Upload folder using huggingface_hub
Browse files- main/README.md +248 -0
- main/adaptive_mask_inpainting.py +1465 -0
main/README.md
CHANGED
@@ -10,6 +10,7 @@ Please also check out our [Community Scripts](https://github.com/huggingface/dif
|
|
10 |
|
11 |
| Example | Description | Code Example | Colab | Author |
|
12 |
|:--------------------------------------------------------------------------------------------------------------------------------------|:---------------------------------------------------------------------------------------------------------------------------------------------------------------------------------------------------------------------------------------------------------------------------------------------------------------------------------------------------------------------------------------------------------------------------------------------------------------------------------------------------------|:------------------------------------------------------------------------------------------|:-------------------------------------------------------------------------------------------------------------------------------------------------------------------------------------------------------------------|--------------------------------------------------------------:|
|
|
|
13 |
|Flux with CFG|[Flux with CFG](https://github.com/ToTheBeginning/PuLID/blob/main/docs/pulid_for_flux.md) provides an implementation of using CFG in [Flux](https://blackforestlabs.ai/announcing-black-forest-labs/).|[Flux with CFG](#flux-with-cfg)|NA|[Linoy Tsaban](https://github.com/linoytsaban), [Apolinário](https://github.com/apolinario), and [Sayak Paul](https://github.com/sayakpaul)|
|
14 |
|Differential Diffusion|[Differential Diffusion](https://github.com/exx8/differential-diffusion) modifies an image according to a text prompt, and according to a map that specifies the amount of change in each region.|[Differential Diffusion](#differential-diffusion)|[](https://huggingface.co/spaces/exx8/differential-diffusion) [](https://colab.research.google.com/github/exx8/differential-diffusion/blob/main/examples/SD2.ipynb)|[Eran Levin](https://github.com/exx8) and [Ohad Fried](https://www.ohadf.com/)|
|
15 |
| HD-Painter | [HD-Painter](https://github.com/Picsart-AI-Research/HD-Painter) enables prompt-faithfull and high resolution (up to 2k) image inpainting upon any diffusion-based image inpainting method. | [HD-Painter](#hd-painter) | [](https://huggingface.co/spaces/PAIR/HD-Painter) | [Manukyan Hayk](https://github.com/haikmanukyan) and [Sargsyan Andranik](https://github.com/AndranikSargsyan) |
|
@@ -73,6 +74,7 @@ Please also check out our [Community Scripts](https://github.com/huggingface/dif
|
|
73 |
| Stable Diffusion BoxDiff Pipeline | Training-free controlled generation with bounding boxes using [BoxDiff](https://github.com/showlab/BoxDiff) | [Stable Diffusion BoxDiff Pipeline](#stable-diffusion-boxdiff) | - | [Jingyang Zhang](https://github.com/zjysteven/) |
|
74 |
| FRESCO V2V Pipeline | Implementation of [[CVPR 2024] FRESCO: Spatial-Temporal Correspondence for Zero-Shot Video Translation](https://arxiv.org/abs/2403.12962) | [FRESCO V2V Pipeline](#fresco) | - | [Yifan Zhou](https://github.com/SingleZombie) |
|
75 |
| AnimateDiff IPEX Pipeline | Accelerate AnimateDiff inference pipeline with BF16/FP32 precision on Intel Xeon CPUs with [IPEX](https://github.com/intel/intel-extension-for-pytorch) | [AnimateDiff on IPEX](#animatediff-on-ipex) | - | [Dan Li](https://github.com/ustcuna/) |
|
|
|
76 |
| HunyuanDiT Differential Diffusion Pipeline | Applies [Differential Diffusion](https://github.com/exx8/differential-diffusion) to [HunyuanDiT](https://github.com/huggingface/diffusers/pull/8240). | [HunyuanDiT with Differential Diffusion](#hunyuandit-with-differential-diffusion) | [](https://colab.research.google.com/drive/1v44a5fpzyr4Ffr4v2XBQ7BajzG874N4P?usp=sharing) | [Monjoy Choudhury](https://github.com/MnCSSJ4x) |
|
77 |
| [🪆Matryoshka Diffusion Models](https://huggingface.co/papers/2310.15111) | A diffusion process that denoises inputs at multiple resolutions jointly and uses a NestedUNet architecture where features and parameters for small scale inputs are nested within those of the large scales. See [original codebase](https://github.com/apple/ml-mdm). | [🪆Matryoshka Diffusion Models](#matryoshka-diffusion-models) | [](https://huggingface.co/spaces/pcuenq/mdm) [](https://colab.research.google.com/gist/tolgacangoz/1f54875fc7aeaabcf284ebde64820966/matryoshka_hf.ipynb) | [M. Tolga Cangöz](https://github.com/tolgacangoz) |
|
78 |
|
@@ -84,6 +86,161 @@ pipe = DiffusionPipeline.from_pretrained("stable-diffusion-v1-5/stable-diffusion
|
|
84 |
|
85 |
## Example usages
|
86 |
|
|
|
|
|
|
|
|
|
|
|
|
|
|
|
|
|
|
|
|
|
|
|
|
|
|
|
|
|
|
|
|
|
|
|
|
|
|
|
|
|
|
|
|
|
|
|
|
|
|
|
|
|
|
|
|
|
|
|
|
|
|
|
|
|
|
|
|
|
|
|
|
|
|
|
|
|
|
|
|
|
|
|
|
|
|
|
|
|
|
|
|
|
|
|
|
|
|
|
|
|
|
|
|
|
|
|
|
|
|
|
|
|
|
|
|
|
|
|
|
|
|
|
|
|
|
|
|
|
|
|
|
|
|
|
|
|
|
|
|
|
|
|
|
|
|
|
|
|
|
|
|
|
|
|
|
|
|
|
|
|
|
|
|
|
|
|
|
|
|
|
|
|
|
|
|
|
|
|
|
|
|
|
|
|
|
|
|
|
|
|
|
|
|
|
|
|
|
|
|
|
|
|
|
|
|
|
|
|
|
|
|
|
|
|
|
|
|
|
|
|
|
|
|
|
|
|
|
|
|
|
|
|
|
|
|
|
|
|
|
|
|
|
|
|
|
|
|
|
|
|
|
|
|
|
|
|
|
|
|
|
|
|
|
|
|
|
|
|
|
|
|
|
|
|
|
|
|
|
|
|
|
|
|
|
|
|
|
|
|
|
|
|
|
|
|
|
|
|
|
|
|
|
|
|
|
|
|
|
|
|
87 |
### Flux with CFG
|
88 |
|
89 |
Know more about Flux [here](https://blackforestlabs.ai/announcing-black-forest-labs/). Since Flux doesn't use CFG, this implementation provides one, inspired by the [PuLID Flux adaptation](https://github.com/ToTheBeginning/PuLID/blob/main/docs/pulid_for_flux.md).
|
@@ -4445,3 +4602,94 @@ grid_image.save(grid_dir + "sample.png")
|
|
4445 |
`pag_scale` : guidance scale of PAG (ex: 5.0)
|
4446 |
|
4447 |
`pag_applied_layers_index` : index of the layer to apply perturbation (ex: ['m0'])
|
|
|
|
|
|
|
|
|
|
|
|
|
|
|
|
|
|
|
|
|
|
|
|
|
|
|
|
|
|
|
|
|
|
|
|
|
|
|
|
|
|
|
|
|
|
|
|
|
|
|
|
|
|
|
|
|
|
|
|
|
|
|
|
|
|
|
|
|
|
|
|
|
|
|
|
|
|
|
|
|
|
|
|
|
|
|
|
|
|
|
|
|
|
|
|
|
|
|
|
|
|
|
|
|
|
|
|
|
|
|
|
|
|
|
|
|
|
|
|
|
|
|
|
|
|
|
|
|
|
|
|
|
|
|
|
|
|
|
|
|
|
|
|
|
|
|
|
|
|
|
|
|
|
|
|
|
|
|
|
|
|
|
|
|
|
|
|
|
|
|
|
|
|
|
|
|
|
|
|
|
|
|
|
10 |
|
11 |
| Example | Description | Code Example | Colab | Author |
|
12 |
|:--------------------------------------------------------------------------------------------------------------------------------------|:---------------------------------------------------------------------------------------------------------------------------------------------------------------------------------------------------------------------------------------------------------------------------------------------------------------------------------------------------------------------------------------------------------------------------------------------------------------------------------------------------------|:------------------------------------------------------------------------------------------|:-------------------------------------------------------------------------------------------------------------------------------------------------------------------------------------------------------------------|--------------------------------------------------------------:|
|
13 |
+
|Adaptive Mask Inpainting|Adaptive Mask Inpainting algorithm from [Beyond the Contact: Discovering Comprehensive Affordance for 3D Objects from Pre-trained 2D Diffusion Models](https://github.com/snuvclab/coma) (ECCV '24, Oral) provides a way to insert human inside the scene image without altering the background, by inpainting with adapting mask.|[Adaptive Mask Inpainting](#adaptive-mask-inpainting)|-|[Hyeonwoo Kim](https://sshowbiz.xyz),[Sookwan Han](https://jellyheadandrew.github.io)|
|
14 |
|Flux with CFG|[Flux with CFG](https://github.com/ToTheBeginning/PuLID/blob/main/docs/pulid_for_flux.md) provides an implementation of using CFG in [Flux](https://blackforestlabs.ai/announcing-black-forest-labs/).|[Flux with CFG](#flux-with-cfg)|NA|[Linoy Tsaban](https://github.com/linoytsaban), [Apolinário](https://github.com/apolinario), and [Sayak Paul](https://github.com/sayakpaul)|
|
15 |
|Differential Diffusion|[Differential Diffusion](https://github.com/exx8/differential-diffusion) modifies an image according to a text prompt, and according to a map that specifies the amount of change in each region.|[Differential Diffusion](#differential-diffusion)|[](https://huggingface.co/spaces/exx8/differential-diffusion) [](https://colab.research.google.com/github/exx8/differential-diffusion/blob/main/examples/SD2.ipynb)|[Eran Levin](https://github.com/exx8) and [Ohad Fried](https://www.ohadf.com/)|
|
16 |
| HD-Painter | [HD-Painter](https://github.com/Picsart-AI-Research/HD-Painter) enables prompt-faithfull and high resolution (up to 2k) image inpainting upon any diffusion-based image inpainting method. | [HD-Painter](#hd-painter) | [](https://huggingface.co/spaces/PAIR/HD-Painter) | [Manukyan Hayk](https://github.com/haikmanukyan) and [Sargsyan Andranik](https://github.com/AndranikSargsyan) |
|
|
|
74 |
| Stable Diffusion BoxDiff Pipeline | Training-free controlled generation with bounding boxes using [BoxDiff](https://github.com/showlab/BoxDiff) | [Stable Diffusion BoxDiff Pipeline](#stable-diffusion-boxdiff) | - | [Jingyang Zhang](https://github.com/zjysteven/) |
|
75 |
| FRESCO V2V Pipeline | Implementation of [[CVPR 2024] FRESCO: Spatial-Temporal Correspondence for Zero-Shot Video Translation](https://arxiv.org/abs/2403.12962) | [FRESCO V2V Pipeline](#fresco) | - | [Yifan Zhou](https://github.com/SingleZombie) |
|
76 |
| AnimateDiff IPEX Pipeline | Accelerate AnimateDiff inference pipeline with BF16/FP32 precision on Intel Xeon CPUs with [IPEX](https://github.com/intel/intel-extension-for-pytorch) | [AnimateDiff on IPEX](#animatediff-on-ipex) | - | [Dan Li](https://github.com/ustcuna/) |
|
77 |
+
PIXART-α Controlnet pipeline | Implementation of the controlnet model for pixart alpha and its diffusers pipeline | [PIXART-α Controlnet pipeline](#pixart-α-controlnet-pipeline) | - | [Raul Ciotescu](https://github.com/raulc0399/) |
|
78 |
| HunyuanDiT Differential Diffusion Pipeline | Applies [Differential Diffusion](https://github.com/exx8/differential-diffusion) to [HunyuanDiT](https://github.com/huggingface/diffusers/pull/8240). | [HunyuanDiT with Differential Diffusion](#hunyuandit-with-differential-diffusion) | [](https://colab.research.google.com/drive/1v44a5fpzyr4Ffr4v2XBQ7BajzG874N4P?usp=sharing) | [Monjoy Choudhury](https://github.com/MnCSSJ4x) |
|
79 |
| [🪆Matryoshka Diffusion Models](https://huggingface.co/papers/2310.15111) | A diffusion process that denoises inputs at multiple resolutions jointly and uses a NestedUNet architecture where features and parameters for small scale inputs are nested within those of the large scales. See [original codebase](https://github.com/apple/ml-mdm). | [🪆Matryoshka Diffusion Models](#matryoshka-diffusion-models) | [](https://huggingface.co/spaces/pcuenq/mdm) [](https://colab.research.google.com/gist/tolgacangoz/1f54875fc7aeaabcf284ebde64820966/matryoshka_hf.ipynb) | [M. Tolga Cangöz](https://github.com/tolgacangoz) |
|
80 |
|
|
|
86 |
|
87 |
## Example usages
|
88 |
|
89 |
+
### Adaptive Mask Inpainting
|
90 |
+
|
91 |
+
**Hyeonwoo Kim\*, Sookwan Han\*, Patrick Kwon, Hanbyul Joo**
|
92 |
+
|
93 |
+
**Seoul National University, Naver Webtoon**
|
94 |
+
|
95 |
+
Adaptive Mask Inpainting, presented in the ECCV'24 oral paper [*Beyond the Contact: Discovering Comprehensive Affordance for 3D Objects from Pre-trained 2D Diffusion Models*](https://snuvclab.github.io/coma), is an algorithm designed to insert humans into scene images without altering the background. Traditional inpainting methods often fail to preserve object geometry and details within the masked region, leading to false affordances. Adaptive Mask Inpainting addresses this issue by progressively specifying the inpainting region over diffusion timesteps, ensuring that the inserted human integrates seamlessly with the existing scene.
|
96 |
+
|
97 |
+
Here is the demonstration of Adaptive Mask Inpainting:
|
98 |
+
|
99 |
+
<video controls>
|
100 |
+
<source src="https://snuvclab.github.io/coma/static/videos/adaptive_mask_inpainting_vis.mp4" type="video/mp4">
|
101 |
+
Your browser does not support the video tag.
|
102 |
+
</video>
|
103 |
+
|
104 |
+
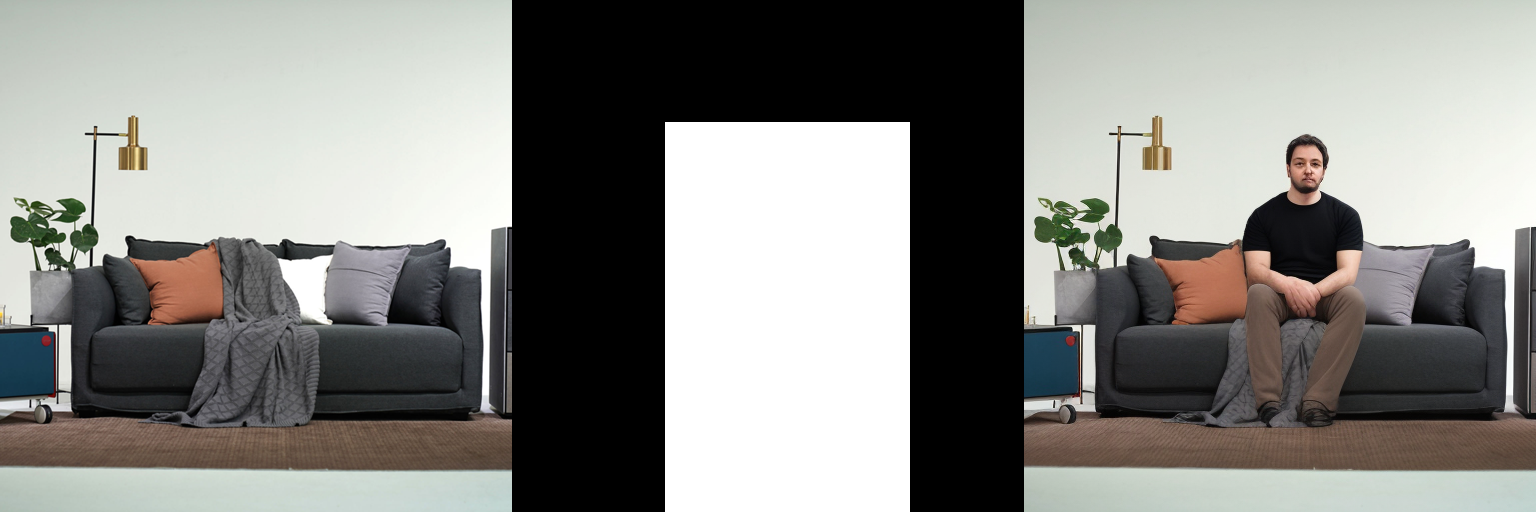
|
105 |
+
|
106 |
+
|
107 |
+
You can find additional information about Adaptive Mask Inpainting in the [paper](https://arxiv.org/pdf/2401.12978) or in the [project website](https://snuvclab.github.io/coma).
|
108 |
+
|
109 |
+
#### Usage example
|
110 |
+
First, clone the diffusers github repository, and run the following command to set environment.
|
111 |
+
```Shell
|
112 |
+
git clone https://github.com/huggingface/diffusers.git
|
113 |
+
cd diffusers
|
114 |
+
|
115 |
+
conda create --name ami python=3.9 -y
|
116 |
+
conda activate ami
|
117 |
+
|
118 |
+
conda install pytorch==1.10.1 torchvision==0.11.2 torchaudio==0.10.1 cudatoolkit=11.3 -c pytorch -c conda-forge -y
|
119 |
+
python -m pip install detectron2==0.6 -f https://dl.fbaipublicfiles.com/detectron2/wheels/cu113/torch1.10/index.html
|
120 |
+
pip install easydict
|
121 |
+
pip install diffusers==0.20.2 accelerate safetensors transformers
|
122 |
+
pip install setuptools==59.5.0
|
123 |
+
pip install opencv-python
|
124 |
+
pip install numpy==1.24.1
|
125 |
+
```
|
126 |
+
Then, run the below code under 'diffusers' directory.
|
127 |
+
```python
|
128 |
+
import numpy as np
|
129 |
+
import torch
|
130 |
+
from PIL import Image
|
131 |
+
|
132 |
+
from diffusers import DDIMScheduler
|
133 |
+
from diffusers import DiffusionPipeline
|
134 |
+
from diffusers.utils import load_image
|
135 |
+
|
136 |
+
from examples.community.adaptive_mask_inpainting import download_file, AdaptiveMaskInpaintPipeline, AMI_INSTALL_MESSAGE
|
137 |
+
|
138 |
+
print(AMI_INSTALL_MESSAGE)
|
139 |
+
|
140 |
+
from easydict import EasyDict
|
141 |
+
|
142 |
+
|
143 |
+
|
144 |
+
if __name__ == "__main__":
|
145 |
+
"""
|
146 |
+
Download Necessary Files
|
147 |
+
"""
|
148 |
+
download_file(
|
149 |
+
url = "https://huggingface.co/datasets/jellyheadnadrew/adaptive-mask-inpainting-test-images/resolve/main/model_final_edd263.pkl?download=true",
|
150 |
+
output_file = "model_final_edd263.pkl",
|
151 |
+
exist_ok=True,
|
152 |
+
)
|
153 |
+
download_file(
|
154 |
+
url = "https://huggingface.co/datasets/jellyheadnadrew/adaptive-mask-inpainting-test-images/resolve/main/pointrend_rcnn_R_50_FPN_3x_coco.yaml?download=true",
|
155 |
+
output_file = "pointrend_rcnn_R_50_FPN_3x_coco.yaml",
|
156 |
+
exist_ok=True,
|
157 |
+
)
|
158 |
+
download_file(
|
159 |
+
url = "https://huggingface.co/datasets/jellyheadnadrew/adaptive-mask-inpainting-test-images/resolve/main/input_img.png?download=true",
|
160 |
+
output_file = "input_img.png",
|
161 |
+
exist_ok=True,
|
162 |
+
)
|
163 |
+
download_file(
|
164 |
+
url = "https://huggingface.co/datasets/jellyheadnadrew/adaptive-mask-inpainting-test-images/resolve/main/input_mask.png?download=true",
|
165 |
+
output_file = "input_mask.png",
|
166 |
+
exist_ok=True,
|
167 |
+
)
|
168 |
+
download_file(
|
169 |
+
url = "https://huggingface.co/datasets/jellyheadnadrew/adaptive-mask-inpainting-test-images/resolve/main/Base-PointRend-RCNN-FPN.yaml?download=true",
|
170 |
+
output_file = "Base-PointRend-RCNN-FPN.yaml",
|
171 |
+
exist_ok=True,
|
172 |
+
)
|
173 |
+
download_file(
|
174 |
+
url = "https://huggingface.co/datasets/jellyheadnadrew/adaptive-mask-inpainting-test-images/resolve/main/Base-RCNN-FPN.yaml?download=true",
|
175 |
+
output_file = "Base-RCNN-FPN.yaml",
|
176 |
+
exist_ok=True,
|
177 |
+
)
|
178 |
+
|
179 |
+
"""
|
180 |
+
Prepare Adaptive Mask Inpainting Pipeline
|
181 |
+
"""
|
182 |
+
# device
|
183 |
+
device = torch.device("cuda") if torch.cuda.is_available() else torch.device("cpu")
|
184 |
+
num_steps = 50
|
185 |
+
|
186 |
+
# Scheduler
|
187 |
+
scheduler = DDIMScheduler(
|
188 |
+
beta_start=0.00085,
|
189 |
+
beta_end=0.012,
|
190 |
+
beta_schedule="scaled_linear",
|
191 |
+
clip_sample=False,
|
192 |
+
set_alpha_to_one=False
|
193 |
+
)
|
194 |
+
scheduler.set_timesteps(num_inference_steps=num_steps)
|
195 |
+
|
196 |
+
## load models as pipelines
|
197 |
+
pipeline = AdaptiveMaskInpaintPipeline.from_pretrained(
|
198 |
+
"Uminosachi/realisticVisionV51_v51VAE-inpainting",
|
199 |
+
scheduler=scheduler,
|
200 |
+
torch_dtype=torch.float16,
|
201 |
+
requires_safety_checker=False
|
202 |
+
).to(device)
|
203 |
+
|
204 |
+
## disable safety checker
|
205 |
+
enable_safety_checker = False
|
206 |
+
if not enable_safety_checker:
|
207 |
+
pipeline.safety_checker = None
|
208 |
+
|
209 |
+
"""
|
210 |
+
Run Adaptive Mask Inpainting
|
211 |
+
"""
|
212 |
+
default_mask_image = Image.open("./input_mask.png").convert("L")
|
213 |
+
init_image = Image.open("./input_img.png").convert("RGB")
|
214 |
+
|
215 |
+
|
216 |
+
seed = 59
|
217 |
+
generator = torch.Generator(device=device)
|
218 |
+
generator.manual_seed(seed)
|
219 |
+
|
220 |
+
image = pipeline(
|
221 |
+
prompt="a man sitting on a couch",
|
222 |
+
negative_prompt="worst quality, normal quality, low quality, bad anatomy, artifacts, blurry, cropped, watermark, greyscale, nsfw",
|
223 |
+
image=init_image,
|
224 |
+
default_mask_image=default_mask_image,
|
225 |
+
guidance_scale=11.0,
|
226 |
+
strength=0.98,
|
227 |
+
use_adaptive_mask=True,
|
228 |
+
generator=generator,
|
229 |
+
enforce_full_mask_ratio=0.0,
|
230 |
+
visualization_save_dir="./ECCV2024_adaptive_mask_inpainting_demo", # DON'T CHANGE THIS!!!
|
231 |
+
human_detection_thres=0.015,
|
232 |
+
).images[0]
|
233 |
+
|
234 |
+
|
235 |
+
image.save(f'final_img.png')
|
236 |
+
```
|
237 |
+
#### [Troubleshooting]
|
238 |
+
|
239 |
+
If you run into an error `cannot import name 'cached_download' from 'huggingface_hub'` (issue [1851](https://github.com/easydiffusion/easydiffusion/issues/1851)), remove `cached_download` from the import line in the file `diffusers/utils/dynamic_modules_utils.py`.
|
240 |
+
|
241 |
+
For example, change the import line from `.../env/lib/python3.8/site-packages/diffusers/utils/dynamic_modules_utils.py`.
|
242 |
+
|
243 |
+
|
244 |
### Flux with CFG
|
245 |
|
246 |
Know more about Flux [here](https://blackforestlabs.ai/announcing-black-forest-labs/). Since Flux doesn't use CFG, this implementation provides one, inspired by the [PuLID Flux adaptation](https://github.com/ToTheBeginning/PuLID/blob/main/docs/pulid_for_flux.md).
|
|
|
4602 |
`pag_scale` : guidance scale of PAG (ex: 5.0)
|
4603 |
|
4604 |
`pag_applied_layers_index` : index of the layer to apply perturbation (ex: ['m0'])
|
4605 |
+
|
4606 |
+
# PIXART-α Controlnet pipeline
|
4607 |
+
|
4608 |
+
[Project](https://pixart-alpha.github.io/) / [GitHub](https://github.com/PixArt-alpha/PixArt-alpha/blob/master/asset/docs/pixart_controlnet.md)
|
4609 |
+
|
4610 |
+
This the implementation of the controlnet model and the pipelne for the Pixart-alpha model, adapted to use the HuggingFace Diffusers.
|
4611 |
+
|
4612 |
+
## Example Usage
|
4613 |
+
|
4614 |
+
This example uses the Pixart HED Controlnet model, converted from the control net model as trained by the authors of the paper.
|
4615 |
+
|
4616 |
+
```py
|
4617 |
+
import sys
|
4618 |
+
import os
|
4619 |
+
import torch
|
4620 |
+
import torchvision.transforms as T
|
4621 |
+
import torchvision.transforms.functional as TF
|
4622 |
+
|
4623 |
+
from pipeline_pixart_alpha_controlnet import PixArtAlphaControlnetPipeline
|
4624 |
+
from diffusers.utils import load_image
|
4625 |
+
|
4626 |
+
from diffusers.image_processor import PixArtImageProcessor
|
4627 |
+
|
4628 |
+
from controlnet_aux import HEDdetector
|
4629 |
+
|
4630 |
+
sys.path.append(os.path.dirname(os.path.dirname(os.path.abspath(__file__))))
|
4631 |
+
from pixart.controlnet_pixart_alpha import PixArtControlNetAdapterModel
|
4632 |
+
|
4633 |
+
controlnet_repo_id = "raulc0399/pixart-alpha-hed-controlnet"
|
4634 |
+
|
4635 |
+
weight_dtype = torch.float16
|
4636 |
+
image_size = 1024
|
4637 |
+
|
4638 |
+
device = torch.device("cuda" if torch.cuda.is_available() else "cpu")
|
4639 |
+
|
4640 |
+
torch.manual_seed(0)
|
4641 |
+
|
4642 |
+
# load controlnet
|
4643 |
+
controlnet = PixArtControlNetAdapterModel.from_pretrained(
|
4644 |
+
controlnet_repo_id,
|
4645 |
+
torch_dtype=weight_dtype,
|
4646 |
+
use_safetensors=True,
|
4647 |
+
).to(device)
|
4648 |
+
|
4649 |
+
pipe = PixArtAlphaControlnetPipeline.from_pretrained(
|
4650 |
+
"PixArt-alpha/PixArt-XL-2-1024-MS",
|
4651 |
+
controlnet=controlnet,
|
4652 |
+
torch_dtype=weight_dtype,
|
4653 |
+
use_safetensors=True,
|
4654 |
+
).to(device)
|
4655 |
+
|
4656 |
+
images_path = "images"
|
4657 |
+
control_image_file = "0_7.jpg"
|
4658 |
+
|
4659 |
+
prompt = "battleship in space, galaxy in background"
|
4660 |
+
|
4661 |
+
control_image_name = control_image_file.split('.')[0]
|
4662 |
+
|
4663 |
+
control_image = load_image(f"{images_path}/{control_image_file}")
|
4664 |
+
print(control_image.size)
|
4665 |
+
height, width = control_image.size
|
4666 |
+
|
4667 |
+
hed = HEDdetector.from_pretrained("lllyasviel/Annotators")
|
4668 |
+
|
4669 |
+
condition_transform = T.Compose([
|
4670 |
+
T.Lambda(lambda img: img.convert('RGB')),
|
4671 |
+
T.CenterCrop([image_size, image_size]),
|
4672 |
+
])
|
4673 |
+
|
4674 |
+
control_image = condition_transform(control_image)
|
4675 |
+
hed_edge = hed(control_image, detect_resolution=image_size, image_resolution=image_size)
|
4676 |
+
|
4677 |
+
hed_edge.save(f"{images_path}/{control_image_name}_hed.jpg")
|
4678 |
+
|
4679 |
+
# run pipeline
|
4680 |
+
with torch.no_grad():
|
4681 |
+
out = pipe(
|
4682 |
+
prompt=prompt,
|
4683 |
+
image=hed_edge,
|
4684 |
+
num_inference_steps=14,
|
4685 |
+
guidance_scale=4.5,
|
4686 |
+
height=image_size,
|
4687 |
+
width=image_size,
|
4688 |
+
)
|
4689 |
+
|
4690 |
+
out.images[0].save(f"{images_path}//{control_image_name}_output.jpg")
|
4691 |
+
|
4692 |
+
```
|
4693 |
+
|
4694 |
+
In the folder examples/pixart there is also a script that can be used to train new models.
|
4695 |
+
Please check the script `train_controlnet_hf_diffusers.sh` on how to start the training.
|
main/adaptive_mask_inpainting.py
ADDED
@@ -0,0 +1,1465 @@
|
|
|
|
|
|
|
|
|
|
|
|
|
|
|
|
|
|
|
|
|
|
|
|
|
|
|
|
|
|
|
|
|
|
|
|
|
|
|
|
|
|
|
|
|
|
|
|
|
|
|
|
|
|
|
|
|
|
|
|
|
|
|
|
|
|
|
|
|
|
|
|
|
|
|
|
|
|
|
|
|
|
|
|
|
|
|
|
|
|
|
|
|
|
|
|
|
|
|
|
|
|
|
|
|
|
|
|
|
|
|
|
|
|
|
|
|
|
|
|
|
|
|
|
|
|
|
|
|
|
|
|
|
|
|
|
|
|
|
|
|
|
|
|
|
|
|
|
|
|
|
|
|
|
|
|
|
|
|
|
|
|
|
|
|
|
|
|
|
|
|
|
|
|
|
|
|
|
|
|
|
|
|
|
|
|
|
|
|
|
|
|
|
|
|
|
|
|
|
|
|
|
|
|
|
|
|
|
|
|
|
|
|
|
|
|
|
|
|
|
|
|
|
|
|
|
|
|
|
|
|
|
|
|
|
|
|
|
|
|
|
|
|
|
|
|
|
|
|
|
|
|
|
|
|
|
|
|
|
|
|
|
|
|
|
|
|
|
|
|
|
|
|
|
|
|
|
|
|
|
|
|
|
|
|
|
|
|
|
|
|
|
|
|
|
|
|
|
|
|
|
|
|
|
|
|
|
|
|
|
|
|
|
|
|
|
|
|
|
|
|
|
|
|
|
|
|
|
|
|
|
|
|
|
|
|
|
|
|
|
|
|
|
|
|
|
|
|
|
|
|
|
|
|
|
|
|
|
|
|
|
|
|
|
|
|
|
|
|
|
|
|
|
|
|
|
|
|
|
|
|
|
|
|
|
|
|
|
|
|
|
|
|
|
|
|
|
|
|
|
|
|
|
|
|
|
|
|
|
|
|
|
|
|
|
|
|
|
|
|
|
|
|
|
|
|
|
|
|
|
|
|
|
|
|
|
|
|
|
|
|
|
|
|
|
|
|
|
|
|
|
|
|
|
|
|
|
|
|
|
|
|
|
|
|
|
|
|
|
|
|
|
|
|
|
|
|
|
|
|
|
|
|
|
|
|
|
|
|
|
|
|
|
|
|
|
|
|
|
|
|
|
|
|
|
|
|
|
|
|
|
|
|
|
|
|
|
|
|
|
|
|
|
|
|
|
|
|
|
|
|
|
|
|
|
|
|
|
|
|
|
|
|
|
|
|
|
|
|
|
|
|
|
|
|
|
|
|
|
|
|
|
|
|
|
|
|
|
|
|
|
|
|
|
|
|
|
|
|
|
|
|
|
|
|
|
|
|
|
|
|
|
|
|
|
|
|
|
|
|
|
|
|
|
|
|
|
|
|
|
|
|
|
|
|
|
|
|
|
|
|
|
|
|
|
|
|
|
|
|
|
|
|
|
|
|
|
|
|
|
|
|
|
|
|
|
|
|
|
|
|
|
|
|
|
|
|
|
|
|
|
|
|
|
|
|
|
|
|
|
|
|
|
|
|
|
|
|
|
|
|
|
|
|
|
|
|
|
|
|
|
|
|
|
|
|
|
|
|
|
|
|
|
|
|
|
|
|
|
|
|
|
|
|
|
|
|
|
|
|
|
|
|
|
|
|
|
|
|
|
|
|
|
|
|
|
|
|
|
|
|
|
|
|
|
|
|
|
|
|
|
|
|
|
|
|
|
|
|
|
|
|
|
|
|
|
|
|
|
|
|
|
|
|
|
|
|
|
|
|
|
|
|
|
|
|
|
|
|
|
|
|
|
|
|
|
|
|
|
|
|
|
|
|
|
|
|
|
|
|
|
|
|
|
|
|
|
|
|
|
|
|
|
|
|
|
|
|
|
|
|
|
|
|
|
|
|
|
|
|
|
|
|
|
|
|
|
|
|
|
|
|
|
|
|
|
|
|
|
|
|
|
|
|
|
|
|
|
|
|
|
|
|
|
|
|
|
|
|
|
|
|
|
|
|
|
|
|
|
|
|
|
|
|
|
|
|
|
|
|
|
|
|
|
|
|
|
|
|
|
|
|
|
|
|
|
|
|
|
|
|
|
|
|
|
|
|
|
|
|
|
|
|
|
|
|
|
|
|
|
|
|
|
|
|
|
|
|
|
|
|
|
|
|
|
|
|
|
|
|
|
|
|
|
|
|
|
|
|
|
|
|
|
|
|
|
|
|
|
|
|
|
|
|
|
|
|
|
|
|
|
|
|
|
|
|
|
|
|
|
|
|
|
|
|
|
|
|
|
|
|
|
|
|
|
|
|
|
|
|
|
|
|
|
|
|
|
|
|
|
|
|
|
|
|
|
|
|
|
|
|
|
|
|
|
|
|
|
|
|
|
|
|
|
|
|
|
|
|
|
|
|
|
|
|
|
|
|
|
|
|
|
|
|
|
|
|
|
|
|
|
|
|
|
|
|
|
|
|
|
|
|
|
|
|
|
|
|
|
|
|
|
|
|
|
|
|
|
|
|
|
|
|
|
|
|
|
|
|
|
|
|
|
|
|
|
|
|
|
|
|
|
|
|
|
|
|
|
|
|
|
|
|
|
|
|
|
|
|
|
|
|
|
|
|
|
|
|
|
|
|
|
|
|
|
|
|
|
|
|
|
|
|
|
|
|
|
|
|
|
|
|
|
|
|
|
|
|
|
|
|
|
|
|
|
|
|
|
|
|
|
|
|
|
|
|
|
|
|
|
|
|
|
|
|
|
|
|
|
|
|
|
|
|
|
|
|
|
|
|
|
|
|
|
|
|
|
|
|
|
|
|
|
|
|
|
|
|
|
|
|
|
|
|
|
|
|
|
|
|
|
|
|
|
|
|
|
|
|
|
|
|
|
|
|
|
|
|
|
|
|
|
|
|
|
|
|
|
|
|
|
|
|
|
|
|
|
|
|
|
|
|
|
|
|
|
|
|
|
|
|
|
|
|
|
|
|
|
|
|
|
|
|
|
|
|
|
|
|
|
|
|
|
|
|
|
|
|
|
|
|
|
|
|
|
|
|
|
|
|
|
|
|
|
|
|
|
|
|
|
|
|
|
|
|
|
|
|
|
|
|
|
|
|
|
|
|
|
|
|
|
|
|
|
|
|
|
|
|
|
|
|
|
|
|
|
|
|
|
|
|
|
|
|
|
|
|
|
|
|
|
|
|
|
|
|
|
|
|
|
|
|
|
|
|
|
|
|
|
|
|
|
|
|
|
|
|
|
|
|
|
|
|
|
|
|
|
|
|
|
|
|
|
|
|
|
|
|
|
|
|
|
|
|
|
|
|
|
|
|
|
|
|
|
|
|
|
|
|
|
|
|
|
|
|
|
|
|
|
|
|
|
|
|
|
|
|
|
|
|
|
|
|
|
|
|
|
|
|
|
|
|
|
|
|
|
|
|
|
|
|
|
|
|
|
|
|
|
|
|
|
|
|
|
|
|
|
|
|
|
|
|
|
|
|
|
|
|
|
|
|
|
|
|
|
|
|
|
|
|
|
|
|
|
|
|
|
|
|
|
|
|
|
|
|
|
|
|
|
|
|
|
|
|
|
|
|
|
|
|
|
|
|
|
|
|
|
|
|
|
|
|
|
|
|
|
|
|
|
|
|
|
|
|
|
|
|
|
|
|
|
|
|
|
|
|
|
|
|
|
|
|
|
|
|
|
|
|
|
|
|
|
|
|
|
|
|
|
|
|
|
|
|
|
|
|
|
|
|
|
|
|
|
|
|
|
|
|
|
|
|
|
|
|
|
|
|
|
|
|
|
|
|
|
|
|
|
|
|
|
|
|
|
|
|
|
|
|
|
|
|
|
|
|
|
|
|
|
|
|
|
|
|
|
|
|
|
|
|
|
|
|
|
|
|
|
|
|
|
|
|
|
|
|
|
|
|
|
|
|
|
|
|
|
|
|
|
|
|
|
|
|
|
|
|
|
|
|
|
|
|
|
|
|
|
|
|
|
|
|
|
|
|
|
|
|
|
|
|
|
|
|
|
|
|
|
|
|
|
|
|
|
|
|
|
|
|
|
|
|
|
|
|
|
|
|
|
|
|
|
|
|
|
|
|
|
|
|
|
|
|
|
|
|
|
|
|
|
|
|
|
|
|
|
|
|
|
|
|
|
|
|
|
|
|
|
|
|
|
|
|
|
|
|
|
|
|
|
|
|
|
|
|
|
|
|
|
|
|
|
|
|
|
|
|
|
|
|
|
|
|
|
|
|
|
|
|
|
|
|
|
|
|
|
|
|
|
|
|
|
|
|
|
|
|
|
|
|
|
|
|
|
|
|
|
|
|
|
|
|
|
|
|
|
|
|
|
|
|
|
|
|
|
|
|
|
|
|
|
|
|
|
|
|
|
|
|
|
|
|
|
|
|
|
|
|
|
|
|
|
|
|
|
|
|
|
|
|
|
|
|
|
|
|
|
|
|
|
|
|
|
|
|
|
|
|
|
|
|
|
|
|
|
|
|
|
|
|
|
|
|
|
|
|
|
|
|
|
|
|
|
|
|
|
|
|
|
|
|
|
|
|
|
|
|
|
|
|
|
|
|
|
|
|
|
|
|
|
|
|
|
|
|
|
|
|
|
|
|
|
|
|
|
|
|
|
|
|
|
|
|
|
|
|
|
|
|
|
|
|
|
|
|
|
|
|
|
|
|
|
|
|
|
|
|
|
|
|
|
|
|
|
|
|
|
|
|
|
|
|
|
|
|
|
|
|
|
|
|
|
|
|
|
|
|
|
|
|
|
|
|
|
|
|
|
|
|
|
|
|
|
|
|
|
|
|
|
|
|
|
|
|
|
|
|
|
|
|
|
|
|
|
|
|
|
|
|
|
|
|
|
|
|
|
|
|
|
|
|
|
|
|
|
|
|
|
|
|
|
|
|
|
|
|
|
|
|
|
|
|
|
|
|
|
|
|
|
|
|
|
|
|
|
|
|
|
|
|
|
|
|
|
|
|
|
|
|
|
|
|
|
|
|
|
|
|
|
|
|
|
|
|
|
|
|
|
|
|
|
|
|
|
|
|
|
|
|
|
|
|
|
|
|
|
|
|
|
|
|
|
|
|
|
|
|
|
|
|
|
|
|
|
|
|
|
|
|
|
|
|
|
|
|
|
|
|
|
|
|
|
|
|
|
|
|
|
|
|
|
|
|
|
|
|
|
|
|
|
|
|
|
|
|
|
|
|
|
|
|
|
|
|
|
|
|
|
|
|
|
|
|
|
|
|
|
|
|
|
|
|
|
|
|
|
|
|
|
|
|
|
|
|
|
|
|
|
|
|
|
|
|
|
|
|
|
|
|
|
|
|
|
|
|
|
|
|
|
|
|
|
|
|
|
|
|
|
|
|
|
|
|
|
|
|
|
|
|
|
|
|
|
|
|
|
|
|
|
|
|
|
|
|
|
|
|
|
|
|
|
|
|
|
|
|
|
|
|
|
|
|
|
|
|
|
|
|
|
|
|
|
|
|
|
|
|
|
|
|
|
|
|
|
|
|
|
|
|
|
|
|
|
|
|
|
|
|
|
|
|
|
|
|
|
|
|
|
|
|
|
|
|
|
|
|
|
|
|
|
|
|
|
|
|
|
|
|
|
|
|
|
|
|
|
|
|
|
|
|
|
|
|
|
|
|
|
|
|
|
|
|
|
|
|
|
|
|
|
|
|
|
|
|
|
|
|
|
|
|
|
|
|
|
|
|
|
|
|
|
|
|
|
|
|
|
|
|
|
|
|
|
|
|
|
|
|
|
|
|
|
|
|
|
|
|
|
|
|
|
|
|
|
|
|
|
|
|
|
|
|
|
|
|
|
|
|
|
|
|
|
|
|
|
|
|
|
|
|
|
|
|
|
|
|
|
|
|
|
|
|
|
|
|
|
|
|
|
|
|
|
|
|
|
|
|
|
|
|
|
|
|
|
|
|
|
|
|
|
|
|
|
|
|
|
|
|
|
|
|
|
|
|
|
|
|
|
|
|
|
|
|
|
|
|
|
|
|
|
|
|
|
|
|
|
|
|
|
|
|
|
|
|
|
|
|
|
|
|
|
|
|
|
|
|
|
|
|
|
|
|
|
|
|
|
|
|
|
|
|
|
|
|
|
|
|
|
|
|
|
|
|
|
|
|
|
|
|
|
|
|
|
|
|
|
|
|
|
|
|
|
|
|
|
|
|
|
|
|
|
|
|
|
|
|
|
|
|
|
|
|
|
|
|
|
|
|
|
|
|
|
|
|
|
|
|
|
|
|
|
|
|
|
|
|
|
|
|
|
|
|
|
|
|
|
|
|
|
|
|
|
|
|
|
|
|
|
|
|
|
|
|
|
|
|
|
|
|
|
|
|
|
|
|
|
|
|
|
|
|
|
|
|
|
|
|
|
|
|
|
|
|
|
|
|
|
|
|
|
|
|
|
|
|
|
|
|
|
|
|
|
|
|
|
|
|
|
|
|
|
|
|
|
|
|
|
|
|
|
|
|
|
|
|
|
|
|
|
|
|
|
|
|
|
|
|
|
|
|
|
|
|
|
|
|
|
|
|
|
|
|
|
|
|
|
|
|
|
|
|
|
|
|
|
|
1 |
+
# Copyright 2023 The HuggingFace Team. All rights reserved.
|
2 |
+
#
|
3 |
+
# Licensed under the Apache License, Version 2.0 (the "License");
|
4 |
+
# you may not use this file except in compliance with the License.
|
5 |
+
# You may obtain a copy of the License at
|
6 |
+
#
|
7 |
+
# http://www.apache.org/licenses/LICENSE-2.0
|
8 |
+
#
|
9 |
+
# Unless required by applicable law or agreed to in writing, software
|
10 |
+
# distributed under the License is distributed on an "AS IS" BASIS,
|
11 |
+
# WITHOUT WARRANTIES OR CONDITIONS OF ANY KIND, either express or implied.
|
12 |
+
# See the License for the specific language governing permissions and
|
13 |
+
# limitations under the License.
|
14 |
+
|
15 |
+
# This model implementation is heavily inspired by https://github.com/haofanwang/ControlNet-for-Diffusers/
|
16 |
+
|
17 |
+
import inspect
|
18 |
+
import os
|
19 |
+
import shutil
|
20 |
+
from glob import glob
|
21 |
+
from typing import Any, Callable, Dict, List, Optional, Union
|
22 |
+
|
23 |
+
import cv2
|
24 |
+
import numpy as np
|
25 |
+
import PIL.Image
|
26 |
+
import requests
|
27 |
+
import torch
|
28 |
+
from detectron2.config import get_cfg
|
29 |
+
from detectron2.data import MetadataCatalog
|
30 |
+
from detectron2.engine import DefaultPredictor
|
31 |
+
from detectron2.projects import point_rend
|
32 |
+
from detectron2.structures.instances import Instances
|
33 |
+
from detectron2.utils.visualizer import ColorMode, Visualizer
|
34 |
+
from packaging import version
|
35 |
+
from tqdm import tqdm
|
36 |
+
from transformers import CLIPImageProcessor, CLIPTextModel, CLIPTokenizer
|
37 |
+
|
38 |
+
from diffusers.configuration_utils import FrozenDict
|
39 |
+
from diffusers.image_processor import VaeImageProcessor
|
40 |
+
from diffusers.loaders import FromSingleFileMixin, LoraLoaderMixin, TextualInversionLoaderMixin
|
41 |
+
from diffusers.models import AsymmetricAutoencoderKL, AutoencoderKL, UNet2DConditionModel
|
42 |
+
from diffusers.pipelines.pipeline_utils import DiffusionPipeline
|
43 |
+
from diffusers.pipelines.stable_diffusion import StableDiffusionPipelineOutput
|
44 |
+
from diffusers.schedulers import KarrasDiffusionSchedulers
|
45 |
+
from diffusers.utils import (
|
46 |
+
deprecate,
|
47 |
+
is_accelerate_available,
|
48 |
+
is_accelerate_version,
|
49 |
+
logging,
|
50 |
+
randn_tensor,
|
51 |
+
)
|
52 |
+
|
53 |
+
|
54 |
+
logger = logging.get_logger(__name__) # pylint: disable=invalid-name
|
55 |
+
|
56 |
+
|
57 |
+
AMI_INSTALL_MESSAGE = """
|
58 |
+
|
59 |
+
Example Demo of Adaptive Mask Inpainting
|
60 |
+
|
61 |
+
Beyond the Contact: Discovering Comprehensive Affordance for 3D Objects from Pre-trained 2D Diffusion Models
|
62 |
+
Kim et al.
|
63 |
+
ECCV-2024 (Oral)
|
64 |
+
|
65 |
+
|
66 |
+
Please prepare the environment via
|
67 |
+
|
68 |
+
```
|
69 |
+
conda create --name ami python=3.9 -y
|
70 |
+
conda activate ami
|
71 |
+
|
72 |
+
conda install pytorch==1.10.1 torchvision==0.11.2 torchaudio==0.10.1 cudatoolkit=11.3 -c pytorch -c conda-forge -y
|
73 |
+
python -m pip install detectron2==0.6 -f https://dl.fbaipublicfiles.com/detectron2/wheels/cu113/torch1.10/index.html
|
74 |
+
pip install easydict
|
75 |
+
pip install diffusers==0.20.2 accelerate safetensors transformers
|
76 |
+
pip install setuptools==59.5.0
|
77 |
+
pip install opencv-python
|
78 |
+
pip install numpy==1.24.1
|
79 |
+
```
|
80 |
+
|
81 |
+
|
82 |
+
Put the code inside the root of diffusers library (e.g., as '/home/username/diffusers/adaptive_mask_inpainting_example.py') and run the python code.
|
83 |
+
|
84 |
+
|
85 |
+
|
86 |
+
|
87 |
+
"""
|
88 |
+
|
89 |
+
|
90 |
+
EXAMPLE_DOC_STRING = """
|
91 |
+
Examples:
|
92 |
+
```py
|
93 |
+
>>> # !pip install transformers accelerate
|
94 |
+
>>> from diffusers import StableDiffusionControlNetInpaintPipeline, ControlNetModel, DDIMScheduler
|
95 |
+
>>> from diffusers.utils import load_image
|
96 |
+
>>> import numpy as np
|
97 |
+
>>> import torch
|
98 |
+
|
99 |
+
>>> init_image = load_image(
|
100 |
+
... "https://huggingface.co/datasets/diffusers/test-arrays/resolve/main/stable_diffusion_inpaint/boy.png"
|
101 |
+
... )
|
102 |
+
>>> init_image = init_image.resize((512, 512))
|
103 |
+
|
104 |
+
>>> generator = torch.Generator(device="cpu").manual_seed(1)
|
105 |
+
|
106 |
+
>>> mask_image = load_image(
|
107 |
+
... "https://huggingface.co/datasets/diffusers/test-arrays/resolve/main/stable_diffusion_inpaint/boy_mask.png"
|
108 |
+
... )
|
109 |
+
>>> mask_image = mask_image.resize((512, 512))
|
110 |
+
|
111 |
+
|
112 |
+
>>> def make_inpaint_condition(image, image_mask):
|
113 |
+
... image = np.array(image.convert("RGB")).astype(np.float32) / 255.0
|
114 |
+
... image_mask = np.array(image_mask.convert("L")).astype(np.float32) / 255.0
|
115 |
+
|
116 |
+
... assert image.shape[0:1] == image_mask.shape[0:1], "image and image_mask must have the same image size"
|
117 |
+
... image[image_mask > 0.5] = -1.0 # set as masked pixel
|
118 |
+
... image = np.expand_dims(image, 0).transpose(0, 3, 1, 2)
|
119 |
+
... image = torch.from_numpy(image)
|
120 |
+
... return image
|
121 |
+
|
122 |
+
|
123 |
+
>>> control_image = make_inpaint_condition(init_image, mask_image)
|
124 |
+
|
125 |
+
>>> controlnet = ControlNetModel.from_pretrained(
|
126 |
+
... "lllyasviel/control_v11p_sd15_inpaint", torch_dtype=torch.float16
|
127 |
+
... )
|
128 |
+
>>> pipe = StableDiffusionControlNetInpaintPipeline.from_pretrained(
|
129 |
+
... "runwayml/stable-diffusion-v1-5", controlnet=controlnet, torch_dtype=torch.float16
|
130 |
+
... )
|
131 |
+
|
132 |
+
>>> pipe.scheduler = DDIMScheduler.from_config(pipe.scheduler.config)
|
133 |
+
>>> pipe.enable_model_cpu_offload()
|
134 |
+
|
135 |
+
>>> # generate image
|
136 |
+
>>> image = pipe(
|
137 |
+
... "a handsome man with ray-ban sunglasses",
|
138 |
+
... num_inference_steps=20,
|
139 |
+
... generator=generator,
|
140 |
+
... eta=1.0,
|
141 |
+
... image=init_image,
|
142 |
+
... mask_image=mask_image,
|
143 |
+
... control_image=control_image,
|
144 |
+
... ).images[0]
|
145 |
+
```
|
146 |
+
"""
|
147 |
+
|
148 |
+
|
149 |
+
def download_file(url, output_file, exist_ok: bool):
|
150 |
+
if exist_ok and os.path.exists(output_file):
|
151 |
+
return
|
152 |
+
|
153 |
+
response = requests.get(url, stream=True)
|
154 |
+
|
155 |
+
with open(output_file, "wb") as file:
|
156 |
+
for chunk in tqdm(response.iter_content(chunk_size=8192), desc=f"Downloading '{output_file}'..."):
|
157 |
+
if chunk:
|
158 |
+
file.write(chunk)
|
159 |
+
|
160 |
+
|
161 |
+
def generate_video_from_imgs(images_save_directory, fps=15.0, delete_dir=True):
|
162 |
+
# delete videos if exists
|
163 |
+
if os.path.exists(f"{images_save_directory}.mp4"):
|
164 |
+
os.remove(f"{images_save_directory}.mp4")
|
165 |
+
if os.path.exists(f"{images_save_directory}_before_process.mp4"):
|
166 |
+
os.remove(f"{images_save_directory}_before_process.mp4")
|
167 |
+
|
168 |
+
# assume there are "enumerated" images under "images_save_directory"
|
169 |
+
assert os.path.isdir(images_save_directory)
|
170 |
+
ImgPaths = sorted(glob(f"{images_save_directory}/*"))
|
171 |
+
|
172 |
+
if len(ImgPaths) == 0:
|
173 |
+
print("\tSkipping, since there must be at least one image to create mp4\n")
|
174 |
+
else:
|
175 |
+
# mp4 configuration
|
176 |
+
video_path = images_save_directory + "_before_process.mp4"
|
177 |
+
|
178 |
+
# Get height and width config
|
179 |
+
images = sorted([ImgPath.split("/")[-1] for ImgPath in ImgPaths if ImgPath.endswith(".png")])
|
180 |
+
frame = cv2.imread(os.path.join(images_save_directory, images[0]))
|
181 |
+
height, width, channels = frame.shape
|
182 |
+
|
183 |
+
# create mp4 video writer
|
184 |
+
fourcc = cv2.VideoWriter_fourcc(*"mp4v")
|
185 |
+
video = cv2.VideoWriter(video_path, fourcc, fps, (width, height))
|
186 |
+
for image in images:
|
187 |
+
video.write(cv2.imread(os.path.join(images_save_directory, image)))
|
188 |
+
cv2.destroyAllWindows()
|
189 |
+
video.release()
|
190 |
+
|
191 |
+
# generated video is not compatible with HTML5. Post-process and change codec of video, so that it is applicable to HTML.
|
192 |
+
os.system(
|
193 |
+
f'ffmpeg -i "{images_save_directory}_before_process.mp4" -vcodec libx264 -f mp4 "{images_save_directory}.mp4" '
|
194 |
+
)
|
195 |
+
|
196 |
+
# remove group of images, and remove video before post-process.
|
197 |
+
if delete_dir and os.path.exists(images_save_directory):
|
198 |
+
shutil.rmtree(images_save_directory)
|
199 |
+
# remove 'before-process' video
|
200 |
+
if os.path.exists(f"{images_save_directory}_before_process.mp4"):
|
201 |
+
os.remove(f"{images_save_directory}_before_process.mp4")
|
202 |
+
|
203 |
+
|
204 |
+
# Copied from diffusers.pipelines.stable_diffusion.pipeline_stable_diffusion_inpaint.prepare_mask_and_masked_image
|
205 |
+
def prepare_mask_and_masked_image(image, mask, height, width, return_image=False):
|
206 |
+
"""
|
207 |
+
Prepares a pair (image, mask) to be consumed by the Stable Diffusion pipeline. This means that those inputs will be
|
208 |
+
converted to ``torch.Tensor`` with shapes ``batch x channels x height x width`` where ``channels`` is ``3`` for the
|
209 |
+
``image`` and ``1`` for the ``mask``.
|
210 |
+
|
211 |
+
The ``image`` will be converted to ``torch.float32`` and normalized to be in ``[-1, 1]``. The ``mask`` will be
|
212 |
+
binarized (``mask > 0.5``) and cast to ``torch.float32`` too.
|
213 |
+
|
214 |
+
Args:
|
215 |
+
image (Union[np.array, PIL.Image, torch.Tensor]): The image to inpaint.
|
216 |
+
It can be a ``PIL.Image``, or a ``height x width x 3`` ``np.array`` or a ``channels x height x width``
|
217 |
+
``torch.Tensor`` or a ``batch x channels x height x width`` ``torch.Tensor``.
|
218 |
+
mask (_type_): The mask to apply to the image, i.e. regions to inpaint.
|
219 |
+
It can be a ``PIL.Image``, or a ``height x width`` ``np.array`` or a ``1 x height x width``
|
220 |
+
``torch.Tensor`` or a ``batch x 1 x height x width`` ``torch.Tensor``.
|
221 |
+
|
222 |
+
|
223 |
+
Raises:
|
224 |
+
ValueError: ``torch.Tensor`` images should be in the ``[-1, 1]`` range. ValueError: ``torch.Tensor`` mask
|
225 |
+
should be in the ``[0, 1]`` range. ValueError: ``mask`` and ``image`` should have the same spatial dimensions.
|
226 |
+
TypeError: ``mask`` is a ``torch.Tensor`` but ``image`` is not
|
227 |
+
(ot the other way around).
|
228 |
+
|
229 |
+
Returns:
|
230 |
+
tuple[torch.Tensor]: The pair (mask, masked_image) as ``torch.Tensor`` with 4
|
231 |
+
dimensions: ``batch x channels x height x width``.
|
232 |
+
"""
|
233 |
+
|
234 |
+
if image is None:
|
235 |
+
raise ValueError("`image` input cannot be undefined.")
|
236 |
+
|
237 |
+
if mask is None:
|
238 |
+
raise ValueError("`mask_image` input cannot be undefined.")
|
239 |
+
|
240 |
+
if isinstance(image, torch.Tensor):
|
241 |
+
if not isinstance(mask, torch.Tensor):
|
242 |
+
raise TypeError(f"`image` is a torch.Tensor but `mask` (type: {type(mask)} is not")
|
243 |
+
|
244 |
+
# Batch single image
|
245 |
+
if image.ndim == 3:
|
246 |
+
assert image.shape[0] == 3, "Image outside a batch should be of shape (3, H, W)"
|
247 |
+
image = image.unsqueeze(0)
|
248 |
+
|
249 |
+
# Batch and add channel dim for single mask
|
250 |
+
if mask.ndim == 2:
|
251 |
+
mask = mask.unsqueeze(0).unsqueeze(0)
|
252 |
+
|
253 |
+
# Batch single mask or add channel dim
|
254 |
+
if mask.ndim == 3:
|
255 |
+
# Single batched mask, no channel dim or single mask not batched but channel dim
|
256 |
+
if mask.shape[0] == 1:
|
257 |
+
mask = mask.unsqueeze(0)
|
258 |
+
|
259 |
+
# Batched masks no channel dim
|
260 |
+
else:
|
261 |
+
mask = mask.unsqueeze(1)
|
262 |
+
|
263 |
+
assert image.ndim == 4 and mask.ndim == 4, "Image and Mask must have 4 dimensions"
|
264 |
+
assert image.shape[-2:] == mask.shape[-2:], "Image and Mask must have the same spatial dimensions"
|
265 |
+
assert image.shape[0] == mask.shape[0], "Image and Mask must have the same batch size"
|
266 |
+
|
267 |
+
# Check image is in [-1, 1]
|
268 |
+
if image.min() < -1 or image.max() > 1:
|
269 |
+
raise ValueError("Image should be in [-1, 1] range")
|
270 |
+
|
271 |
+
# Check mask is in [0, 1]
|
272 |
+
if mask.min() < 0 or mask.max() > 1:
|
273 |
+
raise ValueError("Mask should be in [0, 1] range")
|
274 |
+
|
275 |
+
# Binarize mask
|
276 |
+
mask[mask < 0.5] = 0
|
277 |
+
mask[mask >= 0.5] = 1
|
278 |
+
|
279 |
+
# Image as float32
|
280 |
+
image = image.to(dtype=torch.float32)
|
281 |
+
elif isinstance(mask, torch.Tensor):
|
282 |
+
raise TypeError(f"`mask` is a torch.Tensor but `image` (type: {type(image)} is not")
|
283 |
+
else:
|
284 |
+
# preprocess image
|
285 |
+
if isinstance(image, (PIL.Image.Image, np.ndarray)):
|
286 |
+
image = [image]
|
287 |
+
if isinstance(image, list) and isinstance(image[0], PIL.Image.Image):
|
288 |
+
# resize all images w.r.t passed height an width
|
289 |
+
image = [i.resize((width, height), resample=PIL.Image.LANCZOS) for i in image]
|
290 |
+
image = [np.array(i.convert("RGB"))[None, :] for i in image]
|
291 |
+
image = np.concatenate(image, axis=0)
|
292 |
+
elif isinstance(image, list) and isinstance(image[0], np.ndarray):
|
293 |
+
image = np.concatenate([i[None, :] for i in image], axis=0)
|
294 |
+
|
295 |
+
image = image.transpose(0, 3, 1, 2)
|
296 |
+
image = torch.from_numpy(image).to(dtype=torch.float32) / 127.5 - 1.0
|
297 |
+
|
298 |
+
# preprocess mask
|
299 |
+
if isinstance(mask, (PIL.Image.Image, np.ndarray)):
|
300 |
+
mask = [mask]
|
301 |
+
|
302 |
+
if isinstance(mask, list) and isinstance(mask[0], PIL.Image.Image):
|
303 |
+
mask = [i.resize((width, height), resample=PIL.Image.LANCZOS) for i in mask]
|
304 |
+
mask = np.concatenate([np.array(m.convert("L"))[None, None, :] for m in mask], axis=0)
|
305 |
+
mask = mask.astype(np.float32) / 255.0
|
306 |
+
elif isinstance(mask, list) and isinstance(mask[0], np.ndarray):
|
307 |
+
mask = np.concatenate([m[None, None, :] for m in mask], axis=0)
|
308 |
+
|
309 |
+
mask[mask < 0.5] = 0
|
310 |
+
mask[mask >= 0.5] = 1
|
311 |
+
mask = torch.from_numpy(mask)
|
312 |
+
|
313 |
+
masked_image = image * (mask < 0.5)
|
314 |
+
|
315 |
+
# n.b. ensure backwards compatibility as old function does not return image
|
316 |
+
if return_image:
|
317 |
+
return mask, masked_image, image
|
318 |
+
|
319 |
+
return mask, masked_image
|
320 |
+
|
321 |
+
|
322 |
+
class AdaptiveMaskInpaintPipeline(
|
323 |
+
DiffusionPipeline, TextualInversionLoaderMixin, LoraLoaderMixin, FromSingleFileMixin
|
324 |
+
):
|
325 |
+
r"""
|
326 |
+
Pipeline for text-guided image inpainting using Stable Diffusion.
|
327 |
+
|
328 |
+
This model inherits from [`DiffusionPipeline`]. Check the superclass documentation for the generic methods
|
329 |
+
implemented for all pipelines (downloading, saving, running on a particular device, etc.).
|
330 |
+
|
331 |
+
The pipeline also inherits the following loading methods:
|
332 |
+
- [`~loaders.TextualInversionLoaderMixin.load_textual_inversion`] for loading textual inversion embeddings
|
333 |
+
- [`~loaders.LoraLoaderMixin.load_lora_weights`] for loading LoRA weights
|
334 |
+
- [`~loaders.LoraLoaderMixin.save_lora_weights`] for saving LoRA weights
|
335 |
+
|
336 |
+
Args:
|
337 |
+
vae ([`AutoencoderKL`, `AsymmetricAutoencoderKL`]):
|
338 |
+
Variational Auto-Encoder (VAE) Model to encode and decode images to and from latent representations.
|
339 |
+
text_encoder ([`CLIPTextModel`]):
|
340 |
+
Frozen text-encoder ([clip-vit-large-patch14](https://huggingface.co/openai/clip-vit-large-patch14)).
|
341 |
+
tokenizer ([`~transformers.CLIPTokenizer`]):
|
342 |
+
A `CLIPTokenizer` to tokenize text.
|
343 |
+
unet ([`UNet2DConditionModel`]):
|
344 |
+
A `UNet2DConditionModel` to denoise the encoded image latents.
|
345 |
+
scheduler ([`SchedulerMixin`]):
|
346 |
+
A scheduler to be used in combination with `unet` to denoise the encoded image latents. Can be one of
|
347 |
+
[`DDIMScheduler`], [`LMSDiscreteScheduler`], or [`PNDMScheduler`].
|
348 |
+
safety_checker ([`StableDiffusionSafetyChecker`]):
|
349 |
+
Classification module that estimates whether generated images could be considered offensive or harmful.
|
350 |
+
Please refer to the [model card](https://huggingface.co/runwayml/stable-diffusion-v1-5) for more details
|
351 |
+
about a model's potential harms.
|
352 |
+
feature_extractor ([`~transformers.CLIPImageProcessor`]):
|
353 |
+
A `CLIPImageProcessor` to extract features from generated images; used as inputs to the `safety_checker`.
|
354 |
+
"""
|
355 |
+
|
356 |
+
_optional_components = ["safety_checker", "feature_extractor"]
|
357 |
+
|
358 |
+
def __init__(
|
359 |
+
self,
|
360 |
+
vae: Union[AutoencoderKL, AsymmetricAutoencoderKL],
|
361 |
+
text_encoder: CLIPTextModel,
|
362 |
+
tokenizer: CLIPTokenizer,
|
363 |
+
unet: UNet2DConditionModel,
|
364 |
+
scheduler: KarrasDiffusionSchedulers,
|
365 |
+
# safety_checker: StableDiffusionSafetyChecker,
|
366 |
+
safety_checker,
|
367 |
+
feature_extractor: CLIPImageProcessor,
|
368 |
+
requires_safety_checker: bool = True,
|
369 |
+
):
|
370 |
+
super().__init__()
|
371 |
+
|
372 |
+
self.register_adaptive_mask_model()
|
373 |
+
self.register_adaptive_mask_settings()
|
374 |
+
|
375 |
+
if hasattr(scheduler.config, "steps_offset") and scheduler.config.steps_offset != 1:
|
376 |
+
deprecation_message = (
|
377 |
+
f"The configuration file of this scheduler: {scheduler} is outdated. `steps_offset`"
|
378 |
+
f" should be set to 1 instead of {scheduler.config.steps_offset}. Please make sure "
|
379 |
+
"to update the config accordingly as leaving `steps_offset` might led to incorrect results"
|
380 |
+
" in future versions. If you have downloaded this checkpoint from the Hugging Face Hub,"
|
381 |
+
" it would be very nice if you could open a Pull request for the `scheduler/scheduler_config.json`"
|
382 |
+
" file"
|
383 |
+
)
|
384 |
+
deprecate("steps_offset!=1", "1.0.0", deprecation_message, standard_warn=False)
|
385 |
+
new_config = dict(scheduler.config)
|
386 |
+
new_config["steps_offset"] = 1
|
387 |
+
scheduler._internal_dict = FrozenDict(new_config)
|
388 |
+
|
389 |
+
if hasattr(scheduler.config, "skip_prk_steps") and scheduler.config.skip_prk_steps is False:
|
390 |
+
deprecation_message = (
|
391 |
+
f"The configuration file of this scheduler: {scheduler} has not set the configuration"
|
392 |
+
" `skip_prk_steps`. `skip_prk_steps` should be set to True in the configuration file. Please make"
|
393 |
+
" sure to update the config accordingly as not setting `skip_prk_steps` in the config might lead to"
|
394 |
+
" incorrect results in future versions. If you have downloaded this checkpoint from the Hugging Face"
|
395 |
+
" Hub, it would be very nice if you could open a Pull request for the"
|
396 |
+
" `scheduler/scheduler_config.json` file"
|
397 |
+
)
|
398 |
+
deprecate("skip_prk_steps not set", "1.0.0", deprecation_message, standard_warn=False)
|
399 |
+
new_config = dict(scheduler.config)
|
400 |
+
new_config["skip_prk_steps"] = True
|
401 |
+
scheduler._internal_dict = FrozenDict(new_config)
|
402 |
+
|
403 |
+
if safety_checker is None and requires_safety_checker:
|
404 |
+
logger.warning(
|
405 |
+
f"You have disabled the safety checker for {self.__class__} by passing `safety_checker=None`. Ensure"
|
406 |
+
" that you abide to the conditions of the Stable Diffusion license and do not expose unfiltered"
|
407 |
+
" results in services or applications open to the public. Both the diffusers team and Hugging Face"
|
408 |
+
" strongly recommend to keep the safety filter enabled in all public facing circumstances, disabling"
|
409 |
+
" it only for use-cases that involve analyzing network behavior or auditing its results. For more"
|
410 |
+
" information, please have a look at https://github.com/huggingface/diffusers/pull/254 ."
|
411 |
+
)
|
412 |
+
|
413 |
+
if safety_checker is not None and feature_extractor is None:
|
414 |
+
raise ValueError(
|
415 |
+
"Make sure to define a feature extractor when loading {self.__class__} if you want to use the safety"
|
416 |
+
" checker. If you do not want to use the safety checker, you can pass `'safety_checker=None'` instead."
|
417 |
+
)
|
418 |
+
|
419 |
+
is_unet_version_less_0_9_0 = hasattr(unet.config, "_diffusers_version") and version.parse(
|
420 |
+
version.parse(unet.config._diffusers_version).base_version
|
421 |
+
) < version.parse("0.9.0.dev0")
|
422 |
+
is_unet_sample_size_less_64 = hasattr(unet.config, "sample_size") and unet.config.sample_size < 64
|
423 |
+
if is_unet_version_less_0_9_0 and is_unet_sample_size_less_64:
|
424 |
+
deprecation_message = (
|
425 |
+
"The configuration file of the unet has set the default `sample_size` to smaller than"
|
426 |
+
" 64 which seems highly unlikely .If you're checkpoint is a fine-tuned version of any of the"
|
427 |
+
" following: \n- CompVis/stable-diffusion-v1-4 \n- CompVis/stable-diffusion-v1-3 \n-"
|
428 |
+
" CompVis/stable-diffusion-v1-2 \n- CompVis/stable-diffusion-v1-1 \n- runwayml/stable-diffusion-v1-5"
|
429 |
+
" \n- runwayml/stable-diffusion-inpainting \n you should change 'sample_size' to 64 in the"
|
430 |
+
" configuration file. Please make sure to update the config accordingly as leaving `sample_size=32`"
|
431 |
+
" in the config might lead to incorrect results in future versions. If you have downloaded this"
|
432 |
+
" checkpoint from the Hugging Face Hub, it would be very nice if you could open a Pull request for"
|
433 |
+
" the `unet/config.json` file"
|
434 |
+
)
|
435 |
+
deprecate("sample_size<64", "1.0.0", deprecation_message, standard_warn=False)
|
436 |
+
new_config = dict(unet.config)
|
437 |
+
new_config["sample_size"] = 64
|
438 |
+
unet._internal_dict = FrozenDict(new_config)
|
439 |
+
|
440 |
+
# Check shapes, assume num_channels_latents == 4, num_channels_mask == 1, num_channels_masked == 4
|
441 |
+
if unet.config.in_channels != 9:
|
442 |
+
logger.info(f"You have loaded a UNet with {unet.config.in_channels} input channels which.")
|
443 |
+
|
444 |
+
self.register_modules(
|
445 |
+
vae=vae,
|
446 |
+
text_encoder=text_encoder,
|
447 |
+
tokenizer=tokenizer,
|
448 |
+
unet=unet,
|
449 |
+
scheduler=scheduler,
|
450 |
+
safety_checker=safety_checker,
|
451 |
+
feature_extractor=feature_extractor,
|
452 |
+
)
|
453 |
+
self.vae_scale_factor = 2 ** (len(self.vae.config.block_out_channels) - 1)
|
454 |
+
self.image_processor = VaeImageProcessor(vae_scale_factor=self.vae_scale_factor)
|
455 |
+
self.register_to_config(requires_safety_checker=requires_safety_checker)
|
456 |
+
|
457 |
+
""" Preparation for Adaptive Mask inpainting """
|
458 |
+
|
459 |
+
# Copied from diffusers.pipelines.stable_diffusion.pipeline_stable_diffusion.StableDiffusionPipeline.enable_model_cpu_offload
|
460 |
+
def enable_model_cpu_offload(self, gpu_id=0):
|
461 |
+
r"""
|
462 |
+
Offload all models to CPU to reduce memory usage with a low impact on performance. Moves one whole model at a
|
463 |
+
time to the GPU when its `forward` method is called, and the model remains in GPU until the next model runs.
|
464 |
+
Memory savings are lower than using `enable_sequential_cpu_offload`, but performance is much better due to the
|
465 |
+
iterative execution of the `unet`.
|
466 |
+
"""
|
467 |
+
if is_accelerate_available() and is_accelerate_version(">=", "0.17.0.dev0"):
|
468 |
+
from accelerate import cpu_offload_with_hook
|
469 |
+
else:
|
470 |
+
raise ImportError("`enable_model_cpu_offload` requires `accelerate v0.17.0` or higher.")
|
471 |
+
|
472 |
+
device = torch.device(f"cuda:{gpu_id}")
|
473 |
+
|
474 |
+
if self.device.type != "cpu":
|
475 |
+
self.to("cpu", silence_dtype_warnings=True)
|
476 |
+
torch.cuda.empty_cache() # otherwise we don't see the memory savings (but they probably exist)
|
477 |
+
|
478 |
+
hook = None
|
479 |
+
for cpu_offloaded_model in [self.text_encoder, self.unet, self.vae]:
|
480 |
+
_, hook = cpu_offload_with_hook(cpu_offloaded_model, device, prev_module_hook=hook)
|
481 |
+
|
482 |
+
if self.safety_checker is not None:
|
483 |
+
_, hook = cpu_offload_with_hook(self.safety_checker, device, prev_module_hook=hook)
|
484 |
+
|
485 |
+
# We'll offload the last model manually.
|
486 |
+
self.final_offload_hook = hook
|
487 |
+
|
488 |
+
# Copied from diffusers.pipelines.stable_diffusion.pipeline_stable_diffusion.StableDiffusionPipeline._encode_prompt
|
489 |
+
def _encode_prompt(
|
490 |
+
self,
|
491 |
+
prompt,
|
492 |
+
device,
|
493 |
+
num_images_per_prompt,
|
494 |
+
do_classifier_free_guidance,
|
495 |
+
negative_prompt=None,
|
496 |
+
prompt_embeds: Optional[torch.FloatTensor] = None,
|
497 |
+
negative_prompt_embeds: Optional[torch.FloatTensor] = None,
|
498 |
+
lora_scale: Optional[float] = None,
|
499 |
+
):
|
500 |
+
r"""
|
501 |
+
Encodes the prompt into text encoder hidden states.
|
502 |
+
|
503 |
+
Args:
|
504 |
+
prompt (`str` or `List[str]`, *optional*):
|
505 |
+
prompt to be encoded
|
506 |
+
device: (`torch.device`):
|
507 |
+
torch device
|
508 |
+
num_images_per_prompt (`int`):
|
509 |
+
number of images that should be generated per prompt
|
510 |
+
do_classifier_free_guidance (`bool`):
|
511 |
+
whether to use classifier free guidance or not
|
512 |
+
negative_prompt (`str` or `List[str]`, *optional*):
|
513 |
+
The prompt or prompts not to guide the image generation. If not defined, one has to pass
|
514 |
+
`negative_prompt_embeds` instead. Ignored when not using guidance (i.e., ignored if `guidance_scale` is
|
515 |
+
less than `1`).
|
516 |
+
prompt_embeds (`torch.FloatTensor`, *optional*):
|
517 |
+
Pre-generated text embeddings. Can be used to easily tweak text inputs, *e.g.* prompt weighting. If not
|
518 |
+
provided, text embeddings will be generated from `prompt` input argument.
|
519 |
+
negative_prompt_embeds (`torch.FloatTensor`, *optional*):
|
520 |
+
Pre-generated negative text embeddings. Can be used to easily tweak text inputs, *e.g.* prompt
|
521 |
+
weighting. If not provided, negative_prompt_embeds will be generated from `negative_prompt` input
|
522 |
+
argument.
|
523 |
+
lora_scale (`float`, *optional*):
|
524 |
+
A lora scale that will be applied to all LoRA layers of the text encoder if LoRA layers are loaded.
|
525 |
+
"""
|
526 |
+
# set lora scale so that monkey patched LoRA
|
527 |
+
# function of text encoder can correctly access it
|
528 |
+
if lora_scale is not None and isinstance(self, LoraLoaderMixin):
|
529 |
+
self._lora_scale = lora_scale
|
530 |
+
|
531 |
+
if prompt is not None and isinstance(prompt, str):
|
532 |
+
batch_size = 1
|
533 |
+
elif prompt is not None and isinstance(prompt, list):
|
534 |
+
batch_size = len(prompt)
|
535 |
+
else:
|
536 |
+
batch_size = prompt_embeds.shape[0]
|
537 |
+
|
538 |
+
if prompt_embeds is None:
|
539 |
+
# textual inversion: procecss multi-vector tokens if necessary
|
540 |
+
if isinstance(self, TextualInversionLoaderMixin):
|
541 |
+
prompt = self.maybe_convert_prompt(prompt, self.tokenizer)
|
542 |
+
|
543 |
+
text_inputs = self.tokenizer(
|
544 |
+
prompt,
|
545 |
+
padding="max_length",
|
546 |
+
max_length=self.tokenizer.model_max_length,
|
547 |
+
truncation=True,
|
548 |
+
return_tensors="pt",
|
549 |
+
)
|
550 |
+
text_input_ids = text_inputs.input_ids
|
551 |
+
untruncated_ids = self.tokenizer(prompt, padding="longest", return_tensors="pt").input_ids
|
552 |
+
|
553 |
+
if untruncated_ids.shape[-1] >= text_input_ids.shape[-1] and not torch.equal(
|
554 |
+
text_input_ids, untruncated_ids
|
555 |
+
):
|
556 |
+
removed_text = self.tokenizer.batch_decode(
|
557 |
+
untruncated_ids[:, self.tokenizer.model_max_length - 1 : -1]
|
558 |
+
)
|
559 |
+
logger.warning(
|
560 |
+
"The following part of your input was truncated because CLIP can only handle sequences up to"
|
561 |
+
f" {self.tokenizer.model_max_length} tokens: {removed_text}"
|
562 |
+
)
|
563 |
+
|
564 |
+
if hasattr(self.text_encoder.config, "use_attention_mask") and self.text_encoder.config.use_attention_mask:
|
565 |
+
attention_mask = text_inputs.attention_mask.to(device)
|
566 |
+
else:
|
567 |
+
attention_mask = None
|
568 |
+
|
569 |
+
prompt_embeds = self.text_encoder(
|
570 |
+
text_input_ids.to(device),
|
571 |
+
attention_mask=attention_mask,
|
572 |
+
)
|
573 |
+
prompt_embeds = prompt_embeds[0]
|
574 |
+
|
575 |
+
if self.text_encoder is not None:
|
576 |
+
prompt_embeds_dtype = self.text_encoder.dtype
|
577 |
+
elif self.unet is not None:
|
578 |
+
prompt_embeds_dtype = self.unet.dtype
|
579 |
+
else:
|
580 |
+
prompt_embeds_dtype = prompt_embeds.dtype
|
581 |
+
|
582 |
+
prompt_embeds = prompt_embeds.to(dtype=prompt_embeds_dtype, device=device)
|
583 |
+
|
584 |
+
bs_embed, seq_len, _ = prompt_embeds.shape
|
585 |
+
# duplicate text embeddings for each generation per prompt, using mps friendly method
|
586 |
+
prompt_embeds = prompt_embeds.repeat(1, num_images_per_prompt, 1)
|
587 |
+
prompt_embeds = prompt_embeds.view(bs_embed * num_images_per_prompt, seq_len, -1)
|
588 |
+
|
589 |
+
# get unconditional embeddings for classifier free guidance
|
590 |
+
if do_classifier_free_guidance and negative_prompt_embeds is None:
|
591 |
+
uncond_tokens: List[str]
|
592 |
+
if negative_prompt is None:
|
593 |
+
uncond_tokens = [""] * batch_size
|
594 |
+
elif prompt is not None and type(prompt) is not type(negative_prompt):
|
595 |
+
raise TypeError(
|
596 |
+
f"`negative_prompt` should be the same type to `prompt`, but got {type(negative_prompt)} !="
|
597 |
+
f" {type(prompt)}."
|
598 |
+
)
|
599 |
+
elif isinstance(negative_prompt, str):
|
600 |
+
uncond_tokens = [negative_prompt]
|
601 |
+
elif batch_size != len(negative_prompt):
|
602 |
+
raise ValueError(
|
603 |
+
f"`negative_prompt`: {negative_prompt} has batch size {len(negative_prompt)}, but `prompt`:"
|
604 |
+
f" {prompt} has batch size {batch_size}. Please make sure that passed `negative_prompt` matches"
|
605 |
+
" the batch size of `prompt`."
|
606 |
+
)
|
607 |
+
else:
|
608 |
+
uncond_tokens = negative_prompt
|
609 |
+
|
610 |
+
# textual inversion: procecss multi-vector tokens if necessary
|
611 |
+
if isinstance(self, TextualInversionLoaderMixin):
|
612 |
+
uncond_tokens = self.maybe_convert_prompt(uncond_tokens, self.tokenizer)
|
613 |
+
|
614 |
+
max_length = prompt_embeds.shape[1]
|
615 |
+
uncond_input = self.tokenizer(
|
616 |
+
uncond_tokens,
|
617 |
+
padding="max_length",
|
618 |
+
max_length=max_length,
|
619 |
+
truncation=True,
|
620 |
+
return_tensors="pt",
|
621 |
+
)
|
622 |
+
|
623 |
+
if hasattr(self.text_encoder.config, "use_attention_mask") and self.text_encoder.config.use_attention_mask:
|
624 |
+
attention_mask = uncond_input.attention_mask.to(device)
|
625 |
+
else:
|
626 |
+
attention_mask = None
|
627 |
+
|
628 |
+
negative_prompt_embeds = self.text_encoder(
|
629 |
+
uncond_input.input_ids.to(device),
|
630 |
+
attention_mask=attention_mask,
|
631 |
+
)
|
632 |
+
negative_prompt_embeds = negative_prompt_embeds[0]
|
633 |
+
|
634 |
+
if do_classifier_free_guidance:
|
635 |
+
# duplicate unconditional embeddings for each generation per prompt, using mps friendly method
|
636 |
+
seq_len = negative_prompt_embeds.shape[1]
|
637 |
+
|
638 |
+
negative_prompt_embeds = negative_prompt_embeds.to(dtype=prompt_embeds_dtype, device=device)
|
639 |
+
|
640 |
+
negative_prompt_embeds = negative_prompt_embeds.repeat(1, num_images_per_prompt, 1)
|
641 |
+
negative_prompt_embeds = negative_prompt_embeds.view(batch_size * num_images_per_prompt, seq_len, -1)
|
642 |
+
|
643 |
+
# For classifier free guidance, we need to do two forward passes.
|
644 |
+
# Here we concatenate the unconditional and text embeddings into a single batch
|
645 |
+
# to avoid doing two forward passes
|
646 |
+
prompt_embeds = torch.cat([negative_prompt_embeds, prompt_embeds])
|
647 |
+
|
648 |
+
return prompt_embeds
|
649 |
+
|
650 |
+
# Copied from diffusers.pipelines.stable_diffusion.pipeline_stable_diffusion.StableDiffusionPipeline.run_safety_checker
|
651 |
+
def run_safety_checker(self, image, device, dtype):
|
652 |
+
if self.safety_checker is None:
|
653 |
+
has_nsfw_concept = None
|
654 |
+
else:
|
655 |
+
if torch.is_tensor(image):
|
656 |
+
feature_extractor_input = self.image_processor.postprocess(image, output_type="pil")
|
657 |
+
else:
|
658 |
+
feature_extractor_input = self.image_processor.numpy_to_pil(image)
|
659 |
+
safety_checker_input = self.feature_extractor(feature_extractor_input, return_tensors="pt").to(device)
|
660 |
+
image, has_nsfw_concept = self.safety_checker(
|
661 |
+
images=image, clip_input=safety_checker_input.pixel_values.to(dtype)
|
662 |
+
)
|
663 |
+
return image, has_nsfw_concept
|
664 |
+
|
665 |
+
# Copied from diffusers.pipelines.stable_diffusion.pipeline_stable_diffusion.StableDiffusionPipeline.prepare_extra_step_kwargs
|
666 |
+
def prepare_extra_step_kwargs(self, generator, eta):
|
667 |
+
# prepare extra kwargs for the scheduler step, since not all schedulers have the same signature
|
668 |
+
# eta (η) is only used with the DDIMScheduler, it will be ignored for other schedulers.
|
669 |
+
# eta corresponds to η in DDIM paper: https://arxiv.org/abs/2010.02502
|
670 |
+
# and should be between [0, 1]
|
671 |
+
|
672 |
+
accepts_eta = "eta" in set(inspect.signature(self.scheduler.step).parameters.keys())
|
673 |
+
extra_step_kwargs = {}
|
674 |
+
if accepts_eta:
|
675 |
+
extra_step_kwargs["eta"] = eta
|
676 |
+
|
677 |
+
# check if the scheduler accepts generator
|
678 |
+
accepts_generator = "generator" in set(inspect.signature(self.scheduler.step).parameters.keys())
|
679 |
+
if accepts_generator:
|
680 |
+
extra_step_kwargs["generator"] = generator
|
681 |
+
return extra_step_kwargs
|
682 |
+
|
683 |
+
def check_inputs(
|
684 |
+
self,
|
685 |
+
prompt,
|
686 |
+
height,
|
687 |
+
width,
|
688 |
+
strength,
|
689 |
+
callback_steps,
|
690 |
+
negative_prompt=None,
|
691 |
+
prompt_embeds=None,
|
692 |
+
negative_prompt_embeds=None,
|
693 |
+
):
|
694 |
+
if strength < 0 or strength > 1:
|
695 |
+
raise ValueError(f"The value of strength should in [0.0, 1.0] but is {strength}")
|
696 |
+
|
697 |
+
if height % 8 != 0 or width % 8 != 0:
|
698 |
+
raise ValueError(f"`height` and `width` have to be divisible by 8 but are {height} and {width}.")
|
699 |
+
|
700 |
+
if (callback_steps is None) or (
|
701 |
+
callback_steps is not None and (not isinstance(callback_steps, int) or callback_steps <= 0)
|
702 |
+
):
|
703 |
+
raise ValueError(
|
704 |
+
f"`callback_steps` has to be a positive integer but is {callback_steps} of type"
|
705 |
+
f" {type(callback_steps)}."
|
706 |
+
)
|
707 |
+
|
708 |
+
if prompt is not None and prompt_embeds is not None:
|
709 |
+
raise ValueError(
|
710 |
+
f"Cannot forward both `prompt`: {prompt} and `prompt_embeds`: {prompt_embeds}. Please make sure to"
|
711 |
+
" only forward one of the two."
|
712 |
+
)
|
713 |
+
elif prompt is None and prompt_embeds is None:
|
714 |
+
raise ValueError(
|
715 |
+
"Provide either `prompt` or `prompt_embeds`. Cannot leave both `prompt` and `prompt_embeds` undefined."
|
716 |
+
)
|
717 |
+
elif prompt is not None and (not isinstance(prompt, str) and not isinstance(prompt, list)):
|
718 |
+
raise ValueError(f"`prompt` has to be of type `str` or `list` but is {type(prompt)}")
|
719 |
+
|
720 |
+
if negative_prompt is not None and negative_prompt_embeds is not None:
|
721 |
+
raise ValueError(
|
722 |
+
f"Cannot forward both `negative_prompt`: {negative_prompt} and `negative_prompt_embeds`:"
|
723 |
+
f" {negative_prompt_embeds}. Please make sure to only forward one of the two."
|
724 |
+
)
|
725 |
+
|
726 |
+
if prompt_embeds is not None and negative_prompt_embeds is not None:
|
727 |
+
if prompt_embeds.shape != negative_prompt_embeds.shape:
|
728 |
+
raise ValueError(
|
729 |
+
"`prompt_embeds` and `negative_prompt_embeds` must have the same shape when passed directly, but"
|
730 |
+
f" got: `prompt_embeds` {prompt_embeds.shape} != `negative_prompt_embeds`"
|
731 |
+
f" {negative_prompt_embeds.shape}."
|
732 |
+
)
|
733 |
+
|
734 |
+
def prepare_latents(
|
735 |
+
self,
|
736 |
+
batch_size,
|
737 |
+
num_channels_latents,
|
738 |
+
height,
|
739 |
+
width,
|
740 |
+
dtype,
|
741 |
+
device,
|
742 |
+
generator,
|
743 |
+
latents=None,
|
744 |
+
image=None,
|
745 |
+
timestep=None,
|
746 |
+
is_strength_max=True,
|
747 |
+
return_noise=False,
|
748 |
+
return_image_latents=False,
|
749 |
+
):
|
750 |
+
shape = (batch_size, num_channels_latents, height // self.vae_scale_factor, width // self.vae_scale_factor)
|
751 |
+
if isinstance(generator, list) and len(generator) != batch_size:
|
752 |
+
raise ValueError(
|
753 |
+
f"You have passed a list of generators of length {len(generator)}, but requested an effective batch"
|
754 |
+
f" size of {batch_size}. Make sure the batch size matches the length of the generators."
|
755 |
+
)
|
756 |
+
|
757 |
+
if (image is None or timestep is None) and not is_strength_max:
|
758 |
+
raise ValueError(
|
759 |
+
"Since strength < 1. initial latents are to be initialised as a combination of Image + Noise."
|
760 |
+
"However, either the image or the noise timestep has not been provided."
|
761 |
+
)
|
762 |
+
|
763 |
+
if return_image_latents or (latents is None and not is_strength_max):
|
764 |
+
image = image.to(device=device, dtype=dtype)
|
765 |
+
image_latents = self._encode_vae_image(image=image, generator=generator)
|
766 |
+
|
767 |
+
if latents is None:
|
768 |
+
noise = randn_tensor(shape, generator=generator, device=device, dtype=dtype)
|
769 |
+
# if strength is 1. then initialise the latents to noise, else initial to image + noise
|
770 |
+
latents = noise if is_strength_max else self.scheduler.add_noise(image_latents, noise, timestep)
|
771 |
+
# if pure noise then scale the initial latents by the Scheduler's init sigma
|
772 |
+
latents = latents * self.scheduler.init_noise_sigma if is_strength_max else latents
|
773 |
+
else:
|
774 |
+
noise = latents.to(device)
|
775 |
+
latents = noise * self.scheduler.init_noise_sigma
|
776 |
+
|
777 |
+
outputs = (latents,)
|
778 |
+
|
779 |
+
if return_noise:
|
780 |
+
outputs += (noise,)
|
781 |
+
|
782 |
+
if return_image_latents:
|
783 |
+
outputs += (image_latents,)
|
784 |
+
|
785 |
+
return outputs
|
786 |
+
|
787 |
+
def _encode_vae_image(self, image: torch.Tensor, generator: torch.Generator):
|
788 |
+
if isinstance(generator, list):
|
789 |
+
image_latents = [
|
790 |
+
self.vae.encode(image[i : i + 1]).latent_dist.sample(generator=generator[i])
|
791 |
+
for i in range(image.shape[0])
|
792 |
+
]
|
793 |
+
image_latents = torch.cat(image_latents, dim=0)
|
794 |
+
else:
|
795 |
+
image_latents = self.vae.encode(image).latent_dist.sample(generator=generator)
|
796 |
+
|
797 |
+
image_latents = self.vae.config.scaling_factor * image_latents
|
798 |
+
|
799 |
+
return image_latents
|
800 |
+
|
801 |
+
def prepare_mask_latents(
|
802 |
+
self, mask, masked_image, batch_size, height, width, dtype, device, generator, do_classifier_free_guidance
|
803 |
+
):
|
804 |
+
# resize the mask to latents shape as we concatenate the mask to the latents
|
805 |
+
# we do that before converting to dtype to avoid breaking in case we're using cpu_offload
|
806 |
+
# and half precision
|
807 |
+
mask = torch.nn.functional.interpolate(
|
808 |
+
mask, size=(height // self.vae_scale_factor, width // self.vae_scale_factor)
|
809 |
+
)
|
810 |
+
mask = mask.to(device=device, dtype=dtype)
|
811 |
+
|
812 |
+
masked_image = masked_image.to(device=device, dtype=dtype)
|
813 |
+
masked_image_latents = self._encode_vae_image(masked_image, generator=generator)
|
814 |
+
|
815 |
+
# duplicate mask and masked_image_latents for each generation per prompt, using mps friendly method
|
816 |
+
if mask.shape[0] < batch_size:
|
817 |
+
if not batch_size % mask.shape[0] == 0:
|
818 |
+
raise ValueError(
|
819 |
+
"The passed mask and the required batch size don't match. Masks are supposed to be duplicated to"
|
820 |
+
f" a total batch size of {batch_size}, but {mask.shape[0]} masks were passed. Make sure the number"
|
821 |
+
" of masks that you pass is divisible by the total requested batch size."
|
822 |
+
)
|
823 |
+
mask = mask.repeat(batch_size // mask.shape[0], 1, 1, 1)
|
824 |
+
if masked_image_latents.shape[0] < batch_size:
|
825 |
+
if not batch_size % masked_image_latents.shape[0] == 0:
|
826 |
+
raise ValueError(
|
827 |
+
"The passed images and the required batch size don't match. Images are supposed to be duplicated"
|
828 |
+
f" to a total batch size of {batch_size}, but {masked_image_latents.shape[0]} images were passed."
|
829 |
+
" Make sure the number of images that you pass is divisible by the total requested batch size."
|
830 |
+
)
|
831 |
+
masked_image_latents = masked_image_latents.repeat(batch_size // masked_image_latents.shape[0], 1, 1, 1)
|
832 |
+
|
833 |
+
mask = torch.cat([mask] * 2) if do_classifier_free_guidance else mask
|
834 |
+
masked_image_latents = (
|
835 |
+
torch.cat([masked_image_latents] * 2) if do_classifier_free_guidance else masked_image_latents
|
836 |
+
)
|
837 |
+
|
838 |
+
# aligning device to prevent device errors when concating it with the latent model input
|
839 |
+
masked_image_latents = masked_image_latents.to(device=device, dtype=dtype)
|
840 |
+
return mask, masked_image_latents
|
841 |
+
|
842 |
+
# Copied from diffusers.pipelines.stable_diffusion.pipeline_stable_diffusion_img2img.StableDiffusionImg2ImgPipeline.get_timesteps
|
843 |
+
def get_timesteps(self, num_inference_steps, strength, device):
|
844 |
+
# get the original timestep using init_timestep
|
845 |
+
init_timestep = min(int(num_inference_steps * strength), num_inference_steps)
|
846 |
+
|
847 |
+
t_start = max(num_inference_steps - init_timestep, 0)
|
848 |
+
timesteps = self.scheduler.timesteps[t_start * self.scheduler.order :]
|
849 |
+
|
850 |
+
return timesteps, num_inference_steps - t_start
|
851 |
+
|
852 |
+
@torch.no_grad()
|
853 |
+
def __call__(
|
854 |
+
self,
|
855 |
+
prompt: Union[str, List[str]] = None,
|
856 |
+
image: Union[torch.FloatTensor, PIL.Image.Image] = None,
|
857 |
+
default_mask_image: Union[torch.FloatTensor, PIL.Image.Image] = None,
|
858 |
+
height: Optional[int] = None,
|
859 |
+
width: Optional[int] = None,
|
860 |
+
strength: float = 1.0,
|
861 |
+
num_inference_steps: int = 50,
|
862 |
+
guidance_scale: float = 7.5,
|
863 |
+
negative_prompt: Optional[Union[str, List[str]]] = None,
|
864 |
+
num_images_per_prompt: Optional[int] = 1,
|
865 |
+
eta: float = 0.0,
|
866 |
+
generator: Optional[Union[torch.Generator, List[torch.Generator]]] = None,
|
867 |
+
latents: Optional[torch.FloatTensor] = None,
|
868 |
+
prompt_embeds: Optional[torch.FloatTensor] = None,
|
869 |
+
negative_prompt_embeds: Optional[torch.FloatTensor] = None,
|
870 |
+
output_type: Optional[str] = "pil",
|
871 |
+
return_dict: bool = True,
|
872 |
+
callback: Optional[Callable[[int, int, torch.FloatTensor], None]] = None,
|
873 |
+
callback_steps: int = 1,
|
874 |
+
cross_attention_kwargs: Optional[Dict[str, Any]] = None,
|
875 |
+
use_adaptive_mask: bool = True,
|
876 |
+
enforce_full_mask_ratio: float = 0.5,
|
877 |
+
human_detection_thres: float = 0.008,
|
878 |
+
visualization_save_dir: str = None,
|
879 |
+
):
|
880 |
+
r"""
|
881 |
+
The call function to the pipeline for generation.
|
882 |
+
|
883 |
+
Args:
|
884 |
+
prompt (`str` or `List[str]`, *optional*):
|
885 |
+
The prompt or prompts to guide image generation. If not defined, you need to pass `prompt_embeds`.
|
886 |
+
image (`PIL.Image.Image`):
|
887 |
+
`Image` or tensor representing an image batch to be inpainted (which parts of the image to be masked
|
888 |
+
out with `default_mask_image` and repainted according to `prompt`).
|
889 |
+
default_mask_image (`PIL.Image.Image`):
|
890 |
+
`Image` or tensor representing an image batch to mask `image`. White pixels in the mask are repainted
|
891 |
+
while black pixels are preserved. If `default_mask_image` is a PIL image, it is converted to a single channel
|
892 |
+
(luminance) before use. If it's a tensor, it should contain one color channel (L) instead of 3, so the
|
893 |
+
expected shape would be `(B, H, W, 1)`.
|
894 |
+
height (`int`, *optional*, defaults to `self.unet.config.sample_size * self.vae_scale_factor`):
|
895 |
+
The height in pixels of the generated image.
|
896 |
+
width (`int`, *optional*, defaults to `self.unet.config.sample_size * self.vae_scale_factor`):
|
897 |
+
The width in pixels of the generated image.
|
898 |
+
strength (`float`, *optional*, defaults to 1.0):
|
899 |
+
Indicates extent to transform the reference `image`. Must be between 0 and 1. `image` is used as a
|
900 |
+
starting point and more noise is added the higher the `strength`. The number of denoising steps depends
|
901 |
+
on the amount of noise initially added. When `strength` is 1, added noise is maximum and the denoising
|
902 |
+
process runs for the full number of iterations specified in `num_inference_steps`. A value of 1
|
903 |
+
essentially ignores `image`.
|
904 |
+
num_inference_steps (`int`, *optional*, defaults to 50):
|
905 |
+
The number of denoising steps. More denoising steps usually lead to a higher quality image at the
|
906 |
+
expense of slower inference. This parameter is modulated by `strength`.
|
907 |
+
guidance_scale (`float`, *optional*, defaults to 7.5):
|
908 |
+
A higher guidance scale value encourages the model to generate images closely linked to the text
|
909 |
+
`prompt` at the expense of lower image quality. Guidance scale is enabled when `guidance_scale > 1`.
|
910 |
+
negative_prompt (`str` or `List[str]`, *optional*):
|
911 |
+
The prompt or prompts to guide what to not include in image generation. If not defined, you need to
|
912 |
+
pass `negative_prompt_embeds` instead. Ignored when not using guidance (`guidance_scale < 1`).
|
913 |
+
num_images_per_prompt (`int`, *optional*, defaults to 1):
|
914 |
+
The number of images to generate per prompt.
|
915 |
+
eta (`float`, *optional*, defaults to 0.0):
|
916 |
+
Corresponds to parameter eta (η) from the [DDIM](https://arxiv.org/abs/2010.02502) paper. Only applies
|
917 |
+
to the [`~schedulers.DDIMScheduler`], and is ignored in other schedulers.
|
918 |
+
generator (`torch.Generator` or `List[torch.Generator]`, *optional*):
|
919 |
+
A [`torch.Generator`](https://pytorch.org/docs/stable/generated/torch.Generator.html) to make
|
920 |
+
generation deterministic.
|
921 |
+
latents (`torch.FloatTensor`, *optional*):
|
922 |
+
Pre-generated noisy latents sampled from a Gaussian distribution, to be used as inputs for image
|
923 |
+
generation. Can be used to tweak the same generation with different prompts. If not provided, a latents
|
924 |
+
tensor is generated by sampling using the supplied random `generator`.
|
925 |
+
prompt_embeds (`torch.FloatTensor`, *optional*):
|
926 |
+
Pre-generated text embeddings. Can be used to easily tweak text inputs (prompt weighting). If not
|
927 |
+
provided, text embeddings are generated from the `prompt` input argument.
|
928 |
+
negative_prompt_embeds (`torch.FloatTensor`, *optional*):
|
929 |
+
Pre-generated negative text embeddings. Can be used to easily tweak text inputs (prompt weighting). If
|
930 |
+
not provided, `negative_prompt_embeds` are generated from the `negative_prompt` input argument.
|
931 |
+
output_type (`str`, *optional*, defaults to `"pil"`):
|
932 |
+
The output format of the generated image. Choose between `PIL.Image` or `np.array`.
|
933 |
+
return_dict (`bool`, *optional*, defaults to `True`):
|
934 |
+
Whether or not to return a [`~pipelines.stable_diffusion.StableDiffusionPipelineOutput`] instead of a
|
935 |
+
plain tuple.
|
936 |
+
callback (`Callable`, *optional*):
|
937 |
+
A function that calls every `callback_steps` steps during inference. The function is called with the
|
938 |
+
following arguments: `callback(step: int, timestep: int, latents: torch.FloatTensor)`.
|
939 |
+
callback_steps (`int`, *optional*, defaults to 1):
|
940 |
+
The frequency at which the `callback` function is called. If not specified, the callback is called at
|
941 |
+
every step.
|
942 |
+
cross_attention_kwargs (`dict`, *optional*):
|
943 |
+
A kwargs dictionary that if specified is passed along to the [`AttentionProcessor`] as defined in
|
944 |
+
[`self.processor`](https://github.com/huggingface/diffusers/blob/main/src/diffusers/models/attention_processor.py).
|
945 |
+
|
946 |
+
Examples:
|
947 |
+
|
948 |
+
```py
|
949 |
+
>>> import PIL
|
950 |
+
>>> import requests
|
951 |
+
>>> import torch
|
952 |
+
>>> from io import BytesIO
|
953 |
+
|
954 |
+
>>> from diffusers import AdaptiveMaskInpaintPipeline
|
955 |
+
|
956 |
+
|
957 |
+
>>> def download_image(url):
|
958 |
+
... response = requests.get(url)
|
959 |
+
... return PIL.Image.open(BytesIO(response.content)).convert("RGB")
|
960 |
+
|
961 |
+
|
962 |
+
>>> img_url = "https://raw.githubusercontent.com/CompVis/latent-diffusion/main/data/inpainting_examples/overture-creations-5sI6fQgYIuo.png"
|
963 |
+
>>> mask_url = "https://raw.githubusercontent.com/CompVis/latent-diffusion/main/data/inpainting_examples/overture-creations-5sI6fQgYIuo_mask.png"
|
964 |
+
|
965 |
+
>>> init_image = download_image(img_url).resize((512, 512))
|
966 |
+
>>> default_mask_image = download_image(mask_url).resize((512, 512))
|
967 |
+
|
968 |
+
>>> pipe = AdaptiveMaskInpaintPipeline.from_pretrained(
|
969 |
+
... "runwayml/stable-diffusion-inpainting", torch_dtype=torch.float16
|
970 |
+
... )
|
971 |
+
>>> pipe = pipe.to("cuda")
|
972 |
+
|
973 |
+
>>> prompt = "Face of a yellow cat, high resolution, sitting on a park bench"
|
974 |
+
>>> image = pipe(prompt=prompt, image=init_image, default_mask_image=default_mask_image).images[0]
|
975 |
+
```
|
976 |
+
|
977 |
+
Returns:
|
978 |
+
[`~pipelines.stable_diffusion.StableDiffusionPipelineOutput`] or `tuple`:
|
979 |
+
If `return_dict` is `True`, [`~pipelines.stable_diffusion.StableDiffusionPipelineOutput`] is returned,
|
980 |
+
otherwise a `tuple` is returned where the first element is a list with the generated images and the
|
981 |
+
second element is a list of `bool`s indicating whether the corresponding generated image contains
|
982 |
+
"not-safe-for-work" (nsfw) content.
|
983 |
+
"""
|
984 |
+
# 0. Default height and width to unet
|
985 |
+
width, height = image.size
|
986 |
+
# height = height or self.unet.config.sample_size * self.vae_scale_factor
|
987 |
+
# width = width or self.unet.config.sample_size * self.vae_scale_factor
|
988 |
+
|
989 |
+
# 1. Check inputs
|
990 |
+
self.check_inputs(
|
991 |
+
prompt,
|
992 |
+
height,
|
993 |
+
width,
|
994 |
+
strength,
|
995 |
+
callback_steps,
|
996 |
+
negative_prompt,
|
997 |
+
prompt_embeds,
|
998 |
+
negative_prompt_embeds,
|
999 |
+
)
|
1000 |
+
|
1001 |
+
# 2. Define call parameters
|
1002 |
+
if prompt is not None and isinstance(prompt, str):
|
1003 |
+
batch_size = 1
|
1004 |
+
elif prompt is not None and isinstance(prompt, list):
|
1005 |
+
batch_size = len(prompt)
|
1006 |
+
else:
|
1007 |
+
batch_size = prompt_embeds.shape[0]
|
1008 |
+
|
1009 |
+
device = self._execution_device
|
1010 |
+
# here `guidance_scale` is defined analog to the guidance weight `w` of equation (2)
|
1011 |
+
# of the Imagen paper: https://arxiv.org/pdf/2205.11487.pdf . `guidance_scale = 1`
|
1012 |
+
# corresponds to doing no classifier free guidance.
|
1013 |
+
do_classifier_free_guidance = guidance_scale > 1.0
|
1014 |
+
|
1015 |
+
# 3. Encode input prompt
|
1016 |
+
text_encoder_lora_scale = (
|
1017 |
+
cross_attention_kwargs.get("scale", None) if cross_attention_kwargs is not None else None
|
1018 |
+
)
|
1019 |
+
prompt_embeds = self._encode_prompt(
|
1020 |
+
prompt,
|
1021 |
+
device,
|
1022 |
+
num_images_per_prompt,
|
1023 |
+
do_classifier_free_guidance,
|
1024 |
+
negative_prompt,
|
1025 |
+
prompt_embeds=prompt_embeds,
|
1026 |
+
negative_prompt_embeds=negative_prompt_embeds,
|
1027 |
+
lora_scale=text_encoder_lora_scale,
|
1028 |
+
)
|
1029 |
+
|
1030 |
+
# 4. set timesteps
|
1031 |
+
self.scheduler.set_timesteps(num_inference_steps, device=device)
|
1032 |
+
timesteps, num_inference_steps = self.get_timesteps(
|
1033 |
+
num_inference_steps=num_inference_steps, strength=strength, device=device
|
1034 |
+
)
|
1035 |
+
# check that number of inference steps is not < 1 - as this doesn't make sense
|
1036 |
+
if num_inference_steps < 1:
|
1037 |
+
raise ValueError(
|
1038 |
+
f"After adjusting the num_inference_steps by strength parameter: {strength}, the number of pipeline"
|
1039 |
+
f"steps is {num_inference_steps} which is < 1 and not appropriate for this pipeline."
|
1040 |
+
)
|
1041 |
+
# at which timestep to set the initial noise (n.b. 50% if strength is 0.5)
|
1042 |
+
latent_timestep = timesteps[:1].repeat(batch_size * num_images_per_prompt)
|
1043 |
+
# create a boolean to check if the strength is set to 1. if so then initialise the latents with pure noise
|
1044 |
+
is_strength_max = strength == 1.0
|
1045 |
+
|
1046 |
+
# 5. Preprocess mask and image (will be used later, once again)
|
1047 |
+
mask, masked_image, init_image = prepare_mask_and_masked_image(
|
1048 |
+
image, default_mask_image, height, width, return_image=True
|
1049 |
+
)
|
1050 |
+
default_mask_image_np = np.array(default_mask_image).astype(np.uint8) / 255
|
1051 |
+
mask_condition = mask.clone()
|
1052 |
+
|
1053 |
+
# 6. Prepare latent variables
|
1054 |
+
num_channels_latents = self.vae.config.latent_channels
|
1055 |
+
num_channels_unet = self.unet.config.in_channels
|
1056 |
+
return_image_latents = num_channels_unet == 4
|
1057 |
+
|
1058 |
+
latents_outputs = self.prepare_latents(
|
1059 |
+
batch_size * num_images_per_prompt,
|
1060 |
+
num_channels_latents,
|
1061 |
+
height,
|
1062 |
+
width,
|
1063 |
+
prompt_embeds.dtype,
|
1064 |
+
device,
|
1065 |
+
generator,
|
1066 |
+
latents,
|
1067 |
+
image=init_image,
|
1068 |
+
timestep=latent_timestep,
|
1069 |
+
is_strength_max=is_strength_max,
|
1070 |
+
return_noise=True,
|
1071 |
+
return_image_latents=return_image_latents,
|
1072 |
+
)
|
1073 |
+
|
1074 |
+
if return_image_latents:
|
1075 |
+
latents, noise, image_latents = latents_outputs
|
1076 |
+
else:
|
1077 |
+
latents, noise = latents_outputs
|
1078 |
+
|
1079 |
+
# 7. Prepare mask latent variables
|
1080 |
+
mask, masked_image_latents = self.prepare_mask_latents(
|
1081 |
+
mask,
|
1082 |
+
masked_image,
|
1083 |
+
batch_size * num_images_per_prompt,
|
1084 |
+
height,
|
1085 |
+
width,
|
1086 |
+
prompt_embeds.dtype,
|
1087 |
+
device,
|
1088 |
+
generator,
|
1089 |
+
do_classifier_free_guidance,
|
1090 |
+
)
|
1091 |
+
|
1092 |
+
# 8. Check that sizes of mask, masked image and latents match
|
1093 |
+
if num_channels_unet == 9:
|
1094 |
+
# default case for runwayml/stable-diffusion-inpainting
|
1095 |
+
num_channels_mask = mask.shape[1]
|
1096 |
+
num_channels_masked_image = masked_image_latents.shape[1]
|
1097 |
+
if num_channels_latents + num_channels_mask + num_channels_masked_image != self.unet.config.in_channels:
|
1098 |
+
raise ValueError(
|
1099 |
+
f"Incorrect configuration settings! The config of `pipeline.unet`: {self.unet.config} expects"
|
1100 |
+
f" {self.unet.config.in_channels} but received `num_channels_latents`: {num_channels_latents} +"
|
1101 |
+
f" `num_channels_mask`: {num_channels_mask} + `num_channels_masked_image`: {num_channels_masked_image}"
|
1102 |
+
f" = {num_channels_latents+num_channels_masked_image+num_channels_mask}. Please verify the config of"
|
1103 |
+
" `pipeline.unet` or your `default_mask_image` or `image` input."
|
1104 |
+
)
|
1105 |
+
elif num_channels_unet != 4:
|
1106 |
+
raise ValueError(
|
1107 |
+
f"The unet {self.unet.__class__} should have either 4 or 9 input channels, not {self.unet.config.in_channels}."
|
1108 |
+
)
|
1109 |
+
|
1110 |
+
# 9. Prepare extra step kwargs. TODO: Logic should ideally just be moved out of the pipeline
|
1111 |
+
extra_step_kwargs = self.prepare_extra_step_kwargs(generator, eta)
|
1112 |
+
|
1113 |
+
# 10. Denoising loop
|
1114 |
+
mask_image_np = default_mask_image_np
|
1115 |
+
num_warmup_steps = len(timesteps) - num_inference_steps * self.scheduler.order
|
1116 |
+
with self.progress_bar(total=num_inference_steps) as progress_bar:
|
1117 |
+
for i, t in enumerate(timesteps):
|
1118 |
+
# expand the latents if we are doing classifier free guidance
|
1119 |
+
latent_model_input = torch.cat([latents] * 2) if do_classifier_free_guidance else latents
|
1120 |
+
|
1121 |
+
# concat latents, mask, masked_image_latents in the channel dimension
|
1122 |
+
latent_model_input = self.scheduler.scale_model_input(latent_model_input, t)
|
1123 |
+
|
1124 |
+
if num_channels_unet == 9:
|
1125 |
+
latent_model_input = torch.cat([latent_model_input, mask, masked_image_latents], dim=1)
|
1126 |
+
else:
|
1127 |
+
raise NotImplementedError
|
1128 |
+
|
1129 |
+
# predict the noise residual
|
1130 |
+
noise_pred = self.unet(
|
1131 |
+
latent_model_input,
|
1132 |
+
t,
|
1133 |
+
encoder_hidden_states=prompt_embeds,
|
1134 |
+
cross_attention_kwargs=cross_attention_kwargs,
|
1135 |
+
return_dict=False,
|
1136 |
+
)[0]
|
1137 |
+
|
1138 |
+
# perform guidance
|
1139 |
+
if do_classifier_free_guidance:
|
1140 |
+
noise_pred_uncond, noise_pred_text = noise_pred.chunk(2)
|
1141 |
+
noise_pred = noise_pred_uncond + guidance_scale * (noise_pred_text - noise_pred_uncond)
|
1142 |
+
|
1143 |
+
# compute the previous noisy sample x_t -> x_t-1 & predicted original sample x_0
|
1144 |
+
outputs = self.scheduler.step(noise_pred, t, latents, **extra_step_kwargs, return_dict=True)
|
1145 |
+
latents = outputs["prev_sample"] # x_t-1
|
1146 |
+
pred_orig_latents = outputs["pred_original_sample"] # x_0
|
1147 |
+
|
1148 |
+
# run segmentation
|
1149 |
+
if use_adaptive_mask:
|
1150 |
+
if enforce_full_mask_ratio > 0.0:
|
1151 |
+
use_default_mask = t < self.scheduler.config.num_train_timesteps * enforce_full_mask_ratio
|
1152 |
+
elif enforce_full_mask_ratio == 0.0:
|
1153 |
+
use_default_mask = False
|
1154 |
+
else:
|
1155 |
+
raise NotImplementedError
|
1156 |
+
|
1157 |
+
pred_orig_image = self.decode_to_npuint8_image(pred_orig_latents)
|
1158 |
+
dilate_num = self.adaptive_mask_settings.dilate_scheduler(i)
|
1159 |
+
do_adapt_mask = self.adaptive_mask_settings.provoke_scheduler(i)
|
1160 |
+
if do_adapt_mask:
|
1161 |
+
mask, masked_image_latents, mask_image_np, vis_np = self.adapt_mask(
|
1162 |
+
init_image,
|
1163 |
+
pred_orig_image,
|
1164 |
+
default_mask_image_np,
|
1165 |
+
dilate_num=dilate_num,
|
1166 |
+
use_default_mask=use_default_mask,
|
1167 |
+
height=height,
|
1168 |
+
width=width,
|
1169 |
+
batch_size=batch_size,
|
1170 |
+
num_images_per_prompt=num_images_per_prompt,
|
1171 |
+
prompt_embeds=prompt_embeds,
|
1172 |
+
device=device,
|
1173 |
+
generator=generator,
|
1174 |
+
do_classifier_free_guidance=do_classifier_free_guidance,
|
1175 |
+
i=i,
|
1176 |
+
human_detection_thres=human_detection_thres,
|
1177 |
+
mask_image_np=mask_image_np,
|
1178 |
+
)
|
1179 |
+
|
1180 |
+
if self.adaptive_mask_model.use_visualizer:
|
1181 |
+
import matplotlib.pyplot as plt
|
1182 |
+
|
1183 |
+
# mask_image_new_colormap = np.clip(0.6 + (1.0 - mask_image_np), a_min=0.0, a_max=1.0) * 255
|
1184 |
+
|
1185 |
+
os.makedirs(visualization_save_dir, exist_ok=True)
|
1186 |
+
|
1187 |
+
# Image.fromarray(mask_image_new_colormap).convert("L").save(f"{visualization_save_dir}/masks/{i:05}.png")
|
1188 |
+
plt.axis("off")
|
1189 |
+
plt.subplot(1, 2, 1)
|
1190 |
+
plt.imshow(mask_image_np)
|
1191 |
+
plt.subplot(1, 2, 2)
|
1192 |
+
plt.imshow(pred_orig_image)
|
1193 |
+
plt.savefig(f"{visualization_save_dir}/{i:05}.png", bbox_inches="tight")
|
1194 |
+
plt.close("all")
|
1195 |
+
|
1196 |
+
if num_channels_unet == 4:
|
1197 |
+
init_latents_proper = image_latents[:1]
|
1198 |
+
init_mask = mask[:1]
|
1199 |
+
|
1200 |
+
if i < len(timesteps) - 1:
|
1201 |
+
noise_timestep = timesteps[i + 1]
|
1202 |
+
init_latents_proper = self.scheduler.add_noise(
|
1203 |
+
init_latents_proper, noise, torch.tensor([noise_timestep])
|
1204 |
+
)
|
1205 |
+
|
1206 |
+
latents = (1 - init_mask) * init_latents_proper + init_mask * latents
|
1207 |
+
|
1208 |
+
# call the callback, if provided
|
1209 |
+
if i == len(timesteps) - 1 or ((i + 1) > num_warmup_steps and (i + 1) % self.scheduler.order == 0):
|
1210 |
+
progress_bar.update()
|
1211 |
+
if callback is not None and i % callback_steps == 0:
|
1212 |
+
callback(i, t, latents)
|
1213 |
+
|
1214 |
+
if not output_type == "latent":
|
1215 |
+
condition_kwargs = {}
|
1216 |
+
if isinstance(self.vae, AsymmetricAutoencoderKL):
|
1217 |
+
init_image = init_image.to(device=device, dtype=masked_image_latents.dtype)
|
1218 |
+
init_image_condition = init_image.clone()
|
1219 |
+
init_image = self._encode_vae_image(init_image, generator=generator)
|
1220 |
+
mask_condition = mask_condition.to(device=device, dtype=masked_image_latents.dtype)
|
1221 |
+
condition_kwargs = {"image": init_image_condition, "mask": mask_condition}
|
1222 |
+
image = self.vae.decode(latents / self.vae.config.scaling_factor, return_dict=False, **condition_kwargs)[0]
|
1223 |
+
image, has_nsfw_concept = self.run_safety_checker(image, device, prompt_embeds.dtype)
|
1224 |
+
else:
|
1225 |
+
image = latents
|
1226 |
+
has_nsfw_concept = None
|
1227 |
+
|
1228 |
+
if has_nsfw_concept is None:
|
1229 |
+
do_denormalize = [True] * image.shape[0]
|
1230 |
+
else:
|
1231 |
+
do_denormalize = [not has_nsfw for has_nsfw in has_nsfw_concept]
|
1232 |
+
|
1233 |
+
image = self.image_processor.postprocess(image, output_type=output_type, do_denormalize=do_denormalize)
|
1234 |
+
|
1235 |
+
# Offload last model to CPU
|
1236 |
+
if hasattr(self, "final_offload_hook") and self.final_offload_hook is not None:
|
1237 |
+
self.final_offload_hook.offload()
|
1238 |
+
|
1239 |
+
if self.adaptive_mask_model.use_visualizer:
|
1240 |
+
generate_video_from_imgs(images_save_directory=visualization_save_dir, fps=10, delete_dir=True)
|
1241 |
+
|
1242 |
+
if not return_dict:
|
1243 |
+
return (image, has_nsfw_concept)
|
1244 |
+
|
1245 |
+
return StableDiffusionPipelineOutput(images=image, nsfw_content_detected=has_nsfw_concept)
|
1246 |
+
|
1247 |
+
def decode_to_npuint8_image(self, latents):
|
1248 |
+
image = self.vae.decode(latents / self.vae.config.scaling_factor, return_dict=False, **{})[
|
1249 |
+
0
|
1250 |
+
] # torch, float32, -1.~1.
|
1251 |
+
image = self.image_processor.postprocess(image, output_type="pt", do_denormalize=[True] * image.shape[0])
|
1252 |
+
image = (image.squeeze().permute(1, 2, 0).detach().cpu().numpy() * 255).astype(np.uint8) # np, uint8, 0~255
|
1253 |
+
return image
|
1254 |
+
|
1255 |
+
def register_adaptive_mask_settings(self):
|
1256 |
+
from easydict import EasyDict
|
1257 |
+
|
1258 |
+
num_steps = 50
|
1259 |
+
|
1260 |
+
step_num = int(num_steps * 0.1)
|
1261 |
+
final_step_num = num_steps - step_num * 7
|
1262 |
+
# adaptive mask settings
|
1263 |
+
self.adaptive_mask_settings = EasyDict(
|
1264 |
+
dilate_scheduler=MaskDilateScheduler(
|
1265 |
+
max_dilate_num=20,
|
1266 |
+
num_inference_steps=num_steps,
|
1267 |
+
schedule=[20] * step_num
|
1268 |
+
+ [10] * step_num
|
1269 |
+
+ [5] * step_num
|
1270 |
+
+ [4] * step_num
|
1271 |
+
+ [3] * step_num
|
1272 |
+
+ [2] * step_num
|
1273 |
+
+ [1] * step_num
|
1274 |
+
+ [0] * final_step_num,
|
1275 |
+
),
|
1276 |
+
dilate_kernel=np.ones((3, 3), dtype=np.uint8),
|
1277 |
+
provoke_scheduler=ProvokeScheduler(
|
1278 |
+
num_inference_steps=num_steps,
|
1279 |
+
schedule=list(range(2, 10 + 1, 2)) + list(range(12, 40 + 1, 2)) + [45],
|
1280 |
+
is_zero_indexing=False,
|
1281 |
+
),
|
1282 |
+
)
|
1283 |
+
|
1284 |
+
def register_adaptive_mask_model(self):
|
1285 |
+
# declare segmentation model used for mask adaptation
|
1286 |
+
use_visualizer = True
|
1287 |
+
# assert not use_visualizer, \
|
1288 |
+
# """
|
1289 |
+
# If you plan to 'use_visualizer', USE WITH CAUTION.
|
1290 |
+
# It creates a directory of images and masks, which is used for merging into a video.
|
1291 |
+
# The procedure involves deleting the directory of images, which means that
|
1292 |
+
# if you set the directory wrong you can have other important files blown away.
|
1293 |
+
# """
|
1294 |
+
|
1295 |
+
self.adaptive_mask_model = PointRendPredictor(
|
1296 |
+
# pointrend_thres=0.2,
|
1297 |
+
pointrend_thres=0.9,
|
1298 |
+
device="cuda" if torch.cuda.is_available() else "cpu",
|
1299 |
+
use_visualizer=use_visualizer,
|
1300 |
+
config_pth="pointrend_rcnn_R_50_FPN_3x_coco.yaml",
|
1301 |
+
weights_pth="model_final_edd263.pkl",
|
1302 |
+
)
|
1303 |
+
|
1304 |
+
def adapt_mask(self, init_image, pred_orig_image, default_mask_image, dilate_num, use_default_mask, **kwargs):
|
1305 |
+
## predict mask to use for adaptation
|
1306 |
+
adapt_output = self.adaptive_mask_model(pred_orig_image) # vis can be None if 'use_visualizer' is False
|
1307 |
+
mask = adapt_output["mask"]
|
1308 |
+
vis = adapt_output["vis"]
|
1309 |
+
|
1310 |
+
## if mask is empty or too small, use default_mask_image. else, use dilate and intersect with default_mask_image
|
1311 |
+
if use_default_mask or mask.sum() < 512 * 512 * kwargs["human_detection_thres"]: # 0.005
|
1312 |
+
# set mask as default mask
|
1313 |
+
mask = default_mask_image # HxW
|
1314 |
+
|
1315 |
+
else:
|
1316 |
+
## timestep-adaptive mask
|
1317 |
+
mask = cv2.dilate(
|
1318 |
+
mask, self.adaptive_mask_settings.dilate_kernel, iterations=dilate_num
|
1319 |
+
) # dilate_kernel: np.ones((3,3), np.uint8)
|
1320 |
+
mask = np.logical_and(mask, default_mask_image) # HxW
|
1321 |
+
|
1322 |
+
## prepare mask as pt tensor format
|
1323 |
+
mask = torch.tensor(mask, dtype=torch.float32).to(kwargs["device"])[None, None] # 1 x 1 x H x W
|
1324 |
+
mask, masked_image = prepare_mask_and_masked_image(
|
1325 |
+
init_image.to(kwargs["device"]), mask, kwargs["height"], kwargs["width"], return_image=False
|
1326 |
+
)
|
1327 |
+
|
1328 |
+
mask_image_np = mask.clone().squeeze().detach().cpu().numpy()
|
1329 |
+
|
1330 |
+
mask, masked_image_latents = self.prepare_mask_latents(
|
1331 |
+
mask,
|
1332 |
+
masked_image,
|
1333 |
+
kwargs["batch_size"] * kwargs["num_images_per_prompt"],
|
1334 |
+
kwargs["height"],
|
1335 |
+
kwargs["width"],
|
1336 |
+
kwargs["prompt_embeds"].dtype,
|
1337 |
+
kwargs["device"],
|
1338 |
+
kwargs["generator"],
|
1339 |
+
kwargs["do_classifier_free_guidance"],
|
1340 |
+
)
|
1341 |
+
|
1342 |
+
return mask, masked_image_latents, mask_image_np, vis
|
1343 |
+
|
1344 |
+
|
1345 |
+
def seg2bbox(seg_mask: np.ndarray):
|
1346 |
+
nonzero_i, nonzero_j = seg_mask.nonzero()
|
1347 |
+
min_i, max_i = nonzero_i.min(), nonzero_i.max()
|
1348 |
+
min_j, max_j = nonzero_j.min(), nonzero_j.max()
|
1349 |
+
|
1350 |
+
return np.array([min_j, min_i, max_j + 1, max_i + 1])
|
1351 |
+
|
1352 |
+
|
1353 |
+
def merge_bbox(bboxes: list):
|
1354 |
+
assert len(bboxes) > 0
|
1355 |
+
|
1356 |
+
all_bboxes = np.stack(bboxes, axis=0) # shape: N_bbox X 4
|
1357 |
+
merged_bbox = np.zeros_like(all_bboxes[0]) # shape: 4,
|
1358 |
+
|
1359 |
+
merged_bbox[0] = all_bboxes[:, 0].min()
|
1360 |
+
merged_bbox[1] = all_bboxes[:, 1].min()
|
1361 |
+
merged_bbox[2] = all_bboxes[:, 2].max()
|
1362 |
+
merged_bbox[3] = all_bboxes[:, 3].max()
|
1363 |
+
|
1364 |
+
return merged_bbox
|
1365 |
+
|
1366 |
+
|
1367 |
+
class PointRendPredictor:
|
1368 |
+
def __init__(
|
1369 |
+
self,
|
1370 |
+
cat_id_to_focus=0,
|
1371 |
+
pointrend_thres=0.9,
|
1372 |
+
device="cuda",
|
1373 |
+
use_visualizer=False,
|
1374 |
+
merge_mode="merge",
|
1375 |
+
config_pth=None,
|
1376 |
+
weights_pth=None,
|
1377 |
+
):
|
1378 |
+
super().__init__()
|
1379 |
+
|
1380 |
+
# category id to focus (default: 0, which is human)
|
1381 |
+
self.cat_id_to_focus = cat_id_to_focus
|
1382 |
+
|
1383 |
+
# setup coco metadata
|
1384 |
+
self.coco_metadata = MetadataCatalog.get("coco_2017_val")
|
1385 |
+
self.cfg = get_cfg()
|
1386 |
+
|
1387 |
+
# get segmentation model config
|
1388 |
+
point_rend.add_pointrend_config(self.cfg) # --> Add PointRend-specific config
|
1389 |
+
self.cfg.merge_from_file(config_pth)
|
1390 |
+
self.cfg.MODEL.WEIGHTS = weights_pth
|
1391 |
+
self.cfg.MODEL.ROI_HEADS.SCORE_THRESH_TEST = pointrend_thres
|
1392 |
+
self.cfg.MODEL.DEVICE = device
|
1393 |
+
|
1394 |
+
# get segmentation model
|
1395 |
+
self.pointrend_seg_model = DefaultPredictor(self.cfg)
|
1396 |
+
|
1397 |
+
# settings for visualizer
|
1398 |
+
self.use_visualizer = use_visualizer
|
1399 |
+
|
1400 |
+
# mask-merge mode
|
1401 |
+
assert merge_mode in ["merge", "max-confidence"], f"'merge_mode': {merge_mode} not implemented."
|
1402 |
+
self.merge_mode = merge_mode
|
1403 |
+
|
1404 |
+
def merge_mask(self, masks, scores=None):
|
1405 |
+
if self.merge_mode == "merge":
|
1406 |
+
mask = np.any(masks, axis=0)
|
1407 |
+
elif self.merge_mode == "max-confidence":
|
1408 |
+
mask = masks[np.argmax(scores)]
|
1409 |
+
return mask
|
1410 |
+
|
1411 |
+
def vis_seg_on_img(self, image, mask):
|
1412 |
+
if type(mask) == np.ndarray:
|
1413 |
+
mask = torch.tensor(mask)
|
1414 |
+
v = Visualizer(image, self.coco_metadata, scale=0.5, instance_mode=ColorMode.IMAGE_BW)
|
1415 |
+
instances = Instances(image_size=image.shape[:2], pred_masks=mask if len(mask.shape) == 3 else mask[None])
|
1416 |
+
vis = v.draw_instance_predictions(instances.to("cpu")).get_image()
|
1417 |
+
return vis
|
1418 |
+
|
1419 |
+
def __call__(self, image):
|
1420 |
+
# run segmentation
|
1421 |
+
outputs = self.pointrend_seg_model(image)
|
1422 |
+
instances = outputs["instances"]
|
1423 |
+
|
1424 |
+
# merge instances for the category-id to focus
|
1425 |
+
is_class = instances.pred_classes == self.cat_id_to_focus
|
1426 |
+
masks = instances.pred_masks[is_class]
|
1427 |
+
masks = masks.detach().cpu().numpy() # [N, img_size, img_size]
|
1428 |
+
mask = self.merge_mask(masks, scores=instances.scores[is_class])
|
1429 |
+
|
1430 |
+
return {
|
1431 |
+
"asset_mask": None,
|
1432 |
+
"mask": mask.astype(np.uint8),
|
1433 |
+
"vis": self.vis_seg_on_img(image, mask) if self.use_visualizer else None,
|
1434 |
+
}
|
1435 |
+
|
1436 |
+
|
1437 |
+
class MaskDilateScheduler:
|
1438 |
+
def __init__(self, max_dilate_num=15, num_inference_steps=50, schedule=None):
|
1439 |
+
super().__init__()
|
1440 |
+
self.max_dilate_num = max_dilate_num
|
1441 |
+
self.schedule = [num_inference_steps - i for i in range(num_inference_steps)] if schedule is None else schedule
|
1442 |
+
assert len(self.schedule) == num_inference_steps
|
1443 |
+
|
1444 |
+
def __call__(self, i):
|
1445 |
+
return min(self.max_dilate_num, self.schedule[i])
|
1446 |
+
|
1447 |
+
|
1448 |
+
class ProvokeScheduler:
|
1449 |
+
def __init__(self, num_inference_steps=50, schedule=None, is_zero_indexing=False):
|
1450 |
+
super().__init__()
|
1451 |
+
if len(schedule) > 0:
|
1452 |
+
if is_zero_indexing:
|
1453 |
+
assert max(schedule) <= num_inference_steps - 1
|
1454 |
+
else:
|
1455 |
+
assert max(schedule) <= num_inference_steps
|
1456 |
+
|
1457 |
+
# register as self
|
1458 |
+
self.is_zero_indexing = is_zero_indexing
|
1459 |
+
self.schedule = schedule
|
1460 |
+
|
1461 |
+
def __call__(self, i):
|
1462 |
+
if self.is_zero_indexing:
|
1463 |
+
return i in self.schedule
|
1464 |
+
else:
|
1465 |
+
return i + 1 in self.schedule
|