Commit
·
fe663e7
1
Parent(s):
b1722c5
Update parquet files (step 26 of 249)
Browse filesThis view is limited to 50 files because it contains too many changes.
See raw diff
- spaces/101-5/gpt4free/g4f/.v1/gpt4free/aiassist/__init__.py +0 -36
- spaces/1acneusushi/gradio-2dmoleculeeditor/data/Clickteam Fusion 2.5 Developer Upgrade Download] [crack] How to Create Amazing Games with Ease.md +0 -144
- spaces/1acneusushi/gradio-2dmoleculeeditor/data/Download C-Free and Enjoy Multiple Compilers and Features for C and C.md +0 -25
- spaces/1gistliPinn/ChatGPT4/Examples/Ayyappa Songs Lyrics In Tamil Pdf 97.md +0 -84
- spaces/1gistliPinn/ChatGPT4/Examples/Cyberpunk - V3.0 - Core Rules V3.0.pdf [PORTABLE].md +0 -6
- spaces/1pelhydcardo/ChatGPT-prompt-generator/Download Film Yossi And Jagger.md +0 -92
- spaces/1pelhydcardo/ChatGPT-prompt-generator/assets/DJ Smallz 732 - Cupid Pt. 1 The Latest Dance Hit.md +0 -113
- spaces/1phancelerku/anime-remove-background/Burger Please Mod APK Download Make Your Own Burgers and Earn Money.md +0 -109
- spaces/1phancelerku/anime-remove-background/Car Parking Driving How to Master the Open World Multiplayer Mode.md +0 -19
- spaces/4com/README/README.md +0 -16
- spaces/7hao/bingo/src/app/layout.tsx +0 -47
- spaces/801artistry/RVC801/demucs/model.py +0 -202
- spaces/801artistry/RVC801/lib/uvr5_pack/utils.py +0 -120
- spaces/A00001/bingothoo/src/pages/api/kblob.ts +0 -56
- spaces/AI-Dashboards/Topic-Modeling-Clusters-Free-Text/README.md +0 -13
- spaces/AIConsultant/MusicGen/tests/__init__.py +0 -5
- spaces/AIConsultant/MusicGen/tests/modules/test_codebooks_patterns.py +0 -246
- spaces/AIFILMS/audioldm-text-to-audio-generation/audioldm/clap/open_clip/pretrained.py +0 -167
- spaces/AIFILMS/audioldm-text-to-audio-generation/audioldm/clap/open_clip/tokenizer.py +0 -197
- spaces/AIGC-Audio/AudioGPT/text_to_audio/Make_An_Audio/ldm/modules/encoders/__init__.py +0 -0
- spaces/AIGC-Audio/Make_An_Audio/ldm/modules/losses_audio/vggishish/transforms.py +0 -98
- spaces/ATang0729/Forecast4Muses/Model/Model6/Model6_2_ProfileRecogition/mmpretrain/configs/resnet/resnet50_8xb16_cifar10.py +0 -4
- spaces/AgentVerse/agentVerse/ui/src/phaser3-rex-plugins/templates/spinner/custom/Factory.d.ts +0 -5
- spaces/Agusbs98/automatic-ecg-diagnosis/nets/bblocks.py +0 -55
- spaces/Aki004/herta-so-vits/onnxexport/model_onnx_speaker_mix.py +0 -363
- spaces/Alpaca233/SadTalker/src/face3d/models/arcface_torch/backbones/__init__.py +0 -25
- spaces/Alpaca233/SadTalker/src/facerender/modules/dense_motion.py +0 -121
- spaces/Androidonnxfork/CivitAi-to-Diffusers/diffusers/docs/source/en/api/schedulers/repaint.md +0 -23
- spaces/Androidonnxfork/CivitAi-to-Diffusers/diffusers/docs/source/en/using-diffusers/reusing_seeds.md +0 -65
- spaces/Andy1621/uniformer_image_detection/configs/foveabox/fovea_align_r50_fpn_gn-head_mstrain_640-800_4x4_2x_coco.py +0 -25
- spaces/Andy1621/uniformer_image_segmentation/configs/gcnet/gcnet_r101-d8_512x512_40k_voc12aug.py +0 -2
- spaces/AnimalEquality/chatbot/setup.py +0 -57
- spaces/AnnasBlackHat/Image-Downloader/README.md +0 -12
- spaces/Ataturk-Chatbot/HuggingFaceChat/venv/lib/python3.11/site-packages/pip/_vendor/urllib3/fields.py +0 -274
- spaces/Ataturk-Chatbot/HuggingFaceChat/venv/lib/python3.11/site-packages/pkg_resources/__init__.py +0 -0
- spaces/Awiny/Image2Paragraph/models/grit_src/third_party/CenterNet2/docs/notes/compatibility.md +0 -84
- spaces/Awiny/Image2Paragraph/models/segment_models/semantic_segment_anything_model.py +0 -165
- spaces/Ayemos/highlight_text_based_on_surprisals/app.py +0 -102
- spaces/Banbri/zcvzcv/src/app/interface/progress/index.tsx +0 -56
- spaces/Benson/text-generation/Examples/2vd Canciones Mp3 Descargar.md +0 -62
- spaces/Benson/text-generation/Examples/Construir Arte - Elaboracin Amp Construccin De Juegos 3d Apk.md +0 -45
- spaces/Benson/text-generation/Examples/Descargar Dark Bitcoin Minero Pro V7.0 Gratis.md +0 -96
- spaces/BetterAPI/BetterChat/src/lib/utils/share.ts +0 -7
- spaces/BetterAPI/BetterChat_new/postcss.config.js +0 -6
- spaces/CVPR/Dual-Key_Backdoor_Attacks/datagen/detectron2/detectron2/data/datasets/README.md +0 -9
- spaces/CVPR/Dual-Key_Backdoor_Attacks/datagen/detectron2/projects/DensePose/densepose/densepose_coco_evaluation.py +0 -1120
- spaces/CVPR/Example-Echocardiogram-Segmentation/app.py +0 -93
- spaces/CVPR/LIVE/thrust/dependencies/cub/cmake/CubCudaConfig.cmake +0 -133
- spaces/CVPR/LIVE/thrust/thrust/system/cpp/detail/scatter.h +0 -22
- spaces/CVPR/MonoScene/README.md +0 -13
spaces/101-5/gpt4free/g4f/.v1/gpt4free/aiassist/__init__.py
DELETED
@@ -1,36 +0,0 @@
|
|
1 |
-
import urllib.request
|
2 |
-
import json
|
3 |
-
|
4 |
-
|
5 |
-
class Completion:
|
6 |
-
@staticmethod
|
7 |
-
def create(
|
8 |
-
systemMessage: str = "You are a helpful assistant",
|
9 |
-
prompt: str = "",
|
10 |
-
parentMessageId: str = "",
|
11 |
-
temperature: float = 0.8,
|
12 |
-
top_p: float = 1,
|
13 |
-
):
|
14 |
-
json_data = {
|
15 |
-
"prompt": prompt,
|
16 |
-
"options": {"parentMessageId": parentMessageId},
|
17 |
-
"systemMessage": systemMessage,
|
18 |
-
"temperature": temperature,
|
19 |
-
"top_p": top_p,
|
20 |
-
}
|
21 |
-
|
22 |
-
url = "http://43.153.7.56:8080/api/chat-process"
|
23 |
-
headers = {"Content-type": "application/json"}
|
24 |
-
|
25 |
-
data = json.dumps(json_data).encode("utf-8")
|
26 |
-
req = urllib.request.Request(url, data=data, headers=headers)
|
27 |
-
response = urllib.request.urlopen(req)
|
28 |
-
content = response.read().decode()
|
29 |
-
|
30 |
-
return Completion.__load_json(content)
|
31 |
-
|
32 |
-
@classmethod
|
33 |
-
def __load_json(cls, content) -> dict:
|
34 |
-
split = content.rsplit("\n", 1)[1]
|
35 |
-
to_json = json.loads(split)
|
36 |
-
return to_json
|
|
|
|
|
|
|
|
|
|
|
|
|
|
|
|
|
|
|
|
|
|
|
|
|
|
|
|
|
|
|
|
|
|
|
|
|
|
|
|
|
|
|
|
|
|
|
|
|
|
|
|
|
|
|
|
|
|
|
|
|
|
|
|
|
|
|
|
|
|
|
|
|
|
spaces/1acneusushi/gradio-2dmoleculeeditor/data/Clickteam Fusion 2.5 Developer Upgrade Download] [crack] How to Create Amazing Games with Ease.md
DELETED
@@ -1,144 +0,0 @@
|
|
1 |
-
<br />
|
2 |
-
<h1>Clickteam Fusion 2.5 Developer Upgrade: A Powerful Tool for Game and Software Creation</h1>
|
3 |
-
<p>Do you have an idea for a game or software that you want to bring to life? Do you want to create your own applications without coding or programming? Do you want to publish your creations for multiple platforms with ease? If you answered yes to any of these questions, then you might be interested in <strong>Clickteam Fusion 2.5 Developer Upgrade</strong>, a powerful tool that allows you to create games and software with a simple drag-and-drop interface.</p>
|
4 |
-
<h2>Clickteam Fusion 2.5 Developer Upgrade Download] [crack]</h2><br /><p><b><b>Download Zip</b> … <a href="https://byltly.com/2uKz8A">https://byltly.com/2uKz8A</a></b></p><br /><br />
|
5 |
-
<p>Clickteam Fusion 2.5 Developer Upgrade is an enhanced version of Clickteam Fusion 2.5, a game and software creation tool that has been used by thousands of developers around the world. With Clickteam Fusion 2.5 Developer Upgrade, you can access exclusive developer features and logo free use of the runtimes, giving you more freedom and flexibility in your development process.</p>
|
6 |
-
<p>In this article, we will explain how to get Clickteam Fusion 2.5 Developer Upgrade, what are its features, how to use it, and some examples of games and apps made with it. By the end of this article, you will have a better understanding of why Clickteam Fusion 2.5 Developer Upgrade is a powerful tool for game and software creation.</p>
|
7 |
-
<h2>How to get Clickteam Fusion 2.5 Developer Upgrade</h2>
|
8 |
-
<p>If you want to get Clickteam Fusion 2.5 Developer Upgrade, you have two options: purchase the full version or upgrade from the standard version.</p>
|
9 |
-
<p>The full version of Clickteam Fusion 2.5 Developer Upgrade costs $299.99 and can be purchased from <a href="https://www.clickteam.com/clickteam-fusion-2-5-developer">Clickteam's website</a> or from <a href="https://store.steampowered.com/app/267810/Clickteam_Fusion_25_Developer_Upgrade/">Steam</a>. The full version includes the base application Clickteam Fusion 2.5 and all the optional exporters for Windows, Mac, iOS, Android, Flash, XNA (Windows Mobile phone and Xbox) and HTML5.</p>
|
10 |
-
<p>If you already have the standard version of Clickteam Fusion 2.5, you can upgrade to the developer version for $199.99 by submitting a product upgrade request on <a href="https://community.clickteam.com/threads/90385-How-do-you-upgrade-from-Fusion-2-5-Standard-to-Developer">Clickteam's support page</a>. You will need to provide your Clickteam Fusion 2.5 serial number and proof of purchase.</p>
|
11 |
-
<h2>What are the features of Clickteam Fusion 2.5 Developer Upgrade</h2>
|
12 |
-
<p>Clickteam Fusion 2.5 Developer Upgrade has many features that make it a powerful tool for game and software creation. Here are some of the main features that distinguish it from the standard version:</p>
|
13 |
-
<h3>Royalty free, logo and credit free use of the runtimes</h3>
|
14 |
-
<p>One of the biggest benefits of Clickteam Fusion 2.5 Developer Upgrade is that you can use the runtimes without any limitations or requirements. This means that you can publish your games and apps without having to display any logos or credits from Clickteam or pay any royalties to them.</p>
|
15 |
-
<p>How to get Clickteam Fusion 2.5 Developer for free<br />
|
16 |
-
Clickteam Fusion 2.5 Developer full version download<br />
|
17 |
-
Clickteam Fusion 2.5 Developer crack serial keygen<br />
|
18 |
-
Clickteam Fusion 2.5 Developer patch download<br />
|
19 |
-
Clickteam Fusion 2.5 Developer activation code<br />
|
20 |
-
Clickteam Fusion 2.5 Developer license key<br />
|
21 |
-
Clickteam Fusion 2.5 Developer torrent download<br />
|
22 |
-
Clickteam Fusion 2.5 Developer review<br />
|
23 |
-
Clickteam Fusion 2.5 Developer tutorial<br />
|
24 |
-
Clickteam Fusion 2.5 Developer features<br />
|
25 |
-
Clickteam Fusion 2.5 Developer system requirements<br />
|
26 |
-
Clickteam Fusion 2.5 Developer alternatives<br />
|
27 |
-
Clickteam Fusion 2.5 Developer vs GameMaker Studio<br />
|
28 |
-
Clickteam Fusion 2.5 Developer vs Construct 3<br />
|
29 |
-
Clickteam Fusion 2.5 Developer vs Unity<br />
|
30 |
-
Clickteam Fusion 2.5 Developer vs Unreal Engine<br />
|
31 |
-
Clickteam Fusion 2.5 Developer vs Godot Engine<br />
|
32 |
-
Clickteam Fusion 2.5 Developer export options<br />
|
33 |
-
Clickteam Fusion 2.5 Developer extensions<br />
|
34 |
-
Clickteam Fusion 2.5 Developer examples<br />
|
35 |
-
Clickteam Fusion 2.5 Developer games<br />
|
36 |
-
Clickteam Fusion 2.5 Developer tips and tricks<br />
|
37 |
-
Clickteam Fusion 2.5 Developer documentation<br />
|
38 |
-
Clickteam Fusion 2.5 Developer forum<br />
|
39 |
-
Clickteam Fusion 2.5 Developer support<br />
|
40 |
-
Clickteam Fusion 2.5 Developer online course<br />
|
41 |
-
Clickteam Fusion 2.5 Developer cheat sheet<br />
|
42 |
-
Clickteam Fusion 2.5 Developer keyboard shortcuts<br />
|
43 |
-
Clickteam Fusion 2.5 Developer best practices<br />
|
44 |
-
Clickteam Fusion 2.5 Developer bugs and fixes<br />
|
45 |
-
Clickteam Fusion 2.5 Developer roadmap<br />
|
46 |
-
Clickteam Fusion 2.5 Developer update history<br />
|
47 |
-
Clickteam Fusion 2.5 Developer comparison chart<br />
|
48 |
-
Clickteam Fusion 2.5 Developer pros and cons<br />
|
49 |
-
Clickteam Fusion 2.5 Developer discount code<br />
|
50 |
-
Clickteam Fusion 2.5 Developer coupon code<br />
|
51 |
-
Clickteam Fusion 2.5 Developer free trial<br />
|
52 |
-
Clickteam Fusion 2.5 Developer refund policy<br />
|
53 |
-
Clickteam Fusion 2.5 Developer testimonials<br />
|
54 |
-
Clickteam Fusion 2.5 Developer case studies<br />
|
55 |
-
How to make a platformer game with Clickteam Fusion 2.5 Developer <br />
|
56 |
-
How to make a shooter game with Clickteam Fusion 2.5 Developer <br />
|
57 |
-
How to make a puzzle game with Clickteam Fusion 2.5 Developer <br />
|
58 |
-
How to make a RPG game with Clickteam Fusion 2.5 Developer <br />
|
59 |
-
How to make a racing game with Clickteam Fusion 2.5 Developer <br />
|
60 |
-
How to make a strategy game with Clickteam Fusion 2.5 Developer <br />
|
61 |
-
How to make a simulation game with Clickteam Fusion 2.5 Developer <br />
|
62 |
-
How to make a horror game with Clickteam Fusion 2.5 Developer <br />
|
63 |
-
How to make a multiplayer game with Clickteam Fusion 2.5 Developer <br />
|
64 |
-
How to make a mobile game with Clickteam Fusion 2.5 Developer </p>
|
65 |
-
<p>This gives you more control over your branding and monetization strategies, as well as more confidence in your intellectual property rights.</p>
|
66 |
-
<h3>Ability to publish games and apps for multiple platforms</h3>
|
67 |
-
<p>Another feature of Clickteam Fusion 2.5 Developer Upgrade is that you can publish your games and apps for multiple platforms with ease. With the optional exporters included in the full version, you can build your projects for Windows, Mac, iOS, Android, Flash, XNA (Windows Mobile phone and Xbox) and HTML5.</p>
|
68 |
-
<p>This means that you can reach a wider audience and increase your chances of success in different markets.</p>
|
69 |
-
<h3>Exclusive developer only objects</h3>
|
70 |
-
<p>Clickteam Fusion 2.5 Developer Upgrade also gives you access to exclusive developer only objects that provide additional functionality to your projects. These objects include:</p>
|
71 |
-
<ul>
|
72 |
-
<li>Data Grid Object (Windows Only): Allows you to display data in a grid format.</li>
|
73 |
-
<li>Dialog Box Object (Windows Only): Allows you to create custom dialog boxes with buttons.</li>
|
74 |
-
<li>Explorer Object (Windows Only): Allows you to browse files and folders on your computer.</li>
|
75 |
-
<li>List View Object (Windows Only): Allows you to display data in a list format.</li>
|
76 |
-
<li>OS Object (Windows Only): Allows you to access system information and functions.</li>
|
77 |
-
<li>Trial Period Object (Windows Only): Allows you to create trial versions of your software.</li>
|
78 |
-
<li>Camera Functionality (iOS Only): Allows you to access the camera on your device.</li>
|
79 |
-
<li>Game Center Objects (iOS Only): Allows you to integrate Game Center features into your games.</li>
|
80 |
-
<li>In app purchase support (iOS and Android Only): Allows you to implement in-app purchases into your games and apps.</li>
|
81 |
-
<li>Embed Video in App (iOS Only): Allows you to embed video files into your app.</li>
|
82 |
-
<li>Ad Control (XNA Only): Allows you to display ads in your games.</li>
|
83 |
-
<li>Admob support (Android Only): Allows you to display ads from Admob in your games.</li>
|
84 |
-
<li>Chartboost support (Android Only): Allows you to display ads from Chartboost in your games.</li>
|
85 |
-
<li>Leadbolt support (Android Only): Allows you to display ads from Leadbolt in your games.</li>
|
86 |
-
</ul>
|
87 |
-
<h3>Full integrated physics engine</h3>
|
88 |
-
<p>Clickteam Fusion 2.5 Developer Upgrade also takes full advantage of the Box2d physics engine by integrating it into the movement property tab for most objects. This means that you can easily add realistic physics effects such as gravity, friction, collisions, joints, springs and more to your games without coding or programming.</p>
|
89 |
-
<p>This makes your games more fun and immersive for your players.</p>
|
90 |
-
<h3>Hardware accelerated games and apps</h3>
|
91 |
-
<p>Last but not least, Clickteam Fusion 2.5 Developer Upgrade also allows you to make your games and apps faster by using hardware acceleration (subject to runtime used). This means that you can use shaders on powerful Windows machines or OpenGL ES on mobile devices to enhance the graphics quality and performance of your projects.</p>
|
92 |
-
<p>This makes your games and apps more attractive and smooth for your players.</p>
|
93 |
-
<h2>How to use Clickteam Fusion 2.5 Developer Upgrade</h2>
|
94 |
-
<p>with a simple drag-and-drop interface. Here are the basic steps to follow:</p>
|
95 |
-
<h3>Create a new project</h3>
|
96 |
-
<p>The first step is to create a new project in Clickteam Fusion 2.5 Developer Upgrade. You can choose from a variety of templates or start from scratch. You can also customize the project settings such as the name, icon, resolution, frame rate and more.</p>
|
97 |
-
<p>To create a new project, click on the File menu and select New. You will see a window with different options for your project. Choose the one that suits your needs and click OK.</p>
|
98 |
-
<h3>Add objects and events</h3>
|
99 |
-
<p>The next step is to add objects and events to your project. Objects are the elements that make up your game or software, such as sprites, sounds, texts, buttons and more. Events are the actions that define the logic and behavior of your project, such as what happens when you click a button, when you collide with an enemy, when you reach a certain score and more.</p>
|
100 |
-
<p>To add objects and events, you need to use the Frame Editor and the Event Editor. The Frame Editor is where you can drag and drop objects onto the frame (the screen where your game or software runs). The Event Editor is where you can create events using a simple condition-action system.</p>
|
101 |
-
<p>To access the Frame Editor, click on the Frame tab at the bottom of the screen. You will see a toolbar with different categories of objects. To add an object, click on its icon and drag it onto the frame. You can also right-click on an object and select Properties to change its attributes.</p>
|
102 |
-
<p>To access the Event Editor, click on the Event tab at the bottom of the screen. You will see a grid with columns for conditions and actions. To add an event, click on an empty cell in the condition column and select a condition from the list. Then click on an empty cell in the action column and select an action from the list. You can also right-click on an event and select Edit to modify it.</p>
|
103 |
-
<h3>Test and debug</h3>
|
104 |
-
<p>The third step is to test and debug your project. Testing means running your project to see how it works and if there are any errors or bugs. Debugging means finding and fixing those errors or bugs.</p>
|
105 |
-
<p>To test your project, click on the Run menu and select Run Application. You will see your project running in a separate window. You can also use keyboard shortcuts such as F8 to run your project.</p>
|
106 |
-
<p>To debug your project, you can use various tools such as breakpoints, watches, monitors and debug messages. Breakpoints are points in your events where you can pause your project and inspect its state. Watches are variables that you can track during your project's execution. Monitors are windows that display information about your objects and events. Debug messages are texts that you can print to the output window for debugging purposes.</p>
|
107 |
-
<p>To use these tools, you need to enable the Debug mode in Clickteam Fusion 2.5 Developer Upgrade. To do so, click on the Run menu and select Debug Mode On/Off. You will see a green bug icon in the toolbar indicating that Debug mode is on.</p>
|
108 |
-
<h3>Export and publish</h3>
|
109 |
-
<p>The final step is to export and publish your project. Exporting means building your project for a specific platform such as Windows, Mac, iOS, Android, Flash, XNA or HTML5. Publishing means distributing your project to your target audience such as uploading it to a website or app store.</p>
|
110 |
-
<p>To export your project, click on the Build menu and select Build Application or Build HTML5 Application depending on your platform choice. You will see a window with different options for your build such as compression level, encryption key, splash screen and more. Choose the ones that suit your needs and click OK.</p>
|
111 |
-
<p>To publish your project, you need to follow different steps depending on your platform choice such as signing up for a developer account, uploading your files, filling out forms and more. For more details on how to publish your project for each platform, please refer to <a href="https://www.clickteam.com/clickteam-fusion-2-5-developer">Clickteam's website</a> or <a href="https://store.steampowered.com/app/267810/Clickteam_Fusion_25_Developer_Upgrade/">Steam</a>.</p>
|
112 |
-
<h2>Examples of games and apps made with Clickteam Fusion 2.5 Developer Upgrade</h2>
|
113 |
-
<p>Clickteam Fusion 2.5 Developer Upgrade has been used by many developers around the world to create successful games and apps for various platforms. Here are some examples of games and apps made with Clickteam Fusion 2.5 Developer Upgrade:</p>
|
114 |
-
<h3>Five Nights at Freddy's series</h3>
|
115 |
-
<p>Five Nights at Freddy's is a popular horror game series by Scott Cawthon that has spawned several sequels, spin-offs and adaptations. The game puts you in the role of a night guard at a haunted pizzeria where you have to survive five nights against animatronic characters that come to life at night.</p>
|
116 |
-
<p>The game was made with Clickteam Fusion 2.5 Developer Upgrade and has been published for Windows, iOS, Android and other platforms.</p>
|
117 |
-
<h3>The Escapists series</h3>
|
118 |
-
<p>and spin-offs. The game puts you in the role of a prisoner who has to plan and execute an escape from various prisons with different levels of security and difficulty.</p>
|
119 |
-
<p>The game was made with Clickteam Fusion 2.5 Developer Upgrade and has been published for Windows, Mac, iOS, Android and other platforms.</p>
|
120 |
-
<h3>Freedom Planet</h3>
|
121 |
-
<p>Freedom Planet is a retro-style platformer game by GalaxyTrail that pays homage to the classic games of the 16-bit era. The game features four playable characters, each with their own abilities and storylines, who have to save their planet from an evil warlord.</p>
|
122 |
-
<p>The game was made with Clickteam Fusion 2.5 Developer Upgrade and has been published for Windows, Mac, Linux, Wii U, PlayStation 4 and Nintendo Switch.</p>
|
123 |
-
<h1>Conclusion</h1>
|
124 |
-
<p>In conclusion, Clickteam Fusion 2.5 Developer Upgrade is a powerful tool for game and software creation that allows you to create your own applications without coding or programming. With Clickteam Fusion 2.5 Developer Upgrade, you can access exclusive developer features and logo free use of the runtimes, publish your games and apps for multiple platforms with ease, use a full integrated physics engine and hardware acceleration, and more.</p>
|
125 |
-
<p>If you want to get Clickteam Fusion 2.5 Developer Upgrade, you can purchase the full version or upgrade from the standard version from <a href="https://www.clickteam.com/clickteam-fusion-2-5-developer">Clickteam's website</a> or from <a href="https://store.steampowered.com/app/267810/Clickteam_Fusion_25_Developer_Upgrade/">Steam</a>. You can also try the free version first to see if it runs on your system.</p>
|
126 |
-
<p>With Clickteam Fusion 2.5 Developer Upgrade, you can unleash your creativity and make your own games and software with a simple drag-and-drop interface. Whether you are a beginner or a professional, Clickteam Fusion 2.5 Developer Upgrade can help you achieve your development goals.</p>
|
127 |
-
<p>So what are you waiting for? Get Clickteam Fusion 2.5 Developer Upgrade today and start creating!</p>
|
128 |
-
<h2>FAQs</h2>
|
129 |
-
<p>Here are some frequently asked questions about Clickteam Fusion 2.5 Developer Upgrade:</p>
|
130 |
-
<ul>
|
131 |
-
<li><strong>Q: What are the system requirements for Clickteam Fusion 2.5 Developer Upgrade?</strong></li>
|
132 |
-
<li>A: For Windows, you need Windows 10, 8, 7, Vista, XP, 2000 or 98 operating system, 200 Mhz Pentium processor or higher, 32 Mb RAM (256 Mb for XP, Vista, 7, 8 or 10). For Mac, you need OSX 10.9 (Mavericks) to 10.14 (Mojave) - macOS 10.15 (Catalina) is NOT supported as this is a 32-bit application and Apple have removed support for 32-bit. You also need an internet connection for installation, updates and to download the software.</li>
|
133 |
-
<li><strong>Q: How can I learn how to use Clickteam Fusion 2.5 Developer Upgrade?</strong></li>
|
134 |
-
<li>A: You can learn how to use Clickteam Fusion 2.5 Developer Upgrade by following the tutorials and documentation available on <a href="https://www.clickteam.com/clickteam-fusion-2-5-developer">Clickteam's website</a> or on <a href="https://store.steampowered.com/app/267810/Clickteam_Fusion_25_Developer_Upgrade/">Steam</a>. You can also join the community forums and discord server where you can ask questions and get help from other users and developers.</li>
|
135 |
-
<li><strong>Q: How can I get support for Clickteam Fusion 2.5 Developer Upgrade?</strong></li>
|
136 |
-
<li>A: You can get support for Clickteam Fusion 2.5 Developer Upgrade by contacting <a href="https://www.clickteam.com/support">Clickteam's support team</a> via email or ticket system. You can also report bugs or suggest features on <a href="https://community.clickteam.com/bugbox">Clickteam's bugbox</a>.</li>
|
137 |
-
<li><strong>Q: How can I get updates for Clickteam Fusion 2.5 Developer Upgrade?</strong></li>
|
138 |
-
<li>A: You can get updates for Clickteam Fusion 2.5 Developer Upgrade by checking <a href="https://www.clickteam.com/clickteam-fusion-2-5-developer">Clickteam's website</a> or <a href="https://store.steampowered.com/app/267810/Clickteam_Fusion_25_Developer_Upgrade/">Steam</a> for any news or announcements about new versions or patches. You can also enable automatic updates in your Steam settings.</li>
|
139 |
-
<li><strong>Q: How can I share my games and apps made with Clickteam Fusion 2.5 Developer Upgrade?</strong></li>
|
140 |
-
<li>A: You can share your games and apps made with Clickteam Fusion 2.5 Developer Upgrade by publishing them on various platforms such as Windows, Mac, iOS, Android, Flash, XNA or HTML5. You can also upload them to websites such as <a href="https://itch.io/">itch.io</a>, <a href="https://gamejolt.com/">Game Jolt</a>, <a href="https://www.kongregate.com/">Kongregate</a>, <a href="https://www.newgrounds.com/">Newgrounds</a>, <a href="https://store.steampowered.com/">Steam</a>, <a href="https://apps.apple.com/us/genre/ios/id36">App Store</a>, <a href="https://play.google.com/store/apps">Google Play</a>, <a href="https://www.microsoft.com/en-us/store/apps/windows-phone">Windows Phone Store</a>, <a href="https://marketplace.xbox.com/en-US/Games/XboxIndieGames">Xbox Live Indie Games</a> and more.</li>
|
141 |
-
</ul>
|
142 |
-
</p> 0a6ba089eb<br />
|
143 |
-
<br />
|
144 |
-
<br />
|
|
|
|
|
|
|
|
|
|
|
|
|
|
|
|
|
|
|
|
|
|
|
|
|
|
|
|
|
|
|
|
|
|
|
|
|
|
|
|
|
|
|
|
|
|
|
|
|
|
|
|
|
|
|
|
|
|
|
|
|
|
|
|
|
|
|
|
|
|
|
|
|
|
|
|
|
|
|
|
|
|
|
|
|
|
|
|
|
|
|
|
|
|
|
|
|
|
|
|
|
|
|
|
|
|
|
|
|
|
|
|
|
|
|
|
|
|
|
|
|
|
|
|
|
|
|
|
|
|
|
|
|
|
|
|
|
|
|
|
|
|
|
|
|
|
|
|
|
|
|
|
|
|
|
|
|
|
|
|
|
|
|
|
|
|
|
|
|
|
|
|
|
|
|
|
|
|
|
|
|
|
|
|
|
|
|
|
|
|
|
|
|
|
|
|
|
|
|
|
|
|
|
|
|
|
|
|
|
|
|
|
|
|
|
|
|
|
|
|
|
|
|
|
|
|
|
|
|
|
|
|
|
|
|
|
|
|
|
|
|
|
|
|
|
|
|
|
|
|
|
|
|
|
|
|
|
|
|
|
|
|
|
|
|
|
|
|
|
|
|
|
|
|
|
|
|
|
|
|
|
|
|
|
|
|
|
|
|
|
spaces/1acneusushi/gradio-2dmoleculeeditor/data/Download C-Free and Enjoy Multiple Compilers and Features for C and C.md
DELETED
@@ -1,25 +0,0 @@
|
|
1 |
-
|
2 |
-
<h1>How to Download C-Free IDE for Windows</h1>
|
3 |
-
<p>C-Free is a professional C/C++ integrated development environment (IDE) that supports multiple compilers. With this software, you can edit, build, run and debug your C and C++ programs freely. In this article, we will show you how to download and install C-Free IDE for Windows.</p>
|
4 |
-
<h2>c free crack download</h2><br /><p><b><b>Download File</b> ->>->>->> <a href="https://byltly.com/2uKvYb">https://byltly.com/2uKvYb</a></b></p><br /><br />
|
5 |
-
<h2>Step 1: Download C-Free</h2>
|
6 |
-
<p>You can download C-Free from its official website or from other software download sites . The latest version is 5.0, which was released on September 7, 2018. The file size is about 14.6 MB. You can choose either the free trial version or the full version that costs $79.</p>
|
7 |
-
<h2>Step 2: Install C-Free</h2>
|
8 |
-
<p>After downloading the C-Free setup file, double-click it to start the installation process. Follow the instructions on the screen to select the destination folder, the components to install, and the shortcuts to create. You can also choose the default compiler to use among the supported ones, such as MinGW, Cygwin, Borland C++, Microsoft C++, Intel C++, Lcc-Win32, Open Watcom C/C++, Digital Mars C/C++, and Ch Interpreter.</p>
|
9 |
-
<h2>Step 3: Run C-Free</h2>
|
10 |
-
<p>Once the installation is complete, you can launch C-Free from the Start menu or the desktop shortcut. You will see the main interface of C-Free, which consists of several panels, such as the editor, the project explorer, the output window, and the code browser. You can customize the layout and appearance of these panels according to your preferences.</p>
|
11 |
-
<h2>Step 4: Create a New Project</h2>
|
12 |
-
<p>To start coding with C-Free, you need to create a new project first. You can do this by clicking on File > New > Project or by pressing Ctrl+Shift+N. A project wizard will appear, where you can choose the type of project you want to create, such as console application, Windows application, DLL library, static library, or empty project. You can also specify the name and location of your project.</p>
|
13 |
-
<h2>Step 5: Add Source Files</h2>
|
14 |
-
<p>After creating a new project, you need to add source files to it. You can do this by clicking on File > New > File or by pressing Ctrl+N. A file wizard will appear, where you can choose the type of file you want to create, such as C source file (.c), C++ source file (.cpp), header file (.h), or resource file (.rc). You can also specify the name and location of your file.</p>
|
15 |
-
<h2>Step 6: Edit and Build Your Code</h2>
|
16 |
-
<p>Now you can edit your code using the editor panel of C-Free. You can enjoy features such as syntax highlighting, code completion, code parameters, smart input, code folding, bookmarks, breakpoints, and more. You can also use external tools and help files to assist your coding process.</p>
|
17 |
-
<p></p>
|
18 |
-
<p>To build your code, you can click on Build > Build or press F9. This will compile and link your code using the selected compiler and generate an executable file or a library file in the output folder. You can see the build messages in the output window.</p>
|
19 |
-
<h2>Step 7: Run and Debug Your Program</h2>
|
20 |
-
<p>To run your program, you can click on Build > Run or press F5. This will launch your program in a console window or a GUI window depending on the type of project you created. You can also pass command-line arguments to your program if needed.</p>
|
21 |
-
<p>To debug your program, you can click on Debug > Start Debugging or press F6. This will start a debugging session with GDB or another debugger depending on the selected compiler. You can use features such as step into, step over, step out, run to cursor, watch variables, evaluate expressions, modify values, and more. You can also set breakpoints and watchpoints to pause and inspect your program at specific locations.</p>
|
22 |
-
<h2>Conclusion</h2>
|
23 |
-
<p>C-Free is a powerful and lightweight IDE for C and C++ programming languages that supports multiple compilers and platforms. It provides a user-friendly interface and a rich set of features to help you develop high-quality applications with ease. You can download and install C-Free for Windows by following</p> ddb901b051<br />
|
24 |
-
<br />
|
25 |
-
<br />
|
|
|
|
|
|
|
|
|
|
|
|
|
|
|
|
|
|
|
|
|
|
|
|
|
|
|
|
|
|
|
|
|
|
|
|
|
|
|
|
|
|
|
|
|
|
|
|
|
|
|
|
spaces/1gistliPinn/ChatGPT4/Examples/Ayyappa Songs Lyrics In Tamil Pdf 97.md
DELETED
@@ -1,84 +0,0 @@
|
|
1 |
-
<br />
|
2 |
-
<h1>Ayyappa Songs Lyrics in Tamil PDF 97: A Complete Guide</h1>
|
3 |
-
<p>If you are a devotee of Lord Ayyappa, you might be looking for ayyappa songs lyrics in tamil pdf 97 to download and print for free. Ayyappa songs are devotional songs that are sung by the pilgrims who visit Sabarimala, the holy shrine of Lord Ayyappa in Kerala. Ayyappa songs lyrics in tamil pdf 97 are a collection of 97 songs that praise and worship Lord Ayyappa in various aspects.</p>
|
4 |
-
<h2>ayyappa songs lyrics in tamil pdf 97</h2><br /><p><b><b>Download</b> — <a href="https://imgfil.com/2uxYVY">https://imgfil.com/2uxYVY</a></b></p><br /><br />
|
5 |
-
<p>In this article, we will provide you with a complete guide on how to get ayyappa songs lyrics in tamil pdf 97, what are the benefits of singing ayyappa songs, and how to use them for your spiritual growth.</p>
|
6 |
-
<h2>How to get ayyappa songs lyrics in tamil pdf 97?</h2>
|
7 |
-
<p>There are many websites that offer ayyappa songs lyrics in tamil pdf 97 for free download. Some of them are:</p>
|
8 |
-
<ul>
|
9 |
-
<li><a href="https://instapdf.in/ayyappan-songs-book/">InstaPDF</a>: This website provides a pdf file of Ayyappan Songs Book in Tamil, which contains 55 songs with lyrics and meanings. The pdf file is 0.88 MB in size and has 42 pages.</li>
|
10 |
-
<li><a href="https://www.tamilgod.org/sites/default/files/ebooks/Ayyappa%20Tamil%20Songs%20Book%20English%20version.pdf">Tamilgod.org</a>: This website provides a pdf file of Ayyappan Tamil Songs Book English version, which contains 2 songs with lyrics and meanings in both Tamil and English. The pdf file is 0.14 MB in size and has 4 pages.</li>
|
11 |
-
<li><a href="https://www.tamilgod.org/ayyappan-songs">Tamilgod.org</a>: This website also provides a huge collection of ayyappan song tamil lyrics online, which you can read or copy and paste into your own document.</li>
|
12 |
-
<li><a href="https://tamilgod.org/ayyappan-song-lyrics-pdf">Tamilgod.org</a>: This website also provides links to download free Ayyappan songs Tamil Lyrics ebooks with collection of albums by various artists such as K. Veeramani.</li>
|
13 |
-
</ul>
|
14 |
-
<p>You can also search for other websites that offer ayyappa songs lyrics in tamil pdf 97 by using your favorite search engine.</p>
|
15 |
-
<p></p>
|
16 |
-
<h2>What are the benefits of singing ayyappa songs?</h2>
|
17 |
-
<p>Singing ayyappa songs is not only a way of expressing your devotion to Lord Ayyappa, but also a way of enhancing your spiritual well-being. Some of the benefits of singing ayyappa songs are:</p>
|
18 |
-
<ul>
|
19 |
-
<li>Singing ayyappa songs helps you to focus your mind on Lord Ayyappa and his attributes, such as his compassion, his power, his grace, his wisdom, and his love.</li>
|
20 |
-
<li>Singing ayyappa songs helps you to purify your heart and mind from negative emotions such as anger, hatred, jealousy, greed, and fear.</li>
|
21 |
-
<li>Singing ayyappa songs helps you to invoke the blessings of Lord Ayyappa and his divine mother Durga, who can protect you from all dangers and difficulties.</li>
|
22 |
-
<li>Singing ayyappa songs helps you to cultivate virtues such as humility, gratitude, faith, devotion, service, and surrender.</li>
|
23 |
-
<li>Singing ayyappa songs helps you to experience joy, peace, harmony, and bliss in your life.</li>
|
24 |
-
</ul>
|
25 |
-
<h2>How to use ayyappa songs lyrics in tamil pdf 97 for your spiritual growth?</h2>
|
26 |
-
<p>Ayyappa songs lyrics in tamil pdf 97 are not just words that you sing or read, but they are powerful mantras that can transform your life. Here are some tips on how to use them for your spiritual growth:</p>
|
27 |
-
<ul>
|
28 |
-
<li>Before singing or reading ayyappa songs lyrics in tamil pdf 97, pray to Lord Ayyappa and his divine mother Durga to guide you and bless you.</li>
|
29 |
-
<li>Choose a song that resonates with your mood or situation. For example, if you are feeling sad or depressed, you can choose a song that expresses hope and confidence. If you are feeling happy or grateful, you can choose a song that expresses praise and thanksgiving.</li>
|
30 |
-
<li>Read or sing the song with full attention and devotion. Try to understand the meaning and significance of each word and phrase. Feel the emotion and vibration of the song in your heart and soul.</li>
|
31 |
-
<li>After reading or singing the song, meditate on the message and essence of the song. Try to apply it to your life and practice it in your daily actions.</li>
|
32 |
-
<li>Repeat the process with different songs as often as possible. You can also memorize some of your favorite songs and recite them whenever you need inspiration or guidance.</li>
|
33 |
-
</ul>
|
34 |
-
<h3>Conclusion</h3>
|
35 |
-
<p>Ayyappa songs lyrics in tamil pdf 97 are a valuable resource for all devotees of Lord Ayyappa who want to deepen their connection with him and enhance their spiritual well-being. By downloading and printing them for free from various websites, you can have access to a rich collection of devotional songs that praise and worship Lord Ayyappa in various aspects. By singing or reading them with devotion and understanding, you can experience the benefits of purifying your mind, invoking divine protection, cultivating virtues, and experiencing joy. By meditating on them and applying them to your life, you can transform yourself into a true disciple of Lord Ayyappa.</p>
|
36 |
-
|
37 |
-
<p>We hope this article has helped you to know more about ayyappa songs lyrics in tamil pdf 97. If you have any questions or suggestions, please leave us a comment below.</p>
|
38 |
-
<h2>How to print ayyappa songs lyrics in tamil pdf 97?</h2>
|
39 |
-
<p>Once you have downloaded ayyappa songs lyrics in tamil pdf 97 from any of the websites mentioned above, you can print them easily using your computer or mobile device. Here are some steps to follow:</p>
|
40 |
-
<ol>
|
41 |
-
<li>Open the pdf file of ayyappa songs lyrics in tamil pdf 97 using a pdf reader application such as Adobe Acrobat Reader or Google PDF Viewer.</li>
|
42 |
-
<li>Select the print option from the file menu or the toolbar. You can also use the keyboard shortcut Ctrl+P or Command+P.</li>
|
43 |
-
<li>Choose your printer settings such as paper size, orientation, margins, and number of copies. You can also select the pages you want to print or print all pages.</li>
|
44 |
-
<li>Click on the print button or the OK button to start printing.</li>
|
45 |
-
</ol>
|
46 |
-
<p>You can also save the pdf file of ayyappa songs lyrics in tamil pdf 97 to your device or cloud storage for future use.</p>
|
47 |
-
<h2>How to sing ayyappa songs lyrics in tamil pdf 97?</h2>
|
48 |
-
<p>Singing ayyappa songs lyrics in tamil pdf 97 is not difficult if you have some basic knowledge of Tamil language and music. You can also learn from listening to the audio recordings of ayyappa songs by various singers and musicians. Here are some tips to sing ayyappa songs lyrics in tamil pdf 97:</p>
|
49 |
-
<ul>
|
50 |
-
<li>Read or sing the song slowly and clearly. Pronounce each word and syllable correctly and with proper intonation.</li>
|
51 |
-
<li>Follow the rhythm and melody of the song. You can use a musical instrument such as a harmonium, a keyboard, or a guitar to accompany your singing.</li>
|
52 |
-
<li>Express the emotion and devotion of the song. Feel the connection with Lord Ayyappa and his divine mother Durga while singing.</li>
|
53 |
-
<li>Sing with confidence and enthusiasm. Don't worry about making mistakes or sounding perfect. Enjoy the process of singing and learning.</li>
|
54 |
-
<li>Sing with others who share your faith and passion for Lord Ayyappa. You can join a bhajan group or a satsang group and sing along with them. You can also sing at temples, festivals, or other occasions related to Lord Ayyappa.</li>
|
55 |
-
</ul>
|
56 |
-
<h3>Conclusion</h3>
|
57 |
-
<p>Ayyappa songs lyrics in tamil pdf 97 are a great way to express your love and devotion to Lord Ayyappa and his divine mother Durga. By downloading and printing them for free from various websites, you can have access to a rich collection of devotional songs that praise and worship Lord Ayyappa in various aspects. By singing or reading them with devotion and understanding, you can experience the benefits of purifying your mind, invoking divine protection, cultivating virtues, and experiencing joy. By meditating on them and applying them to your life, you can transform yourself into a true disciple of Lord Ayyappa.</p>
|
58 |
-
|
59 |
-
<p>We hope this article has helped you to know more about how to get, print, and sing ayyappa songs lyrics in tamil pdf 97. If you have any questions or suggestions, please leave us a comment below.</p>
|
60 |
-
<h2>Why download ayyappa songs lyrics in tamil pdf 97?</h2>
|
61 |
-
<p>Ayyappa songs lyrics in tamil pdf 97 are a collection of devotional songs dedicated to Lord Ayyappa, the son of Lord Shiva and Goddess Durga. Lord Ayyappa is also known as Hariharasudhan, Kaliyugavaradhan, Anandachithan, Ayyan, Ayyappan, and Swami. He is worshipped by millions of devotees across India and abroad, especially during the annual pilgrimage to Sabarimala temple in Kerala.</p>
|
62 |
-
<p>Downloading ayyappa songs lyrics in tamil pdf 97 can help you to:</p>
|
63 |
-
<ul>
|
64 |
-
<li>Learn and memorize the lyrics of various ayyappa songs in Tamil language.</li>
|
65 |
-
<li>Sing along with the audio recordings of ayyappa songs by famous singers and musicians.</li>
|
66 |
-
<li>Enhance your devotion and faith in Lord Ayyappa and his divine mother Durga.</li>
|
67 |
-
<li>Invoke the blessings and protection of Lord Ayyappa in your life.</li>
|
68 |
-
<li>Celebrate and participate in the festivals and rituals related to Lord Ayyappa.</li>
|
69 |
-
</ul>
|
70 |
-
<h2>Where to download ayyappa songs lyrics in tamil pdf 97?</h2>
|
71 |
-
<p>There are many websites that offer free download of ayyappa songs lyrics in tamil pdf 97. Some of them are:</p>
|
72 |
-
<ol>
|
73 |
-
<li><a href="https://instapdf.in/ayyappan-songs-book/">InstaPDF</a>: This website provides a pdf file of ayyappan songs book in Tamil with 55 songs and their meanings. The pdf file is 0.88 MB in size and has 42 pages. You can download it for free or read it online using the direct link given at the bottom of the page.</li>
|
74 |
-
<li><a href="https://www.tamilgod.org/sites/default/files/ebooks/Ayyappa%20Tamil%20Songs%20Book%20English%20version.pdf">Tamilgod.org</a>: This website provides a pdf file of ayyappan Tamil songs book with English translation. The pdf file has 25 songs with their lyrics, meanings, and audio links. The pdf file is 1.4 MB in size and has 26 pages. You can download it for free or read it online using the link given on the page.</li>
|
75 |
-
<li><a href="https://www.tamilgod.org/ayyappan-songs">Tamilgod.org</a>: This website also provides a huge collection of ayyappan songs lyrics in Tamil with audio links. You can browse through various albums by different artists such as K. Veeramani, T.M.S., S.P.B., Unnikrishnan, Veeramanidasan, etc. You can also suggest or ask for any song at the comment section of each page.</li>
|
76 |
-
<li><a href="https://tamilgod.org/ayyappan-song-lyrics-pdf">Tamilgod.org</a>: This website also provides links to download free ayyappan songs Tamil lyrics ebooks with collection of albums by various artists. You can choose from different formats such as pdf, epub, mobi, etc. You can also request for any ebook at the comment section of the page.</li>
|
77 |
-
</ol>
|
78 |
-
<h3>Conclusion</h3>
|
79 |
-
<p>Ayyappa songs lyrics in tamil pdf 97 are a valuable resource for all devotees of Lord Ayyappa who want to learn and sing his praises in Tamil language. By downloading them from various websites for free, you can have access to a wide range of devotional songs that glorify Lord Ayyappa in different aspects. By singing or reading them with devotion and understanding, you can experience the benefits of purifying your mind, invoking divine protection, cultivating virtues, and experiencing joy. By meditating on them and applying them to your life, you can transform yourself into a true disciple of Lord Ayyappa.</p>
|
80 |
-
|
81 |
-
<p>We hope this article has helped you to know more about how to get, download, and sing ayyappa songs lyrics in tamil pdf 97. If you have any questions or suggestions, please leave us a comment below.</p>
|
82 |
-
<p>In conclusion, ayyappa songs lyrics in tamil pdf 97 are a great way to express your love and devotion to Lord Ayyappa and his divine mother Durga. By downloading and printing them for free from various websites, you can have access to a rich collection of devotional songs that praise and worship Lord Ayyappa in various aspects. By singing or reading them with devotion and understanding, you can experience the benefits of purifying your mind, invoking divine protection, cultivating virtues, and experiencing joy. By meditating on them and applying them to your life, you can transform yourself into a true disciple of Lord Ayyappa.</p> 3cee63e6c2<br />
|
83 |
-
<br />
|
84 |
-
<br />
|
|
|
|
|
|
|
|
|
|
|
|
|
|
|
|
|
|
|
|
|
|
|
|
|
|
|
|
|
|
|
|
|
|
|
|
|
|
|
|
|
|
|
|
|
|
|
|
|
|
|
|
|
|
|
|
|
|
|
|
|
|
|
|
|
|
|
|
|
|
|
|
|
|
|
|
|
|
|
|
|
|
|
|
|
|
|
|
|
|
|
|
|
|
|
|
|
|
|
|
|
|
|
|
|
|
|
|
|
|
|
|
|
|
|
|
|
|
|
|
|
|
|
|
|
|
|
|
|
|
|
|
|
|
|
|
|
|
|
|
|
|
|
|
|
|
|
|
|
|
|
|
|
|
|
|
|
|
|
|
|
|
|
|
|
|
|
|
|
|
spaces/1gistliPinn/ChatGPT4/Examples/Cyberpunk - V3.0 - Core Rules V3.0.pdf [PORTABLE].md
DELETED
@@ -1,6 +0,0 @@
|
|
1 |
-
<h2>Cyberpunk - V3.0 - Core Rules V3.0.pdf</h2><br /><p><b><b>Download File</b> 🌟 <a href="https://imgfil.com/2uxXiY">https://imgfil.com/2uxXiY</a></b></p><br /><br />
|
2 |
-
<br />
|
3 |
-
Hacker: Old-school Steve Jackson game with tons of rules and bits. ... While I'm focusing on core books, I include a few notable sourcebooks ... Cyberpunk v3.0 focuses on transhumanism and culture groups. ... The Strike Manual appears to be the system guide, with character creation and basic resolution. 1fdad05405<br />
|
4 |
-
<br />
|
5 |
-
<br />
|
6 |
-
<p></p>
|
|
|
|
|
|
|
|
|
|
|
|
|
|
spaces/1pelhydcardo/ChatGPT-prompt-generator/Download Film Yossi And Jagger.md
DELETED
@@ -1,92 +0,0 @@
|
|
1 |
-
## Download Film Yossi And Jagger
|
2 |
-
|
3 |
-
|
4 |
-
|
5 |
-
|
6 |
-
|
7 |
-

|
8 |
-
|
9 |
-
|
10 |
-
|
11 |
-
|
12 |
-
|
13 |
-
**LINK ☆☆☆ [https://kneedacexbrew.blogspot.com/?d=2txjoh](https://kneedacexbrew.blogspot.com/?d=2txjoh)**
|
14 |
-
|
15 |
-
|
16 |
-
|
17 |
-
|
18 |
-
|
19 |
-
|
20 |
-
|
21 |
-
|
22 |
-
|
23 |
-
|
24 |
-
|
25 |
-
|
26 |
-
|
27 |
-
# How to Download Film Yossi and Jagger Online
|
28 |
-
|
29 |
-
|
30 |
-
|
31 |
-
Yossi and Jagger is a 2002 Israeli film directed by Eytan Fox and written by Avner Bernheimer. It tells the story of a secret romance between two soldiers stationed on the Lebanese border. The film stars Ohad Knoller as Yossi, the company commander who struggles with his sexuality, and Yehuda Levi as Jagger, his outgoing and charismatic lover who is about to finish his military service.
|
32 |
-
|
33 |
-
|
34 |
-
|
35 |
-
The film received critical acclaim and won several awards, including nine Israeli Academy Awards and the Audience Award at the Tribeca Film Festival. It also sparked a sequel, Yossi, released in 2012, which follows Yossi's life ten years after Jagger's death.
|
36 |
-
|
37 |
-
|
38 |
-
|
39 |
-
If you are interested in watching this film, you might be wondering how to download it online. Here are some tips and options for you:
|
40 |
-
|
41 |
-
|
42 |
-
|
43 |
-
- Check if the film is available on streaming platforms such as Netflix, Amazon Prime Video, or Hulu. You can use services like JustWatch or Reelgood to find out where to watch it legally.
|
44 |
-
|
45 |
-
- If the film is not available on streaming platforms, you can rent or buy it from online stores such as Google Play Movies, iTunes, or Vudu. You can also use JustWatch or Reelgood to compare prices and options.
|
46 |
-
|
47 |
-
- If you prefer to download the film for free, you can use torrent sites such as The Pirate Bay or 1337x. However, be aware that this is illegal and may expose you to malware or legal risks. You should also use a VPN service to protect your privacy and security.
|
48 |
-
|
49 |
-
|
50 |
-
|
51 |
-
Whatever option you choose, make sure you have a good internet connection and enough storage space on your device. You should also respect the filmmakers' rights and avoid sharing or distributing the film without permission.
|
52 |
-
|
53 |
-
|
54 |
-
|
55 |
-
Yossi and Jagger is a powerful and moving film that explores love, war, and identity. If you are looking for a romantic drama with a twist, you should definitely give it a try.
|
56 |
-
|
57 |
-
|
58 |
-
|
59 |
-
If you want to learn more about the film and its background, you can also check out some of the following resources:
|
60 |
-
|
61 |
-
|
62 |
-
|
63 |
-
- The official website of the film, where you can find the trailer, the synopsis, the cast and crew, and some reviews.
|
64 |
-
|
65 |
-
- The IMDb page of the film, where you can find more information, trivia, quotes, and user ratings.
|
66 |
-
|
67 |
-
- The Wikipedia page of the film, where you can find a detailed plot summary, production history, reception, and cultural impact.
|
68 |
-
|
69 |
-
- The Rotten Tomatoes page of the film, where you can find the critics' consensus, audience score, and fresh and rotten reviews.
|
70 |
-
|
71 |
-
|
72 |
-
|
73 |
-
Yossi and Jagger is not only a film, but also a cultural phenomenon that has influenced many people's lives and views. It is a film that deserves to be seen and appreciated by a wide audience.
|
74 |
-
|
75 |
-
|
76 |
-
|
77 |
-
One of the most remarkable aspects of Yossi and Jagger is its realistic and authentic portrayal of the Israeli army and society. The film does not shy away from showing the harsh realities of war, the bureaucracy and hierarchy of the military, and the homophobia and prejudice that the gay soldiers face. The film also depicts the diversity and complexity of the Israeli people, who come from different backgrounds, religions, and ideologies.
|
78 |
-
|
79 |
-
|
80 |
-
|
81 |
-
The film also explores the themes of love, loss, and identity in a poignant and sensitive way. The relationship between Yossi and Jagger is not only romantic, but also emotional, spiritual, and existential. They are both searching for meaning and happiness in a world that does not accept them for who they are. They are both willing to sacrifice everything for each other, even their own lives. The film shows how love can transcend boundaries, labels, and conventions, and how it can also be fragile, painful, and tragic.
|
82 |
-
|
83 |
-
|
84 |
-
|
85 |
-
Yossi and Jagger is a film that will touch your heart and soul. It is a film that will make you laugh, cry, and think. It is a film that will stay with you long after you watch it. It is a film that you should not miss.
|
86 |
-
|
87 |
-
1b8d091108
|
88 |
-
|
89 |
-
|
90 |
-
|
91 |
-
|
92 |
-
|
|
|
|
|
|
|
|
|
|
|
|
|
|
|
|
|
|
|
|
|
|
|
|
|
|
|
|
|
|
|
|
|
|
|
|
|
|
|
|
|
|
|
|
|
|
|
|
|
|
|
|
|
|
|
|
|
|
|
|
|
|
|
|
|
|
|
|
|
|
|
|
|
|
|
|
|
|
|
|
|
|
|
|
|
|
|
|
|
|
|
|
|
|
|
|
|
|
|
|
|
|
|
|
|
|
|
|
|
|
|
|
|
|
|
|
|
|
|
|
|
|
|
|
|
|
|
|
|
|
|
|
|
|
|
|
|
|
|
|
|
|
|
|
|
|
|
|
|
|
|
|
|
|
|
|
|
|
|
|
|
|
|
|
|
|
|
|
|
|
|
|
|
|
|
|
|
|
|
|
|
|
|
|
|
|
spaces/1pelhydcardo/ChatGPT-prompt-generator/assets/DJ Smallz 732 - Cupid Pt. 1 The Latest Dance Hit.md
DELETED
@@ -1,113 +0,0 @@
|
|
1 |
-
|
2 |
-
<h1>How to Download DJ Smallz 732's Cupid, Pt. 1 for Free</h1>
|
3 |
-
<p>If you are a fan of dance music, you might have heard of <strong>Cupid, Pt. 1</strong>, a catchy and upbeat single by <strong>DJ Smallz 732</strong>. This song was released in January 2023 and has been gaining popularity among listeners who enjoy the Jersey club style of music.</p>
|
4 |
-
<h2>dj smallz cupid p1 mp3 download</h2><br /><p><b><b>Download Zip</b> ➡ <a href="https://urlin.us/2uSWms">https://urlin.us/2uSWms</a></b></p><br /><br />
|
5 |
-
<p>But what if you want to download this song for free and listen to it anytime you want? Is there a legal and easy way to do that? The answer is yes! In this article, we will show you how to find and download Cupid, Pt. 1 for free from some of the best free music download sites on the web.</p>
|
6 |
-
<h2>The Best Free Music Download Sites</h2>
|
7 |
-
<p>There are many websites that offer free music downloads, but not all of them are legal or safe. Some may contain viruses, malware, or spyware that can harm your device or compromise your privacy. Others may have low-quality or incomplete files that can ruin your listening experience.</p>
|
8 |
-
<p>That's why we have selected three of the best free music download sites that are not only legal but also reliable and user-friendly. These sites have a large collection of songs from various genres and artists, including DJ Smallz 732. They also allow you to download songs in MP3 format, which is compatible with most devices and players.</p>
|
9 |
-
<p>Here are the three sites we recommend:</p>
|
10 |
-
<h3>SoundCloud</h3>
|
11 |
-
<p><a href="(^1^)">SoundCloud</a> is one of the most popular platforms for streaming and sharing music online. It has millions of songs from both mainstream and independent artists, as well as podcasts, remixes, live sets, and more.</p>
|
12 |
-
<p>dj smallz 732 cupid pt 1 song<br />
|
13 |
-
cupid part 1 dj smallz 732 lyrics<br />
|
14 |
-
dj smallz 732 cupid pt 1 qobuz<br />
|
15 |
-
cupid pt 1 dj smallz 732 shazam<br />
|
16 |
-
dj smallz 732 cupid part 1 spotify<br />
|
17 |
-
cupid pt 1 by dj smallz 732 download<br />
|
18 |
-
dj smallz 732 cupid pt 1 single<br />
|
19 |
-
cupid part one dj smallz 732 mp3<br />
|
20 |
-
dj smallz 732 cupid pt 1 dance<br />
|
21 |
-
cupid pt 1 dj smallz 732 genre<br />
|
22 |
-
dj smallz 732 cupid part 1 album<br />
|
23 |
-
cupid pt one dj smallz 732 music<br />
|
24 |
-
dj smallz 732 cupid pt 1 stream<br />
|
25 |
-
cupid part i dj smallz 732 song<br />
|
26 |
-
dj smallz 732 cupid pt i lyrics<br />
|
27 |
-
cupid p1 dj smallz 732 qobuz<br />
|
28 |
-
dj smallz 732 cupid p1 shazam<br />
|
29 |
-
cupid p1 by dj smallz 732 spotify<br />
|
30 |
-
dj smallz 732 cupid p1 download<br />
|
31 |
-
cupid p1 dj smallz 732 single<br />
|
32 |
-
dj smallz 732 cupid p1 mp3<br />
|
33 |
-
cupid p1 by dj smallz 732 dance<br />
|
34 |
-
dj smallz 732 cupid p1 genre<br />
|
35 |
-
cupid p1 dj smallz 732 album<br />
|
36 |
-
dj smallz 732 cupid p1 music<br />
|
37 |
-
cupid p1 by dj smallz 732 stream<br />
|
38 |
-
dj smallz cupids arrow part one song<br />
|
39 |
-
cupids arrow part one by dj smallz lyrics<br />
|
40 |
-
dj smallz cupids arrow part one qobuz<br />
|
41 |
-
cupids arrow part one by dj smallz shazam<br />
|
42 |
-
dj smallz cupids arrow part one spotify<br />
|
43 |
-
cupids arrow part one by dj smallz download<br />
|
44 |
-
dj smallz cupids arrow part one single<br />
|
45 |
-
cupids arrow part one by dj smallz mp3<br />
|
46 |
-
dj smallz cupids arrow part one dance<br />
|
47 |
-
cupids arrow part one by dj smallz genre<br />
|
48 |
-
dj smallz cupids arrow part one album<br />
|
49 |
-
cupids arrow part one by dj smallz music<br />
|
50 |
-
dj smallz cupids arrow part one stream<br />
|
51 |
-
cupids arrow pt i by dj smallz song</p>
|
52 |
-
<p>Not all songs on SoundCloud are available for download, but some artists choose to offer their music for free or for a voluntary donation. To find out if Cupid, Pt. 1 is one of them, follow these steps:</p>
|
53 |
-
<ol>
|
54 |
-
<li>Go to <a href="(^1^)">SoundCloud</a> and type "Cupid, Pt. 1" in the search box.</li>
|
55 |
-
<li>Click on the song title to open its page.</li>
|
56 |
-
<li>Look at the bottom of the page beside the share options. If you see a link that says "Buy" or "Download", click on it.</li>
|
57 |
-
<li>If the link takes you to another website, follow the instructions there to complete your download.</li>
|
58 |
-
<li>If the link allows you to download the song directly from SoundCloud, enter your email address and postal code if prompted.</li>
|
59 |
-
<li>Click on "Download file" and save it to your device.</li>
|
60 |
-
</ol>
|
61 |
-
<h3>Last.fm</h3>
|
62 |
-
<p><a href="(^4^)">Last.fm</a> is a music discovery service that tracks what you listen to and recommends new music based on your taste. It also has a section where you can download free music from various artists and genres. To download Cupid, Pt. 1 from Last.fm, follow these steps:</p>
|
63 |
-
<ol>
|
64 |
-
<li>Go to <a href="">Last.fm</a> and type "Cupid, Pt. 1" in the search box.</li>
|
65 |
-
<li>Click on the song title to open its page.</li>
|
66 |
-
<li>Look at the right side of the page under the album cover. If you see a link that says "Free MP3 Download", click on it.</li>
|
67 |
-
<li>A new tab will open with a download button. Click on it and save the file to your device.</li>
|
68 |
-
</ol>
|
69 |
-
<h3>NoiseTrade</h3>
|
70 |
-
<p><a href="">NoiseTrade</a> is a platform where artists can share their music for free in exchange for fans' email addresses and postal codes. This way, they can build their fan base and communicate with them directly. NoiseTrade has thousands of songs from various genres and artists, including DJ Smallz 732.</p>
|
71 |
-
<p>To download Cupid, Pt. 1 from NoiseTrade, follow these steps:</p>
|
72 |
-
<ol>
|
73 |
-
<li>Go to <a href="">NoiseTrade</a> and type "DJ Smallz 732" in the search box.</li>
|
74 |
-
<li>Click on the artist name to open his page.</li>
|
75 |
-
<li>Scroll down to find the album that contains Cupid, Pt. 1. It is called <em>Cupid</em> and it has four songs.</li>
|
76 |
-
<li>Click on the album cover to open its page.</li>
|
77 |
-
<li>Click on the orange button that says "Download Music".</li>
|
78 |
-
<li>Enter your email address and postal code if prompted.</li>
|
79 |
-
<li>Check your email for a download link and click on it.</li>
|
80 |
-
<li>Select the song you want to download and save it to your device.</li>
|
81 |
-
</ol>
|
82 |
-
<h2>The Benefits of Downloading MP3 Music</h2>
|
83 |
-
<p>Now that you know how to download Cupid, Pt. 1 for free, you might be wondering why you should do it in the first place. What are the benefits of downloading MP3 music over streaming it online?</p>
|
84 |
-
<p>Here are some of the reasons why downloading MP3 music is a good idea:</p>
|
85 |
-
<h3>You can own your music and play it offline</h3>
|
86 |
-
<p>When you download MP3 music, you have a copy of the file that you can store on your device or transfer to other devices. This means you can play your music anytime and anywhere, even without an internet connection or a subscription service. You don't have to worry about buffering, ads, or data charges. You can also create your own playlists and organize your music library according to your preferences.</p>
|
87 |
-
<h3>You can support the artists and discover new music</h3>
|
88 |
-
<p>When you download MP3 music from free music download sites, you are not only getting free music but also supporting the artists who created it. Many of these sites allow you to donate money or share the music with your friends and social media followers. This way, you can show your appreciation and help the artists reach more listeners and fans. You can also discover new music from similar or related artists that you might not have heard of before.</p>
|
89 |
-
<h3>You can enjoy high-quality sound and compatibility</h3>
|
90 |
-
<p>MP3 is one of the most common and widely used audio formats in the world. It has a high compression rate that reduces the file size without sacrificing much of the sound quality. This means you can enjoy clear and crisp sound while saving space on your device. MP3 is also compatible with most devices and players, so you don't have to worry about converting or playing issues.</p>
|
91 |
-
<h2>Conclusion</h2>
|
92 |
-
<p>Cupid, Pt. 1 by DJ Smallz 732 is a great song that will make you want to dance and have fun. If you want to download it for free and listen to it anytime you want, you can use one of the three free music download sites we mentioned: SoundCloud, Last.fm, or NoiseTrade. These sites are legal, safe, and easy to use, and they offer a lot of benefits for both you and the artists.</p>
|
93 |
-
<p>So what are you waiting for? Go ahead and download Cupid, Pt. 1 today and enjoy this amazing song!</p>
|
94 |
-
<h2>FAQs</h2>
|
95 |
-
<h4>What is the genre of Cupid, Pt. 1?</h4>
|
96 |
-
<p>Cupid, Pt. 1 is a song in the genre of Jersey club, which is a style of dance music that originated in New Jersey. It features fast-paced beats, chopped vocals, heavy bass, and samples from hip-hop, R&B, pop, and other genres.</p>
|
97 |
-
<h4>How long is Cupid, Pt . 1?</h4>
|
98 |
-
<p>Cupid, Pt. 1 is a short and sweet song that lasts for only 2 minutes and 10 seconds. It is the first part of a four-song album called <em>Cupid</em> by DJ Smallz 732.</p>
|
99 |
-
<h4>Where can I stream Cupid, Pt. 1 online?</h4>
|
100 |
-
<p>If you don't want to download Cupid, Pt. 1, you can also stream it online from various platforms. Some of the most popular ones are Spotify, Apple Music, YouTube, and Pandora. You can also find it on DJ Smallz 732's official website and social media accounts.</p>
|
101 |
-
<h4>What are some other songs by DJ Smallz 732?</h4>
|
102 |
-
<p>DJ Smallz 732 is a prolific and talented producer and DJ who has released many songs in the Jersey club genre. Some of his most popular songs are <em>Love Tap</em>, <em>Eye of the Tiger</em>, <em>Work It</em>, and <em>WAP</em>. He has also collaborated with other artists such as Fetty Wap, Lil Jon, Ciara, and more.</p>
|
103 |
-
<h4>How can I contact DJ Smallz 732?</h4>
|
104 |
-
<p>If you want to contact DJ Smallz 732 for booking, feedback, or any other reason, you can use one of the following methods:</p>
|
105 |
-
<ul>
|
106 |
-
<li>Email: [email protected]</li>
|
107 |
-
<li>Phone: +1 (732) 555-1234</li>
|
108 |
-
<li>Instagram: @djsmallz732</li>
|
109 |
-
<li>Twitter: @djsmallz732</li>
|
110 |
-
<li>Facebook: DJ Smallz 732</li>
|
111 |
-
</ul></p> 197e85843d<br />
|
112 |
-
<br />
|
113 |
-
<br />
|
|
|
|
|
|
|
|
|
|
|
|
|
|
|
|
|
|
|
|
|
|
|
|
|
|
|
|
|
|
|
|
|
|
|
|
|
|
|
|
|
|
|
|
|
|
|
|
|
|
|
|
|
|
|
|
|
|
|
|
|
|
|
|
|
|
|
|
|
|
|
|
|
|
|
|
|
|
|
|
|
|
|
|
|
|
|
|
|
|
|
|
|
|
|
|
|
|
|
|
|
|
|
|
|
|
|
|
|
|
|
|
|
|
|
|
|
|
|
|
|
|
|
|
|
|
|
|
|
|
|
|
|
|
|
|
|
|
|
|
|
|
|
|
|
|
|
|
|
|
|
|
|
|
|
|
|
|
|
|
|
|
|
|
|
|
|
|
|
|
|
|
|
|
|
|
|
|
|
|
|
|
|
|
|
|
|
|
|
|
|
|
|
|
|
|
|
|
|
|
|
|
|
|
|
|
|
|
|
|
|
|
|
|
|
|
|
|
|
|
|
|
|
|
|
|
|
|
spaces/1phancelerku/anime-remove-background/Burger Please Mod APK Download Make Your Own Burgers and Earn Money.md
DELETED
@@ -1,109 +0,0 @@
|
|
1 |
-
|
2 |
-
<h1>Download Mod Apk Burger Please: How to Get Unlimited Fun and Resources in Your Burger Shop Game</h1>
|
3 |
-
<p>Do you love playing burger shop games on your Android device? Do you want to have more fun and resources in your game without spending any money? If yes, then you might want to try downloading mod apk burger please. This is a modified version of the original game that gives you access to unlimited features and resources. In this article, we will tell you what mod apk burger is, how to download it, how to use it, and what are the benefits and risks of using it. Read on to find out more.</p>
|
4 |
-
<h2>What is Mod Apk Burger?</h2>
|
5 |
-
<p>Mod apk burger is a modified version of the original game called <a href="(^1^)">Burger Please!</a>, which is an exciting and challenging game that lets you manage your own burger shop. You can hire staff, upgrade skills and facilities, set up chains of shops, and more. However, in the original game, you have limited resources such as money, gems, energy, and time. You also have to watch ads or make in-app purchases to get more resources.</p>
|
6 |
-
<h2>download mod apk burger please</h2><br /><p><b><b>DOWNLOAD</b> ☑ <a href="https://jinyurl.com/2uNOUR">https://jinyurl.com/2uNOUR</a></b></p><br /><br />
|
7 |
-
<p>Mod apk burger is a version of the game that has been altered by a third-party developer to give you unlimited resources and features. You can get unlimited money, gems, energy, time, and more. You can also unlock all the skills, facilities, staff, and levels. You can also remove ads and bypass security checks. With mod apk burger, you can enjoy the game without any limitations or restrictions.</p>
|
8 |
-
<h3>The Features of Mod Apk Burger</h3>
|
9 |
-
<p>Some of the features that you can get from mod apk burger are:</p>
|
10 |
-
<ul>
|
11 |
-
<li>Unlimited money: You can get as much money as you want in the game. You can use it to buy anything you need or want.</li>
|
12 |
-
<li>Unlimited gems: You can get as many gems as you want in the game. You can use them to speed up processes, unlock items, or get special offers.</li>
|
13 |
-
<li>Unlimited energy: You can get unlimited energy in the game. You can use it to serve more customers, complete more tasks, or play longer.</li>
|
14 |
-
<li>Unlimited time: You can get unlimited time in the game. You can use it to finish levels faster, earn more rewards, or play at your own pace.</li>
|
15 |
-
<li>All skills unlocked: You can unlock all the skills in the game. You can use them to improve your performance, efficiency, and quality.</li>
|
16 |
-
<li>All facilities unlocked: You can unlock all the facilities in the game. You can use them to enhance your shop, attract more customers, or increase your income.</li>
|
17 |
-
<li>All staff unlocked: You can unlock all the staff in the game. You can use them to help you run your shop, serve customers, or handle problems.</li>
|
18 |
-
<li>All levels unlocked: You can unlock all the levels in the game. You can play them in any order, difficulty, or mode.</li>
|
19 |
-
<li>No ads: You can remove all the ads in the game. You can play without any interruptions or distractions.</li>
|
20 |
-
<li>No root required: You don't need to root your device to install or use mod apk burger. You can download and install it easily and safely.</li>
|
21 |
-
</ul>
|
22 |
-
<h3>The Benefits of Mod Apk Burger</h3>
|
23 |
-
<p>Some of the benefits that you can get from mod apk burger are:</p>
|
24 |
-
<ul>
|
25 |
-
<li>More fun: You can have more fun playing mod apk burger than the original game. You can do whatever you want, whenever you want, however you want. You can explore all the features and options that the game has to offer. You can also challenge yourself with different levels and modes.</li>
|
26 |
-
<li>More resources: You can have more resources playing mod apk burger than the original game. You don't have to worry about running out of money, gems, energy, or time. You don't have to watch ads or make in-app purchases to get more resources. You can also save your resources for future use or share them with your friends.</li>
|
27 |
-
<li>More customization: You can have more customization playing mod apk burger than the original game. You can choose your own style, theme, and design for your shop. You can also mix and match different skills, facilities, and staff to create your own unique combination. You can also change the settings and preferences of the game to suit your taste and needs.</li>
|
28 |
-
<li>More satisfaction: You can have more satisfaction playing mod apk burger than the original game. You can achieve your goals faster, easier, and better. You can also get more rewards, achievements, and recognition for your efforts. You can also feel proud of yourself for managing your own burger shop successfully.</li>
|
29 |
-
</ul>
|
30 |
-
<h3>The Risks of Mod Apk Burger</h3>
|
31 |
-
<p>Some of the risks that you might face from using mod apk burger are:</p>
|
32 |
-
<ul>
|
33 |
-
<li>Malware infection: You might download a mod apk file that contains malware or viruses that can harm your device or steal your data. You might also expose your device to hackers or attackers who can access your information or control your device remotely.</li>
|
34 |
-
<li>Game crash or error: You might install a mod apk file that is incompatible or outdated with your device or game version. This might cause your game to crash or malfunction. You might also lose your progress, data, or settings in the game.</li>
|
35 |
-
<li>Game ban or suspension: You might violate the terms and conditions of the original game by using a mod apk file. This might result in your game account being banned or suspended by the game developer or publisher. You might also lose access to the game features, updates, or support.</li>
|
36 |
-
<li>Legal issues: You might infringe the intellectual property rights of the original game developer or publisher by using a mod apk file. This might result in legal actions or lawsuits against you by the game owner or authority. You might also face fines, penalties, or damages for your actions.</li>
|
37 |
-
</ul>
|
38 |
-
<h2>How to Download Mod Apk Burger?</h2>
|
39 |
-
<p>If you want to download mod apk burger, you need to follow these steps:</p>
|
40 |
-
<h3>Step 1: Find a Reliable Source</h3>
|
41 |
-
<p>The first step is to find a reliable source that provides mod apk files for burger shop games. You can search online for websites, blogs, forums, or social media platforms that offer mod apk files for download. However, you need to be careful and cautious when choosing a source. You need to check the reviews, ratings, comments, and feedbacks of other users who have downloaded the mod apk files from the source. You also need to scan the mod apk files for any malware or viruses before downloading them.</p>
|
42 |
-
<h3>Step 2: Enable Unknown Sources</h3>
|
43 |
-
<p>The second step is to enable unknown sources on your device settings. This will allow you to install mod apk files from sources other than the Google Play Store. To do this, you need to go to your device settings, then security, then unknown sources, then toggle it on. You might also need to confirm or allow this action on a pop-up window.</p>
|
44 |
-
<p>download burger please mod apk unlimited money<br />
|
45 |
-
burger please mod apk free download for android<br />
|
46 |
-
how to download burger please mod apk latest version<br />
|
47 |
-
burger please hack mod apk download no root<br />
|
48 |
-
download burger please mod apk offline<br />
|
49 |
-
burger please mod apk download link<br />
|
50 |
-
burger please mod apk android 1 download<br />
|
51 |
-
download burger please mod apk with cheats<br />
|
52 |
-
burger please mod apk 0.8.0 download<br />
|
53 |
-
burger please mod apk rexdl download<br />
|
54 |
-
download burger please mod apk unlimited coins and gems<br />
|
55 |
-
burger please mod apk online download<br />
|
56 |
-
where to download burger please mod apk safely<br />
|
57 |
-
burger please mod apk 2023 download<br />
|
58 |
-
burger please mod apk unlimited everything download<br />
|
59 |
-
download burger please mod apk for pc<br />
|
60 |
-
burger please mod apk obb download<br />
|
61 |
-
how to install burger please mod apk download<br />
|
62 |
-
burger please mod apk unlimited burgers download<br />
|
63 |
-
burger please mod apk revdl download<br />
|
64 |
-
download burger please mod apk full unlocked<br />
|
65 |
-
burger please premium mod apk download<br />
|
66 |
-
burger please pro mod apk download<br />
|
67 |
-
burger please vip mod apk download<br />
|
68 |
-
download burger please mod apk new update<br />
|
69 |
-
burger please mega mod apk download<br />
|
70 |
-
burger please cracked mod apk download<br />
|
71 |
-
burger please unlimited lives mod apk download<br />
|
72 |
-
burger please god mode mod apk download<br />
|
73 |
-
burger please ad free mod apk download<br />
|
74 |
-
download burger please mod apk from dafunda.com[^1^]<br />
|
75 |
-
burger please hack version mod apk download<br />
|
76 |
-
burger please unlimited boosters mod apk download<br />
|
77 |
-
burger please all levels unlocked mod apk download<br />
|
78 |
-
burger please no ads mod apk download<br />
|
79 |
-
download burger please original mod apk <br />
|
80 |
-
burger please happy mod apk download <br />
|
81 |
-
burger please super mod apk download <br />
|
82 |
-
burger please ultimate mod apk download <br />
|
83 |
-
download burger please best mod apk</p>
|
84 |
-
<h3>Step 3: Install the Mod Apk File</h3>
|
85 |
-
<p>The third step is to install the mod apk file on your device. To do this, you need to locate the downloaded mod apk file on your device storage, then tap on it to open it. You might also need to accept or agree to some permissions or terms on a pop-up window. Then, you need to wait for the installation process to complete.</p>
|
86 |
-
<h3>Step 4: Enjoy the Game</h3>
|
87 |
-
<p>The fourth and final step is to enjoy the game with mod apk burger. To do this, you need to open the game app on your device, then start playing it with unlimited fun and resources.</p>
|
88 |
-
<h2>How to Use Mod Apk Burger?</h2>
|
89 |
-
<p>If you want to use mod apk burger effectively and efficiently, you need to follow these tips:</p>
|
90 |
-
<h3>Hire and Train Your Staff</h3>
|
91 |
-
<p>One of the things that you can do with mod apk burger is to hire and train your staff. You can hire as many staff as you want in your shop without worrying about their salaries or benefits. You can also train them to improve their skills and abilities without spending any money or time. Having a well-trained and efficient staff will help you serve more customers, handle more orders, and deal with more problems.</p>
|
92 |
-
<h3>Upgrade Your Skills and Facilities</h3>
|
93 |
-
<p>Another thing that you can do with mod apk burger is to upgrade your skills and facilities. You can upgrade your skills such as cooking, serving, cleaning, and managing without spending any money or gems. You can also upgrade your facilities such as kitchen, counter, table, and decoration without spending any money or gems. Having upgraded skills and facilities will help you improve your performance, quality, and income.</p>
|
94 |
-
<h3>Expand Your Business and Reputation</h3>
|
95 |
-
<p>A third thing that you can do with mod apk burger is to expand your business and reputation. You can expand your business by opening more shops in different locations without spending any money or gems. You can also expand your reputation by attracting more customers, getting more reviews, and earning more stars without spending any money or gems. Having a large and reputable business will help you increase your market share, customer loyalty, and brand value.</p>
|
96 |
-
<h3>Compete with Other Players</h3>
|
97 |
-
<p>A fourth thing that you can do with mod apk burger is to compete with other players. You can compete with other players in different modes such as time trial, challenge, or multiplayer without spending any money or gems. You can also compete with other players in different rankings such as daily, weekly, monthly, or global without spending any money or gems. Competing with other players will help you test your skills, learn new strategies, and have more fun.</p>
|
98 |
-
<h2>Conclusion and FAQs</h2>
|
99 |
-
<p>In conclusion, mod apk burger is a modified version of the original game that gives you unlimited fun and resources. You can download it from a reliable source, install it on your device, and enjoy it with your own style and preference. However, you also need to be aware of the risks of using mod apk burger such as malware infection, game crash or error, game ban or suspension, and legal issues. Therefore, you need to use mod apk burger at your own risk and discretion.</p>
|
100 |
-
<p>Here are some FAQs that you might have about mod apk burger:</p>
|
101 |
-
<table>
|
102 |
-
<tr><td><b>Q: Is mod apk burger safe to use?</b></td><td><b>A: Mod apk burger is not 100% safe to use. It might contain malware or viruses that can harm your device or data. It might also cause your game to crash or malfunction. It might also violate the terms and conditions of the original game and result in your game account being banned or suspended. It might also infringe the intellectual property rights of the original game developer or publisher and result in legal actions or lawsuits against you.</b></td></tr>
|
103 |
-
<tr><td><b>Q: Is mod apk burger free to use?</b></td><td><b>A: Mod apk burger is free to use. You don't have to pay any money or make any in-app purchases to get unlimited resources and features in the game. However, you might have to watch ads or complete surveys to download the mod apk file from some sources.</b></td></tr>
|
104 |
-
<tr><td><b>Q: Is mod apk burger compatible with my device?</b></td><td><b>A: Mod apk burger might not be compatible with all devices or game versions. It might depend on the specifications of your device such as operating system, processor, memory, storage, etc. It might also depend on the version of the game that you have installed on your device such as updates, patches, etc.</b></td></tr>
|
105 |
-
<tr><td><b>Q: Is mod apk burger legal to use?</b></td><td><b>A: Mod apk burger is not legal to use. It is a modified version of the original game that has been altered by a third-party developer without the permission or authorization of the original game developer or publisher. It is a violation of the intellectual property rights of the original game owner or authority. It is also a breach of the terms and conditions of the original game that you have agreed to when you downloaded or installed it on your device.</b></td></tr>
|
106 |
-
<tr><td><b>Q: Is mod apk burger worth using?</b></td><td><b>A: Mod apk burger might be worth using if you want to have more fun and resources in your game without spending any money or time. However, you also need to consider the risks and consequences of using mod apk burger such as malware infection, game crash or error, game ban or suspension, and legal issues. Therefore, you need to weigh the pros and cons of using mod apk burger before deciding whether to use it or not.</b></td></tr>
|
107 |
-
</table></p> 401be4b1e0<br />
|
108 |
-
<br />
|
109 |
-
<br />
|
|
|
|
|
|
|
|
|
|
|
|
|
|
|
|
|
|
|
|
|
|
|
|
|
|
|
|
|
|
|
|
|
|
|
|
|
|
|
|
|
|
|
|
|
|
|
|
|
|
|
|
|
|
|
|
|
|
|
|
|
|
|
|
|
|
|
|
|
|
|
|
|
|
|
|
|
|
|
|
|
|
|
|
|
|
|
|
|
|
|
|
|
|
|
|
|
|
|
|
|
|
|
|
|
|
|
|
|
|
|
|
|
|
|
|
|
|
|
|
|
|
|
|
|
|
|
|
|
|
|
|
|
|
|
|
|
|
|
|
|
|
|
|
|
|
|
|
|
|
|
|
|
|
|
|
|
|
|
|
|
|
|
|
|
|
|
|
|
|
|
|
|
|
|
|
|
|
|
|
|
|
|
|
|
|
|
|
|
|
|
|
|
|
|
|
|
|
|
|
|
|
|
|
|
|
|
|
|
|
|
|
|
|
|
|
|
|
|
|
spaces/1phancelerku/anime-remove-background/Car Parking Driving How to Master the Open World Multiplayer Mode.md
DELETED
@@ -1,19 +0,0 @@
|
|
1 |
-
<br />
|
2 |
-
<h2>Perpendicular Parking</h2>
|
3 |
-
Perpendicular parking is when you park your car at a 90-degree angle to the curb or the wall. This is the most common type of parking space in car parks and supermarkets. To park your car in a perpendicular space, follow these steps: - Approach the parking space slowly and keep your car as far to the opposite side as possible. This will give you more room to turn. - Stop your car when your bumper is aligned with the first line of the parking space. You can use your shoulder or your wing mirror as a reference point. - Turn on your indicator to signal your intention to park. - Turn your steering wheel hand over hand in the direction of the space. Aim for the middle or far side of the space so you have room to straighten out. - Check your mirrors and blind spots for any obstacles or pedestrians. If there are any, stop and wait for them to pass. - Straighten out your wheels when the sides of your car are parallel to the lines of the space. Pull forward until your car is centered in the space. - Put your car in park and check that it is completely inside the lines. Make sure you have enough room on each side to open your doors. <h2>Angled Parking</h2>
|
4 |
-
Angled parking is when you park your car at an angle to the curb or the wall. This type of parking space is less common than perpendicular parking, but it can be easier to enter and exit. Angled parking spaces are usually marked with arrows that indicate the direction of traffic flow. To park your car in an angled space, follow these steps: - Approach the parking space slowly and stay in the same lane as the arrows. This will help you align your car with the angle of the space. - Stop your car when the front corner of your car is aligned with the first line of the parking space. You can use your wing mirror or a point on your bonnet as a reference point. - Turn on your indicator to signal your intention to park. - Turn your steering wheel slightly in the direction of the space. Aim for the center of the space so you have room to adjust. - Check your mirrors and blind spots for any obstacles or pedestrians. If there are any, stop and wait for them to pass. - Adjust your position if needed by moving forward or backward until your car is centered in the space. - Put your car in park and check that it is completely inside the lines. Make sure you have enough room on each side to open your doors. <h2>Parallel Parking</h2>
|
5 |
-
Parallel parking is when you park your car parallel to the curb or the wall. This type of parking space is often found on busy streets and can be challenging for beginners. Parallel parking requires good judgment of distance and angle. To park your car in a parallel space, follow these steps: - Find a space that is big enough for your car. A good rule of thumb is to look for a space that is at least one and a half times the length of your car. - Pull up next to the car in front of the space, leaving about one meter of space between them. Align your rear wheels with their rear bumper. - Turn on your indicator to signal your intention to park. - Shift into reverse and turn your steering wheel all the way in the direction of the curb. Start moving backward slowly until you see the rear corner of the car behind you in your side mirror. - Straighten out your wheels and continue moving backward until you are parallel to the curb. You should be about 30 centimeters away from it. - Turn your steering wheel all the way in the opposite direction and move forward slightly until you are centered in the space. - Put your car in park and check that it is completely inside the lines. Make sure you have enough room on each side to open your doors. <h2>How to Use Reference Points</h2>
|
6 |
-
Reference points are visual cues that help you judge the position and size of your car in relation to the parking space and the surroundings. They can be parts of your car, such as mirrors, windows, bumpers, or wheels, or external objects, such as lines, poles, or other cars. Using reference points can help you park your car more accurately and avoid hitting anything. Here are some examples of how to use reference points for different types of parking: - For perpendicular parking, you can use your shoulder or your wing mirror as a reference point to align your bumper with the first line of the space. You can also use the rear window or the rearview mirror as a reference point to center your car in the space. - For angled parking, you can use your wing mirror or a point on your bonnet as a reference point to align your front corner with the first line of the space. You can also use the side window or the side mirror as a reference point to center your car in the space. - For parallel parking, you can use your rear wheels or your rear bumper as a reference point to align your car with the car in front of the space. You can also use your side mirror or your rear corner as a reference point to align your car with the car behind the space. <h2>How to Use Mirrors and Signals</h2>
|
7 |
-
Mirrors and signals are essential tools for car parking driving. They help you see what is behind and around you and communicate your intentions to other drivers and pedestrians. You should always check your mirrors and blind spots before and during any parking maneuver. You should also always use your indicator to signal which way you are turning or which space you are entering. Here are some tips on how to use mirrors and signals for different types of parking: - For perpendicular parking, you should check your rearview mirror and side mirrors before turning into the space. You should also check your blind spots for any obstacles or pedestrians. You should signal in the direction of the space as soon as you stop your car next to it. - For angled parking, you should check your rearview mirror and side mirrors before turning into the space. You should also check your blind spots for any obstacles or pedestrians. You should signal in the direction of the space as soon as you align your front corner with it. - For parallel parking, you should check your rearview mirror and side mirrors before reversing into the space. You should also check your blind spots for any obstacles or pedestrians. You should signal in the direction of the curb as soon as you pull up next to the car in front of the space. <h2>How to Practice Car Parking Driving</h2>
|
8 |
-
Practice makes perfect when it comes to car parking driving. The more you practice, the more confident and skilled you will become. There are many ways to practice car parking driving, such as: - Practicing in an empty car park or a quiet street with plenty of spaces. You can use cones, boxes, or other objects to mark the spaces and practice different types of parking. - Practicing with a friend, a family member, or an instructor who can give you feedback and advice. They can also act as a spotter and help you avoid any collisions. - Practicing with a car parking driving game or simulator that can simulate realistic scenarios and challenges. You can play online or on your phone and improve your skills in a fun and safe way. <h3>Best Car Parking Driving Games and Simulators</h3>
|
9 |
-
There are many car parking driving games and simulators available online or on your phone that can help you practice your skills. Some of them are: - <a href="https://www.crazygames.com/game/real-car-parking">Real Car Parking</a>: This is an online game that lets you park various cars in different environments and levels. You can choose from different camera angles and controls and earn coins to unlock new cars. - <a href="https://play.google.com/store/apps/details?id=com.realcarparking.genetic&hl=en_US&gl=US">Real Car Parking 2</a>: This is an app that lets you park realistic 3D cars in various scenarios and modes. You can customize your car, adjust your settings, and enjoy realistic graphics and sounds. - <a href="https://play.google.com/store/apps/details?id=com.games2win.drivingacademy&hl=en_US&gl=US">Driving Academy - Car School Driver Simulator 2021</a>: This is an app that lets you learn how to drive and park different cars in various situations and rules. You can earn badges, unlock new cars, and test your skills in challenges and tests. <h2>Conclusion</h2>
|
10 |
-
Car parking driving is a skill that every driver needs to master. It can be tricky at first, but with some tips and tricks, it can become easier and more enjoyable . In this article, we have shared some tips and tricks for car parking driving that will help you improve your confidence and accuracy. We have covered different types of parking spaces, such as perpendicular, angled and parallel parking, and how to use reference points, mirrors and signals to park your car smoothly. We have also shown you some of the best car parking driving games and simulators that you can play online or on your phone to practice your skills. We hope you have found this article helpful and informative. Happy parking! <h2>FAQs</h2>
|
11 |
-
<h3>What is the best way to park a car?</h3>
|
12 |
-
There is no definitive answer to this question, as different types of parking spaces require different techniques and skills. However, some general tips that can help you park your car better are: - Approach the parking space slowly and carefully. - Use reference points to align your car with the space and the surroundings. - Use mirrors and signals to check for any obstacles or pedestrians and communicate your intentions. - Adjust your position if needed by moving forward or backward until your car is centered in the space. - Put your car in park and check that it is completely inside the lines. <h3>How do I know if a parking space is big enough for my car?</h3>
|
13 |
-
A good rule of thumb is to look for a space that is at least one and a half times the length of your car. You can also use reference points to estimate the size of the space, such as the lines, the curb, or other cars. If you are not sure, you can always drive past the space and check how much room there is behind and in front of it. <h3>How do I avoid hitting anything when parking?</h3>
|
14 |
-
The best way to avoid hitting anything when parking is to check your mirrors and blind spots before and during any parking maneuver. You should also use signals to alert other drivers and pedestrians of your intentions. If you see any obstacles or pedestrians, stop and wait for them to pass. You can also ask someone to act as a spotter and guide you into the space. <h3>How do I get out of a tight parking space?</h3>
|
15 |
-
To get out of a tight parking space, you need to reverse slowly and carefully until you have enough room to turn. You should check your mirrors and blind spots for any obstacles or pedestrians and use signals to indicate which way you are going. You should also turn your steering wheel hand over hand in the direction you want to go. If you are in a perpendicular or angled space, you should aim for the opposite side of the lane. If you are in a parallel space, you should pull forward until your front bumper clears the rear bumper of the car in front of you. <h3>How do I improve my car parking driving skills?</h3>
|
16 |
-
The best way to improve your car parking driving skills is to practice as much as possible. You can practice in an empty car park or a quiet street with plenty of spaces. You can also practice with a friend, a family member, or an instructor who can give you feedback and advice. Another way to improve your skills is to play car parking driving games or simulators that can simulate realistic scenarios and challenges.</p>
|
17 |
-
<h2>car parking driving</h2><br /><p><b><b>DOWNLOAD</b> ★ <a href="https://jinyurl.com/2uNRnO">https://jinyurl.com/2uNRnO</a></b></p><br /><br /> 401be4b1e0<br />
|
18 |
-
<br />
|
19 |
-
<br />
|
|
|
|
|
|
|
|
|
|
|
|
|
|
|
|
|
|
|
|
|
|
|
|
|
|
|
|
|
|
|
|
|
|
|
|
|
|
|
|
spaces/4com/README/README.md
DELETED
@@ -1,16 +0,0 @@
|
|
1 |
-
---
|
2 |
-
title: README
|
3 |
-
emoji: 📚
|
4 |
-
colorFrom: indigo
|
5 |
-
colorTo: purple
|
6 |
-
sdk: static
|
7 |
-
pinned: false
|
8 |
-
---
|
9 |
-
<h1><center>4COM</center></h1>
|
10 |
-
|
11 |
-
|
12 |
-
<div class="inline-flex flex-none items-center rounded-lg border border-gray-100 bg-gradient-to-b from-purple-50 to-purple-100 px-2 py-0 text-sm text-purple-800 hover:from-purple-50 hover:to-purple-200 dark:from-gray-925 dark:to-gray-950 dark:text-purple-200 dark:hover:from-gray-950 dark:hover:to-gray-950 "><span class="capitalize">companies</span></div>
|
13 |
-
<div class="inline-flex flex-none items-center rounded-lg border border-gray-100 bg-gradient-to-b from-purple-50 to-purple-100 px-2 py-0 text-sm text-purple-800 hover:from-purple-50 hover:to-purple-200 dark:from-gray-925 dark:to-gray-950 dark:text-purple-200 dark:hover:from-gray-950 dark:hover:to-gray-950 "><span class="capitalize">universities</span></div>
|
14 |
-
<div class="inline-flex flex-none items-center rounded-lg border border-gray-100 bg-gradient-to-b from-purple-50 to-purple-100 px-2 py-0 text-sm text-purple-800 hover:from-purple-50 hover:to-purple-200 dark:from-gray-925 dark:to-gray-950 dark:text-purple-200 dark:hover:from-gray-950 dark:hover:to-gray-950 "><span class="capitalize">classrooms</span></div>
|
15 |
-
<div class="inline-flex flex-none items-center rounded-lg border border-gray-100 bg-gradient-to-b from-purple-50 to-purple-100 px-2 py-0 text-sm text-purple-800 hover:from-purple-50 hover:to-purple-200 dark:from-gray-925 dark:to-gray-950 dark:text-purple-200 dark:hover:from-gray-950 dark:hover:to-gray-950 "><span class="capitalize">communities</span></div>
|
16 |
-
<div class="inline-flex flex-none items-center rounded-lg border border-gray-100 bg-gradient-to-b from-purple-50 to-purple-100 px-2 py-0 text-sm text-purple-800 hover:from-purple-50 hover:to-purple-200 dark:from-gray-925 dark:to-gray-950 dark:text-purple-200 dark:hover:from-gray-950 dark:hover:to-gray-950 "><span class="capitalize">non-profit organizations</span></div>
|
|
|
|
|
|
|
|
|
|
|
|
|
|
|
|
|
|
|
|
|
|
|
|
|
|
|
|
|
|
|
|
|
|
spaces/7hao/bingo/src/app/layout.tsx
DELETED
@@ -1,47 +0,0 @@
|
|
1 |
-
import { Metadata } from 'next'
|
2 |
-
import { Toaster } from 'react-hot-toast'
|
3 |
-
import { TailwindIndicator } from '@/components/tailwind-indicator'
|
4 |
-
import { Providers } from '@/components/providers'
|
5 |
-
import { Header } from '@/components/header'
|
6 |
-
|
7 |
-
import '@/app/globals.scss'
|
8 |
-
|
9 |
-
|
10 |
-
export const metadata: Metadata = {
|
11 |
-
title: {
|
12 |
-
default: 'Bing AI Chatbot',
|
13 |
-
template: `%s - Bing AI Chatbot`
|
14 |
-
},
|
15 |
-
description: 'Bing AI Chatbot Web App.',
|
16 |
-
themeColor: [
|
17 |
-
{ media: '(prefers-color-scheme: light)', color: 'white' },
|
18 |
-
{ media: '(prefers-color-scheme: dark)', color: 'dark' }
|
19 |
-
],
|
20 |
-
icons: {
|
21 |
-
icon: '/favicon.ico',
|
22 |
-
shortcut: '../assets/images/logo.svg',
|
23 |
-
apple: '../assets/images/logo.svg'
|
24 |
-
}
|
25 |
-
}
|
26 |
-
|
27 |
-
interface RootLayoutProps {
|
28 |
-
children: React.ReactNode
|
29 |
-
}
|
30 |
-
|
31 |
-
export default function RootLayout({ children }: RootLayoutProps) {
|
32 |
-
return (
|
33 |
-
<html lang="zh-CN" suppressHydrationWarning>
|
34 |
-
<body>
|
35 |
-
<Toaster />
|
36 |
-
<Providers attribute="class" defaultTheme="system" enableSystem>
|
37 |
-
<div className="flex flex-col min-h-screen">
|
38 |
-
{/* @ts-ignore */}
|
39 |
-
<Header />
|
40 |
-
<main className="flex flex-col flex-1">{children}</main>
|
41 |
-
</div>
|
42 |
-
<TailwindIndicator />
|
43 |
-
</Providers>
|
44 |
-
</body>
|
45 |
-
</html>
|
46 |
-
)
|
47 |
-
}
|
|
|
|
|
|
|
|
|
|
|
|
|
|
|
|
|
|
|
|
|
|
|
|
|
|
|
|
|
|
|
|
|
|
|
|
|
|
|
|
|
|
|
|
|
|
|
|
|
|
|
|
|
|
|
|
|
|
|
|
|
|
|
|
|
|
|
|
|
|
|
|
|
|
|
|
|
|
|
|
|
|
|
|
|
|
|
|
|
|
|
|
|
|
|
|
spaces/801artistry/RVC801/demucs/model.py
DELETED
@@ -1,202 +0,0 @@
|
|
1 |
-
# Copyright (c) Facebook, Inc. and its affiliates.
|
2 |
-
# All rights reserved.
|
3 |
-
#
|
4 |
-
# This source code is licensed under the license found in the
|
5 |
-
# LICENSE file in the root directory of this source tree.
|
6 |
-
|
7 |
-
import math
|
8 |
-
|
9 |
-
import julius
|
10 |
-
from torch import nn
|
11 |
-
|
12 |
-
from .utils import capture_init, center_trim
|
13 |
-
|
14 |
-
|
15 |
-
class BLSTM(nn.Module):
|
16 |
-
def __init__(self, dim, layers=1):
|
17 |
-
super().__init__()
|
18 |
-
self.lstm = nn.LSTM(bidirectional=True, num_layers=layers, hidden_size=dim, input_size=dim)
|
19 |
-
self.linear = nn.Linear(2 * dim, dim)
|
20 |
-
|
21 |
-
def forward(self, x):
|
22 |
-
x = x.permute(2, 0, 1)
|
23 |
-
x = self.lstm(x)[0]
|
24 |
-
x = self.linear(x)
|
25 |
-
x = x.permute(1, 2, 0)
|
26 |
-
return x
|
27 |
-
|
28 |
-
|
29 |
-
def rescale_conv(conv, reference):
|
30 |
-
std = conv.weight.std().detach()
|
31 |
-
scale = (std / reference)**0.5
|
32 |
-
conv.weight.data /= scale
|
33 |
-
if conv.bias is not None:
|
34 |
-
conv.bias.data /= scale
|
35 |
-
|
36 |
-
|
37 |
-
def rescale_module(module, reference):
|
38 |
-
for sub in module.modules():
|
39 |
-
if isinstance(sub, (nn.Conv1d, nn.ConvTranspose1d)):
|
40 |
-
rescale_conv(sub, reference)
|
41 |
-
|
42 |
-
|
43 |
-
class Demucs(nn.Module):
|
44 |
-
@capture_init
|
45 |
-
def __init__(self,
|
46 |
-
sources,
|
47 |
-
audio_channels=2,
|
48 |
-
channels=64,
|
49 |
-
depth=6,
|
50 |
-
rewrite=True,
|
51 |
-
glu=True,
|
52 |
-
rescale=0.1,
|
53 |
-
resample=True,
|
54 |
-
kernel_size=8,
|
55 |
-
stride=4,
|
56 |
-
growth=2.,
|
57 |
-
lstm_layers=2,
|
58 |
-
context=3,
|
59 |
-
normalize=False,
|
60 |
-
samplerate=44100,
|
61 |
-
segment_length=4 * 10 * 44100):
|
62 |
-
"""
|
63 |
-
Args:
|
64 |
-
sources (list[str]): list of source names
|
65 |
-
audio_channels (int): stereo or mono
|
66 |
-
channels (int): first convolution channels
|
67 |
-
depth (int): number of encoder/decoder layers
|
68 |
-
rewrite (bool): add 1x1 convolution to each encoder layer
|
69 |
-
and a convolution to each decoder layer.
|
70 |
-
For the decoder layer, `context` gives the kernel size.
|
71 |
-
glu (bool): use glu instead of ReLU
|
72 |
-
resample_input (bool): upsample x2 the input and downsample /2 the output.
|
73 |
-
rescale (int): rescale initial weights of convolutions
|
74 |
-
to get their standard deviation closer to `rescale`
|
75 |
-
kernel_size (int): kernel size for convolutions
|
76 |
-
stride (int): stride for convolutions
|
77 |
-
growth (float): multiply (resp divide) number of channels by that
|
78 |
-
for each layer of the encoder (resp decoder)
|
79 |
-
lstm_layers (int): number of lstm layers, 0 = no lstm
|
80 |
-
context (int): kernel size of the convolution in the
|
81 |
-
decoder before the transposed convolution. If > 1,
|
82 |
-
will provide some context from neighboring time
|
83 |
-
steps.
|
84 |
-
samplerate (int): stored as meta information for easing
|
85 |
-
future evaluations of the model.
|
86 |
-
segment_length (int): stored as meta information for easing
|
87 |
-
future evaluations of the model. Length of the segments on which
|
88 |
-
the model was trained.
|
89 |
-
"""
|
90 |
-
|
91 |
-
super().__init__()
|
92 |
-
self.audio_channels = audio_channels
|
93 |
-
self.sources = sources
|
94 |
-
self.kernel_size = kernel_size
|
95 |
-
self.context = context
|
96 |
-
self.stride = stride
|
97 |
-
self.depth = depth
|
98 |
-
self.resample = resample
|
99 |
-
self.channels = channels
|
100 |
-
self.normalize = normalize
|
101 |
-
self.samplerate = samplerate
|
102 |
-
self.segment_length = segment_length
|
103 |
-
|
104 |
-
self.encoder = nn.ModuleList()
|
105 |
-
self.decoder = nn.ModuleList()
|
106 |
-
|
107 |
-
if glu:
|
108 |
-
activation = nn.GLU(dim=1)
|
109 |
-
ch_scale = 2
|
110 |
-
else:
|
111 |
-
activation = nn.ReLU()
|
112 |
-
ch_scale = 1
|
113 |
-
in_channels = audio_channels
|
114 |
-
for index in range(depth):
|
115 |
-
encode = []
|
116 |
-
encode += [nn.Conv1d(in_channels, channels, kernel_size, stride), nn.ReLU()]
|
117 |
-
if rewrite:
|
118 |
-
encode += [nn.Conv1d(channels, ch_scale * channels, 1), activation]
|
119 |
-
self.encoder.append(nn.Sequential(*encode))
|
120 |
-
|
121 |
-
decode = []
|
122 |
-
if index > 0:
|
123 |
-
out_channels = in_channels
|
124 |
-
else:
|
125 |
-
out_channels = len(self.sources) * audio_channels
|
126 |
-
if rewrite:
|
127 |
-
decode += [nn.Conv1d(channels, ch_scale * channels, context), activation]
|
128 |
-
decode += [nn.ConvTranspose1d(channels, out_channels, kernel_size, stride)]
|
129 |
-
if index > 0:
|
130 |
-
decode.append(nn.ReLU())
|
131 |
-
self.decoder.insert(0, nn.Sequential(*decode))
|
132 |
-
in_channels = channels
|
133 |
-
channels = int(growth * channels)
|
134 |
-
|
135 |
-
channels = in_channels
|
136 |
-
|
137 |
-
if lstm_layers:
|
138 |
-
self.lstm = BLSTM(channels, lstm_layers)
|
139 |
-
else:
|
140 |
-
self.lstm = None
|
141 |
-
|
142 |
-
if rescale:
|
143 |
-
rescale_module(self, reference=rescale)
|
144 |
-
|
145 |
-
def valid_length(self, length):
|
146 |
-
"""
|
147 |
-
Return the nearest valid length to use with the model so that
|
148 |
-
there is no time steps left over in a convolutions, e.g. for all
|
149 |
-
layers, size of the input - kernel_size % stride = 0.
|
150 |
-
|
151 |
-
If the mixture has a valid length, the estimated sources
|
152 |
-
will have exactly the same length when context = 1. If context > 1,
|
153 |
-
the two signals can be center trimmed to match.
|
154 |
-
|
155 |
-
For training, extracts should have a valid length.For evaluation
|
156 |
-
on full tracks we recommend passing `pad = True` to :method:`forward`.
|
157 |
-
"""
|
158 |
-
if self.resample:
|
159 |
-
length *= 2
|
160 |
-
for _ in range(self.depth):
|
161 |
-
length = math.ceil((length - self.kernel_size) / self.stride) + 1
|
162 |
-
length = max(1, length)
|
163 |
-
length += self.context - 1
|
164 |
-
for _ in range(self.depth):
|
165 |
-
length = (length - 1) * self.stride + self.kernel_size
|
166 |
-
|
167 |
-
if self.resample:
|
168 |
-
length = math.ceil(length / 2)
|
169 |
-
return int(length)
|
170 |
-
|
171 |
-
def forward(self, mix):
|
172 |
-
x = mix
|
173 |
-
|
174 |
-
if self.normalize:
|
175 |
-
mono = mix.mean(dim=1, keepdim=True)
|
176 |
-
mean = mono.mean(dim=-1, keepdim=True)
|
177 |
-
std = mono.std(dim=-1, keepdim=True)
|
178 |
-
else:
|
179 |
-
mean = 0
|
180 |
-
std = 1
|
181 |
-
|
182 |
-
x = (x - mean) / (1e-5 + std)
|
183 |
-
|
184 |
-
if self.resample:
|
185 |
-
x = julius.resample_frac(x, 1, 2)
|
186 |
-
|
187 |
-
saved = []
|
188 |
-
for encode in self.encoder:
|
189 |
-
x = encode(x)
|
190 |
-
saved.append(x)
|
191 |
-
if self.lstm:
|
192 |
-
x = self.lstm(x)
|
193 |
-
for decode in self.decoder:
|
194 |
-
skip = center_trim(saved.pop(-1), x)
|
195 |
-
x = x + skip
|
196 |
-
x = decode(x)
|
197 |
-
|
198 |
-
if self.resample:
|
199 |
-
x = julius.resample_frac(x, 2, 1)
|
200 |
-
x = x * std + mean
|
201 |
-
x = x.view(x.size(0), len(self.sources), self.audio_channels, x.size(-1))
|
202 |
-
return x
|
|
|
|
|
|
|
|
|
|
|
|
|
|
|
|
|
|
|
|
|
|
|
|
|
|
|
|
|
|
|
|
|
|
|
|
|
|
|
|
|
|
|
|
|
|
|
|
|
|
|
|
|
|
|
|
|
|
|
|
|
|
|
|
|
|
|
|
|
|
|
|
|
|
|
|
|
|
|
|
|
|
|
|
|
|
|
|
|
|
|
|
|
|
|
|
|
|
|
|
|
|
|
|
|
|
|
|
|
|
|
|
|
|
|
|
|
|
|
|
|
|
|
|
|
|
|
|
|
|
|
|
|
|
|
|
|
|
|
|
|
|
|
|
|
|
|
|
|
|
|
|
|
|
|
|
|
|
|
|
|
|
|
|
|
|
|
|
|
|
|
|
|
|
|
|
|
|
|
|
|
|
|
|
|
|
|
|
|
|
|
|
|
|
|
|
|
|
|
|
|
|
|
|
|
|
|
|
|
|
|
|
|
|
|
|
|
|
|
|
|
|
|
|
|
|
|
|
|
|
|
|
|
|
|
|
|
|
|
|
|
|
|
|
|
|
|
|
|
|
|
|
|
|
|
|
|
|
|
|
|
|
|
|
|
|
|
|
|
|
|
|
|
|
|
|
|
|
|
|
|
|
|
|
|
|
|
|
|
|
|
|
|
|
|
|
|
|
|
|
|
|
|
|
|
|
|
|
|
|
|
|
|
|
|
|
|
|
|
|
|
|
|
|
|
|
|
|
|
|
|
|
|
|
|
|
|
|
|
|
|
|
|
|
|
|
|
|
|
|
|
|
|
|
|
|
|
|
|
|
|
|
|
|
|
|
|
|
|
|
|
|
|
|
|
|
|
|
|
|
|
|
|
|
|
|
|
|
|
|
|
|
|
|
|
|
|
|
|
|
|
|
|
|
|
|
spaces/801artistry/RVC801/lib/uvr5_pack/utils.py
DELETED
@@ -1,120 +0,0 @@
|
|
1 |
-
import torch
|
2 |
-
import numpy as np
|
3 |
-
from tqdm import tqdm
|
4 |
-
import json
|
5 |
-
|
6 |
-
|
7 |
-
def load_data(file_name: str = "./lib/uvr5_pack/name_params.json") -> dict:
|
8 |
-
with open(file_name, "r") as f:
|
9 |
-
data = json.load(f)
|
10 |
-
|
11 |
-
return data
|
12 |
-
|
13 |
-
|
14 |
-
def make_padding(width, cropsize, offset):
|
15 |
-
left = offset
|
16 |
-
roi_size = cropsize - left * 2
|
17 |
-
if roi_size == 0:
|
18 |
-
roi_size = cropsize
|
19 |
-
right = roi_size - (width % roi_size) + left
|
20 |
-
|
21 |
-
return left, right, roi_size
|
22 |
-
|
23 |
-
|
24 |
-
def inference(X_spec, device, model, aggressiveness, data):
|
25 |
-
"""
|
26 |
-
data : dic configs
|
27 |
-
"""
|
28 |
-
|
29 |
-
def _execute(
|
30 |
-
X_mag_pad, roi_size, n_window, device, model, aggressiveness, is_half=True
|
31 |
-
):
|
32 |
-
model.eval()
|
33 |
-
with torch.no_grad():
|
34 |
-
preds = []
|
35 |
-
|
36 |
-
iterations = [n_window]
|
37 |
-
|
38 |
-
total_iterations = sum(iterations)
|
39 |
-
for i in tqdm(range(n_window)):
|
40 |
-
start = i * roi_size
|
41 |
-
X_mag_window = X_mag_pad[
|
42 |
-
None, :, :, start : start + data["window_size"]
|
43 |
-
]
|
44 |
-
X_mag_window = torch.from_numpy(X_mag_window)
|
45 |
-
if is_half:
|
46 |
-
X_mag_window = X_mag_window.half()
|
47 |
-
X_mag_window = X_mag_window.to(device)
|
48 |
-
|
49 |
-
pred = model.predict(X_mag_window, aggressiveness)
|
50 |
-
|
51 |
-
pred = pred.detach().cpu().numpy()
|
52 |
-
preds.append(pred[0])
|
53 |
-
|
54 |
-
pred = np.concatenate(preds, axis=2)
|
55 |
-
return pred
|
56 |
-
|
57 |
-
def preprocess(X_spec):
|
58 |
-
X_mag = np.abs(X_spec)
|
59 |
-
X_phase = np.angle(X_spec)
|
60 |
-
|
61 |
-
return X_mag, X_phase
|
62 |
-
|
63 |
-
X_mag, X_phase = preprocess(X_spec)
|
64 |
-
|
65 |
-
coef = X_mag.max()
|
66 |
-
X_mag_pre = X_mag / coef
|
67 |
-
|
68 |
-
n_frame = X_mag_pre.shape[2]
|
69 |
-
pad_l, pad_r, roi_size = make_padding(n_frame, data["window_size"], model.offset)
|
70 |
-
n_window = int(np.ceil(n_frame / roi_size))
|
71 |
-
|
72 |
-
X_mag_pad = np.pad(X_mag_pre, ((0, 0), (0, 0), (pad_l, pad_r)), mode="constant")
|
73 |
-
|
74 |
-
if list(model.state_dict().values())[0].dtype == torch.float16:
|
75 |
-
is_half = True
|
76 |
-
else:
|
77 |
-
is_half = False
|
78 |
-
pred = _execute(
|
79 |
-
X_mag_pad, roi_size, n_window, device, model, aggressiveness, is_half
|
80 |
-
)
|
81 |
-
pred = pred[:, :, :n_frame]
|
82 |
-
|
83 |
-
if data["tta"]:
|
84 |
-
pad_l += roi_size // 2
|
85 |
-
pad_r += roi_size // 2
|
86 |
-
n_window += 1
|
87 |
-
|
88 |
-
X_mag_pad = np.pad(X_mag_pre, ((0, 0), (0, 0), (pad_l, pad_r)), mode="constant")
|
89 |
-
|
90 |
-
pred_tta = _execute(
|
91 |
-
X_mag_pad, roi_size, n_window, device, model, aggressiveness, is_half
|
92 |
-
)
|
93 |
-
pred_tta = pred_tta[:, :, roi_size // 2 :]
|
94 |
-
pred_tta = pred_tta[:, :, :n_frame]
|
95 |
-
|
96 |
-
return (pred + pred_tta) * 0.5 * coef, X_mag, np.exp(1.0j * X_phase)
|
97 |
-
else:
|
98 |
-
return pred * coef, X_mag, np.exp(1.0j * X_phase)
|
99 |
-
|
100 |
-
|
101 |
-
def _get_name_params(model_path, model_hash):
|
102 |
-
data = load_data()
|
103 |
-
flag = False
|
104 |
-
ModelName = model_path
|
105 |
-
for type in list(data):
|
106 |
-
for model in list(data[type][0]):
|
107 |
-
for i in range(len(data[type][0][model])):
|
108 |
-
if str(data[type][0][model][i]["hash_name"]) == model_hash:
|
109 |
-
flag = True
|
110 |
-
elif str(data[type][0][model][i]["hash_name"]) in ModelName:
|
111 |
-
flag = True
|
112 |
-
|
113 |
-
if flag:
|
114 |
-
model_params_auto = data[type][0][model][i]["model_params"]
|
115 |
-
param_name_auto = data[type][0][model][i]["param_name"]
|
116 |
-
if type == "equivalent":
|
117 |
-
return param_name_auto, model_params_auto
|
118 |
-
else:
|
119 |
-
flag = False
|
120 |
-
return param_name_auto, model_params_auto
|
|
|
|
|
|
|
|
|
|
|
|
|
|
|
|
|
|
|
|
|
|
|
|
|
|
|
|
|
|
|
|
|
|
|
|
|
|
|
|
|
|
|
|
|
|
|
|
|
|
|
|
|
|
|
|
|
|
|
|
|
|
|
|
|
|
|
|
|
|
|
|
|
|
|
|
|
|
|
|
|
|
|
|
|
|
|
|
|
|
|
|
|
|
|
|
|
|
|
|
|
|
|
|
|
|
|
|
|
|
|
|
|
|
|
|
|
|
|
|
|
|
|
|
|
|
|
|
|
|
|
|
|
|
|
|
|
|
|
|
|
|
|
|
|
|
|
|
|
|
|
|
|
|
|
|
|
|
|
|
|
|
|
|
|
|
|
|
|
|
|
|
|
|
|
|
|
|
|
|
|
|
|
|
|
|
|
|
|
|
|
|
|
|
|
|
|
|
|
|
|
|
|
|
|
|
|
|
|
|
|
|
|
|
|
|
|
|
|
|
|
|
|
|
|
|
|
|
|
|
|
|
|
|
|
|
|
|
|
|
|
|
spaces/A00001/bingothoo/src/pages/api/kblob.ts
DELETED
@@ -1,56 +0,0 @@
|
|
1 |
-
'use server'
|
2 |
-
|
3 |
-
import { NextApiRequest, NextApiResponse } from 'next'
|
4 |
-
import FormData from 'form-data'
|
5 |
-
import { fetch } from '@/lib/isomorphic'
|
6 |
-
import { KBlobRequest } from '@/lib/bots/bing/types'
|
7 |
-
|
8 |
-
const API_DOMAIN = 'https://bing.vcanbb.top'
|
9 |
-
|
10 |
-
export const config = {
|
11 |
-
api: {
|
12 |
-
bodyParser: {
|
13 |
-
sizeLimit: '10mb' // Set desired value here
|
14 |
-
}
|
15 |
-
}
|
16 |
-
}
|
17 |
-
|
18 |
-
export default async function handler(req: NextApiRequest, res: NextApiResponse) {
|
19 |
-
try {
|
20 |
-
const { knowledgeRequest, imageBase64 } = req.body as KBlobRequest
|
21 |
-
|
22 |
-
const formData = new FormData()
|
23 |
-
formData.append('knowledgeRequest', JSON.stringify(knowledgeRequest))
|
24 |
-
if (imageBase64) {
|
25 |
-
formData.append('imageBase64', imageBase64)
|
26 |
-
}
|
27 |
-
|
28 |
-
const response = await fetch(`${API_DOMAIN}/images/kblob`,
|
29 |
-
{
|
30 |
-
method: 'POST',
|
31 |
-
body: formData.getBuffer(),
|
32 |
-
headers: {
|
33 |
-
"sec-ch-ua": "\"Not/A)Brand\";v=\"99\", \"Google Chrome\";v=\"115\", \"Chromium\";v=\"115\"",
|
34 |
-
"sec-ch-ua-mobile": "?0",
|
35 |
-
"sec-ch-ua-platform": "\"Windows\"",
|
36 |
-
"Referer": `${API_DOMAIN}/web/index.html`,
|
37 |
-
"Referrer-Policy": "origin-when-cross-origin",
|
38 |
-
'x-ms-useragent': 'azsdk-js-api-client-factory/1.0.0-beta.1 core-rest-pipeline/1.10.0 OS/Win32',
|
39 |
-
...formData.getHeaders()
|
40 |
-
}
|
41 |
-
}
|
42 |
-
).then(res => res.text())
|
43 |
-
|
44 |
-
res.writeHead(200, {
|
45 |
-
'Content-Type': 'application/json',
|
46 |
-
})
|
47 |
-
res.end(response || JSON.stringify({ result: { value: 'UploadFailed', message: '请更换 IP 或代理后重试' } }))
|
48 |
-
} catch (e) {
|
49 |
-
return res.json({
|
50 |
-
result: {
|
51 |
-
value: 'UploadFailed',
|
52 |
-
message: `${e}`
|
53 |
-
}
|
54 |
-
})
|
55 |
-
}
|
56 |
-
}
|
|
|
|
|
|
|
|
|
|
|
|
|
|
|
|
|
|
|
|
|
|
|
|
|
|
|
|
|
|
|
|
|
|
|
|
|
|
|
|
|
|
|
|
|
|
|
|
|
|
|
|
|
|
|
|
|
|
|
|
|
|
|
|
|
|
|
|
|
|
|
|
|
|
|
|
|
|
|
|
|
|
|
|
|
|
|
|
|
|
|
|
|
|
|
|
|
|
|
|
|
|
|
|
|
|
|
|
|
|
|
|
|
|
spaces/AI-Dashboards/Topic-Modeling-Clusters-Free-Text/README.md
DELETED
@@ -1,13 +0,0 @@
|
|
1 |
-
---
|
2 |
-
title: Topic Modeling Clusters Free Text
|
3 |
-
emoji: 🐨
|
4 |
-
colorFrom: yellow
|
5 |
-
colorTo: yellow
|
6 |
-
sdk: streamlit
|
7 |
-
sdk_version: 1.17.0
|
8 |
-
app_file: app.py
|
9 |
-
pinned: false
|
10 |
-
license: mit
|
11 |
-
---
|
12 |
-
|
13 |
-
Check out the configuration reference at https://huggingface.co/docs/hub/spaces-config-reference
|
|
|
|
|
|
|
|
|
|
|
|
|
|
|
|
|
|
|
|
|
|
|
|
|
|
|
|
spaces/AIConsultant/MusicGen/tests/__init__.py
DELETED
@@ -1,5 +0,0 @@
|
|
1 |
-
# Copyright (c) Meta Platforms, Inc. and affiliates.
|
2 |
-
# All rights reserved.
|
3 |
-
#
|
4 |
-
# This source code is licensed under the license found in the
|
5 |
-
# LICENSE file in the root directory of this source tree.
|
|
|
|
|
|
|
|
|
|
|
|
spaces/AIConsultant/MusicGen/tests/modules/test_codebooks_patterns.py
DELETED
@@ -1,246 +0,0 @@
|
|
1 |
-
# Copyright (c) Meta Platforms, Inc. and affiliates.
|
2 |
-
# All rights reserved.
|
3 |
-
#
|
4 |
-
# This source code is licensed under the license found in the
|
5 |
-
# LICENSE file in the root directory of this source tree.
|
6 |
-
|
7 |
-
import pytest
|
8 |
-
import torch
|
9 |
-
|
10 |
-
from audiocraft.modules.codebooks_patterns import (
|
11 |
-
DelayedPatternProvider,
|
12 |
-
ParallelPatternProvider,
|
13 |
-
Pattern,
|
14 |
-
UnrolledPatternProvider,
|
15 |
-
)
|
16 |
-
|
17 |
-
|
18 |
-
class TestParallelPatternProvider:
|
19 |
-
|
20 |
-
@pytest.mark.parametrize("n_q", [1, 4, 32])
|
21 |
-
@pytest.mark.parametrize("timesteps", [0, 1, 16, 100])
|
22 |
-
def test_get_pattern(self, n_q: int, timesteps: int):
|
23 |
-
provider = ParallelPatternProvider(n_q)
|
24 |
-
pattern = provider.get_pattern(timesteps)
|
25 |
-
# + 1 to account for 1st step
|
26 |
-
assert len(pattern.layout) == timesteps + 1
|
27 |
-
|
28 |
-
@pytest.mark.parametrize("n_q", [1, 4, 32])
|
29 |
-
@pytest.mark.parametrize("timesteps", [8, 16, 100])
|
30 |
-
def test_pattern_content(self, n_q: int, timesteps: int):
|
31 |
-
provider = ParallelPatternProvider(n_q)
|
32 |
-
pattern = provider.get_pattern(timesteps)
|
33 |
-
for s, v in enumerate(pattern.layout):
|
34 |
-
for i, code in enumerate(v):
|
35 |
-
assert i == code.q
|
36 |
-
assert code.t == s - 1 # account for the 1st empty step
|
37 |
-
|
38 |
-
@pytest.mark.parametrize("n_q", [1, 4, 32])
|
39 |
-
@pytest.mark.parametrize("timesteps", [8, 16, 100])
|
40 |
-
def test_pattern_max_delay(self, n_q: int, timesteps: int):
|
41 |
-
provider = ParallelPatternProvider(n_q)
|
42 |
-
pattern = provider.get_pattern(timesteps)
|
43 |
-
assert pattern.max_delay == 0
|
44 |
-
assert len(pattern.valid_layout) == len(pattern.layout) - pattern.max_delay
|
45 |
-
|
46 |
-
|
47 |
-
class TestDelayedPatternProvider:
|
48 |
-
|
49 |
-
@pytest.mark.parametrize("n_q", [1, 4, 32])
|
50 |
-
@pytest.mark.parametrize("timesteps", [0, 1, 16, 100])
|
51 |
-
def test_get_pattern(self, n_q: int, timesteps: int):
|
52 |
-
delays = [
|
53 |
-
list(range(n_q)),
|
54 |
-
[0] + [1] * (n_q - 1),
|
55 |
-
[0] + [4] * (n_q - 1),
|
56 |
-
]
|
57 |
-
for delay in delays:
|
58 |
-
provider = DelayedPatternProvider(n_q, delay)
|
59 |
-
pattern = provider.get_pattern(timesteps)
|
60 |
-
# + 1 to account for 1st step
|
61 |
-
assert len(pattern.layout) == timesteps + max(delay) + 1
|
62 |
-
|
63 |
-
@pytest.mark.parametrize("n_q", [1, 4, 32])
|
64 |
-
@pytest.mark.parametrize("timesteps", [8, 16, 100])
|
65 |
-
def test_pattern_content(self, n_q: int, timesteps: int):
|
66 |
-
provider = DelayedPatternProvider(n_q)
|
67 |
-
pattern = provider.get_pattern(timesteps)
|
68 |
-
for s, v in enumerate(pattern.layout):
|
69 |
-
for i, code in enumerate(v):
|
70 |
-
assert i == code.q
|
71 |
-
assert code.t == max(0, s - code.q - 1)
|
72 |
-
|
73 |
-
@pytest.mark.parametrize("timesteps", [8, 16, 100])
|
74 |
-
@pytest.mark.parametrize("delay", [[0, 1, 2, 3], [0, 1, 1, 1], [0, 3, 3, 3], [0, 3]])
|
75 |
-
def test_pattern_max_delay(self, timesteps: int, delay: list):
|
76 |
-
provider = DelayedPatternProvider(len(delay), delay)
|
77 |
-
pattern = provider.get_pattern(timesteps)
|
78 |
-
assert pattern.max_delay == max(delay)
|
79 |
-
assert len(pattern.valid_layout) == len(pattern.layout) - pattern.max_delay
|
80 |
-
|
81 |
-
|
82 |
-
class TestUnrolledPatternProvider:
|
83 |
-
|
84 |
-
@pytest.mark.parametrize("timesteps", [0, 1, 16])
|
85 |
-
@pytest.mark.parametrize("flattening", [[0, 1, 2], [0, 1, 1]])
|
86 |
-
@pytest.mark.parametrize("delays", [[0, 0, 0], [0, 5, 5]])
|
87 |
-
def test_get_pattern(self, timesteps: int, flattening: list, delays: list):
|
88 |
-
n_q = len(flattening)
|
89 |
-
max_delay = max(delays)
|
90 |
-
provider = UnrolledPatternProvider(n_q, flattening, delays)
|
91 |
-
pattern = provider.get_pattern(timesteps)
|
92 |
-
assert len(pattern.layout) == provider.num_virtual_steps(timesteps) + max_delay
|
93 |
-
|
94 |
-
@pytest.mark.parametrize("timesteps", [0, 1, 16])
|
95 |
-
@pytest.mark.parametrize("flattening", [[0, 1, 2], [0, 1, 1]])
|
96 |
-
@pytest.mark.parametrize("delays", [[0, 0, 0], [0, 5, 5]])
|
97 |
-
def test_pattern_max_delay(self, timesteps: int, flattening: list, delays: list):
|
98 |
-
n_q = len(flattening)
|
99 |
-
max_delay = max(delays)
|
100 |
-
provider = UnrolledPatternProvider(n_q, flattening, delays)
|
101 |
-
pattern = provider.get_pattern(timesteps)
|
102 |
-
assert pattern.max_delay == max_delay
|
103 |
-
|
104 |
-
|
105 |
-
class TestPattern:
|
106 |
-
|
107 |
-
def ref_build_pattern_sequence(self, z: torch.Tensor, pattern: Pattern, special_token: int):
|
108 |
-
"""Reference method to build the sequence from the pattern without using fancy scatter."""
|
109 |
-
bs, n_q, T = z.shape
|
110 |
-
z = z.cpu().numpy()
|
111 |
-
assert n_q == pattern.n_q
|
112 |
-
assert T <= pattern.timesteps
|
113 |
-
inp = torch.full((bs, n_q, len(pattern.layout)), special_token, dtype=torch.long).numpy()
|
114 |
-
inp[:] = special_token
|
115 |
-
for s, v in enumerate(pattern.layout):
|
116 |
-
for (t, q) in v:
|
117 |
-
if t < T:
|
118 |
-
inp[:, q, s] = z[:, q, t]
|
119 |
-
return torch.from_numpy(inp)
|
120 |
-
|
121 |
-
def ref_revert_pattern_sequence(self, z: torch.Tensor, pattern: Pattern, special_token: int):
|
122 |
-
"""Reference method to revert the sequence from the pattern without using fancy scatter."""
|
123 |
-
z = z.cpu().numpy()
|
124 |
-
bs, n_q, S = z.shape
|
125 |
-
assert pattern.n_q == n_q
|
126 |
-
inp = torch.full((bs, pattern.n_q, pattern.timesteps), special_token, dtype=torch.long).numpy()
|
127 |
-
inp[:] = special_token
|
128 |
-
for s, v in enumerate(pattern.layout):
|
129 |
-
for (t, q) in v:
|
130 |
-
if t < pattern.timesteps:
|
131 |
-
inp[:, q, t] = z[:, q, s]
|
132 |
-
return torch.from_numpy(inp)
|
133 |
-
|
134 |
-
def ref_revert_pattern_logits(self, z: torch.Tensor, pattern: Pattern, special_token: float):
|
135 |
-
"""Reference method to revert the logits from the pattern without using fancy scatter."""
|
136 |
-
z = z.cpu().numpy()
|
137 |
-
bs, card, n_q, S = z.shape
|
138 |
-
assert pattern.n_q == n_q
|
139 |
-
ref_layout = pattern.layout
|
140 |
-
inp = torch.full((bs, card, pattern.n_q, pattern.timesteps), special_token, dtype=torch.float).numpy()
|
141 |
-
inp[:] = special_token
|
142 |
-
for s, v in enumerate(ref_layout[1:]):
|
143 |
-
if s < S:
|
144 |
-
for (t, q) in v:
|
145 |
-
if t < pattern.timesteps:
|
146 |
-
inp[:, :, q, t] = z[:, :, q, s]
|
147 |
-
return torch.from_numpy(inp)
|
148 |
-
|
149 |
-
def _get_pattern_providers(self, n_q: int):
|
150 |
-
pattern_provider_1 = ParallelPatternProvider(n_q)
|
151 |
-
pattern_provider_2 = DelayedPatternProvider(n_q, list(range(n_q)))
|
152 |
-
pattern_provider_3 = DelayedPatternProvider(n_q, [0] + [1] * (n_q - 1))
|
153 |
-
pattern_provider_4 = UnrolledPatternProvider(
|
154 |
-
n_q, flattening=list(range(n_q)), delays=[0] * n_q
|
155 |
-
)
|
156 |
-
pattern_provider_5 = UnrolledPatternProvider(
|
157 |
-
n_q, flattening=[0] + [1] * (n_q - 1), delays=[0] * n_q
|
158 |
-
)
|
159 |
-
pattern_provider_6 = UnrolledPatternProvider(
|
160 |
-
n_q, flattening=[0] + [1] * (n_q - 1), delays=[0] + [5] * (n_q - 1)
|
161 |
-
)
|
162 |
-
return [
|
163 |
-
pattern_provider_1,
|
164 |
-
pattern_provider_2,
|
165 |
-
pattern_provider_3,
|
166 |
-
pattern_provider_4,
|
167 |
-
pattern_provider_5,
|
168 |
-
pattern_provider_6,
|
169 |
-
]
|
170 |
-
|
171 |
-
@pytest.mark.parametrize("n_q", [1, 4, 32])
|
172 |
-
@pytest.mark.parametrize("timesteps", [16, 72])
|
173 |
-
def test_build_pattern_sequence(self, n_q: int, timesteps: int):
|
174 |
-
bs = 2
|
175 |
-
card = 256
|
176 |
-
special_token = card
|
177 |
-
|
178 |
-
pattern_providers = self._get_pattern_providers(n_q)
|
179 |
-
for pattern_provider in pattern_providers:
|
180 |
-
pattern = pattern_provider.get_pattern(timesteps)
|
181 |
-
# we can correctly build the sequence from the pattern
|
182 |
-
z = torch.randint(0, card, (bs, n_q, timesteps))
|
183 |
-
ref_res = self.ref_build_pattern_sequence(z, pattern, special_token)
|
184 |
-
res, indexes, mask = pattern.build_pattern_sequence(z, special_token)
|
185 |
-
assert (res == ref_res).float().mean() == 1.0
|
186 |
-
|
187 |
-
# expected assertion fails on the number of timesteps
|
188 |
-
invalid_timesteps = [timesteps + 1]
|
189 |
-
if pattern.num_sequence_steps != pattern.timesteps:
|
190 |
-
invalid_timesteps.append(pattern.num_sequence_steps)
|
191 |
-
for i_timesteps in invalid_timesteps:
|
192 |
-
z2 = torch.randint(0, card, (bs, n_q, i_timesteps))
|
193 |
-
with pytest.raises(AssertionError):
|
194 |
-
pattern.build_pattern_sequence(z2, special_token)
|
195 |
-
|
196 |
-
# expected assertion fails on the number of codebooks
|
197 |
-
invalid_qs = [0, n_q - 1, n_q + 1]
|
198 |
-
for i_q in invalid_qs:
|
199 |
-
z3 = torch.randint(0, card, (bs, i_q, timesteps))
|
200 |
-
with pytest.raises(AssertionError):
|
201 |
-
pattern.build_pattern_sequence(z3, special_token)
|
202 |
-
|
203 |
-
@pytest.mark.parametrize("n_q", [1, 4, 32])
|
204 |
-
@pytest.mark.parametrize("timesteps", [16, 72])
|
205 |
-
def test_revert_pattern_sequence(self, n_q: int, timesteps: int):
|
206 |
-
bs = 2
|
207 |
-
card = 256
|
208 |
-
special_token = card
|
209 |
-
|
210 |
-
pattern_providers = self._get_pattern_providers(n_q)
|
211 |
-
for pattern_provider in pattern_providers:
|
212 |
-
pattern = pattern_provider.get_pattern(timesteps)
|
213 |
-
# this works assuming previous tests are successful
|
214 |
-
z = torch.randint(0, card, (bs, n_q, timesteps))
|
215 |
-
s = self.ref_build_pattern_sequence(z, pattern, special_token)
|
216 |
-
ref_out = self.ref_revert_pattern_sequence(s, pattern, special_token)
|
217 |
-
# ensure our reference script retrieve the original sequence
|
218 |
-
assert z.shape == ref_out.shape
|
219 |
-
assert (z == ref_out).float().mean() == 1.0
|
220 |
-
# now we can test the scatter version
|
221 |
-
out, indexes, mask = pattern.revert_pattern_sequence(s, special_token)
|
222 |
-
assert out.shape == ref_out.shape
|
223 |
-
assert (out == ref_out).float().mean() == 1.0
|
224 |
-
|
225 |
-
@pytest.mark.parametrize("n_q", [1, 4, 32])
|
226 |
-
@pytest.mark.parametrize("timesteps", [16, 72])
|
227 |
-
@pytest.mark.parametrize("card", [1, 2, 256, 1024])
|
228 |
-
def test_revert_pattern_logits(self, n_q: int, timesteps: int, card: int):
|
229 |
-
bs = 2
|
230 |
-
special_token = card
|
231 |
-
logits_special_token = float('nan')
|
232 |
-
|
233 |
-
pattern_providers = self._get_pattern_providers(n_q)
|
234 |
-
for pattern_provider in pattern_providers:
|
235 |
-
pattern = pattern_provider.get_pattern(timesteps)
|
236 |
-
# this works assuming previous tests are successful
|
237 |
-
z = torch.randint(0, card, (bs, n_q, timesteps))
|
238 |
-
s = self.ref_build_pattern_sequence(z, pattern, special_token)
|
239 |
-
logits = torch.randn((bs, card, n_q, s.shape[-1]))
|
240 |
-
ref_out = self.ref_revert_pattern_logits(logits, pattern, logits_special_token)
|
241 |
-
# ensure our reference script retrieve the original sequence
|
242 |
-
assert ref_out.shape == torch.Size([bs, card, n_q, timesteps])
|
243 |
-
# now we can test the scatter version
|
244 |
-
out, indexes, mask = pattern.revert_pattern_logits(logits, logits_special_token)
|
245 |
-
assert out.shape == ref_out.shape
|
246 |
-
assert (out == ref_out).float().mean() == 1.0
|
|
|
|
|
|
|
|
|
|
|
|
|
|
|
|
|
|
|
|
|
|
|
|
|
|
|
|
|
|
|
|
|
|
|
|
|
|
|
|
|
|
|
|
|
|
|
|
|
|
|
|
|
|
|
|
|
|
|
|
|
|
|
|
|
|
|
|
|
|
|
|
|
|
|
|
|
|
|
|
|
|
|
|
|
|
|
|
|
|
|
|
|
|
|
|
|
|
|
|
|
|
|
|
|
|
|
|
|
|
|
|
|
|
|
|
|
|
|
|
|
|
|
|
|
|
|
|
|
|
|
|
|
|
|
|
|
|
|
|
|
|
|
|
|
|
|
|
|
|
|
|
|
|
|
|
|
|
|
|
|
|
|
|
|
|
|
|
|
|
|
|
|
|
|
|
|
|
|
|
|
|
|
|
|
|
|
|
|
|
|
|
|
|
|
|
|
|
|
|
|
|
|
|
|
|
|
|
|
|
|
|
|
|
|
|
|
|
|
|
|
|
|
|
|
|
|
|
|
|
|
|
|
|
|
|
|
|
|
|
|
|
|
|
|
|
|
|
|
|
|
|
|
|
|
|
|
|
|
|
|
|
|
|
|
|
|
|
|
|
|
|
|
|
|
|
|
|
|
|
|
|
|
|
|
|
|
|
|
|
|
|
|
|
|
|
|
|
|
|
|
|
|
|
|
|
|
|
|
|
|
|
|
|
|
|
|
|
|
|
|
|
|
|
|
|
|
|
|
|
|
|
|
|
|
|
|
|
|
|
|
|
|
|
|
|
|
|
|
|
|
|
|
|
|
|
|
|
|
|
|
|
|
|
|
|
|
|
|
|
|
|
|
|
|
|
|
|
|
|
|
|
|
|
|
|
|
|
|
|
|
|
|
|
|
|
|
|
|
|
|
|
|
|
|
|
|
|
|
|
|
|
|
|
|
|
|
|
|
|
|
|
|
|
|
|
|
|
|
|
|
|
|
|
|
|
|
|
|
|
|
|
|
|
|
|
|
|
|
|
|
|
|
|
|
|
|
|
|
|
|
|
|
|
|
|
|
|
|
|
|
|
|
|
|
|
|
|
|
|
|
|
|
|
|
|
|
|
|
|
|
|
|
|
spaces/AIFILMS/audioldm-text-to-audio-generation/audioldm/clap/open_clip/pretrained.py
DELETED
@@ -1,167 +0,0 @@
|
|
1 |
-
import hashlib
|
2 |
-
import os
|
3 |
-
import urllib
|
4 |
-
import warnings
|
5 |
-
|
6 |
-
from tqdm import tqdm
|
7 |
-
|
8 |
-
_RN50 = dict(
|
9 |
-
openai="https://openaipublic.azureedge.net/clip/models/afeb0e10f9e5a86da6080e35cf09123aca3b358a0c3e3b6c78a7b63bc04b6762/RN50.pt",
|
10 |
-
yfcc15m="https://github.com/mlfoundations/open_clip/releases/download/v0.2-weights/rn50-quickgelu-yfcc15m-455df137.pt",
|
11 |
-
cc12m="https://github.com/mlfoundations/open_clip/releases/download/v0.2-weights/rn50-quickgelu-cc12m-f000538c.pt",
|
12 |
-
)
|
13 |
-
|
14 |
-
_RN50_quickgelu = dict(
|
15 |
-
openai="https://openaipublic.azureedge.net/clip/models/afeb0e10f9e5a86da6080e35cf09123aca3b358a0c3e3b6c78a7b63bc04b6762/RN50.pt",
|
16 |
-
yfcc15m="https://github.com/mlfoundations/open_clip/releases/download/v0.2-weights/rn50-quickgelu-yfcc15m-455df137.pt",
|
17 |
-
cc12m="https://github.com/mlfoundations/open_clip/releases/download/v0.2-weights/rn50-quickgelu-cc12m-f000538c.pt",
|
18 |
-
)
|
19 |
-
|
20 |
-
_RN101 = dict(
|
21 |
-
openai="https://openaipublic.azureedge.net/clip/models/8fa8567bab74a42d41c5915025a8e4538c3bdbe8804a470a72f30b0d94fab599/RN101.pt",
|
22 |
-
yfcc15m="https://github.com/mlfoundations/open_clip/releases/download/v0.2-weights/rn101-quickgelu-yfcc15m-3e04b30e.pt",
|
23 |
-
)
|
24 |
-
|
25 |
-
_RN101_quickgelu = dict(
|
26 |
-
openai="https://openaipublic.azureedge.net/clip/models/8fa8567bab74a42d41c5915025a8e4538c3bdbe8804a470a72f30b0d94fab599/RN101.pt",
|
27 |
-
yfcc15m="https://github.com/mlfoundations/open_clip/releases/download/v0.2-weights/rn101-quickgelu-yfcc15m-3e04b30e.pt",
|
28 |
-
)
|
29 |
-
|
30 |
-
_RN50x4 = dict(
|
31 |
-
openai="https://openaipublic.azureedge.net/clip/models/7e526bd135e493cef0776de27d5f42653e6b4c8bf9e0f653bb11773263205fdd/RN50x4.pt",
|
32 |
-
)
|
33 |
-
|
34 |
-
_RN50x16 = dict(
|
35 |
-
openai="https://openaipublic.azureedge.net/clip/models/52378b407f34354e150460fe41077663dd5b39c54cd0bfd2b27167a4a06ec9aa/RN50x16.pt",
|
36 |
-
)
|
37 |
-
|
38 |
-
_RN50x64 = dict(
|
39 |
-
openai="https://openaipublic.azureedge.net/clip/models/be1cfb55d75a9666199fb2206c106743da0f6468c9d327f3e0d0a543a9919d9c/RN50x64.pt",
|
40 |
-
)
|
41 |
-
|
42 |
-
_VITB32 = dict(
|
43 |
-
openai="https://openaipublic.azureedge.net/clip/models/40d365715913c9da98579312b702a82c18be219cc2a73407c4526f58eba950af/ViT-B-32.pt",
|
44 |
-
laion400m_e31="https://github.com/mlfoundations/open_clip/releases/download/v0.2-weights/vit_b_32-quickgelu-laion400m_e31-d867053b.pt",
|
45 |
-
laion400m_e32="https://github.com/mlfoundations/open_clip/releases/download/v0.2-weights/vit_b_32-quickgelu-laion400m_e32-46683a32.pt",
|
46 |
-
laion400m_avg="https://github.com/mlfoundations/open_clip/releases/download/v0.2-weights/vit_b_32-quickgelu-laion400m_avg-8a00ab3c.pt",
|
47 |
-
)
|
48 |
-
|
49 |
-
_VITB32_quickgelu = dict(
|
50 |
-
openai="https://openaipublic.azureedge.net/clip/models/40d365715913c9da98579312b702a82c18be219cc2a73407c4526f58eba950af/ViT-B-32.pt",
|
51 |
-
laion400m_e31="https://github.com/mlfoundations/open_clip/releases/download/v0.2-weights/vit_b_32-quickgelu-laion400m_e31-d867053b.pt",
|
52 |
-
laion400m_e32="https://github.com/mlfoundations/open_clip/releases/download/v0.2-weights/vit_b_32-quickgelu-laion400m_e32-46683a32.pt",
|
53 |
-
laion400m_avg="https://github.com/mlfoundations/open_clip/releases/download/v0.2-weights/vit_b_32-quickgelu-laion400m_avg-8a00ab3c.pt",
|
54 |
-
)
|
55 |
-
|
56 |
-
_VITB16 = dict(
|
57 |
-
openai="https://openaipublic.azureedge.net/clip/models/5806e77cd80f8b59890b7e101eabd078d9fb84e6937f9e85e4ecb61988df416f/ViT-B-16.pt",
|
58 |
-
)
|
59 |
-
|
60 |
-
_VITL14 = dict(
|
61 |
-
openai="https://openaipublic.azureedge.net/clip/models/b8cca3fd41ae0c99ba7e8951adf17d267cdb84cd88be6f7c2e0eca1737a03836/ViT-L-14.pt",
|
62 |
-
)
|
63 |
-
|
64 |
-
_PRETRAINED = {
|
65 |
-
"RN50": _RN50,
|
66 |
-
"RN50-quickgelu": _RN50_quickgelu,
|
67 |
-
"RN101": _RN101,
|
68 |
-
"RN101-quickgelu": _RN101_quickgelu,
|
69 |
-
"RN50x4": _RN50x4,
|
70 |
-
"RN50x16": _RN50x16,
|
71 |
-
"ViT-B-32": _VITB32,
|
72 |
-
"ViT-B-32-quickgelu": _VITB32_quickgelu,
|
73 |
-
"ViT-B-16": _VITB16,
|
74 |
-
"ViT-L-14": _VITL14,
|
75 |
-
}
|
76 |
-
|
77 |
-
|
78 |
-
def list_pretrained(as_str: bool = False):
|
79 |
-
"""returns list of pretrained models
|
80 |
-
Returns a tuple (model_name, pretrain_tag) by default or 'name:tag' if as_str == True
|
81 |
-
"""
|
82 |
-
return [
|
83 |
-
":".join([k, t]) if as_str else (k, t)
|
84 |
-
for k in _PRETRAINED.keys()
|
85 |
-
for t in _PRETRAINED[k].keys()
|
86 |
-
]
|
87 |
-
|
88 |
-
|
89 |
-
def list_pretrained_tag_models(tag: str):
|
90 |
-
"""return all models having the specified pretrain tag"""
|
91 |
-
models = []
|
92 |
-
for k in _PRETRAINED.keys():
|
93 |
-
if tag in _PRETRAINED[k]:
|
94 |
-
models.append(k)
|
95 |
-
return models
|
96 |
-
|
97 |
-
|
98 |
-
def list_pretrained_model_tags(model: str):
|
99 |
-
"""return all pretrain tags for the specified model architecture"""
|
100 |
-
tags = []
|
101 |
-
if model in _PRETRAINED:
|
102 |
-
tags.extend(_PRETRAINED[model].keys())
|
103 |
-
return tags
|
104 |
-
|
105 |
-
|
106 |
-
def get_pretrained_url(model: str, tag: str):
|
107 |
-
if model not in _PRETRAINED:
|
108 |
-
return ""
|
109 |
-
model_pretrained = _PRETRAINED[model]
|
110 |
-
if tag not in model_pretrained:
|
111 |
-
return ""
|
112 |
-
return model_pretrained[tag]
|
113 |
-
|
114 |
-
|
115 |
-
def download_pretrained(url: str, root: str = os.path.expanduser("~/.cache/clip")):
|
116 |
-
os.makedirs(root, exist_ok=True)
|
117 |
-
filename = os.path.basename(url)
|
118 |
-
|
119 |
-
if "openaipublic" in url:
|
120 |
-
expected_sha256 = url.split("/")[-2]
|
121 |
-
else:
|
122 |
-
expected_sha256 = ""
|
123 |
-
|
124 |
-
download_target = os.path.join(root, filename)
|
125 |
-
|
126 |
-
if os.path.exists(download_target) and not os.path.isfile(download_target):
|
127 |
-
raise RuntimeError(f"{download_target} exists and is not a regular file")
|
128 |
-
|
129 |
-
if os.path.isfile(download_target):
|
130 |
-
if expected_sha256:
|
131 |
-
if (
|
132 |
-
hashlib.sha256(open(download_target, "rb").read()).hexdigest()
|
133 |
-
== expected_sha256
|
134 |
-
):
|
135 |
-
return download_target
|
136 |
-
else:
|
137 |
-
warnings.warn(
|
138 |
-
f"{download_target} exists, but the SHA256 checksum does not match; re-downloading the file"
|
139 |
-
)
|
140 |
-
else:
|
141 |
-
return download_target
|
142 |
-
|
143 |
-
with urllib.request.urlopen(url) as source, open(download_target, "wb") as output:
|
144 |
-
with tqdm(
|
145 |
-
total=int(source.info().get("Content-Length")),
|
146 |
-
ncols=80,
|
147 |
-
unit="iB",
|
148 |
-
unit_scale=True,
|
149 |
-
) as loop:
|
150 |
-
while True:
|
151 |
-
buffer = source.read(8192)
|
152 |
-
if not buffer:
|
153 |
-
break
|
154 |
-
|
155 |
-
output.write(buffer)
|
156 |
-
loop.update(len(buffer))
|
157 |
-
|
158 |
-
if (
|
159 |
-
expected_sha256
|
160 |
-
and hashlib.sha256(open(download_target, "rb").read()).hexdigest()
|
161 |
-
!= expected_sha256
|
162 |
-
):
|
163 |
-
raise RuntimeError(
|
164 |
-
f"Model has been downloaded but the SHA256 checksum does not not match"
|
165 |
-
)
|
166 |
-
|
167 |
-
return download_target
|
|
|
|
|
|
|
|
|
|
|
|
|
|
|
|
|
|
|
|
|
|
|
|
|
|
|
|
|
|
|
|
|
|
|
|
|
|
|
|
|
|
|
|
|
|
|
|
|
|
|
|
|
|
|
|
|
|
|
|
|
|
|
|
|
|
|
|
|
|
|
|
|
|
|
|
|
|
|
|
|
|
|
|
|
|
|
|
|
|
|
|
|
|
|
|
|
|
|
|
|
|
|
|
|
|
|
|
|
|
|
|
|
|
|
|
|
|
|
|
|
|
|
|
|
|
|
|
|
|
|
|
|
|
|
|
|
|
|
|
|
|
|
|
|
|
|
|
|
|
|
|
|
|
|
|
|
|
|
|
|
|
|
|
|
|
|
|
|
|
|
|
|
|
|
|
|
|
|
|
|
|
|
|
|
|
|
|
|
|
|
|
|
|
|
|
|
|
|
|
|
|
|
|
|
|
|
|
|
|
|
|
|
|
|
|
|
|
|
|
|
|
|
|
|
|
|
|
|
|
|
|
|
|
|
|
|
|
|
|
|
|
|
|
|
|
|
|
|
|
|
|
|
|
|
|
|
|
|
|
|
|
|
|
|
|
|
|
|
|
|
|
|
|
|
|
|
|
|
|
|
|
|
|
|
|
|
|
|
|
|
|
|
|
|
|
|
|
|
|
|
|
|
|
|
|
|
|
|
|
|
|
|
|
|
|
|
|
|
|
|
|
|
|
|
|
|
|
|
|
|
|
|
|
|
|
spaces/AIFILMS/audioldm-text-to-audio-generation/audioldm/clap/open_clip/tokenizer.py
DELETED
@@ -1,197 +0,0 @@
|
|
1 |
-
""" CLIP tokenizer
|
2 |
-
|
3 |
-
Copied from https://github.com/openai/CLIP. Originally MIT License, Copyright (c) 2021 OpenAI.
|
4 |
-
"""
|
5 |
-
import gzip
|
6 |
-
import html
|
7 |
-
import os
|
8 |
-
from functools import lru_cache
|
9 |
-
from typing import Union, List
|
10 |
-
|
11 |
-
import ftfy
|
12 |
-
import regex as re
|
13 |
-
import torch
|
14 |
-
|
15 |
-
|
16 |
-
@lru_cache()
|
17 |
-
def default_bpe():
|
18 |
-
return os.path.join(
|
19 |
-
os.path.dirname(os.path.abspath(__file__)), "bpe_simple_vocab_16e6.txt.gz"
|
20 |
-
)
|
21 |
-
|
22 |
-
|
23 |
-
@lru_cache()
|
24 |
-
def bytes_to_unicode():
|
25 |
-
"""
|
26 |
-
Returns list of utf-8 byte and a corresponding list of unicode strings.
|
27 |
-
The reversible bpe codes work on unicode strings.
|
28 |
-
This means you need a large # of unicode characters in your vocab if you want to avoid UNKs.
|
29 |
-
When you're at something like a 10B token dataset you end up needing around 5K for decent coverage.
|
30 |
-
This is a signficant percentage of your normal, say, 32K bpe vocab.
|
31 |
-
To avoid that, we want lookup tables between utf-8 bytes and unicode strings.
|
32 |
-
And avoids mapping to whitespace/control characters the bpe code barfs on.
|
33 |
-
"""
|
34 |
-
bs = (
|
35 |
-
list(range(ord("!"), ord("~") + 1))
|
36 |
-
+ list(range(ord("¡"), ord("¬") + 1))
|
37 |
-
+ list(range(ord("®"), ord("ÿ") + 1))
|
38 |
-
)
|
39 |
-
cs = bs[:]
|
40 |
-
n = 0
|
41 |
-
for b in range(2**8):
|
42 |
-
if b not in bs:
|
43 |
-
bs.append(b)
|
44 |
-
cs.append(2**8 + n)
|
45 |
-
n += 1
|
46 |
-
cs = [chr(n) for n in cs]
|
47 |
-
return dict(zip(bs, cs))
|
48 |
-
|
49 |
-
|
50 |
-
def get_pairs(word):
|
51 |
-
"""Return set of symbol pairs in a word.
|
52 |
-
Word is represented as tuple of symbols (symbols being variable-length strings).
|
53 |
-
"""
|
54 |
-
pairs = set()
|
55 |
-
prev_char = word[0]
|
56 |
-
for char in word[1:]:
|
57 |
-
pairs.add((prev_char, char))
|
58 |
-
prev_char = char
|
59 |
-
return pairs
|
60 |
-
|
61 |
-
|
62 |
-
def basic_clean(text):
|
63 |
-
text = ftfy.fix_text(text)
|
64 |
-
text = html.unescape(html.unescape(text))
|
65 |
-
return text.strip()
|
66 |
-
|
67 |
-
|
68 |
-
def whitespace_clean(text):
|
69 |
-
text = re.sub(r"\s+", " ", text)
|
70 |
-
text = text.strip()
|
71 |
-
return text
|
72 |
-
|
73 |
-
|
74 |
-
class SimpleTokenizer(object):
|
75 |
-
def __init__(self, bpe_path: str = default_bpe(), special_tokens=None):
|
76 |
-
self.byte_encoder = bytes_to_unicode()
|
77 |
-
self.byte_decoder = {v: k for k, v in self.byte_encoder.items()}
|
78 |
-
merges = gzip.open(bpe_path).read().decode("utf-8").split("\n")
|
79 |
-
merges = merges[1 : 49152 - 256 - 2 + 1]
|
80 |
-
merges = [tuple(merge.split()) for merge in merges]
|
81 |
-
vocab = list(bytes_to_unicode().values())
|
82 |
-
vocab = vocab + [v + "</w>" for v in vocab]
|
83 |
-
for merge in merges:
|
84 |
-
vocab.append("".join(merge))
|
85 |
-
if not special_tokens:
|
86 |
-
special_tokens = ["<start_of_text>", "<end_of_text>"]
|
87 |
-
else:
|
88 |
-
special_tokens = ["<start_of_text>", "<end_of_text>"] + special_tokens
|
89 |
-
vocab.extend(special_tokens)
|
90 |
-
self.encoder = dict(zip(vocab, range(len(vocab))))
|
91 |
-
self.decoder = {v: k for k, v in self.encoder.items()}
|
92 |
-
self.bpe_ranks = dict(zip(merges, range(len(merges))))
|
93 |
-
self.cache = {t: t for t in special_tokens}
|
94 |
-
special = "|".join(special_tokens)
|
95 |
-
self.pat = re.compile(
|
96 |
-
special + r"""|'s|'t|'re|'ve|'m|'ll|'d|[\p{L}]+|[\p{N}]|[^\s\p{L}\p{N}]+""",
|
97 |
-
re.IGNORECASE,
|
98 |
-
)
|
99 |
-
|
100 |
-
self.vocab_size = len(self.encoder)
|
101 |
-
self.all_special_ids = [self.encoder[t] for t in special_tokens]
|
102 |
-
|
103 |
-
def bpe(self, token):
|
104 |
-
if token in self.cache:
|
105 |
-
return self.cache[token]
|
106 |
-
word = tuple(token[:-1]) + (token[-1] + "</w>",)
|
107 |
-
pairs = get_pairs(word)
|
108 |
-
|
109 |
-
if not pairs:
|
110 |
-
return token + "</w>"
|
111 |
-
|
112 |
-
while True:
|
113 |
-
bigram = min(pairs, key=lambda pair: self.bpe_ranks.get(pair, float("inf")))
|
114 |
-
if bigram not in self.bpe_ranks:
|
115 |
-
break
|
116 |
-
first, second = bigram
|
117 |
-
new_word = []
|
118 |
-
i = 0
|
119 |
-
while i < len(word):
|
120 |
-
try:
|
121 |
-
j = word.index(first, i)
|
122 |
-
new_word.extend(word[i:j])
|
123 |
-
i = j
|
124 |
-
except:
|
125 |
-
new_word.extend(word[i:])
|
126 |
-
break
|
127 |
-
|
128 |
-
if word[i] == first and i < len(word) - 1 and word[i + 1] == second:
|
129 |
-
new_word.append(first + second)
|
130 |
-
i += 2
|
131 |
-
else:
|
132 |
-
new_word.append(word[i])
|
133 |
-
i += 1
|
134 |
-
new_word = tuple(new_word)
|
135 |
-
word = new_word
|
136 |
-
if len(word) == 1:
|
137 |
-
break
|
138 |
-
else:
|
139 |
-
pairs = get_pairs(word)
|
140 |
-
word = " ".join(word)
|
141 |
-
self.cache[token] = word
|
142 |
-
return word
|
143 |
-
|
144 |
-
def encode(self, text):
|
145 |
-
bpe_tokens = []
|
146 |
-
text = whitespace_clean(basic_clean(text)).lower()
|
147 |
-
for token in re.findall(self.pat, text):
|
148 |
-
token = "".join(self.byte_encoder[b] for b in token.encode("utf-8"))
|
149 |
-
bpe_tokens.extend(
|
150 |
-
self.encoder[bpe_token] for bpe_token in self.bpe(token).split(" ")
|
151 |
-
)
|
152 |
-
return bpe_tokens
|
153 |
-
|
154 |
-
def decode(self, tokens):
|
155 |
-
text = "".join([self.decoder[token] for token in tokens])
|
156 |
-
text = (
|
157 |
-
bytearray([self.byte_decoder[c] for c in text])
|
158 |
-
.decode("utf-8", errors="replace")
|
159 |
-
.replace("</w>", " ")
|
160 |
-
)
|
161 |
-
return text
|
162 |
-
|
163 |
-
|
164 |
-
_tokenizer = SimpleTokenizer()
|
165 |
-
|
166 |
-
|
167 |
-
def tokenize(
|
168 |
-
texts: Union[str, List[str]], context_length: int = 77
|
169 |
-
) -> torch.LongTensor:
|
170 |
-
"""
|
171 |
-
Returns the tokenized representation of given input string(s)
|
172 |
-
|
173 |
-
Parameters
|
174 |
-
----------
|
175 |
-
texts : Union[str, List[str]]
|
176 |
-
An input string or a list of input strings to tokenize
|
177 |
-
context_length : int
|
178 |
-
The context length to use; all CLIP models use 77 as the context length
|
179 |
-
|
180 |
-
Returns
|
181 |
-
-------
|
182 |
-
A two-dimensional tensor containing the resulting tokens, shape = [number of input strings, context_length]
|
183 |
-
"""
|
184 |
-
if isinstance(texts, str):
|
185 |
-
texts = [texts]
|
186 |
-
|
187 |
-
sot_token = _tokenizer.encoder["<start_of_text>"]
|
188 |
-
eot_token = _tokenizer.encoder["<end_of_text>"]
|
189 |
-
all_tokens = [[sot_token] + _tokenizer.encode(text) + [eot_token] for text in texts]
|
190 |
-
result = torch.zeros(len(all_tokens), context_length, dtype=torch.long)
|
191 |
-
|
192 |
-
for i, tokens in enumerate(all_tokens):
|
193 |
-
if len(tokens) > context_length:
|
194 |
-
tokens = tokens[:context_length] # Truncate
|
195 |
-
result[i, : len(tokens)] = torch.tensor(tokens)
|
196 |
-
|
197 |
-
return result
|
|
|
|
|
|
|
|
|
|
|
|
|
|
|
|
|
|
|
|
|
|
|
|
|
|
|
|
|
|
|
|
|
|
|
|
|
|
|
|
|
|
|
|
|
|
|
|
|
|
|
|
|
|
|
|
|
|
|
|
|
|
|
|
|
|
|
|
|
|
|
|
|
|
|
|
|
|
|
|
|
|
|
|
|
|
|
|
|
|
|
|
|
|
|
|
|
|
|
|
|
|
|
|
|
|
|
|
|
|
|
|
|
|
|
|
|
|
|
|
|
|
|
|
|
|
|
|
|
|
|
|
|
|
|
|
|
|
|
|
|
|
|
|
|
|
|
|
|
|
|
|
|
|
|
|
|
|
|
|
|
|
|
|
|
|
|
|
|
|
|
|
|
|
|
|
|
|
|
|
|
|
|
|
|
|
|
|
|
|
|
|
|
|
|
|
|
|
|
|
|
|
|
|
|
|
|
|
|
|
|
|
|
|
|
|
|
|
|
|
|
|
|
|
|
|
|
|
|
|
|
|
|
|
|
|
|
|
|
|
|
|
|
|
|
|
|
|
|
|
|
|
|
|
|
|
|
|
|
|
|
|
|
|
|
|
|
|
|
|
|
|
|
|
|
|
|
|
|
|
|
|
|
|
|
|
|
|
|
|
|
|
|
|
|
|
|
|
|
|
|
|
|
|
|
|
|
|
|
|
|
|
|
|
|
|
|
|
|
|
|
|
|
|
|
|
|
|
|
|
|
|
|
|
|
|
|
|
|
|
|
|
|
|
|
|
|
|
|
|
|
|
|
|
|
|
|
|
|
|
|
|
|
|
|
|
|
|
|
|
|
|
|
|
|
|
|
|
|
|
|
|
|
|
|
|
|
|
|
|
|
|
|
|
|
|
spaces/AIGC-Audio/AudioGPT/text_to_audio/Make_An_Audio/ldm/modules/encoders/__init__.py
DELETED
File without changes
|
spaces/AIGC-Audio/Make_An_Audio/ldm/modules/losses_audio/vggishish/transforms.py
DELETED
@@ -1,98 +0,0 @@
|
|
1 |
-
import logging
|
2 |
-
import os
|
3 |
-
from pathlib import Path
|
4 |
-
|
5 |
-
import albumentations
|
6 |
-
import numpy as np
|
7 |
-
import torch
|
8 |
-
from tqdm import tqdm
|
9 |
-
|
10 |
-
logger = logging.getLogger(f'main.{__name__}')
|
11 |
-
|
12 |
-
|
13 |
-
class StandardNormalizeAudio(object):
|
14 |
-
'''
|
15 |
-
Frequency-wise normalization
|
16 |
-
'''
|
17 |
-
def __init__(self, specs_dir, train_ids_path='./data/vggsound_train.txt', cache_path='./data/'):
|
18 |
-
self.specs_dir = specs_dir
|
19 |
-
self.train_ids_path = train_ids_path
|
20 |
-
# making the stats filename to match the specs dir name
|
21 |
-
self.cache_path = os.path.join(cache_path, f'train_means_stds_{Path(specs_dir).stem}.txt')
|
22 |
-
logger.info('Assuming that the input stats are calculated using preprocessed spectrograms (log)')
|
23 |
-
self.train_stats = self.calculate_or_load_stats()
|
24 |
-
|
25 |
-
def __call__(self, item):
|
26 |
-
# just to generalizat the input handling. Useful for FID, IS eval and training other staff
|
27 |
-
if isinstance(item, dict):
|
28 |
-
if 'input' in item:
|
29 |
-
input_key = 'input'
|
30 |
-
elif 'image' in item:
|
31 |
-
input_key = 'image'
|
32 |
-
else:
|
33 |
-
raise NotImplementedError
|
34 |
-
item[input_key] = (item[input_key] - self.train_stats['means']) / self.train_stats['stds']
|
35 |
-
elif isinstance(item, torch.Tensor):
|
36 |
-
# broadcasts np.ndarray (80, 1) to (1, 80, 1) because item is torch.Tensor (B, 80, T)
|
37 |
-
item = (item - self.train_stats['means']) / self.train_stats['stds']
|
38 |
-
else:
|
39 |
-
raise NotImplementedError
|
40 |
-
return item
|
41 |
-
|
42 |
-
def calculate_or_load_stats(self):
|
43 |
-
try:
|
44 |
-
# (F, 2)
|
45 |
-
train_stats = np.loadtxt(self.cache_path)
|
46 |
-
means, stds = train_stats.T
|
47 |
-
logger.info('Trying to load train stats for Standard Normalization of inputs')
|
48 |
-
except OSError:
|
49 |
-
logger.info('Could not find the precalculated stats for Standard Normalization. Calculating...')
|
50 |
-
train_vid_ids = open(self.train_ids_path)
|
51 |
-
specs_paths = [os.path.join(self.specs_dir, f'{i.rstrip()}_mel.npy') for i in train_vid_ids]
|
52 |
-
means = [None] * len(specs_paths)
|
53 |
-
stds = [None] * len(specs_paths)
|
54 |
-
for i, path in enumerate(tqdm(specs_paths)):
|
55 |
-
spec = np.load(path)
|
56 |
-
means[i] = spec.mean(axis=1)
|
57 |
-
stds[i] = spec.std(axis=1)
|
58 |
-
# (F) <- (num_files, F)
|
59 |
-
means = np.array(means).mean(axis=0)
|
60 |
-
stds = np.array(stds).mean(axis=0)
|
61 |
-
# saving in two columns
|
62 |
-
np.savetxt(self.cache_path, np.vstack([means, stds]).T, fmt='%0.8f')
|
63 |
-
means = means.reshape(-1, 1)
|
64 |
-
stds = stds.reshape(-1, 1)
|
65 |
-
return {'means': means, 'stds': stds}
|
66 |
-
|
67 |
-
class ToTensor(object):
|
68 |
-
|
69 |
-
def __call__(self, item):
|
70 |
-
item['input'] = torch.from_numpy(item['input']).float()
|
71 |
-
# if 'target' in item:
|
72 |
-
item['target'] = torch.tensor(item['target'])
|
73 |
-
return item
|
74 |
-
|
75 |
-
class Crop(object):
|
76 |
-
|
77 |
-
def __init__(self, cropped_shape=None, random_crop=False):
|
78 |
-
self.cropped_shape = cropped_shape
|
79 |
-
if cropped_shape is not None:
|
80 |
-
mel_num, spec_len = cropped_shape
|
81 |
-
if random_crop:
|
82 |
-
self.cropper = albumentations.RandomCrop
|
83 |
-
else:
|
84 |
-
self.cropper = albumentations.CenterCrop
|
85 |
-
self.preprocessor = albumentations.Compose([self.cropper(mel_num, spec_len)])
|
86 |
-
else:
|
87 |
-
self.preprocessor = lambda **kwargs: kwargs
|
88 |
-
|
89 |
-
def __call__(self, item):
|
90 |
-
item['input'] = self.preprocessor(image=item['input'])['image']
|
91 |
-
return item
|
92 |
-
|
93 |
-
|
94 |
-
if __name__ == '__main__':
|
95 |
-
cropper = Crop([80, 848])
|
96 |
-
item = {'input': torch.rand([80, 860])}
|
97 |
-
outputs = cropper(item)
|
98 |
-
print(outputs['input'].shape)
|
|
|
|
|
|
|
|
|
|
|
|
|
|
|
|
|
|
|
|
|
|
|
|
|
|
|
|
|
|
|
|
|
|
|
|
|
|
|
|
|
|
|
|
|
|
|
|
|
|
|
|
|
|
|
|
|
|
|
|
|
|
|
|
|
|
|
|
|
|
|
|
|
|
|
|
|
|
|
|
|
|
|
|
|
|
|
|
|
|
|
|
|
|
|
|
|
|
|
|
|
|
|
|
|
|
|
|
|
|
|
|
|
|
|
|
|
|
|
|
|
|
|
|
|
|
|
|
|
|
|
|
|
|
|
|
|
|
|
|
|
|
|
|
|
|
|
|
|
|
|
|
|
|
|
|
|
|
|
|
|
|
|
|
|
|
|
|
|
|
|
|
|
|
|
|
|
|
|
|
|
|
|
|
|
|
|
|
|
|
|
|
|
|
|
|
|
|
spaces/ATang0729/Forecast4Muses/Model/Model6/Model6_2_ProfileRecogition/mmpretrain/configs/resnet/resnet50_8xb16_cifar10.py
DELETED
@@ -1,4 +0,0 @@
|
|
1 |
-
_base_ = [
|
2 |
-
'../_base_/models/resnet50_cifar.py', '../_base_/datasets/cifar10_bs16.py',
|
3 |
-
'../_base_/schedules/cifar10_bs128.py', '../_base_/default_runtime.py'
|
4 |
-
]
|
|
|
|
|
|
|
|
|
|
spaces/AgentVerse/agentVerse/ui/src/phaser3-rex-plugins/templates/spinner/custom/Factory.d.ts
DELETED
@@ -1,5 +0,0 @@
|
|
1 |
-
import Custom from './Custom';
|
2 |
-
|
3 |
-
export default function Factory(
|
4 |
-
config?: Custom.IConfig
|
5 |
-
): Custom;
|
|
|
|
|
|
|
|
|
|
|
|
spaces/Agusbs98/automatic-ecg-diagnosis/nets/bblocks.py
DELETED
@@ -1,55 +0,0 @@
|
|
1 |
-
|
2 |
-
import os, sys
|
3 |
-
from libs import *
|
4 |
-
from .layers import *
|
5 |
-
from .modules import *
|
6 |
-
|
7 |
-
class LightSEResBlock(nn.Module):
|
8 |
-
def __init__(self,
|
9 |
-
in_channels,
|
10 |
-
downsample = False,
|
11 |
-
):
|
12 |
-
super(LightSEResBlock, self).__init__()
|
13 |
-
if downsample:
|
14 |
-
self.out_channels = in_channels*2
|
15 |
-
self.conv_1 = DSConv1d(
|
16 |
-
in_channels, self.out_channels,
|
17 |
-
kernel_size = 7, padding = 3, stride = 2,
|
18 |
-
)
|
19 |
-
self.identity = nn.Sequential(
|
20 |
-
DSConv1d(
|
21 |
-
in_channels, self.out_channels,
|
22 |
-
kernel_size = 1, padding = 0, stride = 2,
|
23 |
-
),
|
24 |
-
nn.BatchNorm1d(self.out_channels),
|
25 |
-
)
|
26 |
-
else:
|
27 |
-
self.out_channels = in_channels
|
28 |
-
self.conv_1 = DSConv1d(
|
29 |
-
in_channels, self.out_channels,
|
30 |
-
kernel_size = 7, padding = 3, stride = 1,
|
31 |
-
)
|
32 |
-
self.identity = nn.Identity()
|
33 |
-
self.conv_2 = DSConv1d(
|
34 |
-
self.out_channels, self.out_channels,
|
35 |
-
kernel_size = 7, padding = 3, stride = 1,
|
36 |
-
)
|
37 |
-
|
38 |
-
self.convs = nn.Sequential(
|
39 |
-
self.conv_1,
|
40 |
-
nn.BatchNorm1d(self.out_channels),
|
41 |
-
nn.ReLU(),
|
42 |
-
nn.Dropout(0.3),
|
43 |
-
self.conv_2,
|
44 |
-
nn.BatchNorm1d(self.out_channels),
|
45 |
-
LightSEModule(self.out_channels),
|
46 |
-
)
|
47 |
-
self.act_fn = nn.ReLU()
|
48 |
-
|
49 |
-
def forward(self,
|
50 |
-
input,
|
51 |
-
):
|
52 |
-
output = self.convs(input) + self.identity(input)
|
53 |
-
output = self.act_fn(output)
|
54 |
-
|
55 |
-
return output
|
|
|
|
|
|
|
|
|
|
|
|
|
|
|
|
|
|
|
|
|
|
|
|
|
|
|
|
|
|
|
|
|
|
|
|
|
|
|
|
|
|
|
|
|
|
|
|
|
|
|
|
|
|
|
|
|
|
|
|
|
|
|
|
|
|
|
|
|
|
|
|
|
|
|
|
|
|
|
|
|
|
|
|
|
|
|
|
|
|
|
|
|
|
|
|
|
|
|
|
|
|
|
|
|
|
|
|
|
|
|
|
spaces/Aki004/herta-so-vits/onnxexport/model_onnx_speaker_mix.py
DELETED
@@ -1,363 +0,0 @@
|
|
1 |
-
import torch
|
2 |
-
from torch import nn
|
3 |
-
from torch.nn import functional as F
|
4 |
-
import cluster
|
5 |
-
import modules.attentions as attentions
|
6 |
-
import modules.commons as commons
|
7 |
-
import modules.modules as modules
|
8 |
-
|
9 |
-
from torch.nn import Conv1d, ConvTranspose1d, AvgPool1d, Conv2d
|
10 |
-
from torch.nn.utils import weight_norm, remove_weight_norm, spectral_norm
|
11 |
-
|
12 |
-
import utils
|
13 |
-
from modules.commons import init_weights, get_padding
|
14 |
-
from vdecoder.hifigan.models import Generator
|
15 |
-
from utils import f0_to_coarse
|
16 |
-
|
17 |
-
|
18 |
-
class ResidualCouplingBlock(nn.Module):
|
19 |
-
def __init__(self,
|
20 |
-
channels,
|
21 |
-
hidden_channels,
|
22 |
-
kernel_size,
|
23 |
-
dilation_rate,
|
24 |
-
n_layers,
|
25 |
-
n_flows=4,
|
26 |
-
gin_channels=0):
|
27 |
-
super().__init__()
|
28 |
-
self.channels = channels
|
29 |
-
self.hidden_channels = hidden_channels
|
30 |
-
self.kernel_size = kernel_size
|
31 |
-
self.dilation_rate = dilation_rate
|
32 |
-
self.n_layers = n_layers
|
33 |
-
self.n_flows = n_flows
|
34 |
-
self.gin_channels = gin_channels
|
35 |
-
|
36 |
-
self.flows = nn.ModuleList()
|
37 |
-
for i in range(n_flows):
|
38 |
-
self.flows.append(
|
39 |
-
modules.ResidualCouplingLayer(channels, hidden_channels, kernel_size, dilation_rate, n_layers,
|
40 |
-
gin_channels=gin_channels, mean_only=True))
|
41 |
-
self.flows.append(modules.Flip())
|
42 |
-
|
43 |
-
def forward(self, x, x_mask, g=None, reverse=False):
|
44 |
-
if not reverse:
|
45 |
-
for flow in self.flows:
|
46 |
-
x, _ = flow(x, x_mask, g=g, reverse=reverse)
|
47 |
-
else:
|
48 |
-
for flow in reversed(self.flows):
|
49 |
-
x = flow(x, x_mask, g=g, reverse=reverse)
|
50 |
-
return x
|
51 |
-
|
52 |
-
|
53 |
-
class Encoder(nn.Module):
|
54 |
-
def __init__(self,
|
55 |
-
in_channels,
|
56 |
-
out_channels,
|
57 |
-
hidden_channels,
|
58 |
-
kernel_size,
|
59 |
-
dilation_rate,
|
60 |
-
n_layers,
|
61 |
-
gin_channels=0):
|
62 |
-
super().__init__()
|
63 |
-
self.in_channels = in_channels
|
64 |
-
self.out_channels = out_channels
|
65 |
-
self.hidden_channels = hidden_channels
|
66 |
-
self.kernel_size = kernel_size
|
67 |
-
self.dilation_rate = dilation_rate
|
68 |
-
self.n_layers = n_layers
|
69 |
-
self.gin_channels = gin_channels
|
70 |
-
|
71 |
-
self.pre = nn.Conv1d(in_channels, hidden_channels, 1)
|
72 |
-
self.enc = modules.WN(hidden_channels, kernel_size, dilation_rate, n_layers, gin_channels=gin_channels)
|
73 |
-
self.proj = nn.Conv1d(hidden_channels, out_channels * 2, 1)
|
74 |
-
|
75 |
-
def forward(self, x, x_lengths, g=None):
|
76 |
-
# print(x.shape,x_lengths.shape)
|
77 |
-
x_mask = torch.unsqueeze(commons.sequence_mask(x_lengths, x.size(2)), 1).to(x.dtype)
|
78 |
-
x = self.pre(x) * x_mask
|
79 |
-
x = self.enc(x, x_mask, g=g)
|
80 |
-
stats = self.proj(x) * x_mask
|
81 |
-
m, logs = torch.split(stats, self.out_channels, dim=1)
|
82 |
-
z = (m + torch.randn_like(m) * torch.exp(logs)) * x_mask
|
83 |
-
return z, m, logs, x_mask
|
84 |
-
|
85 |
-
|
86 |
-
class TextEncoder(nn.Module):
|
87 |
-
def __init__(self,
|
88 |
-
out_channels,
|
89 |
-
hidden_channels,
|
90 |
-
kernel_size,
|
91 |
-
n_layers,
|
92 |
-
gin_channels=0,
|
93 |
-
filter_channels=None,
|
94 |
-
n_heads=None,
|
95 |
-
p_dropout=None):
|
96 |
-
super().__init__()
|
97 |
-
self.out_channels = out_channels
|
98 |
-
self.hidden_channels = hidden_channels
|
99 |
-
self.kernel_size = kernel_size
|
100 |
-
self.n_layers = n_layers
|
101 |
-
self.gin_channels = gin_channels
|
102 |
-
self.proj = nn.Conv1d(hidden_channels, out_channels * 2, 1)
|
103 |
-
self.f0_emb = nn.Embedding(256, hidden_channels)
|
104 |
-
|
105 |
-
self.enc_ = attentions.Encoder(
|
106 |
-
hidden_channels,
|
107 |
-
filter_channels,
|
108 |
-
n_heads,
|
109 |
-
n_layers,
|
110 |
-
kernel_size,
|
111 |
-
p_dropout)
|
112 |
-
|
113 |
-
def forward(self, x, x_mask, f0=None, z=None):
|
114 |
-
x = x + self.f0_emb(f0).transpose(1, 2)
|
115 |
-
x = self.enc_(x * x_mask, x_mask)
|
116 |
-
stats = self.proj(x) * x_mask
|
117 |
-
m, logs = torch.split(stats, self.out_channels, dim=1)
|
118 |
-
z = (m + z * torch.exp(logs)) * x_mask
|
119 |
-
return z, m, logs, x_mask
|
120 |
-
|
121 |
-
|
122 |
-
class DiscriminatorP(torch.nn.Module):
|
123 |
-
def __init__(self, period, kernel_size=5, stride=3, use_spectral_norm=False):
|
124 |
-
super(DiscriminatorP, self).__init__()
|
125 |
-
self.period = period
|
126 |
-
self.use_spectral_norm = use_spectral_norm
|
127 |
-
norm_f = weight_norm if use_spectral_norm == False else spectral_norm
|
128 |
-
self.convs = nn.ModuleList([
|
129 |
-
norm_f(Conv2d(1, 32, (kernel_size, 1), (stride, 1), padding=(get_padding(kernel_size, 1), 0))),
|
130 |
-
norm_f(Conv2d(32, 128, (kernel_size, 1), (stride, 1), padding=(get_padding(kernel_size, 1), 0))),
|
131 |
-
norm_f(Conv2d(128, 512, (kernel_size, 1), (stride, 1), padding=(get_padding(kernel_size, 1), 0))),
|
132 |
-
norm_f(Conv2d(512, 1024, (kernel_size, 1), (stride, 1), padding=(get_padding(kernel_size, 1), 0))),
|
133 |
-
norm_f(Conv2d(1024, 1024, (kernel_size, 1), 1, padding=(get_padding(kernel_size, 1), 0))),
|
134 |
-
])
|
135 |
-
self.conv_post = norm_f(Conv2d(1024, 1, (3, 1), 1, padding=(1, 0)))
|
136 |
-
|
137 |
-
def forward(self, x):
|
138 |
-
fmap = []
|
139 |
-
|
140 |
-
# 1d to 2d
|
141 |
-
b, c, t = x.shape
|
142 |
-
if t % self.period != 0: # pad first
|
143 |
-
n_pad = self.period - (t % self.period)
|
144 |
-
x = F.pad(x, (0, n_pad), "reflect")
|
145 |
-
t = t + n_pad
|
146 |
-
x = x.view(b, c, t // self.period, self.period)
|
147 |
-
|
148 |
-
for l in self.convs:
|
149 |
-
x = l(x)
|
150 |
-
x = F.leaky_relu(x, modules.LRELU_SLOPE)
|
151 |
-
fmap.append(x)
|
152 |
-
x = self.conv_post(x)
|
153 |
-
fmap.append(x)
|
154 |
-
x = torch.flatten(x, 1, -1)
|
155 |
-
|
156 |
-
return x, fmap
|
157 |
-
|
158 |
-
|
159 |
-
class DiscriminatorS(torch.nn.Module):
|
160 |
-
def __init__(self, use_spectral_norm=False):
|
161 |
-
super(DiscriminatorS, self).__init__()
|
162 |
-
norm_f = weight_norm if use_spectral_norm == False else spectral_norm
|
163 |
-
self.convs = nn.ModuleList([
|
164 |
-
norm_f(Conv1d(1, 16, 15, 1, padding=7)),
|
165 |
-
norm_f(Conv1d(16, 64, 41, 4, groups=4, padding=20)),
|
166 |
-
norm_f(Conv1d(64, 256, 41, 4, groups=16, padding=20)),
|
167 |
-
norm_f(Conv1d(256, 1024, 41, 4, groups=64, padding=20)),
|
168 |
-
norm_f(Conv1d(1024, 1024, 41, 4, groups=256, padding=20)),
|
169 |
-
norm_f(Conv1d(1024, 1024, 5, 1, padding=2)),
|
170 |
-
])
|
171 |
-
self.conv_post = norm_f(Conv1d(1024, 1, 3, 1, padding=1))
|
172 |
-
|
173 |
-
def forward(self, x):
|
174 |
-
fmap = []
|
175 |
-
|
176 |
-
for l in self.convs:
|
177 |
-
x = l(x)
|
178 |
-
x = F.leaky_relu(x, modules.LRELU_SLOPE)
|
179 |
-
fmap.append(x)
|
180 |
-
x = self.conv_post(x)
|
181 |
-
fmap.append(x)
|
182 |
-
x = torch.flatten(x, 1, -1)
|
183 |
-
|
184 |
-
return x, fmap
|
185 |
-
|
186 |
-
|
187 |
-
class F0Decoder(nn.Module):
|
188 |
-
def __init__(self,
|
189 |
-
out_channels,
|
190 |
-
hidden_channels,
|
191 |
-
filter_channels,
|
192 |
-
n_heads,
|
193 |
-
n_layers,
|
194 |
-
kernel_size,
|
195 |
-
p_dropout,
|
196 |
-
spk_channels=0):
|
197 |
-
super().__init__()
|
198 |
-
self.out_channels = out_channels
|
199 |
-
self.hidden_channels = hidden_channels
|
200 |
-
self.filter_channels = filter_channels
|
201 |
-
self.n_heads = n_heads
|
202 |
-
self.n_layers = n_layers
|
203 |
-
self.kernel_size = kernel_size
|
204 |
-
self.p_dropout = p_dropout
|
205 |
-
self.spk_channels = spk_channels
|
206 |
-
|
207 |
-
self.prenet = nn.Conv1d(hidden_channels, hidden_channels, 3, padding=1)
|
208 |
-
self.decoder = attentions.FFT(
|
209 |
-
hidden_channels,
|
210 |
-
filter_channels,
|
211 |
-
n_heads,
|
212 |
-
n_layers,
|
213 |
-
kernel_size,
|
214 |
-
p_dropout)
|
215 |
-
self.proj = nn.Conv1d(hidden_channels, out_channels, 1)
|
216 |
-
self.f0_prenet = nn.Conv1d(1, hidden_channels, 3, padding=1)
|
217 |
-
self.cond = nn.Conv1d(spk_channels, hidden_channels, 1)
|
218 |
-
|
219 |
-
def forward(self, x, norm_f0, x_mask, spk_emb=None):
|
220 |
-
x = torch.detach(x)
|
221 |
-
if spk_emb is not None:
|
222 |
-
x = x + self.cond(spk_emb)
|
223 |
-
x += self.f0_prenet(norm_f0)
|
224 |
-
x = self.prenet(x) * x_mask
|
225 |
-
x = self.decoder(x * x_mask, x_mask)
|
226 |
-
x = self.proj(x) * x_mask
|
227 |
-
return x
|
228 |
-
|
229 |
-
|
230 |
-
class SynthesizerTrn(nn.Module):
|
231 |
-
"""
|
232 |
-
Synthesizer for Training
|
233 |
-
"""
|
234 |
-
|
235 |
-
def __init__(self,
|
236 |
-
spec_channels,
|
237 |
-
segment_size,
|
238 |
-
inter_channels,
|
239 |
-
hidden_channels,
|
240 |
-
filter_channels,
|
241 |
-
n_heads,
|
242 |
-
n_layers,
|
243 |
-
kernel_size,
|
244 |
-
p_dropout,
|
245 |
-
resblock,
|
246 |
-
resblock_kernel_sizes,
|
247 |
-
resblock_dilation_sizes,
|
248 |
-
upsample_rates,
|
249 |
-
upsample_initial_channel,
|
250 |
-
upsample_kernel_sizes,
|
251 |
-
gin_channels,
|
252 |
-
ssl_dim,
|
253 |
-
n_speakers,
|
254 |
-
sampling_rate=44100,
|
255 |
-
**kwargs):
|
256 |
-
super().__init__()
|
257 |
-
self.spec_channels = spec_channels
|
258 |
-
self.inter_channels = inter_channels
|
259 |
-
self.hidden_channels = hidden_channels
|
260 |
-
self.filter_channels = filter_channels
|
261 |
-
self.n_heads = n_heads
|
262 |
-
self.n_layers = n_layers
|
263 |
-
self.kernel_size = kernel_size
|
264 |
-
self.p_dropout = p_dropout
|
265 |
-
self.resblock = resblock
|
266 |
-
self.resblock_kernel_sizes = resblock_kernel_sizes
|
267 |
-
self.resblock_dilation_sizes = resblock_dilation_sizes
|
268 |
-
self.upsample_rates = upsample_rates
|
269 |
-
self.upsample_initial_channel = upsample_initial_channel
|
270 |
-
self.upsample_kernel_sizes = upsample_kernel_sizes
|
271 |
-
self.segment_size = segment_size
|
272 |
-
self.gin_channels = gin_channels
|
273 |
-
self.ssl_dim = ssl_dim
|
274 |
-
self.emb_g = nn.Embedding(n_speakers, gin_channels)
|
275 |
-
|
276 |
-
self.pre = nn.Conv1d(ssl_dim, hidden_channels, kernel_size=5, padding=2)
|
277 |
-
|
278 |
-
self.enc_p = TextEncoder(
|
279 |
-
inter_channels,
|
280 |
-
hidden_channels,
|
281 |
-
filter_channels=filter_channels,
|
282 |
-
n_heads=n_heads,
|
283 |
-
n_layers=n_layers,
|
284 |
-
kernel_size=kernel_size,
|
285 |
-
p_dropout=p_dropout
|
286 |
-
)
|
287 |
-
hps = {
|
288 |
-
"sampling_rate": sampling_rate,
|
289 |
-
"inter_channels": inter_channels,
|
290 |
-
"resblock": resblock,
|
291 |
-
"resblock_kernel_sizes": resblock_kernel_sizes,
|
292 |
-
"resblock_dilation_sizes": resblock_dilation_sizes,
|
293 |
-
"upsample_rates": upsample_rates,
|
294 |
-
"upsample_initial_channel": upsample_initial_channel,
|
295 |
-
"upsample_kernel_sizes": upsample_kernel_sizes,
|
296 |
-
"gin_channels": gin_channels,
|
297 |
-
}
|
298 |
-
self.dec = Generator(h=hps)
|
299 |
-
self.enc_q = Encoder(spec_channels, inter_channels, hidden_channels, 5, 1, 16, gin_channels=gin_channels)
|
300 |
-
self.flow = ResidualCouplingBlock(inter_channels, hidden_channels, 5, 1, 4, gin_channels=gin_channels)
|
301 |
-
self.f0_decoder = F0Decoder(
|
302 |
-
1,
|
303 |
-
hidden_channels,
|
304 |
-
filter_channels,
|
305 |
-
n_heads,
|
306 |
-
n_layers,
|
307 |
-
kernel_size,
|
308 |
-
p_dropout,
|
309 |
-
spk_channels=gin_channels
|
310 |
-
)
|
311 |
-
self.emb_uv = nn.Embedding(2, hidden_channels)
|
312 |
-
self.predict_f0 = False
|
313 |
-
cluster_model_path="kmeans_10000.pt"
|
314 |
-
if os.path.exists(cluster_model_path):
|
315 |
-
self.cluster_model = cluster.get_cluster_model(cluster_model_path)
|
316 |
-
else:
|
317 |
-
self.cluster_model = None
|
318 |
-
self.speaker_map = []
|
319 |
-
self.export_mix = False
|
320 |
-
|
321 |
-
def export_chara_mix(self, n_speakers_mix):
|
322 |
-
spkmap = []
|
323 |
-
for i in range(n_speakers_mix):
|
324 |
-
spkmap.append(self.emb_g(torch.LongTensor([[i]])).transpose(1, 2).detach().numpy())
|
325 |
-
self.speaker_map = torch.tensor(spkmap)
|
326 |
-
self.export_mix = True
|
327 |
-
|
328 |
-
def forward(self, c, f0, mel2ph, uv, noise=None, g=None, cluster_infer_ratio=0.1):
|
329 |
-
|
330 |
-
decoder_inp = F.pad(c, [0, 0, 1, 0])
|
331 |
-
mel2ph_ = mel2ph.unsqueeze(2).repeat([1, 1, c.shape[-1]])
|
332 |
-
c = torch.gather(decoder_inp, 1, mel2ph_).transpose(1, 2) # [B, T, H]
|
333 |
-
|
334 |
-
if self.cluster_model is not None:
|
335 |
-
predict = self.cluster_model[speaker].predict(c.transpose(0, 1))
|
336 |
-
model[speaker].cluster_centers_[predict]
|
337 |
-
cluster_c = cluster.get_cluster_center_result(self.cluster_model, c.cpu().numpy().T, speaker).T
|
338 |
-
cluster_c = torch.FloatTensor(cluster_c).to(self.dev)
|
339 |
-
c = cluster_infer_ratio * cluster_c + (1 - cluster_infer_ratio) * c
|
340 |
-
|
341 |
-
c_lengths = (torch.ones(c.size(0)) * c.size(-1)).to(c.device)
|
342 |
-
|
343 |
-
if self.export_mix:
|
344 |
-
spk_mix = spk_mix.unsqueeze(-1).unsqueeze(-1).unsqueeze(-1)
|
345 |
-
g = torch.sum(spk_mix * self.speaker_map, dim=0).transpose(1, 2)
|
346 |
-
else:
|
347 |
-
g = g.unsqueeze(0)
|
348 |
-
g = self.emb_g(g).transpose(1, 2)
|
349 |
-
|
350 |
-
|
351 |
-
x_mask = torch.unsqueeze(commons.sequence_mask(c_lengths, c.size(2)), 1).to(c.dtype)
|
352 |
-
x = self.pre(c) * x_mask + self.emb_uv(uv.long()).transpose(1, 2)
|
353 |
-
|
354 |
-
if self.predict_f0:
|
355 |
-
lf0 = 2595. * torch.log10(1. + f0.unsqueeze(1) / 700.) / 500
|
356 |
-
norm_lf0 = utils.normalize_f0(lf0, x_mask, uv, random_scale=False)
|
357 |
-
pred_lf0 = self.f0_decoder(x, norm_lf0, x_mask, spk_emb=g)
|
358 |
-
f0 = (700 * (torch.pow(10, pred_lf0 * 500 / 2595) - 1)).squeeze(1)
|
359 |
-
|
360 |
-
z_p, m_p, logs_p, c_mask = self.enc_p(x, x_mask, f0=f0_to_coarse(f0), z=noise)
|
361 |
-
z = self.flow(z_p, c_mask, g=g, reverse=True)
|
362 |
-
o = self.dec(z * c_mask, g=g, f0=f0)
|
363 |
-
return o
|
|
|
|
|
|
|
|
|
|
|
|
|
|
|
|
|
|
|
|
|
|
|
|
|
|
|
|
|
|
|
|
|
|
|
|
|
|
|
|
|
|
|
|
|
|
|
|
|
|
|
|
|
|
|
|
|
|
|
|
|
|
|
|
|
|
|
|
|
|
|
|
|
|
|
|
|
|
|
|
|
|
|
|
|
|
|
|
|
|
|
|
|
|
|
|
|
|
|
|
|
|
|
|
|
|
|
|
|
|
|
|
|
|
|
|
|
|
|
|
|
|
|
|
|
|
|
|
|
|
|
|
|
|
|
|
|
|
|
|
|
|
|
|
|
|
|
|
|
|
|
|
|
|
|
|
|
|
|
|
|
|
|
|
|
|
|
|
|
|
|
|
|
|
|
|
|
|
|
|
|
|
|
|
|
|
|
|
|
|
|
|
|
|
|
|
|
|
|
|
|
|
|
|
|
|
|
|
|
|
|
|
|
|
|
|
|
|
|
|
|
|
|
|
|
|
|
|
|
|
|
|
|
|
|
|
|
|
|
|
|
|
|
|
|
|
|
|
|
|
|
|
|
|
|
|
|
|
|
|
|
|
|
|
|
|
|
|
|
|
|
|
|
|
|
|
|
|
|
|
|
|
|
|
|
|
|
|
|
|
|
|
|
|
|
|
|
|
|
|
|
|
|
|
|
|
|
|
|
|
|
|
|
|
|
|
|
|
|
|
|
|
|
|
|
|
|
|
|
|
|
|
|
|
|
|
|
|
|
|
|
|
|
|
|
|
|
|
|
|
|
|
|
|
|
|
|
|
|
|
|
|
|
|
|
|
|
|
|
|
|
|
|
|
|
|
|
|
|
|
|
|
|
|
|
|
|
|
|
|
|
|
|
|
|
|
|
|
|
|
|
|
|
|
|
|
|
|
|
|
|
|
|
|
|
|
|
|
|
|
|
|
|
|
|
|
|
|
|
|
|
|
|
|
|
|
|
|
|
|
|
|
|
|
|
|
|
|
|
|
|
|
|
|
|
|
|
|
|
|
|
|
|
|
|
|
|
|
|
|
|
|
|
|
|
|
|
|
|
|
|
|
|
|
|
|
|
|
|
|
|
|
|
|
|
|
|
|
|
|
|
|
|
|
|
|
|
|
|
|
|
|
|
|
|
|
|
|
|
|
|
|
|
|
|
|
|
|
|
|
|
|
|
|
|
|
|
|
|
|
|
|
|
|
|
|
|
|
|
|
|
|
|
|
|
|
|
|
|
|
|
|
|
|
|
|
|
|
|
|
|
|
|
|
|
|
|
|
|
|
|
|
|
|
|
|
|
|
|
|
|
|
|
|
|
|
|
|
|
|
|
|
|
|
|
|
|
|
|
|
|
|
|
|
|
|
|
|
|
|
|
|
|
|
|
|
|
|
|
|
|
|
|
|
|
|
|
|
|
|
|
|
|
|
|
|
|
|
|
|
|
|
|
|
|
|
|
|
|
|
|
|
|
|
|
|
|
|
|
|
|
|
|
|
|
|
|
|
|
|
|
|
|
|
|
|
|
|
|
|
|
|
|
|
|
|
|
|
|
|
|
|
|
|
|
|
|
|
|
|
|
|
|
|
|
|
|
|
|
|
|
|
|
|
|
|
|
|
spaces/Alpaca233/SadTalker/src/face3d/models/arcface_torch/backbones/__init__.py
DELETED
@@ -1,25 +0,0 @@
|
|
1 |
-
from .iresnet import iresnet18, iresnet34, iresnet50, iresnet100, iresnet200
|
2 |
-
from .mobilefacenet import get_mbf
|
3 |
-
|
4 |
-
|
5 |
-
def get_model(name, **kwargs):
|
6 |
-
# resnet
|
7 |
-
if name == "r18":
|
8 |
-
return iresnet18(False, **kwargs)
|
9 |
-
elif name == "r34":
|
10 |
-
return iresnet34(False, **kwargs)
|
11 |
-
elif name == "r50":
|
12 |
-
return iresnet50(False, **kwargs)
|
13 |
-
elif name == "r100":
|
14 |
-
return iresnet100(False, **kwargs)
|
15 |
-
elif name == "r200":
|
16 |
-
return iresnet200(False, **kwargs)
|
17 |
-
elif name == "r2060":
|
18 |
-
from .iresnet2060 import iresnet2060
|
19 |
-
return iresnet2060(False, **kwargs)
|
20 |
-
elif name == "mbf":
|
21 |
-
fp16 = kwargs.get("fp16", False)
|
22 |
-
num_features = kwargs.get("num_features", 512)
|
23 |
-
return get_mbf(fp16=fp16, num_features=num_features)
|
24 |
-
else:
|
25 |
-
raise ValueError()
|
|
|
|
|
|
|
|
|
|
|
|
|
|
|
|
|
|
|
|
|
|
|
|
|
|
|
|
|
|
|
|
|
|
|
|
|
|
|
|
|
|
|
|
|
|
|
|
|
|
|
|
spaces/Alpaca233/SadTalker/src/facerender/modules/dense_motion.py
DELETED
@@ -1,121 +0,0 @@
|
|
1 |
-
from torch import nn
|
2 |
-
import torch.nn.functional as F
|
3 |
-
import torch
|
4 |
-
from src.facerender.modules.util import Hourglass, make_coordinate_grid, kp2gaussian
|
5 |
-
|
6 |
-
from src.facerender.sync_batchnorm import SynchronizedBatchNorm3d as BatchNorm3d
|
7 |
-
|
8 |
-
|
9 |
-
class DenseMotionNetwork(nn.Module):
|
10 |
-
"""
|
11 |
-
Module that predicting a dense motion from sparse motion representation given by kp_source and kp_driving
|
12 |
-
"""
|
13 |
-
|
14 |
-
def __init__(self, block_expansion, num_blocks, max_features, num_kp, feature_channel, reshape_depth, compress,
|
15 |
-
estimate_occlusion_map=False):
|
16 |
-
super(DenseMotionNetwork, self).__init__()
|
17 |
-
# self.hourglass = Hourglass(block_expansion=block_expansion, in_features=(num_kp+1)*(feature_channel+1), max_features=max_features, num_blocks=num_blocks)
|
18 |
-
self.hourglass = Hourglass(block_expansion=block_expansion, in_features=(num_kp+1)*(compress+1), max_features=max_features, num_blocks=num_blocks)
|
19 |
-
|
20 |
-
self.mask = nn.Conv3d(self.hourglass.out_filters, num_kp + 1, kernel_size=7, padding=3)
|
21 |
-
|
22 |
-
self.compress = nn.Conv3d(feature_channel, compress, kernel_size=1)
|
23 |
-
self.norm = BatchNorm3d(compress, affine=True)
|
24 |
-
|
25 |
-
if estimate_occlusion_map:
|
26 |
-
# self.occlusion = nn.Conv2d(reshape_channel*reshape_depth, 1, kernel_size=7, padding=3)
|
27 |
-
self.occlusion = nn.Conv2d(self.hourglass.out_filters*reshape_depth, 1, kernel_size=7, padding=3)
|
28 |
-
else:
|
29 |
-
self.occlusion = None
|
30 |
-
|
31 |
-
self.num_kp = num_kp
|
32 |
-
|
33 |
-
|
34 |
-
def create_sparse_motions(self, feature, kp_driving, kp_source):
|
35 |
-
bs, _, d, h, w = feature.shape
|
36 |
-
identity_grid = make_coordinate_grid((d, h, w), type=kp_source['value'].type())
|
37 |
-
identity_grid = identity_grid.view(1, 1, d, h, w, 3)
|
38 |
-
coordinate_grid = identity_grid - kp_driving['value'].view(bs, self.num_kp, 1, 1, 1, 3)
|
39 |
-
|
40 |
-
# if 'jacobian' in kp_driving:
|
41 |
-
if 'jacobian' in kp_driving and kp_driving['jacobian'] is not None:
|
42 |
-
jacobian = torch.matmul(kp_source['jacobian'], torch.inverse(kp_driving['jacobian']))
|
43 |
-
jacobian = jacobian.unsqueeze(-3).unsqueeze(-3).unsqueeze(-3)
|
44 |
-
jacobian = jacobian.repeat(1, 1, d, h, w, 1, 1)
|
45 |
-
coordinate_grid = torch.matmul(jacobian, coordinate_grid.unsqueeze(-1))
|
46 |
-
coordinate_grid = coordinate_grid.squeeze(-1)
|
47 |
-
|
48 |
-
|
49 |
-
driving_to_source = coordinate_grid + kp_source['value'].view(bs, self.num_kp, 1, 1, 1, 3) # (bs, num_kp, d, h, w, 3)
|
50 |
-
|
51 |
-
#adding background feature
|
52 |
-
identity_grid = identity_grid.repeat(bs, 1, 1, 1, 1, 1)
|
53 |
-
sparse_motions = torch.cat([identity_grid, driving_to_source], dim=1) #bs num_kp+1 d h w 3
|
54 |
-
|
55 |
-
# sparse_motions = driving_to_source
|
56 |
-
|
57 |
-
return sparse_motions
|
58 |
-
|
59 |
-
def create_deformed_feature(self, feature, sparse_motions):
|
60 |
-
bs, _, d, h, w = feature.shape
|
61 |
-
feature_repeat = feature.unsqueeze(1).unsqueeze(1).repeat(1, self.num_kp+1, 1, 1, 1, 1, 1) # (bs, num_kp+1, 1, c, d, h, w)
|
62 |
-
feature_repeat = feature_repeat.view(bs * (self.num_kp+1), -1, d, h, w) # (bs*(num_kp+1), c, d, h, w)
|
63 |
-
sparse_motions = sparse_motions.view((bs * (self.num_kp+1), d, h, w, -1)) # (bs*(num_kp+1), d, h, w, 3) !!!!
|
64 |
-
sparse_deformed = F.grid_sample(feature_repeat, sparse_motions)
|
65 |
-
sparse_deformed = sparse_deformed.view((bs, self.num_kp+1, -1, d, h, w)) # (bs, num_kp+1, c, d, h, w)
|
66 |
-
return sparse_deformed
|
67 |
-
|
68 |
-
def create_heatmap_representations(self, feature, kp_driving, kp_source):
|
69 |
-
spatial_size = feature.shape[3:]
|
70 |
-
gaussian_driving = kp2gaussian(kp_driving, spatial_size=spatial_size, kp_variance=0.01)
|
71 |
-
gaussian_source = kp2gaussian(kp_source, spatial_size=spatial_size, kp_variance=0.01)
|
72 |
-
heatmap = gaussian_driving - gaussian_source
|
73 |
-
|
74 |
-
# adding background feature
|
75 |
-
zeros = torch.zeros(heatmap.shape[0], 1, spatial_size[0], spatial_size[1], spatial_size[2]).type(heatmap.type())
|
76 |
-
heatmap = torch.cat([zeros, heatmap], dim=1)
|
77 |
-
heatmap = heatmap.unsqueeze(2) # (bs, num_kp+1, 1, d, h, w)
|
78 |
-
return heatmap
|
79 |
-
|
80 |
-
def forward(self, feature, kp_driving, kp_source):
|
81 |
-
bs, _, d, h, w = feature.shape
|
82 |
-
|
83 |
-
feature = self.compress(feature)
|
84 |
-
feature = self.norm(feature)
|
85 |
-
feature = F.relu(feature)
|
86 |
-
|
87 |
-
out_dict = dict()
|
88 |
-
sparse_motion = self.create_sparse_motions(feature, kp_driving, kp_source)
|
89 |
-
deformed_feature = self.create_deformed_feature(feature, sparse_motion)
|
90 |
-
|
91 |
-
heatmap = self.create_heatmap_representations(deformed_feature, kp_driving, kp_source)
|
92 |
-
|
93 |
-
input_ = torch.cat([heatmap, deformed_feature], dim=2)
|
94 |
-
input_ = input_.view(bs, -1, d, h, w)
|
95 |
-
|
96 |
-
# input = deformed_feature.view(bs, -1, d, h, w) # (bs, num_kp+1 * c, d, h, w)
|
97 |
-
|
98 |
-
prediction = self.hourglass(input_)
|
99 |
-
|
100 |
-
|
101 |
-
mask = self.mask(prediction)
|
102 |
-
mask = F.softmax(mask, dim=1)
|
103 |
-
out_dict['mask'] = mask
|
104 |
-
mask = mask.unsqueeze(2) # (bs, num_kp+1, 1, d, h, w)
|
105 |
-
|
106 |
-
zeros_mask = torch.zeros_like(mask)
|
107 |
-
mask = torch.where(mask < 1e-3, zeros_mask, mask)
|
108 |
-
|
109 |
-
sparse_motion = sparse_motion.permute(0, 1, 5, 2, 3, 4) # (bs, num_kp+1, 3, d, h, w)
|
110 |
-
deformation = (sparse_motion * mask).sum(dim=1) # (bs, 3, d, h, w)
|
111 |
-
deformation = deformation.permute(0, 2, 3, 4, 1) # (bs, d, h, w, 3)
|
112 |
-
|
113 |
-
out_dict['deformation'] = deformation
|
114 |
-
|
115 |
-
if self.occlusion:
|
116 |
-
bs, c, d, h, w = prediction.shape
|
117 |
-
prediction = prediction.view(bs, -1, h, w)
|
118 |
-
occlusion_map = torch.sigmoid(self.occlusion(prediction))
|
119 |
-
out_dict['occlusion_map'] = occlusion_map
|
120 |
-
|
121 |
-
return out_dict
|
|
|
|
|
|
|
|
|
|
|
|
|
|
|
|
|
|
|
|
|
|
|
|
|
|
|
|
|
|
|
|
|
|
|
|
|
|
|
|
|
|
|
|
|
|
|
|
|
|
|
|
|
|
|
|
|
|
|
|
|
|
|
|
|
|
|
|
|
|
|
|
|
|
|
|
|
|
|
|
|
|
|
|
|
|
|
|
|
|
|
|
|
|
|
|
|
|
|
|
|
|
|
|
|
|
|
|
|
|
|
|
|
|
|
|
|
|
|
|
|
|
|
|
|
|
|
|
|
|
|
|
|
|
|
|
|
|
|
|
|
|
|
|
|
|
|
|
|
|
|
|
|
|
|
|
|
|
|
|
|
|
|
|
|
|
|
|
|
|
|
|
|
|
|
|
|
|
|
|
|
|
|
|
|
|
|
|
|
|
|
|
|
|
|
|
|
|
|
|
|
|
|
|
|
|
|
|
|
|
|
|
|
|
|
|
|
|
|
|
|
|
|
|
|
|
|
|
|
|
|
|
|
|
|
|
|
|
|
|
|
|
|
|
spaces/Androidonnxfork/CivitAi-to-Diffusers/diffusers/docs/source/en/api/schedulers/repaint.md
DELETED
@@ -1,23 +0,0 @@
|
|
1 |
-
<!--Copyright 2023 The HuggingFace Team. All rights reserved.
|
2 |
-
|
3 |
-
Licensed under the Apache License, Version 2.0 (the "License"); you may not use this file except in compliance with
|
4 |
-
the License. You may obtain a copy of the License at
|
5 |
-
|
6 |
-
http://www.apache.org/licenses/LICENSE-2.0
|
7 |
-
|
8 |
-
Unless required by applicable law or agreed to in writing, software distributed under the License is distributed on
|
9 |
-
an "AS IS" BASIS, WITHOUT WARRANTIES OR CONDITIONS OF ANY KIND, either express or implied. See the License for the
|
10 |
-
specific language governing permissions and limitations under the License.
|
11 |
-
-->
|
12 |
-
|
13 |
-
# RePaint scheduler
|
14 |
-
|
15 |
-
## Overview
|
16 |
-
|
17 |
-
DDPM-based inpainting scheduler for unsupervised inpainting with extreme masks.
|
18 |
-
Intended for use with [`RePaintPipeline`].
|
19 |
-
Based on the paper [RePaint: Inpainting using Denoising Diffusion Probabilistic Models](https://arxiv.org/abs/2201.09865)
|
20 |
-
and the original implementation by Andreas Lugmayr et al.: https://github.com/andreas128/RePaint
|
21 |
-
|
22 |
-
## RePaintScheduler
|
23 |
-
[[autodoc]] RePaintScheduler
|
|
|
|
|
|
|
|
|
|
|
|
|
|
|
|
|
|
|
|
|
|
|
|
|
|
|
|
|
|
|
|
|
|
|
|
|
|
|
|
|
|
|
|
|
|
|
|
spaces/Androidonnxfork/CivitAi-to-Diffusers/diffusers/docs/source/en/using-diffusers/reusing_seeds.md
DELETED
@@ -1,65 +0,0 @@
|
|
1 |
-
<!--Copyright 2023 The HuggingFace Team. All rights reserved.
|
2 |
-
|
3 |
-
Licensed under the Apache License, Version 2.0 (the "License"); you may not use this file except in compliance with
|
4 |
-
the License. You may obtain a copy of the License at
|
5 |
-
|
6 |
-
http://www.apache.org/licenses/LICENSE-2.0
|
7 |
-
|
8 |
-
Unless required by applicable law or agreed to in writing, software distributed under the License is distributed on
|
9 |
-
an "AS IS" BASIS, WITHOUT WARRANTIES OR CONDITIONS OF ANY KIND, either express or implied. See the License for the
|
10 |
-
specific language governing permissions and limitations under the License.
|
11 |
-
-->
|
12 |
-
|
13 |
-
# Improve image quality with deterministic generation
|
14 |
-
|
15 |
-
[[open-in-colab]]
|
16 |
-
|
17 |
-
A common way to improve the quality of generated images is with *deterministic batch generation*, generate a batch of images and select one image to improve with a more detailed prompt in a second round of inference. The key is to pass a list of [`torch.Generator`](https://pytorch.org/docs/stable/generated/torch.Generator.html#generator)'s to the pipeline for batched image generation, and tie each `Generator` to a seed so you can reuse it for an image.
|
18 |
-
|
19 |
-
Let's use [`runwayml/stable-diffusion-v1-5`](runwayml/stable-diffusion-v1-5) for example, and generate several versions of the following prompt:
|
20 |
-
|
21 |
-
```py
|
22 |
-
prompt = "Labrador in the style of Vermeer"
|
23 |
-
```
|
24 |
-
|
25 |
-
Instantiate a pipeline with [`DiffusionPipeline.from_pretrained`] and place it on a GPU (if available):
|
26 |
-
|
27 |
-
```python
|
28 |
-
>>> from diffusers import DiffusionPipeline
|
29 |
-
|
30 |
-
>>> pipe = DiffusionPipeline.from_pretrained("runwayml/stable-diffusion-v1-5", torch_dtype=torch.float16)
|
31 |
-
>>> pipe = pipe.to("cuda")
|
32 |
-
```
|
33 |
-
|
34 |
-
Now, define four different `Generator`'s and assign each `Generator` a seed (`0` to `3`) so you can reuse a `Generator` later for a specific image:
|
35 |
-
|
36 |
-
```python
|
37 |
-
>>> import torch
|
38 |
-
|
39 |
-
>>> generator = [torch.Generator(device="cuda").manual_seed(i) for i in range(4)]
|
40 |
-
```
|
41 |
-
|
42 |
-
Generate the images and have a look:
|
43 |
-
|
44 |
-
```python
|
45 |
-
>>> images = pipe(prompt, generator=generator, num_images_per_prompt=4).images
|
46 |
-
>>> images
|
47 |
-
```
|
48 |
-
|
49 |
-
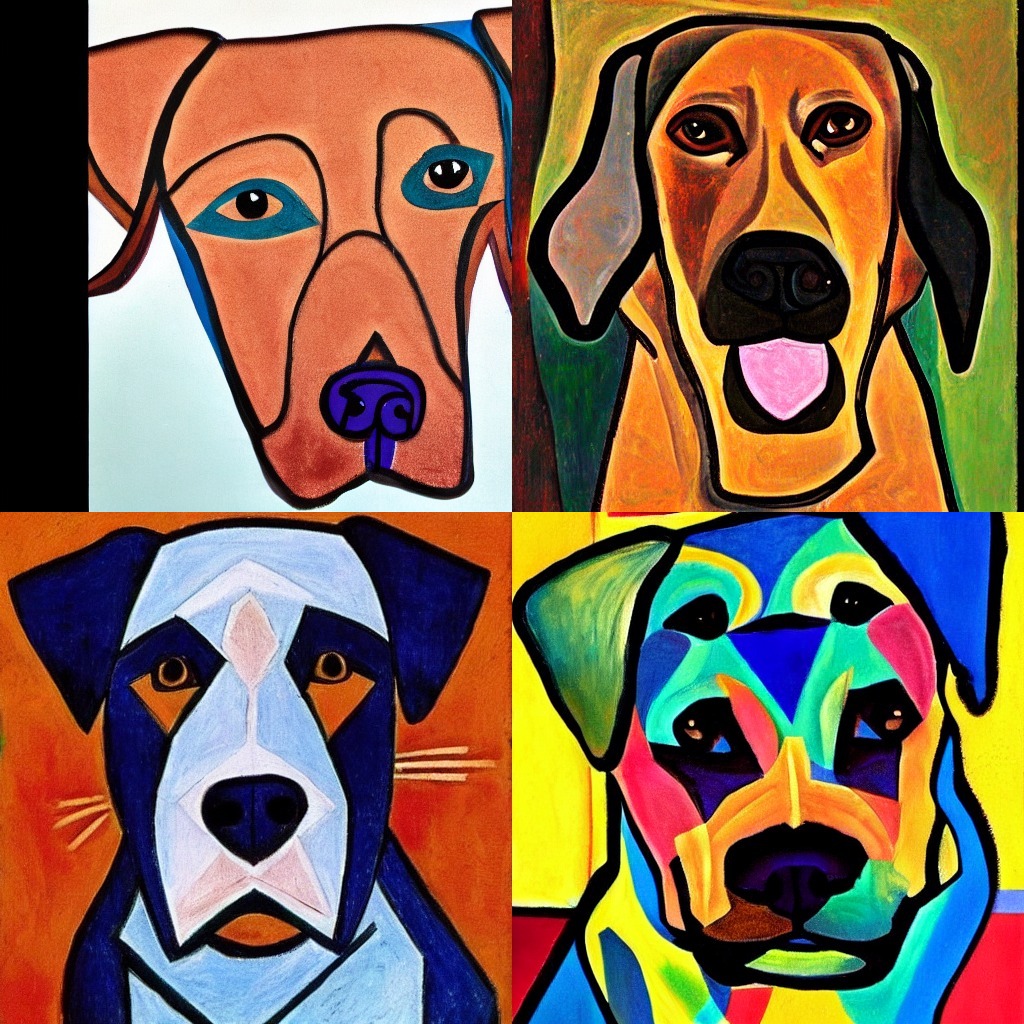
|
50 |
-
|
51 |
-
In this example, you'll improve upon the first image - but in reality, you can use any image you want (even the image with double sets of eyes!). The first image used the `Generator` with seed `0`, so you'll reuse that `Generator` for the second round of inference. To improve the quality of the image, add some additional text to the prompt:
|
52 |
-
|
53 |
-
```python
|
54 |
-
prompt = [prompt + t for t in [", highly realistic", ", artsy", ", trending", ", colorful"]]
|
55 |
-
generator = [torch.Generator(device="cuda").manual_seed(0) for i in range(4)]
|
56 |
-
```
|
57 |
-
|
58 |
-
Create four generators with seed `0`, and generate another batch of images, all of which should look like the first image from the previous round!
|
59 |
-
|
60 |
-
```python
|
61 |
-
>>> images = pipe(prompt, generator=generator).images
|
62 |
-
>>> images
|
63 |
-
```
|
64 |
-
|
65 |
-
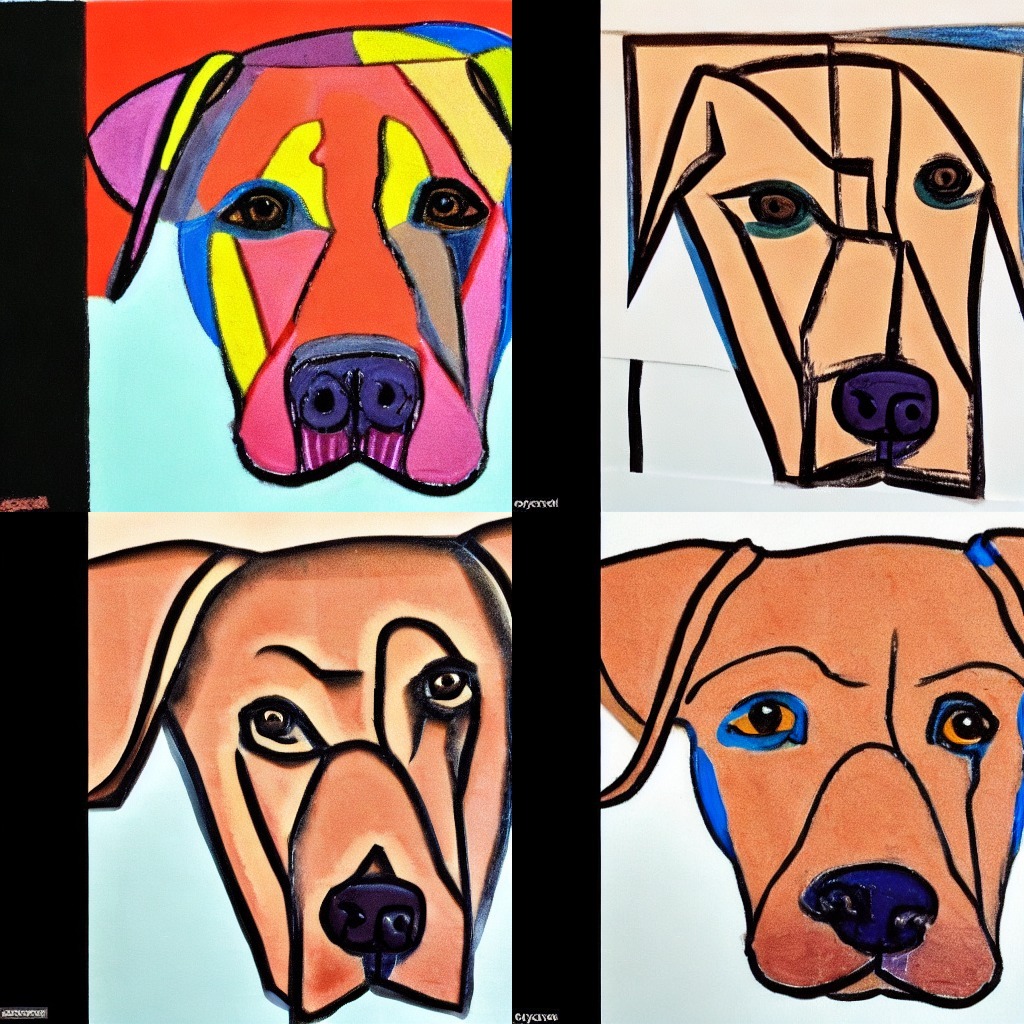
|
|
|
|
|
|
|
|
|
|
|
|
|
|
|
|
|
|
|
|
|
|
|
|
|
|
|
|
|
|
|
|
|
|
|
|
|
|
|
|
|
|
|
|
|
|
|
|
|
|
|
|
|
|
|
|
|
|
|
|
|
|
|
|
|
|
|
|
|
|
|
|
|
|
|
|
|
|
|
|
|
|
|
|
|
|
|
|
|
|
|
|
|
|
|
|
|
|
|
|
|
|
|
|
|
|
|
|
|
|
|
|
|
|
|
|
|
|
|
|
|
|
|
|
|
|
|
|
|
|
|
|
spaces/Andy1621/uniformer_image_detection/configs/foveabox/fovea_align_r50_fpn_gn-head_mstrain_640-800_4x4_2x_coco.py
DELETED
@@ -1,25 +0,0 @@
|
|
1 |
-
_base_ = './fovea_r50_fpn_4x4_1x_coco.py'
|
2 |
-
model = dict(
|
3 |
-
bbox_head=dict(
|
4 |
-
with_deform=True,
|
5 |
-
norm_cfg=dict(type='GN', num_groups=32, requires_grad=True)))
|
6 |
-
img_norm_cfg = dict(
|
7 |
-
mean=[123.675, 116.28, 103.53], std=[58.395, 57.12, 57.375], to_rgb=True)
|
8 |
-
train_pipeline = [
|
9 |
-
dict(type='LoadImageFromFile'),
|
10 |
-
dict(type='LoadAnnotations', with_bbox=True),
|
11 |
-
dict(
|
12 |
-
type='Resize',
|
13 |
-
img_scale=[(1333, 640), (1333, 800)],
|
14 |
-
multiscale_mode='value',
|
15 |
-
keep_ratio=True),
|
16 |
-
dict(type='RandomFlip', flip_ratio=0.5),
|
17 |
-
dict(type='Normalize', **img_norm_cfg),
|
18 |
-
dict(type='Pad', size_divisor=32),
|
19 |
-
dict(type='DefaultFormatBundle'),
|
20 |
-
dict(type='Collect', keys=['img', 'gt_bboxes', 'gt_labels']),
|
21 |
-
]
|
22 |
-
data = dict(train=dict(pipeline=train_pipeline))
|
23 |
-
# learning policy
|
24 |
-
lr_config = dict(step=[16, 22])
|
25 |
-
runner = dict(type='EpochBasedRunner', max_epochs=24)
|
|
|
|
|
|
|
|
|
|
|
|
|
|
|
|
|
|
|
|
|
|
|
|
|
|
|
|
|
|
|
|
|
|
|
|
|
|
|
|
|
|
|
|
|
|
|
|
|
|
|
|
spaces/Andy1621/uniformer_image_segmentation/configs/gcnet/gcnet_r101-d8_512x512_40k_voc12aug.py
DELETED
@@ -1,2 +0,0 @@
|
|
1 |
-
_base_ = './gcnet_r50-d8_512x512_40k_voc12aug.py'
|
2 |
-
model = dict(pretrained='open-mmlab://resnet101_v1c', backbone=dict(depth=101))
|
|
|
|
|
|
spaces/AnimalEquality/chatbot/setup.py
DELETED
@@ -1,57 +0,0 @@
|
|
1 |
-
from pkg_resources import parse_version
|
2 |
-
from configparser import ConfigParser
|
3 |
-
import setuptools, shlex
|
4 |
-
assert parse_version(setuptools.__version__)>=parse_version('36.2')
|
5 |
-
|
6 |
-
# note: all settings are in settings.ini; edit there, not here
|
7 |
-
config = ConfigParser(delimiters=['='])
|
8 |
-
config.read('settings.ini', encoding='utf-8')
|
9 |
-
cfg = config['DEFAULT']
|
10 |
-
|
11 |
-
cfg_keys = 'version description keywords author author_email'.split()
|
12 |
-
expected = cfg_keys + "lib_name user branch license status min_python audience language".split()
|
13 |
-
for o in expected: assert o in cfg, "missing expected setting: {}".format(o)
|
14 |
-
setup_cfg = {o:cfg[o] for o in cfg_keys}
|
15 |
-
|
16 |
-
licenses = {
|
17 |
-
'apache2': ('Apache Software License 2.0','OSI Approved :: Apache Software License'),
|
18 |
-
'mit': ('MIT License', 'OSI Approved :: MIT License'),
|
19 |
-
'gpl2': ('GNU General Public License v2', 'OSI Approved :: GNU General Public License v2 (GPLv2)'),
|
20 |
-
'gpl3': ('GNU General Public License v3', 'OSI Approved :: GNU General Public License v3 (GPLv3)'),
|
21 |
-
'bsd3': ('BSD License', 'OSI Approved :: BSD License'),
|
22 |
-
}
|
23 |
-
statuses = [ '1 - Planning', '2 - Pre-Alpha', '3 - Alpha',
|
24 |
-
'4 - Beta', '5 - Production/Stable', '6 - Mature', '7 - Inactive' ]
|
25 |
-
py_versions = '3.6 3.7 3.8 3.9 3.10'.split()
|
26 |
-
|
27 |
-
requirements = shlex.split(cfg.get('requirements', ''))
|
28 |
-
if cfg.get('pip_requirements'): requirements += shlex.split(cfg.get('pip_requirements', ''))
|
29 |
-
min_python = cfg['min_python']
|
30 |
-
lic = licenses.get(cfg['license'].lower(), (cfg['license'], None))
|
31 |
-
dev_requirements = (cfg.get('dev_requirements') or '').split()
|
32 |
-
|
33 |
-
setuptools.setup(
|
34 |
-
name = cfg['lib_name'],
|
35 |
-
license = lic[0],
|
36 |
-
classifiers = [
|
37 |
-
'Development Status :: ' + statuses[int(cfg['status'])],
|
38 |
-
'Intended Audience :: ' + cfg['audience'].title(),
|
39 |
-
'Natural Language :: ' + cfg['language'].title(),
|
40 |
-
] + ['Programming Language :: Python :: '+o for o in py_versions[py_versions.index(min_python):]] + (['License :: ' + lic[1] ] if lic[1] else []),
|
41 |
-
url = cfg['git_url'],
|
42 |
-
packages = setuptools.find_packages(),
|
43 |
-
include_package_data = True,
|
44 |
-
install_requires = requirements,
|
45 |
-
extras_require={ 'dev': dev_requirements },
|
46 |
-
dependency_links = cfg.get('dep_links','').split(),
|
47 |
-
python_requires = '>=' + cfg['min_python'],
|
48 |
-
long_description = open('README.md', encoding='utf-8').read(),
|
49 |
-
long_description_content_type = 'text/markdown',
|
50 |
-
zip_safe = False,
|
51 |
-
entry_points = {
|
52 |
-
'console_scripts': cfg.get('console_scripts','').split(),
|
53 |
-
'nbdev': [f'{cfg.get("lib_path")}={cfg.get("lib_path")}._modidx:d']
|
54 |
-
},
|
55 |
-
**setup_cfg)
|
56 |
-
|
57 |
-
|
|
|
|
|
|
|
|
|
|
|
|
|
|
|
|
|
|
|
|
|
|
|
|
|
|
|
|
|
|
|
|
|
|
|
|
|
|
|
|
|
|
|
|
|
|
|
|
|
|
|
|
|
|
|
|
|
|
|
|
|
|
|
|
|
|
|
|
|
|
|
|
|
|
|
|
|
|
|
|
|
|
|
|
|
|
|
|
|
|
|
|
|
|
|
|
|
|
|
|
|
|
|
|
|
|
|
|
|
|
|
|
|
|
|
|
spaces/AnnasBlackHat/Image-Downloader/README.md
DELETED
@@ -1,12 +0,0 @@
|
|
1 |
-
---
|
2 |
-
title: Image Downloader
|
3 |
-
emoji: 🐠
|
4 |
-
colorFrom: green
|
5 |
-
colorTo: red
|
6 |
-
sdk: gradio
|
7 |
-
sdk_version: 3.1.7
|
8 |
-
app_file: app.py
|
9 |
-
pinned: false
|
10 |
-
---
|
11 |
-
|
12 |
-
Check out the configuration reference at https://huggingface.co/docs/hub/spaces-config-reference
|
|
|
|
|
|
|
|
|
|
|
|
|
|
|
|
|
|
|
|
|
|
|
|
|
|
spaces/Ataturk-Chatbot/HuggingFaceChat/venv/lib/python3.11/site-packages/pip/_vendor/urllib3/fields.py
DELETED
@@ -1,274 +0,0 @@
|
|
1 |
-
from __future__ import absolute_import
|
2 |
-
|
3 |
-
import email.utils
|
4 |
-
import mimetypes
|
5 |
-
import re
|
6 |
-
|
7 |
-
from .packages import six
|
8 |
-
|
9 |
-
|
10 |
-
def guess_content_type(filename, default="application/octet-stream"):
|
11 |
-
"""
|
12 |
-
Guess the "Content-Type" of a file.
|
13 |
-
|
14 |
-
:param filename:
|
15 |
-
The filename to guess the "Content-Type" of using :mod:`mimetypes`.
|
16 |
-
:param default:
|
17 |
-
If no "Content-Type" can be guessed, default to `default`.
|
18 |
-
"""
|
19 |
-
if filename:
|
20 |
-
return mimetypes.guess_type(filename)[0] or default
|
21 |
-
return default
|
22 |
-
|
23 |
-
|
24 |
-
def format_header_param_rfc2231(name, value):
|
25 |
-
"""
|
26 |
-
Helper function to format and quote a single header parameter using the
|
27 |
-
strategy defined in RFC 2231.
|
28 |
-
|
29 |
-
Particularly useful for header parameters which might contain
|
30 |
-
non-ASCII values, like file names. This follows
|
31 |
-
`RFC 2388 Section 4.4 <https://tools.ietf.org/html/rfc2388#section-4.4>`_.
|
32 |
-
|
33 |
-
:param name:
|
34 |
-
The name of the parameter, a string expected to be ASCII only.
|
35 |
-
:param value:
|
36 |
-
The value of the parameter, provided as ``bytes`` or `str``.
|
37 |
-
:ret:
|
38 |
-
An RFC-2231-formatted unicode string.
|
39 |
-
"""
|
40 |
-
if isinstance(value, six.binary_type):
|
41 |
-
value = value.decode("utf-8")
|
42 |
-
|
43 |
-
if not any(ch in value for ch in '"\\\r\n'):
|
44 |
-
result = u'%s="%s"' % (name, value)
|
45 |
-
try:
|
46 |
-
result.encode("ascii")
|
47 |
-
except (UnicodeEncodeError, UnicodeDecodeError):
|
48 |
-
pass
|
49 |
-
else:
|
50 |
-
return result
|
51 |
-
|
52 |
-
if six.PY2: # Python 2:
|
53 |
-
value = value.encode("utf-8")
|
54 |
-
|
55 |
-
# encode_rfc2231 accepts an encoded string and returns an ascii-encoded
|
56 |
-
# string in Python 2 but accepts and returns unicode strings in Python 3
|
57 |
-
value = email.utils.encode_rfc2231(value, "utf-8")
|
58 |
-
value = "%s*=%s" % (name, value)
|
59 |
-
|
60 |
-
if six.PY2: # Python 2:
|
61 |
-
value = value.decode("utf-8")
|
62 |
-
|
63 |
-
return value
|
64 |
-
|
65 |
-
|
66 |
-
_HTML5_REPLACEMENTS = {
|
67 |
-
u"\u0022": u"%22",
|
68 |
-
# Replace "\" with "\\".
|
69 |
-
u"\u005C": u"\u005C\u005C",
|
70 |
-
}
|
71 |
-
|
72 |
-
# All control characters from 0x00 to 0x1F *except* 0x1B.
|
73 |
-
_HTML5_REPLACEMENTS.update(
|
74 |
-
{
|
75 |
-
six.unichr(cc): u"%{:02X}".format(cc)
|
76 |
-
for cc in range(0x00, 0x1F + 1)
|
77 |
-
if cc not in (0x1B,)
|
78 |
-
}
|
79 |
-
)
|
80 |
-
|
81 |
-
|
82 |
-
def _replace_multiple(value, needles_and_replacements):
|
83 |
-
def replacer(match):
|
84 |
-
return needles_and_replacements[match.group(0)]
|
85 |
-
|
86 |
-
pattern = re.compile(
|
87 |
-
r"|".join([re.escape(needle) for needle in needles_and_replacements.keys()])
|
88 |
-
)
|
89 |
-
|
90 |
-
result = pattern.sub(replacer, value)
|
91 |
-
|
92 |
-
return result
|
93 |
-
|
94 |
-
|
95 |
-
def format_header_param_html5(name, value):
|
96 |
-
"""
|
97 |
-
Helper function to format and quote a single header parameter using the
|
98 |
-
HTML5 strategy.
|
99 |
-
|
100 |
-
Particularly useful for header parameters which might contain
|
101 |
-
non-ASCII values, like file names. This follows the `HTML5 Working Draft
|
102 |
-
Section 4.10.22.7`_ and matches the behavior of curl and modern browsers.
|
103 |
-
|
104 |
-
.. _HTML5 Working Draft Section 4.10.22.7:
|
105 |
-
https://w3c.github.io/html/sec-forms.html#multipart-form-data
|
106 |
-
|
107 |
-
:param name:
|
108 |
-
The name of the parameter, a string expected to be ASCII only.
|
109 |
-
:param value:
|
110 |
-
The value of the parameter, provided as ``bytes`` or `str``.
|
111 |
-
:ret:
|
112 |
-
A unicode string, stripped of troublesome characters.
|
113 |
-
"""
|
114 |
-
if isinstance(value, six.binary_type):
|
115 |
-
value = value.decode("utf-8")
|
116 |
-
|
117 |
-
value = _replace_multiple(value, _HTML5_REPLACEMENTS)
|
118 |
-
|
119 |
-
return u'%s="%s"' % (name, value)
|
120 |
-
|
121 |
-
|
122 |
-
# For backwards-compatibility.
|
123 |
-
format_header_param = format_header_param_html5
|
124 |
-
|
125 |
-
|
126 |
-
class RequestField(object):
|
127 |
-
"""
|
128 |
-
A data container for request body parameters.
|
129 |
-
|
130 |
-
:param name:
|
131 |
-
The name of this request field. Must be unicode.
|
132 |
-
:param data:
|
133 |
-
The data/value body.
|
134 |
-
:param filename:
|
135 |
-
An optional filename of the request field. Must be unicode.
|
136 |
-
:param headers:
|
137 |
-
An optional dict-like object of headers to initially use for the field.
|
138 |
-
:param header_formatter:
|
139 |
-
An optional callable that is used to encode and format the headers. By
|
140 |
-
default, this is :func:`format_header_param_html5`.
|
141 |
-
"""
|
142 |
-
|
143 |
-
def __init__(
|
144 |
-
self,
|
145 |
-
name,
|
146 |
-
data,
|
147 |
-
filename=None,
|
148 |
-
headers=None,
|
149 |
-
header_formatter=format_header_param_html5,
|
150 |
-
):
|
151 |
-
self._name = name
|
152 |
-
self._filename = filename
|
153 |
-
self.data = data
|
154 |
-
self.headers = {}
|
155 |
-
if headers:
|
156 |
-
self.headers = dict(headers)
|
157 |
-
self.header_formatter = header_formatter
|
158 |
-
|
159 |
-
@classmethod
|
160 |
-
def from_tuples(cls, fieldname, value, header_formatter=format_header_param_html5):
|
161 |
-
"""
|
162 |
-
A :class:`~urllib3.fields.RequestField` factory from old-style tuple parameters.
|
163 |
-
|
164 |
-
Supports constructing :class:`~urllib3.fields.RequestField` from
|
165 |
-
parameter of key/value strings AND key/filetuple. A filetuple is a
|
166 |
-
(filename, data, MIME type) tuple where the MIME type is optional.
|
167 |
-
For example::
|
168 |
-
|
169 |
-
'foo': 'bar',
|
170 |
-
'fakefile': ('foofile.txt', 'contents of foofile'),
|
171 |
-
'realfile': ('barfile.txt', open('realfile').read()),
|
172 |
-
'typedfile': ('bazfile.bin', open('bazfile').read(), 'image/jpeg'),
|
173 |
-
'nonamefile': 'contents of nonamefile field',
|
174 |
-
|
175 |
-
Field names and filenames must be unicode.
|
176 |
-
"""
|
177 |
-
if isinstance(value, tuple):
|
178 |
-
if len(value) == 3:
|
179 |
-
filename, data, content_type = value
|
180 |
-
else:
|
181 |
-
filename, data = value
|
182 |
-
content_type = guess_content_type(filename)
|
183 |
-
else:
|
184 |
-
filename = None
|
185 |
-
content_type = None
|
186 |
-
data = value
|
187 |
-
|
188 |
-
request_param = cls(
|
189 |
-
fieldname, data, filename=filename, header_formatter=header_formatter
|
190 |
-
)
|
191 |
-
request_param.make_multipart(content_type=content_type)
|
192 |
-
|
193 |
-
return request_param
|
194 |
-
|
195 |
-
def _render_part(self, name, value):
|
196 |
-
"""
|
197 |
-
Overridable helper function to format a single header parameter. By
|
198 |
-
default, this calls ``self.header_formatter``.
|
199 |
-
|
200 |
-
:param name:
|
201 |
-
The name of the parameter, a string expected to be ASCII only.
|
202 |
-
:param value:
|
203 |
-
The value of the parameter, provided as a unicode string.
|
204 |
-
"""
|
205 |
-
|
206 |
-
return self.header_formatter(name, value)
|
207 |
-
|
208 |
-
def _render_parts(self, header_parts):
|
209 |
-
"""
|
210 |
-
Helper function to format and quote a single header.
|
211 |
-
|
212 |
-
Useful for single headers that are composed of multiple items. E.g.,
|
213 |
-
'Content-Disposition' fields.
|
214 |
-
|
215 |
-
:param header_parts:
|
216 |
-
A sequence of (k, v) tuples or a :class:`dict` of (k, v) to format
|
217 |
-
as `k1="v1"; k2="v2"; ...`.
|
218 |
-
"""
|
219 |
-
parts = []
|
220 |
-
iterable = header_parts
|
221 |
-
if isinstance(header_parts, dict):
|
222 |
-
iterable = header_parts.items()
|
223 |
-
|
224 |
-
for name, value in iterable:
|
225 |
-
if value is not None:
|
226 |
-
parts.append(self._render_part(name, value))
|
227 |
-
|
228 |
-
return u"; ".join(parts)
|
229 |
-
|
230 |
-
def render_headers(self):
|
231 |
-
"""
|
232 |
-
Renders the headers for this request field.
|
233 |
-
"""
|
234 |
-
lines = []
|
235 |
-
|
236 |
-
sort_keys = ["Content-Disposition", "Content-Type", "Content-Location"]
|
237 |
-
for sort_key in sort_keys:
|
238 |
-
if self.headers.get(sort_key, False):
|
239 |
-
lines.append(u"%s: %s" % (sort_key, self.headers[sort_key]))
|
240 |
-
|
241 |
-
for header_name, header_value in self.headers.items():
|
242 |
-
if header_name not in sort_keys:
|
243 |
-
if header_value:
|
244 |
-
lines.append(u"%s: %s" % (header_name, header_value))
|
245 |
-
|
246 |
-
lines.append(u"\r\n")
|
247 |
-
return u"\r\n".join(lines)
|
248 |
-
|
249 |
-
def make_multipart(
|
250 |
-
self, content_disposition=None, content_type=None, content_location=None
|
251 |
-
):
|
252 |
-
"""
|
253 |
-
Makes this request field into a multipart request field.
|
254 |
-
|
255 |
-
This method overrides "Content-Disposition", "Content-Type" and
|
256 |
-
"Content-Location" headers to the request parameter.
|
257 |
-
|
258 |
-
:param content_type:
|
259 |
-
The 'Content-Type' of the request body.
|
260 |
-
:param content_location:
|
261 |
-
The 'Content-Location' of the request body.
|
262 |
-
|
263 |
-
"""
|
264 |
-
self.headers["Content-Disposition"] = content_disposition or u"form-data"
|
265 |
-
self.headers["Content-Disposition"] += u"; ".join(
|
266 |
-
[
|
267 |
-
u"",
|
268 |
-
self._render_parts(
|
269 |
-
((u"name", self._name), (u"filename", self._filename))
|
270 |
-
),
|
271 |
-
]
|
272 |
-
)
|
273 |
-
self.headers["Content-Type"] = content_type
|
274 |
-
self.headers["Content-Location"] = content_location
|
|
|
|
|
|
|
|
|
|
|
|
|
|
|
|
|
|
|
|
|
|
|
|
|
|
|
|
|
|
|
|
|
|
|
|
|
|
|
|
|
|
|
|
|
|
|
|
|
|
|
|
|
|
|
|
|
|
|
|
|
|
|
|
|
|
|
|
|
|
|
|
|
|
|
|
|
|
|
|
|
|
|
|
|
|
|
|
|
|
|
|
|
|
|
|
|
|
|
|
|
|
|
|
|
|
|
|
|
|
|
|
|
|
|
|
|
|
|
|
|
|
|
|
|
|
|
|
|
|
|
|
|
|
|
|
|
|
|
|
|
|
|
|
|
|
|
|
|
|
|
|
|
|
|
|
|
|
|
|
|
|
|
|
|
|
|
|
|
|
|
|
|
|
|
|
|
|
|
|
|
|
|
|
|
|
|
|
|
|
|
|
|
|
|
|
|
|
|
|
|
|
|
|
|
|
|
|
|
|
|
|
|
|
|
|
|
|
|
|
|
|
|
|
|
|
|
|
|
|
|
|
|
|
|
|
|
|
|
|
|
|
|
|
|
|
|
|
|
|
|
|
|
|
|
|
|
|
|
|
|
|
|
|
|
|
|
|
|
|
|
|
|
|
|
|
|
|
|
|
|
|
|
|
|
|
|
|
|
|
|
|
|
|
|
|
|
|
|
|
|
|
|
|
|
|
|
|
|
|
|
|
|
|
|
|
|
|
|
|
|
|
|
|
|
|
|
|
|
|
|
|
|
|
|
|
|
|
|
|
|
|
|
|
|
|
|
|
|
|
|
|
|
|
|
|
|
|
|
|
|
|
|
|
|
|
|
|
|
|
|
|
|
|
|
|
|
|
|
|
|
|
|
|
|
|
|
|
|
|
|
|
|
|
|
|
|
|
|
|
|
|
|
|
|
|
|
|
|
|
|
|
|
|
|
|
|
|
|
|
|
|
|
|
|
|
|
|
|
|
|
|
|
|
|
|
|
|
|
|
|
|
|
|
|
|
|
|
|
|
|
|
|
|
|
|
|
|
|
|
|
|
|
|
|
|
|
|
|
|
|
|
|
|
|
|
|
|
|
|
|
|
|
|
|
|
|
|
|
|
|
|
|
|
|
|
|
|
|
|
|
|
|
|
|
|
|
|
|
|
|
|
|
|
|
|
|
|
|
|
|
|
|
|
|
|
|
|
|
|
|
|
|
|
|
|
|
|
|
|
|
|
|
|
|
|
|
|
|
|
spaces/Ataturk-Chatbot/HuggingFaceChat/venv/lib/python3.11/site-packages/pkg_resources/__init__.py
DELETED
The diff for this file is too large to render.
See raw diff
|
|
spaces/Awiny/Image2Paragraph/models/grit_src/third_party/CenterNet2/docs/notes/compatibility.md
DELETED
@@ -1,84 +0,0 @@
|
|
1 |
-
# Compatibility with Other Libraries
|
2 |
-
|
3 |
-
## Compatibility with Detectron (and maskrcnn-benchmark)
|
4 |
-
|
5 |
-
Detectron2 addresses some legacy issues left in Detectron. As a result, their models
|
6 |
-
are not compatible:
|
7 |
-
running inference with the same model weights will produce different results in the two code bases.
|
8 |
-
|
9 |
-
The major differences regarding inference are:
|
10 |
-
|
11 |
-
- The height and width of a box with corners (x1, y1) and (x2, y2) is now computed more naturally as
|
12 |
-
width = x2 - x1 and height = y2 - y1;
|
13 |
-
In Detectron, a "+ 1" was added both height and width.
|
14 |
-
|
15 |
-
Note that the relevant ops in Caffe2 have [adopted this change of convention](https://github.com/pytorch/pytorch/pull/20550)
|
16 |
-
with an extra option.
|
17 |
-
So it is still possible to run inference with a Detectron2-trained model in Caffe2.
|
18 |
-
|
19 |
-
The change in height/width calculations most notably changes:
|
20 |
-
- encoding/decoding in bounding box regression.
|
21 |
-
- non-maximum suppression. The effect here is very negligible, though.
|
22 |
-
|
23 |
-
- RPN now uses simpler anchors with fewer quantization artifacts.
|
24 |
-
|
25 |
-
In Detectron, the anchors were quantized and
|
26 |
-
[do not have accurate areas](https://github.com/facebookresearch/Detectron/issues/227).
|
27 |
-
In Detectron2, the anchors are center-aligned to feature grid points and not quantized.
|
28 |
-
|
29 |
-
- Classification layers have a different ordering of class labels.
|
30 |
-
|
31 |
-
This involves any trainable parameter with shape (..., num_categories + 1, ...).
|
32 |
-
In Detectron2, integer labels [0, K-1] correspond to the K = num_categories object categories
|
33 |
-
and the label "K" corresponds to the special "background" category.
|
34 |
-
In Detectron, label "0" means background, and labels [1, K] correspond to the K categories.
|
35 |
-
|
36 |
-
- ROIAlign is implemented differently. The new implementation is [available in Caffe2](https://github.com/pytorch/pytorch/pull/23706).
|
37 |
-
|
38 |
-
1. All the ROIs are shifted by half a pixel compared to Detectron in order to create better image-feature-map alignment.
|
39 |
-
See `layers/roi_align.py` for details.
|
40 |
-
To enable the old behavior, use `ROIAlign(aligned=False)`, or `POOLER_TYPE=ROIAlign` instead of
|
41 |
-
`ROIAlignV2` (the default).
|
42 |
-
|
43 |
-
1. The ROIs are not required to have a minimum size of 1.
|
44 |
-
This will lead to tiny differences in the output, but should be negligible.
|
45 |
-
|
46 |
-
- Mask inference function is different.
|
47 |
-
|
48 |
-
In Detectron2, the "paste_mask" function is different and should be more accurate than in Detectron. This change
|
49 |
-
can improve mask AP on COCO by ~0.5% absolute.
|
50 |
-
|
51 |
-
There are some other differences in training as well, but they won't affect
|
52 |
-
model-level compatibility. The major ones are:
|
53 |
-
|
54 |
-
- We fixed a [bug](https://github.com/facebookresearch/Detectron/issues/459) in
|
55 |
-
Detectron, by making `RPN.POST_NMS_TOPK_TRAIN` per-image, rather than per-batch.
|
56 |
-
The fix may lead to a small accuracy drop for a few models (e.g. keypoint
|
57 |
-
detection) and will require some parameter tuning to match the Detectron results.
|
58 |
-
- For simplicity, we change the default loss in bounding box regression to L1 loss, instead of smooth L1 loss.
|
59 |
-
We have observed that this tends to slightly decrease box AP50 while improving box AP for higher
|
60 |
-
overlap thresholds (and leading to a slight overall improvement in box AP).
|
61 |
-
- We interpret the coordinates in COCO bounding box and segmentation annotations
|
62 |
-
as coordinates in range `[0, width]` or `[0, height]`. The coordinates in
|
63 |
-
COCO keypoint annotations are interpreted as pixel indices in range `[0, width - 1]` or `[0, height - 1]`.
|
64 |
-
Note that this affects how flip augmentation is implemented.
|
65 |
-
|
66 |
-
|
67 |
-
[This article](https://ppwwyyxx.com/blog/2021/Where-are-Pixels/)
|
68 |
-
explains more details on the above mentioned issues
|
69 |
-
about pixels, coordinates, and "+1"s.
|
70 |
-
|
71 |
-
|
72 |
-
## Compatibility with Caffe2
|
73 |
-
|
74 |
-
As mentioned above, despite the incompatibilities with Detectron, the relevant
|
75 |
-
ops have been implemented in Caffe2.
|
76 |
-
Therefore, models trained with detectron2 can be converted in Caffe2.
|
77 |
-
See [Deployment](../tutorials/deployment.md) for the tutorial.
|
78 |
-
|
79 |
-
## Compatibility with TensorFlow
|
80 |
-
|
81 |
-
Most ops are available in TensorFlow, although some tiny differences in
|
82 |
-
the implementation of resize / ROIAlign / padding need to be addressed.
|
83 |
-
A working conversion script is provided by [tensorpack Faster R-CNN](https://github.com/tensorpack/tensorpack/tree/master/examples/FasterRCNN/convert_d2)
|
84 |
-
to run a standard detectron2 model in TensorFlow.
|
|
|
|
|
|
|
|
|
|
|
|
|
|
|
|
|
|
|
|
|
|
|
|
|
|
|
|
|
|
|
|
|
|
|
|
|
|
|
|
|
|
|
|
|
|
|
|
|
|
|
|
|
|
|
|
|
|
|
|
|
|
|
|
|
|
|
|
|
|
|
|
|
|
|
|
|
|
|
|
|
|
|
|
|
|
|
|
|
|
|
|
|
|
|
|
|
|
|
|
|
|
|
|
|
|
|
|
|
|
|
|
|
|
|
|
|
|
|
|
|
|
|
|
|
|
|
|
|
|
|
|
|
|
|
|
|
|
|
|
|
|
|
|
|
|
|
|
|
|
|
|
|
|
|
|
|
|
|
|
|
|
|
|
|
|
|
|
|
|
spaces/Awiny/Image2Paragraph/models/segment_models/semantic_segment_anything_model.py
DELETED
@@ -1,165 +0,0 @@
|
|
1 |
-
from transformers import (CLIPProcessor, CLIPModel, AutoProcessor, CLIPSegForImageSegmentation,
|
2 |
-
OneFormerProcessor, OneFormerForUniversalSegmentation,
|
3 |
-
BlipProcessor, BlipForConditionalGeneration)
|
4 |
-
import torch
|
5 |
-
import mmcv
|
6 |
-
import torch.nn.functional as F
|
7 |
-
import numpy as np
|
8 |
-
import spacy
|
9 |
-
from PIL import Image
|
10 |
-
import pycocotools.mask as maskUtils
|
11 |
-
from models.segment_models.configs.ade20k_id2label import CONFIG as CONFIG_ADE20K_ID2LABEL
|
12 |
-
from models.segment_models.configs.coco_id2label import CONFIG as CONFIG_COCO_ID2LABEL
|
13 |
-
from utils.util import resize_long_edge, resize_long_edge_cv2
|
14 |
-
# from mmdet.core.visualization.image import imshow_det_bboxes # comment this line if you don't use mmdet
|
15 |
-
|
16 |
-
nlp = spacy.load('en_core_web_sm')
|
17 |
-
|
18 |
-
class SemanticSegment():
|
19 |
-
def __init__(self, device):
|
20 |
-
self.device = device
|
21 |
-
self.model_init()
|
22 |
-
|
23 |
-
def model_init(self):
|
24 |
-
self.init_clip()
|
25 |
-
self.init_oneformer_ade20k()
|
26 |
-
self.init_oneformer_coco()
|
27 |
-
self.init_blip()
|
28 |
-
self.init_clipseg()
|
29 |
-
|
30 |
-
def init_clip(self):
|
31 |
-
# model_name = "openai/clip-vit-large-patch14"
|
32 |
-
model_name = "openai/clip-vit-base-patch32"
|
33 |
-
self.clip_processor = CLIPProcessor.from_pretrained(model_name)
|
34 |
-
self.clip_model = CLIPModel.from_pretrained(model_name).to(self.device)
|
35 |
-
|
36 |
-
def init_oneformer_ade20k(self):
|
37 |
-
# model_name = "shi-labs/oneformer_ade20k_swin_large"
|
38 |
-
model_name = "shi-labs/oneformer_ade20k_swin_tiny"
|
39 |
-
self.oneformer_ade20k_processor = OneFormerProcessor.from_pretrained(model_name)
|
40 |
-
self.oneformer_ade20k_model = OneFormerForUniversalSegmentation.from_pretrained(model_name).to(self.device)
|
41 |
-
|
42 |
-
def init_oneformer_coco(self):
|
43 |
-
model_name = "shi-labs/oneformer_coco_swin_large"
|
44 |
-
self.oneformer_coco_processor = OneFormerProcessor.from_pretrained(model_name)
|
45 |
-
self.oneformer_coco_model = OneFormerForUniversalSegmentation.from_pretrained(model_name).to(self.device)
|
46 |
-
|
47 |
-
def init_blip(self):
|
48 |
-
model_name = "Salesforce/blip-image-captioning-base"
|
49 |
-
# model_name = "Salesforce/blip-image-captioning-large"
|
50 |
-
self.blip_processor = BlipProcessor.from_pretrained(model_name)
|
51 |
-
self.blip_model = BlipForConditionalGeneration.from_pretrained(model_name).to(self.device)
|
52 |
-
|
53 |
-
def init_clipseg(self):
|
54 |
-
model_name = "CIDAS/clipseg-rd64-refined"
|
55 |
-
self.clipseg_processor = AutoProcessor.from_pretrained(model_name)
|
56 |
-
self.clipseg_model = CLIPSegForImageSegmentation.from_pretrained(model_name).to(self.device)
|
57 |
-
self.clipseg_processor.image_processor.do_resize = False
|
58 |
-
|
59 |
-
@staticmethod
|
60 |
-
def get_noun_phrases(text):
|
61 |
-
doc = nlp(text)
|
62 |
-
return [chunk.text for chunk in doc.noun_chunks]
|
63 |
-
|
64 |
-
def open_vocabulary_classification_blip(self, raw_image):
|
65 |
-
captioning_inputs = self.blip_processor(raw_image, return_tensors="pt").to(self.device)
|
66 |
-
out = self.blip_model.generate(**captioning_inputs)
|
67 |
-
caption = self.blip_processor.decode(out[0], skip_special_tokens=True)
|
68 |
-
return SemanticSegment.get_noun_phrases(caption)
|
69 |
-
|
70 |
-
def oneformer_segmentation(self, image, processor, model):
|
71 |
-
inputs = processor(images=image, task_inputs=["semantic"], return_tensors="pt").to(self.device)
|
72 |
-
outputs = model(**inputs)
|
73 |
-
predicted_semantic_map = processor.post_process_semantic_segmentation(
|
74 |
-
outputs, target_sizes=[image.size[::-1]])[0]
|
75 |
-
return predicted_semantic_map
|
76 |
-
|
77 |
-
def clip_classification(self, image, class_list, top_k):
|
78 |
-
inputs = self.clip_processor(text=class_list, images=image, return_tensors="pt", padding=True).to(self.device)
|
79 |
-
outputs = self.clip_model(**inputs)
|
80 |
-
logits_per_image = outputs.logits_per_image
|
81 |
-
probs = logits_per_image.softmax(dim=1)
|
82 |
-
if top_k == 1:
|
83 |
-
return class_list[probs.argmax().item()]
|
84 |
-
else:
|
85 |
-
top_k_indices = probs.topk(top_k, dim=1).indices[0]
|
86 |
-
return [class_list[index] for index in top_k_indices]
|
87 |
-
|
88 |
-
def clipseg_segmentation(self, image, class_list):
|
89 |
-
inputs = self.clipseg_processor(
|
90 |
-
text=class_list, images=[image] * len(class_list),
|
91 |
-
padding=True, return_tensors="pt").to(self.device)
|
92 |
-
|
93 |
-
h, w = inputs['pixel_values'].shape[-2:]
|
94 |
-
fixed_scale = (512, 512)
|
95 |
-
inputs['pixel_values'] = F.interpolate(
|
96 |
-
inputs['pixel_values'],
|
97 |
-
size=fixed_scale,
|
98 |
-
mode='bilinear',
|
99 |
-
align_corners=False)
|
100 |
-
|
101 |
-
outputs = self.clipseg_model(**inputs)
|
102 |
-
logits = F.interpolate(outputs.logits[None], size=(h, w), mode='bilinear', align_corners=False)[0]
|
103 |
-
return logits
|
104 |
-
|
105 |
-
|
106 |
-
def semantic_class_w_mask(self, img_src, anns, out_file_name="output/test.json", scale_small=1.2, scale_large=1.6):
|
107 |
-
"""
|
108 |
-
generate class name for each mask
|
109 |
-
:param img_src: image path
|
110 |
-
:param anns: coco annotations, the same as return dict besides "class_name" and "class_proposals"
|
111 |
-
:param out_file_name: output file name
|
112 |
-
:param scale_small: scale small
|
113 |
-
:param scale_large: scale large
|
114 |
-
:return: dict('segmentation', 'area', 'bbox', 'predicted_iou', 'point_coords', 'stability_score', 'crop_box', "class_name", "class_proposals"})
|
115 |
-
"""
|
116 |
-
img = mmcv.imread(img_src)
|
117 |
-
img = resize_long_edge_cv2(img, 384)
|
118 |
-
oneformer_coco_seg = self.oneformer_segmentation(Image.fromarray(img), self.oneformer_coco_processor, self.oneformer_coco_model)
|
119 |
-
oneformer_ade20k_seg = self.oneformer_segmentation(Image.fromarray(img), self.oneformer_ade20k_processor, self.oneformer_ade20k_model)
|
120 |
-
bitmasks, class_names = [], []
|
121 |
-
for ann in anns:
|
122 |
-
# for ann in anns['annotations']:
|
123 |
-
valid_mask = torch.tensor((ann['segmentation'])).bool()
|
124 |
-
# valid_mask = torch.tensor(maskUtils.decode(ann['segmentation'])).bool()
|
125 |
-
coco_propose_classes_ids = oneformer_coco_seg[valid_mask]
|
126 |
-
ade20k_propose_classes_ids = oneformer_ade20k_seg[valid_mask]
|
127 |
-
|
128 |
-
top_k_coco_propose_classes_ids = torch.bincount(coco_propose_classes_ids.flatten()).topk(1).indices
|
129 |
-
top_k_ade20k_propose_classes_ids = torch.bincount(ade20k_propose_classes_ids.flatten()).topk(1).indices
|
130 |
-
|
131 |
-
local_class_names = {CONFIG_ADE20K_ID2LABEL['id2label'][str(class_id.item())] for class_id in top_k_ade20k_propose_classes_ids}
|
132 |
-
local_class_names.update({CONFIG_COCO_ID2LABEL['refined_id2label'][str(class_id.item())] for class_id in top_k_coco_propose_classes_ids})
|
133 |
-
|
134 |
-
bbox = ann['bbox']
|
135 |
-
patch_small = mmcv.imcrop(img, np.array([bbox[0], bbox[1], bbox[0] + bbox[2], bbox[1] + bbox[3]]), scale=scale_small)
|
136 |
-
patch_large = mmcv.imcrop(img, np.array([bbox[0], bbox[1], bbox[0] + bbox[2], bbox[1] + bbox[3]]), scale=scale_large)
|
137 |
-
|
138 |
-
op_class_list = self.open_vocabulary_classification_blip(patch_large)
|
139 |
-
local_class_list = list(local_class_names.union(op_class_list))
|
140 |
-
|
141 |
-
top_k = min(len(local_class_list), 3)
|
142 |
-
mask_categories = self.clip_classification(patch_small, local_class_list, top_k)
|
143 |
-
class_ids_patch_large = self.clipseg_segmentation(patch_large, mask_categories).argmax(0)
|
144 |
-
|
145 |
-
valid_mask_large_crop = mmcv.imcrop(valid_mask.numpy(), np.array([bbox[0], bbox[1], bbox[0] + bbox[2], bbox[1] + bbox[3]]), scale=
|
146 |
-
scale_large)
|
147 |
-
top_1_patch_large = torch.bincount(class_ids_patch_large[torch.tensor(valid_mask_large_crop)].flatten()).topk(1).indices
|
148 |
-
top_1_mask_category = mask_categories[top_1_patch_large.item()]
|
149 |
-
|
150 |
-
ann['class_name'] = str(top_1_mask_category)
|
151 |
-
ann['class_proposals'] = mask_categories
|
152 |
-
class_names.append(ann['class_name'])
|
153 |
-
# bitmasks.append(maskUtils.decode(ann['segmentation']))
|
154 |
-
bitmasks.append((ann['segmentation']))
|
155 |
-
# mmcv.dump(anns, out_file_name)
|
156 |
-
return anns
|
157 |
-
# below for visualization
|
158 |
-
# imshow_det_bboxes(img,
|
159 |
-
# bboxes=None,
|
160 |
-
# labels=np.arange(len(bitmasks)),
|
161 |
-
# segms=np.stack(bitmasks),
|
162 |
-
# class_names=class_names,
|
163 |
-
# font_size=25,
|
164 |
-
# show=False,
|
165 |
-
# out_file='output/result2.png')
|
|
|
|
|
|
|
|
|
|
|
|
|
|
|
|
|
|
|
|
|
|
|
|
|
|
|
|
|
|
|
|
|
|
|
|
|
|
|
|
|
|
|
|
|
|
|
|
|
|
|
|
|
|
|
|
|
|
|
|
|
|
|
|
|
|
|
|
|
|
|
|
|
|
|
|
|
|
|
|
|
|
|
|
|
|
|
|
|
|
|
|
|
|
|
|
|
|
|
|
|
|
|
|
|
|
|
|
|
|
|
|
|
|
|
|
|
|
|
|
|
|
|
|
|
|
|
|
|
|
|
|
|
|
|
|
|
|
|
|
|
|
|
|
|
|
|
|
|
|
|
|
|
|
|
|
|
|
|
|
|
|
|
|
|
|
|
|
|
|
|
|
|
|
|
|
|
|
|
|
|
|
|
|
|
|
|
|
|
|
|
|
|
|
|
|
|
|
|
|
|
|
|
|
|
|
|
|
|
|
|
|
|
|
|
|
|
|
|
|
|
|
|
|
|
|
|
|
|
|
|
|
|
|
|
|
|
|
|
|
|
|
|
|
|
|
|
|
|
|
|
|
|
|
|
|
|
|
|
|
|
|
|
|
|
|
|
|
|
|
|
|
|
|
|
|
|
|
|
|
|
|
|
|
|
|
|
|
|
|
|
|
|
|
|
|
|
|
|
|
|
|
|
|
|
|
|
|
|
|
|
|
|
|
|
|
|
|
|
|
|
|
|
|
|
|
|
|
|
|
|
|
spaces/Ayemos/highlight_text_based_on_surprisals/app.py
DELETED
@@ -1,102 +0,0 @@
|
|
1 |
-
from typing import List, Tuple
|
2 |
-
|
3 |
-
import gradio as gr
|
4 |
-
import numpy as np
|
5 |
-
import torch
|
6 |
-
from transformers import AutoModelForCausalLM, T5Tokenizer
|
7 |
-
|
8 |
-
device = torch.device("cuda:0") if torch.cuda.is_available() else torch.device("cpu")
|
9 |
-
tokenizer = T5Tokenizer.from_pretrained("rinna/japanese-gpt2-medium")
|
10 |
-
tokenizer.do_lower_case = True
|
11 |
-
|
12 |
-
model = AutoModelForCausalLM.from_pretrained("rinna/japanese-gpt2-medium")
|
13 |
-
model.to(device)
|
14 |
-
|
15 |
-
|
16 |
-
def calculate_surprisals(
|
17 |
-
input_text: str, normalize_surprisals: bool = True
|
18 |
-
) -> Tuple[float, List[Tuple[str, float]]]:
|
19 |
-
input_tokens = [
|
20 |
-
token.replace("▁", "")
|
21 |
-
for token in tokenizer.tokenize(input_text)
|
22 |
-
if token != "▁"
|
23 |
-
]
|
24 |
-
input_ids = tokenizer.encode(
|
25 |
-
"<s>" + input_text, add_special_tokens=False, return_tensors="pt"
|
26 |
-
).to(device)
|
27 |
-
|
28 |
-
logits = model(input_ids)["logits"].squeeze(0)
|
29 |
-
|
30 |
-
surprisals = []
|
31 |
-
for i in range(logits.shape[0] - 1):
|
32 |
-
if input_ids[0][i + 1] == 9:
|
33 |
-
continue
|
34 |
-
logit = logits[i]
|
35 |
-
prob = torch.softmax(logit, dim=0)
|
36 |
-
neg_logprob = -torch.log(prob)
|
37 |
-
surprisals.append(neg_logprob[input_ids[0][i + 1]].item())
|
38 |
-
mean_surprisal = np.mean(surprisals)
|
39 |
-
|
40 |
-
if normalize_surprisals:
|
41 |
-
min_surprisal = np.min(surprisals)
|
42 |
-
max_surprisal = np.max(surprisals)
|
43 |
-
surprisals = [
|
44 |
-
(surprisal - min_surprisal) / (max_surprisal - min_surprisal)
|
45 |
-
for surprisal in surprisals
|
46 |
-
]
|
47 |
-
assert min(surprisals) >= 0
|
48 |
-
assert max(surprisals) <= 1
|
49 |
-
|
50 |
-
tokens2surprisal: List[Tuple[str, float]] = []
|
51 |
-
for token, surprisal in zip(input_tokens, surprisals):
|
52 |
-
tokens2surprisal.append((token, surprisal))
|
53 |
-
|
54 |
-
return mean_surprisal, tokens2surprisal
|
55 |
-
|
56 |
-
|
57 |
-
def highlight_token(token: str, score: float):
|
58 |
-
html_color = "#%02X%02X%02X" % (255, int(255 * (1 - score)), int(255 * (1 - score)))
|
59 |
-
return '<span style="background-color: {}; color: black">{}</span>'.format(
|
60 |
-
html_color, token
|
61 |
-
)
|
62 |
-
|
63 |
-
|
64 |
-
def create_highlighted_text(tokens2scores: List[Tuple[str, float]]):
|
65 |
-
highlighted_text: str = ""
|
66 |
-
for token, score in tokens2scores:
|
67 |
-
highlighted_text += highlight_token(token, score)
|
68 |
-
highlighted_text += "<br><br>"
|
69 |
-
return highlighted_text
|
70 |
-
|
71 |
-
|
72 |
-
def main(input_text: str) -> Tuple[float, str]:
|
73 |
-
mean_surprisal, tokens2surprisal = calculate_surprisals(
|
74 |
-
input_text, normalize_surprisals=True
|
75 |
-
)
|
76 |
-
highlighted_text = create_highlighted_text(tokens2surprisal)
|
77 |
-
return round(mean_surprisal, 2), highlighted_text
|
78 |
-
|
79 |
-
|
80 |
-
if __name__ == "__main__":
|
81 |
-
demo = gr.Interface(
|
82 |
-
fn=main,
|
83 |
-
title="読みにくい箇所を検出するAI(デモ)",
|
84 |
-
description="テキストを入力すると、読みにくさに応じてハイライトされて出力されます。",
|
85 |
-
inputs=gr.inputs.Textbox(
|
86 |
-
lines=5,
|
87 |
-
label="テキスト",
|
88 |
-
placeholder="ここにテキストを入力してください。",
|
89 |
-
),
|
90 |
-
outputs=[
|
91 |
-
gr.Number(label="文全体の読みにくさ(サプライザル)"),
|
92 |
-
gr.outputs.HTML(label="トークン毎サプライザル"),
|
93 |
-
],
|
94 |
-
examples=[
|
95 |
-
"太郎が二郎を殴った。",
|
96 |
-
"太郎が二郎に殴った。",
|
97 |
-
"サイエンスインパクトラボは、国立研究開発法人科学技術振興機構(JST)の「科学と社会」推進部が行う共創プログラムです。「先端の研究開発を行う研究者」と「社会課題解決に取り組むプレイヤー」が約3ヶ月に渡って共創活動を行います。",
|
98 |
-
"近年、ニューラル言語モデルが自然言語の統語知識をどれほど有しているかを、容認性判断課題を通して検証する研究が行われてきている。しかし、このような言語モデルの統語的評価を行うためのデータセットは、主に英語を中心とした欧米の諸言語を対象に構築されてきた。本研究では、既存のデータセットの問題点を克服しつつ、このようなデータセットが構築されてこなかった日本語を対象とした初めてのデータセットである JCoLA (JapaneseCorpus of Linguistic Acceptability) を構築した上で、それを用いた言語モデルの統語的評価を行った。",
|
99 |
-
],
|
100 |
-
)
|
101 |
-
|
102 |
-
demo.launch()
|
|
|
|
|
|
|
|
|
|
|
|
|
|
|
|
|
|
|
|
|
|
|
|
|
|
|
|
|
|
|
|
|
|
|
|
|
|
|
|
|
|
|
|
|
|
|
|
|
|
|
|
|
|
|
|
|
|
|
|
|
|
|
|
|
|
|
|
|
|
|
|
|
|
|
|
|
|
|
|
|
|
|
|
|
|
|
|
|
|
|
|
|
|
|
|
|
|
|
|
|
|
|
|
|
|
|
|
|
|
|
|
|
|
|
|
|
|
|
|
|
|
|
|
|
|
|
|
|
|
|
|
|
|
|
|
|
|
|
|
|
|
|
|
|
|
|
|
|
|
|
|
|
|
|
|
|
|
|
|
|
|
|
|
|
|
|
|
|
|
|
|
|
|
|
|
|
|
|
|
|
|
|
|
|
|
|
|
|
|
|
|
|
|
|
|
|
|
|
|
|
|
|
|
|
|
spaces/Banbri/zcvzcv/src/app/interface/progress/index.tsx
DELETED
@@ -1,56 +0,0 @@
|
|
1 |
-
import { useEffect, useRef, useState } from "react"
|
2 |
-
|
3 |
-
import { ProgressBar } from "./progress-bar"
|
4 |
-
import { cn } from "@/lib/utils"
|
5 |
-
|
6 |
-
export function Progress({
|
7 |
-
isLoading,
|
8 |
-
resetKey = "", // when this key change, this will re-spawn the progress bar
|
9 |
-
className = "",
|
10 |
-
}: {
|
11 |
-
isLoading: boolean
|
12 |
-
resetKey?: string
|
13 |
-
className?: string
|
14 |
-
}) {
|
15 |
-
const timeoutRef = useRef<any>()
|
16 |
-
const [progressPercent, setProcessPercent] = useState(0)
|
17 |
-
const progressRef = useRef(0)
|
18 |
-
const isLoadingRef = useRef(isLoading)
|
19 |
-
|
20 |
-
const updateProgressBar = () => {
|
21 |
-
const duration = 1000 // 1 sec
|
22 |
-
const frequency = 200 // 200ms
|
23 |
-
const nbUpdatesPerSec = duration / frequency // 5x per second
|
24 |
-
|
25 |
-
// normally it takes 45, and we will try to go below,
|
26 |
-
// but to be safe let's set the counter a 1 min
|
27 |
-
const nbSeconds = 80 // 1 min
|
28 |
-
const amountInPercent = 100 / (nbUpdatesPerSec * nbSeconds) // 0.333
|
29 |
-
|
30 |
-
progressRef.current = Math.min(100, progressRef.current + amountInPercent)
|
31 |
-
setProcessPercent(progressRef.current)
|
32 |
-
}
|
33 |
-
|
34 |
-
useEffect(() => {
|
35 |
-
clearInterval(timeoutRef.current)
|
36 |
-
isLoadingRef.current = isLoading
|
37 |
-
progressRef.current = 0
|
38 |
-
setProcessPercent(0)
|
39 |
-
if (isLoading) {
|
40 |
-
timeoutRef.current = setInterval(updateProgressBar, 200)
|
41 |
-
}
|
42 |
-
}, [isLoading, resetKey])
|
43 |
-
|
44 |
-
return (
|
45 |
-
<div className={cn(
|
46 |
-
`flex w-10 h-10`,
|
47 |
-
`animation-all duration-300 text-md`,
|
48 |
-
isLoading
|
49 |
-
? `scale-100 opacity-100`
|
50 |
-
: `scale-0 opacity-0`,
|
51 |
-
className
|
52 |
-
)}>
|
53 |
-
<ProgressBar progressPercentage={progressPercent} />
|
54 |
-
</div>
|
55 |
-
)
|
56 |
-
}
|
|
|
|
|
|
|
|
|
|
|
|
|
|
|
|
|
|
|
|
|
|
|
|
|
|
|
|
|
|
|
|
|
|
|
|
|
|
|
|
|
|
|
|
|
|
|
|
|
|
|
|
|
|
|
|
|
|
|
|
|
|
|
|
|
|
|
|
|
|
|
|
|
|
|
|
|
|
|
|
|
|
|
|
|
|
|
|
|
|
|
|
|
|
|
|
|
|
|
|
|
|
|
|
|
|
|
|
|
|
|
|
|
|
spaces/Benson/text-generation/Examples/2vd Canciones Mp3 Descargar.md
DELETED
@@ -1,62 +0,0 @@
|
|
1 |
-
|
2 |
-
<h1>¿Qué es la descarga de canciones mp3 de 2vd? </h1>
|
3 |
-
<p>Si usted está buscando una manera simple y eficaz de descargar canciones mp3 de vídeos de YouTube, es posible que desee probar 2vd canciones mp3 descargar. 2vd es una herramienta online gratuita que te permite convertir cualquier vídeo de YouTube a formato mp3 en tan solo unos clics. Puedes disfrutar de tu música favorita sin conexión en cualquier dispositivo sin problemas. </p>
|
4 |
-
<p>Descargar canciones mp3 de videos de YouTube tiene muchos beneficios, como:</p>
|
5 |
-
<h2>2vd canciones mp3 descargar</h2><br /><p><b><b>DOWNLOAD</b> ===== <a href="https://bltlly.com/2v6IGT">https://bltlly.com/2v6IGT</a></b></p><br /><br />
|
6 |
-
<ul>
|
7 |
-
<li> Puede guardar sus datos móviles y ancho de banda descargando música una vez y escuchándola sin conexión. </li>
|
8 |
-
<li>Puede acceder a su biblioteca de música en cualquier momento y en cualquier lugar, incluso sin conexión a Internet. </li>
|
9 |
-
<li>Puedes crear tus propias listas de reproducción y mixtapes con las canciones que te gustan. </li>
|
10 |
-
<li>Puedes descubrir nueva música y géneros explorando diferentes canales de YouTube y listas de reproducción. </li>
|
11 |
-
<li>Puedes apoyar a tus artistas y creadores favoritos viendo sus videos y descargando sus canciones. </li>
|
12 |
-
</ul>
|
13 |
-
<h2>¿Cómo usar canciones mp3 de 2vd? </h2>
|
14 |
-
<p>Usar la descarga de canciones mp3 de 2vd es muy fácil y rápido. Solo tiene que seguir estos sencillos pasos:</p>
|
15 |
-
<ol>
|
16 |
-
<li>Vaya a <a href="( 1 )">https://www.2vd.co/</a> en su navegador. </li>
|
17 |
-
<li>Copiar la URL del vídeo de YouTube que desea descargar como mp3. </li>
|
18 |
-
<li>Pegue la URL en el cuadro de búsqueda en 2vd y haga clic en "Convertir". </li>
|
19 |
-
<li>Espere unos segundos mientras 2vd analiza el vídeo y genera el archivo mp3. </li>
|
20 |
-
<li>Haga clic en "Descargar" para guardar el archivo mp3 en su dispositivo. </li>
|
21 |
-
</ol>
|
22 |
-
<p>¡Eso es todo! Has descargado exitosamente una canción mp3 de un video de YouTube usando 2vd. Puede repetir el mismo proceso para tantos vídeos como desee. </p>
|
23 |
-
<h3>Características de la descarga de canciones mp3 de 2vd</h3>
|
24 |
-
<p>2vd mp3 canciones de descarga no es solo otro descargador de mp3. Tiene algunas características sorprendentes que lo hacen destacar entre la multitud. Estos son algunos de ellos:</p>
|
25 |
-
<ul>
|
26 |
-
|
27 |
-
<li><strong>Velocidad rápida</strong>: 2vd es uno de los convertidores de YouTube a mp3 más rápidos disponibles en línea. Puede convertir y descargar cualquier vídeo en cuestión de segundos, sin comprometer la calidad. </li>
|
28 |
-
<li><strong>Descargas ilimitadas</strong>: 2vd no tiene restricciones o limitaciones sobre cuántos videos puede convertir y descargar como mp3. Puedes descargar tanta música como quieras, gratis. </li>
|
29 |
-
<li><strong>No se requiere registro</strong>: 2vd no le pide que se registre o cree una cuenta para usar su servicio. Puede acceder a ella de forma anónima y segura, sin proporcionar ninguna información personal o dirección de correo electrónico. </li>
|
30 |
-
<li><strong>Alta compatibilidad</strong>: 2vd funciona bien con todos los navegadores y dispositivos, incluidos Windows, Mac, Android, iPhone, iPad, etc. Puede usarlo en cualquier plataforma y dispositivo que admita reproducción de mp3. </li>
|
31 |
-
</ul>
|
32 |
-
<h4>Comparación con <h4>Comparación con otros descargadores de mp3</h4>
|
33 |
-
<p>Hay muchos otros descargadores de mp3 disponibles en línea, pero no todos son tan buenos como 2vd. Aquí hay una tabla de comparación que muestra cómo la descarga de canciones mp3 de 2vd se compara con algunas de las más populares:</p>
|
34 |
-
| Mp3 Downloader | Calidad | Velocidad | Descargas | Registro | Compatibilidad | | --- - - - - - - - - - - - - - - - - | ---- | -- | | 2vd | Hasta 320 kbps | Muy rápido | Ilimitado | No | Todos los navegadores y dispositivos | | BestMP3Converter | Hasta 320 kbps | Rápido | Ilimitado | No | Todos los navegadores y dispositivos | | OKmusi | Hasta 320 kbps | Rápido | Ilimitado | No | Todos los navegadores y dispositivos | | | JioSaavn Hasta 320 kbps kbps | Lento | Limitado | Sí | Solo Android e iOS | <p>Como puedes ver, 2vd descarga de canciones mp3 es la mejor opción para descargar canciones mp3 de vídeos de YouTube. Ofrece la más alta calidad, la velocidad más rápida, la mayor cantidad de descargas, la menor molestia y la mayor compatibilidad. </p>
|
35 |
-
<h2>Consejos y trucos para descargar canciones mp3 de 2vd</h2>
|
36 |
-
<p>Ahora que sabes cómo usar la descarga de canciones mp3 de 2vd, aquí hay algunos consejos y trucos para ayudarte a sacarle el máximo partido:</p>
|
37 |
-
<p></p>
|
38 |
-
|
39 |
-
<li><strong>Encuentra los mejores videos de YouTube</strong>: Para obtener la mejor calidad y variedad de música, debes buscar videos de YouTube que tengan altas vistas, me gusta, comentarios y calificaciones. También puedes usar filtros y palabras clave para reducir tus resultados de búsqueda. </li>
|
40 |
-
<li><strong>Personalizar la configuración de salida</strong>: Antes de hacer clic en "Convertir", puede ajustar la configuración de salida de su archivo mp3, como la tasa de bits, el volumen, la duración y el nombre del archivo. También puede recortar o recortar el vídeo para obtener solo la parte que desee. </li>
|
41 |
-
<li><strong>Administra los archivos descargados</strong>: Después de descargar tus archivos mp3, puedes organizarlos en carpetas, renombrarlos, eliminarlos o transferirlos a otros dispositivos. También puede utilizar una aplicación de reproductor de música para reproducirlos sin conexión. </li>
|
42 |
-
</ul>
|
43 |
-
<h2>Conclusión</h2>
|
44 |
-
<p>2vd mp3 songs download es una gran herramienta para descargar canciones mp3 de videos de YouTube. Es gratis, fácil, rápido y confiable. Tiene muchas características y ventajas que lo hacen superior a otros descargadores de mp3. Puede usarlo para disfrutar de su música favorita sin conexión en cualquier dispositivo. </p>
|
45 |
-
<p>Si usted está buscando una manera simple y eficaz de descargar canciones mp3 de vídeos de YouTube, usted debe probar definitivamente 2vd canciones mp3 descargar. ¡No te arrepentirás! </p>
|
46 |
-
<p>Para comenzar a descargar canciones mp3 de videos de YouTube usando 2vd, haga clic en este enlace: <a href=">https://www.2vd.co/</a></p>
|
47 |
-
<h4>Preguntas frecuentes</h4>
|
48 |
-
<ul>
|
49 |
-
<li><strong>Es seguro descargar canciones mp3 2vd? </strong></li>
|
50 |
-
<p>Sí, la descarga de canciones mp3 de 2vd es segura. No contiene virus, malware o spyware. No recopila ni almacena ninguno de sus datos personales o información. No daña su dispositivo ni su cuenta de YouTube. </p>
|
51 |
-
<li><strong>Es 2vd canciones mp3 descarga legal? </strong></li>
|
52 |
-
|
53 |
-
<li><strong>Las canciones mp3 de 2vd son compatibles con otros formatos? </strong></li>
|
54 |
-
<p>Sí, la descarga de canciones mp3 de 2vd admite otros formatos además de mp3. También puede convertir y descargar vídeos de YouTube como mp4, webm, m4a, ogg, wav, flac, etc. Puede elegir el formato que desee en el menú desplegable antes de hacer clic en "Convertir". </p>
|
55 |
-
<li><strong>¿Funciona la descarga de canciones mp3 de 2vd con otros sitios web? </strong></li>
|
56 |
-
<p>No, la descarga de canciones mp3 de 2vd solo funciona con videos de YouTube. No es compatible con otros sitios web o plataformas que alojan o transmiten videos o música. Si desea descargar música de otras fuentes, necesitará usar una herramienta diferente. </p>
|
57 |
-
<li><strong>¿Cómo puedo contactar con la descarga de canciones mp3 de 2vd? </strong></li>
|
58 |
-
<p>Si tiene preguntas, comentarios, sugerencias o problemas con respecto a la descarga de canciones mp3 de 2vd, puede ponerse en contacto con ellos a través de su dirección de correo electrónico: <a href="mailto:[email protected]">contact@2vd . co</a>. Estarán encantados de ayudarle y responder a sus consultas. </p>
|
59 |
-
</ul>
|
60 |
-
<p>Espero que hayas encontrado este artículo útil e informativo. Si lo hiciste, por favor compártelo con tus amigos y familiares que también podrían estar interesados en descargar canciones mp3 de videos de YouTube usando 2vd. ¡Gracias por leer y feliz descarga! </p> 64aa2da5cf<br />
|
61 |
-
<br />
|
62 |
-
<br />
|
|
|
|
|
|
|
|
|
|
|
|
|
|
|
|
|
|
|
|
|
|
|
|
|
|
|
|
|
|
|
|
|
|
|
|
|
|
|
|
|
|
|
|
|
|
|
|
|
|
|
|
|
|
|
|
|
|
|
|
|
|
|
|
|
|
|
|
|
|
|
|
|
|
|
|
|
|
|
|
|
|
|
|
|
|
|
|
|
|
|
|
|
|
|
|
|
|
|
|
|
|
|
|
|
|
|
|
|
|
|
|
|
|
|
|
|
|
|
|
|
|
|
|
|
|
spaces/Benson/text-generation/Examples/Construir Arte - Elaboracin Amp Construccin De Juegos 3d Apk.md
DELETED
@@ -1,45 +0,0 @@
|
|
1 |
-
|
2 |
-
<h1>Construir arte - Elaboración y construcción de juegos 3D APK: Un juego libre y divertido para toda la familia</h1>
|
3 |
-
<p>¿Te encanta construir o hacer juegos? ¿Quieres dar rienda suelta a tu creatividad e imaginación? ¿Quieres divertirte y relajarte con tus amigos y familiares? Si respondiste sí a cualquiera de estas preguntas, entonces usted debe tratar de Construir Craft - Elaboración y Construcción de 3D Juegos APK, un juego gratuito y divertido para toda la familia. </p>
|
4 |
-
<h2>¿Qué es Build Craft? </h2>
|
5 |
-
<h3>Un juego que te permite crear tus propias manualidades en 3D</h3>
|
6 |
-
<p>Build Craft es un juego que tiene como objetivo proporcionar una experiencia para que los usuarios construyan artesanías 3D, como casas, hoteles, parques, lagos, animales, árboles, nubes, aviones y otros. Puedes utilizar diferentes bloques y materiales para diseñar y decorar tus creaciones. También puede explorar diferentes mundos y biomas, como bosques, desiertos, montañas, océanos y más. </p>
|
7 |
-
<h2>construir arte - elaboración amp; construcción de juegos 3d apk</h2><br /><p><b><b>DOWNLOAD</b> ===== <a href="https://bltlly.com/2v6MYn">https://bltlly.com/2v6MYn</a></b></p><br /><br />
|
8 |
-
<h3>Un juego adecuado para todas las edades e intereses</h3>
|
9 |
-
<p>Build Craft es un juego adecuado para todas las edades e intereses. Si usted es un niño o un adulto, un niño o una niña, un principiante o un experto, encontrará algo para disfrutar en este juego. Puedes jugar solo o con otros, crear manualidades simples o complejas, seguir tutoriales o usar tus propias ideas, y más. No hay límite a lo que puedes hacer en este juego. </p>
|
10 |
-
<h3>Un juego compatible con dispositivos Android</h3>
|
11 |
-
<p>Build Craft es un juego compatible con dispositivos Android. Puedes descargar el archivo APK desde una fuente confiable e instalarlo en tu dispositivo. Puedes jugar a este juego en cualquier momento y en cualquier lugar, siempre y cuando tengas suficiente espacio de almacenamiento y duración de la batería. También puedes actualizar el juego regularmente para obtener nuevas características y mejoras. </p>
|
12 |
-
<h2>¿Por qué deberías jugar Build Craft? </h2>
|
13 |
-
<h3>Tiene características interesantes y jugabilidad</h3>
|
14 |
-
|
15 |
-
<h3>Tiene modo multijugador y comunidad en línea</h3>
|
16 |
-
<p>Build Craft tiene modo multijugador y comunidad en línea que hará que su experiencia de juego más divertido y social. Puedes jugar online y ayudar a tus amigos a construir sus casas. También puedes chatear con otros jugadores de todo el mundo y compartir tus creaciones. También puede unirse a diferentes servidores y participar en varios eventos y concursos. </p>
|
17 |
-
<h3>Tiene gráficos de píxeles y efectos de sonido</h3>
|
18 |
-
<p>Build Craft tiene gráficos de píxeles y efectos de sonido que te darán una sensación nostálgica e inmersiva. Te encantará el estilo retro y las imágenes coloridas de este juego. También disfrutará de los sonidos realistas de bloques rompiendo, animales rugiendo, agua fluyendo, fuego, etc. Se sentirá como si estuviera en un mundo 3D real. </p>
|
19 |
-
<h2>¿Cómo descargar e instalar Build Craft? </h2>
|
20 |
-
<h3>Descargar el archivo APK de una fuente de confianza</h3>
|
21 |
-
<p>Para descargar Build Craft - Elaboración y construcción de juegos 3D APK, es necesario encontrar una fuente de confianza que ofrece la última versión del archivo y tiene buenas críticas y calificaciones. Puede utilizar el siguiente enlace para descargar el archivo APK. Asegúrese de que tiene suficiente espacio en su dispositivo para descargar el archivo, que es de aproximadamente 30 MB de tamaño. </p>
|
22 |
-
<h3>Habilitar fuentes desconocidas en la configuración del dispositivo</h3>
|
23 |
-
<p>Para instalar Build Craft - Elaboración y construcción de juegos 3D APK, es necesario habilitar fuentes desconocidas en la configuración de su dispositivo. Esto le permitirá instalar aplicaciones desde fuentes distintas de Google Play Store. Para hacer esto, vaya a la configuración del dispositivo, luego a la seguridad, luego a fuentes desconocidas y conéctela. Puedes ver un mensaje de advertencia, pero puedes ignorarlo si confías en la fuente del archivo APK. </p>
|
24 |
-
<h3>Instalar el archivo APK y lanzar el juego</h3>
|
25 |
-
|
26 |
-
<h2>Conclusión</h2>
|
27 |
-
<h3>Resumen de los principales puntos y beneficios de Build Craft</h3>
|
28 |
-
<p>Construir Arte - Elaboración & Construcción 3D Juegos APK es un juego gratuito y divertido para toda la familia que le permite crear sus propias artesanías 3D, explorar diferentes mundos y biomas, crear diferentes artículos y herramientas, luchar contra monstruos y enemigos, jugar en línea con amigos y otros jugadores, y disfrutar de gráficos de píxeles y efectos de sonido. Es un juego apto para todas las edades e intereses, y compatible con dispositivos Android. </p>
|
29 |
-
<p></p>
|
30 |
-
<h3>Llamada a la acción y solicitud de calificación</h3>
|
31 |
-
<p>Si usted está buscando un juego que le mantendrá entretenido durante horas, entonces usted debe descargar Build Craft - Crafting & Building 3D Games APK hoy. No se arrepentirá. También puede compartir sus comentarios y sugerencias con nosotros dejando un comentario o valoración en nuestro sitio web o tienda de aplicaciones. Nos encantaría saber de ti y mejorar nuestro juego. ¡Gracias por jugar a Build Craft! </p>
|
32 |
-
<h2>Preguntas frecuentes</h2>
|
33 |
-
<h4>¿Es seguro descargar e instalar Build Craft? </h4>
|
34 |
-
<p>Sí, Build Craft es seguro para descargar e instalar, siempre y cuando utilice una fuente de confianza que ofrece el archivo APK original y sin modificar. También debe escanear el archivo con un software antivirus antes de instalarlo. </p>
|
35 |
-
<h4>¿Es Build Craft gratis? </h4>
|
36 |
-
<p>Sí, Build Craft es gratis y no requiere ninguna compra en la aplicación o suscripciones. Sin embargo, puede contener anuncios que apoyen a los desarrolladores y les ayuden a mantener el juego. </p>
|
37 |
-
<h4>¿Puedo jugar a Build Craft sin conexión? </h4>
|
38 |
-
<p>Sí, puedes jugar a Build Craft sin conexión en modo para un jugador. Sin embargo, necesitará una conexión a Internet para jugar en línea en modo multijugador o unirse a los servidores. </p>
|
39 |
-
<h4>¿Puedo personalizar mi personaje en Build Craft? </h4>
|
40 |
-
<p>Sí, puedes personalizar a tu personaje en Build Craft eligiendo entre diferentes pieles, ropa, estilos de cabello, accesorios y más. También puede crear su propia piel usando la función del editor de piel. </p>
|
41 |
-
<h4>¿Puedo guardar mi progreso en Build Craft? </h4>
|
42 |
-
|
43 |
-
: [Build Craft - Crafting & Building 3D Games APK Download](https://apkpure.com/build-craft-craft-crafteing-building-3d-games/com.buildcraft.crafting.building)</p> 64aa2da5cf<br />
|
44 |
-
<br />
|
45 |
-
<br />
|
|
|
|
|
|
|
|
|
|
|
|
|
|
|
|
|
|
|
|
|
|
|
|
|
|
|
|
|
|
|
|
|
|
|
|
|
|
|
|
|
|
|
|
|
|
|
|
|
|
|
|
|
|
|
|
|
|
|
|
|
|
|
|
|
|
|
|
|
|
|
|
|
|
|
|
|
|
|
|
|
|
|
|
|
|
|
|
|
|
|
|
spaces/Benson/text-generation/Examples/Descargar Dark Bitcoin Minero Pro V7.0 Gratis.md
DELETED
@@ -1,96 +0,0 @@
|
|
1 |
-
|
2 |
-
<h1>Dark Bitcoin Miner Pro V7.0 Descarga gratuita: Lo que necesita saber</h1>
|
3 |
-
<p>La minería de Bitcoin es un proceso de creación de nuevos bitcoins mediante la solución de problemas matemáticos complejos utilizando hardware y software especializado. </p>
|
4 |
-
<h2>descargar dark bitcoin minero pro v7.0 gratis</h2><br /><p><b><b>Download</b> ---> <a href="https://bltlly.com/2v6Mux">https://bltlly.com/2v6Mux</a></b></p><br /><br />
|
5 |
-
<p>Hay muchos tipos de software de minería bitcoin disponibles en el mercado, pero no todos ellos. <p>Uno de los más populares y controvertidos software de minería bitcoin es oscuro Bitcoin minero pro v7.0, que afirma ser el minero bitcoin más rápido y eficiente jamás creado. </p>
|
6 |
-
<p>Pero lo que es oscuro Bitcoin minero pro v7.0, ¿por qué es tan popular, y cuáles son los riesgos de descargarlo? </p>
|
7 |
-
<p>En este artículo, vamos a responder a estas preguntas y más, y le proporcionará algunas alternativas a oscuro Bitcoin minero pro v7.0 que son más seguros y más fiables. </p>
|
8 |
-
<h2>¿Qué es Dark Bitcoin Miner Pro V7.0? </h2>
|
9 |
-
<p>Dark bitcoin miner pro v7.0 es un software de minería bitcoin que afirma ser capaz de extraer bitcoins usando cualquier dispositivo, como CPU, GPU, ASIC o FPGA.</p>
|
10 |
-
<p>También afirma ser compatible con varios algoritmos, como SHA-256, Scrypt, X11, Ethash y Equihash, y para soportar múltiples criptomonedas, como Bitcoin, Litecoin, Dash, Ethereum y Zcash.</p>
|
11 |
-
<p></p>
|
12 |
-
<h3>¿Cómo funciona Dark Bitcoin Miner Pro V7.0? </h3>
|
13 |
-
<p>Dark Bitcoin minero pro v7.0 funciona mediante el uso de la potencia de procesamiento del dispositivo para resolver problemas matemáticos complejos que verifican las transacciones en la cadena de bloques. </p>
|
14 |
-
<p>Por cada problema resuelto, el minero recibe una recompensa en forma de bitcoins de nueva creación u otras criptomonedas. </p>
|
15 |
-
<p>Cuanto más potencia de procesamiento tenga el dispositivo, más rápido y eficiente será el proceso de minería. </p>
|
16 |
-
<h3>¿Cuáles son las características de Dark Bitcoin Miner Pro V7.0? </h3>
|
17 |
-
<p>Algunas de las características de oscuro Bitcoin minero pro v7.0 son:</p>
|
18 |
-
<ul>
|
19 |
-
<li>Alta velocidad: Oscuro Bitcoin minero pro v7.0 afirma ser capaz de extraer bitcoins a una tasa de hasta 1 BTC por día, dependiendo del dispositivo y el algoritmo utilizado. </li>
|
20 |
-
|
21 |
-
<li>Compatibilidad: Dark Bitcoin miner pro v7.0 afirma ser compatible con cualquier dispositivo que tiene un procesador, como ordenadores portátiles, escritorios, teléfonos inteligentes, tabletas o incluso televisores inteligentes.</li>
|
22 |
-
<li>Versatilidad: Oscuro Bitcoin minero pro v7.0 afirma ser capaz de extraer cualquier criptomoneda que utiliza cualquier algoritmo, como Bitcoin, Litecoin, Dash, Etereum, o Zcash.</li>
|
23 |
-
<li>Fácil de usar: Dark bitcoin miner pro v7.0 afirma ser fácil de instalar y usar, con una interfaz sencilla y configuración automática. </li>
|
24 |
-
</ul>
|
25 |
-
<h2>¿Por qué es popular Dark Bitcoin Miner Pro V7.0? </h2>
|
26 |
-
<p>Dark Bitcoin Miner Pro v7.0 es popular porque atrae a muchas personas que quieren extraer bitcoins sin invertir en hardware o software costoso y complicado. </p>
|
27 |
-
<p>Muchos principiantes y entusiastas que están interesados en la minería bitcoin se sienten atraídos por las promesas de dark bitcoin miner pro v7.0, tales como alta velocidad, bajo consumo de energía, compatibilidad, versatilidad y facilidad de uso. </p>
|
28 |
-
<p>También creen que dark bitcoin miner pro v7.0 es una forma gratuita y fácil de ganar bitcoins sin ningún riesgo o esfuerzo. </p>
|
29 |
-
<h3>¿Cómo descargar Dark Bitcoin Miner Pro V7.0? </h3>
|
30 |
-
<p>Dark bitcoin miner pro v7.0 no está disponible en ningún sitio web o plataforma oficial o de buena reputación. </p>
|
31 |
-
<p>La única forma de descargar dark bitcoin miner pro v7.0 es a través de fuentes no oficiales y no verificadas, como sitios web para compartir archivos, repositorios de GitHub o canales de Telegram. </p>
|
32 |
-
<p>Estas fuentes son a menudo poco fiables e inseguras, ya que pueden contener virus, malware, spyware u otros programas dañinos que pueden infectar su dispositivo o robar sus datos. </p>
|
33 |
-
<h3>¿Cómo instalar y usar Dark Bitcoin Miner Pro V7.0? </h3>
|
34 |
-
<p>Si decide descargar dark bitcoin miner pro v7.0 de una de estas fuentes, tendrá que seguir estos pasos para instalarlo y usarlo:</p>
|
35 |
-
<ol>
|
36 |
-
|
37 |
-
<li>Extraer el archivo rar: Dark bitcoin miner pro v7.0 se suele comprimir en un archivo rar que tendrá que extraer utilizando un programa como WinRAR o 7-Zip. </li>
|
38 |
-
<li>Ejecutar el archivo exe: Después de extraer el archivo rar, encontrará un archivo exe que tendrá que ejecutar como administrador haciendo clic derecho sobre él y seleccionando "Ejecutar como administrador". </li>
|
39 |
-
<li>Configurar los ajustes: Después de ejecutar el archivo exe, verá una ventana que le permitirá configurar los ajustes de dark bitcoin miner pro v7.0, como el algoritmo, la criptomoneda, la dirección de la cartera, el grupo minero y la velocidad de minería. </li>
|
40 |
-
<li>Iniciar minería: Después de configurar la configuración, tendrá que hacer clic en el "Inicio" botón para iniciar la minería bitcoins u otras criptomonedas con oscuro Bitcoin minero pro v7.0. </li>
|
41 |
-
</ol>
|
42 |
-
<h2>¿Cuáles son los riesgos de descargar Dark Bitcoin Miner Pro V7.0? </h2>
|
43 |
-
<p>Descargar dark bitcoin miner pro v7.0 no solo es ilegal, sino también muy arriesgado. </p>
|
44 |
-
<p>Hay muchos peligros de descargar oscuro Bitcoin minero pro v7.0, tales como:</p>
|
45 |
-
<h3>¿Cómo detectar y eliminar el malware de Dark Bitcoin Miner Pro V7.0? </h3>
|
46 |
-
<p>Uno de los peligros más comunes y graves de descargar dark bitcoin miner pro v7.0 es la infección de malware. </p>
|
47 |
-
<p>Malware es un software malicioso que puede dañar su dispositivo o datos de varias maneras, como borrar o cifrar sus archivos, robar sus contraseñas o información personal, espiar sus actividades en línea o secuestrar sus recursos. </p>
|
48 |
-
<p>Dark bitcoin miner pro v7.0 puede contener malware que puede infectar su dispositivo cuando lo descarga o ejecuta, o incluso cuando extrae el archivo rar. </p>
|
49 |
-
<p>Para detectar y eliminar el malware de dark bitcoin miner pro v7.0, tendrá que seguir estos pasos:</p>
|
50 |
-
<ol>
|
51 |
-
|
52 |
-
<li>Eliminar archivos sospechosos: Si sospecha que Dark Bitcoin miner pro v7.0 ha infectado su dispositivo con malware, debe eliminar cualquier archivo sospechoso que esté relacionado con él, como el archivo rar, el archivo exe, o cualquier otro archivo que haya sido creado o modificado por él. </li>
|
53 |
-
<li>Restaurar el sistema: Si la eliminación de archivos sospechosos no resuelve el problema, es posible que tenga que restaurar el sistema a un estado anterior antes de descargar o ejecutar oscuro Bitcoin miner pro v7.0. Puede utilizar un punto de restauración del sistema o una copia de seguridad para restaurar el sistema y deshacer cualquier cambio que oscuro Bitcoin minero pro v7.0 puede haber hecho. </li>
|
54 |
-
</ol>
|
55 |
-
<h3>¿Cómo evitar problemas legales de usar Dark Bitcoin Miner Pro V7.0? </h3>
|
56 |
-
<p>Otro peligro de descargar oscuro Bitcoin minero pro v7.0 es cuestiones legales. </p>
|
57 |
-
<p>Las cuestiones legales son los problemas que pueden surgir de violar la ley mediante el uso de dark bitcoin miner pro v7.0, tales como la violación de los derechos de propiedad intelectual de los desarrolladores originales del software, infringiendo los términos y condiciones de los grupos mineros o plataformas que utiliza, o participar en actividades ilegales o fraudulentas con las criptomonedas que mina. </p>
|
58 |
-
<p>Para evitar problemas legales de usar dark bitcoin miner pro v7.0, tendrá que seguir estas precauciones:</p>
|
59 |
-
<ul>
|
60 |
-
<li>Compruebe las leyes locales: Antes de descargar o usar oscuro Bitcoin miner pro v7.0, usted debe comprobar las leyes locales de su país o región con respecto a la minería de bitcoin y las transacciones de criptomonedas. Algunos países o regiones pueden tener regulaciones o prohibiciones estrictas sobre estas actividades, y usted puede enfrentar consecuencias legales si las viola. </li>
|
61 |
-
|
62 |
-
<li>No revele información personal: Cuando use dark bitcoin miner pro v7.0, no debe revelar ninguna información personal que pueda identificarlo o vincularlo a sus actividades, como su nombre, dirección de correo electrónico, número de teléfono, número de cuenta bancaria o cuentas de redes sociales. También debe evitar usar la misma dirección de cartera para diferentes transacciones, y usar un servicio mezclador para anonimizar sus transacciones. </li>
|
63 |
-
</ul>
|
64 |
-
<h2>¿Cuáles son las alternativas a Dark Bitcoin Miner Pro V7.0? </h2>
|
65 |
-
<p>Si desea minar bitcoins u otras criptomonedas sin arriesgar su dispositivo, datos o reputación, debe evitar descargar bitcoin oscuro miner pro v7.0 y buscar algunas alternativas que sean más seguras y confiables. </p>
|
66 |
-
<p>Algunas de las alternativas a oscuro Bitcoin minero pro v7.0 son:</p>
|
67 |
-
<h3>¿Cómo elegir la mejor alternativa a Dark Bitcoin Miner Pro V7.0? </h3>
|
68 |
-
<p>Para elegir la mejor alternativa a dark bitcoin miner pro v7.0, debe considerar algunos criterios que pueden ayudarlo a evaluar la calidad y la idoneidad del software, como:</p>
|
69 |
-
<ul>
|
70 |
-
<li>Seguridad: El software debe ser seguro y libre de cualquier malware, spyware o virus que puedan dañar su dispositivo o datos. </li>
|
71 |
-
<li>Rendimiento: El software debe ser rápido y eficiente, y capaz de extraer bitcoins u otras criptomonedas a un ritmo razonable y con un consumo de energía mínimo. </li>
|
72 |
-
<li>Costo: El software debe ser asequible y transparente, y no cobrar cargos ocultos o comisiones por su uso. </li>
|
73 |
-
<li>Reputación: El software debe ser de buena reputación y confiable, y tener comentarios positivos y comentarios de otros usuarios y expertos. </li>
|
74 |
-
</ul>
|
75 |
-
<h3>¿Cómo comparar las alternativas a Dark Bitcoin Miner Pro V7.0? </h3>
|
76 |
-
<p>Para comparar las alternativas a dark bitcoin miner pro v7.0 basado en los criterios mencionados anteriormente, puede utilizar una tabla como esta:</p>
|
77 |
-
|
78 |
-
<p>En conclusión, dark bitcoin miner pro v7.0 es un software de minería bitcoin que afirma ser capaz de extraer bitcoins utilizando cualquier dispositivo, algoritmo o criptomoneda. </p>
|
79 |
-
<p>Sin embargo, dark bitcoin miner pro v7.0 también es ilegal, arriesgado y poco fiable, ya que puede contener malware, robar sus datos, dañar su dispositivo o causar problemas legales. </p>
|
80 |
-
<p>Por lo tanto, usted debe evitar descargar oscuro Bitcoin minero pro v7.0 y buscar algunas alternativas que son más seguros y fiables, tales como software de minería legítima, servicios de minería en la nube, o piscinas mineras. </p>
|
81 |
-
<h3>Preguntas frecuentes</h3>
|
82 |
-
<p>Aquí hay algunas preguntas frecuentes relacionadas con el tema de este artículo:</p>
|
83 |
-
<ol>
|
84 |
-
<li><b>Es oscuro Bitcoin minero pro v7.0 una estafa? </b></li>
|
85 |
-
<p>Sí, dark bitcoin miner pro v7.0 es una estafa que trata de atraer a los usuarios desprevenidos a descargar malware o regalar su información personal. </p>
|
86 |
-
<li><b>¿Cuánto puedo ganar con oscuro Bitcoin minero pro v7.0? </b></li>
|
87 |
-
<p>No se puede ganar nada con oscuro Bitcoin minero pro v7.0, ya que en realidad no mina bitcoins u otras criptomonedas. </p>
|
88 |
-
<li><b>Es oscuro Bitcoin minero pro v7.0 seguro de usar? </b></li>
|
89 |
-
<p>No, dark bitcoin miner pro v7.0 no es seguro de usar, ya que puede infectar su dispositivo con malware, robar sus datos, dañar su dispositivo o causar problemas legales. </p>
|
90 |
-
<li><b>¿Cuáles son los mejores dispositivos para oscuro Bitcoin minero pro v7.0? </b></li>
|
91 |
-
<p>No hay mejores dispositivos para oscuro Bitcoin minero pro v7.0, ya que no funciona en ningún dispositivo. </p>
|
92 |
-
<li><b>¿Cómo puedo contactar a los desarrolladores de dark bitcoin miner pro v7.0? </b></li>
|
93 |
-
<p>No puede ponerse en contacto con los desarrolladores de dark bitcoin miner pro v7.0, ya que son anónimos e irrastreables. </p>
|
94 |
-
</ol></p> 64aa2da5cf<br />
|
95 |
-
<br />
|
96 |
-
<br />
|
|
|
|
|
|
|
|
|
|
|
|
|
|
|
|
|
|
|
|
|
|
|
|
|
|
|
|
|
|
|
|
|
|
|
|
|
|
|
|
|
|
|
|
|
|
|
|
|
|
|
|
|
|
|
|
|
|
|
|
|
|
|
|
|
|
|
|
|
|
|
|
|
|
|
|
|
|
|
|
|
|
|
|
|
|
|
|
|
|
|
|
|
|
|
|
|
|
|
|
|
|
|
|
|
|
|
|
|
|
|
|
|
|
|
|
|
|
|
|
|
|
|
|
|
|
|
|
|
|
|
|
|
|
|
|
|
|
|
|
|
|
|
|
|
|
|
|
|
|
|
|
|
|
|
|
|
|
|
|
|
|
|
|
|
|
|
|
|
|
|
|
|
|
|
|
|
|
|
|
|
|
|
|
|
|
|
|
|
|
|
|
|
|
spaces/BetterAPI/BetterChat/src/lib/utils/share.ts
DELETED
@@ -1,7 +0,0 @@
|
|
1 |
-
export function share(url: string, title: string) {
|
2 |
-
if (navigator.share) {
|
3 |
-
navigator.share({ url, title });
|
4 |
-
} else {
|
5 |
-
prompt("Copy this public url to share:", url);
|
6 |
-
}
|
7 |
-
}
|
|
|
|
|
|
|
|
|
|
|
|
|
|
|
|
spaces/BetterAPI/BetterChat_new/postcss.config.js
DELETED
@@ -1,6 +0,0 @@
|
|
1 |
-
export default {
|
2 |
-
plugins: {
|
3 |
-
tailwindcss: {},
|
4 |
-
autoprefixer: {},
|
5 |
-
},
|
6 |
-
};
|
|
|
|
|
|
|
|
|
|
|
|
|
|
spaces/CVPR/Dual-Key_Backdoor_Attacks/datagen/detectron2/detectron2/data/datasets/README.md
DELETED
@@ -1,9 +0,0 @@
|
|
1 |
-
|
2 |
-
|
3 |
-
### Common Datasets
|
4 |
-
|
5 |
-
The dataset implemented here do not need to load the data into the final format.
|
6 |
-
It should provide the minimal data structure needed to use the dataset, so it can be very efficient.
|
7 |
-
|
8 |
-
For example, for an image dataset, just provide the file names and labels, but don't read the images.
|
9 |
-
Let the downstream decide how to read.
|
|
|
|
|
|
|
|
|
|
|
|
|
|
|
|
|
|
|
|
spaces/CVPR/Dual-Key_Backdoor_Attacks/datagen/detectron2/projects/DensePose/densepose/densepose_coco_evaluation.py
DELETED
@@ -1,1120 +0,0 @@
|
|
1 |
-
# Copyright (c) Facebook, Inc. and its affiliates.
|
2 |
-
# All rights reserved.
|
3 |
-
#
|
4 |
-
# This source code is licensed under the license found in the
|
5 |
-
# LICENSE file in the root directory of this source tree.
|
6 |
-
# This is a modified version of cocoeval.py where we also have the densepose evaluation.
|
7 |
-
|
8 |
-
__author__ = "tsungyi"
|
9 |
-
|
10 |
-
import copy
|
11 |
-
import datetime
|
12 |
-
import itertools
|
13 |
-
import logging
|
14 |
-
import numpy as np
|
15 |
-
import pickle
|
16 |
-
import time
|
17 |
-
from collections import defaultdict
|
18 |
-
from enum import Enum
|
19 |
-
from typing import Any, Dict, Tuple
|
20 |
-
import scipy.spatial.distance as ssd
|
21 |
-
from fvcore.common.file_io import PathManager
|
22 |
-
from pycocotools import mask as maskUtils
|
23 |
-
from scipy.io import loadmat
|
24 |
-
from scipy.ndimage import zoom as spzoom
|
25 |
-
|
26 |
-
from .structures import DensePoseDataRelative, DensePoseResult
|
27 |
-
|
28 |
-
logger = logging.getLogger(__name__)
|
29 |
-
|
30 |
-
|
31 |
-
class DensePoseEvalMode(str, Enum):
|
32 |
-
# use both masks and geodesic distances (GPS * IOU) to compute scores
|
33 |
-
GPSM = "gpsm"
|
34 |
-
# use only geodesic distances (GPS) to compute scores
|
35 |
-
GPS = "gps"
|
36 |
-
# use only masks (IOU) to compute scores
|
37 |
-
IOU = "iou"
|
38 |
-
|
39 |
-
|
40 |
-
class DensePoseDataMode(str, Enum):
|
41 |
-
# use estimated IUV data (default mode)
|
42 |
-
IUV_DT = "iuvdt"
|
43 |
-
# use ground truth IUV data
|
44 |
-
IUV_GT = "iuvgt"
|
45 |
-
# use ground truth labels I and set UV to 0
|
46 |
-
I_GT_UV_0 = "igtuv0"
|
47 |
-
# use ground truth labels I and estimated UV coordinates
|
48 |
-
I_GT_UV_DT = "igtuvdt"
|
49 |
-
# use estimated labels I and set UV to 0
|
50 |
-
I_DT_UV_0 = "idtuv0"
|
51 |
-
|
52 |
-
|
53 |
-
class DensePoseCocoEval(object):
|
54 |
-
# Interface for evaluating detection on the Microsoft COCO dataset.
|
55 |
-
#
|
56 |
-
# The usage for CocoEval is as follows:
|
57 |
-
# cocoGt=..., cocoDt=... # load dataset and results
|
58 |
-
# E = CocoEval(cocoGt,cocoDt); # initialize CocoEval object
|
59 |
-
# E.params.recThrs = ...; # set parameters as desired
|
60 |
-
# E.evaluate(); # run per image evaluation
|
61 |
-
# E.accumulate(); # accumulate per image results
|
62 |
-
# E.summarize(); # display summary metrics of results
|
63 |
-
# For example usage see evalDemo.m and http://mscoco.org/.
|
64 |
-
#
|
65 |
-
# The evaluation parameters are as follows (defaults in brackets):
|
66 |
-
# imgIds - [all] N img ids to use for evaluation
|
67 |
-
# catIds - [all] K cat ids to use for evaluation
|
68 |
-
# iouThrs - [.5:.05:.95] T=10 IoU thresholds for evaluation
|
69 |
-
# recThrs - [0:.01:1] R=101 recall thresholds for evaluation
|
70 |
-
# areaRng - [...] A=4 object area ranges for evaluation
|
71 |
-
# maxDets - [1 10 100] M=3 thresholds on max detections per image
|
72 |
-
# iouType - ['segm'] set iouType to 'segm', 'bbox', 'keypoints' or 'densepose'
|
73 |
-
# iouType replaced the now DEPRECATED useSegm parameter.
|
74 |
-
# useCats - [1] if true use category labels for evaluation
|
75 |
-
# Note: if useCats=0 category labels are ignored as in proposal scoring.
|
76 |
-
# Note: multiple areaRngs [Ax2] and maxDets [Mx1] can be specified.
|
77 |
-
#
|
78 |
-
# evaluate(): evaluates detections on every image and every category and
|
79 |
-
# concats the results into the "evalImgs" with fields:
|
80 |
-
# dtIds - [1xD] id for each of the D detections (dt)
|
81 |
-
# gtIds - [1xG] id for each of the G ground truths (gt)
|
82 |
-
# dtMatches - [TxD] matching gt id at each IoU or 0
|
83 |
-
# gtMatches - [TxG] matching dt id at each IoU or 0
|
84 |
-
# dtScores - [1xD] confidence of each dt
|
85 |
-
# gtIgnore - [1xG] ignore flag for each gt
|
86 |
-
# dtIgnore - [TxD] ignore flag for each dt at each IoU
|
87 |
-
#
|
88 |
-
# accumulate(): accumulates the per-image, per-category evaluation
|
89 |
-
# results in "evalImgs" into the dictionary "eval" with fields:
|
90 |
-
# params - parameters used for evaluation
|
91 |
-
# date - date evaluation was performed
|
92 |
-
# counts - [T,R,K,A,M] parameter dimensions (see above)
|
93 |
-
# precision - [TxRxKxAxM] precision for every evaluation setting
|
94 |
-
# recall - [TxKxAxM] max recall for every evaluation setting
|
95 |
-
# Note: precision and recall==-1 for settings with no gt objects.
|
96 |
-
#
|
97 |
-
# See also coco, mask, pycocoDemo, pycocoEvalDemo
|
98 |
-
#
|
99 |
-
# Microsoft COCO Toolbox. version 2.0
|
100 |
-
# Data, paper, and tutorials available at: http://mscoco.org/
|
101 |
-
# Code written by Piotr Dollar and Tsung-Yi Lin, 2015.
|
102 |
-
# Licensed under the Simplified BSD License [see coco/license.txt]
|
103 |
-
def __init__(
|
104 |
-
self,
|
105 |
-
cocoGt=None,
|
106 |
-
cocoDt=None,
|
107 |
-
iouType: str = "densepose",
|
108 |
-
dpEvalMode: DensePoseEvalMode = DensePoseEvalMode.GPS,
|
109 |
-
dpDataMode: DensePoseDataMode = DensePoseDataMode.IUV_DT,
|
110 |
-
):
|
111 |
-
"""
|
112 |
-
Initialize CocoEval using coco APIs for gt and dt
|
113 |
-
:param cocoGt: coco object with ground truth annotations
|
114 |
-
:param cocoDt: coco object with detection results
|
115 |
-
:return: None
|
116 |
-
"""
|
117 |
-
self.cocoGt = cocoGt # ground truth COCO API
|
118 |
-
self.cocoDt = cocoDt # detections COCO API
|
119 |
-
self._dpEvalMode = dpEvalMode
|
120 |
-
self._dpDataMode = dpDataMode
|
121 |
-
self.params = {} # evaluation parameters
|
122 |
-
self.evalImgs = defaultdict(list) # per-image per-category eval results [KxAxI]
|
123 |
-
self.eval = {} # accumulated evaluation results
|
124 |
-
self._gts = defaultdict(list) # gt for evaluation
|
125 |
-
self._dts = defaultdict(list) # dt for evaluation
|
126 |
-
self.params = Params(iouType=iouType) # parameters
|
127 |
-
self._paramsEval = {} # parameters for evaluation
|
128 |
-
self.stats = [] # result summarization
|
129 |
-
self.ious = {} # ious between all gts and dts
|
130 |
-
if cocoGt is not None:
|
131 |
-
self.params.imgIds = sorted(cocoGt.getImgIds())
|
132 |
-
self.params.catIds = sorted(cocoGt.getCatIds())
|
133 |
-
self.ignoreThrBB = 0.7
|
134 |
-
self.ignoreThrUV = 0.9
|
135 |
-
|
136 |
-
def _loadGEval(self):
|
137 |
-
smpl_subdiv_fpath = PathManager.get_local_path(
|
138 |
-
"https://dl.fbaipublicfiles.com/densepose/data/SMPL_subdiv.mat"
|
139 |
-
)
|
140 |
-
pdist_transform_fpath = PathManager.get_local_path(
|
141 |
-
"https://dl.fbaipublicfiles.com/densepose/data/SMPL_SUBDIV_TRANSFORM.mat"
|
142 |
-
)
|
143 |
-
pdist_matrix_fpath = PathManager.get_local_path(
|
144 |
-
"https://dl.fbaipublicfiles.com/densepose/data/Pdist_matrix.pkl"
|
145 |
-
)
|
146 |
-
SMPL_subdiv = loadmat(smpl_subdiv_fpath)
|
147 |
-
self.PDIST_transform = loadmat(pdist_transform_fpath)
|
148 |
-
self.PDIST_transform = self.PDIST_transform["index"].squeeze()
|
149 |
-
UV = np.array([SMPL_subdiv["U_subdiv"], SMPL_subdiv["V_subdiv"]]).squeeze()
|
150 |
-
ClosestVertInds = np.arange(UV.shape[1]) + 1
|
151 |
-
self.Part_UVs = []
|
152 |
-
self.Part_ClosestVertInds = []
|
153 |
-
for i in np.arange(24):
|
154 |
-
self.Part_UVs.append(UV[:, SMPL_subdiv["Part_ID_subdiv"].squeeze() == (i + 1)])
|
155 |
-
self.Part_ClosestVertInds.append(
|
156 |
-
ClosestVertInds[SMPL_subdiv["Part_ID_subdiv"].squeeze() == (i + 1)]
|
157 |
-
)
|
158 |
-
|
159 |
-
with open(pdist_matrix_fpath, "rb") as hFile:
|
160 |
-
arrays = pickle.load(hFile, encoding="latin1")
|
161 |
-
self.Pdist_matrix = arrays["Pdist_matrix"]
|
162 |
-
self.Part_ids = np.array(SMPL_subdiv["Part_ID_subdiv"].squeeze())
|
163 |
-
# Mean geodesic distances for parts.
|
164 |
-
self.Mean_Distances = np.array([0, 0.351, 0.107, 0.126, 0.237, 0.173, 0.142, 0.128, 0.150])
|
165 |
-
# Coarse Part labels.
|
166 |
-
self.CoarseParts = np.array(
|
167 |
-
[0, 1, 1, 2, 2, 3, 3, 4, 4, 4, 4, 5, 5, 5, 5, 6, 6, 6, 6, 7, 7, 7, 7, 8, 8]
|
168 |
-
)
|
169 |
-
|
170 |
-
def _prepare(self):
|
171 |
-
"""
|
172 |
-
Prepare ._gts and ._dts for evaluation based on params
|
173 |
-
:return: None
|
174 |
-
"""
|
175 |
-
|
176 |
-
def _toMask(anns, coco):
|
177 |
-
# modify ann['segmentation'] by reference
|
178 |
-
for ann in anns:
|
179 |
-
rle = coco.annToRLE(ann)
|
180 |
-
ann["segmentation"] = rle
|
181 |
-
|
182 |
-
def _getIgnoreRegion(iid, coco):
|
183 |
-
img = coco.imgs[iid]
|
184 |
-
|
185 |
-
if "ignore_regions_x" not in img.keys():
|
186 |
-
return None
|
187 |
-
|
188 |
-
if len(img["ignore_regions_x"]) == 0:
|
189 |
-
return None
|
190 |
-
|
191 |
-
rgns_merged = []
|
192 |
-
for region_x, region_y in zip(img["ignore_regions_x"], img["ignore_regions_y"]):
|
193 |
-
rgns = [iter(region_x), iter(region_y)]
|
194 |
-
rgns_merged.append([next(it) for it in itertools.cycle(rgns)])
|
195 |
-
rles = maskUtils.frPyObjects(rgns_merged, img["height"], img["width"])
|
196 |
-
rle = maskUtils.merge(rles)
|
197 |
-
return maskUtils.decode(rle)
|
198 |
-
|
199 |
-
def _checkIgnore(dt, iregion):
|
200 |
-
if iregion is None:
|
201 |
-
return True
|
202 |
-
|
203 |
-
bb = np.array(dt["bbox"]).astype(np.int)
|
204 |
-
x1, y1, x2, y2 = bb[0], bb[1], bb[0] + bb[2], bb[1] + bb[3]
|
205 |
-
x2 = min([x2, iregion.shape[1]])
|
206 |
-
y2 = min([y2, iregion.shape[0]])
|
207 |
-
|
208 |
-
if bb[2] * bb[3] == 0:
|
209 |
-
return False
|
210 |
-
|
211 |
-
crop_iregion = iregion[y1:y2, x1:x2]
|
212 |
-
|
213 |
-
if crop_iregion.sum() == 0:
|
214 |
-
return True
|
215 |
-
|
216 |
-
if "densepose" not in dt.keys(): # filtering boxes
|
217 |
-
return crop_iregion.sum() / bb[2] / bb[3] < self.ignoreThrBB
|
218 |
-
|
219 |
-
# filtering UVs
|
220 |
-
ignoremask = np.require(crop_iregion, requirements=["F"])
|
221 |
-
mask = self._extract_mask(dt)
|
222 |
-
uvmask = np.require(np.asarray(mask > 0), dtype=np.uint8, requirements=["F"])
|
223 |
-
uvmask_ = maskUtils.encode(uvmask)
|
224 |
-
ignoremask_ = maskUtils.encode(ignoremask)
|
225 |
-
uviou = maskUtils.iou([uvmask_], [ignoremask_], [1])[0]
|
226 |
-
return uviou < self.ignoreThrUV
|
227 |
-
|
228 |
-
p = self.params
|
229 |
-
|
230 |
-
if p.useCats:
|
231 |
-
gts = self.cocoGt.loadAnns(self.cocoGt.getAnnIds(imgIds=p.imgIds, catIds=p.catIds))
|
232 |
-
dts = self.cocoDt.loadAnns(self.cocoDt.getAnnIds(imgIds=p.imgIds, catIds=p.catIds))
|
233 |
-
else:
|
234 |
-
gts = self.cocoGt.loadAnns(self.cocoGt.getAnnIds(imgIds=p.imgIds))
|
235 |
-
dts = self.cocoDt.loadAnns(self.cocoDt.getAnnIds(imgIds=p.imgIds))
|
236 |
-
|
237 |
-
imns = self.cocoGt.loadImgs(p.imgIds)
|
238 |
-
self.size_mapping = {}
|
239 |
-
for im in imns:
|
240 |
-
self.size_mapping[im["id"]] = [im["height"], im["width"]]
|
241 |
-
|
242 |
-
# if iouType == 'uv', add point gt annotations
|
243 |
-
if p.iouType == "densepose":
|
244 |
-
self._loadGEval()
|
245 |
-
|
246 |
-
# convert ground truth to mask if iouType == 'segm'
|
247 |
-
if p.iouType == "segm":
|
248 |
-
_toMask(gts, self.cocoGt)
|
249 |
-
_toMask(dts, self.cocoDt)
|
250 |
-
|
251 |
-
# set ignore flag
|
252 |
-
for gt in gts:
|
253 |
-
gt["ignore"] = gt["ignore"] if "ignore" in gt else 0
|
254 |
-
gt["ignore"] = "iscrowd" in gt and gt["iscrowd"]
|
255 |
-
if p.iouType == "keypoints":
|
256 |
-
gt["ignore"] = (gt["num_keypoints"] == 0) or gt["ignore"]
|
257 |
-
if p.iouType == "densepose":
|
258 |
-
gt["ignore"] = ("dp_x" in gt) == 0
|
259 |
-
|
260 |
-
self._gts = defaultdict(list) # gt for evaluation
|
261 |
-
self._dts = defaultdict(list) # dt for evaluation
|
262 |
-
self._igrgns = defaultdict(list)
|
263 |
-
|
264 |
-
for gt in gts:
|
265 |
-
iid = gt["image_id"]
|
266 |
-
if iid not in self._igrgns.keys():
|
267 |
-
self._igrgns[iid] = _getIgnoreRegion(iid, self.cocoGt)
|
268 |
-
if _checkIgnore(gt, self._igrgns[iid]):
|
269 |
-
self._gts[iid, gt["category_id"]].append(gt)
|
270 |
-
for dt in dts:
|
271 |
-
if _checkIgnore(dt, self._igrgns[dt["image_id"]]):
|
272 |
-
self._dts[dt["image_id"], dt["category_id"]].append(dt)
|
273 |
-
|
274 |
-
self.evalImgs = defaultdict(list) # per-image per-category evaluation results
|
275 |
-
self.eval = {} # accumulated evaluation results
|
276 |
-
|
277 |
-
def evaluate(self):
|
278 |
-
"""
|
279 |
-
Run per image evaluation on given images and store results (a list of dict) in self.evalImgs
|
280 |
-
:return: None
|
281 |
-
"""
|
282 |
-
tic = time.time()
|
283 |
-
logger.info("Running per image DensePose evaluation... {}".format(self.params.iouType))
|
284 |
-
p = self.params
|
285 |
-
# add backward compatibility if useSegm is specified in params
|
286 |
-
if p.useSegm is not None:
|
287 |
-
p.iouType = "segm" if p.useSegm == 1 else "bbox"
|
288 |
-
logger.info("useSegm (deprecated) is not None. Running DensePose evaluation")
|
289 |
-
p.imgIds = list(np.unique(p.imgIds))
|
290 |
-
if p.useCats:
|
291 |
-
p.catIds = list(np.unique(p.catIds))
|
292 |
-
p.maxDets = sorted(p.maxDets)
|
293 |
-
self.params = p
|
294 |
-
|
295 |
-
self._prepare()
|
296 |
-
# loop through images, area range, max detection number
|
297 |
-
catIds = p.catIds if p.useCats else [-1]
|
298 |
-
|
299 |
-
if p.iouType in ["segm", "bbox"]:
|
300 |
-
computeIoU = self.computeIoU
|
301 |
-
elif p.iouType == "keypoints":
|
302 |
-
computeIoU = self.computeOks
|
303 |
-
elif p.iouType == "densepose":
|
304 |
-
computeIoU = self.computeOgps
|
305 |
-
if self._dpEvalMode == DensePoseEvalMode.GPSM:
|
306 |
-
self.real_ious = {
|
307 |
-
(imgId, catId): self.computeDPIoU(imgId, catId)
|
308 |
-
for imgId in p.imgIds
|
309 |
-
for catId in catIds
|
310 |
-
}
|
311 |
-
|
312 |
-
self.ious = {
|
313 |
-
(imgId, catId): computeIoU(imgId, catId) for imgId in p.imgIds for catId in catIds
|
314 |
-
}
|
315 |
-
|
316 |
-
evaluateImg = self.evaluateImg
|
317 |
-
maxDet = p.maxDets[-1]
|
318 |
-
self.evalImgs = [
|
319 |
-
evaluateImg(imgId, catId, areaRng, maxDet)
|
320 |
-
for catId in catIds
|
321 |
-
for areaRng in p.areaRng
|
322 |
-
for imgId in p.imgIds
|
323 |
-
]
|
324 |
-
self._paramsEval = copy.deepcopy(self.params)
|
325 |
-
toc = time.time()
|
326 |
-
logger.info("DensePose evaluation DONE (t={:0.2f}s).".format(toc - tic))
|
327 |
-
|
328 |
-
def getDensePoseMask(self, polys):
|
329 |
-
maskGen = np.zeros([256, 256])
|
330 |
-
for i in range(1, 15):
|
331 |
-
if polys[i - 1]:
|
332 |
-
currentMask = maskUtils.decode(polys[i - 1])
|
333 |
-
maskGen[currentMask > 0] = i
|
334 |
-
return maskGen
|
335 |
-
|
336 |
-
def _generate_rlemask_on_image(self, mask, imgId, data):
|
337 |
-
bbox_xywh = np.array(data["bbox"])
|
338 |
-
x, y, w, h = bbox_xywh
|
339 |
-
im_h, im_w = self.size_mapping[imgId]
|
340 |
-
im_mask = np.zeros((im_h, im_w), dtype=np.uint8)
|
341 |
-
if mask is not None:
|
342 |
-
x0 = max(int(x), 0)
|
343 |
-
x1 = min(int(x + w), im_w, int(x) + mask.shape[1])
|
344 |
-
y0 = max(int(y), 0)
|
345 |
-
y1 = min(int(y + h), im_h, int(y) + mask.shape[0])
|
346 |
-
y = int(y)
|
347 |
-
x = int(x)
|
348 |
-
im_mask[y0:y1, x0:x1] = mask[y0 - y : y1 - y, x0 - x : x1 - x]
|
349 |
-
im_mask = np.require(np.asarray(im_mask > 0), dtype=np.uint8, requirements=["F"])
|
350 |
-
rle_mask = maskUtils.encode(np.array(im_mask[:, :, np.newaxis], order="F"))[0]
|
351 |
-
return rle_mask
|
352 |
-
|
353 |
-
def computeDPIoU(self, imgId, catId):
|
354 |
-
p = self.params
|
355 |
-
if p.useCats:
|
356 |
-
gt = self._gts[imgId, catId]
|
357 |
-
dt = self._dts[imgId, catId]
|
358 |
-
else:
|
359 |
-
gt = [_ for cId in p.catIds for _ in self._gts[imgId, cId]]
|
360 |
-
dt = [_ for cId in p.catIds for _ in self._dts[imgId, cId]]
|
361 |
-
if len(gt) == 0 and len(dt) == 0:
|
362 |
-
return []
|
363 |
-
inds = np.argsort([-d["score"] for d in dt], kind="mergesort")
|
364 |
-
dt = [dt[i] for i in inds]
|
365 |
-
if len(dt) > p.maxDets[-1]:
|
366 |
-
dt = dt[0 : p.maxDets[-1]]
|
367 |
-
|
368 |
-
gtmasks = []
|
369 |
-
for g in gt:
|
370 |
-
if DensePoseDataRelative.S_KEY in g.keys():
|
371 |
-
mask = self.getDensePoseMask(g[DensePoseDataRelative.S_KEY])
|
372 |
-
_, _, w, h = g["bbox"]
|
373 |
-
scale_x = float(max(w, 1)) / mask.shape[1]
|
374 |
-
scale_y = float(max(h, 1)) / mask.shape[0]
|
375 |
-
mask = spzoom(mask, (scale_y, scale_x), order=1, prefilter=False)
|
376 |
-
mask = np.array(mask > 0.5, dtype=np.uint8)
|
377 |
-
else:
|
378 |
-
mask = None
|
379 |
-
rle_mask = self._generate_rlemask_on_image(mask, imgId, g)
|
380 |
-
gtmasks.append(rle_mask)
|
381 |
-
|
382 |
-
dtmasks = []
|
383 |
-
for d in dt:
|
384 |
-
mask = self._extract_mask(d)
|
385 |
-
mask = np.require(np.asarray(mask > 0), dtype=np.uint8, requirements=["F"])
|
386 |
-
rle_mask = self._generate_rlemask_on_image(mask, imgId, d)
|
387 |
-
dtmasks.append(rle_mask)
|
388 |
-
|
389 |
-
# compute iou between each dt and gt region
|
390 |
-
iscrowd = [int(o["iscrowd"]) for o in gt]
|
391 |
-
iousDP = maskUtils.iou(dtmasks, gtmasks, iscrowd)
|
392 |
-
return iousDP
|
393 |
-
|
394 |
-
def computeIoU(self, imgId, catId):
|
395 |
-
p = self.params
|
396 |
-
if p.useCats:
|
397 |
-
gt = self._gts[imgId, catId]
|
398 |
-
dt = self._dts[imgId, catId]
|
399 |
-
else:
|
400 |
-
gt = [_ for cId in p.catIds for _ in self._gts[imgId, cId]]
|
401 |
-
dt = [_ for cId in p.catIds for _ in self._dts[imgId, cId]]
|
402 |
-
if len(gt) == 0 and len(dt) == 0:
|
403 |
-
return []
|
404 |
-
inds = np.argsort([-d["score"] for d in dt], kind="mergesort")
|
405 |
-
dt = [dt[i] for i in inds]
|
406 |
-
if len(dt) > p.maxDets[-1]:
|
407 |
-
dt = dt[0 : p.maxDets[-1]]
|
408 |
-
|
409 |
-
if p.iouType == "segm":
|
410 |
-
g = [g["segmentation"] for g in gt]
|
411 |
-
d = [d["segmentation"] for d in dt]
|
412 |
-
elif p.iouType == "bbox":
|
413 |
-
g = [g["bbox"] for g in gt]
|
414 |
-
d = [d["bbox"] for d in dt]
|
415 |
-
else:
|
416 |
-
raise Exception("unknown iouType for iou computation")
|
417 |
-
|
418 |
-
# compute iou between each dt and gt region
|
419 |
-
iscrowd = [int(o["iscrowd"]) for o in gt]
|
420 |
-
ious = maskUtils.iou(d, g, iscrowd)
|
421 |
-
return ious
|
422 |
-
|
423 |
-
def computeOks(self, imgId, catId):
|
424 |
-
p = self.params
|
425 |
-
# dimension here should be Nxm
|
426 |
-
gts = self._gts[imgId, catId]
|
427 |
-
dts = self._dts[imgId, catId]
|
428 |
-
inds = np.argsort([-d["score"] for d in dts], kind="mergesort")
|
429 |
-
dts = [dts[i] for i in inds]
|
430 |
-
if len(dts) > p.maxDets[-1]:
|
431 |
-
dts = dts[0 : p.maxDets[-1]]
|
432 |
-
# if len(gts) == 0 and len(dts) == 0:
|
433 |
-
if len(gts) == 0 or len(dts) == 0:
|
434 |
-
return []
|
435 |
-
ious = np.zeros((len(dts), len(gts)))
|
436 |
-
sigmas = (
|
437 |
-
np.array(
|
438 |
-
[
|
439 |
-
0.26,
|
440 |
-
0.25,
|
441 |
-
0.25,
|
442 |
-
0.35,
|
443 |
-
0.35,
|
444 |
-
0.79,
|
445 |
-
0.79,
|
446 |
-
0.72,
|
447 |
-
0.72,
|
448 |
-
0.62,
|
449 |
-
0.62,
|
450 |
-
1.07,
|
451 |
-
1.07,
|
452 |
-
0.87,
|
453 |
-
0.87,
|
454 |
-
0.89,
|
455 |
-
0.89,
|
456 |
-
]
|
457 |
-
)
|
458 |
-
/ 10.0
|
459 |
-
)
|
460 |
-
vars = (sigmas * 2) ** 2
|
461 |
-
k = len(sigmas)
|
462 |
-
# compute oks between each detection and ground truth object
|
463 |
-
for j, gt in enumerate(gts):
|
464 |
-
# create bounds for ignore regions(double the gt bbox)
|
465 |
-
g = np.array(gt["keypoints"])
|
466 |
-
xg = g[0::3]
|
467 |
-
yg = g[1::3]
|
468 |
-
vg = g[2::3]
|
469 |
-
k1 = np.count_nonzero(vg > 0)
|
470 |
-
bb = gt["bbox"]
|
471 |
-
x0 = bb[0] - bb[2]
|
472 |
-
x1 = bb[0] + bb[2] * 2
|
473 |
-
y0 = bb[1] - bb[3]
|
474 |
-
y1 = bb[1] + bb[3] * 2
|
475 |
-
for i, dt in enumerate(dts):
|
476 |
-
d = np.array(dt["keypoints"])
|
477 |
-
xd = d[0::3]
|
478 |
-
yd = d[1::3]
|
479 |
-
if k1 > 0:
|
480 |
-
# measure the per-keypoint distance if keypoints visible
|
481 |
-
dx = xd - xg
|
482 |
-
dy = yd - yg
|
483 |
-
else:
|
484 |
-
# measure minimum distance to keypoints in (x0,y0) & (x1,y1)
|
485 |
-
z = np.zeros((k))
|
486 |
-
dx = np.max((z, x0 - xd), axis=0) + np.max((z, xd - x1), axis=0)
|
487 |
-
dy = np.max((z, y0 - yd), axis=0) + np.max((z, yd - y1), axis=0)
|
488 |
-
e = (dx ** 2 + dy ** 2) / vars / (gt["area"] + np.spacing(1)) / 2
|
489 |
-
if k1 > 0:
|
490 |
-
e = e[vg > 0]
|
491 |
-
ious[i, j] = np.sum(np.exp(-e)) / e.shape[0]
|
492 |
-
return ious
|
493 |
-
|
494 |
-
def _extract_mask(self, dt: Dict[str, Any]) -> np.ndarray:
|
495 |
-
(densepose_shape, densepose_data_encoded), densepose_bbox_xywh = dt["densepose"]
|
496 |
-
densepose_data = DensePoseResult.decode_png_data(densepose_shape, densepose_data_encoded)
|
497 |
-
return densepose_data[0]
|
498 |
-
|
499 |
-
def _extract_iuv(
|
500 |
-
self, densepose_data: np.ndarray, py: np.ndarray, px: np.ndarray, gt: Dict[str, Any]
|
501 |
-
) -> Tuple[np.ndarray, np.ndarray, np.ndarray]:
|
502 |
-
"""
|
503 |
-
Extract arrays of I, U and V values at given points as numpy arrays
|
504 |
-
given the data mode stored in self._dpDataMode
|
505 |
-
"""
|
506 |
-
if self._dpDataMode == DensePoseDataMode.IUV_DT:
|
507 |
-
# estimated labels and UV (default)
|
508 |
-
ipoints = densepose_data[0, py, px]
|
509 |
-
upoints = densepose_data[1, py, px] / 255.0 # convert from uint8 by /255.
|
510 |
-
vpoints = densepose_data[2, py, px] / 255.0
|
511 |
-
elif self._dpDataMode == DensePoseDataMode.IUV_GT:
|
512 |
-
# ground truth
|
513 |
-
ipoints = np.array(gt["dp_I"])
|
514 |
-
upoints = np.array(gt["dp_U"])
|
515 |
-
vpoints = np.array(gt["dp_V"])
|
516 |
-
elif self._dpDataMode == DensePoseDataMode.I_GT_UV_0:
|
517 |
-
# ground truth labels, UV = 0
|
518 |
-
ipoints = np.array(gt["dp_I"])
|
519 |
-
upoints = upoints * 0.0
|
520 |
-
vpoints = vpoints * 0.0
|
521 |
-
elif self._dpDataMode == DensePoseDataMode.I_GT_UV_DT:
|
522 |
-
# ground truth labels, estimated UV
|
523 |
-
ipoints = np.array(gt["dp_I"])
|
524 |
-
upoints = densepose_data[1, py, px] / 255.0 # convert from uint8 by /255.
|
525 |
-
vpoints = densepose_data[2, py, px] / 255.0
|
526 |
-
elif self._dpDataMode == DensePoseDataMode.I_DT_UV_0:
|
527 |
-
# estimated labels, UV = 0
|
528 |
-
ipoints = densepose_data[0, py, px]
|
529 |
-
upoints = upoints * 0.0
|
530 |
-
vpoints = vpoints * 0.0
|
531 |
-
else:
|
532 |
-
raise ValueError(f"Unknown data mode: {self._dpDataMode}")
|
533 |
-
return ipoints, upoints, vpoints
|
534 |
-
|
535 |
-
def computeOgps(self, imgId, catId):
|
536 |
-
p = self.params
|
537 |
-
# dimension here should be Nxm
|
538 |
-
g = self._gts[imgId, catId]
|
539 |
-
d = self._dts[imgId, catId]
|
540 |
-
inds = np.argsort([-d_["score"] for d_ in d], kind="mergesort")
|
541 |
-
d = [d[i] for i in inds]
|
542 |
-
if len(d) > p.maxDets[-1]:
|
543 |
-
d = d[0 : p.maxDets[-1]]
|
544 |
-
# if len(gts) == 0 and len(dts) == 0:
|
545 |
-
if len(g) == 0 or len(d) == 0:
|
546 |
-
return []
|
547 |
-
ious = np.zeros((len(d), len(g)))
|
548 |
-
# compute opgs between each detection and ground truth object
|
549 |
-
# sigma = self.sigma #0.255 # dist = 0.3m corresponds to ogps = 0.5
|
550 |
-
# 1 # dist = 0.3m corresponds to ogps = 0.96
|
551 |
-
# 1.45 # dist = 1.7m (person height) corresponds to ogps = 0.5)
|
552 |
-
for j, gt in enumerate(g):
|
553 |
-
if not gt["ignore"]:
|
554 |
-
g_ = gt["bbox"]
|
555 |
-
for i, dt in enumerate(d):
|
556 |
-
#
|
557 |
-
dy = int(dt["bbox"][3])
|
558 |
-
dx = int(dt["bbox"][2])
|
559 |
-
dp_x = np.array(gt["dp_x"]) * g_[2] / 255.0
|
560 |
-
dp_y = np.array(gt["dp_y"]) * g_[3] / 255.0
|
561 |
-
py = (dp_y + g_[1] - dt["bbox"][1]).astype(np.int)
|
562 |
-
px = (dp_x + g_[0] - dt["bbox"][0]).astype(np.int)
|
563 |
-
#
|
564 |
-
pts = np.zeros(len(px))
|
565 |
-
pts[px >= dx] = -1
|
566 |
-
pts[py >= dy] = -1
|
567 |
-
pts[px < 0] = -1
|
568 |
-
pts[py < 0] = -1
|
569 |
-
if len(pts) < 1:
|
570 |
-
ogps = 0.0
|
571 |
-
elif np.max(pts) == -1:
|
572 |
-
ogps = 0.0
|
573 |
-
else:
|
574 |
-
px[pts == -1] = 0
|
575 |
-
py[pts == -1] = 0
|
576 |
-
(densepose_shape, densepose_data_encoded), densepose_bbox_xywh = dt[
|
577 |
-
"densepose"
|
578 |
-
]
|
579 |
-
densepose_data = DensePoseResult.decode_png_data(
|
580 |
-
densepose_shape, densepose_data_encoded
|
581 |
-
)
|
582 |
-
assert densepose_data.shape[2] == dx, (
|
583 |
-
"DensePoseData width {} should be equal to "
|
584 |
-
"detection bounding box width {}".format(densepose_data.shape[2], dx)
|
585 |
-
)
|
586 |
-
assert densepose_data.shape[1] == dy, (
|
587 |
-
"DensePoseData height {} should be equal to "
|
588 |
-
"detection bounding box height {}".format(densepose_data.shape[1], dy)
|
589 |
-
)
|
590 |
-
ipoints, upoints, vpoints = self._extract_iuv(densepose_data, py, px, gt)
|
591 |
-
ipoints[pts == -1] = 0
|
592 |
-
# Find closest vertices in subsampled mesh.
|
593 |
-
cVerts, cVertsGT = self.findAllClosestVerts(gt, upoints, vpoints, ipoints)
|
594 |
-
# Get pairwise geodesic distances between gt and estimated mesh points.
|
595 |
-
dist = self.getDistances(cVertsGT, cVerts)
|
596 |
-
# Compute the Ogps measure.
|
597 |
-
# Find the mean geodesic normalization distance for
|
598 |
-
# each GT point, based on which part it is on.
|
599 |
-
Current_Mean_Distances = self.Mean_Distances[
|
600 |
-
self.CoarseParts[self.Part_ids[cVertsGT[cVertsGT > 0].astype(int) - 1]]
|
601 |
-
]
|
602 |
-
# Compute gps
|
603 |
-
ogps_values = np.exp(-(dist ** 2) / (2 * (Current_Mean_Distances ** 2)))
|
604 |
-
#
|
605 |
-
if len(dist) > 0:
|
606 |
-
ogps = np.sum(ogps_values) / len(dist)
|
607 |
-
ious[i, j] = ogps
|
608 |
-
|
609 |
-
gbb = [gt["bbox"] for gt in g]
|
610 |
-
dbb = [dt["bbox"] for dt in d]
|
611 |
-
|
612 |
-
# compute iou between each dt and gt region
|
613 |
-
iscrowd = [int(o["iscrowd"]) for o in g]
|
614 |
-
ious_bb = maskUtils.iou(dbb, gbb, iscrowd)
|
615 |
-
return ious, ious_bb
|
616 |
-
|
617 |
-
def evaluateImg(self, imgId, catId, aRng, maxDet):
|
618 |
-
"""
|
619 |
-
perform evaluation for single category and image
|
620 |
-
:return: dict (single image results)
|
621 |
-
"""
|
622 |
-
|
623 |
-
p = self.params
|
624 |
-
if p.useCats:
|
625 |
-
gt = self._gts[imgId, catId]
|
626 |
-
dt = self._dts[imgId, catId]
|
627 |
-
else:
|
628 |
-
gt = [_ for cId in p.catIds for _ in self._gts[imgId, cId]]
|
629 |
-
dt = [_ for cId in p.catIds for _ in self._dts[imgId, cId]]
|
630 |
-
if len(gt) == 0 and len(dt) == 0:
|
631 |
-
return None
|
632 |
-
|
633 |
-
for g in gt:
|
634 |
-
# g['_ignore'] = g['ignore']
|
635 |
-
if g["ignore"] or (g["area"] < aRng[0] or g["area"] > aRng[1]):
|
636 |
-
g["_ignore"] = True
|
637 |
-
else:
|
638 |
-
g["_ignore"] = False
|
639 |
-
|
640 |
-
# sort dt highest score first, sort gt ignore last
|
641 |
-
gtind = np.argsort([g["_ignore"] for g in gt], kind="mergesort")
|
642 |
-
gt = [gt[i] for i in gtind]
|
643 |
-
dtind = np.argsort([-d["score"] for d in dt], kind="mergesort")
|
644 |
-
dt = [dt[i] for i in dtind[0:maxDet]]
|
645 |
-
iscrowd = [int(o["iscrowd"]) for o in gt]
|
646 |
-
# load computed ious
|
647 |
-
if p.iouType == "densepose":
|
648 |
-
# print('Checking the length', len(self.ious[imgId, catId]))
|
649 |
-
# if len(self.ious[imgId, catId]) == 0:
|
650 |
-
# print(self.ious[imgId, catId])
|
651 |
-
ious = (
|
652 |
-
self.ious[imgId, catId][0][:, gtind]
|
653 |
-
if len(self.ious[imgId, catId]) > 0
|
654 |
-
else self.ious[imgId, catId]
|
655 |
-
)
|
656 |
-
ioubs = (
|
657 |
-
self.ious[imgId, catId][1][:, gtind]
|
658 |
-
if len(self.ious[imgId, catId]) > 0
|
659 |
-
else self.ious[imgId, catId]
|
660 |
-
)
|
661 |
-
if self._dpEvalMode == DensePoseEvalMode.GPSM:
|
662 |
-
iousM = (
|
663 |
-
self.real_ious[imgId, catId][:, gtind]
|
664 |
-
if len(self.real_ious[imgId, catId]) > 0
|
665 |
-
else self.real_ious[imgId, catId]
|
666 |
-
)
|
667 |
-
else:
|
668 |
-
ious = (
|
669 |
-
self.ious[imgId, catId][:, gtind]
|
670 |
-
if len(self.ious[imgId, catId]) > 0
|
671 |
-
else self.ious[imgId, catId]
|
672 |
-
)
|
673 |
-
|
674 |
-
T = len(p.iouThrs)
|
675 |
-
G = len(gt)
|
676 |
-
D = len(dt)
|
677 |
-
gtm = np.zeros((T, G))
|
678 |
-
dtm = np.zeros((T, D))
|
679 |
-
gtIg = np.array([g["_ignore"] for g in gt])
|
680 |
-
dtIg = np.zeros((T, D))
|
681 |
-
if np.all(gtIg) and p.iouType == "densepose":
|
682 |
-
dtIg = np.logical_or(dtIg, True)
|
683 |
-
|
684 |
-
if len(ious) > 0: # and not p.iouType == 'densepose':
|
685 |
-
for tind, t in enumerate(p.iouThrs):
|
686 |
-
for dind, d in enumerate(dt):
|
687 |
-
# information about best match so far (m=-1 -> unmatched)
|
688 |
-
iou = min([t, 1 - 1e-10])
|
689 |
-
m = -1
|
690 |
-
for gind, _g in enumerate(gt):
|
691 |
-
# if this gt already matched, and not a crowd, continue
|
692 |
-
if gtm[tind, gind] > 0 and not iscrowd[gind]:
|
693 |
-
continue
|
694 |
-
# if dt matched to reg gt, and on ignore gt, stop
|
695 |
-
if m > -1 and gtIg[m] == 0 and gtIg[gind] == 1:
|
696 |
-
break
|
697 |
-
if p.iouType == "densepose":
|
698 |
-
if self._dpEvalMode == DensePoseEvalMode.GPSM:
|
699 |
-
new_iou = np.sqrt(iousM[dind, gind] * ious[dind, gind])
|
700 |
-
elif self._dpEvalMode == DensePoseEvalMode.IOU:
|
701 |
-
new_iou = iousM[dind, gind]
|
702 |
-
elif self._dpEvalMode == DensePoseEvalMode.GPS:
|
703 |
-
new_iou = ious[dind, gind]
|
704 |
-
else:
|
705 |
-
new_iou = ious[dind, gind]
|
706 |
-
if new_iou < iou:
|
707 |
-
continue
|
708 |
-
if new_iou == 0.0:
|
709 |
-
continue
|
710 |
-
# if match successful and best so far, store appropriately
|
711 |
-
iou = new_iou
|
712 |
-
m = gind
|
713 |
-
# if match made store id of match for both dt and gt
|
714 |
-
if m == -1:
|
715 |
-
continue
|
716 |
-
dtIg[tind, dind] = gtIg[m]
|
717 |
-
dtm[tind, dind] = gt[m]["id"]
|
718 |
-
gtm[tind, m] = d["id"]
|
719 |
-
|
720 |
-
if p.iouType == "densepose":
|
721 |
-
if not len(ioubs) == 0:
|
722 |
-
for dind, d in enumerate(dt):
|
723 |
-
# information about best match so far (m=-1 -> unmatched)
|
724 |
-
if dtm[tind, dind] == 0:
|
725 |
-
ioub = 0.8
|
726 |
-
m = -1
|
727 |
-
for gind, _g in enumerate(gt):
|
728 |
-
# if this gt already matched, and not a crowd, continue
|
729 |
-
if gtm[tind, gind] > 0 and not iscrowd[gind]:
|
730 |
-
continue
|
731 |
-
# continue to next gt unless better match made
|
732 |
-
if ioubs[dind, gind] < ioub:
|
733 |
-
continue
|
734 |
-
# if match successful and best so far, store appropriately
|
735 |
-
ioub = ioubs[dind, gind]
|
736 |
-
m = gind
|
737 |
-
# if match made store id of match for both dt and gt
|
738 |
-
if m > -1:
|
739 |
-
dtIg[:, dind] = gtIg[m]
|
740 |
-
if gtIg[m]:
|
741 |
-
dtm[tind, dind] = gt[m]["id"]
|
742 |
-
gtm[tind, m] = d["id"]
|
743 |
-
# set unmatched detections outside of area range to ignore
|
744 |
-
a = np.array([d["area"] < aRng[0] or d["area"] > aRng[1] for d in dt]).reshape((1, len(dt)))
|
745 |
-
dtIg = np.logical_or(dtIg, np.logical_and(dtm == 0, np.repeat(a, T, 0)))
|
746 |
-
# store results for given image and category
|
747 |
-
# print('Done with the function', len(self.ious[imgId, catId]))
|
748 |
-
return {
|
749 |
-
"image_id": imgId,
|
750 |
-
"category_id": catId,
|
751 |
-
"aRng": aRng,
|
752 |
-
"maxDet": maxDet,
|
753 |
-
"dtIds": [d["id"] for d in dt],
|
754 |
-
"gtIds": [g["id"] for g in gt],
|
755 |
-
"dtMatches": dtm,
|
756 |
-
"gtMatches": gtm,
|
757 |
-
"dtScores": [d["score"] for d in dt],
|
758 |
-
"gtIgnore": gtIg,
|
759 |
-
"dtIgnore": dtIg,
|
760 |
-
}
|
761 |
-
|
762 |
-
def accumulate(self, p=None):
|
763 |
-
"""
|
764 |
-
Accumulate per image evaluation results and store the result in self.eval
|
765 |
-
:param p: input params for evaluation
|
766 |
-
:return: None
|
767 |
-
"""
|
768 |
-
logger.info("Accumulating evaluation results...")
|
769 |
-
tic = time.time()
|
770 |
-
if not self.evalImgs:
|
771 |
-
logger.info("Please run evaluate() first")
|
772 |
-
# allows input customized parameters
|
773 |
-
if p is None:
|
774 |
-
p = self.params
|
775 |
-
p.catIds = p.catIds if p.useCats == 1 else [-1]
|
776 |
-
T = len(p.iouThrs)
|
777 |
-
R = len(p.recThrs)
|
778 |
-
K = len(p.catIds) if p.useCats else 1
|
779 |
-
A = len(p.areaRng)
|
780 |
-
M = len(p.maxDets)
|
781 |
-
precision = -np.ones((T, R, K, A, M)) # -1 for the precision of absent categories
|
782 |
-
recall = -np.ones((T, K, A, M))
|
783 |
-
|
784 |
-
# create dictionary for future indexing
|
785 |
-
logger.info("Categories: {}".format(p.catIds))
|
786 |
-
_pe = self._paramsEval
|
787 |
-
catIds = _pe.catIds if _pe.useCats else [-1]
|
788 |
-
setK = set(catIds)
|
789 |
-
setA = set(map(tuple, _pe.areaRng))
|
790 |
-
setM = set(_pe.maxDets)
|
791 |
-
setI = set(_pe.imgIds)
|
792 |
-
# get inds to evaluate
|
793 |
-
k_list = [n for n, k in enumerate(p.catIds) if k in setK]
|
794 |
-
m_list = [m for n, m in enumerate(p.maxDets) if m in setM]
|
795 |
-
a_list = [n for n, a in enumerate(map(lambda x: tuple(x), p.areaRng)) if a in setA]
|
796 |
-
i_list = [n for n, i in enumerate(p.imgIds) if i in setI]
|
797 |
-
I0 = len(_pe.imgIds)
|
798 |
-
A0 = len(_pe.areaRng)
|
799 |
-
# retrieve E at each category, area range, and max number of detections
|
800 |
-
for k, k0 in enumerate(k_list):
|
801 |
-
Nk = k0 * A0 * I0
|
802 |
-
for a, a0 in enumerate(a_list):
|
803 |
-
Na = a0 * I0
|
804 |
-
for m, maxDet in enumerate(m_list):
|
805 |
-
E = [self.evalImgs[Nk + Na + i] for i in i_list]
|
806 |
-
E = [e for e in E if e is not None]
|
807 |
-
if len(E) == 0:
|
808 |
-
continue
|
809 |
-
dtScores = np.concatenate([e["dtScores"][0:maxDet] for e in E])
|
810 |
-
|
811 |
-
# different sorting method generates slightly different results.
|
812 |
-
# mergesort is used to be consistent as Matlab implementation.
|
813 |
-
inds = np.argsort(-dtScores, kind="mergesort")
|
814 |
-
|
815 |
-
dtm = np.concatenate([e["dtMatches"][:, 0:maxDet] for e in E], axis=1)[:, inds]
|
816 |
-
dtIg = np.concatenate([e["dtIgnore"][:, 0:maxDet] for e in E], axis=1)[:, inds]
|
817 |
-
gtIg = np.concatenate([e["gtIgnore"] for e in E])
|
818 |
-
npig = np.count_nonzero(gtIg == 0)
|
819 |
-
if npig == 0:
|
820 |
-
continue
|
821 |
-
tps = np.logical_and(dtm, np.logical_not(dtIg))
|
822 |
-
fps = np.logical_and(np.logical_not(dtm), np.logical_not(dtIg))
|
823 |
-
tp_sum = np.cumsum(tps, axis=1).astype(dtype=np.float)
|
824 |
-
fp_sum = np.cumsum(fps, axis=1).astype(dtype=np.float)
|
825 |
-
for t, (tp, fp) in enumerate(zip(tp_sum, fp_sum)):
|
826 |
-
tp = np.array(tp)
|
827 |
-
fp = np.array(fp)
|
828 |
-
nd = len(tp)
|
829 |
-
rc = tp / npig
|
830 |
-
pr = tp / (fp + tp + np.spacing(1))
|
831 |
-
q = np.zeros((R,))
|
832 |
-
|
833 |
-
if nd:
|
834 |
-
recall[t, k, a, m] = rc[-1]
|
835 |
-
else:
|
836 |
-
recall[t, k, a, m] = 0
|
837 |
-
|
838 |
-
# numpy is slow without cython optimization for accessing elements
|
839 |
-
# use python array gets significant speed improvement
|
840 |
-
pr = pr.tolist()
|
841 |
-
q = q.tolist()
|
842 |
-
|
843 |
-
for i in range(nd - 1, 0, -1):
|
844 |
-
if pr[i] > pr[i - 1]:
|
845 |
-
pr[i - 1] = pr[i]
|
846 |
-
|
847 |
-
inds = np.searchsorted(rc, p.recThrs, side="left")
|
848 |
-
try:
|
849 |
-
for ri, pi in enumerate(inds):
|
850 |
-
q[ri] = pr[pi]
|
851 |
-
except Exception:
|
852 |
-
pass
|
853 |
-
precision[t, :, k, a, m] = np.array(q)
|
854 |
-
logger.info(
|
855 |
-
"Final: max precision {}, min precision {}".format(np.max(precision), np.min(precision))
|
856 |
-
)
|
857 |
-
self.eval = {
|
858 |
-
"params": p,
|
859 |
-
"counts": [T, R, K, A, M],
|
860 |
-
"date": datetime.datetime.now().strftime("%Y-%m-%d %H:%M:%S"),
|
861 |
-
"precision": precision,
|
862 |
-
"recall": recall,
|
863 |
-
}
|
864 |
-
toc = time.time()
|
865 |
-
logger.info("DONE (t={:0.2f}s).".format(toc - tic))
|
866 |
-
|
867 |
-
def summarize(self):
|
868 |
-
"""
|
869 |
-
Compute and display summary metrics for evaluation results.
|
870 |
-
Note this function can *only* be applied on the default parameter setting
|
871 |
-
"""
|
872 |
-
|
873 |
-
def _summarize(ap=1, iouThr=None, areaRng="all", maxDets=100):
|
874 |
-
p = self.params
|
875 |
-
iStr = " {:<18} {} @[ {}={:<9} | area={:>6s} | maxDets={:>3d} ] = {:0.3f}"
|
876 |
-
titleStr = "Average Precision" if ap == 1 else "Average Recall"
|
877 |
-
typeStr = "(AP)" if ap == 1 else "(AR)"
|
878 |
-
measure = "IoU"
|
879 |
-
if self.params.iouType == "keypoints":
|
880 |
-
measure = "OKS"
|
881 |
-
elif self.params.iouType == "densepose":
|
882 |
-
measure = "OGPS"
|
883 |
-
iouStr = (
|
884 |
-
"{:0.2f}:{:0.2f}".format(p.iouThrs[0], p.iouThrs[-1])
|
885 |
-
if iouThr is None
|
886 |
-
else "{:0.2f}".format(iouThr)
|
887 |
-
)
|
888 |
-
|
889 |
-
aind = [i for i, aRng in enumerate(p.areaRngLbl) if aRng == areaRng]
|
890 |
-
mind = [i for i, mDet in enumerate(p.maxDets) if mDet == maxDets]
|
891 |
-
if ap == 1:
|
892 |
-
# dimension of precision: [TxRxKxAxM]
|
893 |
-
s = self.eval["precision"]
|
894 |
-
# IoU
|
895 |
-
if iouThr is not None:
|
896 |
-
t = np.where(np.abs(iouThr - p.iouThrs) < 0.001)[0]
|
897 |
-
s = s[t]
|
898 |
-
s = s[:, :, :, aind, mind]
|
899 |
-
else:
|
900 |
-
# dimension of recall: [TxKxAxM]
|
901 |
-
s = self.eval["recall"]
|
902 |
-
if iouThr is not None:
|
903 |
-
t = np.where(iouThr == p.iouThrs)[0]
|
904 |
-
s = s[t]
|
905 |
-
s = s[:, :, aind, mind]
|
906 |
-
if len(s[s > -1]) == 0:
|
907 |
-
mean_s = -1
|
908 |
-
else:
|
909 |
-
mean_s = np.mean(s[s > -1])
|
910 |
-
logger.info(iStr.format(titleStr, typeStr, measure, iouStr, areaRng, maxDets, mean_s))
|
911 |
-
return mean_s
|
912 |
-
|
913 |
-
def _summarizeDets():
|
914 |
-
stats = np.zeros((12,))
|
915 |
-
stats[0] = _summarize(1)
|
916 |
-
stats[1] = _summarize(1, iouThr=0.5, maxDets=self.params.maxDets[2])
|
917 |
-
stats[2] = _summarize(1, iouThr=0.75, maxDets=self.params.maxDets[2])
|
918 |
-
stats[3] = _summarize(1, areaRng="small", maxDets=self.params.maxDets[2])
|
919 |
-
stats[4] = _summarize(1, areaRng="medium", maxDets=self.params.maxDets[2])
|
920 |
-
stats[5] = _summarize(1, areaRng="large", maxDets=self.params.maxDets[2])
|
921 |
-
stats[6] = _summarize(0, maxDets=self.params.maxDets[0])
|
922 |
-
stats[7] = _summarize(0, maxDets=self.params.maxDets[1])
|
923 |
-
stats[8] = _summarize(0, maxDets=self.params.maxDets[2])
|
924 |
-
stats[9] = _summarize(0, areaRng="small", maxDets=self.params.maxDets[2])
|
925 |
-
stats[10] = _summarize(0, areaRng="medium", maxDets=self.params.maxDets[2])
|
926 |
-
stats[11] = _summarize(0, areaRng="large", maxDets=self.params.maxDets[2])
|
927 |
-
return stats
|
928 |
-
|
929 |
-
def _summarizeKps():
|
930 |
-
stats = np.zeros((10,))
|
931 |
-
stats[0] = _summarize(1, maxDets=20)
|
932 |
-
stats[1] = _summarize(1, maxDets=20, iouThr=0.5)
|
933 |
-
stats[2] = _summarize(1, maxDets=20, iouThr=0.75)
|
934 |
-
stats[3] = _summarize(1, maxDets=20, areaRng="medium")
|
935 |
-
stats[4] = _summarize(1, maxDets=20, areaRng="large")
|
936 |
-
stats[5] = _summarize(0, maxDets=20)
|
937 |
-
stats[6] = _summarize(0, maxDets=20, iouThr=0.5)
|
938 |
-
stats[7] = _summarize(0, maxDets=20, iouThr=0.75)
|
939 |
-
stats[8] = _summarize(0, maxDets=20, areaRng="medium")
|
940 |
-
stats[9] = _summarize(0, maxDets=20, areaRng="large")
|
941 |
-
return stats
|
942 |
-
|
943 |
-
def _summarizeUvs():
|
944 |
-
stats = np.zeros((10,))
|
945 |
-
stats[0] = _summarize(1, maxDets=self.params.maxDets[0])
|
946 |
-
stats[1] = _summarize(1, maxDets=self.params.maxDets[0], iouThr=0.5)
|
947 |
-
stats[2] = _summarize(1, maxDets=self.params.maxDets[0], iouThr=0.75)
|
948 |
-
stats[3] = _summarize(1, maxDets=self.params.maxDets[0], areaRng="medium")
|
949 |
-
stats[4] = _summarize(1, maxDets=self.params.maxDets[0], areaRng="large")
|
950 |
-
stats[5] = _summarize(0, maxDets=self.params.maxDets[0])
|
951 |
-
stats[6] = _summarize(0, maxDets=self.params.maxDets[0], iouThr=0.5)
|
952 |
-
stats[7] = _summarize(0, maxDets=self.params.maxDets[0], iouThr=0.75)
|
953 |
-
stats[8] = _summarize(0, maxDets=self.params.maxDets[0], areaRng="medium")
|
954 |
-
stats[9] = _summarize(0, maxDets=self.params.maxDets[0], areaRng="large")
|
955 |
-
return stats
|
956 |
-
|
957 |
-
def _summarizeUvsOld():
|
958 |
-
stats = np.zeros((18,))
|
959 |
-
stats[0] = _summarize(1, maxDets=self.params.maxDets[0])
|
960 |
-
stats[1] = _summarize(1, maxDets=self.params.maxDets[0], iouThr=0.5)
|
961 |
-
stats[2] = _summarize(1, maxDets=self.params.maxDets[0], iouThr=0.55)
|
962 |
-
stats[3] = _summarize(1, maxDets=self.params.maxDets[0], iouThr=0.60)
|
963 |
-
stats[4] = _summarize(1, maxDets=self.params.maxDets[0], iouThr=0.65)
|
964 |
-
stats[5] = _summarize(1, maxDets=self.params.maxDets[0], iouThr=0.70)
|
965 |
-
stats[6] = _summarize(1, maxDets=self.params.maxDets[0], iouThr=0.75)
|
966 |
-
stats[7] = _summarize(1, maxDets=self.params.maxDets[0], iouThr=0.80)
|
967 |
-
stats[8] = _summarize(1, maxDets=self.params.maxDets[0], iouThr=0.85)
|
968 |
-
stats[9] = _summarize(1, maxDets=self.params.maxDets[0], iouThr=0.90)
|
969 |
-
stats[10] = _summarize(1, maxDets=self.params.maxDets[0], iouThr=0.95)
|
970 |
-
stats[11] = _summarize(1, maxDets=self.params.maxDets[0], areaRng="medium")
|
971 |
-
stats[12] = _summarize(1, maxDets=self.params.maxDets[0], areaRng="large")
|
972 |
-
stats[13] = _summarize(0, maxDets=self.params.maxDets[0])
|
973 |
-
stats[14] = _summarize(0, maxDets=self.params.maxDets[0], iouThr=0.5)
|
974 |
-
stats[15] = _summarize(0, maxDets=self.params.maxDets[0], iouThr=0.75)
|
975 |
-
stats[16] = _summarize(0, maxDets=self.params.maxDets[0], areaRng="medium")
|
976 |
-
stats[17] = _summarize(0, maxDets=self.params.maxDets[0], areaRng="large")
|
977 |
-
return stats
|
978 |
-
|
979 |
-
if not self.eval:
|
980 |
-
raise Exception("Please run accumulate() first")
|
981 |
-
iouType = self.params.iouType
|
982 |
-
if iouType in ["segm", "bbox"]:
|
983 |
-
summarize = _summarizeDets
|
984 |
-
elif iouType in ["keypoints"]:
|
985 |
-
summarize = _summarizeKps
|
986 |
-
elif iouType in ["densepose"]:
|
987 |
-
summarize = _summarizeUvs
|
988 |
-
self.stats = summarize()
|
989 |
-
|
990 |
-
def __str__(self):
|
991 |
-
self.summarize()
|
992 |
-
|
993 |
-
# ================ functions for dense pose ==============================
|
994 |
-
def findAllClosestVerts(self, gt, U_points, V_points, Index_points):
|
995 |
-
#
|
996 |
-
I_gt = np.array(gt["dp_I"])
|
997 |
-
U_gt = np.array(gt["dp_U"])
|
998 |
-
V_gt = np.array(gt["dp_V"])
|
999 |
-
#
|
1000 |
-
# print(I_gt)
|
1001 |
-
#
|
1002 |
-
ClosestVerts = np.ones(Index_points.shape) * -1
|
1003 |
-
for i in np.arange(24):
|
1004 |
-
#
|
1005 |
-
if sum(Index_points == (i + 1)) > 0:
|
1006 |
-
UVs = np.array(
|
1007 |
-
[U_points[Index_points == (i + 1)], V_points[Index_points == (i + 1)]]
|
1008 |
-
)
|
1009 |
-
Current_Part_UVs = self.Part_UVs[i]
|
1010 |
-
Current_Part_ClosestVertInds = self.Part_ClosestVertInds[i]
|
1011 |
-
D = ssd.cdist(Current_Part_UVs.transpose(), UVs.transpose()).squeeze()
|
1012 |
-
ClosestVerts[Index_points == (i + 1)] = Current_Part_ClosestVertInds[
|
1013 |
-
np.argmin(D, axis=0)
|
1014 |
-
]
|
1015 |
-
#
|
1016 |
-
ClosestVertsGT = np.ones(Index_points.shape) * -1
|
1017 |
-
for i in np.arange(24):
|
1018 |
-
if sum(I_gt == (i + 1)) > 0:
|
1019 |
-
UVs = np.array([U_gt[I_gt == (i + 1)], V_gt[I_gt == (i + 1)]])
|
1020 |
-
Current_Part_UVs = self.Part_UVs[i]
|
1021 |
-
Current_Part_ClosestVertInds = self.Part_ClosestVertInds[i]
|
1022 |
-
D = ssd.cdist(Current_Part_UVs.transpose(), UVs.transpose()).squeeze()
|
1023 |
-
ClosestVertsGT[I_gt == (i + 1)] = Current_Part_ClosestVertInds[np.argmin(D, axis=0)]
|
1024 |
-
#
|
1025 |
-
return ClosestVerts, ClosestVertsGT
|
1026 |
-
|
1027 |
-
def getDistances(self, cVertsGT, cVerts):
|
1028 |
-
|
1029 |
-
ClosestVertsTransformed = self.PDIST_transform[cVerts.astype(int) - 1]
|
1030 |
-
ClosestVertsGTTransformed = self.PDIST_transform[cVertsGT.astype(int) - 1]
|
1031 |
-
#
|
1032 |
-
ClosestVertsTransformed[cVerts < 0] = 0
|
1033 |
-
ClosestVertsGTTransformed[cVertsGT < 0] = 0
|
1034 |
-
#
|
1035 |
-
cVertsGT = ClosestVertsGTTransformed
|
1036 |
-
cVerts = ClosestVertsTransformed
|
1037 |
-
#
|
1038 |
-
n = 27554
|
1039 |
-
dists = []
|
1040 |
-
for d in range(len(cVertsGT)):
|
1041 |
-
if cVertsGT[d] > 0:
|
1042 |
-
if cVerts[d] > 0:
|
1043 |
-
i = cVertsGT[d] - 1
|
1044 |
-
j = cVerts[d] - 1
|
1045 |
-
if j == i:
|
1046 |
-
dists.append(0)
|
1047 |
-
elif j > i:
|
1048 |
-
ccc = i
|
1049 |
-
i = j
|
1050 |
-
j = ccc
|
1051 |
-
i = n - i - 1
|
1052 |
-
j = n - j - 1
|
1053 |
-
k = (n * (n - 1) / 2) - (n - i) * ((n - i) - 1) / 2 + j - i - 1
|
1054 |
-
k = (n * n - n) / 2 - k - 1
|
1055 |
-
dists.append(self.Pdist_matrix[int(k)][0])
|
1056 |
-
else:
|
1057 |
-
i = n - i - 1
|
1058 |
-
j = n - j - 1
|
1059 |
-
k = (n * (n - 1) / 2) - (n - i) * ((n - i) - 1) / 2 + j - i - 1
|
1060 |
-
k = (n * n - n) / 2 - k - 1
|
1061 |
-
dists.append(self.Pdist_matrix[int(k)][0])
|
1062 |
-
else:
|
1063 |
-
dists.append(np.inf)
|
1064 |
-
return np.array(dists).squeeze()
|
1065 |
-
|
1066 |
-
|
1067 |
-
class Params:
|
1068 |
-
"""
|
1069 |
-
Params for coco evaluation api
|
1070 |
-
"""
|
1071 |
-
|
1072 |
-
def setDetParams(self):
|
1073 |
-
self.imgIds = []
|
1074 |
-
self.catIds = []
|
1075 |
-
# np.arange causes trouble. the data point on arange is slightly larger than the true value
|
1076 |
-
self.iouThrs = np.linspace(0.5, 0.95, np.round((0.95 - 0.5) / 0.05) + 1, endpoint=True)
|
1077 |
-
self.recThrs = np.linspace(0.0, 1.00, np.round((1.00 - 0.0) / 0.01) + 1, endpoint=True)
|
1078 |
-
self.maxDets = [1, 10, 100]
|
1079 |
-
self.areaRng = [
|
1080 |
-
[0 ** 2, 1e5 ** 2],
|
1081 |
-
[0 ** 2, 32 ** 2],
|
1082 |
-
[32 ** 2, 96 ** 2],
|
1083 |
-
[96 ** 2, 1e5 ** 2],
|
1084 |
-
]
|
1085 |
-
self.areaRngLbl = ["all", "small", "medium", "large"]
|
1086 |
-
self.useCats = 1
|
1087 |
-
|
1088 |
-
def setKpParams(self):
|
1089 |
-
self.imgIds = []
|
1090 |
-
self.catIds = []
|
1091 |
-
# np.arange causes trouble. the data point on arange is slightly larger than the true value
|
1092 |
-
self.iouThrs = np.linspace(0.5, 0.95, np.round((0.95 - 0.5) / 0.05) + 1, endpoint=True)
|
1093 |
-
self.recThrs = np.linspace(0.0, 1.00, np.round((1.00 - 0.0) / 0.01) + 1, endpoint=True)
|
1094 |
-
self.maxDets = [20]
|
1095 |
-
self.areaRng = [[0 ** 2, 1e5 ** 2], [32 ** 2, 96 ** 2], [96 ** 2, 1e5 ** 2]]
|
1096 |
-
self.areaRngLbl = ["all", "medium", "large"]
|
1097 |
-
self.useCats = 1
|
1098 |
-
|
1099 |
-
def setUvParams(self):
|
1100 |
-
self.imgIds = []
|
1101 |
-
self.catIds = []
|
1102 |
-
self.iouThrs = np.linspace(0.5, 0.95, int(np.round((0.95 - 0.5) / 0.05)) + 1, endpoint=True)
|
1103 |
-
self.recThrs = np.linspace(0.0, 1.00, int(np.round((1.00 - 0.0) / 0.01)) + 1, endpoint=True)
|
1104 |
-
self.maxDets = [20]
|
1105 |
-
self.areaRng = [[0 ** 2, 1e5 ** 2], [32 ** 2, 96 ** 2], [96 ** 2, 1e5 ** 2]]
|
1106 |
-
self.areaRngLbl = ["all", "medium", "large"]
|
1107 |
-
self.useCats = 1
|
1108 |
-
|
1109 |
-
def __init__(self, iouType="segm"):
|
1110 |
-
if iouType == "segm" or iouType == "bbox":
|
1111 |
-
self.setDetParams()
|
1112 |
-
elif iouType == "keypoints":
|
1113 |
-
self.setKpParams()
|
1114 |
-
elif iouType == "densepose":
|
1115 |
-
self.setUvParams()
|
1116 |
-
else:
|
1117 |
-
raise Exception("iouType not supported")
|
1118 |
-
self.iouType = iouType
|
1119 |
-
# useSegm is deprecated
|
1120 |
-
self.useSegm = None
|
|
|
|
|
|
|
|
|
|
|
|
|
|
|
|
|
|
|
|
|
|
|
|
|
|
|
|
|
|
|
|
|
|
|
|
|
|
|
|
|
|
|
|
|
|
|
|
|
|
|
|
|
|
|
|
|
|
|
|
|
|
|
|
|
|
|
|
|
|
|
|
|
|
|
|
|
|
|
|
|
|
|
|
|
|
|
|
|
|
|
|
|
|
|
|
|
|
|
|
|
|
|
|
|
|
|
|
|
|
|
|
|
|
|
|
|
|
|
|
|
|
|
|
|
|
|
|
|
|
|
|
|
|
|
|
|
|
|
|
|
|
|
|
|
|
|
|
|
|
|
|
|
|
|
|
|
|
|
|
|
|
|
|
|
|
|
|
|
|
|
|
|
|
|
|
|
|
|
|
|
|
|
|
|
|
|
|
|
|
|
|
|
|
|
|
|
|
|
|
|
|
|
|
|
|
|
|
|
|
|
|
|
|
|
|
|
|
|
|
|
|
|
|
|
|
|
|
|
|
|
|
|
|
|
|
|
|
|
|
|
|
|
|
|
|
|
|
|
|
|
|
|
|
|
|
|
|
|
|
|
|
|
|
|
|
|
|
|
|
|
|
|
|
|
|
|
|
|
|
|
|
|
|
|
|
|
|
|
|
|
|
|
|
|
|
|
|
|
|
|
|
|
|
|
|
|
|
|
|
|
|
|
|
|
|
|
|
|
|
|
|
|
|
|
|
|
|
|
|
|
|
|
|
|
|
|
|
|
|
|
|
|
|
|
|
|
|
|
|
|
|
|
|
|
|
|
|
|
|
|
|
|
|
|
|
|
|
|
|
|
|
|
|
|
|
|
|
|
|
|
|
|
|
|
|
|
|
|
|
|
|
|
|
|
|
|
|
|
|
|
|
|
|
|
|
|
|
|
|
|
|
|
|
|
|
|
|
|
|
|
|
|
|
|
|
|
|
|
|
|
|
|
|
|
|
|
|
|
|
|
|
|
|
|
|
|
|
|
|
|
|
|
|
|
|
|
|
|
|
|
|
|
|
|
|
|
|
|
|
|
|
|
|
|
|
|
|
|
|
|
|
|
|
|
|
|
|
|
|
|
|
|
|
|
|
|
|
|
|
|
|
|
|
|
|
|
|
|
|
|
|
|
|
|
|
|
|
|
|
|
|
|
|
|
|
|
|
|
|
|
|
|
|
|
|
|
|
|
|
|
|
|
|
|
|
|
|
|
|
|
|
|
|
|
|
|
|
|
|
|
|
|
|
|
|
|
|
|
|
|
|
|
|
|
|
|
|
|
|
|
|
|
|
|
|
|
|
|
|
|
|
|
|
|
|
|
|
|
|
|
|
|
|
|
|
|
|
|
|
|
|
|
|
|
|
|
|
|
|
|
|
|
|
|
|
|
|
|
|
|
|
|
|
|
|
|
|
|
|
|
|
|
|
|
|
|
|
|
|
|
|
|
|
|
|
|
|
|
|
|
|
|
|
|
|
|
|
|
|
|
|
|
|
|
|
|
|
|
|
|
|
|
|
|
|
|
|
|
|
|
|
|
|
|
|
|
|
|
|
|
|
|
|
|
|
|
|
|
|
|
|
|
|
|
|
|
|
|
|
|
|
|
|
|
|
|
|
|
|
|
|
|
|
|
|
|
|
|
|
|
|
|
|
|
|
|
|
|
|
|
|
|
|
|
|
|
|
|
|
|
|
|
|
|
|
|
|
|
|
|
|
|
|
|
|
|
|
|
|
|
|
|
|
|
|
|
|
|
|
|
|
|
|
|
|
|
|
|
|
|
|
|
|
|
|
|
|
|
|
|
|
|
|
|
|
|
|
|
|
|
|
|
|
|
|
|
|
|
|
|
|
|
|
|
|
|
|
|
|
|
|
|
|
|
|
|
|
|
|
|
|
|
|
|
|
|
|
|
|
|
|
|
|
|
|
|
|
|
|
|
|
|
|
|
|
|
|
|
|
|
|
|
|
|
|
|
|
|
|
|
|
|
|
|
|
|
|
|
|
|
|
|
|
|
|
|
|
|
|
|
|
|
|
|
|
|
|
|
|
|
|
|
|
|
|
|
|
|
|
|
|
|
|
|
|
|
|
|
|
|
|
|
|
|
|
|
|
|
|
|
|
|
|
|
|
|
|
|
|
|
|
|
|
|
|
|
|
|
|
|
|
|
|
|
|
|
|
|
|
|
|
|
|
|
|
|
|
|
|
|
|
|
|
|
|
|
|
|
|
|
|
|
|
|
|
|
|
|
|
|
|
|
|
|
|
|
|
|
|
|
|
|
|
|
|
|
|
|
|
|
|
|
|
|
|
|
|
|
|
|
|
|
|
|
|
|
|
|
|
|
|
|
|
|
|
|
|
|
|
|
|
|
|
|
|
|
|
|
|
|
|
|
|
|
|
|
|
|
|
|
|
|
|
|
|
|
|
|
|
|
|
|
|
|
|
|
|
|
|
|
|
|
|
|
|
|
|
|
|
|
|
|
|
|
|
|
|
|
|
|
|
|
|
|
|
|
|
|
|
|
|
|
|
|
|
|
|
|
|
|
|
|
|
|
|
|
|
|
|
|
|
|
|
|
|
|
|
|
|
|
|
|
|
|
|
|
|
|
|
|
|
|
|
|
|
|
|
|
|
|
|
|
|
|
|
|
|
|
|
|
|
|
|
|
|
|
|
|
|
|
|
|
|
|
|
|
|
|
|
|
|
|
|
|
|
|
|
|
|
|
|
|
|
|
|
|
|
|
|
|
|
|
|
|
|
|
|
|
|
|
|
|
|
|
|
|
|
|
|
|
|
|
|
|
|
|
|
|
|
|
|
|
|
|
|
|
|
|
|
|
|
|
|
|
|
|
|
|
|
|
|
|
|
|
|
|
|
|
|
|
|
|
|
|
|
|
|
|
|
|
|
|
|
|
|
|
|
|
|
|
|
|
|
|
|
|
|
|
|
|
|
|
|
|
|
|
|
|
|
|
|
|
|
|
|
|
|
|
|
|
|
|
|
|
|
|
|
|
|
|
|
|
|
|
|
|
|
|
|
|
|
|
|
|
|
|
|
|
|
|
|
|
|
|
|
|
|
|
|
|
|
|
|
|
|
|
|
|
|
|
|
|
|
|
|
|
|
|
|
|
|
|
|
|
|
|
|
|
|
|
|
|
|
|
|
|
|
|
|
|
|
|
|
|
|
|
|
|
|
|
|
|
|
|
|
|
|
|
|
|
|
|
|
|
|
|
|
|
|
|
|
|
|
|
|
|
|
|
|
|
|
|
|
|
|
|
|
|
|
|
|
|
|
|
|
|
|
|
|
|
|
|
|
|
|
|
|
|
|
|
|
|
|
|
|
|
|
|
|
|
|
|
|
|
|
|
|
|
|
|
|
|
|
|
|
|
|
|
|
|
|
|
|
|
|
|
|
|
|
|
|
|
|
|
|
|
|
|
|
|
|
|
|
|
|
|
|
|
|
|
|
|
|
|
|
|
|
|
|
|
|
|
|
|
|
|
|
|
|
|
|
|
|
|
|
|
|
|
|
|
|
|
|
|
|
|
|
|
|
|
|
|
|
|
|
|
|
|
|
|
|
|
|
|
|
|
|
|
|
|
|
|
|
|
|
|
|
|
|
|
|
|
|
|
|
|
|
|
|
|
|
|
|
|
|
|
|
|
|
|
|
|
|
|
|
|
|
|
|
|
|
|
|
|
|
|
|
|
|
|
|
|
|
|
|
|
|
|
|
|
|
|
|
|
|
|
|
|
|
|
|
|
|
|
|
|
|
|
|
|
|
|
|
|
|
|
|
|
|
|
|
|
|
|
|
|
|
|
|
|
|
|
|
|
|
|
|
|
|
|
|
|
|
|
|
|
|
|
|
|
|
|
|
|
|
|
|
|
|
|
|
|
|
|
|
|
|
|
|
|
|
|
|
|
|
|
|
|
|
|
|
|
|
|
|
|
|
|
|
|
|
|
|
|
|
|
|
|
|
|
|
|
|
|
|
|
|
|
|
|
|
|
|
|
|
|
|
|
|
|
|
|
|
|
|
|
|
|
|
|
|
|
|
|
|
|
|
|
|
|
|
|
|
|
|
|
|
|
|
|
|
|
|
|
|
|
|
|
|
|
|
|
|
|
|
|
|
|
|
|
|
|
|
|
|
|
|
|
|
|
|
|
|
|
|
|
|
|
|
|
|
|
|
|
|
|
|
|
|
|
|
|
|
|
|
|
|
|
|
|
|
|
|
|
|
|
|
|
|
|
|
|
|
|
|
|
|
|
|
|
|
|
|
|
|
|
|
|
|
|
|
|
|
|
|
|
|
|
|
|
|
|
|
|
|
|
|
|
|
|
|
|
|
|
|
|
|
|
|
|
|
|
|
|
|
|
|
|
|
|
|
|
|
|
|
|
|
|
|
|
|
|
|
|
|
|
|
|
|
|
|
|
|
|
|
|
|
|
|
|
|
|
|
|
|
|
|
|
|
|
|
|
|
|
|
|
|
|
|
|
|
|
|
|
|
|
|
|
|
|
|
|
|
|
|
|
|
|
|
|
|
|
|
|
|
|
|
|
|
|
|
|
|
|
|
|
|
|
|
|
|
|
|
|
|
|
|
|
|
|
|
|
|
|
|
|
|
|
|
|
|
|
|
|
|
|
|
|
|
|
|
|
|
|
|
|
|
|
|
|
|
|
|
|
|
|
|
|
|
|
|
|
|
|
|
|
|
|
|
|
|
|
|
|
|
|
|
|
|
|
|
|
|
|
|
|
|
|
|
|
|
|
|
|
|
|
|
|
|
|
|
|
|
|
|
|
|
|
|
|
|
|
|
|
|
|
|
|
|
|
|
|
|
|
|
|
|
|
|
|
|
|
|
|
|
|
|
|
|
|
|
|
|
|
|
|
|
|
|
|
|
|
|
|
|
|
|
|
|
|
|
|
|
|
|
|
|
|
|
|
|
|
|
|
|
|
|
|
|
|
|
|
|
|
|
|
|
|
|
|
|
|
|
|
|
|
|
|
|
|
|
spaces/CVPR/Example-Echocardiogram-Segmentation/app.py
DELETED
@@ -1,93 +0,0 @@
|
|
1 |
-
import os, os.path
|
2 |
-
from os.path import splitext
|
3 |
-
import numpy as np
|
4 |
-
import sys
|
5 |
-
import matplotlib.pyplot as plt
|
6 |
-
import torch
|
7 |
-
import torchvision
|
8 |
-
import wget
|
9 |
-
|
10 |
-
|
11 |
-
destination_folder = "output"
|
12 |
-
destination_for_weights = "weights"
|
13 |
-
|
14 |
-
if os.path.exists(destination_for_weights):
|
15 |
-
print("The weights are at", destination_for_weights)
|
16 |
-
else:
|
17 |
-
print("Creating folder at ", destination_for_weights, " to store weights")
|
18 |
-
os.mkdir(destination_for_weights)
|
19 |
-
|
20 |
-
segmentationWeightsURL = 'https://github.com/douyang/EchoNetDynamic/releases/download/v1.0.0/deeplabv3_resnet50_random.pt'
|
21 |
-
|
22 |
-
if not os.path.exists(os.path.join(destination_for_weights, os.path.basename(segmentationWeightsURL))):
|
23 |
-
print("Downloading Segmentation Weights, ", segmentationWeightsURL," to ",os.path.join(destination_for_weights, os.path.basename(segmentationWeightsURL)))
|
24 |
-
filename = wget.download(segmentationWeightsURL, out = destination_for_weights)
|
25 |
-
else:
|
26 |
-
print("Segmentation Weights already present")
|
27 |
-
|
28 |
-
torch.cuda.empty_cache()
|
29 |
-
|
30 |
-
def collate_fn(x):
|
31 |
-
x, f = zip(*x)
|
32 |
-
i = list(map(lambda t: t.shape[1], x))
|
33 |
-
x = torch.as_tensor(np.swapaxes(np.concatenate(x, 1), 0, 1))
|
34 |
-
return x, f, i
|
35 |
-
|
36 |
-
model = torchvision.models.segmentation.deeplabv3_resnet50(pretrained=False, aux_loss=False)
|
37 |
-
model.classifier[-1] = torch.nn.Conv2d(model.classifier[-1].in_channels, 1, kernel_size=model.classifier[-1].kernel_size)
|
38 |
-
|
39 |
-
print("loading weights from ", os.path.join(destination_for_weights, "deeplabv3_resnet50_random"))
|
40 |
-
|
41 |
-
if torch.cuda.is_available():
|
42 |
-
print("cuda is available, original weights")
|
43 |
-
device = torch.device("cuda")
|
44 |
-
model = torch.nn.DataParallel(model)
|
45 |
-
model.to(device)
|
46 |
-
checkpoint = torch.load(os.path.join(destination_for_weights, os.path.basename(segmentationWeightsURL)))
|
47 |
-
model.load_state_dict(checkpoint['state_dict'])
|
48 |
-
else:
|
49 |
-
print("cuda is not available, cpu weights")
|
50 |
-
device = torch.device("cpu")
|
51 |
-
checkpoint = torch.load(os.path.join(destination_for_weights, os.path.basename(segmentationWeightsURL)), map_location = "cpu")
|
52 |
-
state_dict_cpu = {k[7:]: v for (k, v) in checkpoint['state_dict'].items()}
|
53 |
-
model.load_state_dict(state_dict_cpu)
|
54 |
-
|
55 |
-
model.eval()
|
56 |
-
|
57 |
-
def segment(inp):
|
58 |
-
x = inp.transpose([2, 0, 1]) # channels-first
|
59 |
-
x = np.expand_dims(x, axis=0) # adding a batch dimension
|
60 |
-
|
61 |
-
mean = x.mean(axis=(0, 2, 3))
|
62 |
-
std = x.std(axis=(0, 2, 3))
|
63 |
-
x = x - mean.reshape(1, 3, 1, 1)
|
64 |
-
x = x / std.reshape(1, 3, 1, 1)
|
65 |
-
|
66 |
-
with torch.no_grad():
|
67 |
-
x = torch.from_numpy(x).type('torch.FloatTensor').to(device)
|
68 |
-
output = model(x)
|
69 |
-
|
70 |
-
y = output['out'].numpy()
|
71 |
-
y = y.squeeze()
|
72 |
-
|
73 |
-
out = y>0
|
74 |
-
|
75 |
-
mask = inp.copy()
|
76 |
-
mask[out] = np.array([0, 0, 255])
|
77 |
-
|
78 |
-
return mask
|
79 |
-
|
80 |
-
import gradio as gr
|
81 |
-
|
82 |
-
i = gr.inputs.Image(shape=(112, 112))
|
83 |
-
o = gr.outputs.Image()
|
84 |
-
|
85 |
-
examples = [["img1.jpg"], ["img2.jpg"]]
|
86 |
-
title = "Example: Echocardiogram Segmentation" #"Left Ventricle Segmentation"
|
87 |
-
description = "This semantic segmentation model identifies the left ventricle in echocardiogram images. Read more at the links below."
|
88 |
-
# videos. Accurate evaluation of the motion and size of the left ventricle is crucial for the assessment of cardiac function and ejection fraction. In this interface, the user inputs apical-4-chamber images from echocardiography videos and the model will output a prediction of the localization of the left ventricle in blue. This model was trained on the publicly released EchoNet-Dynamic dataset of 10k echocardiogram videos with 20k expert annotations of the left ventricle and published as part of ‘Video-based AI for beat-to-beat assessment of cardiac function’ by Ouyang et al. in Nature, 2020."
|
89 |
-
thumbnail = "https://raw.githubusercontent.com/gradio-app/hub-echonet/master/thumbnail.png"
|
90 |
-
|
91 |
-
article = "<div style='text-align: center;'><a href='https://pubmed.ncbi.nlm.nih.gov/31993508/' target='_blank'>Deep learning interpretation of echocardiograms</a><center><img src='https://visitor-badge.glitch.me/badge?page_id=example_space_cvpr' alt='visitor badge'></center></div>"
|
92 |
-
gr.Interface(segment, i, o, examples=examples, allow_flagging=False, analytics_enabled=False,
|
93 |
-
title=title, description=description, thumbnail=thumbnail,article=article).launch(enable_queue=True)
|
|
|
|
|
|
|
|
|
|
|
|
|
|
|
|
|
|
|
|
|
|
|
|
|
|
|
|
|
|
|
|
|
|
|
|
|
|
|
|
|
|
|
|
|
|
|
|
|
|
|
|
|
|
|
|
|
|
|
|
|
|
|
|
|
|
|
|
|
|
|
|
|
|
|
|
|
|
|
|
|
|
|
|
|
|
|
|
|
|
|
|
|
|
|
|
|
|
|
|
|
|
|
|
|
|
|
|
|
|
|
|
|
|
|
|
|
|
|
|
|
|
|
|
|
|
|
|
|
|
|
|
|
|
|
|
|
|
|
|
|
|
|
|
|
|
|
|
|
|
|
|
|
|
|
|
|
|
|
|
|
|
|
|
|
|
|
|
|
|
|
|
|
|
|
|
|
|
|
|
|
|
|
|
|
|
|
|
spaces/CVPR/LIVE/thrust/dependencies/cub/cmake/CubCudaConfig.cmake
DELETED
@@ -1,133 +0,0 @@
|
|
1 |
-
if (NOT ("${CMAKE_CUDA_HOST_COMPILER}" STREQUAL "" OR
|
2 |
-
"${CMAKE_CUDA_HOST_COMPILER}" STREQUAL "${CMAKE_CXX_COMPILER}"))
|
3 |
-
message(FATAL_ERROR
|
4 |
-
"CUB tests and examples require the C++ compiler and the CUDA host "
|
5 |
-
"compiler to be the same; to set this compiler, please use the "
|
6 |
-
"CMAKE_CXX_COMPILER variable, not the CMAKE_CUDA_HOST_COMPILER variable."
|
7 |
-
)
|
8 |
-
endif()
|
9 |
-
set(CMAKE_CUDA_HOST_COMPILER "${CMAKE_CXX_COMPILER}")
|
10 |
-
|
11 |
-
#
|
12 |
-
# Architecture options:
|
13 |
-
#
|
14 |
-
|
15 |
-
set(all_archs 35 37 50 52 53 60 61 62 70 72 75 80)
|
16 |
-
set(arch_message "CUB: Enabled CUDA architectures:")
|
17 |
-
set(enabled_archs)
|
18 |
-
|
19 |
-
# Thrust sets up the architecture flags in CMAKE_CUDA_FLAGS already. Just
|
20 |
-
# reuse them if possible. After we transition to CMake 3.18 CUDA_ARCHITECTURE
|
21 |
-
# target properties this will need to be updated.
|
22 |
-
if (CUB_IN_THRUST)
|
23 |
-
# Configure to use all flags from thrust:
|
24 |
-
set(CMAKE_CUDA_FLAGS "${THRUST_CUDA_FLAGS_BASE} ${THRUST_CUDA_FLAGS_NO_RDC}")
|
25 |
-
|
26 |
-
# Update the enabled architectures list from thrust
|
27 |
-
foreach (arch IN LISTS all_archs)
|
28 |
-
if (THRUST_ENABLE_COMPUTE_${arch})
|
29 |
-
set(CUB_ENABLE_COMPUTE_${arch} True)
|
30 |
-
list(APPEND enabled_archs ${arch})
|
31 |
-
string(APPEND arch_message " sm_${arch}")
|
32 |
-
else()
|
33 |
-
set(CUB_ENABLE_COMPUTE_${arch} False)
|
34 |
-
endif()
|
35 |
-
endforeach()
|
36 |
-
|
37 |
-
# Otherwise create cache options and build the flags ourselves:
|
38 |
-
else() # NOT CUB_IN_THRUST
|
39 |
-
|
40 |
-
# Find the highest arch:
|
41 |
-
list(SORT all_archs)
|
42 |
-
list(LENGTH all_archs max_idx)
|
43 |
-
math(EXPR max_idx "${max_idx} - 1")
|
44 |
-
list(GET all_archs ${max_idx} highest_arch)
|
45 |
-
|
46 |
-
option(CUB_DISABLE_ARCH_BY_DEFAULT
|
47 |
-
"If ON, then all CUDA architectures are disabled on the initial CMake run."
|
48 |
-
OFF
|
49 |
-
)
|
50 |
-
|
51 |
-
set(option_init ON)
|
52 |
-
if (CUB_DISABLE_ARCH_BY_DEFAULT)
|
53 |
-
set(option_init OFF)
|
54 |
-
endif()
|
55 |
-
|
56 |
-
set(arch_flags)
|
57 |
-
foreach (arch IN LISTS all_archs)
|
58 |
-
option(CUB_ENABLE_COMPUTE_${arch}
|
59 |
-
"Enable code generation for sm_${arch}."
|
60 |
-
${option_init}
|
61 |
-
)
|
62 |
-
if (CUB_ENABLE_COMPUTE_${arch})
|
63 |
-
list(APPEND enabled_archs ${arch})
|
64 |
-
string(APPEND arch_flags " -gencode arch=compute_${arch},code=sm_${arch}")
|
65 |
-
string(APPEND arch_message " sm_${arch}")
|
66 |
-
endif()
|
67 |
-
endforeach()
|
68 |
-
|
69 |
-
option(CUB_ENABLE_COMPUTE_FUTURE
|
70 |
-
"Enable code generation for tests for compute_${highest_arch}"
|
71 |
-
${option_init}
|
72 |
-
)
|
73 |
-
if (CUB_ENABLE_COMPUTE_FUTURE)
|
74 |
-
string(APPEND arch_flags
|
75 |
-
" -gencode arch=compute_${highest_arch},code=compute_${highest_arch}"
|
76 |
-
)
|
77 |
-
string(APPEND arch_message " compute_${highest_arch}")
|
78 |
-
endif()
|
79 |
-
|
80 |
-
# TODO Once CMake 3.18 is required, use the CUDA_ARCHITECTURE target props
|
81 |
-
string(APPEND CMAKE_CUDA_FLAGS "${arch_flags}")
|
82 |
-
endif()
|
83 |
-
|
84 |
-
message(STATUS ${arch_message})
|
85 |
-
|
86 |
-
# Create a variable containing the minimal target arch for tests
|
87 |
-
list(SORT enabled_archs)
|
88 |
-
list(GET enabled_archs 0 CUB_MINIMAL_ENABLED_ARCH)
|
89 |
-
|
90 |
-
#
|
91 |
-
# RDC options:
|
92 |
-
#
|
93 |
-
|
94 |
-
option(CUB_ENABLE_TESTS_WITH_RDC
|
95 |
-
"Build all CUB tests with RDC; tests that require RDC are not affected by this option."
|
96 |
-
OFF
|
97 |
-
)
|
98 |
-
|
99 |
-
option(CUB_ENABLE_EXAMPLES_WITH_RDC
|
100 |
-
"Build all CUB examples with RDC; examples which require RDC are not affected by this option."
|
101 |
-
OFF
|
102 |
-
)
|
103 |
-
|
104 |
-
# Check for RDC/SM compatibility and error/warn if necessary
|
105 |
-
set(no_rdc_archs 53 62 72)
|
106 |
-
set(rdc_supported True)
|
107 |
-
foreach (arch IN LISTS no_rdc_archs)
|
108 |
-
if (CUB_ENABLE_COMPUTE_${arch})
|
109 |
-
set(rdc_supported False)
|
110 |
-
break()
|
111 |
-
endif()
|
112 |
-
endforeach()
|
113 |
-
|
114 |
-
set(rdc_opts
|
115 |
-
CUB_ENABLE_TESTS_WITH_RDC
|
116 |
-
CUB_ENABLE_EXAMPLES_WITH_RDC
|
117 |
-
)
|
118 |
-
set(rdc_requested False)
|
119 |
-
foreach (rdc_opt IN LISTS rdc_opts)
|
120 |
-
if (${rdc_opt})
|
121 |
-
set(rdc_requested True)
|
122 |
-
break()
|
123 |
-
endif()
|
124 |
-
endforeach()
|
125 |
-
|
126 |
-
if (rdc_requested AND NOT rdc_supported)
|
127 |
-
string(JOIN ", " no_rdc ${no_rdc_archs})
|
128 |
-
string(JOIN "\n" opts ${rdc_opts})
|
129 |
-
message(FATAL_ERROR
|
130 |
-
"Architectures {${no_rdc}} do not support RDC and are incompatible with "
|
131 |
-
"these options:\n${opts}"
|
132 |
-
)
|
133 |
-
endif()
|
|
|
|
|
|
|
|
|
|
|
|
|
|
|
|
|
|
|
|
|
|
|
|
|
|
|
|
|
|
|
|
|
|
|
|
|
|
|
|
|
|
|
|
|
|
|
|
|
|
|
|
|
|
|
|
|
|
|
|
|
|
|
|
|
|
|
|
|
|
|
|
|
|
|
|
|
|
|
|
|
|
|
|
|
|
|
|
|
|
|
|
|
|
|
|
|
|
|
|
|
|
|
|
|
|
|
|
|
|
|
|
|
|
|
|
|
|
|
|
|
|
|
|
|
|
|
|
|
|
|
|
|
|
|
|
|
|
|
|
|
|
|
|
|
|
|
|
|
|
|
|
|
|
|
|
|
|
|
|
|
|
|
|
|
|
|
|
|
|
|
|
|
|
|
|
|
|
|
|
|
|
|
|
|
|
|
|
|
|
|
|
|
|
|
|
|
|
|
|
|
|
|
|
|
|
|
|
|
|
|
|
|
|
|
|
|
|
|
|
|
|
|
|
|
|
|
|
|
|
|
|
|
|
|
|
|
|
|
|
|
|
|
|
|
|
|
|
|
|
|
|
|
|
|
|
|
|
|
|
|
|
|
|
|
|
|
|
spaces/CVPR/LIVE/thrust/thrust/system/cpp/detail/scatter.h
DELETED
@@ -1,22 +0,0 @@
|
|
1 |
-
/*
|
2 |
-
* Copyright 2008-2013 NVIDIA Corporation
|
3 |
-
*
|
4 |
-
* Licensed under the Apache License, Version 2.0 (the "License");
|
5 |
-
* you may not use this file except in compliance with the License.
|
6 |
-
* You may obtain a copy of the License at
|
7 |
-
*
|
8 |
-
* http://www.apache.org/licenses/LICENSE-2.0
|
9 |
-
*
|
10 |
-
* Unless required by applicable law or agreed to in writing, software
|
11 |
-
* distributed under the License is distributed on an "AS IS" BASIS,
|
12 |
-
* WITHOUT WARRANTIES OR CONDITIONS OF ANY KIND, either express or implied.
|
13 |
-
* See the License for the specific language governing permissions and
|
14 |
-
* limitations under the License.
|
15 |
-
*/
|
16 |
-
|
17 |
-
#pragma once
|
18 |
-
|
19 |
-
#include <thrust/detail/config.h>
|
20 |
-
|
21 |
-
// this system has no special version of this algorithm
|
22 |
-
|
|
|
|
|
|
|
|
|
|
|
|
|
|
|
|
|
|
|
|
|
|
|
|
|
|
|
|
|
|
|
|
|
|
|
|
|
|
|
|
|
|
|
|
|
|
spaces/CVPR/MonoScene/README.md
DELETED
@@ -1,13 +0,0 @@
|
|
1 |
-
---
|
2 |
-
title: MonoScene
|
3 |
-
emoji: 🚘🏙️
|
4 |
-
colorFrom: purple
|
5 |
-
colorTo: pink
|
6 |
-
sdk: gradio
|
7 |
-
sdk_version: 3.0.20
|
8 |
-
app_file: app.py
|
9 |
-
pinned: true
|
10 |
-
license: apache-2.0
|
11 |
-
---
|
12 |
-
|
13 |
-
Check out the configuration reference at https://huggingface.co/docs/hub/spaces-config-reference
|
|
|
|
|
|
|
|
|
|
|
|
|
|
|
|
|
|
|
|
|
|
|
|
|
|
|
|