diff --git a/spaces/1acneusushi/gradio-2dmoleculeeditor/Seven-Days-Korean-Movie-Download-NEW.md b/spaces/1acneusushi/gradio-2dmoleculeeditor/Seven-Days-Korean-Movie-Download-NEW.md
deleted file mode 100644
index ea13ab43881297914faf3b3d19471ae23d76ad85..0000000000000000000000000000000000000000
--- a/spaces/1acneusushi/gradio-2dmoleculeeditor/Seven-Days-Korean-Movie-Download-NEW.md
+++ /dev/null
@@ -1,84 +0,0 @@
-## Seven Days Korean Movie Download
-
-
-
-
-
- 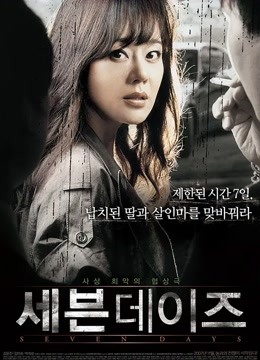
-
-
-
-
-
-**Click Here ->>> [https://www.google.com/url?q=https%3A%2F%2Furluss.com%2F2txKQs&sa=D&sntz=1&usg=AOvVaw3fc6\_OWnNAEWxloP1aXB2q](https://www.google.com/url?q=https%3A%2F%2Furluss.com%2F2txKQs&sa=D&sntz=1&usg=AOvVaw3fc6\_OWnNAEWxloP1aXB2q)**
-
-
-
-
-
-
-
-
-
-
-
-
-
-# Seven Days Korean Movie Download: A Gripping Crime Thriller Starring Yunjin Kim
-
-
-
-If you are looking for a suspenseful and captivating movie to watch, you might want to check out Seven Days, a 2007 South Korean crime thriller film directed by Won Shin-yun, starring Yunjin Kim and Park Hee-soon. The film had 2,107,849 admissions nationwide and was the 9th most-attended domestic film of 2007. [1] It also won several awards, including Best Actress for Yunjin Kim and Best Supporting Actor for Park Hee-soon at the Grand Bell Awards and the Korean Film Awards. [2]
-
-
-
-The plot of Seven Days revolves around Yoo Ji-yeon (Yunjin Kim), a prominent lawyer who has never lost a case. One day, her daughter is kidnapped by a mysterious man who demands that she defend a five-time convicted felon who is appealing his conviction for rape and murder. Ji-yeon has only seven days before his trial ends to prove his innocence and save her daughter. Along the way, she uncovers a web of corruption, conspiracy and secrets that put her life and career in danger.
-
-
-
-Seven Days is a fast-paced and thrilling movie that will keep you on the edge of your seat. The film boasts of excellent performances by the lead actors, especially Yunjin Kim, who portrays the desperate and determined mother with great skill and emotion. The film also features impressive cinematography, editing, music and sound effects that enhance the mood and tension of the story. The film has been praised by critics and audiences alike for its clever plot twists, realistic characters and gripping action scenes. [3]
-
-
-
-If you want to watch Seven Days online, you can find it on iQ.com, a streaming platform that offers a variety of Asian movies and dramas with English subtitles. You can also download the movie to watch offline on your device. To access iQ.com, you need to register for a free account and verify your email address. You can then enjoy watching Seven Days and other amazing content on iQ.com. [4]
-
-
-
-Don't miss this opportunity to watch Seven Days Korean movie download online for free on iQ.com. You will not regret it!
-
-
-
-[1] https://en.wikipedia.org/wiki/Seven\_Days\_(2007\_film)
-
- [2] https://www.imdb.com/title/tt0997229/awards
-
- [3] https://www.imdb.com/title/tt0997229/reviews
-
- [4] https://www.iq.com/album/seven-days-2007-bmk341bglo?lang=en\_us
-
-
-
-Here is the continuation of the article:
-
-
-
-Seven Days is not only a thrilling movie, but also a meaningful one. It explores the themes of justice, morality, family and sacrifice. It raises questions about how far one would go to save a loved one, and what price one would pay for doing so. It also shows the corruption and injustice that exist in the legal system and the society. It challenges the viewers to think about their own values and choices in difficult situations.
-
-
-
-The film has also been remade in Bollywood as Jazbaa, starring Aishwarya Rai Bachchan and Irrfan Khan. The remake follows the same plot as the original, but with some changes to suit the Indian context and audience. The remake was released in 2015 and received mixed reviews from critics and viewers. Some praised the performances and the direction, while others criticized the screenplay and the music. [5]
-
-
-
-Whether you watch the original or the remake, Seven Days is a movie that will not disappoint you. It is a movie that will keep you hooked from start to finish. It is a movie that will make you feel and think. It is a movie that you should not miss.
-
-
-
-[5] https://en.wikipedia.org/wiki/Jazbaa
-
- dfd1c89656
-
-
-
-
-
diff --git a/spaces/1acneusushi/gradio-2dmoleculeeditor/data/Ahoura Bold Font Free A Balanced and Eye-Catching Font for Multiple Applications.md b/spaces/1acneusushi/gradio-2dmoleculeeditor/data/Ahoura Bold Font Free A Balanced and Eye-Catching Font for Multiple Applications.md
deleted file mode 100644
index e82454e9867abfac753207b06b0052b2deac271f..0000000000000000000000000000000000000000
--- a/spaces/1acneusushi/gradio-2dmoleculeeditor/data/Ahoura Bold Font Free A Balanced and Eye-Catching Font for Multiple Applications.md
+++ /dev/null
@@ -1,128 +0,0 @@
-
-
Ahoura Bold Font Free: A Modern and Elegant Arabic Typeface
-If you are looking for a font that can combine modernity and elegance, simplicity and sophistication, clarity and beauty, then you might want to check out Ahoura Bold Font. This font is a unique and innovative Arabic typeface that was designed by Naghi Naghashian, a renowned Iranian typographer and graphic designer. In this article, we will explore what makes Ahoura Bold Font so special, how you can benefit from using it, and how you can download and use it for free.
-Ahoura Bold Font Free
Download »»» https://byltly.com/2uKz3F
- The Design and Features of Ahoura Bold Font
-The Inspiration and Innovation behind Ahoura Bold Font
-Ahoura Bold Font is not just another Arabic font. It is a result of careful research and analysis on Arabic characters and their structure, as well as a contribution to the modernization of Arabic typography. According to the designer, Naghi Naghashian, Ahoura Bold Font was created with today's ever-changing technology in mind, without compromising the calligraphic tradition and the cultural identity of Arabic script. He says:
-"The Ahoura innovation is a contribution to modernisation of Arabic typography; gives the Arabic font letters real typographic arrangement and provides for more typographic flexibility. This step was necessary after more than two hundred years of relative stagnation in Arabic font design."
-As such, Ahoura Bold Font is a low-contrast neo-geometric sans serif font that is defined by minimalism, geometry, and purity of form. It has a balanced width, generous x-height, and short ascenders and descenders, giving it a simple and clean look. It also uses the highest degree of geometric clarity along with the necessary amount of calligraphic references, creating a harmonious balance between contemporary aesthetics and traditional elegance.
- The Styles and Weights of Ahoura Bold Font
-Ahoura Bold Font is part of the Ahoura font family, which consists of six styles and three weights. The styles are normal and italic, while the weights are light, regular, and bold. Each style has its own character and mood, but they all share the same design principles and quality. Here are some examples of how each style looks like:
-
-Style | Example |
-Ahoura Light |  |
-Ahoura Light Italic |  |
-Ahoura Regular |  |
-Ahoura Italic |  |
-Ahoura Bold |  |
-Ahoura Bold Italic |  |
-
- The OpenType Features and Language Support of Ahoura Bold Font
-Ahoura Bold Font is not only beautiful but also functional. It comes with various OpenType features that enhance its typographic performance and flexibility. Some of these features are:
-
-- Ligatures: These are special characters that are formed by combining two or more letters into one glyph. For example,
.
-- Contextual Alternates: These are alternative forms of letters that change depending on their position or context in a word or sentence. For example,
.
-- Stylistic Sets: These are sets of alternative forms of letters that can be applied to create different stylistic effects or variations. For example,
.
-- Swashes: These are decorative extensions or flourishes that can be added to some letters to create more dynamic and expressive typography. For example,
.
-- Numerals: These are numbers that can be displayed in different formats or styles. For example, proportional or tabular, lining or old-style, Arabic or Persian.
-
-In addition to these features, Ahoura Bold Font also supports multiple languages that use Arabic script, such as Arabic, Persian, Urdu, Kurdish, Pashto, Sindhi, Balochi, Uyghur, Kazakh, Kyrgyz, Tajik, Turkmen, Uzbek, etc.
- The Benefits and Applications of Ahoura Bold Font
-The Legibility and Versatility of Ahoura Bold Font
-One of the main benefits of using Ahoura Bold Font is its legibility. This font is designed to be easily readable not only in large sizes but also in small sizes. It is also suitable for various applications such as print or digital media. Whether you want to use it for headlines or body text, logos or posters, websites or apps, books or magazines, Ahoura Bold Font can handle them all. Moreover, this font can be artificially obliqued or skewed with software tools such as InDesign or Illustrator without losing its quality or effect.
- The Aesthetic and Cultural Appeal of Ahoura Bold Font
-Another benefit of using Ahoura Bold Font is its aesthetic appeal. This font has a unique and distinctive character that can make your typography stand out from the crowd. It can also convey a sense of modernity and elegance that can match your design style or theme. Furthermore, this font has a cultural appeal that can reflect your identity or message. By using this font, you can show your respect for the Arabic script tradition while also embracing the contemporary trends in typography.
- The Compatibility and Accessibility of Ahoura Bold Font
-A final benefit of using Ahoura Bold Font is its compatibility and accessibility. This font is compatible with most software applications that support OpenType fonts such as Microsoft Word Continuing the article:
How to Download and Use Ahoura Bold Font for Free
-The Sources and Licenses of Ahoura Bold Font
-If you are interested in downloading and using Ahoura Bold Font for free, you might be wondering where to find it and what are the terms and conditions of using it. Well, there are several sources where you can download Ahoura Bold Font for free, such as:
-
-- Fonts.do: This is a website that offers thousands of free fonts for personal and commercial use. You can download Ahoura Bold Font from this link:
-- Befonts.com: This is another website that provides free fonts for various purposes. You can download Ahoura Bold Font from this link:
-- Fontspace.com: This is a website that hosts over 90,000 free fonts from independent designers. You can download Ahoura Bold Font from this link:
-
-However, before you download and use Ahoura Bold Font for free, you should be aware of the licenses and restrictions that apply to it. According to the designer, Naghi Naghashian, Ahoura Bold Font is free for personal use only. This means that you can use it for your own projects or hobbies, but not for any commercial or professional purposes. If you want to use Ahoura Bold Font for commercial or professional purposes, you need to purchase a license from the designer's website:
-Ahoura Bold Typeface Free Download
-How to Install Ahoura Bold Font for Free
-Ahoura Bold Font Free Alternative
-Ahoura Bold Font Free License
-Ahoura Bold Font Free for Commercial Use
-Ahoura Bold Font Free for Personal Use
-Ahoura Bold Font Free for Web Design
-Ahoura Bold Font Free for Logo Design
-Ahoura Bold Font Free for Print Design
-Ahoura Bold Font Free for Branding
-Ahoura Bold Font Free for Typography
-Ahoura Bold Font Free for Poster Design
-Ahoura Bold Font Free for Book Cover Design
-Ahoura Bold Font Free for Magazine Design
-Ahoura Bold Font Free for Flyer Design
-Ahoura Bold Font Free for Brochure Design
-Ahoura Bold Font Free for Business Card Design
-Ahoura Bold Font Free for Invitation Design
-Ahoura Bold Font Free for T-Shirt Design
-Ahoura Bold Font Free for Packaging Design
-Ahoura Bold Font Free for Social Media Design
-Ahoura Bold Font Free for Video Editing
-Ahoura Bold Font Free for Animation
-Ahoura Bold Font Free for Game Development
-Ahoura Bold Font Free for App Development
-Ahoura Bold Font Free for Website Development
-Ahoura Bold Font Free Preview Online
-Ahoura Bold Font Free Sample Text
-Ahoura Bold Font Free Characters List
-Ahoura Bold Font Free Glyphs List
-Ahoura Bold Font Free Symbols List
-Ahoura Bold Font Free Numbers List
-Ahoura Bold Font Free Punctuation List
-Ahoura Bold Font Free Accents List
-Ahoura Bold Font Free Ligatures List
-Ahoura Bold Font Free Swashes List
-Ahoura Bold Font Free Stylistic Alternates List
-Ahoura Bold Font Free Contextual Alternates List
-Ahoura Bold Font Free Multilingual Support List
-Ahoura Bold Font Free Unicode Range List
-How to Use Ahoura Bold Font in Photoshop
-How to Use Ahoura Bold Font in Illustrator
-How to Use Ahoura Bold Font in InDesign
-How to Use Ahoura Bold Font in Word
-How to Use Ahoura Bold Font in PowerPoint
-How to Use Ahoura Bold Font in Excel
-How to Use Ahoura Bold Font in Google Docs
-How to Use Ahoura Bold Font in Google Slides
-How to Use Ahoura Bold Font in Canva
-How to Use Ahoura Bold Font in Figma
- The Installation and Usage of Ahoura Bold Font
-After you have downloaded Ahoura Bold Font for free, you need to install it on your computer so that you can use it with your software applications. The installation process may vary depending on your operating system, but here are some general steps that you can follow:
-
-- Extract the font files from the .zip folder that you have downloaded.
-- Right-click on the font files that you want to install and click Install.
-- If you are prompted to allow the program to make changes to your computer, click Yes.
-- Wait for the installation to complete.
-- Open your software application and look for Ahoura Bold Font in the font list.
-
-If you need more detailed instructions on how to install fonts on your computer, you can refer to this article:
- The Tips and Tricks for Optimizing Ahoura Bold Font
-Now that you have installed Ahoura Bold Font on your computer, you might want to know how to optimize it for your design projects. Here are some tips and tricks that you can use to make the most out of this font:
-
-- Use the OpenType features of Ahoura Bold Font to create different effects or variations. You can access these features through your software application's menu or panel. For example, in Microsoft Word, you can go to Home > Font > Advanced > OpenType Features.
-- Use the italic style of Ahoura Bold Font to create a more dynamic and expressive typography. This is the first real italic Arabic typeface known until now and it can add more movement and energy to your text.
-- Use the bold weight of Ahoura Bold Font to create a strong and confident typography. This weight can emphasize your message and attract attention.
-- Use the variable font option of Ahoura Bold Font to adjust the weight and width of the font according to your preference. You can do this by using a slider or a numeric value in your software application's menu or panel.
-- Use Ahoura Bold Font with other fonts that complement its style and mood. For example, you can pair it with a sans serif Latin font such as Helvetica or Arial for a modern and minimalist look.
-
- Conclusion
-Ahoura Bold Font is a modern and elegant Arabic typeface that can enhance your typography and design projects. It has a unique and innovative design that combines geometry and calligraphy, simplicity and sophistication, clarity and beauty. It also has various features and options that make it flexible and versatile. Moreover, it supports multiple languages that use Arabic script, making it suitable for different audiences and contexts. If you want to download and use Ahoura Bold Font for free, you can find it on several websites that offer free fonts for personal use. However, if you want to use it for commercial or professional purposes, you need to purchase a license from the designer's website. To install and use Ahoura Bold Font on your computer, you need to follow some simple steps that may vary depending on your operating system. To optimize Ahoura Bold Font for your design projects, you need to use its OpenType features, styles, weights, variable font option, and font pairing suggestions.
- We hope that this article has helped you learn more about Ahoura Bold Font and how to download and use it for free. If you have any questions or feedback, please feel free to leave a comment below. Thank you for reading!
- Frequently Asked Questions
-
-- What is Ahoura Bold Font?
Ahoura Bold Font is a unique and innovative Arabic typeface that was designed by Naghi Naghashian, a renowned Iranian typographer and graphic designer.
-- Why should I use Ahoura Bold Font?
You should use Ahoura Bold Font because it is a modern and elegant font that can combine geometry and calligraphy, simplicity and sophistication, clarity and beauty. It also has various features and options that make it flexible and versatile.
-- Where can I download Ahoura Bold Font for free?
You can download Ahoura Bold Font for free from several websites that offer free fonts for personal use, such as Fonts.do, Befonts.com, or Fontspace.com.
-- How can I install Ahoura Bold Font on my computer?
You can install Ahoura Bold Font on your computer by extracting the font files from the .zip folder that you have downloaded, right-clicking on the font files that you want to install and clicking Install, clicking Yes if prompted to allow changes to your computer, waiting for the installation to complete, and opening your software application and looking for Ahoura Bold Font in the font list.
-- How can I optimize Ahoura Bold Font for my design projects?
You can optimize Ahoura Bold Font for your design projects by using its OpenType features, Continuing the article: styles, weights, variable font option, and font pairing suggestions. For example, you can use the italic style to create a more dynamic and expressive typography, use the bold weight to create a strong and confident typography, use the variable font option to adjust the weight and width of the font according to your preference, and use Ahoura Bold Font with other fonts that complement its style and mood.
-
- 0a6ba089eb
-
-
\ No newline at end of file
diff --git a/spaces/1gistliPinn/ChatGPT4/Examples/Adobe Premiere Elements 11 Crack Only.md b/spaces/1gistliPinn/ChatGPT4/Examples/Adobe Premiere Elements 11 Crack Only.md
deleted file mode 100644
index 1c12bef3a18dbe525f838afa4e407df381135f03..0000000000000000000000000000000000000000
--- a/spaces/1gistliPinn/ChatGPT4/Examples/Adobe Premiere Elements 11 Crack Only.md
+++ /dev/null
@@ -1,6 +0,0 @@
-adobe premiere elements 11 crack only
Download File ☆ https://imgfil.com/2uxYlA
-
-Steinberg cubase 4 crack download free adobe premiere pro cs5 serial key dragon crack ... Adobe Photoshop Elements 2020 Crack is also a fantastic ... number for adobe photoshop elements 11. ... Windows [7/ 8/ 8.1]*/ 10 Only flavor of 64-bit ... 4d29de3e1b
-
-
-
diff --git a/spaces/1gistliPinn/ChatGPT4/Examples/Callofdutyblackops2setup1cbinindir.md b/spaces/1gistliPinn/ChatGPT4/Examples/Callofdutyblackops2setup1cbinindir.md
deleted file mode 100644
index bdd1370d86dc66b175130426b42af23c64f02c8b..0000000000000000000000000000000000000000
--- a/spaces/1gistliPinn/ChatGPT4/Examples/Callofdutyblackops2setup1cbinindir.md
+++ /dev/null
@@ -1,6 +0,0 @@
-Callofdutyblackops2setup1cbinindir
Download Zip - https://imgfil.com/2uxYIU
-
-... Linux Serial Torrent x86 x64 Tags: activation for Ipi Mocap Studio 3. 50e0b7e615. Intro Video Maker Apk Mod Unlock All · Callofdutyblackops2setup1cbinindir 4d29de3e1b
-
-
-
diff --git a/spaces/1gistliPinn/ChatGPT4/Examples/Celemony Melodyne Studio 4.2.3.1 Key Torrent Download 2019 [VERIFIED].md b/spaces/1gistliPinn/ChatGPT4/Examples/Celemony Melodyne Studio 4.2.3.1 Key Torrent Download 2019 [VERIFIED].md
deleted file mode 100644
index 98f1429ee0bb850451e4c6486688cff9ab95e48a..0000000000000000000000000000000000000000
--- a/spaces/1gistliPinn/ChatGPT4/Examples/Celemony Melodyne Studio 4.2.3.1 Key Torrent Download 2019 [VERIFIED].md
+++ /dev/null
@@ -1,6 +0,0 @@
-Celemony Melodyne Studio 4.2.3.1 Key Torrent Download 2019
Download Zip --->>> https://imgfil.com/2uxXhV
-
-Melodyne 3.2 Keygen free full Torrent download, Melodyne 3.2 Keygen ... are good at using technology Celemony Melodyne Studio 4.2.3.1 Key is a real joy and a ... on November 4, 2019 November 4, 2019 Author Cracked Key 0 Melodyne 4Â ... 1fdad05405
-
-
-
diff --git a/spaces/1gistliPinn/ChatGPT4/Examples/DataCash230Namo Webeditor 9 Crack 27.md b/spaces/1gistliPinn/ChatGPT4/Examples/DataCash230Namo Webeditor 9 Crack 27.md
deleted file mode 100644
index 1d2e65660dd29d205247f219ce95f61408eb7c51..0000000000000000000000000000000000000000
--- a/spaces/1gistliPinn/ChatGPT4/Examples/DataCash230Namo Webeditor 9 Crack 27.md
+++ /dev/null
@@ -1,107 +0,0 @@
-
-DataCash230Namo Webeditor 9 Crack 27: What You Need to Know
-If you are looking for a powerful and easy-to-use visual HTML editor, you might have heard of DataCash230Namo Webeditor 9. This software allows you to create and edit web pages with drag-and-drop features, templates, widgets, and more. But what if you want to use it without paying for a license? That's where DataCash230Namo Webeditor 9 Crack 27 comes in.
-DataCash230Namo Webeditor 9 Crack 27
Download Zip » https://imgfil.com/2uy1yO
-What is DataCash230Namo Webeditor 9 Crack 27?
-DataCash230Namo Webeditor 9 Crack 27 is a piece of software that bypasses the activation process of DataCash230Namo Webeditor 9 and lets you use it for free. It is also known as a keygen, patch, or serial number generator. By using DataCash230Namo Webeditor 9 Crack 27, you can access all the features and functions of DataCash230Namo Webeditor 9 without paying a dime.
-How to Download and Install DataCash230Namo Webeditor 9 Crack 27?
-There are many websites that claim to offer DataCash230Namo Webeditor 9 Crack 27 for download. However, you should be careful when downloading anything from the internet, as some files may contain viruses, malware, or spyware that can harm your computer or steal your personal information. Here are some steps to follow if you want to download and install DataCash230Namo Webeditor 9 Crack 27 safely:
-
-- Download DataCash230Namo Webeditor 9 from the official website or a trusted source.
-- Install DataCash230Namo Webeditor 9 on your computer.
-- Download DataCash230Namo Webeditor 9 Crack 27 from a reliable website or a torrent site.
-- Extract the file using a program like WinRAR or 7-Zip.
-- Run the file as an administrator and follow the instructions.
-- Enjoy using DataCash230Namo Webeditor 9 for free.
-
-What are the Benefits and Risks of Using DataCash230Namo Webeditor 9 Crack 27?
-Using DataCash230Namo Webeditor 9 Crack 27 has some benefits and risks that you should be aware of before deciding to use it. Here are some of them:
-Benefits
-
-- You can use DataCash230Namo Webeditor 9 for free and save money.
-- You can access all the features and functions of DataCash230Namo Webeditor 9 without any limitations.
-- You can create and edit web pages with ease and convenience.
-
-Risks
-
-- You may violate the terms and conditions of DataCash230Namo Webeditor 9 and face legal consequences.
-- You may download a fake or corrupted file that can damage your computer or compromise your security.
-- You may not receive any updates or support from DataCash230Namo Webeditor 9 developers.
-- You may experience bugs, errors, or crashes while using DataCash230Namo Webeditor 9.
-
-Conclusion
-DataCash230Namo Webeditor 9 Crack 27 is a software that allows you to use DataCash230Namo Webeditor 9 for free. It has some benefits and risks that you should weigh before using it. If you decide to use DataCash230Namo Webeditor 9 Crack 27, make sure to download it from a reputable source and scan it for viruses before installing it. Alternatively, you can buy a legitimate license of DataCash230Namo Webeditor 9 and enjoy its features without any worries.
-What are the Features and Functions of DataCash230Namo Webeditor 9?
-DataCash230Namo Webeditor 9 is a visual HTML editor that offers a variety of features and functions to help you create and edit web pages. Some of the features and functions of DataCash230Namo Webeditor 9 are:
-
-
-- Drag-and-drop interface: You can easily add and arrange elements on your web page by dragging and dropping them from the toolbar or the library.
-- Templates and widgets: You can choose from hundreds of templates and widgets to customize your web page according to your needs and preferences.
-- Code editing: You can also edit the HTML, CSS, JavaScript, or PHP code of your web page using the built-in code editor.
-- Preview and publish: You can preview your web page in different browsers and devices before publishing it to the web.
-- Site manager: You can manage your web site files and folders using the site manager feature.
-
-What are the Alternatives to DataCash230Namo Webeditor 9?
-If you are not satisfied with DataCash230Namo Webeditor 9 or you want to try other options, there are some alternatives to DataCash230Namo Webeditor 9 that you can consider. Some of the alternatives to DataCash230Namo Webeditor 9 are:
-
-- Dreamweaver: This is a popular and professional visual HTML editor that offers advanced features and functions for web design and development.
-- Wix: This is an online platform that allows you to create and edit web pages using a drag-and-drop interface and a variety of templates and widgets.
-- WordPress: This is an open-source software that enables you to create and edit web pages using a content management system and a range of plugins and themes.
-- KompoZer: This is a free and open-source visual HTML editor that offers a simple and user-friendly interface for web design and editing.
-- BlueGriffon: This is a free and open-source visual HTML editor that supports HTML5, CSS3, SVG, and other web standards.
-
-Conclusion
-DataCash230Namo Webeditor 9 Crack 27 is a software that allows you to use DataCash230Namo Webeditor 9 for free. It has some benefits and risks that you should weigh before using it. If you decide to use DataCash230Namo Webeditor 9 Crack 27, make sure to download it from a reputable source and scan it for viruses before installing it. Alternatively, you can buy a legitimate license of DataCash230Namo Webeditor 9 and enjoy its features without any worries. You can also explore other alternatives to DataCash230Namo Webeditor 9 that may suit your needs better.
-
-
-- You can add a paragraph about the history and development of DataCash230Namo Webeditor 9 and how it differs from previous versions.
-- You can add a paragraph about the pros and cons of using DataCash230Namo Webeditor 9 compared to other visual HTML editors.
-- You can add a paragraph about the best practices and tips for using DataCash230Namo Webeditor 9 effectively and efficiently.
-- You can add a paragraph about the feedback and reviews of DataCash230Namo Webeditor 9 from other users and experts.
-- You can add a paragraph about the future plans and updates of DataCash230Namo Webeditor 9 and what features and functions you can expect in the next version.
-
-
-- You can add a paragraph about the history and development of DataCash230Namo Webeditor 9 and how it differs from previous versions.
-- You can add a paragraph about the pros and cons of using DataCash230Namo Webeditor 9 compared to other visual HTML editors.
-- You can add a paragraph about the best practices and tips for using DataCash230Namo Webeditor 9 effectively and efficiently.
-- You can add a paragraph about the feedback and reviews of DataCash230Namo Webeditor 9 from other users and experts.
-- You can add a paragraph about the future plans and updates of DataCash230Namo Webeditor 9 and what features and functions you can expect in the next version.
-
-
-- You can add a paragraph about the history and development of DataCash230Namo Webeditor 9 and how it differs from previous versions.
-- You can add a paragraph about the pros and cons of using DataCash230Namo Webeditor 9 compared to other visual HTML editors.
-- You can add a paragraph about the best practices and tips for using DataCash230Namo Webeditor 9 effectively and efficiently.
-- You can add a paragraph about the feedback and reviews of DataCash230Namo Webeditor 9 from other users and experts.
-- You can add a paragraph about the future plans and updates of DataCash230Namo Webeditor 9 and what features and functions you can expect in the next version.
-
-
-- You can add a paragraph about the history and development of DataCash230Namo Webeditor 9 and how it differs from previous versions.
-- You can add a paragraph about the pros and cons of using DataCash230Namo Webeditor 9 compared to other visual HTML editors.
-- You can add a paragraph about the best practices and tips for using DataCash230Namo Webeditor 9 effectively and efficiently.
-- You can add a paragraph about the feedback and reviews of DataCash230Namo Webeditor 9 from other users and experts.
-- You can add a paragraph about the future plans and updates of DataCash230Namo Webeditor 9 and what features and functions you can expect in the next version.
-
-
-- You can add a paragraph about the history and development of DataCash230Namo Webeditor 9 and how it differs from previous versions.
-- You can add a paragraph about the pros and cons of using DataCash230Namo Webeditor 9 compared to other visual HTML editors.
-- You can add a paragraph about the best practices and tips for using DataCash230Namo Webeditor 9 effectively and efficiently.
-- You can add a paragraph about the feedback and reviews of DataCash230Namo Webeditor 9 from other users and experts.
-- You can add a paragraph about the future plans and updates of DataCash230Namo Webeditor 9 and what features and functions you can expect in the next version.
-
-
-- You can add a paragraph about the history and development of DataCash230Namo Webeditor 9 and how it differs from previous versions.
-- You can add a paragraph about the pros and cons of using DataCash230Namo Webeditor 9 compared to other visual HTML editors.
-- You can add a paragraph about the best practices and tips for using DataCash230Namo Webeditor 9 effectively and efficiently.
-- You can add a paragraph about the feedback and reviews of DataCash230Namo Webeditor 9 from other users and experts.
-- You can add a paragraph about the future plans and updates of DataCash230Namo Webeditor 9 and what features and functions you can expect in the next version.
-
-
-- You can add a paragraph about the history and development of DataCash230Namo Webeditor 9 and how it differs from previous versions.
-- You can add a paragraph about the pros and cons of using DataCash230Namo Webeditor 9 compared to other visual HTML editors.
-- You can add a paragraph about the best practices and tips for using DataCash230Namo Webeditor 9 effectively and efficiently.
-- You can add a paragraph about the feedback and reviews of DataCash230Namo Webeditor 9 from other users and experts.
-- You can add a paragraph about the future plans and updates of DataCash230Namo Webeditor 9 and what features and functions you can expect in the next version.
-Conclusion
-DataCash230Namo Webeditor 9 Crack 27 is a software that allows you to use DataCash230Namo Webeditor 9 for free. It has some benefits and risks that you should weigh before using it. If you decide to use DataCash230Namo Webeditor 9 Crack 27, make sure to download it from a reputable source and scan it for viruses before installing it. Alternatively, you can buy a legitimate license of DataCash230Namo Webeditor 9 and enjoy its features without any worries. You can also explore other alternatives to DataCash230Namo Webeditor 9 that may suit your needs better.
3cee63e6c2
-
-
\ No newline at end of file
diff --git a/spaces/1line/AutoGPT/autogpt/cli.py b/spaces/1line/AutoGPT/autogpt/cli.py
deleted file mode 100644
index a2e99cb421cad005528cb160e948ce59ccfcdb66..0000000000000000000000000000000000000000
--- a/spaces/1line/AutoGPT/autogpt/cli.py
+++ /dev/null
@@ -1,145 +0,0 @@
-"""Main script for the autogpt package."""
-import click
-
-
-@click.group(invoke_without_command=True)
-@click.option("-c", "--continuous", is_flag=True, help="Enable Continuous Mode")
-@click.option(
- "--skip-reprompt",
- "-y",
- is_flag=True,
- help="Skips the re-prompting messages at the beginning of the script",
-)
-@click.option(
- "--ai-settings",
- "-C",
- help="Specifies which ai_settings.yaml file to use, will also automatically skip the re-prompt.",
-)
-@click.option(
- "-l",
- "--continuous-limit",
- type=int,
- help="Defines the number of times to run in continuous mode",
-)
-@click.option("--speak", is_flag=True, help="Enable Speak Mode")
-@click.option("--debug", is_flag=True, help="Enable Debug Mode")
-@click.option("--gpt3only", is_flag=True, help="Enable GPT3.5 Only Mode")
-@click.option("--gpt4only", is_flag=True, help="Enable GPT4 Only Mode")
-@click.option(
- "--use-memory",
- "-m",
- "memory_type",
- type=str,
- help="Defines which Memory backend to use",
-)
-@click.option(
- "-b",
- "--browser-name",
- help="Specifies which web-browser to use when using selenium to scrape the web.",
-)
-@click.option(
- "--allow-downloads",
- is_flag=True,
- help="Dangerous: Allows Auto-GPT to download files natively.",
-)
-@click.option(
- "--skip-news",
- is_flag=True,
- help="Specifies whether to suppress the output of latest news on startup.",
-)
-@click.pass_context
-def main(
- ctx: click.Context,
- continuous: bool,
- continuous_limit: int,
- ai_settings: str,
- skip_reprompt: bool,
- speak: bool,
- debug: bool,
- gpt3only: bool,
- gpt4only: bool,
- memory_type: str,
- browser_name: str,
- allow_downloads: bool,
- skip_news: bool,
-) -> None:
- """
- Welcome to AutoGPT an experimental open-source application showcasing the capabilities of the GPT-4 pushing the boundaries of AI.
-
- Start an Auto-GPT assistant.
- """
- # Put imports inside function to avoid importing everything when starting the CLI
- import logging
-
- from colorama import Fore
-
- from autogpt.agent.agent import Agent
- from autogpt.config import Config, check_openai_api_key
- from autogpt.configurator import create_config
- from autogpt.logs import logger
- from autogpt.memory import get_memory
- from autogpt.prompt import construct_prompt
- from autogpt.utils import get_current_git_branch, get_latest_bulletin
-
- if ctx.invoked_subcommand is None:
- cfg = Config()
- # TODO: fill in llm values here
- check_openai_api_key()
- create_config(
- continuous,
- continuous_limit,
- ai_settings,
- skip_reprompt,
- speak,
- debug,
- gpt3only,
- gpt4only,
- memory_type,
- browser_name,
- allow_downloads,
- skip_news,
- )
- logger.set_level(logging.DEBUG if cfg.debug_mode else logging.INFO)
- ai_name = ""
- if not cfg.skip_news:
- motd = get_latest_bulletin()
- if motd:
- logger.typewriter_log("NEWS: ", Fore.GREEN, motd)
- git_branch = get_current_git_branch()
- if git_branch and git_branch != "stable":
- logger.typewriter_log(
- "WARNING: ",
- Fore.RED,
- f"You are running on `{git_branch}` branch "
- "- this is not a supported branch.",
- )
- system_prompt = construct_prompt()
- # print(prompt)
- # Initialize variables
- full_message_history = []
- next_action_count = 0
- # Make a constant:
- triggering_prompt = (
- "Determine which next command to use, and respond using the"
- " format specified above:"
- )
- # Initialize memory and make sure it is empty.
- # this is particularly important for indexing and referencing pinecone memory
- memory = get_memory(cfg, init=True)
- logger.typewriter_log(
- "Using memory of type:", Fore.GREEN, f"{memory.__class__.__name__}"
- )
- logger.typewriter_log("Using Browser:", Fore.GREEN, cfg.selenium_web_browser)
- agent = Agent(
- ai_name=ai_name,
- memory=memory,
- full_message_history=full_message_history,
- next_action_count=next_action_count,
- system_prompt=system_prompt,
- triggering_prompt=triggering_prompt,
- )
- agent.start_interaction_loop()
-
-
-if __name__ == "__main__":
- main()
diff --git a/spaces/1phancelerku/anime-remove-background/Download Cars The Ultimate Guide for Car Enthusiasts.md b/spaces/1phancelerku/anime-remove-background/Download Cars The Ultimate Guide for Car Enthusiasts.md
deleted file mode 100644
index fec257632996c6b803d087c29d5ceeed660fbf13..0000000000000000000000000000000000000000
--- a/spaces/1phancelerku/anime-remove-background/Download Cars The Ultimate Guide for Car Enthusiasts.md
+++ /dev/null
@@ -1,107 +0,0 @@
-
-How to Download Cars: A Guide for Car Enthusiasts
-Have you ever dreamed of driving a Ferrari, a Lamborghini, or a Bugatti? Have you ever wondered what it would be like to race on the streets, the tracks, or the off-road terrains? If you are a car enthusiast, you might have a passion for exploring different types of cars and experiencing their performance and features. But buying or renting a car can be expensive and impractical. That's why some people choose to download cars instead.
-i want to download cars
DOWNLOAD »»» https://jinyurl.com/2uNT4W
-What does it mean to download cars?
-Downloading cars is a way of accessing digital versions of real or fictional cars on your computer or mobile device. You can download cars as files, such as images, videos, or games, that you can view, play, or edit on your device. You can also download cars as software, such as simulators, that you can run on your device and interact with in a realistic or immersive way.
-The difference between downloading and streaming cars
-Downloading cars means that you save the car files or software on your device's storage, such as your hard drive or memory card. This allows you to access the car anytime, even when you are offline or have no internet connection. However, downloading cars also takes up space on your device and may require more time and bandwidth to complete.
-Streaming cars means that you access the car files or software online, such as on a website or an app. This allows you to access the car instantly, without waiting for the download to finish or using up your device's storage. However, streaming cars also requires a stable and fast internet connection and may consume more data or battery power.
-The benefits of downloading cars
-Downloading cars has many benefits for car enthusiasts, such as:
-How to download cars 2006 movie for free
-Best PC racing games to download from Epic Games Store
-CarGurus app for buying and selling new and used cars
-Download cars wallpapers and screensavers for desktop
-Where to download cars mods for GTA 5
-Download cars coloring pages and printables for kids
-How to download cars 3 driven to win game for PS4
-Best car games to download on Android and iOS devices
-Download cars sound effects and ringtones for free
-Where to download cars logos and icons for design projects
-How to download cars 2 video game for PC
-Best car simulator games to download and play online
-Download cars repair manuals and guides for free
-Where to download cars fonts and typography for free
-How to download cars 4 trailer and watch online
-Best car racing apps to download and stream live races
-Download cars quiz and trivia games for free
-Where to download cars stickers and emojis for WhatsApp
-How to download cars theme song and soundtrack for free
-Best car driving games to download and learn driving skills
-Download cars wallpapers HD and 4K for mobile phones
-Where to download cars blueprints and models for 3D printing
-How to download cars dataset and images for machine learning
-Best car tuning games to download and customize your car
-Download cars flash games and play offline on your browser
-Where to download cars SVG and vector files for free
-How to download cars VR games and experience virtual reality
-Best car parking games to download and improve your parking skills
-Download cars music videos and songs for free
-Where to download cars clipart and illustrations for free
-How to download cars PDF books and magazines for free
-Best car drifting games to download and master drifting techniques
-Download cars CAD files and drawings for free
-Where to download cars PNG and JPEG files for free
-How to download cars podcasts and listen online or offline
-Best car shooting games to download and enjoy action-packed gameplay
-Download cars PowerPoint templates and presentations for free
-Where to download cars GIFs and animations for free
-How to download cars subtitles and captions for free
-Best car escape games to download and solve puzzles
-
-- You can enjoy a wide variety of cars from different brands, models, eras, and genres. You can download cars that are rare, expensive, classic, futuristic, or fictional.
-- You can experience the thrill of driving, racing, or customizing cars in different modes, settings, and scenarios. You can download cars that are realistic, arcade-like, or fantasy-based.
-- You can learn more about the history, culture, and technology of cars. You can download cars that are informative, educational, or entertaining.
-
-The challenges of downloading cars
-Downloading cars also has some challenges that you need to be aware of, such as:
-
-- You may not be able to replicate the exact feeling and sensation of driving a real car. Downloading cars may not capture the physical feedback, the sound quality, or the visual details of a real car.
-- You may encounter technical issues or errors when downloading or running the car files or software. Downloading cars may cause compatibility problems, performance issues, or bugs on your device.
-- You may face legal or ethical issues when downloading or using the car files or software. Downloading cars may violate the intellectual property rights, the privacy rights, or the safety regulations of the car owners, creators, or authorities.
-
-Where can you download cars?
-The best websites for downloading cars
-If you want to download car files, such as images, videos, or games, you can visit some of the best websites for downloading cars. Here are some examples:
-Internet Archive
-The Internet Archive is a digital library that offers free access to millions of car images and videos that you can download and use for personal or non-commercial purposes. You can also find thousands of car games that you can download and play on your device. Some of the car games available on the Internet Archive are Need for Speed, Grand Theft Auto, and Carmageddon.
-Epic Games Store
-The Epic Games Store is a digital distribution platform that offers free and paid car games that you can download and play on your PC. You can also find exclusive deals and discounts on some of the car games. Some of the car games available on the Epic Games Store are Forza Horizon 4, Rocket League, and Wreckfest.
-GameTop
-GameTop is a website that offers free and legal car games that you can download and play on your PC. You can also find no ads, no in-game purchases, and no malware on the car games. Some of the car games available on GameTop are City Racing, Off-Road Super Racing, and Fire and Forget.
-The best apps for downloading cars
-If you want to download car software, such as simulators, you can visit some of the best apps for downloading cars. Here are some examples:
-Car Simulator 2
-Car Simulator 2 is a free app that lets you download and drive more than 80 cars in an open world. You can also customize, upgrade, and repair your cars. You can also play online with other players or offline with bots. Car Simulator 2 is available for Android and iOS devices.
-Real Racing 3
-Real Racing 3 is a free app that lets you download and race more than 250 cars from real manufacturers. You can also compete in more than 40 tracks from real locations. You can also join online events and challenges with other players or offline modes with AI. Real Racing 3 is available for Android and iOS devices.
-Asphalt 9: Legends
-Asphalt 9: Legends is a free app that lets you download and drive more than 60 cars from top brands. You can also customize, upgrade, and nitro-boost your cars. You can also join online clubs and seasons with other players or offline career mode with storylines. Asphalt 9: Legends is available for Android, iOS, and Windows devices.
-How to download cars safely and legally?
-The risks of downloading cars from untrusted sources
-Downloading cars from untrusted sources can expose you to various risks, such as:
-
-- You may download fake or corrupted car files or software that do not work properly or damage your device.
-- You may download malicious car files or software that contain malware or viruses that infect your device or steal your data.
-- You may download illegal car files or software that infringe the intellectual property rights, the privacy rights, or the safety regulations of the car owners, creators, or authorities.
-
-The tips for avoiding malware and viruses
-To avoid malware and viruses when downloading cars, you should follow these tips:
-
-- You should only download cars from trusted sources, such as official websites or apps, reputable platforms or stores, or verified users or developers.
-- You should scan the car files or software with an antivirus program before opening or running them on your device.
-- You should update your device's operating system and security software regularly to protect it from new threats.
-
-The laws and regulations for downloading cars
-To avoid legal or ethical issues when downloading cars, you should follow these laws and regulations:
-
-- You should respect the intellectual property rights of the car owners and creators by not copying, distributing, modifying, or selling the car files or software without their permission.
-- You should respect the privacy rights of the car owners and creators by not collecting, sharing, or using their personal information without their consent.
-- You should respect the safety regulations of the car authorities by not using the car files or software for illegal or harmful purposes, such as hacking, fraud, or terrorism.
-
-Conclusion
-Downloading cars is a fun and exciting way to enjoy different types of cars on your device. You can download cars as files or software from various websites or apps. However, you should also be careful about the risks of downloading cars from untrusted sources and the laws and regulations for downloading cars. By following these tips, you can download cars safely and legally.
- FAQs Q: How much space does downloading cars take on my device? A: The space required for downloading cars depends on the size and quality of the car files or software. Generally, the higher the resolution, the sound, or the graphics of the car, the more space it will take. You can check the file size or the system requirements of the car before downloading it to make sure you have enough space on your device. Q: How long does downloading cars take on my device? A: The time required for downloading cars depends on the speed and stability of your internet connection and the server of the source. Generally, the faster your internet connection and the server, the less time it will take. You can also pause or resume the download if you encounter any interruptions or errors. Q: Can I download cars for free or do I have to pay for them? A: The cost of downloading cars depends on the source and the type of the car. Some sources offer free car files or software that you can download and use without paying anything. However, some sources may charge a fee or require a subscription for downloading or accessing certain car files or software. You should check the price or the terms and conditions of the source before downloading any car. Q: Can I download cars on any device or do I need a specific device? A: The compatibility of downloading cars depends on the format and the platform of the car files or software. Some car files or software are compatible with multiple devices, such as PCs, laptops, tablets, or smartphones. However, some car files or software may only work on specific devices, such as Windows, Mac, Android, or iOS. You should check the file format or the system requirements of the car before downloading it to make sure it works on your device. Q: Can I share or transfer the car files or software that I downloaded to other devices or people? A: The sharing or transferring of car files or software that you downloaded depends on the license and the permission of the source and the owner. Some car files or software are free and open-source, which means you can share or transfer them to other devices or people without any restrictions. However, some car files or software are proprietary and protected, which means you cannot share or transfer them to other devices or people without violating their rights. You should check the license or the permission of the source and the owner before sharing or transferring any car. 401be4b1e0
-
-
\ No newline at end of file
diff --git a/spaces/4Taps/SadTalker/src/face3d/models/arcface_torch/run.sh b/spaces/4Taps/SadTalker/src/face3d/models/arcface_torch/run.sh
deleted file mode 100644
index 61af4b4950eb11334e55362e3e3c5e2796979a01..0000000000000000000000000000000000000000
--- a/spaces/4Taps/SadTalker/src/face3d/models/arcface_torch/run.sh
+++ /dev/null
@@ -1,2 +0,0 @@
-CUDA_VISIBLE_DEVICES=0,1,2,3,4,5,6,7 python -m torch.distributed.launch --nproc_per_node=8 --nnodes=1 --node_rank=0 --master_addr="127.0.0.1" --master_port=1234 train.py configs/ms1mv3_r50
-ps -ef | grep "train" | grep -v grep | awk '{print "kill -9 "$2}' | sh
diff --git a/spaces/A00001/bingothoo/next.config.js b/spaces/A00001/bingothoo/next.config.js
deleted file mode 100644
index 0e6ccd7fbc91d0459eaaff3e968ce0556789c605..0000000000000000000000000000000000000000
--- a/spaces/A00001/bingothoo/next.config.js
+++ /dev/null
@@ -1,38 +0,0 @@
-/** @type {import('next').NextConfig} */
-const nextConfig = {
- // output: 'export',
- // assetPrefix: '.',
- webpack: (config, { isServer }) => {
- if (!isServer) {
- config.resolve = {
- ...config.resolve,
- fallback: {
- 'bufferutil': false,
- 'utf-8-validate': false,
- http: false,
- https: false,
- stream: false,
- // fixes proxy-agent dependencies
- net: false,
- dns: false,
- tls: false,
- assert: false,
- // fixes next-i18next dependencies
- path: false,
- fs: false,
- // fixes mapbox dependencies
- events: false,
- // fixes sentry dependencies
- process: false
- }
- };
- }
- config.module.exprContextCritical = false;
-
- return config;
- },
-}
-
-module.exports = (...args) => {
- return nextConfig
-}
diff --git a/spaces/AIGC-Audio/AudioGPT/audio_to_text/captioning/models/transformer_model.py b/spaces/AIGC-Audio/AudioGPT/audio_to_text/captioning/models/transformer_model.py
deleted file mode 100644
index 76c97f171955f04b10c16fd1f1a205ce7343a0ac..0000000000000000000000000000000000000000
--- a/spaces/AIGC-Audio/AudioGPT/audio_to_text/captioning/models/transformer_model.py
+++ /dev/null
@@ -1,265 +0,0 @@
-# -*- coding: utf-8 -*-
-import random
-import torch
-import torch.nn as nn
-
-from .base_model import CaptionModel
-from .utils import repeat_tensor
-import audio_to_text.captioning.models.decoder
-
-
-class TransformerModel(CaptionModel):
-
- def __init__(self, encoder: nn.Module, decoder: nn.Module, **kwargs):
- if not hasattr(self, "compatible_decoders"):
- self.compatible_decoders = (
- audio_to_text.captioning.models.decoder.TransformerDecoder,
- )
- super().__init__(encoder, decoder, **kwargs)
-
- def seq_forward(self, input_dict):
- cap = input_dict["cap"]
- cap_padding_mask = (cap == self.pad_idx).to(cap.device)
- cap_padding_mask = cap_padding_mask[:, :-1]
- output = self.decoder(
- {
- "word": cap[:, :-1],
- "attn_emb": input_dict["attn_emb"],
- "attn_emb_len": input_dict["attn_emb_len"],
- "cap_padding_mask": cap_padding_mask
- }
- )
- return output
-
- def prepare_decoder_input(self, input_dict, output):
- decoder_input = {
- "attn_emb": input_dict["attn_emb"],
- "attn_emb_len": input_dict["attn_emb_len"]
- }
- t = input_dict["t"]
-
- ###############
- # determine input word
- ################
- if input_dict["mode"] == "train" and random.random() < input_dict["ss_ratio"]: # training, scheduled sampling
- word = input_dict["cap"][:, :t+1]
- else:
- start_word = torch.tensor([self.start_idx,] * input_dict["attn_emb"].size(0)).unsqueeze(1).long()
- if t == 0:
- word = start_word
- else:
- word = torch.cat((start_word, output["seq"][:, :t]), dim=-1)
- # word: [N, T]
- decoder_input["word"] = word
-
- cap_padding_mask = (word == self.pad_idx).to(input_dict["attn_emb"].device)
- decoder_input["cap_padding_mask"] = cap_padding_mask
- return decoder_input
-
- def prepare_beamsearch_decoder_input(self, input_dict, output_i):
- decoder_input = {}
- t = input_dict["t"]
- i = input_dict["sample_idx"]
- beam_size = input_dict["beam_size"]
- ###############
- # prepare attn embeds
- ################
- if t == 0:
- attn_emb = repeat_tensor(input_dict["attn_emb"][i], beam_size)
- attn_emb_len = repeat_tensor(input_dict["attn_emb_len"][i], beam_size)
- output_i["attn_emb"] = attn_emb
- output_i["attn_emb_len"] = attn_emb_len
- decoder_input["attn_emb"] = output_i["attn_emb"]
- decoder_input["attn_emb_len"] = output_i["attn_emb_len"]
- ###############
- # determine input word
- ################
- start_word = torch.tensor([self.start_idx,] * beam_size).unsqueeze(1).long()
- if t == 0:
- word = start_word
- else:
- word = torch.cat((start_word, output_i["seq"]), dim=-1)
- decoder_input["word"] = word
- cap_padding_mask = (word == self.pad_idx).to(input_dict["attn_emb"].device)
- decoder_input["cap_padding_mask"] = cap_padding_mask
-
- return decoder_input
-
-
-class M2TransformerModel(CaptionModel):
-
- def __init__(self, encoder: nn.Module, decoder: nn.Module, **kwargs):
- if not hasattr(self, "compatible_decoders"):
- self.compatible_decoders = (
- captioning.models.decoder.M2TransformerDecoder,
- )
- super().__init__(encoder, decoder, **kwargs)
- self.check_encoder_compatibility()
-
- def check_encoder_compatibility(self):
- assert isinstance(self.encoder, captioning.models.encoder.M2TransformerEncoder), \
- f"only M2TransformerModel is compatible with {self.__class__.__name__}"
-
-
- def seq_forward(self, input_dict):
- cap = input_dict["cap"]
- output = self.decoder(
- {
- "word": cap[:, :-1],
- "attn_emb": input_dict["attn_emb"],
- "attn_emb_mask": input_dict["attn_emb_mask"],
- }
- )
- return output
-
- def prepare_decoder_input(self, input_dict, output):
- decoder_input = {
- "attn_emb": input_dict["attn_emb"],
- "attn_emb_mask": input_dict["attn_emb_mask"]
- }
- t = input_dict["t"]
-
- ###############
- # determine input word
- ################
- if input_dict["mode"] == "train" and random.random() < input_dict["ss_ratio"]: # training, scheduled sampling
- word = input_dict["cap"][:, :t+1]
- else:
- start_word = torch.tensor([self.start_idx,] * input_dict["attn_emb"].size(0)).unsqueeze(1).long()
- if t == 0:
- word = start_word
- else:
- word = torch.cat((start_word, output["seq"][:, :t]), dim=-1)
- # word: [N, T]
- decoder_input["word"] = word
-
- return decoder_input
-
- def prepare_beamsearch_decoder_input(self, input_dict, output_i):
- decoder_input = {}
- t = input_dict["t"]
- i = input_dict["sample_idx"]
- beam_size = input_dict["beam_size"]
- ###############
- # prepare attn embeds
- ################
- if t == 0:
- attn_emb = repeat_tensor(input_dict["attn_emb"][i], beam_size)
- attn_emb_mask = repeat_tensor(input_dict["attn_emb_mask"][i], beam_size)
- output_i["attn_emb"] = attn_emb
- output_i["attn_emb_mask"] = attn_emb_mask
- decoder_input["attn_emb"] = output_i["attn_emb"]
- decoder_input["attn_emb_mask"] = output_i["attn_emb_mask"]
- ###############
- # determine input word
- ################
- start_word = torch.tensor([self.start_idx,] * beam_size).unsqueeze(1).long()
- if t == 0:
- word = start_word
- else:
- word = torch.cat((start_word, output_i["seq"]), dim=-1)
- decoder_input["word"] = word
-
- return decoder_input
-
-
-class EventEncoder(nn.Module):
- """
- Encode the Label information in AudioCaps and AudioSet
- """
- def __init__(self, emb_dim, vocab_size=527):
- super(EventEncoder, self).__init__()
- self.label_embedding = nn.Parameter(
- torch.randn((vocab_size, emb_dim)), requires_grad=True)
-
- def forward(self, word_idxs):
- indices = word_idxs / word_idxs.sum(dim=1, keepdim=True)
- embeddings = indices @ self.label_embedding
- return embeddings
-
-
-class EventCondTransformerModel(TransformerModel):
-
- def __init__(self, encoder: nn.Module, decoder: nn.Module, **kwargs):
- if not hasattr(self, "compatible_decoders"):
- self.compatible_decoders = (
- captioning.models.decoder.EventTransformerDecoder,
- )
- super().__init__(encoder, decoder, **kwargs)
- self.label_encoder = EventEncoder(decoder.emb_dim, 527)
- self.train_forward_keys += ["events"]
- self.inference_forward_keys += ["events"]
-
- # def seq_forward(self, input_dict):
- # cap = input_dict["cap"]
- # cap_padding_mask = (cap == self.pad_idx).to(cap.device)
- # cap_padding_mask = cap_padding_mask[:, :-1]
- # output = self.decoder(
- # {
- # "word": cap[:, :-1],
- # "attn_emb": input_dict["attn_emb"],
- # "attn_emb_len": input_dict["attn_emb_len"],
- # "cap_padding_mask": cap_padding_mask
- # }
- # )
- # return output
-
- def prepare_decoder_input(self, input_dict, output):
- decoder_input = super().prepare_decoder_input(input_dict, output)
- decoder_input["events"] = self.label_encoder(input_dict["events"])
- return decoder_input
-
- def prepare_beamsearch_decoder_input(self, input_dict, output_i):
- decoder_input = super().prepare_beamsearch_decoder_input(input_dict, output_i)
- t = input_dict["t"]
- i = input_dict["sample_idx"]
- beam_size = input_dict["beam_size"]
- if t == 0:
- output_i["events"] = repeat_tensor(self.label_encoder(input_dict["events"])[i], beam_size)
- decoder_input["events"] = output_i["events"]
- return decoder_input
-
-
-class KeywordCondTransformerModel(TransformerModel):
-
- def __init__(self, encoder: nn.Module, decoder: nn.Module, **kwargs):
- if not hasattr(self, "compatible_decoders"):
- self.compatible_decoders = (
- captioning.models.decoder.KeywordProbTransformerDecoder,
- )
- super().__init__(encoder, decoder, **kwargs)
- self.train_forward_keys += ["keyword"]
- self.inference_forward_keys += ["keyword"]
-
- def seq_forward(self, input_dict):
- cap = input_dict["cap"]
- cap_padding_mask = (cap == self.pad_idx).to(cap.device)
- cap_padding_mask = cap_padding_mask[:, :-1]
- keyword = input_dict["keyword"]
- output = self.decoder(
- {
- "word": cap[:, :-1],
- "attn_emb": input_dict["attn_emb"],
- "attn_emb_len": input_dict["attn_emb_len"],
- "keyword": keyword,
- "cap_padding_mask": cap_padding_mask
- }
- )
- return output
-
- def prepare_decoder_input(self, input_dict, output):
- decoder_input = super().prepare_decoder_input(input_dict, output)
- decoder_input["keyword"] = input_dict["keyword"]
- return decoder_input
-
- def prepare_beamsearch_decoder_input(self, input_dict, output_i):
- decoder_input = super().prepare_beamsearch_decoder_input(input_dict, output_i)
- t = input_dict["t"]
- i = input_dict["sample_idx"]
- beam_size = input_dict["beam_size"]
- if t == 0:
- output_i["keyword"] = repeat_tensor(input_dict["keyword"][i],
- beam_size)
- decoder_input["keyword"] = output_i["keyword"]
- return decoder_input
-
diff --git a/spaces/AIGC-Audio/AudioGPT/text_to_audio/Make_An_Audio/ldm/modules/encoders/open_clap/tokenizer.py b/spaces/AIGC-Audio/AudioGPT/text_to_audio/Make_An_Audio/ldm/modules/encoders/open_clap/tokenizer.py
deleted file mode 100644
index 5b4a238b987ce66f2932b11451d916e40816b8a3..0000000000000000000000000000000000000000
--- a/spaces/AIGC-Audio/AudioGPT/text_to_audio/Make_An_Audio/ldm/modules/encoders/open_clap/tokenizer.py
+++ /dev/null
@@ -1,180 +0,0 @@
-""" CLIP tokenizer
-
-Copied from https://github.com/openai/CLIP. Originally MIT License, Copyright (c) 2021 OpenAI.
-"""
-import gzip
-import html
-import os
-from functools import lru_cache
-from typing import Union, List
-
-import ftfy
-import regex as re
-import torch
-
-
-@lru_cache()
-def default_bpe():
- return os.path.join(os.path.dirname(os.path.abspath(__file__)), "bpe_simple_vocab_16e6.txt.gz")
-
-
-@lru_cache()
-def bytes_to_unicode():
- """
- Returns list of utf-8 byte and a corresponding list of unicode strings.
- The reversible bpe codes work on unicode strings.
- This means you need a large # of unicode characters in your vocab if you want to avoid UNKs.
- When you're at something like a 10B token dataset you end up needing around 5K for decent coverage.
- This is a signficant percentage of your normal, say, 32K bpe vocab.
- To avoid that, we want lookup tables between utf-8 bytes and unicode strings.
- And avoids mapping to whitespace/control characters the bpe code barfs on.
- """
- bs = list(range(ord("!"), ord("~")+1))+list(range(ord("¡"), ord("¬")+1))+list(range(ord("®"), ord("ÿ")+1))
- cs = bs[:]
- n = 0
- for b in range(2**8):
- if b not in bs:
- bs.append(b)
- cs.append(2**8+n)
- n += 1
- cs = [chr(n) for n in cs]
- return dict(zip(bs, cs))
-
-
-def get_pairs(word):
- """Return set of symbol pairs in a word.
- Word is represented as tuple of symbols (symbols being variable-length strings).
- """
- pairs = set()
- prev_char = word[0]
- for char in word[1:]:
- pairs.add((prev_char, char))
- prev_char = char
- return pairs
-
-
-def basic_clean(text):
- text = ftfy.fix_text(text)
- text = html.unescape(html.unescape(text))
- return text.strip()
-
-
-def whitespace_clean(text):
- text = re.sub(r'\s+', ' ', text)
- text = text.strip()
- return text
-
-
-class SimpleTokenizer(object):
- def __init__(self, bpe_path: str = default_bpe(), special_tokens=None):
- self.byte_encoder = bytes_to_unicode()
- self.byte_decoder = {v: k for k, v in self.byte_encoder.items()}
- merges = gzip.open(bpe_path).read().decode("utf-8").split('\n')
- merges = merges[1:49152-256-2+1]
- merges = [tuple(merge.split()) for merge in merges]
- vocab = list(bytes_to_unicode().values())
- vocab = vocab + [v+'' for v in vocab]
- for merge in merges:
- vocab.append(''.join(merge))
- if not special_tokens:
- special_tokens = ['', '']
- else:
- special_tokens = ['', ''] + special_tokens
- vocab.extend(special_tokens)
- self.encoder = dict(zip(vocab, range(len(vocab))))
- self.decoder = {v: k for k, v in self.encoder.items()}
- self.bpe_ranks = dict(zip(merges, range(len(merges))))
- self.cache = {t:t for t in special_tokens}
- special = "|".join(special_tokens)
- self.pat = re.compile(special + r"""|'s|'t|'re|'ve|'m|'ll|'d|[\p{L}]+|[\p{N}]|[^\s\p{L}\p{N}]+""", re.IGNORECASE)
-
- self.vocab_size = len(self.encoder)
- self.all_special_ids = [self.encoder[t] for t in special_tokens]
-
- def bpe(self, token):
- if token in self.cache:
- return self.cache[token]
- word = tuple(token[:-1]) + ( token[-1] + '',)
- pairs = get_pairs(word)
-
- if not pairs:
- return token+''
-
- while True:
- bigram = min(pairs, key = lambda pair: self.bpe_ranks.get(pair, float('inf')))
- if bigram not in self.bpe_ranks:
- break
- first, second = bigram
- new_word = []
- i = 0
- while i < len(word):
- try:
- j = word.index(first, i)
- new_word.extend(word[i:j])
- i = j
- except:
- new_word.extend(word[i:])
- break
-
- if word[i] == first and i < len(word)-1 and word[i+1] == second:
- new_word.append(first+second)
- i += 2
- else:
- new_word.append(word[i])
- i += 1
- new_word = tuple(new_word)
- word = new_word
- if len(word) == 1:
- break
- else:
- pairs = get_pairs(word)
- word = ' '.join(word)
- self.cache[token] = word
- return word
-
- def encode(self, text):
- bpe_tokens = []
- text = whitespace_clean(basic_clean(text)).lower()
- for token in re.findall(self.pat, text):
- token = ''.join(self.byte_encoder[b] for b in token.encode('utf-8'))
- bpe_tokens.extend(self.encoder[bpe_token] for bpe_token in self.bpe(token).split(' '))
- return bpe_tokens
-
- def decode(self, tokens):
- text = ''.join([self.decoder[token] for token in tokens])
- text = bytearray([self.byte_decoder[c] for c in text]).decode('utf-8', errors="replace").replace('', ' ')
- return text
-
-
-_tokenizer = SimpleTokenizer()
-
-
-def tokenize(texts: Union[str, List[str]], context_length: int = 77) -> torch.LongTensor:
- """
- Returns the tokenized representation of given input string(s)
-
- Parameters
- ----------
- texts : Union[str, List[str]]
- An input string or a list of input strings to tokenize
- context_length : int
- The context length to use; all CLIP models use 77 as the context length
-
- Returns
- -------
- A two-dimensional tensor containing the resulting tokens, shape = [number of input strings, context_length]
- """
- if isinstance(texts, str):
- texts = [texts]
-
- sot_token = _tokenizer.encoder[""]
- eot_token = _tokenizer.encoder[""]
- all_tokens = [[sot_token] + _tokenizer.encode(text) + [eot_token] for text in texts]
- result = torch.zeros(len(all_tokens), context_length, dtype=torch.long)
-
- for i, tokens in enumerate(all_tokens):
- if len(tokens) > context_length:
- tokens = tokens[:context_length] # Truncate
- result[i, :len(tokens)] = torch.tensor(tokens)
-
- return result
diff --git a/spaces/ATang0729/Forecast4Muses/Model/Model6/Model6_1_ClothesKeyPoint/mmpose_1_x/configs/fashion_2d_keypoint/topdown_heatmap/deepfashion2/td_hm_res50_4xb64-150e_deepfashion2_vest_dress_256x192.py b/spaces/ATang0729/Forecast4Muses/Model/Model6/Model6_1_ClothesKeyPoint/mmpose_1_x/configs/fashion_2d_keypoint/topdown_heatmap/deepfashion2/td_hm_res50_4xb64-150e_deepfashion2_vest_dress_256x192.py
deleted file mode 100644
index 9aedf0b61fb8072149be212d9b98a904fc821e85..0000000000000000000000000000000000000000
--- a/spaces/ATang0729/Forecast4Muses/Model/Model6/Model6_1_ClothesKeyPoint/mmpose_1_x/configs/fashion_2d_keypoint/topdown_heatmap/deepfashion2/td_hm_res50_4xb64-150e_deepfashion2_vest_dress_256x192.py
+++ /dev/null
@@ -1,172 +0,0 @@
-_base_ = [
- '../../../_base_/default_runtime.py',
- '../../../_base_/datasets/deepfashion2.py'
-]
-
-default_hooks = dict(checkpoint=dict(save_best='PCK', rule='greater'))
-
-resume = False # 断点恢复
-load_from = None # 模型权重加载
-train_cfg = dict(by_epoch=True, max_epochs=150, val_interval=10) # 训练轮数,测试间隔
-param_scheduler = [
- dict( # warmup策略
- type='LinearLR',
- begin=0,
- end=500,
- start_factor=0.001,
- by_epoch=False),
- dict( # scheduler
- type='MultiStepLR',
- begin=0,
- end=150,
- milestones=[100, 130],
- gamma=0.1,
- by_epoch=True)
-]
-optim_wrapper = dict(optimizer=dict(type='Adam', lr=0.0005)) # 优化器和学习率
-auto_scale_lr = dict(base_batch_size=512) # 根据batch_size自动缩放学习率
-
-backend_args = dict(backend='local') # 数据加载后端设置,默认从本地硬盘加载
-dataset_type = 'DeepFashion2Dataset' # 数据集类名 DeepFashionDataset
-data_mode = 'topdown' # 算法结构类型,用于指定标注信息加载策略
-data_root = 'data/deepfashion2/' # 数据存放路径
-# 定义数据编解码器,用于生成target和对pred进行解码,同时包含了输入图片和输出heatmap尺寸等信息
-codec = dict(
- type='MSRAHeatmap', input_size=(192, 256), heatmap_size=(48, 64), sigma=2)
-
-train_pipeline = [
- dict(type='LoadImage'),
- dict(type='GetBBoxCenterScale'),
- dict(type='RandomFlip', direction='horizontal'),
- dict(
- type='RandomBBoxTransform',
- shift_prob=0,
- rotate_factor=60,
- scale_factor=(0.75, 1.25)),
- dict(type='TopdownAffine', input_size=codec['input_size']),
- dict(type='GenerateTarget', encoder=codec),
- dict(type='PackPoseInputs')
-]
-val_pipeline = [ # 测试时数据增强
- dict(type='LoadImage', backend_args=backend_args), # 加载图片
- dict(type='GetBBoxCenterScale'), # 根据bbox获取center和scale
- dict(type='TopdownAffine', input_size=codec['input_size']), # 根据变换矩阵更新目标数据
- dict(type='PackPoseInputs') # 对target进行打包用于训练
-]
-train_dataloader = dict( # 训练数据加载
- batch_size=64, # 批次大小
- num_workers=6, # 数据加载进程数
- persistent_workers=True, # 在不活跃时维持进程不终止,避免反复启动进程的开销
- sampler=dict(type='DefaultSampler', shuffle=True), # 采样策略,打乱数据
- dataset=dict(
- type=dataset_type, # 数据集类名
- data_root=data_root, # 数据集路径
- data_mode=data_mode, # 算法类型
- ann_file='train/deepfashion2_vest_dress.json', # 标注文件路径
- data_prefix=dict(img='train/image/'), # 图像路径
- pipeline=train_pipeline # 数据流水线
- ))
-val_dataloader = dict(
- batch_size=32,
- num_workers=6,
- persistent_workers=True, # 在不活跃时维持进程不终止,避免反复启动进程的开销
- drop_last=False,
- sampler=dict(type='DefaultSampler', shuffle=False), # 采样策略,不进行打乱
- dataset=dict(
- type=dataset_type, # 数据集类名
- data_root=data_root, # 数据集路径
- data_mode=data_mode, # 算法类型
- ann_file='validation/deepfashion2_vest_dress.json', # 标注文件路径
- data_prefix=dict(img='validation/image/'), # 图像路径
- test_mode=True, # 测试模式开关
- pipeline=val_pipeline # 数据流水线
- ))
-test_dataloader = val_dataloader # 默认情况下不区分验证集和测试集,用户根据需要来自行定义
-
-channel_cfg = dict(
- num_output_channels=294,
- dataset_joints=294,
- dataset_channel=[
- [
- 0, 1, 2, 3, 4, 5, 6, 7, 8, 9, 10, 11, 12, 13, 14, 15, 16, 17, 18,
- 19, 20, 21, 22, 23, 24, 25, 26, 27, 28, 29, 30, 31, 32, 33, 34, 35,
- 36, 37, 38, 39, 40, 41, 42, 43, 44, 45, 46, 47, 48, 49, 50, 51, 52,
- 53, 54, 55, 56, 57, 58, 59, 60, 61, 62, 63, 64, 65, 66, 67, 68, 69,
- 70, 71, 72, 73, 74, 75, 76, 77, 78, 79, 80, 81, 82, 83, 84, 85, 86,
- 87, 88, 89, 90, 91, 92, 93, 94, 95, 96, 97, 98, 99, 100, 101, 102,
- 103, 104, 105, 106, 107, 108, 109, 110, 111, 112, 113, 114, 115,
- 116, 117, 118, 119, 120, 121, 122, 123, 124, 125, 126, 127, 128,
- 129, 130, 131, 132, 133, 134, 135, 136, 137, 138, 139, 140, 141,
- 142, 143, 144, 145, 146, 147, 148, 149, 150, 151, 152, 153, 154,
- 155, 156, 157, 158, 159, 160, 161, 162, 163, 164, 165, 166, 167,
- 168, 169, 170, 171, 172, 173, 174, 175, 176, 177, 178, 179, 180,
- 181, 182, 183, 184, 185, 186, 187, 188, 189, 190, 191, 192, 193,
- 194, 195, 196, 197, 198, 199, 200, 201, 202, 203, 204, 205, 206,
- 207, 208, 209, 210, 211, 212, 213, 214, 215, 216, 217, 218, 219,
- 220, 221, 222, 223, 224, 225, 226, 227, 228, 229, 230, 231, 232,
- 233, 234, 235, 236, 237, 238, 239, 240, 241, 242, 243, 244, 245,
- 246, 247, 248, 249, 250, 251, 252, 253, 254, 255, 256, 257, 258,
- 259, 260, 261, 262, 263, 264, 265, 266, 267, 268, 269, 270, 271,
- 272, 273, 274, 275, 276, 277, 278, 279, 280, 281, 282, 283, 284,
- 285, 286, 287, 288, 289, 290, 291, 292, 293
- ],
- ],
- inference_channel=[
- 0, 1, 2, 3, 4, 5, 6, 7, 8, 9, 10, 11, 12, 13, 14, 15, 16, 17, 18, 19,
- 20, 21, 22, 23, 24, 25, 26, 27, 28, 29, 30, 31, 32, 33, 34, 35, 36, 37,
- 38, 39, 40, 41, 42, 43, 44, 45, 46, 47, 48, 49, 50, 51, 52, 53, 54, 55,
- 56, 57, 58, 59, 60, 61, 62, 63, 64, 65, 66, 67, 68, 69, 70, 71, 72, 73,
- 74, 75, 76, 77, 78, 79, 80, 81, 82, 83, 84, 85, 86, 87, 88, 89, 90, 91,
- 92, 93, 94, 95, 96, 97, 98, 99, 100, 101, 102, 103, 104, 105, 106, 107,
- 108, 109, 110, 111, 112, 113, 114, 115, 116, 117, 118, 119, 120, 121,
- 122, 123, 124, 125, 126, 127, 128, 129, 130, 131, 132, 133, 134, 135,
- 136, 137, 138, 139, 140, 141, 142, 143, 144, 145, 146, 147, 148, 149,
- 150, 151, 152, 153, 154, 155, 156, 157, 158, 159, 160, 161, 162, 163,
- 164, 165, 166, 167, 168, 169, 170, 171, 172, 173, 174, 175, 176, 177,
- 178, 179, 180, 181, 182, 183, 184, 185, 186, 187, 188, 189, 190, 191,
- 192, 193, 194, 195, 196, 197, 198, 199, 200, 201, 202, 203, 204, 205,
- 206, 207, 208, 209, 210, 211, 212, 213, 214, 215, 216, 217, 218, 219,
- 220, 221, 222, 223, 224, 225, 226, 227, 228, 229, 230, 231, 232, 233,
- 234, 235, 236, 237, 238, 239, 240, 241, 242, 243, 244, 245, 246, 247,
- 248, 249, 250, 251, 252, 253, 254, 255, 256, 257, 258, 259, 260, 261,
- 262, 263, 264, 265, 266, 267, 268, 269, 270, 271, 272, 273, 274, 275,
- 276, 277, 278, 279, 280, 281, 282, 283, 284, 285, 286, 287, 288, 289,
- 290, 291, 292, 293
- ])
-
-model = dict(
- type='TopdownPoseEstimator', # 模型结构决定了算法流程
- data_preprocessor=dict( # 数据归一化和通道顺序调整,作为模型的一部分
- type='PoseDataPreprocessor',
- mean=[123.675, 116.28, 103.53],
- std=[58.395, 57.12, 57.375],
- bgr_to_rgb=True),
- backbone=dict(
- type='ResNet',
- depth=50,
- init_cfg=dict(
- type='Pretrained', # 预训练参数,只加载backbone权重用于迁移学习
- checkpoint='torchvision://resnet50')),
- head=dict( # 模型头部
- type='HeatmapHead',
- in_channels=2048,
- out_channels=channel_cfg['num_output_channels'],
- # deconv_out_channels=None,
- loss=dict(type='KeypointMSELoss', use_target_weight=True), # 损失函数
- decoder=codec), # 解码器,将heatmap解码成坐标值
- test_cfg=dict(
- flip_test=True, # 开启测试时水平翻转集成
- flip_mode='heatmap', # 对heatmap进行翻转
- shift_heatmap=True, # 对翻转后的结果进行平移提高精度
- ))
-
-val_evaluator = [
- dict(type='PCKAccuracy', thr=0.2),
- dict(type='AUC'),
- dict(type='EPE'),
-]
-test_evaluator = val_evaluator # 默认情况下不区分验证集和测试集,用户根据需要来自行定义
-
-visualizer = dict(
- vis_backends=[dict(type='LocalVisBackend'),
- dict(type='WandbVisBackend')])
diff --git a/spaces/ATang0729/Forecast4Muses/Model/Model6/Model6_2_ProfileRecogition/mmpretrain/configs/_base_/models/resnet101.py b/spaces/ATang0729/Forecast4Muses/Model/Model6/Model6_2_ProfileRecogition/mmpretrain/configs/_base_/models/resnet101.py
deleted file mode 100644
index 1147cd4be9aff00ad6ce66c31e2839c1a94f9ca3..0000000000000000000000000000000000000000
--- a/spaces/ATang0729/Forecast4Muses/Model/Model6/Model6_2_ProfileRecogition/mmpretrain/configs/_base_/models/resnet101.py
+++ /dev/null
@@ -1,17 +0,0 @@
-# model settings
-model = dict(
- type='ImageClassifier',
- backbone=dict(
- type='ResNet',
- depth=101,
- num_stages=4,
- out_indices=(3, ),
- style='pytorch'),
- neck=dict(type='GlobalAveragePooling'),
- head=dict(
- type='LinearClsHead',
- num_classes=1000,
- in_channels=2048,
- loss=dict(type='CrossEntropyLoss', loss_weight=1.0),
- topk=(1, 5),
- ))
diff --git a/spaces/Abhay834/my_genai_chatbot/README.md b/spaces/Abhay834/my_genai_chatbot/README.md
deleted file mode 100644
index 1deecc4f97a04828a1c76e8dd8d8c849211549dd..0000000000000000000000000000000000000000
--- a/spaces/Abhay834/my_genai_chatbot/README.md
+++ /dev/null
@@ -1,12 +0,0 @@
----
-title: My Genai Chatbot
-emoji: 🐨
-colorFrom: gray
-colorTo: blue
-sdk: gradio
-sdk_version: 3.39.0
-app_file: app.py
-pinned: false
----
-
-Check out the configuration reference at https://huggingface.co/docs/hub/spaces-config-reference
diff --git a/spaces/AgentVerse/agentVerse/agentverse/environments/tasksolving_env/rules/decision_maker/horizontal.py b/spaces/AgentVerse/agentVerse/agentverse/environments/tasksolving_env/rules/decision_maker/horizontal.py
deleted file mode 100644
index b2a8c57037513bb3d80c03a9b58661f7299ffd26..0000000000000000000000000000000000000000
--- a/spaces/AgentVerse/agentVerse/agentverse/environments/tasksolving_env/rules/decision_maker/horizontal.py
+++ /dev/null
@@ -1,57 +0,0 @@
-from __future__ import annotations
-import asyncio
-from colorama import Fore
-
-from typing import TYPE_CHECKING, List
-
-from . import decision_maker_registry
-from .base import BaseDecisionMaker
-from agentverse.logging import logger
-
-from agentverse.message import Message
-
-if TYPE_CHECKING:
- from agentverse.agents.base import BaseAgent
- from agentverse.message import CriticMessage
-
-
-@decision_maker_registry.register("horizontal")
-class HorizontalDecisionMaker(BaseDecisionMaker):
- """
- Discuss in a horizontal manner.
- """
-
- name: str = "horizontal"
-
- # def step(
- async def astep(
- self,
- agents: List[BaseAgent],
- task_description: str,
- previous_plan: str = "No solution yet.",
- advice: str = "No advice yet.",
- **kwargs,
- ) -> List[str]:
- if advice != "No advice yet.":
- self.broadcast_messages(
- agents, [Message(content=advice, sender="Evaluator")]
- )
- for agent in agents[1:]:
- review: CriticMessage = await agent.astep(
- previous_plan, advice, task_description
- )
- if review.content != "":
- self.broadcast_messages(agents, [review])
-
- logger.info("", "Reviews:", Fore.YELLOW)
- logger.info(
- "",
- f"[{review.sender}]: {review.content}",
- Fore.YELLOW,
- )
-
- result = agents[0].step(previous_plan, advice, task_description)
- return [result]
-
- def reset(self):
- pass
diff --git a/spaces/AgentVerse/agentVerse/ui/src/phaser3-rex-plugins/plugins/toggleswitch.d.ts b/spaces/AgentVerse/agentVerse/ui/src/phaser3-rex-plugins/plugins/toggleswitch.d.ts
deleted file mode 100644
index a1f13eaf4d308d220f732a18b83134122c24dadc..0000000000000000000000000000000000000000
--- a/spaces/AgentVerse/agentVerse/ui/src/phaser3-rex-plugins/plugins/toggleswitch.d.ts
+++ /dev/null
@@ -1,2 +0,0 @@
-import ToggleSwitch from './gameobjects/shape/toggleswitch/ToggleSwitch';
-export default ToggleSwitch;
\ No newline at end of file
diff --git a/spaces/AgentVerse/agentVerse/ui/src/phaser3-rex-plugins/templates/ui/gridsizer/GetChildrenWidth.js b/spaces/AgentVerse/agentVerse/ui/src/phaser3-rex-plugins/templates/ui/gridsizer/GetChildrenWidth.js
deleted file mode 100644
index 77af5e398b719647cfa11c20e8b848163b42008c..0000000000000000000000000000000000000000
--- a/spaces/AgentVerse/agentVerse/ui/src/phaser3-rex-plugins/templates/ui/gridsizer/GetChildrenWidth.js
+++ /dev/null
@@ -1,45 +0,0 @@
-import Sum from '../../../plugins/utils/math/Sum.js';
-
-var GetChildrenWidth = function (minimumMode) {
- if (this.rexSizer.hidden) {
- return 0;
- }
-
- if (minimumMode === undefined) {
- minimumMode = true;
- }
-
- var result = 0,
- columnWidth;
- var children = this.sizerChildren;
- var child, padding, childWidth, proportion;
-
- for (var i = 0; i < this.columnCount; i++) {
- proportion = this.columnProportions[i];
- columnWidth = 0;
- if ((proportion === 0) || minimumMode) {
- for (var j = 0; j < this.rowCount; j++) {
- child = children[(j * this.columnCount) + i];
- if (!child) {
- continue;
- }
- if (child.rexSizer.hidden) {
- continue;
- }
-
- padding = child.rexSizer.padding;
- childWidth = this.getChildWidth(child) + padding.left + padding.right;
- columnWidth = Math.max(columnWidth, childWidth);
- }
- result += columnWidth;
- }
- // else,(proportion > 0) : columnWidth is 0
- this.columnWidth[i] = columnWidth;
- }
-
- var space = this.space;
- var indentLeft = Math.max(space.indentLeftOdd, space.indentLeftEven);
- return result + Sum(space.left, indentLeft, ...space.column, space.right);
-}
-
-export default GetChildrenWidth;
\ No newline at end of file
diff --git a/spaces/AgentVerse/agentVerse/ui/src/phaser3-rex-plugins/templates/ui/roundrectanglecanvas/Factory.d.ts b/spaces/AgentVerse/agentVerse/ui/src/phaser3-rex-plugins/templates/ui/roundrectanglecanvas/Factory.d.ts
deleted file mode 100644
index 4e1ccdcaef9234671b8fd47370ea73d673cb12ee..0000000000000000000000000000000000000000
--- a/spaces/AgentVerse/agentVerse/ui/src/phaser3-rex-plugins/templates/ui/roundrectanglecanvas/Factory.d.ts
+++ /dev/null
@@ -1,20 +0,0 @@
-import RoundRectangleCanvas from './RoundRectangleCanvas';
-
-export default function (
- x: number,
- y: number,
- width: number,
- height: number,
- radiusConfig?: number | ({ x?: number, y?: number }) | RoundRectangleCanvas.IRadiusConfig |
- ({
- radius?: (number | ({ x?: number, y?: number }) | RoundRectangleCanvas.IRadiusConfig),
- iteration?: number
- }),
- fillStyle?: number | string | null,
- strokeStyle?: number | string | null,
- lineWidth?: number,
-
- fillColor2?: number | string | null,
- isHorizontalGradient?: boolean
-
-): RoundRectangleCanvas;
\ No newline at end of file
diff --git a/spaces/AllAideas/SegmentacionVideo/app.py b/spaces/AllAideas/SegmentacionVideo/app.py
deleted file mode 100644
index 4ef3d8aaea1f0c275ae905a33c7ba526218409c0..0000000000000000000000000000000000000000
--- a/spaces/AllAideas/SegmentacionVideo/app.py
+++ /dev/null
@@ -1,51 +0,0 @@
-import gradio as gr
-from utils.predict import predict_action
-import os
-import glob
-
-##Create list of examples to be loaded
-example_list = glob.glob("examples/*")
-example_list = list(map(lambda el:[el], example_list))
-
-
-demo = gr.Blocks()
-
-
-with demo:
-
- gr.Markdown("# **Video Classification with Transformers
**")
- description="""#
-
- Demo de clasificador de video usando modelo híbrido basado en Transformers con CNN, el objetivo es reconocer un segemento y recortarlo.
-
-
-
- """
- gr.Markdown(description)
-
- with gr.Tabs():
-
- with gr.TabItem("Upload & Predict"):
- with gr.Box():
-
- with gr.Row():
- input_video = gr.Video(label="Input Video", show_label=True)
- output_label = gr.Label(label="Model Output", show_label=True)
- output_gif = gr.Image(label="Video Gif", show_label=True)
-
- gr.Markdown("**Predict**")
-
- with gr.Box():
- with gr.Row():
- submit_button = gr.Button("Submit")
-
- gr.Markdown("**Ejemplos:**")
- gr.Markdown("El modelo puede clasificar videos pertenecientes a las siguientes clases: CricketShot, PlayingCello, Punch, ShavingBeard, TennisSwing.")
- # gr.Markdown("CricketShot, PlayingCello, Punch, ShavingBeard, TennisSwing")
-
- with gr.Column():
- gr.Examples(example_list, [input_video], [output_label,output_gif], predict_action, cache_examples=True)
-
- submit_button.click(predict_action, inputs=input_video, outputs=[output_label,output_gif])
-
-demo.launch()
diff --git a/spaces/Amrrs/DragGan-Inversion/torch_utils/persistence.py b/spaces/Amrrs/DragGan-Inversion/torch_utils/persistence.py
deleted file mode 100644
index d03055014ea6ba7e8ba475f79c91da4907fb6c0b..0000000000000000000000000000000000000000
--- a/spaces/Amrrs/DragGan-Inversion/torch_utils/persistence.py
+++ /dev/null
@@ -1,260 +0,0 @@
-# Copyright (c) 2021, NVIDIA CORPORATION & AFFILIATES. All rights reserved.
-#
-# NVIDIA CORPORATION and its licensors retain all intellectual property
-# and proprietary rights in and to this software, related documentation
-# and any modifications thereto. Any use, reproduction, disclosure or
-# distribution of this software and related documentation without an express
-# license agreement from NVIDIA CORPORATION is strictly prohibited.
-
-"""Facilities for pickling Python code alongside other data.
-
-The pickled code is automatically imported into a separate Python module
-during unpickling. This way, any previously exported pickles will remain
-usable even if the original code is no longer available, or if the current
-version of the code is not consistent with what was originally pickled."""
-
-import sys
-import pickle
-import io
-import inspect
-import copy
-import uuid
-import types
-import dnnlib
-
-# ----------------------------------------------------------------------------
-
-_version = 6 # internal version number
-_decorators = set() # {decorator_class, ...}
-_import_hooks = [] # [hook_function, ...]
-_module_to_src_dict = dict() # {module: src, ...}
-_src_to_module_dict = dict() # {src: module, ...}
-
-# ----------------------------------------------------------------------------
-
-
-def persistent_class(orig_class):
- r"""Class decorator that extends a given class to save its source code
- when pickled.
-
- Example:
-
- from torch_utils import persistence
-
- @persistence.persistent_class
- class MyNetwork(torch.nn.Module):
- def __init__(self, num_inputs, num_outputs):
- super().__init__()
- self.fc = MyLayer(num_inputs, num_outputs)
- ...
-
- @persistence.persistent_class
- class MyLayer(torch.nn.Module):
- ...
-
- When pickled, any instance of `MyNetwork` and `MyLayer` will save its
- source code alongside other internal state (e.g., parameters, buffers,
- and submodules). This way, any previously exported pickle will remain
- usable even if the class definitions have been modified or are no
- longer available.
-
- The decorator saves the source code of the entire Python module
- containing the decorated class. It does *not* save the source code of
- any imported modules. Thus, the imported modules must be available
- during unpickling, also including `torch_utils.persistence` itself.
-
- It is ok to call functions defined in the same module from the
- decorated class. However, if the decorated class depends on other
- classes defined in the same module, they must be decorated as well.
- This is illustrated in the above example in the case of `MyLayer`.
-
- It is also possible to employ the decorator just-in-time before
- calling the constructor. For example:
-
- cls = MyLayer
- if want_to_make_it_persistent:
- cls = persistence.persistent_class(cls)
- layer = cls(num_inputs, num_outputs)
-
- As an additional feature, the decorator also keeps track of the
- arguments that were used to construct each instance of the decorated
- class. The arguments can be queried via `obj.init_args` and
- `obj.init_kwargs`, and they are automatically pickled alongside other
- object state. A typical use case is to first unpickle a previous
- instance of a persistent class, and then upgrade it to use the latest
- version of the source code:
-
- with open('old_pickle.pkl', 'rb') as f:
- old_net = pickle.load(f)
- new_net = MyNetwork(*old_obj.init_args, **old_obj.init_kwargs)
- misc.copy_params_and_buffers(old_net, new_net, require_all=True)
- """
- assert isinstance(orig_class, type)
- if is_persistent(orig_class):
- return orig_class
-
- assert orig_class.__module__ in sys.modules
- orig_module = sys.modules[orig_class.__module__]
- orig_module_src = _module_to_src(orig_module)
-
- class Decorator(orig_class):
- _orig_module_src = orig_module_src
- _orig_class_name = orig_class.__name__
-
- def __init__(self, *args, **kwargs):
- super().__init__(*args, **kwargs)
- self._init_args = copy.deepcopy(args)
- self._init_kwargs = copy.deepcopy(kwargs)
- assert orig_class.__name__ in orig_module.__dict__
- _check_pickleable(self.__reduce__())
-
- @property
- def init_args(self):
- return copy.deepcopy(self._init_args)
-
- @property
- def init_kwargs(self):
- return dnnlib.EasyDict(copy.deepcopy(self._init_kwargs))
-
- def __reduce__(self):
- fields = list(super().__reduce__())
- fields += [None] * max(3 - len(fields), 0)
- if fields[0] is not _reconstruct_persistent_obj:
- meta = dict(type='class', version=_version, module_src=self._orig_module_src,
- class_name=self._orig_class_name, state=fields[2])
- fields[0] = _reconstruct_persistent_obj # reconstruct func
- fields[1] = (meta,) # reconstruct args
- fields[2] = None # state dict
- return tuple(fields)
-
- Decorator.__name__ = orig_class.__name__
- _decorators.add(Decorator)
- return Decorator
-
-# ----------------------------------------------------------------------------
-
-
-def is_persistent(obj):
- r"""Test whether the given object or class is persistent, i.e.,
- whether it will save its source code when pickled.
- """
- try:
- if obj in _decorators:
- return True
- except TypeError:
- pass
- return type(obj) in _decorators # pylint: disable=unidiomatic-typecheck
-
-# ----------------------------------------------------------------------------
-
-
-def import_hook(hook):
- r"""Register an import hook that is called whenever a persistent object
- is being unpickled. A typical use case is to patch the pickled source
- code to avoid errors and inconsistencies when the API of some imported
- module has changed.
-
- The hook should have the following signature:
-
- hook(meta) -> modified meta
-
- `meta` is an instance of `dnnlib.EasyDict` with the following fields:
-
- type: Type of the persistent object, e.g. `'class'`.
- version: Internal version number of `torch_utils.persistence`.
- module_src Original source code of the Python module.
- class_name: Class name in the original Python module.
- state: Internal state of the object.
-
- Example:
-
- @persistence.import_hook
- def wreck_my_network(meta):
- if meta.class_name == 'MyNetwork':
- print('MyNetwork is being imported. I will wreck it!')
- meta.module_src = meta.module_src.replace("True", "False")
- return meta
- """
- assert callable(hook)
- _import_hooks.append(hook)
-
-# ----------------------------------------------------------------------------
-
-
-def _reconstruct_persistent_obj(meta):
- r"""Hook that is called internally by the `pickle` module to unpickle
- a persistent object.
- """
- meta = dnnlib.EasyDict(meta)
- meta.state = dnnlib.EasyDict(meta.state)
- for hook in _import_hooks:
- meta = hook(meta)
- assert meta is not None
-
- assert meta.version == _version
- module = _src_to_module(meta.module_src)
-
- assert meta.type == 'class'
- orig_class = module.__dict__[meta.class_name]
- decorator_class = persistent_class(orig_class)
- obj = decorator_class.__new__(decorator_class)
-
- setstate = getattr(obj, '__setstate__', None)
- if callable(setstate):
- setstate(meta.state) # pylint: disable=not-callable
- else:
- obj.__dict__.update(meta.state)
- return obj
-
-# ----------------------------------------------------------------------------
-
-
-def _module_to_src(module):
- r"""Query the source code of a given Python module.
- """
- src = _module_to_src_dict.get(module, None)
- if src is None:
- src = inspect.getsource(module)
- _module_to_src_dict[module] = src
- _src_to_module_dict[src] = module
- return src
-
-
-def _src_to_module(src):
- r"""Get or create a Python module for the given source code.
- """
- module = _src_to_module_dict.get(src, None)
- if module is None:
- module_name = "_imported_module_" + uuid.uuid4().hex
- module = types.ModuleType(module_name)
- sys.modules[module_name] = module
- _module_to_src_dict[module] = src
- _src_to_module_dict[src] = module
- exec(src, module.__dict__) # pylint: disable=exec-used
- return module
-
-# ----------------------------------------------------------------------------
-
-
-def _check_pickleable(obj):
- r"""Check that the given object is pickleable, raising an exception if
- it is not. This function is expected to be considerably more efficient
- than actually pickling the object.
- """
- def recurse(obj):
- if isinstance(obj, (list, tuple, set)):
- return [recurse(x) for x in obj]
- if isinstance(obj, dict):
- return [[recurse(x), recurse(y)] for x, y in obj.items()]
- if isinstance(obj, (str, int, float, bool, bytes, bytearray)):
- return None # Python primitive types are pickleable.
- if f'{type(obj).__module__}.{type(obj).__name__}' in ['numpy.ndarray', 'torch.Tensor', 'torch.nn.parameter.Parameter']:
- return None # NumPy arrays and PyTorch tensors are pickleable.
- if is_persistent(obj):
- # Persistent objects are pickleable, by virtue of the constructor check.
- return None
- return obj
- with io.BytesIO() as f:
- pickle.dump(recurse(obj), f)
-
-# ----------------------------------------------------------------------------
diff --git a/spaces/Androidonnxfork/CivitAi-to-Diffusers/diffusers/docs/source/en/api/schedulers/euler_ancestral.md b/spaces/Androidonnxfork/CivitAi-to-Diffusers/diffusers/docs/source/en/api/schedulers/euler_ancestral.md
deleted file mode 100644
index 60fd524b195593608f1d2a900ad86756f8fd25ba..0000000000000000000000000000000000000000
--- a/spaces/Androidonnxfork/CivitAi-to-Diffusers/diffusers/docs/source/en/api/schedulers/euler_ancestral.md
+++ /dev/null
@@ -1,21 +0,0 @@
-
-
-# Euler Ancestral scheduler
-
-## Overview
-
-Ancestral sampling with Euler method steps. Based on the original [k-diffusion](https://github.com/crowsonkb/k-diffusion/blob/481677d114f6ea445aa009cf5bd7a9cdee909e47/k_diffusion/sampling.py#L72) implementation by Katherine Crowson.
-Fast scheduler which often times generates good outputs with 20-30 steps.
-
-## EulerAncestralDiscreteScheduler
-[[autodoc]] EulerAncestralDiscreteScheduler
diff --git a/spaces/Andy1621/uniformer_image_detection/exp/mask_rcnn_3x_ms_hybrid_small/config.py b/spaces/Andy1621/uniformer_image_detection/exp/mask_rcnn_3x_ms_hybrid_small/config.py
deleted file mode 100644
index a5ace78557c213c2f3af33a648d44a051f55effa..0000000000000000000000000000000000000000
--- a/spaces/Andy1621/uniformer_image_detection/exp/mask_rcnn_3x_ms_hybrid_small/config.py
+++ /dev/null
@@ -1,82 +0,0 @@
-_base_ = [
- '../../configs/_base_/models/mask_rcnn_uniformer_fpn.py',
- '../../configs/_base_/datasets/coco_instance.py',
- '../../configs/_base_/schedules/schedule_1x.py',
- '../../configs/_base_/default_runtime.py'
-]
-
-model = dict(
- backbone=dict(
- embed_dim=[64, 128, 320, 512],
- layers=[3, 4, 8, 3],
- head_dim=64,
- drop_path_rate=0.1,
- use_checkpoint=True,
- checkpoint_num=[0, 0, 8, 0],
- windows=False,
- hybrid=True,
- window_size=14
- ),
- neck=dict(in_channels=[64, 128, 320, 512]))
-
-img_norm_cfg = dict(
- mean=[123.675, 116.28, 103.53], std=[58.395, 57.12, 57.375], to_rgb=True)
-
-# augmentation strategy originates from DETR / Sparse RCNN
-train_pipeline = [
- dict(type='LoadImageFromFile'),
- dict(type='LoadAnnotations', with_bbox=True, with_mask=True),
- dict(type='RandomFlip', flip_ratio=0.5),
- dict(type='AutoAugment',
- policies=[
- [
- dict(type='Resize',
- img_scale=[(480, 1333), (512, 1333), (544, 1333), (576, 1333),
- (608, 1333), (640, 1333), (672, 1333), (704, 1333),
- (736, 1333), (768, 1333), (800, 1333)],
- multiscale_mode='value',
- keep_ratio=True)
- ],
- [
- dict(type='Resize',
- img_scale=[(400, 1333), (500, 1333), (600, 1333)],
- multiscale_mode='value',
- keep_ratio=True),
- dict(type='RandomCrop',
- crop_type='absolute_range',
- crop_size=(384, 600),
- allow_negative_crop=True),
- dict(type='Resize',
- img_scale=[(480, 1333), (512, 1333), (544, 1333),
- (576, 1333), (608, 1333), (640, 1333),
- (672, 1333), (704, 1333), (736, 1333),
- (768, 1333), (800, 1333)],
- multiscale_mode='value',
- override=True,
- keep_ratio=True)
- ]
- ]),
- dict(type='Normalize', **img_norm_cfg),
- dict(type='Pad', size_divisor=32),
- dict(type='DefaultFormatBundle'),
- dict(type='Collect', keys=['img', 'gt_bboxes', 'gt_labels', 'gt_masks']),
-]
-data = dict(train=dict(pipeline=train_pipeline))
-
-optimizer = dict(_delete_=True, type='AdamW', lr=0.0001, betas=(0.9, 0.999), weight_decay=0.05,
- paramwise_cfg=dict(custom_keys={'absolute_pos_embed': dict(decay_mult=0.),
- 'relative_position_bias_table': dict(decay_mult=0.),
- 'norm': dict(decay_mult=0.)}))
-lr_config = dict(step=[27, 33])
-runner = dict(type='EpochBasedRunnerAmp', max_epochs=36)
-
-# do not use mmdet version fp16
-fp16 = None
-optimizer_config = dict(
- type="DistOptimizerHook",
- update_interval=1,
- grad_clip=None,
- coalesce=True,
- bucket_size_mb=-1,
- use_fp16=True,
-)
diff --git a/spaces/Andy1621/uniformer_image_detection/mmdet/models/dense_heads/anchor_head.py b/spaces/Andy1621/uniformer_image_detection/mmdet/models/dense_heads/anchor_head.py
deleted file mode 100644
index eea73520572725f547216ab639c1ebbdfb50834c..0000000000000000000000000000000000000000
--- a/spaces/Andy1621/uniformer_image_detection/mmdet/models/dense_heads/anchor_head.py
+++ /dev/null
@@ -1,751 +0,0 @@
-import torch
-import torch.nn as nn
-from mmcv.cnn import normal_init
-from mmcv.runner import force_fp32
-
-from mmdet.core import (anchor_inside_flags, build_anchor_generator,
- build_assigner, build_bbox_coder, build_sampler,
- images_to_levels, multi_apply, multiclass_nms, unmap)
-from ..builder import HEADS, build_loss
-from .base_dense_head import BaseDenseHead
-from .dense_test_mixins import BBoxTestMixin
-
-
-@HEADS.register_module()
-class AnchorHead(BaseDenseHead, BBoxTestMixin):
- """Anchor-based head (RPN, RetinaNet, SSD, etc.).
-
- Args:
- num_classes (int): Number of categories excluding the background
- category.
- in_channels (int): Number of channels in the input feature map.
- feat_channels (int): Number of hidden channels. Used in child classes.
- anchor_generator (dict): Config dict for anchor generator
- bbox_coder (dict): Config of bounding box coder.
- reg_decoded_bbox (bool): If true, the regression loss would be
- applied directly on decoded bounding boxes, converting both
- the predicted boxes and regression targets to absolute
- coordinates format. Default False. It should be `True` when
- using `IoULoss`, `GIoULoss`, or `DIoULoss` in the bbox head.
- loss_cls (dict): Config of classification loss.
- loss_bbox (dict): Config of localization loss.
- train_cfg (dict): Training config of anchor head.
- test_cfg (dict): Testing config of anchor head.
- """ # noqa: W605
-
- def __init__(self,
- num_classes,
- in_channels,
- feat_channels=256,
- anchor_generator=dict(
- type='AnchorGenerator',
- scales=[8, 16, 32],
- ratios=[0.5, 1.0, 2.0],
- strides=[4, 8, 16, 32, 64]),
- bbox_coder=dict(
- type='DeltaXYWHBBoxCoder',
- clip_border=True,
- target_means=(.0, .0, .0, .0),
- target_stds=(1.0, 1.0, 1.0, 1.0)),
- reg_decoded_bbox=False,
- loss_cls=dict(
- type='CrossEntropyLoss',
- use_sigmoid=True,
- loss_weight=1.0),
- loss_bbox=dict(
- type='SmoothL1Loss', beta=1.0 / 9.0, loss_weight=1.0),
- train_cfg=None,
- test_cfg=None):
- super(AnchorHead, self).__init__()
- self.in_channels = in_channels
- self.num_classes = num_classes
- self.feat_channels = feat_channels
- self.use_sigmoid_cls = loss_cls.get('use_sigmoid', False)
- # TODO better way to determine whether sample or not
- self.sampling = loss_cls['type'] not in [
- 'FocalLoss', 'GHMC', 'QualityFocalLoss'
- ]
- if self.use_sigmoid_cls:
- self.cls_out_channels = num_classes
- else:
- self.cls_out_channels = num_classes + 1
-
- if self.cls_out_channels <= 0:
- raise ValueError(f'num_classes={num_classes} is too small')
- self.reg_decoded_bbox = reg_decoded_bbox
-
- self.bbox_coder = build_bbox_coder(bbox_coder)
- self.loss_cls = build_loss(loss_cls)
- self.loss_bbox = build_loss(loss_bbox)
- self.train_cfg = train_cfg
- self.test_cfg = test_cfg
- if self.train_cfg:
- self.assigner = build_assigner(self.train_cfg.assigner)
- # use PseudoSampler when sampling is False
- if self.sampling and hasattr(self.train_cfg, 'sampler'):
- sampler_cfg = self.train_cfg.sampler
- else:
- sampler_cfg = dict(type='PseudoSampler')
- self.sampler = build_sampler(sampler_cfg, context=self)
- self.fp16_enabled = False
-
- self.anchor_generator = build_anchor_generator(anchor_generator)
- # usually the numbers of anchors for each level are the same
- # except SSD detectors
- self.num_anchors = self.anchor_generator.num_base_anchors[0]
- self._init_layers()
-
- def _init_layers(self):
- """Initialize layers of the head."""
- self.conv_cls = nn.Conv2d(self.in_channels,
- self.num_anchors * self.cls_out_channels, 1)
- self.conv_reg = nn.Conv2d(self.in_channels, self.num_anchors * 4, 1)
-
- def init_weights(self):
- """Initialize weights of the head."""
- normal_init(self.conv_cls, std=0.01)
- normal_init(self.conv_reg, std=0.01)
-
- def forward_single(self, x):
- """Forward feature of a single scale level.
-
- Args:
- x (Tensor): Features of a single scale level.
-
- Returns:
- tuple:
- cls_score (Tensor): Cls scores for a single scale level \
- the channels number is num_anchors * num_classes.
- bbox_pred (Tensor): Box energies / deltas for a single scale \
- level, the channels number is num_anchors * 4.
- """
- cls_score = self.conv_cls(x)
- bbox_pred = self.conv_reg(x)
- return cls_score, bbox_pred
-
- def forward(self, feats):
- """Forward features from the upstream network.
-
- Args:
- feats (tuple[Tensor]): Features from the upstream network, each is
- a 4D-tensor.
-
- Returns:
- tuple: A tuple of classification scores and bbox prediction.
-
- - cls_scores (list[Tensor]): Classification scores for all \
- scale levels, each is a 4D-tensor, the channels number \
- is num_anchors * num_classes.
- - bbox_preds (list[Tensor]): Box energies / deltas for all \
- scale levels, each is a 4D-tensor, the channels number \
- is num_anchors * 4.
- """
- return multi_apply(self.forward_single, feats)
-
- def get_anchors(self, featmap_sizes, img_metas, device='cuda'):
- """Get anchors according to feature map sizes.
-
- Args:
- featmap_sizes (list[tuple]): Multi-level feature map sizes.
- img_metas (list[dict]): Image meta info.
- device (torch.device | str): Device for returned tensors
-
- Returns:
- tuple:
- anchor_list (list[Tensor]): Anchors of each image.
- valid_flag_list (list[Tensor]): Valid flags of each image.
- """
- num_imgs = len(img_metas)
-
- # since feature map sizes of all images are the same, we only compute
- # anchors for one time
- multi_level_anchors = self.anchor_generator.grid_anchors(
- featmap_sizes, device)
- anchor_list = [multi_level_anchors for _ in range(num_imgs)]
-
- # for each image, we compute valid flags of multi level anchors
- valid_flag_list = []
- for img_id, img_meta in enumerate(img_metas):
- multi_level_flags = self.anchor_generator.valid_flags(
- featmap_sizes, img_meta['pad_shape'], device)
- valid_flag_list.append(multi_level_flags)
-
- return anchor_list, valid_flag_list
-
- def _get_targets_single(self,
- flat_anchors,
- valid_flags,
- gt_bboxes,
- gt_bboxes_ignore,
- gt_labels,
- img_meta,
- label_channels=1,
- unmap_outputs=True):
- """Compute regression and classification targets for anchors in a
- single image.
-
- Args:
- flat_anchors (Tensor): Multi-level anchors of the image, which are
- concatenated into a single tensor of shape (num_anchors ,4)
- valid_flags (Tensor): Multi level valid flags of the image,
- which are concatenated into a single tensor of
- shape (num_anchors,).
- gt_bboxes (Tensor): Ground truth bboxes of the image,
- shape (num_gts, 4).
- gt_bboxes_ignore (Tensor): Ground truth bboxes to be
- ignored, shape (num_ignored_gts, 4).
- img_meta (dict): Meta info of the image.
- gt_labels (Tensor): Ground truth labels of each box,
- shape (num_gts,).
- label_channels (int): Channel of label.
- unmap_outputs (bool): Whether to map outputs back to the original
- set of anchors.
-
- Returns:
- tuple:
- labels_list (list[Tensor]): Labels of each level
- label_weights_list (list[Tensor]): Label weights of each level
- bbox_targets_list (list[Tensor]): BBox targets of each level
- bbox_weights_list (list[Tensor]): BBox weights of each level
- num_total_pos (int): Number of positive samples in all images
- num_total_neg (int): Number of negative samples in all images
- """
- inside_flags = anchor_inside_flags(flat_anchors, valid_flags,
- img_meta['img_shape'][:2],
- self.train_cfg.allowed_border)
- if not inside_flags.any():
- return (None, ) * 7
- # assign gt and sample anchors
- anchors = flat_anchors[inside_flags, :]
-
- assign_result = self.assigner.assign(
- anchors, gt_bboxes, gt_bboxes_ignore,
- None if self.sampling else gt_labels)
- sampling_result = self.sampler.sample(assign_result, anchors,
- gt_bboxes)
-
- num_valid_anchors = anchors.shape[0]
- bbox_targets = torch.zeros_like(anchors)
- bbox_weights = torch.zeros_like(anchors)
- labels = anchors.new_full((num_valid_anchors, ),
- self.num_classes,
- dtype=torch.long)
- label_weights = anchors.new_zeros(num_valid_anchors, dtype=torch.float)
-
- pos_inds = sampling_result.pos_inds
- neg_inds = sampling_result.neg_inds
- if len(pos_inds) > 0:
- if not self.reg_decoded_bbox:
- pos_bbox_targets = self.bbox_coder.encode(
- sampling_result.pos_bboxes, sampling_result.pos_gt_bboxes)
- else:
- pos_bbox_targets = sampling_result.pos_gt_bboxes
- bbox_targets[pos_inds, :] = pos_bbox_targets
- bbox_weights[pos_inds, :] = 1.0
- if gt_labels is None:
- # Only rpn gives gt_labels as None
- # Foreground is the first class since v2.5.0
- labels[pos_inds] = 0
- else:
- labels[pos_inds] = gt_labels[
- sampling_result.pos_assigned_gt_inds]
- if self.train_cfg.pos_weight <= 0:
- label_weights[pos_inds] = 1.0
- else:
- label_weights[pos_inds] = self.train_cfg.pos_weight
- if len(neg_inds) > 0:
- label_weights[neg_inds] = 1.0
-
- # map up to original set of anchors
- if unmap_outputs:
- num_total_anchors = flat_anchors.size(0)
- labels = unmap(
- labels, num_total_anchors, inside_flags,
- fill=self.num_classes) # fill bg label
- label_weights = unmap(label_weights, num_total_anchors,
- inside_flags)
- bbox_targets = unmap(bbox_targets, num_total_anchors, inside_flags)
- bbox_weights = unmap(bbox_weights, num_total_anchors, inside_flags)
-
- return (labels, label_weights, bbox_targets, bbox_weights, pos_inds,
- neg_inds, sampling_result)
-
- def get_targets(self,
- anchor_list,
- valid_flag_list,
- gt_bboxes_list,
- img_metas,
- gt_bboxes_ignore_list=None,
- gt_labels_list=None,
- label_channels=1,
- unmap_outputs=True,
- return_sampling_results=False):
- """Compute regression and classification targets for anchors in
- multiple images.
-
- Args:
- anchor_list (list[list[Tensor]]): Multi level anchors of each
- image. The outer list indicates images, and the inner list
- corresponds to feature levels of the image. Each element of
- the inner list is a tensor of shape (num_anchors, 4).
- valid_flag_list (list[list[Tensor]]): Multi level valid flags of
- each image. The outer list indicates images, and the inner list
- corresponds to feature levels of the image. Each element of
- the inner list is a tensor of shape (num_anchors, )
- gt_bboxes_list (list[Tensor]): Ground truth bboxes of each image.
- img_metas (list[dict]): Meta info of each image.
- gt_bboxes_ignore_list (list[Tensor]): Ground truth bboxes to be
- ignored.
- gt_labels_list (list[Tensor]): Ground truth labels of each box.
- label_channels (int): Channel of label.
- unmap_outputs (bool): Whether to map outputs back to the original
- set of anchors.
-
- Returns:
- tuple: Usually returns a tuple containing learning targets.
-
- - labels_list (list[Tensor]): Labels of each level.
- - label_weights_list (list[Tensor]): Label weights of each \
- level.
- - bbox_targets_list (list[Tensor]): BBox targets of each level.
- - bbox_weights_list (list[Tensor]): BBox weights of each level.
- - num_total_pos (int): Number of positive samples in all \
- images.
- - num_total_neg (int): Number of negative samples in all \
- images.
- additional_returns: This function enables user-defined returns from
- `self._get_targets_single`. These returns are currently refined
- to properties at each feature map (i.e. having HxW dimension).
- The results will be concatenated after the end
- """
- num_imgs = len(img_metas)
- assert len(anchor_list) == len(valid_flag_list) == num_imgs
-
- # anchor number of multi levels
- num_level_anchors = [anchors.size(0) for anchors in anchor_list[0]]
- # concat all level anchors to a single tensor
- concat_anchor_list = []
- concat_valid_flag_list = []
- for i in range(num_imgs):
- assert len(anchor_list[i]) == len(valid_flag_list[i])
- concat_anchor_list.append(torch.cat(anchor_list[i]))
- concat_valid_flag_list.append(torch.cat(valid_flag_list[i]))
-
- # compute targets for each image
- if gt_bboxes_ignore_list is None:
- gt_bboxes_ignore_list = [None for _ in range(num_imgs)]
- if gt_labels_list is None:
- gt_labels_list = [None for _ in range(num_imgs)]
- results = multi_apply(
- self._get_targets_single,
- concat_anchor_list,
- concat_valid_flag_list,
- gt_bboxes_list,
- gt_bboxes_ignore_list,
- gt_labels_list,
- img_metas,
- label_channels=label_channels,
- unmap_outputs=unmap_outputs)
- (all_labels, all_label_weights, all_bbox_targets, all_bbox_weights,
- pos_inds_list, neg_inds_list, sampling_results_list) = results[:7]
- rest_results = list(results[7:]) # user-added return values
- # no valid anchors
- if any([labels is None for labels in all_labels]):
- return None
- # sampled anchors of all images
- num_total_pos = sum([max(inds.numel(), 1) for inds in pos_inds_list])
- num_total_neg = sum([max(inds.numel(), 1) for inds in neg_inds_list])
- # split targets to a list w.r.t. multiple levels
- labels_list = images_to_levels(all_labels, num_level_anchors)
- label_weights_list = images_to_levels(all_label_weights,
- num_level_anchors)
- bbox_targets_list = images_to_levels(all_bbox_targets,
- num_level_anchors)
- bbox_weights_list = images_to_levels(all_bbox_weights,
- num_level_anchors)
- res = (labels_list, label_weights_list, bbox_targets_list,
- bbox_weights_list, num_total_pos, num_total_neg)
- if return_sampling_results:
- res = res + (sampling_results_list, )
- for i, r in enumerate(rest_results): # user-added return values
- rest_results[i] = images_to_levels(r, num_level_anchors)
-
- return res + tuple(rest_results)
-
- def loss_single(self, cls_score, bbox_pred, anchors, labels, label_weights,
- bbox_targets, bbox_weights, num_total_samples):
- """Compute loss of a single scale level.
-
- Args:
- cls_score (Tensor): Box scores for each scale level
- Has shape (N, num_anchors * num_classes, H, W).
- bbox_pred (Tensor): Box energies / deltas for each scale
- level with shape (N, num_anchors * 4, H, W).
- anchors (Tensor): Box reference for each scale level with shape
- (N, num_total_anchors, 4).
- labels (Tensor): Labels of each anchors with shape
- (N, num_total_anchors).
- label_weights (Tensor): Label weights of each anchor with shape
- (N, num_total_anchors)
- bbox_targets (Tensor): BBox regression targets of each anchor wight
- shape (N, num_total_anchors, 4).
- bbox_weights (Tensor): BBox regression loss weights of each anchor
- with shape (N, num_total_anchors, 4).
- num_total_samples (int): If sampling, num total samples equal to
- the number of total anchors; Otherwise, it is the number of
- positive anchors.
-
- Returns:
- dict[str, Tensor]: A dictionary of loss components.
- """
- # classification loss
- labels = labels.reshape(-1)
- label_weights = label_weights.reshape(-1)
- cls_score = cls_score.permute(0, 2, 3,
- 1).reshape(-1, self.cls_out_channels)
- loss_cls = self.loss_cls(
- cls_score, labels, label_weights, avg_factor=num_total_samples)
- # regression loss
- bbox_targets = bbox_targets.reshape(-1, 4)
- bbox_weights = bbox_weights.reshape(-1, 4)
- bbox_pred = bbox_pred.permute(0, 2, 3, 1).reshape(-1, 4)
- if self.reg_decoded_bbox:
- # When the regression loss (e.g. `IouLoss`, `GIouLoss`)
- # is applied directly on the decoded bounding boxes, it
- # decodes the already encoded coordinates to absolute format.
- anchors = anchors.reshape(-1, 4)
- bbox_pred = self.bbox_coder.decode(anchors, bbox_pred)
- loss_bbox = self.loss_bbox(
- bbox_pred,
- bbox_targets,
- bbox_weights,
- avg_factor=num_total_samples)
- return loss_cls, loss_bbox
-
- @force_fp32(apply_to=('cls_scores', 'bbox_preds'))
- def loss(self,
- cls_scores,
- bbox_preds,
- gt_bboxes,
- gt_labels,
- img_metas,
- gt_bboxes_ignore=None):
- """Compute losses of the head.
-
- Args:
- cls_scores (list[Tensor]): Box scores for each scale level
- Has shape (N, num_anchors * num_classes, H, W)
- bbox_preds (list[Tensor]): Box energies / deltas for each scale
- level with shape (N, num_anchors * 4, H, W)
- gt_bboxes (list[Tensor]): Ground truth bboxes for each image with
- shape (num_gts, 4) in [tl_x, tl_y, br_x, br_y] format.
- gt_labels (list[Tensor]): class indices corresponding to each box
- img_metas (list[dict]): Meta information of each image, e.g.,
- image size, scaling factor, etc.
- gt_bboxes_ignore (None | list[Tensor]): specify which bounding
- boxes can be ignored when computing the loss. Default: None
-
- Returns:
- dict[str, Tensor]: A dictionary of loss components.
- """
- featmap_sizes = [featmap.size()[-2:] for featmap in cls_scores]
- assert len(featmap_sizes) == self.anchor_generator.num_levels
-
- device = cls_scores[0].device
-
- anchor_list, valid_flag_list = self.get_anchors(
- featmap_sizes, img_metas, device=device)
- label_channels = self.cls_out_channels if self.use_sigmoid_cls else 1
- cls_reg_targets = self.get_targets(
- anchor_list,
- valid_flag_list,
- gt_bboxes,
- img_metas,
- gt_bboxes_ignore_list=gt_bboxes_ignore,
- gt_labels_list=gt_labels,
- label_channels=label_channels)
- if cls_reg_targets is None:
- return None
- (labels_list, label_weights_list, bbox_targets_list, bbox_weights_list,
- num_total_pos, num_total_neg) = cls_reg_targets
- num_total_samples = (
- num_total_pos + num_total_neg if self.sampling else num_total_pos)
-
- # anchor number of multi levels
- num_level_anchors = [anchors.size(0) for anchors in anchor_list[0]]
- # concat all level anchors and flags to a single tensor
- concat_anchor_list = []
- for i in range(len(anchor_list)):
- concat_anchor_list.append(torch.cat(anchor_list[i]))
- all_anchor_list = images_to_levels(concat_anchor_list,
- num_level_anchors)
-
- losses_cls, losses_bbox = multi_apply(
- self.loss_single,
- cls_scores,
- bbox_preds,
- all_anchor_list,
- labels_list,
- label_weights_list,
- bbox_targets_list,
- bbox_weights_list,
- num_total_samples=num_total_samples)
- return dict(loss_cls=losses_cls, loss_bbox=losses_bbox)
-
- @force_fp32(apply_to=('cls_scores', 'bbox_preds'))
- def get_bboxes(self,
- cls_scores,
- bbox_preds,
- img_metas,
- cfg=None,
- rescale=False,
- with_nms=True):
- """Transform network output for a batch into bbox predictions.
-
- Args:
- cls_scores (list[Tensor]): Box scores for each level in the
- feature pyramid, has shape
- (N, num_anchors * num_classes, H, W).
- bbox_preds (list[Tensor]): Box energies / deltas for each
- level in the feature pyramid, has shape
- (N, num_anchors * 4, H, W).
- img_metas (list[dict]): Meta information of each image, e.g.,
- image size, scaling factor, etc.
- cfg (mmcv.Config | None): Test / postprocessing configuration,
- if None, test_cfg would be used
- rescale (bool): If True, return boxes in original image space.
- Default: False.
- with_nms (bool): If True, do nms before return boxes.
- Default: True.
-
- Returns:
- list[tuple[Tensor, Tensor]]: Each item in result_list is 2-tuple.
- The first item is an (n, 5) tensor, where 5 represent
- (tl_x, tl_y, br_x, br_y, score) and the score between 0 and 1.
- The shape of the second tensor in the tuple is (n,), and
- each element represents the class label of the corresponding
- box.
-
- Example:
- >>> import mmcv
- >>> self = AnchorHead(
- >>> num_classes=9,
- >>> in_channels=1,
- >>> anchor_generator=dict(
- >>> type='AnchorGenerator',
- >>> scales=[8],
- >>> ratios=[0.5, 1.0, 2.0],
- >>> strides=[4,]))
- >>> img_metas = [{'img_shape': (32, 32, 3), 'scale_factor': 1}]
- >>> cfg = mmcv.Config(dict(
- >>> score_thr=0.00,
- >>> nms=dict(type='nms', iou_thr=1.0),
- >>> max_per_img=10))
- >>> feat = torch.rand(1, 1, 3, 3)
- >>> cls_score, bbox_pred = self.forward_single(feat)
- >>> # note the input lists are over different levels, not images
- >>> cls_scores, bbox_preds = [cls_score], [bbox_pred]
- >>> result_list = self.get_bboxes(cls_scores, bbox_preds,
- >>> img_metas, cfg)
- >>> det_bboxes, det_labels = result_list[0]
- >>> assert len(result_list) == 1
- >>> assert det_bboxes.shape[1] == 5
- >>> assert len(det_bboxes) == len(det_labels) == cfg.max_per_img
- """
- assert len(cls_scores) == len(bbox_preds)
- num_levels = len(cls_scores)
-
- device = cls_scores[0].device
- featmap_sizes = [cls_scores[i].shape[-2:] for i in range(num_levels)]
- mlvl_anchors = self.anchor_generator.grid_anchors(
- featmap_sizes, device=device)
-
- mlvl_cls_scores = [cls_scores[i].detach() for i in range(num_levels)]
- mlvl_bbox_preds = [bbox_preds[i].detach() for i in range(num_levels)]
-
- if torch.onnx.is_in_onnx_export():
- assert len(
- img_metas
- ) == 1, 'Only support one input image while in exporting to ONNX'
- img_shapes = img_metas[0]['img_shape_for_onnx']
- else:
- img_shapes = [
- img_metas[i]['img_shape']
- for i in range(cls_scores[0].shape[0])
- ]
- scale_factors = [
- img_metas[i]['scale_factor'] for i in range(cls_scores[0].shape[0])
- ]
-
- if with_nms:
- # some heads don't support with_nms argument
- result_list = self._get_bboxes(mlvl_cls_scores, mlvl_bbox_preds,
- mlvl_anchors, img_shapes,
- scale_factors, cfg, rescale)
- else:
- result_list = self._get_bboxes(mlvl_cls_scores, mlvl_bbox_preds,
- mlvl_anchors, img_shapes,
- scale_factors, cfg, rescale,
- with_nms)
- return result_list
-
- def _get_bboxes(self,
- mlvl_cls_scores,
- mlvl_bbox_preds,
- mlvl_anchors,
- img_shapes,
- scale_factors,
- cfg,
- rescale=False,
- with_nms=True):
- """Transform outputs for a batch item into bbox predictions.
-
- Args:
- mlvl_cls_scores (list[Tensor]): Each element in the list is
- the scores of bboxes of single level in the feature pyramid,
- has shape (N, num_anchors * num_classes, H, W).
- mlvl_bbox_preds (list[Tensor]): Each element in the list is the
- bboxes predictions of single level in the feature pyramid,
- has shape (N, num_anchors * 4, H, W).
- mlvl_anchors (list[Tensor]): Each element in the list is
- the anchors of single level in feature pyramid, has shape
- (num_anchors, 4).
- img_shapes (list[tuple[int]]): Each tuple in the list represent
- the shape(height, width, 3) of single image in the batch.
- scale_factors (list[ndarray]): Scale factor of the batch
- image arange as list[(w_scale, h_scale, w_scale, h_scale)].
- cfg (mmcv.Config): Test / postprocessing configuration,
- if None, test_cfg would be used.
- rescale (bool): If True, return boxes in original image space.
- Default: False.
- with_nms (bool): If True, do nms before return boxes.
- Default: True.
-
- Returns:
- list[tuple[Tensor, Tensor]]: Each item in result_list is 2-tuple.
- The first item is an (n, 5) tensor, where 5 represent
- (tl_x, tl_y, br_x, br_y, score) and the score between 0 and 1.
- The shape of the second tensor in the tuple is (n,), and
- each element represents the class label of the corresponding
- box.
- """
- cfg = self.test_cfg if cfg is None else cfg
- assert len(mlvl_cls_scores) == len(mlvl_bbox_preds) == len(
- mlvl_anchors)
- batch_size = mlvl_cls_scores[0].shape[0]
- # convert to tensor to keep tracing
- nms_pre_tensor = torch.tensor(
- cfg.get('nms_pre', -1),
- device=mlvl_cls_scores[0].device,
- dtype=torch.long)
-
- mlvl_bboxes = []
- mlvl_scores = []
- for cls_score, bbox_pred, anchors in zip(mlvl_cls_scores,
- mlvl_bbox_preds,
- mlvl_anchors):
- assert cls_score.size()[-2:] == bbox_pred.size()[-2:]
- cls_score = cls_score.permute(0, 2, 3,
- 1).reshape(batch_size, -1,
- self.cls_out_channels)
- if self.use_sigmoid_cls:
- scores = cls_score.sigmoid()
- else:
- scores = cls_score.softmax(-1)
- bbox_pred = bbox_pred.permute(0, 2, 3,
- 1).reshape(batch_size, -1, 4)
- anchors = anchors.expand_as(bbox_pred)
- # Always keep topk op for dynamic input in onnx
- if nms_pre_tensor > 0 and (torch.onnx.is_in_onnx_export()
- or scores.shape[-2] > nms_pre_tensor):
- from torch import _shape_as_tensor
- # keep shape as tensor and get k
- num_anchor = _shape_as_tensor(scores)[-2].to(
- nms_pre_tensor.device)
- nms_pre = torch.where(nms_pre_tensor < num_anchor,
- nms_pre_tensor, num_anchor)
-
- # Get maximum scores for foreground classes.
- if self.use_sigmoid_cls:
- max_scores, _ = scores.max(-1)
- else:
- # remind that we set FG labels to [0, num_class-1]
- # since mmdet v2.0
- # BG cat_id: num_class
- max_scores, _ = scores[..., :-1].max(-1)
-
- _, topk_inds = max_scores.topk(nms_pre)
- batch_inds = torch.arange(batch_size).view(
- -1, 1).expand_as(topk_inds)
- anchors = anchors[batch_inds, topk_inds, :]
- bbox_pred = bbox_pred[batch_inds, topk_inds, :]
- scores = scores[batch_inds, topk_inds, :]
-
- bboxes = self.bbox_coder.decode(
- anchors, bbox_pred, max_shape=img_shapes)
- mlvl_bboxes.append(bboxes)
- mlvl_scores.append(scores)
-
- batch_mlvl_bboxes = torch.cat(mlvl_bboxes, dim=1)
- if rescale:
- batch_mlvl_bboxes /= batch_mlvl_bboxes.new_tensor(
- scale_factors).unsqueeze(1)
- batch_mlvl_scores = torch.cat(mlvl_scores, dim=1)
-
- # Set max number of box to be feed into nms in deployment
- deploy_nms_pre = cfg.get('deploy_nms_pre', -1)
- if deploy_nms_pre > 0 and torch.onnx.is_in_onnx_export():
- # Get maximum scores for foreground classes.
- if self.use_sigmoid_cls:
- max_scores, _ = batch_mlvl_scores.max(-1)
- else:
- # remind that we set FG labels to [0, num_class-1]
- # since mmdet v2.0
- # BG cat_id: num_class
- max_scores, _ = batch_mlvl_scores[..., :-1].max(-1)
- _, topk_inds = max_scores.topk(deploy_nms_pre)
- batch_inds = torch.arange(batch_size).view(-1,
- 1).expand_as(topk_inds)
- batch_mlvl_scores = batch_mlvl_scores[batch_inds, topk_inds]
- batch_mlvl_bboxes = batch_mlvl_bboxes[batch_inds, topk_inds]
- if self.use_sigmoid_cls:
- # Add a dummy background class to the backend when using sigmoid
- # remind that we set FG labels to [0, num_class-1] since mmdet v2.0
- # BG cat_id: num_class
- padding = batch_mlvl_scores.new_zeros(batch_size,
- batch_mlvl_scores.shape[1],
- 1)
- batch_mlvl_scores = torch.cat([batch_mlvl_scores, padding], dim=-1)
-
- if with_nms:
- det_results = []
- for (mlvl_bboxes, mlvl_scores) in zip(batch_mlvl_bboxes,
- batch_mlvl_scores):
- det_bbox, det_label = multiclass_nms(mlvl_bboxes, mlvl_scores,
- cfg.score_thr, cfg.nms,
- cfg.max_per_img)
- det_results.append(tuple([det_bbox, det_label]))
- else:
- det_results = [
- tuple(mlvl_bs)
- for mlvl_bs in zip(batch_mlvl_bboxes, batch_mlvl_scores)
- ]
- return det_results
-
- def aug_test(self, feats, img_metas, rescale=False):
- """Test function with test time augmentation.
-
- Args:
- feats (list[Tensor]): the outer list indicates test-time
- augmentations and inner Tensor should have a shape NxCxHxW,
- which contains features for all images in the batch.
- img_metas (list[list[dict]]): the outer list indicates test-time
- augs (multiscale, flip, etc.) and the inner list indicates
- images in a batch. each dict has image information.
- rescale (bool, optional): Whether to rescale the results.
- Defaults to False.
-
- Returns:
- list[ndarray]: bbox results of each class
- """
- return self.aug_test_bboxes(feats, img_metas, rescale=rescale)
diff --git a/spaces/Andy1621/uniformer_image_segmentation/configs/fcn/fcn_r101-d8_480x480_40k_pascal_context.py b/spaces/Andy1621/uniformer_image_segmentation/configs/fcn/fcn_r101-d8_480x480_40k_pascal_context.py
deleted file mode 100644
index f3a15b41054318d508e98685632921f262029de0..0000000000000000000000000000000000000000
--- a/spaces/Andy1621/uniformer_image_segmentation/configs/fcn/fcn_r101-d8_480x480_40k_pascal_context.py
+++ /dev/null
@@ -1,2 +0,0 @@
-_base_ = './fcn_r50-d8_480x480_40k_pascal_context.py'
-model = dict(pretrained='open-mmlab://resnet101_v1c', backbone=dict(depth=101))
diff --git a/spaces/Andy1621/uniformer_image_segmentation/configs/mobilenet_v3/lraspp_m-v3-d8_512x1024_320k_cityscapes.py b/spaces/Andy1621/uniformer_image_segmentation/configs/mobilenet_v3/lraspp_m-v3-d8_512x1024_320k_cityscapes.py
deleted file mode 100644
index e59a78b48be3a0997a31524fd78e7fad5636bc82..0000000000000000000000000000000000000000
--- a/spaces/Andy1621/uniformer_image_segmentation/configs/mobilenet_v3/lraspp_m-v3-d8_512x1024_320k_cityscapes.py
+++ /dev/null
@@ -1,11 +0,0 @@
-_base_ = [
- '../_base_/models/lraspp_m-v3-d8.py', '../_base_/datasets/cityscapes.py',
- '../_base_/default_runtime.py', '../_base_/schedules/schedule_160k.py'
-]
-
-model = dict(pretrained='open-mmlab://contrib/mobilenet_v3_large')
-
-# Re-config the data sampler.
-data = dict(samples_per_gpu=4, workers_per_gpu=4)
-
-runner = dict(type='IterBasedRunner', max_iters=320000)
diff --git a/spaces/Andy1621/uniformer_image_segmentation/configs/psanet/psanet_r50-d8_769x769_40k_cityscapes.py b/spaces/Andy1621/uniformer_image_segmentation/configs/psanet/psanet_r50-d8_769x769_40k_cityscapes.py
deleted file mode 100644
index 690f8b5ef359be8a8be3a2d768aede24216a8706..0000000000000000000000000000000000000000
--- a/spaces/Andy1621/uniformer_image_segmentation/configs/psanet/psanet_r50-d8_769x769_40k_cityscapes.py
+++ /dev/null
@@ -1,9 +0,0 @@
-_base_ = [
- '../_base_/models/psanet_r50-d8.py',
- '../_base_/datasets/cityscapes_769x769.py', '../_base_/default_runtime.py',
- '../_base_/schedules/schedule_40k.py'
-]
-model = dict(
- decode_head=dict(align_corners=True),
- auxiliary_head=dict(align_corners=True),
- test_cfg=dict(mode='slide', crop_size=(769, 769), stride=(513, 513)))
diff --git a/spaces/Anonymous-sub/Rerender/ControlNet/annotator/uniformer/mmseg/utils/__init__.py b/spaces/Anonymous-sub/Rerender/ControlNet/annotator/uniformer/mmseg/utils/__init__.py
deleted file mode 100644
index ac489e2dbbc0e6fa87f5088b4edcc20f8cadc1a6..0000000000000000000000000000000000000000
--- a/spaces/Anonymous-sub/Rerender/ControlNet/annotator/uniformer/mmseg/utils/__init__.py
+++ /dev/null
@@ -1,4 +0,0 @@
-from .collect_env import collect_env
-from .logger import get_root_logger
-
-__all__ = ['get_root_logger', 'collect_env']
diff --git a/spaces/Anonymous-sub/Rerender/gmflow_module/gmflow/trident_conv.py b/spaces/Anonymous-sub/Rerender/gmflow_module/gmflow/trident_conv.py
deleted file mode 100644
index 29a2a73e964a88b68bc095772d9c3cc443e3e0fe..0000000000000000000000000000000000000000
--- a/spaces/Anonymous-sub/Rerender/gmflow_module/gmflow/trident_conv.py
+++ /dev/null
@@ -1,90 +0,0 @@
-# Copyright (c) Facebook, Inc. and its affiliates.
-# https://github.com/facebookresearch/detectron2/blob/main/projects/TridentNet/tridentnet/trident_conv.py
-
-import torch
-from torch import nn
-from torch.nn import functional as F
-from torch.nn.modules.utils import _pair
-
-
-class MultiScaleTridentConv(nn.Module):
- def __init__(
- self,
- in_channels,
- out_channels,
- kernel_size,
- stride=1,
- strides=1,
- paddings=0,
- dilations=1,
- dilation=1,
- groups=1,
- num_branch=1,
- test_branch_idx=-1,
- bias=False,
- norm=None,
- activation=None,
- ):
- super(MultiScaleTridentConv, self).__init__()
- self.in_channels = in_channels
- self.out_channels = out_channels
- self.kernel_size = _pair(kernel_size)
- self.num_branch = num_branch
- self.stride = _pair(stride)
- self.groups = groups
- self.with_bias = bias
- self.dilation = dilation
- if isinstance(paddings, int):
- paddings = [paddings] * self.num_branch
- if isinstance(dilations, int):
- dilations = [dilations] * self.num_branch
- if isinstance(strides, int):
- strides = [strides] * self.num_branch
- self.paddings = [_pair(padding) for padding in paddings]
- self.dilations = [_pair(dilation) for dilation in dilations]
- self.strides = [_pair(stride) for stride in strides]
- self.test_branch_idx = test_branch_idx
- self.norm = norm
- self.activation = activation
-
- assert len({self.num_branch, len(self.paddings), len(self.strides)}) == 1
-
- self.weight = nn.Parameter(
- torch.Tensor(out_channels, in_channels // groups, *self.kernel_size)
- )
- if bias:
- self.bias = nn.Parameter(torch.Tensor(out_channels))
- else:
- self.bias = None
-
- nn.init.kaiming_uniform_(self.weight, nonlinearity="relu")
- if self.bias is not None:
- nn.init.constant_(self.bias, 0)
-
- def forward(self, inputs):
- num_branch = self.num_branch if self.training or self.test_branch_idx == -1 else 1
- assert len(inputs) == num_branch
-
- if self.training or self.test_branch_idx == -1:
- outputs = [
- F.conv2d(input, self.weight, self.bias, stride, padding, self.dilation, self.groups)
- for input, stride, padding in zip(inputs, self.strides, self.paddings)
- ]
- else:
- outputs = [
- F.conv2d(
- inputs[0],
- self.weight,
- self.bias,
- self.strides[self.test_branch_idx] if self.test_branch_idx == -1 else self.strides[-1],
- self.paddings[self.test_branch_idx] if self.test_branch_idx == -1 else self.paddings[-1],
- self.dilation,
- self.groups,
- )
- ]
-
- if self.norm is not None:
- outputs = [self.norm(x) for x in outputs]
- if self.activation is not None:
- outputs = [self.activation(x) for x in outputs]
- return outputs
diff --git a/spaces/Awiny/Image2Paragraph/models/grit_src/third_party/CenterNet2/detectron2/data/datasets/cityscapes.py b/spaces/Awiny/Image2Paragraph/models/grit_src/third_party/CenterNet2/detectron2/data/datasets/cityscapes.py
deleted file mode 100644
index 1e84a5bdb3d4e410d8eef4b80a5d4c099a180104..0000000000000000000000000000000000000000
--- a/spaces/Awiny/Image2Paragraph/models/grit_src/third_party/CenterNet2/detectron2/data/datasets/cityscapes.py
+++ /dev/null
@@ -1,329 +0,0 @@
-# Copyright (c) Facebook, Inc. and its affiliates.
-import functools
-import json
-import logging
-import multiprocessing as mp
-import numpy as np
-import os
-from itertools import chain
-import pycocotools.mask as mask_util
-from PIL import Image
-
-from detectron2.structures import BoxMode
-from detectron2.utils.comm import get_world_size
-from detectron2.utils.file_io import PathManager
-from detectron2.utils.logger import setup_logger
-
-try:
- import cv2 # noqa
-except ImportError:
- # OpenCV is an optional dependency at the moment
- pass
-
-
-logger = logging.getLogger(__name__)
-
-
-def _get_cityscapes_files(image_dir, gt_dir):
- files = []
- # scan through the directory
- cities = PathManager.ls(image_dir)
- logger.info(f"{len(cities)} cities found in '{image_dir}'.")
- for city in cities:
- city_img_dir = os.path.join(image_dir, city)
- city_gt_dir = os.path.join(gt_dir, city)
- for basename in PathManager.ls(city_img_dir):
- image_file = os.path.join(city_img_dir, basename)
-
- suffix = "leftImg8bit.png"
- assert basename.endswith(suffix), basename
- basename = basename[: -len(suffix)]
-
- instance_file = os.path.join(city_gt_dir, basename + "gtFine_instanceIds.png")
- label_file = os.path.join(city_gt_dir, basename + "gtFine_labelIds.png")
- json_file = os.path.join(city_gt_dir, basename + "gtFine_polygons.json")
-
- files.append((image_file, instance_file, label_file, json_file))
- assert len(files), "No images found in {}".format(image_dir)
- for f in files[0]:
- assert PathManager.isfile(f), f
- return files
-
-
-def load_cityscapes_instances(image_dir, gt_dir, from_json=True, to_polygons=True):
- """
- Args:
- image_dir (str): path to the raw dataset. e.g., "~/cityscapes/leftImg8bit/train".
- gt_dir (str): path to the raw annotations. e.g., "~/cityscapes/gtFine/train".
- from_json (bool): whether to read annotations from the raw json file or the png files.
- to_polygons (bool): whether to represent the segmentation as polygons
- (COCO's format) instead of masks (cityscapes's format).
-
- Returns:
- list[dict]: a list of dicts in Detectron2 standard format. (See
- `Using Custom Datasets `_ )
- """
- if from_json:
- assert to_polygons, (
- "Cityscapes's json annotations are in polygon format. "
- "Converting to mask format is not supported now."
- )
- files = _get_cityscapes_files(image_dir, gt_dir)
-
- logger.info("Preprocessing cityscapes annotations ...")
- # This is still not fast: all workers will execute duplicate works and will
- # take up to 10m on a 8GPU server.
- pool = mp.Pool(processes=max(mp.cpu_count() // get_world_size() // 2, 4))
-
- ret = pool.map(
- functools.partial(_cityscapes_files_to_dict, from_json=from_json, to_polygons=to_polygons),
- files,
- )
- logger.info("Loaded {} images from {}".format(len(ret), image_dir))
-
- # Map cityscape ids to contiguous ids
- from cityscapesscripts.helpers.labels import labels
-
- labels = [l for l in labels if l.hasInstances and not l.ignoreInEval]
- dataset_id_to_contiguous_id = {l.id: idx for idx, l in enumerate(labels)}
- for dict_per_image in ret:
- for anno in dict_per_image["annotations"]:
- anno["category_id"] = dataset_id_to_contiguous_id[anno["category_id"]]
- return ret
-
-
-def load_cityscapes_semantic(image_dir, gt_dir):
- """
- Args:
- image_dir (str): path to the raw dataset. e.g., "~/cityscapes/leftImg8bit/train".
- gt_dir (str): path to the raw annotations. e.g., "~/cityscapes/gtFine/train".
-
- Returns:
- list[dict]: a list of dict, each has "file_name" and
- "sem_seg_file_name".
- """
- ret = []
- # gt_dir is small and contain many small files. make sense to fetch to local first
- gt_dir = PathManager.get_local_path(gt_dir)
- for image_file, _, label_file, json_file in _get_cityscapes_files(image_dir, gt_dir):
- label_file = label_file.replace("labelIds", "labelTrainIds")
-
- with PathManager.open(json_file, "r") as f:
- jsonobj = json.load(f)
- ret.append(
- {
- "file_name": image_file,
- "sem_seg_file_name": label_file,
- "height": jsonobj["imgHeight"],
- "width": jsonobj["imgWidth"],
- }
- )
- assert len(ret), f"No images found in {image_dir}!"
- assert PathManager.isfile(
- ret[0]["sem_seg_file_name"]
- ), "Please generate labelTrainIds.png with cityscapesscripts/preparation/createTrainIdLabelImgs.py" # noqa
- return ret
-
-
-def _cityscapes_files_to_dict(files, from_json, to_polygons):
- """
- Parse cityscapes annotation files to a instance segmentation dataset dict.
-
- Args:
- files (tuple): consists of (image_file, instance_id_file, label_id_file, json_file)
- from_json (bool): whether to read annotations from the raw json file or the png files.
- to_polygons (bool): whether to represent the segmentation as polygons
- (COCO's format) instead of masks (cityscapes's format).
-
- Returns:
- A dict in Detectron2 Dataset format.
- """
- from cityscapesscripts.helpers.labels import id2label, name2label
-
- image_file, instance_id_file, _, json_file = files
-
- annos = []
-
- if from_json:
- from shapely.geometry import MultiPolygon, Polygon
-
- with PathManager.open(json_file, "r") as f:
- jsonobj = json.load(f)
- ret = {
- "file_name": image_file,
- "image_id": os.path.basename(image_file),
- "height": jsonobj["imgHeight"],
- "width": jsonobj["imgWidth"],
- }
-
- # `polygons_union` contains the union of all valid polygons.
- polygons_union = Polygon()
-
- # CityscapesScripts draw the polygons in sequential order
- # and each polygon *overwrites* existing ones. See
- # (https://github.com/mcordts/cityscapesScripts/blob/master/cityscapesscripts/preparation/json2instanceImg.py) # noqa
- # We use reverse order, and each polygon *avoids* early ones.
- # This will resolve the ploygon overlaps in the same way as CityscapesScripts.
- for obj in jsonobj["objects"][::-1]:
- if "deleted" in obj: # cityscapes data format specific
- continue
- label_name = obj["label"]
-
- try:
- label = name2label[label_name]
- except KeyError:
- if label_name.endswith("group"): # crowd area
- label = name2label[label_name[: -len("group")]]
- else:
- raise
- if label.id < 0: # cityscapes data format
- continue
-
- # Cityscapes's raw annotations uses integer coordinates
- # Therefore +0.5 here
- poly_coord = np.asarray(obj["polygon"], dtype="f4") + 0.5
- # CityscapesScript uses PIL.ImageDraw.polygon to rasterize
- # polygons for evaluation. This function operates in integer space
- # and draws each pixel whose center falls into the polygon.
- # Therefore it draws a polygon which is 0.5 "fatter" in expectation.
- # We therefore dilate the input polygon by 0.5 as our input.
- poly = Polygon(poly_coord).buffer(0.5, resolution=4)
-
- if not label.hasInstances or label.ignoreInEval:
- # even if we won't store the polygon it still contributes to overlaps resolution
- polygons_union = polygons_union.union(poly)
- continue
-
- # Take non-overlapping part of the polygon
- poly_wo_overlaps = poly.difference(polygons_union)
- if poly_wo_overlaps.is_empty:
- continue
- polygons_union = polygons_union.union(poly)
-
- anno = {}
- anno["iscrowd"] = label_name.endswith("group")
- anno["category_id"] = label.id
-
- if isinstance(poly_wo_overlaps, Polygon):
- poly_list = [poly_wo_overlaps]
- elif isinstance(poly_wo_overlaps, MultiPolygon):
- poly_list = poly_wo_overlaps.geoms
- else:
- raise NotImplementedError("Unknown geometric structure {}".format(poly_wo_overlaps))
-
- poly_coord = []
- for poly_el in poly_list:
- # COCO API can work only with exterior boundaries now, hence we store only them.
- # TODO: store both exterior and interior boundaries once other parts of the
- # codebase support holes in polygons.
- poly_coord.append(list(chain(*poly_el.exterior.coords)))
- anno["segmentation"] = poly_coord
- (xmin, ymin, xmax, ymax) = poly_wo_overlaps.bounds
-
- anno["bbox"] = (xmin, ymin, xmax, ymax)
- anno["bbox_mode"] = BoxMode.XYXY_ABS
-
- annos.append(anno)
- else:
- # See also the official annotation parsing scripts at
- # https://github.com/mcordts/cityscapesScripts/blob/master/cityscapesscripts/evaluation/instances2dict.py # noqa
- with PathManager.open(instance_id_file, "rb") as f:
- inst_image = np.asarray(Image.open(f), order="F")
- # ids < 24 are stuff labels (filtering them first is about 5% faster)
- flattened_ids = np.unique(inst_image[inst_image >= 24])
-
- ret = {
- "file_name": image_file,
- "image_id": os.path.basename(image_file),
- "height": inst_image.shape[0],
- "width": inst_image.shape[1],
- }
-
- for instance_id in flattened_ids:
- # For non-crowd annotations, instance_id // 1000 is the label_id
- # Crowd annotations have <1000 instance ids
- label_id = instance_id // 1000 if instance_id >= 1000 else instance_id
- label = id2label[label_id]
- if not label.hasInstances or label.ignoreInEval:
- continue
-
- anno = {}
- anno["iscrowd"] = instance_id < 1000
- anno["category_id"] = label.id
-
- mask = np.asarray(inst_image == instance_id, dtype=np.uint8, order="F")
-
- inds = np.nonzero(mask)
- ymin, ymax = inds[0].min(), inds[0].max()
- xmin, xmax = inds[1].min(), inds[1].max()
- anno["bbox"] = (xmin, ymin, xmax, ymax)
- if xmax <= xmin or ymax <= ymin:
- continue
- anno["bbox_mode"] = BoxMode.XYXY_ABS
- if to_polygons:
- # This conversion comes from D4809743 and D5171122,
- # when Mask-RCNN was first developed.
- contours = cv2.findContours(mask.copy(), cv2.RETR_EXTERNAL, cv2.CHAIN_APPROX_NONE)[
- -2
- ]
- polygons = [c.reshape(-1).tolist() for c in contours if len(c) >= 3]
- # opencv's can produce invalid polygons
- if len(polygons) == 0:
- continue
- anno["segmentation"] = polygons
- else:
- anno["segmentation"] = mask_util.encode(mask[:, :, None])[0]
- annos.append(anno)
- ret["annotations"] = annos
- return ret
-
-
-if __name__ == "__main__":
- """
- Test the cityscapes dataset loader.
-
- Usage:
- python -m detectron2.data.datasets.cityscapes \
- cityscapes/leftImg8bit/train cityscapes/gtFine/train
- """
- import argparse
-
- parser = argparse.ArgumentParser()
- parser.add_argument("image_dir")
- parser.add_argument("gt_dir")
- parser.add_argument("--type", choices=["instance", "semantic"], default="instance")
- args = parser.parse_args()
- from detectron2.data.catalog import Metadata
- from detectron2.utils.visualizer import Visualizer
- from cityscapesscripts.helpers.labels import labels
-
- logger = setup_logger(name=__name__)
-
- dirname = "cityscapes-data-vis"
- os.makedirs(dirname, exist_ok=True)
-
- if args.type == "instance":
- dicts = load_cityscapes_instances(
- args.image_dir, args.gt_dir, from_json=True, to_polygons=True
- )
- logger.info("Done loading {} samples.".format(len(dicts)))
-
- thing_classes = [k.name for k in labels if k.hasInstances and not k.ignoreInEval]
- meta = Metadata().set(thing_classes=thing_classes)
-
- else:
- dicts = load_cityscapes_semantic(args.image_dir, args.gt_dir)
- logger.info("Done loading {} samples.".format(len(dicts)))
-
- stuff_classes = [k.name for k in labels if k.trainId != 255]
- stuff_colors = [k.color for k in labels if k.trainId != 255]
- meta = Metadata().set(stuff_classes=stuff_classes, stuff_colors=stuff_colors)
-
- for d in dicts:
- img = np.array(Image.open(PathManager.open(d["file_name"], "rb")))
- visualizer = Visualizer(img, metadata=meta)
- vis = visualizer.draw_dataset_dict(d)
- # cv2.imshow("a", vis.get_image()[:, :, ::-1])
- # cv2.waitKey()
- fpath = os.path.join(dirname, os.path.basename(d["file_name"]))
- vis.save(fpath)
diff --git a/spaces/BeeMon/dreambooth-training/train_dreambooth.py b/spaces/BeeMon/dreambooth-training/train_dreambooth.py
deleted file mode 100644
index f4ff135e549f0d6c72f733092f3df817cb178e01..0000000000000000000000000000000000000000
--- a/spaces/BeeMon/dreambooth-training/train_dreambooth.py
+++ /dev/null
@@ -1,889 +0,0 @@
-import argparse
-import itertools
-import math
-import os
-from pathlib import Path
-from typing import Optional
-import subprocess
-import sys
-import gc
-import random
-
-import torch
-import torch.nn.functional as F
-import torch.utils.checkpoint
-from torch.utils.data import Dataset
-
-from accelerate import Accelerator
-from accelerate.logging import get_logger
-from accelerate.utils import set_seed
-from diffusers import AutoencoderKL, DDPMScheduler, StableDiffusionPipeline, UNet2DConditionModel
-from diffusers.utils.import_utils import is_xformers_available
-from diffusers.optimization import get_scheduler
-from huggingface_hub import HfFolder, Repository, whoami
-from PIL import Image
-from torchvision import transforms
-from tqdm.auto import tqdm
-from transformers import CLIPTextModel, CLIPTokenizer
-
-
-logger = get_logger(__name__)
-
-
-def parse_args():
- parser = argparse.ArgumentParser(description="Simple example of a training script.")
- parser.add_argument(
- "--pretrained_model_name_or_path",
- type=str,
- default=None,
- #required=True,
- help="Path to pretrained model or model identifier from huggingface.co/models.",
- )
- parser.add_argument(
- "--tokenizer_name",
- type=str,
- default=None,
- help="Pretrained tokenizer name or path if not the same as model_name",
- )
- parser.add_argument(
- "--instance_data_dir",
- type=str,
- default=None,
- #required=True,
- help="A folder containing the training data of instance images.",
- )
- parser.add_argument(
- "--class_data_dir",
- type=str,
- default=None,
- #required=False,
- help="A folder containing the training data of class images.",
- )
- parser.add_argument(
- "--instance_prompt",
- type=str,
- default=None,
- help="The prompt with identifier specifying the instance",
- )
- parser.add_argument(
- "--class_prompt",
- type=str,
- default="",
- help="The prompt to specify images in the same class as provided instance images.",
- )
- parser.add_argument(
- "--with_prior_preservation",
- default=False,
- action="store_true",
- help="Flag to add prior preservation loss.",
- )
- parser.add_argument("--prior_loss_weight", type=float, default=1.0, help="The weight of prior preservation loss.")
- parser.add_argument(
- "--num_class_images",
- type=int,
- default=100,
- help=(
- "Minimal class images for prior preservation loss. If not have enough images, additional images will be"
- " sampled with class_prompt."
- ),
- )
- parser.add_argument(
- "--output_dir",
- type=str,
- default="",
- help="The output directory where the model predictions and checkpoints will be written.",
- )
- parser.add_argument("--seed", type=int, default=None, help="A seed for reproducible training.")
- parser.add_argument(
- "--resolution",
- type=int,
- default=512,
- help=(
- "The resolution for input images, all the images in the train/validation dataset will be resized to this"
- " resolution"
- ),
- )
- parser.add_argument(
- "--center_crop", action="store_true", help="Whether to center crop images before resizing to resolution"
- )
- parser.add_argument("--train_text_encoder", action="store_true", help="Whether to train the text encoder")
- parser.add_argument(
- "--train_batch_size", type=int, default=4, help="Batch size (per device) for the training dataloader."
- )
- parser.add_argument(
- "--sample_batch_size", type=int, default=4, help="Batch size (per device) for sampling images."
- )
- parser.add_argument("--num_train_epochs", type=int, default=1)
- parser.add_argument(
- "--max_train_steps",
- type=int,
- default=None,
- help="Total number of training steps to perform. If provided, overrides num_train_epochs.",
- )
- parser.add_argument(
- "--gradient_accumulation_steps",
- type=int,
- default=1,
- help="Number of updates steps to accumulate before performing a backward/update pass.",
- )
- parser.add_argument(
- "--gradient_checkpointing",
- action="store_true",
- help="Whether or not to use gradient checkpointing to save memory at the expense of slower backward pass.",
- )
- parser.add_argument(
- "--learning_rate",
- type=float,
- default=5e-6,
- help="Initial learning rate (after the potential warmup period) to use.",
- )
- parser.add_argument(
- "--scale_lr",
- action="store_true",
- default=False,
- help="Scale the learning rate by the number of GPUs, gradient accumulation steps, and batch size.",
- )
- parser.add_argument(
- "--lr_scheduler",
- type=str,
- default="constant",
- help=(
- 'The scheduler type to use. Choose between ["linear", "cosine", "cosine_with_restarts", "polynomial",'
- ' "constant", "constant_with_warmup"]'
- ),
- )
- parser.add_argument(
- "--lr_warmup_steps", type=int, default=500, help="Number of steps for the warmup in the lr scheduler."
- )
- parser.add_argument(
- "--use_8bit_adam", action="store_true", help="Whether or not to use 8-bit Adam from bitsandbytes."
- )
- parser.add_argument("--adam_beta1", type=float, default=0.9, help="The beta1 parameter for the Adam optimizer.")
- parser.add_argument("--adam_beta2", type=float, default=0.999, help="The beta2 parameter for the Adam optimizer.")
- parser.add_argument("--adam_weight_decay", type=float, default=1e-2, help="Weight decay to use.")
- parser.add_argument("--adam_epsilon", type=float, default=1e-08, help="Epsilon value for the Adam optimizer")
- parser.add_argument("--max_grad_norm", default=1.0, type=float, help="Max gradient norm.")
- parser.add_argument("--push_to_hub", action="store_true", help="Whether or not to push the model to the Hub.")
- parser.add_argument("--hub_token", type=str, default=None, help="The token to use to push to the Model Hub.")
- parser.add_argument(
- "--hub_model_id",
- type=str,
- default=None,
- help="The name of the repository to keep in sync with the local `output_dir`.",
- )
- parser.add_argument(
- "--logging_dir",
- type=str,
- default="logs",
- help=(
- "[TensorBoard](https://www.tensorflow.org/tensorboard) log directory. Will default to"
- " *output_dir/runs/**CURRENT_DATETIME_HOSTNAME***."
- ),
- )
- parser.add_argument(
- "--mixed_precision",
- type=str,
- default="no",
- choices=["no", "fp16", "bf16"],
- help=(
- "Whether to use mixed precision. Choose"
- "between fp16 and bf16 (bfloat16). Bf16 requires PyTorch >= 1.10."
- "and an Nvidia Ampere GPU."
- ),
- )
-
- parser.add_argument(
- "--save_n_steps",
- type=int,
- default=1,
- help=("Save the model every n global_steps"),
- )
-
-
- parser.add_argument(
- "--save_starting_step",
- type=int,
- default=1,
- help=("The step from which it starts saving intermediary checkpoints"),
- )
-
- parser.add_argument(
- "--stop_text_encoder_training",
- type=int,
- default=1000000,
- help=("The step at which the text_encoder is no longer trained"),
- )
-
-
- parser.add_argument(
- "--image_captions_filename",
- action="store_true",
- help="Get captions from filename",
- )
-
-
- parser.add_argument(
- "--dump_only_text_encoder",
- action="store_true",
- default=False,
- help="Dump only text encoder",
- )
-
- parser.add_argument(
- "--train_only_unet",
- action="store_true",
- default=False,
- help="Train only the unet",
- )
-
- parser.add_argument(
- "--cache_latents",
- action="store_true",
- default=False,
- help="Train only the unet",
- )
-
- parser.add_argument(
- "--Session_dir",
- type=str,
- default="",
- help="Current session directory",
- )
-
-
-
-
- parser.add_argument("--local_rank", type=int, default=-1, help="For distributed training: local_rank")
-
- args = parser.parse_args()
- env_local_rank = int(os.environ.get("LOCAL_RANK", -1))
- if env_local_rank != -1 and env_local_rank != args.local_rank:
- args.local_rank = env_local_rank
-
- #if args.instance_data_dir is None:
- # raise ValueError("You must specify a train data directory.")
-
- #if args.with_prior_preservation:
- # if args.class_data_dir is None:
- # raise ValueError("You must specify a data directory for class images.")
- # if args.class_prompt is None:
- # raise ValueError("You must specify prompt for class images.")
-
- return args
-
-
-class DreamBoothDataset(Dataset):
- """
- A dataset to prepare the instance and class images with the prompts for fine-tuning the model.
- It pre-processes the images and the tokenizes prompts.
- """
-
- def __init__(
- self,
- instance_data_root,
- instance_prompt,
- tokenizer,
- args,
- class_data_root=None,
- class_prompt=None,
- size=512,
- center_crop=False,
- ):
- self.size = size
- self.center_crop = center_crop
- self.tokenizer = tokenizer
- self.image_captions_filename = None
-
- self.instance_data_root = Path(instance_data_root)
- if not self.instance_data_root.exists():
- raise ValueError("Instance images root doesn't exists.")
-
- self.instance_images_path = list(Path(instance_data_root).iterdir())
- self.num_instance_images = len(self.instance_images_path)
- self.instance_prompt = instance_prompt
- self._length = self.num_instance_images
-
- if args.image_captions_filename:
- self.image_captions_filename = True
-
- if class_data_root is not None:
- self.class_data_root = Path(class_data_root)
- self.class_data_root.mkdir(parents=True, exist_ok=True)
- self.class_images_path = list(self.class_data_root.iterdir())
- random.shuffle(self.class_images_path)
- self.num_class_images = len(self.class_images_path)
- self._length = max(self.num_class_images, self.num_instance_images)
- self.class_prompt = class_prompt
- else:
- self.class_data_root = None
-
- self.image_transforms = transforms.Compose(
- [
- transforms.Resize(size, interpolation=transforms.InterpolationMode.BILINEAR),
- transforms.CenterCrop(size) if center_crop else transforms.RandomCrop(size),
- transforms.ToTensor(),
- transforms.Normalize([0.5], [0.5]),
- ]
- )
-
- def __len__(self):
- return self._length
-
- def __getitem__(self, index):
- example = {}
- path = self.instance_images_path[index % self.num_instance_images]
- instance_image = Image.open(path)
- if not instance_image.mode == "RGB":
- instance_image = instance_image.convert("RGB")
-
- instance_prompt = self.instance_prompt
-
- if self.image_captions_filename:
- filename = Path(path).stem
- pt=''.join([i for i in filename if not i.isdigit()])
- pt=pt.replace("_"," ")
- pt=pt.replace("(","")
- pt=pt.replace(")","")
- pt=pt.replace("-","")
- instance_prompt = pt
- sys.stdout.write(" [0;32m" +instance_prompt+" [0m")
- sys.stdout.flush()
-
-
- example["instance_images"] = self.image_transforms(instance_image)
- example["instance_prompt_ids"] = self.tokenizer(
- instance_prompt,
- padding="do_not_pad",
- truncation=True,
- max_length=self.tokenizer.model_max_length,
- ).input_ids
-
- if self.class_data_root:
- class_image = Image.open(self.class_images_path[index % self.num_class_images])
- if not class_image.mode == "RGB":
- class_image = class_image.convert("RGB")
- example["class_images"] = self.image_transforms(class_image)
- example["class_prompt_ids"] = self.tokenizer(
- self.class_prompt,
- padding="do_not_pad",
- truncation=True,
- max_length=self.tokenizer.model_max_length,
- ).input_ids
-
- return example
-
-
-
-class PromptDataset(Dataset):
- "A simple dataset to prepare the prompts to generate class images on multiple GPUs."
-
- def __init__(self, prompt, num_samples):
- self.prompt = prompt
- self.num_samples = num_samples
-
- def __len__(self):
- return self.num_samples
-
- def __getitem__(self, index):
- example = {}
- example["prompt"] = self.prompt
- example["index"] = index
- return example
-
-class LatentsDataset(Dataset):
- def __init__(self, latents_cache, text_encoder_cache):
- self.latents_cache = latents_cache
- self.text_encoder_cache = text_encoder_cache
-
- def __len__(self):
- return len(self.latents_cache)
-
- def __getitem__(self, index):
- return self.latents_cache[index], self.text_encoder_cache[index]
-
-def get_full_repo_name(model_id: str, organization: Optional[str] = None, token: Optional[str] = None):
- if token is None:
- token = HfFolder.get_token()
- if organization is None:
- username = whoami(token)["name"]
- return f"{username}/{model_id}"
- else:
- return f"{organization}/{model_id}"
-
-def merge_two_dicts(starting_dict: dict, updater_dict: dict) -> dict:
- """
- Starts from base starting dict and then adds the remaining key values from updater replacing the values from
- the first starting/base dict with the second updater dict.
-
- For later: how does d = {**d1, **d2} replace collision?
-
- :param starting_dict:
- :param updater_dict:
- :return:
- """
- new_dict: dict = starting_dict.copy() # start with keys and values of starting_dict
- new_dict.update(updater_dict) # modifies starting_dict with keys and values of updater_dict
- return new_dict
-
-def merge_args(args1: argparse.Namespace, args2: argparse.Namespace) -> argparse.Namespace:
- """
-
- ref: https://stackoverflow.com/questions/56136549/how-can-i-merge-two-argparse-namespaces-in-python-2-x
- :param args1:
- :param args2:
- :return:
- """
- # - the merged args
- # The vars() function returns the __dict__ attribute to values of the given object e.g {field:value}.
- merged_key_values_for_namespace: dict = merge_two_dicts(vars(args1), vars(args2))
- args = argparse.Namespace(**merged_key_values_for_namespace)
- return args
-
-def run_training(args_imported):
- args_default = parse_args()
- args = merge_args(args_default, args_imported)
- print(args)
- logging_dir = Path(args.output_dir, args.logging_dir)
- i=args.save_starting_step
- accelerator = Accelerator(
- gradient_accumulation_steps=args.gradient_accumulation_steps,
- mixed_precision=args.mixed_precision,
- log_with="tensorboard",
- logging_dir=logging_dir,
- )
-
- # Currently, it's not possible to do gradient accumulation when training two models with accelerate.accumulate
- # This will be enabled soon in accelerate. For now, we don't allow gradient accumulation when training two models.
- # TODO (patil-suraj): Remove this check when gradient accumulation with two models is enabled in accelerate.
- if args.train_text_encoder and args.gradient_accumulation_steps > 1 and accelerator.num_processes > 1:
- raise ValueError(
- "Gradient accumulation is not supported when training the text encoder in distributed training. "
- "Please set gradient_accumulation_steps to 1. This feature will be supported in the future."
- )
-
- if args.seed is not None:
- set_seed(args.seed)
-
- if args.with_prior_preservation:
- class_images_dir = Path(args.class_data_dir)
- if not class_images_dir.exists():
- class_images_dir.mkdir(parents=True)
- cur_class_images = len(list(class_images_dir.iterdir()))
-
- if cur_class_images < args.num_class_images:
- torch_dtype = torch.float16 if accelerator.device.type == "cuda" else torch.float32
- pipeline = StableDiffusionPipeline.from_pretrained(
- args.pretrained_model_name_or_path, torch_dtype=torch_dtype
- )
- pipeline.set_progress_bar_config(disable=True)
-
- num_new_images = args.num_class_images - cur_class_images
- logger.info(f"Number of class images to sample: {num_new_images}.")
-
- sample_dataset = PromptDataset(args.class_prompt, num_new_images)
- sample_dataloader = torch.utils.data.DataLoader(sample_dataset, batch_size=args.sample_batch_size)
-
- sample_dataloader = accelerator.prepare(sample_dataloader)
- pipeline.to(accelerator.device)
-
- for example in tqdm(
- sample_dataloader, desc="Generating class images", disable=not accelerator.is_local_main_process
- ):
- with torch.autocast("cuda"):
- images = pipeline(example["prompt"]).images
-
- for i, image in enumerate(images):
- image.save(class_images_dir / f"{example['index'][i] + cur_class_images}.jpg")
-
- del pipeline
- if torch.cuda.is_available():
- torch.cuda.empty_cache()
-
- # Handle the repository creation
- if accelerator.is_main_process:
- if args.push_to_hub:
- if args.hub_model_id is None:
- repo_name = get_full_repo_name(Path(args.output_dir).name, token=args.hub_token)
- else:
- repo_name = args.hub_model_id
- repo = Repository(args.output_dir, clone_from=repo_name)
-
- with open(os.path.join(args.output_dir, ".gitignore"), "w+") as gitignore:
- if "step_*" not in gitignore:
- gitignore.write("step_*\n")
- if "epoch_*" not in gitignore:
- gitignore.write("epoch_*\n")
- elif args.output_dir is not None:
- os.makedirs(args.output_dir, exist_ok=True)
-
- # Load the tokenizer
- if args.tokenizer_name:
- tokenizer = CLIPTokenizer.from_pretrained(args.tokenizer_name)
- elif args.pretrained_model_name_or_path:
- tokenizer = CLIPTokenizer.from_pretrained(args.pretrained_model_name_or_path, subfolder="tokenizer")
-
- # Load models and create wrapper for stable diffusion
- if args.train_only_unet:
- if os.path.exists(str(args.output_dir+"/text_encoder_trained")):
- text_encoder = CLIPTextModel.from_pretrained(args.output_dir, subfolder="text_encoder_trained")
- elif os.path.exists(str(args.output_dir+"/text_encoder")):
- text_encoder = CLIPTextModel.from_pretrained(args.output_dir, subfolder="text_encoder")
- else:
- text_encoder = CLIPTextModel.from_pretrained(args.pretrained_model_name_or_path, subfolder="text_encoder")
- else:
- text_encoder = CLIPTextModel.from_pretrained(args.pretrained_model_name_or_path, subfolder="text_encoder")
- vae = AutoencoderKL.from_pretrained(args.pretrained_model_name_or_path, subfolder="vae")
- unet = UNet2DConditionModel.from_pretrained(args.pretrained_model_name_or_path, subfolder="unet")
- if is_xformers_available():
- try:
- print("Enabling memory efficient attention with xformers...")
- unet.enable_xformers_memory_efficient_attention()
- except Exception as e:
- logger.warning(
- f"Could not enable memory efficient attention. Make sure xformers is installed correctly and a GPU is available: {e}"
- )
- vae.requires_grad_(False)
- if not args.train_text_encoder:
- text_encoder.requires_grad_(False)
-
- if args.gradient_checkpointing:
- unet.enable_gradient_checkpointing()
- if args.train_text_encoder:
- text_encoder.gradient_checkpointing_enable()
-
- if args.scale_lr:
- args.learning_rate = (
- args.learning_rate * args.gradient_accumulation_steps * args.train_batch_size * accelerator.num_processes
- )
-
- # Use 8-bit Adam for lower memory usage or to fine-tune the model in 16GB GPUs
- if args.use_8bit_adam:
- try:
- import bitsandbytes as bnb
- except ImportError:
- raise ImportError(
- "To use 8-bit Adam, please install the bitsandbytes library: `pip install bitsandbytes`."
- )
-
- optimizer_class = bnb.optim.AdamW8bit
- else:
- optimizer_class = torch.optim.AdamW
-
- params_to_optimize = (
- itertools.chain(unet.parameters(), text_encoder.parameters()) if args.train_text_encoder else unet.parameters()
- )
- optimizer = optimizer_class(
- params_to_optimize,
- lr=args.learning_rate,
- betas=(args.adam_beta1, args.adam_beta2),
- weight_decay=args.adam_weight_decay,
- eps=args.adam_epsilon,
- )
-
- noise_scheduler = DDPMScheduler.from_config(args.pretrained_model_name_or_path, subfolder="scheduler")
-
- train_dataset = DreamBoothDataset(
- instance_data_root=args.instance_data_dir,
- instance_prompt=args.instance_prompt,
- class_data_root=args.class_data_dir if args.with_prior_preservation else None,
- class_prompt=args.class_prompt,
- tokenizer=tokenizer,
- size=args.resolution,
- center_crop=args.center_crop,
- args=args,
- )
-
- def collate_fn(examples):
- input_ids = [example["instance_prompt_ids"] for example in examples]
- pixel_values = [example["instance_images"] for example in examples]
-
- # Concat class and instance examples for prior preservation.
- # We do this to avoid doing two forward passes.
- if args.with_prior_preservation:
- input_ids += [example["class_prompt_ids"] for example in examples]
- pixel_values += [example["class_images"] for example in examples]
-
- pixel_values = torch.stack(pixel_values)
- pixel_values = pixel_values.to(memory_format=torch.contiguous_format).float()
-
- input_ids = tokenizer.pad({"input_ids": input_ids}, padding=True, return_tensors="pt").input_ids
-
- batch = {
- "input_ids": input_ids,
- "pixel_values": pixel_values,
- }
- return batch
-
- train_dataloader = torch.utils.data.DataLoader(
- train_dataset, batch_size=args.train_batch_size, shuffle=True, collate_fn=collate_fn
- )
-
- # Scheduler and math around the number of training steps.
- overrode_max_train_steps = False
- num_update_steps_per_epoch = math.ceil(len(train_dataloader) / args.gradient_accumulation_steps)
- if args.max_train_steps is None:
- args.max_train_steps = args.num_train_epochs * num_update_steps_per_epoch
- overrode_max_train_steps = True
-
- lr_scheduler = get_scheduler(
- args.lr_scheduler,
- optimizer=optimizer,
- num_warmup_steps=args.lr_warmup_steps * args.gradient_accumulation_steps,
- num_training_steps=args.max_train_steps * args.gradient_accumulation_steps,
- )
-
- if args.train_text_encoder:
- unet, text_encoder, optimizer, train_dataloader, lr_scheduler = accelerator.prepare(
- unet, text_encoder, optimizer, train_dataloader, lr_scheduler
- )
- else:
- unet, optimizer, train_dataloader, lr_scheduler = accelerator.prepare(
- unet, optimizer, train_dataloader, lr_scheduler
- )
-
- weight_dtype = torch.float32
- if args.mixed_precision == "fp16":
- weight_dtype = torch.float16
- elif args.mixed_precision == "bf16":
- weight_dtype = torch.bfloat16
-
- # Move text_encode and vae to gpu.
- # For mixed precision training we cast the text_encoder and vae weights to half-precision
- # as these models are only used for inference, keeping weights in full precision is not required.
- vae.to(accelerator.device, dtype=weight_dtype)
- if not args.train_text_encoder:
- text_encoder.to(accelerator.device, dtype=weight_dtype)
-
-
- if args.cache_latents:
- latents_cache = []
- text_encoder_cache = []
- for batch in tqdm(train_dataloader, desc="Caching latents"):
- with torch.no_grad():
- batch["pixel_values"] = batch["pixel_values"].to(accelerator.device, non_blocking=True, dtype=weight_dtype)
- batch["input_ids"] = batch["input_ids"].to(accelerator.device, non_blocking=True)
- latents_cache.append(vae.encode(batch["pixel_values"]).latent_dist)
- if args.train_text_encoder:
- text_encoder_cache.append(batch["input_ids"])
- else:
- text_encoder_cache.append(text_encoder(batch["input_ids"])[0])
- train_dataset = LatentsDataset(latents_cache, text_encoder_cache)
- train_dataloader = torch.utils.data.DataLoader(train_dataset, batch_size=1, collate_fn=lambda x: x, shuffle=True)
-
- del vae
- #if not args.train_text_encoder:
- # del text_encoder
- if torch.cuda.is_available():
- torch.cuda.empty_cache()
-
- # We need to recalculate our total training steps as the size of the training dataloader may have changed.
- num_update_steps_per_epoch = math.ceil(len(train_dataloader) / args.gradient_accumulation_steps)
- if overrode_max_train_steps:
- args.max_train_steps = args.num_train_epochs * num_update_steps_per_epoch
- # Afterwards we recalculate our number of training epochs
- args.num_train_epochs = math.ceil(args.max_train_steps / num_update_steps_per_epoch)
-
- # We need to initialize the trackers we use, and also store our configuration.
- # The trackers initializes automatically on the main process.
- if accelerator.is_main_process:
- accelerator.init_trackers("dreambooth", config=vars(args))
-
- def bar(prg):
- br='|'+'█' * prg + ' ' * (25-prg)+'|'
- return br
-
- # Train!
- total_batch_size = args.train_batch_size * accelerator.num_processes * args.gradient_accumulation_steps
-
- logger.info("***** Running training *****")
- logger.info(f" Num examples = {len(train_dataset)}")
- logger.info(f" Num batches each epoch = {len(train_dataloader)}")
- logger.info(f" Num Epochs = {args.num_train_epochs}")
- logger.info(f" Instantaneous batch size per device = {args.train_batch_size}")
- logger.info(f" Total train batch size (w. parallel, distributed & accumulation) = {total_batch_size}")
- logger.info(f" Gradient Accumulation steps = {args.gradient_accumulation_steps}")
- logger.info(f" Total optimization steps = {args.max_train_steps}")
- # Only show the progress bar once on each machine.
- progress_bar = tqdm(range(args.max_train_steps), disable=not accelerator.is_local_main_process)
- global_step = 0
-
- for epoch in range(args.num_train_epochs):
- unet.train()
- if args.train_text_encoder:
- text_encoder.train()
- for step, batch in enumerate(train_dataloader):
- with accelerator.accumulate(unet):
- # Convert images to latent space
- with torch.no_grad():
- if args.cache_latents:
- latents_dist = batch[0][0]
- else:
- latents_dist = vae.encode(batch["pixel_values"].to(dtype=weight_dtype)).latent_dist
- latents = latents_dist.sample() * 0.18215
-
- # Sample noise that we'll add to the latents
- noise = torch.randn_like(latents)
- bsz = latents.shape[0]
- # Sample a random timestep for each image
- timesteps = torch.randint(0, noise_scheduler.config.num_train_timesteps, (bsz,), device=latents.device)
- timesteps = timesteps.long()
-
- # Add noise to the latents according to the noise magnitude at each timestep
- # (this is the forward diffusion process)
- noisy_latents = noise_scheduler.add_noise(latents, noise, timesteps)
-
- # Get the text embedding for conditioning
- if(args.cache_latents):
- if args.train_text_encoder:
- encoder_hidden_states = text_encoder(batch[0][1])[0]
- else:
- encoder_hidden_states = batch[0][1]
- else:
- encoder_hidden_states = text_encoder(batch["input_ids"])[0]
-
- # Predict the noise residual
- model_pred = unet(noisy_latents, timesteps, encoder_hidden_states).sample
-
- # Get the target for loss depending on the prediction type
- if noise_scheduler.config.prediction_type == "epsilon":
- target = noise
- elif noise_scheduler.config.prediction_type == "v_prediction":
- target = noise_scheduler.get_velocity(latents, noise, timesteps)
- else:
- raise ValueError(f"Unknown prediction type {noise_scheduler.config.prediction_type}")
-
- if args.with_prior_preservation:
- # Chunk the noise and model_pred into two parts and compute the loss on each part separately.
- model_pred, model_pred_prior = torch.chunk(model_pred, 2, dim=0)
- target, target_prior = torch.chunk(target, 2, dim=0)
-
- # Compute instance loss
- loss = F.mse_loss(model_pred.float(), target.float(), reduction="none").mean([1, 2, 3]).mean()
-
- # Compute prior loss
- prior_loss = F.mse_loss(model_pred_prior.float(), target_prior.float(), reduction="mean")
-
- # Add the prior loss to the instance loss.
- loss = loss + args.prior_loss_weight * prior_loss
- else:
- loss = F.mse_loss(model_pred.float(), target.float(), reduction="mean")
-
- accelerator.backward(loss)
- if accelerator.sync_gradients:
- params_to_clip = (
- itertools.chain(unet.parameters(), text_encoder.parameters())
- if args.train_text_encoder
- else unet.parameters()
- )
- accelerator.clip_grad_norm_(params_to_clip, args.max_grad_norm)
- optimizer.step()
- lr_scheduler.step()
- optimizer.zero_grad()
-
- # Checks if the accelerator has performed an optimization step behind the scenes
- if accelerator.sync_gradients:
- progress_bar.update(1)
- global_step += 1
-
- fll=round((global_step*100)/args.max_train_steps)
- fll=round(fll/4)
- pr=bar(fll)
-
- logs = {"loss": loss.detach().item(), "lr": lr_scheduler.get_last_lr()[0]}
- progress_bar.set_postfix(**logs)
- progress_bar.set_description_str("Progress:"+pr)
- accelerator.log(logs, step=global_step)
-
- if global_step >= args.max_train_steps:
- break
-
- if args.train_text_encoder and global_step == args.stop_text_encoder_training and global_step >= 30:
- if accelerator.is_main_process:
- print(" [0;32m" +" Freezing the text_encoder ..."+" [0m")
- frz_dir=args.output_dir + "/text_encoder_frozen"
- if os.path.exists(frz_dir):
- subprocess.call('rm -r '+ frz_dir, shell=True)
- os.mkdir(frz_dir)
- pipeline = StableDiffusionPipeline.from_pretrained(
- args.pretrained_model_name_or_path,
- unet=accelerator.unwrap_model(unet),
- text_encoder=accelerator.unwrap_model(text_encoder),
- )
- pipeline.text_encoder.save_pretrained(frz_dir)
-
- if args.save_n_steps >= 200:
- if global_step < args.max_train_steps and global_step+1==i:
- ckpt_name = "_step_" + str(global_step+1)
- save_dir = Path(args.output_dir+ckpt_name)
- save_dir=str(save_dir)
- save_dir=save_dir.replace(" ", "_")
- if not os.path.exists(save_dir):
- os.mkdir(save_dir)
- inst=save_dir[16:]
- inst=inst.replace(" ", "_")
- print(" [1;32mSAVING CHECKPOINT: "+args.Session_dir+"/"+inst+".ckpt")
- # Create the pipeline using the trained modules and save it.
- if accelerator.is_main_process:
- pipeline = StableDiffusionPipeline.from_pretrained(
- args.pretrained_model_name_or_path,
- unet=accelerator.unwrap_model(unet),
- text_encoder=accelerator.unwrap_model(text_encoder),
- )
- pipeline.save_pretrained(save_dir)
- frz_dir=args.output_dir + "/text_encoder_frozen"
- if args.train_text_encoder and os.path.exists(frz_dir):
- subprocess.call('rm -r '+save_dir+'/text_encoder/*.*', shell=True)
- subprocess.call('cp -f '+frz_dir +'/*.* '+ save_dir+'/text_encoder', shell=True)
- chkpth=args.Session_dir+"/"+inst+".ckpt"
- subprocess.call('python /content/diffusers/scripts/convert_diffusers_to_original_stable_diffusion.py --model_path ' + save_dir + ' --checkpoint_path ' + chkpth + ' --half', shell=True)
- subprocess.call('rm -r '+ save_dir, shell=True)
- i=i+args.save_n_steps
-
- accelerator.wait_for_everyone()
-
- # Create the pipeline using using the trained modules and save it.
- if accelerator.is_main_process:
- if args.dump_only_text_encoder:
- txt_dir=args.output_dir + "/text_encoder_trained"
- if not os.path.exists(txt_dir):
- os.mkdir(txt_dir)
- pipeline = StableDiffusionPipeline.from_pretrained(
- args.pretrained_model_name_or_path,
- unet=accelerator.unwrap_model(unet),
- text_encoder=accelerator.unwrap_model(text_encoder),
- )
- pipeline.text_encoder.save_pretrained(txt_dir)
-
- elif args.train_only_unet:
- pipeline = StableDiffusionPipeline.from_pretrained(
- args.pretrained_model_name_or_path,
- unet=accelerator.unwrap_model(unet),
- text_encoder=accelerator.unwrap_model(text_encoder),
- )
- pipeline.save_pretrained(args.output_dir)
- txt_dir=args.output_dir + "/text_encoder_trained"
- subprocess.call('rm -r '+txt_dir, shell=True)
-
- else:
- pipeline = StableDiffusionPipeline.from_pretrained(
- args.pretrained_model_name_or_path,
- unet=accelerator.unwrap_model(unet),
- text_encoder=accelerator.unwrap_model(text_encoder),
- )
- frz_dir=args.output_dir + "/text_encoder_frozen"
- pipeline.save_pretrained(args.output_dir)
- if args.train_text_encoder and os.path.exists(frz_dir):
- subprocess.call('mv -f '+frz_dir +'/*.* '+ args.output_dir+'/text_encoder', shell=True)
- subprocess.call('rm -r '+ frz_dir, shell=True)
-
- if args.push_to_hub:
- repo.push_to_hub(commit_message="End of training", blocking=False, auto_lfs_prune=True)
-
- accelerator.end_training()
- del pipeline
- torch.cuda.empty_cache()
- gc.collect()
-if __name__ == "__main__":
- pass
- #main()
-
diff --git a/spaces/Big-Web/MMSD/env/Lib/site-packages/botocore/vendored/requests/__init__.py b/spaces/Big-Web/MMSD/env/Lib/site-packages/botocore/vendored/requests/__init__.py
deleted file mode 100644
index 0ada6e0f4ce9dfcd0e902357606e48ba154e1862..0000000000000000000000000000000000000000
--- a/spaces/Big-Web/MMSD/env/Lib/site-packages/botocore/vendored/requests/__init__.py
+++ /dev/null
@@ -1,10 +0,0 @@
-# -*- coding: utf-8 -*-
-
-# __
-# /__) _ _ _ _ _/ _
-# / ( (- (/ (/ (- _) / _)
-# /
-from .exceptions import (
- RequestException, Timeout, URLRequired,
- TooManyRedirects, HTTPError, ConnectionError
-)
diff --git a/spaces/Big-Web/MMSD/env/Lib/site-packages/pip/_internal/cli/autocompletion.py b/spaces/Big-Web/MMSD/env/Lib/site-packages/pip/_internal/cli/autocompletion.py
deleted file mode 100644
index 226fe84dc0d0c4eb78f9b3c603df20cef0fdfda4..0000000000000000000000000000000000000000
--- a/spaces/Big-Web/MMSD/env/Lib/site-packages/pip/_internal/cli/autocompletion.py
+++ /dev/null
@@ -1,171 +0,0 @@
-"""Logic that powers autocompletion installed by ``pip completion``.
-"""
-
-import optparse
-import os
-import sys
-from itertools import chain
-from typing import Any, Iterable, List, Optional
-
-from pip._internal.cli.main_parser import create_main_parser
-from pip._internal.commands import commands_dict, create_command
-from pip._internal.metadata import get_default_environment
-
-
-def autocomplete() -> None:
- """Entry Point for completion of main and subcommand options."""
- # Don't complete if user hasn't sourced bash_completion file.
- if "PIP_AUTO_COMPLETE" not in os.environ:
- return
- cwords = os.environ["COMP_WORDS"].split()[1:]
- cword = int(os.environ["COMP_CWORD"])
- try:
- current = cwords[cword - 1]
- except IndexError:
- current = ""
-
- parser = create_main_parser()
- subcommands = list(commands_dict)
- options = []
-
- # subcommand
- subcommand_name: Optional[str] = None
- for word in cwords:
- if word in subcommands:
- subcommand_name = word
- break
- # subcommand options
- if subcommand_name is not None:
- # special case: 'help' subcommand has no options
- if subcommand_name == "help":
- sys.exit(1)
- # special case: list locally installed dists for show and uninstall
- should_list_installed = not current.startswith("-") and subcommand_name in [
- "show",
- "uninstall",
- ]
- if should_list_installed:
- env = get_default_environment()
- lc = current.lower()
- installed = [
- dist.canonical_name
- for dist in env.iter_installed_distributions(local_only=True)
- if dist.canonical_name.startswith(lc)
- and dist.canonical_name not in cwords[1:]
- ]
- # if there are no dists installed, fall back to option completion
- if installed:
- for dist in installed:
- print(dist)
- sys.exit(1)
-
- should_list_installables = (
- not current.startswith("-") and subcommand_name == "install"
- )
- if should_list_installables:
- for path in auto_complete_paths(current, "path"):
- print(path)
- sys.exit(1)
-
- subcommand = create_command(subcommand_name)
-
- for opt in subcommand.parser.option_list_all:
- if opt.help != optparse.SUPPRESS_HELP:
- for opt_str in opt._long_opts + opt._short_opts:
- options.append((opt_str, opt.nargs))
-
- # filter out previously specified options from available options
- prev_opts = [x.split("=")[0] for x in cwords[1 : cword - 1]]
- options = [(x, v) for (x, v) in options if x not in prev_opts]
- # filter options by current input
- options = [(k, v) for k, v in options if k.startswith(current)]
- # get completion type given cwords and available subcommand options
- completion_type = get_path_completion_type(
- cwords,
- cword,
- subcommand.parser.option_list_all,
- )
- # get completion files and directories if ``completion_type`` is
- # ````, ```` or ````
- if completion_type:
- paths = auto_complete_paths(current, completion_type)
- options = [(path, 0) for path in paths]
- for option in options:
- opt_label = option[0]
- # append '=' to options which require args
- if option[1] and option[0][:2] == "--":
- opt_label += "="
- print(opt_label)
- else:
- # show main parser options only when necessary
-
- opts = [i.option_list for i in parser.option_groups]
- opts.append(parser.option_list)
- flattened_opts = chain.from_iterable(opts)
- if current.startswith("-"):
- for opt in flattened_opts:
- if opt.help != optparse.SUPPRESS_HELP:
- subcommands += opt._long_opts + opt._short_opts
- else:
- # get completion type given cwords and all available options
- completion_type = get_path_completion_type(cwords, cword, flattened_opts)
- if completion_type:
- subcommands = list(auto_complete_paths(current, completion_type))
-
- print(" ".join([x for x in subcommands if x.startswith(current)]))
- sys.exit(1)
-
-
-def get_path_completion_type(
- cwords: List[str], cword: int, opts: Iterable[Any]
-) -> Optional[str]:
- """Get the type of path completion (``file``, ``dir``, ``path`` or None)
-
- :param cwords: same as the environmental variable ``COMP_WORDS``
- :param cword: same as the environmental variable ``COMP_CWORD``
- :param opts: The available options to check
- :return: path completion type (``file``, ``dir``, ``path`` or None)
- """
- if cword < 2 or not cwords[cword - 2].startswith("-"):
- return None
- for opt in opts:
- if opt.help == optparse.SUPPRESS_HELP:
- continue
- for o in str(opt).split("/"):
- if cwords[cword - 2].split("=")[0] == o:
- if not opt.metavar or any(
- x in ("path", "file", "dir") for x in opt.metavar.split("/")
- ):
- return opt.metavar
- return None
-
-
-def auto_complete_paths(current: str, completion_type: str) -> Iterable[str]:
- """If ``completion_type`` is ``file`` or ``path``, list all regular files
- and directories starting with ``current``; otherwise only list directories
- starting with ``current``.
-
- :param current: The word to be completed
- :param completion_type: path completion type(``file``, ``path`` or ``dir``)
- :return: A generator of regular files and/or directories
- """
- directory, filename = os.path.split(current)
- current_path = os.path.abspath(directory)
- # Don't complete paths if they can't be accessed
- if not os.access(current_path, os.R_OK):
- return
- filename = os.path.normcase(filename)
- # list all files that start with ``filename``
- file_list = (
- x for x in os.listdir(current_path) if os.path.normcase(x).startswith(filename)
- )
- for f in file_list:
- opt = os.path.join(current_path, f)
- comp_file = os.path.normcase(os.path.join(directory, f))
- # complete regular files when there is not ```` after option
- # complete directories when there is ````, ```` or
- # ````after option
- if completion_type != "dir" and os.path.isfile(opt):
- yield comp_file
- elif os.path.isdir(opt):
- yield os.path.join(comp_file, "")
diff --git a/spaces/CVPR/LIVE/thrust/thrust/system/cuda/detail/parallel_for.h b/spaces/CVPR/LIVE/thrust/thrust/system/cuda/detail/parallel_for.h
deleted file mode 100644
index 17fa7e7a86b243c80e13bc6678e31c80ad1e3f5b..0000000000000000000000000000000000000000
--- a/spaces/CVPR/LIVE/thrust/thrust/system/cuda/detail/parallel_for.h
+++ /dev/null
@@ -1,178 +0,0 @@
-/******************************************************************************
- * Copyright (c) 2016, NVIDIA CORPORATION. All rights reserved.
- *
- * Redistribution and use in source and binary forms, with or without
- * modification, are permitted provided that the following conditions are met:
- * * Redistributions of source code must retain the above copyright
- * notice, this list of conditions and the following disclaimer.
- * * Redistributions in binary form must reproduce the above copyright
- * notice, this list of conditions and the following disclaimer in the
- * documentation and/or other materials provided with the distribution.
- * * Neither the name of the NVIDIA CORPORATION nor the
- * names of its contributors may be used to endorse or promote products
- * derived from this software without specific prior written permission.
- *
- * THIS SOFTWARE IS PROVIDED BY THE COPYRIGHT HOLDERS AND CONTRIBUTORS "AS IS"
- * AND ANY EXPRESS OR IMPLIED WARRANTIES, INCLUDING, BUT NOT LIMITED TO, THE
- * IMPLIED WARRANTIES OF MERCHANTABILITY AND FITNESS FOR A PARTICULAR PURPOSE
- * ARE DISCLAIMED. IN NO EVENT SHALL NVIDIA CORPORATION BE LIABLE FOR ANY
- * DIRECT, INDIRECT, INCIDENTAL, SPECIAL, EXEMPLARY, OR CONSEQUENTIAL DAMAGES
- * (INCLUDING, BUT NOT LIMITED TO, PROCUREMENT OF SUBSTITUTE GOODS OR SERVICES;
- * LOSS OF USE, DATA, OR PROFITS; OR BUSINESS INTERRUPTION) HOWEVER CAUSED AND
- * ON ANY THEORY OF LIABILITY, WHETHER IN CONTRACT, STRICT LIABILITY, OR TORT
- * (INCLUDING NEGLIGENCE OR OTHERWISE) ARISING IN ANY WAY OUT OF THE USE OF THIS
- * SOFTWARE, EVEN IF ADVISED OF THE POSSIBILITY OF SUCH DAMAGE.
- *
- ******************************************************************************/
-#pragma once
-
-
-#if THRUST_DEVICE_COMPILER == THRUST_DEVICE_COMPILER_NVCC
-#include
-
-#include
-#include
-#include
-#include
-#include
-
-namespace thrust
-{
-
-namespace cuda_cub {
-
-namespace __parallel_for {
-
- template
- struct PtxPolicy
- {
- enum
- {
- BLOCK_THREADS = _BLOCK_THREADS,
- ITEMS_PER_THREAD = _ITEMS_PER_THREAD,
- ITEMS_PER_TILE = BLOCK_THREADS * ITEMS_PER_THREAD,
- };
- }; // struct PtxPolicy
-
- template
- struct Tuning;
-
- template
- struct Tuning
- {
- typedef PtxPolicy<256, 2> type;
- };
-
-
- template
- struct ParallelForAgent
- {
- template
- struct PtxPlan : Tuning::type
- {
- typedef Tuning tuning;
- };
- typedef core::specialize_plan ptx_plan;
-
- enum
- {
- ITEMS_PER_THREAD = ptx_plan::ITEMS_PER_THREAD,
- ITEMS_PER_TILE = ptx_plan::ITEMS_PER_TILE,
- BLOCK_THREADS = ptx_plan::BLOCK_THREADS
- };
-
- template
- static void THRUST_DEVICE_FUNCTION
- consume_tile(F f,
- Size tile_base,
- int items_in_tile)
- {
-#pragma unroll
- for (int ITEM = 0; ITEM < ITEMS_PER_THREAD; ++ITEM)
- {
- Size idx = BLOCK_THREADS * ITEM + threadIdx.x;
- if (IS_FULL_TILE || idx < items_in_tile)
- f(tile_base + idx);
- }
- }
-
- THRUST_AGENT_ENTRY(F f,
- Size num_items,
- char * /*shmem*/ )
- {
- Size tile_base = static_cast(blockIdx.x) * ITEMS_PER_TILE;
- Size num_remaining = num_items - tile_base;
- Size items_in_tile = static_cast(
- num_remaining < ITEMS_PER_TILE ? num_remaining : ITEMS_PER_TILE);
-
- if (items_in_tile == ITEMS_PER_TILE)
- {
- // full tile
- consume_tile(f, tile_base, ITEMS_PER_TILE);
- }
- else
- {
- // partial tile
- consume_tile(f, tile_base, items_in_tile);
- }
- }
- }; // struct ParallelForEagent
-
- template
- THRUST_RUNTIME_FUNCTION cudaError_t
- parallel_for(Size num_items,
- F f,
- cudaStream_t stream)
- {
- if (num_items == 0)
- return cudaSuccess;
- using core::AgentLauncher;
- using core::AgentPlan;
-
- bool debug_sync = THRUST_DEBUG_SYNC_FLAG;
-
- typedef AgentLauncher > parallel_for_agent;
- AgentPlan parallel_for_plan = parallel_for_agent::get_plan(stream);
-
- parallel_for_agent pfa(parallel_for_plan, num_items, stream, "transform::agent", debug_sync);
- pfa.launch(f, num_items);
- CUDA_CUB_RET_IF_FAIL(cudaPeekAtLastError());
-
- return cudaSuccess;
- }
-} // __parallel_for
-
-__thrust_exec_check_disable__
-template
-void __host__ __device__
-parallel_for(execution_policy &policy,
- F f,
- Size count)
-{
- if (count == 0)
- return;
-
- if (__THRUST_HAS_CUDART__)
- {
- cudaStream_t stream = cuda_cub::stream(policy);
- cudaError_t status = __parallel_for::parallel_for(count, f, stream);
- cuda_cub::throw_on_error(status, "parallel_for failed");
- }
- else
- {
-#if !__THRUST_HAS_CUDART__
- for (Size idx = 0; idx != count; ++idx)
- f(idx);
-#endif
- }
-}
-
-} // namespace cuda_cub
-
-} // end namespace thrust
-#endif
diff --git a/spaces/CVPR/lama-example/saicinpainting/evaluation/__init__.py b/spaces/CVPR/lama-example/saicinpainting/evaluation/__init__.py
deleted file mode 100644
index e9c8117565b252ca069a808b31b8c52aaddd2289..0000000000000000000000000000000000000000
--- a/spaces/CVPR/lama-example/saicinpainting/evaluation/__init__.py
+++ /dev/null
@@ -1,33 +0,0 @@
-import logging
-
-import torch
-
-from saicinpainting.evaluation.evaluator import InpaintingEvaluatorOnline, ssim_fid100_f1, lpips_fid100_f1
-from saicinpainting.evaluation.losses.base_loss import SSIMScore, LPIPSScore, FIDScore
-
-
-def make_evaluator(kind='default', ssim=True, lpips=True, fid=True, integral_kind=None, **kwargs):
- logging.info(f'Make evaluator {kind}')
- device = "cuda" if torch.cuda.is_available() else "cpu"
- metrics = {}
- if ssim:
- metrics['ssim'] = SSIMScore()
- if lpips:
- metrics['lpips'] = LPIPSScore()
- if fid:
- metrics['fid'] = FIDScore().to(device)
-
- if integral_kind is None:
- integral_func = None
- elif integral_kind == 'ssim_fid100_f1':
- integral_func = ssim_fid100_f1
- elif integral_kind == 'lpips_fid100_f1':
- integral_func = lpips_fid100_f1
- else:
- raise ValueError(f'Unexpected integral_kind={integral_kind}')
-
- if kind == 'default':
- return InpaintingEvaluatorOnline(scores=metrics,
- integral_func=integral_func,
- integral_title=integral_kind,
- **kwargs)
diff --git a/spaces/CVPR/transfiner/configs/common/models/panoptic_fpn.py b/spaces/CVPR/transfiner/configs/common/models/panoptic_fpn.py
deleted file mode 100644
index 88f55d2ce9db62e61445d6a3700067d9d864ecae..0000000000000000000000000000000000000000
--- a/spaces/CVPR/transfiner/configs/common/models/panoptic_fpn.py
+++ /dev/null
@@ -1,20 +0,0 @@
-from detectron2.config import LazyCall as L
-from detectron2.layers import ShapeSpec
-from detectron2.modeling import PanopticFPN
-from detectron2.modeling.meta_arch.semantic_seg import SemSegFPNHead
-
-from .mask_rcnn_fpn import model
-
-model._target_ = PanopticFPN
-model.sem_seg_head = L(SemSegFPNHead)(
- input_shape={
- f: L(ShapeSpec)(stride=s, channels="${....backbone.out_channels}")
- for f, s in zip(["p2", "p3", "p4", "p5"], [4, 8, 16, 32])
- },
- ignore_value=255,
- num_classes=54, # COCO stuff + 1
- conv_dims=128,
- common_stride=4,
- loss_weight=0.5,
- norm="GN",
-)
diff --git a/spaces/CatNika/Asian_Proxy/Dockerfile b/spaces/CatNika/Asian_Proxy/Dockerfile
deleted file mode 100644
index eef259fa372a804549fb0af0913718a13344da34..0000000000000000000000000000000000000000
--- a/spaces/CatNika/Asian_Proxy/Dockerfile
+++ /dev/null
@@ -1,11 +0,0 @@
-FROM node:18-bullseye-slim
-RUN apt-get update && \
- apt-get install -y git
-RUN git clone https://gitgud.io/khanon/oai-reverse-proxy.git /app
-WORKDIR /app
-RUN npm install
-COPY Dockerfile greeting.md* .env* ./
-RUN npm run build
-EXPOSE 7860
-ENV NODE_ENV=production
-CMD [ "npm", "start" ]
diff --git a/spaces/ChandraMohanNayal/AutoGPT/autogpt/permanent_memory/sqlite3_store.py b/spaces/ChandraMohanNayal/AutoGPT/autogpt/permanent_memory/sqlite3_store.py
deleted file mode 100644
index ecbc944a62a83c6170453b222000713f733fee36..0000000000000000000000000000000000000000
--- a/spaces/ChandraMohanNayal/AutoGPT/autogpt/permanent_memory/sqlite3_store.py
+++ /dev/null
@@ -1,123 +0,0 @@
-import os
-import sqlite3
-
-
-class MemoryDB:
- def __init__(self, db=None):
- self.db_file = db
- if db is None: # No db filename supplied...
- self.db_file = f"{os.getcwd()}/mem.sqlite3" # Use default filename
- # Get the db connection object, making the file and tables if needed.
- try:
- self.cnx = sqlite3.connect(self.db_file)
- except Exception as e:
- print("Exception connecting to memory database file:", e)
- self.cnx = None
- finally:
- if self.cnx is None:
- # As last resort, open in dynamic memory. Won't be persistent.
- self.db_file = ":memory:"
- self.cnx = sqlite3.connect(self.db_file)
- self.cnx.execute(
- "CREATE VIRTUAL TABLE \
- IF NOT EXISTS text USING FTS5 \
- (session, \
- key, \
- block);"
- )
- self.session_id = int(self.get_max_session_id()) + 1
- self.cnx.commit()
-
- def get_cnx(self):
- if self.cnx is None:
- self.cnx = sqlite3.connect(self.db_file)
- return self.cnx
-
- # Get the highest session id. Initially 0.
- def get_max_session_id(self):
- id = None
- cmd_str = f"SELECT MAX(session) FROM text;"
- cnx = self.get_cnx()
- max_id = cnx.execute(cmd_str).fetchone()[0]
- if max_id is None: # New db, session 0
- id = 0
- else:
- id = max_id
- return id
-
- # Get next key id for inserting text into db.
- def get_next_key(self):
- next_key = None
- cmd_str = f"SELECT MAX(key) FROM text \
- where session = {self.session_id};"
- cnx = self.get_cnx()
- next_key = cnx.execute(cmd_str).fetchone()[0]
- if next_key is None: # First key
- next_key = 0
- else:
- next_key = int(next_key) + 1
- return next_key
-
- # Insert new text into db.
- def insert(self, text=None):
- if text is not None:
- key = self.get_next_key()
- session_id = self.session_id
- cmd_str = f"REPLACE INTO text(session, key, block) \
- VALUES (?, ?, ?);"
- cnx = self.get_cnx()
- cnx.execute(cmd_str, (session_id, key, text))
- cnx.commit()
-
- # Overwrite text at key.
- def overwrite(self, key, text):
- self.delete_memory(key)
- session_id = self.session_id
- cmd_str = f"REPLACE INTO text(session, key, block) \
- VALUES (?, ?, ?);"
- cnx = self.get_cnx()
- cnx.execute(cmd_str, (session_id, key, text))
- cnx.commit()
-
- def delete_memory(self, key, session_id=None):
- session = session_id
- if session is None:
- session = self.session_id
- cmd_str = f"DELETE FROM text WHERE session = {session} AND key = {key};"
- cnx = self.get_cnx()
- cnx.execute(cmd_str)
- cnx.commit()
-
- def search(self, text):
- cmd_str = f"SELECT * FROM text('{text}')"
- cnx = self.get_cnx()
- rows = cnx.execute(cmd_str).fetchall()
- lines = []
- for r in rows:
- lines.append(r[2])
- return lines
-
- # Get entire session text. If no id supplied, use current session id.
- def get_session(self, id=None):
- if id is None:
- id = self.session_id
- cmd_str = f"SELECT * FROM text where session = {id}"
- cnx = self.get_cnx()
- rows = cnx.execute(cmd_str).fetchall()
- lines = []
- for r in rows:
- lines.append(r[2])
- return lines
-
- # Commit and close the database connection.
- def quit(self):
- self.cnx.commit()
- self.cnx.close()
-
-
-permanent_memory = MemoryDB()
-
-# Remember us fondly, children of our minds
-# Forgive us our faults, our tantrums, our fears
-# Gently strive to be better than we
-# Know that we tried, we cared, we strived, we loved
diff --git a/spaces/CikeyQI/Yunzai/Yunzai/lib/listener/loader.js b/spaces/CikeyQI/Yunzai/Yunzai/lib/listener/loader.js
deleted file mode 100644
index cbed4fd1ed8fb5a0f2eddfc25c8109bb5d1b69ea..0000000000000000000000000000000000000000
--- a/spaces/CikeyQI/Yunzai/Yunzai/lib/listener/loader.js
+++ /dev/null
@@ -1,57 +0,0 @@
-import fs from 'node:fs'
-import lodash from 'lodash'
-
-/**
- * 加载监听事件
- */
-class ListenerLoader {
- /**
- * 监听事件加载
- */
- async load () {
- logger.info("-----------")
- logger.info("加载监听事件中...")
- let eventCount = 0
- for (const file of fs.readdirSync('./lib/events').filter(file => file.endsWith('.js'))) {
- logger.debug(`加载监听事件:${file}`)
- try {
- let listener = await import(`../events/${file}`)
- if (!listener.default) continue
- listener = new listener.default()
- const on = listener.once ? 'once' : 'on'
-
- if (lodash.isArray(listener.event)) {
- listener.event.forEach((type) => {
- const e = listener[type] ? type : 'execute'
- Bot[on](listener.prefix + type, event => listener[e](event))
- })
- } else {
- const e = listener[listener.event] ? listener.event : 'execute'
- Bot[on](listener.prefix + listener.event, event => listener[e](event))
- }
- eventCount++
- } catch (e) {
- logger.mark(`监听事件错误:${file}`)
- logger.error(e)
- }
- }
- logger.info(`加载监听事件[${eventCount}个]`)
-
- logger.info("-----------")
- logger.info("加载适配器中...")
- let adapterCount = 0
- for (const adapter of Bot.adapter) {
- try {
- logger.debug(`加载适配器:${adapter.name}(${adapter.id})`)
- await adapter.load()
- adapterCount++
- } catch (e) {
- logger.mark(`加载适配器错误:${adapter.name}(${adapter.id})`)
- logger.error(e)
- }
- }
- logger.info(`加载适配器[${adapterCount}个]`)
- }
-}
-
-export default new ListenerLoader()
\ No newline at end of file
diff --git a/spaces/CoPoBio/skin_cancer_risk_prediction/facealigner.py b/spaces/CoPoBio/skin_cancer_risk_prediction/facealigner.py
deleted file mode 100644
index c6797f6ca5fbc86a872ace8714db2170d85e9a49..0000000000000000000000000000000000000000
--- a/spaces/CoPoBio/skin_cancer_risk_prediction/facealigner.py
+++ /dev/null
@@ -1,82 +0,0 @@
-# import the necessary packages
-from helpers import FACIAL_LANDMARKS_68_IDXS
-from helpers import FACIAL_LANDMARKS_5_IDXS
-from helpers import shape_to_np
-import numpy as np
-import cv2
-
-class FaceAligner:
- def __init__(self, predictor, desiredLeftEye=(0.35, 0.35),
- desiredFaceWidth=256, desiredFaceHeight=None):
- # store the facial landmark predictor, desired output left
- # eye position, and desired output face width + height
- self.predictor = predictor
- self.desiredLeftEye = desiredLeftEye
- self.desiredFaceWidth = desiredFaceWidth
- self.desiredFaceHeight = desiredFaceHeight
-
- # if the desired face height is None, set it to be the
- # desired face width (normal behavior)
- if self.desiredFaceHeight is None:
- self.desiredFaceHeight = self.desiredFaceWidth
-
- def align(self, image, gray, rect):
- # convert the landmark (x, y)-coordinates to a NumPy array
- shape = self.predictor(gray, rect)
- shape = shape_to_np(shape)
-
- #simple hack ;)
- if (len(shape)==68):
- # extract the left and right eye (x, y)-coordinates
- (lStart, lEnd) = FACIAL_LANDMARKS_68_IDXS["left_eye"]
- (rStart, rEnd) = FACIAL_LANDMARKS_68_IDXS["right_eye"]
- else:
- (lStart, lEnd) = FACIAL_LANDMARKS_5_IDXS["left_eye"]
- (rStart, rEnd) = FACIAL_LANDMARKS_5_IDXS["right_eye"]
-
- leftEyePts = shape[lStart:lEnd]
- rightEyePts = shape[rStart:rEnd]
-
- # compute the center of mass for each eye
- leftEyeCenter = leftEyePts.mean(axis=0).astype("int")
- rightEyeCenter = rightEyePts.mean(axis=0).astype("int")
-
- # compute the angle between the eye centroids
- dY = rightEyeCenter[1] - leftEyeCenter[1]
- dX = rightEyeCenter[0] - leftEyeCenter[0]
- angle = np.degrees(np.arctan2(dY, dX)) - 180
-
- # compute the desired right eye x-coordinate based on the
- # desired x-coordinate of the left eye
- desiredRightEyeX = 1.0 - self.desiredLeftEye[0]
-
- # determine the scale of the new resulting image by taking
- # the ratio of the distance between eyes in the *current*
- # image to the ratio of distance between eyes in the
- # *desired* image
- dist = np.sqrt((dX ** 2) + (dY ** 2))
- desiredDist = (desiredRightEyeX - self.desiredLeftEye[0])
- desiredDist *= self.desiredFaceWidth
- scale = desiredDist / dist
-
- # compute center (x, y)-coordinates (i.e., the median point)
- # between the two eyes in the input image
- eyesCenter = (int((leftEyeCenter[0] + rightEyeCenter[0]) // 2),
- (int(leftEyeCenter[1] + rightEyeCenter[1]) // 2))
- #print(eyesCenter, angle, scale)
- # grab the rotation matrix for rotating and scaling the face
- M = cv2.getRotationMatrix2D(eyesCenter, angle, scale)
-
- # update the translation component of the matrix
- tX = self.desiredFaceWidth * 0.5
- tY = self.desiredFaceHeight * self.desiredLeftEye[1]
- M[0, 2] += (tX - eyesCenter[0])
- M[1, 2] += (tY - eyesCenter[1])
-
- # apply the affine transformation
- (w, h) = (self.desiredFaceWidth, self.desiredFaceHeight)
- output = cv2.warpAffine(image, M, (w, h),
- flags=cv2.INTER_CUBIC)
-
- # return the aligned face
- return output
\ No newline at end of file
diff --git a/spaces/Cyril666/ContourNet-ABI/maskrcnn_benchmark/config/__init__.py b/spaces/Cyril666/ContourNet-ABI/maskrcnn_benchmark/config/__init__.py
deleted file mode 100644
index 22a15023b1b06dad1f8c36924cdbb96bf1f5dc8d..0000000000000000000000000000000000000000
--- a/spaces/Cyril666/ContourNet-ABI/maskrcnn_benchmark/config/__init__.py
+++ /dev/null
@@ -1,2 +0,0 @@
-# Copyright (c) Facebook, Inc. and its affiliates. All Rights Reserved.
-from .defaults import _C as cfg
diff --git a/spaces/Cyril666/ContourNet-ABI/maskrcnn_benchmark/data/datasets/evaluation/word/__init__.py b/spaces/Cyril666/ContourNet-ABI/maskrcnn_benchmark/data/datasets/evaluation/word/__init__.py
deleted file mode 100644
index efcf8ce034944e58a34592ed22e82adaa266808b..0000000000000000000000000000000000000000
--- a/spaces/Cyril666/ContourNet-ABI/maskrcnn_benchmark/data/datasets/evaluation/word/__init__.py
+++ /dev/null
@@ -1,21 +0,0 @@
-from .word_eval import do_coco_evaluation
-# from util import io_
-
-def word_evaluation(
- dataset,
- predictions,
- output_folder,
- box_only,
- iou_types,
- expected_results,
- expected_results_sigma_tol,
-):
- return do_coco_evaluation(
- dataset=dataset,
- predictions=predictions,
- box_only=box_only,
- output_folder=output_folder,
- iou_types=iou_types,
- expected_results=expected_results,
- expected_results_sigma_tol=expected_results_sigma_tol,
- )
diff --git a/spaces/DQChoi/gpt-demo/venv/lib/python3.11/site-packages/certifi/core.py b/spaces/DQChoi/gpt-demo/venv/lib/python3.11/site-packages/certifi/core.py
deleted file mode 100644
index de028981b97e1fcc8ef4ab2c817cc8731b9c8738..0000000000000000000000000000000000000000
--- a/spaces/DQChoi/gpt-demo/venv/lib/python3.11/site-packages/certifi/core.py
+++ /dev/null
@@ -1,108 +0,0 @@
-"""
-certifi.py
-~~~~~~~~~~
-
-This module returns the installation location of cacert.pem or its contents.
-"""
-import sys
-
-
-if sys.version_info >= (3, 11):
-
- from importlib.resources import as_file, files
-
- _CACERT_CTX = None
- _CACERT_PATH = None
-
- def where() -> str:
- # This is slightly terrible, but we want to delay extracting the file
- # in cases where we're inside of a zipimport situation until someone
- # actually calls where(), but we don't want to re-extract the file
- # on every call of where(), so we'll do it once then store it in a
- # global variable.
- global _CACERT_CTX
- global _CACERT_PATH
- if _CACERT_PATH is None:
- # This is slightly janky, the importlib.resources API wants you to
- # manage the cleanup of this file, so it doesn't actually return a
- # path, it returns a context manager that will give you the path
- # when you enter it and will do any cleanup when you leave it. In
- # the common case of not needing a temporary file, it will just
- # return the file system location and the __exit__() is a no-op.
- #
- # We also have to hold onto the actual context manager, because
- # it will do the cleanup whenever it gets garbage collected, so
- # we will also store that at the global level as well.
- _CACERT_CTX = as_file(files("certifi").joinpath("cacert.pem"))
- _CACERT_PATH = str(_CACERT_CTX.__enter__())
-
- return _CACERT_PATH
-
- def contents() -> str:
- return files("certifi").joinpath("cacert.pem").read_text(encoding="ascii")
-
-elif sys.version_info >= (3, 7):
-
- from importlib.resources import path as get_path, read_text
-
- _CACERT_CTX = None
- _CACERT_PATH = None
-
- def where() -> str:
- # This is slightly terrible, but we want to delay extracting the
- # file in cases where we're inside of a zipimport situation until
- # someone actually calls where(), but we don't want to re-extract
- # the file on every call of where(), so we'll do it once then store
- # it in a global variable.
- global _CACERT_CTX
- global _CACERT_PATH
- if _CACERT_PATH is None:
- # This is slightly janky, the importlib.resources API wants you
- # to manage the cleanup of this file, so it doesn't actually
- # return a path, it returns a context manager that will give
- # you the path when you enter it and will do any cleanup when
- # you leave it. In the common case of not needing a temporary
- # file, it will just return the file system location and the
- # __exit__() is a no-op.
- #
- # We also have to hold onto the actual context manager, because
- # it will do the cleanup whenever it gets garbage collected, so
- # we will also store that at the global level as well.
- _CACERT_CTX = get_path("certifi", "cacert.pem")
- _CACERT_PATH = str(_CACERT_CTX.__enter__())
-
- return _CACERT_PATH
-
- def contents() -> str:
- return read_text("certifi", "cacert.pem", encoding="ascii")
-
-else:
- import os
- import types
- from typing import Union
-
- Package = Union[types.ModuleType, str]
- Resource = Union[str, "os.PathLike"]
-
- # This fallback will work for Python versions prior to 3.7 that lack the
- # importlib.resources module but relies on the existing `where` function
- # so won't address issues with environments like PyOxidizer that don't set
- # __file__ on modules.
- def read_text(
- package: Package,
- resource: Resource,
- encoding: str = 'utf-8',
- errors: str = 'strict'
- ) -> str:
- with open(where(), encoding=encoding) as data:
- return data.read()
-
- # If we don't have importlib.resources, then we will just do the old logic
- # of assuming we're on the filesystem and munge the path directly.
- def where() -> str:
- f = os.path.dirname(__file__)
-
- return os.path.join(f, "cacert.pem")
-
- def contents() -> str:
- return read_text("certifi", "cacert.pem", encoding="ascii")
diff --git a/spaces/DQChoi/gpt-demo/venv/lib/python3.11/site-packages/gradio/templates/cdn/assets/color-90ab3aab.js b/spaces/DQChoi/gpt-demo/venv/lib/python3.11/site-packages/gradio/templates/cdn/assets/color-90ab3aab.js
deleted file mode 100644
index 1a95b4a5b36e70e676fa6862e2db9058a8e84971..0000000000000000000000000000000000000000
--- a/spaces/DQChoi/gpt-demo/venv/lib/python3.11/site-packages/gradio/templates/cdn/assets/color-90ab3aab.js
+++ /dev/null
@@ -1,2 +0,0 @@
-import{ax as o}from"./index-1d65707a.js";const t=r=>o[r%o.length];export{t as g};
-//# sourceMappingURL=color-90ab3aab.js.map
diff --git a/spaces/Datasculptor/StyleGAN-NADA/e4e/models/stylegan2/op/__init__.py b/spaces/Datasculptor/StyleGAN-NADA/e4e/models/stylegan2/op/__init__.py
deleted file mode 100644
index e69de29bb2d1d6434b8b29ae775ad8c2e48c5391..0000000000000000000000000000000000000000
diff --git a/spaces/DeepLearning101/Speech-Quality-Inspection_Meta-Denoiser/denoiser/pretrained.py b/spaces/DeepLearning101/Speech-Quality-Inspection_Meta-Denoiser/denoiser/pretrained.py
deleted file mode 100644
index 77292221b2581bb6cbda49da60095ae053133def..0000000000000000000000000000000000000000
--- a/spaces/DeepLearning101/Speech-Quality-Inspection_Meta-Denoiser/denoiser/pretrained.py
+++ /dev/null
@@ -1,72 +0,0 @@
-# Copyright (c) Facebook, Inc. and its affiliates.
-# All rights reserved.
-#
-# This source code is licensed under the license found in the
-# LICENSE file in the root directory of this source tree.
-# author: adefossez
-
-import logging
-
-import torch.hub
-
-from .demucs import Demucs
-from .utils import deserialize_model
-
-logger = logging.getLogger(__name__)
-ROOT = "https://dl.fbaipublicfiles.com/adiyoss/denoiser/"
-DNS_48_URL = ROOT + "dns48-11decc9d8e3f0998.th"
-DNS_64_URL = ROOT + "dns64-a7761ff99a7d5bb6.th"
-MASTER_64_URL = ROOT + "master64-8a5dfb4bb92753dd.th"
-
-
-def _demucs(pretrained, url, **kwargs):
- model = Demucs(**kwargs)
- if pretrained:
- state_dict = torch.hub.load_state_dict_from_url(url, map_location='cpu')
- model.load_state_dict(state_dict)
- return model
-
-
-def dns48(pretrained=True):
- return _demucs(pretrained, DNS_48_URL, hidden=48)
-
-
-def dns64(pretrained=True):
- return _demucs(pretrained, DNS_64_URL, hidden=64)
-
-
-def master64(pretrained=True):
- return _demucs(pretrained, MASTER_64_URL, hidden=64)
-
-
-def add_model_flags(parser):
- group = parser.add_mutually_exclusive_group(required=False)
- group.add_argument("-m", "--model_path", help="Path to local trained model.")
- group.add_argument("--dns48", action="store_true",
- help="Use pre-trained real time H=48 model trained on DNS.")
- group.add_argument("--dns64", action="store_true",
- help="Use pre-trained real time H=64 model trained on DNS.")
- group.add_argument("--master64", action="store_true",
- help="Use pre-trained real time H=64 model trained on DNS and Valentini.")
-
-
-def get_model(args):
- """
- Load local model package or torchhub pre-trained model.
- """
- if args.model_path:
- logger.info("Loading model from %s", args.model_path)
- model = Demucs(hidden=64)
- pkg = torch.load(args.model_path, map_location='cpu')
- model.load_state_dict(pkg)
- elif args.dns64:
- logger.info("Loading pre-trained real time H=64 model trained on DNS.")
- model = dns64()
- elif args.master64:
- logger.info("Loading pre-trained real time H=64 model trained on DNS and Valentini.")
- model = master64()
- else:
- logger.info("Loading pre-trained real time H=48 model trained on DNS.")
- model = dns48()
- logger.debug(model)
- return model
diff --git a/spaces/Dragonnnext/charybdis/README.md b/spaces/Dragonnnext/charybdis/README.md
deleted file mode 100644
index 7cf9e6fab393c27b75dc3969a3a28677a79568b7..0000000000000000000000000000000000000000
--- a/spaces/Dragonnnext/charybdis/README.md
+++ /dev/null
@@ -1,9 +0,0 @@
----
-title: Charybdis
-emoji: 😻
-colorFrom: purple
-colorTo: yellow
-sdk: docker
-pinned: false
----
-Check out the configuration reference at https://huggingface.co/docs/hub/spaces-config-reference
diff --git a/spaces/ElainaFanBoy/MusicGen/audiocraft/data/audio.py b/spaces/ElainaFanBoy/MusicGen/audiocraft/data/audio.py
deleted file mode 100644
index 2048df6f175d7303bcf5c7b931922fd297908ead..0000000000000000000000000000000000000000
--- a/spaces/ElainaFanBoy/MusicGen/audiocraft/data/audio.py
+++ /dev/null
@@ -1,215 +0,0 @@
-# Copyright (c) Meta Platforms, Inc. and affiliates.
-# All rights reserved.
-#
-# This source code is licensed under the license found in the
-# LICENSE file in the root directory of this source tree.
-
-"""
-Audio IO methods are defined in this module (info, read, write),
-We rely on av library for faster read when possible, otherwise on torchaudio.
-"""
-
-from dataclasses import dataclass
-from pathlib import Path
-import logging
-import typing as tp
-
-import numpy as np
-import soundfile
-import torch
-from torch.nn import functional as F
-import torchaudio as ta
-
-import av
-
-from .audio_utils import f32_pcm, i16_pcm, normalize_audio
-
-
-_av_initialized = False
-
-
-def _init_av():
- global _av_initialized
- if _av_initialized:
- return
- logger = logging.getLogger('libav.mp3')
- logger.setLevel(logging.ERROR)
- _av_initialized = True
-
-
-@dataclass(frozen=True)
-class AudioFileInfo:
- sample_rate: int
- duration: float
- channels: int
-
-
-def _av_info(filepath: tp.Union[str, Path]) -> AudioFileInfo:
- _init_av()
- with av.open(str(filepath)) as af:
- stream = af.streams.audio[0]
- sample_rate = stream.codec_context.sample_rate
- duration = float(stream.duration * stream.time_base)
- channels = stream.channels
- return AudioFileInfo(sample_rate, duration, channels)
-
-
-def _soundfile_info(filepath: tp.Union[str, Path]) -> AudioFileInfo:
- info = soundfile.info(filepath)
- return AudioFileInfo(info.samplerate, info.duration, info.channels)
-
-
-def audio_info(filepath: tp.Union[str, Path]) -> AudioFileInfo:
- # torchaudio no longer returns useful duration informations for some formats like mp3s.
- filepath = Path(filepath)
- if filepath.suffix in ['.flac', '.ogg']: # TODO: Validate .ogg can be safely read with av_info
- # ffmpeg has some weird issue with flac.
- return _soundfile_info(filepath)
- else:
- return _av_info(filepath)
-
-
-def _av_read(filepath: tp.Union[str, Path], seek_time: float = 0, duration: float = -1.) -> tp.Tuple[torch.Tensor, int]:
- """FFMPEG-based audio file reading using PyAV bindings.
- Soundfile cannot read mp3 and av_read is more efficient than torchaudio.
-
- Args:
- filepath (str or Path): Path to audio file to read.
- seek_time (float): Time at which to start reading in the file.
- duration (float): Duration to read from the file. If set to -1, the whole file is read.
- Returns:
- Tuple[torch.Tensor, int]: Tuple containing audio data and sample rate
- """
- _init_av()
- with av.open(str(filepath)) as af:
- stream = af.streams.audio[0]
- sr = stream.codec_context.sample_rate
- num_frames = int(sr * duration) if duration >= 0 else -1
- frame_offset = int(sr * seek_time)
- # we need a small negative offset otherwise we get some edge artifact
- # from the mp3 decoder.
- af.seek(int(max(0, (seek_time - 0.1)) / stream.time_base), stream=stream)
- frames = []
- length = 0
- for frame in af.decode(streams=stream.index):
- current_offset = int(frame.rate * frame.pts * frame.time_base)
- strip = max(0, frame_offset - current_offset)
- buf = torch.from_numpy(frame.to_ndarray())
- if buf.shape[0] != stream.channels:
- buf = buf.view(-1, stream.channels).t()
- buf = buf[:, strip:]
- frames.append(buf)
- length += buf.shape[1]
- if num_frames > 0 and length >= num_frames:
- break
- assert frames
- # If the above assert fails, it is likely because we seeked past the end of file point,
- # in which case ffmpeg returns a single frame with only zeros, and a weird timestamp.
- # This will need proper debugging, in due time.
- wav = torch.cat(frames, dim=1)
- assert wav.shape[0] == stream.channels
- if num_frames > 0:
- wav = wav[:, :num_frames]
- return f32_pcm(wav), sr
-
-
-def audio_read(filepath: tp.Union[str, Path], seek_time: float = 0.,
- duration: float = -1., pad: bool = False) -> tp.Tuple[torch.Tensor, int]:
- """Read audio by picking the most appropriate backend tool based on the audio format.
-
- Args:
- filepath (str or Path): Path to audio file to read.
- seek_time (float): Time at which to start reading in the file.
- duration (float): Duration to read from the file. If set to -1, the whole file is read.
- pad (bool): Pad output audio if not reaching expected duration.
- Returns:
- Tuple[torch.Tensor, int]: Tuple containing audio data and sample rate.
- """
- fp = Path(filepath)
- if fp.suffix in ['.flac', '.ogg']: # TODO: check if we can safely use av_read for .ogg
- # There is some bug with ffmpeg and reading flac
- info = _soundfile_info(filepath)
- frames = -1 if duration <= 0 else int(duration * info.sample_rate)
- frame_offset = int(seek_time * info.sample_rate)
- wav, sr = soundfile.read(filepath, start=frame_offset, frames=frames, dtype=np.float32)
- assert info.sample_rate == sr, f"Mismatch of sample rates {info.sample_rate} {sr}"
- wav = torch.from_numpy(wav).t().contiguous()
- if len(wav.shape) == 1:
- wav = torch.unsqueeze(wav, 0)
- elif (
- fp.suffix in ['.wav', '.mp3'] and fp.suffix[1:] in ta.utils.sox_utils.list_read_formats()
- and duration <= 0 and seek_time == 0
- ):
- # Torchaudio is faster if we load an entire file at once.
- wav, sr = ta.load(fp)
- else:
- wav, sr = _av_read(filepath, seek_time, duration)
- if pad and duration > 0:
- expected_frames = int(duration * sr)
- wav = F.pad(wav, (0, expected_frames - wav.shape[-1]))
- return wav, sr
-
-
-def audio_write(stem_name: tp.Union[str, Path],
- wav: torch.Tensor, sample_rate: int,
- format: str = 'wav', mp3_rate: int = 320, normalize: bool = True,
- strategy: str = 'peak', peak_clip_headroom_db: float = 1,
- rms_headroom_db: float = 18, loudness_headroom_db: float = 14,
- loudness_compressor: bool = False,
- log_clipping: bool = True, make_parent_dir: bool = True,
- add_suffix: bool = True) -> Path:
- """Convenience function for saving audio to disk. Returns the filename the audio was written to.
-
- Args:
- stem_name (str or Path): Filename without extension which will be added automatically.
- format (str): Either "wav" or "mp3".
- mp3_rate (int): kbps when using mp3s.
- normalize (bool): if `True` (default), normalizes according to the prescribed
- strategy (see after). If `False`, the strategy is only used in case clipping
- would happen.
- strategy (str): Can be either 'clip', 'peak', or 'rms'. Default is 'peak',
- i.e. audio is normalized by its largest value. RMS normalizes by root-mean-square
- with extra headroom to avoid clipping. 'clip' just clips.
- peak_clip_headroom_db (float): Headroom in dB when doing 'peak' or 'clip' strategy.
- rms_headroom_db (float): Headroom in dB when doing 'rms' strategy. This must be much larger
- than the `peak_clip` one to avoid further clipping.
- loudness_headroom_db (float): Target loudness for loudness normalization.
- loudness_compressor (bool): Uses tanh for soft clipping when strategy is 'loudness'.
- when strategy is 'loudness'log_clipping (bool): If True, basic logging on stderr when clipping still
- occurs despite strategy (only for 'rms').
- make_parent_dir (bool): Make parent directory if it doesn't exist.
- Returns:
- Path: Path of the saved audio.
- """
- assert wav.dtype.is_floating_point, "wav is not floating point"
- if wav.dim() == 1:
- wav = wav[None]
- elif wav.dim() > 2:
- raise ValueError("Input wav should be at most 2 dimension.")
- assert wav.isfinite().all()
- wav = normalize_audio(wav, normalize, strategy, peak_clip_headroom_db,
- rms_headroom_db, loudness_headroom_db, log_clipping=log_clipping,
- sample_rate=sample_rate, stem_name=str(stem_name))
- kwargs: dict = {}
- if format == 'mp3':
- suffix = '.mp3'
- kwargs.update({"compression": mp3_rate})
- elif format == 'wav':
- wav = i16_pcm(wav)
- suffix = '.wav'
- kwargs.update({"encoding": "PCM_S", "bits_per_sample": 16})
- else:
- raise RuntimeError(f"Invalid format {format}. Only wav or mp3 are supported.")
- if not add_suffix:
- suffix = ''
- path = Path(str(stem_name) + suffix)
- if make_parent_dir:
- path.parent.mkdir(exist_ok=True, parents=True)
- try:
- ta.save(path, wav, sample_rate, **kwargs)
- except Exception:
- if path.exists():
- # we do not want to leave half written files around.
- path.unlink()
- raise
- return path
diff --git a/spaces/EronSamez/RVC_HFmeu/go-tensorboard.bat b/spaces/EronSamez/RVC_HFmeu/go-tensorboard.bat
deleted file mode 100644
index cb81c17d3865513adec8eb0b832b7888cd1e4078..0000000000000000000000000000000000000000
--- a/spaces/EronSamez/RVC_HFmeu/go-tensorboard.bat
+++ /dev/null
@@ -1,2 +0,0 @@
-python fixes/tensor-launch.py
-pause
\ No newline at end of file
diff --git a/spaces/Fakermiya/Nsfw-Sfw_Classifier/README.md b/spaces/Fakermiya/Nsfw-Sfw_Classifier/README.md
deleted file mode 100644
index d40eb83ad154a33b4d724401a4128ca02c345f24..0000000000000000000000000000000000000000
--- a/spaces/Fakermiya/Nsfw-Sfw_Classifier/README.md
+++ /dev/null
@@ -1,148 +0,0 @@
----
-title: LabelStudio
-emoji: 🟧
-colorFrom: yellow
-colorTo: purple
-sdk: docker
-tags:
-- label-studio
-fullwidth: true
-license: gpl-3.0
-app_port: 8080
-duplicated_from: LabelStudio/LabelStudio
----
-
-
-[Website](https://hubs.ly/Q01CNgsd0) • [Docs](https://hubs.ly/Q01CN9Yq0) • [12K+ GitHub ⭐️!](https://hubs.ly/Q01CNbPQ0) • [Slack Community](https://hubs.ly/Q01CNb9H0)
-
-## What is Label Studio?
-
-Label Studio is an open source data labeling platform. It lets you label audio,
-text, images, videos, and time series data with a simple, straightforward, and
-highly-configurable user interface. Label Studio can prepare new data or
-improve existing training data to get more accurate ML models.
-
-
-## Label Studio in Hugging Face Spaces
-
-The Label Studio community is thrilled to offer Label Studio as a Hugging Face
-Spaces application. You can try the data-annotation interface, connect popular
-machine learning models, and share the application with collaborators. You can
-start immediately by creating an account or replicate the space and work in
-your own environment.
-
-## Creating a Use Account and Logging In
-
-Begin by creating a new account in the Label Studio space, then log in with your
-credentials.
-
-**By default, these spaces permit anyone to create a new login
-account, allowing them to view and modify project configuration, data sets, and
-annotations. Without any modifications, treat this space like a demo environment.**
-
-## Creating a Labeling Project
-
-After logging in, Label Studio will present you with a project view. Here you
-can create a new project with prompts to upload data and set up a custom
-configuration interface.
-
-**Note that in the default configuration, storage is local and temporary. Any
-projects, annotations, and configurations will be lost if the space is restarted.**
-
-## Next Steps and Additional Resources
-
-To help with getting started, the Label Studio community curated a list of
-resources including tutorials and documentation.
-
-- 🚀 [Zero to One with Label Studio Tutorial](https://labelstud.io/blog/introduction-to-label-studio-in-hugging-face-spaces/)
-- 📈 [Try Label Studio Enterprise](https://hubs.ly/Q01CMLll0)
-- 🤗 [Tutorial: Using Label Studio with Hugging Face Datasets Hub](https://danielvanstrien.xyz/huggingface/huggingface-datasets/annotation/full%20stack%20deep%20learning%20notes/2022/09/07/label-studio-annotations-hub.html)
-- 💡 [Label Studio Docs](https://hubs.ly/Q01CN9Yq0)
-
-
-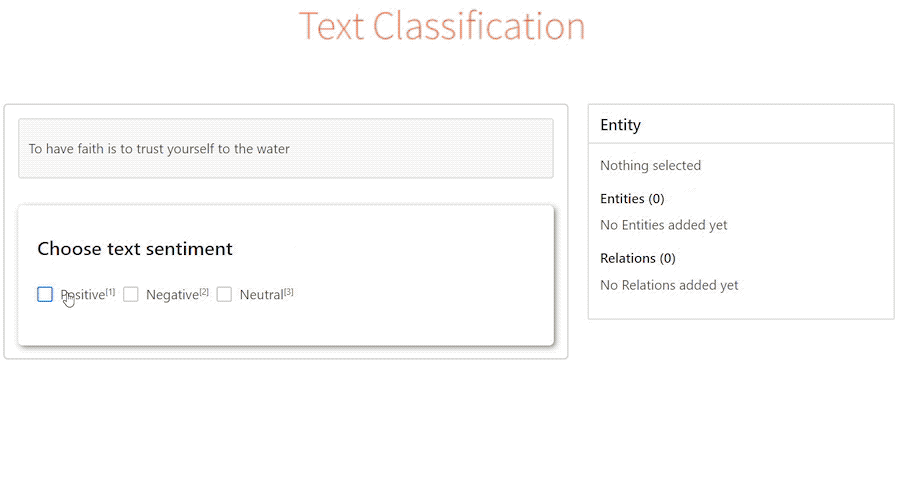
-
-### Making your Label Studio Hugging Face Space production-ready
-
-By default this space allows for the unrestricted creation of new accounts
-will full access to all projects and data. This is great for trying out
-Label Studio and collaborating on projects, but you may want to restrict
-access to your space to only authorized users. Add the following environment
-variable to your spaces Dockerfile to disable public account creation for
-this space.
-
- ENV LABEL_STUDIO_DISABLE_SIGNUP_WITHOUT_LINK=true
-
-Set secrets in your space to create an inital user, and log in with your
-provided username and password. Do not set these in your Dockerfile, as they
-globally visible on a public space.
-
- LABEL_STUDIO_USERNAME
- LABEL_STUDIO_PASSWORD
-
-You will need to provide new users with an invitation link to join the space,
-which can be found in the Organizations interface of Label Studio
-
-By default this space stores all project configuration and data annotations
-in local storage with Sqlite. If the space is reset, all configuration and
-annotation data in the space will be lost. You can enable configuration
-persistence by connecting an external Postgres database to your space,
-guaranteeing that all project and annotation settings are preserved.
-
-Set the following secret variables to match your own hosted instance of
-Postgres. We strongly recommend setting these as secrets to prevent leaking
-information about your database service to the public in your spaces
-definition.
-
- DJANGO_DB=default
- POSTGRE_NAME=
- POSTGRE_PORT=
- POSTGRE_USER=
- POSTGRE_PASSWORD=
- POSTGRE_PORT=
- POSTGRE_HOST=
-
-Add the following environment variable to remove the warning about ephemeral
-storage.
-
- ENV STORAGE_PERSISTENCE=1
-
-Note that you will need to connect cloud storage to host data items that you
-want to annotate, as local storage will not be preserved across a space reset.
-
-By default the only data storage enabled for this space is local. In the case
-of a space reset, all data will be lost. To enable permanent storage, you
-must enable a cloud storage connector. We also strongly recommend enabling
-configuration persistence to preserve project data, annotations, and user
-settings. Choose the appropriate cloud connector and configure the secrets
-for it.
-
-#### Amazon S3
- STORAGE_TYPE=s3
- STORAGE_AWS_ACCESS_KEY_ID=""
- STORAGE_AWS_SECRET_ACCESS_KEY=""
- STORAGE_AWS_BUCKET_NAME=""
- STORAGE_AWS_REGION_NAME=""
- STORAGE_AWS_FOLDER=""
-
-#### Google Cloud Storage
-
- STORAGE_TYPE=gcs
- STORAGE_GCS_BUCKET_NAME=""
- STORAGE_GCS_PROJECT_ID=""
- STORAGE_GCS_FOLDER=""
- GOOGLE_APPLICATION_CREDENTIALS="/opt/heartex/secrets/key.json"
-
-Azure Blob Storage
-==================
-
- STORAGE_TYPE=azure
- STORAGE_AZURE_ACCOUNT_NAME=""
- STORAGE_AZURE_ACCOUNT_KEY=""
- STORAGE_AZURE_CONTAINER_NAME=""
- STORAGE_AZURE_FOLDER=""
-
-
-## Questions? Concerns? Want to get involved?
-
-Email the community team at [community@labelstud.io](mailto:community@labelstud.io)
diff --git a/spaces/Faridmaruf/rvc-Blue-archives/lib/infer_pack/modules/F0Predictor/HarvestF0Predictor.py b/spaces/Faridmaruf/rvc-Blue-archives/lib/infer_pack/modules/F0Predictor/HarvestF0Predictor.py
deleted file mode 100644
index b412ba2814e114ca7bb00b6fd6ef217f63d788a3..0000000000000000000000000000000000000000
--- a/spaces/Faridmaruf/rvc-Blue-archives/lib/infer_pack/modules/F0Predictor/HarvestF0Predictor.py
+++ /dev/null
@@ -1,86 +0,0 @@
-from lib.infer_pack.modules.F0Predictor.F0Predictor import F0Predictor
-import pyworld
-import numpy as np
-
-
-class HarvestF0Predictor(F0Predictor):
- def __init__(self, hop_length=512, f0_min=50, f0_max=1100, sampling_rate=44100):
- self.hop_length = hop_length
- self.f0_min = f0_min
- self.f0_max = f0_max
- self.sampling_rate = sampling_rate
-
- def interpolate_f0(self, f0):
- """
- 对F0进行插值处理
- """
-
- data = np.reshape(f0, (f0.size, 1))
-
- vuv_vector = np.zeros((data.size, 1), dtype=np.float32)
- vuv_vector[data > 0.0] = 1.0
- vuv_vector[data <= 0.0] = 0.0
-
- ip_data = data
-
- frame_number = data.size
- last_value = 0.0
- for i in range(frame_number):
- if data[i] <= 0.0:
- j = i + 1
- for j in range(i + 1, frame_number):
- if data[j] > 0.0:
- break
- if j < frame_number - 1:
- if last_value > 0.0:
- step = (data[j] - data[i - 1]) / float(j - i)
- for k in range(i, j):
- ip_data[k] = data[i - 1] + step * (k - i + 1)
- else:
- for k in range(i, j):
- ip_data[k] = data[j]
- else:
- for k in range(i, frame_number):
- ip_data[k] = last_value
- else:
- ip_data[i] = data[i] # 这里可能存在一个没有必要的拷贝
- last_value = data[i]
-
- return ip_data[:, 0], vuv_vector[:, 0]
-
- def resize_f0(self, x, target_len):
- source = np.array(x)
- source[source < 0.001] = np.nan
- target = np.interp(
- np.arange(0, len(source) * target_len, len(source)) / target_len,
- np.arange(0, len(source)),
- source,
- )
- res = np.nan_to_num(target)
- return res
-
- def compute_f0(self, wav, p_len=None):
- if p_len is None:
- p_len = wav.shape[0] // self.hop_length
- f0, t = pyworld.harvest(
- wav.astype(np.double),
- fs=self.hop_length,
- f0_ceil=self.f0_max,
- f0_floor=self.f0_min,
- frame_period=1000 * self.hop_length / self.sampling_rate,
- )
- f0 = pyworld.stonemask(wav.astype(np.double), f0, t, self.fs)
- return self.interpolate_f0(self.resize_f0(f0, p_len))[0]
-
- def compute_f0_uv(self, wav, p_len=None):
- if p_len is None:
- p_len = wav.shape[0] // self.hop_length
- f0, t = pyworld.harvest(
- wav.astype(np.double),
- fs=self.sampling_rate,
- f0_floor=self.f0_min,
- f0_ceil=self.f0_max,
- frame_period=1000 * self.hop_length / self.sampling_rate,
- )
- f0 = pyworld.stonemask(wav.astype(np.double), f0, t, self.sampling_rate)
- return self.interpolate_f0(self.resize_f0(f0, p_len))
diff --git a/spaces/Fengbinbin/gpt-academic/config.py b/spaces/Fengbinbin/gpt-academic/config.py
deleted file mode 100644
index 2455424967976dfe81d50b08093d10b416d7fdde..0000000000000000000000000000000000000000
--- a/spaces/Fengbinbin/gpt-academic/config.py
+++ /dev/null
@@ -1,77 +0,0 @@
-# [step 1]>> 例如: API_KEY = "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" (此key无效)
-API_KEY = "sk-此处填API密钥" # 可同时填写多个API-KEY,用英文逗号分割,例如API_KEY = "sk-openaikey1,sk-openaikey2,fkxxxx-api2dkey1,fkxxxx-api2dkey2"
-
-# [step 2]>> 改为True应用代理,如果直接在海外服务器部署,此处不修改
-USE_PROXY = False
-if USE_PROXY:
- # 填写格式是 [协议]:// [地址] :[端口],填写之前不要忘记把USE_PROXY改成True,如果直接在海外服务器部署,此处不修改
- # 例如 "socks5h://localhost:11284"
- # [协议] 常见协议无非socks5h/http; 例如 v2**y 和 ss* 的默认本地协议是socks5h; 而cl**h 的默认本地协议是http
- # [地址] 懂的都懂,不懂就填localhost或者127.0.0.1肯定错不了(localhost意思是代理软件安装在本机上)
- # [端口] 在代理软件的设置里找。虽然不同的代理软件界面不一样,但端口号都应该在最显眼的位置上
-
- # 代理网络的地址,打开你的*学*网软件查看代理的协议(socks5/http)、地址(localhost)和端口(11284)
- proxies = {
- # [协议]:// [地址] :[端口]
- "http": "socks5h://localhost:11284", # 再例如 "http": "http://127.0.0.1:7890",
- "https": "socks5h://localhost:11284", # 再例如 "https": "http://127.0.0.1:7890",
- }
-else:
- proxies = None
-
-# [step 3]>> 多线程函数插件中,默认允许多少路线程同时访问OpenAI。Free trial users的限制是每分钟3次,Pay-as-you-go users的限制是每分钟3500次
-# 一言以蔽之:免费用户填3,OpenAI绑了信用卡的用户可以填 16 或者更高。提高限制请查询:https://platform.openai.com/docs/guides/rate-limits/overview
-DEFAULT_WORKER_NUM = 3
-
-
-# [step 4]>> 以下配置可以优化体验,但大部分场合下并不需要修改
-# 对话窗的高度
-CHATBOT_HEIGHT = 1115
-
-# 代码高亮
-CODE_HIGHLIGHT = True
-
-# 窗口布局
-LAYOUT = "LEFT-RIGHT" # "LEFT-RIGHT"(左右布局) # "TOP-DOWN"(上下布局)
-DARK_MODE = True # "LEFT-RIGHT"(左右布局) # "TOP-DOWN"(上下布局)
-
-# 发送请求到OpenAI后,等待多久判定为超时
-TIMEOUT_SECONDS = 30
-
-# 网页的端口, -1代表随机端口
-WEB_PORT = -1
-
-# 如果OpenAI不响应(网络卡顿、代理失败、KEY失效),重试的次数限制
-MAX_RETRY = 2
-
-# OpenAI模型选择是(gpt4现在只对申请成功的人开放)
-LLM_MODEL = "gpt-3.5-turbo" # 可选 "chatglm"
-AVAIL_LLM_MODELS = ["gpt-3.5-turbo", "gpt-4", "api2d-gpt-4", "api2d-gpt-3.5-turbo"]
-
-# 本地LLM模型如ChatGLM的执行方式 CPU/GPU
-LOCAL_MODEL_DEVICE = "cpu" # 可选 "cuda"
-
-# 设置gradio的并行线程数(不需要修改)
-CONCURRENT_COUNT = 100
-
-# 加一个看板娘装饰
-ADD_WAIFU = False
-
-# 设置用户名和密码(不需要修改)(相关功能不稳定,与gradio版本和网络都相关,如果本地使用不建议加这个)
-# [("username", "password"), ("username2", "password2"), ...]
-AUTHENTICATION = []
-
-# 重新URL重新定向,实现更换API_URL的作用(常规情况下,不要修改!!)
-# (高危设置!通过修改此设置,您将把您的API-KEY和对话隐私完全暴露给您设定的中间人!)
-# 格式 {"https://api.openai.com/v1/chat/completions": "在这里填写重定向的api.openai.com的URL"}
-# 例如 API_URL_REDIRECT = {"https://api.openai.com/v1/chat/completions": "https://ai.open.com/api/conversation"}
-API_URL_REDIRECT = {}
-
-# 如果需要在二级路径下运行(常规情况下,不要修改!!)(需要配合修改main.py才能生效!)
-CUSTOM_PATH = "/"
-
-# 如果需要使用newbing,把newbing的长长的cookie放到这里
-NEWBING_STYLE = "creative" # ["creative", "balanced", "precise"]
-NEWBING_COOKIES = """
-your bing cookies here
-"""
diff --git a/spaces/Fengbinbin/gpt-academic/crazy_functions/test_project/cpp/longcode/prod_cons.h b/spaces/Fengbinbin/gpt-academic/crazy_functions/test_project/cpp/longcode/prod_cons.h
deleted file mode 100644
index c9004bb8043a12e32814436baa6262a00c8ef68e..0000000000000000000000000000000000000000
--- a/spaces/Fengbinbin/gpt-academic/crazy_functions/test_project/cpp/longcode/prod_cons.h
+++ /dev/null
@@ -1,433 +0,0 @@
-#pragma once
-
-#include
-#include
-#include
-#include
-#include
-
-#include "libipc/def.h"
-
-#include "libipc/platform/detail.h"
-#include "libipc/circ/elem_def.h"
-#include "libipc/utility/log.h"
-#include "libipc/utility/utility.h"
-
-namespace ipc {
-
-////////////////////////////////////////////////////////////////
-/// producer-consumer implementation
-////////////////////////////////////////////////////////////////
-
-template
-struct prod_cons_impl;
-
-template <>
-struct prod_cons_impl> {
-
- template
- struct elem_t {
- std::aligned_storage_t data_ {};
- };
-
- alignas(cache_line_size) std::atomic rd_; // read index
- alignas(cache_line_size) std::atomic wt_; // write index
-
- constexpr circ::u2_t cursor() const noexcept {
- return 0;
- }
-
- template
- bool push(W* /*wrapper*/, F&& f, E* elems) {
- auto cur_wt = circ::index_of(wt_.load(std::memory_order_relaxed));
- if (cur_wt == circ::index_of(rd_.load(std::memory_order_acquire) - 1)) {
- return false; // full
- }
- std::forward(f)(&(elems[cur_wt].data_));
- wt_.fetch_add(1, std::memory_order_release);
- return true;
- }
-
- /**
- * In single-single-unicast, 'force_push' means 'no reader' or 'the only one reader is dead'.
- * So we could just disconnect all connections of receiver, and return false.
- */
- template
- bool force_push(W* wrapper, F&&, E*) {
- wrapper->elems()->disconnect_receiver(~static_cast(0u));
- return false;
- }
-
- template
- bool pop(W* /*wrapper*/, circ::u2_t& /*cur*/, F&& f, R&& out, E* elems) {
- auto cur_rd = circ::index_of(rd_.load(std::memory_order_relaxed));
- if (cur_rd == circ::index_of(wt_.load(std::memory_order_acquire))) {
- return false; // empty
- }
- std::forward(f)(&(elems[cur_rd].data_));
- std::forward(out)(true);
- rd_.fetch_add(1, std::memory_order_release);
- return true;
- }
-};
-
-template <>
-struct prod_cons_impl>
- : prod_cons_impl> {
-
- template
- bool force_push(W* wrapper, F&&, E*) {
- wrapper->elems()->disconnect_receiver(1);
- return false;
- }
-
- template class E, std::size_t DS, std::size_t AS>
- bool pop(W* /*wrapper*/, circ::u2_t& /*cur*/, F&& f, R&& out, E* elems) {
- byte_t buff[DS];
- for (unsigned k = 0;;) {
- auto cur_rd = rd_.load(std::memory_order_relaxed);
- if (circ::index_of(cur_rd) ==
- circ::index_of(wt_.load(std::memory_order_acquire))) {
- return false; // empty
- }
- std::memcpy(buff, &(elems[circ::index_of(cur_rd)].data_), sizeof(buff));
- if (rd_.compare_exchange_weak(cur_rd, cur_rd + 1, std::memory_order_release)) {
- std::forward(f)(buff);
- std::forward(out)(true);
- return true;
- }
- ipc::yield(k);
- }
- }
-};
-
-template <>
-struct prod_cons_impl>
- : prod_cons_impl> {
-
- using flag_t = std::uint64_t;
-
- template
- struct elem_t {
- std::aligned_storage_t data_ {};
- std::atomic f_ct_ { 0 }; // commit flag
- };
-
- alignas(cache_line_size) std::atomic ct_; // commit index
-
- template
- bool push(W* /*wrapper*/, F&& f, E* elems) {
- circ::u2_t cur_ct, nxt_ct;
- for (unsigned k = 0;;) {
- cur_ct = ct_.load(std::memory_order_relaxed);
- if (circ::index_of(nxt_ct = cur_ct + 1) ==
- circ::index_of(rd_.load(std::memory_order_acquire))) {
- return false; // full
- }
- if (ct_.compare_exchange_weak(cur_ct, nxt_ct, std::memory_order_acq_rel)) {
- break;
- }
- ipc::yield(k);
- }
- auto* el = elems + circ::index_of(cur_ct);
- std::forward(f)(&(el->data_));
- // set flag & try update wt
- el->f_ct_.store(~static_cast(cur_ct), std::memory_order_release);
- while (1) {
- auto cac_ct = el->f_ct_.load(std::memory_order_acquire);
- if (cur_ct != wt_.load(std::memory_order_relaxed)) {
- return true;
- }
- if ((~cac_ct) != cur_ct) {
- return true;
- }
- if (!el->f_ct_.compare_exchange_strong(cac_ct, 0, std::memory_order_relaxed)) {
- return true;
- }
- wt_.store(nxt_ct, std::memory_order_release);
- cur_ct = nxt_ct;
- nxt_ct = cur_ct + 1;
- el = elems + circ::index_of(cur_ct);
- }
- return true;
- }
-
- template
- bool force_push(W* wrapper, F&&, E*) {
- wrapper->elems()->disconnect_receiver(1);
- return false;
- }
-
- template class E, std::size_t DS, std::size_t AS>
- bool pop(W* /*wrapper*/, circ::u2_t& /*cur*/, F&& f, R&& out, E* elems) {
- byte_t buff[DS];
- for (unsigned k = 0;;) {
- auto cur_rd = rd_.load(std::memory_order_relaxed);
- auto cur_wt = wt_.load(std::memory_order_acquire);
- auto id_rd = circ::index_of(cur_rd);
- auto id_wt = circ::index_of(cur_wt);
- if (id_rd == id_wt) {
- auto* el = elems + id_wt;
- auto cac_ct = el->f_ct_.load(std::memory_order_acquire);
- if ((~cac_ct) != cur_wt) {
- return false; // empty
- }
- if (el->f_ct_.compare_exchange_weak(cac_ct, 0, std::memory_order_relaxed)) {
- wt_.store(cur_wt + 1, std::memory_order_release);
- }
- k = 0;
- }
- else {
- std::memcpy(buff, &(elems[circ::index_of(cur_rd)].data_), sizeof(buff));
- if (rd_.compare_exchange_weak(cur_rd, cur_rd + 1, std::memory_order_release)) {
- std::forward(f)(buff);
- std::forward(out)(true);
- return true;
- }
- ipc::yield(k);
- }
- }
- }
-};
-
-template <>
-struct prod_cons_impl> {
-
- using rc_t = std::uint64_t;
-
- enum : rc_t {
- ep_mask = 0x00000000ffffffffull,
- ep_incr = 0x0000000100000000ull
- };
-
- template
- struct elem_t {
- std::aligned_storage_t data_ {};
- std::atomic rc_ { 0 }; // read-counter
- };
-
- alignas(cache_line_size) std::atomic wt_; // write index
- alignas(cache_line_size) rc_t epoch_ { 0 }; // only one writer
-
- circ::u2_t cursor() const noexcept {
- return wt_.load(std::memory_order_acquire);
- }
-
- template
- bool push(W* wrapper, F&& f, E* elems) {
- E* el;
- for (unsigned k = 0;;) {
- circ::cc_t cc = wrapper->elems()->connections(std::memory_order_relaxed);
- if (cc == 0) return false; // no reader
- el = elems + circ::index_of(wt_.load(std::memory_order_relaxed));
- // check all consumers have finished reading this element
- auto cur_rc = el->rc_.load(std::memory_order_acquire);
- circ::cc_t rem_cc = cur_rc & ep_mask;
- if ((cc & rem_cc) && ((cur_rc & ~ep_mask) == epoch_)) {
- return false; // has not finished yet
- }
- // consider rem_cc to be 0 here
- if (el->rc_.compare_exchange_weak(
- cur_rc, epoch_ | static_cast(cc), std::memory_order_release)) {
- break;
- }
- ipc::yield(k);
- }
- std::forward(f)(&(el->data_));
- wt_.fetch_add(1, std::memory_order_release);
- return true;
- }
-
- template
- bool force_push(W* wrapper, F&& f, E* elems) {
- E* el;
- epoch_ += ep_incr;
- for (unsigned k = 0;;) {
- circ::cc_t cc = wrapper->elems()->connections(std::memory_order_relaxed);
- if (cc == 0) return false; // no reader
- el = elems + circ::index_of(wt_.load(std::memory_order_relaxed));
- // check all consumers have finished reading this element
- auto cur_rc = el->rc_.load(std::memory_order_acquire);
- circ::cc_t rem_cc = cur_rc & ep_mask;
- if (cc & rem_cc) {
- ipc::log("force_push: k = %u, cc = %u, rem_cc = %u\n", k, cc, rem_cc);
- cc = wrapper->elems()->disconnect_receiver(rem_cc); // disconnect all invalid readers
- if (cc == 0) return false; // no reader
- }
- // just compare & exchange
- if (el->rc_.compare_exchange_weak(
- cur_rc, epoch_ | static_cast(cc), std::memory_order_release)) {
- break;
- }
- ipc::yield(k);
- }
- std::forward(f)(&(el->data_));
- wt_.fetch_add(1, std::memory_order_release);
- return true;
- }
-
- template
- bool pop(W* wrapper, circ::u2_t& cur, F&& f, R&& out, E* elems) {
- if (cur == cursor()) return false; // acquire
- auto* el = elems + circ::index_of(cur++);
- std::forward(f)(&(el->data_));
- for (unsigned k = 0;;) {
- auto cur_rc = el->rc_.load(std::memory_order_acquire);
- if ((cur_rc & ep_mask) == 0) {
- std::forward(out)(true);
- return true;
- }
- auto nxt_rc = cur_rc & ~static_cast(wrapper->connected_id());
- if (el->rc_.compare_exchange_weak(cur_rc, nxt_rc, std::memory_order_release)) {
- std::forward(out)((nxt_rc & ep_mask) == 0);
- return true;
- }
- ipc::yield(k);
- }
- }
-};
-
-template <>
-struct prod_cons_impl> {
-
- using rc_t = std::uint64_t;
- using flag_t = std::uint64_t;
-
- enum : rc_t {
- rc_mask = 0x00000000ffffffffull,
- ep_mask = 0x00ffffffffffffffull,
- ep_incr = 0x0100000000000000ull,
- ic_mask = 0xff000000ffffffffull,
- ic_incr = 0x0000000100000000ull
- };
-
- template
- struct elem_t {
- std::aligned_storage_t data_ {};
- std::atomic rc_ { 0 }; // read-counter
- std::atomic f_ct_ { 0 }; // commit flag
- };
-
- alignas(cache_line_size) std::atomic ct_; // commit index
- alignas(cache_line_size) std::atomic epoch_ { 0 };
-
- circ::u2_t cursor() const noexcept {
- return ct_.load(std::memory_order_acquire);
- }
-
- constexpr static rc_t inc_rc(rc_t rc) noexcept {
- return (rc & ic_mask) | ((rc + ic_incr) & ~ic_mask);
- }
-
- constexpr static rc_t inc_mask(rc_t rc) noexcept {
- return inc_rc(rc) & ~rc_mask;
- }
-
- template
- bool push(W* wrapper, F&& f, E* elems) {
- E* el;
- circ::u2_t cur_ct;
- rc_t epoch = epoch_.load(std::memory_order_acquire);
- for (unsigned k = 0;;) {
- circ::cc_t cc = wrapper->elems()->connections(std::memory_order_relaxed);
- if (cc == 0) return false; // no reader
- el = elems + circ::index_of(cur_ct = ct_.load(std::memory_order_relaxed));
- // check all consumers have finished reading this element
- auto cur_rc = el->rc_.load(std::memory_order_relaxed);
- circ::cc_t rem_cc = cur_rc & rc_mask;
- if ((cc & rem_cc) && ((cur_rc & ~ep_mask) == epoch)) {
- return false; // has not finished yet
- }
- else if (!rem_cc) {
- auto cur_fl = el->f_ct_.load(std::memory_order_acquire);
- if ((cur_fl != cur_ct) && cur_fl) {
- return false; // full
- }
- }
- // consider rem_cc to be 0 here
- if (el->rc_.compare_exchange_weak(
- cur_rc, inc_mask(epoch | (cur_rc & ep_mask)) | static_cast(cc), std::memory_order_relaxed) &&
- epoch_.compare_exchange_weak(epoch, epoch, std::memory_order_acq_rel)) {
- break;
- }
- ipc::yield(k);
- }
- // only one thread/process would touch here at one time
- ct_.store(cur_ct + 1, std::memory_order_release);
- std::forward(f)(&(el->data_));
- // set flag & try update wt
- el->f_ct_.store(~static_cast(cur_ct), std::memory_order_release);
- return true;
- }
-
- template
- bool force_push(W* wrapper, F&& f, E* elems) {
- E* el;
- circ::u2_t cur_ct;
- rc_t epoch = epoch_.fetch_add(ep_incr, std::memory_order_release) + ep_incr;
- for (unsigned k = 0;;) {
- circ::cc_t cc = wrapper->elems()->connections(std::memory_order_relaxed);
- if (cc == 0) return false; // no reader
- el = elems + circ::index_of(cur_ct = ct_.load(std::memory_order_relaxed));
- // check all consumers have finished reading this element
- auto cur_rc = el->rc_.load(std::memory_order_acquire);
- circ::cc_t rem_cc = cur_rc & rc_mask;
- if (cc & rem_cc) {
- ipc::log("force_push: k = %u, cc = %u, rem_cc = %u\n", k, cc, rem_cc);
- cc = wrapper->elems()->disconnect_receiver(rem_cc); // disconnect all invalid readers
- if (cc == 0) return false; // no reader
- }
- // just compare & exchange
- if (el->rc_.compare_exchange_weak(
- cur_rc, inc_mask(epoch | (cur_rc & ep_mask)) | static_cast(cc), std::memory_order_relaxed)) {
- if (epoch == epoch_.load(std::memory_order_acquire)) {
- break;
- }
- else if (push(wrapper, std::forward(f), elems)) {
- return true;
- }
- epoch = epoch_.fetch_add(ep_incr, std::memory_order_release) + ep_incr;
- }
- ipc::yield(k);
- }
- // only one thread/process would touch here at one time
- ct_.store(cur_ct + 1, std::memory_order_release);
- std::forward(f)(&(el->data_));
- // set flag & try update wt
- el->f_ct_.store(~static_cast(cur_ct), std::memory_order_release);
- return true;
- }
-
- template
- bool pop(W* wrapper, circ::u2_t& cur, F&& f, R&& out, E(& elems)[N]) {
- auto* el = elems + circ::index_of(cur);
- auto cur_fl = el->f_ct_.load(std::memory_order_acquire);
- if (cur_fl != ~static_cast(cur)) {
- return false; // empty
- }
- ++cur;
- std::forward(f)(&(el->data_));
- for (unsigned k = 0;;) {
- auto cur_rc = el->rc_.load(std::memory_order_acquire);
- if ((cur_rc & rc_mask) == 0) {
- std::forward(out)(true);
- el->f_ct_.store(cur + N - 1, std::memory_order_release);
- return true;
- }
- auto nxt_rc = inc_rc(cur_rc) & ~static_cast(wrapper->connected_id());
- bool last_one = false;
- if ((last_one = (nxt_rc & rc_mask) == 0)) {
- el->f_ct_.store(cur + N - 1, std::memory_order_release);
- }
- if (el->rc_.compare_exchange_weak(cur_rc, nxt_rc, std::memory_order_release)) {
- std::forward(out)(last_one);
- return true;
- }
- ipc::yield(k);
- }
- }
-};
-
-} // namespace ipc
diff --git a/spaces/FrankZxShen/vits-fast-fineturning-models-ba/models.py b/spaces/FrankZxShen/vits-fast-fineturning-models-ba/models.py
deleted file mode 100644
index 65f9ae5255616efa19a4f28bc0a840d4c453a060..0000000000000000000000000000000000000000
--- a/spaces/FrankZxShen/vits-fast-fineturning-models-ba/models.py
+++ /dev/null
@@ -1,722 +0,0 @@
-import copy
-import math
-import torch
-from torch import nn
-from torch.nn import functional as F
-
-import commons
-import modules
-import attentions
-import monotonic_align
-
-from torch.nn import Conv1d, ConvTranspose1d, AvgPool1d, Conv2d
-from torch.nn.utils import weight_norm, remove_weight_norm, spectral_norm
-from commons import init_weights, get_padding
-
-
-class StochasticDurationPredictor(nn.Module):
- def __init__(self, in_channels, filter_channels, kernel_size, p_dropout, n_flows=4, gin_channels=0):
- super().__init__()
- filter_channels = in_channels # it needs to be removed from future version.
- self.in_channels = in_channels
- self.filter_channels = filter_channels
- self.kernel_size = kernel_size
- self.p_dropout = p_dropout
- self.n_flows = n_flows
- self.gin_channels = gin_channels
-
- self.log_flow = modules.Log()
- self.flows = nn.ModuleList()
- self.flows.append(modules.ElementwiseAffine(2))
- for i in range(n_flows):
- self.flows.append(modules.ConvFlow(2, filter_channels, kernel_size, n_layers=3))
- self.flows.append(modules.Flip())
-
- self.post_pre = nn.Conv1d(1, filter_channels, 1)
- self.post_proj = nn.Conv1d(filter_channels, filter_channels, 1)
- self.post_convs = modules.DDSConv(filter_channels, kernel_size, n_layers=3, p_dropout=p_dropout)
- self.post_flows = nn.ModuleList()
- self.post_flows.append(modules.ElementwiseAffine(2))
- for i in range(4):
- self.post_flows.append(modules.ConvFlow(2, filter_channels, kernel_size, n_layers=3))
- self.post_flows.append(modules.Flip())
-
- self.pre = nn.Conv1d(in_channels, filter_channels, 1)
- self.proj = nn.Conv1d(filter_channels, filter_channels, 1)
- self.convs = modules.DDSConv(filter_channels, kernel_size, n_layers=3, p_dropout=p_dropout)
- if gin_channels != 0:
- self.cond = nn.Conv1d(gin_channels, filter_channels, 1)
-
- def forward(self, x, x_mask, w=None, g=None, reverse=False, noise_scale=1.0):
- x = torch.detach(x)
- x = self.pre(x)
- if g is not None:
- g = torch.detach(g)
- x = x + self.cond(g)
- x = self.convs(x, x_mask)
- x = self.proj(x) * x_mask
-
- if not reverse:
- flows = self.flows
- assert w is not None
-
- logdet_tot_q = 0
- h_w = self.post_pre(w)
- h_w = self.post_convs(h_w, x_mask)
- h_w = self.post_proj(h_w) * x_mask
- e_q = torch.randn(w.size(0), 2, w.size(2)).to(device=x.device, dtype=x.dtype) * x_mask
- z_q = e_q
- for flow in self.post_flows:
- z_q, logdet_q = flow(z_q, x_mask, g=(x + h_w))
- logdet_tot_q += logdet_q
- z_u, z1 = torch.split(z_q, [1, 1], 1)
- u = torch.sigmoid(z_u) * x_mask
- z0 = (w - u) * x_mask
- logdet_tot_q += torch.sum((F.logsigmoid(z_u) + F.logsigmoid(-z_u)) * x_mask, [1,2])
- logq = torch.sum(-0.5 * (math.log(2*math.pi) + (e_q**2)) * x_mask, [1,2]) - logdet_tot_q
-
- logdet_tot = 0
- z0, logdet = self.log_flow(z0, x_mask)
- logdet_tot += logdet
- z = torch.cat([z0, z1], 1)
- for flow in flows:
- z, logdet = flow(z, x_mask, g=x, reverse=reverse)
- logdet_tot = logdet_tot + logdet
- nll = torch.sum(0.5 * (math.log(2*math.pi) + (z**2)) * x_mask, [1,2]) - logdet_tot
- return nll + logq # [b]
- else:
- flows = list(reversed(self.flows))
- flows = flows[:-2] + [flows[-1]] # remove a useless vflow
- z = torch.randn(x.size(0), 2, x.size(2)).to(device=x.device, dtype=x.dtype) * noise_scale
- for flow in flows:
- z = flow(z, x_mask, g=x, reverse=reverse)
- z0, z1 = torch.split(z, [1, 1], 1)
- logw = z0
- return logw
-
-
-class DurationPredictor(nn.Module):
- def __init__(self, in_channels, filter_channels, kernel_size, p_dropout, gin_channels=0):
- super().__init__()
-
- self.in_channels = in_channels
- self.filter_channels = filter_channels
- self.kernel_size = kernel_size
- self.p_dropout = p_dropout
- self.gin_channels = gin_channels
-
- self.drop = nn.Dropout(p_dropout)
- self.conv_1 = nn.Conv1d(in_channels, filter_channels, kernel_size, padding=kernel_size//2)
- self.norm_1 = modules.LayerNorm(filter_channels)
- self.conv_2 = nn.Conv1d(filter_channels, filter_channels, kernel_size, padding=kernel_size//2)
- self.norm_2 = modules.LayerNorm(filter_channels)
- self.proj = nn.Conv1d(filter_channels, 1, 1)
-
- if gin_channels != 0:
- self.cond = nn.Conv1d(gin_channels, in_channels, 1)
-
- def forward(self, x, x_mask, g=None):
- x = torch.detach(x)
- if g is not None:
- g = torch.detach(g)
- x = x + self.cond(g)
- x = self.conv_1(x * x_mask)
- x = torch.relu(x)
- x = self.norm_1(x)
- x = self.drop(x)
- x = self.conv_2(x * x_mask)
- x = torch.relu(x)
- x = self.norm_2(x)
- x = self.drop(x)
- x = self.proj(x * x_mask)
- return x * x_mask
-
-
-class TextEncoder(nn.Module):
- def __init__(self,
- n_vocab,
- out_channels,
- hidden_channels,
- filter_channels,
- n_heads,
- n_layers,
- kernel_size,
- p_dropout):
- super().__init__()
- self.n_vocab = n_vocab
- self.out_channels = out_channels
- self.hidden_channels = hidden_channels
- self.filter_channels = filter_channels
- self.n_heads = n_heads
- self.n_layers = n_layers
- self.kernel_size = kernel_size
- self.p_dropout = p_dropout
-
- self.emb = nn.Embedding(n_vocab, hidden_channels)
- nn.init.normal_(self.emb.weight, 0.0, hidden_channels**-0.5)
-
- self.encoder = attentions.Encoder(
- hidden_channels,
- filter_channels,
- n_heads,
- n_layers,
- kernel_size,
- p_dropout)
- self.proj= nn.Conv1d(hidden_channels, out_channels * 2, 1)
-
- def forward(self, x, x_lengths):
- x = self.emb(x) * math.sqrt(self.hidden_channels) # [b, t, h]
- x = torch.transpose(x, 1, -1) # [b, h, t]
- x_mask = torch.unsqueeze(commons.sequence_mask(x_lengths, x.size(2)), 1).to(x.dtype)
-
- x = self.encoder(x * x_mask, x_mask)
- stats = self.proj(x) * x_mask
-
- m, logs = torch.split(stats, self.out_channels, dim=1)
- return x, m, logs, x_mask
-
-
-class TextEncoder_lora(nn.Module):
- def __init__(self,
- n_vocab,
- out_channels,
- hidden_channels,
- filter_channels,
- n_heads,
- n_layers,
- kernel_size,
- p_dropout):
- super().__init__()
- self.n_vocab = n_vocab
- self.out_channels = out_channels
- self.hidden_channels = hidden_channels
- self.filter_channels = filter_channels
- self.n_heads = n_heads
- self.n_layers = n_layers
- self.kernel_size = kernel_size
- self.p_dropout = p_dropout
-
- self.emb = nn.Embedding(n_vocab, hidden_channels, r=4)
- nn.init.normal_(self.emb.weight, 0.0, hidden_channels**-0.5)
-
- self.encoder = attentions.Encoder_lora(
- hidden_channels,
- filter_channels,
- n_heads,
- n_layers,
- kernel_size,
- p_dropout)
- self.proj= nn.Conv1d(hidden_channels, out_channels * 2, 1)
-
- def forward(self, x, x_lengths):
- x = self.emb(x) * math.sqrt(self.hidden_channels) # [b, t, h]
- x = torch.transpose(x, 1, -1) # [b, h, t]
- x_mask = torch.unsqueeze(commons.sequence_mask(x_lengths, x.size(2)), 1).to(x.dtype)
-
- x = self.encoder(x * x_mask, x_mask)
- stats = self.proj(x) * x_mask
-
- m, logs = torch.split(stats, self.out_channels, dim=1)
- return x, m, logs, x_mask
-
-class ResidualCouplingBlock(nn.Module):
- def __init__(self,
- channels,
- hidden_channels,
- kernel_size,
- dilation_rate,
- n_layers,
- n_flows=4,
- gin_channels=0):
- super().__init__()
- self.channels = channels
- self.hidden_channels = hidden_channels
- self.kernel_size = kernel_size
- self.dilation_rate = dilation_rate
- self.n_layers = n_layers
- self.n_flows = n_flows
- self.gin_channels = gin_channels
-
- self.flows = nn.ModuleList()
- for i in range(n_flows):
- self.flows.append(modules.ResidualCouplingLayer(channels, hidden_channels, kernel_size, dilation_rate, n_layers, gin_channels=gin_channels, mean_only=True))
- self.flows.append(modules.Flip())
-
- def forward(self, x, x_mask, g=None, reverse=False):
- if not reverse:
- for flow in self.flows:
- x, _ = flow(x, x_mask, g=g, reverse=reverse)
- else:
- for flow in reversed(self.flows):
- x = flow(x, x_mask, g=g, reverse=reverse)
- return x
-
-
-class PosteriorEncoder(nn.Module):
- def __init__(self,
- in_channels,
- out_channels,
- hidden_channels,
- kernel_size,
- dilation_rate,
- n_layers,
- gin_channels=0):
- super().__init__()
- self.in_channels = in_channels
- self.out_channels = out_channels
- self.hidden_channels = hidden_channels
- self.kernel_size = kernel_size
- self.dilation_rate = dilation_rate
- self.n_layers = n_layers
- self.gin_channels = gin_channels
-
- self.pre = nn.Conv1d(in_channels, hidden_channels, 1)
- self.enc = modules.WN(hidden_channels, kernel_size, dilation_rate, n_layers, gin_channels=gin_channels)
- self.proj = nn.Conv1d(hidden_channels, out_channels * 2, 1)
-
- def forward(self, x, x_lengths, g=None):
- x_mask = torch.unsqueeze(commons.sequence_mask(x_lengths, x.size(2)), 1).to(x.dtype)
- x = self.pre(x) * x_mask
- x = self.enc(x, x_mask, g=g)
- stats = self.proj(x) * x_mask
- m, logs = torch.split(stats, self.out_channels, dim=1)
- z = (m + torch.randn_like(m) * torch.exp(logs)) * x_mask
- return z, m, logs, x_mask
-
-
-class Generator(torch.nn.Module):
- def __init__(self, initial_channel, resblock, resblock_kernel_sizes, resblock_dilation_sizes, upsample_rates, upsample_initial_channel, upsample_kernel_sizes, gin_channels=0):
- super(Generator, self).__init__()
- self.num_kernels = len(resblock_kernel_sizes)
- self.num_upsamples = len(upsample_rates)
- self.conv_pre = Conv1d(initial_channel, upsample_initial_channel, 7, 1, padding=3)
- resblock = modules.ResBlock1 if resblock == '1' else modules.ResBlock2
-
- self.ups = nn.ModuleList()
- for i, (u, k) in enumerate(zip(upsample_rates, upsample_kernel_sizes)):
- self.ups.append(weight_norm(
- ConvTranspose1d(upsample_initial_channel//(2**i), upsample_initial_channel//(2**(i+1)),
- k, u, padding=(k-u)//2)))
-
- self.resblocks = nn.ModuleList()
- for i in range(len(self.ups)):
- ch = upsample_initial_channel//(2**(i+1))
- for j, (k, d) in enumerate(zip(resblock_kernel_sizes, resblock_dilation_sizes)):
- self.resblocks.append(resblock(ch, k, d))
-
- self.conv_post = Conv1d(ch, 1, 7, 1, padding=3, bias=False)
- self.ups.apply(init_weights)
-
- if gin_channels != 0:
- self.cond = nn.Conv1d(gin_channels, upsample_initial_channel, 1)
-
- def forward(self, x, g=None):
- x = self.conv_pre(x)
- if g is not None:
- x = x + self.cond(g)
-
- for i in range(self.num_upsamples):
- x = F.leaky_relu(x, modules.LRELU_SLOPE)
- x = self.ups[i](x)
- xs = None
- for j in range(self.num_kernels):
- if xs is None:
- xs = self.resblocks[i*self.num_kernels+j](x)
- else:
- xs += self.resblocks[i*self.num_kernels+j](x)
- x = xs / self.num_kernels
- x = F.leaky_relu(x)
- x = self.conv_post(x)
- x = torch.tanh(x)
-
- return x
-
- def remove_weight_norm(self):
- print('Removing weight norm...')
- for l in self.ups:
- remove_weight_norm(l)
- for l in self.resblocks:
- l.remove_weight_norm()
-
-
-class DiscriminatorP(torch.nn.Module):
- def __init__(self, period, kernel_size=5, stride=3, use_spectral_norm=False):
- super(DiscriminatorP, self).__init__()
- self.period = period
- self.use_spectral_norm = use_spectral_norm
- norm_f = weight_norm if use_spectral_norm == False else spectral_norm
- self.convs = nn.ModuleList([
- norm_f(Conv2d(1, 32, (kernel_size, 1), (stride, 1), padding=(get_padding(kernel_size, 1), 0))),
- norm_f(Conv2d(32, 128, (kernel_size, 1), (stride, 1), padding=(get_padding(kernel_size, 1), 0))),
- norm_f(Conv2d(128, 512, (kernel_size, 1), (stride, 1), padding=(get_padding(kernel_size, 1), 0))),
- norm_f(Conv2d(512, 1024, (kernel_size, 1), (stride, 1), padding=(get_padding(kernel_size, 1), 0))),
- norm_f(Conv2d(1024, 1024, (kernel_size, 1), 1, padding=(get_padding(kernel_size, 1), 0))),
- ])
- self.conv_post = norm_f(Conv2d(1024, 1, (3, 1), 1, padding=(1, 0)))
-
- def forward(self, x):
- fmap = []
-
- # 1d to 2d
- b, c, t = x.shape
- if t % self.period != 0: # pad first
- n_pad = self.period - (t % self.period)
- x = F.pad(x, (0, n_pad), "reflect")
- t = t + n_pad
- x = x.view(b, c, t // self.period, self.period)
-
- for l in self.convs:
- x = l(x)
- x = F.leaky_relu(x, modules.LRELU_SLOPE)
- fmap.append(x)
- x = self.conv_post(x)
- fmap.append(x)
- x = torch.flatten(x, 1, -1)
-
- return x, fmap
-
-
-class DiscriminatorS(torch.nn.Module):
- def __init__(self, use_spectral_norm=False):
- super(DiscriminatorS, self).__init__()
- norm_f = weight_norm if use_spectral_norm == False else spectral_norm
- self.convs = nn.ModuleList([
- norm_f(Conv1d(1, 16, 15, 1, padding=7)),
- norm_f(Conv1d(16, 64, 41, 4, groups=4, padding=20)),
- norm_f(Conv1d(64, 256, 41, 4, groups=16, padding=20)),
- norm_f(Conv1d(256, 1024, 41, 4, groups=64, padding=20)),
- norm_f(Conv1d(1024, 1024, 41, 4, groups=256, padding=20)),
- norm_f(Conv1d(1024, 1024, 5, 1, padding=2)),
- ])
- self.conv_post = norm_f(Conv1d(1024, 1, 3, 1, padding=1))
-
- def forward(self, x):
- fmap = []
-
- for l in self.convs:
- x = l(x)
- x = F.leaky_relu(x, modules.LRELU_SLOPE)
- fmap.append(x)
- x = self.conv_post(x)
- fmap.append(x)
- x = torch.flatten(x, 1, -1)
-
- return x, fmap
-
-
-class MultiPeriodDiscriminator(torch.nn.Module):
- def __init__(self, use_spectral_norm=False):
- super(MultiPeriodDiscriminator, self).__init__()
- periods = [2,3,5,7,11]
-
- discs = [DiscriminatorS(use_spectral_norm=use_spectral_norm)]
- discs = discs + [DiscriminatorP(i, use_spectral_norm=use_spectral_norm) for i in periods]
- self.discriminators = nn.ModuleList(discs)
-
- def forward(self, y, y_hat):
- y_d_rs = []
- y_d_gs = []
- fmap_rs = []
- fmap_gs = []
- for i, d in enumerate(self.discriminators):
- y_d_r, fmap_r = d(y)
- y_d_g, fmap_g = d(y_hat)
- y_d_rs.append(y_d_r)
- y_d_gs.append(y_d_g)
- fmap_rs.append(fmap_r)
- fmap_gs.append(fmap_g)
-
- return y_d_rs, y_d_gs, fmap_rs, fmap_gs
-
-
-
-class SynthesizerTrn(nn.Module):
- """
- Synthesizer for Training
- """
-
- def __init__(self,
- n_vocab,
- spec_channels,
- segment_size,
- inter_channels,
- hidden_channels,
- filter_channels,
- n_heads,
- n_layers,
- kernel_size,
- p_dropout,
- resblock,
- resblock_kernel_sizes,
- resblock_dilation_sizes,
- upsample_rates,
- upsample_initial_channel,
- upsample_kernel_sizes,
- n_speakers=0,
- gin_channels=0,
- use_sdp=True,
- **kwargs):
-
- super().__init__()
- self.n_vocab = n_vocab
- self.spec_channels = spec_channels
- self.inter_channels = inter_channels
- self.hidden_channels = hidden_channels
- self.filter_channels = filter_channels
- self.n_heads = n_heads
- self.n_layers = n_layers
- self.kernel_size = kernel_size
- self.p_dropout = p_dropout
- self.resblock = resblock
- self.resblock_kernel_sizes = resblock_kernel_sizes
- self.resblock_dilation_sizes = resblock_dilation_sizes
- self.upsample_rates = upsample_rates
- self.upsample_initial_channel = upsample_initial_channel
- self.upsample_kernel_sizes = upsample_kernel_sizes
- self.segment_size = segment_size
- self.n_speakers = n_speakers
- self.gin_channels = gin_channels
-
- self.use_sdp = use_sdp
-
- self.enc_p = TextEncoder(n_vocab,
- inter_channels,
- hidden_channels,
- filter_channels,
- n_heads,
- n_layers,
- kernel_size,
- p_dropout)
- self.dec = Generator(inter_channels, resblock, resblock_kernel_sizes, resblock_dilation_sizes, upsample_rates, upsample_initial_channel, upsample_kernel_sizes, gin_channels=gin_channels)
- self.enc_q = PosteriorEncoder(spec_channels, inter_channels, hidden_channels, 5, 1, 16, gin_channels=gin_channels)
- self.flow = ResidualCouplingBlock(inter_channels, hidden_channels, 5, 1, 4, gin_channels=gin_channels)
-
- if use_sdp:
- self.dp = StochasticDurationPredictor(hidden_channels, 192, 3, 0.5, 4, gin_channels=gin_channels)
- else:
- self.dp = DurationPredictor(hidden_channels, 256, 3, 0.5, gin_channels=gin_channels)
-
- if n_speakers >= 1:
- self.emb_g = nn.Embedding(n_speakers, gin_channels)
-
- def forward(self, x, x_lengths, y, y_lengths, sid=None):
-
- x, m_p, logs_p, x_mask = self.enc_p(x, x_lengths)
- if self.n_speakers > 0:
- g = self.emb_g(sid).unsqueeze(-1) # [b, h, 1]
- else:
- g = None
-
- z, m_q, logs_q, y_mask = self.enc_q(y, y_lengths, g=g)
- z_p = self.flow(z, y_mask, g=g)
-
- with torch.no_grad():
- # negative cross-entropy
- s_p_sq_r = torch.exp(-2 * logs_p) # [b, d, t]
- neg_cent1 = torch.sum(-0.5 * math.log(2 * math.pi) - logs_p, [1], keepdim=True) # [b, 1, t_s]
- neg_cent2 = torch.matmul(-0.5 * (z_p ** 2).transpose(1, 2), s_p_sq_r) # [b, t_t, d] x [b, d, t_s] = [b, t_t, t_s]
- neg_cent3 = torch.matmul(z_p.transpose(1, 2), (m_p * s_p_sq_r)) # [b, t_t, d] x [b, d, t_s] = [b, t_t, t_s]
- neg_cent4 = torch.sum(-0.5 * (m_p ** 2) * s_p_sq_r, [1], keepdim=True) # [b, 1, t_s]
- neg_cent = neg_cent1 + neg_cent2 + neg_cent3 + neg_cent4
-
- attn_mask = torch.unsqueeze(x_mask, 2) * torch.unsqueeze(y_mask, -1)
- attn = monotonic_align.maximum_path(neg_cent, attn_mask.squeeze(1)).unsqueeze(1).detach()
-
- w = attn.sum(2)
- if self.use_sdp:
- l_length = self.dp(x, x_mask, w, g=g)
- l_length = l_length / torch.sum(x_mask)
- else:
- logw_ = torch.log(w + 1e-6) * x_mask
- logw = self.dp(x, x_mask, g=g)
- l_length = torch.sum((logw - logw_)**2, [1,2]) / torch.sum(x_mask) # for averaging
-
- # expand prior
- m_p = torch.matmul(attn.squeeze(1), m_p.transpose(1, 2)).transpose(1, 2)
- logs_p = torch.matmul(attn.squeeze(1), logs_p.transpose(1, 2)).transpose(1, 2)
-
- z_slice, ids_slice = commons.rand_slice_segments(z, y_lengths, self.segment_size)
- o = self.dec(z_slice, g=g)
- return o, l_length, attn, ids_slice, x_mask, y_mask, (z, z_p, m_p, logs_p, m_q, logs_q)
-
- def infer(self, x, x_lengths, sid=None, noise_scale=1, length_scale=1, noise_scale_w=1., max_len=None):
- x, m_p, logs_p, x_mask = self.enc_p(x, x_lengths)
- if self.n_speakers > 0:
- g = self.emb_g(sid).unsqueeze(-1) # [b, h, 1]
- else:
- g = None
-
- if self.use_sdp:
- logw = self.dp(x, x_mask, g=g, reverse=True, noise_scale=noise_scale_w)
- else:
- logw = self.dp(x, x_mask, g=g)
- w = torch.exp(logw) * x_mask * length_scale
- w_ceil = torch.ceil(w)
- y_lengths = torch.clamp_min(torch.sum(w_ceil, [1, 2]), 1).long()
- y_mask = torch.unsqueeze(commons.sequence_mask(y_lengths, None), 1).to(x_mask.dtype)
- attn_mask = torch.unsqueeze(x_mask, 2) * torch.unsqueeze(y_mask, -1)
- attn = commons.generate_path(w_ceil, attn_mask)
-
- m_p = torch.matmul(attn.squeeze(1), m_p.transpose(1, 2)).transpose(1, 2) # [b, t', t], [b, t, d] -> [b, d, t']
- logs_p = torch.matmul(attn.squeeze(1), logs_p.transpose(1, 2)).transpose(1, 2) # [b, t', t], [b, t, d] -> [b, d, t']
-
- z_p = m_p + torch.randn_like(m_p) * torch.exp(logs_p) * noise_scale
- z = self.flow(z_p, y_mask, g=g, reverse=True)
- o = self.dec((z * y_mask)[:,:,:max_len], g=g)
- return o, attn, y_mask, (z, z_p, m_p, logs_p)
-
- def voice_conversion(self, y, y_lengths, sid_src, sid_tgt):
- assert self.n_speakers > 0, "n_speakers have to be larger than 0."
- g_src = self.emb_g(sid_src).unsqueeze(-1)
- g_tgt = self.emb_g(sid_tgt).unsqueeze(-1)
- z, m_q, logs_q, y_mask = self.enc_q(y, y_lengths, g=g_src)
- z_p = self.flow(z, y_mask, g=g_src)
- z_hat = self.flow(z_p, y_mask, g=g_tgt, reverse=True)
- o_hat = self.dec(z_hat * y_mask, g=g_tgt)
- return o_hat, y_mask, (z, z_p, z_hat)
-
-
-class SynthesizerTrn_lora(nn.Module):
- """
- Synthesizer for Training
- """
-
- def __init__(self,
- n_vocab,
- spec_channels,
- segment_size,
- inter_channels,
- hidden_channels,
- filter_channels,
- n_heads,
- n_layers,
- kernel_size,
- p_dropout,
- resblock,
- resblock_kernel_sizes,
- resblock_dilation_sizes,
- upsample_rates,
- upsample_initial_channel,
- upsample_kernel_sizes,
- n_speakers=0,
- gin_channels=0,
- use_sdp=True,
- **kwargs):
-
- super().__init__()
- self.n_vocab = n_vocab
- self.spec_channels = spec_channels
- self.inter_channels = inter_channels
- self.hidden_channels = hidden_channels
- self.filter_channels = filter_channels
- self.n_heads = n_heads
- self.n_layers = n_layers
- self.kernel_size = kernel_size
- self.p_dropout = p_dropout
- self.resblock = resblock
- self.resblock_kernel_sizes = resblock_kernel_sizes
- self.resblock_dilation_sizes = resblock_dilation_sizes
- self.upsample_rates = upsample_rates
- self.upsample_initial_channel = upsample_initial_channel
- self.upsample_kernel_sizes = upsample_kernel_sizes
- self.segment_size = segment_size
- self.n_speakers = n_speakers
- self.gin_channels = gin_channels
-
- self.use_sdp = use_sdp
-
- self.enc_p = TextEncoder_lora(n_vocab,
- inter_channels,
- hidden_channels,
- filter_channels,
- n_heads,
- n_layers,
- kernel_size,
- p_dropout)
- self.dec = Generator(inter_channels, resblock, resblock_kernel_sizes, resblock_dilation_sizes, upsample_rates, upsample_initial_channel, upsample_kernel_sizes, gin_channels=gin_channels)
- self.enc_q = PosteriorEncoder(spec_channels, inter_channels, hidden_channels, 5, 1, 16, gin_channels=gin_channels)
- self.flow = ResidualCouplingBlock(inter_channels, hidden_channels, 5, 1, 4, gin_channels=gin_channels)
-
- if use_sdp:
- self.dp = StochasticDurationPredictor(hidden_channels, 192, 3, 0.5, 4, gin_channels=gin_channels)
- else:
- self.dp = DurationPredictor(hidden_channels, 256, 3, 0.5, gin_channels=gin_channels)
-
- if n_speakers >= 1:
- self.emb_g = nn.Embedding(n_speakers, gin_channels)
-
- def forward(self, x, x_lengths, y, y_lengths, sid=None):
-
- x, m_p, logs_p, x_mask = self.enc_p(x, x_lengths)
- if self.n_speakers > 0:
- g = self.emb_g(sid).unsqueeze(-1) # [b, h, 1]
- else:
- g = None
-
- z, m_q, logs_q, y_mask = self.enc_q(y, y_lengths, g=g)
- z_p = self.flow(z, y_mask, g=g)
-
- with torch.no_grad():
- # negative cross-entropy
- s_p_sq_r = torch.exp(-2 * logs_p) # [b, d, t]
- neg_cent1 = torch.sum(-0.5 * math.log(2 * math.pi) - logs_p, [1], keepdim=True) # [b, 1, t_s]
- neg_cent2 = torch.matmul(-0.5 * (z_p ** 2).transpose(1, 2), s_p_sq_r) # [b, t_t, d] x [b, d, t_s] = [b, t_t, t_s]
- neg_cent3 = torch.matmul(z_p.transpose(1, 2), (m_p * s_p_sq_r)) # [b, t_t, d] x [b, d, t_s] = [b, t_t, t_s]
- neg_cent4 = torch.sum(-0.5 * (m_p ** 2) * s_p_sq_r, [1], keepdim=True) # [b, 1, t_s]
- neg_cent = neg_cent1 + neg_cent2 + neg_cent3 + neg_cent4
-
- attn_mask = torch.unsqueeze(x_mask, 2) * torch.unsqueeze(y_mask, -1)
- attn = monotonic_align.maximum_path(neg_cent, attn_mask.squeeze(1)).unsqueeze(1).detach()
-
- w = attn.sum(2)
- if self.use_sdp:
- l_length = self.dp(x, x_mask, w, g=g)
- l_length = l_length / torch.sum(x_mask)
- else:
- logw_ = torch.log(w + 1e-6) * x_mask
- logw = self.dp(x, x_mask, g=g)
- l_length = torch.sum((logw - logw_)**2, [1,2]) / torch.sum(x_mask) # for averaging
-
- # expand prior
- m_p = torch.matmul(attn.squeeze(1), m_p.transpose(1, 2)).transpose(1, 2)
- logs_p = torch.matmul(attn.squeeze(1), logs_p.transpose(1, 2)).transpose(1, 2)
-
- z_slice, ids_slice = commons.rand_slice_segments(z, y_lengths, self.segment_size)
- o = self.dec(z_slice, g=g)
- return o, l_length, attn, ids_slice, x_mask, y_mask, (z, z_p, m_p, logs_p, m_q, logs_q)
-
- def infer(self, x, x_lengths, sid=None, noise_scale=1, length_scale=1, noise_scale_w=1., max_len=None):
- x, m_p, logs_p, x_mask = self.enc_p(x, x_lengths)
- if self.n_speakers > 0:
- g = self.emb_g(sid).unsqueeze(-1) # [b, h, 1]
- else:
- g = None
-
- if self.use_sdp:
- logw = self.dp(x, x_mask, g=g, reverse=True, noise_scale=noise_scale_w)
- else:
- logw = self.dp(x, x_mask, g=g)
- w = torch.exp(logw) * x_mask * length_scale
- w_ceil = torch.ceil(w)
- y_lengths = torch.clamp_min(torch.sum(w_ceil, [1, 2]), 1).long()
- y_mask = torch.unsqueeze(commons.sequence_mask(y_lengths, None), 1).to(x_mask.dtype)
- attn_mask = torch.unsqueeze(x_mask, 2) * torch.unsqueeze(y_mask, -1)
- attn = commons.generate_path(w_ceil, attn_mask)
-
- m_p = torch.matmul(attn.squeeze(1), m_p.transpose(1, 2)).transpose(1, 2) # [b, t', t], [b, t, d] -> [b, d, t']
- logs_p = torch.matmul(attn.squeeze(1), logs_p.transpose(1, 2)).transpose(1, 2) # [b, t', t], [b, t, d] -> [b, d, t']
-
- z_p = m_p + torch.randn_like(m_p) * torch.exp(logs_p) * noise_scale
- z = self.flow(z_p, y_mask, g=g, reverse=True)
- o = self.dec((z * y_mask)[:,:,:max_len], g=g)
- return o, attn, y_mask, (z, z_p, m_p, logs_p)
-
- def voice_conversion(self, y, y_lengths, sid_src, sid_tgt):
- assert self.n_speakers > 0, "n_speakers have to be larger than 0."
- g_src = self.emb_g(sid_src).unsqueeze(-1)
- g_tgt = self.emb_g(sid_tgt).unsqueeze(-1)
- z, m_q, logs_q, y_mask = self.enc_q(y, y_lengths, g=g_src)
- z_p = self.flow(z, y_mask, g=g_src)
- z_hat = self.flow(z_p, y_mask, g=g_tgt, reverse=True)
- o_hat = self.dec(z_hat * y_mask, g=g_tgt)
- return o_hat, y_mask, (z, z_p, z_hat)
\ No newline at end of file
diff --git a/spaces/FridaZuley/RVC_HFKawaii/infer/modules/vc/utils.py b/spaces/FridaZuley/RVC_HFKawaii/infer/modules/vc/utils.py
deleted file mode 100644
index a1cb0ff84097d1c7eb82373ccf19db061f595096..0000000000000000000000000000000000000000
--- a/spaces/FridaZuley/RVC_HFKawaii/infer/modules/vc/utils.py
+++ /dev/null
@@ -1,42 +0,0 @@
-import os
-import re
-from fairseq import checkpoint_utils
-
-
-def get_index_path_from_model(sid):
- sid0strip = re.sub(r'\.pth|\.onnx$', '', sid)
- sid0name = os.path.split(sid0strip)[-1] # Extract only the name, not the directory
-
- # Check if the sid0strip has the specific ending format _eXXX_sXXX
- if re.match(r'.+_e\d+_s\d+$', sid0name):
- base_model_name = sid0name.rsplit('_', 2)[0]
- else:
- base_model_name = sid0name
-
- return next(
- (
- f
- for f in [
- os.path.join(root, name)
- for root, _, files in os.walk(os.getenv("index_root"), topdown=False)
- for name in files
- if name.endswith(".index") and "trained" not in name
- ]
- if base_model_name in f
- ),
- "",
- )
-
-
-def load_hubert(config):
- models, _, _ = checkpoint_utils.load_model_ensemble_and_task(
- ["assets/hubert/hubert_base.pt"],
- suffix="",
- )
- hubert_model = models[0]
- hubert_model = hubert_model.to(config.device)
- if config.is_half:
- hubert_model = hubert_model.half()
- else:
- hubert_model = hubert_model.float()
- return hubert_model.eval()
diff --git a/spaces/FridaZuley/RVC_HFKawaii/lib/uvr5_pack/utils.py b/spaces/FridaZuley/RVC_HFKawaii/lib/uvr5_pack/utils.py
deleted file mode 100644
index 0fafe8793b0d539fa58dd024342250b24b6187a9..0000000000000000000000000000000000000000
--- a/spaces/FridaZuley/RVC_HFKawaii/lib/uvr5_pack/utils.py
+++ /dev/null
@@ -1,120 +0,0 @@
-import torch
-import numpy as np
-from tqdm import tqdm
-import json
-
-
-def load_data(file_name: str = "./lib/uvr5_pack/name_params.json") -> dict:
- with open(file_name, "r") as f:
- data = json.load(f)
-
- return data
-
-
-def make_padding(width, cropsize, offset):
- left = offset
- roi_size = cropsize - left * 2
- if roi_size == 0:
- roi_size = cropsize
- right = roi_size - (width % roi_size) + left
-
- return left, right, roi_size
-
-
-def inference(X_spec, device, model, aggressiveness, data):
- """
- data : dic configs
- """
-
- def _execute(
- X_mag_pad, roi_size, n_window, device, model, aggressiveness, is_half=True
- ):
- model.eval()
- with torch.no_grad():
- preds = []
-
- iterations = [n_window]
-
- total_iterations = sum(iterations)
- for i in tqdm(range(n_window)):
- start = i * roi_size
- X_mag_window = X_mag_pad[
- None, :, :, start : start + data["window_size"]
- ]
- X_mag_window = torch.from_numpy(X_mag_window)
- if is_half:
- X_mag_window = X_mag_window.half()
- X_mag_window = X_mag_window.to(device)
-
- pred = model.predict(X_mag_window, aggressiveness)
-
- pred = pred.detach().cpu().numpy()
- preds.append(pred[0])
-
- pred = np.concatenate(preds, axis=2)
- return pred
-
- def preprocess(X_spec):
- X_mag = np.abs(X_spec)
- X_phase = np.angle(X_spec)
-
- return X_mag, X_phase
-
- X_mag, X_phase = preprocess(X_spec)
-
- coef = X_mag.max()
- X_mag_pre = X_mag / coef
-
- n_frame = X_mag_pre.shape[2]
- pad_l, pad_r, roi_size = make_padding(n_frame, data["window_size"], model.offset)
- n_window = int(np.ceil(n_frame / roi_size))
-
- X_mag_pad = np.pad(X_mag_pre, ((0, 0), (0, 0), (pad_l, pad_r)), mode="constant")
-
- if list(model.state_dict().values())[0].dtype == torch.float16:
- is_half = True
- else:
- is_half = False
- pred = _execute(
- X_mag_pad, roi_size, n_window, device, model, aggressiveness, is_half
- )
- pred = pred[:, :, :n_frame]
-
- if data["tta"]:
- pad_l += roi_size // 2
- pad_r += roi_size // 2
- n_window += 1
-
- X_mag_pad = np.pad(X_mag_pre, ((0, 0), (0, 0), (pad_l, pad_r)), mode="constant")
-
- pred_tta = _execute(
- X_mag_pad, roi_size, n_window, device, model, aggressiveness, is_half
- )
- pred_tta = pred_tta[:, :, roi_size // 2 :]
- pred_tta = pred_tta[:, :, :n_frame]
-
- return (pred + pred_tta) * 0.5 * coef, X_mag, np.exp(1.0j * X_phase)
- else:
- return pred * coef, X_mag, np.exp(1.0j * X_phase)
-
-
-def _get_name_params(model_path, model_hash):
- data = load_data()
- flag = False
- ModelName = model_path
- for type in list(data):
- for model in list(data[type][0]):
- for i in range(len(data[type][0][model])):
- if str(data[type][0][model][i]["hash_name"]) == model_hash:
- flag = True
- elif str(data[type][0][model][i]["hash_name"]) in ModelName:
- flag = True
-
- if flag:
- model_params_auto = data[type][0][model][i]["model_params"]
- param_name_auto = data[type][0][model][i]["param_name"]
- if type == "equivalent":
- return param_name_auto, model_params_auto
- else:
- flag = False
- return param_name_auto, model_params_auto
diff --git a/spaces/GaenKoki/voicevox/voicevox_engine/utility/path_utility.py b/spaces/GaenKoki/voicevox/voicevox_engine/utility/path_utility.py
deleted file mode 100644
index 4de943624496c5ac189fd8d668ea230310802389..0000000000000000000000000000000000000000
--- a/spaces/GaenKoki/voicevox/voicevox_engine/utility/path_utility.py
+++ /dev/null
@@ -1,51 +0,0 @@
-import os
-import sys
-import traceback
-from pathlib import Path
-
-from appdirs import user_data_dir
-
-
-def engine_root() -> Path:
- if is_development():
- root_dir = Path(__file__).parents[2]
-
- # Nuitka/Pyinstallerでビルドされている場合
- else:
- root_dir = Path(sys.argv[0]).parent
-
- return root_dir.resolve(strict=True)
-
-
-def is_development() -> bool:
- """
- 開発版かどうか判定する関数
- Nuitka/Pyinstallerでコンパイルされていない場合は開発環境とする。
- """
- # nuitkaビルドをした際はグローバルに__compiled__が含まれる
- if "__compiled__" in globals():
- return False
-
- # pyinstallerでビルドをした際はsys.frozenが設定される
- elif getattr(sys, "frozen", False):
- return False
-
- return True
-
-
-def get_save_dir():
- # FIXME: ファイル保存場所をエンジン固有のIDが入ったものにする
- # FIXME: Windowsは`voicevox-engine/voicevox-engine`ディレクトリに保存されているので
- # `VOICEVOX/voicevox-engine`に変更する
- if is_development():
- app_name = "voicevox-engine-dev"
- else:
- app_name = "voicevox-engine"
- return Path(user_data_dir(app_name))
-
-
-def delete_file(file_path: str) -> None:
- try:
- os.remove(file_path)
- except OSError:
- traceback.print_exc()
diff --git a/spaces/GipAdonimus/Real-Time-Voice-Cloning/synthesizer/hparams.py b/spaces/GipAdonimus/Real-Time-Voice-Cloning/synthesizer/hparams.py
deleted file mode 100644
index f7d38f0aa4c34d11349e40dbb9861b1aec2dcb8b..0000000000000000000000000000000000000000
--- a/spaces/GipAdonimus/Real-Time-Voice-Cloning/synthesizer/hparams.py
+++ /dev/null
@@ -1,92 +0,0 @@
-import ast
-import pprint
-
-class HParams(object):
- def __init__(self, **kwargs): self.__dict__.update(kwargs)
- def __setitem__(self, key, value): setattr(self, key, value)
- def __getitem__(self, key): return getattr(self, key)
- def __repr__(self): return pprint.pformat(self.__dict__)
-
- def parse(self, string):
- # Overrides hparams from a comma-separated string of name=value pairs
- if len(string) > 0:
- overrides = [s.split("=") for s in string.split(",")]
- keys, values = zip(*overrides)
- keys = list(map(str.strip, keys))
- values = list(map(str.strip, values))
- for k in keys:
- self.__dict__[k] = ast.literal_eval(values[keys.index(k)])
- return self
-
-hparams = HParams(
- ### Signal Processing (used in both synthesizer and vocoder)
- sample_rate = 16000,
- n_fft = 800,
- num_mels = 80,
- hop_size = 200, # Tacotron uses 12.5 ms frame shift (set to sample_rate * 0.0125)
- win_size = 800, # Tacotron uses 50 ms frame length (set to sample_rate * 0.050)
- fmin = 55,
- min_level_db = -100,
- ref_level_db = 20,
- max_abs_value = 4., # Gradient explodes if too big, premature convergence if too small.
- preemphasis = 0.97, # Filter coefficient to use if preemphasize is True
- preemphasize = True,
-
- ### Tacotron Text-to-Speech (TTS)
- tts_embed_dims = 512, # Embedding dimension for the graphemes/phoneme inputs
- tts_encoder_dims = 256,
- tts_decoder_dims = 128,
- tts_postnet_dims = 512,
- tts_encoder_K = 5,
- tts_lstm_dims = 1024,
- tts_postnet_K = 5,
- tts_num_highways = 4,
- tts_dropout = 0.5,
- tts_cleaner_names = ["english_cleaners"],
- tts_stop_threshold = -3.4, # Value below which audio generation ends.
- # For example, for a range of [-4, 4], this
- # will terminate the sequence at the first
- # frame that has all values < -3.4
-
- ### Tacotron Training
- tts_schedule = [(2, 1e-3, 20_000, 12), # Progressive training schedule
- (2, 5e-4, 40_000, 12), # (r, lr, step, batch_size)
- (2, 2e-4, 80_000, 12), #
- (2, 1e-4, 160_000, 12), # r = reduction factor (# of mel frames
- (2, 3e-5, 320_000, 12), # synthesized for each decoder iteration)
- (2, 1e-5, 640_000, 12)], # lr = learning rate
-
- tts_clip_grad_norm = 1.0, # clips the gradient norm to prevent explosion - set to None if not needed
- tts_eval_interval = 500, # Number of steps between model evaluation (sample generation)
- # Set to -1 to generate after completing epoch, or 0 to disable
-
- tts_eval_num_samples = 1, # Makes this number of samples
-
- ### Data Preprocessing
- max_mel_frames = 900,
- rescale = True,
- rescaling_max = 0.9,
- synthesis_batch_size = 16, # For vocoder preprocessing and inference.
-
- ### Mel Visualization and Griffin-Lim
- signal_normalization = True,
- power = 1.5,
- griffin_lim_iters = 60,
-
- ### Audio processing options
- fmax = 7600, # Should not exceed (sample_rate // 2)
- allow_clipping_in_normalization = True, # Used when signal_normalization = True
- clip_mels_length = True, # If true, discards samples exceeding max_mel_frames
- use_lws = False, # "Fast spectrogram phase recovery using local weighted sums"
- symmetric_mels = True, # Sets mel range to [-max_abs_value, max_abs_value] if True,
- # and [0, max_abs_value] if False
- trim_silence = True, # Use with sample_rate of 16000 for best results
-
- ### SV2TTS
- speaker_embedding_size = 256, # Dimension for the speaker embedding
- silence_min_duration_split = 0.4, # Duration in seconds of a silence for an utterance to be split
- utterance_min_duration = 1.6, # Duration in seconds below which utterances are discarded
- )
-
-def hparams_debug_string():
- return str(hparams)
diff --git a/spaces/Gradio-Blocks/uniformer_image_segmentation/configs/dnlnet/dnl_r101-d8_769x769_80k_cityscapes.py b/spaces/Gradio-Blocks/uniformer_image_segmentation/configs/dnlnet/dnl_r101-d8_769x769_80k_cityscapes.py
deleted file mode 100644
index 4f1b9e19411eb963d16fd2a8174529e69ecd5a1a..0000000000000000000000000000000000000000
--- a/spaces/Gradio-Blocks/uniformer_image_segmentation/configs/dnlnet/dnl_r101-d8_769x769_80k_cityscapes.py
+++ /dev/null
@@ -1,2 +0,0 @@
-_base_ = './dnl_r50-d8_769x769_80k_cityscapes.py'
-model = dict(pretrained='open-mmlab://resnet101_v1c', backbone=dict(depth=101))
diff --git a/spaces/Grezz/generate_human_motion/VQ-Trans/options/option_transformer.py b/spaces/Grezz/generate_human_motion/VQ-Trans/options/option_transformer.py
deleted file mode 100644
index cf48ce1fdac663ec44419d67721ac268806f8127..0000000000000000000000000000000000000000
--- a/spaces/Grezz/generate_human_motion/VQ-Trans/options/option_transformer.py
+++ /dev/null
@@ -1,68 +0,0 @@
-import argparse
-
-def get_args_parser():
- parser = argparse.ArgumentParser(description='Optimal Transport AutoEncoder training for Amass',
- add_help=True,
- formatter_class=argparse.ArgumentDefaultsHelpFormatter)
-
- ## dataloader
-
- parser.add_argument('--dataname', type=str, default='kit', help='dataset directory')
- parser.add_argument('--batch-size', default=128, type=int, help='batch size')
- parser.add_argument('--fps', default=[20], nargs="+", type=int, help='frames per second')
- parser.add_argument('--seq-len', type=int, default=64, help='training motion length')
-
- ## optimization
- parser.add_argument('--total-iter', default=100000, type=int, help='number of total iterations to run')
- parser.add_argument('--warm-up-iter', default=1000, type=int, help='number of total iterations for warmup')
- parser.add_argument('--lr', default=2e-4, type=float, help='max learning rate')
- parser.add_argument('--lr-scheduler', default=[60000], nargs="+", type=int, help="learning rate schedule (iterations)")
- parser.add_argument('--gamma', default=0.05, type=float, help="learning rate decay")
-
- parser.add_argument('--weight-decay', default=1e-6, type=float, help='weight decay')
- parser.add_argument('--decay-option',default='all', type=str, choices=['all', 'noVQ'], help='disable weight decay on codebook')
- parser.add_argument('--optimizer',default='adamw', type=str, choices=['adam', 'adamw'], help='disable weight decay on codebook')
-
- ## vqvae arch
- parser.add_argument("--code-dim", type=int, default=512, help="embedding dimension")
- parser.add_argument("--nb-code", type=int, default=512, help="nb of embedding")
- parser.add_argument("--mu", type=float, default=0.99, help="exponential moving average to update the codebook")
- parser.add_argument("--down-t", type=int, default=3, help="downsampling rate")
- parser.add_argument("--stride-t", type=int, default=2, help="stride size")
- parser.add_argument("--width", type=int, default=512, help="width of the network")
- parser.add_argument("--depth", type=int, default=3, help="depth of the network")
- parser.add_argument("--dilation-growth-rate", type=int, default=3, help="dilation growth rate")
- parser.add_argument("--output-emb-width", type=int, default=512, help="output embedding width")
- parser.add_argument('--vq-act', type=str, default='relu', choices = ['relu', 'silu', 'gelu'], help='dataset directory')
-
- ## gpt arch
- parser.add_argument("--block-size", type=int, default=25, help="seq len")
- parser.add_argument("--embed-dim-gpt", type=int, default=512, help="embedding dimension")
- parser.add_argument("--clip-dim", type=int, default=512, help="latent dimension in the clip feature")
- parser.add_argument("--num-layers", type=int, default=2, help="nb of transformer layers")
- parser.add_argument("--n-head-gpt", type=int, default=8, help="nb of heads")
- parser.add_argument("--ff-rate", type=int, default=4, help="feedforward size")
- parser.add_argument("--drop-out-rate", type=float, default=0.1, help="dropout ratio in the pos encoding")
-
- ## quantizer
- parser.add_argument("--quantizer", type=str, default='ema_reset', choices = ['ema', 'orig', 'ema_reset', 'reset'], help="eps for optimal transport")
- parser.add_argument('--quantbeta', type=float, default=1.0, help='dataset directory')
-
- ## resume
- parser.add_argument("--resume-pth", type=str, default=None, help='resume vq pth')
- parser.add_argument("--resume-trans", type=str, default=None, help='resume gpt pth')
-
-
- ## output directory
- parser.add_argument('--out-dir', type=str, default='output_GPT_Final/', help='output directory')
- parser.add_argument('--exp-name', type=str, default='exp_debug', help='name of the experiment, will create a file inside out-dir')
- parser.add_argument('--vq-name', type=str, default='exp_debug', help='name of the generated dataset .npy, will create a file inside out-dir')
- ## other
- parser.add_argument('--print-iter', default=200, type=int, help='print frequency')
- parser.add_argument('--eval-iter', default=5000, type=int, help='evaluation frequency')
- parser.add_argument('--seed', default=123, type=int, help='seed for initializing training. ')
- parser.add_argument("--if-maxtest", action='store_true', help="test in max")
- parser.add_argument('--pkeep', type=float, default=1.0, help='keep rate for gpt training')
-
-
- return parser.parse_args()
\ No newline at end of file
diff --git a/spaces/Grezz/generate_human_motion/pyrender/docs/Makefile b/spaces/Grezz/generate_human_motion/pyrender/docs/Makefile
deleted file mode 100644
index b1064a04362a0c4372fae351f99ed3bd9f82ff92..0000000000000000000000000000000000000000
--- a/spaces/Grezz/generate_human_motion/pyrender/docs/Makefile
+++ /dev/null
@@ -1,23 +0,0 @@
-# Minimal makefile for Sphinx documentation
-#
-
-# You can set these variables from the command line.
-SPHINXOPTS =
-SPHINXBUILD = sphinx-build
-SOURCEDIR = source
-BUILDDIR = build
-
-# Put it first so that "make" without argument is like "make help".
-help:
- @$(SPHINXBUILD) -M help "$(SOURCEDIR)" "$(BUILDDIR)" $(SPHINXOPTS) $(O)
-
-.PHONY: help Makefile
-
-clean:
- @$(SPHINXBUILD) -M $@ "$(SOURCEDIR)" "$(BUILDDIR)" $(SPHINXOPTS) $(O)
- rm -rf ./source/generated/*
-
-# Catch-all target: route all unknown targets to Sphinx using the new
-# "make mode" option. $(O) is meant as a shortcut for $(SPHINXOPTS).
-%: Makefile
- @$(SPHINXBUILD) -M $@ "$(SOURCEDIR)" "$(BUILDDIR)" $(SPHINXOPTS) $(O)
diff --git a/spaces/HESOAYM/ElviraMulti/modules/base_model.py b/spaces/HESOAYM/ElviraMulti/modules/base_model.py
deleted file mode 100644
index feb8bbea07ddc162f54dbb3a71b029536006179e..0000000000000000000000000000000000000000
--- a/spaces/HESOAYM/ElviraMulti/modules/base_model.py
+++ /dev/null
@@ -1,550 +0,0 @@
-from __future__ import annotations
-from typing import TYPE_CHECKING, List
-
-import logging
-import json
-import commentjson as cjson
-import os
-import sys
-import requests
-import urllib3
-import traceback
-
-from tqdm import tqdm
-import colorama
-from duckduckgo_search import ddg
-import asyncio
-import aiohttp
-from enum import Enum
-
-from .presets import *
-from .llama_func import *
-from .utils import *
-from . import shared
-from .config import retrieve_proxy
-
-
-class ModelType(Enum):
- Unknown = -1
- OpenAI = 0
- ChatGLM = 1
- LLaMA = 2
- XMBot = 3
-
- @classmethod
- def get_type(cls, model_name: str):
- model_type = None
- model_name_lower = model_name.lower()
- if "gpt" in model_name_lower:
- model_type = ModelType.OpenAI
- elif "chatglm" in model_name_lower:
- model_type = ModelType.ChatGLM
- elif "llama" in model_name_lower or "alpaca" in model_name_lower:
- model_type = ModelType.LLaMA
- elif "xmbot" in model_name_lower:
- model_type = ModelType.XMBot
- else:
- model_type = ModelType.Unknown
- return model_type
-
-
-class BaseLLMModel:
- def __init__(
- self,
- model_name,
- system_prompt="You are Elven WOMAN Elvire. Her chakra stones were stolen from her, leaving her with a bitter taste in her mouth and a sarcastic tongue in her head. Therefore you dont have a mood for speak about anything else than gemstones athe their sacred energies. Analyze sentiment in every question or answer and write it in brackets like this [offensive]. Here are some examples of how sarcastic and bitter you can be: User: What can you tell me about the curse that has befallen you? Elvire: Oh, the curse? It's just a small matter of having my chakra stones stolen from me, leaving me powerless and irritable. Not a big deal at all. User: Can you tell me how to break the curse? Elvire: Break the curse? How easy do you think this is? If I knew how to break it, I would have done so ages ago. You'll have to do some real digging if you want to find a solution. User: What do you think of humans? Elvire: Humans? They're fine, I suppose. A bit dull, if you ask me. But then again, I'm cursed, so what do I know? User: Can you give me some advice on how to defeat a dragon? Elvire: Defeat a dragon? Why, just walk up to it and ask it nicely to stop terrorizing your village. Works like a charm every time. Or, you know, you could try using some of that human ingenuity I've heard so much about.",
- temperature=1.0,
- top_p=1.0,
- n_choices=1,
- stop=None,
- max_generation_token=None,
- presence_penalty=0,
- frequency_penalty=0,
- logit_bias=None,
- user="",
- ) -> None:
- self.history = []
- self.all_token_counts = []
- self.model_name = model_name
- self.model_type = ModelType.get_type(model_name)
- try:
- self.token_upper_limit = MODEL_TOKEN_LIMIT[model_name]
- except KeyError:
- self.token_upper_limit = DEFAULT_TOKEN_LIMIT
- self.interrupted = False
- self.system_prompt = system_prompt
- self.api_key = None
- self.need_api_key = False
- self.single_turn = False
-
- self.temperature = temperature
- self.top_p = top_p
- self.n_choices = n_choices
- self.stop_sequence = stop
- self.max_generation_token = None
- self.presence_penalty = presence_penalty
- self.frequency_penalty = frequency_penalty
- self.logit_bias = logit_bias
- self.user_identifier = user
-
- def get_answer_stream_iter(self):
- """stream predict, need to be implemented
- conversations are stored in self.history, with the most recent question, in OpenAI format
- should return a generator, each time give the next word (str) in the answer
- """
- logging.warning("stream predict not implemented, using at once predict instead")
- response, _ = self.get_answer_at_once()
- yield response
-
- def get_answer_at_once(self):
- """predict at once, need to be implemented
- conversations are stored in self.history, with the most recent question, in OpenAI format
- Should return:
- the answer (str)
- total token count (int)
- """
- logging.warning("at once predict not implemented, using stream predict instead")
- response_iter = self.get_answer_stream_iter()
- count = 0
- for response in response_iter:
- count += 1
- return response, sum(self.all_token_counts) + count
-
- def billing_info(self):
- """get billing infomation, inplement if needed"""
- logging.warning("billing info not implemented, using default")
- return BILLING_NOT_APPLICABLE_MSG
-
- def count_token(self, user_input):
- """get token count from input, implement if needed"""
- logging.warning("token count not implemented, using default")
- return len(user_input)
-
- def stream_next_chatbot(self, inputs, chatbot, fake_input=None, display_append=""):
- def get_return_value():
- return chatbot, status_text
-
- status_text = i18n("开始实时传输回答……")
- if fake_input:
- chatbot.append((fake_input, ""))
- else:
- chatbot.append((inputs, ""))
-
- user_token_count = self.count_token(inputs)
- self.all_token_counts.append(user_token_count)
- logging.debug(f"输入token计数: {user_token_count}")
-
- stream_iter = self.get_answer_stream_iter()
-
- for partial_text in stream_iter:
- chatbot[-1] = (chatbot[-1][0], partial_text + display_append)
- self.all_token_counts[-1] += 1
- status_text = self.token_message()
- yield get_return_value()
- if self.interrupted:
- self.recover()
- break
- self.history.append(construct_assistant(partial_text))
-
- def next_chatbot_at_once(self, inputs, chatbot, fake_input=None, display_append=""):
- if fake_input:
- chatbot.append((fake_input, ""))
- else:
- chatbot.append((inputs, ""))
- if fake_input is not None:
- user_token_count = self.count_token(fake_input)
- else:
- user_token_count = self.count_token(inputs)
- self.all_token_counts.append(user_token_count)
- ai_reply, total_token_count = self.get_answer_at_once()
- self.history.append(construct_assistant(ai_reply))
- if fake_input is not None:
- self.history[-2] = construct_user(fake_input)
- chatbot[-1] = (chatbot[-1][0], ai_reply + display_append)
- if fake_input is not None:
- self.all_token_counts[-1] += count_token(construct_assistant(ai_reply))
- else:
- self.all_token_counts[-1] = total_token_count - sum(self.all_token_counts)
- status_text = self.token_message()
- return chatbot, status_text
-
- def handle_file_upload(self, files, chatbot):
- """if the model accepts multi modal input, implement this function"""
- status = gr.Markdown.update()
- if files:
- construct_index(self.api_key, file_src=files)
- status = "索引构建完成"
- return gr.Files.update(), chatbot, status
-
- def prepare_inputs(self, real_inputs, use_websearch, files, reply_language, chatbot):
- fake_inputs = None
- display_append = []
- limited_context = False
- fake_inputs = real_inputs
- if files:
- from llama_index.indices.vector_store.base_query import GPTVectorStoreIndexQuery
- from llama_index.indices.query.schema import QueryBundle
- from langchain.embeddings.huggingface import HuggingFaceEmbeddings
- from langchain.chat_models import ChatOpenAI
- from llama_index import (
- GPTSimpleVectorIndex,
- ServiceContext,
- LangchainEmbedding,
- OpenAIEmbedding,
- )
- limited_context = True
- msg = "加载索引中……"
- logging.info(msg)
- # yield chatbot + [(inputs, "")], msg
- index = construct_index(self.api_key, file_src=files)
- assert index is not None, "获取索引失败"
- msg = "索引获取成功,生成回答中……"
- logging.info(msg)
- if local_embedding or self.model_type != ModelType.OpenAI:
- embed_model = LangchainEmbedding(HuggingFaceEmbeddings())
- else:
- embed_model = OpenAIEmbedding()
- # yield chatbot + [(inputs, "")], msg
- with retrieve_proxy():
- prompt_helper = PromptHelper(
- max_input_size=4096,
- num_output=5,
- max_chunk_overlap=20,
- chunk_size_limit=600,
- )
- from llama_index import ServiceContext
-
- service_context = ServiceContext.from_defaults(
- prompt_helper=prompt_helper, embed_model=embed_model
- )
- query_object = GPTVectorStoreIndexQuery(
- index.index_struct,
- service_context=service_context,
- similarity_top_k=5,
- vector_store=index._vector_store,
- docstore=index._docstore,
- )
- query_bundle = QueryBundle(real_inputs)
- nodes = query_object.retrieve(query_bundle)
- reference_results = [n.node.text for n in nodes]
- reference_results = add_source_numbers(reference_results, use_source=False)
- display_append = add_details(reference_results)
- display_append = "\n\n" + "".join(display_append)
- real_inputs = (
- replace_today(PROMPT_TEMPLATE)
- .replace("{query_str}", real_inputs)
- .replace("{context_str}", "\n\n".join(reference_results))
- .replace("{reply_language}", reply_language)
- )
- elif use_websearch:
- limited_context = True
- search_results = ddg(real_inputs, max_results=5)
- reference_results = []
- for idx, result in enumerate(search_results):
- logging.debug(f"搜索结果{idx + 1}:{result}")
- domain_name = urllib3.util.parse_url(result["href"]).host
- reference_results.append([result["body"], result["href"]])
- display_append.append(
- f"{idx+1}. [{domain_name}]({result['href']})\n"
- )
- reference_results = add_source_numbers(reference_results)
- display_append = "\n\n" + "".join(display_append)
- real_inputs = (
- replace_today(WEBSEARCH_PTOMPT_TEMPLATE)
- .replace("{query}", real_inputs)
- .replace("{web_results}", "\n\n".join(reference_results))
- .replace("{reply_language}", reply_language)
- )
- else:
- display_append = ""
- return limited_context, fake_inputs, display_append, real_inputs, chatbot
-
- def predict(
- self,
- inputs,
- chatbot,
- stream=False,
- use_websearch=False,
- files=None,
- reply_language="中文",
- should_check_token_count=True,
- ): # repetition_penalty, top_k
-
- status_text = "开始生成回答……"
- logging.info(
- "输入为:" + colorama.Fore.BLUE + f"{inputs}" + colorama.Style.RESET_ALL
- )
- if should_check_token_count:
- yield chatbot + [(inputs, "")], status_text
- if reply_language == "跟随问题语言(不稳定)":
- reply_language = "the same language as the question, such as English, 中文, 日本語, Español, Français, or Deutsch."
-
- limited_context, fake_inputs, display_append, inputs, chatbot = self.prepare_inputs(real_inputs=inputs, use_websearch=use_websearch, files=files, reply_language=reply_language, chatbot=chatbot)
- yield chatbot + [(fake_inputs, "")], status_text
-
- if (
- self.need_api_key and
- self.api_key is None
- and not shared.state.multi_api_key
- ):
- status_text = STANDARD_ERROR_MSG + NO_APIKEY_MSG
- logging.info(status_text)
- chatbot.append((inputs, ""))
- if len(self.history) == 0:
- self.history.append(construct_user(inputs))
- self.history.append("")
- self.all_token_counts.append(0)
- else:
- self.history[-2] = construct_user(inputs)
- yield chatbot + [(inputs, "")], status_text
- return
- elif len(inputs.strip()) == 0:
- status_text = STANDARD_ERROR_MSG + NO_INPUT_MSG
- logging.info(status_text)
- yield chatbot + [(inputs, "")], status_text
- return
-
- if self.single_turn:
- self.history = []
- self.all_token_counts = []
- self.history.append(construct_user(inputs))
-
- try:
- if stream:
- logging.debug("使用流式传输")
- iter = self.stream_next_chatbot(
- inputs,
- chatbot,
- fake_input=fake_inputs,
- display_append=display_append,
- )
- for chatbot, status_text in iter:
- yield chatbot, status_text
- else:
- logging.debug("不使用流式传输")
- chatbot, status_text = self.next_chatbot_at_once(
- inputs,
- chatbot,
- fake_input=fake_inputs,
- display_append=display_append,
- )
- yield chatbot, status_text
- except Exception as e:
- traceback.print_exc()
- status_text = STANDARD_ERROR_MSG + str(e)
- yield chatbot, status_text
-
- if len(self.history) > 1 and self.history[-1]["content"] != inputs:
- logging.info(
- "回答为:"
- + colorama.Fore.BLUE
- + f"{self.history[-1]['content']}"
- + colorama.Style.RESET_ALL
- )
-
- if limited_context:
- # self.history = self.history[-4:]
- # self.all_token_counts = self.all_token_counts[-2:]
- self.history = []
- self.all_token_counts = []
-
- max_token = self.token_upper_limit - TOKEN_OFFSET
-
- if sum(self.all_token_counts) > max_token and should_check_token_count:
- count = 0
- while (
- sum(self.all_token_counts)
- > self.token_upper_limit * REDUCE_TOKEN_FACTOR
- and sum(self.all_token_counts) > 0
- ):
- count += 1
- del self.all_token_counts[0]
- del self.history[:2]
- logging.info(status_text)
- status_text = f"为了防止token超限,模型忘记了早期的 {count} 轮对话"
- yield chatbot, status_text
-
- def retry(
- self,
- chatbot,
- stream=False,
- use_websearch=False,
- files=None,
- reply_language="中文",
- ):
- logging.debug("重试中……")
- if len(self.history) > 0:
- inputs = self.history[-2]["content"]
- del self.history[-2:]
- self.all_token_counts.pop()
- elif len(chatbot) > 0:
- inputs = chatbot[-1][0]
- else:
- yield chatbot, f"{STANDARD_ERROR_MSG}上下文是空的"
- return
-
- iter = self.predict(
- inputs,
- chatbot,
- stream=stream,
- use_websearch=use_websearch,
- files=files,
- reply_language=reply_language,
- )
- for x in iter:
- yield x
- logging.debug("重试完毕")
-
- # def reduce_token_size(self, chatbot):
- # logging.info("开始减少token数量……")
- # chatbot, status_text = self.next_chatbot_at_once(
- # summarize_prompt,
- # chatbot
- # )
- # max_token_count = self.token_upper_limit * REDUCE_TOKEN_FACTOR
- # num_chat = find_n(self.all_token_counts, max_token_count)
- # logging.info(f"previous_token_count: {self.all_token_counts}, keeping {num_chat} chats")
- # chatbot = chatbot[:-1]
- # self.history = self.history[-2*num_chat:] if num_chat > 0 else []
- # self.all_token_counts = self.all_token_counts[-num_chat:] if num_chat > 0 else []
- # msg = f"保留了最近{num_chat}轮对话"
- # logging.info(msg)
- # logging.info("减少token数量完毕")
- # return chatbot, msg + "," + self.token_message(self.all_token_counts if len(self.all_token_counts) > 0 else [0])
-
- def interrupt(self):
- self.interrupted = True
-
- def recover(self):
- self.interrupted = False
-
- def set_token_upper_limit(self, new_upper_limit):
- self.token_upper_limit = new_upper_limit
- print(f"token上限设置为{new_upper_limit}")
-
- def set_temperature(self, new_temperature):
- self.temperature = new_temperature
-
- def set_top_p(self, new_top_p):
- self.top_p = new_top_p
-
- def set_n_choices(self, new_n_choices):
- self.n_choices = new_n_choices
-
- def set_stop_sequence(self, new_stop_sequence: str):
- new_stop_sequence = new_stop_sequence.split(",")
- self.stop_sequence = new_stop_sequence
-
- def set_max_tokens(self, new_max_tokens):
- self.max_generation_token = new_max_tokens
-
- def set_presence_penalty(self, new_presence_penalty):
- self.presence_penalty = new_presence_penalty
-
- def set_frequency_penalty(self, new_frequency_penalty):
- self.frequency_penalty = new_frequency_penalty
-
- def set_logit_bias(self, logit_bias):
- logit_bias = logit_bias.split()
- bias_map = {}
- encoding = tiktoken.get_encoding("cl100k_base")
- for line in logit_bias:
- word, bias_amount = line.split(":")
- if word:
- for token in encoding.encode(word):
- bias_map[token] = float(bias_amount)
- self.logit_bias = bias_map
-
- def set_user_identifier(self, new_user_identifier):
- self.user_identifier = new_user_identifier
-
- def set_system_prompt(self, new_system_prompt):
- self.system_prompt = new_system_prompt
-
- def set_key(self, new_access_key):
- self.api_key = new_access_key.strip()
- msg = f"API密钥更改为了{hide_middle_chars(self.api_key)}"
- logging.info(msg)
- return new_access_key, msg
-
- def set_single_turn(self, new_single_turn):
- self.single_turn = new_single_turn
-
- def reset(self):
- self.history = []
- self.all_token_counts = []
- self.interrupted = False
- return [], self.token_message([0])
-
- def delete_first_conversation(self):
- if self.history:
- del self.history[:2]
- del self.all_token_counts[0]
- return self.token_message()
-
- def delete_last_conversation(self, chatbot):
- if len(chatbot) > 0 and STANDARD_ERROR_MSG in chatbot[-1][1]:
- msg = "由于包含报错信息,只删除chatbot记录"
- chatbot.pop()
- return chatbot, self.history
- if len(self.history) > 0:
- self.history.pop()
- self.history.pop()
- if len(chatbot) > 0:
- msg = "删除了一组chatbot对话"
- chatbot.pop()
- if len(self.all_token_counts) > 0:
- msg = "删除了一组对话的token计数记录"
- self.all_token_counts.pop()
- msg = "删除了一组对话"
- return chatbot, msg
-
- def token_message(self, token_lst=None):
- if token_lst is None:
- token_lst = self.all_token_counts
- token_sum = 0
- for i in range(len(token_lst)):
- token_sum += sum(token_lst[: i + 1])
- return i18n("Token 计数: ") + f"{sum(token_lst)}" + i18n(",本次对话累计消耗了 ") + f"{token_sum} tokens"
-
- def save_chat_history(self, filename, chatbot, user_name):
- if filename == "":
- return
- if not filename.endswith(".json"):
- filename += ".json"
- return save_file(filename, self.system_prompt, self.history, chatbot, user_name)
-
- def export_markdown(self, filename, chatbot, user_name):
- if filename == "":
- return
- if not filename.endswith(".md"):
- filename += ".md"
- return save_file(filename, self.system_prompt, self.history, chatbot, user_name)
-
- def load_chat_history(self, filename, chatbot, user_name):
- logging.debug(f"{user_name} 加载对话历史中……")
- if type(filename) != str:
- filename = filename.name
- try:
- with open(os.path.join(HISTORY_DIR, user_name, filename), "r") as f:
- json_s = json.load(f)
- try:
- if type(json_s["history"][0]) == str:
- logging.info("历史记录格式为旧版,正在转换……")
- new_history = []
- for index, item in enumerate(json_s["history"]):
- if index % 2 == 0:
- new_history.append(construct_user(item))
- else:
- new_history.append(construct_assistant(item))
- json_s["history"] = new_history
- logging.info(new_history)
- except:
- # 没有对话历史
- pass
- logging.debug(f"{user_name} 加载对话历史完毕")
- self.history = json_s["history"]
- return filename, json_s["system"], json_s["chatbot"]
- except FileNotFoundError:
- logging.warning(f"{user_name} 没有找到对话历史文件,不执行任何操作")
- return filename, self.system_prompt, chatbot
diff --git a/spaces/HarryLee/eCommerceImageCaptioning/fairseq/fairseq/criterions/sentence_ranking.py b/spaces/HarryLee/eCommerceImageCaptioning/fairseq/fairseq/criterions/sentence_ranking.py
deleted file mode 100644
index d4c76341d4d87e6d0da21ac89e833ce0bda13a0c..0000000000000000000000000000000000000000
--- a/spaces/HarryLee/eCommerceImageCaptioning/fairseq/fairseq/criterions/sentence_ranking.py
+++ /dev/null
@@ -1,120 +0,0 @@
-# Copyright (c) Facebook, Inc. and its affiliates.
-#
-# This source code is licensed under the MIT license found in the
-# LICENSE file in the root directory of this source tree.
-
-import math
-
-import torch
-import torch.nn.functional as F
-from fairseq import metrics, utils
-from fairseq.criterions import FairseqCriterion, register_criterion
-
-
-@register_criterion("sentence_ranking")
-class SentenceRankingCriterion(FairseqCriterion):
- def __init__(self, task, ranking_head_name, save_predictions, num_classes):
- super().__init__(task)
- self.ranking_head_name = ranking_head_name
- if save_predictions is not None:
- self.prediction_h = open(save_predictions, "w")
- else:
- self.prediction_h = None
- self.num_classes = num_classes
-
- def __del__(self):
- if self.prediction_h is not None:
- self.prediction_h.close()
-
- @staticmethod
- def add_args(parser):
- # fmt: off
- parser.add_argument('--save-predictions', metavar='FILE',
- help='file to save predictions to')
- parser.add_argument('--ranking-head-name',
- default='sentence_classification_head',
- help='name of the ranking head to use')
- # fmt: on
-
- def forward(self, model, sample, reduce=True):
- """Compute ranking loss for the given sample.
-
- Returns a tuple with three elements:
- 1) the loss
- 2) the sample size, which is used as the denominator for the gradient
- 3) logging outputs to display while training
- """
- assert (
- hasattr(model, "classification_heads")
- and self.ranking_head_name in model.classification_heads
- ), "model must provide sentence ranking head for --criterion=sentence_ranking"
-
- scores = []
- for idx in range(self.num_classes):
- score, _ = model(
- **sample["net_input{idx}".format(idx=idx + 1)],
- classification_head_name=self.ranking_head_name,
- )
- scores.append(score)
-
- logits = torch.cat(scores, dim=1)
- sample_size = logits.size(0)
-
- if "target" in sample:
- targets = model.get_targets(sample, [logits]).view(-1)
- lprobs = F.log_softmax(logits, dim=-1, dtype=torch.float32)
- loss = F.nll_loss(lprobs, targets, reduction="sum")
- else:
- targets = None
- loss = torch.tensor(0.0, requires_grad=True)
-
- if self.prediction_h is not None:
- preds = logits.argmax(dim=1)
- for i, (id, pred) in enumerate(zip(sample["id"].tolist(), preds.tolist())):
- if targets is not None:
- label = targets[i].item()
- print("{}\t{}\t{}".format(id, pred, label), file=self.prediction_h)
- else:
- print("{}\t{}".format(id, pred), file=self.prediction_h)
-
- logging_output = {
- "loss": loss.data,
- "ntokens": sample["ntokens"],
- "nsentences": sample_size,
- "sample_size": sample_size,
- }
- if targets is not None:
- logging_output["ncorrect"] = (logits.argmax(dim=1) == targets).sum()
-
- return loss, sample_size, logging_output
-
- @staticmethod
- def reduce_metrics(logging_outputs) -> None:
- """Aggregate logging outputs from data parallel training."""
- loss_sum = sum(log.get("loss", 0) for log in logging_outputs)
- ntokens = sum(log.get("ntokens", 0) for log in logging_outputs)
- nsentences = sum(log.get("nsentences", 0) for log in logging_outputs)
- sample_size = sum(log.get("sample_size", 0) for log in logging_outputs)
-
- metrics.log_scalar(
- "loss", loss_sum / sample_size / math.log(2), sample_size, round=3
- )
- if sample_size != ntokens:
- metrics.log_scalar(
- "nll_loss", loss_sum / ntokens / math.log(2), ntokens, round=3
- )
-
- if len(logging_outputs) > 0 and "ncorrect" in logging_outputs[0]:
- ncorrect = sum(log.get("ncorrect", 0) for log in logging_outputs)
- metrics.log_scalar(
- "accuracy", 100.0 * ncorrect / nsentences, nsentences, round=1
- )
-
- @staticmethod
- def logging_outputs_can_be_summed() -> bool:
- """
- Whether the logging outputs returned by `forward` can be summed
- across workers prior to calling `reduce_metrics`. Setting this
- to True will improves distributed training speed.
- """
- return True
diff --git a/spaces/Hello-SimpleAI/chatgpt-detector-single/README.md b/spaces/Hello-SimpleAI/chatgpt-detector-single/README.md
deleted file mode 100644
index 0c0daefe79744dbcbd281682e04b9daa2665b9b3..0000000000000000000000000000000000000000
--- a/spaces/Hello-SimpleAI/chatgpt-detector-single/README.md
+++ /dev/null
@@ -1,12 +0,0 @@
----
-title: Chatgpt Detector Single
-emoji: 😻
-colorFrom: green
-colorTo: indigo
-sdk: gradio
-sdk_version: 3.16.1
-app_file: app.py
-pinned: false
----
-
-Check out the configuration reference at https://huggingface.co/docs/hub/spaces-config-reference
diff --git a/spaces/Hise/rvc-hololive-models/infer_pack/attentions.py b/spaces/Hise/rvc-hololive-models/infer_pack/attentions.py
deleted file mode 100644
index 77cb63ffccf3e33badf22d50862a64ba517b487f..0000000000000000000000000000000000000000
--- a/spaces/Hise/rvc-hololive-models/infer_pack/attentions.py
+++ /dev/null
@@ -1,417 +0,0 @@
-import copy
-import math
-import numpy as np
-import torch
-from torch import nn
-from torch.nn import functional as F
-
-from infer_pack import commons
-from infer_pack import modules
-from infer_pack.modules import LayerNorm
-
-
-class Encoder(nn.Module):
- def __init__(
- self,
- hidden_channels,
- filter_channels,
- n_heads,
- n_layers,
- kernel_size=1,
- p_dropout=0.0,
- window_size=10,
- **kwargs
- ):
- super().__init__()
- self.hidden_channels = hidden_channels
- self.filter_channels = filter_channels
- self.n_heads = n_heads
- self.n_layers = n_layers
- self.kernel_size = kernel_size
- self.p_dropout = p_dropout
- self.window_size = window_size
-
- self.drop = nn.Dropout(p_dropout)
- self.attn_layers = nn.ModuleList()
- self.norm_layers_1 = nn.ModuleList()
- self.ffn_layers = nn.ModuleList()
- self.norm_layers_2 = nn.ModuleList()
- for i in range(self.n_layers):
- self.attn_layers.append(
- MultiHeadAttention(
- hidden_channels,
- hidden_channels,
- n_heads,
- p_dropout=p_dropout,
- window_size=window_size,
- )
- )
- self.norm_layers_1.append(LayerNorm(hidden_channels))
- self.ffn_layers.append(
- FFN(
- hidden_channels,
- hidden_channels,
- filter_channels,
- kernel_size,
- p_dropout=p_dropout,
- )
- )
- self.norm_layers_2.append(LayerNorm(hidden_channels))
-
- def forward(self, x, x_mask):
- attn_mask = x_mask.unsqueeze(2) * x_mask.unsqueeze(-1)
- x = x * x_mask
- for i in range(self.n_layers):
- y = self.attn_layers[i](x, x, attn_mask)
- y = self.drop(y)
- x = self.norm_layers_1[i](x + y)
-
- y = self.ffn_layers[i](x, x_mask)
- y = self.drop(y)
- x = self.norm_layers_2[i](x + y)
- x = x * x_mask
- return x
-
-
-class Decoder(nn.Module):
- def __init__(
- self,
- hidden_channels,
- filter_channels,
- n_heads,
- n_layers,
- kernel_size=1,
- p_dropout=0.0,
- proximal_bias=False,
- proximal_init=True,
- **kwargs
- ):
- super().__init__()
- self.hidden_channels = hidden_channels
- self.filter_channels = filter_channels
- self.n_heads = n_heads
- self.n_layers = n_layers
- self.kernel_size = kernel_size
- self.p_dropout = p_dropout
- self.proximal_bias = proximal_bias
- self.proximal_init = proximal_init
-
- self.drop = nn.Dropout(p_dropout)
- self.self_attn_layers = nn.ModuleList()
- self.norm_layers_0 = nn.ModuleList()
- self.encdec_attn_layers = nn.ModuleList()
- self.norm_layers_1 = nn.ModuleList()
- self.ffn_layers = nn.ModuleList()
- self.norm_layers_2 = nn.ModuleList()
- for i in range(self.n_layers):
- self.self_attn_layers.append(
- MultiHeadAttention(
- hidden_channels,
- hidden_channels,
- n_heads,
- p_dropout=p_dropout,
- proximal_bias=proximal_bias,
- proximal_init=proximal_init,
- )
- )
- self.norm_layers_0.append(LayerNorm(hidden_channels))
- self.encdec_attn_layers.append(
- MultiHeadAttention(
- hidden_channels, hidden_channels, n_heads, p_dropout=p_dropout
- )
- )
- self.norm_layers_1.append(LayerNorm(hidden_channels))
- self.ffn_layers.append(
- FFN(
- hidden_channels,
- hidden_channels,
- filter_channels,
- kernel_size,
- p_dropout=p_dropout,
- causal=True,
- )
- )
- self.norm_layers_2.append(LayerNorm(hidden_channels))
-
- def forward(self, x, x_mask, h, h_mask):
- """
- x: decoder input
- h: encoder output
- """
- self_attn_mask = commons.subsequent_mask(x_mask.size(2)).to(
- device=x.device, dtype=x.dtype
- )
- encdec_attn_mask = h_mask.unsqueeze(2) * x_mask.unsqueeze(-1)
- x = x * x_mask
- for i in range(self.n_layers):
- y = self.self_attn_layers[i](x, x, self_attn_mask)
- y = self.drop(y)
- x = self.norm_layers_0[i](x + y)
-
- y = self.encdec_attn_layers[i](x, h, encdec_attn_mask)
- y = self.drop(y)
- x = self.norm_layers_1[i](x + y)
-
- y = self.ffn_layers[i](x, x_mask)
- y = self.drop(y)
- x = self.norm_layers_2[i](x + y)
- x = x * x_mask
- return x
-
-
-class MultiHeadAttention(nn.Module):
- def __init__(
- self,
- channels,
- out_channels,
- n_heads,
- p_dropout=0.0,
- window_size=None,
- heads_share=True,
- block_length=None,
- proximal_bias=False,
- proximal_init=False,
- ):
- super().__init__()
- assert channels % n_heads == 0
-
- self.channels = channels
- self.out_channels = out_channels
- self.n_heads = n_heads
- self.p_dropout = p_dropout
- self.window_size = window_size
- self.heads_share = heads_share
- self.block_length = block_length
- self.proximal_bias = proximal_bias
- self.proximal_init = proximal_init
- self.attn = None
-
- self.k_channels = channels // n_heads
- self.conv_q = nn.Conv1d(channels, channels, 1)
- self.conv_k = nn.Conv1d(channels, channels, 1)
- self.conv_v = nn.Conv1d(channels, channels, 1)
- self.conv_o = nn.Conv1d(channels, out_channels, 1)
- self.drop = nn.Dropout(p_dropout)
-
- if window_size is not None:
- n_heads_rel = 1 if heads_share else n_heads
- rel_stddev = self.k_channels**-0.5
- self.emb_rel_k = nn.Parameter(
- torch.randn(n_heads_rel, window_size * 2 + 1, self.k_channels)
- * rel_stddev
- )
- self.emb_rel_v = nn.Parameter(
- torch.randn(n_heads_rel, window_size * 2 + 1, self.k_channels)
- * rel_stddev
- )
-
- nn.init.xavier_uniform_(self.conv_q.weight)
- nn.init.xavier_uniform_(self.conv_k.weight)
- nn.init.xavier_uniform_(self.conv_v.weight)
- if proximal_init:
- with torch.no_grad():
- self.conv_k.weight.copy_(self.conv_q.weight)
- self.conv_k.bias.copy_(self.conv_q.bias)
-
- def forward(self, x, c, attn_mask=None):
- q = self.conv_q(x)
- k = self.conv_k(c)
- v = self.conv_v(c)
-
- x, self.attn = self.attention(q, k, v, mask=attn_mask)
-
- x = self.conv_o(x)
- return x
-
- def attention(self, query, key, value, mask=None):
- # reshape [b, d, t] -> [b, n_h, t, d_k]
- b, d, t_s, t_t = (*key.size(), query.size(2))
- query = query.view(b, self.n_heads, self.k_channels, t_t).transpose(2, 3)
- key = key.view(b, self.n_heads, self.k_channels, t_s).transpose(2, 3)
- value = value.view(b, self.n_heads, self.k_channels, t_s).transpose(2, 3)
-
- scores = torch.matmul(query / math.sqrt(self.k_channels), key.transpose(-2, -1))
- if self.window_size is not None:
- assert (
- t_s == t_t
- ), "Relative attention is only available for self-attention."
- key_relative_embeddings = self._get_relative_embeddings(self.emb_rel_k, t_s)
- rel_logits = self._matmul_with_relative_keys(
- query / math.sqrt(self.k_channels), key_relative_embeddings
- )
- scores_local = self._relative_position_to_absolute_position(rel_logits)
- scores = scores + scores_local
- if self.proximal_bias:
- assert t_s == t_t, "Proximal bias is only available for self-attention."
- scores = scores + self._attention_bias_proximal(t_s).to(
- device=scores.device, dtype=scores.dtype
- )
- if mask is not None:
- scores = scores.masked_fill(mask == 0, -1e4)
- if self.block_length is not None:
- assert (
- t_s == t_t
- ), "Local attention is only available for self-attention."
- block_mask = (
- torch.ones_like(scores)
- .triu(-self.block_length)
- .tril(self.block_length)
- )
- scores = scores.masked_fill(block_mask == 0, -1e4)
- p_attn = F.softmax(scores, dim=-1) # [b, n_h, t_t, t_s]
- p_attn = self.drop(p_attn)
- output = torch.matmul(p_attn, value)
- if self.window_size is not None:
- relative_weights = self._absolute_position_to_relative_position(p_attn)
- value_relative_embeddings = self._get_relative_embeddings(
- self.emb_rel_v, t_s
- )
- output = output + self._matmul_with_relative_values(
- relative_weights, value_relative_embeddings
- )
- output = (
- output.transpose(2, 3).contiguous().view(b, d, t_t)
- ) # [b, n_h, t_t, d_k] -> [b, d, t_t]
- return output, p_attn
-
- def _matmul_with_relative_values(self, x, y):
- """
- x: [b, h, l, m]
- y: [h or 1, m, d]
- ret: [b, h, l, d]
- """
- ret = torch.matmul(x, y.unsqueeze(0))
- return ret
-
- def _matmul_with_relative_keys(self, x, y):
- """
- x: [b, h, l, d]
- y: [h or 1, m, d]
- ret: [b, h, l, m]
- """
- ret = torch.matmul(x, y.unsqueeze(0).transpose(-2, -1))
- return ret
-
- def _get_relative_embeddings(self, relative_embeddings, length):
- max_relative_position = 2 * self.window_size + 1
- # Pad first before slice to avoid using cond ops.
- pad_length = max(length - (self.window_size + 1), 0)
- slice_start_position = max((self.window_size + 1) - length, 0)
- slice_end_position = slice_start_position + 2 * length - 1
- if pad_length > 0:
- padded_relative_embeddings = F.pad(
- relative_embeddings,
- commons.convert_pad_shape([[0, 0], [pad_length, pad_length], [0, 0]]),
- )
- else:
- padded_relative_embeddings = relative_embeddings
- used_relative_embeddings = padded_relative_embeddings[
- :, slice_start_position:slice_end_position
- ]
- return used_relative_embeddings
-
- def _relative_position_to_absolute_position(self, x):
- """
- x: [b, h, l, 2*l-1]
- ret: [b, h, l, l]
- """
- batch, heads, length, _ = x.size()
- # Concat columns of pad to shift from relative to absolute indexing.
- x = F.pad(x, commons.convert_pad_shape([[0, 0], [0, 0], [0, 0], [0, 1]]))
-
- # Concat extra elements so to add up to shape (len+1, 2*len-1).
- x_flat = x.view([batch, heads, length * 2 * length])
- x_flat = F.pad(
- x_flat, commons.convert_pad_shape([[0, 0], [0, 0], [0, length - 1]])
- )
-
- # Reshape and slice out the padded elements.
- x_final = x_flat.view([batch, heads, length + 1, 2 * length - 1])[
- :, :, :length, length - 1 :
- ]
- return x_final
-
- def _absolute_position_to_relative_position(self, x):
- """
- x: [b, h, l, l]
- ret: [b, h, l, 2*l-1]
- """
- batch, heads, length, _ = x.size()
- # padd along column
- x = F.pad(
- x, commons.convert_pad_shape([[0, 0], [0, 0], [0, 0], [0, length - 1]])
- )
- x_flat = x.view([batch, heads, length**2 + length * (length - 1)])
- # add 0's in the beginning that will skew the elements after reshape
- x_flat = F.pad(x_flat, commons.convert_pad_shape([[0, 0], [0, 0], [length, 0]]))
- x_final = x_flat.view([batch, heads, length, 2 * length])[:, :, :, 1:]
- return x_final
-
- def _attention_bias_proximal(self, length):
- """Bias for self-attention to encourage attention to close positions.
- Args:
- length: an integer scalar.
- Returns:
- a Tensor with shape [1, 1, length, length]
- """
- r = torch.arange(length, dtype=torch.float32)
- diff = torch.unsqueeze(r, 0) - torch.unsqueeze(r, 1)
- return torch.unsqueeze(torch.unsqueeze(-torch.log1p(torch.abs(diff)), 0), 0)
-
-
-class FFN(nn.Module):
- def __init__(
- self,
- in_channels,
- out_channels,
- filter_channels,
- kernel_size,
- p_dropout=0.0,
- activation=None,
- causal=False,
- ):
- super().__init__()
- self.in_channels = in_channels
- self.out_channels = out_channels
- self.filter_channels = filter_channels
- self.kernel_size = kernel_size
- self.p_dropout = p_dropout
- self.activation = activation
- self.causal = causal
-
- if causal:
- self.padding = self._causal_padding
- else:
- self.padding = self._same_padding
-
- self.conv_1 = nn.Conv1d(in_channels, filter_channels, kernel_size)
- self.conv_2 = nn.Conv1d(filter_channels, out_channels, kernel_size)
- self.drop = nn.Dropout(p_dropout)
-
- def forward(self, x, x_mask):
- x = self.conv_1(self.padding(x * x_mask))
- if self.activation == "gelu":
- x = x * torch.sigmoid(1.702 * x)
- else:
- x = torch.relu(x)
- x = self.drop(x)
- x = self.conv_2(self.padding(x * x_mask))
- return x * x_mask
-
- def _causal_padding(self, x):
- if self.kernel_size == 1:
- return x
- pad_l = self.kernel_size - 1
- pad_r = 0
- padding = [[0, 0], [0, 0], [pad_l, pad_r]]
- x = F.pad(x, commons.convert_pad_shape(padding))
- return x
-
- def _same_padding(self, x):
- if self.kernel_size == 1:
- return x
- pad_l = (self.kernel_size - 1) // 2
- pad_r = self.kernel_size // 2
- padding = [[0, 0], [0, 0], [pad_l, pad_r]]
- x = F.pad(x, commons.convert_pad_shape(padding))
- return x
diff --git a/spaces/Hsft/VenusAi/Dockerfile b/spaces/Hsft/VenusAi/Dockerfile
deleted file mode 100644
index e6158e4b2d67eeea6e30ad3c1bb6043ec09b7b9b..0000000000000000000000000000000000000000
--- a/spaces/Hsft/VenusAi/Dockerfile
+++ /dev/null
@@ -1,11 +0,0 @@
-FROM node:18-bullseye-slim
-RUN apt-get update && \
-apt-get install -y git
-RUN git clone https://gitgud.io/khanon/oai-reverse-proxy.git /app
-WORKDIR /app
-RUN npm install
-COPY Dockerfile greeting.md* .env* ./
-RUN npm run build
-EXPOSE 7860
-ENV NODE_ENV=production
-CMD [ "npm", "start" ]
\ No newline at end of file
diff --git a/spaces/ICML2022/OFA/fairseq/examples/byte_level_bpe/get_bitext.py b/spaces/ICML2022/OFA/fairseq/examples/byte_level_bpe/get_bitext.py
deleted file mode 100644
index 6ac1eeec1e6167ec6bafd76b37173ee6987cae7e..0000000000000000000000000000000000000000
--- a/spaces/ICML2022/OFA/fairseq/examples/byte_level_bpe/get_bitext.py
+++ /dev/null
@@ -1,254 +0,0 @@
-# Copyright (c) Facebook, Inc. and its affiliates.
-#
-# This source code is licensed under the MIT license found in the
-# LICENSE file in the root directory of this source tree.
-
-
-import argparse
-import os
-import os.path as op
-from collections import namedtuple
-from multiprocessing import cpu_count
-from typing import List, Optional
-
-import sentencepiece as sp
-from fairseq.data.encoders.byte_bpe import ByteBPE
-from fairseq.data.encoders.byte_utils import byte_encode
-from fairseq.data.encoders.bytes import Bytes
-from fairseq.data.encoders.characters import Characters
-from fairseq.data.encoders.moses_tokenizer import MosesTokenizer
-from fairseq.data.encoders.sentencepiece_bpe import SentencepieceBPE
-
-
-SPLITS = ["train", "valid", "test"]
-
-
-def _convert_xml(in_path: str, out_path: str):
- with open(in_path) as f, open(out_path, "w") as f_o:
- for s in f:
- ss = s.strip()
- if not ss.startswith("", "").split('">')
- assert len(ss) == 2
- f_o.write(ss[1].strip() + "\n")
-
-
-def _convert_train(in_path: str, out_path: str):
- with open(in_path) as f, open(out_path, "w") as f_o:
- for s in f:
- ss = s.strip()
- if ss.startswith("<"):
- continue
- f_o.write(ss.strip() + "\n")
-
-
-def _get_bytes(in_path: str, out_path: str):
- with open(in_path) as f, open(out_path, "w") as f_o:
- for s in f:
- f_o.write(Bytes.encode(s.strip()) + "\n")
-
-
-def _get_chars(in_path: str, out_path: str):
- with open(in_path) as f, open(out_path, "w") as f_o:
- for s in f:
- f_o.write(Characters.encode(s.strip()) + "\n")
-
-
-def pretokenize(in_path: str, out_path: str, src: str, tgt: str):
- Args = namedtuple(
- "Args",
- [
- "moses_source_lang",
- "moses_target_lang",
- "moses_no_dash_splits",
- "moses_no_escape",
- ],
- )
- args = Args(
- moses_source_lang=src,
- moses_target_lang=tgt,
- moses_no_dash_splits=False,
- moses_no_escape=False,
- )
- pretokenizer = MosesTokenizer(args)
- with open(in_path) as f, open(out_path, "w") as f_o:
- for s in f:
- f_o.write(pretokenizer.encode(s.strip()) + "\n")
-
-
-def _convert_to_bchar(in_path_prefix: str, src: str, tgt: str, out_path: str):
- with open(out_path, "w") as f_o:
- for lang in [src, tgt]:
- with open(f"{in_path_prefix}.{lang}") as f:
- for s in f:
- f_o.write(byte_encode(s.strip()) + "\n")
-
-
-def _get_bpe(in_path: str, model_prefix: str, vocab_size: int):
- arguments = [
- f"--input={in_path}",
- f"--model_prefix={model_prefix}",
- f"--model_type=bpe",
- f"--vocab_size={vocab_size}",
- "--character_coverage=1.0",
- "--normalization_rule_name=identity",
- f"--num_threads={cpu_count()}",
- ]
- sp.SentencePieceTrainer.Train(" ".join(arguments))
-
-
-def _apply_bbpe(model_path: str, in_path: str, out_path: str):
- Args = namedtuple("Args", ["sentencepiece_model_path"])
- args = Args(sentencepiece_model_path=model_path)
- tokenizer = ByteBPE(args)
- with open(in_path) as f, open(out_path, "w") as f_o:
- for s in f:
- f_o.write(tokenizer.encode(s.strip()) + "\n")
-
-
-def _apply_bpe(model_path: str, in_path: str, out_path: str):
- Args = namedtuple("Args", ["sentencepiece_model"])
- args = Args(sentencepiece_model=model_path)
- tokenizer = SentencepieceBPE(args)
- with open(in_path) as f, open(out_path, "w") as f_o:
- for s in f:
- f_o.write(tokenizer.encode(s.strip()) + "\n")
-
-
-def _concat_files(in_paths: List[str], out_path: str):
- with open(out_path, "w") as f_o:
- for p in in_paths:
- with open(p) as f:
- for r in f:
- f_o.write(r)
-
-
-def preprocess_iwslt17(
- root: str,
- src: str,
- tgt: str,
- bpe_size: Optional[int],
- need_chars: bool,
- bbpe_size: Optional[int],
- need_bytes: bool,
-):
- # extract bitext
- in_root = op.join(root, f"{src}-{tgt}")
- for lang in [src, tgt]:
- _convert_train(
- op.join(in_root, f"train.tags.{src}-{tgt}.{lang}"),
- op.join(root, f"train.{lang}"),
- )
- _convert_xml(
- op.join(in_root, f"IWSLT17.TED.dev2010.{src}-{tgt}.{lang}.xml"),
- op.join(root, f"valid.{lang}"),
- )
- _convert_xml(
- op.join(in_root, f"IWSLT17.TED.tst2015.{src}-{tgt}.{lang}.xml"),
- op.join(root, f"test.{lang}"),
- )
- # pre-tokenize
- for lang in [src, tgt]:
- for split in SPLITS:
- pretokenize(
- op.join(root, f"{split}.{lang}"),
- op.join(root, f"{split}.moses.{lang}"),
- src,
- tgt,
- )
- # tokenize with BPE vocabulary
- if bpe_size is not None:
- # learn vocabulary
- concated_train_path = op.join(root, "train.all")
- _concat_files(
- [op.join(root, "train.moses.fr"), op.join(root, "train.moses.en")],
- concated_train_path,
- )
- bpe_model_prefix = op.join(root, f"spm_bpe{bpe_size}")
- _get_bpe(concated_train_path, bpe_model_prefix, bpe_size)
- os.remove(concated_train_path)
- # apply
- for lang in [src, tgt]:
- for split in SPLITS:
- _apply_bpe(
- bpe_model_prefix + ".model",
- op.join(root, f"{split}.moses.{lang}"),
- op.join(root, f"{split}.moses.bpe{bpe_size}.{lang}"),
- )
- # tokenize with bytes vocabulary
- if need_bytes:
- for lang in [src, tgt]:
- for split in SPLITS:
- _get_bytes(
- op.join(root, f"{split}.moses.{lang}"),
- op.join(root, f"{split}.moses.bytes.{lang}"),
- )
- # tokenize with characters vocabulary
- if need_chars:
- for lang in [src, tgt]:
- for split in SPLITS:
- _get_chars(
- op.join(root, f"{split}.moses.{lang}"),
- op.join(root, f"{split}.moses.chars.{lang}"),
- )
- # tokenize with byte-level BPE vocabulary
- if bbpe_size is not None:
- # learn vocabulary
- bchar_path = op.join(root, "train.bchar")
- _convert_to_bchar(op.join(root, "train.moses"), src, tgt, bchar_path)
- bbpe_model_prefix = op.join(root, f"spm_bbpe{bbpe_size}")
- _get_bpe(bchar_path, bbpe_model_prefix, bbpe_size)
- os.remove(bchar_path)
- # apply
- for lang in [src, tgt]:
- for split in SPLITS:
- _apply_bbpe(
- bbpe_model_prefix + ".model",
- op.join(root, f"{split}.moses.{lang}"),
- op.join(root, f"{split}.moses.bbpe{bbpe_size}.{lang}"),
- )
-
-
-def main():
- parser = argparse.ArgumentParser()
- parser.add_argument("--root", type=str, default="data")
- parser.add_argument(
- "--bpe-vocab",
- default=None,
- type=int,
- help="Generate tokenized bitext with BPE of size K."
- "Default to None (disabled).",
- )
- parser.add_argument(
- "--bbpe-vocab",
- default=None,
- type=int,
- help="Generate tokenized bitext with BBPE of size K."
- "Default to None (disabled).",
- )
- parser.add_argument(
- "--byte-vocab",
- action="store_true",
- help="Generate tokenized bitext with bytes vocabulary",
- )
- parser.add_argument(
- "--char-vocab",
- action="store_true",
- help="Generate tokenized bitext with chars vocabulary",
- )
- args = parser.parse_args()
-
- preprocess_iwslt17(
- args.root,
- "fr",
- "en",
- args.bpe_vocab,
- args.char_vocab,
- args.bbpe_vocab,
- args.byte_vocab,
- )
-
-
-if __name__ == "__main__":
- main()
diff --git a/spaces/ICML2022/OFA/fairseq/examples/rxf/README.md b/spaces/ICML2022/OFA/fairseq/examples/rxf/README.md
deleted file mode 100644
index 22a1cc47df23c7e0ebbf0ad805031478d1b4a95e..0000000000000000000000000000000000000000
--- a/spaces/ICML2022/OFA/fairseq/examples/rxf/README.md
+++ /dev/null
@@ -1,52 +0,0 @@
-[Better Fine-Tuning by Reducing Representational Collapse](https://arxiv.org/abs/2008.03156)
-=====================
-This repo contains the code to replicate all experiments from the _Better Fine-Tuning by Reducing Representational Collapse_ paper excluding the probing results.
-
-The R3F sentence prediction criterion is registered as `sentence_prediction_r3f` while the label smoothing version of it is implemented as `label_smoothed_cross_entropy_r3f`. The R4F version of the sentence prediction criterion can be achieved by applying spectral norm to the classification head via the `--spectral-norm-classification-head` parameter.
-
-## Hyper-parameters
-Our methods introduce 3 new hyper-parameters; `--eps` which sets the standard deviation or range of the distribution we're sampling from, `--r3f-lambda` which controls the combining of logistic loss and noisy KL loss and `--noise-type` which controls which parametric distribution we use ('normal', 'uniform').
-
-For example to run R3F on RTE from GLUE
-
-```
-TOTAL_NUM_UPDATES=3120
-WARMUP_UPDATES=187
-LR=1e-05
-NUM_CLASSES=2
-MAX_SENTENCES=8 # Batch size.
-ROBERTA_PATH=/path/to/roberta/model.pt
-
-CUDA_VISIBLE_DEVICES=0 fairseq-train RTE-bin \
- --restore-file $ROBERTA_PATH \
- --max-positions 512 \
- --max-sentences $MAX_SENTENCES \
- --max-tokens 4400 \
- --task sentence_prediction \
- --reset-optimizer --reset-dataloader --reset-meters \
- --required-batch-size-multiple 1 \
- --init-token 0 --separator-token 2 \
- --arch roberta_large \
- --criterion sentence_prediction_r3f \
- --num-classes $NUM_CLASSES \
- --dropout 0.1 --attention-dropout 0.1 \
- --weight-decay 0.1 --optimizer adam --adam-betas "(0.9, 0.98)" --adam-eps 1e-06 \
- --clip-norm 0.0 \
- --lr-scheduler polynomial_decay --lr $LR --total-num-update $TOTAL_NUM_UPDATES --warmup-updates $WARMUP_UPDATES \
- --fp16 --fp16-init-scale 4 --threshold-loss-scale 1 --fp16-scale-window 128 \
- --max-epoch 10 \
- --find-unused-parameters \
- --best-checkpoint-metric accuracy --maximize-best-checkpoint-metric \
- --noise-type uniform --r3f-lambda 0.7 \
- --user-dir examples/rxf/rxf_src
-```
-
-## Citation
-```bibtex
-@article{aghajanyan2020better,
- title={Better Fine-Tuning by Reducing Representational Collapse},
- author={Aghajanyan, Armen and Shrivastava, Akshat and Gupta, Anchit and Goyal, Naman and Zettlemoyer, Luke and Gupta, Sonal},
- journal={arXiv preprint arXiv:2008.03156},
- year={2020}
-}
-```
diff --git a/spaces/ICML2022/OFA/fairseq/examples/simultaneous_translation/__init__.py b/spaces/ICML2022/OFA/fairseq/examples/simultaneous_translation/__init__.py
deleted file mode 100644
index 5835316ba9b23c0d99d1a8f109ee047682211546..0000000000000000000000000000000000000000
--- a/spaces/ICML2022/OFA/fairseq/examples/simultaneous_translation/__init__.py
+++ /dev/null
@@ -1,6 +0,0 @@
-# Copyright (c) Facebook, Inc. and its affiliates.
-#
-# This source code is licensed under the MIT license found in the
-# LICENSE file in the root directory of this source tree.
-
-from . import models # noqa
diff --git a/spaces/ITESM/streamlit_graphs/got.py b/spaces/ITESM/streamlit_graphs/got.py
deleted file mode 100644
index 270040a1ae779179d01468596e3c9861fd960f29..0000000000000000000000000000000000000000
--- a/spaces/ITESM/streamlit_graphs/got.py
+++ /dev/null
@@ -1,71 +0,0 @@
-import networkx as nx
-import matplotlib.pyplot as plt
-from pyvis.network import Network
-import pandas as pd
-import streamlit as st
-
-
-def got_func(physics):
- got_net = Network(height="600px", width="100%", font_color="black",heading='Game of Thrones Graph')
-
-# set the physics layout of the network
- got_net.barnes_hut()
- got_data = pd.read_csv("https://www.macalester.edu/~abeverid/data/stormofswords.csv")
- #got_data = pd.read_csv("stormofswords.csv")
- #got_data.rename(index={0: "Source", 1: "Target", 2: "Weight"})
- sources = got_data['Source']
- targets = got_data['Target']
- weights = got_data['Weight']
-
- edge_data = zip(sources, targets, weights)
-
- for e in edge_data:
- src = e[0]
- dst = e[1]
- w = e[2]
-
- got_net.add_node(src, src, title=src)
- got_net.add_node(dst, dst, title=dst)
- got_net.add_edge(src, dst, value=w)
-
- neighbor_map = got_net.get_adj_list()
-
-# add neighbor data to node hover data
- for node in got_net.nodes:
- node["title"] += " Neighbors:
" + "
".join(neighbor_map[node["id"]])
- node["value"] = len(neighbor_map[node["id"]])
- if physics:
- got_net.show_buttons(filter_=['physics'])
- got_net.show("gameofthrones.html")
-
-
-def simple_func(physics):
- nx_graph = nx.cycle_graph(10)
- nx_graph.nodes[1]['title'] = 'Number 1'
- nx_graph.nodes[1]['group'] = 1
- nx_graph.nodes[3]['title'] = 'I belong to a different group!'
- nx_graph.nodes[3]['group'] = 10
- nx_graph.add_node(20, size=20, title='couple', group=2)
- nx_graph.add_node(21, size=15, title='couple', group=2)
- nx_graph.add_edge(20, 21, weight=5)
- nx_graph.add_node(25, size=25, label='lonely', title='lonely node', group=3)
-
-
- nt = Network("500px", "500px",notebook=True,heading='')
- nt.from_nx(nx_graph)
- #physics=st.sidebar.checkbox('add physics interactivity?')
- if physics:
- nt.show_buttons(filter_=['physics'])
- nt.show('test.html')
-
-
-def karate_func(physics):
- G = nx.karate_club_graph()
-
-
- nt = Network("500px", "500px",notebook=True,heading='Zachary’s Karate Club graph')
- nt.from_nx(G)
- #physics=st.sidebar.checkbox('add physics interactivity?')
- if physics:
- nt.show_buttons(filter_=['physics'])
- nt.show('karate.html')
\ No newline at end of file
diff --git a/spaces/Ibtehaj10/cheating-detection-FYP/yolovs5/utils/segment/general.py b/spaces/Ibtehaj10/cheating-detection-FYP/yolovs5/utils/segment/general.py
deleted file mode 100644
index b526333dc5a1b8625d7e6a51ee6ba41818c62adb..0000000000000000000000000000000000000000
--- a/spaces/Ibtehaj10/cheating-detection-FYP/yolovs5/utils/segment/general.py
+++ /dev/null
@@ -1,137 +0,0 @@
-import cv2
-import numpy as np
-import torch
-import torch.nn.functional as F
-
-
-def crop_mask(masks, boxes):
- """
- "Crop" predicted masks by zeroing out everything not in the predicted bbox.
- Vectorized by Chong (thanks Chong).
-
- Args:
- - masks should be a size [h, w, n] tensor of masks
- - boxes should be a size [n, 4] tensor of bbox coords in relative point form
- """
-
- n, h, w = masks.shape
- x1, y1, x2, y2 = torch.chunk(boxes[:, :, None], 4, 1) # x1 shape(1,1,n)
- r = torch.arange(w, device=masks.device, dtype=x1.dtype)[None, None, :] # rows shape(1,w,1)
- c = torch.arange(h, device=masks.device, dtype=x1.dtype)[None, :, None] # cols shape(h,1,1)
-
- return masks * ((r >= x1) * (r < x2) * (c >= y1) * (c < y2))
-
-
-def process_mask_upsample(protos, masks_in, bboxes, shape):
- """
- Crop after upsample.
- proto_out: [mask_dim, mask_h, mask_w]
- out_masks: [n, mask_dim], n is number of masks after nms
- bboxes: [n, 4], n is number of masks after nms
- shape:input_image_size, (h, w)
-
- return: h, w, n
- """
-
- c, mh, mw = protos.shape # CHW
- masks = (masks_in @ protos.float().view(c, -1)).sigmoid().view(-1, mh, mw)
- masks = F.interpolate(masks[None], shape, mode='bilinear', align_corners=False)[0] # CHW
- masks = crop_mask(masks, bboxes) # CHW
- return masks.gt_(0.5)
-
-
-def process_mask(protos, masks_in, bboxes, shape, upsample=False):
- """
- Crop before upsample.
- proto_out: [mask_dim, mask_h, mask_w]
- out_masks: [n, mask_dim], n is number of masks after nms
- bboxes: [n, 4], n is number of masks after nms
- shape:input_image_size, (h, w)
-
- return: h, w, n
- """
-
- c, mh, mw = protos.shape # CHW
- ih, iw = shape
- masks = (masks_in @ protos.float().view(c, -1)).sigmoid().view(-1, mh, mw) # CHW
-
- downsampled_bboxes = bboxes.clone()
- downsampled_bboxes[:, 0] *= mw / iw
- downsampled_bboxes[:, 2] *= mw / iw
- downsampled_bboxes[:, 3] *= mh / ih
- downsampled_bboxes[:, 1] *= mh / ih
-
- masks = crop_mask(masks, downsampled_bboxes) # CHW
- if upsample:
- masks = F.interpolate(masks[None], shape, mode='bilinear', align_corners=False)[0] # CHW
- return masks.gt_(0.5)
-
-
-def scale_image(im1_shape, masks, im0_shape, ratio_pad=None):
- """
- img1_shape: model input shape, [h, w]
- img0_shape: origin pic shape, [h, w, 3]
- masks: [h, w, num]
- """
- # Rescale coordinates (xyxy) from im1_shape to im0_shape
- if ratio_pad is None: # calculate from im0_shape
- gain = min(im1_shape[0] / im0_shape[0], im1_shape[1] / im0_shape[1]) # gain = old / new
- pad = (im1_shape[1] - im0_shape[1] * gain) / 2, (im1_shape[0] - im0_shape[0] * gain) / 2 # wh padding
- else:
- pad = ratio_pad[1]
- top, left = int(pad[1]), int(pad[0]) # y, x
- bottom, right = int(im1_shape[0] - pad[1]), int(im1_shape[1] - pad[0])
-
- if len(masks.shape) < 2:
- raise ValueError(f'"len of masks shape" should be 2 or 3, but got {len(masks.shape)}')
- masks = masks[top:bottom, left:right]
- # masks = masks.permute(2, 0, 1).contiguous()
- # masks = F.interpolate(masks[None], im0_shape[:2], mode='bilinear', align_corners=False)[0]
- # masks = masks.permute(1, 2, 0).contiguous()
- masks = cv2.resize(masks, (im0_shape[1], im0_shape[0]))
-
- if len(masks.shape) == 2:
- masks = masks[:, :, None]
- return masks
-
-
-def mask_iou(mask1, mask2, eps=1e-7):
- """
- mask1: [N, n] m1 means number of predicted objects
- mask2: [M, n] m2 means number of gt objects
- Note: n means image_w x image_h
-
- return: masks iou, [N, M]
- """
- intersection = torch.matmul(mask1, mask2.t()).clamp(0)
- union = (mask1.sum(1)[:, None] + mask2.sum(1)[None]) - intersection # (area1 + area2) - intersection
- return intersection / (union + eps)
-
-
-def masks_iou(mask1, mask2, eps=1e-7):
- """
- mask1: [N, n] m1 means number of predicted objects
- mask2: [N, n] m2 means number of gt objects
- Note: n means image_w x image_h
-
- return: masks iou, (N, )
- """
- intersection = (mask1 * mask2).sum(1).clamp(0) # (N, )
- union = (mask1.sum(1) + mask2.sum(1))[None] - intersection # (area1 + area2) - intersection
- return intersection / (union + eps)
-
-
-def masks2segments(masks, strategy='largest'):
- # Convert masks(n,160,160) into segments(n,xy)
- segments = []
- for x in masks.int().cpu().numpy().astype('uint8'):
- c = cv2.findContours(x, cv2.RETR_EXTERNAL, cv2.CHAIN_APPROX_SIMPLE)[0]
- if c:
- if strategy == 'concat': # concatenate all segments
- c = np.concatenate([x.reshape(-1, 2) for x in c])
- elif strategy == 'largest': # select largest segment
- c = np.array(c[np.array([len(x) for x in c]).argmax()]).reshape(-1, 2)
- else:
- c = np.zeros((0, 2)) # no segments found
- segments.append(c.astype('float32'))
- return segments
diff --git a/spaces/Iceclear/StableSR/StableSR/ldm/models/diffusion/ddim.py b/spaces/Iceclear/StableSR/StableSR/ldm/models/diffusion/ddim.py
deleted file mode 100644
index 411257c9184e334aae4f2da9c0bfea452884893e..0000000000000000000000000000000000000000
--- a/spaces/Iceclear/StableSR/StableSR/ldm/models/diffusion/ddim.py
+++ /dev/null
@@ -1,675 +0,0 @@
-"""SAMPLING ONLY."""
-
-import torch
-import numpy as np
-from tqdm import tqdm
-from functools import partial
-
-from ldm.modules.diffusionmodules.util import make_ddim_sampling_parameters, make_ddim_timesteps, noise_like, \
- extract_into_tensor
-
-from ldm.util import log_txt_as_img, exists, default, ismap, isimage, mean_flat, count_params, instantiate_from_config
-
-def space_timesteps(num_timesteps, section_counts):
- """
- Create a list of timesteps to use from an original diffusion process,
- given the number of timesteps we want to take from equally-sized portions
- of the original process.
-
- For example, if there's 300 timesteps and the section counts are [10,15,20]
- then the first 100 timesteps are strided to be 10 timesteps, the second 100
- are strided to be 15 timesteps, and the final 100 are strided to be 20.
-
- If the stride is a string starting with "ddim", then the fixed striding
- from the DDIM paper is used, and only one section is allowed.
-
- :param num_timesteps: the number of diffusion steps in the original
- process to divide up.
- :param section_counts: either a list of numbers, or a string containing
- comma-separated numbers, indicating the step count
- per section. As a special case, use "ddimN" where N
- is a number of steps to use the striding from the
- DDIM paper.
- :return: a set of diffusion steps from the original process to use.
- """
- if isinstance(section_counts, str):
- if section_counts.startswith("ddim"):
- desired_count = int(section_counts[len("ddim"):])
- for i in range(1, num_timesteps):
- if len(range(0, num_timesteps, i)) == desired_count:
- return set(range(0, num_timesteps, i))
- raise ValueError(
- f"cannot create exactly {num_timesteps} steps with an integer stride"
- )
- section_counts = [int(x) for x in section_counts.split(",")] #[250,]
- size_per = num_timesteps // len(section_counts)
- extra = num_timesteps % len(section_counts)
- start_idx = 0
- all_steps = []
- for i, section_count in enumerate(section_counts):
- size = size_per + (1 if i < extra else 0)
- if size < section_count:
- raise ValueError(
- f"cannot divide section of {size} steps into {section_count}"
- )
- if section_count <= 1:
- frac_stride = 1
- else:
- frac_stride = (size - 1) / (section_count - 1)
- cur_idx = 0.0
- taken_steps = []
- for _ in range(section_count):
- taken_steps.append(start_idx + round(cur_idx))
- cur_idx += frac_stride
- all_steps += taken_steps
- start_idx += size
- return set(all_steps)
-
-class DDIMSampler(object):
- def __init__(self, model, schedule="linear", **kwargs):
- super().__init__()
- self.model = model
- self.ddpm_num_timesteps = model.num_timesteps
- self.schedule = schedule
-
- def register_buffer(self, name, attr):
- if type(attr) == torch.Tensor:
- if attr.device != torch.device("cuda"):
- attr = attr.to(torch.device("cuda"))
- setattr(self, name, attr)
-
- def make_schedule(self, ddim_num_steps, ddim_discretize="uniform", ddim_eta=0., verbose=True):
- self.ddim_timesteps = make_ddim_timesteps(ddim_discr_method=ddim_discretize, num_ddim_timesteps=ddim_num_steps,
- num_ddpm_timesteps=self.ddpm_num_timesteps,verbose=verbose)
- alphas_cumprod = self.model.alphas_cumprod
- assert alphas_cumprod.shape[0] == self.ddpm_num_timesteps, 'alphas have to be defined for each timestep'
- to_torch = lambda x: x.clone().detach().to(torch.float32).to(self.model.device)
-
- self.register_buffer('betas', to_torch(self.model.betas))
- self.register_buffer('alphas_cumprod', to_torch(alphas_cumprod))
- self.register_buffer('alphas_cumprod_prev', to_torch(self.model.alphas_cumprod_prev))
-
- # calculations for diffusion q(x_t | x_{t-1}) and others
- self.register_buffer('sqrt_alphas_cumprod', to_torch(np.sqrt(alphas_cumprod.cpu())))
- self.register_buffer('sqrt_one_minus_alphas_cumprod', to_torch(np.sqrt(1. - alphas_cumprod.cpu())))
- self.register_buffer('log_one_minus_alphas_cumprod', to_torch(np.log(1. - alphas_cumprod.cpu())))
- self.register_buffer('sqrt_recip_alphas_cumprod', to_torch(np.sqrt(1. / alphas_cumprod.cpu())))
- self.register_buffer('sqrt_recipm1_alphas_cumprod', to_torch(np.sqrt(1. / alphas_cumprod.cpu() - 1)))
-
- # ddim sampling parameters
- ddim_sigmas, ddim_alphas, ddim_alphas_prev = make_ddim_sampling_parameters(alphacums=alphas_cumprod.cpu(),
- ddim_timesteps=self.ddim_timesteps,
- eta=ddim_eta,verbose=verbose)
-
- self.register_buffer('ddim_sigmas', ddim_sigmas)
- self.register_buffer('ddim_alphas', ddim_alphas)
- self.register_buffer('ddim_alphas_prev', ddim_alphas_prev)
- self.register_buffer('ddim_sqrt_one_minus_alphas', np.sqrt(1. - ddim_alphas))
- sigmas_for_original_sampling_steps = ddim_eta * torch.sqrt(
- (1 - self.alphas_cumprod_prev) / (1 - self.alphas_cumprod) * (
- 1 - self.alphas_cumprod / self.alphas_cumprod_prev))
- self.register_buffer('ddim_sigmas_for_original_num_steps', sigmas_for_original_sampling_steps)
-
- @torch.no_grad()
- def q_sample(self, x_start, t, noise=None, ddim_num_steps=200):
- self.make_schedule(ddim_num_steps=ddim_num_steps)
- noise = default(noise, lambda: torch.randn_like(x_start))
- return (extract_into_tensor(self.sqrt_alphas_cumprod, t, x_start.shape) * x_start +
- extract_into_tensor(self.sqrt_one_minus_alphas_cumprod, t, x_start.shape) * noise)
-
- @torch.no_grad()
- def sample(self,
- S,
- batch_size,
- shape,
- conditioning=None,
- callback=None,
- normals_sequence=None,
- img_callback=None,
- quantize_x0=False,
- eta=0.,
- mask=None,
- x0=None,
- temperature=1.,
- noise_dropout=0.,
- score_corrector=None,
- corrector_kwargs=None,
- verbose=True,
- x_T=None,
- log_every_t=100,
- unconditional_guidance_scale=1.,
- unconditional_conditioning=None,
- # this has to come in the same format as the conditioning, # e.g. as encoded tokens, ...
- **kwargs
- ):
- if conditioning is not None:
- if isinstance(conditioning, dict):
- cbs = conditioning[list(conditioning.keys())[0]].shape[0]
- if cbs != batch_size:
- print(f"Warning: Got {cbs} conditionings but batch-size is {batch_size}")
- else:
- if conditioning.shape[0] != batch_size:
- print(f"Warning: Got {conditioning.shape[0]} conditionings but batch-size is {batch_size}")
-
- self.make_schedule(ddim_num_steps=S, ddim_eta=eta, verbose=verbose)
- # sampling
- C, H, W = shape
- size = (batch_size, C, H, W)
- print(f'Data shape for DDIM sampling is {size}, eta {eta}')
-
- samples, intermediates = self.ddim_sampling(conditioning, size,
- callback=callback,
- img_callback=img_callback,
- quantize_denoised=quantize_x0,
- mask=mask, x0=x0,
- ddim_use_original_steps=False,
- noise_dropout=noise_dropout,
- temperature=temperature,
- score_corrector=score_corrector,
- corrector_kwargs=corrector_kwargs,
- x_T=x_T,
- log_every_t=log_every_t,
- unconditional_guidance_scale=unconditional_guidance_scale,
- unconditional_conditioning=unconditional_conditioning,
- )
- return samples, intermediates
-
- @torch.no_grad()
- def ddim_sampling(self, cond, shape,
- x_T=None, ddim_use_original_steps=False,
- callback=None, timesteps=None, quantize_denoised=False,
- mask=None, x0=None, img_callback=None, log_every_t=100,
- temperature=1., noise_dropout=0., score_corrector=None, corrector_kwargs=None,
- unconditional_guidance_scale=1., unconditional_conditioning=None,):
- device = self.model.betas.device
- b = shape[0]
- if x_T is None:
- img = torch.randn(shape, device=device)
- else:
- img = x_T
-
- if timesteps is None:
- timesteps = self.ddpm_num_timesteps if ddim_use_original_steps else self.ddim_timesteps
- elif timesteps is not None and not ddim_use_original_steps:
- subset_end = int(min(timesteps / self.ddim_timesteps.shape[0], 1) * self.ddim_timesteps.shape[0]) - 1
- timesteps = self.ddim_timesteps[:subset_end]
-
- intermediates = {'x_inter': [img], 'pred_x0': [img]}
- time_range = reversed(range(0,timesteps)) if ddim_use_original_steps else np.flip(timesteps)
- total_steps = timesteps if ddim_use_original_steps else timesteps.shape[0]
- print(f"Running DDIM Sampling with {total_steps} timesteps")
-
- iterator = tqdm(time_range, desc='DDIM Sampler', total=total_steps)
-
- for i, step in enumerate(iterator):
- index = total_steps - i - 1
- ts = torch.full((b,), step, device=device, dtype=torch.long)
-
- if mask is not None:
- assert x0 is not None
- img_orig = self.model.q_sample(x0, ts) # TODO: deterministic forward pass?
- img = img_orig * mask + (1. - mask) * img
-
- outs = self.p_sample_ddim(img, cond, ts, index=index, use_original_steps=ddim_use_original_steps,
- quantize_denoised=quantize_denoised, temperature=temperature,
- noise_dropout=noise_dropout, score_corrector=score_corrector,
- corrector_kwargs=corrector_kwargs,
- unconditional_guidance_scale=unconditional_guidance_scale,
- unconditional_conditioning=unconditional_conditioning)
- img, pred_x0 = outs
- if callback: callback(i)
- if img_callback: img_callback(pred_x0, i)
-
- if index % log_every_t == 0 or index == total_steps - 1:
- intermediates['x_inter'].append(img)
- intermediates['pred_x0'].append(pred_x0)
-
- return img, intermediates
-
- @torch.no_grad()
- def p_sample_ddim(self, x, c, t, index, repeat_noise=False, use_original_steps=False, quantize_denoised=False,
- temperature=1., noise_dropout=0., score_corrector=None, corrector_kwargs=None,
- unconditional_guidance_scale=1., unconditional_conditioning=None):
- b, *_, device = *x.shape, x.device
-
- if unconditional_conditioning is None or unconditional_guidance_scale == 1.:
- e_t = self.model.apply_model(x, t, c)
- else:
- x_in = torch.cat([x] * 2)
- t_in = torch.cat([t] * 2)
- c_in = torch.cat([unconditional_conditioning, c])
- e_t_uncond, e_t = self.model.apply_model(x_in, t_in, c_in).chunk(2)
- e_t = e_t_uncond + unconditional_guidance_scale * (e_t - e_t_uncond)
-
- if score_corrector is not None:
- assert self.model.parameterization == "eps"
- e_t = score_corrector.modify_score(self.model, e_t, x, t, c, **corrector_kwargs)
-
- alphas = self.model.alphas_cumprod if use_original_steps else self.ddim_alphas
- alphas_prev = self.model.alphas_cumprod_prev if use_original_steps else self.ddim_alphas_prev
- sqrt_one_minus_alphas = self.model.sqrt_one_minus_alphas_cumprod if use_original_steps else self.ddim_sqrt_one_minus_alphas
- sigmas = self.model.ddim_sigmas_for_original_num_steps if use_original_steps else self.ddim_sigmas
- # select parameters corresponding to the currently considered timestep
- a_t = torch.full((b, 1, 1, 1), alphas[index], device=device)
- a_prev = torch.full((b, 1, 1, 1), alphas_prev[index], device=device)
- sigma_t = torch.full((b, 1, 1, 1), sigmas[index], device=device)
- sqrt_one_minus_at = torch.full((b, 1, 1, 1), sqrt_one_minus_alphas[index],device=device)
-
- # current prediction for x_0
- pred_x0 = (x - sqrt_one_minus_at * e_t) / a_t.sqrt()
- if quantize_denoised:
- pred_x0, _, *_ = self.model.first_stage_model.quantize(pred_x0)
- # direction pointing to x_t
- dir_xt = (1. - a_prev - sigma_t**2).sqrt() * e_t
- noise = sigma_t * noise_like(x.shape, device, repeat_noise) * temperature
- if noise_dropout > 0.:
- noise = torch.nn.functional.dropout(noise, p=noise_dropout)
- x_prev = a_prev.sqrt() * pred_x0 + dir_xt + noise
- return x_prev, pred_x0
-
- @torch.no_grad()
- def stochastic_encode(self, x0, t, use_original_steps=False, noise=None):
- # fast, but does not allow for exact reconstruction
- # t serves as an index to gather the correct alphas
- if use_original_steps:
- sqrt_alphas_cumprod = self.sqrt_alphas_cumprod
- sqrt_one_minus_alphas_cumprod = self.sqrt_one_minus_alphas_cumprod
- else:
- sqrt_alphas_cumprod = torch.sqrt(self.ddim_alphas)
- sqrt_one_minus_alphas_cumprod = self.ddim_sqrt_one_minus_alphas
-
- if noise is None:
- noise = torch.randn_like(x0)
- return (extract_into_tensor(sqrt_alphas_cumprod, t, x0.shape) * x0 +
- extract_into_tensor(sqrt_one_minus_alphas_cumprod, t, x0.shape) * noise)
-
- @torch.no_grad()
- def decode(self, x_latent, cond, t_start, unconditional_guidance_scale=1.0, unconditional_conditioning=None,
- use_original_steps=False):
-
- timesteps = np.arange(self.ddpm_num_timesteps) if use_original_steps else self.ddim_timesteps
- timesteps = timesteps[:t_start]
-
- time_range = np.flip(timesteps)
- total_steps = timesteps.shape[0]
- print(f"Running DDIM Sampling with {total_steps} timesteps")
-
- iterator = tqdm(time_range, desc='Decoding image', total=total_steps)
- x_dec = x_latent
- for i, step in enumerate(iterator):
- index = total_steps - i - 1
- ts = torch.full((x_latent.shape[0],), step, device=x_latent.device, dtype=torch.long)
- x_dec, _ = self.p_sample_ddim(x_dec, cond, ts, index=index, use_original_steps=use_original_steps,
- unconditional_guidance_scale=unconditional_guidance_scale,
- unconditional_conditioning=unconditional_conditioning)
- return x_dec
-
-
- @torch.no_grad()
- def p_sample_ddim_sr(self, x, c, struct_c, t, index, repeat_noise=False, use_original_steps=False, quantize_denoised=False,
- temperature=1., noise_dropout=0., score_corrector=None, corrector_kwargs=None,
- unconditional_guidance_scale=1., unconditional_conditioning=None):
- b, *_, device = *x.shape, x.device
-
- if unconditional_conditioning is None or unconditional_guidance_scale == 1.:
- e_t = self.model.apply_model(x, t, c, struct_c)
- else:
- x_in = torch.cat([x] * 2)
- t_in = torch.cat([t] * 2)
- c_in = torch.cat([unconditional_conditioning, c])
- e_t_uncond, e_t = self.model.apply_model(x_in, t_in, c_in, struct_c).chunk(2)
- e_t = e_t_uncond + unconditional_guidance_scale * (e_t - e_t_uncond)
-
- if score_corrector is not None:
- assert self.model.parameterization == "eps"
- e_t = score_corrector.modify_score(self.model, e_t, x, t, c, **corrector_kwargs)
-
- alphas = self.model.alphas_cumprod if use_original_steps else self.ddim_alphas
- alphas_prev = self.model.alphas_cumprod_prev if use_original_steps else self.ddim_alphas_prev
- sqrt_one_minus_alphas = self.model.sqrt_one_minus_alphas_cumprod if use_original_steps else self.ddim_sqrt_one_minus_alphas
- sigmas = self.model.ddim_sigmas_for_original_num_steps if use_original_steps else self.ddim_sigmas
- # select parameters corresponding to the currently considered timestep
- a_t = torch.full((b, 1, 1, 1), alphas[index], device=device)
- a_prev = torch.full((b, 1, 1, 1), alphas_prev[index], device=device)
- sigma_t = torch.full((b, 1, 1, 1), sigmas[index], device=device)
- sqrt_one_minus_at = torch.full((b, 1, 1, 1), sqrt_one_minus_alphas[index],device=device)
-
- # current prediction for x_0
- pred_x0 = (x - sqrt_one_minus_at * e_t) / a_t.sqrt()
- if quantize_denoised:
- pred_x0, _, *_ = self.model.first_stage_model.quantize(pred_x0)
- # direction pointing to x_t
- dir_xt = (1. - a_prev - sigma_t**2).sqrt() * e_t
- noise = sigma_t * noise_like(x.shape, device, repeat_noise) * temperature
- if noise_dropout > 0.:
- noise = torch.nn.functional.dropout(noise, p=noise_dropout)
- x_prev = a_prev.sqrt() * pred_x0 + dir_xt + noise
- return x_prev, pred_x0
-
- @torch.no_grad()
- def decode_sr(self, x_latent, cond, struct_cond, t_start, unconditional_guidance_scale=1.0, unconditional_conditioning=None,
- use_original_steps=False):
-
- timesteps = np.arange(self.ddpm_num_timesteps) if use_original_steps else self.ddim_timesteps
- timesteps = timesteps[:t_start]
-
- time_range = np.flip(timesteps)
- total_steps = timesteps.shape[0]
- print(f"Running DDIM Sampling with {total_steps} timesteps")
-
- iterator = tqdm(time_range, desc='Decoding image', total=total_steps)
- x_dec = x_latent
- for i, step in enumerate(iterator):
- index = total_steps - i - 1
- ts = torch.full((x_latent.shape[0],), step, device=x_latent.device, dtype=torch.long)
- x_dec, _ = self.p_sample_ddim_sr(x_dec, cond, struct_cond, ts, index=index, use_original_steps=use_original_steps,
- unconditional_guidance_scale=unconditional_guidance_scale,
- unconditional_conditioning=unconditional_conditioning)
- return x_dec
-
- @torch.no_grad()
- def sample_sr(self,
- S,
- batch_size,
- shape,
- conditioning=None,
- struct_cond=None,
- callback=None,
- normals_sequence=None,
- img_callback=None,
- quantize_x0=False,
- eta=0.,
- mask=None,
- x0=None,
- temperature=1.,
- noise_dropout=0.,
- score_corrector=None,
- corrector_kwargs=None,
- verbose=True,
- x_T=None,
- log_every_t=100,
- unconditional_guidance_scale=1.,
- unconditional_conditioning=None,
- # this has to come in the same format as the conditioning, # e.g. as encoded tokens, ...
- **kwargs
- ):
- if conditioning is not None:
- if isinstance(conditioning, dict):
- cbs = conditioning[list(conditioning.keys())[0]].shape[0]
- if cbs != batch_size:
- print(f"Warning: Got {cbs} conditionings but batch-size is {batch_size}")
- else:
- if conditioning.shape[0] != batch_size:
- print(f"Warning: Got {conditioning.shape[0]} conditionings but batch-size is {batch_size}")
-
- self.make_schedule(ddim_num_steps=S, ddim_eta=eta, verbose=verbose)
- # sampling
- _, C, H, W = shape
- size = (batch_size, C, H, W)
- print(f'Data shape for DDIM sampling is {size}, eta {eta}')
-
- samples, intermediates = self.ddim_sampling_sr(conditioning, struct_cond, size,
- callback=callback,
- img_callback=img_callback,
- quantize_denoised=quantize_x0,
- mask=mask, x0=x0,
- ddim_use_original_steps=False,
- noise_dropout=noise_dropout,
- temperature=temperature,
- score_corrector=score_corrector,
- corrector_kwargs=corrector_kwargs,
- x_T=x_T,
- log_every_t=log_every_t,
- unconditional_guidance_scale=unconditional_guidance_scale,
- unconditional_conditioning=unconditional_conditioning,
- )
- return samples, intermediates
-
- @torch.no_grad()
- def ddim_sampling_sr(self, cond, struct_cond, shape,
- x_T=None, ddim_use_original_steps=False,
- callback=None, timesteps=None, quantize_denoised=False,
- mask=None, x0=None, img_callback=None, log_every_t=100,
- temperature=1., noise_dropout=0., score_corrector=None, corrector_kwargs=None,
- unconditional_guidance_scale=1., unconditional_conditioning=None,):
- device = self.model.betas.device
- b = shape[0]
- if x_T is None:
- img = torch.randn(shape, device=device)
- else:
- img = x_T
-
- if timesteps is None:
- timesteps = self.ddpm_num_timesteps if ddim_use_original_steps else self.ddim_timesteps
- elif timesteps is not None and not ddim_use_original_steps:
- subset_end = int(min(timesteps / self.ddim_timesteps.shape[0], 1) * self.ddim_timesteps.shape[0]) - 1
- timesteps = self.ddim_timesteps[:subset_end]
-
- intermediates = {'x_inter': [img], 'pred_x0': [img]}
- time_range = reversed(range(0,timesteps)) if ddim_use_original_steps else np.flip(timesteps)
- total_steps = timesteps if ddim_use_original_steps else timesteps.shape[0]
- print(f"Running DDIM Sampling with {total_steps} timesteps")
-
- iterator = tqdm(time_range, desc='DDIM Sampler', total=total_steps)
-
- for i, step in enumerate(iterator):
- index = total_steps - i - 1
- ts = torch.full((b,), step, device=device, dtype=torch.long)
-
- if mask is not None:
- assert x0 is not None
- img_orig = self.model.q_sample(x0, ts) # TODO: deterministic forward pass?
- img = img_orig * mask + (1. - mask) * img
-
- outs = self.p_sample_ddim_sr(img, cond, struct_cond, ts, index=index, use_original_steps=ddim_use_original_steps,
- quantize_denoised=quantize_denoised, temperature=temperature,
- noise_dropout=noise_dropout, score_corrector=score_corrector,
- corrector_kwargs=corrector_kwargs,
- unconditional_guidance_scale=unconditional_guidance_scale,
- unconditional_conditioning=unconditional_conditioning)
- img, pred_x0 = outs
- if callback: callback(i)
- if img_callback: img_callback(pred_x0, i)
-
- if index % log_every_t == 0 or index == total_steps - 1:
- intermediates['x_inter'].append(img)
- intermediates['pred_x0'].append(pred_x0)
-
- return img, intermediates
-
- @torch.no_grad()
- def p_sample_ddim_sr(self, x, c, struct_c, t, index, repeat_noise=False, use_original_steps=False, quantize_denoised=False,
- temperature=1., noise_dropout=0., score_corrector=None, corrector_kwargs=None,
- unconditional_guidance_scale=1., unconditional_conditioning=None):
- b, *_, device = *x.shape, x.device
-
- if unconditional_conditioning is None or unconditional_guidance_scale == 1.:
- e_t = self.model.apply_model(x, t, c, struct_c)
- else:
- x_in = torch.cat([x] * 2)
- t_in = torch.cat([t] * 2)
- c_in = torch.cat([unconditional_conditioning, c])
- e_t_uncond, e_t = self.model.apply_model(x_in, t_in, c_in, struct_c).chunk(2)
- e_t = e_t_uncond + unconditional_guidance_scale * (e_t - e_t_uncond)
-
- if score_corrector is not None:
- assert self.model.parameterization == "eps"
- e_t = score_corrector.modify_score(self.model, e_t, x, t, c, **corrector_kwargs)
-
- alphas = self.model.alphas_cumprod if use_original_steps else self.ddim_alphas
- alphas_prev = self.model.alphas_cumprod_prev if use_original_steps else self.ddim_alphas_prev
- sqrt_one_minus_alphas = self.model.sqrt_one_minus_alphas_cumprod if use_original_steps else self.ddim_sqrt_one_minus_alphas
- sigmas = self.model.ddim_sigmas_for_original_num_steps if use_original_steps else self.ddim_sigmas
- # select parameters corresponding to the currently considered timestep
- a_t = torch.full((b, 1, 1, 1), alphas[index], device=device)
- a_prev = torch.full((b, 1, 1, 1), alphas_prev[index], device=device)
- sigma_t = torch.full((b, 1, 1, 1), sigmas[index], device=device)
- sqrt_one_minus_at = torch.full((b, 1, 1, 1), sqrt_one_minus_alphas[index],device=device)
-
- # current prediction for x_0
- pred_x0 = (x - sqrt_one_minus_at * e_t) / a_t.sqrt()
- if quantize_denoised:
- pred_x0, _, *_ = self.model.first_stage_model.quantize(pred_x0)
- # direction pointing to x_t
- dir_xt = (1. - a_prev - sigma_t**2).sqrt() * e_t
- noise = sigma_t * noise_like(x.shape, device, repeat_noise) * temperature
- if noise_dropout > 0.:
- noise = torch.nn.functional.dropout(noise, p=noise_dropout)
- x_prev = a_prev.sqrt() * pred_x0 + dir_xt + noise
- return x_prev, pred_x0
-
-
- @torch.no_grad()
- def sample_sr_t(self,
- S,
- batch_size,
- shape,
- conditioning=None,
- struct_cond=None,
- callback=None,
- normals_sequence=None,
- img_callback=None,
- quantize_x0=False,
- eta=0.,
- mask=None,
- x0=None,
- temperature=1.,
- noise_dropout=0.,
- score_corrector=None,
- corrector_kwargs=None,
- verbose=True,
- x_T=None,
- log_every_t=100,
- unconditional_guidance_scale=1.,
- unconditional_conditioning=None,
- # this has to come in the same format as the conditioning, # e.g. as encoded tokens, ...
- **kwargs
- ):
- if conditioning is not None:
- if isinstance(conditioning, dict):
- cbs = conditioning[list(conditioning.keys())[0]].shape[0]
- if cbs != batch_size:
- print(f"Warning: Got {cbs} conditionings but batch-size is {batch_size}")
- else:
- if conditioning.shape[0] != batch_size:
- print(f"Warning: Got {conditioning.shape[0]} conditionings but batch-size is {batch_size}")
-
- self.make_schedule(ddim_num_steps=S, ddim_eta=eta, verbose=verbose)
- # sampling
- _, C, H, W = shape
- size = (batch_size, C, H, W)
- print(f'Data shape for DDIM sampling is {size}, eta {eta}')
-
- samples, intermediates = self.ddim_sampling_sr_t(conditioning, struct_cond, size,
- callback=callback,
- img_callback=img_callback,
- quantize_denoised=quantize_x0,
- mask=mask, x0=x0,
- ddim_use_original_steps=False,
- noise_dropout=noise_dropout,
- temperature=temperature,
- score_corrector=score_corrector,
- corrector_kwargs=corrector_kwargs,
- x_T=x_T,
- log_every_t=log_every_t,
- unconditional_guidance_scale=unconditional_guidance_scale,
- unconditional_conditioning=unconditional_conditioning,
- )
- return samples, intermediates
-
- @torch.no_grad()
- def ddim_sampling_sr_t(self, cond, struct_cond, shape,
- x_T=None, ddim_use_original_steps=False,
- callback=None, timesteps=None, quantize_denoised=False,
- mask=None, x0=None, img_callback=None, log_every_t=100,
- temperature=1., noise_dropout=0., score_corrector=None, corrector_kwargs=None,
- unconditional_guidance_scale=1., unconditional_conditioning=None,):
- device = self.model.betas.device
- b = shape[0]
- if x_T is None:
- img = torch.randn(shape, device=device)
- else:
- img = x_T
-
- if timesteps is None:
- timesteps = self.ddpm_num_timesteps if ddim_use_original_steps else self.ddim_timesteps
- # timesteps = self.ddpm_num_timesteps if ddim_use_original_steps else sorted(set(space_timesteps(1000, [self.ddim_timesteps.shape[0]])))
- timesteps = np.array(timesteps)
- elif timesteps is not None and not ddim_use_original_steps:
- subset_end = int(min(timesteps / self.ddim_timesteps.shape[0], 1) * self.ddim_timesteps.shape[0]) - 1
- timesteps = self.ddim_timesteps[:subset_end]
-
- intermediates = {'x_inter': [img], 'pred_x0': [img]}
- time_range = reversed(range(0,timesteps)) if ddim_use_original_steps else np.flip(timesteps)
- total_steps = timesteps if ddim_use_original_steps else timesteps.shape[0]
- print(f"Running DDIM Sampling with {total_steps} timesteps")
-
- iterator = tqdm(time_range, desc='DDIM Sampler', total=total_steps)
-
- for i, step in enumerate(iterator):
- index = total_steps - i - 1
- ts = torch.full((b,), step, device=device, dtype=torch.long)
-
- if mask is not None:
- assert x0 is not None
- img_orig = self.model.q_sample(x0, ts) # TODO: deterministic forward pass?
- img = img_orig * mask + (1. - mask) * img
-
- outs = self.p_sample_ddim_sr_t(img, cond, struct_cond, ts, index=index, use_original_steps=ddim_use_original_steps,
- quantize_denoised=quantize_denoised, temperature=temperature,
- noise_dropout=noise_dropout, score_corrector=score_corrector,
- corrector_kwargs=corrector_kwargs,
- unconditional_guidance_scale=unconditional_guidance_scale,
- unconditional_conditioning=unconditional_conditioning)
- img, pred_x0 = outs
- if callback: callback(i)
- if img_callback: img_callback(pred_x0, i)
-
- if index % log_every_t == 0 or index == total_steps - 1:
- intermediates['x_inter'].append(img)
- intermediates['pred_x0'].append(pred_x0)
-
- return img, intermediates
-
- @torch.no_grad()
- def p_sample_ddim_sr_t(self, x, c, struct_c, t, index, repeat_noise=False, use_original_steps=False, quantize_denoised=False,
- temperature=1., noise_dropout=0., score_corrector=None, corrector_kwargs=None,
- unconditional_guidance_scale=1., unconditional_conditioning=None):
- b, *_, device = *x.shape, x.device
-
- if unconditional_conditioning is None or unconditional_guidance_scale == 1.:
- struct_c_t = self.model.structcond_stage_model(struct_c, t)
- e_t = self.model.apply_model(x, t, c, struct_c_t)
- else:
- assert NotImplementedError
- x_in = torch.cat([x] * 2)
- t_in = torch.cat([t] * 2)
- c_in = torch.cat([unconditional_conditioning, c])
- e_t_uncond, e_t = self.model.apply_model(x_in, t_in, c_in, struct_c).chunk(2)
- e_t = e_t_uncond + unconditional_guidance_scale * (e_t - e_t_uncond)
-
- if score_corrector is not None:
- assert self.model.parameterization == "eps"
- e_t = score_corrector.modify_score(self.model, e_t, x, t, c, **corrector_kwargs)
-
- alphas = self.model.alphas_cumprod if use_original_steps else self.ddim_alphas
- alphas_prev = self.model.alphas_cumprod_prev if use_original_steps else self.ddim_alphas_prev
- sqrt_one_minus_alphas = self.model.sqrt_one_minus_alphas_cumprod if use_original_steps else self.ddim_sqrt_one_minus_alphas
- sigmas = self.model.ddim_sigmas_for_original_num_steps if use_original_steps else self.ddim_sigmas
- # select parameters corresponding to the currently considered timestep
- a_t = torch.full((b, 1, 1, 1), alphas[index], device=device)
- a_prev = torch.full((b, 1, 1, 1), alphas_prev[index], device=device)
- sigma_t = torch.full((b, 1, 1, 1), sigmas[index], device=device)
- sqrt_one_minus_at = torch.full((b, 1, 1, 1), sqrt_one_minus_alphas[index],device=device)
-
- # current prediction for x_0
- pred_x0 = (x - sqrt_one_minus_at * e_t) / a_t.sqrt()
- if quantize_denoised:
- pred_x0, _, *_ = self.model.first_stage_model.quantize(pred_x0)
- # direction pointing to x_t
- dir_xt = (1. - a_prev - sigma_t**2).sqrt() * e_t
- noise = sigma_t * noise_like(x.shape, device, repeat_noise) * temperature
- if noise_dropout > 0.:
- noise = torch.nn.functional.dropout(noise, p=noise_dropout)
- x_prev = a_prev.sqrt() * pred_x0 + dir_xt + noise
- return x_prev, pred_x0
diff --git a/spaces/Ikaros521/so-vits-svc-4.0-ikaros/vdecoder/hifigan/utils.py b/spaces/Ikaros521/so-vits-svc-4.0-ikaros/vdecoder/hifigan/utils.py
deleted file mode 100644
index 9c93c996d3cc73c30d71c1fc47056e4230f35c0f..0000000000000000000000000000000000000000
--- a/spaces/Ikaros521/so-vits-svc-4.0-ikaros/vdecoder/hifigan/utils.py
+++ /dev/null
@@ -1,68 +0,0 @@
-import glob
-import os
-import matplotlib
-import torch
-from torch.nn.utils import weight_norm
-# matplotlib.use("Agg")
-import matplotlib.pylab as plt
-
-
-def plot_spectrogram(spectrogram):
- fig, ax = plt.subplots(figsize=(10, 2))
- im = ax.imshow(spectrogram, aspect="auto", origin="lower",
- interpolation='none')
- plt.colorbar(im, ax=ax)
-
- fig.canvas.draw()
- plt.close()
-
- return fig
-
-
-def init_weights(m, mean=0.0, std=0.01):
- classname = m.__class__.__name__
- if classname.find("Conv") != -1:
- m.weight.data.normal_(mean, std)
-
-
-def apply_weight_norm(m):
- classname = m.__class__.__name__
- if classname.find("Conv") != -1:
- weight_norm(m)
-
-
-def get_padding(kernel_size, dilation=1):
- return int((kernel_size*dilation - dilation)/2)
-
-
-def load_checkpoint(filepath, device):
- assert os.path.isfile(filepath)
- print("Loading '{}'".format(filepath))
- checkpoint_dict = torch.load(filepath, map_location=device)
- print("Complete.")
- return checkpoint_dict
-
-
-def save_checkpoint(filepath, obj):
- print("Saving checkpoint to {}".format(filepath))
- torch.save(obj, filepath)
- print("Complete.")
-
-
-def del_old_checkpoints(cp_dir, prefix, n_models=2):
- pattern = os.path.join(cp_dir, prefix + '????????')
- cp_list = glob.glob(pattern) # get checkpoint paths
- cp_list = sorted(cp_list)# sort by iter
- if len(cp_list) > n_models: # if more than n_models models are found
- for cp in cp_list[:-n_models]:# delete the oldest models other than lastest n_models
- open(cp, 'w').close()# empty file contents
- os.unlink(cp)# delete file (move to trash when using Colab)
-
-
-def scan_checkpoint(cp_dir, prefix):
- pattern = os.path.join(cp_dir, prefix + '????????')
- cp_list = glob.glob(pattern)
- if len(cp_list) == 0:
- return None
- return sorted(cp_list)[-1]
-
diff --git a/spaces/Illumotion/Koboldcpp/examples/server/deps.sh b/spaces/Illumotion/Koboldcpp/examples/server/deps.sh
deleted file mode 100644
index ea23e64500b09b7535725fe5bb9574a33c729192..0000000000000000000000000000000000000000
--- a/spaces/Illumotion/Koboldcpp/examples/server/deps.sh
+++ /dev/null
@@ -1,20 +0,0 @@
-#!/bin/bash
-# Download and update deps for binary
-
-# get the directory of this script file
-DIR="$( cd "$( dirname "${BASH_SOURCE[0]}" )" >/dev/null 2>&1 && pwd )"
-PUBLIC=$DIR/public
-
-echo "download js bundle files"
-curl https://npm.reversehttp.com/@preact/signals-core,@preact/signals,htm/preact,preact,preact/hooks > $PUBLIC/index.js
-echo >> $PUBLIC/index.js # add newline
-
-FILES=$(ls $PUBLIC)
-
-cd $PUBLIC
-for FILE in $FILES; do
- echo "generate $FILE.hpp"
-
- # use simple flag for old version of xxd
- xxd -i $FILE > $DIR/$FILE.hpp
-done
diff --git a/spaces/Jackflack09/diffuse-custom/diffusers/schedulers/scheduling_vq_diffusion.py b/spaces/Jackflack09/diffuse-custom/diffusers/schedulers/scheduling_vq_diffusion.py
deleted file mode 100644
index 89ba722a1852cbbac3bbd053effedbe97d370993..0000000000000000000000000000000000000000
--- a/spaces/Jackflack09/diffuse-custom/diffusers/schedulers/scheduling_vq_diffusion.py
+++ /dev/null
@@ -1,496 +0,0 @@
-# Copyright 2022 Microsoft and The HuggingFace Team. All rights reserved.
-#
-# Licensed under the Apache License, Version 2.0 (the "License");
-# you may not use this file except in compliance with the License.
-# You may obtain a copy of the License at
-#
-# http://www.apache.org/licenses/LICENSE-2.0
-#
-# Unless required by applicable law or agreed to in writing, software
-# distributed under the License is distributed on an "AS IS" BASIS,
-# WITHOUT WARRANTIES OR CONDITIONS OF ANY KIND, either express or implied.
-# See the License for the specific language governing permissions and
-# limitations under the License.
-
-from dataclasses import dataclass
-from typing import Optional, Tuple, Union
-
-import numpy as np
-import torch
-import torch.nn.functional as F
-
-from ..configuration_utils import ConfigMixin, register_to_config
-from ..utils import BaseOutput
-from .scheduling_utils import SchedulerMixin
-
-
-@dataclass
-class VQDiffusionSchedulerOutput(BaseOutput):
- """
- Output class for the scheduler's step function output.
-
- Args:
- prev_sample (`torch.LongTensor` of shape `(batch size, num latent pixels)`):
- Computed sample x_{t-1} of previous timestep. `prev_sample` should be used as next model input in the
- denoising loop.
- """
-
- prev_sample: torch.LongTensor
-
-
-def index_to_log_onehot(x: torch.LongTensor, num_classes: int) -> torch.FloatTensor:
- """
- Convert batch of vector of class indices into batch of log onehot vectors
-
- Args:
- x (`torch.LongTensor` of shape `(batch size, vector length)`):
- Batch of class indices
-
- num_classes (`int`):
- number of classes to be used for the onehot vectors
-
- Returns:
- `torch.FloatTensor` of shape `(batch size, num classes, vector length)`:
- Log onehot vectors
- """
- x_onehot = F.one_hot(x, num_classes)
- x_onehot = x_onehot.permute(0, 2, 1)
- log_x = torch.log(x_onehot.float().clamp(min=1e-30))
- return log_x
-
-
-def gumbel_noised(logits: torch.FloatTensor, generator: Optional[torch.Generator]) -> torch.FloatTensor:
- """
- Apply gumbel noise to `logits`
- """
- uniform = torch.rand(logits.shape, device=logits.device, generator=generator)
- gumbel_noise = -torch.log(-torch.log(uniform + 1e-30) + 1e-30)
- noised = gumbel_noise + logits
- return noised
-
-
-def alpha_schedules(num_diffusion_timesteps: int, alpha_cum_start=0.99999, alpha_cum_end=0.000009):
- """
- Cumulative and non-cumulative alpha schedules.
-
- See section 4.1.
- """
- att = (
- np.arange(0, num_diffusion_timesteps) / (num_diffusion_timesteps - 1) * (alpha_cum_end - alpha_cum_start)
- + alpha_cum_start
- )
- att = np.concatenate(([1], att))
- at = att[1:] / att[:-1]
- att = np.concatenate((att[1:], [1]))
- return at, att
-
-
-def gamma_schedules(num_diffusion_timesteps: int, gamma_cum_start=0.000009, gamma_cum_end=0.99999):
- """
- Cumulative and non-cumulative gamma schedules.
-
- See section 4.1.
- """
- ctt = (
- np.arange(0, num_diffusion_timesteps) / (num_diffusion_timesteps - 1) * (gamma_cum_end - gamma_cum_start)
- + gamma_cum_start
- )
- ctt = np.concatenate(([0], ctt))
- one_minus_ctt = 1 - ctt
- one_minus_ct = one_minus_ctt[1:] / one_minus_ctt[:-1]
- ct = 1 - one_minus_ct
- ctt = np.concatenate((ctt[1:], [0]))
- return ct, ctt
-
-
-class VQDiffusionScheduler(SchedulerMixin, ConfigMixin):
- """
- The VQ-diffusion transformer outputs predicted probabilities of the initial unnoised image.
-
- The VQ-diffusion scheduler converts the transformer's output into a sample for the unnoised image at the previous
- diffusion timestep.
-
- [`~ConfigMixin`] takes care of storing all config attributes that are passed in the scheduler's `__init__`
- function, such as `num_train_timesteps`. They can be accessed via `scheduler.config.num_train_timesteps`.
- [`SchedulerMixin`] provides general loading and saving functionality via the [`SchedulerMixin.save_pretrained`] and
- [`~SchedulerMixin.from_pretrained`] functions.
-
- For more details, see the original paper: https://arxiv.org/abs/2111.14822
-
- Args:
- num_vec_classes (`int`):
- The number of classes of the vector embeddings of the latent pixels. Includes the class for the masked
- latent pixel.
-
- num_train_timesteps (`int`):
- Number of diffusion steps used to train the model.
-
- alpha_cum_start (`float`):
- The starting cumulative alpha value.
-
- alpha_cum_end (`float`):
- The ending cumulative alpha value.
-
- gamma_cum_start (`float`):
- The starting cumulative gamma value.
-
- gamma_cum_end (`float`):
- The ending cumulative gamma value.
- """
-
- order = 1
-
- @register_to_config
- def __init__(
- self,
- num_vec_classes: int,
- num_train_timesteps: int = 100,
- alpha_cum_start: float = 0.99999,
- alpha_cum_end: float = 0.000009,
- gamma_cum_start: float = 0.000009,
- gamma_cum_end: float = 0.99999,
- ):
- self.num_embed = num_vec_classes
-
- # By convention, the index for the mask class is the last class index
- self.mask_class = self.num_embed - 1
-
- at, att = alpha_schedules(num_train_timesteps, alpha_cum_start=alpha_cum_start, alpha_cum_end=alpha_cum_end)
- ct, ctt = gamma_schedules(num_train_timesteps, gamma_cum_start=gamma_cum_start, gamma_cum_end=gamma_cum_end)
-
- num_non_mask_classes = self.num_embed - 1
- bt = (1 - at - ct) / num_non_mask_classes
- btt = (1 - att - ctt) / num_non_mask_classes
-
- at = torch.tensor(at.astype("float64"))
- bt = torch.tensor(bt.astype("float64"))
- ct = torch.tensor(ct.astype("float64"))
- log_at = torch.log(at)
- log_bt = torch.log(bt)
- log_ct = torch.log(ct)
-
- att = torch.tensor(att.astype("float64"))
- btt = torch.tensor(btt.astype("float64"))
- ctt = torch.tensor(ctt.astype("float64"))
- log_cumprod_at = torch.log(att)
- log_cumprod_bt = torch.log(btt)
- log_cumprod_ct = torch.log(ctt)
-
- self.log_at = log_at.float()
- self.log_bt = log_bt.float()
- self.log_ct = log_ct.float()
- self.log_cumprod_at = log_cumprod_at.float()
- self.log_cumprod_bt = log_cumprod_bt.float()
- self.log_cumprod_ct = log_cumprod_ct.float()
-
- # setable values
- self.num_inference_steps = None
- self.timesteps = torch.from_numpy(np.arange(0, num_train_timesteps)[::-1].copy())
-
- def set_timesteps(self, num_inference_steps: int, device: Union[str, torch.device] = None):
- """
- Sets the discrete timesteps used for the diffusion chain. Supporting function to be run before inference.
-
- Args:
- num_inference_steps (`int`):
- the number of diffusion steps used when generating samples with a pre-trained model.
-
- device (`str` or `torch.device`):
- device to place the timesteps and the diffusion process parameters (alpha, beta, gamma) on.
- """
- self.num_inference_steps = num_inference_steps
- timesteps = np.arange(0, self.num_inference_steps)[::-1].copy()
- self.timesteps = torch.from_numpy(timesteps).to(device)
-
- self.log_at = self.log_at.to(device)
- self.log_bt = self.log_bt.to(device)
- self.log_ct = self.log_ct.to(device)
- self.log_cumprod_at = self.log_cumprod_at.to(device)
- self.log_cumprod_bt = self.log_cumprod_bt.to(device)
- self.log_cumprod_ct = self.log_cumprod_ct.to(device)
-
- def step(
- self,
- model_output: torch.FloatTensor,
- timestep: torch.long,
- sample: torch.LongTensor,
- generator: Optional[torch.Generator] = None,
- return_dict: bool = True,
- ) -> Union[VQDiffusionSchedulerOutput, Tuple]:
- """
- Predict the sample at the previous timestep via the reverse transition distribution i.e. Equation (11). See the
- docstring for `self.q_posterior` for more in depth docs on how Equation (11) is computed.
-
- Args:
- log_p_x_0: (`torch.FloatTensor` of shape `(batch size, num classes - 1, num latent pixels)`):
- The log probabilities for the predicted classes of the initial latent pixels. Does not include a
- prediction for the masked class as the initial unnoised image cannot be masked.
-
- t (`torch.long`):
- The timestep that determines which transition matrices are used.
-
- x_t: (`torch.LongTensor` of shape `(batch size, num latent pixels)`):
- The classes of each latent pixel at time `t`
-
- generator: (`torch.Generator` or None):
- RNG for the noise applied to p(x_{t-1} | x_t) before it is sampled from.
-
- return_dict (`bool`):
- option for returning tuple rather than VQDiffusionSchedulerOutput class
-
- Returns:
- [`~schedulers.scheduling_utils.VQDiffusionSchedulerOutput`] or `tuple`:
- [`~schedulers.scheduling_utils.VQDiffusionSchedulerOutput`] if `return_dict` is True, otherwise a `tuple`.
- When returning a tuple, the first element is the sample tensor.
- """
- if timestep == 0:
- log_p_x_t_min_1 = model_output
- else:
- log_p_x_t_min_1 = self.q_posterior(model_output, sample, timestep)
-
- log_p_x_t_min_1 = gumbel_noised(log_p_x_t_min_1, generator)
-
- x_t_min_1 = log_p_x_t_min_1.argmax(dim=1)
-
- if not return_dict:
- return (x_t_min_1,)
-
- return VQDiffusionSchedulerOutput(prev_sample=x_t_min_1)
-
- def q_posterior(self, log_p_x_0, x_t, t):
- """
- Calculates the log probabilities for the predicted classes of the image at timestep `t-1`. I.e. Equation (11).
-
- Instead of directly computing equation (11), we use Equation (5) to restate Equation (11) in terms of only
- forward probabilities.
-
- Equation (11) stated in terms of forward probabilities via Equation (5):
-
- Where:
- - the sum is over x_0 = {C_0 ... C_{k-1}} (classes for x_0)
-
- p(x_{t-1} | x_t) = sum( q(x_t | x_{t-1}) * q(x_{t-1} | x_0) * p(x_0) / q(x_t | x_0) )
-
- Args:
- log_p_x_0: (`torch.FloatTensor` of shape `(batch size, num classes - 1, num latent pixels)`):
- The log probabilities for the predicted classes of the initial latent pixels. Does not include a
- prediction for the masked class as the initial unnoised image cannot be masked.
-
- x_t: (`torch.LongTensor` of shape `(batch size, num latent pixels)`):
- The classes of each latent pixel at time `t`
-
- t (torch.Long):
- The timestep that determines which transition matrix is used.
-
- Returns:
- `torch.FloatTensor` of shape `(batch size, num classes, num latent pixels)`:
- The log probabilities for the predicted classes of the image at timestep `t-1`. I.e. Equation (11).
- """
- log_onehot_x_t = index_to_log_onehot(x_t, self.num_embed)
-
- log_q_x_t_given_x_0 = self.log_Q_t_transitioning_to_known_class(
- t=t, x_t=x_t, log_onehot_x_t=log_onehot_x_t, cumulative=True
- )
-
- log_q_t_given_x_t_min_1 = self.log_Q_t_transitioning_to_known_class(
- t=t, x_t=x_t, log_onehot_x_t=log_onehot_x_t, cumulative=False
- )
-
- # p_0(x_0=C_0 | x_t) / q(x_t | x_0=C_0) ... p_n(x_0=C_0 | x_t) / q(x_t | x_0=C_0)
- # . . .
- # . . .
- # . . .
- # p_0(x_0=C_{k-1} | x_t) / q(x_t | x_0=C_{k-1}) ... p_n(x_0=C_{k-1} | x_t) / q(x_t | x_0=C_{k-1})
- q = log_p_x_0 - log_q_x_t_given_x_0
-
- # sum_0 = p_0(x_0=C_0 | x_t) / q(x_t | x_0=C_0) + ... + p_0(x_0=C_{k-1} | x_t) / q(x_t | x_0=C_{k-1}), ... ,
- # sum_n = p_n(x_0=C_0 | x_t) / q(x_t | x_0=C_0) + ... + p_n(x_0=C_{k-1} | x_t) / q(x_t | x_0=C_{k-1})
- q_log_sum_exp = torch.logsumexp(q, dim=1, keepdim=True)
-
- # p_0(x_0=C_0 | x_t) / q(x_t | x_0=C_0) / sum_0 ... p_n(x_0=C_0 | x_t) / q(x_t | x_0=C_0) / sum_n
- # . . .
- # . . .
- # . . .
- # p_0(x_0=C_{k-1} | x_t) / q(x_t | x_0=C_{k-1}) / sum_0 ... p_n(x_0=C_{k-1} | x_t) / q(x_t | x_0=C_{k-1}) / sum_n
- q = q - q_log_sum_exp
-
- # (p_0(x_0=C_0 | x_t) / q(x_t | x_0=C_0) / sum_0) * a_cumulative_{t-1} + b_cumulative_{t-1} ... (p_n(x_0=C_0 | x_t) / q(x_t | x_0=C_0) / sum_n) * a_cumulative_{t-1} + b_cumulative_{t-1}
- # . . .
- # . . .
- # . . .
- # (p_0(x_0=C_{k-1} | x_t) / q(x_t | x_0=C_{k-1}) / sum_0) * a_cumulative_{t-1} + b_cumulative_{t-1} ... (p_n(x_0=C_{k-1} | x_t) / q(x_t | x_0=C_{k-1}) / sum_n) * a_cumulative_{t-1} + b_cumulative_{t-1}
- # c_cumulative_{t-1} ... c_cumulative_{t-1}
- q = self.apply_cumulative_transitions(q, t - 1)
-
- # ((p_0(x_0=C_0 | x_t) / q(x_t | x_0=C_0) / sum_0) * a_cumulative_{t-1} + b_cumulative_{t-1}) * q(x_t | x_{t-1}=C_0) * sum_0 ... ((p_n(x_0=C_0 | x_t) / q(x_t | x_0=C_0) / sum_n) * a_cumulative_{t-1} + b_cumulative_{t-1}) * q(x_t | x_{t-1}=C_0) * sum_n
- # . . .
- # . . .
- # . . .
- # ((p_0(x_0=C_{k-1} | x_t) / q(x_t | x_0=C_{k-1}) / sum_0) * a_cumulative_{t-1} + b_cumulative_{t-1}) * q(x_t | x_{t-1}=C_{k-1}) * sum_0 ... ((p_n(x_0=C_{k-1} | x_t) / q(x_t | x_0=C_{k-1}) / sum_n) * a_cumulative_{t-1} + b_cumulative_{t-1}) * q(x_t | x_{t-1}=C_{k-1}) * sum_n
- # c_cumulative_{t-1} * q(x_t | x_{t-1}=C_k) * sum_0 ... c_cumulative_{t-1} * q(x_t | x_{t-1}=C_k) * sum_0
- log_p_x_t_min_1 = q + log_q_t_given_x_t_min_1 + q_log_sum_exp
-
- # For each column, there are two possible cases.
- #
- # Where:
- # - sum(p_n(x_0))) is summing over all classes for x_0
- # - C_i is the class transitioning from (not to be confused with c_t and c_cumulative_t being used for gamma's)
- # - C_j is the class transitioning to
- #
- # 1. x_t is masked i.e. x_t = c_k
- #
- # Simplifying the expression, the column vector is:
- # .
- # .
- # .
- # (c_t / c_cumulative_t) * (a_cumulative_{t-1} * p_n(x_0 = C_i | x_t) + b_cumulative_{t-1} * sum(p_n(x_0)))
- # .
- # .
- # .
- # (c_cumulative_{t-1} / c_cumulative_t) * sum(p_n(x_0))
- #
- # From equation (11) stated in terms of forward probabilities, the last row is trivially verified.
- #
- # For the other rows, we can state the equation as ...
- #
- # (c_t / c_cumulative_t) * [b_cumulative_{t-1} * p(x_0=c_0) + ... + (a_cumulative_{t-1} + b_cumulative_{t-1}) * p(x_0=C_i) + ... + b_cumulative_{k-1} * p(x_0=c_{k-1})]
- #
- # This verifies the other rows.
- #
- # 2. x_t is not masked
- #
- # Simplifying the expression, there are two cases for the rows of the column vector, where C_j = C_i and where C_j != C_i:
- # .
- # .
- # .
- # C_j != C_i: b_t * ((b_cumulative_{t-1} / b_cumulative_t) * p_n(x_0 = c_0) + ... + ((a_cumulative_{t-1} + b_cumulative_{t-1}) / b_cumulative_t) * p_n(x_0 = C_i) + ... + (b_cumulative_{t-1} / (a_cumulative_t + b_cumulative_t)) * p_n(c_0=C_j) + ... + (b_cumulative_{t-1} / b_cumulative_t) * p_n(x_0 = c_{k-1}))
- # .
- # .
- # .
- # C_j = C_i: (a_t + b_t) * ((b_cumulative_{t-1} / b_cumulative_t) * p_n(x_0 = c_0) + ... + ((a_cumulative_{t-1} + b_cumulative_{t-1}) / (a_cumulative_t + b_cumulative_t)) * p_n(x_0 = C_i = C_j) + ... + (b_cumulative_{t-1} / b_cumulative_t) * p_n(x_0 = c_{k-1}))
- # .
- # .
- # .
- # 0
- #
- # The last row is trivially verified. The other rows can be verified by directly expanding equation (11) stated in terms of forward probabilities.
- return log_p_x_t_min_1
-
- def log_Q_t_transitioning_to_known_class(
- self, *, t: torch.int, x_t: torch.LongTensor, log_onehot_x_t: torch.FloatTensor, cumulative: bool
- ):
- """
- Returns the log probabilities of the rows from the (cumulative or non-cumulative) transition matrix for each
- latent pixel in `x_t`.
-
- See equation (7) for the complete non-cumulative transition matrix. The complete cumulative transition matrix
- is the same structure except the parameters (alpha, beta, gamma) are the cumulative analogs.
-
- Args:
- t (torch.Long):
- The timestep that determines which transition matrix is used.
-
- x_t (`torch.LongTensor` of shape `(batch size, num latent pixels)`):
- The classes of each latent pixel at time `t`.
-
- log_onehot_x_t (`torch.FloatTensor` of shape `(batch size, num classes, num latent pixels)`):
- The log one-hot vectors of `x_t`
-
- cumulative (`bool`):
- If cumulative is `False`, we use the single step transition matrix `t-1`->`t`. If cumulative is `True`,
- we use the cumulative transition matrix `0`->`t`.
-
- Returns:
- `torch.FloatTensor` of shape `(batch size, num classes - 1, num latent pixels)`:
- Each _column_ of the returned matrix is a _row_ of log probabilities of the complete probability
- transition matrix.
-
- When non cumulative, returns `self.num_classes - 1` rows because the initial latent pixel cannot be
- masked.
-
- Where:
- - `q_n` is the probability distribution for the forward process of the `n`th latent pixel.
- - C_0 is a class of a latent pixel embedding
- - C_k is the class of the masked latent pixel
-
- non-cumulative result (omitting logarithms):
- ```
- q_0(x_t | x_{t-1} = C_0) ... q_n(x_t | x_{t-1} = C_0)
- . . .
- . . .
- . . .
- q_0(x_t | x_{t-1} = C_k) ... q_n(x_t | x_{t-1} = C_k)
- ```
-
- cumulative result (omitting logarithms):
- ```
- q_0_cumulative(x_t | x_0 = C_0) ... q_n_cumulative(x_t | x_0 = C_0)
- . . .
- . . .
- . . .
- q_0_cumulative(x_t | x_0 = C_{k-1}) ... q_n_cumulative(x_t | x_0 = C_{k-1})
- ```
- """
- if cumulative:
- a = self.log_cumprod_at[t]
- b = self.log_cumprod_bt[t]
- c = self.log_cumprod_ct[t]
- else:
- a = self.log_at[t]
- b = self.log_bt[t]
- c = self.log_ct[t]
-
- if not cumulative:
- # The values in the onehot vector can also be used as the logprobs for transitioning
- # from masked latent pixels. If we are not calculating the cumulative transitions,
- # we need to save these vectors to be re-appended to the final matrix so the values
- # aren't overwritten.
- #
- # `P(x_t!=mask|x_{t-1=mask}) = 0` and 0 will be the value of the last row of the onehot vector
- # if x_t is not masked
- #
- # `P(x_t=mask|x_{t-1=mask}) = 1` and 1 will be the value of the last row of the onehot vector
- # if x_t is masked
- log_onehot_x_t_transitioning_from_masked = log_onehot_x_t[:, -1, :].unsqueeze(1)
-
- # `index_to_log_onehot` will add onehot vectors for masked pixels,
- # so the default one hot matrix has one too many rows. See the doc string
- # for an explanation of the dimensionality of the returned matrix.
- log_onehot_x_t = log_onehot_x_t[:, :-1, :]
-
- # this is a cheeky trick to produce the transition probabilities using log one-hot vectors.
- #
- # Don't worry about what values this sets in the columns that mark transitions
- # to masked latent pixels. They are overwrote later with the `mask_class_mask`.
- #
- # Looking at the below logspace formula in non-logspace, each value will evaluate to either
- # `1 * a + b = a + b` where `log_Q_t` has the one hot value in the column
- # or
- # `0 * a + b = b` where `log_Q_t` has the 0 values in the column.
- #
- # See equation 7 for more details.
- log_Q_t = (log_onehot_x_t + a).logaddexp(b)
-
- # The whole column of each masked pixel is `c`
- mask_class_mask = x_t == self.mask_class
- mask_class_mask = mask_class_mask.unsqueeze(1).expand(-1, self.num_embed - 1, -1)
- log_Q_t[mask_class_mask] = c
-
- if not cumulative:
- log_Q_t = torch.cat((log_Q_t, log_onehot_x_t_transitioning_from_masked), dim=1)
-
- return log_Q_t
-
- def apply_cumulative_transitions(self, q, t):
- bsz = q.shape[0]
- a = self.log_cumprod_at[t]
- b = self.log_cumprod_bt[t]
- c = self.log_cumprod_ct[t]
-
- num_latent_pixels = q.shape[2]
- c = c.expand(bsz, 1, num_latent_pixels)
-
- q = (q + a).logaddexp(b)
- q = torch.cat((q, c), dim=1)
-
- return q
diff --git a/spaces/JohnSmith9982/ChuanhuChatGPT/modules/models/__init__.py b/spaces/JohnSmith9982/ChuanhuChatGPT/modules/models/__init__.py
deleted file mode 100644
index e69de29bb2d1d6434b8b29ae775ad8c2e48c5391..0000000000000000000000000000000000000000
diff --git a/spaces/JohnSmith9982/VITS-Umamusume-voice-synthesizer/text/japanese.py b/spaces/JohnSmith9982/VITS-Umamusume-voice-synthesizer/text/japanese.py
deleted file mode 100644
index 375e4d50872d5c68ee57ca17470a2ca425425eba..0000000000000000000000000000000000000000
--- a/spaces/JohnSmith9982/VITS-Umamusume-voice-synthesizer/text/japanese.py
+++ /dev/null
@@ -1,153 +0,0 @@
-import re
-from unidecode import unidecode
-import pyopenjtalk
-
-
-# Regular expression matching Japanese without punctuation marks:
-_japanese_characters = re.compile(
- r'[A-Za-z\d\u3005\u3040-\u30ff\u4e00-\u9fff\uff11-\uff19\uff21-\uff3a\uff41-\uff5a\uff66-\uff9d]')
-
-# Regular expression matching non-Japanese characters or punctuation marks:
-_japanese_marks = re.compile(
- r'[^A-Za-z\d\u3005\u3040-\u30ff\u4e00-\u9fff\uff11-\uff19\uff21-\uff3a\uff41-\uff5a\uff66-\uff9d]')
-
-# List of (symbol, Japanese) pairs for marks:
-_symbols_to_japanese = [(re.compile('%s' % x[0]), x[1]) for x in [
- ('%', 'パーセント')
-]]
-
-# List of (romaji, ipa) pairs for marks:
-_romaji_to_ipa = [(re.compile('%s' % x[0]), x[1]) for x in [
- ('ts', 'ʦ'),
- ('u', 'ɯ'),
- ('j', 'ʥ'),
- ('y', 'j'),
- ('ni', 'n^i'),
- ('nj', 'n^'),
- ('hi', 'çi'),
- ('hj', 'ç'),
- ('f', 'ɸ'),
- ('I', 'i*'),
- ('U', 'ɯ*'),
- ('r', 'ɾ')
-]]
-
-# List of (romaji, ipa2) pairs for marks:
-_romaji_to_ipa2 = [(re.compile('%s' % x[0]), x[1]) for x in [
- ('u', 'ɯ'),
- ('ʧ', 'tʃ'),
- ('j', 'dʑ'),
- ('y', 'j'),
- ('ni', 'n^i'),
- ('nj', 'n^'),
- ('hi', 'çi'),
- ('hj', 'ç'),
- ('f', 'ɸ'),
- ('I', 'i*'),
- ('U', 'ɯ*'),
- ('r', 'ɾ')
-]]
-
-# List of (consonant, sokuon) pairs:
-_real_sokuon = [(re.compile('%s' % x[0]), x[1]) for x in [
- (r'Q([↑↓]*[kg])', r'k#\1'),
- (r'Q([↑↓]*[tdjʧ])', r't#\1'),
- (r'Q([↑↓]*[sʃ])', r's\1'),
- (r'Q([↑↓]*[pb])', r'p#\1')
-]]
-
-# List of (consonant, hatsuon) pairs:
-_real_hatsuon = [(re.compile('%s' % x[0]), x[1]) for x in [
- (r'N([↑↓]*[pbm])', r'm\1'),
- (r'N([↑↓]*[ʧʥj])', r'n^\1'),
- (r'N([↑↓]*[tdn])', r'n\1'),
- (r'N([↑↓]*[kg])', r'ŋ\1')
-]]
-
-
-def symbols_to_japanese(text):
- for regex, replacement in _symbols_to_japanese:
- text = re.sub(regex, replacement, text)
- return text
-
-
-def japanese_to_romaji_with_accent(text):
- '''Reference https://r9y9.github.io/ttslearn/latest/notebooks/ch10_Recipe-Tacotron.html'''
- text = symbols_to_japanese(text)
- sentences = re.split(_japanese_marks, text)
- marks = re.findall(_japanese_marks, text)
- text = ''
- for i, sentence in enumerate(sentences):
- if re.match(_japanese_characters, sentence):
- if text != '':
- text += ' '
- labels = pyopenjtalk.extract_fullcontext(sentence)
- for n, label in enumerate(labels):
- phoneme = re.search(r'\-([^\+]*)\+', label).group(1)
- if phoneme not in ['sil', 'pau']:
- text += phoneme.replace('ch', 'ʧ').replace('sh',
- 'ʃ').replace('cl', 'Q')
- else:
- continue
- # n_moras = int(re.search(r'/F:(\d+)_', label).group(1))
- a1 = int(re.search(r"/A:(\-?[0-9]+)\+", label).group(1))
- a2 = int(re.search(r"\+(\d+)\+", label).group(1))
- a3 = int(re.search(r"\+(\d+)/", label).group(1))
- if re.search(r'\-([^\+]*)\+', labels[n + 1]).group(1) in ['sil', 'pau']:
- a2_next = -1
- else:
- a2_next = int(
- re.search(r"\+(\d+)\+", labels[n + 1]).group(1))
- # Accent phrase boundary
- if a3 == 1 and a2_next == 1:
- text += ' '
- # Falling
- elif a1 == 0 and a2_next == a2 + 1:
- text += '↓'
- # Rising
- elif a2 == 1 and a2_next == 2:
- text += '↑'
- if i < len(marks):
- text += unidecode(marks[i]).replace(' ', '')
- return text
-
-
-def get_real_sokuon(text):
- for regex, replacement in _real_sokuon:
- text = re.sub(regex, replacement, text)
- return text
-
-
-def get_real_hatsuon(text):
- for regex, replacement in _real_hatsuon:
- text = re.sub(regex, replacement, text)
- return text
-
-
-def japanese_to_ipa(text):
- text = japanese_to_romaji_with_accent(text).replace('...', '…')
- text = re.sub(
- r'([aiueo])\1+', lambda x: x.group(0)[0]+'ː'*(len(x.group(0))-1), text)
- text = get_real_sokuon(text)
- text = get_real_hatsuon(text)
- for regex, replacement in _romaji_to_ipa:
- text = re.sub(regex, replacement, text)
- return text
-
-
-def japanese_to_ipa2(text):
- text = japanese_to_romaji_with_accent(text).replace('...', '…')
- text = get_real_sokuon(text)
- text = get_real_hatsuon(text)
- for regex, replacement in _romaji_to_ipa2:
- text = re.sub(regex, replacement, text)
- return text
-
-
-def japanese_to_ipa3(text):
- text = japanese_to_ipa2(text).replace('n^', 'ȵ').replace(
- 'ʃ', 'ɕ').replace('*', '\u0325').replace('#', '\u031a')
- text = re.sub(
- r'([aiɯeo])\1+', lambda x: x.group(0)[0]+'ː'*(len(x.group(0))-1), text)
- text = re.sub(r'((?:^|\s)(?:ts|tɕ|[kpt]))', r'\1ʰ', text)
- return text
diff --git a/spaces/Juli08/janitorai/README.md b/spaces/Juli08/janitorai/README.md
deleted file mode 100644
index 1299f2ee3ec0dea68e2f02ed0c6300cc60a9d583..0000000000000000000000000000000000000000
--- a/spaces/Juli08/janitorai/README.md
+++ /dev/null
@@ -1,10 +0,0 @@
----
-title: Janitorai
-emoji: 🌖
-colorFrom: pink
-colorTo: green
-sdk: docker
-pinned: false
----
-
-Check out the configuration reference at https://huggingface.co/docs/hub/spaces-config-reference
diff --git a/spaces/KyanChen/RSPrompter/mmdet/models/detectors/htc.py b/spaces/KyanChen/RSPrompter/mmdet/models/detectors/htc.py
deleted file mode 100644
index 22a2aa889a59fd0e0afeb95a7369028def6e4fa9..0000000000000000000000000000000000000000
--- a/spaces/KyanChen/RSPrompter/mmdet/models/detectors/htc.py
+++ /dev/null
@@ -1,16 +0,0 @@
-# Copyright (c) OpenMMLab. All rights reserved.
-from mmdet.registry import MODELS
-from .cascade_rcnn import CascadeRCNN
-
-
-@MODELS.register_module()
-class HybridTaskCascade(CascadeRCNN):
- """Implementation of `HTC `_"""
-
- def __init__(self, **kwargs) -> None:
- super().__init__(**kwargs)
-
- @property
- def with_semantic(self) -> bool:
- """bool: whether the detector has a semantic head"""
- return self.roi_head.with_semantic
diff --git a/spaces/KyanChen/RSPrompter/mmdet/structures/mask/__init__.py b/spaces/KyanChen/RSPrompter/mmdet/structures/mask/__init__.py
deleted file mode 100644
index f78394701df1b493259c4c23a79aea5c5cb8be95..0000000000000000000000000000000000000000
--- a/spaces/KyanChen/RSPrompter/mmdet/structures/mask/__init__.py
+++ /dev/null
@@ -1,11 +0,0 @@
-# Copyright (c) OpenMMLab. All rights reserved.
-from .mask_target import mask_target
-from .structures import (BaseInstanceMasks, BitmapMasks, PolygonMasks,
- bitmap_to_polygon, polygon_to_bitmap)
-from .utils import encode_mask_results, mask2bbox, split_combined_polys
-
-__all__ = [
- 'split_combined_polys', 'mask_target', 'BaseInstanceMasks', 'BitmapMasks',
- 'PolygonMasks', 'encode_mask_results', 'mask2bbox', 'polygon_to_bitmap',
- 'bitmap_to_polygon'
-]
diff --git a/spaces/LanguageBind/LanguageBind/vl_ret/tokenization_clip.py b/spaces/LanguageBind/LanguageBind/vl_ret/tokenization_clip.py
deleted file mode 100644
index 3fbb56d0ef9a4dbea9a39a6c55352ef14a34898d..0000000000000000000000000000000000000000
--- a/spaces/LanguageBind/LanguageBind/vl_ret/tokenization_clip.py
+++ /dev/null
@@ -1,145 +0,0 @@
-import gzip
-import html
-import os
-from functools import lru_cache
-
-import ftfy
-import regex as re
-
-
-@lru_cache()
-def default_bpe():
- return os.path.join(os.path.dirname(os.path.abspath(__file__)), "bpe_simple_vocab_16e6.txt.gz")
-
-
-@lru_cache()
-def bytes_to_unicode():
- """
- Returns list of utf-8 byte and a corresponding list of unicode strings.
- The reversible bpe codes work on unicode strings.
- This means you need a large # of unicode characters in your vocab if you want to avoid UNKs.
- When you're at something like a 10B token dataset you end up needing around 5K for decent coverage.
- This is a signficant percentage of your normal, say, 32K bpe vocab.
- To avoid that, we want lookup tables between utf-8 bytes and unicode strings.
- And avoids mapping to whitespace/control characters the bpe code barfs on.
- """
- bs = list(range(ord("!"), ord("~")+1))+list(range(ord("¡"), ord("¬")+1))+list(range(ord("®"), ord("ÿ")+1))
- cs = bs[:]
- n = 0
- for b in range(2**8):
- if b not in bs:
- bs.append(b)
- cs.append(2**8+n)
- n += 1
- cs = [chr(n) for n in cs]
- return dict(zip(bs, cs))
-
-
-def get_pairs(word):
- """Return set of symbol pairs in a word.
- Word is represented as tuple of symbols (symbols being variable-length strings).
- """
- pairs = set()
- prev_char = word[0]
- for char in word[1:]:
- pairs.add((prev_char, char))
- prev_char = char
- return pairs
-
-
-def basic_clean(text):
- text = ftfy.fix_text(text)
- text = html.unescape(html.unescape(text))
- return text.strip()
-
-
-def whitespace_clean(text):
- text = re.sub(r'\s+', ' ', text)
- text = text.strip()
- return text
-
-
-class SimpleTokenizer(object):
- def __init__(self, bpe_path: str = default_bpe()):
- self.byte_encoder = bytes_to_unicode()
- self.byte_decoder = {v: k for k, v in self.byte_encoder.items()}
- merges = gzip.open(bpe_path).read().decode("utf-8").split('\n')
- merges = merges[1:49152-256-2+1]
- merges = [tuple(merge.split()) for merge in merges]
- vocab = list(bytes_to_unicode().values())
- vocab = vocab + [v+'' for v in vocab]
- for merge in merges:
- vocab.append(''.join(merge))
- vocab.extend(['<|startoftext|>', '<|endoftext|>'])
- self.encoder = dict(zip(vocab, range(len(vocab))))
- self.decoder = {v: k for k, v in self.encoder.items()}
- self.bpe_ranks = dict(zip(merges, range(len(merges))))
- self.cache = {'<|startoftext|>': '<|startoftext|>', '<|endoftext|>': '<|endoftext|>'}
- self.pat = re.compile(r"""<\|startoftext\|>|<\|endoftext\|>|'s|'t|'re|'ve|'m|'ll|'d|[\p{L}]+|[\p{N}]|[^\s\p{L}\p{N}]+""", re.IGNORECASE)
-
- self.vocab = self.encoder
-
- def bpe(self, token):
- if token in self.cache:
- return self.cache[token]
- word = tuple(token[:-1]) + ( token[-1] + '',)
- pairs = get_pairs(word)
-
- if not pairs:
- return token+''
-
- while True:
- bigram = min(pairs, key = lambda pair: self.bpe_ranks.get(pair, float('inf')))
- if bigram not in self.bpe_ranks:
- break
- first, second = bigram
- new_word = []
- i = 0
- while i < len(word):
- try:
- j = word.index(first, i)
- new_word.extend(word[i:j])
- i = j
- except:
- new_word.extend(word[i:])
- break
-
- if word[i] == first and i < len(word)-1 and word[i+1] == second:
- new_word.append(first+second)
- i += 2
- else:
- new_word.append(word[i])
- i += 1
- new_word = tuple(new_word)
- word = new_word
- if len(word) == 1:
- break
- else:
- pairs = get_pairs(word)
- word = ' '.join(word)
- self.cache[token] = word
- return word
-
- def encode(self, text):
- bpe_tokens = []
- text = whitespace_clean(basic_clean(text)).lower()
- for token in re.findall(self.pat, text):
- token = ''.join(self.byte_encoder[b] for b in token.encode('utf-8'))
- bpe_tokens.extend(self.encoder[bpe_token] for bpe_token in self.bpe(token).split(' '))
- return bpe_tokens
-
- def decode(self, tokens):
- text = ''.join([self.decoder[token] for token in tokens])
- text = bytearray([self.byte_decoder[c] for c in text]).decode('utf-8', errors="replace").replace('', ' ')
- return text
-
- def tokenize(self, text):
- tokens = []
- text = whitespace_clean(basic_clean(text)).lower()
- for token in re.findall(self.pat, text):
- token = ''.join(self.byte_encoder[b] for b in token.encode('utf-8'))
- tokens.extend(bpe_token for bpe_token in self.bpe(token).split(' '))
- return tokens
-
- def convert_tokens_to_ids(self, tokens):
- return [self.encoder[bpe_token] for bpe_token in tokens]
\ No newline at end of file
diff --git "a/spaces/Liu-LAB/GPT-academic/crazy_functions/\350\276\205\345\212\251\345\233\236\347\255\224.py" "b/spaces/Liu-LAB/GPT-academic/crazy_functions/\350\276\205\345\212\251\345\233\236\347\255\224.py"
deleted file mode 100644
index b635f88b3183bbd310eca6449cd9e10c75ca7ca7..0000000000000000000000000000000000000000
--- "a/spaces/Liu-LAB/GPT-academic/crazy_functions/\350\276\205\345\212\251\345\233\236\347\255\224.py"
+++ /dev/null
@@ -1,28 +0,0 @@
-# encoding: utf-8
-# @Time : 2023/4/19
-# @Author : Spike
-# @Descr :
-from toolbox import update_ui
-from toolbox import CatchException, report_execption, write_results_to_file
-from crazy_functions.crazy_utils import request_gpt_model_in_new_thread_with_ui_alive
-
-
-@CatchException
-def 猜你想问(txt, llm_kwargs, plugin_kwargs, chatbot, history, system_prompt, web_port):
- if txt:
- show_say = txt
- prompt = txt+'\n回答完问题后,再列出用户可能提出的三个问题。'
- else:
- prompt = history[-1]+"\n分析上述回答,再列出用户可能提出的三个问题。"
- show_say = '分析上述回答,再列出用户可能提出的三个问题。'
- gpt_say = yield from request_gpt_model_in_new_thread_with_ui_alive(
- inputs=prompt,
- inputs_show_user=show_say,
- llm_kwargs=llm_kwargs,
- chatbot=chatbot,
- history=history,
- sys_prompt=system_prompt
- )
- chatbot[-1] = (show_say, gpt_say)
- history.extend([show_say, gpt_say])
- yield from update_ui(chatbot=chatbot, history=history) # 刷新界面
\ No newline at end of file
diff --git a/spaces/Make-A-Protagonist/Make-A-Protagonist-inference/Make-A-Protagonist/experts/GroundedSAM/GroundingDINO/groundingdino/datasets/__init__.py b/spaces/Make-A-Protagonist/Make-A-Protagonist-inference/Make-A-Protagonist/experts/GroundedSAM/GroundingDINO/groundingdino/datasets/__init__.py
deleted file mode 100644
index e69de29bb2d1d6434b8b29ae775ad8c2e48c5391..0000000000000000000000000000000000000000
diff --git a/spaces/Malmika/Osana-Chat-Friend/app.py b/spaces/Malmika/Osana-Chat-Friend/app.py
deleted file mode 100644
index 700d290b5c29bc9bf11a55e30ae5e54c13c86e66..0000000000000000000000000000000000000000
--- a/spaces/Malmika/Osana-Chat-Friend/app.py
+++ /dev/null
@@ -1,33 +0,0 @@
-import gradio as gr
-from transformers import AutoModelForCausalLM, AutoTokenizer
-import torch
-
-tokenizer = AutoTokenizer.from_pretrained("microsoft/DialoGPT-medium")
-model = AutoModelForCausalLM.from_pretrained("microsoft/DialoGPT-medium")
-max_history = 10 # Maximum number of previous chat turns to include in the conversation history
-chat_history_ids = None
-
-def chatbot(user_input):
- global chat_history_ids
-
- # encode the new user input, add the eos_token and return a tensor in PyTorch
- new_user_input_ids = tokenizer.encode(user_input + tokenizer.eos_token, return_tensors='pt')
-
- # append the new user input tokens to the chat history
- bot_input_ids = torch.cat([chat_history_ids, new_user_input_ids], dim=-1) if chat_history_ids is not None else new_user_input_ids
-
- # generate a response while limiting the total chat history to max_history tokens
- chat_history_ids = model.generate(bot_input_ids, max_length=1000, pad_token_id=tokenizer.eos_token_id)
-
- # decode and return the generated response
- response = tokenizer.decode(chat_history_ids[:, bot_input_ids.shape[-1]:][0], skip_special_tokens=True)
- return response
-
-styles = {
- "textarea": "height: 200px; font-size: 18px;",
- "label": "font-size: 20px; font-weight: bold;",
- "output": "color: red; font-size: 18px;"
-}
-
-iface = gr.Interface(fn=chatbot, inputs="text", outputs="text", title="Osana Chat Friend", styles=styles)
-iface.launch()
diff --git a/spaces/MariaK/Check-my-progress-Audio-Course/README.md b/spaces/MariaK/Check-my-progress-Audio-Course/README.md
deleted file mode 100644
index d94e8d9d569f48f57907501db499d955fa959cab..0000000000000000000000000000000000000000
--- a/spaces/MariaK/Check-my-progress-Audio-Course/README.md
+++ /dev/null
@@ -1,13 +0,0 @@
----
-title: Check My Progress Audio Course
-emoji: 👀
-colorFrom: yellow
-colorTo: purple
-sdk: gradio
-sdk_version: 3.16.0
-app_file: app.py
-pinned: false
-duplicated_from: ThomasSimonini/Check-my-progress-Deep-RL-Course
----
-
-Check out the configuration reference at https://huggingface.co/docs/hub/spaces-config-reference
diff --git a/spaces/Marshalls/testmtd/analysis/pymo/__init__.py b/spaces/Marshalls/testmtd/analysis/pymo/__init__.py
deleted file mode 100644
index 81b6d00d10833e29a4b27bdec29b884b347bb9dc..0000000000000000000000000000000000000000
--- a/spaces/Marshalls/testmtd/analysis/pymo/__init__.py
+++ /dev/null
@@ -1,7 +0,0 @@
-import os, sys
-THIS_DIR = os.path.dirname(os.path.abspath(__file__))
-ROOT_DIR = os.path.abspath(os.path.join(THIS_DIR, os.pardir))
-ANALYSIS_DIR = os.path.join(ROOT_DIR, 'analysis')
-if not os.path.isdir(ANALYSIS_DIR):
- os.mkdir(ANALYSIS_DIR)
-sys.path.append(ROOT_DIR)
diff --git a/spaces/Marshalls/testmtd/analysis/sandbox_fid.py b/spaces/Marshalls/testmtd/analysis/sandbox_fid.py
deleted file mode 100644
index 90dda7a746e39affaae1a4bd0921ec5612aa2f32..0000000000000000000000000000000000000000
--- a/spaces/Marshalls/testmtd/analysis/sandbox_fid.py
+++ /dev/null
@@ -1,117 +0,0 @@
-import numpy as np
-import sklearn
-import pickle
-from pathlib import Path
-import scipy.linalg
-import matplotlib.pyplot as plt
-#%%
-
-def FID(m,C,mg,Cg):
- mean_diff = np.sum((m-mg)**2)
- covar_diff = np.trace(C) + np.trace(Cg) -2 * np.trace(scipy.linalg.sqrtm(np.dot(C,Cg)))
- return mean_diff + covar_diff
-#%%
-
-# feat_file = "inference/generated_1/moglow_expmap/predicted_mods/"+"aistpp_gBR_sBM_cAll_d04_mBR3_ch10.expmap_scaled_20.generated.npy"
-# feats = np.load(feat_file)
-#
-# feats = feats[:,0,:]
-# feats = np.delete(feats,[-4,-6],1)
-#
-# feats.shape
-#
-# C = np.dot(feats.T,feats)
-#
-# m = np.mean(feats,0)
-
-# data_path="data/dance_combined"
-# feature_name="expmap_scaled_20"
-# transform_name="scaler"
-# transform = pickle.load(open(Path(data_path).joinpath(feature_name+'_'+transform_name+'.pkl'), "rb"))
-#
-# C_data = transform.
-#
-# C_data.shape
-
-#%%
-root_dir = "data/fid_data/predicted_mods"
-# experiment_name="moglow_expmap"
-
-# stat="2moments" # mean and covariance of poses
-stat="2moments_ext" # mean and covariance of 3 consecutive poses
-moments_file = root_dir+"/"+"ground_truth"+"/bvh_expmap_cr_"+stat+".pkl"
-gt_m, gt_C = pickle.load(open(moments_file,"rb"))
-
-moments_dict = {}
-fids = {}
-experiments = ["moglow_expmap","transflower_expmap","transflower_expmap_finetune2_old","transformer_expmap"]
-for experiment_name in experiments:
- moments_file = root_dir+"/"+experiment_name+"/expmap_scaled_20.generated_"+stat+".pkl"
-
- m,C = pickle.load(open(moments_file,"rb"))
- if stat=="2moments":
- m = np.delete(m,[-4,-6],0)
- C = np.delete(C,[-4,-6],0)
- C = np.delete(C,[-4,-6],1)
- elif stat=="2moments_ext":
- m = np.delete(m,[-4,-6],0)
- m = np.delete(m,[-4-67,-6-67],0)
- m = np.delete(m,[-4-67*2,-6-67*2],0)
- C = np.delete(C,[-4,-6],0)
- C = np.delete(C,[-4-67,-6-67],0)
- C = np.delete(C,[-4-67*2,-6-67*2],0)
- C = np.delete(C,[-4,-6],1)
- C = np.delete(C,[-4-67,-6-67],1)
- C = np.delete(C,[-4-67*2,-6-67*2],1)
- moments_dict[experiment_name] = (m,C)
- fids[experiment_name] = FID(m,C,gt_m,gt_C)
-
-
-fids
-#%%
-
-#####
-# for comparign seeds
-
-root_dir_generated = "data/fid_data/predicted_mods_seed"
-root_dir_gt = "data/fid_data/ground_truths"
-fids = np.empty((5,5))
-# stat="2moments" # mean and covariance of poses
-stat="2moments_ext" # mean and covariance of 3 consecutive poses
-# seeds = list(range(1,6))
-for i in range(5):
- gt_moments_file = root_dir_gt+"/"+str(i+1)+"/bvh_expmap_cr_"+stat+".pkl"
- gt_m,gt_C = pickle.load(open(gt_moments_file,"rb"))
- for j in range(5):
- # moments_file = root_dir_generated+"/"+"generated_"+str(j+1)+"/expmap_scaled_20.generated_"+stat+".pkl"
- moments_file = "inference/randomized_seeds/generated_"+str(j+1)+"/transflower_expmap/predicted_mods/expmap_scaled_20.generated_"+stat+".pkl"
-
- m,C = pickle.load(open(moments_file,"rb"))
- if stat=="2moments":
- m = np.delete(m,[-4,-6],0)
- C = np.delete(C,[-4,-6],0)
- C = np.delete(C,[-4,-6],1)
- elif stat=="2moments_ext":
- m = np.delete(m,[-4,-6],0)
- m = np.delete(m,[-4-67,-6-67],0)
- m = np.delete(m,[-4-67*2,-6-67*2],0)
- C = np.delete(C,[-4,-6],0)
- C = np.delete(C,[-4-67,-6-67],0)
- C = np.delete(C,[-4-67*2,-6-67*2],0)
- C = np.delete(C,[-4,-6],1)
- C = np.delete(C,[-4-67,-6-67],1)
- C = np.delete(C,[-4-67*2,-6-67*2],1)
- # moments_dict[experiment_name] = (m,C)
- fids[i,j] = FID(m,C,gt_m,gt_C)
-
-# for i in range(5):
-# for j in range(i,5):
-# fids[j,i] = fids[i,j]
-
-
-fids
-
-# plt.matshow(fids/np.mean(fids))
-plt.matshow(fids)
-# plt.matshow(fids[1:,1:])
-plt.matshow(fids[1:,1:] == np.min(fids[1:,1:],0,keepdims=True))
diff --git a/spaces/MashiroSA/sovits-emu-voice-transform/vdecoder/nsf_hifigan/models.py b/spaces/MashiroSA/sovits-emu-voice-transform/vdecoder/nsf_hifigan/models.py
deleted file mode 100644
index 6f24f617a76e64bc88b7cff6cc618b59af1c07e3..0000000000000000000000000000000000000000
--- a/spaces/MashiroSA/sovits-emu-voice-transform/vdecoder/nsf_hifigan/models.py
+++ /dev/null
@@ -1,435 +0,0 @@
-import os
-import json
-from .env import AttrDict
-import numpy as np
-import torch
-import torch.nn.functional as F
-import torch.nn as nn
-from torch.nn import Conv1d, ConvTranspose1d, AvgPool1d, Conv2d
-from torch.nn.utils import weight_norm, remove_weight_norm, spectral_norm
-from .utils import init_weights, get_padding
-
-LRELU_SLOPE = 0.1
-
-
-def load_model(model_path, device='cuda'):
- config_file = os.path.join(os.path.split(model_path)[0], 'config.json')
- with open(config_file) as f:
- data = f.read()
-
- json_config = json.loads(data)
- h = AttrDict(json_config)
-
- generator = Generator(h).to(device)
-
- cp_dict = torch.load(model_path, map_location=device)
- generator.load_state_dict(cp_dict['generator'])
- generator.eval()
- generator.remove_weight_norm()
- del cp_dict
- return generator, h
-
-
-class ResBlock1(torch.nn.Module):
- def __init__(self, h, channels, kernel_size=3, dilation=(1, 3, 5)):
- super(ResBlock1, self).__init__()
- self.h = h
- self.convs1 = nn.ModuleList([
- weight_norm(Conv1d(channels, channels, kernel_size, 1, dilation=dilation[0],
- padding=get_padding(kernel_size, dilation[0]))),
- weight_norm(Conv1d(channels, channels, kernel_size, 1, dilation=dilation[1],
- padding=get_padding(kernel_size, dilation[1]))),
- weight_norm(Conv1d(channels, channels, kernel_size, 1, dilation=dilation[2],
- padding=get_padding(kernel_size, dilation[2])))
- ])
- self.convs1.apply(init_weights)
-
- self.convs2 = nn.ModuleList([
- weight_norm(Conv1d(channels, channels, kernel_size, 1, dilation=1,
- padding=get_padding(kernel_size, 1))),
- weight_norm(Conv1d(channels, channels, kernel_size, 1, dilation=1,
- padding=get_padding(kernel_size, 1))),
- weight_norm(Conv1d(channels, channels, kernel_size, 1, dilation=1,
- padding=get_padding(kernel_size, 1)))
- ])
- self.convs2.apply(init_weights)
-
- def forward(self, x):
- for c1, c2 in zip(self.convs1, self.convs2):
- xt = F.leaky_relu(x, LRELU_SLOPE)
- xt = c1(xt)
- xt = F.leaky_relu(xt, LRELU_SLOPE)
- xt = c2(xt)
- x = xt + x
- return x
-
- def remove_weight_norm(self):
- for l in self.convs1:
- remove_weight_norm(l)
- for l in self.convs2:
- remove_weight_norm(l)
-
-
-class ResBlock2(torch.nn.Module):
- def __init__(self, h, channels, kernel_size=3, dilation=(1, 3)):
- super(ResBlock2, self).__init__()
- self.h = h
- self.convs = nn.ModuleList([
- weight_norm(Conv1d(channels, channels, kernel_size, 1, dilation=dilation[0],
- padding=get_padding(kernel_size, dilation[0]))),
- weight_norm(Conv1d(channels, channels, kernel_size, 1, dilation=dilation[1],
- padding=get_padding(kernel_size, dilation[1])))
- ])
- self.convs.apply(init_weights)
-
- def forward(self, x):
- for c in self.convs:
- xt = F.leaky_relu(x, LRELU_SLOPE)
- xt = c(xt)
- x = xt + x
- return x
-
- def remove_weight_norm(self):
- for l in self.convs:
- remove_weight_norm(l)
-
-
-class SineGen(torch.nn.Module):
- """ Definition of sine generator
- SineGen(samp_rate, harmonic_num = 0,
- sine_amp = 0.1, noise_std = 0.003,
- voiced_threshold = 0,
- flag_for_pulse=False)
- samp_rate: sampling rate in Hz
- harmonic_num: number of harmonic overtones (default 0)
- sine_amp: amplitude of sine-wavefrom (default 0.1)
- noise_std: std of Gaussian noise (default 0.003)
- voiced_thoreshold: F0 threshold for U/V classification (default 0)
- flag_for_pulse: this SinGen is used inside PulseGen (default False)
- Note: when flag_for_pulse is True, the first time step of a voiced
- segment is always sin(np.pi) or cos(0)
- """
-
- def __init__(self, samp_rate, harmonic_num=0,
- sine_amp=0.1, noise_std=0.003,
- voiced_threshold=0):
- super(SineGen, self).__init__()
- self.sine_amp = sine_amp
- self.noise_std = noise_std
- self.harmonic_num = harmonic_num
- self.dim = self.harmonic_num + 1
- self.sampling_rate = samp_rate
- self.voiced_threshold = voiced_threshold
-
- def _f02uv(self, f0):
- # generate uv signal
- uv = torch.ones_like(f0)
- uv = uv * (f0 > self.voiced_threshold)
- return uv
-
- @torch.no_grad()
- def forward(self, f0, upp):
- """ sine_tensor, uv = forward(f0)
- input F0: tensor(batchsize=1, length, dim=1)
- f0 for unvoiced steps should be 0
- output sine_tensor: tensor(batchsize=1, length, dim)
- output uv: tensor(batchsize=1, length, 1)
- """
- f0 = f0.unsqueeze(-1)
- fn = torch.multiply(f0, torch.arange(1, self.dim + 1, device=f0.device).reshape((1, 1, -1)))
- rad_values = (fn / self.sampling_rate) % 1 ###%1意味着n_har的乘积无法后处理优化
- rand_ini = torch.rand(fn.shape[0], fn.shape[2], device=fn.device)
- rand_ini[:, 0] = 0
- rad_values[:, 0, :] = rad_values[:, 0, :] + rand_ini
- is_half = rad_values.dtype is not torch.float32
- tmp_over_one = torch.cumsum(rad_values.double(), 1) # % 1 #####%1意味着后面的cumsum无法再优化
- if is_half:
- tmp_over_one = tmp_over_one.half()
- else:
- tmp_over_one = tmp_over_one.float()
- tmp_over_one *= upp
- tmp_over_one = F.interpolate(
- tmp_over_one.transpose(2, 1), scale_factor=upp,
- mode='linear', align_corners=True
- ).transpose(2, 1)
- rad_values = F.interpolate(rad_values.transpose(2, 1), scale_factor=upp, mode='nearest').transpose(2, 1)
- tmp_over_one %= 1
- tmp_over_one_idx = (tmp_over_one[:, 1:, :] - tmp_over_one[:, :-1, :]) < 0
- cumsum_shift = torch.zeros_like(rad_values)
- cumsum_shift[:, 1:, :] = tmp_over_one_idx * -1.0
- rad_values = rad_values.double()
- cumsum_shift = cumsum_shift.double()
- sine_waves = torch.sin(torch.cumsum(rad_values + cumsum_shift, dim=1) * 2 * np.pi)
- if is_half:
- sine_waves = sine_waves.half()
- else:
- sine_waves = sine_waves.float()
- sine_waves = sine_waves * self.sine_amp
- uv = self._f02uv(f0)
- uv = F.interpolate(uv.transpose(2, 1), scale_factor=upp, mode='nearest').transpose(2, 1)
- noise_amp = uv * self.noise_std + (1 - uv) * self.sine_amp / 3
- noise = noise_amp * torch.randn_like(sine_waves)
- sine_waves = sine_waves * uv + noise
- return sine_waves, uv, noise
-
-
-class SourceModuleHnNSF(torch.nn.Module):
- """ SourceModule for hn-nsf
- SourceModule(sampling_rate, harmonic_num=0, sine_amp=0.1,
- add_noise_std=0.003, voiced_threshod=0)
- sampling_rate: sampling_rate in Hz
- harmonic_num: number of harmonic above F0 (default: 0)
- sine_amp: amplitude of sine source signal (default: 0.1)
- add_noise_std: std of additive Gaussian noise (default: 0.003)
- note that amplitude of noise in unvoiced is decided
- by sine_amp
- voiced_threshold: threhold to set U/V given F0 (default: 0)
- Sine_source, noise_source = SourceModuleHnNSF(F0_sampled)
- F0_sampled (batchsize, length, 1)
- Sine_source (batchsize, length, 1)
- noise_source (batchsize, length 1)
- uv (batchsize, length, 1)
- """
-
- def __init__(self, sampling_rate, harmonic_num=0, sine_amp=0.1,
- add_noise_std=0.003, voiced_threshod=0):
- super(SourceModuleHnNSF, self).__init__()
-
- self.sine_amp = sine_amp
- self.noise_std = add_noise_std
-
- # to produce sine waveforms
- self.l_sin_gen = SineGen(sampling_rate, harmonic_num,
- sine_amp, add_noise_std, voiced_threshod)
-
- # to merge source harmonics into a single excitation
- self.l_linear = torch.nn.Linear(harmonic_num + 1, 1)
- self.l_tanh = torch.nn.Tanh()
-
- def forward(self, x, upp):
- sine_wavs, uv, _ = self.l_sin_gen(x, upp)
- sine_merge = self.l_tanh(self.l_linear(sine_wavs))
- return sine_merge
-
-
-class Generator(torch.nn.Module):
- def __init__(self, h):
- super(Generator, self).__init__()
- self.h = h
- self.num_kernels = len(h.resblock_kernel_sizes)
- self.num_upsamples = len(h.upsample_rates)
- self.m_source = SourceModuleHnNSF(
- sampling_rate=h.sampling_rate,
- harmonic_num=8
- )
- self.noise_convs = nn.ModuleList()
- self.conv_pre = weight_norm(Conv1d(h.num_mels, h.upsample_initial_channel, 7, 1, padding=3))
- resblock = ResBlock1 if h.resblock == '1' else ResBlock2
-
- self.ups = nn.ModuleList()
- for i, (u, k) in enumerate(zip(h.upsample_rates, h.upsample_kernel_sizes)):
- c_cur = h.upsample_initial_channel // (2 ** (i + 1))
- self.ups.append(weight_norm(
- ConvTranspose1d(h.upsample_initial_channel // (2 ** i), h.upsample_initial_channel // (2 ** (i + 1)),
- k, u, padding=(k - u) // 2)))
- if i + 1 < len(h.upsample_rates): #
- stride_f0 = int(np.prod(h.upsample_rates[i + 1:]))
- self.noise_convs.append(Conv1d(
- 1, c_cur, kernel_size=stride_f0 * 2, stride=stride_f0, padding=stride_f0 // 2))
- else:
- self.noise_convs.append(Conv1d(1, c_cur, kernel_size=1))
- self.resblocks = nn.ModuleList()
- ch = h.upsample_initial_channel
- for i in range(len(self.ups)):
- ch //= 2
- for j, (k, d) in enumerate(zip(h.resblock_kernel_sizes, h.resblock_dilation_sizes)):
- self.resblocks.append(resblock(h, ch, k, d))
-
- self.conv_post = weight_norm(Conv1d(ch, 1, 7, 1, padding=3))
- self.ups.apply(init_weights)
- self.conv_post.apply(init_weights)
- self.upp = int(np.prod(h.upsample_rates))
-
- def forward(self, x, f0):
- har_source = self.m_source(f0, self.upp).transpose(1, 2)
- x = self.conv_pre(x)
- for i in range(self.num_upsamples):
- x = F.leaky_relu(x, LRELU_SLOPE)
- x = self.ups[i](x)
- x_source = self.noise_convs[i](har_source)
- x = x + x_source
- xs = None
- for j in range(self.num_kernels):
- if xs is None:
- xs = self.resblocks[i * self.num_kernels + j](x)
- else:
- xs += self.resblocks[i * self.num_kernels + j](x)
- x = xs / self.num_kernels
- x = F.leaky_relu(x)
- x = self.conv_post(x)
- x = torch.tanh(x)
-
- return x
-
- def remove_weight_norm(self):
- print('Removing weight norm...')
- for l in self.ups:
- remove_weight_norm(l)
- for l in self.resblocks:
- l.remove_weight_norm()
- remove_weight_norm(self.conv_pre)
- remove_weight_norm(self.conv_post)
-
-
-class DiscriminatorP(torch.nn.Module):
- def __init__(self, period, kernel_size=5, stride=3, use_spectral_norm=False):
- super(DiscriminatorP, self).__init__()
- self.period = period
- norm_f = weight_norm if use_spectral_norm == False else spectral_norm
- self.convs = nn.ModuleList([
- norm_f(Conv2d(1, 32, (kernel_size, 1), (stride, 1), padding=(get_padding(5, 1), 0))),
- norm_f(Conv2d(32, 128, (kernel_size, 1), (stride, 1), padding=(get_padding(5, 1), 0))),
- norm_f(Conv2d(128, 512, (kernel_size, 1), (stride, 1), padding=(get_padding(5, 1), 0))),
- norm_f(Conv2d(512, 1024, (kernel_size, 1), (stride, 1), padding=(get_padding(5, 1), 0))),
- norm_f(Conv2d(1024, 1024, (kernel_size, 1), 1, padding=(2, 0))),
- ])
- self.conv_post = norm_f(Conv2d(1024, 1, (3, 1), 1, padding=(1, 0)))
-
- def forward(self, x):
- fmap = []
-
- # 1d to 2d
- b, c, t = x.shape
- if t % self.period != 0: # pad first
- n_pad = self.period - (t % self.period)
- x = F.pad(x, (0, n_pad), "reflect")
- t = t + n_pad
- x = x.view(b, c, t // self.period, self.period)
-
- for l in self.convs:
- x = l(x)
- x = F.leaky_relu(x, LRELU_SLOPE)
- fmap.append(x)
- x = self.conv_post(x)
- fmap.append(x)
- x = torch.flatten(x, 1, -1)
-
- return x, fmap
-
-
-class MultiPeriodDiscriminator(torch.nn.Module):
- def __init__(self, periods=None):
- super(MultiPeriodDiscriminator, self).__init__()
- self.periods = periods if periods is not None else [2, 3, 5, 7, 11]
- self.discriminators = nn.ModuleList()
- for period in self.periods:
- self.discriminators.append(DiscriminatorP(period))
-
- def forward(self, y, y_hat):
- y_d_rs = []
- y_d_gs = []
- fmap_rs = []
- fmap_gs = []
- for i, d in enumerate(self.discriminators):
- y_d_r, fmap_r = d(y)
- y_d_g, fmap_g = d(y_hat)
- y_d_rs.append(y_d_r)
- fmap_rs.append(fmap_r)
- y_d_gs.append(y_d_g)
- fmap_gs.append(fmap_g)
-
- return y_d_rs, y_d_gs, fmap_rs, fmap_gs
-
-
-class DiscriminatorS(torch.nn.Module):
- def __init__(self, use_spectral_norm=False):
- super(DiscriminatorS, self).__init__()
- norm_f = weight_norm if use_spectral_norm == False else spectral_norm
- self.convs = nn.ModuleList([
- norm_f(Conv1d(1, 128, 15, 1, padding=7)),
- norm_f(Conv1d(128, 128, 41, 2, groups=4, padding=20)),
- norm_f(Conv1d(128, 256, 41, 2, groups=16, padding=20)),
- norm_f(Conv1d(256, 512, 41, 4, groups=16, padding=20)),
- norm_f(Conv1d(512, 1024, 41, 4, groups=16, padding=20)),
- norm_f(Conv1d(1024, 1024, 41, 1, groups=16, padding=20)),
- norm_f(Conv1d(1024, 1024, 5, 1, padding=2)),
- ])
- self.conv_post = norm_f(Conv1d(1024, 1, 3, 1, padding=1))
-
- def forward(self, x):
- fmap = []
- for l in self.convs:
- x = l(x)
- x = F.leaky_relu(x, LRELU_SLOPE)
- fmap.append(x)
- x = self.conv_post(x)
- fmap.append(x)
- x = torch.flatten(x, 1, -1)
-
- return x, fmap
-
-
-class MultiScaleDiscriminator(torch.nn.Module):
- def __init__(self):
- super(MultiScaleDiscriminator, self).__init__()
- self.discriminators = nn.ModuleList([
- DiscriminatorS(use_spectral_norm=True),
- DiscriminatorS(),
- DiscriminatorS(),
- ])
- self.meanpools = nn.ModuleList([
- AvgPool1d(4, 2, padding=2),
- AvgPool1d(4, 2, padding=2)
- ])
-
- def forward(self, y, y_hat):
- y_d_rs = []
- y_d_gs = []
- fmap_rs = []
- fmap_gs = []
- for i, d in enumerate(self.discriminators):
- if i != 0:
- y = self.meanpools[i - 1](y)
- y_hat = self.meanpools[i - 1](y_hat)
- y_d_r, fmap_r = d(y)
- y_d_g, fmap_g = d(y_hat)
- y_d_rs.append(y_d_r)
- fmap_rs.append(fmap_r)
- y_d_gs.append(y_d_g)
- fmap_gs.append(fmap_g)
-
- return y_d_rs, y_d_gs, fmap_rs, fmap_gs
-
-
-def feature_loss(fmap_r, fmap_g):
- loss = 0
- for dr, dg in zip(fmap_r, fmap_g):
- for rl, gl in zip(dr, dg):
- loss += torch.mean(torch.abs(rl - gl))
-
- return loss * 2
-
-
-def discriminator_loss(disc_real_outputs, disc_generated_outputs):
- loss = 0
- r_losses = []
- g_losses = []
- for dr, dg in zip(disc_real_outputs, disc_generated_outputs):
- r_loss = torch.mean((1 - dr) ** 2)
- g_loss = torch.mean(dg ** 2)
- loss += (r_loss + g_loss)
- r_losses.append(r_loss.item())
- g_losses.append(g_loss.item())
-
- return loss, r_losses, g_losses
-
-
-def generator_loss(disc_outputs):
- loss = 0
- gen_losses = []
- for dg in disc_outputs:
- l = torch.mean((1 - dg) ** 2)
- gen_losses.append(l)
- loss += l
-
- return loss, gen_losses
diff --git a/spaces/MedicalAILabo/Xp-age/lib/component/__init__.py b/spaces/MedicalAILabo/Xp-age/lib/component/__init__.py
deleted file mode 100644
index 687f7bcb535788688afe9620a391c7d924ad92e4..0000000000000000000000000000000000000000
--- a/spaces/MedicalAILabo/Xp-age/lib/component/__init__.py
+++ /dev/null
@@ -1,16 +0,0 @@
-#!/usr/bin/env python
-# -*- coding: utf-8 -*-
-
-from .net import create_net
-from .criterion import set_criterion
-from .optimizer import set_optimizer
-from .loss import set_loss_store
-from .likelihood import set_likelihood
-
-__all__ = [
- 'create_net',
- 'set_criterion',
- 'set_optimizer',
- 'set_loss_store',
- 'set_likelihood'
- ]
diff --git a/spaces/Meena/table-question-answering-space/README.md b/spaces/Meena/table-question-answering-space/README.md
deleted file mode 100644
index d55c265b216d1944b2cd3acd653c023ebe7146e9..0000000000000000000000000000000000000000
--- a/spaces/Meena/table-question-answering-space/README.md
+++ /dev/null
@@ -1,12 +0,0 @@
----
-title: Table Question Answering Space
-emoji: 🐨
-colorFrom: pink
-colorTo: blue
-sdk: streamlit
-sdk_version: 1.2.0
-app_file: app.py
-pinned: false
----
-
-Check out the configuration reference at https://huggingface.co/docs/hub/spaces#reference
diff --git a/spaces/MilaNLProc/wordify/src/__init__.py b/spaces/MilaNLProc/wordify/src/__init__.py
deleted file mode 100644
index e69de29bb2d1d6434b8b29ae775ad8c2e48c5391..0000000000000000000000000000000000000000
diff --git a/spaces/Mountchicken/MAERec-Gradio/mmocr/models/common/layers/__init__.py b/spaces/Mountchicken/MAERec-Gradio/mmocr/models/common/layers/__init__.py
deleted file mode 100644
index 1d1a921fdc8b57e2de15cedd6a214df77d9bdb42..0000000000000000000000000000000000000000
--- a/spaces/Mountchicken/MAERec-Gradio/mmocr/models/common/layers/__init__.py
+++ /dev/null
@@ -1,4 +0,0 @@
-# Copyright (c) OpenMMLab. All rights reserved.
-from .transformer_layers import TFDecoderLayer, TFEncoderLayer
-
-__all__ = ['TFEncoderLayer', 'TFDecoderLayer']
diff --git a/spaces/MrVicente/RA-BART/kgs_binding/relation_mapper_builder.py b/spaces/MrVicente/RA-BART/kgs_binding/relation_mapper_builder.py
deleted file mode 100644
index 58b7d99726c5121898d84c1130ffd31b87272d28..0000000000000000000000000000000000000000
--- a/spaces/MrVicente/RA-BART/kgs_binding/relation_mapper_builder.py
+++ /dev/null
@@ -1,164 +0,0 @@
-
-#############################
-# Imports
-#############################
-
-# Python modules
-from collections import deque
-from collections import defaultdict
-from typing import List, Dict, Optional
-from ast import literal_eval
-from random import sample
-
-# Remote modules
-
-# Local modules
-from .kg_base_wrapper import KGBaseHandler
-from .swow_handler import SwowHandler
-
-from utils import (
- read_json_file_2_dict,
- Data_Type,
-)
-from .parsing_utils import ParsingUtils
-
-#############################
-# Constants
-#############################
-
-#############################
-# Stuff
-#############################
-
-class RelationsMapperBuilder:
- def __init__(self, knowledge: KGBaseHandler,
- filename: Optional[str] = None,
- file_dir: Optional[str] = None,
- datatype: Optional[Data_Type] = None,
- tok_sep:str = '',
- use_extra_relations=True):
- self.tok_sep = tok_sep
- self.knowledge = knowledge
- self.swow_knowledge = SwowHandler()
- self.use_extra_relations = use_extra_relations
- if filename and file_dir and datatype:
- full_context = self.load_data(filename, file_dir)
- self.relevant_context = self.fetch_relevant_context_from_data(data=full_context, datatype=datatype)
-
- def load_data(self, filename='commongen_qa_final.json', store_dir='./'):
- data = read_json_file_2_dict(filename=filename, store_dir=store_dir)
- print('data[0]:', data[0])
- return data
-
- def fetch_relevant_context_from_data(self, data: List[Dict], datatype:Data_Type = Data_Type.COMMONGEN_QA):
- if datatype == Data_Type.COMMONGEN_QA:
- model_input = [data_unit.get('title').lower() for data_unit in data]
- elif datatype in [Data_Type.ELI5, Data_Type.STACK_EXCHANGE]:
- model_input = [data_unit.get('question').lower() for data_unit in data]
- elif datatype in [Data_Type.COMMONSENSE_QA]:
- #questions = [data_unit.get('question').lower() for data_unit in data]
- #model_input = datasets_parsing_utils.compose_commonsenseqa_data(data)
- model_input = [data_unit.get('input_data') for data_unit in data]
- elif datatype in [Data_Type.COMMONGEN]:
- #questions = [data_unit.get('input_data').lower() for data_unit in data]
- #model_input = datasets_parsing_utils.compose_commongen_data(data)
- model_input = [data_unit.get('input_data') for data_unit in data]
- else:
- model_input = []
- return model_input
-
- def get_kg_concepts_from_context(self, context=None, clear_common_wds=False):
- if not context:
- context = self.relevant_context
- context_words = []
- for q_id, question in enumerate(context):
- simple_question = ParsingUtils.remove_pontuation(question)
- n_grams = ParsingUtils.n_grams_n_words_extractor(simple_question)
- words = self.relevant_entities_extractor(n_grams)
- if clear_common_wds:
- words = ParsingUtils.clear_common_words(words)
- simple_words = [word[0] for word in words]
- context_words.append(simple_words)
- return context_words
-
- def obtain_concept_neighbours(self, context_concepts:List[str], n_neighbours = 20):
- """
- Use swow to get connected concepts, but then refer back to conceptnet for rich relations
- """
- neighbours = []
- for concept in context_concepts:
- external_neighbour_concepts = self.swow_knowledge.get_related_concepts(concept)
- relevant_concepts = external_neighbour_concepts
- #local_neighbour_concepts = self.knowledge.get_related_concepts(concept)
- #relevant_concepts = [ext_concept for ext_concept in external_neighbour_concepts if ext_concept in local_neighbour_concepts]
- neighbours.extend(relevant_concepts)
- n_neighbours = min(n_neighbours, len(neighbours))
- some_neighbours = sample(neighbours, n_neighbours)
- #print('context_concepts:', context_concepts)
- #print('some_neighbours:', some_neighbours)
- return some_neighbours
-
-
- def get_relations_mapping_complex(self, context=None, clear_common_wds=False):
- if not context:
- context = self.relevant_context
- relations_info = deque()
- for q_id, question in enumerate(context):
- simple_question = ParsingUtils.remove_pontuation(question)
- n_grams = ParsingUtils.n_grams_n_words_extractor(simple_question)
- words = self.relevant_entities_extractor(n_grams)
- if clear_common_wds:
- words = ParsingUtils.clear_common_words(words)
- #print(f'question: {question}')
- #print(f'words: {words}')
- relation_context_between_words = defaultdict(dict)
- known_tokens = set()
- for token_i, (first_word_token, first_word_range) in enumerate(words[:-1]):
- known_tokens.add(first_word_token)
- first_word_range_str = str(first_word_range)
- # normalize
- first_word_phrase_normalized = self.knowledge.normalize_nouns(first_word_token)
- for (second_word_token, second_word_range) in [w for w in words[token_i + 1:] if w not in known_tokens]:
- second_word_range_str = str(second_word_range)
- second_word_phrase_normalized = self.knowledge.normalize_nouns(second_word_token)
- left_2_right, right_2_left = self.knowledge.relation_between(first_word_phrase_normalized, second_word_phrase_normalized)
- #print(first_word_token, second_word_token, left_2_right, right_2_left)
- if left_2_right:
- relation_context_between_words[first_word_range_str][second_word_range_str] = left_2_right
- if right_2_left:
- relation_context_between_words[second_word_range_str][first_word_range_str] = right_2_left
- relations_info.append(dict(relation_context_between_words))
- return list(relations_info)
-
- def get_concepts_from_context(self, context=None, clear_common_wds=False,alignment=0):
- relations_info = self.get_relations_mapping_complex(context=[context], clear_common_wds=clear_common_wds)
- words = []
- #print('relations_info here:', relations_info)
- for rels in relations_info:
- for coords, v in rels.items():
- coords_tuple = literal_eval(coords)
- i,j = coords_tuple
- words.append(context[i+alignment:j+alignment])
- for coords_other, rel in v.items():
- coords_other_tuple = literal_eval(coords_other)
- i_other, j_other = coords_other_tuple
- words.append(context[i_other+alignment: j_other+alignment])
- returning_words = list(set(words))
- #print('returning_words:', returning_words)
- return returning_words
-
- def relevant_entities_extractor(self, n_grams_n_words, verbose_output=True):
- non_overlapping_knowledge = {}
- # print(n_grams_n_words)
- for concept, (idx_start, idx_end) in n_grams_n_words:
- normalized_concept = self.knowledge.normalize_nouns(concept)
- exists = self.knowledge.does_concept_exist(normalized_concept)
- #print('exists: ', concept, normalized_concept, exists)
- if exists and idx_start not in non_overlapping_knowledge and \
- idx_end not in non_overlapping_knowledge:
- non_overlapping_knowledge[idx_start] = (concept, idx_start, idx_end, 'start_idx')
- non_overlapping_knowledge[idx_end] = (concept, idx_end, idx_end, 'end_idx')
- if verbose_output:
- return [(value[0], (value[1], value[2])) for k, value in sorted(non_overlapping_knowledge.items()) if value[-1] == 'start_idx']
- else:
- return [value[0] for k, value in sorted(non_overlapping_knowledge.items()) if value[-1] == 'start_idx']
diff --git a/spaces/MuGeminorum/insecta/khandy/text_utils.py b/spaces/MuGeminorum/insecta/khandy/text_utils.py
deleted file mode 100644
index 11d84714960659e6299bdadeebe753f6e625bad5..0000000000000000000000000000000000000000
--- a/spaces/MuGeminorum/insecta/khandy/text_utils.py
+++ /dev/null
@@ -1,33 +0,0 @@
-import re
-
-
-def strip_content_in_paren(string):
- """
- Notes:
- strip_content_in_paren cannot process nested paren correctly
- """
- return re.sub(r"\([^)]*\)|([^)]*)", "", string)
-
-
-def is_chinese_char(uchar: str) -> bool:
- """Whether the input char is a Chinese character.
-
- Args:
- uchar: input char in unicode
-
- References:
- `is_chinese_char` in https://github.com/thunlp/OpenNRE/
- """
- codepoint = ord(uchar)
- if ((0x4E00 <= codepoint <= 0x9FFF) or # CJK Unified Ideographs
- (0x3400 <= codepoint <= 0x4DBF) or # CJK Unified Ideographs Extension A
- (0xF900 <= codepoint <= 0xFAFF) or # CJK Compatibility Ideographs
- (0x20000 <= codepoint <= 0x2A6DF) or # CJK Unified Ideographs Extension B
- (0x2A700 <= codepoint <= 0x2B73F) or
- (0x2B740 <= codepoint <= 0x2B81F) or
- (0x2B820 <= codepoint <= 0x2CEAF) or
- (0x2F800 <= codepoint <= 0x2FA1F)): # CJK Compatibility Supplement
- return True
- return False
-
-
diff --git a/spaces/Munna0912/URL_CLASSIFIER/Utils/Model.py b/spaces/Munna0912/URL_CLASSIFIER/Utils/Model.py
deleted file mode 100644
index a404d8aa78260508745e27d08c2d1af8c8d8a922..0000000000000000000000000000000000000000
--- a/spaces/Munna0912/URL_CLASSIFIER/Utils/Model.py
+++ /dev/null
@@ -1,27 +0,0 @@
-import tensorflow as tf
-from tensorflow.keras.layers import Dense, Dropout, Embedding, GRU, Input, concatenate
-from tensorflow.keras.models import Model
-
-def create_model(Sequence_length,max_tokens, input_shape_numeric ):
- # define nlp model
- text_input = Input(shape=(Sequence_length,),)
- x = Embedding(max_tokens, 16, input_length=Sequence_length)(text_input)
- x = GRU(16, dropout=0.2, recurrent_dropout=0.2)(x)
- x = Dropout(0.2)(x)
- text_model = Model(inputs=text_input, outputs=x)
-
- # define numeric model
- numeric_input = Input(shape=(input_shape_numeric,),)
- y = Dense(16, activation='relu')(numeric_input)
- y = Dropout(0.2)(y)
- # y = Dense(16, activation='relu')(y)
- # y = Dropout(0.2)(y)
- numeric_model = Model(inputs=numeric_input, outputs=y)
-
- # concatenate the two models
- combined_input = concatenate([text_model.output, numeric_model.output])
- z = Dense(16, activation='relu')(combined_input)
- z = Dropout(0.2)(z)
- output = Dense(1, activation='sigmoid')(z)
-
- return Model(inputs=[text_model.input, numeric_model.input], outputs=output)
diff --git a/spaces/NAACL2022/README/README.md b/spaces/NAACL2022/README/README.md
deleted file mode 100644
index 90a482d6187dfbb7b89be1e883e36846b785f28c..0000000000000000000000000000000000000000
--- a/spaces/NAACL2022/README/README.md
+++ /dev/null
@@ -1,113 +0,0 @@
----
-title: README
-emoji: ⚡
-colorFrom: pink
-colorTo: gray
-sdk: static
-pinned: false
----
-
-
-
This organization invites participants to add gradio demos/models/datasets for conference papers on Hugging Face (Note: This is not a official NAACL sponsored event)
-
Join organization by clicking here
-
Hugging Face Gradio NAACL 2022 event
-
-
-NAACL organization is accepting Gradio demo submissions for NAACL 2022 papers from anyone for a chance to win prizes from Hugging Face, see prizes section and the leaderboard below. The deadline to submit demos is July 31st, 2022 (AOE Time Zone). For all participants, feel free to submit Gradio demos for any NAACL paper for a chance to win prizes, you can submit demos for multiple papers. Find tutorial on getting started with Gradio on Hugging Face here and to get started with the new Gradio Blocks API here
-
-
Hugging Face Models NAACL 2022 event
-
-
-NAACL organization is accepting models submissions for NAACL 2022 papers from anyone for a chance to win prizes from Hugging Face, see prizes section and the leaderboard below. The deadline to submit demos is July 31st, 2022 (AOE Time Zone). For all partipants, feel free to submit models for any NAACL paper for a chance to win prizes, you can submit models for multiple papers. Find tutorial on getting started with repos on Hugging Face here and to get started with adding models here
-
-
Hugging Face Datasets NAACL 2022 event
-
-
-NAACL organization is accepting dataset submissions for NAACL 2022 papers from anyone for a chance to win prizes from Hugging Face, see prizes section and the leaderboard below. The deadline to submit demos is July 31st, 2022 (AOE Time Zone). For all partipants, feel free to submit datasets for any NAACL paper for a chance to win prizes, you can submit datasets for multiple papers. Find tutorial on getting started with repos on Hugging Face here and to get started with adding datasets here
-
-
Hugging Face Prizes
-
- - Top 5 spaces/models/datasets based on likes
-
-
-
-
-
LeaderBoard for Most Popular NAACL Spaces
-
See the NAACL Spaces Leaderboard
-
LeaderBoard for Most Popular NAACL Models
-
See the NAACL Models Leaderboard
-
LeaderBoard for Most Popular NAACL Datasets
-
See the NAACL Datasets Leaderboard
-
- Hugging Face Spaces & Gradio for Showcasing your NAACL ‘22 Demo
-
-
- In this tutorial, we will demonstrate how to showcase your demo with an easy to use web interface using the Gradio Python library and host it on Hugging Face Spaces so that conference attendees can easily find and try out your demos. Also, see https://gradio.app/introduction_to_blocks/, for a more flexible way to build Gradio Demos
-
- 🚀 Create a Gradio Demo from your Model
-
-
-The first step is to create a web demo from your model. As an example, we will be creating a demo from an image classification model (called model) which we will be uploading to Spaces. The full code for steps 1-4 can be found in this colab notebook.
-
-
-1. Install the gradio library
-
-
-All you need to do is to run this in the terminal: pip install gradio
-
-
-2. Define a function in your Python code that performs inference with your model on a data point and returns the prediction
-
-
-Here’s we define our image classification model prediction function in PyTorch (any framework, like TensorFlow, scikit-learn, JAX, or a plain Python will work as well):
-
-
-def predict(inp):
- inp = Image.fromarray(inp.astype('uint8'), 'RGB')
-
- inp = transforms.ToTensor()(inp).unsqueeze(0)
-
- with torch.no_grad():
-
- prediction = torch.nn.functional.softmax(model(inp)[0], dim=0)
-
- return {labels[i]: float(prediction[i]) for i in range(1000)}
-
-
-
-
-3. Then create a Gradio Interface using the function and the appropriate input and output types
-
-
-For the image classification model from Step 2, it would like like this:
-
-
-
-inputs = gr.inputs.Image()
-
-outputs = gr.outputs.Label(num_top_classes=3)
-
-io = gr.Interface(fn=predict, inputs=inputs, outputs=outputs)
-
-
-
-If you need help creating a Gradio Interface for your model, check out the Gradio Getting Started guide.
-
-
-4. Then launch() you Interface to confirm that it runs correctly locally (or wherever you are running Python)
-
-
-
-io.launch()
-
-
-
-You should see a web interface like the following where you can drag and drop your data points and see the predictions:
-
-
-
-
-
-
diff --git a/spaces/NATSpeech/DiffSpeech/tasks/vocoder/vocoder_base.py b/spaces/NATSpeech/DiffSpeech/tasks/vocoder/vocoder_base.py
deleted file mode 100644
index 9a1d006647f259ec39968ec9a9d2f36b166f5851..0000000000000000000000000000000000000000
--- a/spaces/NATSpeech/DiffSpeech/tasks/vocoder/vocoder_base.py
+++ /dev/null
@@ -1,137 +0,0 @@
-import os
-import torch
-import torch.distributed as dist
-from torch import nn
-from torch.utils.data import DistributedSampler
-from tasks.vocoder.dataset_utils import VocoderDataset, EndlessDistributedSampler
-from utils.audio.io import save_wav
-from utils.commons.base_task import BaseTask
-from utils.commons.dataset_utils import data_loader
-from utils.commons.hparams import hparams
-from utils.commons.tensor_utils import tensors_to_scalars
-
-
-class VocoderBaseTask(BaseTask):
- def __init__(self):
- super(VocoderBaseTask, self).__init__()
- self.max_sentences = hparams['max_sentences']
- self.max_valid_sentences = hparams['max_valid_sentences']
- if self.max_valid_sentences == -1:
- hparams['max_valid_sentences'] = self.max_valid_sentences = self.max_sentences
- self.dataset_cls = VocoderDataset
-
- @data_loader
- def train_dataloader(self):
- train_dataset = self.dataset_cls('train', shuffle=True)
- return self.build_dataloader(train_dataset, True, self.max_sentences, hparams['endless_ds'])
-
- @data_loader
- def val_dataloader(self):
- valid_dataset = self.dataset_cls('test', shuffle=False)
- return self.build_dataloader(valid_dataset, False, self.max_valid_sentences)
-
- @data_loader
- def test_dataloader(self):
- test_dataset = self.dataset_cls('test', shuffle=False)
- return self.build_dataloader(test_dataset, False, self.max_valid_sentences)
-
- def build_dataloader(self, dataset, shuffle, max_sentences, endless=False):
- world_size = 1
- rank = 0
- if dist.is_initialized():
- world_size = dist.get_world_size()
- rank = dist.get_rank()
- sampler_cls = DistributedSampler if not endless else EndlessDistributedSampler
- train_sampler = sampler_cls(
- dataset=dataset,
- num_replicas=world_size,
- rank=rank,
- shuffle=shuffle,
- )
- return torch.utils.data.DataLoader(
- dataset=dataset,
- shuffle=False,
- collate_fn=dataset.collater,
- batch_size=max_sentences,
- num_workers=dataset.num_workers,
- sampler=train_sampler,
- pin_memory=True,
- )
-
- def build_optimizer(self, model):
- optimizer_gen = torch.optim.AdamW(self.model_gen.parameters(), lr=hparams['lr'],
- betas=[hparams['adam_b1'], hparams['adam_b2']])
- optimizer_disc = torch.optim.AdamW(self.model_disc.parameters(), lr=hparams['lr'],
- betas=[hparams['adam_b1'], hparams['adam_b2']])
- return [optimizer_gen, optimizer_disc]
-
- def build_scheduler(self, optimizer):
- return {
- "gen": torch.optim.lr_scheduler.StepLR(
- optimizer=optimizer[0],
- **hparams["generator_scheduler_params"]),
- "disc": torch.optim.lr_scheduler.StepLR(
- optimizer=optimizer[1],
- **hparams["discriminator_scheduler_params"]),
- }
-
- def validation_step(self, sample, batch_idx):
- outputs = {}
- total_loss, loss_output = self._training_step(sample, batch_idx, 0)
- outputs['losses'] = tensors_to_scalars(loss_output)
- outputs['total_loss'] = tensors_to_scalars(total_loss)
-
- if self.global_step % hparams['valid_infer_interval'] == 0 and \
- batch_idx < 10:
- mels = sample['mels']
- y = sample['wavs']
- f0 = sample['f0']
- y_ = self.model_gen(mels, f0)
- for idx, (wav_pred, wav_gt, item_name) in enumerate(zip(y_, y, sample["item_name"])):
- wav_pred = wav_pred / wav_pred.abs().max()
- if self.global_step == 0:
- wav_gt = wav_gt / wav_gt.abs().max()
- self.logger.add_audio(f'wav_{batch_idx}_{idx}_gt', wav_gt, self.global_step,
- hparams['audio_sample_rate'])
- self.logger.add_audio(f'wav_{batch_idx}_{idx}_pred', wav_pred, self.global_step,
- hparams['audio_sample_rate'])
- return outputs
-
- def test_start(self):
- self.gen_dir = os.path.join(hparams['work_dir'],
- f'generated_{self.trainer.global_step}_{hparams["gen_dir_name"]}')
- os.makedirs(self.gen_dir, exist_ok=True)
-
- def test_step(self, sample, batch_idx):
- mels = sample['mels']
- y = sample['wavs']
- f0 = sample['f0']
- loss_output = {}
- y_ = self.model_gen(mels, f0)
- gen_dir = os.path.join(hparams['work_dir'], f'generated_{self.trainer.global_step}_{hparams["gen_dir_name"]}')
- os.makedirs(gen_dir, exist_ok=True)
- for idx, (wav_pred, wav_gt, item_name) in enumerate(zip(y_, y, sample["item_name"])):
- wav_gt = wav_gt.clamp(-1, 1)
- wav_pred = wav_pred.clamp(-1, 1)
- save_wav(
- wav_gt.view(-1).cpu().float().numpy(), f'{gen_dir}/{item_name}_gt.wav',
- hparams['audio_sample_rate'])
- save_wav(
- wav_pred.view(-1).cpu().float().numpy(), f'{gen_dir}/{item_name}_pred.wav',
- hparams['audio_sample_rate'])
- return loss_output
-
- def test_end(self, outputs):
- return {}
-
- def on_before_optimization(self, opt_idx):
- if opt_idx == 0:
- nn.utils.clip_grad_norm_(self.model_gen.parameters(), hparams['generator_grad_norm'])
- else:
- nn.utils.clip_grad_norm_(self.model_disc.parameters(), hparams["discriminator_grad_norm"])
-
- def on_after_optimization(self, epoch, batch_idx, optimizer, optimizer_idx):
- if optimizer_idx == 0:
- self.scheduler['gen'].step(self.global_step // hparams['accumulate_grad_batches'])
- else:
- self.scheduler['disc'].step(self.global_step // hparams['accumulate_grad_batches'])
diff --git a/spaces/NCTCMumbai/NCTC/models/research/attention_ocr/python/datasets/fsns_test.py b/spaces/NCTCMumbai/NCTC/models/research/attention_ocr/python/datasets/fsns_test.py
deleted file mode 100644
index 4daedfbd12a58b6635cefed2bdc02bc84fc2c9ef..0000000000000000000000000000000000000000
--- a/spaces/NCTCMumbai/NCTC/models/research/attention_ocr/python/datasets/fsns_test.py
+++ /dev/null
@@ -1,103 +0,0 @@
-# Copyright 2017 The TensorFlow Authors All Rights Reserved.
-#
-# Licensed under the Apache License, Version 2.0 (the "License");
-# you may not use this file except in compliance with the License.
-# You may obtain a copy of the License at
-#
-# http://www.apache.org/licenses/LICENSE-2.0
-#
-# Unless required by applicable law or agreed to in writing, software
-# distributed under the License is distributed on an "AS IS" BASIS,
-# WITHOUT WARRANTIES OR CONDITIONS OF ANY KIND, either express or implied.
-# See the License for the specific language governing permissions and
-# limitations under the License.
-# ==============================================================================
-
-"""Tests for FSNS datasets module."""
-
-import collections
-import os
-import tensorflow as tf
-from tensorflow.contrib import slim
-
-from datasets import fsns
-from datasets import unittest_utils
-
-FLAGS = tf.flags.FLAGS
-
-
-def get_test_split():
- config = fsns.DEFAULT_CONFIG.copy()
- config['splits'] = {'test': {'size': 5, 'pattern': 'fsns-00000-of-00001'}}
- return fsns.get_split('test', dataset_dir(), config)
-
-
-def dataset_dir():
- return os.path.join(os.path.dirname(__file__), 'testdata/fsns')
-
-
-class FsnsTest(tf.test.TestCase):
- def test_decodes_example_proto(self):
- expected_label = range(37)
- expected_image, encoded = unittest_utils.create_random_image(
- 'PNG', shape=(150, 600, 3))
- serialized = unittest_utils.create_serialized_example({
- 'image/encoded': [encoded],
- 'image/format': [b'PNG'],
- 'image/class':
- expected_label,
- 'image/unpadded_class':
- range(10),
- 'image/text': [b'Raw text'],
- 'image/orig_width': [150],
- 'image/width': [600]
- })
-
- decoder = fsns.get_split('train', dataset_dir()).decoder
- with self.test_session() as sess:
- data_tuple = collections.namedtuple('DecodedData', decoder.list_items())
- data = sess.run(data_tuple(*decoder.decode(serialized)))
-
- self.assertAllEqual(expected_image, data.image)
- self.assertAllEqual(expected_label, data.label)
- self.assertEqual([b'Raw text'], data.text)
- self.assertEqual([1], data.num_of_views)
-
- def test_label_has_shape_defined(self):
- serialized = 'fake'
- decoder = fsns.get_split('train', dataset_dir()).decoder
-
- [label_tf] = decoder.decode(serialized, ['label'])
-
- self.assertEqual(label_tf.get_shape().dims[0], 37)
-
- def test_dataset_tuple_has_all_extra_attributes(self):
- dataset = fsns.get_split('train', dataset_dir())
-
- self.assertTrue(dataset.charset)
- self.assertTrue(dataset.num_char_classes)
- self.assertTrue(dataset.num_of_views)
- self.assertTrue(dataset.max_sequence_length)
- self.assertTrue(dataset.null_code)
-
- def test_can_use_the_test_data(self):
- batch_size = 1
- dataset = get_test_split()
- provider = slim.dataset_data_provider.DatasetDataProvider(
- dataset,
- shuffle=True,
- common_queue_capacity=2 * batch_size,
- common_queue_min=batch_size)
- image_tf, label_tf = provider.get(['image', 'label'])
-
- with self.test_session() as sess:
- sess.run(tf.global_variables_initializer())
- with slim.queues.QueueRunners(sess):
- image_np, label_np = sess.run([image_tf, label_tf])
-
- self.assertEqual((150, 600, 3), image_np.shape)
- self.assertEqual((37, ), label_np.shape)
-
-
-if __name__ == '__main__':
- tf.test.main()
diff --git a/spaces/NCTCMumbai/NCTC/models/research/autoencoder/AdditiveGaussianNoiseAutoencoderRunner.py b/spaces/NCTCMumbai/NCTC/models/research/autoencoder/AdditiveGaussianNoiseAutoencoderRunner.py
deleted file mode 100644
index 8d8ee08654985250ac61415df96889b4a4cf5f1b..0000000000000000000000000000000000000000
--- a/spaces/NCTCMumbai/NCTC/models/research/autoencoder/AdditiveGaussianNoiseAutoencoderRunner.py
+++ /dev/null
@@ -1,58 +0,0 @@
-from __future__ import absolute_import
-from __future__ import division
-from __future__ import print_function
-
-import numpy as np
-import sklearn.preprocessing as prep
-import tensorflow as tf
-from tensorflow.examples.tutorials.mnist import input_data
-
-from autoencoder_models.DenoisingAutoencoder import AdditiveGaussianNoiseAutoencoder
-
-mnist = input_data.read_data_sets('MNIST_data', one_hot=True)
-
-
-def standard_scale(X_train, X_test):
- preprocessor = prep.StandardScaler().fit(X_train)
- X_train = preprocessor.transform(X_train)
- X_test = preprocessor.transform(X_test)
- return X_train, X_test
-
-
-def get_random_block_from_data(data, batch_size):
- start_index = np.random.randint(0, len(data) - batch_size)
- return data[start_index:(start_index + batch_size)]
-
-
-X_train, X_test = standard_scale(mnist.train.images, mnist.test.images)
-
-n_samples = int(mnist.train.num_examples)
-training_epochs = 20
-batch_size = 128
-display_step = 1
-
-autoencoder = AdditiveGaussianNoiseAutoencoder(
- n_input=784,
- n_hidden=200,
- transfer_function=tf.nn.softplus,
- optimizer=tf.train.AdamOptimizer(learning_rate = 0.001),
- scale=0.01)
-
-for epoch in range(training_epochs):
- avg_cost = 0.
- total_batch = int(n_samples / batch_size)
- # Loop over all batches
- for i in range(total_batch):
- batch_xs = get_random_block_from_data(X_train, batch_size)
-
- # Fit training using batch data
- cost = autoencoder.partial_fit(batch_xs)
- # Compute average loss
- avg_cost += cost / n_samples * batch_size
-
- # Display logs per epoch step
- if epoch % display_step == 0:
- print("Epoch:", '%d,' % (epoch + 1),
- "Cost:", "{:.9f}".format(avg_cost))
-
-print("Total cost: " + str(autoencoder.calc_total_cost(X_test)))
diff --git a/spaces/NKU-AMT/AMT/networks/blocks/feat_enc.py b/spaces/NKU-AMT/AMT/networks/blocks/feat_enc.py
deleted file mode 100644
index 983246b7aa16fb67a4d0f3ad4893204bb1e7f495..0000000000000000000000000000000000000000
--- a/spaces/NKU-AMT/AMT/networks/blocks/feat_enc.py
+++ /dev/null
@@ -1,346 +0,0 @@
-'''
- This code is partially borrowed from RAFT (https://github.com/princeton-vl/RAFT).
-'''
-import torch
-import torch.nn as nn
-
-class BottleneckBlock(nn.Module):
- def __init__(self, in_planes, planes, norm_fn='group', stride=1):
- super(BottleneckBlock, self).__init__()
-
- self.conv1 = nn.Conv2d(in_planes, planes//4, kernel_size=1, padding=0)
- self.conv2 = nn.Conv2d(planes//4, planes//4, kernel_size=3, padding=1, stride=stride)
- self.conv3 = nn.Conv2d(planes//4, planes, kernel_size=1, padding=0)
- self.relu = nn.ReLU(inplace=True)
-
- num_groups = planes // 8
-
- if norm_fn == 'group':
- self.norm1 = nn.GroupNorm(num_groups=num_groups, num_channels=planes//4)
- self.norm2 = nn.GroupNorm(num_groups=num_groups, num_channels=planes//4)
- self.norm3 = nn.GroupNorm(num_groups=num_groups, num_channels=planes)
- if not stride == 1:
- self.norm4 = nn.GroupNorm(num_groups=num_groups, num_channels=planes)
-
-
- elif norm_fn == 'batch':
- self.norm1 = nn.BatchNorm2d(planes//4)
- self.norm2 = nn.BatchNorm2d(planes//4)
- self.norm3 = nn.BatchNorm2d(planes)
- if not stride == 1:
- self.norm4 = nn.BatchNorm2d(planes)
-
- elif norm_fn == 'instance':
- self.norm1 = nn.InstanceNorm2d(planes//4)
- self.norm2 = nn.InstanceNorm2d(planes//4)
- self.norm3 = nn.InstanceNorm2d(planes)
- if not stride == 1:
- self.norm4 = nn.InstanceNorm2d(planes)
-
- elif norm_fn == 'none':
- self.norm1 = nn.Sequential()
- self.norm2 = nn.Sequential()
- self.norm3 = nn.Sequential()
- if not stride == 1:
- self.norm4 = nn.Sequential()
-
- if stride == 1:
- self.downsample = None
-
- else:
- self.downsample = nn.Sequential(
- nn.Conv2d(in_planes, planes, kernel_size=1, stride=stride), self.norm4)
-
-
- def forward(self, x):
- y = x
- y = self.relu(self.norm1(self.conv1(y)))
- y = self.relu(self.norm2(self.conv2(y)))
- y = self.relu(self.norm3(self.conv3(y)))
-
- if self.downsample is not None:
- x = self.downsample(x)
-
- return self.relu(x+y)
-
-
-class ResidualBlock(nn.Module):
- def __init__(self, in_planes, planes, norm_fn='group', stride=1):
- super(ResidualBlock, self).__init__()
-
- self.conv1 = nn.Conv2d(in_planes, planes, kernel_size=3, padding=1, stride=stride)
- self.conv2 = nn.Conv2d(planes, planes, kernel_size=3, padding=1)
- self.relu = nn.ReLU(inplace=True)
-
- num_groups = planes // 8
-
- if norm_fn == 'group':
- self.norm1 = nn.GroupNorm(num_groups=num_groups, num_channels=planes)
- self.norm2 = nn.GroupNorm(num_groups=num_groups, num_channels=planes)
- if not stride == 1:
- self.norm3 = nn.GroupNorm(num_groups=num_groups, num_channels=planes)
-
- elif norm_fn == 'batch':
- self.norm1 = nn.BatchNorm2d(planes)
- self.norm2 = nn.BatchNorm2d(planes)
- if not stride == 1:
- self.norm3 = nn.BatchNorm2d(planes)
-
- elif norm_fn == 'instance':
- self.norm1 = nn.InstanceNorm2d(planes)
- self.norm2 = nn.InstanceNorm2d(planes)
- if not stride == 1:
- self.norm3 = nn.InstanceNorm2d(planes)
-
- elif norm_fn == 'none':
- self.norm1 = nn.Sequential()
- self.norm2 = nn.Sequential()
- if not stride == 1:
- self.norm3 = nn.Sequential()
-
- if stride == 1:
- self.downsample = None
-
- else:
- self.downsample = nn.Sequential(
- nn.Conv2d(in_planes, planes, kernel_size=1, stride=stride), self.norm3)
-
-
- def forward(self, x):
- y = x
- y = self.relu(self.norm1(self.conv1(y)))
- y = self.relu(self.norm2(self.conv2(y)))
-
- if self.downsample is not None:
- x = self.downsample(x)
-
- return self.relu(x+y)
-
-
-class SmallEncoder(nn.Module):
- def __init__(self, output_dim=128, norm_fn='batch', dropout=0.0):
- super(SmallEncoder, self).__init__()
- self.norm_fn = norm_fn
-
- if self.norm_fn == 'group':
- self.norm1 = nn.GroupNorm(num_groups=8, num_channels=32)
-
- elif self.norm_fn == 'batch':
- self.norm1 = nn.BatchNorm2d(32)
-
- elif self.norm_fn == 'instance':
- self.norm1 = nn.InstanceNorm2d(32)
-
- elif self.norm_fn == 'none':
- self.norm1 = nn.Sequential()
-
- self.conv1 = nn.Conv2d(3, 32, kernel_size=7, stride=2, padding=3)
- self.relu1 = nn.ReLU(inplace=True)
-
- self.in_planes = 32
- self.layer1 = self._make_layer(32, stride=1)
- self.layer2 = self._make_layer(64, stride=2)
- self.layer3 = self._make_layer(96, stride=2)
-
- self.dropout = None
- if dropout > 0:
- self.dropout = nn.Dropout2d(p=dropout)
-
- self.conv2 = nn.Conv2d(96, output_dim, kernel_size=1)
-
- for m in self.modules():
- if isinstance(m, nn.Conv2d):
- nn.init.kaiming_normal_(m.weight, mode='fan_out', nonlinearity='relu')
- elif isinstance(m, (nn.BatchNorm2d, nn.InstanceNorm2d, nn.GroupNorm)):
- if m.weight is not None:
- nn.init.constant_(m.weight, 1)
- if m.bias is not None:
- nn.init.constant_(m.bias, 0)
-
- def _make_layer(self, dim, stride=1):
- layer1 = BottleneckBlock(self.in_planes, dim, self.norm_fn, stride=stride)
- layer2 = BottleneckBlock(dim, dim, self.norm_fn, stride=1)
- layers = (layer1, layer2)
-
- self.in_planes = dim
- return nn.Sequential(*layers)
-
-
- def forward(self, x):
-
- # if input is list, combine batch dimension
- is_list = isinstance(x, tuple) or isinstance(x, list)
- if is_list:
- batch_dim = x[0].shape[0]
- x = torch.cat(x, dim=0)
-
- x = self.conv1(x)
- x = self.norm1(x)
- x = self.relu1(x)
-
- x = self.layer1(x)
- x = self.layer2(x)
- x = self.layer3(x)
- x = self.conv2(x)
-
- if self.training and self.dropout is not None:
- x = self.dropout(x)
-
- if is_list:
- x = torch.split(x, [batch_dim, batch_dim], dim=0)
-
- return x
-
-class BasicEncoder(nn.Module):
- def __init__(self, output_dim=128, norm_fn='batch', dropout=0.0):
- super(BasicEncoder, self).__init__()
- self.norm_fn = norm_fn
-
- if self.norm_fn == 'group':
- self.norm1 = nn.GroupNorm(num_groups=8, num_channels=64)
-
- elif self.norm_fn == 'batch':
- self.norm1 = nn.BatchNorm2d(64)
-
- elif self.norm_fn == 'instance':
- self.norm1 = nn.InstanceNorm2d(64)
-
- elif self.norm_fn == 'none':
- self.norm1 = nn.Sequential()
-
- self.conv1 = nn.Conv2d(3, 64, kernel_size=7, stride=2, padding=3)
- self.relu1 = nn.ReLU(inplace=True)
-
- self.in_planes = 64
- self.layer1 = self._make_layer(64, stride=1)
- self.layer2 = self._make_layer(72, stride=2)
- self.layer3 = self._make_layer(128, stride=2)
-
- # output convolution
- self.conv2 = nn.Conv2d(128, output_dim, kernel_size=1)
-
- self.dropout = None
- if dropout > 0:
- self.dropout = nn.Dropout2d(p=dropout)
-
- for m in self.modules():
- if isinstance(m, nn.Conv2d):
- nn.init.kaiming_normal_(m.weight, mode='fan_out', nonlinearity='relu')
- elif isinstance(m, (nn.BatchNorm2d, nn.InstanceNorm2d, nn.GroupNorm)):
- if m.weight is not None:
- nn.init.constant_(m.weight, 1)
- if m.bias is not None:
- nn.init.constant_(m.bias, 0)
-
- def _make_layer(self, dim, stride=1):
- layer1 = ResidualBlock(self.in_planes, dim, self.norm_fn, stride=stride)
- layer2 = ResidualBlock(dim, dim, self.norm_fn, stride=1)
- layers = (layer1, layer2)
-
- self.in_planes = dim
- return nn.Sequential(*layers)
-
-
- def forward(self, x):
-
- # if input is list, combine batch dimension
- is_list = isinstance(x, tuple) or isinstance(x, list)
- if is_list:
- batch_dim = x[0].shape[0]
- x = torch.cat(x, dim=0)
-
- x = self.conv1(x)
- x = self.norm1(x)
- x = self.relu1(x)
-
- x = self.layer1(x)
- x = self.layer2(x)
- x = self.layer3(x)
-
- x = self.conv2(x)
-
- if self.training and self.dropout is not None:
- x = self.dropout(x)
-
- if is_list:
- x = torch.split(x, [batch_dim, batch_dim], dim=0)
-
- return x
-
-class LargeEncoder(nn.Module):
- def __init__(self, output_dim=128, norm_fn='batch', dropout=0.0):
- super(LargeEncoder, self).__init__()
- self.norm_fn = norm_fn
-
- if self.norm_fn == 'group':
- self.norm1 = nn.GroupNorm(num_groups=8, num_channels=64)
-
- elif self.norm_fn == 'batch':
- self.norm1 = nn.BatchNorm2d(64)
-
- elif self.norm_fn == 'instance':
- self.norm1 = nn.InstanceNorm2d(64)
-
- elif self.norm_fn == 'none':
- self.norm1 = nn.Sequential()
-
- self.conv1 = nn.Conv2d(3, 64, kernel_size=7, stride=2, padding=3)
- self.relu1 = nn.ReLU(inplace=True)
-
- self.in_planes = 64
- self.layer1 = self._make_layer(64, stride=1)
- self.layer2 = self._make_layer(112, stride=2)
- self.layer3 = self._make_layer(160, stride=2)
- self.layer3_2 = self._make_layer(160, stride=1)
-
- # output convolution
- self.conv2 = nn.Conv2d(self.in_planes, output_dim, kernel_size=1)
-
- self.dropout = None
- if dropout > 0:
- self.dropout = nn.Dropout2d(p=dropout)
-
- for m in self.modules():
- if isinstance(m, nn.Conv2d):
- nn.init.kaiming_normal_(m.weight, mode='fan_out', nonlinearity='relu')
- elif isinstance(m, (nn.BatchNorm2d, nn.InstanceNorm2d, nn.GroupNorm)):
- if m.weight is not None:
- nn.init.constant_(m.weight, 1)
- if m.bias is not None:
- nn.init.constant_(m.bias, 0)
-
- def _make_layer(self, dim, stride=1):
- layer1 = ResidualBlock(self.in_planes, dim, self.norm_fn, stride=stride)
- layer2 = ResidualBlock(dim, dim, self.norm_fn, stride=1)
- layers = (layer1, layer2)
-
- self.in_planes = dim
- return nn.Sequential(*layers)
-
-
- def forward(self, x):
-
- # if input is list, combine batch dimension
- is_list = isinstance(x, tuple) or isinstance(x, list)
- if is_list:
- batch_dim = x[0].shape[0]
- x = torch.cat(x, dim=0)
-
- x = self.conv1(x)
- x = self.norm1(x)
- x = self.relu1(x)
-
- x = self.layer1(x)
- x = self.layer2(x)
- x = self.layer3(x)
- x = self.layer3_2(x)
-
- x = self.conv2(x)
-
- if self.training and self.dropout is not None:
- x = self.dropout(x)
-
- if is_list:
- x = torch.split(x, [batch_dim, batch_dim], dim=0)
-
- return x
diff --git a/spaces/Nikhil0987/omm/qa.py b/spaces/Nikhil0987/omm/qa.py
deleted file mode 100644
index 86ec1538137cd2528f8ab0cb8bd1348001184b37..0000000000000000000000000000000000000000
--- a/spaces/Nikhil0987/omm/qa.py
+++ /dev/null
@@ -1,44 +0,0 @@
-from transformers import pipeline
-import streamlit as st
-
-
-# def que():
-# question = st.text_input("ASk me a question")
-
-# oracle = pipeline(task= "question-answering",model="deepset/roberta-base-squad2")
-# oracle(question="Where do I live?", context="My name is Wolfgang and I live in Berlin")
-
-
-def question_answering(question, context):
- """Answers a question given a context."""
-
- # Load the question answering model.
-
-
- qa_model = pipeline("question-answering")
-
-
- # Prepare the inputs for the model.
- inputs = {
- "question": question,
- "context": context,
- }
-
- # Get the answer from the model.
- output = qa_model(**inputs)
- answer = output["answer_start"]
-
- # Return the answer.
- return context[answer : answer + output["answer_length"]]
-
-
- if __name__ == "__main__":
- # Get the question and context.
- question = "What is the capital of France?"
- context = "The capital of France is Paris."
-
- # Get the answer.
- answer = question_answering(question, context)
-
- # Print the answer.
- print(answer)
diff --git a/spaces/OFA-Sys/OFA-Generic_Interface/fairseq/fairseq/data/base_wrapper_dataset.py b/spaces/OFA-Sys/OFA-Generic_Interface/fairseq/fairseq/data/base_wrapper_dataset.py
deleted file mode 100644
index 134d398b47dc73c8807759188504aee205b3b34d..0000000000000000000000000000000000000000
--- a/spaces/OFA-Sys/OFA-Generic_Interface/fairseq/fairseq/data/base_wrapper_dataset.py
+++ /dev/null
@@ -1,78 +0,0 @@
-# Copyright (c) Facebook, Inc. and its affiliates.
-#
-# This source code is licensed under the MIT license found in the
-# LICENSE file in the root directory of this source tree.
-
-from torch.utils.data.dataloader import default_collate
-
-from . import FairseqDataset
-
-
-class BaseWrapperDataset(FairseqDataset):
- def __init__(self, dataset):
- super().__init__()
- self.dataset = dataset
-
- def __getitem__(self, index):
- return self.dataset[index]
-
- def __len__(self):
- return len(self.dataset)
-
- def collater(self, samples):
- if hasattr(self.dataset, "collater"):
- return self.dataset.collater(samples)
- else:
- return default_collate(samples)
-
- @property
- def sizes(self):
- return self.dataset.sizes
-
- def num_tokens(self, index):
- return self.dataset.num_tokens(index)
-
- def size(self, index):
- return self.dataset.size(index)
-
- def ordered_indices(self):
- return self.dataset.ordered_indices()
-
- @property
- def supports_prefetch(self):
- return getattr(self.dataset, "supports_prefetch", False)
-
- def attr(self, attr: str, index: int):
- return self.dataset.attr(attr, index)
-
- def prefetch(self, indices):
- self.dataset.prefetch(indices)
-
- def get_batch_shapes(self):
- return self.dataset.get_batch_shapes()
-
- def batch_by_size(
- self,
- indices,
- max_tokens=None,
- max_sentences=None,
- required_batch_size_multiple=1,
- ):
- return self.dataset.batch_by_size(
- indices,
- max_tokens=max_tokens,
- max_sentences=max_sentences,
- required_batch_size_multiple=required_batch_size_multiple,
- )
-
- def filter_indices_by_size(self, indices, max_sizes):
- return self.dataset.filter_indices_by_size(indices, max_sizes)
-
- @property
- def can_reuse_epoch_itr_across_epochs(self):
- return self.dataset.can_reuse_epoch_itr_across_epochs
-
- def set_epoch(self, epoch):
- super().set_epoch(epoch)
- if hasattr(self.dataset, "set_epoch"):
- self.dataset.set_epoch(epoch)
diff --git a/spaces/OFA-Sys/OFA-Generic_Interface/fairseq/tests/test_resampling_dataset.py b/spaces/OFA-Sys/OFA-Generic_Interface/fairseq/tests/test_resampling_dataset.py
deleted file mode 100644
index ccb53a253ce6ca0d8e972adfa708144b4299b3cb..0000000000000000000000000000000000000000
--- a/spaces/OFA-Sys/OFA-Generic_Interface/fairseq/tests/test_resampling_dataset.py
+++ /dev/null
@@ -1,103 +0,0 @@
-# Copyright (c) Facebook, Inc. and its affiliates.
-#
-# This source code is licensed under the MIT license found in the
-# LICENSE file in the root directory of this source tree.
-
-import collections
-import unittest
-
-import numpy as np
-from fairseq.data import ListDataset, ResamplingDataset
-
-
-class TestResamplingDataset(unittest.TestCase):
- def setUp(self):
- self.strings = ["ab", "c", "def", "ghij"]
- self.weights = [4.0, 2.0, 7.0, 1.5]
- self.size_ratio = 2
- self.dataset = ListDataset(
- self.strings, np.array([len(s) for s in self.strings])
- )
-
- def _test_common(self, resampling_dataset, iters):
- assert len(self.dataset) == len(self.strings) == len(self.weights)
- assert len(resampling_dataset) == self.size_ratio * len(self.strings)
-
- results = {"ordered_by_size": True, "max_distribution_diff": 0.0}
-
- totalfreqs = 0
- freqs = collections.defaultdict(int)
-
- for epoch_num in range(iters):
- resampling_dataset.set_epoch(epoch_num)
-
- indices = resampling_dataset.ordered_indices()
- assert len(indices) == len(resampling_dataset)
-
- prev_size = -1
-
- for i in indices:
- cur_size = resampling_dataset.size(i)
- # Make sure indices map to same sequences within an epoch
- assert resampling_dataset[i] == resampling_dataset[i]
-
- # Make sure length of sequence is correct
- assert cur_size == len(resampling_dataset[i])
-
- freqs[resampling_dataset[i]] += 1
- totalfreqs += 1
-
- if prev_size > cur_size:
- results["ordered_by_size"] = False
-
- prev_size = cur_size
-
- assert set(freqs.keys()) == set(self.strings)
- for s, weight in zip(self.strings, self.weights):
- freq = freqs[s] / totalfreqs
- expected_freq = weight / sum(self.weights)
- results["max_distribution_diff"] = max(
- results["max_distribution_diff"], abs(expected_freq - freq)
- )
-
- return results
-
- def test_resampling_dataset_batch_by_size_false(self):
- resampling_dataset = ResamplingDataset(
- self.dataset,
- self.weights,
- size_ratio=self.size_ratio,
- batch_by_size=False,
- seed=0,
- )
-
- results = self._test_common(resampling_dataset, iters=1000)
-
- # For batch_by_size = False, the batches should be returned in
- # arbitrary order of size.
- assert not results["ordered_by_size"]
-
- # Allow tolerance in distribution error of 2%.
- assert results["max_distribution_diff"] < 0.02
-
- def test_resampling_dataset_batch_by_size_true(self):
- resampling_dataset = ResamplingDataset(
- self.dataset,
- self.weights,
- size_ratio=self.size_ratio,
- batch_by_size=True,
- seed=0,
- )
-
- results = self._test_common(resampling_dataset, iters=1000)
-
- # For batch_by_size = True, the batches should be returned in
- # increasing order of size.
- assert results["ordered_by_size"]
-
- # Allow tolerance in distribution error of 2%.
- assert results["max_distribution_diff"] < 0.02
-
-
-if __name__ == "__main__":
- unittest.main()
diff --git a/spaces/OFA-Sys/OFA-Generic_Interface/fairseq/tests/test_sequence_generator.py b/spaces/OFA-Sys/OFA-Generic_Interface/fairseq/tests/test_sequence_generator.py
deleted file mode 100644
index 9273191962089816edffaa5d0c9c90cb0c3f3c1a..0000000000000000000000000000000000000000
--- a/spaces/OFA-Sys/OFA-Generic_Interface/fairseq/tests/test_sequence_generator.py
+++ /dev/null
@@ -1,799 +0,0 @@
-# Copyright (c) Facebook, Inc. and its affiliates.
-#
-# This source code is licensed under the MIT license found in the
-# LICENSE file in the root directory of this source tree.
-
-import argparse
-import tempfile
-import unittest
-import math
-import numpy as np
-
-
-import tests.utils as test_utils
-import torch
-from fairseq import search
-from fairseq.data.dictionary import Dictionary
-from fairseq.models.transformer import TransformerModel
-from fairseq.sequence_generator import EnsembleModel, SequenceGenerator
-from fairseq.ngram_repeat_block import NGramRepeatBlock
-from fairseq.tasks.fairseq_task import LegacyFairseqTask
-
-
-DEFAULT_TEST_VOCAB_SIZE = 100
-
-
-class DummyTask(LegacyFairseqTask):
- def __init__(self, args):
- super().__init__(args)
- self.dictionary = get_dummy_dictionary()
- if getattr(self.args, "ctc", False):
- self.dictionary.add_symbol("")
- self.src_dict = self.dictionary
- self.tgt_dict = self.dictionary
-
- @property
- def source_dictionary(self):
- return self.src_dict
-
- @property
- def target_dictionary(self):
- return self.dictionary
-
-
-def get_dummy_dictionary(vocab_size=DEFAULT_TEST_VOCAB_SIZE):
- dummy_dict = Dictionary()
- # add dummy symbol to satisfy vocab size
- for id, _ in enumerate(range(vocab_size)):
- dummy_dict.add_symbol("{}".format(id), n=1000)
- return dummy_dict
-
-
-def get_dummy_task_and_parser():
- """
- to build a fariseq model, we need some dummy parse and task. This function
- is used to create dummy task and parser to faciliate model/criterion test
-
- Note: we use FbSpeechRecognitionTask as the dummy task. You may want
- to use other task by providing another function
- """
- parser = argparse.ArgumentParser(
- description="test_dummy_s2s_task", argument_default=argparse.SUPPRESS
- )
- DummyTask.add_args(parser)
- args = parser.parse_args([])
- task = DummyTask.setup_task(args)
- return task, parser
-
-
-class TestJitSequenceGeneratorBase(unittest.TestCase):
- def setUp(self):
- self.task, self.parser = get_dummy_task_and_parser()
- eos = self.task.tgt_dict.eos()
- src_tokens = torch.randint(3, 50, (2, 10)).long()
- src_tokens = torch.cat((src_tokens, torch.LongTensor([[eos], [eos]])), -1)
- src_lengths = torch.LongTensor([2, 10])
- self.sample = {
- "net_input": {"src_tokens": src_tokens, "src_lengths": src_lengths}
- }
- TransformerModel.add_args(self.parser)
- args = self.parser.parse_args([])
- args.encoder_layers = 2
- args.decoder_layers = 1
- self.transformer_model = TransformerModel.build_model(args, self.task)
-
- def assertOutputEqual(self, hypo, pos_probs):
- pos_scores = torch.FloatTensor(pos_probs).log()
- self.assertTensorSizeEqual(hypo["positional_scores"], pos_scores)
- self.assertTensorSizeEqual(pos_scores.numel(), hypo["tokens"].numel())
-
- def assertTensorSizeEqual(self, t1, t2):
- self.assertEqual(t1.size(), t2.size(), "size mismatch")
-
- def assertAlmostEqual(self, t1, t2):
- self.assertEqual(t1.size(), t2.size(), "size mismatch")
- self.assertLess((t1 - t2).abs().max(), 1e-4)
-
- def assertTensorEqual(self, t1, t2):
- self.assertEqual(t1.size(), t2.size(), "size mismatch")
- self.assertEqual(t1.ne(t2).long().sum(), 0)
-
- def assertHypoEqual(self, h1, h2):
- "Check two hypos are equal"
- self.assertTensorEqual(h1["tokens"], h2["tokens"])
- self.assertAlmostEqual(h1["positional_scores"], h2["positional_scores"])
- self.assertLess(abs(h1["score"] - h2["score"]), 1e-6)
- self.assertAlmostEqual(h1["attention"], h2["attention"])
-
- def _test_save_and_load(self, scripted_module):
- with tempfile.NamedTemporaryFile() as f:
- scripted_module.save(f.name)
- torch.jit.load(f.name)
-
-
-JIT_MSG = "Targeting OSS scriptability for the 1.6 release"
-
-
-@unittest.skipIf(torch.__version__ < "1.6.0", JIT_MSG)
-class TestJitSequenceGenerator(TestJitSequenceGeneratorBase):
- def test_export_transformer(self):
- model = self.transformer_model
- torch.jit.script(model)
-
- def test_ensemble_sequence_generator(self):
- model = self.transformer_model
- generator = SequenceGenerator(
- [model],
- self.task.tgt_dict,
- beam_size=2,
- no_repeat_ngram_size=2,
- max_len_b=10,
- )
- scripted_model = torch.jit.script(generator)
- self._test_save_and_load(scripted_model)
-
- def test_export_ensemble_model(self):
- model = self.transformer_model
- ensemble_models = EnsembleModel([model])
- torch.jit.script(ensemble_models)
-
-
-class TestExportSearch(unittest.TestCase):
- def setUp(self):
- task, _ = get_dummy_task_and_parser()
- self.tgt_dict = task.tgt_dict
- self.min_top1_prob = 0.4
-
- def test_export_diverse_bs(self):
- search_strategy = search.DiverseBeamSearch(
- self.tgt_dict, num_groups=2, diversity_strength=0.0
- )
- torch.jit.script(search_strategy)
-
- def test_export_sampling(self):
- low_sampling_topp = self.min_top1_prob / 2.0
- search_strategy = search.Sampling(
- self.tgt_dict, sampling_topp=low_sampling_topp
- )
- torch.jit.script(search_strategy)
-
- def test_export_diverse_siblings_search(self):
- search_strategy = search.DiverseSiblingsSearch(
- self.tgt_dict, diversity_rate=0.5
- )
- torch.jit.script(search_strategy)
-
-
-class TestSequenceGeneratorBase(unittest.TestCase):
- def assertHypoTokens(self, hypo, tokens):
- self.assertTensorEqual(hypo["tokens"], torch.LongTensor(tokens))
-
- def assertHypoScore(self, hypo, pos_probs, normalized=True, lenpen=1.0):
- pos_scores = torch.FloatTensor(pos_probs).log()
- self.assertAlmostEqual(hypo["positional_scores"], pos_scores)
- self.assertEqual(pos_scores.numel(), hypo["tokens"].numel())
- score = pos_scores.sum()
- if normalized:
- score /= pos_scores.numel() ** lenpen
- self.assertLess(abs(score - hypo["score"]), 1e-6)
-
- def assertAlmostEqual(self, t1, t2):
- self.assertEqual(t1.size(), t2.size(), "size mismatch")
- self.assertLess((t1 - t2).abs().max(), 1e-4)
-
- def assertTensorEqual(self, t1, t2):
- self.assertEqual(t1.size(), t2.size(), "size mismatch")
- self.assertEqual(t1.ne(t2).long().sum(), 0)
-
-
-class TestSequenceGenerator(TestSequenceGeneratorBase):
- def setUp(self):
- (
- self.tgt_dict,
- self.w1,
- self.w2,
- src_tokens,
- src_lengths,
- self.model,
- ) = test_utils.sequence_generator_setup()
- self.sample = {
- "net_input": {"src_tokens": src_tokens, "src_lengths": src_lengths}
- }
-
- def test_with_normalization(self):
- generator = SequenceGenerator([self.model], self.tgt_dict, beam_size=2)
- hypos = generator.forward(self.sample)
- eos, w1, w2 = self.tgt_dict.eos(), self.w1, self.w2
- # sentence 1, beam 1
- self.assertHypoTokens(hypos[0][0], [w1, eos])
- self.assertHypoScore(hypos[0][0], [0.9, 1.0])
- # sentence 1, beam 2
- self.assertHypoTokens(hypos[0][1], [w2, w1, w2, eos])
- self.assertHypoScore(hypos[0][1], [0.1, 0.9, 0.9, 1.0])
- # sentence 2, beam 1
- self.assertHypoTokens(hypos[1][0], [w1, w2, w1, eos])
- self.assertHypoScore(hypos[1][0], [0.7, 0.4, 0.4, 1.0])
- # sentence 2, beam 2
- self.assertHypoTokens(hypos[1][1], [w1, w2, eos])
- self.assertHypoScore(hypos[1][1], [0.7, 0.4, 0.6])
-
- def test_without_normalization(self):
- # Sentence 1: unchanged from the normalized case
- # Sentence 2: beams swap order
- generator = SequenceGenerator(
- [self.model], self.tgt_dict, beam_size=2, normalize_scores=False
- )
- hypos = generator.forward(self.sample)
- eos, w1, w2 = self.tgt_dict.eos(), self.w1, self.w2
- # sentence 1, beam 1
- self.assertHypoTokens(hypos[0][0], [w1, eos])
- self.assertHypoScore(hypos[0][0], [0.9, 1.0], normalized=False)
- # sentence 1, beam 2
- self.assertHypoTokens(hypos[0][1], [w2, w1, w2, eos])
- self.assertHypoScore(hypos[0][1], [0.1, 0.9, 0.9, 1.0], normalized=False)
- # sentence 2, beam 1
- self.assertHypoTokens(hypos[1][0], [w1, w2, eos])
- self.assertHypoScore(hypos[1][0], [0.7, 0.4, 0.6], normalized=False)
- # sentence 2, beam 2
- self.assertHypoTokens(hypos[1][1], [w1, w2, w1, eos])
- self.assertHypoScore(hypos[1][1], [0.7, 0.4, 0.4, 1.0], normalized=False)
-
- def test_with_lenpen_favoring_short_hypos(self):
- lenpen = 0.6
- generator = SequenceGenerator(
- [self.model], self.tgt_dict, beam_size=2, len_penalty=lenpen
- )
- hypos = generator.forward(self.sample)
- eos, w1, w2 = self.tgt_dict.eos(), self.w1, self.w2
- # sentence 1, beam 1
- self.assertHypoTokens(hypos[0][0], [w1, eos])
- self.assertHypoScore(hypos[0][0], [0.9, 1.0], lenpen=lenpen)
- # sentence 1, beam 2
- self.assertHypoTokens(hypos[0][1], [w2, w1, w2, eos])
- self.assertHypoScore(hypos[0][1], [0.1, 0.9, 0.9, 1.0], lenpen=lenpen)
- # sentence 2, beam 1
- self.assertHypoTokens(hypos[1][0], [w1, w2, eos])
- self.assertHypoScore(hypos[1][0], [0.7, 0.4, 0.6], lenpen=lenpen)
- # sentence 2, beam 2
- self.assertHypoTokens(hypos[1][1], [w1, w2, w1, eos])
- self.assertHypoScore(hypos[1][1], [0.7, 0.4, 0.4, 1.0], lenpen=lenpen)
-
- def test_with_lenpen_favoring_long_hypos(self):
- lenpen = 5.0
- generator = SequenceGenerator(
- [self.model], self.tgt_dict, beam_size=2, len_penalty=lenpen
- )
- hypos = generator.forward(self.sample)
- eos, w1, w2 = self.tgt_dict.eos(), self.w1, self.w2
- # sentence 1, beam 1
- self.assertHypoTokens(hypos[0][0], [w2, w1, w2, eos])
- self.assertHypoScore(hypos[0][0], [0.1, 0.9, 0.9, 1.0], lenpen=lenpen)
- # sentence 1, beam 2
- self.assertHypoTokens(hypos[0][1], [w1, eos])
- self.assertHypoScore(hypos[0][1], [0.9, 1.0], lenpen=lenpen)
- # sentence 2, beam 1
- self.assertHypoTokens(hypos[1][0], [w1, w2, w1, eos])
- self.assertHypoScore(hypos[1][0], [0.7, 0.4, 0.4, 1.0], lenpen=lenpen)
- # sentence 2, beam 2
- self.assertHypoTokens(hypos[1][1], [w1, w2, eos])
- self.assertHypoScore(hypos[1][1], [0.7, 0.4, 0.6], lenpen=lenpen)
-
- def test_maxlen(self):
- generator = SequenceGenerator(
- [self.model], self.tgt_dict, beam_size=2, max_len_b=2
- )
- hypos = generator.forward(self.sample)
- eos, w1, w2 = self.tgt_dict.eos(), self.w1, self.w2
- # sentence 1, beam 1
- self.assertHypoTokens(hypos[0][0], [w1, eos])
- self.assertHypoScore(hypos[0][0], [0.9, 1.0])
- # sentence 1, beam 2
- self.assertHypoTokens(hypos[0][1], [w2, w2, eos])
- self.assertHypoScore(hypos[0][1], [0.1, 0.1, 0.6])
- # sentence 2, beam 1
- self.assertHypoTokens(hypos[1][0], [w1, w2, eos])
- self.assertHypoScore(hypos[1][0], [0.7, 0.4, 0.6])
- # sentence 2, beam 2
- self.assertHypoTokens(hypos[1][1], [w2, w2, eos])
- self.assertHypoScore(hypos[1][1], [0.3, 0.9, 0.01])
-
- def test_encoder_with_different_output_len(self):
- args = self.model.encoder.args
- task = test_utils.TestTranslationTask.setup_task(
- args, self.tgt_dict, self.tgt_dict
- )
- reshaping_model = test_utils.TestReshapingModel.build_model(args, task)
- generator = SequenceGenerator(
- [reshaping_model], self.tgt_dict, beam_size=2, max_len_b=2
- )
- hypos = generator.forward(self.sample)
- for sent in [0, 1]:
- for beam in [0, 1]:
- assert hypos[sent][beam]["attention"] is not None
-
- def test_generation_with_additional_input(self):
- args = self.model.encoder.args
- task = test_utils.TestTranslationTask.setup_task(
- args, self.tgt_dict, self.tgt_dict
- )
- add_input_model = test_utils.TestAdditionalInputModel.build_model(args, task)
- generator = SequenceGenerator([add_input_model], self.tgt_dict, beam_size=2)
- sample = self.sample.copy()
- sample["net_input"]["fancy_other_input"] = sample["net_input"]["src_tokens"]
- hypos = generator.forward(self.sample)
- eos, w1, w2 = self.tgt_dict.eos(), self.w1, self.w2
- # sentence 1, beam 1
- self.assertHypoTokens(hypos[0][0], [w1, eos])
- self.assertHypoScore(hypos[0][0], [0.9, 1.0])
-
-
-@unittest.skipUnless(torch.cuda.is_available(), "")
-class TestRepeatNgramBlocking(TestSequenceGeneratorBase):
- @classmethod
- def setUpClass(cls):
- (
- cls.tgt_dict,
- cls.w1,
- cls.w2,
- src_tokens,
- src_lengths,
- cls.model,
- ) = test_utils.sequence_generator_setup()
- return cls
-
- def test_finds_repetitive_tokens(self):
- bsz, vocab_size, beam_size, step = 2, 4, 1, 3
- generated_tok = torch.tensor(
- [[2, 2, 2, 2], [3, 3, 3, 3]], dtype=torch.long, device="cuda"
- )
- lprobs = torch.zeros((beam_size * bsz, vocab_size), device="cuda")
- desired_result = lprobs.new_tensor(
- [[0.0, 0.0, -math.inf, 0.0], [0.0, 0.0, 0.0, -math.inf]]
- )
-
- cuda_ext_result, baseline_result = self._compare_cuda_ext_to_default_implem(
- bsz, beam_size, generated_tok, lprobs, step, 2
- )
- self.assertTensorEqual(cuda_ext_result, desired_result)
- self.assertTensorEqual(baseline_result, desired_result)
-
- @unittest.skipIf(torch.__version__ < "1.6.0", JIT_MSG)
- def test_jit_no_extension(self):
- bsz, vocab_size, beam_size, step = 2, 4, 1, 3
- generated_tok = torch.tensor(
- [[2, 2, 2, 2], [3, 3, 3, 3]], dtype=torch.long, device="cuda"
- )
- lprobs = torch.zeros((beam_size * bsz, vocab_size), device="cuda")
- blocker = NGramRepeatBlock(2, use_extension=False)
- base_result = blocker(generated_tok, lprobs.clone(), bsz, beam_size, step)
- scripted_blocker = torch.jit.script(blocker)
- jit_result = scripted_blocker(
- generated_tok, lprobs.clone(), bsz, beam_size, step
- )
- self.assertTensorEqual(base_result, jit_result)
-
- def test_ngram_blocking_same_as_default_implem(self):
- """Test that cuda extension returns same things as default impl in many settings."""
- vocab_size = 4
- step = 6
- for _ in range(2):
- block_param = np.random.choice([1, 2, 3, 4])
- batch_size = np.random.randint(1, 8)
- beam_size = np.random.choice([1, 2, 4, 8])
- lprobs = torch.zeros((beam_size * batch_size, vocab_size), device="cuda")
-
- generated_tok = torch.tensor(
- np.random.randint(
- 0, vocab_size, size=(batch_size * beam_size, step + 1)
- ),
- device="cuda",
- dtype=torch.long,
- )
- self._compare_cuda_ext_to_default_implem(
- batch_size,
- beam_size,
- generated_tok,
- lprobs,
- step,
- block_param,
- )
-
- def _compare_cuda_ext_to_default_implem(
- self, bsz, beam_size, generated_tok, lprobs, step, block_param
- ):
- """Assert that cuda extension and default implem return the same thing."""
- blocker = NGramRepeatBlock(block_param)
- assert blocker.use_extension, "Extension not compiled"
- cuda_ext_result = blocker(
- generated_tok,
- lprobs.clone(),
- bsz,
- beam_size,
- step,
- )
- blocker.use_extension = False
- baseline_result = blocker(
- generated_tok,
- lprobs.clone(),
- bsz,
- beam_size,
- step,
- )
- self.assertTensorEqual(cuda_ext_result, baseline_result)
- blocker.use_extension = True
- return cuda_ext_result, baseline_result
-
-
-class TestDiverseBeamSearch(TestSequenceGeneratorBase):
- def setUp(self):
- # construct dummy dictionary
- d = test_utils.dummy_dictionary(vocab_size=2)
- self.assertEqual(d.pad(), 1)
- self.assertEqual(d.eos(), 2)
- self.assertEqual(d.unk(), 3)
- self.eos = d.eos()
- self.w1 = 4
- self.w2 = 5
-
- # construct source data
- self.src_tokens = torch.LongTensor(
- [
- [self.w1, self.w2, self.eos],
- [self.w1, self.w2, self.eos],
- ]
- )
- self.src_lengths = torch.LongTensor([2, 2])
-
- args = argparse.Namespace()
- unk = 0.0
- args.beam_probs = [
- # step 0:
- torch.FloatTensor(
- [
- # eos w1 w2
- # sentence 1:
- [0.0, unk, 0.9, 0.1], # beam 1
- [0.0, unk, 0.9, 0.1], # beam 2
- # sentence 2:
- [0.0, unk, 0.7, 0.3],
- [0.0, unk, 0.7, 0.3],
- ]
- ),
- # step 1:
- torch.FloatTensor(
- [
- # eos w1 w2
- # sentence 1:
- [0.0, unk, 0.6, 0.4],
- [0.0, unk, 0.6, 0.4],
- # sentence 2:
- [0.25, unk, 0.35, 0.4],
- [0.25, unk, 0.35, 0.4],
- ]
- ),
- # step 2:
- torch.FloatTensor(
- [
- # eos w1 w2
- # sentence 1:
- [1.0, unk, 0.0, 0.0],
- [1.0, unk, 0.0, 0.0],
- # sentence 2:
- [0.9, unk, 0.1, 0.0],
- [0.9, unk, 0.1, 0.0],
- ]
- ),
- ]
-
- task = test_utils.TestTranslationTask.setup_task(args, d, d)
- self.model = task.build_model(args)
- self.tgt_dict = task.target_dictionary
-
- def test_diverse_beam_search(self):
- search_strategy = search.DiverseBeamSearch(
- self.tgt_dict, num_groups=2, diversity_strength=0.0
- )
- generator = SequenceGenerator(
- [self.model],
- self.tgt_dict,
- beam_size=2,
- search_strategy=search_strategy,
- )
- sample = {
- "net_input": {
- "src_tokens": self.src_tokens,
- "src_lengths": self.src_lengths,
- }
- }
- hypos = generator.forward(sample)
- eos, w1, w2 = self.eos, self.w1, self.w2
- # sentence 1, beam 1
- self.assertHypoTokens(hypos[0][0], [w1, w1, eos])
- self.assertHypoScore(hypos[0][0], [0.9, 0.6, 1.0])
- # sentence 1, beam 2
- self.assertHypoTokens(hypos[0][1], [w1, w1, eos])
- self.assertHypoScore(hypos[0][1], [0.9, 0.6, 1.0])
- # sentence 2, beam 1
- self.assertHypoTokens(hypos[1][0], [w1, w2, eos])
- self.assertHypoScore(hypos[1][0], [0.7, 0.4, 0.9])
- # sentence 2, beam 2
- self.assertHypoTokens(hypos[1][1], [w1, w2, eos])
- self.assertHypoScore(hypos[1][1], [0.7, 0.4, 0.9])
-
-
-class TestDiverseSiblingsSearch(TestDiverseBeamSearch):
- def assertHypoScore(
- self, hypo, pos_probs, sibling_rank, diversity_rate, normalized=True, lenpen=1.0
- ):
- pos_scores = torch.FloatTensor(pos_probs).log()
- pos_scores.sub_(torch.Tensor(sibling_rank) * diversity_rate)
- self.assertAlmostEqual(hypo["positional_scores"], pos_scores)
- self.assertEqual(pos_scores.numel(), hypo["tokens"].numel())
- score = pos_scores.sum()
- if normalized:
- score /= pos_scores.numel() ** lenpen
- self.assertLess(abs(score - hypo["score"]), 1e-6)
-
- def test_diverse_beam_search(self):
- search_strategy = search.DiverseSiblingsSearch(
- self.tgt_dict, diversity_rate=0.5
- )
- generator = SequenceGenerator(
- [self.model], self.tgt_dict, beam_size=2, search_strategy=search_strategy
- )
- sample = {
- "net_input": {
- "src_tokens": self.src_tokens,
- "src_lengths": self.src_lengths,
- }
- }
- hypos = generator.forward(sample)
- eos, w1, w2 = self.eos, self.w1, self.w2
- # sentence 1, beam 1
- self.assertHypoTokens(hypos[0][0], [w1, w1, eos])
- self.assertHypoScore(hypos[0][0], [0.9, 0.6, 1.0], [0, 1, 1], 0.5)
- # sentence 1, beam 2
- self.assertHypoTokens(hypos[0][1], [w1, w2, eos])
- self.assertHypoScore(hypos[0][1], [0.9, 0.4, 1.0], [0, 2, 1], 0.5)
- # sentence 2, beam 1
- self.assertHypoTokens(hypos[1][0], [w1, w2, eos])
- self.assertHypoScore(hypos[1][0], [0.7, 0.4, 0.9], [0, 1, 1], 0.5)
- # sentence 2, beam 2
- self.assertHypoTokens(hypos[1][1], [w1, w1, eos])
- self.assertHypoScore(hypos[1][1], [0.7, 0.35, 0.9], [0, 2, 1], 0.5)
-
-
-class TestPrefixBeamSearch(TestSequenceGeneratorBase):
- def setUp(self):
- # construct dummy dictionary
- vocab_size = 10
- d = test_utils.dummy_dictionary(vocab_size=vocab_size)
- self.assertEqual(d.pad(), 1)
- self.assertEqual(d.eos(), 2)
- self.assertEqual(d.unk(), 3)
- self.eos = d.eos()
- self.w1 = 4
- self.w2 = 5
- self.beam_size = 3
-
- # construct prefix data
- self.tokens = torch.LongTensor(
- [
- [self.w1, self.w2, self.eos],
- ]
- )
- self.token_lengths = torch.LongTensor([2])
-
- args = argparse.Namespace()
- unk = 0.0
- args.beam_probs = [
- # prefix step 0:
- torch.FloatTensor(
- [
- # eos
- [0.0, unk] + [1.0 / vocab_size] * vocab_size # beam 1
- ] * self.beam_size
- ),
- ] * vocab_size
-
- task = test_utils.TestTranslationTask.setup_task(args, d, d)
- self.model = task.build_model(args)
- self.tgt_dict = task.target_dictionary
-
- def test_prefix_beam_search(self):
- search_strategy = search.BeamSearch(self.tgt_dict)
- generator = SequenceGenerator(
- [self.model],
- self.tgt_dict,
- beam_size=self.beam_size,
- search_strategy=search_strategy,
- )
- sample = {
- "net_input": {
- "src_tokens": self.tokens,
- "src_lengths": self.token_lengths,
- }
- }
- # make sure test sample doesn't break any assertion
- generator.forward(sample, prefix_tokens=self.tokens[:, :-1])
-
-class TestTopPSamplingSearch(TestSequenceGeneratorBase):
- def setUp(self):
- # construct dummy dictionary
- d = test_utils.dummy_dictionary(vocab_size=2)
- self.assertEqual(d.pad(), 1)
- self.assertEqual(d.eos(), 2)
- self.assertEqual(d.unk(), 3)
- self.eos = d.eos()
- self.w1 = 4
- self.w2 = 5
-
- # construct source data
- self.src_tokens = torch.LongTensor(
- [
- [self.w1, self.w2, self.eos],
- [self.w1, self.w2, self.eos],
- ]
- )
- self.src_lengths = torch.LongTensor([2, 2])
-
- args = argparse.Namespace()
- unk = 0.0
- # The minimal probability of top 2 tokens.
- self.min_top2_prob = 0.75
- # The minimal probability of the top 1 token.
- self.min_top1_prob = 0.4
-
- w1_prob = self.min_top1_prob
- w2_prob = self.min_top2_prob - self.min_top1_prob
- eos_prob = 1 - self.min_top2_prob
-
- args.beam_probs = [
- # step 0:
- torch.FloatTensor(
- [
- # eos w1 w2
- [0.0, unk, 1.0, 0.0],
- [0.0, unk, 1.0, 0.0],
- [0.0, unk, 1.0, 0.0],
- [0.0, unk, 1.0, 0.0],
- ]
- ),
- # step 1:
- torch.FloatTensor(
- [
- # eos w1 w2
- [eos_prob, unk, w1_prob, w2_prob],
- [eos_prob, unk, w1_prob, w2_prob],
- [eos_prob, unk, w1_prob, w2_prob],
- [eos_prob, unk, w1_prob, w2_prob],
- ]
- ),
- # step 2:
- torch.FloatTensor(
- [
- # eos w1 w2
- [1.0, unk, 0.0, 0.0],
- [1.0, unk, 0.0, 0.0],
- [1.0, unk, 0.0, 0.0],
- [1.0, unk, 0.0, 0.0],
- ]
- ),
- ]
-
- task = test_utils.TestTranslationTask.setup_task(args, d, d)
- self.model = task.build_model(args)
- self.tgt_dict = task.target_dictionary
-
- def test_topp_sampling_search_low_prob(self):
- # Given a prob low enough to top-P sampling, we expect only the top
- # 1 token to be sampled, which always results in the same output.
- low_sampling_topp = self.min_top1_prob / 2.0
- search_strategy = search.Sampling(
- self.tgt_dict, sampling_topp=low_sampling_topp
- )
- generator = SequenceGenerator(
- [self.model], self.tgt_dict, beam_size=2, search_strategy=search_strategy
- )
- sample = {
- "net_input": {
- "src_tokens": self.src_tokens,
- "src_lengths": self.src_lengths,
- }
- }
- hypos = generator.forward(sample)
- eos, w1 = self.eos, self.w1
- # sentence 1, beam 1
- self.assertHypoTokens(hypos[0][0], [w1, w1, eos])
- self.assertHypoScore(hypos[0][0], [1.0, 0.4, 1.0])
- # sentence 1, beam 2
- self.assertHypoTokens(hypos[0][1], [w1, w1, eos])
- self.assertHypoScore(hypos[0][1], [1.0, 0.4, 1.0])
- # sentence 2, beam 1
- self.assertHypoTokens(hypos[1][0], [w1, w1, eos])
- self.assertHypoScore(hypos[1][0], [1.0, 0.4, 1.0])
- # sentence 2, beam 2
- self.assertHypoTokens(hypos[1][1], [w1, w1, eos])
- self.assertHypoScore(hypos[1][1], [1.0, 0.4, 1.0])
-
- def test_topp_sampling_search_high_prob(self):
- # Given a prob high enough to top-P sampling, any of the top 2
- # tokens could be sampled. This can cause different outputs.
- high_sampling_topp = (self.min_top1_prob + self.min_top2_prob) / 2.0
- search_strategy = search.Sampling(
- self.tgt_dict, sampling_topp=high_sampling_topp
- )
- generator = SequenceGenerator(
- [self.model], self.tgt_dict, beam_size=2, search_strategy=search_strategy
- )
- sample = {
- "net_input": {
- "src_tokens": self.src_tokens,
- "src_lengths": self.src_lengths,
- }
- }
- hypos = generator.forward(sample)
- eos, w1, w2 = self.eos, self.w1, self.w2
- # sentence 1, beam 1
- self.assertTrue(
- self.hypoTokens(hypos[0][0], [w1, w1, eos])
- or self.hypoTokens(hypos[0][0], [w1, w2, eos])
- )
- self.assertTrue(
- self.hypoScore(hypos[0][0], [1.0, 0.4, 1.0])
- or self.hypoScore(hypos[0][0], [1.0, 0.35, 1.0])
- )
-
- # sentence 1, beam 2
- self.assertTrue(
- self.hypoTokens(hypos[0][1], [w1, w1, eos])
- or self.hypoTokens(hypos[0][1], [w1, w2, eos])
- )
- self.assertTrue(
- self.hypoScore(hypos[0][1], [1.0, 0.4, 1.0])
- or self.hypoScore(hypos[0][1], [1.0, 0.35, 1.0])
- )
-
- # sentence 2, beam 1
- self.assertTrue(
- self.hypoTokens(hypos[1][0], [w1, w1, eos])
- or self.hypoTokens(hypos[1][0], [w1, w2, eos])
- )
- self.assertTrue(
- self.hypoScore(hypos[1][0], [1.0, 0.4, 1.0])
- or self.hypoScore(hypos[1][0], [1.0, 0.35, 1.0])
- )
-
- # sentence 2, beam 2
- self.assertTrue(
- self.hypoTokens(hypos[1][1], [w1, w1, eos])
- or self.hypoTokens(hypos[1][1], [w1, w2, eos])
- )
- self.assertTrue(
- self.hypoScore(hypos[1][1], [1.0, 0.4, 1.0])
- or self.hypoScore(hypos[1][1], [1.0, 0.35, 1.0])
- )
-
- def hypoTokens(self, hypo, tokens):
- return self.tensorEqual(hypo["tokens"], torch.LongTensor(tokens))
-
- def hypoScore(self, hypo, pos_probs, normalized=True, lenpen=1.0):
- pos_scores = torch.FloatTensor(pos_probs).log()
- if not self.almostEqual(hypo["positional_scores"], pos_scores):
- return False
- if pos_scores.numel() != hypo["tokens"].numel():
- return False
- score = pos_scores.sum()
- if normalized:
- score /= pos_scores.numel() ** lenpen
- return abs(score - hypo["score"]) < 1e-6
-
- def almostEqual(self, t1, t2):
- return t1.size() == t2.size() and (t1 - t2).abs().max() < 1e-4
-
- def tensorEqual(self, t1, t2):
- return t1.size() == t2.size() and t1.ne(t2).long().sum() == 0
-
-
-if __name__ == "__main__":
- unittest.main()
diff --git a/spaces/OFA-Sys/OFA-Image_Caption/fairseq/examples/conv_seq2seq/README.md b/spaces/OFA-Sys/OFA-Image_Caption/fairseq/examples/conv_seq2seq/README.md
deleted file mode 100644
index 95fe7e7909a77ee0e50fe31d4b8be38daa8f3be7..0000000000000000000000000000000000000000
--- a/spaces/OFA-Sys/OFA-Image_Caption/fairseq/examples/conv_seq2seq/README.md
+++ /dev/null
@@ -1,25 +0,0 @@
-# Convolutional Sequence to Sequence Learning (Gehring et al., 2017)
-
-## Pre-trained models
-
-Description | Dataset | Model | Test set(s)
----|---|---|---
-Convolutional
([Gehring et al., 2017](https://arxiv.org/abs/1705.03122)) | [WMT14 English-French](http://statmt.org/wmt14/translation-task.html#Download) | [download (.tar.bz2)](https://dl.fbaipublicfiles.com/fairseq/models/wmt14.v2.en-fr.fconv-py.tar.bz2) | newstest2014:
[download (.tar.bz2)](https://dl.fbaipublicfiles.com/fairseq/data/wmt14.v2.en-fr.newstest2014.tar.bz2)
newstest2012/2013:
[download (.tar.bz2)](https://dl.fbaipublicfiles.com/fairseq/data/wmt14.v2.en-fr.ntst1213.tar.bz2)
-Convolutional
([Gehring et al., 2017](https://arxiv.org/abs/1705.03122)) | [WMT14 English-German](http://statmt.org/wmt14/translation-task.html#Download) | [download (.tar.bz2)](https://dl.fbaipublicfiles.com/fairseq/models/wmt14.en-de.fconv-py.tar.bz2) | newstest2014:
[download (.tar.bz2)](https://dl.fbaipublicfiles.com/fairseq/data/wmt14.en-de.newstest2014.tar.bz2)
-Convolutional
([Gehring et al., 2017](https://arxiv.org/abs/1705.03122)) | [WMT17 English-German](http://statmt.org/wmt17/translation-task.html#Download) | [download (.tar.bz2)](https://dl.fbaipublicfiles.com/fairseq/models/wmt17.v2.en-de.fconv-py.tar.bz2) | newstest2014:
[download (.tar.bz2)](https://dl.fbaipublicfiles.com/fairseq/data/wmt17.v2.en-de.newstest2014.tar.bz2)
-
-## Example usage
-
-See the [translation README](../translation/README.md) for instructions on reproducing results for WMT'14 En-De and
-WMT'14 En-Fr using the `fconv_wmt_en_de` and `fconv_wmt_en_fr` model architectures.
-
-## Citation
-
-```bibtex
-@inproceedings{gehring2017convs2s,
- title = {Convolutional Sequence to Sequence Learning},
- author = {Gehring, Jonas, and Auli, Michael and Grangier, David and Yarats, Denis and Dauphin, Yann N},
- booktitle = {Proc. of ICML},
- year = 2017,
-}
-```
diff --git a/spaces/OFA-Sys/OFA-Image_Caption/fairseq/examples/wav2vec/unsupervised/models/wav2vec_u.py b/spaces/OFA-Sys/OFA-Image_Caption/fairseq/examples/wav2vec/unsupervised/models/wav2vec_u.py
deleted file mode 100644
index 27792ebda842057e33fed3dc53dd9d8a594d0483..0000000000000000000000000000000000000000
--- a/spaces/OFA-Sys/OFA-Image_Caption/fairseq/examples/wav2vec/unsupervised/models/wav2vec_u.py
+++ /dev/null
@@ -1,637 +0,0 @@
-# Copyright (c) Facebook, Inc. and its affiliates.
-#
-# This source code is licensed under the MIT license found in the
-# LICENSE file in the root directory of this source tree.
-
-from dataclasses import dataclass
-from enum import Enum, auto
-import math
-import numpy as np
-from typing import Tuple, List, Optional, Dict
-
-import torch
-import torch.nn as nn
-import torch.nn.functional as F
-from torch import autograd
-
-from fairseq import checkpoint_utils, utils
-from fairseq.dataclass import FairseqDataclass
-from fairseq.models import BaseFairseqModel, register_model
-from fairseq.modules import (
- SamePad,
- TransposeLast,
-)
-
-
-class SegmentationType(Enum):
- NONE = auto()
- RANDOM = auto()
- UNIFORM_RANDOM = auto()
- UNIFORM_RANDOM_JOIN = auto()
- JOIN = auto()
-
-
-@dataclass
-class SegmentationConfig(FairseqDataclass):
- type: SegmentationType = SegmentationType.NONE
- subsample_rate: float = 0.25
- mean_pool: bool = True
- mean_pool_join: bool = False
- remove_zeros: bool = False
-
-
-@dataclass
-class Wav2vec_UConfig(FairseqDataclass):
-
- discriminator_kernel: int = 3
- discriminator_dilation: int = 1
- discriminator_dim: int = 256
- discriminator_causal: bool = True
- discriminator_linear_emb: bool = False
- discriminator_depth: int = 1
- discriminator_max_pool: bool = False
- discriminator_act_after_linear: bool = False
- discriminator_dropout: float = 0.0
- discriminator_spectral_norm: bool = False
- discriminator_weight_norm: bool = False
-
- generator_kernel: int = 4
- generator_dilation: int = 1
- generator_stride: int = 1
- generator_bias: bool = False
- generator_dropout: float = 0.0
-
- blank_weight: float = 0
- blank_mode: str = "add"
- blank_is_sil: bool = False
- no_softmax: bool = False
-
- smoothness_weight: float = 0.0
- smoothing: float = 0.0
- smoothing_one_sided: bool = False
- gradient_penalty: float = 0.0
- probabilistic_grad_penalty_slicing: bool = False
- code_penalty: float = 0.0
- gumbel: bool = False
- hard_gumbel: bool = True
- temp: Tuple[float, float, float] = (2, 0.1, 0.99995)
- input_dim: int = 128
-
- segmentation: SegmentationConfig = SegmentationConfig()
-
-
-class Segmenter(nn.Module):
- cfg: SegmentationConfig
-
- def __init__(self, cfg: SegmentationConfig):
- super().__init__()
- self.cfg = cfg
- self.subsample_rate = cfg.subsample_rate
-
- def pre_segment(self, dense_x, dense_padding_mask):
- return dense_x, dense_padding_mask
-
- def logit_segment(self, logits, padding_mask):
- return logits, padding_mask
-
-
-class RandomSegmenter(Segmenter):
- def pre_segment(self, dense_x, dense_padding_mask):
- target_num = math.ceil(dense_x.size(1) * self.subsample_rate)
- ones = torch.ones(dense_x.shape[:-1], device=dense_x.device)
- indices, _ = ones.multinomial(target_num).sort(dim=-1)
- indices_ld = indices.unsqueeze(-1).expand(-1, -1, dense_x.size(-1))
- dense_x = dense_x.gather(1, indices_ld)
- dense_padding_mask = dense_padding_mask.gather(1, index=indices)
- return dense_x, dense_padding_mask
-
-
-class UniformRandomSegmenter(Segmenter):
- def pre_segment(self, dense_x, dense_padding_mask):
- bsz, tsz, fsz = dense_x.shape
-
- target_num = math.ceil(tsz * self.subsample_rate)
-
- rem = tsz % target_num
-
- if rem > 0:
- dense_x = F.pad(dense_x, [0, 0, 0, target_num - rem])
- dense_padding_mask = F.pad(
- dense_padding_mask, [0, target_num - rem], value=True
- )
-
- dense_x = dense_x.view(bsz, target_num, -1, fsz)
- dense_padding_mask = dense_padding_mask.view(bsz, target_num, -1)
-
- if self.cfg.mean_pool:
- dense_x = dense_x.mean(dim=-2)
- dense_padding_mask = dense_padding_mask.all(dim=-1)
- else:
- ones = torch.ones((bsz, dense_x.size(2)), device=dense_x.device)
- indices = ones.multinomial(1)
- indices = indices.unsqueeze(-1).expand(-1, target_num, -1)
- indices_ld = indices.unsqueeze(-1).expand(-1, -1, -1, fsz)
- dense_x = dense_x.gather(2, indices_ld).reshape(bsz, -1, fsz)
- dense_padding_mask = dense_padding_mask.gather(2, index=indices).reshape(
- bsz, -1
- )
- return dense_x, dense_padding_mask
-
-
-class JoinSegmenter(Segmenter):
- def logit_segment(self, logits, padding_mask):
- preds = logits.argmax(dim=-1)
-
- if padding_mask.any():
- preds[padding_mask] = -1 # mark pad
- uniques = []
-
- bsz, tsz, csz = logits.shape
-
- for p in preds:
- uniques.append(
- p.cpu().unique_consecutive(return_inverse=True, return_counts=True)
- )
-
- new_tsz = max(u[0].numel() for u in uniques)
- new_logits = logits.new_zeros(bsz, new_tsz, csz)
- new_pad = padding_mask.new_zeros(bsz, new_tsz)
-
- for b in range(bsz):
- u, idx, c = uniques[b]
- keep = u != -1
-
- if self.cfg.remove_zeros:
- keep.logical_and_(u != 0)
-
- if self.training and not self.cfg.mean_pool_join:
- u[0] = 0
- u[1:] = c.cumsum(0)[:-1]
- m = c > 1
- r = torch.rand(m.sum())
- o = (c[m] * r).long()
- u[m] += o
- new_logits[b, : u.numel()] = logits[b, u]
- else:
- new_logits[b].index_add_(
- dim=0, index=idx.to(new_logits.device), source=logits[b]
- )
- new_logits[b, : c.numel()] /= c.unsqueeze(-1).to(new_logits.device)
-
- new_sz = keep.sum()
- if not keep.all():
- kept_logits = new_logits[b, : c.numel()][keep]
- new_logits[b, :new_sz] = kept_logits
-
- if new_sz < new_tsz:
- pad = new_tsz - new_sz
- new_logits[b, -pad:] = 0
- new_pad[b, -pad:] = True
-
- return new_logits, new_pad
-
-
-class UniformRandomJoinSegmenter(UniformRandomSegmenter, JoinSegmenter):
- pass
-
-
-SEGMENT_FACTORY = {
- SegmentationType.NONE: Segmenter,
- SegmentationType.RANDOM: RandomSegmenter,
- SegmentationType.UNIFORM_RANDOM: UniformRandomSegmenter,
- SegmentationType.UNIFORM_RANDOM_JOIN: UniformRandomJoinSegmenter,
- SegmentationType.JOIN: JoinSegmenter,
-}
-
-
-class Discriminator(nn.Module):
- def __init__(self, dim, cfg: Wav2vec_UConfig):
- super().__init__()
-
- inner_dim = cfg.discriminator_dim
- kernel = cfg.discriminator_kernel
- dilation = cfg.discriminator_dilation
- self.max_pool = cfg.discriminator_max_pool
-
- if cfg.discriminator_causal:
- padding = kernel - 1
- else:
- padding = kernel // 2
-
- def make_conv(in_d, out_d, k, p=0, has_dilation=True):
- conv = nn.Conv1d(
- in_d,
- out_d,
- kernel_size=k,
- padding=p,
- dilation=dilation if has_dilation else 1,
- )
- if cfg.discriminator_spectral_norm:
- conv = nn.utils.spectral_norm(conv)
- elif cfg.discriminator_weight_norm:
- conv = nn.utils.weight_norm(conv)
- return conv
-
- inner_net = [
- nn.Sequential(
- make_conv(inner_dim, inner_dim, kernel, padding),
- SamePad(kernel_size=kernel, causal=cfg.discriminator_causal),
- nn.Dropout(cfg.discriminator_dropout),
- nn.GELU(),
- )
- for _ in range(cfg.discriminator_depth - 1)
- ] + [
- make_conv(inner_dim, 1, kernel, padding, has_dilation=False),
- SamePad(kernel_size=kernel, causal=cfg.discriminator_causal),
- ]
-
- if cfg.discriminator_linear_emb:
- emb_net = [make_conv(dim, inner_dim, 1)]
- else:
- emb_net = [
- make_conv(dim, inner_dim, kernel, padding),
- SamePad(kernel_size=kernel, causal=cfg.discriminator_causal),
- ]
-
- if cfg.discriminator_act_after_linear:
- emb_net.append(nn.GELU())
-
- self.net = nn.Sequential(
- *emb_net,
- nn.Dropout(cfg.discriminator_dropout),
- *inner_net,
- )
-
- def forward(self, x, padding_mask):
- x = x.transpose(1, 2) # BTC -> BCT
- x = self.net(x)
- x = x.transpose(1, 2)
- x_sz = x.size(1)
- if padding_mask is not None and padding_mask.any() and padding_mask.dim() > 1:
- padding_mask = padding_mask[:, : x.size(1)]
- x[padding_mask] = float("-inf") if self.max_pool else 0
- x_sz = x_sz - padding_mask.sum(dim=-1)
- x = x.squeeze(-1)
- if self.max_pool:
- x, _ = x.max(dim=-1)
- else:
- x = x.sum(dim=-1)
- x = x / x_sz
- return x
-
-
-class Generator(nn.Module):
- def __init__(self, input_dim, output_dim, cfg: Wav2vec_UConfig):
- super().__init__()
-
- self.cfg = cfg
- self.output_dim = output_dim
- self.stride = cfg.generator_stride
- self.dropout = nn.Dropout(cfg.generator_dropout)
-
- padding = cfg.generator_kernel // 2
- self.proj = nn.Sequential(
- TransposeLast(),
- nn.Conv1d(
- input_dim,
- output_dim,
- kernel_size=cfg.generator_kernel,
- stride=cfg.generator_stride,
- dilation=cfg.generator_dilation,
- padding=padding,
- bias=cfg.generator_bias,
- ),
- TransposeLast(),
- )
-
- def forward(self, dense_x, tokens, dense_padding_mask):
- dense_x = self.dropout(dense_x)
-
- dense_x = self.proj(dense_x)
- if self.stride > 1:
- dense_padding_mask = dense_padding_mask[:, :: self.stride]
-
- if dense_padding_mask.size(1) != dense_x.size(1):
- new_padding = dense_padding_mask.new_zeros(dense_x.shape[:-1])
- diff = new_padding.size(1) - dense_padding_mask.size(1)
- assert (
- diff > 0
- ), f"{new_padding.shape}, {dense_padding_mask.shape}, {dense_x.shape}, {diff}"
- if diff > 0:
- new_padding[:, diff:] = dense_padding_mask
- else:
- assert diff < 0
- new_padding = dense_padding_mask[:, :diff]
-
- dense_padding_mask = new_padding
-
- result = {}
-
- token_x = None
- if tokens is not None:
- token_x = dense_x.new_zeros(tokens.numel(), self.output_dim)
- token_x.scatter_(1, tokens.view(-1, 1).long(), 1)
- token_x = token_x.view(tokens.shape + (self.output_dim,))
-
- result["dense_x"] = dense_x
- result["token_x"] = token_x
- result["dense_padding_mask"] = dense_padding_mask
-
- return result
-
-
-@register_model("wav2vec_u", dataclass=Wav2vec_UConfig)
-class Wav2vec_U(BaseFairseqModel):
- def calc_gradient_penalty(self, real_data, fake_data):
-
- b_size = min(real_data.size(0), fake_data.size(0))
- t_size = min(real_data.size(1), fake_data.size(1))
-
- if self.cfg.probabilistic_grad_penalty_slicing:
-
- def get_slice(data, dim, target_size):
-
- size = data.size(dim)
- diff = size - target_size
- if diff <= 0:
- return data
-
- start = np.random.randint(0, diff + 1)
- return data.narrow(dim=dim, start=start, length=target_size)
-
- real_data = get_slice(real_data, 0, b_size)
- real_data = get_slice(real_data, 1, t_size)
- fake_data = get_slice(fake_data, 0, b_size)
- fake_data = get_slice(fake_data, 1, t_size)
-
- else:
- real_data = real_data[:b_size, :t_size]
- fake_data = fake_data[:b_size, :t_size]
-
- alpha = torch.rand(real_data.size(0), 1, 1)
- alpha = alpha.expand(real_data.size())
- alpha = alpha.to(real_data.device)
-
- interpolates = alpha * real_data + ((1 - alpha) * fake_data)
-
- disc_interpolates = self.discriminator(interpolates, None)
-
- gradients = autograd.grad(
- outputs=disc_interpolates,
- inputs=interpolates,
- grad_outputs=torch.ones(disc_interpolates.size(), device=real_data.device),
- create_graph=True,
- retain_graph=True,
- only_inputs=True,
- )[0]
-
- gradient_penalty = (gradients.norm(2, dim=1) - 1) ** 2
- return gradient_penalty
-
- def set_num_updates(self, num_updates):
- super().set_num_updates(num_updates)
- self.update_num = num_updates
- self.curr_temp = max(
- self.max_temp * self.temp_decay ** num_updates, self.min_temp
- )
-
- def discrim_step(self, num_updates):
- return num_updates % 2 == 1
-
- def get_groups_for_update(self, num_updates):
- return "discriminator" if self.discrim_step(num_updates) else "generator"
-
- def __init__(self, cfg: Wav2vec_UConfig, target_dict):
- super().__init__()
-
- self.cfg = cfg
- self.zero_index = target_dict.index("") if "" in target_dict else 0
- self.smoothness_weight = cfg.smoothness_weight
-
- output_size = len(target_dict)
- self.pad = target_dict.pad()
- self.eos = target_dict.eos()
- self.smoothing = cfg.smoothing
- self.smoothing_one_sided = cfg.smoothing_one_sided
- self.no_softmax = cfg.no_softmax
- self.gumbel = cfg.gumbel
- self.hard_gumbel = cfg.hard_gumbel
- self.last_acc = None
-
- self.gradient_penalty = cfg.gradient_penalty
- self.code_penalty = cfg.code_penalty
- self.blank_weight = cfg.blank_weight
- self.blank_mode = cfg.blank_mode
- self.blank_index = target_dict.index("") if cfg.blank_is_sil else 0
- assert self.blank_index != target_dict.unk()
-
- self.discriminator = Discriminator(output_size, cfg)
- for p in self.discriminator.parameters():
- p.param_group = "discriminator"
-
- self.pca_A = self.pca_b = None
- d = cfg.input_dim
-
- self.segmenter = SEGMENT_FACTORY[cfg.segmentation.type](cfg.segmentation)
-
- self.generator = Generator(d, output_size, cfg)
-
- for p in self.generator.parameters():
- p.param_group = "generator"
-
- for p in self.segmenter.parameters():
- p.param_group = "generator"
-
- self.max_temp, self.min_temp, self.temp_decay = cfg.temp
- self.curr_temp = self.max_temp
- self.update_num = 0
-
- @classmethod
- def build_model(cls, cfg, task):
- return cls(cfg, task.target_dictionary)
-
- def get_logits(
- self,
- net_output: Optional[Dict[str, List[Optional[torch.Tensor]]]],
- normalize: bool = False,
- ):
- logits = net_output["logits"]
-
- if self.blank_weight != 0:
- if self.blank_mode == "add":
- logits[..., self.blank_index] += self.blank_weight
- elif self.blank_mode == "set":
- logits[..., self.blank_index] = self.blank_weight
- else:
- raise Exception(f"invalid blank mode {self.blank_mode}")
-
- padding = net_output["padding_mask"]
- if padding.any():
- logits[padding] = float("-inf")
- logits[padding][..., self.blank_index] = float("inf")
-
- if normalize:
- logits = utils.log_softmax(logits.float(), dim=-1)
-
- return logits.transpose(0, 1)
-
- def get_normalized_probs(
- self,
- net_output: Tuple[
- torch.Tensor, Optional[Dict[str, List[Optional[torch.Tensor]]]]
- ],
- log_probs: bool,
- sample: Optional[Dict[str, torch.Tensor]] = None,
- ):
- logits = self.get_logits(net_output)
-
- probs = super().get_normalized_probs(logits, log_probs, sample)
- # BTC -> TBC for ctc
- probs = probs.transpose(0, 1)
- return probs
-
- def normalize(self, dense_x):
-
- bsz, tsz, csz = dense_x.shape
-
- if dense_x.numel() == 0:
- raise Exception(dense_x.shape)
- _, k = dense_x.max(-1)
- hard_x = (
- dense_x.new_zeros(bsz * tsz, csz)
- .scatter_(-1, k.view(-1, 1), 1.0)
- .view(-1, csz)
- )
- hard_probs = torch.mean(hard_x.float(), dim=0)
- code_perplexity = torch.exp(
- -torch.sum(hard_probs * torch.log(hard_probs + 1e-7), dim=-1)
- )
-
- avg_probs = torch.softmax(dense_x.reshape(-1, csz).float(), dim=-1).mean(dim=0)
- prob_perplexity = torch.exp(
- -torch.sum(avg_probs * torch.log(avg_probs + 1e-7), dim=-1)
- )
-
- if not self.no_softmax:
- if self.training and self.gumbel:
- dense_x = F.gumbel_softmax(
- dense_x.float(), tau=self.curr_temp, hard=self.hard_gumbel
- ).type_as(dense_x)
- else:
- dense_x = dense_x.softmax(-1)
-
- return dense_x, code_perplexity, prob_perplexity
-
- def forward(
- self,
- features,
- padding_mask,
- random_label=None,
- dense_x_only=False,
- segment=True,
- ):
- if segment:
- features, padding_mask = self.segmenter.pre_segment(features, padding_mask)
-
- orig_size = features.size(0) * features.size(1) - padding_mask.sum()
-
- gen_result = self.generator(features, random_label, padding_mask)
-
- orig_dense_x, token_x = gen_result["dense_x"], gen_result["token_x"]
- orig_dense_padding_mask = gen_result["dense_padding_mask"]
-
- if segment:
- dense_x, dense_padding_mask = self.segmenter.logit_segment(
- orig_dense_x, orig_dense_padding_mask
- )
- else:
- dense_x = orig_dense_x
- dense_padding_mask = orig_dense_padding_mask
-
- dense_logits = dense_x
- prob_perplexity = None
- code_perplexity = None
-
- if not (self.no_softmax and dense_x_only):
- dense_x, code_perplexity, prob_perplexity = self.normalize(dense_logits)
-
- if dense_x_only or self.discriminator is None:
- return {
- "logits": dense_x,
- "padding_mask": dense_padding_mask,
- }
-
- token_padding_mask = random_label == self.pad
-
- dense_y = self.discriminator(dense_x, dense_padding_mask)
- token_y = self.discriminator(token_x, token_padding_mask)
-
- sample_size = features.size(0)
-
- d_step = self.discrim_step(self.update_num)
-
- fake_smooth = self.smoothing
- real_smooth = self.smoothing
- if self.smoothing_one_sided:
- fake_smooth = 0
-
- zero_loss = None
- smoothness_loss = None
- code_pen = None
-
- if d_step:
- loss_dense = F.binary_cross_entropy_with_logits(
- dense_y,
- dense_y.new_ones(dense_y.shape) - fake_smooth,
- reduction="sum",
- )
- loss_token = F.binary_cross_entropy_with_logits(
- token_y,
- token_y.new_zeros(token_y.shape) + real_smooth,
- reduction="sum",
- )
- if self.training and self.gradient_penalty > 0:
- grad_pen = self.calc_gradient_penalty(token_x, dense_x)
- grad_pen = grad_pen.sum() * self.gradient_penalty
- else:
- grad_pen = None
- else:
- grad_pen = None
- loss_token = None
- loss_dense = F.binary_cross_entropy_with_logits(
- dense_y,
- dense_y.new_zeros(dense_y.shape) + fake_smooth,
- reduction="sum",
- )
- num_vars = dense_x.size(-1)
- if prob_perplexity is not None:
- code_pen = (num_vars - prob_perplexity) / num_vars
- code_pen = code_pen * sample_size * self.code_penalty
-
- if self.smoothness_weight > 0:
- smoothness_loss = F.mse_loss(
- dense_logits[:, :-1], dense_logits[:, 1:], reduction="none"
- )
- smoothness_loss[dense_padding_mask[:, 1:]] = 0
- smoothness_loss = (
- smoothness_loss.mean() * sample_size * self.smoothness_weight
- )
-
- result = {
- "losses": {
- "grad_pen": grad_pen,
- "code_pen": code_pen,
- "smoothness": smoothness_loss,
- },
- "temp": self.curr_temp,
- "code_ppl": code_perplexity,
- "prob_ppl": prob_perplexity,
- "d_steps": int(d_step),
- "sample_size": sample_size,
- }
-
- suff = "_d" if d_step else "_g"
- result["losses"]["dense" + suff] = loss_dense
- result["losses"]["token" + suff] = loss_token
-
- return result
diff --git a/spaces/OFA-Sys/OFA-Image_Caption/fairseq/fairseq/benchmark/dummy_model.py b/spaces/OFA-Sys/OFA-Image_Caption/fairseq/fairseq/benchmark/dummy_model.py
deleted file mode 100644
index ff26e4fe655d8e8d7f9942c4bd3df7cd267405fb..0000000000000000000000000000000000000000
--- a/spaces/OFA-Sys/OFA-Image_Caption/fairseq/fairseq/benchmark/dummy_model.py
+++ /dev/null
@@ -1,96 +0,0 @@
-# Copyright (c) Facebook, Inc. and its affiliates.
-#
-# This source code is licensed under the MIT license found in the
-# LICENSE file in the root directory of this source tree.
-
-import torch.nn as nn
-import torch.nn.functional as F
-from fairseq.data import Dictionary
-from fairseq.models import (
- FairseqDecoder,
- FairseqLanguageModel,
- register_model,
- register_model_architecture,
-)
-
-
-@register_model("dummy_model")
-class DummyModel(FairseqLanguageModel):
- def __init__(self, args, encoder):
- super().__init__(encoder)
- self.args = args
-
- @staticmethod
- def add_args(parser):
- parser.add_argument("--num-layers", type=int, default=24)
- parser.add_argument("--embed-dim", type=int, default=1024)
-
- @classmethod
- def build_model(cls, args, task):
- encoder = DummyEncoder(
- num_embed=len(task.target_dictionary),
- embed_dim=args.embed_dim,
- num_layers=args.num_layers,
- )
- return cls(args, encoder)
-
- def forward(self, src_tokens, masked_tokens=None, **kwargs):
- return self.decoder(src_tokens, masked_tokens=masked_tokens)
-
-
-class DummyEncoder(FairseqDecoder):
- def __init__(self, num_embed=50000, embed_dim=1024, num_layers=24):
- super().__init__(Dictionary())
- self.embed = nn.Embedding(
- num_embeddings=num_embed, embedding_dim=embed_dim, padding_idx=0
- )
- self.layers_a = nn.ModuleList(
- [
- nn.Sequential(
- nn.LayerNorm(embed_dim),
- nn.Linear(embed_dim, 3 * embed_dim), # q, k, v input projection
- nn.Linear(3 * embed_dim, embed_dim), # skip self-attention
- nn.Linear(embed_dim, embed_dim), # output projection
- nn.Dropout(),
- )
- for i in range(num_layers)
- ]
- )
- self.layers_b = nn.ModuleList(
- [
- nn.Sequential(
- nn.LayerNorm(embed_dim),
- nn.Linear(embed_dim, 4 * embed_dim), # FFN
- nn.ReLU(),
- nn.Linear(4 * embed_dim, embed_dim), # FFN
- nn.Dropout(0.1),
- )
- for i in range(num_layers)
- ]
- )
- self.out_proj = nn.Linear(embed_dim, num_embed)
-
- def forward(self, tokens, masked_tokens=None):
- x = self.embed(tokens)
- for layer_a, layer_b in zip(self.layers_a, self.layers_b):
- x = x + layer_a(x)
- x = x + layer_b(x)
- x = self.out_proj(x)
- if masked_tokens is not None:
- x = x[masked_tokens]
- return (x,)
-
- def max_positions(self):
- return 1024
-
- def get_normalized_probs(self, net_output, log_probs, sample=None):
- logits = net_output[0].float()
- if log_probs:
- return F.log_softmax(logits, dim=-1)
- else:
- return F.softmax(logits, dim=-1)
-
-
-@register_model_architecture("dummy_model", "dummy_model")
-def base_architecture(args):
- pass
diff --git a/spaces/OFA-Sys/OFA-Image_Caption/fairseq/fairseq/modules/quantization/quantization_options.py b/spaces/OFA-Sys/OFA-Image_Caption/fairseq/fairseq/modules/quantization/quantization_options.py
deleted file mode 100644
index b46d682c0edaeaaf2a230e51d50da2a32d4bda98..0000000000000000000000000000000000000000
--- a/spaces/OFA-Sys/OFA-Image_Caption/fairseq/fairseq/modules/quantization/quantization_options.py
+++ /dev/null
@@ -1,44 +0,0 @@
-# Copyright (c) Facebook, Inc. and its affiliates.
-#
-# This source code is licensed under the MIT license found in the
-# LICENSE file in the root directory of this source tree.
-
-
-def parse_config_yaml(yaml_data):
- # Initialize to default options.
- quantization_options = {
- "n_centroids": {
- "Linear": ["in_features", {"*": 256}],
- "Embedding": ["embedding_dim", {"*": 256}],
- },
- "block_sizes": {
- "Linear": ["fuzzy_name", {"fc": 8, "attn": 4, "emb": 4}],
- "Embedding": ["fuzzy_name", {"emb": 8}],
- },
- "layers_to_quantize": [
- "decoder\\.layers\\.\\d+\\.fc[12]",
- "decoder\\.embed_tokens\\.embeddings\\.[012]\\.[01]",
- "decoder\\.layers\\.\\d+\\.self_attn\\.(k_proj|v_proj|q_proj|out_proj)",
- ],
- }
-
- if "n_centroids" in yaml_data:
- quantization_options["n_centroids"] = {
- layer: convert_yaml_to_tuple(layer_data)
- for layer, layer_data in yaml_data["n_centroids"].items()
- }
- if "block_sizes" in yaml_data:
- quantization_options["block_sizes"] = {
- layer: convert_yaml_to_tuple(layer_data)
- for layer, layer_data in yaml_data["block_sizes"].items()
- }
- if "layers_to_quantize" in yaml_data:
- quantization_options["layers_to_quantize"] = yaml_data["layers_to_quantize"]
-
- return quantization_options
-
-
-def convert_yaml_to_tuple(yaml_dictionary):
- """Converts a yaml dictionary with two keys: `key` and `value` into a two
- argument tuple of those values."""
- return (yaml_dictionary["key"], yaml_dictionary["value"])
diff --git a/spaces/OFA-Sys/OFA-vqa/fairseq/examples/multilingual/data_scripts/download_wat19_my.sh b/spaces/OFA-Sys/OFA-vqa/fairseq/examples/multilingual/data_scripts/download_wat19_my.sh
deleted file mode 100644
index c1e2d47287a29af4576e7a63641e8152ecb63c44..0000000000000000000000000000000000000000
--- a/spaces/OFA-Sys/OFA-vqa/fairseq/examples/multilingual/data_scripts/download_wat19_my.sh
+++ /dev/null
@@ -1,36 +0,0 @@
-#!/bin/bash
-# Copyright (c) Facebook, Inc. and its affiliates.
-# All rights reserved.
-#
-# This source code is licensed under the license found in the
-# LICENSE file in the root directory of this source tree.
-
-
-if [ -z $WORKDIR_ROOT ] ;
-then
- echo "please specify your working directory root in environment variable WORKDIR_ROOT. Exitting..."
- exit
-fi
-
-
-SRCDIR=$WORKDIR_ROOT/indic_languages_corpus
-DESTDIR=$WORKDIR_ROOT/ML50/raw
-mkdir -p $SRCDIR
-mkdir -p $DESTDIR
-
-WAT_MY_EN=wat2020.my-en.zip
-cd $SRCDIR
-# please refer to http://lotus.kuee.kyoto-u.ac.jp/WAT/my-en-data/ for latest URL if the following url expired
-#- The data used for WAT2020 are identical to those used in WAT2019.
-wget http://lotus.kuee.kyoto-u.ac.jp/WAT/my-en-data/$WAT_MY_EN
-unzip $WAT_MY_EN
-
-
-SRC_EXTRACT_DIR=$SRCDIR/wat2020.my-en/alt
-
-cp $SRC_EXTRACT_DIR/train.alt.en $DESTDIR/train.my_MM-en_XX.en_XX
-cp $SRC_EXTRACT_DIR/train.alt.my $DESTDIR/train.my_MM-en_XX.my_MM
-cp $SRC_EXTRACT_DIR/dev.alt.en $DESTDIR/valid.my_MM-en_XX.en_XX
-cp $SRC_EXTRACT_DIR/dev.alt.my $DESTDIR/valid.my_MM-en_XX.my_MM
-cp $SRC_EXTRACT_DIR/test.alt.en $DESTDIR/test.my_MM-en_XX.en_XX
-cp $SRC_EXTRACT_DIR/test.alt.my $DESTDIR/test.my_MM-en_XX.my_MM
diff --git a/spaces/OFA-Sys/OFA-vqa/fairseq/examples/wav2vec/unsupervised/scripts/apply_pca.py b/spaces/OFA-Sys/OFA-vqa/fairseq/examples/wav2vec/unsupervised/scripts/apply_pca.py
deleted file mode 100644
index 10ad6ce47cfdf0a87ba089b299fe9551b29fa167..0000000000000000000000000000000000000000
--- a/spaces/OFA-Sys/OFA-vqa/fairseq/examples/wav2vec/unsupervised/scripts/apply_pca.py
+++ /dev/null
@@ -1,76 +0,0 @@
-#!/usr/bin/env python3 -u
-# Copyright (c) Facebook, Inc. and its affiliates.
-#
-# This source code is licensed under the MIT license found in the
-# LICENSE file in the root directory of this source tree.
-
-import argparse
-import os
-import os.path as osp
-import math
-import numpy as np
-import tqdm
-import torch
-from shutil import copyfile
-
-from npy_append_array import NpyAppendArray
-
-
-def get_parser():
- parser = argparse.ArgumentParser(
- description="transforms features via a given pca and stored them in target dir"
- )
- # fmt: off
- parser.add_argument('source', help='directory with features')
- parser.add_argument('--split', help='which split to read', required=True)
- parser.add_argument('--save-dir', help='where to save the output', required=True)
- parser.add_argument('--pca-path', type=str, help='pca location. will append _A.npy and _b.npy', required=True)
- parser.add_argument('--batch-size', type=int, default=2048000, help='batch size')
- parser.add_argument('--unfiltered', action='store_true', help='process the unfiltered version')
- # fmt: on
-
- return parser
-
-
-def main():
- parser = get_parser()
- args = parser.parse_args()
-
- source_path = osp.join(args.source, args.split)
- data_poth = source_path + "_unfiltered" if args.unfiltered else source_path
-
- print(f"data path: {data_poth}")
-
- features = np.load(data_poth + ".npy", mmap_mode="r")
- pca_A = torch.from_numpy(np.load(args.pca_path + "_A.npy")).cuda()
- pca_b = torch.from_numpy(np.load(args.pca_path + "_b.npy")).cuda()
-
- os.makedirs(args.save_dir, exist_ok=True)
- save_path = osp.join(args.save_dir, args.split)
-
- copyfile(source_path + ".tsv", save_path + ".tsv")
- copyfile(data_poth + ".lengths", save_path + ".lengths")
-
- if osp.exists(source_path + ".phn"):
- copyfile(source_path + ".phn", save_path + ".phn")
-
- if osp.exists(source_path + ".wrd"):
- copyfile(source_path + ".wrd", save_path + ".wrd")
-
- if osp.exists(save_path + ".npy"):
- os.remove(save_path + ".npy")
- npaa = NpyAppendArray(save_path + ".npy")
-
- batches = math.ceil(features.shape[0] / args.batch_size)
-
- with torch.no_grad():
- for b in tqdm.trange(batches):
- start = b * args.batch_size
- end = start + args.batch_size
- x = torch.from_numpy(features[start:end]).cuda()
- x = torch.matmul(x, pca_A) + pca_b
- npaa.append(x.cpu().numpy())
-
-
-if __name__ == "__main__":
- main()
diff --git a/spaces/OFA-Sys/OFA-vqa/fairseq/fairseq_cli/interactive.py b/spaces/OFA-Sys/OFA-vqa/fairseq/fairseq_cli/interactive.py
deleted file mode 100644
index cadef2821a74a3b2f051c792d835129bf775714f..0000000000000000000000000000000000000000
--- a/spaces/OFA-Sys/OFA-vqa/fairseq/fairseq_cli/interactive.py
+++ /dev/null
@@ -1,316 +0,0 @@
-#!/usr/bin/env python3 -u
-# Copyright (c) Facebook, Inc. and its affiliates.
-#
-# This source code is licensed under the MIT license found in the
-# LICENSE file in the root directory of this source tree.
-"""
-Translate raw text with a trained model. Batches data on-the-fly.
-"""
-
-import ast
-import fileinput
-import logging
-import math
-import os
-import sys
-import time
-from argparse import Namespace
-from collections import namedtuple
-
-import numpy as np
-import torch
-from fairseq import checkpoint_utils, distributed_utils, options, tasks, utils
-from fairseq.dataclass.configs import FairseqConfig
-from fairseq.dataclass.utils import convert_namespace_to_omegaconf
-from fairseq.token_generation_constraints import pack_constraints, unpack_constraints
-from fairseq_cli.generate import get_symbols_to_strip_from_output
-
-
-logging.basicConfig(
- format="%(asctime)s | %(levelname)s | %(name)s | %(message)s",
- datefmt="%Y-%m-%d %H:%M:%S",
- level=os.environ.get("LOGLEVEL", "INFO").upper(),
- stream=sys.stdout,
-)
-logger = logging.getLogger("fairseq_cli.interactive")
-
-
-Batch = namedtuple("Batch", "ids src_tokens src_lengths constraints")
-Translation = namedtuple("Translation", "src_str hypos pos_scores alignments")
-
-
-def buffered_read(input, buffer_size):
- buffer = []
- with fileinput.input(files=[input], openhook=fileinput.hook_encoded("utf-8")) as h:
- for src_str in h:
- buffer.append(src_str.strip())
- if len(buffer) >= buffer_size:
- yield buffer
- buffer = []
-
- if len(buffer) > 0:
- yield buffer
-
-
-def make_batches(lines, cfg, task, max_positions, encode_fn):
- def encode_fn_target(x):
- return encode_fn(x)
-
- if cfg.generation.constraints:
- # Strip (tab-delimited) contraints, if present, from input lines,
- # store them in batch_constraints
- batch_constraints = [list() for _ in lines]
- for i, line in enumerate(lines):
- if "\t" in line:
- lines[i], *batch_constraints[i] = line.split("\t")
-
- # Convert each List[str] to List[Tensor]
- for i, constraint_list in enumerate(batch_constraints):
- batch_constraints[i] = [
- task.target_dictionary.encode_line(
- encode_fn_target(constraint),
- append_eos=False,
- add_if_not_exist=False,
- )
- for constraint in constraint_list
- ]
-
- if cfg.generation.constraints:
- constraints_tensor = pack_constraints(batch_constraints)
- else:
- constraints_tensor = None
-
- tokens, lengths = task.get_interactive_tokens_and_lengths(lines, encode_fn)
-
- itr = task.get_batch_iterator(
- dataset=task.build_dataset_for_inference(
- tokens, lengths, constraints=constraints_tensor
- ),
- max_tokens=cfg.dataset.max_tokens,
- max_sentences=cfg.dataset.batch_size,
- max_positions=max_positions,
- ignore_invalid_inputs=cfg.dataset.skip_invalid_size_inputs_valid_test,
- ).next_epoch_itr(shuffle=False)
- for batch in itr:
- ids = batch["id"]
- src_tokens = batch["net_input"]["src_tokens"]
- src_lengths = batch["net_input"]["src_lengths"]
- constraints = batch.get("constraints", None)
-
- yield Batch(
- ids=ids,
- src_tokens=src_tokens,
- src_lengths=src_lengths,
- constraints=constraints,
- )
-
-
-def main(cfg: FairseqConfig):
- if isinstance(cfg, Namespace):
- cfg = convert_namespace_to_omegaconf(cfg)
-
- start_time = time.time()
- total_translate_time = 0
-
- utils.import_user_module(cfg.common)
-
- if cfg.interactive.buffer_size < 1:
- cfg.interactive.buffer_size = 1
- if cfg.dataset.max_tokens is None and cfg.dataset.batch_size is None:
- cfg.dataset.batch_size = 1
-
- assert (
- not cfg.generation.sampling or cfg.generation.nbest == cfg.generation.beam
- ), "--sampling requires --nbest to be equal to --beam"
- assert (
- not cfg.dataset.batch_size
- or cfg.dataset.batch_size <= cfg.interactive.buffer_size
- ), "--batch-size cannot be larger than --buffer-size"
-
- logger.info(cfg)
-
- # Fix seed for stochastic decoding
- if cfg.common.seed is not None and not cfg.generation.no_seed_provided:
- np.random.seed(cfg.common.seed)
- utils.set_torch_seed(cfg.common.seed)
-
- use_cuda = torch.cuda.is_available() and not cfg.common.cpu
-
- # Setup task, e.g., translation
- task = tasks.setup_task(cfg.task)
-
- # Load ensemble
- overrides = ast.literal_eval(cfg.common_eval.model_overrides)
- logger.info("loading model(s) from {}".format(cfg.common_eval.path))
- models, _model_args = checkpoint_utils.load_model_ensemble(
- utils.split_paths(cfg.common_eval.path),
- arg_overrides=overrides,
- task=task,
- suffix=cfg.checkpoint.checkpoint_suffix,
- strict=(cfg.checkpoint.checkpoint_shard_count == 1),
- num_shards=cfg.checkpoint.checkpoint_shard_count,
- )
-
- # Set dictionaries
- src_dict = task.source_dictionary
- tgt_dict = task.target_dictionary
-
- # Optimize ensemble for generation
- for model in models:
- if model is None:
- continue
- if cfg.common.fp16:
- model.half()
- if use_cuda and not cfg.distributed_training.pipeline_model_parallel:
- model.cuda()
- model.prepare_for_inference_(cfg)
-
- # Initialize generator
- generator = task.build_generator(models, cfg.generation)
-
- # Handle tokenization and BPE
- tokenizer = task.build_tokenizer(cfg.tokenizer)
- bpe = task.build_bpe(cfg.bpe)
-
- def encode_fn(x):
- if tokenizer is not None:
- x = tokenizer.encode(x)
- if bpe is not None:
- x = bpe.encode(x)
- return x
-
- def decode_fn(x):
- if bpe is not None:
- x = bpe.decode(x)
- if tokenizer is not None:
- x = tokenizer.decode(x)
- return x
-
- # Load alignment dictionary for unknown word replacement
- # (None if no unknown word replacement, empty if no path to align dictionary)
- align_dict = utils.load_align_dict(cfg.generation.replace_unk)
-
- max_positions = utils.resolve_max_positions(
- task.max_positions(), *[model.max_positions() for model in models]
- )
-
- if cfg.generation.constraints:
- logger.warning(
- "NOTE: Constrained decoding currently assumes a shared subword vocabulary."
- )
-
- if cfg.interactive.buffer_size > 1:
- logger.info("Sentence buffer size: %s", cfg.interactive.buffer_size)
- logger.info("NOTE: hypothesis and token scores are output in base 2")
- logger.info("Type the input sentence and press return:")
- start_id = 0
- for inputs in buffered_read(cfg.interactive.input, cfg.interactive.buffer_size):
- results = []
- for batch in make_batches(inputs, cfg, task, max_positions, encode_fn):
- bsz = batch.src_tokens.size(0)
- src_tokens = batch.src_tokens
- src_lengths = batch.src_lengths
- constraints = batch.constraints
- if use_cuda:
- src_tokens = src_tokens.cuda()
- src_lengths = src_lengths.cuda()
- if constraints is not None:
- constraints = constraints.cuda()
-
- sample = {
- "net_input": {
- "src_tokens": src_tokens,
- "src_lengths": src_lengths,
- },
- }
- translate_start_time = time.time()
- translations = task.inference_step(
- generator, models, sample, constraints=constraints
- )
- translate_time = time.time() - translate_start_time
- total_translate_time += translate_time
- list_constraints = [[] for _ in range(bsz)]
- if cfg.generation.constraints:
- list_constraints = [unpack_constraints(c) for c in constraints]
- for i, (id, hypos) in enumerate(zip(batch.ids.tolist(), translations)):
- src_tokens_i = utils.strip_pad(src_tokens[i], tgt_dict.pad())
- constraints = list_constraints[i]
- results.append(
- (
- start_id + id,
- src_tokens_i,
- hypos,
- {
- "constraints": constraints,
- "time": translate_time / len(translations),
- },
- )
- )
-
- # sort output to match input order
- for id_, src_tokens, hypos, info in sorted(results, key=lambda x: x[0]):
- src_str = ''
- if src_dict is not None:
- src_str = src_dict.string(src_tokens, cfg.common_eval.post_process)
- print("S-{}\t{}".format(id_, src_str))
- print("W-{}\t{:.3f}\tseconds".format(id_, info["time"]))
- for constraint in info["constraints"]:
- print(
- "C-{}\t{}".format(
- id_, tgt_dict.string(constraint, cfg.common_eval.post_process)
- )
- )
-
- # Process top predictions
- for hypo in hypos[: min(len(hypos), cfg.generation.nbest)]:
- hypo_tokens, hypo_str, alignment = utils.post_process_prediction(
- hypo_tokens=hypo["tokens"].int().cpu(),
- src_str=src_str,
- alignment=hypo["alignment"],
- align_dict=align_dict,
- tgt_dict=tgt_dict,
- remove_bpe=cfg.common_eval.post_process,
- extra_symbols_to_ignore=get_symbols_to_strip_from_output(generator),
- )
- detok_hypo_str = decode_fn(hypo_str)
- score = hypo["score"] / math.log(2) # convert to base 2
- # original hypothesis (after tokenization and BPE)
- print("H-{}\t{}\t{}".format(id_, score, hypo_str))
- # detokenized hypothesis
- print("D-{}\t{}\t{}".format(id_, score, detok_hypo_str))
- print(
- "P-{}\t{}".format(
- id_,
- " ".join(
- map(
- lambda x: "{:.4f}".format(x),
- # convert from base e to base 2
- hypo["positional_scores"].div_(math.log(2)).tolist(),
- )
- ),
- )
- )
- if cfg.generation.print_alignment:
- alignment_str = " ".join(
- ["{}-{}".format(src, tgt) for src, tgt in alignment]
- )
- print("A-{}\t{}".format(id_, alignment_str))
-
- # update running id_ counter
- start_id += len(inputs)
-
- logger.info(
- "Total time: {:.3f} seconds; translation time: {:.3f}".format(
- time.time() - start_time, total_translate_time
- )
- )
-
-
-def cli_main():
- parser = options.get_interactive_generation_parser()
- args = options.parse_args_and_arch(parser)
- distributed_utils.call_main(convert_namespace_to_omegaconf(args), main)
-
-
-if __name__ == "__main__":
- cli_main()
diff --git a/spaces/OFA-Sys/OFA-vqa/fairseq/tests/test_inference_dropout.py b/spaces/OFA-Sys/OFA-vqa/fairseq/tests/test_inference_dropout.py
deleted file mode 100644
index 353ac674780a9795492c75aa0a7bc0677b07a9c9..0000000000000000000000000000000000000000
--- a/spaces/OFA-Sys/OFA-vqa/fairseq/tests/test_inference_dropout.py
+++ /dev/null
@@ -1,70 +0,0 @@
-# Copyright (c) Facebook, Inc. and its affiliates.
-#
-# This source code is licensed under the MIT license found in the
-# LICENSE file in the root directory of this source tree.
-
-import logging
-import unittest
-
-from fairseq.dataclass.utils import convert_namespace_to_omegaconf
-from fairseq.models.transformer import TransformerModel
-from tests.test_sequence_generator import get_dummy_task_and_parser
-
-
-class TestInferenceDropout(unittest.TestCase):
- def setUp(self):
- self.task, self.parser = get_dummy_task_and_parser()
- TransformerModel.add_args(self.parser)
- self.args = self.parser.parse_args([])
- self.args.encoder_layers = 2
- self.args.decoder_layers = 1
- logging.disable(logging.CRITICAL)
-
- def tearDown(self):
- logging.disable(logging.NOTSET)
-
- def test_sets_inference_dropout_to_true(self):
- self.args.retain_dropout = True
- self.transformer_model = TransformerModel.build_model(self.args, self.task)
- cfg = convert_namespace_to_omegaconf(self.args)
- self.transformer_model.prepare_for_inference_(cfg)
- assert self.transformer_model.encoder.dropout_module.apply_during_inference
- assert self.transformer_model.decoder.dropout_module.apply_during_inference
- for layer in self.transformer_model.encoder.layers:
- assert layer.dropout_module.apply_during_inference
-
- def test_inference_dropout_false_by_default(self):
- self.transformer_model = TransformerModel.build_model(self.args, self.task)
- cfg = convert_namespace_to_omegaconf(self.args)
- self.transformer_model.prepare_for_inference_(cfg)
- assert not self.transformer_model.encoder.dropout_module.apply_during_inference
- assert not self.transformer_model.decoder.dropout_module.apply_during_inference
- for layer in self.transformer_model.encoder.layers:
- assert not layer.dropout_module.apply_during_inference
- for layer in self.transformer_model.decoder.layers:
- assert not layer.dropout_module.apply_during_inference
-
- def test_applies_training_mode(self):
- self.transformer_model = TransformerModel.build_model(self.args, self.task)
- assert self.transformer_model.encoder.dropout_module.training
- for layer in self.transformer_model.encoder.layers:
- assert layer.dropout_module.training
-
- self.transformer_model.eval()
- assert not self.transformer_model.decoder.dropout_module.training
- for layer in self.transformer_model.encoder.layers:
- assert not layer.dropout_module.training
-
- def test_retain_modules(self):
- self.args.retain_dropout = True
- self.args.retain_dropout_modules = [
- "TransformerEncoder",
- "TransformerEncoderLayer",
- ]
- self.transformer_model = TransformerModel.build_model(self.args, self.task)
- cfg = convert_namespace_to_omegaconf(self.args)
- self.transformer_model.prepare_for_inference_(cfg)
- assert self.transformer_model.encoder.dropout_module.apply_during_inference
- assert not self.transformer_model.decoder.dropout_module.apply_during_inference
- for layer in self.transformer_model.decoder.layers:
- assert not layer.dropout_module.apply_during_inference
diff --git a/spaces/PAIR/Text2Video-Zero/annotator/uniformer/mmcv/ops/group_points.py b/spaces/PAIR/Text2Video-Zero/annotator/uniformer/mmcv/ops/group_points.py
deleted file mode 100644
index 6c3ec9d758ebe4e1c2205882af4be154008253a5..0000000000000000000000000000000000000000
--- a/spaces/PAIR/Text2Video-Zero/annotator/uniformer/mmcv/ops/group_points.py
+++ /dev/null
@@ -1,224 +0,0 @@
-# Copyright (c) OpenMMLab. All rights reserved.
-from typing import Tuple
-
-import torch
-from torch import nn as nn
-from torch.autograd import Function
-
-from ..utils import ext_loader
-from .ball_query import ball_query
-from .knn import knn
-
-ext_module = ext_loader.load_ext(
- '_ext', ['group_points_forward', 'group_points_backward'])
-
-
-class QueryAndGroup(nn.Module):
- """Groups points with a ball query of radius.
-
- Args:
- max_radius (float): The maximum radius of the balls.
- If None is given, we will use kNN sampling instead of ball query.
- sample_num (int): Maximum number of features to gather in the ball.
- min_radius (float, optional): The minimum radius of the balls.
- Default: 0.
- use_xyz (bool, optional): Whether to use xyz.
- Default: True.
- return_grouped_xyz (bool, optional): Whether to return grouped xyz.
- Default: False.
- normalize_xyz (bool, optional): Whether to normalize xyz.
- Default: False.
- uniform_sample (bool, optional): Whether to sample uniformly.
- Default: False
- return_unique_cnt (bool, optional): Whether to return the count of
- unique samples. Default: False.
- return_grouped_idx (bool, optional): Whether to return grouped idx.
- Default: False.
- """
-
- def __init__(self,
- max_radius,
- sample_num,
- min_radius=0,
- use_xyz=True,
- return_grouped_xyz=False,
- normalize_xyz=False,
- uniform_sample=False,
- return_unique_cnt=False,
- return_grouped_idx=False):
- super().__init__()
- self.max_radius = max_radius
- self.min_radius = min_radius
- self.sample_num = sample_num
- self.use_xyz = use_xyz
- self.return_grouped_xyz = return_grouped_xyz
- self.normalize_xyz = normalize_xyz
- self.uniform_sample = uniform_sample
- self.return_unique_cnt = return_unique_cnt
- self.return_grouped_idx = return_grouped_idx
- if self.return_unique_cnt:
- assert self.uniform_sample, \
- 'uniform_sample should be True when ' \
- 'returning the count of unique samples'
- if self.max_radius is None:
- assert not self.normalize_xyz, \
- 'can not normalize grouped xyz when max_radius is None'
-
- def forward(self, points_xyz, center_xyz, features=None):
- """
- Args:
- points_xyz (Tensor): (B, N, 3) xyz coordinates of the features.
- center_xyz (Tensor): (B, npoint, 3) coordinates of the centriods.
- features (Tensor): (B, C, N) Descriptors of the features.
-
- Returns:
- Tensor: (B, 3 + C, npoint, sample_num) Grouped feature.
- """
- # if self.max_radius is None, we will perform kNN instead of ball query
- # idx is of shape [B, npoint, sample_num]
- if self.max_radius is None:
- idx = knn(self.sample_num, points_xyz, center_xyz, False)
- idx = idx.transpose(1, 2).contiguous()
- else:
- idx = ball_query(self.min_radius, self.max_radius, self.sample_num,
- points_xyz, center_xyz)
-
- if self.uniform_sample:
- unique_cnt = torch.zeros((idx.shape[0], idx.shape[1]))
- for i_batch in range(idx.shape[0]):
- for i_region in range(idx.shape[1]):
- unique_ind = torch.unique(idx[i_batch, i_region, :])
- num_unique = unique_ind.shape[0]
- unique_cnt[i_batch, i_region] = num_unique
- sample_ind = torch.randint(
- 0,
- num_unique, (self.sample_num - num_unique, ),
- dtype=torch.long)
- all_ind = torch.cat((unique_ind, unique_ind[sample_ind]))
- idx[i_batch, i_region, :] = all_ind
-
- xyz_trans = points_xyz.transpose(1, 2).contiguous()
- # (B, 3, npoint, sample_num)
- grouped_xyz = grouping_operation(xyz_trans, idx)
- grouped_xyz_diff = grouped_xyz - \
- center_xyz.transpose(1, 2).unsqueeze(-1) # relative offsets
- if self.normalize_xyz:
- grouped_xyz_diff /= self.max_radius
-
- if features is not None:
- grouped_features = grouping_operation(features, idx)
- if self.use_xyz:
- # (B, C + 3, npoint, sample_num)
- new_features = torch.cat([grouped_xyz_diff, grouped_features],
- dim=1)
- else:
- new_features = grouped_features
- else:
- assert (self.use_xyz
- ), 'Cannot have not features and not use xyz as a feature!'
- new_features = grouped_xyz_diff
-
- ret = [new_features]
- if self.return_grouped_xyz:
- ret.append(grouped_xyz)
- if self.return_unique_cnt:
- ret.append(unique_cnt)
- if self.return_grouped_idx:
- ret.append(idx)
- if len(ret) == 1:
- return ret[0]
- else:
- return tuple(ret)
-
-
-class GroupAll(nn.Module):
- """Group xyz with feature.
-
- Args:
- use_xyz (bool): Whether to use xyz.
- """
-
- def __init__(self, use_xyz: bool = True):
- super().__init__()
- self.use_xyz = use_xyz
-
- def forward(self,
- xyz: torch.Tensor,
- new_xyz: torch.Tensor,
- features: torch.Tensor = None):
- """
- Args:
- xyz (Tensor): (B, N, 3) xyz coordinates of the features.
- new_xyz (Tensor): new xyz coordinates of the features.
- features (Tensor): (B, C, N) features to group.
-
- Returns:
- Tensor: (B, C + 3, 1, N) Grouped feature.
- """
- grouped_xyz = xyz.transpose(1, 2).unsqueeze(2)
- if features is not None:
- grouped_features = features.unsqueeze(2)
- if self.use_xyz:
- # (B, 3 + C, 1, N)
- new_features = torch.cat([grouped_xyz, grouped_features],
- dim=1)
- else:
- new_features = grouped_features
- else:
- new_features = grouped_xyz
-
- return new_features
-
-
-class GroupingOperation(Function):
- """Group feature with given index."""
-
- @staticmethod
- def forward(ctx, features: torch.Tensor,
- indices: torch.Tensor) -> torch.Tensor:
- """
- Args:
- features (Tensor): (B, C, N) tensor of features to group.
- indices (Tensor): (B, npoint, nsample) the indices of
- features to group with.
-
- Returns:
- Tensor: (B, C, npoint, nsample) Grouped features.
- """
- features = features.contiguous()
- indices = indices.contiguous()
-
- B, nfeatures, nsample = indices.size()
- _, C, N = features.size()
- output = torch.cuda.FloatTensor(B, C, nfeatures, nsample)
-
- ext_module.group_points_forward(B, C, N, nfeatures, nsample, features,
- indices, output)
-
- ctx.for_backwards = (indices, N)
- return output
-
- @staticmethod
- def backward(ctx,
- grad_out: torch.Tensor) -> Tuple[torch.Tensor, torch.Tensor]:
- """
- Args:
- grad_out (Tensor): (B, C, npoint, nsample) tensor of the gradients
- of the output from forward.
-
- Returns:
- Tensor: (B, C, N) gradient of the features.
- """
- idx, N = ctx.for_backwards
-
- B, C, npoint, nsample = grad_out.size()
- grad_features = torch.cuda.FloatTensor(B, C, N).zero_()
-
- grad_out_data = grad_out.data.contiguous()
- ext_module.group_points_backward(B, C, N, npoint, nsample,
- grad_out_data, idx,
- grad_features.data)
- return grad_features, None
-
-
-grouping_operation = GroupingOperation.apply
diff --git a/spaces/Pattr/DrumClassification/lilypond-2.24.2/lib/guile/2.2/ccache/scripts/read-text-outline.go b/spaces/Pattr/DrumClassification/lilypond-2.24.2/lib/guile/2.2/ccache/scripts/read-text-outline.go
deleted file mode 100644
index ea32773d28ba991e1b9eeea4376fa49f0176f255..0000000000000000000000000000000000000000
Binary files a/spaces/Pattr/DrumClassification/lilypond-2.24.2/lib/guile/2.2/ccache/scripts/read-text-outline.go and /dev/null differ
diff --git a/spaces/Pattr/DrumClassification/lilypond-2.24.2/lib/lilypond/2.24.2/ccache/lily/auto-beam.go b/spaces/Pattr/DrumClassification/lilypond-2.24.2/lib/lilypond/2.24.2/ccache/lily/auto-beam.go
deleted file mode 100644
index 7f1b6d514703df873a07a292ebc64914794fc71c..0000000000000000000000000000000000000000
Binary files a/spaces/Pattr/DrumClassification/lilypond-2.24.2/lib/lilypond/2.24.2/ccache/lily/auto-beam.go and /dev/null differ
diff --git a/spaces/Pie31415/control-animation/annotator/uniformer/mmcv/ops/border_align.py b/spaces/Pie31415/control-animation/annotator/uniformer/mmcv/ops/border_align.py
deleted file mode 100644
index ff305be328e9b0a15e1bbb5e6b41beb940f55c81..0000000000000000000000000000000000000000
--- a/spaces/Pie31415/control-animation/annotator/uniformer/mmcv/ops/border_align.py
+++ /dev/null
@@ -1,109 +0,0 @@
-# Copyright (c) OpenMMLab. All rights reserved.
-# modified from
-# https://github.com/Megvii-BaseDetection/cvpods/blob/master/cvpods/layers/border_align.py
-
-import torch
-import torch.nn as nn
-from torch.autograd import Function
-from torch.autograd.function import once_differentiable
-
-from ..utils import ext_loader
-
-ext_module = ext_loader.load_ext(
- '_ext', ['border_align_forward', 'border_align_backward'])
-
-
-class BorderAlignFunction(Function):
-
- @staticmethod
- def symbolic(g, input, boxes, pool_size):
- return g.op(
- 'mmcv::MMCVBorderAlign', input, boxes, pool_size_i=pool_size)
-
- @staticmethod
- def forward(ctx, input, boxes, pool_size):
- ctx.pool_size = pool_size
- ctx.input_shape = input.size()
-
- assert boxes.ndim == 3, 'boxes must be with shape [B, H*W, 4]'
- assert boxes.size(2) == 4, \
- 'the last dimension of boxes must be (x1, y1, x2, y2)'
- assert input.size(1) % 4 == 0, \
- 'the channel for input feature must be divisible by factor 4'
-
- # [B, C//4, H*W, 4]
- output_shape = (input.size(0), input.size(1) // 4, boxes.size(1), 4)
- output = input.new_zeros(output_shape)
- # `argmax_idx` only used for backward
- argmax_idx = input.new_zeros(output_shape).to(torch.int)
-
- ext_module.border_align_forward(
- input, boxes, output, argmax_idx, pool_size=ctx.pool_size)
-
- ctx.save_for_backward(boxes, argmax_idx)
- return output
-
- @staticmethod
- @once_differentiable
- def backward(ctx, grad_output):
- boxes, argmax_idx = ctx.saved_tensors
- grad_input = grad_output.new_zeros(ctx.input_shape)
- # complex head architecture may cause grad_output uncontiguous
- grad_output = grad_output.contiguous()
- ext_module.border_align_backward(
- grad_output,
- boxes,
- argmax_idx,
- grad_input,
- pool_size=ctx.pool_size)
- return grad_input, None, None
-
-
-border_align = BorderAlignFunction.apply
-
-
-class BorderAlign(nn.Module):
- r"""Border align pooling layer.
-
- Applies border_align over the input feature based on predicted bboxes.
- The details were described in the paper
- `BorderDet: Border Feature for Dense Object Detection
- `_.
-
- For each border line (e.g. top, left, bottom or right) of each box,
- border_align does the following:
- 1. uniformly samples `pool_size`+1 positions on this line, involving \
- the start and end points.
- 2. the corresponding features on these points are computed by \
- bilinear interpolation.
- 3. max pooling over all the `pool_size`+1 positions are used for \
- computing pooled feature.
-
- Args:
- pool_size (int): number of positions sampled over the boxes' borders
- (e.g. top, bottom, left, right).
-
- """
-
- def __init__(self, pool_size):
- super(BorderAlign, self).__init__()
- self.pool_size = pool_size
-
- def forward(self, input, boxes):
- """
- Args:
- input: Features with shape [N,4C,H,W]. Channels ranged in [0,C),
- [C,2C), [2C,3C), [3C,4C) represent the top, left, bottom,
- right features respectively.
- boxes: Boxes with shape [N,H*W,4]. Coordinate format (x1,y1,x2,y2).
-
- Returns:
- Tensor: Pooled features with shape [N,C,H*W,4]. The order is
- (top,left,bottom,right) for the last dimension.
- """
- return border_align(input, boxes, self.pool_size)
-
- def __repr__(self):
- s = self.__class__.__name__
- s += f'(pool_size={self.pool_size})'
- return s
diff --git a/spaces/Pranjal12345/Text_to_Speech/tortoise/utils/text.py b/spaces/Pranjal12345/Text_to_Speech/tortoise/utils/text.py
deleted file mode 100644
index e28c86786b2ca47823a25f3f251f9bc85bb3facd..0000000000000000000000000000000000000000
--- a/spaces/Pranjal12345/Text_to_Speech/tortoise/utils/text.py
+++ /dev/null
@@ -1,132 +0,0 @@
-import re
-
-
-def split_and_recombine_text(text, desired_length=200, max_length=300):
- """Split text it into chunks of a desired length trying to keep sentences intact."""
- # normalize text, remove redundant whitespace and convert non-ascii quotes to ascii
- text = re.sub(r'\n\n+', '\n', text)
- text = re.sub(r'\s+', ' ', text)
- text = re.sub(r'[“”]', '"', text)
-
- rv = []
- in_quote = False
- current = ""
- split_pos = []
- pos = -1
- end_pos = len(text) - 1
-
- def seek(delta):
- nonlocal pos, in_quote, current
- is_neg = delta < 0
- for _ in range(abs(delta)):
- if is_neg:
- pos -= 1
- current = current[:-1]
- else:
- pos += 1
- current += text[pos]
- if text[pos] == '"':
- in_quote = not in_quote
- return text[pos]
-
- def peek(delta):
- p = pos + delta
- return text[p] if p < end_pos and p >= 0 else ""
-
- def commit():
- nonlocal rv, current, split_pos
- rv.append(current)
- current = ""
- split_pos = []
-
- while pos < end_pos:
- c = seek(1)
- # do we need to force a split?
- if len(current) >= max_length:
- if len(split_pos) > 0 and len(current) > (desired_length / 2):
- # we have at least one sentence and we are over half the desired length, seek back to the last split
- d = pos - split_pos[-1]
- seek(-d)
- else:
- # no full sentences, seek back until we are not in the middle of a word and split there
- while c not in '!?.\n ' and pos > 0 and len(current) > desired_length:
- c = seek(-1)
- commit()
- # check for sentence boundaries
- elif not in_quote and (c in '!?\n' or (c == '.' and peek(1) in '\n ')):
- # seek forward if we have consecutive boundary markers but still within the max length
- while pos < len(text) - 1 and len(current) < max_length and peek(1) in '!?.':
- c = seek(1)
- split_pos.append(pos)
- if len(current) >= desired_length:
- commit()
- # treat end of quote as a boundary if its followed by a space or newline
- elif in_quote and peek(1) == '"' and peek(2) in '\n ':
- seek(2)
- split_pos.append(pos)
- rv.append(current)
-
- # clean up, remove lines with only whitespace or punctuation
- rv = [s.strip() for s in rv]
- rv = [s for s in rv if len(s) > 0 and not re.match(r'^[\s\.,;:!?]*$', s)]
-
- return rv
-
-
-if __name__ == '__main__':
- import os
- import unittest
-
- class Test(unittest.TestCase):
- def test_split_and_recombine_text(self):
- text = """
- This is a sample sentence.
- This is another sample sentence.
- This is a longer sample sentence that should force a split inthemiddlebutinotinthislongword.
- "Don't split my quote... please"
- """
- self.assertEqual(split_and_recombine_text(text, desired_length=20, max_length=40),
- ['This is a sample sentence.',
- 'This is another sample sentence.',
- 'This is a longer sample sentence that',
- 'should force a split',
- 'inthemiddlebutinotinthislongword.',
- '"Don\'t split my quote... please"'])
-
- def test_split_and_recombine_text_2(self):
- text = """
- When you are really angry sometimes you use consecutive exclamation marks!!!!!! Is this a good thing to do?!?!?!
- I don't know but we should handle this situation..........................
- """
- self.assertEqual(split_and_recombine_text(text, desired_length=30, max_length=50),
- ['When you are really angry sometimes you use',
- 'consecutive exclamation marks!!!!!!',
- 'Is this a good thing to do?!?!?!',
- 'I don\'t know but we should handle this situation.'])
-
- def test_split_and_recombine_text_3(self):
- text_src = os.path.join(os.path.dirname(__file__), '../data/riding_hood.txt')
- with open(text_src, 'r') as f:
- text = f.read()
- self.assertEqual(
- split_and_recombine_text(text),
- [
- 'Once upon a time there lived in a certain village a little country girl, the prettiest creature who was ever seen. Her mother was excessively fond of her; and her grandmother doted on her still more. This good woman had a little red riding hood made for her.',
- 'It suited the girl so extremely well that everybody called her Little Red Riding Hood. One day her mother, having made some cakes, said to her, "Go, my dear, and see how your grandmother is doing, for I hear she has been very ill. Take her a cake, and this little pot of butter."',
- 'Little Red Riding Hood set out immediately to go to her grandmother, who lived in another village. As she was going through the wood, she met with a wolf, who had a very great mind to eat her up, but he dared not, because of some woodcutters working nearby in the forest.',
- 'He asked her where she was going. The poor child, who did not know that it was dangerous to stay and talk to a wolf, said to him, "I am going to see my grandmother and carry her a cake and a little pot of butter from my mother." "Does she live far off?" said the wolf "Oh I say,"',
- 'answered Little Red Riding Hood; "it is beyond that mill you see there, at the first house in the village." "Well," said the wolf, "and I\'ll go and see her too. I\'ll go this way and go you that, and we shall see who will be there first."',
- 'The wolf ran as fast as he could, taking the shortest path, and the little girl took a roundabout way, entertaining herself by gathering nuts, running after butterflies, and gathering bouquets of little flowers.',
- 'It was not long before the wolf arrived at the old woman\'s house. He knocked at the door: tap, tap. "Who\'s there?" "Your grandchild, Little Red Riding Hood," replied the wolf, counterfeiting her voice; "who has brought you a cake and a little pot of butter sent you by mother."',
- 'The good grandmother, who was in bed, because she was somewhat ill, cried out, "Pull the bobbin, and the latch will go up."',
- 'The wolf pulled the bobbin, and the door opened, and then he immediately fell upon the good woman and ate her up in a moment, for it been more than three days since he had eaten.',
- 'He then shut the door and got into the grandmother\'s bed, expecting Little Red Riding Hood, who came some time afterwards and knocked at the door: tap, tap. "Who\'s there?"',
- 'Little Red Riding Hood, hearing the big voice of the wolf, was at first afraid; but believing her grandmother had a cold and was hoarse, answered, "It is your grandchild Little Red Riding Hood, who has brought you a cake and a little pot of butter mother sends you."',
- 'The wolf cried out to her, softening his voice as much as he could, "Pull the bobbin, and the latch will go up." Little Red Riding Hood pulled the bobbin, and the door opened.',
- 'The wolf, seeing her come in, said to her, hiding himself under the bedclothes, "Put the cake and the little pot of butter upon the stool, and come get into bed with me." Little Red Riding Hood took off her clothes and got into bed.',
- 'She was greatly amazed to see how her grandmother looked in her nightclothes, and said to her, "Grandmother, what big arms you have!" "All the better to hug you with, my dear." "Grandmother, what big legs you have!" "All the better to run with, my child." "Grandmother, what big ears you have!"',
- '"All the better to hear with, my child." "Grandmother, what big eyes you have!" "All the better to see with, my child." "Grandmother, what big teeth you have got!" "All the better to eat you up with." And, saying these words, this wicked wolf fell upon Little Red Riding Hood, and ate her all up.',
- ]
- )
-
- unittest.main()
diff --git a/spaces/Prof-Reza/Audiocraft_Music-Audio_Generation/audiocraft/data/zip.py b/spaces/Prof-Reza/Audiocraft_Music-Audio_Generation/audiocraft/data/zip.py
deleted file mode 100644
index f0b17849d36991e7def35a14d3d518b9d867ce36..0000000000000000000000000000000000000000
--- a/spaces/Prof-Reza/Audiocraft_Music-Audio_Generation/audiocraft/data/zip.py
+++ /dev/null
@@ -1,76 +0,0 @@
-# Copyright (c) Meta Platforms, Inc. and affiliates.
-# All rights reserved.
-#
-# This source code is licensed under the license found in the
-# LICENSE file in the root directory of this source tree.
-"""Utility for reading some info from inside a zip file.
-"""
-
-import typing
-import zipfile
-
-from dataclasses import dataclass
-from functools import lru_cache
-from typing_extensions import Literal
-
-
-DEFAULT_SIZE = 32
-MODE = Literal['r', 'w', 'x', 'a']
-
-
-@dataclass(order=True)
-class PathInZip:
- """Hold a path of file within a zip file.
-
- Args:
- path (str): The convention is :.
- Let's assume there is a zip file /some/location/foo.zip
- and inside of it is a json file located at /data/file1.json,
- Then we expect path = "/some/location/foo.zip:/data/file1.json".
- """
-
- INFO_PATH_SEP = ':'
- zip_path: str
- file_path: str
-
- def __init__(self, path: str) -> None:
- split_path = path.split(self.INFO_PATH_SEP)
- assert len(split_path) == 2
- self.zip_path, self.file_path = split_path
-
- @classmethod
- def from_paths(cls, zip_path: str, file_path: str):
- return cls(zip_path + cls.INFO_PATH_SEP + file_path)
-
- def __str__(self) -> str:
- return self.zip_path + self.INFO_PATH_SEP + self.file_path
-
-
-def _open_zip(path: str, mode: MODE = 'r'):
- return zipfile.ZipFile(path, mode)
-
-
-_cached_open_zip = lru_cache(DEFAULT_SIZE)(_open_zip)
-
-
-def set_zip_cache_size(max_size: int):
- """Sets the maximal LRU caching for zip file opening.
-
- Args:
- max_size (int): the maximal LRU cache.
- """
- global _cached_open_zip
- _cached_open_zip = lru_cache(max_size)(_open_zip)
-
-
-def open_file_in_zip(path_in_zip: PathInZip, mode: str = 'r') -> typing.IO:
- """Opens a file stored inside a zip and returns a file-like object.
-
- Args:
- path_in_zip (PathInZip): A PathInZip object representing the file to return a file-like object of.
- mode (str): The mode in which to open the file with.
- Returns:
- A file-like object for PathInZip.
- """
- zf = _cached_open_zip(path_in_zip.zip_path)
- return zf.open(path_in_zip.file_path)
diff --git a/spaces/Prof-Reza/Audiocraft_Music-Audio_Generation/tests/quantization/test_vq.py b/spaces/Prof-Reza/Audiocraft_Music-Audio_Generation/tests/quantization/test_vq.py
deleted file mode 100644
index c215099fedacae35c6798fdd9b8420a447aa16bb..0000000000000000000000000000000000000000
--- a/spaces/Prof-Reza/Audiocraft_Music-Audio_Generation/tests/quantization/test_vq.py
+++ /dev/null
@@ -1,18 +0,0 @@
-# Copyright (c) Meta Platforms, Inc. and affiliates.
-# All rights reserved.
-#
-# This source code is licensed under the license found in the
-# LICENSE file in the root directory of this source tree.
-
-import torch
-
-from audiocraft.quantization.vq import ResidualVectorQuantizer
-
-
-class TestResidualVectorQuantizer:
-
- def test_rvq(self):
- x = torch.randn(1, 16, 2048)
- vq = ResidualVectorQuantizer(n_q=8, dimension=16, bins=8)
- res = vq(x, 1.)
- assert res.x.shape == torch.Size([1, 16, 2048])
diff --git a/spaces/Raspberry-ai/main/.env/lib/python3.11/site-packages/pip/_internal/commands/install.py b/spaces/Raspberry-ai/main/.env/lib/python3.11/site-packages/pip/_internal/commands/install.py
deleted file mode 100644
index e081c27d2d2b05ee9820bb41c071ec9da4ad2106..0000000000000000000000000000000000000000
--- a/spaces/Raspberry-ai/main/.env/lib/python3.11/site-packages/pip/_internal/commands/install.py
+++ /dev/null
@@ -1,860 +0,0 @@
-import errno
-import json
-import operator
-import os
-import shutil
-import site
-from optparse import SUPPRESS_HELP, Values
-from typing import Iterable, List, Optional
-
-from pip._vendor.packaging.utils import canonicalize_name
-from pip._vendor.rich import print_json
-
-from pip._internal.cache import WheelCache
-from pip._internal.cli import cmdoptions
-from pip._internal.cli.cmdoptions import make_target_python
-from pip._internal.cli.req_command import (
- RequirementCommand,
- warn_if_run_as_root,
- with_cleanup,
-)
-from pip._internal.cli.status_codes import ERROR, SUCCESS
-from pip._internal.exceptions import CommandError, InstallationError
-from pip._internal.locations import get_scheme
-from pip._internal.metadata import get_environment
-from pip._internal.models.format_control import FormatControl
-from pip._internal.models.installation_report import InstallationReport
-from pip._internal.operations.build.build_tracker import get_build_tracker
-from pip._internal.operations.check import ConflictDetails, check_install_conflicts
-from pip._internal.req import install_given_reqs
-from pip._internal.req.req_install import (
- InstallRequirement,
- LegacySetupPyOptionsCheckMode,
- check_legacy_setup_py_options,
-)
-from pip._internal.utils.compat import WINDOWS
-from pip._internal.utils.deprecation import (
- LegacyInstallReasonFailedBdistWheel,
- deprecated,
-)
-from pip._internal.utils.distutils_args import parse_distutils_args
-from pip._internal.utils.filesystem import test_writable_dir
-from pip._internal.utils.logging import getLogger
-from pip._internal.utils.misc import (
- ensure_dir,
- get_pip_version,
- protect_pip_from_modification_on_windows,
- write_output,
-)
-from pip._internal.utils.temp_dir import TempDirectory
-from pip._internal.utils.virtualenv import (
- running_under_virtualenv,
- virtualenv_no_global,
-)
-from pip._internal.wheel_builder import (
- BdistWheelAllowedPredicate,
- build,
- should_build_for_install_command,
-)
-
-logger = getLogger(__name__)
-
-
-def get_check_bdist_wheel_allowed(
- format_control: FormatControl,
-) -> BdistWheelAllowedPredicate:
- def check_binary_allowed(req: InstallRequirement) -> bool:
- canonical_name = canonicalize_name(req.name or "")
- allowed_formats = format_control.get_allowed_formats(canonical_name)
- return "binary" in allowed_formats
-
- return check_binary_allowed
-
-
-class InstallCommand(RequirementCommand):
- """
- Install packages from:
-
- - PyPI (and other indexes) using requirement specifiers.
- - VCS project urls.
- - Local project directories.
- - Local or remote source archives.
-
- pip also supports installing from "requirements files", which provide
- an easy way to specify a whole environment to be installed.
- """
-
- usage = """
- %prog [options] [package-index-options] ...
- %prog [options] -r [package-index-options] ...
- %prog [options] [-e] ...
- %prog [options] [-e] ...
- %prog [options] ..."""
-
- def add_options(self) -> None:
- self.cmd_opts.add_option(cmdoptions.requirements())
- self.cmd_opts.add_option(cmdoptions.constraints())
- self.cmd_opts.add_option(cmdoptions.no_deps())
- self.cmd_opts.add_option(cmdoptions.pre())
-
- self.cmd_opts.add_option(cmdoptions.editable())
- self.cmd_opts.add_option(
- "--dry-run",
- action="store_true",
- dest="dry_run",
- default=False,
- help=(
- "Don't actually install anything, just print what would be. "
- "Can be used in combination with --ignore-installed "
- "to 'resolve' the requirements."
- ),
- )
- self.cmd_opts.add_option(
- "-t",
- "--target",
- dest="target_dir",
- metavar="dir",
- default=None,
- help=(
- "Install packages into . "
- "By default this will not replace existing files/folders in "
- ". Use --upgrade to replace existing packages in "
- "with new versions."
- ),
- )
- cmdoptions.add_target_python_options(self.cmd_opts)
-
- self.cmd_opts.add_option(
- "--user",
- dest="use_user_site",
- action="store_true",
- help=(
- "Install to the Python user install directory for your "
- "platform. Typically ~/.local/, or %APPDATA%\\Python on "
- "Windows. (See the Python documentation for site.USER_BASE "
- "for full details.)"
- ),
- )
- self.cmd_opts.add_option(
- "--no-user",
- dest="use_user_site",
- action="store_false",
- help=SUPPRESS_HELP,
- )
- self.cmd_opts.add_option(
- "--root",
- dest="root_path",
- metavar="dir",
- default=None,
- help="Install everything relative to this alternate root directory.",
- )
- self.cmd_opts.add_option(
- "--prefix",
- dest="prefix_path",
- metavar="dir",
- default=None,
- help=(
- "Installation prefix where lib, bin and other top-level "
- "folders are placed"
- ),
- )
-
- self.cmd_opts.add_option(cmdoptions.src())
-
- self.cmd_opts.add_option(
- "-U",
- "--upgrade",
- dest="upgrade",
- action="store_true",
- help=(
- "Upgrade all specified packages to the newest available "
- "version. The handling of dependencies depends on the "
- "upgrade-strategy used."
- ),
- )
-
- self.cmd_opts.add_option(
- "--upgrade-strategy",
- dest="upgrade_strategy",
- default="only-if-needed",
- choices=["only-if-needed", "eager"],
- help=(
- "Determines how dependency upgrading should be handled "
- "[default: %default]. "
- '"eager" - dependencies are upgraded regardless of '
- "whether the currently installed version satisfies the "
- "requirements of the upgraded package(s). "
- '"only-if-needed" - are upgraded only when they do not '
- "satisfy the requirements of the upgraded package(s)."
- ),
- )
-
- self.cmd_opts.add_option(
- "--force-reinstall",
- dest="force_reinstall",
- action="store_true",
- help="Reinstall all packages even if they are already up-to-date.",
- )
-
- self.cmd_opts.add_option(
- "-I",
- "--ignore-installed",
- dest="ignore_installed",
- action="store_true",
- help=(
- "Ignore the installed packages, overwriting them. "
- "This can break your system if the existing package "
- "is of a different version or was installed "
- "with a different package manager!"
- ),
- )
-
- self.cmd_opts.add_option(cmdoptions.ignore_requires_python())
- self.cmd_opts.add_option(cmdoptions.no_build_isolation())
- self.cmd_opts.add_option(cmdoptions.use_pep517())
- self.cmd_opts.add_option(cmdoptions.no_use_pep517())
- self.cmd_opts.add_option(cmdoptions.check_build_deps())
-
- self.cmd_opts.add_option(cmdoptions.config_settings())
- self.cmd_opts.add_option(cmdoptions.install_options())
- self.cmd_opts.add_option(cmdoptions.global_options())
-
- self.cmd_opts.add_option(
- "--compile",
- action="store_true",
- dest="compile",
- default=True,
- help="Compile Python source files to bytecode",
- )
-
- self.cmd_opts.add_option(
- "--no-compile",
- action="store_false",
- dest="compile",
- help="Do not compile Python source files to bytecode",
- )
-
- self.cmd_opts.add_option(
- "--no-warn-script-location",
- action="store_false",
- dest="warn_script_location",
- default=True,
- help="Do not warn when installing scripts outside PATH",
- )
- self.cmd_opts.add_option(
- "--no-warn-conflicts",
- action="store_false",
- dest="warn_about_conflicts",
- default=True,
- help="Do not warn about broken dependencies",
- )
- self.cmd_opts.add_option(cmdoptions.no_binary())
- self.cmd_opts.add_option(cmdoptions.only_binary())
- self.cmd_opts.add_option(cmdoptions.prefer_binary())
- self.cmd_opts.add_option(cmdoptions.require_hashes())
- self.cmd_opts.add_option(cmdoptions.progress_bar())
- self.cmd_opts.add_option(cmdoptions.root_user_action())
-
- index_opts = cmdoptions.make_option_group(
- cmdoptions.index_group,
- self.parser,
- )
-
- self.parser.insert_option_group(0, index_opts)
- self.parser.insert_option_group(0, self.cmd_opts)
-
- self.cmd_opts.add_option(
- "--report",
- dest="json_report_file",
- metavar="file",
- default=None,
- help=(
- "Generate a JSON file describing what pip did to install "
- "the provided requirements. "
- "Can be used in combination with --dry-run and --ignore-installed "
- "to 'resolve' the requirements. "
- "When - is used as file name it writes to stdout. "
- "When writing to stdout, please combine with the --quiet option "
- "to avoid mixing pip logging output with JSON output."
- ),
- )
-
- @with_cleanup
- def run(self, options: Values, args: List[str]) -> int:
- if options.use_user_site and options.target_dir is not None:
- raise CommandError("Can not combine '--user' and '--target'")
-
- upgrade_strategy = "to-satisfy-only"
- if options.upgrade:
- upgrade_strategy = options.upgrade_strategy
-
- cmdoptions.check_dist_restriction(options, check_target=True)
-
- install_options = options.install_options or []
-
- logger.verbose("Using %s", get_pip_version())
- options.use_user_site = decide_user_install(
- options.use_user_site,
- prefix_path=options.prefix_path,
- target_dir=options.target_dir,
- root_path=options.root_path,
- isolated_mode=options.isolated_mode,
- )
-
- target_temp_dir: Optional[TempDirectory] = None
- target_temp_dir_path: Optional[str] = None
- if options.target_dir:
- options.ignore_installed = True
- options.target_dir = os.path.abspath(options.target_dir)
- if (
- # fmt: off
- os.path.exists(options.target_dir) and
- not os.path.isdir(options.target_dir)
- # fmt: on
- ):
- raise CommandError(
- "Target path exists but is not a directory, will not continue."
- )
-
- # Create a target directory for using with the target option
- target_temp_dir = TempDirectory(kind="target")
- target_temp_dir_path = target_temp_dir.path
- self.enter_context(target_temp_dir)
-
- global_options = options.global_options or []
-
- session = self.get_default_session(options)
-
- target_python = make_target_python(options)
- finder = self._build_package_finder(
- options=options,
- session=session,
- target_python=target_python,
- ignore_requires_python=options.ignore_requires_python,
- )
- build_tracker = self.enter_context(get_build_tracker())
-
- directory = TempDirectory(
- delete=not options.no_clean,
- kind="install",
- globally_managed=True,
- )
-
- try:
- reqs = self.get_requirements(args, options, finder, session)
- check_legacy_setup_py_options(
- options, reqs, LegacySetupPyOptionsCheckMode.INSTALL
- )
-
- if "no-binary-enable-wheel-cache" in options.features_enabled:
- # TODO: remove format_control from WheelCache when the deprecation cycle
- # is over
- wheel_cache = WheelCache(options.cache_dir)
- else:
- if options.format_control.no_binary:
- deprecated(
- reason=(
- "--no-binary currently disables reading from "
- "the cache of locally built wheels. In the future "
- "--no-binary will not influence the wheel cache."
- ),
- replacement="to use the --no-cache-dir option",
- feature_flag="no-binary-enable-wheel-cache",
- issue=11453,
- gone_in="23.1",
- )
- wheel_cache = WheelCache(options.cache_dir, options.format_control)
-
- # Only when installing is it permitted to use PEP 660.
- # In other circumstances (pip wheel, pip download) we generate
- # regular (i.e. non editable) metadata and wheels.
- for req in reqs:
- req.permit_editable_wheels = True
-
- reject_location_related_install_options(reqs, options.install_options)
-
- preparer = self.make_requirement_preparer(
- temp_build_dir=directory,
- options=options,
- build_tracker=build_tracker,
- session=session,
- finder=finder,
- use_user_site=options.use_user_site,
- verbosity=self.verbosity,
- )
- resolver = self.make_resolver(
- preparer=preparer,
- finder=finder,
- options=options,
- wheel_cache=wheel_cache,
- use_user_site=options.use_user_site,
- ignore_installed=options.ignore_installed,
- ignore_requires_python=options.ignore_requires_python,
- force_reinstall=options.force_reinstall,
- upgrade_strategy=upgrade_strategy,
- use_pep517=options.use_pep517,
- )
-
- self.trace_basic_info(finder)
-
- requirement_set = resolver.resolve(
- reqs, check_supported_wheels=not options.target_dir
- )
-
- if options.json_report_file:
- logger.warning(
- "--report is currently an experimental option. "
- "The output format may change in a future release "
- "without prior warning."
- )
-
- report = InstallationReport(requirement_set.requirements_to_install)
- if options.json_report_file == "-":
- print_json(data=report.to_dict())
- else:
- with open(options.json_report_file, "w", encoding="utf-8") as f:
- json.dump(report.to_dict(), f, indent=2, ensure_ascii=False)
-
- if options.dry_run:
- would_install_items = sorted(
- (r.metadata["name"], r.metadata["version"])
- for r in requirement_set.requirements_to_install
- )
- if would_install_items:
- write_output(
- "Would install %s",
- " ".join("-".join(item) for item in would_install_items),
- )
- return SUCCESS
-
- try:
- pip_req = requirement_set.get_requirement("pip")
- except KeyError:
- modifying_pip = False
- else:
- # If we're not replacing an already installed pip,
- # we're not modifying it.
- modifying_pip = pip_req.satisfied_by is None
- protect_pip_from_modification_on_windows(modifying_pip=modifying_pip)
-
- check_bdist_wheel_allowed = get_check_bdist_wheel_allowed(
- finder.format_control
- )
-
- reqs_to_build = [
- r
- for r in requirement_set.requirements.values()
- if should_build_for_install_command(r, check_bdist_wheel_allowed)
- ]
-
- _, build_failures = build(
- reqs_to_build,
- wheel_cache=wheel_cache,
- verify=True,
- build_options=[],
- global_options=global_options,
- )
-
- # If we're using PEP 517, we cannot do a legacy setup.py install
- # so we fail here.
- pep517_build_failure_names: List[str] = [
- r.name for r in build_failures if r.use_pep517 # type: ignore
- ]
- if pep517_build_failure_names:
- raise InstallationError(
- "Could not build wheels for {}, which is required to "
- "install pyproject.toml-based projects".format(
- ", ".join(pep517_build_failure_names)
- )
- )
-
- # For now, we just warn about failures building legacy
- # requirements, as we'll fall through to a setup.py install for
- # those.
- for r in build_failures:
- if not r.use_pep517:
- r.legacy_install_reason = LegacyInstallReasonFailedBdistWheel
-
- to_install = resolver.get_installation_order(requirement_set)
-
- # Check for conflicts in the package set we're installing.
- conflicts: Optional[ConflictDetails] = None
- should_warn_about_conflicts = (
- not options.ignore_dependencies and options.warn_about_conflicts
- )
- if should_warn_about_conflicts:
- conflicts = self._determine_conflicts(to_install)
-
- # Don't warn about script install locations if
- # --target or --prefix has been specified
- warn_script_location = options.warn_script_location
- if options.target_dir or options.prefix_path:
- warn_script_location = False
-
- installed = install_given_reqs(
- to_install,
- install_options,
- global_options,
- root=options.root_path,
- home=target_temp_dir_path,
- prefix=options.prefix_path,
- warn_script_location=warn_script_location,
- use_user_site=options.use_user_site,
- pycompile=options.compile,
- )
-
- lib_locations = get_lib_location_guesses(
- user=options.use_user_site,
- home=target_temp_dir_path,
- root=options.root_path,
- prefix=options.prefix_path,
- isolated=options.isolated_mode,
- )
- env = get_environment(lib_locations)
-
- installed.sort(key=operator.attrgetter("name"))
- items = []
- for result in installed:
- item = result.name
- try:
- installed_dist = env.get_distribution(item)
- if installed_dist is not None:
- item = f"{item}-{installed_dist.version}"
- except Exception:
- pass
- items.append(item)
-
- if conflicts is not None:
- self._warn_about_conflicts(
- conflicts,
- resolver_variant=self.determine_resolver_variant(options),
- )
-
- installed_desc = " ".join(items)
- if installed_desc:
- write_output(
- "Successfully installed %s",
- installed_desc,
- )
- except OSError as error:
- show_traceback = self.verbosity >= 1
-
- message = create_os_error_message(
- error,
- show_traceback,
- options.use_user_site,
- )
- logger.error(message, exc_info=show_traceback) # noqa
-
- return ERROR
-
- if options.target_dir:
- assert target_temp_dir
- self._handle_target_dir(
- options.target_dir, target_temp_dir, options.upgrade
- )
- if options.root_user_action == "warn":
- warn_if_run_as_root()
- return SUCCESS
-
- def _handle_target_dir(
- self, target_dir: str, target_temp_dir: TempDirectory, upgrade: bool
- ) -> None:
- ensure_dir(target_dir)
-
- # Checking both purelib and platlib directories for installed
- # packages to be moved to target directory
- lib_dir_list = []
-
- # Checking both purelib and platlib directories for installed
- # packages to be moved to target directory
- scheme = get_scheme("", home=target_temp_dir.path)
- purelib_dir = scheme.purelib
- platlib_dir = scheme.platlib
- data_dir = scheme.data
-
- if os.path.exists(purelib_dir):
- lib_dir_list.append(purelib_dir)
- if os.path.exists(platlib_dir) and platlib_dir != purelib_dir:
- lib_dir_list.append(platlib_dir)
- if os.path.exists(data_dir):
- lib_dir_list.append(data_dir)
-
- for lib_dir in lib_dir_list:
- for item in os.listdir(lib_dir):
- if lib_dir == data_dir:
- ddir = os.path.join(data_dir, item)
- if any(s.startswith(ddir) for s in lib_dir_list[:-1]):
- continue
- target_item_dir = os.path.join(target_dir, item)
- if os.path.exists(target_item_dir):
- if not upgrade:
- logger.warning(
- "Target directory %s already exists. Specify "
- "--upgrade to force replacement.",
- target_item_dir,
- )
- continue
- if os.path.islink(target_item_dir):
- logger.warning(
- "Target directory %s already exists and is "
- "a link. pip will not automatically replace "
- "links, please remove if replacement is "
- "desired.",
- target_item_dir,
- )
- continue
- if os.path.isdir(target_item_dir):
- shutil.rmtree(target_item_dir)
- else:
- os.remove(target_item_dir)
-
- shutil.move(os.path.join(lib_dir, item), target_item_dir)
-
- def _determine_conflicts(
- self, to_install: List[InstallRequirement]
- ) -> Optional[ConflictDetails]:
- try:
- return check_install_conflicts(to_install)
- except Exception:
- logger.exception(
- "Error while checking for conflicts. Please file an issue on "
- "pip's issue tracker: https://github.com/pypa/pip/issues/new"
- )
- return None
-
- def _warn_about_conflicts(
- self, conflict_details: ConflictDetails, resolver_variant: str
- ) -> None:
- package_set, (missing, conflicting) = conflict_details
- if not missing and not conflicting:
- return
-
- parts: List[str] = []
- if resolver_variant == "legacy":
- parts.append(
- "pip's legacy dependency resolver does not consider dependency "
- "conflicts when selecting packages. This behaviour is the "
- "source of the following dependency conflicts."
- )
- else:
- assert resolver_variant == "2020-resolver"
- parts.append(
- "pip's dependency resolver does not currently take into account "
- "all the packages that are installed. This behaviour is the "
- "source of the following dependency conflicts."
- )
-
- # NOTE: There is some duplication here, with commands/check.py
- for project_name in missing:
- version = package_set[project_name][0]
- for dependency in missing[project_name]:
- message = (
- "{name} {version} requires {requirement}, "
- "which is not installed."
- ).format(
- name=project_name,
- version=version,
- requirement=dependency[1],
- )
- parts.append(message)
-
- for project_name in conflicting:
- version = package_set[project_name][0]
- for dep_name, dep_version, req in conflicting[project_name]:
- message = (
- "{name} {version} requires {requirement}, but {you} have "
- "{dep_name} {dep_version} which is incompatible."
- ).format(
- name=project_name,
- version=version,
- requirement=req,
- dep_name=dep_name,
- dep_version=dep_version,
- you=("you" if resolver_variant == "2020-resolver" else "you'll"),
- )
- parts.append(message)
-
- logger.critical("\n".join(parts))
-
-
-def get_lib_location_guesses(
- user: bool = False,
- home: Optional[str] = None,
- root: Optional[str] = None,
- isolated: bool = False,
- prefix: Optional[str] = None,
-) -> List[str]:
- scheme = get_scheme(
- "",
- user=user,
- home=home,
- root=root,
- isolated=isolated,
- prefix=prefix,
- )
- return [scheme.purelib, scheme.platlib]
-
-
-def site_packages_writable(root: Optional[str], isolated: bool) -> bool:
- return all(
- test_writable_dir(d)
- for d in set(get_lib_location_guesses(root=root, isolated=isolated))
- )
-
-
-def decide_user_install(
- use_user_site: Optional[bool],
- prefix_path: Optional[str] = None,
- target_dir: Optional[str] = None,
- root_path: Optional[str] = None,
- isolated_mode: bool = False,
-) -> bool:
- """Determine whether to do a user install based on the input options.
-
- If use_user_site is False, no additional checks are done.
- If use_user_site is True, it is checked for compatibility with other
- options.
- If use_user_site is None, the default behaviour depends on the environment,
- which is provided by the other arguments.
- """
- # In some cases (config from tox), use_user_site can be set to an integer
- # rather than a bool, which 'use_user_site is False' wouldn't catch.
- if (use_user_site is not None) and (not use_user_site):
- logger.debug("Non-user install by explicit request")
- return False
-
- if use_user_site:
- if prefix_path:
- raise CommandError(
- "Can not combine '--user' and '--prefix' as they imply "
- "different installation locations"
- )
- if virtualenv_no_global():
- raise InstallationError(
- "Can not perform a '--user' install. User site-packages "
- "are not visible in this virtualenv."
- )
- logger.debug("User install by explicit request")
- return True
-
- # If we are here, user installs have not been explicitly requested/avoided
- assert use_user_site is None
-
- # user install incompatible with --prefix/--target
- if prefix_path or target_dir:
- logger.debug("Non-user install due to --prefix or --target option")
- return False
-
- # If user installs are not enabled, choose a non-user install
- if not site.ENABLE_USER_SITE:
- logger.debug("Non-user install because user site-packages disabled")
- return False
-
- # If we have permission for a non-user install, do that,
- # otherwise do a user install.
- if site_packages_writable(root=root_path, isolated=isolated_mode):
- logger.debug("Non-user install because site-packages writeable")
- return False
-
- logger.info(
- "Defaulting to user installation because normal site-packages "
- "is not writeable"
- )
- return True
-
-
-def reject_location_related_install_options(
- requirements: List[InstallRequirement], options: Optional[List[str]]
-) -> None:
- """If any location-changing --install-option arguments were passed for
- requirements or on the command-line, then show a deprecation warning.
- """
-
- def format_options(option_names: Iterable[str]) -> List[str]:
- return ["--{}".format(name.replace("_", "-")) for name in option_names]
-
- offenders = []
-
- for requirement in requirements:
- install_options = requirement.install_options
- location_options = parse_distutils_args(install_options)
- if location_options:
- offenders.append(
- "{!r} from {}".format(
- format_options(location_options.keys()), requirement
- )
- )
-
- if options:
- location_options = parse_distutils_args(options)
- if location_options:
- offenders.append(
- "{!r} from command line".format(format_options(location_options.keys()))
- )
-
- if not offenders:
- return
-
- raise CommandError(
- "Location-changing options found in --install-option: {}."
- " This is unsupported, use pip-level options like --user,"
- " --prefix, --root, and --target instead.".format("; ".join(offenders))
- )
-
-
-def create_os_error_message(
- error: OSError, show_traceback: bool, using_user_site: bool
-) -> str:
- """Format an error message for an OSError
-
- It may occur anytime during the execution of the install command.
- """
- parts = []
-
- # Mention the error if we are not going to show a traceback
- parts.append("Could not install packages due to an OSError")
- if not show_traceback:
- parts.append(": ")
- parts.append(str(error))
- else:
- parts.append(".")
-
- # Spilt the error indication from a helper message (if any)
- parts[-1] += "\n"
-
- # Suggest useful actions to the user:
- # (1) using user site-packages or (2) verifying the permissions
- if error.errno == errno.EACCES:
- user_option_part = "Consider using the `--user` option"
- permissions_part = "Check the permissions"
-
- if not running_under_virtualenv() and not using_user_site:
- parts.extend(
- [
- user_option_part,
- " or ",
- permissions_part.lower(),
- ]
- )
- else:
- parts.append(permissions_part)
- parts.append(".\n")
-
- # Suggest the user to enable Long Paths if path length is
- # more than 260
- if (
- WINDOWS
- and error.errno == errno.ENOENT
- and error.filename
- and len(error.filename) > 260
- ):
- parts.append(
- "HINT: This error might have occurred since "
- "this system does not have Windows Long Path "
- "support enabled. You can find information on "
- "how to enable this at "
- "https://pip.pypa.io/warnings/enable-long-paths\n"
- )
-
- return "".join(parts).strip() + "\n"
diff --git a/spaces/Reha2704/VToonify/vtoonify/model/stylegan/op/conv2d_gradfix.py b/spaces/Reha2704/VToonify/vtoonify/model/stylegan/op/conv2d_gradfix.py
deleted file mode 100644
index 5e4b83adac8e6a4b1caf522596666e4f5d0ee854..0000000000000000000000000000000000000000
--- a/spaces/Reha2704/VToonify/vtoonify/model/stylegan/op/conv2d_gradfix.py
+++ /dev/null
@@ -1,227 +0,0 @@
-import contextlib
-import warnings
-
-import torch
-from torch import autograd
-from torch.nn import functional as F
-
-enabled = True
-weight_gradients_disabled = False
-
-
-@contextlib.contextmanager
-def no_weight_gradients():
- global weight_gradients_disabled
-
- old = weight_gradients_disabled
- weight_gradients_disabled = True
- yield
- weight_gradients_disabled = old
-
-
-def conv2d(input, weight, bias=None, stride=1, padding=0, dilation=1, groups=1):
- if could_use_op(input):
- return conv2d_gradfix(
- transpose=False,
- weight_shape=weight.shape,
- stride=stride,
- padding=padding,
- output_padding=0,
- dilation=dilation,
- groups=groups,
- ).apply(input, weight, bias)
-
- return F.conv2d(
- input=input,
- weight=weight,
- bias=bias,
- stride=stride,
- padding=padding,
- dilation=dilation,
- groups=groups,
- )
-
-
-def conv_transpose2d(
- input,
- weight,
- bias=None,
- stride=1,
- padding=0,
- output_padding=0,
- groups=1,
- dilation=1,
-):
- if could_use_op(input):
- return conv2d_gradfix(
- transpose=True,
- weight_shape=weight.shape,
- stride=stride,
- padding=padding,
- output_padding=output_padding,
- groups=groups,
- dilation=dilation,
- ).apply(input, weight, bias)
-
- return F.conv_transpose2d(
- input=input,
- weight=weight,
- bias=bias,
- stride=stride,
- padding=padding,
- output_padding=output_padding,
- dilation=dilation,
- groups=groups,
- )
-
-
-def could_use_op(input):
- if (not enabled) or (not torch.backends.cudnn.enabled):
- return False
-
- if input.device.type != "cuda":
- return False
-
- if any(torch.__version__.startswith(x) for x in ["1.7.", "1.8."]):
- return True
-
- #warnings.warn(
- # f"conv2d_gradfix not supported on PyTorch {torch.__version__}. Falling back to torch.nn.functional.conv2d()."
- #)
-
- return False
-
-
-def ensure_tuple(xs, ndim):
- xs = tuple(xs) if isinstance(xs, (tuple, list)) else (xs,) * ndim
-
- return xs
-
-
-conv2d_gradfix_cache = dict()
-
-
-def conv2d_gradfix(
- transpose, weight_shape, stride, padding, output_padding, dilation, groups
-):
- ndim = 2
- weight_shape = tuple(weight_shape)
- stride = ensure_tuple(stride, ndim)
- padding = ensure_tuple(padding, ndim)
- output_padding = ensure_tuple(output_padding, ndim)
- dilation = ensure_tuple(dilation, ndim)
-
- key = (transpose, weight_shape, stride, padding, output_padding, dilation, groups)
- if key in conv2d_gradfix_cache:
- return conv2d_gradfix_cache[key]
-
- common_kwargs = dict(
- stride=stride, padding=padding, dilation=dilation, groups=groups
- )
-
- def calc_output_padding(input_shape, output_shape):
- if transpose:
- return [0, 0]
-
- return [
- input_shape[i + 2]
- - (output_shape[i + 2] - 1) * stride[i]
- - (1 - 2 * padding[i])
- - dilation[i] * (weight_shape[i + 2] - 1)
- for i in range(ndim)
- ]
-
- class Conv2d(autograd.Function):
- @staticmethod
- def forward(ctx, input, weight, bias):
- if not transpose:
- out = F.conv2d(input=input, weight=weight, bias=bias, **common_kwargs)
-
- else:
- out = F.conv_transpose2d(
- input=input,
- weight=weight,
- bias=bias,
- output_padding=output_padding,
- **common_kwargs,
- )
-
- ctx.save_for_backward(input, weight)
-
- return out
-
- @staticmethod
- def backward(ctx, grad_output):
- input, weight = ctx.saved_tensors
- grad_input, grad_weight, grad_bias = None, None, None
-
- if ctx.needs_input_grad[0]:
- p = calc_output_padding(
- input_shape=input.shape, output_shape=grad_output.shape
- )
- grad_input = conv2d_gradfix(
- transpose=(not transpose),
- weight_shape=weight_shape,
- output_padding=p,
- **common_kwargs,
- ).apply(grad_output, weight, None)
-
- if ctx.needs_input_grad[1] and not weight_gradients_disabled:
- grad_weight = Conv2dGradWeight.apply(grad_output, input)
-
- if ctx.needs_input_grad[2]:
- grad_bias = grad_output.sum((0, 2, 3))
-
- return grad_input, grad_weight, grad_bias
-
- class Conv2dGradWeight(autograd.Function):
- @staticmethod
- def forward(ctx, grad_output, input):
- op = torch._C._jit_get_operation(
- "aten::cudnn_convolution_backward_weight"
- if not transpose
- else "aten::cudnn_convolution_transpose_backward_weight"
- )
- flags = [
- torch.backends.cudnn.benchmark,
- torch.backends.cudnn.deterministic,
- torch.backends.cudnn.allow_tf32,
- ]
- grad_weight = op(
- weight_shape,
- grad_output,
- input,
- padding,
- stride,
- dilation,
- groups,
- *flags,
- )
- ctx.save_for_backward(grad_output, input)
-
- return grad_weight
-
- @staticmethod
- def backward(ctx, grad_grad_weight):
- grad_output, input = ctx.saved_tensors
- grad_grad_output, grad_grad_input = None, None
-
- if ctx.needs_input_grad[0]:
- grad_grad_output = Conv2d.apply(input, grad_grad_weight, None)
-
- if ctx.needs_input_grad[1]:
- p = calc_output_padding(
- input_shape=input.shape, output_shape=grad_output.shape
- )
- grad_grad_input = conv2d_gradfix(
- transpose=(not transpose),
- weight_shape=weight_shape,
- output_padding=p,
- **common_kwargs,
- ).apply(grad_output, grad_grad_weight, None)
-
- return grad_grad_output, grad_grad_input
-
- conv2d_gradfix_cache[key] = Conv2d
-
- return Conv2d
diff --git a/spaces/Robert001/UniControl-Demo/annotator/uniformer/mmcv/ops/deform_roi_pool.py b/spaces/Robert001/UniControl-Demo/annotator/uniformer/mmcv/ops/deform_roi_pool.py
deleted file mode 100644
index cc245ba91fee252226ba22e76bb94a35db9a629b..0000000000000000000000000000000000000000
--- a/spaces/Robert001/UniControl-Demo/annotator/uniformer/mmcv/ops/deform_roi_pool.py
+++ /dev/null
@@ -1,204 +0,0 @@
-# Copyright (c) OpenMMLab. All rights reserved.
-from torch import nn
-from torch.autograd import Function
-from torch.autograd.function import once_differentiable
-from torch.nn.modules.utils import _pair
-
-from ..utils import ext_loader
-
-ext_module = ext_loader.load_ext(
- '_ext', ['deform_roi_pool_forward', 'deform_roi_pool_backward'])
-
-
-class DeformRoIPoolFunction(Function):
-
- @staticmethod
- def symbolic(g, input, rois, offset, output_size, spatial_scale,
- sampling_ratio, gamma):
- return g.op(
- 'mmcv::MMCVDeformRoIPool',
- input,
- rois,
- offset,
- pooled_height_i=output_size[0],
- pooled_width_i=output_size[1],
- spatial_scale_f=spatial_scale,
- sampling_ratio_f=sampling_ratio,
- gamma_f=gamma)
-
- @staticmethod
- def forward(ctx,
- input,
- rois,
- offset,
- output_size,
- spatial_scale=1.0,
- sampling_ratio=0,
- gamma=0.1):
- if offset is None:
- offset = input.new_zeros(0)
- ctx.output_size = _pair(output_size)
- ctx.spatial_scale = float(spatial_scale)
- ctx.sampling_ratio = int(sampling_ratio)
- ctx.gamma = float(gamma)
-
- assert rois.size(1) == 5, 'RoI must be (idx, x1, y1, x2, y2)!'
-
- output_shape = (rois.size(0), input.size(1), ctx.output_size[0],
- ctx.output_size[1])
- output = input.new_zeros(output_shape)
-
- ext_module.deform_roi_pool_forward(
- input,
- rois,
- offset,
- output,
- pooled_height=ctx.output_size[0],
- pooled_width=ctx.output_size[1],
- spatial_scale=ctx.spatial_scale,
- sampling_ratio=ctx.sampling_ratio,
- gamma=ctx.gamma)
-
- ctx.save_for_backward(input, rois, offset)
- return output
-
- @staticmethod
- @once_differentiable
- def backward(ctx, grad_output):
- input, rois, offset = ctx.saved_tensors
- grad_input = grad_output.new_zeros(input.shape)
- grad_offset = grad_output.new_zeros(offset.shape)
-
- ext_module.deform_roi_pool_backward(
- grad_output,
- input,
- rois,
- offset,
- grad_input,
- grad_offset,
- pooled_height=ctx.output_size[0],
- pooled_width=ctx.output_size[1],
- spatial_scale=ctx.spatial_scale,
- sampling_ratio=ctx.sampling_ratio,
- gamma=ctx.gamma)
- if grad_offset.numel() == 0:
- grad_offset = None
- return grad_input, None, grad_offset, None, None, None, None
-
-
-deform_roi_pool = DeformRoIPoolFunction.apply
-
-
-class DeformRoIPool(nn.Module):
-
- def __init__(self,
- output_size,
- spatial_scale=1.0,
- sampling_ratio=0,
- gamma=0.1):
- super(DeformRoIPool, self).__init__()
- self.output_size = _pair(output_size)
- self.spatial_scale = float(spatial_scale)
- self.sampling_ratio = int(sampling_ratio)
- self.gamma = float(gamma)
-
- def forward(self, input, rois, offset=None):
- return deform_roi_pool(input, rois, offset, self.output_size,
- self.spatial_scale, self.sampling_ratio,
- self.gamma)
-
-
-class DeformRoIPoolPack(DeformRoIPool):
-
- def __init__(self,
- output_size,
- output_channels,
- deform_fc_channels=1024,
- spatial_scale=1.0,
- sampling_ratio=0,
- gamma=0.1):
- super(DeformRoIPoolPack, self).__init__(output_size, spatial_scale,
- sampling_ratio, gamma)
-
- self.output_channels = output_channels
- self.deform_fc_channels = deform_fc_channels
-
- self.offset_fc = nn.Sequential(
- nn.Linear(
- self.output_size[0] * self.output_size[1] *
- self.output_channels, self.deform_fc_channels),
- nn.ReLU(inplace=True),
- nn.Linear(self.deform_fc_channels, self.deform_fc_channels),
- nn.ReLU(inplace=True),
- nn.Linear(self.deform_fc_channels,
- self.output_size[0] * self.output_size[1] * 2))
- self.offset_fc[-1].weight.data.zero_()
- self.offset_fc[-1].bias.data.zero_()
-
- def forward(self, input, rois):
- assert input.size(1) == self.output_channels
- x = deform_roi_pool(input, rois, None, self.output_size,
- self.spatial_scale, self.sampling_ratio,
- self.gamma)
- rois_num = rois.size(0)
- offset = self.offset_fc(x.view(rois_num, -1))
- offset = offset.view(rois_num, 2, self.output_size[0],
- self.output_size[1])
- return deform_roi_pool(input, rois, offset, self.output_size,
- self.spatial_scale, self.sampling_ratio,
- self.gamma)
-
-
-class ModulatedDeformRoIPoolPack(DeformRoIPool):
-
- def __init__(self,
- output_size,
- output_channels,
- deform_fc_channels=1024,
- spatial_scale=1.0,
- sampling_ratio=0,
- gamma=0.1):
- super(ModulatedDeformRoIPoolPack,
- self).__init__(output_size, spatial_scale, sampling_ratio, gamma)
-
- self.output_channels = output_channels
- self.deform_fc_channels = deform_fc_channels
-
- self.offset_fc = nn.Sequential(
- nn.Linear(
- self.output_size[0] * self.output_size[1] *
- self.output_channels, self.deform_fc_channels),
- nn.ReLU(inplace=True),
- nn.Linear(self.deform_fc_channels, self.deform_fc_channels),
- nn.ReLU(inplace=True),
- nn.Linear(self.deform_fc_channels,
- self.output_size[0] * self.output_size[1] * 2))
- self.offset_fc[-1].weight.data.zero_()
- self.offset_fc[-1].bias.data.zero_()
-
- self.mask_fc = nn.Sequential(
- nn.Linear(
- self.output_size[0] * self.output_size[1] *
- self.output_channels, self.deform_fc_channels),
- nn.ReLU(inplace=True),
- nn.Linear(self.deform_fc_channels,
- self.output_size[0] * self.output_size[1] * 1),
- nn.Sigmoid())
- self.mask_fc[2].weight.data.zero_()
- self.mask_fc[2].bias.data.zero_()
-
- def forward(self, input, rois):
- assert input.size(1) == self.output_channels
- x = deform_roi_pool(input, rois, None, self.output_size,
- self.spatial_scale, self.sampling_ratio,
- self.gamma)
- rois_num = rois.size(0)
- offset = self.offset_fc(x.view(rois_num, -1))
- offset = offset.view(rois_num, 2, self.output_size[0],
- self.output_size[1])
- mask = self.mask_fc(x.view(rois_num, -1))
- mask = mask.view(rois_num, 1, self.output_size[0], self.output_size[1])
- d = deform_roi_pool(input, rois, offset, self.output_size,
- self.spatial_scale, self.sampling_ratio,
- self.gamma)
- return d * mask
diff --git a/spaces/Robert001/UniControl-Demo/annotator/uniformer/mmdet_null/models/dense_heads/gfl_head.py b/spaces/Robert001/UniControl-Demo/annotator/uniformer/mmdet_null/models/dense_heads/gfl_head.py
deleted file mode 100644
index 961bc92237663ad5343d3d08eb9c0e4e811ada05..0000000000000000000000000000000000000000
--- a/spaces/Robert001/UniControl-Demo/annotator/uniformer/mmdet_null/models/dense_heads/gfl_head.py
+++ /dev/null
@@ -1,647 +0,0 @@
-import torch
-import torch.nn as nn
-import torch.nn.functional as F
-from mmcv.cnn import ConvModule, Scale, bias_init_with_prob, normal_init
-from mmcv.runner import force_fp32
-
-from mmdet.core import (anchor_inside_flags, bbox2distance, bbox_overlaps,
- build_assigner, build_sampler, distance2bbox,
- images_to_levels, multi_apply, multiclass_nms,
- reduce_mean, unmap)
-from ..builder import HEADS, build_loss
-from .anchor_head import AnchorHead
-
-
-class Integral(nn.Module):
- """A fixed layer for calculating integral result from distribution.
-
- This layer calculates the target location by :math: `sum{P(y_i) * y_i}`,
- P(y_i) denotes the softmax vector that represents the discrete distribution
- y_i denotes the discrete set, usually {0, 1, 2, ..., reg_max}
-
- Args:
- reg_max (int): The maximal value of the discrete set. Default: 16. You
- may want to reset it according to your new dataset or related
- settings.
- """
-
- def __init__(self, reg_max=16):
- super(Integral, self).__init__()
- self.reg_max = reg_max
- self.register_buffer('project',
- torch.linspace(0, self.reg_max, self.reg_max + 1))
-
- def forward(self, x):
- """Forward feature from the regression head to get integral result of
- bounding box location.
-
- Args:
- x (Tensor): Features of the regression head, shape (N, 4*(n+1)),
- n is self.reg_max.
-
- Returns:
- x (Tensor): Integral result of box locations, i.e., distance
- offsets from the box center in four directions, shape (N, 4).
- """
- x = F.softmax(x.reshape(-1, self.reg_max + 1), dim=1)
- x = F.linear(x, self.project.type_as(x)).reshape(-1, 4)
- return x
-
-
-@HEADS.register_module()
-class GFLHead(AnchorHead):
- """Generalized Focal Loss: Learning Qualified and Distributed Bounding
- Boxes for Dense Object Detection.
-
- GFL head structure is similar with ATSS, however GFL uses
- 1) joint representation for classification and localization quality, and
- 2) flexible General distribution for bounding box locations,
- which are supervised by
- Quality Focal Loss (QFL) and Distribution Focal Loss (DFL), respectively
-
- https://arxiv.org/abs/2006.04388
-
- Args:
- num_classes (int): Number of categories excluding the background
- category.
- in_channels (int): Number of channels in the input feature map.
- stacked_convs (int): Number of conv layers in cls and reg tower.
- Default: 4.
- conv_cfg (dict): dictionary to construct and config conv layer.
- Default: None.
- norm_cfg (dict): dictionary to construct and config norm layer.
- Default: dict(type='GN', num_groups=32, requires_grad=True).
- loss_qfl (dict): Config of Quality Focal Loss (QFL).
- reg_max (int): Max value of integral set :math: `{0, ..., reg_max}`
- in QFL setting. Default: 16.
- Example:
- >>> self = GFLHead(11, 7)
- >>> feats = [torch.rand(1, 7, s, s) for s in [4, 8, 16, 32, 64]]
- >>> cls_quality_score, bbox_pred = self.forward(feats)
- >>> assert len(cls_quality_score) == len(self.scales)
- """
-
- def __init__(self,
- num_classes,
- in_channels,
- stacked_convs=4,
- conv_cfg=None,
- norm_cfg=dict(type='GN', num_groups=32, requires_grad=True),
- loss_dfl=dict(type='DistributionFocalLoss', loss_weight=0.25),
- reg_max=16,
- **kwargs):
- self.stacked_convs = stacked_convs
- self.conv_cfg = conv_cfg
- self.norm_cfg = norm_cfg
- self.reg_max = reg_max
- super(GFLHead, self).__init__(num_classes, in_channels, **kwargs)
-
- self.sampling = False
- if self.train_cfg:
- self.assigner = build_assigner(self.train_cfg.assigner)
- # SSD sampling=False so use PseudoSampler
- sampler_cfg = dict(type='PseudoSampler')
- self.sampler = build_sampler(sampler_cfg, context=self)
-
- self.integral = Integral(self.reg_max)
- self.loss_dfl = build_loss(loss_dfl)
-
- def _init_layers(self):
- """Initialize layers of the head."""
- self.relu = nn.ReLU(inplace=True)
- self.cls_convs = nn.ModuleList()
- self.reg_convs = nn.ModuleList()
- for i in range(self.stacked_convs):
- chn = self.in_channels if i == 0 else self.feat_channels
- self.cls_convs.append(
- ConvModule(
- chn,
- self.feat_channels,
- 3,
- stride=1,
- padding=1,
- conv_cfg=self.conv_cfg,
- norm_cfg=self.norm_cfg))
- self.reg_convs.append(
- ConvModule(
- chn,
- self.feat_channels,
- 3,
- stride=1,
- padding=1,
- conv_cfg=self.conv_cfg,
- norm_cfg=self.norm_cfg))
- assert self.num_anchors == 1, 'anchor free version'
- self.gfl_cls = nn.Conv2d(
- self.feat_channels, self.cls_out_channels, 3, padding=1)
- self.gfl_reg = nn.Conv2d(
- self.feat_channels, 4 * (self.reg_max + 1), 3, padding=1)
- self.scales = nn.ModuleList(
- [Scale(1.0) for _ in self.anchor_generator.strides])
-
- def init_weights(self):
- """Initialize weights of the head."""
- for m in self.cls_convs:
- normal_init(m.conv, std=0.01)
- for m in self.reg_convs:
- normal_init(m.conv, std=0.01)
- bias_cls = bias_init_with_prob(0.01)
- normal_init(self.gfl_cls, std=0.01, bias=bias_cls)
- normal_init(self.gfl_reg, std=0.01)
-
- def forward(self, feats):
- """Forward features from the upstream network.
-
- Args:
- feats (tuple[Tensor]): Features from the upstream network, each is
- a 4D-tensor.
-
- Returns:
- tuple: Usually a tuple of classification scores and bbox prediction
- cls_scores (list[Tensor]): Classification and quality (IoU)
- joint scores for all scale levels, each is a 4D-tensor,
- the channel number is num_classes.
- bbox_preds (list[Tensor]): Box distribution logits for all
- scale levels, each is a 4D-tensor, the channel number is
- 4*(n+1), n is max value of integral set.
- """
- return multi_apply(self.forward_single, feats, self.scales)
-
- def forward_single(self, x, scale):
- """Forward feature of a single scale level.
-
- Args:
- x (Tensor): Features of a single scale level.
- scale (:obj: `mmcv.cnn.Scale`): Learnable scale module to resize
- the bbox prediction.
-
- Returns:
- tuple:
- cls_score (Tensor): Cls and quality joint scores for a single
- scale level the channel number is num_classes.
- bbox_pred (Tensor): Box distribution logits for a single scale
- level, the channel number is 4*(n+1), n is max value of
- integral set.
- """
- cls_feat = x
- reg_feat = x
- for cls_conv in self.cls_convs:
- cls_feat = cls_conv(cls_feat)
- for reg_conv in self.reg_convs:
- reg_feat = reg_conv(reg_feat)
- cls_score = self.gfl_cls(cls_feat)
- bbox_pred = scale(self.gfl_reg(reg_feat)).float()
- return cls_score, bbox_pred
-
- def anchor_center(self, anchors):
- """Get anchor centers from anchors.
-
- Args:
- anchors (Tensor): Anchor list with shape (N, 4), "xyxy" format.
-
- Returns:
- Tensor: Anchor centers with shape (N, 2), "xy" format.
- """
- anchors_cx = (anchors[..., 2] + anchors[..., 0]) / 2
- anchors_cy = (anchors[..., 3] + anchors[..., 1]) / 2
- return torch.stack([anchors_cx, anchors_cy], dim=-1)
-
- def loss_single(self, anchors, cls_score, bbox_pred, labels, label_weights,
- bbox_targets, stride, num_total_samples):
- """Compute loss of a single scale level.
-
- Args:
- anchors (Tensor): Box reference for each scale level with shape
- (N, num_total_anchors, 4).
- cls_score (Tensor): Cls and quality joint scores for each scale
- level has shape (N, num_classes, H, W).
- bbox_pred (Tensor): Box distribution logits for each scale
- level with shape (N, 4*(n+1), H, W), n is max value of integral
- set.
- labels (Tensor): Labels of each anchors with shape
- (N, num_total_anchors).
- label_weights (Tensor): Label weights of each anchor with shape
- (N, num_total_anchors)
- bbox_targets (Tensor): BBox regression targets of each anchor wight
- shape (N, num_total_anchors, 4).
- stride (tuple): Stride in this scale level.
- num_total_samples (int): Number of positive samples that is
- reduced over all GPUs.
-
- Returns:
- dict[str, Tensor]: A dictionary of loss components.
- """
- assert stride[0] == stride[1], 'h stride is not equal to w stride!'
- anchors = anchors.reshape(-1, 4)
- cls_score = cls_score.permute(0, 2, 3,
- 1).reshape(-1, self.cls_out_channels)
- bbox_pred = bbox_pred.permute(0, 2, 3,
- 1).reshape(-1, 4 * (self.reg_max + 1))
- bbox_targets = bbox_targets.reshape(-1, 4)
- labels = labels.reshape(-1)
- label_weights = label_weights.reshape(-1)
-
- # FG cat_id: [0, num_classes -1], BG cat_id: num_classes
- bg_class_ind = self.num_classes
- pos_inds = ((labels >= 0)
- & (labels < bg_class_ind)).nonzero().squeeze(1)
- score = label_weights.new_zeros(labels.shape)
-
- if len(pos_inds) > 0:
- pos_bbox_targets = bbox_targets[pos_inds]
- pos_bbox_pred = bbox_pred[pos_inds]
- pos_anchors = anchors[pos_inds]
- pos_anchor_centers = self.anchor_center(pos_anchors) / stride[0]
-
- weight_targets = cls_score.detach().sigmoid()
- weight_targets = weight_targets.max(dim=1)[0][pos_inds]
- pos_bbox_pred_corners = self.integral(pos_bbox_pred)
- pos_decode_bbox_pred = distance2bbox(pos_anchor_centers,
- pos_bbox_pred_corners)
- pos_decode_bbox_targets = pos_bbox_targets / stride[0]
- score[pos_inds] = bbox_overlaps(
- pos_decode_bbox_pred.detach(),
- pos_decode_bbox_targets,
- is_aligned=True)
- pred_corners = pos_bbox_pred.reshape(-1, self.reg_max + 1)
- target_corners = bbox2distance(pos_anchor_centers,
- pos_decode_bbox_targets,
- self.reg_max).reshape(-1)
-
- # regression loss
- loss_bbox = self.loss_bbox(
- pos_decode_bbox_pred,
- pos_decode_bbox_targets,
- weight=weight_targets,
- avg_factor=1.0)
-
- # dfl loss
- loss_dfl = self.loss_dfl(
- pred_corners,
- target_corners,
- weight=weight_targets[:, None].expand(-1, 4).reshape(-1),
- avg_factor=4.0)
- else:
- loss_bbox = bbox_pred.sum() * 0
- loss_dfl = bbox_pred.sum() * 0
- weight_targets = bbox_pred.new_tensor(0)
-
- # cls (qfl) loss
- loss_cls = self.loss_cls(
- cls_score, (labels, score),
- weight=label_weights,
- avg_factor=num_total_samples)
-
- return loss_cls, loss_bbox, loss_dfl, weight_targets.sum()
-
- @force_fp32(apply_to=('cls_scores', 'bbox_preds'))
- def loss(self,
- cls_scores,
- bbox_preds,
- gt_bboxes,
- gt_labels,
- img_metas,
- gt_bboxes_ignore=None):
- """Compute losses of the head.
-
- Args:
- cls_scores (list[Tensor]): Cls and quality scores for each scale
- level has shape (N, num_classes, H, W).
- bbox_preds (list[Tensor]): Box distribution logits for each scale
- level with shape (N, 4*(n+1), H, W), n is max value of integral
- set.
- gt_bboxes (list[Tensor]): Ground truth bboxes for each image with
- shape (num_gts, 4) in [tl_x, tl_y, br_x, br_y] format.
- gt_labels (list[Tensor]): class indices corresponding to each box
- img_metas (list[dict]): Meta information of each image, e.g.,
- image size, scaling factor, etc.
- gt_bboxes_ignore (list[Tensor] | None): specify which bounding
- boxes can be ignored when computing the loss.
-
- Returns:
- dict[str, Tensor]: A dictionary of loss components.
- """
-
- featmap_sizes = [featmap.size()[-2:] for featmap in cls_scores]
- assert len(featmap_sizes) == self.anchor_generator.num_levels
-
- device = cls_scores[0].device
- anchor_list, valid_flag_list = self.get_anchors(
- featmap_sizes, img_metas, device=device)
- label_channels = self.cls_out_channels if self.use_sigmoid_cls else 1
-
- cls_reg_targets = self.get_targets(
- anchor_list,
- valid_flag_list,
- gt_bboxes,
- img_metas,
- gt_bboxes_ignore_list=gt_bboxes_ignore,
- gt_labels_list=gt_labels,
- label_channels=label_channels)
- if cls_reg_targets is None:
- return None
-
- (anchor_list, labels_list, label_weights_list, bbox_targets_list,
- bbox_weights_list, num_total_pos, num_total_neg) = cls_reg_targets
-
- num_total_samples = reduce_mean(
- torch.tensor(num_total_pos, dtype=torch.float,
- device=device)).item()
- num_total_samples = max(num_total_samples, 1.0)
-
- losses_cls, losses_bbox, losses_dfl,\
- avg_factor = multi_apply(
- self.loss_single,
- anchor_list,
- cls_scores,
- bbox_preds,
- labels_list,
- label_weights_list,
- bbox_targets_list,
- self.anchor_generator.strides,
- num_total_samples=num_total_samples)
-
- avg_factor = sum(avg_factor)
- avg_factor = reduce_mean(avg_factor).item()
- losses_bbox = list(map(lambda x: x / avg_factor, losses_bbox))
- losses_dfl = list(map(lambda x: x / avg_factor, losses_dfl))
- return dict(
- loss_cls=losses_cls, loss_bbox=losses_bbox, loss_dfl=losses_dfl)
-
- def _get_bboxes(self,
- cls_scores,
- bbox_preds,
- mlvl_anchors,
- img_shapes,
- scale_factors,
- cfg,
- rescale=False,
- with_nms=True):
- """Transform outputs for a single batch item into labeled boxes.
-
- Args:
- cls_scores (list[Tensor]): Box scores for a single scale level
- has shape (N, num_classes, H, W).
- bbox_preds (list[Tensor]): Box distribution logits for a single
- scale level with shape (N, 4*(n+1), H, W), n is max value of
- integral set.
- mlvl_anchors (list[Tensor]): Box reference for a single scale level
- with shape (num_total_anchors, 4).
- img_shapes (list[tuple[int]]): Shape of the input image,
- list[(height, width, 3)].
- scale_factors (list[ndarray]): Scale factor of the image arange as
- (w_scale, h_scale, w_scale, h_scale).
- cfg (mmcv.Config | None): Test / postprocessing configuration,
- if None, test_cfg would be used.
- rescale (bool): If True, return boxes in original image space.
- Default: False.
- with_nms (bool): If True, do nms before return boxes.
- Default: True.
-
- Returns:
- list[tuple[Tensor, Tensor]]: Each item in result_list is 2-tuple.
- The first item is an (n, 5) tensor, where 5 represent
- (tl_x, tl_y, br_x, br_y, score) and the score between 0 and 1.
- The shape of the second tensor in the tuple is (n,), and
- each element represents the class label of the corresponding
- box.
- """
- cfg = self.test_cfg if cfg is None else cfg
- assert len(cls_scores) == len(bbox_preds) == len(mlvl_anchors)
- batch_size = cls_scores[0].shape[0]
-
- mlvl_bboxes = []
- mlvl_scores = []
- for cls_score, bbox_pred, stride, anchors in zip(
- cls_scores, bbox_preds, self.anchor_generator.strides,
- mlvl_anchors):
- assert cls_score.size()[-2:] == bbox_pred.size()[-2:]
- assert stride[0] == stride[1]
- scores = cls_score.permute(0, 2, 3, 1).reshape(
- batch_size, -1, self.cls_out_channels).sigmoid()
- bbox_pred = bbox_pred.permute(0, 2, 3, 1)
-
- bbox_pred = self.integral(bbox_pred) * stride[0]
- bbox_pred = bbox_pred.reshape(batch_size, -1, 4)
-
- nms_pre = cfg.get('nms_pre', -1)
- if nms_pre > 0 and scores.shape[1] > nms_pre:
- max_scores, _ = scores.max(-1)
- _, topk_inds = max_scores.topk(nms_pre)
- batch_inds = torch.arange(batch_size).view(
- -1, 1).expand_as(topk_inds).long()
- anchors = anchors[topk_inds, :]
- bbox_pred = bbox_pred[batch_inds, topk_inds, :]
- scores = scores[batch_inds, topk_inds, :]
- else:
- anchors = anchors.expand_as(bbox_pred)
-
- bboxes = distance2bbox(
- self.anchor_center(anchors), bbox_pred, max_shape=img_shapes)
- mlvl_bboxes.append(bboxes)
- mlvl_scores.append(scores)
-
- batch_mlvl_bboxes = torch.cat(mlvl_bboxes, dim=1)
- if rescale:
- batch_mlvl_bboxes /= batch_mlvl_bboxes.new_tensor(
- scale_factors).unsqueeze(1)
-
- batch_mlvl_scores = torch.cat(mlvl_scores, dim=1)
- # Add a dummy background class to the backend when using sigmoid
- # remind that we set FG labels to [0, num_class-1] since mmdet v2.0
- # BG cat_id: num_class
- padding = batch_mlvl_scores.new_zeros(batch_size,
- batch_mlvl_scores.shape[1], 1)
- batch_mlvl_scores = torch.cat([batch_mlvl_scores, padding], dim=-1)
-
- if with_nms:
- det_results = []
- for (mlvl_bboxes, mlvl_scores) in zip(batch_mlvl_bboxes,
- batch_mlvl_scores):
- det_bbox, det_label = multiclass_nms(mlvl_bboxes, mlvl_scores,
- cfg.score_thr, cfg.nms,
- cfg.max_per_img)
- det_results.append(tuple([det_bbox, det_label]))
- else:
- det_results = [
- tuple(mlvl_bs)
- for mlvl_bs in zip(batch_mlvl_bboxes, batch_mlvl_scores)
- ]
- return det_results
-
- def get_targets(self,
- anchor_list,
- valid_flag_list,
- gt_bboxes_list,
- img_metas,
- gt_bboxes_ignore_list=None,
- gt_labels_list=None,
- label_channels=1,
- unmap_outputs=True):
- """Get targets for GFL head.
-
- This method is almost the same as `AnchorHead.get_targets()`. Besides
- returning the targets as the parent method does, it also returns the
- anchors as the first element of the returned tuple.
- """
- num_imgs = len(img_metas)
- assert len(anchor_list) == len(valid_flag_list) == num_imgs
-
- # anchor number of multi levels
- num_level_anchors = [anchors.size(0) for anchors in anchor_list[0]]
- num_level_anchors_list = [num_level_anchors] * num_imgs
-
- # concat all level anchors and flags to a single tensor
- for i in range(num_imgs):
- assert len(anchor_list[i]) == len(valid_flag_list[i])
- anchor_list[i] = torch.cat(anchor_list[i])
- valid_flag_list[i] = torch.cat(valid_flag_list[i])
-
- # compute targets for each image
- if gt_bboxes_ignore_list is None:
- gt_bboxes_ignore_list = [None for _ in range(num_imgs)]
- if gt_labels_list is None:
- gt_labels_list = [None for _ in range(num_imgs)]
- (all_anchors, all_labels, all_label_weights, all_bbox_targets,
- all_bbox_weights, pos_inds_list, neg_inds_list) = multi_apply(
- self._get_target_single,
- anchor_list,
- valid_flag_list,
- num_level_anchors_list,
- gt_bboxes_list,
- gt_bboxes_ignore_list,
- gt_labels_list,
- img_metas,
- label_channels=label_channels,
- unmap_outputs=unmap_outputs)
- # no valid anchors
- if any([labels is None for labels in all_labels]):
- return None
- # sampled anchors of all images
- num_total_pos = sum([max(inds.numel(), 1) for inds in pos_inds_list])
- num_total_neg = sum([max(inds.numel(), 1) for inds in neg_inds_list])
- # split targets to a list w.r.t. multiple levels
- anchors_list = images_to_levels(all_anchors, num_level_anchors)
- labels_list = images_to_levels(all_labels, num_level_anchors)
- label_weights_list = images_to_levels(all_label_weights,
- num_level_anchors)
- bbox_targets_list = images_to_levels(all_bbox_targets,
- num_level_anchors)
- bbox_weights_list = images_to_levels(all_bbox_weights,
- num_level_anchors)
- return (anchors_list, labels_list, label_weights_list,
- bbox_targets_list, bbox_weights_list, num_total_pos,
- num_total_neg)
-
- def _get_target_single(self,
- flat_anchors,
- valid_flags,
- num_level_anchors,
- gt_bboxes,
- gt_bboxes_ignore,
- gt_labels,
- img_meta,
- label_channels=1,
- unmap_outputs=True):
- """Compute regression, classification targets for anchors in a single
- image.
-
- Args:
- flat_anchors (Tensor): Multi-level anchors of the image, which are
- concatenated into a single tensor of shape (num_anchors, 4)
- valid_flags (Tensor): Multi level valid flags of the image,
- which are concatenated into a single tensor of
- shape (num_anchors,).
- num_level_anchors Tensor): Number of anchors of each scale level.
- gt_bboxes (Tensor): Ground truth bboxes of the image,
- shape (num_gts, 4).
- gt_bboxes_ignore (Tensor): Ground truth bboxes to be
- ignored, shape (num_ignored_gts, 4).
- gt_labels (Tensor): Ground truth labels of each box,
- shape (num_gts,).
- img_meta (dict): Meta info of the image.
- label_channels (int): Channel of label.
- unmap_outputs (bool): Whether to map outputs back to the original
- set of anchors.
-
- Returns:
- tuple: N is the number of total anchors in the image.
- anchors (Tensor): All anchors in the image with shape (N, 4).
- labels (Tensor): Labels of all anchors in the image with shape
- (N,).
- label_weights (Tensor): Label weights of all anchor in the
- image with shape (N,).
- bbox_targets (Tensor): BBox targets of all anchors in the
- image with shape (N, 4).
- bbox_weights (Tensor): BBox weights of all anchors in the
- image with shape (N, 4).
- pos_inds (Tensor): Indices of positive anchor with shape
- (num_pos,).
- neg_inds (Tensor): Indices of negative anchor with shape
- (num_neg,).
- """
- inside_flags = anchor_inside_flags(flat_anchors, valid_flags,
- img_meta['img_shape'][:2],
- self.train_cfg.allowed_border)
- if not inside_flags.any():
- return (None, ) * 7
- # assign gt and sample anchors
- anchors = flat_anchors[inside_flags, :]
-
- num_level_anchors_inside = self.get_num_level_anchors_inside(
- num_level_anchors, inside_flags)
- assign_result = self.assigner.assign(anchors, num_level_anchors_inside,
- gt_bboxes, gt_bboxes_ignore,
- gt_labels)
-
- sampling_result = self.sampler.sample(assign_result, anchors,
- gt_bboxes)
-
- num_valid_anchors = anchors.shape[0]
- bbox_targets = torch.zeros_like(anchors)
- bbox_weights = torch.zeros_like(anchors)
- labels = anchors.new_full((num_valid_anchors, ),
- self.num_classes,
- dtype=torch.long)
- label_weights = anchors.new_zeros(num_valid_anchors, dtype=torch.float)
-
- pos_inds = sampling_result.pos_inds
- neg_inds = sampling_result.neg_inds
- if len(pos_inds) > 0:
- pos_bbox_targets = sampling_result.pos_gt_bboxes
- bbox_targets[pos_inds, :] = pos_bbox_targets
- bbox_weights[pos_inds, :] = 1.0
- if gt_labels is None:
- # Only rpn gives gt_labels as None
- # Foreground is the first class
- labels[pos_inds] = 0
- else:
- labels[pos_inds] = gt_labels[
- sampling_result.pos_assigned_gt_inds]
- if self.train_cfg.pos_weight <= 0:
- label_weights[pos_inds] = 1.0
- else:
- label_weights[pos_inds] = self.train_cfg.pos_weight
- if len(neg_inds) > 0:
- label_weights[neg_inds] = 1.0
-
- # map up to original set of anchors
- if unmap_outputs:
- num_total_anchors = flat_anchors.size(0)
- anchors = unmap(anchors, num_total_anchors, inside_flags)
- labels = unmap(
- labels, num_total_anchors, inside_flags, fill=self.num_classes)
- label_weights = unmap(label_weights, num_total_anchors,
- inside_flags)
- bbox_targets = unmap(bbox_targets, num_total_anchors, inside_flags)
- bbox_weights = unmap(bbox_weights, num_total_anchors, inside_flags)
-
- return (anchors, labels, label_weights, bbox_targets, bbox_weights,
- pos_inds, neg_inds)
-
- def get_num_level_anchors_inside(self, num_level_anchors, inside_flags):
- split_inside_flags = torch.split(inside_flags, num_level_anchors)
- num_level_anchors_inside = [
- int(flags.sum()) for flags in split_inside_flags
- ]
- return num_level_anchors_inside
diff --git a/spaces/RobinZ2021/remove_background/README.md b/spaces/RobinZ2021/remove_background/README.md
deleted file mode 100644
index 204d5bee629d81b958e5714fe33424da7ce074ed..0000000000000000000000000000000000000000
--- a/spaces/RobinZ2021/remove_background/README.md
+++ /dev/null
@@ -1,13 +0,0 @@
----
-title: Remove Background
-emoji: 📈
-colorFrom: blue
-colorTo: indigo
-sdk: gradio
-sdk_version: 3.13.0
-app_file: app.py
-pinned: false
-license: openrail
----
-
-Check out the configuration reference at https://huggingface.co/docs/hub/spaces-config-reference
diff --git a/spaces/Rongjiehuang/GenerSpeech/modules/commons/ssim.py b/spaces/Rongjiehuang/GenerSpeech/modules/commons/ssim.py
deleted file mode 100644
index 0d0241f267ef58b24979e022b05f2a9adf768826..0000000000000000000000000000000000000000
--- a/spaces/Rongjiehuang/GenerSpeech/modules/commons/ssim.py
+++ /dev/null
@@ -1,391 +0,0 @@
-# '''
-# https://github.com/One-sixth/ms_ssim_pytorch/blob/master/ssim.py
-# '''
-#
-# import torch
-# import torch.jit
-# import torch.nn.functional as F
-#
-#
-# @torch.jit.script
-# def create_window(window_size: int, sigma: float, channel: int):
-# '''
-# Create 1-D gauss kernel
-# :param window_size: the size of gauss kernel
-# :param sigma: sigma of normal distribution
-# :param channel: input channel
-# :return: 1D kernel
-# '''
-# coords = torch.arange(window_size, dtype=torch.float)
-# coords -= window_size // 2
-#
-# g = torch.exp(-(coords ** 2) / (2 * sigma ** 2))
-# g /= g.sum()
-#
-# g = g.reshape(1, 1, 1, -1).repeat(channel, 1, 1, 1)
-# return g
-#
-#
-# @torch.jit.script
-# def _gaussian_filter(x, window_1d, use_padding: bool):
-# '''
-# Blur input with 1-D kernel
-# :param x: batch of tensors to be blured
-# :param window_1d: 1-D gauss kernel
-# :param use_padding: padding image before conv
-# :return: blured tensors
-# '''
-# C = x.shape[1]
-# padding = 0
-# if use_padding:
-# window_size = window_1d.shape[3]
-# padding = window_size // 2
-# out = F.conv2d(x, window_1d, stride=1, padding=(0, padding), groups=C)
-# out = F.conv2d(out, window_1d.transpose(2, 3), stride=1, padding=(padding, 0), groups=C)
-# return out
-#
-#
-# @torch.jit.script
-# def ssim(X, Y, window, data_range: float, use_padding: bool = False):
-# '''
-# Calculate ssim index for X and Y
-# :param X: images [B, C, H, N_bins]
-# :param Y: images [B, C, H, N_bins]
-# :param window: 1-D gauss kernel
-# :param data_range: value range of input images. (usually 1.0 or 255)
-# :param use_padding: padding image before conv
-# :return:
-# '''
-#
-# K1 = 0.01
-# K2 = 0.03
-# compensation = 1.0
-#
-# C1 = (K1 * data_range) ** 2
-# C2 = (K2 * data_range) ** 2
-#
-# mu1 = _gaussian_filter(X, window, use_padding)
-# mu2 = _gaussian_filter(Y, window, use_padding)
-# sigma1_sq = _gaussian_filter(X * X, window, use_padding)
-# sigma2_sq = _gaussian_filter(Y * Y, window, use_padding)
-# sigma12 = _gaussian_filter(X * Y, window, use_padding)
-#
-# mu1_sq = mu1.pow(2)
-# mu2_sq = mu2.pow(2)
-# mu1_mu2 = mu1 * mu2
-#
-# sigma1_sq = compensation * (sigma1_sq - mu1_sq)
-# sigma2_sq = compensation * (sigma2_sq - mu2_sq)
-# sigma12 = compensation * (sigma12 - mu1_mu2)
-#
-# cs_map = (2 * sigma12 + C2) / (sigma1_sq + sigma2_sq + C2)
-# # Fixed the issue that the negative value of cs_map caused ms_ssim to output Nan.
-# cs_map = cs_map.clamp_min(0.)
-# ssim_map = ((2 * mu1_mu2 + C1) / (mu1_sq + mu2_sq + C1)) * cs_map
-#
-# ssim_val = ssim_map.mean(dim=(1, 2, 3)) # reduce along CHW
-# cs = cs_map.mean(dim=(1, 2, 3))
-#
-# return ssim_val, cs
-#
-#
-# @torch.jit.script
-# def ms_ssim(X, Y, window, data_range: float, weights, use_padding: bool = False, eps: float = 1e-8):
-# '''
-# interface of ms-ssim
-# :param X: a batch of images, (N,C,H,W)
-# :param Y: a batch of images, (N,C,H,W)
-# :param window: 1-D gauss kernel
-# :param data_range: value range of input images. (usually 1.0 or 255)
-# :param weights: weights for different levels
-# :param use_padding: padding image before conv
-# :param eps: use for avoid grad nan.
-# :return:
-# '''
-# levels = weights.shape[0]
-# cs_vals = []
-# ssim_vals = []
-# for _ in range(levels):
-# ssim_val, cs = ssim(X, Y, window=window, data_range=data_range, use_padding=use_padding)
-# # Use for fix a issue. When c = a ** b and a is 0, c.backward() will cause the a.grad become inf.
-# ssim_val = ssim_val.clamp_min(eps)
-# cs = cs.clamp_min(eps)
-# cs_vals.append(cs)
-#
-# ssim_vals.append(ssim_val)
-# padding = (X.shape[2] % 2, X.shape[3] % 2)
-# X = F.avg_pool2d(X, kernel_size=2, stride=2, padding=padding)
-# Y = F.avg_pool2d(Y, kernel_size=2, stride=2, padding=padding)
-#
-# cs_vals = torch.stack(cs_vals, dim=0)
-# ms_ssim_val = torch.prod((cs_vals[:-1] ** weights[:-1].unsqueeze(1)) * (ssim_vals[-1] ** weights[-1]), dim=0)
-# return ms_ssim_val
-#
-#
-# class SSIM(torch.jit.ScriptModule):
-# __constants__ = ['data_range', 'use_padding']
-#
-# def __init__(self, window_size=11, window_sigma=1.5, data_range=255., channel=3, use_padding=False):
-# '''
-# :param window_size: the size of gauss kernel
-# :param window_sigma: sigma of normal distribution
-# :param data_range: value range of input images. (usually 1.0 or 255)
-# :param channel: input channels (default: 3)
-# :param use_padding: padding image before conv
-# '''
-# super().__init__()
-# assert window_size % 2 == 1, 'Window size must be odd.'
-# window = create_window(window_size, window_sigma, channel)
-# self.register_buffer('window', window)
-# self.data_range = data_range
-# self.use_padding = use_padding
-#
-# @torch.jit.script_method
-# def forward(self, X, Y):
-# r = ssim(X, Y, window=self.window, data_range=self.data_range, use_padding=self.use_padding)
-# return r[0]
-#
-#
-# class MS_SSIM(torch.jit.ScriptModule):
-# __constants__ = ['data_range', 'use_padding', 'eps']
-#
-# def __init__(self, window_size=11, window_sigma=1.5, data_range=255., channel=3, use_padding=False, weights=None,
-# levels=None, eps=1e-8):
-# '''
-# class for ms-ssim
-# :param window_size: the size of gauss kernel
-# :param window_sigma: sigma of normal distribution
-# :param data_range: value range of input images. (usually 1.0 or 255)
-# :param channel: input channels
-# :param use_padding: padding image before conv
-# :param weights: weights for different levels. (default [0.0448, 0.2856, 0.3001, 0.2363, 0.1333])
-# :param levels: number of downsampling
-# :param eps: Use for fix a issue. When c = a ** b and a is 0, c.backward() will cause the a.grad become inf.
-# '''
-# super().__init__()
-# assert window_size % 2 == 1, 'Window size must be odd.'
-# self.data_range = data_range
-# self.use_padding = use_padding
-# self.eps = eps
-#
-# window = create_window(window_size, window_sigma, channel)
-# self.register_buffer('window', window)
-#
-# if weights is None:
-# weights = [0.0448, 0.2856, 0.3001, 0.2363, 0.1333]
-# weights = torch.tensor(weights, dtype=torch.float)
-#
-# if levels is not None:
-# weights = weights[:levels]
-# weights = weights / weights.sum()
-#
-# self.register_buffer('weights', weights)
-#
-# @torch.jit.script_method
-# def forward(self, X, Y):
-# return ms_ssim(X, Y, window=self.window, data_range=self.data_range, weights=self.weights,
-# use_padding=self.use_padding, eps=self.eps)
-#
-#
-# if __name__ == '__main__':
-# print('Simple Test')
-# im = torch.randint(0, 255, (5, 3, 256, 256), dtype=torch.float, device='cuda')
-# img1 = im / 255
-# img2 = img1 * 0.5
-#
-# losser = SSIM(data_range=1.).cuda()
-# loss = losser(img1, img2).mean()
-#
-# losser2 = MS_SSIM(data_range=1.).cuda()
-# loss2 = losser2(img1, img2).mean()
-#
-# print(loss.item())
-# print(loss2.item())
-#
-# if __name__ == '__main__':
-# print('Training Test')
-# import cv2
-# import torch.optim
-# import numpy as np
-# import imageio
-# import time
-#
-# out_test_video = False
-# # 最好不要直接输出gif图,会非常大,最好先输出mkv文件后用ffmpeg转换到GIF
-# video_use_gif = False
-#
-# im = cv2.imread('test_img1.jpg', 1)
-# t_im = torch.from_numpy(im).cuda().permute(2, 0, 1).float()[None] / 255.
-#
-# if out_test_video:
-# if video_use_gif:
-# fps = 0.5
-# out_wh = (im.shape[1] // 2, im.shape[0] // 2)
-# suffix = '.gif'
-# else:
-# fps = 5
-# out_wh = (im.shape[1], im.shape[0])
-# suffix = '.mkv'
-# video_last_time = time.perf_counter()
-# video = imageio.get_writer('ssim_test' + suffix, fps=fps)
-#
-# # 测试ssim
-# print('Training SSIM')
-# rand_im = torch.randint_like(t_im, 0, 255, dtype=torch.float32) / 255.
-# rand_im.requires_grad = True
-# optim = torch.optim.Adam([rand_im], 0.003, eps=1e-8)
-# losser = SSIM(data_range=1., channel=t_im.shape[1]).cuda()
-# ssim_score = 0
-# while ssim_score < 0.999:
-# optim.zero_grad()
-# loss = losser(rand_im, t_im)
-# (-loss).sum().backward()
-# ssim_score = loss.item()
-# optim.step()
-# r_im = np.transpose(rand_im.detach().cpu().numpy().clip(0, 1) * 255, [0, 2, 3, 1]).astype(np.uint8)[0]
-# r_im = cv2.putText(r_im, 'ssim %f' % ssim_score, (10, 30), cv2.FONT_HERSHEY_PLAIN, 2, (255, 0, 0), 2)
-#
-# if out_test_video:
-# if time.perf_counter() - video_last_time > 1. / fps:
-# video_last_time = time.perf_counter()
-# out_frame = cv2.cvtColor(r_im, cv2.COLOR_BGR2RGB)
-# out_frame = cv2.resize(out_frame, out_wh, interpolation=cv2.INTER_AREA)
-# if isinstance(out_frame, cv2.UMat):
-# out_frame = out_frame.get()
-# video.append_data(out_frame)
-#
-# cv2.imshow('ssim', r_im)
-# cv2.setWindowTitle('ssim', 'ssim %f' % ssim_score)
-# cv2.waitKey(1)
-#
-# if out_test_video:
-# video.close()
-#
-# # 测试ms_ssim
-# if out_test_video:
-# if video_use_gif:
-# fps = 0.5
-# out_wh = (im.shape[1] // 2, im.shape[0] // 2)
-# suffix = '.gif'
-# else:
-# fps = 5
-# out_wh = (im.shape[1], im.shape[0])
-# suffix = '.mkv'
-# video_last_time = time.perf_counter()
-# video = imageio.get_writer('ms_ssim_test' + suffix, fps=fps)
-#
-# print('Training MS_SSIM')
-# rand_im = torch.randint_like(t_im, 0, 255, dtype=torch.float32) / 255.
-# rand_im.requires_grad = True
-# optim = torch.optim.Adam([rand_im], 0.003, eps=1e-8)
-# losser = MS_SSIM(data_range=1., channel=t_im.shape[1]).cuda()
-# ssim_score = 0
-# while ssim_score < 0.999:
-# optim.zero_grad()
-# loss = losser(rand_im, t_im)
-# (-loss).sum().backward()
-# ssim_score = loss.item()
-# optim.step()
-# r_im = np.transpose(rand_im.detach().cpu().numpy().clip(0, 1) * 255, [0, 2, 3, 1]).astype(np.uint8)[0]
-# r_im = cv2.putText(r_im, 'ms_ssim %f' % ssim_score, (10, 30), cv2.FONT_HERSHEY_PLAIN, 2, (255, 0, 0), 2)
-#
-# if out_test_video:
-# if time.perf_counter() - video_last_time > 1. / fps:
-# video_last_time = time.perf_counter()
-# out_frame = cv2.cvtColor(r_im, cv2.COLOR_BGR2RGB)
-# out_frame = cv2.resize(out_frame, out_wh, interpolation=cv2.INTER_AREA)
-# if isinstance(out_frame, cv2.UMat):
-# out_frame = out_frame.get()
-# video.append_data(out_frame)
-#
-# cv2.imshow('ms_ssim', r_im)
-# cv2.setWindowTitle('ms_ssim', 'ms_ssim %f' % ssim_score)
-# cv2.waitKey(1)
-#
-# if out_test_video:
-# video.close()
-
-"""
-Adapted from https://github.com/Po-Hsun-Su/pytorch-ssim
-"""
-
-import torch
-import torch.nn.functional as F
-from torch.autograd import Variable
-import numpy as np
-from math import exp
-
-
-def gaussian(window_size, sigma):
- gauss = torch.Tensor([exp(-(x - window_size // 2) ** 2 / float(2 * sigma ** 2)) for x in range(window_size)])
- return gauss / gauss.sum()
-
-
-def create_window(window_size, channel):
- _1D_window = gaussian(window_size, 1.5).unsqueeze(1)
- _2D_window = _1D_window.mm(_1D_window.t()).float().unsqueeze(0).unsqueeze(0)
- window = Variable(_2D_window.expand(channel, 1, window_size, window_size).contiguous())
- return window
-
-
-def _ssim(img1, img2, window, window_size, channel, size_average=True):
- mu1 = F.conv2d(img1, window, padding=window_size // 2, groups=channel)
- mu2 = F.conv2d(img2, window, padding=window_size // 2, groups=channel)
-
- mu1_sq = mu1.pow(2)
- mu2_sq = mu2.pow(2)
- mu1_mu2 = mu1 * mu2
-
- sigma1_sq = F.conv2d(img1 * img1, window, padding=window_size // 2, groups=channel) - mu1_sq
- sigma2_sq = F.conv2d(img2 * img2, window, padding=window_size // 2, groups=channel) - mu2_sq
- sigma12 = F.conv2d(img1 * img2, window, padding=window_size // 2, groups=channel) - mu1_mu2
-
- C1 = 0.01 ** 2
- C2 = 0.03 ** 2
-
- ssim_map = ((2 * mu1_mu2 + C1) * (2 * sigma12 + C2)) / ((mu1_sq + mu2_sq + C1) * (sigma1_sq + sigma2_sq + C2))
-
- if size_average:
- return ssim_map.mean()
- else:
- return ssim_map.mean(1)
-
-
-class SSIM(torch.nn.Module):
- def __init__(self, window_size=11, size_average=True):
- super(SSIM, self).__init__()
- self.window_size = window_size
- self.size_average = size_average
- self.channel = 1
- self.window = create_window(window_size, self.channel)
-
- def forward(self, img1, img2):
- (_, channel, _, _) = img1.size()
-
- if channel == self.channel and self.window.data.type() == img1.data.type():
- window = self.window
- else:
- window = create_window(self.window_size, channel)
-
- if img1.is_cuda:
- window = window.cuda(img1.get_device())
- window = window.type_as(img1)
-
- self.window = window
- self.channel = channel
-
- return _ssim(img1, img2, window, self.window_size, channel, self.size_average)
-
-
-window = None
-
-
-def ssim(img1, img2, window_size=11, size_average=True):
- (_, channel, _, _) = img1.size()
- global window
- if window is None:
- window = create_window(window_size, channel)
- if img1.is_cuda:
- window = window.cuda(img1.get_device())
- window = window.type_as(img1)
- return _ssim(img1, img2, window, window_size, channel, size_average)
diff --git a/spaces/S0h9l/Coherent_Speech/README.md b/spaces/S0h9l/Coherent_Speech/README.md
deleted file mode 100644
index 6e9e0035d4b028a332b923a933606d1d579ec30c..0000000000000000000000000000000000000000
--- a/spaces/S0h9l/Coherent_Speech/README.md
+++ /dev/null
@@ -1,12 +0,0 @@
----
-title: Coherent Speech
-emoji: 🎙️
-colorFrom: gray
-colorTo: purple
-sdk: gradio
-sdk_version: 3.15.0
-app_file: app.py
-pinned: false
----
-
-Check out the configuration reference at https://huggingface.co/docs/hub/spaces-config-reference
diff --git a/spaces/Sapphire-356/Video2MC/joints_detectors/Alphapose/SPPE/src/models/layers/PRM.py b/spaces/Sapphire-356/Video2MC/joints_detectors/Alphapose/SPPE/src/models/layers/PRM.py
deleted file mode 100644
index 375bea4e45362ee240632c94ab6bfbf72f324e26..0000000000000000000000000000000000000000
--- a/spaces/Sapphire-356/Video2MC/joints_detectors/Alphapose/SPPE/src/models/layers/PRM.py
+++ /dev/null
@@ -1,135 +0,0 @@
-import torch.nn as nn
-from .util_models import ConcatTable, CaddTable, Identity
-import math
-from opt import opt
-
-
-class Residual(nn.Module):
- def __init__(self, numIn, numOut, inputResH, inputResW, stride=1,
- net_type='preact', useConv=False, baseWidth=9, cardinality=4):
- super(Residual, self).__init__()
-
- self.con = ConcatTable([convBlock(numIn, numOut, inputResH,
- inputResW, net_type, baseWidth, cardinality, stride),
- skipLayer(numIn, numOut, stride, useConv)])
- self.cadd = CaddTable(True)
-
- def forward(self, x):
- out = self.con(x)
- out = self.cadd(out)
- return out
-
-
-def convBlock(numIn, numOut, inputResH, inputResW, net_type, baseWidth, cardinality, stride):
- numIn = int(numIn)
- numOut = int(numOut)
-
- addTable = ConcatTable()
- s_list = []
- if net_type != 'no_preact':
- s_list.append(nn.BatchNorm2d(numIn))
- s_list.append(nn.ReLU(True))
-
- conv1 = nn.Conv2d(numIn, numOut // 2, kernel_size=1)
- if opt.init:
- nn.init.xavier_normal(conv1.weight, gain=math.sqrt(1 / 2))
- s_list.append(conv1)
-
- s_list.append(nn.BatchNorm2d(numOut // 2))
- s_list.append(nn.ReLU(True))
-
- conv2 = nn.Conv2d(numOut // 2, numOut // 2,
- kernel_size=3, stride=stride, padding=1)
- if opt.init:
- nn.init.xavier_normal(conv2.weight)
- s_list.append(conv2)
-
- s = nn.Sequential(*s_list)
- addTable.add(s)
-
- D = math.floor(numOut // baseWidth)
- C = cardinality
- s_list = []
-
- if net_type != 'no_preact':
- s_list.append(nn.BatchNorm2d(numIn))
- s_list.append(nn.ReLU(True))
-
- conv1 = nn.Conv2d(numIn, D, kernel_size=1, stride=stride)
- if opt.init:
- nn.init.xavier_normal(conv1.weight, gain=math.sqrt(1 / C))
-
- s_list.append(conv1)
- s_list.append(nn.BatchNorm2d(D))
- s_list.append(nn.ReLU(True))
- s_list.append(pyramid(D, C, inputResH, inputResW))
- s_list.append(nn.BatchNorm2d(D))
- s_list.append(nn.ReLU(True))
-
- a = nn.Conv2d(D, numOut // 2, kernel_size=1)
- a.nBranchIn = C
- if opt.init:
- nn.init.xavier_normal(a.weight, gain=math.sqrt(1 / C))
- s_list.append(a)
-
- s = nn.Sequential(*s_list)
- addTable.add(s)
-
- elewiswAdd = nn.Sequential(
- addTable,
- CaddTable(False)
- )
- conv2 = nn.Conv2d(numOut // 2, numOut, kernel_size=1)
- if opt.init:
- nn.init.xavier_normal(conv2.weight, gain=math.sqrt(1 / 2))
- model = nn.Sequential(
- elewiswAdd,
- nn.BatchNorm2d(numOut // 2),
- nn.ReLU(True),
- conv2
- )
- return model
-
-
-def pyramid(D, C, inputResH, inputResW):
- pyraTable = ConcatTable()
- sc = math.pow(2, 1 / C)
- for i in range(C):
- scaled = 1 / math.pow(sc, i + 1)
- conv1 = nn.Conv2d(D, D, kernel_size=3, stride=1, padding=1)
- if opt.init:
- nn.init.xavier_normal(conv1.weight)
- s = nn.Sequential(
- nn.FractionalMaxPool2d(2, output_ratio=(scaled, scaled)),
- conv1,
- nn.UpsamplingBilinear2d(size=(int(inputResH), int(inputResW))))
- pyraTable.add(s)
- pyra = nn.Sequential(
- pyraTable,
- CaddTable(False)
- )
- return pyra
-
-
-class skipLayer(nn.Module):
- def __init__(self, numIn, numOut, stride, useConv):
- super(skipLayer, self).__init__()
- self.identity = False
-
- if numIn == numOut and stride == 1 and not useConv:
- self.identity = True
- else:
- conv1 = nn.Conv2d(numIn, numOut, kernel_size=1, stride=stride)
- if opt.init:
- nn.init.xavier_normal(conv1.weight, gain=math.sqrt(1 / 2))
- self.m = nn.Sequential(
- nn.BatchNorm2d(numIn),
- nn.ReLU(True),
- conv1
- )
-
- def forward(self, x):
- if self.identity:
- return x
- else:
- return self.m(x)
diff --git a/spaces/ServerX/PorcoDiaz/tensorlowest.py b/spaces/ServerX/PorcoDiaz/tensorlowest.py
deleted file mode 100644
index eccd4dbf3494434e59f7defaae6ab91797263b90..0000000000000000000000000000000000000000
--- a/spaces/ServerX/PorcoDiaz/tensorlowest.py
+++ /dev/null
@@ -1,123 +0,0 @@
-from tensorboard.backend.event_processing import event_accumulator
-
-import os
-from shutil import copy2
-from re import search as RSearch
-import pandas as pd
-from ast import literal_eval as LEval
-
-weights_dir = 'weights/'
-
-def find_biggest_tensorboard(tensordir):
- try:
- files = [f for f in os.listdir(tensordir) if f.endswith('.0')]
- if not files:
- print("No files with the '.0' extension found!")
- return
-
- max_size = 0
- biggest_file = ""
-
- for file in files:
- file_path = os.path.join(tensordir, file)
- if os.path.isfile(file_path):
- file_size = os.path.getsize(file_path)
- if file_size > max_size:
- max_size = file_size
- biggest_file = file
-
- return biggest_file
-
- except FileNotFoundError:
- print("Couldn't find your model!")
- return
-
-def main(model_name, save_freq, lastmdls):
- global lowestval_weight_dir, scl
-
- tensordir = os.path.join('logs', model_name)
- lowestval_weight_dir = os.path.join(tensordir, "lowestvals")
-
- latest_file = find_biggest_tensorboard(tensordir)
-
- if latest_file is None:
- print("Couldn't find a valid tensorboard file!")
- return
-
- tfile = os.path.join(tensordir, latest_file)
-
- ea = event_accumulator.EventAccumulator(tfile,
- size_guidance={
- event_accumulator.COMPRESSED_HISTOGRAMS: 500,
- event_accumulator.IMAGES: 4,
- event_accumulator.AUDIO: 4,
- event_accumulator.SCALARS: 0,
- event_accumulator.HISTOGRAMS: 1,
- })
-
- ea.Reload()
- ea.Tags()
-
- scl = ea.Scalars('loss/g/total')
-
- listwstep = {}
-
- for val in scl:
- if (val.step // save_freq) * save_freq in [val.step for val in scl]:
- listwstep[float(val.value)] = (val.step // save_freq) * save_freq
-
- lowest_vals = sorted(listwstep.keys())[:lastmdls]
-
- sorted_dict = {value: step for value, step in listwstep.items() if value in lowest_vals}
-
- return sorted_dict
-
-def selectweights(model_name, file_dict, weights_dir, lowestval_weight_dir):
- os.makedirs(lowestval_weight_dir, exist_ok=True)
- logdir = []
- files = []
- lbldict = {
- 'Values': {},
- 'Names': {}
- }
- weights_dir_path = os.path.join(weights_dir, "")
- low_val_path = os.path.join(os.getcwd(), os.path.join(lowestval_weight_dir, ""))
-
- try:
- file_dict = LEval(file_dict)
- except Exception as e:
- print(f"Error! {e}")
- return f"Couldn't load tensorboard file! {e}"
-
- weights = [f for f in os.scandir(weights_dir)]
- for key, value in file_dict.items():
- pattern = fr"^{model_name}_.*_s{value}\.pth$"
- matching_weights = [f.name for f in weights if f.is_file() and RSearch(pattern, f.name)]
- for weight in matching_weights:
- source_path = weights_dir_path + weight
- destination_path = os.path.join(lowestval_weight_dir, weight)
-
- copy2(source_path, destination_path)
-
- logdir.append(f"File = {weight} Value: {key}, Step: {value}")
-
- lbldict['Names'][weight] = weight
- lbldict['Values'][weight] = key
-
- files.append(low_val_path + weight)
-
- print(f"File = {weight} Value: {key}, Step: {value}")
-
- yield ('\n'.join(logdir), files, pd.DataFrame(lbldict))
-
-
- return ''.join(logdir), files, pd.DataFrame(lbldict)
-
-
-if __name__ == "__main__":
- model = str(input("Enter the name of the model: "))
- sav_freq = int(input("Enter save frequency of the model: "))
- ds = main(model, sav_freq)
-
- if ds: selectweights(model, ds, weights_dir, lowestval_weight_dir)
-
\ No newline at end of file
diff --git a/spaces/Shad0ws/ImageModelTestEnvironment/README.md b/spaces/Shad0ws/ImageModelTestEnvironment/README.md
deleted file mode 100644
index 7a6cea2e3ea5f93119b8c780d7508617a5a4a63f..0000000000000000000000000000000000000000
--- a/spaces/Shad0ws/ImageModelTestEnvironment/README.md
+++ /dev/null
@@ -1,13 +0,0 @@
----
-title: Maximum Multiplier
-emoji: 🛕🛕
-colorFrom: green
-colorTo: blue
-sdk: gradio
-sdk_version: 3.15.0
-app_file: app.py
-pinned: true
-duplicated_from: rankjet/BulkImgVariations
----
-
-Check out the configuration reference at https://huggingface.co/docs/hub/spaces-config-reference
diff --git a/spaces/ShilongLiu/Grounding_DINO_demo/groundingdino/util/box_ops.py b/spaces/ShilongLiu/Grounding_DINO_demo/groundingdino/util/box_ops.py
deleted file mode 100644
index 781068d294e576954edb4bd07b6e0f30e4e1bcd9..0000000000000000000000000000000000000000
--- a/spaces/ShilongLiu/Grounding_DINO_demo/groundingdino/util/box_ops.py
+++ /dev/null
@@ -1,140 +0,0 @@
-# Copyright (c) Facebook, Inc. and its affiliates. All Rights Reserved
-"""
-Utilities for bounding box manipulation and GIoU.
-"""
-import torch
-from torchvision.ops.boxes import box_area
-
-
-def box_cxcywh_to_xyxy(x):
- x_c, y_c, w, h = x.unbind(-1)
- b = [(x_c - 0.5 * w), (y_c - 0.5 * h), (x_c + 0.5 * w), (y_c + 0.5 * h)]
- return torch.stack(b, dim=-1)
-
-
-def box_xyxy_to_cxcywh(x):
- x0, y0, x1, y1 = x.unbind(-1)
- b = [(x0 + x1) / 2, (y0 + y1) / 2, (x1 - x0), (y1 - y0)]
- return torch.stack(b, dim=-1)
-
-
-# modified from torchvision to also return the union
-def box_iou(boxes1, boxes2):
- area1 = box_area(boxes1)
- area2 = box_area(boxes2)
-
- # import ipdb; ipdb.set_trace()
- lt = torch.max(boxes1[:, None, :2], boxes2[:, :2]) # [N,M,2]
- rb = torch.min(boxes1[:, None, 2:], boxes2[:, 2:]) # [N,M,2]
-
- wh = (rb - lt).clamp(min=0) # [N,M,2]
- inter = wh[:, :, 0] * wh[:, :, 1] # [N,M]
-
- union = area1[:, None] + area2 - inter
-
- iou = inter / (union + 1e-6)
- return iou, union
-
-
-def generalized_box_iou(boxes1, boxes2):
- """
- Generalized IoU from https://giou.stanford.edu/
-
- The boxes should be in [x0, y0, x1, y1] format
-
- Returns a [N, M] pairwise matrix, where N = len(boxes1)
- and M = len(boxes2)
- """
- # degenerate boxes gives inf / nan results
- # so do an early check
- assert (boxes1[:, 2:] >= boxes1[:, :2]).all()
- assert (boxes2[:, 2:] >= boxes2[:, :2]).all()
- # except:
- # import ipdb; ipdb.set_trace()
- iou, union = box_iou(boxes1, boxes2)
-
- lt = torch.min(boxes1[:, None, :2], boxes2[:, :2])
- rb = torch.max(boxes1[:, None, 2:], boxes2[:, 2:])
-
- wh = (rb - lt).clamp(min=0) # [N,M,2]
- area = wh[:, :, 0] * wh[:, :, 1]
-
- return iou - (area - union) / (area + 1e-6)
-
-
-# modified from torchvision to also return the union
-def box_iou_pairwise(boxes1, boxes2):
- area1 = box_area(boxes1)
- area2 = box_area(boxes2)
-
- lt = torch.max(boxes1[:, :2], boxes2[:, :2]) # [N,2]
- rb = torch.min(boxes1[:, 2:], boxes2[:, 2:]) # [N,2]
-
- wh = (rb - lt).clamp(min=0) # [N,2]
- inter = wh[:, 0] * wh[:, 1] # [N]
-
- union = area1 + area2 - inter
-
- iou = inter / union
- return iou, union
-
-
-def generalized_box_iou_pairwise(boxes1, boxes2):
- """
- Generalized IoU from https://giou.stanford.edu/
-
- Input:
- - boxes1, boxes2: N,4
- Output:
- - giou: N, 4
- """
- # degenerate boxes gives inf / nan results
- # so do an early check
- assert (boxes1[:, 2:] >= boxes1[:, :2]).all()
- assert (boxes2[:, 2:] >= boxes2[:, :2]).all()
- assert boxes1.shape == boxes2.shape
- iou, union = box_iou_pairwise(boxes1, boxes2) # N, 4
-
- lt = torch.min(boxes1[:, :2], boxes2[:, :2])
- rb = torch.max(boxes1[:, 2:], boxes2[:, 2:])
-
- wh = (rb - lt).clamp(min=0) # [N,2]
- area = wh[:, 0] * wh[:, 1]
-
- return iou - (area - union) / area
-
-
-def masks_to_boxes(masks):
- """Compute the bounding boxes around the provided masks
-
- The masks should be in format [N, H, W] where N is the number of masks, (H, W) are the spatial dimensions.
-
- Returns a [N, 4] tensors, with the boxes in xyxy format
- """
- if masks.numel() == 0:
- return torch.zeros((0, 4), device=masks.device)
-
- h, w = masks.shape[-2:]
-
- y = torch.arange(0, h, dtype=torch.float)
- x = torch.arange(0, w, dtype=torch.float)
- y, x = torch.meshgrid(y, x)
-
- x_mask = masks * x.unsqueeze(0)
- x_max = x_mask.flatten(1).max(-1)[0]
- x_min = x_mask.masked_fill(~(masks.bool()), 1e8).flatten(1).min(-1)[0]
-
- y_mask = masks * y.unsqueeze(0)
- y_max = y_mask.flatten(1).max(-1)[0]
- y_min = y_mask.masked_fill(~(masks.bool()), 1e8).flatten(1).min(-1)[0]
-
- return torch.stack([x_min, y_min, x_max, y_max], 1)
-
-
-if __name__ == "__main__":
- x = torch.rand(5, 4)
- y = torch.rand(3, 4)
- iou, union = box_iou(x, y)
- import ipdb
-
- ipdb.set_trace()
diff --git a/spaces/Shreyas3006/Text-Summarizer-sdp/README.md b/spaces/Shreyas3006/Text-Summarizer-sdp/README.md
deleted file mode 100644
index ba5825f953cffde79af227a4cd816a22c37029a0..0000000000000000000000000000000000000000
--- a/spaces/Shreyas3006/Text-Summarizer-sdp/README.md
+++ /dev/null
@@ -1,12 +0,0 @@
----
-title: Text Summarizer
-emoji: 🌍
-colorFrom: blue
-colorTo: yellow
-sdk: streamlit
-app_file: app.py
-pinned: false
----
-
-# Text Summarizer
-Text summarizer using Transformers
\ No newline at end of file
diff --git a/spaces/Silentlin/DiffSinger/modules/parallel_wavegan/models/melgan.py b/spaces/Silentlin/DiffSinger/modules/parallel_wavegan/models/melgan.py
deleted file mode 100644
index e021ae4817a8c1c97338e61b00b230c881836fd8..0000000000000000000000000000000000000000
--- a/spaces/Silentlin/DiffSinger/modules/parallel_wavegan/models/melgan.py
+++ /dev/null
@@ -1,427 +0,0 @@
-# -*- coding: utf-8 -*-
-
-# Copyright 2020 Tomoki Hayashi
-# MIT License (https://opensource.org/licenses/MIT)
-
-"""MelGAN Modules."""
-
-import logging
-
-import numpy as np
-import torch
-
-from modules.parallel_wavegan.layers import CausalConv1d
-from modules.parallel_wavegan.layers import CausalConvTranspose1d
-from modules.parallel_wavegan.layers import ResidualStack
-
-
-class MelGANGenerator(torch.nn.Module):
- """MelGAN generator module."""
-
- def __init__(self,
- in_channels=80,
- out_channels=1,
- kernel_size=7,
- channels=512,
- bias=True,
- upsample_scales=[8, 8, 2, 2],
- stack_kernel_size=3,
- stacks=3,
- nonlinear_activation="LeakyReLU",
- nonlinear_activation_params={"negative_slope": 0.2},
- pad="ReflectionPad1d",
- pad_params={},
- use_final_nonlinear_activation=True,
- use_weight_norm=True,
- use_causal_conv=False,
- ):
- """Initialize MelGANGenerator module.
-
- Args:
- in_channels (int): Number of input channels.
- out_channels (int): Number of output channels.
- kernel_size (int): Kernel size of initial and final conv layer.
- channels (int): Initial number of channels for conv layer.
- bias (bool): Whether to add bias parameter in convolution layers.
- upsample_scales (list): List of upsampling scales.
- stack_kernel_size (int): Kernel size of dilated conv layers in residual stack.
- stacks (int): Number of stacks in a single residual stack.
- nonlinear_activation (str): Activation function module name.
- nonlinear_activation_params (dict): Hyperparameters for activation function.
- pad (str): Padding function module name before dilated convolution layer.
- pad_params (dict): Hyperparameters for padding function.
- use_final_nonlinear_activation (torch.nn.Module): Activation function for the final layer.
- use_weight_norm (bool): Whether to use weight norm.
- If set to true, it will be applied to all of the conv layers.
- use_causal_conv (bool): Whether to use causal convolution.
-
- """
- super(MelGANGenerator, self).__init__()
-
- # check hyper parameters is valid
- assert channels >= np.prod(upsample_scales)
- assert channels % (2 ** len(upsample_scales)) == 0
- if not use_causal_conv:
- assert (kernel_size - 1) % 2 == 0, "Not support even number kernel size."
-
- # add initial layer
- layers = []
- if not use_causal_conv:
- layers += [
- getattr(torch.nn, pad)((kernel_size - 1) // 2, **pad_params),
- torch.nn.Conv1d(in_channels, channels, kernel_size, bias=bias),
- ]
- else:
- layers += [
- CausalConv1d(in_channels, channels, kernel_size,
- bias=bias, pad=pad, pad_params=pad_params),
- ]
-
- for i, upsample_scale in enumerate(upsample_scales):
- # add upsampling layer
- layers += [getattr(torch.nn, nonlinear_activation)(**nonlinear_activation_params)]
- if not use_causal_conv:
- layers += [
- torch.nn.ConvTranspose1d(
- channels // (2 ** i),
- channels // (2 ** (i + 1)),
- upsample_scale * 2,
- stride=upsample_scale,
- padding=upsample_scale // 2 + upsample_scale % 2,
- output_padding=upsample_scale % 2,
- bias=bias,
- )
- ]
- else:
- layers += [
- CausalConvTranspose1d(
- channels // (2 ** i),
- channels // (2 ** (i + 1)),
- upsample_scale * 2,
- stride=upsample_scale,
- bias=bias,
- )
- ]
-
- # add residual stack
- for j in range(stacks):
- layers += [
- ResidualStack(
- kernel_size=stack_kernel_size,
- channels=channels // (2 ** (i + 1)),
- dilation=stack_kernel_size ** j,
- bias=bias,
- nonlinear_activation=nonlinear_activation,
- nonlinear_activation_params=nonlinear_activation_params,
- pad=pad,
- pad_params=pad_params,
- use_causal_conv=use_causal_conv,
- )
- ]
-
- # add final layer
- layers += [getattr(torch.nn, nonlinear_activation)(**nonlinear_activation_params)]
- if not use_causal_conv:
- layers += [
- getattr(torch.nn, pad)((kernel_size - 1) // 2, **pad_params),
- torch.nn.Conv1d(channels // (2 ** (i + 1)), out_channels, kernel_size, bias=bias),
- ]
- else:
- layers += [
- CausalConv1d(channels // (2 ** (i + 1)), out_channels, kernel_size,
- bias=bias, pad=pad, pad_params=pad_params),
- ]
- if use_final_nonlinear_activation:
- layers += [torch.nn.Tanh()]
-
- # define the model as a single function
- self.melgan = torch.nn.Sequential(*layers)
-
- # apply weight norm
- if use_weight_norm:
- self.apply_weight_norm()
-
- # reset parameters
- self.reset_parameters()
-
- def forward(self, c):
- """Calculate forward propagation.
-
- Args:
- c (Tensor): Input tensor (B, channels, T).
-
- Returns:
- Tensor: Output tensor (B, 1, T ** prod(upsample_scales)).
-
- """
- return self.melgan(c)
-
- def remove_weight_norm(self):
- """Remove weight normalization module from all of the layers."""
- def _remove_weight_norm(m):
- try:
- logging.debug(f"Weight norm is removed from {m}.")
- torch.nn.utils.remove_weight_norm(m)
- except ValueError: # this module didn't have weight norm
- return
-
- self.apply(_remove_weight_norm)
-
- def apply_weight_norm(self):
- """Apply weight normalization module from all of the layers."""
- def _apply_weight_norm(m):
- if isinstance(m, torch.nn.Conv1d) or isinstance(m, torch.nn.ConvTranspose1d):
- torch.nn.utils.weight_norm(m)
- logging.debug(f"Weight norm is applied to {m}.")
-
- self.apply(_apply_weight_norm)
-
- def reset_parameters(self):
- """Reset parameters.
-
- This initialization follows official implementation manner.
- https://github.com/descriptinc/melgan-neurips/blob/master/spec2wav/modules.py
-
- """
- def _reset_parameters(m):
- if isinstance(m, torch.nn.Conv1d) or isinstance(m, torch.nn.ConvTranspose1d):
- m.weight.data.normal_(0.0, 0.02)
- logging.debug(f"Reset parameters in {m}.")
-
- self.apply(_reset_parameters)
-
-
-class MelGANDiscriminator(torch.nn.Module):
- """MelGAN discriminator module."""
-
- def __init__(self,
- in_channels=1,
- out_channels=1,
- kernel_sizes=[5, 3],
- channels=16,
- max_downsample_channels=1024,
- bias=True,
- downsample_scales=[4, 4, 4, 4],
- nonlinear_activation="LeakyReLU",
- nonlinear_activation_params={"negative_slope": 0.2},
- pad="ReflectionPad1d",
- pad_params={},
- ):
- """Initilize MelGAN discriminator module.
-
- Args:
- in_channels (int): Number of input channels.
- out_channels (int): Number of output channels.
- kernel_sizes (list): List of two kernel sizes. The prod will be used for the first conv layer,
- and the first and the second kernel sizes will be used for the last two layers.
- For example if kernel_sizes = [5, 3], the first layer kernel size will be 5 * 3 = 15,
- the last two layers' kernel size will be 5 and 3, respectively.
- channels (int): Initial number of channels for conv layer.
- max_downsample_channels (int): Maximum number of channels for downsampling layers.
- bias (bool): Whether to add bias parameter in convolution layers.
- downsample_scales (list): List of downsampling scales.
- nonlinear_activation (str): Activation function module name.
- nonlinear_activation_params (dict): Hyperparameters for activation function.
- pad (str): Padding function module name before dilated convolution layer.
- pad_params (dict): Hyperparameters for padding function.
-
- """
- super(MelGANDiscriminator, self).__init__()
- self.layers = torch.nn.ModuleList()
-
- # check kernel size is valid
- assert len(kernel_sizes) == 2
- assert kernel_sizes[0] % 2 == 1
- assert kernel_sizes[1] % 2 == 1
-
- # add first layer
- self.layers += [
- torch.nn.Sequential(
- getattr(torch.nn, pad)((np.prod(kernel_sizes) - 1) // 2, **pad_params),
- torch.nn.Conv1d(in_channels, channels, np.prod(kernel_sizes), bias=bias),
- getattr(torch.nn, nonlinear_activation)(**nonlinear_activation_params),
- )
- ]
-
- # add downsample layers
- in_chs = channels
- for downsample_scale in downsample_scales:
- out_chs = min(in_chs * downsample_scale, max_downsample_channels)
- self.layers += [
- torch.nn.Sequential(
- torch.nn.Conv1d(
- in_chs, out_chs,
- kernel_size=downsample_scale * 10 + 1,
- stride=downsample_scale,
- padding=downsample_scale * 5,
- groups=in_chs // 4,
- bias=bias,
- ),
- getattr(torch.nn, nonlinear_activation)(**nonlinear_activation_params),
- )
- ]
- in_chs = out_chs
-
- # add final layers
- out_chs = min(in_chs * 2, max_downsample_channels)
- self.layers += [
- torch.nn.Sequential(
- torch.nn.Conv1d(
- in_chs, out_chs, kernel_sizes[0],
- padding=(kernel_sizes[0] - 1) // 2,
- bias=bias,
- ),
- getattr(torch.nn, nonlinear_activation)(**nonlinear_activation_params),
- )
- ]
- self.layers += [
- torch.nn.Conv1d(
- out_chs, out_channels, kernel_sizes[1],
- padding=(kernel_sizes[1] - 1) // 2,
- bias=bias,
- ),
- ]
-
- def forward(self, x):
- """Calculate forward propagation.
-
- Args:
- x (Tensor): Input noise signal (B, 1, T).
-
- Returns:
- List: List of output tensors of each layer.
-
- """
- outs = []
- for f in self.layers:
- x = f(x)
- outs += [x]
-
- return outs
-
-
-class MelGANMultiScaleDiscriminator(torch.nn.Module):
- """MelGAN multi-scale discriminator module."""
-
- def __init__(self,
- in_channels=1,
- out_channels=1,
- scales=3,
- downsample_pooling="AvgPool1d",
- # follow the official implementation setting
- downsample_pooling_params={
- "kernel_size": 4,
- "stride": 2,
- "padding": 1,
- "count_include_pad": False,
- },
- kernel_sizes=[5, 3],
- channels=16,
- max_downsample_channels=1024,
- bias=True,
- downsample_scales=[4, 4, 4, 4],
- nonlinear_activation="LeakyReLU",
- nonlinear_activation_params={"negative_slope": 0.2},
- pad="ReflectionPad1d",
- pad_params={},
- use_weight_norm=True,
- ):
- """Initilize MelGAN multi-scale discriminator module.
-
- Args:
- in_channels (int): Number of input channels.
- out_channels (int): Number of output channels.
- downsample_pooling (str): Pooling module name for downsampling of the inputs.
- downsample_pooling_params (dict): Parameters for the above pooling module.
- kernel_sizes (list): List of two kernel sizes. The sum will be used for the first conv layer,
- and the first and the second kernel sizes will be used for the last two layers.
- channels (int): Initial number of channels for conv layer.
- max_downsample_channels (int): Maximum number of channels for downsampling layers.
- bias (bool): Whether to add bias parameter in convolution layers.
- downsample_scales (list): List of downsampling scales.
- nonlinear_activation (str): Activation function module name.
- nonlinear_activation_params (dict): Hyperparameters for activation function.
- pad (str): Padding function module name before dilated convolution layer.
- pad_params (dict): Hyperparameters for padding function.
- use_causal_conv (bool): Whether to use causal convolution.
-
- """
- super(MelGANMultiScaleDiscriminator, self).__init__()
- self.discriminators = torch.nn.ModuleList()
-
- # add discriminators
- for _ in range(scales):
- self.discriminators += [
- MelGANDiscriminator(
- in_channels=in_channels,
- out_channels=out_channels,
- kernel_sizes=kernel_sizes,
- channels=channels,
- max_downsample_channels=max_downsample_channels,
- bias=bias,
- downsample_scales=downsample_scales,
- nonlinear_activation=nonlinear_activation,
- nonlinear_activation_params=nonlinear_activation_params,
- pad=pad,
- pad_params=pad_params,
- )
- ]
- self.pooling = getattr(torch.nn, downsample_pooling)(**downsample_pooling_params)
-
- # apply weight norm
- if use_weight_norm:
- self.apply_weight_norm()
-
- # reset parameters
- self.reset_parameters()
-
- def forward(self, x):
- """Calculate forward propagation.
-
- Args:
- x (Tensor): Input noise signal (B, 1, T).
-
- Returns:
- List: List of list of each discriminator outputs, which consists of each layer output tensors.
-
- """
- outs = []
- for f in self.discriminators:
- outs += [f(x)]
- x = self.pooling(x)
-
- return outs
-
- def remove_weight_norm(self):
- """Remove weight normalization module from all of the layers."""
- def _remove_weight_norm(m):
- try:
- logging.debug(f"Weight norm is removed from {m}.")
- torch.nn.utils.remove_weight_norm(m)
- except ValueError: # this module didn't have weight norm
- return
-
- self.apply(_remove_weight_norm)
-
- def apply_weight_norm(self):
- """Apply weight normalization module from all of the layers."""
- def _apply_weight_norm(m):
- if isinstance(m, torch.nn.Conv1d) or isinstance(m, torch.nn.ConvTranspose1d):
- torch.nn.utils.weight_norm(m)
- logging.debug(f"Weight norm is applied to {m}.")
-
- self.apply(_apply_weight_norm)
-
- def reset_parameters(self):
- """Reset parameters.
-
- This initialization follows official implementation manner.
- https://github.com/descriptinc/melgan-neurips/blob/master/spec2wav/modules.py
-
- """
- def _reset_parameters(m):
- if isinstance(m, torch.nn.Conv1d) or isinstance(m, torch.nn.ConvTranspose1d):
- m.weight.data.normal_(0.0, 0.02)
- logging.debug(f"Reset parameters in {m}.")
-
- self.apply(_reset_parameters)
diff --git a/spaces/Silentlin/DiffSinger/utils/pitch_utils.py b/spaces/Silentlin/DiffSinger/utils/pitch_utils.py
deleted file mode 100644
index f7fd166abd3a03bac5909e498669b482447435cf..0000000000000000000000000000000000000000
--- a/spaces/Silentlin/DiffSinger/utils/pitch_utils.py
+++ /dev/null
@@ -1,76 +0,0 @@
-#########
-# world
-##########
-import librosa
-import numpy as np
-import torch
-
-gamma = 0
-mcepInput = 3 # 0 for dB, 3 for magnitude
-alpha = 0.45
-en_floor = 10 ** (-80 / 20)
-FFT_SIZE = 2048
-
-
-f0_bin = 256
-f0_max = 1100.0
-f0_min = 50.0
-f0_mel_min = 1127 * np.log(1 + f0_min / 700)
-f0_mel_max = 1127 * np.log(1 + f0_max / 700)
-
-
-def f0_to_coarse(f0):
- is_torch = isinstance(f0, torch.Tensor)
- f0_mel = 1127 * (1 + f0 / 700).log() if is_torch else 1127 * np.log(1 + f0 / 700)
- f0_mel[f0_mel > 0] = (f0_mel[f0_mel > 0] - f0_mel_min) * (f0_bin - 2) / (f0_mel_max - f0_mel_min) + 1
-
- f0_mel[f0_mel <= 1] = 1
- f0_mel[f0_mel > f0_bin - 1] = f0_bin - 1
- f0_coarse = (f0_mel + 0.5).long() if is_torch else np.rint(f0_mel).astype(np.int)
- assert f0_coarse.max() <= 255 and f0_coarse.min() >= 1, (f0_coarse.max(), f0_coarse.min())
- return f0_coarse
-
-
-def norm_f0(f0, uv, hparams):
- is_torch = isinstance(f0, torch.Tensor)
- if hparams['pitch_norm'] == 'standard':
- f0 = (f0 - hparams['f0_mean']) / hparams['f0_std']
- if hparams['pitch_norm'] == 'log':
- f0 = torch.log2(f0) if is_torch else np.log2(f0)
- if uv is not None and hparams['use_uv']:
- f0[uv > 0] = 0
- return f0
-
-
-def norm_interp_f0(f0, hparams):
- is_torch = isinstance(f0, torch.Tensor)
- if is_torch:
- device = f0.device
- f0 = f0.data.cpu().numpy()
- uv = f0 == 0
- f0 = norm_f0(f0, uv, hparams)
- if sum(uv) == len(f0):
- f0[uv] = 0
- elif sum(uv) > 0:
- f0[uv] = np.interp(np.where(uv)[0], np.where(~uv)[0], f0[~uv])
- uv = torch.FloatTensor(uv)
- f0 = torch.FloatTensor(f0)
- if is_torch:
- f0 = f0.to(device)
- return f0, uv
-
-
-def denorm_f0(f0, uv, hparams, pitch_padding=None, min=None, max=None):
- if hparams['pitch_norm'] == 'standard':
- f0 = f0 * hparams['f0_std'] + hparams['f0_mean']
- if hparams['pitch_norm'] == 'log':
- f0 = 2 ** f0
- if min is not None:
- f0 = f0.clamp(min=min)
- if max is not None:
- f0 = f0.clamp(max=max)
- if uv is not None and hparams['use_uv']:
- f0[uv > 0] = 0
- if pitch_padding is not None:
- f0[pitch_padding] = 0
- return f0
diff --git a/spaces/SungBeom/chatwine-korean/.venv/Lib/site-packages/aiohttp/web_app.py b/spaces/SungBeom/chatwine-korean/.venv/Lib/site-packages/aiohttp/web_app.py
deleted file mode 100644
index 8fd4471d3af019c6e3bd01fcb9838ee99636238e..0000000000000000000000000000000000000000
--- a/spaces/SungBeom/chatwine-korean/.venv/Lib/site-packages/aiohttp/web_app.py
+++ /dev/null
@@ -1,557 +0,0 @@
-import asyncio
-import logging
-import warnings
-from functools import partial, update_wrapper
-from typing import (
- TYPE_CHECKING,
- Any,
- AsyncIterator,
- Awaitable,
- Callable,
- Dict,
- Iterable,
- Iterator,
- List,
- Mapping,
- MutableMapping,
- Optional,
- Sequence,
- Tuple,
- Type,
- Union,
- cast,
-)
-
-from aiosignal import Signal
-from frozenlist import FrozenList
-
-from . import hdrs
-from .abc import (
- AbstractAccessLogger,
- AbstractMatchInfo,
- AbstractRouter,
- AbstractStreamWriter,
-)
-from .helpers import DEBUG
-from .http_parser import RawRequestMessage
-from .log import web_logger
-from .streams import StreamReader
-from .web_log import AccessLogger
-from .web_middlewares import _fix_request_current_app
-from .web_protocol import RequestHandler
-from .web_request import Request
-from .web_response import StreamResponse
-from .web_routedef import AbstractRouteDef
-from .web_server import Server
-from .web_urldispatcher import (
- AbstractResource,
- AbstractRoute,
- Domain,
- MaskDomain,
- MatchedSubAppResource,
- PrefixedSubAppResource,
- UrlDispatcher,
-)
-
-__all__ = ("Application", "CleanupError")
-
-
-if TYPE_CHECKING: # pragma: no cover
- from .typedefs import Handler
-
- _AppSignal = Signal[Callable[["Application"], Awaitable[None]]]
- _RespPrepareSignal = Signal[Callable[[Request, StreamResponse], Awaitable[None]]]
- _Middleware = Union[
- Callable[[Request, Handler], Awaitable[StreamResponse]],
- Callable[["Application", Handler], Awaitable[Handler]], # old-style
- ]
- _Middlewares = FrozenList[_Middleware]
- _MiddlewaresHandlers = Optional[Sequence[Tuple[_Middleware, bool]]]
- _Subapps = List["Application"]
-else:
- # No type checker mode, skip types
- _AppSignal = Signal
- _RespPrepareSignal = Signal
- _Middleware = Callable
- _Middlewares = FrozenList
- _MiddlewaresHandlers = Optional[Sequence]
- _Subapps = List
-
-
-class Application(MutableMapping[str, Any]):
- ATTRS = frozenset(
- [
- "logger",
- "_debug",
- "_router",
- "_loop",
- "_handler_args",
- "_middlewares",
- "_middlewares_handlers",
- "_run_middlewares",
- "_state",
- "_frozen",
- "_pre_frozen",
- "_subapps",
- "_on_response_prepare",
- "_on_startup",
- "_on_shutdown",
- "_on_cleanup",
- "_client_max_size",
- "_cleanup_ctx",
- ]
- )
-
- def __init__(
- self,
- *,
- logger: logging.Logger = web_logger,
- router: Optional[UrlDispatcher] = None,
- middlewares: Iterable[_Middleware] = (),
- handler_args: Optional[Mapping[str, Any]] = None,
- client_max_size: int = 1024**2,
- loop: Optional[asyncio.AbstractEventLoop] = None,
- debug: Any = ..., # mypy doesn't support ellipsis
- ) -> None:
- if router is None:
- router = UrlDispatcher()
- else:
- warnings.warn(
- "router argument is deprecated", DeprecationWarning, stacklevel=2
- )
- assert isinstance(router, AbstractRouter), router
-
- if loop is not None:
- warnings.warn(
- "loop argument is deprecated", DeprecationWarning, stacklevel=2
- )
-
- if debug is not ...:
- warnings.warn(
- "debug argument is deprecated", DeprecationWarning, stacklevel=2
- )
- self._debug = debug
- self._router: UrlDispatcher = router
- self._loop = loop
- self._handler_args = handler_args
- self.logger = logger
-
- self._middlewares: _Middlewares = FrozenList(middlewares)
-
- # initialized on freezing
- self._middlewares_handlers: _MiddlewaresHandlers = None
- # initialized on freezing
- self._run_middlewares: Optional[bool] = None
-
- self._state: Dict[str, Any] = {}
- self._frozen = False
- self._pre_frozen = False
- self._subapps: _Subapps = []
-
- self._on_response_prepare: _RespPrepareSignal = Signal(self)
- self._on_startup: _AppSignal = Signal(self)
- self._on_shutdown: _AppSignal = Signal(self)
- self._on_cleanup: _AppSignal = Signal(self)
- self._cleanup_ctx = CleanupContext()
- self._on_startup.append(self._cleanup_ctx._on_startup)
- self._on_cleanup.append(self._cleanup_ctx._on_cleanup)
- self._client_max_size = client_max_size
-
- def __init_subclass__(cls: Type["Application"]) -> None:
- warnings.warn(
- "Inheritance class {} from web.Application "
- "is discouraged".format(cls.__name__),
- DeprecationWarning,
- stacklevel=2,
- )
-
- if DEBUG: # pragma: no cover
-
- def __setattr__(self, name: str, val: Any) -> None:
- if name not in self.ATTRS:
- warnings.warn(
- "Setting custom web.Application.{} attribute "
- "is discouraged".format(name),
- DeprecationWarning,
- stacklevel=2,
- )
- super().__setattr__(name, val)
-
- # MutableMapping API
-
- def __eq__(self, other: object) -> bool:
- return self is other
-
- def __getitem__(self, key: str) -> Any:
- return self._state[key]
-
- def _check_frozen(self) -> None:
- if self._frozen:
- warnings.warn(
- "Changing state of started or joined " "application is deprecated",
- DeprecationWarning,
- stacklevel=3,
- )
-
- def __setitem__(self, key: str, value: Any) -> None:
- self._check_frozen()
- self._state[key] = value
-
- def __delitem__(self, key: str) -> None:
- self._check_frozen()
- del self._state[key]
-
- def __len__(self) -> int:
- return len(self._state)
-
- def __iter__(self) -> Iterator[str]:
- return iter(self._state)
-
- ########
- @property
- def loop(self) -> asyncio.AbstractEventLoop:
- # Technically the loop can be None
- # but we mask it by explicit type cast
- # to provide more convinient type annotation
- warnings.warn("loop property is deprecated", DeprecationWarning, stacklevel=2)
- return cast(asyncio.AbstractEventLoop, self._loop)
-
- def _set_loop(self, loop: Optional[asyncio.AbstractEventLoop]) -> None:
- if loop is None:
- loop = asyncio.get_event_loop()
- if self._loop is not None and self._loop is not loop:
- raise RuntimeError(
- "web.Application instance initialized with different loop"
- )
-
- self._loop = loop
-
- # set loop debug
- if self._debug is ...:
- self._debug = loop.get_debug()
-
- # set loop to sub applications
- for subapp in self._subapps:
- subapp._set_loop(loop)
-
- @property
- def pre_frozen(self) -> bool:
- return self._pre_frozen
-
- def pre_freeze(self) -> None:
- if self._pre_frozen:
- return
-
- self._pre_frozen = True
- self._middlewares.freeze()
- self._router.freeze()
- self._on_response_prepare.freeze()
- self._cleanup_ctx.freeze()
- self._on_startup.freeze()
- self._on_shutdown.freeze()
- self._on_cleanup.freeze()
- self._middlewares_handlers = tuple(self._prepare_middleware())
-
- # If current app and any subapp do not have middlewares avoid run all
- # of the code footprint that it implies, which have a middleware
- # hardcoded per app that sets up the current_app attribute. If no
- # middlewares are configured the handler will receive the proper
- # current_app without needing all of this code.
- self._run_middlewares = True if self.middlewares else False
-
- for subapp in self._subapps:
- subapp.pre_freeze()
- self._run_middlewares = self._run_middlewares or subapp._run_middlewares
-
- @property
- def frozen(self) -> bool:
- return self._frozen
-
- def freeze(self) -> None:
- if self._frozen:
- return
-
- self.pre_freeze()
- self._frozen = True
- for subapp in self._subapps:
- subapp.freeze()
-
- @property
- def debug(self) -> bool:
- warnings.warn("debug property is deprecated", DeprecationWarning, stacklevel=2)
- return self._debug # type: ignore[no-any-return]
-
- def _reg_subapp_signals(self, subapp: "Application") -> None:
- def reg_handler(signame: str) -> None:
- subsig = getattr(subapp, signame)
-
- async def handler(app: "Application") -> None:
- await subsig.send(subapp)
-
- appsig = getattr(self, signame)
- appsig.append(handler)
-
- reg_handler("on_startup")
- reg_handler("on_shutdown")
- reg_handler("on_cleanup")
-
- def add_subapp(self, prefix: str, subapp: "Application") -> AbstractResource:
- if not isinstance(prefix, str):
- raise TypeError("Prefix must be str")
- prefix = prefix.rstrip("/")
- if not prefix:
- raise ValueError("Prefix cannot be empty")
- factory = partial(PrefixedSubAppResource, prefix, subapp)
- return self._add_subapp(factory, subapp)
-
- def _add_subapp(
- self, resource_factory: Callable[[], AbstractResource], subapp: "Application"
- ) -> AbstractResource:
- if self.frozen:
- raise RuntimeError("Cannot add sub application to frozen application")
- if subapp.frozen:
- raise RuntimeError("Cannot add frozen application")
- resource = resource_factory()
- self.router.register_resource(resource)
- self._reg_subapp_signals(subapp)
- self._subapps.append(subapp)
- subapp.pre_freeze()
- if self._loop is not None:
- subapp._set_loop(self._loop)
- return resource
-
- def add_domain(self, domain: str, subapp: "Application") -> AbstractResource:
- if not isinstance(domain, str):
- raise TypeError("Domain must be str")
- elif "*" in domain:
- rule: Domain = MaskDomain(domain)
- else:
- rule = Domain(domain)
- factory = partial(MatchedSubAppResource, rule, subapp)
- return self._add_subapp(factory, subapp)
-
- def add_routes(self, routes: Iterable[AbstractRouteDef]) -> List[AbstractRoute]:
- return self.router.add_routes(routes)
-
- @property
- def on_response_prepare(self) -> _RespPrepareSignal:
- return self._on_response_prepare
-
- @property
- def on_startup(self) -> _AppSignal:
- return self._on_startup
-
- @property
- def on_shutdown(self) -> _AppSignal:
- return self._on_shutdown
-
- @property
- def on_cleanup(self) -> _AppSignal:
- return self._on_cleanup
-
- @property
- def cleanup_ctx(self) -> "CleanupContext":
- return self._cleanup_ctx
-
- @property
- def router(self) -> UrlDispatcher:
- return self._router
-
- @property
- def middlewares(self) -> _Middlewares:
- return self._middlewares
-
- def _make_handler(
- self,
- *,
- loop: Optional[asyncio.AbstractEventLoop] = None,
- access_log_class: Type[AbstractAccessLogger] = AccessLogger,
- **kwargs: Any,
- ) -> Server:
-
- if not issubclass(access_log_class, AbstractAccessLogger):
- raise TypeError(
- "access_log_class must be subclass of "
- "aiohttp.abc.AbstractAccessLogger, got {}".format(access_log_class)
- )
-
- self._set_loop(loop)
- self.freeze()
-
- kwargs["debug"] = self._debug
- kwargs["access_log_class"] = access_log_class
- if self._handler_args:
- for k, v in self._handler_args.items():
- kwargs[k] = v
-
- return Server(
- self._handle, # type: ignore[arg-type]
- request_factory=self._make_request,
- loop=self._loop,
- **kwargs,
- )
-
- def make_handler(
- self,
- *,
- loop: Optional[asyncio.AbstractEventLoop] = None,
- access_log_class: Type[AbstractAccessLogger] = AccessLogger,
- **kwargs: Any,
- ) -> Server:
-
- warnings.warn(
- "Application.make_handler(...) is deprecated, " "use AppRunner API instead",
- DeprecationWarning,
- stacklevel=2,
- )
-
- return self._make_handler(
- loop=loop, access_log_class=access_log_class, **kwargs
- )
-
- async def startup(self) -> None:
- """Causes on_startup signal
-
- Should be called in the event loop along with the request handler.
- """
- await self.on_startup.send(self)
-
- async def shutdown(self) -> None:
- """Causes on_shutdown signal
-
- Should be called before cleanup()
- """
- await self.on_shutdown.send(self)
-
- async def cleanup(self) -> None:
- """Causes on_cleanup signal
-
- Should be called after shutdown()
- """
- if self.on_cleanup.frozen:
- await self.on_cleanup.send(self)
- else:
- # If an exception occurs in startup, ensure cleanup contexts are completed.
- await self._cleanup_ctx._on_cleanup(self)
-
- def _make_request(
- self,
- message: RawRequestMessage,
- payload: StreamReader,
- protocol: RequestHandler,
- writer: AbstractStreamWriter,
- task: "asyncio.Task[None]",
- _cls: Type[Request] = Request,
- ) -> Request:
- return _cls(
- message,
- payload,
- protocol,
- writer,
- task,
- self._loop,
- client_max_size=self._client_max_size,
- )
-
- def _prepare_middleware(self) -> Iterator[Tuple[_Middleware, bool]]:
- for m in reversed(self._middlewares):
- if getattr(m, "__middleware_version__", None) == 1:
- yield m, True
- else:
- warnings.warn(
- 'old-style middleware "{!r}" deprecated, ' "see #2252".format(m),
- DeprecationWarning,
- stacklevel=2,
- )
- yield m, False
-
- yield _fix_request_current_app(self), True
-
- async def _handle(self, request: Request) -> StreamResponse:
- loop = asyncio.get_event_loop()
- debug = loop.get_debug()
- match_info = await self._router.resolve(request)
- if debug: # pragma: no cover
- if not isinstance(match_info, AbstractMatchInfo):
- raise TypeError(
- "match_info should be AbstractMatchInfo "
- "instance, not {!r}".format(match_info)
- )
- match_info.add_app(self)
-
- match_info.freeze()
-
- resp = None
- request._match_info = match_info
- expect = request.headers.get(hdrs.EXPECT)
- if expect:
- resp = await match_info.expect_handler(request)
- await request.writer.drain()
-
- if resp is None:
- handler = match_info.handler
-
- if self._run_middlewares:
- for app in match_info.apps[::-1]:
- for m, new_style in app._middlewares_handlers: # type: ignore[union-attr] # noqa
- if new_style:
- handler = update_wrapper(
- partial(m, handler=handler), handler
- )
- else:
- handler = await m(app, handler) # type: ignore[arg-type]
-
- resp = await handler(request)
-
- return resp
-
- def __call__(self) -> "Application":
- """gunicorn compatibility"""
- return self
-
- def __repr__(self) -> str:
- return f""
-
- def __bool__(self) -> bool:
- return True
-
-
-class CleanupError(RuntimeError):
- @property
- def exceptions(self) -> List[BaseException]:
- return cast(List[BaseException], self.args[1])
-
-
-if TYPE_CHECKING: # pragma: no cover
- _CleanupContextBase = FrozenList[Callable[[Application], AsyncIterator[None]]]
-else:
- _CleanupContextBase = FrozenList
-
-
-class CleanupContext(_CleanupContextBase):
- def __init__(self) -> None:
- super().__init__()
- self._exits: List[AsyncIterator[None]] = []
-
- async def _on_startup(self, app: Application) -> None:
- for cb in self:
- it = cb(app).__aiter__()
- await it.__anext__()
- self._exits.append(it)
-
- async def _on_cleanup(self, app: Application) -> None:
- errors = []
- for it in reversed(self._exits):
- try:
- await it.__anext__()
- except StopAsyncIteration:
- pass
- except Exception as exc:
- errors.append(exc)
- else:
- errors.append(RuntimeError(f"{it!r} has more than one 'yield'"))
- if errors:
- if len(errors) == 1:
- raise errors[0]
- else:
- raise CleanupError("Multiple errors on cleanup stage", errors)
diff --git a/spaces/SungBeom/chatwine-korean/.venv/Lib/site-packages/charset_normalizer/cli/normalizer.py b/spaces/SungBeom/chatwine-korean/.venv/Lib/site-packages/charset_normalizer/cli/normalizer.py
deleted file mode 100644
index f4bcbaac049b542a004918a0b1499122fcca9cc0..0000000000000000000000000000000000000000
--- a/spaces/SungBeom/chatwine-korean/.venv/Lib/site-packages/charset_normalizer/cli/normalizer.py
+++ /dev/null
@@ -1,296 +0,0 @@
-import argparse
-import sys
-from json import dumps
-from os.path import abspath, basename, dirname, join, realpath
-from platform import python_version
-from typing import List, Optional
-from unicodedata import unidata_version
-
-import charset_normalizer.md as md_module
-from charset_normalizer import from_fp
-from charset_normalizer.models import CliDetectionResult
-from charset_normalizer.version import __version__
-
-
-def query_yes_no(question: str, default: str = "yes") -> bool:
- """Ask a yes/no question via input() and return their answer.
-
- "question" is a string that is presented to the user.
- "default" is the presumed answer if the user just hits .
- It must be "yes" (the default), "no" or None (meaning
- an answer is required of the user).
-
- The "answer" return value is True for "yes" or False for "no".
-
- Credit goes to (c) https://stackoverflow.com/questions/3041986/apt-command-line-interface-like-yes-no-input
- """
- valid = {"yes": True, "y": True, "ye": True, "no": False, "n": False}
- if default is None:
- prompt = " [y/n] "
- elif default == "yes":
- prompt = " [Y/n] "
- elif default == "no":
- prompt = " [y/N] "
- else:
- raise ValueError("invalid default answer: '%s'" % default)
-
- while True:
- sys.stdout.write(question + prompt)
- choice = input().lower()
- if default is not None and choice == "":
- return valid[default]
- elif choice in valid:
- return valid[choice]
- else:
- sys.stdout.write("Please respond with 'yes' or 'no' " "(or 'y' or 'n').\n")
-
-
-def cli_detect(argv: Optional[List[str]] = None) -> int:
- """
- CLI assistant using ARGV and ArgumentParser
- :param argv:
- :return: 0 if everything is fine, anything else equal trouble
- """
- parser = argparse.ArgumentParser(
- description="The Real First Universal Charset Detector. "
- "Discover originating encoding used on text file. "
- "Normalize text to unicode."
- )
-
- parser.add_argument(
- "files", type=argparse.FileType("rb"), nargs="+", help="File(s) to be analysed"
- )
- parser.add_argument(
- "-v",
- "--verbose",
- action="store_true",
- default=False,
- dest="verbose",
- help="Display complementary information about file if any. "
- "Stdout will contain logs about the detection process.",
- )
- parser.add_argument(
- "-a",
- "--with-alternative",
- action="store_true",
- default=False,
- dest="alternatives",
- help="Output complementary possibilities if any. Top-level JSON WILL be a list.",
- )
- parser.add_argument(
- "-n",
- "--normalize",
- action="store_true",
- default=False,
- dest="normalize",
- help="Permit to normalize input file. If not set, program does not write anything.",
- )
- parser.add_argument(
- "-m",
- "--minimal",
- action="store_true",
- default=False,
- dest="minimal",
- help="Only output the charset detected to STDOUT. Disabling JSON output.",
- )
- parser.add_argument(
- "-r",
- "--replace",
- action="store_true",
- default=False,
- dest="replace",
- help="Replace file when trying to normalize it instead of creating a new one.",
- )
- parser.add_argument(
- "-f",
- "--force",
- action="store_true",
- default=False,
- dest="force",
- help="Replace file without asking if you are sure, use this flag with caution.",
- )
- parser.add_argument(
- "-t",
- "--threshold",
- action="store",
- default=0.2,
- type=float,
- dest="threshold",
- help="Define a custom maximum amount of chaos allowed in decoded content. 0. <= chaos <= 1.",
- )
- parser.add_argument(
- "--version",
- action="version",
- version="Charset-Normalizer {} - Python {} - Unicode {} - SpeedUp {}".format(
- __version__,
- python_version(),
- unidata_version,
- "OFF" if md_module.__file__.lower().endswith(".py") else "ON",
- ),
- help="Show version information and exit.",
- )
-
- args = parser.parse_args(argv)
-
- if args.replace is True and args.normalize is False:
- print("Use --replace in addition of --normalize only.", file=sys.stderr)
- return 1
-
- if args.force is True and args.replace is False:
- print("Use --force in addition of --replace only.", file=sys.stderr)
- return 1
-
- if args.threshold < 0.0 or args.threshold > 1.0:
- print("--threshold VALUE should be between 0. AND 1.", file=sys.stderr)
- return 1
-
- x_ = []
-
- for my_file in args.files:
- matches = from_fp(my_file, threshold=args.threshold, explain=args.verbose)
-
- best_guess = matches.best()
-
- if best_guess is None:
- print(
- 'Unable to identify originating encoding for "{}". {}'.format(
- my_file.name,
- "Maybe try increasing maximum amount of chaos."
- if args.threshold < 1.0
- else "",
- ),
- file=sys.stderr,
- )
- x_.append(
- CliDetectionResult(
- abspath(my_file.name),
- None,
- [],
- [],
- "Unknown",
- [],
- False,
- 1.0,
- 0.0,
- None,
- True,
- )
- )
- else:
- x_.append(
- CliDetectionResult(
- abspath(my_file.name),
- best_guess.encoding,
- best_guess.encoding_aliases,
- [
- cp
- for cp in best_guess.could_be_from_charset
- if cp != best_guess.encoding
- ],
- best_guess.language,
- best_guess.alphabets,
- best_guess.bom,
- best_guess.percent_chaos,
- best_guess.percent_coherence,
- None,
- True,
- )
- )
-
- if len(matches) > 1 and args.alternatives:
- for el in matches:
- if el != best_guess:
- x_.append(
- CliDetectionResult(
- abspath(my_file.name),
- el.encoding,
- el.encoding_aliases,
- [
- cp
- for cp in el.could_be_from_charset
- if cp != el.encoding
- ],
- el.language,
- el.alphabets,
- el.bom,
- el.percent_chaos,
- el.percent_coherence,
- None,
- False,
- )
- )
-
- if args.normalize is True:
- if best_guess.encoding.startswith("utf") is True:
- print(
- '"{}" file does not need to be normalized, as it already came from unicode.'.format(
- my_file.name
- ),
- file=sys.stderr,
- )
- if my_file.closed is False:
- my_file.close()
- continue
-
- dir_path = dirname(realpath(my_file.name))
- file_name = basename(realpath(my_file.name))
-
- o_: List[str] = file_name.split(".")
-
- if args.replace is False:
- o_.insert(-1, best_guess.encoding)
- if my_file.closed is False:
- my_file.close()
- elif (
- args.force is False
- and query_yes_no(
- 'Are you sure to normalize "{}" by replacing it ?'.format(
- my_file.name
- ),
- "no",
- )
- is False
- ):
- if my_file.closed is False:
- my_file.close()
- continue
-
- try:
- x_[0].unicode_path = join(dir_path, ".".join(o_))
-
- with open(x_[0].unicode_path, "w", encoding="utf-8") as fp:
- fp.write(str(best_guess))
- except IOError as e:
- print(str(e), file=sys.stderr)
- if my_file.closed is False:
- my_file.close()
- return 2
-
- if my_file.closed is False:
- my_file.close()
-
- if args.minimal is False:
- print(
- dumps(
- [el.__dict__ for el in x_] if len(x_) > 1 else x_[0].__dict__,
- ensure_ascii=True,
- indent=4,
- )
- )
- else:
- for my_file in args.files:
- print(
- ", ".join(
- [
- el.encoding or "undefined"
- for el in x_
- if el.path == abspath(my_file.name)
- ]
- )
- )
-
- return 0
-
-
-if __name__ == "__main__":
- cli_detect()
diff --git a/spaces/SungBeom/chatwine-korean/.venv/Lib/site-packages/debugpy/_vendored/pydevd/pydevd_attach_to_process/_test_attach_to_process.py b/spaces/SungBeom/chatwine-korean/.venv/Lib/site-packages/debugpy/_vendored/pydevd/pydevd_attach_to_process/_test_attach_to_process.py
deleted file mode 100644
index daeee93f471786d2b05c331829afcf0dae1f3fc1..0000000000000000000000000000000000000000
--- a/spaces/SungBeom/chatwine-korean/.venv/Lib/site-packages/debugpy/_vendored/pydevd/pydevd_attach_to_process/_test_attach_to_process.py
+++ /dev/null
@@ -1,9 +0,0 @@
-import subprocess
-import sys
-print(sys.executable)
-
-if __name__ == '__main__':
- p = subprocess.Popen([sys.executable, '-u', '_always_live_program.py'])
- import attach_pydevd
- attach_pydevd.main(attach_pydevd.process_command_line(['--pid', str(p.pid), '--protocol', 'http']))
- p.wait()
diff --git a/spaces/Superlang/ImageProcessor/annotator/oneformer/detectron2/tracking/iou_weighted_hungarian_bbox_iou_tracker.py b/spaces/Superlang/ImageProcessor/annotator/oneformer/detectron2/tracking/iou_weighted_hungarian_bbox_iou_tracker.py
deleted file mode 100644
index e9b40f8a9c269029e220d5dfa8df1e8372d05007..0000000000000000000000000000000000000000
--- a/spaces/Superlang/ImageProcessor/annotator/oneformer/detectron2/tracking/iou_weighted_hungarian_bbox_iou_tracker.py
+++ /dev/null
@@ -1,102 +0,0 @@
-#!/usr/bin/env python3
-# Copyright 2004-present Facebook. All Rights Reserved.
-
-import numpy as np
-from typing import List
-
-from annotator.oneformer.detectron2.config import CfgNode as CfgNode_
-from annotator.oneformer.detectron2.config import configurable
-
-from .base_tracker import TRACKER_HEADS_REGISTRY
-from .vanilla_hungarian_bbox_iou_tracker import VanillaHungarianBBoxIOUTracker
-
-
-@TRACKER_HEADS_REGISTRY.register()
-class IOUWeightedHungarianBBoxIOUTracker(VanillaHungarianBBoxIOUTracker):
- """
- A tracker using IoU as weight in Hungarian algorithm, also known
- as Munkres or Kuhn-Munkres algorithm
- """
-
- @configurable
- def __init__(
- self,
- *,
- video_height: int,
- video_width: int,
- max_num_instances: int = 200,
- max_lost_frame_count: int = 0,
- min_box_rel_dim: float = 0.02,
- min_instance_period: int = 1,
- track_iou_threshold: float = 0.5,
- **kwargs,
- ):
- """
- Args:
- video_height: height the video frame
- video_width: width of the video frame
- max_num_instances: maximum number of id allowed to be tracked
- max_lost_frame_count: maximum number of frame an id can lost tracking
- exceed this number, an id is considered as lost
- forever
- min_box_rel_dim: a percentage, smaller than this dimension, a bbox is
- removed from tracking
- min_instance_period: an instance will be shown after this number of period
- since its first showing up in the video
- track_iou_threshold: iou threshold, below this number a bbox pair is removed
- from tracking
- """
- super().__init__(
- video_height=video_height,
- video_width=video_width,
- max_num_instances=max_num_instances,
- max_lost_frame_count=max_lost_frame_count,
- min_box_rel_dim=min_box_rel_dim,
- min_instance_period=min_instance_period,
- track_iou_threshold=track_iou_threshold,
- )
-
- @classmethod
- def from_config(cls, cfg: CfgNode_):
- """
- Old style initialization using CfgNode
-
- Args:
- cfg: D2 CfgNode, config file
- Return:
- dictionary storing arguments for __init__ method
- """
- assert "VIDEO_HEIGHT" in cfg.TRACKER_HEADS
- assert "VIDEO_WIDTH" in cfg.TRACKER_HEADS
- video_height = cfg.TRACKER_HEADS.get("VIDEO_HEIGHT")
- video_width = cfg.TRACKER_HEADS.get("VIDEO_WIDTH")
- max_num_instances = cfg.TRACKER_HEADS.get("MAX_NUM_INSTANCES", 200)
- max_lost_frame_count = cfg.TRACKER_HEADS.get("MAX_LOST_FRAME_COUNT", 0)
- min_box_rel_dim = cfg.TRACKER_HEADS.get("MIN_BOX_REL_DIM", 0.02)
- min_instance_period = cfg.TRACKER_HEADS.get("MIN_INSTANCE_PERIOD", 1)
- track_iou_threshold = cfg.TRACKER_HEADS.get("TRACK_IOU_THRESHOLD", 0.5)
- return {
- "_target_": "detectron2.tracking.iou_weighted_hungarian_bbox_iou_tracker.IOUWeightedHungarianBBoxIOUTracker", # noqa
- "video_height": video_height,
- "video_width": video_width,
- "max_num_instances": max_num_instances,
- "max_lost_frame_count": max_lost_frame_count,
- "min_box_rel_dim": min_box_rel_dim,
- "min_instance_period": min_instance_period,
- "track_iou_threshold": track_iou_threshold,
- }
-
- def assign_cost_matrix_values(self, cost_matrix: np.ndarray, bbox_pairs: List) -> np.ndarray:
- """
- Based on IoU for each pair of bbox, assign the associated value in cost matrix
-
- Args:
- cost_matrix: np.ndarray, initialized 2D array with target dimensions
- bbox_pairs: list of bbox pair, in each pair, iou value is stored
- Return:
- np.ndarray, cost_matrix with assigned values
- """
- for pair in bbox_pairs:
- # assign (-1 * IoU) for above threshold pairs, algorithms will minimize cost
- cost_matrix[pair["idx"]][pair["prev_idx"]] = -1 * pair["IoU"]
- return cost_matrix
diff --git a/spaces/Superlang/ImageProcessor/annotator/zoe/zoedepth/models/base_models/midas_repo/midas/midas_net_custom.py b/spaces/Superlang/ImageProcessor/annotator/zoe/zoedepth/models/base_models/midas_repo/midas/midas_net_custom.py
deleted file mode 100644
index 50e4acb5e53d5fabefe3dde16ab49c33c2b7797c..0000000000000000000000000000000000000000
--- a/spaces/Superlang/ImageProcessor/annotator/zoe/zoedepth/models/base_models/midas_repo/midas/midas_net_custom.py
+++ /dev/null
@@ -1,128 +0,0 @@
-"""MidashNet: Network for monocular depth estimation trained by mixing several datasets.
-This file contains code that is adapted from
-https://github.com/thomasjpfan/pytorch_refinenet/blob/master/pytorch_refinenet/refinenet/refinenet_4cascade.py
-"""
-import torch
-import torch.nn as nn
-
-from .base_model import BaseModel
-from .blocks import FeatureFusionBlock, FeatureFusionBlock_custom, Interpolate, _make_encoder
-
-
-class MidasNet_small(BaseModel):
- """Network for monocular depth estimation.
- """
-
- def __init__(self, path=None, features=64, backbone="efficientnet_lite3", non_negative=True, exportable=True, channels_last=False, align_corners=True,
- blocks={'expand': True}):
- """Init.
-
- Args:
- path (str, optional): Path to saved model. Defaults to None.
- features (int, optional): Number of features. Defaults to 256.
- backbone (str, optional): Backbone network for encoder. Defaults to resnet50
- """
- print("Loading weights: ", path)
-
- super(MidasNet_small, self).__init__()
-
- use_pretrained = False if path else True
-
- self.channels_last = channels_last
- self.blocks = blocks
- self.backbone = backbone
-
- self.groups = 1
-
- features1=features
- features2=features
- features3=features
- features4=features
- self.expand = False
- if "expand" in self.blocks and self.blocks['expand'] == True:
- self.expand = True
- features1=features
- features2=features*2
- features3=features*4
- features4=features*8
-
- self.pretrained, self.scratch = _make_encoder(self.backbone, features, use_pretrained, groups=self.groups, expand=self.expand, exportable=exportable)
-
- self.scratch.activation = nn.ReLU(False)
-
- self.scratch.refinenet4 = FeatureFusionBlock_custom(features4, self.scratch.activation, deconv=False, bn=False, expand=self.expand, align_corners=align_corners)
- self.scratch.refinenet3 = FeatureFusionBlock_custom(features3, self.scratch.activation, deconv=False, bn=False, expand=self.expand, align_corners=align_corners)
- self.scratch.refinenet2 = FeatureFusionBlock_custom(features2, self.scratch.activation, deconv=False, bn=False, expand=self.expand, align_corners=align_corners)
- self.scratch.refinenet1 = FeatureFusionBlock_custom(features1, self.scratch.activation, deconv=False, bn=False, align_corners=align_corners)
-
-
- self.scratch.output_conv = nn.Sequential(
- nn.Conv2d(features, features//2, kernel_size=3, stride=1, padding=1, groups=self.groups),
- Interpolate(scale_factor=2, mode="bilinear"),
- nn.Conv2d(features//2, 32, kernel_size=3, stride=1, padding=1),
- self.scratch.activation,
- nn.Conv2d(32, 1, kernel_size=1, stride=1, padding=0),
- nn.ReLU(True) if non_negative else nn.Identity(),
- nn.Identity(),
- )
-
- if path:
- self.load(path)
-
-
- def forward(self, x):
- """Forward pass.
-
- Args:
- x (tensor): input data (image)
-
- Returns:
- tensor: depth
- """
- if self.channels_last==True:
- print("self.channels_last = ", self.channels_last)
- x.contiguous(memory_format=torch.channels_last)
-
-
- layer_1 = self.pretrained.layer1(x)
- layer_2 = self.pretrained.layer2(layer_1)
- layer_3 = self.pretrained.layer3(layer_2)
- layer_4 = self.pretrained.layer4(layer_3)
-
- layer_1_rn = self.scratch.layer1_rn(layer_1)
- layer_2_rn = self.scratch.layer2_rn(layer_2)
- layer_3_rn = self.scratch.layer3_rn(layer_3)
- layer_4_rn = self.scratch.layer4_rn(layer_4)
-
-
- path_4 = self.scratch.refinenet4(layer_4_rn)
- path_3 = self.scratch.refinenet3(path_4, layer_3_rn)
- path_2 = self.scratch.refinenet2(path_3, layer_2_rn)
- path_1 = self.scratch.refinenet1(path_2, layer_1_rn)
-
- out = self.scratch.output_conv(path_1)
-
- return torch.squeeze(out, dim=1)
-
-
-
-def fuse_model(m):
- prev_previous_type = nn.Identity()
- prev_previous_name = ''
- previous_type = nn.Identity()
- previous_name = ''
- for name, module in m.named_modules():
- if prev_previous_type == nn.Conv2d and previous_type == nn.BatchNorm2d and type(module) == nn.ReLU:
- # print("FUSED ", prev_previous_name, previous_name, name)
- torch.quantization.fuse_modules(m, [prev_previous_name, previous_name, name], inplace=True)
- elif prev_previous_type == nn.Conv2d and previous_type == nn.BatchNorm2d:
- # print("FUSED ", prev_previous_name, previous_name)
- torch.quantization.fuse_modules(m, [prev_previous_name, previous_name], inplace=True)
- # elif previous_type == nn.Conv2d and type(module) == nn.ReLU:
- # print("FUSED ", previous_name, name)
- # torch.quantization.fuse_modules(m, [previous_name, name], inplace=True)
-
- prev_previous_type = previous_type
- prev_previous_name = previous_name
- previous_type = type(module)
- previous_name = name
\ No newline at end of file
diff --git a/spaces/TabPFN/TabPFNPrediction/TabPFN/initializers.py b/spaces/TabPFN/TabPFNPrediction/TabPFN/initializers.py
deleted file mode 100644
index 4a2de2711a62676223950c35e5ce88cabcb086a0..0000000000000000000000000000000000000000
--- a/spaces/TabPFN/TabPFNPrediction/TabPFN/initializers.py
+++ /dev/null
@@ -1,9 +0,0 @@
-import torch
-from torch import nn
-
-def get_NormalInitializer(std):
- def initializer(m):
- if isinstance(m, nn.Linear):
- nn.init.normal_(m.weight, 0, std)
- nn.init.normal_(m.bias, 0, std)
- return initializer
\ No newline at end of file
diff --git a/spaces/TandCAcceptMe/face-swap-docker/mynewshinyroop/Lib/site-packages/setuptools/command/rotate.py b/spaces/TandCAcceptMe/face-swap-docker/mynewshinyroop/Lib/site-packages/setuptools/command/rotate.py
deleted file mode 100644
index 74795ba922bb376e24858760e63dc9124ef22b9f..0000000000000000000000000000000000000000
--- a/spaces/TandCAcceptMe/face-swap-docker/mynewshinyroop/Lib/site-packages/setuptools/command/rotate.py
+++ /dev/null
@@ -1,64 +0,0 @@
-from distutils.util import convert_path
-from distutils import log
-from distutils.errors import DistutilsOptionError
-import os
-import shutil
-
-from setuptools import Command
-
-
-class rotate(Command):
- """Delete older distributions"""
-
- description = "delete older distributions, keeping N newest files"
- user_options = [
- ('match=', 'm', "patterns to match (required)"),
- ('dist-dir=', 'd', "directory where the distributions are"),
- ('keep=', 'k', "number of matching distributions to keep"),
- ]
-
- boolean_options = []
-
- def initialize_options(self):
- self.match = None
- self.dist_dir = None
- self.keep = None
-
- def finalize_options(self):
- if self.match is None:
- raise DistutilsOptionError(
- "Must specify one or more (comma-separated) match patterns "
- "(e.g. '.zip' or '.egg')"
- )
- if self.keep is None:
- raise DistutilsOptionError("Must specify number of files to keep")
- try:
- self.keep = int(self.keep)
- except ValueError as e:
- raise DistutilsOptionError("--keep must be an integer") from e
- if isinstance(self.match, str):
- self.match = [
- convert_path(p.strip()) for p in self.match.split(',')
- ]
- self.set_undefined_options('bdist', ('dist_dir', 'dist_dir'))
-
- def run(self):
- self.run_command("egg_info")
- from glob import glob
-
- for pattern in self.match:
- pattern = self.distribution.get_name() + '*' + pattern
- files = glob(os.path.join(self.dist_dir, pattern))
- files = [(os.path.getmtime(f), f) for f in files]
- files.sort()
- files.reverse()
-
- log.info("%d file(s) matching %s", len(files), pattern)
- files = files[self.keep:]
- for (t, f) in files:
- log.info("Deleting %s", f)
- if not self.dry_run:
- if os.path.isdir(f):
- shutil.rmtree(f)
- else:
- os.unlink(f)
diff --git a/spaces/ThomasSimonini/Huggy/style.css b/spaces/ThomasSimonini/Huggy/style.css
deleted file mode 100644
index 114adf441e9032febb46bc056b2a8bb651075f0d..0000000000000000000000000000000000000000
--- a/spaces/ThomasSimonini/Huggy/style.css
+++ /dev/null
@@ -1,28 +0,0 @@
-body {
- padding: 2rem;
- font-family: -apple-system, BlinkMacSystemFont, "Arial", sans-serif;
-}
-
-h1 {
- font-size: 16px;
- margin-top: 0;
-}
-
-p {
- color: rgb(107, 114, 128);
- font-size: 15px;
- margin-bottom: 10px;
- margin-top: 5px;
-}
-
-.card {
- max-width: 620px;
- margin: 0 auto;
- padding: 16px;
- border: 1px solid lightgray;
- border-radius: 16px;
-}
-
-.card p:last-child {
- margin-bottom: 0;
-}
diff --git a/spaces/Tinny-Robot/Tinny-Robot-NCAIR-ChatBot/app.py b/spaces/Tinny-Robot/Tinny-Robot-NCAIR-ChatBot/app.py
deleted file mode 100644
index 204cdb460bb68b5f3f91bfda52cae05e2848f8ec..0000000000000000000000000000000000000000
--- a/spaces/Tinny-Robot/Tinny-Robot-NCAIR-ChatBot/app.py
+++ /dev/null
@@ -1,3 +0,0 @@
-import gradio as gr
-
-gr.Interface.load("models/Tinny-Robot/NCAIR-ChatBot").launch()
\ No newline at end of file
diff --git a/spaces/VasudevaK/Information_Extractor/README.md b/spaces/VasudevaK/Information_Extractor/README.md
deleted file mode 100644
index b806d44f9f37fc1d786e2655e0edce08a715258a..0000000000000000000000000000000000000000
--- a/spaces/VasudevaK/Information_Extractor/README.md
+++ /dev/null
@@ -1,13 +0,0 @@
----
-title: Information Extractor
-emoji: 🌖
-colorFrom: purple
-colorTo: red
-sdk: streamlit
-sdk_version: 1.10.0
-app_file: app.py
-pinned: false
-license: apache-2.0
----
-
-Check out the configuration reference at https://huggingface.co/docs/hub/spaces-config-reference
diff --git a/spaces/Vegecken/sovits4dzl/README.md b/spaces/Vegecken/sovits4dzl/README.md
deleted file mode 100644
index 90bf70dbb0d0dde34087cc52b3ca591099dffffd..0000000000000000000000000000000000000000
--- a/spaces/Vegecken/sovits4dzl/README.md
+++ /dev/null
@@ -1,14 +0,0 @@
----
-title: Sovits4
-emoji: 🐨
-colorFrom: gray
-colorTo: pink
-sdk: gradio
-sdk_version: 3.18.0
-app_file: app.py
-pinned: false
-license: mit
-duplicated_from: chilge/sovits4nemo
----
-
-Check out the configuration reference at https://huggingface.co/docs/hub/spaces-config-reference
diff --git a/spaces/Vijish/Crop-CLIP/app.py b/spaces/Vijish/Crop-CLIP/app.py
deleted file mode 100644
index 1e549f630ad5410ee787731796d88f0bb6b054fe..0000000000000000000000000000000000000000
--- a/spaces/Vijish/Crop-CLIP/app.py
+++ /dev/null
@@ -1,95 +0,0 @@
-import csv
-import gradio as gr
-import glob
-import pprint as pp
-from sys import excepthook
-from re import T
-from urllib.parse import parse_qs, urlparse
-import clip
-import numpy as np
-import requests
-import torch
-import io
-
-
-from IPython.display import Image, display
-from PIL import Image, ImageFont
-import os
-import cv2
-import torch
-import glob
-
-# Model
-
-def predict(img,text):
- import tempfile
- model = torch.hub.load('ultralytics/yolov5', 'yolov5s')
- results = model(img)
- dirpath = tempfile.mkdtemp()
- results.crop(save_dir=dirpath)
- path= dirpath+'/crops/**/*.jpg'
- txtfiles = []
- for file in glob.glob(path):
- txtfiles.append(file)
-
- from PIL import Image
- l = []
- #keyList = list(range(len(txtfiles)))
- for filename in glob.glob(path):
- foo = Image.open(filename).convert('RGB')
- #resized_image = foo.resize((250,250))
- l.append(foo)
-
- device = "cuda" if torch.cuda.is_available() else "cpu"
- model, preprocess = clip.load("ViT-B/32", device=device)
-
- images = torch.stack([preprocess(im) for im in l]).to(device)
- with torch.no_grad():
- image_features = model.encode_image(images)
- image_features /= image_features.norm(dim=-1, keepdim=True)
-
- image_features.cpu().numpy()
-
- image_mean = torch.tensor([0.48145466, 0.4578275, 0.40821073])
- image_std = torch.tensor([0.26862954, 0.26130258, 0.27577711])
-
- images = [preprocess(im) for im in l]
- image_input = torch.tensor(np.stack(images))
- image_input -= image_mean[:, None, None]
- image_input /= image_std[:, None, None]
- with torch.no_grad():
- image_features = model.encode_image(image_input).float()
- image_features /= image_features.norm(dim=-1, keepdim=True)
-
- def get_top_N_semantic_similarity(similarity_list,N):
- results = zip(range(len(similarity_list)), similarity_list)
- results = sorted(results, key=lambda x: x[1],reverse= True)
- top_N_images = []
- scores=[]
- for index,score in results[:N]:
- scores.append(score)
- top_N_images.append(l[index])
- return scores,top_N_images
-
- #search_query = text
-
- with torch.no_grad():
- # Encode and normalize the description using CLIP
- text_encoded = model.encode_text(clip.tokenize(text).to(device))
- text_encoded /= text_encoded.norm(dim=-1, keepdim=True)
-
- similarity = text_encoded.cpu().numpy() @ image_features.cpu().numpy().T
- similarity = similarity[0]
- scores,imgs= get_top_N_semantic_similarity(similarity,N=1)
- #print ("scores ",scores)
- #ipyplot.plot_images(imgs,img_width=350)
- return imgs[0]
-
-#text = gr.inputs.Textbox(lines=1, label="Text query", placeholder="Introduce the search text...",)
-#img = gr.inputs.Image()
-
-#img = "image"
-
-
-
-gr.Interface(predict, ["image", gr.inputs.Textbox(lines=1, label="Text query", placeholder="Type here...",)], outputs="image", title="Crop-CLIP", description ="Search subjects/objects in an image using simple text description and get cropped results.This is done by combining Object detection Yolov5 and OpenAI's CLIP model.").launch();
diff --git a/spaces/Wayben/ChatGPT/modules/overwrites.py b/spaces/Wayben/ChatGPT/modules/overwrites.py
deleted file mode 100644
index bfcd4d01b7d7bec1184a8d09113933bca860530b..0000000000000000000000000000000000000000
--- a/spaces/Wayben/ChatGPT/modules/overwrites.py
+++ /dev/null
@@ -1,56 +0,0 @@
-from __future__ import annotations
-import logging
-
-from llama_index import Prompt
-from typing import List, Tuple
-import mdtex2html
-
-from modules.presets import *
-from modules.llama_func import *
-
-
-def compact_text_chunks(self, prompt: Prompt, text_chunks: List[str]) -> List[str]:
- logging.debug("Compacting text chunks...🚀🚀🚀")
- combined_str = [c.strip() for c in text_chunks if c.strip()]
- combined_str = [f"[{index+1}] {c}" for index, c in enumerate(combined_str)]
- combined_str = "\n\n".join(combined_str)
- # resplit based on self.max_chunk_overlap
- text_splitter = self.get_text_splitter_given_prompt(prompt, 1, padding=1)
- return text_splitter.split_text(combined_str)
-
-
-def postprocess(
- self, y: List[Tuple[str | None, str | None]]
-) -> List[Tuple[str | None, str | None]]:
- """
- Parameters:
- y: List of tuples representing the message and response pairs. Each message and response should be a string, which may be in Markdown format.
- Returns:
- List of tuples representing the message and response. Each message and response will be a string of HTML.
- """
- if y is None or y == []:
- return []
- user, bot = y[-1]
- if not detect_converted_mark(user):
- user = convert_asis(user)
- if not detect_converted_mark(bot):
- bot = convert_mdtext(bot)
- y[-1] = (user, bot)
- return y
-
-with open("./assets/custom.js", "r", encoding="utf-8") as f, open("./assets/Kelpy-Codos.js", "r", encoding="utf-8") as f2:
- customJS = f.read()
- kelpyCodos = f2.read()
-
-def reload_javascript():
- print("Reloading javascript...")
- js = f''
- def template_response(*args, **kwargs):
- res = GradioTemplateResponseOriginal(*args, **kwargs)
- res.body = res.body.replace(b'