Abstract
The goal of diffusion generative models is to align the learned distribution with the real data distribution through gradient score matching. However, inherent limitations in training data quality, modeling strategies, and architectural design lead to inevitable gap between generated outputs and real data. To reduce this gap, we propose Weak-to-Strong Diffusion (W2SD), a novel framework that utilizes the estimated difference between existing weak and strong models (i.e., weak-to-strong difference) to approximate the gap between an ideal model and a strong model. By employing a reflective operation that alternates between denoising and inversion with weak-to-strong difference, we theoretically understand that W2SD steers latent variables along sampling trajectories toward regions of the real data distribution. W2SD is highly flexible and broadly applicable, enabling diverse improvements through the strategic selection of weak-to-strong model pairs (e.g., DreamShaper vs. SD1.5, good experts vs. bad experts in MoE). Extensive experiments demonstrate that W2SD significantly improves human preference, aesthetic quality, and prompt adherence, achieving SOTA performance across various modalities (e.g., image, video), architectures (e.g., UNet-based, DiT-based, MoE), and benchmarks. For example, Juggernaut-XL with W2SD can improve with the HPSv2 winning rate up to 90% over the original results. Moreover, the performance gains achieved by W2SD markedly outweigh its additional computational overhead, while the cumulative improvements from different weak-to-strong difference further solidify its practical utility and deployability.
Community
We propose a reflection framework for the inference process of diffusion models, leveraging the distribution gap between the exising weak and strong models to enhance a diffusion model into an ideal model, thereby optimizing the sampling trajectory. The code is publicly available at github.com/xie-lab-ml/Weak-to-Strong-Diffusion-with-Reflection
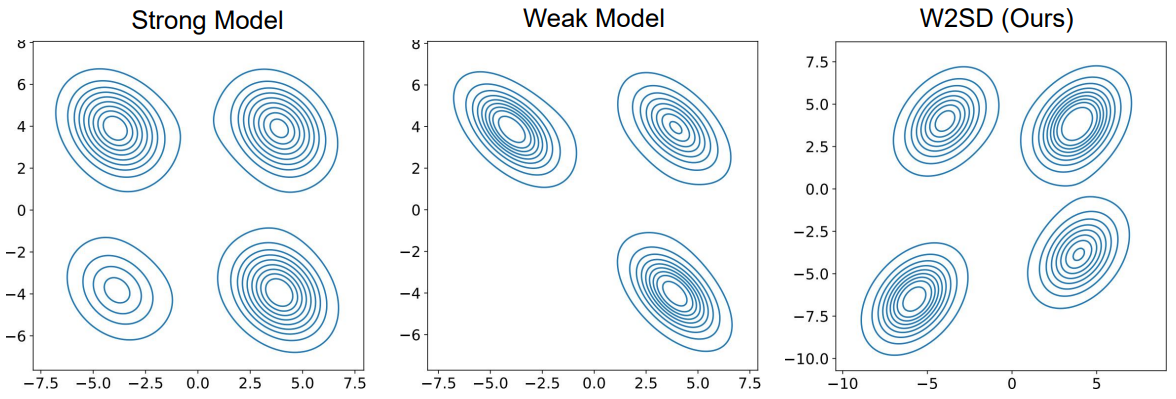
This is an automated message from the Librarian Bot. I found the following papers similar to this paper.
The following papers were recommended by the Semantic Scholar API
- Zigzag Diffusion Sampling: Diffusion Models Can Self-Improve via Self-Reflection (2024)
- E2EDiff: Direct Mapping from Noise to Data for Enhanced Diffusion Models (2024)
- Debate Helps Weak-to-Strong Generalization (2025)
- Representations Shape Weak-to-Strong Generalization: Theoretical Insights and Empirical Predictions (2025)
- Relating Misfit to Gain in Weak-to-Strong Generalization Beyond the Squared Loss (2025)
- A Mutual Information Perspective on Multiple Latent Variable Generative Models for Positive View Generation (2025)
- Exploring Representation-Aligned Latent Space for Better Generation (2025)
Please give a thumbs up to this comment if you found it helpful!
If you want recommendations for any Paper on Hugging Face checkout this Space
You can directly ask Librarian Bot for paper recommendations by tagging it in a comment:
@librarian-bot
recommend
Models citing this paper 0
No model linking this paper
Datasets citing this paper 0
No dataset linking this paper
Spaces citing this paper 0
No Space linking this paper