+
+ st = time.time()
+ print('#' * 25 + 'Start Inference' + '#' * 25)
+ sample_frame = 64 # 32 aud_feat.shape[0]
+
+ for i in range(0, aud_feat.shape[0] - 1, sample_frame):
+ input_mel = torch.Tensor(aud_feat[i: i + sample_frame]).unsqueeze(0).cuda()
+ kp0 = torch.Tensor(outputs[-1])[:, -1].cuda()
+ pred_kp = self.point_diffusion.forward_sample(70, ref_kps=kp0, ori_kps=ori_kp, aud_feat=input_mel,
+ scheduler='ddim', num_inference_steps=50)
+ outputs.append(pred_kp.cpu().numpy())
+
+
+ outputs = np.mean(np.concatenate(outputs, 1)[0], -1)[1:, ]
+ output_dict = self.output_to_dict(outputs)
+ output_dict = self._denorm(output_dict)
+
+ num_frame = output_dict['yaw'].shape[0]
+ x_d_info = {}
+ for key in output_dict:
+ x_d_info[key] = torch.tensor(output_dict[key]).cuda()
+
+ # smooth
+ def smooth(sequence, n_dim_state=1):
+ kf = KalmanFilter(initial_state_mean=sequence[0],
+ transition_covariance=0.05 * np.eye(n_dim_state), # 较小的过程噪声
+ observation_covariance=0.001 * np.eye(n_dim_state)) # 可以增大观测噪声,减少敏感性
+ state_means, _ = kf.smooth(sequence)
+ return state_means
+
+ # scale_data = x_d_info['scale'].cpu().numpy()
+ yaw_data = x_d_info['yaw'].cpu().numpy()
+ pitch_data = x_d_info['pitch'].cpu().numpy()
+ roll_data = x_d_info['roll'].cpu().numpy()
+ t_data = x_d_info['t'].cpu().numpy()
+ exp_data = x_d_info['exp'].cpu().numpy()
+
+ smoothed_pitch = smooth(pitch_data, n_dim_state=1) * smoothed_pitch
+ smoothed_yaw = smooth(yaw_data, n_dim_state=1) * smoothed_yaw
+ smoothed_roll = smooth(roll_data, n_dim_state=1) * smoothed_roll
+ # smoothed_scale = smooth(scale_data, n_dim_state=1)
+ smoothed_t = smooth(t_data, n_dim_state=3) * smoothed_t
+ smoothed_exp = smooth(exp_data, n_dim_state=63)
+
+ # x_d_info['scale'] = torch.Tensor(smoothed_scale).cuda()
+ x_d_info['pitch'] = torch.Tensor(smoothed_pitch).cuda()
+ x_d_info['yaw'] = torch.Tensor(smoothed_yaw).cuda()
+ x_d_info['roll'] = torch.Tensor(smoothed_roll).cuda()
+ x_d_info['t'] = torch.Tensor(smoothed_t).cuda()
+ x_d_info['exp'] = torch.Tensor(smoothed_exp).cuda()
+
+
+
+ template_dct = {'motion': [], 'c_d_eyes_lst': [], 'c_d_lip_lst': []}
+ for i in track(range(num_frame), description='Making motion templates...', total=num_frame):
+ # collect s_d, R_d, δ_d and t_d for inference
+ x_d_i_info = x_d_info
+ R_d_i = get_rotation_matrix(x_d_i_info['pitch'][i], x_d_i_info['yaw'][i], x_d_i_info['roll'][i])
+
+ item_dct = {
+ 'scale': x_d_i_info['scale'][i].cpu().numpy().astype(np.float32),
+ 'R_d': R_d_i.cpu().numpy().astype(np.float32),
+ 'exp': x_d_i_info['exp'][i].reshape(1, 21, -1).cpu().numpy().astype(np.float32),
+ 't': x_d_i_info['t'][i].cpu().numpy().astype(np.float32),
+ }
+
+ template_dct['motion'].append(item_dct)
+ # template_dct['c_d_eyes_lst'].append(x_d_i_info['c_eye'][i])
+ # template_dct['c_d_lip_lst'].append(x_d_i_info['c_lip'][i])
+
+ I_p_lst = []
+ R_d_0, x_d_0_info = None, None
+
+ for i in track(range(num_frame), description='Animating...', total=num_frame):
+ x_d_i_info = template_dct['motion'][i]
+
+ for key in x_d_i_info:
+ x_d_i_info[key] = torch.tensor(x_d_i_info[key]).cuda()
+
+ R_d_i = x_d_i_info['R_d']
+
+ if i == 0:
+ R_d_0 = R_d_i
+ x_d_0_info = x_d_i_info
+
+
+ if self.inf_cfg.flag_relative_motion:
+ R_new = (R_d_i @ R_d_0.permute(0, 2, 1)) @ R_s
+ delta_new = x_s_info['exp'].reshape(1, 21, -1) + (x_d_i_info['exp'] - x_d_0_info['exp'])
+ scale_new = x_s_info['scale'] * (x_d_i_info['scale'] / x_d_0_info['scale'])
+ t_new = x_s_info['t'] + (x_d_i_info['t'] - x_d_0_info['t'])
+ else:
+ R_new = R_d_i
+ delta_new = x_d_i_info['exp']
+ scale_new = x_s_info['scale']
+ t_new = x_d_i_info['t']
+ t_new[..., 2] = 0 # zero tz
+
+ x_d_i_new = scale_new * (x_c_s @ R_new + delta_new) + t_new
+
+
+ # Algorithm 1:
+ if not self.inf_cfg.flag_stitching and not self.inf_cfg.flag_eye_retargeting and not self.inf_cfg.flag_lip_retargeting:
+ # without stitching or retargeting
+ if flag_lip_zero:
+ x_d_i_new += lip_delta_before_animation.reshape(-1, x_s.shape[1], 3)
+ else:
+ pass
+ elif self.inf_cfg.flag_stitching and not self.inf_cfg.flag_eye_retargeting and not self.inf_cfg.flag_lip_retargeting:
+ # with stitching and without retargeting
+ if flag_lip_zero:
+ x_d_i_new = self.live_portrait_pipeline.live_portrait_wrapper.stitching(x_s, x_d_i_new) + lip_delta_before_animation.reshape(-1, x_s.shape[1], 3)
+ else:
+ x_d_i_new = self.live_portrait_pipeline.live_portrait_wrapper.stitching(x_s, x_d_i_new)
+ else:
+ eyes_delta, lip_delta = None, None
+
+ if self.inf_cfg.flag_relative_motion: # use x_s
+ x_d_i_new = x_s + \
+ (eyes_delta.reshape(-1, x_s.shape[1], 3) if eyes_delta is not None else 0) + \
+ (lip_delta.reshape(-1, x_s.shape[1], 3) if lip_delta is not None else 0)
+ else: # use x_d,i
+ x_d_i_new = x_d_i_new + \
+ (eyes_delta.reshape(-1, x_s.shape[1], 3) if eyes_delta is not None else 0) + \
+ (lip_delta.reshape(-1, x_s.shape[1], 3) if lip_delta is not None else 0)
+
+ if self.inf_cfg.flag_stitching:
+ x_d_i_new = self.live_portrait_pipeline.live_portrait_wrapper.stitching(x_s, x_d_i_new)
+
+
+ out = self.live_portrait_pipeline.live_portrait_wrapper.warp_decode(f_s, x_s, x_d_i_new)
+ I_p_i = self.live_portrait_pipeline.live_portrait_wrapper.parse_output(out['out'])[0]
+ I_p_lst.append(I_p_i)
+
+ video_name = os.path.basename(save_path)
+ video_save_dir = os.path.dirname(save_path)
+ path = os.path.join(video_save_dir, video_name)
+
+ imageio.mimsave(path, I_p_lst, fps=float(25))
+
+ audio_name = audio_path.split('/')[-1]
+ new_audio_path = os.path.join(video_save_dir, audio_name)
+ start_time = 0
+ # cog will not keep the .mp3 filename
+ sound = AudioSegment.from_file(audio_path)
+ end_time = start_time + num_frame * 1 / 25 * 1000
+ word1 = sound.set_frame_rate(16000)
+ word = word1[start_time:end_time]
+ word.export(new_audio_path, format="wav")
+
+ save_video_with_watermark(path, new_audio_path, save_path, watermark=False)
+ print(f'The generated video is named {video_save_dir}/{video_name}')
+
+ print('#' * 25 + f'End Inference, cost time {time.time() - st}' + '#' * 25)
+ return save_path
+
+
\ No newline at end of file
diff --git a/difpoint/model/__init__.py b/difpoint/model/__init__.py
new file mode 100644
index 0000000000000000000000000000000000000000..90ee2c77ca69f7700f3fab1838049ec109e7f598
--- /dev/null
+++ b/difpoint/model/__init__.py
@@ -0,0 +1,6 @@
+from .model import ConditionalPointCloudDiffusionModel
+
+def get_model():
+ model = ConditionalPointCloudDiffusionModel()
+ return model
+
diff --git a/difpoint/model/__pycache__/__init__.cpython-310.pyc b/difpoint/model/__pycache__/__init__.cpython-310.pyc
new file mode 100644
index 0000000000000000000000000000000000000000..7dbda5909cb67d57d8206fef030c9293606790a3
Binary files /dev/null and b/difpoint/model/__pycache__/__init__.cpython-310.pyc differ
diff --git a/difpoint/model/__pycache__/__init__.cpython-38.pyc b/difpoint/model/__pycache__/__init__.cpython-38.pyc
new file mode 100644
index 0000000000000000000000000000000000000000..c81f6558d44a623b38e3e09e913bbe783858e353
Binary files /dev/null and b/difpoint/model/__pycache__/__init__.cpython-38.pyc differ
diff --git a/difpoint/model/__pycache__/model.cpython-310.pyc b/difpoint/model/__pycache__/model.cpython-310.pyc
new file mode 100644
index 0000000000000000000000000000000000000000..b046f521c800793280860ad61da55b8122f8c15d
Binary files /dev/null and b/difpoint/model/__pycache__/model.cpython-310.pyc differ
diff --git a/difpoint/model/__pycache__/model.cpython-38.pyc b/difpoint/model/__pycache__/model.cpython-38.pyc
new file mode 100644
index 0000000000000000000000000000000000000000..25fc0e253fdade44f9a2f29e5e0a3c275fb267e9
Binary files /dev/null and b/difpoint/model/__pycache__/model.cpython-38.pyc differ
diff --git a/difpoint/model/__pycache__/model_utils.cpython-310.pyc b/difpoint/model/__pycache__/model_utils.cpython-310.pyc
new file mode 100644
index 0000000000000000000000000000000000000000..9cda42568b20f1ff8f4734a610ecd76bc793cc7f
Binary files /dev/null and b/difpoint/model/__pycache__/model_utils.cpython-310.pyc differ
diff --git a/difpoint/model/__pycache__/model_utils.cpython-38.pyc b/difpoint/model/__pycache__/model_utils.cpython-38.pyc
new file mode 100644
index 0000000000000000000000000000000000000000..257d9542876a1f87547495b57037495c0111bdb1
Binary files /dev/null and b/difpoint/model/__pycache__/model_utils.cpython-38.pyc differ
diff --git a/difpoint/model/__pycache__/point_model.cpython-310.pyc b/difpoint/model/__pycache__/point_model.cpython-310.pyc
new file mode 100644
index 0000000000000000000000000000000000000000..36910474b80090dccd7048363b2bac4e6e8adfe7
Binary files /dev/null and b/difpoint/model/__pycache__/point_model.cpython-310.pyc differ
diff --git a/difpoint/model/__pycache__/point_model.cpython-38.pyc b/difpoint/model/__pycache__/point_model.cpython-38.pyc
new file mode 100644
index 0000000000000000000000000000000000000000..2290381110a1d59a0c2a2d2a0facb50d7eab1f45
Binary files /dev/null and b/difpoint/model/__pycache__/point_model.cpython-38.pyc differ
diff --git a/difpoint/model/model.py b/difpoint/model/model.py
new file mode 100644
index 0000000000000000000000000000000000000000..f87be4c9de12fd0eff5b75a4a67138ac5b275cbe
--- /dev/null
+++ b/difpoint/model/model.py
@@ -0,0 +1,409 @@
+import inspect
+from typing import Optional
+from einops import rearrange
+import torch
+import torch.nn.functional as F
+from diffusers.schedulers.scheduling_ddpm import DDPMScheduler
+from diffusers.schedulers.scheduling_ddim import DDIMScheduler
+from diffusers.schedulers.scheduling_pndm import PNDMScheduler
+
+from torch import Tensor
+from tqdm import tqdm
+from diffusers import ModelMixin
+from .model_utils import get_custom_betas
+from .point_model import PointModel
+
+import copy
+class ConditionalPointCloudDiffusionModel(ModelMixin):
+ def __init__(
+ self,
+ beta_start: float = 1e-5,
+ beta_end: float = 8e-3,
+ beta_schedule: str = 'linear',
+ point_cloud_model: str = 'simple',
+ point_cloud_model_embed_dim: int = 64,
+ ):
+ super().__init__()
+ self.in_channels = 70 # 3 for 3D point positions
+ self.out_channels = 70
+
+ # Checks
+ # Create diffusion model schedulers which define the sampling timesteps
+ scheduler_kwargs = {}
+ if beta_schedule == 'custom':
+ scheduler_kwargs.update(dict(trained_betas=get_custom_betas(beta_start=beta_start, beta_end=beta_end)))
+ else:
+ scheduler_kwargs.update(dict(beta_start=beta_start, beta_end=beta_end, beta_schedule=beta_schedule))
+ self.schedulers_map = {
+ 'ddpm': DDPMScheduler(**scheduler_kwargs, clip_sample=False),
+ 'ddim': DDIMScheduler(**scheduler_kwargs, clip_sample=False),
+ 'pndm': PNDMScheduler(**scheduler_kwargs),
+ }
+ self.scheduler = self.schedulers_map['ddim'] # this can be changed for inference
+
+ # Create point cloud model for processing point cloud at each diffusion step
+ self.point_model = PointModel(
+ model_type=point_cloud_model,
+ embed_dim=point_cloud_model_embed_dim,
+ in_channels=self.in_channels,
+ out_channels=self.out_channels,
+ )
+
+ def forward_train(
+ self,
+ pc: Optional[Tensor],
+ ref_kps: Optional[Tensor],
+ ori_kps: Optional[Tensor],
+ aud_feat: Optional[Tensor],
+ mode: str = 'train',
+ return_intermediate_steps: bool = False
+ ):
+
+ # Normalize colors and convert to tensor
+ x_0 = pc
+ B, Nf, Np, D = x_0.shape# batch, nums of frames, nums of points, 3
+
+
+ x_0=x_0[:,:,:,0]# batch, nums of frames, 70
+
+ # Sample random noise
+ noise = torch.randn_like(x_0)
+
+ # Sample random timesteps for each point_cloud
+ timestep = torch.randint(0, self.scheduler.num_train_timesteps, (B,),
+ device=self.device, dtype=torch.long)
+
+ # Add noise to points
+ x_t = self.scheduler.add_noise(x_0, noise, timestep)
+
+ # Conditioning
+ ref_kps = ref_kps[:, :, 0]
+
+ x_t_input = torch.cat([ori_kps.unsqueeze(1), ref_kps.unsqueeze(1), x_t], dim=1)
+
+ # x_t_input = torch.cat([ref_kps.unsqueeze(1), x_t], dim=1)
+
+ # ori_kps_repeat = torch.repeat_interleave(ori_kps.unsqueeze(1), repeats=Nf+1, dim=1)
+
+ # x_t_input = torch.cat([x_t_input, ori_kps_repeat], dim=-1) #B, 32+1, 51+45
+
+
+ aud_feat = torch.cat([torch.zeros(B, 2, 512).cuda(), aud_feat], 1)
+
+ # Augmentation for audio feature
+ if mode in 'train':
+ if torch.rand(1) > 0.3:
+ mean = torch.mean(aud_feat)
+ std = torch.std(aud_feat)
+ sample = torch.normal(mean=torch.full(aud_feat.shape, mean), std=torch.full(aud_feat.shape, std)).cuda()
+ aud_feat = sample + aud_feat
+ else:
+ pass
+ else:
+ pass
+
+ # Forward
+ noise_pred = self.point_model(x_t_input, timestep, context=aud_feat) #torch.cat([mel_feat,style_embed],-1))
+ noise_pred = noise_pred[:, 2:]
+ #
+ # Check
+ if not noise_pred.shape == noise.shape:
+ raise ValueError(f'{noise_pred.shape=} and {noise.shape=}')
+
+ # Loss
+ loss = F.mse_loss(noise_pred, noise)
+
+ loss_pose = F.mse_loss(noise_pred[:, :, :6], noise[:, :, :6])
+ loss_exp = F.mse_loss(noise_pred[:, :, 6:], noise[:, :, 6:])
+
+
+ # Whether to return intermediate steps
+ if return_intermediate_steps:
+ return loss, (x_0, x_t, noise, noise_pred)
+
+ return loss, loss_exp, loss_pose
+
+ # def forward_train(
+ # self,
+ # pc: Optional[Tensor],
+ # ref_kps: Optional[Tensor],
+ # ori_kps: Optional[Tensor],
+ # aud_feat: Optional[Tensor],
+ # mode: str = 'train',
+ # return_intermediate_steps: bool = False
+ # ):
+ #
+ # # Normalize colors and convert to tensor
+ # x_0 = pc
+ # B, Nf, Np, D = x_0.shape# batch, nums of frames, nums of points, 3
+ #
+ # # ori_kps = torch.repeat_interleave(ori_kps.unsqueeze(1), Nf, dim=1) # B, Nf, 45
+ # #
+ # # ref_kps = ref_kps[:, :, 0]
+ # # ref_kps = torch.repeat_interleave(ref_kps.unsqueeze(1), Nf, dim=1) # B, Nf, 91
+ #
+ # x_0 = x_0[:,:,:,0]
+ #
+ # # Sample random noise
+ # noise = torch.randn_like(x_0)
+ #
+ # # Sample random timesteps for each point_cloud
+ # timestep = torch.randint(0, self.scheduler.num_train_timesteps, (B,),
+ # device=self.device, dtype=torch.long)
+ #
+ # # Add noise to points
+ # x_t = self.scheduler.add_noise(x_0, noise, timestep)
+ #
+ # # Conditioning
+ # ref_kps = ref_kps[:,:,0]
+ #
+ # # x_t_input = torch.cat([ref_kps.unsqueeze(1), x_t], dim=1)
+ #
+ # # x_0 = torch.cat([x_0, ref_kps, ori_kps], dim=2) # B, Nf, 91+91+45
+ #
+ # x_t_input = torch.cat([ref_kps.unsqueeze(1), x_t], dim=1)
+ # # x_t_input = torch.cat([ori_kps.unsqueeze(1), ref_kps.unsqueeze(1), x_t], dim=1)
+ #
+ # aud_feat = torch.cat([torch.zeros(B, 1, 512).cuda(), aud_feat], 1)
+ #
+ # # Augmentation for audio feature
+ # if mode in 'train':
+ # if torch.rand(1) > 0.3:
+ # mean = torch.mean(aud_feat)
+ # std = torch.std(aud_feat)
+ # sample = torch.normal(mean=torch.full(aud_feat.shape, mean), std=torch.full(aud_feat.shape, std)).cuda()
+ # aud_feat = sample + aud_feat
+ # else:
+ # pass
+ # else:
+ # pass
+ #
+ # # Forward
+ # noise_pred = self.point_model(x_t_input, timestep, context=aud_feat)
+ # noise_pred = noise_pred[:, 1:]
+ #
+ # # Check
+ # # if not noise_pred.shape == noise.shape:
+ # # raise ValueError(f'{noise_pred.shape=} and {noise.shape=}')
+ #
+ # # Loss
+ # loss = F.mse_loss(noise_pred, noise)
+ #
+ # # loss_kp = F.mse_loss(noise_pred[:, :, :45], noise[:, :, :45])
+ #
+ # # Whether to return intermediate steps
+ # if return_intermediate_steps:
+ # return loss, (x_0, x_t, noise, noise_pred)
+ #
+ # return loss
+
+ # @torch.no_grad()
+ # def forward_sample(
+ # self,
+ # num_points: int,
+ # ref_kps: Optional[Tensor],
+ # ori_kps: Optional[Tensor],
+ # aud_feat: Optional[Tensor],
+ # # Optional overrides
+ # scheduler: Optional[str] = 'ddpm',
+ # # Inference parameters
+ # num_inference_steps: Optional[int] = 1000,
+ # eta: Optional[float] = 0.0, # for DDIM
+ # # Whether to return all the intermediate steps in generation
+ # return_sample_every_n_steps: int = -1,
+ # # Whether to disable tqdm
+ # disable_tqdm: bool = False,
+ # ):
+ #
+ # # Get scheduler from mapping, or use self.scheduler if None
+ # scheduler = self.scheduler if scheduler is None else self.schedulers_map[scheduler]
+ #
+ # # Get the size of the noise
+ # Np = num_points
+ # Nf = aud_feat.size(1)
+ # B = 1
+ # D = 3
+ # device = self.device
+ #
+ # # Sample noise
+ # x_t = torch.randn(B, Nf, Np, D, device=device)
+ #
+ # x_t = x_t[:, :, :, 0]
+ #
+ # # ori_kps = torch.repeat_interleave(ori_kps.unsqueeze(1), Nf, dim=1) # B, Nf, 45
+ #
+ # ref_kps = ref_kps[:, :, 0]
+ # # ref_kps = torch.repeat_interleave(ref_kps.unsqueeze(1), Nf, dim=1) # B, Nf, 91
+ #
+ # # Set timesteps
+ # accepts_offset = "offset" in set(inspect.signature(scheduler.set_timesteps).parameters.keys())
+ # extra_set_kwargs = {"offset": 1} if accepts_offset else {}
+ # scheduler.set_timesteps(num_inference_steps, **extra_set_kwargs)
+ #
+ # # Prepare extra kwargs for the scheduler step, since not all schedulers have the same signature
+ # # eta (η) is only used with the DDIMScheduler, it will be ignored for other schedulers.
+ # # eta corresponds to η in DDIM paper: https://arxiv.org/abs/2010.02502
+ # # and should be between [0, 1]
+ # accepts_eta = "eta" in set(inspect.signature(scheduler.step).parameters.keys())
+ # extra_step_kwargs = {"eta": eta} if accepts_eta else {}
+ #
+ # # Loop over timesteps
+ # all_outputs = []
+ # return_all_outputs = (return_sample_every_n_steps > 0)
+ # progress_bar = tqdm(scheduler.timesteps.to(device), desc=f'Sampling ({x_t.shape})', disable=disable_tqdm)
+ #
+ # # ori_kps = torch.repeat_interleave(ori_kps[:, 6:].unsqueeze(1), Nf + 1, dim=1)
+ # aud_feat = torch.cat([torch.zeros(B, 1, 512).cuda(), aud_feat], 1)
+ # # aud_feat = torch.cat([ori_kps, aud_feat], -1)
+ #
+ # # aud_feat = torch.cat([torch.zeros(B, 2, 512).cuda(), aud_feat], 1)
+ #
+ # for i, t in enumerate(progress_bar):
+ #
+ # # Conditioning
+ # x_t_input = torch.cat([ref_kps.unsqueeze(1).detach(), x_t], dim=1)
+ # # x_t_input = torch.cat([ori_kps.unsqueeze(1).detach(), ref_kps.unsqueeze(1).detach(), x_t], dim=1)
+ # # x_t_input = torch.cat([x_t, ref_kps, ori_kps], dim=2) # B, Nf, 91+91+45
+ #
+ # # Forward
+ # # noise_pred = self.point_model(x_t_input, t.reshape(1).expand(B), context=aud_feat)[:, 1:]
+ # noise_pred = self.point_model(x_t_input, t.reshape(1).expand(B), context=aud_feat)[:, 1:]
+ #
+ # # noise_pred = noise_pred[:, :, :51]
+ #
+ # # Step
+ # # x_t = x_t[:, :, :51]
+ # x_t = scheduler.step(noise_pred, t, x_t, **extra_step_kwargs).prev_sample
+ #
+ # # Append to output list if desired
+ # if (return_all_outputs and (i % return_sample_every_n_steps == 0 or i == len(scheduler.timesteps) - 1)):
+ # all_outputs.append(x_t)
+ #
+ # # Convert output back into a point cloud, undoing normalization and scaling
+ # output = x_t
+ # output = torch.stack([output, output, output], -1)
+ # if return_all_outputs:
+ # all_outputs = torch.stack(all_outputs, dim=1) # (B, sample_steps, N, D)
+ # return (output, all_outputs) if return_all_outputs else output
+
+
+ @torch.no_grad()
+ def forward_sample(
+ self,
+ num_points: int,
+ ref_kps: Optional[Tensor],
+ ori_kps: Optional[Tensor],
+ aud_feat: Optional[Tensor],
+ # Optional overrides
+ scheduler: Optional[str] = 'ddpm',
+ # Inference parameters
+ num_inference_steps: Optional[int] = 1000,
+ eta: Optional[float] = 0.0, # for DDIM
+ # Whether to return all the intermediate steps in generation
+ return_sample_every_n_steps: int = -1,
+ # Whether to disable tqdm
+ disable_tqdm: bool = False,
+ ):
+
+ # Get scheduler from mapping, or use self.scheduler if None
+ scheduler = self.scheduler if scheduler is None else self.schedulers_map[scheduler]
+
+ # Get the size of the noise
+ Np = num_points
+ Nf = aud_feat.size(1)
+ B = 1
+ D = 3
+ device = self.device
+
+ # Sample noise
+ x_t = torch.randn(B, Nf, Np, D, device=device)
+
+ x_t = x_t[:, :, :, 0]
+
+ ref_kps = ref_kps[:,:,0]
+
+ # Set timesteps
+ accepts_offset = "offset" in set(inspect.signature(scheduler.set_timesteps).parameters.keys())
+ extra_set_kwargs = {"offset": 1} if accepts_offset else {}
+ scheduler.set_timesteps(num_inference_steps, **extra_set_kwargs)
+
+ # Prepare extra kwargs for the scheduler step, since not all schedulers have the same signature
+ # eta (η) is only used with the DDIMScheduler, it will be ignored for other schedulers.
+ # eta corresponds to η in DDIM paper: https://arxiv.org/abs/2010.02502
+ # and should be between [0, 1]
+ accepts_eta = "eta" in set(inspect.signature(scheduler.step).parameters.keys())
+ extra_step_kwargs = {"eta": eta} if accepts_eta else {}
+
+ # Loop over timesteps
+ all_outputs = []
+ return_all_outputs = (return_sample_every_n_steps > 0)
+ progress_bar = tqdm(scheduler.timesteps.to(device), desc=f'Sampling ({x_t.shape})', disable=disable_tqdm)
+
+ # ori_kps = torch.repeat_interleave(ori_kps[:, 6:].unsqueeze(1), Nf + 1, dim=1)
+ # aud_feat = torch.cat([torch.zeros(B, 1, 512).cuda(), aud_feat], 1)
+ # aud_feat = torch.cat([ori_kps, aud_feat], -1)
+
+ aud_feat = torch.cat([torch.zeros(B, 2, 512).cuda(), aud_feat], 1)
+
+ for i, t in enumerate(progress_bar):
+
+ # Conditioning
+ # x_t_input = torch.cat([ref_kps.unsqueeze(1), x_t], dim=1)
+ #
+ # ori_kps_repeat = torch.repeat_interleave(ori_kps.unsqueeze(1), repeats=Nf + 1, dim=1)
+ #
+ # x_t_input = torch.cat([x_t_input.detach(), ori_kps_repeat.detach()], dim=-1) # B, 32+1, 51+45
+
+
+ x_t_input = torch.cat([ori_kps.unsqueeze(1).detach(),ref_kps.unsqueeze(1).detach(), x_t], dim=1)
+ # x_t_input = torch.cat([ref_kps.unsqueeze(1).detach(), x_t], dim=1)
+
+
+ # Forward
+ # noise_pred = self.point_model(x_t_input, t.reshape(1).expand(B), context=aud_feat)[:, 1:]
+ noise_pred = self.point_model(x_t_input, t.reshape(1).expand(B), context=aud_feat)[:, 2:]
+
+
+ # Step
+ x_t = scheduler.step(noise_pred, t, x_t, **extra_step_kwargs).prev_sample
+
+ # Append to output list if desired
+ if (return_all_outputs and (i % return_sample_every_n_steps == 0 or i == len(scheduler.timesteps) - 1)):
+ all_outputs.append(x_t)
+
+ # Convert output back into a point cloud, undoing normalization and scaling
+ output = x_t
+ output = torch.stack([output,output,output],-1)
+ if return_all_outputs:
+ all_outputs = torch.stack(all_outputs, dim=1) # (B, sample_steps, N, D)
+ return (output, all_outputs) if return_all_outputs else output
+
+ def forward(self, batch: dict, mode: str = 'train', **kwargs):
+ """A wrapper around the forward method for training and inference"""
+
+ if mode == 'train':
+ return self.forward_train(
+ pc=batch['sequence_keypoints'],
+ ref_kps=batch['ref_keypoint'],
+ ori_kps=batch['ori_keypoint'],
+ aud_feat=batch['aud_feat'],
+ mode='train',
+ **kwargs)
+ elif mode == 'val':
+ return self.forward_train(
+ pc=batch['sequence_keypoints'],
+ ref_kps=batch['ref_keypoint'],
+ ori_kps=batch['ori_keypoint'],
+ aud_feat=batch['aud_feat'],
+ mode='val',
+ **kwargs)
+ elif mode == 'sample':
+ num_points = 68
+ return self.forward_sample(
+ num_points=num_points,
+ ref_kps=batch['ref_keypoint'],
+ ori_kps=batch['ori_keypoint'],
+ aud_feat=batch['aud_feat'],
+ **kwargs)
+ else:
+ raise NotImplementedError()
\ No newline at end of file
diff --git a/difpoint/model/model_utils.py b/difpoint/model/model_utils.py
new file mode 100644
index 0000000000000000000000000000000000000000..8152fe2cd4082c696cd982e4b0fc6c44aa6d9065
--- /dev/null
+++ b/difpoint/model/model_utils.py
@@ -0,0 +1,35 @@
+import cv2
+import numpy as np
+import torch
+import torch.nn as nn
+
+
+
+def set_requires_grad(module: nn.Module, requires_grad: bool):
+ for p in module.parameters():
+ p.requires_grad_(requires_grad)
+
+
+def compute_distance_transform(mask: torch.Tensor):
+ image_size = mask.shape[-1]
+ distance_transform = torch.stack([
+ torch.from_numpy(cv2.distanceTransform(
+ (1 - m), distanceType=cv2.DIST_L2, maskSize=cv2.DIST_MASK_3
+ ) / (image_size / 2))
+ for m in mask.squeeze(1).detach().cpu().numpy().astype(np.uint8)
+ ]).unsqueeze(1).clip(0, 1).to(mask.device)
+ return distance_transform
+
+
+def default(x, d):
+ return d if x is None else x
+
+def get_custom_betas(beta_start: float, beta_end: float, warmup_frac: float = 0.3, num_train_timesteps: int = 1000):
+ """Custom beta schedule"""
+ betas = np.linspace(beta_start, beta_end, num_train_timesteps, dtype=np.float32)
+ warmup_frac = 0.3
+ warmup_time = int(num_train_timesteps * warmup_frac)
+ warmup_steps = np.linspace(beta_start, beta_end, warmup_time, dtype=np.float64)
+ warmup_time = min(warmup_time, num_train_timesteps)
+ betas[:warmup_time] = warmup_steps[:warmup_time]
+ return betas
diff --git a/difpoint/model/point_model.py b/difpoint/model/point_model.py
new file mode 100644
index 0000000000000000000000000000000000000000..f7156a6be3367d125c4a39d6af621df93e879717
--- /dev/null
+++ b/difpoint/model/point_model.py
@@ -0,0 +1,38 @@
+import torch
+from diffusers.configuration_utils import ConfigMixin, register_to_config
+from diffusers import ModelMixin
+from torch import Tensor
+
+from .temporaltrans.temptrans import SimpleTemperalPointModel, SimpleTransModel
+
+class PointModel(ModelMixin, ConfigMixin):
+ @register_to_config
+ def __init__(
+ self,
+ model_type: str = 'pvcnn',
+ in_channels: int = 3,
+ out_channels: int = 3,
+ embed_dim: int = 64,
+ dropout: float = 0.1,
+ width_multiplier: int = 1,
+ voxel_resolution_multiplier: int = 1,
+ ):
+ super().__init__()
+ self.model_type = model_type
+ if self.model_type == 'simple':
+ self.autocast_context = torch.autocast('cuda', dtype=torch.float32)
+ self.model = SimpleTransModel(
+ embed_dim=embed_dim,
+ num_classes=out_channels,
+ extra_feature_channels=(in_channels - 3),
+ )
+ self.model.output_projection.bias.data.normal_(0, 1e-6)
+ self.model.output_projection.weight.data.normal_(0, 1e-6)
+ else:
+ raise NotImplementedError()
+
+ def forward(self, inputs: Tensor, t: Tensor, context=None) -> Tensor:
+ """ Receives input of shape (B, N, in_channels) and returns output
+ of shape (B, N, out_channels) """
+ with self.autocast_context:
+ return self.model(inputs, t, context)
diff --git a/difpoint/model/temporaltrans/__pycache__/temptrans.cpython-310.pyc b/difpoint/model/temporaltrans/__pycache__/temptrans.cpython-310.pyc
new file mode 100644
index 0000000000000000000000000000000000000000..5ce9589f67ec68a9a2ddd92f10666f7698a3c77f
Binary files /dev/null and b/difpoint/model/temporaltrans/__pycache__/temptrans.cpython-310.pyc differ
diff --git a/difpoint/model/temporaltrans/__pycache__/temptrans.cpython-38.pyc b/difpoint/model/temporaltrans/__pycache__/temptrans.cpython-38.pyc
new file mode 100644
index 0000000000000000000000000000000000000000..2709048a036d7b48eb4320a82843effe5cec860f
Binary files /dev/null and b/difpoint/model/temporaltrans/__pycache__/temptrans.cpython-38.pyc differ
diff --git a/difpoint/model/temporaltrans/__pycache__/transformer_utils.cpython-310.pyc b/difpoint/model/temporaltrans/__pycache__/transformer_utils.cpython-310.pyc
new file mode 100644
index 0000000000000000000000000000000000000000..2d0c2c4e55ab75f2bba8eae62cf432867020f3b6
Binary files /dev/null and b/difpoint/model/temporaltrans/__pycache__/transformer_utils.cpython-310.pyc differ
diff --git a/difpoint/model/temporaltrans/__pycache__/transformer_utils.cpython-38.pyc b/difpoint/model/temporaltrans/__pycache__/transformer_utils.cpython-38.pyc
new file mode 100644
index 0000000000000000000000000000000000000000..5b3d5ac5b5004b4b79dd9b9912e6be0d2c1ea426
Binary files /dev/null and b/difpoint/model/temporaltrans/__pycache__/transformer_utils.cpython-38.pyc differ
diff --git a/difpoint/model/temporaltrans/pointnet_util.py b/difpoint/model/temporaltrans/pointnet_util.py
new file mode 100644
index 0000000000000000000000000000000000000000..8e042a973cf968540bec6f73fed09503617db819
--- /dev/null
+++ b/difpoint/model/temporaltrans/pointnet_util.py
@@ -0,0 +1,311 @@
+import torch
+import torch.nn as nn
+import torch.nn.functional as F
+from time import time
+import numpy as np
+
+
+# reference https://github.com/yanx27/Pointnet_Pointnet2_pytorch, modified by Yang You
+
+
+def timeit(tag, t):
+ print("{}: {}s".format(tag, time() - t))
+ return time()
+
+def pc_normalize(pc):
+ centroid = np.mean(pc, axis=0)
+ pc = pc - centroid
+ m = np.max(np.sqrt(np.sum(pc**2, axis=1)))
+ pc = pc / m
+ return pc
+
+def square_distance(src, dst):
+ """
+ Calculate Euclid distance between each two points.
+ src^T * dst = xn * xm + yn * ym + zn * zm;
+ sum(src^2, dim=-1) = xn*xn + yn*yn + zn*zn;
+ sum(dst^2, dim=-1) = xm*xm + ym*ym + zm*zm;
+ dist = (xn - xm)^2 + (yn - ym)^2 + (zn - zm)^2
+ = sum(src**2,dim=-1)+sum(dst**2,dim=-1)-2*src^T*dst
+ Input:
+ src: source points, [B, N, C]
+ dst: target points, [B, M, C]
+ Output:
+ dist: per-point square distance, [B, N, M]
+ """
+ return torch.sum((src[:, :, None] - dst[:, None]) ** 2, dim=-1)
+
+
+def index_points(points, idx):
+ """
+ Input:
+ points: input points data, [B, N, C]
+ idx: sample index data, [B, S, [K]]
+ Return:
+ new_points:, indexed points data, [B, S, [K], C]
+ """
+ raw_size = idx.size()
+ idx = idx.reshape(raw_size[0], -1)
+ res = torch.gather(points, 1, idx[..., None].expand(-1, -1, points.size(-1)))
+ return res.reshape(*raw_size, -1)
+
+
+def farthest_point_sample(xyz, npoint):
+ """
+ Input:
+ xyz: pointcloud data, [B, N, 3]
+ npoint: number of samples
+ Return:
+ centroids: sampled pointcloud index, [B, npoint]
+ """
+ device = xyz.device
+ B, N, C = xyz.shape
+ centroids = torch.zeros(B, npoint, dtype=torch.long).to(device)
+ distance = torch.ones(B, N).to(device) * 1e10
+ farthest = torch.randint(0, N, (B,), dtype=torch.long).to(device)
+ batch_indices = torch.arange(B, dtype=torch.long).to(device)
+ for i in range(npoint):
+ centroids[:, i] = farthest
+ centroid = xyz[batch_indices, farthest, :].view(B, 1, 3)
+ dist = torch.sum((xyz - centroid) ** 2, -1)
+ distance = torch.min(distance, dist)
+ farthest = torch.max(distance, -1)[1]
+ return centroids
+
+
+def query_ball_point(radius, nsample, xyz, new_xyz):
+ """
+ Input:
+ radius: local region radius
+ nsample: max sample number in local region
+ xyz: all points, [B, N, 3]
+ new_xyz: query points, [B, S, 3]
+ Return:
+ group_idx: grouped points index, [B, S, nsample]
+ """
+ device = xyz.device
+ B, N, C = xyz.shape
+ _, S, _ = new_xyz.shape
+ group_idx = torch.arange(N, dtype=torch.long).to(device).view(1, 1, N).repeat([B, S, 1])
+ sqrdists = square_distance(new_xyz, xyz)
+ group_idx[sqrdists > radius ** 2] = N
+ group_idx = group_idx.sort(dim=-1)[0][:, :, :nsample]
+ group_first = group_idx[:, :, 0].view(B, S, 1).repeat([1, 1, nsample])
+ mask = group_idx == N
+ group_idx[mask] = group_first[mask]
+ return group_idx
+
+
+def sample_and_group(npoint, radius, nsample, xyz, points, returnfps=False, knn=False):
+ """
+ Input:
+ npoint:
+ radius:
+ nsample:
+ xyz: input points position data, [B, N, 3]
+ points: input points data, [B, N, D]
+ Return:
+ new_xyz: sampled points position data, [B, npoint, nsample, 3]
+ new_points: sampled points data, [B, npoint, nsample, 3+D]
+ """
+ B, N, C = xyz.shape
+ S = npoint
+ fps_idx = farthest_point_sample(xyz, npoint) # [B, npoint]
+ torch.cuda.empty_cache()
+ new_xyz = index_points(xyz, fps_idx)
+ torch.cuda.empty_cache()
+ if knn:
+ dists = square_distance(new_xyz, xyz) # B x npoint x N
+ idx = dists.argsort()[:, :, :nsample] # B x npoint x K
+ else:
+ idx = query_ball_point(radius, nsample, xyz, new_xyz)
+ torch.cuda.empty_cache()
+ grouped_xyz = index_points(xyz, idx) # [B, npoint, nsample, C]
+ torch.cuda.empty_cache()
+ grouped_xyz_norm = grouped_xyz - new_xyz.view(B, S, 1, C)
+ torch.cuda.empty_cache()
+
+ if points is not None:
+ grouped_points = index_points(points, idx)
+ new_points = torch.cat([grouped_xyz_norm, grouped_points], dim=-1) # [B, npoint, nsample, C+D]
+ else:
+ new_points = grouped_xyz_norm
+ if returnfps:
+ return new_xyz, new_points, grouped_xyz, fps_idx
+ else:
+ return new_xyz, new_points
+
+
+def sample_and_group_all(xyz, points):
+ """
+ Input:
+ xyz: input points position data, [B, N, 3]
+ points: input points data, [B, N, D]
+ Return:
+ new_xyz: sampled points position data, [B, 1, 3]
+ new_points: sampled points data, [B, 1, N, 3+D]
+ """
+ device = xyz.device
+ B, N, C = xyz.shape
+ new_xyz = torch.zeros(B, 1, C).to(device)
+ grouped_xyz = xyz.view(B, 1, N, C)
+ if points is not None:
+ new_points = torch.cat([grouped_xyz, points.view(B, 1, N, -1)], dim=-1)
+ else:
+ new_points = grouped_xyz
+ return new_xyz, new_points
+
+
+class PointNetSetAbstraction(nn.Module):
+ def __init__(self, npoint, radius, nsample, in_channel, mlp, group_all, knn=False):
+ super(PointNetSetAbstraction, self).__init__()
+ self.npoint = npoint
+ self.radius = radius
+ self.nsample = nsample
+ self.knn = knn
+ self.mlp_convs = nn.ModuleList()
+ self.mlp_bns = nn.ModuleList()
+ last_channel = in_channel
+ for out_channel in mlp:
+ self.mlp_convs.append(nn.Conv2d(last_channel, out_channel, 1))
+ self.mlp_bns.append(nn.BatchNorm2d(out_channel))
+ last_channel = out_channel
+ self.group_all = group_all
+
+ def forward(self, xyz, points):
+ """
+ Input:
+ xyz: input points position data, [B, N, C]
+ points: input points data, [B, N, C]
+ Return:
+ new_xyz: sampled points position data, [B, S, C]
+ new_points_concat: sample points feature data, [B, S, D']
+ """
+ if self.group_all:
+ new_xyz, new_points = sample_and_group_all(xyz, points)
+ else:
+ new_xyz, new_points = sample_and_group(self.npoint, self.radius, self.nsample, xyz, points, knn=self.knn)
+ # new_xyz: sampled points position data, [B, npoint, C]
+ # new_points: sampled points data, [B, npoint, nsample, C+D]
+ new_points = new_points.permute(0, 3, 2, 1) # [B, C+D, nsample,npoint]
+ for i, conv in enumerate(self.mlp_convs):
+ bn = self.mlp_bns[i]
+ new_points = F.relu(bn(conv(new_points)))
+
+ new_points = torch.max(new_points, 2)[0].transpose(1, 2)
+ return new_xyz, new_points
+
+
+class PointNetSetAbstractionMsg(nn.Module):
+ def __init__(self, npoint, radius_list, nsample_list, in_channel, mlp_list, knn=False):
+ super(PointNetSetAbstractionMsg, self).__init__()
+ self.npoint = npoint
+ self.radius_list = radius_list
+ self.nsample_list = nsample_list
+ self.knn = knn
+ self.conv_blocks = nn.ModuleList()
+ self.bn_blocks = nn.ModuleList()
+ for i in range(len(mlp_list)):
+ convs = nn.ModuleList()
+ bns = nn.ModuleList()
+ last_channel = in_channel + 3
+ for out_channel in mlp_list[i]:
+ convs.append(nn.Conv2d(last_channel, out_channel, 1))
+ bns.append(nn.BatchNorm2d(out_channel))
+ last_channel = out_channel
+ self.conv_blocks.append(convs)
+ self.bn_blocks.append(bns)
+
+ def forward(self, xyz, points, seed_idx=None):
+ """
+ Input:
+ xyz: input points position data, [B, C, N]
+ points: input points data, [B, D, N]
+ Return:
+ new_xyz: sampled points position data, [B, C, S]
+ new_points_concat: sample points feature data, [B, D', S]
+ """
+
+ B, N, C = xyz.shape
+ S = self.npoint
+ new_xyz = index_points(xyz, farthest_point_sample(xyz, S) if seed_idx is None else seed_idx)
+ new_points_list = []
+ for i, radius in enumerate(self.radius_list):
+ K = self.nsample_list[i]
+ if self.knn:
+ dists = square_distance(new_xyz, xyz) # B x npoint x N
+ group_idx = dists.argsort()[:, :, :K] # B x npoint x K
+ else:
+ group_idx = query_ball_point(radius, K, xyz, new_xyz)
+ grouped_xyz = index_points(xyz, group_idx)
+ grouped_xyz -= new_xyz.view(B, S, 1, C)
+ if points is not None:
+ grouped_points = index_points(points, group_idx)
+ grouped_points = torch.cat([grouped_points, grouped_xyz], dim=-1)
+ else:
+ grouped_points = grouped_xyz
+
+ grouped_points = grouped_points.permute(0, 3, 2, 1) # [B, D, K, S]
+ for j in range(len(self.conv_blocks[i])):
+ conv = self.conv_blocks[i][j]
+ bn = self.bn_blocks[i][j]
+ grouped_points = F.relu(bn(conv(grouped_points)))
+ new_points = torch.max(grouped_points, 2)[0] # [B, D', S]
+ new_points_list.append(new_points)
+
+ new_points_concat = torch.cat(new_points_list, dim=1).transpose(1, 2)
+ return new_xyz, new_points_concat
+
+
+# NoteL this function swaps N and C
+class PointNetFeaturePropagation(nn.Module):
+ def __init__(self, in_channel, mlp):
+ super(PointNetFeaturePropagation, self).__init__()
+ self.mlp_convs = nn.ModuleList()
+ self.mlp_bns = nn.ModuleList()
+ last_channel = in_channel
+ for out_channel in mlp:
+ self.mlp_convs.append(nn.Conv1d(last_channel, out_channel, 1))
+ self.mlp_bns.append(nn.BatchNorm1d(out_channel))
+ last_channel = out_channel
+
+ def forward(self, xyz1, xyz2, points1, points2):
+ """
+ Input:
+ xyz1: input points position data, [B, C, N]
+ xyz2: sampled input points position data, [B, C, S]
+ points1: input points data, [B, D, N]
+ points2: input points data, [B, D, S]
+ Return:
+ new_points: upsampled points data, [B, D', N]
+ """
+ xyz1 = xyz1.permute(0, 2, 1)
+ xyz2 = xyz2.permute(0, 2, 1)
+
+ points2 = points2.permute(0, 2, 1)
+ B, N, C = xyz1.shape
+ _, S, _ = xyz2.shape
+
+ if S == 1:
+ interpolated_points = points2.repeat(1, N, 1)
+ else:
+ dists = square_distance(xyz1, xyz2)
+ dists, idx = dists.sort(dim=-1)
+ dists, idx = dists[:, :, :3], idx[:, :, :3] # [B, N, 3]
+
+ dist_recip = 1.0 / (dists + 1e-8)
+ norm = torch.sum(dist_recip, dim=2, keepdim=True)
+ weight = dist_recip / norm
+ interpolated_points = torch.sum(index_points(points2, idx) * weight.view(B, N, 3, 1), dim=2)
+
+ if points1 is not None:
+ points1 = points1.permute(0, 2, 1)
+ new_points = torch.cat([points1, interpolated_points], dim=-1)
+ else:
+ new_points = interpolated_points
+
+ new_points = new_points.permute(0, 2, 1)
+ for i, conv in enumerate(self.mlp_convs):
+ bn = self.mlp_bns[i]
+ new_points = F.relu(bn(conv(new_points)))
+ return new_points
\ No newline at end of file
diff --git a/difpoint/model/temporaltrans/pointtransformerv2.py b/difpoint/model/temporaltrans/pointtransformerv2.py
new file mode 100644
index 0000000000000000000000000000000000000000..ce0b37260f23740aba6be722291e260b33bc679f
--- /dev/null
+++ b/difpoint/model/temporaltrans/pointtransformerv2.py
@@ -0,0 +1,250 @@
+from .transformer_utils import BaseTemperalPointModel
+from copy import deepcopy
+import torch
+import einops
+import torch.nn as nn
+import torch.nn.functional as F
+from torch import nn
+from einops import rearrange
+import pointops
+from pointcept.models.utils import offset2batch, batch2offset
+class PointBatchNorm(nn.Module):
+ """
+ Batch Normalization for Point Clouds data in shape of [B*N, C], [B*N, L, C]
+ """
+
+ def __init__(self, embed_channels):
+ super().__init__()
+ self.norm = nn.BatchNorm1d(embed_channels)
+
+ def forward(self, input: torch.Tensor) -> torch.Tensor:
+ if input.dim() == 3:
+ return (
+ self.norm(input.transpose(1, 2).contiguous())
+ .transpose(1, 2)
+ .contiguous()
+ )
+ elif input.dim() == 2:
+ return self.norm(input)
+ else:
+ raise NotImplementedError
+#https://github.com/Pointcept/Pointcept/blob/main/pointcept/models/point_transformer_v2/point_transformer_v2m2_base.py
+class GroupedVectorAttention(nn.Module):
+ def __init__(
+ self,
+ embed_channels,
+ groups,
+ attn_drop_rate=0.0,
+ qkv_bias=True,
+ pe_multiplier=False,
+ pe_bias=True,
+ ):
+ super(GroupedVectorAttention, self).__init__()
+ self.embed_channels = embed_channels
+ self.groups = groups
+ assert embed_channels % groups == 0
+ self.attn_drop_rate = attn_drop_rate
+ self.qkv_bias = qkv_bias
+ self.pe_multiplier = pe_multiplier
+ self.pe_bias = pe_bias
+
+ self.linear_q = nn.Sequential(
+ nn.Linear(embed_channels, embed_channels, bias=qkv_bias),
+ PointBatchNorm(embed_channels),
+ nn.ReLU(inplace=True),
+ )
+ self.linear_k = nn.Sequential(
+ nn.Linear(embed_channels, embed_channels, bias=qkv_bias),
+ PointBatchNorm(embed_channels),
+ nn.ReLU(inplace=True),
+ )
+
+ self.linear_v = nn.Linear(embed_channels, embed_channels, bias=qkv_bias)
+
+ if self.pe_multiplier:
+ self.linear_p_multiplier = nn.Sequential(
+ nn.Linear(3, embed_channels),
+ PointBatchNorm(embed_channels),
+ nn.ReLU(inplace=True),
+ nn.Linear(embed_channels, embed_channels),
+ )
+ if self.pe_bias:
+ self.linear_p_bias = nn.Sequential(
+ nn.Linear(3, embed_channels),
+ PointBatchNorm(embed_channels),
+ nn.ReLU(inplace=True),
+ nn.Linear(embed_channels, embed_channels),
+ )
+ self.weight_encoding = nn.Sequential(
+ nn.Linear(embed_channels, groups),
+ PointBatchNorm(groups),
+ nn.ReLU(inplace=True),
+ nn.Linear(groups, groups),
+ )
+ self.softmax = nn.Softmax(dim=1)
+ self.attn_drop = nn.Dropout(attn_drop_rate)
+
+ def forward(self, feat, coord, reference_index):
+ query, key, value = (
+ self.linear_q(feat),
+ self.linear_k(feat),
+ self.linear_v(feat),
+ )
+ key = pointops.grouping(reference_index, key, coord, with_xyz=True)
+ value = pointops.grouping(reference_index, value, coord, with_xyz=False)
+ pos, key = key[:, :, 0:3], key[:, :, 3:]
+ relation_qk = key - query.unsqueeze(1)
+ if self.pe_multiplier:
+ pem = self.linear_p_multiplier(pos)
+ relation_qk = relation_qk * pem
+ if self.pe_bias:
+ peb = self.linear_p_bias(pos)
+ relation_qk = relation_qk + peb
+ value = value + peb
+
+ weight = self.weight_encoding(relation_qk)
+ weight = self.attn_drop(self.softmax(weight))
+
+ mask = torch.sign(reference_index + 1)
+ weight = torch.einsum("n s g, n s -> n s g", weight, mask)
+ value = einops.rearrange(value, "n ns (g i) -> n ns g i", g=self.groups)
+ feat = torch.einsum("n s g i, n s g -> n g i", value, weight)
+ feat = einops.rearrange(feat, "n g i -> n (g i)")
+ return feat
+
+class BlockSequence(nn.Module):
+ def __init__(
+ self,
+ depth,
+ embed_channels,
+ groups,
+ neighbours=16,
+ qkv_bias=True,
+ pe_multiplier=False,
+ pe_bias=True,
+ attn_drop_rate=0.0,
+ drop_path_rate=0.0,
+ enable_checkpoint=False,
+ ):
+ super(BlockSequence, self).__init__()
+
+ if isinstance(drop_path_rate, list):
+ drop_path_rates = drop_path_rate
+ assert len(drop_path_rates) == depth
+ elif isinstance(drop_path_rate, float):
+ drop_path_rates = [deepcopy(drop_path_rate) for _ in range(depth)]
+ else:
+ drop_path_rates = [0.0 for _ in range(depth)]
+
+ self.neighbours = neighbours
+ self.blocks = nn.ModuleList()
+ for i in range(depth):
+ block = Block(
+ embed_channels=embed_channels,
+ groups=groups,
+ qkv_bias=qkv_bias,
+ pe_multiplier=pe_multiplier,
+ pe_bias=pe_bias,
+ attn_drop_rate=attn_drop_rate,
+ drop_path_rate=drop_path_rates[i],
+ enable_checkpoint=enable_checkpoint,
+ )
+ self.blocks.append(block)
+
+ def forward(self, points):
+ coord, feat, offset = points
+ # reference index query of neighbourhood attention
+ # for windows attention, modify reference index query method
+ reference_index, _ = pointops.knn_query(self.neighbours, coord, offset)
+ for block in self.blocks:
+ points = block(points, reference_index)
+ return points
+
+class GVAPatchEmbed(nn.Module):
+ def __init__(
+ self,
+ depth,
+ in_channels,
+ embed_channels,
+ groups,
+ neighbours=16,
+ qkv_bias=True,
+ pe_multiplier=False,
+ pe_bias=True,
+ attn_drop_rate=0.0,
+ drop_path_rate=0.0,
+ enable_checkpoint=False,
+ ):
+ super(GVAPatchEmbed, self).__init__()
+ self.in_channels = in_channels
+ self.embed_channels = embed_channels
+ self.proj = nn.Sequential(
+ nn.Linear(in_channels, embed_channels, bias=False),
+ PointBatchNorm(embed_channels),
+ nn.ReLU(inplace=True),
+ )
+ self.blocks = BlockSequence(
+ depth=depth,
+ embed_channels=embed_channels,
+ groups=groups,
+ neighbours=neighbours,
+ qkv_bias=qkv_bias,
+ pe_multiplier=pe_multiplier,
+ pe_bias=pe_bias,
+ attn_drop_rate=attn_drop_rate,
+ drop_path_rate=drop_path_rate,
+ enable_checkpoint=enable_checkpoint,
+ )
+
+ def forward(self, points):
+ coord, feat, offset = points
+ feat = self.proj(feat)
+ return self.blocks([coord, feat, offset])
+
+
+class Block(nn.Module):
+ def __init__(
+ self,
+ embed_channels,
+ groups,
+ qkv_bias=True,
+ pe_multiplier=False,
+ pe_bias=True,
+ attn_drop_rate=0.0,
+ drop_path_rate=0.0,
+ enable_checkpoint=False,
+ ):
+ super(Block, self).__init__()
+ self.attn = GroupedVectorAttention(
+ embed_channels=embed_channels,
+ groups=groups,
+ qkv_bias=qkv_bias,
+ attn_drop_rate=attn_drop_rate,
+ pe_multiplier=pe_multiplier,
+ pe_bias=pe_bias,
+ )
+ self.fc1 = nn.Linear(embed_channels, embed_channels, bias=False)
+ self.fc3 = nn.Linear(embed_channels, embed_channels, bias=False)
+ self.norm1 = PointBatchNorm(embed_channels)
+ self.norm2 = PointBatchNorm(embed_channels)
+ self.norm3 = PointBatchNorm(embed_channels)
+ self.act = nn.ReLU(inplace=True)
+ self.enable_checkpoint = enable_checkpoint
+ self.drop_path = (
+ DropPath(drop_path_rate) if drop_path_rate > 0.0 else nn.Identity()
+ )
+
+ def forward(self, points, reference_index):
+ coord, feat, offset = points
+ identity = feat
+ feat = self.act(self.norm1(self.fc1(feat)))
+ feat = (
+ self.attn(feat, coord, reference_index)
+ if not self.enable_checkpoint
+ else checkpoint(self.attn, feat, coord, reference_index)
+ )
+ feat = self.act(self.norm2(feat))
+ feat = self.norm3(self.fc3(feat))
+ feat = identity + self.drop_path(feat)
+ feat = self.act(feat)
+ return [coord, feat, offset]
\ No newline at end of file
diff --git a/difpoint/model/temporaltrans/temptrans.py b/difpoint/model/temporaltrans/temptrans.py
new file mode 100644
index 0000000000000000000000000000000000000000..bf0d853c01470758ef3556f90ec658083b69bcda
--- /dev/null
+++ b/difpoint/model/temporaltrans/temptrans.py
@@ -0,0 +1,347 @@
+import torch
+import torch.nn as nn
+import torch.nn.functional as F
+from torch import nn
+from einops import rearrange
+from .transformer_utils import BaseTemperalPointModel
+import math
+from einops_exts import check_shape, rearrange_many
+from functools import partial
+
+class SinusoidalPosEmb(nn.Module):
+ def __init__(self, dim):
+ super().__init__()
+ self.dim = dim
+
+ def forward(self, x):
+ device = x.device
+ half_dim = self.dim // 2
+ emb = math.log(10000) / (half_dim - 1)
+ emb = torch.exp(torch.arange(half_dim, device=device) * -emb)
+ emb = x[:, None] * emb[None, :]
+ emb = torch.cat((emb.sin(), emb.cos()), dim=-1)
+ return emb
+
+class RelativePositionBias(nn.Module):
+ def __init__(
+ self,
+ heads = 8,
+ num_buckets = 32,
+ max_distance = 128
+ ):
+ super().__init__()
+ self.num_buckets = num_buckets
+ self.max_distance = max_distance
+ self.relative_attention_bias = nn.Embedding(num_buckets, heads)
+
+ @staticmethod
+ def _relative_position_bucket(relative_position, num_buckets = 32, max_distance = 128):
+ ret = 0
+ n = -relative_position
+
+ num_buckets //= 2
+ ret += (n < 0).long() * num_buckets
+ n = torch.abs(n)
+
+ max_exact = num_buckets // 2
+ is_small = n < max_exact
+
+ val_if_large = max_exact + (
+ torch.log(n.float() / max_exact) / math.log(max_distance / max_exact) * (num_buckets - max_exact)
+ ).long()
+ val_if_large = torch.min(val_if_large, torch.full_like(val_if_large, num_buckets - 1))
+
+ ret += torch.where(is_small, n, val_if_large)
+ return ret
+
+ def forward(self, n, device):
+ q_pos = torch.arange(n, dtype = torch.long, device = device)
+ k_pos = torch.arange(n, dtype = torch.long, device = device)
+ rel_pos = rearrange(k_pos, 'j -> 1 j') - rearrange(q_pos, 'i -> i 1')
+ rp_bucket = self._relative_position_bucket(rel_pos, num_buckets = self.num_buckets, max_distance = self.max_distance)
+ values = self.relative_attention_bias(rp_bucket)
+ return rearrange(values, 'i j h -> h i j')
+def exists(x):
+ return x is not None
+
+class Residual(nn.Module):
+ def __init__(self, fn):
+ super().__init__()
+ self.fn = fn
+
+ def forward(self, x, *args, **kwargs):
+ return self.fn(x, *args, **kwargs) + x
+class LayerNorm(nn.Module):
+ def __init__(self, dim, eps = 1e-5):
+ super().__init__()
+ self.eps = eps
+ self.gamma = nn.Parameter(torch.ones(1, 1, dim))
+ self.beta = nn.Parameter(torch.zeros(1, 1, dim))
+
+ def forward(self, x):
+ var = torch.var(x, dim = -1, unbiased = False, keepdim = True)
+ mean = torch.mean(x, dim = -1, keepdim = True)
+ return (x - mean) / (var + self.eps).sqrt() * self.gamma + self.beta
+
+class PreNorm(nn.Module):
+ def __init__(self, dim, fn):
+ super().__init__()
+ self.fn = fn
+ self.norm = LayerNorm(dim)
+
+ def forward(self, x, **kwargs):
+ x = self.norm(x)
+ return self.fn(x, **kwargs)
+
+
+class EinopsToAndFrom(nn.Module):
+ def __init__(self, from_einops, to_einops, fn):
+ super().__init__()
+ self.from_einops = from_einops
+ self.to_einops = to_einops
+ self.fn = fn
+
+ def forward(self, x, **kwargs):
+ shape = x.shape
+ reconstitute_kwargs = dict(tuple(zip(self.from_einops.split(' '), shape)))
+ x = rearrange(x, f'{self.from_einops} -> {self.to_einops}')
+ x = self.fn(x, **kwargs)
+ x = rearrange(x, f'{self.to_einops} -> {self.from_einops}', **reconstitute_kwargs)
+ return x
+
+class Attention(nn.Module):
+ def __init__(
+ self, dim, heads=4, attn_head_dim=None, casual_attn=False,rotary_emb = None):
+ super().__init__()
+ self.num_heads = heads
+ head_dim = dim // heads
+ self.casual_attn = casual_attn
+
+ if attn_head_dim is not None:
+ head_dim = attn_head_dim
+
+ all_head_dim = head_dim * self.num_heads
+ self.scale = head_dim ** -0.5
+ self.to_qkv = nn.Linear(dim, all_head_dim * 3, bias=False)
+ self.proj = nn.Linear(all_head_dim, dim)
+ self.rotary_emb = rotary_emb
+
+ def forward(self, x, pos_bias = None):
+ N, device = x.shape[-2], x.device
+ qkv = self.to_qkv(x).chunk(3, dim = -1)
+
+ q, k, v = rearrange_many(qkv, '... n (h d) -> ... h n d', h=self.num_heads)
+
+ q = q * self.scale
+
+ if exists(self.rotary_emb):
+ q = self.rotary_emb.rotate_queries_or_keys(q)
+ k = self.rotary_emb.rotate_queries_or_keys(k)
+
+ sim = torch.einsum('... h i d, ... h j d -> ... h i j', q, k)
+
+ if exists(pos_bias):
+ sim = sim + pos_bias
+
+ if self.casual_attn:
+ mask = torch.tril(torch.ones(sim.size(-1), sim.size(-2))).to(device)
+ sim = sim.masked_fill(mask[..., :, :] == 0, float('-inf'))
+
+ attn = sim.softmax(dim = -1)
+ x = torch.einsum('... h i j, ... h j d -> ... h i d', attn, v)
+ x = rearrange(x, '... h n d -> ... n (h d)')
+ x = self.proj(x)
+ return x
+
+
+class Block(nn.Module):
+ def __init__(self, dim, dim_out):
+ super().__init__()
+ self.proj = nn.Linear(dim, dim_out)
+ self.norm = LayerNorm(dim)
+ self.act = nn.SiLU()
+
+ def forward(self, x, scale_shift=None):
+ x = self.proj(x)
+
+ if exists(scale_shift):
+ x = self.norm(x)
+ scale, shift = scale_shift
+ x = x * (scale + 1) + shift
+ return self.act(x)
+
+
+class ResnetBlock(nn.Module):
+ def __init__(self, dim, dim_out, cond_dim=None):
+ super().__init__()
+ self.mlp = nn.Sequential(
+ nn.SiLU(),
+ nn.Linear(cond_dim, dim_out * 2)
+ ) if exists(cond_dim) else None
+
+ self.block1 = Block(dim, dim_out)
+ self.block2 = Block(dim_out, dim_out)
+
+ def forward(self, x, cond_emb=None):
+ scale_shift = None
+ if exists(self.mlp):
+ assert exists(cond_emb), 'time emb must be passed in'
+ cond_emb = self.mlp(cond_emb)
+ #cond_emb = rearrange(cond_emb, 'b f c -> b f 1 c')
+ scale_shift = cond_emb.chunk(2, dim=-1)
+
+ h = self.block1(x, scale_shift=scale_shift)
+ h = self.block2(h)
+ return h + x
+
+from rotary_embedding_torch import RotaryEmbedding
+
+
+class SimpleTransModel(BaseTemperalPointModel):
+ """
+ A simple model that processes a point cloud by applying a series of MLPs to each point
+ individually, along with some pooled global features.
+ """
+
+ def get_layers(self):
+
+
+ # self.input_projection = nn.Linear(
+ # in_features=51,
+ # out_features=self.dim
+ # )
+
+ self.input_projection = nn.Linear(
+ in_features=70,
+ out_features=self.dim
+ )
+
+ cond_dim = 512 + self.timestep_embed_dim
+
+ num_head = self.dim//64
+ rotary_emb = RotaryEmbedding(min(32, num_head))
+
+ self.time_rel_pos_bias = RelativePositionBias(heads=num_head, max_distance=128) # realistically will not be able to generate that many frames of video... yet
+
+ temporal_casual_attn = lambda dim: Attention(dim, heads=num_head, casual_attn=False,rotary_emb=rotary_emb)
+
+ cond_block= partial(ResnetBlock,cond_dim=cond_dim)
+
+ layers = nn.ModuleList([])
+
+ for _ in range(self.num_layers):
+ layers.append(nn.ModuleList([
+ cond_block(self.dim,self.dim),
+ cond_block(self.dim,self.dim),
+ Residual(PreNorm(self.dim,temporal_casual_attn(self.dim)))
+ ]))
+
+ return layers
+
+ def forward(self, inputs: torch.Tensor, timesteps: torch.Tensor, context=None):
+ """
+ Apply the model to an input batch.
+ :param x: an [N x C x ...] Tensor of inputs.
+ :param timesteps: a 1-D batch of timesteps.
+ :param context: conditioning plugged in via crossattn
+ """
+ # Prepare inputs
+
+ batch, num_frames, channels = inputs.size()
+
+ device = inputs.device
+ #assert channels==3
+
+ # Positional encoding of point coords
+ # inputs=rearrange(inputs,'b f p c->(b f) p c')
+ # pos_emb=self.positional_encoding(inputs)
+ x = self.input_projection(inputs)
+ #x = rearrange(x,'(b f) p c-> b f p c',b=batch)
+
+ t_emb = self.time_mlp(timesteps) if exists(self.time_mlp) else None
+ t_emb = t_emb[:,None,:].expand(-1, num_frames, -1) # b f c
+ if context is not None:
+ t_emb = torch.cat([t_emb, context],-1)
+
+ time_rel_pos_bias = self.time_rel_pos_bias(num_frames, device=device)
+
+ for block1, block2, temporal_casual_attn in self.layers:
+ x = block1(x, t_emb)
+ x = block2(x, t_emb)
+ x = temporal_casual_attn(x, pos_bias=time_rel_pos_bias)
+
+ # Project
+ x = self.output_projection(x)
+ return x
+
+
+
+class SimpleTemperalPointModel(BaseTemperalPointModel):
+ """
+ A simple model that processes a point cloud by applying a series of MLPs to each point
+ individually, along with some pooled global features.
+ """
+
+ def get_layers(self):
+ audio_dim = 512
+
+ cond_dim = audio_dim + self.timestep_embed_dim
+
+ num_head = 4
+ rotary_emb = RotaryEmbedding(min(32, num_head))
+ self.time_rel_pos_bias = RelativePositionBias(heads=num_head, max_distance=128) # realistically will not be able to generate that many frames of video... yet
+
+ temporal_casual_attn = lambda dim: EinopsToAndFrom('b f p c', 'b p f c', Attention(dim, heads=num_head, casual_attn=False, rotary_emb = rotary_emb))
+
+ spatial_kp_attn= lambda dim: EinopsToAndFrom('b f p c', 'b f p c', Attention(dim, heads=num_head))
+
+ cond_block= partial(ResnetBlock,cond_dim=cond_dim)
+
+ layers = nn.ModuleList([])
+
+ for _ in range(self.num_layers):
+ layers.append(nn.ModuleList([
+ cond_block(self.dim,self.dim),
+ cond_block(self.dim,self.dim),
+ Residual(PreNorm(self.dim,spatial_kp_attn(self.dim))),
+ Residual(PreNorm(self.dim,temporal_casual_attn(self.dim)))
+ ]))
+
+ return layers
+
+ def forward(self, inputs: torch.Tensor, timesteps: torch.Tensor, context=None):
+ """
+ Apply the model to an input batch.
+ :param x: an [N x C x ...] Tensor of inputs.
+ :param timesteps: a 1-D batch of timesteps.
+ :param context: conditioning plugged in via crossattn
+ """
+ # Prepare inputs
+
+ batch, num_frames, num_points, channels = inputs.size()
+ device = inputs.device
+ #assert channels==3
+
+ # Positional encoding of point coords
+ inputs=rearrange(inputs,'b f p c->(b f) p c')
+ pos_emb=self.positional_encoding(inputs)
+ x = self.input_projection(torch.cat([inputs, pos_emb], -1))
+ x = rearrange(x,'(b f) p c-> b f p c',b=batch)
+
+ t_emb = self.time_mlp(timesteps) if exists(self.time_mlp) else None
+ t_emb = t_emb[:,None,:].expand(-1, num_frames, -1) # b f c
+ if context is not None:
+ t_emb = torch.cat([t_emb,context],-1)
+
+ time_rel_pos_bias = self.time_rel_pos_bias(num_frames, device=device)
+
+ for block1, block2, spatial_kp_attn, temporal_casual_attn in self.layers:
+ x = block1(x, t_emb)
+ x = block2(x, t_emb)
+ x = spatial_kp_attn(x)
+ x = temporal_casual_attn(x, pos_bias=time_rel_pos_bias)
+
+ # Project
+ x = self.output_projection(x)
+ return x
+
diff --git a/difpoint/model/temporaltrans/transformer_utils.py b/difpoint/model/temporaltrans/transformer_utils.py
new file mode 100644
index 0000000000000000000000000000000000000000..14a58d0eef0dfbe785b633c66d23cfabfd671b23
--- /dev/null
+++ b/difpoint/model/temporaltrans/transformer_utils.py
@@ -0,0 +1,146 @@
+import torch
+import torch.nn as nn
+import torch.nn.functional as F
+from torch import nn
+from einops import rearrange
+import math
+from einops_exts import check_shape, rearrange_many
+from torch import Size, Tensor, nn
+class SinusoidalPosEmb(nn.Module):
+ def __init__(self, dim):
+ super().__init__()
+ self.dim = dim
+
+ def forward(self, x):
+ device = x.device
+ half_dim = self.dim // 2
+ emb = math.log(10000) / (half_dim - 1)
+ emb = torch.exp(torch.arange(half_dim, device=device) * -emb)
+ emb = x[:, None] * emb[None, :]
+ emb = torch.cat((emb.sin(), emb.cos()), dim=-1)
+ return emb
+
+
+def map_positional_encoding(v: Tensor, freq_bands: Tensor) -> Tensor:
+ """Map v to positional encoding representation phi(v)
+
+ Arguments:
+ v (Tensor): input features (B, IFeatures)
+ freq_bands (Tensor): frequency bands (N_freqs, )
+
+ Returns:
+ phi(v) (Tensor): fourrier features (B, 3 + (2 * N_freqs) * 3)
+ """
+ pe = [v]
+ for freq in freq_bands:
+ fv = freq * v
+ pe += [torch.sin(fv), torch.cos(fv)]
+ return torch.cat(pe, dim=-1)
+
+class FeatureMapping(nn.Module):
+ """FeatureMapping nn.Module
+
+ Maps v to features following transformation phi(v)
+
+ Arguments:
+ i_dim (int): input dimensions
+ o_dim (int): output dimensions
+ """
+
+ def __init__(self, i_dim: int, o_dim: int) -> None:
+ super().__init__()
+ self.i_dim = i_dim
+ self.o_dim = o_dim
+
+ def forward(self, v: Tensor) -> Tensor:
+ """FeratureMapping forward pass
+
+ Arguments:
+ v (Tensor): input features (B, IFeatures)
+
+ Returns:
+ phi(v) (Tensor): mapped features (B, OFeatures)
+ """
+ raise NotImplementedError("Forward pass not implemented yet!")
+
+class PositionalEncoding(FeatureMapping):
+ """PositionalEncoding module
+
+ Maps v to positional encoding representation phi(v)
+
+ Arguments:
+ i_dim (int): input dimension for v
+ N_freqs (int): #frequency to sample (default: 10)
+ """
+
+ def __init__(
+ self,
+ i_dim: int,
+ N_freqs: int = 10,
+ ) -> None:
+ super().__init__(i_dim, 3 + (2 * N_freqs) * 3)
+ self.N_freqs = N_freqs
+
+ a, b = 1, self.N_freqs - 1
+ freq_bands = 2 ** torch.linspace(a, b, self.N_freqs)
+ self.register_buffer("freq_bands", freq_bands)
+
+ def forward(self, v: Tensor) -> Tensor:
+ """Map v to positional encoding representation phi(v)
+
+ Arguments:
+ v (Tensor): input features (B, IFeatures)
+
+ Returns:
+ phi(v) (Tensor): fourrier features (B, 3 + (2 * N_freqs) * 3)
+ """
+ return map_positional_encoding(v, self.freq_bands)
+
+class BaseTemperalPointModel(nn.Module):
+ """ A base class providing useful methods for point cloud processing. """
+
+ def __init__(
+ self,
+ *,
+ num_classes,
+ embed_dim,
+ extra_feature_channels,
+ dim: int = 768,
+ num_layers: int = 6
+ ):
+ super().__init__()
+
+ self.extra_feature_channels = extra_feature_channels
+ self.timestep_embed_dim = 256
+ self.output_dim = num_classes
+ self.dim = dim
+ self.num_layers = num_layers
+
+
+ self.time_mlp = nn.Sequential(
+ SinusoidalPosEmb(dim),
+ nn.Linear(dim, self.timestep_embed_dim ),
+ nn.SiLU(),
+ nn.Linear(self.timestep_embed_dim , self.timestep_embed_dim )
+ )
+
+ self.positional_encoding = PositionalEncoding(i_dim=3, N_freqs=10)
+ positional_encoding_d_out = 3 + (2 * 10) * 3
+
+ # Input projection (point coords, point coord encodings, other features, and timestep embeddings)
+
+ self.input_projection = nn.Linear(
+ in_features=(3 + positional_encoding_d_out),
+ out_features=self.dim
+ )#b f p c
+
+ # Transformer layers
+ self.layers = self.get_layers()
+
+ # Output projection
+ self.output_projection = nn.Linear(self.dim, self.output_dim)
+ def get_layers(self):
+ raise NotImplementedError('This method should be implemented by subclasses')
+
+ def forward(self, inputs: torch.Tensor, t: torch.Tensor):
+ raise NotImplementedError('This method should be implemented by subclasses')
diff --git a/difpoint/src/__init__.py b/difpoint/src/__init__.py
new file mode 100644
index 0000000000000000000000000000000000000000..e063f97ed821a5c502f0e23facbef04f3a6a985d
--- /dev/null
+++ b/difpoint/src/__init__.py
@@ -0,0 +1,5 @@
+# -*- coding: utf-8 -*-
+# @Author : wenshao
+# @Email : wenshaoguo0611@gmail.com
+# @Project : FasterLivePortrait
+# @FileName: __init__.py.py
diff --git a/difpoint/src/__pycache__/__init__.cpython-310.pyc b/difpoint/src/__pycache__/__init__.cpython-310.pyc
new file mode 100644
index 0000000000000000000000000000000000000000..42f715a089d7cb732ea0db9f7648bc40dfe02086
Binary files /dev/null and b/difpoint/src/__pycache__/__init__.cpython-310.pyc differ
diff --git a/difpoint/src/__pycache__/__init__.cpython-38.pyc b/difpoint/src/__pycache__/__init__.cpython-38.pyc
new file mode 100644
index 0000000000000000000000000000000000000000..26e782f213243594b6d85fa456bfd99a6f817471
Binary files /dev/null and b/difpoint/src/__pycache__/__init__.cpython-38.pyc differ
diff --git a/difpoint/src/__pycache__/live_portrait_pipeline.cpython-310.pyc b/difpoint/src/__pycache__/live_portrait_pipeline.cpython-310.pyc
new file mode 100644
index 0000000000000000000000000000000000000000..180ee74ccf1c1a2b26809b7c68fce2f778ad6f23
Binary files /dev/null and b/difpoint/src/__pycache__/live_portrait_pipeline.cpython-310.pyc differ
diff --git a/difpoint/src/__pycache__/live_portrait_wrapper.cpython-310.pyc b/difpoint/src/__pycache__/live_portrait_wrapper.cpython-310.pyc
new file mode 100644
index 0000000000000000000000000000000000000000..5f1725373045f87eb0d8db946a8417aeebb48904
Binary files /dev/null and b/difpoint/src/__pycache__/live_portrait_wrapper.cpython-310.pyc differ
diff --git a/difpoint/src/config/__init__.py b/difpoint/src/config/__init__.py
new file mode 100644
index 0000000000000000000000000000000000000000..e69de29bb2d1d6434b8b29ae775ad8c2e48c5391
diff --git a/difpoint/src/config/__pycache__/__init__.cpython-310.pyc b/difpoint/src/config/__pycache__/__init__.cpython-310.pyc
new file mode 100644
index 0000000000000000000000000000000000000000..7e2eadf3a9d7fae67c2c543e445d858c4f0e9826
Binary files /dev/null and b/difpoint/src/config/__pycache__/__init__.cpython-310.pyc differ
diff --git a/difpoint/src/config/__pycache__/argument_config.cpython-310.pyc b/difpoint/src/config/__pycache__/argument_config.cpython-310.pyc
new file mode 100644
index 0000000000000000000000000000000000000000..1dfd93c802fa677772e3521bd2691432e93c045a
Binary files /dev/null and b/difpoint/src/config/__pycache__/argument_config.cpython-310.pyc differ
diff --git a/difpoint/src/config/__pycache__/base_config.cpython-310.pyc b/difpoint/src/config/__pycache__/base_config.cpython-310.pyc
new file mode 100644
index 0000000000000000000000000000000000000000..6fdec4cb2a7368220cf069cbf88df3fabc0b23c9
Binary files /dev/null and b/difpoint/src/config/__pycache__/base_config.cpython-310.pyc differ
diff --git a/difpoint/src/config/__pycache__/crop_config.cpython-310.pyc b/difpoint/src/config/__pycache__/crop_config.cpython-310.pyc
new file mode 100644
index 0000000000000000000000000000000000000000..9d9da6c684eb87218bad6a897e7567309e18019e
Binary files /dev/null and b/difpoint/src/config/__pycache__/crop_config.cpython-310.pyc differ
diff --git a/difpoint/src/config/__pycache__/inference_config.cpython-310.pyc b/difpoint/src/config/__pycache__/inference_config.cpython-310.pyc
new file mode 100644
index 0000000000000000000000000000000000000000..9cf2d6174c7e55179d8a9c09a2a2572313c1d94f
Binary files /dev/null and b/difpoint/src/config/__pycache__/inference_config.cpython-310.pyc differ
diff --git a/difpoint/src/config/argument_config.py b/difpoint/src/config/argument_config.py
new file mode 100644
index 0000000000000000000000000000000000000000..bacf15abe59b0899f3c4c25e4fcf9ae9b2a7f427
--- /dev/null
+++ b/difpoint/src/config/argument_config.py
@@ -0,0 +1,48 @@
+# coding: utf-8
+
+"""
+All configs for user
+"""
+
+from dataclasses import dataclass
+import tyro
+from typing_extensions import Annotated
+from typing import Optional
+from .base_config import PrintableConfig, make_abs_path
+
+
+@dataclass(repr=False) # use repr from PrintableConfig
+class ArgumentConfig(PrintableConfig):
+ ########## input arguments ##########
+ source_image: Annotated[str, tyro.conf.arg(aliases=["-s"])] = make_abs_path('../../assets/examples/source/s6.jpg') # path to the source portrait
+ driving_info: Annotated[str, tyro.conf.arg(aliases=["-d"])] = make_abs_path('../../assets/examples/driving/d12.mp4') # path to driving video or template (.pkl format)
+ output_dir: Annotated[str, tyro.conf.arg(aliases=["-o"])] = 'animations/' # directory to save output video
+
+ ########## inference arguments ##########
+ flag_use_half_precision: bool = False # whether to use half precision (FP16). If black boxes appear, it might be due to GPU incompatibility; set to False.
+ flag_crop_driving_video: bool = False # whether to crop the driving video, if the given driving info is a video
+ device_id: int = 0 # gpu device id
+ flag_force_cpu: bool = False # force cpu inference, WIP!
+ flag_lip_zero: bool = False # whether let the lip to close state before animation, only take effect when flag_eye_retargeting and flag_lip_retargeting is False
+ flag_eye_retargeting: bool = False # not recommend to be True, WIP
+ flag_lip_retargeting: bool = False # not recommend to be True, WIP
+ flag_stitching: bool = False # recommend to True if head movement is small, False if head movement is large
+ flag_relative_motion: bool = False # whether to use relative motion
+ flag_pasteback: bool = False # whether to paste-back/stitch the animated face cropping from the face-cropping space to the original image space
+ flag_do_crop: bool = False # whether to crop the source portrait to the face-cropping space
+ flag_do_rot: bool = False # whether to conduct the rotation when flag_do_crop is True
+
+ ########## crop arguments ##########
+ scale: float = 2.3 # the ratio of face area is smaller if scale is larger
+ vx_ratio: float = 0 # the ratio to move the face to left or right in cropping space
+ vy_ratio: float = -0.125 # the ratio to move the face to up or down in cropping space
+
+ scale_crop_video: float = 2.2 # scale factor for cropping video
+ vx_ratio_crop_video: float = 0. # adjust y offset
+ vy_ratio_crop_video: float = -0.1 # adjust x offset
+
+ ########## gradio arguments ##########
+ server_port: Annotated[int, tyro.conf.arg(aliases=["-p"])] = 8890 # port for gradio server
+ share: bool = False # whether to share the server to public
+ server_name: Optional[str] = "127.0.0.1" # set the local server name, "0.0.0.0" to broadcast all
+ flag_do_torch_compile: bool = False # whether to use torch.compile to accelerate generation
diff --git a/difpoint/src/config/base_config.py b/difpoint/src/config/base_config.py
new file mode 100644
index 0000000000000000000000000000000000000000..216b8be50aecc8af4b9d1d2a9401e034dd7769e4
--- /dev/null
+++ b/difpoint/src/config/base_config.py
@@ -0,0 +1,29 @@
+# coding: utf-8
+
+"""
+pretty printing class
+"""
+
+from __future__ import annotations
+import os.path as osp
+from typing import Tuple
+
+
+def make_abs_path(fn):
+ return osp.join(osp.dirname(osp.realpath(__file__)), fn)
+
+
+class PrintableConfig: # pylint: disable=too-few-public-methods
+ """Printable Config defining str function"""
+
+ def __repr__(self):
+ lines = [self.__class__.__name__ + ":"]
+ for key, val in vars(self).items():
+ if isinstance(val, Tuple):
+ flattened_val = "["
+ for item in val:
+ flattened_val += str(item) + "\n"
+ flattened_val = flattened_val.rstrip("\n")
+ val = flattened_val + "]"
+ lines += f"{key}: {str(val)}".split("\n")
+ return "\n ".join(lines)
diff --git a/difpoint/src/config/crop_config.py b/difpoint/src/config/crop_config.py
new file mode 100644
index 0000000000000000000000000000000000000000..f7b5a2cc2689dcdf5686cd13ab7a4824da15aec7
--- /dev/null
+++ b/difpoint/src/config/crop_config.py
@@ -0,0 +1,29 @@
+# coding: utf-8
+
+"""
+parameters used for crop faces
+"""
+
+from dataclasses import dataclass
+
+from .base_config import PrintableConfig
+
+
+@dataclass(repr=False) # use repr from PrintableConfig
+class CropConfig(PrintableConfig):
+ insightface_root: str = "./downloaded_repo/pretrained_weights/insightface"
+ landmark_ckpt_path: str = "./downloaded_repo/pretrained_weights/liveportrait/landmark.onnx"
+ device_id: int = 0 # gpu device id
+ flag_force_cpu: bool = False # force cpu inference, WIP
+ ########## source image cropping option ##########
+ dsize: int = 512 # crop size
+ scale: float = 2.0 # scale factor
+ vx_ratio: float = 0 # vx ratio
+ vy_ratio: float = -0.125 # vy ratio +up, -down
+ max_face_num: int = 0 # max face number, 0 mean no limit
+
+ ########## driving video auto cropping option ##########
+ scale_crop_video: float = 2.2 # 2.0 # scale factor for cropping video
+ vx_ratio_crop_video: float = 0.0 # adjust y offset
+ vy_ratio_crop_video: float = -0.1 # adjust x offset
+ direction: str = "large-small" # direction of cropping
diff --git a/difpoint/src/config/inference_config.py b/difpoint/src/config/inference_config.py
new file mode 100644
index 0000000000000000000000000000000000000000..d094ea98bc18ef565d6a2092cbebe2a137f37544
--- /dev/null
+++ b/difpoint/src/config/inference_config.py
@@ -0,0 +1,52 @@
+# coding: utf-8
+
+"""
+config dataclass used for inference
+"""
+
+import os.path as osp
+import cv2
+from numpy import ndarray
+from dataclasses import dataclass
+from typing import Literal, Tuple
+from .base_config import PrintableConfig, make_abs_path
+
+
+@dataclass(repr=False) # use repr from PrintableConfig
+class InferenceConfig(PrintableConfig):
+ # MODEL CONFIG, NOT EXPORTED PARAMS
+ models_config: str = make_abs_path('./models.yaml') # portrait animation config
+ checkpoint_F: str = './downloaded_repo/pretrained_weights/liveportrait/base_models/appearance_feature_extractor.pth' # path to checkpoint of F
+ checkpoint_M: str = './downloaded_repo/pretrained_weights/liveportrait/base_models/motion_extractor.pth' # path to checkpoint pf M
+ checkpoint_G: str = './downloaded_repo/pretrained_weights/liveportrait/base_models/spade_generator.pth' # path to checkpoint of G
+ checkpoint_W: str = './downloaded_repo/pretrained_weights/liveportrait/base_models/warping_module.pth' # path to checkpoint of W
+ checkpoint_S: str = './downloaded_repo/pretrained_weights/liveportrait/base_models/retargeting_models/stitching_retargeting_module.pth' # path to checkpoint to S and R_eyes, R_lip
+
+ # EXPORTED PARAMS
+ flag_use_half_precision: bool = True
+ flag_crop_driving_video: bool = False
+ device_id: int = 0
+ flag_lip_zero: bool = False
+ flag_eye_retargeting: bool = False
+ flag_lip_retargeting: bool = False
+ flag_stitching: bool = False
+ flag_relative_motion: bool = False
+ flag_pasteback: bool = False
+ flag_do_crop: bool = False
+ flag_do_rot: bool = False
+ flag_force_cpu: bool = False
+ flag_do_torch_compile: bool = False
+
+ # NOT EXPORTED PARAMS
+ lip_zero_threshold: float = 0.03 # threshold for flag_lip_zero
+ anchor_frame: int = 0 # TO IMPLEMENT
+
+ input_shape: Tuple[int, int] = (256, 256) # input shape
+ output_format: Literal['mp4', 'gif'] = 'mp4' # output video format
+ crf: int = 15 # crf for output video
+ output_fps: int = 25 # default output fps
+
+ mask_crop: ndarray = cv2.imread(make_abs_path('../utils/resources/mask_template.png'), cv2.IMREAD_COLOR)
+ size_gif: int = 256 # default gif size, TO IMPLEMENT
+ source_max_dim: int = 1280 # the max dim of height and width of source image
+ source_division: int = 2 # make sure the height and width of source image can be divided by this number
diff --git a/difpoint/src/config/models.yaml b/difpoint/src/config/models.yaml
new file mode 100644
index 0000000000000000000000000000000000000000..131d1c65025c31e37af9239e211ea14454128a2e
--- /dev/null
+++ b/difpoint/src/config/models.yaml
@@ -0,0 +1,43 @@
+model_params:
+ appearance_feature_extractor_params: # the F in the paper
+ image_channel: 3
+ block_expansion: 64
+ num_down_blocks: 2
+ max_features: 512
+ reshape_channel: 32
+ reshape_depth: 16
+ num_resblocks: 6
+ motion_extractor_params: # the M in the paper
+ num_kp: 21
+ backbone: convnextv2_tiny
+ warping_module_params: # the W in the paper
+ num_kp: 21
+ block_expansion: 64
+ max_features: 512
+ num_down_blocks: 2
+ reshape_channel: 32
+ estimate_occlusion_map: True
+ dense_motion_params:
+ block_expansion: 32
+ max_features: 1024
+ num_blocks: 5
+ reshape_depth: 16
+ compress: 4
+ spade_generator_params: # the G in the paper
+ upscale: 2 # represents upsample factor 256x256 -> 512x512
+ block_expansion: 64
+ max_features: 512
+ num_down_blocks: 2
+ stitching_retargeting_module_params: # the S in the paper
+ stitching:
+ input_size: 126 # (21*3)*2
+ hidden_sizes: [128, 128, 64]
+ output_size: 65 # (21*3)+2(tx,ty)
+ lip:
+ input_size: 65 # (21*3)+2
+ hidden_sizes: [128, 128, 64]
+ output_size: 63 # (21*3)
+ eye:
+ input_size: 66 # (21*3)+3
+ hidden_sizes: [256, 256, 128, 128, 64]
+ output_size: 63 # (21*3)
diff --git a/difpoint/src/croper.py b/difpoint/src/croper.py
new file mode 100644
index 0000000000000000000000000000000000000000..0ccf5dfc483099ea2112076c0a122cc3e9ecc0c3
--- /dev/null
+++ b/difpoint/src/croper.py
@@ -0,0 +1,299 @@
+import os
+import cv2
+import time
+import glob
+import argparse
+import scipy
+import numpy as np
+from PIL import Image
+from tqdm import tqdm
+from itertools import cycle
+
+from torch.multiprocessing import Pool, Process, set_start_method
+
+
+"""
+brief: face alignment with FFHQ method (https://github.com/NVlabs/ffhq-dataset)
+author: lzhbrian (https://lzhbrian.me)
+date: 2020.1.5
+note: code is heavily borrowed from
+ https://github.com/NVlabs/ffhq-dataset
+ http://dlib.net/face_landmark_detection.py.html
+requirements:
+ apt install cmake
+ conda install Pillow numpy scipy
+ pip install dlib
+ # download face landmark model from:
+ # http://dlib.net/files/shape_predictor_68_face_landmarks.dat.bz2
+"""
+
+import numpy as np
+from PIL import Image
+import dlib
+
+
+class Croper:
+ def __init__(self, path_of_lm):
+ # download model from: http://dlib.net/files/shape_predictor_68_face_landmarks.dat.bz2
+ self.predictor = dlib.shape_predictor(path_of_lm)
+
+ def get_landmark(self, img_np):
+ """get landmark with dlib
+ :return: np.array shape=(68, 2)
+ """
+ detector = dlib.get_frontal_face_detector()
+ dets = detector(img_np, 1)
+ # print("Number of faces detected: {}".format(len(dets)))
+ # for k, d in enumerate(dets):
+ if len(dets) == 0:
+ return None
+ d = dets[0]
+ # Get the landmarks/parts for the face in box d.
+ shape = self.predictor(img_np, d)
+ # print("Part 0: {}, Part 1: {} ...".format(shape.part(0), shape.part(1)))
+ t = list(shape.parts())
+ a = []
+ for tt in t:
+ a.append([tt.x, tt.y])
+ lm = np.array(a)
+ # lm is a shape=(68,2) np.array
+ return lm
+
+ def align_face(self, img, lm, output_size=1024):
+ """
+ :param filepath: str
+ :return: PIL Image
+ """
+ lm_chin = lm[0: 17] # left-right
+ lm_eyebrow_left = lm[17: 22] # left-right
+ lm_eyebrow_right = lm[22: 27] # left-right
+ lm_nose = lm[27: 31] # top-down
+ lm_nostrils = lm[31: 36] # top-down
+ lm_eye_left = lm[36: 42] # left-clockwise
+ lm_eye_right = lm[42: 48] # left-clockwise
+ lm_mouth_outer = lm[48: 60] # left-clockwise
+ lm_mouth_inner = lm[60: 68] # left-clockwise
+
+ # Calculate auxiliary vectors.
+ eye_left = np.mean(lm_eye_left, axis=0)
+ eye_right = np.mean(lm_eye_right, axis=0)
+ eye_avg = (eye_left + eye_right) * 0.5
+ eye_to_eye = eye_right - eye_left
+ mouth_left = lm_mouth_outer[0]
+ mouth_right = lm_mouth_outer[6]
+ mouth_avg = (mouth_left + mouth_right) * 0.5
+ eye_to_mouth = mouth_avg - eye_avg
+
+ # Choose oriented crop rectangle.
+ x = eye_to_eye - np.flipud(eye_to_mouth) * [-1, 1] # Addition of binocular difference and double mouth difference
+ x /= np.hypot(*x) # hypot函数计算直角三角形的斜边长,用斜边长对三角形两条直边做归一化
+ x *= max(np.hypot(*eye_to_eye) * 2.0, np.hypot(*eye_to_mouth) * 1.8) # 双眼差和眼嘴差,选较大的作为基准尺度
+ y = np.flipud(x) * [-1, 1]
+ c = eye_avg + eye_to_mouth * 0.1
+ quad = np.stack([c - x - y, c - x + y, c + x + y, c + x - y]) # 定义四边形,以面部基准位置为中心上下左右平移得到四个顶点
+ qsize = np.hypot(*x) * 2 # 定义四边形的大小(边长),为基准尺度的2倍
+
+ # Shrink.
+ # 如果计算出的四边形太大了,就按比例缩小它
+ shrink = int(np.floor(qsize / output_size * 0.5))
+ if shrink > 1:
+ rsize = (int(np.rint(float(img.size[0]) / shrink)), int(np.rint(float(img.size[1]) / shrink)))
+ img = img.resize(rsize, Image.ANTIALIAS)
+ quad /= shrink
+ qsize /= shrink
+ else:
+ rsize = (int(np.rint(float(img.size[0]))), int(np.rint(float(img.size[1]))))
+
+ # Crop.
+ border = max(int(np.rint(qsize * 0.1)), 3)
+ crop = (int(np.floor(min(quad[:, 0]))), int(np.floor(min(quad[:, 1]))), int(np.ceil(max(quad[:, 0]))),
+ int(np.ceil(max(quad[:, 1]))))
+ crop = (max(crop[0] - border, 0), max(crop[1] - border, 0), min(crop[2] + border, img.size[0]),
+ min(crop[3] + border, img.size[1]))
+ if crop[2] - crop[0] < img.size[0] or crop[3] - crop[1] < img.size[1]:
+ # img = img.crop(crop)
+ quad -= crop[0:2]
+
+ # Pad.
+ pad = (int(np.floor(min(quad[:, 0]))), int(np.floor(min(quad[:, 1]))), int(np.ceil(max(quad[:, 0]))),
+ int(np.ceil(max(quad[:, 1]))))
+ pad = (max(-pad[0] + border, 0), max(-pad[1] + border, 0), max(pad[2] - img.size[0] + border, 0),
+ max(pad[3] - img.size[1] + border, 0))
+ # if enable_padding and max(pad) > border - 4:
+ # pad = np.maximum(pad, int(np.rint(qsize * 0.3)))
+ # img = np.pad(np.float32(img), ((pad[1], pad[3]), (pad[0], pad[2]), (0, 0)), 'reflect')
+ # h, w, _ = img.shape
+ # y, x, _ = np.ogrid[:h, :w, :1]
+ # mask = np.maximum(1.0 - np.minimum(np.float32(x) / pad[0], np.float32(w - 1 - x) / pad[2]),
+ # 1.0 - np.minimum(np.float32(y) / pad[1], np.float32(h - 1 - y) / pad[3]))
+ # blur = qsize * 0.02
+ # img += (scipy.ndimage.gaussian_filter(img, [blur, blur, 0]) - img) * np.clip(mask * 3.0 + 1.0, 0.0, 1.0)
+ # img += (np.median(img, axis=(0, 1)) - img) * np.clip(mask, 0.0, 1.0)
+ # img = Image.fromarray(np.uint8(np.clip(np.rint(img), 0, 255)), 'RGB')
+ # quad += pad[:2]
+
+ # Transform.
+ quad = (quad + 0.5).flatten()
+ lx = max(min(quad[0], quad[2]), 0)
+ ly = max(min(quad[1], quad[7]), 0)
+ rx = min(max(quad[4], quad[6]), img.size[0])
+ ry = min(max(quad[3], quad[5]), img.size[0])
+ # img = img.transform((transform_size, transform_size), Image.QUAD, (quad + 0.5).flatten(),
+ # Image.BILINEAR)
+ # if output_size < transform_size:
+ # img = img.resize((output_size, output_size), Image.ANTIALIAS)
+
+ # Save aligned image.
+ return rsize, crop, [lx, ly, rx, ry]
+
+ # def crop(self, img_np_list):
+ # for _i in range(len(img_np_list)):
+ # img_np = img_np_list[_i]
+ # lm = self.get_landmark(img_np)
+ # if lm is None:
+ # return None
+ # crop, quad = self.align_face(img=Image.fromarray(img_np), lm=lm, output_size=512)
+ # clx, cly, crx, cry = crop
+ # lx, ly, rx, ry = quad
+ # lx, ly, rx, ry = int(lx), int(ly), int(rx), int(ry)
+
+ # _inp = img_np_list[_i]
+ # _inp = _inp[cly:cry, clx:crx]
+ # _inp = _inp[ly:ry, lx:rx]
+ # img_np_list[_i] = _inp
+ # return img_np_list
+
+ def crop(self, img_np_list, still=False, xsize=512): # first frame for all video
+ img_np = img_np_list[0]
+ lm = self.get_landmark(img_np)
+ if lm is None:
+ raise 'can not detect the landmark from source image'
+ rsize, crop, quad = self.align_face(img=Image.fromarray(img_np), lm=lm, output_size=xsize)
+ clx, cly, crx, cry = crop
+ lx, ly, rx, ry = quad
+ lx, ly, rx, ry = int(lx), int(ly), int(rx), int(ry)
+ for _i in range(len(img_np_list)):
+ _inp = img_np_list[_i]
+ _inp = cv2.resize(_inp, (rsize[0], rsize[1]))
+ _inp = _inp[cly:cry, clx:crx]
+ # cv2.imwrite('test1.jpg', _inp)
+ if not still:
+ _inp = _inp[ly:ry, lx:rx]
+ # cv2.imwrite('test2.jpg', _inp)
+ img_np_list[_i] = _inp
+ return img_np_list, crop, quad
+
+
+def read_video(filename, uplimit=100):
+ frames = []
+ cap = cv2.VideoCapture(filename)
+ cnt = 0
+ while cap.isOpened():
+ ret, frame = cap.read()
+ if ret:
+ frame = cv2.resize(frame, (512, 512))
+ frames.append(frame)
+ else:
+ break
+ cnt += 1
+ if cnt >= uplimit:
+ break
+ cap.release()
+ assert len(frames) > 0, f'{filename}: video with no frames!'
+ return frames
+
+
+def create_video(video_name, frames, fps=25, video_format='.mp4', resize_ratio=1):
+ # video_name = os.path.dirname(image_folder) + video_format
+ # img_list = glob.glob1(image_folder, 'frame*')
+ # img_list.sort()
+ # frame = cv2.imread(os.path.join(image_folder, img_list[0]))
+ # frame = cv2.resize(frame, (0, 0), fx=resize_ratio, fy=resize_ratio)
+ # height, width, layers = frames[0].shape
+ height, width, layers = 512, 512, 3
+ if video_format == '.mp4':
+ fourcc = cv2.VideoWriter_fourcc(*'mp4v')
+ elif video_format == '.avi':
+ fourcc = cv2.VideoWriter_fourcc(*'XVID')
+ video = cv2.VideoWriter(video_name, fourcc, fps, (width, height))
+ for _frame in frames:
+ _frame = cv2.resize(_frame, (height, width), interpolation=cv2.INTER_LINEAR)
+ video.write(_frame)
+
+def create_images(video_name, frames):
+ height, width, layers = 512, 512, 3
+ images_dir = video_name.split('.')[0]
+ os.makedirs(images_dir, exist_ok=True)
+ for i, _frame in enumerate(frames):
+ _frame = cv2.resize(_frame, (height, width), interpolation=cv2.INTER_LINEAR)
+ _frame_path = os.path.join(images_dir, str(i)+'.jpg')
+ cv2.imwrite(_frame_path, _frame)
+
+def run(data):
+ filename, opt, device = data
+ os.environ['CUDA_VISIBLE_DEVICES'] = device
+ croper = Croper()
+
+ frames = read_video(filename, uplimit=opt.uplimit)
+ name = filename.split('/')[-1] # .split('.')[0]
+ name = os.path.join(opt.output_dir, name)
+
+ frames = croper.crop(frames)
+ if frames is None:
+ print(f'{name}: detect no face. should removed')
+ return
+ # create_video(name, frames)
+ create_images(name, frames)
+
+
+def get_data_path(video_dir):
+ eg_video_files = ['/apdcephfs/share_1290939/quincheng/datasets/HDTF/backup_fps25/WDA_KatieHill_000.mp4']
+ # filenames = list()
+ # VIDEO_EXTENSIONS_LOWERCASE = {'mp4'}
+ # VIDEO_EXTENSIONS = VIDEO_EXTENSIONS_LOWERCASE.union({f.upper() for f in VIDEO_EXTENSIONS_LOWERCASE})
+ # extensions = VIDEO_EXTENSIONS
+ # for ext in extensions:
+ # filenames = sorted(glob.glob(f'{opt.input_dir}/**/*.{ext}'))
+ # print('Total number of videos:', len(filenames))
+ return eg_video_files
+
+
+def get_wra_data_path(video_dir):
+ if opt.option == 'video':
+ videos_path = sorted(glob.glob(f'{video_dir}/*.mp4'))
+ elif opt.option == 'image':
+ videos_path = sorted(glob.glob(f'{video_dir}/*/'))
+ else:
+ raise NotImplementedError
+ print('Example videos: ', videos_path[:2])
+ return videos_path
+
+
+if __name__ == '__main__':
+ set_start_method('spawn')
+ parser = argparse.ArgumentParser(formatter_class=argparse.ArgumentDefaultsHelpFormatter)
+ parser.add_argument('--input_dir', type=str, help='the folder of the input files')
+ parser.add_argument('--output_dir', type=str, help='the folder of the output files')
+ parser.add_argument('--device_ids', type=str, default='0,1')
+ parser.add_argument('--workers', type=int, default=8)
+ parser.add_argument('--uplimit', type=int, default=500)
+ parser.add_argument('--option', type=str, default='video')
+
+ root = '/apdcephfs/share_1290939/quincheng/datasets/HDTF'
+ cmd = f'--input_dir {root}/backup_fps25_first20s_sync/ ' \
+ f'--output_dir {root}/crop512_stylegan_firstframe_sync/ ' \
+ '--device_ids 0 ' \
+ '--workers 8 ' \
+ '--option video ' \
+ '--uplimit 500 '
+ opt = parser.parse_args(cmd.split())
+ # filenames = get_data_path(opt.input_dir)
+ filenames = get_wra_data_path(opt.input_dir)
+ os.makedirs(opt.output_dir, exist_ok=True)
+ print(f'Video numbers: {len(filenames)}')
+ pool = Pool(opt.workers)
+ args_list = cycle([opt])
+ device_ids = opt.device_ids.split(",")
+ device_ids = cycle(device_ids)
+ for data in tqdm(pool.imap_unordered(run, zip(filenames, args_list, device_ids))):
+ None
diff --git a/difpoint/src/gradio_pipeline.py b/difpoint/src/gradio_pipeline.py
new file mode 100644
index 0000000000000000000000000000000000000000..f7343f7df6b8a6c6815c5af3526ed6dc857a7c0c
--- /dev/null
+++ b/difpoint/src/gradio_pipeline.py
@@ -0,0 +1,117 @@
+# coding: utf-8
+
+"""
+Pipeline for gradio
+"""
+import gradio as gr
+
+from .config.argument_config import ArgumentConfig
+from .live_portrait_pipeline import LivePortraitPipeline
+from .utils.io import load_img_online
+from .utils.rprint import rlog as log
+from .utils.crop import prepare_paste_back, paste_back
+from .utils.camera import get_rotation_matrix
+
+
+def update_args(args, user_args):
+ """update the args according to user inputs
+ """
+ for k, v in user_args.items():
+ if hasattr(args, k):
+ setattr(args, k, v)
+ return args
+
+
+class GradioPipeline(LivePortraitPipeline):
+
+ def __init__(self, inference_cfg, crop_cfg, args: ArgumentConfig):
+ super().__init__(inference_cfg, crop_cfg)
+ # self.live_portrait_wrapper = self.live_portrait_wrapper
+ self.args = args
+
+ def execute_video(
+ self,
+ input_image_path,
+ input_video_path,
+ flag_relative_input,
+ flag_do_crop_input,
+ flag_remap_input,
+ flag_crop_driving_video_input
+ ):
+ """ for video driven potrait animation
+ """
+ if input_image_path is not None and input_video_path is not None:
+ args_user = {
+ 'source_image': input_image_path,
+ 'driving_info': input_video_path,
+ 'flag_relative': flag_relative_input,
+ 'flag_do_crop': flag_do_crop_input,
+ 'flag_pasteback': flag_remap_input,
+ 'flag_crop_driving_video': flag_crop_driving_video_input
+ }
+ # update config from user input
+ self.args = update_args(self.args, args_user)
+ self.live_portrait_wrapper.update_config(self.args.__dict__)
+ self.cropper.update_config(self.args.__dict__)
+ # video driven animation
+ video_path, video_path_concat = self.execute(self.args)
+ gr.Info("Run successfully!", duration=2)
+ return video_path, video_path_concat,
+ else:
+ raise gr.Error("The input source portrait or driving video hasn't been prepared yet 💥!", duration=5)
+
+ def execute_image(self, input_eye_ratio: float, input_lip_ratio: float, input_image, flag_do_crop=True):
+ """ for single image retargeting
+ """
+ # disposable feature
+ f_s_user, x_s_user, source_lmk_user, crop_M_c2o, mask_ori, img_rgb = \
+ self.prepare_retargeting(input_image, flag_do_crop)
+
+ if input_eye_ratio is None or input_lip_ratio is None:
+ raise gr.Error("Invalid ratio input 💥!", duration=5)
+ else:
+ inference_cfg = self.live_portrait_wrapper.inference_cfg
+ x_s_user = x_s_user.to(self.live_portrait_wrapper.device)
+ f_s_user = f_s_user.to(self.live_portrait_wrapper.device)
+ # ∆_eyes,i = R_eyes(x_s; c_s,eyes, c_d,eyes,i)
+ combined_eye_ratio_tensor = self.live_portrait_wrapper.calc_combined_eye_ratio([[input_eye_ratio]], source_lmk_user)
+ eyes_delta = self.live_portrait_wrapper.retarget_eye(x_s_user, combined_eye_ratio_tensor)
+ # ∆_lip,i = R_lip(x_s; c_s,lip, c_d,lip,i)
+ combined_lip_ratio_tensor = self.live_portrait_wrapper.calc_combined_lip_ratio([[input_lip_ratio]], source_lmk_user)
+ lip_delta = self.live_portrait_wrapper.retarget_lip(x_s_user, combined_lip_ratio_tensor)
+ num_kp = x_s_user.shape[1]
+ # default: use x_s
+ x_d_new = x_s_user + eyes_delta.reshape(-1, num_kp, 3) + lip_delta.reshape(-1, num_kp, 3)
+ # D(W(f_s; x_s, x′_d))
+ out = self.live_portrait_wrapper.warp_decode(f_s_user, x_s_user, x_d_new)
+ out = self.live_portrait_wrapper.parse_output(out['out'])[0]
+ out_to_ori_blend = paste_back(out, crop_M_c2o, img_rgb, mask_ori)
+ gr.Info("Run successfully!", duration=2)
+ return out, out_to_ori_blend
+
+ def prepare_retargeting(self, input_image, flag_do_crop=True):
+ """ for single image retargeting
+ """
+ if input_image is not None:
+ # gr.Info("Upload successfully!", duration=2)
+ inference_cfg = self.live_portrait_wrapper.inference_cfg
+ ######## process source portrait ########
+ img_rgb = load_img_online(input_image, mode='rgb', max_dim=1280, n=16)
+ log(f"Load source image from {input_image}.")
+ crop_info = self.cropper.crop_source_image(img_rgb, self.cropper.crop_cfg)
+ if flag_do_crop:
+ I_s = self.live_portrait_wrapper.prepare_source(crop_info['img_crop_256x256'])
+ else:
+ I_s = self.live_portrait_wrapper.prepare_source(img_rgb)
+ x_s_info = self.live_portrait_wrapper.get_kp_info(I_s)
+ R_s = get_rotation_matrix(x_s_info['pitch'], x_s_info['yaw'], x_s_info['roll'])
+ ############################################
+ f_s_user = self.live_portrait_wrapper.extract_feature_3d(I_s)
+ x_s_user = self.live_portrait_wrapper.transform_keypoint(x_s_info)
+ source_lmk_user = crop_info['lmk_crop']
+ crop_M_c2o = crop_info['M_c2o']
+ mask_ori = prepare_paste_back(inference_cfg.mask_crop, crop_info['M_c2o'], dsize=(img_rgb.shape[1], img_rgb.shape[0]))
+ return f_s_user, x_s_user, source_lmk_user, crop_M_c2o, mask_ori, img_rgb
+ else:
+ # when press the clear button, go here
+ raise gr.Error("The retargeting input hasn't been prepared yet 💥!", duration=5)
diff --git a/difpoint/src/live_portrait_pipeline.py b/difpoint/src/live_portrait_pipeline.py
new file mode 100644
index 0000000000000000000000000000000000000000..e20db99a98c8015b99167d9e8e36aef6c615999e
--- /dev/null
+++ b/difpoint/src/live_portrait_pipeline.py
@@ -0,0 +1,285 @@
+# coding: utf-8
+
+"""
+Pipeline of LivePortrait
+"""
+
+import torch
+torch.backends.cudnn.benchmark = True # disable CUDNN_BACKEND_EXECUTION_PLAN_DESCRIPTOR warning
+
+import cv2; cv2.setNumThreads(0); cv2.ocl.setUseOpenCL(False)
+import numpy as np
+import os
+import os.path as osp
+from rich.progress import track
+
+from .config.argument_config import ArgumentConfig
+from .config.inference_config import InferenceConfig
+from .config.crop_config import CropConfig
+from .utils.cropper import Cropper
+from .utils.camera import get_rotation_matrix
+from .utils.video import images2video, concat_frames, get_fps, add_audio_to_video, has_audio_stream
+from .utils.crop import prepare_paste_back, paste_back
+from .utils.io import load_image_rgb, load_driving_info, resize_to_limit, dump, load
+from .utils.helper import mkdir, basename, dct2device, is_video, is_template, remove_suffix
+from .utils.rprint import rlog as log
+# from .utils.viz import viz_lmk
+from .live_portrait_wrapper import LivePortraitWrapper
+
+
+def make_abs_path(fn):
+ return osp.join(osp.dirname(osp.realpath(__file__)), fn)
+
+
+class LivePortraitPipeline(object):
+
+ def __init__(self, inference_cfg: InferenceConfig, crop_cfg: CropConfig):
+ self.live_portrait_wrapper: LivePortraitWrapper = LivePortraitWrapper(inference_cfg=inference_cfg)
+ self.cropper: Cropper = Cropper(crop_cfg=crop_cfg)
+
+ def execute(self, args: ArgumentConfig):
+ # for convenience
+ inf_cfg = self.live_portrait_wrapper.inference_cfg
+ device = self.live_portrait_wrapper.device
+ crop_cfg = self.cropper.crop_cfg
+
+ ######## process source portrait ########
+ img_rgb = load_image_rgb(args.source_image)
+ img_rgb = resize_to_limit(img_rgb, inf_cfg.source_max_dim, inf_cfg.source_division)
+ log(f"Load source image from {args.source_image}")
+
+ crop_info = self.cropper.crop_source_image(img_rgb, crop_cfg)
+ if crop_info is None:
+ raise Exception("No face detected in the source image!")
+ source_lmk = crop_info['lmk_crop']
+ img_crop, img_crop_256x256 = crop_info['img_crop'], crop_info['img_crop_256x256']
+
+ if inf_cfg.flag_do_crop:
+ I_s = self.live_portrait_wrapper.prepare_source(img_crop_256x256)
+ else:
+ img_crop_256x256 = cv2.resize(img_rgb, (256, 256)) # force to resize to 256x256
+ I_s = self.live_portrait_wrapper.prepare_source(img_crop_256x256)
+ x_s_info = self.live_portrait_wrapper.get_kp_info(I_s)
+ x_c_s = x_s_info['kp']
+ R_s = get_rotation_matrix(x_s_info['pitch'], x_s_info['yaw'], x_s_info['roll'])
+ f_s = self.live_portrait_wrapper.extract_feature_3d(I_s)
+ x_s = self.live_portrait_wrapper.transform_keypoint(x_s_info)
+
+ flag_lip_zero = inf_cfg.flag_lip_zero # not overwrite
+ if flag_lip_zero:
+ # let lip-open scalar to be 0 at first
+ c_d_lip_before_animation = [0.]
+ combined_lip_ratio_tensor_before_animation = self.live_portrait_wrapper.calc_combined_lip_ratio(c_d_lip_before_animation, source_lmk)
+ if combined_lip_ratio_tensor_before_animation[0][0] < inf_cfg.lip_zero_threshold:
+ flag_lip_zero = False
+ else:
+ lip_delta_before_animation = self.live_portrait_wrapper.retarget_lip(x_s, combined_lip_ratio_tensor_before_animation)
+ ############################################
+
+ ######## process driving info ########
+ flag_load_from_template = is_template(args.driving_info)
+ driving_rgb_crop_256x256_lst = None
+ wfp_template = None
+
+ if flag_load_from_template:
+ # NOTE: load from template, it is fast, but the cropping video is None
+ log(f"Load from template: {args.driving_info}, NOT the video, so the cropping video and audio are both NULL.", style='bold green')
+ template_dct = load(args.driving_info)
+ n_frames = template_dct['n_frames']
+
+ # set output_fps
+ output_fps = template_dct.get('output_fps', inf_cfg.output_fps)
+ log(f'The FPS of template: {output_fps}')
+
+ if args.flag_crop_driving_video:
+ log("Warning: flag_crop_driving_video is True, but the driving info is a template, so it is ignored.")
+
+ elif osp.exists(args.driving_info) and is_video(args.driving_info):
+ # load from video file, AND make motion template
+ log(f"Load video: {args.driving_info}")
+ if osp.isdir(args.driving_info):
+ output_fps = inf_cfg.output_fps
+ else:
+ output_fps = int(get_fps(args.driving_info))
+ log(f'The FPS of {args.driving_info} is: {output_fps}')
+
+ log(f"Load video file (mp4 mov avi etc...): {args.driving_info}")
+ driving_rgb_lst = load_driving_info(args.driving_info)
+
+ ######## make motion template ########
+ log("Start making motion template...")
+ if inf_cfg.flag_crop_driving_video:
+ ret = self.cropper.crop_driving_video(driving_rgb_lst)
+ log(f'Driving video is cropped, {len(ret["frame_crop_lst"])} frames are processed.')
+ driving_rgb_crop_lst, driving_lmk_crop_lst = ret['frame_crop_lst'], ret['lmk_crop_lst']
+ driving_rgb_crop_256x256_lst = [cv2.resize(_, (256, 256)) for _ in driving_rgb_crop_lst]
+ else:
+ driving_lmk_crop_lst = self.cropper.calc_lmks_from_cropped_video(driving_rgb_lst)
+ driving_rgb_crop_256x256_lst = [cv2.resize(_, (256, 256)) for _ in driving_rgb_lst] # force to resize to 256x256
+
+ c_d_eyes_lst, c_d_lip_lst = self.live_portrait_wrapper.calc_driving_ratio(driving_lmk_crop_lst)
+ # save the motion template
+ I_d_lst = self.live_portrait_wrapper.prepare_driving_videos(driving_rgb_crop_256x256_lst)
+ template_dct = self.make_motion_template(I_d_lst, c_d_eyes_lst, c_d_lip_lst, output_fps=output_fps)
+
+ wfp_template = remove_suffix(args.driving_info) + '.pkl'
+ dump(wfp_template, template_dct)
+ log(f"Dump motion template to {wfp_template}")
+
+ n_frames = I_d_lst.shape[0]
+ else:
+ raise Exception(f"{args.driving_info} not exists or unsupported driving info types!")
+ #########################################
+
+ ######## prepare for pasteback ########
+ I_p_pstbk_lst = None
+ if inf_cfg.flag_pasteback and inf_cfg.flag_do_crop and inf_cfg.flag_stitching:
+ mask_ori_float = prepare_paste_back(inf_cfg.mask_crop, crop_info['M_c2o'], dsize=(img_rgb.shape[1], img_rgb.shape[0]))
+ I_p_pstbk_lst = []
+ log("Prepared pasteback mask done.")
+ #########################################
+
+ I_p_lst = []
+ R_d_0, x_d_0_info = None, None
+
+ for i in track(range(n_frames), description='🚀Animating...', total=n_frames):
+ x_d_i_info = template_dct['motion'][i]
+ x_d_i_info = dct2device(x_d_i_info, device)
+ R_d_i = x_d_i_info['R_d']
+
+ if i == 0:
+ R_d_0 = R_d_i
+ x_d_0_info = x_d_i_info
+
+ if inf_cfg.flag_relative_motion:
+ R_new = (R_d_i @ R_d_0.permute(0, 2, 1)) @ R_s
+ delta_new = x_s_info['exp'] + (x_d_i_info['exp'] - x_d_0_info['exp'])
+ scale_new = x_s_info['scale'] * (x_d_i_info['scale'] / x_d_0_info['scale'])
+ t_new = x_s_info['t'] + (x_d_i_info['t'] - x_d_0_info['t'])
+ else:
+ R_new = R_d_i
+ delta_new = x_d_i_info['exp']
+ scale_new = x_s_info['scale']
+ t_new = x_d_i_info['t']
+
+ t_new[..., 2].fill_(0) # zero tz
+ x_d_i_new = scale_new * (x_c_s @ R_new + delta_new) + t_new
+
+ # Algorithm 1:
+ if not inf_cfg.flag_stitching and not inf_cfg.flag_eye_retargeting and not inf_cfg.flag_lip_retargeting:
+ # without stitching or retargeting
+ if flag_lip_zero:
+ x_d_i_new += lip_delta_before_animation.reshape(-1, x_s.shape[1], 3)
+ else:
+ pass
+ elif inf_cfg.flag_stitching and not inf_cfg.flag_eye_retargeting and not inf_cfg.flag_lip_retargeting:
+ # with stitching and without retargeting
+ if flag_lip_zero:
+ x_d_i_new = self.live_portrait_wrapper.stitching(x_s, x_d_i_new) + lip_delta_before_animation.reshape(-1, x_s.shape[1], 3)
+ else:
+ x_d_i_new = self.live_portrait_wrapper.stitching(x_s, x_d_i_new)
+ else:
+ eyes_delta, lip_delta = None, None
+ if inf_cfg.flag_eye_retargeting:
+ c_d_eyes_i = c_d_eyes_lst[i]
+ combined_eye_ratio_tensor = self.live_portrait_wrapper.calc_combined_eye_ratio(c_d_eyes_i, source_lmk)
+ # ∆_eyes,i = R_eyes(x_s; c_s,eyes, c_d,eyes,i)
+ eyes_delta = self.live_portrait_wrapper.retarget_eye(x_s, combined_eye_ratio_tensor)
+ if inf_cfg.flag_lip_retargeting:
+ c_d_lip_i = c_d_lip_lst[i]
+ combined_lip_ratio_tensor = self.live_portrait_wrapper.calc_combined_lip_ratio(c_d_lip_i, source_lmk)
+ # ∆_lip,i = R_lip(x_s; c_s,lip, c_d,lip,i)
+ lip_delta = self.live_portrait_wrapper.retarget_lip(x_s, combined_lip_ratio_tensor)
+
+ if inf_cfg.flag_relative_motion: # use x_s
+ x_d_i_new = x_s + \
+ (eyes_delta.reshape(-1, x_s.shape[1], 3) if eyes_delta is not None else 0) + \
+ (lip_delta.reshape(-1, x_s.shape[1], 3) if lip_delta is not None else 0)
+ else: # use x_d,i
+ x_d_i_new = x_d_i_new + \
+ (eyes_delta.reshape(-1, x_s.shape[1], 3) if eyes_delta is not None else 0) + \
+ (lip_delta.reshape(-1, x_s.shape[1], 3) if lip_delta is not None else 0)
+
+ if inf_cfg.flag_stitching:
+ x_d_i_new = self.live_portrait_wrapper.stitching(x_s, x_d_i_new)
+
+ out = self.live_portrait_wrapper.warp_decode(f_s, x_s, x_d_i_new)
+ I_p_i = self.live_portrait_wrapper.parse_output(out['out'])[0]
+ I_p_lst.append(I_p_i)
+
+ if inf_cfg.flag_pasteback and inf_cfg.flag_do_crop and inf_cfg.flag_stitching:
+ # TODO: pasteback is slow, considering optimize it using multi-threading or GPU
+ I_p_pstbk = paste_back(I_p_i, crop_info['M_c2o'], img_rgb, mask_ori_float)
+ I_p_pstbk_lst.append(I_p_pstbk)
+
+ mkdir(args.output_dir)
+ wfp_concat = None
+ flag_has_audio = (not flag_load_from_template) and has_audio_stream(args.driving_info)
+
+ ######### build final concact result #########
+ # driving frame | source image | generation, or source image | generation
+ frames_concatenated = concat_frames(driving_rgb_crop_256x256_lst, img_crop_256x256, I_p_lst)
+ wfp_concat = osp.join(args.output_dir, f'{basename(args.source_image)}--{basename(args.driving_info)}_concat.mp4')
+ images2video(frames_concatenated, wfp=wfp_concat, fps=output_fps)
+
+ if flag_has_audio:
+ # final result with concact
+ wfp_concat_with_audio = osp.join(args.output_dir, f'{basename(args.source_image)}--{basename(args.driving_info)}_concat_with_audio.mp4')
+ add_audio_to_video(wfp_concat, args.driving_info, wfp_concat_with_audio)
+ os.replace(wfp_concat_with_audio, wfp_concat)
+ log(f"Replace {wfp_concat} with {wfp_concat_with_audio}")
+
+ # save drived result
+ wfp = osp.join(args.output_dir, f'{basename(args.source_image)}--{basename(args.driving_info)}.mp4')
+ if I_p_pstbk_lst is not None and len(I_p_pstbk_lst) > 0:
+ images2video(I_p_pstbk_lst, wfp=wfp, fps=output_fps)
+ else:
+ images2video(I_p_lst, wfp=wfp, fps=output_fps)
+
+ ######### build final result #########
+ if flag_has_audio:
+ wfp_with_audio = osp.join(args.output_dir, f'{basename(args.source_image)}--{basename(args.driving_info)}_with_audio.mp4')
+ add_audio_to_video(wfp, args.driving_info, wfp_with_audio)
+ os.replace(wfp_with_audio, wfp)
+ log(f"Replace {wfp} with {wfp_with_audio}")
+
+ # final log
+ if wfp_template not in (None, ''):
+ log(f'Animated template: {wfp_template}, you can specify `-d` argument with this template path next time to avoid cropping video, motion making and protecting privacy.', style='bold green')
+ log(f'Animated video: {wfp}')
+ log(f'Animated video with concact: {wfp_concat}')
+
+ return wfp, wfp_concat
+
+ def make_motion_template(self, I_d_lst, c_d_eyes_lst, c_d_lip_lst, **kwargs):
+ n_frames = I_d_lst.shape[0]
+ template_dct = {
+ 'n_frames': n_frames,
+ 'output_fps': kwargs.get('output_fps', 25),
+ 'motion': [],
+ 'c_d_eyes_lst': [],
+ 'c_d_lip_lst': [],
+ }
+
+ for i in track(range(n_frames), description='Making motion templates...', total=n_frames):
+ # collect s_d, R_d, δ_d and t_d for inference
+ I_d_i = I_d_lst[i]
+ x_d_i_info = self.live_portrait_wrapper.get_kp_info(I_d_i)
+ R_d_i = get_rotation_matrix(x_d_i_info['pitch'], x_d_i_info['yaw'], x_d_i_info['roll'])
+
+ item_dct = {
+ 'scale': x_d_i_info['scale'].cpu().numpy().astype(np.float32),
+ 'R_d': R_d_i.cpu().numpy().astype(np.float32),
+ 'exp': x_d_i_info['exp'].cpu().numpy().astype(np.float32),
+ 't': x_d_i_info['t'].cpu().numpy().astype(np.float32),
+ }
+
+ template_dct['motion'].append(item_dct)
+
+ c_d_eyes = c_d_eyes_lst[i].astype(np.float32)
+ template_dct['c_d_eyes_lst'].append(c_d_eyes)
+
+ c_d_lip = c_d_lip_lst[i].astype(np.float32)
+ template_dct['c_d_lip_lst'].append(c_d_lip)
+
+ return template_dct
diff --git a/difpoint/src/live_portrait_wrapper.py b/difpoint/src/live_portrait_wrapper.py
new file mode 100644
index 0000000000000000000000000000000000000000..6c5bd6eebe929d9aa535dc3000c48272e7cdf417
--- /dev/null
+++ b/difpoint/src/live_portrait_wrapper.py
@@ -0,0 +1,318 @@
+# coding: utf-8
+
+"""
+Wrapper for LivePortrait core functions
+"""
+
+import os.path as osp
+import numpy as np
+import cv2
+import torch
+import yaml
+
+from .utils.timer import Timer
+from .utils.helper import load_model, concat_feat
+from .utils.camera import headpose_pred_to_degree, get_rotation_matrix
+from .utils.retargeting_utils import calc_eye_close_ratio, calc_lip_close_ratio
+from .config.inference_config import InferenceConfig
+from .utils.rprint import rlog as log
+
+
+class LivePortraitWrapper(object):
+
+ def __init__(self, inference_cfg: InferenceConfig):
+
+ self.inference_cfg = inference_cfg
+ self.device_id = inference_cfg.device_id
+ self.compile = inference_cfg.flag_do_torch_compile
+ if inference_cfg.flag_force_cpu:
+ self.device = 'cpu'
+ else:
+ self.device = 'cuda:' + str(self.device_id)
+
+ model_config = yaml.load(open(inference_cfg.models_config, 'r'), Loader=yaml.SafeLoader)
+ # init F
+ self.appearance_feature_extractor = load_model(inference_cfg.checkpoint_F, model_config, self.device, 'appearance_feature_extractor')
+ log(f'Load appearance_feature_extractor done.')
+ # init M
+ self.motion_extractor = load_model(inference_cfg.checkpoint_M, model_config, self.device, 'motion_extractor')
+ log(f'Load motion_extractor done.')
+ # init W
+ self.warping_module = load_model(inference_cfg.checkpoint_W, model_config, self.device, 'warping_module')
+ log(f'Load warping_module done.')
+ # init G
+ self.spade_generator = load_model(inference_cfg.checkpoint_G, model_config, self.device, 'spade_generator')
+ log(f'Load spade_generator done.')
+ # init S and R
+ if inference_cfg.checkpoint_S is not None and osp.exists(inference_cfg.checkpoint_S):
+ self.stitching_retargeting_module = load_model(inference_cfg.checkpoint_S, model_config, self.device, 'stitching_retargeting_module')
+ log(f'Load stitching_retargeting_module done.')
+ else:
+ self.stitching_retargeting_module = None
+ # Optimize for inference
+ if self.compile:
+ self.warping_module = torch.compile(self.warping_module, mode='max-autotune')
+ self.spade_generator = torch.compile(self.spade_generator, mode='max-autotune')
+
+ self.timer = Timer()
+
+ def update_config(self, user_args):
+ for k, v in user_args.items():
+ if hasattr(self.inference_cfg, k):
+ setattr(self.inference_cfg, k, v)
+
+ def prepare_source(self, img: np.ndarray) -> torch.Tensor:
+ """ construct the input as standard
+ img: HxWx3, uint8, 256x256
+ """
+ h, w = img.shape[:2]
+ if h != self.inference_cfg.input_shape[0] or w != self.inference_cfg.input_shape[1]:
+ x = cv2.resize(img, (self.inference_cfg.input_shape[0], self.inference_cfg.input_shape[1]))
+ else:
+ x = img.copy()
+
+ if x.ndim == 3:
+ x = x[np.newaxis].astype(np.float32) / 255. # HxWx3 -> 1xHxWx3, normalized to 0~1
+ elif x.ndim == 4:
+ x = x.astype(np.float32) / 255. # BxHxWx3, normalized to 0~1
+ else:
+ raise ValueError(f'img ndim should be 3 or 4: {x.ndim}')
+ x = np.clip(x, 0, 1) # clip to 0~1
+ x = torch.from_numpy(x).permute(0, 3, 1, 2) # 1xHxWx3 -> 1x3xHxW
+ x = x.to(self.device)
+ return x
+
+ def prepare_driving_videos(self, imgs) -> torch.Tensor:
+ """ construct the input as standard
+ imgs: NxBxHxWx3, uint8
+ """
+ if isinstance(imgs, list):
+ _imgs = np.array(imgs)[..., np.newaxis] # TxHxWx3x1
+ elif isinstance(imgs, np.ndarray):
+ _imgs = imgs
+ else:
+ raise ValueError(f'imgs type error: {type(imgs)}')
+
+ y = _imgs.astype(np.float32) / 255.
+ y = np.clip(y, 0, 1) # clip to 0~1
+ y = torch.from_numpy(y).permute(0, 4, 3, 1, 2) # TxHxWx3x1 -> Tx1x3xHxW
+ y = y.to(self.device)
+
+ return y
+
+ def extract_feature_3d(self, x: torch.Tensor) -> torch.Tensor:
+ """ get the appearance feature of the image by F
+ x: Bx3xHxW, normalized to 0~1
+ """
+ with torch.no_grad():
+ with torch.autocast(device_type=self.device[:4], dtype=torch.float16, enabled=self.inference_cfg.flag_use_half_precision):
+ feature_3d = self.appearance_feature_extractor(x)
+
+ return feature_3d.float()
+
+ def get_kp_info(self, x: torch.Tensor, **kwargs) -> dict:
+ """ get the implicit keypoint information
+ x: Bx3xHxW, normalized to 0~1
+ flag_refine_info: whether to trandform the pose to degrees and the dimention of the reshape
+ return: A dict contains keys: 'pitch', 'yaw', 'roll', 't', 'exp', 'scale', 'kp'
+ """
+ with torch.no_grad():
+ with torch.autocast(device_type=self.device[:4], dtype=torch.float16, enabled=self.inference_cfg.flag_use_half_precision):
+ kp_info = self.motion_extractor(x)
+
+ if self.inference_cfg.flag_use_half_precision:
+ # float the dict
+ for k, v in kp_info.items():
+ if isinstance(v, torch.Tensor):
+ kp_info[k] = v.float()
+
+ flag_refine_info: bool = kwargs.get('flag_refine_info', True)
+ if flag_refine_info:
+ bs = kp_info['kp'].shape[0]
+ kp_info['pitch'] = headpose_pred_to_degree(kp_info['pitch'])[:, None] # Bx1
+ kp_info['yaw'] = headpose_pred_to_degree(kp_info['yaw'])[:, None] # Bx1
+ kp_info['roll'] = headpose_pred_to_degree(kp_info['roll'])[:, None] # Bx1
+ kp_info['kp'] = kp_info['kp'].reshape(bs, -1) # B,Nx3
+ kp_info['exp'] = kp_info['exp'].reshape(bs, -1) # B,Nx3
+
+ return kp_info
+
+ def get_pose_dct(self, kp_info: dict) -> dict:
+ pose_dct = dict(
+ pitch=headpose_pred_to_degree(kp_info['pitch']).item(),
+ yaw=headpose_pred_to_degree(kp_info['yaw']).item(),
+ roll=headpose_pred_to_degree(kp_info['roll']).item(),
+ )
+ return pose_dct
+
+ def get_fs_and_kp_info(self, source_prepared, driving_first_frame):
+
+ # get the canonical keypoints of source image by M
+ source_kp_info = self.get_kp_info(source_prepared, flag_refine_info=True)
+ source_rotation = get_rotation_matrix(source_kp_info['pitch'], source_kp_info['yaw'], source_kp_info['roll'])
+
+ # get the canonical keypoints of first driving frame by M
+ driving_first_frame_kp_info = self.get_kp_info(driving_first_frame, flag_refine_info=True)
+ driving_first_frame_rotation = get_rotation_matrix(
+ driving_first_frame_kp_info['pitch'],
+ driving_first_frame_kp_info['yaw'],
+ driving_first_frame_kp_info['roll']
+ )
+
+ # get feature volume by F
+ source_feature_3d = self.extract_feature_3d(source_prepared)
+
+ return source_kp_info, source_rotation, source_feature_3d, driving_first_frame_kp_info, driving_first_frame_rotation
+
+ def transform_keypoint(self, kp_info: dict):
+ """
+ transform the implicit keypoints with the pose, shift, and expression deformation
+ kp: BxNx3
+ """
+ kp = kp_info['kp'] # (bs, k, 3)
+ pitch, yaw, roll = kp_info['pitch'], kp_info['yaw'], kp_info['roll']
+
+ t, exp = kp_info['t'], kp_info['exp']
+ scale = kp_info['scale']
+
+ pitch = headpose_pred_to_degree(pitch)
+ yaw = headpose_pred_to_degree(yaw)
+ roll = headpose_pred_to_degree(roll)
+
+ bs = kp.shape[0]
+ if kp.ndim == 2:
+ num_kp = kp.shape[1] // 3 # Bx(num_kpx3)
+ else:
+ num_kp = kp.shape[1] # Bxnum_kpx3
+
+ rot_mat = get_rotation_matrix(pitch, yaw, roll) # (bs, 3, 3)
+
+ # Eqn.2: s * (R * x_c,s + exp) + t
+ kp_transformed = kp.view(bs, num_kp, 3) @ rot_mat + exp.view(bs, num_kp, 3)
+ kp_transformed *= scale[..., None] # (bs, k, 3) * (bs, 1, 1) = (bs, k, 3)
+ kp_transformed[:, :, 0:2] += t[:, None, 0:2] # remove z, only apply tx ty
+ # kp_transformed[:, :, :] += t[:, None, :]
+
+ return kp_transformed
+
+ def retarget_eye(self, kp_source: torch.Tensor, eye_close_ratio: torch.Tensor) -> torch.Tensor:
+ """
+ kp_source: BxNx3
+ eye_close_ratio: Bx3
+ Return: Bx(3*num_kp+2)
+ """
+ feat_eye = concat_feat(kp_source, eye_close_ratio)
+
+ with torch.no_grad():
+ delta = self.stitching_retargeting_module['eye'](feat_eye)
+
+ return delta
+
+ def retarget_lip(self, kp_source: torch.Tensor, lip_close_ratio: torch.Tensor) -> torch.Tensor:
+ """
+ kp_source: BxNx3
+ lip_close_ratio: Bx2
+ """
+ feat_lip = concat_feat(kp_source, lip_close_ratio)
+
+ with torch.no_grad():
+ delta = self.stitching_retargeting_module['lip'](feat_lip)
+
+ return delta
+
+ def stitch(self, kp_source: torch.Tensor, kp_driving: torch.Tensor) -> torch.Tensor:
+ """
+ kp_source: BxNx3
+ kp_driving: BxNx3
+ Return: Bx(3*num_kp+2)
+ """
+ feat_stiching = concat_feat(kp_source, kp_driving)
+
+ with torch.no_grad():
+ delta = self.stitching_retargeting_module['stitching'](feat_stiching)
+
+ return delta
+
+ def stitching(self, kp_source: torch.Tensor, kp_driving: torch.Tensor) -> torch.Tensor:
+ """ conduct the stitching
+ kp_source: Bxnum_kpx3
+ kp_driving: Bxnum_kpx3
+ """
+
+ if self.stitching_retargeting_module is not None:
+
+ bs, num_kp = kp_source.shape[:2]
+
+ kp_driving_new = kp_driving.clone()
+ delta = self.stitch(kp_source, kp_driving_new)
+
+ delta_exp = delta[..., :3*num_kp].reshape(bs, num_kp, 3) # 1x20x3
+ delta_tx_ty = delta[..., 3*num_kp:3*num_kp+2].reshape(bs, 1, 2) # 1x1x2
+
+ kp_driving_new += delta_exp
+ kp_driving_new[..., :2] += delta_tx_ty
+
+ return kp_driving_new
+
+ return kp_driving
+
+ def warp_decode(self, feature_3d: torch.Tensor, kp_source: torch.Tensor, kp_driving: torch.Tensor) -> torch.Tensor:
+ """ get the image after the warping of the implicit keypoints
+ feature_3d: Bx32x16x64x64, feature volume
+ kp_source: BxNx3
+ kp_driving: BxNx3
+ """
+ # The line 18 in Algorithm 1: D(W(f_s; x_s, x′_d,i))
+ with torch.no_grad():
+ with torch.autocast(device_type=self.device[:4], dtype=torch.float16, enabled=self.inference_cfg.flag_use_half_precision):
+ if self.compile:
+ # Mark the beginning of a new CUDA Graph step
+ torch.compiler.cudagraph_mark_step_begin()
+ # get decoder input
+ ret_dct = self.warping_module(feature_3d, kp_source=kp_source, kp_driving=kp_driving)
+ # decode
+ ret_dct['out'] = self.spade_generator(feature=ret_dct['out'])
+
+ # float the dict
+ if self.inference_cfg.flag_use_half_precision:
+ for k, v in ret_dct.items():
+ if isinstance(v, torch.Tensor):
+ ret_dct[k] = v.float()
+
+ return ret_dct
+
+ def parse_output(self, out: torch.Tensor) -> np.ndarray:
+ """ construct the output as standard
+ return: 1xHxWx3, uint8
+ """
+ out = np.transpose(out.data.cpu().numpy(), [0, 2, 3, 1]) # 1x3xHxW -> 1xHxWx3
+ out = np.clip(out, 0, 1) # clip to 0~1
+ out = np.clip(out * 255, 0, 255).astype(np.uint8) # 0~1 -> 0~255
+
+ return out
+
+ def calc_driving_ratio(self, driving_lmk_lst):
+ input_eye_ratio_lst = []
+ input_lip_ratio_lst = []
+ for lmk in driving_lmk_lst:
+ # for eyes retargeting
+ input_eye_ratio_lst.append(calc_eye_close_ratio(lmk[None]))
+ # for lip retargeting
+ input_lip_ratio_lst.append(calc_lip_close_ratio(lmk[None]))
+ return input_eye_ratio_lst, input_lip_ratio_lst
+
+ def calc_combined_eye_ratio(self, c_d_eyes_i, source_lmk):
+ c_s_eyes = calc_eye_close_ratio(source_lmk[None])
+ c_s_eyes_tensor = torch.from_numpy(c_s_eyes).float().to(self.device)
+ c_d_eyes_i_tensor = torch.Tensor([c_d_eyes_i[0][0]]).reshape(1, 1).to(self.device)
+ # [c_s,eyes, c_d,eyes,i]
+ combined_eye_ratio_tensor = torch.cat([c_s_eyes_tensor, c_d_eyes_i_tensor], dim=1)
+ return combined_eye_ratio_tensor
+
+ def calc_combined_lip_ratio(self, c_d_lip_i, source_lmk):
+ c_s_lip = calc_lip_close_ratio(source_lmk[None])
+ c_s_lip_tensor = torch.from_numpy(c_s_lip).float().to(self.device)
+ c_d_lip_i_tensor = torch.Tensor([c_d_lip_i[0]]).to(self.device).reshape(1, 1) # 1x1
+ # [c_s,lip, c_d,lip,i]
+ combined_lip_ratio_tensor = torch.cat([c_s_lip_tensor, c_d_lip_i_tensor], dim=1) # 1x2
+ return combined_lip_ratio_tensor
diff --git a/difpoint/src/models/XPose/__init__.py b/difpoint/src/models/XPose/__init__.py
new file mode 100644
index 0000000000000000000000000000000000000000..601cd005e85ae5bec92d05bf1e07b136d4fa6ee2
--- /dev/null
+++ b/difpoint/src/models/XPose/__init__.py
@@ -0,0 +1,6 @@
+# -*- coding: utf-8 -*-
+# @Time : 2024/8/5 21:58
+# @Author : shaoguowen
+# @Email : wenshaoguo1026@gmail.com
+# @Project : FasterLivePortrait
+# @FileName: __init__.py.py
diff --git a/difpoint/src/models/XPose/config_model/UniPose_SwinT.py b/difpoint/src/models/XPose/config_model/UniPose_SwinT.py
new file mode 100644
index 0000000000000000000000000000000000000000..64dde5dcc2fd043b3b413d7da3bdc98431cc168c
--- /dev/null
+++ b/difpoint/src/models/XPose/config_model/UniPose_SwinT.py
@@ -0,0 +1,125 @@
+_base_ = ['coco_transformer.py']
+
+use_label_enc = True
+
+num_classes=2
+
+lr = 0.0001
+param_dict_type = 'default'
+lr_backbone = 1e-05
+lr_backbone_names = ['backbone.0']
+lr_linear_proj_names = ['reference_points', 'sampling_offsets']
+lr_linear_proj_mult = 0.1
+ddetr_lr_param = False
+batch_size = 2
+weight_decay = 0.0001
+epochs = 12
+lr_drop = 11
+save_checkpoint_interval = 100
+clip_max_norm = 0.1
+onecyclelr = False
+multi_step_lr = False
+lr_drop_list = [33, 45]
+
+
+modelname = 'UniPose'
+frozen_weights = None
+backbone = 'swin_T_224_1k'
+
+
+dilation = False
+position_embedding = 'sine'
+pe_temperatureH = 20
+pe_temperatureW = 20
+return_interm_indices = [1, 2, 3]
+backbone_freeze_keywords = None
+enc_layers = 6
+dec_layers = 6
+unic_layers = 0
+pre_norm = False
+dim_feedforward = 2048
+hidden_dim = 256
+dropout = 0.0
+nheads = 8
+num_queries = 900
+query_dim = 4
+num_patterns = 0
+pdetr3_bbox_embed_diff_each_layer = False
+pdetr3_refHW = -1
+random_refpoints_xy = False
+fix_refpoints_hw = -1
+dabdetr_yolo_like_anchor_update = False
+dabdetr_deformable_encoder = False
+dabdetr_deformable_decoder = False
+use_deformable_box_attn = False
+box_attn_type = 'roi_align'
+dec_layer_number = None
+num_feature_levels = 4
+enc_n_points = 4
+dec_n_points = 4
+decoder_layer_noise = False
+dln_xy_noise = 0.2
+dln_hw_noise = 0.2
+add_channel_attention = False
+add_pos_value = False
+two_stage_type = 'standard'
+two_stage_pat_embed = 0
+two_stage_add_query_num = 0
+two_stage_bbox_embed_share = False
+two_stage_class_embed_share = False
+two_stage_learn_wh = False
+two_stage_default_hw = 0.05
+two_stage_keep_all_tokens = False
+num_select = 50
+transformer_activation = 'relu'
+batch_norm_type = 'FrozenBatchNorm2d'
+masks = False
+
+decoder_sa_type = 'sa' # ['sa', 'ca_label', 'ca_content']
+matcher_type = 'HungarianMatcher' # or SimpleMinsumMatcher
+decoder_module_seq = ['sa', 'ca', 'ffn']
+nms_iou_threshold = -1
+
+dec_pred_bbox_embed_share = True
+dec_pred_class_embed_share = True
+
+
+use_dn = True
+dn_number = 100
+dn_box_noise_scale = 1.0
+dn_label_noise_ratio = 0.5
+dn_label_coef=1.0
+dn_bbox_coef=1.0
+embed_init_tgt = True
+dn_labelbook_size = 2000
+
+match_unstable_error = True
+
+# for ema
+use_ema = True
+ema_decay = 0.9997
+ema_epoch = 0
+
+use_detached_boxes_dec_out = False
+
+max_text_len = 256
+shuffle_type = None
+
+use_text_enhancer = True
+use_fusion_layer = True
+
+use_checkpoint = False # True
+use_transformer_ckpt = True
+text_encoder_type = 'bert-base-uncased'
+
+use_text_cross_attention = True
+text_dropout = 0.0
+fusion_dropout = 0.0
+fusion_droppath = 0.1
+
+num_body_points=68
+binary_query_selection = False
+use_cdn = True
+ffn_extra_layernorm = False
+
+fix_size=False
diff --git a/difpoint/src/models/XPose/config_model/__init__.py b/difpoint/src/models/XPose/config_model/__init__.py
new file mode 100644
index 0000000000000000000000000000000000000000..601cd005e85ae5bec92d05bf1e07b136d4fa6ee2
--- /dev/null
+++ b/difpoint/src/models/XPose/config_model/__init__.py
@@ -0,0 +1,6 @@
+# -*- coding: utf-8 -*-
+# @Time : 2024/8/5 21:58
+# @Author : shaoguowen
+# @Email : wenshaoguo1026@gmail.com
+# @Project : FasterLivePortrait
+# @FileName: __init__.py.py
diff --git a/difpoint/src/models/XPose/config_model/coco_transformer.py b/difpoint/src/models/XPose/config_model/coco_transformer.py
new file mode 100644
index 0000000000000000000000000000000000000000..39b5f3d04c309f8cbb9893f7229faf2ebb74cc04
--- /dev/null
+++ b/difpoint/src/models/XPose/config_model/coco_transformer.py
@@ -0,0 +1,8 @@
+data_aug_scales = [480, 512, 544, 576, 608, 640, 672, 704, 736, 768, 800]
+data_aug_max_size = 1333
+data_aug_scales2_resize = [400, 500, 600]
+data_aug_scales2_crop = [384, 600]
+
+
+data_aug_scale_overlap = None
+
diff --git a/difpoint/src/models/XPose/models/UniPose/__init__.py b/difpoint/src/models/XPose/models/UniPose/__init__.py
new file mode 100644
index 0000000000000000000000000000000000000000..f03be4ddc6741dd1b45ff39fd0791f213ed1dcac
--- /dev/null
+++ b/difpoint/src/models/XPose/models/UniPose/__init__.py
@@ -0,0 +1,10 @@
+# ------------------------------------------------------------------------
+# Conditional DETR
+# Copyright (c) 2021 Microsoft. All Rights Reserved.
+# Licensed under the Apache License, Version 2.0 [see LICENSE for details]
+# ------------------------------------------------------------------------
+# Copied from DETR (https://github.com/facebookresearch/detr)
+# Copyright (c) Facebook, Inc. and its affiliates. All Rights Reserved.
+# ------------------------------------------------------------------------
+
+from .unipose import build_unipose
diff --git a/difpoint/src/models/XPose/models/UniPose/attention.py b/difpoint/src/models/XPose/models/UniPose/attention.py
new file mode 100644
index 0000000000000000000000000000000000000000..0f8710ea0912d7fa6468be0f1319f61d18d1d0a7
--- /dev/null
+++ b/difpoint/src/models/XPose/models/UniPose/attention.py
@@ -0,0 +1,373 @@
+# ------------------------------------------------------------------------
+# UniPose
+# url: https://github.com/IDEA-Research/UniPose
+# Copyright (c) 2023 IDEA. All Rights Reserved.
+# Licensed under the Apache License, Version 2.0 [see LICENSE for details]
+# ------------------------------------------------------------------------
+# ED-Pose
+# Copyright (c) 2023 IDEA. All Rights Reserved.
+# Licensed under the Apache License, Version 2.0 [see LICENSE for details]
+# ------------------------------------------------------------------------
+# Conditional DETR
+# Copyright (c) 2021 Microsoft. All Rights Reserved.
+# Licensed under the Apache License, Version 2.0 [see LICENSE for details]
+# ------------------------------------------------------------------------
+# Modified from codes in torch.nn
+# ------------------------------------------------------------------------
+
+"""
+MultiheadAttention that support query, key, and value to have different dimensions.
+Query, key, and value projections are removed.
+
+Mostly copy-paste from https://github.com/pytorch/pytorch/blob/master/torch/nn/modules/activation.py#L873
+and https://github.com/pytorch/pytorch/blob/master/torch/nn/functional.py#L4837
+"""
+
+import warnings
+import torch
+from torch.nn.modules.linear import Linear
+from torch.nn.init import constant_
+from torch.nn.modules.module import Module
+from torch._jit_internal import Optional, Tuple
+try:
+ from torch.overrides import has_torch_function, handle_torch_function
+except:
+ from torch._overrides import has_torch_function, handle_torch_function
+from torch.nn.functional import linear, pad, softmax, dropout
+Tensor = torch.Tensor
+
+class MultiheadAttention(Module):
+ r"""Allows the model to jointly attend to information
+ from different representation subspaces.
+ See reference: Attention Is All You Need
+ .. math::
+ \text{MultiHead}(Q, K, V) = \text{Concat}(head_1,\dots,head_h)W^O
+ \text{where} head_i = \text{Attention}(QW_i^Q, KW_i^K, VW_i^V)
+ Args:
+ embed_dim: total dimension of the model.
+ num_heads: parallel attention heads.
+ dropout: a Dropout layer on attn_output_weights. Default: 0.0.
+ bias: add bias as module parameter. Default: True.
+ add_bias_kv: add bias to the key and value sequences at dim=0.
+ add_zero_attn: add a new batch of zeros to the key and
+ value sequences at dim=1.
+ kdim: total number of features in key. Default: None.
+ vdim: total number of features in value. Default: None.
+ Note: if kdim and vdim are None, they will be set to embed_dim such that
+ query, key, and value have the same number of features.
+ Examples::
+ >>> multihead_attn = nn.MultiheadAttention(embed_dim, num_heads)
+ >>> attn_output, attn_output_weights = multihead_attn(query, key, value)
+ """
+ bias_k: Optional[torch.Tensor]
+ bias_v: Optional[torch.Tensor]
+
+ def __init__(self, embed_dim, num_heads, dropout=0., bias=True, add_bias_kv=False, add_zero_attn=False, kdim=None, vdim=None):
+ super(MultiheadAttention, self).__init__()
+ self.embed_dim = embed_dim
+ self.kdim = kdim if kdim is not None else embed_dim
+ self.vdim = vdim if vdim is not None else embed_dim
+ self._qkv_same_embed_dim = self.kdim == embed_dim and self.vdim == embed_dim
+
+ self.num_heads = num_heads
+ self.dropout = dropout
+ self.head_dim = embed_dim // num_heads
+ assert self.head_dim * num_heads == self.embed_dim, "embed_dim must be divisible by num_heads"
+
+ vdim = vdim if vdim is not None else embed_dim
+ self.out_proj = Linear(vdim , vdim)
+
+ self.in_proj_bias = None
+ self.in_proj_weight = None
+ self.bias_k = self.bias_v = None
+ self.q_proj_weight = None
+ self.k_proj_weight = None
+ self.v_proj_weight = None
+
+ self.add_zero_attn = add_zero_attn
+
+ self._reset_parameters()
+
+ def _reset_parameters(self):
+ constant_(self.out_proj.bias, 0.)
+
+ def __setstate__(self, state):
+ # Support loading old MultiheadAttention checkpoints generated by v1.1.0
+ if '_qkv_same_embed_dim' not in state:
+ state['_qkv_same_embed_dim'] = True
+
+ super(MultiheadAttention, self).__setstate__(state)
+
+ def forward(self, query, key, value, key_padding_mask=None,
+ need_weights=True, attn_mask=None):
+ # type: (Tensor, Tensor, Tensor, Optional[Tensor], bool, Optional[Tensor]) -> Tuple[Tensor, Optional[Tensor]]
+ r"""
+ Args:
+ query, key, value: map a query and a set of key-value pairs to an output.
+ See "Attention Is All You Need" for more details.
+ key_padding_mask: if provided, specified padding elements in the key will
+ be ignored by the attention. When given a binary mask and a value is True,
+ the corresponding value on the attention layer will be ignored. When given
+ a byte mask and a value is non-zero, the corresponding value on the attention
+ layer will be ignored
+ need_weights: output attn_output_weights.
+ attn_mask: 2D or 3D mask that prevents attention to certain positions. A 2D mask will be broadcasted for all
+ the batches while a 3D mask allows to specify a different mask for the entries of each batch.
+ Shape:
+ - Inputs:
+ - query: :math:`(L, N, E)` where L is the target sequence length, N is the batch size, E is
+ the embedding dimension.
+ - key: :math:`(S, N, E)`, where S is the source sequence length, N is the batch size, E is
+ the embedding dimension.
+ - value: :math:`(S, N, E)` where S is the source sequence length, N is the batch size, E is
+ the embedding dimension.
+ - key_padding_mask: :math:`(N, S)` where N is the batch size, S is the source sequence length.
+ If a ByteTensor is provided, the non-zero positions will be ignored while the position
+ with the zero positions will be unchanged. If a BoolTensor is provided, the positions with the
+ value of ``True`` will be ignored while the position with the value of ``False`` will be unchanged.
+ - attn_mask: 2D mask :math:`(L, S)` where L is the target sequence length, S is the source sequence length.
+ 3D mask :math:`(N*\text{num_heads}, L, S)` where N is the batch size, L is the target sequence length,
+ S is the source sequence length. attn_mask ensure that position i is allowed to attend the unmasked
+ positions. If a ByteTensor is provided, the non-zero positions are not allowed to attend
+ while the zero positions will be unchanged. If a BoolTensor is provided, positions with ``True``
+ is not allowed to attend while ``False`` values will be unchanged. If a FloatTensor
+ is provided, it will be added to the attention weight.
+ - Outputs:
+ - attn_output: :math:`(L, N, E)` where L is the target sequence length, N is the batch size,
+ E is the embedding dimension.
+ - attn_output_weights: :math:`(N, L, S)` where N is the batch size,
+ L is the target sequence length, S is the source sequence length.
+ """
+ if not self._qkv_same_embed_dim:
+ return multi_head_attention_forward(
+ query, key, value, self.embed_dim, self.num_heads,
+ self.in_proj_weight, self.in_proj_bias,
+ self.bias_k, self.bias_v, self.add_zero_attn,
+ self.dropout, self.out_proj.weight, self.out_proj.bias,
+ training=self.training,
+ key_padding_mask=key_padding_mask, need_weights=need_weights,
+ attn_mask=attn_mask, use_separate_proj_weight=True,
+ q_proj_weight=self.q_proj_weight, k_proj_weight=self.k_proj_weight,
+ v_proj_weight=self.v_proj_weight, out_dim=self.vdim)
+ else:
+ return multi_head_attention_forward(
+ query, key, value, self.embed_dim, self.num_heads,
+ self.in_proj_weight, self.in_proj_bias,
+ self.bias_k, self.bias_v, self.add_zero_attn,
+ self.dropout, self.out_proj.weight, self.out_proj.bias,
+ training=self.training,
+ key_padding_mask=key_padding_mask, need_weights=need_weights,
+ attn_mask=attn_mask, out_dim=self.vdim)
+
+
+def multi_head_attention_forward(query: Tensor,
+ key: Tensor,
+ value: Tensor,
+ embed_dim_to_check: int,
+ num_heads: int,
+ in_proj_weight: Tensor,
+ in_proj_bias: Tensor,
+ bias_k: Optional[Tensor],
+ bias_v: Optional[Tensor],
+ add_zero_attn: bool,
+ dropout_p: float,
+ out_proj_weight: Tensor,
+ out_proj_bias: Tensor,
+ training: bool = True,
+ key_padding_mask: Optional[Tensor] = None,
+ need_weights: bool = True,
+ attn_mask: Optional[Tensor] = None,
+ use_separate_proj_weight: bool = False,
+ q_proj_weight: Optional[Tensor] = None,
+ k_proj_weight: Optional[Tensor] = None,
+ v_proj_weight: Optional[Tensor] = None,
+ static_k: Optional[Tensor] = None,
+ static_v: Optional[Tensor] = None,
+ out_dim: Optional[Tensor] = None
+ ) -> Tuple[Tensor, Optional[Tensor]]:
+ r"""
+ Args:
+ query, key, value: map a query and a set of key-value pairs to an output.
+ See "Attention Is All You Need" for more details.
+ embed_dim_to_check: total dimension of the model.
+ num_heads: parallel attention heads.
+ in_proj_weight, in_proj_bias: input projection weight and bias.
+ bias_k, bias_v: bias of the key and value sequences to be added at dim=0.
+ add_zero_attn: add a new batch of zeros to the key and
+ value sequences at dim=1.
+ dropout_p: probability of an element to be zeroed.
+ out_proj_weight, out_proj_bias: the output projection weight and bias.
+ training: apply dropout if is ``True``.
+ key_padding_mask: if provided, specified padding elements in the key will
+ be ignored by the attention. This is an binary mask. When the value is True,
+ the corresponding value on the attention layer will be filled with -inf.
+ need_weights: output attn_output_weights.
+ attn_mask: 2D or 3D mask that prevents attention to certain positions. A 2D mask will be broadcasted for all
+ the batches while a 3D mask allows to specify a different mask for the entries of each batch.
+ use_separate_proj_weight: the function accept the proj. weights for query, key,
+ and value in different forms. If false, in_proj_weight will be used, which is
+ a combination of q_proj_weight, k_proj_weight, v_proj_weight.
+ q_proj_weight, k_proj_weight, v_proj_weight, in_proj_bias: input projection weight and bias.
+ static_k, static_v: static key and value used for attention operators.
+ Shape:
+ Inputs:
+ - query: :math:`(L, N, E)` where L is the target sequence length, N is the batch size, E is
+ the embedding dimension.
+ - key: :math:`(S, N, E)`, where S is the source sequence length, N is the batch size, E is
+ the embedding dimension.
+ - value: :math:`(S, N, E)` where S is the source sequence length, N is the batch size, E is
+ the embedding dimension.
+ - key_padding_mask: :math:`(N, S)` where N is the batch size, S is the source sequence length.
+ If a ByteTensor is provided, the non-zero positions will be ignored while the zero positions
+ will be unchanged. If a BoolTensor is provided, the positions with the
+ value of ``True`` will be ignored while the position with the value of ``False`` will be unchanged.
+ - attn_mask: 2D mask :math:`(L, S)` where L is the target sequence length, S is the source sequence length.
+ 3D mask :math:`(N*num_heads, L, S)` where N is the batch size, L is the target sequence length,
+ S is the source sequence length. attn_mask ensures that position i is allowed to attend the unmasked
+ positions. If a ByteTensor is provided, the non-zero positions are not allowed to attend
+ while the zero positions will be unchanged. If a BoolTensor is provided, positions with ``True``
+ are not allowed to attend while ``False`` values will be unchanged. If a FloatTensor
+ is provided, it will be added to the attention weight.
+ - static_k: :math:`(N*num_heads, S, E/num_heads)`, where S is the source sequence length,
+ N is the batch size, E is the embedding dimension. E/num_heads is the head dimension.
+ - static_v: :math:`(N*num_heads, S, E/num_heads)`, where S is the source sequence length,
+ N is the batch size, E is the embedding dimension. E/num_heads is the head dimension.
+ Outputs:
+ - attn_output: :math:`(L, N, E)` where L is the target sequence length, N is the batch size,
+ E is the embedding dimension.
+ - attn_output_weights: :math:`(N, L, S)` where N is the batch size,
+ L is the target sequence length, S is the source sequence length.
+ """
+ if not torch.jit.is_scripting():
+ tens_ops = (query, key, value, in_proj_weight, in_proj_bias, bias_k, bias_v,
+ out_proj_weight, out_proj_bias)
+ if any([type(t) is not Tensor for t in tens_ops]) and has_torch_function(tens_ops):
+ return handle_torch_function(
+ multi_head_attention_forward, tens_ops, query, key, value,
+ embed_dim_to_check, num_heads, in_proj_weight, in_proj_bias,
+ bias_k, bias_v, add_zero_attn, dropout_p, out_proj_weight,
+ out_proj_bias, training=training, key_padding_mask=key_padding_mask,
+ need_weights=need_weights, attn_mask=attn_mask,
+ use_separate_proj_weight=use_separate_proj_weight,
+ q_proj_weight=q_proj_weight, k_proj_weight=k_proj_weight,
+ v_proj_weight=v_proj_weight, static_k=static_k, static_v=static_v)
+ tgt_len, bsz, embed_dim = query.size()
+ assert embed_dim == embed_dim_to_check
+ # allow MHA to have different sizes for the feature dimension
+ assert key.size(0) == value.size(0) and key.size(1) == value.size(1)
+
+ head_dim = embed_dim // num_heads
+ v_head_dim = out_dim // num_heads
+ assert head_dim * num_heads == embed_dim, "embed_dim must be divisible by num_heads"
+ scaling = float(head_dim) ** -0.5
+
+ q = query * scaling
+ k = key
+ v = value
+
+ if attn_mask is not None:
+ assert attn_mask.dtype == torch.float32 or attn_mask.dtype == torch.float64 or \
+ attn_mask.dtype == torch.float16 or attn_mask.dtype == torch.uint8 or attn_mask.dtype == torch.bool, \
+ 'Only float, byte, and bool types are supported for attn_mask, not {}'.format(attn_mask.dtype)
+ if attn_mask.dtype == torch.uint8:
+ warnings.warn("Byte tensor for attn_mask in nn.MultiheadAttention is deprecated. Use bool tensor instead.")
+ attn_mask = attn_mask.to(torch.bool)
+
+ if attn_mask.dim() == 2:
+ attn_mask = attn_mask.unsqueeze(0)
+ if list(attn_mask.size()) != [1, query.size(0), key.size(0)]:
+ raise RuntimeError('The size of the 2D attn_mask is not correct.')
+ elif attn_mask.dim() == 3:
+ if list(attn_mask.size()) != [bsz * num_heads, query.size(0), key.size(0)]:
+ raise RuntimeError('The size of the 3D attn_mask is not correct.')
+ else:
+ raise RuntimeError("attn_mask's dimension {} is not supported".format(attn_mask.dim()))
+ # attn_mask's dim is 3 now.
+
+ # convert ByteTensor key_padding_mask to bool
+ if key_padding_mask is not None and key_padding_mask.dtype == torch.uint8:
+ warnings.warn("Byte tensor for key_padding_mask in nn.MultiheadAttention is deprecated. Use bool tensor instead.")
+ key_padding_mask = key_padding_mask.to(torch.bool)
+
+ if bias_k is not None and bias_v is not None:
+ if static_k is None and static_v is None:
+ k = torch.cat([k, bias_k.repeat(1, bsz, 1)])
+ v = torch.cat([v, bias_v.repeat(1, bsz, 1)])
+ if attn_mask is not None:
+ attn_mask = pad(attn_mask, (0, 1))
+ if key_padding_mask is not None:
+ key_padding_mask = pad(key_padding_mask, (0, 1))
+ else:
+ assert static_k is None, "bias cannot be added to static key."
+ assert static_v is None, "bias cannot be added to static value."
+ else:
+ assert bias_k is None
+ assert bias_v is None
+
+ q = q.contiguous().view(tgt_len, bsz * num_heads, head_dim).transpose(0, 1)
+ if k is not None:
+ k = k.contiguous().view(-1, bsz * num_heads, head_dim).transpose(0, 1)
+ if v is not None:
+ v = v.contiguous().view(-1, bsz * num_heads, v_head_dim).transpose(0, 1)
+
+ if static_k is not None:
+ assert static_k.size(0) == bsz * num_heads
+ assert static_k.size(2) == head_dim
+ k = static_k
+
+ if static_v is not None:
+ assert static_v.size(0) == bsz * num_heads
+ assert static_v.size(2) == v_head_dim
+ v = static_v
+
+ src_len = k.size(1)
+
+ if key_padding_mask is not None:
+ assert key_padding_mask.size(0) == bsz
+ assert key_padding_mask.size(1) == src_len
+
+ if add_zero_attn:
+ src_len += 1
+ k = torch.cat([k, torch.zeros((k.size(0), 1) + k.size()[2:], dtype=k.dtype, device=k.device)], dim=1)
+ v = torch.cat([v, torch.zeros((v.size(0), 1) + v.size()[2:], dtype=v.dtype, device=v.device)], dim=1)
+ if attn_mask is not None:
+ attn_mask = pad(attn_mask, (0, 1))
+ if key_padding_mask is not None:
+ key_padding_mask = pad(key_padding_mask, (0, 1))
+
+ attn_output_weights = torch.bmm(q, k.transpose(1, 2))
+ assert list(attn_output_weights.size()) == [bsz * num_heads, tgt_len, src_len]
+
+ if attn_mask is not None:
+ if attn_mask.dtype == torch.bool:
+ attn_output_weights.masked_fill_(attn_mask, float('-inf'))
+ else:
+ attn_output_weights += attn_mask
+
+
+ if key_padding_mask is not None:
+ attn_output_weights = attn_output_weights.view(bsz, num_heads, tgt_len, src_len)
+ attn_output_weights = attn_output_weights.masked_fill(
+ key_padding_mask.unsqueeze(1).unsqueeze(2),
+ float('-inf'),
+ )
+ attn_output_weights = attn_output_weights.view(bsz * num_heads, tgt_len, src_len)
+
+ # attn_output_weights = softmax(
+ # attn_output_weights, dim=-1)
+ attn_output_weights = softmax(
+ attn_output_weights - attn_output_weights.max(dim=-1, keepdim=True)[0], dim=-1)
+ attn_output_weights = dropout(attn_output_weights, p=dropout_p, training=training)
+
+ attn_output = torch.bmm(attn_output_weights, v)
+ assert list(attn_output.size()) == [bsz * num_heads, tgt_len, v_head_dim]
+ attn_output = attn_output.transpose(0, 1).contiguous().view(tgt_len, bsz, out_dim)
+ attn_output = linear(attn_output, out_proj_weight, out_proj_bias)
+
+ if need_weights:
+ # average attention weights over heads
+ attn_output_weights = attn_output_weights.view(bsz, num_heads, tgt_len, src_len)
+ return attn_output, attn_output_weights.sum(dim=1) / num_heads
+ else:
+ return attn_output, None
+
diff --git a/difpoint/src/models/XPose/models/UniPose/backbone.py b/difpoint/src/models/XPose/models/UniPose/backbone.py
new file mode 100644
index 0000000000000000000000000000000000000000..ddf1f18da5a2836fa12c3f5231af5a5d102204f7
--- /dev/null
+++ b/difpoint/src/models/XPose/models/UniPose/backbone.py
@@ -0,0 +1,211 @@
+# ------------------------------------------------------------------------
+# UniPose
+# url: https://github.com/IDEA-Research/UniPose
+# Copyright (c) 2023 IDEA. All Rights Reserved.
+# Licensed under the Apache License, Version 2.0 [see LICENSE for details]
+# ------------------------------------------------------------------------
+# Conditional DETR
+# Copyright (c) 2021 Microsoft. All Rights Reserved.
+# Licensed under the Apache License, Version 2.0 [see LICENSE for details]
+# ------------------------------------------------------------------------
+# Copied from DETR (https://github.com/facebookresearch/detr)
+# Copyright (c) Facebook, Inc. and its affiliates. All Rights Reserved.
+# ------------------------------------------------------------------------
+
+"""
+Backbone modules.
+"""
+
+import torch
+import torch.nn.functional as F
+import torchvision
+from torch import nn
+from torchvision.models._utils import IntermediateLayerGetter
+from typing import Dict, List
+
+from ...util.misc import NestedTensor, is_main_process
+
+from .position_encoding import build_position_encoding
+from .swin_transformer import build_swin_transformer
+
+class FrozenBatchNorm2d(torch.nn.Module):
+ """
+ BatchNorm2d where the batch statistics and the affine parameters are fixed.
+
+ Copy-paste from torchvision.misc.ops with added eps before rqsrt,
+ without which any other models than torchvision.models.resnet[18,34,50,101]
+ produce nans.
+ """
+
+ def __init__(self, n):
+ super(FrozenBatchNorm2d, self).__init__()
+ self.register_buffer("weight", torch.ones(n))
+ self.register_buffer("bias", torch.zeros(n))
+ self.register_buffer("running_mean", torch.zeros(n))
+ self.register_buffer("running_var", torch.ones(n))
+
+ def _load_from_state_dict(
+ self, state_dict, prefix, local_metadata, strict, missing_keys, unexpected_keys, error_msgs
+ ):
+ num_batches_tracked_key = prefix + "num_batches_tracked"
+ if num_batches_tracked_key in state_dict:
+ del state_dict[num_batches_tracked_key]
+
+ super(FrozenBatchNorm2d, self)._load_from_state_dict(
+ state_dict, prefix, local_metadata, strict, missing_keys, unexpected_keys, error_msgs
+ )
+
+ def forward(self, x):
+ # move reshapes to the beginning
+ # to make it fuser-friendly
+ w = self.weight.reshape(1, -1, 1, 1)
+ b = self.bias.reshape(1, -1, 1, 1)
+ rv = self.running_var.reshape(1, -1, 1, 1)
+ rm = self.running_mean.reshape(1, -1, 1, 1)
+ eps = 1e-5
+ scale = w * (rv + eps).rsqrt()
+ bias = b - rm * scale
+ return x * scale + bias
+
+
+class BackboneBase(nn.Module):
+ def __init__(
+ self,
+ backbone: nn.Module,
+ train_backbone: bool,
+ num_channels: int,
+ return_interm_indices: list,
+ ):
+ super().__init__()
+ for name, parameter in backbone.named_parameters():
+ if (
+ not train_backbone
+ or "layer2" not in name
+ and "layer3" not in name
+ and "layer4" not in name
+ ):
+ parameter.requires_grad_(False)
+
+ return_layers = {}
+ for idx, layer_index in enumerate(return_interm_indices):
+ return_layers.update(
+ {"layer{}".format(5 - len(return_interm_indices) + idx): "{}".format(layer_index)}
+ )
+
+ self.body = IntermediateLayerGetter(backbone, return_layers=return_layers)
+ self.num_channels = num_channels
+
+ def forward(self, tensor_list: NestedTensor):
+ xs = self.body(tensor_list.tensors)
+ out: Dict[str, NestedTensor] = {}
+ for name, x in xs.items():
+ m = tensor_list.mask
+ assert m is not None
+ mask = F.interpolate(m[None].float(), size=x.shape[-2:]).to(torch.bool)[0]
+ out[name] = NestedTensor(x, mask)
+ # import ipdb; ipdb.set_trace()
+ return out
+
+
+class Backbone(BackboneBase):
+ """ResNet backbone with frozen BatchNorm."""
+
+ def __init__(
+ self,
+ name: str,
+ train_backbone: bool,
+ dilation: bool,
+ return_interm_indices: list,
+ batch_norm=FrozenBatchNorm2d,
+ ):
+ if name in ["resnet18", "resnet34", "resnet50", "resnet101"]:
+ backbone = getattr(torchvision.models, name)(
+ replace_stride_with_dilation=[False, False, dilation],
+ pretrained=is_main_process(),
+ norm_layer=batch_norm,
+ )
+ else:
+ raise NotImplementedError("Why you can get here with name {}".format(name))
+ # num_channels = 512 if name in ('resnet18', 'resnet34') else 2048
+ assert name not in ("resnet18", "resnet34"), "Only resnet50 and resnet101 are available."
+ assert return_interm_indices in [[0, 1, 2, 3], [1, 2, 3], [3]]
+ num_channels_all = [256, 512, 1024, 2048]
+ num_channels = num_channels_all[4 - len(return_interm_indices) :]
+ super().__init__(backbone, train_backbone, num_channels, return_interm_indices)
+
+
+class Joiner(nn.Sequential):
+ def __init__(self, backbone, position_embedding):
+ super().__init__(backbone, position_embedding)
+
+ def forward(self, tensor_list: NestedTensor):
+ xs = self[0](tensor_list)
+ out: List[NestedTensor] = []
+ pos = []
+ for name, x in xs.items():
+ out.append(x)
+ # position encoding
+ pos.append(self[1](x).to(x.tensors.dtype))
+
+ return out, pos
+
+
+def build_backbone(args):
+ """
+ Useful args:
+ - backbone: backbone name
+ - lr_backbone:
+ - dilation
+ - return_interm_indices: available: [0,1,2,3], [1,2,3], [3]
+ - backbone_freeze_keywords:
+ - use_checkpoint: for swin only for now
+
+ """
+ position_embedding = build_position_encoding(args)
+ train_backbone = True
+ if not train_backbone:
+ raise ValueError("Please set lr_backbone > 0")
+ return_interm_indices = args.return_interm_indices
+ assert return_interm_indices in [[0, 1, 2, 3], [1, 2, 3], [3]]
+ args.backbone_freeze_keywords
+ use_checkpoint = getattr(args, "use_checkpoint", False)
+
+ if args.backbone in ["resnet50", "resnet101"]:
+ backbone = Backbone(
+ args.backbone,
+ train_backbone,
+ args.dilation,
+ return_interm_indices,
+ batch_norm=FrozenBatchNorm2d,
+ )
+ bb_num_channels = backbone.num_channels
+ elif args.backbone in [
+ "swin_T_224_1k",
+ "swin_B_224_22k",
+ "swin_B_384_22k",
+ "swin_L_224_22k",
+ "swin_L_384_22k",
+ ]:
+ pretrain_img_size = int(args.backbone.split("_")[-2])
+ backbone = build_swin_transformer(
+ args.backbone,
+ pretrain_img_size=pretrain_img_size,
+ out_indices=tuple(return_interm_indices),
+ dilation=False,
+ use_checkpoint=use_checkpoint,
+ )
+
+ bb_num_channels = backbone.num_features[4 - len(return_interm_indices) :]
+ else:
+ raise NotImplementedError("Unknown backbone {}".format(args.backbone))
+
+ assert len(bb_num_channels) == len(
+ return_interm_indices
+ ), f"len(bb_num_channels) {len(bb_num_channels)} != len(return_interm_indices) {len(return_interm_indices)}"
+
+ model = Joiner(backbone, position_embedding)
+ model.num_channels = bb_num_channels
+ assert isinstance(
+ bb_num_channels, List
+ ), "bb_num_channels is expected to be a List but {}".format(type(bb_num_channels))
+ return model
diff --git a/difpoint/src/models/XPose/models/UniPose/deformable_transformer.py b/difpoint/src/models/XPose/models/UniPose/deformable_transformer.py
new file mode 100644
index 0000000000000000000000000000000000000000..558d4ffba66692125c164321765f5337096e6797
--- /dev/null
+++ b/difpoint/src/models/XPose/models/UniPose/deformable_transformer.py
@@ -0,0 +1,1230 @@
+# ------------------------------------------------------------------------
+# UniPose
+# url: https://github.com/IDEA-Research/UniPose
+# Copyright (c) 2023 IDEA. All Rights Reserved.
+# Licensed under the Apache License, Version 2.0 [see LICENSE for details]
+# ------------------------------------------------------------------------
+# ED-Pose
+# Copyright (c) 2023 IDEA. All Rights Reserved.
+# Licensed under the Apache License, Version 2.0 [see LICENSE for details]
+# ------------------------------------------------------------------------
+# DINO
+# Copyright (c) 2022 IDEA. All Rights Reserved.
+# Licensed under the Apache License, Version 2.0 [see LICENSE for details]
+# ------------------------------------------------------------------------
+# Modified from DETR (https://github.com/facebookresearch/detr)
+# Copyright (c) Facebook, Inc. and its affiliates. All Rights Reserved.
+# ------------------------------------------------------------------------
+
+import math
+import copy
+import torch
+import torch.utils.checkpoint as checkpoint
+from torch import nn, Tensor
+from typing import Optional
+from ...util.misc import inverse_sigmoid
+
+from .transformer_vanilla import TransformerEncoderLayer
+from .fuse_modules import BiAttentionBlock
+from .utils import gen_encoder_output_proposals, MLP, _get_activation_fn, gen_sineembed_for_position, get_sine_pos_embed
+from .ops.modules import MSDeformAttn
+
+
+class DeformableTransformer(nn.Module):
+
+ def __init__(self, d_model=256, nhead=8,
+ num_queries=300,
+ num_encoder_layers=6,
+ num_unicoder_layers=0,
+ num_decoder_layers=6,
+ dim_feedforward=2048, dropout=0.0,
+ activation="relu", normalize_before=False,
+ return_intermediate_dec=False, query_dim=4,
+ num_patterns=0,
+ modulate_hw_attn=False,
+ # for deformable encoder
+ deformable_encoder=False,
+ deformable_decoder=False,
+ num_feature_levels=1,
+ enc_n_points=4,
+ dec_n_points=4,
+ use_deformable_box_attn=False,
+ box_attn_type='roi_align',
+ # init query
+ learnable_tgt_init=False,
+ decoder_query_perturber=None,
+ add_channel_attention=False,
+ add_pos_value=False,
+ random_refpoints_xy=False,
+ # two stage
+ two_stage_type='no',
+ two_stage_pat_embed=0,
+ two_stage_add_query_num=0,
+ two_stage_learn_wh=False,
+ two_stage_keep_all_tokens=False,
+ # evo of #anchors
+ dec_layer_number=None,
+ rm_enc_query_scale=True,
+ rm_dec_query_scale=True,
+ rm_self_attn_layers=None,
+ key_aware_type=None,
+ # layer share
+ layer_share_type=None,
+ # for detach
+ rm_detach=None,
+ decoder_sa_type='ca',
+ module_seq=['sa', 'ca', 'ffn'],
+ # for dn
+ embed_init_tgt=False,
+
+ use_detached_boxes_dec_out=False,
+ use_text_enhancer=False,
+ use_fusion_layer=False,
+ use_checkpoint=False,
+ use_transformer_ckpt=False,
+ use_text_cross_attention=False,
+ text_dropout=0.1,
+ fusion_dropout=0.1,
+ fusion_droppath=0.0,
+
+ binary_query_selection=False,
+ ffn_extra_layernorm=False,
+ ):
+ super().__init__()
+ self.num_feature_levels = num_feature_levels
+ self.num_encoder_layers = num_encoder_layers
+ self.num_unicoder_layers = num_unicoder_layers
+ self.num_decoder_layers = num_decoder_layers
+ self.deformable_encoder = deformable_encoder
+ self.deformable_decoder = deformable_decoder
+ self.two_stage_keep_all_tokens = two_stage_keep_all_tokens
+ self.num_queries = num_queries
+ self.random_refpoints_xy = random_refpoints_xy
+ self.use_detached_boxes_dec_out = use_detached_boxes_dec_out
+ self.ffn_extra_layernorm = ffn_extra_layernorm
+ assert query_dim == 4
+
+ self.binary_query_selection = binary_query_selection
+ if self.binary_query_selection:
+ self.binary_query_selection_layer = nn.Linear(d_model, 1)
+ # assert not binary_query_selection, 'binary_query_selection not implemented yet'
+
+ if num_feature_levels > 1:
+ assert deformable_encoder, "only support deformable_encoder for num_feature_levels > 1"
+ if use_deformable_box_attn:
+ assert deformable_encoder or deformable_encoder
+
+ assert layer_share_type in [None, 'encoder', 'decoder', 'both']
+ if layer_share_type in ['encoder', 'both']:
+ enc_layer_share = True
+ else:
+ enc_layer_share = False
+ if layer_share_type in ['decoder', 'both']:
+ dec_layer_share = True
+ else:
+ dec_layer_share = False
+ assert layer_share_type is None
+
+ self.decoder_sa_type = decoder_sa_type
+ assert decoder_sa_type in ['sa', 'ca_label', 'ca_content']
+
+ # choose encoder layer type
+ if deformable_encoder:
+ encoder_layer = DeformableTransformerEncoderLayer(d_model, dim_feedforward,
+ dropout, activation,
+ num_feature_levels, nhead, enc_n_points,
+ add_channel_attention=add_channel_attention,
+ use_deformable_box_attn=use_deformable_box_attn,
+ box_attn_type=box_attn_type)
+ else:
+ raise NotImplementedError
+
+ if use_text_enhancer:
+ text_enhance_layer = TransformerEncoderLayer(
+ d_model=d_model,
+ nhead=nhead // 2,
+ dim_feedforward=dim_feedforward // 2,
+ dropout=text_dropout
+ )
+ else:
+ text_enhance_layer = None
+
+ if use_fusion_layer:
+ feature_fusion_layer = BiAttentionBlock(
+ v_dim=d_model,
+ l_dim=d_model,
+ embed_dim=dim_feedforward // 2,
+ num_heads=nhead // 2,
+ dropout=fusion_dropout,
+ drop_path=fusion_droppath
+ )
+ else:
+ feature_fusion_layer = None
+
+ encoder_norm = nn.LayerNorm(d_model) if normalize_before else None
+ assert encoder_norm is None
+ self.encoder = TransformerEncoder(
+ encoder_layer, num_encoder_layers, d_model=d_model,
+ num_queries=num_queries,
+ enc_layer_share=enc_layer_share,
+ text_enhance_layer=text_enhance_layer,
+ feature_fusion_layer=feature_fusion_layer,
+ use_checkpoint=use_checkpoint,
+ use_transformer_ckpt=use_transformer_ckpt,
+ )
+
+ # choose decoder layer type
+ if deformable_decoder:
+ decoder_layer = DeformableTransformerDecoderLayer(d_model, dim_feedforward,
+ dropout, activation,
+ num_feature_levels, nhead, dec_n_points,
+ use_text_cross_attention=use_text_cross_attention,
+ ffn_extra_layernorm=ffn_extra_layernorm, )
+
+ else:
+ raise NotImplementedError
+
+ decoder_norm = nn.LayerNorm(d_model)
+ self.decoder = TransformerDecoder(decoder_layer, num_decoder_layers, decoder_norm,
+ return_intermediate=return_intermediate_dec,
+ d_model=d_model, query_dim=query_dim,
+ modulate_hw_attn=modulate_hw_attn,
+ num_feature_levels=num_feature_levels,
+ deformable_decoder=deformable_decoder,
+ decoder_query_perturber=decoder_query_perturber,
+ dec_layer_number=dec_layer_number, rm_dec_query_scale=rm_dec_query_scale,
+ dec_layer_share=dec_layer_share,
+ use_detached_boxes_dec_out=use_detached_boxes_dec_out
+ )
+
+ self.d_model = d_model
+ self.nhead = nhead
+ self.dec_layers = num_decoder_layers
+ self.num_queries = num_queries # useful for single stage model only
+ self.num_patterns = num_patterns
+ if not isinstance(num_patterns, int):
+ Warning("num_patterns should be int but {}".format(type(num_patterns)))
+ self.num_patterns = 0
+
+ if num_feature_levels > 1:
+ if self.num_encoder_layers > 0:
+ self.level_embed = nn.Parameter(torch.Tensor(num_feature_levels, d_model))
+ else:
+ self.level_embed = None
+
+ self.learnable_tgt_init = learnable_tgt_init
+ assert learnable_tgt_init, "why not learnable_tgt_init"
+ self.embed_init_tgt = embed_init_tgt
+ if (two_stage_type != 'no' and embed_init_tgt) or (two_stage_type == 'no'):
+ self.tgt_embed = nn.Embedding(self.num_queries, d_model)
+ nn.init.normal_(self.tgt_embed.weight.data)
+ else:
+ self.tgt_embed = None
+
+ # for two stage
+ self.two_stage_type = two_stage_type
+ self.two_stage_pat_embed = two_stage_pat_embed
+ self.two_stage_add_query_num = two_stage_add_query_num
+ self.two_stage_learn_wh = two_stage_learn_wh
+ assert two_stage_type in ['no', 'standard'], "unknown param {} of two_stage_type".format(two_stage_type)
+ if two_stage_type == 'standard':
+ # anchor selection at the output of encoder
+ self.enc_output = nn.Linear(d_model, d_model)
+ self.enc_output_norm = nn.LayerNorm(d_model)
+
+ if two_stage_pat_embed > 0:
+ self.pat_embed_for_2stage = nn.Parameter(torch.Tensor(two_stage_pat_embed, d_model))
+ nn.init.normal_(self.pat_embed_for_2stage)
+
+ if two_stage_add_query_num > 0:
+ self.tgt_embed = nn.Embedding(self.two_stage_add_query_num, d_model)
+
+ if two_stage_learn_wh:
+ # import ipdb; ipdb.set_trace()
+ self.two_stage_wh_embedding = nn.Embedding(1, 2)
+ else:
+ self.two_stage_wh_embedding = None
+
+ if two_stage_type == 'no':
+ self.init_ref_points(num_queries) # init self.refpoint_embed
+
+ self.enc_out_class_embed = None
+ self.enc_out_bbox_embed = None
+
+ # evolution of anchors
+ self.dec_layer_number = dec_layer_number
+ if dec_layer_number is not None:
+ if self.two_stage_type != 'no' or num_patterns == 0:
+ assert dec_layer_number[
+ 0] == num_queries, f"dec_layer_number[0]({dec_layer_number[0]}) != num_queries({num_queries})"
+ else:
+ assert dec_layer_number[
+ 0] == num_queries * num_patterns, f"dec_layer_number[0]({dec_layer_number[0]}) != num_queries({num_queries}) * num_patterns({num_patterns})"
+
+ self._reset_parameters()
+
+ self.rm_self_attn_layers = rm_self_attn_layers
+ if rm_self_attn_layers is not None:
+ # assert len(rm_self_attn_layers) == num_decoder_layers
+ print("Removing the self-attn in {} decoder layers".format(rm_self_attn_layers))
+ for lid, dec_layer in enumerate(self.decoder.layers):
+ if lid in rm_self_attn_layers:
+ dec_layer.rm_self_attn_modules()
+
+ self.rm_detach = rm_detach
+ if self.rm_detach:
+ assert isinstance(rm_detach, list)
+ assert any([i in ['enc_ref', 'enc_tgt', 'dec'] for i in rm_detach])
+ self.decoder.rm_detach = rm_detach
+
+ def _reset_parameters(self):
+ for p in self.parameters():
+ if p.dim() > 1:
+ nn.init.xavier_uniform_(p)
+ for m in self.modules():
+ if isinstance(m, MSDeformAttn):
+ m._reset_parameters()
+ if self.num_feature_levels > 1 and self.level_embed is not None:
+ nn.init.normal_(self.level_embed)
+
+ if self.two_stage_learn_wh:
+ nn.init.constant_(self.two_stage_wh_embedding.weight, math.log(0.05 / (1 - 0.05)))
+
+ def get_valid_ratio(self, mask):
+ _, H, W = mask.shape
+ valid_H = torch.sum(~mask[:, :, 0], 1)
+ valid_W = torch.sum(~mask[:, 0, :], 1)
+ valid_ratio_h = valid_H.float() / H
+ valid_ratio_w = valid_W.float() / W
+ valid_ratio = torch.stack([valid_ratio_w, valid_ratio_h], -1)
+ return valid_ratio
+
+ def init_ref_points(self, use_num_queries):
+ self.refpoint_embed = nn.Embedding(use_num_queries, 4)
+
+ if self.random_refpoints_xy:
+ # import ipdb; ipdb.set_trace()
+ self.refpoint_embed.weight.data[:, :2].uniform_(0, 1)
+ self.refpoint_embed.weight.data[:, :2] = inverse_sigmoid(self.refpoint_embed.weight.data[:, :2])
+ self.refpoint_embed.weight.data[:, :2].requires_grad = False
+
+ def forward(self, srcs, masks, refpoint_embed, pos_embeds, tgt, attn_mask=None, attn_mask2=None, text_dict=None,
+ dn_meta=None,targets=None,kpt_embed=None):
+ """
+ Input:
+ - srcs: List of multi features [bs, ci, hi, wi]
+ - masks: List of multi masks [bs, hi, wi]
+ - refpoint_embed: [bs, num_dn, 4]. None in infer
+ - pos_embeds: List of multi pos embeds [bs, ci, hi, wi]
+ - tgt: [bs, num_dn, d_model]. None in infer
+
+ """
+ # if self.two_stage_type != 'no' and self.two_stage_add_query_num == 0:
+ # assert refpoint_embed is None
+
+ # prepare input for encoder
+ src_flatten = []
+ mask_flatten = []
+ lvl_pos_embed_flatten = []
+ spatial_shapes = []
+ for lvl, (src, mask, pos_embed) in enumerate(zip(srcs, masks, pos_embeds)):
+ bs, c, h, w = src.shape
+ spatial_shape = (h, w)
+ spatial_shapes.append(spatial_shape)
+
+ src = src.flatten(2).transpose(1, 2) # bs, hw, c
+ mask = mask.flatten(1) # bs, hw
+ pos_embed = pos_embed.flatten(2).transpose(1, 2) # bs, hw, c
+ if self.num_feature_levels > 1 and self.level_embed is not None:
+ lvl_pos_embed = pos_embed + self.level_embed[lvl].view(1, 1, -1)
+ else:
+ lvl_pos_embed = pos_embed
+ lvl_pos_embed_flatten.append(lvl_pos_embed)
+ src_flatten.append(src)
+ mask_flatten.append(mask)
+ src_flatten = torch.cat(src_flatten, 1) # bs, \sum{hxw}, c
+ mask_flatten = torch.cat(mask_flatten, 1) # bs, \sum{hxw}
+ lvl_pos_embed_flatten = torch.cat(lvl_pos_embed_flatten, 1) # bs, \sum{hxw}, c
+ spatial_shapes = torch.as_tensor(spatial_shapes, dtype=torch.long, device=src_flatten.device)
+ level_start_index = torch.cat((spatial_shapes.new_zeros((1,)), spatial_shapes.prod(1).cumsum(0)[:-1]))
+ valid_ratios = torch.stack([self.get_valid_ratio(m) for m in masks], 1)
+
+ # two stage
+ enc_topk_proposals = enc_refpoint_embed = None
+
+ #########################################################
+ # Begin Encoder
+ #########################################################
+ memory, memory_text = self.encoder(
+ src_flatten,
+ pos=lvl_pos_embed_flatten,
+ level_start_index=level_start_index,
+ spatial_shapes=spatial_shapes,
+ valid_ratios=valid_ratios,
+ key_padding_mask=mask_flatten,
+ memory_text=text_dict['encoded_text'],
+ text_attention_mask=~text_dict['text_token_mask'],
+ # we ~ the mask . False means use the token; True means pad the token
+ position_ids=text_dict['position_ids'],
+ text_self_attention_masks=text_dict['text_self_attention_masks'],
+ )
+ #########################################################
+ # End Encoder
+ # - memory: bs, \sum{hw}, c
+ # - mask_flatten: bs, \sum{hw}
+ # - lvl_pos_embed_flatten: bs, \sum{hw}, c
+ # - enc_intermediate_output: None or (nenc+1, bs, nq, c) or (nenc, bs, nq, c)
+ # - enc_intermediate_refpoints: None or (nenc+1, bs, nq, c) or (nenc, bs, nq, c)
+ #########################################################
+ text_dict['encoded_text'] = memory_text
+
+ if self.two_stage_type == 'standard':
+ if self.two_stage_learn_wh:
+ input_hw = self.two_stage_wh_embedding.weight[0]
+ else:
+ input_hw = None
+ output_memory, output_proposals = gen_encoder_output_proposals(memory, mask_flatten, spatial_shapes,
+ input_hw)
+ output_memory = self.enc_output_norm(self.enc_output(output_memory))
+
+ if self.two_stage_pat_embed > 0:
+ bs, nhw, _ = output_memory.shape
+ # output_memory: bs, n, 256; self.pat_embed_for_2stage: k, 256
+ output_memory = output_memory.repeat(1, self.two_stage_pat_embed, 1)
+ _pats = self.pat_embed_for_2stage.repeat_interleave(nhw, 0)
+ output_memory = output_memory + _pats
+ output_proposals = output_proposals.repeat(1, self.two_stage_pat_embed, 1)
+
+ if self.two_stage_add_query_num > 0:
+ assert refpoint_embed is not None
+ output_memory = torch.cat((output_memory, tgt), dim=1)
+ output_proposals = torch.cat((output_proposals, refpoint_embed), dim=1)
+
+ if self.binary_query_selection:
+ topk_logits = self.binary_query_selection_layer(output_memory).squeeze(-1)
+ else:
+ if text_dict is not None:
+ enc_outputs_class_unselected = self.enc_out_class_embed(output_memory, text_dict)
+ else:
+ enc_outputs_class_unselected = self.enc_out_class_embed(output_memory)
+
+ topk_logits = enc_outputs_class_unselected.max(-1)[0]
+ enc_outputs_coord_unselected = self.enc_out_bbox_embed(
+ output_memory) + output_proposals # (bs, \sum{hw}, 4) unsigmoid
+ topk = self.num_queries
+
+ topk_proposals = torch.topk(topk_logits, topk, dim=1)[1] # bs, nq
+
+ # gather boxes
+ refpoint_embed_undetach = torch.gather(enc_outputs_coord_unselected, 1,
+ topk_proposals.unsqueeze(-1).repeat(1, 1, 4)) # unsigmoid
+ refpoint_embed_ = refpoint_embed_undetach.detach()
+ init_box_proposal = torch.gather(output_proposals, 1,
+ topk_proposals.unsqueeze(-1).repeat(1, 1, 4)).sigmoid() # sigmoid
+
+ # gather tgt
+ tgt_undetach = torch.gather(output_memory, 1, topk_proposals.unsqueeze(-1).repeat(1, 1, self.d_model))
+ if self.embed_init_tgt:
+ tgt_ = self.tgt_embed.weight[:, None, :].repeat(1, bs, 1).transpose(0, 1) # nq, bs, d_model
+ else:
+ tgt_ = tgt_undetach.detach()
+
+ if refpoint_embed is not None:
+ refpoint_embed = torch.cat([refpoint_embed, refpoint_embed_], dim=1)
+ tgt = torch.cat([tgt, tgt_], dim=1)
+ else:
+ refpoint_embed, tgt = refpoint_embed_, tgt_
+
+ elif self.two_stage_type == 'no':
+ tgt_ = self.tgt_embed.weight[:, None, :].repeat(1, bs, 1).transpose(0, 1) # nq, bs, d_model
+ refpoint_embed_ = self.refpoint_embed.weight[:, None, :].repeat(1, bs, 1).transpose(0, 1) # nq, bs, 4
+
+ if refpoint_embed is not None:
+ refpoint_embed = torch.cat([refpoint_embed, refpoint_embed_], dim=1)
+ tgt = torch.cat([tgt, tgt_], dim=1)
+ else:
+ refpoint_embed, tgt = refpoint_embed_, tgt_
+
+ if self.num_patterns > 0:
+ tgt_embed = tgt.repeat(1, self.num_patterns, 1)
+ refpoint_embed = refpoint_embed.repeat(1, self.num_patterns, 1)
+ tgt_pat = self.patterns.weight[None, :, :].repeat_interleave(self.num_queries,
+ 1) # 1, n_q*n_pat, d_model
+ tgt = tgt_embed + tgt_pat
+
+ init_box_proposal = refpoint_embed_.sigmoid()
+
+ else:
+ raise NotImplementedError("unknown two_stage_type {}".format(self.two_stage_type))
+ #########################################################
+ # End preparing tgt
+ # - tgt: bs, NQ, d_model
+ # - refpoint_embed(unsigmoid): bs, NQ, d_model
+ #########################################################
+ # if os.environ.get("SHILONG_AMP_INFNAN_DEBUG") == '1':
+ # if refpoint_embed.isnan().any() | refpoint_embed.isinf().any():
+ # import ipdb; ipdb.set_trace()
+ # if tgt.isnan().any() | tgt.isinf().any():
+ # import ipdb; ipdb.set_trace()
+
+ #########################################################
+ # Begin Decoder
+ #########################################################
+ hs, references = self.decoder(
+ tgt=tgt.transpose(0, 1),
+ memory=memory.transpose(0, 1),
+ memory_key_padding_mask=mask_flatten,
+ pos=lvl_pos_embed_flatten.transpose(0, 1),
+ refpoints_unsigmoid=refpoint_embed.transpose(0, 1),
+ level_start_index=level_start_index,
+ spatial_shapes=spatial_shapes,
+ valid_ratios=valid_ratios, tgt_mask=attn_mask,
+ tgt_mask2=attn_mask2,
+ memory_text=text_dict['encoded_text'],
+ text_attention_mask=~text_dict['text_token_mask'],
+ text_dict=text_dict,
+ dn_meta=dn_meta,
+ targets=targets,
+ kpt_embed=kpt_embed
+ # we ~ the mask . False means use the token; True means pad the token
+ )
+ #########################################################
+ # End Decoder
+ # hs: n_dec, bs, nq, d_model
+ # references: n_dec+1, bs, nq, query_dim
+ #########################################################
+
+ #########################################################
+ # Begin postprocess
+ #########################################################
+ if self.two_stage_type == 'standard':
+ if self.two_stage_keep_all_tokens:
+ hs_enc = output_memory.unsqueeze(0)
+ ref_enc = enc_outputs_coord_unselected.unsqueeze(0)
+ init_box_proposal = output_proposals
+ # import ipdb; ipdb.set_trace()
+ else:
+ hs_enc = tgt_undetach.unsqueeze(0)
+ ref_enc = refpoint_embed_undetach.sigmoid().unsqueeze(0)
+ else:
+ hs_enc = ref_enc = None
+ #########################################################
+ # End postprocess
+ # hs_enc: (n_enc+1, bs, nq, d_model) or (1, bs, nq, d_model) or (n_enc, bs, nq, d_model) or None
+ # ref_enc: (n_enc+1, bs, nq, query_dim) or (1, bs, nq, query_dim) or (n_enc, bs, nq, d_model) or None
+ #########################################################
+
+ return hs, references, hs_enc, ref_enc, init_box_proposal
+ # hs: (n_dec, bs, nq, d_model)
+ # references: sigmoid coordinates. (n_dec+1, bs, bq, 4)
+ # hs_enc: (n_enc+1, bs, nq, d_model) or (1, bs, nq, d_model) or None
+ # ref_enc: sigmoid coordinates. \
+ # (n_enc+1, bs, nq, query_dim) or (1, bs, nq, query_dim) or None
+
+
+class TransformerEncoder(nn.Module):
+
+ def __init__(self,
+ encoder_layer, num_layers, d_model=256,
+ num_queries=300,
+ enc_layer_share=False,
+ text_enhance_layer=None,
+ feature_fusion_layer=None,
+ use_checkpoint=False,
+ use_transformer_ckpt=False,
+ ):
+ """_summary_
+
+ Args:
+ encoder_layer (_type_): _description_
+ num_layers (_type_): _description_
+ norm (_type_, optional): _description_. Defaults to None.
+ d_model (int, optional): _description_. Defaults to 256.
+ num_queries (int, optional): _description_. Defaults to 300.
+ enc_layer_share (bool, optional): _description_. Defaults to False.
+
+ """
+ super().__init__()
+ # prepare layers
+ self.layers = []
+ self.text_layers = []
+ self.fusion_layers = []
+ if num_layers > 0:
+ self.layers = _get_clones(encoder_layer, num_layers, layer_share=enc_layer_share)
+
+ if text_enhance_layer is not None:
+ self.text_layers = _get_clones(text_enhance_layer, num_layers, layer_share=enc_layer_share)
+ if feature_fusion_layer is not None:
+ self.fusion_layers = _get_clones(feature_fusion_layer, num_layers, layer_share=enc_layer_share)
+ else:
+ self.layers = []
+ del encoder_layer
+
+ if text_enhance_layer is not None:
+ self.text_layers = []
+ del text_enhance_layer
+ if feature_fusion_layer is not None:
+ self.fusion_layers = []
+ del feature_fusion_layer
+
+ self.query_scale = None
+ self.num_queries = num_queries
+ self.num_layers = num_layers
+ self.d_model = d_model
+
+ self.use_checkpoint = use_checkpoint
+ self.use_transformer_ckpt = use_transformer_ckpt
+
+ @staticmethod
+ def get_reference_points(spatial_shapes, valid_ratios, device):
+ reference_points_list = []
+ for lvl, (H_, W_) in enumerate(spatial_shapes):
+ ref_y, ref_x = torch.meshgrid(torch.linspace(0.5, H_ - 0.5, H_, dtype=torch.float32, device=device),
+ torch.linspace(0.5, W_ - 0.5, W_, dtype=torch.float32, device=device),)
+ ref_y = ref_y.reshape(-1)[None] / (valid_ratios[:, None, lvl, 1] * H_)
+ ref_x = ref_x.reshape(-1)[None] / (valid_ratios[:, None, lvl, 0] * W_)
+ ref = torch.stack((ref_x, ref_y), -1)
+ reference_points_list.append(ref)
+ reference_points = torch.cat(reference_points_list, 1)
+ reference_points = reference_points[:, :, None] * valid_ratios[:, None]
+ return reference_points
+
+ def forward(self,
+ # for images
+ src: Tensor,
+ pos: Tensor,
+ spatial_shapes: Tensor,
+ level_start_index: Tensor,
+ valid_ratios: Tensor,
+ key_padding_mask: Tensor,
+ # for texts
+ memory_text: Tensor = None,
+ text_attention_mask: Tensor = None,
+ pos_text: Tensor = None,
+ text_self_attention_masks: Tensor = None,
+ position_ids: Tensor = None,
+ ):
+ """
+ Input:
+ - src: [bs, sum(hi*wi), 256]
+ - pos: pos embed for src. [bs, sum(hi*wi), 256]
+ - spatial_shapes: h,w of each level [num_level, 2]
+ - level_start_index: [num_level] start point of level in sum(hi*wi).
+ - valid_ratios: [bs, num_level, 2]
+ - key_padding_mask: [bs, sum(hi*wi)]
+
+ - memory_text: bs, n_text, 256
+ - text_attention_mask: bs, n_text
+ False for no padding; True for padding
+ - pos_text: bs, n_text, 256
+
+ - position_ids: bs, n_text
+ Intermedia:
+ - reference_points: [bs, sum(hi*wi), num_level, 2]
+ Outpus:
+ - output: [bs, sum(hi*wi), 256]
+ """
+
+ output = src
+
+ # preparation and reshape
+ if self.num_layers > 0:
+ reference_points = self.get_reference_points(spatial_shapes, valid_ratios, device=src.device)
+
+ if self.text_layers:
+ # generate pos_text
+ bs, n_text, text_dim = memory_text.shape
+ if pos_text is None and position_ids is None:
+ pos_text = torch.arange(n_text, device=memory_text.device).float().unsqueeze(0).unsqueeze(-1).repeat(bs,
+ 1,
+ 1)
+ pos_text = get_sine_pos_embed(pos_text, num_pos_feats=256, exchange_xy=False)
+ if position_ids is not None:
+ pos_text = get_sine_pos_embed(position_ids[..., None], num_pos_feats=256, exchange_xy=False)
+
+ # main process
+ for layer_id, layer in enumerate(self.layers):
+ # if output.isnan().any() or memory_text.isnan().any():
+ # if os.environ.get('IPDB_SHILONG_DEBUG', None) == 'INFO':
+ # import ipdb; ipdb.set_trace()
+ if self.fusion_layers:
+ if self.use_checkpoint:
+ output, memory_text = checkpoint.checkpoint(
+ self.fusion_layers[layer_id],
+ output,
+ memory_text,
+ key_padding_mask,
+ text_attention_mask
+ )
+ else:
+ output, memory_text = self.fusion_layers[layer_id](v=output, l=memory_text,
+ attention_mask_v=key_padding_mask,
+ attention_mask_l=text_attention_mask)
+
+ if self.text_layers:
+ memory_text = self.text_layers[layer_id](
+ src=memory_text.transpose(0, 1),
+ src_mask=~text_self_attention_masks, # note we use ~ for mask here
+ src_key_padding_mask=text_attention_mask,
+ pos=(pos_text.transpose(0, 1) if pos_text is not None else None)
+ ).transpose(0, 1)
+
+ # main process
+ if self.use_transformer_ckpt:
+ output = checkpoint.checkpoint(
+ layer,
+ output,
+ pos,
+ reference_points,
+ spatial_shapes,
+ level_start_index,
+ key_padding_mask
+ )
+ else:
+ output = layer(src=output, pos=pos, reference_points=reference_points, spatial_shapes=spatial_shapes,
+ level_start_index=level_start_index, key_padding_mask=key_padding_mask)
+
+ return output, memory_text
+
+
+class TransformerDecoder(nn.Module):
+
+ def __init__(self, decoder_layer, num_layers, norm=None,
+ return_intermediate=False,
+ d_model=256, query_dim=4,
+ modulate_hw_attn=False,
+ num_feature_levels=1,
+ deformable_decoder=False,
+ decoder_query_perturber=None,
+ dec_layer_number=None, # number of queries each layer in decoder
+ rm_dec_query_scale=False,
+ dec_layer_share=False,
+ dec_layer_dropout_prob=None,
+ use_detached_boxes_dec_out=False,
+ num_box_decoder_layers=2,
+ num_body_points=68,
+ ):
+ super().__init__()
+ if num_layers > 0:
+ self.layers = _get_clones(decoder_layer, num_layers, layer_share=dec_layer_share)
+ else:
+ self.layers = []
+ self.num_layers = num_layers
+ self.norm = norm
+ self.return_intermediate = return_intermediate
+ assert return_intermediate, "support return_intermediate only"
+ self.query_dim = query_dim
+ assert query_dim in [2, 4], "query_dim should be 2/4 but {}".format(query_dim)
+ self.num_feature_levels = num_feature_levels
+ self.use_detached_boxes_dec_out = use_detached_boxes_dec_out
+
+ self.ref_point_head = MLP(query_dim // 2 * d_model, d_model, d_model, 2)
+ if not deformable_decoder:
+ self.query_pos_sine_scale = MLP(d_model, d_model, d_model, 2)
+ else:
+ self.query_pos_sine_scale = None
+
+ if rm_dec_query_scale:
+ self.query_scale = None
+ else:
+ raise NotImplementedError
+ self.query_scale = MLP(d_model, d_model, d_model, 2)
+ self.bbox_embed = None
+ self.class_embed = None
+ self.pose_embed = None
+ self.pose_hw_embed = None
+ self.d_model = d_model
+ self.modulate_hw_attn = modulate_hw_attn
+ self.deformable_decoder = deformable_decoder
+
+ if not deformable_decoder and modulate_hw_attn:
+ self.ref_anchor_head = MLP(d_model, d_model, 2, 2)
+ else:
+ self.ref_anchor_head = None
+
+ self.decoder_query_perturber = decoder_query_perturber
+ self.box_pred_damping = None
+
+ self.dec_layer_number = dec_layer_number
+ if dec_layer_number is not None:
+ assert isinstance(dec_layer_number, list)
+ assert len(dec_layer_number) == num_layers
+ # assert dec_layer_number[0] ==
+
+ self.dec_layer_dropout_prob = dec_layer_dropout_prob
+ if dec_layer_dropout_prob is not None:
+ assert isinstance(dec_layer_dropout_prob, list)
+ assert len(dec_layer_dropout_prob) == num_layers
+ for i in dec_layer_dropout_prob:
+ assert 0.0 <= i <= 1.0
+
+ self.rm_detach = None
+ self.num_body_points = num_body_points
+
+ self.hw = nn.Embedding(17, 2)
+ self.num_box_decoder_layers = num_box_decoder_layers
+ self.kpt_index = [x for x in range(50 * (self.num_body_points + 1)) if x % (self.num_body_points + 1) != 0]
+ self.hw_append = nn.Embedding(self.num_body_points-17, 2)
+
+ def forward(self, tgt, memory,
+ tgt_mask: Optional[Tensor] = None,
+ tgt_mask2: Optional[Tensor] = None,
+ memory_mask: Optional[Tensor] = None,
+ tgt_key_padding_mask: Optional[Tensor] = None,
+ memory_key_padding_mask: Optional[Tensor] = None,
+ pos: Optional[Tensor] = None,
+ refpoints_unsigmoid: Optional[Tensor] = None, # num_queries, bs, 2
+ # for memory
+ level_start_index: Optional[Tensor] = None, # num_levels
+ spatial_shapes: Optional[Tensor] = None, # bs, num_levels, 2
+ valid_ratios: Optional[Tensor] = None,
+ # for text
+ memory_text: Optional[Tensor] = None,
+ text_attention_mask: Optional[Tensor] = None,
+ text_dict: Optional[Tensor] = None,
+ dn_meta: Optional[Tensor] = None,
+ targets: Optional[Tensor] = None,
+ kpt_embed: Optional[Tensor] = None
+ ):
+ """
+ Input:
+ - tgt: nq, bs, d_model
+ - memory: hw, bs, d_model
+ - pos: hw, bs, d_model
+ - refpoints_unsigmoid: nq, bs, 2/4
+ - valid_ratios/spatial_shapes: bs, nlevel, 2
+ """
+
+ output = tgt
+ output += self.hw.weight[0, 0] * 0.0
+
+
+ intermediate = []
+ reference_points = refpoints_unsigmoid.sigmoid()
+ ref_points = [reference_points]
+ effect_num_dn = dn_meta['pad_size'] if self.training else 0
+ inter_select_number = 50
+ for layer_id, layer in enumerate(self.layers):
+
+ if reference_points.shape[-1] == 4:
+ reference_points_input = reference_points[:, :, None] \
+ * torch.cat([valid_ratios, valid_ratios], -1)[None, :] # nq, bs, nlevel, 4
+ else:
+ assert reference_points.shape[-1] == 2
+ reference_points_input = reference_points[:, :, None] * valid_ratios[None, :]
+ query_sine_embed = gen_sineembed_for_position(reference_points_input[:, :, 0, :]) # nq, bs, 256*2
+
+ # conditional query
+ raw_query_pos = self.ref_point_head(query_sine_embed) # nq, bs, 256
+ pos_scale = self.query_scale(output) if self.query_scale is not None else 1
+ query_pos = pos_scale * raw_query_pos
+ # if os.environ.get("SHILONG_AMP_INFNAN_DEBUG") == '1':
+ # if query_pos.isnan().any() | query_pos.isinf().any():
+ # import ipdb; ipdb.set_trace()
+
+ # main process
+ output = layer(
+ tgt=output,
+ tgt_query_pos=query_pos,
+ tgt_query_sine_embed=query_sine_embed,
+ tgt_key_padding_mask=tgt_key_padding_mask,
+ tgt_reference_points=reference_points_input,
+
+ memory_text=memory_text,
+ text_attention_mask=text_attention_mask,
+
+ memory=memory,
+ memory_key_padding_mask=memory_key_padding_mask,
+ memory_level_start_index=level_start_index,
+ memory_spatial_shapes=spatial_shapes,
+ memory_pos=pos,
+
+ self_attn_mask=tgt_mask,
+ cross_attn_mask=memory_mask
+ )
+ if output.isnan().any() | output.isinf().any():
+ print(f"output layer_id {layer_id} is nan")
+ try:
+ num_nan = output.isnan().sum().item()
+ num_inf = output.isinf().sum().item()
+ print(f"num_nan {num_nan}, num_inf {num_inf}")
+ except Exception as e:
+ print(e)
+
+
+
+
+ intermediate.append(self.norm(output))
+ # iter update
+ if layer_id < self.num_box_decoder_layers:
+ reference_before_sigmoid = inverse_sigmoid(reference_points)
+ delta_unsig = self.bbox_embed[layer_id](output)
+ outputs_unsig = delta_unsig + reference_before_sigmoid
+ new_reference_points = outputs_unsig.sigmoid()
+
+ # select # ref points as anchors
+ if layer_id == self.num_box_decoder_layers - 1:
+ dn_output = output[:effect_num_dn]
+ dn_new_reference_points = new_reference_points[:effect_num_dn]
+ class_unselected = self.class_embed[layer_id](output.transpose(0, 1), text_dict)[:,
+ effect_num_dn:].transpose(0, 1)
+ topk_proposals = torch.topk(class_unselected.max(-1)[0], inter_select_number, dim=0)[1]
+ new_reference_points_for_box = torch.gather(new_reference_points[effect_num_dn:], 0,
+ topk_proposals.unsqueeze(-1).repeat(1, 1, 4))
+ new_output_for_box = torch.gather(output[effect_num_dn:], 0,
+ topk_proposals.unsqueeze(-1).repeat(1, 1, self.d_model))
+ keypoint_embed=kpt_embed.transpose(0, 1)
+
+ new_output_for_keypoint = keypoint_embed[None, :, :, :].repeat(new_output_for_box.shape[0],1,1,1)
+ delta_xy = self.pose_embed[-1](new_output_for_keypoint)[..., :2]
+ keypoint_xy = (inverse_sigmoid(new_reference_points_for_box[..., :2][:, None]) + delta_xy).sigmoid()
+ num_queries, _, bs, _ = keypoint_xy.shape
+ aa = torch.cat((self.hw.weight,self.hw_append.weight),dim=0)
+ keypoint_wh_weight = aa.unsqueeze(0).unsqueeze(-2).repeat(num_queries, 1, bs, 1).sigmoid()
+ keypoint_wh = keypoint_wh_weight * new_reference_points_for_box[..., 2:][:, None]
+ new_reference_points_for_keypoint = torch.cat((keypoint_xy, keypoint_wh), dim=-1)
+ new_reference_points = torch.cat(
+ (new_reference_points_for_box.unsqueeze(1), new_reference_points_for_keypoint), dim=1).flatten(0, 1)
+ output = torch.cat((new_output_for_box.unsqueeze(1), new_output_for_keypoint), dim=1).flatten(0, 1)
+ new_reference_points = torch.cat((dn_new_reference_points, new_reference_points), dim=0)
+ output = torch.cat((dn_output, output), dim=0)
+ tgt_mask = tgt_mask2
+
+ if layer_id >= self.num_box_decoder_layers:
+ reference_before_sigmoid = inverse_sigmoid(reference_points)
+ output_bbox_dn = output[:effect_num_dn]
+ output_bbox_norm = output[effect_num_dn:][0::(self.num_body_points + 1)]
+ reference_before_sigmoid_bbox_dn = reference_before_sigmoid[:effect_num_dn]
+ reference_before_sigmoid_bbox_norm = reference_before_sigmoid[effect_num_dn:][
+ 0::(self.num_body_points + 1)]
+ delta_unsig_dn = self.bbox_embed[layer_id](output_bbox_dn)
+ delta_unsig_norm = self.bbox_embed[layer_id](output_bbox_norm)
+ outputs_unsig_dn = delta_unsig_dn + reference_before_sigmoid_bbox_dn
+ outputs_unsig_norm = delta_unsig_norm + reference_before_sigmoid_bbox_norm
+ new_reference_points_for_box_dn = outputs_unsig_dn.sigmoid()
+ new_reference_points_for_box_norm = outputs_unsig_norm.sigmoid()
+ output_kpt = output[effect_num_dn:].index_select(0, torch.tensor(self.kpt_index, device=output.device))
+ delta_xy_unsig = self.pose_embed[layer_id - self.num_box_decoder_layers](output_kpt)
+ outputs_unsig = reference_before_sigmoid[effect_num_dn:].index_select(0, torch.tensor(self.kpt_index,
+ device=output.device)).clone() ##
+ delta_hw_unsig = self.pose_hw_embed[layer_id - self.num_box_decoder_layers](output_kpt)
+ outputs_unsig[..., :2] += delta_xy_unsig[..., :2]
+ outputs_unsig[..., 2:] += delta_hw_unsig
+ new_reference_points_for_keypoint = outputs_unsig.sigmoid()
+ bs = new_reference_points_for_box_norm.shape[1]
+ new_reference_points_norm = torch.cat((new_reference_points_for_box_norm.unsqueeze(1),
+ new_reference_points_for_keypoint.view(-1, self.num_body_points,
+ bs, 4)), dim=1).flatten(0,
+ 1)
+ new_reference_points = torch.cat((new_reference_points_for_box_dn, new_reference_points_norm), dim=0)
+
+ if self.rm_detach and 'dec' in self.rm_detach:
+ reference_points = new_reference_points
+ else:
+ reference_points = new_reference_points.detach()
+
+ # if layer_id != self.num_layers - 1:
+ if self.use_detached_boxes_dec_out:
+ ref_points.append(reference_points)
+ else:
+ ref_points.append(new_reference_points)
+
+ return [
+ [itm_out.transpose(0, 1) for itm_out in intermediate],
+ [itm_refpoint.transpose(0, 1) for itm_refpoint in ref_points]
+ ]
+
+
+class DeformableTransformerEncoderLayer(nn.Module):
+ def __init__(self,
+ d_model=256, d_ffn=1024,
+ dropout=0.1, activation="relu",
+ n_levels=4, n_heads=8, n_points=4,
+ add_channel_attention=False,
+ use_deformable_box_attn=False,
+ box_attn_type='roi_align',
+ ):
+ super().__init__()
+
+ # self attention
+ self.self_attn = MSDeformAttn(d_model, n_levels, n_heads, n_points)
+ self.dropout1 = nn.Dropout(dropout)
+ self.norm1 = nn.LayerNorm(d_model)
+
+ # ffn
+ self.linear1 = nn.Linear(d_model, d_ffn)
+ self.activation = _get_activation_fn(activation, d_model=d_ffn)
+ self.dropout2 = nn.Dropout(dropout)
+ self.linear2 = nn.Linear(d_ffn, d_model)
+ self.dropout3 = nn.Dropout(dropout)
+ self.norm2 = nn.LayerNorm(d_model)
+
+ # channel attention
+ self.add_channel_attention = add_channel_attention
+ if add_channel_attention:
+ self.activ_channel = _get_activation_fn('dyrelu', d_model=d_model)
+ self.norm_channel = nn.LayerNorm(d_model)
+
+ @staticmethod
+ def with_pos_embed(tensor, pos):
+ return tensor if pos is None else tensor + pos
+
+ def forward_ffn(self, src):
+ src2 = self.linear2(self.dropout2(self.activation(self.linear1(src))))
+ src = src + self.dropout3(src2)
+ src = self.norm2(src)
+ return src
+
+ def forward(self, src, pos, reference_points, spatial_shapes, level_start_index, key_padding_mask=None):
+ # self attention
+ # import ipdb; ipdb.set_trace()
+ src2 = self.self_attn(self.with_pos_embed(src, pos), reference_points, src, spatial_shapes, level_start_index,
+ key_padding_mask)
+ src = src + self.dropout1(src2)
+ src = self.norm1(src)
+
+ # ffn
+ src = self.forward_ffn(src)
+
+ # channel attn
+ if self.add_channel_attention:
+ src = self.norm_channel(src + self.activ_channel(src))
+
+ return src
+
+
+class DeformableTransformerDecoderLayer(nn.Module):
+ def __init__(self, d_model=256, d_ffn=1024,
+ dropout=0.1, activation="relu",
+ n_levels=4, n_heads=8, n_points=4,
+ use_text_feat_guide=False,
+ use_text_cross_attention=False,
+ ffn_extra_layernorm=False
+ ):
+ super().__init__()
+
+ # cross attention
+ # self.cross_attn = MSDeformAttn(d_model, n_levels, n_heads, n_points)
+ self.cross_attn = MSDeformAttn(d_model, n_levels, n_heads, n_points)
+ self.dropout1 = nn.Dropout(dropout) if dropout > 0 else nn.Identity()
+ self.norm1 = nn.LayerNorm(d_model)
+
+ # cross attention text
+ if use_text_cross_attention:
+ self.ca_text = nn.MultiheadAttention(d_model, n_heads, dropout=dropout)
+ self.catext_dropout = nn.Dropout(dropout) if dropout > 0 else nn.Identity()
+ self.catext_norm = nn.LayerNorm(d_model)
+
+ # self attention
+ self.self_attn = nn.MultiheadAttention(d_model, n_heads, dropout=dropout)
+ self.dropout2 = nn.Dropout(dropout) if dropout > 0 else nn.Identity()
+ self.norm2 = nn.LayerNorm(d_model)
+
+ # ffn
+ self.linear1 = nn.Linear(d_model, d_ffn)
+ self.activation = _get_activation_fn(activation, d_model=d_ffn, batch_dim=1)
+ self.dropout3 = nn.Dropout(dropout) if dropout > 0 else nn.Identity()
+ self.linear2 = nn.Linear(d_ffn, d_model)
+ self.dropout4 = nn.Dropout(dropout) if dropout > 0 else nn.Identity()
+ self.norm3 = nn.LayerNorm(d_model)
+ if ffn_extra_layernorm:
+ raise NotImplementedError('ffn_extra_layernorm not implemented')
+ self.norm_ext = nn.LayerNorm(d_ffn)
+ else:
+ self.norm_ext = None
+
+ self.key_aware_proj = None
+ self.use_text_feat_guide = use_text_feat_guide
+ assert not use_text_feat_guide
+ self.use_text_cross_attention = use_text_cross_attention
+
+ def rm_self_attn_modules(self):
+ self.self_attn = None
+ self.dropout2 = None
+ self.norm2 = None
+
+ @staticmethod
+ def with_pos_embed(tensor, pos):
+ return tensor if pos is None else tensor + pos
+
+ def forward_ffn(self, tgt, ipdb_flag=False):
+
+ with torch.cuda.amp.autocast(enabled=False):
+ tgt2 = self.linear2(self.dropout3(self.activation(self.linear1(tgt))))
+
+ tgt = tgt + self.dropout4(tgt2)
+ tgt = self.norm3(tgt)
+ return tgt
+
+ def forward(self,
+ # for tgt
+ tgt: Optional[Tensor], # nq, bs, d_model
+ tgt_query_pos: Optional[Tensor] = None, # pos for query. MLP(Sine(pos))
+ tgt_query_sine_embed: Optional[Tensor] = None, # pos for query. Sine(pos)
+ tgt_key_padding_mask: Optional[Tensor] = None,
+ tgt_reference_points: Optional[Tensor] = None, # nq, bs, 4
+
+ memory_text: Optional[Tensor] = None, # bs, num_token, d_model
+ text_attention_mask: Optional[Tensor] = None, # bs, num_token
+
+ # for memory
+ memory: Optional[Tensor] = None, # hw, bs, d_model
+ memory_key_padding_mask: Optional[Tensor] = None,
+ memory_level_start_index: Optional[Tensor] = None, # num_levels
+ memory_spatial_shapes: Optional[Tensor] = None, # bs, num_levels, 2
+ memory_pos: Optional[Tensor] = None, # pos for memory
+
+ # sa
+ self_attn_mask: Optional[Tensor] = None, # mask used for self-attention
+ cross_attn_mask: Optional[Tensor] = None, # mask used for cross-attention
+ ):
+ """
+ Input:
+ - tgt/tgt_query_pos: nq, bs, d_model
+ -
+ """
+ assert cross_attn_mask is None
+
+ # self attention
+ if self.self_attn is not None:
+ # import ipdb; ipdb.set_trace()
+ q = k = self.with_pos_embed(tgt, tgt_query_pos)
+ tgt2 = self.self_attn(q, k, tgt, attn_mask=self_attn_mask)[0]
+ tgt = tgt + self.dropout2(tgt2)
+ tgt = self.norm2(tgt)
+
+ # if os.environ.get("SHILONG_AMP_INFNAN_DEBUG") == '1':
+ # if tgt.isnan().any() | tgt.isinf().any() :
+ # import ipdb; ipdb.set_trace()
+
+ if self.use_text_cross_attention:
+ tgt2 = self.ca_text(self.with_pos_embed(tgt, tgt_query_pos), memory_text.transpose(0, 1),
+ memory_text.transpose(0, 1), key_padding_mask=text_attention_mask)[0]
+ tgt = tgt + self.catext_dropout(tgt2)
+ tgt = self.catext_norm(tgt)
+
+ # if os.environ.get("SHILONG_AMP_INFNAN_DEBUG") == '1':
+ # if os.environ.get('IPDB_SHILONG_DEBUG', None) == 'INFO':
+ # import ipdb; ipdb.set_trace()
+
+ # if tgt.isnan().any() | tgt.isinf().any() :
+ # import ipdb; ipdb.set_trace()
+
+ tgt2 = self.cross_attn(self.with_pos_embed(tgt, tgt_query_pos).transpose(0, 1),
+ tgt_reference_points.transpose(0, 1).contiguous(),
+ memory.transpose(0, 1), memory_spatial_shapes, memory_level_start_index,
+ memory_key_padding_mask).transpose(0, 1)
+ tgt = tgt + self.dropout1(tgt2)
+ tgt = self.norm1(tgt)
+
+ # if os.environ.get("SHILONG_AMP_INFNAN_DEBUG") == '1':
+ # tgtk = tgt.clone()
+ # if tgt.isnan().any() | tgt.isinf().any() :
+ # import ipdb; ipdb.set_trace()
+
+ # ffn
+ tgt = self.forward_ffn(tgt)
+ # if os.environ.get("SHILONG_AMP_INFNAN_DEBUG") == '1':
+ # if tgt.isnan().any() | tgt.isinf().any() :
+ # tgtk = self.forward_ffn(tgtk, ipdb_flag=True)
+ # import ipdb; ipdb.set_trace()
+
+ return tgt
+
+
+def _get_clones(module, N, layer_share=False):
+ # import ipdb; ipdb.set_trace()
+ if layer_share:
+ return nn.ModuleList([module for i in range(N)])
+ else:
+ return nn.ModuleList([copy.deepcopy(module) for i in range(N)])
+
+
+def build_deformable_transformer(args):
+ decoder_query_perturber = None
+ if args.decoder_layer_noise:
+ from .utils import RandomBoxPerturber
+ decoder_query_perturber = RandomBoxPerturber(
+ x_noise_scale=args.dln_xy_noise, y_noise_scale=args.dln_xy_noise,
+ w_noise_scale=args.dln_hw_noise, h_noise_scale=args.dln_hw_noise)
+
+ use_detached_boxes_dec_out = False
+ try:
+ use_detached_boxes_dec_out = args.use_detached_boxes_dec_out
+ except:
+ use_detached_boxes_dec_out = False
+
+ binary_query_selection = False
+ try:
+ binary_query_selection = args.binary_query_selection
+ except:
+ binary_query_selection = False
+
+ ffn_extra_layernorm = False
+ try:
+ ffn_extra_layernorm = args.ffn_extra_layernorm
+ except:
+ print('ffn_extra_layernorm not found, set to False')
+ ffn_extra_layernorm = False
+
+ return DeformableTransformer(
+ d_model=args.hidden_dim,
+ dropout=args.dropout,
+ nhead=args.nheads,
+ num_queries=args.num_queries,
+ dim_feedforward=args.dim_feedforward,
+ num_encoder_layers=args.enc_layers,
+ num_unicoder_layers=args.unic_layers,
+ num_decoder_layers=args.dec_layers,
+ normalize_before=args.pre_norm,
+ return_intermediate_dec=True,
+ query_dim=args.query_dim,
+ activation=args.transformer_activation,
+ num_patterns=args.num_patterns,
+ modulate_hw_attn=True,
+
+ deformable_encoder=True,
+ deformable_decoder=True,
+ num_feature_levels=args.num_feature_levels,
+ enc_n_points=args.enc_n_points,
+ dec_n_points=args.dec_n_points,
+ use_deformable_box_attn=args.use_deformable_box_attn,
+ box_attn_type=args.box_attn_type,
+
+ learnable_tgt_init=True,
+ decoder_query_perturber=decoder_query_perturber,
+
+ add_channel_attention=args.add_channel_attention,
+ add_pos_value=args.add_pos_value,
+ random_refpoints_xy=args.random_refpoints_xy,
+
+ # two stage
+ two_stage_type=args.two_stage_type, # ['no', 'standard', 'early']
+ two_stage_pat_embed=args.two_stage_pat_embed,
+ two_stage_add_query_num=args.two_stage_add_query_num,
+ two_stage_learn_wh=args.two_stage_learn_wh,
+ two_stage_keep_all_tokens=args.two_stage_keep_all_tokens,
+ dec_layer_number=args.dec_layer_number,
+ rm_self_attn_layers=None,
+ key_aware_type=None,
+ layer_share_type=None,
+
+ rm_detach=None,
+ decoder_sa_type=args.decoder_sa_type,
+ module_seq=args.decoder_module_seq,
+
+ embed_init_tgt=args.embed_init_tgt,
+ use_detached_boxes_dec_out=use_detached_boxes_dec_out,
+ use_text_enhancer=args.use_text_enhancer,
+ use_fusion_layer=args.use_fusion_layer,
+ use_checkpoint=args.use_checkpoint,
+ use_transformer_ckpt=args.use_transformer_ckpt,
+ use_text_cross_attention=args.use_text_cross_attention,
+
+ text_dropout=args.text_dropout,
+ fusion_dropout=args.fusion_dropout,
+ fusion_droppath=args.fusion_droppath,
+
+ binary_query_selection=binary_query_selection,
+ ffn_extra_layernorm=ffn_extra_layernorm,
+ )
diff --git a/difpoint/src/models/XPose/models/UniPose/fuse_modules.py b/difpoint/src/models/XPose/models/UniPose/fuse_modules.py
new file mode 100644
index 0000000000000000000000000000000000000000..0136b8f51d15bba4000983b36260a5647b451bd6
--- /dev/null
+++ b/difpoint/src/models/XPose/models/UniPose/fuse_modules.py
@@ -0,0 +1,276 @@
+import torch
+import torch.nn as nn
+import torch.nn.functional as F
+
+# from timm.models.layers import DropPath
+from src.models.util import DropPath
+
+
+class FeatureResizer(nn.Module):
+ """
+ This class takes as input a set of embeddings of dimension C1 and outputs a set of
+ embedding of dimension C2, after a linear transformation, dropout and normalization (LN).
+ """
+
+ def __init__(self, input_feat_size, output_feat_size, dropout, do_ln=True):
+ super().__init__()
+ self.do_ln = do_ln
+ # Object feature encoding
+ self.fc = nn.Linear(input_feat_size, output_feat_size, bias=True)
+ self.layer_norm = nn.LayerNorm(output_feat_size, eps=1e-12)
+ self.dropout = nn.Dropout(dropout)
+
+ def forward(self, encoder_features):
+ x = self.fc(encoder_features)
+ if self.do_ln:
+ x = self.layer_norm(x)
+ output = self.dropout(x)
+ return output
+
+
+def l1norm(X, dim, eps=1e-8):
+ """L1-normalize columns of X
+ """
+ norm = torch.abs(X).sum(dim=dim, keepdim=True) + eps
+ X = torch.div(X, norm)
+ return X
+
+
+def l2norm(X, dim, eps=1e-8):
+ """L2-normalize columns of X
+ """
+ norm = torch.pow(X, 2).sum(dim=dim, keepdim=True).sqrt() + eps
+ X = torch.div(X, norm)
+ return X
+
+
+def func_attention(query, context, smooth=1, raw_feature_norm="softmax", eps=1e-8):
+ """
+ query: (n_context, queryL, d)
+ context: (n_context, sourceL, d)
+ """
+ batch_size_q, queryL = query.size(0), query.size(1)
+ batch_size, sourceL = context.size(0), context.size(1)
+
+ # Get attention
+ # --> (batch, d, queryL)
+ queryT = torch.transpose(query, 1, 2)
+
+ # (batch, sourceL, d)(batch, d, queryL)
+ # --> (batch, sourceL, queryL)
+ attn = torch.bmm(context, queryT)
+ if raw_feature_norm == "softmax":
+ # --> (batch*sourceL, queryL)
+ attn = attn.view(batch_size * sourceL, queryL)
+ attn = nn.Softmax()(attn)
+ # --> (batch, sourceL, queryL)
+ attn = attn.view(batch_size, sourceL, queryL)
+ elif raw_feature_norm == "l2norm":
+ attn = l2norm(attn, 2)
+ elif raw_feature_norm == "clipped_l2norm":
+ attn = nn.LeakyReLU(0.1)(attn)
+ attn = l2norm(attn, 2)
+ else:
+ raise ValueError("unknown first norm type:", raw_feature_norm)
+ # --> (batch, queryL, sourceL)
+ attn = torch.transpose(attn, 1, 2).contiguous()
+ # --> (batch*queryL, sourceL)
+ attn = attn.view(batch_size * queryL, sourceL)
+ attn = nn.Softmax()(attn * smooth)
+ # --> (batch, queryL, sourceL)
+ attn = attn.view(batch_size, queryL, sourceL)
+ # --> (batch, sourceL, queryL)
+ attnT = torch.transpose(attn, 1, 2).contiguous()
+
+ # --> (batch, d, sourceL)
+ contextT = torch.transpose(context, 1, 2)
+ # (batch x d x sourceL)(batch x sourceL x queryL)
+ # --> (batch, d, queryL)
+ weightedContext = torch.bmm(contextT, attnT)
+ # --> (batch, queryL, d)
+ weightedContext = torch.transpose(weightedContext, 1, 2)
+
+ return weightedContext, attnT
+
+
+class BiMultiHeadAttention(nn.Module):
+ def __init__(self, v_dim, l_dim, embed_dim, num_heads, dropout=0.1, cfg=None):
+ super(BiMultiHeadAttention, self).__init__()
+
+ self.embed_dim = embed_dim
+ self.num_heads = num_heads
+ self.head_dim = embed_dim // num_heads
+ self.v_dim = v_dim
+ self.l_dim = l_dim
+
+ assert (
+ self.head_dim * self.num_heads == self.embed_dim
+ ), f"embed_dim must be divisible by num_heads (got `embed_dim`: {self.embed_dim} and `num_heads`: {self.num_heads})."
+ self.scale = self.head_dim ** (-0.5)
+ self.dropout = dropout
+
+ self.v_proj = nn.Linear(self.v_dim, self.embed_dim)
+ self.l_proj = nn.Linear(self.l_dim, self.embed_dim)
+ self.values_v_proj = nn.Linear(self.v_dim, self.embed_dim)
+ self.values_l_proj = nn.Linear(self.l_dim, self.embed_dim)
+
+ self.out_v_proj = nn.Linear(self.embed_dim, self.v_dim)
+ self.out_l_proj = nn.Linear(self.embed_dim, self.l_dim)
+
+ self.stable_softmax_2d = True
+ self.clamp_min_for_underflow = True
+ self.clamp_max_for_overflow = True
+
+ self._reset_parameters()
+
+ def _shape(self, tensor: torch.Tensor, seq_len: int, bsz: int):
+ return tensor.view(bsz, seq_len, self.num_heads, self.head_dim).transpose(1, 2).contiguous()
+
+ def _reset_parameters(self):
+ nn.init.xavier_uniform_(self.v_proj.weight)
+ self.v_proj.bias.data.fill_(0)
+ nn.init.xavier_uniform_(self.l_proj.weight)
+ self.l_proj.bias.data.fill_(0)
+ nn.init.xavier_uniform_(self.values_v_proj.weight)
+ self.values_v_proj.bias.data.fill_(0)
+ nn.init.xavier_uniform_(self.values_l_proj.weight)
+ self.values_l_proj.bias.data.fill_(0)
+ nn.init.xavier_uniform_(self.out_v_proj.weight)
+ self.out_v_proj.bias.data.fill_(0)
+ nn.init.xavier_uniform_(self.out_l_proj.weight)
+ self.out_l_proj.bias.data.fill_(0)
+
+ def forward(self, v, l, attention_mask_v=None, attention_mask_l=None):
+ """_summary_
+
+ Args:
+ v (_type_): bs, n_img, dim
+ l (_type_): bs, n_text, dim
+ attention_mask_v (_type_, optional): _description_. bs, n_img
+ attention_mask_l (_type_, optional): _description_. bs, n_text
+
+ Returns:
+ _type_: _description_
+ """
+ # if os.environ.get('IPDB_SHILONG_DEBUG', None) == 'INFO':
+ # import ipdb; ipdb.set_trace()
+ bsz, tgt_len, _ = v.size()
+
+ query_states = self.v_proj(v) * self.scale
+ key_states = self._shape(self.l_proj(l), -1, bsz)
+ value_v_states = self._shape(self.values_v_proj(v), -1, bsz)
+ value_l_states = self._shape(self.values_l_proj(l), -1, bsz)
+
+ proj_shape = (bsz * self.num_heads, -1, self.head_dim)
+ query_states = self._shape(query_states, tgt_len, bsz).view(*proj_shape)
+ key_states = key_states.view(*proj_shape)
+ value_v_states = value_v_states.view(*proj_shape)
+ value_l_states = value_l_states.view(*proj_shape)
+
+ src_len = key_states.size(1)
+ attn_weights = torch.bmm(query_states, key_states.transpose(1, 2)) # bs*nhead, nimg, ntxt
+
+ if attn_weights.size() != (bsz * self.num_heads, tgt_len, src_len):
+ raise ValueError(
+ f"Attention weights should be of size {(bsz * self.num_heads, tgt_len, src_len)}, but is {attn_weights.size()}"
+ )
+
+ if self.stable_softmax_2d:
+ attn_weights = attn_weights - attn_weights.max()
+
+ if self.clamp_min_for_underflow:
+ attn_weights = torch.clamp(attn_weights,
+ min=-50000) # Do not increase -50000, data type half has quite limited range
+ if self.clamp_max_for_overflow:
+ attn_weights = torch.clamp(attn_weights,
+ max=50000) # Do not increase 50000, data type half has quite limited range
+
+ attn_weights_T = attn_weights.transpose(1, 2)
+ attn_weights_l = (attn_weights_T - torch.max(attn_weights_T, dim=-1, keepdim=True)[
+ 0])
+ if self.clamp_min_for_underflow:
+ attn_weights_l = torch.clamp(attn_weights_l,
+ min=-50000) # Do not increase -50000, data type half has quite limited range
+ if self.clamp_max_for_overflow:
+ attn_weights_l = torch.clamp(attn_weights_l,
+ max=50000) # Do not increase 50000, data type half has quite limited range
+
+ # mask vison for language
+ if attention_mask_v is not None:
+ attention_mask_v = attention_mask_v[:, None, None, :].repeat(1, self.num_heads, 1, 1).flatten(0, 1)
+ attn_weights_l.masked_fill_(attention_mask_v, float('-inf'))
+
+ attn_weights_l = attn_weights_l.softmax(dim=-1)
+
+ # mask language for vision
+ if attention_mask_l is not None:
+ attention_mask_l = attention_mask_l[:, None, None, :].repeat(1, self.num_heads, 1, 1).flatten(0, 1)
+ attn_weights.masked_fill_(attention_mask_l, float('-inf'))
+ attn_weights_v = attn_weights.softmax(dim=-1)
+
+ attn_probs_v = F.dropout(attn_weights_v, p=self.dropout, training=self.training)
+ attn_probs_l = F.dropout(attn_weights_l, p=self.dropout, training=self.training)
+
+ attn_output_v = torch.bmm(attn_probs_v, value_l_states)
+ attn_output_l = torch.bmm(attn_probs_l, value_v_states)
+
+ if attn_output_v.size() != (bsz * self.num_heads, tgt_len, self.head_dim):
+ raise ValueError(
+ f"`attn_output_v` should be of size {(bsz, self.num_heads, tgt_len, self.head_dim)}, but is {attn_output_v.size()}"
+ )
+
+ if attn_output_l.size() != (bsz * self.num_heads, src_len, self.head_dim):
+ raise ValueError(
+ f"`attn_output_l` should be of size {(bsz, self.num_heads, src_len, self.head_dim)}, but is {attn_output_l.size()}"
+ )
+
+ attn_output_v = attn_output_v.view(bsz, self.num_heads, tgt_len, self.head_dim)
+ attn_output_v = attn_output_v.transpose(1, 2)
+ attn_output_v = attn_output_v.reshape(bsz, tgt_len, self.embed_dim)
+
+ attn_output_l = attn_output_l.view(bsz, self.num_heads, src_len, self.head_dim)
+ attn_output_l = attn_output_l.transpose(1, 2)
+ attn_output_l = attn_output_l.reshape(bsz, src_len, self.embed_dim)
+
+ attn_output_v = self.out_v_proj(attn_output_v)
+ attn_output_l = self.out_l_proj(attn_output_l)
+
+ return attn_output_v, attn_output_l
+
+
+# Bi-Direction MHA (text->image, image->text)
+class BiAttentionBlock(nn.Module):
+ def __init__(self, v_dim, l_dim, embed_dim, num_heads, dropout=0.1,
+ drop_path=.0, init_values=1e-4, cfg=None):
+ """
+ Inputs:
+ embed_dim - Dimensionality of input and attention feature vectors
+ hidden_dim - Dimensionality of hidden layer in feed-forward network
+ (usually 2-4x larger than embed_dim)
+ num_heads - Number of heads to use in the Multi-Head Attention block
+ dropout - Amount of dropout to apply in the feed-forward network
+ """
+ super(BiAttentionBlock, self).__init__()
+
+ # pre layer norm
+ self.layer_norm_v = nn.LayerNorm(v_dim)
+ self.layer_norm_l = nn.LayerNorm(l_dim)
+ self.attn = BiMultiHeadAttention(v_dim=v_dim,
+ l_dim=l_dim,
+ embed_dim=embed_dim,
+ num_heads=num_heads,
+ dropout=dropout)
+
+ # add layer scale for training stability
+ self.drop_path = DropPath(drop_path) if drop_path > 0. else nn.Identity()
+ self.gamma_v = nn.Parameter(init_values * torch.ones((v_dim)), requires_grad=False)
+ self.gamma_l = nn.Parameter(init_values * torch.ones((l_dim)), requires_grad=False)
+
+ def forward(self, v, l, attention_mask_v=None, attention_mask_l=None):
+ v = self.layer_norm_v(v)
+ l = self.layer_norm_l(l)
+ delta_v, delta_l = self.attn(v, l, attention_mask_v=attention_mask_v, attention_mask_l=attention_mask_l)
+ # v, l = v + delta_v, l + delta_l
+ v = v + self.drop_path(self.gamma_v * delta_v)
+ l = l + self.drop_path(self.gamma_l * delta_l)
+ return v, l
diff --git a/difpoint/src/models/XPose/models/UniPose/mask_generate.py b/difpoint/src/models/XPose/models/UniPose/mask_generate.py
new file mode 100644
index 0000000000000000000000000000000000000000..31b566bfb50b2f8df0e1660d5d7997a6d0d8a85c
--- /dev/null
+++ b/difpoint/src/models/XPose/models/UniPose/mask_generate.py
@@ -0,0 +1,56 @@
+import torch
+
+
+def prepare_for_mask(kpt_mask):
+
+
+ tgt_size2 = 50 * 69
+ attn_mask2 = torch.ones(kpt_mask.shape[0], 8, tgt_size2, tgt_size2).to('cuda') < 0
+ group_bbox_kpt = 69
+ num_group=50
+ for matchj in range(num_group * group_bbox_kpt):
+ sj = (matchj // group_bbox_kpt) * group_bbox_kpt
+ ej = (matchj // group_bbox_kpt + 1)*group_bbox_kpt
+ if sj > 0:
+ attn_mask2[:,:,matchj, :sj] = True
+ if ej < num_group * group_bbox_kpt:
+ attn_mask2[:,:,matchj, ej:] = True
+
+
+ bs, length = kpt_mask.shape
+ equal_mask = kpt_mask[:, :, None] == kpt_mask[:, None, :]
+ equal_mask= equal_mask.unsqueeze(1).repeat(1,8,1,1)
+ for idx in range(num_group):
+ start_idx = idx * length
+ end_idx = (idx + 1) * length
+ attn_mask2[:, :,start_idx:end_idx, start_idx:end_idx][equal_mask] = False
+ attn_mask2[:, :,start_idx:end_idx, start_idx:end_idx][~equal_mask] = True
+
+
+
+
+ input_query_label = None
+ input_query_bbox = None
+ attn_mask = None
+ dn_meta = None
+
+ return input_query_label, input_query_bbox, attn_mask, attn_mask2.flatten(0,1), dn_meta
+
+
+def post_process(outputs_class, outputs_coord, dn_meta, aux_loss, _set_aux_loss):
+
+ if dn_meta and dn_meta['pad_size'] > 0:
+
+ output_known_class = [outputs_class_i[:, :dn_meta['pad_size'], :] for outputs_class_i in outputs_class]
+ output_known_coord = [outputs_coord_i[:, :dn_meta['pad_size'], :] for outputs_coord_i in outputs_coord]
+
+ outputs_class = [outputs_class_i[:, dn_meta['pad_size']:, :] for outputs_class_i in outputs_class]
+ outputs_coord = [outputs_coord_i[:, dn_meta['pad_size']:, :] for outputs_coord_i in outputs_coord]
+
+ out = {'pred_logits': output_known_class[-1], 'pred_boxes': output_known_coord[-1]}
+ if aux_loss:
+ out['aux_outputs'] = _set_aux_loss(output_known_class, output_known_coord)
+ dn_meta['output_known_lbs_bboxes'] = out
+ return outputs_class, outputs_coord
+
+
diff --git a/difpoint/src/models/XPose/models/UniPose/ops/__init__.py b/difpoint/src/models/XPose/models/UniPose/ops/__init__.py
new file mode 100644
index 0000000000000000000000000000000000000000..601cd005e85ae5bec92d05bf1e07b136d4fa6ee2
--- /dev/null
+++ b/difpoint/src/models/XPose/models/UniPose/ops/__init__.py
@@ -0,0 +1,6 @@
+# -*- coding: utf-8 -*-
+# @Time : 2024/8/5 21:58
+# @Author : shaoguowen
+# @Email : wenshaoguo1026@gmail.com
+# @Project : FasterLivePortrait
+# @FileName: __init__.py.py
diff --git a/difpoint/src/models/XPose/models/UniPose/ops/functions/__init__.py b/difpoint/src/models/XPose/models/UniPose/ops/functions/__init__.py
new file mode 100644
index 0000000000000000000000000000000000000000..bbb16cd2ed139d1fe15e5ddf2396c2f23d11d2f6
--- /dev/null
+++ b/difpoint/src/models/XPose/models/UniPose/ops/functions/__init__.py
@@ -0,0 +1,10 @@
+# ------------------------------------------------------------------------------------------------
+# Deformable DETR
+# Copyright (c) 2020 SenseTime. All Rights Reserved.
+# Licensed under the Apache License, Version 2.0 [see LICENSE for details]
+# ------------------------------------------------------------------------------------------------
+# Modified from https://github.com/chengdazhi/Deformable-Convolution-V2-PyTorch/tree/pytorch_1.0.0
+# ------------------------------------------------------------------------------------------------
+
+from .ms_deform_attn_func import MSDeformAttnFunction
+
diff --git a/difpoint/src/models/XPose/models/UniPose/ops/functions/ms_deform_attn_func.py b/difpoint/src/models/XPose/models/UniPose/ops/functions/ms_deform_attn_func.py
new file mode 100644
index 0000000000000000000000000000000000000000..b4c4d2b2b16a9af8bee129e1e983820e7df195a4
--- /dev/null
+++ b/difpoint/src/models/XPose/models/UniPose/ops/functions/ms_deform_attn_func.py
@@ -0,0 +1,61 @@
+# ------------------------------------------------------------------------------------------------
+# Deformable DETR
+# Copyright (c) 2020 SenseTime. All Rights Reserved.
+# Licensed under the Apache License, Version 2.0 [see LICENSE for details]
+# ------------------------------------------------------------------------------------------------
+# Modified from https://github.com/chengdazhi/Deformable-Convolution-V2-PyTorch/tree/pytorch_1.0.0
+# ------------------------------------------------------------------------------------------------
+
+from __future__ import absolute_import
+from __future__ import print_function
+from __future__ import division
+
+import torch
+import torch.nn.functional as F
+from torch.autograd import Function
+from torch.autograd.function import once_differentiable
+
+import MultiScaleDeformableAttention as MSDA
+
+
+class MSDeformAttnFunction(Function):
+ @staticmethod
+ def forward(ctx, value, value_spatial_shapes, value_level_start_index, sampling_locations, attention_weights, im2col_step):
+ ctx.im2col_step = im2col_step
+ output = MSDA.ms_deform_attn_forward(
+ value, value_spatial_shapes, value_level_start_index, sampling_locations, attention_weights, ctx.im2col_step)
+ ctx.save_for_backward(value, value_spatial_shapes, value_level_start_index, sampling_locations, attention_weights)
+ return output
+
+ @staticmethod
+ @once_differentiable
+ def backward(ctx, grad_output):
+ value, value_spatial_shapes, value_level_start_index, sampling_locations, attention_weights = ctx.saved_tensors
+ grad_value, grad_sampling_loc, grad_attn_weight = \
+ MSDA.ms_deform_attn_backward(
+ value, value_spatial_shapes, value_level_start_index, sampling_locations, attention_weights, grad_output, ctx.im2col_step)
+
+ return grad_value, None, None, grad_sampling_loc, grad_attn_weight, None
+
+
+def ms_deform_attn_core_pytorch(value, value_spatial_shapes, sampling_locations, attention_weights):
+ # for debug and test only,
+ # need to use cuda version instead
+ N_, S_, M_, D_ = value.shape
+ _, Lq_, M_, L_, P_, _ = sampling_locations.shape
+ value_list = value.split([H_ * W_ for H_, W_ in value_spatial_shapes], dim=1)
+ sampling_grids = 2 * sampling_locations - 1
+ sampling_value_list = []
+ for lid_, (H_, W_) in enumerate(value_spatial_shapes):
+ # N_, H_*W_, M_, D_ -> N_, H_*W_, M_*D_ -> N_, M_*D_, H_*W_ -> N_*M_, D_, H_, W_
+ value_l_ = value_list[lid_].flatten(2).transpose(1, 2).reshape(N_*M_, D_, H_, W_)
+ # N_, Lq_, M_, P_, 2 -> N_, M_, Lq_, P_, 2 -> N_*M_, Lq_, P_, 2
+ sampling_grid_l_ = sampling_grids[:, :, :, lid_].transpose(1, 2).flatten(0, 1)
+ # N_*M_, D_, Lq_, P_
+ sampling_value_l_ = F.grid_sample(value_l_, sampling_grid_l_,
+ mode='bilinear', padding_mode='zeros', align_corners=False)
+ sampling_value_list.append(sampling_value_l_)
+ # (N_, Lq_, M_, L_, P_) -> (N_, M_, Lq_, L_, P_) -> (N_, M_, 1, Lq_, L_*P_)
+ attention_weights = attention_weights.transpose(1, 2).reshape(N_*M_, 1, Lq_, L_*P_)
+ output = (torch.stack(sampling_value_list, dim=-2).flatten(-2) * attention_weights).sum(-1).view(N_, M_*D_, Lq_)
+ return output.transpose(1, 2).contiguous()
diff --git a/difpoint/src/models/XPose/models/UniPose/ops/modules/__init__.py b/difpoint/src/models/XPose/models/UniPose/ops/modules/__init__.py
new file mode 100644
index 0000000000000000000000000000000000000000..ff5ce8300145fc427cfedb3421a70f8defbde7f2
--- /dev/null
+++ b/difpoint/src/models/XPose/models/UniPose/ops/modules/__init__.py
@@ -0,0 +1,9 @@
+# ------------------------------------------------------------------------------------------------
+# Deformable DETR
+# Copyright (c) 2020 SenseTime. All Rights Reserved.
+# Licensed under the Apache License, Version 2.0 [see LICENSE for details]
+# ------------------------------------------------------------------------------------------------
+# Modified from https://github.com/chengdazhi/Deformable-Convolution-V2-PyTorch/tree/pytorch_1.0.0
+# ------------------------------------------------------------------------------------------------
+
+from .ms_deform_attn import MSDeformAttn
diff --git a/difpoint/src/models/XPose/models/UniPose/ops/modules/ms_deform_attn.py b/difpoint/src/models/XPose/models/UniPose/ops/modules/ms_deform_attn.py
new file mode 100644
index 0000000000000000000000000000000000000000..8e53b0ffc3ec8c177901180bb39ba6d8eebaa2eb
--- /dev/null
+++ b/difpoint/src/models/XPose/models/UniPose/ops/modules/ms_deform_attn.py
@@ -0,0 +1,142 @@
+# ------------------------------------------------------------------------------------------------
+# Deformable DETR
+# Copyright (c) 2020 SenseTime. All Rights Reserved.
+# Licensed under the Apache License, Version 2.0 [see LICENSE for details]
+# ------------------------------------------------------------------------------------------------
+# Modified from https://github.com/chengdazhi/Deformable-Convolution-V2-PyTorch/tree/pytorch_1.0.0
+# ------------------------------------------------------------------------------------------------
+
+from __future__ import absolute_import
+from __future__ import print_function
+from __future__ import division
+
+import warnings
+import math, os
+import sys
+sys.path.append(os.path.dirname(os.path.abspath(__file__)))
+
+import torch
+from torch import nn
+import torch.nn.functional as F
+from torch.nn.init import xavier_uniform_, constant_
+
+from src.models.XPose.models.UniPose.ops.functions.ms_deform_attn_func import MSDeformAttnFunction
+
+
+def _is_power_of_2(n):
+ if (not isinstance(n, int)) or (n < 0):
+ raise ValueError("invalid input for _is_power_of_2: {} (type: {})".format(n, type(n)))
+ return (n & (n-1) == 0) and n != 0
+
+
+class MSDeformAttn(nn.Module):
+ def __init__(self, d_model=256, n_levels=4, n_heads=8, n_points=4, use_4D_normalizer=False):
+ """
+ Multi-Scale Deformable Attention Module
+ :param d_model hidden dimension
+ :param n_levels number of feature levels
+ :param n_heads number of attention heads
+ :param n_points number of sampling points per attention head per feature level
+ """
+ super().__init__()
+ if d_model % n_heads != 0:
+ raise ValueError('d_model must be divisible by n_heads, but got {} and {}'.format(d_model, n_heads))
+ _d_per_head = d_model // n_heads
+ # you'd better set _d_per_head to a power of 2 which is more efficient in our CUDA implementation
+ if not _is_power_of_2(_d_per_head):
+ warnings.warn("You'd better set d_model in MSDeformAttn to make the dimension of each attention head a power of 2 "
+ "which is more efficient in our CUDA implementation.")
+
+ self.im2col_step = 64
+
+ self.d_model = d_model
+ self.n_levels = n_levels
+ self.n_heads = n_heads
+ self.n_points = n_points
+
+ self.sampling_offsets = nn.Linear(d_model, n_heads * n_levels * n_points * 2)
+ self.attention_weights = nn.Linear(d_model, n_heads * n_levels * n_points)
+ self.value_proj = nn.Linear(d_model, d_model)
+ self.output_proj = nn.Linear(d_model, d_model)
+
+ self.use_4D_normalizer = use_4D_normalizer
+
+ self._reset_parameters()
+
+ def _reset_parameters(self):
+ constant_(self.sampling_offsets.weight.data, 0.)
+ thetas = torch.arange(self.n_heads, dtype=torch.float32) * (2.0 * math.pi / self.n_heads)
+ grid_init = torch.stack([thetas.cos(), thetas.sin()], -1)
+ grid_init = (grid_init / grid_init.abs().max(-1, keepdim=True)[0]).view(self.n_heads, 1, 1, 2).repeat(1, self.n_levels, self.n_points, 1)
+ for i in range(self.n_points):
+ grid_init[:, :, i, :] *= i + 1
+ with torch.no_grad():
+ self.sampling_offsets.bias = nn.Parameter(grid_init.view(-1))
+ constant_(self.attention_weights.weight.data, 0.)
+ constant_(self.attention_weights.bias.data, 0.)
+ xavier_uniform_(self.value_proj.weight.data)
+ constant_(self.value_proj.bias.data, 0.)
+ xavier_uniform_(self.output_proj.weight.data)
+ constant_(self.output_proj.bias.data, 0.)
+
+ def forward(self, query, reference_points, input_flatten, input_spatial_shapes, input_level_start_index, input_padding_mask=None):
+ """
+ :param query (N, Length_{query}, C)
+ :param reference_points (N, Length_{query}, n_levels, 2), range in [0, 1], top-left (0,0), bottom-right (1, 1), including padding area
+ or (N, Length_{query}, n_levels, 4), add additional (w, h) to form reference boxes
+ :param input_flatten (N, \sum_{l=0}^{L-1} H_l \cdot W_l, C)
+ :param input_spatial_shapes (n_levels, 2), [(H_0, W_0), (H_1, W_1), ..., (H_{L-1}, W_{L-1})]
+ :param input_level_start_index (n_levels, ), [0, H_0*W_0, H_0*W_0+H_1*W_1, H_0*W_0+H_1*W_1+H_2*W_2, ..., H_0*W_0+H_1*W_1+...+H_{L-1}*W_{L-1}]
+ :param input_padding_mask (N, \sum_{l=0}^{L-1} H_l \cdot W_l), True for padding elements, False for non-padding elements
+
+ :return output (N, Length_{query}, C)
+ """
+ N, Len_q, _ = query.shape
+ N, Len_in, _ = input_flatten.shape
+ assert (input_spatial_shapes[:, 0] * input_spatial_shapes[:, 1]).sum() == Len_in
+
+ value = self.value_proj(input_flatten)
+ if input_padding_mask is not None:
+ value = value.masked_fill(input_padding_mask[..., None], float(0))
+ value = value.view(N, Len_in, self.n_heads, self.d_model // self.n_heads)
+ sampling_offsets = self.sampling_offsets(query).view(N, Len_q, self.n_heads, self.n_levels, self.n_points, 2)
+ attention_weights = self.attention_weights(query).view(N, Len_q, self.n_heads, self.n_levels * self.n_points)
+ attention_weights = F.softmax(attention_weights, -1).view(N, Len_q, self.n_heads, self.n_levels, self.n_points)
+ # N, Len_q, n_heads, n_levels, n_points, 2
+
+ # if os.environ.get('IPDB_DEBUG_SHILONG', False) == 'INFO':
+ # import ipdb; ipdb.set_trace()
+
+ if reference_points.shape[-1] == 2:
+ offset_normalizer = torch.stack([input_spatial_shapes[..., 1], input_spatial_shapes[..., 0]], -1)
+ sampling_locations = reference_points[:, :, None, :, None, :] \
+ + sampling_offsets / offset_normalizer[None, None, None, :, None, :]
+ elif reference_points.shape[-1] == 4:
+ if self.use_4D_normalizer:
+ offset_normalizer = torch.stack([input_spatial_shapes[..., 1], input_spatial_shapes[..., 0]], -1)
+ sampling_locations = reference_points[:, :, None, :, None, :2] \
+ + sampling_offsets / offset_normalizer[None, None, None, :, None, :] * reference_points[:, :, None, :, None, 2:] * 0.5
+ else:
+ sampling_locations = reference_points[:, :, None, :, None, :2] \
+ + sampling_offsets / self.n_points * reference_points[:, :, None, :, None, 2:] * 0.5
+ else:
+ raise ValueError(
+ 'Last dim of reference_points must be 2 or 4, but get {} instead.'.format(reference_points.shape[-1]))
+
+
+ # if os.environ.get('IPDB_DEBUG_SHILONG', False) == 'INFO':
+ # import ipdb; ipdb.set_trace()
+
+ # for amp
+ if value.dtype == torch.float16:
+ # for mixed precision
+ output = MSDeformAttnFunction.apply(
+ value.to(torch.float32), input_spatial_shapes, input_level_start_index, sampling_locations.to(torch.float32), attention_weights, self.im2col_step)
+ output = output.to(torch.float16)
+ output = self.output_proj(output)
+ return output
+
+ output = MSDeformAttnFunction.apply(
+ value, input_spatial_shapes, input_level_start_index, sampling_locations, attention_weights, self.im2col_step)
+ output = self.output_proj(output)
+ return output
diff --git a/difpoint/src/models/XPose/models/UniPose/ops/modules/ms_deform_attn_key_aware.py b/difpoint/src/models/XPose/models/UniPose/ops/modules/ms_deform_attn_key_aware.py
new file mode 100644
index 0000000000000000000000000000000000000000..9b5260401d601460f5dbfb2b8997680fd72d8cec
--- /dev/null
+++ b/difpoint/src/models/XPose/models/UniPose/ops/modules/ms_deform_attn_key_aware.py
@@ -0,0 +1,130 @@
+# ------------------------------------------------------------------------------------------------
+# Deformable DETR
+# Copyright (c) 2020 SenseTime. All Rights Reserved.
+# Licensed under the Apache License, Version 2.0 [see LICENSE for details]
+# ------------------------------------------------------------------------------------------------
+# Modified from https://github.com/chengdazhi/Deformable-Convolution-V2-PyTorch/tree/pytorch_1.0.0
+# ------------------------------------------------------------------------------------------------
+
+from __future__ import absolute_import
+from __future__ import print_function
+from __future__ import division
+
+import warnings
+import math, os
+
+import torch
+from torch import nn
+import torch.nn.functional as F
+from torch.nn.init import xavier_uniform_, constant_
+
+try:
+ from src.models.XPose.models.UniPose.ops.functions import MSDeformAttnFunction
+except:
+ warnings.warn('Failed to import MSDeformAttnFunction.')
+
+
+def _is_power_of_2(n):
+ if (not isinstance(n, int)) or (n < 0):
+ raise ValueError("invalid input for _is_power_of_2: {} (type: {})".format(n, type(n)))
+ return (n & (n-1) == 0) and n != 0
+
+
+class MSDeformAttn(nn.Module):
+ def __init__(self, d_model=256, n_levels=4, n_heads=8, n_points=4, use_4D_normalizer=False):
+ """
+ Multi-Scale Deformable Attention Module
+ :param d_model hidden dimension
+ :param n_levels number of feature levels
+ :param n_heads number of attention heads
+ :param n_points number of sampling points per attention head per feature level
+ """
+ super().__init__()
+ if d_model % n_heads != 0:
+ raise ValueError('d_model must be divisible by n_heads, but got {} and {}'.format(d_model, n_heads))
+ _d_per_head = d_model // n_heads
+ # you'd better set _d_per_head to a power of 2 which is more efficient in our CUDA implementation
+ if not _is_power_of_2(_d_per_head):
+ warnings.warn("You'd better set d_model in MSDeformAttn to make the dimension of each attention head a power of 2 "
+ "which is more efficient in our CUDA implementation.")
+
+ self.im2col_step = 64
+
+ self.d_model = d_model
+ self.n_levels = n_levels
+ self.n_heads = n_heads
+ self.n_points = n_points
+
+ self.sampling_offsets = nn.Linear(d_model, n_heads * n_levels * n_points * 2)
+ self.attention_weights = nn.Linear(d_model, n_heads * n_levels * n_points)
+ self.value_proj = nn.Linear(d_model, d_model)
+ self.output_proj = nn.Linear(d_model, d_model)
+
+ self.use_4D_normalizer = use_4D_normalizer
+
+ self._reset_parameters()
+
+ def _reset_parameters(self):
+ constant_(self.sampling_offsets.weight.data, 0.)
+ thetas = torch.arange(self.n_heads, dtype=torch.float32) * (2.0 * math.pi / self.n_heads)
+ grid_init = torch.stack([thetas.cos(), thetas.sin()], -1)
+ grid_init = (grid_init / grid_init.abs().max(-1, keepdim=True)[0]).view(self.n_heads, 1, 1, 2).repeat(1, self.n_levels, self.n_points, 1)
+ for i in range(self.n_points):
+ grid_init[:, :, i, :] *= i + 1
+ with torch.no_grad():
+ self.sampling_offsets.bias = nn.Parameter(grid_init.view(-1))
+ constant_(self.attention_weights.weight.data, 0.)
+ constant_(self.attention_weights.bias.data, 0.)
+ xavier_uniform_(self.value_proj.weight.data)
+ constant_(self.value_proj.bias.data, 0.)
+ xavier_uniform_(self.output_proj.weight.data)
+ constant_(self.output_proj.bias.data, 0.)
+
+ def forward(self, query, key, reference_points, input_flatten, input_spatial_shapes, input_level_start_index, input_padding_mask=None):
+ """
+ :param query (N, Length_{query}, C)
+ :param key (N, 1, C)
+ :param reference_points (N, Length_{query}, n_levels, 2), range in [0, 1], top-left (0,0), bottom-right (1, 1), including padding area
+ or (N, Length_{query}, n_levels, 4), add additional (w, h) to form reference boxes
+ :param input_flatten (N, \sum_{l=0}^{L-1} H_l \cdot W_l, C)
+ :param input_spatial_shapes (n_levels, 2), [(H_0, W_0), (H_1, W_1), ..., (H_{L-1}, W_{L-1})]
+ :param input_level_start_index (n_levels, ), [0, H_0*W_0, H_0*W_0+H_1*W_1, H_0*W_0+H_1*W_1+H_2*W_2, ..., H_0*W_0+H_1*W_1+...+H_{L-1}*W_{L-1}]
+ :param input_padding_mask (N, \sum_{l=0}^{L-1} H_l \cdot W_l), True for padding elements, False for non-padding elements
+
+ :return output (N, Length_{query}, C)
+ """
+ N, Len_q, _ = query.shape
+ N, Len_in, _ = input_flatten.shape
+ assert (input_spatial_shapes[:, 0] * input_spatial_shapes[:, 1]).sum() == Len_in
+
+ value = self.value_proj(input_flatten)
+ if input_padding_mask is not None:
+ value = value.masked_fill(input_padding_mask[..., None], float(0))
+ value = value.view(N, Len_in, self.n_heads, self.d_model // self.n_heads)
+ sampling_offsets = self.sampling_offsets(query).view(N, Len_q, self.n_heads, self.n_levels, self.n_points, 2)
+ attention_weights = self.attention_weights(query).view(N, Len_q, self.n_heads, self.n_levels * self.n_points)
+ attention_weights = F.softmax(attention_weights, -1).view(N, Len_q, self.n_heads, self.n_levels, self.n_points)
+ # N, Len_q, n_heads, n_levels, n_points, 2
+
+ # if os.environ.get('IPDB_DEBUG_SHILONG', False) == 'INFO':
+ # import ipdb; ipdb.set_trace()
+
+ if reference_points.shape[-1] == 2:
+ offset_normalizer = torch.stack([input_spatial_shapes[..., 1], input_spatial_shapes[..., 0]], -1)
+ sampling_locations = reference_points[:, :, None, :, None, :] \
+ + sampling_offsets / offset_normalizer[None, None, None, :, None, :]
+ elif reference_points.shape[-1] == 4:
+ if self.use_4D_normalizer:
+ offset_normalizer = torch.stack([input_spatial_shapes[..., 1], input_spatial_shapes[..., 0]], -1)
+ sampling_locations = reference_points[:, :, None, :, None, :2] \
+ + sampling_offsets / offset_normalizer[None, None, None, :, None, :] * reference_points[:, :, None, :, None, 2:] * 0.5
+ else:
+ sampling_locations = reference_points[:, :, None, :, None, :2] \
+ + sampling_offsets / self.n_points * reference_points[:, :, None, :, None, 2:] * 0.5
+ else:
+ raise ValueError(
+ 'Last dim of reference_points must be 2 or 4, but get {} instead.'.format(reference_points.shape[-1]))
+ output = MSDeformAttnFunction.apply(
+ value, input_spatial_shapes, input_level_start_index, sampling_locations, attention_weights, self.im2col_step)
+ output = self.output_proj(output)
+ return output
diff --git a/difpoint/src/models/XPose/models/UniPose/ops/setup.py b/difpoint/src/models/XPose/models/UniPose/ops/setup.py
new file mode 100644
index 0000000000000000000000000000000000000000..106fecb4eed37d7598bd9373c5366952db2c67b1
--- /dev/null
+++ b/difpoint/src/models/XPose/models/UniPose/ops/setup.py
@@ -0,0 +1,79 @@
+# ------------------------------------------------------------------------------------------------
+# Deformable DETR
+# Copyright (c) 2020 SenseTime. All Rights Reserved.
+# Licensed under the Apache License, Version 2.0 [see LICENSE for details]
+# ------------------------------------------------------------------------------------------------
+# Modified from https://github.com/chengdazhi/Deformable-Convolution-V2-PyTorch/tree/pytorch_1.0.0
+# ------------------------------------------------------------------------------------------------
+
+import os
+import glob
+
+import torch
+
+from torch.utils.cpp_extension import CUDA_HOME
+from torch.utils.cpp_extension import CppExtension
+from torch.utils.cpp_extension import CUDAExtension
+
+from setuptools import find_packages
+from setuptools import setup
+
+requirements = ["torch", "torchvision"]
+
+def get_extensions():
+ this_dir = os.path.dirname(os.path.abspath(__file__))
+ extensions_dir = os.path.join(this_dir, "src")
+
+ main_file = glob.glob(os.path.join(extensions_dir, "*.cpp"))
+ source_cpu = glob.glob(os.path.join(extensions_dir, "cpu", "*.cpp"))
+ source_cuda = glob.glob(os.path.join(extensions_dir, "cuda", "*.cu"))
+
+ sources = main_file + source_cpu
+ extension = CppExtension
+ extra_compile_args = {"cxx": []}
+ define_macros = []
+
+ # import ipdb; ipdb.set_trace()
+
+ if torch.cuda.is_available() and CUDA_HOME is not None:
+ extension = CUDAExtension
+ sources += source_cuda
+ define_macros += [("WITH_CUDA", None)]
+ extra_compile_args["nvcc"] = [
+ "-DCUDA_HAS_FP16=1",
+ "-D__CUDA_NO_HALF_OPERATORS__",
+ "-D__CUDA_NO_HALF_CONVERSIONS__",
+ "-D__CUDA_NO_HALF2_OPERATORS__",
+ # 添加以下行来指定多个 CUDA 架构
+ "-gencode=arch=compute_60,code=sm_60",
+ "-gencode=arch=compute_70,code=sm_70",
+ "-gencode=arch=compute_75,code=sm_75",
+ "-gencode=arch=compute_80,code=sm_80",
+ "-gencode=arch=compute_86,code=sm_86",
+ ]
+ else:
+ raise NotImplementedError('Cuda is not availabel')
+
+ sources = [os.path.join(extensions_dir, s) for s in sources]
+ include_dirs = [extensions_dir]
+ ext_modules = [
+ extension(
+ "MultiScaleDeformableAttention",
+ sources,
+ include_dirs=include_dirs,
+ define_macros=define_macros,
+ extra_compile_args=extra_compile_args,
+ )
+ ]
+ return ext_modules
+
+setup(
+ name="MultiScaleDeformableAttention",
+ version="1.0",
+ author="Weijie Su",
+ url="https://github.com/fundamentalvision/Deformable-DETR",
+ description="PyTorch Wrapper for CUDA Functions of Multi-Scale Deformable Attention",
+ packages=find_packages(exclude=("configs", "tests",)),
+ ext_modules=get_extensions(),
+ cmdclass={"build_ext": torch.utils.cpp_extension.BuildExtension},
+)
diff --git a/difpoint/src/models/XPose/models/UniPose/ops/src/cpu/ms_deform_attn_cpu.cpp b/difpoint/src/models/XPose/models/UniPose/ops/src/cpu/ms_deform_attn_cpu.cpp
new file mode 100644
index 0000000000000000000000000000000000000000..b54a99c30a1837a3bf906007022f90a51abab8d0
--- /dev/null
+++ b/difpoint/src/models/XPose/models/UniPose/ops/src/cpu/ms_deform_attn_cpu.cpp
@@ -0,0 +1,41 @@
+/*!
+**************************************************************************************************
+* Deformable DETR
+* Copyright (c) 2020 SenseTime. All Rights Reserved.
+* Licensed under the Apache License, Version 2.0 [see LICENSE for details]
+**************************************************************************************************
+* Modified from https://github.com/chengdazhi/Deformable-Convolution-V2-PyTorch/tree/pytorch_1.0.0
+**************************************************************************************************
+*/
+
+#include
+
+#include
+#include
+
+
+at::Tensor
+ms_deform_attn_cpu_forward(
+ const at::Tensor &value,
+ const at::Tensor &spatial_shapes,
+ const at::Tensor &level_start_index,
+ const at::Tensor &sampling_loc,
+ const at::Tensor &attn_weight,
+ const int im2col_step)
+{
+ AT_ERROR("Not implement on cpu");
+}
+
+std::vector
+ms_deform_attn_cpu_backward(
+ const at::Tensor &value,
+ const at::Tensor &spatial_shapes,
+ const at::Tensor &level_start_index,
+ const at::Tensor &sampling_loc,
+ const at::Tensor &attn_weight,
+ const at::Tensor &grad_output,
+ const int im2col_step)
+{
+ AT_ERROR("Not implement on cpu");
+}
+
diff --git a/difpoint/src/models/XPose/models/UniPose/ops/src/cpu/ms_deform_attn_cpu.h b/difpoint/src/models/XPose/models/UniPose/ops/src/cpu/ms_deform_attn_cpu.h
new file mode 100644
index 0000000000000000000000000000000000000000..89fad1acdbdd18bb37a3c643813e0eb7c7649789
--- /dev/null
+++ b/difpoint/src/models/XPose/models/UniPose/ops/src/cpu/ms_deform_attn_cpu.h
@@ -0,0 +1,33 @@
+/*!
+**************************************************************************************************
+* Deformable DETR
+* Copyright (c) 2020 SenseTime. All Rights Reserved.
+* Licensed under the Apache License, Version 2.0 [see LICENSE for details]
+**************************************************************************************************
+* Modified from https://github.com/chengdazhi/Deformable-Convolution-V2-PyTorch/tree/pytorch_1.0.0
+**************************************************************************************************
+*/
+
+#pragma once
+#include
+
+at::Tensor
+ms_deform_attn_cpu_forward(
+ const at::Tensor &value,
+ const at::Tensor &spatial_shapes,
+ const at::Tensor &level_start_index,
+ const at::Tensor &sampling_loc,
+ const at::Tensor &attn_weight,
+ const int im2col_step);
+
+std::vector
+ms_deform_attn_cpu_backward(
+ const at::Tensor &value,
+ const at::Tensor &spatial_shapes,
+ const at::Tensor &level_start_index,
+ const at::Tensor &sampling_loc,
+ const at::Tensor &attn_weight,
+ const at::Tensor &grad_output,
+ const int im2col_step);
+
+
diff --git a/difpoint/src/models/XPose/models/UniPose/ops/src/cuda/ms_deform_attn_cuda.cu b/difpoint/src/models/XPose/models/UniPose/ops/src/cuda/ms_deform_attn_cuda.cu
new file mode 100644
index 0000000000000000000000000000000000000000..7c181052f7ac1c40a124d2dda21ed5775814372c
--- /dev/null
+++ b/difpoint/src/models/XPose/models/UniPose/ops/src/cuda/ms_deform_attn_cuda.cu
@@ -0,0 +1,153 @@
+/*!
+**************************************************************************************************
+* Deformable DETR
+* Copyright (c) 2020 SenseTime. All Rights Reserved.
+* Licensed under the Apache License, Version 2.0 [see LICENSE for details]
+**************************************************************************************************
+* Modified from https://github.com/chengdazhi/Deformable-Convolution-V2-PyTorch/tree/pytorch_1.0.0
+**************************************************************************************************
+*/
+
+#include
+#include "cuda/ms_deform_im2col_cuda.cuh"
+
+#include
+#include
+#include
+#include
+
+
+at::Tensor ms_deform_attn_cuda_forward(
+ const at::Tensor &value,
+ const at::Tensor &spatial_shapes,
+ const at::Tensor &level_start_index,
+ const at::Tensor &sampling_loc,
+ const at::Tensor &attn_weight,
+ const int im2col_step)
+{
+ AT_ASSERTM(value.is_contiguous(), "value tensor has to be contiguous");
+ AT_ASSERTM(spatial_shapes.is_contiguous(), "spatial_shapes tensor has to be contiguous");
+ AT_ASSERTM(level_start_index.is_contiguous(), "level_start_index tensor has to be contiguous");
+ AT_ASSERTM(sampling_loc.is_contiguous(), "sampling_loc tensor has to be contiguous");
+ AT_ASSERTM(attn_weight.is_contiguous(), "attn_weight tensor has to be contiguous");
+
+ AT_ASSERTM(value.type().is_cuda(), "value must be a CUDA tensor");
+ AT_ASSERTM(spatial_shapes.type().is_cuda(), "spatial_shapes must be a CUDA tensor");
+ AT_ASSERTM(level_start_index.type().is_cuda(), "level_start_index must be a CUDA tensor");
+ AT_ASSERTM(sampling_loc.type().is_cuda(), "sampling_loc must be a CUDA tensor");
+ AT_ASSERTM(attn_weight.type().is_cuda(), "attn_weight must be a CUDA tensor");
+
+ const int batch = value.size(0);
+ const int spatial_size = value.size(1);
+ const int num_heads = value.size(2);
+ const int channels = value.size(3);
+
+ const int num_levels = spatial_shapes.size(0);
+
+ const int num_query = sampling_loc.size(1);
+ const int num_point = sampling_loc.size(4);
+
+ const int im2col_step_ = std::min(batch, im2col_step);
+
+ AT_ASSERTM(batch % im2col_step_ == 0, "batch(%d) must divide im2col_step(%d)", batch, im2col_step_);
+
+ auto output = at::zeros({batch, num_query, num_heads, channels}, value.options());
+
+ const int batch_n = im2col_step_;
+ auto output_n = output.view({batch/im2col_step_, batch_n, num_query, num_heads, channels});
+ auto per_value_size = spatial_size * num_heads * channels;
+ auto per_sample_loc_size = num_query * num_heads * num_levels * num_point * 2;
+ auto per_attn_weight_size = num_query * num_heads * num_levels * num_point;
+ for (int n = 0; n < batch/im2col_step_; ++n)
+ {
+ auto columns = output_n.select(0, n);
+ AT_DISPATCH_FLOATING_TYPES(value.type(), "ms_deform_attn_forward_cuda", ([&] {
+ ms_deformable_im2col_cuda(at::cuda::getCurrentCUDAStream(),
+ value.data() + n * im2col_step_ * per_value_size,
+ spatial_shapes.data(),
+ level_start_index.data(),
+ sampling_loc.data() + n * im2col_step_ * per_sample_loc_size,
+ attn_weight.data() + n * im2col_step_ * per_attn_weight_size,
+ batch_n, spatial_size, num_heads, channels, num_levels, num_query, num_point,
+ columns.data());
+
+ }));
+ }
+
+ output = output.view({batch, num_query, num_heads*channels});
+
+ return output;
+}
+
+
+std::vector ms_deform_attn_cuda_backward(
+ const at::Tensor &value,
+ const at::Tensor &spatial_shapes,
+ const at::Tensor &level_start_index,
+ const at::Tensor &sampling_loc,
+ const at::Tensor &attn_weight,
+ const at::Tensor &grad_output,
+ const int im2col_step)
+{
+
+ AT_ASSERTM(value.is_contiguous(), "value tensor has to be contiguous");
+ AT_ASSERTM(spatial_shapes.is_contiguous(), "spatial_shapes tensor has to be contiguous");
+ AT_ASSERTM(level_start_index.is_contiguous(), "level_start_index tensor has to be contiguous");
+ AT_ASSERTM(sampling_loc.is_contiguous(), "sampling_loc tensor has to be contiguous");
+ AT_ASSERTM(attn_weight.is_contiguous(), "attn_weight tensor has to be contiguous");
+ AT_ASSERTM(grad_output.is_contiguous(), "grad_output tensor has to be contiguous");
+
+ AT_ASSERTM(value.type().is_cuda(), "value must be a CUDA tensor");
+ AT_ASSERTM(spatial_shapes.type().is_cuda(), "spatial_shapes must be a CUDA tensor");
+ AT_ASSERTM(level_start_index.type().is_cuda(), "level_start_index must be a CUDA tensor");
+ AT_ASSERTM(sampling_loc.type().is_cuda(), "sampling_loc must be a CUDA tensor");
+ AT_ASSERTM(attn_weight.type().is_cuda(), "attn_weight must be a CUDA tensor");
+ AT_ASSERTM(grad_output.type().is_cuda(), "grad_output must be a CUDA tensor");
+
+ const int batch = value.size(0);
+ const int spatial_size = value.size(1);
+ const int num_heads = value.size(2);
+ const int channels = value.size(3);
+
+ const int num_levels = spatial_shapes.size(0);
+
+ const int num_query = sampling_loc.size(1);
+ const int num_point = sampling_loc.size(4);
+
+ const int im2col_step_ = std::min(batch, im2col_step);
+
+ AT_ASSERTM(batch % im2col_step_ == 0, "batch(%d) must divide im2col_step(%d)", batch, im2col_step_);
+
+ auto grad_value = at::zeros_like(value);
+ auto grad_sampling_loc = at::zeros_like(sampling_loc);
+ auto grad_attn_weight = at::zeros_like(attn_weight);
+
+ const int batch_n = im2col_step_;
+ auto per_value_size = spatial_size * num_heads * channels;
+ auto per_sample_loc_size = num_query * num_heads * num_levels * num_point * 2;
+ auto per_attn_weight_size = num_query * num_heads * num_levels * num_point;
+ auto grad_output_n = grad_output.view({batch/im2col_step_, batch_n, num_query, num_heads, channels});
+
+ for (int n = 0; n < batch/im2col_step_; ++n)
+ {
+ auto grad_output_g = grad_output_n.select(0, n);
+ AT_DISPATCH_FLOATING_TYPES(value.type(), "ms_deform_attn_backward_cuda", ([&] {
+ ms_deformable_col2im_cuda(at::cuda::getCurrentCUDAStream(),
+ grad_output_g.data(),
+ value.data() + n * im2col_step_ * per_value_size,
+ spatial_shapes.data(),
+ level_start_index.data(),
+ sampling_loc.data() + n * im2col_step_ * per_sample_loc_size,
+ attn_weight.data() + n * im2col_step_ * per_attn_weight_size,
+ batch_n, spatial_size, num_heads, channels, num_levels, num_query, num_point,
+ grad_value.data() + n * im2col_step_ * per_value_size,
+ grad_sampling_loc.data() + n * im2col_step_ * per_sample_loc_size,
+ grad_attn_weight.data() + n * im2col_step_ * per_attn_weight_size);
+
+ }));
+ }
+
+ return {
+ grad_value, grad_sampling_loc, grad_attn_weight
+ };
+}
\ No newline at end of file
diff --git a/difpoint/src/models/XPose/models/UniPose/ops/src/cuda/ms_deform_attn_cuda.h b/difpoint/src/models/XPose/models/UniPose/ops/src/cuda/ms_deform_attn_cuda.h
new file mode 100644
index 0000000000000000000000000000000000000000..41c0906831e280808dd34c205efdb34731715b7d
--- /dev/null
+++ b/difpoint/src/models/XPose/models/UniPose/ops/src/cuda/ms_deform_attn_cuda.h
@@ -0,0 +1,30 @@
+/*!
+**************************************************************************************************
+* Deformable DETR
+* Copyright (c) 2020 SenseTime. All Rights Reserved.
+* Licensed under the Apache License, Version 2.0 [see LICENSE for details]
+**************************************************************************************************
+* Modified from https://github.com/chengdazhi/Deformable-Convolution-V2-PyTorch/tree/pytorch_1.0.0
+**************************************************************************************************
+*/
+
+#pragma once
+#include
+
+at::Tensor ms_deform_attn_cuda_forward(
+ const at::Tensor &value,
+ const at::Tensor &spatial_shapes,
+ const at::Tensor &level_start_index,
+ const at::Tensor &sampling_loc,
+ const at::Tensor &attn_weight,
+ const int im2col_step);
+
+std::vector ms_deform_attn_cuda_backward(
+ const at::Tensor &value,
+ const at::Tensor &spatial_shapes,
+ const at::Tensor &level_start_index,
+ const at::Tensor &sampling_loc,
+ const at::Tensor &attn_weight,
+ const at::Tensor &grad_output,
+ const int im2col_step);
+
diff --git a/difpoint/src/models/XPose/models/UniPose/ops/src/cuda/ms_deform_im2col_cuda.cuh b/difpoint/src/models/XPose/models/UniPose/ops/src/cuda/ms_deform_im2col_cuda.cuh
new file mode 100644
index 0000000000000000000000000000000000000000..48cbbc3f1f3e78964a99e88f2517aeed2d6e0c9e
--- /dev/null
+++ b/difpoint/src/models/XPose/models/UniPose/ops/src/cuda/ms_deform_im2col_cuda.cuh
@@ -0,0 +1,1327 @@
+/*!
+**************************************************************************
+* Deformable DETR
+* Copyright (c) 2020 SenseTime. All Rights Reserved.
+* Licensed under the Apache License, Version 2.0 [see LICENSE for details]
+**************************************************************************
+* Modified from DCN (https://github.com/msracver/Deformable-ConvNets)
+* Copyright (c) 2018 Microsoft
+**************************************************************************
+*/
+
+#include
+#include
+#include
+
+#include
+#include
+
+#include
+
+#define CUDA_KERNEL_LOOP(i, n) \
+ for (int i = blockIdx.x * blockDim.x + threadIdx.x; \
+ i < (n); \
+ i += blockDim.x * gridDim.x)
+
+const int CUDA_NUM_THREADS = 1024;
+inline int GET_BLOCKS(const int N, const int num_threads)
+{
+ return (N + num_threads - 1) / num_threads;
+}
+
+
+template
+__device__ scalar_t ms_deform_attn_im2col_bilinear(const scalar_t* &bottom_data,
+ const int &height, const int &width, const int &nheads, const int &channels,
+ const scalar_t &h, const scalar_t &w, const int &m, const int &c)
+{
+ const int h_low = floor(h);
+ const int w_low = floor(w);
+ const int h_high = h_low + 1;
+ const int w_high = w_low + 1;
+
+ const scalar_t lh = h - h_low;
+ const scalar_t lw = w - w_low;
+ const scalar_t hh = 1 - lh, hw = 1 - lw;
+
+ const int w_stride = nheads * channels;
+ const int h_stride = width * w_stride;
+ const int h_low_ptr_offset = h_low * h_stride;
+ const int h_high_ptr_offset = h_low_ptr_offset + h_stride;
+ const int w_low_ptr_offset = w_low * w_stride;
+ const int w_high_ptr_offset = w_low_ptr_offset + w_stride;
+ const int base_ptr = m * channels + c;
+
+ scalar_t v1 = 0;
+ if (h_low >= 0 && w_low >= 0)
+ {
+ const int ptr1 = h_low_ptr_offset + w_low_ptr_offset + base_ptr;
+ v1 = bottom_data[ptr1];
+ }
+ scalar_t v2 = 0;
+ if (h_low >= 0 && w_high <= width - 1)
+ {
+ const int ptr2 = h_low_ptr_offset + w_high_ptr_offset + base_ptr;
+ v2 = bottom_data[ptr2];
+ }
+ scalar_t v3 = 0;
+ if (h_high <= height - 1 && w_low >= 0)
+ {
+ const int ptr3 = h_high_ptr_offset + w_low_ptr_offset + base_ptr;
+ v3 = bottom_data[ptr3];
+ }
+ scalar_t v4 = 0;
+ if (h_high <= height - 1 && w_high <= width - 1)
+ {
+ const int ptr4 = h_high_ptr_offset + w_high_ptr_offset + base_ptr;
+ v4 = bottom_data[ptr4];
+ }
+
+ const scalar_t w1 = hh * hw, w2 = hh * lw, w3 = lh * hw, w4 = lh * lw;
+
+ const scalar_t val = (w1 * v1 + w2 * v2 + w3 * v3 + w4 * v4);
+ return val;
+}
+
+
+template
+__device__ void ms_deform_attn_col2im_bilinear(const scalar_t* &bottom_data,
+ const int &height, const int &width, const int &nheads, const int &channels,
+ const scalar_t &h, const scalar_t &w, const int &m, const int &c,
+ const scalar_t &top_grad,
+ const scalar_t &attn_weight,
+ scalar_t* &grad_value,
+ scalar_t* grad_sampling_loc,
+ scalar_t* grad_attn_weight)
+{
+ const int h_low = floor(h);
+ const int w_low = floor(w);
+ const int h_high = h_low + 1;
+ const int w_high = w_low + 1;
+
+ const scalar_t lh = h - h_low;
+ const scalar_t lw = w - w_low;
+ const scalar_t hh = 1 - lh, hw = 1 - lw;
+
+ const int w_stride = nheads * channels;
+ const int h_stride = width * w_stride;
+ const int h_low_ptr_offset = h_low * h_stride;
+ const int h_high_ptr_offset = h_low_ptr_offset + h_stride;
+ const int w_low_ptr_offset = w_low * w_stride;
+ const int w_high_ptr_offset = w_low_ptr_offset + w_stride;
+ const int base_ptr = m * channels + c;
+
+ const scalar_t w1 = hh * hw, w2 = hh * lw, w3 = lh * hw, w4 = lh * lw;
+ const scalar_t top_grad_value = top_grad * attn_weight;
+ scalar_t grad_h_weight = 0, grad_w_weight = 0;
+
+ scalar_t v1 = 0;
+ if (h_low >= 0 && w_low >= 0)
+ {
+ const int ptr1 = h_low_ptr_offset + w_low_ptr_offset + base_ptr;
+ v1 = bottom_data[ptr1];
+ grad_h_weight -= hw * v1;
+ grad_w_weight -= hh * v1;
+ atomicAdd(grad_value+ptr1, w1*top_grad_value);
+ }
+ scalar_t v2 = 0;
+ if (h_low >= 0 && w_high <= width - 1)
+ {
+ const int ptr2 = h_low_ptr_offset + w_high_ptr_offset + base_ptr;
+ v2 = bottom_data[ptr2];
+ grad_h_weight -= lw * v2;
+ grad_w_weight += hh * v2;
+ atomicAdd(grad_value+ptr2, w2*top_grad_value);
+ }
+ scalar_t v3 = 0;
+ if (h_high <= height - 1 && w_low >= 0)
+ {
+ const int ptr3 = h_high_ptr_offset + w_low_ptr_offset + base_ptr;
+ v3 = bottom_data[ptr3];
+ grad_h_weight += hw * v3;
+ grad_w_weight -= lh * v3;
+ atomicAdd(grad_value+ptr3, w3*top_grad_value);
+ }
+ scalar_t v4 = 0;
+ if (h_high <= height - 1 && w_high <= width - 1)
+ {
+ const int ptr4 = h_high_ptr_offset + w_high_ptr_offset + base_ptr;
+ v4 = bottom_data[ptr4];
+ grad_h_weight += lw * v4;
+ grad_w_weight += lh * v4;
+ atomicAdd(grad_value+ptr4, w4*top_grad_value);
+ }
+
+ const scalar_t val = (w1 * v1 + w2 * v2 + w3 * v3 + w4 * v4);
+ *grad_attn_weight = top_grad * val;
+ *grad_sampling_loc = width * grad_w_weight * top_grad_value;
+ *(grad_sampling_loc + 1) = height * grad_h_weight * top_grad_value;
+}
+
+
+template
+__device__ void ms_deform_attn_col2im_bilinear_gm(const scalar_t* &bottom_data,
+ const int &height, const int &width, const int &nheads, const int &channels,
+ const scalar_t &h, const scalar_t &w, const int &m, const int &c,
+ const scalar_t &top_grad,
+ const scalar_t &attn_weight,
+ scalar_t* &grad_value,
+ scalar_t* grad_sampling_loc,
+ scalar_t* grad_attn_weight)
+{
+ const int h_low = floor(h);
+ const int w_low = floor(w);
+ const int h_high = h_low + 1;
+ const int w_high = w_low + 1;
+
+ const scalar_t lh = h - h_low;
+ const scalar_t lw = w - w_low;
+ const scalar_t hh = 1 - lh, hw = 1 - lw;
+
+ const int w_stride = nheads * channels;
+ const int h_stride = width * w_stride;
+ const int h_low_ptr_offset = h_low * h_stride;
+ const int h_high_ptr_offset = h_low_ptr_offset + h_stride;
+ const int w_low_ptr_offset = w_low * w_stride;
+ const int w_high_ptr_offset = w_low_ptr_offset + w_stride;
+ const int base_ptr = m * channels + c;
+
+ const scalar_t w1 = hh * hw, w2 = hh * lw, w3 = lh * hw, w4 = lh * lw;
+ const scalar_t top_grad_value = top_grad * attn_weight;
+ scalar_t grad_h_weight = 0, grad_w_weight = 0;
+
+ scalar_t v1 = 0;
+ if (h_low >= 0 && w_low >= 0)
+ {
+ const int ptr1 = h_low_ptr_offset + w_low_ptr_offset + base_ptr;
+ v1 = bottom_data[ptr1];
+ grad_h_weight -= hw * v1;
+ grad_w_weight -= hh * v1;
+ atomicAdd(grad_value+ptr1, w1*top_grad_value);
+ }
+ scalar_t v2 = 0;
+ if (h_low >= 0 && w_high <= width - 1)
+ {
+ const int ptr2 = h_low_ptr_offset + w_high_ptr_offset + base_ptr;
+ v2 = bottom_data[ptr2];
+ grad_h_weight -= lw * v2;
+ grad_w_weight += hh * v2;
+ atomicAdd(grad_value+ptr2, w2*top_grad_value);
+ }
+ scalar_t v3 = 0;
+ if (h_high <= height - 1 && w_low >= 0)
+ {
+ const int ptr3 = h_high_ptr_offset + w_low_ptr_offset + base_ptr;
+ v3 = bottom_data[ptr3];
+ grad_h_weight += hw * v3;
+ grad_w_weight -= lh * v3;
+ atomicAdd(grad_value+ptr3, w3*top_grad_value);
+ }
+ scalar_t v4 = 0;
+ if (h_high <= height - 1 && w_high <= width - 1)
+ {
+ const int ptr4 = h_high_ptr_offset + w_high_ptr_offset + base_ptr;
+ v4 = bottom_data[ptr4];
+ grad_h_weight += lw * v4;
+ grad_w_weight += lh * v4;
+ atomicAdd(grad_value+ptr4, w4*top_grad_value);
+ }
+
+ const scalar_t val = (w1 * v1 + w2 * v2 + w3 * v3 + w4 * v4);
+ atomicAdd(grad_attn_weight, top_grad * val);
+ atomicAdd(grad_sampling_loc, width * grad_w_weight * top_grad_value);
+ atomicAdd(grad_sampling_loc + 1, height * grad_h_weight * top_grad_value);
+}
+
+
+template
+__global__ void ms_deformable_im2col_gpu_kernel(const int n,
+ const scalar_t *data_value,
+ const int64_t *data_spatial_shapes,
+ const int64_t *data_level_start_index,
+ const scalar_t *data_sampling_loc,
+ const scalar_t *data_attn_weight,
+ const int batch_size,
+ const int spatial_size,
+ const int num_heads,
+ const int channels,
+ const int num_levels,
+ const int num_query,
+ const int num_point,
+ scalar_t *data_col)
+{
+ CUDA_KERNEL_LOOP(index, n)
+ {
+ int _temp = index;
+ const int c_col = _temp % channels;
+ _temp /= channels;
+ const int sampling_index = _temp;
+ const int m_col = _temp % num_heads;
+ _temp /= num_heads;
+ const int q_col = _temp % num_query;
+ _temp /= num_query;
+ const int b_col = _temp;
+
+ scalar_t *data_col_ptr = data_col + index;
+ int data_weight_ptr = sampling_index * num_levels * num_point;
+ int data_loc_w_ptr = data_weight_ptr << 1;
+ const int qid_stride = num_heads * channels;
+ const int data_value_ptr_init_offset = b_col * spatial_size * qid_stride;
+ scalar_t col = 0;
+
+ for (int l_col=0; l_col < num_levels; ++l_col)
+ {
+ const int level_start_id = data_level_start_index[l_col];
+ const int spatial_h_ptr = l_col << 1;
+ const int spatial_h = data_spatial_shapes[spatial_h_ptr];
+ const int spatial_w = data_spatial_shapes[spatial_h_ptr + 1];
+ const scalar_t *data_value_ptr = data_value + (data_value_ptr_init_offset + level_start_id * qid_stride);
+ for (int p_col=0; p_col < num_point; ++p_col)
+ {
+ const scalar_t loc_w = data_sampling_loc[data_loc_w_ptr];
+ const scalar_t loc_h = data_sampling_loc[data_loc_w_ptr + 1];
+ const scalar_t weight = data_attn_weight[data_weight_ptr];
+
+ const scalar_t h_im = loc_h * spatial_h - 0.5;
+ const scalar_t w_im = loc_w * spatial_w - 0.5;
+
+ if (h_im > -1 && w_im > -1 && h_im < spatial_h && w_im < spatial_w)
+ {
+ col += ms_deform_attn_im2col_bilinear(data_value_ptr, spatial_h, spatial_w, num_heads, channels, h_im, w_im, m_col, c_col) * weight;
+ }
+
+ data_weight_ptr += 1;
+ data_loc_w_ptr += 2;
+ }
+ }
+ *data_col_ptr = col;
+ }
+}
+
+template
+__global__ void ms_deformable_col2im_gpu_kernel_shm_blocksize_aware_reduce_v1(const int n,
+ const scalar_t *grad_col,
+ const scalar_t *data_value,
+ const int64_t *data_spatial_shapes,
+ const int64_t *data_level_start_index,
+ const scalar_t *data_sampling_loc,
+ const scalar_t *data_attn_weight,
+ const int batch_size,
+ const int spatial_size,
+ const int num_heads,
+ const int channels,
+ const int num_levels,
+ const int num_query,
+ const int num_point,
+ scalar_t *grad_value,
+ scalar_t *grad_sampling_loc,
+ scalar_t *grad_attn_weight)
+{
+ CUDA_KERNEL_LOOP(index, n)
+ {
+ __shared__ scalar_t cache_grad_sampling_loc[blockSize * 2];
+ __shared__ scalar_t cache_grad_attn_weight[blockSize];
+ unsigned int tid = threadIdx.x;
+ int _temp = index;
+ const int c_col = _temp % channels;
+ _temp /= channels;
+ const int sampling_index = _temp;
+ const int m_col = _temp % num_heads;
+ _temp /= num_heads;
+ const int q_col = _temp % num_query;
+ _temp /= num_query;
+ const int b_col = _temp;
+
+ const scalar_t top_grad = grad_col[index];
+
+ int data_weight_ptr = sampling_index * num_levels * num_point;
+ int data_loc_w_ptr = data_weight_ptr << 1;
+ const int grad_sampling_ptr = data_weight_ptr;
+ grad_sampling_loc += grad_sampling_ptr << 1;
+ grad_attn_weight += grad_sampling_ptr;
+ const int grad_weight_stride = 1;
+ const int grad_loc_stride = 2;
+ const int qid_stride = num_heads * channels;
+ const int data_value_ptr_init_offset = b_col * spatial_size * qid_stride;
+
+ for (int l_col=0; l_col < num_levels; ++l_col)
+ {
+ const int level_start_id = data_level_start_index[l_col];
+ const int spatial_h_ptr = l_col << 1;
+ const int spatial_h = data_spatial_shapes[spatial_h_ptr];
+ const int spatial_w = data_spatial_shapes[spatial_h_ptr + 1];
+ const int value_ptr_offset = data_value_ptr_init_offset + level_start_id * qid_stride;
+ const scalar_t *data_value_ptr = data_value + value_ptr_offset;
+ scalar_t *grad_value_ptr = grad_value + value_ptr_offset;
+
+ for (int p_col=0; p_col < num_point; ++p_col)
+ {
+ const scalar_t loc_w = data_sampling_loc[data_loc_w_ptr];
+ const scalar_t loc_h = data_sampling_loc[data_loc_w_ptr + 1];
+ const scalar_t weight = data_attn_weight[data_weight_ptr];
+
+ const scalar_t h_im = loc_h * spatial_h - 0.5;
+ const scalar_t w_im = loc_w * spatial_w - 0.5;
+ *(cache_grad_sampling_loc+(threadIdx.x << 1)) = 0;
+ *(cache_grad_sampling_loc+((threadIdx.x << 1) + 1)) = 0;
+ *(cache_grad_attn_weight+threadIdx.x)=0;
+ if (h_im > -1 && w_im > -1 && h_im < spatial_h && w_im < spatial_w)
+ {
+ ms_deform_attn_col2im_bilinear(
+ data_value_ptr, spatial_h, spatial_w, num_heads, channels, h_im, w_im, m_col, c_col,
+ top_grad, weight, grad_value_ptr,
+ cache_grad_sampling_loc+(threadIdx.x << 1), cache_grad_attn_weight+threadIdx.x);
+ }
+
+ __syncthreads();
+ if (tid == 0)
+ {
+ scalar_t _grad_w=cache_grad_sampling_loc[0], _grad_h=cache_grad_sampling_loc[1], _grad_a=cache_grad_attn_weight[0];
+ int sid=2;
+ for (unsigned int tid = 1; tid < blockSize; ++tid)
+ {
+ _grad_w += cache_grad_sampling_loc[sid];
+ _grad_h += cache_grad_sampling_loc[sid + 1];
+ _grad_a += cache_grad_attn_weight[tid];
+ sid += 2;
+ }
+
+
+ *grad_sampling_loc = _grad_w;
+ *(grad_sampling_loc + 1) = _grad_h;
+ *grad_attn_weight = _grad_a;
+ }
+ __syncthreads();
+
+ data_weight_ptr += 1;
+ data_loc_w_ptr += 2;
+ grad_attn_weight += grad_weight_stride;
+ grad_sampling_loc += grad_loc_stride;
+ }
+ }
+ }
+}
+
+
+template
+__global__ void ms_deformable_col2im_gpu_kernel_shm_blocksize_aware_reduce_v2(const int n,
+ const scalar_t *grad_col,
+ const scalar_t *data_value,
+ const int64_t *data_spatial_shapes,
+ const int64_t *data_level_start_index,
+ const scalar_t *data_sampling_loc,
+ const scalar_t *data_attn_weight,
+ const int batch_size,
+ const int spatial_size,
+ const int num_heads,
+ const int channels,
+ const int num_levels,
+ const int num_query,
+ const int num_point,
+ scalar_t *grad_value,
+ scalar_t *grad_sampling_loc,
+ scalar_t *grad_attn_weight)
+{
+ CUDA_KERNEL_LOOP(index, n)
+ {
+ __shared__ scalar_t cache_grad_sampling_loc[blockSize * 2];
+ __shared__ scalar_t cache_grad_attn_weight[blockSize];
+ unsigned int tid = threadIdx.x;
+ int _temp = index;
+ const int c_col = _temp % channels;
+ _temp /= channels;
+ const int sampling_index = _temp;
+ const int m_col = _temp % num_heads;
+ _temp /= num_heads;
+ const int q_col = _temp % num_query;
+ _temp /= num_query;
+ const int b_col = _temp;
+
+ const scalar_t top_grad = grad_col[index];
+
+ int data_weight_ptr = sampling_index * num_levels * num_point;
+ int data_loc_w_ptr = data_weight_ptr << 1;
+ const int grad_sampling_ptr = data_weight_ptr;
+ grad_sampling_loc += grad_sampling_ptr << 1;
+ grad_attn_weight += grad_sampling_ptr;
+ const int grad_weight_stride = 1;
+ const int grad_loc_stride = 2;
+ const int qid_stride = num_heads * channels;
+ const int data_value_ptr_init_offset = b_col * spatial_size * qid_stride;
+
+ for (int l_col=0; l_col < num_levels; ++l_col)
+ {
+ const int level_start_id = data_level_start_index[l_col];
+ const int spatial_h_ptr = l_col << 1;
+ const int spatial_h = data_spatial_shapes[spatial_h_ptr];
+ const int spatial_w = data_spatial_shapes[spatial_h_ptr + 1];
+ const int value_ptr_offset = data_value_ptr_init_offset + level_start_id * qid_stride;
+ const scalar_t *data_value_ptr = data_value + value_ptr_offset;
+ scalar_t *grad_value_ptr = grad_value + value_ptr_offset;
+
+ for (int p_col=0; p_col < num_point; ++p_col)
+ {
+ const scalar_t loc_w = data_sampling_loc[data_loc_w_ptr];
+ const scalar_t loc_h = data_sampling_loc[data_loc_w_ptr + 1];
+ const scalar_t weight = data_attn_weight[data_weight_ptr];
+
+ const scalar_t h_im = loc_h * spatial_h - 0.5;
+ const scalar_t w_im = loc_w * spatial_w - 0.5;
+ *(cache_grad_sampling_loc+(threadIdx.x << 1)) = 0;
+ *(cache_grad_sampling_loc+((threadIdx.x << 1) + 1)) = 0;
+ *(cache_grad_attn_weight+threadIdx.x)=0;
+ if (h_im > -1 && w_im > -1 && h_im < spatial_h && w_im < spatial_w)
+ {
+ ms_deform_attn_col2im_bilinear(
+ data_value_ptr, spatial_h, spatial_w, num_heads, channels, h_im, w_im, m_col, c_col,
+ top_grad, weight, grad_value_ptr,
+ cache_grad_sampling_loc+(threadIdx.x << 1), cache_grad_attn_weight+threadIdx.x);
+ }
+
+ __syncthreads();
+
+ for (unsigned int s=blockSize/2; s>0; s>>=1)
+ {
+ if (tid < s) {
+ const unsigned int xid1 = tid << 1;
+ const unsigned int xid2 = (tid + s) << 1;
+ cache_grad_attn_weight[tid] += cache_grad_attn_weight[tid + s];
+ cache_grad_sampling_loc[xid1] += cache_grad_sampling_loc[xid2];
+ cache_grad_sampling_loc[xid1 + 1] += cache_grad_sampling_loc[xid2 + 1];
+ }
+ __syncthreads();
+ }
+
+ if (tid == 0)
+ {
+ *grad_sampling_loc = cache_grad_sampling_loc[0];
+ *(grad_sampling_loc + 1) = cache_grad_sampling_loc[1];
+ *grad_attn_weight = cache_grad_attn_weight[0];
+ }
+ __syncthreads();
+
+ data_weight_ptr += 1;
+ data_loc_w_ptr += 2;
+ grad_attn_weight += grad_weight_stride;
+ grad_sampling_loc += grad_loc_stride;
+ }
+ }
+ }
+}
+
+
+template
+__global__ void ms_deformable_col2im_gpu_kernel_shm_reduce_v1(const int n,
+ const scalar_t *grad_col,
+ const scalar_t *data_value,
+ const int64_t *data_spatial_shapes,
+ const int64_t *data_level_start_index,
+ const scalar_t *data_sampling_loc,
+ const scalar_t *data_attn_weight,
+ const int batch_size,
+ const int spatial_size,
+ const int num_heads,
+ const int channels,
+ const int num_levels,
+ const int num_query,
+ const int num_point,
+ scalar_t *grad_value,
+ scalar_t *grad_sampling_loc,
+ scalar_t *grad_attn_weight)
+{
+ CUDA_KERNEL_LOOP(index, n)
+ {
+ extern __shared__ int _s[];
+ scalar_t* cache_grad_sampling_loc = (scalar_t*)_s;
+ scalar_t* cache_grad_attn_weight = cache_grad_sampling_loc + 2 * blockDim.x;
+ unsigned int tid = threadIdx.x;
+ int _temp = index;
+ const int c_col = _temp % channels;
+ _temp /= channels;
+ const int sampling_index = _temp;
+ const int m_col = _temp % num_heads;
+ _temp /= num_heads;
+ const int q_col = _temp % num_query;
+ _temp /= num_query;
+ const int b_col = _temp;
+
+ const scalar_t top_grad = grad_col[index];
+
+ int data_weight_ptr = sampling_index * num_levels * num_point;
+ int data_loc_w_ptr = data_weight_ptr << 1;
+ const int grad_sampling_ptr = data_weight_ptr;
+ grad_sampling_loc += grad_sampling_ptr << 1;
+ grad_attn_weight += grad_sampling_ptr;
+ const int grad_weight_stride = 1;
+ const int grad_loc_stride = 2;
+ const int qid_stride = num_heads * channels;
+ const int data_value_ptr_init_offset = b_col * spatial_size * qid_stride;
+
+ for (int l_col=0; l_col < num_levels; ++l_col)
+ {
+ const int level_start_id = data_level_start_index[l_col];
+ const int spatial_h_ptr = l_col << 1;
+ const int spatial_h = data_spatial_shapes[spatial_h_ptr];
+ const int spatial_w = data_spatial_shapes[spatial_h_ptr + 1];
+ const int value_ptr_offset = data_value_ptr_init_offset + level_start_id * qid_stride;
+ const scalar_t *data_value_ptr = data_value + value_ptr_offset;
+ scalar_t *grad_value_ptr = grad_value + value_ptr_offset;
+
+ for (int p_col=0; p_col < num_point; ++p_col)
+ {
+ const scalar_t loc_w = data_sampling_loc[data_loc_w_ptr];
+ const scalar_t loc_h = data_sampling_loc[data_loc_w_ptr + 1];
+ const scalar_t weight = data_attn_weight[data_weight_ptr];
+
+ const scalar_t h_im = loc_h * spatial_h - 0.5;
+ const scalar_t w_im = loc_w * spatial_w - 0.5;
+ *(cache_grad_sampling_loc+(threadIdx.x << 1)) = 0;
+ *(cache_grad_sampling_loc+((threadIdx.x << 1) + 1)) = 0;
+ *(cache_grad_attn_weight+threadIdx.x)=0;
+ if (h_im > -1 && w_im > -1 && h_im < spatial_h && w_im < spatial_w)
+ {
+ ms_deform_attn_col2im_bilinear(
+ data_value_ptr, spatial_h, spatial_w, num_heads, channels, h_im, w_im, m_col, c_col,
+ top_grad, weight, grad_value_ptr,
+ cache_grad_sampling_loc+(threadIdx.x << 1), cache_grad_attn_weight+threadIdx.x);
+ }
+
+ __syncthreads();
+ if (tid == 0)
+ {
+ scalar_t _grad_w=cache_grad_sampling_loc[0], _grad_h=cache_grad_sampling_loc[1], _grad_a=cache_grad_attn_weight[0];
+ int sid=2;
+ for (unsigned int tid = 1; tid < blockDim.x; ++tid)
+ {
+ _grad_w += cache_grad_sampling_loc[sid];
+ _grad_h += cache_grad_sampling_loc[sid + 1];
+ _grad_a += cache_grad_attn_weight[tid];
+ sid += 2;
+ }
+
+
+ *grad_sampling_loc = _grad_w;
+ *(grad_sampling_loc + 1) = _grad_h;
+ *grad_attn_weight = _grad_a;
+ }
+ __syncthreads();
+
+ data_weight_ptr += 1;
+ data_loc_w_ptr += 2;
+ grad_attn_weight += grad_weight_stride;
+ grad_sampling_loc += grad_loc_stride;
+ }
+ }
+ }
+}
+
+template
+__global__ void ms_deformable_col2im_gpu_kernel_shm_reduce_v2(const int n,
+ const scalar_t *grad_col,
+ const scalar_t *data_value,
+ const int64_t *data_spatial_shapes,
+ const int64_t *data_level_start_index,
+ const scalar_t *data_sampling_loc,
+ const scalar_t *data_attn_weight,
+ const int batch_size,
+ const int spatial_size,
+ const int num_heads,
+ const int channels,
+ const int num_levels,
+ const int num_query,
+ const int num_point,
+ scalar_t *grad_value,
+ scalar_t *grad_sampling_loc,
+ scalar_t *grad_attn_weight)
+{
+ CUDA_KERNEL_LOOP(index, n)
+ {
+ extern __shared__ int _s[];
+ scalar_t* cache_grad_sampling_loc = (scalar_t*)_s;
+ scalar_t* cache_grad_attn_weight = cache_grad_sampling_loc + 2 * blockDim.x;
+ unsigned int tid = threadIdx.x;
+ int _temp = index;
+ const int c_col = _temp % channels;
+ _temp /= channels;
+ const int sampling_index = _temp;
+ const int m_col = _temp % num_heads;
+ _temp /= num_heads;
+ const int q_col = _temp % num_query;
+ _temp /= num_query;
+ const int b_col = _temp;
+
+ const scalar_t top_grad = grad_col[index];
+
+ int data_weight_ptr = sampling_index * num_levels * num_point;
+ int data_loc_w_ptr = data_weight_ptr << 1;
+ const int grad_sampling_ptr = data_weight_ptr;
+ grad_sampling_loc += grad_sampling_ptr << 1;
+ grad_attn_weight += grad_sampling_ptr;
+ const int grad_weight_stride = 1;
+ const int grad_loc_stride = 2;
+ const int qid_stride = num_heads * channels;
+ const int data_value_ptr_init_offset = b_col * spatial_size * qid_stride;
+
+ for (int l_col=0; l_col < num_levels; ++l_col)
+ {
+ const int level_start_id = data_level_start_index[l_col];
+ const int spatial_h_ptr = l_col << 1;
+ const int spatial_h = data_spatial_shapes[spatial_h_ptr];
+ const int spatial_w = data_spatial_shapes[spatial_h_ptr + 1];
+ const int value_ptr_offset = data_value_ptr_init_offset + level_start_id * qid_stride;
+ const scalar_t *data_value_ptr = data_value + value_ptr_offset;
+ scalar_t *grad_value_ptr = grad_value + value_ptr_offset;
+
+ for (int p_col=0; p_col < num_point; ++p_col)
+ {
+ const scalar_t loc_w = data_sampling_loc[data_loc_w_ptr];
+ const scalar_t loc_h = data_sampling_loc[data_loc_w_ptr + 1];
+ const scalar_t weight = data_attn_weight[data_weight_ptr];
+
+ const scalar_t h_im = loc_h * spatial_h - 0.5;
+ const scalar_t w_im = loc_w * spatial_w - 0.5;
+ *(cache_grad_sampling_loc+(threadIdx.x << 1)) = 0;
+ *(cache_grad_sampling_loc+((threadIdx.x << 1) + 1)) = 0;
+ *(cache_grad_attn_weight+threadIdx.x)=0;
+ if (h_im > -1 && w_im > -1 && h_im < spatial_h && w_im < spatial_w)
+ {
+ ms_deform_attn_col2im_bilinear(
+ data_value_ptr, spatial_h, spatial_w, num_heads, channels, h_im, w_im, m_col, c_col,
+ top_grad, weight, grad_value_ptr,
+ cache_grad_sampling_loc+(threadIdx.x << 1), cache_grad_attn_weight+threadIdx.x);
+ }
+
+ __syncthreads();
+
+ for (unsigned int s=blockDim.x/2, spre=blockDim.x; s>0; s>>=1, spre>>=1)
+ {
+ if (tid < s) {
+ const unsigned int xid1 = tid << 1;
+ const unsigned int xid2 = (tid + s) << 1;
+ cache_grad_attn_weight[tid] += cache_grad_attn_weight[tid + s];
+ cache_grad_sampling_loc[xid1] += cache_grad_sampling_loc[xid2];
+ cache_grad_sampling_loc[xid1 + 1] += cache_grad_sampling_loc[xid2 + 1];
+ if (tid + (s << 1) < spre)
+ {
+ cache_grad_attn_weight[tid] += cache_grad_attn_weight[tid + (s << 1)];
+ cache_grad_sampling_loc[xid1] += cache_grad_sampling_loc[xid2 + (s << 1)];
+ cache_grad_sampling_loc[xid1 + 1] += cache_grad_sampling_loc[xid2 + 1 + (s << 1)];
+ }
+ }
+ __syncthreads();
+ }
+
+ if (tid == 0)
+ {
+ *grad_sampling_loc = cache_grad_sampling_loc[0];
+ *(grad_sampling_loc + 1) = cache_grad_sampling_loc[1];
+ *grad_attn_weight = cache_grad_attn_weight[0];
+ }
+ __syncthreads();
+
+ data_weight_ptr += 1;
+ data_loc_w_ptr += 2;
+ grad_attn_weight += grad_weight_stride;
+ grad_sampling_loc += grad_loc_stride;
+ }
+ }
+ }
+}
+
+template
+__global__ void ms_deformable_col2im_gpu_kernel_shm_reduce_v2_multi_blocks(const int n,
+ const scalar_t *grad_col,
+ const scalar_t *data_value,
+ const int64_t *data_spatial_shapes,
+ const int64_t *data_level_start_index,
+ const scalar_t *data_sampling_loc,
+ const scalar_t *data_attn_weight,
+ const int batch_size,
+ const int spatial_size,
+ const int num_heads,
+ const int channels,
+ const int num_levels,
+ const int num_query,
+ const int num_point,
+ scalar_t *grad_value,
+ scalar_t *grad_sampling_loc,
+ scalar_t *grad_attn_weight)
+{
+ CUDA_KERNEL_LOOP(index, n)
+ {
+ extern __shared__ int _s[];
+ scalar_t* cache_grad_sampling_loc = (scalar_t*)_s;
+ scalar_t* cache_grad_attn_weight = cache_grad_sampling_loc + 2 * blockDim.x;
+ unsigned int tid = threadIdx.x;
+ int _temp = index;
+ const int c_col = _temp % channels;
+ _temp /= channels;
+ const int sampling_index = _temp;
+ const int m_col = _temp % num_heads;
+ _temp /= num_heads;
+ const int q_col = _temp % num_query;
+ _temp /= num_query;
+ const int b_col = _temp;
+
+ const scalar_t top_grad = grad_col[index];
+
+ int data_weight_ptr = sampling_index * num_levels * num_point;
+ int data_loc_w_ptr = data_weight_ptr << 1;
+ const int grad_sampling_ptr = data_weight_ptr;
+ grad_sampling_loc += grad_sampling_ptr << 1;
+ grad_attn_weight += grad_sampling_ptr;
+ const int grad_weight_stride = 1;
+ const int grad_loc_stride = 2;
+ const int qid_stride = num_heads * channels;
+ const int data_value_ptr_init_offset = b_col * spatial_size * qid_stride;
+
+ for (int l_col=0; l_col < num_levels; ++l_col)
+ {
+ const int level_start_id = data_level_start_index[l_col];
+ const int spatial_h_ptr = l_col << 1;
+ const int spatial_h = data_spatial_shapes[spatial_h_ptr];
+ const int spatial_w = data_spatial_shapes[spatial_h_ptr + 1];
+ const int value_ptr_offset = data_value_ptr_init_offset + level_start_id * qid_stride;
+ const scalar_t *data_value_ptr = data_value + value_ptr_offset;
+ scalar_t *grad_value_ptr = grad_value + value_ptr_offset;
+
+ for (int p_col=0; p_col < num_point; ++p_col)
+ {
+ const scalar_t loc_w = data_sampling_loc[data_loc_w_ptr];
+ const scalar_t loc_h = data_sampling_loc[data_loc_w_ptr + 1];
+ const scalar_t weight = data_attn_weight[data_weight_ptr];
+
+ const scalar_t h_im = loc_h * spatial_h - 0.5;
+ const scalar_t w_im = loc_w * spatial_w - 0.5;
+ *(cache_grad_sampling_loc+(threadIdx.x << 1)) = 0;
+ *(cache_grad_sampling_loc+((threadIdx.x << 1) + 1)) = 0;
+ *(cache_grad_attn_weight+threadIdx.x)=0;
+ if (h_im > -1 && w_im > -1 && h_im < spatial_h && w_im < spatial_w)
+ {
+ ms_deform_attn_col2im_bilinear(
+ data_value_ptr, spatial_h, spatial_w, num_heads, channels, h_im, w_im, m_col, c_col,
+ top_grad, weight, grad_value_ptr,
+ cache_grad_sampling_loc+(threadIdx.x << 1), cache_grad_attn_weight+threadIdx.x);
+ }
+
+ __syncthreads();
+
+ for (unsigned int s=blockDim.x/2, spre=blockDim.x; s>0; s>>=1, spre>>=1)
+ {
+ if (tid < s) {
+ const unsigned int xid1 = tid << 1;
+ const unsigned int xid2 = (tid + s) << 1;
+ cache_grad_attn_weight[tid] += cache_grad_attn_weight[tid + s];
+ cache_grad_sampling_loc[xid1] += cache_grad_sampling_loc[xid2];
+ cache_grad_sampling_loc[xid1 + 1] += cache_grad_sampling_loc[xid2 + 1];
+ if (tid + (s << 1) < spre)
+ {
+ cache_grad_attn_weight[tid] += cache_grad_attn_weight[tid + (s << 1)];
+ cache_grad_sampling_loc[xid1] += cache_grad_sampling_loc[xid2 + (s << 1)];
+ cache_grad_sampling_loc[xid1 + 1] += cache_grad_sampling_loc[xid2 + 1 + (s << 1)];
+ }
+ }
+ __syncthreads();
+ }
+
+ if (tid == 0)
+ {
+ atomicAdd(grad_sampling_loc, cache_grad_sampling_loc[0]);
+ atomicAdd(grad_sampling_loc + 1, cache_grad_sampling_loc[1]);
+ atomicAdd(grad_attn_weight, cache_grad_attn_weight[0]);
+ }
+ __syncthreads();
+
+ data_weight_ptr += 1;
+ data_loc_w_ptr += 2;
+ grad_attn_weight += grad_weight_stride;
+ grad_sampling_loc += grad_loc_stride;
+ }
+ }
+ }
+}
+
+
+template
+__global__ void ms_deformable_col2im_gpu_kernel_gm(const int n,
+ const scalar_t *grad_col,
+ const scalar_t *data_value,
+ const int64_t *data_spatial_shapes,
+ const int64_t *data_level_start_index,
+ const scalar_t *data_sampling_loc,
+ const scalar_t *data_attn_weight,
+ const int batch_size,
+ const int spatial_size,
+ const int num_heads,
+ const int channels,
+ const int num_levels,
+ const int num_query,
+ const int num_point,
+ scalar_t *grad_value,
+ scalar_t *grad_sampling_loc,
+ scalar_t *grad_attn_weight)
+{
+ CUDA_KERNEL_LOOP(index, n)
+ {
+ int _temp = index;
+ const int c_col = _temp % channels;
+ _temp /= channels;
+ const int sampling_index = _temp;
+ const int m_col = _temp % num_heads;
+ _temp /= num_heads;
+ const int q_col = _temp % num_query;
+ _temp /= num_query;
+ const int b_col = _temp;
+
+ const scalar_t top_grad = grad_col[index];
+
+ int data_weight_ptr = sampling_index * num_levels * num_point;
+ int data_loc_w_ptr = data_weight_ptr << 1;
+ const int grad_sampling_ptr = data_weight_ptr;
+ grad_sampling_loc += grad_sampling_ptr << 1;
+ grad_attn_weight += grad_sampling_ptr;
+ const int grad_weight_stride = 1;
+ const int grad_loc_stride = 2;
+ const int qid_stride = num_heads * channels;
+ const int data_value_ptr_init_offset = b_col * spatial_size * qid_stride;
+
+ for (int l_col=0; l_col < num_levels; ++l_col)
+ {
+ const int level_start_id = data_level_start_index[l_col];
+ const int spatial_h_ptr = l_col << 1;
+ const int spatial_h = data_spatial_shapes[spatial_h_ptr];
+ const int spatial_w = data_spatial_shapes[spatial_h_ptr + 1];
+ const int value_ptr_offset = data_value_ptr_init_offset + level_start_id * qid_stride;
+ const scalar_t *data_value_ptr = data_value + value_ptr_offset;
+ scalar_t *grad_value_ptr = grad_value + value_ptr_offset;
+
+ for (int p_col=0; p_col < num_point; ++p_col)
+ {
+ const scalar_t loc_w = data_sampling_loc[data_loc_w_ptr];
+ const scalar_t loc_h = data_sampling_loc[data_loc_w_ptr + 1];
+ const scalar_t weight = data_attn_weight[data_weight_ptr];
+
+ const scalar_t h_im = loc_h * spatial_h - 0.5;
+ const scalar_t w_im = loc_w * spatial_w - 0.5;
+ if (h_im > -1 && w_im > -1 && h_im < spatial_h && w_im < spatial_w)
+ {
+ ms_deform_attn_col2im_bilinear_gm(
+ data_value_ptr, spatial_h, spatial_w, num_heads, channels, h_im, w_im, m_col, c_col,
+ top_grad, weight, grad_value_ptr,
+ grad_sampling_loc, grad_attn_weight);
+ }
+ data_weight_ptr += 1;
+ data_loc_w_ptr += 2;
+ grad_attn_weight += grad_weight_stride;
+ grad_sampling_loc += grad_loc_stride;
+ }
+ }
+ }
+}
+
+
+template
+void ms_deformable_im2col_cuda(cudaStream_t stream,
+ const scalar_t* data_value,
+ const int64_t* data_spatial_shapes,
+ const int64_t* data_level_start_index,
+ const scalar_t* data_sampling_loc,
+ const scalar_t* data_attn_weight,
+ const int batch_size,
+ const int spatial_size,
+ const int num_heads,
+ const int channels,
+ const int num_levels,
+ const int num_query,
+ const int num_point,
+ scalar_t* data_col)
+{
+ const int num_kernels = batch_size * num_query * num_heads * channels;
+ const int num_actual_kernels = batch_size * num_query * num_heads * channels;
+ const int num_threads = CUDA_NUM_THREADS;
+ ms_deformable_im2col_gpu_kernel
+ <<>>(
+ num_kernels, data_value, data_spatial_shapes, data_level_start_index, data_sampling_loc, data_attn_weight,
+ batch_size, spatial_size, num_heads, channels, num_levels, num_query, num_point, data_col);
+
+ cudaError_t err = cudaGetLastError();
+ if (err != cudaSuccess)
+ {
+ printf("error in ms_deformable_im2col_cuda: %s\n", cudaGetErrorString(err));
+ }
+
+}
+
+template
+void ms_deformable_col2im_cuda(cudaStream_t stream,
+ const scalar_t* grad_col,
+ const scalar_t* data_value,
+ const int64_t * data_spatial_shapes,
+ const int64_t * data_level_start_index,
+ const scalar_t * data_sampling_loc,
+ const scalar_t * data_attn_weight,
+ const int batch_size,
+ const int spatial_size,
+ const int num_heads,
+ const int channels,
+ const int num_levels,
+ const int num_query,
+ const int num_point,
+ scalar_t* grad_value,
+ scalar_t* grad_sampling_loc,
+ scalar_t* grad_attn_weight)
+{
+ const int num_threads = (channels > CUDA_NUM_THREADS)?CUDA_NUM_THREADS:channels;
+ const int num_kernels = batch_size * num_query * num_heads * channels;
+ const int num_actual_kernels = batch_size * num_query * num_heads * channels;
+ if (channels > 1024)
+ {
+ if ((channels & 1023) == 0)
+ {
+ ms_deformable_col2im_gpu_kernel_shm_reduce_v2_multi_blocks
+ <<>>(
+ num_kernels,
+ grad_col,
+ data_value,
+ data_spatial_shapes,
+ data_level_start_index,
+ data_sampling_loc,
+ data_attn_weight,
+ batch_size,
+ spatial_size,
+ num_heads,
+ channels,
+ num_levels,
+ num_query,
+ num_point,
+ grad_value,
+ grad_sampling_loc,
+ grad_attn_weight);
+ }
+ else
+ {
+ ms_deformable_col2im_gpu_kernel_gm
+ <<>>(
+ num_kernels,
+ grad_col,
+ data_value,
+ data_spatial_shapes,
+ data_level_start_index,
+ data_sampling_loc,
+ data_attn_weight,
+ batch_size,
+ spatial_size,
+ num_heads,
+ channels,
+ num_levels,
+ num_query,
+ num_point,
+ grad_value,
+ grad_sampling_loc,
+ grad_attn_weight);
+ }
+ }
+ else{
+ switch(channels)
+ {
+ case 1:
+ ms_deformable_col2im_gpu_kernel_shm_blocksize_aware_reduce_v1
+ <<>>(
+ num_kernels,
+ grad_col,
+ data_value,
+ data_spatial_shapes,
+ data_level_start_index,
+ data_sampling_loc,
+ data_attn_weight,
+ batch_size,
+ spatial_size,
+ num_heads,
+ channels,
+ num_levels,
+ num_query,
+ num_point,
+ grad_value,
+ grad_sampling_loc,
+ grad_attn_weight);
+ break;
+ case 2:
+ ms_deformable_col2im_gpu_kernel_shm_blocksize_aware_reduce_v1
+ <<>>(
+ num_kernels,
+ grad_col,
+ data_value,
+ data_spatial_shapes,
+ data_level_start_index,
+ data_sampling_loc,
+ data_attn_weight,
+ batch_size,
+ spatial_size,
+ num_heads,
+ channels,
+ num_levels,
+ num_query,
+ num_point,
+ grad_value,
+ grad_sampling_loc,
+ grad_attn_weight);
+ break;
+ case 4:
+ ms_deformable_col2im_gpu_kernel_shm_blocksize_aware_reduce_v1
+ <<>>(
+ num_kernels,
+ grad_col,
+ data_value,
+ data_spatial_shapes,
+ data_level_start_index,
+ data_sampling_loc,
+ data_attn_weight,
+ batch_size,
+ spatial_size,
+ num_heads,
+ channels,
+ num_levels,
+ num_query,
+ num_point,
+ grad_value,
+ grad_sampling_loc,
+ grad_attn_weight);
+ break;
+ case 8:
+ ms_deformable_col2im_gpu_kernel_shm_blocksize_aware_reduce_v1
+ <<>>(
+ num_kernels,
+ grad_col,
+ data_value,
+ data_spatial_shapes,
+ data_level_start_index,
+ data_sampling_loc,
+ data_attn_weight,
+ batch_size,
+ spatial_size,
+ num_heads,
+ channels,
+ num_levels,
+ num_query,
+ num_point,
+ grad_value,
+ grad_sampling_loc,
+ grad_attn_weight);
+ break;
+ case 16:
+ ms_deformable_col2im_gpu_kernel_shm_blocksize_aware_reduce_v1
+ <<>>(
+ num_kernels,
+ grad_col,
+ data_value,
+ data_spatial_shapes,
+ data_level_start_index,
+ data_sampling_loc,
+ data_attn_weight,
+ batch_size,
+ spatial_size,
+ num_heads,
+ channels,
+ num_levels,
+ num_query,
+ num_point,
+ grad_value,
+ grad_sampling_loc,
+ grad_attn_weight);
+ break;
+ case 32:
+ ms_deformable_col2im_gpu_kernel_shm_blocksize_aware_reduce_v1
+ <<>>(
+ num_kernels,
+ grad_col,
+ data_value,
+ data_spatial_shapes,
+ data_level_start_index,
+ data_sampling_loc,
+ data_attn_weight,
+ batch_size,
+ spatial_size,
+ num_heads,
+ channels,
+ num_levels,
+ num_query,
+ num_point,
+ grad_value,
+ grad_sampling_loc,
+ grad_attn_weight);
+ break;
+ case 64:
+ ms_deformable_col2im_gpu_kernel_shm_blocksize_aware_reduce_v2
+ <<>>(
+ num_kernels,
+ grad_col,
+ data_value,
+ data_spatial_shapes,
+ data_level_start_index,
+ data_sampling_loc,
+ data_attn_weight,
+ batch_size,
+ spatial_size,
+ num_heads,
+ channels,
+ num_levels,
+ num_query,
+ num_point,
+ grad_value,
+ grad_sampling_loc,
+ grad_attn_weight);
+ break;
+ case 128:
+ ms_deformable_col2im_gpu_kernel_shm_blocksize_aware_reduce_v2
+ <<>>(
+ num_kernels,
+ grad_col,
+ data_value,
+ data_spatial_shapes,
+ data_level_start_index,
+ data_sampling_loc,
+ data_attn_weight,
+ batch_size,
+ spatial_size,
+ num_heads,
+ channels,
+ num_levels,
+ num_query,
+ num_point,
+ grad_value,
+ grad_sampling_loc,
+ grad_attn_weight);
+ break;
+ case 256:
+ ms_deformable_col2im_gpu_kernel_shm_blocksize_aware_reduce_v2
+ <<>>(
+ num_kernels,
+ grad_col,
+ data_value,
+ data_spatial_shapes,
+ data_level_start_index,
+ data_sampling_loc,
+ data_attn_weight,
+ batch_size,
+ spatial_size,
+ num_heads,
+ channels,
+ num_levels,
+ num_query,
+ num_point,
+ grad_value,
+ grad_sampling_loc,
+ grad_attn_weight);
+ break;
+ case 512:
+ ms_deformable_col2im_gpu_kernel_shm_blocksize_aware_reduce_v2
+ <<>>(
+ num_kernels,
+ grad_col,
+ data_value,
+ data_spatial_shapes,
+ data_level_start_index,
+ data_sampling_loc,
+ data_attn_weight,
+ batch_size,
+ spatial_size,
+ num_heads,
+ channels,
+ num_levels,
+ num_query,
+ num_point,
+ grad_value,
+ grad_sampling_loc,
+ grad_attn_weight);
+ break;
+ case 1024:
+ ms_deformable_col2im_gpu_kernel_shm_blocksize_aware_reduce_v2
+ <<>>(
+ num_kernels,
+ grad_col,
+ data_value,
+ data_spatial_shapes,
+ data_level_start_index,
+ data_sampling_loc,
+ data_attn_weight,
+ batch_size,
+ spatial_size,
+ num_heads,
+ channels,
+ num_levels,
+ num_query,
+ num_point,
+ grad_value,
+ grad_sampling_loc,
+ grad_attn_weight);
+ break;
+ default:
+ if (channels < 64)
+ {
+ ms_deformable_col2im_gpu_kernel_shm_reduce_v1
+ <<>>(
+ num_kernels,
+ grad_col,
+ data_value,
+ data_spatial_shapes,
+ data_level_start_index,
+ data_sampling_loc,
+ data_attn_weight,
+ batch_size,
+ spatial_size,
+ num_heads,
+ channels,
+ num_levels,
+ num_query,
+ num_point,
+ grad_value,
+ grad_sampling_loc,
+ grad_attn_weight);
+ }
+ else
+ {
+ ms_deformable_col2im_gpu_kernel_shm_reduce_v2
+ <<>>(
+ num_kernels,
+ grad_col,
+ data_value,
+ data_spatial_shapes,
+ data_level_start_index,
+ data_sampling_loc,
+ data_attn_weight,
+ batch_size,
+ spatial_size,
+ num_heads,
+ channels,
+ num_levels,
+ num_query,
+ num_point,
+ grad_value,
+ grad_sampling_loc,
+ grad_attn_weight);
+ }
+ }
+ }
+ cudaError_t err = cudaGetLastError();
+ if (err != cudaSuccess)
+ {
+ printf("error in ms_deformable_col2im_cuda: %s\n", cudaGetErrorString(err));
+ }
+
+}
\ No newline at end of file
diff --git a/difpoint/src/models/XPose/models/UniPose/ops/src/ms_deform_attn.h b/difpoint/src/models/XPose/models/UniPose/ops/src/ms_deform_attn.h
new file mode 100644
index 0000000000000000000000000000000000000000..e1928abd9546ee4344ee0f79cdf0e45c7a815e84
--- /dev/null
+++ b/difpoint/src/models/XPose/models/UniPose/ops/src/ms_deform_attn.h
@@ -0,0 +1,62 @@
+/*!
+**************************************************************************************************
+* Deformable DETR
+* Copyright (c) 2020 SenseTime. All Rights Reserved.
+* Licensed under the Apache License, Version 2.0 [see LICENSE for details]
+**************************************************************************************************
+* Modified from https://github.com/chengdazhi/Deformable-Convolution-V2-PyTorch/tree/pytorch_1.0.0
+**************************************************************************************************
+*/
+
+#pragma once
+
+#include "cpu/ms_deform_attn_cpu.h"
+
+#ifdef WITH_CUDA
+#include "cuda/ms_deform_attn_cuda.h"
+#endif
+
+
+at::Tensor
+ms_deform_attn_forward(
+ const at::Tensor &value,
+ const at::Tensor &spatial_shapes,
+ const at::Tensor &level_start_index,
+ const at::Tensor &sampling_loc,
+ const at::Tensor &attn_weight,
+ const int im2col_step)
+{
+ if (value.type().is_cuda())
+ {
+#ifdef WITH_CUDA
+ return ms_deform_attn_cuda_forward(
+ value, spatial_shapes, level_start_index, sampling_loc, attn_weight, im2col_step);
+#else
+ AT_ERROR("Not compiled with GPU support");
+#endif
+ }
+ AT_ERROR("Not implemented on the CPU");
+}
+
+std::vector
+ms_deform_attn_backward(
+ const at::Tensor &value,
+ const at::Tensor &spatial_shapes,
+ const at::Tensor &level_start_index,
+ const at::Tensor &sampling_loc,
+ const at::Tensor &attn_weight,
+ const at::Tensor &grad_output,
+ const int im2col_step)
+{
+ if (value.type().is_cuda())
+ {
+#ifdef WITH_CUDA
+ return ms_deform_attn_cuda_backward(
+ value, spatial_shapes, level_start_index, sampling_loc, attn_weight, grad_output, im2col_step);
+#else
+ AT_ERROR("Not compiled with GPU support");
+#endif
+ }
+ AT_ERROR("Not implemented on the CPU");
+}
+
diff --git a/difpoint/src/models/XPose/models/UniPose/ops/src/vision.cpp b/difpoint/src/models/XPose/models/UniPose/ops/src/vision.cpp
new file mode 100644
index 0000000000000000000000000000000000000000..d37eafbf6698bb0cd0721a217d09090d55492821
--- /dev/null
+++ b/difpoint/src/models/XPose/models/UniPose/ops/src/vision.cpp
@@ -0,0 +1,16 @@
+/*!
+**************************************************************************************************
+* Deformable DETR
+* Copyright (c) 2020 SenseTime. All Rights Reserved.
+* Licensed under the Apache License, Version 2.0 [see LICENSE for details]
+**************************************************************************************************
+* Modified from https://github.com/chengdazhi/Deformable-Convolution-V2-PyTorch/tree/pytorch_1.0.0
+**************************************************************************************************
+*/
+
+#include "ms_deform_attn.h"
+
+PYBIND11_MODULE(TORCH_EXTENSION_NAME, m) {
+ m.def("ms_deform_attn_forward", &ms_deform_attn_forward, "ms_deform_attn_forward");
+ m.def("ms_deform_attn_backward", &ms_deform_attn_backward, "ms_deform_attn_backward");
+}
diff --git a/difpoint/src/models/XPose/models/UniPose/ops/test.py b/difpoint/src/models/XPose/models/UniPose/ops/test.py
new file mode 100644
index 0000000000000000000000000000000000000000..acba8133c08de05fc877c4e09e40610558cdc1fa
--- /dev/null
+++ b/difpoint/src/models/XPose/models/UniPose/ops/test.py
@@ -0,0 +1,89 @@
+# ------------------------------------------------------------------------------------------------
+# Deformable DETR
+# Copyright (c) 2020 SenseTime. All Rights Reserved.
+# Licensed under the Apache License, Version 2.0 [see LICENSE for details]
+# ------------------------------------------------------------------------------------------------
+# Modified from https://github.com/chengdazhi/Deformable-Convolution-V2-PyTorch/tree/pytorch_1.0.0
+# ------------------------------------------------------------------------------------------------
+
+from __future__ import absolute_import
+from __future__ import print_function
+from __future__ import division
+
+import time
+import torch
+import torch.nn as nn
+from torch.autograd import gradcheck
+
+from functions.ms_deform_attn_func import MSDeformAttnFunction, ms_deform_attn_core_pytorch
+
+
+N, M, D = 1, 2, 2
+Lq, L, P = 2, 2, 2
+shapes = torch.as_tensor([(6, 4), (3, 2)], dtype=torch.long).cuda()
+level_start_index = torch.cat((shapes.new_zeros((1, )), shapes.prod(1).cumsum(0)[:-1]))
+S = sum([(H*W).item() for H, W in shapes])
+
+
+torch.manual_seed(3)
+
+
+@torch.no_grad()
+def check_forward_equal_with_pytorch_double():
+ value = torch.rand(N, S, M, D).cuda() * 0.01
+ sampling_locations = torch.rand(N, Lq, M, L, P, 2).cuda()
+ attention_weights = torch.rand(N, Lq, M, L, P).cuda() + 1e-5
+ attention_weights /= attention_weights.sum(-1, keepdim=True).sum(-2, keepdim=True)
+ im2col_step = 2
+ output_pytorch = ms_deform_attn_core_pytorch(value.double(), shapes, sampling_locations.double(), attention_weights.double()).detach().cpu()
+ output_cuda = MSDeformAttnFunction.apply(value.double(), shapes, level_start_index, sampling_locations.double(), attention_weights.double(), im2col_step).detach().cpu()
+ fwdok = torch.allclose(output_cuda, output_pytorch)
+ max_abs_err = (output_cuda - output_pytorch).abs().max()
+ max_rel_err = ((output_cuda - output_pytorch).abs() / output_pytorch.abs()).max()
+
+ print(f'* {fwdok} check_forward_equal_with_pytorch_double: max_abs_err {max_abs_err:.2e} max_rel_err {max_rel_err:.2e}')
+
+
+@torch.no_grad()
+def check_forward_equal_with_pytorch_float():
+ value = torch.rand(N, S, M, D).cuda() * 0.01
+ sampling_locations = torch.rand(N, Lq, M, L, P, 2).cuda()
+ attention_weights = torch.rand(N, Lq, M, L, P).cuda() + 1e-5
+ attention_weights /= attention_weights.sum(-1, keepdim=True).sum(-2, keepdim=True)
+ im2col_step = 2
+ output_pytorch = ms_deform_attn_core_pytorch(value, shapes, sampling_locations, attention_weights).detach().cpu()
+ output_cuda = MSDeformAttnFunction.apply(value, shapes, level_start_index, sampling_locations, attention_weights, im2col_step).detach().cpu()
+ fwdok = torch.allclose(output_cuda, output_pytorch, rtol=1e-2, atol=1e-3)
+ max_abs_err = (output_cuda - output_pytorch).abs().max()
+ max_rel_err = ((output_cuda - output_pytorch).abs() / output_pytorch.abs()).max()
+
+ print(f'* {fwdok} check_forward_equal_with_pytorch_float: max_abs_err {max_abs_err:.2e} max_rel_err {max_rel_err:.2e}')
+
+
+def check_gradient_numerical(channels=4, grad_value=True, grad_sampling_loc=True, grad_attn_weight=True):
+
+ value = torch.rand(N, S, M, channels).cuda() * 0.01
+ sampling_locations = torch.rand(N, Lq, M, L, P, 2).cuda()
+ attention_weights = torch.rand(N, Lq, M, L, P).cuda() + 1e-5
+ attention_weights /= attention_weights.sum(-1, keepdim=True).sum(-2, keepdim=True)
+ im2col_step = 2
+ func = MSDeformAttnFunction.apply
+
+ value.requires_grad = grad_value
+ sampling_locations.requires_grad = grad_sampling_loc
+ attention_weights.requires_grad = grad_attn_weight
+
+ gradok = gradcheck(func, (value.double(), shapes, level_start_index, sampling_locations.double(), attention_weights.double(), im2col_step))
+
+ print(f'* {gradok} check_gradient_numerical(D={channels})')
+
+
+if __name__ == '__main__':
+ check_forward_equal_with_pytorch_double()
+ check_forward_equal_with_pytorch_float()
+
+ for channels in [30, 32, 64, 71, 1025, 2048, 3096]:
+ check_gradient_numerical(channels, True, True, True)
+
+
+
diff --git a/difpoint/src/models/XPose/models/UniPose/position_encoding.py b/difpoint/src/models/XPose/models/UniPose/position_encoding.py
new file mode 100644
index 0000000000000000000000000000000000000000..47a4540635f9b252d0312a9127cb135d133be320
--- /dev/null
+++ b/difpoint/src/models/XPose/models/UniPose/position_encoding.py
@@ -0,0 +1,157 @@
+# ------------------------------------------------------------------------
+# ED-Pose
+# Copyright (c) 2023 IDEA. All Rights Reserved.
+# Licensed under the Apache License, Version 2.0 [see LICENSE for details]
+# ------------------------------------------------------------------------
+# Conditional DETR
+# Copyright (c) 2021 Microsoft. All Rights Reserved.
+# Licensed under the Apache License, Version 2.0 [see LICENSE for details]
+# ------------------------------------------------------------------------
+# Copied from DETR (https://github.com/facebookresearch/detr)
+# Copyright (c) Facebook, Inc. and its affiliates. All Rights Reserved.
+# ------------------------------------------------------------------------
+
+"""
+Various positional encodings for the transformer.
+"""
+import math
+import torch
+from torch import nn
+
+from ...util.misc import NestedTensor
+
+
+class PositionEmbeddingSine(nn.Module):
+ """
+ This is a more standard version of the position embedding, very similar to the one
+ used by the Attention is all you need paper, generalized to work on images.
+ """
+ def __init__(self, num_pos_feats=64, temperature=10000, normalize=False, scale=None):
+ super().__init__()
+ self.num_pos_feats = num_pos_feats
+ self.temperature = temperature
+ self.normalize = normalize
+ if scale is not None and normalize is False:
+ raise ValueError("normalize should be True if scale is passed")
+ if scale is None:
+ scale = 2 * math.pi
+ self.scale = scale
+
+ def forward(self, tensor_list: NestedTensor):
+ x = tensor_list.tensors
+ mask = tensor_list.mask
+ assert mask is not None
+ not_mask = ~mask
+ y_embed = not_mask.cumsum(1, dtype=torch.float32)
+ x_embed = not_mask.cumsum(2, dtype=torch.float32)
+ if self.normalize:
+ eps = 1e-6
+ # if os.environ.get("SHILONG_AMP", None) == '1':
+ # eps = 1e-4
+ # else:
+ # eps = 1e-6
+ y_embed = y_embed / (y_embed[:, -1:, :] + eps) * self.scale
+ x_embed = x_embed / (x_embed[:, :, -1:] + eps) * self.scale
+
+ dim_t = torch.arange(self.num_pos_feats, dtype=torch.float32, device=x.device)
+ dim_t = self.temperature ** (2 * (dim_t // 2) / self.num_pos_feats)
+
+ pos_x = x_embed[:, :, :, None] / dim_t
+ pos_y = y_embed[:, :, :, None] / dim_t
+ pos_x = torch.stack((pos_x[:, :, :, 0::2].sin(), pos_x[:, :, :, 1::2].cos()), dim=4).flatten(3)
+ pos_y = torch.stack((pos_y[:, :, :, 0::2].sin(), pos_y[:, :, :, 1::2].cos()), dim=4).flatten(3)
+ pos = torch.cat((pos_y, pos_x), dim=3).permute(0, 3, 1, 2)
+ return pos
+
+class PositionEmbeddingSineHW(nn.Module):
+ """
+ This is a more standard version of the position embedding, very similar to the one
+ used by the Attention is all you need paper, generalized to work on images.
+ """
+ def __init__(self, num_pos_feats=64, temperatureH=10000, temperatureW=10000, normalize=False, scale=None):
+ super().__init__()
+ self.num_pos_feats = num_pos_feats
+ self.temperatureH = temperatureH
+ self.temperatureW = temperatureW
+ self.normalize = normalize
+ if scale is not None and normalize is False:
+ raise ValueError("normalize should be True if scale is passed")
+ if scale is None:
+ scale = 2 * math.pi
+ self.scale = scale
+
+ def forward(self, tensor_list: NestedTensor):
+ x = tensor_list.tensors
+ mask = tensor_list.mask
+ assert mask is not None
+ not_mask = ~mask
+ y_embed = not_mask.cumsum(1, dtype=torch.float32)
+ x_embed = not_mask.cumsum(2, dtype=torch.float32)
+
+ # import ipdb; ipdb.set_trace()
+
+ if self.normalize:
+ eps = 1e-6
+ y_embed = y_embed / (y_embed[:, -1:, :] + eps) * self.scale
+ x_embed = x_embed / (x_embed[:, :, -1:] + eps) * self.scale
+
+ dim_tx = torch.arange(self.num_pos_feats, dtype=torch.float32, device=x.device)
+ dim_tx = self.temperatureW ** (2 * (dim_tx // 2) / self.num_pos_feats)
+ pos_x = x_embed[:, :, :, None] / dim_tx
+
+ dim_ty = torch.arange(self.num_pos_feats, dtype=torch.float32, device=x.device)
+ dim_ty = self.temperatureH ** (2 * (dim_ty // 2) / self.num_pos_feats)
+ pos_y = y_embed[:, :, :, None] / dim_ty
+
+ pos_x = torch.stack((pos_x[:, :, :, 0::2].sin(), pos_x[:, :, :, 1::2].cos()), dim=4).flatten(3)
+ pos_y = torch.stack((pos_y[:, :, :, 0::2].sin(), pos_y[:, :, :, 1::2].cos()), dim=4).flatten(3)
+ pos = torch.cat((pos_y, pos_x), dim=3).permute(0, 3, 1, 2)
+
+ # import ipdb; ipdb.set_trace()
+
+ return pos
+
+class PositionEmbeddingLearned(nn.Module):
+ """
+ Absolute pos embedding, learned.
+ """
+ def __init__(self, num_pos_feats=256):
+ super().__init__()
+ self.row_embed = nn.Embedding(50, num_pos_feats)
+ self.col_embed = nn.Embedding(50, num_pos_feats)
+ self.reset_parameters()
+
+ def reset_parameters(self):
+ nn.init.uniform_(self.row_embed.weight)
+ nn.init.uniform_(self.col_embed.weight)
+
+ def forward(self, tensor_list: NestedTensor):
+ x = tensor_list.tensors
+ h, w = x.shape[-2:]
+ i = torch.arange(w, device=x.device)
+ j = torch.arange(h, device=x.device)
+ x_emb = self.col_embed(i)
+ y_emb = self.row_embed(j)
+ pos = torch.cat([
+ x_emb.unsqueeze(0).repeat(h, 1, 1),
+ y_emb.unsqueeze(1).repeat(1, w, 1),
+ ], dim=-1).permute(2, 0, 1).unsqueeze(0).repeat(x.shape[0], 1, 1, 1)
+ return pos
+
+
+def build_position_encoding(args):
+ N_steps = args.hidden_dim // 2
+ if args.position_embedding in ('v2', 'sine'):
+ # TODO find a better way of exposing other arguments
+ position_embedding = PositionEmbeddingSineHW(
+ N_steps,
+ temperatureH=args.pe_temperatureH,
+ temperatureW=args.pe_temperatureW,
+ normalize=True
+ )
+ elif args.position_embedding in ('v3', 'learned'):
+ position_embedding = PositionEmbeddingLearned(N_steps)
+ else:
+ raise ValueError(f"not supported {args.position_embedding}")
+
+ return position_embedding
diff --git a/difpoint/src/models/XPose/models/UniPose/swin_transformer.py b/difpoint/src/models/XPose/models/UniPose/swin_transformer.py
new file mode 100644
index 0000000000000000000000000000000000000000..7cb255117747c0001df966fe512fd68e65f44db2
--- /dev/null
+++ b/difpoint/src/models/XPose/models/UniPose/swin_transformer.py
@@ -0,0 +1,701 @@
+
+import torch
+import torch.nn as nn
+import torch.nn.functional as F
+import torch.utils.checkpoint as checkpoint
+import numpy as np
+
+from ...util.misc import NestedTensor
+# from timm.models.layers import DropPath, to_2tuple, trunc_normal_
+from src.models.util import DropPath, to_2tuple, trunc_normal_
+
+
+
+class Mlp(nn.Module):
+ """ Multilayer perceptron."""
+
+ def __init__(self, in_features, hidden_features=None, out_features=None, act_layer=nn.GELU, drop=0.):
+ super().__init__()
+ out_features = out_features or in_features
+ hidden_features = hidden_features or in_features
+ self.fc1 = nn.Linear(in_features, hidden_features)
+ self.act = act_layer()
+ self.fc2 = nn.Linear(hidden_features, out_features)
+ self.drop = nn.Dropout(drop)
+
+ def forward(self, x):
+ x = self.fc1(x)
+ x = self.act(x)
+ x = self.drop(x)
+ x = self.fc2(x)
+ x = self.drop(x)
+ return x
+
+
+def window_partition(x, window_size):
+ """
+ Args:
+ x: (B, H, W, C)
+ window_size (int): window size
+ Returns:
+ windows: (num_windows*B, window_size, window_size, C)
+ """
+ B, H, W, C = x.shape
+ x = x.view(B, H // window_size, window_size, W // window_size, window_size, C)
+ windows = x.permute(0, 1, 3, 2, 4, 5).contiguous().view(-1, window_size, window_size, C)
+ return windows
+
+
+def window_reverse(windows, window_size, H, W):
+ """
+ Args:
+ windows: (num_windows*B, window_size, window_size, C)
+ window_size (int): Window size
+ H (int): Height of image
+ W (int): Width of image
+ Returns:
+ x: (B, H, W, C)
+ """
+ B = int(windows.shape[0] / (H * W / window_size / window_size))
+ x = windows.view(B, H // window_size, W // window_size, window_size, window_size, -1)
+ x = x.permute(0, 1, 3, 2, 4, 5).contiguous().view(B, H, W, -1)
+ return x
+
+
+class WindowAttention(nn.Module):
+ """ Window based multi-head self attention (W-MSA) module with relative position bias.
+ It supports both of shifted and non-shifted window.
+ Args:
+ dim (int): Number of input channels.
+ window_size (tuple[int]): The height and width of the window.
+ num_heads (int): Number of attention heads.
+ qkv_bias (bool, optional): If True, add a learnable bias to query, key, value. Default: True
+ qk_scale (float | None, optional): Override default qk scale of head_dim ** -0.5 if set
+ attn_drop (float, optional): Dropout ratio of attention weight. Default: 0.0
+ proj_drop (float, optional): Dropout ratio of output. Default: 0.0
+ """
+
+ def __init__(self, dim, window_size, num_heads, qkv_bias=True, qk_scale=None, attn_drop=0., proj_drop=0.):
+
+ super().__init__()
+ self.dim = dim
+ self.window_size = window_size # Wh, Ww
+ self.num_heads = num_heads
+ head_dim = dim // num_heads
+ self.scale = qk_scale or head_dim ** -0.5
+
+ # define a parameter table of relative position bias
+ self.relative_position_bias_table = nn.Parameter(
+ torch.zeros((2 * window_size[0] - 1) * (2 * window_size[1] - 1), num_heads)) # 2*Wh-1 * 2*Ww-1, nH
+
+ # get pair-wise relative position index for each token inside the window
+ coords_h = torch.arange(self.window_size[0])
+ coords_w = torch.arange(self.window_size[1])
+ coords = torch.stack(torch.meshgrid([coords_h, coords_w])) # 2, Wh, Ww
+ coords_flatten = torch.flatten(coords, 1) # 2, Wh*Ww
+ relative_coords = coords_flatten[:, :, None] - coords_flatten[:, None, :] # 2, Wh*Ww, Wh*Ww
+ relative_coords = relative_coords.permute(1, 2, 0).contiguous() # Wh*Ww, Wh*Ww, 2
+ relative_coords[:, :, 0] += self.window_size[0] - 1 # shift to start from 0
+ relative_coords[:, :, 1] += self.window_size[1] - 1
+ relative_coords[:, :, 0] *= 2 * self.window_size[1] - 1
+ relative_position_index = relative_coords.sum(-1) # Wh*Ww, Wh*Ww
+ self.register_buffer("relative_position_index", relative_position_index)
+
+ self.qkv = nn.Linear(dim, dim * 3, bias=qkv_bias)
+ self.attn_drop = nn.Dropout(attn_drop)
+ self.proj = nn.Linear(dim, dim)
+ self.proj_drop = nn.Dropout(proj_drop)
+
+ trunc_normal_(self.relative_position_bias_table, std=.02)
+ self.softmax = nn.Softmax(dim=-1)
+
+ def forward(self, x, mask=None):
+ """ Forward function.
+ Args:
+ x: input features with shape of (num_windows*B, N, C)
+ mask: (0/-inf) mask with shape of (num_windows, Wh*Ww, Wh*Ww) or None
+ """
+ B_, N, C = x.shape
+ qkv = self.qkv(x).reshape(B_, N, 3, self.num_heads, C // self.num_heads).permute(2, 0, 3, 1, 4)
+ q, k, v = qkv[0], qkv[1], qkv[2] # make torchscript happy (cannot use tensor as tuple)
+
+ q = q * self.scale
+ attn = (q @ k.transpose(-2, -1))
+
+ relative_position_bias = self.relative_position_bias_table[self.relative_position_index.view(-1)].view(
+ self.window_size[0] * self.window_size[1], self.window_size[0] * self.window_size[1], -1) # Wh*Ww,Wh*Ww,nH
+ relative_position_bias = relative_position_bias.permute(2, 0, 1).contiguous() # nH, Wh*Ww, Wh*Ww
+ attn = attn + relative_position_bias.unsqueeze(0)
+
+ if mask is not None:
+ nW = mask.shape[0]
+ attn = attn.view(B_ // nW, nW, self.num_heads, N, N) + mask.unsqueeze(1).unsqueeze(0)
+ attn = attn.view(-1, self.num_heads, N, N)
+ attn = self.softmax(attn)
+ else:
+ attn = self.softmax(attn)
+
+ attn = self.attn_drop(attn)
+
+ x = (attn @ v).transpose(1, 2).reshape(B_, N, C)
+ x = self.proj(x)
+ x = self.proj_drop(x)
+ return x
+
+
+class SwinTransformerBlock(nn.Module):
+ """ Swin Transformer Block.
+ Args:
+ dim (int): Number of input channels.
+ num_heads (int): Number of attention heads.
+ window_size (int): Window size.
+ shift_size (int): Shift size for SW-MSA.
+ mlp_ratio (float): Ratio of mlp hidden dim to embedding dim.
+ qkv_bias (bool, optional): If True, add a learnable bias to query, key, value. Default: True
+ qk_scale (float | None, optional): Override default qk scale of head_dim ** -0.5 if set.
+ drop (float, optional): Dropout rate. Default: 0.0
+ attn_drop (float, optional): Attention dropout rate. Default: 0.0
+ drop_path (float, optional): Stochastic depth rate. Default: 0.0
+ act_layer (nn.Module, optional): Activation layer. Default: nn.GELU
+ norm_layer (nn.Module, optional): Normalization layer. Default: nn.LayerNorm
+ """
+
+ def __init__(self, dim, num_heads, window_size=7, shift_size=0,
+ mlp_ratio=4., qkv_bias=True, qk_scale=None, drop=0., attn_drop=0., drop_path=0.,
+ act_layer=nn.GELU, norm_layer=nn.LayerNorm):
+ super().__init__()
+ self.dim = dim
+ self.num_heads = num_heads
+ self.window_size = window_size
+ self.shift_size = shift_size
+ self.mlp_ratio = mlp_ratio
+ assert 0 <= self.shift_size < self.window_size, "shift_size must in 0-window_size"
+
+ self.norm1 = norm_layer(dim)
+ self.attn = WindowAttention(
+ dim, window_size=to_2tuple(self.window_size), num_heads=num_heads,
+ qkv_bias=qkv_bias, qk_scale=qk_scale, attn_drop=attn_drop, proj_drop=drop)
+
+ self.drop_path = DropPath(drop_path) if drop_path > 0. else nn.Identity()
+ self.norm2 = norm_layer(dim)
+ mlp_hidden_dim = int(dim * mlp_ratio)
+ self.mlp = Mlp(in_features=dim, hidden_features=mlp_hidden_dim, act_layer=act_layer, drop=drop)
+
+ self.H = None
+ self.W = None
+
+ def forward(self, x, mask_matrix):
+ """ Forward function.
+ Args:
+ x: Input feature, tensor size (B, H*W, C).
+ H, W: Spatial resolution of the input feature.
+ mask_matrix: Attention mask for cyclic shift.
+ """
+ B, L, C = x.shape
+ H, W = self.H, self.W
+ assert L == H * W, "input feature has wrong size"
+
+ shortcut = x
+ x = self.norm1(x)
+ x = x.view(B, H, W, C)
+
+ # pad feature maps to multiples of window size
+ pad_l = pad_t = 0
+ pad_r = (self.window_size - W % self.window_size) % self.window_size
+ pad_b = (self.window_size - H % self.window_size) % self.window_size
+ x = F.pad(x, (0, 0, pad_l, pad_r, pad_t, pad_b))
+ _, Hp, Wp, _ = x.shape
+
+ # cyclic shift
+ if self.shift_size > 0:
+ shifted_x = torch.roll(x, shifts=(-self.shift_size, -self.shift_size), dims=(1, 2))
+ attn_mask = mask_matrix
+ else:
+ shifted_x = x
+ attn_mask = None
+
+ # partition windows
+ x_windows = window_partition(shifted_x, self.window_size) # nW*B, window_size, window_size, C
+ x_windows = x_windows.view(-1, self.window_size * self.window_size, C) # nW*B, window_size*window_size, C
+
+ # W-MSA/SW-MSA
+ attn_windows = self.attn(x_windows, mask=attn_mask) # nW*B, window_size*window_size, C
+
+ # merge windows
+ attn_windows = attn_windows.view(-1, self.window_size, self.window_size, C)
+ shifted_x = window_reverse(attn_windows, self.window_size, Hp, Wp) # B H' W' C
+
+ # reverse cyclic shift
+ if self.shift_size > 0:
+ x = torch.roll(shifted_x, shifts=(self.shift_size, self.shift_size), dims=(1, 2))
+ else:
+ x = shifted_x
+
+ if pad_r > 0 or pad_b > 0:
+ x = x[:, :H, :W, :].contiguous()
+
+ x = x.view(B, H * W, C)
+
+ # FFN
+ x = shortcut + self.drop_path(x)
+ x = x + self.drop_path(self.mlp(self.norm2(x)))
+
+ return x
+
+
+class PatchMerging(nn.Module):
+ """ Patch Merging Layer
+ Args:
+ dim (int): Number of input channels.
+ norm_layer (nn.Module, optional): Normalization layer. Default: nn.LayerNorm
+ """
+ def __init__(self, dim, norm_layer=nn.LayerNorm):
+ super().__init__()
+ self.dim = dim
+ self.reduction = nn.Linear(4 * dim, 2 * dim, bias=False)
+ self.norm = norm_layer(4 * dim)
+
+ def forward(self, x, H, W):
+ """ Forward function.
+ Args:
+ x: Input feature, tensor size (B, H*W, C).
+ H, W: Spatial resolution of the input feature.
+ """
+ B, L, C = x.shape
+ assert L == H * W, "input feature has wrong size"
+
+ x = x.view(B, H, W, C)
+
+ # padding
+ pad_input = (H % 2 == 1) or (W % 2 == 1)
+ if pad_input:
+ x = F.pad(x, (0, 0, 0, W % 2, 0, H % 2))
+
+ x0 = x[:, 0::2, 0::2, :] # B H/2 W/2 C
+ x1 = x[:, 1::2, 0::2, :] # B H/2 W/2 C
+ x2 = x[:, 0::2, 1::2, :] # B H/2 W/2 C
+ x3 = x[:, 1::2, 1::2, :] # B H/2 W/2 C
+ x = torch.cat([x0, x1, x2, x3], -1) # B H/2 W/2 4*C
+ x = x.view(B, -1, 4 * C) # B H/2*W/2 4*C
+
+ x = self.norm(x)
+ x = self.reduction(x)
+
+ return x
+
+
+class BasicLayer(nn.Module):
+ """ A basic Swin Transformer layer for one stage.
+ Args:
+ dim (int): Number of feature channels
+ depth (int): Depths of this stage.
+ num_heads (int): Number of attention head.
+ window_size (int): Local window size. Default: 7.
+ mlp_ratio (float): Ratio of mlp hidden dim to embedding dim. Default: 4.
+ qkv_bias (bool, optional): If True, add a learnable bias to query, key, value. Default: True
+ qk_scale (float | None, optional): Override default qk scale of head_dim ** -0.5 if set.
+ drop (float, optional): Dropout rate. Default: 0.0
+ attn_drop (float, optional): Attention dropout rate. Default: 0.0
+ drop_path (float | tuple[float], optional): Stochastic depth rate. Default: 0.0
+ norm_layer (nn.Module, optional): Normalization layer. Default: nn.LayerNorm
+ downsample (nn.Module | None, optional): Downsample layer at the end of the layer. Default: None
+ use_checkpoint (bool): Whether to use checkpointing to save memory. Default: False.
+ """
+
+ def __init__(self,
+ dim,
+ depth,
+ num_heads,
+ window_size=7,
+ mlp_ratio=4.,
+ qkv_bias=True,
+ qk_scale=None,
+ drop=0.,
+ attn_drop=0.,
+ drop_path=0.,
+ norm_layer=nn.LayerNorm,
+ downsample=None,
+ use_checkpoint=False):
+ super().__init__()
+ self.window_size = window_size
+ self.shift_size = window_size // 2
+ self.depth = depth
+ self.use_checkpoint = use_checkpoint
+
+ # build blocks
+ self.blocks = nn.ModuleList([
+ SwinTransformerBlock(
+ dim=dim,
+ num_heads=num_heads,
+ window_size=window_size,
+ shift_size=0 if (i % 2 == 0) else window_size // 2,
+ mlp_ratio=mlp_ratio,
+ qkv_bias=qkv_bias,
+ qk_scale=qk_scale,
+ drop=drop,
+ attn_drop=attn_drop,
+ drop_path=drop_path[i] if isinstance(drop_path, list) else drop_path,
+ norm_layer=norm_layer)
+ for i in range(depth)])
+
+ # patch merging layer
+ if downsample is not None:
+ self.downsample = downsample(dim=dim, norm_layer=norm_layer)
+ else:
+ self.downsample = None
+
+ def forward(self, x, H, W):
+ """ Forward function.
+ Args:
+ x: Input feature, tensor size (B, H*W, C).
+ H, W: Spatial resolution of the input feature.
+ """
+
+ # calculate attention mask for SW-MSA
+ Hp = int(np.ceil(H / self.window_size)) * self.window_size
+ Wp = int(np.ceil(W / self.window_size)) * self.window_size
+ img_mask = torch.zeros((1, Hp, Wp, 1), device=x.device) # 1 Hp Wp 1
+ h_slices = (slice(0, -self.window_size),
+ slice(-self.window_size, -self.shift_size),
+ slice(-self.shift_size, None))
+ w_slices = (slice(0, -self.window_size),
+ slice(-self.window_size, -self.shift_size),
+ slice(-self.shift_size, None))
+ cnt = 0
+ for h in h_slices:
+ for w in w_slices:
+ img_mask[:, h, w, :] = cnt
+ cnt += 1
+
+ mask_windows = window_partition(img_mask, self.window_size) # nW, window_size, window_size, 1
+ mask_windows = mask_windows.view(-1, self.window_size * self.window_size)
+ attn_mask = mask_windows.unsqueeze(1) - mask_windows.unsqueeze(2)
+ attn_mask = attn_mask.masked_fill(attn_mask != 0, float(-100.0)).masked_fill(attn_mask == 0, float(0.0))
+
+ for blk in self.blocks:
+ blk.H, blk.W = H, W
+ if self.use_checkpoint:
+ x = checkpoint.checkpoint(blk, x, attn_mask)
+ else:
+ x = blk(x, attn_mask)
+ if self.downsample is not None:
+ x_down = self.downsample(x, H, W)
+ Wh, Ww = (H + 1) // 2, (W + 1) // 2
+ return x, H, W, x_down, Wh, Ww
+ else:
+ return x, H, W, x, H, W
+
+
+class PatchEmbed(nn.Module):
+ """ Image to Patch Embedding
+ Args:
+ patch_size (int): Patch token size. Default: 4.
+ in_chans (int): Number of input image channels. Default: 3.
+ embed_dim (int): Number of linear projection output channels. Default: 96.
+ norm_layer (nn.Module, optional): Normalization layer. Default: None
+ """
+
+ def __init__(self, patch_size=4, in_chans=3, embed_dim=96, norm_layer=None):
+ super().__init__()
+ patch_size = to_2tuple(patch_size)
+ self.patch_size = patch_size
+
+ self.in_chans = in_chans
+ self.embed_dim = embed_dim
+
+ self.proj = nn.Conv2d(in_chans, embed_dim, kernel_size=patch_size, stride=patch_size)
+ if norm_layer is not None:
+ self.norm = norm_layer(embed_dim)
+ else:
+ self.norm = None
+
+ def forward(self, x):
+ """Forward function."""
+ # padding
+ _, _, H, W = x.size()
+ if W % self.patch_size[1] != 0:
+ x = F.pad(x, (0, self.patch_size[1] - W % self.patch_size[1]))
+ if H % self.patch_size[0] != 0:
+ x = F.pad(x, (0, 0, 0, self.patch_size[0] - H % self.patch_size[0]))
+
+ x = self.proj(x) # B C Wh Ww
+ if self.norm is not None:
+ Wh, Ww = x.size(2), x.size(3)
+ x = x.flatten(2).transpose(1, 2)
+ x = self.norm(x)
+ x = x.transpose(1, 2).view(-1, self.embed_dim, Wh, Ww)
+
+ return x
+
+
+class SwinTransformer(nn.Module):
+ """ Swin Transformer backbone.
+ A PyTorch impl of : `Swin Transformer: Hierarchical Vision Transformer using Shifted Windows` -
+ https://arxiv.org/pdf/2103.14030
+ Args:
+ pretrain_img_size (int): Input image size for training the pretrained model,
+ used in absolute postion embedding. Default 224.
+ patch_size (int | tuple(int)): Patch size. Default: 4.
+ in_chans (int): Number of input image channels. Default: 3.
+ embed_dim (int): Number of linear projection output channels. Default: 96.
+ depths (tuple[int]): Depths of each Swin Transformer stage.
+ num_heads (tuple[int]): Number of attention head of each stage.
+ window_size (int): Window size. Default: 7.
+ mlp_ratio (float): Ratio of mlp hidden dim to embedding dim. Default: 4.
+ qkv_bias (bool): If True, add a learnable bias to query, key, value. Default: True
+ qk_scale (float): Override default qk scale of head_dim ** -0.5 if set.
+ drop_rate (float): Dropout rate.
+ attn_drop_rate (float): Attention dropout rate. Default: 0.
+ drop_path_rate (float): Stochastic depth rate. Default: 0.2.
+ norm_layer (nn.Module): Normalization layer. Default: nn.LayerNorm.
+ ape (bool): If True, add absolute position embedding to the patch embedding. Default: False.
+ patch_norm (bool): If True, add normalization after patch embedding. Default: True.
+ out_indices (Sequence[int]): Output from which stages.
+ frozen_stages (int): Stages to be frozen (stop grad and set eval mode).
+ -1 means not freezing any parameters.
+ use_checkpoint (bool): Whether to use checkpointing to save memory. Default: False.
+ dilation (bool): if True, the output size if 16x downsample, ow 32x downsample.
+ """
+
+ def __init__(self,
+ pretrain_img_size=224,
+ patch_size=4,
+ in_chans=3,
+ embed_dim=96,
+ depths=[2, 2, 6, 2],
+ num_heads=[3, 6, 12, 24],
+ window_size=7,
+ mlp_ratio=4.,
+ qkv_bias=True,
+ qk_scale=None,
+ drop_rate=0.,
+ attn_drop_rate=0.,
+ drop_path_rate=0.2,
+ norm_layer=nn.LayerNorm,
+ ape=False,
+ patch_norm=True,
+ out_indices=(0, 1, 2, 3),
+ frozen_stages=-1,
+ dilation=False,
+ use_checkpoint=False):
+ super().__init__()
+
+ self.pretrain_img_size = pretrain_img_size
+ self.num_layers = len(depths)
+ self.embed_dim = embed_dim
+ self.ape = ape
+ self.patch_norm = patch_norm
+ self.out_indices = out_indices
+ self.frozen_stages = frozen_stages
+ self.dilation = dilation
+
+ # if use_checkpoint:
+ # print("use_checkpoint!!!!!!!!!!!!!!!!!!!!!!!!")
+
+ # split image into non-overlapping patches
+ self.patch_embed = PatchEmbed(
+ patch_size=patch_size, in_chans=in_chans, embed_dim=embed_dim,
+ norm_layer=norm_layer if self.patch_norm else None)
+
+ # absolute position embedding
+ if self.ape:
+ pretrain_img_size = to_2tuple(pretrain_img_size)
+ patch_size = to_2tuple(patch_size)
+ patches_resolution = [pretrain_img_size[0] // patch_size[0], pretrain_img_size[1] // patch_size[1]]
+
+ self.absolute_pos_embed = nn.Parameter(torch.zeros(1, embed_dim, patches_resolution[0], patches_resolution[1]))
+ trunc_normal_(self.absolute_pos_embed, std=.02)
+
+ self.pos_drop = nn.Dropout(p=drop_rate)
+
+ # stochastic depth
+ dpr = [x.item() for x in torch.linspace(0, drop_path_rate, sum(depths))] # stochastic depth decay rule
+
+ # build layers
+ self.layers = nn.ModuleList()
+ # prepare downsample list
+ downsamplelist = [PatchMerging for i in range(self.num_layers)]
+ downsamplelist[-1] = None
+ num_features = [int(embed_dim * 2 ** i) for i in range(self.num_layers)]
+ if self.dilation:
+ downsamplelist[-2] = None
+ num_features[-1] = int(embed_dim * 2 ** (self.num_layers - 1)) // 2
+ for i_layer in range(self.num_layers):
+ layer = BasicLayer(
+ # dim=int(embed_dim * 2 ** i_layer),
+ dim=num_features[i_layer],
+ depth=depths[i_layer],
+ num_heads=num_heads[i_layer],
+ window_size=window_size,
+ mlp_ratio=mlp_ratio,
+ qkv_bias=qkv_bias,
+ qk_scale=qk_scale,
+ drop=drop_rate,
+ attn_drop=attn_drop_rate,
+ drop_path=dpr[sum(depths[:i_layer]):sum(depths[:i_layer + 1])],
+ norm_layer=norm_layer,
+ # downsample=PatchMerging if (i_layer < self.num_layers - 1) else None,
+ downsample=downsamplelist[i_layer],
+ use_checkpoint=use_checkpoint)
+ self.layers.append(layer)
+
+ # num_features = [int(embed_dim * 2 ** i) for i in range(self.num_layers)]
+ self.num_features = num_features
+
+ # add a norm layer for each output
+ for i_layer in out_indices:
+ layer = norm_layer(num_features[i_layer])
+ layer_name = f'norm{i_layer}'
+ self.add_module(layer_name, layer)
+
+ self._freeze_stages()
+
+ def _freeze_stages(self):
+ if self.frozen_stages >= 0:
+ self.patch_embed.eval()
+ for param in self.patch_embed.parameters():
+ param.requires_grad = False
+
+ if self.frozen_stages >= 1 and self.ape:
+ self.absolute_pos_embed.requires_grad = False
+
+ if self.frozen_stages >= 2:
+ self.pos_drop.eval()
+ for i in range(0, self.frozen_stages - 1):
+ m = self.layers[i]
+ m.eval()
+ for param in m.parameters():
+ param.requires_grad = False
+
+
+
+ def forward_raw(self, x):
+ """Forward function."""
+ x = self.patch_embed(x)
+
+ Wh, Ww = x.size(2), x.size(3)
+ if self.ape:
+ # interpolate the position embedding to the corresponding size
+ absolute_pos_embed = F.interpolate(self.absolute_pos_embed, size=(Wh, Ww), mode='bicubic')
+ x = (x + absolute_pos_embed).flatten(2).transpose(1, 2) # B Wh*Ww C
+ else:
+ x = x.flatten(2).transpose(1, 2)
+ x = self.pos_drop(x)
+
+ outs = []
+ for i in range(self.num_layers):
+ layer = self.layers[i]
+ x_out, H, W, x, Wh, Ww = layer(x, Wh, Ww)
+ # import ipdb; ipdb.set_trace()
+
+ if i in self.out_indices:
+ norm_layer = getattr(self, f'norm{i}')
+ x_out = norm_layer(x_out)
+
+ out = x_out.view(-1, H, W, self.num_features[i]).permute(0, 3, 1, 2).contiguous()
+ outs.append(out)
+ # in:
+ # torch.Size([2, 3, 1024, 1024])
+ # outs:
+ # [torch.Size([2, 192, 256, 256]), torch.Size([2, 384, 128, 128]), \
+ # torch.Size([2, 768, 64, 64]), torch.Size([2, 1536, 32, 32])]
+ return tuple(outs)
+
+
+ def forward(self, tensor_list: NestedTensor):
+ x = tensor_list.tensors
+
+ """Forward function."""
+ x = self.patch_embed(x)
+
+ Wh, Ww = x.size(2), x.size(3)
+ if self.ape:
+ # interpolate the position embedding to the corresponding size
+ absolute_pos_embed = F.interpolate(self.absolute_pos_embed, size=(Wh, Ww), mode='bicubic')
+ x = (x + absolute_pos_embed).flatten(2).transpose(1, 2) # B Wh*Ww C
+ else:
+ x = x.flatten(2).transpose(1, 2)
+ x = self.pos_drop(x)
+
+ outs = []
+ for i in range(self.num_layers):
+ layer = self.layers[i]
+ x_out, H, W, x, Wh, Ww = layer(x, Wh, Ww)
+
+ if i in self.out_indices:
+ norm_layer = getattr(self, f'norm{i}')
+ x_out = norm_layer(x_out)
+
+ out = x_out.view(-1, H, W, self.num_features[i]).permute(0, 3, 1, 2).contiguous()
+ outs.append(out)
+ # in:
+ # torch.Size([2, 3, 1024, 1024])
+ # out:
+ # [torch.Size([2, 192, 256, 256]), torch.Size([2, 384, 128, 128]), \
+ # torch.Size([2, 768, 64, 64]), torch.Size([2, 1536, 32, 32])]
+
+ # collect for nesttensors
+ outs_dict = {}
+ for idx, out_i in enumerate(outs):
+ m = tensor_list.mask
+ assert m is not None
+ mask = F.interpolate(m[None].float(), size=out_i.shape[-2:]).to(torch.bool)[0]
+ outs_dict[idx] = NestedTensor(out_i, mask)
+
+ return outs_dict
+
+
+ def train(self, mode=True):
+ """Convert the model into training mode while keep layers freezed."""
+ super(SwinTransformer, self).train(mode)
+ self._freeze_stages()
+
+
+
+def build_swin_transformer(modelname, pretrain_img_size, **kw):
+ assert modelname in ['swin_T_224_1k', 'swin_B_224_22k', 'swin_B_384_22k', 'swin_L_224_22k', 'swin_L_384_22k']
+
+ model_para_dict = {
+ 'swin_T_224_1k': dict(
+ embed_dim=96,
+ depths=[ 2, 2, 6, 2 ],
+ num_heads=[ 3, 6, 12, 24],
+ window_size=7
+ ),
+ 'swin_B_224_22k': dict(
+ embed_dim=128,
+ depths=[ 2, 2, 18, 2 ],
+ num_heads=[ 4, 8, 16, 32 ],
+ window_size=7
+ ),
+ 'swin_B_384_22k': dict(
+ embed_dim=128,
+ depths=[ 2, 2, 18, 2 ],
+ num_heads=[ 4, 8, 16, 32 ],
+ window_size=12
+ ),
+ 'swin_L_224_22k': dict(
+ embed_dim=192,
+ depths=[ 2, 2, 18, 2 ],
+ num_heads=[ 6, 12, 24, 48 ],
+ window_size=7
+ ),
+ 'swin_L_384_22k': dict(
+ embed_dim=192,
+ depths=[ 2, 2, 18, 2 ],
+ num_heads=[ 6, 12, 24, 48 ],
+ window_size=12
+ ),
+ }
+ kw_cgf = model_para_dict[modelname]
+ kw_cgf.update(kw)
+ model = SwinTransformer(pretrain_img_size=pretrain_img_size, **kw_cgf)
+ return model
+
+if __name__ == "__main__":
+ model = build_swin_transformer('swin_L_384_22k', 384, dilation=True)
+ x = torch.rand(2, 3, 1024, 1024)
+ y = model.forward_raw(x)
+ import ipdb; ipdb.set_trace()
+ x = torch.rand(2, 3, 384, 384)
+ y = model.forward_raw(x)
diff --git a/difpoint/src/models/XPose/models/UniPose/transformer_deformable.py b/difpoint/src/models/XPose/models/UniPose/transformer_deformable.py
new file mode 100644
index 0000000000000000000000000000000000000000..5f321660bb03547cf2a7c93fb59b98b145b10298
--- /dev/null
+++ b/difpoint/src/models/XPose/models/UniPose/transformer_deformable.py
@@ -0,0 +1,595 @@
+# ------------------------------------------------------------------------
+# ED-Pose
+# Copyright (c) 2023 IDEA. All Rights Reserved.
+# Licensed under the Apache License, Version 2.0 [see LICENSE for details]
+# ------------------------------------------------------------------------
+# Deformable DETR
+# Copyright (c) 2020 SenseTime. All Rights Reserved.
+# Licensed under the Apache License, Version 2.0 [see LICENSE for details]
+# ------------------------------------------------------------------------
+# Modified from DETR (https://github.com/facebookresearch/detr)
+# Copyright (c) Facebook, Inc. and its affiliates. All Rights Reserved
+# ------------------------------------------------------------------------
+
+import copy
+import math
+import torch
+from torch import nn, Tensor
+from torch.nn.init import xavier_uniform_, constant_, normal_
+from typing import Optional
+
+from ...util.misc import inverse_sigmoid
+from .ops.modules import MSDeformAttn
+from .utils import MLP, _get_activation_fn, gen_sineembed_for_position
+
+class DeformableTransformer(nn.Module):
+ def __init__(self, d_model=256, nhead=8,
+ num_encoder_layers=6, num_decoder_layers=6, dim_feedforward=1024, dropout=0.1,
+ activation="relu", return_intermediate_dec=False,
+ num_feature_levels=4, dec_n_points=4, enc_n_points=4,
+ two_stage=False, two_stage_num_proposals=300,
+ use_dab=False, high_dim_query_update=False, no_sine_embed=False):
+ super().__init__()
+
+ self.d_model = d_model
+ self.nhead = nhead
+ self.two_stage = two_stage
+ self.two_stage_num_proposals = two_stage_num_proposals
+ self.use_dab = use_dab
+
+ encoder_layer = DeformableTransformerEncoderLayer(d_model, dim_feedforward,
+ dropout, activation,
+ num_feature_levels, nhead, enc_n_points)
+ self.encoder = DeformableTransformerEncoder(encoder_layer, num_encoder_layers)
+
+ decoder_layer = DeformableTransformerDecoderLayer(d_model, dim_feedforward,
+ dropout, activation,
+ num_feature_levels, nhead, dec_n_points)
+ self.decoder = DeformableTransformerDecoder(decoder_layer, num_decoder_layers, return_intermediate_dec,
+ use_dab=use_dab, d_model=d_model, high_dim_query_update=high_dim_query_update, no_sine_embed=no_sine_embed)
+
+ self.level_embed = nn.Parameter(torch.Tensor(num_feature_levels, d_model))
+
+ if two_stage:
+ self.enc_output = nn.Linear(d_model, d_model)
+ self.enc_output_norm = nn.LayerNorm(d_model)
+ self.pos_trans = nn.Linear(d_model * 2, d_model * 2)
+ self.pos_trans_norm = nn.LayerNorm(d_model * 2)
+ else:
+ if not self.use_dab:
+ self.reference_points = nn.Linear(d_model, 2)
+
+ self.high_dim_query_update = high_dim_query_update
+ if high_dim_query_update:
+ assert not self.use_dab, "use_dab must be True"
+
+ self._reset_parameters()
+
+ def _reset_parameters(self):
+ for p in self.parameters():
+ if p.dim() > 1:
+ nn.init.xavier_uniform_(p)
+ for m in self.modules():
+ if isinstance(m, MSDeformAttn):
+ m._reset_parameters()
+ if not self.two_stage and not self.use_dab:
+ xavier_uniform_(self.reference_points.weight.data, gain=1.0)
+ constant_(self.reference_points.bias.data, 0.)
+ normal_(self.level_embed)
+
+ def get_proposal_pos_embed(self, proposals):
+ num_pos_feats = 128
+ temperature = 10000
+ scale = 2 * math.pi
+
+ dim_t = torch.arange(num_pos_feats, dtype=torch.float32, device=proposals.device)
+ dim_t = temperature ** (2 * (dim_t // 2) / num_pos_feats)
+ # N, L, 4
+ proposals = proposals.sigmoid() * scale
+ # N, L, 4, 128
+ pos = proposals[:, :, :, None] / dim_t
+ # N, L, 4, 64, 2
+ pos = torch.stack((pos[:, :, :, 0::2].sin(), pos[:, :, :, 1::2].cos()), dim=4).flatten(2)
+ return pos
+
+ def gen_encoder_output_proposals(self, memory, memory_padding_mask, spatial_shapes):
+ N_, S_, C_ = memory.shape
+ base_scale = 4.0
+ proposals = []
+ _cur = 0
+ for lvl, (H_, W_) in enumerate(spatial_shapes):
+ mask_flatten_ = memory_padding_mask[:, _cur:(_cur + H_ * W_)].view(N_, H_, W_, 1)
+ valid_H = torch.sum(~mask_flatten_[:, :, 0, 0], 1)
+ valid_W = torch.sum(~mask_flatten_[:, 0, :, 0], 1)
+
+ grid_y, grid_x = torch.meshgrid(torch.linspace(0, H_ - 1, H_, dtype=torch.float32, device=memory.device),
+ torch.linspace(0, W_ - 1, W_, dtype=torch.float32, device=memory.device))
+ grid = torch.cat([grid_x.unsqueeze(-1), grid_y.unsqueeze(-1)], -1)
+
+ scale = torch.cat([valid_W.unsqueeze(-1), valid_H.unsqueeze(-1)], 1).view(N_, 1, 1, 2)
+ grid = (grid.unsqueeze(0).expand(N_, -1, -1, -1) + 0.5) / scale
+ wh = torch.ones_like(grid) * 0.05 * (2.0 ** lvl)
+ proposal = torch.cat((grid, wh), -1).view(N_, -1, 4)
+ proposals.append(proposal)
+ _cur += (H_ * W_)
+ output_proposals = torch.cat(proposals, 1)
+ output_proposals_valid = ((output_proposals > 0.01) & (output_proposals < 0.99)).all(-1, keepdim=True)
+ output_proposals = torch.log(output_proposals / (1 - output_proposals))
+ output_proposals = output_proposals.masked_fill(memory_padding_mask.unsqueeze(-1), float('inf'))
+ output_proposals = output_proposals.masked_fill(~output_proposals_valid, float('inf'))
+
+ output_memory = memory
+ output_memory = output_memory.masked_fill(memory_padding_mask.unsqueeze(-1), float(0))
+ output_memory = output_memory.masked_fill(~output_proposals_valid, float(0))
+ output_memory = self.enc_output_norm(self.enc_output(output_memory))
+ return output_memory, output_proposals
+
+ def get_valid_ratio(self, mask):
+ _, H, W = mask.shape
+ valid_H = torch.sum(~mask[:, :, 0], 1)
+ valid_W = torch.sum(~mask[:, 0, :], 1)
+ valid_ratio_h = valid_H.float() / H
+ valid_ratio_w = valid_W.float() / W
+ valid_ratio = torch.stack([valid_ratio_w, valid_ratio_h], -1)
+ return valid_ratio
+
+ def forward(self, srcs, masks, pos_embeds, query_embed=None):
+ """
+ Input:
+ - srcs: List([bs, c, h, w])
+ - masks: List([bs, h, w])
+ """
+ assert self.two_stage or query_embed is not None
+
+ # prepare input for encoder
+ src_flatten = []
+ mask_flatten = []
+ lvl_pos_embed_flatten = []
+ spatial_shapes = []
+ for lvl, (src, mask, pos_embed) in enumerate(zip(srcs, masks, pos_embeds)):
+ bs, c, h, w = src.shape
+ spatial_shape = (h, w)
+ spatial_shapes.append(spatial_shape)
+
+ src = src.flatten(2).transpose(1, 2) # bs, hw, c
+ mask = mask.flatten(1) # bs, hw
+ pos_embed = pos_embed.flatten(2).transpose(1, 2) # bs, hw, c
+ lvl_pos_embed = pos_embed + self.level_embed[lvl].view(1, 1, -1)
+ lvl_pos_embed_flatten.append(lvl_pos_embed)
+ src_flatten.append(src)
+ mask_flatten.append(mask)
+ src_flatten = torch.cat(src_flatten, 1) # bs, \sum{hxw}, c
+ mask_flatten = torch.cat(mask_flatten, 1) # bs, \sum{hxw}
+ lvl_pos_embed_flatten = torch.cat(lvl_pos_embed_flatten, 1)
+ spatial_shapes = torch.as_tensor(spatial_shapes, dtype=torch.long, device=src_flatten.device)
+ level_start_index = torch.cat((spatial_shapes.new_zeros((1, )), spatial_shapes.prod(1).cumsum(0)[:-1]))
+ valid_ratios = torch.stack([self.get_valid_ratio(m) for m in masks], 1)
+
+ # encoder
+ memory = self.encoder(src_flatten, spatial_shapes, level_start_index, valid_ratios, lvl_pos_embed_flatten, mask_flatten)
+ # import ipdb; ipdb.set_trace()
+
+ # prepare input for decoder
+ bs, _, c = memory.shape
+ if self.two_stage:
+ output_memory, output_proposals = self.gen_encoder_output_proposals(memory, mask_flatten, spatial_shapes)
+
+ # hack implementation for two-stage Deformable DETR
+ enc_outputs_class = self.decoder.class_embed[self.decoder.num_layers](output_memory)
+ enc_outputs_coord_unact = self.decoder.bbox_embed[self.decoder.num_layers](output_memory) + output_proposals
+
+ topk = self.two_stage_num_proposals
+ topk_proposals = torch.topk(enc_outputs_class[..., 0], topk, dim=1)[1]
+ topk_coords_unact = torch.gather(enc_outputs_coord_unact, 1, topk_proposals.unsqueeze(-1).repeat(1, 1, 4))
+ topk_coords_unact = topk_coords_unact.detach()
+ reference_points = topk_coords_unact.sigmoid()
+ init_reference_out = reference_points
+ pos_trans_out = self.pos_trans_norm(self.pos_trans(self.get_proposal_pos_embed(topk_coords_unact)))
+ query_embed, tgt = torch.split(pos_trans_out, c, dim=2)
+ elif self.use_dab:
+ reference_points = query_embed[..., self.d_model:].sigmoid()
+ tgt = query_embed[..., :self.d_model]
+ tgt = tgt.unsqueeze(0).expand(bs, -1, -1)
+ init_reference_out = reference_points
+ else:
+ query_embed, tgt = torch.split(query_embed, c, dim=1)
+ query_embed = query_embed.unsqueeze(0).expand(bs, -1, -1)
+ tgt = tgt.unsqueeze(0).expand(bs, -1, -1)
+ reference_points = self.reference_points(query_embed).sigmoid()
+ # bs, num_quires, 2
+ init_reference_out = reference_points
+
+ # decoder
+ # import ipdb; ipdb.set_trace()
+ hs, inter_references = self.decoder(tgt, reference_points, memory,
+ spatial_shapes, level_start_index, valid_ratios,
+ query_pos=query_embed if not self.use_dab else None,
+ src_padding_mask=mask_flatten)
+
+ inter_references_out = inter_references
+ if self.two_stage:
+ return hs, init_reference_out, inter_references_out, enc_outputs_class, enc_outputs_coord_unact
+ return hs, init_reference_out, inter_references_out, None, None
+
+
+class DeformableTransformerEncoderLayer(nn.Module):
+ def __init__(self,
+ d_model=256, d_ffn=1024,
+ dropout=0.1, activation="relu",
+ n_levels=4, n_heads=8, n_points=4,
+ add_channel_attention=False,
+ use_deformable_box_attn=False,
+ box_attn_type='roi_align',
+ ):
+ super().__init__()
+
+ # self attention
+ if use_deformable_box_attn:
+ self.self_attn = MSDeformableBoxAttention(d_model, n_levels, n_heads, n_boxes=n_points, used_func=box_attn_type)
+ else:
+ self.self_attn = MSDeformAttn(d_model, n_levels, n_heads, n_points)
+ self.dropout1 = nn.Dropout(dropout)
+ self.norm1 = nn.LayerNorm(d_model)
+
+ # ffn
+ self.linear1 = nn.Linear(d_model, d_ffn)
+ self.activation = _get_activation_fn(activation, d_model=d_ffn)
+ self.dropout2 = nn.Dropout(dropout)
+ self.linear2 = nn.Linear(d_ffn, d_model)
+ self.dropout3 = nn.Dropout(dropout)
+ self.norm2 = nn.LayerNorm(d_model)
+
+ # channel attention
+ self.add_channel_attention = add_channel_attention
+ if add_channel_attention:
+ self.activ_channel = _get_activation_fn('dyrelu', d_model=d_model)
+ self.norm_channel = nn.LayerNorm(d_model)
+
+ @staticmethod
+ def with_pos_embed(tensor, pos):
+ return tensor if pos is None else tensor + pos
+
+ def forward_ffn(self, src):
+ src2 = self.linear2(self.dropout2(self.activation(self.linear1(src))))
+ src = src + self.dropout3(src2)
+ src = self.norm2(src)
+ return src
+
+ def forward(self, src, pos, reference_points, spatial_shapes, level_start_index, key_padding_mask=None):
+ # self attention
+ # import ipdb; ipdb.set_trace()
+ src2 = self.self_attn(self.with_pos_embed(src, pos), reference_points, src, spatial_shapes, level_start_index, key_padding_mask)
+ src = src + self.dropout1(src2)
+ src = self.norm1(src)
+
+ # ffn
+ src = self.forward_ffn(src)
+
+ # channel attn
+ if self.add_channel_attention:
+ src = self.norm_channel(src + self.activ_channel(src))
+
+ return src
+
+
+class DeformableTransformerEncoder(nn.Module):
+ def __init__(self, encoder_layer, num_layers, norm=None):
+ super().__init__()
+ if num_layers > 0:
+ self.layers = _get_clones(encoder_layer, num_layers)
+ else:
+ self.layers = []
+ del encoder_layer
+ self.num_layers = num_layers
+ self.norm = norm
+
+ @staticmethod
+ def get_reference_points(spatial_shapes, valid_ratios, device):
+ reference_points_list = []
+ for lvl, (H_, W_) in enumerate(spatial_shapes):
+
+ ref_y, ref_x = torch.meshgrid(torch.linspace(0.5, H_ - 0.5, H_, dtype=torch.float32, device=device),
+ torch.linspace(0.5, W_ - 0.5, W_, dtype=torch.float32, device=device))
+ ref_y = ref_y.reshape(-1)[None] / (valid_ratios[:, None, lvl, 1] * H_)
+ ref_x = ref_x.reshape(-1)[None] / (valid_ratios[:, None, lvl, 0] * W_)
+ ref = torch.stack((ref_x, ref_y), -1)
+ reference_points_list.append(ref)
+ reference_points = torch.cat(reference_points_list, 1)
+ reference_points = reference_points[:, :, None] * valid_ratios[:, None]
+ return reference_points
+
+ def forward(self, src, spatial_shapes, level_start_index, valid_ratios, pos=None, padding_mask=None):
+ """
+ Input:
+ - src: [bs, sum(hi*wi), 256]
+ - spatial_shapes: h,w of each level [num_level, 2]
+ - level_start_index: [num_level] start point of level in sum(hi*wi).
+ - valid_ratios: [bs, num_level, 2]
+ - pos: pos embed for src. [bs, sum(hi*wi), 256]
+ - padding_mask: [bs, sum(hi*wi)]
+ Intermedia:
+ - reference_points: [bs, sum(hi*wi), num_lebel, 2]
+ """
+ output = src
+ # bs, sum(hi*wi), 256
+ # import ipdb; ipdb.set_trace()
+ if self.num_layers > 0:
+ reference_points = self.get_reference_points(spatial_shapes, valid_ratios, device=src.device)
+ for _, layer in enumerate(self.layers):
+ output = layer(output, pos, reference_points, spatial_shapes, level_start_index, padding_mask)
+
+ if self.norm is not None:
+ output = self.norm(output)
+
+ return output
+
+
+class DeformableTransformerDecoderLayer(nn.Module):
+ def __init__(self, d_model=256, d_ffn=1024,
+ dropout=0.1, activation="relu",
+ n_levels=4, n_heads=8, n_points=4,
+ use_deformable_box_attn=False,
+ box_attn_type='roi_align',
+ key_aware_type=None,
+ decoder_sa_type='ca',
+ module_seq=['sa', 'ca', 'ffn'],
+ ):
+ super().__init__()
+ self.module_seq = module_seq
+ assert sorted(module_seq) == ['ca', 'ffn', 'sa']
+
+ # cross attention
+ # self.cross_attn = MSDeformAttn(d_model, n_levels, n_heads, n_points)
+ if use_deformable_box_attn:
+ self.cross_attn = MSDeformableBoxAttention(d_model, n_levels, n_heads, n_boxes=n_points, used_func=box_attn_type)
+ else:
+ self.cross_attn = MSDeformAttn(d_model, n_levels, n_heads, n_points)
+ self.dropout1 = nn.Dropout(dropout)
+ self.norm1 = nn.LayerNorm(d_model)
+
+ # self attention
+ self.self_attn = nn.MultiheadAttention(d_model, n_heads, dropout=dropout)
+ self.dropout2 = nn.Dropout(dropout)
+ self.norm2 = nn.LayerNorm(d_model)
+
+ # ffn
+ self.linear1 = nn.Linear(d_model, d_ffn)
+ self.activation = _get_activation_fn(activation, d_model=d_ffn, batch_dim=1)
+ self.dropout3 = nn.Dropout(dropout)
+ self.linear2 = nn.Linear(d_ffn, d_model)
+ self.dropout4 = nn.Dropout(dropout)
+ self.norm3 = nn.LayerNorm(d_model)
+
+ self.key_aware_type = key_aware_type
+ self.key_aware_proj = None
+ self.decoder_sa_type = decoder_sa_type
+ assert decoder_sa_type in ['sa', 'ca_label', 'ca_content']
+
+ if decoder_sa_type == 'ca_content':
+ self.self_attn = MSDeformAttn(d_model, n_levels, n_heads, n_points)
+
+
+
+
+ def rm_self_attn_modules(self):
+ self.self_attn = None
+ self.dropout2 = None
+ self.norm2 = None
+
+
+ @staticmethod
+ def with_pos_embed(tensor, pos):
+ return tensor if pos is None else tensor + pos
+
+ def forward_ffn(self, tgt):
+ tgt2 = self.linear2(self.dropout3(self.activation(self.linear1(tgt))))
+ tgt = tgt + self.dropout4(tgt2)
+ tgt = self.norm3(tgt)
+ return tgt
+
+ def forward_sa(self,
+ # for tgt
+ tgt: Optional[Tensor], # nq, bs, d_model
+ tgt_query_pos: Optional[Tensor] = None, # pos for query. MLP(Sine(pos))
+ tgt_query_sine_embed: Optional[Tensor] = None, # pos for query. Sine(pos)
+ tgt_key_padding_mask: Optional[Tensor] = None,
+ tgt_reference_points: Optional[Tensor] = None, # nq, bs, 4
+
+ # for memory
+ memory: Optional[Tensor] = None, # hw, bs, d_model
+ memory_key_padding_mask: Optional[Tensor] = None,
+ memory_level_start_index: Optional[Tensor] = None, # num_levels
+ memory_spatial_shapes: Optional[Tensor] = None, # bs, num_levels, 2
+ memory_pos: Optional[Tensor] = None, # pos for memory
+
+ # sa
+ self_attn_mask: Optional[Tensor] = None, # mask used for self-attention
+ cross_attn_mask: Optional[Tensor] = None, # mask used for cross-attention
+ ):
+ # self attention
+ if self.self_attn is not None:
+ # import ipdb; ipdb.set_trace()
+ if self.decoder_sa_type == 'sa':
+ q = k = self.with_pos_embed(tgt, tgt_query_pos)
+ tgt2 = self.self_attn(q, k, tgt, attn_mask=self_attn_mask)[0]
+ tgt = tgt + self.dropout2(tgt2)
+ tgt = self.norm2(tgt)
+ elif self.decoder_sa_type == 'ca_label':
+ # import ipdb; ipdb.set_trace()
+ # q = self.with_pos_embed(tgt, tgt_query_pos)
+ bs = tgt.shape[1]
+ k = v = self.label_embedding.weight[:, None, :].repeat(1, bs, 1)
+ tgt2 = self.self_attn(tgt, k, v, attn_mask=self_attn_mask)[0]
+ tgt = tgt + self.dropout2(tgt2)
+ tgt = self.norm2(tgt)
+ elif self.decoder_sa_type == 'ca_content':
+ tgt2 = self.self_attn(self.with_pos_embed(tgt, tgt_query_pos).transpose(0, 1),
+ tgt_reference_points.transpose(0, 1).contiguous(),
+ memory.transpose(0, 1), memory_spatial_shapes, memory_level_start_index, memory_key_padding_mask).transpose(0, 1)
+ tgt = tgt + self.dropout2(tgt2)
+ tgt = self.norm2(tgt)
+ else:
+ raise NotImplementedError("Unknown decoder_sa_type {}".format(self.decoder_sa_type))
+
+ return tgt
+
+ def forward_ca(self,
+ # for tgt
+ tgt: Optional[Tensor], # nq, bs, d_model
+ tgt_query_pos: Optional[Tensor] = None, # pos for query. MLP(Sine(pos))
+ tgt_query_sine_embed: Optional[Tensor] = None, # pos for query. Sine(pos)
+ tgt_key_padding_mask: Optional[Tensor] = None,
+ tgt_reference_points: Optional[Tensor] = None, # nq, bs, 4
+
+ # for memory
+ memory: Optional[Tensor] = None, # hw, bs, d_model
+ memory_key_padding_mask: Optional[Tensor] = None,
+ memory_level_start_index: Optional[Tensor] = None, # num_levels
+ memory_spatial_shapes: Optional[Tensor] = None, # bs, num_levels, 2
+ memory_pos: Optional[Tensor] = None, # pos for memory
+
+ # sa
+ self_attn_mask: Optional[Tensor] = None, # mask used for self-attention
+ cross_attn_mask: Optional[Tensor] = None, # mask used for cross-attention
+ ):
+ # cross attention
+ # import ipdb; ipdb.set_trace()
+ if self.key_aware_type is not None:
+
+ if self.key_aware_type == 'mean':
+ tgt = tgt + memory.mean(0, keepdim=True)
+ elif self.key_aware_type == 'proj_mean':
+ tgt = tgt + self.key_aware_proj(memory).mean(0, keepdim=True)
+ else:
+ raise NotImplementedError("Unknown key_aware_type: {}".format(self.key_aware_type))
+ tgt2 = self.cross_attn(self.with_pos_embed(tgt, tgt_query_pos).transpose(0, 1),
+ tgt_reference_points.transpose(0, 1).contiguous(),
+ memory.transpose(0, 1), memory_spatial_shapes, memory_level_start_index, memory_key_padding_mask).transpose(0, 1)
+ tgt = tgt + self.dropout1(tgt2)
+ tgt = self.norm1(tgt)
+
+ return tgt
+
+ def forward(self,
+ # for tgt
+ tgt: Optional[Tensor], # nq, bs, d_model
+ tgt_query_pos: Optional[Tensor] = None, # pos for query. MLP(Sine(pos))
+ tgt_query_sine_embed: Optional[Tensor] = None, # pos for query. Sine(pos)
+ tgt_key_padding_mask: Optional[Tensor] = None,
+ tgt_reference_points: Optional[Tensor] = None, # nq, bs, 4
+
+ # for memory
+ memory: Optional[Tensor] = None, # hw, bs, d_model
+ memory_key_padding_mask: Optional[Tensor] = None,
+ memory_level_start_index: Optional[Tensor] = None, # num_levels
+ memory_spatial_shapes: Optional[Tensor] = None, # bs, num_levels, 2
+ memory_pos: Optional[Tensor] = None, # pos for memory
+
+ # sa
+ self_attn_mask: Optional[Tensor] = None, # mask used for self-attention
+ cross_attn_mask: Optional[Tensor] = None, # mask used for cross-attention
+ ):
+
+ for funcname in self.module_seq:
+ # if os.environ.get('IPDB_DEBUG_SHILONG') == 'INFO':
+ # import ipdb; ipdb.set_trace()
+ if funcname == 'ffn':
+ tgt = self.forward_ffn(tgt)
+ elif funcname == 'ca':
+ tgt = self.forward_ca(tgt, tgt_query_pos, tgt_query_sine_embed, \
+ tgt_key_padding_mask, tgt_reference_points, \
+ memory, memory_key_padding_mask, memory_level_start_index, \
+ memory_spatial_shapes, memory_pos, self_attn_mask, cross_attn_mask)
+ elif funcname == 'sa':
+ tgt = self.forward_sa(tgt, tgt_query_pos, tgt_query_sine_embed, \
+ tgt_key_padding_mask, tgt_reference_points, \
+ memory, memory_key_padding_mask, memory_level_start_index, \
+ memory_spatial_shapes, memory_pos, self_attn_mask, cross_attn_mask)
+ else:
+ raise ValueError('unknown funcname {}'.format(funcname))
+
+ return tgt
+
+
+
+class DeformableTransformerDecoder(nn.Module):
+ def __init__(self, decoder_layer, num_layers, return_intermediate=False, use_dab=False, d_model=256, query_dim=4):
+ super().__init__()
+ self.layers = _get_clones(decoder_layer, num_layers)
+ self.num_layers = num_layers
+ self.return_intermediate = return_intermediate
+ assert return_intermediate
+ # hack implementation for iterative bounding box refinement and two-stage Deformable DETR
+ self.bbox_embed = None
+ self.class_embed = None
+ self.use_dab = use_dab
+ self.d_model = d_model
+ self.query_dim = query_dim
+ if use_dab:
+ self.query_scale = MLP(d_model, d_model, d_model, 2)
+ self.ref_point_head = MLP(2 * d_model, d_model, d_model, 2)
+
+
+ def forward(self, tgt, reference_points, src, src_spatial_shapes,
+ src_level_start_index, src_valid_ratios,
+ query_pos=None, src_padding_mask=None):
+ output = tgt
+ if self.use_dab:
+ assert query_pos is None
+
+ intermediate = []
+ intermediate_reference_points = [reference_points]
+ for layer_id, layer in enumerate(self.layers):
+ # import ipdb; ipdb.set_trace()
+ if reference_points.shape[-1] == 4:
+ reference_points_input = reference_points[:, :, None] \
+ * torch.cat([src_valid_ratios, src_valid_ratios], -1)[:, None] # bs, nq, 4, 4
+ else:
+ assert reference_points.shape[-1] == 2
+ reference_points_input = reference_points[:, :, None] * src_valid_ratios[:, None]
+
+ if self.use_dab:
+ # import ipdb; ipdb.set_trace()
+ query_sine_embed = gen_sineembed_for_position(reference_points_input[:, :, 0, :]) # bs, nq, 256*2
+ raw_query_pos = self.ref_point_head(query_sine_embed) # bs, nq, 256
+ pos_scale = self.query_scale(output) if layer_id != 0 else 1
+ query_pos = pos_scale * raw_query_pos
+
+ output = layer(output, query_pos, reference_points_input, src, src_spatial_shapes, src_level_start_index, src_padding_mask)
+
+ # hack implementation for iterative bounding box refinement
+ if self.bbox_embed is not None:
+ box_holder = self.bbox_embed(output)
+ box_holder[..., :self.query_dim] += inverse_sigmoid(reference_points)
+ new_reference_points = box_holder[..., :self.query_dim].sigmoid()
+ reference_points = new_reference_points.detach()
+ if layer_id != self.num_layers - 1:
+ intermediate_reference_points.append(new_reference_points)
+
+ intermediate.append(output)
+
+ return torch.stack(intermediate), torch.stack(intermediate_reference_points)
+
+
+def _get_clones(module, N):
+ return nn.ModuleList([copy.deepcopy(module) for i in range(N)])
+
+
+def build_deforamble_transformer(args):
+ return DeformableTransformer(
+ d_model=args.hidden_dim,
+ nhead=args.nheads,
+ num_encoder_layers=args.enc_layers,
+ num_decoder_layers=args.dec_layers,
+ dim_feedforward=args.dim_feedforward,
+ dropout=args.dropout,
+ activation="relu",
+ return_intermediate_dec=True,
+ num_feature_levels=args.ddetr_num_feature_levels,
+ dec_n_points=args.ddetr_dec_n_points,
+ enc_n_points=args.ddetr_enc_n_points,
+ two_stage=args.ddetr_two_stage,
+ two_stage_num_proposals=args.num_queries,
+ use_dab=args.ddetr_use_dab,
+ high_dim_query_update=args.ddetr_high_dim_query_update,
+ no_sine_embed=args.ddetr_no_sine_embed)
diff --git a/difpoint/src/models/XPose/models/UniPose/transformer_vanilla.py b/difpoint/src/models/XPose/models/UniPose/transformer_vanilla.py
new file mode 100644
index 0000000000000000000000000000000000000000..57ad686213a4bd0b2da6ef32142967e8e2bc4cd1
--- /dev/null
+++ b/difpoint/src/models/XPose/models/UniPose/transformer_vanilla.py
@@ -0,0 +1,102 @@
+# Copyright (c) Aishwarya Kamath & Nicolas Carion. Licensed under the Apache License 2.0. All Rights Reserved
+# Copyright (c) Facebook, Inc. and its affiliates. All Rights Reserved
+"""
+DETR Transformer class.
+
+Copy-paste from torch.nn.Transformer with modifications:
+ * positional encodings are passed in MHattention
+ * extra LN at the end of encoder is removed
+ * decoder returns a stack of activations from all decoding layers
+"""
+import torch
+from torch import Tensor, nn
+from typing import List, Optional
+
+from .utils import _get_activation_fn, _get_clones
+
+
+class TextTransformer(nn.Module):
+ def __init__(self, num_layers, d_model=256, nheads=8, dim_feedforward=2048, dropout=0.1):
+ super().__init__()
+ self.num_layers = num_layers
+ self.d_model = d_model
+ self.nheads = nheads
+ self.dim_feedforward = dim_feedforward
+ self.norm = None
+
+ single_encoder_layer = TransformerEncoderLayer(d_model=d_model, nhead=nheads, dim_feedforward=dim_feedforward, dropout=dropout)
+ self.layers = _get_clones(single_encoder_layer, num_layers)
+
+
+ def forward(self, memory_text:torch.Tensor, text_attention_mask:torch.Tensor):
+ """
+
+ Args:
+ text_attention_mask: bs, num_token
+ memory_text: bs, num_token, d_model
+
+ Raises:
+ RuntimeError: _description_
+
+ Returns:
+ output: bs, num_token, d_model
+ """
+
+ output = memory_text.transpose(0, 1)
+
+ for layer in self.layers:
+ output = layer(output, src_key_padding_mask=text_attention_mask)
+
+ if self.norm is not None:
+ output = self.norm(output)
+
+ return output.transpose(0, 1)
+
+
+
+
+class TransformerEncoderLayer(nn.Module):
+ def __init__(self, d_model, nhead, dim_feedforward=2048, dropout=0.1, activation="relu", normalize_before=False):
+ super().__init__()
+ self.self_attn = nn.MultiheadAttention(d_model, nhead, dropout=dropout)
+ # Implementation of Feedforward model
+ self.linear1 = nn.Linear(d_model, dim_feedforward)
+ self.dropout = nn.Dropout(dropout)
+ self.linear2 = nn.Linear(dim_feedforward, d_model)
+
+ self.norm1 = nn.LayerNorm(d_model)
+ self.norm2 = nn.LayerNorm(d_model)
+ self.dropout1 = nn.Dropout(dropout)
+ self.dropout2 = nn.Dropout(dropout)
+
+ self.activation = _get_activation_fn(activation)
+ self.normalize_before = normalize_before
+ self.nhead = nhead
+
+ def with_pos_embed(self, tensor, pos: Optional[Tensor]):
+ return tensor if pos is None else tensor + pos
+
+ def forward(
+ self,
+ src,
+ src_mask: Optional[Tensor] = None,
+ src_key_padding_mask: Optional[Tensor] = None,
+ pos: Optional[Tensor] = None,
+ ):
+ # repeat attn mask
+ if src_mask.dim() == 3 and src_mask.shape[0] == src.shape[1]:
+ # bs, num_q, num_k
+ src_mask = src_mask.repeat(self.nhead, 1, 1)
+
+ q = k = self.with_pos_embed(src, pos)
+
+ src2 = self.self_attn(q, k, value=src, attn_mask=src_mask)[0]
+
+ # src2 = self.self_attn(q, k, value=src, attn_mask=src_mask, key_padding_mask=src_key_padding_mask)[0]
+ src = src + self.dropout1(src2)
+ src = self.norm1(src)
+ src2 = self.linear2(self.dropout(self.activation(self.linear1(src))))
+ src = src + self.dropout2(src2)
+ src = self.norm2(src)
+ return src
+
diff --git a/difpoint/src/models/XPose/models/UniPose/unipose.py b/difpoint/src/models/XPose/models/UniPose/unipose.py
new file mode 100644
index 0000000000000000000000000000000000000000..ca1f1ea7429de6a8bcfb3e9dacef467ecd9353e4
--- /dev/null
+++ b/difpoint/src/models/XPose/models/UniPose/unipose.py
@@ -0,0 +1,621 @@
+# ------------------------------------------------------------------------
+# ED-Pose
+# Copyright (c) 2023 IDEA. All Rights Reserved.
+# Licensed under the Apache License, Version 2.0 [see LICENSE for details]
+# ------------------------------------------------------------------------
+# Modified from Deformable DETR (https://github.com/fundamentalvision/Deformable-DETR)
+# Copyright (c) 2020 SenseTime. All Rights Reserved.
+# ------------------------------------------------------------------------
+import os
+import copy
+import torch
+import torch.nn.functional as F
+from torch import nn
+from typing import List
+
+from ...util.keypoint_ops import keypoint_xyzxyz_to_xyxyzz
+from ...util.misc import NestedTensor, nested_tensor_from_tensor_list,inverse_sigmoid
+
+from .utils import MLP
+from .backbone import build_backbone
+from ..registry import MODULE_BUILD_FUNCS
+from .mask_generate import prepare_for_mask, post_process
+from .deformable_transformer import build_deformable_transformer
+
+
+class UniPose(nn.Module):
+ """ This is the Cross-Attention Detector module that performs object detection """
+
+ def __init__(self, backbone, transformer, num_classes, num_queries,
+ aux_loss=False, iter_update=False,
+ query_dim=2,
+ random_refpoints_xy=False,
+ fix_refpoints_hw=-1,
+ num_feature_levels=1,
+ nheads=8,
+ # two stage
+ two_stage_type='no', # ['no', 'standard']
+ two_stage_add_query_num=0,
+ dec_pred_class_embed_share=True,
+ dec_pred_bbox_embed_share=True,
+ two_stage_class_embed_share=True,
+ two_stage_bbox_embed_share=True,
+ decoder_sa_type='sa',
+ num_patterns=0,
+ dn_number=100,
+ dn_box_noise_scale=0.4,
+ dn_label_noise_ratio=0.5,
+ dn_labelbook_size=100,
+ use_label_enc=True,
+
+ text_encoder_type='bert-base-uncased',
+
+ binary_query_selection=False,
+ use_cdn=True,
+ sub_sentence_present=True,
+ num_body_points=68,
+ num_box_decoder_layers=2,
+ ):
+ """ Initializes the model.
+ Parameters:
+ backbone: torch module of the backbone to be used. See backbone.py
+ transformer: torch module of the transformer architecture. See transformer.py
+ num_classes: number of object classes
+ num_queries: number of object queries, ie detection slot. This is the maximal number of objects
+ Conditional DETR can detect in a single image. For COCO, we recommend 100 queries.
+ aux_loss: True if auxiliary decoding losses (loss at each decoder layer) are to be used.
+
+ fix_refpoints_hw: -1(default): learn w and h for each box seperately
+ >0 : given fixed number
+ -2 : learn a shared w and h
+ """
+ super().__init__()
+ self.num_queries = num_queries
+ self.transformer = transformer
+ self.num_classes = num_classes
+ self.hidden_dim = hidden_dim = transformer.d_model
+ self.num_feature_levels = num_feature_levels
+ self.nheads = nheads
+ self.use_label_enc = use_label_enc
+ if use_label_enc:
+ self.label_enc = nn.Embedding(dn_labelbook_size + 1, hidden_dim)
+ else:
+ raise NotImplementedError
+ self.label_enc = None
+ self.max_text_len = 256
+ self.binary_query_selection = binary_query_selection
+ self.sub_sentence_present = sub_sentence_present
+
+ # setting query dim
+ self.query_dim = query_dim
+ assert query_dim == 4
+ self.random_refpoints_xy = random_refpoints_xy
+ self.fix_refpoints_hw = fix_refpoints_hw
+
+ # for dn training
+ self.num_patterns = num_patterns
+ self.dn_number = dn_number
+ self.dn_box_noise_scale = dn_box_noise_scale
+ self.dn_label_noise_ratio = dn_label_noise_ratio
+ self.dn_labelbook_size = dn_labelbook_size
+ self.use_cdn = use_cdn
+
+
+ self.projection = MLP(512, hidden_dim, hidden_dim, 3)
+
+ self.projection_kpt = MLP(512, hidden_dim, hidden_dim, 3)
+
+
+ device = "cuda" if torch.cuda.is_available() else "cpu"
+ # model, _ = clip.load("ViT-B/32", device=device)
+ # self.clip_model = model
+ # visual_parameters = list(self.clip_model.visual.parameters())
+ # #
+ # for param in visual_parameters:
+ # param.requires_grad = False
+
+ self.pos_proj = nn.Linear(hidden_dim, 768)
+ self.padding = nn.Embedding(1, 768)
+
+ # prepare input projection layers
+ if num_feature_levels > 1:
+ num_backbone_outs = len(backbone.num_channels)
+ input_proj_list = []
+ for _ in range(num_backbone_outs):
+ in_channels = backbone.num_channels[_]
+ input_proj_list.append(nn.Sequential(
+ nn.Conv2d(in_channels, hidden_dim, kernel_size=1),
+ nn.GroupNorm(32, hidden_dim),
+ ))
+ for _ in range(num_feature_levels - num_backbone_outs):
+ input_proj_list.append(nn.Sequential(
+ nn.Conv2d(in_channels, hidden_dim, kernel_size=3, stride=2, padding=1),
+ nn.GroupNorm(32, hidden_dim),
+ ))
+ in_channels = hidden_dim
+ self.input_proj = nn.ModuleList(input_proj_list)
+ else:
+ assert two_stage_type == 'no', "two_stage_type should be no if num_feature_levels=1 !!!"
+ self.input_proj = nn.ModuleList([
+ nn.Sequential(
+ nn.Conv2d(backbone.num_channels[-1], hidden_dim, kernel_size=1),
+ nn.GroupNorm(32, hidden_dim),
+ )])
+
+ self.backbone = backbone
+ self.aux_loss = aux_loss
+ self.box_pred_damping = box_pred_damping = None
+
+ self.iter_update = iter_update
+ assert iter_update, "Why not iter_update?"
+
+ # prepare pred layers
+ self.dec_pred_class_embed_share = dec_pred_class_embed_share
+ self.dec_pred_bbox_embed_share = dec_pred_bbox_embed_share
+ # prepare class & box embed
+ _class_embed = ContrastiveAssign()
+
+
+
+ _bbox_embed = MLP(hidden_dim, hidden_dim, 4, 3)
+ nn.init.constant_(_bbox_embed.layers[-1].weight.data, 0)
+ nn.init.constant_(_bbox_embed.layers[-1].bias.data, 0)
+
+ _pose_embed = MLP(hidden_dim, hidden_dim, 2, 3)
+ _pose_hw_embed = MLP(hidden_dim, hidden_dim, 2, 3)
+ nn.init.constant_(_pose_embed.layers[-1].weight.data, 0)
+ nn.init.constant_(_pose_embed.layers[-1].bias.data, 0)
+
+ if dec_pred_bbox_embed_share:
+ box_embed_layerlist = [_bbox_embed for i in range(transformer.num_decoder_layers)]
+ else:
+ box_embed_layerlist = [copy.deepcopy(_bbox_embed) for i in range(transformer.num_decoder_layers)]
+ if dec_pred_class_embed_share:
+ class_embed_layerlist = [_class_embed for i in range(transformer.num_decoder_layers)]
+ else:
+ class_embed_layerlist = [copy.deepcopy(_class_embed) for i in range(transformer.num_decoder_layers)]
+
+
+ if dec_pred_bbox_embed_share:
+
+ pose_embed_layerlist = [_pose_embed for i in
+ range(transformer.num_decoder_layers - num_box_decoder_layers + 1)]
+ else:
+ pose_embed_layerlist = [copy.deepcopy(_pose_embed) for i in
+ range(transformer.num_decoder_layers - num_box_decoder_layers + 1)]
+
+ pose_hw_embed_layerlist = [_pose_hw_embed for i in
+ range(transformer.num_decoder_layers - num_box_decoder_layers)]
+
+
+ self.num_box_decoder_layers = num_box_decoder_layers
+ self.bbox_embed = nn.ModuleList(box_embed_layerlist)
+ self.class_embed = nn.ModuleList(class_embed_layerlist)
+ self.num_body_points = num_body_points
+ self.pose_embed = nn.ModuleList(pose_embed_layerlist)
+ self.pose_hw_embed = nn.ModuleList(pose_hw_embed_layerlist)
+
+ self.transformer.decoder.bbox_embed = self.bbox_embed
+ self.transformer.decoder.class_embed = self.class_embed
+
+ self.transformer.decoder.pose_embed = self.pose_embed
+ self.transformer.decoder.pose_hw_embed = self.pose_hw_embed
+
+ self.transformer.decoder.num_body_points = num_body_points
+
+
+ # two stage
+ self.two_stage_type = two_stage_type
+ self.two_stage_add_query_num = two_stage_add_query_num
+ assert two_stage_type in ['no', 'standard'], "unknown param {} of two_stage_type".format(two_stage_type)
+ if two_stage_type != 'no':
+ if two_stage_bbox_embed_share:
+ assert dec_pred_class_embed_share and dec_pred_bbox_embed_share
+ self.transformer.enc_out_bbox_embed = _bbox_embed
+ else:
+ self.transformer.enc_out_bbox_embed = copy.deepcopy(_bbox_embed)
+
+ if two_stage_class_embed_share:
+ assert dec_pred_class_embed_share and dec_pred_bbox_embed_share
+ self.transformer.enc_out_class_embed = _class_embed
+ else:
+ self.transformer.enc_out_class_embed = copy.deepcopy(_class_embed)
+
+ self.refpoint_embed = None
+ if self.two_stage_add_query_num > 0:
+ self.init_ref_points(two_stage_add_query_num)
+
+ self.decoder_sa_type = decoder_sa_type
+ assert decoder_sa_type in ['sa', 'ca_label', 'ca_content']
+ # self.replace_sa_with_double_ca = replace_sa_with_double_ca
+ if decoder_sa_type == 'ca_label':
+ self.label_embedding = nn.Embedding(num_classes, hidden_dim)
+ for layer in self.transformer.decoder.layers:
+ layer.label_embedding = self.label_embedding
+ else:
+ for layer in self.transformer.decoder.layers:
+ layer.label_embedding = None
+ self.label_embedding = None
+
+ self._reset_parameters()
+
+ def open_set_transfer_init(self):
+ for name, param in self.named_parameters():
+ if 'fusion_layers' in name:
+ continue
+ if 'ca_text' in name:
+ continue
+ if 'catext_norm' in name:
+ continue
+ if 'catext_dropout' in name:
+ continue
+ if "text_layers" in name:
+ continue
+ if 'bert' in name:
+ continue
+ if 'bbox_embed' in name:
+ continue
+ if 'label_enc.weight' in name:
+ continue
+ if 'feat_map' in name:
+ continue
+ if 'enc_output' in name:
+ continue
+
+ param.requires_grad_(False)
+
+ # import ipdb; ipdb.set_trace()
+
+ def _reset_parameters(self):
+ # init input_proj
+ for proj in self.input_proj:
+ nn.init.xavier_uniform_(proj[0].weight, gain=1)
+ nn.init.constant_(proj[0].bias, 0)
+
+ def init_ref_points(self, use_num_queries):
+ self.refpoint_embed = nn.Embedding(use_num_queries, self.query_dim)
+
+ if self.random_refpoints_xy:
+ # import ipdb; ipdb.set_trace()
+ self.refpoint_embed.weight.data[:, :2].uniform_(0, 1)
+ self.refpoint_embed.weight.data[:, :2] = inverse_sigmoid(self.refpoint_embed.weight.data[:, :2])
+ self.refpoint_embed.weight.data[:, :2].requires_grad = False
+
+ if self.fix_refpoints_hw > 0:
+ print("fix_refpoints_hw: {}".format(self.fix_refpoints_hw))
+ assert self.random_refpoints_xy
+ self.refpoint_embed.weight.data[:, 2:] = self.fix_refpoints_hw
+ self.refpoint_embed.weight.data[:, 2:] = inverse_sigmoid(self.refpoint_embed.weight.data[:, 2:])
+ self.refpoint_embed.weight.data[:, 2:].requires_grad = False
+ elif int(self.fix_refpoints_hw) == -1:
+ pass
+ elif int(self.fix_refpoints_hw) == -2:
+ print('learn a shared h and w')
+ assert self.random_refpoints_xy
+ self.refpoint_embed = nn.Embedding(use_num_queries, 2)
+ self.refpoint_embed.weight.data[:, :2].uniform_(0, 1)
+ self.refpoint_embed.weight.data[:, :2] = inverse_sigmoid(self.refpoint_embed.weight.data[:, :2])
+ self.refpoint_embed.weight.data[:, :2].requires_grad = False
+ self.hw_embed = nn.Embedding(1, 1)
+ else:
+ raise NotImplementedError('Unknown fix_refpoints_hw {}'.format(self.fix_refpoints_hw))
+
+ def forward(self, samples: NestedTensor, targets: List = None, **kw):
+ """ The forward expects a NestedTensor, which consists of:
+ - samples.tensor: batched images, of shape [batch_size x 3 x H x W]
+ - samples.mask: a binary mask of shape [batch_size x H x W], containing 1 on padded pixels
+
+ It returns a dict with the following elements:
+ - "pred_logits": the classification logits (including no-object) for all queries.
+ Shape= [batch_size x num_queries x num_classes]
+ - "pred_boxes": The normalized boxes coordinates for all queries, represented as
+ (center_x, center_y, width, height). These values are normalized in [0, 1],
+ relative to the size of each individual image (disregarding possible padding).
+ See PostProcess for information on how to retrieve the unnormalized bounding box.
+ - "aux_outputs": Optional, only returned when auxilary losses are activated. It is a list of
+ dictionnaries containing the two above keys for each decoder layer.
+ """
+
+ captions = [t['instance_text_prompt'] for t in targets]
+ bs=len(captions)
+ tensor_list = [tgt["object_embeddings_text"] for tgt in targets]
+ max_size = 350
+ padded_tensors = [torch.cat([tensor, torch.zeros(max_size - tensor.size(0), tensor.size(1),device=tensor.device)]) if tensor.size(0) < max_size else tensor for tensor in tensor_list]
+ object_embeddings_text = torch.stack(padded_tensors)
+
+ kpts_embeddings_text = torch.stack([tgt["kpts_embeddings_text"] for tgt in targets])[:, :self.num_body_points]
+ encoded_text=self.projection(object_embeddings_text) # bs, 81, 101, 256
+ kpt_embeddings_specific=self.projection_kpt(kpts_embeddings_text) # bs, 81, 101, 256
+
+
+ kpt_vis = torch.stack([tgt["kpt_vis_text"] for tgt in targets])[:, :self.num_body_points]
+ kpt_mask = torch.cat((torch.ones_like(kpt_vis, device=kpt_vis.device)[..., 0].unsqueeze(-1), kpt_vis), dim=-1)
+
+
+ num_classes = encoded_text.shape[1] # bs, 81, 101, 256
+ text_self_attention_masks = torch.eye(num_classes).unsqueeze(0).expand(bs, -1, -1).bool().to(samples.device)
+ text_token_mask = torch.zeros(samples.shape[0],num_classes).to(samples.device)>0
+ for i in range(bs):
+ text_token_mask[i,:len(captions[i])]=True
+
+ position_ids = torch.zeros(samples.shape[0], num_classes).to(samples.device)
+
+ for i in range(bs):
+ position_ids[i,:len(captions[i])]= 1
+
+
+ text_dict = {
+ 'encoded_text': encoded_text, # bs, 195, d_model
+ 'text_token_mask': text_token_mask, # bs, 195
+ 'position_ids': position_ids, # bs, 195
+ 'text_self_attention_masks': text_self_attention_masks # bs, 195,195
+ }
+
+
+ # import ipdb; ipdb.set_trace()
+
+ if isinstance(samples, (list, torch.Tensor)):
+ samples = nested_tensor_from_tensor_list(samples)
+ features, poss = self.backbone(samples)
+ if os.environ.get("SHILONG_AMP_INFNAN_DEBUG") == '1':
+ import ipdb;
+ ipdb.set_trace()
+
+
+ srcs = []
+ masks = []
+ for l, feat in enumerate(features):
+ src, mask = feat.decompose()
+ srcs.append(self.input_proj[l](src))
+ masks.append(mask)
+ assert mask is not None
+
+ if self.num_feature_levels > len(srcs):
+ _len_srcs = len(srcs)
+ for l in range(_len_srcs, self.num_feature_levels):
+ if l == _len_srcs:
+ src = self.input_proj[l](features[-1].tensors)
+ else:
+ src = self.input_proj[l](srcs[-1])
+ m = samples.mask
+ mask = F.interpolate(m[None].float(), size=src.shape[-2:]).to(torch.bool)[0]
+ pos_l = self.backbone[1](NestedTensor(src, mask)).to(src.dtype)
+ srcs.append(src)
+ masks.append(mask)
+ poss.append(pos_l)
+
+ if self.label_enc is not None:
+ label_enc = self.label_enc
+ else:
+ raise NotImplementedError
+ label_enc = encoded_text
+ if self.dn_number > 0 or targets is not None:
+ input_query_label, input_query_bbox, attn_mask, attn_mask2, dn_meta = \
+ prepare_for_mask(kpt_mask=kpt_mask)
+ else:
+ assert targets is None
+ input_query_bbox = input_query_label = attn_mask = attn_mask2 = dn_meta = None
+
+
+ hs, reference, hs_enc, ref_enc, init_box_proposal = self.transformer(srcs, masks, input_query_bbox, poss,
+ input_query_label, attn_mask, attn_mask2,
+ text_dict, dn_meta,targets,kpt_embeddings_specific)
+
+ # In case num object=0
+ if self.label_enc is not None:
+ hs[0] += self.label_enc.weight[0, 0] * 0.0
+
+ hs[0] += self.pos_proj.weight[0, 0] * 0.0
+ hs[0] += self.pos_proj.bias[0] * 0.0
+ hs[0] += self.padding.weight[0, 0] * 0.0
+
+ num_group = 50
+ effective_dn_number = dn_meta['pad_size'] if self.training else 0
+ outputs_coord_list = []
+ outputs_class = []
+
+
+ for dec_lid, (layer_ref_sig, layer_bbox_embed, layer_cls_embed, layer_hs) in enumerate(
+ zip(reference[:-1], self.bbox_embed, self.class_embed, hs)):
+
+
+ if dec_lid < self.num_box_decoder_layers:
+ layer_delta_unsig = layer_bbox_embed(layer_hs)
+ layer_outputs_unsig = layer_delta_unsig + inverse_sigmoid(layer_ref_sig)
+ layer_outputs_unsig = layer_outputs_unsig.sigmoid()
+ layer_cls = layer_cls_embed(layer_hs, text_dict)
+ outputs_coord_list.append(layer_outputs_unsig)
+ outputs_class.append(layer_cls)
+
+
+ else:
+
+ layer_hs_bbox_dn = layer_hs[:, :effective_dn_number, :]
+ layer_hs_bbox_norm = layer_hs[:, effective_dn_number:, :][:, 0::(self.num_body_points + 1), :]
+ bs = layer_ref_sig.shape[0]
+ reference_before_sigmoid_bbox_dn = layer_ref_sig[:, :effective_dn_number, :]
+ reference_before_sigmoid_bbox_norm = layer_ref_sig[:, effective_dn_number:, :][:,
+ 0::(self.num_body_points + 1), :]
+ layer_delta_unsig_dn = layer_bbox_embed(layer_hs_bbox_dn)
+ layer_delta_unsig_norm = layer_bbox_embed(layer_hs_bbox_norm)
+ layer_outputs_unsig_dn = layer_delta_unsig_dn + inverse_sigmoid(reference_before_sigmoid_bbox_dn)
+ layer_outputs_unsig_dn = layer_outputs_unsig_dn.sigmoid()
+ layer_outputs_unsig_norm = layer_delta_unsig_norm + inverse_sigmoid(reference_before_sigmoid_bbox_norm)
+ layer_outputs_unsig_norm = layer_outputs_unsig_norm.sigmoid()
+ layer_outputs_unsig = torch.cat((layer_outputs_unsig_dn, layer_outputs_unsig_norm), dim=1)
+ layer_cls_dn = layer_cls_embed(layer_hs_bbox_dn, text_dict)
+ layer_cls_norm = layer_cls_embed(layer_hs_bbox_norm, text_dict)
+ layer_cls = torch.cat((layer_cls_dn, layer_cls_norm), dim=1)
+ outputs_class.append(layer_cls)
+ outputs_coord_list.append(layer_outputs_unsig)
+
+ # update keypoints
+ outputs_keypoints_list = []
+ outputs_keypoints_hw = []
+ kpt_index = [x for x in range(num_group * (self.num_body_points + 1)) if x % (self.num_body_points + 1) != 0]
+ for dec_lid, (layer_ref_sig, layer_hs) in enumerate(zip(reference[:-1], hs)):
+ if dec_lid < self.num_box_decoder_layers:
+ assert isinstance(layer_hs, torch.Tensor)
+ bs = layer_hs.shape[0]
+ layer_res = layer_hs.new_zeros((bs, self.num_queries, self.num_body_points * 3))
+ outputs_keypoints_list.append(layer_res)
+ else:
+ bs = layer_ref_sig.shape[0]
+ layer_hs_kpt = layer_hs[:, effective_dn_number:, :].index_select(1, torch.tensor(kpt_index,
+ device=layer_hs.device))
+ delta_xy_unsig = self.pose_embed[dec_lid - self.num_box_decoder_layers](layer_hs_kpt)
+ layer_ref_sig_kpt = layer_ref_sig[:, effective_dn_number:, :].index_select(1, torch.tensor(kpt_index,
+ device=layer_hs.device))
+ layer_outputs_unsig_keypoints = delta_xy_unsig + inverse_sigmoid(layer_ref_sig_kpt[..., :2])
+ vis_xy_unsig = torch.ones_like(layer_outputs_unsig_keypoints,
+ device=layer_outputs_unsig_keypoints.device)
+ xyv = torch.cat((layer_outputs_unsig_keypoints, vis_xy_unsig[:, :, 0].unsqueeze(-1)), dim=-1)
+ xyv = xyv.sigmoid()
+ layer_res = xyv.reshape((bs, num_group, self.num_body_points, 3)).flatten(2, 3)
+ layer_hw = layer_ref_sig_kpt[..., 2:].reshape(bs, num_group, self.num_body_points, 2).flatten(2, 3)
+ layer_res = keypoint_xyzxyz_to_xyxyzz(layer_res)
+ outputs_keypoints_list.append(layer_res)
+ outputs_keypoints_hw.append(layer_hw)
+
+
+ if self.dn_number > 0 and dn_meta is not None:
+ outputs_class, outputs_coord_list = \
+ post_process(outputs_class, outputs_coord_list,
+ dn_meta, self.aux_loss, self._set_aux_loss)
+ out = {'pred_logits': outputs_class[-1], 'pred_boxes': outputs_coord_list[-1],
+ 'pred_keypoints': outputs_keypoints_list[-1]}
+
+ return out
+
+
+@MODULE_BUILD_FUNCS.registe_with_name(module_name='UniPose')
+def build_unipose(args):
+
+ num_classes = args.num_classes
+ device = torch.device(args.device)
+
+ backbone = build_backbone(args)
+
+ transformer = build_deformable_transformer(args)
+
+ try:
+ match_unstable_error = args.match_unstable_error
+ dn_labelbook_size = args.dn_labelbook_size
+ except:
+ match_unstable_error = True
+ dn_labelbook_size = num_classes
+
+ try:
+ dec_pred_class_embed_share = args.dec_pred_class_embed_share
+ except:
+ dec_pred_class_embed_share = True
+ try:
+ dec_pred_bbox_embed_share = args.dec_pred_bbox_embed_share
+ except:
+ dec_pred_bbox_embed_share = True
+
+ binary_query_selection = False
+ try:
+ binary_query_selection = args.binary_query_selection
+ except:
+ binary_query_selection = False
+
+ use_cdn = True
+ try:
+ use_cdn = args.use_cdn
+ except:
+ use_cdn = True
+
+ sub_sentence_present = True
+ try:
+ sub_sentence_present = args.sub_sentence_present
+ except:
+ sub_sentence_present = True
+ # print('********* sub_sentence_present', sub_sentence_present)
+
+ model = UniPose(
+ backbone,
+ transformer,
+ num_classes=num_classes,
+ num_queries=args.num_queries,
+ aux_loss=True,
+ iter_update=True,
+ query_dim=4,
+ random_refpoints_xy=args.random_refpoints_xy,
+ fix_refpoints_hw=args.fix_refpoints_hw,
+ num_feature_levels=args.num_feature_levels,
+ nheads=args.nheads,
+ dec_pred_class_embed_share=dec_pred_class_embed_share,
+ dec_pred_bbox_embed_share=dec_pred_bbox_embed_share,
+ # two stage
+ two_stage_type=args.two_stage_type,
+ # box_share
+ two_stage_bbox_embed_share=args.two_stage_bbox_embed_share,
+ two_stage_class_embed_share=args.two_stage_class_embed_share,
+ decoder_sa_type=args.decoder_sa_type,
+ num_patterns=args.num_patterns,
+ dn_number=args.dn_number if args.use_dn else 0,
+ dn_box_noise_scale=args.dn_box_noise_scale,
+ dn_label_noise_ratio=args.dn_label_noise_ratio,
+ dn_labelbook_size=dn_labelbook_size,
+ use_label_enc=args.use_label_enc,
+
+ text_encoder_type=args.text_encoder_type,
+
+ binary_query_selection=binary_query_selection,
+ use_cdn=use_cdn,
+ sub_sentence_present=sub_sentence_present
+ )
+
+ return model
+
+
+class ContrastiveAssign(nn.Module):
+ def __init__(self, project=False, cal_bias=None, max_text_len=256):
+ """
+ :param x: query
+ :param y: text embed
+ :param proj:
+ :return:
+ """
+ super().__init__()
+ self.project = project
+ self.cal_bias = cal_bias
+ self.max_text_len = max_text_len
+
+ def forward(self, x, text_dict):
+ """_summary_
+
+ Args:
+ x (_type_): _description_
+ text_dict (_type_): _description_
+ {
+ 'encoded_text': encoded_text, # bs, 195, d_model
+ 'text_token_mask': text_token_mask, # bs, 195
+ # True for used tokens. False for padding tokens
+ }
+ Returns:
+ _type_: _description_
+ """
+ assert isinstance(text_dict, dict)
+
+ y = text_dict['encoded_text']
+
+
+ max_text_len = y.shape[1]
+
+
+
+ text_token_mask = text_dict['text_token_mask']
+
+ if self.cal_bias is not None:
+ raise NotImplementedError
+ return x @ y.transpose(-1, -2) + self.cal_bias.weight.repeat(x.shape[0], x.shape[1], 1)
+ res = x @ y.transpose(-1, -2)
+ res.masked_fill_(~text_token_mask[:, None, :], float('-inf'))
+
+ # padding to max_text_len
+ new_res = torch.full((*res.shape[:-1], max_text_len), float('-inf'), device=res.device)
+ new_res[..., :res.shape[-1]] = res
+
+ return new_res
diff --git a/difpoint/src/models/XPose/models/UniPose/utils.py b/difpoint/src/models/XPose/models/UniPose/utils.py
new file mode 100644
index 0000000000000000000000000000000000000000..15ffbd2b34f58b3cb6e4105c470b8e297e78af83
--- /dev/null
+++ b/difpoint/src/models/XPose/models/UniPose/utils.py
@@ -0,0 +1,348 @@
+# ------------------------------------------------------------------------
+# ED-Pose
+# Copyright (c) 2023 IDEA. All Rights Reserved.
+# Licensed under the Apache License, Version 2.0 [see LICENSE for details]
+# ------------------------------------------------------------------------
+
+import copy
+import torch
+import random
+from torch import nn, Tensor
+import os
+import numpy as np
+import math
+import torch.nn.functional as F
+from torch import nn
+
+
+def _get_clones(module, N, layer_share=False):
+ # import ipdb; ipdb.set_trace()
+ if layer_share:
+ return nn.ModuleList([module for i in range(N)])
+ else:
+ return nn.ModuleList([copy.deepcopy(module) for i in range(N)])
+
+
+def get_sine_pos_embed(
+ pos_tensor: torch.Tensor,
+ num_pos_feats: int = 128,
+ temperature: int = 10000,
+ exchange_xy: bool = True,
+):
+ """generate sine position embedding from a position tensor
+ Args:
+ pos_tensor (torch.Tensor): shape: [..., n].
+ num_pos_feats (int): projected shape for each float in the tensor.
+ temperature (int): temperature in the sine/cosine function.
+ exchange_xy (bool, optional): exchange pos x and pos y. \
+ For example, input tensor is [x,y], the results will be [pos(y), pos(x)]. Defaults to True.
+ Returns:
+ pos_embed (torch.Tensor): shape: [..., n*num_pos_feats].
+ """
+ scale = 2 * math.pi
+ dim_t = torch.arange(num_pos_feats, dtype=torch.float32, device=pos_tensor.device)
+ dim_t = temperature ** (2 * torch.div(dim_t, 2, rounding_mode="floor") / num_pos_feats)
+
+ def sine_func(x: torch.Tensor):
+ sin_x = x * scale / dim_t
+ sin_x = torch.stack((sin_x[..., 0::2].sin(), sin_x[..., 1::2].cos()), dim=3).flatten(2)
+ return sin_x
+
+ pos_res = [sine_func(x) for x in pos_tensor.split([1] * pos_tensor.shape[-1], dim=-1)]
+ if exchange_xy:
+ pos_res[0], pos_res[1] = pos_res[1], pos_res[0]
+ pos_res = torch.cat(pos_res, dim=-1)
+ return pos_res
+
+
+def gen_encoder_output_proposals(memory: Tensor, memory_padding_mask: Tensor, spatial_shapes: Tensor, learnedwh=None):
+ """
+ Input:
+ - memory: bs, \sum{hw}, d_model
+ - memory_padding_mask: bs, \sum{hw}
+ - spatial_shapes: nlevel, 2
+ - learnedwh: 2
+ Output:
+ - output_memory: bs, \sum{hw}, d_model
+ - output_proposals: bs, \sum{hw}, 4
+ """
+ N_, S_, C_ = memory.shape
+ base_scale = 4.0
+ proposals = []
+ _cur = 0
+ for lvl, (H_, W_) in enumerate(spatial_shapes):
+ mask_flatten_ = memory_padding_mask[:, _cur:(_cur + H_ * W_)].view(N_, H_, W_, 1)
+ valid_H = torch.sum(~mask_flatten_[:, :, 0, 0], 1)
+ valid_W = torch.sum(~mask_flatten_[:, 0, :, 0], 1)
+
+ # import ipdb; ipdb.set_trace()
+
+ grid_y, grid_x = torch.meshgrid(torch.linspace(0, H_ - 1, H_, dtype=torch.float32, device=memory.device),
+ torch.linspace(0, W_ - 1, W_, dtype=torch.float32, device=memory.device))
+ grid = torch.cat([grid_x.unsqueeze(-1), grid_y.unsqueeze(-1)], -1) # H_, W_, 2
+
+ scale = torch.cat([valid_W.unsqueeze(-1), valid_H.unsqueeze(-1)], 1).view(N_, 1, 1, 2)
+ grid = (grid.unsqueeze(0).expand(N_, -1, -1, -1) + 0.5) / scale
+
+ if learnedwh is not None:
+ # import ipdb; ipdb.set_trace()
+ wh = torch.ones_like(grid) * learnedwh.sigmoid() * (2.0 ** lvl)
+ else:
+ wh = torch.ones_like(grid) * 0.05 * (2.0 ** lvl)
+
+ # scale = torch.cat([W_[None].unsqueeze(-1), H_[None].unsqueeze(-1)], 1).view(1, 1, 1, 2).repeat(N_, 1, 1, 1)
+ # grid = (grid.unsqueeze(0).expand(N_, -1, -1, -1) + 0.5) / scale
+ # wh = torch.ones_like(grid) / scale
+ proposal = torch.cat((grid, wh), -1).view(N_, -1, 4)
+ proposals.append(proposal)
+ _cur += (H_ * W_)
+ # import ipdb; ipdb.set_trace()
+ output_proposals = torch.cat(proposals, 1)
+ output_proposals_valid = ((output_proposals > 0.01) & (output_proposals < 0.99)).all(-1, keepdim=True)
+ output_proposals = torch.log(output_proposals / (1 - output_proposals)) # unsigmoid
+ output_proposals = output_proposals.masked_fill(memory_padding_mask.unsqueeze(-1), float('inf'))
+ output_proposals = output_proposals.masked_fill(~output_proposals_valid, float('inf'))
+
+ output_memory = memory
+ output_memory = output_memory.masked_fill(memory_padding_mask.unsqueeze(-1), float(0))
+ output_memory = output_memory.masked_fill(~output_proposals_valid, float(0))
+
+ # output_memory = output_memory.masked_fill(memory_padding_mask.unsqueeze(-1), float('inf'))
+ # output_memory = output_memory.masked_fill(~output_proposals_valid, float('inf'))
+
+ return output_memory, output_proposals
+
+
+class RandomBoxPerturber():
+ def __init__(self, x_noise_scale=0.2, y_noise_scale=0.2, w_noise_scale=0.2, h_noise_scale=0.2) -> None:
+ self.noise_scale = torch.Tensor([x_noise_scale, y_noise_scale, w_noise_scale, h_noise_scale])
+
+ def __call__(self, refanchors: Tensor) -> Tensor:
+ nq, bs, query_dim = refanchors.shape
+ device = refanchors.device
+
+ noise_raw = torch.rand_like(refanchors)
+ noise_scale = self.noise_scale.to(device)[:query_dim]
+
+ new_refanchors = refanchors * (1 + (noise_raw - 0.5) * noise_scale)
+ return new_refanchors.clamp_(0, 1)
+
+
+def sigmoid_focal_loss(inputs, targets, num_boxes, alpha: float = 0.25, gamma: float = 2, no_reduction=False):
+ """
+ Loss used in RetinaNet for dense detection: https://arxiv.org/abs/1708.02002.
+ Args:
+ inputs: A float tensor of arbitrary shape.
+ The predictions for each example.
+ targets: A float tensor with the same shape as inputs. Stores the binary
+ classification label for each element in inputs
+ (0 for the negative class and 1 for the positive class).
+ alpha: (optional) Weighting factor in range (0,1) to balance
+ positive vs negative examples. Default = -1 (no weighting).
+ gamma: Exponent of the modulating factor (1 - p_t) to
+ balance easy vs hard examples.
+ Returns:
+ Loss tensor
+ """
+ prob = inputs.sigmoid()
+ ce_loss = F.binary_cross_entropy_with_logits(inputs, targets, reduction="none")
+ p_t = prob * targets + (1 - prob) * (1 - targets)
+ loss = ce_loss * ((1 - p_t) ** gamma)
+
+ if alpha >= 0:
+ alpha_t = alpha * targets + (1 - alpha) * (1 - targets)
+ loss = alpha_t * loss
+
+ if no_reduction:
+ return loss
+
+ return loss.mean(1).sum() / num_boxes
+
+
+class MLP(nn.Module):
+ """ Very simple multi-layer perceptron (also called FFN)"""
+
+ def __init__(self, input_dim, hidden_dim, output_dim, num_layers):
+ super().__init__()
+ self.num_layers = num_layers
+ h = [hidden_dim] * (num_layers - 1)
+ self.layers = nn.ModuleList(nn.Linear(n, k) for n, k in zip([input_dim] + h, h + [output_dim]))
+
+ def forward(self, x):
+ for i, layer in enumerate(self.layers):
+ x = F.relu(layer(x)) if i < self.num_layers - 1 else layer(x)
+ return x
+
+
+def _get_activation_fn(activation, d_model=256, batch_dim=0):
+ """Return an activation function given a string"""
+ if activation == "relu":
+ return F.relu
+ if activation == "gelu":
+ return F.gelu
+ if activation == "glu":
+ return F.glu
+ if activation == "prelu":
+ return nn.PReLU()
+ if activation == "selu":
+ return F.selu
+
+ raise RuntimeError(F"activation should be relu/gelu, not {activation}.")
+
+
+def gen_sineembed_for_position(pos_tensor):
+ # n_query, bs, _ = pos_tensor.size()
+ # sineembed_tensor = torch.zeros(n_query, bs, 256)
+ scale = 2 * math.pi
+ dim_t = torch.arange(128, dtype=torch.float32, device=pos_tensor.device)
+ dim_t = 10000 ** (2 * (dim_t // 2) / 128)
+ x_embed = pos_tensor[:, :, 0] * scale
+ y_embed = pos_tensor[:, :, 1] * scale
+ pos_x = x_embed[:, :, None] / dim_t
+ pos_y = y_embed[:, :, None] / dim_t
+ pos_x = torch.stack((pos_x[:, :, 0::2].sin(), pos_x[:, :, 1::2].cos()), dim=3).flatten(2)
+ pos_y = torch.stack((pos_y[:, :, 0::2].sin(), pos_y[:, :, 1::2].cos()), dim=3).flatten(2)
+ if pos_tensor.size(-1) == 2:
+ pos = torch.cat((pos_y, pos_x), dim=2)
+ elif pos_tensor.size(-1) == 4:
+ w_embed = pos_tensor[:, :, 2] * scale
+ pos_w = w_embed[:, :, None] / dim_t
+ pos_w = torch.stack((pos_w[:, :, 0::2].sin(), pos_w[:, :, 1::2].cos()), dim=3).flatten(2)
+
+ h_embed = pos_tensor[:, :, 3] * scale
+ pos_h = h_embed[:, :, None] / dim_t
+ pos_h = torch.stack((pos_h[:, :, 0::2].sin(), pos_h[:, :, 1::2].cos()), dim=3).flatten(2)
+
+ pos = torch.cat((pos_y, pos_x, pos_w, pos_h), dim=2)
+ else:
+ raise ValueError("Unknown pos_tensor shape(-1):{}".format(pos_tensor.size(-1)))
+ return pos
+
+
+def oks_overlaps(kpt_preds, kpt_gts, kpt_valids, kpt_areas, sigmas):
+ sigmas = kpt_preds.new_tensor(sigmas)
+ variances = (sigmas * 2) ** 2
+
+ assert kpt_preds.size(0) == kpt_gts.size(0)
+ kpt_preds = kpt_preds.reshape(-1, kpt_preds.size(-1) // 2, 2)
+ kpt_gts = kpt_gts.reshape(-1, kpt_gts.size(-1) // 2, 2)
+
+ squared_distance = (kpt_preds[:, :, 0] - kpt_gts[:, :, 0]) ** 2 + \
+ (kpt_preds[:, :, 1] - kpt_gts[:, :, 1]) ** 2
+ # import pdb
+ # pdb.set_trace()
+ # assert (kpt_valids.sum(-1) > 0).all()
+ squared_distance0 = squared_distance / (kpt_areas[:, None] * variances[None, :] * 2)
+ squared_distance1 = torch.exp(-squared_distance0)
+ squared_distance1 = squared_distance1 * kpt_valids
+ oks = squared_distance1.sum(dim=1) / (kpt_valids.sum(dim=1) + 1e-6)
+
+ return oks
+
+
+def oks_loss(pred,
+ target,
+ valid=None,
+ area=None,
+ linear=False,
+ sigmas=None,
+ eps=1e-6):
+ """Oks loss.
+ Computing the oks loss between a set of predicted poses and target poses.
+ The loss is calculated as negative log of oks.
+ Args:
+ pred (torch.Tensor): Predicted poses of format (x1, y1, x2, y2, ...),
+ shape (n, 2K).
+ target (torch.Tensor): Corresponding gt poses, shape (n, 2K).
+ linear (bool, optional): If True, use linear scale of loss instead of
+ log scale. Default: False.
+ eps (float): Eps to avoid log(0).
+ Return:
+ torch.Tensor: Loss tensor.
+ """
+ oks = oks_overlaps(pred, target, valid, area, sigmas).clamp(min=eps)
+ if linear:
+ loss = 1 - oks
+ else:
+ loss = -oks.log()
+ return loss
+
+
+class OKSLoss(nn.Module):
+ """IoULoss.
+ Computing the oks loss between a set of predicted poses and target poses.
+ Args:
+ linear (bool): If True, use linear scale of loss instead of log scale.
+ Default: False.
+ eps (float): Eps to avoid log(0).
+ reduction (str): Options are "none", "mean" and "sum".
+ loss_weight (float): Weight of loss.
+ """
+
+ def __init__(self,
+ linear=False,
+ num_keypoints=17,
+ eps=1e-6,
+ reduction='mean',
+ loss_weight=1.0):
+ super(OKSLoss, self).__init__()
+ self.linear = linear
+ self.eps = eps
+ self.reduction = reduction
+ self.loss_weight = loss_weight
+ if num_keypoints == 68:
+ self.sigmas = np.array([
+ .26, .25, .25, .35, .35, .79, .79, .72, .72, .62, .62, 1.07,
+ 1.07, .87, .87, .89, .89, .25, .25, .25, .25, .25, .25, .25, .25,
+ .25, .25, .25, .25, .25, .25, .25, .25, .25, .25, .25, .25, .25,
+ .25, .25, .25, .25, .25, .25, .25, .25, .25, .25, .25, .25, .25, .25, .25, .25,
+ .25, .25, .25, .25, .25, .25, .25, .25, .25, .25, .25, .25, .25, .25,
+ ], dtype=np.float32) / 10.0
+ else:
+ raise ValueError(f'Unsupported keypoints number {num_keypoints}')
+
+ def forward(self,
+ pred,
+ target,
+ valid,
+ area,
+ weight=None,
+ avg_factor=None,
+ reduction_override=None):
+ """Forward function.
+ Args:
+ pred (torch.Tensor): The prediction.
+ target (torch.Tensor): The learning target of the prediction.
+ valid (torch.Tensor): The visible flag of the target pose.
+ area (torch.Tensor): The area of the target pose.
+ weight (torch.Tensor, optional): The weight of loss for each
+ prediction. Defaults to None.
+ avg_factor (int, optional): Average factor that is used to average
+ the loss. Defaults to None.
+ reduction_override (str, optional): The reduction method used to
+ override the original reduction method of the loss.
+ Defaults to None. Options are "none", "mean" and "sum".
+ """
+ assert reduction_override in (None, 'none', 'mean', 'sum')
+ reduction = (
+ reduction_override if reduction_override else self.reduction)
+ if (weight is not None) and (not torch.any(weight > 0)) and (
+ reduction != 'none'):
+ if pred.dim() == weight.dim() + 1:
+ weight = weight.unsqueeze(1)
+ return (pred * weight).sum() # 0
+ if weight is not None and weight.dim() > 1:
+ # TODO: remove this in the future
+ # reduce the weight of shape (n, 4) to (n,) to match the
+ # iou_loss of shape (n,)
+ assert weight.shape == pred.shape
+ weight = weight.mean(-1)
+ loss = self.loss_weight * oks_loss(
+ pred,
+ target,
+ valid=valid,
+ area=area,
+ linear=self.linear,
+ sigmas=self.sigmas,
+ eps=self.eps)
+ return loss
diff --git a/difpoint/src/models/XPose/models/__init__.py b/difpoint/src/models/XPose/models/__init__.py
new file mode 100644
index 0000000000000000000000000000000000000000..4585e55895a9f5d9e33cc6ebc7ac956f135e6928
--- /dev/null
+++ b/difpoint/src/models/XPose/models/__init__.py
@@ -0,0 +1,16 @@
+# ------------------------------------------------------------------------
+# ED-Pose
+# Copyright (c) 2023 IDEA. All Rights Reserved.
+# Licensed under the Apache License, Version 2.0 [see LICENSE for details]
+# ------------------------------------------------------------------------
+# Copyright (c) Facebook, Inc. and its affiliates. All Rights Reserved
+from .UniPose.unipose import build_unipose
+
+def build_model(args):
+ # we use register to maintain models from catdet6 on.
+ from .registry import MODULE_BUILD_FUNCS
+
+ assert args.modelname in MODULE_BUILD_FUNCS._module_dict
+ build_func = MODULE_BUILD_FUNCS.get(args.modelname)
+ model = build_func(args)
+ return model
diff --git a/difpoint/src/models/XPose/models/registry.py b/difpoint/src/models/XPose/models/registry.py
new file mode 100644
index 0000000000000000000000000000000000000000..e3a1c956e7efeba5cc85a7ae8e39d6d1caed2c07
--- /dev/null
+++ b/difpoint/src/models/XPose/models/registry.py
@@ -0,0 +1,58 @@
+# -*- coding: utf-8 -*-
+# @Author: Yihao Chen
+# @Date: 2021-08-16 16:03:17
+# @Last Modified by: Shilong Liu
+# @Last Modified time: 2022-01-23 15:26
+# modified from mmcv
+
+import inspect
+from functools import partial
+
+
+class Registry(object):
+
+ def __init__(self, name):
+ self._name = name
+ self._module_dict = dict()
+
+ def __repr__(self):
+ format_str = self.__class__.__name__ + '(name={}, items={})'.format(
+ self._name, list(self._module_dict.keys()))
+ return format_str
+
+ def __len__(self):
+ return len(self._module_dict)
+
+ @property
+ def name(self):
+ return self._name
+
+ @property
+ def module_dict(self):
+ return self._module_dict
+
+ def get(self, key):
+ return self._module_dict.get(key, None)
+
+ def registe_with_name(self, module_name=None, force=False):
+ return partial(self.register, module_name=module_name, force=force)
+
+ def register(self, module_build_function, module_name=None, force=False):
+ """Register a module build function.
+ Args:
+ module (:obj:`nn.Module`): Module to be registered.
+ """
+ if not inspect.isfunction(module_build_function):
+ raise TypeError('module_build_function must be a function, but got {}'.format(
+ type(module_build_function)))
+ if module_name is None:
+ module_name = module_build_function.__name__
+ if not force and module_name in self._module_dict:
+ raise KeyError('{} is already registered in {}'.format(
+ module_name, self.name))
+ self._module_dict[module_name] = module_build_function
+
+ return module_build_function
+
+MODULE_BUILD_FUNCS = Registry('model build functions')
+
diff --git a/difpoint/src/models/XPose/predefined_keypoints.py b/difpoint/src/models/XPose/predefined_keypoints.py
new file mode 100644
index 0000000000000000000000000000000000000000..c6c11579bd49da39790381a51f253bfda7b8235d
--- /dev/null
+++ b/difpoint/src/models/XPose/predefined_keypoints.py
@@ -0,0 +1,56 @@
+person = {"keypoints":['nose', 'left eye', 'right eye', 'left ear', 'right ear', 'left shoulder', 'right shoulder', 'left elbow', 'right elbow', 'left wrist', 'right wrist', 'left hip', 'right hip', 'left knee', 'right knee', 'left ankle', 'right ankle'],"skeleton": [[16,14],[14,12],[17,15],[15,13],[12,13],[6,12],[7,13],[6,7],[6,8],[7,9],[8,10],[9,11],[2,3],[1,2],[1,3],[2,4],[3,5],[4,6],[5,7]]}
+
+face = {"keypoints": ['right cheekbone 1', 'right cheekbone 2', 'right cheek 1', 'right cheek 2', 'right cheek 3', 'right cheek 4', 'right cheek 5', 'right chin', 'chin center', 'left chin', 'left cheek 5', 'left cheek 4', 'left cheek 3', 'left cheek 2', 'left cheek 1', 'left cheekbone 2', 'left cheekbone 1', 'right eyebrow 1', 'right eyebrow 2', 'right eyebrow 3', 'right eyebrow 4', 'right eyebrow 5', 'left eyebrow 1', 'left eyebrow 2', 'left eyebrow 3', 'left eyebrow 4', 'left eyebrow 5', 'nasal bridge 1', 'nasal bridge 2', 'nasal bridge 3', 'nasal bridge 4', 'right nasal wing 1', 'right nasal wing 2', 'nasal wing center', 'left nasal wing 1', 'left nasal wing 2', 'right eye eye corner 1', 'right eye upper eyelid 1', 'right eye upper eyelid 2', 'right eye eye corner 2', 'right eye lower eyelid 2', 'right eye lower eyelid 1', 'left eye eye corner 1', 'left eye upper eyelid 1', 'left eye upper eyelid 2', 'left eye eye corner 2', 'left eye lower eyelid 2', 'left eye lower eyelid 1', 'right mouth corner', 'upper lip outer edge 1', 'upper lip outer edge 2', 'upper lip outer edge 3', 'upper lip outer edge 4', 'upper lip outer edge 5', 'left mouth corner', 'lower lip outer edge 5', 'lower lip outer edge 4', 'lower lip outer edge 3', 'lower lip outer edge 2', 'lower lip outer edge 1', 'upper lip inter edge 1', 'upper lip inter edge 2', 'upper lip inter edge 3', 'upper lip inter edge 4', 'upper lip inter edge 5', 'lower lip inter edge 3', 'lower lip inter edge 2', 'lower lip inter edge 1'], "skeleton": []}
+
+hand = {"keypoints":['wrist', 'thumb root', "thumb's third knuckle", "thumb's second knuckle", 'thumb’s first knuckle', "forefinger's root", "forefinger's third knuckle", "forefinger's second knuckle", "forefinger's first knuckle", "middle finger's root", "middle finger's third knuckle", "middle finger's second knuckle", "middle finger's first knuckle", "ring finger's root", "ring finger's third knuckle", "ring finger's second knuckle", "ring finger's first knuckle", "pinky finger's root", "pinky finger's third knuckle", "pinky finger's second knuckle", "pinky finger's first knuckle"],"skeleton": []}
+
+animal_in_AnimalKindom = {"keypoints":['head mid top', 'eye left', 'eye right', 'mouth front top', 'mouth back left', 'mouth back right', 'mouth front bottom', 'shoulder left', 'shoulder right', 'elbow left', 'elbow right', 'wrist left', 'wrist right', 'torso mid back', 'hip left', 'hip right', 'knee left', 'knee right', 'ankle left ', 'ankle right', 'tail top back', 'tail mid back', 'tail end back'],"skeleton": [[1, 0], [2, 0], [3, 4], [3, 5], [4, 6], [5, 6], [0, 7], [0, 8], [7, 9], [8, 10], [9, 11], [10, 12], [0, 13], [13, 20], [20, 14], [20, 15], [14, 16], [15, 17], [16, 18], [17, 19], [20, 21], [21, 22]]}
+
+animal_in_AP10K = {"keypoints": ['left eye', 'right eye', 'nose', 'neck', 'root of tail', 'left shoulder', 'left elbow', 'left front paw', 'right shoulder', 'right elbow', 'right front paw', 'left hip', 'left knee', 'left back paw', 'right hip', 'right knee', 'right back paw'], "skeleton": [[1, 2], [1, 3], [2, 3], [3, 4], [4, 5], [4, 6], [6, 7], [7, 8], [4, 9], [9, 10], [10, 11], [5, 12], [12, 13], [13, 14], [5, 15], [15, 16], [16, 17]]}
+
+animal= {"keypoints": ['left eye', 'right eye', 'nose', 'neck', 'root of tail', 'left shoulder', 'left elbow', 'left front paw', 'right shoulder', 'right elbow', 'right front paw', 'left hip', 'left knee', 'left back paw', 'right hip', 'right knee', 'right back paw'], "skeleton": [[1, 2], [1, 3], [2, 3], [3, 4], [4, 5], [4, 6], [6, 7], [7, 8], [4, 9], [9, 10], [10, 11], [5, 12], [12, 13], [13, 14], [5, 15], [15, 16], [16, 17]]}
+
+animal_face = {"keypoints": ['right eye right', 'right eye left', 'left eye right', 'left eye left', 'nose tip', 'lip right', 'lip left', 'upper lip', 'lower lip'], "skeleton": []}
+
+fly = {"keypoints": ['head', 'eye left', 'eye right', 'neck', 'thorax', 'abdomen', 'foreleg right base', 'foreleg right first segment', 'foreleg right second segment', 'foreleg right tip', 'midleg right base', 'midleg right first segment', 'midleg right second segment', 'midleg right tip', 'hindleg right base', 'hindleg right first segment', 'hindleg right second segment', 'hindleg right tip', 'foreleg left base', 'foreleg left first segment', 'foreleg left second segment', 'foreleg left tip', 'midleg left base', 'midleg left first segment', 'midleg left second segment', 'midleg left tip', 'hindleg left base', 'hindleg left first segment', 'hindleg left second segment', 'hindleg left tip', 'wing left', 'wing right'], "skeleton": [[2, 1], [3, 1], [4, 1], [5, 4], [6, 5], [8, 7], [9, 8], [10, 9], [12, 11], [13, 12], [14, 13], [16, 15], [17, 16], [18, 17], [20, 19], [21, 20], [22, 21], [24, 23], [25, 24], [26, 25], [28, 27], [29, 28], [30, 29], [31, 4], [32, 4]]}
+
+locust = {"keypoints": ['head', 'neck', 'thorax', 'abdomen1', 'abdomen2', 'anttip left', 'antbase left', 'eye left', 'foreleg left base', 'foreleg left first segment', 'foreleg left second segment', 'foreleg left tip', 'midleg left base', 'midleg left first segment', 'midleg left second segment', 'midleg left tip', 'hindleg left base', 'hindleg left first segment', 'hindleg left second segment', 'hindleg left tip', 'anttip right', 'antbase right', 'eye right', 'foreleg right base', 'foreleg right first segment', 'foreleg right second segment', 'foreleg right tip', 'midleg right base', 'midleg right first segment', 'midleg right second segment', 'midleg right tip', 'hindleg right base', 'hindleg right first segment', 'hindleg right second segment', 'hindleg right tip'],"skeleton": [[2, 1], [3, 2], [4, 3], [5, 4], [7, 6], [8, 7], [10, 9], [11, 10], [12, 11], [14, 13], [15, 14],[16, 15], [18, 17], [19, 18], [20, 19], [22, 21], [23, 22], [25, 24], [26, 25], [27, 26],[29, 28], [30, 29], [31, 30], [33, 32], [34, 33], [35, 34]]}
+
+car ={"keypoints": ['right front wheel center', 'left front wheel center', 'right rear wheel center', 'left rear wheel center', 'front right', 'front left', 'back right', 'back left', 'none', 'roof front right', 'roof front left', 'roof back right', 'roof back left', 'none'],"skeleton": [[0, 2], [1, 3], [0, 1], [2, 3], [9, 11], [10, 12], [9, 10], [11, 12], [4, 0], [4, 9], [4, 5], [5, 1], [5, 10], [6, 2], [6, 11], [7, 3], [7, 12], [6, 7]]}
+
+short_sleeved_shirt = {'keypoints': ['upper center neckline', 'upper right neckline', 'lower right neckline', 'lower center neckline', 'lower left neckline', 'upper left neckline', 'right sleeve outside 1', 'right sleeve outside 2', 'right cuff outside', 'right cuff inside', 'right sleeve inside 2', 'right sleeve inside 1', 'right side 1', 'right side 2', 'right side 3', 'center hem', 'left side 3', 'left side 2', 'left side 1', 'left sleeve inside 1', 'left sleeve inside 2', 'left cuff inside', 'left cuff outside', 'left sleeve outside 2', 'left sleeve outside 1'], 'skeleton': []}
+
+long_sleeved_outwear={'keypoints': ['upper center neckline', 'lower right center neckline', 'lower right neckline', 'upper right neckline', 'lower left neckline', 'upper left neckline', 'right sleeve outside 1', 'right sleeve outside 2', 'right sleeve outside 3', 'right sleeve outside 4', 'right cuff outside', 'right cuff inside', 'right sleeve inside 1', 'right sleeve inside 2', 'right sleeve inside 3', 'right sleeve inside 4', 'right side outside 1', 'right side outside 2', 'right side outside 3', 'right side inside 3', 'left side outside 3', 'left side outside 2', 'left side outside 1', 'left sleeve inside 4', 'left sleeve inside 3', 'left sleeve inside 2', 'left sleeve inside 1', 'left cuff inside', 'left cuff outside', 'left sleeve outside 4', 'left sleeve outside 3', 'left sleeve outside 2', 'left sleeve outside 1', 'lower left center neckline', 'left side inside 1', 'left side inside 2', 'left side inside 3', 'right side inside 1', 'right side inside 2'], 'skeleton': []}
+
+short_sleeved_outwear={'keypoints': ['upper center neckline', 'lower right center neckline', 'lower right neckline', 'upper right neckline', 'lower left neckline', 'upper left neckline', 'right sleeve outside 1', 'right sleeve outside 2', 'right cuff outside', 'right cuff inside', 'right sleeve inside 2', 'right sleeve inside 1', 'right side outside 1', 'right side outside 2', 'right side outside 3', 'right side inside 3', 'left side outside 3', 'left side outside 2', 'left side outside 1', 'left sleeve inside 1', 'left sleeve inside 2', 'left cuff inside', 'left cuff outside', 'left sleeve outside 2', 'left sleeve outside 1', 'lower left center neckline', 'left side inside 1', 'left side inside 2', 'left side inside 3', 'right side inside 1', 'right side inside 2'], 'skeleton': []}
+
+sling={'keypoints': ['upper center neckline', 'upper right neckline', 'lower right neckline', 'lower center neckline', 'lower left neckline', 'upper left neckline', 'right sleeve', 'right side 1', 'right side 2', 'right side 3', 'center hem', 'left side 3', 'left side 2', 'left side 1', 'left sleeve'], 'skeleton': []}
+
+vest = {'keypoints': ['upper center neckline', 'upper right neckline', 'lower right neckline', 'lower center neckline', 'lower left neckline', 'upper left neckline', 'right sleeve', 'right side 1', 'right side 2', 'right side 3', 'center hem', 'left side 3', 'left side 2', 'left side 1', 'left sleeve'], 'skeleton': []}
+
+long_sleeved_dress={'keypoints': ['upper center neckline', 'upper right neckline', 'lower right neckline', 'lower center neckline', 'lower left neckline', 'upper left neckline', 'right sleeve outside 1', 'right sleeve outside 2', 'right sleeve outside 3', 'right sleeve outside 4', 'right cuff outside', 'right cuff inside', 'right sleeve inside 4', 'right sleeve inside 3', 'right sleeve inside 2', 'right sleeve inside 1', 'right side 1', 'right side 2', 'right side 3', 'right side 4', 'right side 5', 'center hem', 'left side 5', 'left side 4', 'left side 3', 'left side 2', 'left side 1', 'left sleeve inside 1', 'left sleeve inside 2', 'left sleeve inside 3', 'left sleeve inside 4', 'left cuff inside', 'left cuff outside', 'left sleeve outside 4', 'left sleeve outside 3', 'left sleeve outside 2', 'left sleeve outside 1'], 'skeleton': []}
+
+long_sleeved_shirt = {'keypoints': ['upper center neckline', 'upper right neckline', 'lower right neckline', 'lower center neckline', 'lower left neckline', 'upper left neckline', 'right sleeve outside 1', 'right sleeve outside 2', 'right sleeve outside 3', 'right sleeve outside 4', 'right cuff outside', 'right cuff inside', 'right sleeve inside 4', 'right sleeve inside 3', 'right sleeve inside 2', 'right sleeve inside 1', 'right side 1', 'right side 2', 'right side 3', 'center hem', 'left side 3', 'left side 2', 'left side 1', 'left sleeve inside 1', 'left sleeve inside 2', 'left sleeve inside 3', 'left sleeve inside 4', 'left cuff inside', 'left cuff outside', 'left sleeve outside 4', 'left sleeve outside 3', 'left sleeve outside 2', 'left sleeve outside 1'], 'skeleton': []}
+
+trousers = {'keypoints': ['right side outside 1', 'upper center', 'left side outside 1', 'right side outside 2', 'right side outside 3', 'right cuff outside', 'right cuff inside', 'right side inside 1', 'crotch', 'left side inside 1', 'left cuff inside', 'left cuff outside', 'left side outside 3', 'left side outside 2'], 'skeleton': []}
+
+sling_dress = {'keypoints': ['upper center neckline', 'upper right neckline', 'lower right neckline', 'lower center neckline', 'lower left neckline', 'upper left neckline', 'right side 1', 'right side 2', 'right side 3', 'right side 4', 'right side 5', 'right side 6', 'center hem', 'left side 6', 'left side 5', 'left side 4', 'left side 3', 'left side 2', 'left side 1'], 'skeleton': []}
+
+vest_dress = {'keypoints': ['upper center neckline', 'upper right neckline', 'lower right neckline', 'lower center neckline', 'lower left neckline', 'upper left neckline', 'right side 1', 'right side 2', 'right side 3', 'right side 4', 'right side 5', 'right side 6', 'center hem', 'left side 6', 'left side 5', 'left side 4', 'left side 3', 'left side 2', 'left side 1'], 'skeleton': []}
+
+skirt = {'keypoints': ['right side 1', 'upper center', 'left side 1', 'right side 2', 'right side 3', 'center hem', 'left side 3', 'left side 2'], 'skeleton': []}
+
+short_sleeved_dress = {'keypoints': ['upper center neckline', 'upper right neckline', 'lower right neckline', 'lower center neckline', 'lower left neckline', 'upper left neckline', 'right sleeve outside 1', 'right sleeve outside 2', 'right cuff outside', 'right cuff inside', 'right sleeve inside 1', 'right sleeve inside 2', 'left side 1', 'left side 2', 'left side 3', 'left side 4', 'left side 5', 'center hem', 'right side 5', 'right side 4', 'right side 3', 'right side 2', 'right side 1', 'left sleeve inside 2', 'left sleeve inside 1', 'left cuff inside', 'left cuff outside', 'left sleeve outside 2', 'left sleeve outside 1'], 'skeleton': []}
+
+shorts = {'keypoints': ['right side outside 1', 'upper center', 'left side outside 1', 'right side outside 2', 'right cuff outside', 'right cuff inside', 'crotch', 'left cuff inside', 'left cuff outside', 'left side outside 2'], 'skeleton': []}
+
+table = {'keypoints': ['desktop corner 1', 'desktop corner 2', 'desktop corner 3', 'desktop corner 4', 'table leg 1', 'table leg 2', 'table leg 3', 'table leg 4'], 'skeleton': []}
+
+chair = {'keypoints': ['legs righttopcorner', 'legs lefttopcorner', 'legs leftbottomcorner', 'legs rightbottomcorner', 'base righttop', 'base lefttop', 'base leftbottom', 'base rightbottom', 'headboard righttop', 'headboard lefttop'], 'skeleton': []}
+
+bed = {'keypoints': ['legs rightbottomcorner', 'legs righttopcorner', 'base rightbottom', 'base righttop', 'backrest righttop', 'legs leftbottomcorner', 'legs lefttopcorner', 'base leftbottom', 'base lefttop', 'backrest lefttop'], 'skeleton': []}
+
+sofa = {'keypoints': ['legs rightbottomcorner', 'legs righttopcorner', 'base rightbottom', 'base righttop', 'armrests rightbottomcorner', 'armrests righttopcorner', 'backrest righttop', 'legs leftbottomcorner', 'legs lefttopcorner', 'base leftbottom', 'base lefttop', 'armrests leftbottomcorner', 'armrests lefttopcorner', 'backrest lefttop'], 'skeleton': []}
+
+swivelchair = {'keypoints': ['rotatingbase 1', 'rotatingbase 2', 'rotatingbase 3', 'rotatingbase 4', 'rotatingbase 5', 'rotatingbase center', 'base center', 'base righttop', 'base lefttop', 'base leftbottom', 'base rightbottom', 'backrest righttop', 'backrest lefttop'], 'skeleton': []}
+
diff --git a/difpoint/src/models/XPose/transforms.py b/difpoint/src/models/XPose/transforms.py
new file mode 100644
index 0000000000000000000000000000000000000000..5530aa94f3c2bd312daca62546382929683c2df8
--- /dev/null
+++ b/difpoint/src/models/XPose/transforms.py
@@ -0,0 +1,394 @@
+# Copyright (c) Facebook, Inc. and its affiliates. All Rights Reserved
+"""
+Transforms and data augmentation for both image + bbox.
+"""
+import os
+import sys
+import random
+
+import PIL
+import torch
+import torchvision.transforms as T
+import torchvision.transforms.functional as F
+
+sys.path.append(os.path.dirname(os.path.abspath(__file__)))
+from util.box_ops import box_xyxy_to_cxcywh
+from util.misc import interpolate
+
+
+def crop(image, target, region):
+ cropped_image = F.crop(image, *region)
+
+ if target is not None:
+ target = target.copy()
+ i, j, h, w = region
+ id2catname = target["id2catname"]
+ caption_list = target["caption_list"]
+ target["size"] = torch.tensor([h, w])
+
+ fields = ["labels", "area", "iscrowd", "positive_map","keypoints"]
+
+ if "boxes" in target:
+ boxes = target["boxes"]
+ max_size = torch.as_tensor([w, h], dtype=torch.float32)
+ cropped_boxes = boxes - torch.as_tensor([j, i, j, i])
+ cropped_boxes = torch.min(cropped_boxes.reshape(-1, 2, 2), max_size)
+ cropped_boxes = cropped_boxes.clamp(min=0)
+ area = (cropped_boxes[:, 1, :] - cropped_boxes[:, 0, :]).prod(dim=1)
+ target["boxes"] = cropped_boxes.reshape(-1, 4)
+ target["area"] = area
+ fields.append("boxes")
+
+ if "masks" in target:
+ # FIXME should we update the area here if there are no boxes?
+ target['masks'] = target['masks'][:, i:i + h, j:j + w]
+ fields.append("masks")
+
+
+ # remove elements for which the boxes or masks that have zero area
+ if "boxes" in target or "masks" in target:
+ # favor boxes selection when defining which elements to keep
+ # this is compatible with previous implementation
+ if "boxes" in target:
+ cropped_boxes = target['boxes'].reshape(-1, 2, 2)
+ keep = torch.all(cropped_boxes[:, 1, :] > cropped_boxes[:, 0, :], dim=1)
+ else:
+ keep = target['masks'].flatten(1).any(1)
+
+ for field in fields:
+ if field in target:
+ target[field] = target[field][keep]
+
+ if os.environ.get('IPDB_SHILONG_DEBUG', None) == 'INFO':
+ # for debug and visualization only.
+ if 'strings_positive' in target:
+ target['strings_positive'] = [_i for _i, _j in zip(target['strings_positive'], keep) if _j]
+
+
+ if "keypoints" in target:
+ max_size = torch.as_tensor([w, h], dtype=torch.float32)
+ keypoints = target["keypoints"]
+ cropped_keypoints = keypoints.view(-1, 3)[:,:2] - torch.as_tensor([j, i])
+ cropped_keypoints = torch.min(cropped_keypoints, max_size)
+ cropped_keypoints = cropped_keypoints.clamp(min=0)
+ cropped_keypoints = torch.cat([cropped_keypoints, keypoints.view(-1, 3)[:,2].unsqueeze(1)], dim=1)
+ target["keypoints"] = cropped_keypoints.view(target["keypoints"].shape[0], target["keypoints"].shape[1], 3)
+
+ target["id2catname"] = id2catname
+ target["caption_list"] = caption_list
+
+ return cropped_image, target
+
+
+def hflip(image, target):
+ flipped_image = F.hflip(image)
+
+ w, h = image.size
+
+ if target is not None:
+ target = target.copy()
+ if "boxes" in target:
+ boxes = target["boxes"]
+ boxes = boxes[:, [2, 1, 0, 3]] * torch.as_tensor([-1, 1, -1, 1]) + torch.as_tensor([w, 0, w, 0])
+ target["boxes"] = boxes
+
+ if "masks" in target:
+ target['masks'] = target['masks'].flip(-1)
+
+
+ if "keypoints" in target:
+ dataset_name=target["dataset_name"]
+ if dataset_name == "coco_person" or dataset_name == "macaque":
+ flip_pairs = [[1, 2], [3, 4], [5, 6], [7, 8],
+ [9, 10], [11, 12], [13, 14], [15, 16]]
+
+ elif dataset_name=="animalkindom_ak_P1_animal":
+ flip_pairs = [[1, 2], [4, 5],[7,8],[9,10],[11,12],[14,15],[16,17],[18,19]]
+
+ elif dataset_name=="animalweb_animal":
+ flip_pairs = [[0, 3], [1, 2], [5, 6]]
+
+ elif dataset_name=="face":
+ flip_pairs = [
+ [0, 16], [1, 15], [2, 14], [3, 13], [4, 12], [5, 11], [6, 10], [7, 9],
+ [17, 26], [18, 25], [19, 24], [20, 23], [21, 22],
+ [31, 35], [32, 34],
+ [36, 45], [37, 44], [38, 43], [39, 42], [40, 47], [41, 46],
+ [48, 54], [49, 53], [50, 52],
+ [55, 59], [56, 58],
+ [60, 64], [61, 63],
+ [65, 67]
+ ]
+
+ elif dataset_name=="hand":
+ flip_pairs = []
+
+ elif dataset_name=="foot":
+ flip_pairs = []
+
+ elif dataset_name=="locust":
+ flip_pairs = [[5, 20], [6, 21], [7, 22], [8, 23], [9, 24], [10, 25], [11, 26], [12, 27], [13, 28], [14, 29], [15, 30], [16, 31], [17, 32], [18, 33], [19, 34]]
+
+ elif dataset_name=="fly":
+ flip_pairs = [[1, 2], [6, 18], [7, 19], [8, 20], [9, 21], [10, 22], [11, 23], [12, 24], [13, 25], [14, 26], [15, 27], [16, 28], [17, 29], [30, 31]]
+
+ elif dataset_name == "ap_36k_animal" or dataset_name == "ap_10k_animal":
+ flip_pairs = [[0, 1],[5, 8], [6, 9], [7, 10], [11, 14], [12, 15], [13, 16]]
+
+
+
+ keypoints = target["keypoints"]
+ keypoints[:,:,0] = w - keypoints[:,:, 0]-1
+ for pair in flip_pairs:
+ keypoints[:,pair[0], :], keypoints[:,pair[1], :] = keypoints[:,pair[1], :], keypoints[:,pair[0], :].clone()
+ target["keypoints"] = keypoints
+ return flipped_image, target
+
+
+def resize(image, target, size, max_size=None):
+ # size can be min_size (scalar) or (w, h) tuple
+
+ def get_size_with_aspect_ratio(image_size, size, max_size=None):
+ w, h = image_size
+ if max_size is not None:
+ min_original_size = float(min((w, h)))
+ max_original_size = float(max((w, h)))
+ if max_original_size / min_original_size * size > max_size:
+ size = int(round(max_size * min_original_size / max_original_size))
+
+ if (w <= h and w == size) or (h <= w and h == size):
+ return (h, w)
+
+ if w < h:
+ ow = size
+ oh = int(size * h / w)
+ else:
+ oh = size
+ ow = int(size * w / h)
+
+ return (oh, ow)
+
+ def get_size(image_size, size, max_size=None):
+ if isinstance(size, (list, tuple)):
+ return size[::-1]
+ else:
+ return get_size_with_aspect_ratio(image_size, size, max_size)
+
+ size = get_size(image.size, size, max_size)
+ rescaled_image = F.resize(image, size)
+
+ if target is None:
+ return rescaled_image, None
+
+ ratios = tuple(float(s) / float(s_orig) for s, s_orig in zip(rescaled_image.size, image.size))
+ ratio_width, ratio_height = ratios
+
+ target = target.copy()
+ if "boxes" in target:
+ boxes = target["boxes"]
+ scaled_boxes = boxes * torch.as_tensor([ratio_width, ratio_height, ratio_width, ratio_height])
+ target["boxes"] = scaled_boxes
+
+ if "area" in target:
+ area = target["area"]
+ scaled_area = area * (ratio_width * ratio_height)
+ target["area"] = scaled_area
+
+
+ if "keypoints" in target:
+ keypoints = target["keypoints"]
+ scaled_keypoints = keypoints * torch.as_tensor([ratio_width, ratio_height, 1])
+ target["keypoints"] = scaled_keypoints
+
+ h, w = size
+ target["size"] = torch.tensor([h, w])
+
+ if "masks" in target:
+ target['masks'] = interpolate(
+ target['masks'][:, None].float(), size, mode="nearest")[:, 0] > 0.5
+
+ return rescaled_image, target
+
+
+def pad(image, target, padding):
+ # assumes that we only pad on the bottom right corners
+ padded_image = F.pad(image, (0, 0, padding[0], padding[1]))
+ if target is None:
+ return padded_image, None
+ target = target.copy()
+ # should we do something wrt the original size?
+ target["size"] = torch.tensor(padded_image.size[::-1])
+ if "masks" in target:
+ target['masks'] = torch.nn.functional.pad(target['masks'], (0, padding[0], 0, padding[1]))
+ return padded_image, target
+
+
+class ResizeDebug(object):
+ def __init__(self, size):
+ self.size = size
+
+ def __call__(self, img, target):
+ return resize(img, target, self.size)
+
+
+class RandomCrop(object):
+ def __init__(self, size):
+ self.size = size
+
+ def __call__(self, img, target):
+ region = T.RandomCrop.get_params(img, self.size)
+ return crop(img, target, region)
+
+
+class RandomSizeCrop(object):
+ def __init__(self, min_size: int, max_size: int, respect_boxes: bool = False):
+ # respect_boxes: True to keep all boxes
+ # False to tolerence box filter
+ self.min_size = min_size
+ self.max_size = max_size
+ self.respect_boxes = respect_boxes
+
+ def __call__(self, img: PIL.Image.Image, target: dict):
+ init_boxes = len(target["boxes"]) if (target is not None and "boxes" in target) else 0
+ max_patience = 10
+ for i in range(max_patience):
+ w = random.randint(self.min_size, min(img.width, self.max_size))
+ h = random.randint(self.min_size, min(img.height, self.max_size))
+ region = T.RandomCrop.get_params(img, [h, w])
+ result_img, result_target = crop(img, target, region)
+ if target is not None:
+ if not self.respect_boxes or len(result_target["boxes"]) == init_boxes or i == max_patience - 1:
+ return result_img, result_target
+ return result_img, result_target
+
+
+class CenterCrop(object):
+ def __init__(self, size):
+ self.size = size
+
+ def __call__(self, img, target):
+ image_width, image_height = img.size
+ crop_height, crop_width = self.size
+ crop_top = int(round((image_height - crop_height) / 2.))
+ crop_left = int(round((image_width - crop_width) / 2.))
+ return crop(img, target, (crop_top, crop_left, crop_height, crop_width))
+
+
+class RandomHorizontalFlip(object):
+ def __init__(self, p=0.5):
+ self.p = p
+
+ def __call__(self, img, target):
+ if random.random() < self.p:
+ return hflip(img, target)
+ return img, target
+
+
+class RandomResize(object):
+ def __init__(self, sizes, max_size=None):
+ assert isinstance(sizes, (list, tuple))
+ self.sizes = sizes
+ self.max_size = max_size
+
+ def __call__(self, img, target=None):
+ size = random.choice(self.sizes)
+ return resize(img, target, size, self.max_size)
+
+
+class RandomPad(object):
+ def __init__(self, max_pad):
+ self.max_pad = max_pad
+
+ def __call__(self, img, target):
+ pad_x = random.randint(0, self.max_pad)
+ pad_y = random.randint(0, self.max_pad)
+ return pad(img, target, (pad_x, pad_y))
+
+
+class RandomSelect(object):
+ """
+ Randomly selects between transforms1 and transforms2,
+ with probability p for transforms1 and (1 - p) for transforms2
+ """
+ def __init__(self, transforms1, transforms2, p=0.5):
+ self.transforms1 = transforms1
+ self.transforms2 = transforms2
+ self.p = p
+
+ def __call__(self, img, target):
+ if random.random() < self.p:
+ return self.transforms1(img, target)
+ return self.transforms2(img, target)
+
+
+class ToTensor(object):
+ def __call__(self, img, target):
+ return F.to_tensor(img), target
+
+
+class RandomErasing(object):
+
+ def __init__(self, *args, **kwargs):
+ self.eraser = T.RandomErasing(*args, **kwargs)
+
+ def __call__(self, img, target):
+ return self.eraser(img), target
+
+
+class Normalize(object):
+ def __init__(self, mean, std):
+ self.mean = mean
+ self.std = std
+
+ def __call__(self, image, target=None):
+ image = F.normalize(image, mean=self.mean, std=self.std)
+ if target is None:
+ return image, None
+ target = target.copy()
+ h, w = image.shape[-2:]
+ if "boxes" in target:
+ boxes = target["boxes"]
+ boxes = box_xyxy_to_cxcywh(boxes)
+ boxes = boxes / torch.tensor([w, h, w, h], dtype=torch.float32)
+ target["boxes"] = boxes
+
+ if "area" in target:
+ area = target["area"]
+ area = area / (torch.tensor(w, dtype=torch.float32)*torch.tensor(h, dtype=torch.float32))
+ target["area"] = area
+
+ if "keypoints" in target:
+ keypoints = target["keypoints"]
+ V = keypoints[:, :, 2]
+ V[V == 2] = 1
+ Z=keypoints[:, :, :2]
+ Z = Z.contiguous().view(-1, 2 * V.shape[-1])
+ Z = Z / torch.tensor([w, h] * V.shape[-1], dtype=torch.float32)
+ target["valid_kpt_num"] = V.shape[1]
+ Z_pad = torch.zeros(Z.shape[0],68 * 2 - Z.shape[1])
+ V_pad = torch.zeros(V.shape[0],68 - V.shape[1])
+ V=torch.cat([V, V_pad], dim=1)
+ Z=torch.cat([Z, Z_pad], dim=1)
+ all_keypoints = torch.cat([Z, V], dim=1)
+ target["keypoints"] = all_keypoints
+
+
+ return image, target
+
+
+class Compose(object):
+ def __init__(self, transforms):
+ self.transforms = transforms
+
+ def __call__(self, image, target):
+ for t in self.transforms:
+ image, target = t(image, target)
+ return image, target
+
+ def __repr__(self):
+ format_string = self.__class__.__name__ + "("
+ for t in self.transforms:
+ format_string += "\n"
+ format_string += " {0}".format(t)
+ format_string += "\n)"
+ return format_string
diff --git a/difpoint/src/models/XPose/util/__init__.py b/difpoint/src/models/XPose/util/__init__.py
new file mode 100644
index 0000000000000000000000000000000000000000..601cd005e85ae5bec92d05bf1e07b136d4fa6ee2
--- /dev/null
+++ b/difpoint/src/models/XPose/util/__init__.py
@@ -0,0 +1,6 @@
+# -*- coding: utf-8 -*-
+# @Time : 2024/8/5 21:58
+# @Author : shaoguowen
+# @Email : wenshaoguo1026@gmail.com
+# @Project : FasterLivePortrait
+# @FileName: __init__.py.py
diff --git a/difpoint/src/models/XPose/util/addict.py b/difpoint/src/models/XPose/util/addict.py
new file mode 100644
index 0000000000000000000000000000000000000000..2afda4c744255685afcbce82b120f7c7de58238d
--- /dev/null
+++ b/difpoint/src/models/XPose/util/addict.py
@@ -0,0 +1,159 @@
+import copy
+
+
+class Dict(dict):
+
+ def __init__(__self, *args, **kwargs):
+ object.__setattr__(__self, '__parent', kwargs.pop('__parent', None))
+ object.__setattr__(__self, '__key', kwargs.pop('__key', None))
+ object.__setattr__(__self, '__frozen', False)
+ for arg in args:
+ if not arg:
+ continue
+ elif isinstance(arg, dict):
+ for key, val in arg.items():
+ __self[key] = __self._hook(val)
+ elif isinstance(arg, tuple) and (not isinstance(arg[0], tuple)):
+ __self[arg[0]] = __self._hook(arg[1])
+ else:
+ for key, val in iter(arg):
+ __self[key] = __self._hook(val)
+
+ for key, val in kwargs.items():
+ __self[key] = __self._hook(val)
+
+ def __setattr__(self, name, value):
+ if hasattr(self.__class__, name):
+ raise AttributeError("'Dict' object attribute "
+ "'{0}' is read-only".format(name))
+ else:
+ self[name] = value
+
+ def __setitem__(self, name, value):
+ isFrozen = (hasattr(self, '__frozen') and
+ object.__getattribute__(self, '__frozen'))
+ if isFrozen and name not in super(Dict, self).keys():
+ raise KeyError(name)
+ super(Dict, self).__setitem__(name, value)
+ try:
+ p = object.__getattribute__(self, '__parent')
+ key = object.__getattribute__(self, '__key')
+ except AttributeError:
+ p = None
+ key = None
+ if p is not None:
+ p[key] = self
+ object.__delattr__(self, '__parent')
+ object.__delattr__(self, '__key')
+
+ def __add__(self, other):
+ if not self.keys():
+ return other
+ else:
+ self_type = type(self).__name__
+ other_type = type(other).__name__
+ msg = "unsupported operand type(s) for +: '{}' and '{}'"
+ raise TypeError(msg.format(self_type, other_type))
+
+ @classmethod
+ def _hook(cls, item):
+ if isinstance(item, dict):
+ return cls(item)
+ elif isinstance(item, (list, tuple)):
+ return type(item)(cls._hook(elem) for elem in item)
+ return item
+
+ def __getattr__(self, item):
+ return self.__getitem__(item)
+
+ def __missing__(self, name):
+ if object.__getattribute__(self, '__frozen'):
+ raise KeyError(name)
+ return self.__class__(__parent=self, __key=name)
+
+ def __delattr__(self, name):
+ del self[name]
+
+ def to_dict(self):
+ base = {}
+ for key, value in self.items():
+ if isinstance(value, type(self)):
+ base[key] = value.to_dict()
+ elif isinstance(value, (list, tuple)):
+ base[key] = type(value)(
+ item.to_dict() if isinstance(item, type(self)) else
+ item for item in value)
+ else:
+ base[key] = value
+ return base
+
+ def copy(self):
+ return copy.copy(self)
+
+ def deepcopy(self):
+ return copy.deepcopy(self)
+
+ def __deepcopy__(self, memo):
+ other = self.__class__()
+ memo[id(self)] = other
+ for key, value in self.items():
+ other[copy.deepcopy(key, memo)] = copy.deepcopy(value, memo)
+ return other
+
+ def update(self, *args, **kwargs):
+ other = {}
+ if args:
+ if len(args) > 1:
+ raise TypeError()
+ other.update(args[0])
+ other.update(kwargs)
+ for k, v in other.items():
+ if ((k not in self) or
+ (not isinstance(self[k], dict)) or
+ (not isinstance(v, dict))):
+ self[k] = v
+ else:
+ self[k].update(v)
+
+ def __getnewargs__(self):
+ return tuple(self.items())
+
+ def __getstate__(self):
+ return self
+
+ def __setstate__(self, state):
+ self.update(state)
+
+ def __or__(self, other):
+ if not isinstance(other, (Dict, dict)):
+ return NotImplemented
+ new = Dict(self)
+ new.update(other)
+ return new
+
+ def __ror__(self, other):
+ if not isinstance(other, (Dict, dict)):
+ return NotImplemented
+ new = Dict(other)
+ new.update(self)
+ return new
+
+ def __ior__(self, other):
+ self.update(other)
+ return self
+
+ def setdefault(self, key, default=None):
+ if key in self:
+ return self[key]
+ else:
+ self[key] = default
+ return default
+
+ def freeze(self, shouldFreeze=True):
+ object.__setattr__(self, '__frozen', shouldFreeze)
+ for key, val in self.items():
+ if isinstance(val, Dict):
+ val.freeze(shouldFreeze)
+
+ def unfreeze(self):
+ self.freeze(False)
diff --git a/difpoint/src/models/XPose/util/box_ops.py b/difpoint/src/models/XPose/util/box_ops.py
new file mode 100644
index 0000000000000000000000000000000000000000..ab2987cda4a683e40a40b46cb7a914a397fe9f44
--- /dev/null
+++ b/difpoint/src/models/XPose/util/box_ops.py
@@ -0,0 +1,139 @@
+# Copyright (c) Facebook, Inc. and its affiliates. All Rights Reserved
+"""
+Utilities for bounding box manipulation and GIoU.
+"""
+import torch, os
+from torchvision.ops.boxes import box_area
+
+
+def box_cxcywh_to_xyxy(x):
+ x_c, y_c, w, h = x.unbind(-1)
+ b = [(x_c - 0.5 * w), (y_c - 0.5 * h),
+ (x_c + 0.5 * w), (y_c + 0.5 * h)]
+ return torch.stack(b, dim=-1)
+
+
+def box_xyxy_to_cxcywh(x):
+ x0, y0, x1, y1 = x.unbind(-1)
+ b = [(x0 + x1) / 2, (y0 + y1) / 2,
+ (x1 - x0), (y1 - y0)]
+ return torch.stack(b, dim=-1)
+
+
+# modified from torchvision to also return the union
+def box_iou(boxes1, boxes2):
+ area1 = box_area(boxes1)
+ area2 = box_area(boxes2)
+
+ # import ipdb; ipdb.set_trace()
+ lt = torch.max(boxes1[:, None, :2], boxes2[:, :2]) # [N,M,2]
+ rb = torch.min(boxes1[:, None, 2:], boxes2[:, 2:]) # [N,M,2]
+
+ wh = (rb - lt).clamp(min=0) # [N,M,2]
+ inter = wh[:, :, 0] * wh[:, :, 1] # [N,M]
+
+ union = area1[:, None] + area2 - inter
+
+ iou = inter / (union + 1e-6)
+ return iou, union
+
+
+def generalized_box_iou(boxes1, boxes2):
+ """
+ Generalized IoU from https://giou.stanford.edu/
+
+ The boxes should be in [x0, y0, x1, y1] format
+
+ Returns a [N, M] pairwise matrix, where N = len(boxes1)
+ and M = len(boxes2)
+ """
+ # degenerate boxes gives inf / nan results
+ # so do an early check
+ assert (boxes1[:, 2:] >= boxes1[:, :2]).all()
+ assert (boxes2[:, 2:] >= boxes2[:, :2]).all()
+ # except:
+ # import ipdb; ipdb.set_trace()
+ iou, union = box_iou(boxes1, boxes2)
+
+ lt = torch.min(boxes1[:, None, :2], boxes2[:, :2])
+ rb = torch.max(boxes1[:, None, 2:], boxes2[:, 2:])
+
+ wh = (rb - lt).clamp(min=0) # [N,M,2]
+ area = wh[:, :, 0] * wh[:, :, 1]
+
+ return iou - (area - union) / (area + 1e-6)
+
+
+
+# modified from torchvision to also return the union
+def box_iou_pairwise(boxes1, boxes2):
+ area1 = box_area(boxes1)
+ area2 = box_area(boxes2)
+
+ lt = torch.max(boxes1[:, :2], boxes2[:, :2]) # [N,2]
+ rb = torch.min(boxes1[:, 2:], boxes2[:, 2:]) # [N,2]
+
+ wh = (rb - lt).clamp(min=0) # [N,2]
+ inter = wh[:, 0] * wh[:, 1] # [N]
+
+ union = area1 + area2 - inter
+
+ iou = inter / union
+ return iou, union
+
+
+def generalized_box_iou_pairwise(boxes1, boxes2):
+ """
+ Generalized IoU from https://giou.stanford.edu/
+
+ Input:
+ - boxes1, boxes2: N,4
+ Output:
+ - giou: N, 4
+ """
+ # degenerate boxes gives inf / nan results
+ # so do an early check
+ assert (boxes1[:, 2:] >= boxes1[:, :2]).all()
+ assert (boxes2[:, 2:] >= boxes2[:, :2]).all()
+ assert boxes1.shape == boxes2.shape
+ iou, union = box_iou_pairwise(boxes1, boxes2) # N, 4
+
+ lt = torch.min(boxes1[:, :2], boxes2[:, :2])
+ rb = torch.max(boxes1[:, 2:], boxes2[:, 2:])
+
+ wh = (rb - lt).clamp(min=0) # [N,2]
+ area = wh[:, 0] * wh[:, 1]
+
+ return iou - (area - union) / area
+
+def masks_to_boxes(masks):
+ """Compute the bounding boxes around the provided masks
+
+ The masks should be in format [N, H, W] where N is the number of masks, (H, W) are the spatial dimensions.
+
+ Returns a [N, 4] tensors, with the boxes in xyxy format
+ """
+ if masks.numel() == 0:
+ return torch.zeros((0, 4), device=masks.device)
+
+ h, w = masks.shape[-2:]
+
+ y = torch.arange(0, h, dtype=torch.float)
+ x = torch.arange(0, w, dtype=torch.float)
+ y, x = torch.meshgrid(y, x)
+
+ x_mask = (masks * x.unsqueeze(0))
+ x_max = x_mask.flatten(1).max(-1)[0]
+ x_min = x_mask.masked_fill(~(masks.bool()), 1e8).flatten(1).min(-1)[0]
+
+ y_mask = (masks * y.unsqueeze(0))
+ y_max = y_mask.flatten(1).max(-1)[0]
+ y_min = y_mask.masked_fill(~(masks.bool()), 1e8).flatten(1).min(-1)[0]
+
+ return torch.stack([x_min, y_min, x_max, y_max], 1)
+
+if __name__ == '__main__':
+ x = torch.rand(5, 4)
+ y = torch.rand(3, 4)
+ iou, union = box_iou(x, y)
+ import ipdb; ipdb.set_trace()
diff --git a/difpoint/src/models/XPose/util/config.py b/difpoint/src/models/XPose/util/config.py
new file mode 100644
index 0000000000000000000000000000000000000000..913c3cd3084ed553b12027d7a7f58821adb21f39
--- /dev/null
+++ b/difpoint/src/models/XPose/util/config.py
@@ -0,0 +1,425 @@
+# ==========================================================
+# Modified from mmcv
+# ==========================================================
+import sys
+import os.path as osp
+import ast
+import tempfile
+import shutil
+from importlib import import_module
+from argparse import Action
+
+from .addict import Dict
+import os
+
+BASE_KEY = '_base_'
+DELETE_KEY = '_delete_'
+RESERVED_KEYS = ['filename', 'text', 'pretty_text', 'get', 'dump', 'merge_from_dict']
+
+
+def check_file_exist(filename, msg_tmpl='file "{}" does not exist'):
+ if not osp.isfile(filename):
+ raise FileNotFoundError(msg_tmpl.format(filename))
+
+class ConfigDict(Dict):
+
+ def __missing__(self, name):
+ raise KeyError(name)
+
+ def __getattr__(self, name):
+ try:
+ value = super(ConfigDict, self).__getattr__(name)
+ except KeyError:
+ ex = AttributeError(f"'{self.__class__.__name__}' object has no "
+ f"attribute '{name}'")
+ except Exception as e:
+ ex = e
+ else:
+ return value
+ raise ex
+
+
+class Config(object):
+ """
+ config files.
+ only support .py file as config now.
+
+ ref: mmcv.utils.config
+
+ Example:
+ >>> cfg = Config(dict(a=1, b=dict(b1=[0, 1])))
+ >>> cfg.a
+ 1
+ >>> cfg.b
+ {'b1': [0, 1]}
+ >>> cfg.b.b1
+ [0, 1]
+ >>> cfg = Config.fromfile('tests/data/config/a.py')
+ >>> cfg.filename
+ "/home/kchen/projects/mmcv/tests/data/config/a.py"
+ >>> cfg.item4
+ 'test'
+ >>> cfg
+ "Config [path: /home/kchen/projects/mmcv/tests/data/config/a.py]: "
+ "{'item1': [1, 2], 'item2': {'a': 0}, 'item3': True, 'item4': 'test'}"
+ """
+ @staticmethod
+ def _validate_py_syntax(filename):
+ with open(filename) as f:
+ content = f.read()
+ try:
+ ast.parse(content)
+ except SyntaxError:
+ raise SyntaxError('There are syntax errors in config '
+ f'file {filename}')
+
+ @staticmethod
+ def _file2dict(filename):
+ filename = osp.abspath(osp.expanduser(filename))
+ check_file_exist(filename)
+ if filename.lower().endswith('.py'):
+ with tempfile.TemporaryDirectory() as temp_config_dir:
+ # 使用 mkstemp 代替 NamedTemporaryFile
+ fd, temp_path = tempfile.mkstemp(dir=temp_config_dir, suffix='.py')
+ os.close(fd) # 立即关闭文件描述符
+ temp_config_name = os.path.basename(temp_path)
+ shutil.copyfile(filename, os.path.join(temp_config_dir, temp_config_name))
+ temp_module_name = os.path.splitext(temp_config_name)[0]
+ sys.path.insert(0, temp_config_dir)
+ Config._validate_py_syntax(filename)
+ mod = import_module(temp_module_name)
+ sys.path.pop(0)
+ cfg_dict = {
+ name: value
+ for name, value in mod.__dict__.items()
+ if not name.startswith('__')
+ }
+ # delete imported module
+ del sys.modules[temp_module_name]
+ elif filename.lower().endswith(('.yml', '.yaml', '.json')):
+ from .slio import slload
+ cfg_dict = slload(filename)
+ else:
+ raise IOError('Only py/yml/yaml/json type are supported now!')
+
+ cfg_text = filename + '\n'
+ with open(filename, 'r') as f:
+ cfg_text += f.read()
+
+ # parse the base file
+ if BASE_KEY in cfg_dict:
+ cfg_dir = osp.dirname(filename)
+ base_filename = cfg_dict.pop(BASE_KEY)
+ base_filename = base_filename if isinstance(
+ base_filename, list) else [base_filename]
+
+ cfg_dict_list = list()
+ cfg_text_list = list()
+ for f in base_filename:
+ _cfg_dict, _cfg_text = Config._file2dict(osp.join(cfg_dir, f))
+ cfg_dict_list.append(_cfg_dict)
+ cfg_text_list.append(_cfg_text)
+
+ base_cfg_dict = dict()
+ for c in cfg_dict_list:
+ if len(base_cfg_dict.keys() & c.keys()) > 0:
+ raise KeyError('Duplicate key is not allowed among bases')
+ # TODO Allow the duplicate key while warnning user
+ base_cfg_dict.update(c)
+
+ base_cfg_dict = Config._merge_a_into_b(cfg_dict, base_cfg_dict)
+ cfg_dict = base_cfg_dict
+
+ # merge cfg_text
+ cfg_text_list.append(cfg_text)
+ cfg_text = '\n'.join(cfg_text_list)
+
+ return cfg_dict, cfg_text
+
+ @staticmethod
+ def _merge_a_into_b(a, b):
+ """merge dict `a` into dict `b` (non-inplace).
+ values in `a` will overwrite `b`.
+ copy first to avoid inplace modification
+
+ Args:
+ a ([type]): [description]
+ b ([type]): [description]
+
+ Returns:
+ [dict]: [description]
+ """
+ # import ipdb; ipdb.set_trace()
+ if not isinstance(a, dict):
+ return a
+
+ b = b.copy()
+ for k, v in a.items():
+ if isinstance(v, dict) and k in b and not v.pop(DELETE_KEY, False):
+
+ if not isinstance(b[k], dict) and not isinstance(b[k], list):
+ # if :
+ # import ipdb; ipdb.set_trace()
+ raise TypeError(
+ f'{k}={v} in child config cannot inherit from base '
+ f'because {k} is a dict in the child config but is of '
+ f'type {type(b[k])} in base config. You may set '
+ f'`{DELETE_KEY}=True` to ignore the base config')
+ b[k] = Config._merge_a_into_b(v, b[k])
+ elif isinstance(b, list):
+ try:
+ _ = int(k)
+ except:
+ raise TypeError(
+ f'b is a list, '
+ f'index {k} should be an int when input but {type(k)}'
+ )
+ b[int(k)] = Config._merge_a_into_b(v, b[int(k)])
+ else:
+ b[k] = v
+
+ return b
+
+ @staticmethod
+ def fromfile(filename):
+ cfg_dict, cfg_text = Config._file2dict(filename)
+ return Config(cfg_dict, cfg_text=cfg_text, filename=filename)
+
+
+ def __init__(self, cfg_dict=None, cfg_text=None, filename=None):
+ if cfg_dict is None:
+ cfg_dict = dict()
+ elif not isinstance(cfg_dict, dict):
+ raise TypeError('cfg_dict must be a dict, but '
+ f'got {type(cfg_dict)}')
+ for key in cfg_dict:
+ if key in RESERVED_KEYS:
+ raise KeyError(f'{key} is reserved for config file')
+
+ super(Config, self).__setattr__('_cfg_dict', ConfigDict(cfg_dict))
+ super(Config, self).__setattr__('_filename', filename)
+ if cfg_text:
+ text = cfg_text
+ elif filename:
+ with open(filename, 'r') as f:
+ text = f.read()
+ else:
+ text = ''
+ super(Config, self).__setattr__('_text', text)
+
+
+ @property
+ def filename(self):
+ return self._filename
+
+ @property
+ def text(self):
+ return self._text
+
+ @property
+ def pretty_text(self):
+
+ indent = 4
+
+ def _indent(s_, num_spaces):
+ s = s_.split('\n')
+ if len(s) == 1:
+ return s_
+ first = s.pop(0)
+ s = [(num_spaces * ' ') + line for line in s]
+ s = '\n'.join(s)
+ s = first + '\n' + s
+ return s
+
+ def _format_basic_types(k, v, use_mapping=False):
+ if isinstance(v, str):
+ v_str = f"'{v}'"
+ else:
+ v_str = str(v)
+
+ if use_mapping:
+ k_str = f"'{k}'" if isinstance(k, str) else str(k)
+ attr_str = f'{k_str}: {v_str}'
+ else:
+ attr_str = f'{str(k)}={v_str}'
+ attr_str = _indent(attr_str, indent)
+
+ return attr_str
+
+ def _format_list(k, v, use_mapping=False):
+ # check if all items in the list are dict
+ if all(isinstance(_, dict) for _ in v):
+ v_str = '[\n'
+ v_str += '\n'.join(
+ f'dict({_indent(_format_dict(v_), indent)}),'
+ for v_ in v).rstrip(',')
+ if use_mapping:
+ k_str = f"'{k}'" if isinstance(k, str) else str(k)
+ attr_str = f'{k_str}: {v_str}'
+ else:
+ attr_str = f'{str(k)}={v_str}'
+ attr_str = _indent(attr_str, indent) + ']'
+ else:
+ attr_str = _format_basic_types(k, v, use_mapping)
+ return attr_str
+
+ def _contain_invalid_identifier(dict_str):
+ contain_invalid_identifier = False
+ for key_name in dict_str:
+ contain_invalid_identifier |= \
+ (not str(key_name).isidentifier())
+ return contain_invalid_identifier
+
+ def _format_dict(input_dict, outest_level=False):
+ r = ''
+ s = []
+
+ use_mapping = _contain_invalid_identifier(input_dict)
+ if use_mapping:
+ r += '{'
+ for idx, (k, v) in enumerate(input_dict.items()):
+ is_last = idx >= len(input_dict) - 1
+ end = '' if outest_level or is_last else ','
+ if isinstance(v, dict):
+ v_str = '\n' + _format_dict(v)
+ if use_mapping:
+ k_str = f"'{k}'" if isinstance(k, str) else str(k)
+ attr_str = f'{k_str}: dict({v_str}'
+ else:
+ attr_str = f'{str(k)}=dict({v_str}'
+ attr_str = _indent(attr_str, indent) + ')' + end
+ elif isinstance(v, list):
+ attr_str = _format_list(k, v, use_mapping) + end
+ else:
+ attr_str = _format_basic_types(k, v, use_mapping) + end
+
+ s.append(attr_str)
+ r += '\n'.join(s)
+ if use_mapping:
+ r += '}'
+ return r
+
+ cfg_dict = self._cfg_dict.to_dict()
+ text = _format_dict(cfg_dict, outest_level=True)
+ return text
+
+
+ def __repr__(self):
+ return f'Config (path: {self.filename}): {self._cfg_dict.__repr__()}'
+
+ def __len__(self):
+ return len(self._cfg_dict)
+
+ def __getattr__(self, name):
+ # # debug
+ # print('+'*15)
+ # print('name=%s' % name)
+ # print("addr:", id(self))
+ # # print('type(self):', type(self))
+ # print(self.__dict__)
+ # print('+'*15)
+ # if self.__dict__ == {}:
+ # raise ValueError
+
+ return getattr(self._cfg_dict, name)
+
+ def __getitem__(self, name):
+ return self._cfg_dict.__getitem__(name)
+
+ def __setattr__(self, name, value):
+ if isinstance(value, dict):
+ value = ConfigDict(value)
+ self._cfg_dict.__setattr__(name, value)
+
+ def __setitem__(self, name, value):
+ if isinstance(value, dict):
+ value = ConfigDict(value)
+ self._cfg_dict.__setitem__(name, value)
+
+ def __iter__(self):
+ return iter(self._cfg_dict)
+
+ def dump(self, file=None):
+ # import ipdb; ipdb.set_trace()
+ if file is None:
+ return self.pretty_text
+ else:
+ with open(file, 'w') as f:
+ f.write(self.pretty_text)
+
+ def merge_from_dict(self, options):
+ """Merge list into cfg_dict
+
+ Merge the dict parsed by MultipleKVAction into this cfg.
+
+ Examples:
+ >>> options = {'model.backbone.depth': 50,
+ ... 'model.backbone.with_cp':True}
+ >>> cfg = Config(dict(model=dict(backbone=dict(type='ResNet'))))
+ >>> cfg.merge_from_dict(options)
+ >>> cfg_dict = super(Config, self).__getattribute__('_cfg_dict')
+ >>> assert cfg_dict == dict(
+ ... model=dict(backbone=dict(depth=50, with_cp=True)))
+
+ Args:
+ options (dict): dict of configs to merge from.
+ """
+ option_cfg_dict = {}
+ for full_key, v in options.items():
+ d = option_cfg_dict
+ key_list = full_key.split('.')
+ for subkey in key_list[:-1]:
+ d.setdefault(subkey, ConfigDict())
+ d = d[subkey]
+ subkey = key_list[-1]
+ d[subkey] = v
+
+ cfg_dict = super(Config, self).__getattribute__('_cfg_dict')
+ super(Config, self).__setattr__(
+ '_cfg_dict', Config._merge_a_into_b(option_cfg_dict, cfg_dict))
+
+ # for multiprocess
+ def __setstate__(self, state):
+ self.__init__(state)
+
+
+ def copy(self):
+ return Config(self._cfg_dict.copy())
+
+ def deepcopy(self):
+ return Config(self._cfg_dict.deepcopy())
+
+
+class DictAction(Action):
+ """
+ argparse action to split an argument into KEY=VALUE form
+ on the first = and append to a dictionary. List options should
+ be passed as comma separated values, i.e KEY=V1,V2,V3
+ """
+
+ @staticmethod
+ def _parse_int_float_bool(val):
+ try:
+ return int(val)
+ except ValueError:
+ pass
+ try:
+ return float(val)
+ except ValueError:
+ pass
+ if val.lower() in ['true', 'false']:
+ return True if val.lower() == 'true' else False
+ if val.lower() in ['none', 'null']:
+ return None
+ return val
+
+ def __call__(self, parser, namespace, values, option_string=None):
+ options = {}
+ for kv in values:
+ key, val = kv.split('=', maxsplit=1)
+ val = [self._parse_int_float_bool(v) for v in val.split(',')]
+ if len(val) == 1:
+ val = val[0]
+ options[key] = val
+ setattr(namespace, self.dest, options)
+
diff --git a/difpoint/src/models/XPose/util/keypoint_ops.py b/difpoint/src/models/XPose/util/keypoint_ops.py
new file mode 100644
index 0000000000000000000000000000000000000000..de9c43381bec7bcd63a1faf2e3fc89c0dfce6c8c
--- /dev/null
+++ b/difpoint/src/models/XPose/util/keypoint_ops.py
@@ -0,0 +1,29 @@
+import torch, os
+
+def keypoint_xyxyzz_to_xyzxyz(keypoints: torch.Tensor):
+ """_summary_
+
+ Args:
+ keypoints (torch.Tensor): ..., 51
+ """
+ res = torch.zeros_like(keypoints)
+ num_points = keypoints.shape[-1] // 3
+ Z = keypoints[..., :2*num_points]
+ V = keypoints[..., 2*num_points:]
+ res[...,0::3] = Z[..., 0::2]
+ res[...,1::3] = Z[..., 1::2]
+ res[...,2::3] = V[...]
+ return res
+
+def keypoint_xyzxyz_to_xyxyzz(keypoints: torch.Tensor):
+ """_summary_
+
+ Args:
+ keypoints (torch.Tensor): ..., 51
+ """
+ res = torch.zeros_like(keypoints)
+ num_points = keypoints.shape[-1] // 3
+ res[...,0:2*num_points:2] = keypoints[..., 0::3]
+ res[...,1:2*num_points:2] = keypoints[..., 1::3]
+ res[...,2*num_points:] = keypoints[..., 2::3]
+ return res
\ No newline at end of file
diff --git a/difpoint/src/models/XPose/util/misc.py b/difpoint/src/models/XPose/util/misc.py
new file mode 100644
index 0000000000000000000000000000000000000000..57eba72c3c18345e5235ebd36e86e83a2b673930
--- /dev/null
+++ b/difpoint/src/models/XPose/util/misc.py
@@ -0,0 +1,701 @@
+# Copyright (c) Facebook, Inc. and its affiliates. All Rights Reserved
+"""
+Misc functions, including distributed helpers.
+
+Mostly copy-paste from torchvision references.
+"""
+import functools
+import io
+import os
+import random
+import subprocess
+import time
+from collections import OrderedDict, defaultdict, deque
+import datetime
+import pickle
+from typing import Optional, List
+
+import json, time
+import numpy as np
+import torch
+import torch.distributed as dist
+from torch import Tensor
+
+import colorsys
+
+# needed due to empty tensor bug in pytorch and torchvision 0.5
+import torchvision
+__torchvision_need_compat_flag = float(torchvision.__version__.split('.')[1]) < 7
+if __torchvision_need_compat_flag:
+ from torchvision.ops import _new_empty_tensor
+ from torchvision.ops.misc import _output_size
+
+
+class SmoothedValue(object):
+ """Track a series of values and provide access to smoothed values over a
+ window or the global series average.
+ """
+
+ def __init__(self, window_size=20, fmt=None):
+ if fmt is None:
+ fmt = "{median:.4f} ({global_avg:.4f})"
+ self.deque = deque(maxlen=window_size)
+ self.total = 0.0
+ self.count = 0
+ self.fmt = fmt
+
+ def update(self, value, n=1):
+ self.deque.append(value)
+ self.count += n
+ self.total += value * n
+
+ def synchronize_between_processes(self):
+ """
+ Warning: does not synchronize the deque!
+ """
+ if not is_dist_avail_and_initialized():
+ return
+ t = torch.tensor([self.count, self.total], dtype=torch.float64, device='cuda')
+ dist.barrier()
+ dist.all_reduce(t)
+ t = t.tolist()
+ self.count = int(t[0])
+ self.total = t[1]
+
+ @property
+ def median(self):
+ d = torch.tensor(list(self.deque))
+ if d.shape[0] == 0:
+ return 0
+ return d.median().item()
+
+ @property
+ def avg(self):
+ d = torch.tensor(list(self.deque), dtype=torch.float32)
+ return d.mean().item()
+
+ @property
+ def global_avg(self):
+ if os.environ.get("SHILONG_AMP", None) == '1':
+ eps = 1e-4
+ else:
+ eps = 1e-6
+ return self.total / (self.count + eps)
+
+ @property
+ def max(self):
+ return max(self.deque)
+
+ @property
+ def value(self):
+ return self.deque[-1]
+
+ def __str__(self):
+ return self.fmt.format(
+ median=self.median,
+ avg=self.avg,
+ global_avg=self.global_avg,
+ max=self.max,
+ value=self.value)
+
+@functools.lru_cache()
+def _get_global_gloo_group():
+ """
+ Return a process group based on gloo backend, containing all the ranks
+ The result is cached.
+ """
+
+ if dist.get_backend() == "nccl":
+ return dist.new_group(backend="gloo")
+
+ return dist.group.WORLD
+
+def all_gather_cpu(data):
+ """
+ Run all_gather on arbitrary picklable data (not necessarily tensors)
+ Args:
+ data: any picklable object
+ Returns:
+ list[data]: list of data gathered from each rank
+ """
+
+ world_size = get_world_size()
+ if world_size == 1:
+ return [data]
+
+ cpu_group = _get_global_gloo_group()
+
+ buffer = io.BytesIO()
+ torch.save(data, buffer)
+ data_view = buffer.getbuffer()
+ device = "cuda" if cpu_group is None else "cpu"
+ tensor = torch.ByteTensor(data_view).to(device)
+
+ # obtain Tensor size of each rank
+ local_size = torch.tensor([tensor.numel()], device=device, dtype=torch.long)
+ size_list = [torch.tensor([0], device=device, dtype=torch.long) for _ in range(world_size)]
+ if cpu_group is None:
+ dist.all_gather(size_list, local_size)
+ else:
+ print("gathering on cpu")
+ dist.all_gather(size_list, local_size, group=cpu_group)
+ size_list = [int(size.item()) for size in size_list]
+ max_size = max(size_list)
+ assert isinstance(local_size.item(), int)
+ local_size = int(local_size.item())
+
+ # receiving Tensor from all ranks
+ # we pad the tensor because torch all_gather does not support
+ # gathering tensors of different shapes
+ tensor_list = []
+ for _ in size_list:
+ tensor_list.append(torch.empty((max_size,), dtype=torch.uint8, device=device))
+ if local_size != max_size:
+ padding = torch.empty(size=(max_size - local_size,), dtype=torch.uint8, device=device)
+ tensor = torch.cat((tensor, padding), dim=0)
+ if cpu_group is None:
+ dist.all_gather(tensor_list, tensor)
+ else:
+ dist.all_gather(tensor_list, tensor, group=cpu_group)
+
+ data_list = []
+ for size, tensor in zip(size_list, tensor_list):
+ tensor = torch.split(tensor, [size, max_size - size], dim=0)[0]
+ buffer = io.BytesIO(tensor.cpu().numpy())
+ obj = torch.load(buffer)
+ data_list.append(obj)
+
+ return data_list
+
+
+def all_gather(data):
+ """
+ Run all_gather on arbitrary picklable data (not necessarily tensors)
+ Args:
+ data: any picklable object
+ Returns:
+ list[data]: list of data gathered from each rank
+ """
+
+ if os.getenv("CPU_REDUCE") == "1":
+ return all_gather_cpu(data)
+
+
+
+ world_size = get_world_size()
+ if world_size == 1:
+ return [data]
+
+ # serialized to a Tensor
+ buffer = pickle.dumps(data)
+ storage = torch.ByteStorage.from_buffer(buffer)
+ tensor = torch.ByteTensor(storage).to("cuda")
+
+ # obtain Tensor size of each rank
+ local_size = torch.tensor([tensor.numel()], device="cuda")
+ size_list = [torch.tensor([0], device="cuda") for _ in range(world_size)]
+ dist.all_gather(size_list, local_size)
+ size_list = [int(size.item()) for size in size_list]
+ max_size = max(size_list)
+
+ # receiving Tensor from all ranks
+ # we pad the tensor because torch all_gather does not support
+ # gathering tensors of different shapes
+ tensor_list = []
+ for _ in size_list:
+ tensor_list.append(torch.empty((max_size,), dtype=torch.uint8, device="cuda"))
+ if local_size != max_size:
+ padding = torch.empty(size=(max_size - local_size,), dtype=torch.uint8, device="cuda")
+ tensor = torch.cat((tensor, padding), dim=0)
+ dist.all_gather(tensor_list, tensor)
+
+ data_list = []
+ for size, tensor in zip(size_list, tensor_list):
+ buffer = tensor.cpu().numpy().tobytes()[:size]
+ data_list.append(pickle.loads(buffer))
+
+ return data_list
+
+
+def reduce_dict(input_dict, average=True):
+ """
+ Args:
+ input_dict (dict): all the values will be reduced
+ average (bool): whether to do average or sum
+ Reduce the values in the dictionary from all processes so that all processes
+ have the averaged results. Returns a dict with the same fields as
+ input_dict, after reduction.
+ """
+ world_size = get_world_size()
+ if world_size < 2:
+ return input_dict
+ with torch.no_grad():
+ names = []
+ values = []
+ # sort the keys so that they are consistent across processes
+ for k in sorted(input_dict.keys()):
+ names.append(k)
+ values.append(input_dict[k])
+ values = torch.stack(values, dim=0)
+ dist.all_reduce(values)
+ if average:
+ values /= world_size
+ reduced_dict = {k: v for k, v in zip(names, values)}
+ return reduced_dict
+
+
+class MetricLogger(object):
+ def __init__(self, delimiter="\t"):
+ self.meters = defaultdict(SmoothedValue)
+ self.delimiter = delimiter
+
+ def update(self, **kwargs):
+ for k, v in kwargs.items():
+ if isinstance(v, torch.Tensor):
+ v = v.item()
+ assert isinstance(v, (float, int))
+ self.meters[k].update(v)
+
+ def __getattr__(self, attr):
+ if attr in self.meters:
+ return self.meters[attr]
+ if attr in self.__dict__:
+ return self.__dict__[attr]
+ raise AttributeError("'{}' object has no attribute '{}'".format(
+ type(self).__name__, attr))
+
+ def __str__(self):
+ loss_str = []
+ for name, meter in self.meters.items():
+ # print(name, str(meter))
+ # import ipdb;ipdb.set_trace()
+ if meter.count > 0:
+ loss_str.append(
+ "{}: {}".format(name, str(meter))
+ )
+ return self.delimiter.join(loss_str)
+
+ def synchronize_between_processes(self):
+ for meter in self.meters.values():
+ meter.synchronize_between_processes()
+
+ def add_meter(self, name, meter):
+ self.meters[name] = meter
+
+ def log_every(self, iterable, print_freq, header=None, logger=None):
+ if logger is None:
+ print_func = print
+ else:
+ print_func = logger.info
+
+ i = 0
+ if not header:
+ header = ''
+ start_time = time.time()
+ end = time.time()
+ iter_time = SmoothedValue(fmt='{avg:.4f}')
+ data_time = SmoothedValue(fmt='{avg:.4f}')
+ space_fmt = ':' + str(len(str(len(iterable)))) + 'd'
+ if torch.cuda.is_available():
+ log_msg = self.delimiter.join([
+ header,
+ '[{0' + space_fmt + '}/{1}]',
+ 'eta: {eta}',
+ '{meters}',
+ 'time: {time}',
+ 'data: {data}',
+ 'max mem: {memory:.0f}'
+ ])
+ else:
+ log_msg = self.delimiter.join([
+ header,
+ '[{0' + space_fmt + '}/{1}]',
+ 'eta: {eta}',
+ '{meters}',
+ 'time: {time}',
+ 'data: {data}'
+ ])
+ MB = 1024.0 * 1024.0
+ for obj in iterable:
+ data_time.update(time.time() - end)
+ yield obj
+ # import ipdb; ipdb.set_trace()
+ iter_time.update(time.time() - end)
+ if i % print_freq == 0 or i == len(iterable) - 1:
+ eta_seconds = iter_time.global_avg * (len(iterable) - i)
+ eta_string = str(datetime.timedelta(seconds=int(eta_seconds)))
+ if torch.cuda.is_available():
+ print_func(log_msg.format(
+ i, len(iterable), eta=eta_string,
+ meters=str(self),
+ time=str(iter_time), data=str(data_time),
+ memory=torch.cuda.max_memory_allocated() / MB))
+ else:
+ print_func(log_msg.format(
+ i, len(iterable), eta=eta_string,
+ meters=str(self),
+ time=str(iter_time), data=str(data_time)))
+ i += 1
+ end = time.time()
+ total_time = time.time() - start_time
+ total_time_str = str(datetime.timedelta(seconds=int(total_time)))
+ print_func('{} Total time: {} ({:.4f} s / it)'.format(
+ header, total_time_str, total_time / len(iterable)))
+
+
+def get_sha():
+ cwd = os.path.dirname(os.path.abspath(__file__))
+
+ def _run(command):
+ return subprocess.check_output(command, cwd=cwd).decode('ascii').strip()
+ sha = 'N/A'
+ diff = "clean"
+ branch = 'N/A'
+ try:
+ sha = _run(['git', 'rev-parse', 'HEAD'])
+ subprocess.check_output(['git', 'diff'], cwd=cwd)
+ diff = _run(['git', 'diff-index', 'HEAD'])
+ diff = "has uncommited changes" if diff else "clean"
+ branch = _run(['git', 'rev-parse', '--abbrev-ref', 'HEAD'])
+ except Exception:
+ pass
+ message = f"sha: {sha}, status: {diff}, branch: {branch}"
+ return message
+
+
+def collate_fn(batch):
+ # import ipdb; ipdb.set_trace()
+ batch = list(zip(*batch))
+ batch[0] = nested_tensor_from_tensor_list(batch[0])
+ return tuple(batch)
+
+
+def _max_by_axis(the_list):
+ # type: (List[List[int]]) -> List[int]
+ maxes = the_list[0]
+ for sublist in the_list[1:]:
+ for index, item in enumerate(sublist):
+ maxes[index] = max(maxes[index], item)
+ return maxes
+
+
+class NestedTensor(object):
+ def __init__(self, tensors, mask: Optional[Tensor]):
+ self.tensors = tensors
+ self.mask = mask
+ if mask == 'auto':
+ self.mask = torch.zeros_like(tensors).to(tensors.device)
+ if self.mask.dim() == 3:
+ self.mask = self.mask.sum(0).to(bool)
+ elif self.mask.dim() == 4:
+ self.mask = self.mask.sum(1).to(bool)
+ else:
+ raise ValueError("tensors dim must be 3 or 4 but {}({})".format(self.tensors.dim(), self.tensors.shape))
+
+ def imgsize(self):
+ res = []
+ for i in range(self.tensors.shape[0]):
+ mask = self.mask[i]
+ maxH = (~mask).sum(0).max()
+ maxW = (~mask).sum(1).max()
+ res.append(torch.Tensor([maxH, maxW]))
+ return res
+
+ def to(self, device):
+ # type: (Device) -> NestedTensor # noqa
+ cast_tensor = self.tensors.to(device)
+ mask = self.mask
+ if mask is not None:
+ assert mask is not None
+ cast_mask = mask.to(device)
+ else:
+ cast_mask = None
+ return NestedTensor(cast_tensor, cast_mask)
+
+ def to_img_list_single(self, tensor, mask):
+ assert tensor.dim() == 3, "dim of tensor should be 3 but {}".format(tensor.dim())
+ maxH = (~mask).sum(0).max()
+ maxW = (~mask).sum(1).max()
+ img = tensor[:, :maxH, :maxW]
+ return img
+
+ def to_img_list(self):
+ """remove the padding and convert to img list
+
+ Returns:
+ [type]: [description]
+ """
+ if self.tensors.dim() == 3:
+ return self.to_img_list_single(self.tensors, self.mask)
+ else:
+ res = []
+ for i in range(self.tensors.shape[0]):
+ tensor_i = self.tensors[i]
+ mask_i = self.mask[i]
+ res.append(self.to_img_list_single(tensor_i, mask_i))
+ return res
+
+ @property
+ def device(self):
+ return self.tensors.device
+
+ def decompose(self):
+ return self.tensors, self.mask
+
+ def __repr__(self):
+ return str(self.tensors)
+
+ @property
+ def shape(self):
+ return {
+ 'tensors.shape': self.tensors.shape,
+ 'mask.shape': self.mask.shape
+ }
+
+
+def nested_tensor_from_tensor_list(tensor_list: List[Tensor]):
+ # TODO make this more general
+ if tensor_list[0].ndim == 3:
+ if torchvision._is_tracing():
+ # nested_tensor_from_tensor_list() does not export well to ONNX
+ # call _onnx_nested_tensor_from_tensor_list() instead
+ return _onnx_nested_tensor_from_tensor_list(tensor_list)
+
+ # TODO make it support different-sized images
+ max_size = _max_by_axis([list(img.shape) for img in tensor_list])
+ # min_size = tuple(min(s) for s in zip(*[img.shape for img in tensor_list]))
+ batch_shape = [len(tensor_list)] + max_size
+ b, c, h, w = batch_shape
+ dtype = tensor_list[0].dtype
+ device = tensor_list[0].device
+ tensor = torch.zeros(batch_shape, dtype=dtype, device=device)
+ mask = torch.ones((b, h, w), dtype=torch.bool, device=device)
+ for img, pad_img, m in zip(tensor_list, tensor, mask):
+ pad_img[: img.shape[0], : img.shape[1], : img.shape[2]].copy_(img)
+ m[: img.shape[1], :img.shape[2]] = False
+ else:
+ raise ValueError('not supported')
+ return NestedTensor(tensor, mask)
+
+
+# _onnx_nested_tensor_from_tensor_list() is an implementation of
+# nested_tensor_from_tensor_list() that is supported by ONNX tracing.
+@torch.jit.unused
+def _onnx_nested_tensor_from_tensor_list(tensor_list: List[Tensor]) -> NestedTensor:
+ max_size = []
+ for i in range(tensor_list[0].dim()):
+ max_size_i = torch.max(torch.stack([img.shape[i] for img in tensor_list]).to(torch.float32)).to(torch.int64)
+ max_size.append(max_size_i)
+ max_size = tuple(max_size)
+
+ # work around for
+ # pad_img[: img.shape[0], : img.shape[1], : img.shape[2]].copy_(img)
+ # m[: img.shape[1], :img.shape[2]] = False
+ # which is not yet supported in onnx
+ padded_imgs = []
+ padded_masks = []
+ for img in tensor_list:
+ padding = [(s1 - s2) for s1, s2 in zip(max_size, tuple(img.shape))]
+ padded_img = torch.nn.functional.pad(img, (0, padding[2], 0, padding[1], 0, padding[0]))
+ padded_imgs.append(padded_img)
+
+ m = torch.zeros_like(img[0], dtype=torch.int, device=img.device)
+ padded_mask = torch.nn.functional.pad(m, (0, padding[2], 0, padding[1]), "constant", 1)
+ padded_masks.append(padded_mask.to(torch.bool))
+
+ tensor = torch.stack(padded_imgs)
+ mask = torch.stack(padded_masks)
+
+ return NestedTensor(tensor, mask=mask)
+
+
+def setup_for_distributed(is_master):
+ """
+ This function disables printing when not in master process
+ """
+ import builtins as __builtin__
+ builtin_print = __builtin__.print
+
+ def print(*args, **kwargs):
+ force = kwargs.pop('force', False)
+ if is_master or force:
+ builtin_print(*args, **kwargs)
+
+ __builtin__.print = print
+
+
+def is_dist_avail_and_initialized():
+ if not dist.is_available():
+ return False
+ if not dist.is_initialized():
+ return False
+ return True
+
+
+def get_world_size():
+ if not is_dist_avail_and_initialized():
+ return 1
+ return dist.get_world_size()
+
+
+def get_rank():
+ if not is_dist_avail_and_initialized():
+ return 0
+ return dist.get_rank()
+
+
+def is_main_process():
+ return get_rank() == 0
+
+
+def save_on_master(*args, **kwargs):
+ if is_main_process():
+ torch.save(*args, **kwargs)
+
+def init_distributed_mode(args):
+ if 'WORLD_SIZE' in os.environ and os.environ['WORLD_SIZE'] != '': # 'RANK' in os.environ and
+ args.rank = int(os.environ["RANK"])
+ args.world_size = int(os.environ['WORLD_SIZE'])
+ args.gpu = args.local_rank = int(os.environ['LOCAL_RANK'])
+
+ # launch by torch.distributed.launch
+ # Single node
+ # python -m torch.distributed.launch --nproc_per_node=8 main.py --world-size 1 --rank 0 ...
+ # Multi nodes
+ # python -m torch.distributed.launch --nproc_per_node=8 main.py --world-size 2 --rank 0 --dist-url 'tcp://IP_OF_NODE0:FREEPORT' ...
+ # python -m torch.distributed.launch --nproc_per_node=8 main.py --world-size 2 --rank 1 --dist-url 'tcp://IP_OF_NODE0:FREEPORT' ...
+ # args.rank = int(os.environ.get('OMPI_COMM_WORLD_RANK'))
+ # local_world_size = int(os.environ['GPU_PER_NODE_COUNT'])
+ # args.world_size = args.world_size * local_world_size
+ # args.gpu = args.local_rank = int(os.environ['LOCAL_RANK'])
+ # args.rank = args.rank * local_world_size + args.local_rank
+ print('world size: {}, rank: {}, local rank: {}'.format(args.world_size, args.rank, args.local_rank))
+ print(json.dumps(dict(os.environ), indent=2))
+ elif 'SLURM_PROCID' in os.environ:
+ args.rank = int(os.environ['SLURM_PROCID'])
+ args.gpu = args.local_rank = int(os.environ['SLURM_LOCALID'])
+ args.world_size = int(os.environ['SLURM_NPROCS'])
+
+ if os.environ.get('HAND_DEFINE_DIST_URL', 0) == '1':
+ pass
+ else:
+ import util.hostlist as uh
+ nodenames = uh.parse_nodelist(os.environ['SLURM_JOB_NODELIST'])
+ gpu_ids = [int(node[3:]) for node in nodenames]
+ fixid = int(os.environ.get('FIX_DISTRIBUTED_PORT_NUMBER', 0))
+ # fixid += random.randint(0, 300)
+ port = str(3137 + int(min(gpu_ids)) + fixid)
+ args.dist_url = "tcp://{ip}:{port}".format(ip=uh.nodename_to_ip(nodenames[0]), port=port)
+
+ print('world size: {}, world rank: {}, local rank: {}, device_count: {}'.format(args.world_size, args.rank, args.local_rank, torch.cuda.device_count()))
+
+
+ else:
+ print('Not using distributed mode')
+ args.distributed = False
+ args.world_size = 1
+ args.rank = 0
+ args.local_rank = 0
+ return
+
+ print("world_size:{} rank:{} local_rank:{}".format(args.world_size, args.rank, args.local_rank))
+ args.distributed = True
+ torch.cuda.set_device(args.local_rank)
+ args.dist_backend = 'nccl'
+ print('| distributed init (rank {}): {}'.format(args.rank, args.dist_url), flush=True)
+
+ torch.distributed.init_process_group(
+ backend=args.dist_backend,
+ world_size=args.world_size,
+ rank=args.rank,
+ init_method=args.dist_url,
+ )
+
+ print("Before torch.distributed.barrier()")
+ torch.distributed.barrier()
+ print("End torch.distributed.barrier()")
+ setup_for_distributed(args.rank == 0)
+
+
+@torch.no_grad()
+def accuracy(output, target, topk=(1,)):
+ """Computes the precision@k for the specified values of k"""
+ if target.numel() == 0:
+ return [torch.zeros([], device=output.device)]
+ maxk = max(topk)
+ batch_size = target.size(0)
+
+ _, pred = output.topk(maxk, 1, True, True)
+ pred = pred.t()
+ correct = pred.eq(target.view(1, -1).expand_as(pred))
+
+ res = []
+ for k in topk:
+ correct_k = correct[:k].view(-1).float().sum(0)
+ res.append(correct_k.mul_(100.0 / batch_size))
+ return res
+
+@torch.no_grad()
+def accuracy_onehot(pred, gt):
+ """_summary_
+
+ Args:
+ pred (_type_): n, c
+ gt (_type_): n, c
+ """
+ tp = ((pred - gt).abs().sum(-1) < 1e-4).float().sum()
+ acc = tp / gt.shape[0] * 100
+ return acc
+
+
+
+
+
+def interpolate(input, size=None, scale_factor=None, mode="nearest", align_corners=None):
+ # type: (Tensor, Optional[List[int]], Optional[float], str, Optional[bool]) -> Tensor
+ """
+ Equivalent to nn.functional.interpolate, but with support for empty batch sizes.
+ This will eventually be supported natively by PyTorch, and this
+ class can go away.
+ """
+ if __torchvision_need_compat_flag < 0.7:
+ if input.numel() > 0:
+ return torch.nn.functional.interpolate(
+ input, size, scale_factor, mode, align_corners
+ )
+
+ output_shape = _output_size(2, input, size, scale_factor)
+ output_shape = list(input.shape[:-2]) + list(output_shape)
+ return _new_empty_tensor(input, output_shape)
+ else:
+ return torchvision.ops.misc.interpolate(input, size, scale_factor, mode, align_corners)
+
+
+
+class color_sys():
+ def __init__(self, num_colors) -> None:
+ self.num_colors = num_colors
+ colors=[]
+ for i in np.arange(0., 360., 360. / num_colors):
+ hue = i/360.
+ lightness = (50 + np.random.rand() * 10)/100.
+ saturation = (90 + np.random.rand() * 10)/100.
+ colors.append(tuple([int(j*255) for j in colorsys.hls_to_rgb(hue, lightness, saturation)]))
+ self.colors = colors
+
+ def __call__(self, idx):
+ return self.colors[idx]
+
+def inverse_sigmoid(x, eps=1e-3):
+ x = x.clamp(min=0, max=1)
+ x1 = x.clamp(min=eps)
+ x2 = (1 - x).clamp(min=eps)
+ return torch.log(x1/x2)
+
+def clean_state_dict(state_dict):
+ new_state_dict = OrderedDict()
+ for k, v in state_dict.items():
+ if k[:7] == 'module.':
+ k = k[7:] # remove `module.`
+ new_state_dict[k] = v
+ return new_state_dict
\ No newline at end of file
diff --git a/difpoint/src/models/__init__.py b/difpoint/src/models/__init__.py
new file mode 100644
index 0000000000000000000000000000000000000000..1e6d19fbab4ae0cea73b5b82e76b1569ed84d918
--- /dev/null
+++ b/difpoint/src/models/__init__.py
@@ -0,0 +1,13 @@
+# -*- coding: utf-8 -*-
+# @Author : wenshao
+# @Email : wenshaoguo1026@gmail.com
+# @Project : FasterLivePortrait
+# @FileName: __init__.py.py
+
+from .warping_spade_model import WarpingSpadeModel
+from .motion_extractor_model import MotionExtractorModel
+from .appearance_feature_extractor_model import AppearanceFeatureExtractorModel
+from .landmark_model import LandmarkModel
+from .face_analysis_model import FaceAnalysisModel
+from .stitching_model import StitchingModel
+from .mediapipe_face_model import MediaPipeFaceModel
diff --git a/difpoint/src/models/__pycache__/__init__.cpython-310.pyc b/difpoint/src/models/__pycache__/__init__.cpython-310.pyc
new file mode 100644
index 0000000000000000000000000000000000000000..fa9902f8ebc513eb17d3ced0f00b220cb2d77005
Binary files /dev/null and b/difpoint/src/models/__pycache__/__init__.cpython-310.pyc differ
diff --git a/difpoint/src/models/__pycache__/__init__.cpython-38.pyc b/difpoint/src/models/__pycache__/__init__.cpython-38.pyc
new file mode 100644
index 0000000000000000000000000000000000000000..4b28079d58d5c6d5578c968343a043643f3ece69
Binary files /dev/null and b/difpoint/src/models/__pycache__/__init__.cpython-38.pyc differ
diff --git a/difpoint/src/models/__pycache__/appearance_feature_extractor_model.cpython-310.pyc b/difpoint/src/models/__pycache__/appearance_feature_extractor_model.cpython-310.pyc
new file mode 100644
index 0000000000000000000000000000000000000000..0cc9e441ca86a5a7370db5869877ff7fd014b53d
Binary files /dev/null and b/difpoint/src/models/__pycache__/appearance_feature_extractor_model.cpython-310.pyc differ
diff --git a/difpoint/src/models/__pycache__/appearance_feature_extractor_model.cpython-38.pyc b/difpoint/src/models/__pycache__/appearance_feature_extractor_model.cpython-38.pyc
new file mode 100644
index 0000000000000000000000000000000000000000..d3a94b32129502235e6d9a10b881b8a1d310884a
Binary files /dev/null and b/difpoint/src/models/__pycache__/appearance_feature_extractor_model.cpython-38.pyc differ
diff --git a/difpoint/src/models/__pycache__/base_model.cpython-310.pyc b/difpoint/src/models/__pycache__/base_model.cpython-310.pyc
new file mode 100644
index 0000000000000000000000000000000000000000..0da3ec2533a8e2cd8dd191f2568a67d008d790fb
Binary files /dev/null and b/difpoint/src/models/__pycache__/base_model.cpython-310.pyc differ
diff --git a/difpoint/src/models/__pycache__/base_model.cpython-38.pyc b/difpoint/src/models/__pycache__/base_model.cpython-38.pyc
new file mode 100644
index 0000000000000000000000000000000000000000..e612b29f0a90791ab8dc6e9e2f0970f7d62bcc3d
Binary files /dev/null and b/difpoint/src/models/__pycache__/base_model.cpython-38.pyc differ
diff --git a/difpoint/src/models/__pycache__/face_analysis_model.cpython-310.pyc b/difpoint/src/models/__pycache__/face_analysis_model.cpython-310.pyc
new file mode 100644
index 0000000000000000000000000000000000000000..cd1a6fc66d7d9a39465e5194281402ebf0874821
Binary files /dev/null and b/difpoint/src/models/__pycache__/face_analysis_model.cpython-310.pyc differ
diff --git a/difpoint/src/models/__pycache__/face_analysis_model.cpython-38.pyc b/difpoint/src/models/__pycache__/face_analysis_model.cpython-38.pyc
new file mode 100644
index 0000000000000000000000000000000000000000..11effdc40b8e7e17eb239e8cc8520f5f7c5e7551
Binary files /dev/null and b/difpoint/src/models/__pycache__/face_analysis_model.cpython-38.pyc differ
diff --git a/difpoint/src/models/__pycache__/landmark_model.cpython-310.pyc b/difpoint/src/models/__pycache__/landmark_model.cpython-310.pyc
new file mode 100644
index 0000000000000000000000000000000000000000..2fcc7b96f1b89835648f327a3bee5f2913ea4404
Binary files /dev/null and b/difpoint/src/models/__pycache__/landmark_model.cpython-310.pyc differ
diff --git a/difpoint/src/models/__pycache__/landmark_model.cpython-38.pyc b/difpoint/src/models/__pycache__/landmark_model.cpython-38.pyc
new file mode 100644
index 0000000000000000000000000000000000000000..d4252c98eae9a47535f1e4fba3f7cf0428cd89ab
Binary files /dev/null and b/difpoint/src/models/__pycache__/landmark_model.cpython-38.pyc differ
diff --git a/difpoint/src/models/__pycache__/mediapipe_face_model.cpython-310.pyc b/difpoint/src/models/__pycache__/mediapipe_face_model.cpython-310.pyc
new file mode 100644
index 0000000000000000000000000000000000000000..e7965b7f5bff7adf67638b160d66ae30d68ad486
Binary files /dev/null and b/difpoint/src/models/__pycache__/mediapipe_face_model.cpython-310.pyc differ
diff --git a/difpoint/src/models/__pycache__/motion_extractor_model.cpython-310.pyc b/difpoint/src/models/__pycache__/motion_extractor_model.cpython-310.pyc
new file mode 100644
index 0000000000000000000000000000000000000000..3b816477978496f9408b4c3c7b545f153f6c7e39
Binary files /dev/null and b/difpoint/src/models/__pycache__/motion_extractor_model.cpython-310.pyc differ
diff --git a/difpoint/src/models/__pycache__/motion_extractor_model.cpython-38.pyc b/difpoint/src/models/__pycache__/motion_extractor_model.cpython-38.pyc
new file mode 100644
index 0000000000000000000000000000000000000000..e55f5d958c99e6a34ed79a6ad95285b5b00ce5d6
Binary files /dev/null and b/difpoint/src/models/__pycache__/motion_extractor_model.cpython-38.pyc differ
diff --git a/difpoint/src/models/__pycache__/predictor.cpython-310.pyc b/difpoint/src/models/__pycache__/predictor.cpython-310.pyc
new file mode 100644
index 0000000000000000000000000000000000000000..ef4faae4b745c840a202ca307db7d0cf47fb3c3c
Binary files /dev/null and b/difpoint/src/models/__pycache__/predictor.cpython-310.pyc differ
diff --git a/difpoint/src/models/__pycache__/predictor.cpython-38.pyc b/difpoint/src/models/__pycache__/predictor.cpython-38.pyc
new file mode 100644
index 0000000000000000000000000000000000000000..d0386beba624952c24cde0f612621b23d3a54419
Binary files /dev/null and b/difpoint/src/models/__pycache__/predictor.cpython-38.pyc differ
diff --git a/difpoint/src/models/__pycache__/stitching_model.cpython-310.pyc b/difpoint/src/models/__pycache__/stitching_model.cpython-310.pyc
new file mode 100644
index 0000000000000000000000000000000000000000..2246976e3ac7e5fec659e4c810cbe0e23a640d70
Binary files /dev/null and b/difpoint/src/models/__pycache__/stitching_model.cpython-310.pyc differ
diff --git a/difpoint/src/models/__pycache__/warping_spade_model.cpython-310.pyc b/difpoint/src/models/__pycache__/warping_spade_model.cpython-310.pyc
new file mode 100644
index 0000000000000000000000000000000000000000..fc19817b0a380603e0ede37b8e37acee28850f7e
Binary files /dev/null and b/difpoint/src/models/__pycache__/warping_spade_model.cpython-310.pyc differ
diff --git a/difpoint/src/models/__pycache__/warping_spade_model.cpython-38.pyc b/difpoint/src/models/__pycache__/warping_spade_model.cpython-38.pyc
new file mode 100644
index 0000000000000000000000000000000000000000..25343fa7b9747e3b25d9987856357a16696ed8ef
Binary files /dev/null and b/difpoint/src/models/__pycache__/warping_spade_model.cpython-38.pyc differ
diff --git a/difpoint/src/models/appearance_feature_extractor_model.py b/difpoint/src/models/appearance_feature_extractor_model.py
new file mode 100644
index 0000000000000000000000000000000000000000..84d50edb27fc5cac1e584a9c733fc0ceae0116eb
--- /dev/null
+++ b/difpoint/src/models/appearance_feature_extractor_model.py
@@ -0,0 +1,57 @@
+# -*- coding: utf-8 -*-
+# @Author : wenshao
+# @Email : wenshaoguo1026@gmail.com
+# @Project : FasterLivePortrait
+# @FileName: motion_extractor_model.py
+import pdb
+import numpy as np
+from .base_model import BaseModel
+import torch
+from torch.cuda import nvtx
+from .predictor import numpy_to_torch_dtype_dict
+
+
+class AppearanceFeatureExtractorModel(BaseModel):
+ """
+ AppearanceFeatureExtractorModel
+ """
+
+ def __init__(self, **kwargs):
+ super(AppearanceFeatureExtractorModel, self).__init__(**kwargs)
+ self.predict_type = kwargs.get("predict_type", "trt")
+ print(self.predict_type)
+
+ def input_process(self, *data):
+ img = data[0].astype(np.float32)
+ img /= 255.0
+ img = np.transpose(img, (2, 0, 1))
+ return img[None]
+
+ def output_process(self, *data):
+ return data[0]
+
+ def predict_trt(self, *data):
+ nvtx.range_push("forward")
+ feed_dict = {}
+ for i, inp in enumerate(self.predictor.inputs):
+ if isinstance(data[i], torch.Tensor):
+ feed_dict[inp['name']] = data[i]
+ else:
+ feed_dict[inp['name']] = torch.from_numpy(data[i]).to(device=self.device,
+ dtype=numpy_to_torch_dtype_dict[inp['dtype']])
+ preds_dict = self.predictor.predict(feed_dict, self.cudaStream)
+ outs = []
+ for i, out in enumerate(self.predictor.outputs):
+ outs.append(preds_dict[out["name"]].cpu().numpy())
+ nvtx.range_pop()
+ return outs
+
+ def predict(self, *data):
+ #data = self.input_process(*data)
+ data = data[0]
+ if self.predict_type == "trt":
+ preds = self.predict_trt(data)
+ else:
+ preds = self.predictor.predict(data)
+ outputs = self.output_process(*preds)
+ return outputs
diff --git a/difpoint/src/models/base_model.py b/difpoint/src/models/base_model.py
new file mode 100644
index 0000000000000000000000000000000000000000..4795c50565987e4b0e2e6265092aa0b77349d0cc
--- /dev/null
+++ b/difpoint/src/models/base_model.py
@@ -0,0 +1,49 @@
+import copy
+import torch
+from .predictor import get_predictor
+
+
+class BaseModel:
+ """
+ 模型预测的基类
+ """
+
+ def __init__(self, **kwargs):
+ self.kwargs = copy.deepcopy(kwargs)
+ self.predictor = get_predictor(**self.kwargs)
+ self.device = torch.cuda.current_device()
+ self.cudaStream = torch.cuda.current_stream().cuda_stream
+ self.predict_type = kwargs.get("predict_type", "trt")
+
+ if self.predictor is not None:
+ self.input_shapes = self.predictor.input_spec()
+ self.output_shapes = self.predictor.output_spec()
+
+ def input_process(self, *data):
+ """
+ 输入预处理
+ :return:
+ """
+ pass
+
+ def output_process(self, *data):
+ """
+ 输出后处理
+ :return:
+ """
+ pass
+
+ def predict(self, *data):
+ """
+ 预测
+ :return:
+ """
+ pass
+
+ def __del__(self):
+ """
+ 删除实例
+ :return:
+ """
+ if self.predictor is not None:
+ del self.predictor
diff --git a/difpoint/src/models/face_analysis_model.py b/difpoint/src/models/face_analysis_model.py
new file mode 100644
index 0000000000000000000000000000000000000000..5846cbad6b88bf66cebce5e8c0d9d1e8259b0aa3
--- /dev/null
+++ b/difpoint/src/models/face_analysis_model.py
@@ -0,0 +1,326 @@
+# -*- coding: utf-8 -*-
+# @Author : wenshao
+# @Email : wenshaoguo0611@gmail.com
+# @Project : FasterLivePortrait
+# @FileName: face_analysis_model.py
+import pdb
+
+import numpy as np
+from insightface.app.common import Face
+import cv2
+from .predictor import get_predictor
+from ..utils import face_align
+import torch
+from torch.cuda import nvtx
+from .predictor import numpy_to_torch_dtype_dict
+
+
+def sort_by_direction(faces, direction: str = 'large-small', face_center=None):
+ if len(faces) <= 0:
+ return faces
+
+ if direction == 'left-right':
+ return sorted(faces, key=lambda face: face['bbox'][0])
+ if direction == 'right-left':
+ return sorted(faces, key=lambda face: face['bbox'][0], reverse=True)
+ if direction == 'top-bottom':
+ return sorted(faces, key=lambda face: face['bbox'][1])
+ if direction == 'bottom-top':
+ return sorted(faces, key=lambda face: face['bbox'][1], reverse=True)
+ if direction == 'small-large':
+ return sorted(faces, key=lambda face: (face['bbox'][2] - face['bbox'][0]) * (face['bbox'][3] - face['bbox'][1]))
+ if direction == 'large-small':
+ return sorted(faces, key=lambda face: (face['bbox'][2] - face['bbox'][0]) * (face['bbox'][3] - face['bbox'][1]),
+ reverse=True)
+ if direction == 'distance-from-retarget-face':
+ return sorted(faces, key=lambda face: (((face['bbox'][2] + face['bbox'][0]) / 2 - face_center[0]) ** 2 + (
+ (face['bbox'][3] + face['bbox'][1]) / 2 - face_center[1]) ** 2) ** 0.5)
+ return faces
+
+
+def distance2bbox(points, distance, max_shape=None):
+ """Decode distance prediction to bounding box.
+
+ Args:
+ points (Tensor): Shape (n, 2), [x, y].
+ distance (Tensor): Distance from the given point to 4
+ boundaries (left, top, right, bottom).
+ max_shape (tuple): Shape of the image.
+
+ Returns:
+ Tensor: Decoded bboxes.
+ """
+ x1 = points[:, 0] - distance[:, 0]
+ y1 = points[:, 1] - distance[:, 1]
+ x2 = points[:, 0] + distance[:, 2]
+ y2 = points[:, 1] + distance[:, 3]
+ if max_shape is not None:
+ x1 = x1.clamp(min=0, max=max_shape[1])
+ y1 = y1.clamp(min=0, max=max_shape[0])
+ x2 = x2.clamp(min=0, max=max_shape[1])
+ y2 = y2.clamp(min=0, max=max_shape[0])
+ return np.stack([x1, y1, x2, y2], axis=-1)
+
+
+def distance2kps(points, distance, max_shape=None):
+ """Decode distance prediction to bounding box.
+
+ Args:
+ points (Tensor): Shape (n, 2), [x, y].
+ distance (Tensor): Distance from the given point to 4
+ boundaries (left, top, right, bottom).
+ max_shape (tuple): Shape of the image.
+
+ Returns:
+ Tensor: Decoded bboxes.
+ """
+ preds = []
+ for i in range(0, distance.shape[1], 2):
+ px = points[:, i % 2] + distance[:, i]
+ py = points[:, i % 2 + 1] + distance[:, i + 1]
+ if max_shape is not None:
+ px = px.clamp(min=0, max=max_shape[1])
+ py = py.clamp(min=0, max=max_shape[0])
+ preds.append(px)
+ preds.append(py)
+ return np.stack(preds, axis=-1)
+
+
+class FaceAnalysisModel:
+ def __init__(self, **kwargs):
+ self.model_paths = kwargs.get("model_path", [])
+ self.predict_type = kwargs.get("predict_type", "trt")
+ self.device = torch.cuda.current_device()
+ self.cudaStream = torch.cuda.current_stream().cuda_stream
+
+ assert self.model_paths
+ self.face_det = get_predictor(predict_type=self.predict_type, model_path=self.model_paths[0])
+ self.face_det.input_spec()
+ self.face_det.output_spec()
+ self.face_pose = get_predictor(predict_type=self.predict_type, model_path=self.model_paths[1])
+ self.face_pose.input_spec()
+ self.face_pose.output_spec()
+
+ # face det
+ self.input_mean = 127.5
+ self.input_std = 128.0
+ # print(self.output_names)
+ # assert len(outputs)==10 or len(outputs)==15
+ self.use_kps = False
+ self._anchor_ratio = 1.0
+ self._num_anchors = 1
+ self.center_cache = {}
+ self.nms_thresh = 0.4
+ self.det_thresh = 0.5
+ self.input_size = (512, 512)
+ if len(self.face_det.outputs) == 6:
+ self.fmc = 3
+ self._feat_stride_fpn = [8, 16, 32]
+ self._num_anchors = 2
+ elif len(self.face_det.outputs) == 9:
+ self.fmc = 3
+ self._feat_stride_fpn = [8, 16, 32]
+ self._num_anchors = 2
+ self.use_kps = True
+ elif len(self.face_det.outputs) == 10:
+ self.fmc = 5
+ self._feat_stride_fpn = [8, 16, 32, 64, 128]
+ self._num_anchors = 1
+ elif len(self.face_det.outputs) == 15:
+ self.fmc = 5
+ self._feat_stride_fpn = [8, 16, 32, 64, 128]
+ self._num_anchors = 1
+ self.use_kps = True
+
+ self.lmk_dim = 2
+ self.lmk_num = 212 // self.lmk_dim
+
+ def nms(self, dets):
+ thresh = self.nms_thresh
+ x1 = dets[:, 0]
+ y1 = dets[:, 1]
+ x2 = dets[:, 2]
+ y2 = dets[:, 3]
+ scores = dets[:, 4]
+
+ areas = (x2 - x1 + 1) * (y2 - y1 + 1)
+ order = scores.argsort()[::-1]
+
+ keep = []
+ while order.size > 0:
+ i = order[0]
+ keep.append(i)
+ xx1 = np.maximum(x1[i], x1[order[1:]])
+ yy1 = np.maximum(y1[i], y1[order[1:]])
+ xx2 = np.minimum(x2[i], x2[order[1:]])
+ yy2 = np.minimum(y2[i], y2[order[1:]])
+
+ w = np.maximum(0.0, xx2 - xx1 + 1)
+ h = np.maximum(0.0, yy2 - yy1 + 1)
+ inter = w * h
+ ovr = inter / (areas[i] + areas[order[1:]] - inter)
+
+ inds = np.where(ovr <= thresh)[0]
+ order = order[inds + 1]
+
+ return keep
+
+ def detect_face(self, *data):
+ img = data[0] # BGR mode
+ im_ratio = float(img.shape[0]) / img.shape[1]
+ input_size = self.input_size
+ model_ratio = float(input_size[1]) / input_size[0]
+ if im_ratio > model_ratio:
+ new_height = input_size[1]
+ new_width = int(new_height / im_ratio)
+ else:
+ new_width = input_size[0]
+ new_height = int(new_width * im_ratio)
+ det_scale = float(new_height) / img.shape[0]
+ resized_img = cv2.resize(img, (new_width, new_height))
+ det_img = np.zeros((input_size[1], input_size[0], 3), dtype=np.uint8)
+ det_img[:new_height, :new_width, :] = resized_img
+
+ scores_list = []
+ bboxes_list = []
+ kpss_list = []
+ input_size = tuple(img.shape[0:2][::-1])
+
+ det_img = cv2.cvtColor(det_img, cv2.COLOR_BGR2RGB)
+ det_img = np.transpose(det_img, (2, 0, 1))
+ det_img = (det_img - self.input_mean) / self.input_std
+ if self.predict_type == "trt":
+ nvtx.range_push("forward")
+ feed_dict = {}
+ inp = self.face_det.inputs[0]
+ det_img_torch = torch.from_numpy(det_img[None]).to(device=self.device,
+ dtype=numpy_to_torch_dtype_dict[inp['dtype']])
+ feed_dict[inp['name']] = det_img_torch
+ preds_dict = self.face_det.predict(feed_dict, self.cudaStream)
+ outs = []
+ for key in ["448", "471", "494", "451", "474", "497", "454", "477", "500"]:
+ outs.append(preds_dict[key].cpu().numpy())
+ o448, o471, o494, o451, o474, o497, o454, o477, o500 = outs
+ nvtx.range_pop()
+ else:
+ o448, o471, o494, o451, o474, o497, o454, o477, o500 = self.face_det.predict(det_img[None])
+ faces_det = [o448, o471, o494, o451, o474, o497, o454, o477, o500]
+ input_height = det_img.shape[1]
+ input_width = det_img.shape[2]
+ fmc = self.fmc
+ for idx, stride in enumerate(self._feat_stride_fpn):
+ scores = faces_det[idx]
+ bbox_preds = faces_det[idx + fmc]
+ bbox_preds = bbox_preds * stride
+ if self.use_kps:
+ kps_preds = faces_det[idx + fmc * 2] * stride
+ height = input_height // stride
+ width = input_width // stride
+ K = height * width
+ key = (height, width, stride)
+ if key in self.center_cache:
+ anchor_centers = self.center_cache[key]
+ else:
+ # solution-3:
+ anchor_centers = np.stack(np.mgrid[:height, :width][::-1], axis=-1).astype(np.float32)
+ # print(anchor_centers.shape)
+ anchor_centers = (anchor_centers * stride).reshape((-1, 2))
+ if self._num_anchors > 1:
+ anchor_centers = np.stack([anchor_centers] * self._num_anchors, axis=1).reshape((-1, 2))
+ if len(self.center_cache) < 100:
+ self.center_cache[key] = anchor_centers
+
+ pos_inds = np.where(scores >= self.det_thresh)[0]
+ bboxes = distance2bbox(anchor_centers, bbox_preds)
+ pos_scores = scores[pos_inds]
+ pos_bboxes = bboxes[pos_inds]
+ scores_list.append(pos_scores)
+ bboxes_list.append(pos_bboxes)
+ if self.use_kps:
+ kpss = distance2kps(anchor_centers, kps_preds)
+ # kpss = kps_preds
+ kpss = kpss.reshape((kpss.shape[0], -1, 2))
+ pos_kpss = kpss[pos_inds]
+ kpss_list.append(pos_kpss)
+ scores = np.vstack(scores_list)
+ scores_ravel = scores.ravel()
+ order = scores_ravel.argsort()[::-1]
+ bboxes = np.vstack(bboxes_list) / det_scale
+ if self.use_kps:
+ kpss = np.vstack(kpss_list) / det_scale
+ pre_det = np.hstack((bboxes, scores)).astype(np.float32, copy=False)
+ pre_det = pre_det[order, :]
+ keep = self.nms(pre_det)
+ det = pre_det[keep, :]
+ if self.use_kps:
+ kpss = kpss[order, :, :]
+ kpss = kpss[keep, :, :]
+ else:
+ kpss = None
+ return det, kpss
+
+ def estimate_face_pose(self, *data):
+ """
+ 检测脸部关键点
+ :param data:
+ :return:
+ """
+ img, face = data
+ bbox = face.bbox
+ w, h = (bbox[2] - bbox[0]), (bbox[3] - bbox[1])
+ center = (bbox[2] + bbox[0]) / 2, (bbox[3] + bbox[1]) / 2
+ rotate = 0
+ input_size = (192, 192)
+ _scale = input_size[0] / (max(w, h) * 1.5)
+ aimg, M = face_align.transform(img, center, input_size[0], _scale, rotate)
+ input_size = tuple(aimg.shape[0:2][::-1])
+
+ aimg = cv2.cvtColor(aimg, cv2.COLOR_BGR2RGB)
+ aimg = np.transpose(aimg, (2, 0, 1))
+ if self.predict_type == "trt":
+ nvtx.range_push("forward")
+ feed_dict = {}
+ inp = self.face_pose.inputs[0]
+ det_img_torch = torch.from_numpy(aimg[None]).to(device=self.device,
+ dtype=numpy_to_torch_dtype_dict[inp['dtype']])
+ feed_dict[inp['name']] = det_img_torch
+ preds_dict = self.face_pose.predict(feed_dict, self.cudaStream)
+ outs = []
+ for i, out in enumerate(self.face_pose.outputs):
+ outs.append(preds_dict[out["name"]].cpu().numpy())
+ pred = outs[0]
+ nvtx.range_pop()
+ else:
+ pred = self.face_pose.predict(aimg[None])[0]
+ pred = pred.reshape((-1, 2))
+ if self.lmk_num < pred.shape[0]:
+ pred = pred[self.lmk_num * -1:, :]
+ pred[:, 0:2] += 1
+ pred[:, 0:2] *= (input_size[0] // 2)
+ if pred.shape[1] == 3:
+ pred[:, 2] *= (input_size[0] // 2)
+
+ IM = cv2.invertAffineTransform(M)
+ pred = face_align.trans_points(pred, IM)
+ face["landmark"] = pred
+ return pred
+
+ def predict(self, *data, **kwargs):
+ bboxes, kpss = self.detect_face(*data)
+ if bboxes.shape[0] == 0:
+ return []
+ ret = []
+ for i in range(bboxes.shape[0]):
+ bbox = bboxes[i, 0:4]
+ det_score = bboxes[i, 4]
+ kps = kpss[i]
+ face = Face(bbox=bbox, kps=kps, det_score=det_score)
+ self.estimate_face_pose(data[0], face)
+ ret.append(face)
+ ret = sort_by_direction(ret, 'large-small', None)
+ outs = [x.landmark for x in ret]
+ return outs
+
+ def __del__(self):
+ del self.face_det
+ del self.face_pose
diff --git a/difpoint/src/models/landmark_model.py b/difpoint/src/models/landmark_model.py
new file mode 100644
index 0000000000000000000000000000000000000000..77aebfd0d817dc09090cd1b3c398fa204f617159
--- /dev/null
+++ b/difpoint/src/models/landmark_model.py
@@ -0,0 +1,78 @@
+# -*- coding: utf-8 -*-
+# @Author : wenshao
+# @Email : wenshaoguo1026@gmail.com
+# @Project : FasterLivePortrait
+# @FileName: landmark_model.py
+
+from .base_model import BaseModel
+import cv2
+import numpy as np
+from difpoint.src.utils.crop import crop_image, _transform_pts
+import torch
+from torch.cuda import nvtx
+from .predictor import numpy_to_torch_dtype_dict
+
+
+class LandmarkModel(BaseModel):
+ """
+ landmark Model
+ """
+
+ def __init__(self, **kwargs):
+ super(LandmarkModel, self).__init__(**kwargs)
+ self.dsize = 224
+
+ def input_process(self, *data):
+ if len(data) > 1:
+ img_rgb, lmk = data
+ else:
+ img_rgb = data[0]
+ lmk = None
+ if lmk is not None:
+ crop_dct = crop_image(img_rgb, lmk, dsize=self.dsize, scale=1.5, vy_ratio=-0.1)
+ img_crop_rgb = crop_dct['img_crop']
+ else:
+ # NOTE: force resize to 224x224, NOT RECOMMEND!
+ img_crop_rgb = cv2.resize(img_rgb, (self.dsize, self.dsize))
+ scale = max(img_rgb.shape[:2]) / self.dsize
+ crop_dct = {
+ 'M_c2o': np.array([
+ [scale, 0., 0.],
+ [0., scale, 0.],
+ [0., 0., 1.],
+ ], dtype=np.float32),
+ }
+
+ inp = (img_crop_rgb.astype(np.float32) / 255.).transpose(2, 0, 1)[None, ...] # HxWx3 (BGR) -> 1x3xHxW (RGB!)
+ return inp, crop_dct
+
+ def output_process(self, *data):
+ out_pts, crop_dct = data
+ lmk = out_pts[2].reshape(-1, 2) * self.dsize # scale to 0-224
+ lmk = _transform_pts(lmk, M=crop_dct['M_c2o'])
+ return lmk
+
+ def predict_trt(self, *data):
+ nvtx.range_push("forward")
+ feed_dict = {}
+ for i, inp in enumerate(self.predictor.inputs):
+ if isinstance(data[i], torch.Tensor):
+ feed_dict[inp['name']] = data[i]
+ else:
+ feed_dict[inp['name']] = torch.from_numpy(data[i]).to(device=self.device,
+ dtype=numpy_to_torch_dtype_dict[inp['dtype']])
+ preds_dict = self.predictor.predict(feed_dict, self.cudaStream)
+ outs = []
+ for i, out in enumerate(self.predictor.outputs):
+ outs.append(preds_dict[out["name"]].cpu().numpy())
+ nvtx.range_pop()
+ return outs
+
+ def predict(self, *data):
+ input, crop_dct = self.input_process(*data)
+ if self.predict_type == "trt":
+ preds = self.predict_trt(input)
+ else:
+ preds = self.predictor.predict(input)
+ outputs = self.output_process(preds, crop_dct)
+ return outputs
diff --git a/difpoint/src/models/mediapipe_face_model.py b/difpoint/src/models/mediapipe_face_model.py
new file mode 100644
index 0000000000000000000000000000000000000000..6dde091a6e007c345efd3c7da061e7cd56078f43
--- /dev/null
+++ b/difpoint/src/models/mediapipe_face_model.py
@@ -0,0 +1,41 @@
+# -*- coding: utf-8 -*-
+# @Time : 2024/8/7 9:00
+# @Author : shaoguowen
+# @Email : wenshaoguo1026@gmail.com
+# @Project : FasterLivePortrait
+# @FileName: mediapipe_face_model.py
+import cv2
+import mediapipe as mp
+import numpy as np
+
+
+class MediaPipeFaceModel:
+ """
+ MediaPipeFaceModel
+ """
+
+ def __init__(self, **kwargs):
+ mp_face_mesh = mp.solutions.face_mesh
+ self.face_mesh = mp_face_mesh.FaceMesh(
+ static_image_mode=True,
+ max_num_faces=1,
+ refine_landmarks=True,
+ min_detection_confidence=0.5)
+
+ def predict(self, *data):
+ img_bgr = data[0]
+ img_rgb = cv2.cvtColor(img_bgr, cv2.COLOR_BGR2RGB)
+ h, w = img_bgr.shape[:2]
+ results = self.face_mesh.process(cv2.cvtColor(img_rgb, cv2.COLOR_BGR2RGB))
+
+ # Print and draw face mesh landmarks on the image.
+ if not results.multi_face_landmarks:
+ return []
+ outs = []
+ for face_landmarks in results.multi_face_landmarks:
+ landmarks = []
+ for landmark in face_landmarks.landmark:
+ # 提取每个关键点的 x, y, z 坐标
+ landmarks.append([landmark.x * w, landmark.y * h])
+ outs.append(np.array(landmarks))
+ return outs
diff --git a/difpoint/src/models/motion_extractor_model.py b/difpoint/src/models/motion_extractor_model.py
new file mode 100644
index 0000000000000000000000000000000000000000..7550fe30d8bc1a04b35d10540faeb8e992910bda
--- /dev/null
+++ b/difpoint/src/models/motion_extractor_model.py
@@ -0,0 +1,85 @@
+# -*- coding: utf-8 -*-
+# @Author : wenshao
+# @Email : wenshaoguo1026@gmail.com
+# @Project : FasterLivePortrait
+# @FileName: motion_extractor_model.py
+import pdb
+
+import numpy as np
+
+from .base_model import BaseModel
+import torch
+from torch.cuda import nvtx
+from .predictor import numpy_to_torch_dtype_dict
+import torch.nn.functional as F
+
+
+def headpose_pred_to_degree(pred):
+ """
+ pred: (bs, 66) or (bs, 1) or others
+ """
+ if pred.ndim > 1 and pred.shape[1] == 66:
+ # NOTE: note that the average is modified to 97.5
+ idx_array = np.arange(0, 66)
+ pred = np.apply_along_axis(lambda x: np.exp(x) / np.sum(np.exp(x)), 1, pred)
+ degree = np.sum(pred * idx_array, axis=1) * 3 - 97.5
+
+ return degree
+
+ return pred
+
+
+class MotionExtractorModel(BaseModel):
+ """
+ MotionExtractorModel
+ """
+
+ def __init__(self, **kwargs):
+ super(MotionExtractorModel, self).__init__(**kwargs)
+ self.flag_refine_info = kwargs.get("flag_refine_info", True)
+
+ def input_process(self, *data):
+ img = data[0].astype(np.float32)
+ img /= 255.0
+ img = np.transpose(img, (2, 0, 1))
+ return img[None]
+
+ def output_process(self, *data):
+ if self.predict_type == "trt":
+ kp, pitch, yaw, roll, t, exp, scale = data
+ else:
+ pitch, yaw, roll, t, exp, scale, kp = data
+ if self.flag_refine_info:
+ bs = kp.shape[0]
+ pitch = headpose_pred_to_degree(pitch)[:, None] # Bx1
+ yaw = headpose_pred_to_degree(yaw)[:, None] # Bx1
+ roll = headpose_pred_to_degree(roll)[:, None] # Bx1
+ kp = kp.reshape(bs, -1, 3) # BxNx3
+ exp = exp.reshape(bs, -1, 3) # BxNx3
+ return pitch, yaw, roll, t, exp, scale, kp
+
+ def predict_trt(self, *data):
+ nvtx.range_push("forward")
+ feed_dict = {}
+ for i, inp in enumerate(self.predictor.inputs):
+ if isinstance(data[i], torch.Tensor):
+ feed_dict[inp['name']] = data[i]
+ else:
+ feed_dict[inp['name']] = torch.from_numpy(data[i]).to(device=self.device,
+ dtype=numpy_to_torch_dtype_dict[inp['dtype']])
+ preds_dict = self.predictor.predict(feed_dict, self.cudaStream)
+ outs = []
+ for i, out in enumerate(self.predictor.outputs):
+ outs.append(preds_dict[out["name"]].cpu().numpy())
+ nvtx.range_pop()
+ return outs
+
+ def predict(self, *data):
+ #img = self.input_process(*data)
+ img = data[0]
+ if self.predict_type == "trt":
+ preds = self.predict_trt(img)
+ else:
+ preds = self.predictor.predict(img)
+ outputs = self.output_process(*preds)
+ return outputs
diff --git a/difpoint/src/models/predictor.py b/difpoint/src/models/predictor.py
new file mode 100644
index 0000000000000000000000000000000000000000..ab2b76965d902e65e9a07e48dff91d3d083daa61
--- /dev/null
+++ b/difpoint/src/models/predictor.py
@@ -0,0 +1,263 @@
+import pdb
+import threading
+import os
+import time
+
+import numpy as np
+import onnxruntime
+
+import torch
+from torch.cuda import nvtx
+from collections import OrderedDict
+import platform
+
+import spaces
+
+try:
+ import tensorrt as trt
+ import ctypes
+except ModuleNotFoundError:
+ print("No TensorRT Found")
+
+numpy_to_torch_dtype_dict = {
+ np.uint8: torch.uint8,
+ np.int8: torch.int8,
+ np.int16: torch.int16,
+ np.int32: torch.int32,
+ np.int64: torch.int64,
+ np.float16: torch.float16,
+ np.float32: torch.float32,
+ np.float64: torch.float64,
+ np.complex64: torch.complex64,
+ np.complex128: torch.complex128,
+}
+if np.version.full_version >= "1.24.0":
+ numpy_to_torch_dtype_dict[np.bool_] = torch.bool
+else:
+ numpy_to_torch_dtype_dict[np.bool] = torch.bool
+
+
+class TensorRTPredictor:
+ """
+ Implements inference for the EfficientDet TensorRT engine.
+ """
+ @spaces.GPU
+ def __init__(self, **kwargs):
+ """
+ :param engine_path: The path to the serialized engine to load from disk.
+ """
+ if platform.system().lower() == 'linux':
+ ctypes.CDLL("./difpoint/checkpoints/liveportrait_onnx/libgrid_sample_3d_plugin.so", mode=ctypes.RTLD_GLOBAL)
+ else:
+ ctypes.CDLL("./difpoint/checkpoints/liveportrait_onnx/grid_sample_3d_plugin.dll", mode=ctypes.RTLD_GLOBAL)
+ # Load TRT engine
+ self.logger = trt.Logger(trt.Logger.VERBOSE)
+ trt.init_libnvinfer_plugins(self.logger, "")
+ engine_path = os.path.abspath(kwargs.get("model_path", None))
+ print('engine_path', engine_path)
+ self.debug = kwargs.get("debug", False)
+ assert engine_path, f"model:{engine_path} must exist!"
+ print(f"loading trt model:{engine_path}")
+ with open(engine_path, "rb") as f, trt.Runtime(self.logger) as runtime:
+ assert runtime
+ self.engine = runtime.deserialize_cuda_engine(f.read())
+ print('self.engine', self.engine)
+ assert self.engine
+ self.context = self.engine.create_execution_context()
+ assert self.context
+
+ # Setup I/O bindings
+ self.inputs = []
+ self.outputs = []
+ self.tensors = OrderedDict()
+
+ # TODO: 支持动态shape输入
+ for idx in range(self.engine.num_io_tensors):
+ name = self.engine[idx]
+ is_input = self.engine.get_tensor_mode(name).name == "INPUT"
+ shape = self.engine.get_tensor_shape(name)
+ dtype = trt.nptype(self.engine.get_tensor_dtype(name))
+
+ binding = {
+ "index": idx,
+ "name": name,
+ "dtype": dtype,
+ "shape": list(shape)
+ }
+ if is_input:
+ self.inputs.append(binding)
+ else:
+ self.outputs.append(binding)
+
+ assert len(self.inputs) > 0
+ assert len(self.outputs) > 0
+ self.allocate_max_buffers()
+
+ def allocate_max_buffers(self, device="cuda"):
+ nvtx.range_push("allocate_max_buffers")
+ # 目前仅支持 batch 维度的动态处理
+ batch_size = 1
+ for idx in range(self.engine.num_io_tensors):
+ binding = self.engine[idx]
+ shape = self.engine.get_tensor_shape(binding)
+ is_input = self.engine.get_tensor_mode(binding).name == "INPUT"
+ if -1 in shape:
+ if is_input:
+ shape = self.engine.get_tensor_profile_shape(binding, 0)[-1]
+ batch_size = shape[0]
+ else:
+ shape[0] = batch_size
+ dtype = trt.nptype(self.engine.get_tensor_dtype(binding))
+ tensor = torch.empty(
+ tuple(shape), dtype=numpy_to_torch_dtype_dict[dtype]
+ ).to(device=device)
+ self.tensors[binding] = tensor
+ nvtx.range_pop()
+
+ def input_spec(self):
+ """
+ Get the specs for the input tensor of the network. Useful to prepare memory allocations.
+ :return: Two items, the shape of the input tensor and its (numpy) datatype.
+ """
+ specs = []
+ for i, o in enumerate(self.inputs):
+ specs.append((o["name"], o['shape'], o['dtype']))
+ if self.debug:
+ print(f"trt input {i} -> {o['name']} -> {o['shape']}")
+ return specs
+
+ def output_spec(self):
+ """
+ Get the specs for the output tensors of the network. Useful to prepare memory allocations.
+ :return: A list with two items per element, the shape and (numpy) datatype of each output tensor.
+ """
+ specs = []
+ for i, o in enumerate(self.outputs):
+ specs.append((o["name"], o['shape'], o['dtype']))
+ if self.debug:
+ print(f"trt output {i} -> {o['name']} -> {o['shape']}")
+ return specs
+
+ def adjust_buffer(self, feed_dict):
+ nvtx.range_push("adjust_buffer")
+ for name, buf in feed_dict.items():
+ input_tensor = self.tensors[name]
+ current_shape = list(buf.shape)
+ slices = tuple(slice(0, dim) for dim in current_shape)
+ input_tensor[slices].copy_(buf)
+ self.context.set_input_shape(name, current_shape)
+ nvtx.range_pop()
+
+ def predict(self, feed_dict, stream):
+ """
+ Execute inference on a batch of images.
+ :param data: A list of inputs as numpy arrays.
+ :return A list of outputs as numpy arrays.
+ """
+ nvtx.range_push("set_tensors")
+ self.adjust_buffer(feed_dict)
+ for name, tensor in self.tensors.items():
+ self.context.set_tensor_address(name, tensor.data_ptr())
+ nvtx.range_pop()
+ nvtx.range_push("execute")
+ noerror = self.context.execute_async_v3(stream)
+ if not noerror:
+ raise ValueError("ERROR: inference failed.")
+ nvtx.range_pop()
+ return self.tensors
+
+ def __del__(self):
+ del self.engine
+ del self.context
+ del self.inputs
+ del self.outputs
+ del self.tensors
+
+class OnnxRuntimePredictor:
+ """
+ OnnxRuntime Prediction
+ """
+
+ def __init__(self, **kwargs):
+ model_path = kwargs.get("model_path", "") # 用模型路径区分是否是一样的实例
+ assert os.path.exists(model_path), "model path must exist!"
+ # print("loading ort model:{}".format(model_path))
+ self.debug = kwargs.get("debug", False)
+ providers = ['CUDAExecutionProvider', 'CoreMLExecutionProvider', 'CPUExecutionProvider']
+
+ print(f"OnnxRuntime use {providers}")
+ opts = onnxruntime.SessionOptions()
+ # opts.inter_op_num_threads = kwargs.get("num_threads", 4)
+ # opts.intra_op_num_threads = kwargs.get("num_threads", 4)
+ # opts.log_severity_level = 3
+
+ self.onnx_model = onnxruntime.InferenceSession(model_path, providers=providers, sess_options=opts)
+ self.inputs = self.onnx_model.get_inputs()
+ self.outputs = self.onnx_model.get_outputs()
+
+ def input_spec(self):
+ """
+ Get the specs for the input tensor of the network. Useful to prepare memory allocations.
+ :return: Two items, the shape of the input tensor and its (numpy) datatype.
+ """
+ specs = []
+ for i, o in enumerate(self.inputs):
+ specs.append((o.name, o.shape, o.type))
+ if self.debug:
+ print(f"ort {i} -> {o.name} -> {o.shape}")
+ return specs
+
+ def output_spec(self):
+ """
+ Get the specs for the output tensors of the network. Useful to prepare memory allocations.
+ :return: A list with two items per element, the shape and (numpy) datatype of each output tensor.
+ """
+ specs = []
+ for i, o in enumerate(self.outputs):
+ specs.append((o.name, o.shape, o.type))
+ if self.debug:
+ print(f"ort output {i} -> {o.name} -> {o.shape}")
+ return specs
+
+ def predict(self, *data):
+ input_feeds = {}
+ for i in range(len(data)):
+ if self.inputs[i].type == 'tensor(float16)':
+ input_feeds[self.inputs[i].name] = data[i].astype(np.float16)
+ else:
+ input_feeds[self.inputs[i].name] = data[i].astype(np.float32)
+ results = self.onnx_model.run(None, input_feeds)
+ return results
+
+ def __del__(self):
+ del self.onnx_model
+ self.onnx_model = None
+
+
+class OnnxRuntimePredictorSingleton(OnnxRuntimePredictor):
+ """
+ 单例模式,防止模型被加载多次
+ """
+ _instance_lock = threading.Lock()
+ _instance = {}
+
+ def __new__(cls, *args, **kwargs):
+ model_path = kwargs.get("model_path", "") # 用模型路径区分是否是一样的实例
+ assert os.path.exists(model_path), "model path must exist!"
+ # 单例模式,避免重复加载模型
+ with OnnxRuntimePredictorSingleton._instance_lock:
+ if model_path not in OnnxRuntimePredictorSingleton._instance or \
+ OnnxRuntimePredictorSingleton._instance[model_path].onnx_model is None:
+ OnnxRuntimePredictorSingleton._instance[model_path] = OnnxRuntimePredictor(**kwargs)
+
+ return OnnxRuntimePredictorSingleton._instance[model_path]
+
+
+def get_predictor(**kwargs):
+ predict_type = kwargs.get("predict_type", "trt")
+ if predict_type == "ort":
+ return OnnxRuntimePredictorSingleton(**kwargs)
+ elif predict_type == "trt":
+ return TensorRTPredictor(**kwargs)
+ else:
+ raise NotImplementedError
diff --git a/difpoint/src/models/stitching_model.py b/difpoint/src/models/stitching_model.py
new file mode 100644
index 0000000000000000000000000000000000000000..dd0c346f8caa2b2c5b14d22f45525436129b66e5
--- /dev/null
+++ b/difpoint/src/models/stitching_model.py
@@ -0,0 +1,51 @@
+# -*- coding: utf-8 -*-
+# @Author : wenshao
+# @Email : wenshaoguo0611@gmail.com
+# @Project : FasterLivePortrait
+# @FileName: stitching_model.py
+
+from .base_model import BaseModel
+import torch
+from torch.cuda import nvtx
+from .predictor import numpy_to_torch_dtype_dict
+
+
+class StitchingModel(BaseModel):
+ """
+ StitchingModel
+ """
+
+ def __init__(self, **kwargs):
+ super(StitchingModel, self).__init__(**kwargs)
+
+ def input_process(self, *data):
+ input = data[0]
+ return input
+
+ def output_process(self, *data):
+ return data[0]
+
+ def predict_trt(self, *data):
+ nvtx.range_push("forward")
+ feed_dict = {}
+ for i, inp in enumerate(self.predictor.inputs):
+ if isinstance(data[i], torch.Tensor):
+ feed_dict[inp['name']] = data[i]
+ else:
+ feed_dict[inp['name']] = torch.from_numpy(data[i]).to(device=self.device,
+ dtype=numpy_to_torch_dtype_dict[inp['dtype']])
+ preds_dict = self.predictor.predict(feed_dict, self.cudaStream)
+ outs = []
+ for i, out in enumerate(self.predictor.outputs):
+ outs.append(preds_dict[out["name"]].cpu().numpy())
+ nvtx.range_pop()
+ return outs
+
+ def predict(self, *data):
+ data = self.input_process(*data)
+ if self.predict_type == "trt":
+ preds = self.predict_trt(data)
+ else:
+ preds = self.predictor.predict(data)
+ outputs = self.output_process(*preds)
+ return outputs
diff --git a/difpoint/src/models/util.py b/difpoint/src/models/util.py
new file mode 100644
index 0000000000000000000000000000000000000000..b9ea2d620015d9f0cf2a5c3b6a5ba90689a448a4
--- /dev/null
+++ b/difpoint/src/models/util.py
@@ -0,0 +1,452 @@
+# coding: utf-8
+
+"""
+This file defines various neural network modules and utility functions, including convolutional and residual blocks,
+normalizations, and functions for spatial transformation and tensor manipulation.
+"""
+
+from torch import nn
+import torch.nn.functional as F
+import torch
+import torch.nn.utils.spectral_norm as spectral_norm
+import math
+import warnings
+import collections.abc
+from itertools import repeat
+
+def kp2gaussian(kp, spatial_size, kp_variance):
+ """
+ Transform a keypoint into gaussian like representation
+ """
+ mean = kp
+
+ coordinate_grid = make_coordinate_grid(spatial_size, mean)
+ number_of_leading_dimensions = len(mean.shape) - 1
+ shape = (1,) * number_of_leading_dimensions + coordinate_grid.shape
+ coordinate_grid = coordinate_grid.view(*shape)
+ repeats = mean.shape[:number_of_leading_dimensions] + (1, 1, 1, 1)
+ coordinate_grid = coordinate_grid.repeat(*repeats)
+
+ # Preprocess kp shape
+ shape = mean.shape[:number_of_leading_dimensions] + (1, 1, 1, 3)
+ mean = mean.view(*shape)
+
+ mean_sub = (coordinate_grid - mean)
+
+ out = torch.exp(-0.5 * (mean_sub ** 2).sum(-1) / kp_variance)
+
+ return out
+
+
+def make_coordinate_grid(spatial_size, ref, **kwargs):
+ d, h, w = spatial_size
+ x = torch.arange(w).type(ref.dtype).to(ref.device)
+ y = torch.arange(h).type(ref.dtype).to(ref.device)
+ z = torch.arange(d).type(ref.dtype).to(ref.device)
+
+ # NOTE: must be right-down-in
+ x = (2 * (x / (w - 1)) - 1) # the x axis faces to the right
+ y = (2 * (y / (h - 1)) - 1) # the y axis faces to the bottom
+ z = (2 * (z / (d - 1)) - 1) # the z axis faces to the inner
+
+ yy = y.view(1, -1, 1).repeat(d, 1, w)
+ xx = x.view(1, 1, -1).repeat(d, h, 1)
+ zz = z.view(-1, 1, 1).repeat(1, h, w)
+
+ meshed = torch.cat([xx.unsqueeze_(3), yy.unsqueeze_(3), zz.unsqueeze_(3)], 3)
+
+ return meshed
+
+
+class ConvT2d(nn.Module):
+ """
+ Upsampling block for use in decoder.
+ """
+
+ def __init__(self, in_features, out_features, kernel_size=3, stride=2, padding=1, output_padding=1):
+ super(ConvT2d, self).__init__()
+
+ self.convT = nn.ConvTranspose2d(in_features, out_features, kernel_size=kernel_size, stride=stride,
+ padding=padding, output_padding=output_padding)
+ self.norm = nn.InstanceNorm2d(out_features)
+
+ def forward(self, x):
+ out = self.convT(x)
+ out = self.norm(out)
+ out = F.leaky_relu(out)
+ return out
+
+
+class ResBlock3d(nn.Module):
+ """
+ Res block, preserve spatial resolution.
+ """
+
+ def __init__(self, in_features, kernel_size, padding):
+ super(ResBlock3d, self).__init__()
+ self.conv1 = nn.Conv3d(in_channels=in_features, out_channels=in_features, kernel_size=kernel_size, padding=padding)
+ self.conv2 = nn.Conv3d(in_channels=in_features, out_channels=in_features, kernel_size=kernel_size, padding=padding)
+ self.norm1 = nn.BatchNorm3d(in_features, affine=True)
+ self.norm2 = nn.BatchNorm3d(in_features, affine=True)
+
+ def forward(self, x):
+ out = self.norm1(x)
+ out = F.relu(out)
+ out = self.conv1(out)
+ out = self.norm2(out)
+ out = F.relu(out)
+ out = self.conv2(out)
+ out += x
+ return out
+
+
+class UpBlock3d(nn.Module):
+ """
+ Upsampling block for use in decoder.
+ """
+
+ def __init__(self, in_features, out_features, kernel_size=3, padding=1, groups=1):
+ super(UpBlock3d, self).__init__()
+
+ self.conv = nn.Conv3d(in_channels=in_features, out_channels=out_features, kernel_size=kernel_size,
+ padding=padding, groups=groups)
+ self.norm = nn.BatchNorm3d(out_features, affine=True)
+
+ def forward(self, x):
+ out = F.interpolate(x, scale_factor=(1, 2, 2))
+ out = self.conv(out)
+ out = self.norm(out)
+ out = F.relu(out)
+ return out
+
+
+class DownBlock2d(nn.Module):
+ """
+ Downsampling block for use in encoder.
+ """
+
+ def __init__(self, in_features, out_features, kernel_size=3, padding=1, groups=1):
+ super(DownBlock2d, self).__init__()
+ self.conv = nn.Conv2d(in_channels=in_features, out_channels=out_features, kernel_size=kernel_size, padding=padding, groups=groups)
+ self.norm = nn.BatchNorm2d(out_features, affine=True)
+ self.pool = nn.AvgPool2d(kernel_size=(2, 2))
+
+ def forward(self, x):
+ out = self.conv(x)
+ out = self.norm(out)
+ out = F.relu(out)
+ out = self.pool(out)
+ return out
+
+
+class DownBlock3d(nn.Module):
+ """
+ Downsampling block for use in encoder.
+ """
+
+ def __init__(self, in_features, out_features, kernel_size=3, padding=1, groups=1):
+ super(DownBlock3d, self).__init__()
+ '''
+ self.conv = nn.Conv3d(in_channels=in_features, out_channels=out_features, kernel_size=kernel_size,
+ padding=padding, groups=groups, stride=(1, 2, 2))
+ '''
+ self.conv = nn.Conv3d(in_channels=in_features, out_channels=out_features, kernel_size=kernel_size,
+ padding=padding, groups=groups)
+ self.norm = nn.BatchNorm3d(out_features, affine=True)
+ self.pool = nn.AvgPool3d(kernel_size=(1, 2, 2))
+
+ def forward(self, x):
+ out = self.conv(x)
+ out = self.norm(out)
+ out = F.relu(out)
+ out = self.pool(out)
+ return out
+
+
+class SameBlock2d(nn.Module):
+ """
+ Simple block, preserve spatial resolution.
+ """
+
+ def __init__(self, in_features, out_features, groups=1, kernel_size=3, padding=1, lrelu=False):
+ super(SameBlock2d, self).__init__()
+ self.conv = nn.Conv2d(in_channels=in_features, out_channels=out_features, kernel_size=kernel_size, padding=padding, groups=groups)
+ self.norm = nn.BatchNorm2d(out_features, affine=True)
+ if lrelu:
+ self.ac = nn.LeakyReLU()
+ else:
+ self.ac = nn.ReLU()
+
+ def forward(self, x):
+ out = self.conv(x)
+ out = self.norm(out)
+ out = self.ac(out)
+ return out
+
+
+class Encoder(nn.Module):
+ """
+ Hourglass Encoder
+ """
+
+ def __init__(self, block_expansion, in_features, num_blocks=3, max_features=256):
+ super(Encoder, self).__init__()
+
+ down_blocks = []
+ for i in range(num_blocks):
+ down_blocks.append(DownBlock3d(in_features if i == 0 else min(max_features, block_expansion * (2 ** i)), min(max_features, block_expansion * (2 ** (i + 1))), kernel_size=3, padding=1))
+ self.down_blocks = nn.ModuleList(down_blocks)
+
+ def forward(self, x):
+ outs = [x]
+ for down_block in self.down_blocks:
+ outs.append(down_block(outs[-1]))
+ return outs
+
+
+class Decoder(nn.Module):
+ """
+ Hourglass Decoder
+ """
+
+ def __init__(self, block_expansion, in_features, num_blocks=3, max_features=256):
+ super(Decoder, self).__init__()
+
+ up_blocks = []
+
+ for i in range(num_blocks)[::-1]:
+ in_filters = (1 if i == num_blocks - 1 else 2) * min(max_features, block_expansion * (2 ** (i + 1)))
+ out_filters = min(max_features, block_expansion * (2 ** i))
+ up_blocks.append(UpBlock3d(in_filters, out_filters, kernel_size=3, padding=1))
+
+ self.up_blocks = nn.ModuleList(up_blocks)
+ self.out_filters = block_expansion + in_features
+
+ self.conv = nn.Conv3d(in_channels=self.out_filters, out_channels=self.out_filters, kernel_size=3, padding=1)
+ self.norm = nn.BatchNorm3d(self.out_filters, affine=True)
+
+ def forward(self, x):
+ out = x.pop()
+ for up_block in self.up_blocks:
+ out = up_block(out)
+ skip = x.pop()
+ out = torch.cat([out, skip], dim=1)
+ out = self.conv(out)
+ out = self.norm(out)
+ out = F.relu(out)
+ return out
+
+
+class Hourglass(nn.Module):
+ """
+ Hourglass architecture.
+ """
+
+ def __init__(self, block_expansion, in_features, num_blocks=3, max_features=256):
+ super(Hourglass, self).__init__()
+ self.encoder = Encoder(block_expansion, in_features, num_blocks, max_features)
+ self.decoder = Decoder(block_expansion, in_features, num_blocks, max_features)
+ self.out_filters = self.decoder.out_filters
+
+ def forward(self, x):
+ return self.decoder(self.encoder(x))
+
+
+class SPADE(nn.Module):
+ def __init__(self, norm_nc, label_nc):
+ super().__init__()
+
+ self.param_free_norm = nn.InstanceNorm2d(norm_nc, affine=False)
+ nhidden = 128
+
+ self.mlp_shared = nn.Sequential(
+ nn.Conv2d(label_nc, nhidden, kernel_size=3, padding=1),
+ nn.ReLU())
+ self.mlp_gamma = nn.Conv2d(nhidden, norm_nc, kernel_size=3, padding=1)
+ self.mlp_beta = nn.Conv2d(nhidden, norm_nc, kernel_size=3, padding=1)
+
+ def forward(self, x, segmap):
+ normalized = self.param_free_norm(x)
+ segmap = F.interpolate(segmap, size=x.size()[2:], mode='nearest')
+ actv = self.mlp_shared(segmap)
+ gamma = self.mlp_gamma(actv)
+ beta = self.mlp_beta(actv)
+ out = normalized * (1 + gamma) + beta
+ return out
+
+
+class SPADEResnetBlock(nn.Module):
+ def __init__(self, fin, fout, norm_G, label_nc, use_se=False, dilation=1):
+ super().__init__()
+ # Attributes
+ self.learned_shortcut = (fin != fout)
+ fmiddle = min(fin, fout)
+ self.use_se = use_se
+ # create conv layers
+ self.conv_0 = nn.Conv2d(fin, fmiddle, kernel_size=3, padding=dilation, dilation=dilation)
+ self.conv_1 = nn.Conv2d(fmiddle, fout, kernel_size=3, padding=dilation, dilation=dilation)
+ if self.learned_shortcut:
+ self.conv_s = nn.Conv2d(fin, fout, kernel_size=1, bias=False)
+ # apply spectral norm if specified
+ if 'spectral' in norm_G:
+ self.conv_0 = spectral_norm(self.conv_0)
+ self.conv_1 = spectral_norm(self.conv_1)
+ if self.learned_shortcut:
+ self.conv_s = spectral_norm(self.conv_s)
+ # define normalization layers
+ self.norm_0 = SPADE(fin, label_nc)
+ self.norm_1 = SPADE(fmiddle, label_nc)
+ if self.learned_shortcut:
+ self.norm_s = SPADE(fin, label_nc)
+
+ def forward(self, x, seg1):
+ x_s = self.shortcut(x, seg1)
+ dx = self.conv_0(self.actvn(self.norm_0(x, seg1)))
+ dx = self.conv_1(self.actvn(self.norm_1(dx, seg1)))
+ out = x_s + dx
+ return out
+
+ def shortcut(self, x, seg1):
+ if self.learned_shortcut:
+ x_s = self.conv_s(self.norm_s(x, seg1))
+ else:
+ x_s = x
+ return x_s
+
+ def actvn(self, x):
+ return F.leaky_relu(x, 2e-1)
+
+
+def filter_state_dict(state_dict, remove_name='fc'):
+ new_state_dict = {}
+ for key in state_dict:
+ if remove_name in key:
+ continue
+ new_state_dict[key] = state_dict[key]
+ return new_state_dict
+
+
+class GRN(nn.Module):
+ """ GRN (Global Response Normalization) layer
+ """
+
+ def __init__(self, dim):
+ super().__init__()
+ self.gamma = nn.Parameter(torch.zeros(1, 1, 1, dim))
+ self.beta = nn.Parameter(torch.zeros(1, 1, 1, dim))
+
+ def forward(self, x):
+ Gx = torch.norm(x, p=2, dim=(1, 2), keepdim=True)
+ Nx = Gx / (Gx.mean(dim=-1, keepdim=True) + 1e-6)
+ return self.gamma * (x * Nx) + self.beta + x
+
+
+class LayerNorm(nn.Module):
+ r""" LayerNorm that supports two data formats: channels_last (default) or channels_first.
+ The ordering of the dimensions in the inputs. channels_last corresponds to inputs with
+ shape (batch_size, height, width, channels) while channels_first corresponds to inputs
+ with shape (batch_size, channels, height, width).
+ """
+
+ def __init__(self, normalized_shape, eps=1e-6, data_format="channels_last"):
+ super().__init__()
+ self.weight = nn.Parameter(torch.ones(normalized_shape))
+ self.bias = nn.Parameter(torch.zeros(normalized_shape))
+ self.eps = eps
+ self.data_format = data_format
+ if self.data_format not in ["channels_last", "channels_first"]:
+ raise NotImplementedError
+ self.normalized_shape = (normalized_shape, )
+
+ def forward(self, x):
+ if self.data_format == "channels_last":
+ return F.layer_norm(x, self.normalized_shape, self.weight, self.bias, self.eps)
+ elif self.data_format == "channels_first":
+ u = x.mean(1, keepdim=True)
+ s = (x - u).pow(2).mean(1, keepdim=True)
+ x = (x - u) / torch.sqrt(s + self.eps)
+ x = self.weight[:, None, None] * x + self.bias[:, None, None]
+ return x
+
+
+def _no_grad_trunc_normal_(tensor, mean, std, a, b):
+ # Cut & paste from PyTorch official master until it's in a few official releases - RW
+ # Method based on https://people.sc.fsu.edu/~jburkardt/presentations/truncated_normal.pdf
+ def norm_cdf(x):
+ # Computes standard normal cumulative distribution function
+ return (1. + math.erf(x / math.sqrt(2.))) / 2.
+
+ if (mean < a - 2 * std) or (mean > b + 2 * std):
+ warnings.warn("mean is more than 2 std from [a, b] in nn.init.trunc_normal_. "
+ "The distribution of values may be incorrect.",
+ stacklevel=2)
+
+ with torch.no_grad():
+ # Values are generated by using a truncated uniform distribution and
+ # then using the inverse CDF for the normal distribution.
+ # Get upper and lower cdf values
+ l = norm_cdf((a - mean) / std)
+ u = norm_cdf((b - mean) / std)
+
+ # Uniformly fill tensor with values from [l, u], then translate to
+ # [2l-1, 2u-1].
+ tensor.uniform_(2 * l - 1, 2 * u - 1)
+
+ # Use inverse cdf transform for normal distribution to get truncated
+ # standard normal
+ tensor.erfinv_()
+
+ # Transform to proper mean, std
+ tensor.mul_(std * math.sqrt(2.))
+ tensor.add_(mean)
+
+ # Clamp to ensure it's in the proper range
+ tensor.clamp_(min=a, max=b)
+ return tensor
+
+
+def drop_path(x, drop_prob=0., training=False, scale_by_keep=True):
+ """ Drop paths (Stochastic Depth) per sample (when applied in main path of residual blocks).
+
+ This is the same as the DropConnect impl I created for EfficientNet, etc networks, however,
+ the original name is misleading as 'Drop Connect' is a different form of dropout in a separate paper...
+ See discussion: https://github.com/tensorflow/tpu/issues/494#issuecomment-532968956 ... I've opted for
+ changing the layer and argument names to 'drop path' rather than mix DropConnect as a layer name and use
+ 'survival rate' as the argument.
+
+ """
+ if drop_prob == 0. or not training:
+ return x
+ keep_prob = 1 - drop_prob
+ shape = (x.shape[0],) + (1,) * (x.ndim - 1) # work with diff dim tensors, not just 2D ConvNets
+ random_tensor = x.new_empty(shape).bernoulli_(keep_prob)
+ if keep_prob > 0.0 and scale_by_keep:
+ random_tensor.div_(keep_prob)
+ return x * random_tensor
+
+
+class DropPath(nn.Module):
+ """ Drop paths (Stochastic Depth) per sample (when applied in main path of residual blocks).
+ """
+
+ def __init__(self, drop_prob=None, scale_by_keep=True):
+ super(DropPath, self).__init__()
+ self.drop_prob = drop_prob
+ self.scale_by_keep = scale_by_keep
+
+ def forward(self, x):
+ return drop_path(x, self.drop_prob, self.training, self.scale_by_keep)
+
+
+def trunc_normal_(tensor, mean=0., std=1., a=-2., b=2.):
+ return _no_grad_trunc_normal_(tensor, mean, std, a, b)
+
+# From PyTorch internals
+def _ntuple(n):
+ def parse(x):
+ if isinstance(x, collections.abc.Iterable) and not isinstance(x, str):
+ return tuple(x)
+ return tuple(repeat(x, n))
+ return parse
+
+to_2tuple = _ntuple(2)
diff --git a/difpoint/src/models/warping_spade_model.py b/difpoint/src/models/warping_spade_model.py
new file mode 100644
index 0000000000000000000000000000000000000000..6110ca557e43f158890dd1373f027f9edacbd3ad
--- /dev/null
+++ b/difpoint/src/models/warping_spade_model.py
@@ -0,0 +1,58 @@
+# -*- coding: utf-8 -*-
+# @Author : wenshao
+# @Email : wenshaoguo1026@gmail.com
+# @Project : FasterLivePortrait
+# @FileName: warping_spade_model.py
+import pdb
+import numpy as np
+from .base_model import BaseModel
+import torch
+from torch.cuda import nvtx
+from .predictor import numpy_to_torch_dtype_dict
+
+
+class WarpingSpadeModel(BaseModel):
+ """
+ WarpingSpade Model
+ """
+
+ def __init__(self, **kwargs):
+ super(WarpingSpadeModel, self).__init__(**kwargs)
+
+ def input_process(self, *data):
+ feature_3d, kp_source, kp_driving = data
+ return feature_3d, kp_driving, kp_source
+
+ def output_process(self, *data):
+ if self.predict_type != "trt":
+ out = torch.from_numpy(data[0]).to(self.device).float()
+ else:
+ out = data[0]
+ out = out.permute(0, 2, 3, 1)
+ out = torch.clip(out, 0, 1) * 255
+ return out[0]
+
+ def predict_trt(self, *data):
+ nvtx.range_push("forward")
+ feed_dict = {}
+ for i, inp in enumerate(self.predictor.inputs):
+ if isinstance(data[i], torch.Tensor):
+ feed_dict[inp['name']] = data[i]
+ else:
+ feed_dict[inp['name']] = torch.from_numpy(data[i]).to(device=self.device,
+ dtype=numpy_to_torch_dtype_dict[inp['dtype']])
+ preds_dict = self.predictor.predict(feed_dict, self.cudaStream)
+ outs = []
+ for i, out in enumerate(self.predictor.outputs):
+ outs.append(preds_dict[out["name"]].clone())
+ nvtx.range_pop()
+ return outs
+
+ def predict(self, *data):
+ data = self.input_process(*data)
+ if self.predict_type == "trt":
+ preds = self.predict_trt(*data)
+ else:
+ preds = self.predictor.predict(*data)
+ outputs = self.output_process(*preds)
+ return outputs
diff --git a/difpoint/src/modules/__init__.py b/difpoint/src/modules/__init__.py
new file mode 100644
index 0000000000000000000000000000000000000000..e69de29bb2d1d6434b8b29ae775ad8c2e48c5391
diff --git a/difpoint/src/modules/__pycache__/__init__.cpython-310.pyc b/difpoint/src/modules/__pycache__/__init__.cpython-310.pyc
new file mode 100644
index 0000000000000000000000000000000000000000..8a8ee2863db9eac0be1e54551e5a375b2e0f3298
Binary files /dev/null and b/difpoint/src/modules/__pycache__/__init__.cpython-310.pyc differ
diff --git a/difpoint/src/modules/__pycache__/appearance_feature_extractor.cpython-310.pyc b/difpoint/src/modules/__pycache__/appearance_feature_extractor.cpython-310.pyc
new file mode 100644
index 0000000000000000000000000000000000000000..3ed0093668d7c6014f7353554cc971e9dddcd9a2
Binary files /dev/null and b/difpoint/src/modules/__pycache__/appearance_feature_extractor.cpython-310.pyc differ
diff --git a/difpoint/src/modules/__pycache__/convnextv2.cpython-310.pyc b/difpoint/src/modules/__pycache__/convnextv2.cpython-310.pyc
new file mode 100644
index 0000000000000000000000000000000000000000..902759faeeea7d46dcda4433212d11035f8fb9eb
Binary files /dev/null and b/difpoint/src/modules/__pycache__/convnextv2.cpython-310.pyc differ
diff --git a/difpoint/src/modules/__pycache__/dense_motion.cpython-310.pyc b/difpoint/src/modules/__pycache__/dense_motion.cpython-310.pyc
new file mode 100644
index 0000000000000000000000000000000000000000..bdeb5fbffda8b58435abb88ee4cda718421a97e7
Binary files /dev/null and b/difpoint/src/modules/__pycache__/dense_motion.cpython-310.pyc differ
diff --git a/difpoint/src/modules/__pycache__/motion_extractor.cpython-310.pyc b/difpoint/src/modules/__pycache__/motion_extractor.cpython-310.pyc
new file mode 100644
index 0000000000000000000000000000000000000000..6baa333fa78ccdd345a43f41d9232bf4beeeaa50
Binary files /dev/null and b/difpoint/src/modules/__pycache__/motion_extractor.cpython-310.pyc differ
diff --git a/difpoint/src/modules/__pycache__/spade_generator.cpython-310.pyc b/difpoint/src/modules/__pycache__/spade_generator.cpython-310.pyc
new file mode 100644
index 0000000000000000000000000000000000000000..55bbd8ddb3ce4999028450a974841cdfad4a74d9
Binary files /dev/null and b/difpoint/src/modules/__pycache__/spade_generator.cpython-310.pyc differ
diff --git a/difpoint/src/modules/__pycache__/stitching_retargeting_network.cpython-310.pyc b/difpoint/src/modules/__pycache__/stitching_retargeting_network.cpython-310.pyc
new file mode 100644
index 0000000000000000000000000000000000000000..a8c21a9eb90e0f8b1e044ec856cbe47fa367cc29
Binary files /dev/null and b/difpoint/src/modules/__pycache__/stitching_retargeting_network.cpython-310.pyc differ
diff --git a/difpoint/src/modules/__pycache__/util.cpython-310.pyc b/difpoint/src/modules/__pycache__/util.cpython-310.pyc
new file mode 100644
index 0000000000000000000000000000000000000000..1ae4c325ebff0e18b522fbeada3dbbc33cfa314c
Binary files /dev/null and b/difpoint/src/modules/__pycache__/util.cpython-310.pyc differ
diff --git a/difpoint/src/modules/__pycache__/warping_network.cpython-310.pyc b/difpoint/src/modules/__pycache__/warping_network.cpython-310.pyc
new file mode 100644
index 0000000000000000000000000000000000000000..89c3ee92875c9918047414a2531a4c081e9c5e14
Binary files /dev/null and b/difpoint/src/modules/__pycache__/warping_network.cpython-310.pyc differ
diff --git a/difpoint/src/modules/appearance_feature_extractor.py b/difpoint/src/modules/appearance_feature_extractor.py
new file mode 100644
index 0000000000000000000000000000000000000000..8d89e4f18a2fbe58447f52ab4c5e3f2011a4ec80
--- /dev/null
+++ b/difpoint/src/modules/appearance_feature_extractor.py
@@ -0,0 +1,48 @@
+# coding: utf-8
+
+"""
+Appearance extractor(F) defined in paper, which maps the source image s to a 3D appearance feature volume.
+"""
+
+import torch
+from torch import nn
+from .util import SameBlock2d, DownBlock2d, ResBlock3d
+
+
+class AppearanceFeatureExtractor(nn.Module):
+
+ def __init__(self, image_channel, block_expansion, num_down_blocks, max_features, reshape_channel, reshape_depth, num_resblocks):
+ super(AppearanceFeatureExtractor, self).__init__()
+ self.image_channel = image_channel
+ self.block_expansion = block_expansion
+ self.num_down_blocks = num_down_blocks
+ self.max_features = max_features
+ self.reshape_channel = reshape_channel
+ self.reshape_depth = reshape_depth
+
+ self.first = SameBlock2d(image_channel, block_expansion, kernel_size=(3, 3), padding=(1, 1))
+
+ down_blocks = []
+ for i in range(num_down_blocks):
+ in_features = min(max_features, block_expansion * (2 ** i))
+ out_features = min(max_features, block_expansion * (2 ** (i + 1)))
+ down_blocks.append(DownBlock2d(in_features, out_features, kernel_size=(3, 3), padding=(1, 1)))
+ self.down_blocks = nn.ModuleList(down_blocks)
+
+ self.second = nn.Conv2d(in_channels=out_features, out_channels=max_features, kernel_size=1, stride=1)
+
+ self.resblocks_3d = torch.nn.Sequential()
+ for i in range(num_resblocks):
+ self.resblocks_3d.add_module('3dr' + str(i), ResBlock3d(reshape_channel, kernel_size=3, padding=1))
+
+ def forward(self, source_image):
+ out = self.first(source_image) # Bx3x256x256 -> Bx64x256x256
+
+ for i in range(len(self.down_blocks)):
+ out = self.down_blocks[i](out)
+ out = self.second(out)
+ bs, c, h, w = out.shape # ->Bx512x64x64
+
+ f_s = out.view(bs, self.reshape_channel, self.reshape_depth, h, w) # ->Bx32x16x64x64
+ f_s = self.resblocks_3d(f_s) # ->Bx32x16x64x64
+ return f_s
diff --git a/difpoint/src/modules/convnextv2.py b/difpoint/src/modules/convnextv2.py
new file mode 100644
index 0000000000000000000000000000000000000000..83ea12662b607854915df8c7abb160b588d330b1
--- /dev/null
+++ b/difpoint/src/modules/convnextv2.py
@@ -0,0 +1,149 @@
+# coding: utf-8
+
+"""
+This moudle is adapted to the ConvNeXtV2 version for the extraction of implicit keypoints, poses, and expression deformation.
+"""
+
+import torch
+import torch.nn as nn
+# from timm.models.layers import trunc_normal_, DropPath
+from .util import LayerNorm, DropPath, trunc_normal_, GRN
+
+__all__ = ['convnextv2_tiny']
+
+
+class Block(nn.Module):
+ """ ConvNeXtV2 Block.
+
+ Args:
+ dim (int): Number of input channels.
+ drop_path (float): Stochastic depth rate. Default: 0.0
+ """
+
+ def __init__(self, dim, drop_path=0.):
+ super().__init__()
+ self.dwconv = nn.Conv2d(dim, dim, kernel_size=7, padding=3, groups=dim) # depthwise conv
+ self.norm = LayerNorm(dim, eps=1e-6)
+ self.pwconv1 = nn.Linear(dim, 4 * dim) # pointwise/1x1 convs, implemented with linear layers
+ self.act = nn.GELU()
+ self.grn = GRN(4 * dim)
+ self.pwconv2 = nn.Linear(4 * dim, dim)
+ self.drop_path = DropPath(drop_path) if drop_path > 0. else nn.Identity()
+
+ def forward(self, x):
+ input = x
+ x = self.dwconv(x)
+ x = x.permute(0, 2, 3, 1) # (N, C, H, W) -> (N, H, W, C)
+ x = self.norm(x)
+ x = self.pwconv1(x)
+ x = self.act(x)
+ x = self.grn(x)
+ x = self.pwconv2(x)
+ x = x.permute(0, 3, 1, 2) # (N, H, W, C) -> (N, C, H, W)
+
+ x = input + self.drop_path(x)
+ return x
+
+
+class ConvNeXtV2(nn.Module):
+ """ ConvNeXt V2
+
+ Args:
+ in_chans (int): Number of input image channels. Default: 3
+ num_classes (int): Number of classes for classification head. Default: 1000
+ depths (tuple(int)): Number of blocks at each stage. Default: [3, 3, 9, 3]
+ dims (int): Feature dimension at each stage. Default: [96, 192, 384, 768]
+ drop_path_rate (float): Stochastic depth rate. Default: 0.
+ head_init_scale (float): Init scaling value for classifier weights and biases. Default: 1.
+ """
+
+ def __init__(
+ self,
+ in_chans=3,
+ depths=[3, 3, 9, 3],
+ dims=[96, 192, 384, 768],
+ drop_path_rate=0.,
+ **kwargs
+ ):
+ super().__init__()
+ self.depths = depths
+ self.downsample_layers = nn.ModuleList() # stem and 3 intermediate downsampling conv layers
+ stem = nn.Sequential(
+ nn.Conv2d(in_chans, dims[0], kernel_size=4, stride=4),
+ LayerNorm(dims[0], eps=1e-6, data_format="channels_first")
+ )
+ self.downsample_layers.append(stem)
+ for i in range(3):
+ downsample_layer = nn.Sequential(
+ LayerNorm(dims[i], eps=1e-6, data_format="channels_first"),
+ nn.Conv2d(dims[i], dims[i+1], kernel_size=2, stride=2),
+ )
+ self.downsample_layers.append(downsample_layer)
+
+ self.stages = nn.ModuleList() # 4 feature resolution stages, each consisting of multiple residual blocks
+ dp_rates = [x.item() for x in torch.linspace(0, drop_path_rate, sum(depths))]
+ cur = 0
+ for i in range(4):
+ stage = nn.Sequential(
+ *[Block(dim=dims[i], drop_path=dp_rates[cur + j]) for j in range(depths[i])]
+ )
+ self.stages.append(stage)
+ cur += depths[i]
+
+ self.norm = nn.LayerNorm(dims[-1], eps=1e-6) # final norm layer
+
+ # NOTE: the output semantic items
+ num_bins = kwargs.get('num_bins', 66)
+ num_kp = kwargs.get('num_kp', 24) # the number of implicit keypoints
+ self.fc_kp = nn.Linear(dims[-1], 3 * num_kp) # implicit keypoints
+
+ # print('dims[-1]: ', dims[-1])
+ self.fc_scale = nn.Linear(dims[-1], 1) # scale
+ self.fc_pitch = nn.Linear(dims[-1], num_bins) # pitch bins
+ self.fc_yaw = nn.Linear(dims[-1], num_bins) # yaw bins
+ self.fc_roll = nn.Linear(dims[-1], num_bins) # roll bins
+ self.fc_t = nn.Linear(dims[-1], 3) # translation
+ self.fc_exp = nn.Linear(dims[-1], 3 * num_kp) # expression / delta
+
+ def _init_weights(self, m):
+ if isinstance(m, (nn.Conv2d, nn.Linear)):
+ trunc_normal_(m.weight, std=.02)
+ nn.init.constant_(m.bias, 0)
+
+ def forward_features(self, x):
+ for i in range(4):
+ x = self.downsample_layers[i](x)
+ x = self.stages[i](x)
+ return self.norm(x.mean([-2, -1])) # global average pooling, (N, C, H, W) -> (N, C)
+
+ def forward(self, x):
+ x = self.forward_features(x)
+
+ # implicit keypoints
+ kp = self.fc_kp(x)
+
+ # pose and expression deformation
+ pitch = self.fc_pitch(x)
+ yaw = self.fc_yaw(x)
+ roll = self.fc_roll(x)
+ t = self.fc_t(x)
+ exp = self.fc_exp(x)
+ scale = self.fc_scale(x)
+
+ ret_dct = {
+ 'pitch': pitch,
+ 'yaw': yaw,
+ 'roll': roll,
+ 't': t,
+ 'exp': exp,
+ 'scale': scale,
+
+ 'kp': kp, # canonical keypoint
+ }
+
+ return ret_dct
+
+
+def convnextv2_tiny(**kwargs):
+ model = ConvNeXtV2(depths=[3, 3, 9, 3], dims=[96, 192, 384, 768], **kwargs)
+ return model
diff --git a/difpoint/src/modules/dense_motion.py b/difpoint/src/modules/dense_motion.py
new file mode 100644
index 0000000000000000000000000000000000000000..a81bfa09e7d72a11f321705fe25c308ae938b6d6
--- /dev/null
+++ b/difpoint/src/modules/dense_motion.py
@@ -0,0 +1,104 @@
+# coding: utf-8
+
+"""
+The module that predicting a dense motion from sparse motion representation given by kp_source and kp_driving
+"""
+
+from torch import nn
+import torch.nn.functional as F
+import torch
+from .util import Hourglass, make_coordinate_grid, kp2gaussian
+
+
+class DenseMotionNetwork(nn.Module):
+ def __init__(self, block_expansion, num_blocks, max_features, num_kp, feature_channel, reshape_depth, compress, estimate_occlusion_map=True):
+ super(DenseMotionNetwork, self).__init__()
+ self.hourglass = Hourglass(block_expansion=block_expansion, in_features=(num_kp+1)*(compress+1), max_features=max_features, num_blocks=num_blocks) # ~60+G
+
+ self.mask = nn.Conv3d(self.hourglass.out_filters, num_kp + 1, kernel_size=7, padding=3) # 65G! NOTE: computation cost is large
+ self.compress = nn.Conv3d(feature_channel, compress, kernel_size=1) # 0.8G
+ self.norm = nn.BatchNorm3d(compress, affine=True)
+ self.num_kp = num_kp
+ self.flag_estimate_occlusion_map = estimate_occlusion_map
+
+ if self.flag_estimate_occlusion_map:
+ self.occlusion = nn.Conv2d(self.hourglass.out_filters*reshape_depth, 1, kernel_size=7, padding=3)
+ else:
+ self.occlusion = None
+
+ def create_sparse_motions(self, feature, kp_driving, kp_source):
+ bs, _, d, h, w = feature.shape # (bs, 4, 16, 64, 64)
+ identity_grid = make_coordinate_grid((d, h, w), ref=kp_source) # (16, 64, 64, 3)
+ identity_grid = identity_grid.view(1, 1, d, h, w, 3) # (1, 1, d=16, h=64, w=64, 3)
+ coordinate_grid = identity_grid - kp_driving.reshape(bs, self.num_kp, 1, 1, 1, 3)
+
+ k = coordinate_grid.shape[1]
+
+ # NOTE: there lacks an one-order flow
+ driving_to_source = coordinate_grid + kp_source.view(bs, self.num_kp, 1, 1, 1, 3) # (bs, num_kp, d, h, w, 3)
+
+ # adding background feature
+ identity_grid = identity_grid.repeat(bs, 1, 1, 1, 1, 1)
+ sparse_motions = torch.cat([identity_grid, driving_to_source], dim=1) # (bs, 1+num_kp, d, h, w, 3)
+ return sparse_motions
+
+ def create_deformed_feature(self, feature, sparse_motions):
+ bs, _, d, h, w = feature.shape
+ feature_repeat = feature.unsqueeze(1).unsqueeze(1).repeat(1, self.num_kp+1, 1, 1, 1, 1, 1) # (bs, num_kp+1, 1, c, d, h, w)
+ feature_repeat = feature_repeat.view(bs * (self.num_kp+1), -1, d, h, w) # (bs*(num_kp+1), c, d, h, w)
+ sparse_motions = sparse_motions.view((bs * (self.num_kp+1), d, h, w, -1)) # (bs*(num_kp+1), d, h, w, 3)
+ sparse_deformed = F.grid_sample(feature_repeat, sparse_motions, align_corners=False)
+ sparse_deformed = sparse_deformed.view((bs, self.num_kp+1, -1, d, h, w)) # (bs, num_kp+1, c, d, h, w)
+
+ return sparse_deformed
+
+ def create_heatmap_representations(self, feature, kp_driving, kp_source):
+ spatial_size = feature.shape[3:] # (d=16, h=64, w=64)
+ gaussian_driving = kp2gaussian(kp_driving, spatial_size=spatial_size, kp_variance=0.01) # (bs, num_kp, d, h, w)
+ gaussian_source = kp2gaussian(kp_source, spatial_size=spatial_size, kp_variance=0.01) # (bs, num_kp, d, h, w)
+ heatmap = gaussian_driving - gaussian_source # (bs, num_kp, d, h, w)
+
+ # adding background feature
+ zeros = torch.zeros(heatmap.shape[0], 1, spatial_size[0], spatial_size[1], spatial_size[2]).type(heatmap.type()).to(heatmap.device)
+ heatmap = torch.cat([zeros, heatmap], dim=1)
+ heatmap = heatmap.unsqueeze(2) # (bs, 1+num_kp, 1, d, h, w)
+ return heatmap
+
+ def forward(self, feature, kp_driving, kp_source):
+ bs, _, d, h, w = feature.shape # (bs, 32, 16, 64, 64)
+
+ feature = self.compress(feature) # (bs, 4, 16, 64, 64)
+ feature = self.norm(feature) # (bs, 4, 16, 64, 64)
+ feature = F.relu(feature) # (bs, 4, 16, 64, 64)
+
+ out_dict = dict()
+
+ # 1. deform 3d feature
+ sparse_motion = self.create_sparse_motions(feature, kp_driving, kp_source) # (bs, 1+num_kp, d, h, w, 3)
+ deformed_feature = self.create_deformed_feature(feature, sparse_motion) # (bs, 1+num_kp, c=4, d=16, h=64, w=64)
+
+ # 2. (bs, 1+num_kp, d, h, w)
+ heatmap = self.create_heatmap_representations(deformed_feature, kp_driving, kp_source) # (bs, 1+num_kp, 1, d, h, w)
+
+ input = torch.cat([heatmap, deformed_feature], dim=2) # (bs, 1+num_kp, c=5, d=16, h=64, w=64)
+ input = input.view(bs, -1, d, h, w) # (bs, (1+num_kp)*c=105, d=16, h=64, w=64)
+
+ prediction = self.hourglass(input)
+
+ mask = self.mask(prediction)
+ mask = F.softmax(mask, dim=1) # (bs, 1+num_kp, d=16, h=64, w=64)
+ out_dict['mask'] = mask
+ mask = mask.unsqueeze(2) # (bs, num_kp+1, 1, d, h, w)
+ sparse_motion = sparse_motion.permute(0, 1, 5, 2, 3, 4) # (bs, num_kp+1, 3, d, h, w)
+ deformation = (sparse_motion * mask).sum(dim=1) # (bs, 3, d, h, w) mask take effect in this place
+ deformation = deformation.permute(0, 2, 3, 4, 1) # (bs, d, h, w, 3)
+
+ out_dict['deformation'] = deformation
+
+ if self.flag_estimate_occlusion_map:
+ bs, _, d, h, w = prediction.shape
+ prediction_reshape = prediction.view(bs, -1, h, w)
+ occlusion_map = torch.sigmoid(self.occlusion(prediction_reshape)) # Bx1x64x64
+ out_dict['occlusion_map'] = occlusion_map
+
+ return out_dict
diff --git a/difpoint/src/modules/motion_extractor.py b/difpoint/src/modules/motion_extractor.py
new file mode 100644
index 0000000000000000000000000000000000000000..b2982e53c52d9ec1e0bec0453cc05edb51a15d23
--- /dev/null
+++ b/difpoint/src/modules/motion_extractor.py
@@ -0,0 +1,35 @@
+# coding: utf-8
+
+"""
+Motion extractor(M), which directly predicts the canonical keypoints, head pose and expression deformation of the input image
+"""
+
+from torch import nn
+import torch
+
+from .convnextv2 import convnextv2_tiny
+from .util import filter_state_dict
+
+model_dict = {
+ 'convnextv2_tiny': convnextv2_tiny,
+}
+
+
+class MotionExtractor(nn.Module):
+ def __init__(self, **kwargs):
+ super(MotionExtractor, self).__init__()
+
+ # default is convnextv2_base
+ backbone = kwargs.get('backbone', 'convnextv2_tiny')
+ self.detector = model_dict.get(backbone)(**kwargs)
+
+ def load_pretrained(self, init_path: str):
+ if init_path not in (None, ''):
+ state_dict = torch.load(init_path, map_location=lambda storage, loc: storage)['model']
+ state_dict = filter_state_dict(state_dict, remove_name='head')
+ ret = self.detector.load_state_dict(state_dict, strict=False)
+ print(f'Load pretrained model from {init_path}, ret: {ret}')
+
+ def forward(self, x):
+ out = self.detector(x)
+ return out
diff --git a/difpoint/src/modules/spade_generator.py b/difpoint/src/modules/spade_generator.py
new file mode 100644
index 0000000000000000000000000000000000000000..147a9aed0c7707fe6ae3d59ce1a30154ef75afcc
--- /dev/null
+++ b/difpoint/src/modules/spade_generator.py
@@ -0,0 +1,59 @@
+# coding: utf-8
+
+"""
+Spade decoder(G) defined in the paper, which input the warped feature to generate the animated image.
+"""
+
+import torch
+from torch import nn
+import torch.nn.functional as F
+from .util import SPADEResnetBlock
+
+
+class SPADEDecoder(nn.Module):
+ def __init__(self, upscale=1, max_features=256, block_expansion=64, out_channels=64, num_down_blocks=2):
+ for i in range(num_down_blocks):
+ input_channels = min(max_features, block_expansion * (2 ** (i + 1)))
+ self.upscale = upscale
+ super().__init__()
+ norm_G = 'spadespectralinstance'
+ label_num_channels = input_channels # 256
+
+ self.fc = nn.Conv2d(input_channels, 2 * input_channels, 3, padding=1)
+ self.G_middle_0 = SPADEResnetBlock(2 * input_channels, 2 * input_channels, norm_G, label_num_channels)
+ self.G_middle_1 = SPADEResnetBlock(2 * input_channels, 2 * input_channels, norm_G, label_num_channels)
+ self.G_middle_2 = SPADEResnetBlock(2 * input_channels, 2 * input_channels, norm_G, label_num_channels)
+ self.G_middle_3 = SPADEResnetBlock(2 * input_channels, 2 * input_channels, norm_G, label_num_channels)
+ self.G_middle_4 = SPADEResnetBlock(2 * input_channels, 2 * input_channels, norm_G, label_num_channels)
+ self.G_middle_5 = SPADEResnetBlock(2 * input_channels, 2 * input_channels, norm_G, label_num_channels)
+ self.up_0 = SPADEResnetBlock(2 * input_channels, input_channels, norm_G, label_num_channels)
+ self.up_1 = SPADEResnetBlock(input_channels, out_channels, norm_G, label_num_channels)
+ self.up = nn.Upsample(scale_factor=2)
+
+ if self.upscale is None or self.upscale <= 1:
+ self.conv_img = nn.Conv2d(out_channels, 3, 3, padding=1)
+ else:
+ self.conv_img = nn.Sequential(
+ nn.Conv2d(out_channels, 3 * (2 * 2), kernel_size=3, padding=1),
+ nn.PixelShuffle(upscale_factor=2)
+ )
+
+ def forward(self, feature):
+ seg = feature # Bx256x64x64
+ x = self.fc(feature) # Bx512x64x64
+ x = self.G_middle_0(x, seg)
+ x = self.G_middle_1(x, seg)
+ x = self.G_middle_2(x, seg)
+ x = self.G_middle_3(x, seg)
+ x = self.G_middle_4(x, seg)
+ x = self.G_middle_5(x, seg)
+
+ x = self.up(x) # Bx512x64x64 -> Bx512x128x128
+ x = self.up_0(x, seg) # Bx512x128x128 -> Bx256x128x128
+ x = self.up(x) # Bx256x128x128 -> Bx256x256x256
+ x = self.up_1(x, seg) # Bx256x256x256 -> Bx64x256x256
+
+ x = self.conv_img(F.leaky_relu(x, 2e-1)) # Bx64x256x256 -> Bx3xHxW
+ x = torch.sigmoid(x) # Bx3xHxW
+
+ return x
\ No newline at end of file
diff --git a/difpoint/src/modules/stitching_retargeting_network.py b/difpoint/src/modules/stitching_retargeting_network.py
new file mode 100644
index 0000000000000000000000000000000000000000..5f50b7cf5a21cd71c70a7bbaaa4b6b68b4762ea3
--- /dev/null
+++ b/difpoint/src/modules/stitching_retargeting_network.py
@@ -0,0 +1,38 @@
+# coding: utf-8
+
+"""
+Stitching module(S) and two retargeting modules(R) defined in the paper.
+
+- The stitching module pastes the animated portrait back into the original image space without pixel misalignment, such as in
+the stitching region.
+
+- The eyes retargeting module is designed to address the issue of incomplete eye closure during cross-id reenactment, especially
+when a person with small eyes drives a person with larger eyes.
+
+- The lip retargeting module is designed similarly to the eye retargeting module, and can also normalize the input by ensuring that
+the lips are in a closed state, which facilitates better animation driving.
+"""
+from torch import nn
+
+
+class StitchingRetargetingNetwork(nn.Module):
+ def __init__(self, input_size, hidden_sizes, output_size):
+ super(StitchingRetargetingNetwork, self).__init__()
+ layers = []
+ for i in range(len(hidden_sizes)):
+ if i == 0:
+ layers.append(nn.Linear(input_size, hidden_sizes[i]))
+ else:
+ layers.append(nn.Linear(hidden_sizes[i - 1], hidden_sizes[i]))
+ layers.append(nn.ReLU(inplace=True))
+ layers.append(nn.Linear(hidden_sizes[-1], output_size))
+ self.mlp = nn.Sequential(*layers)
+
+ def initialize_weights_to_zero(self):
+ for m in self.modules():
+ if isinstance(m, nn.Linear):
+ nn.init.zeros_(m.weight)
+ nn.init.zeros_(m.bias)
+
+ def forward(self, x):
+ return self.mlp(x)
diff --git a/difpoint/src/modules/util.py b/difpoint/src/modules/util.py
new file mode 100644
index 0000000000000000000000000000000000000000..f83980b24372bee38779ceeb3349fca91735e56e
--- /dev/null
+++ b/difpoint/src/modules/util.py
@@ -0,0 +1,441 @@
+# coding: utf-8
+
+"""
+This file defines various neural network modules and utility functions, including convolutional and residual blocks,
+normalizations, and functions for spatial transformation and tensor manipulation.
+"""
+
+from torch import nn
+import torch.nn.functional as F
+import torch
+import torch.nn.utils.spectral_norm as spectral_norm
+import math
+import warnings
+
+
+def kp2gaussian(kp, spatial_size, kp_variance):
+ """
+ Transform a keypoint into gaussian like representation
+ """
+ mean = kp
+
+ coordinate_grid = make_coordinate_grid(spatial_size, mean)
+ number_of_leading_dimensions = len(mean.shape) - 1
+ shape = (1,) * number_of_leading_dimensions + coordinate_grid.shape
+ coordinate_grid = coordinate_grid.view(*shape)
+ repeats = mean.shape[:number_of_leading_dimensions] + (1, 1, 1, 1)
+ coordinate_grid = coordinate_grid.repeat(*repeats)
+
+ # Preprocess kp shape
+ shape = mean.shape[:number_of_leading_dimensions] + (1, 1, 1, 3)
+ mean = mean.view(*shape)
+
+ mean_sub = (coordinate_grid - mean)
+
+ out = torch.exp(-0.5 * (mean_sub ** 2).sum(-1) / kp_variance)
+
+ return out
+
+
+def make_coordinate_grid(spatial_size, ref, **kwargs):
+ d, h, w = spatial_size
+ x = torch.arange(w).type(ref.dtype).to(ref.device)
+ y = torch.arange(h).type(ref.dtype).to(ref.device)
+ z = torch.arange(d).type(ref.dtype).to(ref.device)
+
+ # NOTE: must be right-down-in
+ x = (2 * (x / (w - 1)) - 1) # the x axis faces to the right
+ y = (2 * (y / (h - 1)) - 1) # the y axis faces to the bottom
+ z = (2 * (z / (d - 1)) - 1) # the z axis faces to the inner
+
+ yy = y.view(1, -1, 1).repeat(d, 1, w)
+ xx = x.view(1, 1, -1).repeat(d, h, 1)
+ zz = z.view(-1, 1, 1).repeat(1, h, w)
+
+ meshed = torch.cat([xx.unsqueeze_(3), yy.unsqueeze_(3), zz.unsqueeze_(3)], 3)
+
+ return meshed
+
+
+class ConvT2d(nn.Module):
+ """
+ Upsampling block for use in decoder.
+ """
+
+ def __init__(self, in_features, out_features, kernel_size=3, stride=2, padding=1, output_padding=1):
+ super(ConvT2d, self).__init__()
+
+ self.convT = nn.ConvTranspose2d(in_features, out_features, kernel_size=kernel_size, stride=stride,
+ padding=padding, output_padding=output_padding)
+ self.norm = nn.InstanceNorm2d(out_features)
+
+ def forward(self, x):
+ out = self.convT(x)
+ out = self.norm(out)
+ out = F.leaky_relu(out)
+ return out
+
+
+class ResBlock3d(nn.Module):
+ """
+ Res block, preserve spatial resolution.
+ """
+
+ def __init__(self, in_features, kernel_size, padding):
+ super(ResBlock3d, self).__init__()
+ self.conv1 = nn.Conv3d(in_channels=in_features, out_channels=in_features, kernel_size=kernel_size, padding=padding)
+ self.conv2 = nn.Conv3d(in_channels=in_features, out_channels=in_features, kernel_size=kernel_size, padding=padding)
+ self.norm1 = nn.BatchNorm3d(in_features, affine=True)
+ self.norm2 = nn.BatchNorm3d(in_features, affine=True)
+
+ def forward(self, x):
+ out = self.norm1(x)
+ out = F.relu(out)
+ out = self.conv1(out)
+ out = self.norm2(out)
+ out = F.relu(out)
+ out = self.conv2(out)
+ out += x
+ return out
+
+
+class UpBlock3d(nn.Module):
+ """
+ Upsampling block for use in decoder.
+ """
+
+ def __init__(self, in_features, out_features, kernel_size=3, padding=1, groups=1):
+ super(UpBlock3d, self).__init__()
+
+ self.conv = nn.Conv3d(in_channels=in_features, out_channels=out_features, kernel_size=kernel_size,
+ padding=padding, groups=groups)
+ self.norm = nn.BatchNorm3d(out_features, affine=True)
+
+ def forward(self, x):
+ out = F.interpolate(x, scale_factor=(1, 2, 2))
+ out = self.conv(out)
+ out = self.norm(out)
+ out = F.relu(out)
+ return out
+
+
+class DownBlock2d(nn.Module):
+ """
+ Downsampling block for use in encoder.
+ """
+
+ def __init__(self, in_features, out_features, kernel_size=3, padding=1, groups=1):
+ super(DownBlock2d, self).__init__()
+ self.conv = nn.Conv2d(in_channels=in_features, out_channels=out_features, kernel_size=kernel_size, padding=padding, groups=groups)
+ self.norm = nn.BatchNorm2d(out_features, affine=True)
+ self.pool = nn.AvgPool2d(kernel_size=(2, 2))
+
+ def forward(self, x):
+ out = self.conv(x)
+ out = self.norm(out)
+ out = F.relu(out)
+ out = self.pool(out)
+ return out
+
+
+class DownBlock3d(nn.Module):
+ """
+ Downsampling block for use in encoder.
+ """
+
+ def __init__(self, in_features, out_features, kernel_size=3, padding=1, groups=1):
+ super(DownBlock3d, self).__init__()
+ '''
+ self.conv = nn.Conv3d(in_channels=in_features, out_channels=out_features, kernel_size=kernel_size,
+ padding=padding, groups=groups, stride=(1, 2, 2))
+ '''
+ self.conv = nn.Conv3d(in_channels=in_features, out_channels=out_features, kernel_size=kernel_size,
+ padding=padding, groups=groups)
+ self.norm = nn.BatchNorm3d(out_features, affine=True)
+ self.pool = nn.AvgPool3d(kernel_size=(1, 2, 2))
+
+ def forward(self, x):
+ out = self.conv(x)
+ out = self.norm(out)
+ out = F.relu(out)
+ out = self.pool(out)
+ return out
+
+
+class SameBlock2d(nn.Module):
+ """
+ Simple block, preserve spatial resolution.
+ """
+
+ def __init__(self, in_features, out_features, groups=1, kernel_size=3, padding=1, lrelu=False):
+ super(SameBlock2d, self).__init__()
+ self.conv = nn.Conv2d(in_channels=in_features, out_channels=out_features, kernel_size=kernel_size, padding=padding, groups=groups)
+ self.norm = nn.BatchNorm2d(out_features, affine=True)
+ if lrelu:
+ self.ac = nn.LeakyReLU()
+ else:
+ self.ac = nn.ReLU()
+
+ def forward(self, x):
+ out = self.conv(x)
+ out = self.norm(out)
+ out = self.ac(out)
+ return out
+
+
+class Encoder(nn.Module):
+ """
+ Hourglass Encoder
+ """
+
+ def __init__(self, block_expansion, in_features, num_blocks=3, max_features=256):
+ super(Encoder, self).__init__()
+
+ down_blocks = []
+ for i in range(num_blocks):
+ down_blocks.append(DownBlock3d(in_features if i == 0 else min(max_features, block_expansion * (2 ** i)), min(max_features, block_expansion * (2 ** (i + 1))), kernel_size=3, padding=1))
+ self.down_blocks = nn.ModuleList(down_blocks)
+
+ def forward(self, x):
+ outs = [x]
+ for down_block in self.down_blocks:
+ outs.append(down_block(outs[-1]))
+ return outs
+
+
+class Decoder(nn.Module):
+ """
+ Hourglass Decoder
+ """
+
+ def __init__(self, block_expansion, in_features, num_blocks=3, max_features=256):
+ super(Decoder, self).__init__()
+
+ up_blocks = []
+
+ for i in range(num_blocks)[::-1]:
+ in_filters = (1 if i == num_blocks - 1 else 2) * min(max_features, block_expansion * (2 ** (i + 1)))
+ out_filters = min(max_features, block_expansion * (2 ** i))
+ up_blocks.append(UpBlock3d(in_filters, out_filters, kernel_size=3, padding=1))
+
+ self.up_blocks = nn.ModuleList(up_blocks)
+ self.out_filters = block_expansion + in_features
+
+ self.conv = nn.Conv3d(in_channels=self.out_filters, out_channels=self.out_filters, kernel_size=3, padding=1)
+ self.norm = nn.BatchNorm3d(self.out_filters, affine=True)
+
+ def forward(self, x):
+ out = x.pop()
+ for up_block in self.up_blocks:
+ out = up_block(out)
+ skip = x.pop()
+ out = torch.cat([out, skip], dim=1)
+ out = self.conv(out)
+ out = self.norm(out)
+ out = F.relu(out)
+ return out
+
+
+class Hourglass(nn.Module):
+ """
+ Hourglass architecture.
+ """
+
+ def __init__(self, block_expansion, in_features, num_blocks=3, max_features=256):
+ super(Hourglass, self).__init__()
+ self.encoder = Encoder(block_expansion, in_features, num_blocks, max_features)
+ self.decoder = Decoder(block_expansion, in_features, num_blocks, max_features)
+ self.out_filters = self.decoder.out_filters
+
+ def forward(self, x):
+ return self.decoder(self.encoder(x))
+
+
+class SPADE(nn.Module):
+ def __init__(self, norm_nc, label_nc):
+ super().__init__()
+
+ self.param_free_norm = nn.InstanceNorm2d(norm_nc, affine=False)
+ nhidden = 128
+
+ self.mlp_shared = nn.Sequential(
+ nn.Conv2d(label_nc, nhidden, kernel_size=3, padding=1),
+ nn.ReLU())
+ self.mlp_gamma = nn.Conv2d(nhidden, norm_nc, kernel_size=3, padding=1)
+ self.mlp_beta = nn.Conv2d(nhidden, norm_nc, kernel_size=3, padding=1)
+
+ def forward(self, x, segmap):
+ normalized = self.param_free_norm(x)
+ segmap = F.interpolate(segmap, size=x.size()[2:], mode='nearest')
+ actv = self.mlp_shared(segmap)
+ gamma = self.mlp_gamma(actv)
+ beta = self.mlp_beta(actv)
+ out = normalized * (1 + gamma) + beta
+ return out
+
+
+class SPADEResnetBlock(nn.Module):
+ def __init__(self, fin, fout, norm_G, label_nc, use_se=False, dilation=1):
+ super().__init__()
+ # Attributes
+ self.learned_shortcut = (fin != fout)
+ fmiddle = min(fin, fout)
+ self.use_se = use_se
+ # create conv layers
+ self.conv_0 = nn.Conv2d(fin, fmiddle, kernel_size=3, padding=dilation, dilation=dilation)
+ self.conv_1 = nn.Conv2d(fmiddle, fout, kernel_size=3, padding=dilation, dilation=dilation)
+ if self.learned_shortcut:
+ self.conv_s = nn.Conv2d(fin, fout, kernel_size=1, bias=False)
+ # apply spectral norm if specified
+ if 'spectral' in norm_G:
+ self.conv_0 = spectral_norm(self.conv_0)
+ self.conv_1 = spectral_norm(self.conv_1)
+ if self.learned_shortcut:
+ self.conv_s = spectral_norm(self.conv_s)
+ # define normalization layers
+ self.norm_0 = SPADE(fin, label_nc)
+ self.norm_1 = SPADE(fmiddle, label_nc)
+ if self.learned_shortcut:
+ self.norm_s = SPADE(fin, label_nc)
+
+ def forward(self, x, seg1):
+ x_s = self.shortcut(x, seg1)
+ dx = self.conv_0(self.actvn(self.norm_0(x, seg1)))
+ dx = self.conv_1(self.actvn(self.norm_1(dx, seg1)))
+ out = x_s + dx
+ return out
+
+ def shortcut(self, x, seg1):
+ if self.learned_shortcut:
+ x_s = self.conv_s(self.norm_s(x, seg1))
+ else:
+ x_s = x
+ return x_s
+
+ def actvn(self, x):
+ return F.leaky_relu(x, 2e-1)
+
+
+def filter_state_dict(state_dict, remove_name='fc'):
+ new_state_dict = {}
+ for key in state_dict:
+ if remove_name in key:
+ continue
+ new_state_dict[key] = state_dict[key]
+ return new_state_dict
+
+
+class GRN(nn.Module):
+ """ GRN (Global Response Normalization) layer
+ """
+
+ def __init__(self, dim):
+ super().__init__()
+ self.gamma = nn.Parameter(torch.zeros(1, 1, 1, dim))
+ self.beta = nn.Parameter(torch.zeros(1, 1, 1, dim))
+
+ def forward(self, x):
+ Gx = torch.norm(x, p=2, dim=(1, 2), keepdim=True)
+ Nx = Gx / (Gx.mean(dim=-1, keepdim=True) + 1e-6)
+ return self.gamma * (x * Nx) + self.beta + x
+
+
+class LayerNorm(nn.Module):
+ r""" LayerNorm that supports two data formats: channels_last (default) or channels_first.
+ The ordering of the dimensions in the inputs. channels_last corresponds to inputs with
+ shape (batch_size, height, width, channels) while channels_first corresponds to inputs
+ with shape (batch_size, channels, height, width).
+ """
+
+ def __init__(self, normalized_shape, eps=1e-6, data_format="channels_last"):
+ super().__init__()
+ self.weight = nn.Parameter(torch.ones(normalized_shape))
+ self.bias = nn.Parameter(torch.zeros(normalized_shape))
+ self.eps = eps
+ self.data_format = data_format
+ if self.data_format not in ["channels_last", "channels_first"]:
+ raise NotImplementedError
+ self.normalized_shape = (normalized_shape, )
+
+ def forward(self, x):
+ if self.data_format == "channels_last":
+ return F.layer_norm(x, self.normalized_shape, self.weight, self.bias, self.eps)
+ elif self.data_format == "channels_first":
+ u = x.mean(1, keepdim=True)
+ s = (x - u).pow(2).mean(1, keepdim=True)
+ x = (x - u) / torch.sqrt(s + self.eps)
+ x = self.weight[:, None, None] * x + self.bias[:, None, None]
+ return x
+
+
+def _no_grad_trunc_normal_(tensor, mean, std, a, b):
+ # Cut & paste from PyTorch official master until it's in a few official releases - RW
+ # Method based on https://people.sc.fsu.edu/~jburkardt/presentations/truncated_normal.pdf
+ def norm_cdf(x):
+ # Computes standard normal cumulative distribution function
+ return (1. + math.erf(x / math.sqrt(2.))) / 2.
+
+ if (mean < a - 2 * std) or (mean > b + 2 * std):
+ warnings.warn("mean is more than 2 std from [a, b] in nn.init.trunc_normal_. "
+ "The distribution of values may be incorrect.",
+ stacklevel=2)
+
+ with torch.no_grad():
+ # Values are generated by using a truncated uniform distribution and
+ # then using the inverse CDF for the normal distribution.
+ # Get upper and lower cdf values
+ l = norm_cdf((a - mean) / std)
+ u = norm_cdf((b - mean) / std)
+
+ # Uniformly fill tensor with values from [l, u], then translate to
+ # [2l-1, 2u-1].
+ tensor.uniform_(2 * l - 1, 2 * u - 1)
+
+ # Use inverse cdf transform for normal distribution to get truncated
+ # standard normal
+ tensor.erfinv_()
+
+ # Transform to proper mean, std
+ tensor.mul_(std * math.sqrt(2.))
+ tensor.add_(mean)
+
+ # Clamp to ensure it's in the proper range
+ tensor.clamp_(min=a, max=b)
+ return tensor
+
+
+def drop_path(x, drop_prob=0., training=False, scale_by_keep=True):
+ """ Drop paths (Stochastic Depth) per sample (when applied in main path of residual blocks).
+
+ This is the same as the DropConnect impl I created for EfficientNet, etc networks, however,
+ the original name is misleading as 'Drop Connect' is a different form of dropout in a separate paper...
+ See discussion: https://github.com/tensorflow/tpu/issues/494#issuecomment-532968956 ... I've opted for
+ changing the layer and argument names to 'drop path' rather than mix DropConnect as a layer name and use
+ 'survival rate' as the argument.
+
+ """
+ if drop_prob == 0. or not training:
+ return x
+ keep_prob = 1 - drop_prob
+ shape = (x.shape[0],) + (1,) * (x.ndim - 1) # work with diff dim tensors, not just 2D ConvNets
+ random_tensor = x.new_empty(shape).bernoulli_(keep_prob)
+ if keep_prob > 0.0 and scale_by_keep:
+ random_tensor.div_(keep_prob)
+ return x * random_tensor
+
+
+class DropPath(nn.Module):
+ """ Drop paths (Stochastic Depth) per sample (when applied in main path of residual blocks).
+ """
+
+ def __init__(self, drop_prob=None, scale_by_keep=True):
+ super(DropPath, self).__init__()
+ self.drop_prob = drop_prob
+ self.scale_by_keep = scale_by_keep
+
+ def forward(self, x):
+ return drop_path(x, self.drop_prob, self.training, self.scale_by_keep)
+
+
+def trunc_normal_(tensor, mean=0., std=1., a=-2., b=2.):
+ return _no_grad_trunc_normal_(tensor, mean, std, a, b)
diff --git a/difpoint/src/modules/warping_network.py b/difpoint/src/modules/warping_network.py
new file mode 100644
index 0000000000000000000000000000000000000000..9191a197055a954272ee8ed86c5e34f3f33f9ad5
--- /dev/null
+++ b/difpoint/src/modules/warping_network.py
@@ -0,0 +1,77 @@
+# coding: utf-8
+
+"""
+Warping field estimator(W) defined in the paper, which generates a warping field using the implicit
+keypoint representations x_s and x_d, and employs this flow field to warp the source feature volume f_s.
+"""
+
+from torch import nn
+import torch.nn.functional as F
+from .util import SameBlock2d
+from .dense_motion import DenseMotionNetwork
+
+
+class WarpingNetwork(nn.Module):
+ def __init__(
+ self,
+ num_kp,
+ block_expansion,
+ max_features,
+ num_down_blocks,
+ reshape_channel,
+ estimate_occlusion_map=False,
+ dense_motion_params=None,
+ **kwargs
+ ):
+ super(WarpingNetwork, self).__init__()
+
+ self.upscale = kwargs.get('upscale', 1)
+ self.flag_use_occlusion_map = kwargs.get('flag_use_occlusion_map', True)
+
+ if dense_motion_params is not None:
+ self.dense_motion_network = DenseMotionNetwork(
+ num_kp=num_kp,
+ feature_channel=reshape_channel,
+ estimate_occlusion_map=estimate_occlusion_map,
+ **dense_motion_params
+ )
+ else:
+ self.dense_motion_network = None
+
+ self.third = SameBlock2d(max_features, block_expansion * (2 ** num_down_blocks), kernel_size=(3, 3), padding=(1, 1), lrelu=True)
+ self.fourth = nn.Conv2d(in_channels=block_expansion * (2 ** num_down_blocks), out_channels=block_expansion * (2 ** num_down_blocks), kernel_size=1, stride=1)
+
+ self.estimate_occlusion_map = estimate_occlusion_map
+
+ def deform_input(self, inp, deformation):
+ return F.grid_sample(inp, deformation, align_corners=False)
+
+ def forward(self, feature_3d, kp_driving, kp_source):
+ if self.dense_motion_network is not None:
+ # Feature warper, Transforming feature representation according to deformation and occlusion
+ dense_motion = self.dense_motion_network(
+ feature=feature_3d, kp_driving=kp_driving, kp_source=kp_source
+ )
+ if 'occlusion_map' in dense_motion:
+ occlusion_map = dense_motion['occlusion_map'] # Bx1x64x64
+ else:
+ occlusion_map = None
+
+ deformation = dense_motion['deformation'] # Bx16x64x64x3
+ out = self.deform_input(feature_3d, deformation) # Bx32x16x64x64
+
+ bs, c, d, h, w = out.shape # Bx32x16x64x64
+ out = out.view(bs, c * d, h, w) # -> Bx512x64x64
+ out = self.third(out) # -> Bx256x64x64
+ out = self.fourth(out) # -> Bx256x64x64
+
+ if self.flag_use_occlusion_map and (occlusion_map is not None):
+ out = out * occlusion_map
+
+ ret_dct = {
+ 'occlusion_map': occlusion_map,
+ 'deformation': deformation,
+ 'out': out,
+ }
+
+ return ret_dct
diff --git a/difpoint/src/pipelines/__init__.py b/difpoint/src/pipelines/__init__.py
new file mode 100644
index 0000000000000000000000000000000000000000..96c9b2de4601fea43e7ffa1d818906ddeb7f5dea
--- /dev/null
+++ b/difpoint/src/pipelines/__init__.py
@@ -0,0 +1,6 @@
+# -*- coding: utf-8 -*-
+# @Time : 2024/7/16 19:22
+# @Author : wenshao
+# @Email : wenshaoguo0611@gmail.com
+# @Project : FasterLivePortrait
+# @FileName: __init__.py.py
diff --git a/difpoint/src/pipelines/__pycache__/__init__.cpython-310.pyc b/difpoint/src/pipelines/__pycache__/__init__.cpython-310.pyc
new file mode 100644
index 0000000000000000000000000000000000000000..009d70b7a8098a6ddc1c22250f62b7c8e6bbbc48
Binary files /dev/null and b/difpoint/src/pipelines/__pycache__/__init__.cpython-310.pyc differ
diff --git a/difpoint/src/pipelines/__pycache__/__init__.cpython-38.pyc b/difpoint/src/pipelines/__pycache__/__init__.cpython-38.pyc
new file mode 100644
index 0000000000000000000000000000000000000000..03c2616a7e05fe928547df4b9c003b84fbdd0531
Binary files /dev/null and b/difpoint/src/pipelines/__pycache__/__init__.cpython-38.pyc differ
diff --git a/difpoint/src/pipelines/__pycache__/faster_live_portrait_pipeline.cpython-310.pyc b/difpoint/src/pipelines/__pycache__/faster_live_portrait_pipeline.cpython-310.pyc
new file mode 100644
index 0000000000000000000000000000000000000000..96006cad4540bde45f3c79eae686e547b24d6312
Binary files /dev/null and b/difpoint/src/pipelines/__pycache__/faster_live_portrait_pipeline.cpython-310.pyc differ
diff --git a/difpoint/src/pipelines/__pycache__/faster_live_portrait_pipeline.cpython-38.pyc b/difpoint/src/pipelines/__pycache__/faster_live_portrait_pipeline.cpython-38.pyc
new file mode 100644
index 0000000000000000000000000000000000000000..e1a88d83ec753730a8df998602a71b6c069dbe9d
Binary files /dev/null and b/difpoint/src/pipelines/__pycache__/faster_live_portrait_pipeline.cpython-38.pyc differ
diff --git a/difpoint/src/pipelines/faster_live_portrait_pipeline.py b/difpoint/src/pipelines/faster_live_portrait_pipeline.py
new file mode 100644
index 0000000000000000000000000000000000000000..c6fda90c45ebb77ff682e24066b3ba8deb8a41cd
--- /dev/null
+++ b/difpoint/src/pipelines/faster_live_portrait_pipeline.py
@@ -0,0 +1,455 @@
+# -*- coding: utf-8 -*-
+# @Author : wenshao
+# @Email : wenshaoguo0611@gmail.com
+# @Project : FasterLivePortrait
+# @FileName: faster_live_portrait_pipeline.py
+
+import copy
+import pdb
+import time
+import traceback
+from PIL import Image
+import cv2
+from tqdm import tqdm
+import numpy as np
+import torch
+
+from .. import models
+from ..utils.crop import crop_image, parse_bbox_from_landmark, crop_image_by_bbox, paste_back, paste_back_pytorch
+from ..utils.utils import resize_to_limit, prepare_paste_back, get_rotation_matrix, calc_lip_close_ratio, \
+ calc_eye_close_ratio, transform_keypoint, concat_feat
+from difpoint.src.utils import utils
+
+
+class FasterLivePortraitPipeline:
+ def __init__(self, cfg, **kwargs):
+ self.cfg = cfg
+ self.init(**kwargs)
+
+ def init(self, **kwargs):
+ self.init_vars(**kwargs)
+ self.init_models(**kwargs)
+
+ def clean_models(self, **kwargs):
+ """
+ clean model
+ :param kwargs:
+ :return:
+ """
+ for key in list(self.model_dict.keys()):
+ del self.model_dict[key]
+ self.model_dict = {}
+
+ def init_models(self, **kwargs):
+ if not kwargs.get("is_animal", False):
+ print("load Human Model >>>")
+ self.is_animal = False
+ self.model_dict = {}
+ for model_name in self.cfg.models:
+ print(f"loading model: {model_name}")
+ print(self.cfg.models[model_name])
+ self.model_dict[model_name] = getattr(models, self.cfg.models[model_name]["name"])(
+ **self.cfg.models[model_name])
+ else:
+ print("load Animal Model >>>")
+ self.is_animal = True
+ self.model_dict = {}
+ from src.utils.animal_landmark_runner import XPoseRunner
+ from src.utils.utils import make_abs_path
+
+ xpose_ckpt_path: str = make_abs_path("../difpoint/checkpoints/liveportrait_animal_onnx/xpose.pth")
+ xpose_config_file_path: str = make_abs_path("models/XPose/config_model/UniPose_SwinT.py")
+ xpose_embedding_cache_path: str = make_abs_path('../difpoint/checkpoints/liveportrait_animal_onnx/clip_embedding')
+ self.model_dict["xpose"] = XPoseRunner(model_config_path=xpose_config_file_path,
+ model_checkpoint_path=xpose_ckpt_path,
+ embeddings_cache_path=xpose_embedding_cache_path,
+ flag_use_half_precision=True)
+ for model_name in self.cfg.animal_models:
+ print(f"loading model: {model_name}")
+ print(self.cfg.animal_models[model_name])
+ self.model_dict[model_name] = getattr(models, self.cfg.animal_models[model_name]["name"])(
+ **self.cfg.animal_models[model_name])
+
+ def init_vars(self, **kwargs):
+ self.mask_crop = cv2.imread(self.cfg.infer_params.mask_crop_path, cv2.IMREAD_COLOR)
+ self.frame_id = 0
+ self.src_lmk_pre = None
+ self.R_d_0 = None
+ self.x_d_0_info = None
+ self.R_d_smooth = utils.OneEuroFilter(4, 1)
+ self.exp_smooth = utils.OneEuroFilter(4, 1)
+
+ ## 记录source的信息
+ self.source_path = None
+ self.src_infos = []
+ self.src_imgs = []
+ self.is_source_video = False
+
+ self.device = torch.device("cuda") if torch.cuda.is_available() else torch.device("cpu")
+
+ def calc_combined_eye_ratio(self, c_d_eyes_i, source_lmk):
+ c_s_eyes = calc_eye_close_ratio(source_lmk[None])
+ c_d_eyes_i = np.array(c_d_eyes_i).reshape(1, 1)
+ # [c_s,eyes, c_d,eyes,i]
+ combined_eye_ratio_tensor = np.concatenate([c_s_eyes, c_d_eyes_i], axis=1)
+ return combined_eye_ratio_tensor
+
+ def calc_combined_lip_ratio(self, c_d_lip_i, source_lmk):
+ c_s_lip = calc_lip_close_ratio(source_lmk[None])
+ c_d_lip_i = np.array(c_d_lip_i).reshape(1, 1) # 1x1
+ # [c_s,lip, c_d,lip,i]
+ combined_lip_ratio_tensor = np.concatenate([c_s_lip, c_d_lip_i], axis=1) # 1x2
+ return combined_lip_ratio_tensor
+
+ def prepare_source(self, source_path, **kwargs):
+ print(f"process source:{source_path} >>>>>>>>")
+ try:
+ if utils.is_image(source_path):
+ self.is_source_video = False
+ elif utils.is_video(source_path):
+ self.is_source_video = True
+ else: # source input is an unknown format
+ raise Exception(f"Unknown source format: {source_path}")
+
+ if self.is_source_video:
+ src_imgs_bgr = []
+ src_vcap = cv2.VideoCapture(source_path)
+ while True:
+ ret, frame = src_vcap.read()
+ if not ret:
+ break
+ src_imgs_bgr.append(frame)
+ src_vcap.release()
+ else:
+ img_bgr = cv2.imread(source_path, cv2.IMREAD_COLOR)
+ src_imgs_bgr = [img_bgr]
+
+ self.src_imgs = []
+ self.src_infos = []
+ self.source_path = source_path
+
+ for ii, img_bgr in tqdm(enumerate(src_imgs_bgr), total=len(src_imgs_bgr)):
+ img_bgr = resize_to_limit(img_bgr, self.cfg.infer_params.source_max_dim,
+ self.cfg.infer_params.source_division)
+ img_rgb = cv2.cvtColor(img_bgr, cv2.COLOR_BGR2RGB)
+ src_faces = []
+ if self.is_animal:
+ with torch.no_grad():
+ img_rgb_pil = Image.fromarray(img_rgb)
+ lmk = self.model_dict["xpose"].run(
+ img_rgb_pil,
+ 'face',
+ 'animal_face',
+ 0,
+ 0
+ )
+ if lmk is None:
+ continue
+ self.src_imgs.append(img_rgb)
+ src_faces.append(lmk)
+ else:
+ src_faces = self.model_dict["face_analysis"].predict(img_bgr)
+ if len(src_faces) == 0:
+ print("No face detected in the this image.")
+ continue
+ self.src_imgs.append(img_rgb)
+ # 如果是实时,只关注最大的那张脸
+ if kwargs.get("realtime", False):
+ src_faces = src_faces[:1]
+
+ crop_infos = []
+ for i in range(len(src_faces)):
+ # NOTE: temporarily only pick the first face, to support multiple face in the future
+ lmk = src_faces[i]
+ # crop the face
+ ret_dct = crop_image(
+ img_rgb, # ndarray
+ lmk, # 106x2 or Nx2
+ dsize=self.cfg.crop_params.src_dsize,
+ scale=self.cfg.crop_params.src_scale,
+ vx_ratio=self.cfg.crop_params.src_vx_ratio,
+ vy_ratio=self.cfg.crop_params.src_vy_ratio,
+ )
+ if self.is_animal:
+ ret_dct["lmk_crop"] = lmk
+ else:
+ lmk = self.model_dict["landmark"].predict(img_rgb, lmk)
+ ret_dct["lmk_crop"] = lmk
+ ret_dct["lmk_crop_256x256"] = ret_dct["lmk_crop"] * 256 / self.cfg.crop_params.src_dsize
+
+ # update a 256x256 version for network input
+ ret_dct["img_crop_256x256"] = cv2.resize(
+ ret_dct["img_crop"], (256, 256), interpolation=cv2.INTER_AREA
+ )
+ crop_infos.append(ret_dct)
+
+ src_infos = [[] for _ in range(len(crop_infos))]
+ for i, crop_info in enumerate(crop_infos):
+ source_lmk = crop_info['lmk_crop']
+ img_crop, img_crop_256x256 = crop_info['img_crop'], crop_info['img_crop_256x256']
+ pitch, yaw, roll, t, exp, scale, kp = self.model_dict["motion_extractor"].predict(
+ img_crop_256x256)
+ x_s_info = {
+ "pitch": pitch,
+ "yaw": yaw,
+ "roll": roll,
+ "t": t,
+ "exp": exp,
+ "scale": scale,
+ "kp": kp
+ }
+ src_infos[i].append(copy.deepcopy(x_s_info))
+ x_c_s = kp
+ R_s = get_rotation_matrix(pitch, yaw, roll)
+ f_s = self.model_dict["app_feat_extractor"].predict(img_crop_256x256)
+ x_s = transform_keypoint(pitch, yaw, roll, t, exp, scale, kp)
+ src_infos[i].extend([source_lmk.copy(), R_s.copy(), f_s.copy(), x_s.copy(), x_c_s.copy()])
+ if not self.is_animal:
+ flag_lip_zero = self.cfg.infer_params.flag_normalize_lip # not overwrite
+ if flag_lip_zero:
+ # let lip-open scalar to be 0 at first
+ c_d_lip_before_animation = [0.]
+ combined_lip_ratio_tensor_before_animation = self.calc_combined_lip_ratio(
+ c_d_lip_before_animation, source_lmk)
+ if combined_lip_ratio_tensor_before_animation[0][
+ 0] < self.cfg.infer_params.lip_normalize_threshold:
+ flag_lip_zero = False
+ src_infos[i].append(None)
+ src_infos[i].append(flag_lip_zero)
+ else:
+ lip_delta_before_animation = self.model_dict['stitching_lip_retarget'].predict(
+ concat_feat(x_s, combined_lip_ratio_tensor_before_animation))
+ src_infos[i].append(lip_delta_before_animation.copy())
+ src_infos[i].append(flag_lip_zero)
+ else:
+ src_infos[i].append(None)
+ src_infos[i].append(flag_lip_zero)
+ else:
+ src_infos[i].append(None)
+ src_infos[i].append(False)
+
+ ######## prepare for pasteback ########
+ if self.cfg.infer_params.flag_pasteback and self.cfg.infer_params.flag_do_crop and self.cfg.infer_params.flag_stitching:
+ mask_ori_float = prepare_paste_back(self.mask_crop, crop_info['M_c2o'],
+ dsize=(img_rgb.shape[1], img_rgb.shape[0]))
+ mask_ori_float = torch.from_numpy(mask_ori_float).to(self.device)
+ src_infos[i].append(mask_ori_float)
+ else:
+ src_infos[i].append(None)
+ M = torch.from_numpy(crop_info['M_c2o']).to(self.device)
+ src_infos[i].append(M)
+ self.src_infos.append(src_infos[:])
+ print(f"finish process source:{source_path} >>>>>>>>")
+ return len(self.src_infos) > 0
+ except Exception as e:
+ traceback.print_exc()
+ return False
+
+ def retarget_eye(self, kp_source, eye_close_ratio):
+ """
+ kp_source: BxNx3
+ eye_close_ratio: Bx3
+ Return: Bx(3*num_kp+2)
+ """
+ feat_eye = concat_feat(kp_source, eye_close_ratio)
+ delta = self.model_dict['stitching_eye_retarget'].predict(feat_eye)
+ return delta
+
+ def retarget_lip(self, kp_source, lip_close_ratio):
+ """
+ kp_source: BxNx3
+ lip_close_ratio: Bx2
+ """
+ feat_lip = concat_feat(kp_source, lip_close_ratio)
+ delta = self.model_dict['stitching_lip_retarget'].predict(feat_lip)
+ return delta
+
+ def stitching(self, kp_source, kp_driving):
+ """ conduct the stitching
+ kp_source: Bxnum_kpx3
+ kp_driving: Bxnum_kpx3
+ """
+
+ bs, num_kp = kp_source.shape[:2]
+
+ kp_driving_new = kp_driving.copy()
+
+ delta = self.model_dict['stitching'].predict(concat_feat(kp_source, kp_driving_new))
+
+ delta_exp = delta[..., :3 * num_kp].reshape(bs, num_kp, 3) # 1x20x3
+ delta_tx_ty = delta[..., 3 * num_kp:3 * num_kp + 2].reshape(bs, 1, 2) # 1x1x2
+
+ kp_driving_new += delta_exp
+ kp_driving_new[..., :2] += delta_tx_ty
+
+ return kp_driving_new
+
+ def run(self, image, img_src, src_info, **kwargs):
+ img_bgr = image
+ img_rgb = cv2.cvtColor(img_bgr, cv2.COLOR_BGR2RGB)
+ I_p_pstbk = torch.from_numpy(img_src).to(self.device).float()
+ realtime = kwargs.get("realtime", False)
+
+ if self.cfg.infer_params.flag_crop_driving_video:
+ if self.src_lmk_pre is None:
+ src_face = self.model_dict["face_analysis"].predict(img_bgr)
+ if len(src_face) == 0:
+ self.src_lmk_pre = None
+ return None, None, None
+ lmk = src_face[0]
+ lmk = self.model_dict["landmark"].predict(img_rgb, lmk)
+ self.src_lmk_pre = lmk.copy()
+ else:
+ lmk = self.model_dict["landmark"].predict(img_rgb, self.src_lmk_pre)
+ self.src_lmk_pre = lmk.copy()
+
+ ret_bbox = parse_bbox_from_landmark(
+ lmk,
+ scale=self.cfg.crop_params.dri_scale,
+ vx_ratio_crop_video=self.cfg.crop_params.dri_vx_ratio,
+ vy_ratio=self.cfg.crop_params.dri_vy_ratio,
+ )["bbox"]
+ global_bbox = [
+ ret_bbox[0, 0],
+ ret_bbox[0, 1],
+ ret_bbox[2, 0],
+ ret_bbox[2, 1],
+ ]
+ ret_dct = crop_image_by_bbox(
+ img_rgb,
+ global_bbox,
+ lmk=lmk,
+ dsize=kwargs.get("dsize", 512),
+ flag_rot=False,
+ borderValue=(0, 0, 0),
+ )
+ lmk_crop = ret_dct["lmk_crop"]
+ img_crop = ret_dct["img_crop"]
+ img_crop = cv2.resize(img_crop, (256, 256))
+ else:
+ if self.src_lmk_pre is None:
+ src_face = self.model_dict["face_analysis"].predict(img_bgr)
+ if len(src_face) == 0:
+ self.src_lmk_pre = None
+ return None, None, None
+ lmk = src_face[0]
+ lmk = self.model_dict["landmark"].predict(img_rgb, lmk)
+ self.src_lmk_pre = lmk.copy()
+ else:
+ lmk = self.model_dict["landmark"].predict(img_rgb, self.src_lmk_pre)
+ self.src_lmk_pre = lmk.copy()
+ lmk_crop = lmk.copy()
+ img_crop = cv2.resize(img_rgb, (256, 256))
+
+ input_eye_ratio = calc_eye_close_ratio(lmk_crop[None])
+ input_lip_ratio = calc_lip_close_ratio(lmk_crop[None])
+ pitch, yaw, roll, t, exp, scale, kp = self.model_dict["motion_extractor"].predict(img_crop)
+ x_d_i_info = {
+ "pitch": pitch,
+ "yaw": yaw,
+ "roll": roll,
+ "t": t,
+ "exp": exp,
+ "scale": scale,
+ "kp": kp
+ }
+ R_d_i = get_rotation_matrix(pitch, yaw, roll)
+
+ if kwargs.get("first_frame", False) or self.R_d_0 is None:
+ self.R_d_0 = R_d_i.copy()
+ self.x_d_0_info = copy.deepcopy(x_d_i_info)
+ # realtime smooth
+ self.R_d_smooth = utils.OneEuroFilter(4, 1)
+ self.exp_smooth = utils.OneEuroFilter(4, 1)
+ R_d_0 = self.R_d_0.copy()
+ x_d_0_info = copy.deepcopy(self.x_d_0_info)
+ out_crop, out_org = None, None
+ for j in range(len(src_info)):
+ x_s_info, source_lmk, R_s, f_s, x_s, x_c_s, lip_delta_before_animation, flag_lip_zero, mask_ori_float, M = \
+ src_info[j]
+ if self.cfg.infer_params.flag_relative_motion:
+ if self.is_source_video:
+ if self.cfg.infer_params.flag_video_editing_head_rotation:
+ R_new = (R_d_i @ np.transpose(R_d_0, (0, 2, 1))) @ R_s
+ R_new = self.R_d_smooth.process(R_new)
+ else:
+ R_new = R_s
+ else:
+ R_new = (R_d_i @ np.transpose(R_d_0, (0, 2, 1))) @ R_s
+ delta_new = x_s_info['exp'] + (x_d_i_info['exp'] - x_d_0_info['exp'])
+ if self.is_source_video:
+ delta_new = self.exp_smooth.process(delta_new)
+ scale_new = x_s_info['scale'] if self.is_source_video else x_s_info['scale'] * (x_d_i_info['scale'] / x_d_0_info['scale'])
+ t_new = x_s_info['t'] if self.is_source_video else x_s_info['t'] + (x_d_i_info['t'] - x_d_0_info['t'])
+ else:
+ if self.is_source_video:
+ if self.cfg.infer_params.flag_video_editing_head_rotation:
+ R_new = R_d_i
+ R_new = self.R_d_smooth.process(R_new)
+ else:
+ R_new = R_s
+ else:
+ R_new = R_d_i
+ delta_new = x_d_i_info['exp'].copy()
+ if self.is_source_video:
+ delta_new = self.exp_smooth.process(delta_new)
+ scale_new = x_s_info['scale'].copy()
+ t_new = x_d_i_info['t'].copy()
+
+ t_new[..., 2] = 0 # zero tz
+ x_d_i_new = scale_new * (x_c_s @ R_new + delta_new) + t_new
+ if not self.is_animal:
+ # Algorithm 1:
+ if not self.cfg.infer_params.flag_stitching and not self.cfg.infer_params.flag_eye_retargeting and not self.cfg.infer_params.flag_lip_retargeting:
+ # without stitching or retargeting
+ if flag_lip_zero:
+ x_d_i_new += lip_delta_before_animation.reshape(-1, x_s.shape[1], 3)
+ else:
+ pass
+ elif self.cfg.infer_params.flag_stitching and not self.cfg.infer_params.flag_eye_retargeting and not self.cfg.infer_params.flag_lip_retargeting:
+ # with stitching and without retargeting
+ if flag_lip_zero:
+ x_d_i_new = self.stitching(x_s, x_d_i_new) + lip_delta_before_animation.reshape(
+ -1, x_s.shape[1], 3)
+ else:
+ x_d_i_new = self.stitching(x_s, x_d_i_new)
+ else:
+ eyes_delta, lip_delta = None, None
+ if self.cfg.infer_params.flag_eye_retargeting:
+ c_d_eyes_i = input_eye_ratio
+ combined_eye_ratio_tensor = self.calc_combined_eye_ratio(c_d_eyes_i,
+ source_lmk)
+ # ∆_eyes,i = R_eyes(x_s; c_s,eyes, c_d,eyes,i)
+ eyes_delta = self.retarget_eye(x_s, combined_eye_ratio_tensor)
+ if self.cfg.infer_params.flag_lip_retargeting:
+ c_d_lip_i = input_lip_ratio
+ combined_lip_ratio_tensor = self.calc_combined_lip_ratio(c_d_lip_i, source_lmk)
+ # ∆_lip,i = R_lip(x_s; c_s,lip, c_d,lip,i)
+ lip_delta = self.retarget_lip(x_s, combined_lip_ratio_tensor)
+
+ if self.cfg.infer_params.flag_relative_motion: # use x_s
+ x_d_i_new = x_s + \
+ (eyes_delta.reshape(-1, x_s.shape[1], 3) if eyes_delta is not None else 0) + \
+ (lip_delta.reshape(-1, x_s.shape[1], 3) if lip_delta is not None else 0)
+ else: # use x_d,i
+ x_d_i_new = x_d_i_new + \
+ (eyes_delta.reshape(-1, x_s.shape[1], 3) if eyes_delta is not None else 0) + \
+ (lip_delta.reshape(-1, x_s.shape[1], 3) if lip_delta is not None else 0)
+
+ if self.cfg.infer_params.flag_stitching:
+ x_d_i_new = self.stitching(x_s, x_d_i_new)
+ else:
+ if self.cfg.infer_params.flag_stitching:
+ x_d_i_new = self.stitching(x_s, x_d_i_new)
+
+ x_d_i_new = x_s + (x_d_i_new - x_s) * self.cfg.infer_params.driving_multiplier
+ out_crop = self.model_dict["warping_spade"].predict(f_s, x_s, x_d_i_new)
+ if not realtime and self.cfg.infer_params.flag_pasteback and self.cfg.infer_params.flag_do_crop and self.cfg.infer_params.flag_stitching:
+ # TODO: pasteback is slow, considering optimize it using multi-threading or GPU
+ # I_p_pstbk = paste_back(out_crop, crop_info['M_c2o'], I_p_pstbk, mask_ori_float)
+ I_p_pstbk = paste_back_pytorch(out_crop, M, I_p_pstbk, mask_ori_float)
+
+ return img_crop, out_crop.to(dtype=torch.uint8).cpu().numpy(), I_p_pstbk.to(dtype=torch.uint8).cpu().numpy()
+
+ def __del__(self):
+ self.clean_models()
diff --git a/difpoint/src/pipelines/gradio_live_portrait_pipeline.py b/difpoint/src/pipelines/gradio_live_portrait_pipeline.py
new file mode 100644
index 0000000000000000000000000000000000000000..1e0ae51bd703de124ad4189e9ed7946ea73c32c6
--- /dev/null
+++ b/difpoint/src/pipelines/gradio_live_portrait_pipeline.py
@@ -0,0 +1,317 @@
+# -*- coding: utf-8 -*-
+# @Author : wenshao
+# @Email : wenshaoguo0611@gmail.com
+# @Project : FasterLivePortrait
+# @FileName: gradio_live_portrait_pipeline.py
+import pdb
+
+import gradio as gr
+import cv2
+import datetime
+import os
+import time
+from tqdm import tqdm
+import subprocess
+import numpy as np
+from .faster_live_portrait_pipeline import FasterLivePortraitPipeline
+from ..utils.utils import video_has_audio
+from ..utils.utils import resize_to_limit, prepare_paste_back, get_rotation_matrix, calc_lip_close_ratio, \
+ calc_eye_close_ratio, transform_keypoint, concat_feat
+from ..utils.crop import crop_image, parse_bbox_from_landmark, crop_image_by_bbox, paste_back, paste_back_pytorch
+from src.utils import utils
+import platform
+import torch
+from PIL import Image
+
+if platform.system().lower() == 'windows':
+ FFMPEG = "third_party/ffmpeg-7.0.1-full_build/bin/ffmpeg.exe"
+else:
+ FFMPEG = "ffmpeg"
+
+
+class GradioLivePortraitPipeline(FasterLivePortraitPipeline):
+ def __init__(self, cfg, **kwargs):
+ super(GradioLivePortraitPipeline, self).__init__(cfg, **kwargs)
+
+ def update_cfg(self, args_user):
+ update_ret = False
+ for key in args_user:
+ if key in self.cfg.infer_params:
+ if self.cfg.infer_params[key] != args_user[key]:
+ update_ret = True
+ print("update infer cfg {} from {} to {}".format(key, self.cfg.infer_params[key], args_user[key]))
+ self.cfg.infer_params[key] = args_user[key]
+ elif key in self.cfg.crop_params:
+ if self.cfg.crop_params[key] != args_user[key]:
+ update_ret = True
+ print("update crop cfg {} from {} to {}".format(key, self.cfg.crop_params[key], args_user[key]))
+ self.cfg.crop_params[key] = args_user[key]
+ else:
+ if key in self.cfg.infer_params and self.cfg.infer_params[key] != args_user[key]:
+ update_ret = True
+ print("add {}:{} to infer cfg".format(key, args_user[key]))
+ self.cfg.infer_params[key] = args_user[key]
+ return update_ret
+
+ def execute_video(
+ self,
+ input_source_image_path=None,
+ input_source_video_path=None,
+ input_driving_video_path=None,
+ flag_relative_input=True,
+ flag_do_crop_input=True,
+ flag_remap_input=True,
+ driving_multiplier=1.0,
+ flag_stitching=True,
+ flag_crop_driving_video_input=True,
+ flag_video_editing_head_rotation=False,
+ flag_is_animal=False,
+ scale=2.3,
+ vx_ratio=0.0,
+ vy_ratio=-0.125,
+ scale_crop_driving_video=2.2,
+ vx_ratio_crop_driving_video=0.0,
+ vy_ratio_crop_driving_video=-0.1,
+ driving_smooth_observation_variance=1e-7,
+ tab_selection=None,
+ ):
+ """ for video driven potrait animation
+ """
+ if tab_selection == 'Image':
+ input_source_path = input_source_image_path
+ elif tab_selection == 'Video':
+ input_source_path = input_source_video_path
+ else:
+ input_source_path = input_source_image_path
+
+ if flag_is_animal != self.is_animal:
+ self.init_models(is_animal=flag_is_animal)
+
+ if input_source_path is not None and input_driving_video_path is not None:
+ args_user = {
+ 'source': input_source_path,
+ 'driving': input_driving_video_path,
+ 'flag_relative_motion': flag_relative_input,
+ 'flag_do_crop': flag_do_crop_input,
+ 'flag_pasteback': flag_remap_input,
+ 'driving_multiplier': driving_multiplier,
+ 'flag_stitching': flag_stitching,
+ 'flag_crop_driving_video': flag_crop_driving_video_input,
+ 'flag_video_editing_head_rotation': flag_video_editing_head_rotation,
+ 'src_scale': scale,
+ 'src_vx_ratio': vx_ratio,
+ 'src_vy_ratio': vy_ratio,
+ 'dri_scale': scale_crop_driving_video,
+ 'dri_vx_ratio': vx_ratio_crop_driving_video,
+ 'dri_vy_ratio': vy_ratio_crop_driving_video,
+ 'driving_smooth_observation_variance': driving_smooth_observation_variance,
+ }
+ # update config from user input
+ update_ret = self.update_cfg(args_user)
+ # video driven animation
+ video_path, video_path_concat, total_time = self.run_local(input_driving_video_path, input_source_path,
+ update_ret=update_ret)
+ gr.Info(f"Run successfully! Cost: {total_time} seconds!", duration=3)
+ return video_path, video_path_concat,
+ else:
+ raise gr.Error("The input source portrait or driving video hasn't been prepared yet 💥!", duration=5)
+
+ def run_local(self, driving_video_path, source_path, **kwargs):
+ t00 = time.time()
+
+ if self.source_path != source_path or kwargs.get("update_ret", False):
+ # 如果不一样要重新初始化变量
+ self.init_vars(**kwargs)
+ ret = self.prepare_source(source_path)
+ if not ret:
+ raise gr.Error(f"Error in processing source:{source_path} 💥!", duration=5)
+
+ vcap = cv2.VideoCapture(driving_video_path)
+ if self.is_source_video:
+ duration, fps = utils.get_video_info(self.source_path)
+ fps = int(fps)
+ else:
+ fps = int(vcap.get(cv2.CAP_PROP_FPS))
+
+ dframe = int(vcap.get(cv2.CAP_PROP_FRAME_COUNT))
+ if self.is_source_video:
+ max_frame = min(dframe, len(self.src_imgs))
+ else:
+ max_frame = dframe
+ h, w = self.src_imgs[0].shape[:2]
+ save_dir = f"./results/{datetime.datetime.now().strftime('%Y-%m-%d-%H%M%S')}"
+ os.makedirs(save_dir, exist_ok=True)
+
+ # render output video
+ fourcc = cv2.VideoWriter_fourcc(*'mp4v')
+ vsave_crop_path = os.path.join(save_dir,
+ f"{os.path.basename(source_path)}-{os.path.basename(driving_video_path)}-crop.mp4")
+ vout_crop = cv2.VideoWriter(vsave_crop_path, fourcc, fps, (512 * 2, 512))
+ vsave_org_path = os.path.join(save_dir,
+ f"{os.path.basename(source_path)}-{os.path.basename(driving_video_path)}-org.mp4")
+ vout_org = cv2.VideoWriter(vsave_org_path, fourcc, fps, (w, h))
+
+ infer_times = []
+ for i in tqdm(range(max_frame)):
+ ret, frame = vcap.read()
+ if not ret:
+ break
+ t0 = time.time()
+ first_frame = i == 0
+ if self.is_source_video:
+ dri_crop, out_crop, out_org = self.run(frame, self.src_imgs[i], self.src_infos[i],
+ first_frame=first_frame)
+ else:
+ dri_crop, out_crop, out_org = self.run(frame, self.src_imgs[0], self.src_infos[0],
+ first_frame=first_frame)
+ if out_crop is None:
+ print(f"no face in driving frame:{i}")
+ continue
+ infer_times.append(time.time() - t0)
+ dri_crop = cv2.resize(dri_crop, (512, 512))
+ out_crop = np.concatenate([dri_crop, out_crop], axis=1)
+ out_crop = cv2.cvtColor(out_crop, cv2.COLOR_RGB2BGR)
+ vout_crop.write(out_crop)
+ out_org = cv2.cvtColor(out_org, cv2.COLOR_RGB2BGR)
+ vout_org.write(out_org)
+ total_time = time.time() - t00
+ vcap.release()
+ vout_crop.release()
+ vout_org.release()
+
+ if video_has_audio(driving_video_path):
+ vsave_crop_path_new = os.path.splitext(vsave_crop_path)[0] + "-audio.mp4"
+ vsave_org_path_new = os.path.splitext(vsave_org_path)[0] + "-audio.mp4"
+ if self.is_source_video:
+ duration, fps = utils.get_video_info(vsave_crop_path)
+ subprocess.call(
+ [FFMPEG, "-i", vsave_crop_path, "-i", driving_video_path,
+ "-b:v", "10M", "-c:v", "libx264", "-map", "0:v", "-map", "1:a",
+ "-c:a", "aac", "-pix_fmt", "yuv420p",
+ "-shortest", # 以最短的流为基准
+ "-t", str(duration), # 设置时长
+ "-r", str(fps), # 设置帧率
+ vsave_crop_path_new, "-y"])
+ subprocess.call(
+ [FFMPEG, "-i", vsave_org_path, "-i", driving_video_path,
+ "-b:v", "10M", "-c:v", "libx264", "-map", "0:v", "-map", "1:a",
+ "-c:a", "aac", "-pix_fmt", "yuv420p",
+ "-shortest", # 以最短的流为基准
+ "-t", str(duration), # 设置时长
+ "-r", str(fps), # 设置帧率
+ vsave_org_path_new, "-y"])
+ else:
+ subprocess.call(
+ [FFMPEG, "-i", vsave_crop_path, "-i", driving_video_path,
+ "-b:v", "10M", "-c:v",
+ "libx264", "-map", "0:v", "-map", "1:a",
+ "-c:a", "aac",
+ "-pix_fmt", "yuv420p", vsave_crop_path_new, "-y", "-shortest"])
+ subprocess.call(
+ [FFMPEG, "-i", vsave_org_path, "-i", driving_video_path,
+ "-b:v", "10M", "-c:v",
+ "libx264", "-map", "0:v", "-map", "1:a",
+ "-c:a", "aac",
+ "-pix_fmt", "yuv420p", vsave_org_path_new, "-y", "-shortest"])
+
+ return vsave_org_path_new, vsave_crop_path_new, total_time
+ else:
+ return vsave_org_path, vsave_crop_path, total_time
+
+ def execute_image(self, input_eye_ratio: float, input_lip_ratio: float, input_image, flag_do_crop=True):
+ """ for single image retargeting
+ """
+ # disposable feature
+ f_s_user, x_s_user, source_lmk_user, crop_M_c2o, mask_ori, img_rgb = \
+ self.prepare_retargeting(input_image, flag_do_crop)
+
+ if input_eye_ratio is None or input_lip_ratio is None:
+ raise gr.Error("Invalid ratio input 💥!", duration=5)
+ else:
+ # ∆_eyes,i = R_eyes(x_s; c_s,eyes, c_d,eyes,i)
+ combined_eye_ratio_tensor = self.calc_combined_eye_ratio([[input_eye_ratio]], source_lmk_user)
+ eyes_delta = self.retarget_eye(x_s_user, combined_eye_ratio_tensor)
+ # ∆_lip,i = R_lip(x_s; c_s,lip, c_d,lip,i)
+ combined_lip_ratio_tensor = self.calc_combined_lip_ratio([[input_lip_ratio]], source_lmk_user)
+ lip_delta = self.retarget_lip(x_s_user, combined_lip_ratio_tensor)
+ num_kp = x_s_user.shape[1]
+ # default: use x_s
+ x_d_new = x_s_user + eyes_delta.reshape(-1, num_kp, 3) + lip_delta.reshape(-1, num_kp, 3)
+ # D(W(f_s; x_s, x′_d))
+ out = self.model_dict["warping_spade"].predict(f_s_user, x_s_user, x_d_new)
+ img_rgb = torch.from_numpy(img_rgb).to(self.device)
+ out_to_ori_blend = paste_back_pytorch(out, crop_M_c2o, img_rgb, mask_ori)
+ gr.Info("Run successfully!", duration=2)
+ return out.to(dtype=torch.uint8).cpu().numpy(), out_to_ori_blend.to(dtype=torch.uint8).cpu().numpy()
+
+ def prepare_retargeting(self, input_image, flag_do_crop=True):
+ """ for single image retargeting
+ """
+ if input_image is not None:
+ ######## process source portrait ########
+ img_bgr = cv2.imread(input_image, cv2.IMREAD_COLOR)
+ img_bgr = resize_to_limit(img_bgr, self.cfg.infer_params.source_max_dim,
+ self.cfg.infer_params.source_division)
+ img_rgb = cv2.cvtColor(img_bgr, cv2.COLOR_BGR2RGB)
+
+ if self.is_animal:
+ raise gr.Error("Animal Model Not Supported in Face Retarget 💥!", duration=5)
+ else:
+ src_faces = self.model_dict["face_analysis"].predict(img_bgr)
+
+ if len(src_faces) == 0:
+ raise gr.Error("No face detect in image 💥!", duration=5)
+ src_faces = src_faces[:1]
+ crop_infos = []
+ for i in range(len(src_faces)):
+ # NOTE: temporarily only pick the first face, to support multiple face in the future
+ lmk = src_faces[i]
+ # crop the face
+ ret_dct = crop_image(
+ img_rgb, # ndarray
+ lmk, # 106x2 or Nx2
+ dsize=self.cfg.crop_params.src_dsize,
+ scale=self.cfg.crop_params.src_scale,
+ vx_ratio=self.cfg.crop_params.src_vx_ratio,
+ vy_ratio=self.cfg.crop_params.src_vy_ratio,
+ )
+
+ lmk = self.model_dict["landmark"].predict(img_rgb, lmk)
+ ret_dct["lmk_crop"] = lmk
+ ret_dct["lmk_crop_256x256"] = ret_dct["lmk_crop"] * 256 / self.cfg.crop_params.src_dsize
+
+ # update a 256x256 version for network input
+ ret_dct["img_crop_256x256"] = cv2.resize(
+ ret_dct["img_crop"], (256, 256), interpolation=cv2.INTER_AREA
+ )
+ ret_dct["lmk_crop_256x256"] = ret_dct["lmk_crop"] * 256 / self.cfg.crop_params.src_dsize
+ crop_infos.append(ret_dct)
+ crop_info = crop_infos[0]
+ if flag_do_crop:
+ I_s = crop_info['img_crop_256x256'].copy()
+ else:
+ I_s = img_rgb.copy()
+ pitch, yaw, roll, t, exp, scale, kp = self.model_dict["motion_extractor"].predict(I_s)
+ x_s_info = {
+ "pitch": pitch,
+ "yaw": yaw,
+ "roll": roll,
+ "t": t,
+ "exp": exp,
+ "scale": scale,
+ "kp": kp
+ }
+ R_s = get_rotation_matrix(x_s_info['pitch'], x_s_info['yaw'], x_s_info['roll'])
+ ############################################
+ f_s_user = self.model_dict["app_feat_extractor"].predict(I_s)
+ x_s_user = transform_keypoint(pitch, yaw, roll, t, exp, scale, kp)
+ source_lmk_user = crop_info['lmk_crop']
+ crop_M_c2o = crop_info['M_c2o']
+ crop_M_c2o = torch.from_numpy(crop_M_c2o).to(self.device)
+ mask_ori = prepare_paste_back(self.mask_crop, crop_info['M_c2o'],
+ dsize=(img_rgb.shape[1], img_rgb.shape[0]))
+ mask_ori = torch.from_numpy(mask_ori).to(self.device).float()
+ return f_s_user, x_s_user, source_lmk_user, crop_M_c2o, mask_ori, img_rgb
+ else:
+ # when press the clear button, go here
+ raise gr.Error("The retargeting input hasn't been prepared yet 💥!", duration=5)
diff --git a/difpoint/src/utils/__init__.py b/difpoint/src/utils/__init__.py
new file mode 100644
index 0000000000000000000000000000000000000000..e69de29bb2d1d6434b8b29ae775ad8c2e48c5391
diff --git a/difpoint/src/utils/__pycache__/__init__.cpython-310.pyc b/difpoint/src/utils/__pycache__/__init__.cpython-310.pyc
new file mode 100644
index 0000000000000000000000000000000000000000..2c477de88cbaeead9f22ccd8f68fb6d5f963f434
Binary files /dev/null and b/difpoint/src/utils/__pycache__/__init__.cpython-310.pyc differ
diff --git a/difpoint/src/utils/__pycache__/__init__.cpython-38.pyc b/difpoint/src/utils/__pycache__/__init__.cpython-38.pyc
new file mode 100644
index 0000000000000000000000000000000000000000..12169feb3ad92ca9d8f0e6503db27cb5b6d7554e
Binary files /dev/null and b/difpoint/src/utils/__pycache__/__init__.cpython-38.pyc differ
diff --git a/difpoint/src/utils/__pycache__/camera.cpython-310.pyc b/difpoint/src/utils/__pycache__/camera.cpython-310.pyc
new file mode 100644
index 0000000000000000000000000000000000000000..e78cac29b0cb2098630763efa5e3deee74b77a66
Binary files /dev/null and b/difpoint/src/utils/__pycache__/camera.cpython-310.pyc differ
diff --git a/difpoint/src/utils/__pycache__/crop.cpython-310.pyc b/difpoint/src/utils/__pycache__/crop.cpython-310.pyc
new file mode 100644
index 0000000000000000000000000000000000000000..d5871b08ad76e67a624aeb06dd3813f9e6f9dfce
Binary files /dev/null and b/difpoint/src/utils/__pycache__/crop.cpython-310.pyc differ
diff --git a/difpoint/src/utils/__pycache__/crop.cpython-38.pyc b/difpoint/src/utils/__pycache__/crop.cpython-38.pyc
new file mode 100644
index 0000000000000000000000000000000000000000..dbda181a73c63be474fdf9b29581a257dd8488e1
Binary files /dev/null and b/difpoint/src/utils/__pycache__/crop.cpython-38.pyc differ
diff --git a/difpoint/src/utils/__pycache__/cropper.cpython-310.pyc b/difpoint/src/utils/__pycache__/cropper.cpython-310.pyc
new file mode 100644
index 0000000000000000000000000000000000000000..66e84bf2c366003b8629f77a6540aa186f8f8865
Binary files /dev/null and b/difpoint/src/utils/__pycache__/cropper.cpython-310.pyc differ
diff --git a/difpoint/src/utils/__pycache__/face_align.cpython-310.pyc b/difpoint/src/utils/__pycache__/face_align.cpython-310.pyc
new file mode 100644
index 0000000000000000000000000000000000000000..9ca905bb1e7eb6bb5c879bb665a4844409bb64cf
Binary files /dev/null and b/difpoint/src/utils/__pycache__/face_align.cpython-310.pyc differ
diff --git a/difpoint/src/utils/__pycache__/face_analysis_diy.cpython-310.pyc b/difpoint/src/utils/__pycache__/face_analysis_diy.cpython-310.pyc
new file mode 100644
index 0000000000000000000000000000000000000000..841e49afd112bb111981381a30346424da8df883
Binary files /dev/null and b/difpoint/src/utils/__pycache__/face_analysis_diy.cpython-310.pyc differ
diff --git a/difpoint/src/utils/__pycache__/helper.cpython-310.pyc b/difpoint/src/utils/__pycache__/helper.cpython-310.pyc
new file mode 100644
index 0000000000000000000000000000000000000000..7c4a7c5f3da6340454df08cc1d2d65457f50d7a3
Binary files /dev/null and b/difpoint/src/utils/__pycache__/helper.cpython-310.pyc differ
diff --git a/difpoint/src/utils/__pycache__/hparams.cpython-310.pyc b/difpoint/src/utils/__pycache__/hparams.cpython-310.pyc
new file mode 100644
index 0000000000000000000000000000000000000000..3781fc8af9d0729cba6907391187e283a23ce719
Binary files /dev/null and b/difpoint/src/utils/__pycache__/hparams.cpython-310.pyc differ
diff --git a/difpoint/src/utils/__pycache__/hparams.cpython-38.pyc b/difpoint/src/utils/__pycache__/hparams.cpython-38.pyc
new file mode 100644
index 0000000000000000000000000000000000000000..c2a7470cfb6350e2b3c54c710b683d886fa092ae
Binary files /dev/null and b/difpoint/src/utils/__pycache__/hparams.cpython-38.pyc differ
diff --git a/difpoint/src/utils/__pycache__/io.cpython-310.pyc b/difpoint/src/utils/__pycache__/io.cpython-310.pyc
new file mode 100644
index 0000000000000000000000000000000000000000..39c6a1f22d7381e1949e624b26ff00517ebd036f
Binary files /dev/null and b/difpoint/src/utils/__pycache__/io.cpython-310.pyc differ
diff --git a/difpoint/src/utils/__pycache__/landmark_runner.cpython-310.pyc b/difpoint/src/utils/__pycache__/landmark_runner.cpython-310.pyc
new file mode 100644
index 0000000000000000000000000000000000000000..018a737d34c3df3fc333ac4ff07f02b59c614d2d
Binary files /dev/null and b/difpoint/src/utils/__pycache__/landmark_runner.cpython-310.pyc differ
diff --git a/difpoint/src/utils/__pycache__/retargeting_utils.cpython-310.pyc b/difpoint/src/utils/__pycache__/retargeting_utils.cpython-310.pyc
new file mode 100644
index 0000000000000000000000000000000000000000..2e8edf55a2329a3da6870bcd476b386978f63cb8
Binary files /dev/null and b/difpoint/src/utils/__pycache__/retargeting_utils.cpython-310.pyc differ
diff --git a/difpoint/src/utils/__pycache__/rprint.cpython-310.pyc b/difpoint/src/utils/__pycache__/rprint.cpython-310.pyc
new file mode 100644
index 0000000000000000000000000000000000000000..b53e7ab10cce9f7ac93b2e045f45895fc43392a9
Binary files /dev/null and b/difpoint/src/utils/__pycache__/rprint.cpython-310.pyc differ
diff --git a/difpoint/src/utils/__pycache__/timer.cpython-310.pyc b/difpoint/src/utils/__pycache__/timer.cpython-310.pyc
new file mode 100644
index 0000000000000000000000000000000000000000..20fcf7fb27c0126445380a423952c3ad6e1f78b3
Binary files /dev/null and b/difpoint/src/utils/__pycache__/timer.cpython-310.pyc differ
diff --git a/difpoint/src/utils/__pycache__/utils.cpython-310.pyc b/difpoint/src/utils/__pycache__/utils.cpython-310.pyc
new file mode 100644
index 0000000000000000000000000000000000000000..49eb53857bf8025332629f259e4e570d3789bba1
Binary files /dev/null and b/difpoint/src/utils/__pycache__/utils.cpython-310.pyc differ
diff --git a/difpoint/src/utils/__pycache__/utils.cpython-38.pyc b/difpoint/src/utils/__pycache__/utils.cpython-38.pyc
new file mode 100644
index 0000000000000000000000000000000000000000..5987b60bde9935ae8ad257974da861a0ffe82a4c
Binary files /dev/null and b/difpoint/src/utils/__pycache__/utils.cpython-38.pyc differ
diff --git a/difpoint/src/utils/__pycache__/video.cpython-310.pyc b/difpoint/src/utils/__pycache__/video.cpython-310.pyc
new file mode 100644
index 0000000000000000000000000000000000000000..3443d761752705ff4abcface7fd7cc045b190ce7
Binary files /dev/null and b/difpoint/src/utils/__pycache__/video.cpython-310.pyc differ
diff --git a/difpoint/src/utils/animal_landmark_runner.py b/difpoint/src/utils/animal_landmark_runner.py
new file mode 100644
index 0000000000000000000000000000000000000000..372d58bb1eff0a6458e77392ae81265a34ccf203
--- /dev/null
+++ b/difpoint/src/utils/animal_landmark_runner.py
@@ -0,0 +1,144 @@
+# coding: utf-8
+
+"""
+face detectoin and alignment using XPose
+"""
+
+import os
+import pickle
+import torch
+import numpy as np
+from PIL import Image
+from torchvision.ops import nms
+from collections import OrderedDict
+
+
+def clean_state_dict(state_dict):
+ new_state_dict = OrderedDict()
+ for k, v in state_dict.items():
+ if k[:7] == 'module.':
+ k = k[7:] # remove `module.`
+ new_state_dict[k] = v
+ return new_state_dict
+
+
+from src.models.XPose import transforms as T
+from src.models.XPose.models import build_model
+from src.models.XPose.predefined_keypoints import *
+from src.models.XPose.util import box_ops
+from src.models.XPose.util.config import Config
+
+
+class XPoseRunner(object):
+ def __init__(self, model_config_path, model_checkpoint_path, embeddings_cache_path=None, cpu_only=False, **kwargs):
+ self.device_id = kwargs.get("device_id", 0)
+ self.flag_use_half_precision = kwargs.get("flag_use_half_precision", True)
+ self.device = f"cuda:{self.device_id}" if not cpu_only else "cpu"
+ self.model = self.load_animal_model(model_config_path, model_checkpoint_path, self.device)
+ # Load cached embeddings if available
+ try:
+ with open(f'{embeddings_cache_path}_9.pkl', 'rb') as f:
+ self.ins_text_embeddings_9, self.kpt_text_embeddings_9 = pickle.load(f)
+ with open(f'{embeddings_cache_path}_68.pkl', 'rb') as f:
+ self.ins_text_embeddings_68, self.kpt_text_embeddings_68 = pickle.load(f)
+ print("Loaded cached embeddings from file.")
+ except Exception:
+ raise ValueError("Could not load clip embeddings from file, please check your file path.")
+
+ def load_animal_model(self, model_config_path, model_checkpoint_path, device):
+ args = Config.fromfile(model_config_path)
+ args.device = device
+ model = build_model(args)
+ checkpoint = torch.load(model_checkpoint_path, map_location=lambda storage, loc: storage)
+ load_res = model.load_state_dict(clean_state_dict(checkpoint["model"]), strict=False)
+ model.eval()
+ return model
+
+ def load_image(self, input_image):
+ image_pil = input_image.convert("RGB")
+ transform = T.Compose([
+ T.RandomResize([800], max_size=1333), # NOTE: fixed size to 800
+ T.ToTensor(),
+ T.Normalize([0.485, 0.456, 0.406], [0.229, 0.224, 0.225]),
+ ])
+ image, _ = transform(image_pil, None)
+ return image_pil, image
+
+ def get_unipose_output(self, image, instance_text_prompt, keypoint_text_prompt, box_threshold, IoU_threshold):
+ instance_list = instance_text_prompt.split(',')
+
+ if len(keypoint_text_prompt) == 9:
+ # torch.Size([1, 512]) torch.Size([9, 512])
+ ins_text_embeddings, kpt_text_embeddings = self.ins_text_embeddings_9, self.kpt_text_embeddings_9
+ elif len(keypoint_text_prompt) == 68:
+ # torch.Size([1, 512]) torch.Size([68, 512])
+ ins_text_embeddings, kpt_text_embeddings = self.ins_text_embeddings_68, self.kpt_text_embeddings_68
+ else:
+ raise ValueError("Invalid number of keypoint embeddings.")
+ target = {
+ "instance_text_prompt": instance_list,
+ "keypoint_text_prompt": keypoint_text_prompt,
+ "object_embeddings_text": ins_text_embeddings.float(),
+ "kpts_embeddings_text": torch.cat(
+ (kpt_text_embeddings.float(), torch.zeros(100 - kpt_text_embeddings.shape[0], 512, device=self.device)),
+ dim=0),
+ "kpt_vis_text": torch.cat((torch.ones(kpt_text_embeddings.shape[0], device=self.device),
+ torch.zeros(100 - kpt_text_embeddings.shape[0], device=self.device)), dim=0)
+ }
+
+ self.model = self.model.to(self.device)
+ image = image.to(self.device)
+
+ with torch.no_grad():
+ with torch.autocast(device_type=self.device[:4], dtype=torch.float16, enabled=self.flag_use_half_precision):
+ outputs = self.model(image[None], [target])
+
+ logits = outputs["pred_logits"].sigmoid()[0]
+ boxes = outputs["pred_boxes"][0]
+ keypoints = outputs["pred_keypoints"][0][:, :2 * len(keypoint_text_prompt)]
+
+ logits_filt = logits.cpu().clone()
+ boxes_filt = boxes.cpu().clone()
+ keypoints_filt = keypoints.cpu().clone()
+ filt_mask = logits_filt.max(dim=1)[0] > box_threshold
+ logits_filt = logits_filt[filt_mask]
+ boxes_filt = boxes_filt[filt_mask]
+ keypoints_filt = keypoints_filt[filt_mask]
+
+ keep_indices = nms(box_ops.box_cxcywh_to_xyxy(boxes_filt), logits_filt.max(dim=1)[0],
+ iou_threshold=IoU_threshold)
+
+ filtered_boxes = boxes_filt[keep_indices]
+ filtered_keypoints = keypoints_filt[keep_indices]
+
+ return filtered_boxes, filtered_keypoints
+
+ def run(self, input_image, instance_text_prompt, keypoint_text_example, box_threshold, IoU_threshold):
+ if keypoint_text_example in globals():
+ keypoint_dict = globals()[keypoint_text_example]
+ elif instance_text_prompt in globals():
+ keypoint_dict = globals()[instance_text_prompt]
+ else:
+ keypoint_dict = globals()["animal"]
+
+ keypoint_text_prompt = keypoint_dict.get("keypoints")
+ keypoint_skeleton = keypoint_dict.get("skeleton")
+
+ image_pil, image = self.load_image(input_image)
+ boxes_filt, keypoints_filt = self.get_unipose_output(image, instance_text_prompt, keypoint_text_prompt,
+ box_threshold, IoU_threshold)
+
+ size = image_pil.size
+ H, W = size[1], size[0]
+ keypoints_filt = keypoints_filt[0].squeeze(0)
+ kp = np.array(keypoints_filt.cpu())
+ num_kpts = len(keypoint_text_prompt)
+ Z = kp[:num_kpts * 2] * np.array([W, H] * num_kpts)
+ Z = Z.reshape(num_kpts * 2)
+ x = Z[0::2]
+ y = Z[1::2]
+ return np.stack((x, y), axis=1)
+
+ def warmup(self):
+ img_rgb = Image.fromarray(np.zeros((512, 512, 3), dtype=np.uint8))
+ self.run(img_rgb, 'face', 'face', box_threshold=0.0, IoU_threshold=0.0)
diff --git a/difpoint/src/utils/camera.py b/difpoint/src/utils/camera.py
new file mode 100644
index 0000000000000000000000000000000000000000..a3dd942697e1f00a96dc3efc75b883d98b52e525
--- /dev/null
+++ b/difpoint/src/utils/camera.py
@@ -0,0 +1,73 @@
+# coding: utf-8
+
+"""
+functions for processing and transforming 3D facial keypoints
+"""
+
+import numpy as np
+import torch
+import torch.nn.functional as F
+
+PI = np.pi
+
+
+def headpose_pred_to_degree(pred):
+ """
+ pred: (bs, 66) or (bs, 1) or others
+ """
+ if pred.ndim > 1 and pred.shape[1] == 66:
+ # NOTE: note that the average is modified to 97.5
+ device = pred.device
+ idx_tensor = [idx for idx in range(0, 66)]
+ idx_tensor = torch.FloatTensor(idx_tensor).to(device)
+ pred = F.softmax(pred, dim=1)
+ degree = torch.sum(pred*idx_tensor, axis=1) * 3 - 97.5
+
+ return degree
+
+ return pred
+
+
+def get_rotation_matrix(pitch_, yaw_, roll_):
+ """ the input is in degree
+ """
+ # transform to radian
+ pitch = pitch_ / 180 * PI
+ yaw = yaw_ / 180 * PI
+ roll = roll_ / 180 * PI
+
+ device = pitch.device
+
+ if pitch.ndim == 1:
+ pitch = pitch.unsqueeze(1)
+ if yaw.ndim == 1:
+ yaw = yaw.unsqueeze(1)
+ if roll.ndim == 1:
+ roll = roll.unsqueeze(1)
+
+ # calculate the euler matrix
+ bs = pitch.shape[0]
+ ones = torch.ones([bs, 1]).to(device)
+ zeros = torch.zeros([bs, 1]).to(device)
+ x, y, z = pitch, yaw, roll
+
+ rot_x = torch.cat([
+ ones, zeros, zeros,
+ zeros, torch.cos(x), -torch.sin(x),
+ zeros, torch.sin(x), torch.cos(x)
+ ], dim=1).reshape([bs, 3, 3])
+
+ rot_y = torch.cat([
+ torch.cos(y), zeros, torch.sin(y),
+ zeros, ones, zeros,
+ -torch.sin(y), zeros, torch.cos(y)
+ ], dim=1).reshape([bs, 3, 3])
+
+ rot_z = torch.cat([
+ torch.cos(z), -torch.sin(z), zeros,
+ torch.sin(z), torch.cos(z), zeros,
+ zeros, zeros, ones
+ ], dim=1).reshape([bs, 3, 3])
+
+ rot = rot_z @ rot_y @ rot_x
+ return rot.permute(0, 2, 1) # transpose
diff --git a/difpoint/src/utils/crop.py b/difpoint/src/utils/crop.py
new file mode 100644
index 0000000000000000000000000000000000000000..065b9f0f9f25be8444b7c9bfca45652f80f5685b
--- /dev/null
+++ b/difpoint/src/utils/crop.py
@@ -0,0 +1,398 @@
+# coding: utf-8
+
+"""
+cropping function and the related preprocess functions for cropping
+"""
+
+import numpy as np
+import os.path as osp
+from math import sin, cos, acos, degrees
+import cv2; cv2.setNumThreads(0); cv2.ocl.setUseOpenCL(False) # NOTE: enforce single thread
+from .rprint import rprint as print
+
+DTYPE = np.float32
+CV2_INTERP = cv2.INTER_LINEAR
+
+def make_abs_path(fn):
+ return osp.join(osp.dirname(osp.realpath(__file__)), fn)
+
+def _transform_img(img, M, dsize, flags=CV2_INTERP, borderMode=None):
+ """ conduct similarity or affine transformation to the image, do not do border operation!
+ img:
+ M: 2x3 matrix or 3x3 matrix
+ dsize: target shape (width, height)
+ """
+ if isinstance(dsize, tuple) or isinstance(dsize, list):
+ _dsize = tuple(dsize)
+ else:
+ _dsize = (dsize, dsize)
+
+ if borderMode is not None:
+ return cv2.warpAffine(img, M[:2, :], dsize=_dsize, flags=flags, borderMode=borderMode, borderValue=(0, 0, 0))
+ else:
+ return cv2.warpAffine(img, M[:2, :], dsize=_dsize, flags=flags)
+
+
+def _transform_pts(pts, M):
+ """ conduct similarity or affine transformation to the pts
+ pts: Nx2 ndarray
+ M: 2x3 matrix or 3x3 matrix
+ return: Nx2
+ """
+ return pts @ M[:2, :2].T + M[:2, 2]
+
+
+def parse_pt2_from_pt101(pt101, use_lip=True):
+ """
+ parsing the 2 points according to the 101 points, which cancels the roll
+ """
+ # the former version use the eye center, but it is not robust, now use interpolation
+ pt_left_eye = np.mean(pt101[[39, 42, 45, 48]], axis=0) # left eye center
+ pt_right_eye = np.mean(pt101[[51, 54, 57, 60]], axis=0) # right eye center
+
+ if use_lip:
+ # use lip
+ pt_center_eye = (pt_left_eye + pt_right_eye) / 2
+ pt_center_lip = (pt101[75] + pt101[81]) / 2
+ pt2 = np.stack([pt_center_eye, pt_center_lip], axis=0)
+ else:
+ pt2 = np.stack([pt_left_eye, pt_right_eye], axis=0)
+ return pt2
+
+
+def parse_pt2_from_pt106(pt106, use_lip=True):
+ """
+ parsing the 2 points according to the 106 points, which cancels the roll
+ """
+ pt_left_eye = np.mean(pt106[[33, 35, 40, 39]], axis=0) # left eye center
+ pt_right_eye = np.mean(pt106[[87, 89, 94, 93]], axis=0) # right eye center
+
+ if use_lip:
+ # use lip
+ pt_center_eye = (pt_left_eye + pt_right_eye) / 2
+ pt_center_lip = (pt106[52] + pt106[61]) / 2
+ pt2 = np.stack([pt_center_eye, pt_center_lip], axis=0)
+ else:
+ pt2 = np.stack([pt_left_eye, pt_right_eye], axis=0)
+ return pt2
+
+
+def parse_pt2_from_pt203(pt203, use_lip=True):
+ """
+ parsing the 2 points according to the 203 points, which cancels the roll
+ """
+ pt_left_eye = np.mean(pt203[[0, 6, 12, 18]], axis=0) # left eye center
+ pt_right_eye = np.mean(pt203[[24, 30, 36, 42]], axis=0) # right eye center
+ if use_lip:
+ # use lip
+ pt_center_eye = (pt_left_eye + pt_right_eye) / 2
+ pt_center_lip = (pt203[48] + pt203[66]) / 2
+ pt2 = np.stack([pt_center_eye, pt_center_lip], axis=0)
+ else:
+ pt2 = np.stack([pt_left_eye, pt_right_eye], axis=0)
+ return pt2
+
+
+def parse_pt2_from_pt68(pt68, use_lip=True):
+ """
+ parsing the 2 points according to the 68 points, which cancels the roll
+ """
+ lm_idx = np.array([31, 37, 40, 43, 46, 49, 55], dtype=np.int32) - 1
+ if use_lip:
+ pt5 = np.stack([
+ np.mean(pt68[lm_idx[[1, 2]], :], 0), # left eye
+ np.mean(pt68[lm_idx[[3, 4]], :], 0), # right eye
+ pt68[lm_idx[0], :], # nose
+ pt68[lm_idx[5], :], # lip
+ pt68[lm_idx[6], :] # lip
+ ], axis=0)
+
+ pt2 = np.stack([
+ (pt5[0] + pt5[1]) / 2,
+ (pt5[3] + pt5[4]) / 2
+ ], axis=0)
+ else:
+ pt2 = np.stack([
+ np.mean(pt68[lm_idx[[1, 2]], :], 0), # left eye
+ np.mean(pt68[lm_idx[[3, 4]], :], 0), # right eye
+ ], axis=0)
+
+ return pt2
+
+
+def parse_pt2_from_pt5(pt5, use_lip=True):
+ """
+ parsing the 2 points according to the 5 points, which cancels the roll
+ """
+ if use_lip:
+ pt2 = np.stack([
+ (pt5[0] + pt5[1]) / 2,
+ (pt5[3] + pt5[4]) / 2
+ ], axis=0)
+ else:
+ pt2 = np.stack([
+ pt5[0],
+ pt5[1]
+ ], axis=0)
+ return pt2
+
+
+def parse_pt2_from_pt_x(pts, use_lip=True):
+ if pts.shape[0] == 101:
+ pt2 = parse_pt2_from_pt101(pts, use_lip=use_lip)
+ elif pts.shape[0] == 106:
+ pt2 = parse_pt2_from_pt106(pts, use_lip=use_lip)
+ elif pts.shape[0] == 68:
+ pt2 = parse_pt2_from_pt68(pts, use_lip=use_lip)
+ elif pts.shape[0] == 5:
+ pt2 = parse_pt2_from_pt5(pts, use_lip=use_lip)
+ elif pts.shape[0] == 203:
+ pt2 = parse_pt2_from_pt203(pts, use_lip=use_lip)
+ elif pts.shape[0] > 101:
+ # take the first 101 points
+ pt2 = parse_pt2_from_pt101(pts[:101], use_lip=use_lip)
+ else:
+ raise Exception(f'Unknow shape: {pts.shape}')
+
+ if not use_lip:
+ # NOTE: to compile with the latter code, need to rotate the pt2 90 degrees clockwise manually
+ v = pt2[1] - pt2[0]
+ pt2[1, 0] = pt2[0, 0] - v[1]
+ pt2[1, 1] = pt2[0, 1] + v[0]
+
+ return pt2
+
+
+def parse_rect_from_landmark(
+ pts,
+ scale=1.5,
+ need_square=True,
+ vx_ratio=0,
+ vy_ratio=0,
+ use_deg_flag=False,
+ **kwargs
+):
+ """parsing center, size, angle from 101/68/5/x landmarks
+ vx_ratio: the offset ratio along the pupil axis x-axis, multiplied by size
+ vy_ratio: the offset ratio along the pupil axis y-axis, multiplied by size, which is used to contain more forehead area
+
+ judge with pts.shape
+ """
+ pt2 = parse_pt2_from_pt_x(pts, use_lip=kwargs.get('use_lip', True))
+
+ uy = pt2[1] - pt2[0]
+ l = np.linalg.norm(uy)
+ if l <= 1e-3:
+ uy = np.array([0, 1], dtype=DTYPE)
+ else:
+ uy /= l
+ ux = np.array((uy[1], -uy[0]), dtype=DTYPE)
+
+ # the rotation degree of the x-axis, the clockwise is positive, the counterclockwise is negative (image coordinate system)
+ # print(uy)
+ # print(ux)
+ angle = acos(ux[0])
+ if ux[1] < 0:
+ angle = -angle
+
+ # rotation matrix
+ M = np.array([ux, uy])
+
+ # calculate the size which contains the angle degree of the bbox, and the center
+ center0 = np.mean(pts, axis=0)
+ rpts = (pts - center0) @ M.T # (M @ P.T).T = P @ M.T
+ lt_pt = np.min(rpts, axis=0)
+ rb_pt = np.max(rpts, axis=0)
+ center1 = (lt_pt + rb_pt) / 2
+
+ size = rb_pt - lt_pt
+ if need_square:
+ m = max(size[0], size[1])
+ size[0] = m
+ size[1] = m
+
+ size *= scale # scale size
+ center = center0 + ux * center1[0] + uy * center1[1] # counterclockwise rotation, equivalent to M.T @ center1.T
+ center = center + ux * (vx_ratio * size) + uy * \
+ (vy_ratio * size) # considering the offset in vx and vy direction
+
+ if use_deg_flag:
+ angle = degrees(angle)
+
+ return center, size, angle
+
+
+def parse_bbox_from_landmark(pts, **kwargs):
+ center, size, angle = parse_rect_from_landmark(pts, **kwargs)
+ cx, cy = center
+ w, h = size
+
+ # calculate the vertex positions before rotation
+ bbox = np.array([
+ [cx-w/2, cy-h/2], # left, top
+ [cx+w/2, cy-h/2],
+ [cx+w/2, cy+h/2], # right, bottom
+ [cx-w/2, cy+h/2]
+ ], dtype=DTYPE)
+
+ # construct rotation matrix
+ bbox_rot = bbox.copy()
+ R = np.array([
+ [np.cos(angle), -np.sin(angle)],
+ [np.sin(angle), np.cos(angle)]
+ ], dtype=DTYPE)
+
+ # calculate the relative position of each vertex from the rotation center, then rotate these positions, and finally add the coordinates of the rotation center
+ bbox_rot = (bbox_rot - center) @ R.T + center
+
+ return {
+ 'center': center, # 2x1
+ 'size': size, # scalar
+ 'angle': angle, # rad, counterclockwise
+ 'bbox': bbox, # 4x2
+ 'bbox_rot': bbox_rot, # 4x2
+ }
+
+
+def crop_image_by_bbox(img, bbox, lmk=None, dsize=512, angle=None, flag_rot=False, **kwargs):
+ left, top, right, bot = bbox
+ if int(right - left) != int(bot - top):
+ print(f'right-left {right-left} != bot-top {bot-top}')
+ size = right - left
+
+ src_center = np.array([(left + right) / 2, (top + bot) / 2], dtype=DTYPE)
+ tgt_center = np.array([dsize / 2, dsize / 2], dtype=DTYPE)
+
+ s = dsize / size # scale
+ if flag_rot and angle is not None:
+ costheta, sintheta = cos(angle), sin(angle)
+ cx, cy = src_center[0], src_center[1] # ori center
+ tcx, tcy = tgt_center[0], tgt_center[1] # target center
+ # need to infer
+ M_o2c = np.array(
+ [[s * costheta, s * sintheta, tcx - s * (costheta * cx + sintheta * cy)],
+ [-s * sintheta, s * costheta, tcy - s * (-sintheta * cx + costheta * cy)]],
+ dtype=DTYPE
+ )
+ else:
+ M_o2c = np.array(
+ [[s, 0, tgt_center[0] - s * src_center[0]],
+ [0, s, tgt_center[1] - s * src_center[1]]],
+ dtype=DTYPE
+ )
+
+ # if flag_rot and angle is None:
+ # print('angle is None, but flag_rotate is True', style="bold yellow")
+
+ img_crop = _transform_img(img, M_o2c, dsize=dsize, borderMode=kwargs.get('borderMode', None))
+ lmk_crop = _transform_pts(lmk, M_o2c) if lmk is not None else None
+
+ M_o2c = np.vstack([M_o2c, np.array([0, 0, 1], dtype=DTYPE)])
+ M_c2o = np.linalg.inv(M_o2c)
+
+ # cv2.imwrite('crop.jpg', img_crop)
+
+ return {
+ 'img_crop': img_crop,
+ 'lmk_crop': lmk_crop,
+ 'M_o2c': M_o2c,
+ 'M_c2o': M_c2o,
+ }
+
+
+def _estimate_similar_transform_from_pts(
+ pts,
+ dsize,
+ scale=1.5,
+ vx_ratio=0,
+ vy_ratio=-0.1,
+ flag_do_rot=True,
+ **kwargs
+):
+ """ calculate the affine matrix of the cropped image from sparse points, the original image to the cropped image, the inverse is the cropped image to the original image
+ pts: landmark, 101 or 68 points or other points, Nx2
+ scale: the larger scale factor, the smaller face ratio
+ vx_ratio: x shift
+ vy_ratio: y shift, the smaller the y shift, the lower the face region
+ rot_flag: if it is true, conduct correction
+ """
+ center, size, angle = parse_rect_from_landmark(
+ pts, scale=scale, vx_ratio=vx_ratio, vy_ratio=vy_ratio,
+ use_lip=kwargs.get('use_lip', True)
+ )
+
+ s = dsize / size[0] # scale
+ tgt_center = np.array([dsize / 2, dsize / 2], dtype=DTYPE) # center of dsize
+
+ if flag_do_rot:
+ costheta, sintheta = cos(angle), sin(angle)
+ cx, cy = center[0], center[1] # ori center
+ tcx, tcy = tgt_center[0], tgt_center[1] # target center
+ # need to infer
+ M_INV = np.array(
+ [[s * costheta, s * sintheta, tcx - s * (costheta * cx + sintheta * cy)],
+ [-s * sintheta, s * costheta, tcy - s * (-sintheta * cx + costheta * cy)]],
+ dtype=DTYPE
+ )
+ else:
+ M_INV = np.array(
+ [[s, 0, tgt_center[0] - s * center[0]],
+ [0, s, tgt_center[1] - s * center[1]]],
+ dtype=DTYPE
+ )
+
+ M_INV_H = np.vstack([M_INV, np.array([0, 0, 1])])
+ M = np.linalg.inv(M_INV_H)
+
+ # M_INV is from the original image to the cropped image, M is from the cropped image to the original image
+ return M_INV, M[:2, ...]
+
+
+def crop_image(img, pts: np.ndarray, **kwargs):
+ dsize = kwargs.get('dsize', 224)
+ scale = kwargs.get('scale', 1.5) # 1.5 | 1.6
+ vy_ratio = kwargs.get('vy_ratio', -0.1) # -0.0625 | -0.1
+
+ M_INV, _ = _estimate_similar_transform_from_pts(
+ pts,
+ dsize=dsize,
+ scale=scale,
+ vy_ratio=vy_ratio,
+ flag_do_rot=kwargs.get('flag_do_rot', True),
+ )
+
+ img_crop = _transform_img(img, M_INV, dsize) # origin to crop
+ pt_crop = _transform_pts(pts, M_INV)
+
+ M_o2c = np.vstack([M_INV, np.array([0, 0, 1], dtype=DTYPE)])
+ M_c2o = np.linalg.inv(M_o2c)
+
+ ret_dct = {
+ 'M_o2c': M_o2c, # from the original image to the cropped image 3x3
+ 'M_c2o': M_c2o, # from the cropped image to the original image 3x3
+ 'img_crop': img_crop, # the cropped image
+ 'pt_crop': pt_crop, # the landmarks of the cropped image
+ }
+
+ return ret_dct
+
+def average_bbox_lst(bbox_lst):
+ if len(bbox_lst) == 0:
+ return None
+ bbox_arr = np.array(bbox_lst)
+ return np.mean(bbox_arr, axis=0).tolist()
+
+def prepare_paste_back(mask_crop, crop_M_c2o, dsize):
+ """prepare mask for later image paste back
+ """
+ mask_ori = _transform_img(mask_crop, crop_M_c2o, dsize)
+ mask_ori = mask_ori.astype(np.float32) / 255.
+ return mask_ori
+
+def paste_back(img_crop, M_c2o, img_ori, mask_ori):
+ """paste back the image
+ """
+ dsize = (img_ori.shape[1], img_ori.shape[0])
+ result = _transform_img(img_crop, M_c2o, dsize=dsize)
+ result = np.clip(mask_ori * result + (1 - mask_ori) * img_ori, 0, 255).astype(np.uint8)
+ return result
diff --git a/difpoint/src/utils/cropper.py b/difpoint/src/utils/cropper.py
new file mode 100644
index 0000000000000000000000000000000000000000..d117fe397beb0fdae1b240b6b70a24a5540f34d9
--- /dev/null
+++ b/difpoint/src/utils/cropper.py
@@ -0,0 +1,196 @@
+# coding: utf-8
+
+import os.path as osp
+from dataclasses import dataclass, field
+from typing import List, Tuple, Union
+
+import cv2; cv2.setNumThreads(0); cv2.ocl.setUseOpenCL(False)
+import numpy as np
+
+from ..config.crop_config import CropConfig
+from .crop import (
+ average_bbox_lst,
+ crop_image,
+ crop_image_by_bbox,
+ parse_bbox_from_landmark,
+)
+from .io import contiguous
+from .rprint import rlog as log
+from .face_analysis_diy import FaceAnalysisDIY
+from .landmark_runner import LandmarkRunner
+
+
+def make_abs_path(fn):
+ return osp.join(osp.dirname(osp.realpath(__file__)), fn)
+
+
+@dataclass
+class Trajectory:
+ start: int = -1 # start frame
+ end: int = -1 # end frame
+ lmk_lst: Union[Tuple, List, np.ndarray] = field(default_factory=list) # lmk list
+ bbox_lst: Union[Tuple, List, np.ndarray] = field(default_factory=list) # bbox list
+
+ frame_rgb_lst: Union[Tuple, List, np.ndarray] = field(default_factory=list) # frame list
+ lmk_crop_lst: Union[Tuple, List, np.ndarray] = field(default_factory=list) # lmk list
+ frame_rgb_crop_lst: Union[Tuple, List, np.ndarray] = field(default_factory=list) # frame crop list
+
+
+class Cropper(object):
+ def __init__(self, **kwargs) -> None:
+ self.crop_cfg: CropConfig = kwargs.get("crop_cfg", None)
+ device_id = kwargs.get("device_id", 0)
+ flag_force_cpu = kwargs.get("flag_force_cpu", False)
+ if flag_force_cpu:
+ device = "cpu"
+ face_analysis_wrapper_provicer = ["CPUExecutionProvider"]
+ else:
+ device = "cuda"
+ face_analysis_wrapper_provicer = ["CUDAExecutionProvider"]
+ self.landmark_runner = LandmarkRunner(
+ ckpt_path=self.crop_cfg.landmark_ckpt_path,
+ onnx_provider=device,
+ device_id=device_id,
+ )
+ self.landmark_runner.warmup()
+
+ self.face_analysis_wrapper = FaceAnalysisDIY(
+ name="buffalo_l",
+ root=make_abs_path(self.crop_cfg.insightface_root),
+ providers=face_analysis_wrapper_provicer,
+ )
+ self.face_analysis_wrapper.prepare(ctx_id=device_id, det_size=(512, 512))
+ self.face_analysis_wrapper.warmup()
+
+ def update_config(self, user_args):
+ for k, v in user_args.items():
+ if hasattr(self.crop_cfg, k):
+ setattr(self.crop_cfg, k, v)
+
+ def crop_source_image(self, img_rgb_: np.ndarray, crop_cfg: CropConfig):
+ # crop a source image and get neccessary information
+ img_rgb = img_rgb_.copy() # copy it
+
+ img_bgr = cv2.cvtColor(img_rgb, cv2.COLOR_RGB2BGR)
+ src_face = self.face_analysis_wrapper.get(
+ img_bgr,
+ flag_do_landmark_2d_106=True,
+ direction=crop_cfg.direction,
+ max_face_num=crop_cfg.max_face_num,
+ )
+
+ if len(src_face) == 0:
+ log("No face detected in the source image.")
+ return None
+ elif len(src_face) > 1:
+ log(f"More than one face detected in the image, only pick one face by rule {crop_cfg.direction}.")
+
+ # NOTE: temporarily only pick the first face, to support multiple face in the future
+ src_face = src_face[0]
+ lmk = src_face.landmark_2d_106 # this is the 106 landmarks from insightface
+
+ # crop the face
+ ret_dct = crop_image(
+ img_rgb, # ndarray
+ lmk, # 106x2 or Nx2
+ dsize=crop_cfg.dsize,
+ scale=crop_cfg.scale,
+ vx_ratio=crop_cfg.vx_ratio,
+ vy_ratio=crop_cfg.vy_ratio,
+ )
+
+ lmk = self.landmark_runner.run(img_rgb, lmk)
+ ret_dct["lmk_crop"] = lmk
+
+ # update a 256x256 version for network input
+ ret_dct["img_crop_256x256"] = cv2.resize(ret_dct["img_crop"], (256, 256), interpolation=cv2.INTER_AREA)
+ ret_dct["lmk_crop_256x256"] = ret_dct["lmk_crop"] * 256 / crop_cfg.dsize
+
+ return ret_dct
+
+ def crop_driving_video(self, driving_rgb_lst, **kwargs):
+ """Tracking based landmarks/alignment and cropping"""
+ trajectory = Trajectory()
+ direction = kwargs.get("direction", "large-small")
+ for idx, frame_rgb in enumerate(driving_rgb_lst):
+ if idx == 0 or trajectory.start == -1:
+ src_face = self.face_analysis_wrapper.get(
+ contiguous(frame_rgb[..., ::-1]),
+ flag_do_landmark_2d_106=True,
+ direction=direction,
+ )
+ if len(src_face) == 0:
+ log(f"No face detected in the frame #{idx}")
+ continue
+ elif len(src_face) > 1:
+ log(f"More than one face detected in the driving frame_{idx}, only pick one face by rule {direction}.")
+ src_face = src_face[0]
+ lmk = src_face.landmark_2d_106
+ lmk = self.landmark_runner.run(frame_rgb, lmk)
+ trajectory.start, trajectory.end = idx, idx
+ else:
+ lmk = self.landmark_runner.run(frame_rgb, trajectory.lmk_lst[-1])
+ trajectory.end = idx
+
+ trajectory.lmk_lst.append(lmk)
+ ret_bbox = parse_bbox_from_landmark(
+ lmk,
+ scale=self.crop_cfg.scale_crop_video,
+ vx_ratio_crop_video=self.crop_cfg.vx_ratio_crop_video,
+ vy_ratio=self.crop_cfg.vy_ratio_crop_video,
+ )["bbox"]
+ bbox = [
+ ret_bbox[0, 0],
+ ret_bbox[0, 1],
+ ret_bbox[2, 0],
+ ret_bbox[2, 1],
+ ] # 4,
+ trajectory.bbox_lst.append(bbox) # bbox
+ trajectory.frame_rgb_lst.append(frame_rgb)
+
+ global_bbox = average_bbox_lst(trajectory.bbox_lst)
+
+ for idx, (frame_rgb, lmk) in enumerate(zip(trajectory.frame_rgb_lst, trajectory.lmk_lst)):
+ ret_dct = crop_image_by_bbox(
+ frame_rgb,
+ global_bbox,
+ lmk=lmk,
+ dsize=kwargs.get("dsize", 512),
+ flag_rot=False,
+ borderValue=(0, 0, 0),
+ )
+ trajectory.frame_rgb_crop_lst.append(ret_dct["img_crop"])
+ trajectory.lmk_crop_lst.append(ret_dct["lmk_crop"])
+
+ return {
+ "frame_crop_lst": trajectory.frame_rgb_crop_lst,
+ "lmk_crop_lst": trajectory.lmk_crop_lst,
+ }
+
+ def calc_lmks_from_cropped_video(self, driving_rgb_crop_lst, **kwargs):
+ """Tracking based landmarks/alignment"""
+ trajectory = Trajectory()
+ direction = kwargs.get("direction", "large-small")
+
+ for idx, frame_rgb_crop in enumerate(driving_rgb_crop_lst):
+ if idx == 0 or trajectory.start == -1:
+ src_face = self.face_analysis_wrapper.get(
+ contiguous(frame_rgb_crop[..., ::-1]), # convert to BGR
+ flag_do_landmark_2d_106=True,
+ direction=direction,
+ )
+ if len(src_face) == 0:
+ log(f"No face detected in the frame #{idx}")
+ raise Exception(f"No face detected in the frame #{idx}")
+ elif len(src_face) > 1:
+ log(f"More than one face detected in the driving frame_{idx}, only pick one face by rule {direction}.")
+ src_face = src_face[0]
+ lmk = src_face.landmark_2d_106
+ lmk = self.landmark_runner.run(frame_rgb_crop, lmk)
+ trajectory.start, trajectory.end = idx, idx
+ else:
+ lmk = self.landmark_runner.run(frame_rgb_crop, trajectory.lmk_lst[-1])
+ trajectory.end = idx
+
+ trajectory.lmk_lst.append(lmk)
+ return trajectory.lmk_lst
diff --git a/difpoint/src/utils/dependencies/insightface/__init__.py b/difpoint/src/utils/dependencies/insightface/__init__.py
new file mode 100644
index 0000000000000000000000000000000000000000..1680083da47850b31da10803c7d255e67dda619a
--- /dev/null
+++ b/difpoint/src/utils/dependencies/insightface/__init__.py
@@ -0,0 +1,20 @@
+# coding: utf-8
+# pylint: disable=wrong-import-position
+"""InsightFace: A Face Analysis Toolkit."""
+from __future__ import absolute_import
+
+try:
+ #import mxnet as mx
+ import onnxruntime
+except ImportError:
+ raise ImportError(
+ "Unable to import dependency onnxruntime. "
+ )
+
+__version__ = '0.7.3'
+
+from . import model_zoo
+from . import utils
+from . import app
+from . import data
+
diff --git a/difpoint/src/utils/dependencies/insightface/__pycache__/__init__.cpython-310.pyc b/difpoint/src/utils/dependencies/insightface/__pycache__/__init__.cpython-310.pyc
new file mode 100644
index 0000000000000000000000000000000000000000..db78d1e4ffab004d18f9994967b3849cfddd3d84
Binary files /dev/null and b/difpoint/src/utils/dependencies/insightface/__pycache__/__init__.cpython-310.pyc differ
diff --git a/difpoint/src/utils/dependencies/insightface/app/__init__.py b/difpoint/src/utils/dependencies/insightface/app/__init__.py
new file mode 100644
index 0000000000000000000000000000000000000000..cc574616885290489798bac5c682e7aaa65a5dad
--- /dev/null
+++ b/difpoint/src/utils/dependencies/insightface/app/__init__.py
@@ -0,0 +1 @@
+from .face_analysis import *
diff --git a/difpoint/src/utils/dependencies/insightface/app/__pycache__/__init__.cpython-310.pyc b/difpoint/src/utils/dependencies/insightface/app/__pycache__/__init__.cpython-310.pyc
new file mode 100644
index 0000000000000000000000000000000000000000..daa7535920bbc0d558e152133249b4ce78c9df70
Binary files /dev/null and b/difpoint/src/utils/dependencies/insightface/app/__pycache__/__init__.cpython-310.pyc differ
diff --git a/difpoint/src/utils/dependencies/insightface/app/__pycache__/common.cpython-310.pyc b/difpoint/src/utils/dependencies/insightface/app/__pycache__/common.cpython-310.pyc
new file mode 100644
index 0000000000000000000000000000000000000000..1e4534e2509f007a211deb006f28a5d4f596c2de
Binary files /dev/null and b/difpoint/src/utils/dependencies/insightface/app/__pycache__/common.cpython-310.pyc differ
diff --git a/difpoint/src/utils/dependencies/insightface/app/__pycache__/face_analysis.cpython-310.pyc b/difpoint/src/utils/dependencies/insightface/app/__pycache__/face_analysis.cpython-310.pyc
new file mode 100644
index 0000000000000000000000000000000000000000..cfd171df6f2bbbf5737d8ab9439e9fee5849772e
Binary files /dev/null and b/difpoint/src/utils/dependencies/insightface/app/__pycache__/face_analysis.cpython-310.pyc differ
diff --git a/difpoint/src/utils/dependencies/insightface/app/common.py b/difpoint/src/utils/dependencies/insightface/app/common.py
new file mode 100644
index 0000000000000000000000000000000000000000..82ca987aeede35510b3aef72b4edf2390ad84e65
--- /dev/null
+++ b/difpoint/src/utils/dependencies/insightface/app/common.py
@@ -0,0 +1,49 @@
+import numpy as np
+from numpy.linalg import norm as l2norm
+#from easydict import EasyDict
+
+class Face(dict):
+
+ def __init__(self, d=None, **kwargs):
+ if d is None:
+ d = {}
+ if kwargs:
+ d.update(**kwargs)
+ for k, v in d.items():
+ setattr(self, k, v)
+ # Class attributes
+ #for k in self.__class__.__dict__.keys():
+ # if not (k.startswith('__') and k.endswith('__')) and not k in ('update', 'pop'):
+ # setattr(self, k, getattr(self, k))
+
+ def __setattr__(self, name, value):
+ if isinstance(value, (list, tuple)):
+ value = [self.__class__(x)
+ if isinstance(x, dict) else x for x in value]
+ elif isinstance(value, dict) and not isinstance(value, self.__class__):
+ value = self.__class__(value)
+ super(Face, self).__setattr__(name, value)
+ super(Face, self).__setitem__(name, value)
+
+ __setitem__ = __setattr__
+
+ def __getattr__(self, name):
+ return None
+
+ @property
+ def embedding_norm(self):
+ if self.embedding is None:
+ return None
+ return l2norm(self.embedding)
+
+ @property
+ def normed_embedding(self):
+ if self.embedding is None:
+ return None
+ return self.embedding / self.embedding_norm
+
+ @property
+ def sex(self):
+ if self.gender is None:
+ return None
+ return 'M' if self.gender==1 else 'F'
diff --git a/difpoint/src/utils/dependencies/insightface/app/face_analysis.py b/difpoint/src/utils/dependencies/insightface/app/face_analysis.py
new file mode 100644
index 0000000000000000000000000000000000000000..aa5128b3f5e02c2c19e7df195cc1c1e7fcf36c4d
--- /dev/null
+++ b/difpoint/src/utils/dependencies/insightface/app/face_analysis.py
@@ -0,0 +1,110 @@
+# -*- coding: utf-8 -*-
+# @Organization : insightface.ai
+# @Author : Jia Guo
+# @Time : 2021-05-04
+# @Function :
+
+
+from __future__ import division
+
+import glob
+import os.path as osp
+
+import numpy as np
+import onnxruntime
+from numpy.linalg import norm
+
+from ..model_zoo import model_zoo
+from ..utils import ensure_available
+from .common import Face
+
+
+DEFAULT_MP_NAME = 'buffalo_l'
+__all__ = ['FaceAnalysis']
+
+class FaceAnalysis:
+ def __init__(self, name=DEFAULT_MP_NAME, root='~/.insightface', allowed_modules=None, **kwargs):
+ onnxruntime.set_default_logger_severity(3)
+ self.models = {}
+ self.model_dir = ensure_available('models', name, root=root)
+ onnx_files = glob.glob(osp.join(self.model_dir, '*.onnx'))
+ onnx_files = sorted(onnx_files)
+ for onnx_file in onnx_files:
+ model = model_zoo.get_model(onnx_file, **kwargs)
+ if model is None:
+ print('model not recognized:', onnx_file)
+ elif allowed_modules is not None and model.taskname not in allowed_modules:
+ print('model ignore:', onnx_file, model.taskname)
+ del model
+ elif model.taskname not in self.models and (allowed_modules is None or model.taskname in allowed_modules):
+ # print('find model:', onnx_file, model.taskname, model.input_shape, model.input_mean, model.input_std)
+ self.models[model.taskname] = model
+ else:
+ print('duplicated model task type, ignore:', onnx_file, model.taskname)
+ del model
+ assert 'detection' in self.models
+ self.det_model = self.models['detection']
+
+
+ def prepare(self, ctx_id, det_thresh=0.5, det_size=(640, 640)):
+ self.det_thresh = det_thresh
+ assert det_size is not None
+ # print('set det-size:', det_size)
+ self.det_size = det_size
+ for taskname, model in self.models.items():
+ if taskname=='detection':
+ model.prepare(ctx_id, input_size=det_size, det_thresh=det_thresh)
+ else:
+ model.prepare(ctx_id)
+
+ def get(self, img, max_num=0):
+ bboxes, kpss = self.det_model.detect(img,
+ max_num=max_num,
+ metric='default')
+ if bboxes.shape[0] == 0:
+ return []
+ ret = []
+ for i in range(bboxes.shape[0]):
+ bbox = bboxes[i, 0:4]
+ det_score = bboxes[i, 4]
+ kps = None
+ if kpss is not None:
+ kps = kpss[i]
+ face = Face(bbox=bbox, kps=kps, det_score=det_score)
+ for taskname, model in self.models.items():
+ if taskname=='detection':
+ continue
+ model.get(img, face)
+ ret.append(face)
+ return ret
+
+ def draw_on(self, img, faces):
+ import cv2
+ dimg = img.copy()
+ for i in range(len(faces)):
+ face = faces[i]
+ box = face.bbox.astype(np.int)
+ color = (0, 0, 255)
+ cv2.rectangle(dimg, (box[0], box[1]), (box[2], box[3]), color, 2)
+ if face.kps is not None:
+ kps = face.kps.astype(np.int)
+ #print(landmark.shape)
+ for l in range(kps.shape[0]):
+ color = (0, 0, 255)
+ if l == 0 or l == 3:
+ color = (0, 255, 0)
+ cv2.circle(dimg, (kps[l][0], kps[l][1]), 1, color,
+ 2)
+ if face.gender is not None and face.age is not None:
+ cv2.putText(dimg,'%s,%d'%(face.sex,face.age), (box[0]-1, box[1]-4),cv2.FONT_HERSHEY_COMPLEX,0.7,(0,255,0),1)
+
+ #for key, value in face.items():
+ # if key.startswith('landmark_3d'):
+ # print(key, value.shape)
+ # print(value[0:10,:])
+ # lmk = np.round(value).astype(np.int)
+ # for l in range(lmk.shape[0]):
+ # color = (255, 0, 0)
+ # cv2.circle(dimg, (lmk[l][0], lmk[l][1]), 1, color,
+ # 2)
+ return dimg
diff --git a/difpoint/src/utils/dependencies/insightface/data/__init__.py b/difpoint/src/utils/dependencies/insightface/data/__init__.py
new file mode 100644
index 0000000000000000000000000000000000000000..665c59ec99b6ebf12822015e0350969c7903e243
--- /dev/null
+++ b/difpoint/src/utils/dependencies/insightface/data/__init__.py
@@ -0,0 +1,2 @@
+from .image import get_image
+from .pickle_object import get_object
diff --git a/difpoint/src/utils/dependencies/insightface/data/__pycache__/__init__.cpython-310.pyc b/difpoint/src/utils/dependencies/insightface/data/__pycache__/__init__.cpython-310.pyc
new file mode 100644
index 0000000000000000000000000000000000000000..2283d991db4936753977da828fbd584273523d76
Binary files /dev/null and b/difpoint/src/utils/dependencies/insightface/data/__pycache__/__init__.cpython-310.pyc differ
diff --git a/difpoint/src/utils/dependencies/insightface/data/__pycache__/image.cpython-310.pyc b/difpoint/src/utils/dependencies/insightface/data/__pycache__/image.cpython-310.pyc
new file mode 100644
index 0000000000000000000000000000000000000000..948b54cca266bd79e161cc1cbdf9af2509aef158
Binary files /dev/null and b/difpoint/src/utils/dependencies/insightface/data/__pycache__/image.cpython-310.pyc differ
diff --git a/difpoint/src/utils/dependencies/insightface/data/__pycache__/pickle_object.cpython-310.pyc b/difpoint/src/utils/dependencies/insightface/data/__pycache__/pickle_object.cpython-310.pyc
new file mode 100644
index 0000000000000000000000000000000000000000..61c3f16d89414f59c3cc6ee6f2118aee9ae6323c
Binary files /dev/null and b/difpoint/src/utils/dependencies/insightface/data/__pycache__/pickle_object.cpython-310.pyc differ
diff --git a/difpoint/src/utils/dependencies/insightface/data/image.py b/difpoint/src/utils/dependencies/insightface/data/image.py
new file mode 100644
index 0000000000000000000000000000000000000000..6d32c4bcb1b13d33bcb0d840cf7b8c08d183b3ea
--- /dev/null
+++ b/difpoint/src/utils/dependencies/insightface/data/image.py
@@ -0,0 +1,27 @@
+import cv2
+import os
+import os.path as osp
+from pathlib import Path
+
+class ImageCache:
+ data = {}
+
+def get_image(name, to_rgb=False):
+ key = (name, to_rgb)
+ if key in ImageCache.data:
+ return ImageCache.data[key]
+ images_dir = osp.join(Path(__file__).parent.absolute(), 'images')
+ ext_names = ['.jpg', '.png', '.jpeg']
+ image_file = None
+ for ext_name in ext_names:
+ _image_file = osp.join(images_dir, "%s%s"%(name, ext_name))
+ if osp.exists(_image_file):
+ image_file = _image_file
+ break
+ assert image_file is not None, '%s not found'%name
+ img = cv2.imread(image_file)
+ if to_rgb:
+ img = img[:,:,::-1]
+ ImageCache.data[key] = img
+ return img
+
diff --git a/difpoint/src/utils/dependencies/insightface/data/images/Tom_Hanks_54745.png b/difpoint/src/utils/dependencies/insightface/data/images/Tom_Hanks_54745.png
new file mode 100644
index 0000000000000000000000000000000000000000..906315d13fa29bb3a5ded3e162592f2c7f041b23
Binary files /dev/null and b/difpoint/src/utils/dependencies/insightface/data/images/Tom_Hanks_54745.png differ
diff --git a/difpoint/src/utils/dependencies/insightface/data/images/mask_black.jpg b/difpoint/src/utils/dependencies/insightface/data/images/mask_black.jpg
new file mode 100644
index 0000000000000000000000000000000000000000..0eab0df555c23f1e033537fe39f3c0c8303dd369
Binary files /dev/null and b/difpoint/src/utils/dependencies/insightface/data/images/mask_black.jpg differ
diff --git a/difpoint/src/utils/dependencies/insightface/data/images/mask_blue.jpg b/difpoint/src/utils/dependencies/insightface/data/images/mask_blue.jpg
new file mode 100644
index 0000000000000000000000000000000000000000..f71336b9a0d3038ebd84e6995ebfbe54946fcbb4
Binary files /dev/null and b/difpoint/src/utils/dependencies/insightface/data/images/mask_blue.jpg differ
diff --git a/difpoint/src/utils/dependencies/insightface/data/images/mask_green.jpg b/difpoint/src/utils/dependencies/insightface/data/images/mask_green.jpg
new file mode 100644
index 0000000000000000000000000000000000000000..ac2ad55f4fc580c915dfa4c157ca3bfc84e453f4
Binary files /dev/null and b/difpoint/src/utils/dependencies/insightface/data/images/mask_green.jpg differ
diff --git a/difpoint/src/utils/dependencies/insightface/data/images/mask_white.jpg b/difpoint/src/utils/dependencies/insightface/data/images/mask_white.jpg
new file mode 100644
index 0000000000000000000000000000000000000000..2148ab2d09fdee6e3f59315470e98ecfc54339e4
Binary files /dev/null and b/difpoint/src/utils/dependencies/insightface/data/images/mask_white.jpg differ
diff --git a/difpoint/src/utils/dependencies/insightface/data/images/t1.jpg b/difpoint/src/utils/dependencies/insightface/data/images/t1.jpg
new file mode 100644
index 0000000000000000000000000000000000000000..8fd6427a177bd01650c0150e9d02457c3a5dcddd
--- /dev/null
+++ b/difpoint/src/utils/dependencies/insightface/data/images/t1.jpg
@@ -0,0 +1,3 @@
+version https://git-lfs.github.com/spec/v1
+oid sha256:47f682e945b659f93a9e490b9c9c4a2a864abe64dace9e1a2893845ddfd69489
+size 128824
diff --git a/difpoint/src/utils/dependencies/insightface/data/objects/meanshape_68.pkl b/difpoint/src/utils/dependencies/insightface/data/objects/meanshape_68.pkl
new file mode 100644
index 0000000000000000000000000000000000000000..d5297e9e8ea5574298ddd287b058252e03aa18c1
--- /dev/null
+++ b/difpoint/src/utils/dependencies/insightface/data/objects/meanshape_68.pkl
@@ -0,0 +1,3 @@
+version https://git-lfs.github.com/spec/v1
+oid sha256:39ffecf84ba73f0d0d7e49380833ba88713c9fcdec51df4f7ac45a48b8f4cc51
+size 974
diff --git a/difpoint/src/utils/dependencies/insightface/data/pickle_object.py b/difpoint/src/utils/dependencies/insightface/data/pickle_object.py
new file mode 100644
index 0000000000000000000000000000000000000000..fbd87030ea15e1d01af1cd4cff1be2bc54cc82dd
--- /dev/null
+++ b/difpoint/src/utils/dependencies/insightface/data/pickle_object.py
@@ -0,0 +1,17 @@
+import cv2
+import os
+import os.path as osp
+from pathlib import Path
+import pickle
+
+def get_object(name):
+ objects_dir = osp.join(Path(__file__).parent.absolute(), 'objects')
+ if not name.endswith('.pkl'):
+ name = name+".pkl"
+ filepath = osp.join(objects_dir, name)
+ if not osp.exists(filepath):
+ return None
+ with open(filepath, 'rb') as f:
+ obj = pickle.load(f)
+ return obj
+
diff --git a/difpoint/src/utils/dependencies/insightface/data/rec_builder.py b/difpoint/src/utils/dependencies/insightface/data/rec_builder.py
new file mode 100644
index 0000000000000000000000000000000000000000..e02abc969da2f882639326f5bad3c7e8d08c1fde
--- /dev/null
+++ b/difpoint/src/utils/dependencies/insightface/data/rec_builder.py
@@ -0,0 +1,71 @@
+import pickle
+import numpy as np
+import os
+import os.path as osp
+import sys
+import mxnet as mx
+
+
+class RecBuilder():
+ def __init__(self, path, image_size=(112, 112)):
+ self.path = path
+ self.image_size = image_size
+ self.widx = 0
+ self.wlabel = 0
+ self.max_label = -1
+ assert not osp.exists(path), '%s exists' % path
+ os.makedirs(path)
+ self.writer = mx.recordio.MXIndexedRecordIO(os.path.join(path, 'train.idx'),
+ os.path.join(path, 'train.rec'),
+ 'w')
+ self.meta = []
+
+ def add(self, imgs):
+ #!!! img should be BGR!!!!
+ #assert label >= 0
+ #assert label > self.last_label
+ assert len(imgs) > 0
+ label = self.wlabel
+ for img in imgs:
+ idx = self.widx
+ image_meta = {'image_index': idx, 'image_classes': [label]}
+ header = mx.recordio.IRHeader(0, label, idx, 0)
+ if isinstance(img, np.ndarray):
+ s = mx.recordio.pack_img(header,img,quality=95,img_fmt='.jpg')
+ else:
+ s = mx.recordio.pack(header, img)
+ self.writer.write_idx(idx, s)
+ self.meta.append(image_meta)
+ self.widx += 1
+ self.max_label = label
+ self.wlabel += 1
+
+
+ def add_image(self, img, label):
+ #!!! img should be BGR!!!!
+ #assert label >= 0
+ #assert label > self.last_label
+ idx = self.widx
+ header = mx.recordio.IRHeader(0, label, idx, 0)
+ if isinstance(label, list):
+ idlabel = label[0]
+ else:
+ idlabel = label
+ image_meta = {'image_index': idx, 'image_classes': [idlabel]}
+ if isinstance(img, np.ndarray):
+ s = mx.recordio.pack_img(header,img,quality=95,img_fmt='.jpg')
+ else:
+ s = mx.recordio.pack(header, img)
+ self.writer.write_idx(idx, s)
+ self.meta.append(image_meta)
+ self.widx += 1
+ self.max_label = max(self.max_label, idlabel)
+
+ def close(self):
+ with open(osp.join(self.path, 'train.meta'), 'wb') as pfile:
+ pickle.dump(self.meta, pfile, protocol=pickle.HIGHEST_PROTOCOL)
+ print('stat:', self.widx, self.wlabel)
+ with open(os.path.join(self.path, 'property'), 'w') as f:
+ f.write("%d,%d,%d\n" % (self.max_label+1, self.image_size[0], self.image_size[1]))
+ f.write("%d\n" % (self.widx))
+
diff --git a/difpoint/src/utils/dependencies/insightface/model_zoo/__init__.py b/difpoint/src/utils/dependencies/insightface/model_zoo/__init__.py
new file mode 100644
index 0000000000000000000000000000000000000000..225623d6142c968b4040f391039bfab88bdd1b2a
--- /dev/null
+++ b/difpoint/src/utils/dependencies/insightface/model_zoo/__init__.py
@@ -0,0 +1,6 @@
+from .model_zoo import get_model
+from .arcface_onnx import ArcFaceONNX
+from .retinaface import RetinaFace
+from .scrfd import SCRFD
+from .landmark import Landmark
+from .attribute import Attribute
diff --git a/difpoint/src/utils/dependencies/insightface/model_zoo/__pycache__/__init__.cpython-310.pyc b/difpoint/src/utils/dependencies/insightface/model_zoo/__pycache__/__init__.cpython-310.pyc
new file mode 100644
index 0000000000000000000000000000000000000000..88176dd94d61d310cb527c497cdec38ae4fe64c2
Binary files /dev/null and b/difpoint/src/utils/dependencies/insightface/model_zoo/__pycache__/__init__.cpython-310.pyc differ
diff --git a/difpoint/src/utils/dependencies/insightface/model_zoo/__pycache__/arcface_onnx.cpython-310.pyc b/difpoint/src/utils/dependencies/insightface/model_zoo/__pycache__/arcface_onnx.cpython-310.pyc
new file mode 100644
index 0000000000000000000000000000000000000000..087e46f7fd829a81f82502b7d5966c5e5311e553
Binary files /dev/null and b/difpoint/src/utils/dependencies/insightface/model_zoo/__pycache__/arcface_onnx.cpython-310.pyc differ
diff --git a/difpoint/src/utils/dependencies/insightface/model_zoo/__pycache__/attribute.cpython-310.pyc b/difpoint/src/utils/dependencies/insightface/model_zoo/__pycache__/attribute.cpython-310.pyc
new file mode 100644
index 0000000000000000000000000000000000000000..09b5bc98cb80172cafac31c4a05572afbff38dd0
Binary files /dev/null and b/difpoint/src/utils/dependencies/insightface/model_zoo/__pycache__/attribute.cpython-310.pyc differ
diff --git a/difpoint/src/utils/dependencies/insightface/model_zoo/__pycache__/inswapper.cpython-310.pyc b/difpoint/src/utils/dependencies/insightface/model_zoo/__pycache__/inswapper.cpython-310.pyc
new file mode 100644
index 0000000000000000000000000000000000000000..df984493302a336747868452a8ef557bb902e76a
Binary files /dev/null and b/difpoint/src/utils/dependencies/insightface/model_zoo/__pycache__/inswapper.cpython-310.pyc differ
diff --git a/difpoint/src/utils/dependencies/insightface/model_zoo/__pycache__/landmark.cpython-310.pyc b/difpoint/src/utils/dependencies/insightface/model_zoo/__pycache__/landmark.cpython-310.pyc
new file mode 100644
index 0000000000000000000000000000000000000000..cb5be17ccaf228136613906e18b6d119de4466ef
Binary files /dev/null and b/difpoint/src/utils/dependencies/insightface/model_zoo/__pycache__/landmark.cpython-310.pyc differ
diff --git a/difpoint/src/utils/dependencies/insightface/model_zoo/__pycache__/model_zoo.cpython-310.pyc b/difpoint/src/utils/dependencies/insightface/model_zoo/__pycache__/model_zoo.cpython-310.pyc
new file mode 100644
index 0000000000000000000000000000000000000000..ca2facf5697ef5ca2a43370a8edfa5e62dbe93f0
Binary files /dev/null and b/difpoint/src/utils/dependencies/insightface/model_zoo/__pycache__/model_zoo.cpython-310.pyc differ
diff --git a/difpoint/src/utils/dependencies/insightface/model_zoo/__pycache__/retinaface.cpython-310.pyc b/difpoint/src/utils/dependencies/insightface/model_zoo/__pycache__/retinaface.cpython-310.pyc
new file mode 100644
index 0000000000000000000000000000000000000000..435e6d994b0090fc5b96391d134d7087534c06cb
Binary files /dev/null and b/difpoint/src/utils/dependencies/insightface/model_zoo/__pycache__/retinaface.cpython-310.pyc differ
diff --git a/difpoint/src/utils/dependencies/insightface/model_zoo/__pycache__/scrfd.cpython-310.pyc b/difpoint/src/utils/dependencies/insightface/model_zoo/__pycache__/scrfd.cpython-310.pyc
new file mode 100644
index 0000000000000000000000000000000000000000..1626e7e8699c7d9f9008f7aa377effb1437aeddd
Binary files /dev/null and b/difpoint/src/utils/dependencies/insightface/model_zoo/__pycache__/scrfd.cpython-310.pyc differ
diff --git a/difpoint/src/utils/dependencies/insightface/model_zoo/arcface_onnx.py b/difpoint/src/utils/dependencies/insightface/model_zoo/arcface_onnx.py
new file mode 100644
index 0000000000000000000000000000000000000000..b537ce2ee15d4a1834d54e185f34e336aab30a77
--- /dev/null
+++ b/difpoint/src/utils/dependencies/insightface/model_zoo/arcface_onnx.py
@@ -0,0 +1,92 @@
+# -*- coding: utf-8 -*-
+# @Organization : insightface.ai
+# @Author : Jia Guo
+# @Time : 2021-05-04
+# @Function :
+
+from __future__ import division
+import numpy as np
+import cv2
+import onnx
+import onnxruntime
+from ..utils import face_align
+
+__all__ = [
+ 'ArcFaceONNX',
+]
+
+
+class ArcFaceONNX:
+ def __init__(self, model_file=None, session=None):
+ assert model_file is not None
+ self.model_file = model_file
+ self.session = session
+ self.taskname = 'recognition'
+ find_sub = False
+ find_mul = False
+ model = onnx.load(self.model_file)
+ graph = model.graph
+ for nid, node in enumerate(graph.node[:8]):
+ #print(nid, node.name)
+ if node.name.startswith('Sub') or node.name.startswith('_minus'):
+ find_sub = True
+ if node.name.startswith('Mul') or node.name.startswith('_mul'):
+ find_mul = True
+ if find_sub and find_mul:
+ #mxnet arcface model
+ input_mean = 0.0
+ input_std = 1.0
+ else:
+ input_mean = 127.5
+ input_std = 127.5
+ self.input_mean = input_mean
+ self.input_std = input_std
+ #print('input mean and std:', self.input_mean, self.input_std)
+ if self.session is None:
+ self.session = onnxruntime.InferenceSession(self.model_file, None)
+ input_cfg = self.session.get_inputs()[0]
+ input_shape = input_cfg.shape
+ input_name = input_cfg.name
+ self.input_size = tuple(input_shape[2:4][::-1])
+ self.input_shape = input_shape
+ outputs = self.session.get_outputs()
+ output_names = []
+ for out in outputs:
+ output_names.append(out.name)
+ self.input_name = input_name
+ self.output_names = output_names
+ assert len(self.output_names)==1
+ self.output_shape = outputs[0].shape
+
+ def prepare(self, ctx_id, **kwargs):
+ if ctx_id<0:
+ self.session.set_providers(['CPUExecutionProvider'])
+
+ def get(self, img, face):
+ aimg = face_align.norm_crop(img, landmark=face.kps, image_size=self.input_size[0])
+ face.embedding = self.get_feat(aimg).flatten()
+ return face.embedding
+
+ def compute_sim(self, feat1, feat2):
+ from numpy.linalg import norm
+ feat1 = feat1.ravel()
+ feat2 = feat2.ravel()
+ sim = np.dot(feat1, feat2) / (norm(feat1) * norm(feat2))
+ return sim
+
+ def get_feat(self, imgs):
+ if not isinstance(imgs, list):
+ imgs = [imgs]
+ input_size = self.input_size
+
+ blob = cv2.dnn.blobFromImages(imgs, 1.0 / self.input_std, input_size,
+ (self.input_mean, self.input_mean, self.input_mean), swapRB=True)
+ net_out = self.session.run(self.output_names, {self.input_name: blob})[0]
+ return net_out
+
+ def forward(self, batch_data):
+ blob = (batch_data - self.input_mean) / self.input_std
+ net_out = self.session.run(self.output_names, {self.input_name: blob})[0]
+ return net_out
+
+
diff --git a/difpoint/src/utils/dependencies/insightface/model_zoo/attribute.py b/difpoint/src/utils/dependencies/insightface/model_zoo/attribute.py
new file mode 100644
index 0000000000000000000000000000000000000000..40c34de3f0995499448cf5779004cc1e5f3564fb
--- /dev/null
+++ b/difpoint/src/utils/dependencies/insightface/model_zoo/attribute.py
@@ -0,0 +1,94 @@
+# -*- coding: utf-8 -*-
+# @Organization : insightface.ai
+# @Author : Jia Guo
+# @Time : 2021-06-19
+# @Function :
+
+from __future__ import division
+import numpy as np
+import cv2
+import onnx
+import onnxruntime
+from ..utils import face_align
+
+__all__ = [
+ 'Attribute',
+]
+
+
+class Attribute:
+ def __init__(self, model_file=None, session=None):
+ assert model_file is not None
+ self.model_file = model_file
+ self.session = session
+ find_sub = False
+ find_mul = False
+ model = onnx.load(self.model_file)
+ graph = model.graph
+ for nid, node in enumerate(graph.node[:8]):
+ #print(nid, node.name)
+ if node.name.startswith('Sub') or node.name.startswith('_minus'):
+ find_sub = True
+ if node.name.startswith('Mul') or node.name.startswith('_mul'):
+ find_mul = True
+ if nid<3 and node.name=='bn_data':
+ find_sub = True
+ find_mul = True
+ if find_sub and find_mul:
+ #mxnet arcface model
+ input_mean = 0.0
+ input_std = 1.0
+ else:
+ input_mean = 127.5
+ input_std = 128.0
+ self.input_mean = input_mean
+ self.input_std = input_std
+ #print('input mean and std:', model_file, self.input_mean, self.input_std)
+ if self.session is None:
+ self.session = onnxruntime.InferenceSession(self.model_file, None)
+ input_cfg = self.session.get_inputs()[0]
+ input_shape = input_cfg.shape
+ input_name = input_cfg.name
+ self.input_size = tuple(input_shape[2:4][::-1])
+ self.input_shape = input_shape
+ outputs = self.session.get_outputs()
+ output_names = []
+ for out in outputs:
+ output_names.append(out.name)
+ self.input_name = input_name
+ self.output_names = output_names
+ assert len(self.output_names)==1
+ output_shape = outputs[0].shape
+ #print('init output_shape:', output_shape)
+ if output_shape[1]==3:
+ self.taskname = 'genderage'
+ else:
+ self.taskname = 'attribute_%d'%output_shape[1]
+
+ def prepare(self, ctx_id, **kwargs):
+ if ctx_id<0:
+ self.session.set_providers(['CPUExecutionProvider'])
+
+ def get(self, img, face):
+ bbox = face.bbox
+ w, h = (bbox[2] - bbox[0]), (bbox[3] - bbox[1])
+ center = (bbox[2] + bbox[0]) / 2, (bbox[3] + bbox[1]) / 2
+ rotate = 0
+ _scale = self.input_size[0] / (max(w, h)*1.5)
+ #print('param:', img.shape, bbox, center, self.input_size, _scale, rotate)
+ aimg, M = face_align.transform(img, center, self.input_size[0], _scale, rotate)
+ input_size = tuple(aimg.shape[0:2][::-1])
+ #assert input_size==self.input_size
+ blob = cv2.dnn.blobFromImage(aimg, 1.0/self.input_std, input_size, (self.input_mean, self.input_mean, self.input_mean), swapRB=True)
+ pred = self.session.run(self.output_names, {self.input_name : blob})[0][0]
+ if self.taskname=='genderage':
+ assert len(pred)==3
+ gender = np.argmax(pred[:2])
+ age = int(np.round(pred[2]*100))
+ face['gender'] = gender
+ face['age'] = age
+ return gender, age
+ else:
+ return pred
+
+
diff --git a/difpoint/src/utils/dependencies/insightface/model_zoo/inswapper.py b/difpoint/src/utils/dependencies/insightface/model_zoo/inswapper.py
new file mode 100644
index 0000000000000000000000000000000000000000..f321c627ee66cceddcab98b561b997441dd4f768
--- /dev/null
+++ b/difpoint/src/utils/dependencies/insightface/model_zoo/inswapper.py
@@ -0,0 +1,114 @@
+import time
+import numpy as np
+import onnxruntime
+import cv2
+import onnx
+from onnx import numpy_helper
+from ..utils import face_align
+
+
+
+
+class INSwapper():
+ def __init__(self, model_file=None, session=None):
+ self.model_file = model_file
+ self.session = session
+ model = onnx.load(self.model_file)
+ graph = model.graph
+ self.emap = numpy_helper.to_array(graph.initializer[-1])
+ self.input_mean = 0.0
+ self.input_std = 255.0
+ #print('input mean and std:', model_file, self.input_mean, self.input_std)
+ if self.session is None:
+ self.session = onnxruntime.InferenceSession(self.model_file, None)
+ inputs = self.session.get_inputs()
+ self.input_names = []
+ for inp in inputs:
+ self.input_names.append(inp.name)
+ outputs = self.session.get_outputs()
+ output_names = []
+ for out in outputs:
+ output_names.append(out.name)
+ self.output_names = output_names
+ assert len(self.output_names)==1
+ output_shape = outputs[0].shape
+ input_cfg = inputs[0]
+ input_shape = input_cfg.shape
+ self.input_shape = input_shape
+ # print('inswapper-shape:', self.input_shape)
+ self.input_size = tuple(input_shape[2:4][::-1])
+
+ def forward(self, img, latent):
+ img = (img - self.input_mean) / self.input_std
+ pred = self.session.run(self.output_names, {self.input_names[0]: img, self.input_names[1]: latent})[0]
+ return pred
+
+ def get(self, img, target_face, source_face, paste_back=True):
+ face_mask = np.zeros((img.shape[0], img.shape[1]), np.uint8)
+ cv2.fillPoly(face_mask, np.array([target_face.landmark_2d_106[[1,9,10,11,12,13,14,15,16,2,3,4,5,6,7,8,0,24,23,22,21,20,19,18,32,31,30,29,28,27,26,25,17,101,105,104,103,51,49,48,43]].astype('int64')]), 1)
+ aimg, M = face_align.norm_crop2(img, target_face.kps, self.input_size[0])
+ blob = cv2.dnn.blobFromImage(aimg, 1.0 / self.input_std, self.input_size,
+ (self.input_mean, self.input_mean, self.input_mean), swapRB=True)
+ latent = source_face.normed_embedding.reshape((1,-1))
+ latent = np.dot(latent, self.emap)
+ latent /= np.linalg.norm(latent)
+ pred = self.session.run(self.output_names, {self.input_names[0]: blob, self.input_names[1]: latent})[0]
+ #print(latent.shape, latent.dtype, pred.shape)
+ img_fake = pred.transpose((0,2,3,1))[0]
+ bgr_fake = np.clip(255 * img_fake, 0, 255).astype(np.uint8)[:,:,::-1]
+ if not paste_back:
+ return bgr_fake, M
+ else:
+ target_img = img
+ fake_diff = bgr_fake.astype(np.float32) - aimg.astype(np.float32)
+ fake_diff = np.abs(fake_diff).mean(axis=2)
+ fake_diff[:2,:] = 0
+ fake_diff[-2:,:] = 0
+ fake_diff[:,:2] = 0
+ fake_diff[:,-2:] = 0
+ IM = cv2.invertAffineTransform(M)
+ img_white = np.full((aimg.shape[0],aimg.shape[1]), 255, dtype=np.float32)
+ bgr_fake = cv2.warpAffine(bgr_fake, IM, (target_img.shape[1], target_img.shape[0]), borderValue=0.0)
+ img_white = cv2.warpAffine(img_white, IM, (target_img.shape[1], target_img.shape[0]), borderValue=0.0)
+ fake_diff = cv2.warpAffine(fake_diff, IM, (target_img.shape[1], target_img.shape[0]), borderValue=0.0)
+ img_white[img_white>20] = 255
+ fthresh = 10
+ fake_diff[fake_diff=fthresh] = 255
+ img_mask = img_white
+ mask_h_inds, mask_w_inds = np.where(img_mask==255)
+ mask_h = np.max(mask_h_inds) - np.min(mask_h_inds)
+ mask_w = np.max(mask_w_inds) - np.min(mask_w_inds)
+ mask_size = int(np.sqrt(mask_h*mask_w))
+ k = max(mask_size//10, 10)
+ #k = max(mask_size//20, 6)
+ #k = 6
+ kernel = np.ones((k,k),np.uint8)
+ img_mask = cv2.erode(img_mask,kernel,iterations = 1)
+ kernel = np.ones((2,2),np.uint8)
+ fake_diff = cv2.dilate(fake_diff,kernel,iterations = 1)
+
+ face_mask = cv2.erode(face_mask,np.ones((11,11),np.uint8),iterations = 1)
+ fake_diff[face_mask==1] = 255
+
+ k = max(mask_size//20, 5)
+ #k = 3
+ #k = 3
+ kernel_size = (k, k)
+ blur_size = tuple(2*i+1 for i in kernel_size)
+ img_mask = cv2.GaussianBlur(img_mask, blur_size, 0)
+ k = 5
+ kernel_size = (k, k)
+ blur_size = tuple(2*i+1 for i in kernel_size)
+ fake_diff = cv2.blur(fake_diff, (11,11), 0)
+ ##fake_diff = cv2.GaussianBlur(fake_diff, blur_size, 0)
+ # print('blur_size: ', blur_size)
+ # fake_diff = cv2.blur(fake_diff, (21, 21), 0) # blur_size
+ img_mask /= 255
+ fake_diff /= 255
+ # img_mask = fake_diff
+ img_mask = img_mask*fake_diff
+ img_mask = np.reshape(img_mask, [img_mask.shape[0],img_mask.shape[1],1])
+ fake_merged = img_mask * bgr_fake + (1-img_mask) * target_img.astype(np.float32)
+ fake_merged = fake_merged.astype(np.uint8)
+ return fake_merged
diff --git a/difpoint/src/utils/dependencies/insightface/model_zoo/landmark.py b/difpoint/src/utils/dependencies/insightface/model_zoo/landmark.py
new file mode 100644
index 0000000000000000000000000000000000000000..598b4b29a2d0674d8bb25b681f921c61460d101c
--- /dev/null
+++ b/difpoint/src/utils/dependencies/insightface/model_zoo/landmark.py
@@ -0,0 +1,114 @@
+# -*- coding: utf-8 -*-
+# @Organization : insightface.ai
+# @Author : Jia Guo
+# @Time : 2021-05-04
+# @Function :
+
+from __future__ import division
+import numpy as np
+import cv2
+import onnx
+import onnxruntime
+from ..utils import face_align
+from ..utils import transform
+from ..data import get_object
+
+__all__ = [
+ 'Landmark',
+]
+
+
+class Landmark:
+ def __init__(self, model_file=None, session=None):
+ assert model_file is not None
+ self.model_file = model_file
+ self.session = session
+ find_sub = False
+ find_mul = False
+ model = onnx.load(self.model_file)
+ graph = model.graph
+ for nid, node in enumerate(graph.node[:8]):
+ #print(nid, node.name)
+ if node.name.startswith('Sub') or node.name.startswith('_minus'):
+ find_sub = True
+ if node.name.startswith('Mul') or node.name.startswith('_mul'):
+ find_mul = True
+ if nid<3 and node.name=='bn_data':
+ find_sub = True
+ find_mul = True
+ if find_sub and find_mul:
+ #mxnet arcface model
+ input_mean = 0.0
+ input_std = 1.0
+ else:
+ input_mean = 127.5
+ input_std = 128.0
+ self.input_mean = input_mean
+ self.input_std = input_std
+ #print('input mean and std:', model_file, self.input_mean, self.input_std)
+ if self.session is None:
+ self.session = onnxruntime.InferenceSession(self.model_file, None)
+ input_cfg = self.session.get_inputs()[0]
+ input_shape = input_cfg.shape
+ input_name = input_cfg.name
+ self.input_size = tuple(input_shape[2:4][::-1])
+ self.input_shape = input_shape
+ outputs = self.session.get_outputs()
+ output_names = []
+ for out in outputs:
+ output_names.append(out.name)
+ self.input_name = input_name
+ self.output_names = output_names
+ assert len(self.output_names)==1
+ output_shape = outputs[0].shape
+ self.require_pose = False
+ #print('init output_shape:', output_shape)
+ if output_shape[1]==3309:
+ self.lmk_dim = 3
+ self.lmk_num = 68
+ self.mean_lmk = get_object('meanshape_68.pkl')
+ self.require_pose = True
+ else:
+ self.lmk_dim = 2
+ self.lmk_num = output_shape[1]//self.lmk_dim
+ self.taskname = 'landmark_%dd_%d'%(self.lmk_dim, self.lmk_num)
+
+ def prepare(self, ctx_id, **kwargs):
+ if ctx_id<0:
+ self.session.set_providers(['CPUExecutionProvider'])
+
+ def get(self, img, face):
+ bbox = face.bbox
+ w, h = (bbox[2] - bbox[0]), (bbox[3] - bbox[1])
+ center = (bbox[2] + bbox[0]) / 2, (bbox[3] + bbox[1]) / 2
+ rotate = 0
+ _scale = self.input_size[0] / (max(w, h)*1.5)
+ #print('param:', img.shape, bbox, center, self.input_size, _scale, rotate)
+ aimg, M = face_align.transform(img, center, self.input_size[0], _scale, rotate)
+ input_size = tuple(aimg.shape[0:2][::-1])
+ #assert input_size==self.input_size
+ blob = cv2.dnn.blobFromImage(aimg, 1.0/self.input_std, input_size, (self.input_mean, self.input_mean, self.input_mean), swapRB=True)
+ pred = self.session.run(self.output_names, {self.input_name : blob})[0][0]
+ if pred.shape[0] >= 3000:
+ pred = pred.reshape((-1, 3))
+ else:
+ pred = pred.reshape((-1, 2))
+ if self.lmk_num < pred.shape[0]:
+ pred = pred[self.lmk_num*-1:,:]
+ pred[:, 0:2] += 1
+ pred[:, 0:2] *= (self.input_size[0] // 2)
+ if pred.shape[1] == 3:
+ pred[:, 2] *= (self.input_size[0] // 2)
+
+ IM = cv2.invertAffineTransform(M)
+ pred = face_align.trans_points(pred, IM)
+ face[self.taskname] = pred
+ if self.require_pose:
+ P = transform.estimate_affine_matrix_3d23d(self.mean_lmk, pred)
+ s, R, t = transform.P2sRt(P)
+ rx, ry, rz = transform.matrix2angle(R)
+ pose = np.array( [rx, ry, rz], dtype=np.float32 )
+ face['pose'] = pose #pitch, yaw, roll
+ return pred
+
+
diff --git a/difpoint/src/utils/dependencies/insightface/model_zoo/model_store.py b/difpoint/src/utils/dependencies/insightface/model_zoo/model_store.py
new file mode 100644
index 0000000000000000000000000000000000000000..50bb85d314f5b7a0ea8211d2cd21186e32791592
--- /dev/null
+++ b/difpoint/src/utils/dependencies/insightface/model_zoo/model_store.py
@@ -0,0 +1,103 @@
+"""
+This code file mainly comes from https://github.com/dmlc/gluon-cv/blob/master/gluoncv/model_zoo/model_store.py
+"""
+from __future__ import print_function
+
+__all__ = ['get_model_file']
+import os
+import zipfile
+import glob
+
+from ..utils import download, check_sha1
+
+_model_sha1 = {
+ name: checksum
+ for checksum, name in [
+ ('95be21b58e29e9c1237f229dae534bd854009ce0', 'arcface_r100_v1'),
+ ('', 'arcface_mfn_v1'),
+ ('39fd1e087a2a2ed70a154ac01fecaa86c315d01b', 'retinaface_r50_v1'),
+ ('2c9de8116d1f448fd1d4661f90308faae34c990a', 'retinaface_mnet025_v1'),
+ ('0db1d07921d005e6c9a5b38e059452fc5645e5a4', 'retinaface_mnet025_v2'),
+ ('7dd8111652b7aac2490c5dcddeb268e53ac643e6', 'genderage_v1'),
+ ]
+}
+
+base_repo_url = 'https://insightface.ai/files/'
+_url_format = '{repo_url}models/{file_name}.zip'
+
+
+def short_hash(name):
+ if name not in _model_sha1:
+ raise ValueError(
+ 'Pretrained model for {name} is not available.'.format(name=name))
+ return _model_sha1[name][:8]
+
+
+def find_params_file(dir_path):
+ if not os.path.exists(dir_path):
+ return None
+ paths = glob.glob("%s/*.params" % dir_path)
+ if len(paths) == 0:
+ return None
+ paths = sorted(paths)
+ return paths[-1]
+
+
+def get_model_file(name, root=os.path.join('~', '.insightface', 'models')):
+ r"""Return location for the pretrained on local file system.
+
+ This function will download from online model zoo when model cannot be found or has mismatch.
+ The root directory will be created if it doesn't exist.
+
+ Parameters
+ ----------
+ name : str
+ Name of the model.
+ root : str, default '~/.mxnet/models'
+ Location for keeping the model parameters.
+
+ Returns
+ -------
+ file_path
+ Path to the requested pretrained model file.
+ """
+
+ file_name = name
+ root = os.path.expanduser(root)
+ dir_path = os.path.join(root, name)
+ file_path = find_params_file(dir_path)
+ #file_path = os.path.join(root, file_name + '.params')
+ sha1_hash = _model_sha1[name]
+ if file_path is not None:
+ if check_sha1(file_path, sha1_hash):
+ return file_path
+ else:
+ print(
+ 'Mismatch in the content of model file detected. Downloading again.'
+ )
+ else:
+ print('Model file is not found. Downloading.')
+
+ if not os.path.exists(root):
+ os.makedirs(root)
+ if not os.path.exists(dir_path):
+ os.makedirs(dir_path)
+
+ zip_file_path = os.path.join(root, file_name + '.zip')
+ repo_url = base_repo_url
+ if repo_url[-1] != '/':
+ repo_url = repo_url + '/'
+ download(_url_format.format(repo_url=repo_url, file_name=file_name),
+ path=zip_file_path,
+ overwrite=True)
+ with zipfile.ZipFile(zip_file_path) as zf:
+ zf.extractall(dir_path)
+ os.remove(zip_file_path)
+ file_path = find_params_file(dir_path)
+
+ if check_sha1(file_path, sha1_hash):
+ return file_path
+ else:
+ raise ValueError(
+ 'Downloaded file has different hash. Please try again.')
+
diff --git a/difpoint/src/utils/dependencies/insightface/model_zoo/model_zoo.py b/difpoint/src/utils/dependencies/insightface/model_zoo/model_zoo.py
new file mode 100644
index 0000000000000000000000000000000000000000..d8366e2a5461d5d6688f23e102a40944330084a4
--- /dev/null
+++ b/difpoint/src/utils/dependencies/insightface/model_zoo/model_zoo.py
@@ -0,0 +1,97 @@
+# -*- coding: utf-8 -*-
+# @Organization : insightface.ai
+# @Author : Jia Guo
+# @Time : 2021-05-04
+# @Function :
+
+import os
+import os.path as osp
+import glob
+import onnxruntime
+from .arcface_onnx import *
+from .retinaface import *
+#from .scrfd import *
+from .landmark import *
+from .attribute import Attribute
+from .inswapper import INSwapper
+from ..utils import download_onnx
+
+__all__ = ['get_model']
+
+
+class PickableInferenceSession(onnxruntime.InferenceSession):
+ # This is a wrapper to make the current InferenceSession class pickable.
+ def __init__(self, model_path, **kwargs):
+ super().__init__(model_path, **kwargs)
+ self.model_path = model_path
+
+ def __getstate__(self):
+ return {'model_path': self.model_path}
+
+ def __setstate__(self, values):
+ model_path = values['model_path']
+ self.__init__(model_path)
+
+class ModelRouter:
+ def __init__(self, onnx_file):
+ self.onnx_file = onnx_file
+
+ def get_model(self, **kwargs):
+ session = PickableInferenceSession(self.onnx_file, **kwargs)
+ # print(f'Applied providers: {session._providers}, with options: {session._provider_options}')
+ inputs = session.get_inputs()
+ input_cfg = inputs[0]
+ input_shape = input_cfg.shape
+ outputs = session.get_outputs()
+
+ if len(outputs)>=5:
+ return RetinaFace(model_file=self.onnx_file, session=session)
+ elif input_shape[2]==192 and input_shape[3]==192:
+ return Landmark(model_file=self.onnx_file, session=session)
+ elif input_shape[2]==96 and input_shape[3]==96:
+ return Attribute(model_file=self.onnx_file, session=session)
+ elif len(inputs)==2 and input_shape[2]==128 and input_shape[3]==128:
+ return INSwapper(model_file=self.onnx_file, session=session)
+ elif input_shape[2]==input_shape[3] and input_shape[2]>=112 and input_shape[2]%16==0:
+ return ArcFaceONNX(model_file=self.onnx_file, session=session)
+ else:
+ #raise RuntimeError('error on model routing')
+ return None
+
+def find_onnx_file(dir_path):
+ if not os.path.exists(dir_path):
+ return None
+ paths = glob.glob("%s/*.onnx" % dir_path)
+ if len(paths) == 0:
+ return None
+ paths = sorted(paths)
+ return paths[-1]
+
+def get_default_providers():
+ return ['CUDAExecutionProvider', 'CPUExecutionProvider']
+
+def get_default_provider_options():
+ return None
+
+def get_model(name, **kwargs):
+ root = kwargs.get('root', '~/.insightface')
+ root = os.path.expanduser(root)
+ model_root = osp.join(root, 'models')
+ allow_download = kwargs.get('download', False)
+ download_zip = kwargs.get('download_zip', False)
+ if not name.endswith('.onnx'):
+ model_dir = os.path.join(model_root, name)
+ model_file = find_onnx_file(model_dir)
+ if model_file is None:
+ return None
+ else:
+ model_file = name
+ if not osp.exists(model_file) and allow_download:
+ model_file = download_onnx('models', model_file, root=root, download_zip=download_zip)
+ assert osp.exists(model_file), 'model_file %s should exist'%model_file
+ assert osp.isfile(model_file), 'model_file %s should be a file'%model_file
+ router = ModelRouter(model_file)
+ providers = kwargs.get('providers', get_default_providers())
+ provider_options = kwargs.get('provider_options', get_default_provider_options())
+ model = router.get_model(providers=providers, provider_options=provider_options)
+ return model
diff --git a/difpoint/src/utils/dependencies/insightface/model_zoo/retinaface.py b/difpoint/src/utils/dependencies/insightface/model_zoo/retinaface.py
new file mode 100644
index 0000000000000000000000000000000000000000..fc4ad91ed70688b38503127137e928dc7e5433e1
--- /dev/null
+++ b/difpoint/src/utils/dependencies/insightface/model_zoo/retinaface.py
@@ -0,0 +1,301 @@
+# -*- coding: utf-8 -*-
+# @Organization : insightface.ai
+# @Author : Jia Guo
+# @Time : 2021-09-18
+# @Function :
+
+from __future__ import division
+import datetime
+import numpy as np
+import onnx
+import onnxruntime
+import os
+import os.path as osp
+import cv2
+import sys
+
+def softmax(z):
+ assert len(z.shape) == 2
+ s = np.max(z, axis=1)
+ s = s[:, np.newaxis] # necessary step to do broadcasting
+ e_x = np.exp(z - s)
+ div = np.sum(e_x, axis=1)
+ div = div[:, np.newaxis] # dito
+ return e_x / div
+
+def distance2bbox(points, distance, max_shape=None):
+ """Decode distance prediction to bounding box.
+
+ Args:
+ points (Tensor): Shape (n, 2), [x, y].
+ distance (Tensor): Distance from the given point to 4
+ boundaries (left, top, right, bottom).
+ max_shape (tuple): Shape of the image.
+
+ Returns:
+ Tensor: Decoded bboxes.
+ """
+ x1 = points[:, 0] - distance[:, 0]
+ y1 = points[:, 1] - distance[:, 1]
+ x2 = points[:, 0] + distance[:, 2]
+ y2 = points[:, 1] + distance[:, 3]
+ if max_shape is not None:
+ x1 = x1.clamp(min=0, max=max_shape[1])
+ y1 = y1.clamp(min=0, max=max_shape[0])
+ x2 = x2.clamp(min=0, max=max_shape[1])
+ y2 = y2.clamp(min=0, max=max_shape[0])
+ return np.stack([x1, y1, x2, y2], axis=-1)
+
+def distance2kps(points, distance, max_shape=None):
+ """Decode distance prediction to bounding box.
+
+ Args:
+ points (Tensor): Shape (n, 2), [x, y].
+ distance (Tensor): Distance from the given point to 4
+ boundaries (left, top, right, bottom).
+ max_shape (tuple): Shape of the image.
+
+ Returns:
+ Tensor: Decoded bboxes.
+ """
+ preds = []
+ for i in range(0, distance.shape[1], 2):
+ px = points[:, i%2] + distance[:, i]
+ py = points[:, i%2+1] + distance[:, i+1]
+ if max_shape is not None:
+ px = px.clamp(min=0, max=max_shape[1])
+ py = py.clamp(min=0, max=max_shape[0])
+ preds.append(px)
+ preds.append(py)
+ return np.stack(preds, axis=-1)
+
+class RetinaFace:
+ def __init__(self, model_file=None, session=None):
+ import onnxruntime
+ self.model_file = model_file
+ self.session = session
+ self.taskname = 'detection'
+ if self.session is None:
+ assert self.model_file is not None
+ assert osp.exists(self.model_file)
+ self.session = onnxruntime.InferenceSession(self.model_file, None)
+ self.center_cache = {}
+ self.nms_thresh = 0.4
+ self.det_thresh = 0.5
+ self._init_vars()
+
+ def _init_vars(self):
+ input_cfg = self.session.get_inputs()[0]
+ input_shape = input_cfg.shape
+ #print(input_shape)
+ if isinstance(input_shape[2], str):
+ self.input_size = None
+ else:
+ self.input_size = tuple(input_shape[2:4][::-1])
+ #print('image_size:', self.image_size)
+ input_name = input_cfg.name
+ self.input_shape = input_shape
+ outputs = self.session.get_outputs()
+ output_names = []
+ for o in outputs:
+ output_names.append(o.name)
+ self.input_name = input_name
+ self.output_names = output_names
+ self.input_mean = 127.5
+ self.input_std = 128.0
+ #print(self.output_names)
+ #assert len(outputs)==10 or len(outputs)==15
+ self.use_kps = False
+ self._anchor_ratio = 1.0
+ self._num_anchors = 1
+ if len(outputs)==6:
+ self.fmc = 3
+ self._feat_stride_fpn = [8, 16, 32]
+ self._num_anchors = 2
+ elif len(outputs)==9:
+ self.fmc = 3
+ self._feat_stride_fpn = [8, 16, 32]
+ self._num_anchors = 2
+ self.use_kps = True
+ elif len(outputs)==10:
+ self.fmc = 5
+ self._feat_stride_fpn = [8, 16, 32, 64, 128]
+ self._num_anchors = 1
+ elif len(outputs)==15:
+ self.fmc = 5
+ self._feat_stride_fpn = [8, 16, 32, 64, 128]
+ self._num_anchors = 1
+ self.use_kps = True
+
+ def prepare(self, ctx_id, **kwargs):
+ if ctx_id<0:
+ self.session.set_providers(['CPUExecutionProvider'])
+ nms_thresh = kwargs.get('nms_thresh', None)
+ if nms_thresh is not None:
+ self.nms_thresh = nms_thresh
+ det_thresh = kwargs.get('det_thresh', None)
+ if det_thresh is not None:
+ self.det_thresh = det_thresh
+ input_size = kwargs.get('input_size', None)
+ if input_size is not None:
+ if self.input_size is not None:
+ print('warning: det_size is already set in detection model, ignore')
+ else:
+ self.input_size = input_size
+
+ def forward(self, img, threshold):
+ scores_list = []
+ bboxes_list = []
+ kpss_list = []
+ input_size = tuple(img.shape[0:2][::-1])
+ blob = cv2.dnn.blobFromImage(img, 1.0/self.input_std, input_size, (self.input_mean, self.input_mean, self.input_mean), swapRB=True)
+ net_outs = self.session.run(self.output_names, {self.input_name : blob})
+
+ input_height = blob.shape[2]
+ input_width = blob.shape[3]
+ fmc = self.fmc
+ for idx, stride in enumerate(self._feat_stride_fpn):
+ scores = net_outs[idx]
+ bbox_preds = net_outs[idx+fmc]
+ bbox_preds = bbox_preds * stride
+ if self.use_kps:
+ kps_preds = net_outs[idx+fmc*2] * stride
+ height = input_height // stride
+ width = input_width // stride
+ K = height * width
+ key = (height, width, stride)
+ if key in self.center_cache:
+ anchor_centers = self.center_cache[key]
+ else:
+ #solution-1, c style:
+ #anchor_centers = np.zeros( (height, width, 2), dtype=np.float32 )
+ #for i in range(height):
+ # anchor_centers[i, :, 1] = i
+ #for i in range(width):
+ # anchor_centers[:, i, 0] = i
+
+ #solution-2:
+ #ax = np.arange(width, dtype=np.float32)
+ #ay = np.arange(height, dtype=np.float32)
+ #xv, yv = np.meshgrid(np.arange(width), np.arange(height))
+ #anchor_centers = np.stack([xv, yv], axis=-1).astype(np.float32)
+
+ #solution-3:
+ anchor_centers = np.stack(np.mgrid[:height, :width][::-1], axis=-1).astype(np.float32)
+ #print(anchor_centers.shape)
+
+ anchor_centers = (anchor_centers * stride).reshape( (-1, 2) )
+ if self._num_anchors>1:
+ anchor_centers = np.stack([anchor_centers]*self._num_anchors, axis=1).reshape( (-1,2) )
+ if len(self.center_cache)<100:
+ self.center_cache[key] = anchor_centers
+
+ pos_inds = np.where(scores>=threshold)[0]
+ bboxes = distance2bbox(anchor_centers, bbox_preds)
+ pos_scores = scores[pos_inds]
+ pos_bboxes = bboxes[pos_inds]
+ scores_list.append(pos_scores)
+ bboxes_list.append(pos_bboxes)
+ if self.use_kps:
+ kpss = distance2kps(anchor_centers, kps_preds)
+ #kpss = kps_preds
+ kpss = kpss.reshape( (kpss.shape[0], -1, 2) )
+ pos_kpss = kpss[pos_inds]
+ kpss_list.append(pos_kpss)
+ return scores_list, bboxes_list, kpss_list
+
+ def detect(self, img, input_size = None, max_num=0, metric='default'):
+ assert input_size is not None or self.input_size is not None
+ input_size = self.input_size if input_size is None else input_size
+
+ im_ratio = float(img.shape[0]) / img.shape[1]
+ model_ratio = float(input_size[1]) / input_size[0]
+ if im_ratio>model_ratio:
+ new_height = input_size[1]
+ new_width = int(new_height / im_ratio)
+ else:
+ new_width = input_size[0]
+ new_height = int(new_width * im_ratio)
+ det_scale = float(new_height) / img.shape[0]
+ resized_img = cv2.resize(img, (new_width, new_height))
+ det_img = np.zeros( (input_size[1], input_size[0], 3), dtype=np.uint8 )
+ det_img[:new_height, :new_width, :] = resized_img
+
+ scores_list, bboxes_list, kpss_list = self.forward(det_img, self.det_thresh)
+
+ scores = np.vstack(scores_list)
+ scores_ravel = scores.ravel()
+ order = scores_ravel.argsort()[::-1]
+ bboxes = np.vstack(bboxes_list) / det_scale
+ if self.use_kps:
+ kpss = np.vstack(kpss_list) / det_scale
+ pre_det = np.hstack((bboxes, scores)).astype(np.float32, copy=False)
+ pre_det = pre_det[order, :]
+ keep = self.nms(pre_det)
+ det = pre_det[keep, :]
+ if self.use_kps:
+ kpss = kpss[order,:,:]
+ kpss = kpss[keep,:,:]
+ else:
+ kpss = None
+ if max_num > 0 and det.shape[0] > max_num:
+ area = (det[:, 2] - det[:, 0]) * (det[:, 3] -
+ det[:, 1])
+ img_center = img.shape[0] // 2, img.shape[1] // 2
+ offsets = np.vstack([
+ (det[:, 0] + det[:, 2]) / 2 - img_center[1],
+ (det[:, 1] + det[:, 3]) / 2 - img_center[0]
+ ])
+ offset_dist_squared = np.sum(np.power(offsets, 2.0), 0)
+ if metric=='max':
+ values = area
+ else:
+ values = area - offset_dist_squared * 2.0 # some extra weight on the centering
+ bindex = np.argsort(
+ values)[::-1] # some extra weight on the centering
+ bindex = bindex[0:max_num]
+ det = det[bindex, :]
+ if kpss is not None:
+ kpss = kpss[bindex, :]
+ return det, kpss
+
+ def nms(self, dets):
+ thresh = self.nms_thresh
+ x1 = dets[:, 0]
+ y1 = dets[:, 1]
+ x2 = dets[:, 2]
+ y2 = dets[:, 3]
+ scores = dets[:, 4]
+
+ areas = (x2 - x1 + 1) * (y2 - y1 + 1)
+ order = scores.argsort()[::-1]
+
+ keep = []
+ while order.size > 0:
+ i = order[0]
+ keep.append(i)
+ xx1 = np.maximum(x1[i], x1[order[1:]])
+ yy1 = np.maximum(y1[i], y1[order[1:]])
+ xx2 = np.minimum(x2[i], x2[order[1:]])
+ yy2 = np.minimum(y2[i], y2[order[1:]])
+
+ w = np.maximum(0.0, xx2 - xx1 + 1)
+ h = np.maximum(0.0, yy2 - yy1 + 1)
+ inter = w * h
+ ovr = inter / (areas[i] + areas[order[1:]] - inter)
+
+ inds = np.where(ovr <= thresh)[0]
+ order = order[inds + 1]
+
+ return keep
+
+def get_retinaface(name, download=False, root='~/.insightface/models', **kwargs):
+ if not download:
+ assert os.path.exists(name)
+ return RetinaFace(name)
+ else:
+ from .model_store import get_model_file
+ _file = get_model_file("retinaface_%s" % name, root=root)
+ return retinaface(_file)
+
+
diff --git a/difpoint/src/utils/dependencies/insightface/model_zoo/scrfd.py b/difpoint/src/utils/dependencies/insightface/model_zoo/scrfd.py
new file mode 100644
index 0000000000000000000000000000000000000000..674db4bba761157592dfb95c5d1638da1099f89c
--- /dev/null
+++ b/difpoint/src/utils/dependencies/insightface/model_zoo/scrfd.py
@@ -0,0 +1,348 @@
+# -*- coding: utf-8 -*-
+# @Organization : insightface.ai
+# @Author : Jia Guo
+# @Time : 2021-05-04
+# @Function :
+
+from __future__ import division
+import datetime
+import numpy as np
+import onnx
+import onnxruntime
+import os
+import os.path as osp
+import cv2
+import sys
+
+def softmax(z):
+ assert len(z.shape) == 2
+ s = np.max(z, axis=1)
+ s = s[:, np.newaxis] # necessary step to do broadcasting
+ e_x = np.exp(z - s)
+ div = np.sum(e_x, axis=1)
+ div = div[:, np.newaxis] # dito
+ return e_x / div
+
+def distance2bbox(points, distance, max_shape=None):
+ """Decode distance prediction to bounding box.
+
+ Args:
+ points (Tensor): Shape (n, 2), [x, y].
+ distance (Tensor): Distance from the given point to 4
+ boundaries (left, top, right, bottom).
+ max_shape (tuple): Shape of the image.
+
+ Returns:
+ Tensor: Decoded bboxes.
+ """
+ x1 = points[:, 0] - distance[:, 0]
+ y1 = points[:, 1] - distance[:, 1]
+ x2 = points[:, 0] + distance[:, 2]
+ y2 = points[:, 1] + distance[:, 3]
+ if max_shape is not None:
+ x1 = x1.clamp(min=0, max=max_shape[1])
+ y1 = y1.clamp(min=0, max=max_shape[0])
+ x2 = x2.clamp(min=0, max=max_shape[1])
+ y2 = y2.clamp(min=0, max=max_shape[0])
+ return np.stack([x1, y1, x2, y2], axis=-1)
+
+def distance2kps(points, distance, max_shape=None):
+ """Decode distance prediction to bounding box.
+
+ Args:
+ points (Tensor): Shape (n, 2), [x, y].
+ distance (Tensor): Distance from the given point to 4
+ boundaries (left, top, right, bottom).
+ max_shape (tuple): Shape of the image.
+
+ Returns:
+ Tensor: Decoded bboxes.
+ """
+ preds = []
+ for i in range(0, distance.shape[1], 2):
+ px = points[:, i%2] + distance[:, i]
+ py = points[:, i%2+1] + distance[:, i+1]
+ if max_shape is not None:
+ px = px.clamp(min=0, max=max_shape[1])
+ py = py.clamp(min=0, max=max_shape[0])
+ preds.append(px)
+ preds.append(py)
+ return np.stack(preds, axis=-1)
+
+class SCRFD:
+ def __init__(self, model_file=None, session=None):
+ import onnxruntime
+ self.model_file = model_file
+ self.session = session
+ self.taskname = 'detection'
+ self.batched = False
+ if self.session is None:
+ assert self.model_file is not None
+ assert osp.exists(self.model_file)
+ self.session = onnxruntime.InferenceSession(self.model_file, None)
+ self.center_cache = {}
+ self.nms_thresh = 0.4
+ self.det_thresh = 0.5
+ self._init_vars()
+
+ def _init_vars(self):
+ input_cfg = self.session.get_inputs()[0]
+ input_shape = input_cfg.shape
+ #print(input_shape)
+ if isinstance(input_shape[2], str):
+ self.input_size = None
+ else:
+ self.input_size = tuple(input_shape[2:4][::-1])
+ #print('image_size:', self.image_size)
+ input_name = input_cfg.name
+ self.input_shape = input_shape
+ outputs = self.session.get_outputs()
+ if len(outputs[0].shape) == 3:
+ self.batched = True
+ output_names = []
+ for o in outputs:
+ output_names.append(o.name)
+ self.input_name = input_name
+ self.output_names = output_names
+ self.input_mean = 127.5
+ self.input_std = 128.0
+ #print(self.output_names)
+ #assert len(outputs)==10 or len(outputs)==15
+ self.use_kps = False
+ self._anchor_ratio = 1.0
+ self._num_anchors = 1
+ if len(outputs)==6:
+ self.fmc = 3
+ self._feat_stride_fpn = [8, 16, 32]
+ self._num_anchors = 2
+ elif len(outputs)==9:
+ self.fmc = 3
+ self._feat_stride_fpn = [8, 16, 32]
+ self._num_anchors = 2
+ self.use_kps = True
+ elif len(outputs)==10:
+ self.fmc = 5
+ self._feat_stride_fpn = [8, 16, 32, 64, 128]
+ self._num_anchors = 1
+ elif len(outputs)==15:
+ self.fmc = 5
+ self._feat_stride_fpn = [8, 16, 32, 64, 128]
+ self._num_anchors = 1
+ self.use_kps = True
+
+ def prepare(self, ctx_id, **kwargs):
+ if ctx_id<0:
+ self.session.set_providers(['CPUExecutionProvider'])
+ nms_thresh = kwargs.get('nms_thresh', None)
+ if nms_thresh is not None:
+ self.nms_thresh = nms_thresh
+ det_thresh = kwargs.get('det_thresh', None)
+ if det_thresh is not None:
+ self.det_thresh = det_thresh
+ input_size = kwargs.get('input_size', None)
+ if input_size is not None:
+ if self.input_size is not None:
+ print('warning: det_size is already set in scrfd model, ignore')
+ else:
+ self.input_size = input_size
+
+ def forward(self, img, threshold):
+ scores_list = []
+ bboxes_list = []
+ kpss_list = []
+ input_size = tuple(img.shape[0:2][::-1])
+ blob = cv2.dnn.blobFromImage(img, 1.0/self.input_std, input_size, (self.input_mean, self.input_mean, self.input_mean), swapRB=True)
+ net_outs = self.session.run(self.output_names, {self.input_name : blob})
+
+ input_height = blob.shape[2]
+ input_width = blob.shape[3]
+ fmc = self.fmc
+ for idx, stride in enumerate(self._feat_stride_fpn):
+ # If model support batch dim, take first output
+ if self.batched:
+ scores = net_outs[idx][0]
+ bbox_preds = net_outs[idx + fmc][0]
+ bbox_preds = bbox_preds * stride
+ if self.use_kps:
+ kps_preds = net_outs[idx + fmc * 2][0] * stride
+ # If model doesn't support batching take output as is
+ else:
+ scores = net_outs[idx]
+ bbox_preds = net_outs[idx + fmc]
+ bbox_preds = bbox_preds * stride
+ if self.use_kps:
+ kps_preds = net_outs[idx + fmc * 2] * stride
+
+ height = input_height // stride
+ width = input_width // stride
+ K = height * width
+ key = (height, width, stride)
+ if key in self.center_cache:
+ anchor_centers = self.center_cache[key]
+ else:
+ #solution-1, c style:
+ #anchor_centers = np.zeros( (height, width, 2), dtype=np.float32 )
+ #for i in range(height):
+ # anchor_centers[i, :, 1] = i
+ #for i in range(width):
+ # anchor_centers[:, i, 0] = i
+
+ #solution-2:
+ #ax = np.arange(width, dtype=np.float32)
+ #ay = np.arange(height, dtype=np.float32)
+ #xv, yv = np.meshgrid(np.arange(width), np.arange(height))
+ #anchor_centers = np.stack([xv, yv], axis=-1).astype(np.float32)
+
+ #solution-3:
+ anchor_centers = np.stack(np.mgrid[:height, :width][::-1], axis=-1).astype(np.float32)
+ #print(anchor_centers.shape)
+
+ anchor_centers = (anchor_centers * stride).reshape( (-1, 2) )
+ if self._num_anchors>1:
+ anchor_centers = np.stack([anchor_centers]*self._num_anchors, axis=1).reshape( (-1,2) )
+ if len(self.center_cache)<100:
+ self.center_cache[key] = anchor_centers
+
+ pos_inds = np.where(scores>=threshold)[0]
+ bboxes = distance2bbox(anchor_centers, bbox_preds)
+ pos_scores = scores[pos_inds]
+ pos_bboxes = bboxes[pos_inds]
+ scores_list.append(pos_scores)
+ bboxes_list.append(pos_bboxes)
+ if self.use_kps:
+ kpss = distance2kps(anchor_centers, kps_preds)
+ #kpss = kps_preds
+ kpss = kpss.reshape( (kpss.shape[0], -1, 2) )
+ pos_kpss = kpss[pos_inds]
+ kpss_list.append(pos_kpss)
+ return scores_list, bboxes_list, kpss_list
+
+ def detect(self, img, input_size = None, max_num=0, metric='default'):
+ assert input_size is not None or self.input_size is not None
+ input_size = self.input_size if input_size is None else input_size
+
+ im_ratio = float(img.shape[0]) / img.shape[1]
+ model_ratio = float(input_size[1]) / input_size[0]
+ if im_ratio>model_ratio:
+ new_height = input_size[1]
+ new_width = int(new_height / im_ratio)
+ else:
+ new_width = input_size[0]
+ new_height = int(new_width * im_ratio)
+ det_scale = float(new_height) / img.shape[0]
+ resized_img = cv2.resize(img, (new_width, new_height))
+ det_img = np.zeros( (input_size[1], input_size[0], 3), dtype=np.uint8 )
+ det_img[:new_height, :new_width, :] = resized_img
+
+ scores_list, bboxes_list, kpss_list = self.forward(det_img, self.det_thresh)
+
+ scores = np.vstack(scores_list)
+ scores_ravel = scores.ravel()
+ order = scores_ravel.argsort()[::-1]
+ bboxes = np.vstack(bboxes_list) / det_scale
+ if self.use_kps:
+ kpss = np.vstack(kpss_list) / det_scale
+ pre_det = np.hstack((bboxes, scores)).astype(np.float32, copy=False)
+ pre_det = pre_det[order, :]
+ keep = self.nms(pre_det)
+ det = pre_det[keep, :]
+ if self.use_kps:
+ kpss = kpss[order,:,:]
+ kpss = kpss[keep,:,:]
+ else:
+ kpss = None
+ if max_num > 0 and det.shape[0] > max_num:
+ area = (det[:, 2] - det[:, 0]) * (det[:, 3] -
+ det[:, 1])
+ img_center = img.shape[0] // 2, img.shape[1] // 2
+ offsets = np.vstack([
+ (det[:, 0] + det[:, 2]) / 2 - img_center[1],
+ (det[:, 1] + det[:, 3]) / 2 - img_center[0]
+ ])
+ offset_dist_squared = np.sum(np.power(offsets, 2.0), 0)
+ if metric=='max':
+ values = area
+ else:
+ values = area - offset_dist_squared * 2.0 # some extra weight on the centering
+ bindex = np.argsort(
+ values)[::-1] # some extra weight on the centering
+ bindex = bindex[0:max_num]
+ det = det[bindex, :]
+ if kpss is not None:
+ kpss = kpss[bindex, :]
+ return det, kpss
+
+ def nms(self, dets):
+ thresh = self.nms_thresh
+ x1 = dets[:, 0]
+ y1 = dets[:, 1]
+ x2 = dets[:, 2]
+ y2 = dets[:, 3]
+ scores = dets[:, 4]
+
+ areas = (x2 - x1 + 1) * (y2 - y1 + 1)
+ order = scores.argsort()[::-1]
+
+ keep = []
+ while order.size > 0:
+ i = order[0]
+ keep.append(i)
+ xx1 = np.maximum(x1[i], x1[order[1:]])
+ yy1 = np.maximum(y1[i], y1[order[1:]])
+ xx2 = np.minimum(x2[i], x2[order[1:]])
+ yy2 = np.minimum(y2[i], y2[order[1:]])
+
+ w = np.maximum(0.0, xx2 - xx1 + 1)
+ h = np.maximum(0.0, yy2 - yy1 + 1)
+ inter = w * h
+ ovr = inter / (areas[i] + areas[order[1:]] - inter)
+
+ inds = np.where(ovr <= thresh)[0]
+ order = order[inds + 1]
+
+ return keep
+
+def get_scrfd(name, download=False, root='~/.insightface/models', **kwargs):
+ if not download:
+ assert os.path.exists(name)
+ return SCRFD(name)
+ else:
+ from .model_store import get_model_file
+ _file = get_model_file("scrfd_%s" % name, root=root)
+ return SCRFD(_file)
+
+
+def scrfd_2p5gkps(**kwargs):
+ return get_scrfd("2p5gkps", download=True, **kwargs)
+
+
+if __name__ == '__main__':
+ import glob
+ detector = SCRFD(model_file='./det.onnx')
+ detector.prepare(-1)
+ img_paths = ['tests/data/t1.jpg']
+ for img_path in img_paths:
+ img = cv2.imread(img_path)
+
+ for _ in range(1):
+ ta = datetime.datetime.now()
+ #bboxes, kpss = detector.detect(img, 0.5, input_size = (640, 640))
+ bboxes, kpss = detector.detect(img, 0.5)
+ tb = datetime.datetime.now()
+ print('all cost:', (tb-ta).total_seconds()*1000)
+ print(img_path, bboxes.shape)
+ if kpss is not None:
+ print(kpss.shape)
+ for i in range(bboxes.shape[0]):
+ bbox = bboxes[i]
+ x1,y1,x2,y2,score = bbox.astype(np.int)
+ cv2.rectangle(img, (x1,y1) , (x2,y2) , (255,0,0) , 2)
+ if kpss is not None:
+ kps = kpss[i]
+ for kp in kps:
+ kp = kp.astype(np.int)
+ cv2.circle(img, tuple(kp) , 1, (0,0,255) , 2)
+ filename = img_path.split('/')[-1]
+ print('output:', filename)
+ cv2.imwrite('./outputs/%s'%filename, img)
+
diff --git a/difpoint/src/utils/dependencies/insightface/utils/__init__.py b/difpoint/src/utils/dependencies/insightface/utils/__init__.py
new file mode 100644
index 0000000000000000000000000000000000000000..6960431b1bd6db38890e391c4c94dd2182f2e1fd
--- /dev/null
+++ b/difpoint/src/utils/dependencies/insightface/utils/__init__.py
@@ -0,0 +1,6 @@
+from __future__ import absolute_import
+
+from .storage import download, ensure_available, download_onnx
+from .filesystem import get_model_dir
+from .filesystem import makedirs, try_import_dali
+from .constant import *
diff --git a/difpoint/src/utils/dependencies/insightface/utils/__pycache__/__init__.cpython-310.pyc b/difpoint/src/utils/dependencies/insightface/utils/__pycache__/__init__.cpython-310.pyc
new file mode 100644
index 0000000000000000000000000000000000000000..24e74977f1ae02a04236842e1f9cf0f141a2d045
Binary files /dev/null and b/difpoint/src/utils/dependencies/insightface/utils/__pycache__/__init__.cpython-310.pyc differ
diff --git a/difpoint/src/utils/dependencies/insightface/utils/__pycache__/constant.cpython-310.pyc b/difpoint/src/utils/dependencies/insightface/utils/__pycache__/constant.cpython-310.pyc
new file mode 100644
index 0000000000000000000000000000000000000000..c096189f16e1ce85a5fa8c699adbd96f38f14b2c
Binary files /dev/null and b/difpoint/src/utils/dependencies/insightface/utils/__pycache__/constant.cpython-310.pyc differ
diff --git a/difpoint/src/utils/dependencies/insightface/utils/__pycache__/download.cpython-310.pyc b/difpoint/src/utils/dependencies/insightface/utils/__pycache__/download.cpython-310.pyc
new file mode 100644
index 0000000000000000000000000000000000000000..9b9577a0427fef59d8745c6626ffa75dc1e0a45f
Binary files /dev/null and b/difpoint/src/utils/dependencies/insightface/utils/__pycache__/download.cpython-310.pyc differ
diff --git a/difpoint/src/utils/dependencies/insightface/utils/__pycache__/face_align.cpython-310.pyc b/difpoint/src/utils/dependencies/insightface/utils/__pycache__/face_align.cpython-310.pyc
new file mode 100644
index 0000000000000000000000000000000000000000..01897963f47b3d100c3b1716c1d1154acdcd2f9f
Binary files /dev/null and b/difpoint/src/utils/dependencies/insightface/utils/__pycache__/face_align.cpython-310.pyc differ
diff --git a/difpoint/src/utils/dependencies/insightface/utils/__pycache__/filesystem.cpython-310.pyc b/difpoint/src/utils/dependencies/insightface/utils/__pycache__/filesystem.cpython-310.pyc
new file mode 100644
index 0000000000000000000000000000000000000000..1b4e0a18af8ef9e4ea9a66034cb4d4f0fc4cafed
Binary files /dev/null and b/difpoint/src/utils/dependencies/insightface/utils/__pycache__/filesystem.cpython-310.pyc differ
diff --git a/difpoint/src/utils/dependencies/insightface/utils/__pycache__/storage.cpython-310.pyc b/difpoint/src/utils/dependencies/insightface/utils/__pycache__/storage.cpython-310.pyc
new file mode 100644
index 0000000000000000000000000000000000000000..144d3bdc89463754c55fa6224b633b14cba17c82
Binary files /dev/null and b/difpoint/src/utils/dependencies/insightface/utils/__pycache__/storage.cpython-310.pyc differ
diff --git a/difpoint/src/utils/dependencies/insightface/utils/__pycache__/transform.cpython-310.pyc b/difpoint/src/utils/dependencies/insightface/utils/__pycache__/transform.cpython-310.pyc
new file mode 100644
index 0000000000000000000000000000000000000000..50f82b4d9b9a97867f95f8c832a99f8d6d088966
Binary files /dev/null and b/difpoint/src/utils/dependencies/insightface/utils/__pycache__/transform.cpython-310.pyc differ
diff --git a/difpoint/src/utils/dependencies/insightface/utils/constant.py b/difpoint/src/utils/dependencies/insightface/utils/constant.py
new file mode 100644
index 0000000000000000000000000000000000000000..8860ff077ae7227235591edfc84c0cdc227a6432
--- /dev/null
+++ b/difpoint/src/utils/dependencies/insightface/utils/constant.py
@@ -0,0 +1,3 @@
+
+DEFAULT_MP_NAME = 'buffalo_l'
+
diff --git a/difpoint/src/utils/dependencies/insightface/utils/download.py b/difpoint/src/utils/dependencies/insightface/utils/download.py
new file mode 100644
index 0000000000000000000000000000000000000000..5cda84dede45b81dcd99161d87792b6c409fa279
--- /dev/null
+++ b/difpoint/src/utils/dependencies/insightface/utils/download.py
@@ -0,0 +1,95 @@
+"""
+This code file mainly comes from https://github.com/dmlc/gluon-cv/blob/master/gluoncv/utils/download.py
+"""
+import os
+import hashlib
+import requests
+from tqdm import tqdm
+
+
+def check_sha1(filename, sha1_hash):
+ """Check whether the sha1 hash of the file content matches the expected hash.
+ Parameters
+ ----------
+ filename : str
+ Path to the file.
+ sha1_hash : str
+ Expected sha1 hash in hexadecimal digits.
+ Returns
+ -------
+ bool
+ Whether the file content matches the expected hash.
+ """
+ sha1 = hashlib.sha1()
+ with open(filename, 'rb') as f:
+ while True:
+ data = f.read(1048576)
+ if not data:
+ break
+ sha1.update(data)
+
+ sha1_file = sha1.hexdigest()
+ l = min(len(sha1_file), len(sha1_hash))
+ return sha1.hexdigest()[0:l] == sha1_hash[0:l]
+
+
+def download_file(url, path=None, overwrite=False, sha1_hash=None):
+ """Download an given URL
+ Parameters
+ ----------
+ url : str
+ URL to download
+ path : str, optional
+ Destination path to store downloaded file. By default stores to the
+ current directory with same name as in url.
+ overwrite : bool, optional
+ Whether to overwrite destination file if already exists.
+ sha1_hash : str, optional
+ Expected sha1 hash in hexadecimal digits. Will ignore existing file when hash is specified
+ but doesn't match.
+ Returns
+ -------
+ str
+ The file path of the downloaded file.
+ """
+ if path is None:
+ fname = url.split('/')[-1]
+ else:
+ path = os.path.expanduser(path)
+ if os.path.isdir(path):
+ fname = os.path.join(path, url.split('/')[-1])
+ else:
+ fname = path
+
+ if overwrite or not os.path.exists(fname) or (
+ sha1_hash and not check_sha1(fname, sha1_hash)):
+ dirname = os.path.dirname(os.path.abspath(os.path.expanduser(fname)))
+ if not os.path.exists(dirname):
+ os.makedirs(dirname)
+
+ print('Downloading %s from %s...' % (fname, url))
+ r = requests.get(url, stream=True)
+ if r.status_code != 200:
+ raise RuntimeError("Failed downloading url %s" % url)
+ total_length = r.headers.get('content-length')
+ with open(fname, 'wb') as f:
+ if total_length is None: # no content length header
+ for chunk in r.iter_content(chunk_size=1024):
+ if chunk: # filter out keep-alive new chunks
+ f.write(chunk)
+ else:
+ total_length = int(total_length)
+ for chunk in tqdm(r.iter_content(chunk_size=1024),
+ total=int(total_length / 1024. + 0.5),
+ unit='KB',
+ unit_scale=False,
+ dynamic_ncols=True):
+ f.write(chunk)
+
+ if sha1_hash and not check_sha1(fname, sha1_hash):
+ raise UserWarning('File {} is downloaded but the content hash does not match. ' \
+ 'The repo may be outdated or download may be incomplete. ' \
+ 'If the "repo_url" is overridden, consider switching to ' \
+ 'the default repo.'.format(fname))
+
+ return fname
diff --git a/difpoint/src/utils/dependencies/insightface/utils/face_align.py b/difpoint/src/utils/dependencies/insightface/utils/face_align.py
new file mode 100644
index 0000000000000000000000000000000000000000..226628b39cf743947df230feffbb97bf5c585e1d
--- /dev/null
+++ b/difpoint/src/utils/dependencies/insightface/utils/face_align.py
@@ -0,0 +1,103 @@
+import cv2
+import numpy as np
+from skimage import transform as trans
+
+
+arcface_dst = np.array(
+ [[38.2946, 51.6963], [73.5318, 51.5014], [56.0252, 71.7366],
+ [41.5493, 92.3655], [70.7299, 92.2041]],
+ dtype=np.float32)
+
+def estimate_norm(lmk, image_size=112,mode='arcface'):
+ assert lmk.shape == (5, 2)
+ assert image_size%112==0 or image_size%128==0
+ if image_size%112==0:
+ ratio = float(image_size)/112.0
+ diff_x = 0
+ else:
+ ratio = float(image_size)/128.0
+ diff_x = 8.0*ratio
+ dst = arcface_dst * ratio
+ dst[:,0] += diff_x
+ tform = trans.SimilarityTransform()
+ tform.estimate(lmk, dst)
+ M = tform.params[0:2, :]
+ return M
+
+def norm_crop(img, landmark, image_size=112, mode='arcface'):
+ M = estimate_norm(landmark, image_size, mode)
+ warped = cv2.warpAffine(img, M, (image_size, image_size), borderValue=0.0)
+ return warped
+
+def norm_crop2(img, landmark, image_size=112, mode='arcface'):
+ M = estimate_norm(landmark, image_size, mode)
+ warped = cv2.warpAffine(img, M, (image_size, image_size), borderValue=0.0)
+ return warped, M
+
+def square_crop(im, S):
+ if im.shape[0] > im.shape[1]:
+ height = S
+ width = int(float(im.shape[1]) / im.shape[0] * S)
+ scale = float(S) / im.shape[0]
+ else:
+ width = S
+ height = int(float(im.shape[0]) / im.shape[1] * S)
+ scale = float(S) / im.shape[1]
+ resized_im = cv2.resize(im, (width, height))
+ det_im = np.zeros((S, S, 3), dtype=np.uint8)
+ det_im[:resized_im.shape[0], :resized_im.shape[1], :] = resized_im
+ return det_im, scale
+
+
+def transform(data, center, output_size, scale, rotation):
+ scale_ratio = scale
+ rot = float(rotation) * np.pi / 180.0
+ #translation = (output_size/2-center[0]*scale_ratio, output_size/2-center[1]*scale_ratio)
+ t1 = trans.SimilarityTransform(scale=scale_ratio)
+ cx = center[0] * scale_ratio
+ cy = center[1] * scale_ratio
+ t2 = trans.SimilarityTransform(translation=(-1 * cx, -1 * cy))
+ t3 = trans.SimilarityTransform(rotation=rot)
+ t4 = trans.SimilarityTransform(translation=(output_size / 2,
+ output_size / 2))
+ t = t1 + t2 + t3 + t4
+ M = t.params[0:2]
+ cropped = cv2.warpAffine(data,
+ M, (output_size, output_size),
+ borderValue=0.0)
+ return cropped, M
+
+
+def trans_points2d(pts, M):
+ new_pts = np.zeros(shape=pts.shape, dtype=np.float32)
+ for i in range(pts.shape[0]):
+ pt = pts[i]
+ new_pt = np.array([pt[0], pt[1], 1.], dtype=np.float32)
+ new_pt = np.dot(M, new_pt)
+ #print('new_pt', new_pt.shape, new_pt)
+ new_pts[i] = new_pt[0:2]
+
+ return new_pts
+
+
+def trans_points3d(pts, M):
+ scale = np.sqrt(M[0][0] * M[0][0] + M[0][1] * M[0][1])
+ #print(scale)
+ new_pts = np.zeros(shape=pts.shape, dtype=np.float32)
+ for i in range(pts.shape[0]):
+ pt = pts[i]
+ new_pt = np.array([pt[0], pt[1], 1.], dtype=np.float32)
+ new_pt = np.dot(M, new_pt)
+ #print('new_pt', new_pt.shape, new_pt)
+ new_pts[i][0:2] = new_pt[0:2]
+ new_pts[i][2] = pts[i][2] * scale
+
+ return new_pts
+
+
+def trans_points(pts, M):
+ if pts.shape[1] == 2:
+ return trans_points2d(pts, M)
+ else:
+ return trans_points3d(pts, M)
+
diff --git a/difpoint/src/utils/dependencies/insightface/utils/filesystem.py b/difpoint/src/utils/dependencies/insightface/utils/filesystem.py
new file mode 100644
index 0000000000000000000000000000000000000000..01e3851975bdcbbf7f5eeb7e68e70a36dc040535
--- /dev/null
+++ b/difpoint/src/utils/dependencies/insightface/utils/filesystem.py
@@ -0,0 +1,157 @@
+"""
+This code file mainly comes from https://github.com/dmlc/gluon-cv/blob/master/gluoncv/utils/filesystem.py
+"""
+import os
+import os.path as osp
+import errno
+
+
+def get_model_dir(name, root='~/.insightface'):
+ root = os.path.expanduser(root)
+ model_dir = osp.join(root, 'models', name)
+ return model_dir
+
+def makedirs(path):
+ """Create directory recursively if not exists.
+ Similar to `makedir -p`, you can skip checking existence before this function.
+
+ Parameters
+ ----------
+ path : str
+ Path of the desired dir
+ """
+ try:
+ os.makedirs(path)
+ except OSError as exc:
+ if exc.errno != errno.EEXIST:
+ raise
+
+
+def try_import(package, message=None):
+ """Try import specified package, with custom message support.
+
+ Parameters
+ ----------
+ package : str
+ The name of the targeting package.
+ message : str, default is None
+ If not None, this function will raise customized error message when import error is found.
+
+
+ Returns
+ -------
+ module if found, raise ImportError otherwise
+
+ """
+ try:
+ return __import__(package)
+ except ImportError as e:
+ if not message:
+ raise e
+ raise ImportError(message)
+
+
+def try_import_cv2():
+ """Try import cv2 at runtime.
+
+ Returns
+ -------
+ cv2 module if found. Raise ImportError otherwise
+
+ """
+ msg = "cv2 is required, you can install by package manager, e.g. 'apt-get', \
+ or `pip install opencv-python --user` (note that this is unofficial PYPI package)."
+
+ return try_import('cv2', msg)
+
+
+def try_import_mmcv():
+ """Try import mmcv at runtime.
+
+ Returns
+ -------
+ mmcv module if found. Raise ImportError otherwise
+
+ """
+ msg = "mmcv is required, you can install by first `pip install Cython --user` \
+ and then `pip install mmcv --user` (note that this is unofficial PYPI package)."
+
+ return try_import('mmcv', msg)
+
+
+def try_import_rarfile():
+ """Try import rarfile at runtime.
+
+ Returns
+ -------
+ rarfile module if found. Raise ImportError otherwise
+
+ """
+ msg = "rarfile is required, you can install by first `sudo apt-get install unrar` \
+ and then `pip install rarfile --user` (note that this is unofficial PYPI package)."
+
+ return try_import('rarfile', msg)
+
+
+def import_try_install(package, extern_url=None):
+ """Try import the specified package.
+ If the package not installed, try use pip to install and import if success.
+
+ Parameters
+ ----------
+ package : str
+ The name of the package trying to import.
+ extern_url : str or None, optional
+ The external url if package is not hosted on PyPI.
+ For example, you can install a package using:
+ "pip install git+http://github.com/user/repo/tarball/master/egginfo=xxx".
+ In this case, you can pass the url to the extern_url.
+
+ Returns
+ -------
+
+ The imported python module.
+
+ """
+ try:
+ return __import__(package)
+ except ImportError:
+ try:
+ from pip import main as pipmain
+ except ImportError:
+ from pip._internal import main as pipmain
+
+ # trying to install package
+ url = package if extern_url is None else extern_url
+ pipmain(['install', '--user',
+ url]) # will raise SystemExit Error if fails
+
+ # trying to load again
+ try:
+ return __import__(package)
+ except ImportError:
+ import sys
+ import site
+ user_site = site.getusersitepackages()
+ if user_site not in sys.path:
+ sys.path.append(user_site)
+ return __import__(package)
+ return __import__(package)
+
+
+def try_import_dali():
+ """Try import NVIDIA DALI at runtime.
+ """
+ try:
+ dali = __import__('nvidia.dali', fromlist=['pipeline', 'ops', 'types'])
+ dali.Pipeline = dali.pipeline.Pipeline
+ except ImportError:
+
+ class dali:
+ class Pipeline:
+ def __init__(self):
+ raise NotImplementedError(
+ "DALI not found, please check if you installed it correctly."
+ )
+
+ return dali
diff --git a/difpoint/src/utils/dependencies/insightface/utils/storage.py b/difpoint/src/utils/dependencies/insightface/utils/storage.py
new file mode 100644
index 0000000000000000000000000000000000000000..5bf37e2d17b28dee2a8839484778815f87fc4a9c
--- /dev/null
+++ b/difpoint/src/utils/dependencies/insightface/utils/storage.py
@@ -0,0 +1,52 @@
+
+import os
+import os.path as osp
+import zipfile
+from .download import download_file
+
+BASE_REPO_URL = 'https://github.com/deepinsight/insightface/releases/download/v0.7'
+
+def download(sub_dir, name, force=False, root='~/.insightface'):
+ _root = os.path.expanduser(root)
+ dir_path = os.path.join(_root, sub_dir, name)
+ if osp.exists(dir_path) and not force:
+ return dir_path
+ print('download_path:', dir_path)
+ zip_file_path = os.path.join(_root, sub_dir, name + '.zip')
+ model_url = "%s/%s.zip"%(BASE_REPO_URL, name)
+ download_file(model_url,
+ path=zip_file_path,
+ overwrite=True)
+ if not os.path.exists(dir_path):
+ os.makedirs(dir_path)
+ with zipfile.ZipFile(zip_file_path) as zf:
+ zf.extractall(dir_path)
+ #os.remove(zip_file_path)
+ return dir_path
+
+def ensure_available(sub_dir, name, root='~/.insightface'):
+ return download(sub_dir, name, force=False, root=root)
+
+def download_onnx(sub_dir, model_file, force=False, root='~/.insightface', download_zip=False):
+ _root = os.path.expanduser(root)
+ model_root = osp.join(_root, sub_dir)
+ new_model_file = osp.join(model_root, model_file)
+ if osp.exists(new_model_file) and not force:
+ return new_model_file
+ if not osp.exists(model_root):
+ os.makedirs(model_root)
+ print('download_path:', new_model_file)
+ if not download_zip:
+ model_url = "%s/%s"%(BASE_REPO_URL, model_file)
+ download_file(model_url,
+ path=new_model_file,
+ overwrite=True)
+ else:
+ model_url = "%s/%s.zip"%(BASE_REPO_URL, model_file)
+ zip_file_path = new_model_file+".zip"
+ download_file(model_url,
+ path=zip_file_path,
+ overwrite=True)
+ with zipfile.ZipFile(zip_file_path) as zf:
+ zf.extractall(model_root)
+ return new_model_file
diff --git a/difpoint/src/utils/dependencies/insightface/utils/transform.py b/difpoint/src/utils/dependencies/insightface/utils/transform.py
new file mode 100644
index 0000000000000000000000000000000000000000..06531d257b694211a0b9a09c9d741b9b2ff53bfe
--- /dev/null
+++ b/difpoint/src/utils/dependencies/insightface/utils/transform.py
@@ -0,0 +1,116 @@
+import cv2
+import math
+import numpy as np
+from skimage import transform as trans
+
+
+def transform(data, center, output_size, scale, rotation):
+ scale_ratio = scale
+ rot = float(rotation) * np.pi / 180.0
+ #translation = (output_size/2-center[0]*scale_ratio, output_size/2-center[1]*scale_ratio)
+ t1 = trans.SimilarityTransform(scale=scale_ratio)
+ cx = center[0] * scale_ratio
+ cy = center[1] * scale_ratio
+ t2 = trans.SimilarityTransform(translation=(-1 * cx, -1 * cy))
+ t3 = trans.SimilarityTransform(rotation=rot)
+ t4 = trans.SimilarityTransform(translation=(output_size / 2,
+ output_size / 2))
+ t = t1 + t2 + t3 + t4
+ M = t.params[0:2]
+ cropped = cv2.warpAffine(data,
+ M, (output_size, output_size),
+ borderValue=0.0)
+ return cropped, M
+
+
+def trans_points2d(pts, M):
+ new_pts = np.zeros(shape=pts.shape, dtype=np.float32)
+ for i in range(pts.shape[0]):
+ pt = pts[i]
+ new_pt = np.array([pt[0], pt[1], 1.], dtype=np.float32)
+ new_pt = np.dot(M, new_pt)
+ #print('new_pt', new_pt.shape, new_pt)
+ new_pts[i] = new_pt[0:2]
+
+ return new_pts
+
+
+def trans_points3d(pts, M):
+ scale = np.sqrt(M[0][0] * M[0][0] + M[0][1] * M[0][1])
+ #print(scale)
+ new_pts = np.zeros(shape=pts.shape, dtype=np.float32)
+ for i in range(pts.shape[0]):
+ pt = pts[i]
+ new_pt = np.array([pt[0], pt[1], 1.], dtype=np.float32)
+ new_pt = np.dot(M, new_pt)
+ #print('new_pt', new_pt.shape, new_pt)
+ new_pts[i][0:2] = new_pt[0:2]
+ new_pts[i][2] = pts[i][2] * scale
+
+ return new_pts
+
+
+def trans_points(pts, M):
+ if pts.shape[1] == 2:
+ return trans_points2d(pts, M)
+ else:
+ return trans_points3d(pts, M)
+
+def estimate_affine_matrix_3d23d(X, Y):
+ ''' Using least-squares solution
+ Args:
+ X: [n, 3]. 3d points(fixed)
+ Y: [n, 3]. corresponding 3d points(moving). Y = PX
+ Returns:
+ P_Affine: (3, 4). Affine camera matrix (the third row is [0, 0, 0, 1]).
+ '''
+ X_homo = np.hstack((X, np.ones([X.shape[0],1]))) #n x 4
+ P = np.linalg.lstsq(X_homo, Y)[0].T # Affine matrix. 3 x 4
+ return P
+
+def P2sRt(P):
+ ''' decompositing camera matrix P
+ Args:
+ P: (3, 4). Affine Camera Matrix.
+ Returns:
+ s: scale factor.
+ R: (3, 3). rotation matrix.
+ t: (3,). translation.
+ '''
+ t = P[:, 3]
+ R1 = P[0:1, :3]
+ R2 = P[1:2, :3]
+ s = (np.linalg.norm(R1) + np.linalg.norm(R2))/2.0
+ r1 = R1/np.linalg.norm(R1)
+ r2 = R2/np.linalg.norm(R2)
+ r3 = np.cross(r1, r2)
+
+ R = np.concatenate((r1, r2, r3), 0)
+ return s, R, t
+
+def matrix2angle(R):
+ ''' get three Euler angles from Rotation Matrix
+ Args:
+ R: (3,3). rotation matrix
+ Returns:
+ x: pitch
+ y: yaw
+ z: roll
+ '''
+ sy = math.sqrt(R[0,0] * R[0,0] + R[1,0] * R[1,0])
+
+ singular = sy < 1e-6
+
+ if not singular :
+ x = math.atan2(R[2,1] , R[2,2])
+ y = math.atan2(-R[2,0], sy)
+ z = math.atan2(R[1,0], R[0,0])
+ else :
+ x = math.atan2(-R[1,2], R[1,1])
+ y = math.atan2(-R[2,0], sy)
+ z = 0
+
+ # rx, ry, rz = np.rad2deg(x), np.rad2deg(y), np.rad2deg(z)
+ rx, ry, rz = x*180/np.pi, y*180/np.pi, z*180/np.pi
+ return rx, ry, rz
+
diff --git a/difpoint/src/utils/face_align.py b/difpoint/src/utils/face_align.py
new file mode 100644
index 0000000000000000000000000000000000000000..88aecf72dce29e777486c42ae677dc1781292cc5
--- /dev/null
+++ b/difpoint/src/utils/face_align.py
@@ -0,0 +1,105 @@
+import cv2
+import numpy as np
+from skimage import transform as trans
+
+arcface_dst = np.array(
+ [[38.2946, 51.6963], [73.5318, 51.5014], [56.0252, 71.7366],
+ [41.5493, 92.3655], [70.7299, 92.2041]],
+ dtype=np.float32)
+
+
+def estimate_norm(lmk, image_size=112, mode='arcface'):
+ assert lmk.shape == (5, 2)
+ assert image_size % 112 == 0 or image_size % 128 == 0
+ if image_size % 112 == 0:
+ ratio = float(image_size) / 112.0
+ diff_x = 0
+ else:
+ ratio = float(image_size) / 128.0
+ diff_x = 8.0 * ratio
+ dst = arcface_dst * ratio
+ dst[:, 0] += diff_x
+ tform = trans.SimilarityTransform()
+ tform.estimate(lmk, dst)
+ M = tform.params[0:2, :]
+ return M
+
+
+def norm_crop(img, landmark, image_size=112, mode='arcface'):
+ M = estimate_norm(landmark, image_size, mode)
+ warped = cv2.warpAffine(img, M, (image_size, image_size), borderValue=0.0)
+ return warped
+
+
+def norm_crop2(img, landmark, image_size=112, mode='arcface'):
+ M = estimate_norm(landmark, image_size, mode)
+ warped = cv2.warpAffine(img, M, (image_size, image_size), borderValue=0.0)
+ return warped, M
+
+
+def square_crop(im, S):
+ if im.shape[0] > im.shape[1]:
+ height = S
+ width = int(float(im.shape[1]) / im.shape[0] * S)
+ scale = float(S) / im.shape[0]
+ else:
+ width = S
+ height = int(float(im.shape[0]) / im.shape[1] * S)
+ scale = float(S) / im.shape[1]
+ resized_im = cv2.resize(im, (width, height))
+ det_im = np.zeros((S, S, 3), dtype=np.uint8)
+ det_im[:resized_im.shape[0], :resized_im.shape[1], :] = resized_im
+ return det_im, scale
+
+
+def transform(data, center, output_size, scale, rotation):
+ scale_ratio = scale
+ rot = float(rotation) * np.pi / 180.0
+ # translation = (output_size/2-center[0]*scale_ratio, output_size/2-center[1]*scale_ratio)
+ t1 = trans.SimilarityTransform(scale=scale_ratio)
+ cx = center[0] * scale_ratio
+ cy = center[1] * scale_ratio
+ t2 = trans.SimilarityTransform(translation=(-1 * cx, -1 * cy))
+ t3 = trans.SimilarityTransform(rotation=rot)
+ t4 = trans.SimilarityTransform(translation=(output_size / 2,
+ output_size / 2))
+ t = t1 + t2 + t3 + t4
+ M = t.params[0:2]
+ cropped = cv2.warpAffine(data,
+ M, (output_size, output_size),
+ borderValue=0.0)
+ return cropped, M
+
+
+def trans_points2d(pts, M):
+ new_pts = np.zeros(shape=pts.shape, dtype=np.float32)
+ for i in range(pts.shape[0]):
+ pt = pts[i]
+ new_pt = np.array([pt[0], pt[1], 1.], dtype=np.float32)
+ new_pt = np.dot(M, new_pt)
+ # print('new_pt', new_pt.shape, new_pt)
+ new_pts[i] = new_pt[0:2]
+
+ return new_pts
+
+
+def trans_points3d(pts, M):
+ scale = np.sqrt(M[0][0] * M[0][0] + M[0][1] * M[0][1])
+ # print(scale)
+ new_pts = np.zeros(shape=pts.shape, dtype=np.float32)
+ for i in range(pts.shape[0]):
+ pt = pts[i]
+ new_pt = np.array([pt[0], pt[1], 1.], dtype=np.float32)
+ new_pt = np.dot(M, new_pt)
+ # print('new_pt', new_pt.shape, new_pt)
+ new_pts[i][0:2] = new_pt[0:2]
+ new_pts[i][2] = pts[i][2] * scale
+
+ return new_pts
+
+
+def trans_points(pts, M):
+ if pts.shape[1] == 2:
+ return trans_points2d(pts, M)
+ else:
+ return trans_points3d(pts, M)
diff --git a/difpoint/src/utils/face_analysis_diy.py b/difpoint/src/utils/face_analysis_diy.py
new file mode 100644
index 0000000000000000000000000000000000000000..f13a659134216958da3c7273aabf3b0f96fb320d
--- /dev/null
+++ b/difpoint/src/utils/face_analysis_diy.py
@@ -0,0 +1,79 @@
+# coding: utf-8
+
+"""
+face detectoin and alignment using InsightFace
+"""
+
+import numpy as np
+from .rprint import rlog as log
+from .dependencies.insightface.app import FaceAnalysis
+from .dependencies.insightface.app.common import Face
+from .timer import Timer
+
+
+def sort_by_direction(faces, direction: str = 'large-small', face_center=None):
+ if len(faces) <= 0:
+ return faces
+
+ if direction == 'left-right':
+ return sorted(faces, key=lambda face: face['bbox'][0])
+ if direction == 'right-left':
+ return sorted(faces, key=lambda face: face['bbox'][0], reverse=True)
+ if direction == 'top-bottom':
+ return sorted(faces, key=lambda face: face['bbox'][1])
+ if direction == 'bottom-top':
+ return sorted(faces, key=lambda face: face['bbox'][1], reverse=True)
+ if direction == 'small-large':
+ return sorted(faces, key=lambda face: (face['bbox'][2] - face['bbox'][0]) * (face['bbox'][3] - face['bbox'][1]))
+ if direction == 'large-small':
+ return sorted(faces, key=lambda face: (face['bbox'][2] - face['bbox'][0]) * (face['bbox'][3] - face['bbox'][1]), reverse=True)
+ if direction == 'distance-from-retarget-face':
+ return sorted(faces, key=lambda face: (((face['bbox'][2]+face['bbox'][0])/2-face_center[0])**2+((face['bbox'][3]+face['bbox'][1])/2-face_center[1])**2)**0.5)
+ return faces
+
+
+class FaceAnalysisDIY(FaceAnalysis):
+ def __init__(self, name='buffalo_l', root='~/.insightface', allowed_modules=None, **kwargs):
+ super().__init__(name=name, root=root, allowed_modules=allowed_modules, **kwargs)
+
+ self.timer = Timer()
+
+ def get(self, img_bgr, **kwargs):
+ max_num = kwargs.get('max_face_num', 0) # the number of the detected faces, 0 means no limit
+ flag_do_landmark_2d_106 = kwargs.get('flag_do_landmark_2d_106', True) # whether to do 106-point detection
+ direction = kwargs.get('direction', 'large-small') # sorting direction
+ face_center = None
+
+ bboxes, kpss = self.det_model.detect(img_bgr, max_num=max_num, metric='default')
+ if bboxes.shape[0] == 0:
+ return []
+ ret = []
+ for i in range(bboxes.shape[0]):
+ bbox = bboxes[i, 0:4]
+ det_score = bboxes[i, 4]
+ kps = None
+ if kpss is not None:
+ kps = kpss[i]
+ face = Face(bbox=bbox, kps=kps, det_score=det_score)
+ for taskname, model in self.models.items():
+ if taskname == 'detection':
+ continue
+
+ if (not flag_do_landmark_2d_106) and taskname == 'landmark_2d_106':
+ continue
+
+ # print(f'taskname: {taskname}')
+ model.get(img_bgr, face)
+ ret.append(face)
+
+ ret = sort_by_direction(ret, direction, face_center)
+ return ret
+
+ def warmup(self):
+ self.timer.tic()
+
+ img_bgr = np.zeros((512, 512, 3), dtype=np.uint8)
+ self.get(img_bgr)
+
+ elapse = self.timer.toc()
+ log(f'FaceAnalysisDIY warmup time: {elapse:.3f}s')
diff --git a/difpoint/src/utils/helper.py b/difpoint/src/utils/helper.py
new file mode 100644
index 0000000000000000000000000000000000000000..0e2af94e137b6447c88ec4df3c7c2c1b1bd94b8a
--- /dev/null
+++ b/difpoint/src/utils/helper.py
@@ -0,0 +1,145 @@
+# coding: utf-8
+
+"""
+utility functions and classes to handle feature extraction and model loading
+"""
+
+import os
+import os.path as osp
+import torch
+from collections import OrderedDict
+
+from ..modules.spade_generator import SPADEDecoder
+from ..modules.warping_network import WarpingNetwork
+from ..modules.motion_extractor import MotionExtractor
+from ..modules.appearance_feature_extractor import AppearanceFeatureExtractor
+from ..modules.stitching_retargeting_network import StitchingRetargetingNetwork
+
+
+def suffix(filename):
+ """a.jpg -> jpg"""
+ pos = filename.rfind(".")
+ if pos == -1:
+ return ""
+ return filename[pos + 1:]
+
+
+def prefix(filename):
+ """a.jpg -> a"""
+ pos = filename.rfind(".")
+ if pos == -1:
+ return filename
+ return filename[:pos]
+
+
+def basename(filename):
+ """a/b/c.jpg -> c"""
+ return prefix(osp.basename(filename))
+
+
+def remove_suffix(filepath):
+ """a/b/c.jpg -> a/b/c"""
+ return osp.join(osp.dirname(filepath), basename(filepath))
+
+
+def is_video(file_path):
+ if file_path.lower().endswith((".mp4", ".mov", ".avi", ".webm")) or osp.isdir(file_path):
+ return True
+ return False
+
+
+def is_template(file_path):
+ if file_path.endswith(".pkl"):
+ return True
+ return False
+
+
+def mkdir(d, log=False):
+ # return self-assined `d`, for one line code
+ if not osp.exists(d):
+ os.makedirs(d, exist_ok=True)
+ if log:
+ print(f"Make dir: {d}")
+ return d
+
+
+def squeeze_tensor_to_numpy(tensor):
+ out = tensor.data.squeeze(0).cpu().numpy()
+ return out
+
+
+def dct2device(dct: dict, device):
+ for key in dct:
+ dct[key] = torch.tensor(dct[key]).to(device)
+ return dct
+
+
+def concat_feat(kp_source: torch.Tensor, kp_driving: torch.Tensor) -> torch.Tensor:
+ """
+ kp_source: (bs, k, 3)
+ kp_driving: (bs, k, 3)
+ Return: (bs, 2k*3)
+ """
+ bs_src = kp_source.shape[0]
+ bs_dri = kp_driving.shape[0]
+ assert bs_src == bs_dri, 'batch size must be equal'
+
+ feat = torch.cat([kp_source.view(bs_src, -1), kp_driving.view(bs_dri, -1)], dim=1)
+ return feat
+
+
+def remove_ddp_dumplicate_key(state_dict):
+ state_dict_new = OrderedDict()
+ for key in state_dict.keys():
+ state_dict_new[key.replace('module.', '')] = state_dict[key]
+ return state_dict_new
+
+
+def load_model(ckpt_path, model_config, device, model_type):
+ model_params = model_config['model_params'][f'{model_type}_params']
+
+ if model_type == 'appearance_feature_extractor':
+ model = AppearanceFeatureExtractor(**model_params).to(device)
+ elif model_type == 'motion_extractor':
+ model = MotionExtractor(**model_params).to(device)
+ elif model_type == 'warping_module':
+ model = WarpingNetwork(**model_params).to(device)
+ elif model_type == 'spade_generator':
+ model = SPADEDecoder(**model_params).to(device)
+ elif model_type == 'stitching_retargeting_module':
+ # Special handling for stitching and retargeting module
+ config = model_config['model_params']['stitching_retargeting_module_params']
+ checkpoint = torch.load(ckpt_path, map_location=lambda storage, loc: storage)
+
+ stitcher = StitchingRetargetingNetwork(**config.get('stitching'))
+ stitcher.load_state_dict(remove_ddp_dumplicate_key(checkpoint['retarget_shoulder']))
+ stitcher = stitcher.to(device)
+ stitcher.eval()
+
+ retargetor_lip = StitchingRetargetingNetwork(**config.get('lip'))
+ retargetor_lip.load_state_dict(remove_ddp_dumplicate_key(checkpoint['retarget_mouth']))
+ retargetor_lip = retargetor_lip.to(device)
+ retargetor_lip.eval()
+
+ retargetor_eye = StitchingRetargetingNetwork(**config.get('eye'))
+ retargetor_eye.load_state_dict(remove_ddp_dumplicate_key(checkpoint['retarget_eye']))
+ retargetor_eye = retargetor_eye.to(device)
+ retargetor_eye.eval()
+
+ return {
+ 'stitching': stitcher,
+ 'lip': retargetor_lip,
+ 'eye': retargetor_eye
+ }
+ else:
+ raise ValueError(f"Unknown model type: {model_type}")
+
+ model.load_state_dict(torch.load(ckpt_path, map_location=lambda storage, loc: storage))
+ model.eval()
+ return model
+
+
+def load_description(fp):
+ with open(fp, 'r', encoding='utf-8') as f:
+ content = f.read()
+ return content
diff --git a/difpoint/src/utils/hparams.py b/difpoint/src/utils/hparams.py
new file mode 100644
index 0000000000000000000000000000000000000000..743c5c7d5a5a9e686f1ccd6fb3c2fb5cb382d62b
--- /dev/null
+++ b/difpoint/src/utils/hparams.py
@@ -0,0 +1,160 @@
+from glob import glob
+import os
+
+class HParams:
+ def __init__(self, **kwargs):
+ self.data = {}
+
+ for key, value in kwargs.items():
+ self.data[key] = value
+
+ def __getattr__(self, key):
+ if key not in self.data:
+ raise AttributeError("'HParams' object has no attribute %s" % key)
+ return self.data[key]
+
+ def set_hparam(self, key, value):
+ self.data[key] = value
+
+
+# Default hyperparameters
+hparams = HParams(
+ num_mels=80, # Number of mel-spectrogram channels and local conditioning dimensionality
+ # network
+ rescale=True, # Whether to rescale audio prior to preprocessing
+ rescaling_max=0.9, # Rescaling value
+
+ # Use LWS (https://github.com/Jonathan-LeRoux/lws) for STFT and phase reconstruction
+ # It"s preferred to set True to use with https://github.com/r9y9/wavenet_vocoder
+ # Does not work if n_ffit is not multiple of hop_size!!
+ use_lws=False,
+
+ n_fft=800, # Extra window size is filled with 0 paddings to match this parameter
+ hop_size=200, # For 16000Hz, 200 = 12.5 ms (0.0125 * sample_rate)
+ win_size=800, # For 16000Hz, 800 = 50 ms (If None, win_size = n_fft) (0.05 * sample_rate)
+ sample_rate=16000, # 16000Hz (corresponding to librispeech) (sox --i )
+
+ frame_shift_ms=None, # Can replace hop_size parameter. (Recommended: 12.5)
+
+ # Mel and Linear spectrograms normalization/scaling and clipping
+ signal_normalization=True,
+ # Whether to normalize mel spectrograms to some predefined range (following below parameters)
+ allow_clipping_in_normalization=True, # Only relevant if mel_normalization = True
+ symmetric_mels=True,
+ # Whether to scale the data to be symmetric around 0. (Also multiplies the output range by 2,
+ # faster and cleaner convergence)
+ max_abs_value=4.,
+ # max absolute value of data. If symmetric, data will be [-max, max] else [0, max] (Must not
+ # be too big to avoid gradient explosion,
+ # not too small for fast convergence)
+ # Contribution by @begeekmyfriend
+ # Spectrogram Pre-Emphasis (Lfilter: Reduce spectrogram noise and helps model certitude
+ # levels. Also allows for better G&L phase reconstruction)
+ preemphasize=True, # whether to apply filter
+ preemphasis=0.97, # filter coefficient.
+
+ # Limits
+ min_level_db=-100,
+ ref_level_db=20,
+ fmin=55,
+ # Set this to 55 if your speaker is male! if female, 95 should help taking off noise. (To
+ # test depending on dataset. Pitch info: male~[65, 260], female~[100, 525])
+ fmax=7600, # To be increased/reduced depending on data.
+
+ ###################### Our training parameters #################################
+ img_size=96,
+ fps=25,
+
+ batch_size=16,
+ initial_learning_rate=1e-4,
+ nepochs=300000, ### ctrl + c, stop whenever eval loss is consistently greater than train loss for ~10 epochs
+ num_workers=20,
+ checkpoint_interval=3000,
+ eval_interval=3000,
+ writer_interval=300,
+ save_optimizer_state=True,
+
+ syncnet_wt=0.0, # is initially zero, will be set automatically to 0.03 later. Leads to faster convergence.
+ syncnet_batch_size=64,
+ syncnet_lr=1e-4,
+ syncnet_eval_interval=1000,
+ syncnet_checkpoint_interval=10000,
+
+ disc_wt=0.07,
+ disc_initial_learning_rate=1e-4,
+)
+
+
+
+# Default hyperparameters
+hparamsdebug = HParams(
+ num_mels=80, # Number of mel-spectrogram channels and local conditioning dimensionality
+ # network
+ rescale=True, # Whether to rescale audio prior to preprocessing
+ rescaling_max=0.9, # Rescaling value
+
+ # Use LWS (https://github.com/Jonathan-LeRoux/lws) for STFT and phase reconstruction
+ # It"s preferred to set True to use with https://github.com/r9y9/wavenet_vocoder
+ # Does not work if n_ffit is not multiple of hop_size!!
+ use_lws=False,
+
+ n_fft=800, # Extra window size is filled with 0 paddings to match this parameter
+ hop_size=200, # For 16000Hz, 200 = 12.5 ms (0.0125 * sample_rate)
+ win_size=800, # For 16000Hz, 800 = 50 ms (If None, win_size = n_fft) (0.05 * sample_rate)
+ sample_rate=16000, # 16000Hz (corresponding to librispeech) (sox --i )
+
+ frame_shift_ms=None, # Can replace hop_size parameter. (Recommended: 12.5)
+
+ # Mel and Linear spectrograms normalization/scaling and clipping
+ signal_normalization=True,
+ # Whether to normalize mel spectrograms to some predefined range (following below parameters)
+ allow_clipping_in_normalization=True, # Only relevant if mel_normalization = True
+ symmetric_mels=True,
+ # Whether to scale the data to be symmetric around 0. (Also multiplies the output range by 2,
+ # faster and cleaner convergence)
+ max_abs_value=4.,
+ # max absolute value of data. If symmetric, data will be [-max, max] else [0, max] (Must not
+ # be too big to avoid gradient explosion,
+ # not too small for fast convergence)
+ # Contribution by @begeekmyfriend
+ # Spectrogram Pre-Emphasis (Lfilter: Reduce spectrogram noise and helps model certitude
+ # levels. Also allows for better G&L phase reconstruction)
+ preemphasize=True, # whether to apply filter
+ preemphasis=0.97, # filter coefficient.
+
+ # Limits
+ min_level_db=-100,
+ ref_level_db=20,
+ fmin=55,
+ # Set this to 55 if your speaker is male! if female, 95 should help taking off noise. (To
+ # test depending on dataset. Pitch info: male~[65, 260], female~[100, 525])
+ fmax=7600, # To be increased/reduced depending on data.
+
+ ###################### Our training parameters #################################
+ img_size=96,
+ fps=25,
+
+ batch_size=2,
+ initial_learning_rate=1e-3,
+ nepochs=100000, ### ctrl + c, stop whenever eval loss is consistently greater than train loss for ~10 epochs
+ num_workers=0,
+ checkpoint_interval=10000,
+ eval_interval=10,
+ writer_interval=5,
+ save_optimizer_state=True,
+
+ syncnet_wt=0.0, # is initially zero, will be set automatically to 0.03 later. Leads to faster convergence.
+ syncnet_batch_size=64,
+ syncnet_lr=1e-4,
+ syncnet_eval_interval=10000,
+ syncnet_checkpoint_interval=10000,
+
+ disc_wt=0.07,
+ disc_initial_learning_rate=1e-4,
+)
+
+
+def hparams_debug_string():
+ values = hparams.values()
+ hp = [" %s: %s" % (name, values[name]) for name in sorted(values) if name != "sentences"]
+ return "Hyperparameters:\n" + "\n".join(hp)
diff --git a/difpoint/src/utils/io.py b/difpoint/src/utils/io.py
new file mode 100644
index 0000000000000000000000000000000000000000..28c2d99f09421fc9eb1f6475419cb1c6e6dcd028
--- /dev/null
+++ b/difpoint/src/utils/io.py
@@ -0,0 +1,125 @@
+# coding: utf-8
+
+import os
+from glob import glob
+import os.path as osp
+import imageio
+import numpy as np
+import pickle
+import cv2; cv2.setNumThreads(0); cv2.ocl.setUseOpenCL(False)
+
+from .helper import mkdir, suffix
+
+
+def load_image_rgb(image_path: str):
+ if not osp.exists(image_path):
+ raise FileNotFoundError(f"Image not found: {image_path}")
+ img = cv2.imread(image_path, cv2.IMREAD_COLOR)
+ return cv2.cvtColor(img, cv2.COLOR_BGR2RGB)
+
+
+def load_driving_info(driving_info):
+ driving_video_ori = []
+
+ def load_images_from_directory(directory):
+ image_paths = sorted(glob(osp.join(directory, '*.png')) + glob(osp.join(directory, '*.jpg')))
+ return [load_image_rgb(im_path) for im_path in image_paths]
+
+ def load_images_from_video(file_path):
+ reader = imageio.get_reader(file_path, "ffmpeg")
+ return [image for _, image in enumerate(reader)]
+
+ if osp.isdir(driving_info):
+ driving_video_ori = load_images_from_directory(driving_info)
+ elif osp.isfile(driving_info):
+ driving_video_ori = load_images_from_video(driving_info)
+
+ return driving_video_ori
+
+
+def contiguous(obj):
+ if not obj.flags.c_contiguous:
+ obj = obj.copy(order="C")
+ return obj
+
+
+def resize_to_limit(img: np.ndarray, max_dim=1920, division=2):
+ """
+ ajust the size of the image so that the maximum dimension does not exceed max_dim, and the width and the height of the image are multiples of n.
+ :param img: the image to be processed.
+ :param max_dim: the maximum dimension constraint.
+ :param n: the number that needs to be multiples of.
+ :return: the adjusted image.
+ """
+ h, w = img.shape[:2]
+
+ # ajust the size of the image according to the maximum dimension
+ if max_dim > 0 and max(h, w) > max_dim:
+ if h > w:
+ new_h = max_dim
+ new_w = int(w * (max_dim / h))
+ else:
+ new_w = max_dim
+ new_h = int(h * (max_dim / w))
+ img = cv2.resize(img, (new_w, new_h))
+
+ # ensure that the image dimensions are multiples of n
+ division = max(division, 1)
+ new_h = img.shape[0] - (img.shape[0] % division)
+ new_w = img.shape[1] - (img.shape[1] % division)
+
+ if new_h == 0 or new_w == 0:
+ # when the width or height is less than n, no need to process
+ return img
+
+ if new_h != img.shape[0] or new_w != img.shape[1]:
+ img = img[:new_h, :new_w]
+
+ return img
+
+
+def load_img_online(obj, mode="bgr", **kwargs):
+ max_dim = kwargs.get("max_dim", 1920)
+ n = kwargs.get("n", 2)
+ if isinstance(obj, str):
+ if mode.lower() == "gray":
+ img = cv2.imread(obj, cv2.IMREAD_GRAYSCALE)
+ else:
+ img = cv2.imread(obj, cv2.IMREAD_COLOR)
+ else:
+ img = obj
+
+ # Resize image to satisfy constraints
+ img = resize_to_limit(img, max_dim=max_dim, division=n)
+
+ if mode.lower() == "bgr":
+ return contiguous(img)
+ elif mode.lower() == "rgb":
+ return contiguous(img[..., ::-1])
+ else:
+ raise Exception(f"Unknown mode {mode}")
+
+
+def load(fp):
+ suffix_ = suffix(fp)
+
+ if suffix_ == "npy":
+ return np.load(fp)
+ elif suffix_ == "pkl":
+ return pickle.load(open(fp, "rb"))
+ else:
+ raise Exception(f"Unknown type: {suffix}")
+
+
+def dump(wfp, obj):
+ wd = osp.split(wfp)[0]
+ if wd != "" and not osp.exists(wd):
+ mkdir(wd)
+
+ _suffix = suffix(wfp)
+ if _suffix == "npy":
+ np.save(wfp, obj)
+ elif _suffix == "pkl":
+ pickle.dump(obj, open(wfp, "wb"))
+ else:
+ raise Exception("Unknown type: {}".format(_suffix))
diff --git a/difpoint/src/utils/landmark_runner.py b/difpoint/src/utils/landmark_runner.py
new file mode 100644
index 0000000000000000000000000000000000000000..7680a2c4a65ebe7f4dadbafc4a35603ab9f90be6
--- /dev/null
+++ b/difpoint/src/utils/landmark_runner.py
@@ -0,0 +1,89 @@
+# coding: utf-8
+
+import os.path as osp
+import cv2; cv2.setNumThreads(0); cv2.ocl.setUseOpenCL(False)
+import torch
+import numpy as np
+import onnxruntime
+from .timer import Timer
+from .rprint import rlog
+from .crop import crop_image, _transform_pts
+
+
+def make_abs_path(fn):
+ return osp.join(osp.dirname(osp.realpath(__file__)), fn)
+
+
+def to_ndarray(obj):
+ if isinstance(obj, torch.Tensor):
+ return obj.cpu().numpy()
+ elif isinstance(obj, np.ndarray):
+ return obj
+ else:
+ return np.array(obj)
+
+
+class LandmarkRunner(object):
+ """landmark runner"""
+
+ def __init__(self, **kwargs):
+ ckpt_path = kwargs.get('ckpt_path')
+ onnx_provider = kwargs.get('onnx_provider', 'cuda') # 默认用cuda
+ device_id = kwargs.get('device_id', 0)
+ self.dsize = kwargs.get('dsize', 224)
+ self.timer = Timer()
+
+ if onnx_provider.lower() == 'cuda':
+ self.session = onnxruntime.InferenceSession(
+ ckpt_path, providers=[
+ ('CUDAExecutionProvider', {'device_id': device_id})
+ ]
+ )
+ else:
+ opts = onnxruntime.SessionOptions()
+ opts.intra_op_num_threads = 4 # 默认线程数为 4
+ self.session = onnxruntime.InferenceSession(
+ ckpt_path, providers=['CPUExecutionProvider'],
+ sess_options=opts
+ )
+
+ def _run(self, inp):
+ out = self.session.run(None, {'input': inp})
+ return out
+
+ def run(self, img_rgb: np.ndarray, lmk=None):
+ if lmk is not None:
+ crop_dct = crop_image(img_rgb, lmk, dsize=self.dsize, scale=1.5, vy_ratio=-0.1)
+ img_crop_rgb = crop_dct['img_crop']
+ else:
+ # NOTE: force resize to 224x224, NOT RECOMMEND!
+ img_crop_rgb = cv2.resize(img_rgb, (self.dsize, self.dsize))
+ scale = max(img_rgb.shape[:2]) / self.dsize
+ crop_dct = {
+ 'M_c2o': np.array([
+ [scale, 0., 0.],
+ [0., scale, 0.],
+ [0., 0., 1.],
+ ], dtype=np.float32),
+ }
+
+ inp = (img_crop_rgb.astype(np.float32) / 255.).transpose(2, 0, 1)[None, ...] # HxWx3 (BGR) -> 1x3xHxW (RGB!)
+
+ out_lst = self._run(inp)
+ out_pts = out_lst[2]
+
+ # 2d landmarks 203 points
+ lmk = to_ndarray(out_pts[0]).reshape(-1, 2) * self.dsize # scale to 0-224
+ lmk = _transform_pts(lmk, M=crop_dct['M_c2o'])
+
+ return lmk
+
+ def warmup(self):
+ self.timer.tic()
+
+ dummy_image = np.zeros((1, 3, self.dsize, self.dsize), dtype=np.float32)
+
+ _ = self._run(dummy_image)
+
+ elapse = self.timer.toc()
+ rlog(f'LandmarkRunner warmup time: {elapse:.3f}s')
diff --git a/difpoint/src/utils/resources/mask_template.png b/difpoint/src/utils/resources/mask_template.png
new file mode 100644
index 0000000000000000000000000000000000000000..bca6ca5977ba820d0d2c05b3793c6231cc82e715
Binary files /dev/null and b/difpoint/src/utils/resources/mask_template.png differ
diff --git a/difpoint/src/utils/retargeting_utils.py b/difpoint/src/utils/retargeting_utils.py
new file mode 100644
index 0000000000000000000000000000000000000000..ae2e5f52effe8107503586c9f5a24f39dfdbbbcf
--- /dev/null
+++ b/difpoint/src/utils/retargeting_utils.py
@@ -0,0 +1,24 @@
+
+"""
+Functions to compute distance ratios between specific pairs of facial landmarks
+"""
+
+import numpy as np
+
+
+def calculate_distance_ratio(lmk: np.ndarray, idx1: int, idx2: int, idx3: int, idx4: int, eps: float = 1e-6) -> np.ndarray:
+ return (np.linalg.norm(lmk[:, idx1] - lmk[:, idx2], axis=1, keepdims=True) /
+ (np.linalg.norm(lmk[:, idx3] - lmk[:, idx4], axis=1, keepdims=True) + eps))
+
+
+def calc_eye_close_ratio(lmk: np.ndarray, target_eye_ratio: np.ndarray = None) -> np.ndarray:
+ lefteye_close_ratio = calculate_distance_ratio(lmk, 6, 18, 0, 12)
+ righteye_close_ratio = calculate_distance_ratio(lmk, 30, 42, 24, 36)
+ if target_eye_ratio is not None:
+ return np.concatenate([lefteye_close_ratio, righteye_close_ratio, target_eye_ratio], axis=1)
+ else:
+ return np.concatenate([lefteye_close_ratio, righteye_close_ratio], axis=1)
+
+
+def calc_lip_close_ratio(lmk: np.ndarray) -> np.ndarray:
+ return calculate_distance_ratio(lmk, 90, 102, 48, 66)
diff --git a/difpoint/src/utils/rprint.py b/difpoint/src/utils/rprint.py
new file mode 100644
index 0000000000000000000000000000000000000000..c43a42f9855bbb019725e6c2b6c6c50e6fa4d0c5
--- /dev/null
+++ b/difpoint/src/utils/rprint.py
@@ -0,0 +1,16 @@
+# coding: utf-8
+
+"""
+custom print and log functions
+"""
+
+__all__ = ['rprint', 'rlog']
+
+try:
+ from rich.console import Console
+ console = Console()
+ rprint = console.print
+ rlog = console.log
+except:
+ rprint = print
+ rlog = print
diff --git a/difpoint/src/utils/timer.py b/difpoint/src/utils/timer.py
new file mode 100644
index 0000000000000000000000000000000000000000..3570fa45d3ff36376471b82a5b3c02efe46eed98
--- /dev/null
+++ b/difpoint/src/utils/timer.py
@@ -0,0 +1,29 @@
+# coding: utf-8
+
+"""
+tools to measure elapsed time
+"""
+
+import time
+
+class Timer(object):
+ """A simple timer."""
+
+ def __init__(self):
+ self.total_time = 0.
+ self.calls = 0
+ self.start_time = 0.
+ self.diff = 0.
+
+ def tic(self):
+ # using time.time instead of time.clock because time time.clock
+ # does not normalize for multithreading
+ self.start_time = time.time()
+
+ def toc(self, average=True):
+ self.diff = time.time() - self.start_time
+ return self.diff
+
+ def clear(self):
+ self.start_time = 0.
+ self.diff = 0.
diff --git a/difpoint/src/utils/transform.py b/difpoint/src/utils/transform.py
new file mode 100644
index 0000000000000000000000000000000000000000..27e49cbf4e60241ce96b04f7d79da46cc3ef62c4
--- /dev/null
+++ b/difpoint/src/utils/transform.py
@@ -0,0 +1,118 @@
+import cv2
+import math
+import numpy as np
+from skimage import transform as trans
+
+
+def transform(data, center, output_size, scale, rotation):
+ scale_ratio = scale
+ rot = float(rotation) * np.pi / 180.0
+ # translation = (output_size/2-center[0]*scale_ratio, output_size/2-center[1]*scale_ratio)
+ t1 = trans.SimilarityTransform(scale=scale_ratio)
+ cx = center[0] * scale_ratio
+ cy = center[1] * scale_ratio
+ t2 = trans.SimilarityTransform(translation=(-1 * cx, -1 * cy))
+ t3 = trans.SimilarityTransform(rotation=rot)
+ t4 = trans.SimilarityTransform(translation=(output_size / 2,
+ output_size / 2))
+ t = t1 + t2 + t3 + t4
+ M = t.params[0:2]
+ cropped = cv2.warpAffine(data,
+ M, (output_size, output_size),
+ borderValue=0.0)
+ return cropped, M
+
+
+def trans_points2d(pts, M):
+ new_pts = np.zeros(shape=pts.shape, dtype=np.float32)
+ for i in range(pts.shape[0]):
+ pt = pts[i]
+ new_pt = np.array([pt[0], pt[1], 1.], dtype=np.float32)
+ new_pt = np.dot(M, new_pt)
+ # print('new_pt', new_pt.shape, new_pt)
+ new_pts[i] = new_pt[0:2]
+
+ return new_pts
+
+
+def trans_points3d(pts, M):
+ scale = np.sqrt(M[0][0] * M[0][0] + M[0][1] * M[0][1])
+ # print(scale)
+ new_pts = np.zeros(shape=pts.shape, dtype=np.float32)
+ for i in range(pts.shape[0]):
+ pt = pts[i]
+ new_pt = np.array([pt[0], pt[1], 1.], dtype=np.float32)
+ new_pt = np.dot(M, new_pt)
+ # print('new_pt', new_pt.shape, new_pt)
+ new_pts[i][0:2] = new_pt[0:2]
+ new_pts[i][2] = pts[i][2] * scale
+
+ return new_pts
+
+
+def trans_points(pts, M):
+ if pts.shape[1] == 2:
+ return trans_points2d(pts, M)
+ else:
+ return trans_points3d(pts, M)
+
+
+def estimate_affine_matrix_3d23d(X, Y):
+ ''' Using least-squares solution
+ Args:
+ X: [n, 3]. 3d points(fixed)
+ Y: [n, 3]. corresponding 3d points(moving). Y = PX
+ Returns:
+ P_Affine: (3, 4). Affine camera matrix (the third row is [0, 0, 0, 1]).
+ '''
+ X_homo = np.hstack((X, np.ones([X.shape[0], 1]))) # n x 4
+ P = np.linalg.lstsq(X_homo, Y)[0].T # Affine matrix. 3 x 4
+ return P
+
+
+def P2sRt(P):
+ ''' decompositing camera matrix P
+ Args:
+ P: (3, 4). Affine Camera Matrix.
+ Returns:
+ s: scale factor.
+ R: (3, 3). rotation matrix.
+ t: (3,). translation.
+ '''
+ t = P[:, 3]
+ R1 = P[0:1, :3]
+ R2 = P[1:2, :3]
+ s = (np.linalg.norm(R1) + np.linalg.norm(R2)) / 2.0
+ r1 = R1 / np.linalg.norm(R1)
+ r2 = R2 / np.linalg.norm(R2)
+ r3 = np.cross(r1, r2)
+
+ R = np.concatenate((r1, r2, r3), 0)
+ return s, R, t
+
+
+def matrix2angle(R):
+ ''' get three Euler angles from Rotation Matrix
+ Args:
+ R: (3,3). rotation matrix
+ Returns:
+ x: pitch
+ y: yaw
+ z: roll
+ '''
+ sy = math.sqrt(R[0, 0] * R[0, 0] + R[1, 0] * R[1, 0])
+
+ singular = sy < 1e-6
+
+ if not singular:
+ x = math.atan2(R[2, 1], R[2, 2])
+ y = math.atan2(-R[2, 0], sy)
+ z = math.atan2(R[1, 0], R[0, 0])
+ else:
+ x = math.atan2(-R[1, 2], R[1, 1])
+ y = math.atan2(-R[2, 0], sy)
+ z = 0
+
+ # rx, ry, rz = np.rad2deg(x), np.rad2deg(y), np.rad2deg(z)
+ rx, ry, rz = x * 180 / np.pi, y * 180 / np.pi, z * 180 / np.pi
+ return rx, ry, rz
diff --git a/difpoint/src/utils/utils.py b/difpoint/src/utils/utils.py
new file mode 100644
index 0000000000000000000000000000000000000000..2a090a138b0af9048ff41c0c675a5e7560096f83
--- /dev/null
+++ b/difpoint/src/utils/utils.py
@@ -0,0 +1,239 @@
+# -*- coding: utf-8 -*-
+import pdb
+
+import cv2
+import numpy as np
+import ffmpeg
+import os
+import os.path as osp
+
+
+def video_has_audio(video_file):
+ try:
+ ret = ffmpeg.probe(video_file, select_streams='a')
+ return len(ret["streams"]) > 0
+ except ffmpeg.Error:
+ return False
+
+
+def get_video_info(video_path):
+ # 使用 ffmpeg.probe 获取视频信息
+ probe = ffmpeg.probe(video_path)
+ video_streams = [stream for stream in probe['streams'] if stream['codec_type'] == 'video']
+
+ if not video_streams:
+ raise ValueError("No video stream found")
+
+ # 获取视频时长
+ duration = float(probe['format']['duration'])
+
+ # 获取帧率 (r_frame_rate),通常是一个分数字符串,如 "30000/1001"
+ fps_string = video_streams[0]['r_frame_rate']
+ numerator, denominator = map(int, fps_string.split('/'))
+ fps = numerator / denominator
+
+ return duration, fps
+
+
+def resize_to_limit(img: np.ndarray, max_dim=1280, division=2):
+ """
+ ajust the size of the image so that the maximum dimension does not exceed max_dim, and the width and the height of the image are multiples of n.
+ :param img: the image to be processed.
+ :param max_dim: the maximum dimension constraint.
+ :param n: the number that needs to be multiples of.
+ :return: the adjusted image.
+ """
+ h, w = img.shape[:2]
+
+ # ajust the size of the image according to the maximum dimension
+ if max_dim > 0 and max(h, w) > max_dim:
+ if h > w:
+ new_h = max_dim
+ new_w = int(w * (max_dim / h))
+ else:
+ new_w = max_dim
+ new_h = int(h * (max_dim / w))
+ img = cv2.resize(img, (new_w, new_h))
+
+ # ensure that the image dimensions are multiples of n
+ division = max(division, 1)
+ new_h = img.shape[0] - (img.shape[0] % division)
+ new_w = img.shape[1] - (img.shape[1] % division)
+
+ if new_h == 0 or new_w == 0:
+ # when the width or height is less than n, no need to process
+ return img
+
+ if new_h != img.shape[0] or new_w != img.shape[1]:
+ img = img[:new_h, :new_w]
+
+ return img
+
+
+def get_rotation_matrix(pitch_, yaw_, roll_):
+ """ the input is in degree
+ """
+ PI = np.pi
+ # transform to radian
+ pitch = pitch_ / 180 * PI
+ yaw = yaw_ / 180 * PI
+ roll = roll_ / 180 * PI
+
+ if pitch.ndim == 1:
+ pitch = np.expand_dims(pitch.cpu(), axis=1)
+ if yaw.ndim == 1:
+ yaw = np.expand_dims(yaw.cpu(), axis=1)
+ if roll.ndim == 1:
+ roll = np.expand_dims(roll.cpu(), axis=1)
+
+ # calculate the euler matrix
+ bs = pitch.shape[0]
+ ones = np.ones([bs, 1])
+ zeros = np.zeros([bs, 1])
+ x, y, z = pitch, yaw, roll
+
+ rot_x = np.concatenate([
+ ones, zeros, zeros,
+ zeros, np.cos(x), -np.sin(x),
+ zeros, np.sin(x), np.cos(x)
+ ], axis=1).reshape([bs, 3, 3])
+
+ rot_y = np.concatenate([
+ np.cos(y), zeros, np.sin(y),
+ zeros, ones, zeros,
+ -np.sin(y), zeros, np.cos(y)
+ ], axis=1).reshape([bs, 3, 3])
+
+ rot_z = np.concatenate([
+ np.cos(z), -np.sin(z), zeros,
+ np.sin(z), np.cos(z), zeros,
+ zeros, zeros, ones
+ ], axis=1).reshape([bs, 3, 3])
+
+ rot = np.matmul(rot_z, np.matmul(rot_y, rot_x))
+ return np.transpose(rot, (0, 2, 1)) # transpose
+
+
+def calculate_distance_ratio(lmk: np.ndarray, idx1: int, idx2: int, idx3: int, idx4: int,
+ eps: float = 1e-6) -> np.ndarray:
+ return (np.linalg.norm(lmk[:, idx1] - lmk[:, idx2], axis=1, keepdims=True) /
+ (np.linalg.norm(lmk[:, idx3] - lmk[:, idx4], axis=1, keepdims=True) + eps))
+
+
+def calc_eye_close_ratio(lmk: np.ndarray, target_eye_ratio: np.ndarray = None) -> np.ndarray:
+ lefteye_close_ratio = calculate_distance_ratio(lmk, 6, 18, 0, 12)
+ righteye_close_ratio = calculate_distance_ratio(lmk, 30, 42, 24, 36)
+ if target_eye_ratio is not None:
+ return np.concatenate([lefteye_close_ratio, righteye_close_ratio, target_eye_ratio], axis=1)
+ else:
+ return np.concatenate([lefteye_close_ratio, righteye_close_ratio], axis=1)
+
+
+def calc_lip_close_ratio(lmk: np.ndarray) -> np.ndarray:
+ return calculate_distance_ratio(lmk, 90, 102, 48, 66)
+
+
+def _transform_img(img, M, dsize, flags=cv2.INTER_LINEAR, borderMode=None):
+ """ conduct similarity or affine transformation to the image, do not do border operation!
+ img:
+ M: 2x3 matrix or 3x3 matrix
+ dsize: target shape (width, height)
+ """
+ if isinstance(dsize, tuple) or isinstance(dsize, list):
+ _dsize = tuple(dsize)
+ else:
+ _dsize = (dsize, dsize)
+
+ if borderMode is not None:
+ return cv2.warpAffine(img, M[:2, :], dsize=_dsize, flags=flags, borderMode=borderMode, borderValue=(0, 0, 0))
+ else:
+ return cv2.warpAffine(img, M[:2, :], dsize=_dsize, flags=flags)
+
+
+def prepare_paste_back(mask_crop, crop_M_c2o, dsize):
+ """prepare mask for later image paste back
+ """
+ mask_ori = _transform_img(mask_crop, crop_M_c2o, dsize)
+ mask_ori = mask_ori.astype(np.float32) / 255.
+ return mask_ori
+
+
+def transform_keypoint(pitch, yaw, roll, t, exp, scale, kp):
+ """
+ transform the implicit keypoints with the pose, shift, and expression deformation
+ kp: BxNx3
+ """
+ bs = kp.shape[0]
+ if kp.ndim == 2:
+ num_kp = kp.shape[1] // 3 # Bx(num_kpx3)
+ else:
+ num_kp = kp.shape[1] # Bxnum_kpx3
+
+ rot_mat = get_rotation_matrix(pitch, yaw, roll) # (bs, 3, 3)
+
+ # Eqn.2: s * (R * x_c,s + exp) + t
+ kp_transformed = kp.reshape(bs, num_kp, 3) @ rot_mat + exp.reshape(bs, num_kp, 3)
+ kp_transformed *= scale[..., None] # (bs, k, 3) * (bs, 1, 1) = (bs, k, 3)
+ kp_transformed[:, :, 0:2] += t[:, None, 0:2] # remove z, only apply tx ty
+
+ return kp_transformed
+
+
+def concat_feat(x, y):
+ bs = x.shape[0]
+ return np.concatenate([x.reshape(bs, -1), y.reshape(bs, -1)], axis=1)
+
+
+def is_image(file_path):
+ image_extensions = ('.jpg', '.jpeg', '.png', '.gif', '.bmp', '.tiff')
+ return file_path.lower().endswith(image_extensions)
+
+
+def is_video(file_path):
+ if file_path.lower().endswith((".mp4", ".mov", ".avi", ".webm")) or os.path.isdir(file_path):
+ return True
+ return False
+
+
+def make_abs_path(fn):
+ return osp.join(os.path.dirname(osp.dirname(osp.realpath(__file__))), fn)
+
+
+class LowPassFilter:
+ def __init__(self):
+ self.prev_raw_value = None
+ self.prev_filtered_value = None
+
+ def process(self, value, alpha):
+ if self.prev_raw_value is None:
+ s = value
+ else:
+ s = alpha * value + (1.0 - alpha) * self.prev_filtered_value
+ self.prev_raw_value = value
+ self.prev_filtered_value = s
+ return s
+
+
+class OneEuroFilter:
+ def __init__(self, mincutoff=1.0, beta=0.0, dcutoff=1.0, freq=30):
+ self.freq = freq
+ self.mincutoff = mincutoff
+ self.beta = beta
+ self.dcutoff = dcutoff
+ self.x_filter = LowPassFilter()
+ self.dx_filter = LowPassFilter()
+
+ def compute_alpha(self, cutoff):
+ te = 1.0 / self.freq
+ tau = 1.0 / (2 * np.pi * cutoff)
+ return 1.0 / (1.0 + tau / te)
+
+ def get_pre_x(self):
+ return self.x_filter.prev_filtered_value
+
+ def process(self, x):
+ prev_x = self.x_filter.prev_raw_value
+ dx = 0.0 if prev_x is None else (x - prev_x) * self.freq
+ edx = self.dx_filter.process(dx, self.compute_alpha(self.dcutoff))
+ cutoff = self.mincutoff + self.beta * np.abs(edx)
+ return self.x_filter.process(x, self.compute_alpha(cutoff))
diff --git a/difpoint/src/utils/video.py b/difpoint/src/utils/video.py
new file mode 100644
index 0000000000000000000000000000000000000000..c62729049e0349c02fb92bdd3149ac21dade294b
--- /dev/null
+++ b/difpoint/src/utils/video.py
@@ -0,0 +1,211 @@
+# coding: utf-8
+
+"""
+Functions for processing video
+
+ATTENTION: you need to install ffmpeg and ffprobe in your env!
+"""
+
+import os.path as osp
+import numpy as np
+import subprocess
+import imageio
+import cv2
+from rich.progress import track
+
+from .rprint import rlog as log
+from .rprint import rprint as print
+from .helper import prefix
+
+
+def exec_cmd(cmd):
+ return subprocess.run(cmd, shell=True, check=True, stdout=subprocess.PIPE, stderr=subprocess.STDOUT)
+
+
+def images2video(images, wfp, **kwargs):
+ fps = kwargs.get('fps', 25)
+ video_format = kwargs.get('format', 'mp4') # default is mp4 format
+ codec = kwargs.get('codec', 'libx264') # default is libx264 encoding
+ quality = kwargs.get('quality') # video quality
+ pixelformat = kwargs.get('pixelformat', 'yuv420p') # video pixel format
+ image_mode = kwargs.get('image_mode', 'rgb')
+ macro_block_size = kwargs.get('macro_block_size', 2)
+ ffmpeg_params = ['-crf', str(kwargs.get('crf', 18))]
+
+ writer = imageio.get_writer(
+ wfp, fps=fps, format=video_format,
+ codec=codec, quality=quality, ffmpeg_params=ffmpeg_params, pixelformat=pixelformat, macro_block_size=macro_block_size
+ )
+
+ n = len(images)
+ for i in track(range(n), description='Writing', transient=True):
+ if image_mode.lower() == 'bgr':
+ writer.append_data(images[i][..., ::-1])
+ else:
+ writer.append_data(images[i])
+
+ writer.close()
+
+
+def video2gif(video_fp, fps=30, size=256):
+ if osp.exists(video_fp):
+ d = osp.split(video_fp)[0]
+ fn = prefix(osp.basename(video_fp))
+ palette_wfp = osp.join(d, 'palette.png')
+ gif_wfp = osp.join(d, f'{fn}.gif')
+ # generate the palette
+ cmd = f'ffmpeg -i "{video_fp}" -vf "fps={fps},scale={size}:-1:flags=lanczos,palettegen" "{palette_wfp}" -y'
+ exec_cmd(cmd)
+ # use the palette to generate the gif
+ cmd = f'ffmpeg -i "{video_fp}" -i "{palette_wfp}" -filter_complex "fps={fps},scale={size}:-1:flags=lanczos[x];[x][1:v]paletteuse" "{gif_wfp}" -y'
+ exec_cmd(cmd)
+ else:
+ print(f'video_fp: {video_fp} not exists!')
+
+
+def merge_audio_video(video_fp, audio_fp, wfp):
+ if osp.exists(video_fp) and osp.exists(audio_fp):
+ cmd = f'ffmpeg -i "{video_fp}" -i "{audio_fp}" -c:v copy -c:a aac "{wfp}" -y'
+ exec_cmd(cmd)
+ print(f'merge {video_fp} and {audio_fp} to {wfp}')
+ else:
+ print(f'video_fp: {video_fp} or audio_fp: {audio_fp} not exists!')
+
+
+def blend(img: np.ndarray, mask: np.ndarray, background_color=(255, 255, 255)):
+ mask_float = mask.astype(np.float32) / 255.
+ background_color = np.array(background_color).reshape([1, 1, 3])
+ bg = np.ones_like(img) * background_color
+ img = np.clip(mask_float * img + (1 - mask_float) * bg, 0, 255).astype(np.uint8)
+ return img
+
+
+def concat_frames(driving_image_lst, source_image, I_p_lst):
+ # TODO: add more concat style, e.g., left-down corner driving
+ out_lst = []
+ h, w, _ = I_p_lst[0].shape
+
+ for idx, _ in track(enumerate(I_p_lst), total=len(I_p_lst), description='Concatenating result...'):
+ I_p = I_p_lst[idx]
+ source_image_resized = cv2.resize(source_image, (w, h))
+
+ if driving_image_lst is None:
+ out = np.hstack((source_image_resized, I_p))
+ else:
+ driving_image = driving_image_lst[idx]
+ driving_image_resized = cv2.resize(driving_image, (w, h))
+ out = np.hstack((driving_image_resized, source_image_resized, I_p))
+
+ out_lst.append(out)
+ return out_lst
+
+
+class VideoWriter:
+ def __init__(self, **kwargs):
+ self.fps = kwargs.get('fps', 30)
+ self.wfp = kwargs.get('wfp', 'video.mp4')
+ self.video_format = kwargs.get('format', 'mp4')
+ self.codec = kwargs.get('codec', 'libx264')
+ self.quality = kwargs.get('quality')
+ self.pixelformat = kwargs.get('pixelformat', 'yuv420p')
+ self.image_mode = kwargs.get('image_mode', 'rgb')
+ self.ffmpeg_params = kwargs.get('ffmpeg_params')
+
+ self.writer = imageio.get_writer(
+ self.wfp, fps=self.fps, format=self.video_format,
+ codec=self.codec, quality=self.quality,
+ ffmpeg_params=self.ffmpeg_params, pixelformat=self.pixelformat
+ )
+
+ def write(self, image):
+ if self.image_mode.lower() == 'bgr':
+ self.writer.append_data(image[..., ::-1])
+ else:
+ self.writer.append_data(image)
+
+ def close(self):
+ if self.writer is not None:
+ self.writer.close()
+
+
+def change_video_fps(input_file, output_file, fps=20, codec='libx264', crf=12):
+ cmd = f'ffmpeg -i "{input_file}" -c:v {codec} -crf {crf} -r {fps} "{output_file}" -y'
+ exec_cmd(cmd)
+
+
+def get_fps(filepath, default_fps=25):
+ try:
+ fps = cv2.VideoCapture(filepath).get(cv2.CAP_PROP_FPS)
+
+ if fps in (0, None):
+ fps = default_fps
+ except Exception as e:
+ log(e)
+ fps = default_fps
+
+ return fps
+
+
+def has_audio_stream(video_path: str) -> bool:
+ """
+ Check if the video file contains an audio stream.
+
+ :param video_path: Path to the video file
+ :return: True if the video contains an audio stream, False otherwise
+ """
+ if osp.isdir(video_path):
+ return False
+
+ cmd = [
+ 'ffprobe',
+ '-v', 'error',
+ '-select_streams', 'a',
+ '-show_entries', 'stream=codec_type',
+ '-of', 'default=noprint_wrappers=1:nokey=1',
+ f'"{video_path}"'
+ ]
+
+ try:
+ # result = subprocess.run(cmd, capture_output=True, text=True)
+ result = exec_cmd(' '.join(cmd))
+ if result.returncode != 0:
+ log(f"Error occurred while probing video: {result.stderr}")
+ return False
+
+ # Check if there is any output from ffprobe command
+ return bool(result.stdout.strip())
+ except Exception as e:
+ log(f"Error occurred while probing video: {video_path}, you may need to install ffprobe! Now set audio to false!", style="bold red")
+ return False
+
+
+def add_audio_to_video(silent_video_path: str, audio_video_path: str, output_video_path: str):
+ cmd = [
+ 'ffmpeg',
+ '-y',
+ '-i', f'"{silent_video_path}"',
+ '-i', f'"{audio_video_path}"',
+ '-map', '0:v',
+ '-map', '1:a',
+ '-c:v', 'copy',
+ '-shortest',
+ f'"{output_video_path}"'
+ ]
+
+ try:
+ exec_cmd(' '.join(cmd))
+ log(f"Video with audio generated successfully: {output_video_path}")
+ except subprocess.CalledProcessError as e:
+ log(f"Error occurred: {e}")
+
+
+def bb_intersection_over_union(boxA, boxB):
+ xA = max(boxA[0], boxB[0])
+ yA = max(boxA[1], boxB[1])
+ xB = min(boxA[2], boxB[2])
+ yB = min(boxA[3], boxB[3])
+ interArea = max(0, xB - xA + 1) * max(0, yB - yA + 1)
+ boxAArea = (boxA[2] - boxA[0] + 1) * (boxA[3] - boxA[1] + 1)
+ boxBArea = (boxB[2] - boxB[0] + 1) * (boxB[3] - boxB[1] + 1)
+ iou = interArea / float(boxAArea + boxBArea - interArea)
+ return iou
diff --git a/difpoint/src/utils/viz.py b/difpoint/src/utils/viz.py
new file mode 100644
index 0000000000000000000000000000000000000000..59443cbf207f3395bee241f63c7acb95b9402530
--- /dev/null
+++ b/difpoint/src/utils/viz.py
@@ -0,0 +1,19 @@
+# coding: utf-8
+
+import cv2; cv2.setNumThreads(0); cv2.ocl.setUseOpenCL(False)
+
+
+def viz_lmk(img_, vps, **kwargs):
+ """可视化点"""
+ lineType = kwargs.get("lineType", cv2.LINE_8) # cv2.LINE_AA
+ img_for_viz = img_.copy()
+ for pt in vps:
+ cv2.circle(
+ img_for_viz,
+ (int(pt[0]), int(pt[1])),
+ radius=kwargs.get("radius", 1),
+ color=(0, 255, 0),
+ thickness=kwargs.get("thickness", 1),
+ lineType=lineType,
+ )
+ return img_for_viz
diff --git a/packages.txt b/packages.txt
new file mode 100644
index 0000000000000000000000000000000000000000..5071c5610b3951db61cf45ba9506c4c1245e12b6
--- /dev/null
+++ b/packages.txt
@@ -0,0 +1 @@
+-y build-essential python3-dev
\ No newline at end of file
diff --git a/requirements.txt b/requirements.txt
new file mode 100644
index 0000000000000000000000000000000000000000..13adc296e04daf8deb28b028372c474eaef3f89a
--- /dev/null
+++ b/requirements.txt
@@ -0,0 +1,55 @@
+torch
+torchvision
+torchaudio
+onnx
+onnxruntime-gpu==1.17.0
+tensorrt
+transformers
+torchgeometry
+pyyaml==6.0.1
+scipy==1.11.4
+imageio==2.34.2
+lmdb==1.4.1
+tqdm==4.64.1
+rich==13.7.1
+ffmpeg-python==0.2.0
+protobuf==3.20.2
+onnx==1.16.1
+scikit-learn
+flask
+pickleshare
+albumentations==1.4.10
+matplotlib==3.7.0
+imageio-ffmpeg==0.5.1
+tyro==0.8.5
+pykalman==0.9.7
+pillow>=10.2.0
+pytorch_fid
+cpbd
+mediapipe
+wandb==0.17.5
+accelerate==0.23.0
+basicsr==1.4.2
+diffusers==0.32.2
+einops==0.6.0
+einops_exts==0.0.4
+hydra-core==1.3.2
+librosa
+moviepy==1.0.3
+omegaconf==2.3.0
+opencv_python_headless>=4.9.0.80
+pydub==0.25.1
+PyYAML==6.0.1
+realesrgan==0.3.0
+rotary_embedding_torch==0.3.0
+timm==0.4.12
+torch_ema==0.3
+warmup_scheduler==0.3
+
+yacs==0.1.8
+numpy==1.26.4
+coqui-tts==0.22.1
+gradio==5.5.0
+dlib-bin
+insightface
+albucore==0.0.16
\ No newline at end of file